Notes
Article history
The research reported in this issue of the journal was funded by the HTA programme as project number 14/151/08. The contractual start date was in June 2016. The draft report began editorial review in August 2017 and was accepted for publication in February 2018. The authors have been wholly responsible for all data collection, analysis and interpretation, and for writing up their work. The HTA editors and publisher have tried to ensure the accuracy of the authors’ report and would like to thank the reviewers for their constructive comments on the draft document. However, they do not accept liability for damages or losses arising from material published in this report.
Declared competing interests of authors
John Stevens is a shareholder of GlaxoSmithKline plc (GSK House, Middlesex, UK), AstraZeneca plc (Cambridge, UK) and Shire Pharmaceuticals Group plc (Shire plc, Dublin, Republic of Ireland).
Permissions
Copyright statement
© Queen’s Printer and Controller of HMSO 2018. This work was produced by Archer et al. under the terms of a commissioning contract issued by the Secretary of State for Health and Social Care. This issue may be freely reproduced for the purposes of private research and study and extracts (or indeed, the full report) may be included in professional journals provided that suitable acknowledgement is made and the reproduction is not associated with any form of advertising. Applications for commercial reproduction should be addressed to: NIHR Journals Library, National Institute for Health Research, Evaluation, Trials and Studies Coordinating Centre, Alpha House, University of Southampton Science Park, Southampton SO16 7NS, UK.
2018 Queen’s Printer and Controller of HMSO
Chapter 1 Background
Description of the health problem
Clinical features of rheumatoid arthritis
Rheumatoid arthritis (RA) is a chronic disease characterised by progressive, irreversible joint damage, impaired joint function, pain, swelling and tenderness of joints. 1 RA is associated with increasing disability and reduced quality of life. 1 Symptoms of RA include pain, morning stiffness, joint swelling, joint tenderness, loss of movement, warmth of the peripheral joints and fatigue. 2,3 RA is associated with substantial costs, both directly (in terms of drug acquisition and hospitalisation) and indirectly, because of reduced productivity. 4 RA has long been linked with increased mortality,5,6 in particular because of cardiovascular events. 7
Epidemiology
It has been estimated that there are approximately 400,000 people in the UK with RA. 8 RA has been reported to have a greater incidence in females (3.6 per 100,000 per year) than in males (1.5 per 100,000 per year). 9 Although the peak age of incidence in the UK is in the eighth decade of life, people of all ages may develop RA. 9
Aetiology
A range of contributing factors, such as genetic and environmental influences, have been implicated as potential causes of RA. The heritability of RA is estimated to be between 53% and 65%,10 with a family history of RA carrying a corresponding risk ratio of 1.6 compared with the general population. 11 Many genes linked with RA susceptibility are concerned with immune regulation. Although infectious agents have been suspected, no consistent relationship with an infective agent has been demonstrated. Sex hormones have also been implicated because of the higher prevalence of RA in women and a tendency for RA to improve during pregnancy. There is no proof of any causal link with lifestyle factors, such as diet, smoking or occupation (but lifestyle factors may increase the risk of developing RA).
Management of rheumatoid arthritis
There are a range of treatment options available for the management of RA, with the aim of alleviating symptoms and of minimising irreversible joint damage that may occur as a result of the disease process. 8
Traditionally, patients have been treated with conventional disease-modifying antirheumatic drugs [(cDMARDs) also known as conventional synthetic disease-modifying antirheumatic drugs], including methotrexate (MTX), sulfasalazine (SSZ), hydroxychloroquine (HCQ), leflunomide (LEF) and gold injections, as well as corticosteroids, analgesics and non-steroidal anti-inflammatory drugs (NSAIDs). However, more recently, a group of biologic immunosuppressant drugs have been developed that specifically modify the disease process by blocking key protein messenger molecules (such as cytokines) or cells (such as B-lymphocytes). 8 These treatments have been termed as biologic disease-modifying antirheumatic drugs (bDMARDs); of these, certolizumab pegol, adalimumab (ADA), etanercept (ETN), golimumab and infliximab (IFX) are tumour necrosis factor (TNF) inhibitors (or antagonists). Of the remaining bDMARDs, tocilizumab is a cytokine interleukin-6 inhibitor, abatacept (ABT) is a selective modulator of the T-lymphocyte activation pathway and rituximab is a monoclonal anti-body against the cluster of differentiation 20 (CD20) protein. For patients who have exhausted all National Institute for Health and Care Excellence (NICE)-recommended treatments, non-biologic final treatment options may be used.
Prompt diagnosis of RA is important in ensuring the appropriate clinical management of patients early in the course of the disease. The 2013 European League Against Rheumatism (EULAR) recommendations for the management of RA state that treatment with disease-modifying antirheumatic drugs (DMARDs) should begin as soon as RA is diagnosed. 12 The lack of sensitivity of the 1987 American College of Rheumatology [(ACR) previously known as the American Rheumatism Association] classification criteria in early disease has been acknowledged and led to the development of the 2010 RA classification criteria. 13 The 2010 criteria for RA require (for classification as definite RA) the confirmed presence of synovitis in at least one joint, the absence of an alternative diagnosis to better explain the synovitis and scoring ≥ 6 (of a maximum of 10) from individual scores across four domains: (1) number and site of affected joints (score range 0–5), (2) serological abnormality (score range 0–3), (3) elevated acute-phase response (score range 0–1) and (4) symptom duration (range 0–1). 13
The EULAR recommended that, if a treatment target is not reached, the use of a bDMARD should be considered in the presence of poor prognostic factors [e.g. high disease activity, positivity to rheumatoid factor (RF) and/or anti-bodies to citrullinated proteins and the early presence of joint damage]. 14
Rheumatoid arthritis is a heterogeneous disease and the disease course can vary considerably between patients. The guideline development group for the NICE guidance on RA [i.e. Clinical Guidance number 79 (CG79)] suggested that it would be useful to clinicians if they could identify at an early stage those RA patients who are most likely to suffer a worse course of disease (or prognosis). 8 These patients could then be closely monitored to ensure that they can receive appropriate treatment to minimise the health problems and joint damage caused by RA. Patients who are considered to be less likely to experience a poor prognosis may require a less intensive follow-up and treatment strategy. The provision of clearer information on the prognosis and prediction of treatment response would be useful to inform the optimal clinical management of patients and to avoid pharmacological overtreatment of patients.
The NICE guidance on RA (i.e. CG79) indicated the potential role of a range of factors in determining the prognosis of patients with early RA. 8 These included RF, anticyclic citrullinated peptide (anti-CCP) positivity, baseline radiological damage, nodules, acute-phase markers, Health Assessment Questionnaire (HAQ) score, grip strength and swollen joint counts (SJCs).
Although there is a large amount of evidence available on the use of tests and assessment tools in determining the prognosis and prediction of treatment response, a recent good-quality evidence synthesis was considered to be lacking. Therefore, there is no clear consensus on which of the available tests and assessment tools used for RA could allow the best assessment of prognosis in people newly diagnosed with RA and whether or not variables, if identified, also predict how well patients respond to different drug treatments.
Measurement of key outcomes in rheumatoid arthritis
Major outcomes in RA include measures of joint destruction (e.g. radiographic progression), disease activity [as assessed via, for example, Disease Activity Score-28 (DAS28)] and disability (as assessed via, for example, HAQ scores).
Radiographic progression is frequently measured in clinical trials and observational research. 15 The two main scoring systems available for measuring radiographic progression are the Larsen et al. 16 system and the Sharp et al. 17 system. The van der Heijde18 modification of the Sharp system includes both hands and feet, erosions, joint-space narrowing and a range of joints.
In the UK, monitoring the progression of RA is often undertaken using the DAS28 in terms of swelling (SW28) and of tenderness to the touch (TEN28). The DAS28 also incorporates erythrocyte sedimentation rate (ESR) and a subjective assessment on a scale of 0–100 made by the patient regarding disease activity in the previous week.
The equation for calculating DAS28 is as follows:19
A second version of the DAS28 using C-reactive protein (CRP) levels instead of ESR exists. The DAS28 can be used to classify both the disease activity of the patient and the level of improvement. Patients with a DAS28 of ≤ 3.2 are classed as having inactive disease, patients with a DAS28 of > 3.2 and ≤ 5.1 are regarded as having moderate disease and those with a DAS28 of > 5.1 are regarded as having very active disease. 19
The HAQ is a key measure of patient functional disability. 20 It is a patient-completed assessment resulting in scores ranging from 0 to 3 (with higher scores indicating greater disability).
Background to prognosis research
Key concepts in prognosis research
Prognosis research describes the investigation of the relationship between future outcomes among people with a given baseline health state in order to improve health. 21,22
The PROGnosis RESearch Strategy (PROGRESS) partnership outlined a framework for prognostic research, with the aim of enhancing the quality and translational impact of prognosis research findings. The framework has four key elements, as outlined below:
-
Fundamental prognosis research – examining the average prognosis of patients (natural course of a disease or condition) with current clinical practice. 21,22
-
Prognostic factor research – studying individual factors that, for patients with a given disease or condition, are associated with the clinical outcome of interest. 21,22
-
Prognostic model research – the use of multiple prognostic factors in combination to provide a clinical prediction model, from which the probability of the outcome can be predicted for individuals. This research theme includes model development, validation and assessing the clinical impact of these models. 23
-
Stratified medicine research – the use of prognostic information to predict an individual’s response to treatment, and hence make treatment decisions that are tailored to individuals. 24
The current assessment focuses on prognostic model research (theme 3) and stratified medicine (theme 4). Briefly, prognostic model research seeks to estimate the absolute response of an outcome for an individual, whereas stratified medicine seeks to target therapy and make the best decisions for groups of similar patients. 23 One approach to stratifying the use of treatments is to consider the absolute response of each individual (as estimated using a prognostic model). Those people with the most severe prognosis are likely to derive the largest absolute benefit from a treatment and may be targeted for intervention. For example, lipid-lowering therapy may be recommended to individuals above a certain threshold for the risk of developing cardiovascular disease. 25 The results from prognostic model research are therefore relevant for guiding stratified medicine research, and the two themes are related.
Clinicians may also stratify medicine because the relative treatment effect is inconsistent across patients. In statistical terms, this means that the patient or disease characteristic is a treatment effect modifier (i.e. there is an interaction between the patient or disease characteristic and the effect of treatment on the outcome). Assessing the presence of these differential treatment effects is important in predicting individual treatment response. Examples include the use of trastuzumab for the treatment of breast cancer in individuals with a positive human epidermal growth factor receptor-2 status. The prediction of treatment response is considered in review 2, and further background on the development of treatment-specific clinical prediction models is given in Background to stratified medicine research. Further examples of stratified treatment decisions in clinical practice, based on the prediction of absolute risks, or differential treatment effects across different subgroups of the population are provided by Hingorani et al. 24
Definition of a prognostic model
Prognostic models are developed to aid health-care providers in their decision-making by estimating the probability that a specific event will occur in the future. 23,26
Moons et al. 27 stated that, because of the complexities in patients and diseases, prognostic studies require a multivariable approach. Indeed, Collins et al. 26 noted that, in practice, the prediction of an individual’s response is not typically based on a single predictor and that clinicians integrate a range of patient characteristics and symptoms in their own estimation of prognosis. 26 We further acknowledge that clinical prediction models would simplify to only one variable if this were the only significant predictor of prognosis.
A prognostic model is a formal combination of multiple predictors from which the probability of a specific outcome can be estimated for individual patients. 23,27 For an individual with a given set of baseline characteristics and assuming that the outcome is binary (e.g. progression/no progression), a prognostic model provides an estimate of the probability of experiencing the outcome within a specific period of time (i.e. an estimate of absolute risk). Measures of relative risk [e.g. odds ratios (ORs), relative risks, hazard ratios] are not relevant when evaluating the performance of prognostic models, other than to obtain an estimate of the absolute risk in conjunction with a baseline response. 28
Clinical prediction model development
Prediction model studies may be classed as model development,29 model validation30 or comprise a combination of both. There are many important statistical considerations to the development process, which we summarise here briefly to aid interpretation of the results. More comprehensive descriptions of model development and validation procedures are given in the provided references.
Clinical prediction models are developed using data from cohorts of patients or clinical trials. The development procedure starts with a preselected set of candidate predictors, and suitable procedures should be used to identify the most important predictors and assign relative weights to the predictors to form the combined clinical prediction model. 31 Important considerations include the following:
-
The selection of candidate predictors. Candidate predictors are variables that are chosen to be studied for their prognostic performance. 31 They should be selected based on subject knowledge and availability in practice, with consideration to the size of the data set. 32 Selection based on univariable analyses could lead to the omission of important predictors and so should be avoided. 33
-
The handling of missing values. It is generally recommended that some form of imputation should be used to account for missing data, because an analysis including only the individuals with completely observed data may lead to biased results. 34 A complete-case analysis may be appropriate, provided that the proportion of missing values is small (i.e. typically < 5%). 33
-
Continuous predictors. Simple transformations of continuous variables to account for non-linearity may be appropriate. 31 The creation of artificial categories leads to a loss of information and power and should therefore be avoided. 35,36
-
Final variable selection. Clinical prediction models are usually developed using multivariable regression. If a full-model approach (containing all candidate variables) is not appropriate, then backwards selection is the preferred method for statistical selection. Selection based purely on statistical significance in univariable analysis should not be used. 31,37,38
-
Interactions between variables should also be considered. Interactions between variables and treatment (treatment effect modification) are considered in review 2.
After a model has been developed, validation is conducted to quantify the performance of the model in the population used to develop the model (internal validation). This is described as apparent validation if the validation is conducted in exactly the same sample as that used for model development. This usually produces the best-possible estimate of model performance, because the model was optimally designed to fit the development data set (described as overfitting) and performs less favourably when applied generally to similar samples of patients. 31 Overfitting is of particular concern when the development data set is small and/or the number of candidate predictors is high. 31
Alternatives to apparent validation should ideally be used. Data splitting involves dividing the development sample into a training data set (used to derive the model) and a validation data set that is used to evaluate model performance. However, this approach is considered to be statistically inefficient, as not all available data are used to formulate the model. 31 Therefore, either bootstrapping or cross-validation is recommended as the preferred method of internal validation. 26 Cross-validation extends the simple data-splitting approach by iterating the procedure over several partitions of the data set. The performance results are averaged to provide an overall measure.
Overfitting can also be avoided or minimised using a procedure called shrinkage. This is a statistical estimation method that preshrinks the model regression coefficients towards zero. 33
Measures of predictive model performance (binary outcomes)
There are several summary measures used to quantify the predictive performance of clinical prediction models in internal and external validation. The following key measures of predictive model performance are considered in the assessment.
Overall model performance statistics
Overall model performance statistics include R2 and the mean-squared error/Brier score. R2 ranges from zero to one and describes the proportion of explained variation in the data. There are several methods for calculating R2, with Nagelkerke’s39 R2 commonly being reported for logistic regression models. The mean-squared error is defined as the average-squared difference between the observed outcome (0 or 1) and the predicted probability of the outcome.
Calibration
Calibration indicates the extent to which expected outcomes (predicted from the clinical prediction model) and observed outcomes agree. Summarising the estimates of calibration performance is challenging, because studies may quantify calibration using different summary statistics. 40 In a recently published guide to systematic review and meta-analysis of prediction model performance, Debray et al. 40 based their assessment of calibration on the total number of observed (O) events compared with the expected number of events (E) predicted by the model. The observed-to-expected (O : E) ratio provides a rough indication of the overall model calibration across the entire range of predicted probabilities. An O : E ratio of close to one would indicate good calibration, whereas values that are < 1 or > 1 indicate a model that either overestimates or underestimates the number of events.
Discrimination
Discrimination refers to the ability of a prediction model to distinguish between patients who do and those who do not experience the outcome of interest. For binary outcomes, discrimination is frequently quantified using the concordance statistic (c-statistic), and it is also known as the area under the receiver operating characteristic curve. For categorical outcomes, Harrell’s c-statistic for ordinal data37 can be used. The c-statistic is also relevant for other outcome measures (e.g. time to event) and is sometimes termed the c-index. A c-statistic of 0.5 indicates no discriminative ability over that that would be expected because of chance, whereas a c-statistic of 1 indicates perfect discriminative ability.
External validation
Demonstrating that a clinical prediction model can successfully predict the outcome of interest in the sample used to derive the model is not, by itself, sufficient to confirm its value. 23,28 A more objective measure of model performance is obtained using external validation, in which the model performance is assessed in a sample of patients who are external to those who were used for the model development.
Model updating or recalibration can be considered if a particular model does not calibrate well in external populations. This is considered to be a better alternative to redeveloping new models in each patient sample as a result of poor performance of existing models. 31 Despite this recommendation, a recent systematic review shows that recalibration is not commonly undertaken. 41
The c-statistic of a clinical prediction model may vary substantially between different validation studies, and a common cause for this heterogeneity is because of differences in the distribution of patient characteristcs. 40 Vergouwe et al. 42 demonstrated in a simulation study that a more heterogeneous sample was related to a higher discriminative ability. It is therefore important to consider the distribution of patient characteristics (case mix) of the included validation studies. This could be done by considering the standard deviation (SD) of key variables or of the combined linear predictor (the weighted sum of regression weights and covariate values in the validation sample).
Background to stratified medicine research
Developing treatment-specific clinical prediction models
Stratified medicine research is concerned with the use of prognostic information to predict an individual’s response (i.e. clinical benefit or adverse events) according to different treatments. The prediction of absolute treatment effects according to different patient and/or disease characteristics for different treatments is based on an analysis of relative treatment effects. The development of treatment-specific clinical prediction models should follow the same process as described in Clinical prediction model development; an assessment of differential treatment effects should be conducted formally, including the use of interaction tests, and not through subgroup analyses.
Issues associated with subgroup analyses
Although it is common for an assessment of differential treatment effects to be based on a series of subgroup analyses, this is not generally recommended. Constructing subgroups based on patient and/or disease characteristics that are continuous (e.g. ESRs of < 25 mm/hour, 25–50 mm/hour and > 50 mm/hour) assumes that treatment effects are constant within categories and have a discontinuity according to each category; in practice, such categorisation is often subjective and not supported by evidence. Some subgroups, including definitions such as early disease, involve other patient and/or disease characteristics that are correlated with such definitions of subgroup, and the resulting estimates of treatment effects may have a more complex interpretation. Absence of evidence is not evidence of absence, and a statistically significant treatment effect in one subgroup but not in another should not be interpreted as there being a true effect in one subgroup but not in another; the result may reflect different degrees of uncertainty or different magnitudes of treatment effect in the subgroups. Assessing the statistical significance of treatment effects in different subgroups does not incorporate an adjustment for other covariates that may be important and assumes that there is no residual differential treatment effect within the subgroup. Treatment effects may be misleading when treatments interact with covariates that were not used to form subgroups, and when the subgrouping variable interacts with a patient and/or disease characteristic that was ignored. 43
Assessing treatment by covariate interactions
Ideally, patient and/or disease characteristics that may affect relative treatment effects should be prespecified. All covariates believed to be prognostic or treatment effect modifiers should be included in the analysis and should be used consistently across data sets irrespective of the statistical significance of the covariates. The main effects should be modelled flexibly and should not assume linearity of response with a change in the value of a covariate; interaction effects should be modelled using the same approach. The statistical significance of all interaction effects included in a model should be assessed using a single test; this controls for multiplicity and will not be affected by potential treatment effect modifiers that are colinear with each other. The absence of a statistically significant interaction effect should not necessarily be interpreted to mean that this is evidence of the absence of an interaction effect. Studies are not typically designed to assess interaction effects, and a lack of statistical significance may simply reflect a lack of power of the test. Similarly, some interaction tests may be statistically significant by chance. Furthermore, it is important when interpreting interaction effects to distinguish between qualitative interactions, whereby the effect of treatment is reversed for some value(s) of the covariate, and quantitative interactions, whereby the treatment effect is in the same direction for different values of the covariate, but the magnitude of the treatment effect is different and may be clinically irrelevant. Finally, in non-linear models, such as the use of logistic regression for binary outcomes, any omitted covariates will result in biased estimates of treatment effect.
Assessing treatment by covariate interactions in this review
Studies used in this assessment to evaluate whether or not different patient and/or disease characteristics are prognostic according to different treatments will include those used in the development of treatment-specific clinical prediction models, as well as randomised controlled trials (RCTs) and observational studies that include patients with differential treatment use at entry. The use of RCTs and observational studies is to acknowledge the evidence that these studies provide about differential treatment effects in the absence of treatment-specific clinical prediction models. Studies that provide information only about the prognostic effect of patient and/or disease characteristics are excluded; nevertheless, studies meeting the inclusion criteria do provide some, albeit selective, information about the prognostic effect of covariates according to treatment. However, the results should be treated with caution for the reasons given above.
The primary parameter of interest is that representing the interaction between treatment and baseline covariate; it is this parameter that quantifies whether or not the response to treatment varies according to the value of the covariate. In practice, such interactions should be assessed in a single regression model incorporating main effects and interaction terms.
As discussed previously, studies may lack sufficient power to detect interaction terms as being statistically significant [i.e. 95% confidence intervals (CIs) not including the null value] and it should not be assumed that absence of evidence is equivalent to evidence of absence. Similarly, in the event that a statistically significant treatment effect modifier is identified, it may not be clinically relevant. In addition, the results should be considered as hypothesis-generating, because studies were generally not designed to detect interaction effects; the potential treatment effect modifiers are assessed univariately in this review, although their relevance may be different when the correlation between multiple covariates is taken into consideration in clinical prediction models, and spurious results may occur by chance given the number of multiple comparisons being performed.
Interpretation of the evidence regarding patient and/or disease characteristics
There are four scenarios that may arise depending on whether a covariate is (or is not) prognostic for at least one treatment and whether a covariate is (or is not) a treatment effect modifier: these are illustrated below for a comparison of two treatments with respect to a single covariate, which may be discrete or continuous.
Scenario 1: prognostic variable for both treatments, but not a treatment effect modifier (Figure 1)
Given two treatments coded such that t1 = 0 and t2 = 1, the mean response (i.e. expected value) for a person with a baseline value of xj who receives treatment t1 is:
For treatment t1 this is:
and for treatment t2 this is:
FIGURE 1.
Scenario 1: prognostic variable for both treatments but not a treatment effect modifier.

In this scenario, the treatment effect is β2, which is constant at each value of the predictor; it is also possible for a baseline variable to be a prognostic and for there to be no treatment effect (in which case, the two regression lines would be superimposed).
Scenario 2: prognostic variable for both treatments and a treatment effect modifier (Figure 2)
Given two treatments coded such that t1 = 0 and t2 = 1, the mean response (i.e. expected value) for a person with a baseline value of xj who receives treatment t1 is:
For treatment t1 this is:
and for treatment t2 this is:
FIGURE 2.
Scenario 2: prognostic variable for both treatments and a treatment effect modifier.
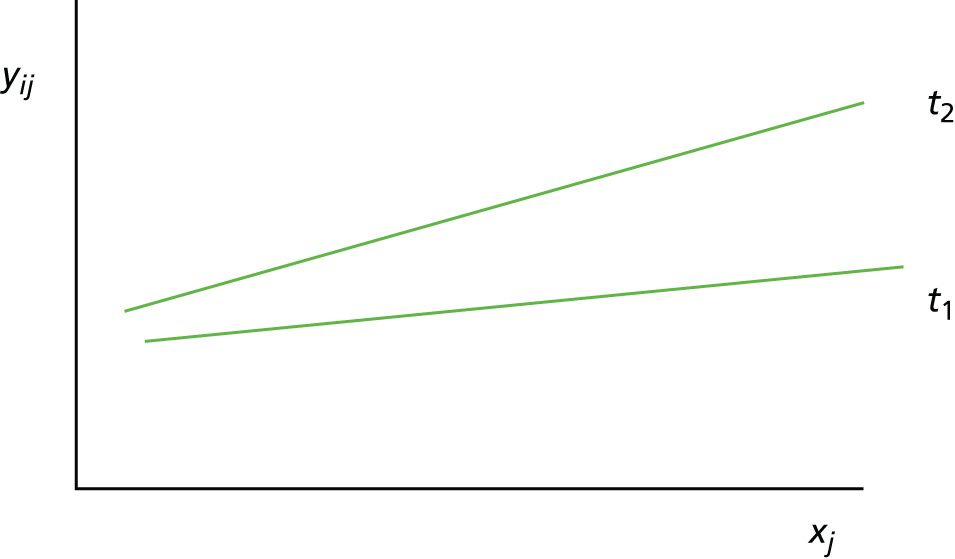
In this scenario, the treatment effect at xj is β2 + β12xj, which depends on the value of the baseline variable; it is also possible that β2 = 0.
Scenario 3: not a prognostic variable for one treatment, but a treatment effect modifier (Figure 3)
Given two treatments coded such that t1 = 0 and t2 = 1, the mean response (i.e. expected value) for a person with a baseline value of xj who receives treatment t1 is:
For treatment t1 this is:
and for treatment t2 this is:
FIGURE 3.
Scenario 3: not a prognostic variable for one treatment, but a treatment effect modifier.

In this scenario, the treatment effect at xj is β2 + β2xj, which depends on the value of the baseline variable; it is also possible that β2 = 0.
Scenario 4: not a prognostic variable for either treatment and not a treatment effect modifier (Figure 4)
Given two treatments coded such that t1 = 0 and t2 = 1, the mean response (i.e. expected value) for a person with a baseline value of xj who receives treatment t1 is:
For treatment t1 this is:
and for treatment t2 this is:
FIGURE 4.
Scenario 4: not a prognostic variable for either treatment and not a treatment effect modifier.

In this scenario, the treatment effect at xj is β2, which is independent of the baseline variable; it is also possible for there to be no treatment effect (in which case, the two regression lines would be superimposed).
Stratified medicine research is concerned with identifying treatment effect modifiers, as illustrated by scenarios 2 and 3.
Chapter 2 Review question and objectives
Review question
The review question as outlined in the Health Technology Assessment (HTA) commissioning brief and final protocol was as follows: what test or combination of clinical, laboratory and imaging tests gives the best assessment of prognosis in RA, and how well do they predict response to treatment?
Assessment structure
The assessment structure consisted of systematic reviews with appropriate predefined subgroup analyses.
It was anticipated in the final protocol that the factors most likely to be of use for prognosis and prediction of treatment response would be assessed through meta-analysis of available aggregate-level data. The de novo development of a specific prediction model and the use of individual participant data (IPD) were outside the remit of this assessment.
Overall objectives of the assessment
The objectives of this work were to undertake systematic reviews to determine the:
-
use of selected tests and assessment tools in the evaluation of prognosis in patients with early RA
-
potential of selected tests and assessment tools as predictive markers of treatment response in patients with early RA.
Chapter 3 Methods for the assessment of prognosis and prediction of treatment response in early rheumatoid arthritis
Assessment structure
This assessment consisted of two related systematic reviews. The first systematic review (review 1, clinical prediction models) investigated the use of assessment tools and tests in the evaluation of prognosis in early RA patients. The second systematic review (review 2, prediction of treatment response) determined the ability of selected assessment tools and tests to predict the response to specific treatments.
The systematic reviews were informed by the Preferred Reporting Items for Systematic Reviews and Meta-Analyses (PRISMA) statement (www.prisma-statement.org/) and current good practice in prognostic reviews, as advocated by the Cochrane Prognosis Methods Group (http://methods.cochrane.org/prognosis/).
A final protocol for this assessment was registered on PROSPERO (as record CRD42016042402).
Scoping of the assessment
The searches for reviews in this assessment were structured in two phases. The phase 1 scoping searches were performed to determine the approximate extent of the evidence base relevant to the assessment and to inform the discussion of prognostic and predictive variables for inclusion in conjunction with clinical advisors (full details are provided in Appendix 1).
Following discussions with two expert clinical advisors who manage patients with early RA in the UK (as described in Acknowledgements), the review team selected variables for inclusion. The selection of prognostic and predictive variables was based on:
-
tests and assessment tools (e.g. selected laboratory tests, imaging tests and clinical assessment measures) the variables being readily available and used in UK clinical practice (and, therefore, genetic markers were not included by the review team)
-
the clinical experience of advisors in evaluating prognosis/treatment response in patients
-
the initial scoping of literature in the area by the review team.
The selected prognostic and predictive variables were used in the development of the full searches for reviews 1 and 2.
Justification of review approach
In view of the anticipated large number of search results, it was necessary for the review team to revise the intended review approach in order to maintain the feasibility of the assessment within the available resources and time scales. Additional details of protocol deviations are provided in Appendix 2.
Therefore, the two related systematic reviews were planned as detailed in the following sections.
Review 1: clinical prediction models
A systematic review of studies that describe the development, external validation or impact of eligible clinical prediction models in early RA was performed. As outlined in Background to prognosis research, prediction model research requires a multivariable analysis approach and, therefore, it was considered methodologically appropriate to restrict review 1 to the study of prognostic variables analysed in combination.
Review 2: prediction of treatment response
A systematic review of primary studies that describe the development, external validation or impact of eligible outcome models to predict treatment response in early RA patients was undertaken. Given that it was anticipated (based on earlier scoping searches) that the availability of outcome models and external validation studies relevant to review 2 would be limited, it was decided that review 2 would also include a review of studies to predict treatment response in patients with early RA. This approach would provide information for researchers who wish to develop outcome models for the prediction of treatment response based on a summary of the key evidence for the variables/tests and assessment tools selected following discussions with our clinical experts.
Methods for review 1 (clinical prediction models)
Identification of studies
Electronic databases
Studies were identified by searching the following electronic databases and research registers:
-
MEDLINE, MEDLINE In-Process & Other Non-Indexed Citations and Epub ahead of print (via Ovid; 1947 to September 2016)
-
EMBASE (via Ovid; 1974 to September 2016)
-
The Cochrane Library (via Wiley Online Library), including Cochrane Database of Systematic Reviews (CDSR), Cochrane Central Register of Controlled Trials (CENTRAL) and Database of Abstracts of Reviews of Effects (DARE), NHS Economic Evaluation Database (NHS EED), HTA databases (inception to September 2016, or 2015 in the case of DARE/NHS EED, which are no longer being updated)
-
Web of Science Conference Proceedings (1990 to September 2016).
Sensitive keyword strategies using free text and, where available, thesaurus terms using Boolean operators and database-specific syntax were developed to search the electronic databases. Synonyms relating to disease (e.g. Arthritis, Rheumatoid/) and prognostic variables were combined with a highly sensitive search filter aimed at restricting results to prognostic studies. 44
No date restrictions were used on any database. However, all searches were restricted to the English language because of time and resource constraints for translation services.
All resources were initially searched from inception to 27 September 2016. An example of the MEDLINE search strategy is provided in Appendix 3.
Research registers and other websites
The following resources were also searched for relevant evidence:
-
The World Health Organization’s trial search portal (http://apps.who.int/trialsearch) and ClinicalTrials.gov [(https://clinicaltrials.gov) records added since 2010 up to the date of the search on 27 September 2016].
-
Arthritis Research UK; British Society for Rheumatology; National Rheumatoid Arthritis Society; Outcome Measures in Rheumatology (OMERACT) Task Force; Royal College of Pathologists; Royal College of Physicians; Royal College of Surgeons; EULAR, American College of Rheumatology; the US Food and Drug Administration (FDA); and the European Medicines Agency (EMA) (no date restrictions).
Results were manually added to EndNote Version X7 [Clarivate Analytics (formerly Thomson Reuters), Philadelphia, PA, USA] for sifting.
Other resources
To identify additional studies, the reference lists of all included studies and key systematic reviews were checked. In addition, key experts in the field were contacted.
Results from the phase 2 full searches were imported into reference management software EndNote and duplicates were removed.
Study selection
Studies were assessed for eligibility for review based on the following criteria.
Inclusion criteria
Population
Adult patients (aged ≥ 18 years) diagnosed with early RA. The final protocol stated that patients would be diagnosed with RA according to established criteria. Patients with early RA were defined in consultation with clinical advisors as being within 2 years of the onset of symptoms. It was noted that studies may report the duration of symptoms or the duration of disease at baseline and so the definitions applied in included studies were noted and tabulated. In the absence of any further information, disease duration at baseline was considered to be equivalent to symptom duration.
Studies investigating mixed populations were included only if ≥ 80% of the study population were early RA patients or if subgroup data were presented for this population.
Externally validated clinical prediction models were included if the external validation population met the inclusion criteria, even if the original development population did not meet the inclusion criteria. The rationale for this was that a clinical prediction model might perform well for the decision problem and it is not important that it was originally developed in a different population. In this case, the study that developed the original clinical prediction model was included even if it did not meet the criteria stated above. In addition, studies proposing clinical prediction models that did not present internal validation were included if they had been externally validated in another study. External validations in populations outside the scope of the assessment were not included in the review, but were referred to in the discussion as appropriate.
Technology
Blood tests, imaging modalities and clinical assessment scores used in the evaluation of prognosis in patients with early RA were included. Specific tests and assessment tools to be included were determined following the phase 1 scoping searches and agreed with clinical advisors. Tests and assessment tools were for the measurement of prognostic variables as described below.
Prognostic variables
Prognostic variables considered in the assessment were informed by the phase 1 scoping searches and agreed following discussion between the review team and clinical advisors. The prognostic variables selected for inclusion for review 1 were:
-
anticitrullinated protein/peptide anti-bodies (ACPAs) status; RF status
-
erosions/joint damage as assessed on radiographs
-
C-reactive protein levels
-
erythrocyte sedimentation rate
-
SJC
-
DAS28
-
early RA untreated for ≥ 12 weeks following the onset of symptoms
-
smoking
-
HAQ scores.
Genetic markers were discussed in the assessment only when these were included in a final clinical prediction model alongside other eligible prognostic factors as selected by the review team in collaboration with the clinical advisors. This was decided on the basis that genetic testing is not currently used in routine UK clinical practice.
Included clinical prediction models contained at least one eligible prognostic variable. Results were presented based on all prognostic variables included in each multivariable model (including those not included in this review).
Outcomes
The selected outcomes considered in this assessment were agreed following discussions between the review team and the clinical advisors. The outcomes below were considered by the review team and the clinical advisors to have clinical relevance and to be important to patients, and are widely reported in RA research. The outcomes selected for inclusion in review 1 were:
-
disease activity as measured by the DAS28
-
physical function as measured by the HAQ
-
joint damage as assessed on radiographs.
Study types
It was anticipated in the final protocol that the study types included in review 1 would be likely to include published reports of cohort studies (and potentially case–control studies), which report the associations between individual prognostic variables and outcomes. However, as described above, in order to maintain the feasibility of the assessment, review 1 was restricted to the inclusion of studies that describe the development, external validation or the impact of eligible clinical prediction models in early RA.
Included studies were categorised in accordance with the Transparent Reporting of a multivariable prediction model for Individual Prognosis Or Diagnosis (TRIPOD) classification, which groups studies according to their methods of determination of performance.
Studies developing clinical prediction models that had not been validated in one of the included external validation studies were included if they presented some form of internal validation to quantify predictive performance [e.g. calibration and discrimination measures, such as the c-statistic or area under the curve (AUC)]. For clinical prediction models that were not externally validated and did not report the c-statistic or AUC or calibration, a study reporting at least two alternative measures of predictive performance [e.g. sensitivity, specificity, positive predictive value (PPV), negative predictive value (NPV), accuracy] was considered to be eligible on the basis of being sufficiently informative to the review. When a study reported only R2 (a measure of the model goodness-of-fit), this was not considered to provide sufficient information regarding predictive performance, and the study was not included (but recorded in the table of excluded full-text studies in Appendix 4).
Exclusion criteria
The following criteria were applied to exclude studies:
-
non-English-language papers
-
reports published as meeting abstracts only, in which insufficient methodological/results details are reported
-
animal models
-
preclinical and biological studies
-
narrative reviews, editorials and opinions.
It was necessary, because of resource and time constraints, to deviate from the final protocol in the applied screening approach (see Appendix 1). An iterative approach to the screening of evidence was undertaken (adapted from Archer et al. 45). Titles and abstracts of search records were examined by one reviewer. Titles and abstracts were searched for terms relating to clinical prediction models. Key terms were identified by consulting publications relating to prediction model research (e.g. Moons et al. ,31 Debray et al. 40). Terms used were risk model*, prognostic model*, prediction model*, predictive model*, risk assessment model*, prediction score*, algorithm*, matrix/matrices, assessment tool*, prediction rule*, decision rule*, and risk score* in order to identify potentially relevant studies for screening at the full-text stage. As described previously, this method was supplemented by the hand-searching of reference lists of included studies, existing key systematic reviews (e.g. Bombardier et al. ,46 Navarro-Compán et al. 47), contact with clinical experts and the searching of grey literature.
Full texts of remaining articles were screened for eligibility before inclusion. Study inclusion based on full-text articles was performed by one reviewer and queries were discussed with a second reviewer. Any discrepancies were resolved by discussion, with the involvement of a third team member when required.
Data extraction
A data extraction form was designed and piloted before use on a minimum of two studies.
Data were extracted by one reviewer. Extracted data were checked for accuracy by a second reviewer. Any discrepancies were discussed and resolved, with reference to a third team member when required.
The CHecklist for critical Appraisal and data extraction for systematic Reviews of prediction Modelling Studies (CHARMS)48 includes guidance on the relevant items for extraction from reports of prediction modelling studies. Data extraction was guided by CHARMS. 48 Predictive performance measures relating to each clinical prediction model’s overall performance, calibration and discriminative ability were extracted from each clinical prediction model development and external validation study. Following advice in Debray et al. ,40 the c-statistic was used as the primary measure of discrimination and the O : E ratio used as the primary measure of calibration. If these measures or the associated variance estimates were not reported for a particular study, they were computed from other information when possible. The results of the Hosmer–Lemeshow test (a measure of model calibration) were also extracted, along with measures of model overall goodness of fit (such as R2). Information regarding the case mix of the development/validation population, which may help to explain the heterogeneity in the results, was also summarised from the available information when possible. Further details relating to the data extraction and associated calculations are provided in Appendix 5.
Quality assessment strategy
The assessment of the study quality characteristics of clinical prediction modelling studies was informed by criteria included in an unpublished draft version of the Prediction model study Risk Of Bias Assessment Tool (PROBAST). 49 The risks of bias for participant selection, predictors and outcomes were discussed narratively and tabulated. Potential key sources of bias in the model development and validation were discussed narratively.
The methodological quality of each included study was assessed by one reviewer and checked with a second reviewer. Disagreements were resolved by discussion between the two reviewers and if agreement could not be reached, a third reviewer was consulted.
Synthesis methods
Data relating to clinical prediction model performance were described in a narrative synthesis, presented separately for internal and external validation studies. An evidence synthesis using meta-analysis was considered for external validation studies.
Variation in predictive performance is expected because of differences in the design and execution of alternative external validation studies and, hence, a random-effects (RE) model that accounts for between-study heterogeneity was used. 40 Results using a fixed-effects (FE) model (i.e. a condition inference given the observed studies) are also presented for comparison.
Results are presented in terms of the summary c-statistic (and 95% CIs) that quantifies the average performance across the included studies. For RE models, it was anticipated that an estimate of the between-study SD (that quantifies the extent of heterogeneity between studies), as well as the 95% prediction intervals (which provide a range for the potential model performance in a new study), would also be provided. However, this was not possible on account of the limited number of studies that validated each prediction model, thereby providing limited information with which to estimate the between-study heterogeneity.
All analyses were conducted in R50 (The R Foundation for Statistical Computing, Vienna, Austria) using the metafor package. 51 A restricted maximum likelihood estimation was used with the Hartung–Knapp–Sidik–Jonkman method (which accounts for uncertainty in the estimated between-study heterogeneity) to estimate the CIs for the pooled estimate and the 95% prediction intervals. 40,52 Analysis was conducted on the logit scale as previously recommended and back-transformed to the original scale for the presentation of results. 40,52
It was stated in the review protocol that meta-analyses would be conducted using a Bayesian RE model. However, this was modified for the final analysis, because there were very few studies that validated each clinical prediction model, thereby providing limited information with which to estimate the between-study heterogeneity. Although it would be possible to implement a Bayesian RE analysis using a weakly informative prior, this was not implemented, because there was a lack of empirical evidence to inform the prior distribution for the heterogeneity parameter and eliciting experts’ beliefs was beyond the scope of this project. Although the analysis deviated slightly from the protocol, the implemented RE model accounts for uncertainty in the between-study heterogeneity and is consistent with methodological recommendations. 40
Given the limited number of studies available, it was not possible to explore heterogeneity in prognostic/predictive effects using metaregression. However, subgroup analyses based on baseline DAS28 were considered in the narrative synthesis of evidence for review 1 and review 2.
Methods for review 2 (prediction of treatment response)
Identification of studies
Electronic databases
Electronic databases, research registers and other websites searched were identical to those for review 1 (see Identification of studies). Sensitive keyword strategies using free text and, when available, thesaurus terms using Boolean operators and database-specific syntax were developed to search the electronic databases. The disease and candidate variable terms used in review 1 were combined with the appropriate search filter for review 2. 53
Research registers and other websites
The resources searched were identical to those used in review 1. The results were manually added to EndNote for sifting.
Other resources
To identify additional studies, the reference lists of all included studies were checked. Hand-searching of key systematic reviews and narrative reviews was also performed. A citation search of included articles [using Google Scholar (Google Inc., Mountain View, CA, USA)] was undertaken. In addition, key experts in the field were contacted.
Results from the phase 2 full searches were imported into reference management software EndNote (version X8) and duplicates were removed.
Study selection
Studies were assessed for eligibility for review 2 based on the following criteria.
Inclusion criteria
Population
Adult RA patients (aged ≥ 18 years) who have:
-
received treatment with cDMARDs/bDMARDs for RA
-
baseline/early disease and follow-up data for selected variables (as defined below).
For the review for the prediction of treatment response (review 2), response to cDMARDs and bDMARDs was studied. It was planned in the final protocol for review 2 that, if data allowed, treatment would be subdivided into cDMARDs and bDMARDs. Response to any other treatments (e.g. steroids) was not assessed for reasons of feasibility. Predictors of treatment response to individual drugs (e.g. specific biologics) would be explored if feasible and if sufficient evidence was available.
Studies were eligible for inclusion in review 2 if they involved at least 6 months’ treatment duration (with the exception of certolizumab pegol, for which the response is largely known at 12 weeks and, therefore, a 12-week treatment duration was considered to be more acceptable by clinical advisors and was applied for this drug).
Technology
The tests and assessment tools for the measurement of predictive variables are as described below.
Predictive variables
The predictive variables considered in the assessment were also informed by phase 1 scoping searches and selected following discussion between the review team and clinical advisors. The predictive variables selected for inclusion in review 2 were the same as those for review 1, with the addition of two variables:
-
body mass index (BMI)
-
vascularity of synovium assessed using power Doppler ultrasound (PDUS).
Outcomes
The outcomes selected for inclusion were the same as for review 1, with the addition of:
-
definitions of response/remission selected in conjunction with clinical advisors (EULAR response; remission: a DAS28 of < 2.6, a Disease Activity Score (DAS) of < 1.6 or ACR/EULAR remission).
Study types
It was anticipated in the protocol that the included study types in review 2 would be cohort studies and RCTs. Following a protocol amendment, in order to maintain the feasibility of the assessment within the available time and resources, review 2 consisted of:
-
a systematic review of studies that describe the development, external validation or impact of eligible clinical prediction models to predict the response to individual treatments in patients with early RA (developed/validated in observational cohorts or experimental data sets)
-
a review of primary studies (experimental or observational) to identify patient characteristics that affect the response to individual treatments in patients with early RA.
The exclusion criteria were as described for review 1.
The titles and abstracts of search records were examined by one reviewer and irrelevant evidence was excluded. The full texts of remaining articles were screened for eligibility before inclusion. Study inclusion based on full-text articles was performed by one reviewer and queries were discussed with a second reviewer. Any discrepancies were resolved by discussion, with the involvement of a third team member when required.
Data extraction
A data extraction form was designed and piloted before use on a minimum of two studies.
Data were extracted by one reviewer. Extracted data were checked for accuracy by a second reviewer. Any discrepancies were discussed and resolved, with reference to a third team member when required.
Quality assessment strategy
Any identified clinical prediction model studies were to be critically appraised, guided by the items included in the PROBAST. 49 Studies of prognostic variables were assessed by criteria informed by the Quality in Prognosis Studies (QUIPS) tool (see Hayden et al. 54), as stated in the final protocol.
Critical appraisal was performed by one reviewer and double-checked by a second reviewer. Discrepancies were resolved by discussion, with the involvement of a third team member if necessary.
Synthesis methods
In the protocol, it was envisaged that a formal meta-analysis comparing all treatments across all studies would be performed with respect to the predictive ability of treatments. However, for specific outcome measures and potential treatment effect modifiers, there were no studies that shared any treatments in common. Consequently, a formal meta-analysis was not performed and the results are presented assessing the predictive ability of treatments by study.
Some authors fitted single regression models to the data from all treatment groups and included only the main effects of treatment and baseline covariates; these models do not allow an assessment of the interaction between treatments and baseline covariates. Other authors fitted separate regression models to the data from each treatment group and presented estimates of treatment effects by covariate; it is these estimates that are used to quantify the extent to which the treatment effect varies by covariate.
For continuous outcomes, the interaction effect is estimated by calculating the difference (and 95% CI for the difference, when possible) in the mean between treatments; departures from zero are indicative of the covariate being a treatment effect modifier. For binary outcomes, the interaction is estimated by calculating the ratio (and 95% CI for the ratio when possible) of the ORs between treatments; departures from one are indicative of the covariate being a treatment effect modifier.
Results indicating that a covariate is not a treatment effect modifier should not be interpreted to mean that a covariate is prognostic, only that the relationship between covariate and response may be the same for both treatments subject to a treatment effect (i.e. the covariate may be prognostic for both treatments or not prognostic for both treatments).
Chapter 4 Results: review 1
Quantity of research available
Searches for evidence (Figure 5) identified 22 model development studies and one combined model development/external validation study, reporting a total of 39 clinical prediction models for the prediction of major outcomes, including radiographically assessed joint damage, the HAQ score and the DAS28 (note that one publication56 considered the development of models for multiple outcomes; however, because of unclear reporting, the individual models could not be summarised and so have been counted as a single entry for the purposes of the assessment). The study by Bombardier et al. 57 was available as a conference abstract only and a full-text publication was not available. Six external validation studies (including the combined model development/external validation study) of eight previously proposed clinical prediction models for radiographically assessed joint damage outcomes were identified. No studies assessing the impact of the use of clinical prediction models in the clinical management of early RA were identified.
Quality of the research available
Potential sources of bias in participant selection, predictors, outcomes, model development and validation are discussed in the following sections and tabulated in Appendix 6.
Types of included prediction model studies
The TRIPOD statement26 was developed with the aim of improving the quality of reporting of prediction model studies. Collins et al. 26 described six categories (listed below) that can be used to classify studies according to their aim (development or/and validation) and the methodology used.
The inclusion criteria for review 1 required that studies developing clinical prediction models apply some form of internal validation to quantify the predictive performance of the developed model (e.g. calibration and discrimination), with the exception of cases in which the clinical prediction model had been subsequently externally validated. In these cases, the external validation results may be considered to be more informative than the omitted internal validation results and it was deemed necessary to include the original development paper for completeness. These studies did not have a category according to the TRIPOD statement, and it was therefore necessary to introduce a new TRIPOD category (Type 0) to allow the description of these studies.
The tailored TRIPOD classification categories used in this review (based on Collins et al. 26) are as follows:
-
Type 0 – development of a clinical prediction model in which the predictive performance is not evaluated in the development paper, but an evaluation of the predictive performance has been considered in a separate publication.
-
Type 1a – development of a clinical prediction model with an evaluation of the predictive performance using the same data set (apparent performance).
-
Type 1b – development of a clinical prediction model using the whole data set and an evaluation of the predictive performance using resampling (e.g. bootstrapping or cross-validation).
-
Type 2a – random splitting of data into two groups, the first for clinical prediction model development and the second for the testing of its predictive performance.
-
Type 2 – non-random splitting of data into two groups, the first for clinical prediction model development and the second for the testing of its predictive performance.
-
Type 3 – development of a clinical prediction model in one data set and an evaluation of the predictive performance on separate data (e.g. from a different study).
-
Type 4 – the evaluation of the predictive performance of an existing clinical prediction model using separate data (external validation).
The TRIPOD classification categories of the 28 included studies are presented in Table 1. The PROBAST49 study-type classifications of the included studies are also presented in Table 1.
First author (year of publication) | Name of clinical prediction modela | Externally validated? (Y/N) | Model presented in useable format? | Category | |
---|---|---|---|---|---|
PROBAST | TRIPODb | ||||
Clinical prediction model development studies | |||||
Bansback (2006)58 | Bansback | N | Y | Development only | 1b |
Berglin (2006)59 | Berglin | N | N | Development only | 1a |
Bombardier (2009)57 | SONORA | Y | N | Development only | 0 |
Brennan (1996)60 | Brennan | N | Y | Development only | 2a |
Centola (2013)61 | Centola | Y | Y | Development only | 0 |
Combe (2001)62 | Combe (A) | N | Y | Development only | 1a |
Combe (2003)63 | Combe (B) | N | N | Development only | 1a |
de Punder (2015)64 | de Punder | N | Y | Development/external validation | 1b/4 |
de Vries-Bouwstra (2006)65 | de Vries-Bouwstra | N | N | Development only | 1a |
Degboé (2015)66 | Degboé | N | N | Development only | 1a |
Dirven (2012)67 | Dirven | N | Y | Development only | 1a |
Dixey (2004)68 | Dixey | N | N | Development only | 2a |
Drossaers-Bakker (2002)56 | Drossaers-Bakker | N | N | Development only | 1a |
Fautrel (2012)69 | ESPOIR | Y | Y | Development only | 1a |
Forslind (2004)70 | Forslind | N | Y | Development only | 1a |
Graell (2009)71 | Graell | N | Y | Development only | 1a |
Houseman (2012)72 | Houseman | N | N | Development only | 1a |
Saevarsdottir (2015)73,74 | SWEFOT | Y | N | Development/external validation | 1a/4 |
Sanmartí (2007)75 | Sanmartí | N | Y | Development only | 1a |
Syversen (2008)76 | Syversen | Y | Y | Development only | 1a |
van Steenbergen (2015)77 | van Steenbergen | N | N | Development only | 1a |
Vastesaeger (2009)78 | ASPIRE | Y | Y | Development and external validation | 3c |
Visser (2010)79 | BeSt | Y | Y | Development only | 1a |
External validation studies | |||||
De Cock (2014)80 | External validation only | 4 | |||
Granger (2016)82 | External validation only | 4 | |||
Hambardzumyan (2015)83 | External validation only | 4 | |||
Heimans (2015)84 | External validation only | 4 | |||
Markusse (2014)85 | External validation only | 4 |
Of the 23 clinical prediction model development studies, the vast majority (n = 16) were TRIPOD type 1a {i.e. Berglin et al. ,59 Visser et al. 79 [Behandelings Strategie (BeSt)], Combe et al. 62 (Combe A), Combe et al. 63 (Combe B), de Vries-Bouwstra et al. ,65 Degboé et al. ,66 Dirven et al. ,67 Drossaers-Bakker et al. ,56 Fautrel et al. 69 [Études et suivi des polyarthrites indifférenciées récentes (ESPOIR)], Forslind et al. ,70 Graell et al. ,71 Houseman et al. ,72 Saevarsdottir et al. 73,74 [Swedish Farmacotherapy (SWEFOT)], Sanmartí et al. ,75 Syversen et al. 76 and van Steenbergen et al. 77}, with validation conducted in exactly the same data as those used for clinical prediction model development. Fewer studies were categorised as type 1b (n = 1; i.e. Bansback et al. 58), using techniques to evaluate performance and optimism of the developed model, type 2a (n = 2; i.e. Brennan et al. 60 and Dixey et al. 68), using a data-splitting approach for development and validation, or type 0 {n = 2; i.e. Bombardier et al. 57 [Study Of New-Onset Rheumatoid Arthritis (SONORA)] and Centola et al. 61}, with no internal validation presented for Bombardier et al. 57 Although internal validation was conducted for Centola et al. ,61 it was developed for a different outcome measure and so the study is categorised as type 0 for the purpose of the current review. One study {n = 1; i.e. Vastesaeger et al. 78 [Active controlled Study of Patients receiving Infliximab for the treatment of Rheumatoid arthritis of Early onset (ASPIRE)]} considered validation in an external population in addition to model development (type 3); however, the external validation population comprised patients with established RA and, therefore, was outside the scope of this assessment. The methods of internal validation and reporting of models in the included development studies are described in greater detail in Model development.
Five additional included studies were external validations of clinical prediction models (TRIPOD class 4). Three of these studies (i.e. De Cock et al. ,80 Granger et al. 82 and Heimans et al. 84) externally validated a total of seven clinical prediction models (i.e. ASPIRE CRP,78 ASPIRE ESR,78 BeSt,79 ESPOIR,69 SONORA,57 SWEFOT73 and Syversen76). The remaining two external validation studies (Hambardzumyan et al. 83 and Markusse et al. 85) evaluated the use of a multibiomarker disease activity (MBDA) test61 (developed as a measure of disease activity) in the prediction of eligible clinical outcomes. In addition to the seven purely external validation studies, the de Punder model development paper64 (TRIPOD category 1b/4) also externally validated the BeSt79 and ESPOIR69 models.
Two additional external validation studies were identified but not included. 73,86 The study reported by Durnez et al. 86 is considered to be a precursor study to the work by De Cock et al. ,80 and so the work by De Cock et al. 80 is utilised in this assessment as being more recent and comprehensive in the coverage of validated models. The application of the BeSt model79 was considered by Saevarsdottir et al. 73 (TRIPOD category 1a/4) using the SWEFOT data set. However, the presented results did not provide sufficient data to be considered as informative to the current review, as no summary statistic of overall performance (e.g. c-statistic) was reported. Saevarsdottir et al. 73 presented the allocation of individuals to risk matrix categories, combined for the whole validation sample rather than separately by treatment group.
Description of data sources for the development of clinical prediction models and external validation
Moons et al. 31 advocated the use of cohort study data in the development of clinical prediction models, which are preferably prospective in design to allow for the greater completeness of data collection. 31 Trial data are also an appropriate data source, although it has been noted that trial eligibility criteria may result in more restricted and less generalisable data than registry cohort studies. 31 All identified clinical prediction models for review 1 were developed in either observational cohort or registry data or in existing cohorts from intervention trials in early RA populations and, therefore, can be considered to have been developed in appropriate data sources. Studies were longitudinal in design, with potential predictors measured in an early RA population at baseline or in an early disease stage before the measurement of a specified outcome at a subsequent time point. There was one main exception to this, Houseman et al.,72 which is discussed further in Description of predictors. The characteristics of the data sources used in the development and external validation of the included clinical prediction models are tabulated in Table 2.
Name of clinical prediction model/external validation | Name and study design of data source | Setting (number of centres) and period of data collection | Key eligibility criteria |
---|---|---|---|
Clinical prediction model development studies | |||
ASPIRE78 |
Active-controlled study of patients receiving infliximab for the treatment of rheumatoid arthritis of early onset (ASPIRE) RCT87 Internally validated in the anti-TNF trial in rheumatoid arthritis with concomitant therapy (ATTRACT)88 [median disease duration, 8.4 years (IQR 4.3–14.7 years); established RA outside scope of assessment] |
Multicentre, multinational study (122 sites in North America and Europe) Patients recruited between 2000 and 2002 |
Aged 18–75 years, met the 1987 revised ACR criteria, persistent synovitis for 3 months to 3 years, ≥ 10 swollen joints and ≥ 12 tender joints. Plus one or more of positive serum RF, radiographically detected erosions of hands/feet or CRP level of ≥ 2.0 mg/dl. Patients excluded if any prior MTX (three or fewer pre-study doses permitted), received other DMARDs within 4 weeks of entry (or LEF within past 6 months) or treated with IFX, ETN, ADA or other anti-TNF Subjects were excluded if they were infected with HIV, hepatitis B or hepatitis C, they had a history of active or past TB, congestive heart failure, lymphoma or other malignancy within the past 5 years (excluding excised skin cancers) |
Bansback58 | Early Rheumatoid Arthritis Study (ERAS) inception cohort |
Unspecified regions of England (nine centres) Cohort established in 1986 |
All consecutive RA patients seen within 2 years of initial symptoms and before second-line drug use. Patients completing 5 years of follow-up included in the analysis |
Berglin59 | Cohort data | Department of Rheumatology, University Hospital, Umeå, Sweden, co-analysed with Northern Sweden Health and Disease Study (NSHDS) cohort and maternity cohort of northern Sweden (Medical Biobank), Umeå, Sweden (number of centres and period of data collection NR) | Department of Rheumatology, University Hospital, Umeå register: early RA (duration of < 1 year) meeting the 1987 revised ACR criteria for RA, with a known date of the onset of symptoms |
BeSt79 | Behandelings Strategie (BeSt) RCT89 |
18 peripheral and two university hospitals in the western Netherlands Patients recruited between 2000 and 2002 |
Early RA defined by the 1987 revised ACR criteria, disease duration of ≤ 2 years, aged ≥ 18 years, active disease with ≥ 6 of 66 swollen joints, ≥ 6 of 68 tender joints and either an ESR of ≥ 28 mm/hour or a global health score of ≥ 20 mm on a 0- to 100-mm VAS (0 = best, 100 = worst) Subjects were excluded if they had previous DMARD treatment other than antimalarials, concomitant treatment with an experimental drug, malignancy within the past 5 years, bone marrow hypoplasia, serum aspartate aminotransferase or alanine aminotransferase (ALT) levels of > 3 times the upper limit of normal level, serum creatinine levels of > 150 µm/l or estimated creatinine clearance of < 75 ml/minute, diabetes mellitus, alcohol or drug abuse, concurrent pregnancy, wish to conceive during study period or inadequate contraception |
Brennan60 | Prospective cohort |
All primary care general practices in Norwich Health Authority, Norfolk, UK (number of centres NR) Recruited between 1990 and 1993 |
Satisfied any subset of the revised 1987 ACR RA criteria and were recruited within 180 days of the onset of symptoms, with complete baseline and 1-year follow-up measurements (and having provided blood for RF testing at baseline) |
Centola61 |
Feasibility studies (stage 2): Oklahoma City cohort and Brigham and Women’s Rheumatoid Arthritis Sequential Study (BRASS) cohort Algorithm training study (stage 3): Brigham and Women’s Rheumatoid Arthritis Sequential Study (BRASS) cohort and Index for Rheumatoid Arthritis Measurement (InFoRM) study |
NR | All patients met ≥ 4 of 7 of the 1987 revised ACR criteria for RA |
Combe (A)62 | Prospective cohort |
France (four centres: Montpellier, Paris-Cochin, Toulouse and Tours) Included March 1993 to October 1994 |
All consecutive outpatients referred from primary care, disease duration of < 1 year, met ACR criteria for RA, DMARD-naive |
Combe (B)63 | Prospective cohort |
France (four centres: Montpellier, Paris-Cochin, Toulouse, Tours) Included March 1993 to October 1994 |
All consecutive outpatients referred from primary care, disease duration of < 1 year, met ACR criteria for RA and were DMARD naive |
de Punder64 | Nijmegen early RA inception cohort |
Radboud University Medical Centre, Nijmegen, the Netherlands Included from 1985 to 2008 |
Satisfied the 1987 ACR criteria for RA, disease duration of ≤ 1 year, no previous DMARD use and aged ≥ 18 years. Patients with radiographs available at inclusion and after 2 or 3 years’ follow-up included in analysis Patients treated with biologic DMARDs within first 3 years were excluded from analysis |
de Vries-Bouwstra65 | Leiden Early Arthritis Clinic cohort |
Early Arthritis Clinic (EAC), Department of Rheumatology, Leiden University Medical Centre, the Netherlands Sample from patients included from 1993 to 1999 |
Arthritis confirmed by rheumatologist, duration of symptoms of < 2 years and patient had not seen another rheumatologist for the same symptoms. All patients presenting with RA/probable RA and in whom a diagnosis of RA was confirmed at 3 months after presentation. Follow-up of ≥ 1-year and radiographs of hands and feet at baseline and after 1 year included in analysis. Definite RA according to the 1987 revised ACR criteria but without the criterion that symptoms must be of 6 weeks’ duration and observed by a physician |
Degboé66 | ESPOIR cohort |
14 regional centres, France Period of data collection NR |
Suspected or confirmed RA diagnosis, aged 18–70 years, ≥ 2 swollen joints for > 6 weeks and < 6 months, no receipt of DMARDs or steroids except for < 2 weeks prior to entry. Included in analysis if satisfied the 2010 ACR/EULAR criteria and with complete radiographs at baseline and year 1 and complete ACPA measurements |
Dirven67 | Behandelings Strategie (BeSt) RCT89 |
The Netherlands Number of centres and period of data collection NR |
DMARD-naive patients, with RA as defined by the 1987 revised ACR criteria |
Dixey68 | Inception cohort |
Rheumatology departments of nine hospitals, country NR, but assumed to be the UK Recruitment of patients from 1986 |
Patients with RA according to the 1987 revised ACR criteria, duration of symptoms of ≤ 2 years, no use of second-line medication. Patients completing 3 years’ follow-up with adequate-quality radiographs for scoring were included in the analysis |
Drossaers-Bakker56 |
Prospective inception cohort Leiden Early Arthritis Clinic cohort |
Rheumatology outpatient clinic of Leiden University Medical Centre, the Netherlands Patients attending from 1982 to 1986 |
All consecutive female RA patients, with symptoms of < 5 years’ duration, aged 20–50 years at first visit |
ESPOIR69 | ESPOIR cohort |
14 regional centres in France (16 university hospital rheumatology departments) Patients referred and included from December 2002 to March 2005 |
Patients recruited from the community, with early arthritis for < 6 months, aged 18–70 years, two or more swollen joints for > 6 weeks and < 6 months, suspected/confirmed RA diagnosis, not receiving any DMARDs or steroids except for < 2 weeks before entry. Current study included ESPOIR patients with RA diagnosis according to the rheumatologist and receipt of first cDMARD with demonstrated efficacy for ≥ 3 months of the first year of follow-up |
Forslind70 | BARFOT (Better Anti-Rheumatic Farmacotherapy) prospective cohort |
Sweden (multicentre observational study, number of centres NR) Cohort recruited from July 1993 to June 1997 |
RA satisfying the 1987 revised ACR criteria, with a disease duration of ≤ 1 year |
Graell71 | Prospective cohort |
Rheumatology units of Hospital Clinical of Barcelona and Hospital Parc Tauli of Sabadell, Spain Patients recruited from 1998 to 2003 |
Patients met the 1987 revised ACR criteria; symptoms for < 24 months, patients previously treated with DMARDs or > 10 mg/day of prednisone were excluded |
Houseman72 | Prospective cohort |
Geographical setting and number of centres NR (general rheumatology clinics) Cohort established between 1998 and 2000 |
Consecutive early RA patients recruited from general rheumatology clinics and from among patients referred for early synovitis. Satisfied the 1987 revised ACR criteria for RA with the onset of persistent symptoms for < 2 years |
Sanmartí75 | Prospective cohort |
Rheumatology units of Hospital Clinical of Barcelona and Hospital Parc Tauli of Sabadell, Spain Patients recruited from 1998 to 2003 |
Patients met the 1987 revised ACR criteria; symptoms for < 24 months, patients previously treated with DMARDs or > 10 mg/day of prednisone were excluded |
SONORA57 | SONORA cohort | North America | Patients diagnosed by rheumatologist with early RA (i.e. a symptom duration ≥ 3 and ≤ 12 months) |
SWEFOT73 | SWEFOT RCT |
15 rheumatology units in Sweden90 Patients screened for inclusion from 2002 to 200590 |
RA according to the 1987 revised ACR criteria, aged ≥ 18 years, with symptom duration for < 1 year, a DAS28 of > 3.2, no previous DMARDs and stable prednisolone dose if present for ≥ 4 weeks before entry and throughout the study at ≤ 10 mg/day |
Syversen76 |
Prospective cohort European Research on Incapacitating Disease and Social Support (EURIDISS) project |
Geographical setting, number of centres NR EURIDISS began in 1992 |
Clinical diagnosis of RA (1987 ACR criteria), aged 20–70 years, with a disease duration of 0–4 years at baseline Patients with Steinbrocker function class IV excluded |
van Steenbergen77 |
Prospective cohort Leiden Early Arthritis Clinic cohort |
Leiden Early Arthritis Clinic Recruited from 1993 to 2006 |
Patients meeting the 1987 ACR criteria and symptom duration of ≤ 2 years |
External validation studies | |||
De Cock80 | Part of observational cohort (Verschueren et al. 200881) |
Department of Rheumatology at University Hospitals of Leuven, Leuven, Belgium Enrolled from 2001 to 2007 |
Consecutive DMARD-naive early RA patients. Patients with radiographs of hands and feet at baseline, year 1 and year 2 included in study Patients in parallel RCTs excluded |
Granger82 | ESPOIR cohort |
France (14 regional centres) Recruited from 2002 to 2005 |
Aged 18–70 years, with two or more swollen joints for > 6 weeks and < 6 months, not taking any DMARDs/steroids except for < 2 weeks before entry. Patients with RA diagnosis from rheumatologist and initiation of first cDMARD |
Hambardzumyan83 | SWEFOT RCT90 |
15 centres, Sweden Patients screened for inclusion from 2002 to 2005 |
Aged > 18 years, DMARD naive, early RA meeting the 1987 revised ACR criteria, with a symptom duration of < 1 year |
Heimans84 | IMPROVED (Induction therapy with MTX and Prednisone in Rheumatoid Or Very Early arthritis Disease) randomised trial |
Multicentre trial; 12 hospitals in the Netherlands Recruited from 2007 to 2010 |
Patients with early RA (ACR/EULAR 2010 criteria, a symptom duration of ≤ 2 years) and UA initially treated with MTX and prednisone |
Markusse85 | Behandelings Strategie (BeSt) RCT89 |
18 peripheral and two university hospitals in the western Netherlands Patients recruited from 2000 to 2002 |
As for BeSt89 |
Four included clinical prediction model development studies used RCT data. 67,73,78,79 The models by Vastesaeger et al. 78 were developed in the ASPIRE RCT. 87 Both the models by Visser et al. 79 and Dirven et al. 67 were generated using data from the BeSt RCT. 89 Data from the SWEFOT RCT90 were used by Saevarsdottir et al. 73 to build the SWEFOT clinical prediction model.
The Leiden Early Arthritis Clinic cohort was a common data source, being used in the development of models by de Vries-Bouwstra et al. ,65 Drossaers-Bakker et al. 56 and van Steenbergen et al. 77 The ESPOIR cohort was used to build models by Degboé et al. 66 and Fautrel et al. 69 Combe et al. developed models for the prediction of radiographic damage/progression (Combe A)62 and HAQ score (Combe B)63 in French cohort data. The Graell71 and Sanmartí75 clinical prediction model development studies used the same Spanish cohort data source. The models by Bansback et al. ,58 Berglin et al. ,59 Brennan et al. ,60 Centola et al. ,61 de Punder et al. ,64 Bombardier et al. 57 (SONORA), Dixey et al. ,68 Forslind et al. ,70 Houseman et al. 72 and Syversen et al. 76 were developed in cohort study data sets.
The five included external validation studies were also performed in appropriate data sources, being either intervention trials {i.e. Hambardzumyan et al. 83 (SWEFOT), Heimans et al. 84 [Induction therapy with MTX and Prednisone in Rheumatoid Or Very Early arthritis Disease (IMPROVED)], Markusse et al. 85 (BeSt)} or cohort study data [i.e. De Cock et al. 80 (Verschueren cohort), Granger et al. 82 (ESPOIR)].
Description of population characteristics
As outlined in Table 3, the majority of clinical prediction models were developed in populations that had satisfied the 1987 ACR criteria for the diagnosis of RA (i.e. ASPIRE,78 Bansback et al. ,58 Berglin et al. ,59 BeSt,79 Brennan et al. ,60 Centola et al. ,61 Combe (A),62 Combe (B),63 de Punder et al. ,64 de Vries-Bouwstra et al. ,65 Dixey et al. ,68 Dirven et al. ,67 Forslind et al. ,70 Graell et al. ,71 Houseman et al. ,72 Sanmartí et al. ,75 SWEFOT,73 Syversen et al. 76 and van Steenbergen et al. 77). Degboé et al. 66 used the 2010 ACR/EULAR criteria in their diagnosis of patients, whereas in the ESPOIR model data source, patients had received a RA diagnosis from their rheumatologist (and the proportion of patients meeting the ACR/EULAR 2010 criteria was reported). The RA diagnosis method used by Drossaers-Bakker et al. 56 was not specified. However, all patients were described as having RA, and two other included studies (i.e. de Vries-Bouwstra et al. 65 and van Steenbergen et al. 77), which were also developed using data from the Leiden Early Arthritis Clinic, both had used the 1987 ACR criteria (and, therefore, the review group has assumed a similar use of the 1987 ACR criteria by Drossaers-Bakker et al. 56). The amount of information available in the conference abstract by Bombardier et al. 57 describing the development of the SONORA clinical prediction model was limited. It was stated that patients included in the SONORA study were diagnosed with RA by a rheumatologist. The populations used in the external validation studies by Hambardzumyan et al. 83 and Markusse et al. 85 were diagnosed with RA according to the 1987 revised ACR criteria. The patients of the ESPOIR cohort, included in the Granger et al. 82 external validation study, were diagnosed with RA by their rheumatologist (and the proportion meeting the ACR/EULAR 2010 criteria was reported). Heimans et al. 84 reported that the ACR/EULAR 2010 criteria were used for the diagnosis of RA in the mixed RA/undifferentiated arthritis population used for their external validation study. 84 In the De Cock et al. 80 external validation study, all patients (in the Verschueren cohort) were described as being newly diagnosed with early RA.
Name of clinical prediction model/external validation study | Diagnosis (and proportion) of RA | Duration of symptoms/disease at baseline | DAS28 at baseline | Treatment |
---|---|---|---|---|
Clinical prediction model development studies | ||||
ASPIRE78 | Satisfied the 1987 revised ACR criteria |
Persistent synovitis for 3 months to 3 years Median disease duration = 0.6 years (IQR 0.4–1.1 years) |
DAS28 NR | MTX-naive patients (three or fewer pre-study doses of MTX permitted) randomised to receive MTX monotherapy or MTX in combination with 3 or 6 mg/kg of infliximab through 46 weeks. IFX and MTX groups were combined in each study |
Bansback58 | Patients meeting the 1987 revised ACR criteria for RA included in the main analysis. Patients not meeting the 1987 ACR criteria followed and considered in the subgroup analysis |
Patients seen within 2 years of initial symptoms The median duration of symptoms (months) reported for patients with none/mild and moderate/severe functional impairment at 5 years was: Mild group = 6 months (IQR 4–11 months) Severe group = 6 months (IQR 4–12 months) |
The median DAS28 reported for patients with none/mild and moderate/severe functional impairment at 5 years was: Mild group = 5.4 (IQR 3.5–7.9) Severe = 6.2 (IQR 3.6–8.1) |
DMARDs used based on physician preference using standard practice of the late 1980s/1990s (i.e. sequential monotherapy and ‘step-up’ combination treatment for more severe RA). A total of 801 patients (81%) received ≥ 1 DMARDs starting at a median of 2 months (68% by 3 months and 87% by 12 months). The remaining 19% of patients received NSAIDs and/or low-dose steroids. 54% of patients received SSZ, 18% received MTX, 13% received intramuscular gold, 9% received D-penicillamine, 4% received antimalarials and 2% received unspecified treatments. A total of 55% of DMARD-treated patients received ≥ 1 drugs. A total of 17% (n = 164) received steroids at ≥ 7.5 mg daily for ≥ 12 months |
Berglin59 | Patients met the 1987 revised ACR classification criteria for RA | Baseline median time after the onset of symptoms = 7.0 months (IQR 5.0–9.0 months) | NR | During the study, 92% of patients were treated with DMARDs for > 6 months (68% received MTX, 30% received SSZ, 14% received oral/parenteral gold, 12% received antimalarials, 0.7% received anti-TNF agents, 12% received other unspecified DMARDs and 30% received combination therapy). A total of 48% of patients received low-dose prednisolone (at ≤ 10 mg/day) for > 6 months during study |
BeSt79 | The 1987 revised ACR criteria |
Symptom duration of ≤ 2 years Baseline symptom duration, median number of weeks: MTX monotherapy = 25 weeks (IQR 14–55 weeks) Combination with prednisone = 24 weeks (IQR 15–56 weeks) Combination with IFX = 22 weeks (IQR 13–44 weeks) |
NR A DAS of 4.3–4.5 across groups |
All patients were DMARD naive and met the 1987 ACR criteria for RA. Patients receiving dynamic treatment strategies (aimed at a low level of disease activity, shown by a DAS of ≤ 2.4). Group 1 (n = 126) and group 2 (n = 121) patients began initial MTX monotherapy which could be switched/extended with other DMARDs. Group 3 (n = 133) patients began combination MTX, SSZ, HCQ and tapered high-dose prednisone. Group 4 (n = 128) patients began a combination of MTX and IFX. Treatment was adjusted every 3 months in accordance with the fixed protocol with the aim of achieving a DAS of ≤ 2.4. Groups 1and 2 were combined for the current analysis. Used data from patients randomised to initial monotherapy or combination therapy |
Brennan60 | Satisfied any subset of the American Rheumatism Association 1987 revised RA criteria |
Patients were recruited within 180 days of the onset of symptoms Baseline disease duration [(days) length of time between patient-reported onset of symptoms and first examination by a metrologist from the study]: 0–90 days, n = 91 (52%) 91–180 days, n = 84 (48%) |
NR |
83 out of 175 patients (47%) were treated with second-line drugs at baseline (SSZ, 66/83 patients) Treated with steroids at baseline, n = 35 (20%) |
Centola61 |
Feasibility studies (stage 2 I–V): all patients met four or more of the seven 1987 revised ACR criteria for RA Algorithm training study (stage 3): all patients met four or more of the seven 1987 revised ACR criteria for RA |
Feasibility studies (stage 2 I–V): NR Algorithm training study (stage 3): NR |
Feasibility studies (stage 2 I–V): DAS28-CRP median = 3.8 (IQR 2.7–5.0) to 5.8 (IQR 4.7–6.5) Algorithm training study (stage 3): DAS28-CRP median = 3.8 (IQR1.6–6.4) |
Feasibility studies (stage 2 I–V): MTX, 48–64%; non-biologic DMARDs, 64–81%; biologics, 43–65%; and corticosteroids, 24–33% Algorithm training study (stage 3) NR |
Combe (A)62 | Patients met the ACR criteria for RA |
Disease duration of < 1 year Mean duration of disease at baseline = 3.3 months (SD 2.6 months) |
NR Mean DAS: 4.1 (SD 0.8) |
Patients were DMARD naive at entry, treated with DMARDs (typically MTX or SSZ, modifiable based on efficacy/side effects). During 3-year study, mean of 1.7 DMARDs used (range 1–5; MTX, n = 166 patients; SSZ, n = 146 patients; gold, n = 32 patients; HCQ, n = 20 patients; D-penicillamine, n = 13 patients; and CsA, n = 1 patients). A total of 63 patients received low-dose prednisone (5–15 mg/day) on more than one occasion during follow-up |
Combe (B)63 | Patients met the ACR criteria for RA |
Disease duration of < 1 year Mean duration of disease at baseline = 3.6 months (SD 2.6 months) |
NR Mean DAS: 4.1 (SD 0.8) |
Patients were DMARD naive at entry, treated with DMARDs (typically MTX or SSZ, modifiable based on efficacy/side effects). During the 5-year study, a mean of 1.95 DMARDs was used (range 1–5; MTX, n = 175 patients; SSZ, n = 147 patients; gold, n = 41 patients; HCQ, n = 25 patients; D-penicillamine, n = 14 patients; and CsA, n = 1 patient). A total of 63 patients received low-dose prednisone (5–15 mg/day) on more than one occasion during follow-up |
de Punder64 | Satisfied the 1987 ACR criteria for RA | Disease duration of ≤ 1 year | Mean baseline DAS28 reported for groups with:
|
Joint damage progression group:
|
de Vries-Bouwstra65 | Definite RA in accordance with the 1987 revised ACR criteria, but without the criterion that symptoms must be of 6 weeks’ duration and observed by a physician |
Duration of symptoms: < 2 years Median symptom duration at first visit = 22 weeks (IQR 11–45 weeks) |
NR | A total of 85% of included patients who received DMARDs during the first year after diagnosis (starting with HCQ for 40% of patients, SSZ for 30% of patients or unspecified other drugs for 30% of patients). A total of 14 patients (9.2%) received combination DMARDs in the first year, nine patients received a combination of antimalarial drugs with other DMARDs (n = 3 patients received antimalarial drugs and MTX; n = 5 patients received antimalarial drugs and ciclosporin; and n = 1 patient received antimalarial drugs and experimental peptide vaccination strategy). Other combinations: SSZ and low-dose prednisone (n = 2); MTX and low-dose prednisone (n = 2); and SSZ and interferon-beta (n = 1). DMARD combination commenced after a mean of 24 weeks after presentation. No patient was treated with combination drugs directly after presentation |
Degboé66 | RA according to the 2010 ACR/EULAR criteria |
Two or more swollen joints for > 6 weeks and < 6 months Median disease duration = 5.0 months (IQR 3.1–7.4 months) |
NR |
Baseline treatment: steroids, n = 71 patients (12.5%) Year 1 treatment: steroids, n = 259 patients (45.8%) Any DMARDs, n = 436 patients (82.4). Biologic DMARDs, n = 40 patients (7.1%) |
Dirven67 | RA according to the 1987 revised ACR criteria |
Median symptom duration (weeks) at baseline: MTX monotherapy group = 25 weeks (IQR 14–55 weeks) Combination + prednisone = 23 weeks (IQR 15–53 weeks) Combination + IFX = 23 weeks (IQR 13–46 weeks) |
Mean DAS at baseline: MTX monotherapy group = 4.5 (SD 0.9) Combination + prednisone = 4.4 (SD 0.9) Combination + IFX = 4.3 (SD 0.9) |
For analysis, strategy groups 1 and 2 combined as patients achieved a HAQ score at 3 months on the same initial treatment, i.e. MTX monotherapy. In strategy 3, patients started on combination MTX, SSZ and tapered high-dose prednisone. In strategy 4, patients started on a combination of MTX and IFX |
Dixey68 | RA according to the 1987 revised ACR criteria |
Duration of symptoms for ≤ 2 years Median duration of RA symptoms prior to presentation to the rheumatologist and study entry was 6 months (4–11 months) |
NR | DMARD selected according to physician preference. A total of 80% of patients received at least one DMARD at a median of 7 weeks from first presentation to a rheumatology clinic. First DMARD:
|
Drossaers-Bakker56 | NR (all RA), but de Vries-Bouwstra et al. (2006)65 and van Steenbergen et al. (2015)77 samples from the Leiden Early Arthritis Clinic both used the 1987 revised ACR criteria (therefore assumed a similar use of the 1987 revised ACR criteria) | Symptoms of < 5 years’ duration (median 1 year) | Median baseline DAS = 2.8 (IQR NR) | NR |
ESPOIR69 | RA diagnosis according to the rheumatologist; n = 316 patients (85.4%) met the ACR/EULAR criteria at baseline |
Early arthritis for < 6 months, two or more swollen joints for > 6 weeks and < 6 months Mean disease duration of 15.2 weeks (SD 15.4 weeks) |
Mean DAS28 = 5.4 (SD 1.2) |
One hundred per cent of patients received MTX or LEF as a first-line agent Treatment based on usual care by rheumatologist. A total of 370 patients started MTX (n = 335, mean dose of 17.5 mg/week) or LEF (n = 35, mean dose of 20 mg/week). A total of 302 patients still receiving MTX at 1 year, 19 patients switched to another cDMARD and 11 patients switched to a bDMARD. Two patients receiving LEF switched to a bDMARD at 1 year |
Forslind70 | RA satisfying the 1987 revised ACR criteria |
Disease duration of ≤ 1 year Median disease duration at baseline = 6 months (IQR 4–8 months) |
Median DAS28 at baseline = 5.10 (IQR 4.22–5.85) | Patients were DMARD naive and corticosteroid naive at entry. At baseline, patients were given: MTX, 36%; SSZ, 51%; other unspecified DMARDs, 13%; and MTX and SSZ, n = 1. At study end, 254 patients received DMARD treatment (MTX, 49%; SSZ, 22%; other unspecified DMARD, 19%; 10% combination therapy). At the start, 167 patients received low-dose prednisolone (mean) at a daily dose of 8.30 mg (SD 2.45 mg) and by study end, 155 patients were receiving prednisolone at a dose of 5.90 mg/day (SD 2.70 mg/day) |
Graell71 | Patients met the 1987 revised ACR criteria |
Symptoms for < 24 months, Baseline mean disease duration = 10 months (SD 6.7 months) |
Mean DAS28 5.66 (SD 0.91) | Patients treated with therapeutic protocol with the early use of DMARDs using a step-up approach. 50 mg/week of intramuscular sodium aurothiomalate prescribed as first-choice DMARD plus 4 mg/day of methylprednisolone. NSAIDs and intra-articular steroids were given at clinical discretion. Introduction of MTX at a dose of 7.5–30 mg if adverse events/lack of efficacy at 6 months (or according to clinical discretion for a high level of disease activity before 6 months). ACR50 responders at 6 months received gold salts every 2 or 3 weeks. ACR20 criteria responders (but not ACR50 criteria responders) at 6 months received a combination of sodium aurothiomalate and MTX. Tapering of oral steroids was done according to clinical judgement. After 1 year, patients were treated using an aggressive approach informed by clinical judgement (with initiation of other DMARDs/bDMARDs when required) |
Houseman72 | Satisfied the 1987 revised ACR criteria for RA |
Onset of persistent symptoms of < 2 years before entry Median disease duration: |
Median baseline DAS28:
|
Treatment according to local practice with sequential DMARD monotherapy or combination therapy. Five patients subsequently received anti-TNF therapy |
Sanmartí75 | Patients met the 1987 revised ACR criteria |
Disease duration of < 2 years Baseline mean disease duration = 10 months (SD 6.7 months) |
Mean DAS28 of 5.7 (SD 0.9); 75.7% of patients had a DAS28 of > 5.1 | Patients treated with therapeutic protocol (as for Graell et al.71) of gold salts and MTX in a step-up approach plus methylprednisolone (4 mg/day) |
SONORA57 | Patients diagnosed with RA by a rheumatologist (method NR) |
Symptom duration of ≥ 3 and ≤ 12 months Mean disease duration 170 days (SD 180 days) |
NR | NR |
SWEFOT73 | RA according to the 1987 revised ACR criteria |
Symptom duration of < 1 year Median symptom duration = 5 months (IQR 4–8 months) |
Median baseline DAS28 = 5.8 (IQR 5.0–6.4) |
Baseline concurrent prednisolone, n = 42 patients (14%) Baseline concurrent NSAIDs, n = 192 patients (62%) MTX monotherapy, combination with IFX, combination with SSZ and HCQ |
Syversen76 | Clinical diagnosis of RA according to the 1987 ACR criteria | Mean disease duration at baseline = 2.3 years (SD 1.2 years) | DAS28 NR |
Patients treated according to rheumatologist preference Baseline (10-year follow-up) treatments:A total of 14.1% of patients received no DMARDs or anti-TNFs during the study |
van Steenbergen77 | Patients meeting the 1987 ACR criteria | Symptom duration of ≤ 2 years | NR | Initial treatment differed based on the inclusion time period:
|
External validation studies | ||||
De Cock80 | All newly diagnosed early RA patients (method NR) |
Mean symptom duration: 8 months (SD 7 months) Mean disease duration: 1 month (SD 1 month) |
Mean DAS28-CR: 4.91 (SD1.22) | ICTS (n = 32 patients) or IMT (n = 42 patients) received based on patient RA severity according to rheumatologist opinion. Evaluated at least every 4 months |
Granger82 | Patients with RA diagnosis from their rheumatologist (n = 316, 85.4% met the 2010 ACR/EULAR criteria) | Disease duration of 15.2 weeks (SD 8.2 weeks) | DAS28: 5.3 (SD 1.2) | ESPOIR treatment strategies were not protocol based. Included patients in this study received MTX or LEF for at least 3 months within the first year of follow-up. Duration of DMARD treatment during the first year of 9.06 months (SD 3.07 months), 26% of patients received concomitant glucocorticoids. Some patients received an ‘insufficient dosage’ of another treatment (cDMARD or bDMARD); this not considered in the analysis |
Hambardzumyan83 | RA in accordance with the 1987 revised ACR criteria | Mean symptom duration: 6.2 months (SD 4.57 months) |
Baseline mean DAS28-ESR: 5.7 (SD 1.01) Baseline mean DAS28-CRP: 6.5 (SD 1.22) |
As for the SWEFOT RCT |
Heimans91 |
Mixed RA/UA population (80.3% of the population for the matrix model had RA) [Patients with early RA (ACR/EULAR 2010 criteria)] |
Symptom duration of ≤ 2 years (Heimans et al. 2014) Median symptom duration: 18 weeks (IQR 9–34 weeks) |
DAS28 NR Mean baseline DAS: 3.34 (SD 0.9) |
IMPROVED study patients initially treated with MTX at a dose of 25 mg/week and prednisone at a dose of 60 mg/day tapered to 7.5 mg/day in 7 weeks. Medication tapered in patients reaching early remission (a DAS of < 1.6 after 4 months). Patients not in early remission after 4 months randomised to either MTX at a dose of 25 mg/week and HCQ at a dose of 400 mg/day and SSZ at a dose of 2000 mg/day and prednisone at a dose of 7.5 mg/day (study arm 1) or to MTX at a dose of 25 mg/week and ADA at a dose of 40 mg/2 weeks (study arm 2). During follow-up, medication was increased or switched in the case of no remission and tapered or stopped in the case of remission |
Markusse85 | 1987 revised ACR criteria | NR, but disease duration of ≤ 2 years in BeSt RCT | Mean baseline DAS28 ranged from 5.82 (SD 1.03) to 5.98 (SD 1.00) | As for BeSt |
As stated in Study selection, early RA was defined in conjunction with clinical experts as being within 2 years of the onset of symptoms. Included studies reported either the duration of symptoms or the duration of disease at baseline (with disease duration at baseline considered by the review team to be equivalent to symptom duration in the absence of further information). The studies set an upper threshold of symptom/disease duration for study eligibility and/or reported the median/mean symptom/disease duration at baseline, as presented in Table 3. Compared across studies, the baseline median/mean symptom/disease duration for included populations was typically approximately 5–6 months. The ESPOIR,69 Combe (A)62 and Combe (B)63 models were derived from patients with earlier RA (mean duration of disease of 15 weeks, 3.3 months and 3.6 months, respectively). The populations in the models by Drossaers-Bakker et al. 56 (median symptom duration of 1 year) and Syversen et al. 76 (mean disease duration of 2.3 years) had disease of a longer duration. It is noted that the population used to develop the Syversen et al. 76 clinical prediction model was outside the 2-year limit used to define early RA. However, this study was eligible for inclusion because it was externally validated in a population that did meet the inclusion criteria (i.e. De Cock et al. 80). The populations in the data sources used for the six included external validation studies were all of ≤ 2-year symptom/disease duration at baseline.
The DAS28 disease severity score at baseline was tabulated when available. A DAS28 of ≥ 5.1 is classed as severe disease, whereas a DAS28 of 3.2–5.1 is regarded as moderate disease severity. 19 Unfortunately, the DAS28 at baseline was not available for the majority of studies. Five clinical prediction models (i.e. ESPOIR,69 Forslind et al. ,70 Graell et al. ,71 Sanmartí et al. 75 and SWEFOT73) were developed in populations that were defined as having severe disease activity based on the DAS28. Two external validation studies were performed in populations with severe DAS28 (i.e. Granger et al. 82 and Hambardzumyan et al. 83). In some studies, the DAS28 at baseline was reported only for subgroups (rather than for the total population), but indicated a severe DAS28. The cohort used in the De Cock et al. 80 external validation was borderline between the moderate and severe DAS28 categories [mean DAS28-CRP of 4.91 (SD 1.22)]. 80 Therefore, unfortunately, sufficient data were not available to evaluate the performance of predictors across moderate and severe DAS28 populations.
The treatment histories and concurrent treatments in included studies are described in Table 3. Additional population characteristics at baseline are presented in Appendix 7, Table 30.
The data sources and participant selection in the included studies were assessed using domain 1 of the PROBAST tool49 (see Appendix 6).
Appropriate data sources were considered to have been used for all model development and external validation studies. Inclusions and exclusions of participants were considered by the review authors to be acceptable overall. However, it was noted that the Drossaers-Bakker model56 included only female participants, which may affect the generalisability of the model. As explained previously, the Syversen model76 was developed in a population with a longer mean disease duration [mean 2.3 years (SD 1.2 years)]. Although the Syversen model76 has been externally validated in a relevant population, this may affect the performance of the model.
It was stated in the final protocol that, for review 1, patients should have been diagnosed with early RA according to established criteria. The methods used for RA diagnosis in included studies are presented in Table 3. The lack of clarity around the method of RA diagnosis used in the populations in the Drossaers-Bakker56 and SONORA57 clinical prediction models and the De Cock external validation study80 may contribute to a potential source of bias. The De Cock external validation study80 was based on a cohort recruited at the University of Leuven, Belgium. The original report for this study cohort described patients as being ‘newly diagnosed with RA.’ Although the specific method used for diagnosis was not reported, the entire cohort was described as having a diagnosis of RA and the duration of symptoms meets our definition of early RA. Therefore, in light of the fact that the De Cock external validation study80 presents key informative evidence comparing the performance of multiple clinical prediction models and is described as being based on an early RA cohort, this study has been included. 80
Three model development studies were rated as having an unclear risk of participant selection bias on PROBAST domain 1 [i.e. Drossaers-Bakker et al. ,56 Bombardier et al. (SONORA)57 and Syversen et al. 76]. All other remaining model development studies were rated as being at a low risk of participant selection bias. Two external validation studies were rated as having an unclear risk of bias for participant selection (i.e. De Cock et al. 80 and Heimans et al. 84). All other external validation studies were categorised as having a low risk of bias for participant selection.
Description of predictors
The candidate predictors considered in the development of clinical prediction models are presented in Table 4. The candidate predictors were interpreted by the review team to be all predictors considered in the model development process (and were not limited to those included in the multivariable analysis) in line with the definition applied in CHARMS (i.e. Moons et al. 48).
Name of the clinical prediction model development study | Definition and measurement of candidate predictors | Timing of measurement |
---|---|---|
ASPIRE78 | TJC, SJC, ESR (Westergren method), CRP level (nephelometry), IgM RF and radiographs of hands and feet. Association tested between ‘all available baseline variables’ from the ASPIRE data set and radiographic progression | Baseline |
Bansback58 | Model development involved the removal of predictors from the full 35-predictor model. Nineteen individual candidate predictors reported: sex, age at onset, months of RA symptoms prior to diagnosis, RF, presence of nodules, Carstairs deprivation index, ACR criteria, number of DMARDs used in the first year, functional grades I–IV, morning stiffness (hours), SJC, TJC, grip strength (0–300 mm), HAQ score, pain score (VAS 0–100), haemoglobin level, ESR, DAS28 and radiographic damage, scored using the Larsen Damage and Erosion Scores | Baseline and 1 year |
Berglin59 | NR, but the multivariable analysis included anti-CCP, IgG, IgM, IgA RF by ELISA, SE via HLA-DRB1 genotyping, ESR (mm/hour), SJC28, Larsen score, therapeutic EULAR response at 6 months | Baseline and therapeutic response at 6 months |
BeSt79 | Age, sex, BMI, symptom duration, smoking, SJC, TJC, DAS, CRP (mg/l), ESR (mm/hour), HAQ score, total SHS, erosion score, IgM RF positivity (measured in participating hospitals according to cut-off point of each laboratory) and ACPA positivity (EuroDiagnostica, Arnhem, the Netherlands and Axis-Shield Diagnostics, Dundee, UK) | Baseline |
Brennan60 | A total of 11 individual candidate predictors reported as being measured at baseline (assumed all were entered into modelling): RF titre (from tube latex test, 1 : 80 cut-off point for positive result), swelling of specific joint areas, number of swollen joints, duration of morning stiffness, presence of rheumatoid nodules, disability score, age, sex, disease duration (length of time between symptom onset and first presentation to register metrologist: < 90 days or 90–180 days), time between onset of disease and radiography and HAQ score | Baseline |
Centola61 | Candidate predictors selected using a multistage approach. A total of 130 candidate biomarkers were tested in feasibility studies and 25 were tested in algorithm development, training and selection | Unclear (assumed baseline for subset of patients) |
Combe (A)62 | Age, sex, BMI, disease duration, duration of morning stiffness, patient VAS pain, SJC, TJC, Ritchie Articular Index, DAS, presence/absence of nodules and extra-articular manifestations, HAQ score, ESR, CRP level, IgA and IgM RF positivity by enzyme-linked immunosorbent assay (ELISA), antikeratin anti-body positivity, antiperinuclear anti-body positivity, antiRA33 anti-body positivity, antiheat shock protein positivity, anticalpastatin anti-body positivity, YKL-40 positivity, antinuclear anti-body positivity, HLA-DRB1 and DQB1 genotyping, SHS | Baseline |
Combe (B)63 | Age, sex, BMI, disease duration, duration of morning stiffness, patient VAS pain, swollen and tender joint counts, Ritchie Articular Index, DAS, presence/absence of nodules and extra-articular manifestations, HAQ score, ESR, CRP level, IgA and IgM RF positivity by ELISA, antikeratin anti-body positivity, antiperinuclear anti-body positivity, antiRA33 anti-body positivity, antiheat shock protein-90 positivity, anticalpastatin anti-body positivity, YKL-40 positivity, antinuclear anti-body positivity, HLA-DRB1 and DQB1 genotyping and radiographic score | Baseline |
de Punder64 | Anti-CCP and/or RF (0, 1 or 2 positive) (positive anti-CCP level of > 25 U/ml, ELISA immunoscan RA mark 2, > 10 U/l for frozen samples, EliA™ CCP (Thermo Fisher Scientific, Waltham, MA, USA; positive RF of > 10 U/ml), SE (positive/negative), smoking status (ever or never), ESR (< 25 , 25–50 or > 50 mm/hour), CRP level (≤ 5, 6–10, ≥ 11 mg/dl), SJC28 (0–5, 6–10, 11–15, > 15), TJC28 (0–5, 6–10, 11–15, > 15), VAS general health (0–29, 30–60, 61–100), VAS pain (0–29, 30–60, 61–100), erosions (0, 1–5, 6–10, > 10 Ratingen points), DAS28 (≤ 3.2, 3.3–5.1, ≥ 5.2), age and sex | Baseline |
de Vries-Bouwstra65 | Age (continuous), sex, duration of symptoms at presentation (> 6 weeks or ≤ 6 weeks), duration of morning stiffness (continuous), patient’s global assessment of disease activity (continuous), HAQ score (continuous), total SJC [maximum 22 (continuous)], Ritchie Articular Index score (continuous), bilateral compression pain in metatarsophalangeals (categorical), IgM RF positivity (≥ 5 U) by ELISA (categorical), ESR (continuous), SE hetero- or homozygosity (categorical), presence of erosions on radiographs (categorical), SHS (continuous) and DMARD therapy received | Baseline |
Degboé66 | Anti-CCPs [anti-CCP2 (DiaSorin, Saluggia, Italy], anti-mutated citrullinated vimentin [positivity of > 20 UA/ml (Orgentec, Mainz, Germany)], anticitrullinated fibrinogen (positivity of > 0.056 AU, in-house ELISA). Categorised as negative, low titre, high titre, IgM RF [(Menarini Diagnostics, Winnersh, UK) positivity of > 9 UI/ml], presence of HLA-DRB1 SE (typing and subtyping using PCR), ESR, CRP level, age, sex, duration of disease course before inclusion, smoking consumption, clinical centre, presence of erosions at baseline and treatment (use of steroids, synthetic or biologic DMARDs within the first year tested in the model) | Baseline |
Dirven67 | Age, sex, symptom duration, DAS, HAQ, pain, Ritchie Articular Index, SJC, TJC, treatment group, presence of RF, anti-CCP, radiological damage (total SHS, erosion score, narrowing score, erosions), SE, smoking status, VAS disease activity, VAS pain, VAS morning stiffness, VAS global health, BMI, CRP level and ESR | Baseline |
Dixey68 | Larsen erosion score, RF, ESR, haemoglobin level, nodules, SJC, grip strength, duration of symptoms, presence of HLA-DR SE, HAQ, DAS. All continuous variables were categorised into quartiles | Baseline and 1 year |
Drossaers-Bakker56 | Disease duration, RF, HLA-DQ and HLA-DR SEs, RA protected, percentage agalactosyl IgG, SJC, Ritchie score, presence of erosions and HAQ score | Baseline |
ESPOIR69 | Age, sex, SJC28 (< 14, 14–20 or ≥ 20), TJC28, ESR, CRP (mg/l, < 4, 4–35 or ≥ 35), elevated ESR or CRP level, DAS28-ESR, ACPA positivity, RF positivity, ACPA or RF positivity, HAQ score, typical RA erosion, disease duration, prednisone at a dose of ≥ 7.5 mg/g, delay before first DMARD initiation for ≥ 6 months after the onset of RA, satisfaction of the 2010 ACR/EULAR criteria. Combe 2007 ESPOIR description: clinical and biological variables recorded at baseline and each visit. Baseline CRP level (number of < 10 mg/l), IgM and IgA RF [ELISA (Menarini, Rungis, France), positive at > 9 U/ml], anti-CCP2 anti-bodies [ELISA (DiaSorin, Antony, France), positive at > 5 U/ml] measured for all patients using the same method in a central laboratory | Baseline |
Forslind70 | Anti-CCP1 [ELISA (EuroDiagnostica, Malmö, Sweden)], positive result titre of ≥ 25 U/ml), RF [Serodia agglutination test (Fujirebio Inc., Tokyo, Japan)], positive result titre of 20 IU/ml), HLA-DRB104 genotyping, DAS28, ESR (mm/hour), CRP (mg/l), VAS global health, VAS pain, HAQ score and Larsen score | Baseline |
Graell71 | Sex, age, disease duration, marital status (widowed), handworkers, education, active work, HLA-DRB104, SE, RF (> 25 U/l), anti-CCP (> 50 U/l), ESR (mm/hour), CRP (mg/dl), haemoglobin level, TJC28, SJC28, patient’s global assessment, physician’s global assessment, VAS pain, DAS28, a DAS28 of > 5.1, MHAQ and Larsen score | Baseline |
Houseman72 | Anti-CCP positivity [titre of > 6 U/ml (Axis-Shield Diagnostics, Dundee, UK)], RF (> 40 U/ml), elevated MMP-3 levels (i.e. > 85.79 ng/ml), C-telopeptide of type II collagen level (> 20 µg/mmol), cartilage oligomeric matrix protein level (> 11.20 U/l), tissue inhibitor of metalloproteinase 1 level(> 688.68 ng/ml,) ESR (> 20 mm/hour), CRP level (> 5 mg/l), radiographic damage (i.e. a SHS of > 7) and a physician’s global assessment on the VAS (> 49.00 mm) | Baseline, anti-CCP taken at the 8.2-year follow-up |
Sanmartí75 | NR, but univariable analysis reported to include significant factors: haemoglobin level, ESR, sex, SE, SE homozygosity, HLA-DRB1*04 genotype, anti-CCP, RF and MHAQ | Baseline |
SONORA57 | Limited reporting of candidate predictors | Baseline |
SWEFOT73 | Sex, age, symptom duration, anti-CCP positivity [Immunoscan-RA mark 2 (ELISA test, EuroDiagnostica, Malmö, Sweden)], RF positivity, (determined using routine methods), RF and/or anti-CCP positivity, smoking status (current, past or never), radiographic erosions, concurrent prednisolone use, DAS28 (per unit increase), SJC (< 10, 10–17 or > 17), TJC (per increase of 10), CRP level (< 10 mg/dl, 10–35 mg/dl, > 35 mg/dl), ESR (< 21 mm/hour, 21–50 mm/hour or > 50 mm/hour), VAS-global health (per increase of 10), HAQ score (per unit increase) and HLA-DRB1 SE | Baseline |
Syversen76 | CRP [positive at > 10 mg/l; phyCardioPhase hsCRP (Dade Behring Inc., Deerfield, IL, USA)], ESR (positive at > 20 mm/hour; Westergren method range 0–140 mm/hour), anti-CCP [negative at < 25 U/ml, 25–200 U/ml (low to moderate) or > 200 U/ml (high), as assessed via second-generation ELISA (Inova Diagnostics, San Diego, CA, USA)], IgA RF and IgM RF (positive at > 25 U/l, in-house ELISA), sex, HAQ score, age and radiographic progression rate | Baseline |
van Steenbergen77 | Age, sex, symptom duration at first visit, localisation of initial joint symptoms, SJC66, ACPA status, RF, ESR, SE, selected SNPs located in/nearby gene(s) (i.e. CD40, IL-15, DKK-1, IL2RA, GRZB, IL4R, SPAG16, C5orf30, MMP-9, rs1465788 and OPG) | Baseline |
The timings of the measurement of candidate predictors in the included model development studies were noted. Measurement was at baseline for all studies, although some studies (i.e. Bansback et al. ,58 Berglin et al. ,59 Dixey et al. 68 and Houseman et al. 72) also measured predictors at later time points. Anti-CCP positivity was measured at the extension visit (at 8.2 years of follow-up) in the Houseman et al. study72 and was also included in the final clinical prediction models. The inclusion of predictors measured at substantially later time points is potentially problematic to the validity of the resulting clinical prediction model. Variables measured at later time points are problematic: they cannot be used to make predictions about outcomes in patients with newly diagnosed RA in clinical practice because the observation will not be available until after the decision has been made to treat the patient. The outcome will depend on the specific sequence of treatments administered, and such observations will bias the model relative to models that use only baseline information. None of these models has been externally validated, which highlights the difficulty in their application.
Given the incomplete reporting in the conference abstract, it was unclear which candidate predictors were considered in the SONORA model. 57
We considered whether or not all relevant candidate predictors were analysed (i.e. whether or not all variables selected for inclusion in conjunction with clinical advisors were considered in the model development studies as candidate predictors). When selected variables of interest were not examined as candidate predictors, these studies were rated as being at an unclear risk of bias (as it was not clear what potential contribution the untested predictors may have had).
Description of outcomes
The outcomes assessed by the included studies are presented in Table 5 for the model development studies and in Table 6 for the external validation studies.
Name of clinical prediction model | Outcome category | Outcome definition and measurement | Predicted time point |
---|---|---|---|
ASPIRE78 | Radiographic progression (modified SHS)a | Radiographs of hands and feet obtained and scored using the van der Heijde modification of the SHS. RRP was defined as a threshold change in the modified Sharp/van der Heijde (SHS) of ≥ 5 U/year | 1 year |
Bansback58 | HAQ score | A HAQ score of ≥ 1.5 was defined as moderate to severe disease. Patients completed the disability index of the modified Stanford HAQ (range 0–3) | 5 years |
Berglin59 | Radiographic progression (Larsen) | Radiographic progression defined as present if the difference in Larsen score at baseline and 2 years was greater than the median value. Posteroanterior radiographs of hands, wrists and feet | 2 years |
BeSt79 | Radiographic progression (SHS)a | Radiographs of hands and feet taken at baseline and after 1 year. RRP (increase in SHS of ≥ 5 after 1 year) | 1 year |
Brennan60 | Presence of radiological erosions (Larsen) | Presence of radiological erosions in hands or feet, or both, at least 12 months after the onset of symptoms. Radiographs scored using the Larsen method and patients dichotomised according to the radiographic evidence of erosions in any joints of the hands/feet (Larsen’s grade ≥ 2) | 1 year |
Centola61 | DAS28 | Low level of disease activity/moderate to high level of disease activity (using cut-off points of DAS28-CRP of 2.67 or median DAS28-CRP) | NR |
Combe (A)62 | Radiographic score and radiographic progression (SHS) | Hand, wrist and foot radiographs taken at baseline and 3 years, scored using the SHS method. Radiographic progression defined by a change in radiographic scores that are greater than the upper boundary of 95% CI of differences (i.e. change of at least 3.2, 2.9 and 3.4 in erosions score, narrowing score and total damage score, respectively) | 3 years |
Combe (B)63 | HAQ score | Functional disability according to the HAQ score (as a continuous variable) | 3 and 5 years |
de Punder64 (extended) de Punder (simplified)64 |
Radiographic progression (Ratingen) | Radiographs at baseline and after 3 years of follow-up scored using the Ratingen method. Modelled change in Ratingen score between baseline and 3 years. Joint damage progression defined as the difference in ≥ 5 Ratingen points between baseline and 36 months’ follow-up | 3 years |
de Vries-Bouwstra65 | Radiographic progression (SHS) | Radiographic progression during the first year, measured as the difference between the SHS score at baseline and 1 year (SHS of > 0). Patients classed as:
|
1 year |
Degboé66 | Radiographic progression (modified SHS)a | Radiographs scored using modified Sharp/van der Heijde method. RRP defined as an increase in a total modified SHS of ≥ 5 per year | 1 year |
Dirven67 | HAQ score | Short-term functional disability (i.e. a HAQ score of ≥ 1) after 3 months of treatment | 3 months |
Dixey68 | Radiographic assessment of joint damage (Larsen) | Radiographs of hands and feet taken at baseline and annually, and scored using the Larsen method. Radiographs digitised and scored randomly by one observer using the Larsen method. Larsen’s erosion score used as outcome | 3 years |
Drossaers-Bakker56 | Outcomes categorised as:
|
Radiographs of hands and feet scored using modified Sharp/van der Heijde method. Radiographs of large joints scored using the Larsen large joint score (0–60). Disease activity measured using the DAS (pooled ESR, SJC and Ritchie Articular Index). Cumulative disease activity measured using the AUC of all DAS assessments during 12 years’ follow-up. A panel of five experienced rheumatologists jointly defined the criteria and classed patients as having a mild or severe RA course. Measured tertiles of radiographic damage (modified Sharp/van der Heijde method), disability (measured using the HAQ) and severe disease course defined as patients with either 33% highest cumulative disease activity (AUC of all observed disease activity scores) or highest tertile of radiographic damage. Identified patients in lowest (mild) and highest (severe) tertiles of each outcome measure | 12 years |
ESPOIR69 | Radiographic progression (modified SHS)a | RRP defined as an increase of ≥ 5 on the modified SHS | 1 year |
Forslind70 | Joint damage and radiological progression (Larsen score) | Posteroanterior radiographs taken of hands, wrists and forefeet at baseline and after 2 years. Radiographs read by one reader. Joint damage defined as present if the end point Larsen score was ≥ 10 (median). Radiological progression was defined as present if the difference between the end point and baseline Larsen score was ≥ 8 (median) | 2 years |
Graell71 | Functional disability measured using the MHAQ (MHAQ score of > 0) | MHAQ (eight questions on activities of daily living, scored 0–3) Final score was the mean score of all eight items. Disability = MHAQ score of > 0 | 2 years |
Houseman72 | Radiographic progression (modified SHS) | Posteroanterior radiographs of hands/wrists and feet taken at baseline and 8.2 years. Radiographic progression measured using the van der Heijde/modified Sharp method. Based on median SHS change from baseline to 8.2 years, patients categorised into low or high progression groups. Additional analyses performed using only total SHS at 8.2 years for ‘absolute’ radiographic outcome and cohort categorised into non-progressors and progressors using the lower quartile value (value = 6) from the distribution of total SHS (median value = 26.5) | 8.2 years |
Sanmartí75 | Radiographic progression (Larsen) | Radiographs obtained of hands and feet scored using the modified Larsen method. Radiographic progression defined as a change of > 4 Larsen units between baseline and 24 months | 2 years |
SONORA57 | Radiographic progression (original Sharp method) | Hand radiographs at baseline, year 1 and year 2 scored using the original Sharp method (range 0–280). Radiographic progression defined as a change of ≥ 3.54 in the TSS (as reported in the Granger et al.82 external validation) | 1 and 2 years |
SWEFOT73 | Radiographic progression (modified SHS method)a | Radiographs of hands and feet at baseline and 1 year scored using the modified SHS method. Radiographic progression defined as an increase in total SHS of ≥ 5 at 1 year | 1 year |
Syversen76 | Radiographic progression (van der Heijde/modified Sharp score) | Anteroposterior radiographs of hands obtained. Radiographs scored using the van der Heijde/modified Sharp score. Radiographic progression defined as a change in the SHS of hands from baseline to 10 years of > 10 and dichotomised as the presence or absence of radiographic progression in the analyses | 10 years |
van Steenbergen77 | Radiographic progression (SHS) | Radiographs of hands and feet obtained. Progression in SHS over 6 years (as a continuous outcome or categorised as no/little progression (ΔSHS of ≤ 6), moderate (ΔSHS of 7–30) or severe (ΔSHS of > 30) progression) | 6 years |
Name of external validation | Outcome definition and measurement | Outcome time point (years) | Externally validated clinical prediction model(s) | Does the outcome definition in the external validation match the outcome definition in the model development? (Y/N) |
---|---|---|---|---|
De Cock80 | RRP defined as TSS progression of ≥ 5 units per yeara | 1, 2 | ASPIRE CRP | Y (two validations) |
ASPIRE ESR | Y (two validations) | |||
BeSt | Y (two validations) | |||
ESPOIR | Y (two validations) | |||
SWEFOT | Y (two validations) | |||
Syversen | Nb (two validations) | |||
De Cock80 | TSS progression of ≥ 10 units from baseline to year 2 also defined as RRP. Radiographs scored using the modified Sharp/van der Heijde method (van der Heijde, 199918) | > 2 | ASPIRE CRP | Na |
ASPIRE ESR | Na | |||
BeSt | Na | |||
ESPOIR | Na | |||
SWEFOT | Na | |||
Syversen | Nb | |||
de Punder64 | See Table 5 | 3 | BeSt | Na |
ESPOIR | Na | |||
Granger82 |
Radiographs scored using van der Heijde/modified Sharp score at 1 year RRP defined as an increase in van der Heijde/modified Sharp score of ≥ 5 in the first yeara |
1 | ASPIRE CRP | Y |
ASPIRE ESR | Y | |||
BeSt | Y | |||
ESPOIR | Y | |||
SONORA | Nc | |||
Hambardzumyan83 | Radiographic progression (increase in van der Heijde/modified Sharp score of ≥ 5 over 1 year)a | 1 | MBDA | Nd |
Heimans84 | Radiographs of hands and feet (blinded for patient identity) scored for the presence of erosions and JSN. c-statistic reported for the ability of the model to predict radiological progression (≥ 0.5-point increase in the SHS) | 1 | BeSt | Ne |
Markusse85 |
Radiographic progression (increase in van der Heijde/modified Sharp score of ≥ 0.5) Radiographic progression (increase in van der Heijde/modified Sharp score of ≥ 5)a |
1, 2 | MBDA | Nd (four validations) |
Among the clinical prediction model development studies, the majority assessed radiographic joint damage as an outcome. Six models were developed to predict radiographic progression [modified Sharp–van der Heijde score (SHS)] at 1 year: ASPIRE,78 BeSt,79 de Vries-Bouwstra,65 Degboé,66 ESPOIR69 and SWEFOT. 73 The SONORA model57 assessed radiographic progression (original Sharp score) at 1 and 2 years. Combe (A)62 predicted radiographic score and radiographic progression (modified SHS) at 3 years. Van Steenbergen et al. 77 and Houseman et al. 72 studied radiographic progression (modified SHS) at 6 and 8.2 years, respectively. The Syversen model76 predicted radiographic progression (modified SHS) over the longest follow-up period (i.e. 10 years). Five studies evaluated radiographic joint damage/progression, measured using the Larsen score (i.e. Brennan et al. 60 at 1 year; Berglin et al. ,59 Forslind et al. 70 and Sanmartí et al. 75 at 2 years; and Dixey et al. 68 at 3 years). De Punder et al. 64 assessed radiographic progression (according to the Ratingen score) at 3 years.
Five other clinical prediction models were designed for the prediction of other eligible outcomes. Dirven et al. 67 assessed short-term functional disability (HAQ score of ≥ 1) after 3 months of treatment. The outcome used in the Graell model71 was the modified HAQ (MHAQ) at 2 years. Bansback et al. 58 and Combe (B)63 both modelled the HAQ score at 5 years [with Combe (B)63 also modelling the 3-year HAQ score]. Drossaers-Bakker et al. 56 included a broad range of outcomes, categorised as (1) body functions and structure (impairment), (2) activities at the individual level (disability), (3) participation in society (handicap) and (4) disease course. However, because of unclear reporting, the performance of the individual models could not be summarised, and so this study was counted as a single model in the combined total of 39 included models.
As summarised above, the majority of studies considered binary outcomes, based on dichotomising an observed continuous variable. Although these outcomes are considered to be standard practice, it is worth noting that dichotomising continuous variables has previously described disadvantages. 92,93 The magnitude of the association will depend on the cut-off point used to dichotomise the variable.
As described in Table 6, all five external validation studies and the study by de Punder et al. ,64 which considered development and external validation, considered radiographic progression at 1 and/or 2 years as the outcome variable.
The external validation studies considered a total of eight previously developed clinical risk prediction models, and conducted a total of 31 external validations. In addition to describing the outcome considered in the external validation study, Table 6 lists which clinical prediction models were considered and whether or not the outcome considered by the external validation study was consistent with that in the original model development paper.
For 17 of the 31 external validations that were conducted, the outcome considered by the external validation was different to that used for the model development. Five of these studies (labelled as Nd in Table 6) externally validated the MBDA clinical prediction model (originally developed to predict the DAS28) for the prediction of radiographic progression outcomes. Ten external validations (labelled as Na and Nb in Table 6) externally validated risk models using the outcomes at a different time point than that defined in the original model development paper. Two external validations (labelled as Nc and Ne in Table 6) externally validated risk models using different thresholds to define events, compared with that used in the original model development study.
The handling of outcomes in included studies was critically appraised using domain 3 of the PROBAST tool (see Appendix 6). The included studies were rated as being at a low or unclear risk of bias.
Model development
The methods used in the development of the included clinical prediction models are summarised in Table 7. Clinical prediction models were typically developed in the included studies using data from patients with complete cases only [i.e. having complete predictor and outcome data (e.g. Bansback,58 Brennan,60 Combe A,62 Combe B,63 de Punder,64 de Vries-Bouwstra,65 Degboé,66 Dirven,67 Dixey,68 Drossaers-Bakker,56 Forslind,70 Graell,71 Sanmartí,75 SWEFOT73 and Syversen76)].
Name of clinical prediction model | Method of | Presentation of model | |
---|---|---|---|
Model development | Internal validation | ||
ASPIRE78 Two models: ESR and CRP |
Correlations of all baseline factors with RRP were explored. Selected factors (selection unclear) included in multivariable logistic regression | None | Risk matrices with predicted probabilities stratified by treatment |
Bansback58 | Logistic regression (multivariable, removal of predictors from full model, including covariables with a p-value of < 0.2) | Bootstrapping resampling to estimate slope shrinkage | Full model regression coefficients, nomogram |
Berglin59 Two models |
Candidate predictors selected using univariable regression or clinical assumptions (description unclear). Logistic multivariable regression (backward stepwise) | Apparent performance in development cohort | OR only |
BeSt79 Two models: ESR and CRP |
Logistic regression. Univariable and multivariable with backwards selection using a p-value of > 0.1. Interactions with treatment explored | Apparent performance in development cohort | OR presented for ESR and CRP models. Risk matrices with predicted probabilities presented for CRP model only, stratified by treatment |
Brennan60 | Logistic regression (multivariable, stepwise removal of predictors with a p-value of > 0.05, model checked by sequential addition of removed variables and sequential removal of remaining variables) | Randomly selected sample (prediction sample 60%, n = 105; validation sample 40%, n = 70) | Tabulation of PR for each risk category |
Centola61 |
Stage 1: candidate predictors prioritised based on univariable and multivariable regression Stage 2: multivariable modelling with forward stepwise selection using three methods (OLS, LASSO, elastic net modelling) Stage 3: multivariable modelling using OLS, LASSO, elastic net method. Curds and whey multivariable response method with OLS or LASSO |
Performance compared in 70/30 cross-validation (repeat training in randomly selected 70% of data and testing in remaining 30%) | Full model regression coefficients |
Combe (A)62 | Univariable analysis of all baseline variables. Logistic regression (stepwise, multivariable, selection from univariable analysis a p-value of ≤ 0.15). Results confirmed with stepwise multilinear model (with continuous random variables instead of dichotomous variables) | Apparent performance in development cohort | Full model regression coefficients |
Combe (B)63 | Prediction of HAQ score (continuous outcome) considered separately at 3 and 5 years. Univariable analysis of all baseline variables. Multivariable linear regression model with continuous and categorical candidate predictors | Apparent performance in development cohort | Coefficients only |
de Punder64 Simplified and extended models |
Logistic regression (univariable multivariable, backwards selection with a p-value of < 0.20, as well as consideration of previously identified factors) | Bootstrapping using 300 samples for each of the five imputed data sets | Full model regression coefficients |
de Vries-Bouwstra65,a | Model considers prediction of ΔSHS (continuous outcome). Developed using linear regression (univariable and multivariable) with categorical and continuous outcomes. Resulting predicted progression score and SD then used to calculate the probability of severe disease (ΔSHS of > 0, binary outcome) for which PPV is reported | Apparent performance in development cohort | OR only |
Degboé66 Four models |
Logistic regression (univariable and multivariable) | Apparent performance in development cohort | OR only |
Dirven67 | Logistic regression. Potential confounders and candidate predictors with a p-value of < 0.1 considered in multivariable model. Backward selection process with a p-value of 0.10. Final regression model then fitted with variables categorised based on tertiles to allow matrix construction | Apparent performance in development cohort | Three matrices according to treatment group:
|
Dixey68 Three models |
Logistic regression (univariable and multivariable) | Randomly selected sample (prediction sample 60%; validation sample 40%) | OR only |
Drossaers-Bakker56 Five outcomes with two models for each |
Logistic regression (multivariable stepwise forward method) | Apparent performance in development cohort | OR only. Basic decision tree for one model |
ESPOIR69 | Logistic regression (univariable, variables with a p-value of ≥ 0.1 included in multivariable forwards and backwards selection procedure with a p-value of < 0.05) | Apparent performance in development cohort | Full model regression coefficients and prediction matrix |
Forslind70 | Logistic regression (univariable analysis used to select candidate predictors a p-value of < 0.05, then forward stepwise selection for final model) | Apparent performance in development cohort | Full model regression coefficients |
Graell71 | Univariable analysis, multivariable logistic regression (forward, stepwise). Clinically relevant interactions were considered | Apparent performance in development cohort | Full model regression coefficients |
Houseman72 | Logistic regression [univariable and multivariable, forward stepwise (entry probability = 0.05, removal probability = 0.1)] | Apparent performance in development cohort | Selected multivariable OR provided in text. Full clinical prediction model regression coefficients not provided |
Sanmartí75 | Univariable analysis used to identify significant (p < 0.25) variables for inclusion in multivariable logistic regression (stepwise) | Apparent performance in development cohort | Full model regression coefficients |
SONORA57 | Univariable models to identify factors, general estimation equation model used for the multivariable model | NR | OR only |
SWEFOT73 Four matrices |
Logistic regression (univariable and multivariable. Parameters showing ‘strongest association’ chosen to make three parameter risk matrices) | None | Risk matrices show observed proportions of events rather than predicted probabilities. De Cock external validation80 based on related conference abstract |
Syversen76 Three models |
Logistic regression (variables with a p-value of < 0.15 in univariable analysis included in multivariable model) | Apparent performance in development cohort | Full model regression coefficients provided for model 1, OR only for models 2 and 3. Histogram |
van Steenbergen77 | Linear mixed-model analysis and linear regression analysis, with continuous and categorical outcomes considered for model development | Apparent performance in development cohort | Presents performance measures only |
Continuous variables were frequently converted to dichotomous/trichotomous variables during the clinical prediction model development process. This was the case for seven of the externally validated models developed for the prediction of radiographic progression (all externally validated models, with the exception of MBDA). This conversion is not considered to be good practice. 31 The categorisations used for the variables included in the final models are described in Table 8.
Name of clinical prediction model | Clinical prediction model variables | |||||
---|---|---|---|---|---|---|
Clinical | Biomarkers | Radiography | Demographic | Genetic | Treatment | |
ASPIRE78 CRP/ESR models |
SJC28 (< 10, 10–17 or > 17) |
CRP level (< 0.6 mg/dl, 0.6–3 mg/dl, > 3 mg/dl) or ESR (< 21 mm/hour, 21–50 mm/hour or > 50 mm/hour) RF (< 80 U/ml, 80–200 U/ml or > 200 U/ml) |
– | – | MTX monotherapy or MTX and IFX | |
Berglin59 Two models |
Model 1: SJC28 Models 1 and 2: therapeutic response (yes/no) (EULAR response criteria no vs. good/moderate response) |
Model 1: anti-CCP (yes/no) Model 2: ESR Model 2: IgA RF (yes/no) |
– | – | – | – |
BeSt79 | – | ACPA and/or RF 0, 1 or 2 positive, a CRP level of < 10 mg/l, 10–35 mg/l or ≥ 35 mg/l | Erosion score of 0, 1–4 or ≥ 4 | – | – | Treatment strategy (initial monotherapy, initial combination with prednisone, initial combination with IFX) |
Brennan60 |
Involvement of ≥ 2 large joints Disease duration of > 90 days (i.e. 12.9 weeks) at first presentation |
RF positive (titre of ≥ 1 : 80) | – | – | – | – |
Combe (A)62 | Model 2 (high > 4 Sharp score): pain at ≥ 59 mm (median value at baseline on a VAS scale of 0–100) |
Model 1 (radiographic progression): an ESR of ≥ 33 mm/hour (median value at baseline) Models 1 (radiographic progression) and 2 (high > 4 Sharp score): IgM RF positivity (≥ 20 IU/ml) (categorical low/absent or high/present) |
Model 1 (radiographic progression): erosions score of ≥ 1 (categorical low/absent or high/present) Model 2 (high > 4 Sharp score): initial Sharp score (categorical low/absent or high/present) |
– | Models 1 (radiographic progression) and 2 (high > 4 SHS): HLA-DRB1*04 positivity | – |
de Punder64 Simplified and extended models |
– |
Extended: anti-CCP and/or RF (0, 1 or 2 positive), an ESR of < 25 mm/hour, 25–50 mm/hour or > 50 mm/hour Simplified: dichotomised anti-CCP level of > 25 U/ml (> 10 U/l in frozen samples) and a dichotomised ESR of > 25 mm/hour |
Extended: erosions (0 Ratingen points, 1–5 Ratingen points, 6–10 Ratingen points or > 10 Ratingen points) Simplified: dichotomised baseline Ratingen score of ≥ 1 point |
Aged < 45 years, 45–64 years, > 64 years at diagnosis and female sex | – | – |
de Vries-Bouwstra65,b | Bilateral compression pain in metatarsophalangeal joints (categorical), duration of symptoms at presentation (> or ≤ 6 weeks), HAQ score (continuous), morning stiffness duration (continuous), Ritchie Articular Index score (continuous), total SJC (max 22) (continuous), VAS disease activity (continuous) | ESR (continuous), IgM RF positivity [≥ 5 U (categorical)] | Presence of erosions on radiographs (categorical), SHS (continuous) | Age (continuous) and sex | SE hetero- or homozygosity (categorical) | – |
Four models |
– |
Model 1: anti-CCP-2 positivity and a CRP level of > 10 mg/l Model 2: anti-MCV positivity, a CRP level of > 10 mg/l and RF positivity Model 3: AhFibA positivity, a CRP level of > 10 mg/l Model 4: high anti-CCP2 or AhFibA titres, high anti-MCV titres and a CRP level of > 10 mg/l Model 2: RF positivity |
Models 1 and 4: erosions at baseline | Model 3: age at RA onset | – | – |
Dixey68 Three models |
All continuous variables categorised into quartiles Model 2: SJC Model 2: nodules |
Models 1 and 3: ESR Model 1: RF positivity |
Model 2: joint score Models 2 and 3: Larsen score |
– | – | – |
Drossaers-Bakker56 |
Mild radiographic damage (all): SJC Mild radiographic damage (selected): SJC Severe radiographic damage (all): SJC Severe radiographic damage (selected): SJC |
Mild radiographic damage (all): RF positivity Mild radiographic damage (selected): RF positivity Severe radiographic damage (all): RF positivity Severe radiographic damage (selected): RF positivity |
Mild radiographic damage (all): erosive at study start Mild radiographic damage (selected): erosive at study start Severe radiographic damage (all): erosive at study start Severe radiographic damage (selected): erosive at study start |
– |
Mild radiographic damage (all): SE Mild radiographic damage (selected): – Severe radiographic damage (all): RAP Severe radiographic damage (selected): – |
– |
ESPOIR69 | SJC28 (< 14, 14–20 or ≥ 20) |
ACPA positive/negative CRP ≥ 35 mg/l, 4 mg/l ≤ CRP < 35 mg/l, CRP < 4 mg/l |
Presence/absence of typical RA erosions on radiographs | – | – | – |
Forslind70 Two models |
– |
(Continuous variables categorised by median baseline value as cut-off point; anti-CCP and RF positivity) Model 1 (radiological damage) and model 2 (radiological progression): anti-CCP positivity Models 1 (radiological damage) and 2 (radiological progression): ESR |
Models 1 (radiological damage) and 2 (radiological progression): Larsen score | – | – | – |
Houseman72 Two models |
– |
Radiographic progression model: anti-CCP positivity (titre of > 6 U/ml) (taken at the 8.2-year follow-up visit) and baseline MMP-3 elevation (> 85.79 ng/ml) Absolute radiographic outcome model: anti-CCP positivity (titre of > 6 U/ml) (taken at the 8.2-year follow-up visit) and baseline MMP-3 level (> 85.79 ng/ml) |
Radiographic progression model: baseline SHS (SHS of > 7) | – | – | – |
Sanmartí75 | – | Anti-CCP positive | – | Sex | HLA-DRB1*04 positive | – |
SONORA57 | DAS28 (≤ 3.2 or > 3.2) | Anti-CCP (≤ 20 UI/l or > 20 UI/l) | SHS (0, 1–5 or 6–10) | – | – | – |
SWEFOT73 Original, sex and treatment models |
– | Original, sex and treatment models: CRP level (< 10 mg/dl, 10–35 mg/dl or > 35 mg/dl) | Original, sex and treatment models: presence of erosions |
Original, sex and treatment models: current smoking Sex model |
– | Treatment model (treatment month 3–12): whole group, MTX monotherapy, combination with IFX, combination with SSZ and HCQ |
Syversen76 | – |
Anti-CCP positivity (> 25 U/ml), ESR (> 20 mm/hour) and IgM RF positivity (> 25 U/ml) |
– | Sex | – | – |
van Steenbergen77 | Traditional factors, including localisation of initial joint symptoms, SJC66 | Traditional factors, including presence of ACPA, presence of ESR and presence of RF | – | Traditional factors, including age, sex and symptom duration at first visit | Single nucleotide polymorphisms/genetic variants as listed in table footnotesd | Treatment effects (initial treatment with NSAIDs; initial treatment with HCZ or SSZ; initial treatment with MTX) |
Models predicting HAQ score or disease course | ||||||
Bansback58 |
DAS28 year 1 HAQ score at baseline and year 1 Functional grade at baseline (I, II, III and IV) and year 1 (I, II, III and IV) |
Haemoglobin level at baseline | Larsen score at baseline | Carstairs deprivation index (1, 2, 3, 4 or 5) | – | – |
Combe (B)63 Model 1: 5-year HAQ score Model 2: 3-year HAQ score |
Model 1 and 2: HAQ score Model 1: Ritchie Articular Index |
Model 1: CRP Model 1: ESR |
– | – | – | – |
Dirven67 |
HAQ score of < 1.38, 1.38–2.00 or > 2.00 Ritchie Articular Index score of < 10, 10–16 or > 16 VAS pain score of < 40, 40–60 or > 60 |
– | – | – | – | Monotherapy, combination therapy with prednisone, combination therapy with IFX |
Drossaers-Bakker56 |
Mild HAQ (all): HAQ score Mild HAQ (selected): HAQ score Severe HAQ (all): HAQ score Severe HAQ (selected): HAQ score Severe disease course (all): SJC and HAQ score Severe disease course (selected): SJC and Ritchie score |
– |
Mild HAQ (all): erosive at study start Mild HAQ (selected): erosive at study start Severe HAQ (all): – Severe HAQ (selected): – Severe disease course (all): – Severe disease course (selected): – |
– |
Mild HAQ (all): – Mild HAQ (selected): – Severe HAQ (all): – Severe HAQ (selected): – Severe disease course (all): SE Severe disease course (selected): – |
– |
Graell71 | Baseline MHAQ score (> 0.5) | RF positivity | – | Age | – | – |
Models developed as a measure of disease activity | ||||||
Centola61 | – | 12 biomarker model:
|
– | – | – | – |
Clinical prediction models were developed using multivariable regression, with the majority of studies using logistic regression [i.e. ASPIRE78, Bansback et al. ,58 Berglin et al. ,59 BeSt,79 Brennan et al. ,60 Combe et al. (A),62 de Punder et al. ,64 Degboé et al. ,66 Dixey et al. ,68 Dirven et al. ,67 Drossaers-Bakker et al. ,56 ESPOIR,69 Forslind et al. ,70 Graell et al. ,71 Houseman et al. ,72 Sanmartí et al. ,75 SWEFOT et al. 73 and Syversen76], as the outcome variables were binary. Backwards selection was reported for three models (i.e. Berglin et al. ,59 de Punder et al. 64 and Dirven et al. 67) as being used in the selection of the final variables in the model, whereas forwards selection was used in four model development studies (i.e. Drossaers-Bakker et al. ,56 Forslind et al. ,70 Graell et al. 71 and Houseman et al. 72). There were variations in how the selection was implemented (e.g. levels of statistical significance and combination with other techniques, such as bootstrapping, see Table 7).
The BeSt model development (by Visser et al. 79) and the model by Graell et al. 71 were the only two model development studies to specifically describe having considered the presence of interactions between variables. As Visser et al. 79 also considered the effect of treatment, this is of relevance to review 2 and is discussed in further detail in Results: synthesis of primary studies.
The majority of the included clinical prediction model development studies used a limited form of internal validation, with apparent predictive performance tested within the original development data set (TRIPOD statement type 1a). Testing the apparent performance of a clinical prediction model using the same data in which the model was developed can lead to optimistic estimates of performance. 26 This is attributed to ‘overfitting’, whereby there are too few outcome events in relation to the number of candidate predictors. Therefore, the performance estimates derived from the included TRIPOD statement type 1a studies should be viewed with caution in the absence of more robust validation.
Ideally, clinical prediction model development studies should include a method of internal validation to quantify any optimism in the predictive performance. Two studies (i.e. Bansback et al. 58 and de Punder et al. 64) used bootstrapping for estimating model performance (i.e. TRIPOD statement type 1b). A further two studies (i.e. Brennan et al. 60 and Dixey et al. 68) used a data-splitting approach.
One study tested the performance of its developed clinical prediction models on separate data from a different study (TRIPOD statement type 3). Vastesaeger et al. 78 tested the performance of their ASPIRE model for the prediction of radiographic progression in data from ATTRACT (Anti-TNF trial in rheumatoid arthritis with concomitant therapy). As stated previously, as the ATTRACT trial was conducted in patients with established RA, this validation is outside the scope of this assessment and so is not considered further.
The form in which the clinical prediction models were presented determines the ease with which they may be used in clinical practice, and not all of the model development studies presented their models in sufficient detail for them to be applied to other populations. In order for clinicians to directly apply a model, one option is to present the full model regression coefficients (including the intercept term), which allows the model to be used to generate absolute risks. Alternatively, the absolute risks for each individual combination of variables (e.g. in a risk matrix format) could be presented. Of the 39 clinical prediction models, 18 were presented in a useable format.
Of the eight externally validated risk models, the majority were presented as risk matrices in the published report: ASPIRE78 (ESR and CRP), BeSt,79 Dirven et al. 67 and ESPOIR. 69 Two externally validated clinical prediction models (MBDA61 and Syversen et al. 76) presented the full model regression coefficients. Syversen et al. 76 also presented the final model graphically using a histogram. The SWEFOT73 models were not presented in a useable format in the model development publication, and for this reason they were not tested in the Granger et al. 82 external validation. However, the main SWEFOT model was considered in the De Cock et al. 80 external validation, based on results that are available in conference proceedings. 73
Some studies were noted as reporting ‘OR only’ in Table 7, indicating that the coefficient of the intercept term in the regression was not provided and, therefore, the model would not be able to be used to provide absolute risks.
The final predictors selected for use in the included clinical prediction models are presented in Table 8. The prognostic variables selected for consideration in the assessment in conjunction with clinical advisors were very commonly incorporated as predictors in the final clinical prediction models. However, the continuous variables were generally categorised, with the models applying a range of differing thresholds in their construction.
Additional clinical predictors
The clinical prediction models also included the following additional clinical predictors:
-
bilateral compression pain (i.e. de Vries-Bouwstra et al. 65)
-
functional grade (i.e. Bansback et al. 58)
-
localisation of initial joint symptoms (i.e. van Steenbergen et al. 77)
-
morning stiffness (i.e. de Vries-Bouwstra et al. 65)
-
nodules [i.e. Dixey et al. (2)68]
-
Ritchie Articular Index [i.e. de Vries-Bouwstra et al. ,65 Combe et al. (B1),63 Dirven et al. ,67 Drossaers-Bakker et al. 56 (severe disease course, selected)]
-
therapeutic response [EULAR response criteria: no vs. good/moderate response; i.e. Berglin et al. (1),59 Berglin et al. (2)59]
-
visual analogue scale disease activity (i.e. de Vries-Bouwstra et al. 65)
-
visual analogue scale pain [i.e. Dirven et al. ,67 Combe et al. (A2)62].
Additional biomarkers
The following biomarkers were also included in the two final clinical prediction models:
Additional demographic predictors
Demographic variables were included in the following final clinical prediction models:
-
age [i.e. de Punder et al. (extended),64 de Vries-Bouwstra et al. ,65 Degboé et al. (3),66 Graell et al. 71 and van Steenbergen et al. 77]
-
Carstairs Deprivation Index (i.e. Bansback et al. 58)
-
sex [i.e. de Vries-Bouwstra et al. ,65 Sanmartí et al. ,75 SWEFOT et al. (sex),73 Syversen et al. ,76 van Steenbergen et al. 77].
Genetic predictors
A minority of clinical prediction models also integrated genetic predictors:
-
RA protected [i.e. Drossaers-Bakker et al. (severe radiographic damage, all)56]
-
shared epitope (SE) [i.e. de Vries-Bouwstra et al. ,65 Drossaers-Bakker et al. (mild radiographic damage, all),56 Drossaers-Bakker et al. (severe disease course, all)56]
-
HLA-DRB1*04 positivity [i.e. Combe et al. (A1),62 Combe et al. (A2),62 Sanmartí et al. 75]
-
single nucleotide polymorphisms located in/near human leucocyte antigen (HLA)-DRB1 (SE), CD40 (cluster of differentiation 40), IL-15 (interleukin 15), DKK-1 (dickkopf WNT signalling pathway inhibitor 1), IL2RA (interleukin 2 receptor alpha chain), GRZB (granzyme B), IL4R (interleukin 4 receptor), SPAG16 (sperm-associated antigen 16), C5orf30 (chromosome 5 open reading frame 30), MMP-9, rs (reference single nucleotide polymorphisms cluster ID) 1465788 and OPG (osteoprotegerin) (i.e. van Steenbergen et al. 77).
Treatment
The ASPIRE CRP,78 ASPIRE ESR,78 BeSt,79 Dirven67 and SWEFOT (treatment)73 clinical prediction models were developed using RCT data and included treatment as a variable in the final model. For all of these studies, the final clinical prediction model was presented using separate risk matrices according to treatment group. Although separate matrices by treatment were presented, the final models did not include treatment by predictor interaction terms (as is considered in review 2). This assumes that all predictors are prognostic for all treatments, and that the magnitude of the prognostic effect of each predictor does not vary by treatment, which is unlikely to be true in practice. As noted in Model development, Visser et al. 79 assessed the interaction between predictors, but found these to be not statistically significant and, therefore, did not include interactions in the final model. It should be noted that the absence of evidence for interaction effects is not evidence of the absence of interaction effects, and such terms should be retained in a model if there is reason to believe them to be real effects, irrespective of their statistical significance.
It was considered in PROBAST domain 2 whether or not all (final) predictors would be available at the time at which the model was intended to be used. The majority of predictors were considered to be widely available. Data for the Carstairs Deprivation Index, SE, HLA-DRB1*04 testing, RA protected, MMP-3 and genetic tests may be less accessible.
The majority of the model development studies were rated as having an unclear risk of bias for domain 2. All external validation studies were classed as being at a low risk of bias.
Performance results: clinical prediction model development studies
The performance of the included clinical prediction models, as reported in the original model development studies, is presented in Table 9. Discrimination (using the c-statistic) was recorded when available, in addition to information relating to the model calibration and overall model goodness of fit. Additional performance measures presented by the studies are shown in Appendix 7. Unless otherwise indicated, sample sizes and the number of events refer to the sample used for the final multivariable prediction models.
Name of clinical prediction model(s) | Outcome definition | Sample size (n) | Total number of observed events | Overall model fit | Discrimination, c-statistic (95% CI) | Calibration/assessment of overfitting |
---|---|---|---|---|---|---|
Prediction of radiographic joint damage (ΔSHS ≥ 5 at 1 year) | ||||||
ASPIRE78 CRP ESR |
ΔSHS of ≥ 5 at 1 year | Total trial population, n = 1049 | ≌ 124 (MTX monotherapy, 22.8%; IFX and MTX, 8.3%) | NR | NR | NR |
BeSt79 CRP ESR |
ΔSHS of ≥ 5 at 1 year | 465 | 102 |
CRP: Nagelkerke’s R2 = 0.31 ESR: Nagelkerke’s R2 = 0.29 |
CRP: 0.81 (0.77 to 0.86) ESR: 0.80 (0.75 to 0.85) |
NR |
Degboé66 Anti-CCP Anti-MCV AhFibA High ACPA titre |
ΔSHS of ≥ 5 at 1 year | 566 | 145 | NR |
Anti-CCP: 0.65 (0.60 to 0.70) Anti-MCV: 0.63 (0.58 to 0.68) AhFibA: 0.65 (0.60 to 0.71) High ACPA titre: 0.65 (0.60 to 0.70) |
NR |
ESPOIR69 | ΔSHS of ≥ 5 at 1 year | 370 | 41 (11.1%) | NR | 0.75 | Hosmer–Lemeshow described but not presented |
SWEFOT73 Four models |
ΔSHS ≥ 5 at 1 year | 269 | 72 | NR | NR | NR |
Prediction of radiographic joint damage (other outcome definitions) | ||||||
de Vries-Bouwstra65 | ΔSHS of > 0 at 1 year | 95 | 69 | R2 = 0.36 | NR | Calibration plot/table presented |
SONORA57 Year 1 Year 2 |
ΔSHS of ≥ 3.54a at 1 year ΔSHS of ≥ 3.54 at 2 years |
994 |
Year 1: 10.4% Year 2: 11.7% |
NR | NR | NR |
Combe (A)62 Total damage Erosions |
Total damage: ΔSHS of ≥ 3.4 Erosions: ΔSHS of ≥ 3.2 at 3 years |
172 |
Total damage: 71 Erosions: 55 |
NR | NR | NR |
van Steenbergen77 Treatment and traditional Treatment and traditional and geneticb |
ΔSHS at 6 years None: ΔSHS of ≤ 6 Moderate: ΔSHS of 7–30 Severe: ΔSHS of > 30 |
239 | 72, 99, 68 (none, moderate, severe) |
Treatment and traditional: R2 = 0.36 Treatment and traditional and genetic R2 = 0.44 |
Treatment and traditional c = 0.78 (0.73 to 0.82) Treatment and traditional and genetic c = 0.82 (0.77 to 0.86) |
NR |
Houseman72 Change in the SHS Absolute SHS |
ΔSHS of > 10.5 Total SHS of > 6 Both at 8.2 years |
58 |
29 44 |
NR NR |
0.87 0.84 |
NR |
Syversen76 | ΔSHS of > 10 at 10 years | 125 | 74 | NR | NR | NR |
Brennan60 | Based on Larsen score at 1 year | 175 |
Prediction: 37/105 Validation: 26/70 Total: 63/175 |
NR | NR | NR |
Berglin59 Model 1 Model 2 |
Based on Larsen score at 2 years | Baseline, n = 138 | NR for total sample |
Model 1: Nagelkerke’s R2 = 0.21 Model 2: Nagelkerke’s R2 = 0.24 |
NR | NR |
Forslind70 Joint damage Progression |
Joint damage: Larsen score ≥ 10 at 2 years Progression: Δ Larsen score ≥ 10 at 2 years |
333 | NR |
Joint damage: Nagelkerke’s R2 ≌ 0.5 Progression: Nagelkerke’s R2 ≌ 0.4 |
NR | NR |
Sanmartí75 | Based on Larsen score at 2 years | 105 | 34 (32%) | NR | NR | NR |
Dixey68 Model 1 Model 2 Model 3 |
Larsen score at 3 years |
Overall (60% development sample): Model 1 – 587 (365) Model 2 – 850 (518) Model 3 – 649 (370) |
Number in development population: Model 1 – 210 erosive Model 2 – 121 severe Model 3 – 62 severe |
NR | NR | NR |
de Punder64 Extended Simplified |
≥ 5 Ratingen points at 3 years | 425 | 175 | NR |
Extended: 0.77 (0.72 to 0.81) Simplified: 0.75 (0.70 to 0.80) |
Extended: Hosmer–Lemeshow, p = 0.41–0.85. Shrinkage factor: 0.90 (95% CI 0.88 to 0.92). Calibration slope = 1.0 (95% CI 0.82 to 1.21) Simplified model: Hosmer–Lemeshow, p = 0.87–0.99. Shrinkage factor: 0.98 (95% CI 0.96 to 1.0). Calibration slope = 1.1 (95% CI 0.89 to 1.33) |
Prediction of HAQ score | ||||||
Dirven67 | ≥ 1 at 3 months | 497 | 199 (40%) | Nagelkerke’s R2 = 0.29 | 0.78 (0.74 to 0.82) | NR |
Bansback58 | ≥ 1.5 at 5 years | 985c | 298 | Nagelkerke’s R2 = 0.39 | 0.82 | Slope shrinkage of 0.89 based on 200 bootstrap samples |
Combe (B)63 | Continuous variable at 5 years (3-year data not shown) | 156 | N/A (continuous variable) | NR | NR | NR |
Prediction of MHAQ score | ||||||
Graell71 | MHAQ score of > 0 at 2 years | 105 | 28 | NR | NR | NR |
Prediction radiographic damage, HAQ score and severe disease course at 12 years | ||||||
Drossaers-Bakker56,d |
Mild/severe SHS at 12 years HAQ score and severe disease course at 12 years |
Total population, n = 112 | Unclear | NR | NR | NR |
Prediction of DAS28 | ||||||
MBDA (Centola et al.)61 | DAS28-CRP ≥ 2.67 at 3 months | 708 (stage 3 algorithm training) | NR | NR | NR for final MBDA model | NR |
As previously discussed, good discriminative ability in the population used to develop the model would be expected. The external validation of all models in the same data set or multiple data sets is required to objectively compare the reported c-statistics. The results of these external validations are presented in Results: external validation studies.
Models predicting radiographic joint damage
Discrimination was reported using the c-statistic by six of the studies,64,66,69,72,77,79 which considered a total of 13 clinical prediction models. c-statistics for models predicting radiographic joint damage are summarised in a forest plot in Figure 6.
FIGURE 6.
Forest plot of predictive performance of the included clinical prediction models for radiographic joint damage based on internal validation. Anti-MCV, antimutated citrullinated vimentin; TT, treatment and traditional factors; TTG, treatment, traditional and genetic factors.

