Notes
Article history
The research reported in this issue of the journal was funded by the HTA programme as project number 13/20/01. The contractual start date was in May 2016. The draft report began editorial review in August 2019 and was accepted for publication in March 2020. The authors have been wholly responsible for all data collection, analysis and interpretation, and for writing up their work. The HTA editors and publisher have tried to ensure the accuracy of the authors’ report and would like to thank the reviewers for their constructive comments on the draft document. However, they do not accept liability for damages or losses arising from material published in this report.
Disclaimer
This report contains transcripts of interviews conducted in the course of the research and contains language that may offend some readers.
Permissions
Copyright statement
© Queen’s Printer and Controller of HMSO 2021. This work was produced by Stone et al. under the terms of a commissioning contract issued by the Secretary of State for Health and Social Care. This issue may be freely reproduced for the purposes of private research and study and extracts (or indeed, the full report) may be included in professional journals provided that suitable acknowledgement is made and the reproduction is not associated with any form of advertising. Applications for commercial reproduction should be addressed to: NIHR Journals Library, National Institute for Health Research, Evaluation, Trials and Studies Coordinating Centre, Alpha House, University of Southampton Science Park, Southampton SO16 7NS, UK.
2021 Queen’s Printer and Controller of HMSO
Chapter 1 Introduction
Background
Patients approaching the end of their lives, as well as their relatives and clinicians, value accurate prognostic information. 1–5 This information is usually obtained by clinicians using their clinical intuition. However, clinician prediction of survival (CPS) is often inaccurate and overoptimistic. 6 The need for more accurate methods of prognostication was one of the issues highlighted by the Neuberger report7 into the shortcomings of the implementation of the Liverpool Care Pathway. 8
Systematic identification of patients approaching the ‘end of life’ is a key recommendation of the Department of Health and Social Care’s end-of-life care strategy. 9 The Gold Standards Framework (GSF) service improvement programmes (widely used in general practice, nursing homes and, increasingly, acute hospitals) have produced proactive identification guidance to identify patients approaching the last year of life. 10 However, many patients who would potentially benefit from inclusion in such programmes are currently unidentified by clinicians. More reliable prognostic estimates may facilitate the identification of such patients and may improve the prioritisation of patients who are referred to palliative care services. Improved prognostication would benefit patients and their carers by providing them with better-quality information to inform their choices about future care. 3 Improved prognostication would also help clinicians to plan services and to ensure that patients are cared for in the most appropriate environment and with the most appropriate treatments. Prognostic scores could also facilitate comparison of services by more accurately describing the case mix of referrals.
The Prognosis in Palliative care Study (PiPS) predictive models of survival were previously developed by members of our research team to provide an objective aid to clinicians’ intuition. 11 The original study prospectively recruited a cohort of 1018 patients with advanced cancer who were no longer undergoing disease-modifying treatment. This was a multicentre study involving 18 specialist palliative care services across England. Separate prognostic models were created for patients without or with available blood results [PiPS-A (Prognosis in Palliative care Study – All) and PiPS-B (Prognosis in Palliative care Study – Blood), respectively]. Logistic regression identified 11 core variables [i.e. pulse rate, general health status, mental test score, performance status, presence of anorexia, presence of any site of metastatic disease, presence of liver metastases, serum C-reactive protein (CRP) concentration, white blood cell count, platelet count and serum urea concentration] that were independently predictive of both 2-week and 2-month survival. Four variables had prognostic significance for 2-week survival only (i.e. dyspnoea, dysphagia, bone metastases and alanine transaminase concentration) and eight variables had prognostic significance for 2-month survival only (i.e. primary breast cancer, male genital cancer, tiredness, weight loss, lymphocyte count, neutrophil count, alkaline phosphatase concentration and albumin concentration). The receiver operating characteristic (ROC) area under the curve (AUC) for all models varied between 0.79 and 0.86.
Each PiPS model consists of two prognostic submodels [PiPS-A 14-day model (PiPS-A14) and PiPS-A 56-day model (PiPS-A56); PiPS-B 14-day model (PiPS-B14) and PiPS-B 56-day model (PiPS-B56)] that are combined using a ‘decision rule’. PiPS-A14 and PiPS-B14 predict whether a patient will die within days (< 14 days) or will live for ≥ 14 days. PiPS-A56 and PiPS-B56 predict whether a patient will live for ‘months+’ (≥ 56 days) or will die ≤ 55 days. The output of the two submodels are combined using the PiPS decision rule, which determines that, if the 14-day model predicts that the probability of survival is < 50%, then the patient is predicted to die within days (0–13 days); if the 56-day model predicts that the probability of survival is > 50%, then the patient is predicted to live for months+ (56 days or more); and, if the probability of 14-day survival is > 50% and the probability of 56-day survival is < 50%, then the patient is predicted to survive for weeks (14–55 days).
Thus, PiPS scores are able to predict whether a patient is likely to live for days (< 14 days), weeks (2–7 weeks) or months+ (≥ 2 months). These survival categories were chosen as they were deemed to have the greatest face validity among clinicians. Both PiPS-A and PiPS-B were shown to perform as well as CPS. The PiPS-B prognostic estimate was found to be significantly better than clinicians’ or nurses’ prognostic estimates but no better than a multidisciplinary agreed prognosis.
Following the publication of the PiPS prognostic tools, the National Institute for Health Research (NIHR) Health Technology Assessment (HTA) programme issued a commissioned call for studies to undertake ‘the validation of models of survival to improve prognostication in advanced cancer care to include the Prognosis in Palliative care Study (PiPS) predictor models’.
The commissioning brief12 from the NIHR was to evaluate the PiPS tools and other prognostic indices. Based on the results of systematic reviews,13,14 four other prognostic models were identified that might also be useful in clinical practice and that were in need of further evaluation. These were the Palliative Prognostic Index (PPI),15 the Palliative Performance Scale (PPS),16 the Palliative Prognostic (PaP)17 score and the Feliu Prognostic Nomogram (FPN). 18
The PPI and the PPS can be calculated without the need for a blood test (like PiPS-A). The PPI model stratifies patients into three groups: survival for < 3 weeks, survival for < 6 weeks and survival for > 6 weeks. 15 The PPI has shown a high level of accuracy in patients with short estimates of survival. 19 The PPS is a measure of functional status and is one of the variables included in the PPI score. Although not specifically designed as a prognostic instrument, and therefore lacking some face validity as a stand-alone prognostic tool, the PPS has been found to have prognostic significance in patients with advanced disease. 20,21 Using retrospective data from large observational studies, the PPS was found to distinguish between groups of patients with different probabilities of dying across a range of survival times. 20
The PaP and the FPN require blood test results (like PiPS-B). The PaP classifies patients into three risk groups based on a 30-day survival probability of < 30%, 30–70% and > 70%. 17 There is increasing evidence to support its validity in a variety of settings. 22–26 The total PaP score was shown in one study to be more accurate than a simple CPS. 27 One practical concern with the PaP score is that it relies on CPS. This can make the PaP challenging to use when clinicians are unsure about survival times or when an ‘objective’ estimate is required that is free from the influence of CPS. The FPN predicts survival at 15, 30 and 60 days. 18 In one study, the FPN was found to be more accurate than the PaP18 and it does not rely on subjective CPS.
Although clinician estimates of survival have been shown to be inaccurate and overoptimistic, it is important that they are included as a comparator in any evaluation of prognostic scores because this is the method by which most clinicians currently form their opinion regarding likely survival. Our own work in this area has suggested that a multidisciplinary estimate of survival is more accurate than a nurse’s estimate of survival. Accordingly, the PiPS2 study compared the accuracy of PiPS-B with a clinician’s, a nurse’s or a multiprofessional estimate of survival.
The NIHR HTA commissioning brief12 also requested that an ‘assessment of the acceptability to patients and clinicians of the use of prognostic models’ should be included. Our research has tackled these questions using qualitative methods. We also assessed the acceptability of the models to the relatives/carers of patients. This is particularly relevant because in clinical practice it is often the relatives and carers who most wish to have access to accurate prognostic information.
As highlighted in the 2013 Neuberger report,7 a key research priority for the NHS is to determine the best ways to communicate uncertainty to patients and families about prognostic estimates. Previous research has shown that the majority of patients (61%) would want to know their prognosis if such information was available. 28 Our qualitative substudy arm was designed to explore with patients and carers the type and extent of prognostic information they require and the best (and most sensitive) way to present this to them. The qualitative substudy also asked clinicians about the acceptability and practical utility of using prognostic indicators to support their subjective estimates and any facilitators of or barriers to their use.
Research objectives
Validation study
Primary objective:
-
to validate the PiPS models and to compare the performance of PiPS-B risk categories with CPS, including both individuals' estimates of survival and agreed multiprofessional estimates of survival (AMPESs).
Secondary objectives:
-
to validate PaP, FPN, PPI and PPS
-
to determine the acceptability of all prognostic models (including PiPS) to patients, carers and clinicians, and to identify potential barriers to clinical use.
Nested qualitative substudy
Primary objectives:
-
to assess the acceptability of the prognostic models to patients, carers and clinicians.
-
to identify barriers to and facilitators of clinical use.
Secondary objectives:
-
to explore clinicians’ views and opinions about the usefulness of prognostic models
-
to identify potential barriers to and facilitators of using prognostic models in clinical practice
-
to understand how clinicians discuss prognostic information with patients and relatives or carers.
Chapter 2 Research methods
The protocol (of both the validation and the nested qualitative studies) has been published elsewhere (Kalpakidou et al. ). 29 Parts of this chapter have been adapted from Kalpakidou et al. 29 This is an Open Access article distributed in accordance with the terms of the Creative Commons Attribution (CC BY 4.0) license, which permits others to distribute, remix, adapt and build upon this work, for commercial use, provided the original work is properly cited. See: http://creativecommons.org/licenses/by/4.0/. The text below includes minor additions and formatting changes to the original text.
In this report, we first describe the involvement of patients and the public in our research. We then describe the methods of validation and the qualitative substudy separately.
Patient and public involvement
We regarded the involvement of patients and the public as an important factor in the design, delivery, interpretation and dissemination of our research.
Involvement in research design
As part of the PiPS development study,11,28 we asked for patients’ views about obtaining prognostic information. The majority (478/778; 61%) of patients with capacity to consent to participate in Prognosis in Palliative care Study I (PiPS1) indicated that, if the information were available, they would want to know their prognosis, 176 (23%) indicated that they would prefer not to know and 124 (16%) were ambivalent (they ‘did not know’ or ‘did not care’ about the issue). This reaffirmed to us the importance of ensuring that prognostic information is not ‘thrust upon’ patients who would rather not receive it and underlined the need to include further qualitative work in PiPS2 to understand the best way to present this information to patients and their families.
In preparation for PiPS2, we undertook a more in-depth consultation with seven individual cancer patients and one focus group of users comprising a further eight carers/patients. All users agreed that the subject was an important area for clinical research. We asked users to reflect on the involvement of patients without capacity in the study and in whom we should undertake blood tests. In the light of service user feedback, we decided to include patients without capacity in the research, but to limit their involvement to observational measurements only.
Two service users (PB and DAF) joined the study team early in the course of the research. They contributed to the design of the topic guide and the interview schedule used in the qualitative substudy and ensured that the content was appropriate and comprehensive. Our service user representatives also contributed to the creation of the patient and carer information sheets used in the quantitative and qualitative studies.
Involvement in research delivery
Three service user representatives, recruited via the Marie Curie (London, UK; www.mariecurie.org.uk) ‘Expert Voices’ users’ group, were actively involved throughout the conduct of the study. One service user representative acted as a member of the Study Steering Committee and had an important role in overseeing the study. The other two service user representatives (PB and DAF) were fully involved in the ongoing management and delivery of the study. They participated as research team members in minuted, monthly Study Management Group meetings (usually by teleconference) and contributed to discussions of issues arising during the trial, thereby ensuring the smooth running of the study. They contributed to the interpretation of the qualitative data arising from the interviews with patients and their carers and throughout data analysis. They were presented with emerging themes and anonymised quotes, and their interpretations were included in the ongoing and final narrative.
Dissemination of research findings
Most of the dissemination activities of the grant are yet to be undertaken. Nonetheless, our service user representatives have already coauthored poster presentations at conferences. PB and DAF, co-authors of this report, attended the Annual Supportive and Palliative care conference (21–22 March 2019) in Harrogate, where they were co-authors on a poster presentation relating to patients’ and carers’ perspectives on the use of the PiPS predictor models. The main results relating to validation of PiPS (both quantitative and qualitative) will be delivered as an oral presentation at the European Association of Palliative Care Congress in 2020 (online) and have been written up, but not yet accepted, for publication in a peer-reviewed journal. Preliminary results relating to recruitment processes were presented in poster format at the European Association of Palliative Care congresses in Madrid (2018) and Berlin (2019). Preliminary results relating to the qualitative subsidy were presented in poster format at the European Association of Palliative Care congress in Bern (2018).
Validation study
Design
This was a multicentre, prospective, observational, cohort study to validate various prognostic models in patients with advanced, incurable cancer. The study involved patients from 27 palliative care services across England and Wales and was sponsored by University College London (UCL), London, UK. A full list of participating units and the principal investigators (PIs) at each site is provided in Appendix 3.
Patients were recruited in three palliative care settings:
-
community palliative care teams (CPCTs) (including day hospice and palliative care outpatients)
-
hospital palliative care teams (HPCTs)
-
hospices [including inpatient palliative care units (IPCUs)].
The recruitment period was from August 2016 to the end of April 2018. Three months after recruitment ended, a list of study participants was sent to NHS Digital to determine dates of death. The accuracy of the studied prognostic models was then compared with actual survival.
Following Medical Research Council guidance,30 a subset of patients who agreed to participate in the main study, their next of kin (informal carers), patients who declined to participate in the main study and health-care professionals was recruited into a nested qualitative substudy to explore views about prognostication and the use of prognostic tools (see Methods of nested qualitative substudy, and Chapters 3 and 4).
Participants
The study involved patients with and without the capacity to consent to participate. Many patients at the end of their lives become confused, semiconscious or comatose, or may have pre-existing cognitive impairment; consequently, they frequently lack the capacity to consent to participate in research. The inclusion of patients who lacked capacity to consent was therefore of great importance because the study population should be representative of those patients commonly seen in palliative care services. Capacity to consent was assessed by the attending clinician using Department of Health and Social Care guidance. 31 If capacity to consent was in doubt, the clinician carried out a capacity test as provided by the Royal College of General Practitioners’ Mental Capacity Act Toolkit for Adults in England and Wales. 32
Inclusion criteria
-
Locally advanced or metastatic incurable cancer (estimated prognosis of survival < 1 year).
