Notes
Article history
The research reported in this issue of the journal was funded by the HTA programme as project number 11/102/03. The contractual start date was in August 2013. The draft report began editorial review in June 2016 and was accepted for publication in January 2017. The authors have been wholly responsible for all data collection, analysis and interpretation, and for writing up their work. The HTA editors and publisher have tried to ensure the accuracy of the authors’ report and would like to thank the reviewers for their constructive comments on the draft document. However, they do not accept liability for damages or losses arising from material published in this report.
Declared competing interests of authors
Maria Pufulete is a Research Fellow who was entirely funded during the project by the British Heart Foundation and hence this fellowship paid for her time spent on this research. Rachel Maishman was a National Institute for Health Research (NIHR) Methodology Research Fellow during the course of the project and hence this fellowship paid for her time spent on this research. Lucy Dabner had a proportion of her salary paid for by the grant from the NIHR Health Technology Assessment (HTA) programme for this research (through her academic employer). Syed Mohiuddin reports receiving a grant from the NIHR HTA programme for this research (which paid for a proportion of his time through his academic employer). William Hollingworth reports receiving a grant from the NIHR HTA programme for this research (which paid for a proportion of his time through his academic employer). Chris A Rogers is a Senior Research Fellow who was entirely funded during the project by the British Heart Foundation and hence this fellowship paid for her time spent on this research. Julian Higgins reports receiving a grant from the NIHR HTA programme for this research (which paid for a proportion of his time through his academic employer). Mark Dayer reports giving a talk sponsored by Roche on point-of-care B-type natriuretic peptide (BNP) testing. John MacLeod reports receiving a grant from the NIHR HTA programme for this research (which paid for a proportion of his time through his academic employer). Sarah Purdy reports receiving a grant from the NIHR HTA programme for this research (which paid for a proportion of her time through her academic employer). Theresa McDonagh is Clinical Audit Lead for the National Heart Failure Audit. Rachael Williams is employed by the Clinical Practice Research Datalink and reports funding from the University of Bristol to her employer, Clinical Practice Research Datalink, for access to the data during the conduct of the study; and funding from various organisations for commissioned research outside the submitted work. Barnaby C Reeves reports receiving a grant from the NIHR HTA programme for this research (which paid for a proportion of his time through his academic employer), and membership of the HTA Commissioning Board (up to 31 March 2016) and of the Systematic Reviews Programme Advisory Group (up to 5 July 2017).
Permissions
Copyright statement
© Queen’s Printer and Controller of HMSO 2017. This work was produced by Pufulete et al. under the terms of a commissioning contract issued by the Secretary of State for Health. This issue may be freely reproduced for the purposes of private research and study and extracts (or indeed, the full report) may be included in professional journals provided that suitable acknowledgement is made and the reproduction is not associated with any form of advertising. Applications for commercial reproduction should be addressed to: NIHR Journals Library, National Institute for Health Research, Evaluation, Trials and Studies Coordinating Centre, Alpha House, University of Southampton Science Park, Southampton SO16 7NS, UK.
Chapter 1 Introduction
Background and definition of the clinical problem
Heart failure (HF) is a complex syndrome in which the heart is unable to pump blood around the body at the right pressure. It affects around 500,000 people in the UK,1 most of whom are older, with an estimated prevalence of 6–10% in those aged > 65 years,2 increasing to 14% in those aged > 85 years. 3 The prevalence is expected to increase as a result of the ageing population and the improved survival of people with ischaemic heart disease. The prognosis of patients with HF is poor; up to 40% of newly diagnosed patients die within 1 year. 4,5 One of the reasons for the poor prognosis is that many patients are not treated in accordance with guidelines and do not receive the optimal doses of available medications. 6
Heart failure markedly impairs quality of life. HF signs and symptoms, which get progressively worse over time, include fluid retention, shortness of breath and fatigue, especially on exertion. 7 HF is one of the most costly conditions to manage in the NHS, accounting for 5% of all emergency medical admissions and consuming about 2% of the annual NHS budget. 8 Global estimates indicate that, annually, HF results in direct care costs of US$65B and lost productivity costs of US$43B owing to morbidity and premature mortality. 9 Health-care costs increase sharply at the end of life and are dominated by hospital care. 10 The most common causes of HF are ischaemic heart disease and high blood pressure. Other causes include congenital heart defects, genetic disease of the heart muscle, cardiac arrhythmia and alcohol misuse.
There are two main types of HF. HF caused by left ventricular systolic dysfunction (LVSD) occurs because the left ventricle of the heart becomes weak and does not contract properly. This type of HF is referred to as heart failure with a reduced ejection fraction (HFrEF). The other type of HF, referred to as heart failure with a preserved ejection fraction (HFpEF), is caused by the left ventricle becoming stiff, which makes it difficult for the heart chamber to fill with blood. Of patients with HF, just over half have predominantly HFrEF and just under half have predominantly HFpEF. However, there is no agreement on the cut-off point that defines low ejection fraction, and a range between < 35% and < 50% has been used in clinical trials to classify patients as having HFrEF or HFpEF. Patients with HFrEF and those with HFpEF have different demographic and clinical characteristics. Patients with HFpEF tend to be older, are more likely to be women and are more likely to have hypertensive heart disease, renal failure, anaemia, atrial fibrillation and obesity. Patients with HFrEF are more likely to have ischaemic heart disease, dilated cardiomyopathy and hyperlipidaemia. Rates of morbidity and mortality are similar in both groups.
Pharmacological treatment for HF is complex, and includes angiotensin-converting enzyme inhibitors (ACEis), angiotensin receptor blockers (ARBs), beta-blockers and mineralocorticoid receptor antagonists. These drugs are currently administered at doses defined by clinical trials. National and international guidelines recommend up-titration of these drugs to target (or maximally tolerated) doses, but this is difficult to achieve in practice given the number of drugs involved and the fact that the sequence of addition and up-titration is based largely on clinician judgement. However, many patients receive suboptimal treatment because some clinicians are reluctant to increase medication doses after the initial clinical improvement because they want to avoid potential side effects such as kidney failure and low blood pressure.
There are significant gaps and variation in medical care of HF patients in the UK. 11 Patients who are discharged from hospital following an acute HF episode are largely managed in primary care. Surveys have highlighted that general practitioners (GPs), cardiologists and HF nurses lack confidence in diagnosing and managing HF (particularly HFpEF), and awareness of the relevant evidence base for care and GPs’ personal preferences and organisational care pathways varies. 12,13
The health technology being assessed: B-type natriuretic peptide-guided therapy
Biomarkers such as natriuretic peptides [B-type natriuretic peptide (BNP) or its derivative N-terminal pro-B-type natriuretic peptide (NT-proBNP), collectively referred to here as BNP] have been used as a more objective means of assessing HF severity and to prompt the appropriate titration of HF therapies. BNP is a hormone secreted in the ventricular myocardium during periods of increased ventricular stretch and wall tension. BNP levels reflect cardiac function. BNP levels are raised in patients with HF, with concentrations rising in line with the severity of symptoms [New York Heart Association (NYHA) class]. BNP is, therefore, useful for ruling out HF14,15 and for risk stratification; for every 100 ng/l increase in BNP, there is a corresponding 35% relative increase in the risk of death. 16 BNP testing is recommended by the National Institute for Health and Care Excellence (NICE) as an essential part of the diagnostic pathway for HF, and it has been shown to be cost-effective for diagnosis in both primary and secondary care. 8
Treating HF with appropriate drugs leads to a reduction in BNP levels. 17,18 Therefore, the use of BNP test results to guide up-titration of medication has been proposed as an objective means of achieving optimal therapy in patients with HF. The NICE 2010 guidelines8 recommended monitoring with BNP for some groups of patients (e.g. those in whom up-titration is problematic and those who have been admitted to hospital). However, it is currently unknown whether or not any HF patient group in the UK receives serial BNP monitoring and, if they do, whether or not implementing serial BNP monitoring in practice has changed patient management and improved clinical outcomes.
Several randomised controlled trials (RCTs) have assessed whether or not the use of serial BNP tests to guide up-titration of medication improves clinical outcomes compared with symptom-guided therapy. The RCTs were heterogeneous in design. Most used a BNP-lowering strategy, for which a BNP target was set (a single target for all patients or an individualised target) and HF therapy was intensified to lower or maintain BNP at the prespecified target. Other RCTs have used a BNP-monitoring strategy, with the treating clinician being allowed to intensify therapy based on serial BNP results or if BNP increases by a certain proportion above a patient’s baseline value, but without setting a BNP target. Data from RCTs using a BNP-lowering strategy have been pooled in six aggregate data meta-analyses19–24 and one individual participant data (IPD) meta-analysis. 25 All of these analyses showed that health outcomes were better in patients in the BNP-lowering group than in patients in the symptom-guided therapy group.
There is uncertainty about the balance of benefit and harm of BNP-guided therapy in the broader spectrum of patients who make up the UK HF population (as opposed to the population included in RCTs). In elderly patients with multiple comorbidities, the risks of adverse outcomes from intensified therapy may outweigh any benefits. For example, up-titration of diuretics, ACEis and beta-blockers may worsen clinical outcomes in elderly patients by causing hypotension and aggravating renal failure.
Rationale for the study
The overall aim of this study was to evaluate the clinical effectiveness and cost-effectiveness of BNP-guided therapy compared with symptom-guided therapy (usual care) in patients with HF. The study included three components: a systematic review and meta-analysis of IPD and aggregate data; an analysis of a cohort of patients with HF that is geographically representative of patients with HF being managed in primary and secondary care in the UK; and a lifetime cost-effectiveness model to evaluate the cost per quality-adjusted life-year (QALY) gained by BNP-guided therapy versus symptom-guided therapy in patients with HF.
The systematic review and meta-analysis included all RCTs, regardless of BNP-monitoring strategy. This differs from all previous meta-analyses, which have focused only on trials that used a BNP-lowering strategy. The meta-analysis was conducted in accordance with the methods recommended by the IPD Meta-analysis Methods Group of Cochrane26 and other published guidelines. 27 We supplemented the systematic review and meta-analysis with a cohort study created by linking data from the Clinical Practice Research Datalink (CPRD), Hospital Episode Statistics (HES) and Office for National Statistics (ONS) mortality register. We were concerned that the RCTs included in meta-analyses did not represent the wider UK HF population. The RCTs had highly selected populations (younger patients; more men than women; patients with HFrEF, high baseline BNP levels and no significant comorbidities), none was conducted in the UK and all but one were conducted in secondary care settings. These features are not representative of the broader UK population with HF (medical outpatients or patients in primary care) or the context in which GPs and clinicians in the UK want to use BNP monitoring.
The NICE 2010 guidelines recommend further research on cost-effectiveness. 8 The evidence on the cost-effectiveness of BNP-guided therapy includes (1) economic evaluations conducted alongside RCTs evaluating cost-effectiveness within the follow-up period of the trials28,29 and (2) model-based analyses based on evidence from one30,31 or more32 RCTs, extrapolating costs and outcomes to the lifetime of patients. We conducted a model-based cost-effectiveness analysis of BNP-guided monitoring in recently hospitalised patients with HF. We aimed to extend previous economic evaluations in two ways. First, we exploited recent IPD meta-analyses,25,33 including the analyses presented in this report, in estimating the relative effect of BNP-guided therapy. Among the advantages of IPD meta-analysis is the opportunity to investigate the (cost-)effectiveness of BNP-guided therapy in subgroups of patients who are not analysed consistently or not reported in the original RCT publications. 34 Second, we used linked data from the CPRD, HES and ONS to inform key parameters of the model. In particular, we used these data to estimate the NHS costs of care for patients with HF who are stable and managed in primary care compared with the costs of those who are admitted to hospital.
Chapter 2 B-type natriuretic peptide-guided therapy for heart failure: systematic review and meta-analysis of individual participant data and aggregate data
Aims and objectives
The main aim of the meta-analysis was to determine the clinical effectiveness of BNP-guided therapy versus standard care. The specific objectives were to:
-
estimate the effect of BNP-guided therapy on clinical outcomes
-
estimate the extent of effect modification for key outcomes including all-cause mortality and hospital admission for clinically important subgroups
-
quantify the extent to which improved outcomes are explained by up-titration of medication and/or reduction in BNP levels
-
combine adverse event and discontinuation data to describe the safety of BNP-guided therapy in patients with HF.
Methods
The protocol for the systematic review and meta-analysis has been reported in detail elsewhere35 and is registered with the PROSPERO register of systematic reviews as CRD42013005335 (www.crd.york.ac.uk/PROSPERO/display_record.asp?ID=CRD42013005335; accessed 1 December 2016).
Study eligibility criteria
The meta-analysis comprised all RCTs of BNP-guided therapy for HF. The study population was all patients aged > 18 years who were being treated for HF in primary or secondary care and who received treatment guided by serial BNP or NT-proBNP measurements (BNP-guided therapy) or treatment guided by clinical assessment (symptom-guided therapy).
Outcomes
The outcomes of interest were all-cause mortality, death related to HF, cardiovascular death, all-cause hospital admission, hospital admission for HF, adverse events and quality of life.
Search methods for identification of studies
The search strategy is shown in Appendix 1. Published systematic reviews19–24 were initially used to identify relevant trials. The following electronic databases were searched: MEDLINE (via Ovid) from 1950 to 9 June 2016; EMBASE (via Ovid) from 1980 to week 23 2016; The Cochrane Library; and Web of Science (Citations Index and Conference Proceedings). The World Health Organization International Clinical Trials Registry Platform (http://apps.who.int/trialsearch/; accessed 1 December 2016) and Current Controlled Trials (www.controlled-trials.com; accessed 1 December 2016) were searched to identify trials in progress. Reference lists of all full-text papers were reviewed and other grey literature was checked (www.opengrey.eu; accessed 8 June 2016) to ensure that no unpublished study was missed.
Study selection
Two members of the review team independently triaged the titles and abstracts identified by the search. The remaining papers had clear inclusion criteria applied to them. Disagreements about study inclusion were resolved by discussion with a third review author. All trials excluded from the review were given reasons for exclusion. No language restriction was applied.
Establishing the individual participant data collaboration
Authors of eligible RCTs were invited to join the collaboration. Corresponding authors were sent the IPD meta-analysis protocol with a cover letter explaining the study. Other RCT investigators were contacted if a corresponding author failed to respond.
Quality assessment
Randomised controlled trials were assessed as having low, unclear or high risk of bias in accordance with recent Cochrane guidelines. 36 For blinding and incomplete outcome data, risk of bias was assessed separately for the prespecified outcome domains (all-cause mortality, cause-specific mortality, adverse events and quality of life). All-cause mortality was separated from cause-specific mortality because cause-specific mortality may have risk of bias depending on whether or not the person assigning the cause of death is blinded to the allocated intervention. RCT authors were asked to provide a study protocol, if available.
Two members of the review team independently assessed the risk of bias in each included RCT from all available information using the domain-based evaluation tool described in the Cochrane Handbook for Systematic Reviews of Interventions. 37 Disagreements were resolved by discussion with a third review author. All authors of relevant unpublished RCTs were contacted to request data.
Data collection
Individual participant data were sought from all included RCTs (see Appendix 2 for the list of variables requested) and collated into a single database. Data were requested for all randomised patients. The formal data dictionary for the data set (a table of information about the data elements) and data collection schedule (time points at which data were collected) were also requested. Detailed study information was collected using a standardised data collection form (see Appendix 3).
Data checking
All data sets were checked for consistency against the original publication reports and discrepancies were discussed and clarified with authors through e-mail communication. When clarification was not provided by the authors, assumptions were made regarding the data and these were documented.
Statistical analysis
All analyses (see the IPD meta-analysis statistical analysis plan, which is available from the authors) were performed on an intention-to-treat basis. The primary outcome of all-cause mortality, defined as the time from randomisation to death from any cause, was analysed by survival methods. A hazard ratio (HR) was estimated using Cox regression modelling for each RCT. The HRs were combined across RCTs using random-effects meta-analysis38 and heterogeneity was assessed using the I2 test statistic. A fixed-effects meta-analysis was also performed as a secondary analysis.
Subgroup effects were determined by estimating a treatment-by-covariate interaction term for each RCT and combining the HRs for the subgroup-specific HRs as for the main analysis. 39 Covariates defining subgroups were age (< 75 years vs. ≥ 75 years), sex, NYHA class (class I/II vs. class III/IV), type of HF [HFrEF vs. HFpEF, based on left ventricular ejection fraction (LVEF), < 40% in studies providing IPD and < 45% in studies providing aggregate data], diabetes status, BNP level (median or lower vs. higher than the median across all trial participants, with separate medians calculated for trials that reported BNP and NT-proBNP; cause of HF (ischaemic/non-ischaemic), previous atrial fibrillation, body mass index (BMI) and systolic blood pressure (SBP). The age cut-off point was chosen for consistency and to allow easy comparison with the meta-analysis by Troughton et al. 25 Participants in recent HF trials focusing specifically on the effect of therapies in the elderly [e.g. Irbesartan in Patients with Heart Failure and PRESERVEd Ejection Fraction (I-PRESERVE); Study of the Effects of Nebivolol Intervention on Outcomes and Rehospitalisation in Seniors with Heart Failure (SENIORS); Cardiac Insufficiency Bisoprolol Study in Elderly (CIBIS-ELD); Evaluation of Losartan in the Elderly Study (ELITE); Perindopril in Elderly People with Chronic Heart Failure (PEP-CHF)]40–44 had a mean age of 73–76 years, and it is generally accepted that patients above this age range represent elderly patients with HF. For the LVEF cut-off point, the clinicians on our team suggested that we use the lower limit of normal LVEF (40%) used in clinical practice. For RCTs that did not provide IPD, HR estimates from published reports (for both the main effects and subgroup effects) were combined with HR estimates derived from the IPD. For the subgroup analyses, the HRs and associated 95% confidence intervals (CIs) for the interaction effect were derived from the subgroup-specific HRs with 95% CIs. Stratum-specific treatment effects for all-cause mortality (age and type of HF) and HF-specific admission (type of HF) were available from a previous IPD meta-analysis33 that included seven RCTs. Interactions were calculated and pooled as an aggregate estimate with additional trials which had contributed IPD for this study. For age and sex, our analysis included an ‘aggregate’ interaction estimate from one RCT [Trial of Intensified versus Standard Medical Therapy in Elderly Patients with Congestive Heart Failure (Time-CHF); subgroup effects reported by the triallists] for outcomes not reported in the previous IPD.
All of the analyses were prespecified and carried out in accordance with the statistical analysis plan, except for the way in which interaction estimates from the previous IPD were combined to estimate some subgroups more precisely. The decision to include the estimates from the previous IPD meta-analysis prioritises greater precision of the revised estimates over exact adherence to our prespecified statistical analysis plan. Two RCTs contributed IPD to the study and were also included in the previous meta-analysis; for one, data to the end of follow-up were used for the overall analysis and data up to 90 days (used for the previous meta-analysis) were used for the previously reported subgroup effects.
The relationship between the size of the treatment effect and the change in BNP values was investigated by plotting the ratio of change in BNP values (in the BNP-guided therapy group compared with the symptom-guided therapy group) against the hazard rate for each study with data available. The ratio of change in BNP values was calculated as:
For the three studies providing IPD, the ratio of change was also calculated using the patient-specific change from baseline; after logarithmic transformation of all BNP values, the median change from baseline was calculated in each treatment group and the ratio of the exponents of medians was calculated. All analyses were conducted using Stata® version 14.0 (StataCorp LP, College Station, TX, USA), using the ‘ipdmetan’ command. 45
Inclusion of aggregate data
For RCTs that did not provide IPD, aggregate data were included. Estimates of HRs from reports of studies not providing IPD46 were combined with estimated HRs derived from the IPD.
Sensitivity analysis
The following sensitivity analyses (SAs) were conducted: restricting the analysis to RCTs that defined a BNP target and restricting the analysis to trials with good allocation concealment, as this has been shown to be an important source of bias in RCTs. 47,48 A SA including only RCTs that had a low risk of bias across all domains was planned; however, no RCT met this condition and therefore these analyses were not carried out.
Checking for publication and data availability bias
Funnel plots were used to investigate association between the precision of the effect size and effect size (which could be because of publication bias or ‘small study effects’),49 including and excluding RCTs for which IPD were unavailable.
Changes to the study from the protocol stage
A major difficulty we faced when establishing the IPD collaboration was that an IPD meta-analysis of BNP-guided therapy had already been published in abstract form by Troughton et al. 50 We sought to establish a collaboration with Professor Troughton and colleagues over a period of 15 months. We initially asked for the data for the RCTs included in their meta-analysis, allowing us to carry out the analyses; then we proposed that they should do the analyses to our analysis plan; tried to reach a compromise over the proposed analyses (with them doing the analyses); and asked for the pooled estimates from subgroup analyses that had already been carried out. 51 None of these proposals was successful and, therefore, we were unable to obtain IPD, or the results of subgroup analyses, from most RCTs included in the IPD meta-analysis by Troughton et al. 25,33 Consequently, we were unable to perform the subgroup analyses specified in the protocol. 35
Other changes to the study from the protocol stage include:
-
A meta-analysis on cause-specific mortality outcomes was not conducted, as there were only two studies (IPD) with data on cause of death.
-
Meta-analyses on adverse event and quality of life were not conducted because no IPD studies provided adverse event data and only one provided quality-of-life data.
-
The following subgroup analyses were specified but could not be performed because the data were not available from most studies: previous atrial fibrillation (there were only two RCTs with data, The Strategies for Tailoring Advanced Heart Failure Regimens in the Outpatient Setting (STARBRITE) trial52 and Anguita et al. 53); BMI [there were only two RCTs with data, Use of PeptideS in Tailoring hEart failure Project (UPSTEP)54 and NorthStar55 (the STARBRITE trial52 also provided BMI but had many missing data)]; SBP (there were only two RCTs with data, NorthStar55 and STARBRITE;52 Shochat et al. 56 also provided SBP but had many missing data); cause of HF (ischaemic/non-ischaemic, there were only two RCTs with data, STARBRITE52 and NorthStar;55 Anguita et al. 53 also provided cause of HF data but correspondence suggested that some of these data were not accurate).
Results
Figure 1 shows the flow of studies through the review process. The literature search identified 2502 abstracts, which were screened for eligibility, 70 of which were screened as full-text articles. There were 23 RCTs eligible for inclusion (one was translated from Spanish):
-
Nineteen RCTs involved long-term BNP monitoring in patients with stable HF, defined as monitoring extended beyond the index hospital admission when a participant was recruited (most RCTs recruited patients following an acute admission to hospital and stabilisation). Five studies provided IPD. Of the 14 RCTs that did not provide IPD, eight provided estimates of HRs for one or more of the outcomes of interest in the published report (or these were available from the analyses of Troughton et al. 25,33), two had not finished recruiting at the time of writing this report and four had not published the results in full so aggregate data were not available. In total, data from 13 RCTs involving long-term BNP monitoring were used in the analysis.
-
Four RCTs involved short-term BNP monitoring, defined as monitoring in hospital during the index admission (in patients hospitalised for an acute HF episode). Only one RCT provided IPD. The remaining three RCTs had been published only as abstracts and estimates of HRs for the outcomes of interest were not available. Therefore, a meta-analysis of RCTs evaluating short-term monitoring could not be carried out.
FIGURE 1.
Preferred Reporting Items for Systematic Reviews and Meta-Analyses (PRISMA) flow diagram. One eligible study,57 published in abstract form in 2015, was identified at a late stage (June 2016) and therefore could not be included.

Study characteristics
Table 1 shows the characteristics of the long-term BNP monitoring RCTs eligible for inclusion in the meta-analysis. Of the 13 studies included in the meta-analysis, eight were conducted in Europe (none in the UK),53–55,59,61–63,65 two were conducted in New Zealand,58,64 two were conducted in North America52,66 and one was conducted in Israel. 56 Only one RCT63 [Swedish Intervention study – Guidelines and NT-proBNP AnaLysis in Heart Failure (SIGNAL-HF)] was conducted in primary care; 12 were conducted in hospital HF clinics, with most of these recruiting patients during or straight after hospitalisation for HF. Two different types of BNP-guided therapy were identified.
-
BNP-lowering strategy: a BNP target (single target or individual target) was set and HF therapy was intensified to lower BNP to the prespecified target.
-
BNP-monitoring strategy: a BNP target was not set; the treating clinician was allowed to intensify therapy using serial BNP information or when BNP increased by a certain proportion from a patient’s baseline value, that is, value at randomisation or previous visit.
Study | Country | Study period | Setting | Duration of follow-up | Follow-up schedule | BNP/NT-proBNP target | Clinical target | Primary end point | Treatment algorithm |
---|---|---|---|---|---|---|---|---|---|
Studies that provided IPD | |||||||||
Anguita et al.53 | Spain | 2006–8 | HF clinic | 18 months | 1, 2, 3, 6, 12 and 18 months | BNP level < 100 pg/ml | Framingham HF score of < 2 | Composite of all-cause mortality or cardiovascular hospital admission | BNP group: therapy intensified to achieve target BNP. Control group: therapy intensified to achieve target congestion score |
NorthStar55 | Denmark | 2005–9 | HF clinic | 2.5 years | Every 1–3 months at the discretion of the investigator | No set target | Clinical assessment | Composite of all-cause mortality or cardiovascular hospital admission | BNP group: checklist to evaluate need for further investigation or intensification of therapy when NT-proBNP was > 30% from randomisation visit. Control group: therapy evaluated and intensified at clinician discretion |
Shochat et al.56 (published as abstract only) | Israel | 2007–10 | HF clinic | Median 11 months (IQR 3–22) | Every 1–2 months | No set target | Not known | All-cause mortality | BNP group: therapy intensified if NT-proBNP was higher by > 30% from previous clinic visit. Control group: not stated in abstract |
STARBRITE trial52 | USA | 2003–5 | HF clinic | 4 months | Week 1 and then 1, 2, 3 and 4 months | Individual BNP at discharge | Individual congestion score | Number of days alive and not hospitalised during the 90 days after the first clinic visit | BNP group: therapy intensified if BNP levels were two times greater than or less than the target BNP. Control group: therapy intensified to achieve target congestion score |
UPSTEP54 | Sweden and Norway | 2006–9 | HF clinic | ≥ 12 months | Weeks 2, 6, 10, 16, 24, 36 and 48 and then every 6 months | < 75 years: BNP level < 150 pg/ml; ≥ 75 years: BNP level < 300 pg/ml | Clinical assessment | Composite of all-cause mortality or hospitalisation or worsening HF | BNP group: therapy intensified according to stepwise algorithm to achieve maximally tolerated or guideline-recommended target doses. Control group: therapy intensified at clinician discretion |
Studies that provided aggregate data | |||||||||
Christchurch pilot58 | New Zealand | 1998–9 | HF clinic | 9.5 months | Every 3 months unless treatment targets not met | NT-proBNP level < 1700 pg/ml | Framingham HF score of < 2 | Total cardiovascular events (mortality, hospital admission, new HF-related outpatient episode) | BNP group: therapy intensified according to stepwise algorithm to achieve target NT-proBNP. Control group: therapy intensified according to stepwise algorithm to achieve target HF score |
Time-CHF59,60 (HFrEF and HFpEF) | Switzerland and Germany | 2003–6 | HF clinic | 18 months | 1, 3, 6, 12 and 18 months | NT-proBNP less than two times upper limit of normal: (< 400 pg/ml for patients < 75 years; < 800 pg/ml for patients ≥ 75 years) | NYHA class ≤ II | Hospital-free survival | BNP group: therapy intensified according to stepwise algorithm to achieve target NT-proBNP. Control group: therapy intensified according to stepwise algorithm to achieve NYHA ≤ II |
Berger et al.61 | Austria | 2003–4 | HF clinic | 15 months | Every 2 weeks until medical therapy optimised or maximum doses prescribed; then scheduled visits at 1, 3, 6 and 12 months | NT-proBNP < 2200 pg/l | Clinical assessment | Composite of all-cause mortality or HF hospitalisation | BNP group: therapy intensified according to set protocol to maintain target NT-proBNP. Control group: therapy intensified at clinician discretion |
PRIMA62 | Netherlands | 2004–7 | HF clinic | 24 months | 2 weeks, 1 month, then 3-monthly for 2 years | Individual NT-proBNP level (lowest level at discharge or at 2 weeks’ follow-up) | Clinical assessment | Number of days alive outside the hospital after index admission | BNP group: therapy intensified according to clinical guidelines to maintain target NT-proBNP. Control group: therapy intensified at clinician discretion |
SIGNAL-HF63 | Sweden | 2006–9 | Primary care | 9 months | 1, 3, 6 and 9 months | Individual NT-proBNP level (reduction of 50% from baseline) | Clinical assessment | Described as ‘a composite end point of days alive, days out of hospital [for cardiovascular (CV) reasons], and symptom score from the Kansas City Cardiomyopathy Questionnaire (KCCQ).’ Reported as composite: time to cardiovascular death or cardiovascular hospitalisation | BNP group: stepwise algorithm to increase therapy to achieve target NT-proBNP. Control group: therapy intensified at clinician discretion |
BATTLESCARRED64 | New Zealand | 2001–6 | HF clinic | 3 years | Every 2 weeks, until treatment target met, then every 3 months | NT-proBNP < 1300 pg/ml | Framingham HF score of < 2 | All-cause mortality | BNP group: therapy intensified according to stepwise algorithm to achieve target NT-proBNP and congestion score of < 2. Control group: therapy intensified to achieve target congestion score of < 2 |
STARS-BNP65 | France | Not stated | HF clinic | 15 months | Months 1, 2 and 3 and then every 3 months | BNP level < 100 pg/ml | Clinical assessment | Composite of HF mortality or HF hospitalisation | BNP group: therapy intensified according to clinical guidelines to maintain target NT-proBNP. Control group: therapy intensified at clinician discretion |
PROTECT66 | USA | 2006–10 | HF clinic | At least 6 months | As required to meet treatment target and then every 3 months | NT-proBNP ≤ 1000 pg/ml | Clinical assessment | Composite of worsening HF or HF hospitalisation or cardiovascular events | BNP group: therapy intensified according to clinical guidelines to maintain target NT-proBNP. Control group: therapy intensified at clinician discretion |
Eligible studies that did not provide IPD or aggregate data | |||||||||
Karavidas et al.67 (published as abstract only) | Greece | Not stated | Not stated | 12 months | Not stated | Not stated but probably no set target | Clinical assessment | Not clear. Composite of all-cause mortality cardiovascular hospitalisation? | Not stated |
aHOME (clinical trial registration only) | Ireland, UK, Australia and Canada | 2011–14 | Not stated | 6 months | 1, 3 and 6 months | Not stated but probably no set target | Not stated | Average number of ‘hard’ events per subject (HF mortality, hospitalisation for HF, unplanned outpatient episodes for decompensated HF (including change in diuretic therapy) | BNP group: therapy intensified at clinician discretion using BNP information. Control group: as above but without the BNP information |
OPTIMA68 (published as poster only) | Czech Republic | Not stated | Not stated | Not stated | Not stated | Not stated but probably a BNP-lowering strategy | Clinical assessment | Composite of cardiovascular mortality or HF hospitalisation or outpatient episodes of worsening HF requiring an increase in diuretic by at least 50% | BNP group: therapy intensified to ‘normalise’ plasma BNP levels. Control group: therapy intensified at clinician discretion in accordance with guidelines |
bKoshkina et al.57 (published as abstract only) | Russian Federation | Not stated | HF clinic | Mean (SD) 10 ± 2.5 months | Not stated | NT-proBNP < 1000 pg/ml or at least 50% of the initial | Clinical assessment | Total cardiovascular events | Not stated |
cEX IMPROVE CHF study (study ongoing) | Canada | 2007–ongoing | HF clinic | Minimum 12 months | Not stated | No set target | Clinical assessment | Composite of all-cause mortality or HF hospitalisation | BNP group: therapy intensified at clinician discretion using BNP information. Control group: As above but without the BNP information |
dGUIDE-IT (study terminated earlye) | USA | 2012–17 | HF clinic | 12 months | Initially every 2 weeks (until optimal therapy achieved) and then every 3 months | NT-proBNP < 1000 pg/ml | Clinical assessment | Composite of cardiovascular mortality or HF hospitalisation | BNP group: therapy intensified according to stepwise algorithm to achieve target NT-proBNP. Control group: therapy intensified according to stepwise algorithm in accordance with clinical guidelines |
Eleven RCTs used a BNP-lowering strategy52–54,58,59,61–66 and two used a BNP-monitoring strategy. 55,56 Of the 11 RCTs that used a BNP-lowering strategy, eight set a single target (BNP 100–300 pg/ml; NT-proBNP 400–2200 pg/ml),53,54,58,59,61,64–66 two of which stratified by age (< 75 years and ≥ 75 years),54,59 and three set an individual BNP target (BNP level at discharge, reduction of 50% from baseline). 52,62,63 Treatment algorithms in the BNP group differed slightly between studies but all were based on stepwise titration of therapy according to clinical guidelines. In the two RCTs that used a BNP-monitoring strategy, clinicians intensified treatment if BNP increased by > 30% from randomisation visit (NorthStar55) or previous clinic visit (Shochat et al. 56). In the control group, five studies52,53,58,59,64 used an algorithm designed to achieve a target HF score based on signs and symptoms (e.g. Framingham HF score and NYHA class), and in six studies61–63,65,66,69 therapy was entirely at the clinician’s discretion.
Risk of bias in included studies
Figure 2 and Table 2 show risk of bias for the included RCTs by risk of bias and outcome domains. None of the RCTs had a low risk of bias across all domains. Ten out of 13 RCTs (77%)52–55,59,61–63,65,66 were rated as having a high risk of bias in at least one domain. Three out of 13 RCTs (23%)56,58,64 were rated as having unclear risk of bias; one of these studies56 provided IPD but no study protocol and was published as an abstract only, so its risk of bias could not be fully assessed. The main factor that contributed to having a high risk of bias was the lack of blinding (of participants and care-giving clinicians), which could lead to differential departure from the intended intervention (performance bias). For some outcomes, outcome assessors were blinded. For risk of bias from incomplete outcome data and selective outcome reporting, we assessed only RCTs that had contributed aggregate data.
FIGURE 2.
Risk-of-bias graph. Review authors’ judgements about each risk-of-bias item presented as a percentage for all included studies by outcome domain. Of the included studies, only 8 out of 13 RCTs (the RCTs that contributed aggregate data) were assessed for risk of bias from incomplete outcome data and selective outcome reporting. All 13 RCTs were assessed for other risk-of-bias domains.

Study | Selection bias | Performance bias | Detection bias | Attrition bias | Reporting bias | Other bias | ||||||||||
---|---|---|---|---|---|---|---|---|---|---|---|---|---|---|---|---|
Blinding of participants and personnel | Blinding of outcome assessment | Incomplete outcome dataa | Selective reportinga | Other sources of bias | ||||||||||||
Random sequence generation | Allocation concealment | All-cause mortality | Cause-specific mortalityb | Harmsc | Quality of life | All-cause mortality | Cause-specific mortalityb | Harmsc | Quality of life | All-cause mortality | Cause-specific mortalityb | Harmsc | Quality of life | |||
Anguita et al.53 | Unclear | Unclear | High | High | High | Unclear | Low | High | High | Unclear | – | – | – | – | – | Low |
NorthStar55 | Low | Unclear | High | High | High | High | Low | Low | Low | High | – | – | – | – | – | Unclear |
Shochat et al.56 (published as abstract only) | Unclear | Unclear | Unclear | Unclear | Unclear | Unclear | Low | Unclear | Unclear | Unclear | – | – | – | – | – | Unclear |
STARBRITE trial52 | Low | Low | High | Unclear | High | Unclear | Low | Unclear | High | Unclear | – | – | – | – | – | Low |
UPSTEP54 | Low | Unclear | High | High | High | Unclear | Low | Low | Low | Unclear | – | – | – | – | – | Unclear |
Christchurch pilot58 | Unclear | Unclear | Low | Unclear | Low | Low | Low | Unclear | Low | Low | Low | Unclear | Low | Unclear | Unclear | Low |
Time-CHF59,60 | Low | Low | High | High | High | Low | Low | Low | Low | Low | Low | Low | Low | Unclear | Low | Low |
Berger et al.61 | Low | Low | High | Unclear | High | Unclear | Low | Unclear | Low | Unclear | Unclear | Unclear | Unclear | Unclear | Unclear | Low |
PRIMA62 | Unclear | Unclear | High | High | High | Unclear | Low | Low | Low | High | Low | Low | Low | Unclear | Low | Low |
SIGNAL-HF63 | Unclear | Unclear | High | High | High | Unclear | Low | High | High | Unclear | Low | Low | Low | Unclear | High | Unclear |
BATTLESCARRED64 | Low | Unclear | Low | Unclear | Low | Low | Low | Unclear | Low | Low | Unclear | Unclear | Unclear | Unclear | Low | Low |
STARS-BNP65 | Unclear | Unclear | High | High | High | Unclear | Low | Unclear | Low | Unclear | Low | Low | Low | Unclear | Unclear | Unclear |
PROTECT66 | Low | Unclear | High | High | High | High | Low | Low | Low | High | Low | Low | Low | Low | Low | Unclear |
Participant characteristics
Patient characteristics are shown in Table 3. In the IPD data set, the average age of participants was 70 years; over one-third were aged ≥ 75 years. Three-quarters of patients were men. Most patients had LVSD (median LVEF, 30%); only 8% of patients had LVEF > 40%. The majority of patients (> 80%) had NYHA class II or III. The patients in studies providing aggregate data had similar characteristics.
Study | Number of patients (BNP/clinical target) | Age (years), mean (SD) | Patients ≥ 75 years, n (%) | Male, n/N (%) | LVEF (%), median (IQR)a | LVEF ≥ 40%b, n (%) | NYHA class I/II/III/IV | Smoking status (non-smoker/ex-smoker/current smoker) | BMI, kg/m2 (mean, SD) | SBP, mmHg (mean, SD) | DBP, mmHg (mean, SD) |
---|---|---|---|---|---|---|---|---|---|---|---|
Studies that provided IPD | |||||||||||
Anguita et al.53 | 60 (30/30) | 69 (10) | 18/54 (33) | 41/60 (68) | 40 (26–65) | 27/55 (49) | 3/38/19/0 | 37/7/16 | – | – | – |
NorthStar55 | 407 (199/208) | 73 (8) | 186/407(46) | 309/407 (76) | 30 (25–35) | 36/402 (9) | 80/268/59/0 | 47/114/110 | 26 (5) | 127 (19) | 73 (12) |
Shochat et al.56 | 120 (60/60) | 70 (11) | 50/120 (42) | 103/120 (86) | 30 (25–35) | 11/75 (15) | 1/55/41/16 | 34/0/40 | – | 125 (21) | 74 (11) |
STARBRITE trial52 | 130 (65/65) | 60 (15) | 24/130 (18) | 91/130 (70) | 20 (15–25) | 0/129 (0) | Not given | – | 29 (8) | 111 (21) | 69 (13) |
UPSTEP54 | 268 (140/128) | 71 (10) | 105/268 (39) | 196/268 (73) | – | 0/268 (0) | 0/83/147/37 | – | 27 (5) | – | – |
All | 985 (494/491) | 70 (11) | 383/979 (39) | 740/985 (75) | 30 (20–35) | 74/929 (8) | 84/444/266/53 | 118/121/166 | 27 (5) | 124 (21) | 73 (12) |
Studies that provided aggregate datac | |||||||||||
Christchurch pilot58 | 69 (33/36) | 70 (10) | 24/69 (35) | 53/69 (7) | 27 (8) | 0/69 (0) | ≈70% in NYHA class II | – | – | 127 (SD not provided) | 76 (SD not provided) |
Time-CHF59 (HFrEF) | 499 (251/248) | 76 (8) | 289/499 (58) | 327/499 (66) | 30 (8) | 0/499 (0) | NYHA class ≥ III: 371/499 (74%) | – | 25 (4) | 119 (19) | – |
Time-CHF60 (HFpEF) | 123 (59/64) | BNP group: 80.3 (6.8). CG: 79.9 (7.2) | Not given | 42/123 (34) | BNP group: 56 (6). CG: 56 (7) | Not given | –/21/82/20 | Not given | BNP group: 26.0 (4.9). CG: 27.4 (5.8) | BNP group: 135 (21). CG: 137 (24) | BNP group: 73 (11). CG: 75 (13) |
Berger et al.61 | 188 (92/96) | 71 (12) | 88/188 (47) | 147/188 (78) | 29 (9) | 11/188 (6) | All patients NYHA class III or IV | – | – | 121 (18) | 72 (12) |
PRIMA62 | 345 (174/171) | 72 (12) | 166/345 (48) | 197/345 (57) | 36 (14) | 93/345 (27) | 37/234/74 | 166/105/74 | – | 118 (21) | 69 (11) |
SIGNAL-HF63 | 252 (127/125) | 78 (7) | 184/252 (73) | 180/252 (71) | 32 (8) | 5/252 (2) | 0/154/96/0 | – | – | 134 (22) | 74 (12) |
BATTLESCARRED64 | 242 (121/121) | 74 (9) | 138/242 (57) | 157/242 (65) | 39 (15) | 90/242 (37) | 24/162/52/4 | – | – | 124 (23) | 71 (13) |
STARS-BNP65 | 220 (110/110) | 66 (5) | – | 127/220 (58) | 31 (8) | – | – | 101/220d | – | – | – |
PROTECT66 | 151 (75/76) | 63 (14) | 38/151 (25) | 127/151 (84) | 27 (9) | 0/151 (0) | NYHA class II or III: 129/151 (85%) | 92/48/11 | 29 (6) | 110 (16) | 66 (9) |
Table 4 shows the baseline concentrations of NT-proBNP, BNP and other biomarkers for patients in included studies. The severity of HF at baseline (as indicated by BNP or NT-proBNP levels) differed between studies: one study recruited patients with mild HF (Anguita et al. ,53 BNP 100 pg/ml) while other studies recruited patients with more severe HF (BNP> 400 pg/ml or NT-proBNP > 2000 pg/ml, e.g. STARBRITE52 and UPSTEP54). Table 5 shows the comorbidities of patients in the included studies. Among the studies providing IPD, about half of all patients had hypertension and a previous myocardial infarction (MI) and just over one-third were diabetic. The proportions of patients with these comorbidities were similar in studies providing aggregate data.
Study | NT-proBNP (pg/ml), median (IQR) | BNP (pg/ml), median (IQR) | Creatinine (mg/dl), median | Sodium (mmol/l), mean (SD) | Potassium (mmol/l), mean (SD) | Haemoglobin (g/dl), mean (SD) |
---|---|---|---|---|---|---|
Studies that provided IPD | ||||||
Anguita et al.53 | – | 24 (6–92) | 1.1 (1.0–1.5 IQR) | 138 (5) | 4.2 (0.5) | 13.3 (1.7) |
NorthStar55 | 1955 (1387–3270) | – | 1.2 (1.0–1.4 IQR) | 139 (4) | 4.3 (0.4) | 13.4 (1.6) |
Shochat et al.56 | 1686 (918–4766) | – | 1.2 (0.9–1.5 IQR) | – | – | 13.2 (1.8) |
STARBRITE trial52 | – | 446 (191–1030) | 1.4 (1.1–1.8 IQR) | 136 (4) | 4.3 (0.5) | 12.7 (2.4) |
UPSTEP54 | – | 609 (356–947) | 1.1 (0.9–1.4 IQR) | – | – | – |
Studies that provided aggregate dataa | ||||||
Christchurch pilot58 | 1980 (1077–2806) | – | – | – | – | – |
Time-CHF59 (HFrEF) | 4194 (2270–7414) | – | 1.3 (0.4 SD) | – | – | – |
Time-CHF60 (HFpEF) | 2210 (1514–4513) | – | 1.2 (0.4 SD) | – | – | 12.2 (1.9) |
Berger et al.61 | 2280 (1255–5192) | – | 16% of patients with creatinine of > 2 mg/dl | – | – | – |
PRIMA62 | 2949 (1318–5445) | – | Not clear from report | 139 (4) | 4.3 (0.5) | 13.7 (2.1) |
SIGNAL-HF63 | 2362 (1372–4039) | – | 1.2 (0.4 SD) | – | – | – |
BATTLESCARRED64 | 2001 (1235–2974) | – | 1.4 (0.5 SD) | – | – | – |
STARS-BNP65 | – | – | 1.1 (0.5 SD) | – | – | – |
PROTECT66 | 2118 (1121–3831) | – | 1.5 (0.5 SD) | 138 (3) | 4.3 (0.4) | – |
Study | Comorbidity | |||||||
---|---|---|---|---|---|---|---|---|
Diabetes | Hypertension | Stroke | COPD | MI | PCI or CABG | Angina | AF | |
Studies providing IPD | ||||||||
Anguita et al.53 | 28/60 (47) | 43/60 (72) | – | – | – | – | 12/60 (20) | 25/60 (42) |
NorthStar55 | 88/407 (22) | 173/400 (43) | 61/407 (15) | 64/407(16) | 201/407 (49) | 167/407 (41) | 29/406 (7) | – |
Shochat et al.56 | 52/89 (58) | 68/89 (76) | – | – | 51/78 (65) | – | – | 19/21 (91) |
STARBRITE trial52 | 54/125 (43) | 83/126 (66) | – | 22/124 (18) | – | – | – | 47/125 (38) |
UPSTEP54 | 85/268 (32) | 116/268 (43) | – | – | – | – | – | – |
Across all studies | 307/949 (32) | 483/943 (51) | 61/407 (15) | 86/531 (16) | 252/485 (52) | 167/407 (41) | 41/466 (9) | 91/206 (44) |
Studies providing aggregate dataa | ||||||||
Christchurch pilot58 | 9/69 (13) | 45/69 (65) | – | – | 21/69 (30) | CABG only: 15/69 (22) | – | – |
Time-CHF59 (HFrEF) | 172/499 (34) | 354/499 (71) | 76/499 (15) | 104/499 (21) | – | – | – | 160/499 (32) |
Time-CHF60 (HFpEF) | 50/123 (41) | 107/123 (87) | Stroke/transient ischaemic attack reported 22/123 (18) | 19/123 (15) | – | – | – | 52/123 (42) |
Berger et al.61 | 86/188 (46) | 126/188 (67) | 21/188 (11) | 30/188 (16) | 86/188 (46) | – | – | 60/188 (32) |
PRIMA62 | 91/345 (26) | 167/345 (48) | 35/345 (10) | 59/345 (17) | 139/345 (40) | PCI: 44/345 (13). CABG: 61/345 (18) | – | Chronic: 58/345 (17); paroxysmal: 54/345 (16) |
SIGNAL-HF63 | 50/250 (20) | 137/250 (55) | – | 27/250 (11) | 112/250 (45) | – | – | 137/250 (55) |
BATTLESCARRED64 | 52/242 (21) | 118/242 (49) | 52/242 (21) | 52/242 (21) | 108/242 (45) | – | – | – |
STARS-BNP65 | 39/220 (18) | 66/220 (30) | – | – | – | – | – | – |
PROTECT66 | 62/151 (41) | 79/151 (52) | – | 31/151 (21) | 58/151 (38) | – | – | 61/151 (40) |
Table 6 summarises the studies that were included in the meta-analysis for each outcome.
Study | Mortality (all cause) | Hospitalisation (all cause) | Hospitalisation for HF |
---|---|---|---|
Studies providing IPD | |||
Anguita et al.53 | ✓ | ✓ | ✓ |
NorthStar55 | ✓ | ✓ | ✓ |
Shochat et al.56 | ✓ | ✓ | ✓ |
STARBRITE trial52 | ✓ | ✓ | |
UPSTEP54 | ✓ | ✓ | ✓ |
Studies providing aggregate data | |||
Christchurch pilot58 | ✓ | ✓ | |
Time-CHF59,60 | ✓ | ✓ | ✓ |
Berger et al.61 | ✓ | ✓ | |
PRIMA62 | ✓ | ✓ | |
SIGNAL-HF63 | ✓ | ✓ | |
BATTLESCARRED64 | ✓ | ✓ | |
STARS-BNP65 | ✓ | ✓ | |
PROTECT66 | ✓ |
Primary outcome
Across all RCTs, 254 out of 1399 patients (18%) in the BNP groups and 290 out of 1399 patients (21%) in the control groups died during follow-up. Median follow-up in the five RCTs52–56 that provided IPD was 18 months [interquartile range (IQR) 8–27 months]. There was a small reduction in the hazard of death from any cause in the BNP-guided therapy group compared with the symptom-guided therapy group (HR 0.87, 95% CI 0.73 to 1.04) (Figure 3). There was no significant heterogeneity between RCTs (see Figure 3). The SA excluding the two RCTs that did not use a BNP-lowering strategy did not substantially alter this finding (HR 0.86, 95% CI 0.71 to 1.04).
FIGURE 3.
All-cause mortality: unadjusted individual HRs with 95% CIs for five studies providing IPD and seven studies providing aggregate data. Meta-analysis HR (95% CI) presented both within IPD and aggregate data sources and overall. Time-CHF reported results separately for patients with HFrEF59 and patients with HFpEF. 60 HR for all-cause mortality was not available for the PROTECT study. 66
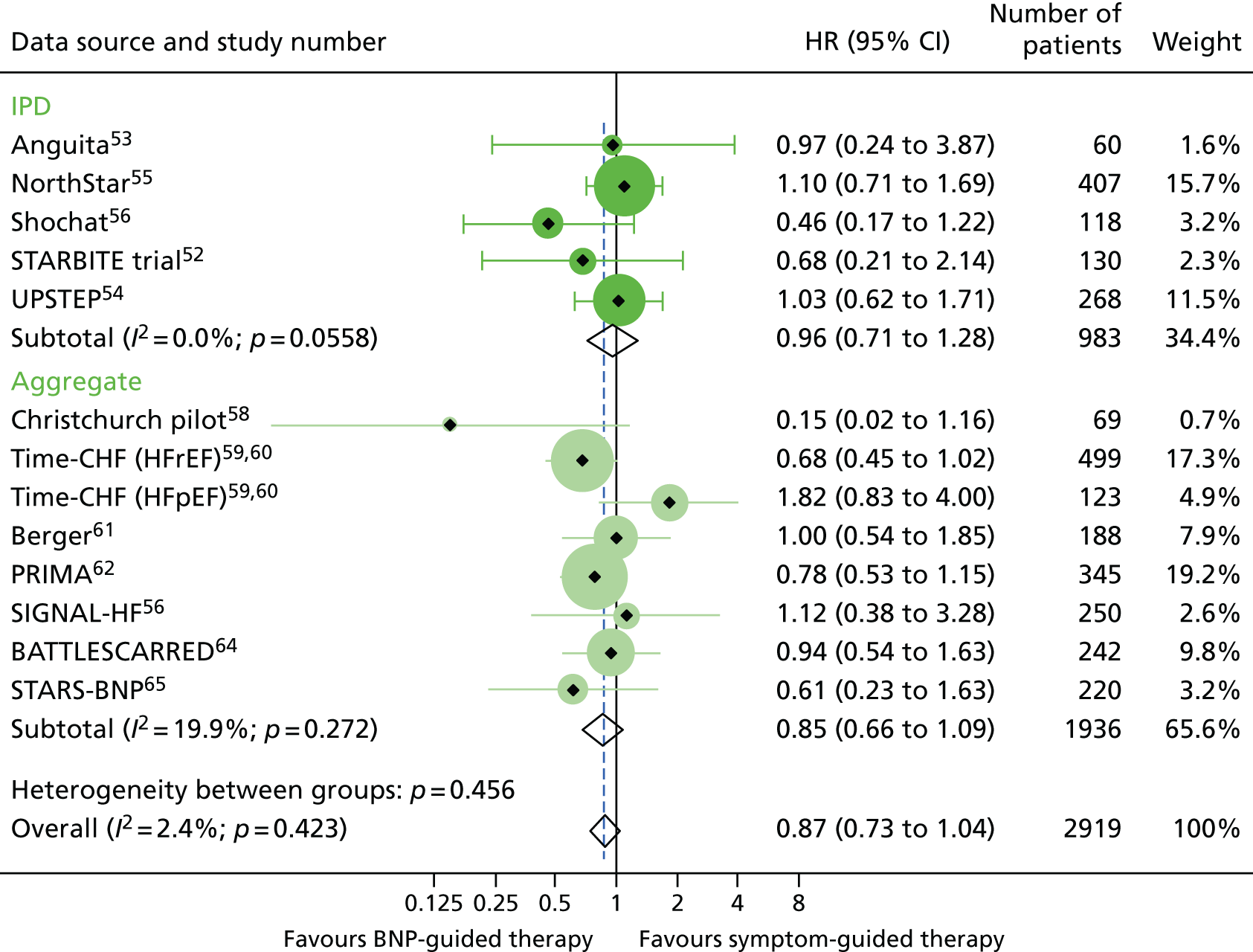
Only three RCTs52,59–61 were found to have good allocation concealment. The SA combining the effect estimates from these three RCTs showed no difference in the hazard of death between groups (HR 0.93, 95% CI 0.60 to 1.44). In all meta-analyses, the results from the fixed-effects meta-analyses did not differ from the reported results for the random-effects meta-analyses.
Secondary outcomes
Across six RCTs52–56,59,60 with data on all-cause hospitalisation, a total of 285 out of 493 patients (58%) in the BNP group had at least one hospital admission, compared with 281 out of 491 patients (57%) in the control group. BNP-guided therapy did not reduce the hazard of hospitalisation from any cause (HR 0.97, 95% CI 0.85 to 1.10) (Figure 4). The results did not differ in the analysis restricted to studies that used a BNP-lowering strategy (HR 0.95, 95% CI 0.81 to 1.11). Of the three studies with good allocation concealment, only two52,59,60 provided data on all-cause hospitalisations and the SA was not performed.
FIGURE 4.
All-cause hospitalisation: unadjusted individual HRs with 95% CIs for five studies providing IPD and one study providing aggregate data. Meta-analysis HR (95% CI) presented both within IPD and aggregate data sources and overall. HR for all-cause hospitalisation was available only for TIME-CHF: HFrEF59 and HFpEF60; no HR could be obtained for the remaining studies that did not provide IPD.
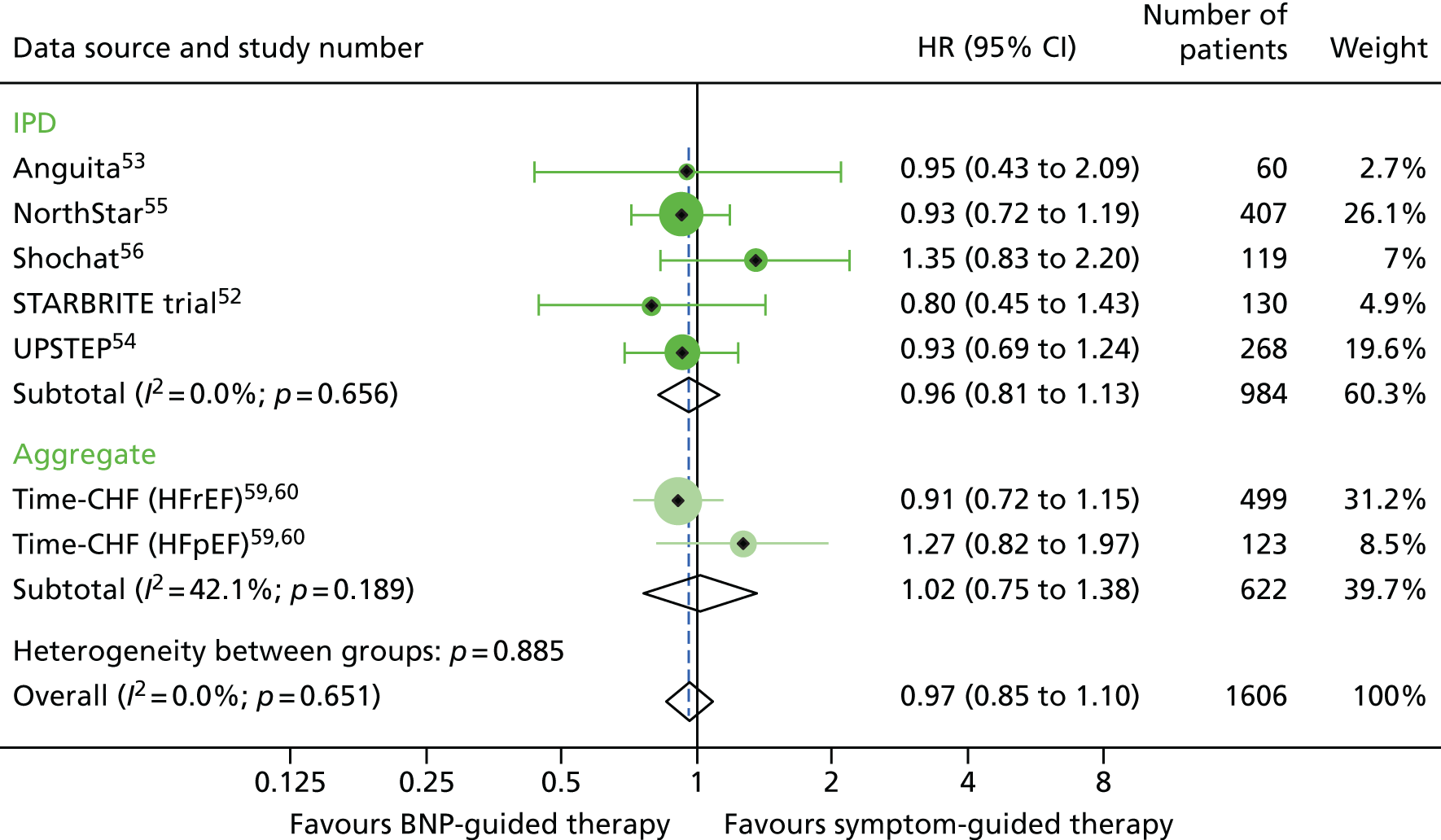
Data on the number of patients with HF-specific hospitalisations were available from only three RCTs providing aggregate data (Time-CHF,59,60 Berger et al. 61 and STARS-BNP65) and four RCTs providing IPD. 53–56 Across these studies, 245 out of 882 patients in the BNP groups (28%) were hospitalised for HF at least once, compared with 311 out of 879 patients (35%) in the symptom-guided therapy groups. In a meta-analysis of the effect of BNP-guided therapy on the hazard of hospitalisation as a result of HF, BNP-guided therapy reduced the hazard (four RCTs provided IPD53–56 and eight provided aggregate data;58–66 HR 0.78, 95% CI 0.65 to 0.95) (Figure 5). The results did not differ in the SA restricted to studies that used a BNP-lowering strategy (HR 0.76, 95% CI 0.60 to 0.96). The SA with respect to allocation concealment was again not performed because there were only two RCTs59–61 classified as having a low risk of bias. No data were available for the other prespecified secondary outcomes (death related to HF, cardiovascular death, adverse events and quality of life).
FIGURE 5.
Heart failure hospitalisation: unadjusted individual HRs with 95% CIs for five studies providing IPD and eight studies providing aggregate data. Meta-analysis HR (95% CI) presented both within IPD and aggregate data sources and overall.