The BeSt model79 for the prediction of radiographic progression at 1 year demonstrated reasonably good discrimination, which was broadly comparable between the CRP and ESR models (CRP model c-statistic 0.81, 95% CI 0.77 to 0.86; ESR model c-statistic 0.80, 95% CI 0.75 to 0.85).
The de Punder extended and simplified models64 predicted radiographic progression at 3 years. The c-statistics obtained indicated moderate predictive performance (extended c-statistic 0.77, 95% CI 0.72 to 0.81; simplified c-statistic 0.75, 95% CI 0.70 to 0.80).
Four clinical prediction models were produced by Degboé66 for the prediction of radiographic progression at 1 year. The apparent performance (TRIPOD statement type 1a) of these models was reported. The c-statistics were lower than for other models predicting radiographic progression over 1 year [anti-CCP c-statistic 0.65, 95% CI 0.60 to 0.70; antimutated citrullinated vimentin (anti-MCV) c-statistic 0.63, 95% CI 0.58 to 0.68; anti-human citrullinated fibrinogen anti-body (AhFibA) c-statistic 0.65, 95% CI 0.60 to 0.71; high-level ACPA titre c-statistic 0.65, 95% CI 0.60 to 0.70].
The ESPOIR clinical prediction model69 for radiographic progression at 1 year yielded a c-statistic of 0.754 [95% CI not reported (TRIPOD statement type 1a)].
Two clinical prediction models were constructed by Houseman et al. 72 The apparent performances (TRIPOD statement type 1a) were reported. The first model (for radiographic progression at 8.2 years) showed good performance (with a c-statistic of 0.87). The second model (for absolute radiographic outcome at 8.2 years) showed similarly good discriminatory abilities (with a c-statistic of 0.84). It was noted that anti-CCP positivity was measured at the 8.2-year follow-up point, as opposed to baseline or in the early stages of disease, as discussed in Description of predictors.
c-Statistics were presented for the clinical prediction models by van Steenbergen et al. 77 for predicting radiographic progression at 6 years. For this study, an outcome variable with three categories was considered and the reported c-statistics were computed using Harrell’s c-statistic for ordinal data. 37 The model that included treatment plus traditional (i.e. selected demographic, clinical and biomarker) variables had a moderate performance (c-statistic 0.78, 95% CI 0.73 to 0.82). The addition of genetic factors to the model (treatment and traditional and genetic) increased the discrimination of the model slightly (c-statistic 0.82, 95% CI 0.77 to 0.86).
The model goodness of fit (R2) was only rarely reported. Low Nagelkerke’s R2 values were obtained for the Berglin models (model 1: 0.21; model 2: 0.24);59 Forslind model 1 (radiological damage)70 yielded a Nagelkerke’s R2 value of 0.5, whereas the fit for model 2 (radiological progression) was slightly poorer at 0.4. For the de Vries-Bouwstra model,65 the fit (R2) was 0.36. The R2 for the van Steenbergen models77 increased when genetic factors were added to treatment plus traditional factors (0.43 from 0.36, an increase of 0.07; p = 0.06, adjusted R2 increase = 0.03). Although Nagelkerke’s R2 was reported in several publications and used to compare the overall model fit of competing models, it does not provide an assessment of the models’ predictive performance. A model may have a low R2 value while still providing a useful prediction tool.
Model calibration was not frequently reported. De Punder et al. 64 assessed calibration using the Hosmer–Lemeshow test, with p-values being large (p > 0.4) for all clinical prediction models, indicating that there was no evidence to suggest a poor model fit. Calibration slopes and shrinkage factors were also presented for the de Punder extended model64 (shrinkage factor 0.90, 95% CI 0.88 to 0.92; calibration slope 1.0, 95% CI 0.82 to 1.21) and the de Punder simplified64 models (shrinkage factor 0.98, 95% CI 0.96 to 1.0; calibration slope 1.1, 95% CI 0.89 to 1.33). For the ESPOIR model,69 the use of the Hosmer–Lemeshow test was described but not presented.
Models predicting Health Assessment Questionnaire score/disease course
The Bansback model58 predicted a HAQ score of ≥ 1 at 5 years. The internal validation of the model resulted in a c-statistic of 0.82.
The goodness of fit of the Bansback model was 0.39 (Nagelkerke’s R2). 58 The reported Nagelkerke’s R2 value for the Dirven model was 0.29. 67
Secondary predictive performance measures (e.g. accuracy, sensitivity, specificity, PPV, NPV) are presented when these were available (see Tables 31 and 32). Several studies presented performance measures based on a specific threshold to define an event, when thresholds were often defined according to the study population, and thus differed across studies. These measures are less informative than overall performance measures, such as the c-statistic. They are presented for completeness but are not discussed further.
Results: external validation studies
The performance results of the external validation studies are summarised in Table 10. Six external validation studies (including one combined model development/external validation study) were identified that tested the performance of a total of eight previously developed clinical prediction models. All studies focused on the prediction of radiographic joint damage.
Name of external validation study (submodels) | Clinical prediction model | Sample size (n) | Total number of observed events | Overall model fit (Nagelkerke’s R2) | Discrimination c-statistic (95% CI) | Calibration/assessment of overfitting |
---|---|---|---|---|---|---|
Prediction of radiographic joint damage (ΔSHS of > 5 at 1 year) | ||||||
De Cock first year80 | ASPIRE CRP | 74a | 4 | NR | 0.68 | O : E ratio:b 0.35 |
ASPIRE ESR | 74 | 0.68 | 0.42 | |||
BeSt | 73 | 0.60 | 0.27 | |||
Syversen | 73 | 0.34 | 0.08 | |||
SWEFOT | 74 | 0.52 | 0.20 | |||
ESPOIR | 73 | 0.35 | 0.31 | |||
De Cock second year80 | ASPIRE CRP | 74 | 5 | NR | 0.65 | NR |
ASPIRE ESR | 0.37 | |||||
BeSt | 0.50 | |||||
Syversen | 0.54 | |||||
SWEFOT | 0.44 | |||||
ESPOIR | 0.50 | |||||
Granger82 | ESPOIR | 370 | 41 | 0.17 | 0.75 (0.70 to 0.84) | Hosmer–Lemeshow test:c 0.96 |
mESPOIR | 0.30 | 0.82 (0.76 to 0.89) | 0.55 | |||
SONORA | 0.00 | 0.76 (0.68 to 0.83) | < 0.01 | |||
SONORA (recalculated) | 0.00 | 0.76 (0.69 to 0.83) | < 0.01 | |||
BeSt | 0.00 | 0.73 (0.65 to 0.81) | < 0.01 | |||
BeSt (recalibrated) | 0.00 | 0.73 (0.64 to 0.80) | < 0.01 | |||
ASPIRE ESR | 0.00 | 0.54 (0.45 to 0.64) | < 0.01 | |||
ASPIRE ESR (recalibrated) | 0.00 | 0.54 (0.46 to 0.64) | < 0.01 | |||
ASPIRE CRP | 0.00 | 0.62 (0.54 to 0.69) | 0.25 | |||
ASPIRE CRP (recalibrated) | 0.00 | 0.62 (0.41 to 0.60)d | < 0.01 | |||
Hambardzumyan83 | MBDA | 235 | 44e | NR | NR (sensitivity, specificity, PPV and NPV presented in Table 32) | NR |
Markusse85 | MBDA | 84 | NR | NR | 0.77 (0.64 to 0.90) | NR |
Prediction of radiographic joint damage (ΔSHS of > 5 at 2 years) | ||||||
Markusse85 | MBDA | 81 | NR | NR | 0.69 (0.453 to 0.93) | NR |
Prediction of radiographic joint damage (ΔSHS of > 10 at 2 years) | ||||||
De Cock over 2 years80 | ASPIRE CRP | 74a | 4 | NR | 0.70 | O : E ratio:b 0.35 |
ASPIRE ESR | 74 | 0.60 | 0.42 | |||
BeSt | 73 | 0.60 | 0.27 | |||
Syversen | 73 | 0.25 | 0.08 | |||
SWEFOT | 74 | 0.41 | 0.20 | |||
ESPOIR | 73 | 0.35 | 0.31 | |||
Prediction of radiographic joint damage (ΔSHS of > 0.5 at 1 year) | ||||||
Heimans84 | BeSt | 537 (431 RA and 106 UA) | 32 | NR | 0.56 (0.45 to 0.68)f | 1.21 |
Markusse | MBDA | 84 | NR | NR | 0.61 (0.48 to 0.73) | NR |
Prediction of radiographic joint damage (ΔSHS > 0.5 at 2 years) | ||||||
Markusse | MBDA | 81 | 0.69 (0.56 to 0.81) | |||
Prediction of radiographic joint damage (≥ 5 Ratingen points at 3 years) | ||||||
de Punder64 |
BeSt ESPOIR |
NR | NR | NR | Range 0.76–0.79 (no further information reported) | NR |
The De Cock et al. 80 external validation study considered six previously developed clinical prediction models (i.e. ASPIRE94 CRP, ASPIRE94 ESR, BeSt,79 Syversen,76 SWEFOT73 and ESPOIR69). Note that the Syversen prediction model76 was referred to as SWEFOT 1 in the De Cock et al. external validation,80 whereas the SWEFOT model by Saevarsdottir et al. 73 was denoted as SWEFOT 2. De Cock et al. 80 presented results for three different time intervals: first year (baseline to year 1); second year (year 1 to year 2); and over 2 years (baseline to year 2). For this final assessment, progression was defined as total Sharp score (TSS) of > 10 over 2 years, as opposed to a TSS of > 5 over 1 year and, as such, differs from the outcome definition used in the model development studies.
The Granger external validation study82 presented results from four clinical prediction models that had been developed in other populations. Results were also presented for the ESPOIR model69 and an updated mESPOIR82 model; however, given that the ESPOIR model69 was initially developed in this population, this should not be considered as an external validation. The results are presented here for completeness, but are not used for the subsequent meta-analysis. Granger et al. 82 considered two versions of each model, with the first being based on the published model and the second recalibrated with a new estimate of the model intercept, but fixing the regression coefficients to be the same. The models were recalibrated on a subsample of one-third of the ESPOIR69 population and then tested on the remaining two-thirds of the population. Details of how the calibration sample was chosen were not provided.
The BeSt79 and ESPOIR69 models were also externally validated in the report of the de Punder models. 64 Heimans et al. 84 externally validated the BeSt model; however, the only version of the c-statistic that was reported was based on a different definition of the outcome (i.e. a SHS of > 0.5 rather than SHS of > 5) than that was used to derive the model and to assess performance in the other external validation studies. Hambardzumyan et al. 83 and Markusse et al. 85 externally validated MBDA.
The application of the BeSt model79 was considered by Saevarsdottir et al. 73 using the SWEFOT data set. However, the presented results did not provide sufficient details to be considered to be informative to the current review, as no summary statistic of overall performance (e.g. the c-statistic) was provided.
Assessment of calibration
In order to assess the calibration of the models, the expected number of events according to each clinical prediction model was calculated in the external validation populations when possible (the De Cock et al. 80 and Heimans et al. 84 populations). Further details of the calculations used are provided in Appendix 5.
In the De Cock et al. 80 study, four events were observed in the first year of follow-up. However, the number of events predicted is substantially higher for all six of the considered clinical prediction models, leading to O : E ratios that are < 1. The O : E ratio ranged from 0.42 for ASPIRE ESR to 0.08 for Syversen et al. ,76 illustrating the poor calibration of the models in this population.
The calibration of the BeSt model79 in the Heimans et al. 84 population appeared to be better, with an O : E of 1.21 (32 observed events, compared with 26.5 events predicted by the model). However, it was noted by the review authors that this external validation study used a different outcome definition of a > 0.5-unit increase in the SHS in order to classify the 32 events. Using the outcome definition of a SHS of > 5 (used in the BeSt model development), only one individual experienced rapid radiographic progression (RRP), which indicates a poor calibration (i.e. an O : E ratio of 0.04). Modification of the outcome definition by Heimans et al. 84 demonstrates that the BeSt model is not suitable for use in this population without recalibration.
In the case of the Granger et al. 82 validation study, it was not possible to compute the expected number of events. However, the study reported p-values from the Hosmer–Lemeshow test in order to assess calibration. This indicated that all models provided a poor fit to the data (p < 0.01) apart from the ASPIRE CRP model (p = 0.25). Contrary to what might be expected, the recalibrated ASPIRE CRP model provided a poorer calibration according to the Hosmer–Lemeshow test (p < 0.01) and also showed a higher value of the Bayesian information criterion (results shown in Granger et al. 82), which raises questions as to the success of the calibration procedure.
Assessment of discrimination
The discriminatory ability of the models based on the c-statistic ranged from 0.344 to 0.675 for the Syversen and ASPIRE models, respectively, in the De Cock et al. 80 validation study assessed at 1 year. It is worth noting that the Syversen model76 was developed in a cohort that included patients with up to 4 years’ disease duration (mean 2.3. years’ disease duration) and so was outside the 2-year limit used to define early RA in this assessment. This may partly explain the model’s poor performance in the early RA external validation. The observed c-statistics ranged from 0.54 to 0.76 for the ASPIRE ESR and SONORA models, respectively, in the Granger et al. validation study. 82 The discrimination of BeSt79 in the Heimans et al. external validation84 (using a TSS of > 0.5 to define progression) was 0.56 (95% CI 0.45 to 0.68), showing poor discrimination with the CI including 0.5, despite calibration appearing to be reasonable in this population.
Assessment of heterogeneity
The predictive performance varied widely across the external validation populations. The two ASPIRE models provided the highest discriminatory ability in the De Cock et al. validation;80 however, these models demonstrated the lowest discriminatory ability in the Granger et al. 82 validation. It has previously been demonstrated that the distribution of patient characteristics could substantially affect the discriminatory ability of a prediction model. When possible, variations in the populations that could be used to explain heterogeneity in the observed predictive performance were quantified by calculating the case mix of the population, using the mean and SD of the linear predictor/risk score as described in Debray et al. 40 Further details on how this was calculated are provided in Appendix 5, along with histograms of the risk scores for the models considered in De Cock et al. 80 and for the BeSt model considered in Heimans et al. 84 The mean risk scores in the De Cock et al. 80 external validation population ranged from a 12.80% (SD 8.71%) predicted risk (PR) of progression, using the ASPIRE ESR model, to a 70.15% (SD 21.01%) PR of progression, using the Syversen model. This shows that, when evaluated in the same external population, the alternative risk models provide substantially different average risk predictions. The mean risk score for the BeSt model in the Heimans et al. external validation84 population was 4.93% (SD 4.54%), compared with 20.59% (SD 16.83%) in the De Cock et al. validation population. 80 Although the De Cock et al. population had a larger case mix (with a greater SD of the linear predictor than obtained in the Heimans population), the observed discriminatory performance was lower. However, the calibration of the BeSt model in the De Cock et al. population was shown to be poor (O : E ratio of 0.27).
Although consideration of the case mix is of interest to illustrate the heterogeneity between alternative risk models, the case mix could not be computed for all of the external validation studies included in this review. There was also a small overall number of external validations. Further consideration of heterogeneity in discriminatory ability using metaregression was therefore not feasible.
Results of the evidence synthesis
In order to provide a comprehensive overview of the available evidence, a meta-analysis was considered for risk models that were externally validated in more than one study. For predicting RRP (SHS of > 5 in 1 year), three clinical prediction models (i.e. BeSt79, ASPIRE CRP78 and ASPIRE ESR78) were each externally validated in two studies (i.e. De Cock et al. 80 and Granger et al. 82) that provided data in a suitable format. A meta-analysis was conducted using the c-statistic as a measure of discrimination. Although it would also be possible to synthesise other performance measures (e.g. the O : E ratio), other outcomes were not widely reported.
The predictive performance results are presented in Table 11 for all external validations that considered RRP (SHS of > 5 in 1 year) as an outcome, along with the results of the FE and RE meta-analysis for the models that were assessed in more than one external validation study.
Risk model | External validation, c-statistic (95% CI) | Pooled estimate, mean (95%CI) | |||
---|---|---|---|---|---|
aDe Cock et al.80 | Granger et al.82 | Markusse et al.85 | RE | FE | |
BeSt | 0.60 (0.31 to 0.83) | 0.73 (0.65 to 0.81) | 0.72 (0.20 to 0.96) | 0.72 (0.63 to 0.79) | |
ASPIRE CRP | 0.68 (0.38 to 0.88) | 0.54 (0.45 to 0.64) | 0.55 (0.13 to 0.91) | 0.55 (0.46 to 0.64) | |
ASPIRE ESR | 0.68 (0.38 to 0.88) | 0.62 (0.54 to 0.69) | 0.62 (0.44 to 0.78) | 0.62 (0.55 to 0.69) | |
Syversen | 0.34 (0.13 to 0.64) | ||||
SWEFOT | 0.52 (0.25 to 0.78) | ||||
ESPOIR | 0.35 (0.14 to 0.65) | ||||
SONORA | 0.76 (0.68 to 0.83) | ||||
MBDA | 0.77 (0.64 to 0.90) |
Of the models that were externally validated in more than one population, the BeSt model79 shows the highest overall predictive performance (overall c-statistic 0.72, 95% CI 0.20 to 0.96), followed by ASPIRE ESR78 and then ASPIRE CRP. 78 However, there is considerable uncertainty in the pooled estimates. For all three clinical prediction models, the 95% CI of the pooled estimate from the RE model contains 0.5, indicating that there is no confidence that the c-statistic is better than would be expected by chance. The 95% prediction intervals are not presented for the RE model as a result of the limited number of studies contributing to the analyses (therefore the prediction intervals returned by the model are the same as the CIs). The highest predictive performance in an individual study assessing the performance of models for the prediction of RRP at 1 year was shown by MBDA, with a c-statistic of 0.77 (95% CI 0.64 to 0.90), followed by SONORA with a c-statistic of 0.76 (95% CI 0.68 to 0.83); however, neither of these models were externally validated in more than one population (using the same outcome definition and presenting a suitable performance measure).
Consideration of results according to baseline disease severity
Given the limited reporting of the DAS28 at baseline in the included model development and external validation studies (see Table 3), it was not possible to draw conclusive inferences regarding the development or performance of clinical prediction models in populations with moderate versus severe disease activity based on the DAS28 at baseline.
Discussion
Twenty-two model development studies and one combined model development/external validation study describing 39 clinical prediction models for the assessment of prognosis in early RA patients were identified across a range of major outcomes (radiographic joint damage, HAQ score and DAS28). The large majority of these model development studies (n = 16) tested apparent performance only (TRIPOD statement type 1a). A total of six external validation studies (including the combined model development/external validation study) were identified that tested the performance of a total of eight previously developed clinical prediction models. All included external validation studies focused on radiographic joint damage outcomes.
Variation was observed in the methods used to develop the included clinical prediction models, such as those used to select or reject candidate predictors from the final model (e.g. informed by univariable analysis, statistical selection or clinical judgement) and handling of continuous predictors (e.g. division into categories). Some studies presented a ‘matrix model’, and so continuous variables were categorised to allow this presentation format, even though the categorisation of continuous covariates is generally not recommended for prognostic model development. 35,36 Model development studies also generally failed to assess interactions between predictors, including interactions with the treatment group, and so did not generate truly treatment-specific models. This is considered in further detail in Chapter 5.
There was also inconsistency in the measures of predictive performance reported from internal validation. The c-statistic was reported for 8 of the 23 model development studies, although sensitivity and specificity (eight studies), accuracy (seven studies) and PPV and/or NPV (12 studies) were also commonly reported. However, even if consistent approaches had been used for internal validation, comparing the performance of clinical prediction models that have been developed and internally validated in different populations would still be limited, as good discriminative ability in the population used to develop the model would be expected. External validation is required to provide an objective comparison.
Of the eight studies that reported predictive performance in internal validation using the c-statistic, the results were variable. The c-statistics for radiographic progression outcomes ranged between 0.63 (i.e. Degboé et al. ,66 predicting a ΔSHS of ≥ 5 at 1 year) and 0.87 (i.e. Houseman et al. ,72 predicting a ΔSHS of ≥ 10.5 at 8.2 years). The Houseman72 models were notable in that they were among the few models that included MMP-3 in their final selection of predictors (MBDA also includes MMP-3). Unfortunately, the Houseman models72 were assessed using apparent performance only and were not externally validated, and so it is not possible to verify the strong apparent predictive performance suggested in the study report. Furthermore, the Houseman model72 included anti-CCP measured at the 8.2-year follow-up visit, which would bias the performance results relative to models that use only baseline information. Two studies predicting the HAQ score reported c-statistics [0.78 (i.e. Dirven et al. ,67 HAQ score of ≥ 1 at 3 months) to 0.82 (i.e. Bansback et al. ,58 HAQ score of ≥ 1.5 at 5 years)], which also showed promising discrimination, albeit derived from internal validation.
Although the 39 included clinical prediction models present predictive performance results based on internal validation, 31 of these have not been externally validated. This could be largely explained by the fact that 21 of the clinical prediction models were not published in a useable format. Of the eight clinical prediction models that were externally validated, the majority were presented as risk matrices in the published report,67,69,78,79 whereas two models were presented using the full model regression coefficients. 61,76 The SWEFOT73 models were not presented in a useable format in the model development publication; however, the main SWEFOT model was considered in the De Cock et al. 80 external validation based on the results that are available in conference proceedings. 73 The SONORA model was externally validated by Granger et al. ,82 despite being available only in a non-useable format in abstracts.
For models that have been externally validated, predictive performance was observed to vary widely. The highest predictive performance of a clinical prediction model for the prediction of radiographic progression (ΔSHS of ≥ 5 at 1 year) in an individual external validation study was shown by MBDA, which was externally validated by Markusse et al. 85 (c-statistic 0.77, 95% CI 0.64 to 0.90), followed by SONORA, which was externally validated by Granger et al. 82 (c-statistic 0.76, 95% CI 0.68 to 0.83). However, neither of these models was externally validated for the prediction of radiographic progression in more than one population. The lowest predictive performance of a clinical prediction model for the prediction of radiographic progression (ΔSHS of ≥ 5 at 1 year) in an individual external validation study was shown by Syversen et al. ,76 which was externally validated by De Cock et al. 80 (c-statistic = 0.34) followed by ESPOIR (c-statistic = 0.35). For both of these models, the external validation results suggest a performance that is poorer than would be expected by chance (c-statistic < 0.5). However, the performance of ESPOIR was much more promising when externally validated by de Punder et al. ,64 in which c-statistics were reported to range from 0.76 to 0.79 using a different outcome definition (≥ 5 Ratingen points at 3 years).
Three clinical prediction models (i.e. ASPIRE CRP, ASPIRE ESR and BeSt) were externally validated in more than one population using the same outcome definition (ΔSHS of ≥ 5 at 1 year). The results of the RE meta-analysis implied that the most favourable performance across external validations was observed using the BeSt model (c-statistic 0.72, 95% CI 0.20 to 0.96), followed by ASPIRE ESR (c-statistic 0.62, 95% CI 0.44 to 0.78) and ASPIRE CRP (c-statistic 0.55, 95% CI 0.13 to 0.91). However, the favourable performance of BeSt was largely informed by the high c-statistic observed in the Granger et al. external validation,82 and the BeSt model performed less favourably than either of the ASPIRE models in the De Cock et al. external validation. 80 There is considerable heterogeneity for all three models, with the wide CIs suggesting substantial uncertainty in the expected predictive performance in a new sample of patients. The 95% CIs of the pooled estimates contain 0.5 for all three clinical prediction models, indicating that there is limited confidence that the performance of the models is better than would be expected by chance.
Further external validations were identified in populations outside the scope of the assessment. These external validations were in populations with established RA. Vastesaeger et al. 78 tested the performance of their ASPIRE model78 in data from the ATTRACT RCT {median disease duration of 8.4 years [interquartile range (IQR) 4.3–14.7 years]}. Lillegraven et al. 95 also tested the performance of the ASPIRE CRP,78 BeSt79 and SWEFOT73 clinical prediction models in patients with established RA [median disease duration of 12 years (IQR 4–23 years)] and reported low predictive performance (c-statistics: ASPIRE, 0.59; BeSt, 0.65; and SWEFOT, 0.57).
No clinical prediction model performed consistently better than any other, and the current evidence therefore does not support the recommendation of one clinical prediction model over another. The inconsistent results generated by the clinical prediction models on external validation indicates that there is heterogeneity in the populations in which the models are being tested that is not explained by the currently proposed models. However, in this assessment, it was not possible to explore the reasons for the observed heterogeneity, because of the small number of external validations.
The external validation of a clinical prediction model may not be straightforward if the treatment regimens used in the external validation population differ from those used in the original model development population. The authors of the external validation studies included in this review aimed to select the published clinical prediction matrices that provided the closest approximation to the treatment strategy in their external validation population. The issue of treatment practices changing over time, with the resulting differences in treatment between model development and external validation populations, was acknowledged by Steyerberg et al. 23 in their contribution to the PROGRESS series of publications. However, these authors also noted that it was important to consider whether or not existing models could be updated and their predictive performance improved by, for example, recalibration or the addition of new predictor variables. Moons et al. 31 also advocated the value of updating existing prediction models in order to improve their predictive performance in new settings or populations. Recalibration was considered only in the external validation by Granger et al. 82 However, there was evidence that recalibration may not have resulted in a better model fit.
In order to assess the comparative performance of the competing models more thoroughly, further external validations would be required. These should be conducted in clinically appropriate populations with early RA, previously untreated and with sufficient variation (or case mix) in the population to ensure that the results are generalisable to the target population.
Although further external validation would help to clarify whether or not selected models are likely to perform better than others, as previously discussed, there were limitations identified in the methods used to develop the clinical prediction models in many of the development studies. Limitations included the absence of potentially important candidate predictors, inconsistent selection procedures, incorrect handling of continuous predictors and failure to test for interactions between predictors. It is therefore likely that the most clinically useful prediction model would contain predictors from across more than one of the reviewed clinical prediction models and/or consider alternative handling of key predictive variables. Access to IPD would allow harmonisation (both in terms of model development and validation) beyond that which is possible in the current assessment, which considers only aggregate-level data. 96 Guidance on IPD meta-analyses of clinical prediction modelling studies, including the advantages and challenges of undertaking such projects, is given by Debray et al. 97 Methods for assessing clinical prediction models using IPD from multiple studies are also described by Pennells et al. 98 Although the provided reference focuses on the prediction of cardiovascular disease outcomes using time-to-event data, the key principles are relevant for the current review.
Therefore, despite the availability of a range of clinical prediction models, uncertainty still remains over the most appropriate clinical prediction model(s) for use in clinical practice. Future research efforts should focus on consolidating understanding from existing clinical prediction models and external validation studies, and ensuring that recommended practice for model development and reporting is adhered to.
The meta-analysis was limited by the small number of external validation studies available for analysis. The synthesised estimates are indicative of performance in the observed studies, but cannot be used to provide a definitive conclusion about the performance in future studies or to explore the reasons for the heterogeneity between studies. A bivariate meta-analysis of calibration and discrimination has also been proposed and could potentially be used to increase the precision of summary estimates and avoid the exclusion of studies for which relevant estimates are missing. 99 However, this was not considered in this assessment because of the other described limitations of the evidence base.
Review 1 conclusions
Despite the availability of a range of clinical prediction models, uncertainty still remains over the most appropriate clinical prediction model(s) for use in clinical practice. In order to assess the comparative performance of the competing models more thoroughly, further external validations would be required. However, limitations were observed in the methods used to develop the clinical prediction models. It is likely that the most clinically useful prediction model would contain predictors from across more than one of the reviewed clinical prediction models and/or consider alternative handling of key predictive variables.
Chapter 5 Results: review 2
Quantity of research available
Only one of the clinical prediction models involving multiple treatments that were reported in review 1 investigated potential interactions between treatments and predictor variables. 79
The study selection process for review 2 is depicted in Figure 7. A total of 12 studies were included,78,100–111 two of which used the same data set. 78,100
It was stated in the final protocol that any potential overlap would be highlighted between this report and the National Institute for Health Research (NIHR) HTA Technology Assessment Review (TAR) report number 14/16/01. One study included in review 2 (i.e. Taylor et al. 101) was also included in the HTA TAR report number 14/16/01. This study examined the effect of baseline synovial vascularity assessed by PDUS on radiographic progression at 54 weeks by MTX versus MTX and IFX. Other studies in the NIHR HTA TAR report number 14/16/01 were screened for relevance to the current review, but were excluded for not examining an early RA population and/or not examining the interaction between baseline patient and/or disease characteristics and treatments.
A summary of the 12 included studies that are used to assess the prediction of treatment response by patient and/or disease characteristics is presented in Table 12. All included studies were post hoc subgroup analyses of RCTs. 78,100–111 The geographical locations of the studies included the Netherlands,103 the USA,105,110 Finland,106 Italy,107 Germany108 and the UK,101,109 with three studies examining data from two multinational trials78,100,104 and one study not reporting location. 102 Sample sizes ranged from 24 to 1004 participants; most studies were funded by the pharmaceutical industry,78,100–105,107,110 two studies reported funding from local or national health-care agencies106,109 and one study did not report the funding source. 108
First author (year of publication), name of trial or cohort (if relevant) | Characteristics | ||||||
---|---|---|---|---|---|---|---|
Study design | Geographical location and period of data collection | Key eligibility criteria | Maximum length of follow-up | Sample size (number of participants) | Assessment of risk of bias (low/moderate/high)a | Funding | |
Garnero (2002)102 | Cohort study [efficacy analyses of patients included in a RCT (i.e. Bathon 2000112)] |
Unclear location 1997–9 |
Early active RA for ≤ 3 years with ≥ 10 swollen joints, ≥ 12 tender joints, aged ≥ 18 years, diagnosed in accordance with the 1987 revised ACR criteria, RF+, serum CRP level of ≥ 2.0 mg/dl, three or more radiographic erosions of the hands, wrists or feet | 12 months | 116 | Moderate | Immunex (Seattle, WA, USA) |
Heimans (2013)103 BeSt |
RCT (post hoc analyses) |
The Netherlands 2000–2 (recruitment) |
Early active RA (1987 revised ACR criteria); disease duration of ≤ 2 years; active disease (≥ 6/66 swollen joints, ≥ 6/68 tender joints, and either an ESR of 28 mm/hour or a global health score of 20 mm on a 0- to 100-mm visual analogue scale), aged ≥ 18 yearsb,c | 10 years | 484 patients (sample size at 8 years’ follow-up; initial cohort = 508 patients) | Moderate | Dutch College of Health Insurance Companies; Centocor Inc. (Horsham, PA, USA); and Schering-Plough (Kenilworth, NJ, USA) |
Huizinga (2015)104 AVERT |
Post hoc analysis of RCT (phase 3b, multicentre, randomised, active-controlled study) |
Multicentre – 72 worldwide sites, including North America, South America, Europe and the rest of the world NR |
Adults (≥ 18 years old) with active clinical synovitis of ≥ 2 joints for ≥ 8 weeks, persistent symptoms for ≤ 2 years, DAS28-CRP of ≥ 3.2 and anti-CCP-2 anti-body positivity, MTX naive or received MTX (≤ 10 mg/week) for ≤ 4 weeks with no MTX for 1 month prior to enrolment | 18 months | 511 patients enrolled; 351 patients randomised | Moderate | Bristol-Myers Squibb (New York City, NY, USA) |
Maska (2012)105 TEAR |
RCT (subgroup analyses) |
USA NR |
Early active RA (1987 revised ACR criteria; disease duration of < 3 years); aged > 18 years, active disease (≥ 4 swollen joints and ≥ 4 tender joints based on DAS28-joint count), RF+ or ACPA+, or ≥ 2 erosions on radiographs of the hands/wrists/feet if RF– or ACPA– | 2 years (102 weeks) | A total of 412 patients (with available serum cotinine data at baseline, week 48 and week 102 from study sample; n = 755) included in the analyses | Moderate | Amgen (Thousand Oaks, CA, USA; provided ETN and placebo); Barr pharmaceuticals (Montvale, NJ, USA; provided MTX); Pharmacia (New Jersey, USA) (SSZ and placebo); and NIH planning grant (PI: Moreland; 1 R34 AR055122) from NIAMS for the TEAR study |
Mustila (2011)106 FIN-RACo |
RCT |
Finland (18 centres) 1993–5 (recruitment) |
Clinically active RA (1987 revised ACR criteria), symptom duration of < 2 years, DMARD naive | 5 years | 129 (of 199 randomised – completing 5 years’ follow-up, with clinical and radiographic data) | Moderate | Competitive research funding of the Pirkanmaa Hospital District, Tampere University Hospital (Tampere, Finland) |
Pasero (1996)107 | RCT (subgroup of patients with or without joint erosions) |
Italy 1991–3 (recruitment) |
Early active RA; disease duration of at least 6 months, but ≤ 4 years; aged 18–65 years, fulfilling 4/7 1987 revised ACR criteria and in Steinbrocker functional class I, previously untreated or treated with a maximum of only one DMARD (an antimalarial agent or auranofin), discontinued because of side effects or lack of efficacy, ESR > 30 mm/hour, morning stiffness for ≥ 45 minutes, and three or more swollen and tender joints, including two or more hand joints | 12 months | 361 patients enrolled; 340 patients available for ITT analysis (complete set of radiographs, available for n = 284 patients) | Moderate | Sandoz PF (Corso Verona, Italy) |
Rau (1998)108 | Randomised, double-blind, parallel-group trial |
Germany (two centres) NR |
Active disease RA (presence of three of:A disease duration of ≥ 4 months, with erosive disease Patients with advanced radiographic changes (Larsen stages III–V) in any joint, prior treatment with MTX or GSTM, treatment with any other DMARD during the previous 3 months, and intra-articular steroid injection within the previous 4 weeks were excluded |
12 months | 174 patients enrolled (complete set of radiographs, available for n = 152 patients) | Moderate | NR |
Seegobin (2014)109 CARDERA |
RCT |
UK (42 centres) 2000–4d |
Early active RA (1987 ACR criteria, < 2 years’ duration), aged ≥ 18 years, with three of three or more swollen joints, six or more tender joints, ≥ 45 minutes’ morning stiffness, ESR of ≥ 28 mm/hour | 2 years | 431 (from 467 recruited – with evaluable baseline sera) | Moderate | Arthritis Research UK and NIHR under its Research for Patient Benefit Programme |
Smolen (2006)100 Vastesaeger (2009)78 ASPIRE |
Exploratory analysis of RCT data |
Multicentre study (122 centres in Europe – Austria, the Netherlands, Germany, the UK and the USA) 2000–2 (recruitment)e |
Early active RA (1987 revised ACR criteria); age 18–75 years, persistent synovitis for ≥ 3 months and ≤ 3 years, ≥ 10 swollen joints, ≥ 12 tender joints, one or more of RF, serum CRP level of ≥ 2.0 mg/dl, radiographic erosions of the hands or feet. No prior treatment with MTX, no other DMARDs within 4 weeks of study entry | 54 weeks | 1004 | Moderate |
Centocor Inc. (Horsham, PA, USA; subsidiary of Johnson & Johnson) and Schering-Plough (Kenilworth, NJ, USA) |
Sokolove (2016)110 AMPLE |
Exploratory analysis of RCT |
USA 2009–12 |
bDMARD-naive patients with active RA (1987 revised ACR criteria) and an inadequate response to MTX; a DAS28-CRP of ≥ 3.2 and a history of ACPA+ or RF+ and/or elevated ESR or CRP levels,f aged > 18 years and disease duration of ≤ 5 years | 729 days (2 years approximately) | 573 patients completed 2 years, with 508 who had serum samples at baseline | Moderate | Bristol-Myers Squibb (New York City, NY, USA) |
Taylor (2004)101 | RCT |
UK NR |
RA diagnosis (1987 revised ACR criteria), symptoms for 6 months to 3 years, two or more swollen MCP joints despite MTX, IgM RF+, receiving MTX at a stable dose of 12.5–17.5 mg/week for ≤ 4 weeks prior to screening, either not taking corticosteroids or receiving a stable dose of ≥ 10 mg of prednisolone per day for ≥ 4 weeks, plus either:
|
54 weeks | 24 | Moderate | Centocor (Horsham, PA, USA; supplied study drug, had a role in analysis and interpretation) and the Rheumatoid Arthritis Campaign |
Overall quality of research available
All included studies were rated as being at a moderate risk of bias, according to the QUIPS assessment tool54 (Table 13). Generally, most studies were rated as being at a moderate risk of bias for study participation. This was mainly because most studies did not report the source of the target population and the methods used to recruit patients to the study, with many not reporting or partially reporting whether or not there was adequate participation in the study. Most studies reported the recruitment period, inclusion and exclusion criteria and baseline characteristics.
First author (year of publication), name of trial or cohort (if relevant) | Risk of bias of | Overall risk of bias summary | |||||
---|---|---|---|---|---|---|---|
Study participation | Study attrition | Prognostic factor measurement | Outcome measurement | Study confounding | Statistical analysis and presentation | ||
Garnero (2002)102 | High | Moderate | Moderate | Low | Moderate | High | Moderate |
Heimans (2013)103 BeSt |
Low | Moderate | Low | Moderate | Moderate to low | Moderate | Moderate |
Huizinga (2015)104 AVERT |
Moderate | Moderate | Moderate | Moderate | Moderate | Moderate | Moderatea |
Maska (2012)105 TEAR |
Low | Moderate | Moderate | Low | Moderate to low | Low | Moderate |
Mustila (2011)106 FIN-RACo |
Moderate | Moderate | Low | Low | High | Moderate | Moderate |
Pasero (1996)107 | Moderate | Moderate | Moderate | Moderate | Moderate | Moderate | Moderate |
Rau (1998)108 | High | Low | Moderate | Low | Moderate | Moderate | Moderate |
Seegobin (2014)109 CARDERA |
Moderate | Moderate | Low | Low | Moderate | Low | Moderate |
Smolen (2006)100 ASPIRE |
Moderate | High | Moderate | Low | Moderate | Moderate | Moderate |
Sokolove (2015)110 AMPLE |
Moderate to high | Moderate | Moderate | Moderate | Moderate | Moderate | Moderate |
Taylor (2004)101 | Moderate | Moderate | Moderate to low | Low | Moderate to high | Moderate | Moderate |
Vastesaeger (2009)78 ASPIRE |
Moderate | High | Moderate | Low | Moderate | High | Moderate |
The majority of studies were also rated as being at a moderate risk of bias for study attrition. This was mainly because most studies did not report the proportion of the baseline sample that provided data for analysis or what attempts were made to collect information on participants who dropped out. Most studies did not report, or partially reported, the key characteristics for participants who dropped out or the comparative characteristics of those who dropped out and those who provided follow-up data. However, reasons for the loss of follow-up data were typically reported.
Most studies were also rated as being at a moderate risk of bias for prognostic factor measurement. This was mainly because of a lack of clarity or reporting surrounding the proportion of data on the prognostic factor that was available for analysis and the method used for dealing with missing data in most studies. The method and setting of prognostic factor measurement was reported by approximately half of the included studies, and most studies reported a definition and also valid and reliable measurement of the prognostic factor.
Most studies were rated as being at a low risk of bias for outcome measurement. This was mainly attributable to most of the studies reporting a clear definition of the outcome, valid and reliable measurement of the outcome and the method and setting of the outcome being the same for all participants.
Study confounding was also rated as being at a moderate risk of bias for most studies. This was mainly attributable to a lack of reporting or partial reporting among the majority of studies on whether or not important confounders were measured and the definition and valid and reliable measurement of these, and also the method and setting of confounding measurement and whether or not important confounders were accounted for in the study design and analysis.
Most studies were also rated as being at a moderate risk of bias for statistical analysis. This was mainly because of uncertainty over whether or not the presentation of the analytical strategy and model development strategy and the avoidance of selective reporting of results were adequate, because of a lack of a clear description in most studies.
Treatments, baseline variables and outcomes examined of the included studies
Table 14 summarises the treatments, patient and/or disease characteristics and outcomes examined. Numerous treatment comparisons were made, including:
-
ETN versus MTX102
-
sequential monotherapy with initial MTX versus step-up monotherapy with initial MTX versus MTX and SSZ and tapered prednisone versus MTX and IFX103
-
ABT (subcutaneous administration) and MTX versus ABT (subcutaneous administration) versus MTX104
-
MTX and ETN immediate therapy versus MTX and SSZ and HCQ immediate therapy versus MTX and ETN step-up therapy versus MTX and SSZ and HCQ step-up therapy105
-
MTX and HCQ and SSZ in a treat-to-target (TTT) regime versus cDMARD sequential monotherapy in a TTT regime, starting with SSZ106
-
ciclosporin A (CsA) versus cDMARDs107
-
MTX versus gold sodium thiomalate (GSTM)108
-
MTX versus MTX and CsA versus MTX and prednisolone versus MTX and CsA and prednisolone (in a factorial design)109
-
MTX and ABT (subcutaneous administration) versus MTX and ADA (subcutaneous administration),110 and
-
MTX versus MTX and IFX. 101
First author (year of publication), name of trial or cohort (if relevant) | Treatment comparison | Variable(s) | Measurement and timing of variable | Outcomes | Measurement and timing of outcomes | Assessment time of outcome measurement |
---|---|---|---|---|---|---|
Garnero (2002)102 |
ETN MTX |
CRP level | Baseline (high levels of CRP were defined as those in the upper tertile of the RA population). No further details reported | Erosion and radiographic progression | Change from baseline in SHS erosion score and SHS total score at 12 months | 1 year |
Heimans (2013)103 BeSt |
Sequential monotherapy (initial MTX) Step-up combination therapy (initial MTX) Combination therapy MTX and SSZ and tapered prednisone Combination therapy MTX and IFX |
BMI | Calculated from height and weight, as assessed at baseline by a research nurse. Dichotomised to < 25 kg/m2 and ≥ 25 kg/m2 | Treatment response | A DAS of > 2.4, failed response to treatment, measured in the first 3 months of treatment and at year 1 | 1 year |
Huizinga (2015)104 AVERT |
ABT (s.c. administration) and MTX ABT (s.c. administration); MTX |
ACPA status (anti-CCP2 status) | Measured at baseline; no further details reported | Remission | A DAS28 of < 2.6 at 12 months | 1 year |
Maska (2012)105 TEAR |
MTX and ETN immediate therapy; MTX and SSZ and HCQ immediate therapy; MTX and ETN step-up therapy; MTX and SSZ and HCQ step-up therapy |
Smoking status | Measured at baseline and week 48: smokers = detectable (serum) cotinine level of > 5 ng/ml at both visits; non-smokers = undetectable cotinine levels at both baseline and week 48 visits | Disease activity |
Mean DAS28 at 48–102 weeks Mean DAS28 (absolute) at 24 weeks, 48 weeks and 102 weeks |
1–2 years 6 months 1 year 2 years |
Mustila (2011)106 FIN-RACo | MTX and HCQ and SSZ in a TTT regime;cDMARD sequential monotherapy in a TTT regime (starting with SSZ) | ACPA status | ACPAs were determined from a serum sample drawn at baseline and at 1, 2 and 5 years; a cut-off value of 25 U/ml. ACPA results of < 25 U/ml were defined as negative | Erosion by radiographic progression | Larsen score obtained from once-yearly radiographs of the hands and feet by one experienced radiologist, blinded to clinical data | 1, 2, 3, 4 and 5 years |
Pasero (1996)107 |
CsA Conventional DMARDs |
Erosions | Presence or absence of erosions at baseline, as determined by the Larsen–Dale method | Erosions/radiographic progression | Progression in eroded joint count (PEJC) and progression in damage score calculated as the difference in eroded joint count and damage score from baseline using the Larsen–Dale method | 12 months |
Rau (1998)108 |
MTX GSTM |
RF | Baseline, after 6 and 12 months | Radiographic progression | Rau-modified Sharp/Larsen score | 6 and 12 months |
ESR | Baseline, after 1, 3, 6, 9 and 12 months | |||||
CRP level | Baseline, after 1, 3, 6, 9 and 12 months | |||||
Seegobin (2014)109 CARDERA |
MTX monotherapy MTX and CsA MTX and prednisolone MTX and CsA and prednisolone (in a factorial design) |
ACPA status | ACPA status (positive or negative), evaluated using an anti-CCP2 test with a cut-off point of > 5 units/ml taken as positive | Radiological progression | Onset of new erosions at 24 months and modified Larsen scores every 6 months | 6, 12, 18 and 24 months |
Disease activity | DAS28 every 6 months | |||||
Physical function | HAQ score every 6 months | |||||
Smolen (2006)100 ASPIRE |
MTX MTX and IFX (3 mg/kg or 6 mg/kg) |
ESR, CRP level, erosions, RF status, SJC | Baseline ESR, CRP level and radiographic erosions of hands and feet (assessed at time of study entry) using SHS | Radiographic progression | Worsening of radiographic joint damage (change in the SHS of > 0) from baseline to week 54 | Week 54 |
Sokolove (2016)110 AMPLE |
MTX and ABT (s.c. administration) MTX and ADA (s.c. administration) |
ACPA status (anti-CCP2 status) |
Baseline anti-CCP2 anti-body status (positive/negative) was determined using an anti-CCP2 IgG ELISA. Patients with a baseline anti-CCP2 IgG concentration of ≥ 25 AU/ml were considered to be positive and were further divided into equal quartiles according to concentration [Q1–Q4 (highest concentration)] Measurement assessed at various time points up to day 729 |
Disease activity | Adjusted mean change from baseline in DAS28-CRP at various time points up to 729 days (2 years) – data extracted only for 6 months, 1 year and 2 years | Various time points up to 729 days (2 years) – data extracted only for 6 months, 1 year and 2 years |
Physical function | Adjusted mean change from baseline in HAQ-DI score at various time points up to 729 days (2 years) | |||||
Taylor (2004)101 |
MTX MTX and IFX |
Baseline synovial vascularity | MCP joints scanned in PD mode (14 MHz) and images demonstrating maximal synovial vascularity were scored by calculating the sum of the individual joint scores, which consisted of the number of colour Doppler pixels in a defined region of interest for each joint. Taken at baseline and week 18 | Radiographic progression | Progression in total SHS scored by blinded, independent observers from radiographs taken at baseline and at week 24 (mean of the scores of the two independent assessors). Change from baseline to week 54 calculated | Week 54 |
Vastesaeger (2009)78 ASPIRE |
MTX MTX and IFX (3 mg/kg or 6 mg/kg) |
ESR, CRP level, RF status, SJC | ESR assessed at baseline via the Westergren method; CRP level measured at baseline by nephelometry; RF status evaluated from baseline samples evaluated at the central laboratory; 66-joint SJC taken at baseline (although 28-joint SJC used) | Radiographic progression | Differences between treatment groups in mean van der Waerden normal scores of the change of ≥ 5 units/year in total SHS | Week 54 |
Eligible baseline variables were ACPA status,104,106,109,110 smoking status,105 erosions,100,107 RF status,100,111 CRP level,100–102 ESR,100,108 SJC,100 BMI103 and vascularity of synovium detected using PDUS. 101
Eligible outcomes were erosions/radiographic progression,100–102,106–109 disease activity,105,109 physical function,109,110 remission103,110 and a DAS of > 2.4. 103 Four studies assessed outcomes at 6 months,105,108–110 11 studies assessed outcomes at 1 year,100–103,106–110 one study assessed outcomes at 18 months,109 four studies assessed outcomes at 2 years,105,106,109,110 and one study assessed outcomes at 3, 4 and 5 years. 106
Description of population characteristics
The key population characteristics are summarised in Table 15 (and additional population characteristics are summarised in Appendix 9, Table 34).
First author (year), name of trial or cohort (if relevant) | Diagnosis of RA | Definition of early RA | Group/arm | Mean/median duration (months) of symptoms/disease at baseline | Mean/median DAS28 at baseline | Treatment history and current treatments |
---|---|---|---|---|---|---|
Garnero (2002)102 | 1987 revised ACR criteria | Active RA for ≤ 3 years | All participants | 12 (SD 12)a | NR | Not previously treated with MTX. DMARDs, including HCQ and SSZ, were discontinued at least 4 weeks before study entry; stable doses of corticosteroids (≤ 10 mg of prednisone or equivalent) and NSAIDs were permitted. A total of 46% of participants in the MTX arm, 39% in the ETN (10 mg) arm and 40% in the ETN (25 mg) arm had previously used DMARDs, with the mean (SD) number of DMARDs being 0.6 (0.7), 0.5 (0.7) and 0.5 (0.7), respectively; 80%, 76% and 86%, respectively, using concomitant NSAIDs at baseline; and 41%, 42% and 39%, respectively, using concomitant glucocorticoids at baselineb |
Heimans (2013)103 BeSt |
1987 revised ACR criteria | Active RA for ≤ 2 years | All participants | NR | DAS: 4.4 (SD 0.9)c | No prior DMARDs |
A BMI of < 25 kg/m2 | 5.3 (IQR 3.0–13.2)d | DAS: 4.4 (SD 0.8) | ||||
A BMI of ≥ 25 kg/m2 | 5.3 (IQR 3.0–10.8)d | DAS: 4.4 (SD 0.9) | ||||
Sequential monotherapy | 5.3 (IQR 3.2–12.5)d | DAS: 4.5 (SD 0.9) | ||||
Step-up combination therapy | 6.0 (IQR 3.2–12.9)d | DAS: 4.5 (SD 0.8) | ||||
MTX + SSZ + tapered prednisone | 5.3 (IQR 3.5–12.2)d | DAS: 4.4 (SD 0.9) | ||||
MTX + IFX | 5.3 (IQR 3.0–10.6)d | DAS: 4.3 (SD 0.9) | ||||
Huizinga (2015)104 AVERT |
NR | Onset of symptoms for ≤ 2 years | All participants | 6.72 (SD 6.0)d,e | 5.4 (SD 1.2)e,f | MTX naive or received MTX (≤ 10 mg/week) for ≤ 4 weeks with no MTX for 1 month prior to enrolment. Patients receiving oral corticosteroids were required to be on a stable dose (i.e. ≤ 10 mg/day for ≥ 4 weeks) at initiation and to maintain that dose until month 12 |
ABT (s.c. administration) and MTX | 6.96 (SD 6.0)d,e | 5.5 (SD 1.3)e,f | ||||
ABT (s.c. administration) | 7.08 (SD 6.24)d,e | 5.5 (SD 1.1)e,f | ||||
MTX | 6.0 (SD 5.88)d,e | 5.3 (SD 1.3)e,f | ||||
Maska (2012)105 TEAR |
1987 revised ACR criteria | Disease duration for < 3 years | All participants (with serum cotinine at baseline and week 48) | 3.7 (SD 6.6)a | 5.8 (1.1) |
bDMARD naiveg On stable corticosteroid treatment and < 10 mg/day of prednisone or equivalent or on a stable dose of NSAIDs Prior MTX use in 157 out of 755 patients (21%)g |
Current smokers | 3.4 (SD 6.9)a | 5.8 (SD 1.1) | ||||
Non-smokers | 3.8 (SD 6.5)a | 5.8 (SD 1.0) | ||||
Mustila (2011)106 FIN-RACo |
1987 revised ACR criteria | Symptom duration for < 2 years | All participants | 7.7 (SD 5.0)a,c | 7.7 (SD 5.0)c | DMARD naive |
ACPA+ | NR | NR | ||||
ACPA– | NR | NR | ||||
MTX and HCQ and SSZ in a TTT regime | 7.3 (SD 4.7)a | 5.5 (SD 1.0) | ||||
cDMARD sequential monotherapy in a TTT regime (starting with SSZ) | 8.2 (SD 5.2)a | 5.6 (SD 1.1) | ||||
Pasero (1996)107 | 1987 revised ACR criteria | Active RA, a duration of at least 6 months but ≤ 4 years | All participants | 1.4 (SD 1.1)a,c | NR |
DMARD naive or treated with a maximum of only one DMARD (an antimalarial agent or auranofin) 94 out of 141 patients receiving CsA and 103 out of 143 patients in the radiologically evaluable ITT subset had previously used NSAIDs; 56 out of 141 and 52 out of 143, respectively, for corticosteroids; 43 out of 141 and 31 out of 143, respectively, for antimalarial agents or auranofin |
Patients with joint erosions at baseline | NR | NR | ||||
Patients without joint erosions at baseline | NR | NR | ||||
CsA arm | 1.4 (SD 1.2)a | NR | ||||
Other cDMARDs arm | 1.3 (SD 1.1)a | NR | ||||
Rau (1998)108 | ACR case criteria | By radiographic definition: patients with advanced disease (higher than Larsen grade III) were excluded | All patients | NR | NR | No prior treatment with MTX or GSTM, no treatment with any other DMARD during the previous 3 months, no intra-articular steroid injection within the previous 4 weeks |
MTX | 11.5a,h | NR | ||||
GSTM | 11.2a,h | NR | ||||
Seegobin (2014)109 CARDERA |
1987 ACR criteria | Active RA for < 2 years | All participants | 4.0 (SD 5.1)a,c | 5.8 (SD 1.3)c | A total of 65 patients (14% of the trial population) had previously received DMARDs |
ACPA+ | 2.00 (IQR 0.00–5.00)a | 5.72 (IQR 4.91–6.73) | ||||
ACPA– | 1.00 (IQR 0.00–4.00)a | 5.96 (IQR 4.92–6.85) | ||||
MTX monotherapyi | 2.7 (SD 3.8)a | 5.8 (SD 1.2) | ||||
CsA and MTX | 4.2 (SD 5.7)a | 5.9 (SD 1.3) | ||||
Prednisolone and MTX | 5.1 (SD 5.8)a | 5.8 (SD 1.4) | ||||
Triple therapy | 3.9 (SD 5.2)a | 5.6 (SD 1.3) | ||||
Smolen (2006)100 Vastesaeger (2009)78 ASPIRE |
1987 revised ACR criteria | Active RA < 3 years | All participants | 10.4 (SD 8.8)a,c | 6.7 (SD 1.0)d | No prior treatment with MTX, no other DMARDs within 4 weeks of study entry |
MTX | 10.8 (SD 8.4)a | 6.7 (SD 1.0)j | ||||
IFX (all doses) | 10.2 (SD 9.0)a,c | 6.7 (SD 1.0)c | ||||
MTX + IFX 3mg/kg | 9.6 (SD 8.4)a | 6.6 (SD 1.1)j | ||||
MTX + IFX 6mg/kg | 10.8 (SD 9.6)a | 6.7 (SD 1.0)j | ||||
Sokolove (2015)110 AMPLE |
1987 revised ACR criteria | Disease duration of ≤ 2 years | All patients | NR | NR | bDMARD-naive patients with an inadequate response to MTX |
Anti-CCP2 |
ABT: 5.5 (range 2.5–7.4)j ADA: 5.3 (range 3.0–7.3)k |
|||||
Anti-CCP2+; Q1 28–235 AU/ml |
ABT: 5.0 (range 3.1–7.6)j ADA: 5.5 (range 3.1–7.3)k |
|||||
Anti-CCP2+; Q2 236–609 AU/ml |
ABT: 5.6 (range 3.5–7.6)j ADA: 6.0 (range 2.8–7.4)k |
|||||
Anti-CCP2+; Q3 613–1046 AU/ml |
ABT: 5.5 (range 2.8–8.1)k ADA: 5.7 (range 3.7–7.9)k |
|||||
Anti-CCP2+; Q4 1060–4894 AU/ml |
ABT: 6.0 (range 2.7–7.8)j ADA: 5.3 (range 1.7–7.8)j |
|||||
Taylor (2004)101 | 1987 revised ACR criteria | 6 months to ≤ 3 years | All | 17.9 (SD 7.6)a,c | 5.3 (SD 1.1)c | Receiving MTX at a stable dose of 12.5–17.5 mg/week for ≤ 4 weeks prior to screening, either not taking corticosteroids or receiving a stable dose of ≥ 10 mg of prednisolone per day for ≥ 4 weeks |
MTX | 19.7 (SD 7.6)a | 5.2 (SD 1.1) | ||||
IFX and MTX | 16.0 (SD 7.7)a | 5.4 (SD 1.1) |
The majority of included studies used the 1987 revised ACR criteria for RA diagnosis. 100–103,105–107,109,110 One study did not report the diagnosis criteria104 and one study reported using the ACR case criteria. 108 Five studies defined early RA as being active RA or the presence of symptoms for a duration of < 2 years,103,104,106,109,110 four studies defined early RA as being active RA or the presence of symptoms for a duration of < 3 years100–102,105 and two studies used other definitions (active RA for ≥ 6 months but ≤ 4 years,107 or by radiographic definition, i.e. patients without advanced disease108); in all cases, baseline mean/median disease/symptom duration met the inclusion criterion for early RA (i.e. being within 2 years of the onset of symptoms) for this review. The duration of RA was reported in some studies as disease duration and in others as symptom duration. Disease duration ranged from a mean of 1.4 months107 to a mean of 17.9 months,101 and from a median of 1.0 month109 to a median of 24.0 months. 110 Symptom duration ranged from a mean of 6.0 months to a mean of 7.1 months,104 and from a median of 5.3 months to a median of 6.0 months. 103 Baseline DAS28, when reported, ranged from a mean of 5.3101,104 to a mean of 6.7,100 and one study reported DAS, with a baseline mean of 4.4,103 indicating severe RA in all populations for which the DAS28 or the DAS at baseline was reported. Three studies did not report the DAS28 or the DAS at baseline. 102,107,108 Participants were DMARD naive in two studies103,106 and MTX naive in three studies. 100,102,108 One study reported that participants were DMARD naive or treated with a maximum of one DMARD, which could be an antimalarial agent (30% of the sample) or auranofin (22% of the sample). 107 One study reported prior DMARD use in 14% of the sample,109 one study reported that participants had to be bDMARD naive with an inadequate response to MTX110 and one study reported that participants had to be receiving a stable dose of MTX. 101 One study reported that participants were bDMARD naive, with prior MTX use in 21% of the sample,105 and one study reported that participants were MTX naive or had received a low dose of MTX previously for ≤ 4 weeks with a 1-month washout period. 104
Results: synthesis of primary studies
Treatment prediction models presents the findings for clinical prediction models developed according to specific treatments. Prediction of treatment effect by anticitrullinated protein/peptide anti-body status at baseline: erosions presents information regarding patient and/or disease characteristics that are potential treatment effect modifiers.
Tables 16 and 17 provide the estimates of the within-treatment responses and interaction effects by baseline variable for continuous outcomes. Appendix 10 presents plots of the estimates of the within-treatment responses by baseline variable. The results are presented by study because the combination of outcomes and predictors generated no studies that shared any treatment in common, so that a formal synthesis was not possible.
First author (year), name of trial or cohort (if relevant), measurement of outcome | Variable | Treatment | Follow-up time point | Sample size (n) | Mean (SD/standard error/95% CI) | p-value for difference between treatment arms | Treatment effect statistic (SD/standard error/95% CI) | p-value for treatment effect |
---|---|---|---|---|---|---|---|---|
Prediction of treatment response by ACPA status at baseline: erosions | ||||||||
Mustila (2011)106 FIN-RACo Larsen score (absolute value) |
ACPA+ | MTX and SSZ and HCQ | 1 year | 46 | 5.91 (3.8 to 8.2)a | NR | NR | NR |
Sequential cDMARDs | 46 | 10.21 (6.98 to 13.46)a | NR | NR | NR | |||
ACPA– | MTX and SSZ and HCQ | 23 | 3.57 (1.52 to 5.93)a | NR | NR | NR | ||
Sequential cDMARDs | 14 | 7.81 (3.21 to 12.56)a | NR | NR | NR | |||
ACPA+ | MTX and SSZ and HCQ | 2 years | 46 | 8.82 (6.54 to 11.52)a | NR | NR | NR | |
Sequential cDMARDs | 46 | 14.61 (11.12 to 18.07)a | NR | NR | NR | |||
ACPA– | MTX and SSZ and HCQ | 23 | 4.09 (1.82 to 6.44)a | NR | NR | NR | ||
Sequential cDMARDs | 14 | 10.87 (5.83 to 16.22)a | NR | NR | NR | |||
ACPA+ | MTX and SSZ and HCQ | 3 years | 46 | 12.57 (8.88 to 16.38)a | NR | NR | NR | |
Sequential cDMARDs | 46 | 18.52 (14.84 to 22.52)a | NR | NR | NR | |||
ACPA– | MTX and SSZ and HCQ | 23 | 4.96 (2.26 to 7.86)a | NR | NR | NR | ||
Sequential cDMARDs | 14 | 16.61 (8.99 to 24.34)a | NR | NR | NR | |||
ACPA+ | MTX and SSZ and HCQ | 4 years | 46 | 14.56 (11.21 to 18.15)a | NR | NR | NR | |
Sequential cDMARDs | 46 | 22.15 (18.01 to 26.48)a | NR | NR | NR | |||
ACPA– | MTX and SSZ and HCQ | 23 | 5.77 (2.9 to 9.1)a | NR | NR | NR | ||
Sequential cDMARDs | 14 | 20.31 (11.13 to 29.82)a | NR | NR | NR | |||
ACPA+ | MTX and SSZ and HCQ | 5 years | 46 | 19.3 (13.69 to 25.26)a | NR | NR | NR | |
Sequential cDMARDs | 46 | 24.66 (20.22 to 29.33)a | NR | NR | NR | |||
ACPA– | MTX and SSZ and HCQ | 23 | 7.62 (3.35 to 12.1)a | NR | NR | NR | ||
Sequential cDMARDs | 14 | 21.06 (11.34 to 30.83)a | NR | NR | NR | |||
Seegobin (2014)109 CARDERA Change in Larsen score from baseline |
ACPA+ | MTX and CsA and prednisolone | 6 months | 79 | 1.25 (0.28b) | 0.003 | NR | NR |
MTX | 73 | 3.86 (0.8b) | NR | NR | ||||
ACPA– | MTX and CsA and prednisolone | 28 | 0.48 (0.35b) | 0.093 | NR | NR | ||
MTX | 34 | 1.6 (0.55b) | NR | NR | NR | |||
ACPA+ | MTX and CsA and prednisolone | 12 months | 79 | 2.41 (0.52b) | < 0.001 | NR | NR | |
MTX | 73 | 6.92 (1.1b) | NR | NR | NR | |||
ACPA– | MTX and CsA and prednisolone | 28 | 1.36 (0.47b) | 0.409 | NR | NR | ||
MTX | 34 | 2.03 (0.66b) | NR | NR | NR | |||
ACPA+ | MTX and CsA and prednisolone | 18 months | 79 | 3.2 (0.66b) | 0.001 | NR | NR | |
MTX | 73 | 8.52 (1.39b) | NR | NR | NR | |||
ACPA– | MTX and CsA and prednisolone | 28 | 1.66 (0.64b) | 0.388 | NR | NR | ||
MTX | 34 | 2.57 (0.79b) | NR | NR | NR | |||
ACPA+ | MTX and CsA and prednisolone | 24 months | 79 | 3.66 (0.7/2.27 to 5.05c) | < 0.001 | NR | NR | |
MTX | 73 | 9.58 (1.41/6.76 to 12.39c) | NR | NR | NR | |||
ACPA– | MTX and CsA and prednisolone | 28 | 1.7 (0.69/0.29 to 3.10c) | 0.335 | NR | NR | ||
MTX | 34 | 2.72 (0.77/1.15 to 4.29c) | NR | NR | NR | |||
N/A | N/A | N/A | N/A | NR | NR | 7.05d | < 0.001 | |
Prediction of treatment response by ACPA status at baseline: disease activity | ||||||||
Seegobin (2014)109 CARDERA Change in DAS28 from baseline |
ACPA+ | MTX and CsA and prednisolone | 6 months | 79 | –1.98 (0.18b) | < 0.001 | NR | NR |
MTX | 73 | –0.99 (0.17b) | NR | NR | NR | |||
ACPA– | MTX and CsA and prednisolone | 28 | –1.43 (0.33b) | 0.792 | NR | NR | ||
MTX | 34 | –1.32 (0.22b) | NR | NR | NR | |||
ACPA+ | MTX and CsA and prednisolone | 12 months | 79 | –1.48 (0.18b) | 0.190 | NR | NR | |
MTX | 73 | –1.14 (0.19b) | NR | NR | NR | |||
ACPA– | MTX and CsA and prednisolone | 28 | –0.73 (0.33b) | 0.186 | NR | NR | ||
MTX | 34 | –1.36 (0.33b) | NR | NR | NR | |||
ACPA+ | MTX and CsA and prednisolone | 18 months | 79 | –1.64 (0.2b) | 0.356 | NR | NR | |
MTX | 73 | –1.37 (0.2b) | NR | NR | NR | |||
ACPA– | MTX and CsA and prednisolone | 28 | –1.27 (0.37b) | 0.462 | NR | NR | ||
MTX | 34 | –1.62 (0.3b) | NR | NR | NR | |||
ACPA+ | MTX and CsA and prednisolone | 24 months | 79 | –1.84 (0.19b) | 0.087 | NR | NR | |
MTX | 73 | –1.36 (0.22b) | NR | NR | NR | |||
ACPA– | MTX and CsA and prednisolone | 28 | –1.27 (0.32b) | 0.464 | NR | NR | ||
MTX | 34 | –1.59 (0.3b) | NR | NR | NR | |||
N/A | N/A | N/A | N/A | N/A | N/A | 3.99d | 0.008 | |
Sokolove (2015)110 AMPLE Mean change from baseline in DAS28-CRP |
Anti-CCP2– | MTX and ABT (s.c. administration) | 6 months | 66 | –1.9 (–1.76 to –2.2)a,b,e | NR | NR | NR |
MTX and ADA (s.c. administration) | 54 | –1.525 (–1.375 to –1.7)a,b,e | NR | NR | NR | |||
Anti-CCP2+; Q1 28–235 AU/ml | MTX and ABT (s.c. administration) | 42 | –2.05 (–1.8 to –2.23)a,b,e | NR | NR | NR | ||
MTX and ADA (s.c. administration) | 51 | –2.425 (unreadable)a,b,e | NR | NR | NR | |||
Anti CCP2+; Q2 236–609 AU/ml | MTX and ABT (s.c. administration) | 51 | –2.1 (–1.9 to –2.3)a,b,e | NR | NR | NR | ||
MTX and ADA (s.c. administration) | 46 | –2.3 [–2.1 to (unreadable)]a,b,e | NR | NR | NR | |||
Anti CCP2+; Q3 613–1046 AU/ml | MTX and ABT (s.c. administration) | 46 | –2.2 (–2.0 to –2.35)a,b,e | NR | NR | NR | ||
MTX and ADA (s.c. administration) | 51 | –2.125 (–1.95 to –2.3)a,b,e | NR | NR | NR | |||
Anti CCP2+; Q4 1060–4894 AU/ml | MTX and ABT (s.c. administration) | 46 | –2.6 (–2.4 to –2.8)a,b,e | NR | NR | NR | ||
MTX and ADA (s.c. administration) | 51 | –2.5 (–2.3 to –2.675)a,b,e | NR | NR | NR | |||
Anti-CCP2– | MTX and ABT (s.c. administration) | 1 year | 66 | –1.9 [–1.75 to (unreadable)]a,b,e | NR | NR | NR | |
MTX and ADA (s.c. administration) | 54 | –1.65 (–1.49 to –1.85)a,b,e | NR | NR | NR | |||
Anti CCP2+; Q1 28–235 AU/ml | MTX and ABT (s.c. administration) | 42 | –2.075 [–1.85 to (unreadable)]a,b,e | NR | NR | NR | ||
MTX and ADA (s.c. administration) | 51 | –2.275 (–2.075 to –2.48)a,b,e | NR | NR | NR | |||
Anti-CCP2+; Q2 236–609 AU/ml | MTX and ABT (s.c. administration) | 51 | –2.45 (–2.225 to –2.625)a,b,e | NR | NR | NR | ||
MTX and ADA (s.c. administration) | 46 | –2.38 (–2.15 to –2.58)a,b,e | NR | NR | NR | |||
Anti-CCP2+; Q3 613–104 AU/ml | MTX and ABT (s.c. administration) | 46 | –2.3 (–2.15 to –2.5)a,b,e | NR | NR | NR | ||
MTX and ADA (s.c. administration) | 51 | –2.43 (–2.25 to –2.5)a,b,e | NR | NR | NR | |||
Anti-CCP2+; Q4 1060–4894 AU/ml | MTX and ABT (s.c. administration) | 46 | –2.825 (–2.625 to –3.0)a,b,e | NR | NR | NR | ||
MTX and ADA (s.c. administration) | 51 | –2.6 (–2.3 to –2.78)a,e | NR | NR | NR | |||
Anti-CCP2– | MTX and ABT (s.c. administration) | 2 years | 66 | –1.7 (–1.56 to –1.9)a,b,e | NR | NR | NR | |
MTX and ADA (s.c. administration) | 54 | –1.625 (–1.4 to –1.85)a,b,e | NR | NR | NR | |||
Anti-CCP2+; Q1 28–235 AU/ml | MTX and ABT (s.c. administration) | 42 | –2.2 [–1.925 to (unreadable)]a,b,e | NR | NR | NR | ||
MTX and ADA (s.c. administration) | 51 | –2.38 (–2.125 to –2.6)a,b,e | NR | NR | NR | |||
Anti-CCP2+; Q2 236–609 AU/ml | MTX and ABT (s.c. administration) | 51 | –2.55 (–2.28 to –2.7)a,b,e | NR | NR | NR | ||
MTX and ADA (s.c. administration) | 46 | –2.65 (–2. 35 to –2.83)a,b,e | NR | NR | NR | |||
Anti-CCP2+; Q3 613–1046 AU/ml | MTX and ABT (s.c. administration) | 46 | –2.5 (–2.25 to –2.7)a,b,e | NR | NR | NR | ||
MTX and ADA (s.c. administration) | 51 | –2.5 (–2.275 to –2.675)a,b,e | NR | NR | NR | |||
Anti-CCP2+; Q4 1060–4894 AU/ml | MTX and ABT (s.c. administration) | 46 | –3.195 (–2.975 to –3.35)a,b,e | NR | NR | NR | ||
MTX and ADA (s.c. administration) | 51 | –2.75 (–2.6 to –2.83)a,b,e | NR | NR | NR | |||
Prediction of treatment response by ACPA status at baseline: physical function (as assessed via the HAQ score) | ||||||||
Seegobin (2014)109 CARDERA Change in HAQ score from baseline |
N/A | N/A | N/A | N/A | N/A | N/A | 0.48d | 0.696 |
Sokolove (2015)110 AMPLE Mean change from baseline in HAQ-DI score |
Anti-CCP2– | MTX and ABT (s.c. administration) | 6 months | 66 | –0.48 (–0.4 to –0.54)a,b,e | NR | NR | NR |
MTX and ADA (s.c. administration) | 54 | –0.25 (–0.18 to –0.32)a,b,e | NR | NR | NR | |||
Anti-CCP2+; Q1 28–235 AU/ml | MTX and ABT (s.c. administration) | 42 | –0.49 (–0.42 to –0.575)a,b,e | NR | NR | NR | ||
MTX and ADA (s.c. administration) | 51 | –0.63 (–0.55 to –0.71)a,b,e | NR | NR | NR | |||
Anti-CCP2+; Q2 236–609 AU/ml | MTX and ABT (s.c. administration) | 51 | –0.7 (–0.62 to –0.78)a,b,e | NR | NR | NR | ||
MTX and ADA (s.c. administration) | 46 | –0.58 (–0.49 to –0.67)a,b,e | NR | NR | NR | |||
Anti-CCP2+; Q3 613–1046 AU/ml | MTX and ABT (s.c. administration) | 46 | –0.72 (–0.63 to –0.815)a,b,e | NR | NR | NR | ||
MTX and ADA (s.c. administration) | 51 | –0.59 (–0.50 to –0.67)a,b,e | NR | NR | NR | |||
Anti-CCP2+; Q4 1060–4894 AU/ml | MTX and ABT (s.c. administration) | 46 | –0.83 (–0.73 to –0.93)a,b,e | NR | NR | NR | ||
MTX and ADA (s.c. administration) | 51 | –0.75 (–0.66 to –0.84)a,b,e | NR | NR | NR | |||
Anti-CCP2– | MTX and ABT (s.c. administration) | 1 year | 66 | –0.45 (–0.385 to –0.52)a,b,e | NR | NR | NR | |
MTX and ADA (s.c. administration) | 54 | –0.3 (–0.23 to –0.37)a,b,e | NR | NR | NR | |||
Anti-CCP2+; Q1 28–235 AU/ml | MTX and ABT (s.c. administration) | 42 | –0.575 (–0.48 to –0.68)a,b,e | NR | NR | NR | ||
MTX and ADA (s.c. administration) | 51 | –0.68 (–0.59 to –0.77)a,b,e | NR | NR | NR | |||
Anti-CCP2+; Q2 236–609 AU/ml | MTX and ABT (s.c. administration) | 51 | –0.69 (–0.62 to –0.77)a,b,e | NR | NR | NR | ||
MTX and ADA (s.c. administration) | 46 | –0.59 (–0.50 to –0.68)a,b,e | NR | NR | NR | |||
Anti-CCP2+; Q3 613–1046 AU/ml | MTX and ABT (s.c. administration) | 46 | –0.63 (–0.53 to –0.73)a,b,e | NR | NR | NR | ||
MTX and ADA (s.c. administration) | 51 | –0.65 (–0.55 to –0.75)a,b,e | NR | NR | NR | |||
Anti-CCP2+; Q4 1060–4894 AU/ml | MTX and ABT (s.c. administration) | 46 | –0.93 (–0.83 to –1.035)a,b,e | NR | NR | NR | ||
MTX and ADA (s.c. administration) | 51 | –0.72 (–0.63 to –0.81)a,b,e | NR | NR | NR | |||
Anti-CCP2– | MTX and ABT (s.c. administration) | 2 years | 66 | –0.45 (–0.39 to –0.515)a,b,e | NR | NR | NR | |
MTX and ADA (s.c. administration) | 54 | –0.32 (–0.25 to –0.38)a,b,e | NR | NR | NR | |||
Anti-CCP2+; Q1 28–235 AU/ml | MTX and ABT (s.c. administration) | 42 | –0.575 (–0.485 to –0.67)a,b,e | NR | NR | NR | ||
MTX and ADA (s.c. administration) | 51 | –0.68 (–0.59 to –0.77)a,b,e | NR | NR | NR | |||
Anti-CCP2+; Q2 236–609 AU/ml | MTX and ABT (s.c. administration) | 51 | –0.66 (–0.57 to –0.75)a,b,e | NR | NR | NR | ||
MTX and ADA (s.c. administration) | 46 | –0.67 (–0.57 to –0.77)a,b,e | NR | NR | NR | |||
Anti-CCP2+; Q3 613–1046 AU/ml | MTX and ABT (s.c. administration) | 2 years | 46 | –0.58 (–0.50 to –0.70)a,b,e | NR | NR | NR | |
MTX and ADA (s.c. administration) | 51 | –0.64 (–0.54 to –0.74)a,b,e | NR | NR | NR | |||
Anti-CCP2+; Q4 1060–4894 AU/ml | MTX and ABT (s.c. administration) | 46 | –0.99 (–0.89 to –1.08)a,b,e | NR | NR | NR | ||
MTX and ADA (s.c. administration) | 51 | –0.81 (–0.71 to –0.90)a,b,e | NR | NR | NR | |||
Prediction of treatment response by smoking status at baseline: disease activity | ||||||||
Maska (2012)105 TEAR Mean DAS28 (absolute value) |
Non-smokers | MTX and ETN IT | 1–2 years (mean) | NR | 3.01 (1.3f) | NR | NR | NR |
MTX and SSZ and HCQ IT | NR | 3.24 (1.3f) | NR | NR | NR | |||
MTX and ETN SUT | NR | 3.31 (1.3f) | NR | NR | NR | |||
MTX and SSZ and HCQ SUT | NR | 3.07 (1.4f) | NR | NR | NR | |||
Current smokers | MTX and ETN IT | NR | 3.07 (1.2f) | NR | NR | NR | ||
MTX and SSZ and HCQ IT | NR | 3.15 (1.9f) | NR | NR | NR | |||
MTX and ETN SUT | NR | 3.2 (1.4f) | NR | NR | NR | |||
MTX and SSZ and HCQ SUT | NR | 3.2 (1.1f) | NR | NR | NR | |||
Non-smokers | ETN and MTX (COM) | 24 weeks | NR | 3.89 | NR | NR | NR | |
MTX and SSZ and HCQ (COM) | NR | 4.01 | NR | NR | NR | |||
Current smokers | ETN and MTX (COM) | NR | 3.81 | NR | NR | NR | ||
MTX and SSZ and HCQ (COM) | NR | 3.66 | NR | NR | NR | |||
Non-smokers | ETN and MTX (COM) | 48 weeks | NR | 3.3 | NR | NR | NR | |
MTX and SSZ and HCQ (COM) | NR | 3.33 | NR | NR | NR | |||
Current smokers | ETN and MTX (COM) | NR | 3.17 | NR | NR | NR | ||
MTX and SSZ and HCQ (COM) | NR | 3.33 | NR | NR | NR | |||
Non-smokers | ETN and MTX (COM) | 102 weeks | NR | 2.93 | NR | NR | NR | |
MTX and SSZ and HCQ (COM) | NR | 3.15 | NR | NR | NR | |||
Current smokers | ETN and MTX (COM) | NR | 2.93 | NR | NR | NR | ||
MTX and SSZ and HCQ (COM) | NR | 3.15 | NR | NR | NR | |||
Prediction of treatment response by erosions at baseline: radiographic progression | ||||||||
Pasero (1996)107 Change from baseline in the Larsen–Dale EJC |
Erosions at baseline | CsA | 12 months | 104 | 1.61 (0.35)a | NR | NR | NR |
Other cDMARDs | 89 | 2.94 (0.30)a | NR | NR | NR | |||
No erosions at baseline | CsA | 37 | 0.46 (0.18)a | NR | NR | NR | ||
Other cDMARDs | 54 | 1.62 (0.32)a | NR | NR | NR | |||
Pasero (1996)107 Change from baseline in the Larsen–Dale damage score |
Erosions at baseline | CsA | 104 | 4.75 (0.89)a | NR | NR | NR | |
Other cDMARDs | 89 | 7.96 (0.93)a | NR | NR | NR | |||
No erosions at baseline | CsA | 37 | 0.48 (0.96)a | NR | NR | NR | ||
Other cDMARDs | 54 | 5.14 (1.28)a | NR | NR | NR | |||
Smolen (2006)100 ASPIRE Change from baseline in the SHS |
SHS of < 2.6 | MTX | Week 54 | 100 | 1.87 | NR | NR | |
MTX and IFX | 231 | 0.83 | < 0.05g | NR | NR | |||
SHS of ≥ 2.6 to < 10.5 | MTX | 87 | 4.98 | NR | NR | |||
MTX and IFX | 246 | 0.84 | < 0.001g | NR | NR | |||
SHS of ≥ 10.5 | MTX | 92 | 3.78 | NR | NR | |||
MTX and IFX | 238 | –0.41 | < 0.001g | NR | NR | |||
Prediction of treatment response by RF status at baseline: radiographic progression | ||||||||
Vastesaeger (2009)78 ASPIRE Increase of ≥ 5/year in the total SHS score |
RF of < 80 U/ml | MTX | Week 54 | 82 | Reference | NR | NR | |
MTX and IFX | 201 | 0.38 (0.13b,h)a,i | 0.0030g | NR | NR | |||
RF of 80–200 U/ml | MTX | 73 | Reference | NR | NR | |||
MTX and IFX | 783 | 0.38 (0.14b,h)a,i | 0.0048g | NR | NR | |||
RF of > 200 U/ml | MTX | 128 | Reference | NR | NR | |||
MTX and IFX | 341 | 0.54 (0.11b,h)a,i | < 0.0001g | NR | NR | |||
Prediction of treatment response by CRP level at baseline: radiographic progression | ||||||||
Smolen (2006)100 ASPIRE Change from baseline in the SHS |
CRP level of < 0.6 mg/dl | MTX | Week 54 | 93 | 1.7a | NR | NR | |
MTX and IFX | 232 | 1.15a | NS | NR | NR | |||
CRP level of ≥ 0.6 to < 3 mg/dl | MTX | 103 | 4.36a | NR | NR | |||
MTX and IFX | 242 | 0.84a | < 0.001g | NR | NR | |||
CRP level of ≥ 3 mg/dl | MTX | 86 | 5.99a | NR | NR | |||
MTX and IFX | 248 | 1.35a | < 0.001g | NR | NR | |||
Vastesaeger 200978 ASPIRE Increase of ≥ 5/year total SHS score |
CRP level of < 0.6 mg/dl | MTX | 93 | Reference | NR | NR | ||
MTX and IFX | 232 | 0.15 (0.08b,h)a,i | 0.2172g | NR | NR | |||
CRP level of 0.6–3.0 mg/dl | MTX | 105 | Reference | NR | NR | |||
MTX and IFX | 252 | 0.51 (0.26b,h)a,i | < 0.0001g | NR | NR | |||
CRP level of > 3.0 mg/dl | MTX | 84 | Reference | NR | NR | |||
MTX and IFX | 238 | 0.65 (0.33b,h)a,i | < 0.0001g | NR | NR | |||
Prediction of treatment response by ESR at baseline: radiographic progression | ||||||||
Smolen (2006)100 ASPIRE Change from baseline in the SHS |
ESR of < 28 mm/hour | MTX | Week 54 | 93 | 2.88a | NR | NR | |
MTX and IFX | 223 | (Unreadable) | < 0.05g | NR | NR | |||
ESR of ≥ 28 to < 52 mm/hour | MTX | 89 | 2.65a | NR | NR | |||
MTX and IFX | 233 | 0.47a | < 0.001g | NR | NR | |||
ESR of ≥ 52 mm/hour | MTX | 87 | 6.13a | NR | NR | |||
MTX and IFX | 234 | 1.64a | < 0.001g | NR | NR | |||
Vastesaeger (2009)78 ASPIRE Increase of ≥ 5/year in the total SHS score |
ESR of < 21 mm/hour | MTX | 57 | Reference | NR | NR | ||
MTX and IFX | 141 | 0.13 (0.16b,h)a,i | 0.3755g | NR | NR | |||
ESR of 21–50 mm/hour | MTX | 125 | Reference | NR | NR | |||
MTX and IFX | 313 | 0.45 (0.12b,h)a,i | < 0.0001g | NR | NR | |||
ESR of > 50 mm/hour | MTX | 87 | Reference | NR | NR | |||
MTX and IFX | 236 | 0.60 (0.12b,h)a,i | < 0.0001g | NR | NR | |||
Prediction of treatment response by SJC at baseline: radiographic progression | ||||||||
Vastesaeger (2009)78 ASPIRE Increase of ≥ 5/year in the total SHS score |
SJC28 of < 10 | MTX | Week 54 | 50 | Reference | NR | NR | |
MTX and IFX | 145 | 0.31 (0.17b,h)a,i | 0.0524g | NR | NR | |||
SJC28 of 10–17 | MTX | 146 | Reference | NR | NR | |||
MTX and IFX | 367 | 0.44 (0.10b,h)a,i | < 0.0001g | NR | NR | |||
SJC28 of > 17 | MTX | 85 | Reference | NR | NR | |||
MTX and IFX | 210 | 0.51 (0.13b,h)a,i | < 0.0001g | NR | NR |
First author (year), name of trial or cohort (if relevant) | Outcome | Variable | Treatment | Follow-up time point | Treatment effect | Interaction effect, mean (95% CI) | Figure | Scenario/summary |
---|---|---|---|---|---|---|---|---|
Prediction of treatment response by ACPA status at baseline: erosions | ||||||||
Mustila (2011)106 FIN-RACo |
Erosions Larsen score (absolute value) |
ACPA+/ACPA– | MTX and SSZ and HCQ vs. sequential cDMARDs | 1 year |
ACPA+: –4.30 ACPA–: –4.24 |
–0.06 (–6.68 to 6.56) | 11 | 1 |
2 years |
ACPA+: –5.79 ACPA–: –6.78 |
0.99 (–6.32 to 8.30) | 12 | 1 | ||||
3 years |
ACPA+: –5.95 ACPA–: –11.65 |
5.70 (–4.23 to 15.63) | 13 | 2a | ||||
4 years |
ACPA+: –7.59 ACPA–: –14.54 |
6.95 (–4.59 to 18.49) | 14 | 2 | ||||
5 years |
ACPA+: –5.36 ACPA–: –13.44 |
8.08 (–5.07 to 21.23) | 15 | 2 | ||||
Seegobin (2014)109 CARDERA |
Erosions Change in the Larsen score from baseline |
ACPA+/ACPA– | MTX and CsA and prednisolone vs. MTX | 6 months |
ACPA+: –2.61 ACPA–: –1.12 |
–1.49 (–3.59 to 0.61) | 16 | 2b |
12 months |
ACPA+: –4.51 ACPA–: –0.67 |
–3.84 (–17.53 to 9.85) | 17 | 2 | ||||
18 months |
ACPA+: –5.32 ACPA–: –0.91 |
–4.41 (–8.02 to –0.80) | 18 | 2, interaction effect statistically significant | ||||
24 months |
ACPA+: –5.92 ACPA–: –1.02 |
–4.90 (–8.59 to –1.21) | 19 | 2, interaction effect statistically significand | ||||
Prediction of treatment response by ACPA status at baseline: disease activity | ||||||||
Seegobin (2014)109 CARDERA |
Disease activity Change in the DAS28 from baseline |
ACPA+/ACPA– | MTX and CsA and prednisolone vs. MTX | 6 months |
ACPA+: –0.99 ACPA–: –0.11 |
–0.88 (–1.80 to 0.04) | 24 | |
12 months |
ACPA+: –0.34 ACPA–: 0.63 |
–0.97 (–2.02 to 0.08) | 25 | Qualitative interaction, not statistically significant | ||||
18 months |
ACPA+: –0.27 ACPA–: 0.35 |
–0.62 (–1.71 to 0.47) | 26 | Qualitative interaction, not statistically significant | ||||
24 months |
ACPA+: –0.48 ACPA–: 0.32 |
–0.80 (–1.83 to 0.23) | 27 | Qualitative interaction, not statistically significant | ||||
Sokolove (2015)110 AMPLE |
Mean change from baseline in DAS28-CRP |
Anti-CCP2– Anti-CCP2+; Q1 28–235 AU/ml Anti CCP2+; Q2 236–609 AU/ml Anti-CCP2+; Q3 613–1046 AU/ml Anti-CCP2+; Q4 1060–4894 AU/ml |
MTX and ABT (s.c. administration) vs. MTX and ADA (s.c. administration) | 6 months |
Anti-CCP2–: –0.38 Anti-CCP2+; Q1: 0.38 Anti-CCP2+; Q2: 0.20 Anti-CCP2+; Q3: –0.08 Anti-CCP2+; Q4: –0.10 |
Interaction with anti-CCP2–: Anti-CCP2+, Q1: 0.75 (Not estimable) Anti-CCP2+, Q2: 0.58 (–0.11 to 1.26) Anti-CCP2+, Q3: 0.30 (–0.36 to 0.96) Anti-CCP2+, Q4: 0.28 (–0.41 to 0.96) |
32 | No evidence of TE or interaction |
1 year |
Anti-CCP2–: –0.25 Anti-CCP2+ Q1: 0.20 Anti-CCP2+ Q2: –0.07 Anti-CCP2+ Q3: 0.13 Anti-CCP2+ Q4: –0.23 |
Interaction with anti-CCP2–: Anti-CCP2+, Q1: 0.45 (–0.28 to 1.18) Anti-CCP2+, Q2: 0.18 (–0.58 to 0.94) Anti-CCP2+, Q3: 0.38 (–0.25 to 1.01) Anti-CCP2+, Q4: 0.02 (–0.80 to 0.85) |
33 | |||||
2 years |
Anti-CCP2–: –0.08 Anti-CCP2+, Q1: 0.18 Anti-CCP2+, Q2: 0.10 Anti-CCP2+, Q3: 0.00 Anti-CCP2+, Q4: –0.45 |
Interaction with anti-CCP2–: Anti-CCP2+, Q1: 0.26 (–0.65 to 1.16) Anti-CCP2+, Q2: 0.18 (–0.77 to 1.12) Anti-CCP2+, Q3: 0.08 (–0.76 to 0.91) Anti-CCP2+ Q4: –0.37 (–1.11 to 0.37) |
34 | |||||
Prediction of treatment response by ACPA status at baseline – physical function (as assessed via the HAQ score) | ||||||||
Seegobin (2014)109 CARDERA |
Change in the HAQ score from baseline | N/A | N/A | N/A | N/A | N/A | ||
Sokolove (2015)110 AMPLE |
Physical function: mean change from baseline in the HAQ-DI score |
Anti-CCP2– Anti-CCP2+; Q1 28–235 AU/ml Anti-CCP2+; Q2 236–609 AU/ml/ml Anti-CCP2+; Q4 1060–4894 AU/ml |
MTX and ABT (s.c. administration) vs. MTX and ADA (s.c. administration) | 6 months |
Anti-CCP2–: –0.23 Anti-CCP2+, Q1: 0.14 Anti-CCP2+, Q2: –0.12 Anti-CCP2+, Q3: –0.13 Anti-CCP2+, Q4: –0.08 |
Interaction with anti-CCP2–: Anti-CCP2+, Q1: 0.37 (0.08 to 0.66) Anti-CCP2+, Q2: 0.11 (–0.20 to 0.42) Anti-CCP2+, Q3: 0.10 (–0.20 to 0.42) Anti-CCP2+, Q4: 0.15 (–0.19 to 0.49) |
35 | No evidence of TE or interaction |
1 year |
Anti-CCP2–: –0.15 Anti-CCP2+, Q1: 1.26 Anti-CCP2+, Q2: –0.10 Anti-CCP2+, Q3: 0.02 Anti-CCP2+, Q4: –0.21 |
Interaction with anti-CCP2–: Anti-CCP2+, Q1: 1.41 (1.09 to 1.72) Anti-CCP2+, Q2: 0.05 (–0.24 to 0.34) Anti-CCP2+, Q3: 0.17 (–0.16 to 0.50) Anti-CCP2+, Q4: –0.06 (–0.38 to 0.26) |
36 | No evidence of TE or interaction | ||||
2 years |
Anti-CCP2–: –0.13 Anti-CCP2+, Q1: 0.11 Anti-CCP2+, Q2: 0.01 Anti-CCP2+, Q3: 0.06 Anti-CCP2+, Q4: –0.18 |
Interaction with anti-CCP2–: Anti-CCP2+, Q1: 0.24 (–0.07 to 0.54) Anti-CCP2+, Q2: 0.14 (–0.15 to 0.43) Anti-CCP2+, Q3: 0.19 (–0.12 to 0.50) Anti-CCP2+, Q4: –0.05 (–0.38 to 0.28) |
37 | No evidence of TE or interaction | ||||
Prediction of treatment response by smoking status at baseline: disease activity | ||||||||
Maska (2012)105 TEAR |
Disease activity Mean DAS28 (absolute value) |
Non-smokers Current smokers |
MTX and ETN IT vs. MTX and SSZ and HCQ IT vs. MTX and ETN SU vs. MTX and SSZ and HCQ SU | 1–2 years (mean) | 38 | Cannot assess statistical significance | ||
ETN and MTX (COM) vs. MTX and SSZ and HCQ (COM) | 24 weeks | 39 | Cannot assess statistical significance. Qualitative interaction | |||||
48 weeks | 40 | 3 | ||||||
102 weeks | 41 | Not prognostic for either treatment | ||||||
Prediction of treatment response by erosions at baseline: radiographic progression | ||||||||
Pasero (1996)107 |
Radiographic progression Change from baseline in the Larsen–Dale EJC |
Erosions at baseline: yes/no | CsA vs. other cDMARDs | 12 months |
Erosions at baseline: –1.33 No erosions at baseline: –1.16 |
–0.17 (–1.33 to 0.99) | 42 | 1, prognostic of response but not a treatment effect modifier |
Pasero (1996)107 | Change from baseline in the Larsen–Dale damage score | Erosions at baseline: yes/no | CsA vs. other cDMARDs | 12 months |
Erosions at baseline: –3.21 No erosions at baseline: –4.66 |
1.45 (–2.57 to 5.47) | 43 | 1 |
Smolen (2006)100 ASPIRE |
Radiographic progression Change from baseline in the SHS |
SHS of < 2.6 SHS of ≥ 2.6 to < 10.5 SHS of ≥ 10.5 |
MTX vs. IFX | Week 54 | 44 | Could not formally assess interaction. Suggest a treatment effect modifier | ||
Prediction of treatment response by RF status at baseline – radiographic progression | ||||||||
Vastesaeger (2009)78 ASPIRE |
Erosions/radiographic progression Increase of ≥ 5/year in the total SHS score |
RF of < 80 U/ml RF of 80–200 U/ml RF of > 200 U/ml |
MTX vs. MTX and IFX | Week 54 |
RF of < 80 U/ml: 0.38 RF of 80–200 U/ml: 0.38 RF of > 200 U/ml: 0.54 |
RF of 80–200 U/ml and RF of < 80 U/ml: 0.00 (–0.38 to 0.38) RF of > 200 U/ml and RF of < 80 U/ml: 0.16 (–0.17 to 0.49) |
||
Prediction of treatment response by CRP at baseline: radiographic progression | ||||||||
Smolen (2006)100 ASPIRE Change in SHS from baseline |
Radiographic progression Change in SHS from baseline |
CRP level of < 0.6 mg/dl CRP level of ≥ 0.6 to < 3 mg/dl CRP level of ≥ 3 mg/dl |
MTX vs. MTX and IFX | Week 54 | 45 | 3, could not formally assess interaction | ||
Vastesaeger (2009)78 ASPIRE |
Increase of ≥ 5/year in the total SHS score |
CRP level of < 0.6 mg/dl CRP level of 0.6–3.0 mg/dl CRP level of > 3.0 mg/dl |
MTX vs. MTX and IFX | Week 54 |
CRP level of < 0.6 mg/dl: 0.15 CRP level of 0.6–3.0 mg/dl: 0.51 CRP level of > 3.0 mg/dl: 0.65 |
CRP level of 0.6–3.0 mg/dl and CRP level of < 0.6 mg/dl: 0.36 (0.02 to 0.70) CRP level of > 3.0 mg/dl and CRP level of < 0.6 mg/dl: 0.50 (0.15 to 0.85) |
||
Prediction of treatment response by ESR at baseline: radiographic progression | ||||||||
Smolen (2006)100 ASPIRE |
Change in SHS from baseline |
ESR of < 28 mm/hour ESR of ≥ 28 to < 52 mm/hour ESR of ≥ 52 mm/hour |
MTX vs. MTX and IFX | Week 54 | 46 | Could not formally assess interaction. Suggest a treatment effect modifier | ||
Vastesaeger (2009)78 ASPIRE |
Increase of ≥ 5/year in the total SHS score |
ESR of < 21 mm/hour ESR of 21–50 mm/hour ESR of > 50 mm/hour |
MTX vs. MTX and IFX | Week 54 |
ESR of < 21 mm/hour: 0.13 ESR of 21–50 mm/hour: 0.45 ESR of > 50 mm/hour: 0.60 |
ESR of 21–50 mm/hour and an ESR of < 21 mm/hour: 0.32 (–0.07 to 0.71) ESR of > 50 mm/hour and an ESR of < 21 mm/hour: 0.47 (0.07 to 0.87) |
||
Prediction of treatment response by SJC at baseline: radiographic progression | ||||||||
Vastesaeger (2009)78 ASPIRE |
Increase of ≥ 5/year in the total SHS score |
SJC28 of < 10 SJC28 of 10–17 SJC28 of > 17 |
MTX vs. MTX and IFX | Week 54 |
SJC28 of < 10: 0.31 SJC28 10–17: 0.44 SJC28 of > 17: 0.51 |
SJC28 of 10–17 and SJC28 of < 10: 0.13 (–0.26 to 0.52) SJC28 of > 17 and SJC28 of < 10: 0.20 (–0.22 to 0.62) |
Treatment prediction models
Only one of the clinical prediction models involving multiple treatments that was reported in review 1 investigated the potential interactions between treatments and baseline variables. 79 Consequently, it was not possible to distinguish between covariates that were prognostic and those that were treatment effect modifiers in most clinical prediction models. Visser et al. 79 investigated the interaction between treatments and baseline variables and reported that there were no statistically significant interactions between covariates (ACPA status, RF status, CRP tertile, erosion score) and treatments (initial MTX monotherapy, initial MTX and prednisone combination therapy and initial MTX and IFX combination therapy), although this may be because the analysis lacked sufficient power to detect such effects as being statistically significant.
Prediction of treatment effect by anticitrullinated protein/peptide anti-body status at baseline: erosions
The results from Mustila et al. 106 suggest that the effect of triple therapy (MTX and SSZ and HCQ) versus sequential monotherapy on erosions at 1 (see Appendix 10, Figure 10) and 2 years (see Appendix 10, Figure 11) was similar in patients who were ACPA positive and ACPA negative at baseline (i.e. scenario 1). However, at 3 (see Appendix 10, Figure 12), 4 (see Appendix 10, Figure 13) and 5 years (see Appendix 10, Figure 14), the observed effect of treatment was less in patients who were ACPA positive than the effect of treatment in patients who were ACPA negative at baseline (i.e. scenario 2), although the results were not statistically significant.
Results from Seegobin et al. 109 (see Appendix 10, Figures 15–18) suggest that the effect of triple therapy (MTX and CsA and prednisolone) versus MTX on erosions was greater in patients who were ACPA positive than the effect of treatment in patients who were ACPA negative at baseline (scenario 2). At 18 (see Appendix 10, Figure 17) and 24 months (see Appendix 10, Figure 18), the interaction effect was statistically significant (–4.41, 95% CI –8.02 to –0.80; –4.9, 95% CI –8.59 to –1.21, respectively).
Seegobin et al. 109 performed a repeated-measures analysis of variance (ANOVA) examining the interaction between treatment and ACPA status at baseline on the change from baseline in Larsen score, and reported a significant treatment × ACPA interaction (F = 7.05; p < 0.001) when treatment was one of the four randomised treatment arms (CsA, prednisolone, MTX monotherapy or MTX and CsA and prednisolone triple therapy).
Prediction of treatment effect by anticitrullinated protein/peptide anti-body status at baseline: disease activity
The results from Seegobin et al. 109 (see Appendix 10, Figures 23–26) suggest that there was a qualitative interaction between treatment and ACPA status at baseline and at 12, 18 and 24 months; patients who were ACPA negative at baseline had a worse change from the baseline DAS28 when treated with triple therapy (MTX and CsA and prednisolone) than the effect of being treated with MTX, whereas patients had a better change from baseline in DAS28 when treated with triple therapy (MTX and CsA and prednisolone) than the effect of being treated with MTX. However, these effects were not statistically significant.
Seegobin et al. 109 performed a repeated measures ANOVA examining the interaction between treatment and ACPA status at baseline on the change from the baseline DAS28, and reported a significant treatment × ACPA interaction (F = 3.99; p = 0.008) when treatment was one of the four randomised treatment arms [(1) CsA, (2) prednisolone, (3) MTX monotherapy or (4) MTX and CsA and prednisolone triple therapy].
Sokolove et al. 110 examined the effect of treatment [MTX and ABT (subcutaneous administration) vs. MTX and ADA (subcutaneous administration)] by anti-CCP2 status (negativity and four quartiles of anti-CCP2 positivity) on the change from baseline in DAS28-CRP at multiple time points up to 2 years (this study examined changes at 6 months, 1 year and 2 years for consistency with other evidence, because it was not feasible to examine all time points). There was no evidence of a difference in the effect of treatments or that the treatment effect varied according to anti-CCP2 status at baseline and at 6 months, and at 1 and 2 years (see Appendix 10, Figures 31–33).
Prediction of treatment effect by anticitrullinated protein/peptide anti-body status at baseline: physical function
Seegobin et al. 109 performed a repeated measures ANOVA examining the interaction between treatment and ACPA status at baseline on the change from the baseline HAQ score, but this was not statistically significant (F = 0.48; p = 0.696) when treatment was one of the four randomised treatment arms [(1) CsA, (2) prednisolone, (3) MTX monotherapy or (4) MTX and CsA and prednisolone triple therapy].
Sokolove et al. 110 examined the effect of treatment [MTX and ABT (subcutaneous administration) vs. MTX and ADA (subcutaneous administration)] by anti-CCP2 status (negativity and four quartiles of anti-CCP2 positivity) on the change from the baseline HAQ – disability index (HAQ-DI) score at multiple time points up to 2 years (we examined changes at 6 months, and at 1 and 2 years for consistency with other evidence, because it was not feasible to examine all time points). There was no evidence of a difference in the effect of treatments or that treatment effect varied according to anti-CCP2 status at baseline at 6 months and at 1 and 2 years (see Appendix 10, Figures 34 and 35).
Prediction of treatment effect by anticitrullinated protein/peptide anti-body status at baseline: remission
Results from Huizinga et al. 104 (Tables 18 and 19) suggest that the effect of ABT versus MTX on DAS28-CRP remission at 1 year was worse for patients who were ACPA positive at baseline than for those who were ACPA negative at baseline (ratio of ORs 0.57); however, this was not statistically significant (95% CI 0.19 to 1.69). The effect of ABT and MTX versus MTX on DAS28-CRP remission at 1 year was better in patients who were ACPA positive at baseline than in those who were ACPA negative at baseline (ratio of odds ratios 1.33), but this was not statistically significant (95% CI 0.45 to 3.90).
First author (year), name of trial or cohort (if relevant), measurement of outcome | Variable | Treatment | Follow-up time point | Sample size (n) | OR (SD/standard error/95% CI) | Number (%) of cases in category group |
---|---|---|---|---|---|---|
Prediction of treatment response by ACPA status at baseline: remission | ||||||
Huizinga (2015)104 AVERT DAS28-CRP level of < 2.6 |
Anti-CCP2 IgM positive | ABT (s.c. administration) and MTX | 12 months | 66 |
2.21a Log-OR: 0.79 (variance: 0.12) |
43b (65.1%) |
ABT (s.c. administration) | 62 |
0.70a Log-OR: –0.36 (variance 0.13) |
23b (37.4%) | |||
MTX | 72 | Reference | 33b (45.6%) | |||
Anti-CCP2 IgM negative | ABT (s.c. administration) and MTX | 52 |
1.67a Log-OR: 0.51 (variance 0.18) |
30b (57.8%) | ||
ABT (s.c. administration) | 50 |
1.22a Log-OR: 0.20 (variance 0.18) |
25b (50.4%) | |||
MTX | 40 | Reference | 18b (45.0%) | |||
Prediction of treatment response by BMI at baseline: disease activity (DAS response) | ||||||
Heimans (2013)103 BeSt DAS of > 2.4 |
BMI of < 25 kg/m2 | MTX initial monotherapy | 1 year | NR | Reference | NR |
BMI of ≥ 25 kg/m2 | NR |
Log-RR: 0.05 (standard error 0.11)e |
NR | |||
BMI of < 25 kg/m2 | MTX and SSZ and tapered prednisone | NR | Reference | NR | ||
BMI of ≥ 25 kg/m2 | NR |
Log-RR: 0.38 (standard error 0.34)d |
NR | |||
BMI of < 25 kg/m2 | MTX and IFX | NR | Reference | NR | ||
BMI of ≥ 25 kg/m2 | NR |
Log-RR: 0.79 (standard error 0.41)e |
NR |
First author (year), name of trial or cohort (if relevant) | Variable | Comparison | Follow-up time point | OR | Interaction effect, ratio of ORs (95% CI) |
---|---|---|---|---|---|
Prediction of treatment response by ACPA status at baseline: remission | |||||
Huizinga (2015)104 DAS28-CRP remission |
ACPA status | ABT vs. MTX | 1 year |
ACPA+: 0.70 ACPA–: 1.22 |
0.57 (95% CI 0.19 to 1.68) |
ABT and MTX vs. MTX |
ACPA+: 2.21 ACPA–: 1.67 |
1.33 (95% CI 0.45 to 3.90) | |||
Prediction of treatment response by BMI at baseline: disease activity (DAS response) | |||||
Heimans (2013)103 BeSt DAS of > 2.4 |
BMI | BMI of ≥ 25 kg/m2 vs. BMI of < 25 kg/m2 | 1 year |
MTX and SSZ and tapered prednisone: 1.46a MTX initial monotherapy: 1.05a |
1.39b (95% CI 0.43 to 2.79) |
MTX and IFX: 2.20a MTX initial monotherapy: 1.05a |
2.10b (95% CI 0.91 to 4.82) | ||||
Prediction of treatment response by erosions at baseline: radiographic progression | |||||
Smolen (2006)100 ASPIRE Worsening radiographic joint damage (change in the SHS of > 0) |
SHS | N/Ac | Week 54 |
MTX and IFX: 0.99d MTX: 1.01d |
0.98 (95% CI 0.96 to 1.00) |
Prediction of treatment response by RF status at baseline: radiographic progression | |||||
Smolen (2006)100 ASPIRE Worsening radiographic joint damage (change in the SHS of > 0) |
RF status | N/Ac | Week 54 |
MTX and IFX: 1.00d MTX: 1.00d |
1.00 (95% CI 1.00 to 1.00) |
Prediction of treatment response by CRP level at baseline: radiographic progression | |||||
Smolen (2006)100 ASPIRE Worsening radiographic joint damage (change in the SHS of > 0) |
CRP level | N/Ac | Week 54 |
MTX and IFX: 1.03d MTX: 1.05d |
0.99 (95% CI 0.86 to 1.13) |
Prediction of treatment response by ESR at baseline: radiographic progression | |||||
Smolen (2006)100 ASPIRE Worsening radiographic joint damage (change in the SHS of > 0) |
ESR | N/Ac | Week 54 |
MTX and IFX: 1.00d MTX: 1.02d |
0.99 (95% CI 0.97 to 1.00) |
Prediction of treatment response by SJC at baseline: radiographic progression | |||||
Smolen (2006)100 ASPIRE Worsening radiographic joint damage (change in the SHS of > 0) |
SJC | N/Ac | Week 54 |
MTX and IFX: 1.00d MTX: 1.04d |
0.96 (95% CI 0.93 to 1.00) |
Prediction of treatment effect by smoking status at baseline: disease activity
It was not possible to formally assess the interaction effect between treatment and smoking status at baseline on DAS28 disease activity in the Maska et al. 105 study because standard errors were not available. The results of the comparison of ETN and MTX with MTX and SSZ and HCQ were inconsistent at 24, 48 and 102 weeks. At 24 weeks, there was a suggestion of a qualitative interaction between treatment and smoking status (see Appendix 10, Figure 38); patients who were non-smokers had a lower mean response when treated with ETN and MTX than those who were treated with MTX and SSZ and HCQ, whereas patients who were current smokers had a higher mean response when treated with ETN and MTX than those who were treated with MTX and SSZ and HCQ. At 48 weeks (see Appendix 10, Figure 39), the results suggested that smoking status was not prognostic in the case of ETN and MTX, whereas patients who were current smokers had a lower mean response when treated with ETN and MTX than non-smokers (i.e. scenario 3). At 102 weeks (see Appendix 10, Figure 40), the results suggested that smoking status was not a prognostic factor for both treatments.
Prediction of treatment effect by erosions at baseline: radiographic progression
The results from Pasero et al. 107 suggest that the presence of erosion versus the absence of erosion was prognostic of response but was not a treatment effect modifier for CsA versus other cDMARDs at 12 months with respect to the change from baseline in the Larsen–Dale eroded joint count (scenario 1; see Appendix 10, Figure 41) and with respect to the change from baseline in the Larsen–Dale damage score (scenario 1; see Appendix 10, Figure 42).
It was not possible to formally assess the interaction effect between treatment and SHS at baseline in the Smolen et al. 100 study, which reported baseline SHS in tertiles because standard errors were not available. The observed results suggest that SHS is a treatment effect modifier of the effect of MTX and IFX versus MTX on radiographic progression, but that SHS might not be prognostic for MTX and IFX (see Appendix 10, Figure 43).
Smolen et al. 100 reported a significant inverse correlation between erosions at baseline and change from baseline in SHS of > 0 at week 54 for the MTX and IFX treatment arm (r = –0.19; p < 0.0001), but not for the MTX arm (r = 0.07; p = 0.25; Table 20); this suggests that erosions may be a treatment effect modifier, but that it is not prognostic for MTX.
First author (year), name of trial or cohort (if relevant) | Treatment | Follow-up time point | Sample size (n) | Correlation coefficient | p-value for correlation coefficient | Regression coefficient | p-value for regression coefficient |
---|---|---|---|---|---|---|---|
Correlations between erosions at baseline and radiographic progression at follow-up | |||||||
Smolen (2006)100 ASPIRE Worsening radiographic joint damage (change in the SHS of > 0) |
MTX | Week 54 | 279 | r = 0.07 | 0.2511 | β = 0.005 | 0.583 |
MTX and IFX | 715 | r = –0.19 | < 0.0001 | β = –0.016 |
0.007 OR 0.99 (95% CI 0.97 to 1.0) |
||
Correlations between RF status at baseline and radiographic progression at follow-up | |||||||
Smolen (2006)100 ASPIRE Worsening radiographic joint damage (change in the SHS of > 0) |
MTX | Week 54 | 282a | r = 0.13 | 0.0324 | β = –0.0003 | 0.491 |