-
Age ≥ 18 years.
-
Recent referral to palliative care services. For community, day hospice or palliative care outpatients, ‘recent’ signifies fewer than three previous contacts with the palliative care service before recruitment to the study. For inpatient palliative care patients (including HPCTs), ‘recent’ signifies an appointment with member of the palliative care team no more than 7 days previously.
-
Sufficient English language skills to read and understand the patient information sheet and undertake study assessments.
Exclusion criterion
-
Receiving (or planning to receive) treatment with curative intent at the time of consent (patients receiving palliative treatment were eligible to participate).
Study assessments
Data were collected at a single time point and were usually obtained from a review of medical notes or a discussion with clinical staff. If patients were able to respond to questions, data could be obtained directly from them. The data required for the calculation of each prognostic score are shown in Table 1. The case report forms (CRFs) that were used are shown in Appendix 4.
Variable | Prognostic model | ||||||
---|---|---|---|---|---|---|---|
Type | Name | PiPS-A | PiPS-B | PaP | FPN | PPI | PPS |
Assessments by clinician | CPS | ✗ | |||||
ECOG | ✗ | ✗ | ✗ | ||||
General health status | ✗ | ✗ | |||||
KPS | ✗ | ||||||
PPS | ✗ | ✗ | |||||
Time to terminal disease | ✗ | ||||||
Blood tests | Albumin concentration | ✗ | ✗ | ||||
Alkaline phosphatase concentration | ✗ | ||||||
Alanine transaminase concentration | ✗ | ||||||
CRP concentration | ✗ | ||||||
Lactate dehydrogenase concentration | ✗ | ||||||
Lymphocyte count | ✗ | ✗ | ✗ | ||||
Neutrophil count | ✗ | ||||||
Platelet count | ✗ | ||||||
Urea concentration | ✗ | ||||||
White blood cell count | ✗ | ✗ | |||||
Clinical signs and symptoms | AMTS | ✗ | ✗ | ||||
Anorexia | ✗ | ✗ | ✗ | ||||
Delirium | ✗ | ||||||
Dysphagia | ✗ | ||||||
Dyspnoea at rest | ✗ | ✗ | ✗ | ||||
Fatigue | ✗ | ||||||
Oedema | ✗ | ||||||
Oral intake | ✗ | ||||||
Pulse rate | ✗ | ✗ | |||||
Weight loss in last month | ✗ | ||||||
Disease extent | Bone metastases | ✗ | ✗ | ||||
Breast cancer | ✗ | ||||||
Distant metastases | ✗ | ✗ | |||||
Liver metastases | ✗ | ||||||
Prostate cancer | ✗ | ✗ |
Demographic-, disease- and treatment-related data and capacity recording
Demographic details of enrolled patients, such as age, gender and current location (e.g. home, hospital or hospice) were recorded. NHS number and date of birth were also recorded to be sent to NHS Digital. Information on the nature and site of primary tumour and sites of metastases (if any) were collected and patients’ capacity to consent to participate in the study was documented.
Key symptoms
The presence or absence of key symptoms was recorded: anorexia, dysphagia, dyspnoea, fatigue and weight loss. This was required for the scoring of the prognostic models.
Abbreviated Mental Test Score
The Abbreviated Mental Test Score (AMTS) assesses cognitive function. 33 Patients who lacked capacity and so could not consent to participate in the study (including those who were unconscious) were attributed scores of zero.
Clinical assessments
The following clinical assessments were undertaken.
Clinical examination
Researchers recorded the presence or absence of ascites, peripheral oedema, delirium and decreased oral intake. Pulse rate was measured over 1 minute.
Measures of performance status
The Eastern Co-operative Oncology Group (ECOG) performance status34 was recorded. This is a measurement of a patient’s of everyday functioning and is required for the calculation of PiPS-A and PiPS-B.
The Karnofsky Performance Scale (KPS)35 was used to assess patients’ functional impairment. The KPS is required for the calculation of the PaP score.
The PPS16 describes a patient’s current ambulatory level, activity level, extent of disease, self-care abilities and intake and conscious level. It is one of the prognostic models being evaluated.
General health status
General health status was rated using a seven-point observer-rated scale with scores ranging from very poor (1) to excellent (7).
Time to terminal disease
The time to terminal disease (TTD) was estimated by clinicians. TTD is defined as the time that elapsed between the diagnosis and development of incurable disease and is required to calculate the FPN.
Blood tests
For patients with capacity to consent, a fresh blood specimen was taken. For patients without capacity to consent, there was no requirement to take a fresh blood specimen, but if a blood sample was being taken for another reason as a part of routine clinical care within 72 hours of study enrolment, then the relevant tests were requested. Even if no new specimen was being taken from a patient without capacity to consent, if relevant results were available within 72 hours of being enrolled in the study then these results were recorded.
The blood test results required were white blood cell count, lymphocyte count, neutrophil count, platelet count, urea concentration, albumin concentration, alkaline phosphatase concentration, alanine transaminase concentration, CRP concentration and lactate dehydrogenase (LDH) concentration. Blood specimens were processed locally in the routine clinical laboratory using usual local arrangements.
Clinicians’ estimates of survival
The attending clinician and nurse estimated survival of study participants independently. When the estimates agreed, this represented the combined multiprofessional prediction. When they were discordant, the clinician and nurse discussed the case and reached a consensus. To characterise the prognosticators in more detail, participants were asked to provide information about themselves (i.e. age, gender, professional training and years of specialist experience). Clinicians were also asked to provide their prognostic estimates using a number of different formats to facilitate comparison with the outputs of the prognostic scores. Clinicians were asked to (1) provide approximate estimates of length of survival: days (0–13 days), weeks (14–55 days) or months+ (≥ 56 days), (2) provide more specific estimates of survival to the nearest week (from < 1 week to > 12 weeks) and (3) estimate the probability of survival at specific time points (1, 3, 7, 15, 30 and 60 days).
Data management
A study database for the storage, management and analyses of identifiable data was developed via a secure web application named REDCap (Research Electronic Data Capture, UCL, London, UK) and using the UCL Data Safe Haven secure system. This system is certified to the ISO27001 information security standard.
The remainder of the (non-identifiable) study data [i.e. electronic case report forms (eCRFs)] were sent to a separate database that had been created and supported by a company named Sealed Envelope (London, UK). Data from the paper CRFs were manually transferred to the eCRFs by the researchers at each participating site.
Ethics approval
The study received ethics approval from the Yorkshire and the Humber – Leeds East Research Ethics Committee (REC) on 12 April 2016 (reference 16/YH/0132).
Ethics/protocol amendments
During the course of the study six amendments were submitted to and approved by the Health Research Authority (HRA): five non-substantial/minor amendments and one substantial amendment. In line with the relevant regulations, the substantial amendment was also submitted to and approved by the Yorkshire and the Humber – Leeds East REC. The REC was notified of the five non-substantial/minor amendments.
The amendments are listed below in chronological order:
-
The first non-substantial/minor amendment was submitted on 24 May 2016, involving the addition of new participating sites. This was approved on 21 July 2016.
-
A second non-substantial/minor amendment was submitted on 20 September 2016, involving minor changes to study documentation and the replacement of the PIs at four participating sites. These changes were approved on 26 September 2016.
-
A substantial amendment was submitted to the HRA and the REC on 30 January 2017, involving two main changes: a change/increase in the sample size and the inclusion of the nested qualitative substudy. These changes were approved by the HRA and REC on 7 February 2017.
-
A third non-substantial/minor amendment was submitted on 12 April 2017, involving the replacement of the PI at one participating site. This was approved on 18 April 2017.
-
A fourth non-substantial/minor amendment was submitted on 3 July 2017, involving the replacement of the PI at one participating site. This was approved on 12 July 2017.
-
A fifth non-substantial/minor amendment to the protocol of our qualitative substudy was submitted on 21 August 2017. The amendment clarified which carers could or could not be included in the qualitative substudy. This was approved on 10 October 2017.
Recruitment procedure
Patient identification
In each participating service, members of the clinical team maintained a screening log of all new referrals to the service. For the patients not eligible to participate, the screening log recorded age group, gender and reason for ineligibility.
Consent procedure
Study procedures differed between patients with and patients without the capacity to consent.
Patients with capacity to consent
Eligible patients were approached by a member of the clinical team about participation in the study. If eligible patients were not approached by a team member then the reason for failure to do so was recorded on the screening log.
Potential participants who had been approached by a member of the clinical team were asked if they were willing to speak to a member of the research team and were handed a patient information sheet (PIS). A member of the research team then discussed the study with the patient and sought their consent to participate. For community patients, this discussion sometimes occurred over the telephone. Written informed consent was usually obtained at least 24 hours after the PIS had been handed out but could occur on the same day if it was more convenient and acceptable for the patient. If the patient declined to participate in the study then the reason for this (if known) was documented on the screening log.
Patients without capacity to consent
For patients without capacity, a personal consultee was sought for advice. For patients with no personal consultee, the advice of a nominated consultee was sought. The nominated consultee was usually another doctor working in the hospital/hospice (who was not involved in the research), a social worker, a chaplain or the patient’s general practitioner (GP).
In a similar manner to the approach adopted for patients with capacity to consent, consultees of patients without capacity to consent were advised that it was usual practice to wait for 24 hours before giving assent. If the consultee gave telephone advice for the patient to be included in the study but they were unable to visit the unit to provide written evidence of assent, then verbal agreement was initially deemed sufficient to allow the research team to enrol the patient in the study and start data collection. However, in these circumstances an assent form was posted to the consultee to be signed and returned to the research team within 2 weeks of the patient being enrolled in the study. If no signed assent form was received then the patient was withdrawn from the study and all data were destroyed.
Patients with fluctuating capacity to consent
If a patient who temporarily lacked and then recovered capacity to consent was included in the study, then, when they recovered capacity, they would be informed about their involvement in the study and would have the opportunity to withdraw or confirm participation.
Quantitative statistical methods
Outcome measures
The primary outcome measures were survival of patients (measured from the date patients consented to participate), the CPS and the predictions of the PiPS-A and PiPS-B prognostic models. The secondary outcome measures were the predictions produced by the PPI, PPS, PaP and FPN.
Sample size
The sample size calculations were based on data collected during the original study. 11
For the comparison between PiPS-B model predictions and clinical prediction of survival
The primary model of interest for this research was PiPS-B. To show at least a 5% improvement in correct predictions using PiPS-B compared with clinicians’ predictions, assuming 80% power and 5% significance level and using a McNemar’s test, 1267 patients with complete PiPS-B data would be required. The formula and the software used for these calculations were based on the work produced by Machin et al. 36 It was estimated that to obtain 1267 complete PiPS-B data sets it would be necessary to recruit 1334 patients with capacity to consent (assuming 5% missing data). Furthermore, to recruit 1334 patients with capacity to consent, it was estimated that it would be necessary to recruit approximately 1778 patients in total (assuming 25% of patients would lack capacity to consent).
For the validation of the PiPS models
To validate predictions from a risk model it has been recommended that the validation data should have at least 100 events. 37 The validation data for PiPS2 involved several centres. There is no guidance on sample size calculation for multicentre prognostic validation data. We expected clustering of patients within centres to be minimal, based on other studies in community care. 38 However, to be conservative, we inflated the number of events required in the validation data to 150. Assuming an event rate of 17.8%, based on the original study, we estimated that we would require 843 patients to validate the PiPS-B model. In fact, to have sufficient participants to compare PiPS-B with CPS, we planned to recruit approximately 1778 patients, most of whom would be able to provide data for the validation of both PiPS-A and PiPS-B. Thus, the proposed sample size was more than adequate to validate both models. Using similar arguments, the proposed sample size was also more than sufficient to validate the other prognostic models (i.e. PPI, PPS, PaP and FPN).
Statistical analyses
Descriptive analysis
Predictors and outcomes were summarised using descriptive analysis. Categorical predictors have been reported as raw numbers and percentages. Continuous variables have been summarised using mean or median and standard deviation (SD) or interquartile range (IQR) as appropriate. The percentage of values missing for each predictor has also been presented. The survival times of patients have been summarised using median and IQRs, and Kaplan–Meier graphs.
Primary analyses
For both PiPS-A and PiPS-B, in the original study,11 two separate models were developed to predict the 2-week (14-day) and 2-month (56-day) survival of patients. The models were then combined using the original PiPS decision rule, thereby generating three prognostic categories (i.e. survival of < 2 weeks, 2 weeks to 2 months and > 2 months).
The performance of prognostic models is typically assessed by calculating measures of model discrimination and calibration. The discrimination of a model refers to how well it differentiates between those at higher risk of having the event of interest and those at lower risk. Calibration refers to the level of agreement between the observed outcomes and the predictions. 39 The discriminatory ability of the PiPS models has been assessed using the c-statistic. Separate c-statistics have been calculated for the 2-week and 2-month survival models. The c-statistic can vary between zero and 1.0, where a value of 0.5 means that the model shows no ability to discriminate. Model performance has also been assessed by plotting Kaplan–Meier survival curves for each of the three risk groups identified by the PiPS models (days, weeks and months+).
Model calibration has been assessed by comparing observed and predicted probabilities by calculation of the calibration in the large (CiL) and calibration slope (CS). 40 For a prognostic model to be well calibrated, the CiL should be close to zero and the CS should be close to 1.0. 41
To compare the accuracy of the model predictions and CPS, the primary analysis focused on the PiPS-B model. McNemar’s test was used to compare the proportion of overall patient deaths predicted correctly by PiPS-B (using the original decision rule11) with the corresponding proportion predicted correctly by clinicians.
Secondary analyses
As part of the secondary analyses, the PiPS models’ predictions for the 2-week and 2-month cut-off points were combined to produce a categorical prediction of survival (days, weeks or months+) and were compared with clinicians’ estimates and the corresponding observed values with respect to their accuracy. Linear-weighted k has also been used to compare the performance of the clinicians with that of the models.
The PPI, PPS, PaP and FPN prognostic models were also assessed as part of the secondary analyses. The calibration of these prognostic models were assessed using the CS,40 based on a logistic model for binary outcomes and the Cox model for survival outcomes. 42 Model discrimination was assessed using the c-statistic for binary outcomes and Harrell’s concordance index for survival outcomes. 41 The performance measures estimated for the various models were compared descriptively. The predictions made by these prognostic models were also compared with the corresponding observed outcomes and clinician predictions (where available or where possible).
