Notes
Article history
The research reported in this issue of the journal was funded by the HTA programme as project number 11/63/01. The contractual start date was in November 2016. The draft report began editorial review in February 2017 and was accepted for publication in April 2018. The authors have been wholly responsible for all data collection, analysis and interpretation, and for writing up their work. The HTA editors and publisher have tried to ensure the accuracy of the authors’ report and would like to thank the reviewers for their constructive comments on the draft document. However, they do not accept liability for damages or losses arising from material published in this report.
Declared competing interests of authors
Andrew Price reports personal fees from Zimmer Biomet, DePuy Synthes and Smith & Nephew plc, and grants from the National Institute for Health Research (NIHR) and Arthritis Research UK, outside the submitted work. Alastair Gray reports grants from NIHR, during the conduct of the study. Rachael Gooberman-Hill reports grants from the NIHR Health Services and Delivery Research programme for this work during the conduct of the study. Helen Dakin reports grants from NIHR during the conduct of the study and consultancy for Halyard Health outside the submitted work. David Beard reports grants from NIHR outside the submitted work. Jonathan Cook was a member of the NIHR Health Technology Assessment (HTA) Efficient Trial Designs Board (2014–16). Jill Dawson reports grants from the NIHR HTA programme during the conduct of the study and royalty payments from Oxford University Innovation (a university technology transfer company) outside the submitted work, and is one of the original developers of the Oxford Hip and Knee Scores. Raymond Fitzpatrick is one of the developers of the Oxford Hip and Knee Scores. Ashley Blom is the principal investigator in a research project funded by Stryker Corporation. Andrew Judge reports personal fees for consultancy from Anthera Pharmaceuticals, Inc., and Freshfields Bruckhaus Deringer LLP, outside the submitted work. Nigel Arden reports grants from Bioibérica and Novartis Pharmaceuticals UK Ltd, and personal fees from Bioventus, Flexion Therapeutics, Freshfields Bruckhaus Deringer LLP, Janssen Pharmaceutica, Merck & Co. Inc. and Regeneron Pharmaceuticals, Inc., outside the submitted work. David Murray reports grants from the NIHR HTA programme and grants and personal fees from Zimmer Biomet outside the submitted work.
Permissions
Copyright statement
© Queen’s Printer and Controller of HMSO 2019. This work was produced by Price et al. under the terms of a commissioning contract issued by the Secretary of State for Health and Social Care. This issue may be freely reproduced for the purposes of private research and study and extracts (or indeed, the full report) may be included in professional journals provided that suitable acknowledgement is made and the reproduction is not associated with any form of advertising. Applications for commercial reproduction should be addressed to: NIHR Journals Library, National Institute for Health Research, Evaluation, Trials and Studies Coordinating Centre, Alpha House, University of Southampton Science Park, Southampton SO16 7NS, UK.
2019 Queen’s Printer and Controller of HMSO
Chapter 1 Introduction
Research questions specified in the National Institute for Health Research Health Technology Assessment research call
-
Can clinical tools for assessment of a patient’s suitability for knee or hip replacement be used to set thresholds for an operation?
-
How does the choice of threshold affect the cost-effectiveness of the procedure and subsequent improvements in patient quality of life?
Background
Hip and knee osteoarthritis is a common musculoskeletal condition causing significant pain and loss of function for patients. Using patient-reported outcome measures (PROMs), joint replacement treatment for end-stage disease has been shown to be an effective treatment. 1 Each year, 150,000 hip and knee replacements are carried out in the UK, with the majority of patients having successful outcomes. 2 However, the nationally collected patient-reported outcome data for hip and knee replacements have identified two striking issues with regard to the provision of joint replacement in the UK. First, there is marked variation in current clinical practice in referring and undertaking surgery in patients with arthritis of the hip and knee. 3 Previous studies from the UK support this observation, with recent evidence showing that access to joint replacement is currently inequitable, with deprived areas associated with greater symptom severity and lower surgery rates. 4–6 A previous large national survey of UK NHS patients undergoing joint surgery7 also concluded that there was no evidence that patients were being prioritised on the basis of the severity of their symptoms and function. Second, the national outcomes data have revealed that 10–15% of patients undergoing hip or knee joint replacement are not satisfied with their treatment, and these findings, particularly for the knee patients, are supported by other recent studies. 8,9 It has been suggested that selecting patients too early in their disease process may play a role in producing dissatisfaction with surgery. 9 Overall, these findings suggest that there is no standardisation to the process by which patients are assessed and selected for hip and knee replacement surgery. This is a particular concern given both the projected increased need for joint replacement over the next decade to accommodate an ageing population and the pressure of potential reductions in NHS funding. 10
Assessing patients for joint replacement surgery within the NHS is generally a two-stage process that begins with the patient presenting to a general practitioner (GP) with hip or knee pain (Figure 1). The assessment process usually takes place over a number of consultations, often including an radiography to confirm osteoarthritis. The GP continues to monitor symptoms and responses to non-operative treatments, eventually deciding when the patient is a candidate for joint replacement surgery, and at this point the patient is referred to secondary care. Currently, there are no widely accepted guidelines within the NHS specifically to help health professionals estimate the level of patient symptoms that warrants referral. 11 In the secondary care setting, the specialist assesses the patient, confirms the diagnosis and radiographic severity, reviews a patient’s symptoms and shares information with them about available treatment options. For each patient, the decision to offer surgery requires a personalised assessment of individual preferences, expectations, functional limitations and requirements, degree of radiographic pathology, comorbidities and predicted outcome. 11 Ideally, the patient and their specialist then make a shared decision, with the patient ultimately deciding whether or not to undergo surgery. We estimate that there are around 1 million GP patient assessments for hip and knee pain each year, with around one-quarter of patients who present being referred to a specialist. Although only a small proportion of these patients receive joint replacement, this amounts to 150,000 procedures a year. 12,13 At the Nuffield Orthopaedic Centre (NOC), our data suggest that approximately 15% of patients who are referred from primary care for possible joint replacement do not have symptoms at a level that requires joint replacement and could have been safely managed without an appointment in secondary care.
FIGURE 1.
Patient pathway framework for the NHS to identify candidates for hip and knee replacement. The selected assessment score may also be used later in the pathway to measure the outcome of surgery and to offer surveillance for joint replacement post surgery. THA, total hip arthroplasty; TKA, total knee arthroplasty.

Given the issues of unwarranted variation and poor outcome in some patients, outlined above, there has been significant interest in trying to standardise the process of referral and selection for joint replacement. The use of certain ‘priority criteria’ (such as the Western Canada Waiting List score,14 the Ontario criteria15 or the New Zealand score16) has been investigated as a more consistent method of selecting patients for referral and treatment. These tools identify candidates for surgery in primary care and are based on estimating a patient’s capacity to benefit from surgery. They are generic and attempt to standardise the patient pathway for joint replacement at the entry point. The New Zealand priority criteria16 have been used in some regions within the NHS but have not reached widespread acceptance, and the current evidence of their reliability and validity is minimal. 17,18 Other tools have been developed but not fully tested in clinical practice within the UK. 19–21 The Osteoarthritis Research Society International (OARSI) Standing Committee for Clinical Trials Response Criteria Initiative and the Outcome Measures in Rheumatology (OMERACT) international initiative has attempted to deliver a standardised approach and has highlighted pain and disability as among the key domains for identifying the capacity to benefit. 22–25 It has therefore been a logical progression to investigate if existing assessment tools used in the joint replacement pathway, that measure pain and disability, could be used as a single score to identify candidates for surgery by referring their preoperative assessment score to a threshold for intervention.
In 2009, the Department of Health and Social Care introduced the routine collection of PROMs for hip and knee surgery to measure the outcome of surgery undertaken in NHS hospitals. 8,26 There has been government support for extending the use of scoring systems preoperatively to create thresholds for referral and candidacy for surgery. 27,28 In fact, many primary care trusts and NHS trusts have already introduced PROM-based severity score thresholds for surgery, although the thresholds used vary widely between regions. 29–36 However, evidence underpinning and endorsing the use of PROMs or any assessment score for thresholds is scant and without validation. This poses a significant risk to patients as an incorrectly set threshold may unfairly restrict access to care or, conversely, inappropriately select patients for joint replacement. 2 The development of a preoperative threshold score to identify candidates for hip and knee replacement offers a significant opportunity to standardise the patient pathway. However, this Health Technology Assessment (HTA) call reflects the pressing need within the NHS to produce evidence to support or refute their use.
A number of scoring systems are used to assess the patients in their care pathway. Many are PROM based, such as the Intermittent and Constant Osteoarthritis Pain Measure (ICOAP),37 the EuroQol-5 Dimensions (EQ-5D) and the Oxford Hip and Knee Scores,38–40 whereas others require a clinician’s involvement (e.g. the New Zealand score16). Some systems were designed to measure the burden of osteoarthritis symptoms [e.g. the Western Ontario and McMaster Universities Arthritis Index (WOMAC®) or ICOAP],41 whereas others were designed to measure the effect of an intervention (e.g. Oxford Hip and Knee Scores). Some scores were produced to measure more general aspects of health status [Short Form questionnaire-36 items (SF-36) or EQ-5D]42 and others aim to prioritise patients for surgery (e.g. the New Zealand priority criteria16). None of these scores has been developed for the specific role of applying thresholds for access to care for joint replacement within the setting of the NHS. It may be that one or more of these scores may be appropriate for such use but evidence is required to validate and justify this role.
To be fit for purpose as a screening device, any candidate score must satisfy a number of requirements.
First, the score must have adequate measurement properties to enable assessment of patients for joint replacement, namely adequate validity. This includes evidence of adequate reliability at an individual level (test–retest and intraclass correlation coefficient), precision [standard error (SE) of the measurement] and responsiveness to change [smallest detectable change and minimally clinically important difference (MCID)]. The effect of comorbidity on the score must also be established.
Second, valid evidence-based thresholds must be produced. The calculation of thresholds is not straightforward, with several different methods available. To generate upper thresholds (i.e. least severity) in preintervention scores, above which patients should not be considered candidates for arthroplasty, methods must account for the likelihood of a patient’s capacity to benefit (i.e. likelihood of achieving a positive change score) and perceive satisfactory improvement following surgery. 43 The measurement properties of the instrument (as described previously), such as the MCID (i.e. the smallest amount of change in a score that patients detect and consider important) and standard error of the mean (SEM) (which relates to the reliability of the instrument and denotes the amount of change that is ‘real’ and beyond measurement error), are also important operational considerations when calculating thresholds. Furthermore, any chosen threshold must distinguish between cases (patients in need of surgery) and non-cases with a consistent level of diagnostic accuracy (discriminative ability).
The process of calculating absolute thresholds will also produce additional and valuable information for patients who are found to be candidates for surgery. By highlighting an individual’s ‘chance’ of benefit following surgery (based on their preoperative score), patients are provided with key information to help with their decision-making, particularly in secondary care. It would provide evidence to support the use of a score embedded within the NHS direct knee/hip osteoarthritis decision aid. Hence, clearly highlighting the risks and benefits may make the decision to have surgery clearer for many patients. This type of information allows patients to more comprehensively participate in the decisions made about their care.
Third, we must understand how the introduction of thresholds for surgery affects the cost-effectiveness of the treatment. Lower-limb joint replacement has previously been shown to be highly cost-effective, costing between €1276 and €18,300 per quality-adjusted life-year (QALY) gained for the average patient,44–48 which is substantially lower than the £20,000–30,000 per QALY range that the National Institute for Health and Care Excellence (NICE) considers to be cost-effective for use within the NHS. 49 However, it is important for commissioners of hip and knee replacement surgery to understand how cost-effectiveness varies between patient and procedure subgroups, and how thresholds for hip and knee surgery affect the cost utility of the interventions. We have recently demonstrated the feasibility of this approach in a pilot study exploring the relationship between costs and improvements in EQ-5D utility and preoperative PROM scores in total knee arthroplasty (TKA). 50
Finally, having identified and validated a clinical tool and calculated valid and evidence-based thresholds for surgery, within the NHS, it must be established whether or not the tools are acceptable to the ‘end-users’. Despite some thresholds for hip and knee replacement having already been introduced to clinical practice in parts of the country, there has been little or no engagement with the wider stakeholders about the appropriateness of this approach or how thresholds should be used in practice. The introduction of thresholds requires the support of patients, health-care professionals and commissioners.
Although the requirements of threshold scores in primary and secondary care may differ, in order to provide consistency for patients and health-care professionals any scoring system would ideally be applicable to both sectors. One aim is to ensure this compatibility by consciously considering the requirements within each setting. In primary care, the requirement is for a simple-to-use patient-based score linked to the patient’s potential to benefit from arthroplasty. This would provide a distinct upper threshold for referral and candidacy for joint replacement. The thresholds calculated for the identified scoring system will be incorporated into a user-friendly knee and hip replacement candidacy assessment tool – the Arthroplasty Candidacy Help Engine (ACHE) tool. Secondary care involves more complex assessments, involving expectation, comorbidity and age-related factors. The ACHE tool would be a starting point for secondary care assessment, linking to other patient decision-support tools. 51,52
In summary, greater standardisation is required in the patient pathway leading to hip or knee joint replacement surgery. The aim of this study is to develop an evidence-based method for identifying patients in primary care who are possible candidates for surgery, using valid thresholds applied to scoring systems that are already available (see Figure 1).
Research objectives
The following research objectives will be met:
-
Create a shortlist of scoring systems that are potentially useful for selecting candidates for arthroplasty surgery.
-
From the literature, establish the scores/instruments available. Published evidence concerning their measurement properties, and their past or projected use in setting thresholds for hip and knee replacement, will be reviewed. This will generate a shortlist of potential scoring systems.
-
Using existing data sets and guidance from users, refine the shortlist by establishing the necessary measurement properties of potential scores/instruments when not available in the literature.
-
-
Identify a scoring system, and a set of threshold values, to be used to select candidates for hip and knee surgery.
-
For each shortlisted instrument, determine score thresholds for candidacy for joint replacement surgery.
-
Determine the relationship between threshold levels and cost-effectiveness of hip and knee arthroplasty surgery.
-
Select the most applicable single score and set of thresholds for incorporation into the ACHE tool.
-
-
Explore the clinical effectiveness and cost-effectiveness of the ACHE tool and determine the potential acceptability of the tool and thresholds to stakeholders and patients.
-
Determine the effect of using the ACHE tool on patterns of referral of hip and knee patients to secondary care.
-
Evaluate user opinion – GPs and patients.
-
Engage with a wider stakeholder group to assess the acceptability of the ACHE tool.
-
The overall aim was to develop a standardised NHS framework for identifying patients for hip and knee replacement surgery using safe and equitable thresholds. This was achieved by creating the ACHE tool, based on a currently available assessment score, with thresholds that take account of patients’ capacity to benefit from surgery and the cost-effectiveness of the treatment. The new system is applicable in both primary and secondary care.
The user group
From the outset of the original design for this project, all service users’ perspectives were considered integral to its success. The ACHE tool was to be designed to help patients, GPs, secondary care personnel, arthroplasty specialists and health-care commissioning staff. It was therefore decided very early on to utilise a ‘user group’ concept, in which representatives of these identified bodies inputted, critiqued and reviewed the progress of the study at appropriate intervals. Importantly, the user group was established as a proactive entity rather than as a passive and disengaged review and agreement exercise. The decisions of the group were critical to the direction of the project and were instrumental in sanctioning various aspects/decisions and vetoing others. The user group was given full autonomy under the direction of the chairperson. Investigators of the study were allowed to be present but did not participate in the meeting unless invited by the chairperson for clarification purposes only. As a result, the final ACHE tool did not reflect the wishes of the researchers, but the group for whom the instrument was designed.
The user group was assembled and provided input at regular and preset intervals during the course of the study. The sequence was predetermined and the role dovetailed with each stage of the project (Figure 2). One option for this report was to describe user group input in separate sections (chapters) in sequence and inserted within the main report at the appropriate temporal intervals. However, some meetings were introductory or had limited remit and do not contain sufficient content to justify separate chapters. A decision was made to report all user group activity in a single separate chapter (see Chapter 10). Readers of the report are required to cross-reference this section and appreciate that user group input took place for each academic section.
FIGURE 2.
Work plan schema highlighting the user group meetings and input. HES, Hospital Episode Statistics; NJR, National Joint Registry; WP, work package.

Chapter 2 Systematic review of existing measures (work package 1)
Background
The aim of this study was to develop an evidence-based system for identifying patients who might be candidates for hip or knee replacement surgery, introducing valid thresholds based on scores that are already available. The first objective in achieving this aim was to create a shortlist of scoring systems that could be used in this way. After discussion within the user group (see Chapter 10), it was established that candidate scores placed on the shortlist would need to meet certain essential criteria:
-
A score must be a patient-reported measure to ensure that patients were engaged in the assessment process and that the score used reflected their perspective on the outcome.
-
A score must demonstrate adequate measurement properties and have been validated within the hip and knee replacement populations. 53–58
Many different scoring systems and outcome measures have been used for assessing the outcomes of hip or knee arthroplasty, but not all measures have evidence of, or reach, even the minimum psychometric standards for their proposed uses. 1,59–61 Therefore, the aims of this work were to use systemic review methodology to identify and evaluate English-language versions of PROMs that have been evaluated with patients undergoing hip or knee replacement surgery and to provide a comprehensive profile of their measurement properties so that a shortlist of candidate scores could be established.
Methods
Identification of studies
The search was conducted in May 2014; it was limited to English-language articles and no time restrictions were set. MEDLINE, EMBASE, PsycINFO and the Allied and Complementary Medicine Database (AMED) were searched using an adjusted methodological filter through Ovid ProQolid, the Oxford PROMs Database, the Database of Abstracts of Reviews of Effects (DARE) and EconLit were also searched using a combination of medical subject heading and free-text terms. 62 Hand-searching of titles of the following key journals in the 6 months preceding the search was also conducted: Health and Quality of Life Outcomes, The Journal of Bone and Joint Surgery (American and British volumes) and The Journal of Arthroplasty.
Screening of articles and instruments
Titles and abstracts of all identified articles were assessed for inclusion/exclusion by two reviewers (KH and EG), with agreement assessed on a screening sample of 313 abstracts. The first round of testing yielded a 77% agreement rate and the second round yielded a 99% agreement rate between reviewers. Full texts of the articles that were to be included in the review were retrieved. Inclusion criteria were:
-
The instrument uses a standard scoring system (representing indices or scales).
-
The instrument is already available and has been used in clinical settings or research to assess adult (aged > 18 years) patients prior to hip or knee replacement.
-
The instrument has been validated for the English-language population.
-
The study design is principle development, concurrent revalidation or a prospective study of a score with information on its measurement properties (e.g. reliability, validity and responsiveness). Retrospective studies (except historical cohort studies) were excluded.
-
The sample size in the study was > 50 subjects/patients.
Titles and abstracts were obtained relating to any tools identified at this stage, and these were scrutinised using the aforementioned inclusion criteria. The same methodology was applied to full-text documents for their inclusion in the review. Selected full-text articles were then screened for all measures that were used in analyses. The aforementioned inclusion criteria were applied to the list of identified measures. Furthermore, the following exclusion criteria were applied to the initial list of measures:
-
The assessment is not patient reported and requires the patient to be assessed on each/every occasion by a clinician.
-
The assessment requires some kind of technical information or equipment [such as a magnetic resonance imaging (MRI) scan or radiographic report], which might not always be available or standardised, or which might not make sense as part of an assessment conducted at both preoperative and postoperative stages.
-
The measure is not capable of demonstrating patients’ ‘capacity to benefit’ because it was not designed to be a health status/outcome measure, and therefore cannot measure change (e.g. purely retrospective measures were excluded).
Instrument-specific search
A specific search was undertaken for each of the identified instruments, with a developmental study and then a population and validation filter applied to the list of citations stemming from the developmental study.
Data extraction
Data were extracted on the psychometric performance and operational characteristics of each PROM. Assessment and evaluation of the methodological quality of PROMs were undertaken independently by three reviewers adapting the London School of Hygiene & Tropical Medicine appraisal criteria outlined in a previous review. 1
Measurement properties assessed
Reliability was assessed by test–retest reliability and internal consistency. Test–retest reliability refers to the stability of a measuring instrument over time, assessed by administering the instrument to respondents on two different occasions and examining the correlation between test and retest scores. Internal consistency refers to the extent to which items constituting a scale measure the same construct (e.g. homogeneity of items in a scale) and is assessed by Cronbach’s alpha and item–total correlations.
Content and construct validity were assessed. Content validity relates to the extent to which the content of a scale is representative of the conceptual domain it is intended to cover and is usually assessed qualitatively during the questionnaire development phase through pretesting with patients, with patients involved in item generation. Construct validity looks at the evidence that the scale is correlated with other measures of the same or similar constructs in the hypothesised direction and is assessed on the basis of correlations between the measure and other similar measures, preferably based on an a priori hypothesis with predicted strength of correlation.
Responsiveness refers to the ability of a scale to detect significant change over time and is assessed by comparing scores before and after an intervention of known efficacy or when other evidence indicates important change on the basis of various methods including paired t-tests, effect sizes (ESs), standardised response mean values or responsiveness statistics. Ideally, evidence of responsiveness will include high correlations between the change scores of the scale and relevant constructs, preferably based on an a priori hypothesis with predicted strength of correlation.
Interpretability relates to the degree to which one can assign qualitative meaning – that is, clinical or commonly understood connotations – to an instrument’s quantitative change in score. It can be assessed by estimating the precision of the measure when used at an individual patient level, by multiplying the SE of measurement with the standard score (z-value). In addition, MCIDs changes can be calculated by relating change to an external anchor, using either mean change or the receiver operating characteristic (ROC) curve method.
Floor and ceiling effects relate to the ability of an instrument to accurately measure across the full spectrum of a construct. If a measure has > 15% of participants achieving a top or bottom score, this is indicative of a ceiling/floor effect.
Acceptability is a practical property of an instrument and reflects respondents’ willingness to complete it without feeling unduly burdened, indicated by, for example, response rates and completion rates.
Measurement properties for each instrument were assessed separately for hip, knee and mixed hip and knee populations (depending on the availability of published studies). The information was then summarised into the appraisal summary tables, which rated the overall quality of evidence for each of the measurement properties. Three authors (KH, EG and JD) reviewed their own respective sections, following which the results were cross-checked to ensure consistency of assessment and scoring across the reviewers.
Results
Identification of studies
The initial search in Ovid yielded 3774 abstracts. After the removal of duplicates, the number of abstracts for assessment was 2887. In addition, keyword searches (combination of knee, hip and orthopaedics) in EconLit yielded 162 results, the PROMs database identified 454 results and DARE had no results (Figure 3).
FIGURE 3.
Instrument flow diagram. AMED, Allied and Complementary Medicine Database; EconLit, American Economic Association’s electronic bibliography; OU, University of Oxford. Reproduced with permission from Harris et al. 63 This is an Open Access article distributed in accordance with the terms of the Creative Commons Attribution (CC BY 3.0) license, which permits others to distribute, remix, adapt and build upon this work, for commercial use, provided the original work is properly cited. See: http://creativecommons.org/licenses/by/3.0/.
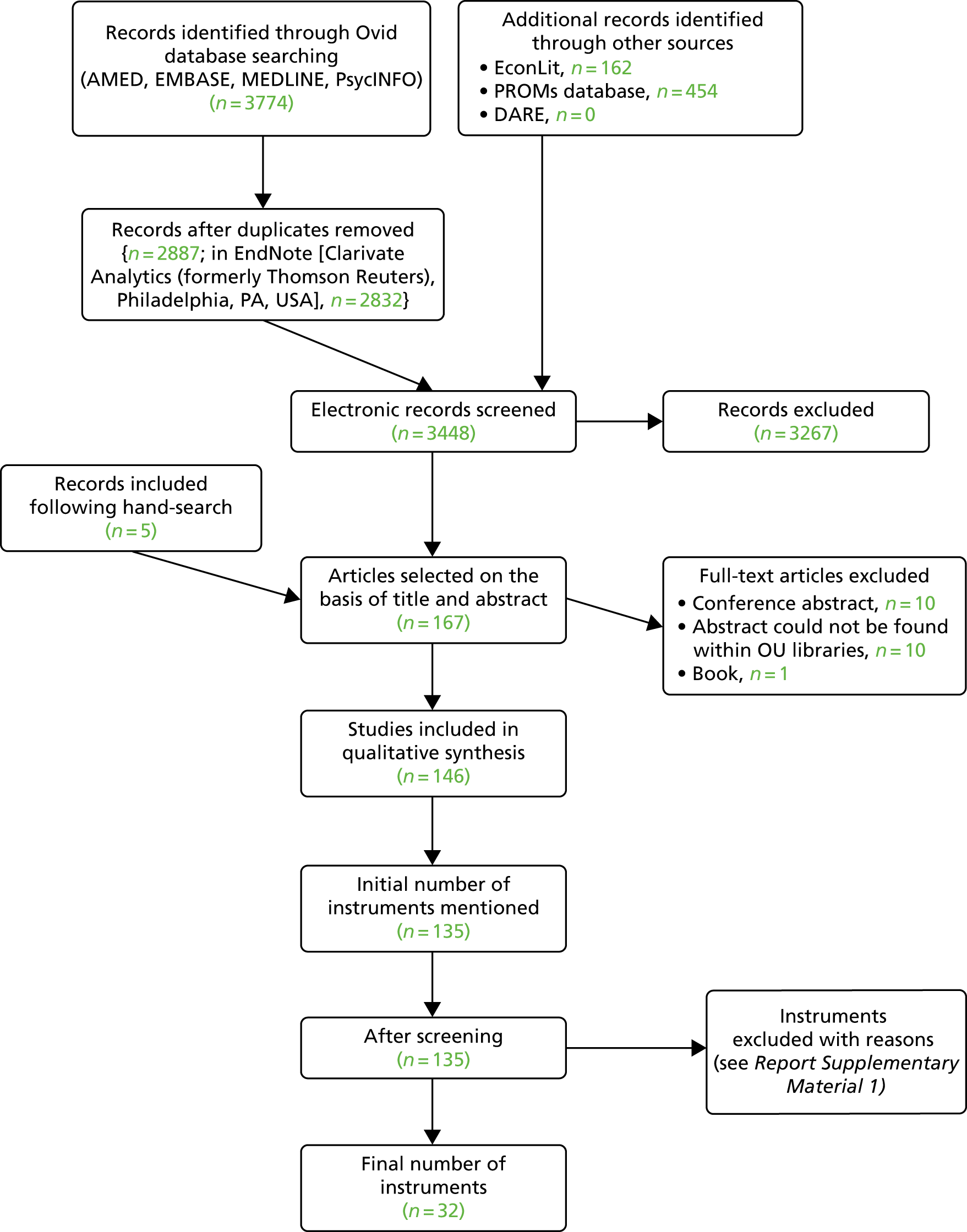
Hand-searching of titles of the following key journals in the 6 months preceding the search was conducted:
-
Health and Quality of Life Outcomes (number of articles, one)
-
The Journal of Bone and Joint Surgery (American and British volumes; number of articles, one)
-
The Journal of Arthroplasty (number of articles, three).
Screening of articles and instruments
Out of the 167 selected abstracts, 146 eligible full-text articles were then screened for all PROMs that were analysed, identifying 135 instruments. If the instrument was not validated (developed for or subsequently validated) for use in a population of patients undergoing hip or knee replacement surgery, it was excluded, leaving 67 instruments. A reliability exercise was undertaken for 16 full-text articles between two reviewers, and the agreement was 95% (38/40 questionnaires identified). An instrument-specific search was then undertaken for each of the 67 identified instruments. By this method, 21 new validation papers (in addition to 42 developmental papers) in the targeted population were identified. Furthermore, on closer examination of shortlisted instruments, 21 initially identified instruments were additionally excluded.
Data extraction
Relevant data on the psychometric performance and operational characteristics were extracted for each PROM. The summary texts were sent to corresponding authors from the developmental study of each respective PROM, and further information was added as a result of this exercise. The appraisal summaries are presented in Tables 1–4.
Instrument (groups tested) | Score | ||||||||
---|---|---|---|---|---|---|---|---|---|
Hip | Knee | ||||||||
HOOS | HRQ | PSI | OHS | Knee disorders subjective history (VAS) | KOOS | KOOS-PS | OKS | OKS-APQ | |
Number of studies | 5 | 1 | 4 | 20 | 1 | 3 | 2 | 23 | 1 |
Reproducibility | ++ | + | + | ++ | 0 | + | 0 | +++ | +++ |
Internal consistency | + | 0 | 0 | ++ | 0 | 0 | +++ | +++ | +++ |
Validity: content | 0 | 0 | ++ | ++ | + | + | + | +++ | +++ |
Construct | ++ | + | ++ | +++ | + | + | ++ | +++ | +++ |
Responsiveness | + | + | ++ | +++ | 0 | 0 | ++ | +++ | +++ |
Interpretability | 0 | 0 | 0 | +++ | 0 | 0 | 0 | ++ | 0 |
Floor and ceiling/precision | + | 0 | 0 | –/+ | 0 | + | 0 | ++ | ++ |
Acceptability | 0 | 0 | 0 | +++ | – | 0 | 0 | +++ | +++ |
Instrument (group tested) | Score | |||||||||
---|---|---|---|---|---|---|---|---|---|---|
Lower limb | Pain | |||||||||
LEFS (h/k) | WOMAC (h/k) | WOMAC (h) | WOMAC (k) | WOMAC SF (h/k) | Lower limb core score (h/k) | MODEMS-HK (AAOS) hip and knee core score (h/k) | ICOAP (h/k) | P4 (h/k) | McGill pain-short form (h/k) | |
Number of studies | 5 | 25 | N/A | N/A | N/A | 1 | 1 | 2 | 1 | 2 |
Reproducibility | + | ++ | ++ | + | 0 | 0 | 0 | + | 0 | ++ |
Internal consistency | + | + | 0 | 0 | + | 0 | 0 | + | ++ | 0 |
Validity: content | + | + | + | + | + | + | + | ++ | + | 0 |
Construct | ++ | +++ | + | ++ | ++ | 0 | + | + | + | + |
Responsiveness | ++ | +++ | ++ | ++ | + | 0 | ++ | – | 0 | – |
Interpretability | + | ++ | ++ | ++ | 0 | 0 | 0 | 0 | 0 | 0 |
Floor and ceiling/precision | 0 | –/+ | – | 0 | 0 | 0 | ++ | 0 | 0 | 0 |
Acceptability | 0 | ++ | + | + | 0 | 0 | + | 0 | 0 | 0 |
Instrument (group tested) | Instrument group | |||||||||||
---|---|---|---|---|---|---|---|---|---|---|---|---|
Utility | Generic | |||||||||||
SF-6D (h) | HUI2 and HUI3 (h) | EQ-5D (h/k) | EQ-5D (h) | EQ-5D (k) | SF-36 (h/k) | SF-36 (h) | SF-36 (k) | SF-12 (h/k) | SF-12 (h) | SF-12 (k) | SIP (h) | |
Number of studies | 1 | 4 | 9 | N/A | N/A | 14 | N/A | N/A | 3 | N/A | N/A | 2 |
Reproducibility | 0 | 0 | 0 | 0 | 0 | 0 | 0 | 0 | ++ | 0 | 0 | 0 |
Internal consistency | 0 | 0 | N/A | N/A | N/A | 0 | 0 | – | 0 | 0 | 0 | 0 |
Validity: content | 0 | 0 | 0 | 0 | 0 | 0 | 0 | 0 | 0 | 0 | 0 | + |
Construct | 0 | ++ | + | 0 | + | + | 0 | + | 0 | 0 | + | + |
Responsiveness | ++ | + | 0 | 0 | + | 0 | ++ | + | 0 | + | + | – |
Interpretability | 0 | 0 | 0 | ++ | ++ | 0 | + | + | 0 | + | + | 0 |
Floor and ceiling/precision | – | 0 | 0 | 0 | ++ | 0 | – | 0 | +++ | 0 | 0 | – |
Acceptability | 0 | 0 | 0 | 0 | ++ | 0 | 0 | 0 | 0 | 0 | 0 | 0 |
Instrument (group tested) | Instrument | |||||||||
---|---|---|---|---|---|---|---|---|---|---|
WHOQOL-BREF (h/k) | Aberdeen IAP (h/k) | Aberdeen IAP (modified) (h/k) | NEADL (h) | AQOL (h/k) | MSK functional limitations index (k) | HAQ (k) | MHAQ (h/k) | MHAQ (h) | K10 (h/k) | |
Number of studies | 1 | 1 | 1 | 1 | 2 | 1 | 2 | 2 | N/A | 1 |
Reproducibility | 0 | 0 | 0 | ++ | 0 | 0 | 0 | 0 | 0 | 0 |
Internal consistency | ++ | + | ++ | ++ | 0 | 0 | – | 0 | 0 | 0 |
Validity: content | + | + | 0 | – | 0 | 0 | 0 | 0 | + | – |
Construct | 0 | + | + | + | + | + | ++ | + | + | + |
Responsiveness | + | 0 | 0 | – | ++ | 0 | – | – | + | – |
Interpretability | 0 | 0 | 0 | 0 | 0 | 0 | 0 | 0 | 0 | 0 |
Floor and ceiling/precision | ++ | + | 0 | + | 0 | 0 | + | ++ | 0 | ++ |
Acceptability | 0 | 0 | 0 | 0 | 0 | + | 0 | 0 | 0 | 0 |
Table 1 summarises the evidence of measurement and operational performance applying the adapted appraisal criteria for the hip PROMs identified in this review. On the basis of the volume and quality of evidence, the Oxford Hip Score (OHS) clearly has the best evidence of measurement properties within the hip-specific PROM category. Within the ‘knee scores’ subgroup (see Table 1), the Oxford Knee Score (OKS) [with the OKS – Activity and Participation Questionnaire (OKS-APQ)] demonstrated best evidence of its measurement properties within the knee-specific PROM category. The Knee injury and Osteoarthritis Outcome Score (KOOS) and the KOOS – Physical Score (KOOS-PS) have some favourable evidence of their measurement properties, although compared with the OKS, the evidence is lacking and further evaluations are needed.
Table 2 summarises the evidence of measurement and operational performance by applying the adapted appraisal criteria to the lower-limb and pain PROMs identified in these reviews. The best-performing lower-limb measure for hip/knee patients is the WOMAC, followed by the Lower Extremity Functional Scale. The WOMAC also performed best when applied to separate hip or knee groups. Satisfactory evidence of measurement properties was generally lacking for all of the three identified pain measures (ICOAP, P4 and the McGill Pain-Short Form). ICOAP and McGill Pain-Short Form had no evidence in favour of their responsiveness and P4 did not have any reported evidence of its responsiveness. Three utility and generic measures identified in the review are listed in Table 3. As with the pain scores, the evidence for utility PROMs was generally lacking, with the EQ-5D scoring worse on construct validity and responsiveness than the Short Form questionnaire-6 Dimensions (SF-6D) and the Health Utilities Index Mark 2 (HUI2) and Mark 3 (HUI3). On the basis of the volume and quality of evidence, among all identified generic measures, the Short Form questionnaire-12 items (SF-12) is clearly the most promising one.
Nine measures identified in the review were categorised as ‘other’ scales. Table 4 summarises evidence of their measurement properties. The World Health Organization Quality of Life (WHOQOL)-BREF instrument, Aberdeen Impairment, activity limitation and participation restriction [Aberdeen Impairment, Activity Limitation, and Participation Restriction (Aberdeen IAP)] and assessment of quality of life had the best overall evidence in this subcategory (on a mixed hip/knee population). However, the overall evidence of their validity was generally lacking.
Discussion
Our review has identified the WOMAC, OHS and OKS to be the most promising disease-/site-specific scores that perhaps provide best coverage of the construct of interest and better responsiveness. The best-performing generic measure was the SF-12. However, further research on some of the missing measurement properties in these measures is required. For the WOMAC, further evidence on ceiling/floor effect, content validity and acceptability is required in both the hip and the knee groups of patients. The OHS is currently lacking evidence on its ceiling/floor effects. Many other PROMs do not have sufficient measurement property validation to recommend their use. Given its widespread use in this clinical area (e.g. national PROMs data), it was disappointing that the EQ-5D score did not perform better.
Our findings are supported by existing literature. Alviar et al. 60 published a systematic review of measurement properties of 28 PROMs used in hip/knee arthroplasty based on published evidence up to December 2009 and found the WOMAC, OKS and SF-36 to be the most comprehensively tested measures at that time, although the need for more rigorous evaluation of reliability, responsiveness and interpretability was noted. Our review has updated this evidence, both in breadth (we have assessed 67 instruments) and time period (our search was until May 2014). Browne et al. 64 identified the OHS and OKS (used alongside the EQ-5D) as primary outcome measures of choice to be used in the UK PROMs programme for hip and knee replacement.
It should be noted that the standards (and indeed scope/tolerance) for reporting details of qualitative procedures and psychometric analysis have changed over the past 20 years (very much so in the musculoskeletal literature), so that although measures that were devised earlier in that period have had a longer time in which to accrue evidence of their measurement properties, they can frequently lack relevant detail specifically in relation to the development of the instrument. Reporting has improved, probably as a consequence of the evolving methods and the recognition that minimum standards are required [e.g. Streiner et al. ,65 COSMIN (COnsensus-based Standards for the selection of health Measurement INstruments)54 and the US Food and Drug Administration55].
Further detail and supplementary material can be found in the publication based on this work by Harris et al. 63
Chapter 3 Calculation of measurement properties (work package 1)
Background
The systematic review of the measurement properties reported the properties for the EuroQol-5 Dimensions, three-level version (EQ-5D-3L), SF-12, OHS, OKS and WOMAC tools based on the existing literature. The OHS (20 studies) and OKS (23 studies) are good in terms of reproducibility, internal consistency, validity (content), construct, responsiveness, interpretability, floor and ceiling effects, precision (except OHS) and acceptability. WOMAC (25 studies) was reported as good in terms of reproducibility, validity (content), construct, responsiveness, interpretability and acceptability. Only fair outcomes for knee for EQ-5D-3L (nine studies) were reported in terms of interpretability and acceptability when construct and responsiveness were not applicable. SF-12 (three studies) was poor in terms of construct, responsiveness and interpretability. There were a number of outcomes for which there was no or little available evidence on one of more of the measurement properties. To be fit for purpose, any candidate score to be used as a screening instrument must satisfy a number of requirements, one being that the score must have adequate measurement properties to enable assessment of patients for joint replacement {i.e. adequate validity [ACHE protocol version 4, Nuffield Department of Orthopaedics, Rheumatology and Musculoskeletal Sciences (NDORMS), 2015]}.
Methods
General approach
Following the review of the evidence on the measurement properties of the possible instruments for measuring outcome after knee and hip replacement operations, a number of gaps in the evidence base were identified. Using available data sets, before we refined the shortlist of candidate tools (Table 5), the missing measurement properties were calculated when possible using available data sets.
Data set | Instrument | ||||
---|---|---|---|---|---|
EQ-5D-3L index | SF-12/SF-36 | OKS | OHS | WOMAC | |
Knee | |||||
KAT | ✓ | ✓ | ✓ | ||
ADAPT | ✓ | ✓ | |||
APEX | ✓ | ✓ | |||
Hip | |||||
EUROHIP | ✓ | ✓ | ✓ | ||
EPOS | ✓ | ✓ | |||
ADAPT | ✓ | ✓ | |||
APEX | ✓ | ✓ |
Research aim and objectives
Patient-level data were available from a number of data sets, which included data on several relevant scoring systems. As reported in Chapter 1 and summarised in Tables 1–4, most of the instruments identified in the systematic review lacked evidence on one or more measurement properties. Using the available data sets, missing measurement properties were calculated. The instruments covered varied across the data sets (see Table 5). No data sets were available that included SF-6D, SF-36, ICOAP, KOOS or KOOS-PS, among others.
Data sets
A brief description of the data sets used is provided in the following sections.
The Knee Arthroplasty Trial
Summary
The Knee Arthroplasty Trial (KAT)66 is a National Institute for Health Research (NIHR) HTA programme-funded study that has examined the outcome of 2352 total knee arthroplasties (TKAs) over a median of 10 years, and includes data on costs and resource use. Continued development of TKA systems has seen improvement in quality of life and increased duration of prosthetic survival. It was a pragmatic, multicentre (116 surgeons in 34 centres in the UK) randomised controlled trial (RCT). A total of 2352 participants were randomly allocated to be treated with or without a metal backing of the tibial component (n = 409), a patellar resurfacing (n = 1715) or a mobile bearing (n = 539); in total, 2318 patients took part.
The trial is registered as ISRCTN45837371.
Available outcome measures of interest
The available outcome measures of interest were the OKS, SF-12 [predominantly version 2 (n = 2091), although a small number of version 1 questionnaires were initially used (n = 126)] and the EQ-5D-3L descriptive system (referred to as the ‘EQ-5D-3L index’ in this report).
The European Collaborative Database of Cost and Practice Patterns of Total Hip Replacement
Summary
This used a cohort of people having primary hip replacement for primary osteoarthritis from the UK and other European countries. The European Collaborative Database of Cost and Practice Patterns of Total Hip Replacement (EUROHIP) consortium includes 20 orthopaedic centres in 12 different European countries. 67 The cohort was comprised of 1051 people having primary hip replacement for primary hip osteoarthritis. Originally, 147 patients in the cohort came from the UK (143 remained for the analysis for the OHS) and the remaining 1373 patients were from other European countries (1184 remained for the analysis). In total, 1327 participants were used for analyses of WOMAC and the EQ-5D-3L index. A total of 908 participants (68.4%) completed the 12-month follow-up questionnaire. A minimum of 50 consecutive, consenting patients receiving primary total hip arthroplasty (THA) for hip osteoarthritis in each of the 20 participating orthopaedic centres entered the study. Preoperative data included demographics, employment and educational attainment, drug utilisation and involvement of other joints. 67
Available outcome measures of interest
The available outcome measures of interest were the OHS, EQ-5D-3L index and WOMAC (a five-point Likert version).
The Exeter Prosthesis Outcome Study
Summary
The Exeter Primary Outcome Study (EPOS) recruited 1590 patients who had undergone Exeter hip replacement implants between March 1999 and February 2002. 68 There were 1375 patients (1431 hips) with a primary diagnosis of osteoarthritis. The unit of analysis was the implant rather than the patient, of whom 56 had bilateral procedures. A total of 1431 THRs were undertaken by consultant and non-consultant surgeons using anterolateral or posterior approaches. 69
Available outcome measures of interest
The available outcome measures of interest were the OHS and SF-36.
After Partial and Total Joint Replacement
Between February 2010 and November 2011, 125 patients undergoing THA and 128 patients undergoing TKA were recruited to the cohort. The protocol and full details of the research design and findings have been published. 70 The studies were approved by the Southampton and South West Hampshire Research Ethics Committee (09/H0102/72) and all participants provided informed, written consent. 71 The patients recruited were due to undergo a range of hip and knee replacement procedures, meaning that functional measures could be investigated across a range of people with diverse indications for surgery and degrees of functional impairment.
Available outcome measures of interest
The available outcome measures of interest were the SF-12 version 1 and WOMAC.
Arthroplasty Pain Experience
Summary
Between 2009 and 2012, 322 patients undergoing THA and 316 patients undergoing TKA were recruited. The inclusion criteria were waiting for a primary unilateral THA or TKA for osteoarthritis. The published protocol and clinical results paper for the Arthroplasty Pain EXperience (APEX) study provide full details of the research design and findings. 70,72 The exclusion criteria were the inability to provide informed consent or complete questionnaires and medical comorbidity precluding the use of spinal anaesthesia, regional blocks or strong analgesics postoperatively.
Available outcome measures of interest
The available outcome measures of interest were the EQ-5D-3L index and WOMAC.
Approvals
We successfully sought direct approval from the data controller of each data set to obtain access to the anonymised data.
Available data by data set
Tables 6 and 7 show the number of individuals who responded to each hip and knee measurement tool for the respective data sets. The percentages of items missing in those with an incomplete measurement tool were about 6–24% for the EQ-5D-3L index, 15–41% for the OHS and 12% for the OKS and 21–36% for the WOMAC total score post operation (see Report Supplementary Material 1, Online Supplement 1, for further details. Please note that all further citations to ‘online supplements’ refer to those within Report Supplementary Material 1).
Measurement tool | Pre operation | Post operation | ||||
---|---|---|---|---|---|---|
Individuals who responded to any question in the measurement tool (n) | Individuals who fully completed the measurement tool (n) | Items missing for those with an incomplete measurement tool response (%) | Individuals who responded to any question in the measurement tool (n) | Individuals who fully completed the measurement tool (n) | Items missing for those with an incomplete measurement tool response (%) | |
APEX | ||||||
EQ-5D-3L index | 309 | 302 | 86.8 | 271 | 266 | 94.8 |
EUROHIP | ||||||
EQ-5D-3L index | 1266 | 1228 | 72.9 | 903 | 883 | 96.9 |
ADAPT | ||||||
SF-12 MCS/PCS | 125 | 119 | 53.8 | 112 | 104 | 76.0 |
EPOS | ||||||
OHS | 1534 | 1517 | 79.9 | 1262 | 1239 | 94.9 |
EUROHIP | ||||||
OHS | 140 | 127 | 33.9 | 123 | 114 | 74.7 |
OHS pain | 140 | 133 | 51.7 | 123 | 120 | 92.0 |
OHS function | 139 | 131 | 47.2 | 123 | 115 | 39.6 |
ADAPT | ||||||
WOMAC total | 125 | 112 | 34.5 | 111 | 102 | 70.4 |
WOMAC pain | 125 | 122 | 77.5 | 111 | 109 | 93.3 |
WOMAC function | 125 | 115 | 42.4 | 111 | 104 | 75.8 |
WOMAC stiffness | 125 | 125 | – | 111 | 109 | 95.2 |
APEX | ||||||
WOMAC total | 324 | 261 | 42.7 | 283 | 234 | 65.7 |
WOMAC pain | 324 | 323 | 96.0 | 283 | 279 | 95.0 |
WOMAC function | 308 | 270 | 53.7 | 273 | 242 | 74.0 |
WOMAC stiffness | 309 | 300 | 89.5 | 273 | 268 | 96.7 |
EUROHIP | ||||||
WOMAC total | 1272 | 1243 | 73.4 | 902 | 865 | 94.3 |
WOMAC pain | 1268 | 1255 | 91.1 | 886 | 875 | 98.8 |
WOMAC function | 1270 | 1253 | 86.7 | 901 | 888 | 98.4 |
WOMAC stiffness | 1266 | 1266 | – | 888 | 888 | – |
Measurement tool | Pre operation | Post operation | ||||
---|---|---|---|---|---|---|
Individuals who responded to any question of the measurement tool (n) | Patients who fully completed the measurement tool (n) | Items missing in those with incomplete measurement tool (%) | Individuals who responded to any question of the measurement tool (n) | Patients who fully completed the measurement tool (n) | Items missing in those with incomplete measurement tool (%) | |
APEX | ||||||
EQ-5D-3L index | 302 | 298 | 92.2 | 263 | 261 | 97.9 |
KAT | ||||||
EQ-5D-3L index | 2156 | 2120 | 71.6 | 1995 | 1939 | 84.2 |
ADAPT | ||||||
SF-12 MCS/PCS | 128 | 116 | 43.2 | 110 | 96 | 68.5 |
KAT | ||||||
SF-12 MCS/PCS | 2156 | 2087 | 58.3 | – | 1904 | – |
KAT | ||||||
OKS | 2159 | 2112 | 59.9 | 1996 | 1691 | 47.9 |
OKS pain | 2159 | 2136 | 76.4 | 1996 | 1906 | 76.2 |
OKS function | 2159 | 2132 | 75.8 | 1996 | 1753 | 58.8 |
ADAPT | ||||||
WOMAC total | 128 | 118 | 41.4 | 110 | 102 | 74.6 |
WOMAC pain | 127 | 123 | 64.4 | 110 | 109 | 96.5 |
WOMAC function | 127 | 120 | 49.0 | 110 | 103 | 77.5 |
WOMAC stiffness | 127 | 127 | – | 110 | 110 | – |
APEX | ||||||
WOMAC total | 318 | 246 | 41.6 | 277 | 214 | 59.0 |
WOMAC pain | 318 | 318 | 0.0 | 277 | 269 | 90.9 |
WOMAC function | 301 | 253 | 50.5 | 268 | 224 | 66.6 |
WOMAC stiffness | 301 | 293 | 91.3 | 268 | 260 | 94.9 |
Statistical analysis
The statistical methods used to calculate the five measurement properties of interest are described in the following sections.
Internal consistency
The corresponding Cronbach’s alpha was calculated using preoperation and postoperation data for the SF-12 [physical component score (PCS) and mental component score (MCS)] and OKS (pain, function and total scores). For the OKS total scores and subscales, the actual internal consistency can be assessed (as these summary scores are simple summations of the individual items). For the EQ-5D-3L index and the SF-12 summary scores, the respective Cronbach’s alpha relates to a summary score of the five domains, and, therefore, 12 items were carried out at the best indirectly assessed internal consistency. The SF-12 uses 12-item response values to generate two summary scores: physical and mental. This was carried out for the baseline data only and by version. The analyses were carried out in Stata® (version 14; StataCorp LP, College Station, TX, USA) using the alpha command. The Cronbach’s alpha including all standard items, with each of the constituent items dropped in turn, was calculated along with the correlation between each individual item and the sum of all the other items.
Construct validity
A priori hypotheses about the magnitude and direction of correlations between primary outcomes at pre operation have been proposed (see Analysis plan document). Spearman’s and Pearson’s correlations were calculated for each pair of measures. These were calculated in Stata® using the ci2 command with 95% confidence intervals (CIs). The CI for Pearson’s correlation was calculated in two ways: using Fisher’s r-to-z transformation and using bootstrapping with 1000 replications in Stata® using the bootstrap command. Cohen’s convention is to interpret ES as follows: a correlation coefficient of 0.10 is thought to represent a weak or small association, a correlation coefficient of 0.30 is considered a moderate correlation and a correlation coefficient of 0.50 or larger is thought to represent a strong or large correlation. 73
Responsiveness
Responsiveness was assessed by examining the magnitude and direction of correlations of the change scores (pre to post operation) between the primary outcomes.
Floor and ceiling effects
The proportion of patients responding with the highest and lowest possible scores at pre and post operation were calculated to assess the possibility of floor/ceiling effects. Jette et al. 74 considered that for the measurement of a stage to be useful, no more than 20% of patients’ measurements should show floor and ceiling effects. More values close to the extremes of the instrument’s range suggest more limited ability of a measurement to discriminate among patients’ function at the minimum or maximum possible scale. 74 The proportion specified to designate a floor/ceiling effect is arbitrary, with 20% used for the current study.
Interpretability
The ACHE project methodology aims to calculate the minimal detectable change (MDC), minimally important change (MIC) and minimally important difference (MID). Various approaches can be used to assess these properties. Minor variations in these definitions exist. The definitions used for the estimation of these attributes are given in the following sections.
The MDC was calculated as stated in the following section. The MIC was calculated in three ways: the ROC MIC, MIC (group) and MIC (ES). The MID was calculated in two ways: using a patient-reported global transition item MID (anchor) and using an ES approach MID. The specific methods used were in accordance with the methodology proposed by Beard et al. 75
Minimal detectable change (90% significance level)
This is often called a distribution method for calculating an important change. The SE of the measurement can be defined as:
where SD is the standard deviation and R is a reliability parameter [e.g. test–retest reliability or intracluster correlation coefficient (ICC)]. In this analysis, test–retest reliability was used. 76,77 Applying a 90% significance level for z-distribution (z-statistics value of 1.645), a range for the possible difference between two observations under the same conditions (test–retest scenario) was calculated to define the MDC:77
To calculate an estimate of the MDC, the ICC (test–retest reliability) should be imputed given repeatability data because it is not possible to calculate an estimate of the ICC (i.e. the outcome measured at the same time point multiple times) in the KAT data set. A previous study has estimated ICCs of 0.84 and 0.80 for the SF-12 physical and mental scores, respectively, on a mixed sample of patients undergoing hip and knee replacement surgery, comparing scores at 3 weeks and 1 week pre operation. 78 Although these estimates are not optimal (the ICC would preferably be estimated separately for hip and knee replacement surgery), it is thought that they will be sufficiently close to be used to enable the respective MDCs to be calculated. A previous study79 has reported ICCs of 0.73, 0.78 and 0.53 for WOMAC pain, physical function and stiffness, respectively, at pre operation for patients undergoing THA. In this analysis, the MDCs were calculated for the SF-12 physical and mental scores pre operation using the preoperative ICCs given above. 78
Minimally important change
Receiver operating characteristic minimally important change
The previous MIC approach can be modified by using ROC curve methodology (using the anchor definition as the reference standard for an important change) in order to determine the optimal cut-point. The optimal cut-point can be defined in various ways. In this analysis, Youden’s Index80 is used by maximising (sensitivity + specificity – 1), and shortest distance by minimising:
The area under the corresponding curve was calculated using a non-parametric ROC approach in Stata® (roctab command) to generate an associated 95% CI.
Minimally important change (group)
The MIC can be calculated as the mean change score for patients who identify themselves as having a ‘minimal’ (e.g. ‘a little’) difference on a patient-reported global transition item (anchor). An anchor-based MID was calculated using ‘somewhat satisfied’ versus ‘somewhat dissatisfied’ groups when there was no neutral scale.
Minimally important change (effect size)
An ES for the MIC can be calculated as follows:
where pooled SD is:
and SDs are group-specific SDs and Ns are study sample sizes. An ES of 0.5 has been proposed as an estimate of a MIC; the MIC (ES). 81 The MICs for health-related quality of life instruments have been noted to be close to half a SD in other studies. 77
Minimally important difference
Minimally important difference (group)
The MID (group) can be calculated as the difference in the mean change score for patients who identify themselves as having a ‘minimal’ difference (e.g. ‘a little better’) and those who identify themselves as having no change (e.g. ‘about the same’) on a patient-reported global transition item (anchor). EPOS and EUROHIP data sets both had a satisfaction Likert scale (Table 8). An anchor-based MID was calculated using ‘somewhat satisfied’ versus ‘somewhat dissatisfied’ groups when there was no neutral scale.
Satisfaction | Data set | |||
---|---|---|---|---|
EUROHIP (12 months) | EPOS (24 months) | |||
n | % | n | % | |
Very satisfied | 165 | 68 | 829 | 79 |
Somewhat satisfied | 59 | 24 | 167 | 16 |
Somewhat dissatisfied | 13 | 5 | 39 | 4 |
Very dissatisfied | 7 | 3 | 18 | 2 |
Total | 244 | 100 | 1053 | 100 |
Minimally important difference (effect size)
An ES estimate of the MID can be calculated using an anchor (e.g. satisfaction after the operation):
where pooled SD is:
and the SD is the group-specific SD and N is the study sample size. 82 Half of a SD (ES approach) has been proposed as an estimate of a MID. 83 The MIDs for health-related quality of life instruments had previously been suggested to be close to a half of a SD. 84
Results
Internal consistency
Internal consistency results for hip and knee scores are shown in Table 9.
Measurement tool (total) | Time point | |||
---|---|---|---|---|
Pre operation | Post operationa | |||
n | α | n | α | |
Hip | ||||
EQ-5D-3L index | ||||
APEX | 302 | 0.66 | 266 | 0.82 |
EUROHIP | 1228 | 0.66 | 883 | 0.81 |
SF-12 | ||||
ADAPT | 119 | 0.86 | 104 | 0.89 |
OHS | ||||
EPOS | 1517 | 0.88 | 1239 | 0.93 |
EUROHIP | 127 | 0.89 | 114 | 0.93 |
WOMAC | ||||
ADAPT | 112 | 0.97 | 102 | 0.98 |
APEX | 261 | 0.96 | 234 | 0.98 |
EUROHIP | 1243 | 0.95 | 865 | 0.98 |
Knee | ||||
EQ-5D-3L index | ||||
APEX | 298 | 0.66 | 261 | 0.80 |
KAT | 2120 | 0.55 | 1939 | 0.79 |
SF-12a | ||||
ADAPT | 116 | 0.81 | 96 | 0.89 |
KAT | ||||
Version 1 | 116 | –0.40 | – | |
Version 2 | 1791 | 0.38 | – | |
OKS | ||||
KAT | 2112 | 0.86 | 1691 | 0.93 |
WOMAC | ||||
ADAPT | 118 | 0.96 | 102 | 0.98 |
Hip
The internal consistency of all the instruments demonstrated that the total score can be adequately considered as one scale for hip [except the preoperative EQ-5D-3L Index (Cronbach’s alpha = 0.66)], with Cronbach’s alphas in the range of 0.88–0.89 and 0.93 at pre and post operation, respectively, for the OHS and no improvement obtained by removal of any item. Likewise, no significant improvement was obtained by removal of any item in the EQ-5D-3L index, SF-12 and WOMAC. Cronbach’s alpha values for each individual item were similarly high and are provided in Appendix 1. The postoperation data set showed slightly higher Cronbach’s alphas.
Knee
The internal consistency of all of the instruments demonstrated that the total score can be adequately considered as one scale for knee [except the preoperative EQ-5D-3L Index (Cronbach’s alpha = 0.55–0.66) and the SF-12 (Cronbach’s alpha = 0.38 and 0.40 with KAT)], with Cronbach’s alphas in the range of 0.86 and 0.93 at pre and post operation, respectively, for the OKS and no improvement obtained by removal of any item. No significant improvement was obtained by the removal of any item in the EQ-5D-3L index, SF-12 and WOMAC. Alpha values for each individual item were similarly high and are provided in Appendix 1. The postoperation data set showed slightly higher Cronbach’s alphas.
Construct validity
Construct validity results for hip and knee data sets are shown in Tables 10 and 11.
Comparator | Time point | |||
---|---|---|---|---|
Baseline | 12 months | |||
n | Spearman’s correlation (95% CI) | n | Spearman’s correlation (95% CI) | |
EQ-5D index | ||||
APEX | ||||
WOMAC pain | 301 | 0.59 (0.51 to 0.66) | 262 | 0.67 (0.60 to 0.73) |
WOMAC function | 266 | 0.69 (0.62 to 0.75) | 233 | 0.68 (0.60 to 0.74) |
WOMAC stiffness | 294 | 0.53 (0.44 to 0.61) | 261 | 0.54 (0.44 to 0.62) |
WOMAC total | 259 | 0.70 (0.63 to 0.76) | 228 | 0.70 (0.63 to 0.76) |
EUROHIP | ||||
OHS | 124 | 0.75 (0.66 to 0.82) | 113 | 0.78 (0.70 to 0.84) |
OHS function | 128 | 0.71 (0.62 to 0.79) | 114 | 0.78 (0.70 to 0.84) |
OHS pain | 130 | 0.70 (0.59 to 0.78) | 119 | 0.62 (0.50 to 0.72) |
SF-12 PCS | ||||
ADAPT | ||||
WOMAC total | 118 | 0.57 (0.44 to 0.68) | 75 | 0.70 (0.56 to 0.80) |
WOMAC pain | 118 | 0.45 (0.29 to 0.58) | 75 | 0.71 (0.58 to 0.81) |
WOMAC function | 118 | 0.58 (0.45 to 0.69) | 75 | 0.72 (0.59 to 0.82) |
WOMAC stiffness | 118 | 0.38 (0.21 to 0.52) | 75 | 0.52 (0.33 to 0.67) |
SF-12 MCS | 118 | –0.11 (–0.28 to 0.08) | 75 | –0.13 (–0.35 to 0.09) |
SF-12 MCS | ||||
WOMAC total | 118 | 0.28 (0.10 to 0.44) | 75 | 0.18 (–0.05 to 0.39) |
WOMAC pain | 118 | 0.30 (0.12 to 0.45) | 75 | 0.15 (–0.08 to 0.36) |
WOMAC function | 118 | 0.27 (0.09 to 0.43) | 75 | 0.17 (–0.06 to 0.38) |
WOMAC stiffness | 118 | 0.24 (0.06 to 0.40) | 75 | 0.24 (0.02 to 0.45) |
SF-12 PCS | 118 | –0.11 (–0.28 to 0.08) | 75 | –0.13 (–0.35 to 0.09) |
OHS | ||||
EPOS | ||||
SF-36 general health | 1043 | 0.26 (0.21 to 0.32) | 765 | 0.50 (0.44 to 0.55) |
OHS function | ||||
SF-36 physical function | 1042 | 0.71 (0.68 to 0.74) | 773 | 0.79 (0.76 to 0.81) |
SF-36 role physical | 1038 | 0.37 (0.32 to 0.43) | 785 | 0.63 (0.59 to 0.67) |
SF-36 role emotional | 1043 | 0.26 (0.20 to 0.31) | 781 | 0.44 (0.38 to 0.49) |
SF-36 pain | 1048 | 0.65 (0.61 to 0.68) | 800 | 0.65 (0.61 to 0.69) |
SF-36 vitality | 1033 | 0.42 (0.37 to 0.47) | 780 | 0.60 (0.55 to 0.64) |
SF-36 mental health | 1034 | 0.30 (0.24 to 0.35) | 780 | 0.41 (0.35 to 0.47) |
SF-36 social function | 1042 | 0.58 (0.54 to 0.62) | 783 | 0.64 (0.58 to 0.67) |
OHS pain | ||||
SF-36 physical function | 1042 | 0.53 (0.49 to 0.58) | 771 | 0.57 (0.52 to 0.62) |
SF-36 role physical | 1038 | 0.32 (0.26 to 0.37) | 783 | 0.49 (0.43 to 0.54) |
SF-36 pain | 1048 | 0.69 (0.66 to 0.72) | 800 | 0.61 (0.56 to 0.65) |
WOMAC total | ||||
ADAPT | ||||
WOMAC pain | 125 | 0.88 (0.83 to 0.91) | 111 | 0.81 (0.74 to 0.87) |
WOMAC function | 125 | 0.99 (0.98 to 0.99) | 111 | 0.97 (0.96 to 0.98) |
WOMAC stiffness | 125 | 0.85 (0.79 to 0.89) | 111 | 0.74 (0.64 to 0.81) |
SF-12 MCS | 118 | 0.28 (0.10 to 0.44) | 75 | 0.18 (–0.05 to 0.39) |
SF-12 PCS | 118 | 0.57 (0.44 to 0.68) | 75 | 0.70 (0.56 to 0.80) |
APEX | ||||
EQ-5D score | 301 | 0.59 (0.51 to 0.66) | 262 | 0.67 (0.60 to 0.73) |
WOMAC function | 269 | 0.77 (0.72 to 0.81) | 241 | 0.70 (0.63 to 0.76) |
WOMAC stiffness | 299 | 0.63 (0.56 to 0.70) | 264 | 0.58 (0.49 to 0.65) |
WOMAC total | 261 | 0.85 (0.81 to 0.88) | 234 | 0.79 (0.74 to 0.84) |
WOMAC function | ||||
EUROHIP | ||||
OHS function | 115 | 0.84 (0.78 to 0.89) | 114 | 0.85 (0.79 to 0.89) |
WOMAC pain | ||||
EUROHIP | ||||
OHS pain | 131 | 0.78 (0.70 to 0.84) | 118 | 0.66 (0.54 to 0.75) |
Comparator | Time point | |||
---|---|---|---|---|
Baseline | 12 months | |||
n | Spearman’s correlation (95% CI) | n | Spearman’s correlation (95% CI) | |
EQ-5D index | ||||
KAT | ||||
OKS | 2073 | 0.70 (0.67 to 0.72) | 1647 | 0.78 (0.76 to 0.80) |
OKS function | 2097 | 0.65 (0.63 to 0.68) | 1702 | 0.74 (0.72 to 0.76) |
OKS pain | 2093 | 0.62 (0.59 to 0.65) | 1857 | 0.74 (0.72 to 0.76) |
SF-12 PCS | 2055 | 0.43 (0.40 to 0.47) | 1857 | 0.72 (0.70 to 0.74) |
SF-12 MCS | 2055 | 0.42 (0.38 to 0.45) | 1857 | 0.46 (0.43 to 0.50) |
SF-12 PCS | ||||
ADAPT | ||||
WOMAC total | 114 | 0.36 (0.19 to 0.51) | 74 | 0.75 (0.64 to 0.84) |
WOMAC pain | 115 | 0.38 (0.21 to 0.52) | 74 | 0.65 (0.49 to 0.76) |
WOMAC function | 115 | 0.35 (0.18 to 0.50) | 74 | 0.74 (0.61 to 0.83) |
WOMAC stiffness | 115 | 0.20 (0.02 to 0.37) | 74 | 0.57 (0.39 to 0.70) |
SF-12 MCS | 116 | –0.36 (–0.51 to –0.19) | 74 | 0.0 (–0.23 to 0.23) |
KAT | ||||
OKS function | 2062 | 0.50 (0.47 to 0.53) | 1678 | 0.71 (0.68 to 0.73) |
OKS pain | 2066 | 0.50 (0.47 to 0.54) | 1831 | 0.62 (0.59 to 0.65) |
SF-12 MCS | ||||
ADAPT | ||||
WOMAC total | 114 | 0.11 (–0.07 to 0.29) | 74 | 0.25 (0.02 to 0.45) |
WOMAC pain | 115 | 0.02 (–0.16 to 0.21) | 74 | 0.19 (–0.04 to 0.40) |
WOMAC function | 115 | 0.12 (–0.07 to 0.29) | 74 | 0.26 (0.03 to 0.46) |
WOMAC stiffness | 115 | 0.19 (0.01 to 0.36) | 74 | 0.23 (0.01 to 0.44) |
SF-12 PCS | 116 | –0.36 (–0.51 to –0.19) | 74 | 0 (–0.23 to 0.23) |
KAT | ||||
OKS function | 2062 | 0.41 (0.37 to 0.44) | 1678 | 0.43 (0.39 to 0.47) |
WOMAC total | ||||
ADAPT | ||||
WOMAC pain | 126 | 0.86 (0.81 to 0.90) | 110 | 0.92 (0.88 to 0.94) |
WOMAC function | 126 | 0.98 (0.97 to 0.98) | 110 | 0.99 (0.98 to 0.99) |
WOMAC stiffness | 126 | 0.69 (0.59 to 0.77) | 110 | 0.81 (0.73 to 0.87) |
SF-12 MCS | 114 | 0.11 (–0.07 to 0.29) | 74 | 0.25 (0.02 to 0.45) |
SF-12 PCS | 114 | 0.36 (0.19 to 0.51) | 74 | 0.75 (0.64 to 0.84) |
APEX | ||||
EQ-5D index | 244 | 0.72 (0.65 to 0.77) | 209 | 0.80 (0.74 to 0.84) |
WOMAC pain | 246 | 0.85 (0.81 to 0.88) | 214 | 0.89 (0.86 to 0.92) |
WOMAC function | 246 | 0.98 (0.98 to 0.99) | 214 | 0.99 (0.98 to 0.99) |
WOMAC stiffness | 246 | 0.70 (0.63 to 0.76) | 214 | 0.79 (0.73 to 0.84) |
Hip
Construct validity (based on Spearman’s correlation) was generally supported with moderate to strong correlations between the instruments, except for correlations involving the SF-12 PCS, SF-12 MCS and SF-36. Correlations between the instruments at pre operation versus post operation were generally similar.
Knee
For the knee instruments, the pattern was broadly similar to the hip instruments. Construct validity (based on Spearman’s correlation) was generally supported with moderate to strong correlations between the instruments, except for correlations involving the SF-12 PCS and SF-12 MCS. Correlations between the instruments at post operation tended to be higher than those at pre operation.
Responsiveness
Responsiveness results for hip and knee scores are shown in Tables 12 and 13, respectively.
Comparator | n | Correlation (95% CI) | |
---|---|---|---|
Spearman’s | Pearson’s | ||
EQ-5D-3L index | |||
APEX | |||
WOMAC pain | 247 | 0.53 (0.44 to 0.62) | 0.55 (0.46 to 0.63) |
WOMAC function | 198 | 0.58 (0.48 to 0.66) | 0.59 (0.49 to 0.67) |
WOMAC stiffness | 243 | 0.46 (0.36 to 0.56) | 0.48 (0.38 to 0.57) |
WOMAC total | 193 | 0.58 (0.48 to 0.67) | 0.59 (0.49 to 0.67) |
Change SF-12 PCS | |||
ADAPT | |||
SF-12 MCS | 72 | –0.27 (–0.47 to –0.04) | –0.21 (–0.42 to 0.02) |
WOMAC pain | 72 | 0.47 (0.26 to 0.63) | 0.44 (0.23 to 0.61) |
WOMAC function | 72 | 0.46 (0.25 to 0.62) | 0.48 (0.28 to 0.64) |
WOMAC stiffness | 72 | 0.18 (–0.06 to 0.39) | 0.22 (–0.01 to 0.43) |
WOMAC total | 72 | 0.45 (0.25 to 0.62) | 0.47 (0.26 to 0.63) |
Change SF-12 MCS | |||
SF-12 PCS | 72 | –0.27 (–0.47 to –0.04) | –0.21 (–0.42 to 0.02) |
WOMAC pain | 72 | 0.27 (0.04 to 0.47) | 0.35 (0.13 to 0.54) |
WOMAC function | 72 | 0.24 (0.01 to 0.45) | 0.31 (0.09 to 0.51) |
WOMAC stiffness | 72 | 0.34 (0.12 to 0.53) | 0.33 (0.10 to 0.52) |
WOMAC total | 72 | 0.27 (0.04 to 0.47) | 0.33 (0.11 to 0.52) |
Change OHS | |||
EPOS | |||
SF-36 general health | 739 | –0.25 (–0.31 to –0.18) | –0.27 (–0.34 to –0.20) |
EUROHIP | |||
EQ-5D-3L usual activities | 113 | 0.44 (0.28 to 0.58) | 0.44 (0.28 to 0.58) |
EQ-5D-3L pain | 113 | 0.58 (0.44 to 0.69) | 0.57 (0.43 to 0.68) |
EQ-5D-3L self-care | 113 | 0.33 (0.15 to 0.48) | 0.36 (0.18 to 0.51) |
EQ-5D-3L index | 110 | 0.57 (0.43 to 0.68) | 0.56 (0.42 to 0.68) |
WOMAC pain | 110 | 0.63 (0.50 to 0.73) | 0.60 (0.47 to 0.71) |
EUROHIP | |||
EQ-5D-3L index | 107 | 0.54 (0.40 to 0.67) | 0.53 (0.38 to 0.66) |
WOMAC function | 107 | 0.80 (0.71 to 0.86) | 0.80 (0.72 to 0.86) |
Change WOMAC total | |||
ADAPT | |||
SF-12 MCS | 72 | 0.27 (0.04 to 0.47) | 0.33 (0.11 to 0.52) |
SF-12 PCS | 72 | 0.45 (0.25 to 0.62) | 0.47 (0.26 to 0.63) |
WOMAC pain | 110 | 0.89 (0.85 to 0.93) | 0.89 (0.84 to 0.92) |
WOMAC function | 110 | 0.99 (0.99 to 0.99) | 0.99 (0.99 to 0.99) |
WOMAC stiffness | 110 | 0.81 (0.74 to 0.87) | 0.83 (0.77 to 0.88) |
APEX | |||
EQ-5D-3L index | 193 | 0.58 (0.48 to 0.67) | 0.59 (0.49 to 0.67) |
WOMAC pain | 200 | 0.79 (0.73 to 0.84) | 0.84 (0.79 to 0.87) |
WOMAC function | 200 | 0.98 (0.97 to 0.99) | 0.98 (0.98 to 0.99) |
WOMAC stiffness | 200 | 0.70 (0.62 to 0.76) | 0.71 (0.63 to 0.77) |
Comparator | n | Correlation (95% CI) | |
---|---|---|---|
Spearman’s | Pearson’s | ||
Change EQ-5D-3L index | |||
APEX | |||
WOMAC pain | 240 | 0.50 (0.40 to 0.59) | 0.53 (0.43 to 0.62) |
WOMAC function | 180 | 0.53 (0.41 to 0.63) | 0.56 (0.45 to 0.65) |
WOMAC stiffness | 233 | 0.50 (0.40 to 0.59) | 0.53 (0.43 to 0.62) |
WOMAC total | 169 | 0.57 (0.46 to 0.67) | 0.60 (0.49 to 0.69) |
KAT | |||
OKS | 1565 | 0.56 (0.52 to 0.59) | 0.56 (0.53 to 0.60) |
OKS function | 1632 | 0.47 (0.43 to 0.51) | 0.49 (0.45 to 0.53) |
OKS pain | 1784 | 0.55 (0.52 to 0.58) | 0.55 (0.52 to 0.59) |
SF-12 PCS | 1749 | 0.41 (0.37 to 0.45) | 0.42 (0.38 to 0.45) |
SF-12 MCS | 1749 | 0.24 (0.20 to 0.29) | 0.27 (0.22 to 0.31) |
Change SF-12 PCS | |||
ADAPT | |||
SF-12 MCS | 65 | 0.04 (–0.20 to 0.28) | 0.10 (–0.15 to 0.33) |
WOMAC pain | 65 | 0.56 (0.37 to 0.71) | 0.56 (0.37 to 0.71) |
WOMAC function | 65 | 0.63 (0.45 to 0.76) | 0.61 (0.43 to 0.75) |
WOMAC stiffness | 64 | 0.48 (0.26 to 0.65) | 0.41 (0.18 to 0.59) |
WOMAC total | 64 | 0.64 (0.47 to 0.76) | 0.62 (0.44 to 0.75) |
KAT | |||
OKS function | 1581 | 0.54 (0.50 to 0.57) | 0.55 (0.52 to 0.59) |
OKS pain | 1731 | 0.54 (0.50 to 0.57) | 0.55 (0.52 to 0.58) |
Change SF-12 MCS | |||
ADAPT | |||
SF-12 PCS | 65 | 0.04 (–0.20 to 0.28) | 0.10 (–0.15 to 0.33) |
WOMAC pain | 65 | 0.24 (–0.00 to 0.46) | 0.28 (0.04 to 0.49) |
WOMAC function | 65 | 0.27 (0.03 to 0.49) | 0.34 (0.10 to 0.54) |
WOMAC stiffness | 64 | 0.28 (0.03 to 0.49) | 0.33 (0.09 to 0.53) |
WOMAC total | 64 | 0.28 (0.04 to 0.50) | 0.34 (0.11 to 0.54) |
KAT | |||
OKS function | 1581 | 0.27 (0.22 to 0.31) | 0.30 (0.25 to 0.34) |
Change WOMAC total | |||
ADAPT | |||
SF-12 MCS | 64 | 0.28 (0.04 to 0.50) | 0.34 (0.11 to 0.54) |
SF-12 PCS | 64 | 0.64 (0.47 to 0.76) | 0.62 (0.44 to 0.75) |
WOMAC pain | 108 | 0.88 (0.83 to 0.92) | 0.90 (0.86 to 0.93) |
WOMAC function | 108 | 0.98 (0.98 to 0.99) | 0.99 (0.98 to 0.99) |
WOMAC stiffness | 108 | 0.74 (0.65 to 0.82) | 0.73 (0.63 to 0.81) |
APEX | |||
EQ-5D-3L index | 169 | 0.57 (0.46 to 0.67) | 0.60 (0.49 to 0.69) |
WOMAC pain | 174 | 0.84 (0.79 to 0.88) | 0.86 (0.82 to 0.90) |
WOMAC function | 174 | 0.98 (0.97 to 0.98) | 0.98 (0.98 to 0.99) |
WOMAC stiffness | 174 | 0.74 (0.66 to 0.80) | 0.77 (0.70 to 0.82) |
Hip
Overall, correlations between the change scores of the instruments show a moderate (> 0.5) association, except mainly for the correlations involving SF-12 MCS and PCS instruments. Some of the individual EQ-5D-3L domains also had lower correlations. Pearson’s and Spearman’s correlations were similar.
Knee
Overall, correlations between the change scores of the instruments show a moderate (> 0.5) association, except for the correlations involving the SF-12, MCS and PCS instruments. Pearson’s and Spearman’s correlations were similar.
Floor and ceiling effects
Floor and ceiling effect results for the hip and knee scores are shown in Tables 14 and 15, respectively.
Measurement tool | Time point | |||||
---|---|---|---|---|---|---|
Pre operation | Post operation | |||||
n | Floor (%) | Ceiling (%) | n | Floor (%) | Ceiling (%) | |
EUROHIP | ||||||
EQ-5D-3L index | 1228 | 0.1 | 0.7 | 883 | – | 39.0 |
APEX | ||||||
EQ-5D-3L index | 302 | 0.0 | 1.3 | 266 | 0.0 | 46.2 |
ADAPT | ||||||
SF-12 MCS | 118 | 0.0 | 0.0 | 75 | 0.0 | 0.0 |
SF-12 PCS | 118 | 0.0 | 0.0 | 75 | 0.0 | 0.0 |
EPOS | ||||||
OHS total | 1517 | 0.1 | – | 1239 | – | 19.1 |
OHS pain | 1527 | 2.6 | 0.1 | 1247 | – | 33.8 |
OHS function | 1520 | 0.3 | 0.1 | 1248 | 0.2 | 26.4 |
EUROHIP | ||||||
OHS total | 127 | – | – | 114 | – | 14.0 |
OHS pain | 133 | 3.8 | – | 120 | – | 35.8 |
OHS function | 131 | – | – | 115 | – | 19.1 |
ADAPT | ||||||
WOMAC total | 125 | 0 | 0 | 111 | 0 | 20.7 |
WOMAC pain | 125 | 1.6 | 1.6 | 111 | 0 | 55.0 |
WOMAC function | 125 | 0.8 | 1.6 | 111 | 0 | 24.3 |
WOMAC stiffness | 125 | 4.8 | 4.8 | 111 | 0 | 53.2 |
APEX | ||||||
WOMAC total | 261 | 0.4 | 0 | 234 | 0 | 19.7 |
WOMAC pain | 323 | 2.5 | 0.6 | 279 | 0 | 46.6 |
WOMAC function | 270 | 0.7 | 0 | 242 | 0 | 27.7 |
WOMAC stiffness | 300 | 4.3 | 3.3 | 268 | 0 | 44.8 |
EUROHIP | ||||||
WOMAC total | 1243 | 0.2 | – | 865 | 0.1 | 7.2 |
WOMAC pain | 1255 | 1.0 | 0.2 | 875 | 0.1 | 33.3 |
WOMAC function | 1266 | 5.9 | 1.6 | 888 | 0.5 | 26.6 |
WOMAC stiffness | 1253 | 0.6 | – | 888 | – | 9.2 |
Measurement tool | Time point | |||||
---|---|---|---|---|---|---|
Pre operation | Post operation | |||||
n | Floor (%) | Ceiling (%) | n | Floor (%) | Ceiling (%) | |
APEX | ||||||
EQ-5D-3L index | 298 | 0 | 0.7 | 261 | 0.4 | 29.5 |
KAT | ||||||
EQ-5D-3L index | 2120 | – | 0.4 | 1939 | – | 25.4 |
SF-12 MCS | 116 | 0 | 0 | 74 | 0 | 0 |
SF-12 PCS | 116 | 0 | 0 | 74 | 0 | 0 |
KAT | ||||||
OKS total | 2112 | 0.1 | – | 1691 | 0.12 | 2.3 |
OKS pain | 2132 | 0.1 | 0.1 | 1753 | 0.2 | 3.7 |
OKS function | 2136 | 0.9 | 0.1 | 1906 | 0.1 | 17.0 |
ADAPT | ||||||
WOMAC total | 126 | 0.8 | 0.0 | 110 | 0.0 | 3.6 |
WOMAC pain | 127 | 0.8 | 0.0 | 110 | 0.0 | 20.9 |
WOMAC function | 127 | 0.8 | 0.0 | 110 | 0.0 | 9.1 |
WOMAC stiffness | 127 | 3.9 | 2.4 | 110 | 0.9 | 17.3 |
APEX | ||||||
WOMAC total | 246 | 0.0 | 0.0 | 214 | 0.0 | 5.1 |
WOMAC pain | 318 | 2.2 | 0.0 | 269 | 0.4 | 30.5 |
WOMAC function | 253 | 0.0 | 0.0 | 224 | 0.0 | 9.4 |
WOMAC stiffness | 293 | 2.7 | 1.0 | 260 | 0.8 | 17.3 |
Hip
No floor effects were detected at pre operation for any of the instruments. Substantial ceiling effects were noted at post operation only. This was particularly the case for the EQ-5D-3L index (39–46%), although all instruments assessed mostly had a substantial proportion with the highest possible value. The minimum ceiling effect was 7%, which was observed for the WOMAC total in the EUROHIP data set.
Knee
No floor effects were detected at pre operation for any of the instruments. Substantial ceiling effects were noted, but to a lower extent than for hip data sets at post operation only for the EQ-5D-3L index and WOMAC pain scores. Again, this was most strongly the case for the EQ-5D-3L index (25–30%).
Interpretability
Minimal detectable change (90% significance level)
Literature-based minimal detectable change
Minimal detectable change (90% significance) was calculated using the literature review ICC values presented in Table 16. The SF-12 PCS, assuming an ICC of 0.84, had ± 7–8 MDC points. The MDC values were 10–12 points for the SF-12 MCS with an ICC of 0.80 for hip and knee. 78 MDC values for the WOMAC pain, physical function and stiffness for hip and knee using ICCs of 0.73, 0.78 and 0.53, respectively, ranged from 20–27, 20–27 and 23–39, respectively. 79
Measurement tool | Pre operation | ||
---|---|---|---|
n | ICC | MDC 90% | |
Hip | |||
ADAPT | |||
SF-12 PCS | 72 | 0.84 | ±7.94 |
SF-12 MCS | 72 | 0.80 | ±9.55 |
ADAPT | |||
WOMAC pain | 110 | 0.73 | ±26.60 |
WOMAC function | 110 | 0.78 | ±24.27 |
WOMAC stiffness | 110 | 0.53 | ±38.71 |
APEX | |||
WOMAC pain | 278 | 0.73 | ±22.71 |
WOMAC function | 209 | 0.78 | ±20.64 |
WOMAC stiffness | 255 | 0.53 | ±36.60 |
EUROHIP | |||
WOMAC pain | 1255 | 0.73 | ±21.55 |
WOMAC function | 1253 | 0.53 | ±26.67 |
WOMAC stiffness | 1266 | 0.78 | ±22.62 |
Knee | |||
ADAPT | |||
SF-12 PCS | 65 | 0.84 | ±6.67 |
SF-12 MCS | 65 | 0.80 | ±9.54 |
KAT | |||
SF-12 PCS | 2087 | 0.84 | ±7.60 |
SF-12 MCS | 2087 | 0.80 | ±11.99 |
ADAPT | |||
WOMAC pain | 109 | 0.73 | ±22.89 |
WOMAC function | 109 | 0.78 | ±19.87 |
WOMAC stiffness | 108 | 0.53 | ±32.34 |
APEX | |||
WOMAC pain | 269 | 0.73 | ±20.20 |
WOMAC function | 187 | 0.78 | ±19.54 |
WOMAC stiffness | 242 | 0.53 | ±32.21 |
Minimal detectable change using assumed intracluster correlation coefficient values
Reported ICC values from previous studies reflect the limitations of those studies in terms of population and precision. The arbitrary ICC figures of 0.5, 0.7 and 0.9 that were also used to calculate MDCs are reported in Table 17 for hip and knee scores.
Measurement tool | Pre operation | |||
---|---|---|---|---|
n | MDC (90%) | |||
ICC 0.5 | ICC 0.7 | ICC 0.9 | ||
Hip | ||||
EUROHIP | ||||
EQ-5D-3L index | 1228 | ±0.54 | ±0.42 | ±0.24 |
APEX | ||||
EQ-5D-3L index | 250 | ±0.54 | ±0.42 | ±0.24 |
ADAPT | ||||
SF-12 PCS | 72 | ±14.04 | ±10.87 | ±6.28 |
SF-12 MCS | 72 | ±15.11 | ±11.70 | ±6.76 |
EPOS | ||||
OHS | 1517 | ±13.12 | ±10.16 | ±5.87 |
EUROHIP | ||||
OHS | 127 | ±13.10 | ±10.15 | ±5.86 |
ADAPT | ||||
WOMAC total | 110 | ±35.00 | ±27.11 | ±15.65 |
WOMAC pain | 110 | ±36.20 | ±28.04 | ±16.19 |
WOMAC function | 110 | ±36.59 | ±28.34 | ±16.36 |
WOMAC stiffness | 110 | ±39.92 | ±30.92 | ±17.85 |
APEX | ||||
WOMAC total | 200 | ±29.47 | ±22.83 | ±13.18 |
WOMAC pain | 278 | ±30.90 | ±23.93 | ±13.82 |
WOMAC function | 209 | ±31.11 | ±24.10 | ±13.91 |
WOMAC stiffness | 255 | ±37.75 | ±29.24 | ±16.88 |
EUROHIP | ||||
WOMAC total | 1243 | ±26.41 | ±20.46 | ±11.81 |
WOMAC pain | 1255 | ±29.33 | ±22.72 | ±13.12 |
WOMAC function | 1253 | ±27.51 | ±21.31 | ±12.30 |
WOMAC stiffness | 1266 | ±34.10 | ±26.41 | ±15.25 |
Knee | ||||
KAT | ||||
EQ-5D-3L index | 2120 | ±0.51 | ±0.39 | ±0.23 |
APEX | ||||
EQ-5D-3L index | 248 | ±0.51 | ±0.39 | ±0.23 |
KAT | ||||
SF-12 PCS | 2087 | ±13.43 | ±10.40 | ±6.01 |
SF-12 MCS | 2087 | ±18.96 | ±14.68 | ±8.48 |
ADAPT | ||||
SF-12 PCS | 65 | ±11.78 | ±9.13 | ±5.27 |
SF-12 MCS | 65 | ±15.08 | ±11.68 | ±6.74 |
KAT | ||||
OKS | 2112 | ±12.40 | ±9.60 | ±5.54 |
ADAPT | ||||
WOMAC total | 108 | ±28.68 | ±22.22 | ±12.83 |
WOMAC pain | 109 | ±31.15 | ±24.13 | ±13.93 |
WOMAC function | 109 | ±29.96 | ±23.20 | ±13.40 |
WOMAC stiffness | 108 | ±33.36 | ±25.84 | ±14.92 |
APEX | ||||
WOMAC total | 174 | ±27.06 | ±20.96 | ±12.10 |
WOMAC pain | 269 | ±27.49 | ±21.29 | ±12.29 |
WOMAC function | 187 | ±29.45 | ±22.82 | ±13.17 |
WOMAC stiffness | 242 | ±33.23 | ±25.74 | ±14.86 |
Hip
Using an ICC of 0.9 provided MDCs of 0.24 for the EQ-5D-3L index, 6 points for the OHS total score, 6 and 7 for the SF-12 PCS and MCS, respectively, and 12–16 points for the WOMAC total score across the data sets. MDCs with ICCs of 0.5 and 0.7 were substantially larger, as would be anticipated.
Knee
Using an ICC of 0.9 provided MDCs of 0.23 for the EQ-5D-3L index, 5 and 6 for the SF-12 PCS and MCS, respectively, 6 points for the OKS total score and 13 for the WOMAC subscales across the data sets. MDCs with assumed ICCs of 0.5 and 0.7 were substantially larger, as would be anticipated.
Minimally important change and minimally important difference
Hip
A suitable anchor was available in two data sets: EUROHIP (EQ-5D-3L, OHS and WOMAC) and EPOS (OHS) and applied to the total score only. In EUROHIP, 244 patients answered for the satisfaction question after operation for EUROHIP. Of these, 59 patients (24%) answered ‘somewhat satisfied’ and 13 patients (5.3%) answered ‘somewhat dissatisfied’. These ‘somewhat satisfied’ versus ‘somewhat dissatisfied’ groups were used for the anchor-based analyses in this report when there was no neutral scale. In EPOS, 1053 patients answered the satisfaction question after operation. Of these, 167 patients (16%) answered ‘somewhat satisfied’ and 39 patients (4%) answered ‘somewhat dissatisfied’. These ‘somewhat satisfied’ versus ‘somewhat dissatisfied’ groups were used for the anchor-based analyses in this report.
Figure 4 shows the EQ-5D-3L index ROC curve for the EUROHIP data. Table 18 gives the pre and post operative values in accordance with the anchor, and Table 19 gives the area under the curve (AUC), MIC (ROC), MIC (group), MIC (ES), MID (group) and MID (ES) estimates, along with the sensitivity and specificity for the optimal cut-off point. The AUC was 0.69. The MIC ROC values were 0.07 for both the Youden and the shortest distance methods. MIC (group) and MID (group) were much larger, at 0.36 and 0.28 points, respectively. The values for MIC (ES) and MID (ES) were both around 1.00.
FIGURE 4.
The EQ-5D-3L index: EUROHIP data set ROC curve.
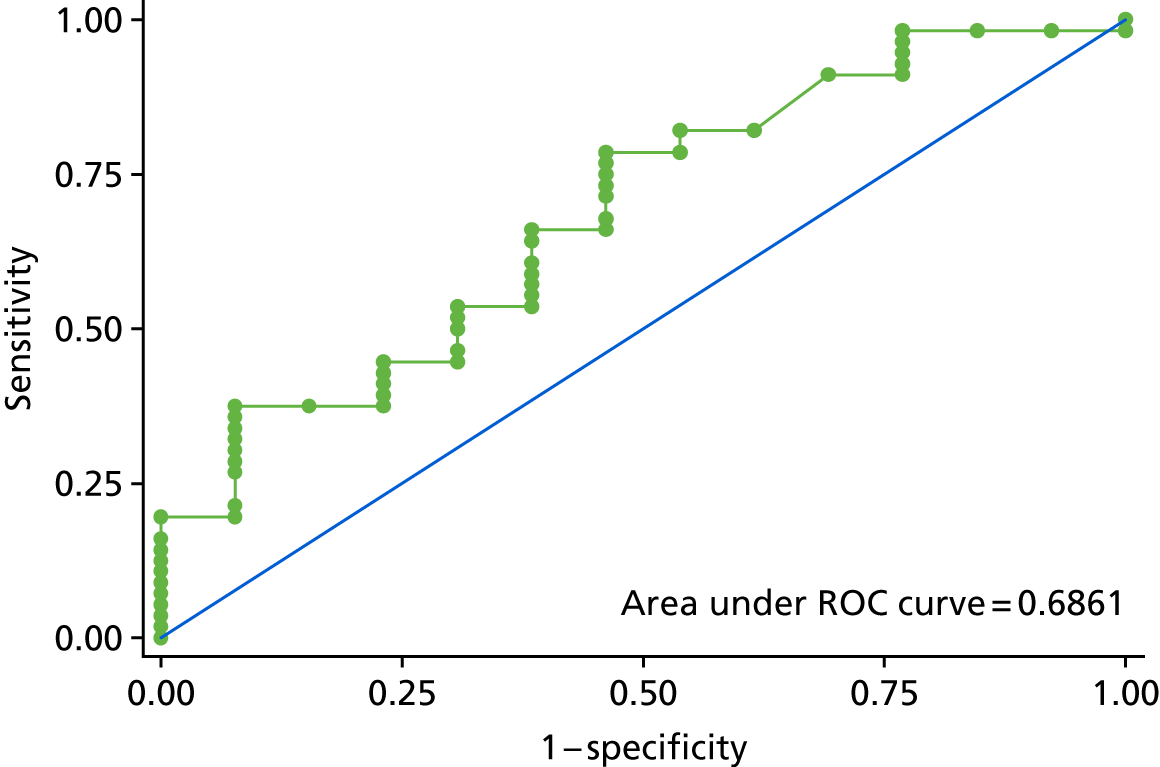
Score | Satisfaction | |||||
---|---|---|---|---|---|---|
Somewhat satisfied | Somewhat dissatisfied | |||||
n | Mean score | SD | n | Mean score | SD | |
Preoperative | 56 | 0.33 | 0.32 | 13 | 0.34 | 0.33 |
Postoperative | 59 | 0.69 | 0.25 | 13 | 0.42 | 0.32 |
Change | 56 | 0.36 | 0.29 | 13 | 0.08 | 0.33 |
MIC | MID | |||||
---|---|---|---|---|---|---|
Individual-level MIC (ROC analysis) | Scale | MIC | ||||
AUC (95% CI) | Optimal cut-off point | Sensitivity | 1 – specificity | |||
0.69 (0.52 to 0.85) | ||||||
Youden’s index | 0.07 | 0.79 | 0.46 | Group | 0.36 | 0.28 |
Shortest distance | 0.07 | 0.79 | 0.46 | ES | 1.26 | 0.97 |
Owing to the low number of OHS observations in the EUROHIP data set (Table 20), in which the satisfaction was ‘somewhat satisfied’ and ‘somewhat dissatisfied’, the MIC ROC was not calculated in this report when there was no neutral scale.
Score | Satisfaction | |||||
---|---|---|---|---|---|---|
Somewhat satisfied | Somewhat dissatisfied | |||||
n | Mean | SD | n | Mean | SD | |
Preoperative | 11 | 14.36 | 3.61 | 3 | 13.33 | 10.50 |
Postoperative | 11 | 30.50 | 7.99 | 4 | 26.50 | 3.32 |
Change | 10 | 16.14 | 6.09 | 3 | 13.17 | 7.12 |
Figure 5 shows the OHS ROC curve for the EPOS data set. Table 21 gives the pre and post operative values in accordance with the anchor, and Table 22 gives the corresponding AUC, MIC (ROC), MIC (group), MIC (ES), MID (group) and MID (ES) estimates, along with the sensitivity and specificity for the optimal cut-off point. The AUC was 0.69. The MIC ROC values were 7 and 14 points for the Youden and shortest distance methods, respectively. The MIC (group) was larger, at 16 points, but the MID (group) was similar, at 6 points. The MIC (ES) and MID (ES) were 2 and 1, respectively.
FIGURE 5.
The total OHS: EPOS data set ROC curve.
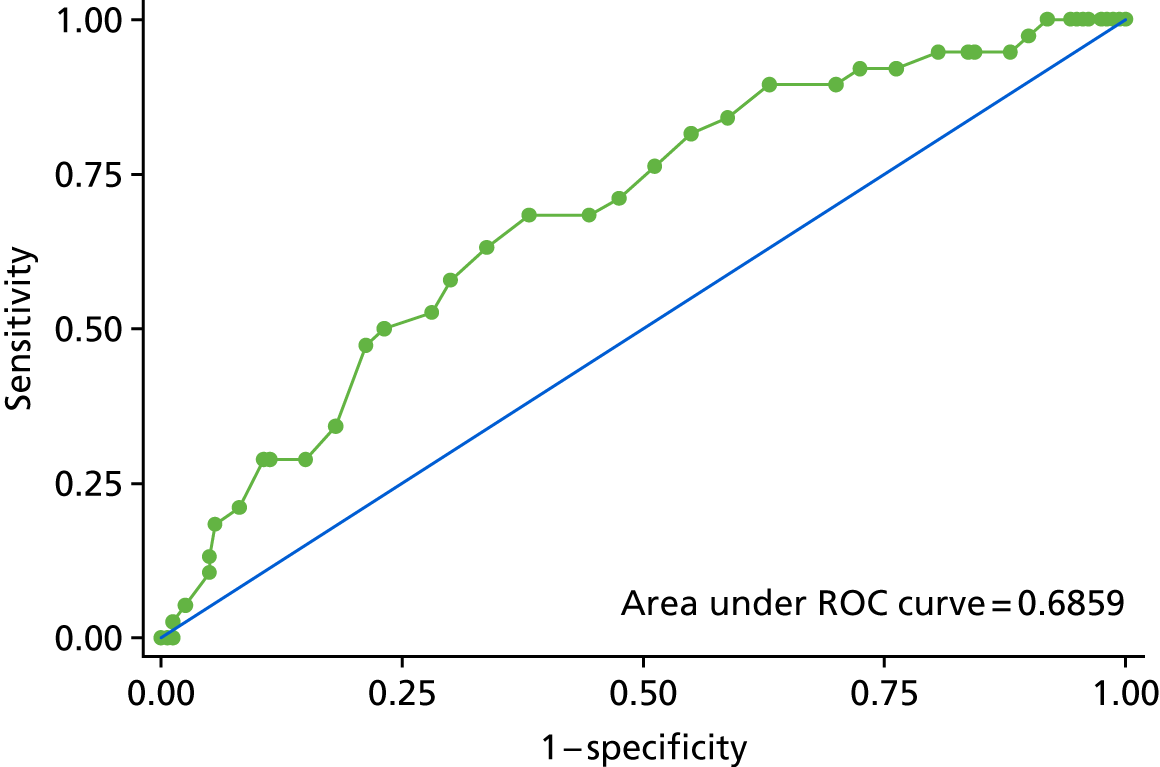
Score | Satisfaction | |||||
---|---|---|---|---|---|---|
Somewhat satisfied | Somewhat dissatisfied | |||||
n | Mean | SD | n | Mean | SD | |
Preoperative | 163 | 14.52 | 7.10 | 39 | 14.80 | 8.00 |
Postoperative | 164 | 30.85 | 9.09 | 38 | 25.05 | 8.13 |
Change | 163 | 16.33 | 8.16 | 39 | 10.25 | 8.06 |
MIC | MID | |||||
---|---|---|---|---|---|---|
Individual-level MIC (ROC analysis) | Scale | MID | ||||
AUC (95% CI) | Optimal cut-off point | Sensitivity | 1 – specificity | |||
0.69 (0.59 to 0.78) | ||||||
Youden’s index | 7 | 0.01 | 1 | Group | 16.33 | 6.08 |
Shortest distance | 14 | 0.44 | 0.68 | ES | 2 | 0.75 |
Figure 6 shows the WOMAC total ROC curve for the EUROHIP data. Table 23 gives the preoperative and postoperative values in accordance with the anchor, and Table 24 gives the AUC, MIC (ROC), MIC (group), MIC (ES), MID (group) and MID (ES) estimates, along with the sensitivity and specificity for the optimal cut-off point. The AUC was 0.76. The MIC ROC optimal cut-off point was the same for the Youden and shortest distance methods: 26 points. The MIC (group) and MID (group) were larger, at 31 and 19 points, respectively. The MIC (ES) and MID (ES) were 2 and 1 points, respectively.
FIGURE 6.
The total WOMAC score: EUROHIP data set ROC curve.