MTX and IFX | 722a | r = 0.05 | 0.1906 | β = 0.00 | 0.695 | ||
Correlations between CRP level at baseline and radiographic progression at follow-up | |||||||
Garnero (2002)102 Change from baseline in the SHS erosion score |
MTX | 12 months | 39 | r = 0.18 | NR (NS) | NR | NR |
ETN | 77 | r = 0.09 | NR (NS) | NR | NR | ||
Garnero 2002102 Change from baseline in the SHS total score |
MTX | 12 months | 39 | r = 0.28 | NR (NS) | NR | NR |
ETN | 77 | r = 0.18 | NR (NS) | NR | NR | ||
Smolen (2006)100 ASPIRE Worsening radiographic joint damage (change in the SHS of > 0) |
MTX | Week 54 | 282 | r = 0.24 | < 0.0001 | β = 0.048 | 0.43 |
MTX and IFX | 722 | r = 0.03 | 0.4643 | β = 0.033 | 0.291 | ||
Taylor (2004)101 Change from baseline in the SHS |
MTX | 54 weeks | 12 | r = 0.58 | 0.077 | NR | NR |
MTX and IFX | 12 | r = –0.19 | 0.562 | NR | NR | ||
Correlations between ESR at baseline and radiographic progression at follow-up | |||||||
Smolen (2006)100 ASPIRE Worsening radiographic joint damage (change in the SHS of > 0) |
MTX | Week 54 | 269 | r = 0.27 | < 0.0001 | β = 0.018 |
0.003 OR 1.02 (95% CI 1.01 to 1.03) |
MTX and IFX | 690 | r = 0.05 | 0.2356 | β = 0.004 | 0.335 | ||
Rau (1998)108 Radiographic progression (change in the Rau-modified Larsen/Sharp score) |
MTX | 6 months | 87 | r = 0.41 | < 0.001 | NR | NR |
GSTM | 87 | r = 0.24 | 0.04 | NR | NR | ||
MTX | 12 months | 87 | r = 0.41 | < 0.001 | NR | NR | |
GSTM | 87 | r = 0.25 | < 0.03 | NR | NR | ||
Correlations between SJC at baseline and radiographic progression at follow-up | |||||||
Smolen (2006)100 ASPIRE Worsening radiographic joint damage (change in the SHS of > 0) |
MTX | Week 54 | 282a | r = 0.16 | 0.0088 | β = 0.039 |
0.010 OR 1.04 (95% CI 1.01 to 1.07) |
MTX and IFX | 722a | r = –0.01 | 0.7291 | β = 0.002 | 0.866 | ||
Correlations between vascularity of synovium using PDUS at baseline and radiographic progression at follow-up | |||||||
Taylor (2004)101 Change from baseline in the SHS |
MTX | 54 weeks | 12 | r = 0.78 | 0.005 | NR | NR |
MTX and IFX | 12 | r = –0.28 | 0.372 | NR | NR |
Smolen et al. 100 also performed separate within-treatment logistic regression analyses of worsening joint damage at week 54, adjusting for baseline characteristics on their continuous scales. The estimated ORs per unit change in covariate were generally small and were only statistically significant for the SHS for patients treated with MTX and IFX. In spite of this finding, the results suggest that the SHS may have little prognostic value in predicting the response for both MTX and IFX and MTX alone. When formally comparing the ORs between treatments, the results suggest that there were small differences in the effect of MTX and IFX versus MTX on worsening joint damage at week 54 by baseline SHS (ratio of ORs 0.98, 95% CI 0.96 to 1.00).
Prediction of treatment effect by rheumatoid factor status at baseline: erosions/radiographic progression
Smolen et al. 100 (see Table 20) reported a significant correlation between RF at baseline and change in the SHS of > 0 at week 54 for the MTX treatment arm (r = 0.13; p = 0.03), but not for the MTX and IFX arm (r = 0.05; p = 0.19). However, RF at baseline was not associated with a change in the SHS of > 0 from baseline to week 54 in separate logistic regression analyses by treatment group, adjusting for baseline demographic and other clinical characteristics (no further details reported) in the MTX and IFX arm (β = 0.00; p = 0.70) or the MTX arm (β = –0.0003; p = 0.49) (see Table 20).
Vastesaeger et al. 78 analysed the change from baseline in RRP (≥ 5/year in the total modified SHS score) using an ANOVA on the van der Waerden score, and presented the results of the effect of MTX and IFX versus MTX by RF subgroups. There was a suggestion of a treatment (i.e. MTX and IFX vs. MTX) by RF subgroup interaction, with patients who had > 200 U/ml of RF having a greater effect when treated with MTX and IFX versus MTX than patients with a lower RF score, although this was not statistically significant.
Rau et al. 108 performed separate multivariable within-treatment linear regression analyses for MTX and GSTM, including covariates for tender joint count, SJC, Activities of Daily Living (ADL) score, ESR, CRP level and RF status on radiographic change between months 0–6 and 0–12. Of these, the authors found only ESR to be statistically significant (β = 0.49, p = 0.001 for progression between 0 and 6 months; β = 0.39, p = 0.007 for progression between 0 and 12 months) for MTX. None of the covariates was statistically significant for GSTM. Thus, RF status was not found to be prognostic for MTX and GSTM (scenario 4).
Prediction of treatment effect by C-reactive protein level at baseline: radiographic progression
It was not possible to formally assess the interaction between treatment and CRP level at baseline in the Smolen et al. 100 study, which reported baseline CRP level in tertiles because standard errors were not available. The observed results suggest an increasing effect of MTX versus MTX, with higher levels of baseline CRP. The combination of MTX and IFX seems to have an effect on radiographic progression at all levels of CRP at baseline; this effect was not observed in the case of MTX. Baseline CRP level appears to be a treatment modifier with respect to MTX and IFX and MTX alone, but does not appear to be prognostic for MTX and IFX (scenario 3) (see Appendix 10, Figure 44).
Smolen et al. 100 reported a significant correlation between CRP level at baseline and change in the SHS of > 0 at week 54 for the MTX treatment arm (r = 0.24; p < 0.0001), but not for the MTX and IFX arm (r = 0.03; p = 0.46); this supports the assertion that MTX and IFX treatment has an effect irrespective of CRP level at baseline and that CRP level at baseline is a treatment effect modifier. However, CRP level at baseline was not associated with a change in the SHS of > 0 from baseline to week 54 in separate logistic regression analyses, adjusting for baseline demographic and other clinical characteristics (no further details reported) in the MTX and IFX arm (β = 0.033; p = 0.29) or the MTX arm (β = 0.048; p = 0.43) (see Table 20).
Vastesaeger et al. 78 analysed the change from baseline in RRP (≥ 5/year in the total modified SHS score) using an ANOVA on the van der Waerden score, and presented the results of the effect of MTX and IFX versus MTX by CRP subgroups. There was a suggestion of a treatment interaction (i.e. MTX and IFX vs. MTX) by CRP subgroup, with the effect of MTX and IFX being greater with higher baseline CRP levels, although the results were not statistically significant.
Garnero et al. 102 (see Table 19) reported no significant correlations between CRP level at baseline and change from baseline in the SHS erosion score in either the MTX or the ETN treatment arm at 12 months (r = 0.18 and r = 0.09, respectively; p-values not reported). Likewise, no significant associations were reported between CRP level at baseline and change from baseline in the SHS total score in either the MTX or ETN arm at 12 months (r = 0.28 and r = 0.18, respectively; p-values not reported). However, in both cases the observed association was greater for patients treated with MTX, which may indicate that CRP level at baseline is a treatment effect modifier with respect to the effect of MTX versus ETN.
Taylor et al. 101 (see Table 19) reported no significant associations between CRP level at baseline and change from baseline in the SHS score in either the MTX treatment arm or the MTX and IFX treatment arm at 54 weeks (r = 0.58, p = 0.08; and r = –0.19, p = 0.56, respectively). However, in both cases the observed association was greater for patients treated with MTX, which may indicate that CRP level at baseline is a treatment effect modifier with respect to the effect of MTX and IFX versus MTX.
Rau et al. 108 performed separate multivariable within-treatment linear regression analyses for MTX and GSTM, including covariates for tender joint count, SJC, ADL score, ESR, CRP level and RF status on radiographic change between months 0–6 and 0–12. Of these, the authors found only ESR to be statistically significant (data previously shown), with no predictive effect of CRP level for either treatment (data not shown) for MTX. None of the covariates was statistically significant for GSTM. Thus, CRP level was not found to be prognostic for MTX and GSTM (scenario 4).
Prediction of treatment effect by erythrocyte sedimentation rate at baseline: radiographic progression
It was not possible to formally assess the interaction between treatment and ESR at baseline in the Smolen et al. 100 study, which reported baseline ESR in tertiles, because standard errors were not available, and it was not possible to extract the mean from the published graph for patients treated with IFX and MTX and with a baseline ESR of < 28 mm/hour. Nevertheless, the observed results suggest that a baseline ESR of < 28 mm/hour and ≥ 28 to < 52 mm/hour may not be prognostic of response for MTX and IFX and MTX alone, but that it may be a treatment effect modifier for patients with a baseline ESR of ≥ 52 mm/hour (see Appendix 10, Figure 45).
Smolen et al. 100 (see Table 20) reported a significant correlation between ESR at baseline and the change from baseline in the SHS of > 0 for the MTX treatment arm (r = 0.27; p < 0.0001) but not for the MTX and IFX treatment arm (r = 0.05; p = 0.24); this supports the assertion that treatment with MTX and IFX has an effect irrespective of ESR at baseline and that ESR at baseline is a treatment effect modifier. ESR at baseline was found to be significantly associated with a change in the SHS of > 0 from baseline to week 54 in separate logistic regression analyses, adjusting for baseline demographic characteristics and other clinical characteristics (no further details reported) in the MTX arm (β = 0.018; p = 0.003), with no significant association in the MTX and IFX arm (β = 0.004; p = 0.34) (see Table 20). Again, baseline ESR may be prognostic for MTX but not for MTX and IFX.
Vastesaeger et al. 78 analysed the change from baseline in RRP (≥ 5/year in the total modified SHS score) using an ANOVA on the van der Waerden score, and presented the results of the effect of MTX and IFX versus MTX by ESR subgroup. There was a suggestion of a treatment (i.e. MTX + IFX vs. MTX) by ESR subgroup interaction. The treatment effect increased with increasing baseline ESR and was statistically significant when comparing the treatment effect in patients with baseline ESR of ≥ 52 mm/hour against the treatment effect in patients with a baseline ESR of < 21 mm/hour (difference in the treatment effects between subgroups 0.47 mm/hour, 95% CI 0.07 to 0.87 mm/hour).
Rau et al. 108 reported a significant correlation between ESR at baseline and Rau-modified Larsen/Sharp score for both MTX and GSTM at 6 months (r = 0.41, p < 0.0001; r = 0.24, p = 0.04, respectively) and 12 months (r = 0.41, p < 0.001; r = 0.25, p < 0.03, respectively). The association was greater in patients treated with MTX, which may indicate that baseline ESR is a treatment effect modifier.
Rau et al. 108 performed separate multivariable within-treatment linear regression analyses for MTX and GSTM, including covariates for tender joint count, SJC, ADL score, ESR, CRP level and RF status on radiographic change between months 0–6 and 0–12. Of these, ESR was the only statistically significant covariate (radiographic progression over 0–6 months, regression coefficient 0.49; p = 0.001; radiographic progression over 0–12 months, regression coefficient 0.39; p = 0.007) for MTX. None of the covariates was statistically significant for GSTM. Thus, ESR may be a treatment effect modifier with respect to MTX and GSTM, but it is not prognostic with respect to GSTM (scenario 3).
Prediction of treatment effect by swollen joint count at baseline: radiographic progression
Smolen et al. 100 reported a significant correlation between SJC at baseline and the change from baseline in SHS of > 0 for the MTX treatment arm (r = 0.16; p = 0.0088), but not for the MTX and IFX treatment arm (r = –0.01; p = 0.73). Although the association in the case of MTX is weak, the results suggest that baseline SJC may be a treatment effect modifier (i.e. it is prognostic for MTX but not for MTX and IFN). SJC at baseline was found to be significantly associated with a change in the SHS of > 0 from baseline to week 54 in separate logistic regression analyses, adjusting for baseline demographic characteristics and other clinical characteristics (no further details reported) in the MTX treatment arm (β = 0.039; p = 0.010), with no significant association in the MTX and IFX treatment arm (β = 0.002; p = 0.87). Again, baseline SJC may be a treatment effect modifier (i.e. it is prognostic for MTX but not for MTX and IFX).
Vastesaeger et al. 78 analysed the change from baseline in RRP (≥ 5/year in total modified SHS score) using an ANOVA on the van der Waerden score and presented the results of the effect of MTX and IFX versus MTX by 28 swollen joint count (SJC28) subgroups. There was a suggestion of a treatment (i.e. MTX and IFX vs. MTX) by SJC28 subgroup interaction; the observed treatment effects increased with increasing baseline SJC28, although these were not statistically significant.
Rau et al. 108 performed separate multivariable within-treatment linear regression analyses for MTX and GSTM, including covariates for tender joint count, SJC, ADL score, ESR, CRP level and RF on radiographic change between months 0–6 and 0–12. Of these, ESR was the only statistically significant covariate (radiographic progression over 0–6 months, regression-coefficient 0.49, p = 0.001; radiographic progression over 0–12 months, regression-coefficient 0.39, p = 0.007) for MTX. None of the covariates was statistically significant for GSTM. Thus, SJC was not found to be prognostic for MTX and GSTM (scenario 4).
Prediction of treatment effect by body mass index at baseline: disease activity (Disease Activity Score response)
The results from Heimans et al. 103 suggest that the relative risk of MTX and SSZ and tapered prednisone versus initial MTX monotherapy on treatment non-response (i.e. a DAS of > 2.4) at 1 year was greater for patients with a BMI of ≥ 25 kg/m2 at baseline than for those with a BMI of < 25 kg/m2 at baseline (ratio of relative risks 1.39); however, this was not statistically significant (95% CI 0.43 to 2.79). The relative risk of MTX and IFX versus initial MTX monotherapy on treatment non-response (i.e. a DAS of > 2.4) at 1 year was approximately twice that for patients with a BMI of ≥ 25 kg/m2 at baseline compared with those with a BMI of < 25 kg/m2 at baseline (ratio of relative risks 2.10); however, this was not statistically significant (95% CI 0.91 to 4.82).
Prediction of treatment effect by power Doppler ultrasound at baseline: radiographic progression
Taylor et al. 101 reported a significant correlation between the vascularity of synovium using PDUS at baseline and the change from baseline in SHS score at 54 weeks for the MTX treatment arm (r = 0.78; p = 0.005), but not for the MTX and IFX treatment arm (r = –0.28; p = 0.37). The difference in the strength of the association suggests that baseline vascularity of synovium may be a treatment effect modifier.
Discussion
Twelve primary studies were identified with which to assess the prediction of treatment response in terms of baseline covariates. Covariates examined included ACPA status, smoking status, erosions, RF status, CRP level, ESR, SJC, BMI and vascularity of synovium on PDUS. Outcomes examined included erosions/radiographic progression, disease activity, physical function and DAS28 remission.
There is no evidence, from the current review, that the end point (i.e. remission or low disease activity) had an impact on the results. One study (i.e. Huizinga et al. 104) examined remission as an outcome with ACPA status as the baseline covariate, and found no significant interaction between treatment and covariate. Similarly, one study (i.e. Heimans et al. 103) looked at low disease activity as an outcome, with BMI as the baseline covariate, and found no significant interaction between treatment and covariate. In order to explore the question of how end points of remission and low disease activity affect the prediction of treatment response, further research is needed to examine both end points and the same baseline covariates, preferably within the same treatment regime.
Considerable variation was found within the evidence examining the prediction of treatment response in terms of baseline covariates, in terms of covariates examined, treatments compared, outcomes assessed and statistical methodology. Most of the studies performed within-treatment analyses and examined the effects of baseline patient and/or disease characteristics using subgroup analyses, and rarely adjusted for other covariates. More importantly, no consideration was given to more flexible relationships between covariates and response and their interaction effects. It was rare for studies to perform a formal assessment of any treatment by covariate interactions; when these were performed, it is likely that the test lacked sufficient power to detect interaction effects as being statistically significant.
We have compared treatment effects across values of baseline variables in an attempt to identify potential treatment effect modifiers using data presented in the included papers (when possible). Given the heterogeneity between studies in the outcomes assessed, the differences in modelling approaches and the lack of information on the correlation between covariates, a formal quantitative synthesis of the evidence was not conducted. The conclusions were limited to a comparison between treatments at the study level and should be treated as hypothesis-generating for certain patient and/or disease characteristics being modifiers of treatment effect. Such predictors should be formally evaluated in treatment-specific clinical prediction models.
Review 2 conclusions
There was statistical evidence to suggest that ACPA status, SJC28 and PDUS status at baseline may be treatment effect modifiers, but not necessarily that they are prognostic of response for all treatments. Most of the results were subject to considerable uncertainty and were not statistically significant. In general, there was insufficient evidence that the effect of treatment depended on baseline characteristics. However, it should be noted that insufficient evidence for a covariate by treatment interaction does not mean that there is no interaction or that baseline covariates are prognostic for both treatments. The inability to reject the null hypothesis of no interaction effect is likely to reflect the power of the test being low for meaningful interaction effects.
Chapter 6 Assessment of factors relevant to the NHS and other parties
Predictors included in the clinical prediction models identified in this assessment may be useful in supplementing clinicians’ decision-making regarding patient prognosis. However, because of uncertainties and limitations in the evidence base, no single clinical prediction model can currently be recommended over any other for use in clinical practice.
The prognostic factors selected for consideration in the assessment are readily available in clinical practice. However, the optimal prediction model(s) may include other biomarkers/genetic tests that are not currently available. The use of these tests may have associated practical and cost implications that will need to be considered before potential future implementation.
Collaboration between professionals within the clinical and research community would facilitate further model development and external validation research.
Chapter 7 Discussion
Statement of principal findings
Review 1 (clinical prediction models)
A total of 28 studies were identified that investigated the use of assessment tools and tests in the evaluation of prognosis in early RA patients. Twenty-two model development studies and one combined model development/external validation study reported the development and/or internal validation of a total of 39 clinical prediction models for outcomes, including radiographic joint damage, HAQ score and DAS28. Six external validation studies (including the combined model development/external validation study) were included, which tested the performance of eight clinical prediction models57,61,69,73,76,78,79 for radiographic joint damage outcomes.
The predictive performance in internal validation was reported using the c-statistic in eight studies with variable results. c-Statistics for radiographic progression ranged between 0.63 (Degboé et al. 66 predicting a ΔSHS of ≥ 5 at 1 year) and 0.87 (Houseman et al. 72 predicting a ΔSHS of ≥ 10.5 at 8.2 years). However, many of the included models had not been externally validated. For the eight models that had been externally validated, predictive performance varied considerably. Five clinical prediction models (i.e. Syversen,76 SWEFOT,73 ESPOIR,69 MBDA61 and SONORA57) were externally validated in only one population per outcome. Three clinical prediction models (i.e. ASPIRE CRP,78 ASPIRE ESR78 and BeSt79) were externally validated using the same outcome definition in more than one population. The results of the RE meta-analysis indicated that the most favourable performance across external validations was for the BeSt model79 (c-statistic 0.72, 95% CI 0.20 to 0.96), followed by ASPIRE ESR78 (c-statistic 0.62, 95% CI 0.44 to 0.78) and ASPIRE CRP78 (c-statistic 0.55, 95% CI 0.13 to 0.91). However, there is considerable heterogeneity for all three models, with the wide CIs suggesting substantial uncertainty in the expected predictive performance in a new sample of patients. The 95% CIs of the pooled estimates contain 0.5 for all three clinical prediction models, indicating that we cannot be confident that the performance of the models is better than would be expected by chance.
Limitations were observed in the methods used to develop the included clinical prediction models, such as the absence of potentially important candidate predictors and the incorrect handling of continuous predictors. For models developed using RCT data with patients assigned to alternative treatment strategies, the model development generally failed to assess the interactions between predictors and treatment group, and so did not generate truly treatment-specific models.
There was no evidence to suggest that a single clinical prediction model performs well in all patients. Further research is required to determine the optimal clinical prediction model(s) for use in clinical practice and to determine the value of any emerging, currently untested candidate predictors in the development of future prediction models.
Review 2 (prediction of treatment response)
Twelve primary studies were identified with which to assess the prediction of treatment response according to baseline covariates. Covariates examined included ACPA status, smoking status, erosions, RF status, CRP level, ESR, SJC, BMI and vascularity of synovium on PDUS. Outcomes examined included erosions/radiographic progression, disease activity, physical function and DAS28 remission.
There was statistical evidence to suggest that ACPA status, SJC28 and PDUS status at baseline may be treatment effect modifiers, but not necessarily that they are prognostic of response for all treatments. Most of the results were subject to considerable uncertainty and were not statistically significant. In general, there was insufficient evidence that the effect of treatment depended on baseline characteristics.
Strengths and limitations of the assessment
This assessment has been undertaken according to current good practice in prognostic systematic reviews. 26,48,118 A comprehensive range of predictors readily available in clinical practice and key outcomes were selected for review. However, in order to maintain the feasibility of the assessment within the resources and time scales available, a limited number of protocol amendments were necessary, as described in Appendix 2. It was also necessary to limit the inclusion of studies to those published in the English language, because resources were not available to allow for the translation of non-English-language articles.
The evaluation of prognostic models and the evidence regarding differential treatment effects according to different values of predictor variables was limited to published aggregate data; published models typically involved different predictor variables and did not include interaction terms with treatment. In both cases, the ideal scenario would be to fit common models to the IPD. Access to IPD would allow harmonisation (both in terms of model development and validation). However, analyses using IPD were beyond the scope of this assessment.
Uncertainties
Despite the availability of a range of clinical prediction models, uncertainty remains over which (if any) is the most appropriate for use in clinical practice. In order to assess the comparative performance of the competing models more thoroughly, further external validations would be required. However, limitations were observed in the methods used to develop the clinical prediction models. It is therefore likely that the most clinically useful prediction model would contain predictors from across more than one of the reviewed clinical prediction models and would consider alternative handling of key predictive variables. Interactions between predictors and treatment group were rarely considered, and so there is limited evidence regarding differential treatment effects according to different values of predictor variables.
Future research should seek to demonstrate the predictive value of existing variables that are currently included in available clinical prediction models and should also aim to identify new, potentially valuable variables for testing in future clinical prediction models. The development of new clinical prediction models should conform to recommended good practice in model development and reporting (e.g. adherence to the TRIPOD statement). Developed models should be externally validated in order to demonstrate their usefulness. External validations should be conducted in clinically appropriate populations with previously untreated early RA, and with sufficient variation (or case mix) in the population to ensure that the results are generalisable to the target clinical population.
Chapter 8 Conclusions
Implications for service provision
Review 1: clinical prediction models
No single clinical prediction model can currently be recommended in preference to any other model for use in clinical practice because of uncertainties and limitations in the existing evidence base. The optimal prediction model(s) may include variables (e.g. biomarkers/genetic tests) that are not routinely or currently available. Their potential use may have associated practical and cost implications that will need to be evaluated before future implementation.
Review 2: prediction of treatment response
There was limited evidence with which to assess whether or not specific baseline variables can predict differential effects according to the treatment administered. Nevertheless, the available evidence suggested that some baseline variables do affect relative treatment effects and that not all baseline variables may be prognostic of response for all treatments.
There was statistical evidence to suggest that ACPA status, SJC28 and PDUS status at baseline may be treatment effect modifiers, but not necessarily that these are prognostic of response for all treatments. Most of the results were subject to considerable uncertainty and were not statistically significant.
In general, there was insufficient evidence that the effect of treatment depended on baseline characteristics. This may be a real effect or may be because studies lacked statistical power to detect interaction effects. The true effect of baseline variables should be evaluated in single multivariable models adjusting for all relevant confounders.
Suggested research priorities
Review 1: clinical prediction models
-
Collaborative research, including the use of IPD, for further (1) development/internal validation and (2) external validation of clinical prediction model(s) with improved predictive performance.
-
Adherence to good reporting standards of future clinical prediction model studies (e.g. in accordance with the TRIPOD statement26).
Review 2: prediction of treatment response
-
Clinical prediction models should be developed and validated with respect to individual treatments.
-
The assessment of treatment by covariate interactions should follow good statistical practice: subgroup analyses should be avoided; categorising continuous baseline covariates should be avoided; and the interactions between treatments and baseline variables should be specifically modelled.
-
The results of multivariable analyses presented in published reports should include estimates of the main effects of covariates and any interaction effects, together with their standard errors and covariances for secondary research purposes.
Acknowledgements
We would like to thank Dr Frank McKenna (Consultant Rheumatologist, Central Manchester University Hospitals NHS Foundation Trust, Manchester, UK) and Dr Patrick Kiely (Consultant Rheumatologist, St George’s University Hospitals NHS Foundation Trust, London, UK) for providing clinical advice. The team would also like to acknowledge the input of two patient and public involvement representatives in providing comments on the plain English summary and the draft assessment report. We thank Gill Rooney (Programme Manager, School of Health and Related Research, University of Sheffield, Sheffield, UK) for providing administrative support and for preparing and formatting the report. We would like to thank Robert Wolff for sharing an unpublished version of the PROBAST quality assessment tool. We would also like to thank Diederik De Cock for responding to queries relating to his published study.
About the School of Health and Related Research
The School of Health and Related Research (ScHARR) is one of the twelve departments that comprise the Faculty of Medicine, Dentistry and Health at the University of Sheffield. ScHARR specialises in health services and public health research, and the application of health economics and decision science to the development of health services and the improvement of public health.
The ScHARR Technology Assessment Group (ScHARR-TAG) synthesises research on the clinical effectiveness and cost-effectiveness of health-care interventions for the NIHR HTA programme on behalf of a range of policy-makers, including the National Institute for Health and Care Excellence (NICE). ScHARR-TAG is part of a wider collaboration of a number of units from other regions including Southampton Health Technology Assessment Centre (SHTAC), University of Southampton; Aberdeen HTA Group, University of Aberdeen; Liverpool Reviews & Implementation Group (LRiG), University of Liverpool; Peninsular Technology Assessment Group (PenTAG), University of Exeter; Centre for Reviews and Dissemination (CRD) and Centre for Health Economics (CHE), University of York; Warwick Evidence, University of Warwick; the BMJ Group and Kleijnen Systematic Reviews.
Contributions of authors
Rachel Archer (Research Fellow; area of specialty: systematic reviewing) was the lead for the overall assessment and the lead systematic reviewer for review 1.
Emma Hock (Research Fellow; area of specialty: systematic reviewing) was the lead systematic reviewer for review 2.
Jean Hamilton (Research Fellow; area of specialty: statistical methods in health economics) was involved in study selection, the checking of data extraction, data analysis and interpretation and performing the meta-analysis of external validation studies in review 1, and provided statistical advice to the overall assessment.
John Stevens (Reader in Decision Science; area of specialty: medical statistics and evidence synthesis) was involved in study selection, data analysis and the interpretation of results for review 2, and provided statistical advice to the overall assessment.
Munira Essat (Research Fellow; area of specialty: systematic reviewing) and Edith Poku (Research Fellow; area of specialty: systematic reviewing and evidence-based medicine) were involved with the double-checking of the extracted data and the quality assessment results for reviews 1 and 2.
Mark Clowes (Information Specialist; area of specialty: information retrieval) performed the scoping and full searches.
Abdullah Pandor (Senior Research Fellow, area of specialty: systematic reviewing) and Matt Stevenson (Professor of Health Technology Assessment; area of specialty: HTA) provided advice on the methods and conduct of the assessment.
All authors were involved in drafting and commenting on the final report.
Data-sharing statement
All data requests should be submitted to the corresponding author for consideration. Access to anonymised data may be granted following review.
Disclaimers
This report presents independent research funded by the National Institute for Health Research (NIHR). The views and opinions expressed by authors in this publication are those of the authors and do not necessarily reflect those of the NHS, the NIHR, NETSCC, the HTA programme or the Department of Health and Social Care. If there are verbatim quotations included in this publication the views and opinions expressed by the interviewees are those of the interviewees and do not necessarily reflect those of the authors, those of the NHS, the NIHR, NETSCC, the HTA programme or the Department of Health and Social Care.
References
- Scott DL, Steer S. The course of established rheumatoid arthritis. Best Pract Res Clin Rheumatol 2007;21:943-67. https://doi.org/10.1016/j.berh.2007.05.006.
- Pincus T, Fuchs HA, Callahan LF, Nance EP, Kaye JJ. Early radiographic joint space narrowing and erosion and later malalignment in rheumatoid arthritis: a longitudinal analysis. J Rheumatol 1998;25:636-40.
- Drossaers-Bakker KW, Kroon HM, Zwinderman AH, Breedveld FC, Hazes JM. Radiographic damage of large joints in long-term rheumatoid arthritis and its relation to function. Rheumatology 2000;39:998-1003. https://doi.org/10.1093/rheumatology/39.9.998.
- Allaire S, Wolfe F, Niu J, LaValley MP, Zhang B, Reisine S. Current risk factors for work disability associated with rheumatoid arthritis: recent data from a US national cohort. Arthritis Rheum 2009;61:321-8. https://doi.org/10.1002/art.24281.
- Naz SM, Symmons DP. Mortality in established rheumatoid arthritis. Best Pract Res Clin Rheumatol 2007;21:871-83. https://doi.org/10.1016/j.berh.2007.05.003.
- Dadoun S, Zeboulon-Ktorza N, Combescure C, Elhai M, Rozenberg S, Gossec L, et al. Mortality in rheumatoid arthritis over the last fifty years: systematic review and meta-analysis. Joint Bone Spine 2013;80:29-33. https://doi.org/10.1016/j.jbspin.2012.02.005.
- Meune C, Touzé E, Trinquart L, Allanore Y. Trends in cardiovascular mortality in patients with rheumatoid arthritis over 50 years: a systematic review and meta-analysis of cohort studies. Rheumatology 2009;48:1309-13. https://doi.org/10.1093/rheumatology/kep252.
- Rheumatoid Arthritis: National Clinical Guideline for Management and Treatment in Adults. London: Royal College of Physicians; 2009.
- Symmons DP, Barrett EM, Bankhead CR, Scott DG, Silman AJ. The incidence of rheumatoid arthritis in the United Kingdom: results from the Norfolk Arthritis Register. Br J Rheumatol 1994;33:735-9. https://doi.org/10.1093/rheumatology/33.8.735.
- MacGregor AJ, Snieder H, Rigby AS, Koskenvuo M, Kaprio J, Aho K, et al. Characterizing the quantitative genetic contribution to rheumatoid arthritis using data from twins. Arthritis Rheum 2000;43:30-7. https://doi.org/10.1002/1529-0131(200001)43:1<30::AID-ANR5>3.0.CO;2-B.
- Jones MA, Silman AJ, Whiting S, Barrett EM, Symmons DPM. Occurrence of rheumatoid arthritis is not increased in the first degree relatives of a population based inception cohort of inflammatory polyarthritis. Ann Rheum Dis 1996;55:89-93. https://doi.org/10.1136/ard.55.2.89.
- Smolen JS, Kay J, Landewe R, Matteson EK, Gaylis N, Wollenhaupt J, et al. Final 5-year safety and efficacy results of a phase 3, randomized, placebo-controlled trial of golimumab in patients with active rheumatoid arthritis despite previous anti-tumor necrosis factor therapy. Rheumatology 2014;53:i87-8. https://doi.org/10.1093/rheumatology/keu101.006.
- Aletaha D, Neogi T, Silman AJ, Funovits J, Felson DT, Bingham CO, et al. 2010 Rheumatoid arthritis classification criteria: an American College of Rheumatology/European League Against Rheumatism collaborative initiative. Arthritis Rheum 2010;62:2569-81. https://doi.org/10.1002/art.27584.
- Smolen JS, Landewe R, Breedveld FC, Buch M, Burmester G, Chatzidionysiou K, et al. EULAR recommendations for the management of rheumatoid arthritis with synthetic and biological disease-modifying antirheumatic drugs: 2013 update. Ann Rheum Dis 2014;73:492-509. https://doi.org/10.1136/annrheumdis-2013-204573.
- Landewe R, van der Heijde D. Radiographic progression in rheumatoid arthritis. Clin Exp Rheumatol 2005;23:S63-S8.
- Larsen A, Dale K, Eek M. Radiographic evaluation of rheumatoid arthritis and related conditions by standard reference films. Acta Radiol Diagn 1977;18:481-91. https://doi.org/10.1177/028418517701800415.
- Sharp JT, Lidsky MD, Collins LC, Moreland J. Methods of scoring the progression of radiologic changes in rheumatoid arthritis. Correlation of radiologic, clinical and laboratory abnormalities. Arthritis Rheum 1971;14:706-20. https://doi.org/10.1002/art.1780140605.
- van der Heijde D. How to read radiographs according to the Sharp/van der Heijde method. J Rheumatol 2000;27:261-3.
- Prevoo ML, van ‘t Hof MA, Kuper HH, van Leeuwen MA, van de Putte LB, van Riel PL. Modified disease activity scores that include twenty-eight-joint counts. Development and validation in a prospective longitudinal study of patients with rheumatoid arthritis. Arthritis Rheum 1995;38:44-8. https://doi.org/10.1002/art.1780380107.
- Bruce B, Fries JF. The Health Assessment Questionnaire (HAQ). Clin Exp Rheumatol 2005;23:S14-8.
- Hemingway H, Croft P, Perel P, Hayden JA, Abrams K, Timmis A, et al. Prognosis research strategy (PROGRESS) 1: a framework for researching clinical outcomes. BMJ 2013;346. https://doi.org/10.1136/bmj.e5595.
- Riley RD, Hayden JA, Steyerberg EW, Moons KG, Abrams K, Kyzas PA, et al. Prognosis Research Strategy (PROGRESS) 2: prognostic factor research. PLOS Med 2013;10. https://doi.org/10.1371/journal.pmed.1001380.
- Steyerberg EW, Moons KG, van der Windt DA, Hayden JA, Perel P, Schroter S, et al. Prognosis Research Strategy (PROGRESS) 3: prognostic model research. PLOS Med 2013;10. https://doi.org/10.1371/journal.pmed.1001381.
- Hingorani AD, Windt DA, Riley RD, Abrams K, Moons KG, Steyerberg EW, et al. Prognosis research strategy (PROGRESS) 4: stratified medicine research. BMJ 2013;346. https://doi.org/10.1136/bmj.e5793.
- Hingorani AD, Hemingway H. How should we balance individual and population benefits of statins for preventing cardiovascular disease?. BMJ 2010;342. https://doi.org/10.1136/bmj.c6244.
- Collins GS, Reitsma JB, Altman DG, Moons KGM. Transparent reporting of a multivariable prediction model for individual prognosis or diagnosis (TRIPOD): the TRIPOD statement. Ann Intern Med 2015;162:55-6. https://doi.org/10.7326/M14-0697.
- Moons KG, Royston P, Vergouwe Y, Grobbee DE, Altman DG. Prognosis and prognostic research: what, why, and how?. BMJ 2009;338. https://doi.org/10.1136/bmj.b375.
- Moons KG, Altman DG, Vergouwe Y, Royston P. Prognosis and prognostic research: application and impact of prognostic models in clinical practice. BMJ 2009;338. https://doi.org/10.1136/bmj.b606.
- Royston P, Moons KG, Altman DG, Vergouwe Y. Prognosis and prognostic research: developing a prognostic model. BMJ 2009;338. https://doi.org/10.1136/bmj.b604.
- Altman DG, Vergouwe Y, Royston P, Moons KGM. Prognosis and prognostic research: validating a prognostic model. BMJ 2009;338. https://doi.org/10.1136/bmj.b605.
- Moons KG, Kengne AP, Woodward M, Royston P, Vergouwe Y, Altman DG, et al. Risk prediction models: I. Development, internal validation, and assessing the incremental value of a new (bio)marker. Heart 2012;98:683-90. https://doi.org/10.1136/heartjnl-2011-301246.
- Peduzzi P, Concato J, Kemper E, Holford TR, Feinstein AR. A simulation study of the number of events per variable in logistic regression analysis. J Clin Epidemiol 1996;49:1373-9. https://doi.org/10.1016/S0895-4356(96)00236-3.
- Harrell F. Regression Modelling Strategies with Applications to Linear Models, Logistic Regression and Survival Analysis. New York, NY: Springer; 2001.
- White IR, Royston P, Wood AM. Multiple imputation using chained equations: issues and guidance for practice. Stat Med 2011;30:377-99. https://doi.org/10.1002/sim.4067.
- Royston P, Altman DG, Sauerbrei W. Dichotomizing continuous predictors in multiple regression: a bad idea. Stat Med 2006;25:127-41. https://doi.org/10.1002/sim.2331.
- Altman DG, Lausen B, Sauerbrei W, Schumacher M. Dangers of using ‘optimal’ cutpoints in the evaluation of prognostic factors. J Natl Cancer Inst 1994;86:829-35. https://doi.org/10.1093/jnci/86.11.829.
- Harrell FE, Lee KL, Mark DB. Multivariable prognostic models: issues in developing models, evaluating assumptions and adequacy, and measuring and reducing errors. Stat Med 1996;15:361-87.
- Steyerberg EW, Eijkemans MJ, Harrell FE, Habbema JD. Prognostic modeling with logistic regression analysis: in search of a sensible strategy in small data sets. Med Decis Making 2001;21:45-56. https://doi.org/10.1177/0272989X0102100106.
- Nagelkerke NJD. A note on a general definition of the coefficient of determination. Biometrika 1991;78:691-2. https://doi.org/10.1093/biomet/78.3.691.
- Debray TP, Damen JA, Snell KI, Ensor J, Hooft L, Reitsma JB, et al. A guide to systematic review and meta-analysis of prediction model performance. BMJ 2017;356. https://doi.org/10.1136/bmj.i6460.
- Collins GS, de Groot JA, Dutton S, Omar O, Shanyinde M, Tajar A, et al. External validation of multivariable prediction models: a systematic review of methodological conduct and reporting. BMC Med Res Methodol 2014;14. https://doi.org/10.1186/1471-2288-14-40.
- Vergouwe Y, Moons KG, Steyerberg EW. External validity of risk models: use of benchmark values to disentangle a case-mix effect from incorrect coefficients. Am J Epidemiol 2010;172:971-80. https://doi.org/10.1093/aje/kwq223.
- Harrell FEJ, Slaughter JC. Biostatistics for Biomedical Research. Nashville, TN: Department of Biostatistics, Vanderbilt University School of Medicine; 2017.
- Wilczynski NL, Haynes RB. Hedges Team . Developing optimal search strategies for detecting clinically sound prognostic studies in MEDLINE: an analytic survey. BMC Med 2004;2. https://doi.org/10.1186/1741-7015-2-23.
- Archer R, Paisley S, Essat M, Preston L, Thornhill M. Iterative sifting in the selection of research evidence: implications for reviews and other decision problems. Int J Technol Assess Health Care 2015;31:54-8. https://doi.org/10.1017/S0266462315000112.
- Bombardier C, Barbieri M, Parthan A, Zack DJ, Walker V, Macarios D, et al. The relationship between joint damage and functional disability in rheumatoid arthritis: a systematic review. Ann Rheum Dis 2012;71:836-44. https://doi.org/10.1136/annrheumdis-2011-200343.
- Navarro-Compán V, Smolen JS, Huizinga TW, Landewé R, Ferraccioli G, da Silva JA, et al. Quality indicators in rheumatoid arthritis: results from the METEOR database. Rheumatology 2015;54:1630-9. https://doi.org/10.1093/rheumatology/kev108.
- Moons KG, de Groot JA, Bouwmeester W, Vergouwe Y, Mallett S, Altman DG, et al. Critical appraisal and data extraction for systematic reviews of prediction modelling studies: the CHARMS checklist. PLOS Med 2014;11. https://doi.org/10.1371/journal.pmed.1001744.
- Wolff RF, Moons KGM, Riley RD, Whiting PF, Westwood M, Collins G, et al. PROBAST – A Risk-Of-Bias Tool for Prediction-Modelling Studies 2017;9.
- R: A Language and Environment for Statistical Computing. Vienna: R Foundation for Statistical Computing; 2004.
- Viechtbauer W. Conducting meta-analyses in R with the metafor package. J Stat Softw 2010;36:1-48. https://doi.org/10.18637/jss.v036.i03.
- Snell KI, Ensor J, Debray TP, Moons KG, Riley RD. Meta-analysis of prediction model performance across multiple studies: which scale helps ensure between-study normality for the C-statistic and calibration measures?. Stat Methods Med Res 2018;27. https://doi.org/10.1177/0962280217705678.
- Wong SS-L, Wilczynski NL, Haynes RB, Ramkissoonsingh R. Hedges Team . Developing optimal search strategies for detecting sound clinical prediction studies in MEDLINE. AMIA Annu Symp Proc 2003:728-32.
- Hayden JA, van der Windt DA, Cartwright JL, Côté P, Bombardier C. Assessing bias in studies of prognostic factors. Ann Intern Med 2013;158:280-6. https://doi.org/10.7326/0003-4819-158-4-201302190-00009.
- Moher D, Liberati A, Tetzlaff J, Altman DG. PRISMA Group . Preferred Reporting Items for Systematic Reviews and Meta-Analyses: the PRISMA statement. PLOS Med 2009;6. https://doi.org/10.1371/journal.pmed.1000097.
- Drossaers-Bakker KW, Zwinderman AH, Vliet Vlieland TP, Van Zeben D, Vos K, Breedveld FC, et al. Long-term outcome in rheumatoid arthritis: a simple algorithm of baseline parameters can predict radiographic damage, disability, and disease course at 12-year followup. Arthritis Rheum 2002;47:383-90. https://doi.org/10.1002/art.10513.
- Bombardier C, Li X, Gregersen P, Van der Heijde D, Chen M. A risk model for the prediction of radiographic progression: results from SONORA study. Arthritis Rheum 2009;61.
- Bansback N, Young A, Brennan A, Dixey J. A prognostic model for functional outcome in early rheumatoid arthritis. J Rheumatol 2006;33:1503-10.
- Berglin E, Johansson T, Sundin U, Jidell E, Wadell G, Hallmans G, et al. Radiological outcome in rheumatoid arthritis is predicted by presence of antibodies against cyclic citrullinated peptide before and at disease onset, and by IgA-RF at disease onset. Ann Rheum Dis 2006;65:453-8. https://doi.org/10.1136/ard.2005.041376.
- Brennan P, Harrison B, Barrett E, Chakravarty K, Scott D, Silman A, et al. A simple algorithm to predict the development of radiological erosions in patients with early rheumatoid arthritis: prospective cohort study. BMJ 1996;313:471-6. https://doi.org/10.1136/bmj.313.7055.471.
- Centola M, Cavet G, Shen Y, Ramanujan S, Knowlton N, Swan KA, et al. Development of a multi-biomarker disease activity test for rheumatoid arthritis. PLOS ONE 2013;8. https://doi.org/10.1371/journal.pone.0060635.
- Combe B, Dougados M, Goupille P, Cantagrel A, Eliaou JF, Sibilia J, et al. Prognostic factors for radiographic damage in early rheumatoid arthritis: a multiparameter prospective study. Arthritis Rheum 2001;44:1736-43. https://doi.org/10.1002/1529-0131(200108)44:8<1736::AID-ART308>3.0.CO;2-I.
- Combe B, Cantagrel A, Goupille P, Bozonnat MC, Sibilia J, Eliaou JF, et al. Predictive factors of 5-year health assessment questionnaire disability in early rheumatoid arthritis. J Rheumatol 2003;30:2344-9.
- de Punder YM, van Riel PL, Fransen J. A simplified baseline prediction model for joint damage progression in rheumatoid arthritis: a step toward personalized medicine. J Rheumatol 2015;42:391-7. https://doi.org/10.3899/jrheum.140327.
- de Vries-Bouwstra J, Le Cessie S, Allaart C, Breedveld F, Huizinga T. Using predicted disease outcome to provide differentiated treatment of early rheumatoid arthritis. J Rheumatol 2006;33:1747-53.
- Degboé Y, Constantin A, Nigon D, Tobon G, Cornillet M, Schaeverbeke T, et al. Predictive value of autoantibodies from anti-CCP2, anti-MCV and anti-human citrullinated fibrinogen tests, in early rheumatoid arthritis patients with rapid radiographic progression at 1 year: results from the ESPOIR cohort. RMD Open 2015;1. https://doi.org/10.1136/rmdopen-2015-000180.
- Dirven L, Visser K, Klarenbeek NB, Ewals JA, Han KH, Peeters AJ, et al. Towards personalized treatment: predictors of short-term HAQ response in recent-onset active rheumatoid arthritis are different from predictors of rapid radiological progression. Scand J Rheumatol 2012;41:15-9. https://doi.org/10.3109/03009742.2011.594964.
- Dixey J, Solymossy C, Young A, Early RA. Study. Is it possible to predict radiological damage in early rheumatoid arthritis (RA)? A report on the occurrence, progression, and prognostic factors of radiological erosions over the first 3 years in 866 patients from the Early RA Study (ERAS). J Rheumatol Suppl 2004;69:48-54.
- Fautrel B, Granger B, Combe B, Saraux A, Guillemin F, Le Loet X. Matrix to predict rapid radiographic progression of early rheumatoid arthritis patients from the community treated with methotrexate or leflunomide: results from the ESPOIR cohort. Arthritis Res Ther 2012;14. https://doi.org/10.1186/ar4092.
- Forslind K, Ahlmén M, Eberhardt K, Hafström I, Svensson B. BARFOT Study Group . Prediction of radiological outcome in early rheumatoid arthritis in clinical practice: role of antibodies to citrullinated peptides (anti-CCP). Ann Rheum Dis 2004;63:1090-5. https://doi.org/10.1136/ard.2003.014233.
- Graell E, Vazquez I, Larrosa M, Rodríguez-Cros JR, Hernández MV, Gratacós J, et al. Disability measured by the modified health assessment questionnaire in early rheumatoid arthritis: prognostic factors after two years of follow-up. Clin Exp Rheumatol 2009;27:284-91.
- Houseman M, Potter C, Marshall N, Lakey R, Cawston T, Griffiths I, et al. Baseline serum MMP-3 levels in patients with Rheumatoid Arthritis are still independently predictive of radiographic progression in a longitudinal observational cohort at 8 years follow up. Arthritis Res Ther 2012;14. https://doi.org/10.1186/ar3734.
- Saevarsdottir S, Rezaei H, Geborek P, Petersson I, Ernestam S, Albertsson K, et al. Current smoking status is a strong predictor of radiographic progression in early rheumatoid arthritis: results from the SWEFOT trial. Ann Rheum Dis 2015;74:1509-14. https://doi.org/10.1136/annrheumdis-2013-204601.
- Saevarsdottir S, Forslind K, Albertsson K, Rezaei H, Engstrom A, Geborek P, et al. Development of a Matrix Risk Model to Predict Rapid Radiographic Progression in Early Rheumatoid Arthritis. Results from a Randomized Trial Population n.d.
- Sanmartí R, Gómez-Centeno A, Ercilla G, Larrosa M, Viñas O, Vazquez I, et al. Prognostic factors of radiographic progression in early rheumatoid arthritis: a two year prospective study after a structured therapeutic strategy using DMARDs and very low doses of glucocorticoids. Clin Rheumatol 2007;26:1111-18. https://doi.org/10.1007/s10067-006-0462-4.
- Syversen SW, Gaarder PI, Goll GL, Ødegård S, Haavardsholm EA, Mowinckel P, et al. High anti-cyclic citrullinated peptide levels and an algorithm of four variables predict radiographic progression in patients with rheumatoid arthritis: results from a 10-year longitudinal study. Ann Rheum Dis 2008;67:212-17. https://doi.org/10.1136/ard.2006.068247.
- van Steenbergen HW, Tsonaka R, Huizinga TW, le Cessie S, van der Helm-van Mil AH. Predicting the severity of joint damage in rheumatoid arthritis; the contribution of genetic factors. Ann Rheum Dis 2015;74:876-82. https://doi.org/10.1136/annrheumdis-2013-204277.
- Vastesaeger N, Xu S, Aletaha D, St Clair EW, Smolen JS. A pilot risk model for the prediction of rapid radiographic progression in rheumatoid arthritis. Rheumatology 2009;48:1114-21. https://doi.org/10.1093/rheumatology/kep155.
- Visser K, Goekoop-Ruiterman YP, de Vries-Bouwstra JK, Ronday HK, Seys PE, Kerstens PJ, et al. A matrix risk model for the prediction of rapid radiographic progression in patients with rheumatoid arthritis receiving different dynamic treatment strategies: post hoc analyses from the BeSt study. Ann Rheum Dis 2010;69:1333-7. https://doi.org/10.1136/ard.2009.121160.
- De Cock D, Vanderschueren G, Meyfroidt S, Joly J, Van der Elst K, Westhovens R, et al. The performance of matrices in daily clinical practice to predict rapid radiologic progression in patients with early RA. Semin Arthritis Rheum 2014;43:627-31. https://doi.org/10.1016/j.semarthrit.2013.09.004.
- Verschueren P, Esselens G, Westhovens R. Daily practice effectiveness of a step-down treatment in comparison with a tight step-up for early rheumatoid arthritis. Rheumatology 2008;47:59-64.
- Granger B, Combe B, Le Loet X, Saraux A, Guillemin F, Fautrel B. Performance of matrices developed to identify patients with early rheumatoid arthritis with rapid radiographic progression despite methotrexate therapy: an external validation study based on the ESPOIR cohort data. RMD Open 2016;2. https://doi.org/10.1136/rmdopen-2016-000245.
- Hambardzumyan K, Bolce R, Saevarsdottir S, Cruickshank SE, Sasso EH, Chernoff D, et al. Pretreatment multi-biomarker disease activity score and radiographic progression in early RA: results from the SWEFOT trial. Ann Rheum Dis 2015;74:1102-9. https://doi.org/10.1136/annrheumdis-2013-204986.
- Heimans L, Wevers-deBoer KVC, Ronday HK, Collee G, de Sonnaville PBJ, Grillet BAM, et al. Can we prevent rapid radiological progression in patients with early rheumatoid arthritis?. Clin Rheumatol 2015;34:163-6. https://doi.org/10.1007/s10067-014-2815-8.
- Markusse IM, Dirven L, van den Broek M, Bijkerk C, Han KH, Ronday HK, et al. A multibiomarker disease activity score for rheumatoid arthritis predicts radiographic joint damage in the BeSt study. J Rheumatol 2014;41:2114-19. https://doi.org/10.3899/jrheum.131412.
- Durnez A, Vanderschueren G, Lateur L, Westhovens R, Verschueren P. Effectiveness of initial treatment allocation based on expert opinion for prevention of rapid radiographic progression in daily practice of an early RA cohort. Ann Rheum Dis 2011;70:634-7. https://doi.org/10.1136/ard.2010.135319.
- St Clair EW, van der Heijde DM, Smolen JS, Maini RN, Bathon JM, Emery P, et al. Combination of infliximab and methotrexate therapy for early rheumatoid arthritis: a randomized, controlled trial. Arthritis Rheum 2004;50:3432-43. https://doi.org/10.1002/art.20568.
- Lipsky PE, van der Heijde DM, St Clair EW, Furst DE, Breedveld FC, Kalden JR, et al. Infliximab and methotrexate in the treatment of rheumatoid arthritis. Anti-Tumor Necrosis Factor Trial in Rheumatoid Arthritis with Concomitant Therapy Study Group. N Engl J Med 2000;343:1594-602. https://doi.org/10.1056/NEJM200011303432202.
- Goekoop-Ruiterman YP, de Vries-Bouwstra JK, Allaart CF, van Zeben D, Kerstens PJ, Hazes JM, et al. Clinical and radiographic outcomes of four different treatment strategies in patients with early rheumatoid arthritis (the BeSt study): a randomized, controlled trial. Arthritis Rheum 2005;52:3381-90. https://doi.org/10.1002/art.21405.
- van Vollenhoven RF, Ernestam S, Geborek P, Petersson IF, Cöster L, Waltbrand E, et al. Addition of infliximab compared with addition of sulfasalazine and hydroxychloroquine to methotrexate in patients with early rheumatoid arthritis (SWEFOT trial): 1-year results of a randomised trial. Lancet 2009;374:459-66. https://doi.org/10.1016/S0140-6736(09)60944-2.
- Heimans L, Wevers-de Boer KV, Visser K, Goekoop RJ, van Oosterhout M, Harbers JB, et al. A two-step treatment strategy trial in patients with early arthritis aimed at achieving remission: the IMPROVED study. Ann Rheum Dis 2014;73:1356-61. https://doi.org/10.1136/annrheumdis-2013-203243.
- Anderson DR, Meyers MJ, Vernier WF, Mahoney MW, Kurumbail RG, Caspers N, et al. Pyrrolopyridine inhibitors of mitogen-activated protein kinase-activated protein kinase 2 (MK-2). J Med Chem 2007;50:2647-54. https://doi.org/10.1021/jm0611004.
- Senn S, Julious S. Measurement in clinical trials: a neglected issue for statisticians?. Stat Med 2009;28:3189-209. https://doi.org/10.1002/sim.3603.
- Vastesaeger N, Xu S, Aletaha D, Clair EWS, Smolen J. Matrix risk model for prediction of rapid radiographic progression in rheumatoid arthritis. Arthritis Rheumatol 2008;58:S755-S6.
- Lillegraven S, Paynter N, Prince FH, Shadick NA, Haavardsholm EA, Frits ML, et al. Performance of matrix-based risk models for rapid radiographic progression in a cohort of patients with established rheumatoid arthritis. Arthritis Care Res 2013;65:526-33. https://doi.org/10.1002/acr.21870.
- Thompson S, Kaptoge S, White I, Wood A, Perry P, Danesh J. Emerging Risk Factors Collaboration . Statistical methods for the time-to-event analysis of individual participant data from multiple epidemiological studies. Int J Epidemiol 2010;39:1345-59. https://doi.org/10.1093/ije/dyq063.
- Debray TP, Riley RD, Rovers MM, Reitsma JB, Moons KG. Cochrane IPD Meta-analysis Methods group . Individual participant data (IPD) meta-analyses of diagnostic and prognostic modeling studies: guidance on their use. PLOS Med 2015;12. https://doi.org/10.1371/journal.pmed.1001886.
- Pennells L, Kaptoge S, White IR, Thompson SG, Wood AM. Emerging Risk Factors Collaboration . Assessing risk prediction models using individual participant data from multiple studies. Am J Epidemiol 2014;179:621-32. https://doi.org/10.1093/aje/kwt298.
- Snell KI, Hua H, Debray TP, Ensor J, Look MP, Moons KG, et al. Multivariate meta-analysis of individual participant data helped externally validate the performance and implementation of a prediction model. J Clin Epidemiol 2016;69:40-5. https://doi.org/10.1016/j.jclinepi.2015.05.009.
- Smolen JS, van der Heijde DM, St Clair EW, Emery P, Bathon JM, Keystone E, et al. Predictors of joint damage in patients with early rheumatoid arthritis treated with high-dose methotrexate with or without concomitant infliximab: results from the ASPIRE trial. Arthritis Rheum 2006;54:702-10. https://doi.org/10.1002/art.21678.
- Taylor PC, Steuer A, Gruber J, Cosgrove DO, Blomley MJ, Marsters PA, et al. Comparison of ultrasonographic assessment of synovitis and joint vascularity with radiographic evaluation in a randomized, placebo-controlled study of infliximab therapy in early rheumatoid arthritis. Arthritis Rheum 2004;50:1107-16. https://doi.org/10.1002/art.20123.
- Garnero P, Gineyts E, Christgau S, Finck B, Delmas PD. Association of baseline levels of urinary glucosyl-galactosyl-pyridinoline and type II collagen C-telopeptide with progression of joint destruction in patients with early rheumatoid arthritis. Arthritis Rheum 2002;46:21-30. https://doi.org/10.1002/1529-0131(200201)46:1<21::AID-ART10061>3.0.CO;2-Q.
- Heimans L, van den Broek M, le Cessie S, Siegerink B, Riyazi N, Han KH, et al. Association of high body mass index with decreased treatment response to combination therapy in recent-onset rheumatoid arthritis patients. Arthritis Care Res 2013;65:1235-42. https://doi.org/10.1002/acr.21978.
- Huizinga TWJ, Connolly SE, Johnsen A, Zhu J, Furst DE, Bykerk VP, et al. Effect of anti-cyclic citrullinated peptide 2 immunoglobulin m serostatus on efficacy outcomes following treatment with abatacept plus methotrexate in the AVERT trial. Ann Rheum Dis 2015;74:234-5. https://doi.org/10.1136/annrheumdis-2015-eular.1983.
- Maska LB, Sayles HR, O’Dell JR, Curtis JR, Bridges SL, Moreland LW, et al. Serum cotinine as a biomarker of tobacco exposure and the association with treatment response in early rheumatoid arthritis. Arthritis Care Res 2012;64:1804-10. https://doi.org/10.1002/acr.21758.
- Mustila A, Korpela M, Haapala AM, Kautiainen H, Laasonen L, Möttönen T, et al. Anti-citrullinated peptide antibodies and the progression of radiographic joint erosions in patients with early rheumatoid arthritis treated with FIN-RACo combination and single disease-modifying antirheumatic drug strategies. Clin Exp Rheumatol 2011;29:500-5.
- Pasero G, Priolo F, Marubini E, Fantini F, Ferraccioli G, Magaro M, et al. Slow progression of joint damage in early rheumatoid arthritis treated with cyclosporin A. Arthritis Rheum 1996;39:1006-15. https://doi.org/10.1002/art.1780390618.
- Rau R, Herborn G, Menninger H, Sangha O. Progression in early erosive rheumatoid arthritis: 12 month results from a randomized controlled trial comparing methotrexate and gold sodium thiomalate. Br J Rheumatol 1998;37:1220-6. https://doi.org/10.1093/rheumatology/37.11.1220.
- Seegobin SD, Ma MH, Dahanayake C, Cope AP, Scott DL, Lewis CM, et al. ACPA-positive and ACPA-negative rheumatoid arthritis differ in their requirements for combination DMARDs and corticosteroids: secondary analysis of a randomized controlled trial. Arthritis Res Ther 2014;16. https://doi.org/10.1186/ar4439.
- Sokolove J, Schiff M, Fleischmann R, Weinblatt ME, Connolly SE, Johnsen A, et al. Impact of baseline anti-cyclic citrullinated peptide-2 antibody concentration on efficacy outcomes following treatment with subcutaneous abatacept or adalimumab: 2-year results from the AMPLE trial. Ann Rheum Dis 2016;75:709-14. https://doi.org/10.1136/annrheumdis-2015-207942.
- Matteson EL, Weyand CM, Fulbright JW, Christianson TJ, McClelland RL, Goronzy JJ. How aggressive should initial therapy for rheumatoid arthritis be? Factors associated with response to ‘non-aggressive’ DMARD treatment and perspective from a 2-yr open label trial. Rheumatology 2004;43:619-25. https://doi.org/10.1093/rheumatology/keh135.
- Bathon JM, Martin RW, Fleischmann RM, Tesser JR, Schiff MH, Keystone EC, et al. A comparison of etanercept and methotrexate in patients with early rheumatoid arthritis. N Engl J Med 2000;343:1586-93. https://doi.org/10.1056/NEJM200011303432201.
- van der Kooij SM, Goekoop-Ruiterman YP, de Vries-Bouwstra JK, Güler-Yüksel M, Zwinderman AH, Kerstens PJ, et al. Drug-free remission, functioning and radiographic damage after 4 years of response-driven treatment in patients with recent-onset rheumatoid arthritis. Ann Rheum Dis 2009;68:914-21. https://doi.org/10.1136/ard.2008.092254.
- Choy EH, Smith CM, Farewell V, Walker D, Hassell A, Chau L, et al. CARDERA (Combination Anti-Rheumatic Drugs in Early Rheumatoid Arthritis) Trial Group . Factorial randomised controlled trial of glucocorticoids and combination disease modifying drugs in early rheumatoid arthritis. Ann Rheum Dis 2008;67:656-63. https://doi.org/10.1136/ard.2007.076299.
- Weinblatt ME, Schiff M, Valente R, van der Heijde D, Citera G, Zhao C, et al. Head-to-head comparison of subcutaneous abatacept versus adalimumab for rheumatoid arthritis: findings of a phase IIIb, multinational, prospective, randomized study. Arthritis Rheum 2013;65:28-3. https://doi.org/10.1002/art.37711.
- Emery P, Burmester GR, Bykerk VP, Combe BG, Furst DE, Barré E, et al. Evaluating drug-free remission with abatacept in early rheumatoid arthritis: results from the phase 3b, multicentre, randomised, active-controlled AVERT study of 24 months, with a 12-month, double-blind treatment period. Ann Rheum Dis 2015;74:19-26. https://doi.org/10.1136/annrheumdis-2014-206106.
- Moreland LW, O’Dell JR, Paulus HE, Curtis JR, Bathon JM, St Clair EW, et al. A randomized comparative effectiveness study of oral triple therapy versus etanercept plus methotrexate in early aggressive rheumatoid arthritis: the treatment of Early Aggressive Rheumatoid Arthritis Trial. Arthritis Rheum 2012;64:2824-35. https://doi.org/10.1002/art.34498.
- Moons KGM, Wolff RF, Riley RD, Whiting PF, Westwood M, Collins GS, et al. PROBAST: Prediction Model Risk of Bias Assessment Tool 2017. www.systematic-reviews.com/probast (accessed 20 February 2017).
- Chatterley T, Dennett L. Utilisation of search filters in systematic reviews of prognosis questions. Health Info Libr J 2012;29:309-22. https://doi.org/10.1111/hir.12004.
- Geersing GJ, Bouwmeester W, Zuithoff P, Spijker R, Leeflang M, Moons KG, et al. Search filters for finding prognostic and diagnostic prediction studies in Medline to enhance systematic reviews. PLOS ONE 2012;7. https://doi.org/10.1371/journal.pone.0032844.
- van Eck NJ, Waltman L. Text mining and visualization using VOS Viewer. ISSI Newsletter 2011;7:50-4.
- Akhavan P, Chen M, Li X, Bombardier C. Prevalence of and predictive factors for sustained remission in early RA: results from SONORA study. Reumatol Clin Suplementos 2011;7.
- Alemao E, Joo S, Allison P, Al M, Rutten-van Molken M, L’Italien G, et al. Development and validation of a prognostic clinical model for rapid radiographic progression in patients with RA. Ann Rheum Dis 2014;73. https://doi.org/10.1136/annrheumdis-2014-eular.2233.
- Aletaha D, Alasti F, Smolen JS. Rheumatoid factor determines structural progression of rheumatoid arthritis dependent and independent of disease activity. Ann Rheum Dis 2013;72:875-80. https://doi.org/10.1136/annrheumdis-2012-201517.
- Alishiri GH, Bayat N, Fathi Ashtiani A, Tavallaii SA, Assari S, Moharamzad Y. Logistic regression models for predicting physical and mental health-related quality of life in rheumatoid arthritis patients. Mod Rheumatol 2008;18:601-8. https://doi.org/10.1007/s10165-008-0092-6.
- Allaart CF, Dirven L, Hirata S, Kerstens PJSM, Dijkmans BAC, Chernoff D, et al. A Multi-Biomarker Disease Activity (Vectra DA) Algorithm Score for Rheumatoid Arthritis Predicts Radiographic Progression in the BeSt Study n.d.
- Alves C, Luime J, Van Zeben J, Huisman M, Weel A, Barendregt P, et al. The ACR/EULAR 2010 criteria as well as other predictive algorithms for rheumatoid arthritis show good diagnostic performance. Arthritis Rheum 2010;62.
- Anderson JJ, Wells G, Verhoeven AC, Felson DT. Factors predicting response to treatment in rheumatoid arthritis: the importance of disease duration. Arthritis Rheum 2000;43:22-9. https://doi.org/10.1002/1529-0131(200001)43:1<22::AID-ANR4>3.0.CO;2-9.
- Balsa A, Del Amo J, Blanco F, Caliz R, Silva L, Sanmartí R, et al. Prediction of functional impairment and remission in rheumatoid arthritis patients by biochemical variables and genetic polymorphisms. Rheumatology 2010;49:458-66. https://doi.org/10.1093/rheumatology/kep380.
- Bakker MF, Shen Y, Bijlsma JWJ, Jacobs JWG, Lafeber FPJG, Cavet G, et al. Development of a multi-biomarker test for rheumatoid arthritis (RA) disease activity (Vectra DA). Arthritis Rheum 2010;62.
- Bakker MF, Cavet G, Jacobs JW, Bijlsma JW, Haney DJ, Shen Y, et al. Performance of a multi-biomarker score measuring rheumatoid arthritis disease activity in the CAMERA tight control study. Ann Rheum Dis 2012;71:1692-7. https://doi.org/10.1136/annrheumdis-2011-200963.
- Barnabe C, Huang WT, Sun Y, Boire G, Hitchon CA, Keystone EC, et al. Socio-demographic and health status characteristics explain clinical outcome trajectories in Early Inflammatory Arthritis (EIA). Ann Rheum Dis 2014;73. https://doi.org/10.1136/annrheumdis-2014-eular.2946.
- Bedran Z, Quiroz C, Rosa J, Catoggio LJ, Soriano ER. Validation of a prediction rule for the diagnosis of rheumatoid arthritis in patients with recent onset undifferentiated arthritis. Int J Rheumatol 2013;2013. https://doi.org/10.1155/2013/548502.
- Belghomari H, Saraux A, Allain J, Guedes C, Youinou P, Le Goff P. Risk factors for radiographic articular destruction of hands and wrists in rheumatoid arthritis. J Rheumatol 1999;26:2534-8.
- Bombardier C. Which Subgroups Are at Higher Risk of Rapid Radiographic Progression in Early Rheumatoid Arthritis: Results from SONORA Study n.d.
- Bombardier C, Chen M, Li X, van der Heijde D. Radiographic damage and radiographic progression are predictors for physical function: results from SONORA study. J Rheumatol 2010;37:1321-2.
- Bombardier C, Akhavan P, Chen M, Li X. Sustained remission in early RA: results from SONORA study. Arthritis Rheum 2010;62.
- Bombardier C, Chen M, Li X, van der Heijde D, Gregersen P. Clinical prognostic factors for radiographic damage in early rheumatoid arthritis: results from SONORA study. J Rheumatol 2010;37.
- Bøyesen P, Hoff M, Odegård S, Haugeberg G, Syversen SW, Gaarder PI, et al. Antibodies to cyclic citrullinated protein and erythrocyte sedimentation rate predict hand bone loss in patients with rheumatoid arthritis of short duration: a longitudinal study. Arthritis Res Ther 2009;11. https://doi.org/10.1186/ar2749.
- Breedveld F. Multiple faces of rheumatoid arthritis: diagnostic and therapeutic algorithms. Autoimmun Rev 2004;3.
- Britsemmer K, Ursum J, Gerritsen M, van Tuyl LH, van Tuyl L, van Schaardenburg D. Validation of the 2010 ACR/EULAR classification criteria for rheumatoid arthritis: slight improvement over the 1987 ACR criteria. Ann Rheum Dis 2011;70:1468-70. https://doi.org/10.1136/ard.2010.148619.
- Brown DA, Moore J, Johnen H, Smeets TJ, Bauskin AR, Kuffner T, et al. Serum macrophage inhibitory cytokine 1 in rheumatoid arthritis: a potential marker of erosive joint destruction. Arthritis Rheum 2007;56:753-64. https://doi.org/10.1002/art.22410.
- Bruynesteyn K, van der Heijde D, Boers M, Saudan A, Peloso P, Paulus H, et al. Detecting radiological changes in rheumatoid arthritis that are considered important by clinical experts: influence of reading with or without known sequence. J Rheumatol 2002;29:2306-12.
- Bukhari M, Lunt M, Harrison BJ, Scott DG, Symmons DP, Silman AJ. Rheumatoid factor is the major predictor of increasing severity of radiographic erosions in rheumatoid arthritis: results from the Norfolk Arthritis Register Study, a large inception cohort. Arthritis Rheum 2002;46:906-12. https://doi.org/10.1002/art.10167.
- Carrier N, Marotta A, de Brum-Fernandes AJ, Liang P, Masetto A, Ménard HA, et al. Serum levels of 14-3-3η protein supplement C-reactive protein and rheumatoid arthritis-associated antibodies to predict clinical and radiographic outcomes in a prospective cohort of patients with recent-onset inflammatory polyarthritis. Arthritis Res Ther 2016;18. https://doi.org/10.1186/s13075-016-0935-z.
- Caruso I, Santandrea S, Sarzi Puttini P, Boccassini L, Montrone F, Cazzola M, et al. Clinical, laboratory and radiographic features in early rheumatoid arthritis. J Rheumatol 1990;17:1263-7.
- Chalan P, Kroesen BJ, Van Der Geest KSM, Huitema MG, Abdulahad WH, Brouwer E, et al. Circulating CD4+CD161+ T lymphocytes are increased in seropositive arthralgia patients but decreased in patients with newly diagnosed rheumatoid arthritis. Arthritis Rheum 2013;65. https://doi.org/10.1371/journal.pone.0079370.
- Chibnik LB, Keenan BT, Cui J, Liao KP, Costenbader KH, Plenge RM, et al. Genetic risk score predicting risk of rheumatoid arthritis phenotypes and age of symptom onset. PLOS ONE 2011;6. https://doi.org/10.1371/journal.pone.0024380.
- Ciurtin C, Wyszynski K, Clarke R, Mouyis M, Manson J, Marra G. Ultrasound-detected subclinical inflammation was better reflected by the disease activity score (DAS-28) in patients with suspicion of inflammatory arthritis compared to established rheumatoid arthritis. Clin Rheumatol 2016;35:2411-19. https://doi.org/10.1007/s10067-016-3326-6.
- Conaghan PG, Hensor EMA, Keenan AM, Morgan AW, Emery P. Persistently moderate DAS-28 is not benign: loss of function occurs in early RA despite step-up DMARD therapy. Rheumatology 2010;49:1894-9. https://doi.org/10.1093/rheumatology/keq178.
- Conaghan PG. Predicting outcomes in rheumatoid arthritis. Clin Rheumatol 2011;30:41-7. https://doi.org/10.1007/s10067-010-1639-4.
- Corbett M, Young A. The Middlesex Hospital prospective study of early rheumatoid disease. Br J Rheumatol 1988;27:171-2. https://doi.org/10.1093/rheumatology/XXVII.suppl_2.171.
- Corbett M, Dalton S, Young A, Silman A, Shipley M. Factors predicting death, survival and functional outcome in a prospective study of early rheumatoid disease over fifteen years. Br J Rheumatol 1993;32:717-23. https://doi.org/10.1093/rheumatology/32.8.717.
- Courvoisier N, Dougados M, Cantagrel A, Goupille P, Meyer O, Sibilia J, et al. Prognostic factors of 10-year radiographic outcome in early rheumatoid arthritis: a prospective study. Arthritis Res Ther 2008;10. https://doi.org/10.1186/ar2498.
- Curtis JR, Haney D, Van Der Helm AHM, Shen Y, Knevel R, Cavet G, et al. Validation of a multi-biomarker test for rheumatoid arthritis (RA) disease activity (Vectra DA) in a multi-cohort study. Arthritis Rheum 2010;62.
- Curtis JR, van der Helm-van Mil AH, Knevel R, Huizinga TW, Haney DJ, Shen Y, et al. Validation of a novel multibiomarker test to assess rheumatoid arthritis disease activity. Arthritis Care Res 2012;64:1794-803. https://doi.org/10.1002/acr.21767.
- Curtis JR, Churchill M, Kivitz A, Samad A, Gauer L, Gervitz L, et al. A randomized trial comparing disease activity measures for the assessment and prediction of response in rheumatoid arthritis patients initiating certolizumab pegol. Arthritis Rheumatol 2015;67:3104-12. https://doi.org/10.1002/art.39322.
- Dawes PT, Fowler PD, Jackson R, Collins M, Shadforth MF, Stone R, et al. Prediction of progressive joint damage in patients with rheumatoid arthritis receiving gold or D-penicillamine therapy. Ann Rheum Dis 1986;45:945-9. https://doi.org/10.1136/ard.45.11.945.
- de Carvalho A, Graudal H. Radiographic progression of rheumatoid arthritis related to some clinical and laboratory parameters. Acta Radiol Diagn 1980;21:551-5. https://doi.org/10.1177/028418518002100419.
- de Punder YM, Jansen TL, van Ede AE, den Broeder AA, van Riel PL, Fransen J. Personalizing treatment targets in rheumatoid arthritis by using a simple prediction model. J Rheumatol 2015;42:398-404. https://doi.org/10.3899/jrheum.140085.
- de Vries-Bouwstra JK, Goekoop-Ruiterman YP, Verpoort KN, Schreuder GM, Ewals JA, Terwiel JP, et al. Progression of joint damage in early rheumatoid arthritis: association with HLA-DRB1, rheumatoid factor, and anti-citrullinated protein antibodies in relation to different treatment strategies. Arthritis Rheum 2008;58:1293-8. https://doi.org/10.1002/art.23439.
- Desai RJ, Solomon DH, Weinblatt ME, Shadick N, Kim SC. An external validation study reporting poor correlation between the claims-based index for rheumatoid arthritis severity and the disease activity score. Arthritis Res Ther 2015;17. https://doi.org/10.1186/s13075-015-0599-0.
- Eastman PS, Manning WC, Qureshi F, Haney D, Cavet G, Alexander C, et al. Characterization of a multiplex, 12-biomarker test for rheumatoid arthritis. J Pharm Biomed Anal 2012;70:415-24. https://doi.org/10.1016/j.jpba.2012.06.003.
- Eberhardt KB, Rydgren LC, Pettersson H, Wollheim FA. Early rheumatoid arthritis – onset, course, and outcome over 2 years. Rheumatol Int 1990;10:135-42. https://doi.org/10.1007/BF02274837.
- Eberhardt K, Fex E, Johnson U, Wollheim FA. Associations of HLA-DRB and -DQB genes with two and five year outcome in rheumatoid arthritis. Ann Rheum Dis 1996;55:34-9. https://doi.org/10.1136/ard.55.1.34.
- Elshafie AI, Nourein S, Manivel VA, Sohrabian A, Elidrisi MIE, Elagib EM, et al. IgA rheumatoid factor is more predominant than anti-CCP in Sudanese rheumatoid arthritis patients, whereas IgG RF is a strong prognostic marker and associate D with early onset. Ann Rheum Dis 2013;72. https://doi.org/10.1136/annrheumdis-2013-203224.15.
- Emery P, Gough A, Devlin J, Green M. Algorithm to predict radiological erosions in early rheumatoid arthritis. Messages from paper are incorrect. BMJ 1996;313. https://doi.org/10.1136/bmj.313.7069.1398.
- Emery P, McInnes IB, van Vollenhoven R, Kraan MC. Clinical identification and treatment of a rapidly progressing disease state in patients with rheumatoid arthritis. Rheumatology 2008;47:392-8. https://doi.org/10.1093/rheumatology/kem257.
- Forslind K, Hafström I, Ahlmén M, Svensson B. BARFOT Study Group . Sex: a major predictor of remission in early rheumatoid arthritis?. Ann Rheum Dis 2007;66:46-52. https://doi.org/10.1136/ard.2006.056937.
- Fautrel B, Granger B, Combe BG, Le Loet XX. Identification of Patients Who Could Require Early Biologic Therapy by Developing a Matrix Predicting Rapid Radiographic Progression in Early Rheumatoid Arthritis Patients Treated by Methotrexate. A Study Based on the ESPOIR Cohort Data n.d.
- Fautrel B, Granger B, Combe B, Guillemin F, Saraux A, Loet XL. Value of matrices developed to identify early rheumatoid arthritis patients with rapid radiographic progression despite methotrexate therapy: a comparison of their performance in the early rheumatoid arthritis ESPOIR cohort. Arthritis Rheumatol 2012;64:S786-S7.
- Fautrel B, Granger B, Combe B, Le Loet X. Performance of rapid radiographic progression prediction matrices in the early rheumatoid arthritis patients of the ESPOIR cohort. Ann Rheum Dis 2013;71. https://doi.org/10.1136/annrheumdis-2012-eular.2526.
- Fautrel B, Nab HW, Brault Y, Gallo G. Identifying patients with rheumatoid arthritis with moderate disease activity at risk of significant radiographic progression despite methotrexate treatment. RMD Open 2015;1. https://doi.org/10.1136/rmdopen-2014-000018.
- Fransen J, Hazes M, Visser H. Validity of the revised ACR/EULAR classification criteria for rheumatoid arthritis: predicting persistent arthritis and joint erosions after 2 years in patients with early undifferentiated arthritis. Arthritis Rheumatol 2010;62.
- Gardiner J, Su B, Ellis B, Soljak M. Estimating Under-Diagnosis of Rheumatoid Arthritis in Primary Care Data from the UK Clinical Practice Research Datalink n.d.
- Goronzy JJ, Matteson EL, Fulbright JW, Warrington KJ, Chang-Miller A, Hunder GG, et al. Prognostic markers of radiographic progression in early rheumatoid arthritis. Arthritis Rheum 2004;50:43-54. https://doi.org/10.1002/art.11445.
- Graudal N. The natural history and prognosis of rheumatoid arthritis: association of radiographic outcome with process variables, joint motion and immune proteins. Scand J Rheumatol Suppl 2004;118:1-38. https://doi.org/10.1080/03009740310004847.
- Graudal N. Scandinavian Journal of Rheumatology: preface. Scand J Rheumatol 2004;33:i-37.
- Green MJ, Gough AK, Devlin J, Smith J, Astin P, Taylor D, et al. Serum MMP-3 and MMP-1 and progression of joint damage in early rheumatoid arthritis. Rheumatology 2003;42:83-8. https://doi.org/10.1093/rheumatology/keg037.
- Haji Y, Kishimoto M, Rokutanda R, Ohde S, Deshpande GA, Ohara Y, et al. Can sustained remission of rheumatoid arthritis be predicted? An analysis from the Japanese national database of rheumatic disease (NinJa). Arthritis Rheumatol 2012;64.
- Haji Y, Kishimoto M, Rokutanda R, Min C, Ohara Y, Suyama Y, et al. A prediction rule for sustained remission of rheumatoid arthritis. Ann Rheum Dis 2013;72. https://doi.org/10.1136/annrheumdis-2013-eular.1765.
- Hambardzumyan K, Bolce R, Cavet G, Chernoff D, Haney D, Forslind K, et al. A multi-biomarker disease activity blood test (Vectra DA) correlates with radiographic progression in early rheumatoid arthritis: results from the SWEFOT trial. Ann Rheum Dis 2013;72. https://doi.org/10.1136/annrheumdis-2013-eular.1187.
- Hambardzumyan K, Bolce R, Cruickshank SE, Sasso EH, Chernoff D, Forslind K, et al. A multi-biomarker disease activity score correlates with radiographic progression in early rheumatoid arthritis: results from a randomized trial. Arthritis Rheumatol 2013;65.
- Hambardzumyan K, Saevarsdottir S, Bolce R, Forslind K, Ernestam S, Petersson I, et al. Multi-biomarker disease activity (MBDA) score and the 12 individual biomarkers in early rheumatoid arthritis patients relate differentially to clinical response and radiographic progression: results from the SWEFOT trial. Ann Rheum Dis 2013;72:A388-9. https://doi.org/10.1136/annrheumdis-2013-eular.1188.
- Hambardzumyan K, Saevarsdottir S, Bolce R, Forslind K, Ernestam S, Petersson IF, et al. In early rheumatoid arthritis, the 12 individual biomarkers that comprise the multiple biomarker disease activity score relate differentially to clinical response and radiographic progression: results from a randomized trial. Arthritis Rheumatol 2013;65.
- Harrison B, Thomson W, Symmons D, Ollier B, Wiles N, Payton T, et al. The influence of HLA-DRB1 alleles and rheumatoid factor on disease outcome in an inception cohort of patients with early inflammatory arthritis. Arthritis Rheum 1999;42:2174-83. https://doi.org/10.1002/1529-0131(199910)42:10<2174::AID-ANR19>3.0.CO;2-G.
- Harrison BJ, Silman AJ, Wiles NJ, Scott DG, Symmons DP. The association of cigarette smoking with disease outcome in patients with early inflammatory polyarthritis. Arthritis Rheum 2001;44:323-30. https://doi.org/10.1002/1529-0131(200102)44:2<323::AID-ANR49>3.0.CO;2-C.
- Hazes JM, Luime JJ. The epidemiology of early inflammatory arthritis. Nat Rev Rheumatol 2011;7:381-90. https://doi.org/10.1038/nrrheum.2011.78.
- Hendrikx J, Fransen J, Toniolo A, Van Riel PLCM. Moving towards personalized healthcare: a patient reported outcome based algorithm can aid rheumatologists and patients in monitoring rheumatoid arthritis in daily clinical practice. RMD Open 2013;65:S1135-S6.
- Hendrikx J, Fransen J, van Riel PL. Monitoring rheumatoid arthritis using an algorithm based on patient-reported outcome measures: a first step towards personalised healthcare. RMD Open 2015;1. https://doi.org/10.1136/rmdopen-2015-000114.
- Hirata S, Dirven L, Shen Y, Centola M, Cavet G, Lems WF, et al. A multi-biomarker score measures rheumatoid arthritis disease activity in the BeSt study. Rheumatology 2013;52:1202-7. https://doi.org/10.1093/rheumatology/kes362.
- Hirata S, Tanaka Y. Assessment of disease activity in rheumatoid arthritis by multi-biomarker disease activity (MBDA) score. Nihon Rinsho Meneki Gakkai Kaishi 2016;39:37-41. https://doi.org/10.2177/jsci.39.37.
- Houssien DA, Jónsson T, Davies E, Scott DL. Rheumatoid factor isotypes, disease activity and the outcome of rheumatoid arthritis: comparative effects of different antigens. Scand J Rheumatol 1998;27:46-53. https://doi.org/10.1080/030097498441173.
- James D, Young A, Kulinskaya E, Knight E, Thompson W, Ollier W, et al. Orthopaedic intervention in early rheumatoid arthritis. Occurrence and predictive factors in an inception cohort of 1064 patients followed for 5 years. Rheumatology 2004;43:369-76. https://doi.org/10.1093/rheumatology/keh059.
- Jessome M, Rodrigues I, Tomizza M, Barbosa J, Koh MXP, Beattie K, et al. Assessing the validity and reliability of a novel MRI semi-automated algorithm for quantifying bone loss in the hand. Arthritis Rheumatol 2014;66.
- Jessome M, Tomizza M, Beattie K, Bensen W, Bobba R, Cividino A, et al. Early erosions in rheumatoid arthritis (EERA) software reliably measures erosive damage on MRI in the metacarpophalangeal joints of rheumatoid arthritis patients. J Rheumatol 2015;42:1320-1.
- Kaarela K. Prognostic factors and diagnostic criteria in early rheumatoid arthritis. Scand J Rheumatol Suppl 1985;57:1-54. https://doi.org/10.3109/03009748509104317.
- Kapoor SR, Filer A, Buckley C, Young SP, Raza K. The impact of inflammation on metabolomic profiles in patients with arthritis. Ann Rheum Dis 2013;72. https://doi.org/10.1136/annrheumdis-2013-eular.2428.
- Kastbom A, Forslind K, Ernestam S, Geborek P, Karlsson JA, Petersson IF, et al. Changes in the anticitrullinated peptide antibody response in relation to therapeutic outcome in early rheumatoid arthritis: results from the SWEFOT trial. Ann Rheum Dis 2016;75:356-61. https://doi.org/10.1136/annrheumdis-2014-205698.
- Kaufmann J, Seel S, Roske AE. Comparison between several prediction scores and the new EULAR/ACR criteria for diagnosis and prognosis of rheumatoid arthritis. Ann Rheum Dis 2012;71. https://doi.org/10.1136/annrheumdis-2012-eular.294.
- Keller SD, Ware JE, Hatoum HT, Kong SX. The SF-36 Arthritis-Specific Health Index (ASHI): II. Tests of validity in four clinical trials. Med Care 1999;37:M51-60. https://doi.org/10.1097/00005650-199905001-00005.
- Kent JW. Analysis of multiple phenotypes. Genet Epidemiol 2009;33:33-9. https://doi.org/10.1002/gepi.20470.
- Kirino Y, Hama M, Takase-Minegishi K, Kunishita Y, Kishimoto D, Yoshimi R, et al. Predicting joint destruction in rheumatoid arthritis with power Doppler, anti-citrullinated peptide antibody, and joint swelling. Mod Rheumatol 2015;25:842-8. https://doi.org/10.3109/14397595.2015.1026025.
- Kita J, Kawakami A, Tamai M, Iwamoto N, Kawashiri SY, Arima K, et al. MRI-proven bone edema of wrist and finger joints at entry is the strongest predictor toward further radiographic progression in patients with undifferentiated arthritis: results from the prospective cohort at Nagasaki University. Arthritis Rheumatol 2010;62.
- Knudsen LS, Klarlund M, Skjødt H, Jensen T, Ostergaard M, Jensen KE, et al. Biomarkers of inflammation in patients with unclassified polyarthritis and early rheumatoid arthritis. Relationship to disease activity and radiographic outcome. J Rheumatol 2008;35:1277-87.
- Kojima M, Kojima T, Suzuki S, Takahashi N, Funahashi K, Asai S, et al. Patient-reported outcomes as assessment tools and predictors of long-term prognosis: a 7-year follow-up study of patients with rheumatoid arthritis. Int J Rheum Dis 2017;20:1193-200. https://doi.org/10.1111/1756-185X.12789.
- Kokebie R, Aggarwal R, Lidder S, Hakimiyan AA, Rueger DC, Block JA, et al. The role of synovial fluid markers of catabolism and anabolism in osteoarthritis, rheumatoid arthritis and asymptomatic organ donors. Arthritis Res Ther 2011;13. https://doi.org/10.1186/ar3293.
- Krabben A, Abhishek A, Britsemmer K, Filer A, Huizinga TW, Raza K, et al. Risk of rheumatoid arthritis development in patients with unclassified arthritis according to the 2010 ACR/EULAR criteria for rheumatoid arthritis. Rheumatology 2013;52:1265-70. https://doi.org/10.1093/rheumatology/ket011.
- Kroot EJ, de Jong BA, van Leeuwen MA, Swinkels H, van den Hoogen FH, van’t Hof M, et al. The prognostic value of anti-cyclic citrullinated peptide antibody in patients with recent-onset rheumatoid arthritis. Arthritis Rheum 2000;43:1831-5. https://doi.org/10.1002/1529-0131(200008)43:8<1831::AID-ANR19>3.0.CO;2-6.
- Kvien TK, Haugeberg G, Uhlig T, Falch JA, Halse JI, Lems WF, et al. Data driven attempt to create a clinical algorithm for identification of women with rheumatoid arthritis at high risk of osteoporosis. Ann Rheum Dis 2000;59:805-11. https://doi.org/10.1136/ard.59.10.805.
- Lahiri M, Luben RN, Morgan C, Bunn DK, Marshall T, Lunt M, et al. Using lifestyle factors to identify individuals at higher risk of inflammatory polyarthritis (results from the European Prospective Investigation of Cancer-Norfolk and the Norfolk Arthritis Register – the EPIC-2-NOAR Study). Ann Rheum Dis 2014;73:219-26. https://doi.org/10.1136/annrheumdis-2012-202481.
- Lahiri M, Morgan C, Luben RN, Bunn DK, Lunt M, Verstappen SM, et al. A risk score to identify individuals at higher risk of inflammatory polyarthritis: results from the European prospective investigation of cancer (Norfolk) and the Norfolk arthritis register (the EPIC-2-NOAR study). Ann Rheum Dis 2014;73:219-26. https://doi.org/10.1136/annrheumdis-2012-eular.1729.
- Landewé R. Predictive markers in rapidly progressing rheumatoid arthritis. J Rheumatol Suppl 2007;80:8-15.
- Lanfant K, Michot C, Daveau R, Milliez PY, Auquit-Auckbur I, Fardellonne P, et al. An algorithm including the intrasynovial expression of CD20 and serum biomarkers (rheumatoid factors, Anti-CCP2 and RANK-ligand) able to predict the progression of bone erosions in very early arthritis. Arthritis Rheumatol 2008;58:S759-S60.
- Lanfant-Weybel K, Michot C, Daveau R, Milliez PY, Auquit-Auckbur I, Fardellone P, et al. Synovium CD20 expression is a potential new predictor of bone erosion progression in very-early arthritis treated by sequential DMARDs monotherapy – a pilot study from the VErA cohort. Joint Bone Spine 2012;79:574-80. https://doi.org/10.1016/j.jbspin.2011.11.006.
- Lauwerys BR, Hernández-Lobato D, Gramme P, Ducreux J, Dessy A, Focant I, et al. Heterogeneity of synovial molecular patterns in patients with arthritis. PLOS ONE 2015;10. https://doi.org/10.1371/journal.pone.0122104.
- Le Loët X, Brazier M, Mejjad O, Boumier P, Daragon A, Gayet A, et al. Serum IgA rheumatoid factor and pyridinoline in very early arthritis as predictors of erosion(s) at two years: a simple model of prediction from a conservatively treated community-based inception cohort. Arthritis Care Res 2010;62:1739-47. https://doi.org/10.1002/acr.20321.
- Li W, Sasso EH, van der Helm-van Mil AH, Huizinga TW. Relationship of multi-biomarker disease activity score and other risk factors with radiographic progression in an observational study of patients with rheumatoid arthritis. Rheumatology 2016;55:357-66. https://doi.org/10.1093/rheumatology/kev341.
- Li W, Van Der Helm-Van Mil A, Knevel R, Cavet G, Huizinga T, Haney D. Biomarker-based estimates of risk of radiographic progression in the Leiden early arthritis cohort. Ann Rheum Dis 2013;72. https://doi.org/10.1136/annrheumdis-2013-eular.1225.
- Li W, Sasso EH, Emerling D, Cavet G, Ford K. Impact of a multi-biomarker disease activity test on rheumatoid arthritis treatment decisions and therapy use. Curr Med Res Opin 2013;29:85-92. https://doi.org/10.1185/03007995.2012.753042.
- Liao KP, Weinblatt ME, Cui J, Yoshioka H, Iannaccone C, Chibnik L, et al. Clinical factors that predict erosion-free status in rheumatoid arthritis. Arthritis Rheumatol 2009;60.
- Liao KP, Weinblatt ME, Cui J, Iannaccone C, Chibnik LB, Lu B, et al. Clinical predictors of erosion-free status in rheumatoid arthritis: a prospective cohort study. Rheumatology 2011;50:1473-9. https://doi.org/10.1093/rheumatology/ker129.
- Lillegraven S, Paynter N, Prince FH, Shadick NA, Haavardsholm EA, Frits ML, et al. The performance of matrix-based risk models for rapid radiographic progression in an observational cohort of established rheumatoid arthritis patients. Ann Rheum Dis 2013;71. https://doi.org/10.1136/annrheumdis-2012-eular.2902.
- Lindqvist E, Saxne T, Geborek P, Eberhardt K. Ten year outcome in a cohort of patients with early rheumatoid arthritis: health status, disease process, and damage. Ann Rheum Dis 2002;61:1055-9. https://doi.org/10.1136/ard.61.12.1055.
- Lindqvist E, Eberhardt K, Bendtzen K, Heinegård D, Saxne T. Prognostic laboratory markers of joint damage in rheumatoid arthritis. Ann Rheum Dis 2005;64:196-201. https://doi.org/10.1136/ard.2003.019992.
- Linn-Rasker SP, van der Helm-van Mil AH, Breedveld FC, Huizinga TW. Arthritis of the large joints - in particular, the knee – at first presentation is predictive for a high level of radiological destruction of the small joints in rheumatoid arthritis. Ann Rheum Dis 2007;66:646-50. https://doi.org/10.1136/ard.2006.066704.
- Lisitsyna TA, Veltishchev D, Gerasimov A, Seravina O, Kovalevskaya O, Zeltyn A, et al. Factors associated with major depressive disorder in rheumatoid arthritis patients. Ann Rheum Dis 2014;73:416-17. https://doi.org/10.1136/annrheumdis-2014-eular.2151.
- Lisitsyna TA, Veltishchev D, Gerasimov A, Seravina O, Kovalevskaya O, Zeltyn A, et al. Depression as a risk factor for joints destruction in rheumatoid arthritis patients. Ann Rheum Dis 2015;74. https://doi.org/10.1136/annrheumdis-2015-eular.2488.
- Liu Z, Sokka T, Maas K, Olsen NJ, Aune TM. Prediction of disease severity in patients with early rheumatoid arthritis by gene expression profiling. Hum Genomics Proteomics 2009;2009. https://doi.org/10.4061/2009/484351.
- Løppenthin K, Esbensen BA, Østergaard M, Jennum P, Tolver A, Aadahl M, et al. Physical activity and the association with fatigue and sleep in Danish patients with rheumatoid arthritis. Rheumatol Int 2015;35:1655-64. https://doi.org/10.1007/s00296-015-3274-5.
- Luukkainen R, Kaarela K, Isomäki H, Martio J, Kiviniemi P, Räsänen J, et al. The prediction of radiological destruction during the early stage of rheumatoid arthritis. Clin Exp Rheumatol 1983;1:295-8.
- Ma MH, Garrood T, Li W, Defranoux NA, Kingsley GH, Cope AP, et al. Multi-biomarker disease activity score is associated with power Doppler ultrasound in patients with rheumatoid arthritis in low disease activity state. Arthritis Rheum 2014;66.
- Ma MH, Ramanujan S, Cavet G, Haney D, Kingsley GH, Scott D, et al. Biomarker signature in rheumatoid arthritis patients with low disease activity: the REMIRA study. Rheumatology 2012;51.
- Ma MH, Ramanujan S, Cavet G, Haney DJ, Zhao X, Eastman PS, et al. Investigation of a multi-biomarker disease activity (Vectra DA) signature and algorithm score in rheumatoid arthritis patients with low disease activity: the REMIRA study. Arthritis Rheum 2011;63.
- Ma MH, Scott IC, Dahanayake C, Cope AP, Scott DL. Clinical and serological predictors of remission in rheumatoid arthritis are dependent on treatment regimen. J Rheumatol 2014;41:1298-303. https://doi.org/10.3899/jrheum.131401.
- Ma MH, Ibrahim F, Walker D, Hassell A, Choy EH, Kiely PD, et al. Remission in early rheumatoid arthritis: predicting treatment response. J Rheumatol 2012;39:470-5. https://doi.org/10.3899/jrheum.110169.
- Machold KP, Stamm TA, Eberl GJ, Nell VK, Dunky A, Uffmann M, et al. Very recent onset arthritis – clinical, laboratory, and radiological findings during the first year of disease. J Rheumatol 2002;29:2278-87.
- Mamehara A, Sugimoto T, Sugiyama D, Morinobu S, Tsuji G, Kawano S, et al. Serum matrix metalloproteinase-3 as predictor of joint destruction in rheumatoid arthritis, treated with non-biological disease modifying anti-rheumatic drugs. Kobe J Med Sci 2010;56:E98-107.
- Mathsson L, Mullazehi M, Wick MC, Sjöberg O, van Vollenhoven R, Klareskog L, et al. Antibodies against citrullinated vimentin in rheumatoid arthritis: higher sensitivity and extended prognostic value concerning future radiographic progression as compared with antibodies against cyclic citrullinated peptides. Arthritis Rheum 2008;58:36-45. https://doi.org/10.1002/art.23188.
- Mei L, Li X, Yang K, Cui J, Fang B, Guo X, et al. Evaluating gene × gene and gene × smoking interaction in rheumatoid arthritis using candidate genes in GAW15. BMC Proc 2007;1. https://doi.org/10.1186/1753-6561-1-s1-s17.
- Miller A, Nightingale AL, Sammon CJ, Holt T, Mahtani KR, McHugh N, et al. The diagnostic accuracy of rheumatoid factor testing in primary Care. Arthritis Rheum 2013;65:S964-S5.
- Miller A, Nightingale AL, Sammon CJ, Mahtani KR, Holt T, McHugh N, et al. Negative rheumatoid factor in primary care delays referral of patients with rheumatoid arthritis. Rheumatology 2014;53. https://doi.org/10.1093/rheumatology/keu086.002.
- Mierau R, Genth E. Diagnosis and prognosis of early rheumatoid arthritis, with special emphasis on laboratory analysis. Clin Chem Lab Med 2006;44:138-43. https://doi.org/10.1515/CCLM.2006.026.
- Möller B, Scherer A, Förger F, Villiger PM, Finckh A. Swiss Clinical Quality Management Program for Rheumatic Diseases . Anaemia may add information to standardised disease activity assessment to predict radiographic damage in rheumatoid arthritis: a prospective cohort study. Ann Rheum Dis 2014;73:691-6. https://doi.org/10.1136/annrheumdis-2012-202709.
- Morel J, Combe B. How to predict prognosis in early rheumatoid arthritis. Best Pract Res Clin Rheumatol 2005;19:137-46. https://doi.org/10.1016/j.berh.2004.08.008.
- Möttönen TT. Prediction of erosiveness and rate of development of new erosions in early rheumatoid arthritis. Ann Rheum Dis 1988;47:648-53. https://doi.org/10.1136/ard.47.8.648.
- Möttönen T, Paimela L, Leirisalo-Repo M, Kautiainen H, Ilonen J, Hannonen P. Only high disease activity and positive rheumatoid factor indicate poor prognosis in patients with early rheumatoid arthritis treated with ‘sawtooth’ strategy. Ann Rheum Dis 1998;57:533-9. https://doi.org/10.1136/ard.57.9.533.
- Nakashima Y, Tamai M, Kita J, Michitsuji T, Shimizu T, Fukui S, et al. Magnetic resonance imaging bone edema at enrollment predicts rapid radiographic progression in patients with early RA: results from the Nagasaki University early arthritis cohort. J Rheumatol 2016;43:1278-84. https://doi.org/10.3899/jrheum.150988.
- Nell VP, Machold KP, Stamm TA, Eberl G, Heinzl H, Uffmann M, et al. Autoantibody profiling as early diagnostic and prognostic tool for rheumatoid arthritis. Ann Rheum Dis 2005;64:1731-6. https://doi.org/10.1136/ard.2005.035691.
- Nielen MM, van der Horst AR, van Schaardenburg D, van der Horst-Bruinsma IE, van de Stadt RJ, Aarden L, et al. Antibodies to citrullinated human fibrinogen (ACF) have diagnostic and prognostic value in early arthritis. Ann Rheum Dis 2005;64:1199-204. https://doi.org/10.1136/ard.2004.029389.
- Nishiguchi S, Ito H, Yamada M, Yoshitomi H, Furu M, Ito T, et al. Self-assessment tool of disease activity of rheumatoid arthritis by using a smartphone application. Telemed J E Health 2014;20:235-40. https://doi.org/10.1089/tmj.2013.0162.
- Nishiguchi S, Ito H, Yamada M, Yoshitomi H, Furu M, Ito T, et al. Self-assessment of rheumatoid arthritis disease activity using a smartphone application. Development and 3-month feasibility study. Methods Inf Med 2016;55:65-9. https://doi.org/10.3414/ME14-01-0106.
- Norton S, Sacker A, Dixey J, Done J, Williams P, Young A. Early Rheumatoid Arthritis Study . Trajectories of functional limitation in early rheumatoid arthritis and their association with mortality. Rheumatology 2013;52:2016-24. https://doi.org/10.1093/rheumatology/ket253.
- Norton S, Koduri G, Nikiphorou E, Dixey J, Williams P, Young A. A study of baseline prevalence and cumulative incidence of comorbidity and extra-articular manifestations in RA and their impact on outcome. Rheumatology 2013;52:99-110. https://doi.org/10.1093/rheumatology/kes262.
- Norton S, Fu B, Scott DL, Deighton C, Symmons DP, Wailoo AJ, et al. Health Assessment Questionnaire disability progression in early rheumatoid arthritis: systematic review and analysis of two inception cohorts. Semin Arthritis Rheum 2014;44:131-44. https://doi.org/10.1016/j.semarthrit.2014.05.003.
- Ødegård S, Landewé R, van der Heijde D, Kvien TK, Mowinckel P, Uhlig T. Association of early radiographic damage with impaired physical function in rheumatoid arthritis: a ten-year, longitudinal observational study in 238 patients. Arthritis Rheum 2006;54:68-75. https://doi.org/10.1002/art.21548.
- Papadopoulos NG, Alamanos Y, Voulgari PV, Epagelis EK, Tsifetaki N, Drosos AA. Does cigarette smoking influence disease expression, activity and severity in early rheumatoid arthritis patients?. Clin Exp Rheumatol 2005;23:861-6.
- Park GS, Wong WK, Elashoff DA, Khanna D, Gold RH, Paulus HE. Patterns of radiographic outcomes in early, seropositive rheumatoid arthritis: a baseline analysis. Contemp Clin Trials 2011;32:160-8. https://doi.org/10.1016/j.cct.2010.11.009.
- Park GS, Wong WK, Khanna D, Gold RH, Paulus HE. Examining radiographic outcomes over time. Rheumatol Int 2014;34:271-9. https://doi.org/10.1007/s00296-013-2879-9.
- Plant MJ, Saklatvala J, Borg AA, Jones PW, Dawes PT. Measurement and prediction of radiological progression in early rheumatoid arthritis. J Rheumatol 1994;21:1808-13.
- Plant MJ, Williams AL, O’Sullivan MM, Lewis PA, Coles EC, Jessop JD. Relationship between time-integrated C-reactive protein levels and radiologic progression in patients with rheumatoid arthritis. Arthritis Rheum 2000;43:1473-7. https://doi.org/10.1002/1529-0131(200007)43:7<1473::AID-ANR9>3.0.CO;2-N.
- Posthumus MD, Limburg PC, Westra J, van Leeuwen MA, van Rijswijk MH. Serum matrix metalloproteinase 3 in early rheumatoid arthritis is correlated with disease activity and radiological progression. J Rheumatol 2000;27:2761-8.
- Putrik P, Ramiro S, Lie E, Keszei AP, Kvien TK, van der Heijde D, et al. Less educated and older patients have reduced access to biologic DMARDs even in a country with highly developed social welfare (Norway): results from Norwegian cohort study NOR-DMARD. Rheumatology 2016;55:1217-24. https://doi.org/10.1093/rheumatology/kew048.
- Quinn MA, Green MJ, Marzo-Ortega H, Proudman S, Karim Z, Wakefield RJ, et al. Prognostic factors in a large cohort of patients with early undifferentiated inflammatory arthritis after application of a structured management protocol. Arthritis Rheum 2003;48:3039-45. https://doi.org/10.1002/art.11269.