As multiple comparisons are performed in this report, a Bonferroni correction43 has been used to adjust the significance level for the secondary analyses. The amended significance level of 0.05/7 (0.0071) has been used for the secondary analyses.
Bias owing to missing data was investigated and multiple imputation based on chained equations44 was used to impute missing predictor values.
Data checking
Before analysis, basic checks were performed to confirm the quality of the data. Incomplete or inconsistent data included missing data, data outside the expected range and other inconsistencies. If any inconsistencies were found, the corresponding values were double checked with the researchers and corrected if necessary. All changes were documented by the study statistician. Analyses were performed after dates of death had been obtained from NHS Digital, 3 months after completion of the study. Prior to the final analysis, all relevant data were entered, checked and locked, the analysis plan was finalised and approved and the analysis programs were prepared. The primary analysis was performed independently by two statisticians (VV and RO) to ensure accuracy.
Software
Data were downloaded from the study-specific online database provided by Sealed Envelope into a format suitable for Stata® (StataCorp LP, College Station, TX, USA). Statistical analyses were performed using Stata version 14.
Methods of nested qualitative substudy
A purposive sample of patients, carers and clinicians who had been asked to participate in the quantitative study in the Manchester and Derby areas (hospice, community and hospital sites) were also asked to consider participation in the qualitative substudy. Semistructured, face-to-face interviews were conducted with patients, carers and clinicians.
Sample size
The final sample size was determined by data saturation.
Study setting
For pragmatic reasons the setting for the qualitative substudy was mostly the Greater Manchester area. The demographic diversity of this area permitted us to recruit from a wide range of backgrounds. However, following advice from the Study Steering Committee, we extended the sampling frame to Derby, to recruit a small number of palliative home care patients.
Characteristics of patient and carer sample
The patient and carer sample comprised patients with capacity to consent and carers of patients with or without capacity to consent, who had agreed to participate in the quantitative study. We also approached patients and carers who had declined to participate in the quantitative study. We purposively sampled patients and carers according to prespecified characteristics so that our sample was as varied as possible and represented the views and experiences of a wide range of patients and carers. Gender and age are known examples of factors that may influence the decision to receive prognostic information45–49 and, therefore, were characteristics for which we purposively sampled.
Characteristics of health-care professional sample
The clinician sample was pragmatic and comprised health-care professionals who routinely cared for patients with advanced cancer and made prognostic predictions, such as palliative care specialists, oncologists, nurses and GPs.
Recruitment procedure
Patients and carers
Patients or carers in the qualitative substudy were initially approached by a member of the clinical team after they had been approached about the quantitative study. At this point they were handed the PIS for the qualitative substudy and asked if they would be happy to speak to a member of the research team about the study in more detail. Because interviewing patients/carers about prognosis is sensitive and could potentially cause distress, we employed a researcher experienced in interviewing palliative patients/discussing sensitive topics.
Health-care professionals
Health-care professionals were given at least 24 hours to decide whether or not they wanted to take part and were told that they were free to withdraw at any time. Written consent was taken prior to the interview.
Scope and nature of the interviews
Interviews were interactive and explored the acceptability of PiPS and other models. Interviews used topic guides (see Appendices 1 and 2) that were based on reviews of the literature, results of previous consultations with service users and the MORECare (Methods Of Researching End of life Care) recommendations for conducting research at the end of life. 50 The topic guides were iterative to allow new themes that emerged during interviews to be explored with future participants. Interview duration was mindful of participants’ needs to ensure that they were not overburdened and were expected to last < 1 hour. Interviews took place at a venue of the participant’s choice.
Topics explored in patient/carer interviews
Patient/carer interview content included the following topic areas: experiences of being approached to take part in the PiPS2 prognostic study, perspectives on the development and use of prognostic indicators, views and opinions of how best, and the most sensitive way, to present prognostic information to patients and/or relatives/carers, and opinions about the usefulness of such an indicator/tool.
Topics explored in health-care professional interviews
Clinicians were shown the prognostic models, tried them out during the interview and commented on their perceived clinical usefulness (e.g. ease of completion and interpretability of outputs). Clinician interview content included the following topic areas: experiences of making predictions of survival length to patients/carers, the information currently shared with patients/carers about prognosis and how it is conveyed, opinions on the development and use in clinical practice of prognostic indicators/tools, and barriers to and facilitators of clinical use of prognostic indicators/tools.
Conduct of the interviews
Face-to-face interviews were conducted by the researcher at a location to suit the participant. Generally this was a private side room of the hospice/hospital ward. However, some patients preferred the interview to take place at their bedside, especially if they were feeling particularly unwell. Some participants wished to be interviewed in their own homes.
Qualitative data analysis
Interview data were entered into NVivo 10 (QSR International, Warrington, UK) and analysed using the five stages of framework analysis:51 familiarisation, developing a thematic framework, indexing, charting, and mapping and interpretation. During the first stage (familiarisation), the research team became immersed in the data52 by reading and rereading the transcripts and discussing emerging themes. A thematic framework was then developed based on the topic guide. 53 After this, transcripts were indexed (coded) line by line using the thematic framework but we remained open to new themes that emerged. 54 Next, the data were entered into a chart so that coded extracts could be attributed to individual participants. Finally, participants’ views were compared and contrasted and the data were presented schematically (mapping). Rival explanations were explored.
An iterative and inductive approach to analysis was followed so that data analysis started alongside data collection. Themes and issues identified from interviews informed further questions and probing in both ongoing and future interviews. Memoranda and notes were written about emerging themes to summarise or analyse a point and to relate emerging ideas and concepts to existing literature. The qualitative research team met on a regular basis to discuss the development of codes, themes, categories and theories about the phenomenon being studied.
Emotional/physical distress during qualitative interviews
Owing to the sensitive nature of the research relating to discussions of prognostic information of palliative care patients, we developed a distress policy for the researcher completing face-to-face interviews to consider. For the qualitative substudy we collected data from patients, their relatives/carers and clinical staff shortly (a few days) after patients had been approached to take part in PiPS2. If the patient/carer became upset during the interview, the researcher asked if they would like to stop the interview and, if the interview was stopped, whether or not they wished to resume. If further support was needed, the researcher linked participants to existing support mechanisms in the hospice/organisation. All patients had already been referred to palliative care services and the relevant clinical services were notified of any distress detected during the study for follow-up.
Chapter 3 Research results
The results of the validation study and the qualitative substudy are presented separately.
Results of validation study
Recruitment
Between 1 August 2016 and 30 April 2018, 1833 patients (with capacity to consent, n = 1610; without capacity to consent, n = 223) were recruited at 27 different sites in England and Wales. One site failed to correctly follow study procedures and recruited only one participant. This site and this participant were withdrawn from the study and have not been included in any of the analyses. The numbers of patients recruited at each stage of the recruitment process are shown in Figure 1.
FIGURE 1.
Flow diagram of patient recruitment to the PiPS2 validation study. a, For two patients there was more than one reason for being ineligible; b, nine patients were not approached for more than one reason; c, other reasons include patients’ extreme fatigue, distress and feeling too unwell; d, the original paper CRFs had been misplaced for two patients at two distinct participating sites and data had not been entered in the eCRF. Both patients were included in the analysis and data were treated as missing; e, 61 of the participants without capacity to consent had blood taken as part of routine assessment and therefore were included in the primary analysis of the PiPS-B model.
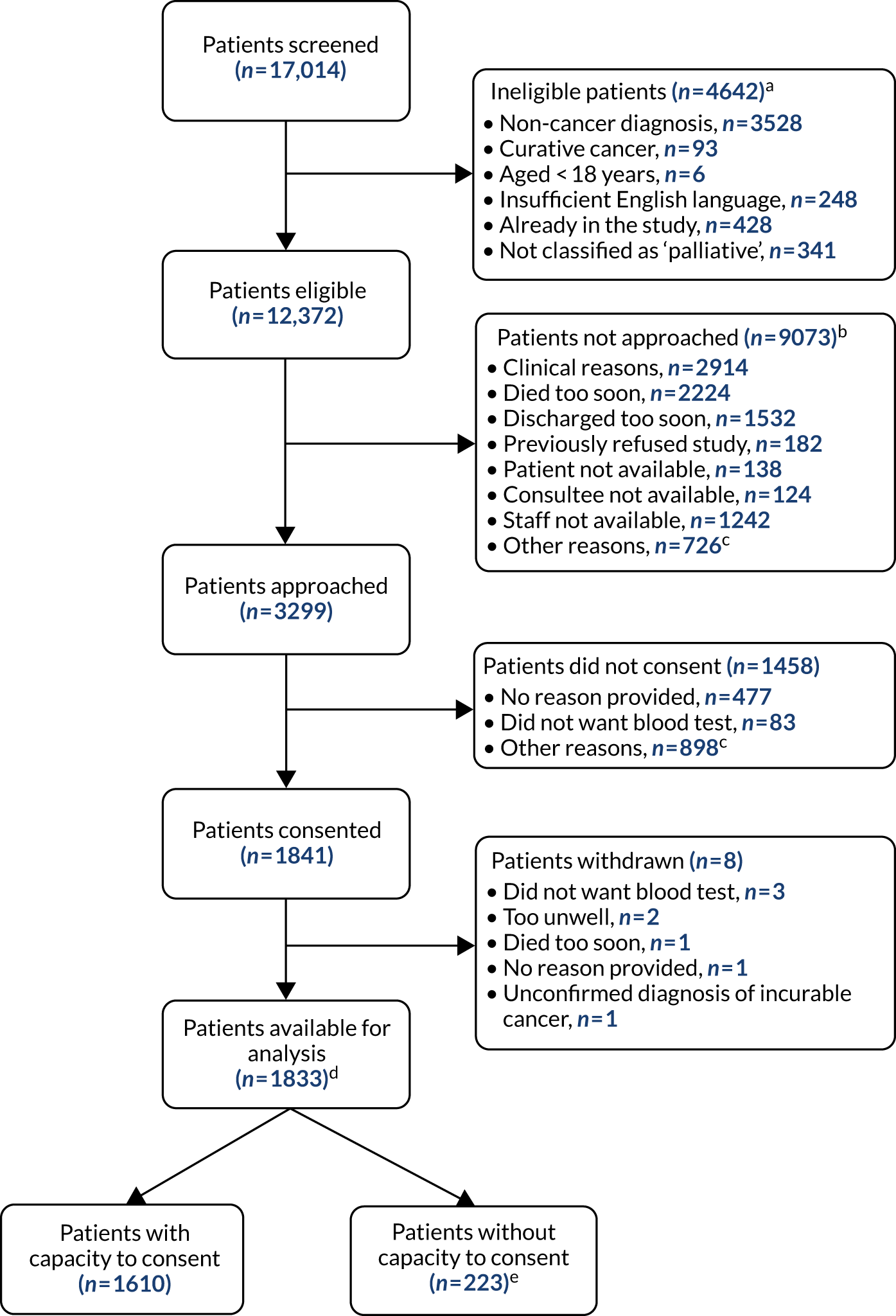
Ineligible patients
Of 17,014 patients screened, 4642 (27.3%) were ineligible for the study. The most common reason for not being eligible was a non-cancer diagnosis.
Patients who were not approached
Of 12,372 eligible patients, 9073 (73.3%) were not approached by a member of the research team to be considered for study enrolment. The most common reasons for failure to approach patients about the study were:
-
clinical reasons (i.e. the clinician judged that involvement in the research study would not be appropriate for that patient)
-
premature death of the patient (i.e. before there was time for them to be approached)
-
early discharge from the service
-
because staff were not available to discuss the study with the potential participant.
Patients who did not provide consent
Of 3299 patients (with capacity, n = 2969; without capacity, n = 330) who were approached about the study by a member of the research team, 1458 (44.2%) did not provide consent (or their relatives did not agree to their involvement, in the case of patients lacking capacity). Patients (or relatives) who declined consent were not obliged to provide any reason for doing so. Those who did so provided a wide variety of reasons. The most common reasons were that they were experiencing extreme fatigue, distress or malaise, or they had too many other competing priorities.
Recruitment by site
The number of patients recruited at each site is summarised in Appendix 5. The median number of participants recruited at each site was 47.
Patient participant characteristics
Participant characteristics are shown in Tables 2 and 3. These summaries are based on actual observations only (no data have been imputed).