Score | Satisfaction | |||||
---|---|---|---|---|---|---|
Somewhat satisfied | Somewhat dissatisfied | |||||
n | Mean | SD | n | Mean | SD | |
Preoperative | 58 | 63.37 | 14.41 | 13 | 61.23 | 13.33 |
Postoperative | 58 | 32.10 | 18.63 | 13 | 48.64 | 20.55 |
Change | 55 | –31.27 | 16.60 | 13 | –12.59 | 17.32 |
MIC | MID | |||||
---|---|---|---|---|---|---|
Individual-level MIC (ROC analysis) | Scale | MID | ||||
AUC (95% CI) | Optimal cut-off point | Sensitivity | 1 – specificity | |||
0.76 (0.62 to 0.90) | ||||||
Youden’s Index | 26 | 0.63 | 0.15 | Group | 31.27 | 18.68 |
Shortest distance | 26 | 0.63 | 0.15 | ES | 1.88 | 1.13 |
Knee
None of the available knee data sets had a suitable anchor for which interpretability properties could be assessed.
Discussion
We calculated the measurement properties of the candidate scores using various statistical methods, using multiple hip and knee replacement data sets for the candidate instruments. This enabled us to obtain estimates for some of the properties for which population-specific estimates had not been previously reported. Estimates for most of the missing measurement properties could be calculated given the available data sets for the measurement tools of interest.
The OHS (EUROHIP and EPOS) and OKS (KAT) showed generally positive additional evidence regarding the measurement properties (internal consistency, construct validity, responsiveness and interpretability). Similarly, the WOMAC [ADAPT (Assessing Disability After Partial and Total Joint Replacement), the APEX study and EUROHIP] showed generally positive additional evidence regarding the measurement properties. For the SF-12 PCS and MCS, the evidence was more mixed and the lack of agreement between MCS and PCS was noteworthy and, although perhaps unsurprising, is problematic in terms of use in the proposed context.
There was no sign of a flooring effect in any instruments. The OHS showed high ceiling effects at post operation, whereas the OKS had a ceiling effect of only 2% at post operation. The high ceiling effects of the OHS after the arthroplasty surgery could be examined in relation to patient-reported satisfaction and/or assessments measuring success. The EQ-5D-3L index showed high ceiling effects for both hip and knee data sets, in keeping with previous evidence. 85 In respect of the systematic review, there is a substantial ceiling effect for patient ratings in the EQ-5D-3L measurement tool. Different versions of SF-12 questionnaires were used in different studies, illustrating how it can be difficult to obtain unified data sets for one measurement tool. Further research is still required for responsiveness, between the generic questionnaires [e.g. the EQ-5D-3L/EuroQol-5 Dimensions, five-level version (EQ-5D-5L) and SF-12] and disease-specific questionnaires. Taken overall, there was generally a reasonable amount of positive evidence of the measurement properties of the OHS, OKS and WOMAC.
Although the WOMAC physical function, pain and stiffness scales were reported as reliable in the systematic review of measurement properties presented in Chapter 2, the pain scale was highly related to physical function including item scales. 86 In this study, we focused on the relationship between the WOMAC total score and WOMAC subscales; further research may be required regarding the WOMAC subscales and their use as instruments for developing thresholds. Measurement properties of the SF-12 PCS were more positive than the MCS, which is similar to some previous studies. 87,88 Further research may be required to clarify what values of the SF-12 MCS are plausible as thresholds for referral and candidacy for the joint replacement surgery and the role, if any, it can play in this context.
The study had a number of limitations. Most important were the variations between available data sets in terms of size and also the collection of relevant instruments and variables (e.g. anchor questions). Evidence on a number of properties for a number of instruments is still lacking or limited. None of the data sets was ideal and they only contained a subset of relevant instruments, which made comparison between instruments difficult. For the WOMAC score, only relatively small data sets were available. Imbalance between data sets and outcomes collected makes direct comparison between the instruments very problematic, and, therefore, we have restricted reporting to the individual properties as opposed to the contrasting instruments. Similarly, the version of the instrument used varied and in some cases (e.g. the EQ-5D-3L) a more recent version of the tool has been proposed (the EQ-5D-5L) for which no data sets were available. The methods used to assess the measurement properties were relatively simplistic, although commonly used in the literature and in general do not provide definitive answers, only suggestive findings.
Conclusion
From the data sets available, additional data on measurement properties were calculated for the EQ-5D-3L, OHS, OKS, WOMAC, SF-12 (PCS and MCS) and SF-36. These results were added to the information identified from the systematic review to produce a summary table of the measurement properties of each of the 36 instruments originally identified. This additional information was used to update the summary of instrument measurement property evidence produced as part of the systematic review in Chapter 2. This updated summary, shown in Tables 25–28, was presented to the user group to inform the choice of candidate instrument to take forward.
Criteria | Instrument | |||||||||||||||||
---|---|---|---|---|---|---|---|---|---|---|---|---|---|---|---|---|---|---|
HOOS | HRQ | PSI | OHS | Knee disorders subjective history (VAS) | KOOS | KOOS-PS | OKS | OKS-APQ | LEFS (h/k) | LEFS (h) | LEFS (K) | WOMAC (h/k) | WOMAC (h) | WOMAC (K) | WOMAC SF (h/k) | WOMAC SF (h) | WOMAC SF (k) | |
Number of studies | 5 | 1 | 4 | 20 | 1 | 3 | 2 | 23 | 1 | 5 | 0 | 0 | 25 | 0 | 0 | |||
Reproducibility | ++ | + | + | ++ | 0 | + | 0 | +++ | +++ | + | 0 | 0 | ++ | ++ | + | 0 | 0 | 0 |
Internal consistency | + | 0 | 0 | +++ | 0 | 0 | +++ | +++ | +++ | + | 0 | 0 | + | +++ | +++ | + | 0 | 0 |
Validity: content | 0 | 0 | ++ | ++ | + | + | + | +++ | +++ | + | 0 | 0 | + | + | + | + | 0 | 0 |
Construct | ++ | + | ++ | +++ | + | + | ++ | +++ | +++ | ++ | 0 | 0 | +++ | + | ++ | ++ | 0 | 0 |
Responsiveness | + | + | ++ | +++ | 0 | 0 | ++ | +++ | +++ | ++ | 0 | 0 | +++ | ++ | ++ | + | 0 | 0 |
Interpretability | 0 | 0 | 0 | +++ | 0 | 0 | 0 | ++ | 0 | + | 0 | 0 | ++ | ++ | ++ | 0 | 0 | 0 |
Floor and ceiling/precision | + | 0 | 0 | –/+ | 0 | + | 0 | ++ | ++ | 0 | 0 | 0 | –/+ | –/+ | –/+ | 0 | 0 | 0 |
Acceptability | 0 | 0 | 0 | +++ | – | 0 | 0 | +++ | +++ | 0 | 0 | 0 | ++ | + | + | 0 | 0 | 0 |
Data accessible | N | N | N | Y | N | N | N | Y | N | N | N | N | N | N | N | N | N | N |
Criteria | Instrument | ||||||||||||||
---|---|---|---|---|---|---|---|---|---|---|---|---|---|---|---|
Lower limb core score (h/k) | Lower limb core score (h) | Lower limb core score (k) | MODEMS-HK/(AAOS) hip and knee core score (h/k) | MODEMS-HK/(AAOS) hip and knee core score (h) | MODEMS-HK/(AAOS) hip and knee core score (k) | ICOAP (h/k) | ICOAP (h) | ICOAP (k) | P4 (h/k) | P4 (h) | P4 (k) | McGill Pain-SF (h/k) | McGill Pain-SF (h) | McGill Pain-SF (k) | |
Number of studies | 1 | 0 | 0 | 1 | 0 | 0 | 2 | 0 | 0 | 1 | 0 | 0 | 2 | 0 | 0 |
Reproducibility | 0 | 0 | 0 | 0 | 0 | 0 | + | 0 | 0 | 0 | 0 | 0 | ++ | 0 | 0 |
Internal consistency | 0 | 0 | 0 | 0 | 0 | 0 | + | +++ | +++ | ++ | 0 | 0 | 0 | 0 | 0 |
Validity: content | + | 0 | 0 | + | 0 | 0 | ++ | 0 | 0 | + | 0 | 0 | 0 | 0 | 0 |
Construct | 0 | 0 | 0 | + | 0 | 0 | + | 0 | 0 | + | 0 | 0 | + | 0 | 0 |
Responsiveness | 0 | 0 | 0 | ++ | 0 | 0 | – | 0 | 0 | 0 | 0 | 0 | – | 0 | 0 |
Interpretability | 0 | 0 | 0 | 0 | 0 | 0 | 0 | 0 | 0 | 0 | 0 | 0 | 0 | 0 | 0 |
Floor and ceiling/precision | 0 | 0 | 0 | ++ | 0 | 0 | 0 | – | – | 0 | 0 | 0 | 0 | 0 | 0 |
Acceptability | 0 | 0 | 0 | + | 0 | 0 | 0 | 0 | 0 | 0 | 0 | 0 | 0 | 0 | 0 |
Data accessible | N | N | N | N | N | N | N | Y | Y | N | N | N | N | N | N |
Criteria | Instrument | |||||||||||||||||
---|---|---|---|---|---|---|---|---|---|---|---|---|---|---|---|---|---|---|
SF-6D (h) | SF-6D (k) | SF-6D (h/k) | HUI2 and HUI3 (h) | HUI2 and HUI3 (h) | HUI2 and HUI3 (h) | EQ-5D (h/k) | EQ-5D (h) | EQ-5D (k) | SF-36 (h/k) | SF-36 (h) | SF-36 (k) | SF-12 (h/k) | SF-12 (h) | SF-12 (k) | SIP (h) | SIP (k) | SIP (h/k) | |
Number of studies | 1 | 0 | 0 | 4 | 0 | 0 | 9 | 14 | 3 | 2 | 0 | 0 | ||||||
Reproducibility | 0 | 0 | 0 | 0 | 0 | 0 | 0 | 0 | 0 | 0 | 0 | 0 | ++ | 0 | 0 | 0 | 0 | 0 |
Internal consistency | 0 | 0 | 0 | 0 | 0 | 0 | N/A | N/A | N/A | 0 | 0 | – | 0 | +++ | +++ | 0 | 0 | 0 |
Validity: content | 0 | 0 | 0 | 0 | 0 | 0 | 0 | 0 | 0 | 0 | 0 | 0 | 0 | 0 | 0 | + | 0 | 0 |
Construct | 0 | 0 | 0 | ++ | 0 | 0 | + | – | – | + | + | + | 0 | + | –/+ | + | 0 | 0 |
Responsiveness | ++ | 0 | 0 | + | 0 | 0 | 0 | – | – | 0 | +/– | + | 0 | + | –/+ | – | 0 | 0 |
Interpretability | + | 0 | 0 | 0 | 0 | 0 | 0 | ++ | ++ | 0 | + | + | 0 | + | + | 0 | 0 | 0 |
Floor and ceiling/precision | 0 | 0 | 0 | 0 | 0 | 0 | 0 | – | + | 0 | – | 0 | +++ | +++ | +++ | 0 | 0 | 0 |
Acceptability | 0 | 0 | 0 | 0 | 0 | 0 | 0 | 0 | ++ | 0 | 0 | 0 | 0 | 0 | 0 | 0 | 0 | 0 |
Data accessible | N | N | N | N | N | N | Y | Y | Y | N | Y | N | Y | Y | Y | N | N | N |
Criteria | Instrument | ||||||||||||||||||||||||||
---|---|---|---|---|---|---|---|---|---|---|---|---|---|---|---|---|---|---|---|---|---|---|---|---|---|---|---|
WHOQOL-BREF (h/k) | WHOQOL-BREF (h) | WHOQOL-BREF (k) | Aberdeen IAP (h/k) | Aberdeen IAP (h) | Aberdeen IAP (k) | Aberdeen IAP (modified) (h/k) | Aberdeen IAP (modified) (h) | Aberdeen IAP (modified) (k) | NEADL (h/k) | NEADL (h) | NEADL (k) | AQOL (h/k) | AQOL (h) | AQOL (k) | MSK Functional Limitations Index (h/k) | MSK Functional Limitations Index (h) | MSK Functional Limitations Index (k) | HAQ (h/k) | HAQ (h) | HAQ (k) | MHAQ (h/K) | MHAQ (h) | MHAQ (k) | K10 (h/k) | K10 (h) | K10 (k) | |
Number of studies | 1 | 0 | 0 | 1 | 0 | 0 | 1 | 0 | 0 | 0 | 1 | 0 | 2 | 0 | 0 | 0 | 0 | 1 | 0 | 0 | 2 | 2 | 0 | 1 | 0 | 0 | |
Reproducibility | 0 | 0 | 0 | 0 | 0 | 0 | 0 | 0 | 0 | 0 | ++ | 0 | 0 | 0 | 0 | 0 | 0 | 0 | 0 | 0 | 0 | 0 | 0 | 0 | 0 | 0 | 0 |
Internal consistency | ++ | 0 | 0 | + | 0 | 0 | ++ | 0 | 0 | 0 | ++ | 0 | 0 | 0 | 0 | 0 | 0 | 0 | 0 | 0 | – | 0 | 0 | 0 | 0 | 0 | 0 |
Validity: content | + | 0 | 0 | + | 0 | 0 | 0 | 0 | 0 | 0 | – | 0 | 0 | 0 | 0 | 0 | 0 | 0 | 0 | 0 | 0 | 0 | + | 0 | – | 0 | 0 |
Construct | 0 | 0 | 0 | + | 0 | 0 | + | 0 | 0 | 0 | + | 0 | + | 0 | 0 | 0 | 0 | + | 0 | 0 | ++ | + | + | 0 | + | 0 | 0 |
Responsiveness | + | 0 | 0 | 0 | 0 | 0 | 0 | 0 | 0 | 0 | – | 0 | ++ | 0 | 0 | 0 | 0 | 0 | 0 | 0 | – | – | + | 0 | – | 0 | 0 |
Interpretability | 0 | 0 | 0 | 0 | 0 | 0 | 0 | 0 | 0 | 0 | 0 | 0 | 0 | 0 | 0 | 0 | 0 | 0 | 0 | 0 | 0 | 0 | 0 | 0 | 0 | 0 | 0 |
Floor and ceiling/precision | ++ | 0 | 0 | + | 0 | 0 | 0 | 0 | 0 | 0 | + | 0 | 0 | 0 | 0 | 0 | 0 | 0 | 0 | 0 | + | ++ | 0 | 0 | ++ | 0 | 0 |
Acceptability | 0 | 0 | 0 | 0 | 0 | 0 | 0 | 0 | 0 | 0 | 0 | 0 | 0 | 0 | 0 | 0 | 0 | + | 0 | 0 | 0 | 0 | 0 | 0 | 0 | 0 | 0 |
Data accessible | N | N | N | N | N | N | N | N | N | N | N | N | N | N | N | N | N | N | N | N | N | N | N | N | N | N | N |
Chapter 4 Calculation of threshold values (work package 2)
Background
The selected candidate tools are shown in Table 29. The OKS and OHS consist of 12 questions with 0–48 integer score ranges, with higher scores indicating better health status. The SF-12 has two component scores: PCS, with a theoretical range of 4.3 to 76.4 (US version 2), and MCS, with a theoretical range of –1.1 to 79.6 (US version 2), with higher scores also indicating better health status (see Appendix 2). The WOMAC total score consists of 24 questions: the pain subsection has five questions, the physical function subsection has 17 questions and the stiffness subsection has two questions (all have a score range of 0–100). The WOMAC scores were converted to higher scores indicating better health status. The KOOS-PS consists of seven questions (score range of 0–100); no data were available for the KOOS-PS for evaluating in this study.
Tool | Subscale |
---|---|
Hip | |
OHS | |
Knee | |
KOOS-PS (no data) | |
OKS | |
Hip and knee | |
SF-12 | PCS |
MCS | |
WOMAC | Total |
Pain | |
Physical function | |
Stiffness |
Methods
Data sets
Multiple data sets were used to calculate the thresholds of individual candidate scores when possible (Table 30). We used the 2012–15 web-based NHS PROMs data together with the KAT and EPOS data sets for the analyses of the OHS and OKS, SF-12 PCS and MCS scores. The EUROHIP data set was used for WOMAC scores. The ADAPT and APEX studies were used for the SF-12 PCS and MCS (ADAPT) scores and the WOMAC scores (for both hip and knee) analyses. Postoperative scores were assessed at 6 months post surgery for NHS PROMs; at 12 months for the KAT, EUROHIP, ADAPT and APEX studies; and at 2 years for EPOS. The inclusion criterion was that patients had to have received primary knee or hip replacement surgery (no revision). Cross-validation has been completed when multiple data sets were used for both development and validation.
PROM tool | Data set | ||
---|---|---|---|
1 | 2 | 3 | |
Hip | |||
OHS | NHS PROMs | EPOS | – |
SF-12 | |||
PCS | ADAPT | – | – |
MCS | ADAPT | – | – |
Total | ADAPT | APEX | EUROHIP |
WOMAC | |||
Pain | ADAPT | APEX | EUROHIP |
Physical function | ADAPT | APEX | EUROHIP |
Stiffness | ADAPT | APEX | EUROHIP |
KOOS-PS (no data) | – | – | – |
Knee | |||
OKS | NHS PROMs | KAT | – |
SF-12 | PCS | KAT | ADAPT |
MCS | KAT | ADAPT | |
Total | ADAPT | APEX | |
WOMAC | Pain | ADAPT | APEX |
Physical function | ADAPT | APEX | |
Stiffness | ADAPT | APEX | |
KOOS-PS (no data) | – | – | – |
Improvement criteria
Patient benefit can be defined in various ways. We restricted the definition in this study to improvement and used approaches that were applicable to all candidate scores (limited by data; see Online Supplement 2). Four definitions of improvement were applied:
-
any increase after surgery from before surgery (change score of > 0)
-
medium ES (0.5) × SD of change score (MCID)
-
minimal detectable change (MDC90 and ICC of 0.7)
-
minimal detectable change (MDC90 and ICC of 0.9).
In this study, the MCID is derived from the assumption that the mean change score needed to obtain a medium or large ES to be clinically meaningful. 89 Clinically meaningful refers to a change indicating the efficacy of an intervention (i.e. hip and knee replacement surgery in this study) in domains of health-related functional status tools. 90 For clinical evaluation studies such as this one, the usefulness of the measurement candidate tools will depend on their ability to detect a change that is clinically meaningful. 90 Applying a 0.5 ES (medium/moderate practical importance), classified by Cohen,73 using the variability of the change scores is ideal in this context. It was calculated using the SD of the change score of the candidate tools multiplied by the medium ES (i.e. 0.5 × the SD of the change score (b). For the remainder of this chapter, results based on criterion B are presented.
The MDC was defined as the minimal change that falls beyond the measurement error in the score of a candidate tool measuring a symptom. 91 In this study, the fixed (arbitrary) reliability parameter (e.g. test–retest reliability or ICC) values of 0.7 and 0.9 were applied. We applied the 90% confidence level with z-distribution (z-statistics value of 1.645), a range for the possible difference between the two observations under the same conditions (test–retest scenario) to define the MDC as ± 1.645 × √2 × SE of the measurement, where the SE of the measurement is defined as the SD of the preoperative score × √((1 – R)) and R is the reliability parameter of 0.7 and 0.9 (improvement criteria C and D). 92 Stata® version 14 was used for all statistical analyses.
Statistical analysis
Absolute threshold
We estimated an absolute threshold in the preoperative score, using each data set above, at a level in which an individual could not improve. We examined the theoretical thresholds for the different definitions of improvement (B, C and D) with the method of subtracting each improvement score from the maximum possible score of the candidate tools. The specificity (i.e. true negative) of each threshold was calculated for all data sets. Sensitivity was 100% by definition.
Relative threshold
We calculated the preoperative value (relative threshold) in which individuals are more likely to improve than in others, using each improvement criterion (A–D). We used two modelling approaches and assessed model properties for the four different definitions of improvement (A–D).
Linear regression
Linear regressions including the best-fit third-degree polynomials of the change score (postoperative to preoperative score) were used to estimate at what preoperative score the predicted change is likely to fall below each criterion.
The equation of the linear regression is:
where y^1=(y1−x1) and ei ∼ N(0, σ2), and y1 and x1 indicate the postoperative and preoperative scores for the ith observation, respectively.
Logistic regression
Logistic regressions with dichotomised change score (postoperative to preoperative score) by each improvement criterion were used to estimate the preoperative score at which the probability of improving fell below 50% and 75%.
where p is the probability that p^ of improved/N of preoperative score, yi ∼ B(ni, p), and yi and xi indicate the postoperative and preoperative scores for the ith observation, respectively.
Model performance for the relative threshold
The area under the ROC curve with 95% binomial exact CIs was calculated to examine discriminative ability with each improvement criterion using the observed (rather than estimated) postoperative score. In addition, goodness of fit incorporated inspection using plots of observed versus fitted values, plots of residuals versus fitted values and mean/SD of residuals by decile (or quintile). The predictive models’ performance for the logistic regression was assessed using calibration graphs: an illustration of the Hosmer–Lemeshow goodness-of-fit test, by decile (or quintile) of the predicted probabilities. 93
Threshold reporting
Thresholds for the OHS, OKS, SF-12 and WOMAC were reported, always rounding up the estimated value (e.g. 89.1 to 90.1) to avoid excluding any individuals who can benefit through rounding down. Sensitivity and specificity values were calculated using these thresholds and rounded to the nearest integer except when < 1%.
Percentages of population coverage
Percentages of study population coverage, which is the cumulative percentage up to the estimated absolute and relative thresholds and indicates the percentage of those who achieved the improvements, were calculated for each data set.
Results
Characteristics of the data sets
The mean ages of participants were 65–70 years (SD 8–14 years), the mean body mass index (BMI) values were 27–32 kg/m2 (SD 4–6 kg/m2) and the percentage of females was between 52% and 62% (Table 31).
Characteristic | Data set | ||||||||
---|---|---|---|---|---|---|---|---|---|
NHS PROMsa | KAT | EPOS | EUROHIP | ADAPT | APEX | ||||
Hip | Knee | Knee | Hip | Hip | Hip | Knee | Hip | Knee | |
Age (years) | |||||||||
n | 95,890 | 103,519 | 1634 | 1580 | 1298 | 125 | 128 | 343 | 339 |
Mean | – | – | 69.8 | 68.3 | 65.7 | 64.5 | 66.6 | 67.0 | 69.8 |
SD (< 60%) | 13.7 | 9.5 | 8.1 | 10.8 | 10.9 | 11.7 | 9.7 | 11.0 | 8.6 |
IQR: 25 (60–80%) | 73.2 | 78.3 | 65.0 | 62.6 | 59.0 | 57 | 60.1 | 60.0 | 63.3 |
IQR: 75 (≥ 80%) | 13.1 | 12.2 | 76.0 | 75.8 | 73.8 | 72.5 | 73.5 | 75.0 | 75.9 |
BMI (kg/m2) | |||||||||
n | – | – | 1584 | 1487 | 1225 | 130 | 132 | 338 | 334 |
Mean | – | – | 29.7 | 27.2 | 27.5 | 27.5 | 31.5 | 29.0 | 32.4 |
SD | – | – | 5.5 | 4.9 | 4.4 | 4.4 | 5.9 | 5.5 | 6.4 |
IQR: 25 | – | – | 26.1 | 24.1 | 24.5 | 24.5 | 27.5 | 26.0 | 27.9 |
IQR: 75 | – | – | 32.5 | 30.0 | 29.8 | 29.8 | 35.3 | 32.0 | 36.1 |
Sex | |||||||||
n | 95,816 | 103,412 | 1634 | 1581 | 1267 | 130 | 132 | 343 | 339 |
Female (%) | 61.4 | 58.3 | 55.9 | 62.2 | 55.9 | 49.2 | 53.0 | 59.0 | 52.2 |
Male (%) | 38.7 | 41.8 | 44.1 | 37.8 | 44.1 | 50.8 | 47.0 | 41.0 | 47.8 |
Minimally clinically important difference and minimally detectable change (90%) values
Hip
The MCID was 5 (range 5.0–5.1) units for the OHS; 5.4 and 4.5 for the SF-12 PCS and MCS, respectively; and 9.5–11.1 for the WOMAC total score across the data sets. Using an ICC of 0.9 provided MDCs of 6 (range 5.9–6.0) units for the OHS; 6.3 and 6.8 for the SF-12 PCS and MCS, respectively; and 11.8–15.7 for the WOMAC total score (Table 32).
Data set | Candidate tools | |||||||||||||
---|---|---|---|---|---|---|---|---|---|---|---|---|---|---|
OKS | OHS | SF-12 | WOMAC | |||||||||||
PCS | MCS | Total | Pain | Function | Stiffness | |||||||||
K | H | K | H | K | H | K | H | K | H | K | H | K | H | |
PROMs | ||||||||||||||
MCID 0.5 SD | 4.9 | 5 | ||||||||||||
MDC (±) | ||||||||||||||
L | ||||||||||||||
F | ||||||||||||||
0.7 | 9.8 | 10.3 | ||||||||||||
0.9 | 5.7 | 6 | ||||||||||||
KAT | ||||||||||||||
MCID 0.5 SD | 5.1 | 5.4 | 5.8 | |||||||||||
MDC (±) | ||||||||||||||
L | 7.6 | 12 | ||||||||||||
F | ||||||||||||||
0.7 | 9.6 | 10 | 15 | |||||||||||
0.9 | 5.5 | 6 | 8.5 | |||||||||||
EPOS | ||||||||||||||
MCID 0.5 SD | 5.1 | |||||||||||||
MDC (±) | ||||||||||||||
L | ||||||||||||||
F | ||||||||||||||
0.7 | 10 | |||||||||||||
0.9 | 5.9 | |||||||||||||
EUROHIP | ||||||||||||||
MCID 0.5 SD | 10.7 | 11.6 | 10.9 | 14.3 | ||||||||||
MDC (±) | ||||||||||||||
L | 21.6 | 18.3 | 33.1 | |||||||||||
F | ||||||||||||||
0.7 | 20.5 | 22.7 | 21.3 | 26.4 | ||||||||||
0.9 | 11.8 | 13.1 | 12.3 | 15.3 | ||||||||||
ADAPT | ||||||||||||||
MCID 0.5 SD | 5.3 | 5.4 | 4.6 | 4.5 | 10 | 11.1 | 11.2 | 10.9 | 10.2 | 11.7 | 12.6 | 12.5 | ||
MDC (±) | ||||||||||||||
L | 6.7 | 7.9 | 9.5 | 9.6 | 22.9 | 26.6 | 19.9 | 24.3 | 32.3 | 38.7 | ||||
F | ||||||||||||||
0.7 | 9.1 | 10.9 | 11.7 | 11.7 | 22.2 | 27.1 | 24.1 | 28 | 23.2 | 28.3 | 25.8 | 11.7 | ||
0.9 | 5.3 | 6.3 | 6.7 | 6.8 | 12.8 | 15.7 | 13.9 | 16.9 | 13.4 | 16.4 | 14.9 | 6.8 | ||
APEX | ||||||||||||||
MCID 0.5 SD | 9.8 | 9.5 | 10.5 | 11 | 9.9 | 10.4 | 12.7 | 12.3 | ||||||
MDC (±) | ||||||||||||||
L | 20.2 | 22.7 | 19.5 | 20.6 | 32.2 | 36.6 | ||||||||
F | ||||||||||||||
0.7 | 21 | 22.8 | 21.3 | 23.9 | 22.8 | 24.1 | 25.7 | 29.2 | ||||||
0.9 | 12.1 | 13.2 | 12.3 | 13.8 | 13.2 | 13.9 | 14.9 | 16.9 |
Knee
The MCID was 5 (range 4.9–5.1) units for the OKS, 5.3–5.4 for the SF-12 PCS, 4.6–5.8 for the SF-12 MCS, and 9.8–10.0 for the WOMAC total score. Using an ICC of 0.9 provided MDCs of 6 (range 5.5–5.7) units for the OKS; 5.3–6.0 and 6.7–8.5 for the SF-12 PCS and MCS, respectively; and 12.1–12.8 for the WOMAC total score (see Table 32).
Percentage of population improving
Using the MCID 0.5 improvement criterion B, the OHS shows a 94% improvement and the OKS shows an 85–88% improvement. The WOMAC total scores show an 84–86% improvement for hip score and a 75–88% improvement for knee score (Tables 33 and 34). The SF-12 has some theoretically possible scores, which are unrealistic to obtain from the postoperative quality-of-life or health status outcome.
Candidate tools | Data sets | ||||||||||||||||||||||||
---|---|---|---|---|---|---|---|---|---|---|---|---|---|---|---|---|---|---|---|---|---|---|---|---|---|
PROMs | EPOS | EUROHIP | ADAPT | APEX | |||||||||||||||||||||
n | Improvement criteria | n | Improvement criteria | n | Improvement criteria | n | Improvement criteria | n | Improvement criteria | ||||||||||||||||
A | B | C | D | A | B | C | D | A | B | C | D | A | B | C | D | A | B | C | D | ||||||
OHS | 102,404 | 97 | 94 | 86 | 93 | 1179 | 98 | 94 | 90 | 94 | |||||||||||||||
SF-12 | |||||||||||||||||||||||||
PCS | 118 | 86 | 65 | 49 | 61 | ||||||||||||||||||||
MCS | 118 | 47 | 26 | 15 | 21 | ||||||||||||||||||||
WOMAC | |||||||||||||||||||||||||
Total | 845 | 93 | 86 | 76 | 85 | 125 | 96 | 84 | 56 | 75 | 261 | 98 | 95 | 87 | 95 | ||||||||||
Pain | 865 | 93 | 83 | 72 | 83 | 125 | 96 | 87 | 61 | 76 | 323 | 97 | 94 | 86 | 94 | ||||||||||
Physical function | 874 | 93 | 85 | 73 | 84 | 125 | 95 | 81 | 55 | 69 | 270 | 97 | 94 | 82 | 94 | ||||||||||
Stiffness | 883 | 71 | 53 | 71 | 83 | 125 | 96 | 89 | 56 | 73 | 300 | 98 | 89 | 60 | 77 |
Candidate tools | Data sets | |||||||||||||||||||
---|---|---|---|---|---|---|---|---|---|---|---|---|---|---|---|---|---|---|---|---|
PROMs | KAT | ADAPT | APEX | |||||||||||||||||
n | Improvement criteria | n | Improvement criteria | n | Improvement criteria | n | Improvement criteria | |||||||||||||
A | B | C | D | A | B | C | D | A | B | C | D | A | B | C | D | |||||
OHS | 108,832 | 94 | 88 | 76 | 86 | 1634 | 91 | 85 | 74 | 85 | ||||||||||
SF-12 | ||||||||||||||||||||
PCS | 1518 | 60 | 46 | 62 | 80 | 127 | 77 | 51 | 40 | 51 | ||||||||||
MCS | 1518 | 54 | 33 | 12 | 25 | 127 | 55 | 35 | 12 | 22 | ||||||||||
WOMAC | ||||||||||||||||||||
Total | 126 | 90 | 75 | 52 | 69 | 246 | 93 | 88 | 73 | 86 | ||||||||||
Pain | 127 | 93 | 82 | 59 | 82 | 318 | 97 | 89 | 78 | 89 | ||||||||||
Physical function | 127 | 88 | 78 | 47 | 63 | 253 | 93 | 86 | 70 | 82 | ||||||||||
Stiffness | 127 | 91 | 64 | 36 | 64 | 293 | 94 | 67 | 45 | 67 |
Absolute threshold using criterion B
In this section, results based on improvement in criterion B are provided (see Online Supplement 3 for results based on the other criteria). Tables 35 and 36 describe the absolute threshold with specificity (95% CIs) and the study population coverage (%) by the thresholds for hips and knees, respectively.
Candidate tools | Preoperative threshold | Specificity (%) (95% CI) | Population coverage (%) |
---|---|---|---|
OHS | |||
NHS PROMs | 43 | 2 (1 to 2) | 100 |
EPOS | 43 | 2 (< 0.1 to 9) | 100 |
SF-12 PCS | |||
ADAPT | 66 | 0 (0 to 14) | 100 |
SF-12 MCS | |||
ADAPT | 65 | 0 (0 to 7) | 100 |
WOMAC total | |||
ADAPT | 89 | 22 (6 to 48) | 96 |
APEX | 91 | 0 (0 to 31) | 100 |
EUROHIP | 90 | 0 (0 to 2) | 100 |
WOMAC pain | |||
ADAPT | 90 | 21 (5 to 51) | 96 |
APEX | 89 | 18 (4 to 43) | 99 |
EUROHIP | 89 | 4.2 (1 to 9) | 100 |
WOMAC physical function | |||
ADAPT | 89 | 33 (15 to 57) | 94 |
APEX | 90 | 17 (2 to 48) | 99 |
EUROHIP | 90 | 0.8 (0.0 to 5) | 100 |
WOMAC stiffness | |||
ADAPT | 88 | 42 (15 to 72) | 95 |
APEX | 88 | 29 (13 to 49) | 97 |
EUROHIP | 86 | 8.1 (5 to 12) | 99 |
Candidate tools | Preoperative threshold | Specificity (%) (95% CI) | Population coverage (%) |
---|---|---|---|
OKS | |||
NHS PROMs | 43 | 0.5 (0.4 to 0.7) | 100 |
KAT | 43 | 0.9 (0.1 to 3) | 100 |
SF-12 PCS | |||
ADAPT | 66 | 0 (0 to 11) | 100 |
KAT | 71 | 0 (0 to 1) | 100 |
SF-12 MCS | |||
ADAPT | 65 | 2 (0 to 13) | 100 |
KAT | 74 | 0.3 (0.1 to 0.9) | 100 |
WOMAC total | |||
ADAPT | 90 | 7 (1 to 24) | 100 |
APEX | 91 | 0 (0 to 16) | 100 |
WOMAC pain | |||
ADAPT | 89 | 5 (0 to 25) | 100 |
APEX | 90 | 0 (0 to 12) | 100 |
WOMAC physical function | |||
ADAPT | 90 | 8 (1 to 27) | 99 |
APEX | 91 | 7 (1 to 24) | 100 |
WOMAC stiffness | |||
ADAPT | 88 | 8 (2 to 21) | 98 |
APEX | 88 | 3 (0 to 9) | 99 |
Hip
The absolute threshold was 43 for the OHS. The preoperative scores of the SF-12 PCS and MCS were 66 and 65, respectively. We found the threshold range of scores to be 89–91 for the WOMAC total, 89–90 for the WOMAC pain, 89–90 for the WOMAC physical function and 86–88 for the WOMAC stiffness. Study population coverages were 100% for the OHS and SF-12 and ≥ 94% for the WOMAC (see Table 35).
Knee
The absolute threshold was 43 for the OKS. The ranges of the preoperative scores for the SF-12 PCS and MCS were 66–71 and 65–74, respectively. The threshold range was 90–91 for the WOMAC total, 89–90 for the WOMAC pain, 90–91 for the WOMAC physical function and 88 for the WOMAC stiffness. The study population coverages were 100% for the OHS and SF-12 and ≥ 98% for the WOMAC (see Table 36).
Relative threshold using criterion B
Tables 37 and 38 show the relative threshold with sensitivity and specificity (95% CIs) (see Online Supplement 4 for full results) and the study population coverage (%) by each threshold. The AUC (95% CI) for the OHS, OKS, SF-12 PCS and WOMAC total showed poor (< 0.7) discrimination abilities overall.
Candidate tools | Probability level | Preoperative threshold | Specificity (%) (95% CIs) | AUC (95% CI) | Population coverage (%) |
---|---|---|---|---|---|
OHS | |||||
NHS PROMs | |||||
Model 1 | 40 | 4 (4 to 5) | 100 | ||
Model 2 | 0.5 | 43 | 2 (1 to 2) | 0.65 (0.65 to 0.66) | 100 |
0.75 | 38 | 6 (6 to 7) | 99 | ||
EPOS | |||||
Model 1 | 40 | 3 (0 to 12) | 100 | ||
Model 2 | 0.5 | 42 | 2 (0 to 9) | 0.62 (0.59 to 0.64) | 100 |
0.75 | 39 | 3 (0 to 12) | 99 | ||
SF-12 PCS | |||||
ADAPT | |||||
Model 1 | 46 | 24 (9 to 45) | 91 | ||
Model 2 | 0.5 | 47 | 20 (7 to 41) | 0.58 (0.46 to 0.7) | 92 |
0.75 | 35 | 48 (28 to 69) | 70 | ||
SF-12 MCS | |||||
ADAPT | |||||
Model 1 | 42 | 91 (79 to 97) | 26 | ||
Model 2 | 0.5 | 39 | 96 (87 to 100) | 0.93 (0.85 to 0.98) | 19 |
0.75 | 37 | 100 (93 to 100) | 16 | ||
WOMAC total | |||||
ADAPT | |||||
Model 1 | 81 | 50 (26 to 74) | 90 | ||
Model 2 | 0.5 | 85 | 44 (22 to 69) | 0.76 (0.67 to 0.84) | 94 |
0.75 | 78 | 56 (31 to 78) | 86 | ||
APEX | |||||
Model 1 | 86 | 20 (3 to 56) | 99 | ||
Model 2 | 0.5 | 86 | 20 (3 to 56) | 0.61 (0.53 to 0.67) | 99 |
0.75 | 82 | 20 (3 to 56) | 98 | ||
EUROHIP | |||||
Model 1 | 83 | 3 (1 to 8) | 99 | ||
Model 2 | 0.5 | 87 | 1 (0 to 5) | 0.56 (0.53 to 0.59) | 100 |
0.75 | 80 | 4 (1 to 9) | 99 | ||
WOMAC pain | |||||
ADAPT | |||||
Model 1 | 83 | 36 (13 to 65) | 94 | ||
Model 2 | 0.5 | 89 | 36 (13 to 65) | 0.66 (0.57 to 0.75) | 96 |
0.75 | 82 | 36 (13 to 65) | 94 | ||
APEX | |||||
Model 1 | 83 | 18 (4 to 43) | 99 | ||
Model 2 | 0.5 | 88 | 18 (4 to 43) | 0.65 (0.59 to 0.7) | 99 |
0.75 | 83 | 18 (4 to 43) | 99 | ||
EUROHIP | |||||
Model 1 | 84 | 6 (3 to 12) | 99 | ||
Model 2 | 0.5 | 89 | 4 (2 to 9) | 0.58 (0.55 to 0.62) | 100 |
0.75 | 78 | 8 (4 to 14) | 98 | ||
WOMAC physical function | |||||
ADAPT | |||||
Model 1 | 81 | 48 (26 to 70) | 86 | ||
Model 2 | 0.5 | 86 | 43 (22 to 66) | 0.82 (0.73 to 0.89) | 91 |
0.75 | 78 | 52 (30 to 74) | 82 | ||
APEX | |||||
Model 1 | 86 | 17 (2 to 48) | 99 | ||
Model 2 | 0.5 | 88 | 17 (2 to 48) | 0.65 (0.58 to 0.72) | 99 |
0.75 | 83 | 17 (2 to 48) | 99 | ||
EUROHIP | |||||
Model 1 | 82 | 5 (2 to 10) | 99 | ||
Model 2 | 0.5 | 86 | 3 (1 to 8) | 0.55 (0.52 to 0.58) | 100 |
0.75 | 79 | 7 (3 to 13) | 99 | ||
WOMAC stiffness | |||||
ADAPT | |||||
Model 1 | 82 | 50 (21 to 79) | 95 | ||
Model 2 | 0.5 | 91 | 42 (15 to 72) | 0.81 (0.72 to 0.88) | 100 |
0.75 | 86 | 50 (21 to 79) | 95 | ||
APEX | |||||
Model 1 | 82 | 32 (16 to 52) | 97 | ||
Model 2 | 0.5 | 90 | 29 (13 to 49) | 0.77 (0.71 to 0.82) | 100 |
0.75 | 83 | 32 (16 to 52) | 97 | ||
EUROHIP | |||||
Model 1 | 65 | 20 (15 to 25) | 98 | ||
Model 2 | 0.5 | 67 | 20 (15 to 25) | 0.71 (0.68 to 0.74) | 98 |
0.75 | 36 | 81 (75 to 85) | 61 |
Candidate tools | Probability level | Preoperative threshold | Specificity (%) (95% CI) | AUC (95% CI) | Population coverage (%) |
---|---|---|---|---|---|
OKS | |||||
NHS PROMs | |||||
Model 1 | 37 | 4 (4 to 5) | 99 | ||
Model 2 | 0.5 | 40 | 2 (2 to 2) | 0.62 (0.61 to 0.62) | 100 |
0.75 | 33 | 11 (10 to 11) | 96 | ||
KAT | |||||
Model 1 | 35 | 5 (3 to 9) | 98 | ||
Model 2 | 0.5 | 39 | 3 (1 to 6) | 0.62 (0.60 to 0.65) | 100 |
0.75 | 29 | 14 (10 to 20) | 93 | ||
SF-12 PCS | |||||
ADAPT | |||||
Model 1 | 36 | 25 (11 to 43) | 87 | ||
Model 2 | 0.5 | 34 | 31 (16 to 50) | 0.64 (0.5 to 0.75) | 78 |
0.75 | – | – | – | ||
KAT | |||||
Model 1 | 39 | 27 (23 to 31) | 86 | ||
Model 2 | 0.5 | 43 | 16 (13 to 19) | 0.65 (0.63 to 0.68) | 92 |
0.75 | 22 | 94 (92 to 96) | 13 | ||
SF-12 MCS | |||||
ADAPT | |||||
Model 1 | 40 | 93 (81 to 99) | 22 | ||
Model 2 | 0.5 | 49 | 74 (58 to 86) | 0.81 (0.7 to 0.9) | 51 |
0.75 | 34 | 100 (92 to 100) | 8 | ||
KAT | |||||
Model 1 | 43 | 85 (83 to 88) | 28 | ||
Model 2 | 0.5 | 49 | 72 (69 to 75) | 0.78 (0.75 to 0.80) | 45 |
0.75 | 26 | 100 (99 to 100) | 2 | ||
WOMAC total | |||||
ADAPT | |||||
Model 1 | 86 | 7 (1 to 24) | 100 | ||
Model 2 | 0.5 | 81 | 15 (4 to 34) | 0.55 (0.46 to 0.65) | 97 |
0.75 | 71 | 19 (6 to 38) | 92 | ||
APEX | |||||
Model 1 | 81 | 10 (1 to 30) | 99 | ||
Model 2 | 0.5 | 85 | 5 (0 to 24) | 0.60 (0.53 to 0.68) | 100 |
0.75 | 75 | 10 (1 to 30) | 97 | ||
WOMAC pain | |||||
ADAPT | |||||
Model 1 | 78 | 5 (0 to 25) | 98 | ||
Model 2 | 0.5 | 82 | 5 (0 to 25) | 0.56 (0.46 to 0.65) | 100 |
0.75 | 71 | 10 (1 to 32) | 98 | ||
APEX | |||||
Model 1 | 81 | 0 (0 to 12) | 100 | ||
Model 2 | 0.5 | – | – | 0.59 (0.53 to 0.65) | – |
0.75 | 85 | 0 (0 to 12) | 100 | ||
WOMAC physical function | |||||
ADAPT | |||||
Model 1 | 87 | 13 (3 to 32) | 98 | ||
Model 2 | 0.5 | 89 | 8 (1 to 27) | 0.47 (0.37 to 0.57) | 99 |
0.75 | 82 | 13 (3 to 32) | 96 | ||
APEX | |||||
Model 1 | 82 | 15 (4 to 34) | 98 | ||
Model 2 | 0.5 | 86 | 11 (2 to 29) | 0.62 (0.55 to 0.69) | 99 |
0.75 | 75 | 19 (6 to 38) | 94 | ||
WOMAC stiffness | |||||
ADAPT | |||||
Model 1 | 72 | 18 (8 to 34) | 97 | ||
Model 2 | 0.5 | 65 | 18 (8 to 34) | 0.69 (0.59 to 0.77) | 97 |
0.75 | 24 | 97 (87 to 100) | 24 | ||
APEX | |||||
Model 1 | 68 | 28 (18 to 39) | 97 | ||
Model 2 | 0.5 | 65 | 28 (18 to 39) | 0.71 (0.65 to 0.77) | 97 |
0.75 | 34 | 86 (76 to 93) | 58 |
Hip
The range of relative thresholds was 38–43 with specificity of 2–6 for the OHS. A histogram of the preoperative OHS distribution for the NHS PROMs data sets is given in Figure 7. Threshold ranges of the SF-12 PCS and MCS were 35–47 (specificity 20–48%) and 37–42 (specificity 91–100%), respectively. The threshold ranges were 78–87 (specificity 1–56%) for the WOMAC total, 78–89 (specificity 4–36%) for the WOMAC pain, 78–88 (specificity 3–52%) for the WOMAC physical function and 36–91 (specificity 20–50%, EUROHIP 81) for the WOMAC stiffness. Study population coverages for the thresholds of a 50% probability level were 100% for the OHS, 19% for the SF-12 PCS, 92% for the SF-12 MCS and 91–100% for the WOMAC. Figures 8 and 9 give the linear regression model, absolute threshold and logistic regression model estimates. The CI bands (see Figure 8) show the lack of fit for the variation in the OHS outcome for the linear model, although the point estimate seems reasonable. Linear regression models for the other outcomes (not shown) showed a similar lack of fit.
FIGURE 7.
The OHS: NHS PROMs preoperative histogram with the absolute and linear relative thresholds using criterion B.