- Quinn MA, Gough AK, Green MJ, Devlin J, Hensor EM, Greenstein A, et al. Anti-CCP antibodies measured at disease onset help identify seronegative rheumatoid arthritis and predict radiological and functional outcome. Rheumatology 2006;45:478-80. https://doi.org/10.1093/rheumatology/kei203.
- Rech J, Hueber AJ, Finzel S, Englbrecht M, Haschka J, Manger B, et al. Prediction of disease relapses by multibiomarker disease activity and autoantibody status in patients with rheumatoid arthritis on tapering DMARD treatment. Ann Rheum Dis 2016;75:1637-44. https://doi.org/10.1136/annrheumdis-2015-207900.
- Rezaei H, Saevarsdottir S, Forslind K, Albertsson K, Wallin H, Bratt J, et al. In early rheumatoid arthritis, patients with a good initial response to methotrexate have excellent 2-year clinical outcomes, but radiological progression is not fully prevented: data from the methotrexate responders population in the SWEFOT trial. Ann Rheum Dis 2012;71:186-91. https://doi.org/10.1136/annrheumdis-2011-200038.
- Rhodes B, Merriman ME, Harrison A, Nissen MJ, Smith M, Stamp L, et al. A genetic association study of serum acute-phase C-reactive protein levels in rheumatoid arthritis: implications for clinical interpretation. PLOS Med 2010;7. https://doi.org/10.1371/journal.pmed.1000341.
- Saevarsdottir S, Wallin H, Seddighzadeh M, Ernestam S, Geborek P, Petersson IF, et al. Predictors of response to methotrexate in early DMARD naive rheumatoid arthritis: results from the initial open-label phase of the SWEFOT trial. Ann Rheum Dis 2011;70:469-75. https://doi.org/10.1136/ard.2010.139212.
- Saevarsdottir S, Wedrén S, Seddighzadeh M, Bengtsson C, Wesley A, Lindblad S, et al. Patients with early rheumatoid arthritis who smoke are less likely to respond to treatment with methotrexate and tumor necrosis factor inhibitors: observations from the Epidemiological Investigation of Rheumatoid Arthritis and the Swedish Rheumatology Register cohorts. Arthritis Rheum 2011;63:26-3. https://doi.org/10.1002/art.27758.
- Salaffi F, Ciapetti A, Gasparini S, Filippucci E, Carotti M, Grassi W. A predictive algorithm combining routine assessment and power Doppler ultrasonography for the development of rheumatoid arthritis from an early-onset undifferentiated arthritis. Arthritis Rheum 2009;60.
- Scherer HU, van der Woude D, Willemze A, Trouw LA, Knevel R, Syversen SW, et al. Distinct ACPA fine specificities, formed under the influence of HLA shared epitope alleles, have no effect on radiographic joint damage in rheumatoid arthritis. Ann Rheum Dis 2011;70:1461-4. https://doi.org/10.1136/ard.2010.146506.
- Scott DL. Prognostic factors in early rheumatoid arthritis. Rheumatology 2000;39:24-9. https://doi.org/10.1093/oxfordjournals.rheumatology.a031490.
- Scott IC, Steer S, Tan R, Forabosco P, Morgan AW, Hinks A, et al. Prediction model for rheumatoid arthritis: modelling 46 genetic risk variants with smoking. Lancet 2013;381. https://doi.org/10.1016/S0140-6736(13)60537-1.
- Semb AG. Development of a transatlantic cardiovascular risk calculator for rheumatoid arthritis (ATACC-RA). Circulation 2014;130.
- Semb AG. Development of a transatlantic cardiovascular risk calculator for rheumatoid arthritis. Eur J Prev Cardiol 2015;22.
- Shen Y, Dirven L, Cavet G, Centola M, Dijkmans BAC, Hesterberg LK, et al. Serum biomarkers predict progressive structural damage in the BeSt study. Arthritis Rheum 2010;62.
- Shen Y, Liao KP, Cai T. Sparse kernel machine regression for ordinal outcomes. Biometrics 2015;71:63-70. https://doi.org/10.1111/biom.12223.
- Sjöblom KG, Saxne T, Pettersson H, Wollheim FA. Factors related to the progression of joint destruction in rheumatoid arthritis. Scand J Rheumatol 1984;13:21-7. https://doi.org/10.3109/03009748409102663.
- Smolen JS, Aletaha D. Forget personalised medicine and focus on abating disease activity. Ann Rheum Dis 2013;72:3-6. https://doi.org/10.1136/annrheumdis-2012-202361.
- Solomon DH, Greenberg J, Curtis JR, Liu M, Farkouh ME, Tsao P, et al. Derivation and internal validation of an expanded cardiovascular risk prediction score for rheumatoid arthritis: a Consortium of Rheumatology Researchers of North America Registry Study. Arthritis Rheumatol 2015;67:1995-2003. https://doi.org/10.1002/art.39195.
- Sparks JA, Chen CY, Jiang X, Hiraki LT, Klareskog L, Alfredsson L, et al. Performance of prediction models for rheumatoid arthritis serologic phenotypes among women using family history, genetics and environmental factors. Arthritis Rheum 2013;65:S1131-S2.
- Stucki G, Langenegger T. Management of rheumatoid arthritis. Curr Opin Rheumatol 1997;9:229-35. https://doi.org/10.1097/00002281-199705000-00009.
- Suarez-Almazor ME, Soskolne CL, Saunders LD, Russell AS. Outcome in rheumatoid arthritis. A 1985 inception cohort study. J Rheumatol 1994;21:1438-46.
- Syversen SW, Goll GL, van der Heijde D, Landewé R, Lie BA, Odegård S, et al. Prediction of radiographic progression in rheumatoid arthritis and the role of antibodies against mutated citrullinated vimentin: results from a 10-year prospective study. Ann Rheum Dis 2010;69:345-51. https://doi.org/10.1136/ard.2009.113092.
- Tchetverikov I, Lard LR, DeGroot J, Verzijl N, TeKoppele JM, Breedveld FC, et al. Matrix metalloproteinases-3, -8, -9 as markers of disease activity and joint damage progression in early rheumatoid arthritis. Ann Rheum Dis 2003;62:1094-9. https://doi.org/10.1136/ard.62.11.1094.
- Teitsson I, Withrington RH, Seifert MH, Valdimarsson H. Prospective study of early rheumatoid arthritis. I. Prognostic value of IgA rheumatoid factor. Ann Rheum Dis 1984;43:673-8. https://doi.org/10.1136/ard.43.5.673.
- Ting G, Schneeweiss S, Scranton R, Katz JN, Weinblatt ME, Young M, et al. Development of a health care utilisation data-based index for rheumatoid arthritis severity: a preliminary study. Arthritis Res Ther 2008;10. https://doi.org/10.1186/ar2482.
- Tobón G, Saraux A, Lukas C, Gandjbakhch F, Gottenberg JE, Mariette X, et al. First-year radiographic progression as a predictor of further progression in early arthritis: results of a large national French cohort. Arthritis Care Res 2013;65:1907-15. https://doi.org/10.1002/acr.22078.
- Uhlig T, Smedstad LM, Vaglum P, Moum T, Gérard N, Kvien TK. The course of rheumatoid arthritis and predictors of psychological, physical and radiographic outcome after 5 years of follow-up. Rheumatology 2000;39:732-41. https://doi.org/10.1093/rheumatology/39.7.732.
- van Beers-Tas MH, Turk SA, van Schaardenburg D. How does established rheumatoid arthritis develop, and are there possibilities for prevention?. Best Pract Res Clin Rheumatol 2015;29:527-42. https://doi.org/10.1016/j.berh.2015.09.001.
- van Den Broek M, Dirven L, De Vries-Bouwstra J, Dehpoor A, Goekoop-Ruiterman Y, Han K, et al. The clinical relevance of rapid radiological progression in the first year of treatment during 8 years of follow-up of early rheumatoid arthritis patients. Ann Rheum Dis 2013;71. https://doi.org/10.1136/annrheumdis-2012-eular.2516.
- van den Broek M, Dirven L, de Vries-Bouwstra JK, Dehpoor AJ, Goekoop-Ruiterman YP, Gerards AH, et al. Rapid radiological progression in the first year of early rheumatoid arthritis is predictive of disability and joint damage progression during 8 years of follow-up. Ann Rheum Dis 2012;71:1530-3. https://doi.org/10.1136/annrheumdis-2011-201212.
- van der Heijde DM, van Riel PL, van Leeuwen MA, van ‘t Hof MA, van Rijswijk MH, van de Putte LB. Prognostic factors for radiographic damage and physical disability in early rheumatoid arthritis. A prospective follow-up study of 147 patients. Br J Rheumatol 1992;31:519-25. https://doi.org/10.1093/rheumatology/31.8.519.
- van der Heide A, Remme CA, Hofman DM, Jacobs JW, Bijlsma JW. Prediction of progression of radiologic damage in newly diagnosed rheumatoid arthritis. Arthritis Rheum 1995;38:1466-74. https://doi.org/10.1002/art.1780381013.
- van der Helm-van Mil AH, Verpoort KN, Breedveld FC, Toes RE, Huizinga TW. Antibodies to citrullinated proteins and differences in clinical progression of rheumatoid arthritis. Arthritis Res Ther 2005;7:R949-58. https://doi.org/10.1186/ar1767.
- van der Helm-van Mil AH, Detert J, le Cessie S, Filer A, Bastian H, Burmester GR, et al. Validation of a prediction rule for disease outcome in patients with recent-onset undifferentiated arthritis: moving toward individualized treatment decision-making. Arthritis Rheum 2008;58:2241-7. https://doi.org/10.1002/art.23681.
- van der Helm-van Mil AH, Toes RE, Huizinga TW. Genetic variants in the prediction of rheumatoid arthritis. Ann Rheum Dis 2010;69:1694-6. https://doi.org/10.1136/ard.2009.123828.
- van der Helm-van Mil AH, Knevel R, Cavet G, Huizinga TW, Haney DJ. An evaluation of molecular and clinical remission in rheumatoid arthritis by assessing radiographic progression. Rheumatology 2013;52:839-46. https://doi.org/10.1093/rheumatology/kes378.
- van Jaarsveld CH, ter Borg EJ, Jacobs JW, Schellekens GA, Gmelig-Meyling FH, van Booma-Frankfort C, et al. The prognostic value of the antiperinuclear factor, anti-citrullinated peptide antibodies and rheumatoid factor in early rheumatoid arthritis. Clin Exp Rheumatol 1999;17:689-97.
- van Nies JA, van Steenbergen HW, Krabben A, Stomp W, Huizinga TW, Reijnierse M, et al. Evaluating processes underlying the predictive value of baseline erosions for future radiological damage in early rheumatoid arthritis. Ann Rheum Dis 2015;74:883-9. https://doi.org/10.1136/annrheumdis-2013-204659.
- van Steenbergen HW, van Nies JA, van der Helm-van Mil AH. Anaemia to predict radiographic progression in rheumatoid arthritis. Ann Rheum Dis 2013;72. https://doi.org/10.1136/annrheumdis-2013-203718.
- van Zeben D, Hazes JM, Zwinderman AH, Cats A, Schreuder GM, D’Amaro J, et al. Association of HLA-DR4 with a more progressive disease course in patients with rheumatoid arthritis. Results of a followup study. Arthritis Rheum 1991;34:822-30. https://doi.org/10.1002/art.1780340707.
- van Zeben D, Hazes JM, Zwinderman AH, Vandenbroucke JP, Breedveld FC. Factors predicting outcome of rheumatoid arthritis: results of a followup study. J Rheumatol 1993;20:1288-96.
- Varache S, Cornec D, Morvan J, Devauchelle-Pensec V, Berthelot JM, Le Henaff-Bourhis C, et al. Diagnostic accuracy of ACR/EULAR 2010 criteria for rheumatoid arthritis in a 2-year cohort. J Rheumatol 2011;38:1250-7. https://doi.org/10.3899/jrheum.101227.
- Versteegh MM, Rowen D, Brazier JE, Stolk EA. Mapping onto Eq-5 D for patients in poor health. Health Qual Life Outcomes 2010;8. https://doi.org/10.1186/1477-7525-8-141.
- Vesperini V, Lukas C, Fautrel B, Le Loet X, Rincheval N, Combe B. Association of tobacco exposure and reduction of radiographic progression in early rheumatoid arthritis: results from a French multicenter cohort. Arthritis Care Res 2013;65:1899-906. https://doi.org/10.1002/acr.22057.
- Visser H, le Cessie S, Vos K, Breedveld FC, Hazes JM. How to diagnose rheumatoid arthritis early: a prediction model for persistent (erosive) arthritis. Arthritis Rheum 2002;46:357-65. https://doi.org/10.1002/art.10117.
- Visser K, Goekoop-Ruiterman YPM, De Vries-Bouwstra JK, Ronday HK, Seys PEH, Kerstens P, et al. The BeSt matrix model in recent-onset rheumatoid arthritis patients: individual prediction of rapid radiographic progression and numbers-needed-to-treat with initial combination therapy. Arthritis Rheumatol 2009;60.
- Visser H, Hazes JM, Luime J. The clinical relevance of a prediction rule for disease outcome in patients with undifferentiated arthritis: comment on the article by van der Helm-van Mil et al. Arthritis Rheum 2009;60:2208-9. https://doi.org/10.1002/art.24623.
- Visvanathan S, Marini JC, Smolen JS, Clair EW, Pritchard C, Shergy W, et al. Changes in biomarkers of inflammation and bone turnover and associations with clinical efficacy following infliximab plus methotrexate therapy in patients with early rheumatoid arthritis. J Rheumatol 2007;34:1465-74.
- Wessels JA, van der Kooij SM, le Cessie S, Kievit W, Barerra P, Allaart CF, et al. A clinical pharmacogenetic model to predict the efficacy of methotrexate monotherapy in recent-onset rheumatoid arthritis. Arthritis Rheum 2007;56:1765-75. https://doi.org/10.1002/art.22640.
- Westedt ML, Daha MR, Baldwin WM, Stijnen T, Cats A. Serum immune complexes containing IgA appear to predict erosive arthritis in a longitudinal study in rheumatoid arthritis. Ann Rheum Dis 1986;45:809-15. https://doi.org/10.1136/ard.45.10.809.
- Weyand CM, Hicok KC, Conn DL, Goronzy JJ. The influence of HLA-DRB1 genes on disease severity in rheumatoid arthritis. Ann Intern Med 1992;117:801-6. https://doi.org/10.7326/0003-4819-117-10-801.
- Widdifield J, Bernatsky S, Paterson JM, Tu K, Ng R, Thorne JC, et al. Accuracy of Canadian health administrative databases in identifying patients with rheumatoid arthritis: a validation study using the medical records of rheumatologists. Arthritis Care Res 2013;65:1582-91. https://doi.org/10.1002/acr.22031.
- Wolfe F, Sharp JT. Radiographic outcome of recent-onset rheumatoid arthritis: a 19-year study of radiographic progression. Arthritis Rheum 1998;41:1571-82. https://doi.org/10.1002/1529-0131(199809)41:9<1571::AID-ART7>3.0.CO;2-R.
- Wolfe F. A reappraisal of HAQ disability in rheumatoid arthritis. Arthritis Rheum 2000;43:2751-61. https://doi.org/10.1002/1529-0131(200012)43:12<2751::AID-ANR15>3.0.CO;2-6.
- Wolfe F. The effect of smoking on clinical, laboratory, and radiographic status in rheumatoid arthritis. J Rheumatol 2000;27:630-7.
- Wong AL, Harker JO, Mittman BS, Levy GD, Bulpitt KJ, Colburn KK, et al. Development and evaluation of a patient self-report case-finding method for rheumatoid arthritis. Semin Arthritis Rheum 2004;34:484-99. https://doi.org/10.1016/j.semarthrit.2003.12.002.
- Yamanaka H, Matsuda Y, Tanaka M, Sendo W, Nakajima H, Taniguchi A, et al. Serum matrix metalloproteinase 3 as a predictor of the degree of joint destruction during the six months after measurement, in patients with early rheumatoid arthritis. Arthritis Rheum 2000;43:852-8. https://doi.org/10.1002/1529-0131(200004)43:4<852::AID-ANR16>3.0.CO;2-7.
- Young A, Bielawska C, Corbett M, Roitt I. A prospective study of early onset rheumatoid arthritis over fifteen years: prognostic features and outcome. Clin Rheumatol 1987;6:12-9. https://doi.org/10.1007/BF02203380.
- Young A, Corbett M, Winfield J, Jaqueremada D, Williams P, Papasavvas G, et al. A prognostic index for erosive changes in the hands, feet, and cervical spines in early rheumatoid arthritis. Br J Rheumatol 1988;27:94-101. https://doi.org/10.1093/rheumatology/27.2.94.
- Young A, van der Heijde DM. Can we predict aggressive disease?. Baillieres Clin Rheumatol 1997;11:27-48. https://doi.org/10.1016/S0950-3579(97)80031-3.
- Young A, Emery P, Gough A, James D, Prouse P, Williams P, et al. Socioeconomic deprivation and rheumatoid disease: what lessons for the health service?. Ann Rheum Dis 2000;59:794-9. https://doi.org/10.1136/ard.59.10.794.
- Young-Min S, Cawston T, Marshall N, Coady D, Christgau S, Saxne T, et al. Biomarkers predict radiographic progression in early rheumatoid arthritis and perform well compared with traditional markers. Arthritis Rheum 2007;56:3236-47. https://doi.org/10.1002/art.22923.
- Addimanda O, Possemato N, Macchioni P, Salvarani C. Efficacy and safety of tocilizumab in refractory rheumatoid arthritis: a real life cohort from a single centre. Clin Exp Rheumatol 2014;32:460-4.
- Aga AB, Lie E, Uhlig T, Kvien TK, Haavardsholm E. Clinical predictors of response to methotrexate treatment in DMARD naive patients with early rheumatoid arthritis: results from a longitudinal observational study. Ann Rheum Dis 2013;71. https://doi.org/10.1136/annrheumdis-2012-eular.3028.
- Ajeganova S, Andersson ML, Hafström I. BARFOT Study Group . Association of obesity with worse disease severity in rheumatoid arthritis as well as with comorbidities: a long-term followup from disease onset. Arthritis Care Res 2013;65:78-87. https://doi.org/10.1002/acr.21710.
- Akdemir G, Heimans L, Wevers-de Boer KVC, Verheul MK, Schouffoer AA, Van Oosterhout M, et al. Radiological outcomes after two years of remission steered treatment in early arthritis patients. Arthritis Rheumatol 2014;66.
- Akdemir G, Markusse I, Dirven L, Broek M, Molenaar E, Schouffoer A, et al. ACPA-negative RA patients benefit from initial combination therapy with early clinical improvement-a sub-analysis of the best study. Ann Rheum Dis 2014;73:271-2. https://doi.org/10.1136/annrheumdis-2014-eular.2142.
- Akdemir G, Verheul MK, Heimans L, Wevers-de Boer KV, Goekoop-Ruiterman YP, van Oosterhout M, et al. Predictive factors of radiological progression after 2 years of remission-steered treatment in early arthritis patients: a post hoc analysis of the IMPROVED study. RMD Open 2016;2. https://doi.org/10.1136/rmdopen-2015-000172.
- Akhavan P, Tomlinson G, Fortin P, Bombardier C. The impact of reaching low disease activity in the first year on future disability and damage in patients with early rheumatoid arthritis. J Rheumatol 2013;40.
- Akhavan P, Jacob B, Fortin P, Tomlinson G, Bombardier C. Predictive validity of low disease activity using patient reported measures on long-term outcomes in early rheumatoid arthritis-results from study of new onset rheumatoid arthritis and Ontario best practices initiative. J Rheumatol 2014;41:1435-6.
- Alemao E, Joo S, Kawabata H, Al MJ, Allison PD, Rutten-van Mölken MP, et al. Effects of achieving target measures in rheumatoid arthritis on functional status, quality of life, and resource utilization: analysis of clinical practice data. Arthritis Care Res 2016;68:308-17. https://doi.org/10.1002/acr.22678.
- Alessandri C, Bombardieri M, Papa N, Cinquini M, Magrini L, Tincani A, et al. Decrease of anti-cyclic citrullinated peptide antibodies and rheumatoid factor following anti-TNFalpha therapy (infliximab) in rheumatoid arthritis is associated with clinical improvement. Ann Rheum Dis 2004;63:1218-21. https://doi.org/10.1136/ard.2003.014647.
- Aletaha D, Funovits J, Keystone EC, Smolen JS. Disease activity early in the course of treatment predicts response to therapy after one year in rheumatoid arthritis patients. Arthritis Rheum 2007;56:3226-35. https://doi.org/10.1002/art.22943.
- Aletaha D, Funovits J, Breedveld FC, Sharp J, Segurado O, Smolen JS. Rheumatoid arthritis joint progression in sustained remission is determined by disease activity levels preceding the period of radiographic assessment. Arthritis Rheum 2009;60:1242-9. https://doi.org/10.1002/art.24433.
- Aletaha D, Alasti F, Smolen JS. Rituximab dissociates the tight link between disease activity and joint damage in rheumatoid arthritis patients. Ann Rheum Dis 2013;72:7-12. https://doi.org/10.1136/annrheumdis-2012-201970.
- Alivernini S, Peluso G, Correra M, Fedele AL, Gremese E, Ferraccioli G. Ultrasonography as useful tool to identify rheumatoid arthritis patients in clinical remission for tapering or withdrawal TNFa blockers without disease relapse. Ann Rheum Dis 2014;73. https://doi.org/10.1136/annrheumdis-2014-eular.4474.
- Alivernini S, Peluso G, Fedele AL, Tolusso B, Gremese E, Ferraccioli G. Tapering and discontinuation of TNF-α blockers without disease relapse using ultrasonography as a tool to identify patients with rheumatoid arthritis in clinical and histological remission. Arthritis Res Ther 2016;18. https://doi.org/10.1186/s13075-016-0927-z.
- Allaart CF, Goekoop-Ruiterman YP, de Vries-Bouwstra JK, Breedveld FC, Dijkmans BA. FARR study group . Aiming at low disease activity in rheumatoid arthritis with initial combination therapy or initial monotherapy strategies: the BeSt study. Clin Exp Rheumatol 2006;24:77-82.
- Allaart CF. When to start which DMARDs in rheumatoid arthritis?. Scand J Rheumatol 2010;39.
- Ammitzbøll CG, Jensenius JC, Ellingsen T, Thiel S, Horslev-Petersen K, Hetland M, et al. M-ficolin, an activator of the complement system, predicts DAS28 remission in early DMARD-naive rheumatoid arthritis. Scand J Rheumatol 2012;41.
- Ammitzbøll CG, Jensenius JC, Ellingsen T, Thiel S, Kim HP, Hetland ML, et al. M-ficolin, an activator of the complement system, is the strongest predictor of both DAS28 remission and low disease activity in a cohort of 180 early DMARD naive rheumatoid arthritis patients followed in the opera-study. Scand J Rheumatol 2012;64.
- Ammitzbøll CG, Jensenius JC, Ellingsen T, Thiel S, Horslev-Petersen K, Hetland M, et al. M-ficolin, an activator of the complement system, predicts DAS28 remission in early DMARD naive rheumatoid arthritis. Ann Rheum Dis 2012;71. https://doi.org/10.1136/annrheumdis-2012-eular.1906.
- Ammitzbøll CG, Thiel S, Jensenius JC, Ellingsen T, Hørslev-Petersen K, Hetland ML, et al. M-ficolin levels reflect disease activity and predict remission in early rheumatoid arthritis. Rheum Arthritis 2013;65:3045-50. https://doi.org/10.1002/art.38179.
- Ancuta I, Codreanu C, Ionescu R, Balanescu A, Rezus E, Suta M, et al. Correlation between time to switch and clinical response amplitude to rituximab in second line treatment in rheumatoid arthritis patients with treatment failure to tumor necrosis factor inhibitors: 3-year data from repeat observational study. Ann Rheum Dis 2014;73. https://doi.org/10.1136/annrheumdis-2014-eular.2613.
- Angwin J, Boers M, Van der Heijde D, Landewe R, James MF, Binks M. Radiographic progression in the Cobra study: computer measurements of joint space, a comparison with scoring. Ann Rheum Dis 2006;65:578-9.
- Atzeni F, Antivalle M, Pallavicini FB, Caporali R, Bazzani C, Gorla R, et al. Predicting response to anti-TNF treatment in rheumatoid arthritis patients. Autoimmun Rev 2009;8:431-7. https://doi.org/10.1016/j.autrev.2009.01.005.
- Atzeni F, Bongiovanni S, Marchesoni A, Filippini M, Caporali R, Gorla R, et al. Predictors of response to anti-TNF therapy in RA patients with moderate or high DAS28 scores. Joint Bone Spine 2014;81:37-40. https://doi.org/10.1016/j.jbspin.2013.04.005.
- Baddoura R, Haddad S, Awada H, Al-Masri AF, Merheb G, Attoui S, et al. Severity of rheumatoid arthritis: the SEVERA study. Clin Rheumatol 2006;25:700-4. https://doi.org/10.1007/s10067-005-0136-7.
- Baer P, Bensen W, Chow A, Faraawi R, Choquette D, Fortin I, et al. Does low disease activity at six months predict remission at 12 months in rheumatoid arthritis patients treated with biologics in a real-world setting?. J Rheumatol 2014;41.
- Baillet A, Trocme C, Marotte H, Soubrier M, Tebib J, Thomas T, et al. Biomarker sets predict therapeutic response to TNF-inhibitors in rheumatoid arthritis and spondyloarthritis patients: a theragnostic approach in a multicenter cohort. Ann Rheum Dis 2014;73. https://doi.org/10.1136/annrheumdis-2014-eular.5750.
- Balogh E, Madruga Dias J, Mullan R, Harty L, Gallagher P, Molloy M, et al. Comparison of DAS-28 and 2011-ACR/EULAR remission criteria in a biologic-treated rheumatoid arthritis patient cohort. Ir J Med Sci 2013;182.
- Balogh E, Madruga Dias J, Orr C, Mullan R, Harty L, FitzGerald O, et al. Comparison of remission criteria in a tumour necrosis factor inhibitor treated rheumatoid arthritis longitudinal cohort: patient global health is a confounder. Arthritis Res Ther 2013;15. https://doi.org/10.1186/ar4421.
- Barnabe CCM, Homik J, Barr SG, Maksymowych WP, Martin L. Predictors for Remission in Rheumatoid Arthritis Are Affected by Remission Definition n.d.
- Barnabe C, Homik J, Barr S, Maksymowych W, Martin L. Identified predictors for remission in rheumatoid arthritis depend on remission definition. Ann Rheum Dis 2012;39:1734-5.
- Barnabe C, Homik J, Barr SG, Martin L, Maksymowych WP. The effect of different remission definitions on identification of predictors of both point and sustained remission in rheumatoid arthritis treated with anti-TNF therapy. J Rheumatol 2014;41:1607-13. https://doi.org/10.3899/jrheum.131451.
- Baruth M, Wilcox S, Schoffman DE, Becofsky K. Factors associated with disability in a sample of adults with arthritis. Disabil Health J 2013;6:377-84. https://doi.org/10.1016/j.dhjo.2013.04.008.
- Bathon JM, Martin RW, Fleischmann RM, Bingham CO, Whitmore JB, Eickenhorst T. Disease activity scores using CRP versus ESR and the relationship between EULAR and ACR responses in patients with early rheumatoid arthritis. Ann Rheum Dis 2005;64.
- Baumgartner SW, Fleischmann RM, Moreland LW, Schiff MH, Markenson J, Whitmore JB. Etanercept (Enbrel) in patients with rheumatoid arthritis with recent onset versus established disease: improvement in disability. J Rheumatol 2004;31:1532-7.
- Bay-Jensen AC, Byrjalsen I, Siebuhr AS, Christiansen C, Platt A, Karsdal MA. Serological biomarkers of joint tissue turnover predict tocilizumab response at baseline. J Clin Rheumatol 2014;20:332-5. https://doi.org/10.1097/RHU.0000000000000150.
- Bejarano V, Hensor E, Green M, Haugeberg G, Brown AK, Buch MH, et al. Relationship between early bone mineral density changes and long-term function and radiographic progression in rheumatoid arthritis. Arthritis Care Res 2012;64:66-70. https://doi.org/10.1002/acr.20553.
- Bellis E, Scire CA, Carrara G, Adinolfi A, Batticciotto A, Bortoluzzi A, et al. Ultrasound-detected synovitis and tenosynovitis independently associate with flare in patients with rheumatoid arthritis in clinical remission. Ann Rheum Dis 2015;74. https://doi.org/10.1136/annrheumdis-2015-eular.4927.
- Ben Slama I, Allali F, El Kabbaj S, Lakhdar T, Medrare L, Ngeuleu A, et al. Comparison of activity score DAS28-ESR and DAS28-CRP in patients with rheumatoid arthritis. Ann Rheum Dis 2014;73. https://doi.org/10.1136/annrheumdis-2014-eular.5807.
- Benedict A, Vanness DJ, Roy S, Cifaldi MA. Comparative effectiveness of biologic therapies for treating rheumatoid arthritis (RA) in patients who failed an anti tumor necrosis factor agent: a meta-regression analysis. Arthritis Rheumatol 2010;62.
- Bentzon MW, Gad I, Halberg P, Halskov O, Jacobsen BK, Lorenzen I, et al. Influence of previous gold treatment and other patient variables on outcome of treatment with disease modifying anti-rheumatic drugs (DMARD) in patients with rheumatoid arthritis. Clin Rheumatol 1986;5:39-48. https://doi.org/10.1007/BF02030966.
- Benucci M, Manfredi M, Puttini PS, Atzeni F. Predictive factors of response to rituximab therapy in rheumatoid arthritis: what do we know today?. Autoimmun Rev 2010;9:801-3. https://doi.org/10.1016/j.autrev.2010.07.006.
- Bijlsma JW, Weinblatt ME. Optimal use of methotrexate: the advantages of tight control. Ann Rheum Dis 2007;66:1409-10. https://doi.org/10.1136/ard.2007.076463.
- Bingham ICO, Weinblatt M, Mendelsohn A, Kim L, Mack M, Lu J, et al. Predictors of significant Disease Activity Score-28 (using C-reactive protein) remission achieved with intravenous golimumab in patients with active rheumatoid arthritis despite methotrexate therapy: results of the phase 3, multicenter, double-blind, placebo-controlled trial. Ann Rheum Dis 2012;64.
- Bingham CO, Weinblatt ME, Mendelsohn A, Kim L, Mack M, Lu JD, et al. Predictors of significant Disease Activity Score-28 (using C-reactive protein) remission achieved with intravenous golimumab in patients with active rheumatoid arthritis despite methotrexate therapy: results of the phase iii, multicentre, double-blind, placebo-controlled trial. Rheumatology 2013;52:82-3.
- Bobbio-Pallavicini F, Caporali R, Alpini C, Avalle S, Epis OM, Klersy C, et al. High IgA rheumatoid factor levels are associated with poor clinical response to tumour necrosis factor alpha inhibitors in rheumatoid arthritis. Ann Rheum Dis 2007;66:302-7. https://doi.org/10.1136/ard.2006.060608.
- Boers M, Kostense PJ, Verhoeven AC, van der Linden S. COBRA Trial Group . Combinatietherapie Bij Reumatoide Artritis. Inflammation and damage in an individual joint predict further damage in that joint in patients with early rheumatoid arthritis. Arthritis Rheum 2001;44:2242-6. https://doi.org/10.1002/1529-0131(200110)44:10<2242::AID-ART386>3.0.CO;2-F.
- Borangiu A, Gudu T, Opris D, Mazilu D, Abobului M, Moldoveanu V, et al. Ultrasound active synovitis can be predicted by using clinical measures. Clin Exp Rheumatol 2014;32.
- Bouman C, Van Der Maas A, Segurado OG, Sasso EH, Van Den Hoogen F, Den Broeder A. Associations of a multi-biomarker disease activity score with clinical and radiographic parameters in rheumatoid arthritis. Ann Rheum Dis 2015;74. https://doi.org/10.1136/annrheumdis-2015-eular.1932.
- Braun J, Kästner P, Flaxenberg P, Währisch J, Hanke P, Demary W, et al. Comparison of the clinical efficacy and safety of subcutaneous versus oral administration of methotrexate in patients with active rheumatoid arthritis: results of a six-month, multicenter, randomized, double-blind, controlled, phase IV trial. Arthritis Rheum 2008;58:73-81. https://doi.org/10.1002/art.23144.
- Breedveld FC, Han C, Bala M, van der Heijde D, Baker D, Kavanaugh AF, et al. Association between baseline radiographic damage and improvement in physical function after treatment of patients with rheumatoid arthritis. Ann Rheum Dis 2005;64:52-5. https://doi.org/10.1136/ard.2003.017160.
- Brown AK, Quinn MA, Karim Z, Wakefield RJ, Conaghan PG, Pollard ASE, et al. Baseline clinical, functional and ultrasonographic assessment can be used to predict subsequent remission in rheumatoid arthritis. Arthritis Rheumatol 2002;46.
- Buch MH, English A, Cunnane G, Henshaw K, Bingham SJ, Seto Y, et al. Does synovial cytokine expression predict response to tumour necrosis factor-alpha blockade in patients with rheumatoid arthritis?. Arthritis Rheumatol 2003;48.
- Buchanan R, Will R, Koenig A, Szumski A, Jones H, Thirunavukkarasu K, et al. Basal metabolic rate as an indicator of rheumatoid arthritis disease activity and predictor of remission: Australian results from period 1 of the preserve trial. Intern Med 2013;43.
- Burmester GR, Ferraccioli G, Flipo RM, Monteagudo-Saez I, Unnebrink K, Kary S, et al. Clinical remission and/or minimal disease activity in patients receiving adalimumab treatment in a multinational, open-label, twelve-week study. Arthritis Care Res 2008;59:32-41. https://doi.org/10.1002/art.23247.
- Burmester GR, Kivitz AJ, Kupper H, Arulmani U, Florentinus S, Goss SL, et al. Efficacy and safety of ascending methotrexate dose in combination with adalimumab: the randomised CONCERTO trial. Ann Rheum Dis 2015;74:1037-44. https://doi.org/10.1136/annrheumdis-2013-204769.
- Bykerk VP, Burmester GR, Combe BG, Furst DE, Huizinga TWJ, Wong DA, et al. On drug and drug-free remission by baseline disease duration in the avert trial: abatacept versus methotrexate comparison in patients with early rheumatoid arthritis. Ann Rheum Dis 2015;74:477-8. https://doi.org/10.1136/annrheumdis-2015-eular.2071.
- Callaghan CA, Boyter AC, Mullen AB, McRorie ER. Biological therapy for rheumatoid arthritis: is personalised medicine possible?. Eur J Hosp Pharm Sci Pract 2014;21:229-37. https://doi.org/10.1136/ejhpharm-2013-000386.
- Cañete JD, Albaladejo C, Hernández MV, Laínez B, Pinto JA, Ramírez J, et al. Clinical significance of high levels of soluble tumour necrosis factor-α receptor-2 produced by alternative splicing in rheumatoid arthritis: a longitudinal prospective cohort study. Rheumatology 2011;50:721-8. https://doi.org/10.1093/rheumatology/keq381.
- Canhão H, Rodrigues AM, Mourão AF, Martins F, Santos MJ, Canas-Silva J, et al. Comparative effectiveness and predictors of response to tumour necrosis factor inhibitor therapies in rheumatoid arthritis. Rheumatology 2012;51:2020-6. https://doi.org/10.1093/rheumatology/kes184.
- Cantini F, Niccoli L, Nannini C, Cassarà E, Kaloudi O, Giulio Favalli E, et al. Tailored first-line biologic therapy in patients with rheumatoid arthritis, spondyloarthritis, and psoriatic arthritis. Semin Arthritis Rheum 2016;45:519-32. https://doi.org/10.1016/j.semarthrit.2015.10.001.
- Capell HA, Porter DR, Madhok R, Hunter JA. Second line (disease modifying) treatment in rheumatoid arthritis: which drug for which patient?. Ann Rheum Dis 1993;52:423-8. https://doi.org/10.1136/ard.52.6.423.
- Castrejón I, Dougados M, Combe B, Fautrel B, Guillemin F, Pincus T. Prediction of remission in a French early arthritis cohort by RAPID3 and other core data set measures, but not by the absence of rheumatoid factor, anticitrullinated protein antibodies, or radiographic erosions. J Rheumatol 2016;43:1285-91. https://doi.org/10.3899/jrheum.141586.
- Casu C, Bruschi E, Alpini C, Marceglia S, Giacomelli L, Epis O. Clinical and serological response to tocilizumab in patients with rheumatoid arthritis. Ann Rheum Dis 2013;72. https://doi.org/10.1136/annrheumdis-2013-eular.2626.
- Cavet G, Shen Y, Abraham S, Chernoff D, Centola M, Taylor P. Predicting radiographic progression in rheumatoid arthritis with ultrasound and biomarkers. Arthritis Rheumatol 2009;60.
- Cazzato M, Bazzichi IL, Bombardieri S, Giacomelli C. Early response indicator early predicts clinical response to certolizumab in rheumatoid arthritis patients. Arthritis Rheumatol 2014;66.
- Chandrashekara S, Rajendran A, Bai Jaganath A, Krishnamurthy R. Neutrophil-lymphocyte ratio, pain perception, and disease activity score may serve as important predictive markers for sustained remission in rheumatoid arthritis. Reumatismo 2015;67:109-15. https://doi.org/10.4081/reumatismo.2015.838.
- Chatzidionysiou K, Lie E, Nasonov E. Seropositivity and in particular ACPA positivity is a strong predictor of response to treatment with rituximab: pooled data from 10 European registries. Ann Rheum Dis 2011;69.
- Chatzidionysiou K, Lie E, Nasonov E, Lukina G, Hetland M, Tarp U, et al. Seropositivity and response to RTX: data from the cererra collaboration. Ann Rheum Dis 2013;71. https://doi.org/10.1136/annrheumdis-2012-eular.2051.
- Chatzidionysiou K, Lie E, Nasonov E, Lukina G, Hetland ML, Hauge E, et al. Smoking and response to rituximab in anti-CCP positive and negative rheumatoid arthritis-results from an international European collaboration. Ann Rheum Dis 2015;74:484-5. https://doi.org/10.1136/annrheumdis-2015-eular.5225.
- Chen Y, Yu Z, Packham JC, Mattey DL. Influence of adult height on rheumatoid arthritis: association with disease activity, impairment of joint function and overall disability. PLOS ONE 2013;8. https://doi.org/10.1371/journal.pone.0064862.
- Chen LF, Mo YQ, Ma JD, Luo L, Zheng DH, Dai L. Elevated serum IgG4 defines specific clinical phenotype of rheumatoid arthritis. Mediators Inflamm 2014;2014. https://doi.org/10.1155/2014/635293.
- Cheung P, Mari K, Devauchelle V, Bentin J, Jousse-Joulin S, D’Agostino MA, et al. Are tender joints better than synovitis to predict structural damage in rheumatoid arthritis?. Arthritis Rheumatol 2014;66.
- Chitale S, Estrach C, Moots RJ, Goodson NJ. Initial triple DMARD therapy predicts ACR EULAR remission in an early rheumatoid arthritis inception cohort. Rheumatology 2012;51.
- Cho SK, Sung YK, Choi CB, Cha HS, Choe JY, Chung WT, et al. Do patients with elderly-onset rheumatoid arthritis have severe functional disability?. Semin Arthritis Rheum 2012;42:23-31. https://doi.org/10.1016/j.semarthrit.2012.02.004.
- Choquette D, Bensen W, Khalil H, Sampalis J. Impact of disease duration on the outcome of RA patients treated with infliximab in Canada. Ann Rheum Dis 2010;37:1323-4.
- Choquette D, Bensen WG, Sheriff MK, Kelsall JT, Baker MF, Sampalis JS, et al. Comparison of Disease Characteristics of Rheumatoid Arthritis Patients in Remission According to the DAS Criteria Versus the New ACR EULAR Criteria in a Real-World Patient Population n.d.
- Choquette D, Bensen W, Sheriff M, Kelsall J, Baker M, Sampalis J, et al. Comparison of disease characteristics of RA patients in remission according to the DAS criteria versus the new ACR/EULAR criteria in a real-world patient population. Ann Rheum Dis 2013;71. https://doi.org/10.1136/annrheumdis-2012-eular.2520.
- Choquette D, Faraawi R, Zummer M, Chow A, Kelsall J, Rampakakis E, et al. What is the impact of rheumatoid factor positivity on the real-world effectiveness of infliximab treatment in rheumatoid arthritis?. J Rheumatol 2013;40.
- Choquette D, Bessette L, Haraoui B, Massicotte F, Pelletier JP, Raynauld JP, et al. Use of rituximab compared to anti-TNF agents as second and third line therapy in patients with rheumatoid arthritis: 6-year follow-up report from the rhumadata clinical database and registry. Arthritis Rheumatol 2015;67.
- Choquette D, Coupal L, Laliberte MC, Desjardins O. Predictors of clinical response to biologics in rheumatoid arthritis: experience from a Canadian clinic. J Rheumatol 2015;42.
- Chow A, Arendse R, Olszynski W, Kelsall J, Baker M, Bensen W, et al. What is the effect of TNF inhibitors on employment status in rheumatoid arthritis patients and what are the predictors of progression to unemployment?. J Rheumatol 2015;42. https://doi.org/10.1136/annrheumdis-2015-eular.6199.
- Choy E. Does the Health Assessment Questionnaire predict 5-year quality of life in early RA?. Nat Clin Pract Rheumatol 2007;3:380-1. https://doi.org/10.1038/ncprheum0533.
- Choy T, Bykerk VP, Haraoui BP, Boire G, Hitchon C, Thorne C, et al. Physician global assessment at three months is strongly predictive of disease activity at 12 months in early rheumatoid arthritis. Results from the catch cohort. Ann Rheum Dis 2013;72:A384-5. https://doi.org/10.1136/annrheumdis-2013-eular.1177.
- Christensen AW, Rifbjerg-Madsen S, Christensen R, Amris K, Taylor PC, Locht H, et al. Temporal summation of pain and ultrasound Doppler activity as predictors of treatment response in patients with rheumatoid arthritis: protocol for the Frederiksberg hospitals Rheumatoid Arthritis, pain assessment and Medical Evaluation (FRAME-cohort) study. BMJ Open 2014;4. https://doi.org/10.1136/bmjopen-2013-004313.
- Christensen AW, Rifbjerg-Madsen S, Christensen R, Dreyer L, Boesen M, Ellegaard K, et al. Ultrasound Doppler but not temporal summation of pain predicts DAS28 response in rheumatoid arthritis: a prospective cohort study. Rheumatology (Oxford) 2016;55:1091-8. https://doi.org/10.1093/rheumatology/kew034.
- Ciurtin C, Wyszynski K, Manson J, Marra G. Evaluating impact of risk associated outcomes on ultrasound Doppler score of patients with inflammatory hand joint pain using a beta-binomial model. Ann Rheum Dis 2015;74. https://doi.org/10.1136/annrheumdis-2015-eular.1886.
- Coburn B, Thiele GM, Duryee MJ, Yu F, Sayles H, Sokolove J, et al. Anti-Citrullinated Protein Antibody and Radiographic Disease Progression in Rheumatoid Arthritis n.d.
- Cohen SB, Kavanaugh AF, Emery P, Keystone EC, Segurado OG. C-reactive protein predicts treatment response to adalimumab (HUMIRA®) in patients with rheumatoid arthritis. Ann Rheum Dis 2005;64.
- Colmegna I, Hitchon CA, Bardales MC, Puri L, Bartlett SJ. High rates of obesity and greater associated disability among people with rheumatoid arthritis in Canada. Clin Rheumatol 2016;35:457-60. https://doi.org/10.1007/s10067-015-3154-0.
- Couderc M, Mathieu S, Pereira B, Glace B, Soubrier M. Predictive factors of rituximab response in rheumatoid arthritis: results from a French university hospital. Arthritis Care Res 2013;65:648-52. https://doi.org/10.1002/acr.21865.
- Courvoisier DS, Alpizar-Rodriguez D, Gottenberg JE, Hernandez MV, Iannone F, Lie E, et al. Rheumatoid arthritis patients after initiation of a new biologic agent: trajectories of disease activity in a large multinational cohort study. EBioMedicine 2016;11:302-6. https://doi.org/10.1016/j.ebiom.2016.08.024.
- Cuchacovich M, Catalan D, Wainstein E, Gatica H, Soto L, Aravena O, et al. Basal anti-cyclic citrullinated peptide (anti-CCP) antibody levels and a decrease in anti-CCP titres are associated with clinical response to adalimumab in rheumatoid arthritis. Clin Exp Rheumatol 2008;26:1067-73.
- Cuppen BV, Welsing PM, Sprengers JJ, Bijlsma JW, Marijnissen AC, Van Laar JM, et al. Personalized biological treatment for rheumatoid arthritis: a systematic review with a focus on clinical applicability. Ann Rheum Dis 2015;74. https://doi.org/10.1136/annrheumdis-2015-eular.3815.
- Cuppen BV, Jacobs JW, Marijnissen AC, Van Laar JM, Lafeber FP. Towards individualized risk determination in RA: a prediction model for TNFi discontinuation within the first year after start. Ann Rheum Dis 2015;74. https://doi.org/10.1136/annrheumdis-2015-eular.3839.
- Cuppen BV, Fu J, van Wietmarschen HA, Harms AC, Koval S, Marijnissen AC, et al. Exploring the inflammatory metabolomic profile to predict response to TNF-α inhibitors in rheumatoid arthritis. PLOS ONE 2016;11. https://doi.org/10.1371/journal.pone.0163087.
- Curtis JR, Yang S, Chen L, Park GS, Bitman B, Wang BC, et al. Prediction of one-year response to etanercept and methotrexate in rheumatoid arthritis patients in TEMPO. Arthritis Rheumatol 2010;62.
- Curtis JR, Baddley JW, Yang S, Patkar N, Chen L, Delzell E, et al. Derivation and preliminary validation of an administrative claims-based algorithm for the effectiveness of medications for rheumatoid arthritis. Arthritis Res Ther 2011;13. https://doi.org/10.1186/ar3471.
- Curtis JR, Baddley JW, Yang S, Patkar N, Chen L, Delzell ES, et al. Validation of a preliminary administrative claims-based algorithm for the effectiveness of medications for rheumatoid arthritis. Pharmacoepidemiol Drug Saf 2011;20:S69-70.
- Curtis JR, Luijtens K, Kavanaugh A. Predicting future response to certolizumab pegol in rheumatoid arthritis patients: features at 12 weeks associated with low disease activity at 1 year. Arthritis Care Res 2012;64:658-67. https://doi.org/10.1002/acr.21600.
- Curtis JR, McVie T, Mikuls TR, Reynolds RJ, Navarro-Millán I, O’Dell J, et al. Clinical response within 12 weeks as a predictor of future low disease activity in patients with early RA: results from the TEAR Trial. J Rheumatol 2013;40:572-8. https://doi.org/10.3899/jrheum.120715.
- D’Agostino MA, Boers M, Wakefield RJ, Hammer HB, Vittecoq O, Galeazzi M, et al. Do ultrasound (power Doppler ultrasonography) and Disease Activity Score-28 measure different aspects of disease activity? Analyses from an open-label study of power Doppler ultrasonography response patients with rheumatoid arthritis patients starting abatacept. Rheumatology 2015;54:81-2.
- D’Agostino MA, Le Bars M, Taylor M, Chou B, Zhu J, Ranganath VK. In patients with rheumatoid arthritis and an inadequate response to methotrexate, does body mass index influence the efficacy of abatacept on inflammation when measured by power Doppler ultrasonography? Results from the appraise study. Ann Rheum Dis 2015;74:231-2. https://doi.org/10.1136/annrheumdis-2015-eular.1727.
- Dahl LL, Geborek P, Englund M, Olofsson T, Petersson IF. TNF-inhibitors in established rheumatoid arthritis: the effect of age on treatment response and predictors of remission. Arthritis Rheumatol 2009;60.
- Dai L, Mo YQ, Zheng DH, Wei XN, Zhang BY, Zhu LJ. The clinical response to etanercept in rheumatoid arthritis is in part dependent on pretreatment tumour necrosis factor-alpha expression in the synovium. Int J Rheum Dis 2010;13.
- Daïen CI, Morel J. Predictive factors of response to biological disease modifying antirheumatic drugs: towards personalized medicine. Mediators Inflamm 2014;2014. https://doi.org/10.1155/2014/386148.
- Dames SR, Mikuls TR, Kerr G, Richards JS, Hooker R, Kazi S, et al. Utility of Disease Activity Score-28 (DAS-28) for determining need for DMARD change in veterans affairs rheumatoid arthritis (VARA) patients: an analysis of discrepancies between DAS-28 scores and clinical stability status. Arthritis Rheumatol 2006;54:S187-S8.
- da Mota LM, Dos Santos Neto LL, Oliveira AC, Pereira IA, Burlingame RW, Ménard HA, et al. Baseline HAQ and SF-36 questionnaire scores cannot predict clinical remission, radiographic progression or the need for biological therapy in a three-year prospective study of a Brazilian early rheumatoid arthritis cohort. Rheumatol Int 2012;32:3937-43. https://doi.org/10.1007/s00296-011-2261-8.
- Danve A, Sayles H, Mikuls TR, O’Dell JR. Male gender and higher hemoglobin predict response to methotrexate in rheumatoid arthritis. Arthritis Rheumatol 2015;67.
- Darawankul B, Chaiamnuay S, Pakchotanon R, Asavatanabodee P, Narongroeknawin P. The good EULAR response at the first year is strongly predictive of clinical remission in rheumatoid arthritis: results from the TARAC cohort. Clin Rheumatol 2015;34:43-9. https://doi.org/10.1007/s10067-014-2749-1.
- Dasgupta B, Combe B, Durez P, Schulze-Koops H, Louw I, Wollenhaupt J, et al. Predictors of low disease activity and remission after one dose of golimumab in patients with rheumatoid arthritis. Ann Rheum Dis 2014;73:233-4. https://doi.org/10.1136/annrheumdis-2014-eular.2258.
- Dejaco C, Duftner C, Klotz W, Schirmer M, Herold M. Third generation anti-cyclic citrullinated peptide antibodies do not predict anti-TNF-alpha treatment response in rheumatoid arthritis. Rheumatol Int 2010;30:451-4. https://doi.org/10.1007/s00296-009-0978-4.
- de Jong PH, Quax RA, Huisman M, Gerards AH, Feelders RA, de Sonnaville PB, et al. Response to glucocorticoids at 2 weeks predicts the effectiveness of DMARD induction therapy at 3 months: post hoc analyses from the tREACH study. Ann Rheum Dis 2013;72:1659-63. https://doi.org/10.1136/annrheumdis-2012-202152.
- de Jong TD, Vosslamber S, Blits M, Wolbink G, Nurmohamed MT, van der Laken CJ, et al. Effect of prednisone on type I interferon signature in rheumatoid arthritis: consequences for response prediction to rituximab. Arthritis Res Ther 2015;17. https://doi.org/10.1186/s13075-015-0564-y.
- De Miguel E, De Vicente E, Diaz Alcazar F, De La Iglesia JL, Garcia Vivar ML, Uson Jaeger J, et al. Doppler ultrasound better predicts X-Ray progression in rheumatoid arthritis than any definition of clinical remission. Ann Rheum Dis 2015;74. https://doi.org/10.1136/annrheumdis-2015-eular.5223.
- de Rooy DPC, van der Linden MPM, Knevel R, Huizinga TWJ, van der Helm-van Mil AHM. Predicting arthritis outcomes – what can be learned from the Leiden Early Arthritis Clinic?. Rheumatology (Oxford) 2011;50:93-100. https://doi.org/10.1093/rheumatology/keq230.
- Demir F, Gok H, Dincer G, Kosucu P, Salih M. Value of wrist ultrasound in prediction of disease activity in patients with rheumatoid arthritis. Ann Rheum Dis 2003;62.
- Di Cicco M, Kelly S, Humby F, Ng N, Hands RE, Rocher V, et al. Synovial ectopic lymphoneogenesis predicts primary clinical response to certolizumab pegol in patients with rheumatoid arthritis. Rheumatology (Oxford) 2014;53. https://doi.org/10.1093/rheumatology/keu101.022.
- Di Cicco M, Humby FC, Kelly S, Ng N, Mahto A, Hands R, et al. Presence of synovial lymphocyte aggregates predicts clinical response to DMARD therapy in patients with early rheumatoid arthritis. Ann Rheum Dis 2014;73. https://doi.org/10.1136/annrheumdis-2014-eular.5365.
- Ding R, Li P, Song D, Zhang X, Bi L. Predictors of response to TNF-α antagonist therapy in Chinese rheumatoid arthritis. Clin Rheumatol 2015;34:1203-10. https://doi.org/10.1007/s10067-015-2973-3.
- Dirven L, Ewals JAPM, Huizinga TWJ, Kerstens PJSM, Peeters AJ, Dijkmans BAC, et al. Predictors of HAQ response after 3 months of treatment with different strategies in recent onset active RA. Arthritis Rheumatol 2009;60.
- Dougados M, Schiff M, Luggen M, Becker JC, Aranda R, Li T, et al. Sustained efficacy along with improvements in disease activity score 28 (DAS 28) and patient (Pt)-reported outcomes (PROs) with abatacept (Aba) in rheumatoid arthritis (RA) pts with an inadequate response to anti-tumor necrosis factor (TNF) therapy: the long-term extension (LTE) of the attain trial. Rheumatology 2007;46.
- Drouin J, Haraoui B. 3e Initiative Group . Predictors of clinical response and radiographic progression in patients with rheumatoid arthritis treated with methotrexate monotherapy. J Rheumatol 2010;37:1405-10. https://doi.org/10.3899/jrheum.090838.
- Durez P, Pavelka K, Lazaro M, Garcia Kutzbach A, Moots R, Amital H, et al. Baseline predictors of remission rates during golimumab treatment for rheumatoid arthritis in the go-more study. Ann Rheum Dis 2014;73. https://doi.org/10.1136/annrheumdis-2014-eular.1699.
- Ellingsen T, Hansen I, Thorsen J, Moller BK, Tarp U, Hetland ML, et al. The increased cc chemokine ligand 19 (CCL19) at baseline is an independent predictor of the 5-year radiographic progression in early steroid and DMARD-naive rheumatoid arthritis (RA) patients. Arthritis Rheumatol 2009;60.
- Emery P, Westhovens R, Russell A, Moreland L, Kremer J, Becker JC, et al. Abatacept inhibits structural damage progression, as assessed by the Genant-modified Sharp scoring system, in rheumatoid arthritis (RA) patients with an inadequate response to methotrexate (MTX): the aim (abatacept in inadequate responders to MTX) trial – a sub-analysis by disease duration. Rheumatology 2006;45:I41-I2.
- Emery P, van der Heijde D, Ostergaard M, Conaghan PG, Genovese MC, Keystone EC, et al. Exploratory analyses of the association of MRI with clinical, laboratory and radiographic findings in patients with rheumatoid arthritis. Ann Rheum Dis 2011;70:2126-30. https://doi.org/10.1136/ard.2011.154500.
- Emery P, Dörner T. Optimising treatment in rheumatoid arthritis: a review of potential biological markers of response. Ann Rheum Dis 2011;70:2063-70. https://doi.org/10.1136/ard.2010.148015.
- Emery P, Matteson EL, Genovese M, Sague S, Hsia EC, Doyle MK, et al. Post-treatment changes in serum C-reactive protein levels and clinical response in rheumatoid arthritis. Ann Rheum Dis 2011;50:iii113-iii4.
- Emery P, Kvien TK, Combe B, Freundlich B, Robertson D, Ferdousi T, et al. Combination etanercept and methotrexate provides better disease control in very early (≤ 4 months) versus early rheumatoid arthritis (> 4 months and < 2 years): post hoc analyses from the COMET study. Ann Rheum Dis 2012;71:989-92. https://doi.org/10.1136/annrheumdis-2011-201066.
- Emery P, Baker D, Hsia E, Fleischmann R, Xu S, Xu W. Golimumab’s efficacy in patients with very active disease in methotrexate-naive rheumatoid arthritis. Int J Rheum Dis 2012;15.
- Emery P, Fleischmann R, Han C, van der Heijde D, Conaghan P, Xu W, et al. Predictors of radiographic progression in methotrexate-naive patients with rheumatoid arthritis based on one-year radiographic data from the go-before golimumab clinical trial. Rheumatology 2012;51:iii68-iii9.
- Emery P, Szumski A, Jones H. Radiographic progression in patients with early rheumatoid arthritis treated with etanercept: results from the prize study. Ann Rheum Dis 2013;72:A399-400. https://doi.org/10.1136/annrheumdis-2013-eular.1217.
- Emery P, Pedersen R, Bukowski J, Marshall L. Early response to etanercept-methotrexate induction therapy predicts sustained remission with reduced-dose combination regimen in the prize study. Ann Rheum Dis 2014;73. https://doi.org/10.1136/annrheumdis-2014-eular.1704.
- Emery P, Pedersen R, Bukowski J, Marshall L. Early response to full-dose etanercept-plus-methotrexate induction therapy predicts sustained remission with reduced-dose combination therapy in early rheumatoid arthritis patients. Arthritis Rheumatol 2014;66.
- Emery P, Fleischmann RM, Hsia EC, Xu S, Zhou Y, Baker D. Efficacy of golimumab plus methotrexate in methotrexate-naïve patients with severe active rheumatoid arthritis. Clin Rheumatol 2014;33:1239-46. https://doi.org/10.1007/s10067-014-2731-y.
- Emery P, Burmester G, Bykerk VP, Combe B, Furst DE, Barre E, et al. Predictors of drug-free remission following treatment with abatacept (in combination with methotrexate or as monotherapy) in early rheumatoid arthritis. Arthritis Rheumatol 2014;66.
- Emery P, Burmester G, Bykerk VP, Combe B, Furst DE, Maldonado M, et al. Abatacept plus methotrexate can effectively and safely regain the target of remission following re-treatment for flares after drug-free withdrawal in patients with early rheumatoid arthritis. Arthritis Rheumatol 2015;67.
- Emery P, Solem C, Majer I, Cappelleri JC, Tarallo M. A European chart review study on early rheumatoid arthritis treatment patterns, clinical outcomes, and healthcare utilization. Rheumatol Int 2015;35:1837-49. https://doi.org/10.1007/s00296-015-3312-3.
- Erickson A, Rybin D, Brophy M, Lew R, Mikuls TR, Moore T, et al. Predictors of radiologic disease progression during the rheumatoid arthritis comparison of active therapies trial. Arthritis Rheumatol 2014;66.
- Eser F, Garip Y, Bodur H. Extraarticular manifestations in Turkish patients with rheumatoid arthritis: impact of EAMs on the health-related quality of life in terms of disease activity, functional status, severity of pain, and social and emotional functioning. Rheumatol Int 2012;32:1521-5. https://doi.org/10.1007/s00296-011-1822-1.
- Estrach C, Mpofu S, Thompson RN, Williams E, Abernethy VE, Moots RJ, et al. Swollen joint count as a predictor of response to anti-tumor necrosis factor alpha therapy in rheumatoid arthritis: comment on the article by Sokka and Pincus (multiple letters) [3]. Arthritis Rheumatol 2003;48:3611-5. https://doi.org/10.1002/art.11335.
- Fabricio EO, Sifuentes C, Mariana M, Arce-Salinas CA. Predictability to achieve low activity and/or remission with leflunomide use in patients with rheumatoid arthritis. Ann Rheum Dis 2015;74. https://doi.org/10.1136/annrheumdis-2015-eular.2100.
- Fabris M, Quartuccio L, Lombardi S, Benucci M, Manfredi M, Saracco M, et al. Study on the possible role of the –174G>C IL-6 promoter polymorphism in predicting response to rituximab in rheumatoid arthritis. Reumatismo 2010;62:253-8.
- Fautrel B, Gallo G, Brault Y, Nab H. Moderate rheumatoid arthritis despite methotrexate treatment: risk of radiographic progression. Ann Rheum Dis 2012;64.
- Fautrel B, Pham T, Alfaiate T, Gandjbakhch F, Foltz V, Morel J, et al. Step-down strategy of spacing TNF-blocker injections for established rheumatoid arthritis in remission: results of the multicentre non-inferiority randomised open-label controlled trial (STRASS: Spacing of TNF-blocker injections in Rheumatoid ArthritiS Study). Ann Rheum Dis 2016;75:59-67. https://doi.org/10.1136/annrheumdis-2014-206696.
- Favalli EG, Biggioggero M, Pregnolato F, Meroni PL. Disease duration and year of publication affect the results of studies on rheumatoid arthritis damage progression by biologic agents: a systematic review and meta-analysis. Ann Rheum Dis 2013;72. https://doi.org/10.1136/annrheumdis-2013-eular.679.
- Favalli EG, Pregnolato F, Biggioggero M, Meroni PL. The comparison of effects of biologic agents on rheumatoid arthritis damage progression is biased by period of enrollment: data from a systematic review and meta-analysis. Semin Arthritis Rheum 2014;43:730-7. https://doi.org/10.1016/j.semarthrit.2013.11.006.
- Fedele AL, Tolusso B, Gremese E, Canestri S, Di Mario C, Nowik M, et al. Clinical parameters and B cell subsets as biomarkers of response to tocilizumab in rheumatoid arthritis. Arthritis Rheumatol 2015;67.
- Fedorenko E, Lukina G, Sigidin Y, Karateev D, Smirnov A, Nasonov E. Effects of four different treatment regimens on radiologic progression in early rheumatoid arthritis (RA). Ann Rheum Dis 2014;73. https://doi.org/10.1136/annrheumdis-2013-205124.36.
- Feist E, Rubbert-Roth A, Braun J, Kaufmann J, Kellner HL, Kurthen R, et al. A study to evaluate the effectiveness and safety of the interleukin-6 (IL-6) receptor antagonist tocilizumab (TCZ) after 4 and 24 weeks in patients with active rheumatoid arthritis (RA) final effectiveness results of the TAMARA study. Arthritis Rheumatol 2010;62.
- Ferdousi T, Koenig A, Jones T. Disease Duration As a Determinate Factor of Disease Activity and Radiographic Progression in Rheumatoid Arthritis n.d.
- Fernández-Nebro A, Irigoyen MV, Ureña I, Belmonte-López MA, Coret V, Jiménez-Núñez FG, et al. Effectiveness, predictive response factors, and safety of anti-tumor necrosis factor (TNF) therapies in anti-TNF-naive rheumatoid arthritis. J Rheumatol 2007;34:2334-42.
- Filer A, De Pablo P, Allen G, Nightingale P, Jordan A, Jobanputra P, et al. Utility of ultrasound joint counts as predictors of outcome in patients with very early arthritis. Rheumatology 2010;49:i162-i3.
- Filippi N, Lukas C, Morel J, Combe B, Mouterde G. Predictors of persistence of power Doppler ultrasound synovitis in rheumatoid arthritis patients in clinical remission. Ann Rheum Dis 2015;74. https://doi.org/10.1136/annrheumdis-2015-eular.1908.
- Finckh A, Dehler S, Costenbader KH, Gabay C. Swiss Clinical Quality Management project for RA . Cigarette smoking and radiographic progression in rheumatoid arthritis. Ann Rheum Dis 2007;66:1066-71. https://doi.org/10.1136/ard.2006.065060.
- Finckh A, Ciurea A, Brulhart L, Möller B, Walker UA, Courvoisier D, et al. Which subgroup of patients with rheumatoid arthritis benefits from switching to rituximab versus alternative anti-tumour necrosis factor (TNF) agents after previous failure of an anti-TNF agent?. Ann Rheum Dis 2010;69:387-93. https://doi.org/10.1136/ard.2008.105064.
- Finckh A, Gottenberg J, Hernandez MV, Iannone F, Lie E, Canhao H, et al. The impact of tobacco smoking on the effectiveness of abatacept in rheumatoid arthritis: data from a pan European analysis of RA registries. Arthritis Rheumatol 2015;67.
- Fisher B, Plant D, Lundberg K, P JC, Barton A, Venables P. Fine specificity of anti-citrullinated peptide antibodies is associated with response to anti-TNF agents in rheumatoid arthritis: results from the British society of rheumatology biologics register. Rheumatology 2011;50.
- Fisher BA, Plant D, Lundberg K, Charles PJ, Barton A, Venables P. Biologics in Rheumatoid Arthritis Genetics and Genomics Study Syndicate (BRAGGSS) . Heterogeneity of anticitrullinated peptide antibodies and response to anti-tumor necrosis factor agents in rheumatoid arthritis. J Rheumatol 2012;39:929-32. https://doi.org/10.3899/jrheum.111315.
- Fleischmann R, Koenig AS, Szumski A, Nab HW, Marshall L, Bananis E. Short-term efficacy of etanercept plus methotrexate vs combinations of disease-modifying anti-rheumatic drugs with methotrexate in established rheumatoid arthritis. Rheumatology 2014;53:1984-93. https://doi.org/10.1093/rheumatology/keu235.
- Folkersen L, Brynedal B, Diaz-Gallo LM, Ramskold D, Shchetynsky K, Westerlind H, et al. Integration of known DNA, RNA and protein biomarkers provides prediction of anti-TNF response in rheumatoid arthritis: results from the COMBINE study. Mol Med 2016;22:322-8. https://doi.org/10.2119/molmed.2016.00078.
- Foltz V, Loeuille D, Etchepare F, Chary-Valckenaere I, Rosenberg C, Tanguy M-L, et al. Ultrasound and magnetic resonance imaging did not provide early assessment of biotherapy response in patients with rheumatoid arthritis. Joint Bone Spine 2009;76:113-14. https://doi.org/10.1016/j.jbspin.2008.06.006.
- Forsblad-d’Elia H, Bengtsson K, Kristensen LE, Jacobsson LT. Drug adherence, response and predictors thereof for tocilizumab in patients with rheumatoid arthritis: results from the Swedish biologics register. Rheumatology 2015;54:1186-93. https://doi.org/10.1093/rheumatology/keu455.
- Fransen J, Kooloos WM, Wessels JA, Huizinga TW, Guchelaar HJ, van Riel PL, et al. Clinical pharmacogenetic model to predict response of MTX monotherapy in patients with established rheumatoid arthritis after DMARD failure. Pharmacogenomics 2012;13:1087-94. https://doi.org/10.2217/pgs.12.83.
- Fujimura T, Fujimoto T, Hara R, Kondo S, Shimmyo N, Kobata Y, et al. Predicting future response to tumor necrosis factor inhibitors by the distribution of affected joints in rheumatoid arthritis patients. Ann Rheum Dis 2014;73:483-4. https://doi.org/10.1136/annrheumdis-2014-eular.1980.
- Furst DE, Bykerk VP, Burmester G, Combe BG, Huizinga TWJ, Alemao E, et al. Evaluation of patient-reported outcomes by baseline disease duration: 6-month data from two clinical trials of patients with early rheumatoid arthritis treated with abatacept. Ann Rheum Dis 2015;67.
- Furst DE, Kavanaugh A, Florentinus S, Kupper H, Karunaratne M, Birbara CA. Final 10-year effectiveness and safety results from study DE020: adalimumab treatment in patients with rheumatoid arthritis and an inadequate response to standard therapy. Rheumatology 2015;54:2188-97. https://doi.org/10.1093/rheumatology/kev249.
- Furuya H, Kasama T, Isozaki T, Umemura M, Otsuka K, Isojima S, et al. Effect of TNF antagonists on the productivity of daily work of patients with rheumatoid arthritis. J Multidiscip Healthc 2013;6:25-30.
- Gardette A, Ottaviani S, Tubach F, Roy C, Nicaise-Roland P, Palazzo E, et al. High anti-CCP antibody titres predict good response to rituximab in patients with active rheumatoid arthritis. Joint Bone Spine 2014;81:416-20. https://doi.org/10.1016/j.jbspin.2014.06.001.
- Gherghe AM, Ramiro S, Landewé R, Mihai C, van der Heijde D. Association of the different types of radiographic damage with physical function in patients with rheumatoid arthritis: analysis of the RAPID trials. RMD Open 2016;2. https://doi.org/10.1136/rmdopen-2015-000219.
- Giacomelli C, Ferrari C, Stagnaro C, Talarico R, Consensi A, Sernissi F, et al. Mathematical model to predict the early responders in a monocentric cohort of patients with rheumatoid arthritis treated by anti TNFalpha. Arthritis Rheumatol 2013;65:S562-S3.
- Glave-Testino C, Cardiel MH, Arce-Salinas A, Alarcón-Segovia D. Factors associated with disease severity in Mexican patients with rheumatoid arthritis. Clin Exp Rheumatol 1994;12:589-94.
- Goetz I, Carter GC, Lucero M, Zarotsky V, Alatorre CI, Cantrell RA, et al. Review of treatment response in rheumatoid arthritis: assessment of heterogeneity. Curr Med Res Opin 2011;27:697-711. https://doi.org/10.1185/03007995.2010.549805.
- Gomez A, Vinas O, Ercilla G, Salvador G, Canete JD, Rodriguez JR, et al. High titers of anti-CCP antibodies at baseline and after one year of DMARDs may predict a poor clinical response in patients with early rheumatoid arthritis. Ann Rheum Dis 2004;63:201-2.
- González-Alvaro I, Ortiz AM, Tomero EG, Balsa A, Orte J, Laffon A, et al. Baseline serum RANKL levels may serve to predict remission in rheumatoid arthritis patients treated with TNF antagonists. Ann Rheum Dis 2007;66:1675-8. https://doi.org/10.1136/ard.2007.071910.
- Gonzalez-Lopez L, Rocha-Muñoz AD, Ponce-Guarneros M, Flores-Chavez A, Salazar-Paramo M, Nava A, et al. Anti-cyclic citrullinated peptide (anti-CCP) and anti-mutated citrullinated vimentin (anti-MCV) relation with extra-articular manifestations in rheumatoid arthritis. J Immunol Res 2014;2014. https://doi.org/10.1155/2014/536050.
- Goodman SM, Ma Y, Zhang W, Schulman E, Bartlett SJ, Andersen KM, et al. Body mass index is an independent risk factor for not achieving sustained remission in early rheumatoid arthritis: results from the catch observational study. Ann Rheum Dis 2015;74:138-9. https://doi.org/10.1136/annrheumdis-2015-eular.1973.
- Gottenberg JE, Dayer JM, Lukas C, Ducot B, Chiocchia G, Cantagrel A, et al. Serum IL-6 and IL-21 are associated with markers of B cell activation and structural progression in early rheumatoid arthritis: results from the ESPOIR cohort. Ann Rheum Dis 2012;71:1243-8. https://doi.org/10.1136/annrheumdis-2011-200975.
- Graudal N, Tarp U, Jurik AG, Galløe AM, Garred P, Milman N, et al. Inflammatory patterns in rheumatoid arthritis estimated by the number of swollen and tender joints, the erythrocyte sedimentation rate, and hemoglobin: longterm course and association to radiographic progression. J Rheumatol 2000;27:47-5.
- Gremese E, Salaffi F, Bosello SL, Ciapetti A, Bobbio-Pallavicini F, Caporali R, et al. Very early rheumatoid arthritis as a predictor of remission: a multicentre real life prospective study. Ann Rheum Dis 2013;72:858-62. https://doi.org/10.1136/annrheumdis-2012-201456.
- Gremese E, Tolusso B, Fedele AL, Nowik M, Canestri S, Ferraccioli G. Clinical and B cell subsets biomarkers of response to tocilizumab in rheumatoid arthritis. Ann Rheum Dis 2015;74. https://doi.org/10.1136/annrheumdis-2015-eular.4712.
- Gullick NJ, Young A, Choy EH, Scott DL. Predicting ongoing active disease in early rheumatoid arthritis using clinical measures. Rheumatology 2012;51.
- Hall GM, Studnicke A, Huskisson EC, Scott DL. Rheumatoid nodules do not predict response to treatment with slow-acting anti-rheumatic drugs. J Clin Rheumtol 1991;10:384-7. https://doi.org/10.1007/BF02206657.
- Hama M, Uehara T, Takase K, Ihata A, Ueda A, Takeno M, et al. Power Doppler ultrasonography is useful for assessing disease activity and predicting joint destruction in rheumatoid arthritis patients receiving tocilizumab – preliminary data. Rheumatol Int 2012;32:1327-33. https://doi.org/10.1007/s00296-011-1802-5.
- Hama M, Sugiyama Y, Tsuchida N, Kunishita Y, Kishimoto D, Kamiyama R, et al. PD signal detected by ultrasonography relates to joint destruction in rheumatoid arthritis under biologics therapy in real world. Arthritis Rheumatol 2014;66:S53-S4.
- Hama M, Kirino Y, Toyota Y, Minegishi K, Yoshimi R, Takeno M, et al. Wrist PD signal detected by ultrasonography relates to joint destruction in rheumatoid arthritis under biologics therapy in real world. Ann Rheum Dis 2015;74:885-6. https://doi.org/10.1136/annrheumdis-2015-eular.2542.
- Hamann P, Holland R, Hyrich K, Pauling JD, Shaddick G, Nightingale A, et al. Factors associated with sustained remission in rheumatoid arthritis in patients treated with anti-tumour necrosis factor (anti-TNF). Arthritis Care Res 2017;69:783-93.
- Hambardzumyan K, Bolce RJ, Saevarsdottir S, Forslind K, Karlsson JA, Van Vollenhoven RF. Predictive biomarkers for response or non-response to MTX monotherapy in early RA. Arthritis Rheumatol 2015;67.
- Hammer HB, Sveinsson M, Kongtorp AK, Kvien TK. A 78-joints ultrasonographic assessment is associated with clinical assessments and is highly responsive to improvement in a longitudinal study of patients with rheumatoid arthritis starting adalimumab treatment. Ann Rheum Dis 2010;69:1349-51. https://doi.org/10.1136/ard.2009.126995.
- Hammer HB, Birkelund B, Kvien TK. Patients With Rheumatoid Arthritis on Anti-TNF Therapy; Responders With Major Reduction in Power Doppler Activity Can Be Identified After One Month n.d.
- Haney DJ, Cavet G, Durez P, Alten R, Burmester GR, Tak PP, et al. Correlation of a multi-biomarker disease activity response assessment to disease activity score 28 (C-reactive protein) response assessment and OMERACT RAMRIS scores in a placebo-controlled rheumatoid arthritis clinical trial with abatacept (ASSET). Arthritis Rheumatol 2012;64.
- Haroon N, Misra R, Aggarwal A. Tailor-made therapy in rheumatoid arthritis: fact or fiction?. Isr Med Assoc J 2008;10:139-41.
- Harris J, Bykerk VP, Hitchon CA, Keystone E, Thorne JC, Boire G, et al. Can we improve outcomes in early rheumatoid arthritis by determining best practices? An analysis of the Canadian early rheumatoid arthritis cohort (CATCH). Arthritis Rheumatol 2012;64:S175-S6.
- Harris J, Bykerk V, Hitchon C, Keystone E, Thorne C, Boire G, et al. Can we improve outcomes in early rheumatoid arthritis (ERA) by determining best practices? An analysis of the Canadian era cohort (CATCH). Ann Rheum Dis 2013;71. https://doi.org/10.1136/annrheumdis-2012-eular.2535.
- Hazlewood GS, Barnabe C, Boire G, Hitchon C, Keystone EC, Haraoui B, et al. Enhancing comparative effectiveness research by combining observational and randomized trial data to personalize the choice between methotrexate and triple therapy for methotrexate-naive patients with early rheumatoid arthritis. Arthritis Rheumatol 2015;67.
- Heidari B, Heidari P, Tayebi ME. The value of changes in CRP and ESR for predicting treatment response in rheumatoid arthritis. APLAR J Rheumatol 2007;10:23-8. https://doi.org/10.1111/j.1479-8077.2007.00250.x.
- Heimans L, van Den Broek M, Dirven L, Schouffoer AA, Speyer I, Kerstens PJSM, et al. Body mass index is associated with decreased response to initial and delayed treatment with dose escalated infliximab in patients with recent onset rheumatoid arthritis. Arthritis Rheumatol 2011;63.
- Hernandez MV, Narvaez J, Sanmartí R, Reina D, Diaz-Torne C, Magallares B, et al. Predictive factors of response to tocilizumab in patients with active rheumatoid arthritis. Clin Rheumatolo 2013;65.
- Herold M, Fasching P, Graninger W, Lunzer R, Zamani O, Leeb B. Efficacy of tocilizumab monotherapy in patients with RA is not influenced by ACPA positivity. Arthritis Rheumatol 2015;67.
- Hetland ML, Stengaard-Pedersen K, Junker P, Østergaard M, Ejbjerg BJ, Jacobsen S, et al. Radiographic progression and remission rates in early rheumatoid arthritis – MRI bone oedema and anti-CCP predicted radiographic progression in the 5-year extension of the double-blind randomised CIMESTRA trial. Ann Rheum Dis 2010;69:1789-95. https://doi.org/10.1136/ard.2009.125534.
- Hirano Y, Hayashi M, Hirabara S, Takahashi N, Kanayama Y, Kaneko A, et al. Predictors of effectiveness in golimumab treatment and efficacy of dose-escalation of golimumab in patients with rheumatoid arthritis–a multicenter registry study TBCR. Ann Rheum Dis 2015;74. https://doi.org/10.1136/annrheumdis-2015-eular.1247.
- Hirata S, Li WY, Defranoux NA, Bolce R, Sasso EH, Kubo S, et al. Repeated high or low multi-biomarker disease activity (Vectra® DA algorithm) scores associated with radiographic outcomes in patients with rheumatoid arthritis treated with tumor necrosis factor inhibitors. Arthritis Rheumatol 2013;65:S616-S7.
- Hirata S, Marotta A, Gui Y, Hanami K, Tanaka Y. Serum 14-3-3η level is associated with severity and clinical outcomes of rheumatoid arthritis, and its pretreatment level is predictive of DAS28 remission with tocilizumab. Arthritis Res Ther 2015;17. https://doi.org/10.1186/s13075-015-0799-7.
- Hoekstra M, van Ede AE, Haagsma CJ, van de Laar MA, Huizinga TW, Kruijsen MW, et al. Factors associated with toxicity, final dose, and efficacy of methotrexate in patients with rheumatoid arthritis. Ann Rheum Dis 2003;62:423-6. https://doi.org/10.1136/ard.62.5.423.
- Horton SC, Tan AL, Freeston JE, Wakefield RJ, Buch MH, Emery P. Discordance between the predictors of clinical and imaging remission in patients with early rheumatoid arthritis in clinical practice: implications for the use of ultrasound within a treatment-to-target strategy. Rheumatology 2016;55:1177-87. https://doi.org/10.1093/rheumatology/kew037.
- Hoshi D, Shidara K, Seto Y, Tanaka E, Nakajima A, Momohara S, et al. Greater physical dysfunction is a negative predictor for achieving Boolean-based remission in patients with rheumatoid arthritis treated with tocilizumab. Arthritis Rheumatol 2011;63:S482-3.
- Hu C, Xu Z, Zhang Y, Rahman MU, Davis HM, Zhou H. Population approach for exposure-response modeling of golimumab in patients with rheumatoid arthritis. J Clin Pharmacol 2011;51:639-48. https://doi.org/10.1177/0091270010372520.
- Huizinga T, Nigrovic P, Ruderman E, Schulze-Koops H. Very early rheumatoid arthritis is the major predictor of major outcomes: clinical ACR remission and radiographic non-progression – Commentary. Int J Adv Rheumatol 2011;9:140-1.
- Iagnocco A, Finucci A, Ceccarelli F, Perricone C, Iorgoveanu V, Valesini G. Power Doppler ultrasound monitoring of response to anti-tumour necrosis factor alpha treatment in patients with rheumatoid arthritis. Rheumatology 2015;54:1890-6. https://doi.org/10.1093/rheumatology/kev211.
- Iannaccone CK, Lee YC, Cui J, Frits ML, Glass RJ, Plenge RM, et al. Using genetic and clinical data to understand response to disease-modifying anti-rheumatic drug therapy: data from the Brigham and Women’s Hospital Rheumatoid Arthritis Sequential Study. Rheumatology (Oxford) 2011;50:40-6. https://doi.org/10.1093/rheumatology/keq263.
- Iannone F, Ferraccioli G, Ferri C, Galeazzi M, Gerli R, Giacomelli R, et al. Etanercept therapy in rheumatoid arthritis patients with moderate or severe disease activity. Ann Rheum Dis 2013;71. https://doi.org/10.1136/annrheumdis-2012-eular.465.
- Iannone F, Carlino G, Marchesoni A, Sarzi-Puttini P, Gorla R, Salaffi F, et al. Early good EULAR response predicts low/remission disease state in rheumatoid arthritis patients on treatment with certolizumab in real life settings. Data from Italian registry GISEA. Ann Rheum Dis 2015;74. https://doi.org/10.1136/annrheumdis-2015-eular.2487.
- Ickinger C, Musenge E, Tikly M. Predictors of joint damage in South Africans with rheumatoid arthritis. Rheumatology (Oxford) 2011;50. https://doi.org/10.1093/rheumatology/ker029.
- Ideguchi H, Ohno S, Hattori H, Senuma A, Ishigatsubo Y. Bone erosions in rheumatoid arthritis can be repaired through reduction in disease activity with conventional disease-modifying antirheumatic drugs. Arthritis Res Ther 2006;8. https://doi.org/10.1186/ar1943.
- Inanc N, Ozen G, Direskeneli H. Can ultrasonographic findings predict response to tumor necrosis factor-alpha inhibitor treatment in rheumatoid arthritis?. Arthritis Rheumatol 2013;65.
- Inanc N, Ozen G, Direskeneli H. Ultrasonographic assessment of joint inflammation in rheumatoid arthritis: predictive value in response to tumor necrosis factor-a inhibitor treatment. Clin Exp Rheumatol 2014;32. https://doi.org/10.1136/annrheumdis-2014-eular.1918.
- Inanc N, Ozen G, Direskeneli H. Ultrasonographic assessment of joint inflammation in rheumatoid arthritis: predictive value in response to tumor necrosis factor-alpha inhibitor treatment. Ann Rheum Dis 2013;72:A544-5.
- Inanc N, Ozen G, Direskeneli H. Predictive value of ultrasonographic assessment of disease activity in response to tumor necrosis factor-α inhibitor treatment in rheumatoid arthritis: a prospective cohort study. Clin Exp Rheumatol 2016;34.
- Inoue S, Hashiguchi M, Takagi K, Kawai S, Mochizuki M. Preliminary study to identify the predictive factors for the response to methotrexate therapy in patients with rheumatoid arthritis. Yakugaku Zasshi 2009;129:843-9. https://doi.org/10.1248/yakushi.129.843.
- Inoue Y, Nakajima A, Tanaka E, Inoue E, Kobayashi A, Hoshi D, et al. Effect of smoking on remission proportions differs between male and female patients with rheumatoid arthritis: a study based on the IORRA Survey. J Rheumatol 2015;42:1083-9. https://doi.org/10.3899/jrheum.140376.
- Isaacs J, Olech E, Tak P, Deodhar A, Keystone E, Emery P. Autoantibody-positive rheumatoid arthritis (RA) patients (pts) have enhanced clinical response to rituximab (RTX) when compared with seronegative patients. Ann Rheum Dis 2009;68.
- Isaacs JD, Cohen SB, Emery P, Tak PP, Wang J, Lei G, et al. Effect of baseline rheumatoid factor and anticitrullinated peptide antibody serotype on rituximab clinical response: a meta-analysis. Ann Rheum Dis 2013;72:329-36. https://doi.org/10.1136/annrheumdis-2011-201117.
- Ishiguro N, Atsumi T, Harigai M, Mimori T, Nishimoto N, Sumida T, et al. Effectiveness and safety of tocilizumab in achieving clinical and functional remission, and sustaining efficacy in biologics-naive patients with rheumatoid arthritis: The FIRST Bio study. Mod Rheumatol 2017;27:217-26.
- Iwamoto N, Kawakami A, Fujikawa K, Aramaki T, Kawashiri SY, Tamai M, et al. Prediction of DAS28-ESR remission at 6 months by baseline variables in patients with rheumatoid arthritis treated with etanercept in Japanese population. Mod Rheumatol 2009;19:488-92. https://doi.org/10.1007/s10165-009-0187-8.
- Iwamoto N, Fukui S, Umeda M, Nishino A, Nakashima Y, Suzuki T, et al. Evaluation of switching from intravenous to subcutaneous formulation of tocilizumab in patients with rheumatoid arthritis. Mod Rheumatol 2016;26:662-6. https://doi.org/10.3109/14397595.2015.1129692.
- Izumi K, Kaneko Y, Hashizume M, Yoshimoto K, Takeuchi T. Baseline serum osteopontin levels predict the clinical effectiveness of tocilizumab but not infliximab in biologic-naive patients with rheumatoid arthritis: a single-center prospective study at 1 year (the Keio First-Bio Cohort Study). PLOS ONE 2015;10. https://doi.org/10.1371/journal.pone.0145468.
- Jansen LM, van der Horst-Bruinsma IE, van Schaardenburg D, Bezemer PD, Dijkmans BA. Predictors of radiographic joint damage in patients with early rheumatoid arthritis. Ann Rheum Dis 2001;60:924-7. https://doi.org/10.1136/ard.60.10.924.
- Jawaheer D, Olsen J, Hetland ML. Sex differences in response to anti-tumor necrosis factor therapy in early and established rheumatoid arthritis – results from the DANBIO registry. J Rheumatol 2012;39:46-53. https://doi.org/10.3899/jrheum.110548.
- Jayakumar K, Norton S, Dixey J, James D, Gough A, Williams P, et al. Sustained clinical remission in rheumatoid arthritis: prevalence and prognostic factors in an inception cohort of patients treated with conventional DMARDS. Rheumatology 2012;51:169-75. https://doi.org/10.1093/rheumatology/ker250.
- Jessome M, Tomizza M, Beattie KA, Bensen WG, Bobba R, Cividino A, et al. Does computerized segmentation of early erosions on magnetic resonance imaging predict functional ability in rheumatoid arthritis?. Arthritis Rheumatol 2015;67.
- Jilani AA, Mackworth-Young CG. The role of citrullinated protein antibodies in predicting erosive disease in rheumatoid arthritis: a systematic literature review and meta-analysis. Int J Rheumatol 2015;2015. https://doi.org/10.1155/2015/728610.
- Jones JG. Factors predicting outcome in rheumatoid arthritis. J Rheumatol 1994;21.
- Joo YB, Bang SY, Ryu JA, Lee S, Choi J, Lee HS, et al. Clinical predictors of severe radiographic damage in Korean patients with rheumatoid arthritis. Ann Rheum Dis 2015;74. https://doi.org/10.1136/annrheumdis-2015-eular.5737.
- Jurgens MS, Overman CL, Jacobs JW, Geenen R, Cuppen BV, Marijnissen AC, et al. Contribution of the individual components of the disease activity score (DAS28) to the total DAS28 score among responders and non-responders to biological therapy for rheumatoid arthritis. Ann Rheum Dis 2014;73:389-90. https://doi.org/10.1136/annrheumdis-2014-eular.4125.
- Jurgens MS, Overman CL, Jacobs JW, Geenen R, Cuppen BV, Marijnissen AC, et al. Contribution of the subjective components of the disease activity score to the response to biologic treatment in rheumatoid arthritis. Arthritis Care Res 2015;67:923-8. https://doi.org/10.1002/acr.22532.
- Kameda H, Kanbe K, Sato E, Ueki Y, Saito K, Nagaoka S, et al. Continuation/discontinuation of methotrexate and clinical response to etanercept determine the radiographic progression/repair in patients with rheumatoid arthritis: a subanalysis of 52-week results from the JESMR study. Ann Rheum Dis 2013;71. https://doi.org/10.1136/annrheumdis-2012-eular.3078.
- Kameda H, Kanbe K, Sato E, Ueki Y, Saito K, Nagaoka S, et al. A merged presentation of clinical and radiographic data using probability plots in a clinical trial, the JESMR study. Ann Rheum Dis 2013;72:310-12. https://doi.org/10.1136/annrheumdis-2012-201804.
- Kaneko A, Takahashi N, Kida D, Hirano Y, Ishikawa H, Kojima T, et al. Predictors of clinical remission (DAS28CRP<2.6) in patients with RA who were treated with abatacept. Ann Rheum Dis 2013;71. https://doi.org/10.1136/annrheumdis-2012-eular.515.
- Kaneshita S, Min C, Fumio O, Suda M, Rokutanda R, Shimizu H, et al. Highly elevated rheumatoid factor is a risk factor for abatacept treatment failure in Japanese patients with rheumatoid arthritis. Arthritis Rheumatol 2015;67.
- Kanters S, Druyts E, Mills EJ, Thorlund K. What drives the comparative effectiveness of biologics vs. methotrexate in rheumatoid arthritis? Meta-regression and graphical inspection of suspected clinical factors. Rheumatology 2014;53:1264-73. https://doi.org/10.1093/rheumatology/ket492.
- Karateev D, Luchikhina E, Demidova N, Loukina G, Kanonirova M, Olyunin Y, et al. Dynamics of disease activity scores during the first 12 weeks allow to predict the necessity in combination therapy with methotrexate and biologics within T2T strategy in patients with early and established rheumatoid arthritis (REMARCA study). Ann Rheum Dis 2015;74:976-7. https://doi.org/10.1136/annrheumdis-2015-eular.5277.
- Katchamart W, Johnson S, Lin HJ, Phumethum V, Salliot C, Bombardier C. Predictors for remission in rheumatoid arthritis patients: a systematic review. Arthritis Care Res 2010;62:1128-43. https://doi.org/10.1002/acr.20188.
- Kawada T. Predictors of biological antirheumatic drug discontinuation in patients with rheumatoid arthritis while in remission. J Rheumatol 2016;43. https://doi.org/10.3899/jrheum.151462.
- Kawasaki Y, Hashimoto T, Okano T, Sakai Y, Yamane T, Shiozawa K, et al. Shorter disease duration is important for tocilizumab to achieve Boolean remission. Mod Rheumatol 2013;23:1192-7. https://doi.org/10.1007/s10165-012-0822-7.
- Kawashiri SY, Ueki Y, Migita K, Matsuoka N, Mizokami A, Mine M, et al. Evaluation of the efficacy of tocilizumab toward the patients with active rheumatoid arthritis of Nagasaki Prefecture, Japan. Ann Rheum Dis 2013;71. https://doi.org/10.1136/annrheumdis-2012-eular.574.
- Kawashiri S, Ueki Y, Migita K, Nakamura H, Aoyagi K, Kawakami A. Baseline low modified health assessment questionnaire (MHAQ) predicts the state of remission estimated by clinical disease activity index and MHAQ at 1 year in tocilizumab-treated rheumatoid arthritis patients. Clin Exp Rheumatol 2014;32.
- Kay J, Morgacheva O, Messing SP, Kremer JM, Greenberg JD, Reed GW, et al. Clinical disease activity and acute phase reactant levels are discordant among patients with active rheumatoid arthritis: acute phase reactant levels contribute separately to predicting outcome at one year. Arthritis Res Ther 2014;16. https://doi.org/10.1186/ar4469.
- Kekow J, Muller-Ladner U, Schulze-Koops H, Link R, Breuer S, Kobialko M. Real life treatment with rituximab in TNF blocker non-responders is superior to treatment with a second TNF blocker. Arthritis Rheumatol 2010;62.
- Khan A, Scott DL. Smoking and rheumatoid factor status in predicting responses to biologics. Rheumatology 2012;51:iii41-iii2.
- Kim SK, Choe JY, Park SH, Lee H. No predictive effect of body mass index on clinical response in patients with rheumatoid arthritis after 24 weeks of biological disease-modifying antirheumatic drugs: a single-center study. Clin Rheumatol 2016;35:1129-36. https://doi.org/10.1007/s10067-016-3220-2.
- Kimura N, Suzuki K, Takeuchi T. Time lag between the initiation of adalimumab after methotrexate correlates with the efficacy of adalimumab in rheumatoid arthritis patients. Mod Rheumatol 2016;26:676-80. https://doi.org/10.3109/14397595.2015.1132952.
- Klaasen R, Wijbrandts CA, Gerlag DM, Tak PP. Is the response to infliximab influenced by body mass index in rheumatoid arthritis patients?. Arthritis Rheumatol 2009;60.
- Klaasen R, Thurlings RM, Wijbrandts CA, van Kuijk AW, Baeten D, Gerlag DM, et al. The relationship between synovial lymphocyte aggregates and the clinical response to infliximab in rheumatoid arthritis: a prospective study. Arthritis Rheum 2009;60:3217-24. https://doi.org/10.1002/art.24913.
- Klaasen R, Wijbrandts CA, Gerlag DM, Tak PP. Body mass index and clinical response to infliximab in rheumatoid arthritis. Arthritis Rheum 2011;63:359-64. https://doi.org/10.1002/art.30136.
- Koike T, Harigai M, Inokuma S, Ishiguro N, Ryu J, Takeuchi T, et al. Safety and effectiveness responses to etanercept for rheumatoid arthritis in Japan: a sub-analysis of a post-marketing surveillance study focusing on the duration of rheumatoid arthritis. Rheumatol Int 2012;32:1511-19. https://doi.org/10.1007/s00296-010-1784-8.
- Koike T, Harigai M, Inokuma S, Ishiguro N, Ryu J, Takeuchi T, et al. Effectiveness and safety of tocilizumab: postmarketing surveillance of 7901 patients with rheumatoid arthritis in Japan. J Rheumatol 2014;41:15-23. https://doi.org/10.3899/jrheum.130466.
- Kojima T, Kaneko A, Hirano Y, Ishikawa H, Yabe Y, Takagi H, et al. Early aggressive intervention for rheumatoid arthritis increases rate of remission defined using a Boolean approach in clinical practice with tocilizumab. Mod Rheumatol 2012;22:370-5.
- Kojima T, Takahashi N, Funahashi K, Kato D, Hattori Y, Hanabayashi M, et al. Importance of concomitant MTX use during treatment with tocilizumab in patients with rheumatoid arthritis. Ann Rheum Dis 2013;72:A628-9. https://doi.org/10.1136/annrheumdis-2013-eular.1865.
- Kojima T, Takahashi N, Funahashi K, Asai S, Hanabayashi M, Hirabara S, et al. Relationship between physicians’ decision to use concomitant glucocorticoid and remission during treatment with tocilizumab in patients with background of limited dose of MTX. Ann Rheum Dis 2013;65.
- Kojima T, Yabe Y, Kaneko A, Takahashi N, Funahashi K, Kato D, et al. Importance of methotrexate therapy concomitant with tocilizumab treatment in achieving better clinical outcomes for rheumatoid arthritis patients with high disease activity: an observational cohort study. Rheumatology 2015;54:113-20. https://doi.org/10.1093/rheumatology/keu302.
- Kojima T, Takahashi N, Kaneko A, Kida D, Hirano Y, Fujibayashi T, et al. Importance of both disease activity at 12 weeks and clinical response up to 12 weeks to predict achievement of low disease activity at 52 weeks during abatacept treatment in biologics-switching patients with rheumatoid arthritis: a multicenter observational cohort study in Japan. Ann Rheum Dis 2015;74. https://doi.org/10.1136/annrheumdis-2015-eular.1318.
- Kojima T, Takahashi N, Funahashi K, Asai S, Takemoto T, Asai N, et al. Predictive factors for achieving low disease activity at 52 weeks after switching from tumor necrosis factor inhibitors to abatacept: Results from a multicenter observational cohort study of Japanese patients. Clin Rheumatol 2016;35:219-25.
- Kotak S, Koenig A, Collier D, Saunders K, He P, Kremer J, et al. Characteristics of a moderate rheumatoid arthritis patient population who lost remission or low disease activity: data from the Consortium of Rheumatology Researchers of North America, Inc. (CORRONA). Ann Rheum Dis 2013;71. https://doi.org/10.1136/annrheumdis-2012-eular.330.
- Kovalchik SA, Charles-Schoeman C, Khanna D, Paulus HE. An association study of disease activity score components and patient satisfaction with overall health for early RA patients on non-biologic DMARD therapy. Rheumatol Int 2012;32:2725-9. https://doi.org/10.1007/s00296-011-2037-1.
- Kristensen LE, Kapetanovic MC, Gülfe A, Söderlin M, Saxne T, Geborek P. Predictors of response to anti-TNF therapy according to ACR and EULAR criteria in patients with established RA: results from the South Swedish Arthritis Treatment Group Register. Rheumatology 2008;47:495-9. https://doi.org/10.1093/rheumatology/ken002.
- Kronisch C, McLernon DJ, Dale J, Paterson C, Ralston SH, Reid DM, et al. Brief report: predicting functional disability: one-year results from the Scottish early rheumatoid arthritis inception cohort. Arthritis Rheumatol 2016;68:1596-602. https://doi.org/10.1002/art.39627.
- Krüger K, Wollenhaupt J, Lorenz HM, Röther E, Wittig BM. Reduction in sickness absence in patients with rheumatoid arthritis receiving adalimumab: data from a German noninterventional study. Rheumatol Int 2012;32:3977-83. https://doi.org/10.1007/s00296-011-2317-9.
- Kumar A. Rheumatoid arthritis: clinical spectrum, diagnosis and management. Int Med Sci Acad 2002;15:13-9.
- Kume K, Yamada S, Amano K, Hatta K, Ohta H, Kuwaba N. Anti-cyclic citrullinated protein antibodies as a predictor of response to tocilizumab in patients with rheumatoid arthritis a prospective study. Arthritis Rheumatol 2012;64.
- Kuriya B, Xiong J, Boire G, Haraoui B, Hitchon C, Pope J, et al. Earlier time to remission predicts sustained clinical remission in early rheumatoid arthritis – results from the Canadian Early Arthritis Cohort (CATCH). J Rheumatol 2014;41:2161-6. https://doi.org/10.3899/jrheum.140137.
- Kuriya B, Lin D, Barnabe C, Boire G, Hitchon C, Jamal S, et al. Working status and improvements in work productivity over time in an early rheumatoid arthritis (ERA) cohort. J Rheumatol 2015;42:1272-3.
- Kynde I, Sorensen J, Hetland ML, Hjelmborg JVB. Delay in RA diagnosis of more than 12 months is associated with deteriorated functional status in patients in bDMARD treatment-results from a prospective study. Ann Rheum Dis 2014;73. https://doi.org/10.1136/annrheumdis-2014-eular.4286.
- Lard LR, Visser H, Speyer I, vander Horst-Bruinsma IE, Zwinderman AH, Breedveld FC, et al. Early versus delayed treatment in patients with recent-onset rheumatoid arthritis: comparison of two cohorts who received different treatment strategies. Am J Med 2001;111:446-51. https://doi.org/10.1016/S0002-9343(01)00872-5.
- Le Blay P, Rubenstein E, Ferreira Lopez R, Jorgensen C, Pers YM. Progressive spacing tocilizumab infusions after remission in rheumatoid arthritis: the absence of erosion would be determinant in choosing this strategy. Ann Rheum Dis 2014;73:955-6. https://doi.org/10.1136/annrheumdis-2014-eular.3292.
- Lee R, Yazici Y, Pincus T. Disease Activity Score (DAS) and Health Assessment Questionnaire (HAQ) values show similar changes from baseline to endpoint in clinical trials of biological agents in patients with rheumatoid arthritis (RA). Arthritis Rheumatol 2008;58:S773-S4.
- Lee YC, Hackett J, Alexander C, Frits MA, Iannaccone CK, Shadick NA, et al. Application of a multi-biomarker disease activity (Vectra® DA) score for assessing rheumatoid arthritis patients with fibromyalgia or low c-reactive protein. Arthritis Rheumatol 2013;65:S1145-S6.
- Li Z, Zhang F, Kay J, Fei K, Han C, Zhuang Y, et al. Efficacy and safety results from a Phase 3, randomized, placebo-controlled trial of subcutaneous golimumab in Chinese patients with active rheumatoid arthritis despite methotrexate therapy. Int J Rheum Dis 2013;72.
- Lie E, Heiberg M, Nordvag B, Rodevand E, Kaufmann C, Mikkelsen K, et al. Predictors of response to methotrexate treatment: results from a longitudinal observational study of 876 patients with RA. Ann Rheum Dis 2006;65.
- Lie E, Chatzidionysiou K, Nasonov EL, Lukina G, Pavelka K, Nordstrom DC, et al. Characterization of long-term responders to first treatment course of rituximab (RTX) results from the CERERRA collaboration. Arthritis Rheumatol 2011;63.
- Lie E, Chatzidionysiou K, Nasonov EL, Lukina G, Pavelka K, Nordstrom DC, et al. Early versus delayed retreatment with rituximab (RTX) in relation to long term clinical response data from the CERERRA collaboration. Arthritis Rheumatol 2011;63.
- Lie E, Uhlig T, van der Heijde D, Rødevand E, Kalstad S, Kaufmann C, et al. Effectiveness of sulfasalazine and methotrexate in 1102 DMARD-naive patients with early RA. Rheumatology 2012;51:670-8. https://doi.org/10.1093/rheumatology/ker356.
- Lima A, Bernardes M, Monteiro J, Azevedo R, Costa L, Ventura F, et al. The influence of clinical and genetic variables on methotrexate effectiveness in Portuguese rheumatoid arthritis patients. Ann Rheum Dis 2013;72. https://doi.org/10.1136/annrheumdis-2013-eular.2486.
- Liu Y, Kaplan G, Eksteen B, Barnabe C. Impact of obesity on the disease course of rheumatoid arthritis. Arthritis Rheumatol 2015;67.
- Ljung L, Egerer K, Bang H, Feist E, Burmester GR, Dahlqvist SR. New assay generation for antibodies against modified and citrullinated peptides predicts poor response to TNF inhibitor therapy. Arthritis Rheumatol 2011;63:S330-1.
- Loppin E, Garlantezec R, Solau-Gervais E. Low rate of rheumatoid arthritis remission in real life: might predictive factors explain?. Arthritis Rheumatol 2010;62.
- Lukas C, van der Heijde D, Fatenajad S, Landewé R. Repair of erosions occurs almost exclusively in damaged joints without swelling. Ann Rheum Dis 2010;69:851-5. https://doi.org/10.1136/ard.2009.119156.
- Lv Q, Yin Y, Li X, Shan G, Wu X, Liang D, et al. The status of rheumatoid factor and anti-cyclic citrullinated peptide antibody are not associated with the effect of anti-TNFα agent treatment in patients with rheumatoid arthritis: a meta-analysis. PLOS ONE 2014;9. https://doi.org/10.1371/journal.pone.0089442.
- Ma MH, Scott DL, Kiely P, Williams R, Walsh D, Young A. HAQ and gender predict remission in early RA: experience in the Early Rheumatoid Arthritis Network (ERAN). Rheumatology 2009;48.
- Ma MH, Walker D, Hassell A, Choy EH, Scott DL. Predicting remission in trial of intensive therapy in early RA: HAQ and gender are key factors. Ann Rheum Dis 2009;48.
- Ma MH, Li W, Defranoux N, Kingsley GH, Scott DL, Cope AP. A multi-biomarker disease activity (Vectra™ DA algorithm) score and components are associated with sustained clinical remission in rheumatoid arthritis: the REMIRA study. Ann Rheum Dis 2013;72.
- Ma MH, Garrood T, Li W, Defranoux N, Kingsley GH, Scott DL, et al. Multi-biomarker disease activity (Vectra® DA algorithm) score is associated with power Doppler ultrasound in patients with rheumatoid arthritis in low disease activity state: the REMIRA cohort. Arthritis Rheum 2014;73. https://doi.org/10.1136/annrheumdis-2014-eular.2757.
- Macchioni P, Magnani M, Mulè R, Galletti S, Catanoso M, Pignotti E, et al. Ultrasonographic predictors for the development of joint damage in rheumatoid arthritis patients: a single joint prospective study. Clin Exp Rheumatol 2013;31:8-17.
- Machado FS, Furtado RNV, Takahashi RD, de Buosi ALP, Natour J. Predictors of rheumatoid arthritis: quantitative and semiquantitative sonographic measurements of peripheral joints. Arthritis Rheumatol 2012;64.
- Makinen H, Hannonen P, Sokka T. Sex: a major predictor of remission as measured by 28-joint Disease Activity Score (DAS28) in early rheumatoid arthritis?. Ann Rheum Dis 2008;67:1052-3. https://doi.org/10.1136/ard.2007.084897.
- Mancarella L, Bobbio-Pallavicini F, Ceccarelli F, Falappone PC, Ferrante A, Malesci D, et al. Good clinical response, remission, and predictors of remission in rheumatoid arthritis patients treated with tumor necrosis factor-alpha blockers: the GISEA study. J Rheumatol 2007;34:1670-3.
- Manders S, Kievit W, Braakman A, Hendriks L, Brus H, Fransen J, et al. Are TNF blocking agents associated with changes in work participation in patients with RA?. Ann Rheum Dis 2013;71. https://doi.org/10.1136/annrheumdis-2012-eular.3427.
- Manders SH, Kievit W, Braakman-Jansen AL, Brus HL, Hendriks L, Fransen J, et al. Determinants associated with work participation in patients with established rheumatoid arthritis taking tumor necrosis factor inhibitors. J Rheumatol 2014;41:1263-9. https://doi.org/10.3899/jrheum.130878.
- Maneiro R, Salgado E, Carmona L, Gomez-Reino J. Rheumatoid factor as predictor of response to abatacept, rituximab and tocilizumab in rheumatoid arthritis: systematic review and meta-analysis (Provisional abstract). Semin Arthritis Rheum 2013;43:9-17. https://doi.org/10.1016/j.semarthrit.2012.11.007.
- Maneiro JR, Salgado E, Carmona L, Gomez-Reino JJ. Rheumatoid factor as predictor of response to non TNF antagonist biologic therapies in rheumatoid arthritis: systematic review and meta-analysis. Ann Rheum Dis 2013;71. https://doi.org/10.1136/annrheumdis-2012-eular.2536.
- Markusse I, Han K, Peeters A, Ronday H, Kerstens P, Huizinga T, et al. Initial Combination Therapy in Early Rheumatoid Arthritis: Which Patients Benefit? n.d.
- Marotte H, Miossec P. Biomarkers for prediction of TNFalpha blockers response in rheumatoid arthritis. Joint Bone Spine 2010;77:297-305. https://doi.org/10.1016/j.jbspin.2010.02.026.
- Martin WJ, Shim M, Paulus HE, Chaudhari S, Feng J, Elashoff D, et al. Older age at rheumatoid arthritis onset and comorbidities correlate with less Health Assessment Questionnaire-Disability Index and Clinical Disease Activity Index response to etanercept in the RADIUS 2 registry. J Clin Rheumatol 2014;20:301-5. https://doi.org/10.1097/RHU.0000000000000152.
- Martin-Mola E, Balsa A, García-Vicuna R, Gómez-Reino J, González-Gay MA, Sanmartí R, et al. Anti-citrullinated peptide antibodies and their value for predicting responses to biologic agents: a review. Rheumatol Int 2016;36:1043-63. https://doi.org/10.1007/s00296-016-3506-3.
- Matsushita I, Motomura H, Seki E, Kimura T. Radiographic changes and factors associated with subsequent progression of damage in weight-bearing joints of patients with rheumatoid arthritis under TNF-blocking therapies. Three-year observational study. Mod Rheumatol 2017;4:570-5.
- Mattey DL, Brownfield A, Dawes PT. Relationship between pack-year history of smoking and response to tumor necrosis factor antagonists in patients with rheumatoid arthritis. J Rheumatol 2009;36:1180-7. https://doi.org/10.3899/jrheum.081096.
- Miceli-Richard C, Verstuyft C, Comets E, Loiseau P, Tamouza R, Contreras L, et al. Analysis of the shared epitope and selected pro- and anti-inflammatory cytokine genes polymorphism as predictive factors of response to adalimumab in rheumatoid arthritis patients treated in the ReAct study. Arthritis Rheumatol 2006;54.
- Mirpourian M, Salesi M, Abdolahi H, Farajzadegan Z, Karimzadeh H. The association of body mass index with disease activity and clinical response to combination therapy in patients with rheumatoid arthritis. J Res Med Sci 2014;19:509-14.
- Miwa Y, Furuya H, Yanai R, Ohtsuka K, Sato M, Takahashi R, et al. Factors leading to HAQ remission after beginning biologics treatment in patients with rheumatoid arthritis. Ann Rheum Dis 2013;71. https://doi.org/10.1136/annrheumdis-2012-eular.448.
- Miyoshi F, Honne K, Minota S, Okada M, Ogawa N, Mimura T. A novel method predicting good response using only background clinical data in RA patients treated with infliximab. Arthritis Rheumatol 2013;65:S627-S8.
- Molina E, Del Rincon I, Restrepo JF, Battafarano DF, Escalante A. Association of socioeconomic status with treatment delays, disease activity, joint damage, and disability in rheumatoid arthritis. Arthritis Care Res 2015;67:940-6. https://doi.org/10.1002/acr.22542.
- Moorthy A, Fisher J, Duncan A, Taylor J. Does smoking predict poor response to anti-TNF therapy for rheumatoid arthritis?. Rheumatology 2007;46:I31-I.
- Möttönen T, Hannonen P, Korpela M, Nissilä M, Kautiainen H, Ilonen J, et al. Delay to institution of therapy and induction of remission using single-drug or combination-disease-modifying antirheumatic drug therapy in early rheumatoid arthritis. Arthritis Rheum 2002;46:894-8. https://doi.org/10.1002/art.10135.
- Munro R, Capell H. C-reactive protein levels correlate with functional outcome. J Rheumatol 1997;24:1852-3.
- Nagasawa H, Kameda H, Sekiguchi N, Amano K, Takeuchi T. Improvement of the HAQ score by infliximab treatment in patients with RA: its association with disease activity and joint destruction. Mod Rheumatol 2009;19:166-72. https://doi.org/10.1007/s10165-008-0142-0.
- Nair SC, Welsing PM, Choi IY, Roth J, Holzinger D, Bijlsma JW, et al. A personalized approach to biological therapy using prediction of clinical response based on MRP8/14 serum complex levels in rheumatoid arthritis patients. PLOS ONE 2016;11. https://doi.org/10.1371/journal.pone.0152362.
- Narvaez J, Torne CD, Ruiz JM, Hernandez MV, Torrente V, Ros S, et al. Predictors of response to rituximab in patients with active rheumatoid arthritis and inadequate response to anti-TNF agents or traditional DMARD. Ann Rheum Dis 2010;62.
- Narvaez J, Díaz-Torné C, Ruiz JM, Hernandez MV, Torrente-Segarra V, Ros S, et al. Predictors of response to rituximab in patients with active rheumatoid arthritis and inadequate response to anti-TNF agents or traditional DMARDs. Clin Exp Rheumatol 2011;29:991-7.
- Navarro-Millán I, Chen L, Greenberg JD, Pappas DA, Curtis JR. Predictors and persistence of new-onset clinical remission in rheumatoid arthritis patients. Semin Arthritis Rheum 2013;43:137-43. https://doi.org/10.1016/j.semarthrit.2013.02.002.
- Nawata M, Saito K, Nakayamada S, Tanaka Y. Discontinuation of infliximab in rheumatoid arthritis patients in clinical remission. Mod Rheumatol 2008;18:460-4. https://doi.org/10.1007/s10165-008-0089-1.
- Nguyen H, Ruyssen-Witrand A, Constantin AL, Foltz V, Gandjbakhch F, Cantagrel AG. The prevalence of the ultrasonographic positive power Doppler synovitis is high and predicts the risk of relapse and structural progression in rheumatoid arthritis in clinical remission: a systematic literature review and meta analysis. Arthritis Rheumatol 2012;64.