Variable | Total (N = 1833) |
---|---|
Age (years), mean (SD) (N = 1832)a | 70.2 (11.9) |
Gender, n (%) (N = 1832)a | |
Male | 938 (51.2) |
Female | 894 (48.8) |
Location, n (%) | |
IPCU | 1241 (67.7) |
CPCT | 468 (25.5) |
HPCT | 124 (6.8) |
Site of primary tumour,b n (%) | |
Lung | 362 (19.8) |
Upper gastrointestinal tract | 337 (18.4) |
Lower gastrointestinal tract | 280 (15.3) |
Prostate | 160 (8.7) |
Breast | 146 (8.0) |
Gynaecological | 133 (7.3) |
Other | 123 (6.7) |
Urological (bladder, testes, renal) | 112 (6.1) |
Head and neck | 81 (4.4) |
Haematological | 70 (3.8) |
Unknown | 45 (2.5) |
Neurological | 38 (2.1) |
Rare tumour | 27 (1.5) |
Site of metastatic diseases, n (%) | |
Bone | 555 (30.3) |
Liver | 538 (29.4) |
Nodal | 516 (28.2) |
Lung | 477 (26.0) |
Other | 353 (19.3) |
None | 279 (15.2) |
Brain | 134 (7.3) |
Pleural effusion | 98 (5.4) |
Ascites | 95 (5.2) |
Adrenal | 79 (4.3) |
Unknown | 60 (3.3) |
Skin | 36 (2.0) |
Renal | 20 (1.1) |
Currently receiving tumour therapy, n (%) | 391 (21.3) |
Chemotherapy | 190 (48.6) |
Radiotherapy | 118 (30.2) |
Hormone therapy | 76 (19.4) |
Other tumour directed therapy (e.g. immunotherapy) | 42 (10.7) |
Capacity to consent, n (%) | 1610 (87.8) |
Time between diagnosis and date became incurable (months) (N = 1821) | |
Mean (SD) | 13.2 (32.8) |
Median (IQR) | 0 (0–12) |
AMTS, n (%) (N = 1826) | |
< 4 | 208 (11.4) |
≥ 4 | 1618 (88.6) |
Presence of key symptoms, n (%) | |
Anorexia (N = 1830) | 968 (52.9) |
Dysphagia (N = 1830) | 554 (30.3) |
Dyspnoea (N = 1831) | 652 (35.6) |
Fatigue (N = 1831) | 1617 (88.3) |
Weight loss (N = 1831) | 1194 (65.2) |
Clinical assessments, n (%) | |
Ascites (N = 1830) | 245 (13.4) |
Presence of peripheral oedema (N = 1831) | 685 (37.4) |
Pulse rate (b.p.m.); mean (SD) (N = 1817) | 82.2 (14.7) |
Presence of delirium (N = 1830) | 66 (3.6) |
Considered to be caused by a single medication | 2 (3.0) |
Oral intake (N = 1830), n (%) | |
Normal | 587 (32.1) |
Moderately reduced | 666 (36.4) |
Severely reduced | 577 (31.5) |
ECOG performance status grade, n (%) (N = 1831) | |
0 | 15 (0.8) |
1 | 202 (11.0) |
2 | 520 (28.4) |
3 | 822 (44.9) |
4 | 272 (14.9) |
General health status (overall health), n (%) (N = 1823) | |
1 (Very poor) | 144 (7.9) |
2 | 414 (22.7) |
3 | 680 (37.3) |
4 | 348 (19.1) |
5 | 180 (9.9) |
6 | 49 (2.7) |
7 (Excellent) | 8 (0.4) |
KPS, n (%) (N = 1830) | |
10 | 63 (3.4) |
20 | 108 (5.9) |
30 | 136 (7.4) |
40 | 229 (12.5) |
50 | 465 (25.4) |
60 | 404 (22.1) |
70 | 276 (15.1) |
80 | 114 (6.2) |
90 | 33 (1.8) |
100 | 2 (0.1) |
Results | N | Mean (SD) | Median (IQR) |
---|---|---|---|
Full blood count | |||
White blood cell count (×109/l) | 1602 | 11.3 (11.2) | 9.5 (6.8–12.9) |
Lymphocyte count (×109/l) | 1596 | 1.2 (2.0) | 1.1 (0.7–1.45) |
Neutrophil count (×109/l) | 1600 | 8.8 (6.2) | 7.4 (4.9–10.6) |
Platelet count (×109/l) | 1601 | 312.9 (147.6) | 289 (210–391) |
Biochemistry | |||
Urea concentration (mmol/l) | 1601 | 8.0 (6.4) | 6.4 (4.7–9.2) |
Albumin concentration (g/l) | 1600 | 30.1 (7.0) | 30 (25–35) |
Alkaline phosphatase concentration (U/l) | 1587 | 231.7 (319.9) | 122 (86–232) |
Alanine transaminase concentration (U/l) | 1581 | 33.3 (71.7) | 19 (11–32) |
CRP concentration (mg/l) | 1565 | 68.6 (73.5) | 45 (14.4–96) |
LDH concentration (mmol/l) | 1467 | 505.4 (446.0) | 381 (251–572) |
The mean age of participants was 70.2 years and 51.2% were men. The majority of participants were recruited from IPCUs. The most common tumour types were lung, head and neck and upper gastrointestinal cancers and the most common sites of metastases were bone, liver and lymph nodes. Most patients were no longer receiving tumour-directed therapies, although, consistent with the trend to initiate palliative care earlier in the course of the disease trajectory, 21.3% of patients were still receiving active oncological therapies. Most participants had a poor ECOG performance status, with 59.8% having a score of 3 or 4 (i.e. in bed for > 50% of the day, or completely bed-bound).
Characteristics of the doctors
Doctors who participated in the research by providing prognostic estimates were also asked to provide some data about themselves, including age, gender, specialty, years working as a doctor, years of experience in palliative medicine and their professional grade. However, doctors were not individually identified and many doctors participated in the research on more than one occasion, thus making it difficult to summarise the characteristics of the individual doctors. To provide insight into the characteristics of the physician prognosticators, we assumed that doctors working in the same institution who were of the same gender and age and had the same professional grade, years qualified and years working in palliative care were the same individual. We allowed for an increase of up to 2 years over the duration of the study for age, years qualified and years working in palliative care. On this basis, the characteristics of the estimated 431 individual doctor participants are shown in Table 4.
Variable | n (%) | Mean (SD) | Median (IQR) |
---|---|---|---|
Estimated number of individual doctors (N = 431) | – | – | – |
Specialty (N = 420) | |||
Palliative care | 360 (85.7) | – | – |
Oncology | 13 (3.1) | – | – |
GP | 24 (5.7) | – | – |
Medicine | 12 (2.9) | – | – |
Other | 15 (3.6) | – | – |
Professional grade (N = 419) | |||
Foundation years | 33 (7.9) | – | – |
Core medical trainee | 44 (10.5) | – | – |
Specialist trainee | 118 (28.2) | – | – |
Consultant | 110 (26.3) | – | – |
Non-consultant, non-trainee doctor | 87 (20.8) | ||
Other | 27 (6.4) | – | – |
Years working as a doctor since qualified (N = 415) | – | 12.8 (9.7) | 9 (5–20) |
Years working in palliative medicine (N = 412) | – | 6.2 (7.1) | 3 (0–10.5) |
Gender (N = 418) | |||
Male | 117 (28.0) | – | – |
Female | 301 (72.0) | – | – |
Age (years) (N = 351) | – | 37.3 (9.0) | 35 (30–44) |
Characteristics of the doctor–patient relationship
When doctors provided a prognostic estimate for patient participants in the validation study they were also asked to indicate how long they had been caring for the patient and when they had last assessed them. The summary statistics for each of these prognostic estimates are shown in Table 5. Most commonly, the doctor providing the prognostic estimate had known the patient for < 1 week. However, most of the estimates were made soon after the doctor had last reviewed the patient, with 75.8% of estimates occurring after the doctor had reviewed the patient in the previous 3 days and more than half occurring on the same day.
Variable | n (%) |
---|---|
Length of relationship with patient | |
< 1 week | 1211 (67.0) |
< 1 month | 223 (12.3) |
< 3 months | 95 (5.3) |
≥ 3 months | 38 (2.1) |
Never met patient | 241 (13.3) |
Last assessed patient | |
Today | 963 (53.2) |
Within last 3 days | 409 (22.6) |
Within last week | 110 (6.1) |
Within last month | 72 (4.0) |
> 1 month ago | 15 (0.8) |
Never met patient | 240 (13.3) |
Characteristics of nurses
Nurses who participated in the research by providing prognostic estimates were also asked to provide data about themselves, including age, gender, specialty, years working as a nurse, years of experience in palliative care and professional grade. However, nurses were not individually identified, and many nurses participated in the research on more than one occasion, making it difficult to summarise the characteristics of the individual nurses. To provide insight into the characteristics of the nurse prognosticators, we assumed that nurses working in the same institution who were of the same gender and age and had the same professional grade, years qualified and years working in palliative care were the same individual. We allowed for an increase of up to 2 years over the duration of the study for age, years qualified and years working in palliative care. On this basis, the estimated characteristics of the individual nurse participants are shown in Table 6.
Variable | n (%) | Mean (SD) | Median (IQR) |
---|---|---|---|
Estimated number of individual nurses (N = 777) | – | – | – |
Specialty (N = 768) | |||
Palliative care | 755 (98.3) | – | – |
Oncology | 8 (1.0) | – | – |
Other | 5 (0.7) | – | – |
Professional grade (N = 1066) | – | ||
Band 5 | 529 (68.6) | – | – |
Band 6 | 102 (13.2) | – | – |
Band 7 | 120 (15.6) | – | – |
Band 8 | 10 (1.3) | – | – |
Nurse consultant | 2 (0.3) | – | – |
Other | 8 (1.0) | – | – |
Years worked as a nurse since qualified (N = 768) | – | 19.4 (11.8) | 19 (9–30) |
Years working in palliative care (N = 770) | – | 8.5 (7.6) | 6 (2–12) |
Gender (N = 768) | |||
Male | 36 (4.7) | – | – |
Female | 732 (95.3) | – | – |
Age (years) (N = 652) | – | 45.6 (10.5) | 47.5 (37–54) |
Characteristics of the nurse–patient relationship
Nurses providing a prognostic estimate of survival for patient participants in the validation study were also asked to indicate how long they had been caring for the patient and when they had last assessed them. The summary statistics for each of these prognostic estimates are shown in Table 7. Most commonly, the nurse providing the prognostic estimate had known the patient for < 1 week. However, most of the estimates were made soon after the nurse had last reviewed the patient, with 82.6% of estimates occurring after the nurse had reviewed the patient in the previous 3 days and nearly three-quarters occurring on the same day.
Variable | n (%) |
---|---|
Length of relationship with patient | |
< 1 week | 1246 (68.3) |
< 1 month | 362 (19.9) |
< 3 months | 83 (4.6) |
≥ 3 months | 47 (2.6) |
Never met patient | 86 (4.7) |
Last assessed patient (N = 1809) | |
Today | 1295 (71) |
Within last three days | 211 (11.6) |
Within last week | 96 (5.3) |
Within last month | 118 (6.5) |
> 1 month ago | 17 (0.9) |
Never met patient | 87 (4.8) |
Overall survival of the patient participants
The median survival of the patients was 45 days (IQR 16–140 days). The survival time of patients was categorised into days (0–13 days), weeks (14–55 days) and months+ (≥ 56 days) because this corresponded to the predictions provided by the PiPS models. The number of participants in the days category was 405 (22.1%), the weeks category 601 (32.8%) and the months+ category 827 (45.1%).
The survival curve for the study population is shown in Figure 2.
FIGURE 2.
Kaplan–Meier curve displaying survival for all participants.
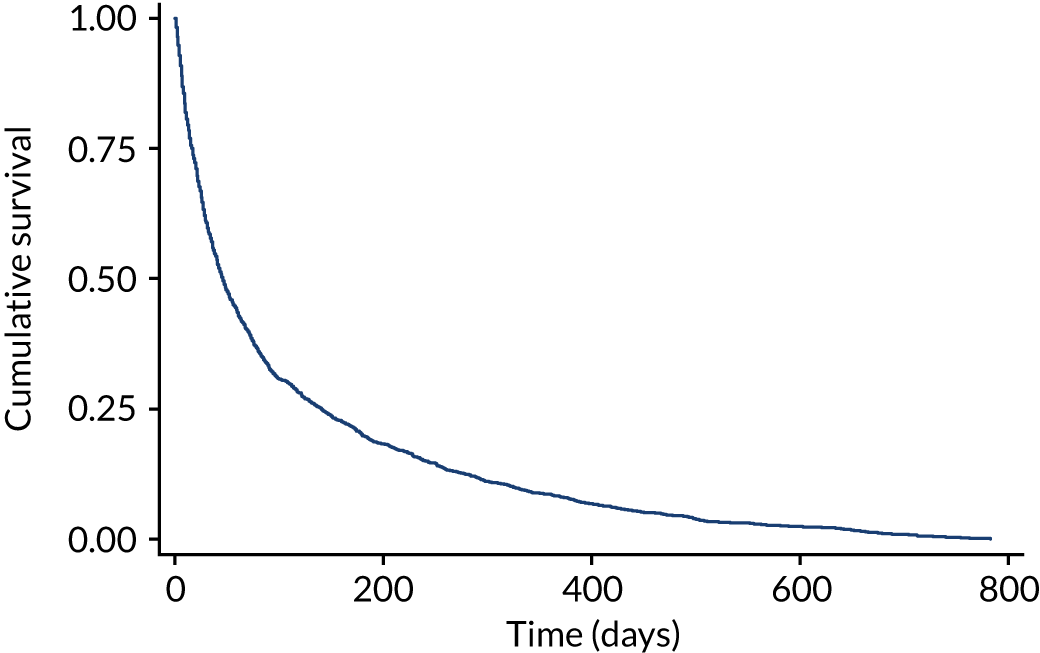
The median survival time of all patients (n = 1833) was 45 days (IQR 16–140 days). The median survival time of patients recruited from IPCU (n = 1241) was 29 days (IQR 11–72 days). The median survival time of community palliative care patients (n = 468) was 171 days (IQR 73–418 days). The median survival time of patients recruited from HPCTs (n = 124) was 43 days (IQR 15–129 days). The results are shown in Figure 3.
FIGURE 3.
Kaplan–Meier curve displaying survival of participants by setting of recruitment.
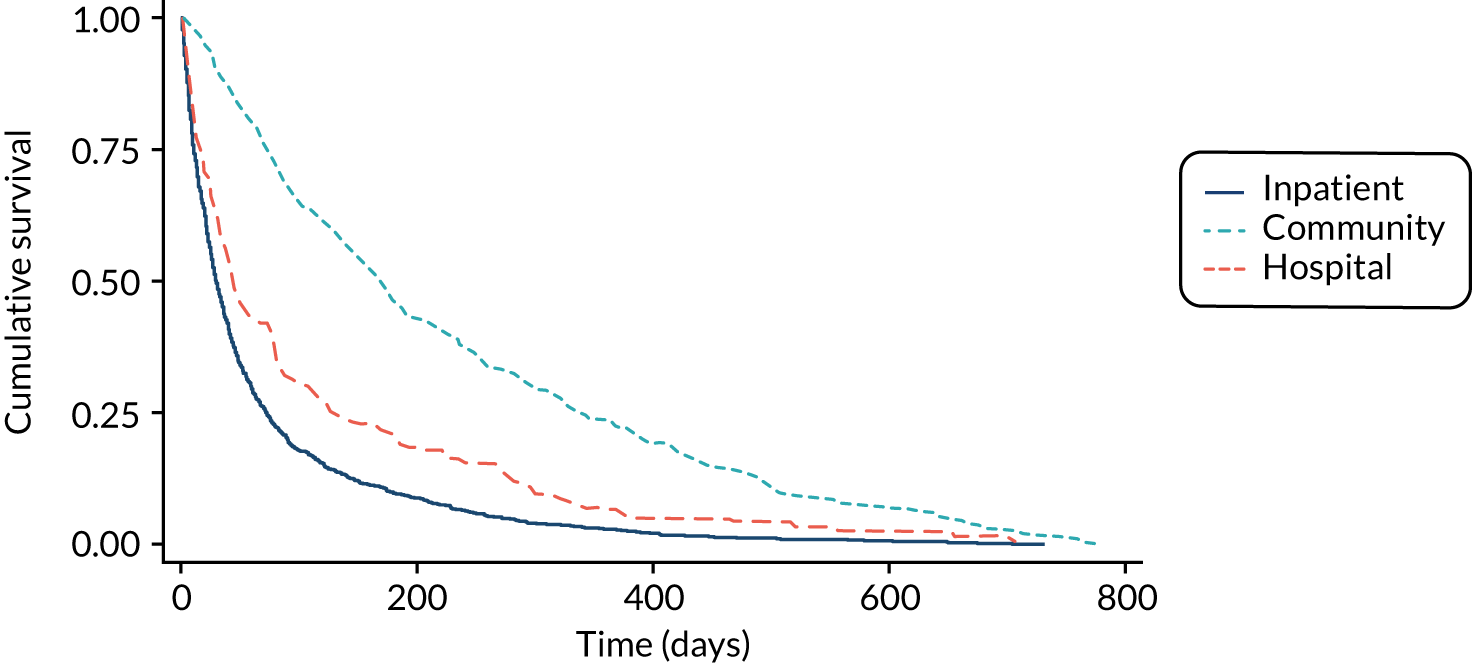
Predicted survival time of patients by doctors and nurses and an agreed multiprofessional estimate of survival
The predicted survival time of patients in the study are shown in Table 8. Slightly more nurses than clinicians were available (and able/willing) to provide a survival estimate for participants. Health-care professionals were initially asked to provide a broad survival estimate according to the PiPS categories (days, weeks or months+) and were then asked for a more specific prognosis in terms of weeks. Finally, they were asked to estimate the probability of patients surviving to different time points.