In all meta-analyses, the results from the fixed-effects meta-analyses did not differ from the results from the random-effects meta-analyses.
Subgroup analyses
Subgroup analyses for age and LVEF included RCTs for which we had IPD combined with estimates reported by Brunner-La Rocca et al. 33 on the basis of the IPD that were available to them (ensuring that data for no trial were included twice). Otherwise, the subgroup analyses were restricted to the three RCTs that provided IPD and, for sex, one RCT that provided aggregate data (Time-CHF59,60).
There was a significant interaction between treatment and age (< 75 vs. ≥ 75 years) for all-cause mortality (based on four RCTs52,53,55,56 for which we had IPD and seven RCTs54,58–64 contributing to the estimates reported by Brunner-La Rocca et al. ,33 z = 2.119; p = 0.034) (Figure 6). BNP-guided therapy was beneficial for trial participants who were < 75 years old (HR 0.70, 95% CI 0.53 to 0.92) but not for trial participants who were ≥ 75 years old (HR 1.07, 95% CI 0.84 to 1.37). Interactions were not significant for any of the other outcomes investigated. This is likely to be because Brunner-La Rocca et al. 33 did not fully report age-specific estimates for other outcomes; consequently, our estimates of the interaction for other outcomes were less precise. Nevertheless, age-specific estimates for all outcomes were consistent, with younger patients benefiting more from BNP-guided therapy than older patients for all outcomes (Figures 7 and 8; see also Figure 16).
FIGURE 6.
All-cause mortality subgroup analysis: (a) younger vs. (b) older age (< 75 vs. ≥ 75 years). All-cause mortality: unadjusted individual HRs with 95% CI for four studies providing IPD and seven studies included in a previous IPD meta-analysis (aggregate).

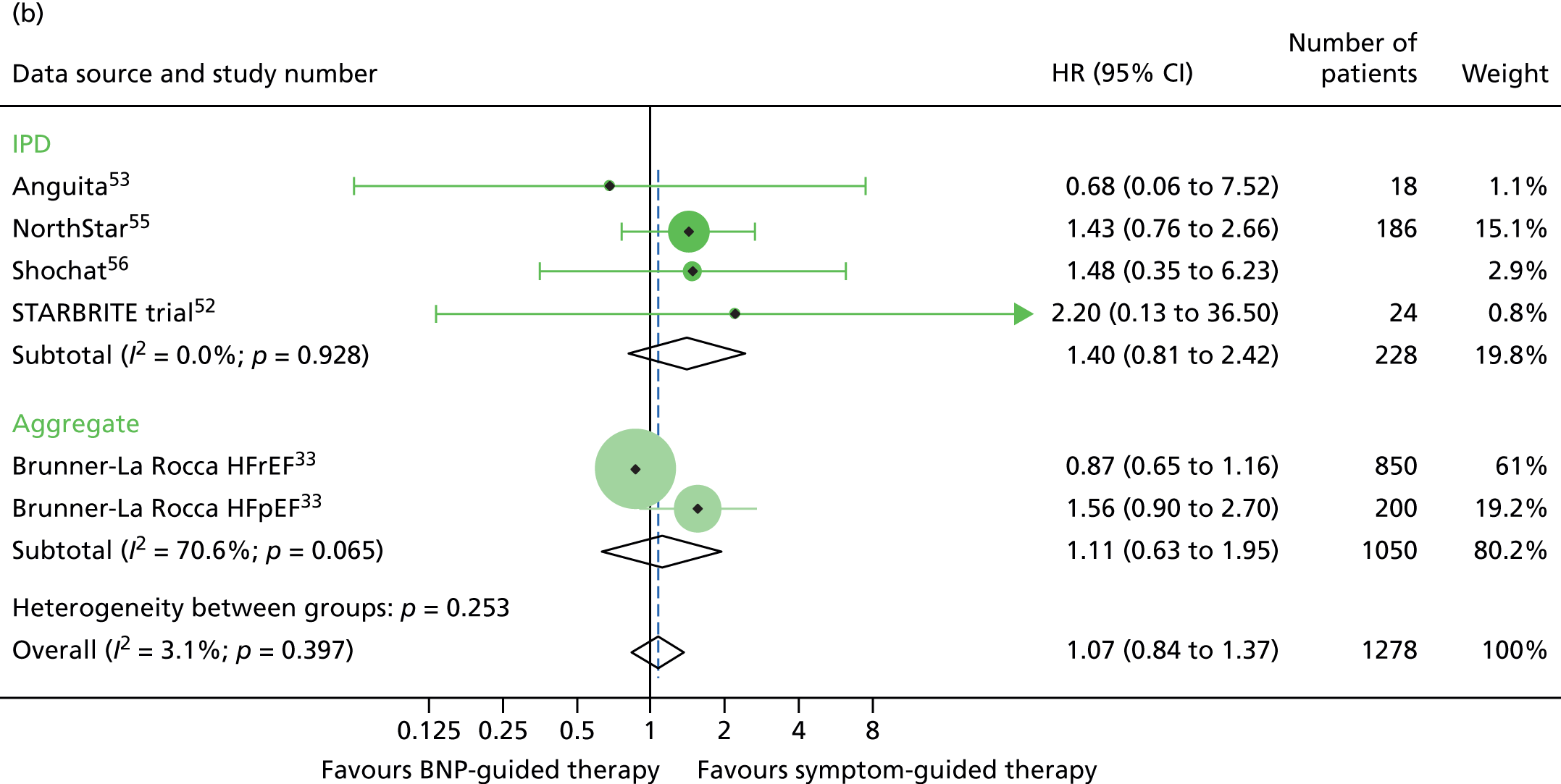
FIGURE 7.
All-cause hospitalisation subgroup analysis: (a) younger vs. (b) older age (< 75 vs. ≥ 75 years). All-cause hospitalisation: unadjusted individual HRs with 95% CIs for five studies providing IPD and one study providing aggregate data. Meta-analysis HR (95% CI) presented both within IPD and aggregate data sources and overall.


FIGURE 8.
Heart failure hospitalisation subgroup analysis: (a) younger vs. (b) older age (< 75 vs. ≥ 75 years). HF hospitalisation: unadjusted individual HRs with 95% CIs for five studies providing IPD and one study providing aggregate data. Meta-analysis HR (95% CI) presented both within IPD and aggregate data sources and overall.


There was also a significant interaction between treatment strategy and LVEF for all-cause mortality (based on four RCTs for which we had IPD, including one available to Brunner-La Rocca et al. 33 and six additional RCTs contributing to the estimates reported by Brunner-La Rocca et al. ;33 z = 2.228; p = 0.026). BNP-guided therapy appeared to be beneficial for trial participants with HFrEF (HR 0.83, 95% CI 0.68 to 1.01) but not for trial participants with HFpEF (HR 1.33, 95% CI 0.83 to 2.11). There was no evidence of an interaction between treatment strategy and LVEF for HF-related hospitalisation (based on two RCTs for which we had IPD53,55 and seven additional RCTs contributing to the estimates reported by Brunner-La Rocca et al. ;33 z = 1.246; p = 0.213) or for other outcomes. Stratum-specific estimates for HF-related hospitalisation were consistent with those for all-cause mortality, suggesting benefit of BNP-guided therapy for trial participants with HFrEF (HR 0.81, 95% CI 0.68 to 0.96) but not for trial participants with HFpEF (HR 1.07, 95% CI 0.73 to 1.57) (see Figure 6).
There were no significant interactions between the treatment strategy and any of the other covariates investigated in the subgroup analyses for all-cause mortality (sex, p = 0.29, six RCTs;52–56,59 NYHA class, p = 0.98, three RCTs;54–56 diabetes, p = 0.55, five RCTs;52–55,59 baseline BNP/NT-proBNP, p = 0.60, five RCTs52,54–56,59) or all-cause hospitalisation (age, p = 0.31, six RCTs;52–56,59 sex, p = 0.10, six RCTs;52–56,59 NYHA class, p = 0.95, four RCTs;53,54,56,59 LVEF, p = 0.89, three RCTs;53,55,60 diabetes, p = 0.35, five RCTs;52–55,59 baseline BNP/NT-proBNP, p = 0.81, five RCTs). 52,54–56,59
There were no significant interactions between treatment strategy and any of the other covariates investigated in the subgroup analyses for HF hospitalisation (sex, p = 0.80, five RCTs;53–56,59 NYHA class, p = 0.59, four RCTs;53–56 diabetes, p = 0.27, four RCTs;53–55,59 baseline BNP, p = 0.75, four RCTs54–56,59).
For sex, the treatment effect estimates for all three outcomes investigated were of a similar magnitude in men and women (Figures 9–11).
FIGURE 9.
All-cause mortality subgroup analysis: (a) men vs. (b) women. All-cause mortality: unadjusted individual HRs with 95% CIs for five studies providing IPD and one study providing aggregate data. Meta-analysis HR (95% CI) presented both within IPD and aggregate data sources and overall.


FIGURE 10.
All-cause hospitalisation subgroup analysis: (a) men vs. (b) women. All-cause hospitalisation: unadjusted individual HRs with 95% CIs for five studies providing IPD and one study providing aggregate data. Meta-analysis HR (95% CI) presented both within IPD and aggregate data sources and overall.


FIGURE 11.
Heart failure hospitalisation subgroup analysis: (a) men vs. (b) women. HF hospitalisation: unadjusted individual HRs with 95% CIs for four studies providing IPD and one study providing aggregate data. Meta-analysis HR (95% CI) presented both within IPD and aggregate data sources and overall.


For NYHA class (class I/II vs. class III/IV), the treatment effect estimates were similar in the two NYHA class strata for all outcomes investigated (Figures 12–14).
FIGURE 12.
All-cause mortality subgroup analysis: (a) good vs. (b) poor NYHA class (class I/II vs. class III/IV). All-cause mortality: unadjusted individual HRs with 95% CIs for three studies providing IPD, with meta-analysis HR and 95% CI.


FIGURE 13.
All-cause hospitalisation subgroup analysis: (a) good vs. (b) poor NYHA class (class I/II vs. class III/IV). All-cause hospitalisation: unadjusted individual HRs with 95% CIs for four studies providing IPD, with meta-analysis HR and 95% CI.
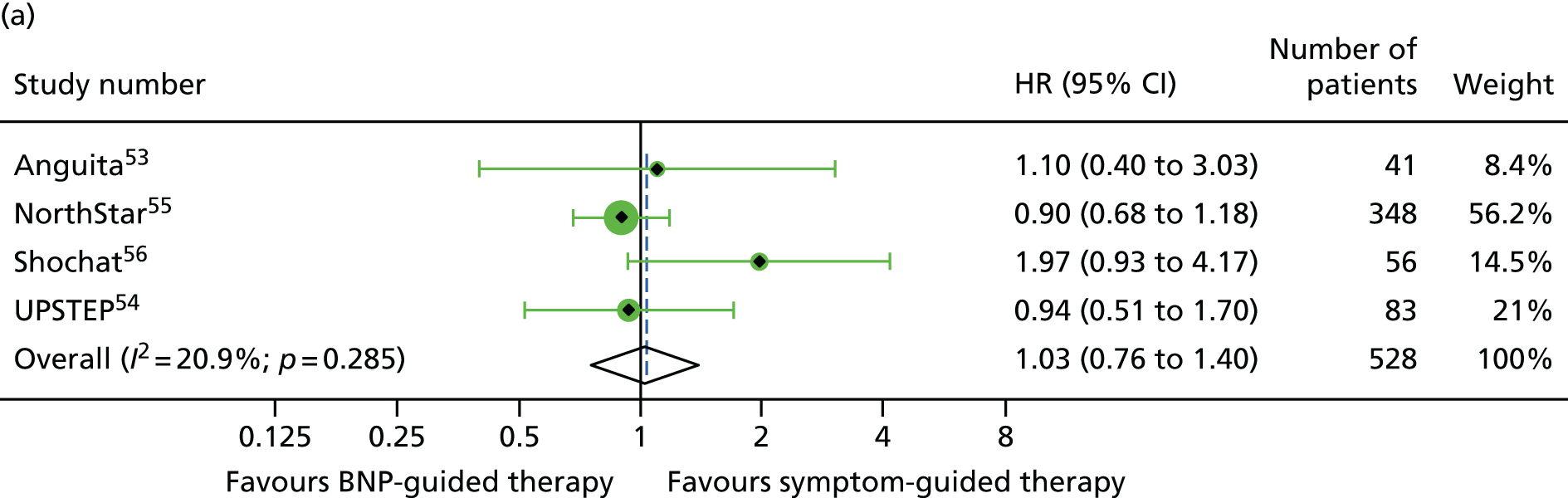

FIGURE 14.
Heart failure hospitalisation subgroup analysis: (a) good vs. (b) poor NYHA class (class I/II vs. class III/IV). HF hospitalisation: unadjusted individual HRs with 95% CIs for four studies providing IPD, with meta-analysis HR and 95% CI.


Of the five studies providing IPD, only two (Anguita et al. 53 and NorthStar55) included patients with LVEF > 40%, with 63 patients across the two trials. LVEF subgroup estimates for all-cause mortality were available for seven studies54,58–64 providing aggregate data from the recently published subgroup analysis by Brunner-La Rocca et al. 33 Figures 15–17 show the treatment effect estimates in the LVEF strata for all outcomes investigated. For all-cause mortality, the treatment effect estimate for the lower LVEF subgroup (< 40% in studies providing IPD and < 45% in studies providing aggregate data) suggested a protective effect of BNP-guided therapy (HR 0.83, 95% CI 0.68 to 1.01), which was not evident in the higher LVEF subgroup (HR 1.33, 95% CI 0.83 to 2.11). This effect was largely driven by one RCT (Time-CHF59,60); when this RCT was excluded from the analysis, the protective effect in the lower LVEF subgroup was attenuated (HR 0.89, 95% CI 0.71 to 1.11). For HF hospitalisation, the treatment effect suggested a protective effect of BNP-guided therapy in the lower LVEF subgroup (HR 0.78, 95% CI 0.64 to 0.96) but no protective effect in the higher LVEF subgroup (HR 1.61, 95% CI 0.95 to 2.71). For diabetic status, only one study59 providing aggregate data included estimates for any outcome by diabetes status (non-diabetic vs. diabetic). Diabetes status was available from four studies providing IPD. 52–55 The treatment effect estimates for the two strata are shown in Figures 18–20. Although the interaction between treatment strategy and diabetes status was not significant, Figure 20 suggests a protective effect of BNP-guided therapy on HF hospitalisations in non-diabetic patients.
FIGURE 15.
All-cause mortality subgroup analysis: (a) reduced vs. (b) preserved LVEF (≤ 40% vs. > 40% for IPD studies and ≤ 45% vs. > 45% for aggregate studies). All-cause mortality: unadjusted individual HR with 95% CIs for four studies providing IPD and six studies included in a previous IPD meta-analysis (aggregate). (a) Reduced LVEF; and (b) preserved LVEF. The individual estimates shown in the aggregate subgraph were reported previously. 60 This previous IPD meta-analysis also included estimates for STARBRITE trial and UPSTEP but IPD were available for these RCTs.
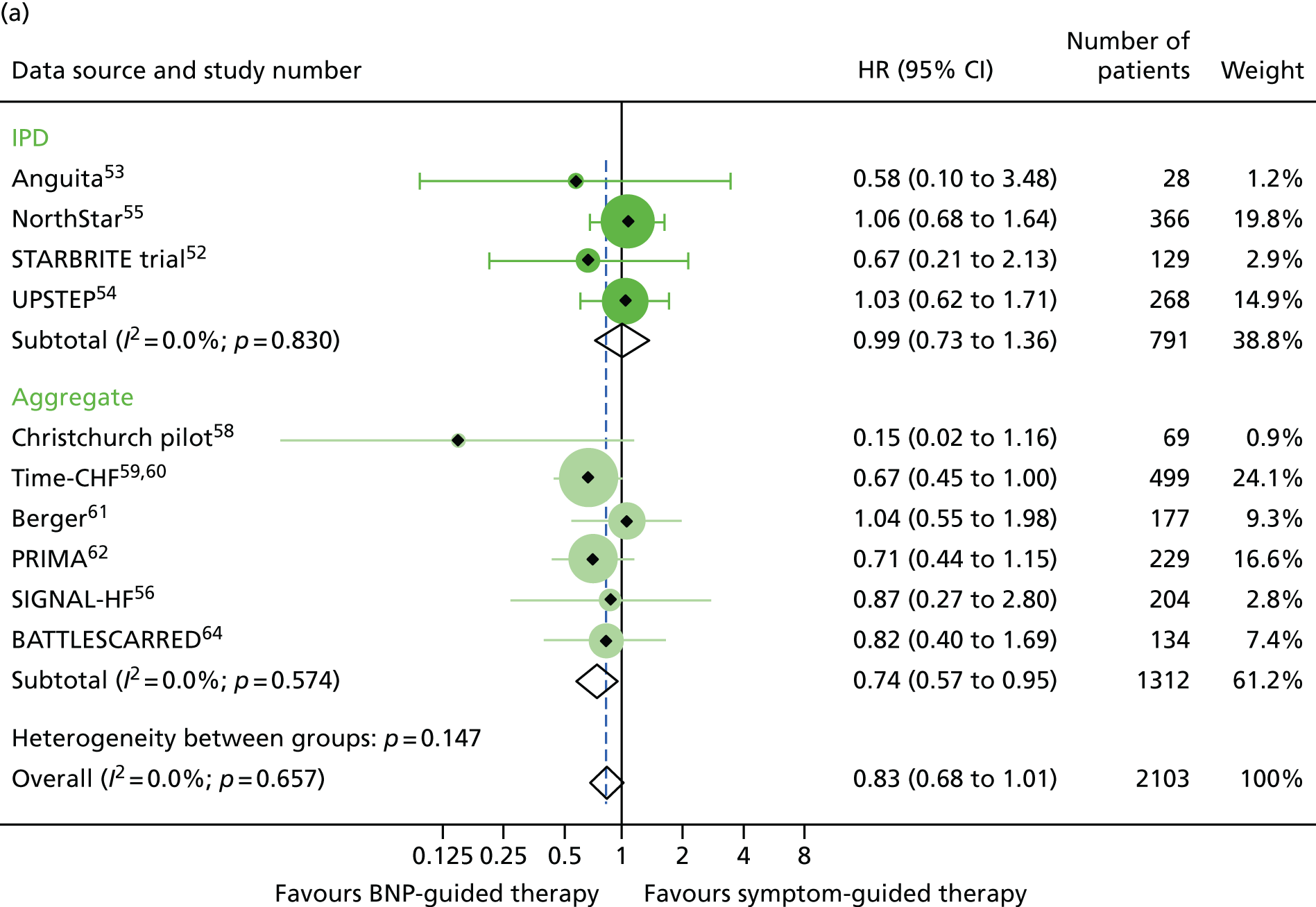

FIGURE 16.
All-cause hospitalisation subgroup analysis: (a) reduced vs. (b) preserved LVEF (≤ 40% vs. > 40% for IPD studies and ≤ 45% vs. > 45% for aggregate studies). All-cause hospitalisation: unadjusted individual HRs with 95% CIs for four studies providing IPD, with meta-analysis HR and 95% CI.
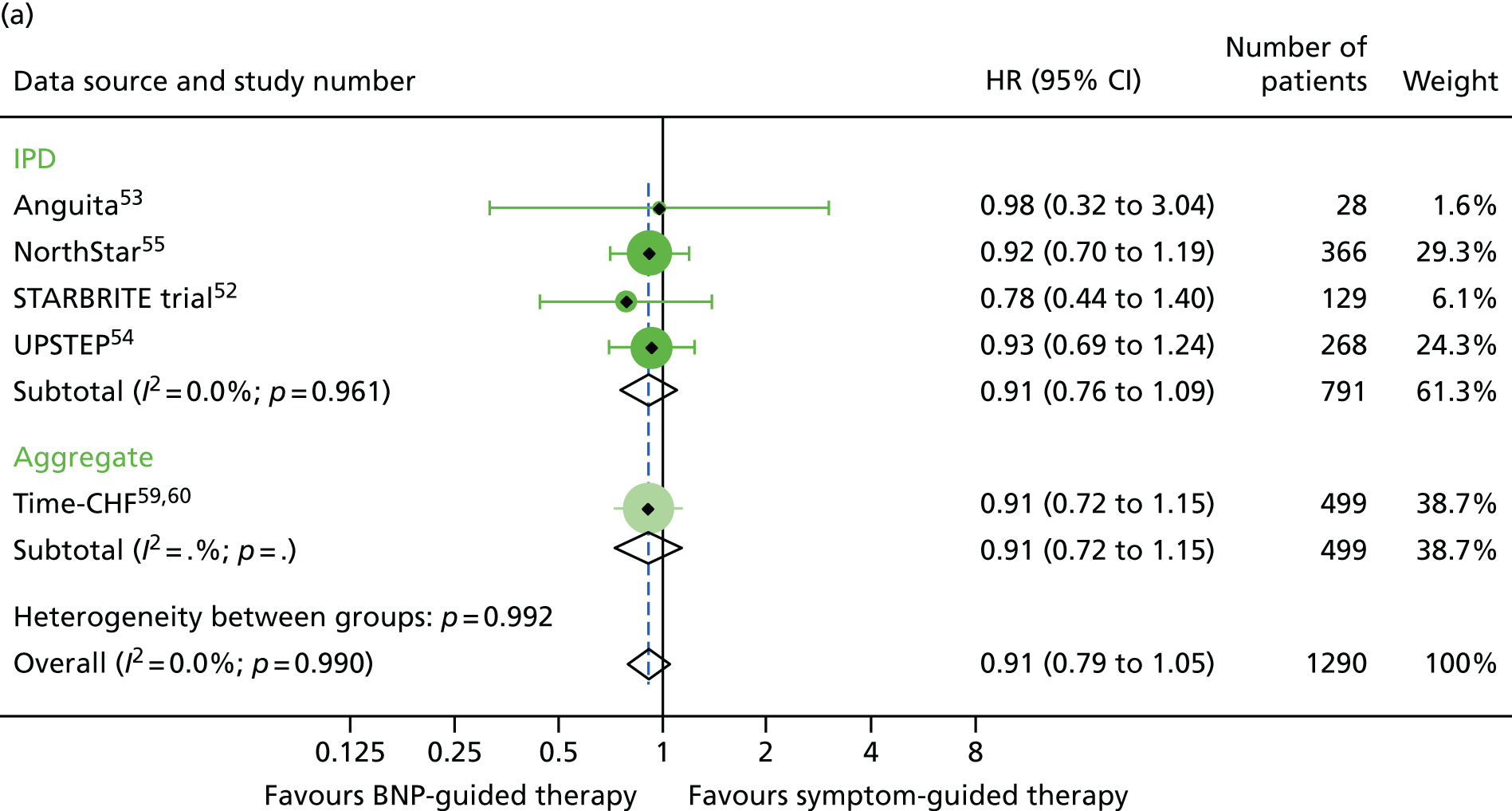

FIGURE 17.
Heart failure hospitalisation subgroup analysis: (a) reduced vs. (b) preserved LVEF (≤ 40% vs. > 40% for IPD studies and ≤ 45% vs. > 45% for aggregate studies). HF hospitalisation: unadjusted individual HRs with 95% CIs for two studies providing IPD and seven studies included in a previous IPD meta-analysis (aggregate).


FIGURE 18.
All-cause mortality subgroup analysis: (a) diabetic vs. (b) non-diabetic. All-cause mortality: unadjusted individual HRs with 95% CIs for four studies providing IPD and one study providing aggregate data. Meta-analysis HR (95% CI) presented both within IPD and aggregate data sources and overall.
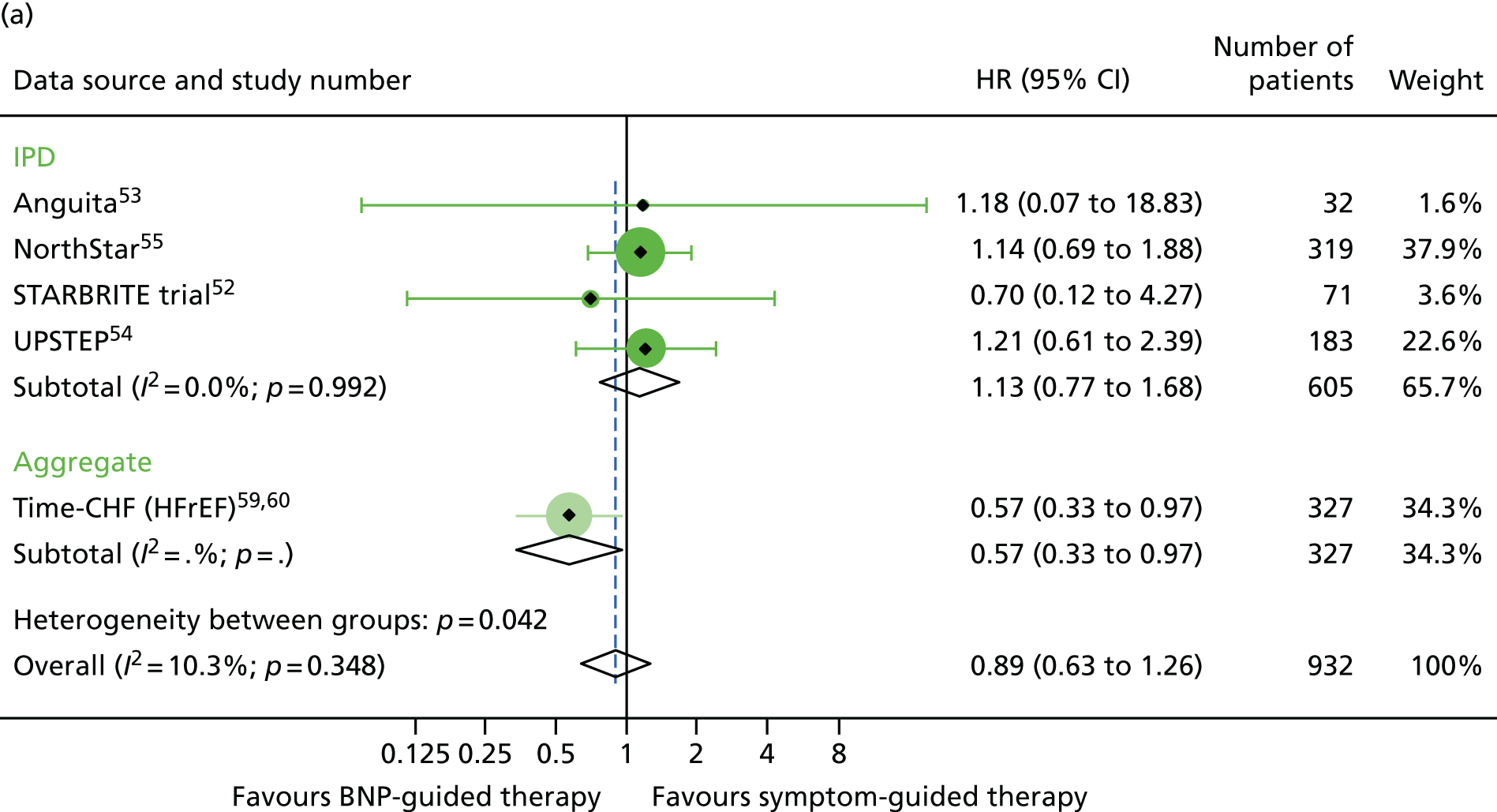

FIGURE 19.
All-cause hospitalisation subgroup analysis: (a) diabetic vs. (b) non-diabetic. All-cause hospitalisation: unadjusted individual HRs with 95% CIs for four studies providing IPD and one study providing aggregate data. Meta-analysis HR (95% CI) presented both within IPD and aggregate data sources and overall.
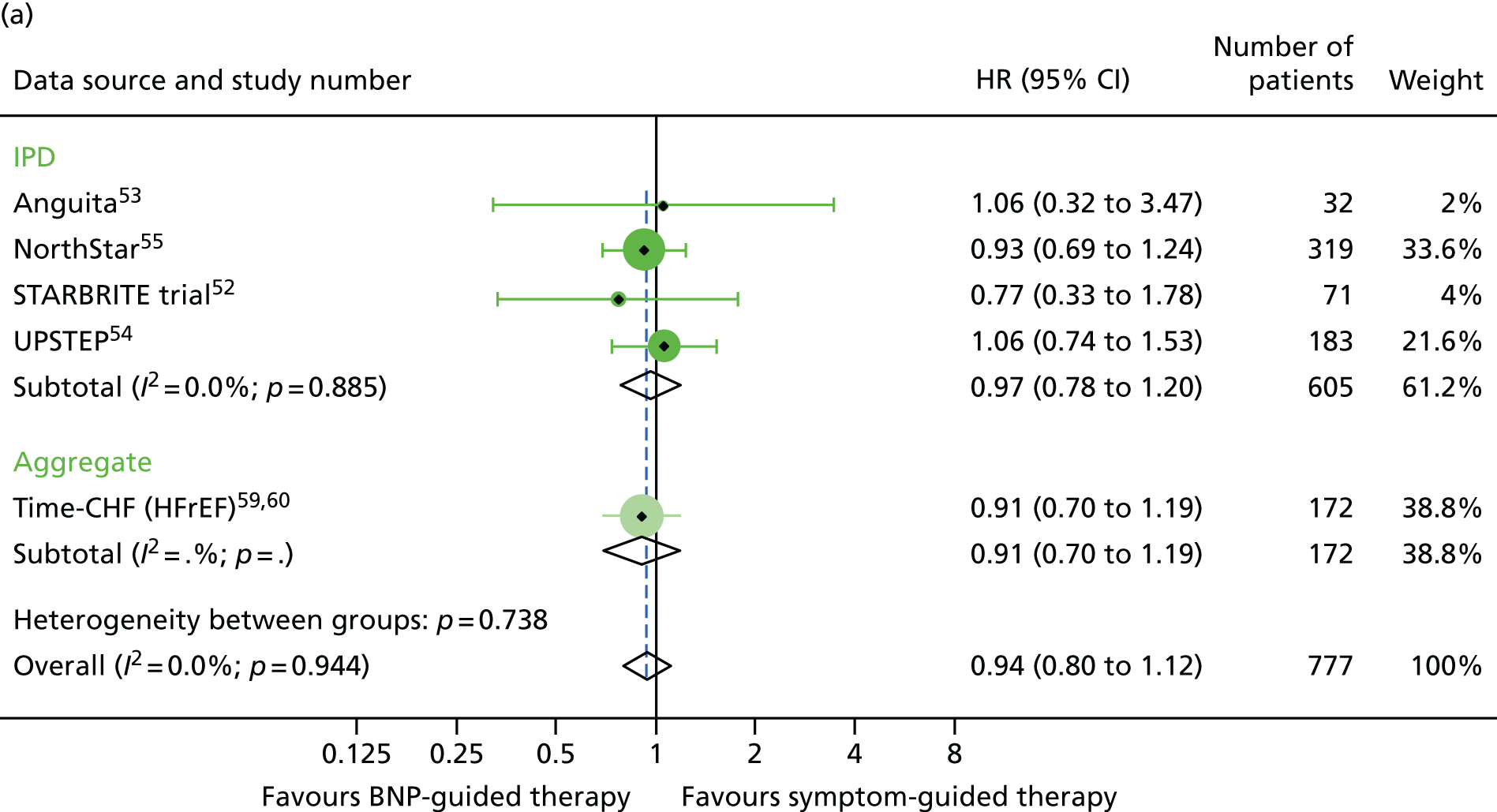

FIGURE 20.
Heart failure hospitalisation subgroup analysis: (a) diabetic vs. (b) non-diabetic. HF hospitalisation: unadjusted individual HRs with 95% CIs for four studies providing IPD and one study providing aggregate data. Meta-analysis HR (95% CI) presented both within IPD and aggregate data sources and overall.


For baseline BNP subgroups (above vs. below the median BNP at baseline), data were combined for four studies providing IPD52,54–56 and one study providing aggregate data. 59 The treatment effect estimates were of a similar magnitude in the low- and high-baseline-BNP groups for all outcomes investigated (Figures 21–23).
FIGURE 21.
All-cause mortality subgroup analysis: (a) high vs. (b) low BNP at baseline (≤ median vs. > median). All-cause mortality: unadjusted individual HRs with 95% CIs for four studies providing IPD and one study providing aggregate data. Meta-analysis HR (95% CIs) presented both within IPD and aggregate data sources and overall.


FIGURE 22.
All-cause hospitalisation subgroup analysis: (a) high vs. (b) low BNP at baseline (≤ median vs. > median). All-cause hospitalisation: unadjusted individual HRs with 95% CIs for four studies providing IPD and one study providing aggregate data. Meta-analysis HR (95% CI) presented both within IPD and aggregate data sources and overall.