- Nguyen H, Ruyssen-Witrand A, Gandjbakhch F, Constantin A, Foltz V, Cantagrel A. Prevalence of ultrasound-detected residual synovitis and risk of relapse and structural progression in rheumatoid arthritis patients in clinical remission: a systematic review and meta-analysis. Rheumatology 2014;53:2110-18. https://doi.org/10.1093/rheumatology/keu217.
- Nishimoto N, Amano K, Hirabayashi Y, Horiuchi T, Ishii T, Iwahashi M, et al. Drug free REmission/low disease activity after cessation of tocilizumab (Actemra) Monotherapy (DREAM) study. Mod Rheumatol 2013:1-9. https://doi.org/10.1007/s10165-013-0894-z.
- Nishino A, Kawashiri SY, Kawakami A, Yoshitama T, Eiraku N, Matsuoka N, et al. Ultrasound evaluation of the efficacy of biologic and targeted synthetic DMARDs toward rheumatoid arthritis patients: Kyushu multicenter rheumatoid arthritis ultrasound prospective observational cohort in japan. Arthritis Rheumatol 2015;67.
- Nishiyama S, Ohashi K, Aita T, Yoshinaga Y, Miyawaki S, Nishino J, et al. To develop a regression model for predicting damage-related HAQ: a nationwide study based on the NinJa (national database of rheumatic diseases by iR-net in Japan) 2011. Ann Rheum Dis 2013;72:A845-6. https://doi.org/10.1136/annrheumdis-2013-eular.2518.
- Nixon RM, Bansback N, Brennan A. Using mixed treatment comparisons and meta-regression to perform indirect comparisons to estimate the efficacy of biologic treatments in rheumatoid arthritis. Stat Med 2007;26:1237-54. https://doi.org/10.1002/sim.2624.
- Nordberg LB, Lillegraven S, Lie E, Aga A-B, Olsen IC, Hammer HB, et al. Patients with seronegative RA have more inflammatory activity compared with patients with seropositive RA in an inception cohort of DMARD-naive patients classified according to the 2010 ACR/EULAR criteria. Ann Rheum Dis 2016.
- Ogawa Y, Takahashi N, Funahashi K, Asai S, Takemoto T, Watanabe T, et al. Titer of anti-citrullinated peptide antibody affects the efficacy of first biological treatment in rheumatoid arthritis. Arthritis Rheumatol 2015;67.
- Oguma A, Takahashi S, Sawabe T. (F) good outcomes at week 24 is lead by early introduction of tocilizumab and favorable treatment response up to week 12. Ann Rheum Dis 2013;71. https://doi.org/10.1136/annrheumdis-2012-eular.513.
- Okada A, Kawakami A, Fukuda T, Hidaka T, Ishii T, Ueki Y, et al. Characteristic of the Japanese patients with rheumatoid arthritis (RA) of rapid radiographic progression (RRP) treated with synthetic disease modifying anti-rheumatic drugs (DMARDs) in daily practice: a large-scale prospective longitudinal cohort study (the 1st report of apple survey). Arthritis Rheumatol 2012;64.
- Okada A, Kawakami A, Fukuda T, Hidaka T, Ishii T, Ueki Y, et al. Characteristic of the Japanese patients with rheumatoid arthritis (RA) of rapid radiographic progression (RRP) treated with synthetic disease modifying anti-rheumatic drugs (DMARDs) in daily practice: a large-scale prospective longitudinal cohort study. Ann Rheum Dis 2013;72. https://doi.org/10.1136/annrheumdis-2013-eular.649.
- Okada A, Kawakami A, Fukuda T, Hidaka T, Ishii T, Ueki Y, et al. Evaluation of the Japanese patients with rheumatoid arthritis (RA) of rapid radiographic progression (RRP) treated with synthetic disease modifying anti-rheumatic drugs (DMARDs) in daily practice: a large-scale prospective longitudinal cohort study (an interim report of Apple Survey). Arthritis Rheumatol 2013;65.
- Okada A, Kawakami A, Fukuda T, Hidaka T, Ishii T, Ueki Y, et al. Evaluation of the Japanese patients with rheumatoid arthritis (RA) of rapid radiographic progression (RRP) treated with synthetic disease modifying anti-rheumatic drugs (DMARDs) in daily practice: a large-scale prospective longitudinal cohort study. Ann Rheum Dis 2014;73. https://doi.org/10.1136/annrheumdis-2014-eular.4035.
- Ometto F, Raffeiner B, Bernardi L, Botsios K, Punzi L, Doria A. Self-reported flares predict radiographic progression in rheumatoid arthritis patients in remission undergoing etanercept tapering. Ann Rheum Dis 2015;74. https://doi.org/10.1136/annrheumdis-2015-eular.6075.
- Ono K, Ohashi S, Oka H, Kadono Y, Yasui T, Isawa K, et al. The impacts of disease of the joints on modified health assessment questionnaire scores in rheumatoid arthritis patients: a retrospective study using the national database of rheumatic diseases by iR-net in Japan. Ann Rheum Dis 2014;73. https://doi.org/10.1136/annrheumdis-2014-eular.3642.
- Onuora S. Rheumatoid arthritis. How bad is obesity for RA?. Nat Rev Rheumatol 2012;8. https://doi.org/10.1038/nrrheum.2012.71.
- Opris D, Diana M, Gainaru C, Iliuta M, Groseanu L, Saulescu I, et al. Serum drug level and anti-citrullinated peptide antibodies as biomarkers that predict EULAR response in rheumatoid arthritis-a new step to personalized medicine. Ann Rheum Dis 2014;73:946-7. https://doi.org/10.1136/annrheumdis-2014-eular.4812.
- Opris D, Borangiu A, Gudu T, Saulescu I, Groseanu L, Predeteanu D, et al. Active synovitis in rheumatoid arthritis patients while being on SDAI remission. Ann Rheum Dis 2015;74. https://doi.org/10.1136/annrheumdis-2015-eular.5684.
- Ortiz AM, Rosario Lozano MP, Martinez Fernandez C, Gonzalez-Alvaro I. Is there a difference in the effectiveness in the treatment of rheumatoid arthritis with rituximab in patients with rheumatoid factor positive and negative? A systematic review. Ann Rheum Dis 2014;73. https://doi.org/10.1136/annrheumdis-2014-eular.4000.
- Osipyants R, Karateev D, Panasyuk E, Smirnov A, Lukina G, Glukhova S, et al. Associations between functional status and ultrasound-detected synovitis and joint damage in rheumatoid arthritis during tocilizumab treatment. Ann Rheum Dis 2013;72:A755-6. https://doi.org/10.1136/annrheumdis-2013-eular.2238.
- Osipyants R, Karateev D, Panasyuk E, Smirnov A, Lukina G, Glukhova S, et al. Imaging rather than clinical inflammation is associated with radiographic progression in tocilizumab-treated rheumatoid arthritis patients. Ann Rheum Dis 2013;72. https://doi.org/10.1136/annrheumdis-2013-eular.2237.
- Ottaviani S, Gardette A, Tubach F, Roy C, Palazzo E, Gill G, et al. Body mass index and response to infliximab in rheumatoid arthritis. Clin Exp Rheumatol 2015;33:478-83.
- Pamuk ON, Cetin P, Solmaz D, Ertenli I, Akar S, Karaaslan Y, et al. Work productivity in rheumatoid arthritis: analysis from a multicenter Turkish study. Ann Rheum Dis 2015;74. https://doi.org/10.1136/annrheumdis-2015-eular.5501.
- Pascual-Ramos V, Contreras-Yañez I, Cabiedes-Contreras J, Rull-Gabayet M, Villa AR, Vázquez-Lamadrid J, et al. Hypervascular synovitis and American College of Rheumatology Classification Criteria as predictors of radiographic damage in early rheumatoid arthritis. Ultrasound Q 2009;25:31-8. https://doi.org/10.1097/RUQ.0b013e3181981df0.
- Pavelka K, Gatterova J. Prediction of radiographic progression of rheumatoid arthritis. Ceska Revmatologie 2010;18:19-25.
- Peltea A, Mazilu D, Gudu T, Opris D, Ionescu R. Predictive factors for the EULAR response in RA patients with rituximab treatment. Ann Rheum Dis 2014;73. https://doi.org/10.1136/annrheumdis-2013-205124.4.
- Peluso G, Michelutti A, Bosello S, Gremese E, Tolusso B, Ferraccioli G. Clinical and ultrasonographic remission determines different chances of relapse in early and long standing rheumatoid arthritis. Ann Rheum Dis 2011;70:172-5. https://doi.org/10.1136/ard.2010.129924.
- Pers YM, Fortunet C, Constant E, Lambert J, Mazouyes A, Valnet M, et al. Multicenter retrospective study: response to tocilizumab in clinical practice is not influenced by the number of previous biotherapy or by association with a DMARD. Ann Rheum Dis 2013;71. https://doi.org/10.1136/annrheumdis-2012-eular.2091.
- Pers YM, Fortunet C, Constant E, Lambert J, Godfrin-Valnet M, De Jong A, et al. Predictors of response and remission in a large cohort of rheumatoid arthritis patients treated with tocilizumab in clinical practice. Rheumatology 2014;53:76-84. https://doi.org/10.1093/rheumatology/ket301.
- Pers YM, Cadart D, Rittore C, Ravel P, Daïen V, Fabre S, et al. TNFRII polymorphism is associated with response to TNF blockers in rheumatoid arthritis patients seronegative for ACPA. Joint Bone Spine 2014;81:370-2. https://doi.org/10.1016/j.jbspin.2013.12.005.
- Pers YM, Godfrin-Valnet M, Lambert J, Fortunet C, Constant E, Mura T, et al. Response to tocilizumab in rheumatoid arthritis is not influenced by the body mass index of the patient. J Rheumatol 2015;42:580-4. https://doi.org/10.3899/jrheum.140673.
- Peterfy CG, Haraoui B, Kavanaugh A, Smolen JS, Santra S, Kupper H, et al. Baseline levels of the inflammatory biomarker C-reactive protein are significantly correlated with magnetic resonance imaging measures of synovitis at baseline and after 26 weeks of treatment in patients with early rheumatoid arthritis. Arthritis Rheumatol 2011;63.
- Peterfy C, Haraoui B, Kavanaugh A, Smolen J, Santra S, Kupper H, et al. Baseline levels of the inflammatory biomarker CRP are significantly correlated with MRI measures of synovitis at baseline and after 26 weeks of treatment in patients with early rheumatoid arthritis. J Rheumatol 2012;39.
- Plant MJ, O’Sullivan MM, Lewis PA, Camilleri JP, Coles EC, Jessop JD. What factors influence functional ability in patients with rheumatoid arthritis. Do they alter over time?. Rheumatology 2005;44:1181-5. https://doi.org/10.1093/rheumatology/keh707.
- Pomirleanu C, Ancuta C, Miu S, Chirieac R. A predictive model for remission and low disease activity in patients with established rheumatoid arthritis receiving TNF blockers. Clin Rheumatol 2013;32:665-70. https://doi.org/10.1007/s10067-012-2146-6.
- Pope JE, Thorne C, Haraoui BP, Truong D, Psaradellis E, Sampalis JS. Does C-reactive protein add value in active rheumatoid arthritis? Results from the Optimization of Humira Trial. Scand J Rheumatol 2011;40:232-3. https://doi.org/10.3109/03009742.2010.517548.
- Puolakka K, Kautiainen H, Mottonen T, Hannonen P, Korpela M, Julkunen H, et al. High self-esteem is associated with low HAQ score in patients with rheumatoid arthritis. Arthritis Rheumatol 2004;50:S391-S2.
- Quartuccio L, Fabris M, Salvin S, Atzeni F, Saracco M, Benucci M, et al. Rheumatoid factor positivity rather than anti-CCP positivity, a lower disability and a lower number of anti-TNF agents failed are associated with response to rituximab in rheumatoid arthritis. Rheumatology 2009;48:1557-9. https://doi.org/10.1093/rheumatology/kep314.
- Quintana-Duque MA, Rondon-Herrera F, Mantilla RD, Calvo-Paramo E, Yunis JJ, Varela-Nariño A, et al. Predictors of remission, erosive disease and radiographic progression in a Colombian cohort of early onset rheumatoid arthritis: a 3-year follow-up study. Clin Rheumatol 2016;35:1463-73. https://doi.org/10.1007/s10067-016-3246-5.
- Radovits BJ, Fransen J, van Riel P, Laan R. Influence of age and gender on the interpretation of the Disease Activity Score-28 (DAS28) in rheumatoid arthritis. Ann Rheum Dis 2007;66.
- Ranganath VK, Ben-Artzi A, Duffy E, Brook J, Taylor M, Choi S, et al. Elevated baseline power Doppler discriminates an RA subgroup highly responsive to therapy. Rheumatology 2015;54:2285-6. https://doi.org/10.1093/rheumatology/kev301.
- Rantalaiho V, Leirisalo-Repo M, Kautiainen H, Mankinen P, Martikainen J, Puolakka K. Vitality, presenteeism, and their determinants in patients with early rheumatoid arthritis treated with a 6-month induction infliximab therapy added on a triple combination therapy. Arthritis Rheumatol 2015;67.
- Rashid N, Aranda G, Li Z, Guerrero V, Nadkarni A, Patel C. Factors and reasons associated with switching in rheumatoid arthritis patients newly initiated on biologic DMARDs and impact on health care resource utilization in a managed care organization. Value Health 2015;18. https://doi.org/10.1016/j.jval.2015.03.889.
- Rashid N, Patel C, Li Z, Guerrero V, Nadkarni A, Aranda G. Factors and reasons associated with switching in rheumatoid arthritis patients experienced on biologic DMARDs and impact on health care resource utilization in an integrated healthcare system. Value Health 2015;18. https://doi.org/10.1016/j.jval.2015.03.888.
- Rech J, Hueber AJ, Englbrecht M, Finzel S, Haschka J, Manger B, et al. Prediction of disease relapses by multi-biomarker disease test activity in rheumatoid arthritis patients tapering DMARD treatment. Arthritis Rheumatol 2016;75:1637-44.
- Reina D, Narvaez J, Garcia-Diaz S, Diaz Torne C, Magallares B, Hernandez MV, et al. Predictive factors of response to tocilizumab in patients with active rheumatoid arthritis. Ann Rheum Dis 2013;72. https://doi.org/10.1136/annrheumdis-2013-eular.1351.
- Rich E, Moreland LW, Alarcón GS. Paucity of radiographic progression in rheumatoid arthritis treated with methotrexate as the first disease modifying antirheumatic drug. J Rheumatol 1999;26:259-61.
- Rkain H, Aktaou S, Raissouni H, Hajjaj-Hassouni N. Clinical and ultrasonographic assessment of evolution in patients with rheumatoid arthritis treated with tocilizumab. Osteoporos Int 2011;22.
- Rodrigues AM, Reis JE, Santos C, Pereira MP, Loureiro C, Martins F, et al. Obesity is a risk factor for worse treatment response in rheumatoid arthritis patients – results from reuma.pt. Ann Rheum Dis 2014;73. https://doi.org/10.1136/annrheumdis-2013-205124.1.
- Romao VC, DiCicco M, Mahto A, Lazarou I, Fonseca JE, Kelly S, et al. Clinical and pathological differences of elderly- and younger-onset rheumatoid arthritis in an early arthritis cohort. Ann Rheum Dis 2015;74:A76-A7. https://doi.org/10.1136/annrheumdis-2015-eular.3399.
- Röther E, Schochat T, Peter HH. Antineutrophil cytoplasmic antibodies (ANCA) in rheumatoid arthritis: a prospective study. Rheumatol Int 1996;15:231-7. https://doi.org/10.1007/BF00290376.
- Ruiz-Esquide V, Gómez-Puerta JA, Cañete JD, Graell E, Vazquez I, Ercilla MG, et al. Effects of smoking on disease activity and radiographic progression in early rheumatoid arthritis. J Rheumatol 2011;38:2536-9. https://doi.org/10.3899/jrheum.110410.
- Russell A, Dougados M, Khraishi M, Flipo R, Loet X, Smolen J, et al. Efficacy with abatacept in patients with earlier versus more longstanding disease: Insights from the AIM trial. J Rheumatol 2012;39.
- Saevarsdottir S. Predicting response to treatment in patients with rheumatoid arthritis. European Musculoskeletal Review 2011;6:228-33.
- Saevarsdottir S, Holmqvist M, Askling J, Alfredsson L, Klareskog L. Predictive value of anti-CCP positivity on disease course and response to therapy in early rheumatoid arthritis. Results from the Swedish EIRA study. Arthritis Rheumatol 2012;64.
- Sagawa A, Akira S. Long-term treatment with tocilizumab (TCZ) strongly suppresses joint destruction in biologic-naive patients with rheumatoid arthritis (RA) regardless of inflammation status. Arthritis Rheumatol 2014;66.
- Sakthiswary R, Shaharir SS, Mohd Said MS, Asrul AW, Shahril NS. IgA rheumatoid factor as a serological predictor of poor response to tumour necrosis factor α inhibitors in rheumatoid arthritis. Int J Rheum Dis 2014;17:872-7. https://doi.org/10.1111/1756-185X.12443.
- Salgado E, Maneiro JR, Carmona L, Gomez-Reino JJ. Influence of Baseline Rheumatoid Factor on the Response to Tumor Necrosis Factor Antagonists of Rheumatoid Arthritis Patients: A Systematic Review and Meta-Analysis n.d.
- Salgado E, Maneiro JR, Carmona L, Gomez-Reino JJ. Rheumatoid factor and response to TNF antagonists in rheumatoid arthritis: systematic review and meta-analysis of observational studies. Ann Rheum Dis 2013;71. https://doi.org/10.1136/annrheumdis-2012-eular.279.
- Salgado E, Campos J, Moreira-Navarrete V, Mulero J, Navarro F, Perez-Pampin E, et al. Rheumatoid factor does not predict response to TNF antagonists in rheumatoid arthritis: three centers experience. Joint Bone Spine 2013;80:438-40. https://doi.org/10.1016/j.jbspin.2013.01.004.
- Salgado E, Campos J, Moreira-Navarrete V, Mulero J, Navarro F, Perez-Pampin E, et al. The effect of rheumatoid factor titer on the response to TNF antagonists. Ann Rheum Dis 2013;71. https://doi.org/10.1136/annrheumdis-2012-eular.278.
- Salgado E, Maneiro J, Carmona L, Gomez-Reino J. Rheumatoid factor and response to TNF antagonists in rheumatoid arthritis: systematic review and meta-analysis of observational studies (provisional abstract). Joint Bone Spine 2014;81:41-50. https://doi.org/10.1016/j.jbspin.2013.04.004.
- Salgado E, Maneiro JR, Gomez-Reino JJ. Predictors of response to TNF antagonists. Curr Pharm Des 2015;21:221-32. https://doi.org/10.2174/1381612820666140825124152.
- Sanmartí R, Gomez A, Ercilla G, Gratacos J, Larrosa M, Suris X, et al. Radiological progression in early rheumatoid arthritis after DMARDS: a one-year follow-up study in a clinical setting. Rheumatology 2003;42:1044-9. https://doi.org/10.1093/rheumatology/keg284.
- Sasso EH, Wu G, Hwang CC, Weinblatt ME, Shadick NA, Alexander C, et al. The multi-biomarker disease activity score as a predictor of radiographic progression in a registry of patients with rheumatoid arthritis. Arthritis Rheumatol 2014;66:S1299-S300.
- Sauer BC, Teng CC, He T, Leng J, Lu CC, Curtis JR, et al. Effectiveness and costs of biologics in veterans with rheumatoid arthritis. Am J Pharm Benefits 2015;7:280-9.
- Schulman E, Andersen K, Zhang M, Goodman SM, Lin D, Boire G, et al. High body mass index negatively impacts time to achieving sustained remission in early rheumatoid arthritis: results from a multicenter early arthritis cohort study. Arthritis Rheumatol 2015;67.
- Segaud N, Haffner C, Philippe P, Deprez X, Houvenagel E, Coquerel P, et al. Therapeutic response to tocilizumab in rheumatoid arthritis: does body weight have an influence?. Ann Rheum Dis 2014;73:679-80. https://doi.org/10.1136/annrheumdis-2014-eular.3855.
- Sekiguchi M, Fujii T, Kitano M, Matsui K, Hashimoto H, Yokota A, et al. Predicting factors associated with sustained clinical remission by abatacept are different between in younger and elderly patients with biologic-naive rheumatoid arthritis (ABROAD study). Ann Rheum Dis 2015;74. https://doi.org/10.1136/annrheumdis-2015-eular.2693.
- Sekiguchi M, Fujii T, Matsui K, Murakami K, Morita S, Ohmura K, et al. Differences in predictive factors for sustained clinical remission with abatacept between younger and elderly patients with biologic-naive rheumatoid arthritis: results from the ABROAD study. J Rheumatol 2016;43:1974-83. https://doi.org/10.3899/jrheum.160051.
- Sellam J, Hendel-Chavez H, Rouanet S, Abbed K, Combe B, Le Loët X, et al. B cell activation biomarkers as predictive factors for the response to rituximab in rheumatoid arthritis: a six-month, national, multicenter, open-label study. Arthritis Rheum 2011;63:933-8. https://doi.org/10.1002/art.30233.
- Sergeant JC, Hope HF, Anderson J, Barton A, Hyrich KL, Verstappen SMM. Lifestyle, clinical and psychosocial predictors of good response to methotrexate therapy in the rheumatoid arthritis medication study (RAMS). Ann Rheum Dis 2015;74:783-4. https://doi.org/10.1136/annrheumdis-2015-eular.5305.
- Shah BG, Quershi HJ, Zafar U. Serum anti-CCP antibody and its correlation with disease activity in local Pakistani rheumatoid arthritis patients. Pak J Med Health Sci 2014;8:1041-4.
- Sharma TS, Kirillova L, Berger A, Newman ED. Biologic de-escalation in rheumatoid arthritis: cost savings and clinical success. Arthritis Rheumatol 2014;66.
- Sherrer YS, Bloch DA, Mitchell DM, Roth SH, Wolfe F, Fries JF. Disability in rheumatoid arthritis: comparison of prognostic factors across three populations. J Rheumatol 1987;14:705-9.
- Shiozawa K, Tanaka Y, Yoshihara R, Murata M, Yamane T, Tanaka C, et al. MMP-3 as a predictor that identifies a subgroup with potential radiographic progression requiring additional biologics to halt future progression among the rheumatoid arthritis patients succeedingly treated with methotrexate (MTX) alone. Ann Rheum Dis 2013;72. https://doi.org/10.1136/annrheumdis-2013-eular.2499.
- Shrestha P, Batley M, Koduri G, Scott DL. Effect of body mass index on clinical response to anti-TNF therapies in rheumatoid arthritis: a retrospective study. Rheumatology 2013;52:i103-i4.
- Shrestha P, Koduri G, Scott DL, Batley M. Effect of body mass index on clinical response to anti-TNF therapy in rheumatoid arthritis. Ann Rheum Dis 2013;72. https://doi.org/10.1136/annrheumdis-2013-eular.1215.
- Shu J, Bykerk V, Haraoui B, Thorne C, Keystone E, Boire G, et al. Impact of missing anti-cyclic citrullinated peptide (CCP) antibody serology on clinical outcomes in early rheumatoid arthritis: results from catch (Canadian early arthritis cohort). J Rheumatol 2014;41:1534-5.
- Sibilia J, Flipo R, Combe B, Gaillez C, Bars M, Poncet C, et al. Abatacept confers clinical efficacy regardless of baseline CRP status in patients with rheumatoid arthritis and inadequate response to methotrexate in the aim trial. Rheumatology 2012;51:iii124-iii5.
- Simone D, Canestri S, Nowik M, Messuti L, Miceli MC, Privitera R, et al. Genetic and clinical predictors of response to TNF-alpha therapy in an Italian axial-SPA cohort. Ann Rheum Dis 2014;73. https://doi.org/10.1136/annrheumdis-2014-eular.4272.
- Simone D, Canestri S, Nowik M, Messuti L, Miceli MC, Gremese E, et al. Genetic and clinical predictors of response to TNF blocker in an Italian axial-SPA cohort. Clin Exp Rheumatol 2014;32.
- Smith SL, Plant D, Eyre S, Yarwood A, Hyrich KL, Morgan AW, et al. Can CD11C expression successfully predict response to etanercept in rheumatoid arthritis patients?. Ann Rheum Dis 2013;72. https://doi.org/10.1136/annrheumdis-2013-eular.2611.
- Smolen J, Szumski A, Marshall L, Koenig AS. Baseline predictors of remission with combination etanercept-methotrexate therapy in moderately active rheumatoid arthritis: Interim results of the preserve trial. Arthritis Rheumatol 2011;63.
- Smolen JS, Avila JC, Aletaha D. Tocilizumab inhibits progression of joint damage in rheumatoid arthritis irrespective of its anti-inflammatory effects: disassociation of the link between inflammation and destruction. Ann Rheum Dis 2012;71:687-93. https://doi.org/10.1136/annrheumdis-2011-200395.
- Smolen JS, Aletaha D, Chen S, Florentinus S. The effect of prior disease duration and prior DMARD use on treatment outcomes in patients with early or established rheumatoid arthritis. Arthritis Rheumatol 2015;67.
- Sockalingam S, Khuan CS, Sthaneshwar P. Prevalence of anti cyclic citrullinated peptide antibodies in Malaysian rheumatoid arthritis patients and its correlation with disease activity. Int J Rheum Dis 2009;12:211-15. https://doi.org/10.1111/j.1756-185X.2009.01412.x.
- Söderlin MK, Bergman S. BARFOT Study Group . Absent ‘Window of Opportunity’ in smokers with short disease duration. Data from BARFOT, a multicenter study of early rheumatoid arthritis. J Rheumatol 2011;38:2160-8. https://doi.org/10.3899/jrheum.100991.
- Söderlin MK, Petersson IF, Geborek P. The effect of smoking on response and drug survival in rheumatoid arthritis patients treated with their first anti-TNF drug. Scand J Rheumatol 2012;41:1-9. https://doi.org/10.3109/03009742.2011.599073.
- Söderlin MK, Andersson M, Bergman S. BARFOT study group . Second-hand exposure to tobacco smoke and its effect on disease activity in Swedish rheumatoid arthritis patients. Data from BARFOT, a multicenter study of RA. Clin Exp Rheumatol 2013;31:122-4.
- Sokka T, Kankainen A, Hannonen P. Scores for functional disability in patients with rheumatoid arthritis are correlated at higher levels with pain scores than with radiographic scores. Arthritis Rheum 2000;43:386-9. https://doi.org/10.1002/1529-0131(200002)43:2<386::AID-ANR19>3.0.CO;2-Z.
- Solau-Gervais E, Prudhomme C, Philippe P, Duhamel A, Dupont-Créteur C, Legrand JL, et al. Efficacy of rituximab in the treatment of rheumatoid arthritis. Influence of serologic status, coprescription of methotrexate and prior TNF-alpha inhibitors exposure. Joint Bone Spine 2012;79:281-4. https://doi.org/10.1016/j.jbspin.2011.05.002.
- Soliman MM, Ashcroft DM, Watson KD, Lunt M, Symmons D, Hyrich KL. Predictors of response to rituximab in patients with rheumatoid arthritis: results from the British Society for Rheumatology Biologics Register (BSRBR). Rheumatology 2011;50. https://doi.org/10.1093/rheumatology/ker073.
- Srirangan S, Kirkham B, Scott DL. Functional outcome in response to treatment with DMARDs compared to anti-TNF agents in a cohort with rheumatoid arthritis. Ann Rheum Dis 2013;71. https://doi.org/10.1136/annrheumdis-2012-eular.495.
- Stagnaro C, Ferrari C, Talarico R, Giacomelli C, Bombardieri S, Bazzichi L. A useful mathematical model able to predict the early response to tocilizumab in rheumatoid arthritis. Arthritis Rheumatol 2013;65.
- Stavropoulos-Kalinoglou A, Metsios GS, Koutedakis Y, Nevill AM, Douglas KM, Jamurtas A, et al. Redefining overweight and obesity in rheumatoid arthritis patients. Ann Rheum Dis 2007;66:1316-21. https://doi.org/10.1136/ard.2006.060319.
- Steunebrink LMM, Versteeg GA, Vonkeman HE, Ten Klooster PM, Kuper IH, Van Der Bijl AE, et al. Superiority of initial combination-over step up therapy in treatment to the target of remission in daily clinical practice in early rheumatoid arthritis patients: results from the DREAM registry. Arthritis Rheumatol 2015;67.
- Strand V, Jones TV, Li W, Koenig AS, Kotak S. Factors that impact work productivity in the preserve trial: a randomized controlled trial of combination etanercept–methotrexate therapy in patients with moderately active rheumatoid arthritis. Arthritis Rheumatol 2012;64.
- Strand V, Jones TV, Li W, Koenig AS, Kotak S. Predictors of work impairment in a randomized controlled trial of etanercept–methotrexate therapy in patients with moderate rheumatoid arthritis. Ann Rheum Dis 2013;71. https://doi.org/10.1136/annrheumdis-2012-eular.1385.
- Strand V, Jones TV, Li W, Koenig AS, Kotak S. The impact of rheumatoid arthritis on work and predictors of overall work impairment from three therapeutic scenarios. Int J Clin Rheumatol 2015;10:317-28. https://doi.org/10.2217/ijr.15.40.
- Szekanecz Z, Pulai J, Drescher E, Varga T, Kiss C, Gal J, et al. Analysis of the association between cigarette smoking and clinical response to certolizumab pegol treatment in Hungarian patients with rheumatoid arthritis. Ann Rheum Dis 2015;74. https://doi.org/10.1136/annrheumdis-2015-eular.1603.
- Tada M, Koike T, Okano T, Sugioka Y, Mamoto K, Wakitani S, et al. Predictive factors for radiographic progression in low- and standard-dose etanercept therapy for rheumatoid arthritis from the prevention of cartilage destruction by etanercept (precept) study. Ann Rheum Dis 2013;71. https://doi.org/10.1136/annrheumdis-2012-eular.3088.
- Tak PP. A personalized medicine approach to biologic treatment of rheumatoid arthritis: a preliminary treatment algorithm. Rheumatology 2012;51:600-9. https://doi.org/10.1093/rheumatology/ker300.
- Takeuchi T, Tanaka Y, Amano K, Hoshi D, Nawata M, Nagasawa H, et al. Clinical, radiographic and functional effectiveness of tocilizumab for rheumatoid arthritis patients – REACTION 52-week study. Rheumatology 2011;50:1908-15. https://doi.org/10.1093/rheumatology/ker221.
- Takeuchi T, Ishii Y, Tanaka K, Ukyo Y, Sekine H. Analysis on predictors for long-term clinical efficacies of golimumab in patients with rheumatoid arthritis. Arthritis Rheumatol 2014;66.
- Takeuchi T, Shinmura Y, Nakajima R, Hiramatsu K, Kubo T, Kimoto A, et al. Impact of disease duration before starting adalimumab treatment on work productivity in Japanese patients with rheumatoid arthritis; analysis of 24-weeks data from the ANOUVEAU study. Ann Rheum Dis 2014;73. https://doi.org/10.1136/annrheumdis-2014-eular.2192.
- Tam LS, Griffith JF, Yu AB, Li TK, Li EK. Rapid improvement in rheumatoid arthritis patients on combination of methotrexate and infliximab: clinical and magnetic resonance imaging evaluation. Clin Rheumatol 2007;26:941-6. https://doi.org/10.1007/s10067-006-0372-5.
- Tamai M, Nakashima Y, Suzuki T, Horai Y, Okada A, Kita J, et al. Magnetic resonance imaging-proven osteitis at baseline predicts the early rheumatoid arthritis patients who will develop rapid radiographic progression: MRI is beneficial to find the window of opportunity in early RA. Arthritis Rheumatol 2012;64:S906-S7.
- Tamai M, Arima K, Nakashima Y, Umeda M, Fukui S, Nishino A, et al. Investigation of MRI bone changes in early-stage RA patients achieved in sustained clinical good response: sub-analysis from Nagasaki University early arthritis cohort. Arthritis Rheumatol 2014;66.
- Tanaka E, Mannalithara A, Inoue E, Hara M, Tomatsu T, Kamatani N, et al. Efficient management of rheumatoid arthritis significantly reduces long-term functional disability. Ann Rheum Dis 2008;67:1153-8. https://doi.org/10.1136/ard.2007.072751.
- Tanaka Y, Harigai M, Takeuchi T, Yamanaka H, Ishiguro N, Yamamoto K, et al. Prevention of joint destruction in patients with high disease activity or high C-reactive protein. Arthritis Rheumatol 2012;64.
- Tanaka Y, Yamanaka H, Saito K, Iwata S, Miyagawa I, Seto Y, et al. Structural damages disturb functional improvement in patients with rheumatoid arthritis treated with etanercept. Mod Rheumatol 2012;22:186-94. https://doi.org/10.1007/s10165-011-0510-z.
- Tanaka H, Kanazawa M, Kawakami T, Kido K, Kifune M, Kubo M, et al. Efficacy and safety of tocilizumab therapy in rheumatoid arthritis: prevalence and predictive factors of sustained remission. Ann Rheum Dis 2013;72. https://doi.org/10.1136/annrheumdis-2013-eular.1363.
- Tanaka E, Inoue E, Yamaguchi R, Shimizu Y, Sugimoto N, Hoshi D, et al. A longitudinal study of factors contributing to the worsening of absenteeism in patients with rheumatoid arthritis based on the IORRA cohort. Ann Rheum Dis 2015;74:672-3. https://doi.org/10.1136/annrheumdis-2015-eular.3665.
- Tanaka Y, Harigai M, Takeuchi T, Yamanaka H, Ishiguro N, Yamamoto K, et al. Prevention of joint destruction in patients with high disease activity or high C-reactive protein levels: post hoc analysis of the GO-FORTH study. Mod Rheumatol 2016;26:323-30. https://doi.org/10.3109/14397595.2015.1086041.
- Teitsma X, Jacobs J, Welsing P, Woodworth T, Petho-Schramm A, Borm M, et al. Predicting the need for additional treatment in early rheumatoid arthritis patients treated to target on methotrexate monotherapy. Arthritis Rheumatol 2015;67.
- Terao C, Yamakawa N, Yano K, Markusse IM, Ikari K, Yoshida S, et al. Rheumatoid factor is associated with the distribution of hand joint destruction in rheumatoid arthritis. Arthritis Rheumatol 2015;67:3113-23. https://doi.org/10.1002/art.39306.
- Thorne C, Bensen WG, Choquette D, Chow A, Khraishi M, Atkins CJ, et al. Effectiveness and safety of infliximab in rheumatoid arthritis: analysis from a Canadian multicenter prospective observational registry. Arthritis Care Res 2014;66:1142-51. https://doi.org/10.1002/acr.22290.
- Tolusso B, Di Pietro F, Pallavicini FB, Ravagnani V, Benucci M, Podesta E, et al. Analysis of the association of CDA (cytidin deaminase) (K27Q), TNF-(–308G>A) and PTPN22 R620W genetic polymorphisms with auto-antibody seropositive RA and the response to B cell depletion. Arthritis Rheumatol 2010;62.
- Tony H, Roll P, Mei H, Gnuegge L, Kobialko M, Dörner T. Predictive factors for response to rituximab in patients with rheumatoid arthritis (FIRST). Ann Rheum Dis 2011;70.
- Torrente-Segarra V, Urruticoechea A, Corominas H, Sanchez A, Victor Tovar J, Munoz A, et al. Assessment of 12-month efficacy and safety of 168 certolizumab pegol rheumatoid arthritis treated patients from a multicenter retrospective national study in Spain. Arthritis Rheumatol 2014;66:S203-S4.
- Torrente-Segarra V, Urruticoechea Arana A, Sánchez-Andrade Fernández A, Tovar Beltrán JV, Muñoz Jiménez A, Martínez-Cristóbal A, et al. RENACER study: assessment of 12-month efficacy and safety of 168 certolizumab pegol rheumatoid arthritis-treated patients from a Spanish multicenter national database. Mod Rheumatol 2015;26:336-41. https://doi.org/10.3109/14397595.2015.1101200.
- Troelsen LN, Jacobsen S, Abrahams JL, Royle L, Rudd PM, Narvestad E, et al. IgG glycosylation changes and MBL2 polymorphisms: associations with markers of systemic inflammation and joint destruction in rheumatoid arthritis. J Rheumatol 2012;39:463-9. https://doi.org/10.3899/jrheum.110584.
- Tsuji S, Yura A, Katayama M, Watanabe A, Teshigawara S, Yoshimura M, et al. Baseline procalcitonin (PCT) level as a predictive marker for clinical remission (DAS28-ESR, CDAI) at 52 weeks in biologic naive rheumatoid arthritis (RA) patients treated by tocilizumab (TCZ); a single center retrospective study. Ann Rheum Dis 2014;73:956-7. https://doi.org/10.1136/annrheumdis-2014-eular.2437.
- Ursum J, Bos WH, van Dillen N, Dijkmans BA, van Schaardenburg D. Levels of anti-citrullinated protein antibodies and IgM rheumatoid factor are not associated with outcome in early arthritis patients: a cohort study. Arthritis Res Ther 2010;12. https://doi.org/10.1186/ar2907.
- van den Broek M, Dirven L, Klarenbeek NB, Molenaar TH, Han KH, Kerstens PJ, et al. The association of treatment response and joint damage with ACPA-status in recent-onset RA: a subanalysis of the 8-year follow-up of the BeSt study. Ann Rheum Dis 2012;71:245-8. https://doi.org/10.1136/annrheumdis-2011-200379.
- van den Broek M, Visser K, Allaart CF, Huizinga TW. Personalized medicine: predicting responses to therapy in patients with RA. Curr Opin Pharmacol 2013;13:463-9. https://doi.org/10.1016/j.coph.2013.03.006.
- van der Heijde D, Landewe R, Sharp J, Bathon J, Patra K, Spencer-Green G. Baseline CRP concentrations predict radiographic progression in MTX-naive patients with early RA: subanalysis of the PREMIER study. Ann Rheum Dis 2005;58648.
- van der Helm-van Mil AH, van der Kooij SM, Allaart CF, Toes RE, Huizinga TW. A high body mass index has a protective effect on the amount of joint destruction in small joints in early rheumatoid arthritis. Ann Rheum Dis 2008;67:769-74. https://doi.org/10.1136/ard.2007.078832.
- van der Horst-Bruinsma I . Inclusion criteria based on DAS28 score: strength of improvement is less dependent on baseline disease activity than expected. J Rheumatol 2011;38. https://doi.org/10.3899/jrheum.110928.
- Van Der Maas A, Van Herwaarden N, Woodworth T, Minten M, Furst D, Christensen R, et al. Validity of OMERACT preliminary flare questions in a randomized controlled trial, that assesses impact of disease activity guided down-titration of anti-TNF treatment in rheumatoid arthritis patients in low disease activity. Ann Rheum Dis 2014;73:260-1. https://doi.org/10.1136/annrheumdis-2014-eular.2337.
- van der Woude D, Young A, Jayakumar K, Mertens BJ, Toes RE, van der Heijde D, et al. Prevalence of and predictive factors for sustained disease-modifying antirheumatic drug-free remission in rheumatoid arthritis: results from two large early arthritis cohorts. Arthritis Rheum 2009;60:2262-71. https://doi.org/10.1002/art.24661.
- van Gaalen FA, van Aken J, Huizinga TW, Schreuder GM, Breedveld FC, Zanelli E, et al. Association between HLA class II genes and autoantibodies to cyclic citrullinated peptides (CCPs) influences the severity of rheumatoid arthritis. Arthritis Rheum 2004;50:2113-21. https://doi.org/10.1002/art.20316.
- van Laar JM. Do high levels of IgA rheumatoid factor indicate a poor response to treatment with TNF inhibitors in patients with RA?. Nat Clin Pract Rheumatol 2007;3:544-5. https://doi.org/10.1038/ncprheum0596.
- van Nies JA, Krabben A, Schoones JW, Huizinga TW, Kloppenburg M, van der Helm-van Mil AH. What is the evidence for the presence of a therapeutic window of opportunity in rheumatoid arthritis? A systematic literature review. Ann Rheum Dis 2014;73:861-70. https://doi.org/10.1136/annrheumdis-2012-203130.
- Van Sijl AM, Tsang ASMWP, Raterman HG, Nurmohamed MT, Voskuyl AE. A novel and effective prediction model of response to rituximab in rheumatoid arthritis. Arthritis Rheumatol 2013;65.
- Van Vollenhoven RF, Chatzidionysiou K, Nasonov E, Lukina G, Hetland ML, Tarp U, et al. Six-month results from the collaborative European registries for rituximab in rheumatoid arthritis (cererra). Efficacy of rituximab is highest in RF-positive patients and in those who failed at most one prior anti-TNF. Arthritis Rheumatol 2009;60.
- Van Vollenhoven RF, Betts K, Signorovitch J, Bao C, Shaw JW, Ganguli A. A prediction model that identifies patients most likely to benefit from first-line therapy with adalimumab plus methotrexate in early rheumatoid arthritis. Ann Rheum Dis 2014;73. https://doi.org/10.1136/annrheumdis-2014-206383.
- Vastesaeger N, Kutzbach AG, Amital H, Pavelka K, Lazaro MA, Moots RJ, et al. Prediction of remission and low disease activity in disease-modifying anti-rheumatic drug-refractory patients with rheumatoid arthritis treated with golimumab. Rheumatology 2016;55:1466-76. https://doi.org/10.1093/rheumatology/kew179.
- Vázquez I, Graell E, Gratacós J, Cañete JD, Viñas O, Ercilla MG, et al. Prognostic markers of clinical remission in early rheumatoid arthritis after two years of DMARDs in a clinical setting. Clin Exp Rheumatol 2007;25:231-8.
- Velloso Feijoo ML, Martinez Perez R, Mayordomo Gonzalez L, Marenco De La Fuente JL. B cell depletion therapy in a cohort of patients with seropositive and seronegative rheumatoid arthritis. Ann Rheum Dis 2013;72:A32-A3.
- Ven M, Kuijper M, Gerards A, Tchetverikov I, Weel A, Zeben J, et al. Can we use ultrasound to identify rheumatoid arthritis patients in remission who can taper their medication?. Ann Rheum Dis 2014;73.
- Verschueren P, Esselens G, Westhovens R. Predictors of remission, normalized physical function, and changes in the working situation during followup of patients with early rheumatoid arthritis an observational study. Scand J Rheumatol 2009;38:166-72. https://doi.org/10.1080/03009740802484846.
- Verstappen SM, Boonen A, Bijlsma JW, Buskens E, Verkleij H, Schenk Y, et al. Working status among Dutch patients with rheumatoid arthritis: work disability and working conditions. Rheumatology 2005;44:202-6. https://doi.org/10.1093/rheumatology/keh400.
- Verstappen SMM, Bakker MF, Heurkens AHM, Van Der Veen MJ, Kruize AA, Geurts M, et al. Adverse events and factors associated with toxicity in patients with early rheumatoid arthritis treated with methotrexate (the CAMERA study). Arthritis Rheumatol 2009;60.
- Verstappen SM, Watson KD, Lunt M, McGrother K, Symmons DP, Hyrich KL. BSR Biologics Register . Working status in patients with rheumatoid arthritis, ankylosing spondylitis and psoriatic arthritis: results from the British Society for Rheumatology Biologics Register. Rheumatology 2010;49:1570-7. https://doi.org/10.1093/rheumatology/keq131.
- Vidal C, Barnetche T, Morel J, Combe B, Daien C. Influence of body mass index on disease activity and radiographic joint damage in rheumatoid arthritis: a systematic review and meta-analysis. Arthritis Rheumatol 2014;66:S167-S8.
- Visman IM, Bartelds GM, Ouwerkerk W, Ravelli AC, Peelen LM, Dijkmans BA, et al. Effect of the application of trial inclusion criteria on the efficacy of adalimumab therapy in a rheumatoid arthritis cohort. J Rheumatol 2011;38:1884-90. https://doi.org/10.3899/jrheum.101283.
- Visser K, Verpoort KN, van Dongen H, van der Kooij SM, Allaart CF, Toes RE, et al. Pretreatment serum levels of anti-cyclic citrullinated peptide antibodies are associated with the response to methotrexate in recent-onset arthritis. Ann Rheum Dis 2008;67:1194-5. https://doi.org/10.1136/ard.2008.088070.
- Vital EM, Cuthbert RJ, Dass S, Parmar R, Rawstron AC, Ponchel FC, et al. Serum cytokine profile predicts response to rituximab therapy in rheumatoid arthritis. Arthritis Rheumatol 2010;62.
- Volkov A, Osipyants R, Panasyuk E, Smirnov A, Lukina G, Gluchova S, et al. Monitoring anti-interleukin 6 receptor antibody treatment in rheumatoid arthritis and prediction progressive structural damage of the wrist joints by ultrasonography. Ann Rheum Dis 2013;71. https://doi.org/10.1136/annrheumdis-2012-eular.1915.
- Vreju FA, Filippucci E, Gutierrez M, Di Geso L, Ciapetti A, Ciurea ME, et al. Subclinical ultrasound synovitis in a particular joint is associated with ultrasound evidence of bone erosions in that same joint in rheumatoid patients in clinical remission. Clin Exp Rheumatol 2016;34:673-8.
- Wagner C, Rahman MU, Hsia EC. Serum markers associated with clinical response in methotrexate naïve rheumatoid arthritis patients treated with golimumab, a human anti-TNFα monoclonal antibody. Arthritis Rheumatol 2009;60.
- Wagner C, Rahman M, Genovese M. Association of serum markers with clinical response measures in rheumatoid arthritis patients treated with golimumab, a human anti-TNF alpha monoclonal antibody. Ann Rheum Dis 2010;69.
- Wang Y, Zhu R, Xiao J, Davis JC, Mandema JW, Jin JY, et al. Short-term efficacy reliably predicts long-term clinical benefit in rheumatoid arthritis clinical trials as demonstrated by model-based meta-analysis. J Clin Pharmacol 2016;56:835-44. https://doi.org/10.1002/jcph.668.
- Wang J, Devenport J, Low JM, Yu D, Hitraya E. Relationship between baseline and early changes in C-reactive protein and interleukin-6 levels and clinical response to tocilizumab in rheumatoid arthritis. Arthritis Care Res 2016;68:882-5. https://doi.org/10.1002/acr.22765.
- Watanabe R, Okuno H, Ishii T, Hirabayashi Y, Harigae H. Tocilizumab efficiently halts radiographic progression in patients with rheumatoid arthritis and swollen joint counts within a year predict long-term radiographic outcomes: three year results from Michinoku Tocilizumab Study Group. Arthritis Rheumatol 2013;65:S1012-S3.
- Weinblatt ME, Keystone EC, Cohen MD, Freundlich B, Li J, Chon Y, et al. Predictors of poor radiographic response in rheumatoid arthritis subjects treated with methotrexate: preliminary analysis from the ERA and TEMPO studies. Arthritis Rheumatol 2006;54:S181-S2.
- Weinblatt ME, Keystone EC, Cohen MD, Freundlich B, Li J, Chon Y, et al. Factors associated with radiographic progression in patients with rheumatoid arthritis who were treated with methotrexate. J Rheumatol 2011;38:242-6. https://doi.org/10.3899/jrheum.091446.
- Westhoff G, Callhoff J, Schneider M, Zink A. Indicators of depression are stronger predictors of work disability in early arthritis than disease activity or response to therapy. Ann Rheum Dis 2013;72. https://doi.org/10.1136/annrheumdis-2013-eular.297.
- Wevers-De Boer KVC, Heimans L, Visser K, Ronday HK, Molenaar THE, Seys PEH, et al. Remission induction therapy with methotrexate and prednisone in patients with early rheumatoid and undifferentiated arthritis. Arthritis Rheumatol 2011;63.
- Wevers-De Boer K, Visser K, Heimans L, Ronday HK, Molenaar E, Groenendael JHLM, et al. Extended report: remission induction therapy with methotrexate and prednisone in patients with early rheumatoid and undifferentiated arthritis (the IMPROVED study). Ann Rheum Dis 2012;71:1472-7. https://doi.org/10.1136/annrheumdis-2011-200736.
- Wevers-De Boer KVC, Heimans L, Visser K, Schouffoer AA, Molenaar THE, Harbers JB, et al. Remission after one year in ACPA positive and ACPA negative patients with early arthritis. Arthritis Rheumatol 2012;64.
- Wevers-De Boer KVC, Heimans L, Visser K, Kalvesten J, Goekoop R, Molenaar E, et al. Early metacarpal bone mineral density loss is predictive for radiologic joint damage progression after 1 year in patients with early arthritis. Ann Rheum Dis 2013;72. https://doi.org/10.1136/annrheumdis-2013-eular.1644.
- White D, Chen YS, Duggan E, Pahau H, Thomas R. Clinical features of disease severity and body mass index predict outcome at 6 months in a cohort of patients with early rheumatoid arthritis. Intern Med 2009;39.
- Wolfe F, Michaud K. Effect of body mass index on mortality and clinical status in rheumatoid arthritis. Arthritis Care Res 2012;64:1471-9. https://doi.org/10.1002/acr.21627.
- Xibille DX, Hernandez Gongora SE, Bustos Bahena C, Ortiz Panozo E, Montiel Hernandez JL. Plasma adiponectin level and body mass index (BMI) predict response to treatment in patients with rheumatoid arthritis. Ann Rheum Dis 2013;72. https://doi.org/10.1136/annrheumdis-2013-eular.668.
- Xibille DX, Hernandez Gongora SE, Sandoval Rios M, Ortiz Panozo JE, Bustos Rivera Bahena C, Montiel Hernandez JL. Leptin and adiponectin serum levels as predictors of treatment response in patients with rheumatoid arthritis. Ann Rheum Dis 2013;71. https://doi.org/10.1136/annrheumdis-2012-eular.3033.
- Yamanaka H, Tanaka Y, Sekiguchi N, Inoue E, Saito K, Kameda H, et al. Retrospective clinical study on the notable efficacy and related factors of infliximab therapy in a rheumatoid arthritis management group in Japan (RECONFIRM). Mod Rheumatol 2007;17:28-32. https://doi.org/10.1007/s10165-006-0532-0.
- Yamanaka H, Harigai M, Ishiguro N, Inokuma S, Takei S, Takeuchi T, et al. Trend of patient characteristics and its impact on the response to adalimumab in patients with rheumatoid arthritis: post hoc time-course analysis of an all-case PMS in Japan. Mod Rheumatol 2015;25:495-502. https://doi.org/10.3109/14397595.2014.994263.
- Yamasaki M. Predictive marker for the long-term discontinuation of infliximab in rheumatoid arthritis with clinical remission. Ann Rheum Dis 2014;73. https://doi.org/10.1136/annrheumdis-2014-eular.4147.
- Yazici Y, Moniz Reed D, Klem C, Rosenblatt L, Wu G, Kremer JM. Greater remission rates in patients with early versus long-standing disease in biologic-naive rheumatoid arthritis patients treated with abatacept: a post hoc analysis of randomized clinical trial data. Clin Exp Rheumatol 2011;29:494-9.
- Yoshida K, Radner H, Mjaavatten MD, Greenberg JD, Kavanaugh A, Kishimoto M, et al. Incidence and predictors of biological antirheumatic drug discontinuation attempts among patients with rheumatoid arthritis in remission: a CORRONA and NinJa collaborative cohort study. J Rheumatol 2015;42:2238-46. https://doi.org/10.3899/jrheum.150240.
- Yoshimi R, Hama M, Takase K, Ihata A, Kishimoto D, Terauchi K, et al. Ultrasonography is a potent tool for the prediction of progressive joint destruction during clinical remission of rheumatoid arthritis. Mod Rheumatol 2013;23:456-65. https://doi.org/10.1007/s10165-012-0690-1.
- Yuasa S, Yamaguchi H, Nakanishi Y, Kawaminami S, Tabata R, Shimizu N, et al. Treatment responses and their predictors in patients with rheumatoid arthritis treated with biological agents. J Med Invest 2013;60:77-90. https://doi.org/10.2152/jmi.60.77.
- Zeidler H. Reply to: very early rheumatoid arthritis as a predictor of remission: a multicentre real life prospective study. Ann Rheum Dis 2012;71. https://doi.org/10.1136/annrheumdis-2012-202480.
- Zhang B, Lavalley M, Felson DT. The sensitivity to change for lower disease activity is greater than for higher disease activity in rheumatoid arthritis trials. Ann Rheum Dis 2009;68:1255-9. https://doi.org/10.1136/ard.2008.092460.
- Zheng G, Wang L, Jia X, Li F, Yan Y, Yu Z, et al. Application of high frequency color Doppler ultrasound in the monitoring of rheumatoid arthritis treatment. Exp Ther Med 2014;8:1807-12. https://doi.org/10.3892/etm.2014.2001.
- Zhilyaev EV. Efficacy of Biological Treatment in Cohort of Rheumatoid Arthritis Patients in Moscow n.d.
- Zufferey P, Dudler J, Scherer A, Finckh A. Disease activity in rheumatoid arthritis patients at initiation of biologic agents and 1 year of treatment: results from the Swiss SCQM registry. Joint Bone Spine 2013;80:160-4. https://doi.org/10.1016/j.jbspin.2012.05.003.
Appendix 1 Additional details of the scoping of the assessment
Appropriate methods for the identification of prognostic and predictive studies were considered by the review team. Although various filters are available, published validation studies suggested that none of these can be completely relied on. 119,120 Therefore, the review team consulted the website of the Cochrane Prognosis Methods Group [http://methods.cochrane.org/prognosis/our-publications (accessed July 2016)] and judged that the ‘sensitivity-maximising’ search strategies, devised by the Hedges’ team at McMaster University for identifying prognostic and clinical prediction studies, would be most suitable for use in this assessment. 44,53
The initial phase 1 scoping searches of MEDLINE were conducted to assess the size of the available literature. The numbers of results retrieved were multiplied by 2.5 for an approximate estimate of the total numbers to be found across other databases. The search terms for RA were initially combined with the Hedges’ team filter to retrieve as broad as possible a set of prognostic studies. This was estimated to yield approximately 40,000 records, if the searches were extended to all included databases.
According to a validation study by Geersing et al. ,120 the Haynes’ filter results in a very low number of missed studies; however, Geersing et al. 120 recommended its use for scoping purposes only, suggesting that, if a review is interested in only one or two specific variables, it is best to search for these without the application of any filter.
Potential candidate prognostic and predictive variables were identified to inform discussion with clinical experts.
A separate methodological exercise was undertaken to examine a sample of the records that were retrieved by the Haynes’ filter but did not contain any of the terms relating to our candidate variables. The free-data visualisation tool VOSviewer version 1.6.5 (Centre for Science and Technology Studies, Leiden University, the Netherlands)121 was used to display frequently occurring words and phrases in a sample of the discarded records in an attempt to reveal any emerging variables that were unknown to the team. No new potential candidate variables for discussion were revealed through this process.
Following discussions with two expert clinical advisors who manage patients with early RA in the UK (see Acknowledgements), the review team selected variables for inclusion based on:
-
tests and assessment tools (e.g. selected laboratory tests, imaging tests and clinical assessment measures) being readily available and used in UK clinical practice (and, therefore, genetic markers were not included by the review team)
-
the clinical experience of advisors in evaluating prognosis/treatment response in patients
-
the initial scoping of literature in the area by the review team.
Appendix 2 Additional details of deviations from the final protocol
Review 1
-
It was anticipated that, in the final protocol, the study types included in review 1 would probably include published reports of cohort studies (and potentially case–control studies) that report the associations between individual prognostic variables and outcomes. In order to maintain the feasibility of the assessment, review 1 was restricted to the inclusion of studies that describe the development, external validation or impact of eligible clinical prediction models in early RA. Therefore, in line with the multivariable approach employed in prediction model research, individual prognostic factors were not studied.
-
It was necessary, because of resource and time constraints, to adopt an iterative approach to the screening of evidence during the review 1 study selection (as described in Chapter 3, Study selection). It was also originally intended in the final protocol that a randomly selected sample of titles/abstracts would be checked by a second reviewer. However, in light of the iterative screening approach applied in review 1, it was no longer considered appropriate to undertake this stage.
-
It was originally intended in the final protocol that all studies would be assessed by criteria informed by the QUIPS tool. 54 However, it was necessary to revise this approach in order to allow for the quality assessment of the included clinical prediction model and external validation studies using the most methodologically appropriate tool (PROBAST). 49
-
It was stated in the review protocol that meta-analyses would be conducted using a Bayesian RE model. However, this was modified for the final analysis because there were very few studies that validated each clinical prediction model, thereby providing limited information with which to estimate the between-study heterogeneity. Although it would be possible to implement a Bayesian RE analysis using a weakly informative prior, this was not implemented because there was a lack of empirical evidence to inform the prior distribution for the heterogeneity parameter and eliciting experts’ beliefs was beyond the scope of this project. Although the analysis deviated slightly from the protocol, the implemented RE model accounts for uncertainty in the between-study heterogeneity and is consistent with the methodological recommendations. 40
Review 2
Following a protocol amendment, to maintain the feasibility of the assessment, review 2 comprised:
-
a systematic review of studies that describe the development, external validation or impact of eligible clinical prediction models to predict the response to individual treatments in patients with early RA (developed/validated in observational cohorts or experimental data sets)
-
a review of primary studies (experimental or observational) to identify patient characteristics that affect the response to individual treatments in patients with early RA.
Appendix 3 Sample search strategy (MEDLINE)
Database: Ovid MEDLINE (R) Epub Ahead of Print, MEDLINE In-Process & Other Non-Indexed Citations, Ovid MEDLINE (R) Daily, Ovid MEDLINE and Versions (R).
Date range searched: 1947 to September 2016.
Date searched: 27 September 2016.
Search strategy
-
1. exp rheumatoid arthritis/ (101,628)
-
2. rheumatoid arthritis.tw. (89,399)
-
3. 1 or 2 (129,354)
-
4. incidence.sh. or exp mortality/ or follow-up studies.sh. or prognos:.tw. or predict:.tw. or course:.tw. (2,798,958)
-
5. exp animals/ not exp humans/ (4,320,871)
-
6. 4 not 5 (2,590,653)
-
7. predict:.mp. or scor:.tw. or observ:.mp. (4,442,632)
-
8. 7 not 5 (3,608,545)
-
9. 3 and 6 (17,646)
-
10. (3 and 8) not 9 (17,584)
-
11. (ACPA or ACPAs or anti-CCP* or antiCCP* or anti-CPA or antiCPA or ACCP*).mp. (3078)
-
12. (anti-cyclic citrullinated or anticyclic citrullinated).mp. (1271)
-
13. (anticitrullinated or anti-citrullinated).mp. (924)
-
14. 11 or 12 or 13 (3733)
-
15. (rheumat* factor* or RF).mp. (38,768)
-
16. exp radiography/ (707,925)
-
17. (radiologic* or radiograph* or x-ray* or xray*).mp. (1,010,600)
-
18. ((erosi* or erode*) adj3 (bone* or joint*)).mp. (3790)
-
19. erosion progression.mp. (57)
-
20. sharp score.mp. (340)
-
21. joint space narrowing.mp. (1373)
-
22. JSN.mp. (405)
-
23. 16 or 17 or 18 or 19 or 20 or 21 or 22 (1,262,189)
-
24. (disease activity score or DAS).mp. (51,082)
-
25. (28 joint* or twenty eight joint*).mp. (1665)
-
26. 24 and 25 (1509)
-
27. (DAS28 or DAS 28).mp. [mp=title, abstract, original title, name of substance word, subject heading word, keyword heading word, protocol supplementary concept word, rare disease supplementary concept word, unique identifier, synonyms] (2897)
-
28. 26 or 27 (3287)
-
29. (ESR or (erythrocyte* adj3 sediment*)).mp. or erythrocyte sedimentation rate/ (30,112)
-
30. C-Reactive Protein/ or (c reactive protein* or CRP or C-RP).mp. (699,592)
-
31. (SJC or (sw#ll* adj3 joint* adj2 (count* or number*))).mp. (1375)
-
32. 28 or 29 or 30 or 31 (94,961)
-
33. (smok* or tobacco or nicotine or cigarette* or ecig* or vape*).mp. (335,894)
-
34. Delayed Diagnosis/ (3879)
-
35. ((late or delay*) adj3 (treat* or present* or diagnos* or help-seeking or visit* or doctor* or GP* or report* or consult* or assess*)).mp. (70,970)
-
36. ("time to presentation" or untreated or un-treated).mp. (154,236)
-
37. disease duration.mp. (14,321)
-
38. 34 or 35 or 36 or 37 (250,927)
-
39. (BMI or body mass ind* or obes* or overweight or over-weight).mp. (410,712)
-
40. haq* or health assessment questionnaire*).mp. (4175)
-
41. exp ultrasonography/ (280,410)
-
42. (ultrasound or ultrasonogra* or sonogra* or doppler).mp. (408,038)
-
43. 41 or 42 (495,613)
-
44. exp Antirheumatic Agents/ (384,372)
-
45. (Disease-modifying antirheumatic* or Disease-modifying anti-rheumatic*).mp. (4245)
-
46. DMARD* or bDMARD* or cDMARD*).mp. [mp=title, abstract, original title, name of substance word, subject heading word, keyword heading word, protocol supplementary concept word, rare disease supplementary concept word, unique identifier, synonyms] (3486)
-
47. exp Biological Therapy/ or biological therap*.mp. (395,939)
-
48. (biologic or biologics).mp. (57,465)
-
49. ciclosporin or cyclosporine or cyclophosphamide or cytophosphane or (gold adj2 (inject* or intraven*)) or myocrisin or sodium aurothiomalate or gold sodium thiomolate or hydroxychloroquine or HCQ or Plaquenil or leflunomide or arava or methotrexate or amethopterin or mycophenolate or Mycophenolic acid or CellCept or Myfortic or sulfasalazine or sulphasalazine or salazopyrin or sulazine or azulfidine or abatacept or CTLA41g or orencia or rituximab or mabthera or tocilizumab or Actemra or RoActemra or adalimumab or humira or certolizumab pegol or cimzia or etanercept or enbrel or golimumab or simponi or infliximab or remicade).af. (187,989)
-
50. (anti-TNF or antiTNF or ((TNF* or tumo?r necros#s factor*) adj3 (inhibit* or block* or antagonist* or anti* or alpha)) or interleukin 1 or IL-1 or monoclonal antibod* or costimulation blocker* or co-stimulation blocker* or interleukin 6 or IL-6 or ("T lymphocyt*" adj2 activat*) or B lymphocyt* or Biosimilar*).mp. (525,731)
-
51. (adalimumab or humira or d 2e7 or d2e7).af. or 331731-18-1.rn. or etanercept.af. or enbrel.af. or 185243-69-0.rn. or infliximab.af. or remicade.af. or 170277-31-3.rn. or ta650.af. or ta 650.af. or certolizumab pegol.af. or cimzia.af. or cdp870.af. or 428863-50-7.rn. or 1132819-27-2.rn. or czp.af. or abatacept.af. (20,564)
-
52. (orencia or 213252-14-3 or 332348-12-6 or bms188667 or bms 188667 or ctla4ig or ctla 4ig or golimumab or cnto148 or cnto 148 or simponi or 476181-74-5 or tocilizumab or atlizumab or actemra or roactemra or 375823-41-9 or tofacitinib or xeljanz or tasocitinib or cp690550 or cp 690550 or 540737-29-9 or rituximab or rituxan or mabthera).af. or 174722-31-7.rn. (19,971)
-
53. atacicept.af. or 845264-92-8.rn. or unii-k3d9a0icq3.af. or uniik3d9a0icq3.af. or taci-fc5.af. or tacifc5.af. or taci-ig.af. or taciig.af. (107)
-
54. 44 or 45 or 46 or 47 or 48 or 49 or 50 or 51 or 52 or 53 (1,313,135)
-
55. 14 or 15 or 23 or 32 or 33 or 38 or 39 or 40 (2,253,817)
-
56. 9 and 55 (7258)
-
57. limit 56 to english language (6321)
-
58. 55 or 43 (2,627,621)
-
59. 3 and 8 and 58 (12,425)
-
60. 54 and 59 (5264)
-
61. limit 60 to english language (4943)
Appendix 4 Review 1 excluded full-text studies
First author (date of publication) | Title of publication | Justification for exclusion |
---|---|---|
Akhavan (2011)122 | Prevalence of and predictive factors for sustained remission in early RA: results from SONORA study | No measure of predictive performance reported |
Alemao (2014)123 | Development and validation of a prognostic clinical model for rapid radiographic progression in patients with RA | Not early RA |
Aletaha (2013)124 | Rheumatoid factor determines structural progression in rheumatoid arthritis dependent and independent of disease activity | No measure of predictive performance reported |
Alishiri (2008)125 | Logistic regression models for predicting physical and mental health-related quality of life in rheumatoid arthritis patients | Not early RA |
Allaart (2011)126 | A multi-biomarker disease activity (Vectra DA) algorithm score for rheumatoid arthritis predicts radiographic progression in the BeSt study | Preliminary report. No further relevant data reported |
Alves (2010)127 | The ACR/EULAR 2010 criteria as well as other predictive algorithms for rheumatoid arthritis show good diagnostic performance | Study design |
Anderson (2000)128 | Factors predicting response to treatment in rheumatoid arthritis: the importance of disease duration | Study design |
Balsa (2010)129 | Prediction of functional impairment and remission in rheumatoid arthritis patients by biochemical variables and genetic polymorphisms | Not early RA |
Bakker (2010)130 | Development of a multi-biomarker test for rheumatoid arthritis (RA) disease activity (Vectra DA) | Preliminary report. No further relevant data reported |
Bakker (2012)131 | Performance of a multi-biomarker score measuring rheumatoid arthritis disease activity in the CAMERA tight control study | No measure of predictive performance reported for radiographic progression. Associations with DAS28 reported (not used in prediction) |
Barnabe (2014)132 | Socio-demographic and health status characteristics explain clinical outcome trajectories in early inflammatory arthritis (EIA) | Conference abstract with no definition of early RA. Early inflammatory arthritis |
Bedran (2013)133 | Validation of a prediction rule for the diagnosis of rheumatoid arthritis in patients with recent onset undifferentiated arthritis | Diagnostic study |
Belghomari (1999)134 | Risk factors for radiographic articular destruction of hands and wrists in rheumatoid arthritis | Study design |
Bombardier (2009b)135 | Which subgroups are at higher risk of rapid radiographic progression in early rheumatoid arthritis: results from SONORA study | Not handled as primary report of SONORA radiographic risk model |
Bombardier (2010)136 | Radiographic damage and radiographic progression are predictors for physical function: results from SONORA study | Limited reporting and no measure of predictive performance |
Bombardier (2010)137 | Sustained remission in early RA: results from SONORA study | Limited reporting and no measure of predictive performance |
Bombardier (2010)138 | Clinical prognostic factors for radiographic damage in early rheumatoid arthritis: results from SONORA study | Not handled as primary report of SONORA radiographic risk model |
Bøyesen (2009)139 | Antibodies to cyclic citrullinated protein and erythrocyte sedimentation rate predict hand bone loss in patients with rheumatoid arthritis of short duration: a longitudinal study | Study design |
Breedveld (2004)140 | Multiple faces of rheumatoid arthritis: diagnostic and therapeutic algorithms | Study design |
Britsemmer (2011)141 | Validation of the 2010 ACR/EULAR classification criteria for rheumatoid arthritis: slight improvement over the 1987 ACR criteria | Study design |
Brown (2007)142 | Serum macrophage inhibitory cytokine 1 in rheumatoid arthritis: a potential marker of erosive joint destruction | Not early RA |
Bruynesteyn (2002)143 | Detecting radiological changes in rheumatoid arthritis that are considered important by clinical experts: influence of reading with or without known sequence | Study design |
Bukhari (2002)144 | Rheumatoid factor is the major predictor of increasing severity of radiographic erosions in rheumatoid arthritis: results from the Norfolk Arthritis Register Study, a large inception cohort | No measure of predictive performance reported |
Carrier (2016)145 | Serum levels of 14-3-3η protein supplement C-reactive protein and rheumatoid arthritis-associated antibodies to predict clinical and radiographic outcomes in a prospective cohort of patients with recent-onset inflammatory polyarthritis | No measure of predictive performance reported |
Caruso (1990)146 | Clinical, laboratory and radiographic features in early rheumatoid arthritis | No measure of predictive performance reported |
Chalan (2013)147 | Circulating CD4+CD161+ T lymphocytes are increased in seropositive arthralgia patients but decreased in patients with newly diagnosed rheumatoid arthritis | No prognostic/predictive factors under assessment |
Chibnik (2011)148 | Genetic risk score predicting risk of rheumatoid arthritis phenotypes and age of symptom onset | Unclear reporting of duration of disease at baseline |
Ciurtin (2016)149 | Ultrasound-detected subclinical inflammation was better reflected by the disease activity score (DAS-28) in patients with suspicion of inflammatory arthritis compared to established rheumatoid arthritis | Not early RA |
Conaghan (2010)150 | Persistently moderate DAS28 is not benign: loss of function occurs in early RA despite step-up DMARD therapy | Study design |
Conaghan (2011)151 | Predicting outcomes in rheumatoid arthritis | Literature review |
Corbett (1988)152 | The Middlesex hospital prospective study of early rheumatoid disease | Insufficient details available on population characteristics and methods |
Corbett (1993)153 | Factors predicting death, survival and functional outcome in a prospective study of early rheumatoid disease over fifteen years | Not eligible outcome |
Courvoisier (2008)154 | Prognostic factors of 10-year radiographic outcome in early rheumatoid arthritis: a prospective study | No measure of predictive performance reported |
Curtis (2010)155 | Validation of a multi-biomarker test for rheumatoid arthritis (RA) disease activity (Vectra DA) in a multi-cohort study | Preliminary report of Curtis et al.156 |
Curtis (2012)156 | Validation of a novel multibiomarker test to assess rheumatoid arthritis disease activity | Symptom duration at baseline not reported |
Curtis (2015)157 | A randomized trial comparing disease activity measures for the assessment and prediction of response in rheumatoid arthritis patients initiating certolizumab pegol | Not early RA |
Dawes (1986)158 | Prediction of progressive joint damage in patients with rheumatoid arthritis receiving gold or D-penicillamine therapy | Duration of symptoms for total group not reported, but data available indicate not early RA |
de Carvalho (1980)159 | Radiographic progression of rheumatoid arthritis related to some clinical and laboratory parameters | Study design |
de Punder (2015)160 | Personalising treatment targets in rheumatoid arthritis by using a simple prediction model | Prediction model includes variables measured outside early RA definition |
de Vries-Bouwstra (2008)161 | Progression of joint damage in early rheumatoid arthritis: association with HLA-DRB1, rheumatoid factor, and anti-citrullinated protein antibodies in relation to different treatment strategies | No measure of predictive performance reported |
Desai (2015)162 | An external validation study reporting poor correlation between the claims-based index for rheumatoid arthritis severity and the disease activity score | Duration of symptoms not reported |
Eastman (2012)163 | Characterisation of a multiplex, 12-biomarker test for rheumatoid arthritis | Study design |
Eberhardt (1990)164 | Early rheumatoid arthritis – onset, course and outcome over 2 years | Limited reporting of predictive performance |
Eberhardt (1996)165 | Associations of HLA-DRB and -DBQ genes with two and 5 year outcome in rheumatoid arthritis | Not eligible factor |
Elshafie (2013)166 | IgA rheumatoid factor is more predominant than anti-CCP in Sudanese rheumatoid arthritis patients, whereas IgG RF is a strong prognostic marker and associated with early onset | Study design |
Emery (1996)167 | Algorithm to predict radiological erosions in early rheumatoid arthritis. Messages from paper are incorrect | Letter to editor |
Emery (2008)168 | Clinical identification and treatment of a rapidly progressing disease state in patients with rheumatoid arthritis | Review |
Forslind (2007)169 | Sex: a major predictor of remission in early rheumatoid arthritis? | No measure of predictive performance reported |
Fautrel (2011)170 | Identification of patients who could require early biologic therapy by developing a matrix predicting rapid radiographic progression in early rheumatoid arthritis patients treated by methotrexate. A study based on the ESPOIR cohort data | Preliminary report of included study. No further relevant data reported |
Fautrel (2012)171 | Value of matrices developed to identify early rheumatoid arthritis patients with rapid radiographic progression despite methotrexate therapy: a comparison of their performance in the early rheumatoid arthritis ESPOIR cohort | Preliminary report of included study. No further relevant data reported |
Fautrel (2013)172 | Performance of rapid radiographic progression prediction matrices in the early rheumatoid arthritis patients of the ESPOIR cohort | Preliminary report of included study. No further relevant data reported |
Fautrel (2015)173 | Identifying patients with rheumatoid arthritis with moderate disease activity at risk of significant radiographic progression despite methotrexate treatment | Not early RA |
Fransen (2010)174 | Validity of the revised ACR/EULAR classification criteria for rheumatoid arthritis: predicting persistent arthritis and joint erosions after 2 years in patients with early undifferentiated arthritis | Diagnostic study |
Gardiner (2015)175 | Estimating under-diagnosis of rheumatoid arthritis in primary care data from the UK clinical practice research datalink | Diagnostic study |
Garnero (2002)102 | Association of baseline levels of urinary glucosyl-galactosyl-pyridinoline and type II collagen C-telopeptide with progression of joint destruction in patients with early rheumatoid arthritis | No measure of predictive performance reported |
Goronzy (2004)176 | Prognostic markers of radiographic progression in early rheumatoid arthritis | No measure of predictive performance reported |
Graudal (2004)177 | The natural history and prognosis of rheumatoid arthritis: association of radiographic outcome with process variables, joint motion and immune proteins | Not eligible risk model with measure of predictive performance |
Graudal (2004)178 | Scandinavian Journal of Rheumatology: preface | No further data to Graudal et al.177 |
Green (2003)179 | Serum MMP-3 and MMP-1 and progression of joint damage in early rheumatoid arthritis | Limited reporting of predictive performance |
Haji (2012)180 | Can sustained remission of rheumatoid arthritis be predicted? An analysis from the Japanese national database of rheumatic disease (NinJa) | Disease duration at baseline not reported |
Haji (2013)181 | A prediction rule for sustained remission of rheumatoid arthritis | Disease duration at baseline not reported |
Hambardzumyan (2013)182 | A multi-biomarker disease activity blood test (Vectra DA) correlates with radiographic progression in early rheumatoid arthritis: results from the SWEFOT trial | Preliminary report of included study. No further relevant data reported |
Hambardzumyan (2013)183 | A multi-biomarker disease activity score correlates with radiographic progression in early rheumatoid arthritis: results from a randomised trial | Preliminary report of included study. No further relevant data reported |
Hambardzumyan (2013)184 | Multi-biomarker disease activity (MBDA) score and the 12 individual biomarkers in early rheumatoid arthritis patients relate differentially to clinical response and radiographic progression: results from the SWEFOT trial | Preliminary report of included study. No further relevant data reported |
Hambardzumyan (2013)185 | In early rheumatoid arthritis, the 12 individual biomarkers that comprise the multiple biomarker disease activity score relate differentially to clinical response and radiographic progression: results from a randomised trial | Preliminary report of included study. No further relevant data reported |
Harrison (1999)186 | The influence of HLA-DRB1 alleles and rheumatoid factor on disease outcome in an inception cohort of patients with early inflammatory arthritis | Study design |
Harrison (2001)187 | The association of cigarette smoking with disease outcome in patients with early inflammatory polyarthritis | Mixed arthritis population (< 80% were people with RA) and no subgroup. No measure of predictive performance reported |
Hazes (2011)188 | The epidemiology of early inflammatory arthritis | Literature review |
Heimans 201491 | A two-step treatment strategy trial in patients with early arthritis aimed at achieving remission: the IMPROVED study | Study design |
Hendrikx (2013)189 | Moving towards personalized healthcare: a patient reported outcome based algorithm can aid rheumatologists and patients in monitoring rheumatoid arthritis in daily clinical practice | Study design |
Hendrikx (2015)190 | Monitoring rheumatoid arthritis using an algorithm based on patient-reported outcome measures: a first step towards personalised healthcare | Study design |
Hirata (2013)191 | A multi-biomarker score measures rheumatoid arthritis disease activity in the BeSt study | Outcome measurement time point unclear |
Hirata (2016)192 | Assessment of disease activity in rheumatoid arthritis by multi-biomarker disease activity (MBDA) score | Full text not in the English language |
Houssien (1998)193 | Rheumatoid factor isotypes, disease activity and the outcome of rheumatoid arthritis: comparative effects of different antigens | Not early RA. Study design |
James (2004)194 | Orthopaedic intervention in early rheumatoid arthritis. Occurrence and predictive factors in an inception cohort and 1064 patients followed for 5 years | Not eligible outcome. Study design |
Jessome (2014)195 | Assessing the validity and reliability of a novel MRI semi-automated algorithm for quantifying bone loss in the hand | Study design |
Jessome (2015)196 | Early erosions in rheumatoid arthritis (EERA) software reliably measures erosive damage on MRI in the metacarpophalangeal joints of rheumatoid arthritis patients | Study design |
Kaarela (1985)197 | Prognostic factors and diagnostic criteria in early rheumatoid arthritis | Mixed early arthritis population and diagnostic focus of study |
Kapoor (2013)198 | The impact of inflammation on metabolomic profiles in patients with arthritis | Study design |
Kastbom (2016)199 | Changes in the anticitrullinated peptide antibody response in relation to therapeutic outcome in early rheumatoid arthritis: results from the SWEFOT trial | No measure of predictive performance |
Kaufmann (2013)200 | Comparison between several prediction scores and the new EULAR/ACR criteria for diagnosis and prognosis of rheumatoid arthritis | Study design. Comparison of criteria for development of RA in early arthritis cohort |
Keller (1999)201 | The SF-36 Arthritis-Specific Health Index (ASHI): II. Tests of validity in four clinical trials | Study design |
Kent (2009)202 | Analysis of multiple phenotypes | Literature review |
Kirino (2015)203 | Predicting joint destruction in rheumatoid arthritis with power Doppler, anti-citrullinated peptide antibody, and joint swelling | Not early RA |
Kita (2010)204 | MRI-proven bone edema of wrist and finger joints at entry is the strongest predictor toward further radiographic progression in patients with undifferentiated arthritis: results from the prospective cohort at Nagasaki university | Insufficient results reported |
Knudsen (2008)205 | Biomarkers of inflammation in patients with unclassified polyarthritis and early rheumatoid arthritis. Relationship to disease activity and radiographic outcome | Study design |
Kojima (2015)206 | Patient-reported outcomes as assessment tools and predictors of long-term prognosis: a 7-year follow-up study of patients with rheumatoid arthritis | Not early RA |
Kokebie (2010)207 | The role of synovial fluid markers of catabolism and anabolism in osteoarthritis, rheumatoid arthritis and asymptomatic organ donors | Study design |
Kooloos WM, Huizinga TWJ, Guchelaar HJ, Klareskog L, Padyukov L, Wessels JAM, van Vollenhoven RF. Department of Clinical Pharmacy and Toxicology, and Department of Rheumatology, Leiden University Medical Center, Leiden, The Netherlands; 2009 | External validation of the clinical pharmacogenetic model for predicting MTX monotherapy efficacy using a Swedish cohort of patients with recent-onset rheumatoid arthritis | Not eligible outcome |
Krabben (2013)208 | Risk of rheumatoid arthritis development in patients with unclassified arthritis according to the 2010 ACR/EULAR criteria for rheumatoid arthritis | Not early RA. Study evaluating progression to RA in unclassified arthritis cohort |
Kroot (2000)209 | The prognostic value of anti-cyclic citrullinated peptide antibody in patients with recent-onset rheumatoid arthritis | Limited reporting of predictive performance |
Kvien (2000)210 | Data driven attempt to create a clinical algorithm for identification of women with rheumatoid arthritis at high risk of osteoporosis | Not early RA |
Lahiri (2014)211 | Using lifestyle factors to identify individuals at higher risk of inflammatory polyarthritis (results from the European Prospective Investigation of Cancer-Norfolk and the Norfolk Arthritis Register-the EPIC-2-NOAR Study) | Not eligible end point. Risk of developing inflammatory polyarthritis or RA |
Lahiri (2013)212 | A risk score to identify individuals at higher risk of inflammatory polyarthritis: results from the European prospective investigation of cancer (Norfolk) and the Norfolk arthritis register (the EPIC-2-NOAR study) | Not eligible end point. Risk of developing inflammatory polyarthritis or RA |
Landewe (2007)213 | Predictive markers in rapidly progressing rheumatoid arthritis | Review |
Lanfant (2008)214 | An algorithm including the intrasynovial expression of CD20 and serum biomarkers (rheumatoid factors, anti-CCP2 and RANK-ligand) able to predict the progression of bone erosions in very early arthritis | Insufficient details reported |
Lanfant-Weybel (2012)215 | Synovium CD20 expression is a potential new predictor of bone erosion progression in very early arthritis treated by sequential DMARDs monotherapy – a pilot study from the VErA cohort | Study design |
Lauwerys (2015)216 | Heterogeneity of synovial molecular patterns in patients with arthritis | Study design |
Le Loët (2010)217 | Serum IgA rheumatoid factor and pyridinoline in very early arthritis as predictors of erosion(s) at two years: a simple model of prediction from a conservatively treated community-based inception cohort | Study design. Study population: ≤ 80% with RA |
Li (2016)218 | Relationship of multi-biomarker disease activity score and other risk factors with radiographic progression in an observational study of patients with rheumatoid arthritis | Not early RA |
Li (2013)219 | Biomarker-based estimates of risk of radiographic progression in the Leiden early arthritis cohort | Conference abstract of Li et al.218 |
Li (2013)220 | Impact of a multi-biomarker disease activity test on rheumatoid arthritis treatment decisions and therapy use | Not available |
Liao (2009)221 | Clinical factors that predict erosion-free status in rheumatoid arthritis | Not early RA |
Liao 2011222 | Clinical predictors of erosion-free status in rheumatoid arthritis: a prospective cohort study | Available data indicate not early RA |
Lillegraven (2013)223 | The performance of matrix-based risk models for rapid radiographic progression in an observational cohort of established rheumatoid arthritis patients | External validation cohort not early RA |
Lillegraven (2013)95 | Performance of matrix-based risk models for rapid radiographic progression in a cohort of patients with established rheumatoid arthritis | External validation cohort not early RA |
Lindqvist (2002)224 | Ten year outcome in a cohort of patients with early rheumatoid arthritis: health status, disease process, and damage | Limited reporting of predictive performance |
Lindqvist (2005)225 | Prognostic laboratory markers of joint damage in rheumatoid arthritis | No measure of predictive performance reported |
Linn-Rasker (2007)226 | Arthritis of the large joints – in particular, the knee, at first presentation is predictive for a high level of radiological destruction of the small joints in rheumatoid arthritis | No measure of predictive performance reported |
Lisitsyna (2014)227 | Factors associated with major depressive disorder in rheumatoid arthritis patients | No eligible end point |
Lisitsyna (2015)228 | Depression as a risk factor for joints destruction in rheumatoid arthritis patients | Insufficient details reported |
Liu (2009)229 | Prediction of disease severity in patients with early rheumatoid arthritis by gene expression profiling | Study design |
Løppenthin (2015)230 | Physical activity and the association with fatigue and sleep in Danish patients with rheumatoid arthritis | Not early RA |
Luukkainen (1983)231 | The prediction of radiological destruction during the early stage of rheumatoid arthritis | Not available |
Ma (2014)232 | Multi-biomarker disease activity score is associated with Power Doppler ultrasound in patients with rheumatoid arthritis in low disease activity state | Duration of RA at baseline not reported |
Ma (2012)233 | Biomarker signature in rheumatoid arthritis patients with low disease activity: the REMIRA study | Not available |
Ma (2011)234 | Investigation of a multi-biomarker disease activity (Vectra DA) signature and algorithm score in rheumatoid arthritis patients with low disease activity: the REMIRA study | Not early RA |
Ma (2014)235 | Clinical and serological predictors of remission in rheumatoid arthritis are dependent on treatment regimen | Insufficient reporting of predictive performance |
Ma (2012)236 | Remission in early rheumatoid arthritis: predicting treatment response | No eligible factor included in final model |
Machold (2007)237 | Very recent onset rheumatoid arthritis: clinical and serological patient characteristics associated with radiographic progression over the first years of disease | Limited reporting of predictive performance |
Mamehara (2010)238 | Serum matrix metalloproteinase-3 as predictor of joint destruction in rheumatoid arthritis, treated with non-biological disease modifying anti-rheumatic drugs | Not early RA |
Mathsson (2008)239 | Antibodies against citrullinated vimentin in rheumatoid arthritis | Not eligible risk model with measure of predictive performance |
Mei (2007)240 | Evaluating gene × gene and gene × smoking interaction in rheumatoid arthritis using candidate genes in GAW15 | Study design |
Miller (2013)241 | The diagnostic accuracy of rheumatoid factor testing in primary care | Diagnostic study |
Miller (2014)242 | Negative rheumatoid factor in primary care delays referral of patients with rheumatoid arthritis | Study design |
Mierau (2006)243 | Diagnosis and prognosis of early rheumatoid arthritis, with special emphasis on laboratory analysis | Review |
Möller (2014)244 | Anaemia may add information to standardised disease activity assessment to predict radiographic damage in rheumatoid arthritis: a prospective cohort study | Duration of symptoms at baseline not reported for total population but available data indicate not early RA |
Morel (2005)245 | How to predict prognosis in early rheumatoid arthritis | Review |
Möttönen (1988)246 | Prediction of erosiveness and rate of development of new erosions in early rheumatoid arthritis | Limited reporting of predictive performance |
Möttönen (1998)247 | Only high disease activity and positive rheumatoid factor indicate poor prognosis in patients with early rheumatoid arthritis | No measure of predictive performance reported |
Nakashima (2016)248 | Magnetic resonance imaging bone oedema at enrolment predicts rapid radiographic progression in patients with early RA: results from the Nagasaki University early arthritis cohort | Unclear reporting of predictive performance |
Nell (2005)249 | Autoantibody profiling as early diagnostic and prognostic tool for rheumatoid arthritis | Limited reporting of predictive performance |
Nielen (2005)250 | Antibodies to citrullinated human fibrinogen (ACF) have diagnostic and prognostic value in early arthritis | Mixed population. Available data indicate that < 80% of the study population had RA and no early RA subgroup |
Nishiguchi (2014)251 | Self-assessment tool of disease activity of rheumatoid arthritis by using a smartphone application | Study design |
Nishiguchi (2016)252 | Self-assessment of rheumatoid arthritis disease activity using a smartphone application. Development and 3-month feasibility study | Study design |
Norton (2013)253 | Trajectories of functional limitation in early rheumatoid arthritis and their association with mortality | No measure of predictive performance reported |
Norton (2013)254 | A study of baseline prevalence and cumulative incidence of comorbidity and extra-articular manifestations in RA and their impact on outcome | Not eligible risk model with measure of predictive performance |
Norton (2014)255 | Health Assessment Questionnaire disability progression in early rheumatoid arthritis: systematic review and analysis of two inception cohorts | No measure of predictive performance |
Ødegård (2006) 256 | Association of early radiographic damage with impaired physical function in rheumatoid arthritis: a ten-year, longitudinal observational study in 238 patients | Not early RA |
Papadopoulos (2005)257 | Does cigarette smoking influence disease expression, activity and severity in early rheumatoid arthritis? | No measure of predictive performance reported |
Park (2011)258 | Patterns of radiographic outcomes in early, seropositive rheumatoid arthritis: a baseline analysis | Study design |
Park (2014)259 | Examining radiographic outcomes over time | Study design |
Plant (1994)260 | Measurement and prediction of radiological progression in early rheumatoid arthritis | Study design |
Plant (2000)261 | Relationship between time-integrated C-reactive protein levels and radiologic progression in patients with rheumatoid arthritis | No measure of predictive performance reported |
Posthumus (2000)262 | Serum matrix metalloproteinase 3 in early rheumatoid arthritis is correlated with disease activity and radiological progression | Study design |
Putrik (2016)263 | Less educated and older patients have reduced access to biologic DMARDs even in a country with highly developed social welfare (Norway): results from Norwegian cohort study NOR-DMARD | Not an eligible end point |
Quinn (2003)264 | Prognostic factors in a large cohort of patients with early undifferentiated inflammatory arthritis after application of a structured management protocol | Not early RA |
Quinn (2006)265 | Anti-CCP antibodies measured at disease onset help identify seronegative rheumatoid arthritis and predict radiological and functional outcome | Limited reporting of predictive performance |
Rech (2016)266 | Prediction of disease relapses by multibiomarker disease activity and autoantibody status in patients with rheumatoid arthritis on tapering DMARD treatment | Not early RA |
Rezaei (2012)267 | In early rheumatoid arthritis, patients with a good initial response to methotrexate have excellent 2-year clinical outcomes, but radiological progression is not fully prevented: data from the methotrexate responders population in the SWEFOT trial | Study design |
Rhodes (2010)268 | A genetic association study of serum acute-phase C-reactive protein levels in rheumatoid arthritis: implications for clinical interpretation | Study design |
Saevarsdottir (2011)269 | Predictors of response to methotrexate in early DMARD naive rheumatoid arthritis: results from the initial open-label phase of the SWEFOT trial | No measure of predictive performance reported |
Saevarsdottir (2011)270 | Patients with early rheumatoid arthritis who smoke are less likely to respond to treatment with methotrexate and tumor necrosis factor inhibitors: observations from the Epidemiological Investigation of Rheumatoid Arthritis and the Swedish Rheumatology Register cohorts | No measure of predictive performance reported |
Salaffi (2009)271 | A predictive algorithm combining routine assessment and power Doppler ultrasonography for the development of rheumatoid arthritis from an early-onset undifferentiated arthritis | Not early RA |
Scherer (2011)272 | Distinct ACPA fine specificities, formed under the influence of HLA shared epitope alleles, have no effect on radiographic joint damage in rheumatoid arthritis | Study design |
Scott (2000)273 | Prognostic factors in early rheumatoid arthritis | Review |
Scott (2013)274 | Prediction model for rheumatoid arthritis: modelling 46 genetic risk variants with smoking | Study design |
Semb (2014)275 | Development of a transatlantic cardiovascular risk calculator for rheumatoid arthritis (ATACC-RA) | Study design |
Semb (2015)276 | Development of a transatlantic cardiovascular risk calculator for rheumatoid arthritis | Study design |
Shen (2010)277 | Serum biomarkers predict progressive structural damage in the BeSt study | Limited reporting of methods/results |
Shen (2015)278 | Sparse kernel machine regression for ordinal outcomes | No measure of predictive performance reported |
Sjoblom (1984)279 | Factors related to the progression and joint destruction in rheumatoid arthritis | Not early RA |
Smolen (2006)100 | Predictors of joint damage in patients with early rheumatoid arthritis treated with high-dose methotrexate with or without concomitant infliximab: results from the ASPIRE trial | Study design |
Smolen (2013)280 | Forget personalised medicine and focus on abating disease activity | Commentary |
Solomon (2015)281 | Derivation and internal validation of an expanded cardiovascular risk prediction score for rheumatoid arthritis: a Consortium of Rheumatology Researchers of North America Registry Study | Not eligible outcome |
Sparks (2013)282 | Performance of prediction models for rheumatoid arthritis serological phenotypes among women using family history, genetics and environmental factors | Modelling risk of RA |
Stucki (1997)283 | Management of rheumatoid arthritis | Literature review |
Suarez-Almazor (1994)284 | Outcome in rheumatoid arthritis. A 1985 inception cohort | Study design |
Syversen (2010)285 | Prediction of radiographic progression in rheumatoid arthritis and the role of antibodies against mutated citrullinated vimentin: results from a 10-year prospective study | No measure of predictive performance reported |
Tchetverikov (2003)286 | Matrix metalloproteinases-3, -8, –9 as markers of disease activity and joint damage progression in early rheumatoid arthritis | No measure of predictive performance reported |
Teitsson (1984)287 | Prospective study of early rheumatoid arthritis. I. Prognostic value of IgA rheumatoid factor | No measure of predictive performance reported |
Ting (2008)288 | Development of a health care utilisation data-based index for rheumatoid arthritis severity: a preliminary study | Duration of symptoms at baseline not reported. Not an eligible outcome |
Tobón (2013)289 | First-year radiographic progression as a predictor of further progression in early arthritis: results of a large national French cohort | ≤ 80% of the study population had RA and no early RA subgroup available |
Uhlig (2000)290 | The course of rheumatoid arthritis and predictors of psychological, physical and radiographic outcome after 5 years of follow-up | Does not meet early RA definition |
van Beers-Tas (2015)291 | How does established rheumatoid arthritis develop, and are there possibilities for prevention? | Literature review |
van den Broek (2013)292 | The clinical relevance of rapid radiological progression in the first year of treatment during 8 years of follow-up of early rheumatoid arthritis patients | Conference abstract. Appears to be linked with van den Broek et al.293 |
van den Broek (2012)293 | Rapid radiological progression in the first year of early rheumatoid arthritis is predictive of disability and joint damage progression during 8 years of follow-up | Study design |
van der Heijde (1992)294 | Prognostic factors for radiographic damage and physical disability in early rheumatoid arthritis. A prospective follow-up study of 147 patients | Limited reporting of predictive performance |
van der Heide (1995)295 | Prediction of progression of radiological damage in newly diagnosed rheumatoid arthritis | Limited reporting of predictive performance |
van der Helm-van Mil (2005)296 | Antibodies to citrullinated proteins and differences in clinical progression of rheumatoid arthritis | Study design |
van der Helm-van Mil (2008)297 | Validation of a prediction rule for disease outcome in patients with recent-onset undifferentiated arthritis: moving towards individualised treatment decision-making | Not early RA |
van der Helm-van Mil (2010)298 | Genetic variants in the prediction of rheumatoid arthritis | Modelling risk of RA |
van der Helm-van Mil (2013)299 | An evaluation of molecular and clinical remission in rheumatoid arthritis by assessing radiographic progression | Measure of predictive performance not appropriate to review question |
van Jaarsveld (1999)300 | The prognostic value of the antiperinuclear factor, anti-citrullinated peptide antibodies and rheumatoid factor in early rheumatoid arthritis | Limited reporting of predictive performance |
van Nies (2015)301 | Evaluating processes underlying the predictive value of baseline erosions for future radiological damage in early rheumatoid arthritis | No measure of predictive performance |
van Steenbergen (2013)302 | Anaemia to predict radiographic progression in rheumatoid arthritis | No measure of predictive performance reported |
van Zeben (1991)303 | Association of HLA-DR4 with a more progressive disease course in patients with rheumatoid arthritis | Study design |
van Zeben (1993)304 | Factors predicting outcome of rheumatoid arthritis: results of a follow up study | Symptom duration at baseline unclear |
Varache (2011)305 | Diagnostic accuracy of ACR/EULAR 2010 criteria for rheumatoid arthritis in a 2-year cohort | Study design |
Vastesaeger (2008)94 | Matrix risk model for prediction of rapid radiographic progression in rheumatoid arthritis | Preliminary report of included study. No further relevant data reported |
Versteegh (2010)306 | Mapping onto Eq-5 D for patients in poor health | Study design |
Vesperini (2013)307 | Association of tobacco exposure and reduction of radiographic progression in early rheumatoid arthritis: results from a French multicentre cohort | No measure of predictive performance reported |
Visser 2002308 | How to diagnose rheumatoid arthritis early: a prediction model for persistent (erosive) arthritis | Study design. Mixed early arthritis population |
Visser (2009a)309 | The BeSt matrix model in recent-onset rheumatoid arthritis patients: individual prediction of rapid radiographic progression and numbers-needed-to-treat with initial combination therapy | Preliminary report of included study. No further relevant data reported |
Visser (2009b)310 | The clinical relevance of a prediction rule for disease outcome in patients with undifferentiated arthritis: comment on the article by van der Helm-van Mil et al. | Letter to editor |
Visvanathan (2007)311 | Changes in biomarkers of inflammation and bone turnover and associations with clinical efficacy following infliximab plus methotrexate therapy in patients with early rheumatoid arthritis | No measure of predictive performance reported |
Wessels (2007)312 | A clinical pharmacogenetic model to predict the efficacy of methotrexate monotherapy in recent-onset rheumatoid arthritis | Not an eligible outcome. DAS not DAS28 (borderline exclusion) |
Westedt (1986)313 | Serum immune complexes containing IgA appear to predict erosive arthritis in a longitudinal study in rheumatoid arthritis | No measure of predictive performance reported |
Weyand (1992)314 | The influence of HLA-DRB1 genes on disease severity in rheumatoid arthritis | Not early RA |
Widdifield (2013)315 | Accuracy of Canadian health administrative databases in identifying patients with rheumatoid arthritis: a validation study using the medical records of rheumatologists | Study design |
Wolfe (1998)316 | Radiographic outcome of recent-onset RA: a 19-year study of radiographic progression | Limited reporting of predictive performance |
Wolfe (2000)317 | A reappraisal of HAQ disability in rheumatoid arthritis | Study design. No measure of predictive performance reported |
Wolfe (2000)318 | The effect of smoking on clinical, laboratory, and radiographic status in rheumatoid arthritis | Not early RA |
Wong (2004)319 | Development and evaluation of a patient self-report case-finding method for rheumatoid arthritis | Study design |
Yamanaka (2000)320 | Serum matrix metalloproteinase 3 as a predictor of the degree of joint destruction during the six months after measurement, in patients with early rheumatoid arthritis | Study design. No measure of predictive performance reported |
Young (1987)321 | A prospective study of early onset rheumatoid arthritis over fifteen years: prognostic features and outcome | Limited reporting of predictive performance |
Young (1988)322 | A prognostic index for erosive changes in the hands, feet and cervical spines in early rheumatoid arthritis | Limited reporting of predictive performance |
Young (1997)323 | Can we predict aggressive disease? | Review |
Young (2000)324 | Socioeconomic deprivation and rheumatoid disease: what lessons for the health service? | No measure of predictive performance reported |
Young-Min (2007)325 | Biomarkers predict radiographic progression in early rheumatoid arthritis and perform well compared with traditional markers | No eligible factor included in baseline model for radiographic progression. Longitudinal model time frame outside 2-year early RA definition |
Appendix 5 Additional calculations for data extractions
Obtaining estimates of logit(C) and standard error
External validation studies that reported the c-statistic were considered. The logit transform is used to put the reported value on a suitable scale for analysis. Granger et al. 82 and Heimans et al. 84 report the associated CIs that can be used to obtain the standard error as follows:
No appropriate estimates of uncertainty are provided in the De Cock et al. 80 validation, but the standard error was approximated from the total number of observed events, expected events and the sample size, as described in Debray et al. 40 (Appendix 6):
in which O is the total number of observed events, N is the total sample size and:
Calculating the expected number of events
When possible, the expected number of events was calculated for each risk model evaluated in each external validation population. Here, we give an example of calculating the number of expected events for one risk model only, the Syversen model,76 chosen as it has a smaller number of risk categories than other externally validated risk models.
For each risk category, the PR is provided in the published risk development study. The number of individuals in the external validation study who are assigned to each risk category (N) is provided in the external validation study (De Cock et al. 80 in this example). The expected number of events in each risk category (E) is computed by multiplying the PR and the number of individuals. The total number of expected events is then found by summing these expected numbers over all risk categories (Table 22).
Risk category | PR | De Cock et al.80 population | ||
---|---|---|---|---|
N | E | O | ||
1 | 0.0928 | 0 | 0 | 0 |
2 | 0.2535 | 1 | 0.2535 | 0 |
3 | 0.2891 | 0 | 0 | 0 |
4 | 0.5744 | 2 | 1.1488 | 1 |
5 | 0.2460 | 5 | 1.23 | 1 |
6 | 0.5200 | 5 | 2.6 | 0 |
7 | 0.5646 | 3 | 1.6938 | 0 |
8 | 0.8115 | 5 | 4.0575 | 0 |
9 | 0.2387 | 1 | 0.2387 | 0 |
10 | 0.5100 | 4 | 2.04 | 1 |
11 | 0.5548 | 6 | 3.3288 | 0 |
12 | 0.8053 | 9 | 7.2477 | 0 |
13 | 0.5000 | 1 | 0.5 | 0 |
14 | 0.7685 | 4 | 3.074 | 0 |
15 | 0.7990 | 10 | 7.99 | 1 |
16 | 0.9296 | 17 | 15.8032 | |
Total | 73 | 51.206 | 4 |
Calculating distribution of linear predictor (case mix)
For each risk category, the PR is provided in the published risk development study and the number of individuals in the external validation study who are assigned to each risk category (N) is provided in the external validation study. These can be used to work out the distribution of risks (Figures 8 and 9).
FIGURE 8.
Distribution of linear predictor (case mix) for risk models in the De Cock et al. 80 external validation population. (a) ASPIRE CRP; (b) ASPIRE ESR; (c) BeSt; (d) ESPOIR; (e) SWEFOT 2; and (f) Syversen.