Variable | Doctors’ estimates, n (%) (N = 1809) | Nurses’ estimates, n (%) (N = 1824) | ||
---|---|---|---|---|
Estimated survival by PiPS categories | ||||
Days (0–13 days) | 197 (10.9) | 178 (9.8) | ||
Weeks (14–55 days) | 670 (37.0) | 672 (36.8) | ||
Months (≥ 56 days) | 942 (52.1) | 974 (53.4) | ||
More specific survival estimates (weeks) | ||||
≤ 1 | 122 (6.7) | 111 (6.1) | ||
2 | 114 (6.3) | 101 (5.5) | ||
3 | 99 (5.5) | 79 (4.3) | ||
4 | 135 (7.5) | 128 (7.0) | ||
5 | 47 (2.6) | 64 (3.5) | ||
6 | 159 (8.8) | 133 (7.3) | ||
7–8 | 208 (11.5) | 247 (13.5) | ||
9–10 | 153 (8.5) | 112 (6.1) | ||
11–12 | 218 (12.1) | 210 (11.5) | ||
> 12 | 554 (30.6) | 639 (35.0) | ||
Estimated survival time (days) | Mean (SD) | Median (IQR) | Mean (SD) | Median (IQR) |
1 | 95.5 (11.1) | 100 (95–100) | 96.4 (11.8) | 100 (99–100) |
3 | 90.6 (17.3) | 98 (90–100) | 91.6 (17.9) | 100 (90–100) |
7 | 83.2 (22.7) | 90 (80–99) | 84.5 (23.6) | 95 (80–100) |
15 | 73.1 (26.7) | 80 (60–93) | 74.1 (28.0) | 80 (60–100) |
30 | 58.2 (29.2) | 60 (40–80) | 59.8 (30.8) | 70 (40–80) |
60 | 43.1 (29.8) | 50 (20–70) | 45.0 (31.6) | 50 (20–70) |
If the clinician and the nurse independently agreed on the length of survival (using the PiPS categories) then this was regarded as representing the AMPES. If they disagreed, then they were asked to confer with each other and come to an agreed response. The AMPES was days on 188 occasions (10.3%), weeks on 696 occasions (38.1%) and months+ on 947 occasions (51.7%).
Accuracy of survival predictions by health-care professionals
The accuracy of clinicians’ survival predictions (using the PiPS prognostic categories of days, weeks and months+) was assessed by comparing the predictions with the observed outcomes.
Accuracy of nurses’ predictions of survival
A comparison between the nurses’ predictions and actual survival is shown in Table 9. The overall accuracy of the nurses’ predictions was 1080 out of 1824 (59.2%).
Nurses’ predictions | Observed survival | Total | ||
---|---|---|---|---|
Days | Weeks | Months+ | ||
Days | 142 | 29 | 7 | 178 |
Weeks | 195 | 299 | 178 | 672 |
Months+ | 62 | 273 | 639 | 974 |
Total | 399 | 601 | 824 | 1824 |
Accuracy of clinicians’ predictions of survival
A comparison between the clinicians’ predictions and actual survival is shown in Table 10. The overall accuracy of the clinicians’ predictions was 1094 out of 1809 (60.5%).
Clinicians’ predictions | Observed survival | Total | ||
---|---|---|---|---|
Days | Weeks | Months+ | ||
Days | 153 | 35 | 9 | 197 |
Weeks | 194 | 302 | 174 | 670 |
Months+ | 44 | 259 | 639 | 942 |
Total | 391 | 596 | 822 | 1809 |
Accuracy of agreed multiprofessional estimates of survival
A comparison between the AMPES and actual survival is shown in Table 11. The overall accuracy of AMPESs was 1134 out of 1831 (61.9%).
AMPES | Observed survival | Total | ||
---|---|---|---|---|
Days | Weeks | Months+ | ||
Days | 154 | 28 | 6 | 188 |
Weeks | 215 | 321 | 160 | 696 |
Months+ | 36 | 252 | 659 | 947 |
Total | 405 | 601 | 825 | 1831 |
Validation of the PiPS models
In the development study, for both PiPS-A and PiPS-B two separate models were developed to predict 2-week (14-day) and 2-month (56-day) survival, thus generating three prognostic categories: days (< 14 days), weeks (14–55 days) and months+ (> 55 days). The weeks and months+ models included different sets of predictors. The variables included in PiPS-A and PiPS-B are shown in Appendix 6. The regression equations for calculating the PiPS-A and PiPS-B scores are shown in Appendix 7. To combine the outputs from the 14-day and the 56-day models to provide a unitary prediction about survival, the PiPS investigators combined the outputs of each model using a simple decision rule. The decision rule (see Appendix 7) was that, for both models (weeks and months+), if the predicted probability of the event (i.e. survival) exceeded 50% for a patient then the patient was classified to have the event (i.e. survived at least 14 or 56 days for each model). Otherwise, it was assumed that the patient did not have the event (i.e. did not survive 14 or 56 days for each model). Thus, if the models predicted that a patient would survive 2 weeks, but predicted that the patient would die within 2 months then the PiPS prediction would be that the patient would die in weeks.
The first analyses were undertaken using complete data sets only (without imputation). They are described below.
Analysis without imputation of missing data
Performance of the 14-day and 56-day models
Before considering the global performance of the PiPS-A and PiPS-B models (which involved assessing how well the combined 14-day and 56-day models performed using the decision rule), each model was assessed independently.
The discriminatory ability of the models was assessed using the c-statistic. Separate c-statistics [with 95% confidence intervals (CIs)] were calculated for the weeks and months+ models. For the PiPS-A 14-day model (n = 1802) the c-statistic was 0.825 (95% CI 0.803 to 0.848) and for the PiPS-A 56-day model (n = 1803) the c-statistic was 0.776 (95% CI 0.755 to 0.797). For the PiPS-B 14-day model (n = 1484) the c-statistic was 0.837 (95% CI 0.810 to 0.863) and for the PiPS-B 56-day model (n = 1498) the c-statistic was 0.810 (95% CI 0.788 to 0.832). The discrimination of the models was good or excellent. 55
The calibration of the prognostic models was assessed using the CiL and CS based on a logistic regression model fitted to the validation data using the predicted log-odds as the only predictor. The CiL and CS are shown in Table 12.
Variable | CiL (95% CI) | CS (95% CI) |
---|---|---|
PiPS-A | ||
14 days (‘2 weeks’) | –0.037 (–0.168 to 0.095) | 0.981 (0.872 to 1.09) |
56 days (‘2 months’) | 0.109 (0.002 to 0.215) | 0.946 (0.842 to 1.05) |
PiPS-B | ||
14 days (‘2 weeks’) | –0.202 (–0.364 to –0.039) | 0.840 (0.730 to 0.950) |
56 days (‘2 months’) | 0.152 (0.030 to 0.273) | 0.914 (0.808 to 1.02) |
A risk model is considered to be well calibrated if the CiL is close to zero and the CS is close to 1. The PiPS-A14 and PiPS-A56 were both well calibrated. The PiPS-B56 model was well calibrated. PiPS-B14 showed some degree of overfitting (CS 0.840), with predictions slightly higher for the 57–74% risk group.
The calibration of both the PiPS-B14 and PiPS-B56 models has also been assessed by comparing the observed and the predicted proportions of events (Figures 4 and 5, respectively).
FIGURE 4.
Observed and predicted proportion of events using PiPS-B14 model. n = 1497; proportion of events = 1238 out of 1498 (82.7%). One participant was removed from the analysis because their PiPS-B prognostic index was an outlier. The data have been divided into 10 equal-sized groups (deciles) to produce the graph.
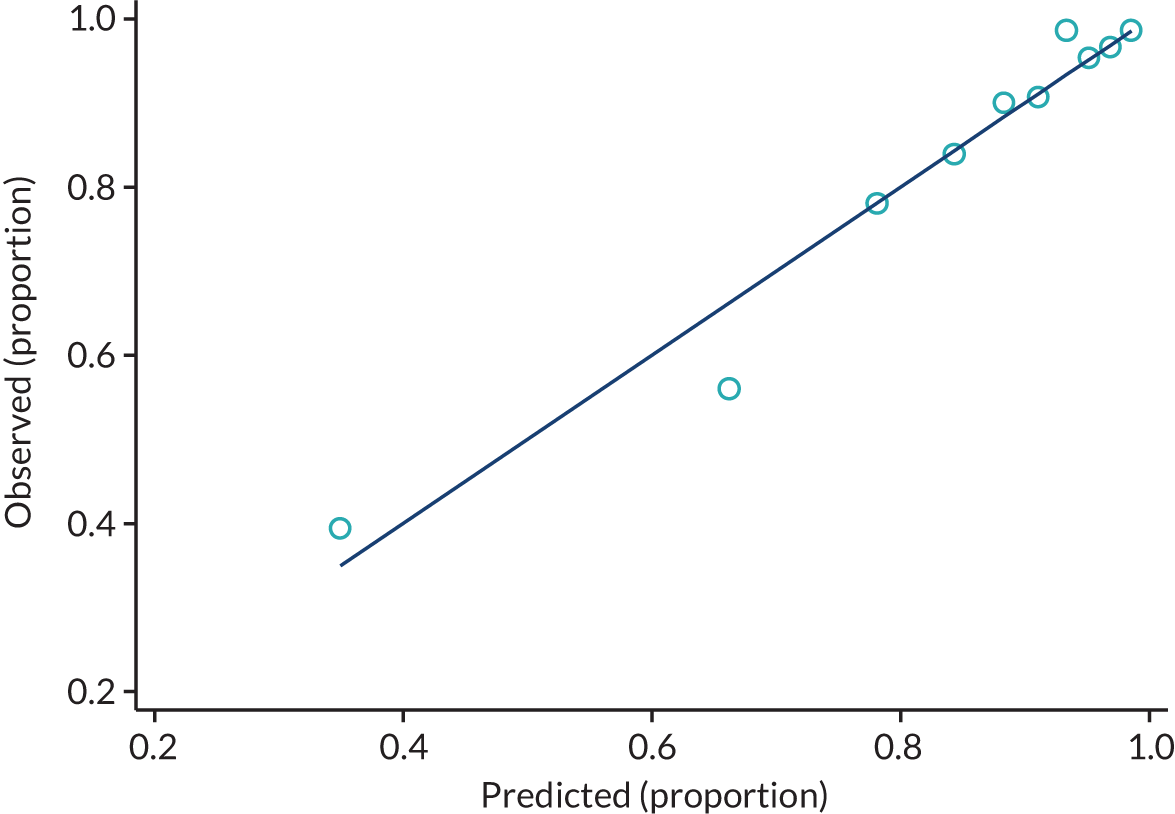
FIGURE 5.
Observed and predicted proportion of events using PiPS-B56 model. n = 1498; proportion of events = 727 out of 1498 (48.5%).
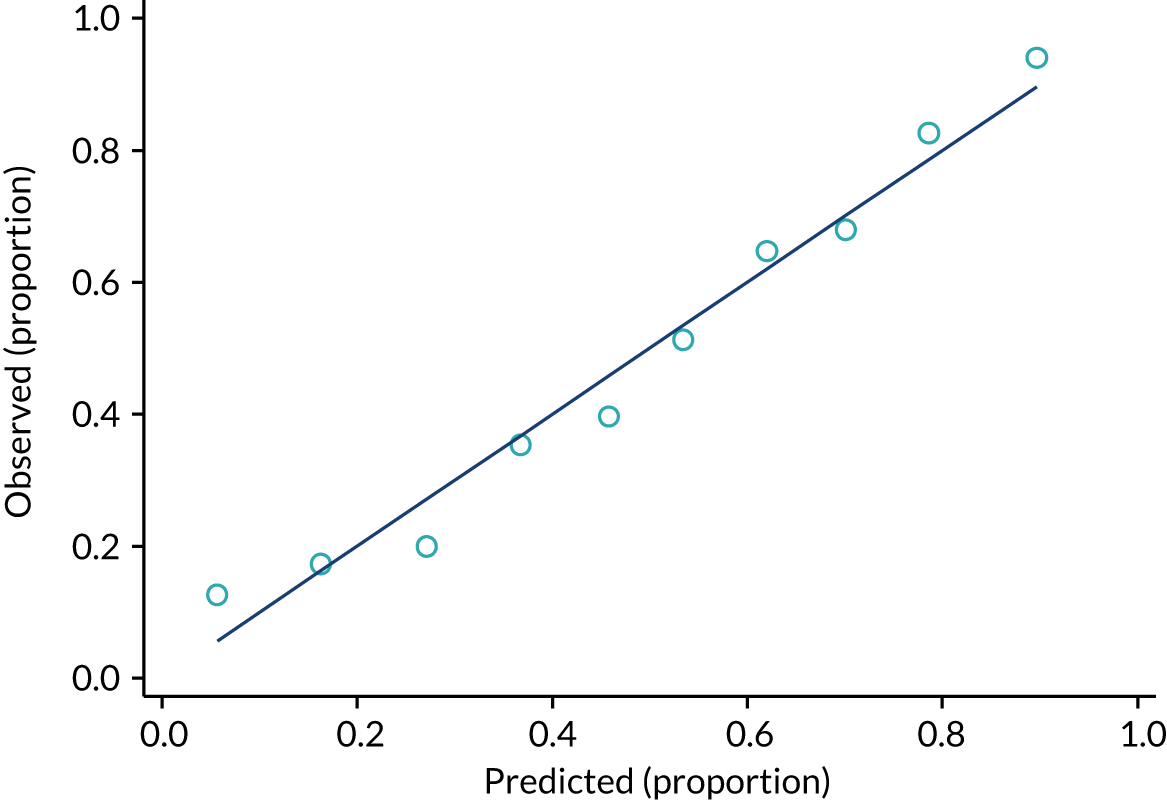
Performance of the combined PiPS-A and PiPS-B models using the decision rule
When the outputs from the 14- and 56-day models were combined using the decision rule previously described (see also Appendix 7), the result was a prediction about whether the patient would survive for days (0–13 days), weeks (14–55 days) or months+ (> 55 days).