FIGURE 23.
Heart failure hospitalisation subgroup analysis: (a) high vs. (b) low BNP at baseline (≤ median vs. > median). HF hospitalisation: unadjusted individual HRs with 95% CIs for four studies providing IPD and one study providing aggregate data. Meta-analysis HR (95% CI) presented both within IPD and aggregate data sources and overall.
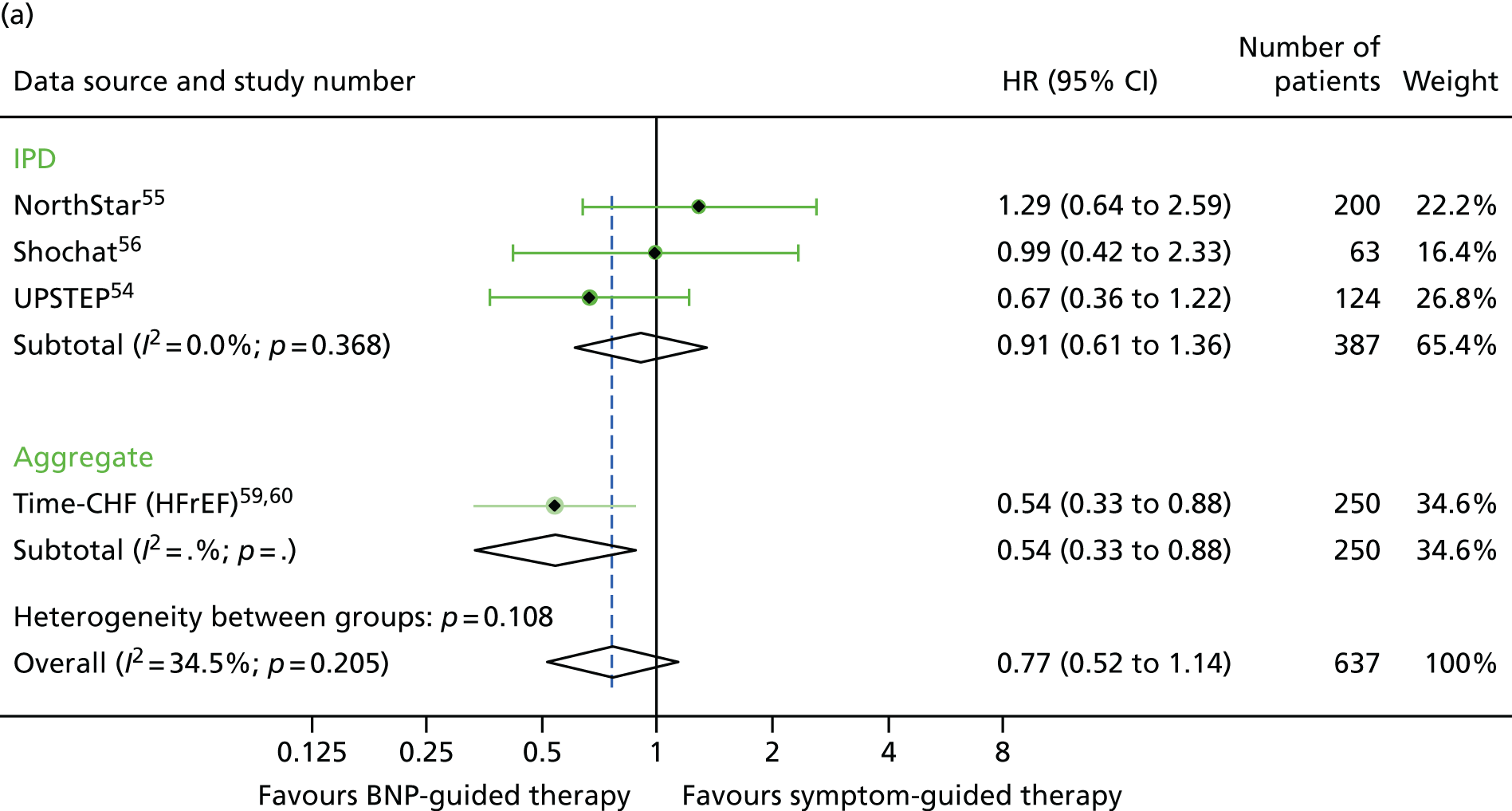
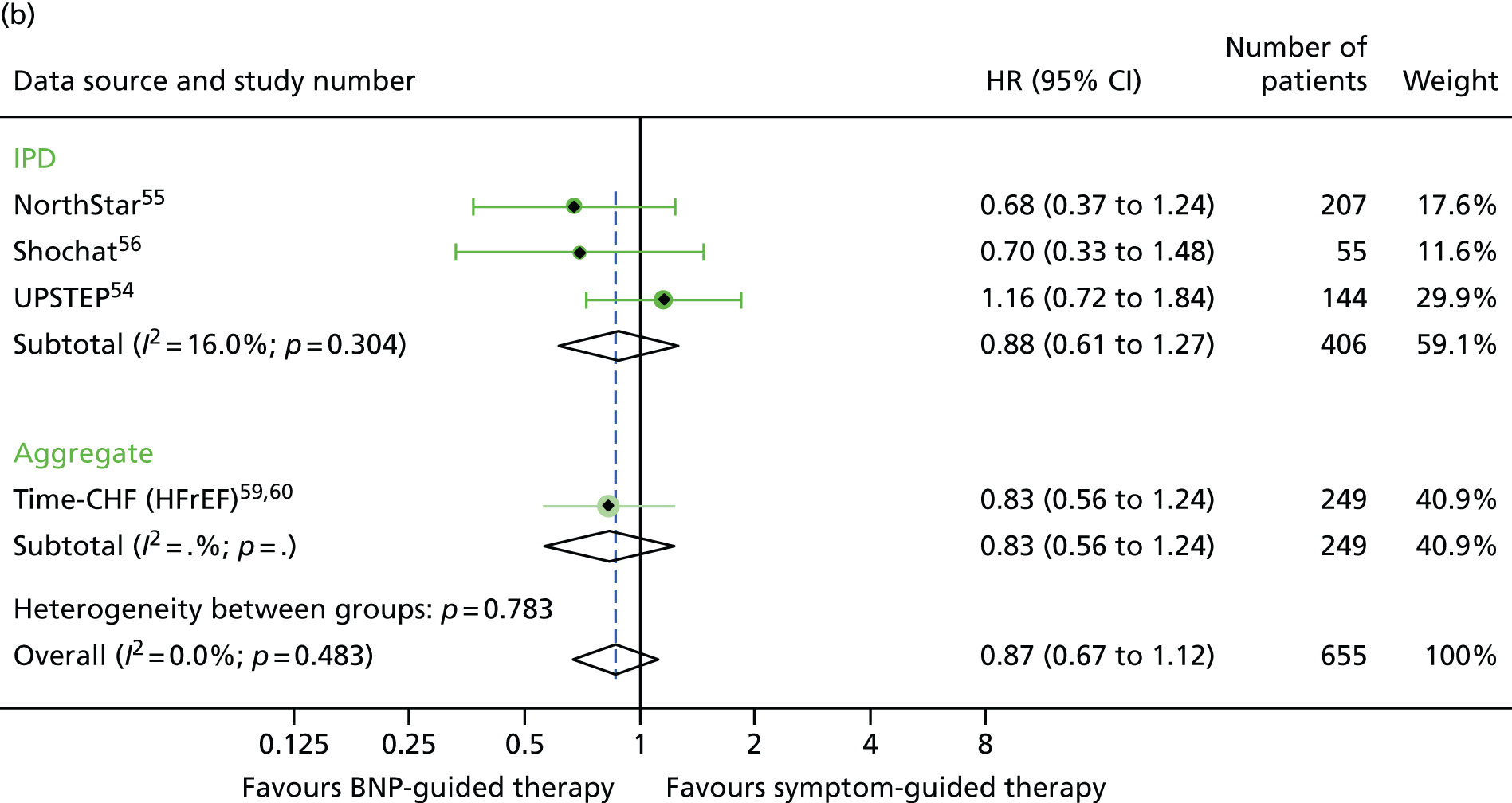
Changes in B-type natriuretic peptide/N-terminal pro-B-type natriuretic peptide from baseline to end of follow-up
B-type natriuretic peptide/NT-BNP levels at baseline and end of follow-up were available for 8 out of 13 RCTs (three IPD52,53,56 and five aggregate,58,62–64,66 Table 7). In five of these BNP/NT-BNP levels fell in both groups. 58,62–64,66 In three RCTs,62–64 the decrease in BNP/NT-BNP was greater in the symptom-guided therapy group than in the BNP-guided therapy group. In one RCT (Shochat et al. 56), NT-BNP levels had increased by the end of follow-up in both groups.
Group | Studies that provided IPD | Studies that provided aggregate data | |||||||||||
---|---|---|---|---|---|---|---|---|---|---|---|---|---|
Anguita et al.53 (n = 60), median (IQR) | NorthStar55 (n = 407), median (IQR) | Shochat et al.56 (n = 120), median (IQR) | STARBRITE trial52 (n = 130), median (IQR) | UPSTEP69 (n = 258), median (IQR) | Christchurch pilot58 (n = 69)a | Time-CHF59,60 (n = 499), median (IQR) | Berger et al.61 (n = 278), median (IQR) | PRIMA62 (n = 345), median (IQR) | SIGNAL-HF63 (n = 252), geometric mean | BATTLESCARRED64 (n = 364), median (IQR) | STARS-BNP65 (n = 220), mean reported | PROTECT66 (n = 151), median | |
BNP group | |||||||||||||
Baselinea | 34 (7 to 83) | 1884 (1385 to 2955) | 1905 (1099 to 4488) | 453 (221 to 1135) | 601 (346 to 946) | 1839 | HFrEF, 3998 (2075 to 7220); HFpEF, 2210 (1514 to 4081) | 2216 (355 to 9649) | 2961 (discharge) (1383 to 5144) | 2661 (2.1) | 2012 (516 to 10,233) | 352 (260) | 2344 |
End of follow-up | 8 (3 to 83) | – | 1765 (476 to 3966) | 413 (111 to 894) | – | 1169 | – | – | 2529 | 2360 | 1610 (6 months) | 284 (180) (3 months) | 1125 |
Difference | 2 (–31 to 28) | – | –81 (–1273 to 512) | –14 (–461 to 248) | – | –670 | – | – | –432 (–1392 to 297) | –301 | –402 | –68 | 1219 |
% change from baseline | 6 | – | –4 | –3 | – | –36 | – | – | –15 | –11 | –20 | –19 | –52 |
Control group | |||||||||||||
Baselinea | 22 (5 to 104) | 2042 (1390 to 3560) | 1569 (784 to 4919) | 441 (189 to 981) | 609 (376 to 952) | 2127 | HFrEF, 4657 (2455 to 7520); HFpEF, 2191 (1478 to 4890) | 2469 (355 to 18,487) | 2936 (discharge) (1291 to 5525) | 2429 (2.1) | 1996 (425 to 6588) | – | 1946 |
End of follow-up | 39 (6 to 104) | – | 1822 (618 to 4489) | 471 (236 to 1180) | – | 2102 | – | – | 2364 | 2067 | 1537 (6 months) | – | 1844 |
Difference | 4 (–20 to 46) | – | 73 (–554 to 1245) | 51 (–130 to 288) | – | –25 | – | – | –572(–1329 to 434) | –362 | –459 | – | 102 |
% change from baseline | 18 | – | 5 | 12 | – | –1 | – | – | –19.5 | –15 | –23 | – | –5 |
Figure 24 shows a scatterplot of the HR (and 95% CI) for all-cause mortality versus the ratio of the change in BNP/NT-proBNP from baseline between BNP-guided therapy and symptom-guided therapy groups for all studies. There was no consistent relationship between the change in BNP from baseline between groups and the HR for all-cause mortality for the same trial. The studies that provided evidence for a relationship (i.e. studies with the most extreme HRs for mortality favouring BNP guided-therapy and in which BNP fell substantially more in the BNP-guided group than in the symptom-guided group) provided least weight in the meta-analysis. Calculating the relative change between groups using IPD (for studies that provided IPD) provided even less evidence for a relationship.
FIGURE 24.
Relationship between HRs for all-cause mortality and the ratio of the change in BNP/NT-proBNP from baseline between the BNP-guided therapy group and symptom-guided therapy group. Filled circles represent the ratio of the change calculated using aggregate data and open circles represent the ratio of the change calculated using IPD when available; the change in the position on the x-axis for three trials shows how the effect estimates change with the two analyses methods, while the position on the y-axis remains the same.

Medication changes
The numbers of patients on each medication at baseline were available for all but one RCT that provided IPD (Shochat et al. 56) (Table 8). Two RCTs providing IPD (Anguita et al. 53 and NorthStar55) and one RCT providing aggregate data [Can PRo-brain-natriuretic peptide guided therapy of chronic heart failure IMprove heart fAilure morbidity and mortality? (PRIMA)62] also provided numbers of patients on different medications at the end of follow-up. In these three RCTs, the proportion of patients on different medications at baseline and end of follow-up were similar in the BNP-guided therapy group and control group. Medication doses were provided in only one RCT (Anguita et al. 53). Therefore, it was not possible to carry out an analysis investigating the association between the changes in medication and outcomes.
Medications | Studies that provided IPD, n (%) | Studies that provided aggregate data, n (%) | |||||||||||
---|---|---|---|---|---|---|---|---|---|---|---|---|---|
Anguita et al.53 | STARBRITE trial52 | UPSTEP69 | NorthStar55 | Shochat et al.56 | Christchurch pilot58 | Time-CHF59,60 | Berger et al.61 | PRIMA62 | SIGNAL-HF63 | BATTLESCARRED64 | STARS-BNP65 | PROTECT66 | |
ACEis | |||||||||||||
BNP guided | |||||||||||||
Baseline | 21/30 (70) | 49/65 (75) | 108/140 (77) | – | – | – | – | – | 112/174 (64) | 89/126 (71) | – | – | 53/75 (71) |
End of follow-up | 22/30 (73) | – | – | – | – | – | – | – | – | – | – | – | – |
Control | |||||||||||||
Baseline | 18/30 (60) | 52/65 (80) | 88/128 (69) | – | – | – | – | – | 111/171 (64) | 76/124 (61) | – | – | 47/76 (62) |
End of follow-up | 15/29 (52) | – | – | – | – | – | – | – | – | – | – | – | – |
ARBs | |||||||||||||
BNP guided | |||||||||||||
Baseline | 7/30 (23) | 8/63 (13) | 49/140 (35) | – | – | – | – | – | 31/174 (18) | 33/126 (26) | – | – | 8/75 (11) |
End of follow-up | 9/30 (30) | – | – | – | – | – | – | – | – | – | – | – | – |
Control | |||||||||||||
Baseline | 9/30 (30) | 9/64 (14) | 46/128 (36) | – | – | – | – | – | 34/171 (20) | 36/124 (29) | – | – | 15/76 (20) |
End of follow-up | 11/29 (38) | – | – | – | – | – | – | – | – | – | – | – | – |
ACEis or ARBs | |||||||||||||
BNP guided | |||||||||||||
Baseline | – | – | – | 165/199 (83) | – | – | HFrEF: 238/251 (95). HFpEF: 52/59 (88) | 84/92 (91) | – | – | 102/121 (84) | 109/110 (99) | – |
End of follow-up | – | – | – | 178/199 (89) | – | – | – | – | – | – | – | – | – |
Control | |||||||||||||
Baseline | – | – | – | 172/208 (83) | – | – | HFrEF: 235/248 (95). HFpEF: 54/64 (84) | 84/96 (88) | – | – | 102/121 (84) | 109/110 (99) | – |
End of follow-up | – | – | – | 175/208 (84) | – | – | – | – | – | – | – | – | – |
Beta-blocker | |||||||||||||
BNP guided | |||||||||||||
Baseline | 13/30 (43) | 56/65 (86) | 131/140 (94) | 168/199 (84) | – | 4/33 (12) | HFrEF: 191/251 (76). HFpEF: 40/59 (68) | 75/92 (82) | 139/174 (80) | 100/126 (79) | 79/121 (65) | 109/110 (99) | 74/75 (99) |
End of follow-up | 17/30 (57) | – | – | 169/199 (85) | – | – | – | – | – | – | – | – | – |
Control | |||||||||||||
Baseline | 20/30 (67) | 51/65 (78) | 121/128 (95) | 181/208 (87) | – | 1/36 (3) | HFrEF: 201/248 (81). HFpEF: 44/64 (69) | 73/96 (76) | 126/171 (74) | 94/124 (76) | 85/121 (70) | 107/110 (97) | 71/76 (93) |
End of follow-up | 20/29 (69) | – | – | 188/208 (90) | – | – | – | – | – | – | – | – | – |
Loop diuretic | |||||||||||||
BNP guided | |||||||||||||
Baseline | – | 62/65 (95) | 117/140 (84) | 142/199 (71) | – | – | HFrEF: 232/251 (92). HFpEF: 52/59 (88) | 78/92 (85) | 169/174 (97) | – | 114/121 (94) | 110/110 (100) | 67/75 (89) |
End of follow-up | – | – | – | 156/199 (78) | – | – | – | – | – | – | – | – | – |
Control | |||||||||||||
Baseline | – | 60/65 (92) | 115/128 (90) | 155/208 (75) | – | – | HFrEF: 234/248 (94). HFpEF: 57/64 (89) | 75/96 (78) | 162/171 (95) | – | 119/121 (98) | 110/110 (100) | 71/76 (93) |
End of follow-up | – | – | – | 166/208 (80) | – | – | – | – | – | – | – | – | – |
Thiazide diuretics | |||||||||||||
BNP guided | |||||||||||||
Baseline | – | 0/65 (0) | – | 6/199 (3) | – | – | – | – | – | – | – | – | 5/75 (7) |
End of follow-up | – | – | – | – | – | – | – | – | – | – | – | – | – |
Control | |||||||||||||
Baseline | – | 1/65 (2) | – | 17/208 (8) | – | – | – | – | – | – | – | – | 3/76 (4) |
End of follow-up | – | – | – | – | – | – | – | – | – | – | – | – | – |
Diuretics (non-specific) | |||||||||||||
BNP guided | |||||||||||||
Baseline | 28/30 (93) | – | – | – | – | – | – | – | – | 93/126 (74) | – | – | – |
End of follow-up | 29/30 (97) | – | – | – | – | – | – | – | – | – | – | – | – |
Control | |||||||||||||
Baseline | 29/30 (97) | – | – | – | – | – | – | – | – | 78/124 (63) | – | – | – |
End of follow-up | 29/29 (100) | – | – | – | – | – | – | – | – | – | – | – | – |
Aldosterone antagonist (e.g. spironolactone) | |||||||||||||
BNP guided | |||||||||||||
Baseline | 24/30 (80) | 46/65 (71) | 77/140 (55) | – | – | – | HFrEF: 102/251 (41). HFpEF: 18/59 (31) | 41/92 (45) | 92/174 (53) | 28/126 (22) | 15/121 (12) | 28/110 (25) | 37/75 (49) |
End of follow-up | 24/30 (80) | – | – | – | – | – | – | – | – | – | – | – | – |
Control | |||||||||||||
Baseline | 19/30 (63) | 42/65 (65) | 74/128 (58) | – | – | – | HFrEF: 100/248 (40). HFpEF: 14/64 (22) | 40/96 (42) | 95/171 (56) | 22/124 (18) | 15/121 (12) | 24/110 (22) | 26/76 (34) |
End of follow-up | 18/29 (62) | – | – | – | – | – | – | – | – | – | – | – | – |
Digoxin | |||||||||||||
BNP guided | |||||||||||||
Baseline | 6/30 (20) | 20/65 (31) | – | 35/199 (18) | – | – | HFrEF: not given. HFpEF: 8/59 (14) | – | – | 18/126 (14) | – | – | 22/75 (29) |
End of follow-up | 9/30 (30) | – | – | – | – | – | – | – | – | – | – | – | – |
Control | |||||||||||||
Baseline | 12/30 (40) | 20/65 (31) | – | 42/208 (20) | – | – | HFrEF: not given. HFpEF: 9/64 (14) | – | – | 11/124 (9) | – | – | 25/76 (33) |
End of follow-up | 11/29 (38) | – | – | – | – | – | – | – | – | – | – | – | – |
Calcium channel blockers | |||||||||||||
BNP guided | |||||||||||||
Baseline | 7/30 (23) | – | – | – | – | – | – | – | – | – | – | – | – |
End of follow-up | 6/30 (20) | – | – | – | – | – | – | – | – | – | – | – | – |
Control | |||||||||||||
Baseline | 7/30 (23) | – | – | – | – | – | – | – | – | – | – | – | – |
End of follow-up | 6/29 (21) | – | – | – | – | – | – | – | – | – | – | – | – |
Adverse events
None of the IPD studies or aggregate data studies provided data on adverse events. Therefore, it was not possible to assess the safety of BNP-guided therapy. However, six out of eight studies58,59,62,63,65,66 providing aggregate data reported that there were no significant differences in adverse events between the BNP-guided and symptom-guided treatment groups.
Quality of life
Only one IPD study55 provided quality-of-life data at baseline. However, the study publication reported no differences in quality of life (using the Minnesota Living with Heart Failure questionnaire) between BNP-guided therapy and symptom-guided therapy groups (p = 0.09). None of the other IPD studies provided any data on quality of life. Five out of eight aggregate data studies58,59,62,64,66 reported quality of life; four of these58,59,62,64 showed no difference between BNP-guided therapy and symptom-guided therapy groups, although one66 found greater improvement in quality-of-life scores among patients in the BNP-guided therapy group.
Funnel plots to assess small study effects
Funnel plots were generated for each outcome to visualise the risk of small study effects arising from differential risk of reporting biases and methodological quality by sample size (Figures 25–27). Formal statistical tests of funnel plot asymmetry were not carried out, given the small number of RCTs contributing to each plot. None of the plots suggested marked asymmetry.
FIGURE 25.
Funnel plot with pseudo 95% confidence limits for the primary outcome of all-cause mortality.

FIGURE 26.
Funnel plot with pseudo 95% confidence limits for the secondary outcome of all-cause hospitalisation.

FIGURE 27.
Funnel plot with pseudo 95% confidence limits for the secondary outcome of HF hospitalisation.
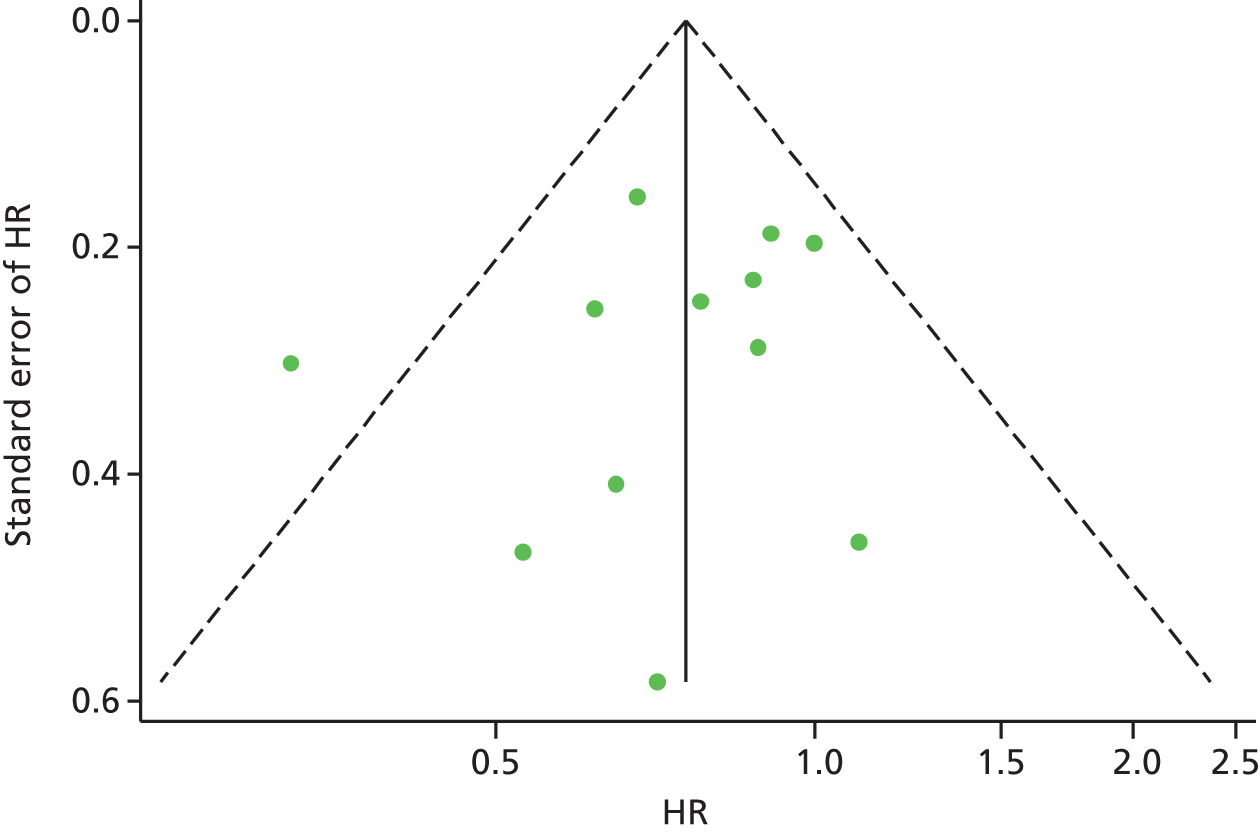
Summary of findings
Our meta-analyses, including up to 3074 patients with HF (1536 randomised to BNP-guided therapy and 1538 randomised to symptom-guided therapy), showed that BNP-guided therapy reduced the hazard of death from any cause by 13% (HR 0.87, 95% CI 0.73 to 1.04) and the hazard of hospital admission for HF by 22% (HR 0.78, 95% CI 0.65 to 0.95). These results are consistent with those of a previous IPD meta-analysis by Troughton et al. 25 (including 11 trials, 2000 patients, also in the present analysis, but excluding HFpEF participants recruited to the TIME-CHF) RCT,60 which showed an 18% reduction in the hazard of death from any cause (HR 0.82, 95% CI 0.67 to 1.00) and a 26% reduction in the hazard of hospital admission for HF (HR 0.74, 95% CI 0.60 to 0.90).
The results from our subgroup analyses showed more benefit of BNP-guided therapy in patients < 75 years old and patients with HFrEF, which is consistent with the analyses reported by Troughton et al. 25 and Brunner-La Rocca et al. 33
There were significant interactions between treatment strategy and age, and between treatment strategy and type of HF, for all-cause mortality. These interactions were not significant for any of the other outcomes investigated, although age-specific and type of HF-specific estimates for all outcomes were consistent with the findings for all-cause mortality. The interaction between treatment strategy and type of HF was largely driven by one RCT (Time-CHF); when this RCT was excluded from the analysis, the protective effect in the HFrEF subgroup was considerably attenuated.
There also appeared to be a protective effect of BNP-guided therapy on HF hospitalisations in non-diabetic patients (HR 0.76, 95% CI 0.58 to 0.98), which was not evident in diabetic patients (HR 0.93, 95% CI 0.67 to 1.30), although the test of interaction for this analysis was not statistically significant.
Unlike Brunner-La Rocca et al. ,33 we could not investigate the interaction effect of treatment strategy and age controlling for the interaction effect of treatment strategy and type of HF because we had IPD for a minority of the RCTs. Brunner-La Rocca et al. 33 reported that the interactions were independent but noted that the interaction of treatment strategy with type of HF was explained by comorbidities, in particular renal failure.
Chapter 3 B-type natriuretic peptide (or N-terminal pro-B-type natriuretic peptide) testing and monitoring in patients with chronic heart failure: a population-based cohort study in the Clinical Practice Research Datalink
Aims and objectives
The aim of the cohort study was to characterise a representative cohort of English patients with HF and to evaluate the clinical effectiveness of BNP-guided therapy compared with usual care in patients with chronic HF. The specific objectives were to:
-
create a cohort of HF patients from routinely collected data sets [CPRD, National Heart Failure Audit (NHFA), ONS, HES] and profile their longitudinal care pathway, from diagnosis, through treatment, to outcome
-
classify patients in the cohort according to their history of BNP testing
-
estimate the effect of BNP-guided therapy on clinical outcomes compared with usual care
-
estimate the effect of BNP-guided therapy in predefined subgroups of HF patients (e.g. by age group, sex, type of HF, severity of HF, baseline BNP levels)
-
derive summary resource use statistics to characterise the cohort to be used to inform parameter estimates in a cost-effectiveness model of BNP monitoring in primary and secondary care in the UK.
Methods
This was a retrospective population-based longitudinal cohort study. The cohort was created by linking data from the CPRD, HES and ONS mortality.
Data sources
The CPRD includes all data from the General Practice Research Database, which was established in 1987. It contains anonymised longitudinal primary care records, usable for research purposes, from over 13 million patients in the UK (from 684 GP practices in England, Wales, Scotland and Northern Ireland). The geographical distribution of the GP practices participating in the General Practice Research Database is representative of the UK population (www.cprd.com/home; accessed 1 December 2016). The CPRD GOLD data set contains details of consultations, diagnoses, interventions, test results, prescriptions and referrals. Coded data are provided as several data sets (clinical, referral, test and therapy data sets). The data set includes a combination of coded data (coded at the time of entry, usually by the GP) and free text associated with the coded entries: the free text has been either typed by the GP or obtained from hospital letters. CPRD GOLD includes data from about 8% of the UK population. GP practices that contribute data to the CPRD are each assigned an up-to-standard (UTS) date, that is, the date after which the data submitted by the practice are considered to meet assigned data quality standards. Only data submitted after the UTS date were used for analysis.
CPRD GOLD is linked with the HES inpatient data set, which contains details of all hospital admissions in England, including dates of admission and discharge and main diagnoses. The CPRD recently established linkage with the HES outpatient data set, which contains details of outpatient appointments and the clinical diagnoses these relate to. CPRD GOLD is also linked with the ONS mortality data set, which contains the date and cause of death (from the death certificate) for the population of England and Wales. The linkage is performed via NHS Digital (a trusted third party). GP practices submit patient data directly to NHS Digital, which links IPD with HES and ONS via the patient’s NHS number, sex and partial date of birth. Only about 40% of GP practices that contribute to the CPRD have given approval for their data to be linked with HES. NHS Digital submits patient data to the CPRD with pseudonym identifiers, either linked with HES/ONS or not linked (for GP practices that have not given approval for linkage).
Additional planned linkage
The original application also proposed linking the CPRD GOLD data source with the NHFA (January 2007 and March 2013), which contains data on the care and treatment of patients who have an unscheduled admission to a participating hospital resulting from HF. The NHFA provides information that is not captured in HES, including detailed clinical information, test results, medications and diagnoses during admission. The data items are collected by NHFA in order to audit adherence to national guidance on the care and treatment of patients with HF by participating hospitals. The planned flow of data is shown in Figure 28.
FIGURE 28.
Flow of data for the planned linked cohort. NICOR, National Institute for Cardiovascular Outcomes Research; UoB, University of Bristol. a, GP practices submit pseudo patient identifiers and patient identifiers to NHS Digital; b, NICOR will not provide HES data on patients linked with the CPRD.

Study population
The study population was identified in CPRD GOLD. Patients were included in the study if they had a HF diagnosis between 1 April 2005 and 31 March 2013 with no HF diagnosis in the preceding year (i.e. incident HF), had at least 1 year of UTS follow-up before HF diagnosis and had a linked HES and/or ONS record. HF patients from GP practices that did not participate in the HES/ONS linkage programme were excluded.
Incident HF was defined from Read Codes, which are a hierarchical clinical coding system of over 80,000 terms that are used in general practice in the UK. In the absence of any established algorithm for identifying incident HF from Read Codes, three incidence algorithms were defined, which were organised hierarchically to have decreasing sensitivity but increasing specificity, to identify patients with HF:
-
Specific HF diagnosis codes OR symptom-specific codes, which included codes for a variety of heart conditions that could potentially represent HF (e.g. oedema). This was the most sensitive algorithm (cohort 1).
-
Same codes as in cohort 1 AND the presence of a BNP test or echocardiography test within 6 months of the HF code AND more than one HF medication prescription within 6 months of HF code (cohort 2).
-
Specific HF diagnosis codes only AND the presence of a BNP test or echocardiography test within 6 months of the HF code AND more than one HF medication prescription within 6 months of HF code. This was the most specific algorithm (cohort 3).
Algorithm 3 was used to define the main study cohort. The specific HF diagnosis codes used for algorithm 3 were based on the published Quality and Outcomes Framework guidance for coding HF in the CPRD (www.pcc-cic.org.uk/article/qof-read-codes-v30/; accessed 22 June 2017) (Read Code lists for algorithms 1–3 together with BNP test codes, echocardiography test codes and qualifying HF medications are shown in the statistical analysis plan, which is available from the authors, for the cohort study).
Exposures (B-type natriuretic peptide testing and B-type natriuretic peptide monitoring)
Patients were classified as BNP monitored, BNP tested or never tested based on the rate of BNP testing. We planned to compare outcomes for the BNP-monitored and BNP-tested groups with the never-tested group. The groups were defined as follows.
-
Never tested (reference group): no BNP tests recorded in the CPRD.
-
BNP tested: one or more BNP test (irrespective of the duration of observation for a patient in the cohort) but not meeting criteria for BNP monitored.
-
BNP monitored: ≥ 6 months of observation time AND three or more BNP tests carried out AND two or more tests per year.
These definitions were developed by examining the data set for serial BNP testing but before any analyses that described outcomes by group. Different definitions were developed and the clinical members of the team reached consensus about the definition that was most likely to represent some form of monitoring (based on the frequency of tests per year).
Outcomes
The primary outcome measure of the study was time to all-cause mortality from diagnosis of HF. Patients were censored either at date of death or on 1 May 2014 (the last date on which ONS data were available). Patients who had a diagnosis of HF recorded for the first time on the same date as death were given a survival time of 0.5 days to enable them to be included in the analysis population. The secondary outcomes were time from diagnosis of HF to emergency in hospital admission(s)/unscheduled readmission(s), length of hospital stay following all unscheduled admissions for HF, prescribed medications on discharge after all unscheduled admissions for HF, new medication/s started, current medication(s) stopped, time on each medication, annual rate of outpatient clinic and GP attendance and annual rate of HF-related investigations (e.g. echocardiography).
Patients’ observation time was censored either at date of death or on 1 April 2014 (the last date at which ONS data were available). Patients who experienced a secondary outcome before diagnosis of HF were censored at that point, that is, their observation time did not contribute to measures of the frequencies of outcomes after diagnosis.
Emergency/unscheduled hospital admissions and HF hospital admissions were identified from HES, the latter using International Classification of Diseases, Tenth Edition,70 codes (I11.0, I13.0, I13.2, I50, I50.0, I50.1, I50.9) recorded against the hospital spells. Length of hospital stay for each admission was provided in HES; admissions were grouped into continuous inpatient spells (full details are given in the statistical analysis plan for the cohort study, which is available from the authors). Outpatient appointments were identified from the HES outpatient data set. Multiple appointments on the same day under different treatment specialties were treated as separate appointments; multiple appointments on the same day under the same treatment specialty were treated as a single appointment. Outpatient appointments in which the patient was treated under the cardiology specialty were identified. Medications were grouped into classes (e.g. ACEis, ARBs, beta-blockers). Within each medication class, ‘courses’ of medications were identified. A new course of medication was defined as a prescription for any medication within the class with no prescription in the previous 6 months. The period of time for each course of medication was calculated as the difference between the dates of the last and first prescription within the course plus 28 days (assuming an average prescription length of 28 days for all medication classes). HF-related investigations referred to echocardiograms and BNP tests.
Comorbidities were identified from previously defined Read Codes in the CPRD (https://clinicalcodes.rss.mhs.man.ac.uk; accessed 1 May 2016)71 in the year prior to incidence date (cohort entry). The absence of a comorbidity Read Code was assumed to mean the patient did not have that comorbidity. The Charlson Comorbidity Index is calculated as the sum of weights for all comorbidities recorded for the patient in the year prior to cohort entry. 72
Covariates
Confounders were specified a priori. Two groups of potential confounders were specified: patient-level confounders (age, sex, severity of HF, degree of comorbidity classified using the Charlson Comorbidity Index) and practice-level confounding (practice deprivation index). Severity of HF was assessed using the NYHA classification; for patients without NYHA classification recorded in the CPRD, the severity was treated as unknown.
Statistical analysis
The main study cohort used for the analysis was defined by algorithm 3 (HF-specific Read Codes only). We summarised the baseline characteristics of the HF patients identified using algorithm 3 and algorithm 2 (more sensitive). We used means and standard deviations (SDs) to summarise normally distributed continuous data and medians and IQRs to summarise continuous data that were not normally distributed. Categorical variables were summarised as the number and percentage of patients within each category. We also determined the baseline characteristics stratified by BNP testing groups (not tested, BNP tested and BNP monitored).
Kaplan–Meier curves were used to compare time to all-cause mortality among the three BNP-testing groups. For all other time-to-event outcomes, we compared the cumulative incidence function between the BNP-testing groups, accounting for the competing risk of mortality before the event of interest.
For all secondary outcomes, including the number of events during follow-up (e.g. number of attended GP appointments) or number of days when a particular event was experienced (e.g. time on medications, length of hospital stay following unscheduled admissions), we calculated event rates as the number of events experienced/number of days event experienced divided by the duration of follow-up. These data were summarised as the number of patients with at least one event (e.g. the number of patients with a rate over zero) and the median (IQR) rate among those with a non-zero rate. For the outcomes of unexpected hospital admission (all cause and HF specific), we summarised the number of patients with at least one admission, the median (IQR) number of admissions and the median (IQR) total number of days hospitalised following all admissions.
We summarised medication use in 6-monthly intervals from incidence to 4 years post incidence and in the remaining follow-up time > 4 years. Within each time period, we summarised the number of patients taking each medication class at any point and the proportion of time during the time period in which the patient was taking the medication (calculated as the number of days taking medication during time period divided by the number of days of follow-up within the time period). We carried out a SA for the primary outcome in the cohort of patients defined by algorithm 2.
We characterised the cohort of HF patients in the NHFA data set but made no formal comparisons with the cohort identified in the CPRD. Prior to analyses, we performed basic checks on the data set and excluded any entries for which the patient identification number was unknown. When patients had multiple admissions within the same month (the NHFA data set included only month and year of admission), we treated the admission with the longest time to censoring as the first admission. We defined incident HF in NHFA as an admission with no admission recorded in the previous year; if a patient met the incidence criteria for more than one admission, the first admission at which the criteria were met was taken as the incident admission. We summarised demographic data at the ‘incidence admission’ in tables, the number of patients with more than one admission and the admission rate among patients with HF incidence in NHFA. We also summarised median (IQR) time from HF incidence to mortality; patients whose status at the end of follow-up was unknown were excluded from this analysis. Follow-up time has also been summarised separately for the patients who were alive and deceased at the end of follow-up. All analyses were prespecified and described in the statistical analysis plan for the cohort study.
Deviation from the planned analyses
There were two main changes to the planned cohort study, the first affecting the planned linkage for the cohort and the second relating to the planned analyses.
It was not possible to link CPRD GOLD with the NHFA data set for this project. The CPRD’s Independent Scientific Advisory Committee granted approval for the study, including the proposed linkage, in August 2014. However, at that time linkage agreements with specific data sets and disease registries were not in place. The CPRD secured generic agreement from the Ethics and Confidentiality Committee to link audit and other data sets to CPRD GOLD. It then started working on a generic agreement with relevant organisations and data controllers for permission to link to its data sets [including but not restricted to the National Institute for Cardiovascular Outcomes Research (NICOR) and the Healthcare Quality Improvement Partnership, which manage and commission the NHFA, respectively].
The approval process was too slow for the timeline of the study. Therefore, the CPRD attempted to expedite it by applying for study-specific approval outside of the generic agreement to fast track our study for bespoke approval. Healthcare Quality Improvement Partnership granted the study-specific approval and the final application for linkage was made to NHS Digital, the trusted third party that was to perform the linkage. However, this coincided with the moratorium on processing of applications and the implementation of new governance procedures by NHS Digital and the standard agreements used by the CPRD and Healthcare Quality Improvement Partnership did not meet NHS Digital’s new governance requirements. Further clarification and amendments of existing agreements were required. Given that the process of seeking approval for the planned linkage had been ongoing for almost 2 years, that there was no indication from the CPRD or NHS Digital about how long approvals would take and that the project was overdue, we decided not to pursue the linkage between CPRD GOLD and the NHFA.
We had already applied to NICOR to use the NHFA data set for the study and requested the data set while the applications for linkage between NHFA and CPRD GOLD were ongoing. NICOR provided the unlinked NHFA data set to the study team.
As described previously, we explored different definitions for classifying patients to BNP exposure groups. The final classification resulted in a very small number of patients being classified as BNP monitored, relative to the size of the overall cohort. Therefore, the formal analyses comparing outcomes between groups (detailed in the statistical analysis plan, which is available from the authors) were not conducted. We decided that these analyses would be misleading: the treatment effects for the BNP-monitored cohort would be extremely imprecise, the treatment effects for the larger BNP-tested cohort were not relevant to the objectives of the study and the characteristics of exposure groups suggest that the BNP-monitored group is highly selected for reasons that we were not able to identify.
Results
Study population and characteristics
Figure 29 shows the total number of patients with HF identified in the CPRD using the three HF incidence algorithms. A total of 40,073 patients met the inclusion criteria for incident HF using algorithm 1. Of these, 20,367 patients met the inclusion criteria for incident HF using algorithm 2 and 17,095 met the inclusion criteria for incident HF using algorithm 3 (main study cohort). There was a steady increase in the cumulative number of patients identified with incident HF in the CPRD over time (Figure 30), indicating that the rate at which new patients accrued to the cohort was constant. The number of BNP tests increased slightly faster over time than the number of patients (Figure 31).
FIGURE 29.
Flow diagram of the study population with HF defined using three incidence algorithms. a, Includes 52 patients who met incidence definition 1 but were aged < 18 years at time of incidence; b, There were 47 patients who had incident HF in the main cohort but did not meet the criteria for incident HF using algorithms 1 and 2. These patients had HF symptom Read Codes in the year prior to the HF-specific Read Code, preventing them from having 1 year free from Read Codes when including both the HF symptom and HF-specific Read Codes.

FIGURE 30.
Cumulative number of patients with HF over the study period in the CPRD.