FIGURE 8.
The OHS: NHS PROMs change scores.

FIGURE 9.
The OHS: NHS PROMs percentage improved using criterion B.

Knee
The range of the relative (observed) threshold was 29–40 with a specificity of 2–14 for the OKS. A histogram of the preoperative OHS distribution for the NHS PROMs data sets is given in Figure 10. Threshold ranges of the preoperative score of the SF-12 PCS and MCS were 22–43 (specificity 16–31%, KAT 94) and 26–49 (specificity 72–100%), respectively. The threshold ranges were 71–86 (specificity 5–19%) for the WOMAC total, 71–85 (specificity 0–10%) for the WOMAC pain, 75–89 (specificity 8–19%) for the WOMAC physical function and 24–72 (specificity 18–28%, ADAPT 97 and APEX 86) for the WOMAC stiffness. Study population coverages for the thresholds of a 50% probability level were 100% for the OHS, 78–92% for the SF-12 PCS, 45–51% for the SF-12 MCS and 97–100% for the WOMAC. Figures 11 and 12 give the linear regression model, absolute threshold and logistic regression model estimates. The CI bands (see Figure 11) show the lack of fit for the variation in the OHS outcome for the linear model, although the point estimate seems reasonable. Linear regression models for the other outcomes (not shown) showed a similar lack of fit.
FIGURE 10.
The OKS: NHS PROMs preoperative histogram with the absolute and linear relative thresholds using criterion B.

FIGURE 11.
The OKS: NHS PROMs change scores.

FIGURE 12.
The OKS: NHS PROMs percentage improved using criterion B.

Discussion
Main findings
We examined the percentage of the population who improved in accordance with each improvement criterion. Based on the MCID 0.5 improvement criterion B, all the candidate tools show > 75% improvement for the OHS, OKS and WOMAC scores (except the WOMAC stiffness: 53–64%) (see Tables 33 and 34).
The ability to predict postoperative quality-of-life or health status outcome from the preoperative score has limitations; it is apparent from the AUC values that although the preoperative score is informative, it does not fully determine what the postoperative outcome will be. Other factors may improve the prediction (this was explored for the OHS and OKS in Chapter 5); however, it is worth noting that the variability in postoperative quality of life or health status is substantial. Related to this, the linear regression model fitted poorly in terms of representing the variability, although, as it was being used as a best guess, this is not problematic per se to the estimation of the relative threshold based on the average response. Regression analysis techniques that can deal with this are available, such as the use of transformations, modelling the variability separately and quantile regression.
The estimated absolute threshold for the OHS, OKS, SF-12 and WOMAC, when applied retrospectively, only excluded a very small proportion of the patients within our data sets who received hip and knee replacement surgery, which is reassuring. Use of this threshold is reliant on the definition of improvement used, not on the predictive performance. Although a small proportion of patients did receive surgery that may not have been suitable, it is possible that other benefits not detected with these outcome tools could explain and justify the operations for these individuals.
There was a suggestion of ceiling effects in the postoperative OHS, although this may to some degree reflect the real positive postoperative results. However, we need to be cautious about the ability of the OHS to distinguish postoperative results at the very top of the range. The OKS seemed to show a ceiling effect only for the function subscale in work package 1; it may be that function after the surgery may not be as well differentiated as pain by the instrument. For the OHS and OKS, we used the web-based NHS PROMs data set (2012–15), which was also used for work package 1. Interpreting with the specificity (false-negative) scores suggests that the NHS PROMs data set could be a more reliable source to develop the tools than other data sets.
The SF-12 PCS and, especially, the SF-12 MCS showed very high specificity (very poor false-negative) scores for relative thresholds (hip ranges of 20–48 for PCS and 91–100 for MCS, and knee ranges of 16–94 for PCS and 72–100 for MCS). The SF-12 PCS and MCS did not well cover the range of possible scores in the population (see Appendix 1). This may suggest that the SF-12 may not be an ideal tool for developing the standardised thresholds for hip or knee replacement surgeries. Use of the SF-12 PCS and MCS individually was problematic given that they are negatively correlated. The SF-12 MCS, perhaps unsurprisingly, did not perform well in terms of face validity of the threshold impact.
Strengths and limitations
The main strengths of our study were (1) the use of diverse methods to define the thresholds and assess prognostic performance and impact, (2) the inclusion of multiple candidate instruments that were selected after a comprehensive search and selection process and (3) the use of multiple data sets including a number of large cohorts in musculoskeletal populations (e.g. NHS PROMs data).
The difficulties of defining a clinically important improvement, particularly for measuring quality of life (whether it is generic or disease-specific), are well known. 90,94 Furthermore, criteria had to be applicable to all of the candidate tools. Given the variation in available information on this despite the work carried out in work package 1, the choice was restricted to definitions that could be applied on data sets and were not reliant on pre-existing work (e.g. the anchor estimate of the MIC in the relevant population), which was not available for all the measures. We used four methods, of which most emphasis was given to the medium ES (0.5)-based definition, in which a medium ES is of moderate clinical importance. 95 It is worth noting that these are relatively simple approaches, for instance, which do not take into account the preoperative score level, which itself may have influence on the clinically important improvement. We also applied the following approaches: any increase in the change score (> 0) and MDC defined as the minimal change beyond the measurement error. None of these is without criticism as a definition of clinically important improvement, although the need to compare evenly across measurement tools limited the options. Nevertheless, we believe that the findings have value, particularly the medium ES definition.
The study had a number of limitations. Potential sources of bias or limitations in the study methodology were (1) the improvement criteria used reflected limitations in the literature and data sets available for the candidate tools; (2) estimation did not control for the baseline characteristic information, such as patient age, gender and comorbidities, which could be prognostically important; (3) complete-case analyses were undertaken (without imputation of the missing data), which could influence the findings; (4) the thresholds will be influenced by the measurement tool’s properties, such as responsiveness, which may mean that there is a failure to reflect meaning impact in some patients; and (5) only point threshold estimations were reported in this study.
Further research needed
Our definition of improvement could be more rigorous. Additional benefit could be extended beyond ‘improvement’ to incorporate other concepts (e.g. patient satisfaction) or impacts of treatment (i.e. other relevant measures of benefit will be needed, especially for relative threshold). The MCID approaches can be defined and calculated in various ways, for example the approaches could be defined subjectively based on a patient’s satisfaction after the surgery (an anchor-based approach). The MIC using the mean change score (pre and post operation) is another potential alternative.
The NHS PROMs data were from a very large data set but only had a 6-month follow-up period, whereas most other data sets used 12 months of follow-up (except EPOS, which used 24 months). This may have somewhat influenced the magnitude of findings. 96 It would have been advantageous to have access to larger data sets to assess the other tools (i.e. non-Oxford scores), particularly with regard to the WOMAC score, for which more precise estimates of relative thresholds could have been achieved. The impact of the baseline characteristics (i.e. main prognostic variables) on the estimation of the relative threshold will be applied in work package 3.
Conclusion
In this study, various improvement definitions and analytical methods were used systemically to calculate threshold levels for the candidate tools in various data sets. The results demonstrated that thresholds of three candidate tools (the OHS, OKS and WOMAC), which suggested promising initial cross-sectional psychometric properties (from work package 1), were consistent across data sets (except for the SF-12).
Chapter 5 Health economic evaluation of thresholds values (work package 2)
Background
This chapter aims to answer the following two inter-related questions:
-
What is the economic threshold for each clinical tool (i.e. what is the highest score at which arthroplasty is cost-effective)?
-
How do the incremental costs, QALYs and cost-effectiveness of arthroplasty vary depending on the threshold and clinical tool used?
We addressed these questions in a UK setting from a NHS perspective by conducting a series of cost–utility analyses, comparing the incremental cost-effectiveness of TKA and THA with no arthroplasty in men and women of different ages with different preoperative scores on each clinical tool.
The analyses presented in this chapter focused on total joint arthroplasty (TJA) because this type of surgery constitutes 92% of knee and 99% of hip arthroplasty procedures conducted in the UK. 97 We compared immediate TJA with having no arthroplasty surgery during the 10-year time horizon used in the analysis. This enabled us to calculate the economic threshold for each clinical tool by comparing the cost-effectiveness in different groups with one another and with the £20,000-per-QALY ceiling ratio typically used in NHS decision-making. 98 The economic threshold simply comprised the highest clinical tool score at which the incremental cost-effectiveness ratio (ICER) is < £20,000 per QALY gained. In practice, patients who are not deemed to warrant immediate surgery may have treatment later, after their condition has worsened. However, there are relatively limited data on how clinical tool scores change over time without surgery,99–102 and modelling the referral pathway and outcomes for patients who undergo surgery at different times would have greatly complicated the analysis. Instead, by directly comparing immediate TJA with no arthroplasty over 10 years, we made the most of existing UK data sets and directly assessed the cost-effectiveness of arthroplasty.
We focused on NHS and Personal Social Services costs in line with current UK guidelines,103 but have narrowed the perspective further to focus on NHS costs because only one of the available data sets (APEX) included non-NHS costs. Health benefits were measured in terms of QALYs, in line with guidelines and in order to capture the effect of surgery on both quality of life and mortality. 103 The cost-effectiveness of TJA versus no arthroplasty was therefore calculated as the difference in cost (between patients undergoing TJA and those having no arthroplasty) divided by the difference in QALYs.
The analysis primarily concerned patients aged between 50 and 90 years and with an ASA (American Society of Anesthesiologists) grade of 1–3 who were undergoing unilateral TKA or THA for osteoarthritis. However, patients not meeting these criteria were not explicitly excluded from the regression analyses used to estimate input parameters to ensure consistency with the clinical analyses. Because the model begins when patients undergo (or do not undergo) TJA, the analyses presented in this chapter would apply regardless of whether the thresholds are applied in the setting of general practice, musculoskeletal hubs or secondary care.
Methods
Costs and QALYs for hypothetical patients with different clinical tool scores and demographic characteristics were estimated using decision-analytic models built in Microsoft Excel® 2010 (Microsoft Corporation, Redmond, WA, USA). Model-based economic evaluation enabled us to synthesise data from different data sets and extrapolate beyond the end of the available data. Separate models were built for TKA and THA for each of the three clinical tools. Model parameters (e.g. costs, utilities and the probability of death or revision) were based on regression models estimated using patient-level data from existing data sets; such models were used to estimate model parameters for patients with different preoperative characteristics. Hypothetical patients of different ages and genders with a wide range of clinical tool scores were run through the models separately and the costs and QALYs with and without TJA were calculated for each hypothetical patient. The models were probabilistic and took account of uncertainty around regression parameters.
Like the analyses described in Chapter 4, we considered hypothetical patients with different total OKSs or total OHSs on a 0–48 scale. For the WOMAC, for simplicity we focused on total scores, rather than considering pain, physical functioning and stiffness separately. As for the clinical analyses, the scale on the total WOMAC score (the sum of the three subscores ranging from 0 to 96) was multiplied by a factor of 10096 to rescale it, and then reversed by subtracting it from 100, such that 0 indicates severe problems and 100 indicates no problems. However, because there is no single summary score for the SF-12, we considered the physical and mental scores as two separate patient characteristics and evaluated the cost-effectiveness of different combinations of physical and mental scores, estimating threshold SF-12 physical scores for patients with different mental scores.
Model
Literature reviews on modelling approach
A comprehensive literature review was conducted to identify previous decision-analytic models that assessed the cost-effectiveness of arthroplasty, specific types of surgery or prostheses or changes to the timing of surgery (see Online Supplement 7). This review identified 41 previous model-based economic evaluations. Almost all of the published studies used Markov models to allow for repeating cycles. Models typically allowed for a proportion of patients having one or more revision operations, which were sometimes separated into one- and two-stage revisions, revisions for infection or other causes, or total and partial revisions. Some models used separate health states to differentiate between patients with good and poor outcomes or quality of life after surgery. Some of the models differentiated between patient groups based on age, sex, comorbidities, ASA grade and/or obesity, and one analysis estimated results for patients with different Kellgren and Lawrence grades with and without symptoms. 104 However, no model-based evaluations calculated how costs and QALYs varied with clinical tool scores. Five studies using patient-level data assessed how costs and/or QALYs varied with the Oxford Hip and Knee Scores or the WOMAC. 2,68,105–107 One study that was published after our search date assessed how cost-effectiveness varied with the SF-12. 108
Description of the Markov model
We used the results of this literature review to inform the design of our model. Like most studies in the literature, we used a Markov model with annual cycles to allow for the fact that patients are at risk of death or needing a revision each year. We estimated costs and QALYs using cohort simulation, because modelling thousands of individual patient trajectories for up to 1410 hypothetical patients using patient-level simulation would have been too computationally time-consuming. Revision rates and mortality varied depending on the time since primary arthroplasty and the age and sex of the hypothetical individual. Costs and EQ-5D utilities also varied depending on the time since primary arthroplasty, age, sex and clinical tool score.
The model started at the point at which patients in the arthroplasty arm underwent primary TKA or THA (Figure 13). Revision was defined in the same way as in the National Joint Registry (NJR), namely an:
. . . operation performed to remove (and usually replace) one or more components of a total joint prosthesis for whatever reason.
FIGURE 13.
State transition diagram for the Markov model.

The base-case analysis used a 10-year time horizon because it was considered clinically unrealistic to assume that patients in the no arthroplasty arm would never have surgery. This also approximated the longest follow-up time available in the data sets used to estimate model parameters. However, different time horizons were examined in the sensitivity analysis. Costs, QALYs and life-years accrued beyond year 1 were discounted at 3.5% per annum. 103,109
The model did not apply a standard half-cycle correction to all health states, because the cost of primary TJA was assumed to be at the start of the year regardless of what subsequently happened to the patient and is not evenly distributed across the first 12-month period. We assumed that patients who die in the same year as having a primary TJA or revision surgery will incur the entire cost of the hospital stay in which the TJA or revision surgery was conducted. The cost of the admission for primary or revision surgery was assumed to be the same regardless of whether patients died during or soon after surgery. Other costs were assumed to be evenly distributed across the year, such that patients who die in any given year were assumed to accrue half of the cost and half of the number of QALYs that they would have accrued if they had lived for the whole year.
The model used a probabilistic sensitivity analysis (PSA) to propagate the uncertainty around all uncertain parameters (see Presentation of results and analysis of uncertainty).
Data sets
Data inputs
Model input parameters were estimated using regression models predicting the model parameter as a function of clinical tool score, age and sex. Each of the six Markov models required regression models informing 19 sets of model parameters (Table 39). As described in Literature reviews on model inputs, we began by conducting literature searches to identify any published regression models that could be directly used in the model. If no suitable regression model was identified, the following data sets (described in Chapter 3, Data sets) were considered as candidates for estimating the regression models conducted before the second user group meeting:
-
PROMs data freely available at www.hscic.gov.uk/proms (accessed 7 October 2015)
-
the KAT66
-
the EUROHIP132
-
Belfast.
Parameter | Data sources: knees | Data sources: hips | ||||
---|---|---|---|---|---|---|
OKS | SF-12 | WOMAC | OHS | SF-12 | WOMAC | |
Preoperative EQ-5D utility (mapping) |
|
|
|
|
|
|
Postoperative EQ-5D utility 3–12 months after arthroplasty |
|
|
|
|
|
|
Annual change in EQ-5D at > 3–6 months after arthroplasty, in patients with and without revision |
|
|
|
|
|
|
EQ-5D utility before revision |
|
|
|
|
||
EQ-5D utility after revision |
|
|
|
|
||
Cost of the initial arthroplasty procedure and hospital stay |
|
|
|
|
|
|
Community, outpatient and re-admission costs beyond the initial hospital stay: year 1 |
|
|
|
|
|
|
Community, outpatient and re-admission costs beyond the initial hospital stay: > 1 year after TJA |
|
|
|
|
|
|
Cost of revision arthroplasty procedure and hospital stay |
|
|
|
|
|
|
Community, outpatient and re-admission costs beyond the initial hospital stay for revision (estimated separately for the year of revision and > 1 year after revision) |
|
|
|
|
||
Community, outpatient and inpatient costs without arthroplasty |
|
|
|
|||
Annual change in clinical tools without arthroplasty | ||||||
Annual change in EQ-5D utility without arthroplasty | ||||||
Probability of revision surgery | ||||||
Probability of re-revision |
|
|
||||
Operative mortality: primary arthroplasty |
|
|
||||
Operative mortality: revision arthroplasty |
|
|
||||
Healthy patient effect |
|
|
||||
All-cause mortality |
|
The COASt is a prospective, dual-centre longitudinal cohort study recruiting patients across two hospitals: the University Hospital Southampton NHS Foundation Trust and NOC as part of Oxford University Hospitals NHS Foundation Trust. The COASt was established in 2010, and patients who were placed on the waiting list for knee or hip replacement surgery were recruited to the study. 131 The data sets extracted for the ACHE tool contained observations for 810 hip surgery patients and 858 patients undergoing knee surgery. After excluding patients who underwent procedures other than TKA/THA (e.g. hip resurfacing), the data sets contained 806 observations on THA patients and 484 observations for TKA patients. Data were collected prior to surgery as well as at 6 weeks and then annually for 5 years thereafter. COASt is funded by NIHR under its Programme Grants for Applied Research programme (reference number RP-PG-0407–10064). The study obtained ethics approval from Oxford Research Ethics Committee (reference number 10/H0604/91) and written consent was obtained from each participant.
As detailed in Chapter 7 and Table 39, nine of the parameters within the OKS/OHS models were re-estimated after the second user group meeting and after a new linked extract of Hospital Episode Statistics (HES) and PROMs data became available. This provides a much larger sample of more recent data, including patients’ exact age, and can be directly used to calculate the payment by results tariff applicable to each patient.
Data sets were not pooled, because they differed substantially in age and data collected and there was little advantage in pooling a small data set with a much larger one (e.g. PROMs). Instead, we selected the most appropriate data set among those reporting the tool and outcome of interest based on size, age of the data set and consistency with other analyses. When possible, we aimed to use the same data set for several time points, tools or arms of the model and aimed to use data sets that measured quality of life at the same time point.
Utility was measured using the EQ-5D-3L using the UK time trade-off tariff133 to ensure consistency across models and with NICE guidelines. 103
Literature reviews on model inputs
We began by conducting literature searches to identify any previous studies that reported regression models that could be used directly in our analysis. Five specific searches were conducted:
-
A review of previous economic evaluations and costing studies using patient-level data (see Online Supplement 7) aimed to inform the model structure and identify data for all model parameters.
-
A review of studies reporting changes in clinical tool scores over time for osteoarthritis patients without arthroplasty surgery (see Online Supplement 9) aimed to inform assumptions about how clinical tool scores change over time.
-
A review of studies reporting long-term changes in clinical scores, mortality and risk of revision after arthroplasty surgery (see Online Supplement 9) aimed to identify any data for long-term costs, utilities and transition probabilities.
-
A review of studies mapping from any of the clinical tools onto the EQ-5D, or between any of the clinical tools (see Online Supplement 11) aimed to identify models predicting baseline EQ-5D utility, because several such studies were identified in a previous systematic review. 134
-
A review of studies reporting mortality after primary or revision knee/hip arthroplasty (see Online Supplement 10) aimed to identify studies on mortality, because none of the available data sets provided data on mortality for a large sample.
The second literature review aimed to inform a key assumption in the model by identifying studies that reported changes in clinical tool scores over time for patients without arthroplasty; this is the comparator for the analysis and therefore has a strong influence on the results. A total of 22 such studies were identified. 99–101,115–117,135–150 However, the reported results were ambiguous. Most studies focused only on changes in WOMAC subscores and only reported data over a 2- to 5-year follow-up period. Only two studies reported results for the OHS or SF-12. 100,115 Overall, the results indicated that patients’ clinical tool scores might either improve or worsen, with several studies reporting approximately equal probabilities for both. 101,142,145 We therefore assumed that, in the absence of arthroplasty, clinical tool scores remain constant over the 10-year time horizon (see Other model assumptions and inputs). However, we did allow for reductions in EQ-5D utility with age. The review of economic evaluations identified three previous estimates of the rate of change in utility with age;2,112,118 we used the Ara and Brazier112 model 1 in our analysis because it is based on patient-level UK data and is not specific to patients with certain comorbid conditions; the variance–covariance matrix for the model was obtained from the authors.
The reviews identified nine studies estimating how mortality varied with age, sex and/or other characteristics. However, only one series of studies reported the full set of model coefficients, or considered mortality beyond 90 days after surgery;118,119,121,123 therefore, we used the most recent of these studies in our model. 119,121
Four studies were identified that mapped from the OKS, OHS and WOMAC total scores or from the SF-12 version 2 physical and domain scores onto the EQ-5D. 50,110,111,126 It was necessary to focus on models mapping from total scores or from physical/mental scores, to match the way in which clinical tool scores are modelled in the rest of the model. However, the study mapping from the WOMAC used a small sample [348 observations vs. 978 (knees)/1067 (hips) available in the APEX study] and presented no measures of uncertainty around model coefficients. 111 The study on the SF-12 version 2 was based on general public samples, rather than patients with arthritis, and mapped onto the US EQ-5D tariff, rather than the UK EQ-5D tariff. 110 Furthermore, variance–covariance matrices were only available for the studies mapping from the OKS and OHS. We therefore used the published studies mapping from the OKS and OHS for the economic evaluations described in this chapter and estimated new mapping algorithms for the WOMAC and SF-12 using the available data sets. 50,126 For the further analyses, we subsequently re-estimated mapping algorithms for the OKS and OHS using PROMs data providing patients’ exact ages.
An analysis conducted as part of the COASt work programme using data from HES and the Clinical Practice Research Datalink (CPRD) was used to provide estimates of ambulatory costs accrued > 1 year after primary THA and the cost of revision surgery, because none of the available data sets provided data on these parameters. 113,131
However, the literature reviews identified no studies predicting any of our model parameters conditional on any of the clinical tools under consideration. Therefore, we used the individual patient data available to us to estimate new regression models.
Costing analyses
The reference year for costs was 2014. Within the data sets giving individual patient data on resource use, the cost of primary TJA was estimated based on the national payment by results tariff for relevant Healthcare Resource Groups (HRGs), whereas other health-care resources were valued using unit costs (see Online Supplement 8). Costs taken from Pinedo Villanueva113 were inflated from 2010–11 to 2013–14 using the Hospital and Community Health Services Index. 151
The analysis focused on resource use associated with the joint that was replaced, because resource use unrelated to the joint in question was excluded from all of the available data sets. The analyses estimating the community, outpatient and re-admission costs after hospital discharge also excluded medications, personal care, nursing homes, convalescence care, equipment, home modifications, alternative practitioners, etc. These costs were available for certain data sets (e.g. the APEX study), but not others (e.g. KAT). Excluding such costs greatly reduced the analysis time and made it easier to make fair comparisons between clinical tools. We assumed that all physiotherapy was paid for by the NHS.
None of the data sets available before the second user group meeting provided sufficient data to put through the NHS Payment Grouper,152 a computer program that allocates individual hospital episodes to HRGs based on procedures, diagnoses and patient characteristics. In the analyses presented in this chapter, we, therefore, manually synthesised an estimate of the cost of the initial hospital stay for primary TJA using information from the national tariff 2014–15153 and the NHS Payment Grouper152 and excess bed-days using the NHS tariff. Under the payment by results scheme, hospitals are paid a fixed amount for each HRG unless the patient remains in hospital for longer than the ‘trim point’. For hospital stays lasting beyond the trim point, hospitals are paid an excess bed-day tariff for each additional day beyond the trim point. For primary arthroplasty, there are separate HRGs for patients with no complications, minor complications and major complications; these HRGs differ in their tariff price and trim point, whereas the excess bed-day price is the same across the relevant HRGs. Because it is difficult to establish defensible and consistent methods for identifying which patients would have had minor or major complications based on the comorbidity and/or complication fields in other data sets, we used national data to estimate weighted average costs for every possible length of stay, taking into account the trim point, tariff price and excess bed-day price, and applied this to all patients with that length of stay (see Online Supplement 12). This approach implicitly assumes that the incidence of complications is unrelated to clinical tool score and ignores the association between age and complications.
Changes in guidelines and waiting time targets have halved the average length of stay for arthroplasty in the last 15 years. 154,155 Consequently, length-of-stay data from trials that started in the early 2000s (e.g. KAT and EPOS) do not accurately reflect current practice. However, because KAT and EPOS are the only data sets containing the SF-12 instrument that also report resource use, we relied on these two data sets to estimate how the cost of primary arthroplasty varies with preoperative SF-12 scores. Applying the current national tariff to these older data sets would have systematically overestimated costs, because the share of patients with excess bed-days would be considerably higher than the share for more recent studies. We addressed this problem by adjusting the length-of-stay data for primary TJA using data from the COASt study, which was conducted in 2011 (see Online Supplement 12). 131
A simple approach was used to value re-admissions occurring after patients were discharged from hospital following primary TJA. Such re-admissions were costed up by estimating a (weighted) mean cost per orthopaedic bed-day for those HRGs with the word ‘hip’ or ‘knee’ in the Department of Health and Social Care reference costs HRG description, and multiplying this by the length of stay. 156 A similar method was used to value day cases, which was applied to all admissions in which the admission and discharge dates were the same. No adjustment was made for the calendar year in which the re-admission or revision took place, because any such adjustment would have been extremely complex as the re-admissions observed in the KAT data set were spread over a 12-year period. The COASt questionnaires on costs before arthroplasty provide no data on length of stay for re-admissions, only the number of such re-admissions. In these cases, we therefore applied to each admission the (weighted) mean cost per orthopaedic admission for HRGs with the word ‘hip’ or ‘knee’ in the description from NHS Reference Costs 2013 to 2014. 156 In order to avoid underestimating costs by excluding patients with missing length of stay data for re-admissions, we applied the same weighted average cost to all patients with missing data on length of stay unless the providers of the data set also provided clear guidance on how to impute missing length of stay data. For KAT, we used the same mean imputation that was already applied in the KAT data set, whereby a length of stay of about 9 days for washout procedures with missing length of stay (mean imputation) was applied, and a length of stay of 14.25 days for the second stage of a two-stage revision with missing length of stay. However, these simple analyses were superseded by the HES data used to calculate re-admissions in the analyses presented in Chapter 7.
Owing to lack of data, the cost of re-admissions other than revisions that took place > 1 year after THA were excluded from the analyses presented in this chapter. No patient-level data giving costs > 1 year after THA were available at that time and the only available cost estimates cover only ambulatory consultations, not re-admissions. 113 The analyses presented within this chapter may, therefore, slightly underestimate costs for patients who have had THA. The cost of re-admissions was therefore added to the analyses conducted after the second user group meeting once HES data became available.
Regression analyses
Regression models predicting each model parameter conditional on preoperative clinical tool score, age and sex were estimated on the individual patient data using Stata® version 14.
A complete-case analysis was conducted to avoid overcomplicating the analysis with multiple imputed data sets. Observations with missing data on the clinical tool or the model parameter in question would have provided very little information to inform model estimation, and there is no reason to expect the relationship between clinical tool and outcome to differ between people with and without missing data on these variables. Each individual analysis excluded patients with missing data on age, sex or either the clinical tool score or the outcome variable being estimated in that regression analysis. As a result, sample sizes differed between regression analyses using the same data set.
The EPOS was the only data set providing information on THA patients beyond year 1. However, EPOS participants who did not complete the EQ-5D and SF-6D utilities cannot be directly compared with those measured using the EQ-5D because the SF-6D values health states using standard gamble rather than time trade-off and tends to produce non-comparable utilities (e.g. higher utilities for patients with poor health states). 157 We therefore mapped participants’ SF-12 responses onto the EQ-5D before analysis. Based on the literature review on mapping studies (see Online Supplement 11), we selected a response-mapping algorithm mapping from the SF-12 version 1 item responses that was slightly modified from the one estimated by Gray et al. 158 and estimated predictions using the expected value method. 159 This algorithm was chosen as it had better prediction accuracy than other algorithms based on the same version of the SF-12 that was used in the EPOS (see Online Supplement 11). Postoperative EQ-5D utilities calculated in this way were used in subsequent regression models in the same way as observed EQ-5D utilities, ignoring the uncertainty around the mapping model (which is in any case likely to be small owing to the large sample size used in the mapping study).
For each model parameter, we began by conducting exploratory data analysis to identify the distribution of the dependent variable and the shape of the relationship between the dependent variable and the clinical tool score, age and (when appropriate) time since primary arthroplasty. Exploratory data analysis was used to identify the most appropriate model specifications for each dependent variable. We then estimated regression models on each of the parameters listed in Table 39 and selected the model specification best predicting each model parameter using mean squared error (MSE). MSE was chosen in preference to information criteria in order to focus on model prediction and because information criteria cannot easily be calculated for some model types (e.g. two-part models) or necessarily be compared between linear and non-linear models.
We used K-fold cross-validation to reduce overfitting. For each of the below steps for each model parameter, each data set was divided into 10 parts of approximately equal size using pseudo-randomly generated numbers. Unless otherwise stated, for the analyses estimated on long format data sets (i.e. costs and EQ-5D utility before/after revision and long-term trends in EQ-5D utility), patients were divided into 10 groups and all observations for the same patient were included in the same part. For the analyses based on KAT and EPOS, we also stratified patients based on whether or not they had a revision ≥ 12 months after primary TJA when dividing patients between the 10 parts; no such stratification was done for PROMs/HES when conducting the analyses described in Chapter 7, because the sample size was markedly larger. Candidate models being considered in any given step were estimated on 9 of the 10 parts and validated on the 10th part; estimation and validation were repeated for each of the 10 validation samples, resulting in an estimate of the squared error (i.e. the squared difference between predicted and observed values in the validation sample) for each candidate model for each observation. MSE was calculated as the crude mean across the squared errors and the model specification with the lowest MSE was chosen for use in the next step.
Regression analyses on each of the parameters listed in Table 39 were conducted using the following steps.
Step 1. Functional form of the outcome variable:
-
Choose a number of candidate regression functions [e.g. ordinary least squares (OLS), generalised linear model (GLM), two-part model] based on the exploratory data analysis.
-
Define a simple model with the following covariates, with no polynomials or interactions –
-
– Clinical tool score.
-
– Age (this comprised dummies for 10-year age bands for the freely available PROMs data set, and continuous age for other data sets).
-
– Sex.
-
– For analyses including RCT data, we added a covariate for treatment effect if the treatment allocation was very different from current practice and the trial found significant differences between treatments. A dummy for treatment effect was therefore included in analyses on the APEX study, but not in KAT. However, no dummy was included in the mapping models predicting EQ-5D utility conditional on patients’ contemporaneous clinical tool scores.
-
– For certain model parameters, we also included time since primary arthroplasty and indicators of previous revision surgery as covariates (see Online Supplement 12).
-
-
Estimate the simple model using the candidate regression functions.
-
Calculate MSE for each model.
-
Choose functional form with lowest MSE to use in step 2
-
Optional step 1b. Functional form for time:
-
For the models of long-term EQ-5D utility, re-admission costs beyond year 1, and the cost utility of revisions, step 1(b) then identified the functional form for time since primary operation based on MSE.
-
Step 2. Functional form of the clinical tool:
-
Based on the exploratory data analysis, we chose candidates for the parameterisation of the clinical tool scores. Depending on the exploratory data analysis results, these included logarithms, polynomials, splines and/or interactions between different domain scores. For the SF-12, we chose the parameterisation for the physical domain first, then the mental domain, then assessed whether or not an interaction between physical and mental domains improved MSE. If non-logarithmic forms were chosen for the SF-12, we only considered the interaction between the absolute physical and absolute mental domain scores (i.e. no splines, quadratic or cubic terms for the interaction); if logarithmic forms were chosen for one (or both) SF-12 domains, we also considered interactions between linear and log scores, or between log-physical and log-mental scores. The model with the lowest MSE was used in step 3.
Step 3. Functional form of covariates:
-
Based on the exploratory data analysis, we chose a limited range of candidates for the parameterisation of age, estimated the candidate models and selected the model with the lowest MSE to use in step 3(b). Interactions between the clinical tool and other covariates were not considered. We also considered models that dropped age. For the models of re-admission costs beyond year 1 and the cost or utility of revisions, we first compared MSE with age at the time of primary surgery with MSE with age at the time of revision/year of data and selected the parameterisation for age based on the variable with the lowest MSE. Analyses of costs > 1 year after revisions used age in the current year throughout (owing to the Markovian assumption inherent within the model).
-
We assessed whether or not dropping the sex variable reduces the MSE. The model with the lowest MSE from step 3(b) was chosen for step 4.
Step 4. Final regression models and their variance–covariance matrices:
For two-part models, the selection of variables in steps 2 and 3 was done simultaneously for both parts of the model, such that the same variables were included in both parts of the model unless one of the final selected models was unstable (e.g. SEs of > 600 for a logit model); in such cases, we reran model selection for the problematic part of the model, leaving the other part unchanged. Online Supplement 12 describes how models for specific parameters were estimated, specific data cleaning and processing steps were conducted on specific data sets and model parameters, and specific assumptions were used when applying the parameters in the Markov models.
Other model assumptions and inputs
The model made the following assumptions:
-
Patients in the no arthroplasty arm were assumed to undergo no knee or hip arthroplasty during the 10-year time horizon. In practice, patients whose symptoms are not currently severe enough to warrant arthroplasty may have surgery later, once their symptoms have deteriorated. However, modelling arthroplasty procedures conducted at different time points as well as allowing for changes in revision rates, mortality, utilities and costs over time would have greatly complicated the model structure and required six patient-level simulation models, each following up to 1410 hypothetical individuals. Furthermore, there were very limited data on the proportion of patients whose clinical tool scores would deteriorate in the absence of arthroplasty, or on the rate at which scores are likely to deteriorate.
-
As discussed in Literature reviews on model inputs, the studies identified in the literature review suggested that some patients’ symptoms worsened over time in the absence of arthroplasty, whereas symptoms for other patients improved or remained the same (see Online Supplement 9). Although the literature review identified several US studies using WOMAC, we identified only one UK study using the WOMAC physical functioning subscale144 and one study showing how OHS100 and SF-12,115 respectively, change over time in the absence of arthroplasty. It is also unclear whether or not all of the patients in such data sets would be considered candidates for TJA. We therefore assumed that clinical tool scores remain unchanged for the time horizon of the model in patients who do not have arthroplasty, but assumed that EQ-5D utility decreases with age following a published model. 112 If patients’ osteoarthritis symptoms did, on average, deteriorate in the absence of arthroplasty, this would mean that our analyses overestimate the QALYs accrued in the no arthroplasty arm and, therefore, overestimate ICERs and underestimate the economic threshold.
-
We placed no restrictions on the number of revisions that patients could have within the Markov models, although patients could not have more than one revision operation per year.
-
Patients in the no arthroplasty arm were assumed to accrue a fixed cost each year. This cost was based on the costs accrued by patients in the COASt study in the year before arthroplasty, because no UK data were available on patients who were potentially eligible for arthroplasty but did not have surgery.
-
For certain parameters (e.g. community, outpatient and re-admission costs or utility > 1 year after TJA), there were no data for WOMAC. Furthermore, the only UK data set providing information on costs before or without arthroplasty (COASt) did not include the SF-12. 131 In these cases, we estimated regression models that used preoperative EQ-5D utility as an explanatory variable in place of the WOMAC or SF-12. Mapping models were used to calculate preoperative EQ-5D utility from preoperative clinical tool scores. For those parameters that were estimated as a function of EQ-5D, we calculated predicted costs/utilities by multiplying mapped preoperative EQ-5D utility by the regression coefficient for preoperative EQ-5D utility.
-
In all analyses, we assumed that costs and quality-of-life questionnaires were completed at the designated time. For example, if a patient had a revision 1.99 years after primary TJA, we assumed that the year 2 EQ-5D questionnaires were completed after the revision (rather than before).
-
Mortality rates incorporated a healthy patient effect estimated by Pennington et al. 119,121 using NJR data that allows for the fact that patients selected to undergo TJA have lower mortality for around 8 years after surgery than people who are not considered candidates for TJA. This was operationalised as a multiple of the annual all-cause mortality risk for individuals in the UK of the relevant age and sex, in which the multiple varies with age, sex and time since TJA. 125 Because the patients in the no arthroplasty arm were assumed to be identical to those patients in the arthroplasty arm, the healthy patient effect was also applied for the first 8 years of the no arthroplasty arm.
-
Mortality associated with revision surgery was excluded from the analyses described in this chapter, unless the models developed by Pennington et al. 119,121 predicted that mortality would be > 10% higher than would be expected in the absence of revision surgery (in which case mortality was assumed to be 10% higher than without revision surgery). Furthermore, no excess mortality was applied to revisions taking place within 12 months of primary arthroplasty. However, the published models of mortality associated with revision surgery were applied to all revision procedures in Chapter 7. This assumption is unlikely to have any significant effect on the conclusions because only a small minority of patients have revisions and revision rates are assumed to not vary with clinical tool score.
-
The cost of re-admissions and ambulatory costs were excluded for those patients who were revised in year 1 in the analyses described in this chapter. This assumption is unlikely to have any significant effect on the conclusions because only a small minority of patients have revisions and revision rates are assumed to not vary with clinical tool score.
-
After a patient’s 100th birthday, we assumed that the all-cause mortality for patients’ 100th year applies for all subsequent years. This assumption was only used in sensitivity analyses extending the time horizon beyond 10 years.
-
We assumed that revision rates do not vary with clinical tool score, because the PROMs/HES extract was not linked to NJR and the available data sets included very few revisions that could be linked to clinical scores measured before primary procedures. We therefore used published models estimated using NJR data that predict revision rates conditional on age, sex, time since primary TJA and other variables. 119,121 In these models, revision rates have a non-linear relationship with several variables that are not explicitly captured as patient characteristics in the model.
-
Postoperative EQ-5D utility and the cost of re-admissions and community/outpatient consultations in year 1 were estimated for all patients, regardless of whether or not they had been revised. This simplification was made because it was not possible to reliably identify the patients who were revised within 12 months of primary surgery in the freely available PROMs data set. This assumption should not affect estimates of economic thresholds, because revision rates were assumed to not vary with clinical tool score.
-
When modelling the QALY profile in the first year after arthroplasty, we assumed that EQ-5D utility at 3 months is the same as EQ-5D utility at 6 months and we assumed a linear change in utilities in the first 3 months after primary arthroplasty. Exploratory data analysis on the APEX study suggested that this approach gives a good approximation of the QALYs that we would calculate for the first year after primary arthroplasty if we had more frequent measurements, and performs at least as well as more complex methods. A recent study on SF-6D utilities after TKA confirmed this finding. 160
-
In all other cases, we assumed that utility changes linearly during the year; therefore, the QALYs accrued during the year equal the crude average of utility at the start of the year and utility at the end of the year. For simplicity, this assumption was also applied in the year of revision surgery, because for some data sets (e.g. KAT) revision surgery may take place at any point in the year. Furthermore, patients undergoing revision are likely to have had a quality of life similar to the pre-revision utility in the months leading up to revision surgery and experience utility similar to that observed 6 months after revision later in the year.
-
Because the > 1 year after revision state includes people with revision operations that took place anywhere between 1 and 9 years earlier and the Markov model cannot differentiate between people with respect to time since revision, we assumed that the utility in this state was equal to the post-revision utility that would have occurred if the revision had taken place in the present year relative to the annual EQ-5D questionnaire. As a result, any effect of ageing on utility after revision is assumed to be captured in the post-revision utility model.
-
For THA, the cost of community and outpatient care > 1 year after hospital discharge was based on an analysis of CPRD data done as part of the COASt study because no individual patient data were available (see Online Supplement 12 for a description of the assumptions made when applying these costs). 131
-
In PSA, all utilities were constrained to be between –0.594 and 1. Utilities that would otherwise be < –0.594 were set to –0.594 and those that would otherwise be > 1 were set to 1. With the exception of the community costs taken from Pinedo Villanueva,113 all costs were constrained to be ≥ £0 and values that would otherwise be < £0 were set to £0. The community costs taken from Pinedo Villanueva113 represent differences between resource use in patients with osteoarthritis and resource use in those without osteoarthritis and were, therefore, permitted to be negative, in line with how they were used in the original study.
Presentation of results and analysis of uncertainty
Hypothetical individuals with different combinations of age, sex and clinical tool score were run through the model sequentially, both using mean values for all parameters and using parameter values sampled from their distributions (PSA). PSA was run separately on the six Markov models representing TKA and THA with each of the three tools. However, within each model, the same set of sampled values was used for all hypothetical individuals to ensure that differences between hypothetical individuals were not masked by differences between sampled values.
All uncertain parameters, including all regression coefficients, were varied in PSA. We allowed for correlations between coefficients estimated in the same regression model by assuming a multivariate normal distribution. 161 Variance–covariance matrices for published models were obtained from the authors;50,112,119,121,126 those for the models estimated on patient-level data were estimated in Stata® and are available on request. However, for simplicity, we did not allow for correlations between the coefficients from different regression models, or between the coefficients for the logit and OLS/GLM parts of two-part models. Differences in the cost of ambulatory consultations after THA were assumed to follow independent normal distributions, whereas the cost of hip revision surgery in different patient subgroups was assumed to follow independent gamma distributions. 113
We ran all six models using men and women aged exactly 50, 60, 70, 80 and 90 years. It should be noted that several data sets include relatively few people at the upper and lower ends of this age range and that the published models of mortality and revision rates excluded individuals aged < 55 or > 85 years. 119,121 Results for patients aged 50 or 90 years should, therefore, be interpreted with caution, but may give an indication of incremental cost-effectiveness for these patients. A wide range of integer values for the OKS, OHS, WOMAC and SF-12 physical score were selected to cover the range of possible scores for the instrument, with greater concentration of scores in the region where preliminary analyses had shown the threshold to be. For the SF-12, results were also repeated for patients with SF-12 mental scores of 30, 50 or 70; although 30 and 70 represent very extreme values that were observed for very few patients, they were chosen to give an indication of the range of possible values. In total, between 490 and 1410 hypothetical individuals were analysed using point estimates for all model parameters and between 220 and 780 hypothetical individuals were analysed in PSA. For the WOMAC, OKS and OHS models, 2000 PSA runs were conducted; for the SF-12 models, 1000 PSA runs were conducted, because there was insufficient computing time for 2000 runs for each of the three mental scores.
We also present the weighted average across men and women and across ages; unless otherwise stated, all figures averaging across sexes and/or ages are weighted by the proportion of people in each group. The proportion of men and women in different age groups was calculated using the number of procedures per 100,000 people aged ≥ 10 years by age and sex published in the final PROMs report for 2013–14162 and the corresponding population numbers from the Office for National Statistics’ Mid-2013 Population Estimates in England163 (see Online Supplement 12). These proportions were multiplied by the total number of primary arthroplasty procedures conducted solely for osteoarthritis in England in 2014–15 (76,617 knee replacements and 69,313 hip replacements) to give patient numbers. The total number of primary arthroplasty procedures (79,726 knee replacements and 77,880 hip replacements) was calculated from HES based on the number of finished consultant episodes for the OPCS (Office of Population Censuses and Surveys Classification of Surgical Operations and Procedures) codes beginning with O or W that the NJR used to identify primary hip/knee replacement and was multiplied by the proportion of all primary arthroplasty procedures conducted solely for osteoarthritis (96% for knees and 89% for hips). 154,164,165 Data from PROMs/HES, the APEX study, KAT and EPOS were used to calculate the proportion of people with different clinical tool scores (see Online Supplement 12). We allowed for the fact that the age distribution varies between men and women and the fact that the distribution of SF-12 mental scores varies with physical scores. However, for simplicity and to ensure consistency between models on different clinical tools, we assumed that the distribution of clinical tool scores was independent of age and sex.
The base-case results represent the point estimates, keeping all parameters at their mean values. This approach was used because it was not feasible to run PSA for all analyses in time for the second user group meeting. Allowing for non-linearities by taking the expected value from PSA had very little effect on ICERs or thresholds (results not shown). 161
The costs and QALYs with and without TJA were calculated for each hypothetical individual and were used to calculate the cost per QALY gained for TJA versus no TJA; these ICERs are displayed in the decision grids shown in Results. The threshold clinical tool score was defined as the highest clinical tool score at which the ICER for TJA versus no arthroplasty was < £20,000 per QALY gained for patients of any given age, sex and, when applicable, SF-12 mental score.
We also used PSA results to calculate 95% credible intervals (CrIs) around the threshold clinical score. These intervals were calculated by first examining the results of each individual PSA replicate to identify the threshold clinical tool score for that PSA draw (within each age/sex group). The 95% CrI limits for the threshold were assumed to equal the 2.5th percentile and the 97.5th percentile across the sets of PSA results. However, because PSA was run for only a finite number of hypothetical individuals owing to the long simulation time, only even-numbered SF-12 physical scores were evaluated and, for this reason, the 95% CrI around the SF-12 thresholds consider only even-numbered scores. Similarly, we only conducted PSA on WOMAC scores that were multiples of five or > 95. We also averaged incremental net benefits (INBs) (INB = Rc • ΔQALYs – ΔCost) across men and women at a £20,000-per-QALY ceiling ratio (Rc), and also across ages and calculated 95% CrIs across thresholds based on these averaged results.
We also used data on the distribution of patients by age, sex and clinical tool score to calculate the number of people who currently undergo arthroplasty but would no longer have access to surgery if different threshold tool scores were introduced. We also calculated the net health benefit (or net harm) of stratifying access to TJA using different thresholds using published methods. 166,167 These estimates explicitly exclude patients who do not currently have arthroplasty but might gain access to surgery if national guidelines were to introduce a threshold that was higher than that currently used by local commissioners or primary health-care services; estimates of the impact of different thresholds that include this population of people are described in Chapter 8.
The expected value of stratifying access to TJA based on different clinical tool thresholds, age and sex (ValueofStratifying) was calculated by first multiplying the number of people in England of age (a), sex (s) and clinical tool score (c) (Nasc) by the incremental net (health) benefit of TJA calculated in the model for that patient group (INBasc = Rc • ΔQALYsasc – ΔCostasc). The expected value of introducing a specific threshold was calculated by summing these figures across all clinical tool scores less than or equal to the threshold (T) and subtracting that sum from 0:
For example, if the economic threshold was estimated to be 1 for tool (c) for people of age group (a) and sex (s) and the INB was 2 for the 100 patients with a score of 0 and 1 for the 200 patients with a score of 1, we would calculate the value of stratifying by clinical tool score in this demographic group as:
We would then add these values across all demographic groups.
Because there may be practical and equity arguments against rationing access to surgery by age or sex, we also calculated the weighted average incremental costs and incremental QALYs for TJA versus no arthroplasty at different clinical tool scores, weighting by the proportion of people of different ages and sexes (see Online Supplement 12). These values were used to calculate the ICER for different clinical tool scores averaged across sexes or across all age/sex groups. We then calculated the value of stratifying only by clinical tool score, and the value of stratifying only by clinical tool score and age:
The proportion of PSA draws that find TJA to be cost-effective compared with no TJA (i.e. the proportion that have positive INB) was identified for each hypothetical patient group at different ceiling ratios. The probability that TKA is cost-effective was plotted against clinical tool score. For brevity, we present figures averaged over men and women by calculating the weighted average probability that TKA is cost-effective, weighted by the proportion of patients who are female.
We also conducted four sensitivity analyses on each of the six Markov models:
-
Taking a 5-year time horizon (cf. 10 years in the base-case analysis).
-
Taking a 60-year (lifetime) time horizon.
-
Assuming that EQ-5D utility without TJA worsens by 0.025 per year (cf. a 0.0036–0.0069 decrease per year, depending on age in the base-case analysis). The figure of 0.025 was based on the smallest measurable difference in the original study used to estimate the EQ-5D time trade-off tariff (3 months in 10 years). 168
-
Assuming that EQ-5D without TJA increases by 0.115 per year in the first year of the model and then follows an age-related decline after that. The figure of 0.115 was based on the increase in EQ-5D in the non-surgical treatment arm of a recent RCT that compared TKA against 12 weeks’ non-surgical treatment comprising exercise, education, dietary advice, insoles and pain medication. 169 This figure is conservative because the non-surgical treatment used in the trial was relatively intensive and many NHS patients may have already received similar conservative management before being listed for surgery.
For brevity, and to reduce computation time, we present point estimates only for sensitivity analyses, not PSA. Furthermore, sensitivity analyses were run using the same reduced set of clinical tool score values used for PSA; subsequently, the thresholds estimated for sensitivity analyses on the SF-12 and WOMAC are approximate and may slightly overestimate the true threshold, because costs and QALYs for hypothetical individuals that were not simulated were assumed to equal those for patients with a score one point higher than the score that was not simulated.
Results
Effect of scores on costs and utilities
Regression analyses demonstrated that the preoperative OKS, OHS, WOMAC and SF-12 physical and mental scores had a statistically significant effect on preoperative EQ-5D utility, EQ-5D utility 3–12 months after primary arthroplasty, EQ-5D utility > 12 months after primary arthroplasty and EQ-5D utility after knee revision surgery (p < 0.05; see Online Supplement 12). The OKS, OHS and SF-12, but not the WOMAC, had a significant effect on the cost of primary arthroplasty surgery (p < 0.05). The OHS had a significant effect on costs in the absence of hip arthroplasty (p = 0.003), but allowing for preoperative EQ-5D worsened prediction accuracy. However, within the analyses conducted before the second user group meeting, the clinical tool score was found to have no significant impact on EQ-5D utility before revision surgery, the cost of revision surgery and costs in the absence of knee arthroplasty (p > 0.05; see Online Supplement 12). The results of all regression models are shown in Online Supplement 12.
There was a strong relationship between preoperative clinical tool score and both preoperative and postoperative utility that varied slightly with age (Figures 14–19). The magnitude of the difference between preoperative and postoperative utility was, in turn, the main driver determining the QALYs gained from TJA, the ICERs and the threshold clinical tool scores.
FIGURE 14.
Effect of the preoperative OKS and age on preoperative and 6-month EQ-5D utility.

FIGURE 15.
Effect of the preoperative OHS and age on preoperative and 6-month EQ-5D utility, based on a published mapping algorithm and a two-part model of the relationship between log-OHS, age and sex estimated on PROMs data. 126

FIGURE 16.
Effect of the preoperative WOMAC score and age on preoperative and 6-month EQ-5D utility in TKA patients, based on the APEX study data. Preoperative utilities were predicted based on a Tobit model that included quadratic and cubic terms, whereas postoperative utilities were modelled using linear regression.

FIGURE 17.
Effect of the preoperative WOMAC score and age on preoperative and 6-month EQ-5D utility in THA patients, based on linear regression on the APEX study data. Models of preoperative utility included quadratic and cubic terms for WOMAC.

FIGURE 18.
Effect of the preoperative SF-12 physical score on preoperative and 6-month EQ-5D utility in 70-year-old TKA patients at SF-12 mental scores of (a) 30, (b) 50 or (c) 70, based on Tobit models on KAT data that included polynomial terms for SF-12 scores.
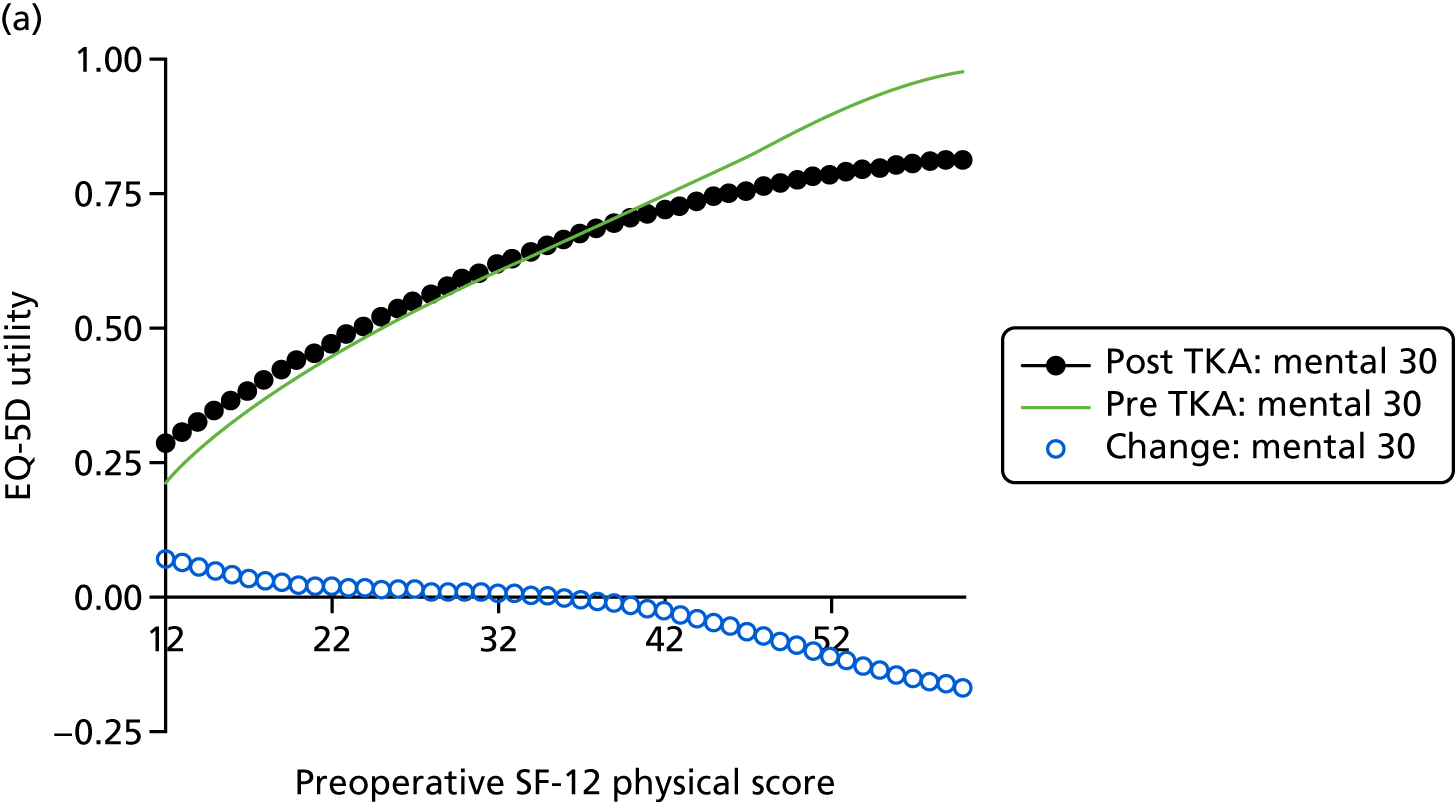
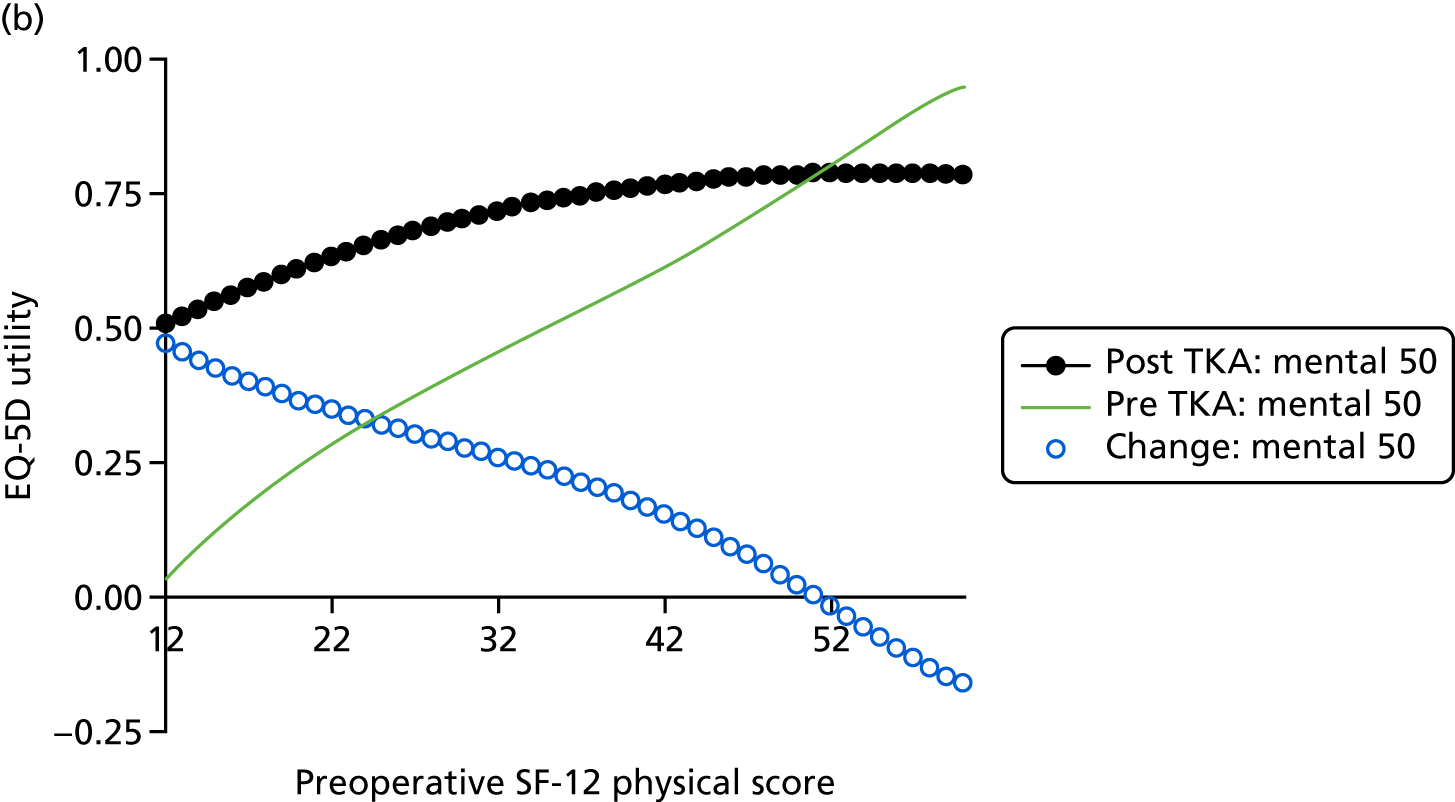

FIGURE 19.
Effect of the preoperative SF-12 physical score on preoperative and 6-month EQ-5D utility in 70-year-old THA patients at SF-12 mental scores of (a) 30, (b) 50 or (c) 70. Postoperative utility was based on a GLM with log-link estimated on EPOS.