The accuracy of the resulting predictions of the combined PiPS-A and PiPS-B models are shown in Tables 13 and 14. The overall accuracy of the PiPS-A combined model was 1012 out of 1802 (56.2%). The overall accuracy of the PiPS-B combined model was 910 out of 1484 (61.3%).
PiPS-A predictions | Observed survival | Total | ||
---|---|---|---|---|
Days | Weeks | Months+ | ||
Days | 159 | 45 | 15 | 219 |
Weeks | 199 | 377 | 324 | 900 |
Months+ | 37 | 170 | 476 | 683 |
Total | 395 | 592 | 815 | 1802 |
PiPS-B predictions | Observed survival | Total | ||
---|---|---|---|---|
Days | Weeks | Months+ | ||
Days | 75 | 26 | 16 | 117 |
Weeks | 157 | 340 | 209 | 706 |
Months+ | 22 | 144 | 495 | 661 |
Total | 254 | 510 | 720 | 1484 |
The discriminatory performance of the 14-day and 56-day models using the decision rule was calculated using the c-statistic. The discrimination of all models is reduced after applying the decision rule. The c-statistic for the PiPS-A 14-day model (n = 1802) using the decision rule was 0.680 (95% CI 0.655 to 0.705). The c-statistic for the PiPS-A 56-day model (n = 1802) using the decision rule was 0.687 (95% CI 0.666 to 0.708). The c-statistic for the PiPS-B 14-day model (n = 1484) using the decision rule was 0.631 (95% CI 0.602 to 0.659). The c-statistic for the PiPS-B 56- day model (n = 1484) using the decision rule was 0.735 (95% CI 0.713 to 0.757).
The performance of the PiPS-A and PiPS-B models (using the decision rule for combining the 14-day and 56-day models) was further assessed by plotting Kaplan–Meier survival curves for each of the three risk groups: days, weeks and months+. The results are shown in Figures 6 and 7. The Kaplan–Meier curves were also redrawn (Figures 8 and 9) to illustrate the ability of the PiPS-A and PiPS-B models to discriminate between the three risk categories at the clinically relevant time points of 14 and 56 days.
FIGURE 6.
Kaplan–Meier curve by PiPS-A predictions.
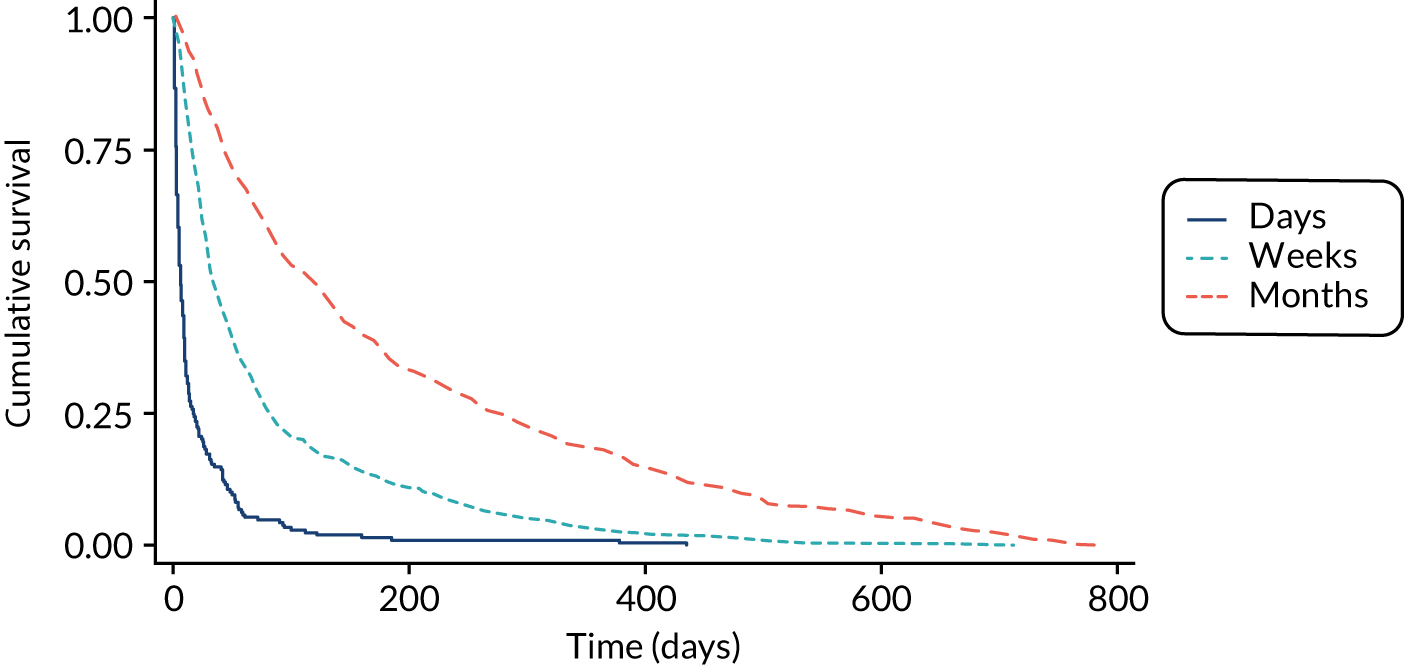
FIGURE 7.
Kaplan–Meier curve by PiPS-B predictions.
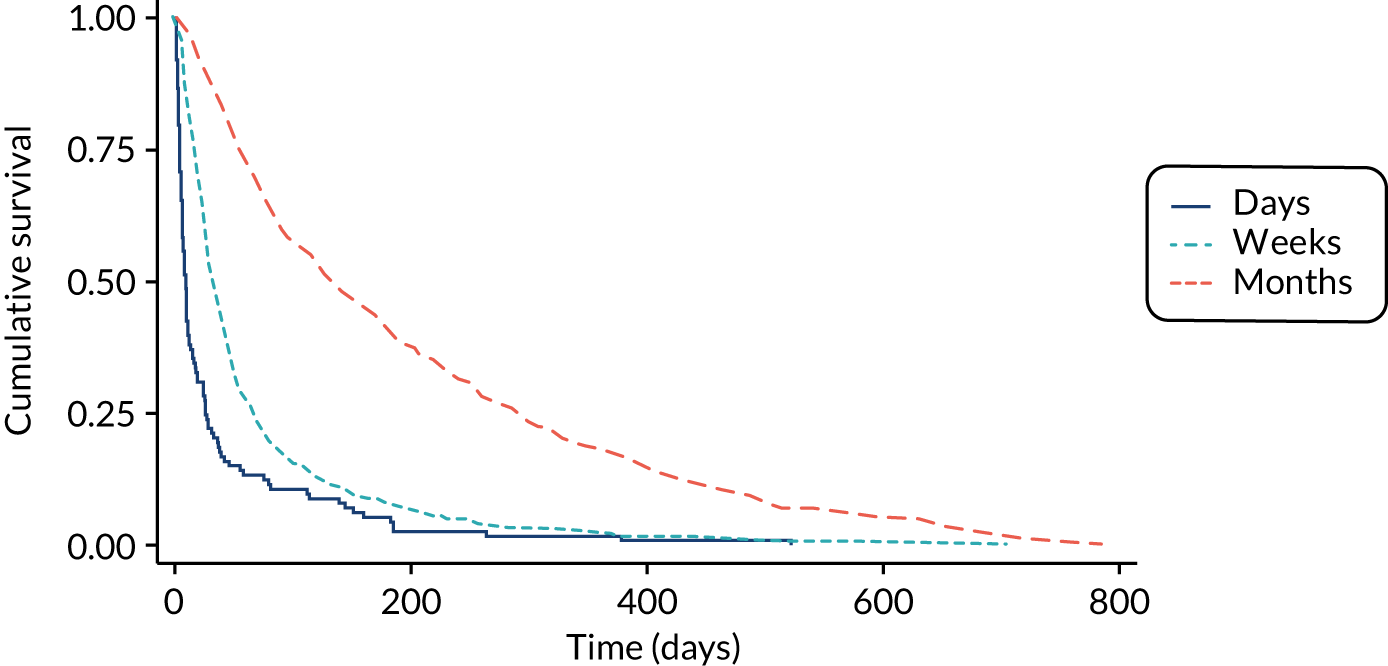
FIGURE 8.
Kaplan–Meier curve by PiPS-A predictions, censored at 56 days. Reference lines show day 14 and day 56.
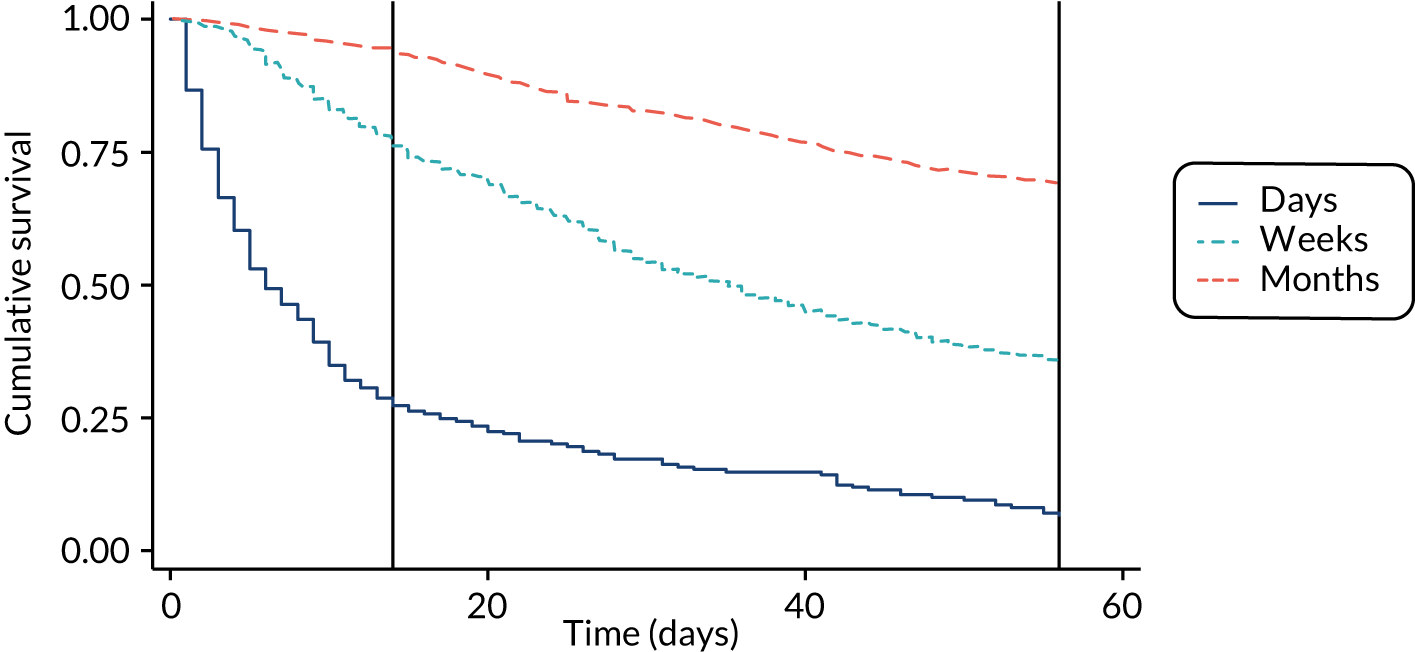
FIGURE 9.
Kaplan–Meier curve by PiPS-B predictions, censored at 56 days. Reference lines show day 14 and day 56.
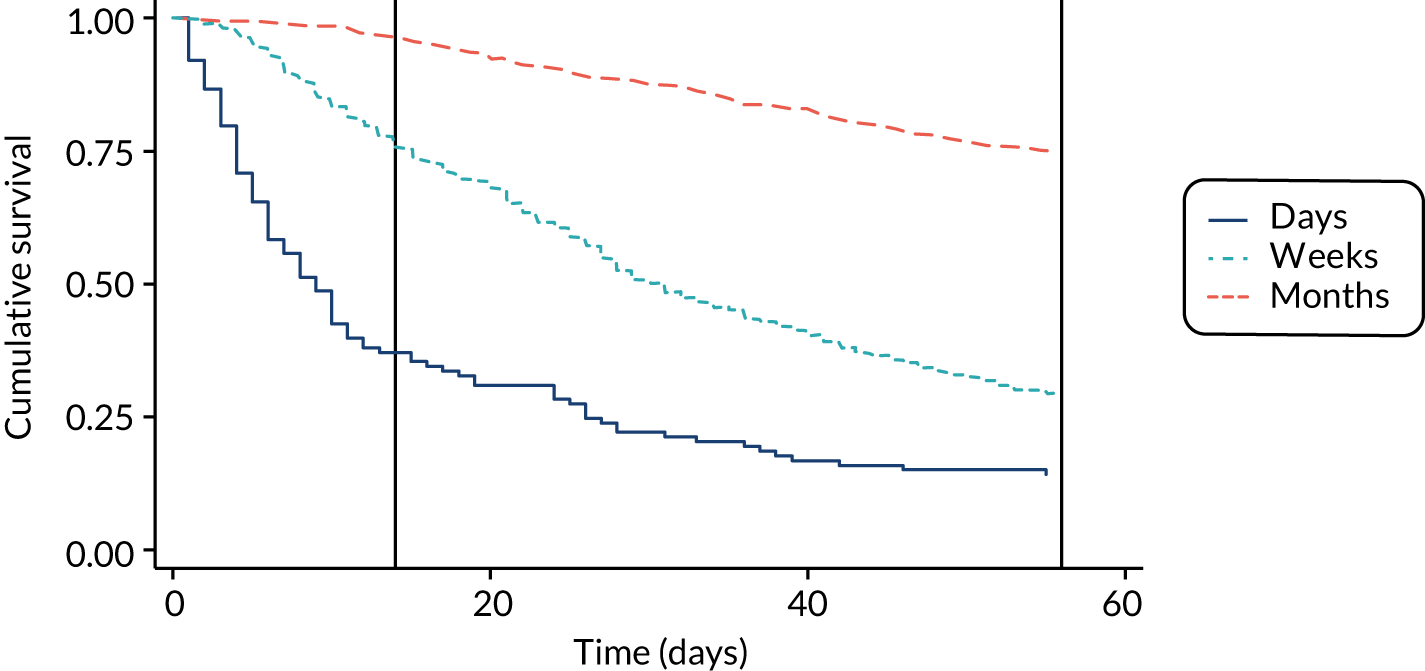
The summary statistics of the survival times for the participants separated by PiPS-A and PiPS-B predictions are shown in Table 15.