FIGURE 31.
Number of BNP tests over time during the study period in the CPRD.
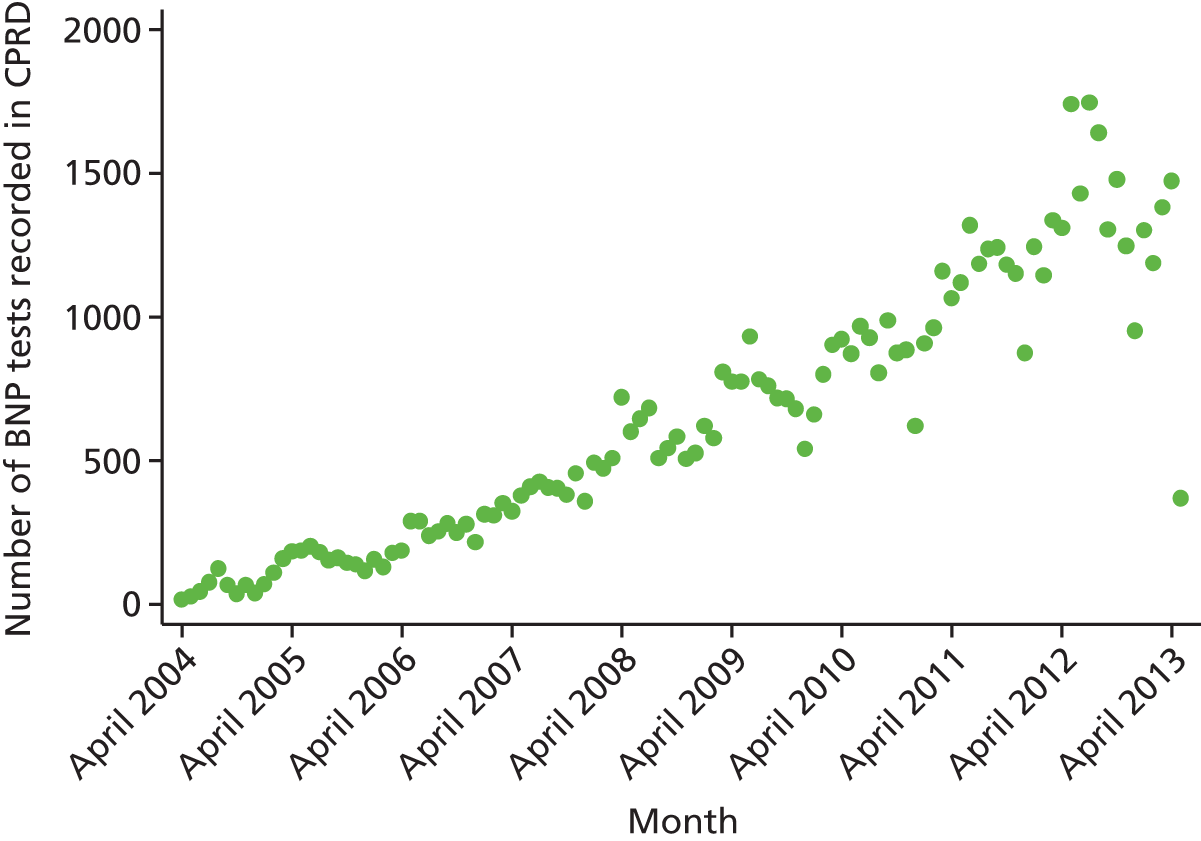
Table 9 shows the characteristics of the main study cohort (overall and stratified by BNP testing) and the cohort defined using incidence algorithm 2. The characteristics of the cohorts defined by algorithms 2 and 3 were very similar. There were extreme differences in the sizes of the exposure groups; 13,632 were classified as never tested, 3392 as BNP tested and only 71 as BNP monitored. Compared with patients who were never tested, patients who were tested were on average older and more likely to be female. Patients who were monitored were even older and more likely to be female; in addition, a lower proportion were classified as overweight or obese (BMI ≥ 25 kg/m2). Based on the Charlson Comorbidity Index, comorbidities appeared to be similar between exposure groups but there were some marked differences in proportions with specific morbidities between groups.
Baseline demographic and clinical characteristics and medical history | Never tested (n = 13,632) | BNP tested (n = 3392) | BNP monitored (n = 71) | Overall (N = 17,095) | Cohort using incidence algorithm 2 (N = 20,367) (%) |
---|---|---|---|---|---|
Age at incidence (years), median (IQR) | 77.4 (68.8–83.9) | 79.8 (72.6–85.2) | 82.0 (75.0–85.7) | 77.9 (69.7–84.2) | 77.5 (69.2–83.9) |
Aged ≥ 75 years, n/N (%) | 7994/13,632 (58.6) | 2273/3392 (67.0) | 54/71 (76.1) | 10,321/17,095 (60.4) | 12,014/20,367 (59.0) |
Male, n/N (%) | 7798/13,632 (57.2) | 1759/3392 (51.9) | 29/71 (40.8) | 9586/17,095 (56.1) | 11,488/20,367 (56.4) |
BMI (kg/m2), median (IQR) | 28.2 (24.8–32.6) | 28.5 (25.0–33.2) | 27.1 (24.1–31.1) | 28.3 (24.8–32.7) | 28.4 (24.9–32.6) |
Score, n/N (%) | |||||
< 20 | 228/6055 (3.8) | 56/1613 (3.5) | 3/40 (7.5) | 287/7708 (3.7) | 367/9360 (3.9) |
≥ 20 and < 25 | 1338/6055 (22.1) | 342/1613 (21.2) | 11/40 (27.5) | 1691/7708 (21.9) | 1987/9360 (21.2) |
≥ 25 and < 30 | 2101/6055 (34.7) | 553/1613 (34.3) | 13/40 (32.5) | 2667/7708 (34.6) | 3281/9360 (35.1) |
≥ 30 | 2388/6055 (39.4) | 662/1613 (41.0) | 13/40 (32.5) | 3063/7708 (39.7) | 3725/9360 (39.8) |
SBP, mmHg (mean, SD) | 134 (20) | 135 (20) | 134 (20) | 134 (20) | 134 (20) |
DBP, mmHg (mean, SD) | 75.4 (12) | 75.6 (12) | 74.7 (12) | 75.5 (12) | 75.5 (12) |
Number of patients with baseline BNP or NT-proBNP, n/N (%) | 0/1363 (2) | 2719/3392 (80) | 61/71 (86) | 2780/17,095 (16) | 3491/20,367 (17) |
BNP (pg/ml),a median (IQR) | – | 730 (286–2042) | 866 (408–1682) | 734 (289–2032) | 668 (249–1898) |
NT-proBNP (pg/ml),b median (IQR) | – | 1633 (681–3245) | – | 1633 (681–3245) | 1547 (626–3247) |
Deprivation score (quintile), n/N (%) | |||||
1 | 1893/13,632 (13.9) | 492/3392 (14.5) | 7/71 (9.9) | 2392/17,095 (14.0) | 2824/20,367 (13.9) |
2 | 3009/13,632 (22.1) | 767/3392 (22.6) | 17/71 (23.9) | 3793/17,095 (22.2) | 4626/20,367 (22.7) |
3 | 2938/13,632 (21.6) | 755/3392 (22.3) | 23/71 (32.4) | 3716/17,095 (21.7) | 4289/20,367 (21.1) |
4 | 3194/13,632 (23.4) | 771/3392 (22.7) | 8/71 (11.3) | 3973/17,095 (23.2) | 4746/20,367 (23.3) |
5 | 2598/13,632 (19.1) | 607/3392 (17.9) | 16/71 (22.5) | 3221/17,095 (18.8) | 3882/20,367 (19.1) |
Medical history (in the year prior to HF incidence), n/N (%) | |||||
Previous MI | 1890/13,632 (13.9) | 175/3392 (5.2) | 1/71 (1.4) | 2066/17,095 (12.1) | 2479/20,367 (12.2) |
Previous PCI | 441/13,632 (3.2) | 42/3392 (1.2) | 0/71 (0.0) | 483/17,095 (2.8) | 640/20,367 (3.1) |
Previous CABG | 205/13,632 (1.5) | 32/3392 (0.9) | 2/71 (2.8) | 239/17,095 (1.4) | 300/20,367 (1.5) |
Medical history – other | |||||
Previous AF, n/N (%) | 2767/13,632 (20.3) | 581/3392 (17.1) | 8/71 (11.3) | 3356/17,095 (19.6) | 3811/20,367 (18.7) |
Ischaemic heart disease, n/N (%) | 2572/13,632 (18.9) | 280/3392 (8.3) | 4/71 (5.6) | 2856/17,095 (16.7) | 3403/20,367 (16.7) |
Arrhythmias, n/N (%) | 3068/13,632 (22.5) | 629/3392 (18.5) | 11/71 (15.5) | 3708/17,095 (21.7) | 4239/20,367 (20.8) |
Stroke, n/N (%) | 260/13,632 (1.9) | 40/3392 (1.2) | 0/71 (0.0) | 300/17,095 (1.8) | 339/20,367 (1.7) |
Diabetes, n/N (%) | 557/13,632 (4.1) | 124/3392 (3.7) | 6/71 (8.5) | 687/17,095 (4.0) | 808/20,367 (4.0) |
Renal disease, n/N (%) | 1380/13,632 (10.1) | 386/3392 (11.4) | 10/71 (14.1) | 1776/17,095 (10.4) | 2034/20,367 (10.0) |
COPD, n/N (%) | 854/13,632 (6.3) | 209/3392 (6.2) | 1/71 (1.4) | 1064/17,095 (6.2) | 1236/20,367 (6.1) |
Charlson Comorbidity Index, median (IQR) | 0.0 (0.0–1.0) | 0.0 (0.0–1.0) | 0.0 (0.0–1.0) | 0.0 (0.0–1.0) | 0.0 (0.0–1.0) |
Score, n/N (%) | |||||
0 | 8449/13,632 (62.0) | 2186/3392 (64.4) | 44/71 (62.0) | 10,679/17,095 (62.5) | 12,944/20,367 (63.6) |
1 | 2942/13,632 (21.6) | 629/3392 (18.5) | 15/71 (21.1) | 3586/17,095 (21.0) | 4179/20,367 (20.5) |
2 | 1466/13,632 (10.8) | 378/3392 (11.1) | 8/71 (11.3) | 1852/17,095 (10.8) | 2140/20,367 (10.5) |
3 | 576/13,632 (4.2) | 157/3392 (4.6) | 3/71 (4.2) | 736/17,095 (4.3) | 836/20,367 (4.1) |
4 | 125/13,632 (0.9) | 30/3392 (0.9) | 1/71 (1.4) | 156/17,095 (0.9) | 165/20,367 (0.8) |
5 | 53/13,632 (0.4) | 11/3392 (0.3) | 0/71 (0.0) | 64/17,095 (0.4) | 73/20,367 (0.4) |
≥ 6 | 21/13,632 (0.2) | 1/3392 (0.0) | 0/71 (0.0) | 22/17,095 (0.1) | 30/20,367 (0.1) |
Figure 32 shows the timing of BNP tests in the 71 patients identified as BNP monitored. There was no obvious pattern in the timing or frequency of BNP tests: in some patients testing was carried out monthly and in other patients testing was the minimum required frequency of tests that allowed them to be classified as monitored.
FIGURE 32.
Timing of BNP tests in the BNP monitored group. Crosses represent BNP tests; filled black circles represent the end of follow-up for each patient.
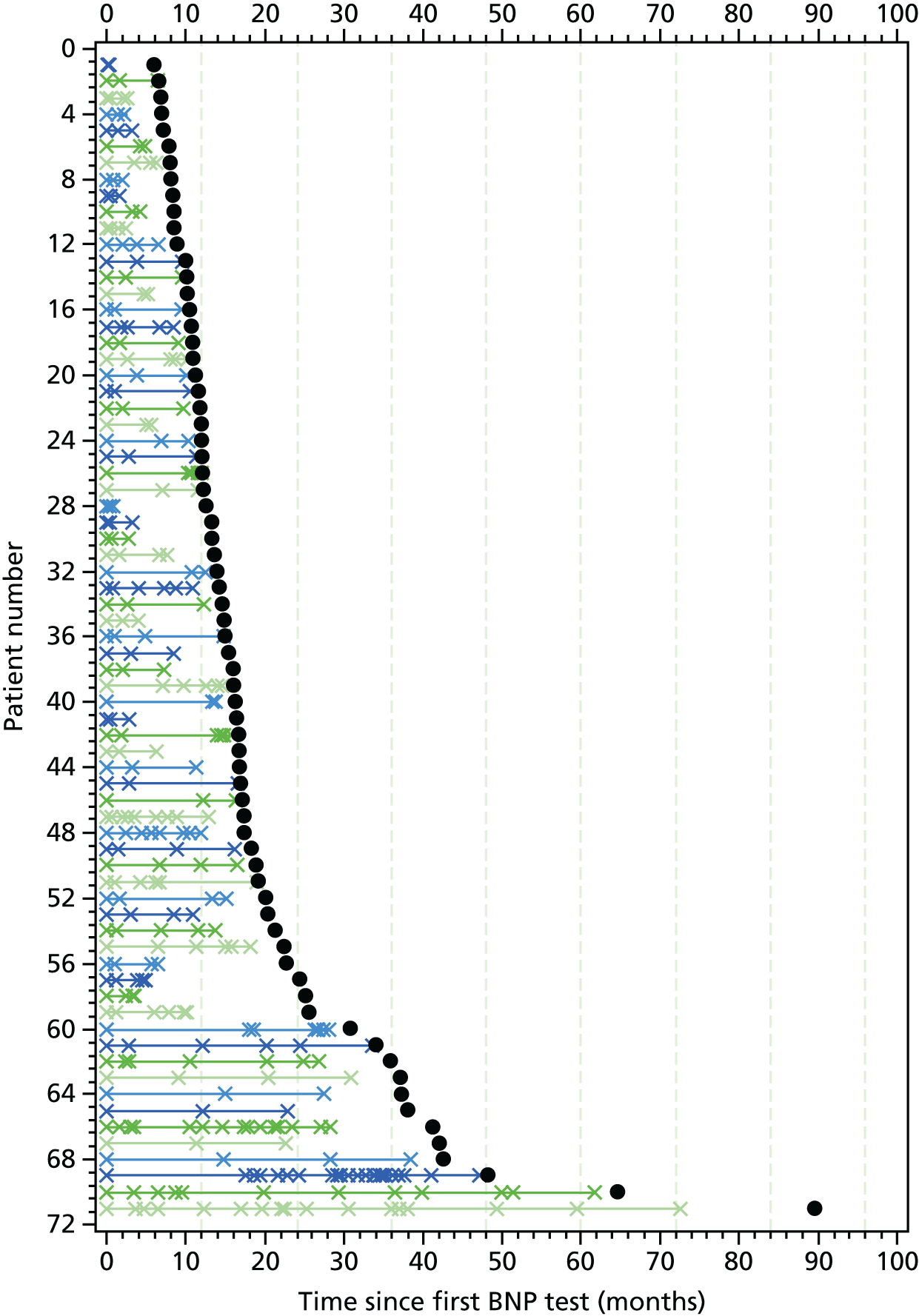
Primary outcome: all-cause mortality
Of the 17,095 patients in the main study cohort, 164 (1%) were assigned a HF diagnosis only at the time of death.
Table 10 shows the rates of death from any cause for the cohorts defined using incidence algorithms 2 and 3 (main study cohort), overall and stratified by BNP testing. Overall, in the main study cohort, 8311 out of 17,095 (49%) patients died during follow-up (median 3.0 years, IQR 1.5–5.1 years), generating a crude death rate of 141.5 (95% CI 138.5 to 144.6) per 1000 person-years. For patients who died, the median time (IQR) between incident HF and death was 1.9 years (0.7–3.5 years). The death rate was higher in the small group of patients who were defined as BNP monitored than in those who were classified as BNP tested or never tested (186.5 vs. 130.6 and 186.5 vs. 143.9 per 1000 patient-years, respectively). The results were similar for the cohort defined using incidence algorithm 2. Death rates were slightly higher in men than women across all age groups (Table 11).
Death from any cause by BNP exposure group | Number of deaths, n/N (%) | Patient-years of observation | Number of deaths | Death rate per 1000 patient-years (95% CI) |
---|---|---|---|---|
Main study cohort (defined using incidence algorithm 3) | ||||
Overall | 8311/17,095 (49) | 58,721 | 8311 | 141.5 (138.5 to 144.6) |
Never tested | 6844/13,632 (50) | 47,577 | 6844 | 143.9 (140.5 to 147.3) |
BNP tested | 1430/3392 (42) | 10,946 | 1430 | 130.6 (124.0 to 137.6) |
BNP monitored | 37/71 (52) | 198 | 37 | 186.5 (135.1 to 257.4) |
Cohort (defined using incidence algorithm 2) | ||||
Overall | 9469/20,367 (46) | 70,855 | 9469 | 133.6 (131.0 to 136.4) |
Never tested | 7706/16,074 (48) | 57,072 | 7706 | 135.0 (132.0 to 138.1) |
BNP tested | 1716/4205 (41) | 13,533 | 1716 | 126.8 (120.9 to 132.9) |
BNP monitored | 47/88 (53) | 249 | 47 | 188.6 (141.7 to 251.0) |
Sex | Age group (years) | ||||
---|---|---|---|---|---|
< 50 | 50–60 | 60–70 | 70–80 | > 80 | |
Male | 37 (28 to 49) | 47 (40 to 54) | 71 (65 to 76) | 113 (108 to 119) | 229 (221 to 237) |
Female | 32 (25 to 43) | 41 (35 to 48) | 62 (57 to 67) | 99 (94 to 105) | 201 (194 to 208) |
Figure 33 shows the Kaplan–Meier survival curves for death from any cause in the main study cohort, stratified by BNP testing. In the never tested group, 84% of patients were alive at 1 year after HF diagnosis, 74% at 2 years, 64% at 3 years and 56% at 4 years. In the BNP-tested group, 85%, 76%, 67% and 60% of patients were alive at 1, 2, 3 and 4 years after HF diagnosis, respectively. In the BNP-monitored group, 86%, 72%, 57% and 44% of patients were alive at 1, 2, 3 and 4 years post incidence, respectively. The results of the SA using the cohort defined using algorithm 2 (more sensitive) are consistent with those for the main study cohort.
FIGURE 33.
Kaplan–Meier survival curves for death from any cause in the main study cohort, stratified by BNP testing.

Secondary outcome: mortality caused by heart failure and cardiovascular causes
Table 12 shows the rates of death due to HF and cardiovascular causes in the main study cohort, overall and stratified by BNP testing. Rates of death due to HF and cardiovascular causes were higher in patients who were monitored than in patients who were never tested (100.8 vs. 61.9 for death due to HF; 166.4 vs. 114.2 for death due to cardiovascular causes) and patients who were tested (100.8 vs. 59.1 for death due to HF; 166.4 vs. 104.9 for death due to cardiovascular causes). The cumulative incidence of death due to HF and death due to cardiovascular causes, stratified by BNP testing, are shown in Figures 34 and 35, respectively. Patients who were monitored had higher cumulative incidences of deaths.
Cause of death by BNP exposure group | Number of deaths, n/N (%) | Death rate per 1000 patient-years (95% CI) |
---|---|---|
Death due to HF | ||
Overall | 3633/17,095 (21) | 61.9 (59.9 to 63.9) |
Never tested | 2966/13,632 (22) | 62.3 (60.1 to 64.6) |
BNP tested | 647/3392 (19) | 59.1 (54.7 to 63.8) |
BNP monitored | 20/71 (28) | 100.8 (65.0 to 156.3) |
Death due to cardiovascular causes | ||
Overall | 6707/17,095 (39) | 114.2 (111.5 to 117.0) |
Never tested | 5526/13,632 (41) | 116.1 (113.1 to 119.3) |
BNP tested | 1148/3392 (34) | 104.9 (99.0 to 111.1) |
BNP monitored | 33/71 (46) | 166.4 (118.3 to 234.0) |
FIGURE 34.
Cumulative incidence of death due to HF in the main study cohort stratified by BNP testing.

FIGURE 35.
Cumulative incidence of death due to cardiovascular causes in the main study cohort stratified by BNP testing.
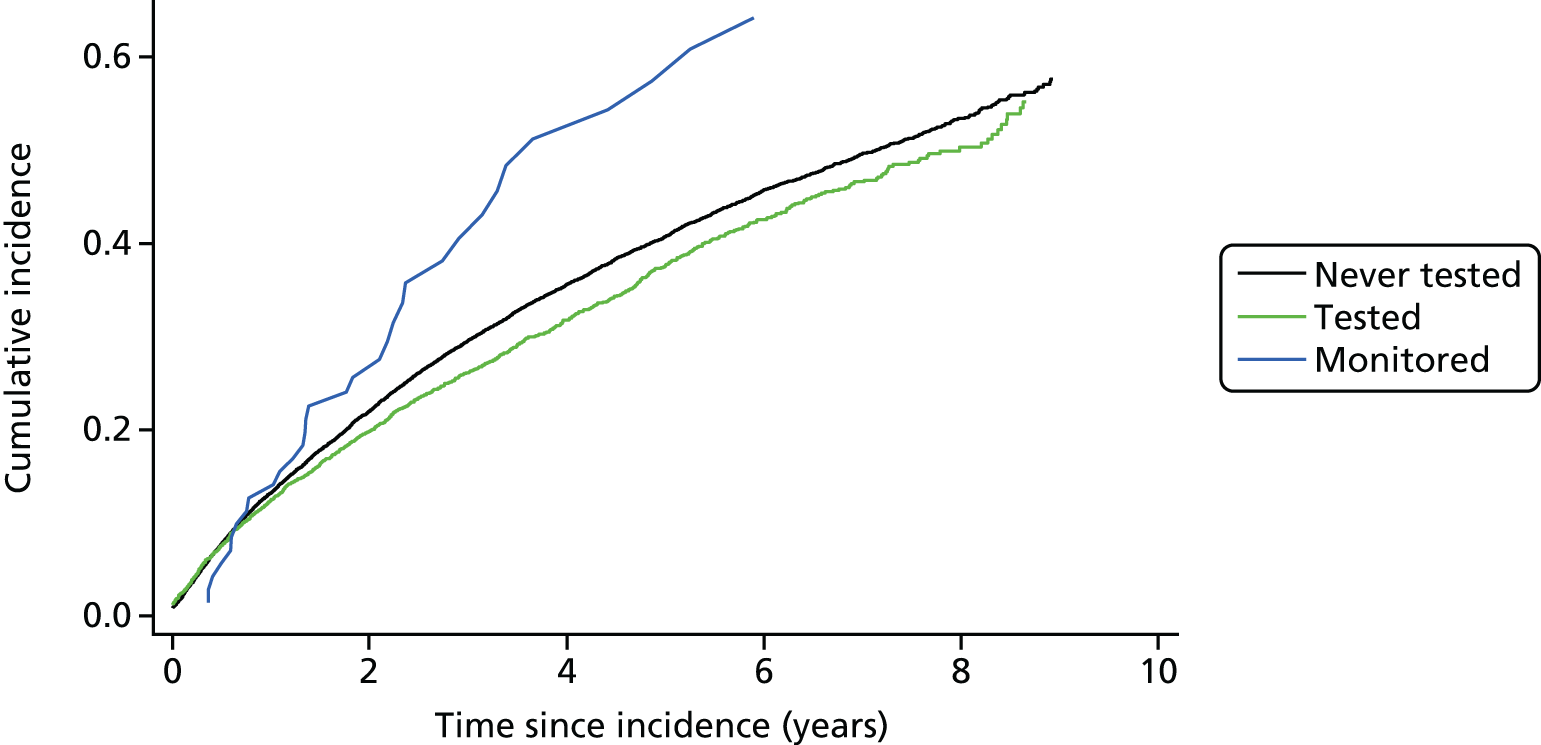
Secondary outcome: unscheduled hospital admissions
Table 13 shows the proportions of patients who were admitted to hospital during follow-up in the main study cohort. Overall, admission rates were worst for the BNP-monitored group and best for the BNP-tested group; the rates of admission were highest in the BNP-monitored group (for all-cause hospitalisation, 651 per 1000 patient-years; for HF-related hospitalisation, 345 per 1000 patient-years) and lowest in the BNP-tested group (438 per 1000 patient-years and 218 per 1000 patient-years, respectively, for all-cause and HF-related admissions).
Unscheduled hospital admissions by BNP exposure group | Number of patients with ≥ 1 admission, n/N (%) | Rate of patients with ≥ 1 admission per 1000 patient-years (95% CI) | Number of admissionsa (median, IQR) | Total number of days in hospital after all admissionsa (median, IQR) | Number of days hospitalised per yeara (median, IQR) |
---|---|---|---|---|---|
Unscheduled admissions for any cause | |||||
Overall | 13,180/17,095 (77) | 502.1 (493.6 to 510.7) | 2 (1–4) | 23 (8–51) | 8.8 (2.8–25.4) |
Never tested | 10,703/13,632 (79) | 518.5 (508.7 to 528.4) | 2 (1–4) | 23 (9–53) | 9.1 (2.9–26.1) |
BNP tested | 2422/3392 (71) | 438.4 (421.3 to 456.2) | 2 (1–4) | 19 (7–44) | 7.7 (2.4–21.6) |
BNP monitored | 55/71 (77) | 650.8 (499.7 to 847.7) | 2 (1–4) | 22 (9–58) | 8.8 (2.7–27.5) |
Unscheduled admissions for HF | |||||
Overall | 9611/17,095 (56) | 253.4 (248.4 to 258.5) | 2 (1–3) | 19 (8–42) | 7.7 (2.5–22.8) |
Never tested | 7887/13,632 (58) | 262.1 (256.4 to 267.9) | 2 (1–3) | 20 (8–43) | 7.9 (2.6–23.4) |
BNP tested | 1682/3392 (50) | 217.9 (207.8 to 228.6) | 2 (1–3) | 16 (7–37) | 6.8 (2.3–20.4) |
BNP monitored | 42/71 (59) | 345.3 (255.2 to 467.2) | 1.5 (1–3) | 18.5 (5–54) | 8.6 (2.0–26.7) |
Other statistics are shown in Table 13. The pattern across groups for the number of patients hospitalised for any cause was similar to that described above but less obviously so for hospitalisations because of HF. Statistics involving number of days admitted are difficult to interpret because some admissions will have resulted in death in hospital, potentially reducing the average length of admissions in the BNP-monitored group, which had a higher death rate.
Figures 36 and 37 show the cumulative incidence of unscheduled hospital admission for all causes and unscheduled hospital admissions for HF, respectively. Patients who were monitored were more likely to be admitted to hospital than patients who were tested and never tested. There was a slightly lower incidence of both all-cause and HF-related hospital admission among those who were tested than among those who were never tested.
FIGURE 36.
Cumulative incidence of unscheduled hospital admission (all cause) in the main study cohort stratified by BNP exposure.