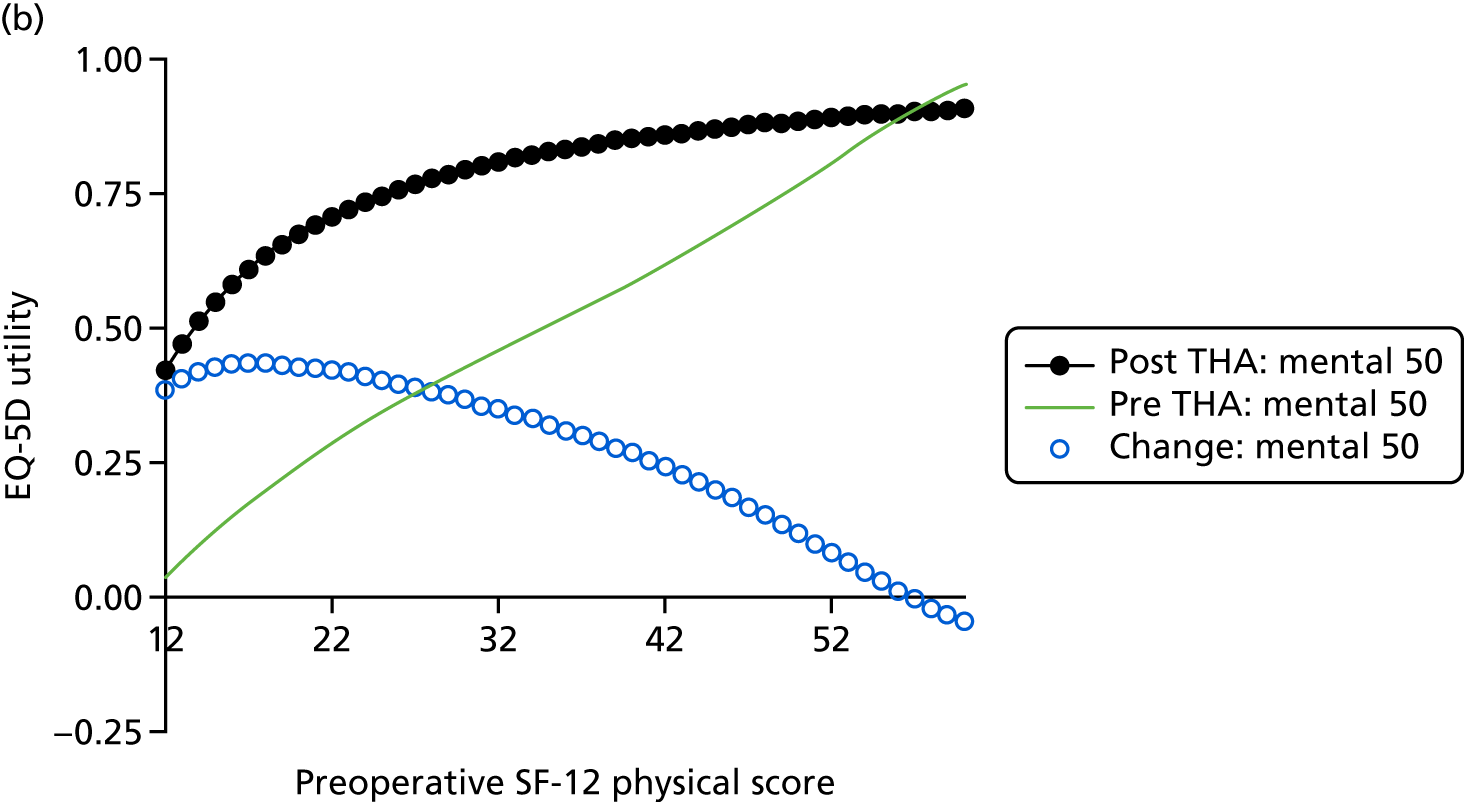
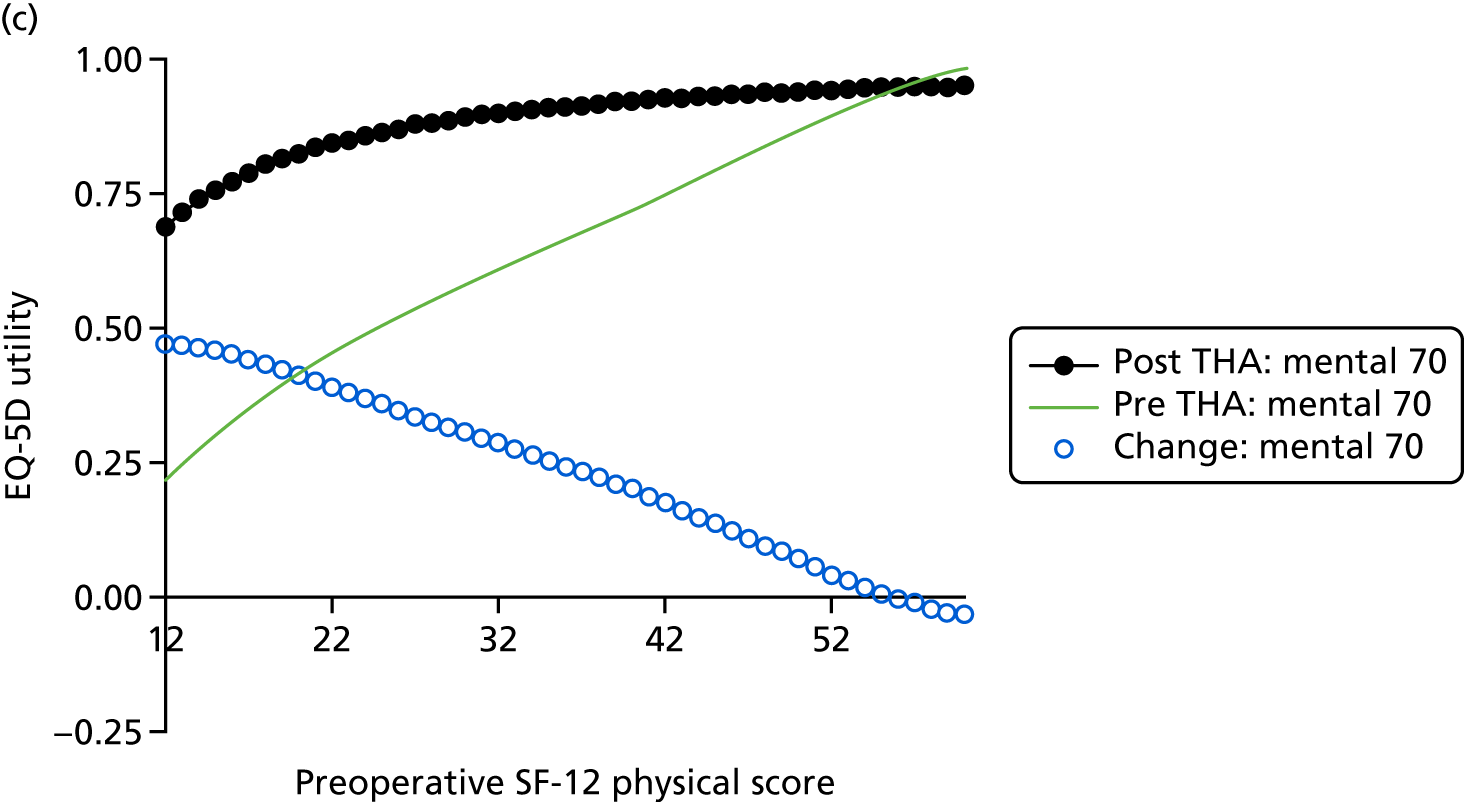
Figure 14 shows the relationship between the preoperative OKS and preoperative and postoperative EQ-5D utility estimated using regression equations used in the Markov model. Preoperative EQ-5D utility was estimated using a published linear mapping model,50 whereas 6-month utility was estimated on freely available PROMs data using a Tobit model that included quadratic and cubic terms for OKS. Both preoperative and postoperative utility increase sharply with preoperative OKS; however, the relationship between the preoperative OKS and 6-month EQ-5D utility is non-linear and is markedly less steep for patients with a baseline OKS of > 20. As a result, the change in utility following TKA is greatest at an OKS of 9 or 10, and declines steadily at higher scores until the difference becomes negative (i.e. TKA is expected to reduce quality of life) at an OKS of 41–44 or higher. Age had a relatively modest effect, with the postoperative utility being between 0.02 and 0.08 lower for 50-year-old patients than for those aged 70–90 years. After adjusting for age and OKS, gender was found to have no significant impact on utility after TKA and was dropped from the final model. Results for the OHS were similar (see Figure 15).
The relationship between preoperative WOMAC and preoperative and postoperative utility was similar to that for the OKS and OHS (see Figures 16 and 17). However, the models for WOMAC predicted that postoperative utility would always be higher than preoperative utility at all baseline WOMAC scores. The linear models predicted postoperative EQ-5D utility to be > 1 for patients with high baseline WOMAC; such predictions were set to 1 in the model.
The relationship between preoperative SF-12 physical score and preoperative and postoperative EQ-5D utility varied with SF-12 mental score (see Figure 18). At a mental score of 30, the change in utility following TKA was very small and was negative for patients with physical scores of ≥ 36, whereas the change in utility was markedly larger for patients with high mental scores and declined more sharply with SF-12 physical score. The way in which SF-12 is calculated means that patients cannot simultaneously get very low scores on both the physical and mental scales, or get very high scores on both. In particular, the highest possible physical score for patients with a mental score of 70 is 42.13, which is lower than the physical score at which the models predict that the change in utility following TKA becomes negative; TKA is therefore predicted to be beneficial for all patients with high mental scores.
For THA, the relationship between SF-12 physical score and change in utility following THA (see Figure 19) was similar to that of TKA at high mental scores. However, for patients with a SF-12 mental score of 30, the curve for utility after THA crossed the curve for utility before THA in two places. As a result, the change in utility was negative for patients with low SF-12 physical scores as well as for those with high scores. For 70-year-olds, the physical scores at which the change in utility is negative are below the minimum possible score for patients with SF-12 mental scores of 30, although the models predict that for 90-year-olds there are some attainable combinations of low physical and mental scores at which the change in utility following THA is negative.
Because operative mortality is rare, relatively few patients have revision surgery and patients’ utility remains relatively constant after 6 months (see Online Supplement 12), the change in utility in the first 6 months after TKA is approximately proportional to the QALY gain from TKA. 119 Figures 14–19 therefore indicate the relationships that are driving the ICERs and clinical tool thresholds shown in the next six sections.
Effect of the Oxford Knee Score on the cost-effectiveness of knee replacement
The Markov models used the regression models predicting EQ-5D utilities, costs, mortality and revision rates shown in Online Supplement 12 to calculate the costs and QALYs that different patient subgroups would accrue over the 10-year period after TKA or after the decision was made not to operate. These results were used to calculate the incremental cost-effectiveness of TKA versus no arthroplasty in people of different ages with different preoperative OKSs (Table 40).
Preoperative OKS (selected values only) | Cost | |||||
---|---|---|---|---|---|---|
Age (years) | ||||||
50 | 60 | 70 | 80 | 90 | Average | |
0 | £2059 | £1643 | £1978 | £2781 | £4762 | £2155 |
10 | £33 | £221 | £566 | £1230 | £2778 | £655 |
20 | Dominant | £211 | £616 | £1377 | £3154 | £710 |
21 | £53 | £266 | £680 | £1460 | £3291 | £778 |
24 | £378 | £508 | £956 | £1812 | £3858 | £1071 |
28 | £1167 | £1061 | £1597 | £2627 | £5151 | £1748 |
29 | £1457 | £1254 | £1828 | £2924 | £5624 | £1990 |
30 | £1799 | £1475 | £2099 | £3276 | £6187 | £2274 |
31 | £2207 | £1730 | £2418 | £3696 | £6863 | £2608 |
32 | £2697 | £2025 | £2800 | £4204 | £7689 | £3006 |
33 | £3296 | £2370 | £3263 | £4830 | £8717 | £3485 |
34 | £4044 | £2778 | £3834 | £5619 | £10,027 | £4073 |
35 | £5003 | £3267 | £4556 | £6641 | £11,752 | £4811 |
36 | £6277 | £3865 | £5499 | £8020 | £14,125 | £5765 |
37 | £8058 | £4614 | £6786 | £9981 | £17,593 | £7049 |
38 | £10,733 | £5582 | £8652 | £12,998 | £23,145 | £8874 |
39 | £15,226 | £6888 | £11,616 | £18,258 | £33,482 | £11,687 |
40 | £24,418 | £8761 | £17,084 | £29,791 | £59,554 | £16,616 |
41 | £54,138 | £11,690 | £30,672 | £75,796 | £253,711 | £27,568 |
42 | Dominated | £16,983 | £124,962 | Dominated | Dominated | £73,754 |
43 | Dominated | £29,746 | Dominated | Dominated | Dominated | Dominated |
44 | Dominated | £99,241 | Dominated | Dominated | Dominated | Dominated |
45 | Dominated | Dominated | Dominated | Dominated | Dominated | Dominated |
46 | Dominated | Dominated | Dominated | Dominated | Dominated | Dominated |
47 | Dominated | Dominated | Dominated | Dominated | Dominated | Dominated |
48 | Dominated | Dominated | Dominated | Dominated | Dominated | Dominated |
Threshold (95% CrI) | 39 (37 to 43) | 42 (41 to 45) | 40 (39 to 42) | 39 (38 to 40) | 37 (24 to 41) | 40 (39 to 42) |
The results show that, as expected, TKA is highly cost-effective for most patients who currently undergo surgery (see Table 40). The light blue area in Table 40 and subsequent decision grids indicates patient groups for which TKA is cost-effective (i.e. costs < £20,000 per QALY gained compared with no arthroplasty). Table 40 shows only selected OKS values, focusing on values close to the threshold; for the OKS values not shown in the decision grids, TKA costs < £20,000 per QALY gained. Based on the distribution of the preoperative OKS, age and sex within the final PROMs/HES extract (see Online Supplement 12), TKA costs < £20,000 per QALY gained compared with no arthroplasty in 99.7% of patients who currently undergo surgery and it costs < £5000 per QALY gained in 97.4% of patients. TKA was most cost-effective (i.e. has the lowest cost/QALY) for those patients with low preoperative OKSs.
The QALY gains from TKA are highest for patients with a preoperative OKS of around 10 and decrease steadily as the OKS increases (mean incremental QALYs: 2.570 at OKS of 0, 3.301 at OKS of 9 and –0.355 at OKS of 48, averaged over all age and sex groups). This follows naturally from the difference in EQ-5D utility before and after TKA shown in Figure 14. The difference in cost between patients undergoing TKA and those having non-operative management was highest for patients with very low and very high OKS, and lowest for patients with scores between 14 and 21 (mean incremental cost: £5539 at OKS of 0, £6055 at OKS of 48 and £1710 at OKS of 16, averaged over all age and sex groups).
Cost-effectiveness results were primarily driven by the difference in QALYs, and the ICER (calculated as the difference in cost divided by the difference in QALYs) increased sharply as the difference in QALYs approached zero. For any given age group, the ICER for TKA versus no arthroplasty was very low for patients with an OKS of below around 35, but increased sharply as the OKS increases and the difference in quality of life between patients with and without TKA approached zero. For those patient subgroups shown in dark or medium green in Table 40, TKA costs > £20,000 per QALY gained; although arthroplasty improves quality of life, it is not cost-effective compared with the cost-effectiveness threshold that is generally used in NHS decision-making. For patient subgroups with very high OKSs (the dominated area, shown in white, in Table 40), TKA is dominated: it increases costs to the NHS and, on average, people in these groups will have fewer QALYs than those having no arthroplasty because their EQ-5D utility is expected to be lower with TKA than without and (to a lesser extent) because of surgical mortality.
For any given age group, we can identify the economic threshold as the score at the bottom of the light blue area in Table 40. The economic threshold varies with age, being highest for 60-year-olds [42 (95% CrI 41 to 45)] and lowest for 90-year-olds [37 (95% CrI 24 to 41)]. The economic threshold is slightly lower for older patients because they have lower life expectancy and therefore enjoy the quality of life improvements for a shorter period of time. 125 Similarly, thresholds were somewhat lower for 50-year-olds as they have higher revision rates and lower postoperative EQ-5D utility than patients aged 60–80 years. 119
Cost-effectiveness also varied slightly between men and women, with all ICERs being slightly lower for women than for men (see Online Supplement 12). However, the threshold OKS did not differ.
Averaging costs and QALYs across all age and sex groups suggests that if a single threshold were to be applied for patients of all ages, the threshold OKS would be 40 (95% CrI 39 to 42).
However, there was uncertainty around the results, particularly for 90-year-olds (see Table 40) and patients with an OKS close to the economic threshold. Figure 20 shows how the probability that TKA is cost-effective compared with no arthroplasty (i.e. costs < £20,000 per QALY gained) changes with the amount that the NHS is willing or able to pay to gain 1 QALY. For 70-year-olds with preoperative OKSs of < 40, we can be > 99% confident that TKA is good value for money. However, this decreases to 73% at an OKS of 40 and to 18% at an OKS of 41.
FIGURE 20.
Effect of the OKS on the probability that TKA is cost-effective at a £20,000-per-QALY ceiling ratio.
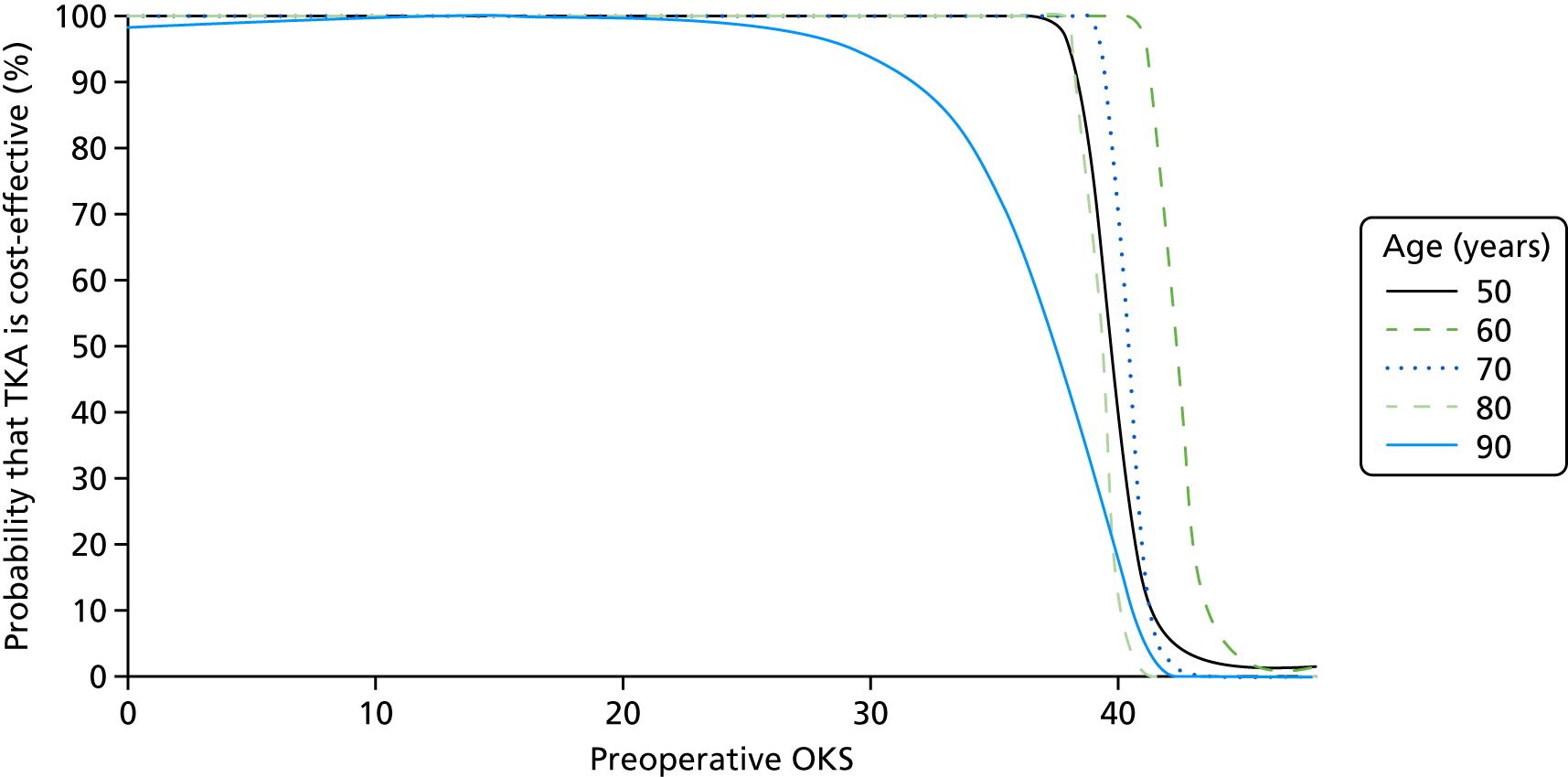
Effect of the Western Ontario and McMaster Universities Arthritis Index on the cost-effectiveness of total knee arthroplasty
To facilitate the comparison of results across the different clinical tools, the results presented here are based on a reversed and rescaled WOMAC total score, in which 0 represents the worst possible score and 100 represents the best possible score. As in the previous section, incremental cost-effectiveness was calculated using the Markov model, in which EQ-5D utility, costs, revision rates and mortality were predicted based on a patient’s preoperative characteristics.
For almost all WOMAC total scores, TKA is highly cost-effective (Table 41). The QALY gains were highest for patients with low WOMAC scores (mean incremental QALYs at a score of 0 = 6.428) and decreased steadily with WOMAC score (mean incremental QALYs at a score of 50 = 1.509; at a score of 100 = 0.109). Similarly, incremental costs for patients with TKA compared with patients without TKA were lowest for patients with WOMAC scores of 5 (mean incremental cost = £2) and highest for patients with high scores (mean incremental costs for a score of 100 = £3361).
WOMAC score (rescaled to 0–100; 0 indicates poor function) | Cost | |||||
---|---|---|---|---|---|---|
Age (years) | ||||||
50 | 60 | 70 | 80 | 90 | Average | |
0 | Dominant | Dominant | £57 | £354 | £949 | £83 |
10 | Dominant | Dominant | £14 | £460 | £1350 | £68 |
20 | Dominant | £28 | £313 | £940 | £2276 | £401 |
30 | £262 | £362 | £713 | £1588 | £3574 | £851 |
40 | £647 | £706 | £1131 | £2312 | £5168 | £1329 |
50 | £989 | £996 | £1489 | £2968 | £6816 | £1743 |
60 | £1265 | £1220 | £1768 | £3490 | £8321 | £2064 |
70 | £1522 | £1427 | £2026 | £3993 | £9895 | £2366 |
80 | £1827 | £1677 | £2345 | £4707 | £12,190 | £2755 |
85 | £2289 | £2055 | £2873 | £6029 | £17,305 | £3408 |
86 | £2472 | £2201 | £3085 | £6609 | £19,984 | £3674 |
87 | £2689 | £2371 | £3331 | £7295 | £23,561 | £3983 |
88 | £2943 | £2566 | £3617 | £8118 | £28,555 | £4343 |
89 | £3236 | £2788 | £3944 | £9117 | £35,977 | £4759 |
90 | £3578 | £3040 | £4321 | £10,350 | £48,101 | £5244 |
91 | £3978 | £3327 | £4758 | £11,901 | £71,295 | £5813 |
92 | £4451 | £3656 | £5267 | £13,899 | £132,863 | £6486 |
93 | £5014 | £4033 | £5863 | £16,549 | £763,143 | £7289 |
94 | £5688 | £4466 | £6565 | £20,207 | Dominated | £8256 |
95 | £6504 | £4965 | £7396 | £25,534 | Dominated | £9432 |
96 | £7503 | £5538 | £8387 | £33,931 | Dominated | £10,881 |
97 | £8739 | £6198 | £9578 | £48,964 | Dominated | £12,693 |
98 | £10,294 | £6957 | £11,019 | £83,197 | Dominated | £14,997 |
99 | £12,286 | £7829 | £12,780 | £235,097 | Dominated | £17,997 |
100 | £14,902 | £8830 | £14,958 | Dominated | Dominated | £22,018 |
Threshold (95% CrI) | 100 (80 to 100) | 100 (85 to 100) | 100 (80 to 100) | 93 (65 to 99) | 86 (40 to 90) | 99 (80 to 100) |
The economic threshold averaged across age and gender was identified as 99 (95% CrI 80 to 100; see Table 41), which is very close to the maximum value of 100. It should be noted that the highest observed WOMAC score in the APEX study data was 87; the identified threshold value of 99 lies out with the sample. However, there was considerable variation with age. For patients aged ≤ 70 years, TKA is cost-effective at all WOMAC scores. TKA ceases to be cost-effective at a WOMAC score of 93 (95% CrI 65 to 99) for patients aged 80 years, and the threshold is 86 (95% CrI 40 to 90) for those aged 90 years. Again, this reflects the lower life expectancy at these ages, which means that the small benefits of TKA for patients with a relatively good clinical score (see Figure 16) are not accrued for as long a period as younger patients.
There is considerable uncertainty around these threshold values, as shown by the wide 95% CrI. For example, the 95% CI for the threshold averaged over all age and gender groups (99) ranges from 80 to 100. Similarly, the 95% CrI for patients aged 90 years ranges from 40 to 90, covering half of all possible scores. This is likely because of the relatively small sample sizes of the data sets used for the regression models (the regressions of WOMAC on EQ-5D utility were based on 221 patients whereas those on costs of the primary operation were based on 272 patients).
There was slight variation in cost-effectiveness between men and women. At higher ages (80 and 90 years), the threshold for men was (in both cases) 3 points higher than for women (95 vs. 92 at age 80 years and 87 vs. 84 at age 90 years).
The PSA demonstrated that there is greater uncertainty around the results for the WOMAC than for the OKS (Figure 21). For 70-year-olds, the probability that TKA was cost-effective was > 99% for patients with WOMAC scores of ≤ 65 and fell to 96% for patients with a score of 90, 78% for a score of 98 and 62% for a score of 100. For 90-year-olds, the probability that TKA was cost-effective was < 99% for scores of ≥ 40 and was < 0.1% for scores of ≥ 96.
FIGURE 21.
Effect of the WOMAC on the probability that TKA is cost-effective at a £20,000-per-QALY ceiling ratio.
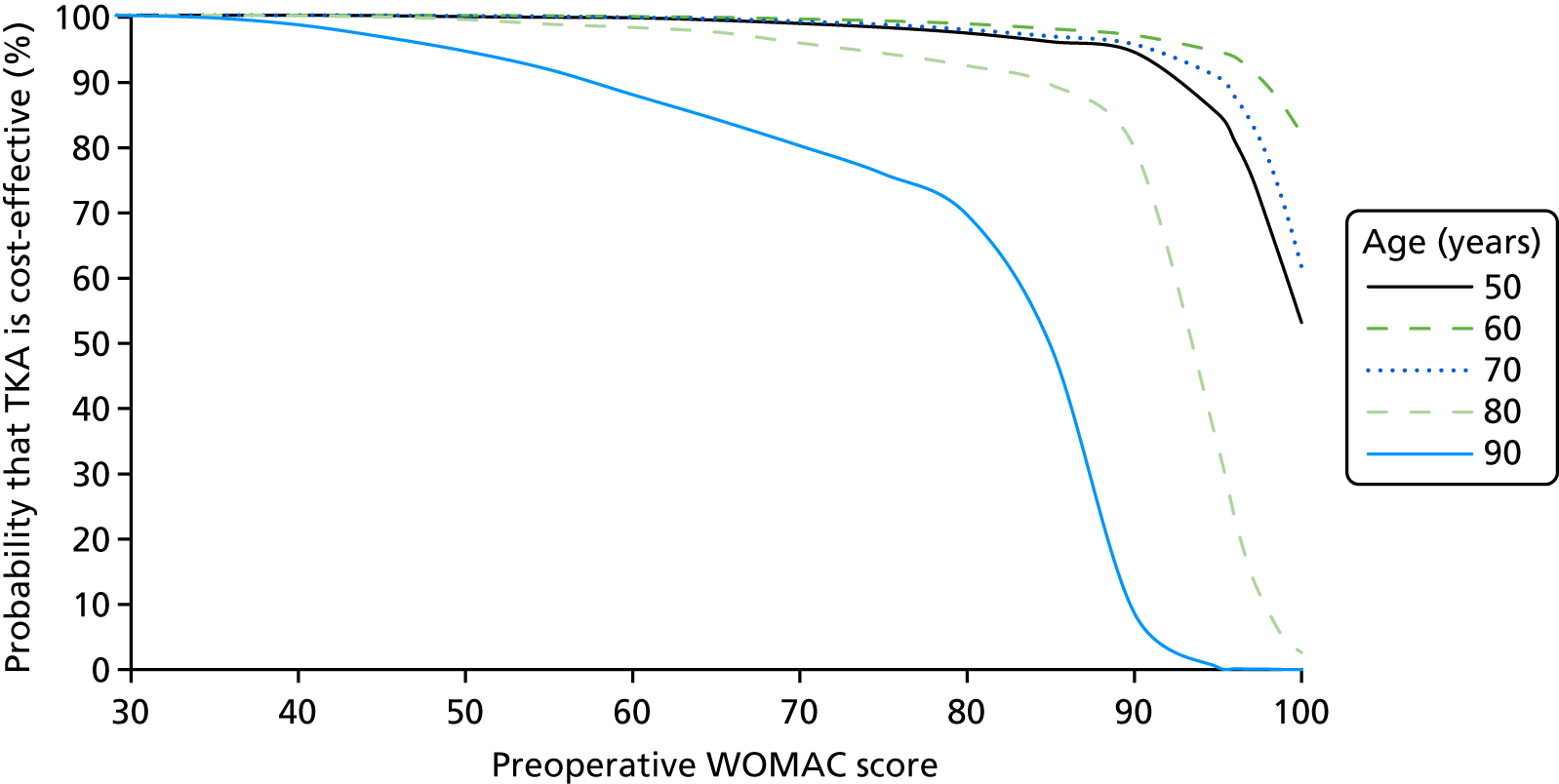
Effect of the Short Form questionnaire-12 items on the cost-effectiveness of total knee arthroplasty
For the SF-12, results were estimated for a range of physical scores at each of three mental scores (30, 50 and 70). The SF-12 scoring system decreases the physical scores for patients who have good mental health or good social function and decreases the mental scores for patients with poor physical or general health. As a result, it is not possible for the same person to have the lowest (or highest) scores on both the physical domain and the mental domain. Results are only presented for those combinations of scores that are possible on the SF-12 scoring system.
For all three mental scores, the incremental cost of TKA versus no arthroplasty changed very little with SF-12 physical score, whereas QALYs decreased very sharply. For example, for a mental score of 50, the average incremental cost increased from £1954 per patient at a physical score of 15 to £3169 per patient at a physical score of 60, whereas incremental QALYs decreased from 3.591 at a physical score of 12 to –1.388 at a physical score of 60.
At a mental score of 30 (indicating very poor mental health), the threshold physical score was 58 (95% CrI 54 to 60), averaging across all ages (Table 42). For patients with a mental score of 50 (indicating average mental health), thresholds were generally markedly lower than they were at a mental score of 30, and the effect of age was more pronounced. Averaging across ages, the threshold physical score at a mental score of 50 was 50 (95% CrI 48 to 52) (Table 43).
SF-12 physical score | Cost | |||||
---|---|---|---|---|---|---|
Age (years) | ||||||
50 | 60 | 70 | 80 | 90 | Average | |
18 | £367 | £440 | £682 | £1273 | £3586 | £789 |
19 | £321 | £400 | £652 | £1257 | £3620 | £760 |
20 | £287 | £370 | £631 | £1247 | £3658 | £739 |
25 | £236 | £314 | £594 | £1249 | £3860 | £708 |
30 | £279 | £334 | £616 | £1297 | £4088 | £738 |
35 | £367 | £391 | £672 | £1389 | £4440 | £807 |
40 | £509 | £490 | £774 | £1562 | £5164 | £931 |
45 | £769 | £669 | £969 | £1919 | £7018 | £1172 |
49 | £1206 | £953 | £1282 | £2544 | £12,030 | £1573 |
50 | £1391 | £1065 | £1404 | £2806 | £15,284 | £1734 |
51 | £1633 | £1204 | £1557 | £3145 | £21,478 | £1939 |
52 | £1966 | £1384 | £1752 | £3600 | £37,694 | £2206 |
53 | £2450 | £1624 | £2007 | £4236 | £186,187 | £2566 |
54 | £3216 | £1957 | £2355 | £5181 | Dominated | £3078 |
55 | £4610 | £2449 | £2852 | £6722 | Dominated | £3853 |
56 | £7924 | £3247 | £3618 | £9648 | Dominated | £5161 |
57 | £25,718 | £4757 | £4937 | £17,221 | Dominated | £7803 |
58 | Dominated | £8646 | £7707 | £80,227 | Dominated | £15,819 |
59 | Dominated | £40,402 | £17,051 | Dominated | Dominated | Dominated |
60 | Dominated | Dominated | Dominated | Dominated | Dominated | Dominated |
Threshold (95% CrI) | 56 (52 to 60) | 58 (54 to 60) | 59 (56 to 60) | 57 (53.95 to 60) | 50 (44 to 54.05) | 58 (54 to 60) |
SF-12 physical score | Cost | |||||
---|---|---|---|---|---|---|
Age (years) | ||||||
50 | 60 | 70 | 80 | 90 | Average | |
12 | Dominant | £198 | £506 | £1101 | £3255 | £581 |
15 | Dominant | £175 | £516 | £1182 | £3736 | £601 |
20 | Dominant | £225 | £598 | £1377 | £4736 | £707 |
25 | £108 | £322 | £718 | £1614 | £6028 | £855 |
30 | £263 | £447 | £866 | £1909 | £7970 | £1039 |
35 | £483 | £619 | £1075 | £2351 | £12,162 | £1303 |
38 | £692 | £775 | £1265 | £2784 | £19,302 | £1551 |
39 | £787 | £843 | £1347 | £2981 | £24,634 | £1661 |
40 | £902 | £923 | £1444 | £3219 | £34,769 | £1791 |
42 | £1229 | £1133 | £1696 | £3877 | £326,196 | £2139 |
43 | £1473 | £1276 | £1864 | £4349 | Dominated | £2380 |
47 | £4823 | £2490 | £3202 | £9381 | Dominated | £4550 |
48 | £9637 | £3230 | £3938 | £13,673 | Dominated | £5978 |
49 | £193,008 | £4562 | £5129 | £25,787 | Dominated | £8763 |
50 | Dominated | £7654 | £7374 | £272,840 | Dominated | £16,538 |
51 | Dominated | £22,689 | £13,137 | Dominated | Dominated | £152,507 |
52 | Dominated | Dominated | £59,560 | Dominated | Dominated | Dominated |
53 | Dominated | Dominated | Dominated | Dominated | Dominated | Dominated |
60 | Dominated | Dominated | Dominated | Dominated | Dominated | Dominated |
Threshold (95% CrI) | 48 (44 to 52) | 50 (48 to 52) | 51 (48 to 52) | 48 (46 to 50) | 38 (25 to 44) | 50 (48 to 52) |
For patients with a mental score of 70 (indicating very good mental health), the highest possible physical score is 42. The threshold physical score is above the maximum that can be achieved at this mental score for all groups other than 90-year-olds (Table 44) and 50- or 80-year-old men (see Online Supplement 13). Averaging across all ages, the threshold physical score is 44 (95% CrI 42 to 46) (see Table 44); this is 2 points higher than the maximum achievable.
SF-12 physical score | Cost | |||||
---|---|---|---|---|---|---|
Age (years) | ||||||
50 | 60 | 70 | 80 | 90 | Average | |
12 | Dominant | Dominant | £274 | £845 | £2630 | £314 |
15 | Dominant | Dominant | £353 | £1000 | £3189 | £407 |
20 | Dominant | £81 | £508 | £1309 | £4485 | £589 |
25 | Dominant | £209 | £695 | £1706 | £6689 | £813 |
30 | Dominant | £373 | £940 | £2282 | £11,907 | £1118 |
31 | £5 | £414 | £1002 | £2437 | £14,066 | £1197 |
32 | £44 | £459 | £1070 | £2614 | £17,205 | £1285 |
33 | £89 | £511 | £1147 | £2818 | £22,217 | £1386 |
34 | £141 | £569 | £1235 | £3057 | £31,542 | £1501 |
35 | £204 | £637 | £1336 | £3342 | £55,118 | £1637 |
36 | £282 | £717 | £1454 | £3690 | £233,756 | £1798 |
37 | £382 | £814 | £1594 | £4126 | Dominated | £1994 |
38 | £516 | £932 | £1763 | £4687 | Dominated | £2238 |
39 | £703 | £1083 | £1973 | £5442 | Dominated | £2551 |
40 | £986 | £1279 | £2241 | £6509 | Dominated | £2967 |
41 | £1461 | £1546 | £2594 | £8136 | Dominated | £3548 |
42 | £2421 | £1930 | £3080 | £10,919 | Dominated | £4416 |
Threshold (95% CrI) | 43 (38 to 46) | 44 (42 to 48) | 44 (44 to 48) | 43 (40 to 46) | 32 (25 to 38) | 44 (42 to 46) |
The ICERs and threshold SF-12 physical scores varied with age, with 70-year-olds having the highest threshold SF-12 physical score and (generally) the lowest ICERs. Thresholds were markedly lower for 90-year-olds. There was also substantially more uncertainty around thresholds for older patients, shown by the wider 95% CrI. Thresholds were between 0 and 5 points higher for women than for men for subgroups aged 50–80 years, with large differences between sexes for 90-year-olds (see Online Supplement 13).
The PSA demonstrated that we can be > 99% confident that TKA is cost-effective for 70-year-olds with SF-12 mental scores of 50 and SF-12 physical scores of ≤ 48 at a £20,000-per-QALY ceiling ratio (Figure 22). However, as would be expected, there is substantially more uncertainty around cost-effectiveness for patients around the threshold: at a mental score of 50, the probability that TKA is cost-effective for patients with a SF-12 physical score of 50 is 75%, which decreases to 40% for patients with a physical score of 52 and to 0% for patients with a score of 56. The level of uncertainty was similar across the range of mental scores, but was markedly greater for 90-year-olds.
FIGURE 22.
Effect of the SF-12 score on the probability that TKA is cost-effective at a £20,000-per-QALY ceiling ratio.

Effect of the Oxford Hip Score on cost-effectiveness of total hip arthroplasty
The incremental cost of THA compared with no arthroplasty increased with the OHS, from £779 per patient at an OHS of 0 up to £4258 at an OHS of 48 (averaged across men and women of all ages). THA was less costly than conducting no arthroplasty for 50- and 60-year-old patients with an OHS of 0 or 1, because the cost of non-surgical management was highest for patients with very low OHSs. The QALY gains from surgery were highest for patients with an OHS of 6 or 7: THA produced an increase of 3.68 QALYs for the average patient with an OHS of 0, 4.47 QALYs at an OHS of 6 and –0.61 QALYs at an OHS of 48. Therefore, conducting THA dominated no arthroplasty for 50- and 60-year-olds with an OHS of 0 or 1, given that it was less costly and generated more QALYs.
The threshold OHS above which THA ceased to be cost-effective was 43 (95% CrI 42 to 43) for 70-year-olds, but fell to 38 (95% CrI 34 to 41) for 90-year-olds (Table 45). The analysis averaging across all age groups suggested that if it was not acceptable to have different thresholds for different age groups, a single threshold of 42 (95% CrI 42 to 42) would be most appropriate. PSA demonstrated that there is very little uncertainty around the economic threshold, with 95% of the 2000 PSA replicates indicating a threshold of 42 when results were averaged across men and women of all age groups. The difference between men and women was negligible: the threshold was 1 point higher for 80-year-old men and 1 point lower for 70-year-old women, although thresholds for men and women were otherwise the same as those shown in Table 45 (see Online Supplement 13).
Preoperative OHS (selected values only) | Cost | |||||
---|---|---|---|---|---|---|
Age (years) | ||||||
50 | 60 | 70 | 80 | 90 | Average | |
0 | Dominant | Dominant | £326 | £571 | £1208 | £212 |
10 | £630 | £616 | £737 | £958 | £1885 | £779 |
20 | £1055 | £966 | £1029 | £1273 | £2171 | £1105 |
30 | £1936 | £1699 | £1762 | £2214 | £4101 | £1928 |
35 | £3080 | £2600 | £2737 | £3556 | £7682 | £3027 |
36 | £3480 | £2902 | £3071 | £4042 | £9310 | £3410 |
37 | £3994 | £3280 | £3497 | £4680 | £11,816 | £3901 |
38 | £4682 | £3769 | £4058 | £5556 | £16,172 | £4554 |
39 | £5648 | £4426 | £4830 | £6832 | £25,631 | £5467 |
40 | £7105 | £5355 | £5960 | £8865 | £61,836 | £6832 |
41 | £9557 | £6769 | £7775 | £12,616 | Dominated | £9097 |
42 | £14,554 | £9187 | £11,169 | £21,853 | Dominated | £13,592 |
43 | £30,346 | £14,265 | £19,787 | £81,441 | Dominated | £26,810 |
44 | Dominated | £31,771 | £86,085 | Dominated | Dominated | £910,589 |
45 | Dominated | Dominated | Dominated | Dominated | Dominated | Dominated |
46 | Dominated | Dominated | Dominated | Dominated | Dominated | Dominated |
47 | Dominated | Dominated | Dominated | Dominated | Dominated | Dominated |
48 | Dominated | Dominated | Dominated | Dominated | Dominated | Dominated |
Threshold (95% CrI) | 42 (41 to 43) | 43 (43 to 43) | 43 (42 to 43) | 41 (41 to 42) | 38 (34 to 41) | 42 (42 to 42) |
The PSA demonstrated that there was relatively little uncertainty around cost-effectiveness for patients aged ≤ 80 years (Figure 23). For 70-year-olds, the probability that THA cost < £20,000 per QALY gained was > 99% for patients with an OHS of ≤ 42 and fell to 47% for patients with an OHS of 43 and to < 0.1% for patients with an OHS of ≥ 44. Uncertainty was markedly greater for 90-year-olds over a wide range of OHSs.
FIGURE 23.
Effect of the OHS on the probability that THA is cost-effective at a £20,000-per-QALY ceiling ratio.
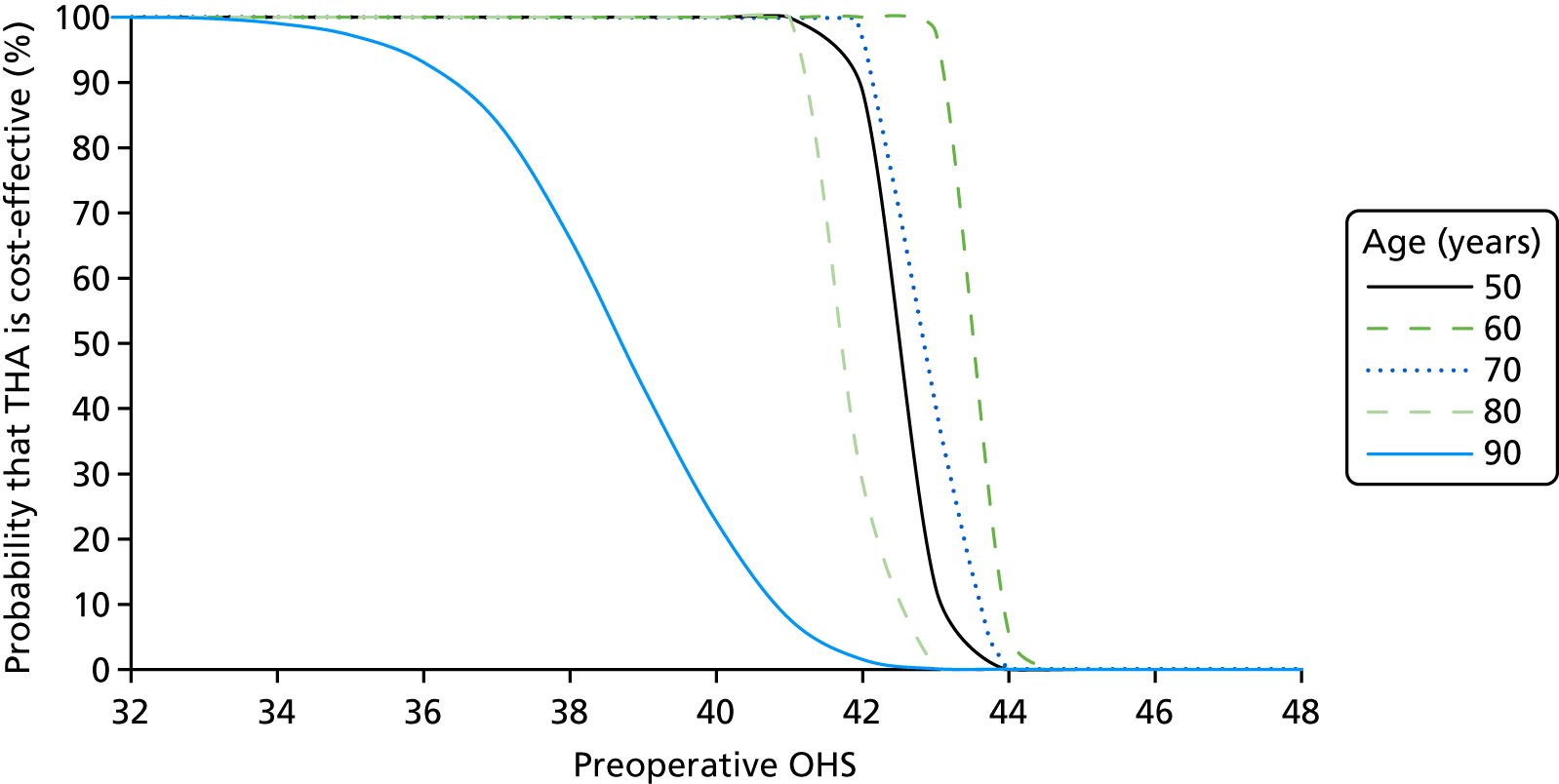
Effect of the Western Ontario and McMaster Universities Arthritis Index on the cost-effectiveness of total hip arthroplasty
The QALY gains from THA were highest for patients with low WOMAC scores (mean incremental QALYs at a score of 0 = 8.228) and decreased for patients with higher scores (mean incremental QALYs at a score of 50 = 3.054, and at a score of 100 = 0.289). In contrast, the model results suggest that WOMAC scores do not predict costs of THA compared with no arthroplasty. The mean incremental costs were around £3200, regardless of WOMAC score.
Total hip arthroplasty was cost-effective at almost all WOMAC scores (Table 46). The only exception was for patients aged 90 years and those with a WOMAC score of 100 (the best possible score). In contrast to the results for TKA, the 95% CrIs indicate little uncertainty about the threshold; for almost all age groups, the CrIs range from 98 to 100. There are almost no differences in cost-effectiveness between men and women, the only exception being patients aged 90 years with a clinical score of 99, among whom THA is cost-effective for women but not for men. Again, it should be noted that there were no patients observed at these scores in the available data sets.
Preoperative WOMAC score (rescaled to 0–100; 0 = poor function; selected values only) | Cost | |||||
---|---|---|---|---|---|---|
Age (years) | ||||||
50 | 60 | 70 | 80 | 90 | Average | |
0 | £372 | £366 | £399 | £499 | £743 | £424 |
10 | £427 | £418 | £454 | £574 | £878 | £485 |
20 | £510 | £496 | £537 | £683 | £1060 | £577 |
30 | £624 | £604 | £652 | £829 | £1294 | £702 |
40 | £762 | £735 | £790 | £1005 | £1570 | £852 |
50 | £922 | £885 | £949 | £1205 | £1885 | £1024 |
60 | £1115 | £1065 | £1137 | £1441 | £2257 | £1229 |
70 | £1395 | £1323 | £1406 | £1777 | £2791 | £1522 |
80 | £1915 | £1796 | £1899 | £2392 | £3774 | £2060 |
85 | £2391 | £2222 | £2342 | £2944 | £4663 | £2545 |
90 | £3227 | £2952 | £3094 | £3879 | £6188 | £3373 |
95 | £5069 | £4484 | £4650 | £5804 | £9402 | £5104 |
96 | £5742 | £5019 | £5186 | £6465 | £10,529 | £5706 |
97 | £6631 | £5707 | £5870 | £7306 | £11,980 | £6478 |
98 | £7861 | £6623 | £6771 | £8411 | £13,919 | £7504 |
99 | £9672 | £7906 | £8016 | £9929 | £16,644 | £8933 |
100 | £12,610 | £9830 | £9844 | £12,148 | £20,753 | £11,064 |
Threshold (95% CrI) | 100 (98 to 100) | 100 (99 to 100) | 100 (99 to 100) | 100 (98 to 100) | 99 (96 to 100) | 100 (99 to 100) |
The PSA suggested that there was relatively little parameter uncertainty within the model: the probability that THA was cost-effective at a £20,000-per-QALY ceiling ratio was ≥ 99% for all patients with WOMAC scores of ≤ 90 (Figure 24).
FIGURE 24.
Effect of the WOMAC on the probability that THA is cost-effective at a £20,000-per-QALY ceiling ratio.
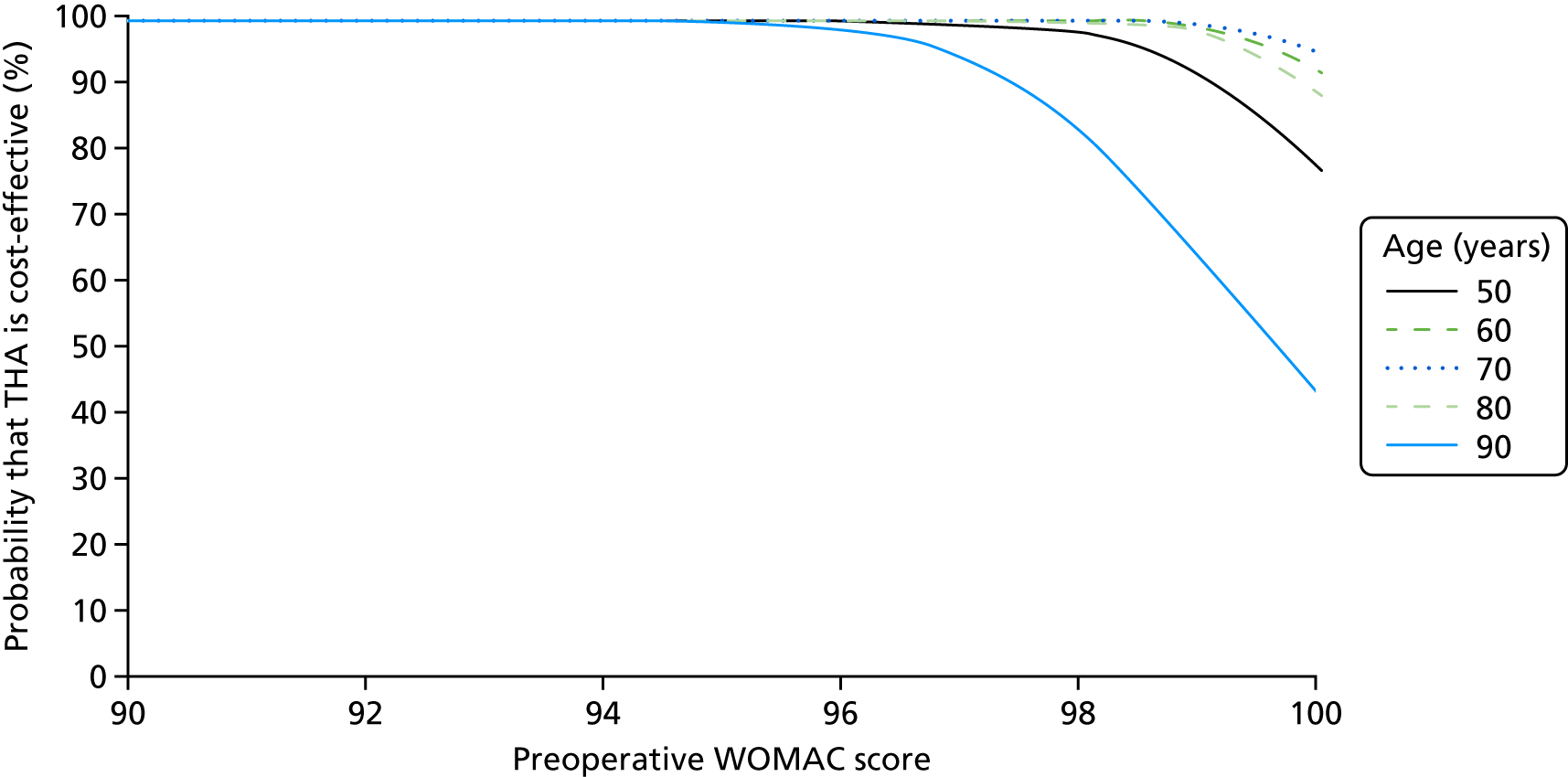
Effect of the Short Form questionnaire-12 items on the cost-effectiveness of total hip arthroplasty
For patients with SF-12 mental scores of 30 or 50, incremental QALYs were highest at physical scores of between 18 and 28 and were markedly lower for patients with higher or lower scores. By contrast, for patients with a mental score of 70, incremental QALYs were highest at a physical score of 12 and declined steadily with an increasing physical score. For all three mental scores, the incremental cost of TKA versus no arthroplasty changed very little with SF-12 physical score. For example, for a mental score of 50, the average incremental cost (across men and women of all ages) decreased from £4267 per patient at a physical score of 19 to £3058 per patient at a physical score of 60, whereas incremental QALYs decreased from 3.42 to –0.26. As was the case for TKA, THA was dominated by no arthroplasty (generating fewer QALYs at a higher cost) for patients with very high physical scores. However, THA was also dominated for 90-year-old patients with extremely low physical scores and below-average mental scores. For patients with very poor mental health (a mental score of 30), the threshold physical score was 59 (95% CrI 56 to 60), averaging across all ages (Table 47). Thresholds were slightly lower for patients with a mental score of 50 (i.e. average mental health), for whom the threshold physical score was 56 (95% CrI 54 to 58), averaging across ages (Table 48).
Preoperative SF-12 physical score (selected values only) | Cost | |||||
---|---|---|---|---|---|---|
Age (years) | ||||||
50 | 60 | 70 | 80 | 90 | Average | |
18 | £1268 | £1096 | £1204 | £2051 | Dominated | £1439 |
19 | £1205 | £1057 | £1166 | £1930 | Dominated | £1376 |
20 | £1159 | £1028 | £1138 | £1843 | £103,982 | £1329 |
21 | £1124 | £1007 | £1117 | £1778 | £23,002 | £1294 |
22 | £1098 | £991 | £1102 | £1730 | £13,811 | £1268 |
30 | £1043 | £977 | £1106 | £1650 | £5200 | £1236 |
40 | £1094 | £827 | £936 | £1659 | £5086 | £1151 |
50 | £1293 | £1245 | £1467 | £2448 | £11,212 | £1690 |
51 | £1392 | £1340 | £1590 | £2708 | £13,914 | £1837 |
52 | £1516 | £1456 | £1745 | £3049 | £18,758 | £2022 |
53 | £1672 | £1603 | £1945 | £3514 | £29,816 | £2262 |
54 | £1877 | £1794 | £2209 | £4180 | £79,333 | £2585 |
55 | £2154 | £2050 | £2573 | £5208 | Dominated | £3029 |
56 | £2547 | £2407 | £3104 | £6981 | Dominated | £3694 |
57 | £3144 | £2938 | £3941 | £10,715 | Dominated | £4781 |
58 | £4148 | £3801 | £5435 | £23,396 | Dominated | £6853 |
59 | £6164 | £5423 | £8798 | Dominated | Dominated | £12,230 |
60 | £12,140 | £9504 | £22,862 | Dominated | Dominated | £56,760 |
Threshold (95% CrI) | 60 (58 to 60) | 60 (58 to 60) | 59 (58 to 60) | 57 (54 to 60) | 52 (11 to 56) | 59 (56 to 60) |
Preoperative SF-12 physical score (selected values only) | Cost | |||||
---|---|---|---|---|---|---|
Age (years) | ||||||
50 | 60 | 70 | 80 | 90 | Average | |
12 | £1226 | £1091 | £1214 | £1987 | £580,230 | £1419 |
15 | £1079 | £996 | £1120 | £1711 | £7280 | £1268 |
20 | £1045 | £993 | £1050 | £1670 | £4454 | £1222 |
30 | £864 | £849 | £976 | £1488 | £4257 | £1103 |
40 | £1110 | £1105 | £1310 | £2051 | £4913 | £1455 |
50 | £2060 | £2056 | £2625 | £4737 | £17,623 | £2933 |
51 | £2311 | £2304 | £2990 | £5621 | £26,282 | £3351 |
52 | £2643 | £2630 | £3485 | £6936 | £52,926 | £3923 |
53 | £3103 | £3075 | £4189 | £9078 | Dominated | £4748 |
54 | £3772 | £3715 | £5257 | £13,126 | Dominated | £6027 |
55 | £4826 | £4699 | £7046 | £23,438 | Dominated | £8244 |
56 | £6702 | £6382 | £10,592 | £99,530 | Dominated | £12,941 |
57 | £10,892 | £9850 | £20,671 | Dominated | Dominated | £29,016 |
58 | £27,878 | £20,768 | £218,915 | Dominated | Dominated | Dominated |
59 | Dominated | Dominated | Dominated | Dominated | Dominated | Dominated |
60 | Dominated | Dominated | Dominated | Dominated | Dominated | Dominated |
Threshold (95% CrI) | 57 (54 to 58) | 57 (56 to 58) | 56 (54 to 58) | 54 (52 to 56) | 50 (40 to 54) | 56 (54 to 58) |
The threshold physical score for patients with a mental score of 70 (i.e. very good mental health) was substantially higher than the maximum that can be achieved at this mental score for all age groups (Table 49). Averaging across all ages, the models predicted that the threshold physical score is 55 (95% CrI 52 to 56) for a mental score of 70: 13 points higher than the maximum achievable score (42).
Preoperative SF-12 physical score (selected values only) | Cost | |||||
---|---|---|---|---|---|---|
Age (years) | ||||||
50 | 60 | 70 | 80 | 90 | Average | |
12 | £940 | £855 | £817 | £1419 | £3356 | £1020 |
15 | £691 | £662 | £730 | £1061 | £2976 | £826 |
20 | £749 | £726 | £809 | £1168 | £2572 | £898 |
25 | £836 | £816 | £921 | £1333 | £2709 | £1014 |
30 | £946 | £929 | £1063 | £1552 | £3094 | £1165 |
35 | £1096 | £1081 | £1256 | £1861 | £3700 | £1373 |
40 | £1328 | £1317 | £1562 | £2375 | £4822 | £1703 |
41 | £1393 | £1383 | £1649 | £2524 | £5169 | £1797 |
42 | £1467 | £1458 | £1748 | £2698 | £5586 | £1904 |
Threshold (95% CrI) | 57 (54 to 58) | 57 (56 to 58) | 56 (54 to 58) | 54 (52 to 56) | 50 (40 to 54) | 56 (54 to 58) |
The PSA suggested that there was very little uncertainty around the results for 70-year-olds (Figure 25). The probability that THA costs < £20,000 per QALY gained was > 99% for 70-year-olds with SF-12 physical scores of ≥ 52 and fell sharply as physical scores increased. However, there was markedly greater uncertainty for 90-year-olds across the range of physical scores.
FIGURE 25.
Effect of the SF-12 physical score on the probability that THA is cost-effective at a £20,000-per-QALY ceiling ratio.

Sensitivity analysis
Sensitivity analyses demonstrated that the results are reasonably robust to large changes in two key assumptions (Table 50). Reducing the time horizon from 10 to 5 years reduced thresholds slightly, because it reduces the duration for which patients are assumed to experience the postarthroplasty EQ-5D utility. Conversely, increasing the time horizon to 60 years had minimal impact on thresholds.
Sensitivity analysis | Economic threshold for TKA ignoring age and sex | Economic threshold for THA ignoring age and sex | ||||||||
---|---|---|---|---|---|---|---|---|---|---|
OKS | WOMAC score | SF-12 physical and mental scores of: | OHS | WOMAC score | SF-12 physical and mental scores of: | |||||
30 | 50 | 70 | 30 | 50 | 70 | |||||
Base-case analysis | 40 | 98 | 58 | 49 | 44 | 42 | 100 | 59 | 56 | 56 |
5-year time horizon | 38 | 94 | 57 | 48 | 43 | 41 | 99 | 59 | 55 | 53 |
60-year (lifetime) time horizon | 40 | 98 | 57 | 49 | 44 | 42 | 100 | 60 | 57 | 55 |
EQ-5D utility without TJA worsens by 0.025 per year | 46 | 100 | 59 | 54 | 50 | 47 | 100 | 60 | 60 | 60 |
EQ-5D utility without TJA increases by 0.115 in the first year and follows age-related decline thereafter | 33 | 80 | 52 | 43 | 37 | 36 | 89 | 55 | 51 | 47 |
In the base-case analysis, we assumed that patients’ osteoarthritis symptoms would remain at a constant level in the absence of arthroplasty, with EQ-5D utility reducing by around 0.0036–0.0069 per year because of ageing. Increasing the rate at which EQ-5D utility decreases to an arbitrary rate of 0.025 per year (the smallest difference in valuations that was possible in the EQ-5D valuation study168) substantially increased all thresholds, because it reduced the number of QALYs accrued in the no arthroplasty arm. We also conducted an analysis that assumed that EQ-5D utility for patients in the no arthroplasty arm would increase by 0.115 in year 1 (the mean EQ-5D change in a trial comparing arthroplasty against an intensive non-surgical management intervention)169 and then decline at the same age-dependent rate assumed in the base-case analysis; this analysis substantially reduced thresholds.
Discussion
Summary of the results
We were able to calculate thresholds for TKA and THA for the OKS, OHS, WOMAC and SF-12 physical scores that represent the highest score at which arthroplasty is cost-effective if the NHS is willing or able to pay £20,000 per QALY gained (Table 51). These thresholds were generally somewhat higher than the relative thresholds calculated in Chapter 4, but a little lower than the absolute thresholds. The economic thresholds are more similar to the absolute thresholds than relative thresholds as they are based on the score at which there is zero difference in net benefits, rather than a MID. However, unlike the clinical analyses, the economic evaluation measures outcomes using the EQ-5D rather than the score in question and takes account of mortality, revisions, complications and costs. The economic evaluation also explicitly compares costs and outcomes with arthroplasty against the costs and outcomes that are expected to be accrued without arthroplasty. Unlike the relative thresholds described in Chapter 4, the economic thresholds also evaluate outcomes on a continuous scale (being more like the linear regression models described in Chapter 4 than the logistic regression models) and are also based on the average (mean) benefit, rather than the probability of benefit. No MID is imposed on the data, and the analysis directly takes account of operative mortality and the cost of surgery and subsequent follow-up and revision surgery. Because arthroplasty is relatively cheap and produces such large QALY gains for those people who benefit, it is cost-effective for almost all patients who are expected to have any improvement in quality of life.
Clinical tool | Threshold (95% CrI) | QALYs gained from stratifyinga | Number of operations avoideda |
---|---|---|---|
Knees | |||
OKS | |||
Economic thresholdb | 40 (39 to 42) | 53 | 219 |
Clinical relative thresholdc | 32 | –2020 | 3342 |
WOMAC | |||
Economic thresholdb | 99 (80 to 100) | 0 | 0 |
Clinical relative thresholdc | 71 | –4234 | 4813 |
SF-12d | |||
Economic thresholdb | 50 (48 to 52) | 867 | 1584 |
Clinical relative thresholdc | 37 | –15,655 | 17,326 |
Hips | |||
OHS | |||
Economic thresholdb | 42 (42 to 42) | 25 | 100 |
Clinical relative thresholdc | 37 | –314 | 814 |
WOMAC | |||
Economic thresholdb | 100 (99 to 100) | 0 | 0 |
Clinical relative thresholdc | 80 | –1775 | 1411 |
SF-12d | |||
Economic thresholdb | 56 (54 to 58) | 54 | 224 |
Clinical relative thresholdc | 35 | –22,389 | 11,566 |
We also calculated how the number of operations currently done in England would change using each of the six economic thresholds and the corresponding clinical relative thresholds, based on the distribution of scores in the best available data sets (see Online Supplement 12) and assuming that 76,617 knee replacements and 69,313 hip replacements are done each year (see Presentation of results and analysis of uncertainty). 154,165 This calculation has two parts: (1) operations currently done that would be avoided (based on those patients currently receiving operations who are above the threshold) and (2) operations not currently done that might be considered (based on patients who are below the threshold but at present are not listed for arthroplasty). The calculations presented in this chapter focused only on the first part (i.e. the number of current operations conducted on patients above the threshold).
If the economic threshold of 40 (ignoring age and sex) was applied to all 76,617 knee replacements done in England each year,154,165 then 219 operations could be avoided, because only 219 patients (0.29%) having knee replacement operations have an OKS of > 40 (see Table 51). If the NHS stopped doing knee arthroplasty on those 219 patients, the equivalent of 53 QALYs would be gained from avoiding undertaking surgery that would reduce quality of life in some of these 219 patients, and by saving money that could be spent on other patients who would gain additional health benefits. Similarly, applying the economic threshold of 42 would avoid 100 of the 69,313 hip replacements currently done each year,154,165 gaining 25 QALYs.
For comparison, the clinical analysis suggested a relative threshold for an OKS of 32; using this threshold, we would avoid 3342 operations, but this policy would be expected to reduce the amount of health benefits gained from the NHS budget by 2020 QALYs, by avoiding treatment in patients for whom knee replacement would improve quality of life. Similarly, the clinical relative threshold for hip replacement (37) would avoid 731 operations but reduce health by 284 QALYs.
The economic thresholds estimated for the WOMAC were extremely close to the top of the scale and WOMAC did not identify any patients aged < 90 years for whom THA is not cost-effective, nor any patients aged 60 or 70 years for whom TKA is not cost-effective. No patients recruited to the studies used to estimate EQ-5D utility and costs after TKA had WOMAC scores of > 87, whereas the highest score observed among THA patients was 93. Taken at face value, this implies that no patients who currently undergo surgery would be denied treatment based on the economic thresholds estimated in the analysis. However, owing to the lack of data in patients with high WOMAC scores, the results for patients with high WOMAC scores and the estimated WOMAC thresholds should be interpreted with caution as they are based on predictions extrapolated outside the range of the observed data. In particular, it seems implausible that TJA would be cost-effective in patients with a WOMAC score of 100, who would have no pain, stiffness or loss of function. Conversely, the clinical thresholds estimated for WOMAC are low relative to those for the OKS and OHS and would, therefore, be expected to avoid more operations, but with greater loss of patients’ health. Therefore, more research on larger data sets including patients with scores of > 85 is needed to estimate economic thresholds for the WOMAC.
The threshold SF-12 physical score varied markedly with SF-12 mental scores. As would be expected, thresholds were lower for patients with mental scores of 30 (poor mental health) than for patients with mental scores of 50 (average mental health). However, other than for TKA patients aged 90 years, the threshold physical score for patients with SF-12 mental scores of 70 (indicating very good mental health) was higher than the maximum score that can be achieved at that mental score. As a result, SF-12 cannot distinguish between those people who would benefit from arthroplasty and those who would not among those with very good mental health. Furthermore, the scoring of the SF-12 is such that people with very poor physical scores are assigned better mental scores than people who have good physical scores but the same level of mental health.
The model also suggested that THA (but not TKA) is poor value for money in 90-year-old patients with extremely low SF-12 physical scores and SF-12 mental scores of either 30 or 50. One possible explanation for this unexpected finding is that the generic SF-12 instrument captures a wide range of health problems, including comorbid conditions, whereas the OHS and WOMAC focus on hip or musculoskeletal symptoms. It is possible that these comorbid conditions are worsened by arthroplasty or that, in patients who have other conditions limiting their quality of life, THA produces relatively limited improvements in EQ-5D utility because patients still experience severe pain and limited function because of their other health problems. However, this result could also be an artefact of the way that SF-12 scores are calculated or the shortage of data in this patient group; only nine patients in EPOS were aged ≥ 90 years and no patients (of any age) had SF-12 mental scores of < 35 and SF-12 physical scores of < 22.
The results for the OKS are similar to those estimated previously for KAT by Dakin et al. :2 we estimate the economic threshold to be 40 (95% CrI 39 to 42), and Dakin et al. 2 estimated a threshold of 39 for patients with an ASA grade of 1 or 2 and a threshold of 34 for patients with an ASA grade of 3. However, the difference between men and women appears to be smaller in the current analysis than was suggested by Dakin et al. 2 These differences appear to be largely attributable to the differences in time horizon (5 years vs. 10 years in the current analysis), because the sensitivity analysis using a 5-year time horizon estimated the OKS threshold to be 38. Furthermore, the additional data sets available in this analysis (particularly COASt) enabled us to allow for the costs and quality-of-life changes likely to arise without arthroplasty. Although we used KAT data for costs and long-term outcomes, we also used data from published studies,50,112,119,125 COASt and national PROMs and used very different analytical methods, synthesising data from different sources in a Markov model rather than estimating total costs and total QALYs for individual trial participants in a within-trial analysis. In particular, PROMs provided a much larger sample of patients with very high OKSs. The current study also allowed for a wide range of non-linear models, which suggested that it may be appropriate to have lower thresholds for patients aged < 60 years. Given the differences in analytical approach and data inputs, the similarity of the thresholds in the two studies appears to suggest that the thresholds estimated are relatively robust.
Jenkins et al. 105 also estimated the relationship between the Oxford Hip and Knee Scores and QALYs for both TKA and THA, and Fordham et al. 68 assessed how cost-effectiveness varied with the OHS. Lavernia et al. 106 assessed how cost-effectiveness varied with preoperative WOMAC score and, unlike the current analysis, found that patients in the top quartile for WOMAC scores (with worst function and pain) had the largest QALY gains and lowest ICERs for THA. Whereas these previous studies had found arthroplasty to be highly cost-effective for most patients, Ferket et al. 108 found TKA to cost more than US$100,000 per QALY gained unless treatment was restricted to patients with SF-12 physical scores of ≤ 20. The high ICERs in the Ferket et al. 108 study appear to largely arise from the small estimates of the improvements in utility achieved through arthroplasty (0.008), which appear to contradict the findings of a RCT (which found the difference in EQ-5D utility between patients with and without TKA to be 0.078)169 and English National PROMs data (which observe a mean increase in EQ-5D utility of 0.310). The difference could reflect differences between EQ-5D and SF-12 utilities, use of propensity weights in the Ferket et al. 108 study or the small sample of US patients with mild osteoarthritis who were analysed by Ferket et al. 108 None of these studies estimated thresholds that can be directly compared with the current analysis.
Limitations
The economic evaluation compared immediate TJA with conducting no arthroplasty for 10 years. In practice, arthroplasty may simply be delayed in those patients who have high PROM scores. Although the choice of a 10-year time horizon is arbitrary, sensitivity analyses suggested that extending or shortening the time horizon had minimal effect on the results.
Whereas the NHS PROMs data provided Oxford Hip and Knee Scores for > 80% of all arthroplasty operations conducted in England,162 the data sets available for the WOMAC and SF-12 were < 2% of the size and included very few patients with very high scores, which meant that the economic threshold for the WOMAC could only be estimated by extrapolating beyond the observed data. Furthermore, the only data set providing preoperative costs (COASt) did not use the WOMAC or SF-12 and no data sets with preoperative WOMAC scores provided more than 1 year’s follow-up; as a result, it was necessary to estimate the relationship between the WOMAC and SF-12 and these outcomes indirectly via EQ-5D utilities. KAT was the only study using both the SF-12 and the EQ-5D. All estimates of improvements in EQ-5D utility and long-term changes in utility for the SF-12 in THA are therefore based on mapped utilities, which introduces additional errors not propagated through the analysis, and this could introduce bias. Unlike the Oxford Hip and Knee Scores, there are two available versions of the SF-12; in this analysis, we assumed that versions 1 and 2 are equivalent.
Equity implications
At present, GPs, commissioning groups and hospitals use a wide variety of different tools and mechanisms to determine eligibility for arthroplasty. 170–176 As a result, patients’ access to surgery depends on where they live, not just their clinical need. Furthermore, the thresholds used by commissioning groups, such as 19 or 24,170,171,176 are far below the score at which arthroplasty ceases to be cost-effective and, therefore, thousands of patients who would get substantial benefit from surgery are denied treatment. In principle, introducing an evidence-based threshold across the country could reduce postcode rationing and improve equity of access.
The results suggest that a modest amount of population health could be gained by setting different thresholds for patients of different ages. Focusing on those patients currently undergoing surgery and ignoring the large numbers of patients who are currently not being referred for treatment suggests that society could avoid 219 operations and gain 53 QALYs by stopping all operations on patients with OKSs of > 40, but could avoid 247 operations and gain 62 QALYs by having different thresholds for patients of different ages. Similarly, for the OHS, we could avoid 100 operations and gain 25 QALYs using a fixed threshold of 42, and could avoid 114 operations and gain 29 QALYs by having different thresholds for patients of different ages. However, restricting access to care by age as well as clinical need raises equity and ethics concerns. NICE guidance may refer only to age if age is a good indicator of health status or response to treatment and/or adverse effects, or if there is no practical way of identifying patients other than by age. 98 Our regression models and those identified in our literature review demonstrate that age does influence mortality, revision rates, quality of life and the cost of surgery, suggesting that setting different thresholds by age could be clinically justifiable. However, we found the effect of age on the estimates of the economic thresholds to be generally quite small and most noticeable in the highest age groups (e.g. ≥ 90 years) in which very few data were available. Moreover, the effect of age is likely to arise largely through physical activity, general health and comorbidities, which have not been taken into account in this analysis, and it may be possible to identify those patients likely to have minimal benefit or greatest risk based on these characteristics, rather than relying on age.
In principle, thresholds could also vary by gender; thresholds for the OHS were 1 point lower for women aged 70 years and 1 point higher for men aged 80 years, although OKS thresholds were the same for men and women. However, a policy varying thresholds by sex would avoid no knee operations and only three hip operations and gain 0.25 QALYs across England compared with thresholds that varied only by age. These modest gains are unlikely to be sufficient to justify the additional complexity or the equity implications of rationing access to care by gender.
Implementation issues
Our results suggest that population health could be improved by introducing thresholds that are markedly higher than those used by most hospitals and commissioning groups. If such thresholds were introduced, it is highly likely that more patients would come forward for surgery and be referred for surgical assessment. This would require additional funding for arthroplasty procedures. Our results suggest that health would be improved by increasing spending on arthroplasty in preference to less cost-effective interventions. However, to achieve these health gains, less cost-effective interventions would have to be identified and deprioritised. Furthermore, capacity constraints of trained surgeons and operating theatres would have to be addressed.
The current analysis is based on UK data and may not necessarily be generalisable to other countries, particularly those in which the cost of TJA or non-surgical management are very different. The analysis also focuses on TJA for osteoarthritis; the costs and benefits of unicompartmental knee arthroplasty or hip resurfacing may also differ from those of total arthroplasty, and the risks and benefits of arthroplasty may be very different for patients with conditions other than osteoarthritis.
Additional findings
The models developed to map from the WOMAC and SF-12 to EQ-5D utilities could also be applied in other settings in which WOMAC or SF-12 data are available, but EQ-5D utilities are not. Coefficients for these models are shown in Online Supplement 12. However, owing to the nature of our analysis, we focused on models based on the WOMAC total score and the SF-12 physical and mental scores; more accurate models could have been obtained by exploring the effect of subdomain scores or dummy variables for individual questions. Our analyses suggested that published models mapping from the SF-12 onto EQ-5D utility that were estimated on general public samples performed poorly in knee arthroplasty candidates,110,158,177 probably because these patients have low utility compared with the general public. This highlights the importance of selecting a mapping model that has been developed or validated in the sample of interest.
Conclusion
In conclusion, economic thresholds have been estimated for the OKS, OHS, WOMAC and SF-12 physical score, which reflect the incremental costs and benefits of total hip/knee arthroplasty compared with conducting no arthroplasty. However, there is a shortage of data on the WOMAC, particularly from patients with very high scores, which means that we cannot identify patients for whom arthroplasty is not cost-effective based on the available data. The threshold SF-12 physical score varies with mental score and, among those patients with very good mental health, the SF-12 physical score cannot identify any patients for whom arthroplasty is not cost-effective. The economic thresholds for the Oxford Hip and Knee Scores are somewhat higher than the relative clinical thresholds estimated in Chapter 4 and are markedly higher than those currently used in clinical practice. There is some evidence that modest additional health gains could be achieved by using different thresholds for patients of different ages, but setting different thresholds for men and women is unlikely to be justifiable.
Chapter 6 Further analysis of threshold values (work package 2)
Background
Based on the work presented in the previous chapters and after input from the user group (see Chapter 10), the Oxford Hip and Knee Scores were selected as the candidate tools. In this chapter, relative thresholds for the OHS and OKS were estimated through more advanced modelling approaches using the NHS PROMs data set (2009–15) linked to HES. It was agreed in the third user group meeting to develop the ACHE tool based on the principle of the capacity of a patient to benefit from surgery. To do this, more detailed information was required on the effect of the patient’s preoperative score on the potential range of outcomes. The data could then be incorporated into delivering the ACHE tool.
Research aims
The aims of this work were to:
-
estimate the postoperative Oxford score using the respective hip and knee preoperative scores and the respective relative thresholds using an improvement criterion based on an individual-level ROC estimate
-
assess the influence and prognostic value of candidate baseline covariates to predict postoperative improvement in the Oxford Hip and Knee Scores.
Methods
General approach
Two modelling approaches were used for analyses of both of the Oxford scores. First, third-degree polynomial-based quantile regression models were used to estimate the change score (postoperative to preoperative) using the preoperative Oxford score. Quantile regression seeks to extend the ideas, that the population could be divided into several segments using quantiles or percentiles, to develop the models in which the quantiles of the conditional distribution of the response variable are expressed as functions of observed covariates. 178 The 10th, 20th, 30th and 50th percentile models were produced and the corresponding accuracy was assessed by visually comparing the estimated values, again with the observed percentile values across the preoperative score range. From these relative threshold values, the preoperative score for which the respective percentile matches the respective improvement criteria was estimated (see Improvement criteria for further details). In addition, we compared the percentile model (from 1st to 99th) of those achieving an important difference against the observed proportion of individuals who improved in accordance with the improvement criteria. Given the very large size of the available data set, modelling performance was internally validated by assessing sensitivity to key factors (time period, gender and age) through carrying out subset analyses and not by selecting a random sample or bootstrapping approach.
The second approach used fractional polynomial logistic regressions. Using this approach, the benefit of the baseline covariates on the capacity of benefit was investigated. Fractional polynomial regression was introduced in terms of parsimonious modelling. 179
The baseline covariates investigated in the logistic regression models were age (as a continuous variable), gender, the presence or absence of 12 patient-reported comorbidities (heart disease, high blood pressure, problems caused by stroke, leg pain when walking owing to poor circulation, lung disease, diabetes, kidney disease, disease of the nervous system, liver disease, cancer, depression and arthritis) and symptom period (up to 5 years or 6 to ≥ 10 years). For age, the optimal relationship was assessed individually between the variables using the fractional polynomial logistic regression when the default model included the preoperative score. Comorbidities were assessed simultaneously with only those significant and with a log-odds ratio (OR) of ≥ 0.8 remaining a candidate. For each of the candidate covariates, the discriminative performance with only that covariate in addition to the preoperative score was calculated. Covariates were selected for inclusion in the final model if the AUC with only the candidate covariate was ≥ 0.01 greater than the AUC for the model with the preoperative score included. The performance of the final model was assessed in terms of discrimination and calibration by calculating the AUC and producing calibration plots. Sensitivity and specificity values for the estimated relative threshold were calculated with corresponding 95% CIs. Complete-case analyses were conducted throughout. Stata® version 14 was used for all of the further statistical analyses looking at the threshold.
Data set
The NHS PROMs linked to HES (2009–15) was used. The inclusion criterion was that patients must have undergone a primary procedure and those undergoing revision procedures were excluded. Using PROMs and HES variables, primary only were selected. First, patients who have the same procedure [as identified by ‘PROMS_PROC_CODE’ (a code identifying the type of procedure that the patient underwent)] carried out on the same side of the body more than once (e.g. two primary hip replacements of the left hip) were excluded based on the PROMs data. Using the variables ‘EPISODE_MATCH_RANK’ [a score is attributed to each part of the linking process, in which the quality of the match is denoted by the rank with the lowest rank (i.e. 1) being the highest-quality match; the scores for each possible match are compared and the highest match is chosen] and ‘EPIKEY’, the PROMs and HES data were linked and duplicate entries were identified and removed. The former variable ensured that the highest-quality match between HES and PROMs is listed first, and the latter variable ensures that a unique sorting is produced. Based on this sorting, we dropped the second observations from the duplicate pairs. In addition, patients who answered that they have not had previous surgery of the type they were going to undergo were included, using the ‘Q1_PREVIOUS_SURGERY’ variable.
Approval
We successfully applied for access to the NHS PROMs/HES-linked data from NHS Digital (reference NIC-392690-F7H2Q).
Improvement criteria
Patient benefit can be defined in various ways. We restricted the definition to improvement in OHS and OKS patient-reported scores using standard approaches. Definitions of improvement were applied in this chapter as follows:
-
B. medium ES (0.5) × SD of change score (MCID) – 5 (OHS and OKS)
-
E. anchor-based best cut-off point using the individual-level ROC approach from the literature – 8 (OHS) or 7 (OKS).
A previously reported anchor-based best cut-off point was applied (using the NHS PROMs data set from 2009 to 2011). 90 This was a ROC curve analysis with the probability of correctly identifying patients based on an patient-reported anchor, ‘a little better’ versus ‘the same’ from the post-surgery evaluation in the questionnaire. When it was used at an individual level (MIC from ROC analysis), any changes in the OHS and OKS beyond 8 and 7 points, respectively, were considered as ‘clinically relevant’ changes. 75 These values lead (under improvement criterion E) to absolute threshold values for the OHS and OKS of 40 and 41 points, respectively. Corresponding values for definition B were as previously: 43 for both scores.
In addition, we also applied a 0.5 (medium, moderate practical importance) ES approach [as classified by Cohen180] using the variability of the change scores as an approach consistent with work package 2. It was calculated simply by using the SD of change scores of the candidate tools multiplied by the medium ES (0.5): 0.5 × SD of change score (criterion B). 181
Results
Descriptive statistics
A total of 644,656 observations were available for PROMs data linked to HES (2009–15). After dropping the identified duplicates from the data set linked to HES, there were 602,287 observations remaining (289,808 for hip and 312,479 for knee). Excluding those patients who had not confirmed in the PROMs questionnaire whether or not they had undergone previous surgery on the respective side reduced the data set further (to 575,980; 277,786 for hip and 298,194 for knee). Of these, only a subset of patients who had completed both the preoperative and the postoperative questionnaires were included, using the ‘Q1 and Q2 Complete’ variables (443,262; 214,038 for hip and 229,224 for knee). In addition, patients who had submitted both the preoperative and the postoperative hip questionnaires with sufficient procedure-specific data to derive scores were included, using the ‘Q1 and Q2 Complete’ variables (209,761 for hip and 222,933 operations) (Figure 26).
FIGURE 26.
Flow diagrams for hip and knee PROMs linked to HES data sets. (a) Hip; and (b) knee.