Variable | n | Survival time (days) | ||
---|---|---|---|---|
25% | 50% | 75% | ||
PiPS-A | ||||
Days | 219 | 2 | 6 | 16 |
Weeks | 900 | 15 | 35 | 82 |
Months | 683 | 43 | 120 | 300 |
Total | 1802 | 16 | 46 | 142 |
PiPS-B | ||||
Days | 117 | 4 | 8 | 26 |
Weeks | 706 | 15 | 31 | 65 |
Months | 661 | 55 | 135 | 326 |
Total | 1484 | 21 | 52 | 161 |
The median survival of the patients predicted to die within days (0–13 days), weeks (14–55 days) or months (≥ 56 days) was in the required range for both PiPS-A and PiPS-B predictions.
The accuracy of the PiPS-A and PiPS-B models (using the decision rule to combine the 14-day and 56-day models) was compared with the accuracy of AMPESs. These results are shown in Tables 16 and 17 for PiPS-A and PiPS-B, respectively.
PiPS-A risk categories compared with observed deaths | AMPES compared with observed deaths | Total (n) | |
---|---|---|---|
Number (%) of patients when AMPES was correct | Number (%) of patients when AMPES was incorrect | ||
Number (%) of patients when PiPS-A risk category was correct | 762 (42.3) | 250 (13.9) | 1012 |
Number (%) of patients when PiPS-A risk category was incorrect | 355 (19.7) | 435 (23.6) | 790 |
Total | 1117 | 685 | 1802 |
PiPS-B risk categories compared with observed deaths | AMPES compared with observed deaths | Total (n) | |
---|---|---|---|
Number (%) of patients when AMPES was correct | Number (%) of patients when AMPES was incorrect | ||
Number (%) of patients when PiPS-B risk category was correct | 685 (46.2) | 225 (15.2) | 910 |
Number (%) of patients when PiPS-B risk category was incorrect | 229 (15.4) | 345 (23.2) | 574 |
Total | 914 | 570 | 1484 |
A McNemar’s test was used to compare the proportion of overall patient deaths predicted correctly by PiPS-A with the corresponding proportion predicted correctly by using an AMPES. There was a statistically significant (p < 0.001) difference between the percentage of correct AMPESs and the percentage of correct PiPS-A risk model predictions when compared with the observed deaths. The percentage of correct AMPESs was higher than the percentage of correct PiPS-A risk categories (using the decision rule).
A McNemar’s test was used to compare the proportion of overall patient deaths predicted correctly by PiPS-B with the corresponding proportion predicted correctly by an AMPES. There was no statistically significant (p = 0.851) difference between the percentage of correct AMPESs and the percentage of correct PiPS-B risk categories when compared with the observed deaths. Odds ratios have been calculated to compare the proportion of overall patient deaths predicted correctly by PiPS-B with the corresponding proportion predicted correctly by the AMPES. The odds of correctly predicting the patient death is similar for PiPS-B compared with AMPESs (OR 0.983, 95% CI 0.814 to 1.186). Given that the clinically important difference (OR 1.28) is not included in this CI, we can conclude that AMPESs are no better than PiPS-B.
In addition to comparing the accuracy of the PiPS-A and B risk categories with the AMPES, we compared the performance of the risk categories with doctors’ and nurses’ uni-professional survival estimates. For these secondary analyses the threshold for statistical significance level was corrected for multiple statistical testing using a Bonferroni adjustment. 43 The threshold for comparison was set at a p-value of 0.007 (i.e. 0.05/7).
Comparison with doctors’ survival estimates
The comparison between the doctors’ survival estimates and those provided by PiPS-A and PiPS-B risk categories (using the decision rule) is shown in Tables 18 and 19, respectively.
PiPS-A risk categories compared with observed deaths | Doctors’ predictions compared with observed deaths | Total (n) | |
---|---|---|---|
Number (%) of patients when doctors’ prediction was correct | Number (%) of patients when doctors’ prediction was incorrect | ||
Number (%) of patients when PiPS-A risk category was correct | 738 (41.5) | 263 (14.8) | 1001 |
Number (%) of patients when PiPS-A risk category was incorrect | 338 (19.0) | 441 (24.8) | 779 |
Total | 1076 | 704 | 1780 |
PiPS-B risk categories compared with observed deaths | Doctors’ predictions compared with observed deaths | Total (n) | |
---|---|---|---|
Number (%) of patients when doctors’ prediction was correct | Number (%) of patients when doctors’ prediction was incorrect | ||
Number (%) of patients when PiPS-B risk category was correct | 662 (45.1) | 243 (16.5) | 905 |
Number (%) of patients when PiPS-B risk category was incorrect | 215 (14.6) | 349 (23.8) | 564 |
Total | 877 | 592 | 1469 |
A McNemar’s test was used to compare the proportion of overall patient deaths predicted correctly by PiPS-A risk categories with the corresponding proportion predicted correctly by the doctors. There was a statistically significant (p = 0.002) difference between the percentage of correct predictions made by doctors and the percentage of correct PiPS-A risk categories when compared with the observed deaths. The percentage of doctors’ overall correct predictions was higher than that of the combined PiPS-A model risk categories (using the decision rule).
A McNemar’s test was used to compare the proportion of overall patient deaths predicted correctly by PiPS-B risk categories with the corresponding proportion predicted correctly by the doctors. The percentage of correct predictions made by PiPS-B risk categories and the doctors were similar and there was no statistically significant (p = 0.191) difference between the percentage of correct predictions made by doctors and the percentage of correct PiPS-B risk categories when compared with the observed deaths.
Comparison with nurses’ survival estimates
The comparison between the nurses’ survival estimates and those provided by PiPS-A and PiPS-B risk categories (using the decision rule) is shown in Tables 20 and 21, respectively.
PiPS-A risk categories compared with observed deaths | Nurses’ predictions compared with observed deaths | Total (n) | |
---|---|---|---|
Number (%) of patients when nurses’ prediction was correct | Number (%) of patients when nurses’ prediction was incorrect | ||
Number (%) of patients when PiPS-A risk category was correct | 705 (39.3) | 304 (16.9) | 1009 |
Number (%) of patients when PiPS-A risk category was incorrect | 358 (19.9) | 428 (23.8) | 786 |
Total | 1063 | 732 | 1795 |
PiPS-B risk categories compared with observed deaths | Nurses’ predictions compared with observed deaths | Total (n) | |
---|---|---|---|
Number (%) of patients when nurses’ prediction was correct | Number (%) of patients when nurses’ prediction was incorrect | ||
Number (%) of patients when PiPS-B risk category was correct | 644 (43.5) | 263 (17.8) | 907 |
Number (%) of patients when PiPS-B risk category was incorrect | 225 (15.2) | 347 (23.5) | 572 |
Total | 869 | 610 | 1479 |
A McNemar’s test was used to compare the proportion of overall patient deaths predicted correctly by PiPS-A risk categories with the corresponding proportion predicted correctly by the nurses. There was no statistically significant (p = 0.036) difference between the percentage of correct predictions made by nurses and the percentage of correct PiPS-A risk categories when compared with the observed deaths.
A McNemar’s test was used to compare the proportion of overall patient deaths predicted correctly by PiPS-B with the corresponding proportion predicted correctly by the nurses. There was no statistically significant (p = 0.085) difference between the percentage of correct predictions made by nurses and the percentage of correct PiPS-B risk model predictions when compared with the observed deaths.
Analysis using multiple imputation for missing data in the PiPS-B model
Parts of this section have been reproduced with permission from Stone et al. 56 This is an Open Access article distributed in accordance with the terms of the Creative Commons Attribution (CC BY 4.0) license, which permits others to distribute, remix, adapt and build upon this work, for commercial use, provided the original work is properly cited. See: http://creativecommons.org/licenses/by/4.0/. The text below includes minor additions and formatting changes to the original text.
The PiPS-B model requires blood test results for its calculation. For patients with capacity to consent, a blood sample was obtained. For patients without capacity to consent, blood tests results were available only if the patient had had a blood specimen collected as part of their routine clinical care within 72 hours of study entry. A total of 1610 patients with capacity to consent consented to participate in the study. A further 61 participants, without capacity, had blood results obtained as part of routine clinical care. Consequently, we were potentially able to calculate PiPS-B values for a maximum of 1671 participants. In fact, we had the complete data required to calculate PiPS-B model values on only 1484 patients (1423 participants with capacity to consent and 61 participants without capacity to consent). Consequently, we had complete PiPS-B data on 89% (1484/1671) of those participants who were potentially available to have a PiPS-B value calculated.
In the statistical analysis plan we had prespecified that if the proportion of missing data to calculate the PiPS-B model was > 10% then we would use multiple imputation for the primary analysis of the PiPS-B model. Given that the proportion of missing data was 11%, we used multiple imputation based on chained equations to impute missing predictor values.
The imputation model for missing values included the predictors in the PiPS-B risk model, AMTS (the only predictor of missingness) and centre (given that there was variation in the proportion of missing data across the centres). Additionally, the intracluster correlation coefficient for the setting was 0.21 (95% CI 0.05 to 0.57). We used Rubin’s rules to combine the performance measures for the c-statistic, CiL and CS. For the McNemar’s test using the imputed data sets it was necessary to pool the p-values. We used the methods proposed by Rubin57 and extended by Li et al. 58
We used 10 imputed data sets. Predictive mean matching imputation was used for the continuous and ordinal values and logistic regression was used for the binary outcomes. To represent the centres we used the setting (inpatient, community or hospital).
Discriminatory ability of PiPS-B using imputed data
The discriminatory ability of the PiPS-B models was assessed using the c-statistic. Separate c-statistics were calculated for the weeks and the months+ models. For the PiPS-B 14-day model the c-statistic was 0.836 (95% CI 0.810 to 0.861). For the PiPS-B 56-day model the c-statistic was 0.808 (95% CI 0.787 to 0.829). The results are very similar to those obtained using the complete data sets without imputation. The discrimination of both models was excellent. 55
The calibration of the prognostic models was assessed using the CiL and CS based on a logistic regression model fitted to the validation data using the predicted log-odds as the only predictor. The CiL and CS are shown in Table 22.
Variable | CiL (95% CI) | CS (95% CI) |
---|---|---|
14 days (‘2 weeks’) | –0.266 (–0.419 to –0.114) | 0.792 (0.689 to 0.895) |
56 days (‘2 months’) | 0.145 (0.030 to 0.261) | 0.900 (0.798 to 1.00) |
Accuracy of PiPS-B risk categories (using the decision rule and with imputed data) against agreed multiprofessional estimates of survival
McNemar’s test was used to compare the proportion of overall patient deaths predicted correctly by PiPS-B risk categories (in the imputed data set) with the corresponding proportion predicted correctly by AMPES. There was no statistically significant (p = 0.875) difference between the proportion of patient deaths predicted correctly by PiPS-B risk categories (using the decision rule) and the corresponding proportion predicted correctly by AMPES. The 2 × 2 tables are not presented because the results are based on the calculation of the p-value across 10 imputed data sets.
Secondary analysis of the validation study
As part of the secondary analyses, we investigated the agreement between each PiPS model’s predictions, the AMPES and actual survival (using linearly weighted Cohen’s kappa). We also validated the other risk models (PaP, FPN, PPI and PPS).
Secondary analysis of PiPS models
Table 23 provides a comparison of the AMPES and the model risk categories with observed survival. This shows that AMPESs were correct on 1134 out of 1831 (61.9%) occasions and that incorrect AMPESs tended to be overoptimistic (503 estimates; 27.5%). PiPS-A risk categories were correct on 1012 out of 1802 (56.2%) occasions and incorrect PiPS-A risk categories also tended to be overoptimistic (406 estimates; 22.5%). PiPS-B model risk categories were correct on 910 out of 1484 (61.3%) occasions and incorrect PiPS-B risk categories also tended to be overoptimistic (323 estimates; 21.8%).
Variable | Observed survival, n (%) | Total (n) | ||
---|---|---|---|---|
Days | Weeks | Months | ||
AMPES | ||||
Days | 154 (81.9) | 28 (14.9) | 6 (3.2) | 188 |
Weeks | 215 (30.9) | 321 (46.1) | 160 (23) | 696 |
Months | 36 (3.8) | 252 (26.6) | 659 (69.6) | 947 |
Total | 405 (22.1) | 601 (32.8) | 825 (45.1) | 1831 |
PiPS-A model risk categories | ||||
Days | 159 (72.6) | 45 (20.6) | 15 (6.9) | 219 |
Weeks | 199 (22.1) | 377 (41.9) | 324 (36) | 900 |
Months | 37 (5.4) | 170 (24.9) | 476 (69.7) | 683 |
Total | 395 (21.9) | 592 (32.9) | 815 (45.2) | 1802 |
PiPS-B model risk categories | ||||
Days | 75 (64.1) | 26 (22.2) | 16 (13.7) | 117 |
Weeks | 157 (22.2) | 340 (48.2) | 209 (29.6) | 706 |
Months | 22 (3.3) | 144 (21.8) | 495 (74.9) | 661 |
Total | 254 (17.1) | 510 (34.4) | 720 (48.5) | 1484 |
Linear weighted kappa has been used to compare the performance of the AMPES against actual survival with that of the PiPS-A and PiPS-B risk categories against actual survival. For the PiPS-A risk categories, the agreement with actual survival was 76.6%, the expected agreement was 61.2% and the kappa was 0.398 [standard error (SE) 0.018]. For the PiPS-B risk categories, the agreement with actual survival was 79.4%, the expected agreement was 63.9% and the kappa was 0.43 (SE 0.02). For the AMPES, the agreement with actual survival was 79.8%, the expected agreement was 61.0% and the kappa was 0.48 (SE 0.02).