FIGURE 37.
Cumulative incidence of unscheduled hospital admission (HF related) in the main study cohort stratified by BNP exposure.
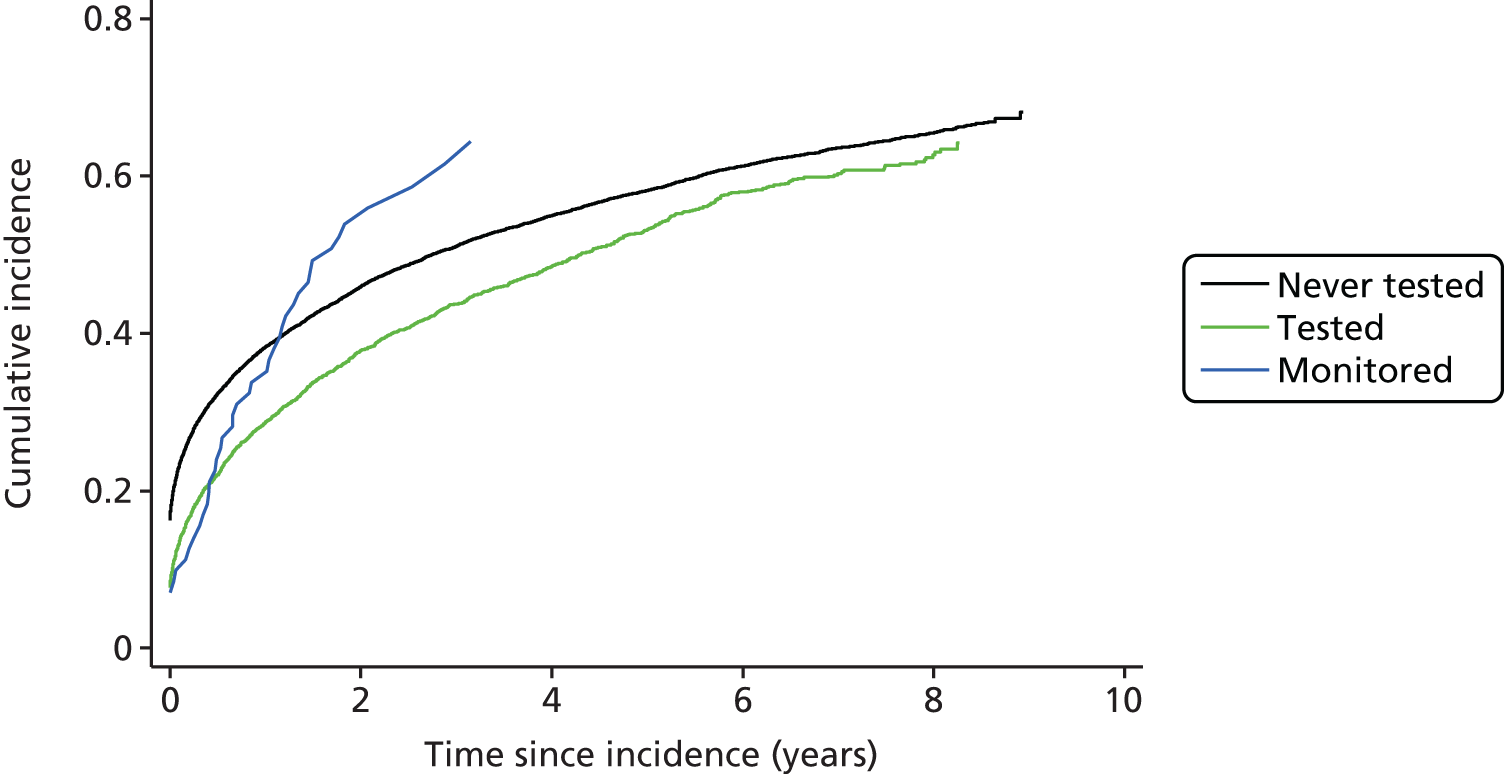
Secondary outcome: general practice consultations and outpatient appointments
Across the cohort, 98% of patients had at least one GP consultation (face to face or telephone). There were an average of 17 GP consultations per year (Table 14). Patients in the BNP-tested and never tested groups had a similar number of GP consultations (17 per year); this number was higher for patients in the BNP-monitored group (22 per year). Only 40% of patients in the cohort had GP consultations linked to a specific- or symptom-based HF code. The proportion of patients with at least one GP consultation linked to HF was higher in the BNP-monitored group (66%). The rate of GP consultations among those who had at least one consultation for HF was also higher in the monitored group (two consultations per year compared with less than one in the never tested and tested groups).
GP consultations by BNP exposure group | Number of patients with one or more GP consultation, n/N (%) | Rate of GP consultationsa (median, IQR) | Number of patients with one or more GP consultation for HF, n/N (%) | Rate of GP consultations for HF (median, IQR) (specific or symptom based) |
---|---|---|---|---|
Overall | 16,818/17,095 (98) | 16.7 (10.4–27.2) | 6886/17,095 (40) | 0.6 (0.3–1.4) |
Never tested | 13,428/13,632 (99) | 16.6 (10.3–27.3) | 5437/13,632 (40) | 0.6 (0.3–1.3) |
Blood pressure tested | 3319/3392 (98) | 16.7 (10.7–26.7) | 1402/3392 (41) | 0.7 (0.4–1.7) |
BNP monitored | 71/71 (100) | 22.4 (15.7–35.7) | 47/71 (66) | 1.8 (0.9–2.6) |
The majority of patients in the main study cohort had at least one outpatient appointment for any specialty (92% of patients) (Table 15), with similar proportions in each of the BNP groups. Similar yearly outpatient appointment rates were observed in the never tested and tested groups, with a slightly higher rate in the monitored group (5.9 tests per year vs. 4.4 and 4.3, respectively). There were similar proportions of patients in the three groups having outpatient appointments for the cardiology specialty.
OP appointments by BNP exposure group | Number of patients with one or more OP appointment (all treatment specialties), n/N (%) | Rate of OP appointmentsa (all treatment specialties), median (IQR) | Number of patients with one or more OP appointment (cardiology specialty), n/N (%) | Rate of OP appointmentsa (cardiology specialty), median, (IQR) |
---|---|---|---|---|
Overall | 15,648/17,095 (92) | 4.4 (2.1–8.3) | 11,090/17,095 (65) | 1.3 (0.6–2.6) |
Never tested | 12,509/13,632 (92) | 4.4 (2.1–8.4) | 8872/13,632 (65) | 1.3 (0.6–2.6) |
BNP tested | 3073/3392 (91) | 4.3 (2.1–7.7) | 2171/3392 (64) | 1.3 (0.6–2.5) |
BNP monitored | 66/71 (93) | 5.9 (2.9–11.2) | 47/71 (66) | 1.8 (0.7–3.7) |
Secondary outcome: heart failure-related investigations (B-type natriuretic peptide and echocardiography tests)
The annual rates of HF-related investigations are shown in Table 16. The rate of HF-related investigations was low, with only 52% of patients having one or more echocardiogram or BNP test recorded after cohort entry. Only 49% of patients in the never tested group had a record of a HF-related investigation after incidence, compared with 63% of patients in the tested group and 97% of patients in the monitored group.
HF-related investigations by BNP exposure group | Number of patients with one or more test (BNP or echocardiography), n/N (%) | Rate of any testa (BNP or echocardiography), median (IQR) | Number of patients with one or more BNP test, n/N (%) | Rate of BNP tests,a median (IQR) | Number of patients with one or more echocardiography test, n/N (%) | Rate of echocardiography,a median (IQR) |
---|---|---|---|---|---|---|
Overall | 8934/17,095 (52) | 0.6 (0.3–1.1) | 1535/17,095 (9) | 0.4 (0.3–0.9) | 8290/17,095 (4) | 0.5 (0.3–1.0) |
Never tested | 6718/13,632 (49) | 0.5 (0.3–1.1) | 0/13,632 (0) | – | 6718/13,632 (4) | 0.5 (0.3–1.1) |
Tested | 2147/3392 (63) | 0.7 (0.4–1.3) | 1468/3392 (43) | 0.4 (0.3–0.9) | 1539/3392 (4) | 0.5 (0.3–1.0) |
Monitored | 69/71 (97) | 2.4 (1.6–3.6) | 67/71 (94) | 2.0 (1.4–2.7) | 33/71 (46) | 0.8 (0.4–1.5) |
Table 17 shows the proportion of patients on different medications at the time of incident HF, overall and stratified by BNP exposure group.
HF medications | Never tested (n = 13,632), n/N (%) | BNP tested (n = 3392), n/N (%) | BNP monitored (n = 71), n/N (%) | Overall (N = 17,095), n/N (%) |
---|---|---|---|---|
ACEis | 6042/13,632 (44.3) | 1544/3392 (45.5) | 33/71 (46.5) | 7619/17,095 (44.6) |
ARBs | 2088/13,632 (15.3) | 582/3392 (17.2) | 21/71 (29.6) | 2691/17,095 (15.7) |
Beta-blockers | 3501/13,632 (25.7) | 826/3392 (24.4) | 25/71 (35.2) | 4352/17,095 (25.5) |
Digoxin | 1927/13,632 (14.1) | 500/3392 (14.7) | 17/71 (23.9) | 2444/17,095 (14.3) |
Loop diuretics | 7280/13,632 (53.4) | 2110/3392 (62.2) | 49/71 (69.0) | 9439/17,095 (55.2) |
Thiazide diuretics | 1197/13,632 (8.8) | 370/3392 (10.9) | 7/71 (9.9) | 1574/17,095 (9.2) |
Hydralazine | 35/13,632 (0.3) | 12/3392 (0.4) | 0/71 (0.0) | 47/17,095 (0.3) |
Isosorbide | 1647/13,632 (12.1) | 341/3392 (10.1) | 8/71 (11.3) | 1996/17,095 (11.7) |
Spironolactone | 1028/13,632 (7.5) | 236/3392 (7.0) | 9/71 (12.7) | 1273/17,095 (7.4) |
Eplerenone | 69/13,632 (0.5) | 15/3392 (0.4) | 2/71 (2.8) | 86/17,095 (0.5) |
Amiloride | 304/13,632 (2.2) | 96/3392 (2.8) | 0/71 (0.0) | 400/17,095 (2.3) |
Triamterene | 11/13,632 (0.1) | 2/3392 (0.1) | 0/71 (0.0) | 13/17,095 (0.1) |
Calcium channel blockers | 2600/13,632 (19.1) | 794/3392 (23.4) | 17/71 (23.9) | 3411/17,095 (20.0) |
Statins | 6835/13,632 (50.1) | 1674/3392 (49.4) | 41/71 (57.7) | 8550/17,095 (50.0) |
Anticoagulants | 2650/13,632 (19.4) | 720/3392 (21.2) | 24/71 (33.8) | 3394/17,095 (19.9) |
Aspirin | 5590/13,632 (41.0) | 1360/3392 (40.1) | 30/71 (42.3) | 6980/17,095 (40.8) |
Table 18 shows how these proportions changed during time since diagnosis of incident HF. The denominators decrease with increasing time since diagnosis of incident HF because the observation time for an increasing proportion of cohort participants stopped because of death or loss to follow-up.
HF medication | Never tested (n = 13,632) | BNP tested (n = 3392) | BNP monitored (n = 71) | Overall (N = 17,095) |
---|---|---|---|---|
ACEis | ||||
0–6 months | ||||
Number of patients, n/N (%) | 10,087/13,632 (74.0) | 2454/3392 (72.3) | 45/71 (63.4) | 12,586/17,095 (73.6) |
Proportion of time on medication, median (IQR) | 1.0 (0.8–1.0) | 1.0 (0.8–1.0) | 1.0 (0.9–1.0) | 1.0 (0.8–1.0) |
6–12 months | ||||
Number of patients, n/N (%) | 7706/11,673 (66.0) | 1770/2793 (63.4) | 34/66 (51.5) | 9510/14,532 (65.4) |
Proportion of time on medication, median (IQR) | 1.0 (1.0–1.0) | 1.0 (1.0–1.0) | 1.0 (0.9–1.0) | 1.0 (1.0–1.0) |
12–18 months | ||||
Number of patients, n/N (%) | 6275/9958 (63.0) | 1423/2343 (60.7) | 20/50 (40.0) | 7718/12,351 (62.5) |
Proportion of time on medication, median (IQR) | 1.0 (1.0–1.0) | 1.0 (1.0–1.0) | 1.0 (1.0–1.0) | 1.0 (1.0–1.0) |
18–24 months | ||||
Number of patients, n/N (%) | 5272/8508 (62.0) | 1188/1990 (59.7) | 13/31 (41.9) | 6473/10,529 (61.5) |
Proportion of time on medication, median (IQR) | 1.0 (1.0–1.0) | 1.0 (1.0–1.0) | 1.0 (1.0–1.0) | 1.0 (1.0–1.0) |
24–30 months | ||||
Number of patients, n/N (%) | 4420/7260 (60.9) | 973/1659 (58.6) | 12/24 (50.0) | 5405/8943 (60.4) |
Proportion of time on medication, median (IQR) | 1.0 (1.0–1.0) | 1.0 (1.0–1.0) | 1.0 (1.0–1.0) | 1.0 (1.0–1.0) |
30–36 months | ||||
Number of patients, n/N (%) | 3702/6127 (60.4) | 793/1380 (57.5) | 9/18 (50.0) | 4504/7525 (59.9) |
Proportion of time on medication, median (IQR) | 1.0 (1.0–1.0) | 1.0 (1.0–1.0) | 1.0 (1.0–1.0) | 1.0 (1.0–1.0) |
36–42 months | ||||
Number of patients, n/N (%) | 3055/5084 (60.1) | 654/1149 (56.9) | 3/13 (23.1) | 3712/6246 (59.4) |
Proportion of time on medication, median (IQR) | 1.0 (1.0–1.0) | 1.0 (1.0–1.0) | 1.0 (0.8–1.0) | 1.0 (1.0–1.0) |
42–48 months | ||||
Number of patients, n/N (%) | 2505/4229 (59.2) | 519/930 (55.8) | 1/8 (12.5) | 3025/5167 (58.5) |
Proportion of time on medication, median (IQR) | 1.0 (1.0–1.0) | 1.0 (1.0–1.0) | 1.0 (1.0–1.0) | 1.0 (1.0–1.0) |
> 48 months | ||||
Number of patients, n/N (%) | 2094/3478 (60.2) | 413/730 (56.6) | 2/7 (28.6) | 2509/4215 (59.5) |
Proportion of time on medication, median (IQR) | 1.0 (1.0–1.0) | 1.0 (0.9–1.0) | 0.5 (0.0–1.0) | 1.0 (0.9–1.0) |
ARBs | ||||
0–6 months | ||||
Number of patients, n/N (%) | 2992/13,632 (21.9) | 827/3392 (24.4) | 26/71 (36.6) | 3845/17,095 (22.5) |
Proportion of time on medication, median (IQR) | 1.0 (0.6–1.0) | 1.0 (0.7–1.0) | 1.0 (0.5–1.0) | 1.0 (0.6–1.0) |
6–12 months | ||||
Number of patients, n/N (%) | 2627/11,673 (22.5) | 699/2793 (25.0) | 21/66 (31.8) | 3347/14,532 (23.0) |
Proportion of time on medication, median (IQR) | 1.0 (1.0–1.0) | 1.0 (1.0–1.0) | 1.0 (1.0–1.0) | 1.0 (1.0–1.0) |
12–18 months | ||||
Number of patients, n/N (%) | 2323/9958 (23.3) | 612/2343 (26.1) | 17/50 (34.0) | 2952/12,351 (23.9) |
Proportion of time on medication, median (IQR) | 1.0 (1.0–1.0) | 1.0 (1.0–1.0) | 1.0 (1.0–1.0) | 1.0 (1.0–1.0) |
18–24 months | ||||
Number of patients, n/N (%) | 2053/8508 (24.1) | 527/1990 (26.5) | 11/31 (35.5) | 2591/10,529 (24.6) |
Proportion of time on medication, median (IQR) | 1.0 (1.0–1.0) | 1.0 (1.0–1.0) | 1.0 (1.0–1.0) | 1.0 (1.0–1.0) |
24–30 months | ||||
Number of patients, n/N (%) | 1790/7260 (24.7) | 463/1659 (27.9) | 9/24 (37.5) | 2262/8943 (25.3) |
Proportion of time on medication, median (IQR) | 1.0 (1.0–1.0) | 1.0 (1.0–1.0) | 1.0 (1.0–1.0) | 1.0 (1.0–1.0) |
30–36 months | ||||
Number of patients, n/N (%) | 1559/6127 (25.4) | 383/1380 (27.8) | 8/18 (44.4) | 1950/7525 (25.9) |
Proportion of time on medication, median (IQR) | 1.0 (1.0–1.0) | 1.0 (1.0–1.0) | 1.0 (1.0–1.0) | 1.0 (1.0–1.0) |
36–42 months | ||||
Number of patients, n/N (%) | 1312/5084 (25.8) | 316/1149 (27.5) | 7/13 (53.8) | 1635/6246 (26.2) |
Proportion of time on medication, median (IQR) | 1.0 (1.0–1.0) | 1.0 (1.0–1.0) | 1.0 (0.7–1.0) | 1.0 (1.0–1.0) |
42–48 months | ||||
Number of patients, n/N (%) | 1123/4229 (26.6) | 275/930 (29.6) | 4/8 (50.0) | 1402/5167 (27.1) |
Proportion of time on medication, median (IQR) | 1.0 (1.0–1.0) | 1.0 (1.0–1.0) | 1.0 (1.0–1.0) | 1.0 (1.0–1.0) |
> 48 months | ||||
Number of patients, n/N (%) | 982/3478 (28.2) | 232/730 (31.8) | 4/7 (57.1) | 1218/4215 (28.9) |
Proportion of time on medication, median (IQR) | 1.0 (0.9–1.0) | 1.0 (0.9–1.0) | 1.0 (0.8–1.0) | 1.0 (0.9–1.0) |
Beta-blockers | ||||
0–6 months | ||||
Number of patients, n/N (%) | 7864/13632 (57.7) | 1783/3392 (52.6) | 37/71 (52.1) | 9684/17,095 (56.6) |
Proportion of time on medication, median (IQR) | 0.9 (0.7–1.0) | 1.0 (0.7–1.0) | 1.0 (1.0–1.0) | 0.9 (0.7–1.0) |
6–12 months | ||||
Number of patients, n/N (%) | 6631/11,673 (56.8) | 1421/2793 (50.9) | 34/66 (51.5) | 8086/14,532 (55.6) |
Proportion of time on medication, median (IQR) | 1.0 (1.0–1.0) | 1.0 (1.0–1.0) | 1.0 (0.9–1.0) | 1.0 (1.0–1.0) |
12–18 months | ||||
Number of patients, n/N (%) | 5640/9958 (56.6) | 1198/2343 (51.1) | 23/50 (46.0) | 6861/12,351 (55.6) |
Proportion of time on medication, median (IQR) | 1.0 (1.0–1.0) | 1.0 (1.0–1.0) | 1.0 (1.0–1.0) | 1.0 (1.0–1.0) |
18–24 months | ||||
Number of patients, n/N (%) | 4853/8508 (57.0) | 1006/1990 (50.6) | 15/31 (48.4) | 5874/10,529 (55.8) |
Proportion of time on medication, median (IQR) | 1.0 (1.0–1.0) | 1.0 (1.0–1.0) | 1.0 (1.0–1.0) | 1.0 (1.0–1.0) |
24–30 months | ||||
Number of patients, n/N (%) | 4136/7260 (57.0) | 840/1659 (50.6) | 14/24 (58.3) | 4990/8943 (55.8) |
Proportion of time on medication, median (IQR) | 1.0 (1.0–1.0) | 1.0 (1.0–1.0) | 1.0 (1.0–1.0) | 1.0 (1.0–1.0) |
30–36 months | ||||
Number of patients, n/N (%) | 3473/6127 (56.7) | 690/1380 (50.0) | 13/18 (72.2) | 4176/7525 (55.5) |
Proportion of time on medication, median (IQR) | 1.0 (1.0–1.0) | 1.0 (1.0–1.0) | 1.0 (0.8–1.0) | 1.0 (1.0–1.0) |
36–42 months | ||||
Number of patients, n/N (%) | 2898/5084 (57.0) | 586/1149 (51.0) | 11/13 (84.6) | 3495/6246 (56.0) |
Proportion of time on medication, median (IQR) | 1.0 (1.0–1.0) | 1.0 (1.0–1.0) | 1.0 (0.5–1.0) | 1.0 (1.0–1.0) |
42–48 months | ||||
Number of patients, n/N (%) | 2407/4229 (56.9) | 485/930 (52.2) | 5/8 (62.5) | 2897/5167 (56.1) |
Proportion of time on medication, median (IQR) | 1.0 (1.0–1.0) | 1.0 (1.0–1.0) | 1.0 (1.0–1.0) | 1.0 (1.0–1.0) |
> 48 months | ||||
Number of patients, n/N (%) | 2129/3478 (61.2) | 409/730 (56.0) | 6/7 (85.7) | 2544/4215 (60.4) |
Proportion of time on medication, median (IQR) | 1.0 (0.9–1.0) | 1.0 (0.9–1.0) | 1.0 (1.0–1.0) | 1.0 (0.9–1.0) |
Digoxin | ||||
0–6 months | ||||
Number of patients, n/N (%) | 3739/13,632 (27.4) | 853/3392 (25.1) | 22/71 (31.0) | 4614/17,095 (27.0) |
Proportion of time on medication, median (IQR) | 1.0 (0.7–1.0) | 1.0 (0.7–1.0) | 1.0 (0.8–1.0) | 1.0 (0.7–1.0) |
6–12 months | ||||
Number of patients, n/N (%) | 2981/11,673 (25.5) | 666/2793 (23.8) | 23/66 (34.8) | 3670/14,532 (25.3) |
Proportion of time on medication, median (IQR) | 1.0 (1.0–1.0) | 1.0 (1.0–1.0) | 1.0 (0.9–1.0) | 1.0 (1.0–1.0) |
12–18 months | ||||
Number of patients, n/N (%) | 2501/9958 (25.1) | 556/2343 (23.7) | 18/50 (36.0) | 3075/12,351 (24.9) |
Proportion of time on medication, median (IQR) | 1.0 (1.0–1.0) | 1.0 (1.0–1.0) | 1.0 (1.0–1.0) | 1.0 (1.0–1.0) |
18–24 months | ||||
Number of patients, n/N (%) | 2103/8508 (24.7) | 477/1990 (24.0) | 9/31 (29.0) | 2589/10,529 (24.6) |
Proportion of time on medication, median (IQR) | 1.0 (1.0–1.0) | 1.0 (1.0–1.0) | 1.0 (1.0–1.0) | 1.0 (1.0–1.0) |
24–30 months | ||||
Number of patients, n/N (%) | 1776/7260 (24.5) | 389/1659 (23.4) | 8/24 (33.3) | 2173/8943 (24.3) |
Proportion of time on medication, median (IQR) | 1.0 (1.0–1.0) | 1.0 (1.0–1.0) | 1.0 (1.0–1.0) | 1.0 (1.0–1.0) |
30–36 months | ||||
Number of patients, n/N (%) | 1480/6127 (24.2) | 316/1380 (22.9) | 6/18 (33.3) | 1802/7525 (23.9) |
Proportion of time on medication, median (IQR) | 1.0 (1.0–1.0) | 1.0 (1.0–1.0) | 1.0 (1.0–1.0) | 1.0 (1.0–1.0) |
36–42 months | ||||
Number of patients, n/N (%) | 1227/5084 (24.1) | 250/1149 (21.8) | 5/13 (38.5) | 1482/6246 (23.7) |
Proportion of time on medication, median (IQR) | 1.0 (1.0–1.0) | 1.0 (1.0–1.0) | 1.0 (1.0–1.0) | 1.0 (1.0–1.0) |
42–48 months | ||||
Number of patients, n/N (%) | 1046/4229 (24.7) | 205/930 (22.0) | 3/8 (37.5) | 1254/5167 (24.3) |
Proportion of time on medication, median (IQR) | 1.0 (1.0–1.0) | 1.0 (1.0–1.0) | 1.0 (0.6–1.0) | 1.0 (1.0–1.0) |
> 48 months | ||||
Number of patients, n/N (%) | 931/3478 (26.8) | 178/730 (24.4) | 2/7 (28.6) | 1111/4215 (26.4) |
Proportion of time on medication, median (IQR) | 1.0 (0.9–1.0) | 1.0 (0.9–1.0) | 1.0 (1.0–1.0) | 1.0 (0.9–1.0) |
Loop diuretics | ||||
0–6 months | ||||
Number of patients, n/N (%) | 11,516/13,632 (84.5) | 2971/3392 (87.6) | 65/71 (91.5) | 14552/17,095 (85.1) |
Proportion of time on medication, median (IQR) | 1.0 (0.9–1.0) | 1.0 (0.9–1.0) | 1.0 (0.9–1.0) | 1.0 (0.9–1.0) |
6–12 months | ||||
Number of patients, n/N (%) | 9243/11,673 (79.2) | 2261/2793 (81.0) | 55/66 (83.3) | 11,559/14,532 (79.5) |
Proportion of time on medication, median (IQR) | 1.0 (1.0–1.0) | 1.0 (1.0–1.0) | 1.0 (0.9–1.0) | 1.0 (1.0–1.0) |
12–18 months | ||||
Number of patients, n/N (%) | 7612/9958 (76.4) | 1835/2343 (78.3) | 41/50 (82.0) | 9488/12,351 (76.8) |
Proportion of time on medication, median (IQR) | 1.0 (1.0–1.0) | 1.0 (1.0–1.0) | 1.0 (1.0–1.0) | 1.0 (1.0–1.0) |
18–24 months | ||||
Number of patients, n/N (%) | 6392/8508 (75.1) | 1531/1990 (76.9) | 24/31 (77.4) | 7947/10,529 (75.5) |
Proportion of time on medication, median (IQR) | 1.0 (1.0–1.0) | 1.0 (1.0–1.0) | 1.0 (1.0–1.0) | 1.0 (1.0–1.0) |
24–30 months | ||||
Number of patients, n/N (%) | 5322/7260 (73.3) | 1276/1659 (76.9) | 21/24 (87.5) | 6619/8943 (74.0) |
Proportion of time on medication, median (IQR) | 1.0 (1.0–1.0) | 1.0 (1.0–1.0) | 1.0 (1.0–1.0) | 1.0 (1.0–1.0) |
30–36 months | ||||
Number of patients, n/N (%) | 4423/6127 (72.2) | 1045/1380 (75.7) | 17/18 (94.4) | 5485/7525 (72.9) |
Proportion of time on medication, median (IQR) | 1.0 (1.0–1.0) | 1.0 (1.0–1.0) | 1.0 (1.0–1.0) | 1.0 (1.0–1.0) |
36–42 months | ||||
Number of patients, n/N (%) | 3609/5084 (71.0) | 869/1149 (75.6) | 11/13 (84.6) | 4489/6246 (71.9) |
Proportion of time on medication, median (IQR) | 1.0 (1.0–1.0) | 1.0 (1.0–1.0) | 1.0 (0.4–1.0) | 1.0 (1.0–1.0) |
42–48 months | ||||
Number of patients, n/N (%) | 2970/4229 (70.2) | 708/930 (76.1) | 7/8 (87.5) | 3685/5167 (71.3) |
Proportion of time on medication, median (IQR) | 1.0 (1.0–1.0) | 1.0 (1.0–1.0) | 1.0 (0.4–1.0) | 1.0 (1.0–1.0) |
> 48 months | ||||
Number of patients, n/N (%) | 2567/3478 (73.8) | 582/730 (79.7) | 7/7 (100) | 3156/4215 (74.9) |
Proportion of time on medication, median (IQR) | 1.0 (0.9–1.0) | 1.0 (0.9–1.0) | 0.6 (0.1–1.0) | 1.0 (0.9–1.0) |
Thiazide diuretics | ||||
0–6 months | ||||
Number of patients, n/N (%) | 1630/13,632 (12.0) | 459/3392 (13.5) | 10/71 (14.1) | 2099/17,095 (12.3) |
Proportion of time on medication, median (IQR) | 0.4 (0.1–1.0) | 0.5 (0.2–1.0) | 0.2 (0.1–0.6) | 0.4 (0.1–1.0) |
6–12 months | ||||
Number of patients, n/N (%) | 810/11,673 (6.9) | 234/2793 (8.4) | 5/66 (7.6) | 1049/14,532 (7.2) |
Proportion of time on medication, median (IQR) | 1.0 (0.4–1.0) | 1.0 (0.5–1.0) | 0.4 (0.2–0.4) | 1.0 (0.4–1.0) |
12–18 months | ||||
Number of patients, n/N (%) | 650/9958 (6.5) | 197/2343 (8.4) | 2/50 (4.0) | 849/12,351 (6.9) |
Proportion of time on medication, median (IQR) | 1.0 (0.5–1.0) | 1.0 (0.6–1.0) | 0.7 (0.3–1.0) | 1.0 (0.5–1.0) |
18–24 months | ||||
Number of patients, n/N (%) | 525/8508 (6.2) | 155/1990 (7.8) | 2/31 (6.5) | 682/10,529 (6.5) |
Proportion of time on medication, median (IQR) | 1.0 (0.5–1.0) | 1.0 (0.7–1.0) | 0.2 (0.2–0.3) | 1.0 (0.5–1.0) |
24–30 months | ||||
Number of patients, n/N (%) | 444/7260 (6.1) | 115/1659 (6.9) | 1/24 (4.2) | 560/8943 (6.3) |
Proportion of time on medication, median (IQR) | 1.0 (0.6–1.0) | 1.0 (0.7–1.0) | 0.3 (0.3–0.3) | 1.0 (0.6–1.0) |
30–36 months | ||||
Number of patients, n/N (%) | 364/6127 (5.9) | 88/1380 (6.4) | 2/18 (11.1) | 454/7525 (6.0) |
Proportion of time on medication, median (IQR) | 1.0 (0.6–1.0) | 1.0 (0.8–1.0) | 0.5 (0.1–0.9) | 1.0 (0.7–1.0) |
36–42 months | ||||
Number of patients, n/N (%) | 298/5084 (5.9) | 74/1149 (6.4) | 1/13 (7.7) | 373/6246 (6.0) |
Proportion of time on medication, median (IQR) | 1.0 (0.7–1.0) | 1.0 (0.5–1.0) | 1.0 (1.0–1.0) | 1.0 (0.7–1.0) |
42–48 months | ||||
Number of patients, n/N (%) | 250/4229 (5.9) | 65/930 (7.0) | 1/8 (12.5) | 316/5167 (6.1) |
Proportion of time on medication, median (IQR) | 1.0 (0.7–1.0) | 1.0 (0.7–1.0) | 0.4 (0.4–0.4) | 1.0 (0.7–1.0) |
> 48 months | ||||
Number of patients, n/N (%) | 273/3478 (7.8) | 75/730 (10.3) | 0/7 (0.0) | 348/4215 (8.3) |
Proportion of time on medication, median (IQR) | 0.7 (0.2–1.0) | 0.6 (0.2–1.0) | 0.7 (0.2–1.0) | |
Hydralazine | ||||
0–6 months | ||||
Number of patients, n/N (%) | 96/13,632 (0.7) | 30/3392 (0.9) | 0/71 (0.0) | 126/17,095 (0.7) |
Proportion of time on medication, median (IQR) | 0.7 (0.4–1.0) | 0.9 (0.4–1.0) | 0.7 (0.4–1.0) | |
6–12 months | ||||
Number of patients, n/N (%) | 82/11,673 (0.7) | 27/2793 (1.0) | 0/66 (0.0) | 109/14,532 (0.8) |
Proportion of time on medication, median (IQR) | 1.0 (0.5–1.0) | 1.0 (0.6–1.0) | 1.0 (0.6–1.0) | |
12–18 months | ||||
Number of patients, n/N (%) | 55/9958 (0.6) | 19/2343 (0.8) | 1/50 (2.0) | 75/12,351 (0.6) |
Proportion of time on medication, median (IQR) | 1.0 (1.0–1.0) | 1.0 (1.0–1.0) | 0.3 (0.3–0.3) | 1.0 (1.0–1.0) |
18–24 months | ||||
Number of patients, n/N (%) | 54/8508 (0.6) | 17/1990 (0.9) | 0/31 (0.0) | 71/10,529 (0.7) |
Proportion of time on medication, median (IQR) | 1.0 (1.0–1.0) | 1.0 (1.0–1.0) | 1.0 (1.0–1.0) | |
24–30 months | ||||
Number of patients, n/N (%) | 52/7260 (0.7) | 10/1659 (0.6) | 0/24 (0.0) | 62/8943 (0.7) |
Proportion of time on medication, median (IQR) | 1.0 (0.9–1.0) | 1.0 (1.0–1.0) | 1.0 (1.0–1.0) | |
30–36 months | ||||
Number of patients, n/N (%) | 36/6127 (0.6) | 5/1380 (0.4) | 0/18 (0.0) | 41/7525 (0.5) |
Proportion of time on medication, median (IQR) | 1.0 (0.9–1.0) | 1.0 (1.0–1.0) | 1.0 (1.0–1.0) | |
36–42 months | ||||
Number of patients, n/N (%) | 31/5084 (0.6) | 6/1149 (0.5) | 0/13 (0.0) | 37/6246 (0.6) |
Proportion of time on medication, median (IQR) | 1.0 (0.8–1.0) | 1.0 (0.6–1.0) | 1.0 (0.8–1.0) | |
42–48 months | ||||
Number of patients, n/N (%) | 25/4229 (0.6) | 4/930 (0.4) | 0/8 (0.0) | 29/5167 (0.6) |
Proportion of time on medication, median (IQR) | 1.0 (1.0–1.0) | 1.0 (0.8–1.0) | 1.0 (0.9–1.0) | |
> 48 months | ||||
Number of patients, n/N (%) | 35/3478 (1.0) | 5/730 (0.7) | 0/7 (0.0) | 40/4215 (0.9) |
Proportion of time on medication, median (IQR) | 0.7 (0.1–1.0) | 0.4 (0.3–1.0) | 0.6 (0.2–1.0) | |
Isosorbide | ||||
0–6 months | ||||
Number of patients, n/N (%) | 2287/13,632 (16.8) | 455/3392 (13.4) | 11/71 (15.5) | 2753/17,095 (16.1) |
Proportion of time on medication, median (IQR) | 1.0 (0.7–1.0) | 1.0 (0.8–1.0) | 1.0 (0.4–1.0) | 1.0 (0.7–1.0) |
6–12 months | ||||
Number of patients, n/N (%) | 1846/11,673 (15.8) | 368/2793 (13.2) | 9/66 (13.6) | 2223/14,532 (15.3) |
Proportion of time on medication, median (IQR) | 1.0 (1.0–1.0) | 1.0 (1.0–1.0) | 1.0 (0.7–1.0) | 1.0 (1.0–1.0) |
12–18 months | ||||
Number of patients, n/N (%) | 1543/9958 (15.5) | 314/2343 (13.4) | 4/50 (8.0) | 1861/12,351 (15.1) |
Proportion of time on medication, median (IQR) | 1.0 (1.0–1.0) | 1.0 (1.0–1.0) | 1.0 (1.0–1.0) | 1.0 (1.0–1.0) |
18–24 months | ||||
Number of patients, n/N (%) | 1301/8508 (15.3) | 271/1990 (13.6) | 1/31 (3.2) | 1573/10,529 (14.9) |
Proportion of time on medication, median (IQR) | 1.0 (1.0–1.0) | 1.0 (1.0–1.0) | 1.0 (1.0–1.0) | 1.0 (1.0–1.0) |
24–30 months | ||||
Number of patients, n/N (%) | 1096/7260 (15.1) | 226/1659 (13.6) | 2/24 (8.3) | 1324/8943 (14.8) |
Proportion of time on medication, median (IQR) | 1.0 (1.0–1.0) | 1.0 (1.0–1.0) | 0.6 (0.2–1.0) | 1.0 (1.0–1.0) |
30–36 months | ||||
Number of patients, n/N (%) | 936/6127 (15.3) | 192/1380 (13.9) | 2/18 (11.1) | 1130/7525 (15.0) |
Proportion of time on medication, median (IQR) | 1.0 (1.0–1.0) | 1.0 (1.0–1.0) | 1.0 (1.0–1.0) | 1.0 (1.0–1.0) |
36–42 months | ||||
Number of patients, n/N (%) | 757/5084 (14.9) | 158/1149 (13.8) | 2/13 (15.4) | 917/6246 (14.7) |
Proportion of time on medication, median (IQR) | 1.0 (1.0–1.0) | 1.0 (1.0–1.0) | 0.6 (0.2–1.0) | 1.0 (1.0–1.0) |
42–48 months | ||||
Number of patients, n/N (%) | 617/4229 (14.6) | 126/930 (13.5) | 1/8 (12.5) | 744/5167 (14.4) |
Proportion of time on medication, median (IQR) | 1.0 (1.0–1.0) | 1.0 (1.0–1.0) | 1.0 (1.0–1.0) | 1.0 (1.0–1.0) |
> 48 months | ||||
Number of patients, n/N (%) | 572/3478 (16.4) | 114/730 (15.6) | 0/7 (0.0) | 686/4215 (16.3) |
Proportion of time on medication, median (IQR) | 1.0 (0.7–1.0) | 1.0 (0.7–1.0) | 1.0 (0.7–1.0) | |
Spironolactone | ||||
0–6 months | ||||
Number of patients, n/N (%) | 3894/13,632 (28.6) | 817/3392 (24.1) | 32/71 (45.1) | 4743/17,095 (27.7) |
Proportion of time on medication, median (IQR) | 0.8 (0.5–1.0) | 0.8 (0.4–1.0) | 0.7 (0.2–1.0) | 0.8 (0.4–1.0) |
6–12 months | ||||
Number of patients, n/N (%) | 3126/11,673 (26.8) | 663/2793 (23.7) | 27/66 (40.9) | 3816/14,532 (26.3) |
Proportion of time on medication, median (IQR) | 1.0 (0.7–1.0) | 1.0 (0.6–1.0) | 1.0 (0.6–1.0) | 1.0 (0.7–1.0) |
12–18 months | ||||
Number of patients, n/N (%) | 2496/9958 (25.1) | 546/2343 (23.3) | 16/50 (32.0) | 3058/12,351 (24.8) |
Proportion of time on medication, median (IQR) | 1.0 (0.9–1.0) | 1.0 (0.9–1.0) | 1.0 (0.7–1.0) | 1.0 (0.9–1.0) |
18–24 months | ||||
Number of patients, n/N (%) | 2072/8508 (24.4) | 446/1990 (22.4) | 12/31 (38.7) | 2530/10,529 (24.0) |
Proportion of time on medication, median (IQR) | 1.0 (1.0–1.0) | 1.0 (0.9–1.0) | 0.9 (0.3–1.0) | 1.0 (0.9–1.0) |
24–30 months | ||||
Number of patients, n/N (%) | 1696/7260 (23.4) | 353/1659 (21.3) | 9/24 (37.5) | 2058/8943 (23.0) |
Proportion of time on medication, median (IQR) | 1.0 (1.0–1.0) | 1.0 (0.9–1.0) | 1.0 (1.0–1.0) | 1.0 (1.0–1.0) |
30–36 months | ||||
Number of patients, n/N (%) | 1353/6127 (22.1) | 281/1380 (20.4) | 8/18 (44.4) | 1642/7525 (21.8) |
Proportion of time on medication, median (IQR) | 1.0 (1.0–1.0) | 1.0 (1.0–1.0) | 1.0 (0.8–1.0) | 1.0 (1.0–1.0) |
36–42 months | ||||
Number of patients, n/N (%) | 1142/5084 (22.5) | 243/1149 (21.1) | 6/13 (46.2) | 1391/6246 (22.3) |
Proportion of time on medication, median (IQR) | 1.0 (1.0–1.0) | 1.0 (1.0–1.0) | 0.9 (0.5–1.0) | 1.0 (1.0–1.0) |
42–48 months | ||||
Number of patients, n/N (%) | 946/4229 (22.4) | 195/930 (21.0) | 3/8 (37.5) | 1144/5167 (22.1) |
Proportion of time on medication, median (IQR) | 1.0 (1.0–1.0) | 1.0 (1.0–1.0) | 1.0 (0.2–1.0) | 1.0 (1.0–1.0) |
> 48 months | ||||
Number of patients, n/N (%) | 905/3478 (26.0) | 200/730 (27.4) | 4/7 (57.1) | 1109/4215 (26.3) |
Proportion of time on medication, median (IQR) | 1.0 (0.5–1.0) | 0.8 (0.3–1.0) | 1.0 (0.9–1.0) | 1.0 (0.5–1.0) |
Eplerenone | ||||
0–6 months | ||||
Number of patients, n/N (%) | 309/13,632 (2.3) | 61/3392 (1.8) | 3/71 (4.2) | 373/17,095 (2.2) |
Proportion of time on medication, median (IQR) | 0.8 (0.4–1.0) | 0.8 (0.3–1.0) | 0.2 (0.2–1.0) | 0.8 (0.3–1.0) |
6–12 months | ||||
Number of patients, n/N (%) | 307/11,673 (2.6) | 51/2793 (1.8) | 0/66 (0.0) | 358/14532 (2.5) |
Proportion of time on medication, median (IQR) | 1.0 (0.6– 1.0) | 1.0 (0.8–1.0) | 1.0 (0.7–1.0) | |
12–18 months | ||||
Number of patients, n/N (%) | 270/9958 (2.7) | 58/2343 (2.5) | 0/50 (0.0) | 328/12,351 (2.7) |
Proportion of time on medication, median (IQR) | 1.0 (1.0–1.0) | 1.0 (0.4–1.0) | 1.0 (0.9–1.0) | |
18–24 months | ||||
Number of patients, n/N (%) | 221/8508 (2.6) | 42/1990 (2.1) | 1/31 (3.2) | 264/10,529 (2.5) |
Proportion of time on medication, median (IQR) | 1.0 (1.0–1.0) | 1.0 (1.0–1.0) | 0.6 (0.6–0.6) | 1.0 (1.0–1.0) |
24–30 months | ||||
Number of patients, n/N (%) | 197/7260 (2.7) | 30/1659 (1.8) | 2/24 (8.3) | 229/8943 (2.6) |
Proportion of time on medication, median (IQR) | 1.0 (1.0–1.0) | 1.0 (0.8–1.0) | 0.8 (0.5–1.0) | 1.0 (1.0–1.0) |
30–36 months | ||||
Number of patients, n/N (%) | 171/6127 (2.8) | 28/1380 (2.0) | 1/18 (5.6) | 200/7525 (2.7) |
Proportion of time on medication, median (IQR) | 1.0 (1.0–1.0) | 1.0 (0.9–1.0) | 1.0 (1.0–1.0) | 1.0 (1.0–1.0) |
36–42 months | ||||
Number of patients, n/N (%) | 137/5084 (2.7) | 30/1149 (2.6) | 0/13 (0.0) | 167/6246 (2.7) |
Proportion of time on medication, median (IQR) | 1.0 (1.0–1.0) | 1.0 (0.6–1.0) | 1.0 (1.0–1.0) | |
42–48 months | ||||
Number of patients, n/N (%) | 115/4229 (2.7) | 24/930 (2.6) | 0/8 (0.0) | 139/5167 (2.7) |
Proportion of time on medication, median (IQR) | 1.0 (1.0–1.0) | 1.0 (1.0–1.0) | 1.0 (1.0–1.0) | |
> 48 months | ||||
Number of patients, n/N (%) | 120/3478 (3.5) | 26/730 (3.6) | 0/7 (0.0) | 146/4215 (3.5) |
Proportion of time on medication, median (IQR) | 1.0 (0.6–1.0) | 0.8 (0.2–1.0) | 1.0 (0.5–1.0) | |
Amiloride | ||||
0–6 months | ||||
Number of patients, n/N (%) | 483/13632 (3.5) | 147/3392 (4.3) | 0/71 (0.0) | 630/17,095 (3.7) |
Proportion of time on medication, median (IQR) | 0.4 (0.2–1.0) | 0.5 (0.2–1.0) | 0.5 (0.2–1.0) | |
6–12 months | ||||
Number of patients, n/N (%) | 267/11,673 (2.3) | 79/2793 (2.8) | 1/66 (1.5) | 347/14,532 (2.4) |
Proportion of time on medication, median (IQR) | 1.0 (0.4–1.0) | 1.0 (0.3–1.0) | 0.8 (0.8–0.8) | 1.0 (0.4–1.0) |
12–18 months | ||||
Number of patients, n/N (%) | 202/9958 (2.0) | 57/2343 (2.4) | 2/50 (4.0) | 261/12,351 (2.1) |
Proportion of time on medication, median (IQR) | 1.0 (0.7–1.0) | 1.0 (0.2–1.0) | 0.9 (0.9–1.0) | 1.0 (0.6–1.0) |
18–24 months | ||||
Number of patients, n/N (%) | 177/8508 (2.1) | 40/1990 (2.0) | 2/31 (6.5) | 219/10,529 (2.1) |
Proportion of time on medication, median (IQR) | 1.0 (0.7–1.0) | 1.0 (0.4–1.0) | 0.6 (0.2–1.0) | 1.0 (0.6–1.0) |
24–30 months | ||||
Number of patients, n/N (%) | 143/7260 (2.0) | 35/1659 (2.1) | 0/24 (0.0) | 178/8943 (2.0) |
Proportion of time on medication, median (IQR) | 1.0 (0.8–1.0) | 1.0 (0.3–1.0) | 1.0 (0.7–1.0) | |
30–36 months | ||||
Number of patients, n/N (%) | 117/6127 (1.9) | 24/1380 (1.7) | 1/18 (5.6) | 142/7525 (1.9) |
Proportion of time on medication, median (IQR) | 1.0 (1.0–1.0) | 1.0 (0.9–1.0) | 0.2 (0.2–0.2) | 1.0 (1.0–1.0) |
36–42 months | ||||
Number of patients, n/N (%) | 95/5084 (1.9) | 19/1149 (1.7) | 0/13 (0.0) | 114/6246 (1.8) |
Proportion of time on medication, median (IQR) | 1.0 (0.8–1.0) | 1.0 (0.6–1.0) | 1.0 (0.8–1.0) | |
42–48 months | ||||
Number of patients, n/N (%) | 72/4229 (1.7) | 21/930 (2.3) | 0/8 (0.0) | 93/5167 (1.8) |
Proportion of time on medication, median (IQR) | 1.0 (1.0–1.0) | 0.6 (0.2–1.0) | 1.0 (0.7–1.0) | |
> 48 months | ||||
Number of patients, n/N (%) | 76/3478 (2.2) | 18/730 (2.5) | 0/7 (0.0) | 94/4215 (2.2) |
Proportion of time on medication, median (IQR) | 0.9 (0.3–1.0) | 0.4 (0.1–1.0) | 0.9 (0.2–1.0) | |
Triamterene | ||||
0–6 months | ||||
Number of patients, n/N (%) | 15/13,632 (0.1) | 2/3392 (0.1) | 0/71 (0.0) | 17/17,095 (0.1) |
Proportion of time on medication, median (IQR) | 0.2 (0.1–1.0) | 0.1 (0.1–0.1) | 0.2 (0.1–0.4) | |
6–12 months | ||||
Number of patients, n/N (%) | 5/11,673 (0.0) | 0/2793 (0.0) | 0/66 (0.0) | 5/14,532 (0.0) |
Proportion of time on medication, median (IQR) | 1.0 (0.6–1.0) | 1.0 (0.6–1.0) | ||
12–18 months | ||||
Number of patients, n/N (%) | 5/9958 (0.1) | 0/2343 (0.0) | 0/50 (0.0) | 5/12,351 (0.0) |
Proportion of time on medication, median (IQR) | 1.0 (0.6–1.0) | 1.0 (0.6–1.0) | ||
18–24 months | ||||
Number of patients, n/N (%) | 3/8508 (0.0) | 0/1990 (0.0) | 0/31 (0.0) | 3/10,529 (0.0) |
Proportion of time on medication, median (IQR) | 1.0 (1.0–1.0) | 1.0 (1.0–1.0) | ||
24–30 months | ||||
Number of patients, n/N (%) | 3/7260 (0.0) | 0/1659 (0.0) | 0/24 (0.0) | 3/8943 (0.0) |
Proportion of time on medication, median (IQR) | 1.0 (0.3–1.0) | 1.0 (0.3–1.0) | ||
30–36 months | ||||
Number of patients, n/N (%) | 3/6127 (0.0) | 0/1380 (0.0) | 0/18 (0.0) | 3/7525 (0.0) |
Proportion of time on medication, median (IQR) | 1.0 (0.6–1.0) | 1.0 (0.6–1.0) | ||
36–42 months | ||||
Number of patients, n/N (%) | 3/5084 (0.1) | 0/1149 (0.0) | 0/13 (0.0) | 3/6246 (0.0) |
Proportion of time on medication, median (IQR) | 1.0 (1.0–1.0) | 1.0 (1.0–1.0) | ||
42–48 months | ||||
Number of patients, n/N (%) | 2/4229 (0.0) | 0/930 (0.0) | 0/8 (0.0) | 2/5167 (0.0) |
Proportion of time on medication, median (IQR) | 0.7 (0.4–1.0) | 0.7 (0.4–1.0) | ||
> 48 months | ||||
Number of patients, n/N (%) | 1/3478 (0.0) | 0/730 (0.0) | 0/7 (0.0) | 1/4215 (0.0) |
Proportion of time on medication, median (IQR) | 1.0 (1.0–1.0) | 1.0 (1.0–1.0) | ||
Calcium channel blockers | ||||
0–6 months | ||||
Number of patients, n/N (%) | 3091/13,632 (22.7) | 889/3392 (26.2) | 17/71 (23.9) | 3997/17,095 (23.4) |
Proportion of time on medication, median (IQR) | 1.0 (0.3–1.0) | 1.0 (0.4–1.0) | 1.0 (1.0–1.0) | 1.0 (0.3–1.0) |
6–12 months | ||||
Number of patients, n/N (%) | 1950/11,673 (16.7) | 561/2793 (20.1) | 15/66 (22.7) | 2526/14,532 (17.4) |
Proportion of time on medication, median (IQR) | 1.0 (0.9–1.0) | 1.0 (1.0–1.0) | 1.0 (0.9–1.0) | 1.0 (0.9–1.0) |
12–18 months | ||||
Number of patients, n/N (%) | 1682/9958 (16.9) | 463/2343 (19.8) | 11/50 (22.0) | 2156/12,351 (17.5) |
Proportion of time on medication, median (IQR) | 1.0 (1.0–1.0) | 1.0 (1.0–1.0) | 1.0 (1.0–1.0) | 1.0 (1.0–1.0) |
18–24 months | ||||
Number of patients, n/N (%) | 1447/8508 (17.0) | 390/1990 (19.6) | 8/31 (25.8) | 1845/10,529 (17.5) |
Proportion of time on medication, median (IQR) | 1.0 (1.0–1.0) | 1.0 (1.0–1.0) | 1.0 (0.8–1.0) | 1.0 (1.0–1.0) |
24–30 months | ||||
Number of patients, n/N (%) | 1237/7260 (17.0) | 338/1659 (20.4) | 5/24 (20.8) | 1580/8943 (17.7) |
Proportion of time on medication, median (IQR) | 1.0 (1.0–1.0) | 1.0 (1.0–1.0) | 1.0 (1.0–1.0) | 1.0 (1.0–1.0) |
30–36 months | ||||
Number of patients, n/N (%) | 1049/6127 (17.1) | 278/1380 (20.1) | 4/18 (22.2) | 1331/7525 (17.7) |
Proportion of time on medication, median (IQR) | 1.0 (1.0–1.0) | 1.0 (1.0–1.0) | 1.0 (0.5–1.0) | 1.0 (1.0–1.0) |
36–42 months | ||||
Number of patients, n/N (%) | 896/5084 (17.6) | 222/1149 (19.3) | 3/13 (23.1) | 1121/6246 (17.9) |
Proportion of time on medication, median (IQR) | 1.0 (1.0–1.0) | 1.0 (1.0–1.0) | 1.0 (1.0–1.0) | 1.0 (1.0–1.0) |
42–48 months | ||||
Number of patients, n/N (%) | 765/4229 (18.1) | 175/930 (18.8) | 2/8 (25.0) | 942/5167 (18.2) |
Proportion of time on medication, median (IQR) | 1.0 (1.0–1.0) | 1.0 (1.0–1.0) | 0.6 (0.2–1.0) | 1.0 (1.0–1.0) |
> 48 months | ||||
Number of patients, n/N (%) | 746/3478 (21.4) | 176/730 (24.1) | 3/7 (42.9) | 925/4215 (21.9) |
Proportion of time on medication, median (IQR) | 1.0 (0.6–1.0) | 1.0 (0.6–1.0) | 0.3 (0.3–0.4) | 1.0 (0.6–1.0) |
Statins | ||||
0–6 months | ||||
Number of patients, n/N (%) | 8412/13,632 (61.7) | 1930/3392 (56.9) | 43/71 (60.6) | 10,385/17,095 (60.7) |
Proportion of time on medication, median (IQR) | 1.0 (1.0–1.0) | 1.0 (1.0–1.0) | 1.0 (1.0–1.0) | 1.0 (1.0–1.0) |
6–12 months | ||||
Number of patients, n/N (%) | 7200/11,673 (61.7) | 1633/2793 (58.5) | 39/66 (59.1) | 8872/14,532 (61.1) |
Proportion of time on medication, median (IQR) | 1.0 (1.0–1.0) | 1.0 (1.0–1.0) | 1.0 (1.0–1.0) | 1.0 (1.0–1.0) |
12–18 months | ||||
Number of patients, n/N (%) | 6230/9958 (62.6) | 1392/2343 (59.4) | 28/50 (56.0) | 7650/12,351 (61.9) |
Proportion of time on medication, median (IQR) | 1.0 (1.0–1.0) | 1.0 (1.0–1.0) | 1.0 (1.0–1.0) | 1.0 (1.0–1.0) |
18–24 months | ||||
Number of patients, n/N (%) | 5382/8508 (63.3) | 1199/1990 (60.3) | 18/31 (58.1) | 6599/10,529 (62.7) |
Proportion of time on medication, median (IQR) | 1.0 (1.0–1.0) | 1.0 (1.0–1.0) | 1.0 (1.0–1.0) | 1.0 (1.0–1.0) |
24–30 months | ||||
Number of patients, n/N (%) | 4631/7260 (63.8) | 1008/1659 (60.8) | 17/24 (70.8) | 5656/8943 (63.2) |
Proportion of time on medication, median (IQR) | 1.0 (1.0–1.0) | 1.0 (1.0–1.0) | 1.0 (1.0–1.0) | 1.0 (1.0–1.0) |
30–36 months | ||||
Number of patients, n/N (%) | 3907/6127 (63.8) | 853/1380 (61.8) | 12/18 (66.7) | 4772/7525 (63.4) |
Proportion of time on medication, median (IQR) | 1.0 (1.0–1.0) | 1.0 (1.0–1.0) | 1.0 (1.0–1.0) | 1.0 (1.0–1.0) |
36–42 months | ||||
Number of patients, n/N (%) | 3300/5084 (64.9) | 700/1149 (60.9) | 9/13 (69.2) | 4009/6246 (64.2) |
Proportion of time on medication, median (IQR) | 1.0 (1.0–1.0) | 1.0 (1.0–1.0) | 1.0 (1.0–1.0) | 1.0 (1.0–1.0) |
42–48 months | ||||
Number of patients, n/N (%) | 2780/4229 (65.7) | 572/930 (61.5) | 5/8 (62.5) | 3357/5167 (65.0) |
Proportion of time on medication, median (IQR) | 1.0 (1.0–1.0) | 1.0 (1.0–1.0) | 1.0 (1.0–1.0) | 1.0 (1.0–1.0) |
> 48 months | ||||
Number of patients, n/N (%) | 2407/3478 (69.2) | 474/730 (64.9) | 4/7 (57.1) | 2885/4215 (68.4) |
Proportion of time on medication, median (IQR) | 1.0 (0.9–1.0) | 1.0 (0.9–1.0) | 1.0 (1.0–1.0) | 1.0 (0.9–1.0) |
Anticoagulants | ||||
0–6 months | ||||
Number of patients, n/N (%) | 4444/13,632 (32.6) | 1091/3392 (32.2) | 26/71 (36.6) | 5561/17,095 (32.5) |
Proportion of time on medication, median (IQR) | 1.0 (0.7–1.0) | 1.0 (0.8–1.0) | 1.0 (1.0–1.0) | 1.0 (0.7–1.0) |
6–12 months | ||||
Number of patients, n/N (%) | 3816/11,673 (32.7) | 927/2793 (33.2) | 23/66 (34.8) | 4766/14,532 (32.8) |
Proportion of time on medication, median (IQR) | 1.0 (1.0–1.0) | 1.0 (1.0–1.0) | 1.0 (0.9–1.0) | 1.0 (1.0–1.0) |
12–18 months | ||||
Number of patients, n/N (%) | 3259/9958 (32.7) | 782/2343 (33.4) | 17/50 (34.0) | 4058/12,351 (32.9) |
Proportion of time on medication, median (IQR) | 1.0 (1.0–1.0) | 1.0 (1.0–1.0) | 1.0 (1.0–1.0) | 1.0 (1.0–1.0) |
18–24 months | ||||
Number of patients, n/N (%) | 2770/8508 (32.6) | 628/1990 (31.6) | 10/31 (32.3) | 3408/10,529 (32.4) |
Proportion of time on medication, median (IQR) | 1.0 (1.0–1.0) | 1.0 (1.0–1.0) | 1.0 (1.0–1.0) | 1.0 (1.0–1.0) |
24–30 months | ||||
Number of patients, n/N (%) | 2368/7260 (32.6) | 533/1659 (32.1) | 8/24 (33.3) | 2909/8943 (32.5) |
Proportion of time on medication, median (IQR) | 1.0 (1.0–1.0) | 1.0 (1.0–1.0) | 1.0 (1.0–1.0) | 1.0 (1.0–1.0) |
30–36 months | ||||
Number of patients, n/N (%) | 1998/6127 (32.6) | 425/1380 (30.8) | 6/18 (33.3) | 2429/7525 (32.3) |
Proportion of time on medication, median (IQR) | 1.0 (1.0–1.0) | 1.0 (1.0–1.0) | 1.0 (1.0–1.0) | 1.0 (1.0–1.0) |
36–42 months | ||||
Number of patients, n/N (%) | 1687/5084 (33.2) | 357/1149 (31.1) | 4/13 (30.8) | 2048/6246 (32.8) |
Proportion of time on medication, median (IQR) | 1.0 (1.0–1.0) | 1.0 (1.0–1.0) | 1.0 (0.6–1.0) | 1.0 (1.0–1.0) |
42–48 months | ||||
Number of patients, n/N (%) | 1423/4229 (33.6) | 306/930 (32.9) | 2/8 (25.0) | 1731/5167 (33.5) |
Proportion of time on medication, median (IQR) | 1.0 (1.0–1.0) | 1.0 (1.0–1.0) | 1.0 (1.0–1.0) | 1.0 (1.0–1.0) |
> 48 months | ||||
Number of patients, n/N (%) | 1245/3478 (35.8) | 263/730 (36.0) | 2/7 (28.6) | 1510/4215 (35.8) |
Proportion of time on medication, median (IQR) | 1.0 (0.8–1.0) | 1.0 (0.8–1.0) | 1.0 (1.0–1.0) | 1.0 (0.8–1.0) |
Aspirin | ||||
0–6 months | ||||
Number of patients, n/N (%) | 7695/13,632 (56.4) | 1701/3392 (50.1) | 34/71 (47.9) | 9430/17,095 (55.2) |
Proportion of time on medication, median (IQR) | 1.0 (0.8–1.0) | 1.0 (0.8–1.0) | 1.0 (1.0–1.0) | 1.0 (0.8–1.0) |
6–12 months | ||||
Number of patients, n/N (%) | 5995/11,673 (51.4) | 1285/2793 (46.0) | 29/66 (43.9) | 7309/14,532 (50.3) |
Proportion of time on medication, median (IQR) | 1.0 (1.0–1.0) | 1.0 (1.0–1.0) | 1.0 (0.9–1.0) | 1.0 (1.0–1.0) |
12–18 months | ||||
Number of patients, n/N (%) | 5068/9958 (50.9) | 1070/2343 (45.7) | 20/50 (40.0) | 6158/12,351 (49.9) |
Proportion of time on medication, median (IQR) | 1.0 (1.0–1.0) | 1.0 (1.0–1.0) | 1.0 (1.0–1.0) | 1.0 (1.0–1.0) |
18–24 months | ||||
Number of patients, n/N (%) | 4311/8508 (50.7) | 907/1990 (45.6) | 16/31 (51.6) | 5234/10,529 (49.7) |
Proportion of time on medication, median (IQR) | 1.0 (1.0–1.0) | 1.0 (1.0–1.0) | 1.0 (1.0–1.0) | 1.0 (1.0–1.0) |
24–30 months | ||||
Number of patients, n/N (%) | 3650/7260 (50.3) | 764/1659 (46.1) | 14/24 (58.3) | 4428/8943 (49.5) |
Proportion of time on medication, median (IQR) | 1.0 (1.0–1.0) | 1.0 (1.0–1.0) | 1.0 (1.0–1.0) | 1.0 (1.0–1.0) |
30–36 months | ||||
Number of patients, n/N (%) | 3024/6127 (49.4) | 659/1380 (47.8) | 10/18 (55.6) | 3693/7525 (49.1) |
Proportion of time on medication, median (IQR) | 1.0 (1.0–1.0) | 1.0 (1.0–1.0) | 1.0 (1.0–1.0) | 1.0 (1.0–1.0) |
36–42 months | ||||
Number of patients, n/N (%) | 2467/5084 (48.5) | 527/1149 (45.9) | 8/13 (61.5) | 3002/6246 (48.1) |
Proportion of time on medication, median (IQR) | 1.0 (1.0–1.0) | 1.0 (1.0–1.0) | 1.0 (0.9–1.0) | 1.0 (1.0–1.0) |
42–48 months | ||||
Number of patients, n/N (%) | 2029/4229 (48.0) | 430/930 (46.2) | 5/8 (62.5) | 2464/5167 (47.7) |
Proportion of time on medication, median (IQR) | 1.0 (1.0–1.0) | 1.0 (1.0–1.0) | 1.0 (1.0–1.0) | 1.0 (1.0–1.0) |
> 48 months | ||||
Number of patients, n/N (%) | 1797/3478 (51.7) | 364/730 (49.9) | 4/7 (57.1) | 2165/4215 (51.4) |
Proportion of time on medication, median (IQR) | 1.0 (0.8–1.0) | 1.0 (0.7–1.0) | 1.0 (1.0–1.0) | 1.0 (0.8–1.0) |
Figure 38 shows the proportion of patients taking each class of medication during 6-monthly intervals of follow-up. There are no obvious differences between the groups in prescription of any of the classes of medications. Although there are higher proportions of patients on medications in the monitored group, the number of patients in this group is too small to reach definitive conclusions (note that, at the later time points, follow-up data are available for only a very small number of patients).
FIGURE 38.
Proportion of patients taking each class of medication at any point during each 6-month time period, stratified by BNP testing. (a) ACEis; (b) ARBs; (c) beta-blockers; (d) digoxin; (e) loop diuretics; (f) thiazide diuretics; (g) spironolactone; and (h) calcium channel blockers. Solid lines, never tested group; dashed lines, tested group; dotted lines, monitored group. Numbers of patients with follow-up during each time period in the never tested, tested and monitored groups are: 0–6 months – 13,632, 3392, 471; 6–12 months – 11,673, 2793, 66; 12–18 months – 9958, 2343, 50; 18–24 months – 8508, 1990, 31; 24–30 months – 7260, 1659, 24; 30–36 months – 6127, 1380, 18; 36–42 months – 5084, 1149, 13; 42–48 months – 4229, 930, 8; > 48 months – 3478, 730, 7.
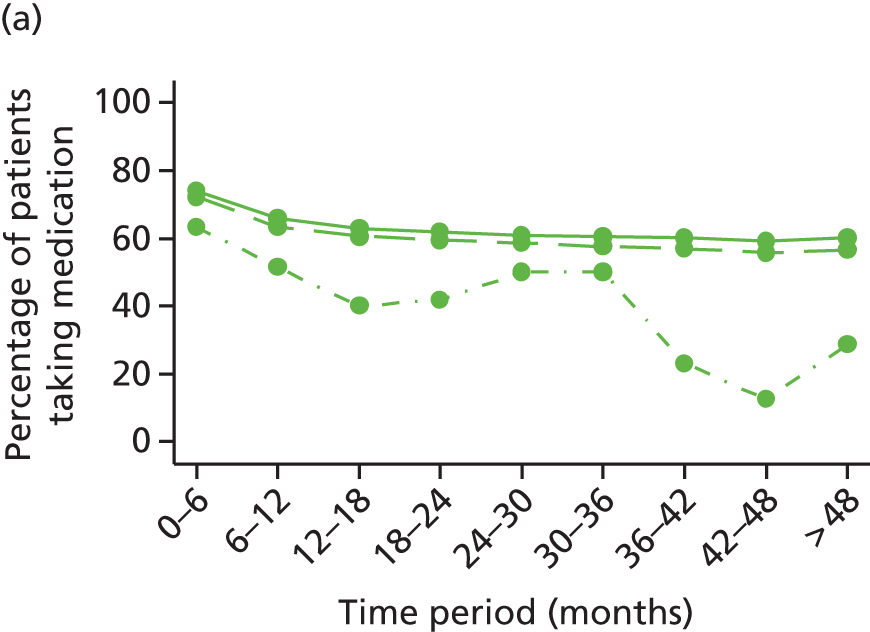
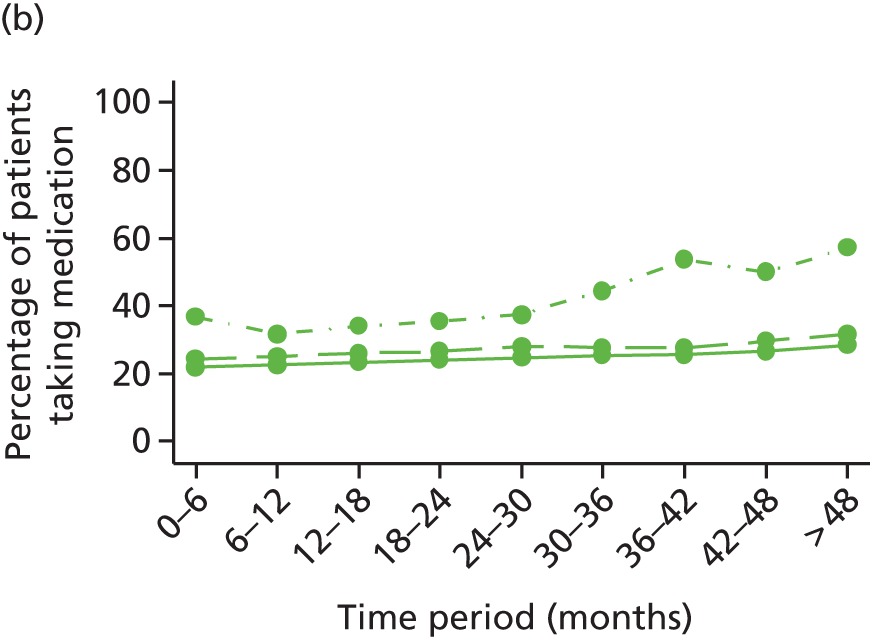






National Heart Failure Audit
A total of 163,244 admissions were recorded across 130,433 patients in the NHFA between January 2007 and March 2013. Of the patients in the NHFA, 126,686 (97%) met the definition of incident HF admission. The cumulative number of patients identified as having incident HF in the NHFA is shown in Figure 39. The number of admissions recorded each month increased from 298 in January 2007 to > 3100 in 2013 (with almost 3700 in January 2013) (Figure 40). The admission rate in patients with an incident HF admission was 1.1 per year (IQR 0.5–3.5); 17% were readmitted during follow-up, with a median of 1 readmission (IQR 1–2).
FIGURE 39.
Cumulative number of patients identified as having incident HF in NHFA cohort.

FIGURE 40.
Number of admissions recorded in the NHFA data set per month.

B-type natriuretic peptide tests were carried out in 10,114 admissions (6%). The proportion of visits at which a BNP test was carried out increased from 0% at the start of the audit to 10% by March 2013 (Figure 41).
FIGURE 41.
Percentage of admissions at which a BNP test was recorded per month in the NHFA data set.

Table 19 shows demographic data and clinical characteristics of patients in the NHFA. Sex distribution, BMI and blood pressure appear similar between patients in the NHFA cohort and those in the CPRD cohort. The patients in the NHFA cohort appear to be older (67% of patients aged ≥ 75 years vs. 60% in the CPRD cohort) and have more comorbidities or previous events, such as myocardial infarction, percutaneous coronary intervention (PCI) or coronary artery bypass grafting (CABG) surgery. However, to some extent this is likely to be because the NHFA audit collects information on any medical history prior to the admission, whereas the CPRD cohort reports such data only for the year prior to incident HF.
Patient characteristics | Incident HF admission (N = 126,686) |
---|---|
Age at incidence (median, IQR) | 80 (71–86) |
Aged ≥ 75 years, n (%) | 84,936/126,632 (67) |
Male, n (%) | 69,765/126,564 (55) |
BMI (median, IQR) | 26.7 (22.8–31.2) |
< 20 kg/m2, n (%) | 1738/14,573 (12) |
≥ 20 and < 25 kg/m2, n (%) | 3923/14,573 (27) |
≥ 25 and < 30 kg/m2, n (%) | 4344/14,573 (30) |
≥ 30 kg/m2, n (%) | 4568/14,573 (31) |
SBP (mean, SD) | 128 (22) |
DBP (mean, SD) | 74 (17) |
Medical history, n (%) | |
Previous MI | 34976/115,940 (30) |
Previous PCI | 691/8007 (9) |
Previous CABG | 997/8166 (12) |
Medical history – other | |
Arrhythmias, n (%) | 2865/8049 (36) |
CVA, n (%) | 940/8523 (11) |
Diabetes, n (%) | 34723/119,833 (29) |
Peripheral vascular disease, n (%) | 559/7947 (7) |
COPD, n (%) | 7170/42,161 (17) |
BNP/NT-proBNP measurement, n (%) | 7723/126,686 (6) |
BNP (median, IQR) | 672 (304–1478) |
NT-proBNP (median, IQR) | 2727.5 (626–7572.5) |
Patients had a median follow-up time of 1.1 years (IQR 0.3–2.2 years). A total of 60,677 out of 125,719 patients with incident HF were reported to have died at the end of follow-up.
Figure 42 shows the Kaplan–Meier curve for the survival time in the NHFA cohort. The median survival time was shorter in the NHFA cohort (2.2 years) than in the CPRD cohort (5 years).
FIGURE 42.
Kaplan–Meier survival curves for death in the NHFA cohort.