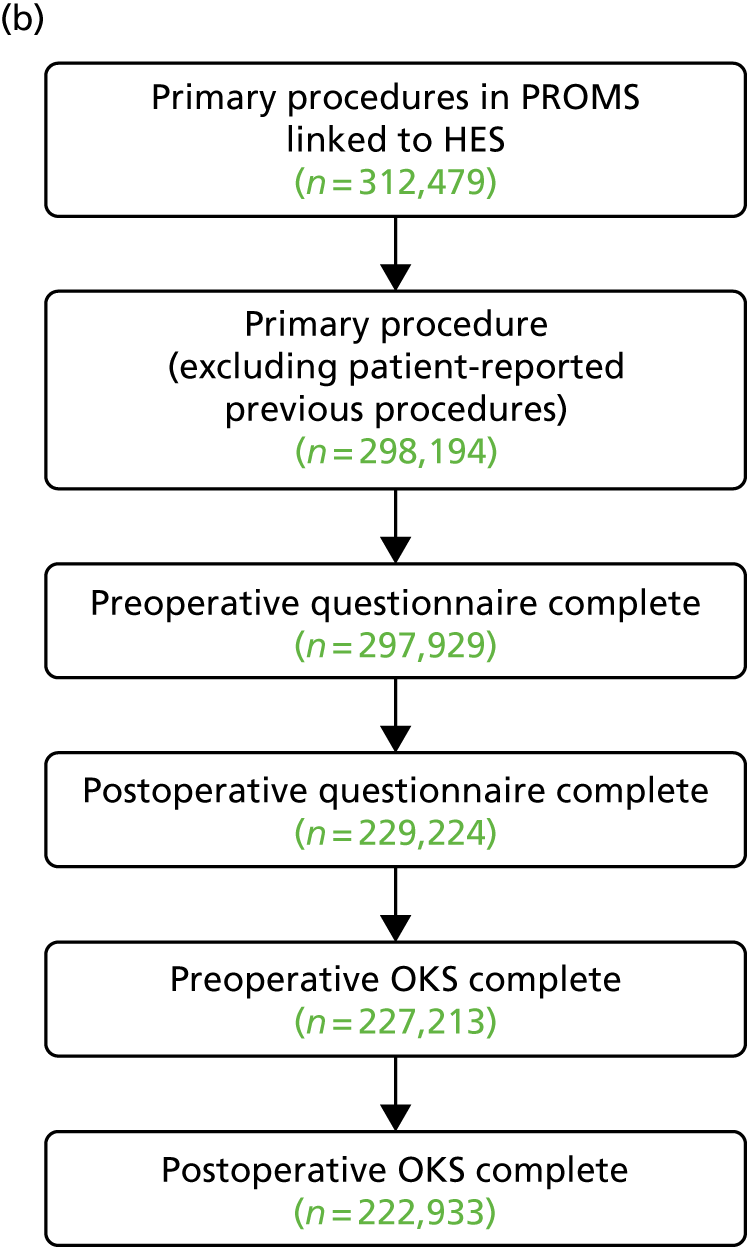
Demographics
The patient characteristics for those included in the matched hip and knee data sets is provided in Table 52. In total, 209,761 hip replacement surgeries were included; over half (125,058; 59.6%) were female, with ages ranging from 13 to 100 years, with a mean age of 69 years. In total, 96,041 NHS PROMs linked to HES (45.8%) were measured in 2009–11. In total, 222,933 knee replacement surgeries were included; over half (126,885; 56.9%) were female, with ages ranging from 18 to 99 years, with a mean age of 69.6 years. In total, 96,041 NHS PROMs linked to HES (45.8%) were measured for hip replacements and 102,448 (50.0%) were measured for knee replacements in 2009–11. In total, 113,720 (54.2%) post-operation scores were completed for hip and 120,485 (54.1%) for knee in 2012–15. Figure 27 shows histograms of the OHS and OKS scores pre and post surgery.
Characteristic | Hip replacement (N = 209,760) | Knee replacement (N = 222,933) |
---|---|---|
Age (years) | ||
Mean | 68.37 | 69.52 |
SD | 10.46 | 8.92 |
Minimum | 13 | 16 |
Maximum | 100 | 102 |
Age category (years), n (%) | ||
< 60 | 37,904 (18.07) | 29,349 (13.16) |
60–79 | 144,064 (68.68) | 164,132 (73.62) |
≥ 80 | 27,793 (13.25) | 29,452 (13.21) |
Gender, n (%) | ||
Male | 84,673 (40.37) | 96,006 (43.06) |
Female | 125,058 (59.62) | 126,885 (56.92) |
Comorbidity, n (%) | ||
Heart disease | 19,679 (9.38) | 23,340 (10.47) |
High blood pressure | 82,428 (39.30) | 102,542 (46.00) |
Stroke | 2912 (1.39) | 3733 (1.67) |
Circulation | 11,968 (5.71) | 16,464 (7.39) |
Lung disease | 15,592 (7.43) | 18,571 (8.33) |
Diabetes | 18,449 (8.80) | 27,789 (12.47) |
Kidney | 3550 (1.69) | 4022 (1.80) |
Nervous system | 1566 (0.75) | 2155 (0.97) |
Liver disease | 1081 (0.52) | 1199 (0.54) |
Cancer | 10,085 (4.81) | 10,416 (4.67) |
Depression | 15,264 (7.28) | 18,375 (8.24) |
Arthritis | 151,331 (72.14) | 174,391 (78.23) |
Comorbidity group, n (%) | ||
0 | 29,933 (14.27) | 22,121 (9.92) |
1 | 79,168 (37.74) | 73,599 (33.01) |
2 | 63,076 (30.07) | 74,649 (33.48) |
≥ 3 | 37,584 (17.92) | 52,564 (23.58) |
Year of NHS PROMs, n (%) | ||
2009–11 | 96,041 (45.79) | 102,448 (45.95) |
2012–15 | 113,720 (54.21) | 120,485 (54.05) |
Living arrangement, n (%) | ||
I live with someone | 151,669 (72.31) | 164,451 (73.77) |
I live alone | 53,318 (25.42) | 52,985 (23.77) |
I live in a care home, etc. | 259 (0.12) | 219 (0.10) |
Other | 769 (0.37) | 802 (0.36) |
Symptom period (years), n (%) | ||
< 1 | 29,053 (13.85) | 11,041 (4.95) |
1–5 | 142,960 (68.15) | 116,195 (52.12) |
6–10 | 23,108 (11.02) | 48,340 (21.68) |
> 10 | 13,588 (6.48) | 46,273 (20.76) |
FIGURE 27.
The NHS PROMs histograms. (a) OHS; and (b) OKS.
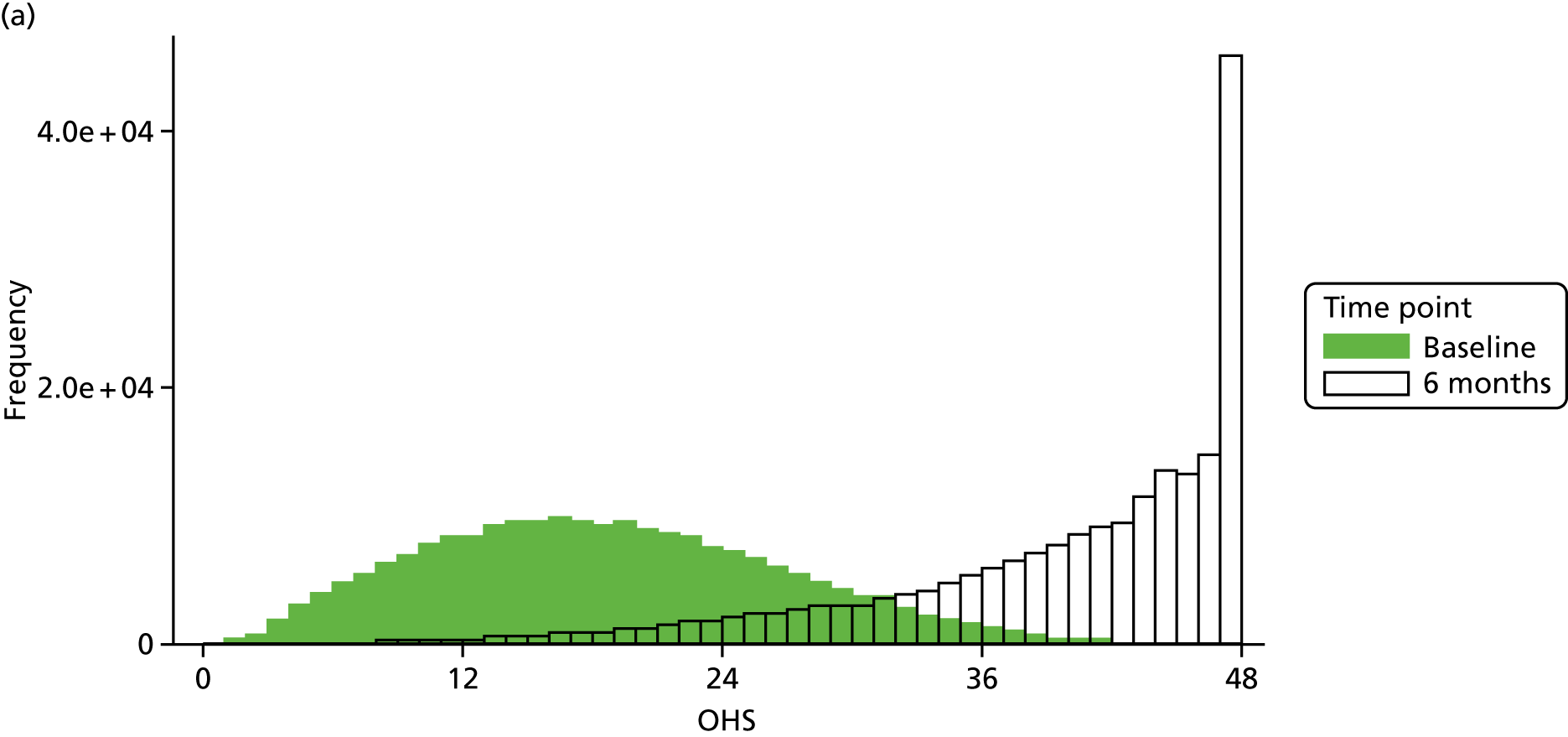

Relative thresholds
Hip
Quantile regression was used to estimate the shape of the 10th, 20th, 30th and 50th percentile change in OHS. For example, the prediction of the third-degree polynomial 50th quantile regression model was:
where x is the preoperative score, εi i. i. d. ∼N(0,σ2) and i refers to the ith patient.
The observed postoperative change in the OHS against the preoperative score along with the observed and estimated percentiles using the quantile regression model are shown in Figure 28. Estimated relative thresholds using the E improvement criterion for the 10th, 20th, 30th and 50th percentiles are presented in Table 53 for the whole data set (‘Total’) and also by key factors (age, gender and time period). There was no clear sign of a different threshold value in accordance with the covariates, with some suggestion that the group of patients aged ≥ 80 years had slightly lower estimates. Results based on the B improvement criterion are available in Online Supplement 14.
FIGURE 28.
The OHS: NHS PROMs. (a) Observed percentiles and 10th, 20th, 30th and 50th quantile regression curves (coloured dots indicate each observational percentile and grey dots indicate the actual weighted observations); and (b) proportion improved using improvement criterion E (observed and quantile regression model).


Baseline covariate | n | Percentiles | |||||||
---|---|---|---|---|---|---|---|---|---|
Predicted | Observed | ||||||||
10th | 20th | 30th | 50th | 10th | 20th | 30th | 50th | ||
Total | 209, 761 | 23 | 32 | 35 | 38 | 24 | 31 | 35 | 38 |
Age category (years) | |||||||||
< 60 | 37,904 | 26 | 33 | 36 | 38 | 27 | 32 | 38 | 38 |
60–79 | 144,064 | 24 | 32 | 35 | 38 | 24 | 32 | 35 | 38 |
≥80 | 27,793 | 19 | 27 | 32 | 36 | 19 | 26 | 31 | 36 |
Gender | |||||||||
Male | 84,673 | 25 | 32 | 35 | 38 | 26 | 33 | 35 | 38 |
Female | 125,058 | 22 | 31 | 34 | 38 | 23 | 31 | 35 | 38 |
Year of NHS PROMs | |||||||||
2009–11 | 96,041 | 22 | 31 | 34 | 38 | 20 | 31 | 35 | 38 |
2012–15 | 113,720 | 25 | 32 | 35 | 38 | 25 | 31 | 35 | 38 |
Figure 28b shows the percentages improved using the A improvement criterion against preoperative score observed and estimated using the predictions from the quantile regression models. Corresponding figures presenting the findings in the six population subsets by age and gender are given in Online Supplement 14.
Knee
Quantile regression was used to estimate the shape of the 10th, 20th, 30th and 50th percentile change in the OKS. For example, the prediction of the third-degree polynomial 50th quantile regression model was:
where x is the preoperative score, εi i. i. d. ∼N(0,σ2) and i refers to the ith observation.
The observed postoperative change in score against preoperative score along with the observed and estimated percentiles using the quantile regression model are shown in Figure 29a. Estimated relative thresholds using the E improvement criterion for 10th, 20th, 30th and 50th percentiles are presented in the Table 54 for the whole data set (‘Total’) and also by key factors (age, gender and time period). There was no clear sign of different threshold values in accordance with the covariates. Results based on the B improvement criterion are available in Online Supplement 15.
FIGURE 29.
The OKS: NHS PROMs. (a) Observed percentiles and 10th, 20th, 30th and 50th quantile regression curves (coloured dots indicate each observational percentile and grey dots indicate the actual weighted observations; and (b) proportion improved using improvement criterion E against preoperative score (observed and quantile regression model).
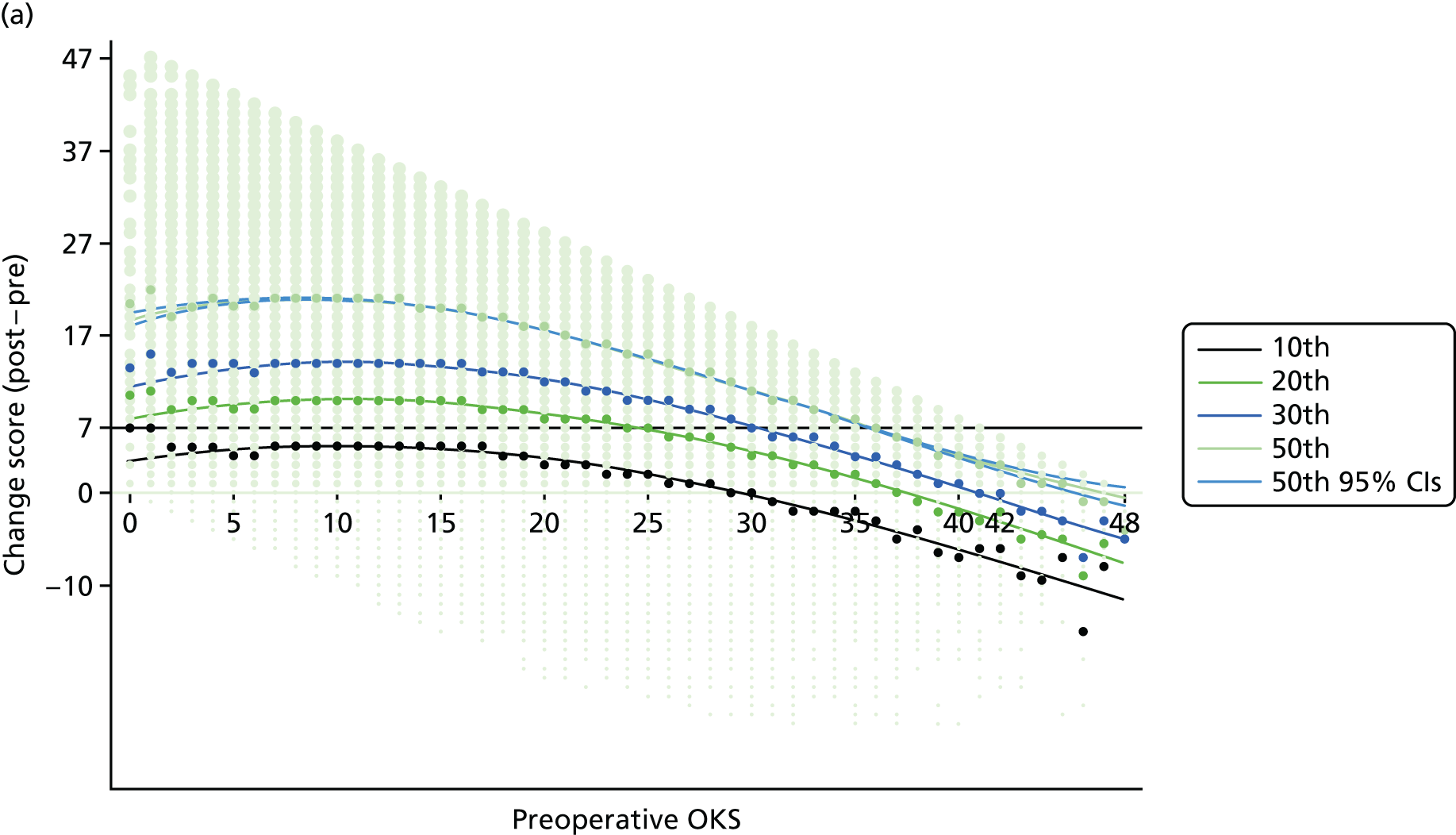

Baseline covariate | n | Percentiles | |||||||
---|---|---|---|---|---|---|---|---|---|
Predicted | Observed | ||||||||
10th | 20th | 30th | 50th | 10th | 20th | 30th | 50th | ||
Total | 222,933 | N/A | 25 | 31 | 36 | 1 | 25 | 30 | 36 |
Age category (years) | |||||||||
< 60 | 29,349 | N/A | 18 | 29 | 35 | 1 | 21 | 30 | 35 |
60–79 | 164,132 | N/A | 26 | 31 | 36 | 1 | 26 | 31 | 36 |
≥ 80 | 29,452 | 13 | 24 | 30 | 35 | 15 | 26 | 30 | 36 |
Gender | |||||||||
Male | 96,006 | N/A | 26 | 31 | 36 | 0 | 26 | 32 | 36 |
Female | 126,885 | N/A | 25 | 30 | 35 | 1 | 25 | 30 | 36 |
Year of NHS PROMs | |||||||||
2009–11 | 102,448 | N/A | 24 | 30 | 35 | 1 | 23 | 30 | 36 |
2012–15 | 120,485 | N/A | 27 | 31 | 36 | 1 | 26 | 32 | 36 |
Internal validation
Internal validation on the quantile regression estimates was carried out by applying quantile regression to population subsets based on age, gender and year of operation (time period). The data set was split into the 2009–11 and 2012–15 time period subsets to internally validate the estimates and check for sensitivity within the data set to the time period. The two time period subpopulation analyses did not show distinct shapes (Figures 30 and 31 provide the OHS and OKS results, respectively).
FIGURE 30.
The OHS: NHS PROMs. (a) Age; (b) gender; and (c) time period subset populations (observed change and 50th quantile regression model).
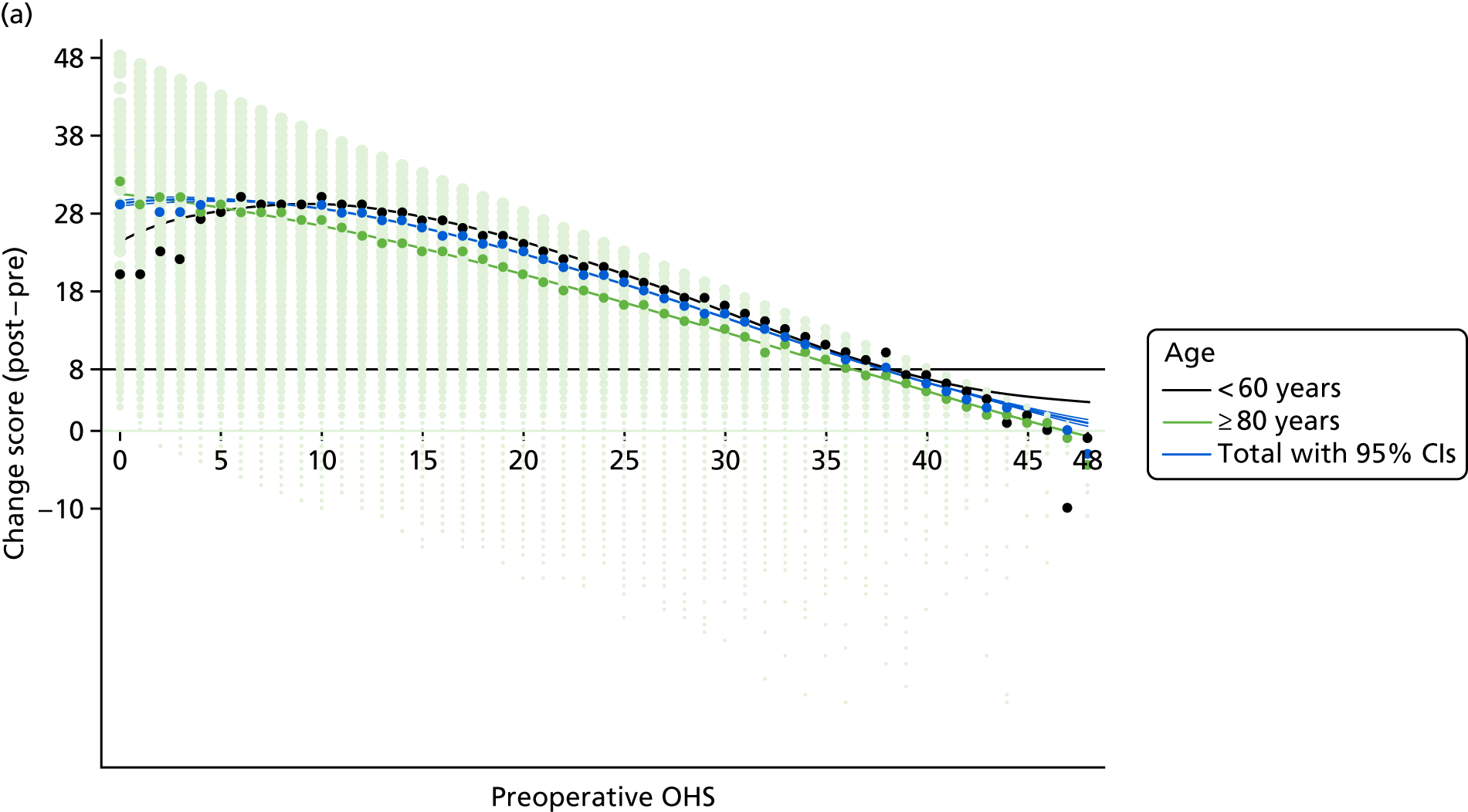


FIGURE 31.
The OKS: NHS PROMs. (a) Age; (b) gender; and (c) time period subset populations (observed change and 50th quantile regression model).


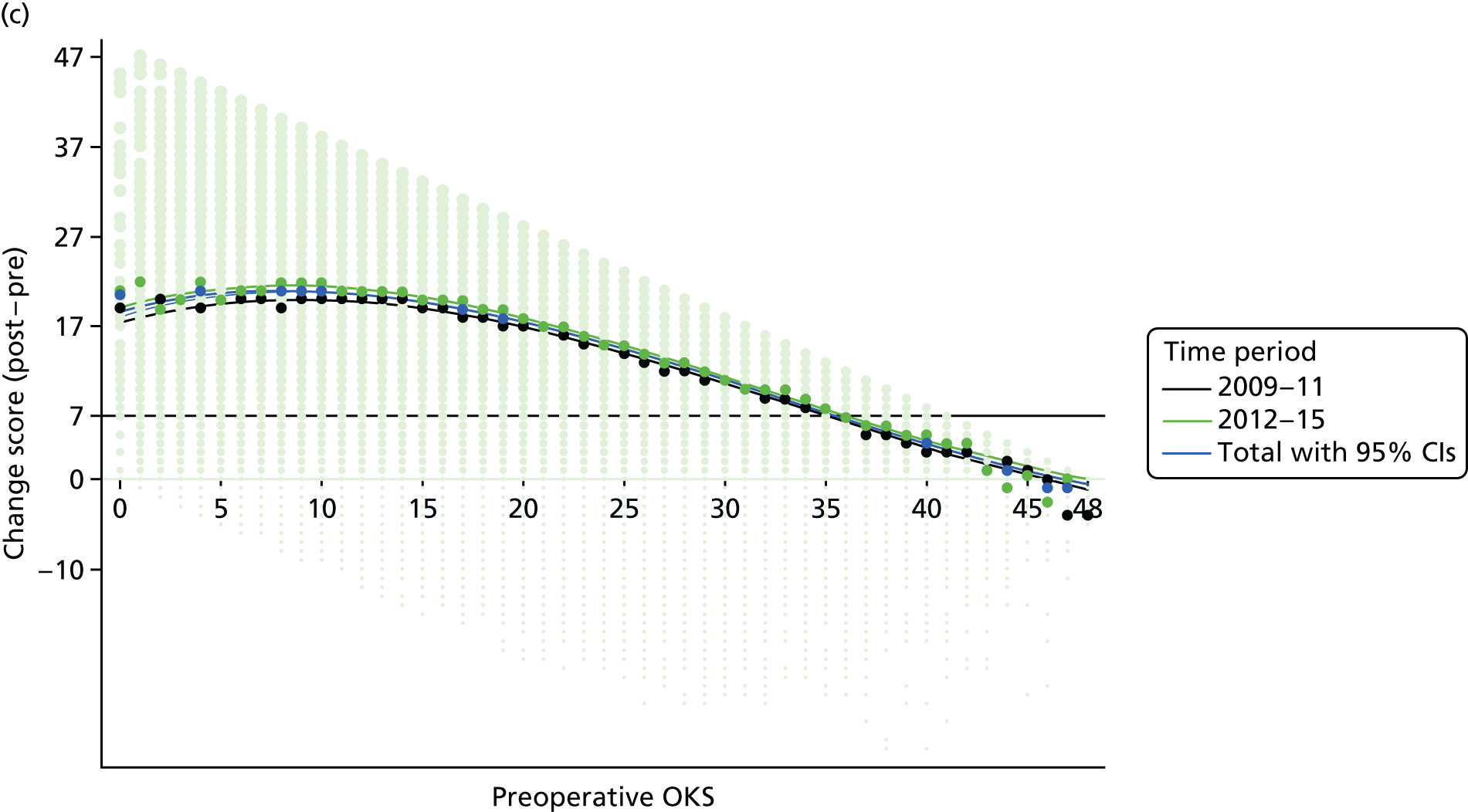
Figures are shown in Online Supplement 14 and the subsets showed some different patterns around the relative threshold values for each percentile, particularly around low scores (see Figures 30 and 31).
The gender subpopulation analyses did not show distinct shapes (see Figures 30 and 31). Corresponding figures presenting the findings in the six age-by-gender population subsets for both the OHS and the OKS are given in Online Supplement 14.
Model performance
Sensitivity and specificity were calculated using the E improvement criterion as the ‘gold standard’ regarding the OHS and OKS relative thresholds for each of the quantile model. The OHS and OKS results are shown in Tables 55 and 56, respectively. Relative threshold values from the 20th quantile regression showed relatively good sensitivity and poor specificity outcomes compared with other thresholds from the 10th, 30th and 50th quantile regressions. Specificity outcomes for the 80% population coverage relative threshold (i.e. 20th percentile) were 19 (95% CI 18 to 19) for the OHS and 35 (95% CI 34 to 35) for the OKS. Specificity outcomes for the 50% population coverage relative threshold (i.e. 50th percentile) were 6 (95% CI 5 to 6) for the OHS and 5 (95% CI 5 to 6) for the OKS (see Table 4).
Baseline covariate | Percentiles | |||||||||||
---|---|---|---|---|---|---|---|---|---|---|---|---|
10th | 20th | 30th | 50th | |||||||||
Threshold score | Sensitivity (95% CI) | Specificity (95% CI) | Threshold score | Sensitivity (95% CI) | Specificity (95% CI) | Threshold score | Sensitivity (95% CI) | Specificity (95% CI) | Threshold score | Sensitivity (95% CI) | Specificity (95% CI) | |
Total | 23 | 77 (77 to 77) | 47 (47 to 48) | 32 | 97 (97 to 97) | 19 (18 to 19) | 35 | 99 (99 to 99) | 12 (12 to 12) | 38 | 100 (100 to 100) | 6 (6 to 7) |
Age category (years) | ||||||||||||
< 60 | 26 | 87 (86 to 87) | 37 (36 to 37) | 33 | 98 (98 to 98) | 16 (16 to 17) | 36 | 100 (99 to 100) | 10 (10 to 10) | 38 | 100 (100 to 100) | 6 (6 to 7) |
60–80 | 24 | 81 (80 to 81) | 44 (43 to 44) | 32 | 97 (97 to 97) | 19 (18 to 19) | 35 | 99 (99 to 99) | 12 (12 to 12) | 38 | 100 (100 to 100) | 6 (6 to 7) |
≥ 80 | 19 | 61 (61 to 61) | 61 (61 to 62) | 27 | 89 (89 to 89) | 34 (33 to 34) | 32 | 97 (97 to 97) | 19 (18 to 19) | 36 | 100 (99 to 100) | 10 (10 to 10) |
Gender | ||||||||||||
Male | 25 | 84 (84 to 84) | 40 (40 to 41) | 32 | 97 (97 to 97) | 19 (18 to 19) | 35 | 99 (99 to 99) | 12 (12 to 12) | 38 | 100 (100 to 100) | 6 (6 to 7) |
Female | 22 | 74 (73 to 74) | 51 (50 to 51) | 31 | 96 (96 to 96) | 22 (21 to 22) | 34 | 99 (99 to 99) | 14 (14 to 15) | 38 | 100 (100 to 100) | 6 (6 to 7) |
Year of NHS PROMs | ||||||||||||
2009–11 | 22 | 74 (73 to 74) | 51 (50 to 51) | 31 | 96 (96 to 96) | 22 (21 to 22) | 34 | 99 (99 to 99) | 14 (14 to 15) | 38 | 100 (100 to 100) | 6 (6 to 7) |
2012–15 | 25 | 84 (84 to 84) | 40 (40 to 41) | 32 | 97 (97 to 97) | 19 (18 to 19) | 35 | 99 (99 to 99) | 12 (12 to 12) | 38 | 100 (100 to 100) | 6 (6 to 7) |
Baseline covariate | Percentile | |||||||||||
---|---|---|---|---|---|---|---|---|---|---|---|---|
10th | 20th | 30th | 50th | |||||||||
Threshold score | Sensitivity (95% CI) | Specificity (95% CI) | Threshold score | Sensitivity (95% CI) | Specificity (95% CI) | Threshold score | Sensitivity (95% CI) | Specificity (95% CI) | Threshold score | Sensitivity (95% CI) | Specificity (95% CI) | |
Total | – | – | – | 25 | 83 (83 to 83) | 35 (34 to 35) | 31 | 96 (96 to 96) | 15 (15 to 15) | 36 | 100 (100 to 100) | 5 (5 to 6) |
Age category (years) | ||||||||||||
< 60 | – | – | – | 18 | 52 (52 to 53) | 63 (63 to 64) | 29 | 93 (93 to 93) | 21 (20 to 21) | 35 | 99 (99 to 99) | 7 (6 to 7) |
60–80 | – | – | – | 26 | 86 (86 to 87) | 31 (30 to 31) | 31 | 96 (96 to 96) | 15 (15 to 15) | 36 | 100 (100 to 100) | 5 (5 to 6) |
≥ 80 | 13 | 28 (28 to 28) | 81 (80 to 81) | 24 | 80 (79 to 80) | 38 (38 to 39) | 30 | 95 (95 to 95) | 18 (18 to 18) | 35 | 99 (99 to 99) | 7 (6 to 7) |
Gender | ||||||||||||
Male | – | – | – | 26 | 86 (86 to 87) | 31 (30 to 31) | 31 | 96 (96 to 96) | 15 (15 to 15) | 36 | 100 (100 to 100) | 5 (5 to 6) |
Female | – | – | – | 25 | 83 (83 to 83) | 35 (34 to 35) | 30 | 95 (95 to 95) | 18 (18 to 18) | 35 | 99 (99 to 99) | 7 (6 to 7) |
Year of NHS PROMs | ||||||||||||
2009–11 | – | – | – | 24 | 80 (79 to 80) | 38 (38 to 39) | 30 | 95 (95 to 95) | 18 (18 to 18) | 35 | 99 (99 to 99) | 7 (6 to 7) |
2012–15 | – | – | – | 27 | 89 (89 to 89) | 27 (27 to 28) | 31 | 96 (96 to 96) | 15 (15 to 15) | 36 | 100 (100 to 100) | 5 (5 to 6) |
Figures 30 and 31 show close agreement with the estimated observed proportions across the range of preoperative Oxford Hip and Knee Scores, both for the overall data set and by subpopulation.
Influence of covariates
Fourth-degree fractional polynomial logistic regressions with dichotomised change scores (postoperative to preoperative score) by the improvement criterion (A) were used to examine the benefit of the baseline covariates on the capacity of benefit.
Hip
The selected fractional model that was selected had the following form:
where x is preoperative score, p^i=N of improved/N of preoperative score and i refers to the ith patient.
Predicted probabilities with 95% CIs using the logistic regressions in comparison with the observational proportion with 95% CIs are presented in Figure 32a. Generally, the fit was good, with the exception of the lowest handful of preoperative scores. The AUC was 0.65 (95% CI 0.64 to 0.65). Model performance was also examined using the calibration graphs in Figure 32, which showed a good level of calibration.
FIGURE 32.
The OHS: NHS PROMs. (a) Proportion improved (improvement criterion E) and observed proportion (logistic regression); and (b) calibration graph.
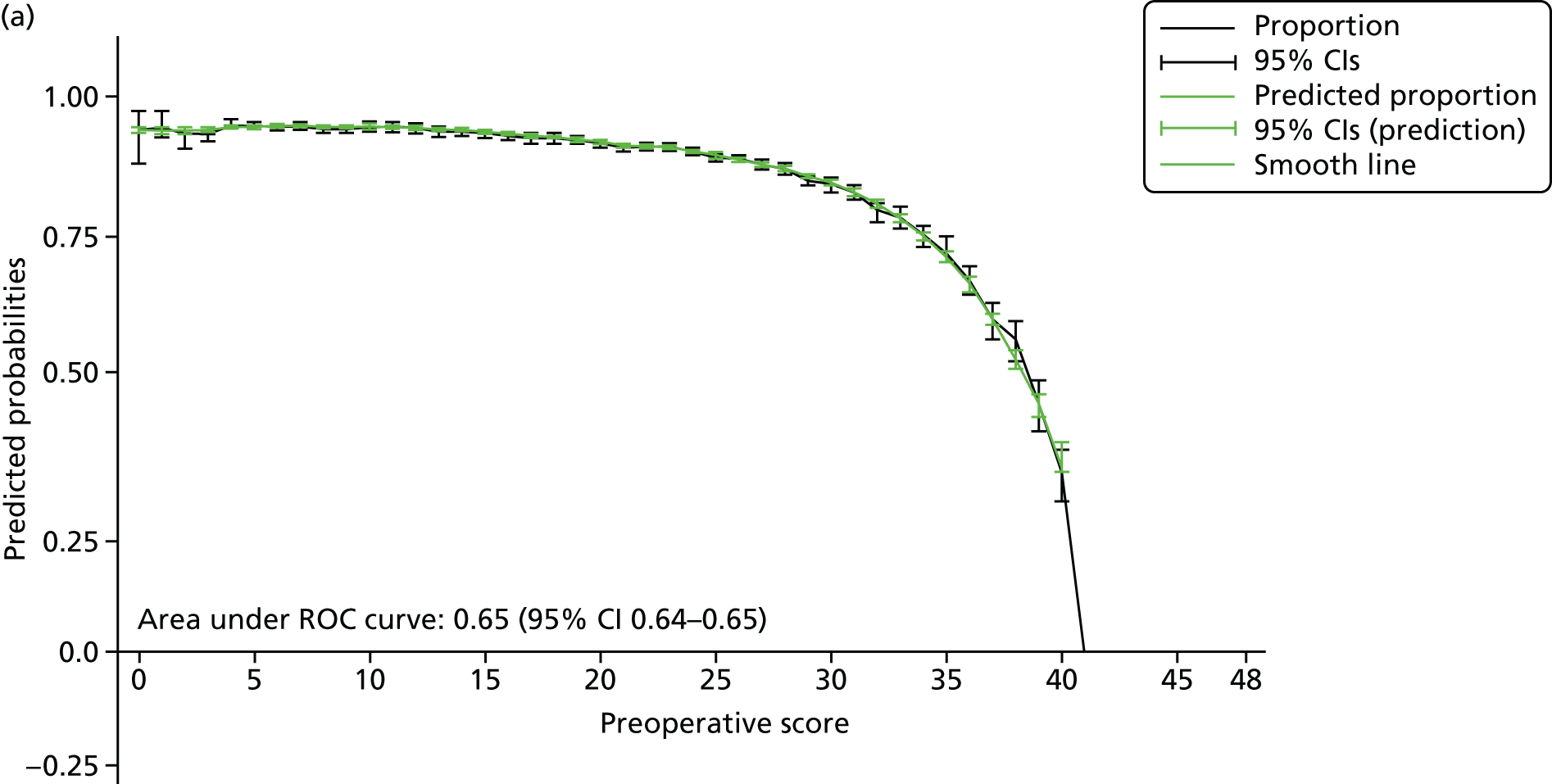
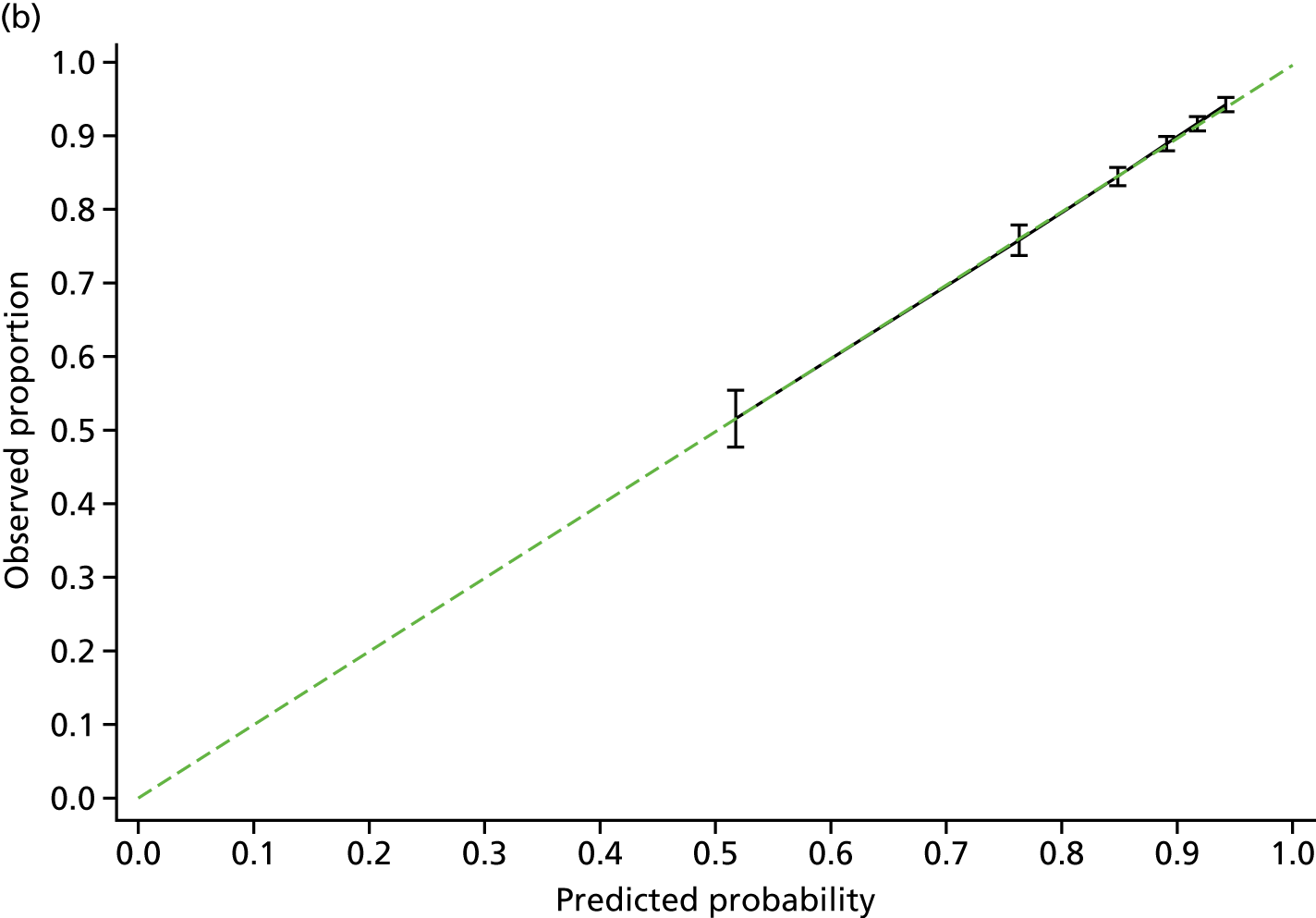
Knee
The selected fractional model had the following form:
where x is the preoperative score, p^i=N of improved/N of preoperative score and i refers to the ith patient.
Predicted probabilities with 95% CIs using the logistic regressions in comparison with the observational proportion with 95% CIs are presented in Figure 33a. Generally, the fit was good, with the exception of the lowest handful of preoperative scores. The AUC was 0.61 (95% CI 0.61 to 0.62). Model performance was also examined using the calibration graphs in Figure 33b, which showed a good level of calibration.
FIGURE 33.
The OKS: NHS PROMs. (a) Proportion improved (improvement criterion E) and observed proportion (logistic regression); and (b) calibration graph.


Selected models with baseline covariates
Hip
Following the model-building process, the selected model with covariates including circulation and depression showed meaningful but marginal impacts on the OHS. The model had the following form:
where x is preoperative score, p^=N of improvedN of preoperative score and i refers to the ith observation.
The AUC was 0.68 (95% CI 0.67 to 0.68). The proportion improving and the calibration graphs were very similar to the model without the additional covariates and, therefore, are not provided here.
Knee
Following the model-building process, the selected model including age, circulation, diabetes and depression showed meaningful but marginal impacts on the OKS. It had the following form:
where x is preoperative score, p^=N of improvedN of preoperative score and i refers to the ith observation.
The area under the ROC curve, for the final model was 0.65 (95% CI 0.65 to 0.66). The proportion improving and the calibration graphs were very similar to the model without the additional covariates and, therefore, are not provided here.
Discussion
The postoperative score and the probability of improvement were modelled for both the OHS and the OKS. In addition, we calculated threshold estimates using both of these estimates and assessed performance.
Strengths and limitations
Two different modelling approaches were used to get the robust and consistent estimated thresholds: logistic and quantile regression. Unlike standard regression models, the quantile regression deals with the asymmetric spread of outcomes and enables estimates of the corresponding proportion of individuals to be estimates. Performance showed excellent agreement between the observed and estimated results for this approach, with only the highest values showing some signs of an inadequate fit. This reflects both the regression models used and also the very data sets that they were applied to. The results showed that the estimated relative thresholds by percentiles and predicted probabilities were equal or similar to the thresholds from the logistic regressions that the dichotomised outcomes (i.e. improved or not improved) used. In this study, the relative thresholds were based on the quantile regressions and were reported for the 10th, 20th, 30th and 50th percentiles.
In addition, the baseline covariates were examined in terms of their impact on the patient’s capacity of benefit, in addition to their impact on preoperative score. In terms of the potential threshold value, they did not generally seem to have much influence, perhaps with the exception of the 80-years age group.
The PROMs data set linked to HES provided a very large and rich data source, which enabled robust and reliable estimates. Internal validation was undertaken, dividing the data set into two time periods. Even the data subsets were very large and there were no clear differences of threshold estimates between the two internal data sets. The model diagnoses and validations that were performed suggested that the quantile models were a good fit, except for the extremes of the Oxford Hip and Knee Scores.
This study defined improvement (and, by implication, the capacity of benefit) using a literature-reported anchor-based best cut-off point. 75 This score was calculated using the patient-reported anchor-based question; however, there are various approaches to define the improvement. One of the approaches applied in Chapters 4 and 6 was a MDC-based approach. We explored another definition (medium ES) in this chapter and provided the results in Online Supplement 13. It is worth noting that even the anchor-based approach is still somewhat crude and does not account for the preoperative state, which has been shown in some settings to influence the magnitude of a difference (change) that would be considered important by patients. Measurement errors are problematic in the repeatedly measured patient-reported outcomes, and there will be several ways to control the measurement errors (e.g. using the adjusted improvement score and applying advance statistical inference approaches such as Bayesian models with computational methods). It is the applicability of the finding to an individual that is problematic; population estimates should be fair. The impact of measurement errors in prognostic modelling is an area of active research. In terms of performance, the estimated proportion improvement should accurately reflect the population. Based on Chapter 3, those within 6 points of a cut-off point (MDCs using an ICC of 0.9) could plausibly have a value sufficient to meet the cut-off point. Further research may be required; care is needed when applying to use the estimated relative thresholds in terms of this aspect. A related issue was that the data set reflected patients who received arthroplasty and, therefore, it may not fully represent the population of those who were considering arthroplasty. A further limitation of our analysis was the substantial amount of missing data, which reflected the passive nature of data collection. Missing data analyses that utilised available information on the missing data (which was very limited) are, in our view, very unlikely to substantively alter the findings.
Conclusion
We estimated the postoperative scores and the probability of improvement based on both the OHS and the OKS. From these, we calculated relative thresholds for the OHS and OKS. The model generally showed good performance for predicting the probability of improvement for individuals at each preoperative score level. The results clearly reflect both the substantial improvement in score from pre to post operation, but also the uncertainty about where an individual patient will end up. The quantile regression nicely models differing proportions of individuals and their expected outcomes.
A very large data set was used, which enabled a data-intensive approach (quantile regression) to be successfully used, with only the extreme handful of values at each end of the scale showing uncertainty. The NHS PROMs data set is arguably the best representation of the typical NHS patient available. Sensitivity to other factors was also assessed; there was some suggestion that the ≥ 80-years age group were somewhat different. Overall, the explanatory value of the available factors (collected routinely) were limited and a number showed no value. There was a substantial amount of variability between the individuals’ postoperative outcomes, which is unexplained.
As previously stated, it should be borne in mind that all of these analyses reflect the change in pain and function estimated by the Oxford Hip and Knee Scores only. Other justifications for arthroplasty surgery exist that would not be fully reflected in these data. Similarly, these analyses do not take into account the potential risk of infection and other problems that may require further treatment (e.g. revision surgery).
Chapter 7 Further health economic evaluation of threshold values (work package 2)
Background
We extended and improved the analyses described in Chapter 5 to obtain more accurate estimates of preoperative economic thresholds for the OKS and OHS using the NHS PROMs data linked to HES. In particular, the PROMs/HES data set provides:
-
Sufficient data to estimate the cost of admissions for primary surgery, revision or subsequent re-admissions using the NHS Payment Grouper.
-
The ability to link revisions and re-admissions with patients’ primary operations.
-
Additional years of data with indicators of which operations were revisions that were not available in the freely available PROMs data.
-
Patients’ exact ages, rather than 10-year age bands.
-
Data on age and sex for all patients. By contrast, the extract of PROMs data freely available online omitted age and sex data for individuals (generally the very old and very young) to avoid the potential for patients to be identified.
We did not evaluate the impact of additional covariates other than age and sex, because the variables available in the PROMs/HES data extract had very little effect on prediction accuracy and have not been included in the ACHE tool intended for general practice. The effect of BMI was not evaluated because this variable is not included within PROMs/HES and it was not possible to obtain NJR data (in which BMI is recorded) linked to PROMs/HES; subsequently, the only data sets providing BMI data were small and likely to be underpowered.
Like the analyses described in Chapter 5, the economic evaluation comprised a cost–utility analysis evaluating the cost-effectiveness of TKA or THA compared with no arthroplasty over a 10-year time horizon in a UK setting from the perspective of the UK NHS (see Chapter 5, Background). However, it is worth noting that, in the absence of linked NJR data, it is not possible to distinguish TKA and THA from other types of knee and hip arthroplasty (e.g. hip resurfacing) or to reliably identify the indication for arthroplasty, and, therefore, all analyses based on PROMs and/or HES data include all types of primary knee and hip arthroplasty conducted for any indication.
Method
We conducted additional regression analyses on the new extract of PROMs/HES linked data and adapted the Markov models described in Chapter 5 to accommodate the new regression models. The Markov model structure and assumptions were generally identical to those described in Chapter 5, Model. In particular, the analysis used a 10-year time horizon and used 2014 as the reference year for costs. We also took a NHS perspective and focused on the costs of hospital admissions and consultations with health-care professionals that were associated with the joint in question.
The model used the same assumptions described in Chapter 5, Other model assumptions and inputs, with the following exceptions:
-
The analyses described in this chapter allowed for mortality associated with all revision procedures. By contrast, in Chapter 5, mortality associated with revisions was only included for all revisions > 12 months after primary arthroplasty and only if the mortality associated with revisions was expected to be > 10% above all-cause mortality. In the analyses described in this chapter, the probability of dying in the year of hip revision surgery was based on the mortality in the year of revision estimated by Pennington et al. 121 However, we followed Pennington et al. 119 in using the same model to predict mortality after revision and primary knee arthroplasty.
-
For both TKA and THA, we followed Pennington et al. 119,121 by capping mortality in the year of revision at a maximum of 10% above all-cause mortality to avoid extrapolating very high mortality rates to very old patients, who were generally outside the sample used to estimate mortality rates. We also allowed for the additional mortality associated with revisions taking place within 12 months of primary arthroplasty (see Online Supplement 15).
-
The cost of re-admissions and ambulatory consultations were taken into account for all health states and in all years of the model.
-
We followed the assumption used in Chapter 5 that QALYs in the year of revision were equal to the average of the before-revision utility and the after-revision utility. Although PROMs specifically includes data before revision and 6 months afterwards, calculating QALYs as the average of these utilities allows for the fact that people are likely to have had a quality of life similar to the prerevision utility in the months leading up to revision surgery and experience utility similar to that observed 6 months after revision later in the year.
Revision rates, costs in the no arthroplasty arm and the rate of change in EQ-5D utility with age or over time were the same as those used in Chapter 5, and mortality rates were also based on those by Pennington et al. 119,121 (see Online Supplement 12). In the hip model, the cost of ambulatory consultations was also based on the same models used in Chapter 5. 113 The following parameters were re-estimated (see Table 39):
-
Mapping models predicting preoperative EQ-5D score based on preoperative Oxford Hip and Knee Scores were re-estimated using the PROMs/HES extract, replacing the published algorithms that were used previously. 126,134 Re-estimating the mapping equations enabled us to consider non-linear relationships between EQ-5D scores and Oxford Hip and Knee Scores and evaluate how EQ-5D utility varies with patient’s age and sex.
-
Models predicting EQ-5D utility 6 months after primary arthroplasty were re-estimated using PROMs/HES data because this updated data set includes a larger sample and patients’ exact ages.
-
The EQ-5D utilities before and after revision were re-estimated using PROMs/HES data because this data set enables utility before and after revisions to be linked to the Oxford Hip and Knee Scores measured before patients’ primary arthroplasty procedures and provides a far larger number of revisions than KAT or EPOS. 66
-
The costs of primary and revision arthroplasty were re-estimated using PROMs/HES data because this data set is very large and up to date and provides sufficient information to use the NHS Payment Grouper,152 thereby avoiding the additional assumptions that were necessary to estimate the cost of re-admissions using KAT, COASt or published estimates. 66,113,131
-
The cost of re-admissions was re-estimated using PROMs/HES data because this large, up-to-date data set enables the Oxford Hip and Knee Scores measured before patients’ primary arthroplasty procedures to be linked to all subsequent re-admissions. This enabled the cost of re-admissions to be included in the hip model; such costs were excluded from the analyses described in Chapter 5 as there were no available data. Although re-admission costs following TKA could be estimated from KAT and COASt, PROMs/HES provided a substantially larger and more up-to-date data set.
-
The cost of community and outpatient visits were re-estimated for TKA in all years and for THA in year 1 to exclude the cost of re-admissions, which were now captured separately. The cost of community and outpatient visits beyond year 1 in THA patients continued to be based on published estimates. 113 The methods and assumptions used to estimate the cost of community and outpatient visits were the same as those described in Chapter 5, Costing analyses.
The next section describes how the PROMs/HES data were manipulated prior to conducting these regression analyses. Additional details on the methods and results of each regression analysis are given in Online Supplement 15.
Methods for manipulating and analysing NHS Patient-Reported Outcome Measures/Hospital Episode Statistics linked data
Outline of the approach
We obtained the complete NHS PROMs data set for all patients undergoing hip and knee arthroplasty between April 2009 and October 2015, as well as admitted patient care (APC) data from HES. These two data sets were linked and manipulated to create data sets on preoperative and 6-month utility, costs of primary arthroplasty, costs of revision arthroplasty, utility before and after revisions, and the costs of re-admissions related to arthroplasty. Hospital re-admissions were defined as related to arthroplasty if they either took place within 30 days of primary arthroplasty or the patient had a primary diagnosis for hip or knee arthritis, had a procedure code relating to the hip or knee joint or if the patient had a primary diagnosis of infections commonly associated with hip or knee arthroplasty.
We used the NHS Local Payment Grouper for 2014/15152 and the corresponding tariffs from the National Schedule 2014/15153 to derive the costs for primary and revision arthroplasty as well as for relevant re-admissions. We assumed that all primary and revision arthroplasty procedures were elective. For re-admissions, we distinguished between elective and non-elective admissions. Further details on data cleaning, data manipulation and handling of missing data are provided in Online Supplement 15.
The resulting data sets were used to estimate inputs for the Markov models. They included 309,001 primary knee arthroplasty procedures, 286,812 primary hip arthroplasty procedures, 3403 knee revisions and 2346 hip revisions. We also identified 171,459 relevant admissions, of which 75,803 took place within the first 30 days, 41,583 were for patients who had a relevant primary diagnosis, 6613 had a relevant procedure code and 83,774 were for patients who had a diagnosis of infection.
Regression analyses
Regression models were estimated in Stata® version 14 using the same methods as were described in Chapter 5, Regression analyses. Details of specific methods used for each regression analyses are described in Online Supplement 15. Variance–covariance matrices for the regression models are available from the corresponding author on request.
Presentation of results and analysis of uncertainty
The same set of hypothetical individuals with different combinations of age, sex and clinical tool score were run through the revised models using mean values for all parameters and using PSA. PSA and calculation of 95% CrIs around the threshold were conducted in the same way as for Chapter 5, generating 2000 estimates of costs and QALYs with and without arthroplasty for each of the 260 hypothetical individuals in both the knee and the hip models. Plots of the probability that arthroplasty is cost-effective against the OHSs and OKSs were generated as described previously (see Chapter 5, Presentation of results and analysis of uncertainty). The cost-effectiveness acceptability curves (CEACs) shown in Online Supplement 16 were generated using the same methods but varying the ceiling ratio.
We conducted the following sensitivity analyses:
-
taking a 5-, 20- or 60-year time horizon (cf. 10 years in the base-case analysis)
-
assuming that EQ-5D utility without TJA worsens by 0.025 per year (see Chapter 5, Presentation of results and analysis of uncertainty)168
-
assuming that EQ-5D without TJA increases by 0.115 per year in the first year of the model and then follows an age-related decline after that169
-
assuming that patients accrued no costs in the absence of arthroplasty
-
halving the cost in the absence of arthroplasty
-
doubling the cost in the absence of arthroplasty
-
discounting QALYs at 1.5% and costs at 3.5%
-
no discounting.
Results
Effect of Oxford Knee Score on cost-effectiveness of total knee arthroplasty
Regression analyses demonstrated that the preoperative OKS had a statistically significant effect on preoperative EQ-5D utility, EQ-5D utility 6 months after surgery, EQ-5D utility before and after revision surgery, the cost of primary arthroplasty, re-admission costs in year 1 and subsequent years, ambulatory costs in year 1 and in subsequent years, and re-admission costs and ambulatory costs > 1 year after revision surgery (p < 0.05) (see Online Supplement 15). However, the OKS had no significant impact on the cost of revision surgery or the cost of re-admissions or ambulatory consultations in the year of revision surgery (p > 0.05) (see Online Supplement 15 for results of all regression models).
The regression models predicting preoperative and 6-month EQ-5D utility were re-estimated using the PROMs/HES extract (Figure 34 and see Online Supplement 15). The model of 6-month EQ-5D produced extremely similar predictions as that used in Chapter 5 (see Figures 14 and 34). However, whereas Chapter 5 used a published linear model of the relationship between the OKS and EQ-5D that included baseline and postoperative measurements,134 the analyses described in this chapter used the PROMs/HES extract to assess how preoperative EQ-5D varies with age and gender as well as preoperative OKS and to explore non-linear functions. The model selection process suggested that prediction accuracy was optimised by a Tobit model including polynomials for OKS and age. This model predicted that EQ-5D utility would rise steeply as OKSs rose from 40 to 48 (see Figure 34). The difference between preoperative and 6-month EQ-5D utility was predicted to be negative for patients with a preoperative OKS of 45–46 or higher (depending on age). In contrast, the regression functions used in Chapter 5 predicted the change in EQ-5D utility to be negative for patients with an OKS of 41–44 or higher (see Figure 14). Although the observed data suggest that EQ-5D utility rises more slowly as the OKS increases from 40 to 48 than is predicted by the Tobit model, the models used in this chapter accurately predict that the change in EQ-5D after knee arthroplasty is negative for patients with a preoperative OKS of 47, but positive for patients with a preoperative OKS of ≤ 44 (see Figure 34).
FIGURE 34.
Comparison of observed mean EQ-5D utility in PROMs/HES for patients with different preoperative OKSs against the predictions for the Tobit regression functions used in the Markov model.

The Markov model predicted the costs and QALYs that each hypothetical individual would accrue over a 10-year period with and without arthroplasty, taking into account the change in EQ-5D utility predicted in the models shown in Figure 34, operative mortality, the cost of primary and revision surgery, inpatient and ambulatory care required by patients with and without arthroplasty and the changes in quality of life that occur before/after revisions and as patients age.
The difference in QALYs between patients with and without arthroplasty was highest for patients with a preoperative OKS of 6 or 7 and declined steadily with increasing OKSs; averaged across all ages, arthroplasty gained 2.99 QALYs per patient with an OKS of 0, 4.05 QALYs per patient with an OKS of 6 and 0.19 QALYs per patient with an OKS of 44. TKA was predicted to increase QALYs for all patients with an OKS of below 44–46, depending on age.
The difference in cost between patients with and without arthroplasty was lowest for patients with an OKS of 16–19, but was markedly higher for patients with lower or higher than average OKSs. The model predicted that TKA was less costly than no surgery for 50-year-old men with an OKS of between 15 and 18 and for 50-year-old women with an OKS of between 10 and 21. Averaged across all ages, the difference in cost was £6457 per patient with an OKS of 0, £1898 per patient with an OKS of 16 and £6481 per patient with an OKS of 48.
As was demonstrated in Chapter 5, the results demonstrate that TKA is highly cost-effective for the vast majority of patients who currently undergo surgery (Table 57), although ICERs rose as the OKS increased from 4 to 48. In particular, the decision grid shows only selected OKS values, focusing on values in the region of the threshold; TKA costs < £20,000 per QALY gained for all age groups at the OKS values omitted from the grid. As a result of re-estimating models using PROMs/HES data, TKA is more cost-effective (i.e. has lower ICERs) in almost all groups compared with the analyses described in Chapter 5 (see Table 40). In particular, TKA was dominated by no arthroplasty (i.e. produced fewer QALYs at a greater cost) only for patients with an OKS of 44–46 or greater (cf. an OKS of 42–45 in Chapter 5). The current analysis also estimated that there was only a narrow band of one or two OKS values at which TKA improves patients’ health but is not cost-effective (the areas shown in dark and medium green in Table 57).
Preoperative OKS (selected values only) | Cost | |||||
---|---|---|---|---|---|---|
Age (years) | ||||||
50 | 60 | 70 | 80 | 90 | Average | |
0 | £1979 | £1693 | £1939 | £2808 | £5257 | £2156 |
10 | £41 | £247 | £577 | £1195 | £2656 | £646 |
20 | Dominant | £278 | £911 | £2094 | £4986 | £1015 |
21 | Dominant | £355 | £1035 | £2325 | £5523 | £1150 |
24 | £288 | £711 | £1543 | £3190 | £7473 | £1693 |
28 | £1158 | £1412 | £2393 | £4401 | £9907 | £2582 |
29 | £1404 | £1596 | £2589 | £4625 | £10,252 | £2782 |
30 | £1643 | £1771 | £2765 | £4802 | £10,460 | £2962 |
31 | £1870 | £1936 | £2922 | £4934 | £10,547 | £3119 |
32 | £2083 | £2090 | £3060 | £5033 | £10,547 | £3256 |
33 | £2285 | £2236 | £3187 | £5111 | £10,505 | £3382 |
34 | £2479 | £2378 | £3311 | £5189 | £10,467 | £3504 |
35 | £2675 | £2523 | £3446 | £5288 | £10,485 | £3637 |
36 | £2887 | £2683 | £3605 | £5433 | £10,618 | £3796 |
37 | £3132 | £2871 | £3811 | £5658 | £10,935 | £4003 |
38 | £3437 | £3109 | £4095 | £6014 | £11,547 | £4291 |
39 | £3846 | £3428 | £4509 | £6582 | £12,642 | £4710 |
40 | £4439 | £3885 | £5146 | £7523 | £14,615 | £5357 |
41 | £5377 | £4590 | £6205 | £9199 | £18,462 | £6427 |
42 | £7061 | £5787 | £8197 | £12,653 | £27,668 | £8421 |
43 | £10,813 | £8158 | £12,934 | £22,592 | £68,515 | £13,060 |
44 | £25,078 | £14,537 | £34,786 | £172,396 | Dominated | £32,707 |
45 | Dominated | £67,573 | Dominated | Dominated | Dominated | Dominated |
46 | Dominated | Dominated | Dominated | Dominated | Dominated | Dominated |
47 | Dominated | Dominated | Dominated | Dominated | Dominated | Dominated |
48 | Dominated | Dominated | Dominated | Dominated | Dominated | Dominated |
Threshold (95% CrI) | 43 (43 to 48) | 44 (43 to 48) | 43 (43 to 44) | 42 (42 to 43) | 41 (40 to 42) | 43 (43 to 44) |
The economic threshold OKS (i.e. the highest OKS at which TKA costs < £20,000 per QALY gained, shown in light blue in Table 57) was 44 (95% CrI 43 to 48) for 60-year-olds and 41 (95% CrI 40 to 42) for 90-year-olds (see Table 57). If a single threshold were to be set across all ages, a threshold of 43 (95% CrI 43 to 44) would be the most cost-effective value to choose. This is somewhat higher than the threshold estimated in Chapter 5 (40, 95% CrI 39 to 42) (see Table 40). Overall, TKA costs < £20,000 per QALY gained compared with no arthroplasty for 99.9% of patients who currently undergo surgery and costs < £5000 per QALY gained for 96.6% of patients who currently undergo surgery.
The effect of age on cost-effectiveness and thresholds was markedly less pronounced than in the analyses described in Chapter 5. In addition, although ICERs were generally slightly lower for women than for men (see Online Supplement 16), the economic threshold was identical for men and women.
However, there was a modest amount of uncertainty around the economic thresholds. The 95% CrI demonstrated that we can be 95% confident that the economic threshold for all ages combined lies between 43 and 44. The probability that TKA is cost-effective varied with age, OKS (Figure 35) and how much the NHS is willing or able to pay per QALY gained (see Online Supplement 16). For 70-year-olds, we can be > 99% confident that TKA is cost-effective at a £20,000-per-QALY ceiling ratio at an OKS of ≤ 42, although this falls to 98% for patients with an OKS of 43, 18% for patients with an OKS of 44 and 2% for patients with an OKS of 45. Substantially greater uncertainty is observed for patients aged 50 or 90. CEACs for men and women at different ages are shown in Online Supplement 16.
FIGURE 35.
Effect of preoperative OKS on the probability that TKA is cost-effective at a £20,000-per-QALY ceiling ratio.