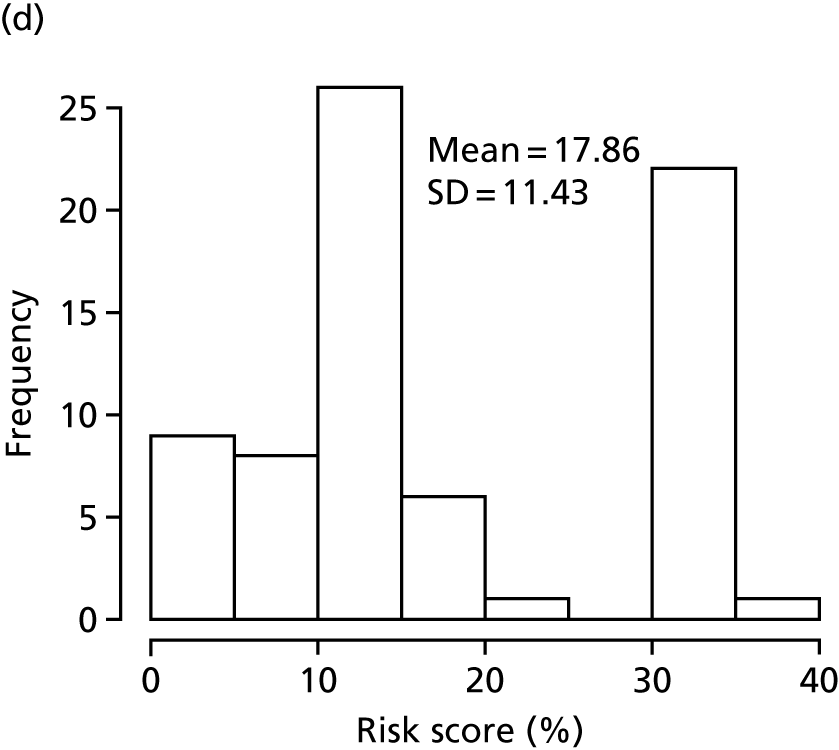

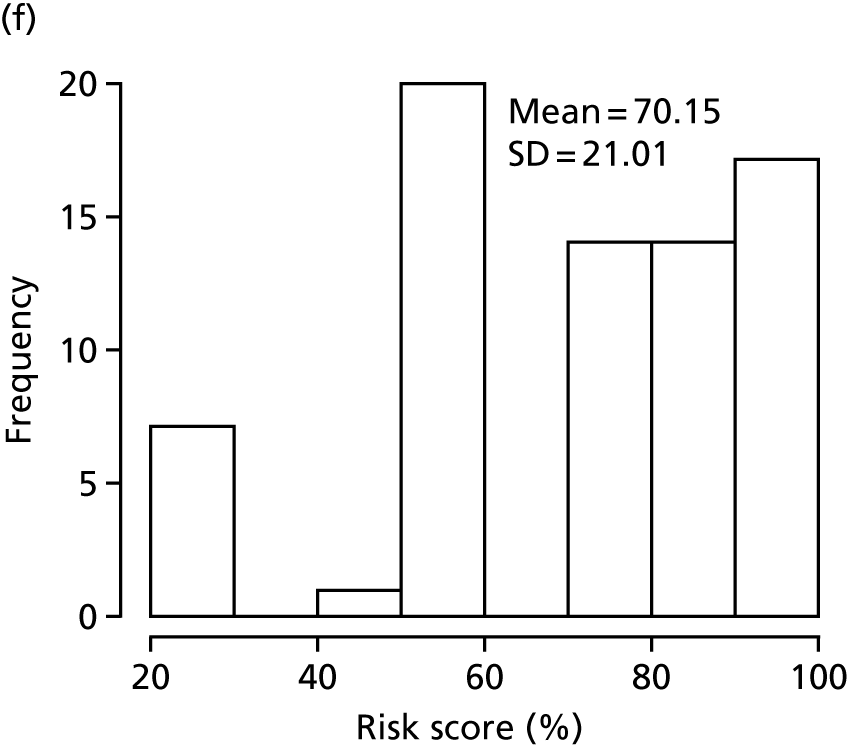
FIGURE 9.
Distribution of linear predictor (case mix) for the BeSt models in Heimans et al. 84 external validation population.