Table 20 shows the proportion of patients on each medication class on admission and at discharge from the incident HF admission. Data were more complete for medications at discharge, but it can be seen that the proportion of patients taking ACEis, beta-blockers, loop diuretics and spironolactone/eplerenone was higher at discharge than at admission.
Medications for HF | Incident HF admission (N = 126,686), n (%) |
---|---|
ACEis | |
Admission | 4732/10,335 (46) |
Discharge | 64,155/119,936 (53) |
ARBs | |
Admission | 1477/10,085 (15) |
Discharge | 15,177/120,951 (13) |
Beta-blockers | |
Admission | 4641/10,006 (46) |
Discharge | 61,390/114,682 (54) |
Loop diuretics | |
Admission | 6646/10,375 (64) |
Discharge | 100,225/121,890 (82) |
Thiazide diuretics | |
Admission | 626/9851 (6) |
Discharge | 4331/112,302 (4) |
Digoxin | |
Admission | 1653/10,299 (16) |
Discharge | 23142/112,022 (21) |
Spironolactone or eplerenone | |
Incidence | 1952/10,108 (19) |
End of follow-up | 37,227/121,102 (31) |
Antiplatelets | |
Incidence | 3661/7087 (52) |
End of follow-up | 5160/8697 (59) |
Calcium channel blockers | |
Incidence | 1313/7328 (18) |
End of follow-up | 1037/10,342 (10) |
Statins | |
Incidence | 3929/7388 (53) |
End of follow-up | 5337/10,818 (49) |
Readmissions were recorded in the NHFA for 20,949 patients (17%). Patients were readmitted once on average (IQR 1–2), with a yearly admission rate of 1.1 admissions per year (IQR 0.5–3.5 admissions per year) in patients who had at least one readmission.
Summary of main findings
We conducted a population-based cohort study of 17,095 patients with incident HF followed for up to 9 years (median 3 years), half of whom died during follow-up. Only 71 out of 17,095 (0.4%) of patients were identified as having had BNP monitoring during their follow-up after diagnosis of HF. Patients identified as ‘BNP monitored’ had higher rates of death from any cause and death related to HF or cardiovascular causes. These patients were also more likely to be admitted to hospital, had more GP and outpatient consultations and more HF-related investigations (echocardiography) than patients who were identified as BNP tested and never tested. These results indicate that patients identified as BNP monitored were a sicker group of HF patients.
This finding does not appear consistent with the findings from our meta-analysis or that of Troughton et al. ,25 both of which suggest a benefit of BNP monitoring on clinical outcomes. However, given the very small size of the BNP-monitored group (0.4% of the study cohort), patients classified as having been BNP monitored may have been highly selected on grounds that were not characterised in the cohort. We also had no definitive evidence that the patients we identified as ‘BNP monitored’ were in fact monitored (i.e. had serial BNP tests in order to guide up-titration of medication). Therefore, we have not reported analyses comparing groups of patients defined as BNP tested, BNP monitored and never tested.
The cohort of HF patients in NHFA was broadly comparable with the cohort identified in the CPRD but was, on average, older and had more comorbidities and previous medical events. These differences are consistent with NHFA patients, on average, having progressed to a more advanced stage of HF at the time of entry into the cohort, supported by them having shorter median survival (2.2 vs. 5 years). The proportion of admissions during which a BNP test was carried out increased from 0% at the start of the audit to 10% by March 2013.
Chapter 4 A model-based cost-effectiveness analysis of B-type natriuretic peptide monitoring in patients with chronic heart failure in primary and secondary care
Aims and objectives
Our objective was to synthesise the evidence on the cost-effectiveness of BNP-guided monitoring in patient subgroups defined by age and LVEF status, thereby helping clinicians and policy-makers decide which patients may benefit from BNP-guided therapy.
The aim of this cost-effectiveness analysis was to model the cost-effectiveness of BNP guided-therapy in primary and secondary care in the UK using efficacy data derived from the IPD meta-analysis and resource use data derived from the cohort study to inform key parameters of the model. The specific objectives were to:
-
identify the relative contribution of different types of care (i.e. inpatient, outpatient, primary care and medications) to the overall cost of HF
-
determine how cost differs by age (< 75 vs. ≥ 75 years), proximity to death and underlying cause of death (circulatory vs. other).
Methods
Overview of the model
We developed a decision-analytical model to assess the cost-effectiveness of BNP-guided strategy compared with standard clinically guided (CG) strategy for optimising medical therapy in patients with HF and who have recently been discharged from hospital following an acute episode. We considered recently hospitalised patients with HF because the data that we used from the IPD meta-analyses25,33 are based on RCTs that recruited patients who had recently been hospitalised because of HF. One exception, the SIGNAL-HF trial,63 which recruited patients with stable HF from primary care, found no improvements in outcomes as a result of BNP-guided monitoring. We compared specialist-led BNP-guided therapy with specialist-led CG therapy. Although two RCTs61,64 included a third arm in which usual care was provided by a primary care physician, in common with the IPD meta-analyses,25,33 we focused on whether or not BNP-guided therapy is a cost-effective addition to specialist-led care.
We followed the criteria for selecting an appropriate modelling approach set out by Barton et al. 73 A Markov cohort model was considered to be appropriate for this decision problem, as HF is a chronic disease affecting patients over their remaining lifetime, patient prognoses could adequately be represented with a relatively small number of health states and, as a non-communicable disease, interaction between patients could be ignored. Markov models segregate patients’ possible prognoses into a series of discrete health states over time divided into periods or cycles of equal duration. Transition probabilities determine the movement of patients between health states at the end of each cycle. Each health state is associated with a cost, representing NHS care costs, and a ‘utility’, representing patient health-related quality of life. The model tracks the patient cohort over a large number of cycles to estimate cumulative costs and health benefits based on the length of time patients spend in each health state.
Goehler et al. ,74 in a review of HF decision-analytical models, found similar numbers of models using NYHA class (n = 9), rehospitalisation (n = 6) or alive/dead health states (n = 10) to track disease progression and survival. They note that the choice of model structure is dependent on, among other factors, data availability. Our choice was limited to a simplified model structure as the majority of RCTs do not measure or report changes in NYHA class at follow-up. Our model is similar to the structure of a previous cost-effectiveness model that was used to develop NICE clinical guidelines on the management of HF8 and later updated with additional RCT evidence. 32 We used a simple Markov process consisting of two health states: alive and dead (Figure 43). The probability of death (pt) varied with time since initial hospitalisation and care (BNP/CG) received. We tracked the probability of hospitalisation (rt) among survivors, which also varied with time since initial hospitalisation and care (BNP/CG) received. This was important because BNP-guided monitoring may reduce acute episodes and the need for hospitalisation, which would affect the cost of care and quality of life. However, we did not model the interaction between the number of hospitalisations and the subsequent risk of death. This was a pragmatic decision, as RCTs do not report mortality hazard rates conditional on the number of hospitalisations. The simplicity of the two-state Markov model is a potential limitation, which we return to in the discussion.
FIGURE 43.
Markov model of disease progression.
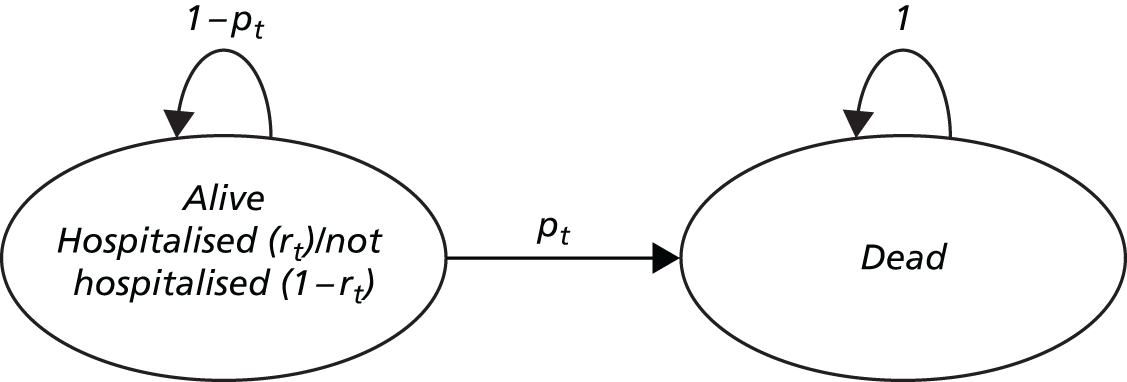
We estimated the average costs and health benefits of a hypothetical cohort of 1000 patients with HF over the course of their lifetime. 10 The recent literature25,33 and our own work in this project have used IPD meta-analysis of RCTs to explore the relative effectiveness of BNP-guided monitoring in various subgroups of the HF population including age (< 75/≥ 75 years) and LVEF (≤ 45%/> 45%) status. The evidence that BNP-guided therapy is effective is strongest in younger patients and in those with HFrEF. The evidence is weaker in older patients with HFpEF. Initially, we used the model to explore the cost-effectiveness of BNP-guided therapy in two patient subgroups: (1) all HF patients aged < 75 years; and (2) all HF patients aged ≥ 75 years. Based on evidence published in September 2015,33 we then extended the model to explore three further subgroups: (3) HFrEF patients aged < 75 years; (4) HFpEF patients aged < 75 years; and (5) HFrEF patients aged ≥ 75 years. We excluded older patients with HFpEF, as there is no evidence of benefit in this subgroup of patients (HR 1.56, 95% CI 0.90 to 2.70). 33
We chose a cycle length of 3 months to track changes in health. In many RCTs,58,59,64 BNP was monitored every 3 months during follow-up and mortality differences have emerged by 3 months in IPD meta-analyses. 25,33 To estimate costs and health benefits, we assumed that transitions between health states occur halfway through each cycle (i.e. a half-cycle correction). We assumed that the mean age of the age subgroups (< 75 and ≥ 75 years) at the inception of treatment was 65 and 81 years, respectively. 33 We assumed that age at inception was also 65 years in the ‘HFrEF < 75 years’ and ‘HFpEF < 75 years’ subgroups and 81 years in the ‘HFrEF ≥ 75 years’ subgroup. We tracked outcomes for a period of 30 years for all patient subgroups with age < 75 years and for a period of 15 years for all patient subgroups with age ≥ 75 years. In all cases, this equated to a period when more than 99% of patients died in both monitoring strategies.
We chose a UK NHS perspective for costs. HF undoubtedly has a large impact on social care, patients and carers. However, there are no suitable data on the broader economic burden of HF in the UK. Health benefits were quantified in terms of QALYs,10 calculated by multiplying the utility value for each health state with the time spent in that state. 75 A utility value represents a patient’s health-related quality of life on a scale anchored at 0 (= death) and 1 (= perfect health). All costs and QALYs were discounted at an annual rate of 3.5%, as recommended by NICE. 76 We compared the cost-effectiveness of monitoring strategies based on incremental net monetary benefits (iNMB). The iNMB of BNP-guided versus CG strategies is expressed as:
where λ represents the maximum amount that the NHS is ‘willing to pay’ to gain one QALY. NICE typically uses λ values of between £20,000 and £30,000 per QALY to identify technologies that are cost-effective enough for use in the NHS. 77 In our analyses, we used the lower figure (λ = £20,000) in calculating iNMB. An iNMB value greater than zero indicates that BNP-guided monitoring is cost-effective. We also present cost-effectiveness acceptability curves (CEACs) to demonstrate how the NHS ‘willingness-to-pay’ threshold affects the probability that BNP-guided therapy is considered to be cost-effective. 78
Model parameters
The model used four sets of parameters: (1) baseline probabilities, which determine the probability of being hospitalised or of dying at each cycle in the CG group; (2) relative treatment effects expressed as HRs and relative risks (RRs), which determine how the baseline probabilities differ in the BNP-guided monitoring group; (3) utilities, which represent the health-related quality of life of patients in each state; and (4) costs incurred by the NHS in each state. All model parameters are listed in Tables 21 and 22. The sources of these data are described in the following sections.
Parameter | Estimate | Distribution | Source |
---|---|---|---|
Baseline monthly hazard rate of all-cause mortality for the first 8 years of the model (< 75 years) | 0.009 | LN (–4.718, 0.012 SE) | CPRD–ONSa |
HR (≥ 75 years vs. < 75 years) of all-cause mortality for the first 8 years of the model | 2.80 | LN (1.030, 0.014 SE) | CPRD–ONSa |
3 monthly risk of all-cause mortality in general population | Age variant | Fixed | ONS79 |
RR (HF patients vs. general population) of all-cause mortality | 3.14 | Beta (199, 94) HF/ Beta (176, 410) general population | van Jaarsveld et al.80 |
RR (HFpEF patients vs. HFrEF patients) of all-cause mortality | 0.78 | Beta (766, 2865) HFpEF/ Beta (584, 1621) HFrEF | Nichols et al.81 |
BNP HR of all-cause mortality for all HF patients aged < 75 years | 0.62 | LN (–0.478, 0.162 SE) | Troughton et al.25 |
BNP HR of all-cause mortality for all HF patients aged ≥ 75 years | 0.98 | LN (–0.020, 0.140 SE) | Troughton et al.25 |
BNP HR of all-cause mortality for HFrEF patients aged < 75 years | 0.68 | LN (–0.386, 0.177 SE) | Brunner-La Rocca et al.33 |
BNP HR of all-cause mortality for HFpEF patients aged < 75 years | 0.76 | LN (–0.274, 0.487 SE) | Brunner-La Rocca et al.33 |
BNP HR of all-cause mortality for HFrEF patients aged ≥ 75 years | 0.87 | LN (–0.139, 0.148 SE) | Brunner-La Rocca et al.33 |
Baseline hazard rate per month of all-cause hospitalisation (< 75 years) | 0.066 | LN (–2.711, 0.008 SE) | CPRD–HESa |
HR (≥ 75 years vs. < 75 years) of all-cause hospitalisation | 1.248 | LN (0.222, 0.010 SE) | CPRD–HESa |
BNP HR of all-cause hospitalisation | 0.94 | LN (–0.062, 0.062 SE) | Troughton et al.25 |
Parameter | Estimate | Distribution | Source |
---|---|---|---|
HF utility score when hospitalised | 0.66 | Beta (7321, 3772) | Reed et al.82 |
HF utility score when not hospitalised | 0.77 | Beta (7978, 2383) | Reed et al.82 |
Duration of hospitalisation (days) | 13.21 | Gamma (1148.29, 0.01) | CPRD–HES10 |
3-monthly cost when hospitalised (aged < 75 years), (£) | 9104 | Gamma (678.06, 13.43) | CPRD–HES10 |
3-monthly cost when not hospitalised (aged < 75 years), (£) | 682 | Gamma (827.17, 0.82) | CPRD–HES10 |
3-monthly cost when hospitalised (aged ≥ 75 years), (£) | 8057 | Gamma (1746.96, 4.61) | CPRD–HES10 |
3-monthly cost when not hospitalised (aged ≥ 75 years), (£) | 569 | Gamma (1536.51, 0.37) | CPRD–HES10 |
CG unscheduled outpatient visits (24 months) | 1.1 | Gamma (71.60, 0.02) | PRIMA62 |
BNP-guided unscheduled outpatient visits (24 months) | 1.4 | Gamma (94.52, 0.02) | PRIMA62 |
BNP-guided additional cost of medications (18 months), (£) | 58.32 | Gamma (88.42, 0.66) | TIME-CHF29 |
Unit cost of an outpatient visit, (£) | 123 | Fixed | DoH83 |
Unit cost of a BNP test, (£) | 25 | Fixed | NICE84 |
Baseline probabilities: mortality
There are a number of potential sources of information on the baseline probability of all-cause mortality in patients who receive CG therapy. The CG arms of the RCTs pooled in the IPD meta-analyses25,33 provide one potential data source. A drawback of these data is that they provide evidence on survival only over the relatively short follow-up period of the RCTs, typically up to 24 months. Survival beyond that period must be extrapolated or estimated from other sources. A further drawback is that the largest IPD meta-analysis25 available at the start of this project provided a Kaplan–Meier survival curve but not the number of events and number at risk at each follow-up time point. Therefore, we used a software (Version 3.9, WebPlot Digitizer, Austin, TX, USA) to estimate, from this published survival curve, the mortality hazard in the RCT CG arms. This process is approximate and most suited to fitting simple constant hazard functions (e.g. exponential).
As an alternative, we used routinely collected CPRD–HES–ONS linked data from April 2005 up to the censoring date of April 2014, including 52,122 patients, to estimate the monthly mortality rate in clinical practice. This provides an opportunity to estimate survival over a longer time period than is available from RCTs and to compare the fit of different parametric survival models. Arguably, it may also provide a better estimation of the ‘real-world’ effectiveness of BNP-guided monitoring if used outside a RCT setting. However, this requires the strong assumption that the treatment effect, derived from RCT participants, can be generalised to the patients with HF in the CPRD–HES–ONS linked data. In our primary analysis, we used the exponential distribution using the all-cause mortality rate obtained from the CPRD–HES–ONS linked data to estimate survival for the first 8 years of the model. In SAs, we used two alternative survival models to evaluate the robustness of our results: (1) the Weibull distribution for the first 8 years of the model based on the CPRD–HES–ONS linked data as described above (SA1); and (2) the exponential distribution for the first 2 years of the model based on data extracted from the Kaplan–Meier curve25 (SA2).
Beyond the initial period, we used age- and sex-specific ONS 2011–13 population life tables for the UK to estimate survival,79 assuming that two-thirds of patients were male, as reported in the published IPD meta-analysis,25 and inflating general population mortality to mortality for the HF patient population using a RR derived from an observational study. 80 Van Jaarsveld et al. 80 report 32% survival at 7 years for 293 incident HF cases diagnosed in the Netherlands between 1993 and 1998, compared with 70% survival among 586 age- and sex-matched control subjects without HF. These 7-year survival probabilities were converted to 3-monthly survival probabilities.
The majority of patients recruited to trials have HFrEF. After adjusting for age, sex and other covariates, mortality has been demonstrated to be lower in patients with HFpEF. 81,85 Therefore, we adjusted survival in the HFpEF subgroup evaluated in our model, using results from a cohort of more than 6500 patients hospitalised for HF, which reported an adjusted 1-year mortality RR of 1.25 (95% CI 1.12 to 1.41) in patients with HFrEF versus HFpEF. 81
Relative effects: mortality
Troughton et al. 25 conducted a systematic review and IPD meta-analysis to evaluate the effect of BNP-guided monitoring on a primary outcome of all-cause mortality and secondary outcomes, including all-cause and HF-specific hospitalisation. For the primary outcome, their meta-analysis was based on eight RCTs,52,54,58,59,61–64 which provided IPD on 2000 patients, 994 of whom were randomised to the CG group and 1006 were randomised to the BNP-guided group. This meta-analysis explored the interaction between age and relative effectiveness. There were 982 patients in the younger (< 75 years) subgroup and 1018 in the older (≥ 75 years) subgroup. The authors estimated Kaplan–Meier survival curves and HRs for all-cause mortality in both subgroups. BNP-guided monitoring reduced all-cause mortality in the younger subgroup (HR 0.62, 95% CI 0.45 to 0.85) but there was no evidence of a beneficial effect in the older subgroup (HR 0.98, 95% CI 0.75 to 1.3). These findings are qualitatively quite similar to our age subgroup findings, reported in previous sections of this report, based on six RCTs52–56,59 including 1476 patients combined with the estimate of Brunner-La Rocca et al. :33 greater benefit of BNP-guided therapy was observed in the younger subgroup (HR 0.70, 95% CI 0.53 to 0.92) than in the older subgroup (HR 1.07, 95% CI 0.84 to 1.37).
In a recent IPD meta-analysis, Brunner-La Rocca et al. 33 explored additional age and LVEF status subgroups based on a subset of seven RCTs54,58,59,61–64 in patients with HFrEF (n = 1580) and four RCTs60–62,64 in patients with HFpEF (n = 296). They found the strongest evidence of a beneficial effect of BNP-guided monitoring among younger patients (< 75 years) with HFrEF (HR 0.68, 95% CI 0.48 to 0.96, n = 881), although the evidence was weaker among the smaller number of younger patients with HFpEF (HR 0.76, 95% CI 0.29 to 1.96, n = 96). The evidence was not conclusive in older (≥ 75 years) patients with HFrEF (HR 0.87, 95% CI 0.65 to 1.16, n = 850) but did not exclude the possibility of a clinically important effect.
In our primary analyses, we used the relative effects reported in the Troughton et al. 25 and Brunner-La Rocca et al. 33 subgroup meta-analyses to estimate cost-effectiveness, as they represent the largest IPD meta-analyses to date. In SAs, we used relative effects from our IPD meta-analysis to assess the sensitivity of results to the subset of RCTs selected (SA3). Long-term follow-up is not available for most RCTs. In the TIME-CHF,86 BNP-guided therapy ceased at 18 months; over a 5-year follow-up period, the study found a non-significant trend for continued improved survival in younger HFrEF patients with treatment guided by BNP (HR 0.62, 95% CI 0.37 to 1.03). Very few patients were followed up for the full 5 years in TIME-CHF. Therefore, in our primary analysis, we assumed that BNP-guided therapy would cease after 18 months and that the relative treatment effect would end after 4 years. However, it is plausible that BNP-guided care becomes ineffective or less effective before 4 years if, for example, compliance with BNP-guided therapy decreases or the efficacy of BNP-guided therapy decreases with age. Equally, patients may benefit from longer periods of BNP-guided therapy and the relative effect may extend beyond 4 years. To test the importance of these assumptions, we performed SAs assuming that the relative effect and cost of BNP-guided therapy ceased at 2 years (SA4) or that the relative effect and cost of BNP-guided therapy extend for the lifetime of patients (SA5).
Baseline probabilities and relative effects: hospitalisation
For patients surviving each cycle of the model, we estimated the probability of all-cause hospitalisation. In the CG group we used the CPRD–HES–ONS linked data, as described earlier, to estimate the monthly hazard rate of all-cause hospitalisation. We then applied this hazard rate throughout the lifetime of patients in the model. Troughton et al. 25 found no strong evidence that all-cause hospitalisation was reduced in the BNP-guided monitoring arm (HR 0.94, 95% CI 0.84 to 1.07). However, HF-specific hospitalisation was lower in the BNP-guided therapy arm (HR 0.80, 95% CI 0.67 to 0.94). In our analyses, we modelled all-cause hospitalisation. We selected all-cause hospitalisations because this outcome is important to patients and costly for the NHS. This is consistent with our focus on all-cause mortality and allows for the possibility that savings through reduced HF readmissions may be partially offset by more admissions for concomitant disorders or side effects of more intensive HF pharmacotherapy.
Neither Troughton et al. 25 nor Brunner-La Rocca et al. 33 reported HRs for all-cause hospitalisation stratified by LVEF status. In the absence of evidence, we used a HR of 0.94 (0.84 to 1.07) for patients with any type of HF reported by Troughton et al. 25 to estimate the relative effect of BNP-guided monitoring on all-cause hospitalisation since, after adjustment for covariates, there is negligible difference in the risk of all-cause hospitalisation at 1 year (RR 1.02, 95% CI 0.95 to 1.10). 81 Therefore, we used the same risk of all-cause hospitalisation for patients with HFrEF and HFpEF.
Utility parameters
We used two utility parameters to distinguish between patients with stable HF managed in the community and patients who are hospitalised with HF. We conducted a brief literature search to identify studies which reported utility scores, preferably using the EuroQol-5 Dimensions, in patients with HF stratified by hospitalisation status. The Acute Study of Clinical Effectiveness of Nesiritide in Decompensated Heart Failure (ASCEND-HF) multinational trial82 reported utility scores among more than 6000 patients hospitalised with acute decompensated HF and randomised to Nesiritide or placebo. EuroQol-5 Dimensions, three levels (EQ-5D-3L) scores were collected at baseline, 24 hours, discharge and 30 days. In the placebo arm, mean EQ-5D-3L utility scores increased from 0.55 (SD 0.29) at admission to 0.66 (SD 0.26) at 24 hours and 0.77 (SD 0.23) at discharge. EQ-5D-3L scores did not change substantially post discharge: 0.74 (SD 0.25). In our model, we assumed that the utility score at 24 hours was representative of the average utility score (Uh) of patients with acute decompensation during hospitalisation and that the utility score at discharge was representative of the average utility score (Unh) among patients with stable HF who were not hospitalised. We assumed that these two utility values were independent of monitoring strategy; therefore any improvement in quality of life from BNP-guided monitoring strategy in the model is the result of reducing the risk of readmission. Evidence from most RCTs59,62–64 that measured quality of life indicates no difference between patients with BNP-guided and those with CG treatment. We assumed that utility values did not decline with age or differ by LVEF status.
Using the CPRD–HES linked data, we estimated that patients hospitalised with HF would have a mean (standard error) length of stay of 13.21 (0.39) days within a 3-month cycle. Therefore, the mean QALYs gained during a 3-month (i.e. 91.31 days) cycle which included a hospitalisation (QALYh) and a 3-month cycle with stable HF not hospitalised (QALYnh) are:
Cost parameters
The model includes several cost parameters: (1) the cost of BNP and renal testing, (2) up-titration of pharmacotherapy related to BNP monitoring, (3) unscheduled outpatient appointments, (4) the ongoing cost of managing patients with HF in the community and (5) the costs of treating patients with HF in hospital. BNP-guided therapy is likely to initially increase costs due to BNP testing and up-titration of medications but this may be offset in the longer term through reduced hospital admissions. BNP-guided therapy will also increase NHS costs if it extends survival.
The cost of a BNP blood test is £15–25. 84 We used the top end of this range (£25) in our primary analysis to include the costs of additional renal function tests. The cumulative cost will depend on how frequently and for how long monitoring continues. Several RCTs58,59,64 used BNP testing at approximately 3-monthly intervals, whereas others54,62 used testing more frequently initially and then at tapered intervals. Our default assumption was that BNP-guided monitoring would cease at 18 months.
There is mixed evidence of the effect of BNP-guided monitoring on drug utilisation. Some trials58,59,62 reported increases in doses of some drugs in the BNP-guided therapy arm, whereas others54,63,64 did not. In the economic evaluation conducted alongside TIME-CHF,29 medication costs were 12% higher [US$747 vs. US$668; p = 0.04 (2006 values)] in the BNP-guided therapy arm over an 18-month follow-up period. We used this incremental cost (US$79), inflated to 2013/14 values and converted to £(GBP), to estimate the potential increase in medication costs. BNP-guided therapy may also increase the number of unscheduled outpatient visits due to increased side effects of pharmacotherapy; however, most trials do not report this outcome. The PRIMA trial62 found inconclusive evidence of a higher mean number of unscheduled outpatient appointments in the BNP-guided monitoring arm than the CG arm at 2 years (1.4 vs. 1.1; p = 0.06). We used this estimate and a unit cost of £123 per outpatient appointment. 83
We estimated the costs of managing patients hospitalised with HF and with stable HF in the community, stratified by age group, based on the CPRD–HES–ONS linked data. 10 In brief, we identified 1555 adults in England who died with HF in 2012/13. We used the CPRD–HES linked data to estimate the cost of medications, primary and hospital health care during each 90 day period in the 5 years before death. The mean cost of NHS care (hospital admissions, outpatient clinics, primary care consultations, medications and investigations), for patients hospitalised and not-hospitalised, stratified by age group, are reported in Table 22. These analyses found no strong evidence of additional NHS costs for patients with left ventricular dysfunction (mean incremental cost £234, 95% CI –£113 to £580) and therefore we used the same estimates in HFrEF and HFpEF subgroup analyses. All costs were estimated in 2013/14 £(GBP).
Probabilistic sensitivity analysis
We used probabilistic sensitivity analysis (PSA) to propagate the probabilistic uncertainty about each model parameter and estimate 95% CIs around the cost-effectiveness results. 87 Monte Carlo simulation was used to draw a randomly selected estimate of each model parameter from the distribution described in Tables 21 and 22 and calculate the iNMB. We used beta distributions to represent the uncertainty in the probability and utility parameters because these values are typically bounded at zero and one. We used log-normal distributions to estimate uncertainty in hazard rates and ratios. We used gamma distributions to represent the uncertainty in the cost parameters because these values are constrained to be non-negative but can have skewed distributions. The model was built in Microsoft Excel® 2013 (Microsoft Corporation, Redmond, WA, USA) and programmed using Microsoft Visual Basic for Applications® 2013 (Microsoft Corporation, Redmond, WA, USA) to run the PSA. We used a conventional number of iterations (n = 10,000) to empirically estimate the uncertainty surrounding the mean iNMBs calculated from the model.
Deterministic sensitivity analyses
Probabilistic sensitivity analysis on model parameters does not account for all uncertainties underlying the model. There are a number of structural assumptions that are more difficult to parameterise and test in the PSA. Therefore, we conducted a number of deterministic SAs to evaluate the robustness of our results to several of the assumptions made within the model. In addition to the SAs described previously, we also tested the sensitivity of model results to a 50% decrease (SA6) and 50% increase (SA7) in the cost of BNP testing.
Results
Younger patients (aged < 75 years) with heart failure
Our results indicate that BNP-guided therapy is more costly but also more effective than CG therapy over the lifetime of younger patients with any type of HF (see Table 23). If the relative reduction in mortality is sustained for 4 years, then median survival is approximately 1.5 years longer in patients with BNP-guided therapy (7.98 years vs. 6.46 years; Figure 44). The difference in mean QALYs is smaller (5.68 vs. 5.02; see Table 23), reflecting the imperfect health of survivors and the discounting of health gained in future years. Lifetime costs are substantially higher in patients with BNP-guided therapy (£64,777 vs. £58,139; Table 23), as the potential for decreased hospitalisation observed in RCTs is more than offset by BNP testing, medications and the costs of health care during the extended survival period.
FIGURE 44.
Survival curves for all HF patients aged < 75 years.

Subgroup | CG | BNP | iNMB (95% CI)b (£) | ||
---|---|---|---|---|---|
Costa (£) | QALYsa | Costa (£) | QALYsa | ||
Patients aged < 75 years | |||||
All HF | 58,139 | 5.02 | 64,777 | 5.68 | 6426 (2401 to 10,075) |
HFrEF | 58,139 | 5.02 | 63,527 | 5.57 | 5424 (987 to 9469) |
HFpEF | 67,694 | 5.86 | 71,097 | 6.23 | 3155 (–10,307 to 11,613) |
Patients aged ≥ 75 years | |||||
All HF | 26,093 | 2.20 | 25,802 | 2.23 | 869 (–2814 to 4606) |
HFrEF | 26,093 | 2.20 | 27,676 | 2.39 | 2267 (–1524 to 6074) |
The positive iNMB statistic (£6426, 95% CI £2401 to £10,075; see Table 23) indicates that BNP-guided therapy is cost-effective in this patient subgroup at the £20,000 per QALY threshold used by NICE. The CI is broad, primarily because of the uncertainty around the mortality HR from the RCT meta-analysis; however, it does not include zero. Therefore, there is a high probability (0.99) that BNP-guided therapy is cost-effective for this patient subgroup at the NICE £20,000 per QALY threshold (Figure 45).
FIGURE 45.
Cost-effectiveness acceptability curves for all HF patients aged < 75 years.

Younger patients (aged < 75 years) with heart failure; stratified by left ventricular ejection fraction status
The findings in the subgroup of younger patients with HFrEF are broadly similar to those described above for younger patients with any HF. This is unsurprising, as the majority of younger patients (90%)33 recruited to RCTs have HFrEF. The positive iNMB statistic (£5424, 95% CI £987 to £9469; see Table 23) indicates that BNP-guided therapy is cost-effective in this patient subgroup at the £20,000 per QALY threshold used by NICE.
The evidence of cost-effectiveness of BNP-guided therapy is less strong for the subgroup of younger patients with HFpEF. Median survival (9.54 vs. 8.43 years; Figure 46) and mean QALYs (6.23 vs. 5.86 QALYs; see Table 23) are estimated to be higher in patients with BNP-guided monitoring. However, the iNMB is relatively small with a broad CI spanning zero (£3155, 95% CI –£10,307 to £11,613; see Table 23). Nevertheless, there is a relatively high probability (0.75) that BNP-guided therapy is cost-effective for this patient subgroup at the NICE £20,000 per QALY threshold (Figure 47).
FIGURE 46.
Survival curves for HFpEF patients aged < 75 years.

FIGURE 47.
Cost-effectiveness acceptability curves for HFpEF patients aged < 75 years.

Older patients (aged ≥ 75 years) with any heart failure and heart failure reduced ejection fraction
There is little evidence of meaningful health benefits or NHS cost savings of BNP-guided therapy among older patients with any type of HF. The iNMB is small, with a broad CI spanning zero (£869, 95% CI –£2814 to £4606; see Table 23). There is some evidence that BNP-guided therapy has the potential to be cost-effective among older patients with HFrEF. However, life expectancy is much lower in this subgroup than in younger patients, and the estimated gain in QALYs (2.39 vs. 2.20 QALYs) is therefore relatively small. Likewise, the iNMB is relatively small and the CI spans zero (£2267, 95% CI –£1524 to £6074; see Table 23). There is a relatively high probability (0.88) that BNP-guided therapy is cost-effective for this patient subgroup at the NICE £20,000 per QALY threshold (Figure 48).
FIGURE 48.
Cost-effectiveness acceptability curves for HFrEF patients aged ≥ 75 years.

Sensitivity analyses
The estimated benefit of BNP-guided therapy is sensitive to assumptions about its sustained effect (Table 24). If the relative effect and cost of BNP-guided monitoring ceases at 2 years (SA4), the estimated gains in survival (Figure 49) and QALYs (5.39 vs. 5.02 QALYs; see Table 24) are smaller. However, because the costs of BNP-guided monitoring also fall when survival decreases, the conclusion that BNP-guided monitoring is probably cost-effective in younger patients (< 75 years) with HF does not change (iNMB £3395, 95% CI £1137 to £5368; see Table 24).
SA | CG | BNP | iNMB (95% CI)b (£) | ||
---|---|---|---|---|---|
Costa (£) | QALYsa | Costa (£) | QALYsa | ||
SA1: survival based on the Weibull distribution | 59,025 | 5.10 | 66,293 | 5.81 | 6838 (2512 to 10,825) |
SA2: survival based on the Kaplan–Meier curve from Troughton et al.25 | 62,270 | 5.38 | 69,640 | 6.10 | 6914 (2632 to 10,847) |
SA3: BNP HR based on our own IPD meta-analysis | 58,139 | 5.02 | 63,117 | 5.53 | 5138 (1242 to 8680) |
SA4: BNP-guided monitoring ceases at 2 years | 58,139 | 5.02 | 62,008 | 5.39 | 3395 (1137 to 5368) |
SA5: BNP-guided monitoring continues for lifetime | 58,139 | 5.02 | 74,686 | 6.60 | 15,033 (4330 to 26,556) |
SA6: low cost (£12.50) of a BNP test | 58,139 | 5.02 | 64,708 | 5.68 | 6438 (2391 to 10,142) |
SA7: high cost (£37.50) of a BNP test | 58,139 | 5.02 | 64,846 | 5.68 | 6358 (2319 to 10,027) |
FIGURE 49.
Sensitivity analysis 4: survival curves if BNP-guided monitoring ceases at 2 years.