Effect of Oxford Hip Score on the cost-effectiveness of total hip arthroplasty
Regression analyses demonstrated that the preoperative OHS had a statistically significant effect on preoperative EQ-5D utility, EQ-5D utility 6 months after surgery, EQ-5D utility before and after revision surgery, cost of primary arthroplasty and revision surgery, re-admission costs in year 1 and subsequent years, ambulatory costs in year 1 and re-admission costs > 1 year after revision surgery (p < 0.05) (see Online Supplement 15). However, the OHS had no significant impact on re-admission costs in the year of revision (p > 0.05) (see Online Supplement 15 for the results of all regression models).
The Tobit models predicting EQ-5D before and 6 months after hip arthroplasty showed similar trends to those for knee arthroplasty (Figure 36). In particular, the polynomial function predicting how preoperative EQ-5D utility varies with the preoperative OHS predicted a sharp increase in EQ-5D as the OHS increases from 40 to 48. The models predicted that arthroplasty would increase EQ-5D utility for patients with a preoperative OHS of ≤ 45–46 or less (depending on age and gender) and would decrease EQ-5D utility for patients with an OHS of ≥ 46–47. By contrast, within the observed data, the mean EQ-5D change was positive for patients with an OHS of ≤ 47 and negative for patients with an OHS of 48, which suggests that the estimated Tobit models may underestimate thresholds for THA (see Figure 36).
FIGURE 36.
Comparison of observed mean EQ-5D utility in PROMs/HES for patients with different preoperative OHSs against the predictions for the Tobit regression functions used in the Markov model.

Following the trends observed for change in EQ-5D utility (see Figure 36), the QALY gain from THA was greatest for patients with an OHS of 5 or 6 and declined steadily with increasing OHSs; the average patient with an OHS of 5 gained 5.28 QALYs, compared with 4.33 for patients with an OHS of 0 and 0.05 for patients with an OHS of 46. The model predicted that, on average, THA would worsen health by up to 0.44 QALYs for patients with an OHS of 47 or 48, and for 80- or 90-year-olds with an OHS of 46.
The difference in costs between patients with and without THA was smallest for patients with an OHS of 1 (£1975 per patient, averaged across all ages). THA was predicted to be less costly than conducting no arthroplasty surgery for 50-year-old women with an OHS of 1, but was more costly for all other groups. The incremental cost of THA rose gradually as OHS increased from 1 to 48; the average incremental cost across all ages was £5113 for patients with an OHS of 48.
The results demonstrate that THA is highly cost-effective for the vast majority of patients who currently undergo surgery (Table 58). As was observed for TKA, ICERs for patients with high Oxford Hip and Knee Scores were markedly lower than in the analyses described in Chapter 5 (see Table 45). For patients aged ≤ 70 years, THA cost < £20,000 per QALY gained when the OHS was ≤ 45. The economic threshold reduced to 43 (95% CrI 43 to 44) for 90-year-olds. The economic threshold ignoring age and gender was 45 (95% CrI 44 to 45), markedly higher than the threshold of 42 estimated in Chapter 5 (see Table 45).
Preoperative OHS (selected values only) | Cost | |||||
---|---|---|---|---|---|---|
Age (years) | ||||||
50 | 60 | 70 | 80 | 90 | Average | |
0 | £92 | £327 | £501 | £926 | £1945 | £533 |
10 | £809 | £792 | £820 | £1187 | £2011 | £923 |
18 | £1413 | £1330 | £1368 | £1776 | £2899 | £1491 |
20 | £1640 | £1537 | £1580 | £2058 | £3369 | £1724 |
21 | £1760 | £1651 | £1696 | £2214 | £3632 | £1852 |
24 | £2148 | £2009 | £2065 | £2710 | £4479 | £2257 |
28 | £2584 | £2409 | £2472 | £3253 | £5410 | £2705 |
29 | £2656 | £2474 | £2537 | £3334 | £5548 | £2776 |
30 | £2711 | £2524 | £2586 | £3391 | £5646 | £2828 |
35 | £2833 | £2634 | £2683 | £3474 | £5752 | £2932 |
40 | £3412 | £3169 | £3228 | £4188 | £6991 | £3529 |
41 | £3786 | £3515 | £3590 | £4695 | £7911 | £3929 |
42 | £4397 | £4079 | £4186 | £5557 | £9533 | £4590 |
43 | £5474 | £5066 | £5247 | £7173 | £12,765 | £5777 |
44 | £7636 | £7027 | £7421 | £10,842 | £21,196 | £8244 |
45 | £13,344 | £12,059 | £13,428 | £24,427 | £76,368 | £15,330 |
46 | £50,387 | £40,160 | £64,676 | Dominated | Dominated | £97,787 |
47 | Dominated | Dominated | Dominated | Dominated | Dominated | Dominated |
48 | Dominated | Dominated | Dominated | Dominated | Dominated | Dominated |
Threshold (95% CrI) | 45 (44 to 46) | 45 (44 to 46) | 45 (44 to 46) | 44 (44 to 45) | 43 (43 to 44) | 45 (44 to 45) |
Age had little impact on ICERs and economic threshold compared with the estimates from Chapter 5. Furthermore, gender had very little impact on ICERs and the economic threshold was the same for men and women (see Online Supplement 16).
Total hip arthroplasty was found to cost < £20,000 per QALY gained compared with no arthroplasty for 99.96% of patients who currently undergo arthroplasty surgery and to cost < £5000 per QALY gained for 99.16% of patients.
The PSA demonstrated that we can be 95% confident that the economic threshold, ignoring age and sex, is between 44 and 45. For 70-year-olds, the probability that THA is cost-effective was > 95% at an OHS of ≤ 44, 90% at an OHS of 45 and 5% at an OHS of 46 (Figure 37). There was markedly greater uncertainty for patients aged 80 and 90 years.
FIGURE 37.
Effect of the Oxford Hip and Knee Scores on the probability that THA is cost-effective at a £20,000-per-QALY ceiling ratio.

Sensitivity analyses
Ten sensitivity analyses were conducted to evaluate the sensitivity of the results to changes in time horizon and discount rates and to the assumptions made around EQ-5D utility and costs for patients who do not undergo TJA (Table 59 and see Online Supplement 16). This demonstrated that the results are reasonably robust to changes in all of the key assumptions. Halving the time horizon from 10 to 5 years reduced the economic threshold by 1 point, whereas extending the time horizon had no impact. Reducing the discount rates used to adjust for time preference or drastically changing the costs assumed to be accrued by patients in the absence of arthroplasty had little or no impact.
Sensitivity analysis | Economic threshold ignoring age and sex | |
---|---|---|
Knee arthroplasty | Hip arthroplasty | |
Base-case analysis | 43 | 45 |
5-year time horizon | 42 | 44 |
20-year time horizon | 43 | 45 |
60-year (lifetime) time horizon | 43 | 45 |
EQ-5D utility without TJA worsens by 0.025 per year | 46 | 48 |
EQ-5D utility without TJA increases by 0.115 in the first year and follows age-related decline thereafter | 39 | 41 |
Assuming that patients accrued no costs in the absence of arthroplasty | 43 | 44 |
Halving the cost in the absence of arthroplasty | 43 | 45 |
Doubling the cost in the absence of arthroplasty | 43 | 45 |
Discounting QALYs at 1.5% and costs at 3.5% | 43 | 45 |
No discounting | 43 | 45 |
Assuming that EQ-5D utility will decrease by 0.025 per year (a decrease 4–7 times larger than was assumed in the base-case analysis) markedly increased the threshold Oxford Hip or Knee Score at which TJA ceases to be cost-effective; indeed, in this analysis, THA was cost-effective for all patients < 80 years of age, regardless of OHS. Conversely, making an extremely optimistic assumption about the efficacy of the first year of non-operative management (assuming that EQ-5D utility would increase by 0.115 in the first year)169 reduced the economic threshold for TKA to 39 and reduced the economic for THA to 41. As discussed previously, this figure is conservative because the non-surgical treatment used by Skou et al. 169 was relatively intensive.
Discussion
Summary of findings
Findings of the economic evaluation
The results presented in this chapter suggest that TJA is cost-effective for > 99.9% of patients who currently undergo surgery if the NHS is willing to pay £20,000 per QALY gained. Averaging across men and women of all ages, it is cost-effective to conduct TKA on patients with an OKS of ≤ 43 (95% CrI 43 to 44) and to conduct THA on patients with an OHS of ≤ 45 (95% CrI 44 to 45).
These thresholds are slightly higher than those estimated in Chapter 5, largely owing to re-estimation of the models of preoperative EQ-5D utility to take account of age and gender and to allow for non-linear relationships between Oxford Hip and Knee Scores and EQ-5D utility. The values presented in this chapter make full use of the PROMs/HES linked data, which cover 608,170 knee and hip arthroplasty procedures, including around 67.7% of the 897,619 operations conducted in England between April 2009 and October 2015. 162,182–184 Because the analyses described in Chapter 5 used small data sets for several key parameters (including the cost of primary arthroplasty), the economic thresholds and cost-effectiveness results presented in this chapter should be used in decision-making in preference to the results described in Chapter 5 for the OKS and OHS.
The thresholds for TKA were also substantially higher than those estimated previously by Dakin et al. ,2 who used KAT data and estimated the economic threshold to be 39 for patients of ASA grades 1 and 2 and a threshold of 34 for patients of ASA grade 3. As discussed in Chapter 5, Summary of the results, this difference may arise from the substantially larger number of patients with high OKSs in PROMs data, which enabled us to take account of non-linear relationships between OKS, costs and quality of life. Nonetheless, the analyses described in this chapter confirm the earlier findings described in Chapter 5 and by Dakin et al. 2 that suggest that TKA is cost-effective for patients with an OKS of ≥ 30–39.
The economic thresholds are slightly higher than the absolute thresholds owing to differences in the aims and principles of each analysis. The clinical thresholds are based on the probability that patients will achieve a MIC in Oxford Hip or Knee Score following surgery, which was defined as 7 for the OHS and 8 for the OKS. By contrast, the economic threshold is based on the difference in mean QALYs and mean cost between patients who undergo arthroplasty and those who do not. Although the economic analyses took account of patients’ life expectancy, surgical mortality and the cost of primary and revision arthroplasty, the results are primarily driven by the mean change in EQ-5D utility following arthroplasty. The PROMs/HES data demonstrate that, on average, arthroplasty increases patients’ EQ-5D utility at all Oxford Hip and Knee Scores of ≤ 46. Although patients with Oxford Hip and Knee Scores between 42 and 46 cannot have a 7-point increase in score following surgery, 73.5% (845/1149) of these patients nonetheless had an increase in Oxford Hip or Knee Score and 55.4% (453/817) of such patients had an increase in EQ-5D utility; among patients with improvements in EQ-5D, the mean improvement was 0.215 following arthroplasty. Only 16.9% (138/817) had a decrease in EQ-5D utility; among these patients, the mean change was –0.181. Because TKA costs around £5642,153 providing there are no complications and the benefits of surgery last 10 years, EQ-5D utility needs to improve by only 0.032 after arthroplasty in order for TKA to cost < £20,000 per QALY gained [0.032 = £5642/£20,000, all divided by 8.72 years (10 years, discounted at 3.5% per annum)].
However, even though TJA is cost-effective for patients with high Oxford Hip and Knee Scores, many patients may choose not to undergo surgery as they feel that the risks of surgery may outweigh the benefits. In particular, most patients are likely to be risk averse and prefer to maintain their current level of quality of life rather than undergo surgery that will, on average, improve their health but that carries a risk that their joint will deteriorate or that they will die or have a serious cardiovascular complication as a result of surgery. By contrast, the NHS as a whole spreads the risk across the population of patients (including the majority who benefit and the minority who are worse off after surgery), and so may be able to ignore risk aversity in their resource allocation decisions.
Nonetheless, the analyses described in this chapter demonstrate that there is no economic justification for restricting access to TKA for patients with Oxford Hip and Knee Scores of ≤ 43, or restricting access to THA for patients with Oxford Hip and Knee Scores of ≤ 45. The results also demonstrate that there is no economic justification for using different thresholds for men and women and suggest that thresholds based on cost-effectiveness vary little with age.
Strengths/limitations
Strengths and limitations of the economic modelling
The economic evaluation used the best available UK evidence, including PROMs/HES data on > 608,170 primary arthroplasty procedures and trial data sets following patients for up to 12 years after primary arthroplasty. However, some model inputs, including ambulatory costs and the long-term changes in EQ-5D utility, were based on smaller data sets such as KAT and COASt.
The biggest shortcoming of the analysis is the limited data on patients who have not undergone arthroplasty. No UK longitudinal data following patients who have not undergone arthroplasty were available and the only international data sets identified used the WOMAC. 185,186 Within this analysis, we therefore assumed that the costs accrued in the absence of arthroplasty would be the same as those accrued by COASt participants in the year before surgery, and assumed that EQ-5D utility would decrease at the same rate as is observed in the general population samples. In practice, many osteoarthritis patients are likely to experience a marked worsening of symptoms in the absence of arthroplasty; taking account of this trend would raise the economic threshold further. A sensitivity analysis allowing for EQ-5D utility worsening in patients who have not undergone arthroplasty estimated economic thresholds to be 2–3 points higher than the base case. However, other sensitivity analyses showed that thresholds would be substantially lower if non-surgical management markedly improved patients’ quality of life and showed that even substantial changes to the assumptions about the costs accrued by patients without arthroplasty had a negligible impact on the conclusions.
For simplicity, the economic evaluation compared immediate TJA with a strategy of no arthroplasty for 10 years. The choice of a 10-year time horizon is arbitrary, although sensitivity analyses demonstrated that this had little impact on the results. In practice, many patients whose symptoms are considered not sufficiently severe to warrant surgery at the present time may have surgery in the future. However, given the shortage of data on changes in Oxford Hip and Knee Scores in the absence of arthroplasty, the current analysis provides a convenient assessment of the cost-effectiveness of arthroplasty that enables economic thresholds to be estimated. We also excluded the cost of assessing patients to determine whether or not they are appropriate candidates for surgery; the impact of including these costs is evaluated in Chapter 8, which evaluates the cost-effectiveness of referral to surgical assessment compared with no referral.
The analyses took a NHS perspective and excluded the cost of medications, personal care, nursing homes, convalescence, equipment, home modifications, lost productivity and informal care. It is likely that broadening the perspective to include these wider costs and taking account of any deterioration in health in the absence of arthroplasty would improve the cost-effectiveness of TJA and increase the economic threshold. Indeed, one economic evaluation187 evaluating TKA in patients aged ≥ 90 years argued that TKA would be cost-saving owing to reductions in the cost of nursing home placement.
The PSA suggested that there was very little parameter uncertainty around the economic threshold. The 95% CrIs take account of uncertainty around all model inputs and allow for correlations between regression coefficients from the same regression model; these CrIs are narrow because most of the parameters are estimated on PROMs/HES data on around 300,000 operations. However, the PSA does not take account of uncertainty around the choice of regression function, uncertainty around model assumptions/structure or methodological uncertainty. 188
This analysis also cannot evaluate the impact of BMI on thresholds because it was not possible to obtain NJR data linked to PROMs/HES within the time frame of this project, and the only available BMI data came from comparatively small studies that may be underpowered to assess the impact of BMI over and above the impact of the Oxford Hip and Knee Scores, age and sex. In the absence of NJR data, we also assumed that the Oxford Hip and Knee Scores had no impact on revision rates.
Although the PROMs/HES extract includes data on > 608,018 primary arthroplasty procedures, only 0.5% (2884/608,018) of the sample had Oxford Hip or Knee Scores of > 40. Furthermore, only 0.5% (3250/608,018) of those in the sample were aged ≥ 90 years and 5.0% (30,437/608,018) were aged ≤ 50 years, and the published estimates of mortality and revision rates excluded patients aged ≤ 55 or > 84 years. 119,121 Results for 50- and 90-year-olds should therefore be interpreted with caution. Furthermore, the model selection process described in Chapter 5, Regression analyses, is likely to select models that give best prediction accuracy for patients with an OKS close to the mean, and place less importance on prediction accuracy for patients with high Oxford Hip and Knee Scores. This may introduce additional uncertainty around the economic threshold that is not captured within the PSA and the reported 95% CrIs. In particular, the Tobit models predicting preoperative EQ-5D utility may overestimate EQ-5D utility for those patients with Oxford Hip and Knee Scores of > 43 (see Figures 34 and 36), although the models appear to predict change in EQ-5D utility accurately.
As described in Chapter 5, the current analyses are based on UK data and may not generalise to other countries. Analyses conducted on KAT and COASt data sets and the published studies providing mortality and revision rates excluded patients who did not undergo TJA. 119,121 However, in the absence of NJR data, it was not possible to reliably identify which primary arthroplasty procedures within the PROMs/HES data set were TJA and which comprised unicompartmental knee replacement or hip resurfacing. Costs, QALYs and cost-effectiveness may differ between different types of arthroplasty and between different indications in ways that cannot be assessed using the current data.
Further research needed for economic modelling
Further research is needed on the impact of BMI and to assess whether or not the Oxford Hip and Knee Scores affect revision rates. Additional analyses using NJR data linked to PROMs and HES could also be used to assess whether or not the Oxford Hip and Knee Scores affect the rate of revision surgery.
Further research is needed on the costs accrued in patients who do not undergo arthroplasty and on how costs, Oxford Hip and Knee Scores and EQ-5D utility change over time in the absence of surgery.
Additional findings
We also developed additional models to map from the Oxford Hip and Knee Scores to EQ-5D utilities, which could also be applied in other settings in which OKS or OHS data are available but EQ-5D utilities are not. Coefficients for these models are given in Online Supplement 15. However, owing to the nature of the economic evaluation, we considered only models based on OKS/OHS total score, rather than responses to individual questions. As a result, these models have substantially worse prediction accuracy than those developed by Dakin et al. 134 and Pinedo-Villanueva et al. ,126 which mapped from dummy variables for individual questions. However, our OKS mapping model had better prediction accuracy than the simple OLS model mapping from total OKS to EQ-5D utility developed by Dakin134 (MSE 0.047 vs. 0.052 in the preoperative estimation sample). The models shown in Online Supplement 15 were estimated only on preoperative data and may not perform as well in data sets that include postoperative scores. These analyses suggest that there is a non-linear relationship between OKS/OHS total score and EQ-5D utility and that age and gender have a significant effect on EQ-5D utility that is not explained by OKS/OHS. The models give good prediction accuracy, although they may overestimate utility for patients with Oxford Hip or Knee Scores of > 43.
Conclusion
The economic evaluation demonstrates that TKA is cost-effective for patients with an OKS of ≤ 43, whereas THA is cost-effective for patients with an OHS of ≤ 45. Therefore, it is not appropriate to restrict access to arthroplasty for patients with Oxford Hip or Knee Scores below these limits on cost-effectiveness grounds. The analysis also suggests that it is not cost-effective to set separate thresholds for men and women and that age has little impact on economic thresholds.
Chapter 8 Determining the outcome of using the Arthroplasty Candidacy Help Engine tool in the NHS (work package 3)
Background
Over the past decade, many clinical commissioning groups (CCGs) have used PROMs such as the Oxford Hip and Knee Scores to set thresholds for arthroplasty. A 2014 review by the Royal College of Surgeons170 found that 31% (16/52) of CCGs that they reviewed imposed an OHS threshold. The thresholds used have frequently been relatively low, such as 19 and 24 on the OKS and OHSs, respectively. 170,171,176 The work, described in Chapters 6 and 7 have shown that these thresholds are inappropriate, because patients with an OKS or OHS of 19 or 24, respectively, have a ≥ 80% chance of a good outcome, and TKA and THA would each cost < £10,000 per QALY gained for these patients.
Whereas a threshold of 24 would exclude 21% of current arthroplasty operations, avoiding referrals for patients with a < 70% chance of a good outcome would exclude only 3% of patients who currently have hip arthroplasty and 7% of those having knee arthroplasty. This demonstrates that the vast majority of arthroplasty operations that are currently done in the UK are appropriate and are on patients who have a high capacity to benefit. Furthermore, some of the patients with high Oxford Hip or Knee Scores may need arthroplasty regardless of the referral thresholds (e.g. for indications other than osteoarthritis, or severe deformity in the absence of other symptoms). However, there are likely to be many patients currently managed in general practice or at musculoskeletal hubs who have high capacity to benefit who do not currently undergo surgery; if the thresholds estimated in Chapters 6 and 7 were introduced, numbers of referrals from this population might increase.
There is a shortage of published studies and national data on the patients who do not currently have surgery, and there are no data on OKSs/OHSs for patients managed in primary care. We therefore obtained data from the musculoskeletal hub that is run from the NOC in Oxford to get an initial estimate of how the introduction of the ACHE tool and the choice of threshold may affect the number of referrals and operations, costs and health benefits.
In the Thames Valley CCGs, osteoarthritis patients cannot be referred for consideration of joint surgery unless their symptoms have a substantial impact on their quality of life and are refractory to non-surgical treatment, including advice, activity and exercise. 172,173 All patients with a BMI of ≥ 25 kg/m2 must also be offered, and be strongly encouraged to participate in, a weight-loss programme. Until October 2016, patients with an OKS of > 32 could only be listed for knee surgery if approved by two consultants. 189 In practice, nearly all patients attending the NOC hub with knee or hip symptoms are asked to complete the OKS or OHS and patients with a BMI of ≥ 40 kg/m2 cannot be listed for surgery until they have first completed a monitored weight-loss programme.
This analysis aims to:
-
Evaluate how the probability of referral and undergoing surgery varies with preoperative characteristics in current clinical practice using an audit of a musculoskeletal hub.
-
Estimate the number of patients who might be expected to be referred for surgical assessment or undergo arthroplasty if the ACHE tool were introduced into NHS practice.
-
Assess how the economic thresholds estimated in Chapter 7 change when we take into account the additional numbers of surgical assessments that would be required if the ACHE tool were introduced into routine clinical practice and evaluate the cost-effectiveness of referring patients for surgical assessment compared with no referral (rather than the cost-effectiveness of arthroplasty vs. no arthroplasty, as was evaluated in Chapters 5 and 7).
-
Assess the cost-effectiveness of using the ACHE tool with different thresholds or different probabilities of benefiting from surgery to assess osteoarthritis patients attending the musculoskeletal hub compared with current practice, from the perspective of the UK NHS.
The predictions of the impact the ACHE tool might have if it were introduced into clinical practice are, by their nature, speculative. In particular, they rely on assumptions around how the ACHE tool would be used in practice and what impact it would have on referrals. In addition, the analysis excludes patients who are not currently referred by their GPs but might, in some circumstances, be candidates for arthroplasty, because there are no data on this population. We also assumed that referral patterns across England are the same as those in Oxfordshire, because this comprised the only data set available for analysis. The figures presented in this chapter must therefore be interpreted with caution and represent initial estimates of the direction and possible magnitude of changes that could be brought about by the ACHE tool.
Methods
General approach
We conducted an audit of medical records for patients who were referred to the NOC hub with knee or hip pain. We used the results to estimate the probability that patients with different baseline characteristics are referred from the hub to an orthopaedic surgeon and the probability that such patients then undergo arthroplasty. These probabilities were then used to estimate the number of patients of different ages, genders and with Oxford Hip and Knee Scores who may be referred nationally each year. The costs and QALYs estimated for each patient group within the economic evaluation described in Chapter 7 were multiplied by the number of patients anticipated to come forward based on the hub data to predict the potential impact that the ACHE tool may have on cost and health benefits, and how such costs and benefits may vary with the threshold.
In principle, the ACHE tool could be used at several points in the referral pathway. It could be used by GPs to decide if or when to refer the patient to secondary care. Oxford Hip and Knee Scores or the ACHE probability of benefit could be stated on the GP’s referral letter to help hub staff identify the next course of action, or the tool could be used during a hub consultation to inform decisions about whether or not the patient should be referred for surgical assessment. The ACHE tool could also be used at the surgical assessment to guide discussions between the surgeon and the patient about whether or not arthroplasty is appropriate. In this chapter, we assumed that the ACHE tool would be used only during face-to-face consultations at the hub for three reasons. First, discussions with GPs and hub staff suggested that it is likely to be most practical to use the ACHE tool at the hub rather than at the general practice. Second, there are no reliable data on the total number of patients consulting their GPs about hip or knee pain who are not currently referred, and no information on the distribution of Oxford Hip and Knee Scores for such patients. By contrast, we were able to obtain a set of data for patients attending the NOC hub. Third, although the ACHE tool may also be used to inform joint decision-making by the surgeon and patient during the surgical assessment, we did not attempt to model the impact of using the ACHE tool during the surgical assessment because the final decision to proceed or not with surgery is a more complex, personalised, shared decision and cannot be easily modelled given current data.
Approval
We successfully sought permission to analyse anonymised data from the Oxford musculoskeletal hub under direction of the lead and clinical director of Oxford University Hospitals NHS Foundation Trust (Oxford University Hospitals research and development reference number 11603).
Analysis of data from the Nuffield Orthopaedic Centre musculoskeletal hub
The primary analysis aimed to estimate how the probability that patients who attend face-to-face consultations at the hub will be referred to secondary care varies with OKS/OHS, age and gender. Following a well-established rule of thumb,190 we aimed to collect sufficient data to have at least 10 referrals from the hub to secondary care for each of these three explanatory variables.
Two medically-qualified surgical research fellows extracted data on patients’ gender, age and OKS/OHS at the time of each patient’s first face-to-face attendance at the hub into a pre-prepared data extraction table, as well as details on the date of attendance and whether or not the patient was referred to secondary care from the hub’s electronic medical records database. The date of any surgical assessment visit, whether or not patients were listed for knee/hip arthroplasty and the date of surgery were also recorded. Free-text fields were also used to record additional information on imaging, referrals to other clinics, other surgery and other diagnoses. Additional data on OKSs were extracted from the clinical pathway database for patients who had been referred directly for surgical assessment. Data on BMI were not extracted because BMI is not part of the ACHE tool.
The following exclusion criteria were used to exclude from the analysis patients who are unlikely to use the ACHE tool in clinical practice:
-
Patients aged < 50 years because knee/hip pain in younger patients is unlikely to be caused by osteoarthritis.
-
Patients for whom it was clear from the records that the symptoms were attributable to a condition other than osteoarthritis. In particular, the analysis excluded patients with rheumatoid arthritis, fracture (or arthritis secondary to fracture), gout, chondroid lesions, sports or other injuries, bone/joint infections, bursitis or quadriceps rupture. Patients requiring limb reconstruction, those with hip pain caused by previous spinal surgery and those recorded as having no mechanical symptoms were also excluded.
-
Patients who had previously had arthroplasty on the joint in question.
-
Patients who had attended the hub or surgical assessment before July 2015, and patients for whom medical records were inaccessible for research.
Patients referred for radiography, MRI or physiotherapy were in the analysis, regardless of whether or not they attended a face-to-face hub assessment.
Modelling the treatment pathway
Decision tree model
We constructed a decision tree model of the treatment pathway based on a preliminary examination of the data and consultation with hub staff (Figure 38). GPs’ letters referring patients to secondary care are first reviewed by senior hub staff, who examine the patient’s radiographs, and take into account the GP’s description of the patient’s symptoms, their BMI and what treatments have been previously tried.
FIGURE 38.
Schematic of the decision tree model. P(x) indicates the probability of x occurring; M indicates the Markov model that is used to calculate payoffs for the relevant branch (green for the arthroplasty Markov model and blue for the no-arthroplasty Markov model); and # indicates the residual probability (i.e. 1 minus the probabilities of the other branches leading from the preceding chance node).
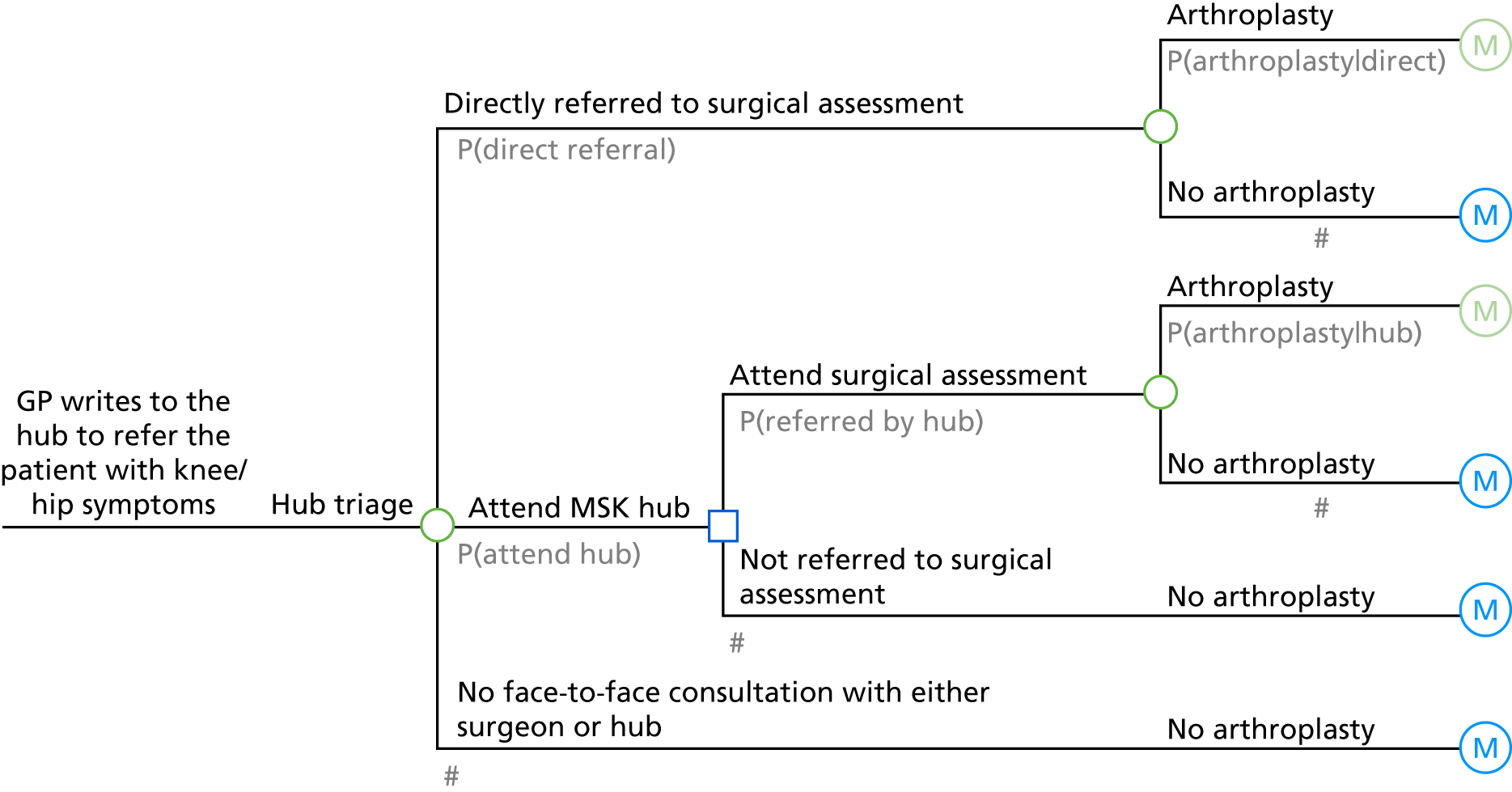
As a result of this triage process, some patients are referred directly to surgical assessment, including those who have severe symptoms and those who have previously been referred to secondary care but chose not to have surgery at that time.
Triage also identifies some patients who can be managed in primary care and therefore do not need to attend a face-to-face consultation at the hub or with an orthopaedic surgeon. These patients may have mild symptoms, or may not have exhausted all of the non-surgical treatment options (e.g. advice and information, activity and exercise, and, if appropriate weight loss). 191–193 In other cases, it may be clear from the referral letter that the patient is unfit for surgery or has a recent injury that needs more time to heal before a secondary care assessment is necessary. Other patients are sent referral letters, but do not call to book an appointment or miss booked appointments. A small minority of patients opt for non-NHS care. In some cases, it is necessary to conduct radiography or other imaging before the patient can be assessed.
The remaining patients attend the hub for a face-to-face assessment. This group tends to be those with moderate symptoms, those for whom there is uncertainty around the clinical diagnosis and those patients with BMIs of ≥ 40 kg/m2 who must be referred for a weight-loss programme before surgery. At the hub, consultant physiotherapists or orthopaedic fellows assess the patient to confirm diagnosis. Although the CCG no longer specifies a threshold Oxford Hip or Knee Score for referral,172,173 patients complete the OKS or OHS to assess whether or not symptoms are sufficiently severe to warrant surgery and to guide discussions about patients’ symptom profiles. Staff discuss with the patient what arthroplasty involves, including the potential need for revision surgery, recovery times and the need for support at home after hospital discharge, and how these might be affected by patients’ comorbidities and living arrangements. Landmark injections, injections for trochanteric bursitis and diagnostic imaging may also be done during the hub consultation. Obese patients are referred for monitored weight-loss programmes that must be followed for 12 months before surgery. Based on the hub visit, patients may be referred to an assessment consultation with a hip or knee surgeon if it is considered that they may be appropriate candidates for arthroplasty, or for other interventions such as arthroscopy, interventional radiology or anterior cruciate ligament repair. Other patients may be referred to other outpatient clinics, such as a sports injury clinic, or rheumatology. Patients may also be referred for physiotherapy or other non-surgical management, or may choose not to be referred because they have decided that they would prefer not to have surgery.
Patients attending a surgical assessment consultation will discuss the risks and benefits of surgery with an orthopaedic surgeon and make an informed decision about whether or not to undergo arthroplasty or another type of surgery, taking account of the severity of their symptoms and comorbidities.
Methods for estimating patient numbers
The decision tree model required estimation of five probabilities, shown in grey in Figure 38. We also evaluated whether or not the probability of being referred by the hub to surgical assessment and the probability of subsequently undergoing arthroplasty varied with Oxford Hip or Knee Score, age and sex. From these figures, we estimated the probability that men and women aged 50, 60, 70, 80 and 90 years with different Oxford Hip and Knee Scores will undergo arthroplasty after being referred by their GP with hip/knee symptoms.
We then calculated how many patients in each of these groups would need to be referred with knee/hip symptoms to account for the number of patients undergoing arthroplasty surgery across England each year. The number of operations in each group was first calculated by multiplying the proportion of patients in different age and sex groups (see Online Supplement 15) by the proportion of different Oxford Hip and Knee Scores (see Online Supplement 12) and by the number of primary knee/hip replacements conducted solely for osteoarthritis in England in 2014–15 (76,617 knee replacements and 69,313 hip replacements;154,165 see Chapter 5, Presentation of results and analysis of uncertainty). The number of patients in each group who are likely to have been referred with knee/hip symptoms was then calculated by dividing the number of operations in each group by the probability that each group of patients will undergo surgery.
We then estimated what impact using the ACHE tool at the hub might have on the number of referrals to surgical assessment and on the number of operations. In practice, many patients who are not referred to surgical assessment after their hub visit would not be considered candidates for arthroplasty regardless of their Oxford Hip or Knee Scores. In particular, some patients may decide after their hub visit that they do not want arthroplasty surgery, whereas others may be unsuitable owing to comorbid conditions. For the purposes of estimating the potential impact of the ACHE tool, we therefore assumed that half of the patients who are not currently referred from the hub to surgical assessment would not go on to surgical assessment even if the ACHE tool were introduced, whereas the remaining patients will be referred only if the ACHE tool predicts them to have capacity to benefit. We modelled the likely patient numbers if the ACHE tool were used to restrict referral to patients with at least a 50%, 60%, 70%, 80% or 85% probability of achieving a MID in Oxford Hip or Knee Score based on the data.
These patient numbers were used to assess the cost of the referral pathway by applying the costs described in Cost inputs. The impact of changing the number of arthroplasty operations on total costs and QALYs was then calculated by applying the costs and QALYs with and without arthroplasty that were estimated in Chapter 7 to the number of patients in each group who were expected to undergo arthroplasty or not have surgery within each scenario. The net health benefit of stratifying patients using the ACHE tool was also evaluated using the methods described in Chapter 5, Presentation of results and analysis of uncertainty, assuming a £20,000-per-QALY ceiling ratio. 166,167
We also estimated the total costs and QALYs that would be accrued if all hub attendees were referred for surgical assessment and those that would be accrued if no hub attendees were referred. From these estimates, we calculated the cost-effectiveness of referring hub attendees of different ages, genders and Oxford Scores to surgical assessment compared with no referral and produced alternative estimates of the decision grids and economic thresholds that take account of the cost of surgical assessment. We also used the ‘Goal Seek’ function in Microsoft Excel® 2010 to estimate the ICER at which the number of knee/hip arthroplasty procedures that would be conducted using the economic threshold equal to a specific target.
The analysis made the following assumptions:
-
Because there were no data on OKSs/OHSs patients who did not attend the hub, the probability of attending a face-to-face visit at the hub and the probability that patients are referred directly to surgery were assumed to be independent of Oxford Hip or Knee Score, age and sex. In practice, it is likely that those patients referred directly to surgical assessment will have more-severe symptoms, whereas those who attend neither the surgical assessment nor the hub may have less-severe symptoms. There may also be differences in the probability of referral by age and sex. However, because we evaluated the impact of the ACHE tool only in those patients attending the hub, allowing for variations in these parameters by age and sex would have had little or no impact on the conclusions. These probabilities were therefore estimated as the proportion of the osteoarthritis patients included in the analyses who attended the hub or were referred directly to surgical assessment without attending the hub.
-
For patients who were directly referred to surgical assessment, we assumed that the probability of undergoing surgery was independent of Oxford Hip or Knee Score, age and sex under current practice. As described above, this assumption is unlikely to have affected the estimates of the impact of the ACHE tool on hub attendees.
-
The five probabilities estimated based on the NOC hub data were assumed to be representative of clinical practice across the UK. Clinical practice at this hub is likely to differ from practices in other areas in several respects (see Strengths, limitations and further research requirements). However, this assumption was necessary as no other data were available.
-
All patients were assumed to attend a surgical assessment visit before surgery, as was observed in the audit.
-
We assumed that the ACHE tool would not be used at the surgical assessment and that the probability of undergoing surgery after attending surgical assessment (conditional on Oxford Hip or Knee Score, age and sex) will be unaffected by the threshold used.
-
Because there were insufficient data to evaluate non-linear functions, age and Oxford Hip and Knee Score were assumed to have linear effects on the log-odds of being referred for surgical assessment and on the log-odds of having surgery if referred.
-
Patients who were on the waiting list for arthroplasty surgery or for whom surgery had been delayed because of comorbidities (including high BMI scores) after being referred at the surgical assessment were counted as having been referred for surgery.
Owing to time and resource constraints, we did not attempt to quantify the uncertainty around the results presented in this chapter using PSA.
Regression methods
Logistic regression models were used to assess how patients’ Oxford Hip and Knee Scores, age and sex affected the odds of being referred for surgical assessment and the odds of subsequently undergoing surgery. Regression analyses and two-sample tests of proportions were conducted in Stata® version 14. Comparisons of Oxford Hip or Knee Scores between patient groups were conducted using unpaired t-tests conducted in Stata®; an F-test for equal variances was first conducted, which determined whether the t-test assumed equal or unequal variance. Oxford Hip and Knee Scores in the hub sample were compared against the population mean (nationally or in Oxfordshire) using a one-sample t-test in Stata®. Population means, nationally and in Oxfordshire, were based on NHS PROMs reports,162,182–184,194,195 which provide average preoperative OKSs and OHSs at the provider and commissioner level; the national average OKSs and OHSs were based on 2014–15 data, although owing to be smaller numbers of operations, those for the NHS Oxfordshire CCG comprise weighted average scores over all years from April 2009 to March 2015, and these averages were weighted by the number of procedures conducted in each year.
Oxford Hip and Knee Scores, age and sex were initially considered as potential explanatory variables, but were dropped from the regression analyses if they were found to be poor predictors. Given the sample of around 100 patients attending the hub, it was not possible to follow the 10-fold cross-validation approach used to select the regression specifications used in the Markov models. We therefore selected regression models based on Akaike information criterion (AIC), testing variables in a prespecified sequence. We began with a model including just the constant term and evaluated whether or not adding OHS or OKS to the model reduced the AIC; if it did, we added in the Oxford Hip or Knee Score in the next step, and if not, we kept the model as just constant. In the next step, we assessed whether or not adding age into the model reduced the AIC, and in the final step, we assessed whether or not adding sex into the model reduced AIC. The order in which variables were considered for the models (OHS or OKS, then age and then sex) was specified in advance of data analysis; we hypothesised that disease severity (i.e. OHS and OKS) would be most important, followed by age (because it is a proxy for comorbidities) and finally sex (because we had no prior hypotheses about how this variable would affect referrals).
The data for patients attending the hub were also used to obtain two measures of the current threshold OHS and OKS for referral. First, the absolute threshold was determined simply as the highest OHS and OKS observed among the patients who were referred for surgical assessment. Second, the logistic threshold was estimated from the regression output for the model selected using the process described in the previous paragraph: the model coefficients were used to calculate the OHS or OKS at which the probability of being referred for surgical assessment is 50%.
Cost inputs
We assumed that each 40-minute hub attendance cost £58, whereas surgical assessment visits cost £132 (see Online Supplement 8). The costs of GP consultations and the hub triage process were excluded from the analysis because they occurred before the hub attendance at which the ACHE tool is assumed to be used. Similarly, the cost of radiography, imaging, physiotherapy, injections, weight-loss programmes, missed appointments and referrals to other clinics was excluded because there is no reason to expect the introduction of the ACHE tool to affect the proportion of patients requiring these resources.
Results
Results of the musculoskeletal hub audit
Results of the audit for knee replacement
Records were reviewed for 616 patients referred to the hub with knee symptoms, of whom 315 osteoarthritis patients aged ≥ 50 years were included in the analysis (Figure 39). Of the 18 patients aged ≥ 50 years who were excluded because of conditions other than osteoarthritis, five had rheumatoid arthritis, four had bone or joint infections, two had injuries, one had gout, one had a chondroid lesion, one had no mechanical symptoms, one had quadriceps rupture, one needed limb reconstruction and two had arthritis secondary to fracture.
FIGURE 39.
Patient flow diagram for patients referred with knee symptoms.
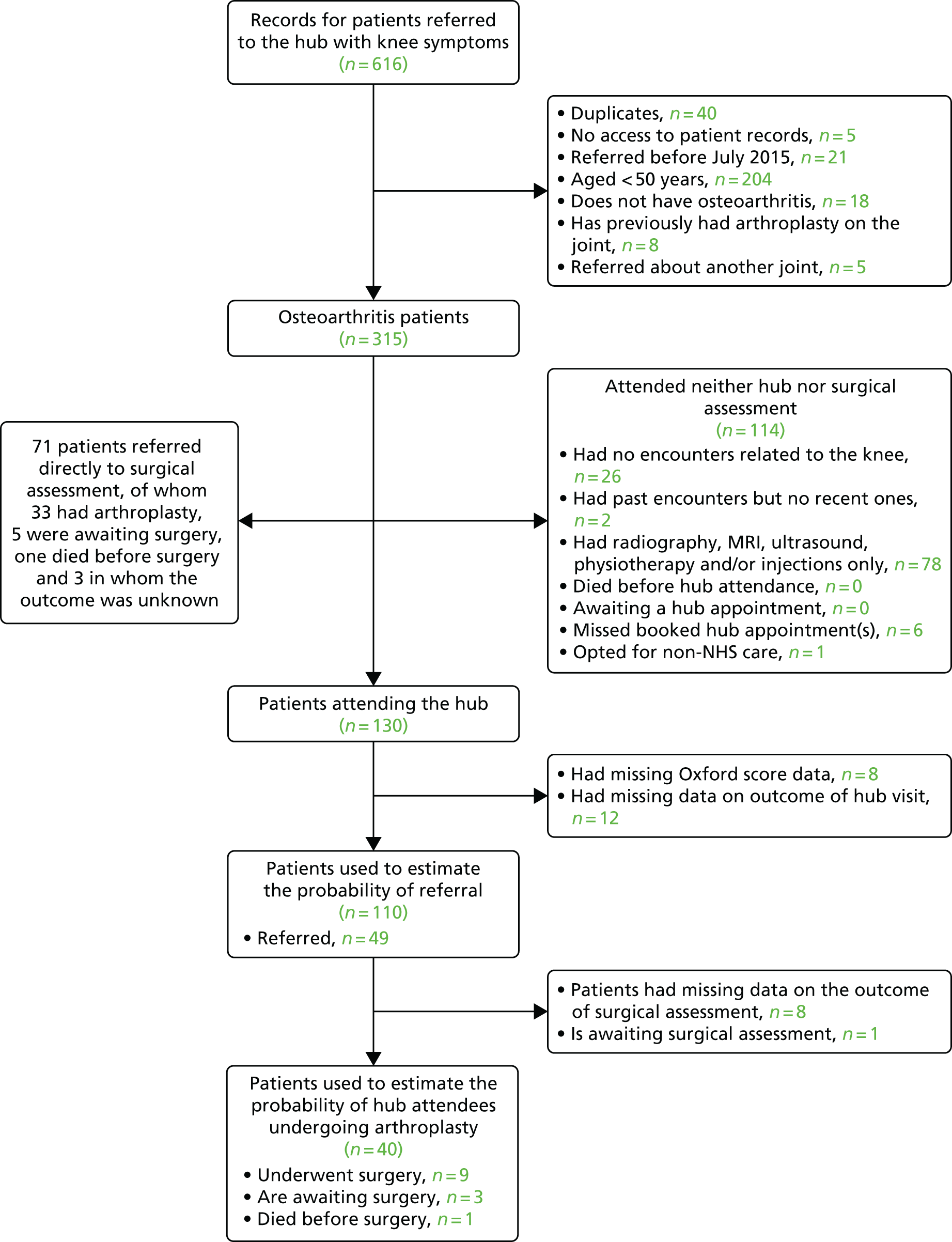
Of the patients analysed, 44% (130/315) attended face-to-face consultations at the hub and 23% (71/315) were referred directly to surgical assessment based on the hub triage.
Among the 68 patients who were referred directly to surgical assessment and had outcomes recorded, 56% (38/68) underwent or were awaiting surgery. Data on the OKS measured at the surgical assessment visit were available for 27 of these patients registered in the Javlin study (URL: www.hra.nhs.uk/planning-and-improving-research/application-summaries/research-summaries/local-javlin-registry-v10/; accessed 6 February 2019) who had a mean OKS of 15 (range 1–30). Among the 22 of these patients who went on to have knee arthroplasty, the mean OKS was 14 (range 1–28), non-significantly higher than the average for the five patients who did not have arthroplasty (mean score 21, range 10–30; p = 0.08).
Five of the 114 patients who did not attend face-to-face consultations at the hub or surgical assessment were referred for physiotherapy, 60 had radiography, 16 had MRI and two had ultrasounds. Ten patients had two of these contacts.
Among the 110 patients attending the hub who had complete data, the average OKS was 21 (range 1–48), which was significantly higher than the average for patients undergoing arthroplasty nationally (mean score 18.43; p = 0.015) (Figure 40), but not significantly different from that in Oxfordshire (mean score 19.6; p = 0.134). 162,182–184,194,195 Of the 110 hub attendees, 49 (45%) were referred. OKS values were significantly lower for those patients who were referred (mean score 18, range 3–41) than for those who were not referred (mean score 23, range 1–48; p = 0.013). The highest OKS at which patients were referred to surgical assessment was 41, although the second-highest score was 32. Logistic regression analyses suggested that the odds of being referred for surgical assessment varied with OKS, although allowing for age and gender worsened model fit. This analysis suggested that the odds of being referred to surgical assessment decreased by 4.7% for each 1-point increase in OKS (p = 0.019), and suggested that the OKS at which patients have a 50% chance of being referred is just 16.
FIGURE 40.
Distribution of OKSs for patients attending the hub with knee pain compared with the distribution of patients undergoing knee arthroplasty in England in PROMs/HES data (2009–15).

Among the 40 hub attendees who were referred to surgical assessment and had data on the outcome of that consultation, 30% (12/40) underwent or were awaiting arthroplasty surgery: significantly fewer than among those patients directly referred to surgical assessment [56% (38/68); p = 0.0092]. Among the sample of hub attendees, OKS, age and gender did not predict which patients would undergo arthroplasty. This may reflect the role of comorbidities and patient choice in the decisions made at the surgical assessment. For two patients (who both attended the hub), the medical notes indicated that it was necessary for the patients to reduce weight before knee replacement could be conducted.
Results of the audit for hip replacement
We reviewed records for 1022 patients referred to the hub with hip symptoms, of whom 607 osteoarthritis patients aged ≥ 50 years were included in the analysis (Figure 41). Among the 19 patients aged ≥ 50 years who were excluded because they had conditions other than osteoarthritis, nine had rheumatoid arthritis, three had fractures, two had bone or joint infections, two needed limb reconstruction, one had a sports injury, one had no mechanical symptoms and one had hip symptoms caused by previous spinal surgery.
FIGURE 41.
Patient flow diagram for patients referred with hip symptoms.
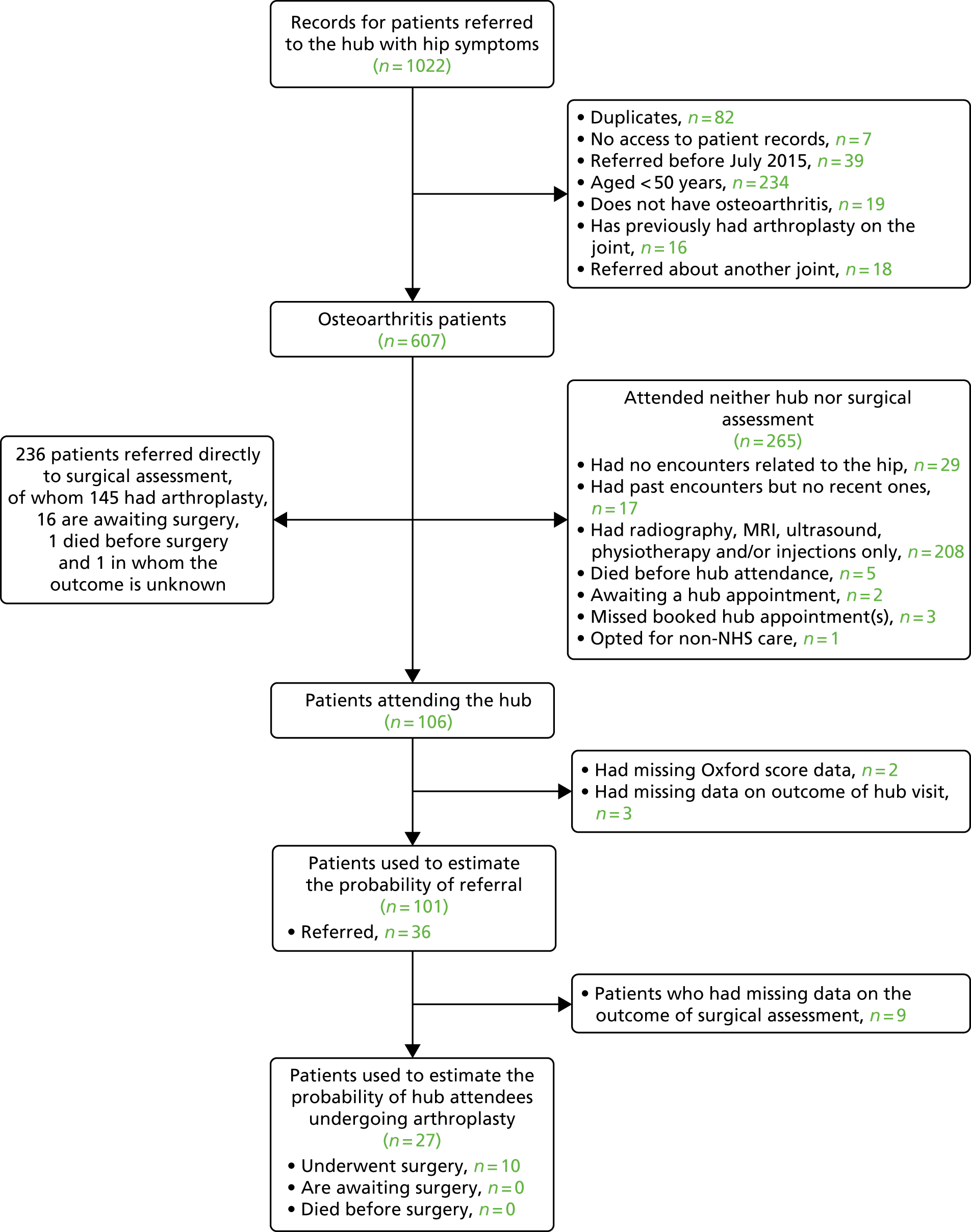
Face-to-face consultations at the hub were attended by 17% of patients (106/607), whereas 39% (236/607) were referred directly to surgical assessment. Of the 235 patients who were directly referred to surgical assessment and had known outcomes, 69% (161) had hip arthroplasty or were awaiting surgery. Because Javlin recruited only knee patients in the period covered by our audit, no OHS data were available on this patient group.
Of the 265 patients (44% of the total) who attended neither the hub nor the surgical assessment, six had physiotherapy, four were referred to other clinics, 10 had hip injections, 186 had radiography, eight had MRI and nine had ultrasound. Twenty-nine patients had two or more of these contacts.
Across the 101 hub attendees with complete data, the mean OHS was 23.7 (range 5–46), which was significantly higher than the average for hip arthroplasty patients nationally (mean score 17.5; p < 0.001) (Figure 42) and in Oxfordshire (mean score 18.7; p < 0.0001). 162,182–184,194,195 Overall, 36% of hub attendees (36/101) were referred for surgical assessment.
FIGURE 42.
Distribution of OHSs for patients attending the hub with hip pain compared with the distribution of patients undergoing hip arthroplasty in England in PROMs/HES data (2009–15).

The mean OHS was non-significantly lower for patients who were referred (mean score 21, range: 5–44) than for patients who were not referred (mean score 25, range 5–46; p = 0.057). One patient was referred with a score of 44, although the second-highest score was 35. Logistic regression analysis suggested that the odds of referral varied with OHS and gender but not age. Each 1-point increase in OHS reduced the odds of referral by 3.9% (p = 0.062). The analysis also suggested that men attending the hub were twice as likely to be referred than women, although the difference did not reach statistical significance (p = 0.147). Women were more likely to attend the hub.
Among the hub attendees who were referred for surgical assessment, 37% (10/27) underwent hip arthroplasty. Logistic regression analysis suggested that OHS, age and gender did not predict which patients would undergo arthroplasty.
Medical records for one patient attending the hub and eight patients who were directly referred to surgical assessment indicated that the patients needed to reduce weight. Of these, two patients had surgery delayed because they needed to lose weight, after they had been directly referred to surgical assessment and then referred for hip arthroplasty.
Anticipated patient numbers, budget impact and cost-effectiveness of the Arthroplasty Candidacy Help Engine
Knee arthroplasty
We extrapolated the hub data to estimate the number of patients who may be referred to secondary care with knee pain in England by dividing the number of knee arthroplasty procedures conducted on patients with different OKSs, ages and genders by the probability of hub attendees undergoing surgery. This suggested that GPs across England refer > 400,000 patients aged ≥ 50 years to secondary care with knee osteoarthritis symptoms each year (Figure 43). Of these, > 170,000 patients might be expected to attend the hub if all CCGs followed a treatment pathway similar to that in Oxfordshire. Among the hub attendees, there may be around 80,000 referrals and 24,000 arthroplasty procedures, suggesting that around 53,000 of the 76,600 primary knee arthroplasty operations conducted for osteoarthritis each year are done on patients who were directly referred to surgical assessment.
FIGURE 43.
Number of patients predicted to be referred with knee osteoarthritis symptoms in England.

We also modelled the impact that the introduction of the ACHE tool might have on patient numbers, costs and QALYs (Table 60). As discussed in Methods, we assumed that the ACHE tool would only be used in face-to-face hub consultations and would not influence decisions about referrals or surgery in other settings. We also assumed that 26% of all hub attendees (half of the 53% of hub attendees who are not currently referred to surgical assessment) would not be referred regardless of the ACHE tool.
Outcome | Current practice | The ACHE tool referring patients with a specified probability of achieving a good outcome | Optimal health economic threshold | |||||
---|---|---|---|---|---|---|---|---|
85% | 80% | 70% | 60% | 50% | Taking into account assessment pathway costs | Ignoring assessment pathway costs | ||
Range of threshold OKSs across age and gender groups | N/A | 1–20 | 2–27 | 27–32 | 32–35 | 34–37 | 40–44 | 41–44 |
Number of attendances at the surgical outpatient visit | 80,211 | 69,702 | 103,731 | 121,507 | 127,545 | 130,019 | 131,965 | 131,967 |
Number of arthroplasty procedures conducted | 24,063 | 20,911 | 31,119 | 36,452 | 38,263 | 39,006 | 39,589 | 39,590 |
Total cost of the assessment pathway (£) | 20,397 | 19,005 | 23,511,404 | 25,865,310 | 26,664,801 | 26,992,441 | 27,250,072 | 27,250,414 |
10-year cost excluding the assessment pathway (£) | 1,077,817 | 1,067,131 | 1,091,969 | 1,105,648 | 1,112,839 | 1,116,290 | 1,119,389 | 1,119,394 |
Total cost (£) | 1,098,213 | 1,086,137 | 1,115,481 | 1,131,513 | 1,139,503 | 1,143,282 | 1,146,639 | 1,146,645 |
Difference in cost vs. current practice (£) | N/A | –12,077 | 17,268 | 33,300 | 41,290 | 45,069 | 48,426 | 48,431 |
10-year QALYs | 564,744 | 568,033 | 585,592 | 594,130 | 596,303 | 597,212 | 597,855 | 597,855 |
Difference in QALYs vs. current practice | N/A | 3289 | 20,848 | 29,385 | 31,559 | 32,468 | 33,111 | 33,111 |
Net health benefit (QALYs) | 509,834 | 513,726 | 529,818 | 537,554 | 539,328 | 540,048 | 540,523 | 540,523.21 |
Difference in net health benefit vs. current practice | N/A | 3892 | 19,984 | 27,720 | 29,494 | 30,214 | 30,689 | 30,689 |
ICER vs. current practice | N/A | Dominant | £828 | £1133 | £1308 | £1388 | £1463 | £1463 |
ICER vs. next best non-dominated option | N/A | Dominant | £1671 | £1878 | £3677 | £4157 | £5220 | £20,470 |
This analysis suggested that introducing the ACHE tool is likely to substantially increase the number of referrals, the number of arthroplasty procedures, costs and health benefits. The ACHE tool was predicted to be cost-effective compared with current practice if the value the NHS places on each QALY is £20,000; for example, using the ACHE tool to identify patients with ≥ 70% of a good outcome (which is equivalent to an OKS threshold of 32 for 60- to 79-year-old men and 30 for 60- to 79-year-old women) would lead to an additional 122,000 surgical assessments and 36,000 additional knee arthroplasty procedures. Taking account of the patients who were directly referred to surgical assessment (and for whom it is assumed that the ACHE tool would not be used), this equates to a 24% increase in the number of surgical assessments and a 16% increase in the number of knee arthroplasty procedures. Conducting these additional operations would cost an additional £33 million compared with current practice, but would gain > 29,000 healthy years or QALYs over the 10-year time horizon. Compared with current practice, this strategy costs £1133 per QALY gained: well below the £20,000–30,000 that the NHS is typically willing to pay to gain 1 QALY. 98 Taking into account the improved health for knee arthroplasty patients and the health forgone by spending additional NHS money on knee arthroplasty rather than other services, using the ACHE tool with a 70% cut-off point would gain the equivalent of 28,000 QALYs.
The number of referrals, procedures, costs and benefits increase as the capacity-to-benefit threshold is decreased. Because the probability of a good outcome from TKA is < 85% for patients aged < 60 years who have an OKS of ≥ 2 and for older patients with an OKS of 19–21 and above, referring only patients with ≥ 85% chance of a good outcome would reduce the number of referrals and operations and save money but would also reduce the health benefits.
Applying the health economic thresholds estimated in Chapter 7 would increase the number of referrals and operations further, but would gain 33,000 QALYs compared with current practice and gain 3700 more QALYs than referring patients who had a ≥ 70% chance of a good outcome. After taking into account the health benefits that are forgone by spending money on knee arthroplasty rather than other NHS services, using the health economic thresholds would gain around 31,000 QALYs compared with current practice.
Hip arthroplasty
Applying the same methods to hip data suggests that GPs in England refer around 235,000 patients with hip osteoarthritis symptoms each year (Figure 44). Of these, around 41,000 would be expected to attend the musculoskeletal hub if all CCGs followed the same referral pathway as that followed in Oxfordshire. Around 18,000 hub attendees may be referred for surgical assessment, of whom around 7000 undergo hip arthroplasty. However, the NOC hub data suggest that around 90% of hip arthroplasty procedures (around 63,000 operations nationally) are conducted on patients who were referred directly to surgical assessment without attending the hub.
FIGURE 44.
Number of patients predicted to be referred with hip symptoms in England.