Calculating risk probabilities for the Syversen model
The final risk model provided in Syversen et al. 76 is given as:
This results in the following risk matrix, which provides the risks for each combination of factors (Table 23).
RF status | ESR | ACPA status | |||
---|---|---|---|---|---|
ACPA– | ACPA+ | ||||
Male | Female | Male | Female | ||
RF positive | High | 0.5 | 0.7685 | 0.799 | 0.9296 |
Low | 0.2387 | 0.51 | 0.5548 | 0.8053 | |
RF negative | High | 0.246 | 0.52 | 0.5646 | 0.8115 |
Low | 0.0928 | 0.2535 | 0.2891 | 0.5744 |
Appendix 6 Quality assessment results for Prediction model study Risk Of Bias Assessment Tool domains 1, 2 and 3 (review 1)
Prediction model study Risk Of Bias Assessment Tool domain 1: participant selection
Name of risk model | Were appropriate data sources used? | Were all inclusions and exclusions of participants appropriate? | Risk of bias introduced by the selection of participants |
---|---|---|---|
ASPIRE78 | Y | Y | Low |
Bansback58 | Y | Y | Low |
Berglin59 | Y | Y | Low |
BeSt79 | Y | Y | Low |
Brennan60 | Y | Y | Low |
Centola61 | Y | Y | Low |
Combe (A)62 | Y | Y | Low |
Combe (B)63 | Y | Y | Low |
de Punder64 | Y | Y | Low |
de Vries-Bouwstra65 | Y | Y | Low |
Degboé66 | Y | Y | Low |
Dirven67 | Y | Y | Low |
Dixey68 | Y | Y | Low |
Drossaers-Bakker56 | Y | PYa,b | Unclear |
ESPOIR69 | Y | Y | Low |
Forslind70 | Y | Y | Low |
Graell71 | Y | Y | Low |
Houseman72 | Y | Y | Low |
Sanmartí75 | Y | Y | Low |
SONORA57 | Y | NIb | Unclear |
SWEFOT73 | Y | Y | Low |
Syversen76 | Y | PYc | Unclear |
van Steenbergen77 | Y | Y | Low |
Prediction model study Risk Of Bias Assessment Tool domain 1: participant selection
First author of external validation study | Were appropriate data sources used? | Were all inclusions and exclusions of participants appropriate? | Risk of bias introduced by the selection of participants |
---|---|---|---|
De Cock80 | Y | PYa | Unclear |
Granger82 | Y | Y | Low |
Hambardzumyan83 | Y | Y | Low |
Heimans84 | Y | PYb | Unclear |
Markusse85 | Y | Y | Low |
Prediction model study Risk Of Bias Assessment Tool domain 2: predictors
Name of risk model | Were predictors defined and assessed in a similar way for all participants?a | Were predictor assessments made without knowledge of outcome data?b | Were all (final) predictors available at the time at which the model was intended to be used? | Were all relevant predictors analysed (i.e. all selected prognostic factors analysed as candidate predictors)? | Risk of bias introduced by predictors or their assessment (low/high/unclear)c |
---|---|---|---|---|---|
ASPIRE78 | PY | Y | Y | No ACPA status, DAS28, smoking status, HAQ score or early RA untreated for ≥ 12 weeks following the onset of symptoms | Unclear |
Bansback58 | PY | Y | PY (dependent on availability of the Carstairs index) | No ACPA status, CRP levels or smoking status | Unclear |
Berglin59 | PY | Y | Y | No early RA untreated for ≥ 12 weeks following the onset of symptoms, HAQ score or smoking status | Unclear |
BeSt79 | PY | Y | Y | No DAS28 (DAS only) | Low |
Brennan60 | PY | Y | Y | No ACPA status, erosions/joint damage on radiographic analysis, smoking status, ESR or DAS28 | Unclear |
Centola61 | PY | PY | PY [dependent on the availability of biomarker assay(s)] | Biomarker tests only | Uncleard |
Combe (A)62 | PY | Y | PY (dependent on the availability of SE testing) | No ACPA status, DAS28 or smoking status | Unclear |
Combe (B)63 | PY | Y | Y | No ACPA status, DAS28 or smoking status | Unclear |
de Punder64 | PY | Y | Y | No early RA untreated for ≥ 12 weeks following the onset of symptoms or HAQ score | Unclear |
de Vries-Bouwstra65 | PY | Y | PY (dependent on the availability of SE testing) | No ACPA status, CRP levels, DAS28 or smoking status | Unclear |
Degboé66 | PY | Y | PY (dependent on the availability of anti-MCV, AhFibA testing) | No SJC, ESR, DAS28, smoking status or HAQ score | Unclear |
Dirven67 | PY | Y | Y | No DAS28 (DAS only) | Low |
Dixey68 | PY | Y | Y | No ACPA status, CRP levels, DAS28 or smoking status | Unclear |
Drossaers-Bakker56 | PY | Y | PY (alternative models provided with available predictors) | No ACPA status, CRP levels, DAS28 or smoking status | Unclear |
ESPOIR69 | PY | Y | Y | No smoking status | Low |
Forslind70 | PY | Y | Y | No SJC, smoking status or early RA untreated for ≥ 12 weeks following the onset of symptoms | Unclear |
Graell71 | PY | Y | Y | No smoking status | Low |
Houseman72 | PY | PY (anti-CCP measured at 8.2 years) | PY (dependent on the availability of MMP-3 testing) | No SJC, DAS28, smoking status, HAQ score or early RA untreated for ≥ 12 weeks following the onset of symptoms | Unclear |
Sanmartí75 | PY | Y | PY (dependent on the availability of HLA-DRB1*04 test) | Assumed same as for Graell et al.71 and, therefore, no smoking status | Low |
SONORA57 | NI | Y | Y | NI | Unclear |
SWEFOT73 | PY | Y | Y | Y | Low |
Syversen76 | PY | Y | Y | No SJC, DAS28, smoking status or early RA untreated for ≥ 12 weeks following the onset of symptoms | Unclear |
van Steenbergen77 | PY | Y | PY (dependent on the availability of genetic tests) | No erosions at baseline, CRP levels, DAS28, smoking status or HAQ score | Unclear |
First author of external validation study | Were predictors defined and assessed in a similar way for all participants?a | Were predictor assessments made without knowledge of the outcome data?b | Risk of bias introduced by predictors or their assessment (low/high/unclear) |
---|---|---|---|
De Cock80 | PY | Y | Low |
Granger82 | PY | Y | Low |
Hambardzumyan83 | PY | Y | Low |
Heimans84 | PY | Y | Low |
Markusse85 | PY | Y | Low |
Prediction model study Risk Of Bias Assessment Tool domain 3: outcomes
Name of risk model | Was a prespecified outcome definition used?a | Was the outcome defined and determined in a similar way for all participants?b | Was the outcome determined without knowledge of predictor information? | Risk of bias introduced by outcome or its determination (low/high/unclear) |
---|---|---|---|---|
ASPIRE78 | Y | PY | PY | Low |
Bansback58 | PY | PY | NI | Unclear |
Berglin59 | PY | PY | Y | Low |
BeSt79 | Y | PY | PY | Low |
Brennan60 | PY | PY | NI | Unclear |
Centola61 | PY | PY | NI | Unclear |
Combe (A)62 | Y | PY | Y | Low |
Combe (B)63 | PY | PY | NI | Unclear |
de Punder64 | Y | PY | NI | Unclear |
de Vries-Bouwstra65 | PY | PY | Y | Low |
Degboé66 | PY | PY | Y | Low |
Dirven67 | Y | PY | PY | Low |
Dixey68 | PY | PY | NI | Unclear |
Drossaers-Bakker56 | PY | PY | NI | Unclear |
ESPOIR69 | Y | PY | Y | Low |
Forslind70 | PY | PY | Y | Low |
Graell71 | PY | PY | NI | Unclear |
Houseman72 | PY | PY | PY | Low |
Sanmartí75 | Y | PY | NI | Unclear |
SONORA57 | PY | PY | NI | Unclear |
SWEFOT73 | Y | PY | NI | Unclear |
Syversen76 | PY | PY | Y | Low |
van Steenbergen77 | PY | PY | Y | Low |
Name of external validation study | Was a prespecified outcome definition used?a | Was the outcome defined and determined in a similar way for all participants?b | Was the outcome determined without knowledge of predictor information? | Risk of bias introduced by outcome or its determination (low/high/unclear) |
---|---|---|---|---|
De Cock80 | Y | PY | NI | Unclear |
Granger82 | Y | PY | Y (blinded to clinical evaluation) | Low |
Hambardzumyan83 | Y | PY | NI | Unclear |
Heimans84 | Y | PY | PY (blinded to patient identity) | Lowc |
Markusse85 | PY | PY | PY (blinded to patient identity) | Low |
Appendix 7 Additional evidence tables: review 1
Name of risk model/external validation study (number of baseline characteristics reported) | Characteristic | ||||||||||
---|---|---|---|---|---|---|---|---|---|---|---|
Age | Sex | HAQ score | Radiographic joint damage | ACPA/anti-CCP status | RF status | SJC | TJC | ESR | CRP level | Smoking status | |
Risk model development studies | |||||||||||
ASPIRE78 (n = 1049) |
Median age: 51 years (IQR 41–60 years) | 71.1% female | Median (scale 0–3): 1.5 (IQR 1.0–1.9) |
Median total modified SHS: 5.0 (IQR 1.5–14.0) 82% (n = 854) of patients with joint erosion 65.9% (n = 687) of patients with JSN 0.3% of patients (n = 3) with prior joint surgery |
NR | Median: = 175 U/ml (IQR 30–357 U/ml) | Median: 19 (IQR 14–26) | Median: 31 (IQR 22–44) | Median: 40 mm/hour (IQR 23–61 mm/hour) | Median:1.4 mg/dl (IQR 0.4–4.1 mg/dl) | NR |
Bansback58 (n = 985, NR across total sample) |
Aged 17–93 years at disease onset (median 55 years, IQR NR) | Female: n = 654 (66%) | Median: 1.00 (IQR NR) | n = 241 (24%) had erosions at baseline | NR | 74% (n = 730) of patients were seropositive at baseline | NR | NR | NR | NR | NR |
Berglin59 | NR [mean age at onset of symptoms was 54 years (range 23–73 years)] | NR | NR | NR | Anti-CCP: 74.8% |
IgM RF: 85.7% IgA RF: 78.6% IgG RF: 46.8% |
NR | NR | NR | NR | NR |
BeSt79 (Reported for 465/508; NR for whole sample, reported by treatment group) |
Mean age: 54 years across groups (SD 13–14 years) | Female sex: 66–70% across groups (SD 66–70%) | HAQ score of 1.4 across groups (0.6 to 0.7) |
Median erosions: 1.8–2.0 across groups (IQR 0.5–4.5) Total median SHS: 3.3–4.0 across groups (IQR 1.5–8.5) |
ACPA+: 55–64% | RF positive: 62.66% | Median: 13–14 across groups | Median: 11–14 | Median: 35–38 mm/hour across groups | Median: 21–22 mg/l across groups | NR |
Brennan60 (Total population, n = 175) |
18–51 years: 32% 52–66 years: 34% > 66 years: 34% |
71% female | HAQ score ≥ 1 (scale 0–3): 59% | NR | NR | NR | NR | NR | NR | NR | NR |
Centola61 (Feasibility studies I–IV, n = 652; algorithm training study stage 3, n = 249 samples) |
Feasibility studies I–IV (stage 2), mean age: 59 years Algorithm training study (stage 3), median age: 58 years (IQR 49–67 years) |
Feasibility studies I–IV (stage 2): 77–91% female Algorithm training study (stage 3): 75% female |
Feasibility studies I–IV (stage 2): NR Algorithm training study (stage 3): NR |
Feasibility studies I–IV (stage 2): NR Algorithm training study (stage 3): NR |
Feasibility studies I–IV (stage 2): 61–63% CCP positive Algorithm training study (stage 3): 58% anti-CCP positive |
Feasibility studies I–IV (stage 2): 64–97% positive Algorithm training study (stage 3): 61% positive |
Feasibility studies I–IV (stage 2), median SJC28: 2.0 (IQR 0.0–10) to 16 (IQR 12–21) Algorithm training study (stage 3), median: 4 (IQR 0–17) |
Feasibility studies I–IV (stage 2), median TJC28: 2.0 (IQR 0–8.3) to 12 (IQR 4.8–20) Algorithm training study (stage 3), median: 5 (IQR 0–18) |
Feasibility studies I–IV (stage 2): NR Algorithm training study (stage 3): NR |
Feasibility studies I–IV (stage 2), median: 14 mg/l (IQR 4.0–32 mg/l) to 18 mg/l (IQR 6.9–47 mg/l) Algorithm training study (stage 3), median: 3.8 mg/l (IQR 1.3–20.5 mg/l) |
Feasibility studies I–IV (stage 2): NR Algorithm training study (stage 3): NR |
Combe A62 (n = 191) |
Mean age at diagnosis: 50.5 years (SD 14.7 years) | 73.3% female | Mean: 1.3 (SD 0.7) | Mean Sharp score: 3.6 (SD 7.7) | NR |
RF positive: 80.6% IgM RF: 68.0% IgA RF: 75.4% |
Mean: 9 (SD 5.9) | Mean: 21 (SD 10) | Mean: 40.2 mm/hour (SD 28.5 mm/hour) | Mean: 34.1 mg/l (SD 43.2 mg/l) | NR |
Combe B63 (n = 191) |
Mean age: 50.5 years (SD 14.7 years) | 73% female |
NR Mean: 1.3 (range 0–2.75) |
NR Erosion score, mean: 1.7 (range 0–33.0) |
NR | IgA or IgM RF positive: 80.8% | Mean: 9.0 (SD 5.9) | Mean: 21.7 (SD 10.5) | Mean: 40.2 mm/hour (SD 28.5 mm/hour) | Mean: 34.1 mg/l (SD 43.2mg/l) | NR |
de Punder64 (n = 425; NR for total group) |
Mean age:
|
Joint damage progressors: 59% Non-progressors: 66% |
NR | Erosions:
|
Anti-CCP positive:
|
RF positive:
|
SJC28:
|
TJC28: Joint damage progressors, median: 7 (IQR4–13) Non-progressors, median: 6 (IQR 2–12) |
Joint damage progressors, median: 40 mm/hour (IQR 21–59 mm/hour) Non-progressors, median: 21 mm/hour (IQR 9–38 mm/hour) |
Joint damage progressors, median: 22 mg/l (IQR 7–52 mg/l) Non-progressors, median: 3 mg/l (IQR 0–23 mg/l) |
Smoker status (ever):
|
de Vries-Bouwstra65 (n = 152) |
Median age: 66 years (IQR 55–76 years) | 68% female | NR | Patients with erosions in hands or feet: 57 (38%) | NR | IgM RF positive: 93 (61%) | Median number swollen joints: 6 (IQR 4–8) | NR | NR | NR | NR |
Degboé66 (n = 566) |
Median age: 50.5 years (IQR 40.2–57.0 years) | 78.6% female | NR | mTSS, median: 3 (IQR 0–7) | ACPA+: 333 | RF positive: 54.2% | NR | NR | Median: 22 mm/hour (IQR 12–37 mm/hour) | Median: 9 mg/l (IQR 0–21 mg/l) | Smokers: 48.1% |
Dirven67 (NR for total group; MTX mono, n = 239; combination and prednisone, n = 131; combination and IFX, n = 127) |
Mean age:
|
MTX monotherapy: 70% Combination and prednisone: 66% Combination and IFX: 66% |
Mean: 1.4 (SD 0.6–0.7) across groups | Median total SHS:
|
ACPA positive:
|
RF positive:
|
|
Median:
|
Median:
|
Median:
|
Smoking status (yes/no):
|
Dixey68 (n = 866) |
|
66% female | NR | Erosion score:
|
NR | RF negative: 27% | NR | NR | NR | NR | NR |
Drossaers-Bakker56 (n = 112) |
Mean age: 37 years (SD 8.4 years) | 100% female | Median: 0.75 (IQR NR) (range 0–2.88) |
Median SHS: 12 (IQR NR) Presence of erosions: 22% |
NR | RF positive: 55% | Median: 3.5 (IQR NR) | NR | Median: 27 mm/hour (IQR NR) | NR | NR |
ESPOIR69 (n = 370) |
Mean age: 49.4 years (SD 11.4 years) | 73.2% female | Mean HAQ score: 1.03 (SD 0.7) |
18% with typical RA erosion on radiographs Mean vSHS 6.02 (SD 9.7) |
ACPA positivity: 50% | IgM RF positivity: 55.1% | Mean SJC28: 7.9 (SD 5.4) | Mean TJC28: 8.7 (SD 6.9) | Mean ESR: 32.7 mm/first hour (SD 25 mm/first hour) | Mean CRP level: 24.8 mg/l (SD 37.7 mg/l) | NR |
Forslind70 (n = 379) |
Median age: 55 years (IQR 45–67 years) | 65% female | HAQ score (scale 0–3), median: 0.90 (IQR 0.50–1.38) | Larsen score scale range (0–200), median: 4 (IQR 0–10) | NR | RF positive: 61% | NR | NR | Median: 29 mm/hour (IQR 14–50 mm/hour) | Median: 19 mg/l (IQR 6–43 mg/l) | Current/previous smoker: 60% |
Graell71 (n = 105) |
Mean age: 55 years (SD 14.9 years) | 81% female | MHAQ, mean score: 0.97 (SD 0.56) | Larsen score, mean: 1.2 (SD 2.7) | Anti-CCP positive: 70.4% | RF positive: 73.3% | SJC28, mean: 8.3 (SD 4.1) | TJC28, mean: 10.1 (SD 5.9) | Mean: 39.5 mm/hour (SD 24.5 mm/hour) | Mean: 2.8 mg/dl (SD 2.9 mg/dl) | NR |
Houseman72 (n = 58) |
Mean age: 53 years (SD 11 years) | 64% female | Median: 1.4 (IQR 0.9–2.1) | Larsen score, median: 1 (IQR 0–2.5) | NR | RF positive: 77% | Median: 9 (IQR 4–14) | Median: 15 (IQR 6–18) | Median: 28 mm/hour (IQR 14–45 mm/hour) | Median: 17 mg/l (IQR 5–40 mg/l) | NR |
Sanmartí75 (n = 105) |
Mean age: 55 years (SD 14.9 years) | 81% female | MHAQ, mean score: 1 (SD 0.6) |
Larsen score, mean: 1.2 (SD 2.7) Erosion joint count, mean: 0.4 (SD 0.9) |
Anti-CCP positive: 70.4% | RF positive: 74.3% | SJC28, mean: 8.3 (SD 4.1) | TJC28, mean: 10.1 (SD5.9) | Mean: 39.6 mm/hour (SD 24.5 mm/hour) | Mean: 2.8 mg/dl (SD 2.9 mg/dl) | NR |
SONORA57 (n = 994) | Mean age: 53 years (SD 14.81 years) | 72% female | NR | Sharp score, mean: 5.49 (SD 7.85) (n = 746) | NR | NR | NR | NR | NR | NR | NR |
SWEFOT73 (n = 269) |
Median age: 57 years (IQR 46–63 years) | 28% male | HAQ score: 1.1 (IQR 0.9–1.6) |
SHS, median: 2 (IQR 0–6) JSN score, median: 0 (IQR 0–3) Erosion score, median: 0 (IQR 0–2), 42% erosions |
Anti-CCP positive: 62% |
RF positive: 67% RF and ACPA+: 49% |
SJC28, median: 10 (IQR 6–14) | TJC28, median: 8 (IQR 5–13) | Median: 34 mm/hour (IQR 22–54 mm/hour) | CRP level, median: 19 mg/l (IQR 9–47 mg/l) |
Current smokers: 24% Past smokers: 37% Never smoked: 39% |
Syversen76 (n = 238) |
Mean age: 51.9 years (SD 13.0 years) | 73.5% female | Mean: 0.9 (SD 0.6) | Erosive disease: 55.2% | Anti-CCP positive: 60.5% |
IgA RF positive: 37.8% IgM RF positive: 47.9% |
NR | NR | Median: 20.5 mm/hour (IQR 10.0–36.0 mm/hour) | Median: 5.3 mg/l (IQR 1.7–13.8 mg/l) | NR |
van Steenbergen77 (n = 426) |
Mean age : 56.6 years (SD 15.3 years) | 68.1% female | NR | SHS, median: 5.0 (IQR 2.0–10.0) | ACPA+: 53.3% | IgM RF positive: 58.2% | SJC66, median: 8 (IQR 4–14) | NR | Median: 33.0 mm/hour (18.0–55.0 mm/hour) | NR | NR |
External validation studies | |||||||||||
De Cock80 (n = 74) |
Mean age: 52 years (SD 16 years) | 65% female | Mean HAQ score (scale 0–3): 1.10 (SD 0.76) | NR | ACPA+: 71% |
RF positive: 71% RF: 224.7 U/ml (281.1 U/ml) |
Total SJC, mean 12.45 (SD 8.19) SJC28, mean: 8.01 (SD 5.35) |
Total TJC, mean: 14.35 (SD 10.59) TJC28, mean: 8.28 (SD 6.38) |
Mean ESR: 36.66 mm/hour (SD 24.06 mm/hour) | Mean CRP level: 28.80 mg/l (33.78 mg/l) | Smokers: 28% |
Granger82 (n = 370) |
Mean age: 49.4 years (SD 11.4 years) | 73.2% female | Mean (HAQ score: 1.0 (SD 0.7) |
Typical RA erosion: 17.8% vSHS baseline total score: 4.5 (SD 6.8) |
ACPA+: 50% | IgM RF positive: 55.1% | SJC, mean: 287.9 (SD 5.4) | TJC, mean: 288.7 (SD 6.9) | ESR, mean: 32.7 mm/first hour (SD 25 mm/first hour) | Mean CRP level: 24.8 mg/l (SD 37.7 mg/l) | NR |
Hambardzumyan83 (n = 487) |
NR | 70% female | NR | Mean SHS: 4.5 (median 2) | Anti-CCP positive: 57% | RF positive: 68% | SJC28, mean: 10.8 (SD 5.28) | TJC28, mean: 9.6 (SD 6.07) | Mean: 39.9 mm/hour (SD 25.9 mm/hour) | Mean: 33.8 mg/l (SD 36.81 mg/l) | NR |
Heimans84 (RA n = 479) |
Mean age: 52 years (SD 13 years) | 70% female | NR |
Median, total SHS: 0 (IQR 0–0) Erosive: 15% |
ACPA+: 68% | RF positive: 69% | Median SJC: 7 (IQR 3–11) | Median TJC: 7 (IQR 4–10) | NR | NR | NR |
Markusse85 (n = 508) |
NR for total group | NR for total group | NR for total group | NR for total group | NR for total group | NR for total group | NR for total group | NR for total group | NR for total group | NR for total group | NR for total group |
Name of clinical prediction model (submodels) | Other performance measures (e.g. sensitivity, specificity, PPV, NPV, accuracy) |
---|---|
Models predicting radiographic joint damage | |
ASPIRE78 ESR CRP |
NR |
Berglin59 |
Model 1: accuracy 73% Model 2: accuracy 67% |
BeSt79 ESR CRP |
PPV and NPV reported for a range of PRs. At 60% PR (100% patients classified), PPV = 75% and NPV = 69% |
Brennan60 |
Threshold of 0.5 PR Prediction sample: accuracy = 76/105 (72%) Validation sample: PPV = 76%; NPV = 80%; and accuracy = 79% Addition of second-line drugs and/or steroids tested: PPV = 72%; NPV = 82%; and accuracy = 79% |
Combe (A)62 Two models |
p = 0.50 as probability cut-off point Model 1 (radiographic progression): sensitivity, 0.71; and specificity, 0.74 Model 2 (high Sharp score of > 4): sensitivity, 0.78; and specificity, 0.85 |
de Punder64 Extended Simplified |
NR |
de Vries-Bouwstra65 | Presence of progression of radiological damage PPV = 75.3% |
Degboé66 Four models Anti-CCP Anti-MCV AhFibA High ACPA level |
Anti-CCP2 (25 U/ml, 95% diagnosis specificity): PPV = 63.5%; and NPV, 57.5% Anti-CCP2 (40 U/ml, 98% diagnosis specificity): PPV = 62.8%; and NPV, 58.4% Anti-MCV (20 UA/ml, 95% diagnosis specificity): PPV = 64.8%; and NPV, 51.5% Anti-MCV (35 UA/ml, 98% diagnosis specificity): PPV = 62.1%; and NPV, 57.5% AhFibA (0.056 AU, 95% diagnosis specificity): PPV = 67.6%; and NPV, 53.4%a AhFibA (0.119 AU, 98% diagnosis specificity): PPV = 62.8%; and NPV, 56.3%a |
Dixey68 Three models |
Non-erosive group at baseline (68%): correctly predicted ERO or not by 3 years in 67% (sensitivity, 52%; specificity, 78%; and PPV, 68%). Severity of ERO predicted correctly in 82% (sensitivity, 96%; specificity, 42%; and PPV, 77%) and Larsen score and ESR at 1 year (90% correct; sensitivity, 98%; specificity, 52%; and PPV, 84%). Validity of each model tested in a random 40% subset of the cohort not used in the analysis and ‘confirmed the prognostic value of these variables’ (overall: 67.30% 79.20% and 87.20% in the test sample for the three models) |
Drossaers-Bakker56 |
Mild radiographic damage: all (SJC, RF, ERO, SE), all-over correct, 88%; PPV, 80%; and NPV, 91% Selected (SJC, RF, ERO): all-over correct, 87%; PPV, 87%; and NPV, 86% Severe radiographic damage: all (SJC, RF, ERO, RAP), all-over correct, 85%; PPV, 91%; and NPV, 80% Selected (SJC, RF, ERO): all-over correct, 84%; PPV, 85%; and NPV, 74% |
ESPOIR69 | NR |
Forslind70 |
Model 1 (radiological damage): accuracy, 78%; sensitivity, 0.79; specificity, 0.77; PPV, 0.79; and NPV, 0.77 Model 2 (radiological progression): accuracy, 75%; sensitivity, 0.75; specificity, 0.75; PPV, 0.77; and NPV, 0.73 |
Houseman72 |
Model for radiographic progression PPV = 81%, NPV = 85% Model for absolute radiographic outcome PPV = 87%, NPV 64% |
Sanmartí75 | Sensitivity, 53.9%; specificity, 81.7%; PPV, 56%; and NPV, 80.3% |
SONORA57 | NR |
SWEFOT73 Whole group Whole group stratified Anti-CCP Sex |
NR |
Syversen76 |
Model 1 (externally validated in De Cock et al. 80): sensitivity, 89.2%; specificity, 51%; and accuracy, 73.6% Model 2 (adjusted for baseline radiographic score): sensitivity, 81.1%; specificity, 76.5%; and accuracy, 76.5% Model 3 (anti-CCP as a continuous variable): sensitivity, 86.5%; specificity, 64.7%; and accuracy, 77.6% |
van Steenbergen77 | NR |
Models predicting HAQ/disease course | |
Bansback58 | NR |
Combe (B)63 | p = 0.408 (probability of 5-year HAQ score of > 1). PPV = 46.15%, NPV = 92.71% |
Dirven67 | 67% patients reliably classified using cut-off points of < 35% (low risk) and > 60% (high risk); PPV = 71%; and NPV = 74% |
Drossaers-Bakker56 |
Mild HAQ score: all (HAQ score, ERO), all-over correct, 88%; PPV, 89%; and NPV, 85% Selected (HAQ score, ERO), all-over correct, 88%; PPV, 89%; and NPV, 85% Severe HAQ score: all (HAQ score), all-over correct, 84%; PPV, 91%; and NPV, 72% Selected (HAQ score): all-over correct, 84%; PPV, 91%; and NPV, 72% Severe disease course: all (SJC, HAQ, SE), all-over correct, 80%; PPV, 84%; and NPV, 76% Selected (SJC, Ritchie score): all-over correct, 83%; PPV, 91%; and NPV, 76% |
Graell71 | Sensitivity, 70.1%; specificity, 64%; PPV, 83.9%; and NPV, 44.4% |
Models predicting DAS28 | |
Centola61 | NR |
Name of clinical prediction model (submodels) | Other performance measures (e.g. sensitivity, specificity, PPV, NPV, accuracy) |
---|---|
De Cock80 | NR |
Hambardzumyan83 | Sensitivity, 0.98; specificity, 0.17; PPV = 0.21; and NPV = 0.97 |
Heimans84 | RA (progression of ≥ 0.5 on the SHS, based on intermediate or higher risk for progression) PPV = 15.4%, NPV for no progression = 98.8% |
Markusse85 | NR |
Appendix 8 Review 2 excluded studies
First author (date) | Title | Justification for exclusion |
---|---|---|
Addimanda (2014)326 | Efficacy and safety of tocilizumab in refractory rheumatoid arthritis: a real life cohort from a single centre | Not early RA |
Aga (2013)327 | Clinical predictors of response to methotrexate treatment in DMARD naive patients with early rheumatoid arthritis: results from a longitudinal observational study | Treatment duration of < 6 months |
Ajeganova (2013)328 | Association of obesity with worse disease severity in rheumatoid arthritis as well as with comorbidities: a long-term followup from disease onset | Not prediction of treatment response |
Akdemir (2014a)329 | Radiological outcomes after two years of remission steered treatment in early arthritis patients | Early RA and UA patients, results not separated |
Akdemir (2014b)330 | ACPA-negative RA patients benefit from initial combination therapy with early clinical improvement-a sub-analysis of the best study | Not prediction of treatment response |
Akdemir (2016)331 | Predictive factors of radiological progression after two years of remission steered treatment in early arthritis patients | Early RA and UA patients, results not separated |
Akhavan (2011)122 | Prevalence of and predictive factors for sustained remission in early RA: Results from SONORA study | Not prediction of treatment response |
Akhavan (2013)332 | The impact of reaching low disease activity in the first year on future disability and damage in patients with early rheumatoid arthritis | Not prediction of treatment response |
Akhavan (2014)333 | Predictive validity of low disease activity using patient reported measures on long-term outcomes in early rheumatoid arthritis-results from study of new onset rheumatoid arthritis and Ontario best practices initiative | Not prediction of treatment response |
Alemao (2016)334 | Effects of achieving target measures in rheumatoid arthritis on functional status, quality of life, and resource utilization: analysis of clinical practice data | Not early RA |
Alessandri (2004)335 | Decrease of anti-cyclic citrullinated peptide antibodies and rheumatoid factor following anti-TNFalpha therapy (infliximab) in rheumatoid arthritis is associated with clinical improvement | Disease duration at baseline not reported |
Aletaha (2007)336 | Disease activity early in the course of treatment predicts response to therapy after one year in rheumatoid arthritis patients | No eligible factor/end point (DAS28 data not reported) |
Aletaha (2009a)337 | Rheumatoid arthritis joint progression in sustained remission is determined by disease activity levels preceding the period of radiographic assessment | No baseline predictors |
Aletaha (2013)338 | Rituximab dissociates the tight link between disease activity and joint damage in rheumatoid arthritis patients | Not prediction of treatment response |
Aletaha (2013)124 | Rheumatoid factor determines structural progression of rheumatoid arthritis dependent and independent of disease activity | Not prediction of treatment response |
Alivernini (2014)339 | Ultrasonography as useful tool to identify rheumatoid arthritis patients in clinical remission for tapering or withdrawal TNFa blockers without disease relapse | Disease duration at baseline not reported |
Alivernini (2016)340 | Tapering and discontinuation of TNF-alpha blockers without disease relapse using ultrasonography as a tool to identify patients with rheumatoid arthritis in clinical and histological remission | Not early RA |
Allaart (2006)341 | Aiming at low disease activity in rheumatoid arthritis with initial combination therapy or initial monotherapy strategies: the BeSt study | No data on prediction of treatment response |
Allaart (2010)342 | When to start which DMARDs in rheumatoid arthritis? | Not possible to obtain paper |
Allaart (2011)126 | A multi-biomarker disease activity (Vectra DA) algorithm score for rheumatoid arthritis predicts radiographic progression in the BeSt study | Not prediction of treatment response |
Ammitzbøll (2012)343 | M-ficolin, an activator of the complement system, predicts DAS28 remission in early DMARD-naive rheumatoid arthritis | Not prediction of treatment response |
Ammitzbøll (2012)344 | M-ficolin, an activator of the complement system, is the strongest predictor of both DAS28 remission and low disease activity in a cohort of 180 early DMARD naive rheumatoid arthritis patients followed in the opera-study | Not prediction of treatment response |
Ammitzbøll (2013)345 | M-ficolin, an activator of the complement system, predicts DAS28 remission in early DMARD naive rheumatoid arthritis | Not prediction of treatment response |
Ammitzbøll (2013)346 | M-ficolin levels reflect disease activity and predict remission in early rheumatoid arthritis | Not prediction of treatment response |
Ancuta (2014)347 | Correlation between time to switch and clinical response amplitude to rituximab in second line treatment in rheumatoid arthritis patients with treatment failure to tumor necrosis factor inhibitors: 3-year data from repeat observational study | No relevant predictive factors |
Anderson (2000)128 | Factors predicting response to treatment in rheumatoid arthritis: the importance of disease duration | Not early RA |
Angwin (2006)348 | Radiographic progression in the Cobra study: computer measurements of joint space, a comparison with scoring | Not possible to obtain paper |
Atzeni (2009)349 | Predicting response to anti-TNF treatment in rheumatoid arthritis patients | Not early RA |
Atzeni (2014)350 | Predictors of response to anti-TNF therapy in RA patients with moderate or high DAS28 scores | Not early RA |
Baddoura (2006)351 | Severity of rheumatoid arthritis: the SEVERA study | Not prediction of treatment response and no eligible predictive factor |
Baer (2014)352 | Does low disease activity at six months predict remission at 12 months in rheumatoid arthritis patients treated with biologics in a real-world setting? | Not prediction of treatment response |
Baillet (2014)353 | Biomarker sets predict therapeutic response to TNF-inhibitors in rheumatoid arthritis and spondyloarthritis patients: a theragnostic approach in a multicenter cohort | Results not reported separately for RA patients on relevant predictors |
Bakker (2010)130 | Development of a multi-biomarker test for rheumatoid arthritis (RA) disease activity (Vectra DA) | Not prediction of treatment response |
Balogh (2013)354 | Comparison of DAS-28 and 2011-ACR/EULAR remission criteria in a biologic-treated rheumatoid arthritis patient cohort | Not early RA |
Balogh (2013)355 | Comparison of remission criteria in a tumour necrosis factor inhibitor treated rheumatoid arthritis longitudinal cohort: patient global health is a confounder | Not early RA |
Balsa (2010)129 | Prediction of functional impairment and remission in rheumatoid arthritis patients by biochemical variables and genetic polymorphisms | Not early RA |
Barnabe (2011)356 | Predictors for remission in rheumatoid arthritis are affected by remission definition | Not early RA |
Barnabe (2012)357 | Identified predictors for remission in rheumatoid arthritis depend on remission definition | Not early RA |
Barnabe (2014)358 | The effect of different remission definitions on identification of predictors of both point and sustained remission in rheumatoid arthritis treated with anti-TNF therapy | Not early RA |
Baruth (2013)359 | Factors associated with disability in a sample of adults with arthritis | Not early RA |
Bathon (2005)360 | Disease activity scores using CRP versus ESR and the relationship between EULAR and ACR responses in patients with early rheumatoid arthritis | Not prediction of treatment response |
Baumgartner (2004)361 | Etanercept (Enbrel) in patients with rheumatoid arthritis with recent onset versus established disease: improvement in disability | Not early RA |
Bay-Jensen (2014)362 | Serological biomarkers of joint tissue turnover predict tocilizumab response at baseline | Not early RA |
Bejarano (2012)363 | Relationship between early bone mineral density changes and long-term function and radiographic progression in rheumatoid arthritis | No relevant predictive factors |
Bellis (2015)364 | Ultrasound-detected synovitis and tenosynovitis independently associate with flare in patients with rheumatoid arthritis in clinical remission | Not early RA |
Ben Slama (2014)365 | Comparison of activity score DAS28-ESR and DAS28-CRP in patients with rheumatoid arthritis | Not early RA |
Benedict (2010)366 | Comparative effectiveness of biologic therapies for treating rheumatoid arthritis (RA) in patients who failed an anti tumor necrosis factor agent: a meta-regression analysis | Not early RA |
Bentzon (1986)367 | Influence of previous gold treatment and other patient variables on outcome of treatment with disease modifying anti-rheumatic drugs (DMARD) in patients with rheumatoid arthritis | Not early RA |
Benucci (2010)368 | Predictive factors of response to rituximab therapy in rheumatoid arthritis: what do we know today? | Review (not systematic) |
Bijlsma (2007)369 | Optimal use of methotrexate: the advantages of tight control | Editorial |
Bingham (2012)370 | Predictors of significant Disease Activity Score-28 (using CRP) remission achieved with intravenous golimumab in patients with active rheumatoid arthritis despite methotrexate therapy: results of the phase 3, multicenter, double-blind, placebo-controlled trial | Not early RA |
Bingham (2013)371 | Predictors of significant Disease Activity Score-28 (using C-reactive protein) remission achieved with intravenous golimumab in patients with active rheumatoid arthritis despite methotrexate therapy: results of the phase III, multicentre, double-blind, placebo-controlled trial | Not early RA |
Bobbio-Pallavicini (2007)372 | High IgA rheumatoid factor levels are associated with poor clinical response to tumour necrosis factor alpha inhibitors in rheumatoid arthritis | Not early RA |
Boers (2001)373 | Inflammation and damage in an individual joint predict further damage in that joint in patients with early rheumatoid arthritis | Not prediction of treatment response |
Borangiu (2014)374 | Ultrasound active synovitis can be predicted by using clinical measures | Disease duration at baseline not reported |
Bouman (2015)375 | Associations of a multi-biomarker disease activity score with clinical and radiographic parameters in rheumatoid arthritis | Not prediction of treatment effect |
Braun (2008)376 | Comparison of the clinical efficacy and safety of subcutaneous versus oral administration of methotrexate in patients with active rheumatoid arthritis: results of a six-month, multicenter, randomised, double-blind, controlled, phase IV trial | No relevant outcomes |
Breedveld (2005)377 | Association between baseline radiographic damage and improvement in physical function after treatment of patients with rheumatoid arthritis | Not early RA |
Brown (2002)378 | Baseline clinical, functional and ultrasonographic assessment can be used to predict subsequent remission in rheumatoid arthritis | Disease duration at baseline not reported |
Buch (2003)379 | Does synovial cytokine expression predict response to tumour necrosis factor-alpha blockade in patients with rheumatoid arthritis? | Not prediction of treatment response |
Buchanan (2013)380 | Basal metabolic rate as an indicator of rheumatoid arthritis disease activity and predictor of remission: Australian results from period 1 of the preserve trial | Disease duration at baseline not reported |
Burmester (2008)381 | Clinical remission and/or minimal disease activity in patients receiving adalimumab treatment in a multinational, open-label, twelve-week study | Not early RA |
Burmester (2015)382 | Efficacy and safety of ascending methotrexate dose in combination with adalimumab: the randomised CONCERTO trial | Not prediction of treatment effect |
Bykerk (2015)383 | On drug and drug-free remission by baseline disease duration in the avert trial: abatacept versus methotrexate comparison in patients with early rheumatoid arthritis | Insufficient detail of baseline characteristics |
Callaghan (2014)384 | Biological therapy for rheumatoid arthritis: is personalised medicine possible? | Not early RA |
Cañete (2011)385 | Clinical significance of high levels of soluble tumour necrosis factor-alpha receptor-2 produced by alternative splicing in rheumatoid arthritis: a longitudinal prospective cohort study | No relevant predictive factors |
Canhão (2012)386 | Comparative effectiveness and predictors of response to tumour necrosis factor inhibitor therapies in rheumatoid arthritis | Not early RA |
Cantini (2016)387 | Tailored first-line biologic therapy in patients with rheumatoid arthritis, spondyloarthritis, and psoriatic arthritis | Not early RA |
Capell (1993)388 | Second line (disease modifying) treatment in rheumatoid arthritis: which drug for which patient? | Not prediction of treatment response |
Castrejón (2016)389 | Prediction of remission in a French early arthritis cohort by RAPID3 and other core data set measures, but not by the absence of rheumatoid factor, anticitrullinated protein antibodies, or radiographic erosions | Not prediction of treatment response |
Casu (2013)390 | Clinical and serological response to tocilizumab in patients with rheumatoid arthritis | Not early RA |
Cavet (2009)391 | Predicting radiographic progression in rheumatoid arthritis with ultrasound and biomarkers | Disease duration at baseline not reported |
Cazzato (2014)392 | Early response indicator early predicts clinical response to certolizumab in rheumatoid arthritis patients | Disease duration at baseline not reported |
Chandrashekara (2015)393 | Neutrophil–lymphocyte ratio, pain perception, and disease activity score may serve as important predictive markers for sustained remission in rheumatoid arthritis | Not prediction of treatment response |
Chatzidionysiou (2011)394 | Seropositivity and in particular ACPA positivity is a strong predictor of response to treatment with rituximab: pooled data from 10 European registries | Not possible to obtain paper |
Chatzidionysiou (2013)395 | Seropositivity and response to RTX: data from the cererra collaboration | Disease duration at baseline not reported |
Chatzidionysiou (2015)396 | Smoking and response to rituximab in anti-CCP positive and negative rheumatoid arthritis-results from an international European collaboration | Not early RA |
Chen (2013)397 | Influence of adult height on rheumatoid arthritis: association with disease activity, impairment of joint function and overall disability | No relevant predictive factors |
Chen (2014)398 | Elevated serum IgG4 defines specific clinical phenotype of rheumatoid arthritis | Not early RA |
Cheung (2014)399 | Are tender joints better than synovitis to predict structural damage in rheumatoid arthritis? | Not early RA |
Chitale (2012)400 | Initial triple DMARD therapy predicts ACR EULAR remission in an early rheumatoid arthritis inception cohort | Not prediction of treatment response |
Cho (2012)401 | Do patients with elderly-onset rheumatoid arthritis have severe functional disability? | Not early RA |
Choquette (2010)402 | Impact of disease duration on the outcome of RA patients treated with infliximab in Canada | Not early RA |
Choquette (2011)403 | Comparison of disease characteristics of rheumatoid arthritis patients in remission according to the DAS criteria versus the new ACR/EULAR criteria in a real-world patient population | Not possible to obtain paper |
Choquette (2013)404 | Comparison of disease characteristics of RA patients in remission according to the DAS criteria versus the new ACR/EULAR criteria in a real-world patient population | Not early RA |
Choquette (2013)405 | What is the impact of rheumatoid factor positivity on the real-world effectiveness of infliximab treatment in rheumatoid arthritis? | Disease duration at baseline not reported |
Choquette (2015a)406 | Use of rituximab compared to anti-TNF agents as second and third line therapy in patients with rheumatoid arthritis: 6-year follow-up report from the rhumadata clinical database and registry | No relevant predictive factors or outcomes |
Choquette (2015b)407 | Predictors of clinical response to biologics in rheumatoid arthritis: experience from a Canadian clinic | Not early RA |
Chow (2015)408 | What is the effect of TNF inhibitors on employment status in rheumatoid arthritis patients and what are the predictors of progression to unemployment? | Disease duration at baseline not reported |
Choy (2007)409 | Does the Health Assessment Questionnaire predict 5-year quality of life in early RA? | Commentary |
Choy (2013)410 | Physician global assessment at three months is strongly predictive of disease activity at 12 months in early rheumatoid arthritis. Results from the catch cohort | Not early RA |
Christensen (2014)411 | Temporal summation of pain and ultrasound Doppler activity as predictors of treatment response in patients with rheumatoid arthritis: protocol for the Frederiksberg hospitals Rheumatoid Arthritis, pain assessment and Medical Evaluation (FRAME-cohort) study | Protocol |
Christensen (2016)412 | Ultrasound Doppler but not temporal summation of pain predicts DAS28 response in rheumatoid arthritis: a prospective cohort study | Not early RA |
Ciurtin (2015)413 | Evaluating impact of risk associated outcomes on ultrasound Doppler score of patients with inflammatory hand joint pain using a beta-binomial model | Insufficient detail reported |
Ciurtin (2016)149 | Ultrasound-detected subclinical inflammation was better reflected by the disease activity score (DAS-28) in patients with suspicion of inflammatory arthritis compared to established rheumatoid arthritis | Not prediction of treatment response |
Coburn (2015)414 | Anti-citrullinated protein antibody and radiographic disease progression in rheumatoid arthritis | Disease duration at baseline not reported |
Cohen (2005)415 | C-reactive protein predicts treatment response to adalimumab (HUMIRA (R)) in patients with rheumatoid arthritis | Not possible to obtain paper |
Colmegna (2016)416 | High rates of obesity and greater associated disability among people with rheumatoid arthritis in Canada | Not early RA |
Combe (2001)62 | Prognostic factors for radiographic damage in early rheumatoid arthritis: a multiparameter prospective study | Not prediction of treatment response |
Couderc (2013)417 | Predictive factors of rituximab response in rheumatoid arthritis: results from a French university hospital | Not early RA |
Couderc (2013)417 | Predictive factors for the response to rituximab in rheumatoid arthritis: results from a French university hospital | Not early RA |
Courvoisier (2016)418 | Rheumatoid arthritis patients after initiation of a new biologic agent: trajectories of disease activity in a large multinational cohort study | Not early RA |
Cuchacovich (2008)419 | Basal anti-cyclic citrullinated peptide (anti-CCP) antibody levels and a decrease in anti-CCP titres are associated with clinical response to adalimumab in rheumatoid arthritis | Not early RA |
Cuppen (2015a)420 | Personalized biological treatment for rheumatoid arthritis: a systematic review with a focus on clinical applicability | Not early RA |
Cuppen (2015b)421 | Towards individualized risk determination in RA: a prediction model for TNFi discontinuation within the first year after start | Insufficient detail reported |
Cuppen (2016)422 | Exploring the inflammatory metabolomic profile to predict response to TNF-alpha inhibitors in rheumatoid arthritis | Not early RA |
Curtis (2010)155 | Validation of a multi-biomarker test for rheumatoid arthritis (RA) disease activity (Vectra DA) in a multi-cohort study | Insufficient information to determine treatment response |
Curtis (2010)423 | Prediction of one-year response to etanercept and methotrexate in rheumatoid arthritis patients in TEMPO | Not early RA |
Curtis (2011)424 | Derivation and preliminary validation of an administrative claims-based algorithm for the effectiveness of medications for rheumatoid arthritis | No relevant predictors |
Curtis (2011)425 | Validation of a preliminary administrative claims-based algorithm for the effectiveness of medications for rheumatoid arthritis | No relevant predictors |
Curtis (2012)426 | Predicting future response to certolizumab pegol in rheumatoid arthritis patients: features at 12 weeks associated with low disease activity at 1 year | Not early RA |
Curtis (2012)156 | Validation of a novel multibiomarker test to assess rheumatoid arthritis disease activity | Treatment duration of < 6 months |
Curtis (2013)427 | Clinical response within 12 weeks as a predictor of future low disease activity in patients with early RA: results from the TEAR Trial | No relevant predictors |
Curtis (2015)157 | A randomized trial comparing disease activity measures for the assessment and prediction of response in rheumatoid arthritis patients initiating certolizumab pegol | Not early RA |
D’Agostino (2015a)428 | Do ultrasound (power Doppler ultrasonography) and Disease Activity Score-28 measure different aspects of disease activity? Analyses from an open-label study of power Doppler ultrasonography response patients with rheumatoid arthritis patients starting abatacept | Nothing on prediction of treatment effect |
D’Agostino (2015b)429 | In patients with rheumatoid arthritis and an inadequate response to methotrexate, does body mass index influence the efficacy of abatacept on inflammation when measured by power Doppler ultrasonography? Results from the appraise study | Not early RA |
Dahl (2009)430 | TNF-inhibitors in established rheumatoid arthritis: the effect of age on treatment response and predictors of remission | No baseline disease duration reported |
Dai (2010)431 | The clinical response to etanercept in rheumatoid arthritis is in part dependent on pretreatment tumour necrosis factor-alpha expression in the synovium | Not possible to obtain paper |
Daïen (2014)432 | Predictive factors of response to biological disease modifying antirheumatic drugs: towards personalized medicine | Review (not systematic) |
Dames (2006)433 | Utility of Disease Activity Score-28 (DAS-28) for determining need for DMARD change in veterans affairs rheumatoid arthritis (VARA) patients: an analysis of discrepancies between DAS-28 scores and clinical stability status | Not prediction of treatment response |
da Mota (2012)434 | Baseline HAQ and SF-36 questionnaire scores cannot predict clinical remission, radiographic progression or the need for biological therapy in a three-year prospective study of a Brazilian early rheumatoid arthritis cohort | Not prediction of treatment response |
Danve (2015)435 | Male gender and higher hemoglobin predict response to methotrexate in rheumatoid arthritis | No relevant predictive factors or outcomes |
Darawankul (2015)436 | The good EULAR response at the first year is strongly predictive of clinical remission in rheumatoid arthritis: results from the TARAC cohort | Not early RA |
Dasgupta (2014)437 | Predictors of low disease activity and remission after one dose of golimumab in patients with rheumatoid arthritis | Not early RA |
Dejaco (2010)438 | Third generation anti-cyclic citrullinated peptide antibodies do not predict anti-TNF-alpha treatment response in rheumatoid arthritis | Disease duration unclear |
de Jong (2013)439 | Response to glucocorticoids at 2 weeks predicts the effectiveness of DMARD induction therapy at 3 months: post hoc analyses from the tREACH study | Treatment duration of < 6 months at time of analysis |
de Jong (2015)440 | Effect of prednisone on type I interferon signature in rheumatoid arthritis: consequences for response prediction to rituximab | Not early RA |
De Miguel (2015)441 | Doppler ultrasound better predicts X-Ray progression in rheumatoid arthritis than any definition of clinical remission | Not early RA |
de Rooy (2011)442 | Predicting arthritis outcomes-what can be learned from the Leiden Early Arthritis Clinic? | Not prediction of treatment response |
De Vries-Bouwstra (2006)65 | Using predicted disease outcome to provide differentiated treatment of early rheumatoid arthritis | Not prediction of treatment response |
De Vries-Bouwstra (2008)161 | Progression of joint damage in early rheumatoid arthritis: association with HLA–DRB1, rheumatoid factor, and anti–citrullinated protein antibodies in relation to different treatment strategies | Time of predictor measurement not stated and unclear whether or not it was within the 2-year early RA window |
Demir (2003)443 | Value of wrist ultrasound in prediction of disease activity in patients with rheumatoid arthritis | Not possible to obtain paper |
Di Cicco (2014a)444 | Synovial ectopic lymphoneogenesis predicts primary clinical response to certolizumab pegol in patients with rheumatoid arthritis | Not early RA |
Di Cicco (2014b)445 | Presence of synovial lymphocyte aggregates predicts clinical response to DMARD therapy in patients with early rheumatoid arthritis | Not prediction of treatment response |
Ding (2015)446 | Predictors of response to TNF-α antagonist therapy in Chinese rheumatoid arthritis | No relevant outcomes |
Dirven (2009)447 | Predictors of HAQ response after 3 months of treatment with different strategies in recent onset active RA | Not prediction of treatment response |
Dougados (2007)448 | Sustained efficacy along with improvements in disease activity score 28 (DAS 28) and patient (Pt)-reported outcomes (PROs) with abatacept (Aba) in rheumatoid arthritis (RA) pts with an inadequate response to anti-tumor necrosis factor (TNF) therapy: the long-term extension (LTE) of the attain trial | Not prediction of treatment response |
Drouin (2010)449 | Predictors of clinical response and radiographic progression in patients with rheumatoid arthritis treated with methotrexate monotherapy | Not early RA |
Durez (2014)450 | Baseline predictors of remission rates during golimumab treatment for rheumatoid arthritis in the go-more study | Not early RA |
Ellingsen (2009)451 | The increased cc chemokine ligand 19 (CCL19) at baseline is an independent predictor of the 5-year radiographic progression in early steroid and DMARD-naive rheumatoid arthritis (RA) patients | Insufficient information to determine treatment response |
Emery (2006)452 | Abatacept inhibits structural damage progression, as assessed by the Genant-modified Sharp scoring system, in Rheumatoid Arthritis (RA) patients with an inadequate response to Methotrexate (MTX): the aim (abatacept in inadequate responders to MTX) trial – a sub-analysis by disease duration | Not possible to obtain paper |
Emery (2011)453 | Exploratory analyses of the association of MRI with clinical, laboratory and radiographic findings in patients with rheumatoid arthritis | Not prediction of treatment effect |
Emery (2011)454 | Optimising treatment in rheumatoid arthritis: a review of potential biological markers of response | Review (not systematic) |
Emery (2011)455 | Post-treatment changes in serum C-reactive protein levels and clinical response in rheumatoid arthritis | Not early RA |
Emery (2012)456 | Combination etanercept and methotrexate provides better disease control in very early (≤ 4 months) versus early rheumatoid arthritis (> 4 months and < 2 years): post hoc analyses from the COMET study | Does not meet criteria for the window of opportunity covariate (not all patients DMARD naive) |
Emery (2012)457 | Golimumab’s efficacy in patients with very active disease in methotrexate-naive rheumatoid arthritis | Not early RA |
Emery (2012)458 | Predictors of radiographic progression in methotrexate-naive patients with rheumatoid arthritis based on one-year radiographic data from the go-before golimumab clinical trial | Not early RA |
Emery (2013)459 | Radiographic progression in patients with early rheumatoid arthritis treated with etanercept: results from the prize study | Not prediction of treatment response |
Emery (2014a)460 | Early response to etanercept-methotrexate induction therapy predicts sustained remission with reduced–dose combination regimen in the prize study | Insufficient methodological details reported |
Emery (2014b)461 | Early response to full-dose etanercept-plus-methotrexate induction therapy predicts sustained remission with reduced-dose combination therapy in early rheumatoid arthritis patients | Insufficient methodological details reported |
Emery (2014c)462 | Efficacy of golimumab plus methotrexate in methotrexate-naive patients with severe active rheumatoid arthritis | Not early RA |
Emery (2014d)463 | Predictors of drug-free remission following treatment with abatacept (in combination with methotrexate or as monotherapy) in early rheumatoid arthritis | No relevant outcomes |
Emery (2015a)464 | Abatacept plus methotrexate can effectively and safely regain the target of remission following re-treatment for flares after drug-free withdrawal in patients with early rheumatoid arthritis | Not prediction of treatment effect |
Emery (2015b)465 | A European chart review study on early rheumatoid arthritis treatment patterns, clinical outcomes, and healthcare utilization | Not early RA |
Emery (2015c)116 | Evaluating drug-free remission with abatacept in early rheumatoid arthritis: results from the phase 3b, multicentre, randomised, active-controlled AVERT study of 24 months, with a 12-month, double-blind treatment period | No relevant outcome |
Erickson (2014)466 | Predictors of radiologic disease progression during the rheumatoid arthritis comparison of active therapies trial | Not early RA |
Eser (2012)467 | Extraarticular manifestations in Turkish patients with rheumatoid arthritis: impact of EAMs on the health-related quality of life in terms of disease activity, functional status, severity of pain, and social and emotional functioning |
Not early RA Not prediction of treatment effect |
Estrach (2003)468 | Swollen joint count as a predictor of response to anti-tumor necrosis factor alpha therapy in rheumatoid arthritis: comment on the article by Sokka and Pincus (multiple letters) [3] | Not early RA |
Fabricio (2015)469 | Predictability to achieve low activity and/or remission with leflunomide use in patients with rheumatoid arthritis | Insufficient methodological details reported |
Fabris (2010)470 | Study on the possible role of the –174G>C IL-6 promoter polymorphism in predicting response to rituximab in rheumatoid arthritis | Not early RA |
Fautrel (2012)471 | Moderate rheumatoid arthritis despite methotrexate treatment: risk of radiographic progression | Not early RA |
Fautrel (2016)472 | Step-down strategy of spacing TNF-blocker injections for established rheumatoid arthritis in remission: results of the multicentre non-inferiority randomised open-label controlled trial (STRASS: Spacing of TNF-blocker injections in Rheumatoid ArthritiS Study) | Not early RA |
Favalli (2013)473 | Disease duration and year of publication affect the results of studies on rheumatoid arthritis damage progression by biologic agents: a systematic review and meta-analysis | Not prediction of treatment response |
Favalli (2014)474 | The comparison of effects of biologic agents on rheumatoid arthritis damage progression is biased by period of enrolment: data from a systematic review and meta-analysis | Not prediction of treatment response |
Fedele (2015)475 | Clinical parameters and B cell subsets as biomarkers of response to tocilizumab in rheumatoid arthritis | Insufficient detail reported |
Fedorenko (2014)476 | Effects of four different treatment regimens on radiologic progression in early rheumatoid arthritis (RA) | Insufficient detail reported on methods and results relating to prediction of treatment response |
Feist (2010)477 | A study to evaluate the effectiveness and safety of the interleukin-6 (IL-6) receptor antagonist tocilizumab (TCZ) after 4 and 24 weeks in patients with active rheumatoid arthritis (RA) final effectiveness results of the TAMARA study | Not prediction of treatment response |
Ferdousi (2011)478 | Disease duration as a determinate factor of disease activity and radiographic progression in rheumatoid arthritis | Pooled data from 2 trials (not risk model) |
Fernández-Nebro (2007)479 | Effectiveness, predictive response factors, and safety of anti-tumor necrosis factor (TNF) therapies in anti-TNF-naive rheumatoid arthritis | Not early RA |
Filer (2010)480 | Utility of ultrasound joint counts as predictors of outcome in patients with very early arthritis | Not prediction of treatment response |
Filippi (2015)481 | Predictors of persistence of power Doppler ultrasound synovitis in rheumatoid arthritis patients in clinical remission | Not early RA |
Finckh (2007)482 | Cigarette smoking and radiographic progression in rheumatoid arthritis | Not early RA |
Finckh (2010)483 | Which subgroup of patients with rheumatoid arthritis benefits from switching to rituximab versus alternative anti-tumor necrosis factor (TNF) agents after previous failure of an anti-TNF agent? | Not early RA |
Finckh (2015)484 | The impact of tobacco smoking on the effectiveness of abatacept in rheumatoid arthritis: data from a pan European analysis of RA registries | Not early RA |
Fisher (2011)485 | Fine specificity of anti-citrullinated peptide antibodies is associated with response to anti-TNF agents in rheumatoid arthritis: results from the British Society of Rheumatology biologics register | Disease duration at baseline not reported |
Fisher (2012)486 | Heterogeneity of anticitrullinated peptide antibodies and response to anti-tumour necrosis factor agents in rheumatoid arthritis | Not early RA |
Fleischmann (2014)487 | Short-term efficacy of etanercept plus methotrexate vs. combinations of disease-modifying anti-rheumatic drugs with methotrexate in established rheumatoid arthritis | Not early RA |
Folkersen (2016)488 | Integration of known DNA, RNA and protein biomarkers provides prediction of anti-TNF response in rheumatoid arthritis: results from the COMBINE study | No relevant predictors |
Foltz (2009)489 | Ultrasound and magnetic resonance imaging did not provide early assessment of biotherapy response in patients with rheumatoid arthritis | Not prediction of treatment response |
Forsblad-d’Elia (2015)490 | Drug adherence, response and predictors thereof for tocilizumab in patients with rheumatoid arthritis: results from the Swedish biologics register | Not early RA |
Forslind (2007)169 | Sex: a major predictor of remission in early rheumatoid arthritis? | Not prediction of treatment response |
Fransen (2012)491 | Clinical pharmacogenetic model to predict response of MTX monotherapy in patients with established rheumatoid arthritis after DMARD failure | Not early RA |
Fujimura (2014)492 | Predicting future response to tumor necrosis factor inhibitors by the distribution of affected joints in rheumatoid arthritis patients | Not early RA |
Furst (2015a)493 | Evaluation of patient-reported outcomes by baseline disease duration: 6-month data from two clinical trials of patients with early rheumatoid arthritis treated with abatacept | No relevant predictive factors |
Furst (2015b)494 | Final 10-year effectiveness and safety results from study DE020: adalimumab treatment in patients with rheumatoid arthritis and an inadequate response to standard therapy | Not early RA |
Furuya (2013)495 | Effect of TNF antagonists on the productivity of daily work of patients with rheumatoid arthritis | Not early RA |
Gardette (2013)496 | High level of anti-CCP antibodies is predictive of good response to rituximab in patients with active rheumatoid arthritis | Not early RA |
Gherghe (2016)497 | Association of the different types of radiographic damage with physical function in patients with rheumatoid arthritis: analysis of the RAPID trials | Not early RA |
Giacomelli (2013)498 | Mathematical model to predict the early responders in a monocentric cohort of patients with rheumatoid arthritis treated by anti TNFalpha | Insufficient detail reported |
Glave-Testino (1994)499 | Factors associated with disease severity in Mexican patients with rheumatoid arthritis | Not early RA |
Goetz (2011)500 | Review of treatment response in rheumatoid arthritis: assessment of heterogeneity | Not early RA |
Gomez (2004)501 | High titres of anti-CCP antibodies at baseline and after one year of DMARDs may predict a poor clinical response in patients with early rheumatoid arthritis | Not possible to obtain paper |
González-Alvaro (2007)502 | Baseline serum RANKL levels may serve to predict remission in rheumatoid arthritis patients treated with TNF antagonists | Not early RA |
Gonzalez-Lopez (2014)503 | Anti-cyclic citrullinated peptide (anti-CCP) and anti-mutated citrullinated vimentin (anti-MCV) relation with extra-articular manifestations in rheumatoid arthritis |
Not early RA No relevant outcomes |
Goodman (2015)504 | Body mass index is an independent risk factor for not achieving sustained remission in early rheumatoid arthritis: results from the catch observational study | Insufficient methods detail on assessment of prediction of treatment response |
Goronzy (2004)176 | Prognostic markers of radiographic progression in early rheumatoid arthritis | Not prediction of treatment response |
Gottenberg (2012)505 | Serum IL-6 and IL-21 are associated with markers of B cell activation and structural progression in early rheumatoid arthritis: results from the ESPOIR cohort | No relevant predictive factors |
Graudal (2000)506 | Inflammatory patterns in rheumatoid arthritis estimated by the number of swollen and tender joints, the erythrocyte sedimentation rate, and hemoglobin: long term course and association to radiographic progression | Not prediction of treatment response |
Gremese (2013)507 | Very early rheumatoid arthritis as a predictor of remission: a multicentre real life prospective study | Not prediction of treatment response |
Gremese (2015)508 | Clinical and B cell subsets biomarkers of response to tocilizumab in rheumatoid arthritis | Not early RA |
Gullick (2012)509 | Predicting ongoing active disease in early rheumatoid arthritis using clinical measures | Pooled data from two trials (not risk model) |
Hall (1991)510 | Rheumatoid nodules do not predict response to treatment with slow-acting anti-rheumatic drugs | Not early RA |
Hama (2012)511 | Power Doppler ultrasonography is useful for assessing disease activity and predicting joint destruction in rheumatoid arthritis patients receiving tocilizumab-preliminary data | Not early RA |
Hama (2014)512 | PD signal detected by ultrasonography relates to joint destruction in rheumatoid arthritis under biologics therapy in real world | Not early RA |
Hama (2015)513 | Wrist PD signal detected by ultrasonography relates to joint destruction in rheumatoid arthritis under biologics therapy in real world | Not early RA |
Hamann (2016)514 | Factors associated with sustained remission in rheumatoid arthritis in patients treated with anti-tumor necrosis factor (anti-TNF) | Not early RA |
Hambardzumyan (2013)183 | A multi-biomarker disease activity score correlates with radiographic progression in early rheumatoid arthritis: results from a randomized trial | Not prediction of treatment response |
Hambardzumyan (2013)182 | A multi-biomarker disease activity blood test (Vectra DA) correlates with radiographic progression in early rheumatoid arthritis: results from the SWEFOT trial | Not prediction of treatment response |
Hambardzumyan (2013)185 | In early rheumatoid arthritis, the 12 individual biomarkers that comprise the multiple biomarker disease activity score relate differentially to clinical response and radiographic progression: results from a randomized trial | Not prediction of treatment response |
Hambardzumyan (2013)184 | Multi-biomarker disease activity (MBDA) score and the 12 individual biomarkers in early rheumatoid arthritis patients relate differentially to clinical response and radiographic progression: results from the SWEFOT trial | Not prediction of treatment response |
Hambardzumyan (2015)515 | Predictive biomarkers for response or non-response to MTX monotherapy in early RA | No relevant predictive factors |
Hammer (2010)516 | A 78-joints ultrasonographic assessment is associated with clinical assessments and is highly responsive to improvement in a longitudinal study of patients with rheumatoid arthritis starting adalimumab treatment | Not early RA |
Hammer (2011)517 | Patients with rheumatoid arthritis on anti-TNF therapy; responders with major reduction in power Doppler activity can be identified after one month | Not early RA |
Haney (2012)518 | Correlation of a multi-biomarker disease activity response assessment to disease activity score 28 (C-reactive protein) response assessment and OMERACT RAMRIS scores in a placebo-controlled rheumatoid arthritis clinical trial with abatacept (ASSET) | Treatment duration of < 6 months |
Haroon (2008)519 | Tailor-made therapy in rheumatoid arthritis: fact or fiction? | Review (not systematic) |
Harris (2012)520 | Can we improve outcomes in early rheumatoid arthritis by determining best practices? An analysis of the Canadian early rheumatoid arthritis cohort (CATCH) | Not prediction of treatment response |
Harris (2013)521 | Can we improve outcomes in early rheumatoid arthritis (ERA) by determining best practices? An analysis of the Canadian era cohort (CATCH) | Not prediction of treatment response |
Hazlewood (2015)522 | Enhancing comparative effectiveness research by combining observational and randomized trial data to personalize the choice between methotrexate and triple therapy for methotrexate-naive patients with early rheumatoid arthritis | No relevant outcome |
Heidari (2007)523 | The value of changes in CRP and ESR for predicting treatment response in rheumatoid arthritis | Not early RA |
Heimans (2011)524 | Body mass index is associated with decreased response to initial and delayed treatment with dose escalated infliximab in patients with recent onset rheumatoid arthritis | Insufficient detail reported on methods and results relating to prediction of treatment response |
Heimans (2014)91 | A two-step treatment strategy trial in patients with early arthritis aimed at achieving remission: the IMPROVED study | Not prediction of treatment response |
Hernandez (2013)525 | Predictive factors of response to tocilizumab in patients with active rheumatoid arthritis | Not early RA |
Herold (2015)526 | Efficacy of tocilizumab monotherapy in patients with RA is not influenced by ACPA positivity | Insufficient reporting of results |
Hetland (2010)527 | Radiographic progression and remission rates in early rheumatoid arthritis – MRI bone oedema and anti-CCP predicted radiographic progression in the 5-year extension of the double-blind randomised CIMESTRA trial | Not prediction of treatment response |
Hirano (2015)528 | Predictors of effectiveness in golimumab treatment and efficacy of dose-escalation of golimumab in patients with rheumatoid arthritis-a multicenter registry study TBCR | Not early RA |
Hirata (2013)529 | Repeated high or low multi-biomarker disease activity (Vectra® DA algorithm) scores associated with radiographic outcomes in patients with rheumatoid arthritis treated with tumor necrosis factor inhibitors | Not early RA |
Hirata (2015)530 | Serum 14–3–η level is associated with severity and clinical outcomes of rheumatoid arthritis, and its pretreatment level is predictive of DAS28 remission with tocilizumab | Not early RA |
Hoekstra (2003)531 | Factors associated with toxicity, final dose, and efficacy of methotrexate in patients with rheumatoid arthritis | Not early RA |
Horton (2016)532 | Discordance between the predictors of clinical and imaging remission in patients with early rheumatoid arthritis in clinical practice: implications for the use of ultrasound within a treatment-to-target strategy | Not prediction of treatment response |
Hoshi (2011)533 | Greater physical dysfunction is a negative predictor for achieving Boolean-based remission in patients with rheumatoid arthritis treated with tocilizumab | Not early RA |
Hu (2011)534 | Population approach for exposure–response modelling of golimumab in patients with rheumatoid arthritis | Not early RA |
Huizinga (2011)535 | Very early rheumatoid arthritis is the major predictor of major outcomes: clinical ACR remission and radiographic non-progression – commentary | Not possible to obtain paper |
Iagnocco (2015)536 | Power Doppler ultrasound monitoring of response to anti-tumor necrosis factor alpha treatment in patients with rheumatoid arthritis | Not early RA |
Iannaccone (2011)537 | Using genetic and clinical data to understand response to disease-modifying anti-rheumatic drug therapy: data from the Brigham and Women’s Hospital Rheumatoid Arthritis Sequential Study | Not early RA |
Iannone (2013)538 | Etanercept therapy in rheumatoid arthritis patients with moderate or severe disease activity | Not early RA |
Iannone (2015)539 | Early good EULAR response predicts low/remission disease state in rheumatoid arthritis patients on treatment with certolizumab in real life settings. Data from Italian registry GISEA | Not early RA |
Ickinger (2011)540 | Predictors of joint damage in South Africans with rheumatoid arthritis | Not early RA |
Ideguchi (2006)541 | Bone erosions in rheumatoid arthritis can be repaired through reduction in disease activity with conventional disease-modifying antirheumatic drugs | Not early RA |
Inanc (2013)542 | Can ultrasonographic findings predict response to tumor necrosis factor-alpha inhibitor treatment in rheumatoid arthritis? | Not early RA |
Inanc (2014a)543 | Ultrasonographic assessment of joint inflammation in rheumatoid arthritis: predictive value in response to tumor necrosis factor-a inhibitor treatment | Not early RA |
Inanc (2014b)544 | Ultrasonographic assessment of joint inflammation in rheumatoid arthritis: predictive value in response to tumor necrosis factor-alpha inhibitor treatment | Not early RA |
Inanc (2016)545 | Predictive value of ultrasonographic assessment of disease activity in response to tumor necrosis factor-alpha inhibitor treatment in rheumatoid arthritis: a prospective cohort study | Not early RA |
Inoue (2009)546 | Preliminary study to identify the predictive factors for the response to methotrexate therapy in patients with rheumatoid arthritis | Not early RA |
Inoue (2015)547 | Effect of smoking on remission proportions differs between male and female patients with rheumatoid arthritis: a study based on the IORRA survey | Not early RA |
Isaacs (2009)548 | Autoantibody-positive rheumatoid arthritis (RA) patients (PTS) have enhanced clinical response to rituximab (RTX) when compared with seronegative patients | Not possible to obtain paper |
Isaacs (2013)549 | Effect of baseline rheumatoid factor and anticitrullinated peptide antibody serotype on rituximab clinical response: a meta-analysis | Not early RA |
Ishiguro (2016)550 | Effectiveness and safety of tocilizumab in achieving clinical and functional remission, and sustaining efficacy in biologics-naive patients with rheumatoid arthritis: the FIRST Bio study | Not early RA |
Iwamoto (2009)551 | Prediction of DAS28-ESR remission at 6 months by baseline variables in patients with rheumatoid arthritis treated with etanercept in Japanese population | Not early RA |
Iwamoto (2016)552 | Evaluation of switching from intravenous to subcutaneous formulation of tocilizumab in patients with rheumatoid arthritis | Not possible to obtain paper |
Izumi (2015)553 | Baseline serum osteopontin levels predict the clinical effectiveness of tocilizumab but not infliximab in biologic-naive patients with rheumatoid arthritis: a single-centre prospective study at 1 year (the Keio First-Bio Cohort Study) | Not early RA |
Jansen (2001)554 | Predictors of radiographic joint damage in patients with early rheumatoid arthritis | Not prediction of treatment response |
Jawaheer (2012)555 | Sex differences in response to anti-tumor necrosis factor therapy in early and established rheumatoid arthritis – results from the DANBIO Registry | Not prediction of treatment response |
Jayakumar (2012)556 | Sustained clinical remission in rheumatoid arthritis: prevalence and prognostic factors in an inception cohort of patients treated with conventional DMARDS | Not prediction of treatment response |
Jessome (2015)557 | Does computerized segmentation of early erosions on magnetic resonance imaging predict functional ability in rheumatoid arthritis? | Not early RA |
Jilani (2015)558 | The role of citrullinated protein antibodies in predicting erosive disease in rheumatoid arthritis: a systematic literature review and meta-analysis | Not prediction of treatment response |
Jones (1994)559 | Factors predicting outcome in rheumatoid arthritis | Insufficient detail reported |
Joo (2015)560 | Clinical predictors of severe radiographic damage in Korean patients with rheumatoid arthritis | Insufficient details reported on methods and results for prediction of treatment response and model construction |
Jurgens (2014)561 | Contribution of the individual components of the disease activity score (DAS28) to the total DAS28 score among responders and non-responders to biological therapy for rheumatoid arthritis | No eligible predictive factors |
Jurgens (2015)562 | Contribution of the subjective components of the disease activity score to the response to biologic treatment in rheumatoid arthritis | No eligible predictive factors |
Kameda (2013)563 | Continuation/discontinuation of methotrexate and clinical response to etanercept determine the radiographic progression/repair in patients with rheumatoid arthritis: a subanalysis of 52-week results from the JESMR study | No relevant outcomes |
Kameda (2013)564 | A merged presentation of clinical and radiographic data using probability plots in a clinical trial, the JESMR study | Not early RA |
Kaneko (2013)565 | Predictors of clinical remission (DAS28CRP < 2.6) in patients with RA who were treated with abatacept | Disease duration at baseline not reported |
Kaneshita (2015)566 | Highly elevated rheumatoid factor is a risk factor for abatacept treatment failure in Japanese patients with rheumatoid arthritis | Not early RA |
Kanters (2014)567 | What drives the comparative effectiveness of biologics vs. methotrexate in rheumatoid arthritis? Meta-regression and graphical inspection of suspected clinical factors | No relevant outcome |
Karateev (2015)568 | Dynamics of disease activity scores during the first 12 weeks allow to predict the necessity in combination therapy with methotrexate and biologics within T2T strategy in patients with early and established rheumatoid arthritis (REMARCA study) | Not prediction of treatment effect |
Kastbom (2016)199 | Changes in the anticitrullinated peptide antibody response in relation to therapeutic outcome in early rheumatoid arthritis: results from the SWEFOT trial | Not prediction of treatment response |
Katchamart (2010)569 | Predictors for remission in rheumatoid arthritis patients: a systematic review | Not prediction of treatment response |
Kawada (2016)570 | Predictors of biological antirheumatic drug discontinuation in patients with rheumatoid arthritis while in remission | Comment (not study findings) |
Kawasaki (2013)571 | Shorter disease duration is important for tocilizumab to achieve Boolean remission | Not early RA |
Kawashiri (2013)572 | Evaluation of the efficacy of tocilizumab toward the patients with active rheumatoid arthritis of Nagasaki Prefecture, Japan | Not early RA |
Kawashiri (2014)573 | Baseline low modified health assessment questionnaire (MHAQ) predicts the state of remission estimated by clinical disease activity index and MHAQ at 1 year in tocilizumab-treated rheumatoid arthritis patients | Not early RA |
Kay (2014)574 | Clinical disease activity and acute phase reactant levels are discordant among patients with active rheumatoid arthritis: acute phase reactant levels contribute separately to predicting outcome at one year | Not early RA |
Kekow (2010)575 | Real life treatment with rituximab in TNF blocker non-responders is superior to treatment with a second TNF blocker | Not early RA |
Khan (2012)576 | Smoking and rheumatoid factor status in predicting responses to biologics | Not early RA |
Kim (2016)577 | No predictive effect of body mass index on clinical response in patients with rheumatoid arthritis after 24 weeks of biological disease-modifying antirheumatic drugs: a single-centre study | Not early RA |
Kimura (2016)578 | Time lag between the initiation of adalimumab after methotrexate correlates with the efficacy of adalimumab in rheumatoid arthritis patients | Not early RA |
Klaasen (2009)579 | Is the response to infliximab influenced by body mass index in rheumatoid arthritis patients? | Not early RA |
Klaasen (2009)580 | The relationship between synovial lymphocyte aggregates and the clinical response to infliximab in rheumatoid arthritis: a prospective study | Not early RA |
Klaasen (2011)581 | Body mass index and clinical response to infliximab in rheumatoid arthritis | Not early RA |
Knudsen (2008)205 | Biomarkers of inflammation in patients with unclassified polyarthritis and early rheumatoid arthritis. Relationship to disease activity and radiographic outcome | Not prediction of treatment response |
Koike (2012)582 | Safety and effectiveness responses to etanercept for rheumatoid arthritis in Japan: a sub-analysis of a post-marketing surveillance study focusing on the duration of rheumatoid arthritis | Not early RA |
Koike (2014)583 | Effectiveness and safety of tocilizumab: postmarketing surveillance of 7901 patients with rheumatoid arthritis in Japan | Not early RA |
Kojima (2011)584 | Early aggressive intervention for rheumatoid arthritis increases rate of remission defined using a Boolean approach in clinical practice with tocilizumab | Not early RA |
Kojima (2013)585 | Importance of concomitant MTX use during treatment with tocilizumab in patients with rheumatoid arthritis | Not early RA |
Kojima (2013)586 | Relationship between physicians’ decision to use concomitant glucocorticoid and remission during treatment with tocilizumab in patients with background of limited dose of MTX | Not early RA |
Kojima (2014)587 | Importance of methotrexate therapy concomitant with tocilizumab treatment in achieving better clinical outcomes for rheumatoid arthritis patients with high disease activity: an observational cohort study | Not early RA |
Kojima (2015a)588 | Importance of both disease activity at 12 weeks and clinical response up to 12 weeks to predict achievement of low disease activity at 52 weeks during abatacept treatment in biologics-switching patients with rheumatoid arthritis: a multicenter observational cohort study in Japan | Not early RA |
Kojima (2015b)589 | Predictive factors for achieving low disease activity at 52 weeks after switching from tumor necrosis factor inhibitors to abatacept: results from a multicenter observational cohort study of Japanese patients | Not early RA |
Kotak (2013)590 | Characteristics of a moderate rheumatoid arthritis patient population who lost remission or low disease activity: data from the Consortium of Rheumatology Researchers of North America, Inc. (CORRONA) | Not early RA |
Kovalchik (2012)591 | An association study of disease activity score components and patient satisfaction with overall health for early RA patients on non-biologic DMARD therapy | No relevant outcome measure |
Kristensen (2008)592 | Predictors of response to anti-TNF therapy according to ACR and EULAR criteria in patients with established RA: results from the South Swedish Arthritis Treatment Group Register | Not early RA |
Kronisch (2016)593 | Brief report: predicting functional disability: one-year results from the Scottish early rheumatoid arthritis inception cohort | Mixed RA and UA population with no separate analyses |
Kruger (2012)594 | Reduction in sickness absence in patients with rheumatoid arthritis receiving adalimumab: data from a German noninterventional study | Not early RA |
Kumar (2002)595 | Validation of an Indian version of the Health Assessment Questionnaire in patients with rheumatoid arthritis | Not prediction of treatment effect |
Kume (2012)596 | Anti-cyclic citrullinated protein antibodies as a predictor of response to tocilizumab in patients with rheumatoid arthritis a prospective study | No definition of duration of RA and no full text identified |
Kuriya (2014)597 | Earlier time to remission predicts sustained clinical remission in early rheumatoid arthritis – results from the Canadian Early Arthritis Cohort (CATCH). Predictors of sustained clinical remission in early rheumatoid arthritis-results from the Canadian early arthritis cohort | Not prediction of treatment response |
Kuriya (2015)598 | Working status and improvements in work productivity over time in an early rheumatoid arthritis (ERA) cohort | No baseline predictors (6 months) |
Kynde (2014)599 | Delay in RA diagnosis of more than 12 months is associated with deteriorated functional status in patients in bDMARD treatment-results from a prospective study | No definition of duration of RA |
Lard (200)600 | Early versus delayed treatment in patients with recent-onset rheumatoid arthritis: comparison of two cohorts who received different treatment strategies | Not prediction of treatment response |
Le Blay (2014)601 | Progressive spacing tocilizumab infusions after remission in rheumatoid arthritis: the absence of erosion would be determinant in choosing this strategy | Not early RA |
Lee (2008)602 | Disease Activity Score (DAS) and Health Assessment Questionnaire (HAQ) values show similar changes from baseline to endpoint in clinical trials of biological agents in patients with rheumatoid arthritis (RA) | Not prediction of treatment response |
Lee (2013)603 | Application of a multi-biomarker disease activity (Vectra® DA) score for assessing rheumatoid arthritis patients with fibromyalgia or low C-reactive protein | Not prediction of treatment effect |
Li (2015a)604 | Efficacy and safety results from a Phase 3, randomized, placebo-controlled trial of subcutaneous golimumab in Chinese patients with active rheumatoid arthritis despite methotrexate therapy | Not early RA |
Li (2015b)218 | Relationship of multi-biomarker disease activity score and other risk factors with radiographic progression in an observational study of patients with rheumatoid arthritis | Not prediction of treatment response [and median disease duration suggests not early RA – although it was an early RA (< 2 years) cohort] |
Lie (2006)605 | Predictors of response to methotrexate treatment: Results from a longitudinal observational study of 876 patients with RA [abstract] | Not prediction of treatment response |
Lie (2011)606 | Characterization of long-term responders to first treatment course of rituximab (RTX) results from the CERERRA collaboration | Not early RA |
Lie (2011)607 | Early versus delayed retreatment with rituximab (RTX) in relation to long term clinical response data from the CERERRA collaboration | No definition of duration of RA |
Lie (2012)608 | Effectiveness of sulfasalazine and methotrexate in 1102 DMARD-naive patients with early RA | Not prediction of treatment effect |
Lima (2013)609 | The influence of clinical and genetic variables on methotrexate effectiveness in Portuguese rheumatoid arthritis patients | Not early RA |
Liu (2015)610 | Impact of obesity on the disease course of rheumatoid arthritis | Insufficient detail reported |
Ljung (2011)611 | New assay generation for antibodies against modified and citrullinated peptides predicts poor response to TNF inhibitor therapy | Not early RA |
Loppin (2010)612 | Low rate of rheumatoid arthritis remission in real life: might predictive factors explain? | Incomplete definition of duration of disease and no full text identified |
Lukas (2010)613 | Repair of erosions occurs almost exclusively in damaged joints without swelling | Not early RA |
Lv (2014)614 | The status of rheumatoid factor and anti-cyclic citrullinated peptide antibody are not associated with the effect of anti-TNFA agent treatment in patients with rheumatoid arthritis: a meta-analysis | Not early RA |
Ma (2009a)615 | HAQ and gender predict remission in early RA: experience in the Early Rheumatoid Arthritis Network (ERAN) | Insufficient reporting of duration of disease at baseline |
Ma (2009b)616 | Predicting remission in trial of intensive therapy in early RA: HAQ and gender are key factors | Insufficient details reported on methods |
Ma (2012)236 | Remission in early rheumatoid arthritis: predicting treatment response | Not prediction of treatment response |
Ma (2013)617 | A multi-biomarker disease activity (Vectra™ DA algorithm) score and components are associated with sustained clinical remission in rheumatoid arthritis: the REMIRA study | Not early RA |
Ma (2014a)235 | Clinical and serological predictors of remission in rheumatoid arthritis are dependent on treatment regimen | Not prediction of treatment response |
Ma (2014b)618 | Multi-biomarker disease activity (Vectra® DA algorithm) score is associated with power Doppler ultrasound in patients with rheumatoid arthritis in low disease activity state: the REMIRA cohort | Not early RA |
Ma (2014c)618 | Multi-biomarker disease activity (Vectra DA algorithm) score is associated with power Doppler ultrasound in patients with rheumatoid arthritis in low disease activity state: The REMIRA cohort | Not early RA |
Macchioni (2013)619 | Ultrasonographic predictors for the development of joint damage in rheumatoid arthritis patients: a single joint prospective study | Not prediction of treatment response |
Machado (2012)620 | Predictors of rheumatoid arthritis: quantitative and semiquantitative sonographic measurements of peripheral joints | Not prediction of treatment response |
Makinen (2008)621 | Sex: a major predictor of remission as measured by 28-joint Disease Activity Score (DAS28) in early rheumatoid arthritis? | Not prediction of treatment response |
Mancarella (2007)622 | Good clinical response, remission, and predictors of remission in rheumatoid arthritis patients treated with tumor necrosis factor-alpha blockers: the GISEA study | Not early RA |
Manders (2013)623 | Are TNF blocking agents associated with changes in work participation in patients with RA? | Not early RA |
Manders (2014)624 | Determinants associated with work participation in patients with established rheumatoid arthritis taking tumor necrosis factor inhibitors | Not early RA |
Maneiro (2013)625 | Rheumatoid factor as predictor of response to abatacept, rituximab and tocilizumab in rheumatoid arthritis: systematic review and meta-analysis (Provisional abstract) | Not early RA |
Maneiro (2013)625 | Rheumatoid factor as predictor of response to abatacept, rituximab and tocilizumab in rheumatoid arthritis: systematic review and meta-analysis | Not early RA |
Maneiro (2013) 626 | Rheumatoid factor as predictor of response to non TNF antagonist biologic therapies in rheumatoid arthritis: systematic review and meta-analysis | Insufficient detail |
Markusse (2013)627 | Initial combination therapy in early rheumatoid arthritis: which patients benefit? | No relevant predictors |
Marotte (2010)628 | Biomarkers for prediction of TNFalpha blockers response in rheumatoid arthritis | Review (not systematic) |
Martin (2014)629 | Older age at rheumatoid arthritis onset and comorbidities correlate with less health assessment questionnaire-disability index and clinical disease activity index response to etanercept in the RADIUS 2 registry | Not early RA |
Martin-Mola (2016)630 | Anti-citrullinated peptide antibodies and their value for predicting responses to biologic agents: a review | Review (not systematic) |
Matsushita (2016)631 | Radiographic changes and factors associated with subsequent progression of damage in weight-bearing joints of patients with rheumatoid arthritis under TNF-blocking therapies. Three-year observational study | Not possible to obtain paper |
Matteson (2004)111 | How aggressive should initial therapy for rheumatoid arthritis be? Factors associated with response to ‘non-aggressive’ DMARD treatment and perspective from a 2-yr open label trial | Single-arm study (not able to assess prediction of treatment response) |
Mattey (2009)632 | Relationship between pack-year history of smoking and response to tumor necrosis factor antagonists in patients with rheumatoid arthritis | Not early RA |
Miceli-Richard (2006)633 | Analysis of the shared epitope and selected pro- and anti-inflammatory cytokine genes polymorphism as predictive factors of response to adalimumab in rheumatoid arthritis patients treated in the ReAct study | No baseline disease duration reported; no relevant predictor; no relevant outcome |
Mirpourian (2014)634 | The association of body mass index with disease activity and clinical response to combination therapy in patients with rheumatoid arthritis | Not early RA |
Miwa (2013)635 | Factors leading to HAQ remission after beginning biologics treatment in patients with rheumatoid arthritis | No definition of duration of RA reported and no full text identified |
Miyoshi (2013)636 | A novel method predicting good response using only background clinical data in RA patients treated with infliximab | Insufficient detail |
Molina (2015)637 | Association of socioeconomic status with treatment delays, disease activity, joint damage, and disability in rheumatoid arthritis | Not early RA |
Moorthy (2007)638 | Does smoking predict poor response to anti-TNF therapy for rheumatoid arthritis? | Not early RA |
Mottonen (2002)639 | Delay to institution of therapy and induction of remission using single drug or combination–disease-modifying antirheumatic drug therapy in early rheumatoid arthritis | No relevant outcomes |
Munro (1997)640 | C-reactive protein levels correlate with functional outcome | Not early RA |
Nagasawa (2009)641 | Improvement of the HAQ score by infliximab treatment in patients with RA: its association with disease activity and joint destruction | Not early RA |
Nair (2016)642 | A personalized approach to biological therapy using prediction of clinical response based on MRP8/14 serum complex levels in rheumatoid arthritis patients | Not early RA |
Narvaez (2010)643 | Predictors of response to rituximab in patients with active rheumatoid arthritis and inadequate response to anti-TNF agents or traditional DMARD | Not early RA |
Narvaez (2011)644 | Predictors of response to rituximab in patients with active rheumatoid arthritis and inadequate response to anti-TNF agents or traditional DMARDs | Not early RA |
Navarro-Millan (2013)645 | Predictors and persistence of new-onset clinical remission in rheumatoid arthritis patients | Not early RA |
Nawata (2008)646 | Discontinuation of infliximab in rheumatoid arthritis patients in clinical remission | Not early RA |
Nguyen (2012)647 | The prevalence of the ultrasonographic positive power Doppler synovitis is high and predicts the risk of relapse and structural progression in rheumatoid arthritis in clinical remission: a systematic literature review and meta analysis | Not prediction of treatment response |
Nguyen (2014)648 | Prevalence of ultrasound-detected residual synovitis and risk of relapse and structural progression in rheumatoid arthritis patients in clinical remission: a systematic review and meta-analysis | Not prediction of treatment response |
Nishimoto (2013)649 | Drug free REmission/low disease activity after cessation of tocilizumab (Actemra) Monotherapy (DREAM) study | Not early RA |
Nishino (2015)650 | Ultrasound evaluation of the efficacy of biologic and targeted synthetic DMARDs toward rheumatoid arthritis patients: Kyushu multicenter rheumatoid arthritis ultrasound prospective observational cohort in Japan | No definition of duration of RA reported and no full text identified |
Nishiyama (2013)651 | To develop a regression model for predicting damage-related HAQ: a nationwide study based on the ninja (national database of rheumatic diseases by iR-net in Japan) 2011 | Not prediction of treatment response |
Nixon (2007)652 | Using mixed treatment comparisons and meta-regression to perform indirect comparisons to estimate the efficacy of biologic treatments in rheumatoid arthritis | Insufficient detail reported |
Nordberg (2016)653 | Patients with seronegative RA have more inflammatory activity compared with patients with seropositive RA in an inception cohort of DMARD-naive patients classified according to the 2010 ACR/EULAR criteria | Not prediction of treatment response |
Ogawa (2015)654 | Titre of anti-citrullinated peptide antibody affects the efficacy of first biological treatment in rheumatoid arthritis | Not early RA |
Oguma (2013)655 | (F) good outcomes at week 24 is lead by early introduction of tocilizumab and favorable treatment response up to week 12 | Not early RA |
Okada (2012)656 | Characteristic of the Japanese patients with rheumatoid arthritis (RA) of rapid radiographic progression (RRP) treated with synthetic disease modifying anti-rheumatic drugs (DMARDs) in daily practice: a large-scale prospective longitudinal cohort study (the 1st report of apple survey) | No definition of duration of RA reported and no full text identified |
Okada (2013)657 | Characteristic of the Japanese patients with rheumatoid arthritis (RA) of rapid radiographic progression (RRP) treated with synthetic disease modifying anti-rheumatic drugs (DMARDs) in daily practice: a large-scale prospective longitudinal cohort study | No definition of duration of RA reported and no full text identified |
Okada (2013)658 | Evaluation of the Japanese patients with rheumatoid arthritis (RA) of rapid radiographic progression (RRP) treated with synthetic disease modifying anti-rheumatic drugs (DMARDs) in daily practice: a large-scale prospective longitudinal cohort study (an interim report of Apple Survey) | No definition of duration of RA reported and no full text identified |
Okada (2014)659 | Evaluation of the Japanese patients with rheumatoid arthritis (RA) of rapid radiographic progression (RRP) treated with synthetic disease modifying anti-rheumatic drugs (DMARDs) in daily practice: a large-scale prospective longitudinal cohort study | No definition of duration of RA reported and no full text identified |
Ometto (2015)660 | Self-reported flares predict radiographic progression in rheumatoid arthritis patients in remission undergoing etanercept tapering | Duration of disease at baseline not reported in EN abstract |
Ono (2014)661 | The impacts of disease of the joints on modified health assessment questionnaire scores in rheumatoid arthritis patients: a retrospective study using the national database of rheumatic diseases by iR-net in Japan | Not early RA |
Onuora (2012)662 | Rheumatoid arthritis: how bad is obesity for RA? | Review (not systematic) |
Opris (2014)663 | Serum drug level and anti-citrullinated peptide antibodies as biomarkers that predict EULAR response in rheumatoid arthritis-a new step to personalized medicine | Not prediction of treatment response |
Opris (2015)664 | Active synovitis in rheumatoid arthritis patients while being on SDAI remission | Not early RA |
Ortiz (2014)665 | Is there a difference in the effectiveness in the treatment of rheumatoid arthritis with rituximab in patients with rheumatoid factor positive and negative? A systematic review | Insufficient detail |
Osipyants (2013)666 | Associations between functional status and ultrasound-detected synovitis and joint damage in rheumatoid arthritis during tocilizumab treatment | Not early RA |
Osipyants (2013)667 | Imaging rather than clinical inflammation is associated with radiographic progression in tocilizumab-treated rheumatoid arthritis patients | Not early RA |
Ottaviani (2015)668 | Body mass index and response to infliximab in rheumatoid arthritis | Not early RA |
Pamuk (2015)669 | Work productivity in rheumatoid arthritis: analysis from multicenter Turkish study | Not early RA |
Papadopoulos (2005)257 | Does cigarette smoking influence disease expression, activity and severity in early rheumatoid arthritis patients? | Not prediction of treatment response |
Pascual-Ramos (2009)670 | Hypervascular synovitis and American College of Rheumatology Classification Criteria as predictors of radiographic damage in early rheumatoid arthritis | Not prediction of treatment response |
Pavelka (2010)671 | Prediction of radiographic progression of rheumatoid arthritis | Language not English |
Peltea (2014)672 | Predictive factors for the EULAR response in RA patients with rituximab treatment | No definition of duration of RA and no full text identified |
Peluso (2011)673 | Clinical and ultrasonographic remission determines different chances of relapse in early and long standing rheumatoid arthritis | No relevant outcomes |
Pers (2013)674 | Multicenter retrospective study: response to tocilizumab in clinical practice is not influenced by the number of previous biotherapy or by association with a DMARD | Not early RA |
Pers (2014a)675 | Predictors of response and remission in a large cohort of rheumatoid arthritis patients treated with tocilizumab in clinical practice | Not early RA |
Pers (2014b)676 | TNFRII polymorphism is associated with response to TNF blockers in rheumatoid arthritis patients seronegative for ACPA | Not early RA |
Pers (2015)677 | Response to tocilizumab in rheumatoid arthritis is not influenced by the body mass index of the patient | Not early RA |
Peterfy (2011)678 | Baseline levels of the inflammatory biomarker C-reactive protein are significantly correlated with magnetic resonance imaging measures of synovitis at baseline and after 26 weeks of treatment in patients with early rheumatoid arthritis | No eligible end point |
Peterfy (2012)679 | Baseline levels of the inflammatory biomarker CRP are significantly correlated with MRI measures of synovitis at baseline and after 26 weeks of treatment in patients with early rheumatoid arthritis | No eligible end point |
Plant (2000)261 | Relationship between time-integrated C-reactive protein levels and radiologic progression in patients with rheumatoid arthritis | Not prediction of treatment response |
Plant (2005)680 | What factors influence functional ability in patients with rheumatoid arthritis. Do they alter over time? | Not early RA |
Pomirleanu (2013)681 | A predictive model for remission and low disease activity in patients with established rheumatoid arthritis receiving TNF blockers | Not early RA |
Pope (2011)682 | Does C-reactive protein add value in active rheumatoid arthritis? Results from the Optimisation of Humira Trial | Not early RA |
Puolakka (2004)683 | High self-esteem is associated with low HAQ score in patients with rheumatoid arthritis | Not prediction of treatment response |
Quartuccio (2009)684 | Rheumatoid factor positivity rather than anti-CCP positivity, a lower disability and a lower number of anti-TNF agents failed are associated with response to rituximab in rheumatoid arthritis | Not early RA |
Quintana-Duque (2016)685 | Predictors of remission, erosive disease and radiographic progression in a Colombian cohort of early onset rheumatoid arthritis: a 3-year follow-up study | Not prediction of treatment response |
Radovits (2007)686 | Influence of age and gender on the interpretation of the Disease Activity Score-28 (DAS28) in rheumatoid arthritis | Disease duration at baseline not reported |
Ranganath (2015)687 | Elevated baseline power Doppler discriminates an RA subgroup highly responsive to therapy | No definition of duration of RA and no full text identified |
Rantalaiho (2015)688 | Vitality, presenteeism, and their determinants in patients with early rheumatoid arthritis treated with a 6-month induction infliximab therapy added on a triple combination therapy | No eligible end point |
Rashid (2015a)689 | Factors and reasons associated with switching in rheumatoid arthritis patients newly initiated on biologic DMARDs and impact on health care resource utilization in a managed care organization | No relevant outcomes |
Rashid (2015b)690 | Factors and reasons associated with switching in rheumatoid arthritis patients experienced on biologic DMARDs and impact on health care resource utilization in an integrated healthcare system | No relevant outcomes |
Rech (2015)691 | Prediction of disease relapses by multi-biomarker disease test activity in rheumatoid arthritis patients tapering DMARD treatment | Not early RA |
Reina (2013)692 | Predictive factors of response to tocilizumab in patients with active rheumatoid arthritis | Not early RA |
Rezaei (2012)267 | In early rheumatoid arthritis, patients with a good initial response to methotrexate have excellent 2-year clinical outcomes, but radiological progression is not fully prevented: data from the methotrexate responders population in the SWEFOT trial | Not prediction of treatment response |
Rich (1999)693 | Paucity of radiographic progression in rheumatoid arthritis treated with methotrexate as the first disease modifying antirheumatic drug | Not prediction of treatment response |
Rkain (2011)694 | Clinical and ultrasonographic assessment of evolution in patients with rheumatoid arthritis treated with tocilizumab | Not early RA |
Rodrigues (2014)695 | Obesity is a risk factor for worse treatment response in rheumatoid arthritis patients – results from reuma.pt | Not prediction of treatment response |
Romao (2015)696 | Clinical and pathological differences of elderly- and younger-onset rheumatoid arthritis in an early arthritis cohort | Insufficient detail reported on duration of RA and no full text identified |
Rother (1996)697 | Antineutrophil cytoplasmic antibodies (ANCA) in rheumatoid arthritis: a prospective study | Not early RA |
Ruiz-Esquide (2011)698 | Effects of smoking on disease activity and radiographic progression in early rheumatoid arthritis | Not prediction of treatment response |
Russell (2012)699 | Efficacy with abatacept in patients with earlier versus more longstanding disease: insights from the AIM trial | Not early RA |
Saevarsdottir (2011)74 | Development of a matrix risk model to predict rapid radiographic progression in early rheumatoid arthritis. Results from a randomized trial population | Not prediction of treatment response |
Saevarsdottir (2011)270 | Patients with early rheumatoid arthritis who smoke are less likely to respond to treatment with methotrexate and tumor necrosis factor inhibitors: observations from the Epidemiological Investigation of Rheumatoid Arthritis and the Swedish Rheumatology Register cohorts | Low methodological quality (overlap in treatment arms) |
Saevarsdottir (2011)700 | Predicting response to treatment in patients with rheumatoid arthritis | Not possible to obtain paper |
Saevarsdottir (2011)269 | Predictors of response to methotrexate in early DMARD naïve rheumatoid arthritis: results from the initial open-label phase of the SWEFOT trial | Not prediction of treatment response |
Saevarsdottir (2012)701 | Predictive value of anti-CCP positivity on disease course and response to therapy in early rheumatoid arthritis. Results from the Swedish EIRA study | Insufficient detail on treatment response |
Sagawa (2014)702 | Long-term treatment with tocilizumab (TCZ) strongly suppresses joint destruction in biologic-naive patients with rheumatoid arthritis (RA) regardless of inflammation status | Not early RA |
Sakthiswary (2014)703 | IgA rheumatoid factor as a serological predictor of poor response to tumour necrosis factor alpha inhibitors in rheumatoid arthritis | Not early RA |
Salgado (2011)704 | Influence of baseline rheumatoid factor on the response to tumor necrosis factor antagonists of rheumatoid arthritis patients: a systematic review and meta-analysis | Not early RA |
Salgado (2013)705 | Rheumatoid factor and response to TNF antagonists in rheumatoid arthritis: systematic review and meta-analysis of observational studies | Not early RA |
Salgado (2013)706 | Rheumatoid factor does not predict response to TNF antagonists in rheumatoid arthritis: three centers experience | Not early RA |
Salgado (2013)707 | The effect of rheumatoid factor titre on the response to TNF antagonists | Not early RA |
Salgado (2014)708 | Rheumatoid factor and response to TNF antagonists in rheumatoid arthritis: systematic review and meta-analysis of observational studies (provisional abstract) | Not early RA |
Salgado (2015)709 | Predictors of response to TNF antagonists | Review (not systematic) |
Sanmartí (2003)710 | Radiological progression in early rheumatoid arthritis after DMARDS: a one-year follow-up study in a clinical setting | Not prediction of treatment response |
Sanmartí (2007)75 | Prognostic factors of radiographic progression in early rheumatoid arthritis: a two year prospective study after a structured therapeutic strategy using DMARDs and very low doses of glucocorticoids | Not prediction of treatment response |
Sasso (2014)711 | The multi-biomarker disease activity score as a predictor of radiographic progression in a registry of patients with rheumatoid arthritis | Not early RA |
Sauer (2015)712 | Effectiveness and costs of biologics in veterans with rheumatoid arthritis | Disease duration at baseline not reported |
Schulman (2015)713 | High body mass index negatively impacts time to achieving sustained remission in early rheumatoid arthritis: results from a multicenter early arthritis cohort study | Insufficient details reported on assessment of prediction of treatment response |
Segaud (2014)714 | Therapeutic response to tocilizumab in rheumatoid arthritis: does body weight have an influence? | No definition of duration of RA at baseline and no full text identified |
Sekiguchi (2015)715 | Predicting factors associated with sustained clinical remission by abatacept are different between in younger and elderly patients with biologic-naive rheumatoid arthritis (ABROAD study) | Not early RA; no relevant predictive factors |
Sekiguchi (2016)716 | Differences in predictive factors for sustained clinical remission with abatacept between younger and elderly patients with biologic-naive rheumatoid arthritis: results from the ABROAD study | Not early RA |
Sellam (2011)717 | B cell activation biomarkers as predictive factors for the response to rituximab in rheumatoid arthritis: a six-month, national, multicenter, open-label study | Not early RA |
Sergeant (2015)718 | Lifestyle, clinical and psychosocial predictors of good response to methotrexate therapy in the rheumatoid arthritis medication study (RAMS) | Single-arm study (not able to assess prediction of treatment response) |
Shah (2014)719 | Serum anti-CCP antibody and its correlation with disease activity in local Pakistani rheumatoid arthritis patients | Not early RA |
Sharma (2014)720 | Biologic de-escalation in rheumatoid arthritis: cost savings and clinical success | No relevant outcomes |
Shen (2010)277 | Serum biomarkers predict progressive structural damage in the BeSt study | Not prediction of treatment response |
Sherrer (1987)721 | Disability in rheumatoid arthritis: comparison of prognostic factors across three populations | Not early RA |
Shiozawa (2013)722 | MMP-3 as a predictor that identifies a subgroup with potential radiographic progression requiring additional biologics to halt future progression among the rheumatoid arthritis patients succeedingly treated with methotrexate (MTX) alone | Not early RA |
Shrestha (2013)723 | Effect of body mass index on clinical response to anti-TNF therapies in rheumatoid arthritis: a retrospective study | No definition of duration of RA at baseline and no full text identified |
Shrestha (2013)724 | Effect of body mass index on clinical response to anti-TNF therapy in rheumatoid arthritis | No definition of duration of RA at baseline and no full text identified |
Shu (2014)725 | Impact of missing anti-cyclic citrullinated peptide (CCP) antibody serology on clinical outcomes in early rheumatoid arthritis: results from catch (Canadian early arthritis cohort) | Not prediction of treatment response |
Sibilia (2012)726 | Abatacept confers clinical efficacy regardless of baseline CRP status in patients with rheumatoid arthritis and inadequate response to methotrexate in the aim trial | Not early RA |
Simone (2014a)727 | Genetic and clinical predictors of response to TNF-alpha therapy in an Italian axial-SPA cohort | Not RA |
Simone (2014b)728 | Genetic and clinical predictors of response to TNF blocker in an Italian axial-SPA cohort | Not RA |
Smith (2013)729 | Can CD11C expression successfully predict response to etanercept in rheumatoid arthritis patients? | Not early RA |
Smolen (2011)730 | Baseline predictors of remission with combination etanercept-methotrexate therapy in moderately active rheumatoid arthritis: Interim results of the preserve trial | Not early RA |
Smolen (2012)731 | Tocilizumab inhibits progression of joint damage in rheumatoid arthritis irrespective of its anti-inflammatory effects: disassociation of the link between inflammation and destruction | Not early RA |
Smolen (2015)732 | The effect of prior disease duration and prior DMARD use on treatment outcomes in patients with early or established rheumatoid arthritis | No eligible factor |
Sockalingam (2009)733 | Prevalence of anti cyclic citrullinated peptide antibodies in Malaysian rheumatoid arthritis patients and its correlation with disease activity | Not prediction of treatment response |
Soderlin (2011)734 | Absent ‘Window of Opportunity’ in smokers with short disease duration. Data from BARFOT, a multicenter study of early rheumatoid arthritis | Insufficient details reported on assessment of prediction of treatment response |
Soderlin (2012)735 | The effect of smoking on response and drug survival in rheumatoid arthritis patients treated with their first anti-TNF drug | Not early RA |
Soderlin (2013)736 | Second-hand exposure to tobacco smoke and its effect on disease activity in Swedish rheumatoid arthritis patients. Data from BARFOT, a multicenter study of RA | No relevant predictors |
Sokka (2000)737 | Scores for functional disability in patients with rheumatoid arthritis are correlated at higher levels with pain scores than with radiographic scores | Not early RA |
Solau-Gervais (2012)738 | Efficacy of rituximab in the treatment of rheumatoid arthritis. Influence of serological status, coprescription of methotrexate and prior TNF-alpha inhibitors exposure | Not early RA |
Soliman (2011)739 | Predictors of response to rituximab in patients with rheumatoid arthritis: results from the British Society for Rheumatology Biologics Register (BSRBR) | Not early RA |
Srirangan (2013)740 | Functional outcome in response to treatment with DMARDs compared to anti-TNF agents in a cohort with rheumatoid arthritis | Not early RA |
Stagnaro (2013)741 | A useful mathematical model able to predict the early response to tocilizumab in rheumatoid arthritis | Insufficient detail reported |
Stavropoulos-Kalinoglou (2007)742 | Redefining overweight and obesity in rheumatoid arthritis patients | Not prediction of treatment response |
Steunebrink (2015)743 | Superiority of initial combination-over step up therapy in treatment to the target of remission in daily clinical practice in early rheumatoid arthritis patients: results from the DREAM registry | Disease duration at baseline not reported |
Strand (2012)744 | Factors that impact work productivity in the preserve trial: a randomized controlled trial of combination etanercept-methotrexate therapy in patients with moderately active rheumatoid arthritis | Not early RA |
Strand (2013)745 | Predictors of work impairment in a randomized controlled trial of etanercept-methotrexate therapy in patients with moderate rheumatoid arthritis | Not early RA |
Strand (2015)746 | The impact of rheumatoid arthritis on work and predictors of overall work impairment from three therapeutic scenarios | Not early RA |
Sauer (2015)712 | Effectiveness and costs of biologics in veterans with rheumatoid arthritis | Disease duration at baseline not reported |
Szekanecz (2015)747 | Analysis of the association between cigarette smoking and clinical response to certolizumab pegol treatment in Hungarian patients with rheumatoid arthritis | No baseline disease duration reported and no full text identified |
Tada (2013)748 | Predictive factors for radiographic progression in low- and standard-dose etanercept therapy for rheumatoid arthritis from the prevention of cartilage destruction by etanercept (precept) study | Not early RA |
Tak (2012)749 | A personalised medicine approach to biologic treatment of rheumatoid arthritis: a preliminary treatment algorithm | Not a prediction model or primary study |
Takeuchi (2011)750 | Clinical, radiographic and functional effectiveness of tocilizumab for rheumatoid arthritis patients-REACTION 52-week study | Not early RA |
Takeuchi (2014a)751 | Analysis on predictors for long-term clinical efficacies of golimumab in patients with rheumatoid arthritis | Not early RA |
Takeuchi (2014b)752 | Impact of disease duration before starting adalimumab treatment on work productivity in Japanese patients with rheumatoid arthritis; analysis of 24-weeks data from the ANOUVEAU study | Not early RA |
Tam (2007)753 | Rapid improvement in rheumatoid arthritis patients on combination of methotrexate and infliximab: clinical and magnetic resonance imaging evaluation | Not early RA |
Tamai (2012)754 | Magnetic resonance imaging-proven osteitis at baseline predicts the early rheumatoid arthritis patients who will develop rapid radiographic progression: MRI is beneficial to find the window of opportunity in early RA | No relevant predictors |
Tamai (2014)755 | Investigation of MRI bone changes in early-stage RA patients achieved in sustained clinical good response: sub-analysis from Nagasaki University early arthritis cohort | No relevant predictors |
Tanaka (2008)756 | Efficient management of rheumatoid arthritis significantly reduces long-term functional disability | Not early RA |
Tanaka (2012)757 | Prevention of joint destruction in patients with high disease activity or high C-reactive protein | Not early RA |
Tanaka (2012)758 | Structural damages disturb functional improvement in patients with rheumatoid arthritis treated with etanercept | Not early RA |
Tanaka (2013)759 | Efficacy and safety of tocilizumab therapy in rheumatoid arthritis: prevalence and predictive factors of sustained remission | Not early RA |
Tanaka (2015)760 | A longitudinal study of factors contributing to the worsening of absenteeism in patients with rheumatoid arthritis based on the IORRA cohort | Not early RA |
Tanaka (2016)761 | Prevention of joint destruction in patients with high disease activity or high C-reactive protein levels: post hoc analysis of the GO-FORTH study | Not possible to obtain paper |
Teitsma (2015)762 | Predicting the need for additional treatment in early rheumatoid arthritis patients treated to target on methotrexate monotherapy | Insufficient details reported on assessment of prediction of treatment response |
Terao (2015)763 | Rheumatoid factor is associated with the distribution of hand joint destruction in rheumatoid arthritis | Not early RA |
Thorne (2014)764 | Effectiveness and safety of infliximab in rheumatoid arthritis: analysis from a Canadian multicenter prospective observational registry | Not early RA |
Tolusso (2010)765 | Analysis of the association of CDA (cytidin deaminase) (K27Q), TNF-(–308G>A) and PTPN22 R620W genetic polymorphisms with auto-antibody seropositive RA and the response to B cell depletion | No baseline disease duration reported (Also no relevant predictors) |
Tony (2011)766 | Predictive factors for response to rituximab in patients with rheumatoid arthritis (FIRST) | Not possible to obtain paper |
Torrente-Segarra (2014)767 | Assessment of 12-month efficacy and safety of 168 certolizumab pegol rheumatoid arthritis treated patients from a multicenter retrospective national study in Spain | Not early RA |
Torrente-Segarra (2016)768 | RENACER study: assessment of 12-month efficacy and safety of 168 certolizumab PEGol rheumatoid arthritis-treated patients from a Spanish multicenter national database | Not early RA |
Troelsen (2012)769 | IgG glycosylation changes and MBL2 polymorphisms: associations with markers of systemic inflammation and joint destruction in rheumatoid arthritis | Not early RA |
Tsuji (2014)770 | Baseline procalcitonin (PCT) level as a predictive marker for clinical remission (DAS28-ESR, CDAI) at 52 weeks in biologic naive rheumatoid arthritis (RA) patients treated by tocilizumab (TCZ); a single centre retrospective study | Disease duration at baseline not reported |
Ursum (2010)771 | Levels of anti-citrullinated protein antibodies and IgM rheumatoid factor are not associated with outcome in early arthritis patients: a cohort study | Not early RA |
van den Broek (2012)772 | The association of treatment response and joint damage with ACPA-status in recent-onset RA: a subanalysis of the 8-year follow-up of the BeSt study | Not prediction of treatment response |
van den Broek (2013)773 | Personalized medicine: predicting responses to therapy in patients with RA | Review (not systematic) |
van der Heijde (2005)774 | Baseline CRP concentrations predict radiographic progression in MTX-naive patients with recent-onset RA: subanalysis of the PREMIER study [abstract] | Not prediction of treatment response |
van der Helm-van Mil (2005)296 | Antibodies to citrullinated proteins and differences in clinical progression of rheumatoid arthritis | Not prediction of treatment response |
van der Helm-van Mil (2008)775 | A high body mass index has a protective effect on the amount of joint destruction in small joints in early rheumatoid arthritis | Not prediction of treatment response |
van Der Horst-Bruinsma (2011)776 | Inclusion criteria based on DAS28 score: strength of improvement is less dependent on baseline disease activity than expected | Commentary (not a study) |
van der Maas (2014)777 | Validity of OMERACT preliminary flare questions in a randomized controlled trial, that assesses impact of disease activity guided down-titration of anti-TNF treatment in rheumatoid arthritis patients in low disease activity | Not early RA |
van der Woude (2009)778 | Prevalence of and predictive factors for sustained disease-modifying antirheumatic drug-free remission in rheumatoid arthritis: results from two large early arthritis cohorts | No relevant outcome |
van Gaalen (2004)779 | Association between HLA class II genes and autoantibodies to cyclic citrullinated peptides (CCPs) influences the severity of rheumatoid arthritis | Insufficient information on treatment response |
van Jaarsveld (1999)300 | The prognostic value of the antiperinuclear factor, anti-citrullinated peptide antibodies and rheumatoid factor in early rheumatoid arthritis | Not prediction of treatment response |
van Laar (2007)780 | Do high levels of IgA rheumatoid factor indicate a poor response to treatment with TNF inhibitors in patients with RA? | Commentary |
van Nies (2014)781 | What is the evidence for the presence of a therapeutic window of opportunity in rheumatoid arthritis? A systematic literature review | Review – cross-checked |
van Sijl (2013)782 | A novel and effective prediction model of response to rituximab in rheumatoid arthritis | Insufficient detail reported |
van Vollenhoven (2009)783 | Six-month results from the collaborative European registries for rituximab in rheumatoid arthritis (CERERRA). Efficacy of rituximab is highest in RF-positive patients and in those who failed at most one prior anti-TNF | Not early RA |
van Vollenhoven (2014)784 | A prediction model that identifies patients most likely to benefit from first-line therapy with adalimumab plus methotrexate in early rheumatoid arthritis | Insufficient detail reported |
Vastesaeger (2009)78 | Matrix risk model for prediction of rapid radiographic progression in rheumatoid arthritis | Not prediction of treatment response |
Vastesaeger (2016)785 | Prediction of remission and low disease activity in disease-modifying anti-rheumatic drug-refractory patients with rheumatoid arthritis treated with golimumab | Not early RA |
Vazquez (2007)786 | Prognostic markers of clinical remission in early rheumatoid arthritis after two years of DMARDs in a clinical setting | Not prediction of treatment response |
Velloso Feijoo (2013)787 | B cell depletion therapy in a cohort of patients with seropositive and seronegative rheumatoid arthritis | Not early RA |
Ven (2014)788 | Can we use ultrasound to identify rheumatoid arthritis patients in remission who can taper their medication? | Disease duration at baseline not reported |
Verschueren (2009)789 | Predictors of remission, normalized physical function, and changes in the working situation during follow-up of patients with early rheumatoid arthritis: an observational study | Not prediction of treatment response |
Verstappen (2005)790 | Working status among Dutch patients with rheumatoid arthritis: work disability and working conditions | Not early RA |
Verstappen (2009)791 | Adverse events and factors associated with toxicity in patients with early rheumatoid arthritis treated with methotrexate (the CAMERA study) | No relevant outcomes |
Verstappen (2010)792 | Working status in patients with rheumatoid arthritis, ankylosing spondylitis and psoriatic arthritis: results from the British Society for Rheumatology Biologics Register | Not early RA |
Vesperini (2013)307 | Association of tobacco exposure and reduction of radiographic progression in early rheumatoid arthritis: results from a French multicenter cohort | Not prediction of treatment response |
Vidal (2014)793 | Influence of body mass index on disease activity and radiographic joint damage in rheumatoid arthritis: a systematic review and meta-analysis | Not early RA |
Visman (2011)794 | Effect of the application of trial inclusion criteria on the efficacy of adalimumab therapy in a rheumatoid arthritis cohort | Not early RA |
Visser (2008)795 | Pretreatment serum levels of anti-cyclic citrullinated peptide antibodies are associated with the response to methotrexate in recent-onset arthritis | Population (UA) |
Visser (2010)79 | A matrix risk model for the prediction of rapid radiographic progression in patients with rheumatoid arthritis receiving different dynamic treatment strategies: post hoc analyses from the BeSt study | Not prediction of treatment response |
Visvanathan (2007)311 | Changes in biomarkers of inflammation and bone turnover and associations with clinical efficacy following infliximab plus methotrexate therapy in patients with early rheumatoid arthritis | No relevant predictors |
Vital (2010)796 | Serum cytokine profile predicts response to rituximab therapy in rheumatoid arthritis | Not prediction of treatment response |
Volkov (2013)797 | Monitoring anti-interleukin 6 receptor antibody treatment in rheumatoid arthritis and prediction progressive structural damage of the wrist joints by ultrasonography | Not early RA |
Vreju (2016)798 | Subclinical ultrasound synovitis in a particular joint is associated with ultrasound evidence of bone erosions in that same joint in rheumatoid patients in clinical remission | Not early RA |
Wagner (2009)799 | Serum markers associated with clinical response in methotrexate naïve rheumatoid arthritis patients treated with golimumab, a human anti-TNFα monoclonal antibody | Not possible to obtain paper |
Wagner (2010)800 | Association of serum markers with clinical response measures in rheumatoid arthritis patients treated with golimumab, a human anti-TNF alpha monoclonal antibody | Not possible to obtain paper |
Wang (2016)801 | Short-term efficacy reliably predicts long-term clinical benefit in rheumatoid arthritis clinical trials as demonstrated by model-based meta-analysis | No relevant outcomes |
Wang (2016)802 | Relationship between baseline and early changes in C-reactive protein and interleukin-6 levels and clinical response to tocilizumab in rheumatoid arthritis | Not early RA |
Watanabe (2013)803 | Tocilizumab efficiently halts radiographic progression in patients with rheumatoid arthritis and swollen joint counts within a year predict long-term radiographic outcomes: three year results from Michinoku Tocilizumab Study Group | Not early RA |
Weinblatt (2006)804 | Predictors of poor radiographic response in rheumatoid arthritis subjects treated with methotrexate: preliminary analysis from the era and tempo studies | Not prediction of treatment response |
Weinblatt (2011)805 | Factors associated with radiographic progression in patients with rheumatoid arthritis who were treated with methotrexate | Not early RA |
Wessels (2007)312 | A clinical pharmacogenetic model to predict the efficacy of methotrexate monotherapy in recent-onset rheumatoid arthritis | Not prediction of treatment response |
Westhoff (2013)806 | Indicators of depression are stronger predictors of work disability in early arthritis than disease activity or response to therapy | No relevant outcomes |
Wevers-De Boer (2011)807 | Remission induction therapy with methotrexate and prednisone in patients with early rheumatoid and undifferentiated arthritis | Treatment duration of < 6 months |
Wevers-De Boer (2012)808 | Extended report: remission induction therapy with methotrexate and prednisone in patients with early rheumatoid and undifferentiated arthritis (the IMPROVED study) | Treatment duration of < 6 months |
Wevers-De Boer (2012)809 | Remission after one year in ACPA positive and ACPA negative patients with early arthritis | Treatment duration of < 6 months |
Wevers-De Boer (2013)810 | Early metacarpal bone mineral density loss is predictive for radiologic joint damage progression after 1 year in patients with early arthritis | Combined sample of RA and UA, analyses not reported separately in this abstract |
White (2009)811 | Clinical features of disease severity and body mass index predict outcome at 6 months in a cohort of patients with early rheumatoid arthritis | Insufficient detail reported on methods and treatment |
Wolfe (2000)318 | The effect of smoking on clinical, laboratory, and radiographic status in rheumatoid arthritis | Not early RA |
Wolfe (2012)812 | Effect of body mass index on mortality and clinical status in rheumatoid arthritis | Not early RA |
Xibille (2013)813 | Plasma adiponectin level and body mass index (BMI) predict response to treatment in patients with rheumatoid arthritis | Not early RA |
Xibille (2013)814 | Leptin and adiponectin serum levels as predictors of treatment response in patients with rheumatoid arthritis | Not early RA |
Yamanaka (2007)815 | Retrospective clinical study on the notable efficacy and related factors of infliximab therapy in a rheumatoid arthritis management group in Japan (RECONFIRM) | Not early RA |
Yamanaka (2015)816 | Trend of patient characteristics and its impact on the response to adalimumab in patients with rheumatoid arthritis: post hoc time-course analysis of an all-case pms in Japan | Not possible to obtain paper |
Yamasaki (2014)817 | Predictive marker for the long-term discontinuation of infliximab in rheumatoid arthritis with clinical remission | Disease duration at baseline not reported |
Yazici (2011)818 | Greater remission rates in patients with early versus long-standing disease in biologic-naive rheumatoid arthritis patients treated with abatacept: a post hoc analysis of randomized clinical trial data | Not early RA |
Yoshida (2015)819 | Incidence and predictors of biological antirheumatic drug discontinuation attempts among patients with rheumatoid arthritis in remission: a CORRONA and NinJa collaborative cohort study | Not early RA |
Yoshimi (2013)820 | Ultrasonography is a potent tool for the prediction of progressive joint destruction during clinical remission of rheumatoid arthritis | Not early RA |
Yuasa (2013)821 | Treatment responses and their predictors in patients with rheumatoid arthritis treated with biological agents | Not early RA |
Zeidler (2012)822 | Reply to: very early rheumatoid arthritis as a predictor of remission: a multicentre real life prospective study | No data (comment) |
Zhang (2009)823 | The sensitivity to change for lower disease activity is greater than for higher disease activity in rheumatoid arthritis trials | No relevant outcomes |
Zheng (2014)824 | Application of high frequency color Doppler ultrasound in the monitoring of rheumatoid arthritis treatment | Not early RA |
Zhilyaev (2014)825 | Efficacy of biological treatment in cohort of rheumatoid arthritis patients in Moscow | Not early RA |
Zufferey (2013)826 | Disease activity in rheumatoid arthritis patients at initiation of biologic agents and 1 year of treatment: results from the Swiss SCQM registry | Not early RA |
Appendix 9 Additional evidence tables: review 2
Author (year), name of trial or cohort (if relevant) | Characteristic | |||||||||||
---|---|---|---|---|---|---|---|---|---|---|---|---|
Group/treatment arm | Mean/median age (years) | Sex (% female) | Mean/median HAQ score at baseline | Mean/median baseline erosions on radiograph or percentage of erosions | ACPA+/anti-CCP-positive at baseline (%) | RF positive at baseline (%) | Mean/median SJC at baseline | Mean/median ESR at baseline | Mean/median CRP levels at baseline | Baseline smoking status | Vascularity of synovium using PD ultrasound | |
Garnero (2002)102 | All participants | 49 (SD 12) | 80 | 1.49 (SD 0.67) |
SHS erosion score: 6.3 (SD 9.5) SHS total score: 11.9 (SD 16.6) |
NR | 88 | 23 (SD 11) | NR | 4.2 mg/dl (SD 6.0) | NR | NR |
Heimans (2013)103 BeSt |
All participants | 55 (SD 14)a | 68a | 1.4 (SD 0.7)a |
SHS erosion score: 3.7 (SD 5.1) SHS total score: 6.6 (SD 8.2) |
57a | 65a | NR | NR | NR | Smoker, n: 177 (35%)a | NR |
BMI of < 25 kg/m2 | 53 (SD 15) | 72 | 1.4 (SD 0.6) | NR | 65 | 69 | 13 (IQR 10–19) | 38 mm/hour (IQR 20–58 mm/hour) | 20 mg/l (IQR 8–55 mg/l) | Smoker, n: 88 (41%) | NR | |
BMI of ≥ 25 kg/m2 | 56 (SD 13) | 64 | 1.4 (SD 0.7) | NR | 59 | 62 | 14 (IQR 9–18) | 34 mm/hour (IQR 18–56 mm/hour) | 21 mg/l (IQR 9–50 mg/l) | Smoker, n: 89 (31%) | NR | |
Sequential monotherapy | 54 (SD 13) | 68 | 1.4 (SD 0.7)b |
SHS erosion score: 4.1 (SD 6.2) SHS total score: 7.3 (SD 9.5) |
NR | 67 | NR | NR | NR | NR | NR | |
Step-up combination therapy | 54 (SD 13) | 71 | 1.4 (SD 0.6)b |
SHS erosion score: 3.5 (SD 4.3) SHS total score: 6.3 (SD 6.9) |
NR | 64 | NR | NR | NR | NR | NR | |
MTX and SSZ and tapered prednisone | 55 (SD 14) | 65 | 1.4 (SD 0.7)b |
SHS erosion score: 3.3 (SD 4.3) SHS total score: 5.9 (SD 6.5) |
NR | 65 | NR | NR | NR | NR | NR | |
MTX and IFX | 54 (SD 14) | 66 | 1.4 (SD 0.7)b |
SHS erosion score: 3.9 (SD 5.8) SHS total score: 7.0 (SD 10.0) |
NR | 64 | NR | NR | NR | NR | NR | |
Huizinga (2015)104 AVERT |
All participants | 47.0 (SD 12.6)c | 77.8 | 1.4 (SD 0.66) | NR | 58 | 95.2c | NR | NR | 17.5 mg/l (SD 25.0 mg/l)c | NR | NR |
ABT s.c. and MTX | 46.4 (SD 13.2)c | 79.8c | 1.5 (SD 0.68)c | NR | 56 | 95c | NR | NR | 18.1 mg/l (SD 28.4 mg/l)c | NR | NR | |
ABT s.c. | 45.4 (SD 11.9)c | 76.7c | 1.4 (SD 0.66)c | NR | 55 | 95.7c | NR | NR | 16.9 mg/l (SD 23.9 mg/l)c | NR | NR | |
MTX | 49.1 (SD 12.4)c | 76.7c | 1.4 (SD 0.65)c | NR | 64 | 94.8c | NR | NR | 17.3 mg/l (SD 22.4 mg/l)c | NR | NR | |
Maska (2012)105 TEAR |
All participants (with serum cotinine at baseline and week 48) | 49.6 (SD 12.2) | 73 | 1.2 (SD 0.4) | NR | NR | NR | 12.5 (SD 5.7) | 32.8 mm/hour (SD 24.0 mm/hour) | NR | See below | NR |
Current smokers | 50.1 (SD 10.8) | 67 | 1.3 (SD 0.4) | NR | NR | NR | 12.5 (SD 5.8) | 32.6 mm/hour (SD 24.3 mm/hour) | NR | Current smokers: 119 (29%) | NR | |
Non-smokers | 49.4 (SD 12.7) | 75 | 1.2 (SD 0.4) | NR | NR | NR | 12.4 (SD 5.7) | 32.9 mm/hour (SD 23.9 mm/hour) | NR | Non-smokers: 293 (71%) | NR | |
Mustila (2011)106 FIN-RACo |
All participants | 47 (SD 10)a | 63a | 0.87 (SD 0.58)a | Erosive disease: 45%a | 71a | 65a | 13 (SD 7)a | 37 mm/hour (SD 23 mm/hour)a | NR | NR | NR |
ACPA+ | NR | NR | NR | Erosive disease: 54% | 100 | 83 | NR | NR | NR | NR | NR | |
ACPA– | NR | NR | NR | Erosive disease: 22% | 0 | 22 | NR | NR | NR | NR | NR | |
MTX and HCQ and SSZ in a TTT regime | 46 (SD 10) | 61 | 0.84 (SD 0.54) | Erosions in hand or foot radiographs: 42% | NR | 68 | 13 (SD 6) | 37 mm/hour (SD 24 mm/hour) | NR | NR | NR | |
cDMARD sequential monotherapy in a TTT regime (starting with SSZ) | 48 (SD 11) | 65 | 0.91 (SD 0.63) | Erosions in hand or foot radiographs: 48% | NR | 62 | 14 (SD 7) | 37 mm/hour (SD 21 mm/hour) | NR | NR | NR | |
Pasero (1996)107 | All participants | 49.6 (SD 11.6)a | 78 | NR |
Number of patients with erosions: 193a Number of patients without erosions: 91a |
NR | NR | 14.8 (SD 7.7)a | 47.2 mm/hour (SD 28.0 mm/hour)a | 8.4 mg/dl (SD 17.1 mg/dl)a | NR | NR |
Patients with joint erosions at baseline | NR | NR | NR | NR | NR | NR | NR | NR | NR | NR | NR | |
Patients without joint erosions at baseline | NR | NR | NR | NR | NR | NR | NR | NR | NR | NR | NR | |
CsA arm | 48.4 (SD 11.6) | 77 | NR |
Number of patients with erosions: 104 Number of patients without erosions: 37 |
NR | NR | 14.9 (SD 7.5) | 46.9 mm/hour (SD 25.2 mm/hour) | 8.3 mg/dl (SD 16.7 mg/dl) | NR | NR | |
Other cDMARDs arm | 50.8 (SD 11.5) | 78 | NR |
Number of patients with erosions: 89 Number of patients without erosions: 54 |
NR | NR | 14.7 (SD 7.8) | 47.5 mm/hour (SD 30.8 mm/hour) | 8.5 mg/dl (SD 17.5 mg/dl) | NR | NR | |
Rau (1998)108 | All participants | 55.5 (SD 9.5)a | 66 | NR |
Mean radiographic score (0–190): 5.2a Number of eroded joints (0–38): 4.4a |
NR | 61 | 15.2 (SD 6.9)a | 40.9 mm/hour (SD 24.0 mm/hour)a | 4.4 mg/dl (SD 4.0 mg/dl)a | NR | NR |
MTX | 54.2 (SD 8.6) | 60 | NR |
Mean radiographic score (0–190): 5.8 Number of eroded joints (0–38): 4.2 |
NR | 68 | 15.3 (± 6.6) | 41.1 mm/hour (± 24.5 mm/hour) | 4.1 mg/100 ml (± 3.6 mg/100 ml) | NR | NR | |
GSTM | 56.8 (SD 10.4) | 72 | NR |
Mean radiographic score (0–190): 4.6 Number of eroded joints (0–38): 4.6 |
NR | 54 | 15.1 (± 7.2) | 40.6 mm/hour (± 23.6 mm/hour) | 4.6 mg/100 ml (± 4.4 mg/100 ml) | NR | NR | |
Seegobin (2014)109 CARDERA |
All participants | 54 (IQR 20–89)a,d | 69 | 1.6 (SD 0.7) | NR | 48 | 68 | NR | NR | NR | NR | NR |
ACPA+ | 54.0 (IQR 46.0–64.0) | 67 | 1.62 (IQR 1.00–2.12) | Larsen score: 7.50 (IQR 2.50–21.25) | 100 | 79e | NR | NR | NR | NR | NR | |
ACPA– | 55.0 (IQR 47.0–62.0) | 74 | 1.62 (IQR 1.12–2.12) | Larsen score: 4.50 (IQR 1.00–9.50) | 0 | 39 | NR | NR | NR | NR | NR | |
MTX monotherapy | 54 (range 21–80)d,f | 67f | 1.5 (SD 0.7)f | Larsen score: 7 (IQR 3–15)f | NR | 66f | NR | NR | NR | NR | NR | |
CsA and MTX | 53 (range 20–89)d,f | 66f | 1.7 (SD 0.7)f | Larsen score: 8 (IQR 3–23)f | NR | 65f | NR | NR | NR | NR | NR | |
Prednisolone and MTX | 54 (range 27–84)d,f | 66f | 1.6 (0.7)f | Larsen score: 6 (IQR 2–20)f | NR | 66f | NR | NR | NR | NR | NR | |
Triple therapy | 55 (range 20–78)d,f | 67f | 1.6 (SD 0.7) | Larsen score: 5 (IQR 2–14)f | NR | 72f | NR | NR | NR | NR | NR | |
Smolen (2006)100 Vastesaeger (2009)78 ASPIRE |
All participants | 50 (SD 13)a | 71 | 1.5 (SD 0.6) | 82.5% with erosionsa | NR | 72a | 22 (SD 10)a | 44 mm/hour (SD 28 mm/hour)a | 3.0 mg/dl (SD 3.1 mg/dl)a | NR | NR |
MTX | 50 (SD 13) | 75 | 1.5 (SD 0.6)a | 80% with erosions | NR | 71 | 22 (SD 11) | 43 mm/hour (SD 28 mm/hour) | 2.6 mg/dl (SD 2.9 mg/dl) | NR | NR | |
IFX (all doses) | 50 (SD 13)a | 70 | 1.5 (SD 0.6) | 83.5% with erosionsa | NR | 72a | 22 (SD 10)a | 44 mm/hour (SD 28 mm/hour)a | 3.0 mg/dl (SD 3.3 mg/dl)a | NR | NR | |
MTX and IFX 3 mg/kg | 51 (SD 12) | 71 | 1.5 (SD 0.7)a | 84% with erosions | NR | 71 | 21 (SD 10) | 45 mm/hour (SD 29 mm/hour) | 2.9 mg/dl (SD 3.3 mg/dl) | NR | NR | |
MTX and IFX 6 mg/kg | 50 (SD 13) | 68 | 1.5 (SD 0.6) | 83% with erosions | NR | 73 | 22 (SD 11) | 44 mm/hour (SD 27 mm/hour) | 3.0 mg/l (SD 3.4 mg/dl) | NR | NR | |
Sokolove (2015)110 AMPLE |
All patients | NR | NR | NR | NR | 76 | NR | NR | NR | NR | NR | NR |
Anti-CCP2 negative |
ABT: 52 (IQR 24.0–80.0) ADA: 58 (IQR 21.0–83.0) |
ABT: 84.8 ADA: 85.2 |
ABT: 1.3 (IQR 0.0–2.9) ADA: 1.4 (IQR 0.0–2.6) |
NR | 0 |
ABT: 42.4 ADA: 51.9 |
NR | NR |
ABT: 0.6 mg/dl (IQR 0.0–10.4 mg/dl) ADA: 0.6 mg/dl (IQR 0.0–42 mg/dl) |
ABT:
|
NR | |
Anti-CCP2 positive; Q1 |
ABT: 50 (IQR 22.0–70.0) ADA: 50 (IQR 19.0–78.0) |
ABT: 88.1 ADA: 83.6 |
ABT: 1.4 (IQR 0.0–2.5) ADA: 1.3 (IQR 0.0–2.5) |
NR | 100 |
ABT: 85.7 ADA: 92.7 |
NR | NR |
ABT: 0.8 mg/dl (IQR 0.1–8.4 mg/dl) ADA: 0.6 mg/dl (IQR 0.0–4.8 mg/dl) |
ABT:
|
NR | |
Anti-CCP2 positive; Q2 |
ABT: 52 (IQR 21.0–78.0) ADA: 49 (IQR 22.0–73.0) |
ABT: 80.4 ADA: 87.0 |
ABT: 1.7 (IQR 0.0–2.8) ADA: 1.6 (IQR 0.0–2.9) |
NR | 100 |
ABT: 98.0 ADA: 93.5 |
NR | NR |
ABT: 0.9 mg/dl (IQR 0.0–9.4 mg/dl) ADA: 1.3 mg/dl (IQR 0.1–5.8 mg/dl) |
ABT:
|
NR | |
Anti-CCP2 positive; Q3 |
ABT: 47.5 (IQR 25.0–73.0) ADA: 52 (IQR 26.0–78.0) |
ABT: 82.6 ADA: 80.4 |
ABT: 1.4 (IQR 0.0–2.8) ADA: 1.6 (IQR 0.0–3.0) |
NR | 100 |
ABT: 100.0 ADA: 96.1 |
NR | NR |
ABT: 0.9 mg/dl (IQR 0.1–11.3 mg/dl) ADA: 1.0 mg/dl (IQR 0.0–9.0 mg/dl) |
ABT:
|
NR | |
Anti-CCP2 positive; Q4 |
ABT: 51.5 (IQR 19.0–70.0) ADA: 52 (IQR 27.0–85.0) |
ABT: 78.3 ADA: 72.5 |
ABT: 1.6 (IQR 0.0–2.9) ADA: 1.8 (IQR 0.0–2.8) |
NR | 100 |
ABT: 95.7 ADA: 100.0 |
NR | NR |
ABT: 0.9 mg/dl (IQR 0.0–13.9 mg/dl) ADA: 0.7 mg/dl (IQR 0.0–11.8 mg/dl) |
ABT:
|
NR | |
Taylor (2004)101 | All | 53.3 (SD 12.9)a | 75a | NR | Total SHS: 8.1 (11.8)a | NR | NR | 9.2 (SD 3.2)a | 31.6 mm/hour (SD 20.3 mm/hour)a | 18.9 mg/dl (SD 23.0 mg/dl)a | NR | Total CDA: 8597 pixels (SD 6644)a |
MTX | 51.4 (SD 14.0) | 67a | NR | Total SHS: 7.1 (7.8) | NR | NR | 8.8 (SD 2.7) | 35.7 mm/hour (SD 21.2) | 25.0 mg/dl (SD 25.4 mg/dl) | NR | Total CDA: 8212 pixels (SD 6479) | |
IFX and MTX | 55.2 (SD 11.8) | 83a | NR | Total SHS: 9.0 (15.9) | NR | NR | 9.5 (SD 3.8) | 27.4 mm/hour (SD 19.5 mm/hour) | 12.7 mg/dl (SD 20.7 mg/dl) | NR | Total CDA: 9072 pixels (SD 6718) |
Appendix 10 Interaction between baseline predictor and treatment figures
FIGURE 10.
Anticitrullinated protein/peptide anti-body status–treatment interaction on erosions at 1 year from Mustila et al. 106 ACPA–, anticitrullinated protein/peptide anti-body negative; ACPA+, anticitrullinated protein/peptide anti-body positive.