If the benefit of BNP-guided therapy is sustained over patients’ lifetimes (SA5), the estimated benefit and CI width both increase greatly (iNMB £15,033, 95% CI £4330 to £26,556; see Table 24). Plausible changes in the unit cost of the BNP test have minimal impact on conclusions about cost-effectiveness in this patient group (SA6, SA7; see Table 24). Different assumptions about the function form of survival, based on the Weibull distribution (SA1) or Kaplan–Meier curve from Troughton et al. 25 (SA2), also had negligible impact on conclusions about cost-effectiveness (see Table 24). The use of the HR derived from our IPD meta-analysis did not change the iNMB estimate greatly (SA3; see Table 24).
Summary of main findings
We found strong evidence that BNP-guided therapy is a cost-effective alternative to CG therapy strategy in younger (< 75 years) patients with HFrEF and who have recently been discharged from hospital after an acute episode. This conclusion holds even if the impact of BNP-guided therapy on mortality is assumed to dissipate after 2 years. The additional upfront costs of BNP-guided therapy are justified by improvements in survival in this patient subgroup. The costs of BNP-guided monitoring may be offset by fewer hospitalisations but there is no strong evidence that BNP-guided therapy reduces all-cause hospitalisation.
We also found that BNP-guided therapy has the potential to be cost-effective in younger patients with HFpEF and older patients (≥ 75 years) with HFrEF. However, more evidence is required before any firm conclusions can be drawn. There were relatively few younger patients with HFpEF included in RCTs; therefore, conclusions about clinical effectiveness and cost-effectiveness in this subgroup are tentative. Although a larger number of older patients with HFrEF have participated in RCTs, the effectiveness of BNP-guided therapy appears to be attenuated in this subgroup compared with younger patients with HFrEF and cost-effectiveness remains unproven.
Chapter 5 Discussion
Main findings: study conduct
The main findings with respect to study conduct relate to challenges with data sources and data management.
We had extreme difficulty in obtaining the IPD from RCTs that we identified for the systematic review. We followed established recommendations for establishing an IPD collaboration, taking advice from the Cochrane IPD meta-analysis Methods Group as well as from experts within the team. Several triallists/participants in the first meta-analysis of BNP-guided therapy,25 led by Troughton, argued that, contrary to our protocol, trials that did not set a BNP target should be excluded. We had addressed this issue by specifying in our analysis plan that we would carry out a SA, excluding trials that did not set a target. Nevertheless, Troughton et al. declined to participate. Therefore, we estimated the main effect of BNP-guided therapy by combining IPD and aggregate data, including ‘aggregate’ estimates for key subgroups from a previous meta-analysis in order to maximise the power of these analyses. Regrettably, we could carry out only very limited other subgroup analyses, which had low power.
The process for obtaining the linked cohort data was complex and took much longer than expected. The organisations responsible were not as seamlessly integrated as we had expected and the information they provided was not always clear; ultimately, the process for gaining approval to link the CPRD data with the NHFA data set was so protracted that we proceeded without this aspect of the linked cohort. There were two main reasons for delay we experienced: when we started the project the linkage agreements between CPRD GOLD and specific disease registries were not in place and later the reorganisation of the governance arrangements in NHS Digital halted all applications for data and data linkages. When we obtained the CPRD GOLD data, the principle of creating the longitudinal cohort worked satisfactorily.
In relation to the cohort study, in the absence of any established algorithms to identify patients with HF and classify patients as exposed to BNP monitoring or not, we developed our own algorithms. We found that Read Codes for HF are diverse but reasonably consistently used. We chose the most specific algorithm for incident HF in order to have the greatest confidence that the cohort truly comprised patients with HF; it was reassuring that a relatively small number of patients were excluded by this algorithm, compared with the next most sensitive algorithm, and that (when we compared them) the findings for the two algorithms were broadly similar.
Designing an algorithm to identify patients who were exposed to BNP monitoring was more challenging. We were able to quantify the number of BNP tests for each cohort participant and rate of BNP testing. The final algorithm we chose aimed to take into account the total number of tests, the rate of testing and the period of observation, in order to allow for regular but infrequent tests. This algorithm classified very few patients (< 0.5%) as monitored. Although such patients were required to have had three or more BNP tests at a rate of two or more per year, we could not confirm that the test results were used to up-titrate HF medication because medication doses in the CPRD were inconsistently reported. The small number of patients classified as BNP monitored were different from patients who had never had a BNP test or who had had fewer BNP tests: more were female and > 75 years of age and they had a lower BMI and a differing pattern of comorbidity. Given the low frequency of BNP monitoring identified by the algorithm, different nature of the patients classified as BNP monitored and our uncertainty about the success of the algorithm in identifying BNP-monitored patients, we have not reported estimates of the effectiveness of algorithm-determined BNP monitoring in the cohort.
Main findings: study results
Systematic review and meta-analysis
There were four main findings from the systematic review and meta-analysis (the meta-analysis carried out for this project, incorporating evidence from the Troughton et al. 25 and Brunner-La Rocca et al. 33 IPD meta-analyses).
First, BNP-guided therapy appeared efficacious in reducing hospital admissions for HF. Although the meta-analysis by Troughton et al. 25 suggested a protective effect of BNP-guided therapy on all-cause mortality, this excluded the subgroup of patients with HFpEF from the Time-CHF trial. The inclusion of this subgroup in our analysis slightly attenuated the overall effect of BNP-guided therapy on all-cause mortality, which became not statistically significant (p = 0.13).
Second, the benefit of BNP-guided therapy appeared to be greatest in patients < 75 years and in patients with HFrEF. Brunner-La Rocca et al. 33 appear to have considered the independent effects of the interactions between BNP monitoring with age and type of HF, presumably fitting both interactions in one model. They reported the efficacy of BNP monitoring for the four combinations of age and type of HF separately; patients < 75 years with HFrEF benefited the most. They also included comorbidities in the model and concluded that the interaction between BNP-guided therapy and age was explained by the older group having more comorbidities.
Third, the magnitude of treatment effects for clinical outcomes observed in RCTs was not associated with differences in BNP levels between BNP-guided and CG groups or the stringency of the BNP target in trials that set a target. In their meta-analysis, Troughton et al. 25 also showed that there were no major differences between groups in their medications at the end of follow-up.
Fourth, BNP-guided therapy was implemented in diverse ways across trials. This is perhaps not surprising, given that there is no established guideline describing how to monitor BNP or what the BNP target should be. The majority of trials used a target-setting approach (reducing or maintaining the BNP level below a defined target) but with different targets and test frequencies. The heterogeneity between trials and the absence of an established BNP target level were the main factors responsible for our decision to include evidence from all RCTs, regardless of the BNP monitoring approach used.
Finally, none of the studies that provided IPD included individual data for adverse events. Published original reports of each individual study did not identify any significant differences in adverse events between study groups.
These findings pose a paradox. On the one hand, BNP-guided therapy appears to be efficacious in the kinds of patients with HF recruited to the RCTs that have been carried out. On the other hand, there was no strong evidence to support the mechanism by which such a benefit is hypothesised to arise, that is, differences in BNP changes from baseline between BNP-guided and symptom-guided therapy groups and more aggressive up-titration of all medications for HF in the BNP-guided group compared with the symptom-guided therapy group. Some of the key findings are discussed in more detail below.
Which groups of patients benefit from B-type natriuretic peptide-guided therapy?
The results from our subgroup analyses showed more benefit of BNP-guided therapy in patients < 75 years old and patients with HFrEF, with significant interactions between BNP-guided therapy and age and between BNP-guided therapy and type of HF for all-cause mortality. These interactions were also found by Brunner-La Rocca et al. ,33 which is unsurprising, as the latter’s estimates account for over 80% of the weight in our analyses. For type of HF, the effect appeared to be substantially driven by TIME-CHF; when this study was excluded the interaction between the treatment response on mortality and the type of HF was no longer statistically significant (the treatment effect in the two strata converged).
The subgroup analysis by Brunner-La Rocca et al. 33 suggested that the effect of age on treatment response disappeared when interactions between comorbidities (e.g. hypertension, renal failure, chronic obstructive pulmonary disease) and treatment strategy allocation were considered. Similarly, in patients with HFrEF, who formed the majority of the study populations, comorbidities, such as diabetes and chronic obstructive pulmonary disease, attenuated the efficacy of BNP-guided therapy on mortality.
Was the treatment effect a result of decreasing B-type natriuretic peptide and up-titration of medication?
The observed benefit in the BNP-guided therapy group could not be attributed to changes in BNP/NT-proBNP levels during follow-up. In most of the RCTs (5/8)58,62–64,66 that described BNP/NT-proBNP levels at baseline and end of follow-up, levels decreased in both treatment groups at the end of follow-up. The meta-analysis by Troughton et al. 25 also showed that NT-proBNP levels (available from seven studies providing IPD) fell by a similar amount in the two groups, 35% in the BNP-guided therapy group and 32% in the symptom-guided therapy group. There was no consistent relationship between the magnitude of the HR for all-cause mortality and the BNP/NT-proBNP decrease (see Figure 24). The trials that showed the greatest benefit and in which BNP fell substantially more in the BNP-guided group than in the symptom-guided group provided least weight in the meta-analysis.
This interpretation of the lack of relationship between treatment effects and changes in BNP/NT-proBNP levels might be criticised as naive. For example, the RCT populations had different baseline BNP/NT-proBNP levels and the effect of BNP-guided therapy may differ according to HF severity. In addition, the dynamics of BNP levels are complex, influenced not only by HF severity but also a multitude of factors, including demographic (age, sex, BMI),88,89 genetic,90 and a variety of other cardiac and non-cardiac conditions (e.g. atrial fibrillation, pulmonary hypertension, myocardial ischaemia, ischaemic stroke, pulmonary embolism, chronic kidney disease and liver dysfunction). 91 Nevertheless, these important prognostic factors should have been distributed similarly in BNP-guided therapy and symptom-guided therapy groups in all of the RCTs by virtue of randomisation, and proponents of BNP-guided therapy need to explain the absence of a relationship.
The proportion of patients on different HF medications was similar in both the BNP-guided therapy and symptom-guided therapy groups at baseline and at the end of follow-up. It could not be determined whether or not and how HF medication doses changed during follow-up because medication doses were not available for most of the studies providing IPD. The meta-analysis by Troughton et al. 25 showed no differences between groups in the changes in medication doses from baseline to the end of follow-up, except for ACEis and ARBs (higher doses in the BNP-guided therapy group). Treatment with ACEis and ARBs in accordance with guidelines has been shown to reduce the risk of death and hospitalisation in both RCTs and large registries. 92–95
B-type natriuretic peptide-lowering versus B-type natriuretic peptide-monitoring strategy
Our systematic review and meta-analyses aimed to include all RCTs that used serial BNP measurements to guide HF therapy, regardless of the monitoring strategy used. The exclusion of two RCTs that did not use a BNP-lowering strategy did not alter the findings of the meta-analysis. Troughton and Januzzi (R Troughton, University of Otago/Christchurch Hospital and JL Januzzi, Harvard Medical School/Massachusetts General Hospital, 2015, personal communication) have argued that only studies using a similar design should be pooled because including studies that use different monitoring approaches will increase clinical heterogeneity, leaving clinicians uncertain as to which approach to follow. A published protocol is not available and, despite several requests, Troughton et al. were unable to provide one. Therefore, it is unclear whether or not this was an a priori point of view when Troughton et al. carried out their first meta-analysis. It is also unclear whether their meta-analysis was based on a systematic literature search. There is no mention in the published meta-analysis of Troughton et al. 25 of the different BNP-monitoring strategies, although the NorthStar results were presented between Troughton’s conference presentation50 and his published meta-analysis. 25
At the outset, we disagreed with the point of view of Troughton et al. for several reasons. First, we aimed to provide realistic treatment effect estimates given that there is no established guideline describing how to monitor BNP or what the BNP target should be, and therefore clinicians are likely to vary in how they use BNP levels to manage their patients (e.g. some may use BNP to check the status quo and others may take a more stringent approach to lower BNP as much as possible or to a particular target).
Second, it can be argued that the two strategies are not fundamentally dissimilar, as both approaches will prompt a review of the treatment a patient receives, with appropriate intensification to bring BNP down to a set target or within the specified range (and it can be reasonably argued that the latter also represents monitoring to a target).
Third, the RCTs that used a BNP-lowering strategy were themselves highly heterogeneous in design, treatment strategies (in both the BNP group and the control group) and BNP/NT-proBNP cut-off points used. This heterogeneity, combined with the fact that the optimal target is not known and that the effect of BNP-guided therapy appears to be independent of BNP changes over time makes it difficult to argue for the exclusion of any trials, regardless of the BNP-monitoring approach used.
Finally, we prespecified in our protocol that we intended to include all trials of BNP monitoring. We made this decision because we are aware that UK patients with HF are managed mainly in primary care, in which target setting may be less appropriate, and wished to avoid publication-related biases, data availability bias and reviewer selection bias. 26,37,96 These biases can lead to meta-analyses being biased towards more favourable treatment effects96,97 and have been highlighted as a potential problem in meta-analyses that use IPD. 96 We included a SA to investigate the impact of the distinction between BNP-guided strategies that do and do not set a target.
Risk of bias in randomised controlled trials included in meta-analyses and systematic reviews
It is important also to consider whether or not the apparent benefits of BNP-guided therapy could have arisen because of bias, either in the primary RCTs or through the conduct of the reviews, particularly in view of the lack of an apparent mechanism for the benefits.
Most of the included RCTs (10/13) had unclear risk of bias because they did not report whether or not allocation was concealed (see Table 1 and Figure 2). SAs including just the three RCTs52,59,61 that were judged to have had concealed allocation appeared to generate attenuated effects but these analyses had low power.
Most of the included RCTs were considered to have a high risk of bias because there was a lack of blinding of participants and the care team. This lack of blinding means that cointerventions affecting outcomes (including all-cause mortality) could have been initiated by either the doctors or the participants themselves, conditional on their knowledge of the allocation. (By contrast, risk of bias because of blinding of outcome assessors was assumed to be low for all RCTs for all-cause mortality and high or unclear for about 50% of RCTs for other outcomes.) Two RCTs were reported as double-blind58,64 and one reported blinding patients to group allocation. 59 Among the other included RCTs, ten made no attempt to blind investigators52–55,59–63,65,66 and one was unclear,56 and two made no attempt to blind participants55,66 and eight were unclear52–54,56,61–63,65 (see Table 2 and Figure 2).
Blinding of participants required blood samples to be taken (but not acted on) in both groups at all time points when BNP measurements were scheduled to be used to guide management in the BNP-guided group. The investigators of the TIME-CHF RCT described this arrangement: ‘The N-terminal BNP levels were determined centrally at every visit in all patients, but only results of patients in the N-terminal BNP-guided strategy group were sent to the investigators (treating physicians)’. 59 The same was true in the Christchurch pilot58 and BATTLESCARRED RCTs. 64 Although no attempt to assess the success of participant blinding was reported, it seems likely that this was successful in these three trials, meaning the reporting of quality of life had low risk of bias.
Blinding of doctors managing participants required objective criteria to be applied for treatment intensification, with assessment of a participant’s status against the criteria by an independent physician not otherwise involved in managing the participant’s care. This arrangement is described for the Christchurch pilot and for the BATTLESCARRED RCTs: ‘Heart failure scores ≥ 2.0 triggered escalation of drug therapy according to a pre-set algorithm . . . For the NT-proBNP group, adjustments in medications and additional follow-up visits were triggered by a NT-proBNP level > 150 pmol/l and/or a heart failure score ≥ 2.0 according to instructions by 1 investigator (JGL) who did not undertake the clinical assessments’. 64 No attempt to assess the success of investigator blinding was reported.
It is striking that in the Christchurch pilot58 and BATTLESCARRED RCTs,64 both of which blinded both investigators and participants, treatment effects spanned almost the total range of the effects across trials (HR 0.15 and 0.94; these estimates were extracted from a previous IPD meta-analysis),25 accepting that the former estimate was extremely imprecise.
Neither previous meta-analysis reported that it was based on a protocol or carried out a systematic literature search. 25,33 Importantly, without protocols, one cannot be sure that study eligibility criteria, outcomes and analyses were prespecified. Most of the reported effects took no account of multiple testing and were of borderline statistical significance (0.05 > p > 0.01), the exceptions being stratum-specific estimates for HF admission or a composite outcome of HF admission or death. The magnitude of the reported benefits of BNP-guided therapy (HRs around 0.8–0.9) are likely to be clinically important and, based on the cost-effectiveness model we report, very likely to be cost-effective for younger patients with HFrEF. However, there remains considerable uncertainty about the size of the effects and the extent to which they may be a result of bias.
Cohort study of patients with incident heart failure
The cohort of patients with incident HF provided a detailed description of the natural history of English patients with HF and informed the cost-effectiveness model, primarily in relation to the costs of managing patients with HF.
Three nested algorithms, designed to have differing sensitivity and specificity for incident HF, were applied. The main cohort findings were based on the most specific algorithm, which included only patients with one or more specific HF diagnosis codes. This algorithm yielded only slightly fewer patients with incident HF (15%) than the intermediate algorithm, which also included patients with HF symptom codes; both these algorithms also required patients to have a code for an investigation or test for HF and to have been prescribed a medication indicated for HF.
The cohort study provided a comprehensive description of incident HF patients in the UK, through follow-up in primary and secondary care (CPRD GOLD data linked with hospital data). We were able to use the cohort data to estimate the incidence of adverse clinical outcomes in patients with HF and describe patterns of BNP testing in HF patients in the UK. New patients with incident HF accrued to the cohort at a constant rate over time, suggesting that GPs use the Read Codes for HF in a consistent manner. The rate of BNP testing appeared to increase to some extent over time. The median longevity (death from any cause) following diagnosis with HF was about 4.5 years.
Across all follow-up, patients had about one unscheduled admission to hospital every 2 years. In a separate publication10 we used the CPRD data to identify a subset of 1555 adults in England who died with HF in 2012/13 and described the pattern of resource over time; this analysis showed that admissions were not spread evenly during follow-up but increased in frequency substantially as patients’ health deteriorated. In this subset of patients, we also estimated the cost of medications, primary and hospital health care and derived cost estimates for the cost-effectiveness model. We also presented data for the cohort of patients with unscheduled hospital admissions in the NHFA. The cohorts were comparable with respect to demographic and baseline clinical characteristics.
This is the first study which has attempted to identify BNP monitoring in a cohort of HF patients from routinely collected data. The algorithm for classifying patients according to their BNP test exposure classified very few patients (< 0.5%) as monitored. The small number of patients classified as BNP-monitored also appeared to be different from patients who had never had a BNP test or who had had fewer BNP tests: more were female and > 75 years of age and they had a lower BMI and a differing pattern of comorbidity.
There were only five Read Codes that identified a BNP test in the CPRD, all of which are specific for BNP; therefore, it is likely that BNP tests were identified consistently and accurately. The algorithms for defining BNP exposure, in particular BNP-monitored patients, was developed with input from all clinical members of the study team. However, this task was hampered by the fact that there is currently no accepted definition of BNP monitoring on which we could base our algorithm (see Strengths and limitations of the study).
Model-based cost-effectiveness analysis
There were two main findings of the cost-effectiveness analysis:
-
BNP-guided therapy is a cost-effective alternative to symptom-guided therapy in younger (< 75 years) patients with HFrEF and who are recently discharged from hospital after an acute episode. This finding remains true even if the effect of BNP-guided therapy on mortality is limited to 2 years.
-
BNP-guided therapy is cost-effective, despite the costs of BNP-guided monitoring, over a longer period of time, because more QALYs accrue with longer survival. BNP-guided therapy might reduce hospitalisations but there is insufficient evidence to conclude that this is the case.
We compared our own findings with those of previous economic evaluations of BNP-guided monitoring in patients with HF (Table 25). The largest prospective economic evaluation was conducted alongside TIME-CHF. 29 The RCT reported that more patients with HFrEF survived to 18 months (mean age 76 years) with BNP-guided therapy, although the CI included one result (HR 0.68, 95% CI 0.45 to 1.02) that was not statistically significant. 59 The economic evaluation estimated higher costs (US$384, 95% CI –US$3462 to US$4803; after excluding residential costs), longer survival (0.07 life-years, 95% CI 0.00 to 0.14 life-years) and higher QALYs (0.05 QALYs, 95% CI –0.02 to 0.11 QALYs) in patients with BNP-guided therapy. 29 The authors concluded that BNP-guided therapy had a high probability of being cost-effective but note that this probability was lower in older patients.
Author | Study design | Patients | Interventions | Outcomes | Results |
---|---|---|---|---|---|
Sanders-van Wijk et al.29 | CUA alongside TIME-CHF | n = 438; ≥ 60 years; LVEF ≤ 45% | 1. Symptom guided; 2. NT-proBNP guided | QALYs at 18 months; 2006 payer costs | QALYs: 0.91 (BNP), 0.87 (SG), difference 0.05 (95% CI –0.02 to 0.11). Costs: US$16,792 (BNP), US$16,364 (SG), difference US$384 (95% CI –US$3462 to US$4803) |
Adlbrecht et al.28 | CEAs alongside Berger et al.61 trial | n = 190; LVEF < 40% or CTR > 0.5 | 1. UC; 2. HNC; 3. BNP | Mortality at 18 months; 2005 payer costs | Mortality: 45% UC; 24% HNC; 17% BNP. Costs: €12,450 UC; €12,391 HNC; €9674 BNP |
Morimoto et al.31 | CUA Markov model | 35–85 years; LVEF ≤ 40% | 1. CG group; 2. BNP group | QALYs at 18 months; 2002 costs | QALYs: 1.07 (BNP), 0.94 (CG). Costs: US$20,737 (BNP), US$19,723 (CG) |
Moertl et al.30 | CUA Markov model | LVEF < 40% or CTR > 0.5 | 1. UC; 2. HNC; 3. BNP | QALYs up to 20 years; 2010 health-care costs | QALYs: 2.36 (UC); 3.04 (HNC); 3.20 (BNP). Costs: €36,110 (UC); €38,653 (HNC); €35,155 (BNP) |
Laramée et al.32 | CUA Markov model | Chronic HF due to LVSD and other subgroups | 1. Clinical assessment; 2. NP monitoring | QALYs over lifetime; 2011 NHS and social service costs | QALYs: 4.85 (CA); 5.19 (NP). Costs: £12,869 (CA); £13,972 (NP) |
Laramée et al. 32 published the only UK-based economic model of BNP-guided therapy, developing previous work included in the NICE HF clinical guidelines. 8 Their analysis is based on aggregate data, rather than IPD, from six RCTs. 58,59,62,64–66 The structure of their model is similar to ours but the key model parameter estimates differ, particularly those we derived from our analyses of the CPRD data. They concluded that BNP-guided therapy is most probably cost-effective in patients with HFrEF and in younger patients with HF from any cause. An acknowledged limitation of their analysis is that they could not explore cost-effectiveness in patients with HFpEF.
Our study, using pooled IPD across several RCTs, was able to explore patient subgroups further than previous economic evaluations. Our findings are in agreement with previous work that BNP-guided therapy is a cost-effective alternative to CG therapy in younger (< 75 years) patients with HFrEF. Our analysis suggests that BNP-guided therapy has the potential to be cost-effective in younger patients with HFpEF and older patients (≥ 75 years) with HFrEF. However, it would be unreasonable to conclude that BNP-guided therapy may be efficacious in these patients, given the small number of patients with HFpEF and patients ≥ 75 years of age who were recruited into the RCTs and the fact that BNP monitoring can work only through optimising HF therapy but current HF drugs appear not to improve outcomes in HFpEF patients. 98 There is also uncertainty with respect to what constitutes an optimal BNP-monitoring strategy. The trials were heterogeneous in how BNP-guided therapy was administered and to date no group of researchers has defined an optimal strategy. In addition, we have no strong evidence of an association between the magnitude of the change in BNP levels during follow-up between groups and the size of the clinical effect, which suggests that the intervention may not be responsible for the observed effects (as there appears to be no mechanism for the efficacy of the intervention). Most of the RCTs also had a high risk of performance bias.
Patient and public involvement and engagement
Patients and the public were not actively involved in identifying the research question for our project because it had been prioritised by the NHS as a commissioned research programme. Nevertheless, during the later stages of the application, we included Peter Billingham, who is the chairperson of the Avon, Gloucestershire, Wiltshire and Somerset Cardiac and Stroke Network Cardiac Patients and Carers Group, as a co-applicant. Mr Billingham has a history of cardiovascular disease and HF. We invited him to join the study to help us understand the perspective of patients with HF, interpret the findings of the study and disseminate the results.
During the course of the study, the newly appointed patient and public involvement and engagement (PPI&E) lead for the Cardiovascular Biomedical Research Unit co-ordinated further PPI&E for this study. We invited a small group of current HF patients so that we could talk to them about our project and gain their insight and opinions about aspects of HF management that are important to patients with HF.
We enlisted the help of the HF specialist nurse at the University Hospitals Bristol NHS Foundation Trust to identify patients to invite to the group. However, shortly after initiating the work, the specialist nurse left her post and was not immediately replaced. Once we had compiled most of the study findings, we invited patients to a focus group in order to elicit their perspectives on the emerging findings and to discuss their views about disseminating the results of the study.
Patient and participant involvement focus group
Four participants attended the focus group. All four were male and in their late fifties or older. Although each was living with a HF diagnosis, they were in reasonably good health. Three of the participants brought their partners with them and each (partner) fully participated in the group discussion. The discussion was facilitated by the PPI&E lead, one of the authors (Maria Pufulete), a cardiovascular research nurse (Kim Wright) and a second author, whose role was primarily to record minutes of the discussion (Rachel Maishman).
Participants shared the following details of their histories.
-
Participant A: this participant’s pathway started when he fainted and was admitted to hospital for tests. He had a number of follow-ups over the subsequent years to determine what was wrong with him and, gradually, the term ‘heart failure’ started to be used to describe his condition. The participant said that his GP had never really been involved in the management of his HF and that he was usually seen in the pacing clinic or by specialist HF nurses at the hospital.
-
Participant B: the participant said that he had had a bad experience with the GP in relation to his diagnosis. Initially, he was treated for a variety of other conditions (e.g. asthma, hay fever). Eventually, the participant demanded to be referred to hospital, where he was immediately diagnosed. The participant has no regular follow-ups at the GP and said that all of his HF management takes place in the hospital. The participant said that he has only regular follow-up appointments because he is in a drug trial with scheduled visits.
-
Participant C: the participant noticed that he was unwell because his physical fitness was not as good as usual. The participant was then diagnosed after an admission to hospital. He has not been back to the GP since the diagnosis and all follow-up is with a specialist HF nurse at the local health centre. The participant has follow-ups every 2–3 weeks and occasional consultations at the hospital with a cardiologist specialising in HF. He is currently trying different medications to find the best combination for him.
-
Participant D: the participant has been to see the GP only a couple of times about his HF. The participant was diagnosed following a referral by his GP to a cardiologist. He sees the cardiologist every 12 months to check his medications. The participant was also in the same trial as participant B.
Participants’ views on B-type natriuretic peptide-monitoring
Participants were asked whether or not they would be happy having BNP tests at regular follow-ups to guide medication changes.
They said that they would welcome an objective way of saying whether the medication was working or not. One participant said that the blood test would be a good idea and that, in terms of weighing up ‘better heart condition’ versus ‘increased side effects from more intensive drug treatment’, he would like the results of the test in order to guide the recommendation to change treatment. He could then make an informed decision to change treatment or not.
Although all participants were overwhelmingly in favour of BNP monitoring, they did acknowledge that this was in part a reflection of their current health status. They agreed that deteriorating health and potentially severe side effects resulting from more intensive medication may well cause their views to differ. For one of the participants this was a real concern, as he had experienced incapacitating side effects. Nobody else spoke about experiencing adverse side effects: the other three participants had all experienced only benefits from their prescribed HF medications.
All participants in the group were focused on ‘maintaining’ their current, relatively healthy status and prolonging life. BNP testing was viewed in this context, as a tool to achieve this goal. The potential for being prescribed more intensified treatment was not seen as a deterrent, primarily reflecting the fact that they had not experienced side effects with their current medications; rather, BNP testing was perceived as a means of making more informed choices.
Other comments arising from the discussion
-
Participant B said that, since his discharge from hospital, his medications had not been changed.
-
Participant C felt that there had been a pattern of poor communication between the consultants/specialists and the GPs involved in his care.
-
Some of the participants asked if they would be able to go to the GP and ask for the testing to be done.
-
Participant D asked that, if this is a test for diagnosis of HF, why is it not offered to everyone with a history of HF?
-
Demographics of participant groups in studies were discussed in relation to the kinds of patients who would be likely to be most assertive about requesting treatment/tests not routinely offered; it was suggested that patients with a lower level of education would be less likely push for testing. In this context, participants reflected on the composition of the focus group, noting that they perceived themselves to be an intelligent, well-read/informed and mostly healthy group of people. They discussed how their perspectives might have differed had they had a different socioeconomic profile. All participants felt that their demographic characteristics had the potential to impact on their responses to questions, as did their current health status.
-
Subsequently, the group requested that any report resulting from the focus group should be transparent about the health status and demographic composition of the group, particularly that no participant had ‘end-stage’ HF.
Dissemination of the results of the study
The focus group discussed dissemination at two levels, namely with respect to general information level about HF and information specific about the research.
At the general level, it was suggested that the leaflets available in British Heart Foundation (BHF) shops were an excellent resource and that this information was of high quality. Participants talked about the ways in which they used the BHF website and their booklets to keep up to date with news relating to their condition and as a source of general information. All participants were keen to find ways of keeping up to date with literature about HF, treatments and relevant research.
It was pointed out that general awareness of HF is poor. The consequence of this poor awareness is that people are not aware of the signs and symptoms of HF so do not go to their GPs and are not diagnosed. Participants viewed this as a bigger problem than the specific issue of interest, that is BNP testing.
The group pointed out that, apart from information provided by the BHF, it was not easy to find appropriate literature on specific research or literature relating to issues of specific interest. A comment was made that ‘in clinic there are 1000 different leaflets and you don’t know what you are looking for’.
With respect to specific research information, one participant had come with newspaper clippings about HF research that had been published in the media and spoke about being keen to be as informed as possible.
The companions of the participants with HF also played a key role in gaining information about their partner’s conditions. Three of the participants came with a companion and all three companions were very well-informed about their partner’s condition and were clearly keen to learn more. The companions discussed how they kept up to date with literature about HF, treatments and research: this largely involved following leads from the BHF.
Other ways of disseminating information were suggested:
-
Leaflets in GP surgeries, cardiology clinics, etc.
-
Coverage on television, radio and in other media; dissemination in these fora is always popular and is also very achievable, particularly radio and newspapers.
-
Posters were highlighted as a way of increasing general awareness about HF. Participants also suggested that posters are a way to engage with the public about research-specific topics.
-
Public lectures/workshops (group meetings to disseminate research). One participant talked about a conference that they went to where there were stands and professionals presenting their work. He said that the conference was very well-attended by the general public and something that he really enjoyed. Another participant had also attended similar events but felt that the lack of inclusion of the patients had had an impact on its success from their perspective.
Each of the participants fed back that they had enjoyed participating in the group and concluded that they had particularly enjoyed meeting the other participants and talking about their health experiences together.
Next steps
Each participant was sent a thank you letter after the meeting. Participants were also invited to join an ongoing patient advisory group about early-phase research in the Cardiovascular Biomedical Research Unit. One of the participants agreed to join this group.
Dissemination of the findings of the study to patients and the public will draw on the points made by participants and the continuing input of Peter Billingham, who will help us to disseminate our findings through the Cardiac and Stroke Network.
Conclusion
Some key points emerged from focus group participants’ accounts of their medical histories in relation to HF:
-
they reported not being actively managed for HF by their GPs
-
the lack of active management in primary care extended to diagnosis of HF; two of the four participants reported being diagnosed only after referral or admission to hospital
-
they actively sought out specialist hospital-based care, through specialist clinics or by participating in research.
It is difficult to generalise from these accounts, as the participants were not representative and appeared to be very engaged in managing their own conditions. Nevertheless, their accounts are consistent with management in primary care being suboptimal, as reported by the BATTLESCARRED trial. 64
Participants said that the principle of BNP monitoring, with regular blood tests, was acceptable and that they would welcome an objective way of monitoring whether their HF medications were working or not. With respect to an increased risk of side effects, it was felt that more information about HF status could only be helpful in making difficult decisions about whether or not to opt for more intensive medication. BNP testing was perceived as a means of making more informed choices.
Strengths and limitations of the study
Systematic review and meta-analysis
The present meta-analysis has several strengths. The review team systematically identified all RCTs that used BNP-guided therapy in patients with HF and conducted the meta-analysis in accordance with a prespecified protocol35 and published guidelines. All trials identified for which data were available (IPD or aggregate) were included. There was no evidence of bias due to small study effects. The analysis plan was prespecified.
The main weakness was the inability to obtain IPD from most of the trials included in the meta-analysis by Troughton et al. 25 because of the differing view about the appropriateness of combining data for trials when a BNP/NT-proBNP target was not set. This limitation constrained the subgroup analyses that could be conducted. Unfortunately, it was not possible to combine the IPD subgroup estimates in our study with other reported subgroup effects for all subgroups; the meta-analysis by Troughton et al. 25 did not quantify the age subgroup effect and the subgroup analysis based on the same set of trials33 quantified the age subgroup effect within HFrEF and non-HFrEF strata. We asked Troughton et al. for their subgroup estimates to allow us to carry out more detailed analyses but they did not respond.
Other limitations are inherent to the design of the RCTs: heterogeneity in how BNP-guided therapy was administered; the recruitment of certain types of patients with HF (younger patients with HFrEF and without comorbidities), which makes it difficult to generalise the results to the broader population with HF; and the potential for bias, as clinicians and patients were not blinded to treatment allocation in most of the trials. In addition, despite combining results from 13 RCTs, the pooled sample size (up to 3074 patients with HF) was relatively small in comparison with sample sizes in other meta-analyses in this patient population (some of which included > 13,000 patients)99 and multiple estimates have been reported with 95% CIs; therefore, chance may explain some of the apparently significant findings.
Cohort study of patients with incident heart failure
This cohort study had several strengths with respect to the descriptive objectives. We identified a large cohort of patients newly diagnosed with HF, rather than a combination of incident and prevalent patients, reducing the potential for bias and reflecting the most current population diagnosed with HF. We used three different algorithms for identifying HF in the CPRD. Algorithms 2 and 3 included HF Read Codes and the presence of HF-related investigations and HF medications. Although we did not validate our algorithms (e.g. by requesting additional data from GPs for a subset of patients), results for the SA did not find differences between the algorithms with intermediate (2) and least sensitivity (3). Similar findings have been reported in other studies using CPRD data to identify different conditions,100–102 which suggests that CPRD Read Codes can be used to identify a representative sample of patients with a particular condition in the UK. Our study was conducted and reported in accordance with recent guidelines for studies conducted using observational routinely collected health data (REporting of studies Conducted using Observational Routinely collected health Data, RECORD guidelines103), which recognise the additional challenges of conducting research using routinely collected data obtained for administrative and clinical purposes rather than research.
In the context of the overall study, the main limitation was our lack of confidence that the patients identified as BNP monitored were in fact monitored, as there was large diversity in the patterns of BNP tests recorded in the CPRD data set. For example, some patients had numerous tests for a short period of follow-up and no other tests recorded for the remainder of follow-up, there were irregular time intervals between tests for different patients and there was no clear pattern of repeated BNP testing among most of the 71 patients classified as BNP monitored. In addition, a proportion of patients did not have sufficient observation time in the cohort study for the algorithm to discriminate between BNP tested and BNP monitored. Ideally, we should have conducted a validation study (sending questionnaires to GPs for a sample of the cohort to verify the diagnosis and the timing of the diagnosis)100 on the exposure algorithm but this was difficult given the lack of consensus about what BNP monitoring should constitute and the limited time frame of the project.
We were also aware at the outset that BNP monitoring, such as it was implemented in RCTs, is unlikely to exist in ‘real-life’ practice. We were unable to establish if the serial BNP tests in the CPRD represented clinicians ordering these tests and using BNP values to check that patients were stable (or adjust their medications), indicated different clinicians ordering BNP tests at particular points in time to check the severity of HF (in effect, cross-sectional testing), or represented tests ordered around hospital admissions or following a cardiologist’s request after an outpatient appointment. Certainly, given that patients we identified as monitored in the present study had poorer outcomes, it seems likely that BNP tests were ordered for the sickest patients to check the severity of their HF. These patients were on average older and had a lower BMI, factors which are both associated with disease severity and poor outcome in chronic HF. 104
Furthermore, with respect to investigating the mechanism of any effect of BNP monitoring, we could not determine whether or not there was a relationship between BNP testing and changes in medications because medication dose could not be determined accurately in the CPRD therapy data set (there were many missing data on doses of medications). Evidence for such a relationship would have increased our confidence that the serial testing represented monitoring. Collectively, these limitations led to our decision not to report analyses of clinical outcomes according to BNP test exposure (BNP monitored vs. BNP tested vs. never tested).
Other limitations of the cohort study include our inability to distinguish patients with HFrEF and HFpEF because specific codes for the two types of HF were not used consistently. We were also unable to perform the linkage between CPRD GOLD and the NHFA, which would have provided more detailed information on unscheduled hospital admissions for patients in the cohort. A final point is that not all HF patients in the UK are treated by GPs; some are treated in community HF clinics and at home by HF specialist nurses. Community care databases are not linked with CPRD GOLD and, therefore, a substantial proportion of data relating to some patients’ care pathways will be missing from the CPRD.
Model-based cost-effectiveness analysis
Our primary analyses were based on the IPD analyses of Troughton et al. 25 and Brunner-La Rocca et al. 33 including approximately 2000 patients participating in several RCTs. We were able to investigate the cost-effectiveness of BNP-guided therapy in subgroups of patients that were not reported in all the original RCT publications. The use of both previously published IPD meta-analyses25,33 and the meta-analyses conducted in this report using an overlapping set of RCTs provided further confirmation of the probable cost-effectiveness of BNP-guided therapy in younger patients. We conducted a PSA that allowed us to identify patient subgroups (e.g. HFpEF patients aged < 75 years and HFrEF patients aged ≥ 75 years) for which further evidence is needed. We did not extend this to estimate expected value of information. 105 Expected value of information would estimate the costs of benefits of future RCTs in these patient subgroups but was beyond the remit of this research grant. We conducted deterministic SAs to explore the influence of selected assumptions on the model. This allowed us to demonstrate that plausible changes to assumptions about the sustained benefit of BNP-guided therapy do not alter conclusions that BNP-guided monitoring is cost-effective in younger patients with HFrEF but that they are influential in estimating the absolute health benefit for patients.
We used a highly simplified two-state Markov model to track costs and patient outcomes. A more complex model tracking dysfunction (e.g. through NYHA class) and making the probability of hospitalisation and death dependent on dysfunction would provide a more realistic representation of disease progression. For example, Ieva et al. 106 have used routine administrative data to model the decrease in time to readmission with each successive admission and the association between age, sex and readmission. The simplicity of our model might lead to poor estimates of cost-effectiveness if BNP-guided therapy has a large effect (positive or negative) on functional decline among survivors. However, evidence from several RCTs59,62–64 that have measured quality of life indicates no difference between patients with BNP-guided versus CG treatment. One exception is the ProBNP Outpatient Tailored Chronic Heart Failure Therapy (PROTECT) trial,107 which found greater improvement in quality-of-life scores among patients with HFrEF and who received BNP-guided therapy. Our analyses focus on costs to the health service, rather than wider costs falling on social care or patients and families. BNP-guided therapy may be more cost-effective from a broader societal perspective if, for example, it results in fewer admissions to residential or nursing homes.
The conclusions of our study are limited by the quality of available evidence. In some patient subgroups (i.e. HFpEF patients aged < 75 years and HFrEF patients aged ≥ 75 years), too little evidence exists to reach definitive conclusions about cost-effectiveness. There was no evidence on all-cause hospitalisation stratified by patient subgroup, therefore we assumed that the HR reported for all HF patients could be applied to all patient subgroups. Although this parameter was not very influential in our model, additional evidence on this is needed for the patient subgroups (i.e. HFrEF patients aged ≥ 75 years) for which cost-effectiveness is most marginal.
Lessons for the future: implications for clinicians and policy-makers
The present meta-analysis suggests that BNP-guided therapy improves clinical outcomes. However, the applicability of this conclusion to all health settings is not clear. The RCTs included mainly patients < 75 years of age with HFrEF and who were treated by cardiologists in dedicated HF clinics. By contrast, across many European countries, including the UK, cardiologists do not lead the management of patients with HF and about half of all patients have HFpEF. There are significant gaps and variation in the medical care of HF patients and there is evidence that not all patients are receiving optimal treatment according to guidelines. 11
The lack of evidence supporting plausible mechanisms by which BNP guiding might have an effect is also a concern. The reduction in BNP levels and the increase in the doses of most medications in both treatment groups reported in the RCTs suggest that patients in both groups were treated more aggressively (according to trial protocols optimising symptom-guided therapy or clinical guidelines). Thus, our results suggest that the first priority should be to ensure adherence to guidelines for managing HF. Despite the apparent efficacy of BNP monitoring, its usefulness in guiding up-titration of therapy is not clearly established, given the lack of relationship between the change in BNP levels between groups and improved outcome.
B-type natriuretic peptide testing is recommended by NICE as an essential part of the diagnostic pathway for HF. 8 We have shown that ordering of BNP tests has increased to some extent over time, as would be expected, but there is no hard evidence that BNP monitoring is currently happening in the UK, given the small proportion of the cohort who had serial BNP tests. The introduction of a Read Code to indicate that serial BNP testing is being carried out explicitly to guide up-titration of HF medications, and consistent use of the code, would facilitate a future similar investigation using the CPRD data.
The National Institute for Health and Care Excellence also advised clinicians to consider specialist monitoring of BNP in some patients, for example those recently admitted to hospital, but also recommended further research on cost-effectiveness. 8 It has been suggested that the guidelines did not recommend routine use of BNP monitoring because of uncertainties about the impact of this monitoring in older patients who represent the majority of patients with HF. 32 The NICE clinical guidelines are scheduled to be updated in 2018. Our results indicate that BNP-guided therapy conducted by a specialist team is cost-effective in younger patients with HFrEF and who have recently been discharged from hospital after an acute episode. However, more evidence is required to decide whether or not it is cost-effective in younger patients with HFpEF and older patients with HFrEF, particularly with respect to the setting, the frequency, duration and BNP target for monitoring.
Although BNP tests are relatively cheap, there will be logistical and financial challenges to routine implementation in the UK. If, as in most RCTs, BNP monitoring is conducted in an outpatient setting by physicians skilled in HF care, existing gaps between guidelines and current practice need to be bridged: many patients in the UK do not receive follow-up by a cardiologist or HF specialist nurse. 108 The BATTLESCARRED trial64 compared BNP-guided and CG care in a specialist clinic with usual primary care and found that usual primary care resulted in inferior survival at 1 year. Therefore, ensuring that more patients with acute HF episodes get specialist follow-up is a necessary first step to using BNP-guided monitoring to improve care for younger patients with HFrEF in a cost-effective way.
Furthermore, the SIGNAL-HF trial,63 which recruited patients with stable HF in primary care, found no important improvements in clinical outcomes for patients with BNP-guided therapy in primary care. This suggests that the clinical effectiveness and cost-effectiveness results cannot be generalised from specialist to primary care. Another hurdle to implementing BNP-guided treatment is that there is little consensus on the optimal frequency, duration and BNP target for monitoring.
The frequency and duration of BNP-guided therapy and the algorithm used to titrate therapy are important considerations for clinicians and policy-makers. Statistically, there was relatively little variation in the mortality outcomes of RCTs; however, there were important differences in the BNP-guided monitoring protocols used. TIME-CHF,86 which has provided the best evidence to date of a sustained effect in younger patients with HFrEF, scheduled visits at 1, 3, 6, 12 and 18 months with the aim of rapidly up-titrating therapy within 6 months. However, other protocols may be more cost-effective.
Future research recommendations
The present systematic review and meta-analysis have not been able to draw inference of an optimal BNP-monitoring strategy and no group of researchers has defined one. An important area of future research should, therefore, be an initiative to define an optimal BNP monitoring strategy, for example through a formal consensus process involving researchers, cardiologists, GPs and patients. This initiative may also consider which patient subgroups are likely to benefit from BNP monitoring and whether or not a single optimal strategy should apply to all patient subgroups.