The model predicts that introducing the ACHE tool into clinical practice would increase the number of referrals, the number of hip arthroplasty procedures, costs and health benefits (Table 61). Numbers of referrals and operations, costs and health benefits increase as the threshold for referral is relaxed. However, all strategies are cost-effective compared with current practice if the NHS is willing to pay ≥ £4000 per QALY gained.
Outcome | Current practice | The ACHE tool referring patients with a specified probability of achieving a good outcome | Optimal health economic threshold | |||||
---|---|---|---|---|---|---|---|---|
85% | 80% | 70% | 60% | 50% | Taking into account assessment pathway costs | Ignoring assessment pathway costs | ||
Range of threshold OHS values across age and gender groups | N/A | 22–31 | 26–33 | 31–36 | 34–38 | 36–39 | 43–45 | 43–45 |
Number of attendances at the surgical outpatient visit | 17,793 | 27,762 | 29,846 | 31,145 | 31,720 | 31,884 | 32,213 | 32,213 |
Number of arthroplasty procedures conducted | 6590 | 10,282 | 11,054 | 11,535 | 11,748 | 11,809 | 11,931 | 11,931 |
Total cost of the assessment pathway (£) | 4,690,561 | 6,010,730 | 6,286,718 | 6,458,609 | 6,534,762 | 6,556,546 | 6,600,029 | 6,600,029 |
10-year cost excluding the assessment pathway (£) | 163,711,246 | 180,550,234 | 184,214,155 | 186,503,073 | 187,529,122 | 187,827,262 | 188,423,603 | 188,423,603 |
Total cost (£) | 168,401,807 | 186,560,964 | 190,500,872 | 192,961,683 | 194,063,884 | 194,383,808 | 195,023,632 | 195,023,632 |
Difference in cost vs. current practice (£) | N/A | 18,159,157 | 22,099,066 | 24,559,876 | 25,662,078 | 25,982,001 | 26,621,825 | 26,621,825 |
10-year QALYs | 123,674 | 137,629 | 138,976 | 139,718 | 140,038 | 140,129 | 140,288 | 140,288 |
Difference in QALYs vs. current practice | N/A | 13,955 | 15,302 | 16,043 | 16,364 | 16,455 | 16,614 | 16,614 |
Net health benefit (QALYs) | 115,254 | 128,301 | 129,451 | 130,070 | 130,335 | 130,410 | 130,537 | 130,536.95 |
Difference in net health benefit vs. current practice | N/A | 13,047 | 14,197 | 14,815 | 15,080 | 15,156 | 15,283 | 15,283 |
ICER vs. current practice | N/A | £1301 | £1444 | £1531 | £1568 | £1579 | £1602 | £1602 |
ICER vs. next best non-dominated option | N/A | £1301 | £2925 | £3316 | £3444 | £3516 | £4024 | a |
Using the ACHE tool to identify patients with a ≥ 70% chance of a good outcome from hip arthroplasty would result in around 13,000 additional referrals to surgical assessment and around 5000 additional arthroplasty procedures in England each year. However, because we assumed that the ACHE tool would have no impact on the 90% of hip arthroplasty procedures conducted on patients who did not attend the hub, the total number of operations would increase by only 7%. This strategy would increase the total cost of referrals and operations by around £25M, but would gain 16,000 QALYs. Even greater health benefits and greater cost-effectiveness could be achieved using the economic thresholds described in Chapter 7.
Impact of assessment pathway costs on economic thresholds
We also re-estimated the economic thresholds presented in Chapter 7 to allow for the cost of the additional surgical assessments that are conducted as a result of using different thresholds. This demonstrated that taking account of the additional surgical assessments has a negligible impact on the economic thresholds. The threshold for knee replacement reduced by 1 point for men aged 90 years but remained the same for all other demographic groups (Table 62). The threshold for hip replacement was unaffected by taking into account the cost of surgical assessments (Table 63). Applying these slightly revised thresholds rather than those described in Chapter 7 would slightly reduce the number of referrals, operations and costs, slightly increase net benefits and have a negligible impact on health (see Tables 60 and 61).
Preoperative OKS (selected values only) | Cost/QALY | ||||
---|---|---|---|---|---|
Age (years) | |||||
50 | 60 | 70 | 80 | 90 | |
0 | £2169 | £1865 | £2121 | £3029 | £5580 |
1 | £1598 | £1454 | £1721 | £2521 | £4699 |
2 | £1236 | £1182 | £1449 | £2173 | £4100 |
4 | £788 | £833 | £1105 | £1745 | £3386 |
5 | £636 | £714 | £992 | £1612 | £3177 |
6 | £512 | £619 | £905 | £1515 | £3033 |
15 | Dominant | £306 | £765 | £1604 | £3587 |
18 | £19 | £389 | £958 | £2012 | £4549 |
19 | £58 | £446 | £1060 | £2207 | £4995 |
23 | £466 | £873 | £1700 | £3321 | £7488 |
24 | £643 | £1031 | £1916 | £3669 | £8246 |
25 | £848 | £1207 | £2146 | £4026 | £9009 |
27 | £1323 | £1591 | £2618 | £4703 | £10,374 |
28 | £1575 | £1785 | £2841 | £4990 | £10,890 |
29 | £1825 | £1973 | £3044 | £5225 | £11,257 |
30 | £2064 | £2150 | £3224 | £5406 | £11,472 |
31 | £2288 | £2313 | £3379 | £5537 | £11,555 |
37 | £3527 | £3236 | £4260 | £6245 | £11,907 |
38 | £3843 | £3483 | £4560 | £6623 | £12,562 |
39 | £4275 | £3822 | £5003 | £7235 | £13,742 |
40 | £4908 | £4313 | £5692 | £8254 | £15,875 |
41 | £5919 | £5075 | £6845 | £10,077 | £20,041 |
42 | £7742 | £6377 | £9019 | £13,841 | £30,019 |
43 | £11,817 | £8963 | £14,201 | £24,683 | £74,302 |
44 | £27,326 | £15,932 | £38,125 | £188,150 | Dominated |
45 | Dominated | £73,897 | Dominated | Dominated | Dominated |
46 | Dominated | Dominated | Dominated | Dominated | Dominated |
Threshold (see Chapter 8) | 43 | 44 | 43 | 42 | 40 |
Threshold (see Chapter 7) | 43 | 44 | 43 | 42 | 41 |
Preoperative OHS (selected values only) | Cost/QALY | ||||
---|---|---|---|---|---|
Age (years) | |||||
50 | 60 | 70 | 80 | 90 | |
0 | £186 | £420 | £601 | £1048 | £2119 |
3 | £681 | £744 | £843 | £1169 | £1958 |
12 | £1013 | £983 | £1016 | £1324 | £2199 |
13 | £1083 | £1042 | £1078 | £1389 | £2279 |
14 | £1159 | £1110 | £1147 | £1477 | £2395 |
15 | £1243 | £1186 | £1224 | £1576 | £2546 |
16 | £1334 | £1270 | £1310 | £1687 | £2723 |
17 | £1432 | £1359 | £1403 | £1809 | £2922 |
19 | £1660 | £1568 | £1619 | £2094 | £3388 |
20 | £1787 | £1685 | £1739 | £2254 | £3653 |
21 | £1917 | £1809 | £1867 | £2424 | £3939 |
23 | £2197 | £2070 | £2136 | £2786 | £4550 |
24 | £2338 | £2201 | £2271 | £2968 | £4859 |
25 | £2474 | £2327 | £2401 | £3143 | £5158 |
28 | £2810 | £2636 | £2719 | £3562 | £5873 |
29 | £2887 | £2708 | £2790 | £3652 | £6024 |
30 | £2945 | £2761 | £2843 | £3714 | £6129 |
31 | £2986 | £2799 | £2879 | £3752 | £6190 |
40 | £3693 | £3457 | £3539 | £4577 | £7576 |
41 | £4095 | £3832 | £3934 | £5129 | £8570 |
42 | £4755 | £4445 | £4586 | £6069 | £10,327 |
43 | £5917 | £5519 | £5746 | £7832 | £13,828 |
44 | £8252 | £7654 | £8125 | £11,837 | £22,963 |
45 | £14,420 | £13,135 | £14,704 | £26,690 | £82,853 |
46 | £54,573 | £43,824 | £71,107 | Dominated | Dominated |
47 | Dominated | Dominated | Dominated | Dominated | Dominated |
Threshold (see Chapter 8) | 45 | 45 | 45 | 44 | 43 |
Threshold (see Chapter 7) | 45 | 45 | 45 | 44 | 43 |
In practice, the number of arthroplasty procedures that CCGs or hospitals can commission or conduct is likely to be limited by the available budget and the number of surgeons, operating theatres and beds. In this resource-constrained environment, it is therefore extremely useful to be able to prioritise patients, such that the finite number of operations is conducted on the patient groups that have the greatest capacity to benefit and for whom treatment would be most cost-effective. Therefore, we further stratified the patient groups for whom arthroplasty is cost-effective (i.e. costs < £20,000 per QALY gained) to indicate those patient groups that would be the most cost-effective for arthroplasty if we wished to keep the total number of operations the same as in 2014–15 (76,617 knee replacements and 69,313 hip replacements)154,165 or increase or decrease this number by up to 15%. This analysis assumed that the number of operations conducted on patients referred directly to surgical assessment remains the same.
This analysis suggested that if we were to limit the number of primary knee arthroplasty procedures conducted for osteoarthritis to 88,109 (a value 15% greater than the number conducted in 2014–15), it would be most cost-effective to refer those hub attendees for whom arthroplasty costs < £4262 per QALY gained (Table 64). This would equate to a threshold OKS of 39 for 60-year-olds and 16 for 90-year-olds (see Table 62). If we were to apply the ACHE tool at the hub in such a way as to restrict the number of procedures to the same number conducted in 2014–15 (76,617), it would be most cost-effective to refer those for whom arthroplasty costs < £2090 per QALY gained, which would result in restrictions for those patients with very low OKSs as well as those with moderate to high OKSs. This result implies that the value that the NHS currently places on QALYs gained through arthroplasty is £2090 per QALY gained.
Percentage change in the total number of operations compared with 2014–15 | Total number of primary arthroplasty procedures conducted in England each year | Number of such operations conducted on hub attendees | ICER for referral to surgical assessment (vs. no referral) that would produce this number of referrals (£) | Maximum Oxford Hip or Knee Score at which a 70-year-old woman would be treated | The minimum capacity to benefit that is implied by this threshold (%) |
---|---|---|---|---|---|
Knee arthroplasty | |||||
+15% | 88,109 | 35,556 | 4262 | 35 | 58 |
+10% | 84,278 | 31,725 | 3345 | 27 | 78 |
+5% | 80,448 | 27,894 | 2654 | 24 | 81 |
0% | 76,617 | 24,063 | 2090 | 21 | 84 |
–5% | 72,786 | 20,232 | 1792 | 18 | 86 |
–10% | 68,955 | 16,402 | 1527 | 16 | 87 |
Hip arthroplasty | |||||
+5% | 72,779 | 10,055 | 2771 | 29 | 85 |
+2.5% | 71,046 | 8323 | 2186 | 23 | 91 |
0% | 69,313 | 6590 | 1703 | 19 | 92 |
–2.5% | 67,580 | 4857 | 1356 | 16 | 93 |
–5% | 65,848 | 3124 | 1138 | 14 | 94 |
A similar analysis was conducted for hip arthroplasty (see Table 64). However, because only 17% of hip patients attend the hub (vs. 41% of knee patients), we examined only ±5% changes in the number of operations. If we wished to limit the increase in the number of hip arthroplasty procedures conducted to 72,779 (a 5% increase on 2014–15), it would be cost-effective to operate on patients for whom arthroplasty referrals cost < £2771 per QALY gained, which equates to a threshold OHS of 29 for 70-year-old women, or referring only those patients with a ≥ 85% chance of a good outcome.
Discussion
Summary of the findings and the implications for commissioners and hospitals
This analysis demonstrates that using the ACHE tool in a musculoskeletal hub to identify potential candidates for arthroplasty is likely to be cost-effective and to substantially improve population health, but would increase the number of referrals and arthroplasty procedures and increase costs to the NHS.
We also demonstrated that referrals, operations, costs, health improvements and net benefits increase as referral criteria are relaxed to allow patients with a lower probability of achieving a good outcome to be referred. Setting stringent referral criteria, such as requiring patients to have a ≥ 80% chance of a good outcome, would limit the increases in costs and numbers of operations, but have a substantial opportunity cost in terms of the health forgone compared with allowing patients with a lower chance of a good outcome to undergo surgery. The most cost-effective strategy considered was to use the economic thresholds shown in Tables 62 and 63. The economic thresholds shown in Tables 62 and 63 take account of the additional cost of conducting additional surgical assessments and, therefore, provide a more comprehensive assessment of cost-effectiveness than those shown in Tables 57 and 58. However, Tables 62 and 63 incorporate additional assumptions around the proportion of patients attending surgical assessment who will go on to have arthroplasty, which is not propagated through the analysis. Nonetheless, this analysis confirms the robustness of the base-case economic evaluation, with thresholds changing only slightly when we take account of the cost of the surgical assessment.
If it is necessary to limit the number of operations owing to the availability of NHS funds, surgeons, operating theatres or beds, the decision grids shown in Tables 62 and 63 give an indication of which patient groups represent best value for money. However, placing any limitation on the number of operations conducted is likely to reduce the amount of health benefits that can be produced with the available funds. For example, reducing the number of operations conducted by 5% compared with current numbers means that we would only operate on patients for whom knee arthroplasty costs < £2654 per QALY gained and those for whom hip arthroplasty costs < £1138 per QALY gained. By contrast, NICE states that treatments costing < £20,000–30,000 per QALY gained should be considered cost-effective and there is evidence that the threshold used in practice may be closer to £40,000 per QALY gained. 98,196 Consequently, the money saved by restricting access to arthroplasty is likely to be spent on substantially less cost-effective treatments, resulting in a net loss of health.
By contrast, there is no evidence to support the low thresholds used by many CCGs and these thresholds do not represent good value for money. 170 Referring only patients with Oxford Hip and Knee Scores of ≤ 24 would avoid 12,000 operations and save £51M compared with using the economic thresholds shown in Chapter 7, but would lose the NHS 14,000 QALYs, even after taking account of the additional QALYs that could be gained by spending the saved money on other services. Using a threshold of 18 would avoid 25,000 operations and save £92M compared with the economic thresholds, but would lose 38,000 QALYs.
The hub audit also demonstrates that Oxford Hip and Knee Scores are not the only factor taken into account when hubs consider whether or not to refer patients for arthroplasty. First, the hubs play an important role in confirming patients’ diagnoses and directing patients to other NHS services, such as rheumatology clinics or physiotherapy. In Oxfordshire, the hubs also take account of BMI and refer overweight and obese patients for weight-loss programmes. We were not able to assess the cost-effectiveness of these additional aspects of the hub service because it was outside the scope of the study.
The audit also demonstrated that only 60% of the patients referred for surgical assessment underwent arthroplasty [46% (50/108) for knees and 65% (171/262) for hips]. Many patients attended the surgical assessment but decided not to have surgery on the basis of a detailed discussion with the surgeon about the risks and benefits. However, some of the remaining patients had other surgery, such as arthroscopy, meniscectomy and injections. Many patients will be referred to the surgical assessment with the intention of having these other procedures, rather than arthroplasty.
Strengths, limitations and further research requirements
This chapter describes preliminary analyses that are intended to give a first estimate of the impact that the ACHE tool might have in clinical practice and of how this impact may vary with the selected threshold. Obtaining a more accurate estimate of the impact of the ACHE tool would require a tool to be piloted in a representative sample of general practices or hubs. The analysis projects hypothetical scenarios based on a number of assumptions that cannot be tested based on current data.
The analysis assumed that the trends observed at the NOC hub would apply nationally. In practice, there are many reasons why referral patterns may differ geographically. First, in many CCGs, patients are referred directly by GPs to surgical assessment and decision-making by GPs may differ markedly from that within a hub. Second, guidelines for referring patients for arthroplasty and clinical practice in implementing these guidelines differ substantially between CCGs. Some areas still use low threshold Oxford Hip and Knee Scores (e.g. 24),170,171,176 whereas the Thames Valley CCGs used a threshold of 32 for knee arthroplasty during the time period covered by this analysis and had abolished OHS thresholds for hip arthroplasty. 172,189 The Thames Valley CCG also impose stricter guidelines on referring overweight or obese patients for monitored weight-loss programmes before they can be referred for surgery. Oxfordshire CCG is in the highest quintile for expenditure on musculoskeletal disease, but its rate of hip replacement is around or just above the national average. 197 Preoperative EQ-5D utility and the mean change in EQ-5D utility and OHSs or OKSs following primary knee/hip arthroplasty are also similar to the national average. 198 Data from more hubs would be required to get a more accurate picture of how the ACHE tool would affect referral patterns.
Further research is needed to assess the impact and cost-effectiveness of using the ACHE tool in general practice, at the hub triage and in the surgical assessment. The analysis focused on those patients who currently attend the hub, and evaluated the impact and cost-effectiveness of using the ACHE tool during face-to-face hub consultations. There are currently no data on the Oxford Hip or Knee Scores of patients visiting their GP with knee/hip osteoarthritis symptoms, and it is not known how the ACHE tool might affect GPs’ referral decisions. As a result, it is not possible to assess the total impact of the ACHE tool; if the ACHE tool were used widely by patients and/or GPs, it could prompt GPs to refer more patients to hubs and surgical assessment, increasing the number of additional operations still further.
The ACHE tool is also likely to be extremely useful for guiding discussions about the likely risks and benefits of arthroplasty between patients and orthopaedic surgeons and may, therefore, affect the proportion of surgical assessments that result in arthroplasty. However, it is impossible to reliably assess what impact this tool would have had on patients’ and surgeons’ decisions using the current data.
It is unknown to what extent the ACHE tool would change referral patterns. In this analysis, we assumed that 50% of people who do not currently get referred by the hub would not be referred regardless of the ACHE tool, although this figure is arbitrary and a pilot study would be required to obtain evidence on this. Furthermore, although we assumed that patients above the threshold would not be referred, there may be some patients who are currently referred with high Oxford Hip and Knee Scores who would be referred regardless (e.g. patients who have significant deformity but little or no pain).
The audit included only a small sample of patients, with only around 100 hub attendees having complete data for each joint. In particular, the study included only seven patients aged ≥ 90 years and only eight hub attendees with OHSs and OKSs of ≥ 40 across hips and knees combined. Therefore, models may be extrapolating beyond the observed data.
The audit included patients who were referred to the hub during a 12-month period that ended only 1 month before data were extracted. Therefore, we may have underestimated the number of operations conducted within this cohort because some patients may still be in the care pathway and may subsequently go on to have surgery. In particular, patients are discharged from the hub when they are referred for physiotherapy or weight-loss programmes and will be referred back to the hub if their symptoms persist after completing the physiotherapy or weight-loss programme. This is likely to be the case for the 60 patients who were excluded from the analysis as they had attended surgical assessments or hub consultations before July 2015. Underestimating the number of operations within our cohort would mean that we may have overestimated the number of patients being referred to hubs nationally and overestimated the likely impact of the ACHE tool on numbers of operations, costs and QALYs.
It was difficult to reliably identify from medical records all of the patients who had previously had arthroplasty on the joint in question or who had conditions other than osteoarthritis, particularly for those patients who did not attend either the hub or the surgical assessment. Some of the patients included in the analysis may, therefore, have had other conditions or been considering revision surgery. This may have caused us to underestimate the probability that osteoarthritis patients will undergo primary arthroplasty, which would mean that we could have underestimated the likely impact of the ACHE tool.
The audit collected no data on BMI, although obesity is one of the main reasons why patients are not referred for surgery in Oxfordshire. Therefore, BMI is an omitted variable in all regression analyses. It is also unclear to what extent the introduction of the ACHE tool would affect the referral of obese patients compared with non-obese patients.
Equity implications
The results also suggest that the number of arthroplasty procedures conducted in the UK is well below the figure that would be justifiable on cost-effectiveness grounds. Indeed, the number of operations currently conducted would only be justifiable if the maximum the NHS was willing to pay for each QALY gained through arthroplasty was just £1703–2100. Because there is evidence that the NHS routinely pays £20,000 or even £40,000 per QALY gained for patients with other conditions, this suggests that it would be better value for money to reduce spending on other conditions in order to conduct additional arthroplasty procedures. 98,196 However, other factors can also play a role in the funding of health care, such as equity considerations.
As described in Chapter 5, Equity implications, the introduction of the ACHE tool could help make access to surgical assessments more equitable by removing postcode prescribing. We also found that at a £20,000-per-QALY ceiling ratio, economic thresholds do not vary with gender and that there is therefore no economic benefit to setting different thresholds for men and women. However, there were some differences between genders at lower ceiling ratios. Based on the social value judgements used by NICE,98 there is unlikely to be a justification for distinguishing between individuals on the basis of gender, as the benefits and risks are very similar for men and women.
Age also had a relatively small impact on the economic threshold. Setting different economic OKS thresholds for different age groups would result in five fewer knee arthroplasty procedures and increase the net health benefit from the ACHE tool by 1 QALY across England each year. For hip arthroplasty, varying the economic threshold with age would result in three fewer hip arthroplasty procedures, gaining 0.08 QALYs across England each year. Because the benefits of setting different thresholds for different age groups are negligible, it may therefore be simpler and more equitable to set a single threshold across all age groups. Furthermore, as discussed in Chapter 5, Equity implications, there are few data for patients in the oldest age groups, and it may be possible to identify patients who are likely to be at a high risk or have fewer benefits from arthroplasty based on physical activity, general health and comorbidities, rather than age.
Conclusion
The analyses described in this chapter demonstrate that many patients with relatively low Oxford Hip and Knee Scores are not currently referred for surgical assessment. In many cases, this is likely to be appropriate, if the patient is medically unfit or has chosen not to have surgery. However, for other patients, surgery may be appropriate, cost-effective and have a high chance of a good outcome.
The ACHE tool is likely to be cost-effective and improve health, but leads to increases in the number of referrals and operations and a large increase in cost. Setting a high threshold Oxford Hip or Knee Score or allowing patients with a modest probability of achieving a good outcome to be referred for surgical assessment would be cost-effective and improve patient health, but produce a large increase in the number of operations. Conversely, setting a low threshold Oxford Hip or Knee Score or referring only those patients with a high probability (e.g. 80%) of a good outcome would deny cost-effective treatment to patients who are likely to have a good outcome.
Chapter 9 Evaluation of users’ opinions of the Arthroplasty Candidacy Help Engine tool (work package 3)
Overview
The aim of the study was to explore patient and GP opinion regarding the usability of the ACHE tool. To do this, we developed digital materials to demonstrate the tool to patients, the public and GPs, together with online surveys to gather opinions. Despite attempts to disseminate the survey widely, the response rate and final number of completed surveys was very low. The limited information collected did suggest some support for the ACHE tool and highlighted important issues to consider before implementation. However, this work must be viewed as a pilot evaluation and more work is required to test the use of the ACHE tool in practice.
Methods
General practitioner and patient/public survey development
Two surveys were developed: one specifically for GPs and the other for patients and the public. Each survey contained questions relating to the use of the ACHE tool in the UK setting. The surveys were developed, reviewed and revised in collaboration with (1) support from the user group, which included patients and GPs; (2) GPs; (3) quantitative and qualitative researchers; and (4) the Nuffield Patient Liaison Group (PLG), which included patient representatives and health-care professionals who consult, design and conduct surveys for the well-being of patients. The Nuffield PLG has consulted on various research projects, audits and service improvements projects in the trust. From the feedback received, it was recommended that the survey and instructional video needed to give more detail on the ACHE tool.
Participants were approached by e-mail with a request to take part in the surveys. The e-mail contained links to an instructional video regarding the ACHE tool, followed by an online ACHE tool to complete, finally followed by an acceptability/usability survey. By clicking on the usability/acceptability survey link, the patient is presented with a series of forms, using check boxes, drop-down selections and other standard form elements including free text (Table 65).
Tool | Website URL |
---|---|
GP video | https://vimeo.com/angelsharp/review/181495797/8e90d797e7 (accessed 29 January 2019) |
GP ACHE tool | www.ndorms.ox.ac.uk/clinical-trials/current-trials-and-studies/ache-completed/ache-tool/ache-tool-gps (accessed 29 January 2019) |
GP usability survey | https://cview.pro-mapp.com/9500-achegp#/ (accessed 29 January 2019) |
Patient video | https://vimeo.com/angelsharp/review/181484159/472f66f6c1 (accessed 29 January 2019) |
Patient ACHE tool | www.ndorms.ox.ac.uk/clinical-trials/current-trials-and-studies/ache-completed/ache-tool/ache-tool-patients (accessed 29 January 2019) |
Patient usability survey | https://cview.pro-mapp.com/9500-achepatient#/ (accessed 29 January 2019) |
The Arthroplasty Candidacy Help Engine online tool
The online ACHE tool is based on the models developed in Chapter 6, in which a patient’s preoperative Oxford Score is used to establish the probability of achieving at least a minimum level of meaningful benefit. In addition, the tool uses sex and age as additional covariables.
Piloting the surveys
Once the questions were developed, face validity and content validity assessments of the survey were judged by the ACHE user group (including patients and GPs), independent GPs and the PLG. These groups determined content validity in terms of whether or not items were relevant and representative of a usability/acceptability survey. The groups made recommendations regarding changes to the wording of questions, as well as examining the overall survey’s ability in testing the use, usefulness and acceptability of the ACHE tool for doctors and patients. Finally, the survey went to an information technology (IT) specialist for review, who specifically looked at the user experience of having to click on the video, ACHE tool and usability/acceptability survey. From this initial pilot testing, an invitation e-mail and link were sent to both patients and GPs.
Participants
Several organisations were identified from both the user group and the steering group as potential links to reach patients, the public and GPs. The General Medical Council, the Royal College of General Practitioners, Arthritis Research UK (ARUK), the local Patient Advice and Liaison Service, INVOLVE (a national advisory group that supports greater public involvement in NHS; www.invo.org.uk, accessed 6 February 2019) and, for doctors, the NIHR Clinical Research Network (CRN). These groups were all approached to collaborate in the study, allowing potential access to large numbers of doctor and patient representatives. The NIHR CRN agreed to e-mail GPs on our behalf and INVOLVE agreed to e-mail patients on our behalf. Other organisations agreed to advertise our survey and to provide a link to the survey website.
Data analysis
Numerical data were collected from closed questions and were analysed using descriptive statistics. The surveys have also been designed so that participants have the freedom to express in their own words their views in response to their answers.
Ethics approval
Ethics approval was granted for this study (research ethics committee reference 15/NE/0426).
Results
Unfortunately, we received very few completed surveys: 22 out of 271 from patient/public participants (8%) and 10 out of 348 from GPs (3%).
The results of the collected surveys are presented in Tables 66 and 67 (see Appendices 1 and 3 for additional data).
Questions | Responses (%) | |
---|---|---|
Yes | No | |
1. Do you think it would be helpful to have a standard questionnaire (ACHE tool) to help GPs decide whether a patient should see a surgeon to discuss a hip or knee replacement operation? | 100 | 0 |
2. Would you be prepared to fill out a questionnaire (ACHE tool) about your hip or knee condition to help you GP decide whether you should see a surgeon? | 100 | 0 |
3. If the questionnaire (ACHE tool) could tell us who can benefit the most from the operation, would you be happy with surgeons using the results to prioritise the waiting list? | 86 | 14 |
4. If you are not eligible for surgery, would you like to use the questionnaire (ACHE tool) yourself to track your disease at home? | 91 | 9 |
5. Where would you prefer to complete the questionnaire (ACHE tool)? | 73 (at home) | 27 (during consultation) |
6. How would you prefer to use the questionnaire (ACHE tool)? | 90 (electronically) | 10 (on paper) |
Questions | Responses (%) | |
---|---|---|
Yes | No | |
1. In your practice, would you like to use a tool to objectively assess patients need for referral for a surgical assessment? | 90 | 10 |
2. Would it be feasible for you to use the tool in the format we have created? | 90 | 10 |
3. Are your patients going through a musculoskeletal hub? | 90 | 10 |
4. Do you think this tool should be preferably used in the musculoskeletal hub instead of primary care? | 20 | 80 |
5. In what format would you prefer to use the tool? | 90 (electronically) | 10 (on paper) |
6. When do you think it would be best to ask patients to complete the questionnaire (ACHE tool)? | 60 (at home) | 40 (during consultation) |
Patients and the public
We received 22 out of a possible 271 surveys from this group, giving a response rate of 8%. Sixteen were from patients and six were from members of the public. All respondents indicated that the ACHE tool would be useful to aid the referral process. There was some preference to complete the ACHE tool at home before visiting the GP. Electronic use was supported; however, a minority of participants opted for a paper-based approach. One of the most interesting findings was general support (86%) for the tool to be used as a potential way of prioritising patients on a waiting list, although a cautious approach to this was emphasised in general comments. Of those participating, 91% felt that they would use the tool to track their disease state at home. The need for the tool to be used as part of a shared decision-making process was a common theme in comments.
General practitioners
We received very few completed surveys from GPs. Of 348 sent out, there were 10 responses, giving a response rate of 3%. This is obviously a very limited sample of GP opinion.
A majority of GPs felt that the format in which the tool was presented made it feasible for use, although there were a few comments questioning the potential for actual uptake by GPs in practice. In comparison with patients and the public, just under half of those surveyed felt that the tool should be deployed during the GP consultation compared with the tool being used at home. One comment suggested that the tool could be sent to patients as a result of the consultation via e-mail, with the form then being filled out at home. The majority of GPs surveyed were using a musculoskeletal hub for referral, but they felt that the ACHE tool would be best deployed in the primary care setting rather than a hub.
Conclusion
Owing to the very low response rates from patients, the public and GPs, it is difficult to draw strong conclusions from our findings. It was encouraging to note from the limited responses we received that patients, the public and GPs showed some support for use of the ACHE tool as a method of supporting the referral process. Debate remained as to how to deliver the tool to patients in primary care: the principal question centring around whether the tool should be completed by patients at the GP consultation or at home (prior or after the consultation). It is clear that further work is required to test the ACHE tool in practice, with more input from the potential stakeholders involved in its use.
Chapter 10 The user group
It is important to state that the establishment of a user group, and its role within the overall study, was akin to a patient and public involvement and stakeholder engagement process rather than a formal piece of stand-alone qualitative research. For this reason, the methodology and process for the user group meetings leant towards debate, common sense and consensus approach to the synthesis of ideas rather than the utilisation of formal or sophisticated qualitative methodology (i.e. there was no intention to conduct a formal Delphi exercise). The meetings were conducted as focus groups with a semistructured format. There was no intention to report separately or publish the methodology or output of the user group.
Recruitment to the user group was undertaken with care to ensure full representation. A full list of the names of the personnel attending the user group is given in Appendix 2. An emphasis was placed on patient representation. Members included those from a dedicated patient organisation at the NOC: the NOC Network and several patient representatives attended each meeting. The INVOLVE organisation was also engaged in costing patient representatives’ involvement. On completion of the project and construction of the ACHE tool, the research findings will be presented to the Patient & Research Engagement Forum at the NOC. The purpose of this group is to further involve, inform and educate patients and the public in all aspects of musculoskeletal research in Oxford to improve the relevance, quality and appropriateness of that research from the patients’ perspective. The main target audience includes local residents, charities and patient support groups, University of Oxford medical students, Oxford Brookes University health sciences students, sixth form students with an interest in medicine from local schools, patients in local GP surgeries and current/former patients of the NOC.
In addition to patient representatives from the Oxford (NOC) Patient Network and Bristol patient involvement group [Patient Experience Partnership in Research group (PEP-R)], the user group also included GPs from primary care, orthopaedic knee surgeons (also representing the British Association for Surgery of the Knee), an orthopaedic hip surgeon (representing the British Hip Society), extended practitioner physiotherapists and commissioners of hip and knee surgery from various CCGs.
There were four separate user group meetings, which were all timetabled at appropriate times in the study schedule in accordance with the protocol. Although a complete chapter reporting the entire user involvement throughout might be more cohesive and avoid short chapter reports, a decision was made for this report to integrate each user group meeting in temporal sequence in accordance with the remainder of the project. This is deemed more intuitive. Therefore, each of the meetings is reported in turn throughout the manuscript, with some reports being necessarily concise.
User group meeting 1 (work package 1: introductory meeting)
The initial user group meeting (user group meeting 1) was held on 3 April 2014. The purpose of the meeting was to introduce the project and its aims, provide an introduction of each member of the group and provide an explanation of the role and expectations for the group. The meeting also sought to provide an overview of PROMs and the current problem of thresholding for joint replacement within the NHS. Those in attendance are listed in Table 68.
Name | Role |
---|---|
Karen Barker | Clinical Director for Musculoskeletal Services at the NOC and Proposed Lead/Organiser of the Therapy Project |
Matthew Cheetham | GP at Summertown Health Centre |
Anne Clarkson Webb | Extended-scope physiotherapist working with hip and knee team in Oxfordshire |
Gill Dean | GP working with sports injury and rheumatology |
Kristina Harris | Postdoctoral Associate/Lead Co-ordinator of ACHE Project |
Jo Hewanicka | PA to Andrew Price (minute-taking) |
Laura Ingle (attended via teleconference) | GP working with sports and exercise medicine; Musculoskeletal Service, Buckinghamshire |
Kate Jackson (attended via teleconference) | GP working in sports and exercise medicine for MOD and ARUK |
Chip Johnson | Retired GP from Manchester and patient |
John Nolan (attended via teleconference) | Consultant Orthopaedic Surgeon, Norwich; President Elect of the British Hip Society |
Andrew Price | Professor and Consultant Orthopaedic Surgeon; HTA ACHE Grant PI (standing in as Chairperson for David Beard) |
Prior to attending, each member received a concise information pack about the study and its requirements. The agenda included a review of general progress of the project to date. Specifically, the group were given instructions on how to familiarise themselves with some of the various scoring systems and how the voting system would work at subsequent meetings. User group members were informed that before the next meeting the research team intended to appraise the measurement properties of selected PROMs, calculate missing measurement properties using data from existing databases and request the opinion of the user group to shortlist the best-performing instruments. The group was informed that three to four instruments were to be selected to go forward for threshold characteristics or health economic evaluation.
User group meeting 2 (work package 1: instrument shortlisting)
The second user group meeting (user group meeting 2) was held on 14 January 2015. The purpose of the meeting was to review the overall project aims, briefly remind members of the group’s role, briefly provide an overview of the characteristics of PROMs scores and review any pertinent literature from a recent search. The meeting sought, as a main aim, to share the measurement properties discovered for selected scoring systems, identify gaps in the knowledge or information available for some scores and identify which scoring systems should be ‘shortlisted’ for further consideration. A final aim of the investigators was to provide the group with sufficient information for them to assess whether or not full threshold evaluation would be possible for each instrument considering the availability of specific data sets for that specific instrument. The meeting was considered an essential component of the overall study and one in which voting for potential scores was required. The attendees are listed in Table 69.
Name | Role |
---|---|
Karen Barker | Clinical Director for Musculoskeletal Services at the NOC and Proposed Lead/Organiser of the Therapy Project |
David Beard | Professor and Co-applicant of ACHE Project; Chairperson |
Jennifer Bostock | Patient Representative |
Chad Lion Cachet | Patient Representative |
Anne Clarkson Webb | Extended-scope Physiotherapist working with hip and knee team in Oxfordshire |
Gill Dean | GP working with sports injury and rheumatology |
Kristina Harris | Postdoctoral Associate/Lead Co-ordinator of ACHE Project |
Jo Hewanicka | PA to Andrew Price (minute-taking) |
Kate Jackson | GP working in sports and exercise medicine for MOD and ARUK |
Anthony (Chip) Johnson | Retired GP from Manchester and Patient Representative |
Gillian Kempster | Patient Representative |
Jennie Kramer | Patient Representative |
Jiyang Li | PA to David Beard |
Fraser Old | Patient Representative |
Andrew Price | Professor and Consultant Orthopaedic Surgeon; HTA ACHE Grant PI |
Patricia Mary (Polly) Rubery | Patient Representative |
Mary Snow | Patient Representative |
Fiona Watt | Rheumatologist |
The literature review and methods used to screen, categorise and establish measurement characteristics in work package 1 were presented to the group by the chairperson using summary slides. The inclusion criteria for screening each score was reported to the group. The inclusion criteria consisted of scores:
-
that were defined as a standard scoring system
-
that were readily available and presently used in clinical settings or research
-
that were used for hip or knee replacement
-
that were validated for the English-language population
-
that had evidence of an obvious and appropriate validation study (i.e. on prospective data)
-
in which the validation had been carried out on a sample size of > 50 subjects/patients.
The exclusion criteria used were also presented to the group. These were scores that:
-
Were not fully patient reported or required clinician input.
-
Required technical or clinical test information such as a MRI scan or radiographic report.
-
Were not capable of demonstrating patients’ ‘capacity to benefit’ (i.e. cannot measure change). This involved discussing responsiveness with the group.
The number of studies examined and screened were provided and presented to the group in a simple flow diagram. The system and methodology used to identify records through various databases were presented. The reasons for the exclusion of certain records were also presented to the group. It was reported that a specific search was done for 67 identified instruments. Twenty-one new validation papers (in addition to 42 developmental papers) were identified. Twenty-one initially identified instruments were excluded because of failure to meet appropriate criteria. A separate PRISMA (Preferred Reporting Items for Systematic Reviews and Meta-Analyses) diagram was used to convey exclusions to the user group. The group could not use these data to make informed choices or contribute to selection. The purpose was to demonstrate the methodology used and that that it was sufficiently rigorous, and demonstrate how the summary data had been constructed.
The user group was introduced to the concepts required to assess suitability for each instrument and how these criteria had been used to grade each instrument. This included test–retest reliability, internal consistency, content validity, construct validity, responsiveness, interpretability, floor and ceiling effects, acceptability and feasibility/burden. Owing to the variety in expertise and personnel type, this was revision for some members and the introduction of new concepts for others. A simplified approach to explanation was taken by the chairperson. Some understanding of these concepts was sought and confirmed by the majority (by hand show) but members still had limited knowledge of some of the concepts.
The group were then informed of the availability of data sets for several of the scoring systems. Some scores had no available data set and the group were informed of an intention by investigators to contact the custodian of these data sets.
The group were also provided with the coding system used previously by the researchers to quantify psychometric and operational criteria for each of the scores identified in the literature. This ranged from ‘not being reported’ to ‘no evidence to support the psychometric property’ to ‘good evidence’ in favour of the stated criteria. This was a visual exercise to demonstrate summary findings from the literature. The colour-coded system key is provided in Figure 45, which shows how measurement tools could be shown to be lacking evidence of established validity. The range varied from no evidence to strong evidence. When the evidence was considered equivocal, a mixed evidence label was attached. A matrix for all the selected scores was used to present the findings to the user group.
FIGURE 45.
The key showing the colour coding and classification presented to the user group for the validity assessment matrix.
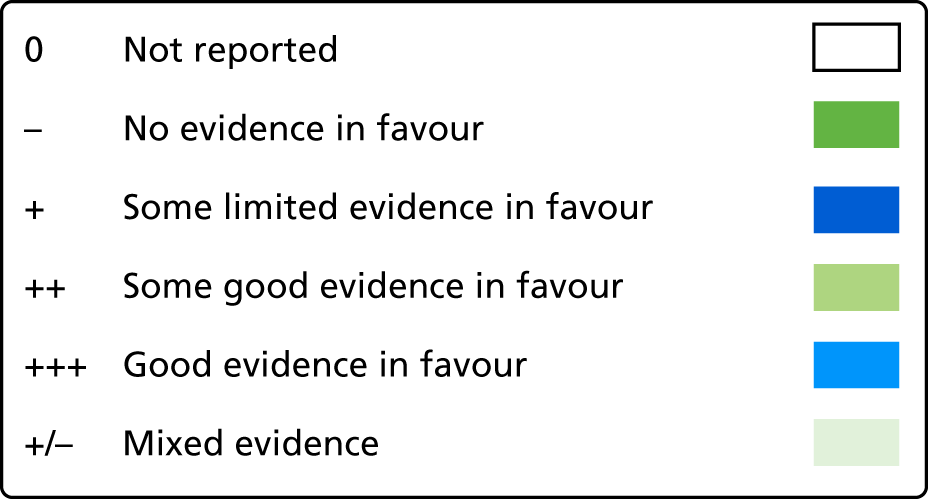
Each score had been assessed and categorised for each listed property. The categorisation was added to the assessment matrix and the allocations for each score were shown to the group. An example is provided in Table 70. Here it can be seen (for the hip scores) that the OHS fulfils many of the required properties, whereas other scores, such as the Hip Rating Questionnaire, fail to reach the required standard. All scores were graded in this way.
Instrument | HOOS | HRQ | PSI | OHS |
---|---|---|---|---|
Number of studies | 5 | 1 | 4 | 20 |
Reproducibility | ++ | + | + | ++ |
Internal consistency | + | 0 | 0 | +++ |
Validity: content | 0 | 0 | ++ | ++ |
Construct | ++ | + | ++ | +++ |
Responsiveness | + | + | ++ | +++ |
Interpretability | 0 | 0 | 0 | +++ |
Floor and ceiling/precision | + | 0 | 0 | –/+ |
Acceptability | 0 | 0 | 0 | +++ |
Data accessible | No | No | No | Yes |
Guidance about the quality of measurement characteristics for each of the instruments was provided by the chairperson. The group members were requested to agree or disagree (by a simple hand-raising exercise to vote) with the provisional decision made for the quality and number of measurement properties of each score by the research team. Opportunities were given for members of the user group to raise any concerns or ask any questions before voting. The nature of the data meant that nearly all propositions were accepted unanimously by the user group. Some clarification was required for a few of the instruments, but once clarified they were easily settled. Again, it should be noted that the exercise was not a formal qualitative study process but a seeking of agreement from the user group.
The scores were designated in accordance with their domain or type of measurement (location). These were hip scores, knee scores, lower limb scores, pain scores, utility scores, generic scores and other scores (non-defined).
At the end of the voting exercise, three scores (from a possible 78) were agreed to have sufficiently high-level evidence to support their validity and use for assessment of hip and knee replacement. These were the WOMAC score, the OHS and the OKS. Four scores were considered to be potential candidates and required further discussion: the Lower Extremity Functional Scale, the EQ-5D, SF-12 and the SF-36. Five scores were considered to be unlikely candidates but some discussion and argument was required by the group: WOMAC short form, Musculoskeletal Outcome Data Evaluation and Management System Hip and Knee Core Scale (MODEMS HK), Aberdeen IAP, WHOQOL and MHAQ.
All others scores were considered to have insufficient evidence to demonstrate validity in the TKA/THA domain.
The chairperson summarised the findings for the group and consensus was achieved for five scores to be taken forward for consideration into work package 2. These were the:
-
Oxford Knee Score (knee score).
-
Oxford Hip Score (hip score).
-
Western Ontario and McMaster Universities Arthritis Index (lower limb score).
-
Short Form questionnaire-12 items (generic score).
-
Knee injury and Osteoarthritis Outcome Score – Physical Score (knee score).
It was revealed that the KOOS had no data set from which to further explore measurement properties. It was agreed that the research team would contact the chief investigator (Dr Ewa Roos, Denmark) to see if data could be supplied to permit this evaluation. The user group were asked if they were satisfied with the process and accepted the outcome. No dissent or concern was registered.
User group meeting 3 (work package 2: threshold decisions)
Prior to the third user group meeting, a pre-user group meeting was held by the academic and analysis personnel to review the progress made and discuss the calculations for clinical and health economic thresholds. This meeting was held on 9 December 2015. The purpose was to ensure that any information conveyed to the user group at the next meeting had been fully reviewed and agreed by the study group. Those who attended are listed in Table 71.
Name | Role |
---|---|
David Beard | Professor and Co-applicant of ACHE Project; Chairperson |
Andrew Price | Professor and Consultant Orthopaedic Surgeon; HTA ACHE Grant PI |
Elena Benedetto | ACHE Project Co-ordinator (Oxford) |
Kristina Harris | Research Fellow (Oxford) |
Alastair Gray | Health Economics (Oxford) |
Helen Dakin | Health Economics (Oxford) |
Peter Eibich | Health Economics (Oxford) |
Ashley Blom | Professor of Orthopaedic Surgery (Bristol) |
Adrian Sayers | Statistician (Bristol) |
Laura Miller | Statistician (Bristol) |
Jonathan Cook | Statistician (Oxford) |
Andrew Judge | Statistician (Oxford) |
Ray Fitzpatrick | Public Health (Oxford) |
Elizabeth Gibbons | Public Health (Oxford) |
Jill Dawson | Public Health (Oxford) |
Jo Hewanicka | PA to Andrew Price |
Jiyang Li | PA to David Beard |
A review of the work to determine clinical thresholds was presented to the user group. It was found and reported that the Oxford Hip and Knee Scores had the most reliable data for estimating thresholds and subsequently these scores (OHS/OKS) were the easiest for which to apply thresholds. The absolute threshold was shown to be the determined theoretical upper limit. The method for calculating a relative threshold was discussed.
Following the review of clinical thresholds, a summary of the economic modelling was provided by Helen Dakin. Using a cost-effectiveness framework, a summary of economic modelling was provided. The economic modelling results for the OHS, OKS, WOMAC and SF-12 were reviewed by the study team. There were several assumptions and limitations and these were discussed by the group. It was agreed that the analysis could be presented to the user group.
The third user group meeting was held on 14 January 2016 (attendees are listed in Table 72). The purpose of this meeting was to review the project and report any progress, present the calculated clinical thresholds, present the newly established health-care economics and agree on the outcome scores to take forward to work package 3 in the light of the new analysis.
Name | Role |
---|---|
David Beard | Professor and Co-applicant of ACHE Project; Chairperson |
Andrew Price | Professor and Consultant Orthopaedic Surgeon; HTA ACHE Grant PI |
Alastair Gray | Director of the Health Economic Research Centre and Professor of Health Economics |
Helen Dakin | Senior Researcher in Health Economics |
Peter Eibich | Senior Researcher in Health Economics |
Jonathan Cook | Associate Professor of Statistics |
Sujin Kang | Senior Statistician |
Elena Benedetto | ACHE Project Co-ordinator |
Karen Barker | Clinical Director for Musculoskeletal Services at the NOC |
Sharon Barrington | OCCG Lead for elective care |
Kate Jackson | GP working in sports and exercise medicine for MOD and ARUK |
Gill Dean | GP working with sports injury and rheumatology |
Laura Ingle | GP in Botley |
Matthew Cheetham | GP in Summertown |
Fraser Old | Patient Representative |
Anthony (Chip) Johnson | Retired GP from Manchester and Patient Representative |
Anne Clarkson Webb | Extended-scope Physiotherapist working with hip and knee team in Oxfordshire |
Fiona Watt | Consultant Rheumatologist |
Patricia Mary (Polly) Rubery | Patient Representative |
Following an update on progress, the group was provided with a conceptual framework on which threshold calculations had been based. The group was informed that the purpose of the meeting was to review the methodology to date, review the choice of instrument/score taken forward, discuss the evidence from the measurement characteristics and health economics and ultimately agree on an absolute threshold. The group was also requested to identify what percentage of people (of the arthroplasty population) improving should be considered adequate for definition of ‘benefit’ for future calculations. A variety of potential thresholds and ranges existed and the pitfalls regarding the choice of these were also mooted.
The group was informed that, despite best efforts, it had proved impossible to obtain the KOOS data from which to establish measurement properties. The KOOS had, therefore, been excluded from further work or inclusion.
The group was informed about how thresholds for clinical benefit were calculated. The ‘absolute threshold’ was defined as the value beyond which we can be very confident that an individual could not improve despite intervention. The ‘relative threshold’ was defined as the range of values for which individuals could still improve but ‘relative’ to their pre-intervention status. Four methods can be used to define the ‘improvement’ after surgery. Each were explained in turn. It was suggested to the user group that that definition D (based on SD 0.5 – ‘medium’ ES) was most suitable for the purpose. The members approved the decision with a show of hands.
Using the adopted ‘improvement descriptor’, the results for each of the four chosen instruments were shown to the group. It was seen that the OHS/OKS had the most reliable data for estimating thresholds and are the easiest scores to apply thresholds to, but with less detail than the other two. The WOMAC and SF-12 were also shown but the calculations are based on small data sets and with a select population (clinical trial data sets). This was considered a shortcoming.
The health-care economics analysis was described to the group following the descriptions of clinical benefit thresholds (HD). In accordance with the pre-user group meeting, a summary of economic modelling was provided for the user group. Preliminary results for the OKS, OHS, WOMAC and SF-12 were shown. Assumptions and limitations were highlighted. A conclusion was that it remained impossible to identify non-cost-effective practices using the WOMAC score because of the lack of available data.
Issues that were raised by the user group included:
-
Why radiography and other tests were not included in the evaluation tool. Explanations were given that the tool had to stand alone and be easily used in primary care.
-
Whether or not the data sets identified were generally adequate for purpose. Reassurance was provided.
-
Whether or not the preoperative and postoperative data had been screened with sufficient granularity to determine the change for each range of preoperative scores. It was explained that this level of analysis could not be carried out for all the candidate scores owing to limitation in some data sets.
-
It was asked if more variables would be included in the model. The group was informed that age and gender would be included. Other factors were to be explored for inclusion if data and time allowed. Some inclusion of other factors was incorporated in the final version.
The user group agreed on the following statements:
That the methodology for the analysis on the clinical and health economics thresholds on the four selected scores is appropriate.
That the OHS/OKS are selected as the scores to be incorporated into the ACHE tool, based on the evidence presented and an understanding that large data sets (national PROM collection) are only available for these instruments.
That the absolute thresholds for OHS/OKS are evidence based and acceptable.
That the ‘relative’ threshold, which describes the percentage of probability to improve after surgery, should not be a specific set value.
The probability to improve should be used to help patients to make a decision on having or not having surgery.
Agreement was requested by a show of hands. The OKS and OHS were the scores chosen to be used for the final ACHE tool, and this was passed unanimously.
User group meeting 4 (work package 3: extended user group – completion)
The final user group meeting was held on 13 October 2016. The aim of this meeting was to demonstrate the constructed tool; identify any specific issues with the current iteration of the ACHE tool, especially from individual representative groups; and achieve project sign-off. Some exploration of how to trial the new tool (in musculoskeletal hubs or general practice) was intended. Those who attended are listed in Table 73.
Name | Role |
---|---|
Sharon Barrington | CCG |
David Beard | Professor of Musculoskeletal Sciences; Co-Director of SITU Oxford; Chairperson |
Chad Lion Cachet | Patient Representative |
Anne Clarkson-Webb | Extended-scope Practitioner |
Jonathan Cook | Senior Statistician |
Helen Dakin | Health Economist |
Gill Dean | GP |
Vida Field | Patient Representative |
Anthony Johnson | Patient Representative |
Jiyang Li | Observer |
Fraser Old | Patient Representative |
Andrew Price | Professor of Orthopaedic Surgery; Consultant Surgeon; Chief Investigator |
James Smith | Study Co-ordinator |
Fiona Watt | Consultant Rheumatologist |
Tim Wilton | President of British Orthopaedic Association |
Following the progress update, the group were shown the development pathway and mock-ups of a potential IT interface that could be used to host the new ACHE scoring system The ACHE system was shown to the user group using a prototype computer-based system to demonstrate potential and facilitate discussion on its utility.
The mock-up tool consisted of a plot of preoperative Oxford Hip and Knee Scores with a distribution of 90,000 patients (with osteoarthritis before they had a knee replacement), showing different regions (colour coded) for areas of different capacity to benefit in accordance with preoperative score (Figure 46). An example of an individual patient’s score was marked on the plot (see Figure 46). The likely capacity to benefit for that patient was shown and, therefore, implied a recommendation for management (refer on to secondary care or not). It was shown that patients with scores of < 38 can almost certainly benefit (85% chance) (green zone) and patients with scores of > 45 cannot benefit (red zone) (absolute threshold). The amber zone (38–45) is an area of more uncertain capacity to benefit.
FIGURE 46.
Distribution of the preoperative OKSs of 90,000 patients with knee osteoarthritis.

For the mock tool, the amber area was extracted and enhanced to show how the capacity to benefit varies within the equivocal range of 38–45 for a particular OKS or OHS (Figure 47).
FIGURE 47.
Demonstration of capacity to benefit when the OKS is in the equivocal range and when the example OKS is 41.

Candidacy was then expressed using three categories:
-
Yes – your score indicates that you are very likely to benefit from knee replacement.
-
No – your score indicates it is highly unlikely that you will benefit from knee replacement.
-
Maybe – your score indicates it is possible that you might benefit from knee replacement. Your clinician will discuss the chance of benefit.
The discussion about the implications of the ACHE tool in practice from a health economics perspective (HD) provoked varied responses. Historically, commissioners have used cut-off thresholds for surgery of 18 or 24 (an arbitrary threshold) and this was found to be inappropriate given the new ACHE data. It would deny cost-effective treatment. A threshold of 24 would exclude 21% of current arthroplasty operations. The work showed that for hip replacement, if a threshold of 35 was set, this might suggest that ≥ 70% of patients have the capacity to benefit from THA. Only 2% of patients currently having surgery would be excluded.
A further exercise with the group explored models for the frequency of referral to secondary care if the ACHE tool were introduced or not. It was shown that, overall, (1) the ACHE tool is likely to be cost-effective and improve health, but may lead to additional operations, (2) setting a high threshold or a low probability of benefit will be cost-effective and improve patient health, (3) the ACHE tool may increase numbers of referrals, numbers of operations and costs, (4) setting a low threshold or high probability of benefit will deny cost-effective surgery to patients who are likely to have a good outcome. These concepts were delivered to the group and discussed.
The user group expressed a variety of opinions and queries following demonstration. These could be defined in accordance with participant type: patients, clinicians (surgeons and physiotherapists) and commissioners. Each groups’ comments are described in brief in the following sections.
Patients
-
Patients were happy with the video example of the ACHE tool. They considered the tool a useful adjunct to decision-making.
-
Patients were concerned about when they would complete the assessment: before seeing the GP or while with the GP. Both are an option and field testing will provide more information. Ideally, the patients complete the tool at home to leave time for discussion with the clinician in the appointment.
-
There were some concerns that the tool could be used to restrict access to care and treatment.
-
Some patients suggested that it would be useful if the tool could be operated in a paper-based system rather than being entirely electronic.
-
There was a concern that not all GPs would use the tool, leaving an inequality of access to care.
-
Patients themselves suggested that it was possible to manipulate or ‘game’ the output to obtain surgical treatment. No solution for gaming could be offered at that time.
-
Most patients were highly satisfied with being in the decision-making process for both the design of the tool and the individual decision-making for themselves.
-
Some patients were worried that they did not fully understand the complexity of the science behind achieving a threshold score.
Clinicians
Clinicians involved in the delivery of the ACHE tool would be surgical staff in secondary care, physiotherapists (perhaps in a musculoskeletal hub) and GPs. Views expressed included the following:
-
The GPs felt that the system was useful and suited their needs providing that it was time efficient.
-
Some GPs felt that it undermined their autonomy.
-
There was some concern that IT integration may be difficult.
-
The GPs asked when and where they could get the ACHE tool, where it would be completed and if they would have sufficient time to engage with it.
-
There were strong opinions expressed in support of the evidence-based format of the tool.
-
Clinicians in secondary care asked if there could be higher levels of integration into more complex decision-making.
-
There was concern among several participants about the use of the ACHE tool for rationing purposes.
-
Several members requested that it should not replace appropriate shared decision-making instruments.
-
It was thought to be of great help for extended-scope physiotherapists working in hub environments.
Commissioners
-
Commissioners were largely supportive. It was thought to be of great help for musculoskeletal hubs.
-
They felt that the evidence-based nature of obtaining threshold values was much more suitable than current practice. This was echoed by CCG representatives.
The next stage of testing and rollout was discussed. It was proposed that a survey evaluation of potential users’ opinions of the ACHE tool (GPs and patients) would be valuable. Furthermore, the determination of GP and patient opinion regarding the content and acceptability of the completed ACHE tool would also be valuable.
On completion of the feedback session, a final summary of the ACHE project was provided to the user group (DB). The initial premise for the study was reviewed, outlining the arbitrary nature of current thresholds for arthroplasty. From the outset, the ACHE tool had the potential to show that (1) thresholding was not possible or appropriate using PROMs scores, (2) the current thresholds were adequate and correctly guiding access to secondary care and arthroplasty and (3) the current thresholds were incorrect and that the population has greater capacity to benefit from arthroplasty. The group agreed that finding 3 is likely to be the output message and this may have resource implications, which are discussed in Chapter 11.
A final round of questions was put to the group by the chairperson and generated the following consensus responses:
Would the ACHE tool (version 1) be a useful addition to the clinical pathway? [All user group members responded ‘Yes, very useful’.]
Do you think there is a role for ACHE to be deployed nationally (in hubs or primary care) to standardise management nationally? [A unanimous ‘Yes’ to this question was received.]
Do you believe that one day a tool like ACHE will be mandated nationally in the patient pathway? [Members also voted ‘yes’ to this question but there was widespread opinion from the members that more research was needed on this subject.]
The user group members reflected on the entire project and were given the opportunity to provide any final comments. They were satisfied that a useful instrument to guide hip and knee patients’ referrals to secondary care had been developed. All gave their support for rollout into clinical practice, but emphasised the requirement for further research to guide deployment and selection of relative thresholds.
Chapter 11 Discussion
The study was carried out in a sequenced way using mixed methodologies and each part of study will be considered in turn in this chapter.
Systematic review
The systematic review that was undertaken was extremely comprehensive, identifying 36 PROMs that have been previously used to assess outcomes for patients undergoing hip or knee replacement. What was notable was the very low levels of supporting literature to define the measurement properties of most of the scores in this population. Three condition- and site-specific scores were identified as the best-performing scores: WOMAC, OKS and OHS. In addition, the SF-12 score was identified as the best-performing general quality-of-life measure. The results did not show strong evidence to support the use of the EQ-5D as an outcome measure for hip and knee replacement patients. This was surprising given the widespread use of the score in the NHS to measure the outcome of hip and knee replacements (national PROMs collection). Further evidence is clearly required to define the validity of using the EQ-5D in this population and, in fact, it was recognised that all of these scores required further study to define missing measurement properties.
Calculation of measurement properties
The data sets available allowed the calculation of most of the missing measurement properties for a number of the scores identified as possible candidate scores from the systematic review. This process was dependent on the nature of the data sets available to the research team. We were able to determine further measurement characteristics on the WOMAC, OHS, OKS, SF-12 and EQ-5D. The work allowed a comprehensive assessment of the scores to be produced for the user group to assess.
Selecting a set of candidate scores
The user group process was used to select a series of candidate scores. The process generated a shortlist of candidate scores to take forward to work package 2: the OKS, OHS, WOMAC and SF-12. The KOOS-PS was also identified as an option but concern about available data was expressed. The OHS and OKS were identified as the condition- and site-specific scores with the most comprehensive evidence for adequate measurement properties. This is perhaps not surprising as they were designed to measure outcome in the specific population under consideration. However, the WOMAC score also had very encouraging properties and was an equally valid score to take forward into the candidate score group. The advantage of this score is its nature as a generic non-site-specific score and it could potentially be used as a single score in the ACHE tool. One generic non-condition-specific score was selected, the SF-12, owing to its profile of measurement properties, greatly enhanced by the calculation of measurement properties in the second part of work package 2. The EQ-5D is widely used in assessing health status change in the NHS and is routinely collected in patients undergoing hip and knee replacement, with a significant number of previous publications. However, from the published literature and our own calculations, its measurement properties, specifically within the hip and knee arthroplasty population, were deemed inadequate and it was not taken forward as a candidate score.
Calculation of threshold values
The aim of this section was to identify, for each shortlisted instrument, a set of thresholds for candidacy for arthroplasty surgery. We developed a model based on calculating thresholds based on an individual’s capacity to benefit from arthroplasty, depending on their preoperative score. This would enable determination of an upper ‘absolute threshold’ in which no meaningful benefit could be achieved by undergoing arthroplasty. In addition, ‘relative thresholds’ could be determined, in which the probability of obtaining benefit could be determined as preoperative scores varied. Prior to determining threshold values, we defined a level of improvement that would indicate that the patient had benefited from surgery. We standardised our approach so that we could accommodate all the candidate scores given the data sets and prior information available to us. We were able to determine absolute and relative thresholds for all the scores as outlined in Chapter 5 in a fair and scientific manner. Both the value of the preoperative score and the variability of change for individuals with the same score were apparent across the multitude of analyses conducted for the different scores. However, the OHS/OKS had the best data available and hence had the most robust findings.
Calculation of economic thresholds
The health economic analysis used the same data sets to assess how the cost-effectiveness of arthroplasty varied with each individual score and estimate economic thresholds. The economic evaluation described in Chapter 5 demonstrated that economic thresholds could be estimated for the OKS and OHS. However, no thresholds could be estimated for the WOMAC because TKA and THA were cost-effective at all WOMAC scores for patients aged 60 or 70 years. Thresholds for other age groups were higher than the scores observed in the available data. Thresholds for the SF-12 physical score varied with mental score and TJA was cost-effective for all patients aged < 90 years with mental scores of 70.
Selection of final score
In the third user group meeting, we were able to display the results of the threshold and health economic analysis together in a comparative manner, with the aim of selecting scores to take forward to work package 3. After considering the data and analysis undertaken, the group was in agreement that the OHS and OKS were the most appropriate scores to select. These scores had demonstrated excellent measurement properties and the data available had allowed a comprehensive assessment of both clinical and health economic thresholds. In addition, the scores are already widely used in the NHS in the assessment of hip and knee replacement, whereas the WOMAC and SF-12 scores are not.
Further analysis of the Oxford Hip and Knee Scores to produce the Arthroplasty Candidacy Help Engine tool
Having identified the OHS and OKS as the chosen scoring systems to use in the ACHE tool, it was then possible to undertake a more detailed analysis of the national NHS PROM–HES linked data set to enable the construction of the ACHE tool. Using a large NHS PROMs database enabled us to model the probability of a good outcome for patients after hip or knee replacement over the full range of preoperative scores. We were also able to determine this for patients of differing ages and genders, to some extent personalising the ACHE probability graph to the individual (Figure 48). However, the majority of factors that we were able to consider in the model did not affect the outcome to a significant degree and any improvements in prognostic value were limited.
FIGURE 48.
Specimen ACHE graphs showing the probability of a good outcome after (a) knee replacement and (b) hip replacement, depending on preoperative Oxford Hip and Knee Scores.
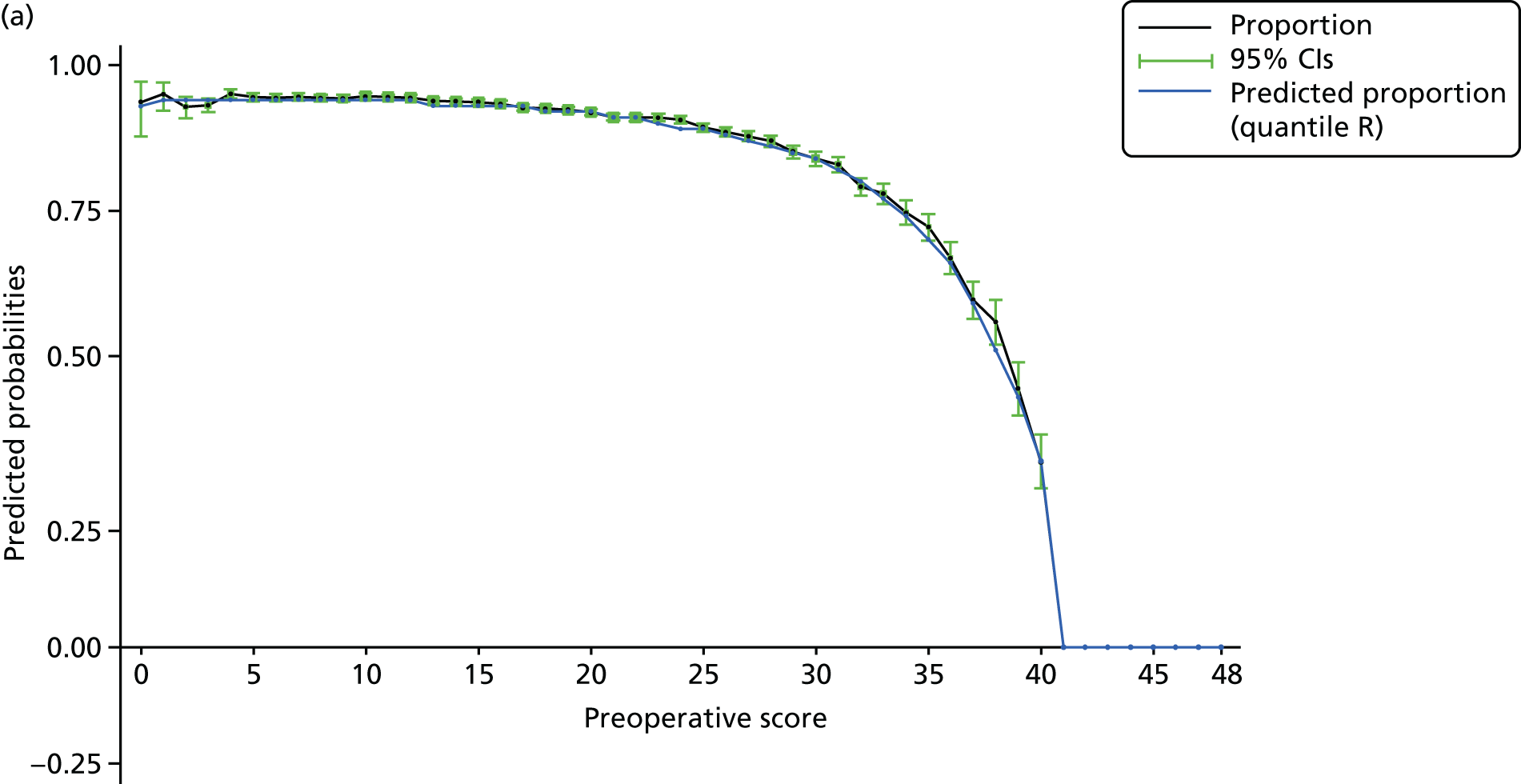