FIGURE 11.
Anticitrullinated protein/peptide anti-body status–treatment interaction on erosions at 2 years from Mustila et al. 106 ACPA–, anticitrullinated protein/peptide anti-body negative; ACPA+, anticitrullinated protein/peptide anti-body positive.
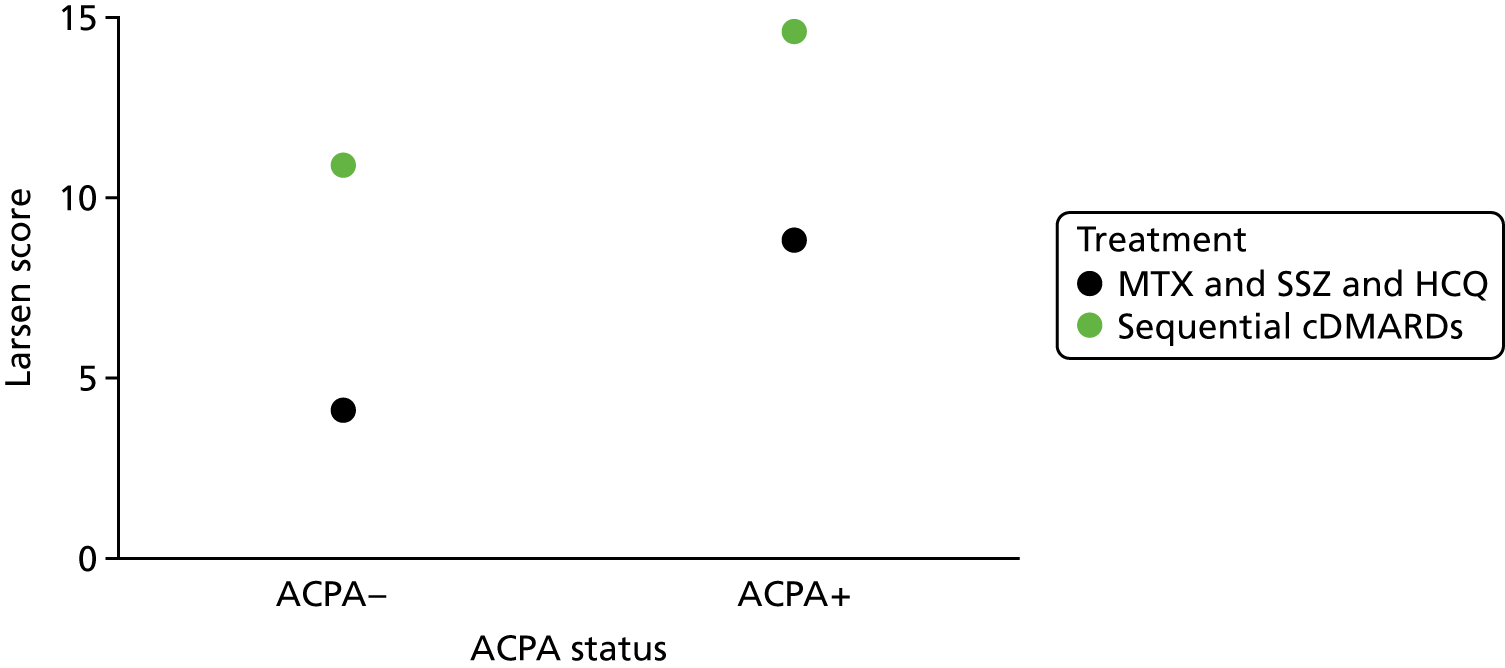
FIGURE 12.
Anticitrullinated protein/peptide anti-body status–treatment interaction on erosions at 3 years from Mustila et al. 106 ACPA–, anticitrullinated protein/peptide anti-body negative; ACPA+, anticitrullinated protein/peptide anti-body positive.

FIGURE 13.
Anticitrullinated protein/peptide anti-body status–treatment interaction on erosions at 4 years from Mustila et al. 106 ACPA–, anticitrullinated protein/peptide anti-body negative; ACPA+, anticitrullinated protein/peptide anti-body positive.

FIGURE 14.
Anticitrullinated protein/peptide anti-body status–treatment interaction on erosions at 5 years from Mustila et al. 106 ACPA–, anticitrullinated protein/peptide anti-body negative; ACPA+, anticitrullinated protein/peptide anti-body positive.
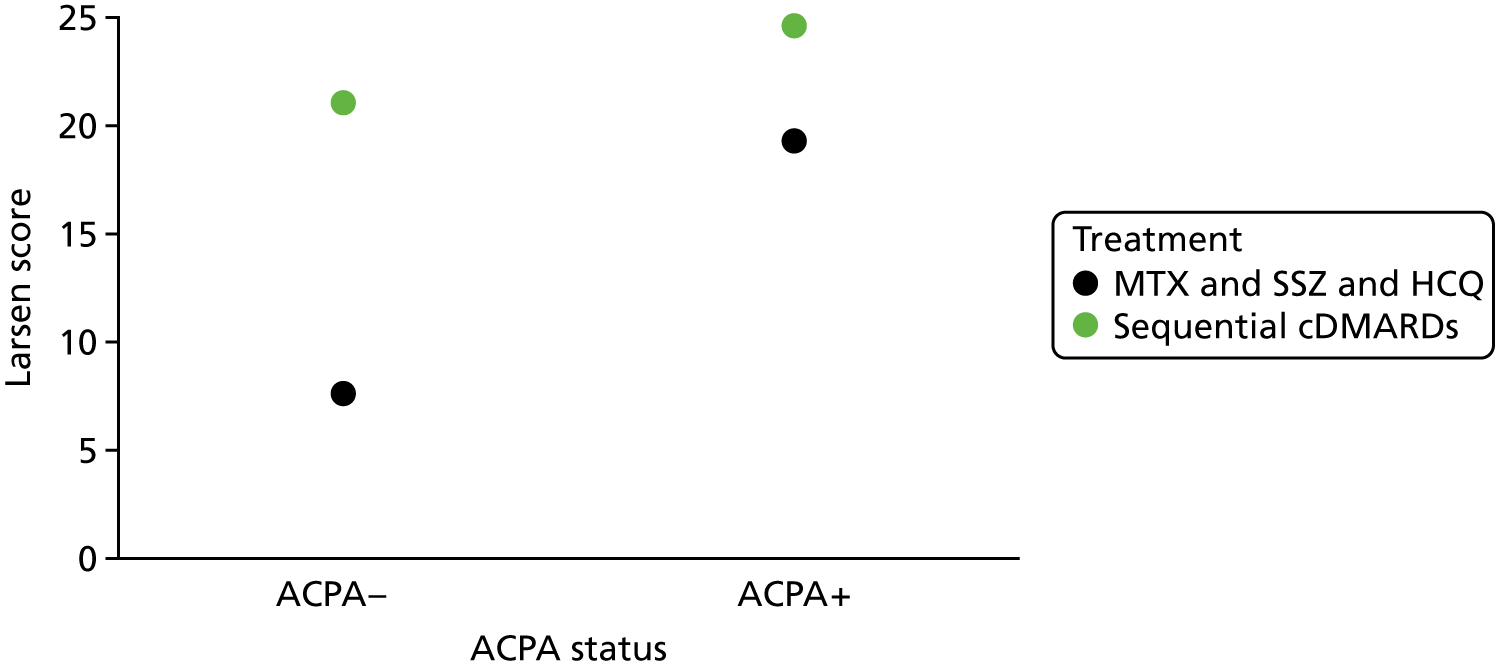
FIGURE 15.
Anticitrullinated protein/peptide anti-body status–treatment interaction on erosions at 6 months from Seegobin et al. 109 ACPA–, anticitrullinated protein/peptide anti-body negative; ACPA+, anticitrullinated protein/peptide anti-body positive.

FIGURE 16.
Anticitrullinated protein/peptide anti-body status–treatment interaction on erosions at 12 months from Seegobin et al. 109 ACPA–, anticitrullinated protein/peptide anti-body negative; ACPA+, anticitrullinated protein/peptide anti-body positive.

FIGURE 17.
Anticitrullinated protein/peptide anti-body status–treatment interaction on erosions at 18 months from Seegobin et al. 109 ACPA–, anticitrullinated protein/peptide anti-body negative; ACPA+, anticitrullinated protein/peptide anti-body positive.
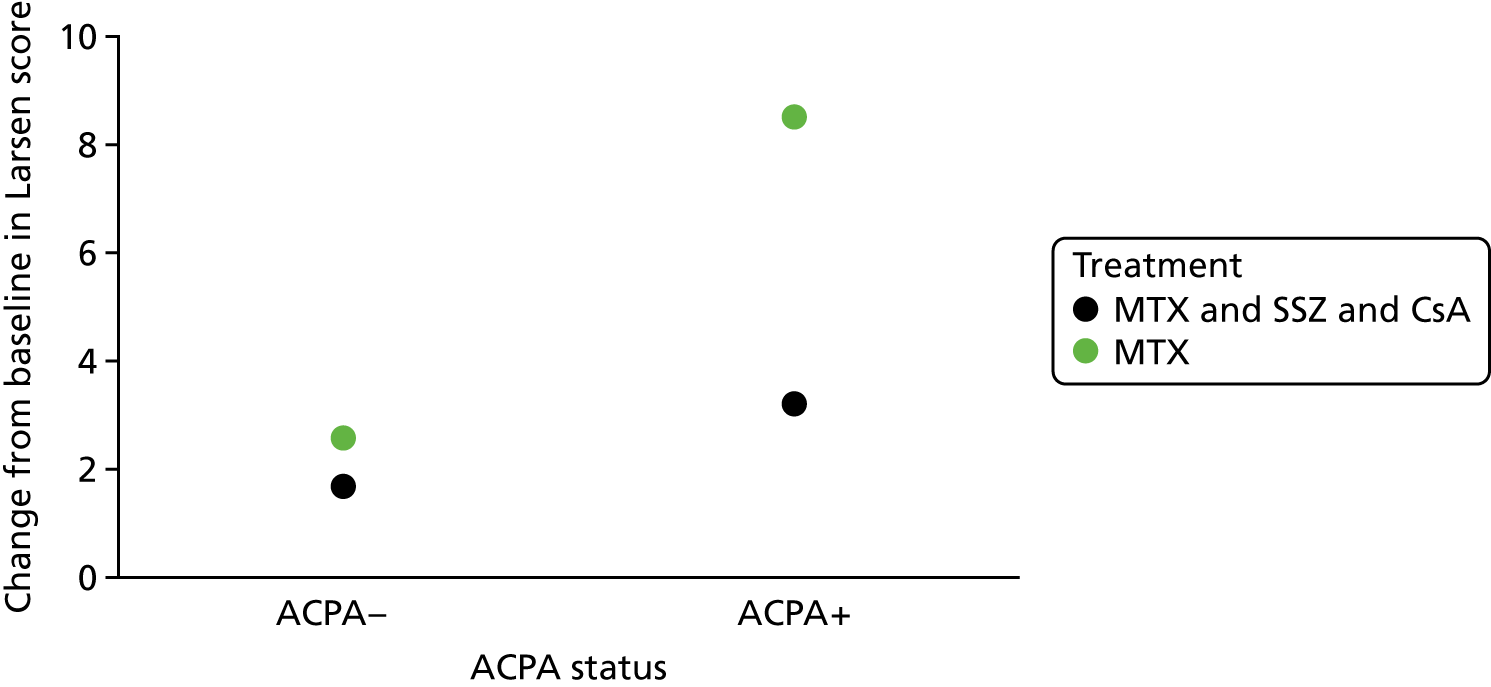
FIGURE 18.
Anticitrullinated protein/peptide anti-body status–treatment interaction on erosions at 24 months from Seegobin et al. 109 ACPA–, anticitrullinated protein/peptide anti-body negative; ACPA+, anticitrullinated protein/peptide anti-body positive.

FIGURE 19.
Anticitrullinated protein/peptide anti-body status–treatment interaction on erosions at 6 months (CsA vs. placebo) from Seegobin et al. 109 ACPA–, anticitrullinated protein/peptide anti-body negative; ACPA+, anticitrullinated protein/peptide anti-body positive.

FIGURE 20.
Anticitrullinated protein/peptide anti-body status–treatment interaction on erosions at 12 months (CsA vs. placebo) from Seegobin et al. 109 ACPA–, anticitrullinated protein/peptide anti-body negative; ACPA+, anticitrullinated protein/peptide anti-body positive.

FIGURE 21.
Anticitrullinated protein/peptide anti-body status–treatment interaction on erosions at 18 months (CsA vs. placebo) from Seegobin et al. 109 ACPA–, anticitrullinated protein/peptide anti-body negative; ACPA+, anticitrullinated protein/peptide anti-body positive.

FIGURE 22.
Anticitrullinated protein/peptide anti-body status–treatment interaction on erosions at 24 months (CsA vs. placebo) from Seegobin et al. 109 ACPA–, anticitrullinated protein/peptide anti-body negative; ACPA+, anticitrullinated protein/peptide anti-body positive.

FIGURE 23.
Anticitrullinated protein/peptide anti-body status–treatment interaction on disease activity at 6 months from Seegobin et al. 109 ACPA–, anticitrullinated protein/peptide anti-body negative; ACPA+, anticitrullinated protein/peptide anti-body positive.

FIGURE 24.
Anticitrullinated protein/peptide anti-body status–treatment interaction on disease activity at 12 months from Seegobin et al. 109 ACPA–, anticitrullinated protein/peptide anti-body negative; ACPA+, anticitrullinated protein/peptide anti-body positive.
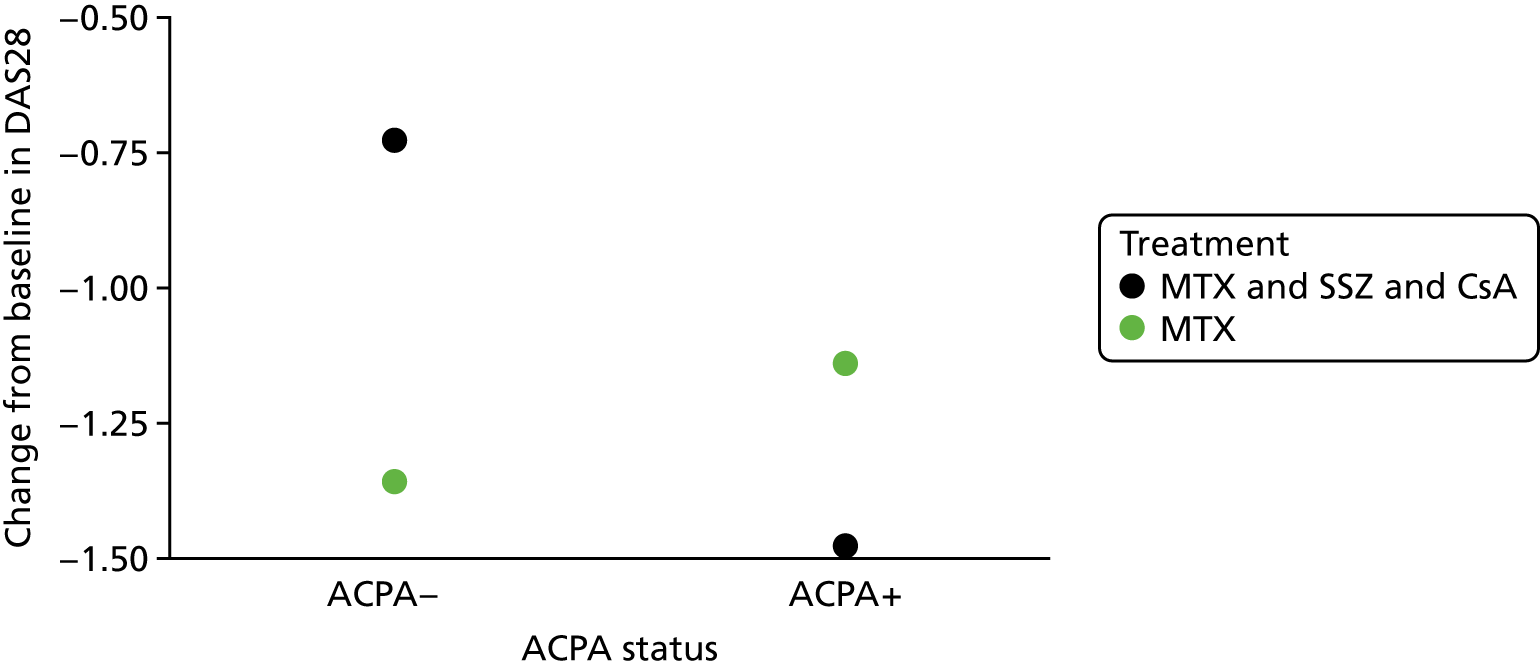
FIGURE 25.
Anticitrullinated protein/peptide anti-body status–treatment interaction on disease activity at 18 months from Seegobin et al. 109 ACPA–, anticitrullinated protein/peptide anti-body negative; ACPA+, anticitrullinated protein/peptide anti-body positive.

FIGURE 26.
Anticitrullinated protein/peptide anti-body status–treatment interaction on disease activity at 24 months from Seegobin et al. 109 ACPA–, anticitrullinated protein/peptide anti-body negative; ACPA+, anticitrullinated protein/peptide anti-body positive.

FIGURE 27.
Anticitrullinated protein/peptide anti-body status–treatment interaction on disease activity at 6 months (CsA vs. placebo) from Seegobin et al. 109 ACPA–, anticitrullinated protein/peptide anti-body negative; ACPA+, anticitrullinated protein/peptide anti-body positive.

FIGURE 28.
Anticitrullinated protein/peptide anti-body status–treatment interaction on disease activity at 12 months (CsA vs. placebo) from Seegobin et al. 109 ACPA–, anticitrullinated protein/peptide anti-body negative; ACPA+, anticitrullinated protein/peptide anti-body positive.

FIGURE 29.
Anticitrullinated protein/peptide anti-body status–treatment interaction on disease activity at 18 months (CsA vs. placebo) from Seegobin et al. 109 ACPA–, anticitrullinated protein/peptide anti-body negative; ACPA+, anticitrullinated protein/peptide anti-body positive.

FIGURE 30.
Anticitrullinated protein/peptide anti-body status–treatment interaction on disease activity at 24 months (CsA vs. placebo) from Seegobin et al. 109 ACPA–, anticitrullinated protein/peptide anti-body negative; ACPA+, anticitrullinated protein/peptide anti-body positive.

FIGURE 31.
Anticitrullinated protein/peptide anti-body status–treatment interaction on disease activity at 6 months from Sokolove et al. 110 ACPA–, anticitrullinated protein/peptide anti-body negative; ACPA+, anticitrullinated protein/peptide anti-body positive; Q, quartile; s.c., subcutaneous.

FIGURE 32.
Anticitrullinated protein/peptide anti-body status–treatment interaction on disease activity at 1 year from Sokolove et al. 110 ACPA–, anticitrullinated protein/peptide anti-body negative; ACPA+, anticitrullinated protein/peptide anti-body positive; Q, quartile; s.c., subcutaneous.

FIGURE 33.
Anticitrullinated protein/peptide anti-body status–treatment interaction on disease activity at 2 years from Sokolove et al. 110 ACPA–, anticitrullinated protein/peptide anti-body negative; ACPA+, anticitrullinated protein/peptide anti-body positive; Q, quartile; s.c., subcutaneous.
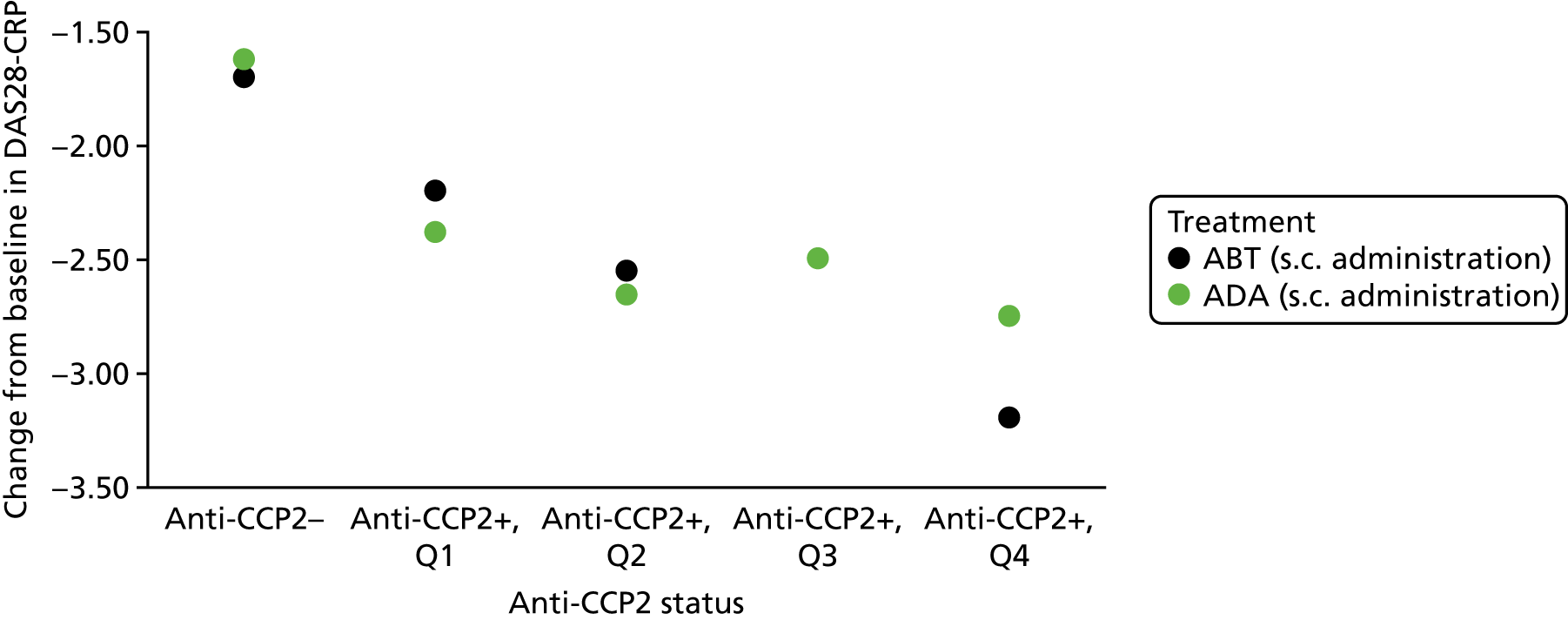
FIGURE 34.
Anticitrullinated protein/peptide anti-body status–treatment interaction on physical function at 6 months from Sokolove et al. 110 ACPA–, anticitrullinated protein/peptide anti-body negative; ACPA+, anticitrullinated protein/peptide anti-body positive; Q, quartile; s.c., subcutaneous.

FIGURE 35.
Anticitrullinated protein/peptide anti-body status–treatment interaction on physical function at 1 year from Sokolove et al. 110 ACPA–, anticitrullinated protein/peptide anti-body negative; ACPA+, anticitrullinated protein/peptide anti-body positive; Q, quartile; s.c., subcutaneous.

FIGURE 36.
Anticitrullinated protein/peptide anti-body status–treatment interaction on physical function at 2 years from Sokolove et al. 110 ACPA–, anticitrullinated protein/peptide anti-body negative; ACPA+, anticitrullinated protein/peptide anti-body positive; Q, quartile; s.c., subcutaneous.

FIGURE 37.
Smoking status–treatment interaction on disease activity at 1–2 years from Maska et al. 105

FIGURE 38.
Smoking status–treatment interaction on disease activity at 24 weeks from Maska et al. 105

FIGURE 39.
Smoking status–treatment interaction on disease activity at 48 weeks from Maska et al. 105

FIGURE 40.
Smoking status–treatment interaction on disease activity at 102 weeks from Maska et al. 105

FIGURE 41.
Erosion status–treatment interaction on radiographic progression (progression in the eroded joint count) at 12 months from Pasero et al. 107 EJC, eroded joint count.

FIGURE 42.
Erosion status–treatment interaction on radiographic progression (progression in the damage score) at 12 months from Pasero et al. 107
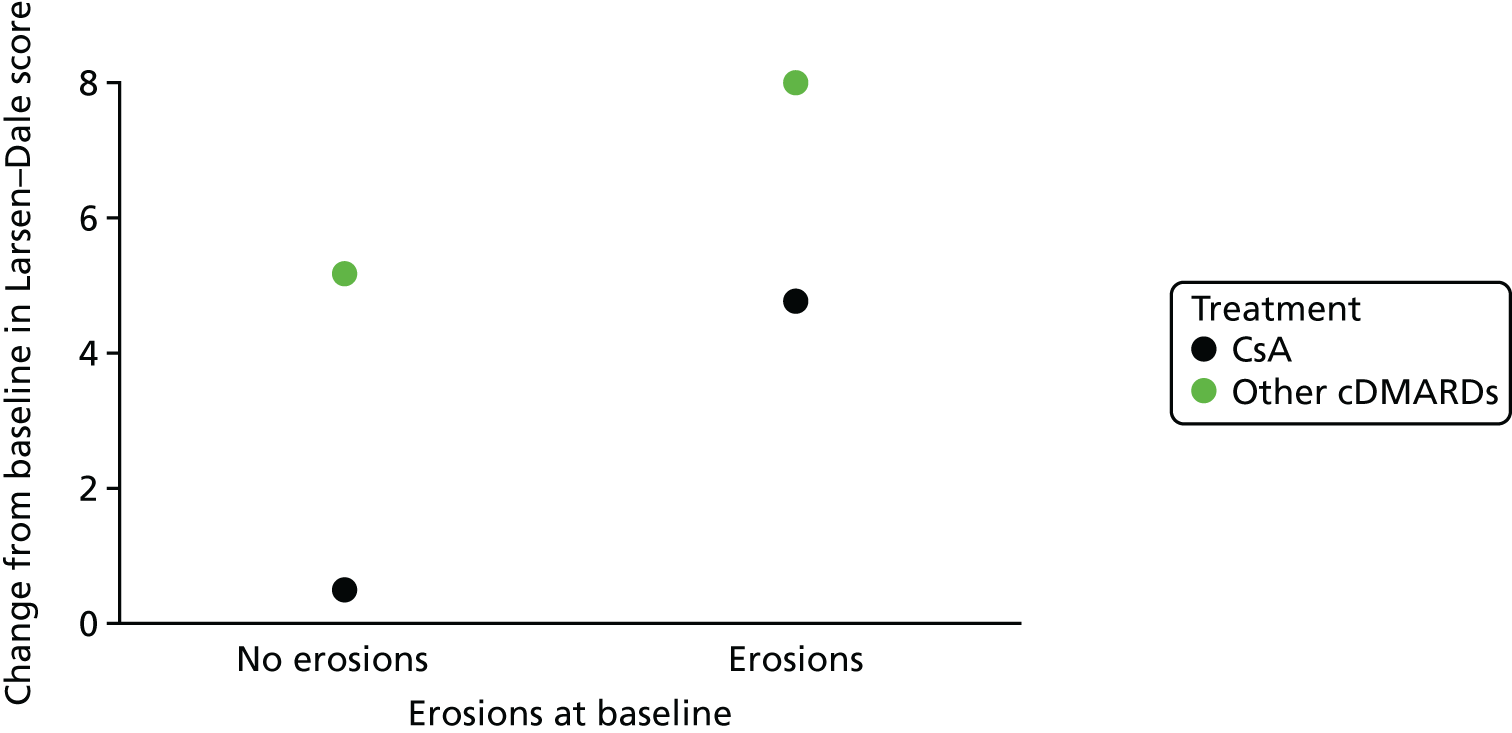
FIGURE 43.
Radiographic score tertile–treatment interaction on radiographic progression at 54 weeks from Smolen et al. 100

FIGURE 44.
C-reactive protein tertile–treatment interaction on radiographic progression at 54 weeks from Smolen et al. 100

FIGURE 45.
Erythrocyte sedimentation rate tertile–treatment interaction on radiographic progression at 54 weeks from Smolen et al. 100

Glossary
- Accuracy
- The degree to which a measurement or estimate agrees with the true value.
- Apparent validation
- A form of internal validation using exactly the same population as that used for the model development.
- Area under the curve
- An alternative term used to describe the c-statistic. In a logistic regression analysis, the c-statistic can be interpreted as the area under the receiver operating characteristic curve.
- Calibration
- This describes the extent to which the expected outcomes (predicted by the model) and observed outcomes agree.
- Clinical prediction model
- A formal combination of multiple predictors, from which the probability of occurrence of a specific clinical outcome can be estimated for individual patients. Commonly developed to aid health-care providers in their decision-making.
- Correlation
- A measure of the statistical relationship, whether or not causal, between two random variables.
- Cross-validation
- A statistical technique commonly used for the internal validation of a clinical prediction model, which aims to limit overfitting. As with data splitting, separate partitions of the data set are used for deriving the model and evaluating the model performance. Cross-validation extends the simple data-splitting approach by iterating the procedure over several partitions of the data set. The performance results are averaged to provide an overall measure.
- Data splitting
- A statistical technique commonly used for the internal validation of a clinical prediction model, which aims to limit overfitting. Data are divided into a training data set (used to derive the model) and a validation data set that is used to evaluate the model’s performance.
- Discrimination
- Describes the ability of the clinical prediction model to distinguish between individuals who do and do not experience the outcome of interest.
- Effect modifier
- A covariate that alters the relative effect of treatments on outcomes, so that the treatment is more or less effective in different subgroups formed by levels of the effect modifier. Effect modifiers are not necessarily also prognostic variables. The effect modifier status is specific to a given scale: the positive status of a covariate as an effect modifier on one scale does not necessarily imply, either positively or negatively, an effect modifier status on another scale; however, a covariate that is not an effect modifier on one scale is guaranteed to be an effect modifier on another.
- External validation
- This is used to quantify the performance of a clinical prediction model in a population that is external to that which was used for the model development.
- Interaction
- An interaction arises between two or more variables when their joint impact on a dependent variable is greater than the sum of their individual contributions.
- Internal validation
- Used to quantify the performance of a clinical prediction model in the population used to develop the model.
- Logistic regression
- A statistical technique used to model the relationship between a binary outcome variable and one or more covariates of interest.
- Main effect
- The effect of an independent variable on a dependent variable averaging across the levels of any other independent variables.
- Meta-analysis
- A statistical method by which the results of a number of studies are aggregated to provide a combined summary statistic.
- Negative predictive value
- For individuals who are not expected to experience the outcome of interest (as predicted by the model), the proportion who do not experience the outcome.
- Overfitting
- Occurs when a model is optimally designed to fit the development data set and performs less favourably in more general situations.
- Positive predictive value
- For individuals who are expected to experience the outcome of interest (as predicted by the model), the proportion who experience the outcome.
- Prognostic variable
- A covariate that affects (or is prognostic of) the outcome. We make the distinction between prognostic variables and effect modifiers; effect modifiers are not necessarily also prognostic variables.
- R2
- A measurement of overall model performance that describes the proportion of explained variation.
- Sensitivity
- For individuals who are known to experience the outcome of interest, the proportion that were correctly predicted (using the clinical prediction model) to experience the outcome.
- Shrinkage
- A statistical estimation procedure that preshrinks regression coefficients towards zero, so that the clinical prediction model will not need to be recalibrated in a new data set. Also linked to the shrinkage factor of a calibration plot, which will be less than one when a clinical prediction model is applied to a new data set, if overfitting has occurred during the model development.
- Specificity
- For individuals who are known not to experience the outcome of interest, this is the proportion that was correctly predicted (using the clinical prediction model) not to experience the outcome.
List of abbreviations
- ABT
- abatacept
- ACPA
- anticitrullinated protein/peptide anti-body
- ACR
- American College of Rheumatology
- ADA
- adalimumab
- ADL
- Activities of Daily Living
- AhFibA
- anti-human citrullinated fibrinogen anti-body
- ANOVA
- analysis of variance
- anti-CCP
- anticyclic citrullinated peptide
- anti-MCV
- antimutated citrullinated vimentin
- ASPIRE
- Active controlled Study of Patients receiving Infliximab for the treatment of Rheumatoid arthritis of Early onset
- ATTRACT
- anti-TNF trial in rheumatoid arthritis with concomitant therapy
- AUC
- area under the curve
- bDMARD
- biologic disease-modifying antirheumatic drug
- BeSt
- Behandel Strategieen
- BMI
- body mass index
- cDMARD
- conventional disease-modifying antirheumatic drug
- CG79
- Clinical Guidance number 79
- CHARMS
- CHecklist for critical Appraisal and data extraction for systematic Reviews of prediction Modelling Studies
- CI
- confidence interval
- CRP
- C-reactive protein
- CsA
- ciclosporin A
- DARE
- Database of Abstracts of Reviews of Effects
- DAS
- Disease Activity Score
- DAS28
- Disease Activity Score-28
- DMARD
- disease-modifying antirheumatic drug
- ERO
- erosive
- ESPOIR
- Étude et suivi des polyarthrites indifférenciées récentes
- ESR
- erythrocyte sedimentation rate
- ETN
- etanercept
- EULAR
- European League Against Rheumatism
- FE
- fixed effects
- GSTM
- gold sodium thiomalate
- HAQ
- Health Assessment Questionnaire
- HAQ-DI
- Health Assessment Questionnaire – disability index
- HCQ
- hydroxychloroquine
- HLA
- human leucocyte antigen
- HTA
- Health Technology Assessment
- IFX
- infliximab
- IMPROVED
- Induction therapy with MTX and Prednisone in Rheumatoid Or Very Early arthritis Disease
- IPD
- individual participant data
- IQR
- interquartile range
- LEF
- leflunomide
- MBDA
- multibiomarker disease activity
- MHAQ
- modified Health Assessment Questionnaire
- MMP
- matrix metalloproteinase
- MTX
- methotrexate
- NHS EED
- NHS Economic Evaluation Database
- NICE
- National Institute for Health and Care Excellence
- NIHR
- National Institute for Health Research
- NPV
- negative predictive value
- NSAID
- non-steroidal anti-inflammatory drug
- O : E
- observed to expected (ratio)
- OR
- odds ratio
- PDUS
- power Doppler ultrasound
- PPV
- positive predictive value
- PR
- predicted risk
- PRISMA
- Preferred Reporting Items for Systematic Reviews and Meta-Analyses
- PROBAST
- Prediction model study Risk Of Bias Assessment Tool
- PROGRESS
- PROGnosis RESearch Strategy
- QUIPS
- Quality in Prognosis Studies
- RA
- rheumatoid arthritis
- RCT
- randomised controlled trial
- RE
- random effects
- RF
- rheumatoid factor
- RRP
- rapid radiographic progression
- ScHARR
- School of Health and Related Research
- SD
- standard deviation
- SE
- shared epitope
- SHS
- Sharp–van der Heijde score
- SJC
- swollen joint count
- SJC28
- 28 swollen joint count
- SONORA
- Study Of New-Onset Rheumatoid Arthritis
- SSZ
- sulfasalazine
- SWEFOT
- Swedish Farmacotherapy
- TAR
- Technology Assessment Review
- TNF
- tumour necrosis factor
- TRIPOD
- Transparent Reporting of a multivariable prediction model for Individual Prognosis Or Diagnosis
- TSS
- total Sharp score
- TTT
- treat to target