A striking feature of the trials is that HF medications increased in both BNP-monitored and CG groups, strongly suggesting that HF management outside of the RCTs was suboptimal; this is also implied by the comparison between participants managed in specialist clinics versus primary care in the BATTLESCARRED trial. 64 This evidence suggests that interventions are needed to optimise management of HF in accordance with current guidelines. 8 It is not clear whether or not this can be achieved through the commissioning process (i.e. implementing incentives for practitioners to implement existing knowledge and expertise in HF management), upskilling of practitioners (i.e. giving practitioners the knowledge and expertise to achieve HF management consistent with the guidelines) and transferring care to a specialist setting (i.e. because the setting of primary care does not allow HF management consistent with the guidelines). Understanding which of these alternative reasons applies at present may require qualitative research with different types of practitioner providing care for HF patients.
Depending on the findings from the two pieces of research described above, there might be a case to commission a large pragmatic RCT of BNP monitoring in the UK in a primary care setting but with improved adherence to existing HF management guidelines.
With respect to the cost-effectiveness model, most of the uncertainty is caused by wide CIs surrounding the effect size of BNP-guided therapy, particularly in patient subgroups that are not well-represented in RCTs. The Guiding Evidence Based Therapy Using Biomarker Intensified Treatment (GUIDE-IT) trial,109 which aimed to randomise 1100 patients with HFrEF to BNP-guided or symptom-guided therapy, was terminated by the Duke Clinical Research Institute on 23 September 2016 (https://dcri.org/dcri-announces-halt-guide-trial/; accessed 19 April 2017) having randomised 894 patients (https://clinicaltrials.gov/ct2/show/NCT01685840?term=guide-it&rank=1; accessed 19 April 2017) because of a lack of difference in the primary outcome between the treatment groups. No results from the trial are available yet but, when published, the trial should contribute important new evidence about BNP-guided therapy in older patients with HFrEF and help to support or refute existing evidence from smaller RCTs for younger patients with HFrEF. Larger numbers of RCT participants would also enable more detailed exploration into further patient subgroups. For instance, it has been suggested that comorbidities may explain the lower efficacy of BNP-guided monitoring in older patients. 33 This could be substantiated in future updated IPD meta-analyses incorporating the GUIDE-IT findings, providing the collaboration of the triallists could be obtained.
The sustainability of any treatment effect guided by BNP is likely to be important in patient subgroups for whom the economic case for monitoring is more marginal. In TIME-CHF,59,86 a non-statistically significant trend for improved survival among older patients with HFrEF at 18 months was no longer evident at 5 years. Similar research collecting routine data on long-term mortality and hospitalisation among participants in other completed and ongoing RCTs would greatly enhance their value to clinicians and policy-makers. Despite the high prevalence of HF, there is surprisingly little research on the economic impact on health systems, families and societies. 10 Future research, particularly on residential care needs, informal care needs and productivity losses due to HF, is needed in order to better judge the economic case for interventions such as BNP-guided monitoring.
Chapter 6 Conclusion
This study has shown that BNP-guided therapy, as implemented in RCTs in specialist HF clinics, appears to be efficacious and cost-effective in patients who have predominantly been recruited to the RCTs, namely those who are < 75 years of age and with HFrEF. The conclusions about the efficacy of BNP-guided therapy are uncertain because the findings are of borderline statistical significance and the majority of trials contributing to the findings were judged to have high risk of bias.
The application of this evidence to HF patients in the UK is also uncertain. First, none of the RCTs on BNP monitoring was conducted in the UK. Second, there is no consensus about the optimal BNP-monitoring strategy. Third, patients with HF in the UK are not predominantly managed in specialist clinics and there is evidence that HF management in primary care has worse clinical outcomes. Finally, the mechanism through which BNP monitoring reduces all-cause mortality is unclear as, in the RCTs, BNP monitoring did not result in notable differences in BNP levels or HF medications between groups.
Acknowledgements
The authors would like to thank the patient and public contributors for sharing their experiences of their care and contributing their opinions in relation to interpretation of the findings.
Contributions of authors
Maria Pufulete (Research Fellow) was lead researcher. She managed the team, established the collaboration, designed the search strategy and extracted data for the systematic review, organised approvals for the CPRD linked cohort and co-ordinated the analyses of the cohort and drafted the final report.
Rachel Maishman (Research Associate) was statistician. She carried out the meta-analyses and cohort study analyses, with advice from Julian Higgins and Chris A Rogers, and drafted some of the results sections for the final report.
Lucy Dabner (Clinical Trial co-ordinator) screened the abstracts and extracted data for the systematic review, liaised with IPD collaborators and drafted some sections of the final report.
Syed Mohiuddin (Research Associate) was health economist. He developed the cost-effectiveness model in collaboration with William Hollingworth.
William Hollingworth (Professor of Health Economics) was senior health economist. He developed the cost-effectiveness model in collaboration with Syed Mohiuddin.
Chris A Rogers (Reader in Medical Statistics) was senior statistician. She advised on meta-analyses and cohort study analyses.
Julian Higgins (Professor of Evidence Synthesis) was senior statistician with expertise in evidence synthesis. He advised on the systematic review and meta-analyses.
Mark Dayer (Consultant Cardiologist) was clinical advisor. He advised about secondary care aspects of HF management and interpretation of the findings.
John Macleod (Professor of Primary Care) was GP advisor with expertise in using CPRD data for research. He advised about analyses of CPRD data, primary care aspects of HF management and interpretation of the findings.
Sarah Purdy (Professor of Primary Care) was GP advisor. She advised about primary care aspects of HF management and interpretation of the findings.
Theresa McDonagh (Professor of Cardiology) was clinical advisor with responsibility for the NHFA. She advised about the NHFA data, secondary care aspects of HF management and interpretation of the findings.
Angus Nightingale (Consultant Cardiologist and Senior Clinical Lecturer in Clinical Science) was clinical advisor. He advised about secondary care aspects of HF management and interpretation of the findings.
Rachael Williams (Senior Researcher, CPRD) assisted with the data specification and advised about the CPRD GOLD and linked HES data sets throughout the project.
Barnaby C Reeves (Professor of Health Services Research) was chief investigator with overall responsibility for the project and guarantor. He provided strategic direction for the systematic review and cohort study and interpretation of the findings and edited the final report.
Publications
Hollingworth W, Biswas M, Maishman RL, Dayer MJ, McDonagh T, Purdy S, et al. The healthcare costs of heart failure during the last five years of life: a retrospective cohort study. Int J Cardiol 2016;224:132–8.
Pufulete M, Higgins JPT, Rogers CA, Dreyer L, Hollingworth W, Dayer M, et al. Protocol for a systematic review and individual participant data meta-analysis of B-type natriuretic peptide-guided therapy for heart failure. Syst Rev 2014;3:41.
Mohiuddin S, Reeves BC, Pufulete M, Maishman R, Dayer M, Macleod J, et al. A model-based cost-effectiveness analysis of B-type natriuretic peptide-guided care in patients with heart failure. BMJ Open 2016;6:e014010.
Data sharing statement
Data for the IPD meta-analysis were requested from triallists under the terms of a data collaboration agreement. Key terms of this agreement prevent further data sharing of the IPD with third parties:
-
to use the data only for the purposes stated in the study protocol and in the analyses set out in the analysis plan
-
to store data submitted for the IPD meta-analysis in password-protected files on a secure University of Bristol computer that will only be accessible by members of the management committee
-
to deposit the anonymised data sets and the final individual patient data set in a data archive in accordance with patient data archiving procedures required by the UK NHS
-
all data will remain the property of the original researchers and can be withdrawn from the analyses at any time, if they so wish
-
all data will be held securely and will not be shared with anyone other than the research team assembled for this project without the permission of the original researchers.
The data for the cohort study from NHS Digital were also obtained under an agreement with the Secretary of State for Health, which stipulated that:
The licence granted in clause 3.1 is subject to the use of the Dataset or any other information obtained by the Customer or its Affiliated Companies (if any) in accordance with this Agreement being restricted to carrying out the Study for Medical and Health Research Purposes and any other use of the Dataset, that is not expressly specified in the Protocol, is strictly prohibited.
We regret that these agreements preclude us from sharing the data sets.
Disclaimers
This report presents independent research funded by the National Institute for Health Research (NIHR). The views and opinions expressed by authors in this publication are those of the authors and do not necessarily reflect those of the NHS, the NIHR, NETSCC, the HTA programme or the Department of Health. If there are verbatim quotations included in this publication the views and opinions expressed by the interviewees are those of the interviewees and do not necessarily reflect those of the authors, those of the NHS, the NIHR, NETSCC, the HTA programme or the Department of Health.
References
- Townsend N, Bhatnagar P, Wilkins E, Wickramasinghe K, Rayner M. Cardiovascular Disease Statistics 2015. London: British Heart Foundation; 2015.
- McMurray JJ, Pfeffer MA. Heart failure. Lancet 2005;365:1877-89. https://doi.org/10.1016/S0140-6736(05)66621-4.
- Davies M, Hobbs F, Davis R, Kenkre J, Roalfe AK, Hare R, et al. Prevalence of left-ventricular systolic dysfunction and heart failure in the Echocardiographic Heart of England Screening study: a population based study. Lancet 2001;358:439-44. https://doi.org/10.1016/S0140-6736(01)05620-3.
- Cowie MR, Wood DA, Coats AJ, Thompson SG, Suresh V, Poole-Wilson PA, et al. Survival of patients with a new diagnosis of heart failure: a population based study. Heart 2000;83:505-10. https://doi.org/10.1136/heart.83.5.505.
- Hobbs FD, Roalfe AK, Davis RC, Davies MK, Hare R. Midlands Research Practices Consortium (MidReC). Prognosis of all-cause heart failure and borderline left ventricular systolic dysfunction: 5 year mortality follow-up of the Echocardiographic Heart of England Screening Study (ECHOES). Eur Heart J 2007;28:1128-34. https://doi.org/10.1093/eurheartj/ehm102.
- Sharma A, Ezekowitz JA. Similarities and differences in patient characteristics between heart failure registries versus clinical trials. Curr Heart Fail Rep 2013;10:373-9. http://dx.doi.org/10.1007/s11897-013-0152-x.
- McMurray JJ, Adamopoulos S, Anker SD, Auricchio A, Böhm M, Dickstein K, et al. ESC guidelines for the diagnosis and treatment of acute and chronic heart failure 2012: The Task Force for the Diagnosis and Treatment of Acute and Chronic Heart Failure 2012 of the European Society of Cardiology. Developed in collaboration with the Heart Failure Association (HFA) of the ESC. Eur Heart J 2012;3:1787-84.
- Chronic Heart Failure. National Clinical Guideline for Diagnosis and Management in Primary and Secondary Care. London: NICE; 2010.
- Cook C, Cole G, Asaria P, Jabbour R, Francis DP. The annual global economic burden of heart failure. Int J Cardiol 2014;171:368-76. http://dx.doi.org/10.1016/j.ijcard.2013.12.028.
- Hollingworth W, Biswas M, Maishman RL, Dayer MJ, McDonagh T, Purdy S, et al. The healthcare costs of heart failure during the last five years of life: a retrospective cohort study. Int J Cardiol 2016;224:132-8. https://doi.org/10.1016/j.ijcard.2016.09.021.
- Pushing The Boundaries: Improving Services For People With Heart Failure. London: Healthcare Commission; 2007.
- Fuat A, Hungin AP, Murphy JJ. Barriers to accurate diagnosis and effective management of heart failure in primary care: qualitative study. BMJ 2003;326. https://doi.org/10.1136/bmj.326.7382.196.
- Hancock HC, Close H, Fuat A, Murphy JJ, Hungin AP, Mason JM. Barriers to accurate diagnosis and effective management of heart failure have not changed in the past 10 years: a qualitative study and national survey. BMJ Open 2014;4. http://dx.doi.org/10.1136/bmjopen-2013-003866.
- Apple FS, Wu AH, Jaffe AS, Panteghini M, Christenson RH, Cannon CP, et al. National Academy of Clinical Biochemistry and IFCC Committee for Standardization of Markers of Cardiac Damage Laboratory Medicine practice guidelines: analytical issues for biomarkers of heart failure. Circulation 2007;116:e95-8. https://doi.org/10.1161/CIRCULATIONAHA.107.185266.
- Maisel AS, Krishnaswamy P, Nowak RM, McCord J, Hollander JE, Duc P, et al. Rapid measurement of B-type natriuretic peptide in the emergency diagnosis of heart failure. N Engl J Med 2002;347:161-7. https://doi.org/10.1056/NEJMoa020233.
- Doust JA, Pietrzak E, Dobson A, Glasziou P. How well does B-type natriuretic peptide predict death and cardiac events in patients with heart failure: systematic review. BMJ 2005;330. https://doi.org/10.1136/bmj.330.7492.625.
- Daniels LB, Maisel AS. Natriuretic peptides. J Am Coll Cardiol 2007;50:2357-68. https://doi.org/10.1016/j.jacc.2007.09.021.
- White M, Lepage S, Lavoie J, De Denus S, Leblanc MH, Gossard D, et al. Effects of combined candesartan and ACE inhibitors on BNP, markers of inflammation and oxidative stress, and glucose regulation in patients with symptomatic heart failure. J Card Fail 2007;13:86-94. https://doi.org/10.1016/j.cardfail.2006.10.013.
- Felker GM, Hasselblad V, Hernandez AF, O’Connor CM. Biomarker-guided therapy in chronic heart failure: a meta-analysis of randomized controlled trials. Am Heart J 2009;158:422-30. http://dx.doi.org/10.1016/j.ahj.2009.06.018.
- Porapakkham P, Porapakkham P, Zimmet H, Billah B, Krum H. B-type natriuretic peptide-guided heart failure therapy: a meta-analysis. Arch Intern Med 2010;170:507-14. http://dx.doi.org/10.1001/archinternmed.2010.35.
- Savarese G, Trimarco B, Dellegrottaglie S, Prastaro M, Gambardella F, Rengo G, et al. Natriuretic peptide-guided therapy in chronic heart failure: a meta-analysis of 2,686 patients in 12 randomized trials. PLOS ONE 2013;8. http://dx.doi.org/10.1371/journal.pone.0058287.
- Li P, Luo Y, Chen YM. B-type natriuretic peptide-guided chronic heart failure therapy: a meta-analysis of 11 randomised controlled trials. Heart Lung Circ 2013;22:852-60. http://dx.doi.org/10.1016/j.hlc.2013.03.077.
- Xin W, Lin Z, Mi S. Does B-type natriuretic peptide-guided therapy improve outcomes in patients with chronic heart failure? A systematic review and meta-analysis of randomized controlled trials. Heart Fail Rev 2015;20:69-80. http://dx.doi.org/10.1007/s10741-014-9437-8.
- De Vecchis R, Esposito C, Di Biase G, Ariano C, Giasi A, Cioppa C. B-type natriuretic peptide-guided versus symptom-guided therapy in outpatients with chronic heart failure: a systematic review with meta-analysis. J Cardiovasc Med 2014;15:122-34. http://dx.doi.org/10.2459/JCM.0b013e328364bde1.
- Troughton RW, Frampton CM, Brunner-La Rocca HP, Pfisterer M, Eurlings LW, Erntell H, et al. Effect of B-type natriuretic peptide-guided treatment of chronic heart failure on total mortality and hospitalization: an individual patient meta-analysis. Eur Heart J 2014;35:1559-67. http://dx.doi.org/10.1093/eurheartj/ehu090.
- Cochrane IPD. Meta-analysis Methods Group . Resources n.d. http://ipdmamg.cochrane.org/resources (accessed 1 December 2016).
- Riley RD, Lambert PC, Abo-Zaid G. Meta-analysis of individual participant data: rationale, conduct, and reporting. BMJ 2010;340. http://dx.doi.org/10.1136/bmj.c221.
- Adlbrecht C, Huelsmann M, Berger R, Moertl D, Strunk G, Oesterle A, et al. Cost analysis and cost-effectiveness of NT-proBNP-guided heart failure specialist care in addition to home-based nurse care. Eur J Clin Invest 2011;41:315-22. http://dx.doi.org/10.1111/j.1365-2362.2010.02412.x.
- Sanders-van Wijk S, van Asselt AD, Rickli H, Estlinbaum W, Erne P, Rickenbacher P, et al. Cost-effectiveness of N-terminal pro-B-type natriuretic-guided therapy in elderly heart failure patients: results from TIME-CHF (Trial of Intensified versus Standard Medical Therapy in Elderly Patients with Congestive Heart Failure). JACC Heart Fail 2013;1:64-71. http://dx.doi.org/10.1016/j.jchf.2012.08.002.
- Moertl D, Steiner S, Coyle D, Berger R. Cost-utility analysis of NT-proBNP-guided multidisciplinary care in chronic heart failure. Int J Technol Assess Health Care 2013;29:3-11. http://dx.doi.org/10.1017/S0266462312000712.
- Morimoto T, Hayashino Y, Shimbo T, Izumi T, Fukui T. Is B-type natriuretic peptide-guided heart failure management cost-effective?. Int J Cardiol 2004;96:177-81. http://dx.doi.org/10.1016/j.ijcard.2003.05.036.
- Laramée P, Wonderling D, Swain S, Al-Mohammad A, Mant J. Cost-effectiveness analysis of serial measurement of circulating natriuretic peptide concentration in chronic heart failure. Heart 2013;99:267-71. http://dx.doi.org/10.1136/heartjnl-2012-302692.
- Brunner-La Rocca HP, Eurlings L, Richards AM, Januzzi JL, Pfisterer ME, Dahlström U, et al. Which heart failure patients profit from natriuretic peptide guided therapy? A meta-analysis from individual patient data of randomized trials. Eur J Heart Fail 2015;17:1252-61. http://dx.doi.org/10.1002/ejhf.401.
- Simmonds MC, Higgins JP, Stewart LA, Tierney JF, Clarke MJ, Thompson SG. Meta-analysis of individual patient data from randomized trials: a review of methods used in practice. Clin Trials 2005;2:209-17. https://doi.org/10.1191/1740774505cn087oa.
- Pufulete M, Higgins JP, Rogers CA, Dreyer L, Hollingworth W, Dayer M, et al. Protocol for a systematic review and individual participant data meta-analysis of B-type natriuretic peptide-guided therapy for heart failure. Syst Rev 2014;3. http://dx.doi.org/10.1186/2046-4053-3-41.
- Higgins JP, Altman DG, Gøtzsche PC, Jüni P, Moher D, Oxman AD, et al. The Cochrane Collaboration’s tool for assessing risk of bias in randomised trials. BMJ 2011;343. http://dx.doi.org/10.1136/bmj.d5928.
- Higgins JPT, Green S. Cochrane Handbook for Systematic Reviews of Interventions 2011. http://handbook.cochrane.org/ (accessed 1 December 2016).
- Higgins JP, Whitehead A, Turner RM, Omar RZ, Thompson SG. Meta-analysis of continuous outcome data from individual patients. Stat Med 2001;20:2219-41. http://dx.doi.org/10.1002/sim.918.
- Thompson SG, Higgins JP. Treating individuals 4: can meta-analysis help target interventions at individuals most likely to benefit?. Lancet 2005;365:341-6. https://doi.org/10.1016/S0140-6736(05)70200-2.
- Cleland JG, Tendera M, Adamus J, Freemantle N, Polonski L, Taylor J. PEP-CHF Investigators. The perindopril in elderly people with chronic heart failure (PEP-CHF) study. Eur Heart J 2006;27:2338-45. https://doi.org/10.1093/eurheartj/ehl250.
- Düngen HD, Apostolovic S, Inkrot S, Tahirovic E, Töpper A, Mehrhof F, et al. Titration to target dose of bisoprolol vs. carvedilol in elderly patients with heart failure: the CIBIS-ELD trial. Eur J Heart Fail 2011;13:670-80. http://dx.doi.org/10.1093/eurjhf/hfr020.
- Flather MD, Shibata MC, Coats AJ, Van Veldhuisen DJ, Parkhomenko A, Borbola J, et al. Randomized trial to determine the effect of nebivolol on mortality and cardiovascular hospital admission in elderly patients with heart failure (SENIORS). Eur Heart J 2005;26:215-25. https://doi.org/10.1093/eurheartj/ehi115.
- Massie BM, Carson PE, McMurray JJ, Komajda M, McKelvie R, Zile MR, et al. Irbesartan in patients with heart failure and preserved ejection fraction. N Engl J Med 2008;359:2456-67. http://dx.doi.org/10.1056/NEJMoa0805450.
- Pitt B, Segal R, Martinez FA, Meurers G, Cowley AJ, Thomas I, et al. Randomised trial of losartan versus captopril in patients over 65 with heart failure (Evaluation of Losartan in the Elderly Study, ELITE). Lancet 1997;349:747-52. https://doi.org/10.1016/S0140-6736(97)01187-2.
- Fisher DJ. Two-stage individual participant data meta-analysis and generalized forest plots. Stata J 2015;15:369-96.
- Parmar MK, Torri V, Stewart L. Extracting summary statistics to perform meta-analyses of the published literature for survival endpoints. Stat Med 1998;17:2815-34. https://doi.org/10.1002/(SICI)1097-0258(19981230)17:24<2815::AID-SIM110>3.0.CO;2-8.
- Moher D, Liberati A, Tetzlaff J, Altman DG. PRISMA Group . Preferred reporting items for systematic reviews and meta-analyses: the PRISMA statement. BMJ 2009;339. https://doi.org/10.1136/bmj.b2535.
- Schulz KF, Chalmers I, Hayes RJ, Altman DG. Empirical evidence of bias. Dimensions of methodological quality associated with estimates of treatment effects in controlled trials. JAMA 1995;273:408-12. https://doi.org/10.1001/jama.1995.03520290060030.
- Sterne JAC, Harbord RM. Funnel plots in meta-analysis. Stata J 2004;4:127-41.
- Troughton R, Brunner-La Rocca HP, Frampton CM, Berger R, Persson H, O’Connor CM, et al. B-Type Natriuretic Peptide Guided Treatment of Heart Failure and All Cause Mortality: An Individual Patient Data Meta-Analysis n.d. http://eurheartj.oxfordjournals.org/content/ehj/32/suppl_1/313.full.pdf (accessed 2 December 2016).
- Brunner-La Rocca HP. Which heart failure patients profit from natriuretic peptide guided therapy? A meta-analysis from individual patient data of randomised trials. Eur Heart J 2014;35. https://eurheartj.oxfordjournals.org/content/ehj/35/suppl_1/local/front-matter.pdf.
- Shah MR, Califf RM, Nohria A, Bhapkar M, Bowers M, Mancini DM, et al. The STARBRITE trial: a randomized, pilot study of B-type natriuretic peptide-guided therapy in patients with advanced heart failure. J Card Fail 2011;17:613-21. http://dx.doi.org/10.1016/j.cardfail.2011.04.012.
- Anguita M, Esteban F, Castillo JC, Mazuelos F, López-Granados A, Arizón JM, et al. Usefulness of brain natriuretic peptide levels, as compared with usual clinical control, for the treatment monitoring of patients with heart failure. Med Clin 2010;135:435-40. http://dx.doi.org/10.1016/j.medcli.2009.11.048.
- Karlstrom P, Alehagen U, Boman K, Ulf D. behalf of the UPSTEP-study group . Brain natriuretic peptide-guided treatment does not improve morbidity and mortality in extensively treated patients with chronic heart failure: responders to treatment have a significantly better outcome. Eur J Heart Fail 2011;13:1096-103. https://doi.org/10.1093/eurjhf/hfr078.
- Schou M, Gustafsson F, Videbaek L, Andersen H, Toft J, Nyvad O, et al. Adding serial N-terminal pro brain natriuretic peptide measurements to optimal clinical management in outpatients with systolic heart failure: a multicentre randomized clinical trial (NorthStar monitoring study). Eur J Heart Fail 2013;15:818-27. http://dx.doi.org/10.1093/eurjhf/hft037.
- Shochat M, Shotan A, Dahan I, Shochat I, Levy Y, Asif A, et al. NT-proBNP-Guided Preemptive Treatment of Outpatients with Chronic Heart Failure Followed in a Out Hospital Clinic. J Card Fail 2012;18. https://doi.org/10.1016/j.cardfail.2012.06.198.
- Koshkina D, Skvortsov A, Narusov O, Protasov V, Nasonova S, Masenko V, et al. NT-proBNP-guided treatment of high risk heart failure patients after acute decompensation. Eur Heart J 2015;36:153-54.
- Troughton RW, Frampton CM, Yandle TG, Espiner EA, Nicholls MG, Richards AM. Treatment of heart failure guided by plasma aminoterminal brain natriuretic peptide (N-BNP) concentrations. Lancet 2000;355:1126-30. https://doi.org/10.1016/S0140-6736(00)02060-2.
- Pfisterer M, Buser P, Rickli H, Gutmann M, Erne P, Rickenbacher P, et al. BNP-guided vs symptom-guided heart failure therapy: the Trial of Intensified vs Standard Medical Therapy in Elderly Patients With Congestive Heart Failure (TIME-CHF) randomized trial. JAMA 2009;301:383-92. http://dx.doi.org/10.1001/jama.2009.2.
- Maeder MT, Rickenbacher P, Rickli H, Abbühl H, Gutmann M, Erne P, et al. N-terminal pro brain natriuretic peptide-guided management in patients with heart failure and preserved ejection fraction: findings from the Trial of Intensified versus standard medical therapy in elderly patients with congestive heart failure (TIME-CHF). Eur J Heart Fail 2013;15:1148-56. http://dx.doi.org/10.1093/eurjhf/hft076.
- Berger R, Moertl D, Peter S, Ahmadi R, Huelsmann M, Yamuti S, et al. N-terminal pro-B-type natriuretic peptide-guided, intensive patient management in addition to multidisciplinary care in chronic heart failure a 3-arm, prospective, randomized pilot study. J Am Coll Cardiol 2010;55:645-53. http://dx.doi.org/10.1016/j.jacc.2009.08.078.
- Eurlings LW, van Pol PE, Kok WE, van Wijk S, Lodewijks-van der Bolt C, Balk AH, et al. Management of chronic heart failure guided by individual N-terminal pro-B-type natriuretic peptide targets: results of the PRIMA (Can PRo-brain-natriuretic peptide guided therapy of chronic heart failure IMprove heart fAilure morbidity and mortality?) study. J Am Coll Cardiol 2010;56:2090-100. http://dx.doi.org/10.1016/j.jacc.2010.07.030.
- Persson H, Erntell H, Eriksson B, Johansson G, Swedberg K, Dahlström U. Improved pharmacological therapy of chronic heart failure in primary care: a randomized Study of NT-proBNP Guided Management of Heart Failure – SIGNAL-HF (Swedish Intervention study – Guidelines and NT-proBNP AnaLysis in Heart Failure). Eur J Heart Fail 2010;12:1300-8. http://dx.doi.org/10.1093/eurjhf/hfq169.
- Lainchbury JG, Troughton RW, Strangman KM, Frampton CM, Pilbrow A, Yandle TG, et al. N-terminal pro-B-type natriuretic peptide-guided treatment for chronic heart failure: results from the BATTLESCARRED (NT-proBNP-Assisted Treatment To Lessen Serial Cardiac Readmissions and Death) trial. J Am Coll Cardiol 2009;55:53-60. http://dx.doi.org/10.1016/j.jacc.2009.02.095.
- Jourdain P, Jondeau G, Funck F, Gueffet P, Le Helloco A, Donal E, et al. Plasma brain natriuretic peptide-guided therapy to improve outcome in heart failure: the STARS-BNP Multicenter Study. J Am Coll Cardiol 2007;49:1733-9. https://doi.org/10.1016/j.jacc.2006.10.081.
- Januzzi JL, Rehman SU, Mohammed AA, Bhardwaj A, Barajas L, Barajas J, et al. Use of amino-terminal pro-B-type natriuretic peptide to guide outpatient therapy of patients with chronic left ventricular systolic dysfunction. J Am Coll Cardiol 2011;58:1881-9. http://dx.doi.org/10.1016/j.jacc.2011.03.072.
- Karavidas A, Konstantinou K, Nikolaou M, Matzaraki V, Papoutsidakis N, Pyrgakis, et al. Guiding decongestion treatment in chronic heart failure patients. Clinical assessment or serial laboratory evaluation?. Eur J Heart Failure 2013;34.
- Krupicka J, Janota T, Hradec J. Optimalization of Heart Failure Therapy Guided by Plasma BNP Concentrations n.d.
- Karlström P, Johansson P, Dahlström U, Boman K, Alehagen U. Can BNP-guided therapy improve health-related quality of life, and do responders to BNP-guided heart failure treatment have improved health-related quality of life? Results from the UPSTEP study. BMC Cardiovasc Disord 2016;16. http://dx.doi.org/10.1186/s12872-016-0221-7.
- International Classification of Diseases. Geneva: World Health Organization; 2016.
- An Online Clinical Codes Repository to Improve Validity and Reproducibility of Medical Database Research. n.d.
- Khan NF, Perera R, Harper S, Rose PW. Adaptation and validation of the Charlson Index for Read/OXMIS coded databases. BMC Fam Pract 2010;11. http://dx.doi.org/10.1186/1471-2296-11-1.
- Barton P, Bryan S, Robinson S. Modelling in the economic evaluation of health care: selecting the appropriate approach. J Health Serv Res Policy 2004;9:110-18. http://dx.doi.org/10.1258/135581904322987535.
- Goehler A, Geisler BP, Manne JM, Jahn B, Conrads-Frank A, Schnell-Inderst P, et al. Decision-analytic models to simulate health outcomes and costs in heart failure: a systematic review. PharmacoEconomics 2011;29:753-69. http://dx.doi.org/10.2165/11585990-000000000-00000.
- Weinstein MC, Torrance G, McGuire A. QALYs: the basics. Value Health 2009;12:5-9. http://dx.doi.org/10.1111/j.1524-4733.2009.00515.x.
- Guide To The Methods Of Technology Appraisal 2013. London: NICE; 2013.
- Rawlins MD, Culyer AJ. National Institute for Clinical Excellence and its value judgments. BMJ 2004;329:224-7. http://dx.doi.org/10.1136/bmj.329.7459.224.
- Fenwick E, Byford S. A guide to cost-effectiveness acceptability curves. Br J Psychiatry 2005;187:106-8. https://doi.org/10.1192/bjp.187.2.106.
- National Life Tables, United Kingdom, 1980-82 to 2012-14. Newport: ONS; 2015.
- van Jaarsveld CH, Ranchor AV, Kempen GI, Coyne JC, van Veldhuisen DJ, Sanderman R. Epidemiology of heart failure in a community-based study of subjects aged > or = 57 years: incidence and long-term survival. Eur J Heart Fail 2006;8:23-30. https://doi.org/10.1016/j.ejheart.2005.04.012.
- Nichols GA, Reynolds K, Kimes TM, Rosales AG, Chan WW. Comparison of risk of re-hospitalization, all-cause mortality, and medical care resource utilization in patients with heart failure and preserved versus reduced ejection fraction. Am J Cardiol 2015;116:1088-92. http://dx.doi.org/10.1016/j.amjcard.2015.07.018.
- Reed SD, Kaul P, Li Y, Eapen ZJ, Davidson-Ray L, Schulman KA, et al. Medical resource use, costs, and quality of life in patients with acute decompensated heart failure: findings from ASCEND-HF. J Card Fail 2013;19:611-20. http://dx.doi.org/10.1016/j.cardfail.2013.07.003.
- NHS Reference Costs 2013 to 2014. London: DH; 2014.
- Chronic Heart Failure Shared Learning: Use of The Scenarios Simulation in the Introduction of Serum Natriuretic Peptide Testing. London: NICE; 2011.
- Meta-analysis Global Group in Chronic Heart Failure . The survival of patients with heart failure with preserved or reduced left ventricular ejection fraction: an individual patient data meta-analysis. Eur Heart J 2012;33:1750-7. https://doi.org/10.1093/eurheartj/ehr254.
- Sanders-van Wijk S, Maeder MT, Nietlispach F, Rickli H, Estlinbaum W, Erne P, et al. Long-term results of intensified, N-terminal-pro-B-type natriuretic peptide-guided versus symptom-guided treatment in elderly patients with heart failure: five-year follow-up from TIME-CHF. Circ Heart Fail 2014;7:131-9. http://dx.doi.org/10.1161/CIRCHEARTFAILURE.113.000527.
- Claxton K. Exploring uncertainty in cost-effectiveness analysis. PharmacoEconomics 2008;26:781-98. https://doi.org/10.2165/00019053-200826090-00008.
- Redfield MM, Rodeheffer RJ, Jacobsen SJ, Mahoney DW, Bailey KR, Burnett JC. Plasma brain natriuretic peptide concentration: impact of age and gender. J Am Coll Cardiol 2002;40:976-82. https://doi.org/10.1016/S0735-1097(02)02059-4.
- Wang TJ, Larson MG, Levy D, Benjamin EJ, Leip EP, Wilson PW, et al. Impact of obesity on plasma natriuretic peptide levels. Circulation 2004;109:594-600. http://dx.doi.org/10.1161/01.CIR.0000112582.16683.EA.
- Newton-Cheh C, Larson MG, Vasan RS, Levy D, Bloch KD, Surti A, et al. Association of common variants in NPPA and NPPB with circulating natriuretic peptides and blood pressure. Nat Genet 2009;41:348-53. http://dx.doi.org/10.1038/ng.328.
- Ponikowski P, Voors AA, Anker SD, Bueno H, Cleland JG, Coats AJ, et al. 2016 ESC Guidelines for the diagnosis and treatment of acute and chronic heart failure: The Task Force for the diagnosis and treatment of acute and chronic heart failure of the European Society of Cardiology (ESC). Developed with the special contribution of the Heart Failure Association (HFA) of the ESC. Eur J Heart Fail 2016;18:891-975. https://doi.org/10.1002/ejhf.592.
- CONSENSUS Trial Study Group . Effects of enalapril on mortality in severe congestive heart failure. Results of the Cooperative North Scandinavian Enalapril Survival Study (CONSENSUS). The CONSENSUS Trial Study Group. N Engl J Med 1987;316:1429-35.
- Yusuf S, Pitt B, Davis CE, Hood WB, Cohn JN. SOLVD Investigators . Effect of enalapril on survival in patients with reduced left ventricular ejection fractions and congestive heart failure. The SOLVD Investigators. N Engl J Med 1991;325:293-302.
- Cohn JN, Tognoni G. Valsartan Heart Failure Trial Investigators. A randomized trial of the angiotensin-receptor blocker valsartan in chronic heart failure. N Engl J Med 2001;345:1667-75. http://dx.doi.org/10.1056/NEJMoa010713.
- Neubauer S, Schilling T, Zeidler J, Lange A, Engel S, Linder R, et al. Impact of guideline adherence on mortality in treatment of left heart failure. Herz 2016;41:614-24. http://dx.doi.org/10.1007/s00059-016-4401-0.
- Ahmed I, Sutton AJ, Riley RD. Assessment of publication bias, selection bias, and unavailable data in meta-analyses using individual participant data: a database survey. BMJ 2012;344. http://dx.doi.org/10.1136/bmj.d7762.
- Burdett S, Stewart LA, Tierney JF. Publication bias and meta-analyses: a practical example. Int J Technol Assess Health Care 2003;19:129-34. https://doi.org/10.1017/S0266462303000126.
- Pitt B, Pfeffer MA, Assmann SF, Boineau R, Anand IS, Claggett B, et al. Spironolactone for heart failure with preserved ejection fraction. N Engl J Med 2014;370:1383-92. http://dx.doi.org/10.1056/NEJMoa1313731.
- Kotecha D, Manzano L, Krum H, Rosano G, Holmes J, Altman DG, et al. Effect of age and sex on efficacy and tolerability of β blockers in patients with heart failure with reduced ejection fraction: individual patient data meta-analysis. BMJ 2016;353. http://dx.doi.org/10.1136/bmj.i1855.
- Kang EM, Pinheiro SP, Hammad TA, Abou-Ali A. Evaluating the validity of clinical codes to identify cataract and glaucoma in the UK Clinical Practice Research Datalink. Pharmacoepidemiol Drug Saf 2015;24:38-44. http://dx.doi.org/10.1002/pds.3726.
- Quint JK, Müllerova H, DiSantostefano RL, Forbes H, Eaton S, Hurst JR, et al. Validation of chronic obstructive pulmonary disease recording in the Clinical Practice Research Datalink (CPRD-GOLD). BMJ Open 2014;4. http://dx.doi.org/10.1136/bmjopen-2014-005540.
- Rothnie KJ, Müllerová H, Hurst JR, Smeeth L, Davis K, Thomas SL, et al. Validation of the Recording of Acute Exacerbations of COPD in UK Primary Care Electronic Healthcare Records. PLOS ONE 2016;11. http://dx.doi.org/10.1371/journal.pone.0151357.
- Benchimol EI, Smeeth L, Guttmann A, Harron K, Moher D, Petersen I, et al. The REporting of studies Conducted using Observational Routinely-collected health Data (RECORD) statement. PLOS Med 2015;12. https://doi.org/10.1371/journal.pmed.1001885.
- Oreopoulos A, Padwal R, Kalantar-Zadeh K, Fonarow GC, Norris CM, McAlister FA. Body mass index and mortality in heart failure: a meta-analysis. Am Heart J 2008;156:13-22. http://dx.doi.org/10.1016/j.ahj.2008.02.014.
- Campbell JD, McQueen RB, Libby AM, Spackman DE, Carlson JJ, Briggs A. Cost-effectiveness uncertainty analysis methods: a comparison of one-way sensitivity, analysis of covariance, and expected value of partial perfect information. Med Decis Making 2015;35:596-607. http://dx.doi.org/10.1177/0272989X14556510.
- Ieva F, Jackson CH, Sharples LD. Multi-state modelling of repeated hospitalisation and death in patients with heart failure: the use of large administrative databases in clinical epidemiology. Stat Methods Med Res 2015;26:1350-72. https://doi.org/10.1177/0962280215578777.
- Bhardwaj A, Rehman SU, Mohammed AA, Gaggin HK, Barajas L, Barajas J, et al. Quality of life and chronic heart failure therapy guided by natriuretic peptides: results from the ProBNP Outpatient Tailored Chronic Heart Failure Therapy (PROTECT) study. Am Heart J 2012;164:793-99.
- National Heart Failure Audit: April 2013 – March 2014. London: British Society for Heart Failure; 2015.
- Felker GM, Ahmad T, Anstrom KJ, Adams KF, Cooper LS, Ezekowitz JA, et al. Rationale and design of the GUIDE-IT study: Guiding Evidence Based Therapy Using Biomarker Intensified Treatment in Heart Failure. JACC Heart Fail 2014;2:457-65. http://dx.doi.org/10.1016/j.jchf.2014.05.007.
Appendix 1 Literature searches
2012 search
Database: MEDLINE (via Ovid) 1950 to present
Search strategy
-
(BNP adj5 (guide$ or monitor$ or target$ or predict$)).tw. (732)
-
(proBNP adj5 (guide or monitor$ or target$ or predict$)).tw. (462)
-
(NTproBNP adj5 (guide$ or monitor$ or target$ or predict$)).tw. (22)
-
((natriuretic peptide or natriuretic propeptide) adj5 (guide$ or monitor$ or target$ or predict$)).tw. (650)
-
((NTproBNP or Natriuretic Peptide or natriuretic propeptide or BNP or proBNP) adj5 (retest$ or serial or series)).tw. (153)
-
((NTproBNP or Natriuretic Peptide or natriuretic propeptide or BNP or proBNP) adj5 (manag$ or tailor$ or treat$ or therap$ or strateg$)).tw. (863)
-
or/1-6 (2266)
-
exp Heart Failure/ (77,650)
-
heart failure.tw. (88,345)
-
cardiac failure.tw. (9104)
-
HF.tw. (15,642)
-
CHF.tw. (9142)
-
or/8-12 (132,395)
-
Natriuretic Peptide, Brain/ (8154)
-
Monitoring, Physiologic/ (42,028)
-
‘Predictive Value of Tests’/ (121,365)
-
‘Health Status Indicators’/ (17,985)
-
or/15-17 (179,642)
-
14 and 18 (1234)
-
(BNP adj5 (guide$ or monitor$ or target$ or predict$)).tw. (732)
-
(proBNP adj5 (guide or monitor$ or target$ or predict$)).tw. (462)
-
(NTproBNP adj5 (guide$ or monitor$ or target$ or predict$)).tw. (22)
-
((natriuretic peptide or natriuretic propeptide) adj5 (guide$ or monitor$ or target$ or predict$)).tw. (650)
-
((NTproBNP or Natriuretic Peptide or natriuretic propeptide or BNP or proBNP) adj5 (retest$ or serial or series)).tw. (153)
-
((NTproBNP or Natriuretic Peptide or natriuretic propeptide or BNP or proBNP) adj5 (manag$ or tailor$ or therap$ or strateg$)).tw. (461)
-
or/20-25 (1923)
-
19 or 26 (2616)
-
13 and 27 (1471)
-
randomized controlled trial.pt. (330,201)
-
controlled clinical trial.pt. (84,375)
-
randomized.ab. (233,876)
-
placebo.ab. (132,230)
-
drug therapy.fs. (1,543,331)
-
randomly.ab. (168,558)
-
trial.ab. (242,070)
-
groups.ab. (1,106,725)
-
or/29-36 (2,867,649)
-
exp animals/ not humans/ (3,736,636)
-
37 not 38 (2,435,187)
-
28 and 39 (506)
Database: EMBASE (via Ovid) 1980 to 2012 Week 26
Search strategy
-
exp heart failure/ (240,304)
-
heart failure.tw. (125,430)
-
cardiac failure.tw. (11,574)
-
CHF.tw. (13,935)
-
HF.tw. (26,271)
-
or/1-5 (284,966)
-
brain natriuretic peptide/ (13,290)
-
monitoring/ (68,421)
-
predictive value/ (18,283)
-
‘disease course’/ (253,029)
-
‘symptom’/ (82,438)
-
disease course/ (253,029)
-
‘pathophysiology’/ (552,261)
-
patient monitoring/ (57,907)
-
biological monitoring/ (11,401)
-
hemodynamic monitoring/ (11,474)
-
8 or 9 or 10 or 11 or 12 or 13 or 14 or 15 or 16 (1,015,916)
-
7 and 17 (2351)
-
(BNP adj5 (guide$ or monitor$ or target$ or predict$)).tw. (1252)
-
(proBNP adj5 (guide or monitor$ or target$ or predict$)).tw. (813)
-
(NTproBNP adj5 (guide$ or monitor$ or target$ or predict$)).tw. (111)
-
((natriuretic peptide or natriuretic propeptide) adj5 (guide$ or monitor$ or target$ or predict$)).tw. (894)
-
((NTproBNP or Natriuretic Peptide or natriuretic propeptide or BNP or proBNP) adj5 (retest$ or serial or series)).tw. (236)
-
((NTproBNP or Natriuretic Peptide or natriuretic propeptide or BNP or proBNP) adj5 (manag$ or tailor$ or treat$ or therap$ or strateg$)).tw. (1373)
-
or/19-24 (3676)
-
18 or 25 (5641)
-
6 and 26 (3599)
-
random$.tw. (734,627)
-
factorial$.tw. (18,994)
-
(crossover$ or cross-over$).tw. (61,441)
-
placebo$.tw. (175,748)
-
(doubl$ adj blind$).tw. (128,507)
-
(singl$ adj blind$).tw. (12,267)
-
assign$.tw. (204,642)
-
allocat$.tw. (68,787)
-
volunteer$.tw. (157,058)
-
Crossover Procedure/ (34,246)
-
Double-blind Procedure/ (109,462)
-
Randomized Controlled Trial/ (324,293)
-
Single-blind Procedure/ (16,047)
-
or/28-40 (1,210,587)
-
(animal/ or nonhuman/) not human/ (4,452,630)
-
41 not 42 (1,063,367)
-
27 and 43 (461)
-
limit 44 to embase (395)
The Cochrane Library
#1 MeSH descriptor Heart Failure explode all trees
#2 ‘heart failure’
#3 ‘cardiac failure’
#4 CHF
#5 HF
#6 (#1 OR #2 OR #2 OR #4 OR #5)
#7 MeSH descriptor Natriuretic Peptide, Brain, this term only
#8 (BNP near/5 (guide* or monitor* or target* or predict*))
#9 (NTproBNP near/5 (guide* or monitor* or target* or predict*))
#10 ((‘natriuretic peptide’) near/5 (guide* or monitor* or target* or predict*))
#11 ((NTproBNP or ‘Natriuretic Peptide’ or ‘natriuretic propeptide’ or BNP or proBNP) near/5 (retest* or serial or series))
#12 (‘natriuretic propeptide’ near/5 (guide* or monitor* or target* or predict*))
#13 (NTproBNP or ‘Natriuretic Peptide’ or ‘natriuretic propeptide’ or BNP or proBNP):ti
#14 (NTproBNP or ‘Natriuretic Peptide’ or ‘natriuretic propeptide’ or BNP or proBNP) near/5 (manag* or tailor* or therap* or strateg*)
#15 (proBNP near/5 (guide* or monitor* or target* or predict*))
#16 (#7 OR #8 OR #9 OR #10 OR #11 OR #12 OR #13 OR #14 OR #15)
#17 (#6 AND #16)
Web of Science (Citations Index and Conference Proceedings)
#18 #17 AND #16
#17 TS=(random* or trial or placebo* or groups (double same blind*) or (single same blind*))
#16 #15 AND #1
#15 #14 OR #13 OR #12 OR #11 OR #10 OR #9 OR #8 OR #7 OR #6 OR #5 OR #4 OR #3 OR #2
#14 TS=((NTproBNP or ‘Natriuretic Peptide’ or ‘natriuretic propeptide’ or BNP or proBNP) NEAR (strateg*))
#13 TS=((NTproBNP or ‘Natriuretic Peptide’ or ‘natriuretic propeptide’ or BNP or proBNP) NEAR (therap*))
#12 TS=((NTproBNP or ‘Natriuretic Peptide’ or ‘natriuretic propeptide’ or BNP or proBNP) NEAR (tailor*))
#11 TS=((NTproBNP or ‘Natriuretic Peptide’ or ‘natriuretic propeptide’ or BNP or proBNP) NEAR (manag*))
#10 TS=((NTproBNP or ‘Natriuretic Peptide’ or ‘natriuretic propeptide’ or BNP or proBNP) NEAR series)
#9 TS=((NTproBNP or ‘Natriuretic Peptide’ or ‘natriuretic propeptide’ or BNP or proBNP) NEAR serial*)
#8 TS=((NTproBNP or ‘Natriuretic Peptide’ or ‘natriuretic propeptide’ or BNP or proBNP) NEAR retest*)
#7 TS=(‘natriuretic propeptide’ NEAR (guide* or monitor* or target* or predict*))
#6 TS=(‘natriuretic peptide’ NEAR (guide* or monitor* or target* or predict*))
#5 TS=(NTproBNP NEAR (guide* or monitor* or target* or predict*))
#4 TS=(proBNP NEAR (guide or monitor* or target* or predict*))
#3 TS=(BNP NEAR (guide* or monitor* or target* or predict*))
#2 TS=(‘natriuretic peptide’ NEAR target*) or TS=(‘natriuretic propeptide’ NEAR target*)
#1 TS=(‘heart failure’ or ‘cardiac failure’ or CHF or HF)
2014 updated search
The original search was rerun from 2012 to January 2014 (date of search 11 January 2014) using the above listed strategies (but please see below for correction to MEDLINE strategy text – there were some duplicated lines in the original MEDLINE search).
Database: MEDLINE 1950 to present
Search strategy
-
(BNP adj5 (guide$ or monitor$ or target$ or predict$)).tw. (875)
-
(proBNP adj5 (guide or monitor$ or target$ or predict$)).tw. (585)
-
(NTproBNP adj5 (guide$ or monitor$ or target$ or predict$)).tw. (30)
-
((natriuretic peptide or natriuretic propeptide) adj5 (guide$ or monitor$ or target$ or predict$)).tw. (770)
-
((NTproBNP or Natriuretic Peptide or natriuretic propeptide or BNP or proBNP) adj5 (retest$ or serial or series)).tw. (177)
-
((NTproBNP or Natriuretic Peptide or natriuretic propeptide or BNP or proBNP) adj5 (manag$ or tailor$ or treat$ or therap$ or strateg$)).tw. (1034)
-
or/1-6 (2734)
-
exp Heart Failure/ (88,614)
-
heart failure.tw. (103,737)
-
cardiac failure.tw. (9777)
-
HF.tw. (19,875)
-
CHF.tw. (10,524)
-
or/8-12 (152,446)
-
Natriuretic Peptide, Brain/ (9880)
-
Monitoring, Physiologic/ (45,222)
-
‘Predictive Value of Tests’/ (145,475)
-
‘Health Status Indicators’/ (21,035)
-
or/15-17 (209,672)
-
14 and 18 (1512)
-
(BNP adj5 (guide$ or monitor$ or target$ or predict$)).tw. (875)
-
(proBNP adj5 (guide or monitor$ or target$ or predict$)).tw. (585)
-
(NTproBNP adj5 (guide$ or monitor$ or target$ or predict$)).tw. (30)
-
((natriuretic peptide or natriuretic propeptide) adj5 (guide$ or monitor$ or target$ or predict$)).tw. (770)
-
((NTproBNP or Natriuretic Peptide or natriuretic propeptide or BNP or proBNP) adj5 (retest$ or serial or series)).tw. (177)
-
((NTproBNP or Natriuretic Peptide or natriuretic propeptide or BNP or proBNP) adj5 (manag$ or tailor$ or therap$ or strateg$)).tw. (540)
-
or/20-25 (2324)
-
19 or 26 (3187)
-
13 and 27 (1767)
-
randomized controlled trial.pt. (390,995)
-
controlled clinical trial.pt. (90,070)
-
randomized.ab. (288,395)
-
placebo.ab. (157,299)
-
drug therapy.fs. (1,772,029)
-
randomly.ab. (200,079)
-
trial.ab. (303,857)
-
groups.ab. (1,280,166)
-
or/29-36 (3,308,511)
-
exp animals/ not humans/ (4,066,609)
-
37 not 38 (2,817,704)
-
28 and 39 (622)
-
(‘2012$’ or ‘2013$’ or ‘2014$’).ed. (1,700,243)
-
40 and 41 (105)
2016 updated search
Search date: 8 June 2016.
Summary of search results (1 January 2014–8 June 2016)
-
MEDLINE, n = 141
-
MEDLINE In-Process & Other Non-Indexed Citations, n = 22
-
EMBASE, n = 160
-
Web of Science (Core Collection), n = 161.
Total = 484.
De-duplicated (against each other and 2014 search results), n = 367
-
The Cochrane Library (all years to 8 June 2016):
-
Cochrane Central Register of Controlled Trials, n = 834 (216)
-
Cochrane Database of Systematic Reviews, n = 6
-
Database of Abstracts of Reviews of Effects, n = 49
-
Health Technology Assessment database, n = 20
-
NHS Economic Evaluation Database, n = 25.
-
All years total = 934.
De-duplicated (against each other and 2014 search results), n = 217.
Total number of ‘new’ references to screen, n = 584.
MEDLINE, MEDLINE & Other Non-Indexed Citations (via Ovid)
Search strategies
-
(BNP adj5 (guide$ or monitor$ or target$ or predict$)).tw.
-
(proBNP adj5 (guide or monitor$ or target$ or predict$)).tw.
-
(NTproBNP adj5 (guide$ or monitor$ or target$ or predict$)).tw.
-
((natriuretic peptide or natriuretic propeptide) adj5 (guide$ or monitor$ or target$ or predict$)).tw.
-
((NTproBNP or Natriuretic Peptide or natriuretic propeptide or BNP or proBNP) adj5 (retest$ or serial or series)).tw.
-
((NTproBNP or Natriuretic Peptide or natriuretic propeptide or BNP or proBNP) adj5 (manag$ or tailor$ or treat$ or therap$ or strateg$)).tw.
-
or/1-6
-
exp Heart Failure/
-
heart failure.tw.
-
cardiac failure.tw.
-
HT.tw.
-
CHF.tw.
-
or/8-12
-
Natriuretic Peptide, Brain/
-
Monitoring, Physiologic/
-
‘Predictive Value of Tests’/
-
‘Health Status Indicators’/
-
or/15-17
-
14 and 18
-
(BNP adj5 (guide$ or monitor$ or target$ or predict$)).tw.
-
(proBNP adj5 (guide or monitor$ or target$ or predict$)).tw.
-
(NTproBNP adj5 (guide$ or monitor$ or target$ or predict$)).tw.
-
((natriuretic peptide or natriuretic propeptide) adj5 (guide$ or monitor$ or target$ or predict$)).tw.
-
((NTproBNP or Natriuretic Peptide or natriuretic propeptide or BNP or proBNP) adj5 (retest$ or serial or series)).tw.
-
((NTproBNP or Natriuretic Peptide or natriuretic propeptide or BNP or proBNP) adj5 (manag$ or tailor$ or therap$ or strateg$)).tw.
-
or/20-25
-
19 or 26
-
13 and 27
-
randomized controlled trial.pt.
-
controlled clinical trial.pt.
-
randomized.ab.
-
placebo.ab.
-
drug therapy.fs.
-
randomly.ab.
-
trial.ab.
-
groups.ab.
-
or/29-36
-
exp animals/ not humans/
-
37 not 38
-
28 and 39
-
(‘2014$’ or ‘2015$’ or ‘2016$’).ed,yr.
-
40 and 41 [n=141] [n=22 (MEDLINE-in-Process)]
EMBASE (via Ovid)
-
exp heart failure/
-
heart failure.tw.
-
cardiac failure.tw.
-
CHF.tw.
-
HF.tw.
-
or/1-5
-
brain natriuretic peptide/
-
monitoring/
-
predictive value/
-
‘disease course’/
-
‘symptom’/
-
‘pathophysiology’/
-
patient monitoring/
-
biological monitoring/
-
hemodynamic monitoring/
-
or/8-15
-
7 and 16
-
(BNP adj5 (guide$ or monitor$ or target$ or predict$)).tw.
-
(proBNP adj5 (guide or monitor$ or target$ or predict$)).tw.
-
(NTproBNP adj5 (guide$ or monitor$ or target$ or predict$)).tw.
-
((natriuretic peptide or natriuretic propeptide) adj5 (guide$ or monitor$ or target$ or predict$)).tw.
-
((NTproBNP or Natriuretic Peptide or natriuretic propeptide or BNP or proBNP) adj5 (retest$ or serial or series)).tw.
-
((NTproBNP or Natriuretic Peptide or natriuretic propeptide or BNP or proBNP) adj5 (manag$ or tailor$ or treat$ or therap$ or strateg$)).tw.
-
or/18-23
-
17 or 24
-
6 and 25
-
random$.tw.
-
factorial$.tw.
-
(crossover$ or cross-over$).tw.
-
placebo$.tw.
-
(doubl$ adj blind$).tw.
-
(singl$ adj blind$).tw.
-
assign$.tw.
-
allocat$.tw.
-
volunteer$.tw.
-
Crossover Procedure/
-
Double-blind Procedure/
-
Randomized Controlled Trial/
-
Single-blind Procedure/
-
or/27-39
-
(animal/ or nonhuman/) not human/
-
40 not 41
-
26 and 42
-
limit 43 to embase
-
(2014$ or 2015$ or 2016$).em,yr.
-
44 and 45 [n=160]
Web of Science Core Collection
Indexes=SCI-EXPANDED, SSCI, A&HCI, CPCI-S, CPCI-SSH, ESCI Timespan=2014-2016 [n=161]
#1 TS=(‘heart failure’ or ‘cardiac failure’ or CHF or HF)
#2 TS=(‘natriuretic peptide’ NEAR target*) or TS=(‘natriuretic propeptide’ NEAR target*)
#3 TS=(BNP NEAR (guide* or monitor* or target* or predict*))
#4 TS=(proBNP NEAR (guide or monitor* or target* or predict*))
#5 TS=(NTproBNP NEAR (guide* or monitor* or target* or predict*))
#6 TS=(‘natriuretic peptide’ NEAR (guide* or monitor* or target* or predict*))
#7 TS=(‘natriuretic propeptide’ NEAR (guide* or monitor* or target* or predict*))
#8 TS=((NTproBNP or ‘Natriuretic Peptide’ or ‘natriuretic propeptide’ or BNP or proBNP) NEAR retest*)
#9 TS=((NTproBNP or ‘Natriuretic Peptide’ or ‘natriuretic propeptide’ or BNP or proBNP) NEAR serial*)
#10 TS=((NTproBNP or ‘Natriuretic Peptide’ or ‘natriuretic propeptide’ or BNP or proBNP) NEAR series)
#11 TS=((NTproBNP or ‘Natriuretic Peptide’ or ‘natriuretic propeptide’ or BNP or proBNP) NEAR (manag*))
#12 TS=((NTproBNP or ‘Natriuretic Peptide’ or ‘natriuretic propeptide’ or BNP or proBNP) NEAR (tailor*))
#13 TS=((NTproBNP or ‘Natriuretic Peptide’ or ‘natriuretic propeptide’ or BNP or proBNP) NEAR (therap*))
#14 TS=((NTproBNP or ‘Natriuretic Peptide’ or ‘natriuretic propeptide’ or BNP or proBNP) NEAR (strateg*))
#15 #14 OR #13 OR #12 OR #11 OR #10 OR #9 OR #8 OR #7 OR #6 OR #5 OR #4 OR #3 OR #2
#16 #15 AND #1
#17 TS=(random* or trial or placebo* or groups (double same blind*) or (single same blind*))
#18 #17 AND #16
#19 TS=(((clinical near trial* or crossover or cross over) or ((single* or doubl* or trebl* or tripl*) near (blind* or mask* or dummy)) or (singleblind* or doubleblind* or trebleblind* or tripleblind* or placebo* or random*))) or TI=(((clinical near trial* or crossover or cross over) or ((single* or doubl* or trebl* or tripl*) near (blind* or mask* or dummy)) or (singleblind* or doubleblind* or trebleblind* or tripleblind* or placebo* or random*)))
The Cochrane Library
#1 MeSH descriptor: [Heart Failure] explode all trees
#2 ‘heart failure’
#3 ‘cardiac failure’
#4 CHF or HF:ab (Word variations have been searched)
#5 #1 or #2 or #3 or #4
#6 MeSH descriptor: [Natriuretic Peptide, Brain] this term only
#7 (BNP near/5 (guide* or monitor* or target* or predict*))
#8 (NTproBNP near/5 (guide* or monitor* or target* or predict*))
#9 ((‘natriuretic peptide’) near/5 (guide* or monitor* or target* or predict*))
#10 ((NTproBNP or ‘Natriuretic Peptide’ or ‘natriuretic propeptide’ or BNP or proBNP) near/5 (retest* or serial or series))
#11 (‘natriuretic propeptide’ near/5 (guide* or monitor* or target* or predict*))
#12 (NTproBNP or ‘Natriuretic Peptide’ or ‘natriuretic propeptide’ or BNP or proBNP):ti
#13 (NTproBNP or ‘Natriuretic Peptide’ or ‘natriuretic propeptide’ or BNP or proBNP) near/5 (manag* or tailor* or therap* or strateg*)
#14 (proBNP near/5 (guide* or monitor* or target* or predict*))
#15 (#6 or #7 or #8 or #9 or #10 or #11 or #12 or #13 or #14)
#16 #5 and #15
#17 (2014 or 2015 or 2016)
#18 #16 and #17
Appendix 2 List of data items requested
Study-level data | |
---|---|
Country in which study was carried out | |
Number of participants randomised | |
Number allocated to the BNP group | |
Number allocated to the standard care group | |
Setting (primary care, hospitals, specialist clinics) | |
Date first patient randomised | |
Date last patient randomised | |
Date of final follow-up | |
Did the study measure quality of life (state tool that was used) | |
Did the study measure HF risk score (state tool that was used) | |
Details of intervention (frequency of testing, actions, etc.) | |
Details of comparator (frequency of review, actions, etc.) | |
IPD | Variables collected at study entry |
Demography | Age |
Sex | |
Weight | |
Height | |
BMI | |
Smoking status | |
Medical history | Cause of HF |
Previous myocardial infarction | |
Previous intervention (PCI/CABG) | |
Previous stroke | |
Previous angina pectoris | |
Previous peripheral artery disease | |
Diabetes status (including type) | |
History of hypertension | |
History of atrial fibrillation | |
History of chronic obstructive pulmonary disease | |
Pacemaker in situ | |
Cardiac resynchronization therapy device in situ | |
Implantable cardioverter defibrillator in situ | |
IPD | Variables collected at each visit – data required for all visits including baseline visits |
Clinical | NYHA class |
LVEF | |
Resting heart rate | |
SBP | |
DBP | |
HF score | |
Laboratory | BNP/NT-proBNP |
Creatinine | |
Sodium | |
Potassium | |
Blood urea nitrogen | |
Haemoglobin | |
Drug treatment | ACEis |
ARBs | |
Beta blockers | |
Mineralocorticoid receptor antagonists | |
Loop diuretic | |
Thiazide diuretics | |
Vasodilator | |
Other potassium sparing diuretic | |
Ivabradine | |
Aspirin | |
Other antiplatelet agent | |
Oral anticoagulant | |
Digoxin | |
Amiodarone | |
Other antiarrhythmiac | |
Calcium-channel blocker | |
Statin | |
Quality of life | |
IPD | Clinical outcomes – data required for all deaths, hospital admissions or cardiovascular events |
Date of death | |
Cause of death | |
Date of hospital admission/cardiovascular event | |
Date of hospital discharge | |
Details of reason for admission/cardiovascular event (e.g. HF, non-fatal myocardial infarction, non-fatal stroke, new atrial fibrillation, fitting of pacemaker/cardiac resynchronization therapy device/implantable cardioverter defibrillator) |
Appendix 3 Data extraction form
B-type natriuretic peptide-guided therapy in heart failure
List of abbreviations
- ACEi
- angiotensin-converting enzyme inhibitor
- ARB
- angiotensin receptor blocker
- BHF
- British Heart Foundation
- BMI
- body mass index
- BNP
- B-type natriuretic peptide
- CABG
- coronary artery bypass grafting
- CG
- clinically guided
- CI
- confidence interval
- CPRD
- Clinical Practice Research Datalink
- EQ-5D-3L
- EuroQol-5 Dimensions, three levels
- GP
- general practitioner
- HES
- Hospital Episode Statistics
- HF
- heart failure
- HFpEF
- heart failure with a preserved ejection fraction
- HFrEF
- heart failure with a reduced ejection fraction
- HR
- hazard ratio
- iNMB
- incremental net monetary benefit
- IPD
- individual participant data
- IQR
- interquartile range
- LVEF
- left ventricular ejection fraction
- LVSD
- left ventricular systolic dysfunction
- NHFA
- National Heart Failure Audit
- NICE
- National Institute for Health and Care Excellence
- NICOR
- National Institute for Cardiovascular Outcomes Research
- NT-proBNP
- N-terminal pro-B-type natriuretic peptide
- NYHA
- New York Heart Association
- ONS
- Office for National Statistics
- PCI
- percutaneous coronary intervention
- PPI&E
- patient and public involvement and engagement
- PROTECT
- ProBNP Outpatient Tailored Chronic Heart Failure Therapy
- PSA
- probabilistic sensitivity analysis
- QALY
- quality-adjusted life-year
- RCT
- randomised controlled trial
- RR
- relative risk
- SA
- sensitivity analysis
- SBP
- systolic blood pressure
- SD
- standard deviation
- SIGNAL-HF
- Swedish Intervention study – Guidelines and NT-proBNP AnaLysis in Heart Failure
- STARBRITE
- The Strategies for Tailoring Advanced Heart Failure Regimens in the Outpatient Setting
- TIME-CHF
- Trial of Intensified versus Standard Medical Therapy in Elderly Patients with Congestive Heart Failure
- UTS
- up to standard