Our model calculates the probability of improvement at each preoperative score. The overall pattern of probability was similar for both hip and knee patients, although hip patients are slightly more likely to do well for any given preoperative score. The peak probability for improvement occurs for both hip and knee replacement when the preoperative score is < 20, with approximately 90% of hip and 85% of knee patients gaining significant benefit. These percentages change as the preoperative score increases, with 75% of patients obtaining benefit at scores of 35 for hip and 30 for knee. For a 50% chance of gaining benefit, the scores are 36 for knee patients and 38 for hip. The absolute thresholds (i.e. when no benefit can be achieved) were estimated to be 40 for hip replacement and 41 for knee replacement. Using this methodology, the absolute thresholds are very slightly less than those calculated earlier in the project (43 for hip and knee) using, in our view, the less useful MCID (B) criterion owing to the limitations in the information available for the other candidate scores.
The final economic evaluation for the OKS and OHS (averaging across demographic groups) demonstrated that TJA is cost-effective for > 99.9% of patients who currently undergo surgery. The analysis demonstrated that it is cost-effective to conduct TKA on patients with an OKS of ≤ 43 (95% CrI 43 to 44) and to conduct THA on patients with an OHS of ≤ 45 (95% CrI 44 to 45) (see Chapter 7). The economic thresholds varied slightly with age, but not with gender. The PSA suggested that there was relatively little parameter uncertainty around the results, whereas sensitivity analyses suggested that the results were robust to changes in key assumptions.
The economic thresholds are slightly higher than the relative thresholds, because the economic thresholds are based on the difference in mean QALYs and mean cost between patients who undergo arthroplasty and those who do not, whereas the absolute clinical thresholds are based on the probability that a patient will achieve a MIC in Oxford Hip or Knee Score following surgery (see Chapter 7, Findings of the economic evaluation). The clinical analyses estimate that patients with an OKS of ≥ 41 have a 0% chance of achieving a good outcome (defined as a 7-point increase in OKS) because the OKS cannot exceed 48. In contrast, because PROMs/HES data demonstrate that the mean change in EQ-5D utility is 0.057 among patients with an OKS of 43, the economic evaluation estimates that these patients will, on average, gain 0.46 QALYs over the 10 years following arthroplasty and that TKA costs £13,617 per QALY gained for this patient group. The apparent discrepancy arises for three main reasons. First, most of the patients who do not achieve a ‘good outcome’ (meet the improvement criterion) nonetheless have some increase in the absolute clinical scores. Second, the clinical analyses do not taken into account any cost implications. Third, the cost-effectiveness analyses are based on the change in EQ-5D utility, whereas the clinical analyses are based on change in Oxford Hip or Knee Score. Piloting and qualitative work may be needed to identify the best way to make the issues clear to patients and clinicians using the ACHE tool in clinical practice.
One additional area of controversy comprises whether or not the ACHE tool should reflect differences in the probability of a good outcome between patients of different ages and genders given the characteristics’ limited apparent prognostic value. This choice has potential implications for equity and fairness, particularly if the ACHE tool were used as part of mandatory referral criteria. When setting national guidelines, NICE is permitted to differentiate between individuals based on age or gender in their guidelines only if these are indicators of the risks or benefits of interventions. 98 Our analyses found that age had produced a limited effect on the probability of a MIC in both Oxford Hip and Knee Scores, perhaps driven by difference in those aged ≥ 80 years. Age had a significant effect on preoperative and postoperative EQ-5D scores, and the cost of primary arthroplasty also influenced the cost-effectiveness. Gender did not seem to influence the probability of a MIC in Oxford Hip and Knee Scores, but had a statistically significant effect on the cost of primary arthroplasty. However, these analyses were based on a very large data set (PROMs/HES data) in which even small differences may be statistically significant. Age and gender had an additional effect on cost-effectiveness by influencing surgical mortality, revision rates and all-cause mortality. However, economic thresholds varied little with age and were the same for men and women. Furthermore, the economic evaluation found that the benefits of setting different thresholds for patients of different ages was negligible, and there was no benefit from setting different thresholds for men and women.
Addressing our stated research questions
Can clinical tools for assessment of a patient’s suitability for knee or hip replacement be used to set thresholds for operation?
This study has demonstrated that the OHS and OKS (both clinical tools previously used for assessment of a patient’s suitability for knee or hip replacement) can be used to set preoperative thresholds for intervention, based on assessing an individual’s capacity to benefit (in terms of PROM score) from surgery. It is important to note that there was substantial individual variation in the change in score by pre score and also that there may be other benefits and risks that are not reflected in the Oxford Hip and Knee Scores.
How does the choice of threshold affect the cost-effectiveness of the procedure and subsequent improvements in patient quality of life?
We have demonstrated that hip and knee replacement is cost-effective for all levels of threshold, up to and including the absolute threshold (a preoperative score above which no meaningful benefit can be gained by a patient), based on the widely used ceiling ratio of £20,000 per QALY gained. Our work does show that, in general, the procedures are more cost-effective at lower preoperative scores, but even as the absolute threshold is approached, arthroplasty remains cost-effective. This reflects the very large health gains made by the many patients who undergo this surgery, which outweigh the cost of surgery and the small decrease in quality of life experienced by a small minority of patients. Age and gender have no meaningful effect on these outcomes.
Using the Arthroplasty Candidacy Help Engine tool in the NHS
To develop the ACHE tool, we determined a way of linking preoperative OHSs and OKSs to the probability of an individual patient having a good outcome after hip or knee replacement. The next stage of the work was to construct a method of delivering the ACHE tool to patients and clinicians involved in the pathway.
The ACHE tool has been designed to support the referral decision that a GP or a musculoskeletal referral hub makes with a patient. The tool is not designed for use in secondary care, in which a more complex package of personalised decision support is required. In addition to being a tool used by GPs and referral hubs, the ACHE tool could also be accessible to patients directly. In this way, the tool and the process of referral can be understood and is transparent. This was discussed in the third user group meeting and we gained support for our approach of developing an electronic web-based delivery vehicle for the ACHE tool. The user group did raise the question of whether or not this may limit access for those patients who do not have access to, or do not use, the internet. The choice of a web-based vehicle was also based on the observed success of other online GP referral aids such as Keele University’s STarT Back Tool (www.keele.ac.uk/sbst/startbacktool/sbtoolonline/), which was developed in, and is widely used, in the NHS.
We believe that the issue of restriction of access to non-internet users could be addressed by the tool being used during the GP consultation, accessed by the GP and filled in together or after the patient had completed a paper version of the OHS and OKS. The development of a web-based ACHE tool also allowed us to show the tool to patients and GPs in work package 3.
Assessing the impact of the Arthroplasty Candidacy Help Engine tool on the NHS pathway
The first part of work package 3 was aimed at modelling the effect of using the ACHE tool in the NHS setting. We investigated the potential impact of using the tool in one NHS referral hub, in which patients who are referred from general practice are assessed for potential referral to secondary care, and then extrapolated this to the NHS through modelling methods. National PROMs data demonstrate that virtually all patients who currently undergo hip and knee replacement lie within the threshold values that we have calculated, so its introduction is unlikely to reduce the current referral rate. Within the example of care we investigated, which we believe is generally reflective of the NHS, we found that a proportion of patients were not referred for surgical assessment despite having scores below the threshold level. We explored the effect of using the ACHE tool set at different threshold levels (1–44 for knee replacement and 22–45 for hip replacement). We accounted for the fact that not all of these patients would consider surgery (and hence would not be referred), but estimated that a proportion of such patients would consider surgery and, therefore, would wish to be referred. This modelling exercise demonstrated that the ACHE tool could potentially increase the number of patients being referred for surgery if a threshold of > 21 for knee and > 19 for hip replacement was used. However, because TJA is highly cost-effective, even a policy of referring patients with a 50% chance of a good outcome is likely to be cost-effective and improve population health, taking account of the health benefits forgone by spending money on arthroplasty rather than other health services.
This study has shown that thresholds for referral based around a preoperative Oxford Hip or Knee Score can be implemented, based on considering an individual’s capacity to benefit. Having established that, the aim of the study was not to set the threshold at any specific level, but rather to explore the effect of different threshold levels being used at an individual and population level.
If the tool were used and threshold values were set at levels previously suggested and used in the NHS (19–24), the effect would be to potentially prevent many patients undergoing appropriate care and not being given access to highly cost-effective treatment.
The economic evaluation demonstrated that setting a threshold close to the absolute threshold (41/42) would be cost-effective on a population level. However, on an individual-level, setting a high threshold would mean that significant numbers of patients would be referred who have only a low probability of having a good outcome. This would seem unrealistic, as treatment would be offered to some patients (with scores approaching 40) for whom there was very low probability of improvement.
Setting the threshold scores at a level at which patients had approximately a 75% chance of a good outcome (around 30 for knee and 35 for hip) would mean that referrals (and in turn total numbers of operations) may increase, but would ensure patients’ access to appropriate, cost-effective care.
In practice, the number of operations may be limited by the availability of NHS funds, surgeons, operating theatres or beds. If such operational constraints prevent the referral of all patient groups for whom TJA is likely to be beneficial and cost-effective, a threshold probability of a good outcome or the decision grids shown in Chapter 8 could be used to identify and prioritise those patient groups for whom TJA is most beneficial or represents the best value for money. However, placing any limitation on the number of operations conducted is likely to reduce the amount of health benefits that can be produced with the available funds.
Patient, public and general practitioners’ views
The low response rate to our patient/public and GP survey means that the results in reality represent a pilot study of patient, public and GP views. Both surveys identified support for the use of the ACHE tool in supporting the referral process around hip and knee replacement. All groups felt that an online electronic vehicle for the tool was best, although 10% of patients stated a preference for a paper-based tool. Patients would mainly like to use the tool at home, before or after a consultation, whereas opinion was split among GPs regarding whether the tool was best filled in outside the consultation or embedded within the discussion in the clinic. Of note was the view from patients regarding the ACHE tool being used to prioritise patients for surgery. The pilot data suggests that more information should be gathered as to the usability of the tool with these groups.
Views from the extended user group meeting
The final extended user group meeting was designed to facilitate a discussion as to the eventual use of the ACHE tool in the NHS. There was overall support for its design and the evidence that supported it. In turn, there was encouraging support to test its use within the NHS in a controlled manner, with some caution expressed about making any decision as to the level of relative threshold that should be used.
Further research
Our work has demonstrated that the OHS and OKS can be used within the ACHE tool to assist patients and clinicians in deciding who should be referred for hip and knee surgery. Within the different threshold levels explored in the study, there is overwhelming evidence as to the cost-effectiveness of hip and knee replacement. However, our work has highlighted the need for more research:
-
What would the level of uptake be by patients, GPs and other health-care workers involved in the referral process for hip and knee replacement if the ACHE tool were introduced to the NHS?
-
What is the impact of the ACHE tool on the numbers of patients referred for hip and knee replacement?
-
What is the best method of delivery for the ACHE tool (i.e. electronic or paper format)?
-
In general practice, how should the ACHE tool be best integrated into the patient consultation?
-
Would the ACHE tool be susceptible to ‘gaming’ if used within the NHS?
-
What probability of success, following hip and knee replacement, is acceptable to patients, and how does this vary between groups (e.g. gender, age and co-morbidity)?
-
Can the ACHE tool be directly linked to secondary care following referral (e.g. forming the basis of a more complex personalised decision support tool in secondary care)?
-
What is the impact of other patient characteristics (e.g. BMI and smoking) on the post-surgery outcome and cost-effectiveness of hip and knee replacement, and do thresholds for arthroplasty vary with other factors?
-
Additional primary data are needed on how clinical tool scores, costs and utility change over time in the absence of arthroplasty and on how patients who do not have arthroplasty are managed in routine clinical practice. These data are essential to fully understand what impact arthroplasty has on disease and provide data to inform future economic evaluations.
-
Can a WOMAC version of the tool be developed? This may require additional primary data collection on the WOMAC.
-
There is a need to assess whether or not revision rates vary with presurgery OKSs and OHSs. We have demonstrated that the preoperative OKS and OHS predict postoperative scores, costs and EQ-5D utility. However, future research should assess whether or not the rate of revisions also varies with Oxford Hip and Knee Scores and whether or not taking account of any variation in revision rates improves shared decision-making or affects the economic threshold.
Chapter 12 Conclusions
We have shown that scoring systems routinely used to assess hip and knee replacement outcomes can be used to provide an individual an estimate of their chances of improving after hip or knee replacement.
We have determined, based on the data available to us at the time of this study, that the Oxford Hip and Knee Scores are the best-suited measures for this purpose within the NHS.
We have identified that the WOMAC is highly likely to be able to fulfil this role as well, but that more data are required to assess it.
We have determined absolute thresholds for the Oxford Hip and Knee Scores (41/48 and 42/48, respectively) at which there is likely to be no benefit from surgery.
We have created a scientific method for calculating a patient’s probability of having a good outcome after hip or knee surgery based on their preoperative level of symptoms (as measured by the Oxford Hip and Knee Scores), age and gender, allowing us the ability to determine the outcome for patients when different ‘relative’ thresholds for referral are set.
We have shown that hip and knee replacements are both highly cost-effective up to the absolute threshold scores.
We have produced a web-based vehicle to deliver the above model to patients and GPs for use in the NHS – the ACHE tool.
We have modelled the effect of the ACHE tool on the NHS, demonstrating that its introduction is likely to be cost-effective. We have determined that at whichever level of relative threshold introduced it may increase referral rates and the numbers of patients undergoing surgery.
We have collected pilot data that suggest that patients and GPs may welcome the use of the ACHE tool into clinical practice; however, more work is required to fully demonstrate this.
We conclude that the ACHE tool should be tested within the NHS to determine its usability, uptake and effect of referral patterns within the NHS.
Acknowledgements
We thank the ADAPT, APEX, Belfast, COASt, EPOS, EUROHIP and KAT study groups for providing access to their data for use in this project, as well as all of the researchers and participants involved in those studies.
The ADAPT study was funded by the NIHR Programme Grants for Applied Research programme (reference number RP-PG-0407–10070).
The Arthroplasty Pain Experience Study was funded by the NIHR Programme Grants for Applied Research programme (reference number RP-PG-0407–10070). In particular, we would like to thank Sian Noble for her work in providing the data on costs and resource use from the APEX study.
The Clinical Outcomes in Arthroplasty Study is funded by NIHR under the Programme Grants for Applied Research programme (reference number RP-PG-0407–10064). We would like to thank all the participants of COASt and the COASt team for their time and dedication, and NIHR for their funding support to the study.
The Belfast data set was funded locally through Mr Beverland’s (Principal Investigator) Research Unit. EPOS was funded by Stryker UK Ltd. The EUROHIP cohort was funded by Bertelsmann Foundation and Centerpulse Orthopedics Ltd (Sulzer Medical Ltd). KAT was funded by the NIHR HTA programme (reference number 95/10/01).
Data on NHS PROMs linked to HES APC data were reused with the permission of NHS Digital, with all rights reserved.
We would like to thank David Beverland, Richard Field, Andrew Chilvers, Fraser Old, Muir Gray, Mary Keenan, Doung Altman and Tim Wilton for their help and support with the ACHE project.
We would like to thank Professor Paul Dieppe and Professor Cheema Valderas for their input in writing the original grant application.
We would like to extend thanks to Reza Mafi and Sarah Dorman who helped with data cleaning of the data set used in Chapter 8.
We would like to say a special thank you to the patient representatives recruited through INVOLVE (the patient liaison group), who helped deliver this research.
We would also like to thank Mark Pennington for providing the model inputs and variance–covariance matrices for his papers on knee and hip replacement, as well as for really helpful responses to questions on his modelling approach.
We would like to thank Rafael Pinedo Villanueva for useful discussions about modelling approaches and for providing variance–covariance matrices and additional information on the model inputs used in his thesis.
We would like to thank Chaudhry Shah and Joaquim Soares do Brito for their help with identifying the relevant codes for hospital readmissions used in the HES data.
We would like to thank all non-research team members of the user group for their essential contribution to the study: Anne Clarkson Webb, Chip Johnson, Chad Lion Cachet, Fiona Watt, Fraser Old, Gill Dean, Gillian Kempster, Jennie Kramer, Jennifer Bostock, Jiyang Li, Jo Hewanicka, John Nolan, Kate Jackson, Laura Ingle, Mary Snow, Matthew Cheetham, Patricia Rubery, Sharon Barrington, Tim Wilton and Vida Field.
Contributions of authors
Professor Andrew Price [Chief Investigator, Professor of Orthopaedic Surgery, Nuffield Department of Orthopaedics, Rheumatology and Musculoskeletal Science (NDORMS), University of Oxford] was responsible for leading the grant application, managing the research programme, co-ordinating analysis teams and writing the report.
Dr James Smith (Research Fellow, NDORMS, University of Oxford) was the research manager for the programme for the last year of the project and helped write the report.
Dr Helen Dakin (Senior Researcher, Health Economics Research Centre, University of Oxford) was a co-applicant, contributed to the design of the research, was the health economic analysis lead for the programme and helped write the report.
Ms Sujin Kang (Medical Statistician, NDORMS, University of Oxford) worked with the statistical analysis team, carried out most of the analyses in Chapters 3, 4 and 6 and drafted these chapters. She also contributed more generally to writing the report.
Dr Peter Eibich (Senior Researcher, Health Economics Research Centre, University of Oxford) worked on the health economic analysis and contributed to writing the report.
Professor Jonathan Cook (Associate Professor, NDORMS, University of Oxford) was a co-applicant, the statistical analysis lead for the programme and helped write the report.
Professor Alastair Gray (Director of the Health Economics Research Centre, University of Oxford) was a co-applicant who helped design the research programme and supervised the heath economic analysis.
Dr Kristina Harris (Research Fellow, NDORMS, University of Oxford) was lead for the systematic review and helped with writing the report.
Mr Robert Middleton (Research Fellow, NDORMS University of Oxford) contributed to managing the study and writing the report.
Dr Elizabeth Gibbons (Senior Researcher, Department of Public Health and Primary Care, University of Oxford) contributed to the systematic review of outcome measures.
Dr Elena Benedetto (Research Fellow, NDORMS, University of Oxford) was research manager for the programme of work, co-ordinating work packages and contributing to writing the report.
Dr Stephanie Smith (Research Fellow, NDORMS, University of Oxford) contributed in planning and delivering work package 3.
Professor Jill Dawson (Associate Professor, Department of Public Health and Primary Care, Oxford) was a co-applicant and helped design and deliver work package 1.
Professor Raymond Fitzpatrick (Professor of Public Health and Primary Care, University of Oxford) was a co-applicant who helped design the research programme and contributed to the systematic review and analysis of outcome measure properties.
Dr Adrian Sayers (Statistician and Senior Researcher, University of Bristol) contributed to the statistical analyses of work packages 1 and 2 and helped draft Chapters 2 and 3.
Dr Laura Miller (Research Fellow, University of Bristol) undertook a substantial number of analyses for work package 2 and contributed to the drafting of Chapter 3.
Dr Elsa Marques (Research Fellow, University of Bristol) contributed to the delivery of work package 1 and prepared the data set of costs and resource use for the APEX study used in work package 2.
Professor Rachael Gooberman-Hill (Professor of Health and Anthropology, University of Bristol) was a co-applicant who helped design the research programme and contributed to the interpretation of results.
Professor Ashley Blom (Professor of Orthopaedics, University of Bristol) was a co-applicant who helped design the research programme.
Professor Andrew Judge (Professor of Medical Statistics, University of Bristol) was a co-applicant who contributed to design of the statistical analysis plans within the study.
Professor Nigel Arden (Professor in Rheumatic Diseases, NDORMS, University of Oxford) was a co-applicant who helped design the research programme.
Professor David Murray (Professor of Orthopaedic Surgery, NDORMS, University of Oxford) was a co-applicant who helped design the research programme.
Professor Sion Glyn-Jones (Professor of Orthopaedic Surgery, NDORMS University of Oxford) was a co-applicant who helped design the research programme.
Professor Karen Barker (Clinical Director, NOC, Oxford University Hospitals NHS Foundation Trust) was a co-applicant who helped design the research programme and was an active member of the user group.
Professor Andrew Carr (Head of Department, NDORMS, University of Oxford) was a co-applicant who helped design the research programme.
Professor David Beard (Professor of Musculoskeletal Science, Nuffield Department of Orthopaedics, Rheumatology and Musculoskeletal Science (NDORMS), University of Oxford) was a co-applicant who contributed to the design of the research, writing the grant application, chairing the user group, the interpretating the results and writing the report.
Publications
Harris K, Dawson J, Gibbons E, Lim CR, Beard DJ, Fitzpatrick R, Price AJ. Systematic review of measurement properties of patient-reported outcome measures used in patients undergoing hip and knee arthroplasty. Patient Relat Outcome Meas 2016;7:101–8.
Eibich P, Dakin HA, Price AJ, Beard D, Arden NK, Gray AM. Associations between preoperative Oxford hip and knee scores and costs and quality of life of patients undergoing primary total joint replacement in the NHS England: an observational study. BMJ Open 2018;8:e019477.
Price A, Kang S, Cook J, Dakin HA, Blom A, Arden N, et al. The use of patient-reported outcome measures to guide referral for hip and knee replacement. Part 1 – the development of evidence-based thresholds. Bone Joint J 2019; submitted.
Dakin HA, Eibich P, Beard D, Gray A, Price A, et al. The use of patient-reported outcome measures to guide referral for hip and knee replacement. Part 2 – a cost-effectiveness analysis. Bone Joint J 2019; submitted.
Dakin HA, Eibich P, Gray A, Smith J, Barker K, Beard D, et al. Who gets referred for knee or hip replacement? A theoretical model of the potential impact of evidence-based referral thresholds using data from a retrospective review of clinic records from an English musculoskeletal referral hub. BMJ Open 2019; submitted.
Data-sharing statement
All data requests should be submitted to the corresponding author for consideration. Access to available anonymised data may be granted following review.
Patient data
This work uses data provided by patients and collected by the NHS as part of their care and support. Using patient data is vital to improve health and care for everyone. There is huge potential to make better use of information from people’s patient records, to understand more about disease, develop new treatments, monitor safety, and plan NHS services. Patient data should be kept safe and secure, to protect everyone’s privacy, and it’s important that there are safeguards to make sure that it is stored and used responsibly. Everyone should be able to find out about how patient data are used. #datasaveslives You can find out more about the background to this citation here: https://understandingpatientdata.org.uk/data-citation.
Disclaimers
This report presents independent research funded by the National Institute for Health Research (NIHR). The views and opinions expressed by authors in this publication are those of the authors and do not necessarily reflect those of the NHS, the NIHR, NETSCC, the HTA programme or the Department of Health and Social Care. If there are verbatim quotations included in this publication the views and opinions expressed by the interviewees are those of the interviewees and do not necessarily reflect those of the authors, those of the NHS, the NIHR, NETSCC, the HTA programme or the Department of Health and Social Care.
References
- Smith SC, Cano S, Lamping DL, Staniszewska S, Browne J, Lewsey J, et al. Patient-Reported Outcome Measures (PROMs) for Routine Use in Treatment Centres: Recommendations Based on a Review of the Scientific Evidence. London: Department of Health and Social Care; 2005.
- Dakin H, Gray A, Fitzpatrick R, Maclennan G, Murray D. KAT Trial Group . Rationing of total knee replacement: a cost-effectiveness analysis on a large trial data set. BMJ Open 2012;2. https://doi.org/10.1136/bmjopen-2011-000332.
- QIPP/Right Care . The NHS Atlas of Variation in Healthcare 2010. https://fingertips.phe.org.uk/profile/atlas-of-variation (accessed 29 January 2019).
- Dixon T, Shaw M, Ebrahim S, Dieppe P. Trends in hip and knee joint replacement: socioeconomic inequalities and projections of need. Ann Rheum Dis 2004;63:825-30. https://doi.org/10.1136/ard.2003.012724.
- Morris RW, Fitzpatrick R, Hajat S, Reeves BC, Murray DW, Hannen D, et al. Primary total hip replacement: variations in patient management in Oxford & Anglia, Trent, Yorkshire & Northern ‘regions’. Ann R Coll Surg Engl 2001;83:190-6.
- Judge A, Welton NJ, Sandhu J, Ben-Shlomo Y. Equity in access to total joint replacement of the hip and knee in England: cross sectional study. BMJ 2010;341. https://doi.org/10.1136/bmj.c4092.
- Fitzpatrick R, Norquist JM, Reeves BC, Morris RW, Murray DW, Gregg PJ. Equity and need when waiting for total hip replacement surgery. J Eval Clin Pract 2004;10:3-9. https://doi.org/10.1111/j.1365-2753.2003.00448.x.
- Provisional Monthly Patient Reported Outcome Measures (PROMs) in England. April 2009 – April 2010 – Pre- and Post-operative Data, Experimental Statistics. Leeds: NHS Digital; 2010.
- Scott CE, Howie CR, MacDonald D, Biant LC. Predicting dissatisfaction following total knee replacement: a prospective study of 1217 patients. J Bone Joint Surg Br 2010;92:1253-8. https://doi.org/10.1302/0301-620X.92B9.24394.
- Price A, Alvand A, Troelsen A, Katz JN, Hooper G, Gray A, et al. Knee replacement. Lancet 2018;392:1672-82.
- Dieppe P, Lim K, Lohmander S. Who should have knee joint replacement surgery for osteoarthritis?. Int J Rheum Dis 2011;14:175-80. https://doi.org/10.1111/j.1756-185X.2011.01611.x.
- Linsell L, Dawson J, Zondervan K, Rose P, Carr A, Randall T, et al. Population survey comparing older adults with hip versus knee pain in primary care. Br J Gen Pract 2005;55:192-8.
- Jüni P, Low N, Reichenbach S, Villiger PM, Williams S, Dieppe PA. Gender inequity in the provision of care for hip disease: population-based cross-sectional study. Osteoarthr Cartil 2010;18:640-5. https://doi.org/10.1016/j.joca.2009.12.010.
- De Coster C, McMillan S, Brant R, McGurran J, Noseworthy T. Primary Care Panel of the Western Canada Waiting List Project. The Western Canada Waiting List Project: development of a priority referral score for hip and knee arthroplasty. J Eval Clin Pract 2007;13:192-6. https://doi.org/10.1111/j.1365-2753.2006.00671.x.
- Naylor CD, Williams JI. Primary hip and knee replacement surgery: Ontario criteria for case selection and surgical priority. Qual Health Care 1996;5:20-3. https://doi.org/10.1136/qshc.5.1.20.
- Hadorn DC, Holmes AC. The New Zealand priority criteria project. Part 1: overview. BMJ 1997;314:131-4. https://doi.org/10.1136/bmj.314.7074.131.
- MacCormick AD, Collecutt WG, Parry BR. Prioritizing patients for elective surgery: a systematic review. ANZ J Surg 2003;73:633-42. https://doi.org/10.1046/j.1445-2197.2003.02605.x.
- Edwards RT. Points for pain: waiting list priority scoring systems. BMJ 1999;318:412-14. https://doi.org/10.1136/bmj.318.7181.412.
- Judge A, Javaid K, Arden NK, Cushnaghan J, Reading I, Croft P, et al. A clinical tool to identify patients who are most likely to receive long term improvement in physical function after total hip arthroplasty. Arthritis Care &Amp; Research 2012;64:881-9. https://doi.org/10.1002/acr.21594.
- Löfvendahl S, Bizjajeva S, Ranstam J, Lidgren L. Indications for hip and knee replacement in Sweden. J Eval Clin Pract 2011;17:251-60. https://doi.org/10.1111/j.1365-2753.2010.01430.x.
- Osborne RH, Haynes K, Edbrook L, de Steiger R, Brand C, Wicks C, et al. Development of a Management and Prioritization Tool (MAPT) for Orthopaedic Waiting Lists: A Model for Healthcare Reform in Victoria n.d.
- Dougados M, Hawker G, Lohmander S, Davis AM, Dieppe P, Maillefert JF, et al. OARSI/OMERACT criteria of being considered a candidate for total joint replacement in knee/hip osteoarthritis as an endpoint in clinical trials evaluating potential disease modifying osteoarthritic drugs. J Rheumatol 2009;36:2097-9. https://doi.org/10.3899/jrheum.090365.
- Gossec L, Hawker G, Davis AM, Maillefert JF, Lohmander LS, Altman R, et al. OMERACT/OARSI initiative to define states of severity and indication for joint replacement in hip and knee osteoarthritis. J Rheumatol 2007;34:1432-5.
- Gossec L, Jordan JM, Lam MA, Fang F, Renner JB, Davis A, et al. Comparative evaluation of three semi-quantitative radiographic grading techniques for hip osteoarthritis in terms of validity and reproducibility in 1404 radiographs: report of the OARSI-OMERACT Task Force. Osteoarthr Cartil 2009;17:182-7. https://doi.org/10.1016/j.joca.2008.06.009.
- Gossec L, Paternotte S, Bingham CO, Clegg DO, Coste P, Conaghan PG, et al. OARSI/OMERACT initiative to define states of severity and indication for joint replacement in hip and knee osteoarthritis. An OMERACT 10 Special Interest Group. J Rheumatol 2011;38:1765-9. https://doi.org/10.3899/jrheum.110403.
- Guidance on the Routine Collection of PROMs. London: DHSC; 2009.
- The Musculoskeletal Services Framework – a Joint Responsibility: Doing It Differently. London: DHSC; 2006.
- Improving Orthopaedic Services: a guide for Clinicians, Managers & Service Commissioners. London: Department of Health and Social Care; 2002.
- Southern Derbyshire Clinical Commissioning Group . Commissioning Policy for Procedures of Limited Clinical Value (PLCV) n.d. www.southernderbyshireccg.nhs.uk/EasySiteWeb/GatewayLink.aspx?alId=3286 (accessed 22 January 2019).
- NHS Harrogate and Rural District Clinical Commissioning Group . Clinical Thresholds n.d. www.harrogateandruraldistrictccg.nhs.uk/data/uploads/rss2/hip-and-knee-arthroplasty.pdf (accessed 24 November 2017).
- Bristol Clinical Commissioning Group . Knee Replacement Surgery (including Partial and Total Knee Replacement With or Without Patella Replacement or Resurfacing) – Criteria Based Access Policy n.d. www.bristolccg.nhs.uk/media/medialibrary/2016/10/Knee_Replacement_Surgery_Policy_6tw3a9e.pdf (accessed 24 November 2017).
- Birmingham Cross City Clinical Commissioning Group . Commissioning Policy – Hip and Knee Replacement for Patients With Osteoarthritis n.d. http://bhamcrosscityccg.nhs.uk/about-us/publication/treatment-policies-reference-library/3198-hip-and-knee-replacement-for-patients-with-osteoarthritis/file (accessed 24 November 2017).
- NHS Oxfordshire . INTERIM Treatment Threshold Statement: Knee Arthroplasty 2010. www.webarchive.org.uk/wayback/archive/20130303121712/http://www.oxfordshirepct.nhs.uk/professional-resources/priority-setting/lavender-statements/documents/PS188Kneereplacement.pdf (accessed 21 November 2017).
- North Derbyshire Clinical Commissioning Group . Hip and Knee Pathway for Patients With Osteoarthritis Requiring Large Joint Arthroplasty Only n.d. www.northderbyshireccg.nhs.uk/assets/Clinical_Guidelines_/Ortho_Rheum/hip_and_knee_pathway_flowchart_09.pdf (accessed 24 November 2017).
- Cambridge and Peterborough Clinical Commissioning Group . Primary Knee Replacement Surgery Policy n.d. www.cambridgeshireandpeterboroughccg.nhs.uk/EasySiteWeb/GatewayLink.aspx?alId=9661 (accessed 24 November 2017).
- South Worcestershire, Redditch & Bromsgrove and Wyre Forest Clinical Commissioning Groups . Musculoskeletal Surgery Interventions n.d. www.worcestershire.nhs.uk/EasySiteWeb/GatewayLink.aspx?alId=30974 (accessed 24 November 2017).
- Hawker GA, Davis AM, French MR, Cibere J, Jordan JM, March L, et al. Development and preliminary psychometric testing of a new OA pain measure – an OARSI/OMERACT initiative. Osteoarthr Cartil 2008;16:409-14. https://doi.org/10.1016/j.joca.2007.12.015.
- Brooks R. EuroQol: the current state of play. Health Policy 1996;37:53-72. https://doi.org/10.1016/0168-8510(96)00822-6.
- Dawson J, Fitzpatrick R, Carr A, Murray D. Questionnaire on the perceptions of patients about total hip replacement. J Bone Joint Surg Br 1996;78:185-90. https://doi.org/10.1302/0301-620X.78B2.0780185.
- Dawson J, Fitzpatrick R, Murray D, Carr A. Questionnaire on the perceptions of patients about total knee replacement. J Bone Joint Surg Br 1998;80:63-9. https://doi.org/10.1302/0301-620X.80B1.7859.
- Bellamy N, Buchanan WW, Goldsmith CH, Campbell J, Stitt LW. Validation study of WOMAC: a health status instrument for measuring clinically important patient relevant outcomes to antirheumatic drug therapy in patients with osteoarthritis of the hip or knee. J Rheumatol 1988;15.
- Ware JE, Kosinski M, Dewey JE, Gandek B. SF-36 Health Survey: Manual and Interpretation Guide. Lincoln, RI: Quality Metric Inc.; 2000.
- Judge A, Arden NK, Price A, Glyn-Jones S, Beard D, Carr AJ, et al. Assessing patients for joint replacement: can pre-operative Oxford hip and knee scores be used to predict patient satisfaction following joint replacement surgery and to guide patient selection?. J Bone Joint Surg Br 2011;93:1660-4. https://doi.org/10.1302/0301-620X.93B12.27046.
- Räsänen P, Paavolainen P, Sintonen H, Koivisto AM, Blom M, Ryynänen OP, et al. Effectiveness of hip or knee replacement surgery in terms of quality-adjusted life years and costs. Acta Orthop 2007;78:108-15. https://doi.org/10.1080/17453670610013501.
- Rissanen P, Aro S, Sintonen H, Asikainen K, Slätis P, Paavolainen P. Costs and cost-effectiveness in hip and knee replacements. A prospective study. Int J Technol Assess Health Care 1997;13:575-88. https://doi.org/10.1017/S0266462300010059.
- Lavernia CJ, Guzman JF, Gachupin-Garcia A. Cost effectiveness and quality of life in knee arthroplasty. Clin Orthop Relat Res 1997;345:134-9. https://doi.org/10.1097/00003086-199712000-00018.
- Losina E, Walensky RP, Kessler CL, Emrani PS, Reichmann WM, Wright EA, et al. Cost-effectiveness of total knee arthroplasty in the United States: patient risk and hospital volume. Arch Intern Med 2009;169:1113-21. https://doi.org/10.1001/archinternmed.2009.136.
- Navarro Espigares JL, Hernández Torres E. Cost-outcome analysis of joint replacement: evidence from a Spanish public hospital. Gac Sanit 2008;22:337-43. https://doi.org/10.1157/13125355.
- Social Value Judgements: Principles for the Development of NICE Guidance. Second Edition. Manchester: NICE; 2008.
- Dakin H, Gray A, Murray D. Mapping analyses to estimate EQ-5D utilities and responses based on Oxford Knee Score. Qual Life Res 2013;22:683-94. https://doi.org/10.1007/s11136-012-0189-4.
- Jayadev C, Khan T, Coulter A, Beard DJ, Price AJ. Patient decision aids in knee replacement surgery. Knee 2012;19:746-50. https://doi.org/10.1016/j.knee.2012.02.001.
- NHS England . Shared Decision Making – Osteoarthritis of the Knee n.d. www.dartfordgraveshamswanleyccg.nhs.uk/wp-content/uploads/sites/3/2017/05/SDM-osteoarthritis-of-the-knee-updated-February-2017.pdf (accessed 6 February 2019).
- Fitzpatrick R, Davey C, Buxton MJ, Jones DR. Evaluating patient-based outcome measures for use in clinical trials. Health Technol Assess 1998;2.
- Mokkink LB, Terwee CB, Patrick DL, Alonso J, Stratford PW, Knol DL, et al. The COSMIN study reached international consensus on taxonomy, terminology, and definitions of measurement properties for health-related patient-reported outcomes. J Clin Epidemiol 2010;63:737-45. https://doi.org/10.1016/j.jclinepi.2010.02.006.
- US Department of Health Human Services . Guidance for industry: patient-reported outcome measures: use in medical product development to support labeling claims: draft guidance. Health Qual Life Outcomes 2006;4. https://doi.org/10.1186/1477-7525-4-79.
- Patrick DL, Burke LB, Gwaltney CJ, Leidy NK, Martin ML, Molsen E, et al. Content validity – establishing and reporting the evidence in newly developed patient-reported outcomes (PRO) instruments for medical product evaluation: ISPOR PRO good research practices task force report: part 1 – eliciting concepts for a new PRO instrument. Value Health 2011;14:967-77. https://doi.org/10.1016/j.jval.2011.06.014.
- Patrick DL, Burke LB, Gwaltney CJ, Leidy NK, Martin ML, Molsen E, et al. Content validity – establishing and reporting the evidence in newly developed patient-reported outcomes (PRO) instruments for medical product evaluation: ISPOR PRO Good Research Practices Task Force report: part 2 – assessing respondent understanding. Value Health 2011;14:978-88. https://doi.org/10.1016/j.jval.2011.06.013.
- Rothman M, Burke L, Erickson P, Leidy NK, Patrick DL, Petrie CD. Use of existing patient-reported outcome (PRO) instruments and their modification: the ISPOR good research practices for evaluating and documenting content validity for the use of existing instruments and their modification PRO task force report. Value Health 2009;12:1075-83. https://doi.org/10.1111/j.1524-4733.2009.00603.x.
- Garratt A, Schmidt L, Mackintosh A, Fitzpatrick R. Quality of life measurement: bibliographic study of patient assessed health outcome measures. BMJ 2002;324. https://doi.org/10.1136/bmj.324.7351.1417.
- Alviar MJ, Olver J, Brand C, Tropea J, Hale T, Pirpiris M, et al. Do patient-reported outcome measures in hip and knee arthroplasty rehabilitation have robust measurement attributes? A systematic review. J Rehabil Med 2011;43:572-83. https://doi.org/10.2340/16501977-0828.
- Garratt AM, Brealey S, Gillespie WJ. DAMASK Trial Team . Patient-assessed health instruments for the knee: a structured review. Rheumatology 2004;43:1414-23. https://doi.org/10.1093/rheumatology/keh362.
- Terwee CB, Jansma EP, Riphagen II, de Vet HC. Development of a methodological PubMed search filter for finding studies on measurement properties of measurement instruments. Qual Life Res 2009;18:1115-23. https://doi.org/10.1007/s11136-009-9528-5.
- Harris K, Dawson J, Gibbons E, Lim CR, Beard DJ, Fitzpatrick R, et al. Systematic review of measurement properties of patient-reported outcome measures used in patients undergoing hip and knee arthroplasty. Patient Relat Outcome Meas 2016;7:101-8. https://doi.org/10.2147/PROM.S97774.
- Browne J, Jamieson L, Lewsey J, van der Meulen J. Patient Reported Outcome Measures (PROMs) in Elective Surgery Report to the Department of Health 2007. www.lshtm.ac.uk/php/departmentofhealthservicesresearchandpolicy/assets/proms_report_12_dec_07.pdf (accessed 3 September 2014).
- Streiner DL, Norman GR, Cairney J. Health Measurement Scales: A Practical Guide to their Development and Use. Oxford: Oxford University Press; 2008.
- Murray DW, MacLennan GS, Breeman S, Dakin HA, Johnston L, Campbell MK, et al. A randomised controlled trial of the clinical effectiveness and cost-effectiveness of different knee prostheses: the Knee Arthroplasty Trial (KAT). Health Technol Assess 2014;18. https://doi.org/10.3310/hta18190.
- Dieppe P, Judge A, Williams S, Ikwueke I, Guenther KP, Floeren M, et al. Variations in the pre-operative status of patients coming to primary hip replacement for osteoarthritis in European orthopaedic centres. BMC Musculoskelet Disord 2009;10. https://doi.org/10.1186/1471-2474-10-19.
- Fordham R, Skinner J, Wang X, Nolan J. Exeter Primary Outcome Study Group . The economic benefit of hip replacement: a 5-year follow-up of costs and outcomes in the Exeter Primary Outcomes Study. BMJ Open 2012;2. https://doi.org/10.1136/bmjopen-2011-000752.
- Judge A, Arden NK, Batra RN, Thomas G, Beard D, Javaid MK, et al. The association of patient characteristics and surgical variables on symptoms of pain and function over 5 years following primary hip-replacement surgery: a prospective cohort study. BMJ Open 2013;3. https://doi.org/10.1136/bmjopen-2012-002453.
- Wylde V, Gooberman-Hill R, Horwood J, Beswick A, Noble S, Brookes S, et al. The effect of local anaesthetic wound infiltration on chronic pain after lower limb joint replacement: a protocol for a double-blind randomised controlled trial. BMC Musculoskelet Disord 2011;12. https://doi.org/10.1186/1471-2474-12-53.
- Wylde V, Blom AW, Bolink S, Brunton L, Dieppe P, Gooberman-Hill R, et al. Assessing function in patients undergoing joint replacement: a study protocol for a cohort study. BMC Musculoskelet Disord 2012;13. https://doi.org/10.1186/1471-2474-13-220.
- Wylde V, Lenguerrand E, Gooberman-Hill R, Beswick AD, Marques E, Noble S, et al. Effect of local anaesthetic infiltration on chronic postsurgical pain after total hip and knee replacement: the APEX randomised controlled trials. Pain 2015;156:1161-70. https://doi.org/10.1097/j.pain.0000000000000114.
- Cohen J. Statistical Power Analysis for the Behavioral Sciences. Hillsdale, NJ: L. Erlbaum Associates; 1988.
- Jette DU, Warren RL, Wirtalla C. Functional independence domains in patients receiving rehabilitation in skilled nursing facilities: evaluation of psychometric properties. Arch Phys Med Rehabil 2005;86:1089-94. https://doi.org/10.1016/j.apmr.2004.11.018.
- Beard DJ, Harris K, Dawson J, Doll H, Murray DW, Carr AJ, et al. Meaningful changes for the Oxford hip and knee scores after joint replacement surgery. J Clin Epidemiol 2015;68:73-9. https://doi.org/10.1016/j.jclinepi.2014.08.009.
- Terwee CB, Bot SD, de Boer MR, van der Windt DA, Knol DL, Dekker J, et al. Quality criteria were proposed for measurement properties of health status questionnaires. J Clin Epidemiol 2007;60:34-42. https://doi.org/10.1016/j.jclinepi.2006.03.012.
- de Vet HC, Terwee CB, Ostelo RW, Beckerman H, Knol DL, Bouter LM. Minimal changes in health status questionnaires: distinction between minimally detectable change and minimally important change. Health Qual Life Outcomes 2006;4. https://doi.org/10.1186/1477-7525-4-54.
- Poitras S, Beaule PE, Dervin GF. Validity of a short-term quality of life questionnaire in patients undergoing joint replacement: the Quality of Recovery–40. J Arthroplasty 2012;27:1604-8.e1. https://doi.org/10.1016/j.arth.2012.03.015.
- Wright JG, Young NL. A comparison of different indices of responsiveness. J Clin Epidemiol 1997;50:239-46. https://doi.org/10.1016/S0895-4356(96)00373-3.
- Yamada C, Moriyama K, Takahashi E. Optimal cut-off point for homeostasis model assessment of insulin resistance to discriminate metabolic syndrome in non-diabetic Japanese subjects. J Diabetes Investig 2012;3:384-7. https://doi.org/10.1111/j.2040-1124.2012.00194.x.
- Sloan JA, Loprinzi CL, Kuross SA, Miser AW, O’Fallon JR, Mahoney MR, et al. Randomized comparison of four tools measuring overall quality of life in patients with advanced cancer. J Clin Oncol 1998;16:3662-73. https://doi.org/10.1200/JCO.1998.16.11.3662.
- Hoare DJ, Kowalkowski VL, Kang S, Hall DA. Systematic review and meta-analyses of randomized controlled trials examining tinnitus management. Laryngoscope 2011;121:1555-64. https://doi.org/10.1002/lary.21825.
- Norman GR, Sloan JA, Wyrwich KW. Interpretation of changes in health-related quality of life: the remarkable universality of half a standard deviation. Med Care 2003;41:582-92. https://doi.org/10.1097/01.MLR.0000062554.74615.4C.
- Farivar SS, Liu H, Hays RD. Half standard deviation estimate of the minimally important difference in HRQOL scores?. Expert Rev Pharmacoecon Outcomes Res 2004;4:515-23. https://doi.org/10.1586/14737167.4.5.515.
- Hounsome N, Orrell M, Edwards RT. EQ-5D as a quality of life measure in people with dementia and their carers: evidence and key issues. Value Health 2011;14:390-9. https://doi.org/10.1016/j.jval.2010.08.002.
- Gandek B. Measurement properties of the Western Ontario and McMaster Universities Osteoarthritis Index: a systematic review. Arthritis Care Res 2015;67:216-29. https://doi.org/10.1002/acr.22415.
- Bohannon RW, Maljanian R, Lee N, Ahlquist M. Measurement properties of the short form (SF)-12 applied to patients with stroke. Int J Rehabil Res 2004;27:151-4. https://doi.org/10.1097/01.mrr.0000127349.25287.de.
- Dorman PJ, Dennis M, Sandercock P. How do scores on the EuroQol relate to scores on the SF-36 after stroke?. Stroke 1999;30:2146-51. https://doi.org/10.1161/01.STR.30.10.2146.
- Portney L, Watkins MP. Foundations of Clinical Research: Applications to Practice. Upper Saddle River, NJ: Prentice Hall, Inc.; 2000.
- Middel B, van Sonderen E. Statistical significant change versus relevant or important change in (quasi) experimental design: some conceptual and methodological problems in estimating magnitude of intervention-related change in health services research. Int J Integr Care 2002;2. https://doi.org/10.5334/ijic.65.
- Kovacs FM, Abraira V, Royuela A, Corcoll J, Alegre L, Tomás M, et al. Minimum detectable and minimal clinically important changes for pain in patients with nonspecific neck pain. BMC Musculoskelet Disord 2008;9. https://doi.org/10.1186/1471-2474-9-43.
- van der Roer N, Ostelo RW, Bekkering GE, van Tulder MW, de Vet HC. Minimal clinically important change for pain intensity, functional status, and general health status in patients with nonspecific low back pain. Spine 2006;31:578-82. https://doi.org/10.1097/01.brs.0000201293.57439.47.
- Steyerberg EW, Vickers AJ, Cook NR, Gerds T, Gonen M, Obuchowski N, et al. Assessing the performance of prediction models: a framework for traditional and novel measures. Epidemiology 2010;21:128-38. https://doi.org/10.1097/EDE.0b013e3181c30fb2.
- Copay AG, Subach BR, Glassman SD, Polly DW, Schuler TC. Understanding the minimum clinically important difference: a review of concepts and methods. Spine J 2007;7:541-6. https://doi.org/10.1016/j.spinee.2007.01.008.
- Hojat M, Xu G. A visitor’s guide to effect sizes: statistical significance versus practical (clinical) importance of research findings. Adv Health Sci Educ Theory Pract 2004;9:241-9. https://doi.org/10.1023/B:AHSE.0000038173.00909.f6.
- Oeffinger D, Bagley A, Rogers S, Gorton G, Kryscio R, Abel M, et al. Outcome tools used for ambulatory children with cerebral palsy: responsiveness and minimum clinically important differences. Dev Med Child Neurol 2008;50:918-25. https://doi.org/10.1111/j.1469-8749.2008.03150.x.
- National Joint Registry for England, Wales, Northern Ireland and the Isle of Man . 12th Annual Report 2015. www.njrcentre.org.uk/njrcentre/Portals/0/Documents/England/Reports/12th%20annual%20report/NJR%20Online%20Annual%20Report%202015.pdf (accessed 21 November 2017).
- Social Value Judgements: Principles for the Development of NICE Guidance. Second Edition. Manchester: NICE; 2008.
- Passey C, Kimko H, Nandy P, Kagan L. Osteoarthritis disease progression model using six year follow-up data from the osteoarthritis initiative. J Clin Pharmacol 2015;55:269-78. https://doi.org/10.1002/jcph.399.
- Ostendorf M, Buskens E, van Stel H, Schrijvers A, Marting L, Dhert W, et al. Waiting for total hip arthroplasty: avoidable loss in quality time and preventable deterioration. J Arthroplasty 2004;19:302-9. https://doi.org/10.1016/j.arth.2003.09.015.
- Holla JF, van der Leeden M, Heymans MW, Roorda LD, Bierma-Zeinstra SM, Boers M, et al. Three trajectories of activity limitations in early symptomatic knee osteoarthritis: a 5-year follow-up study. Ann Rheum Dis 2014;73:1369-75. https://doi.org/10.1136/annrheumdis-2012-202984.
- Anon . UK Prospective Diabetes Study (UKPDS). VIII. Study design, progress and performance. Diabetologia 1991;34:877-90. https://doi.org/10.1007/BF00400195.
- Guide to the Methods of Technology Appraisal 2013. Manchester: NICE; 2013.
- Holt HL, Katz JN, Reichmann WM, Gerlovin H, Wright EA, Hunter DJ, et al. Forecasting the burden of advanced knee osteoarthritis over a 10-year period in a cohort of 60–64 year-old US adults. Osteoarthr Cartil 2011;19:44-50. https://doi.org/10.1016/j.joca.2010.10.009.
- Jenkins PJ, Clement ND, Hamilton DF, Gaston P, Patton JT, Howie CR. Predicting the cost-effectiveness of total hip and knee replacement: a health economic analysis. Bone Joint J 2013;95-B:115-21. https://doi.org/10.1302/0301-620X.95B1.29835.
- Lavernia CJ, Iacobelli DA, Brooks L, Villa JM. The cost–utility of total hip arthroplasty: earlier intervention, improved economics. J Arthroplasty 2015;30:945-9. https://doi.org/10.1016/j.arth.2014.12.028.
- Vogl M, Wilkesmann R, Lausmann C, Plötz W. The impact of preoperative patient characteristics on the cost-effectiveness of total hip replacement: a cohort study. BMC Health Serv Res 2014;14. https://doi.org/10.1186/1472-6963-14-342.
- Ferket BS, Feldman Z, Zhou J, Oei EH, Bierma-Zeinstra SM, Mazumdar M. Impact of total knee replacement practice: cost-effectiveness analysis of data from the Osteoarthritis Initiative. BMJ 2017;356. https://doi.org/10.1136/bmj.j1131.
- The Green Book: Appraisal and Evaluation in Central Government. London: The Stationery Office; 2003.
- Le QA. Probabilistic mapping of the health status measure SF-12 onto the health utility measure EQ-5D using the US-population-based scoring models. Qual Life Res 2014;23:459-66. https://doi.org/10.1007/s11136-013-0517-3.
- Barton GR, Sach TH, Jenkinson C, Avery AJ, Doherty M, Muir KR. Do estimates of cost-utility based on the EQ-5D differ from those based on the mapping of utility scores?. Health Qual Life Outcomes 2008;6. https://doi.org/10.1186/1477-7525-6-51.
- Ara R, Brazier JE. Populating an economic model with health state utility values: moving toward better practice. Value Health 2010;13:509-18. https://doi.org/10.1111/j.1524-4733.2010.00700.x.
- Pinedo Villanueva RA. Total Hip Replacement in the UK: Cost-Effectiveness of a Prediction Tool and Outcomes Mapping 2013.
- Vale L, Wyness L, McCormack K, McKenzie L, Brazzelli M, Stearns SC. A systematic review of the effectiveness and cost-effectiveness of metal-on-metal hip resurfacing arthroplasty for treatment of hip disease. Health Technol Assess 2002;6. https://doi.org/10.3310/hta6150.
- Batsis JA, Zbehlik AJ, Barre LK, Bynum JP, Pidgeon D, Bartels SJ. Impact of obesity on disability, function, and physical activity: data from the Osteoarthritis Initiative. Scand J Rheumatol 2015;44:495-502. https://doi.org/10.3109/03009742.2015.1021376.
- Bruyere O, Cooper C, Pavelka K, Rabenda V, Buckinx F, Beaudart C, et al. Changes in structure and symptoms in knee osteoarthritis and prediction of future knee replacement over 8 years. Calcif Tissue Int 2013;93:502-7. https://doi.org/10.1007/s00223-013-9781-z.
- Kapstad H, Rustøen T, Hanestad BR, Moum T, Langeland N, Stavem K. Changes in pain, stiffness and physical function in patients with osteoarthritis waiting for hip or knee joint replacement surgery. Osteoarthr Cartil 2007;15:837-43. https://doi.org/10.1016/j.joca.2007.01.015.
- Pennington M, Grieve R, Sekhon JS, Gregg P, Black N, van der Meulen JH. Cemented, cementless, and hybrid prostheses for total hip replacement: cost effectiveness analysis. BMJ 2013;346. https://doi.org/10.1136/bmj.f1026.
- Pennington M, Grieve R, Black N, van der Meulen JH. Cost-effectiveness of five commonly used prosthesis brands for total knee replacement in the UK: a study using the NJR dataset. PLOS ONE 2016;11. https://doi.org/10.1371/journal.pone.0150074.
- Sibanda N, Copley LP, Lewsey JD, Borroff M, Gregg P, MacGregor AJ, et al. Revision rates after primary hip and knee replacement in England between 2003 and 2006. PLOS Med 2008;5. https://doi.org/10.1371/journal.pmed.0050179.
- Pennington MW, Grieve R, van der Meulen JH. Lifetime cost-effectiveness of different brands of prosthesis used for total hip arthroplasty: a study using the NJR dataset. Bone Joint J 2015;97–B:762-70. https://doi.org/10.1302/0301-620X.97B6.34806.
- Pulikottil-Jacob R, Connock M, Kandala NB, Mistry H, Grove A, Freeman K, et al. Cost-effectiveness of total hip arthroplasty in osteoarthritis: comparison of devices with differing bearing surfaces and modes of fixation. Bone Joint J 2015;97-B:449-57. https://doi.org/10.1302/0301-620X.97B4.34242.
- Pennington M, Grieve R, Black N, van der Meulen JH. Functional outcome, revision rates and mortality after primary total hip replacement – a national comparison of nine prosthesis brands in England. PLOS ONE 2013;8. https://doi.org/10.1371/journal.pone.0073228.
- Clarke A, Pulikottil-Jacob R, Grove A, Freeman K, Mistry H, Tsertsvadze A, et al. Total hip replacement and surface replacement for the treatment of pain and disability resulting from end-stage arthritis of the hip (review of technology appraisal guidance 2 and 44): systematic review and economic evaluation. Health Technol Assess 2015;19. https://doi.org/10.3310/hta19100.
- National Life Tables, United Kingdom, 1980–82 to 2011–13. Newport: Office for National Statistics; 2014.
- Pinedo-Villanueva RA, Turner D, Judge A, Raftery JP, Arden NK. Mapping the Oxford hip score onto the EQ-5D utility index. Qual Life Res 2013;22:665-75. https://doi.org/10.1007/s11136-012-0174-y.
- Blom AW, Artz N, Beswick AD, Burston A, Dieppe P, Elvers KT, et al. Improving patients’ experience and outcome of total joint replacement: the RESTORE programme. Programme Grants Applied Res 2016;4.
- Marques EM, Blom AW, Lenguerrand E, Wylde V, Noble SM. Local anaesthetic wound infiltration in addition to standard anaesthetic regimen in total hip and knee replacement: long-term cost-effectiveness analyses alongside the APEX randomised controlled trials. BMC Med 2015;13. https://doi.org/10.1186/s12916-015-0389-1.
- Palan J, Gulati A, Andrew JG, Murray DW, Beard DJ. EPOS study group . The trainer, the trainee and the surgeons’ assistant: clinical outcomes following total hip replacement. J Bone Joint Surg Br 2009;91:928-34. https://doi.org/10.1302/0301-620X.91B7.22021.
- Andrew JG, Palan J, Kurup HV, Gibson P, Murray DW, Beard DJ. Obesity in total hip replacement. J Bone Joint Surg Br 2008;90:424-9. https://doi.org/10.1302/0301-620X.90B4.20522.
- Arden N, Altman D, Beard D, Carr A, Clarke N, Collins G, et al. Lower limb arthroplasty: can we predict outcome and failure and is it cost effective? An epidemiological study. Programme Grants for Applied Research 2017;5. https://doi.org/10.3310/pgfar05120.
- Judge A, Cooper C, Williams S, Dreinhoefer K, Dieppe P. Patient-reported outcomes one year after primary hip replacement in a European Collaborative Cohort. Arthritis Care Res 2010;62:480-8. https://doi.org/10.1002/acr.20038.
- Dolan P. Modeling valuations for EuroQol health states. Med Care 1997;35:1095-108. https://doi.org/10.1097/00005650-199711000-00002.
- Dakin H. Review of studies mapping from quality of life or clinical measures to EQ-5D: an online database. Health Qual Life Outcomes 2013;11. https://doi.org/10.1186/1477-7525-11-151.
- Colbert CJ, Almagor O, Chmiel JS, Song J, Dunlop D, Hayes KW, et al. Excess body weight and four-year function outcomes: comparison of African Americans and whites in a prospective study of osteoarthritis. Arthritis Care Res 2013;65:5-14. https://doi.org/10.1002/acr.21811.
- Colbert CJ, Song J, Dunlop D, Chmiel JS, Hayes KW, Cahue S, et al. Knee confidence as it relates to physical function outcome in persons with or at high risk of knee osteoarthritis in the osteoarthritis initiative. Arthritis Rheum 2012;64:1437-46. https://doi.org/10.1002/art.33505.
- Hawker GA, Gignac MA, Badley E, Davis AM, French MR, Li Y, et al. A longitudinal study to explain the pain-depression link in older adults with osteoarthritis. Arthritis Care Res 2011;63:1382-90. https://doi.org/10.1002/acr.20298.
- Holla JF, Steultjens MP, Roorda LD, Heymans MW, Ten Wolde S, Dekker J. Prognostic factors for the two-year course of activity limitations in early osteoarthritis of the hip and/or knee. Arthritis Care Res 2010;62:1415-25. https://doi.org/10.1002/acr.20263.
- Oiestad BE, White DK, Booton R, Niu J, Zhang Y, Torner J, et al. The longitudinal course of physical function in people with symptomatic knee osteoarthritis: data from the MOST study and the OAI. Arthritis Care Res 2015;68:325-31. https://doi.org/10.1002/acr.22674.
- Riddle DL, Kong X, Fitzgerald GK. Psychological health impact on 2-year changes in pain and function in persons with knee pain: data from the Osteoarthritis Initiative. Osteoarthr Cartil 2011;19:1095-101. https://doi.org/10.1016/j.joca.2011.06.003.
- Sanchez-Ramirez DC, van der Leeden M, van der Esch M, Roorda LD, Verschueren S, van Dieën J, et al. Increased knee muscle strength is associated with decreased activity limitations in established knee osteoarthritis: two-year follow-up study in the Amsterdam osteoarthritis cohort. J Rehabil Med 2015;47:647-54. https://doi.org/10.2340/16501977-1973.
- Sharma L, Cahue S, Song J, Hayes K, Pai YC, Dunlop D. Physical functioning over three years in knee osteoarthritis: role of psychosocial, local mechanical, and neuromuscular factors. Arthritis Rheum 2003;48:3359-70. https://doi.org/10.1002/art.11420.
- Stannus OP, Jones G, Blizzard L, Cicuttini FM, Ding C. Associations between serum levels of inflammatory markers and change in knee pain over 5 years in older adults: a prospective cohort study. Ann Rheum Dis 2013;72:535-40. https://doi.org/10.1136/annrheumdis-2011-201047.
- Thomas E, Peat G, Mallen C, Wood L, Lacey R, Duncan R, et al. Predicting the course of functional limitation among older adults with knee pain: do local signs, symptoms and radiographs add anything to general indicators?. Ann Rheum Dis 2008;67:1390-8. https://doi.org/10.1136/ard.2007.080945.
- van Dijk GM, Veenhof C, Spreeuwenberg P, Coene N, Burger BJ, van Schaardenburg D, et al. Prognosis of limitations in activities in osteoarthritis of the hip or knee: a 3-year cohort study. Arch Phys Med Rehabil 2010;91:58-66. https://doi.org/10.1016/j.apmr.2009.08.147.
- White DK, Zhang Y, Felson DT, Niu J, Keysor JJ, Nevitt MC, et al. The independent effect of pain in one versus two knees on the presence of low physical function in a multicenter knee osteoarthritis study. Arthritis Care Res 2010;62:938-43. https://doi.org/10.1002/acr.20166.
- White DK, Keysor JJ, Lavalley MP, Lewis CE, Torner JC, Nevitt MC, et al. Clinically important improvement in function is common in people with or at high risk of knee OA: the MOST study. J Rheumatol 2010;37:1244-51. https://doi.org/10.3899/jrheum.090989.
- Felson DT, Gross KD, Nevitt MC, Yang M, Lane NE, Torner JC, et al. The effects of impaired joint position sense on the development and progression of pain and structural damage in knee osteoarthritis. Arthritis Rheum 2009;61:1070-6. https://doi.org/10.1002/art.24606.
- Riddle DL, Stratford PW. Body weight changes and corresponding changes in pain and function in persons with symptomatic knee osteoarthritis: a cohort study. Arthritis Care Res 2013;65:15-22. https://doi.org/10.1002/acr.21692.
- Collins JE, Katz JN, Dervan EE, Losina E. Trajectories and risk profiles of pain in persons with radiographic, symptomatic knee osteoarthritis: data from the osteoarthritis initiative. Osteoarthr Cartil 2014;22:622-30. https://doi.org/10.1016/j.joca.2014.03.009.
- Curtis L. Unit Costs of Health and Social Care 2014. Canterbury: Personal Social Services Research Unit; 2014.
- HRG4 Grouper Reference Manual Payment 14/15. Leeds: NHS Digital; 2014.
- Payment By Results in the NHS: Tariff for 2014 to 2015: 2014–15 Tariff Information Spreadsheet. London: DHSC; 2014.
- Hospital Episode Statistics . Admitted Patient Care England 2014–15: Procedures and Interventions 2015. www.hscic.gov.uk/catalogue/PUB19124/hosp-epis-stat-admi-proc-2014-15-tab.xlsx (accessed 6 April 2016).
- Hospital Episode Statistics . Hospital Episode Statistics, Admitted Patient Care – England, 2001–02: Main Operations, 4 Character Table 2002. www.hscic.gov.uk/catalogue/PUB03929/hosp-epis-stat-admi-main-ops-4cha-01-02-tab.xls (accessed 6 April 2016).
- NHS Reference Costs 2013 to 2014 – National Schedule of Reference Costs: The Main Schedule. London: DHSC; 2014.
- Gray AM, Clarke PM, Wolstenholme JL, Wordsworth S. Applied Methods of Cost-Effectiveness Analysis in Health Care. Oxford: Oxford University Press; 2011.
- Gray AM, Rivero-Arias O, Clarke PM. Estimating the association between SF-12 responses and EQ-5D utility values by response mapping. Med Decis Making 2006;26:18-29. https://doi.org/10.1177/0272989X05284108.
- Ramos-Goni JM, Rivero-Arias O, Dakin H. Response mapping to translate health outcomes into the generic health-related quality-of-life instrument EQ-5D: introducing the mrs2eq and oks2eq commands. Stata J 2013;13:474-91.
- Schilling C, Dowsey MM, Clarke PM, Choong PF. Using patient-reported outcomes for economic evaluation: getting the timing right. Value Health 2016;19:945-50. https://doi.org/10.1016/j.jval.2016.05.014.
- Briggs A, Sculpher M, Claxton K, Gray A, Briggs A. Decision Modelling for Health Economic Evaluation. Oxford: Oxford University Press; 2006.
- Health and Social Care Information Centre . Finalised Patient Reported Outcome Measures (PROMs) in England, April 2013 to March 2014 2015. www.hscic.gov.uk/catalogue/PUB17876/final-proms-eng-apr13-mar14-fin-report-v1.pdf (accessed 6 April 2016).
- Office for National Statistics . Mid-2013 Population Estimates 2015. www.ons.gov.uk/file?uri=/peoplepopulationandcommunity/populationandmigration/populationestimates/datasets/populationestimatesforukenglandandwalesscotlandandnorthernireland/mid2013/previous/v2/rft---mid-2013-uk-population-estimates(10).zip (accessed 3 December 2016).
- National Joint Registry (NJR) for England and Wales . OPCS Codes Relevant Procedure Is Recorded in the NJR 2013. www.njrcentre.org.uk/njrcentre/Portals/0/Documents/England/Data%20collection%20forms/OPCS%20Procedure%20codes%20relevant%20to%20NJR.pdf (accessed 29 September 2016).
- National Joint Registry for England and Wales . 13th Annual Report 2016. www.njrreports.org.uk/Portals/0/PDFdownloads/NJR%2013th%20Annual%20Report%202016.pdf (accessed 21 November 2017).
- Coyle D, Buxton MJ, O’Brien BJ. Stratified cost-effectiveness analysis: a framework for establishing efficient limited use criteria. Health Econ 2003;12:421-7. https://doi.org/10.1002/hec.788.
- Espinoza MA, Manca A, Claxton K, Sculpher MJ. The value of heterogeneity for cost-effectiveness subgroup analysis: conceptual framework and application. Med Decis Making 2014;34:951-64. https://doi.org/10.1177/0272989X14538705.
- Dolan P, Gudex C, Kind P, Williams A. The time trade-off method: results from a general population study. Health Econ 1996;5:141-54. https://doi.org/10.1002/(SICI)1099-1050(199603)5:2<141::AID-HEC189>3.0.CO;2-N.
- Skou ST, Roos EM, Laursen MB, Rathleff MS, Arendt-Nielsen L, Simonsen O, et al. A randomized, controlled trial of total knee replacement. N Engl J Med 2015;373:1597-606. https://doi.org/10.1056/NEJMoa1505467.
- The Royal College of Surgeons of England . Is Access to Surgery a Postcode Lottery? 2014. www.rcseng.ac.uk/news-and-events/media-centre/press-releases/many-ccgs-are-ignoring-clinical-evidence-in-their-surgical-commissioning-policies/ (accessed 8 November 2016).
- Harrogate and Rural District Clinical Commissioning Group . Clinical Thresholds: Hip and Knee Arthroplasty for Osteoarthritis (Only) 2014. www.harrogateandruraldistrictccg.nhs.uk/data/uploads/rss2/hip-and-knee-arthroplasty.pdf (accessed 8 November 2016).
- Thames Valley Priorities Committee . Thames Valley Priorities Committee Commissioning Policy Statement. Policy No. 187b (TVPC30) Primary Hip Joint Replacement for Patients With Osteoarthritis of the Hip 2015. www.oxfordshireccg.nhs.uk (accessed 20 October 2016).
- Thames Valley Priorities Committee . Thames Valley Priorities Committee Commissioning Policy Statement. Policy No. 188a (TVPC7) Primary Total Knee Replacement Surgery for Patients With Osteoarthritis of the Knee 2016. www.oxfordshireccg.nhs.uk/professional-resources/documents/commissioning-statements/188a-Total-Knee-Joint-Replacement.pdf (accessed 20 October 2016).
- Individual Funding Request Team . Commissioning Policy Individual Funding Request: Hip Replacement Surgery – Including Referral for Surgical Assessment of Osteoarthritis 2016. www.bristolccg.nhs.uk/media/medialibrary/2016/09/hip_replacement_surgery__including_referral_for_surgical_assessment_of_osteoarthritis.pdf (accessed 8 November 2016).
- Individual Funding Request Team . Commissioning Policy Individual Funding Request: Knee Replacement Surgery (Including Partial and Total Knee Replacement With or Without Patella Replacement or Resurfacing) 2016. www.bristolccg.nhs.uk/media/medialibrary/2016/10/Knee_Replacement_Surgery_Policy_6tw3a9e.pdf (accessed 8 November 2016).
- Scarborough and Ryedale Clinical Commissioning Group . Hip Replacement Pathway 2015. www.scarboroughryedaleccg.nhs.uk/data/uploads/rss2/orthopaedics/hip-replacement-march-2015.pdf (accessed 8 November 2016).
- Franks P, Lubetkin EI, Gold MR, Tancredi DJ, Jia H. Mapping the SF-12 to the EuroQol EQ-5D index in a national US sample. Med Decis Making 2004;24:247-54. https://doi.org/10.1177/0272989X04265477.
- Koenker R, Hallock KF. Quantile regression. J Econ Perspect 2001;15:143-56. https://doi.org/10.1257/jep.15.4.143.
- Royston P, Altman DG. Regression using fractional polynomials of continuous covariates: parsimonious parametric modelling. J R Stat Soc Ser C Appl Stat 1994;43:429-67. https://doi.org/10.2307/2986270.
- Cohen J. Statistical Power Analysis for the Behavioral Sciences. Hillsdale, NJ: Erlbaum; 1988.
- Murray DW, Fitzpatrick R, Rogers K, Pandit H, Beard DJ, Carr AJ, et al. The use of the Oxford hip and knee scores. J Bone Joint Surg Br 2007;89:1010-14. https://doi.org/10.1302/0301-620X.89B8.19424.
- Finalised Patient Reported Outcome Measures (PROMS) in England, April 2011 to March 2012 (Annual Report). Leeds: NHS Digital; 2013.
- Finalised Patient Reported Outcome Measures (PROMs) in England, April 2012 to March 2013 (Annual Report). Leeds: NHS Digital; 2014.
- Finalised Patient Reported Outcome Measures (PROMs) in England, April 2014 to March 2015 (Annual Report). Leeds: NHS Digital; 2016.
- Eckstein F, Wirth W, Nevitt MC. Recent advances in osteoarthritis imaging – the osteoarthritis initiative. Nat Rev Rheumatol 2012;8:622-30. https://doi.org/10.1038/nrrheum.2012.113.
- Segal NA, Nevitt MC, Gross KD, Gross KD, Hietpas J, Glass NA, et al. The Multicenter Osteoarthritis Study: opportunities for rehabilitation research. PM R 2013;5:647-54. https://doi.org/10.1016/j.pmrj.2013.04.014.
- Karuppiah SV, Banaszkiewicz PA, Ledingham WM. The mortality, morbidity and cost benefits of elective total knee arthroplasty in the nonagenarian population. Int Orthop 2008;32:339-43. https://doi.org/10.1007/s00264-007-0324-y.
- Gray A, Clarke P, Wolstenholme J, Wordsworth S. Applied Methods of Cost-Effectiveness Analysis in Health Care. Oxford: Oxford University Press; 2011.
- NHS Oxfordshire . INTERIM Treatment Threshold Statement Knee Arthroplasty PS188 2010. www.oxfordshireccg.nhs.uk (accessed 5 October 2016).
- Moons KG, Royston P, Vergouwe Y, Grobbee DE, Altman DG. Prognosis and prognostic research: what, why, and how?. BMJ 2009;338. https://doi.org/10.1136/bmj.b375.
- Osteoarthritis: NICE Quality Standard 87. Manchester: NICE; 2015.
- Osteoarthritis: The Care and Management of Osteoarthritis in Adults: NICE Clinical Guideline 177. Manchester: NICE; 2014.
- Royal College of Surgeons . Commissioning Guide: Painful Osteoarthritis of the Knee, Version 1.1 2014. www.rcseng.ac.uk/-/media/files/rcs/library-and-publications/non-journal-publications/osteoarthritis-of-knee-commissioning-guide.pdf (accessed 21 Nov 2017).
- Finalised Patient Reported Outcome Measures (PROMs) in England, April 2010 to March 2011 (Annual Report). Leeds: NHS Digital; 2012.
- Finalised Patient Reported Outcome Measures (PROMs) in England, April 2009 to March 2010 (Annual Report). Leeds: NHS Digital; 2011.
- Dakin H, Devlin N, Feng Y, Rice N, O’Neill P, Parkin D. The influence of cost-effectiveness and other factors on NICE decisions. Health Econ 2015;24:1256-71. https://doi.org/10.1002/hec.3086.
- The NHS Atlas of Variation in Health Care: Reducing Unwarranted Variation to Increase Value and Improve Quality. Leeds: NHS Digital; 2010.
- NHS Digital . Finalised Patient Reported Outcome Measures in England – April 2014 to March 2015: Score Comparison Tool 2016. http://content.digital.nhs.uk/catalogue/PUB21189 (accessed 11 November 2016).
Appendix 1 Additional data relating to Chapter 9 (unedited general practitioner comments)
As part of the survey carried out with GPs a number of free-text comments were recorded. The free-text comments are listed below in an unedited format:
Looks promising! Will need a lot of publicity, however, to sell to colleagues.
Useful where patient pressure for surgery but not really appropriate.
Too long many GP’s won’t use it in this format. May be better to give patient to take home to answer and bring back at next appointment for inputting into computerised tool. Many GP’s won’t use it in this format. Great idea though.
Hip and knee referrals go to physio for assessment. They then refer onwards to orthopaedics if needed – unless evidence of server OA [osteoarthritis] radiologically (which can be referred directly to orthopaedics.
Excellent tool but there might be exceptions and need to allow for this.
If used by GP would potentially avoid unnecessary referrals. Also acts as a tool to aid discussion with GP about management options.
The MSK [musculoskeletal] hub is not the right environment. In an ideal world the tool would have a threshold that would allow the patient to bypass the MSK hub altogether and be referred directly in to an orthopaedic clinic.
I think it is good to get patients thinking about surgery BEFORE the hub. This might also reduce referrals!
By e-mail preferable – can e-mail them a link during the consult, have them complete it at home and return to me.
Paper at home electronically in practice.
Ideally at home, but in surgery not a disaster, as pretty quick to complete.
The tool seems to require a degree of understanding stats/interpreting risk by both GP and patient.
Too long.
Problem if the patient comes with 2 or 3 other problems! But we can focus on hip/knee and ask them to return to discuss other problems.
Can aid discussion with patients about management options.
But it has to be user-friendly and quick, as the hip/knee problem is likely to be one of 2 or 3 problems the patient brings to the consultation!
Appendix 2 Complete list of user group members
Name | Role |
---|---|
Alastair Gray | Professor of Health Economics |
Andrew Price | Professor and Consultant Orthopaedic Surgeon (Chief Investigator) |
Anne Clarkson Webb | Extended Scope Physiotherapist NOC |
Anthony Johnson | Retired GP and Patient Representative |
Chad Lion Cachet | Patient Representative |
David Beard | Professor of Musculoskeletal Sciences, Co-Director of SITU Oxford, Chairperson |
Elena Benedetto | ACHE Project Co-ordinator |
Fiona Watt | Consultant Rheumatologist |
Fraser Old | Patient Representative |
Gill Dean | GP working with Sports Injury and Rheumatology |
Gillian Kempster | Patient Representative |
Helen Dakin | Senior Researcher in Health Economics |
James Smith | Study Co-ordinator |
Jannie Kramer | Patient Representative |
Jennifer Bostock | Patient Representative |
Jiyang Li | PA to David Beard |
Jo Hewanicka | PA to Andrew Price |
John Nolan | Consultant Orthopaedic Surgeon and President Elect of British Hip Society |
Jonathon Cook | Associate Professor of Statistics |
Karen Barker | Clinical Director for Musculoskeletal Services at the NOC |
Kate Jackson | GP working in Sports and Exercise Medicine for MOD and ARUK |
Kristina Harris | Post Doctoral Associate/Lead Co-ordinator of ACHE Project |
Laura Ingle | GP |
Mary Snow | Patient Representative |
Matthew Cheerham | GP |
Patricia Mary Rubery | Patient Representative |
Peter Eibich | Senior Research in Health Economics |
Sharon Barrington | OCCG Lead for Elective Care |
Sujin Kang | Senior Statistician |
Tim Wilton | President of British Orthopaedic Association |
Vida Field | Patient Representative |
Appendix 3 Additional data relating to Chapter 9 (unedited patient and public comments)
As part of the survey carried out with patients and the public a number of free-text comments were recorded. The free-text comments are listed below in an unedited format:
Allow patient to complete these BEFORE attending the doctor to speed up the consultation time.
As long as it does not become an inflexible tick-box process.
However it is important to stress that a decision for treatment whatever that treatment is, should be made jointly i.e. the GP and the patient. It is not the GP who decides on their own whether a patient should see a consultant/surgeon. The patient may choose to wait; they may wish to continue taking medication.
Useful additional tool for both GPs and patients but clearly both should factor in other relevant information e.g. recovery time, availability of physiotherapy, etc.
If this had existed, I would have welcomed some form of self-assessment.
Complete with GP or at home? Happy with either really although if completing at home it would be good to have a GP discussion after this.
I can always keep a note of my responses, and score to discuss with my GP at an appointment should this be necessary. Is there any way a copy of the questionnaire can be saved on a home computer?
Paper or electronically? Either.
I don’t care either way.
I think a follow-up face-to-face in-depth discussion between patient and surgeon would be essential before making that decision. Ticks in a box cannot express the amount of pain and suffering that a face and voice does.
But only if it was going to make a difference to eligibility.
It is extremely difficult to record the level of pain. Consider more use be made of radiography and scans to determine degree of bone loss.
A good tool for both the GP and the patient.
I think this is a good starting tool but possibly needs a few, more detailed questions, i.e. could do with drilling deeper, otherwise I think it is too open for inaccurate reporting and decision-making. For example, if pain is intolerable, is that sporadically in short bursts of 5–10 minutes or waves of half an hour, does it last all day, or does it get worse as the day goes on or is it relieved by certain movement or action. If it affects work or day-to-day tasks, in what way and what action is taken to relieve it or to be able to carry on. There aren’t any questions about increased or decreased pain or pain management, which I think would be useful and should influence the decision-making.
Shouldn’t occupation and levels and types of activity and medication be taken into consideration? And as mentioned previously, there should be questions to ascertain a benchmark for an individual’s pain threshold and resilience to pain.
I would also like to know how age influences the decision-making and prioritising. My perception is that a joint operation is left until as late as possible, especially hip operations which are synonymous with older patients and giving them a decent quality of later life, but in my humble opinion, a 35-year-old’s quality of life for the next 20 years, while they can be and are still active and having to work, is surely worth prioritising as much as a 75-year-old’s next 20 years?
I realise that if a GP is completing this with the patient, there is the potential risk of rushing it or not giving as accurate information as would be useful because 10 minutes is not a long time; perhaps there could be an advisory note to the effect that a double appointment is required to complete the questionnaire?
Show on a scale the weighting factors based on the input criteria, i.e. if I changed an entry from ‘moderate pain’ to ‘severe pain’ what effect that has on the results – as this can be subjective.
Also – explain how to grade your pain levels as they differ so much from person to person. Also – going up and down stairs – do you hear your knees making a noise? And at other times.
The questionnaire does not really reflect the reality of the condition, or at least mine. It is not a matter of ‘last four weeks’, but of sometimes one, sometimes another. The degree of pain or difficulty in doing something can vary radically over as little as half an hour from bad to better to bad again, so that e.g. little pain and moderate pain are not constants. A ‘sometimes’ choice would make a much more accurate choice.
What are you doing for those patients who do not have access to a computer, and will need time to become familiar with a new process? This primarily I would imagine would be elderly people.
Technology is being heavily invested in as a support tool for clinical decision-making (vs. GPs decision-making!) in a number of areas. Would it be sensible to consider GPs surgeries having a computer in the waiting room set up with all the tools, and a support staff there to help familiarise patients.
List of abbreviations
- ACHE
- Arthroplasty Candidacy Help Engine
- ADAPT
- Assessing Disability After Partial and Total Joint Replacement
- AIC
- Akaike information criterion
- APC
- admitted patient care
- APEX
- Arthroplasty Pain EXperience study
- ARUK
- Arthritis Research UK
- ASA
- American Society of Anesthesiologists
- AUC
- area under the curve
- BMI
- body mass index
- CCG
- clinical commissioning group
- CEAC
- cost-effectiveness acceptability curve
- CI
- confidence interval
- COASt
- Clinical Outcomes in Arthroplasty Study
- CPRD
- Clinical Practice Research Datalink
- CrI
- credible interval
- CRN
- clinical research network
- DARE
- Database of Abstracts of Reviews of Effects
- EPOS
- Exeter Primary Outcome Study
- EQ-5D
- EuroQol-5 Dimensions
- EQ-5D-3L
- EuroQol-5 Dimensions, three-level version
- EQ-5D-5L
- EuroQol-5 Dimensions, five-level version
- ES
- effect size
- EUROHIP
- The European Collaborative Database of Cost and Practice Patterns of Total Hip Replacement
- GLM
- generalised linear model
- GP
- general practitioner
- HES
- Hospital Episode Statistics
- HRG
- Healthcare Resource Group
- HTA
- Health Technology Assessment
- HUI2
- Health Utilities Index Mark 2
- HUI3
- Health Utilities Index Mark 3
- IAP
- Impairment Activity Limitation and Participation Restriction
- ICC
- intracluster correlation coefficient
- ICER
- incremental cost-effectiveness ratio
- ICOAP
- Intermittent and Constant Osteoarthritis Pain Measure
- INB
- incremental net benefit
- IT
- information technology
- KAT
- Knee Arthroplasty Trial
- KOOS
- Knee injury and Osteoarthritis Outcome Score
- KOOS-PS
- Knee injury and Osteoarthritis Outcome Score – Physical Score
- MCID
- minimally clinically important difference
- MCS
- mental component score
- MDC
- minimally detectable change
- MIC
- minimally important change
- MID
- minimally important difference
- MRI
- magnetic resonance imaging
- MSE
- mean squared error
- NDORMS
- Nuffield Department of Orthopaedics, Rheumatology and Musculoskeletal Sciences
- NICE
- National Institute for Health and Care Excellence
- NIHR
- National Institute for Health Research
- NJR
- National Joint Registry
- NOC
- Nuffield Orthopaedic Centre
- OHS
- Oxford Hip Score
- OKS
- Oxford Knee Score
- OKS-APQ
- Oxford Knee Score – Activity and Participation Questionnaire
- OLS
- ordinary least squares
- PCS
- physical component score
- PLG
- patient liaison group
- PROM
- patient-reported outcome measure
- PSA
- probabilistic sensitivity analysis
- QALY
- quality-adjusted life-year
- RCT
- randomised controlled trial
- ROC
- receiver operating characteristic
- SD
- standard deviation
- SE
- standard error
- SEM
- standard error of the mean
- SF-6D
- Short Form questionnaire-6 Dimensions
- SF-12
- Short Form questionnaire-12 items
- SF-36
- Short Form questionnaire-36 items
- THA
- total hip arthroplasty
- TJA
- total joint arthroplasty
- TKA
- total knee arthroplasty
- WHOQOL
- World Health Organization Quality of Life
- WOMAC
- Western Ontario and McMaster Universities Arthritis Index