The kappa value shows that there is a moderate strength of agreement between the PiPS risk categories and actual survival as well as between the AMPES and actual survival.
The c-statistic, CS and CiL were initially estimated without taking into account the potential patient clustering in each setting. In a secondary analysis, these performance measures have been calculated for each setting separately (Tables 24–26).
Model | Setting | |||||
---|---|---|---|---|---|---|
Inpatient | Community | Hospital | ||||
n (number of events) | c-statistic (95% CI) | n (number of events) | c-statistic (95% CI) | n (number of events) | c-statistic (95% CI) | |
PiPS-A14 | 1214 (864) | 0.797 (0.770 to 0.825) | 465 (449) | 0.782 (0.678 to 0.886) | 123 (94) | 0.735 (0.624 to 0.846) |
PiPS-A56 | 1215 (383) | 0.733 (0.704 to 0.762) | 465 (378) | 0.738 (0.676 to 0.799) | 123 (54) | 0.563 (0.46 to 0.665) |
PiPS-B14 | 981 (760) | 0.808 (0.776 to 0.841) | 408 (394) | 0.790 (0.642 to 0.939) | 109 (85) | 0.793 (0.68 to 0.906) |
PiPS-B56 | 977 (341) | 0.755 (0.723 to 0.786) | 409 (337) | 0.810 (0.755 to 0.864) | 112 (49) | 0.684 (0.584 to 0.784) |
Model | Setting | |||||
---|---|---|---|---|---|---|
Inpatient | Community | Hospital | ||||
n (number of events) | c-statistic (95% CI) | n (number of events) | c-statistic (95% CI) | n (number of events) | c-statistic (95% CI) | |
PiPS-A14 | 1214 (864) | 0.625 (0.597 to 0.653) | 465 (449) | 0.677 (0.62 to 0.733) | 123 (94) | 0.56 (0.478 to 0.642) |
PiPS-A56 | 1215 (383) | 0.619 (0.588 to 0.65) | 465 (378) | 0.672 (0.542 to 0.803) | 123 (54) | 0.664 (0.562 to 0.765) |
PiPS-B14 | 981 (760) | 0.679 (0.648 to 0.71) | 408 (394) | 0.720 (0.659 to 0.78) | 109 (85) | 0.623 (0.535 to 0.711) |
PiPS-B56 | 977 (341) | 0.679 (0.652 to 0.706) | 409 (337) | 0.559 (0.475 to 0.643) | 112 (49) | 0.651 (0.561 to 0.742) |
Model | Setting | |||||
---|---|---|---|---|---|---|
Inpatient | Community | Hospital | ||||
n (number of events) | CS (95% CI) | n (number of events) | CS (95% CI) | n (number of events) | CS (95% CI) | |
PiPS-A14 | 1214 (864) | 0.932 (0.808 to 1.056) | 465 (449) | 0.833 (0.427 to 1.239) | 123 (94) | 0.714 (0.357 to 1.07) |
PiPS-A56 | 1215 (383) | 0.809 (0.678 to 0.939) | 465 (378) | 0.865 (0.616 to 1.113) | 123 (54) | 0.265 (–0.055 to 0.586) |
PiPS-B14 | 981 (760) | 0.75 (0.625 to 0.874) | 408 (394) | 0.638 (0.356 to 0.92) | 109 (85) | 0.721 (0.366 to 1.076) |
PiPS-B56 | 977 (341) | 0.749 (0.621 to 0.876) | 409 (337) | 0.94 (0.687 to 1.193) | 112 (49) | 0.489 (0.163 to 0.815) |
For both models, the discriminatory ability is lowest in the hospital setting. The number of non-events (deaths) was small in the community setting. The number of participants was very small in the hospital setting.
A risk model is considered to be well calibrated if the CS is close to 1. The CS varies across the three settings. PIPS-B14 showed a degree of overfitting for all settings. The PiPS-A56 and PiPS-B56 models are not well calibrated in the hospital setting.
The participants’ characteristics in the development and validation sample were compared (Table 27). A greater proportion of participants had capacity to consent in the validation sample than in the development sample (87.8% vs. 76.1%, respectively). A greater proportion of participants in the validation sample than in the development sample were recruited from the community (25.5% vs. 12.1%, respectively) and fewer participants were recruited from the hospital (6.8% vs. 17.9%, respectively). In the validation sample, 10% of the patients were receiving chemotherapy treatment compared with none in the development sample. There was a smaller proportion of participants in the validation sample than in the development sample who died within days (22.1% vs. 27.6%, respectively) and a greater proportion of participants who died within months (45.1% vs. 39.3%, respectively). There was a smaller proportion of participants with anorexia in the validation sample than in the development sample (52.9% vs. 65.6%, respectively). There was a greater proportion of participants with dyspnoea in the validation sample than in the development sample (35.6% vs. 27.6%, respectively). There was a smaller proportion of people with very poor health (as measured by the general health status) in the validation sample than in the development sample (7.9% vs. 12.3%, respectively).
Variable | Development sample (%) | Validation sample (%) |
---|---|---|
Capacity to consent | 76.1 | 87.8 |
Gender: male | 54.7 | 51.2 |
Location | ||
IPCU | 68.9 | 67.7 |
CPCT | 12.1 | 25.5 |
HPCT | 17.9 | 6.8 |
Other | 2.5 | – |
Site of primary tumour | ||
Breast | 7.3 | 8.0 |
Lung | 23.4 | 19.8 |
Prostate | 7.0 | 8.7 |
Urological (bladder, testes, renal) | 6.7 | 6.1 |
Haematological | 3.5 | 3.8 |
Digestive organs (upper/lower gastrointestinal tract) | 30.0 | 33.7 |
Head and neck | 3.2 | 4.4 |
Gynaecological | 5.1 | 7.3 |
Neurological | 3.7 | 2.1 |
Rare tumour/unknown/other | 10.1 | 10.7 |
Site of metastatic diseases | ||
Liver | 27.1 | 29.4 |
Lung | 20.9 | 26.0 |
Bone | 26.3 | 30.3 |
Brain | 8.8 | 7.3 |
Skin | 2.0 | 2.0 |
Nodal | 20.5 | 28.2 |
Adrenal | 2.5 | 4.3 |
Ascites | 6.7 | 5.2 |
Pleural effusion | 4.9 | 5.4 |
Other/unknown | 8.1 | 22.6 |
None | 32.8 | 16.3 |
Currently receiving tumour therapy | ||
Chemotherapy | 0.0 | 10.4 |
Radiotherapy | 3.1 | 6.4 |
Hormone therapy | 9.9 | 4.1 |
Other tumour-directed therapy (e.g. immunotherapy) | 0.0 | 2.3 |
Survival | ||
Days | 27.6 | 22.1 |
Weeks | 33.1 | 32.8 |
Months | 39.3 | 45.1 |
AMTS | ||
< 4 | 23.1 | 11.4 |
≥ 4 | 76.9 | 88.6 |
Anorexia | 65.6 | 52.9 |
Dysphagia | 28.8 | 30.3 |
Dyspnoea | 27.6 | 35.6 |
Fatigue | 86.7 | 88.3 |
Weight loss | 69.4 | 65.2 |
ECOG performance status grade | ||
0 | 0.2 | 0.8 |
1 | 8.0 | 11.0 |
2 | 21.3 | 28.4 |
3 | 42.9 | 44.9 |
4 | 27.7 | 14.9 |
General health status (overall health) | ||
1 (very poor) | 12.3 | 7.9 |
2 | 15.2 | 22.7 |
3 | 33.1 | 37.3 |
4 | 28.6 | 19.1 |
5 | 9.3 | 9.9 |
6 | 1.6 | 2.7 |
7 (excellent) | 0.0 | 0.4 |
Validation of other prognostic models
As part of the secondary analyses we also investigated the prognostic performance of other models and (where possible) compared their performance with the predictions of clinicians.
Palliative Prognostic score
The PaP score categorises patients into one of three risk groups to predict 30-day survival: A (> 70% probability of surviving 30 days), B (30–70% probability of surviving 30 days) and C (< 30% probability of surviving 30 days). Higher scores predict shorter survival. The PaP score is generated by applying a weighted score to each of six variables (i.e. CPS, KPS, anorexia, dyspnoea, total white blood cell count and lymphocyte percentage). There were survival data available for 1592 patients with PaP scores recorded. There were 794 (49.1%) study participants in risk group A, 655 (41.1%) in risk group B and 143 (9.0%) in risk group C.
The discrimination of the PaP was investigated by plotting survival curves for each risk group, which are shown in Figure 10.
FIGURE 10.
Kaplan–Meier survival curves for PaP risk groups. This figure has been reproduced with permission from Stone et al. 59 This is an Open Access article distributed in accordance with the terms of the Creative Commons Attribution (CC BY 4.0) license, which permits others to distribute, remix, adapt and build upon this work, for commercial use, provided the original work is properly cited. See: http://creativecommons.org/licenses/by/4.0/. The figure includes minor additions and formatting changes to the original figure.
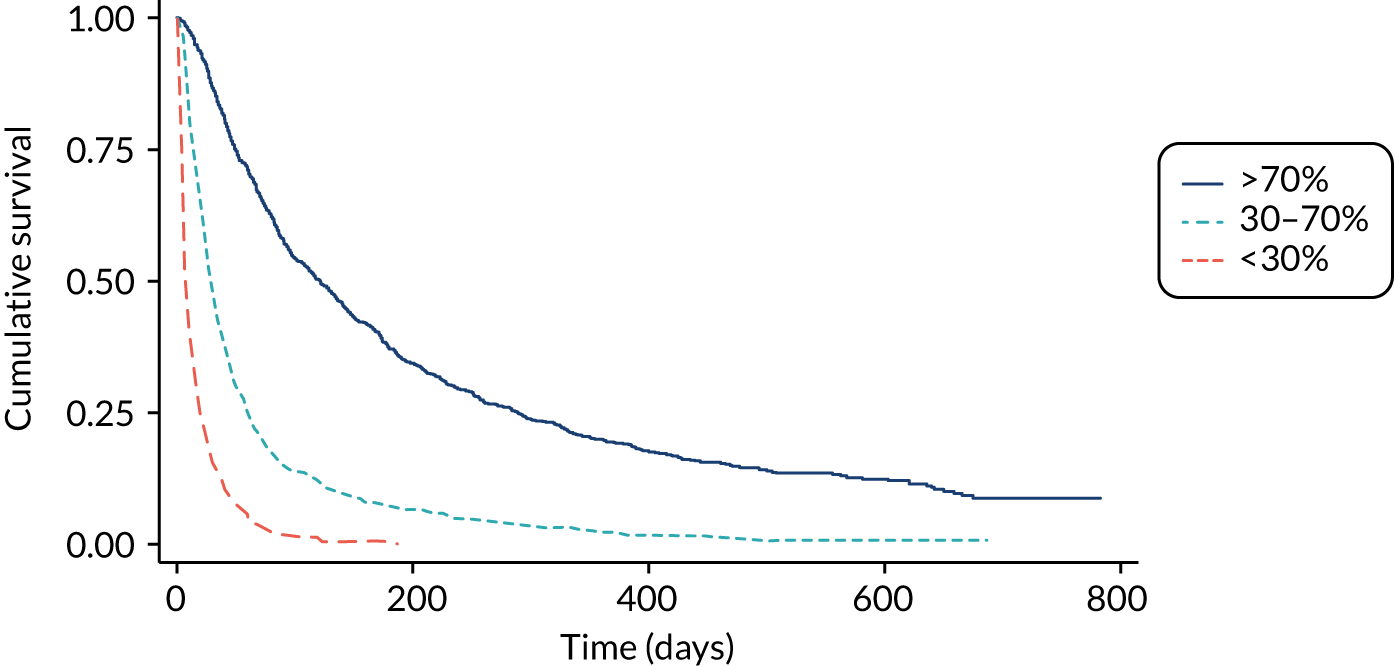
The median survival for patients for whom PaP scores were available was 51 days (IQR 21–151 days). The median survival for risk group A was 121 days (IQR 49–289 days). The median survival for risk group B was 28 days (IQR 14–60 days). The median survival for risk group C was 7 days (IQR 4–19 days).
Because PaP is specifically designed to predict survival at 30 days, we also calculated the number of participants in each risk group who were observed to survive 30 days. For risk group A, the observed proportion of patients actually surviving ≥ 30 days was 86.5% (687/794). For risk group B, the observed proportion of patients actually surviving ≥ 30 days was 46.8% (306/655). For risk group C, the observed proportion of patients actually surviving ≥ 30 days was 15.4% (22/143).
It was not possible to compare PaP predictions against agreed multiprofessional estimates of 30-day survival, because these data were not collected. However, Table 28 provides a comparison of clinicians’ predictions of 30-day survival with the PaP predictions.
PaP risk group | Clinicians’ predictions (n) | Total (n) | ||
---|---|---|---|---|
> 70% | 30–70% | < 30% | ||
> 70% (score 0–5.5) | 627 | 157 | 3 | 787 |
30–70% (score 5.6–11.0) | 156 | 396 | 93 | 645 |
< 30% (score 11.1–17.5) | 1 | 31 | 109 | 141 |
Total | 784 | 584 | 205 | 1573 |
It is not possible to make a straightforward comparison between the accuracy of the PaP score and the accuracy of clinicians’ predictions. This is because, for any individual, the PaP provides a prognostic output that is a broad range of survival probabilities and it does not make a categorical prediction about whether or not the patient will or will not survive for 30 days.
Feliu Prognostic Nomogram
The FPN uses five variables (ECOG performance status, serum albumin concentration, LDH concentration, lymphocyte counts and TTD) to predict the probability of survival at 15, 30 and 60 days.
The discriminatory ability was assessed using Harrell’s c-index. For the FPN model, the c-index was 0.684 (95% CI 0.669 to 0.700; n = 1432), which is somewhat low.
The CS for the FPN model is 1.049 (95% CI 0.939 to 1.158), which is well calibrated.
Because the FPN provides a probabilistic rather than a temporal prediction of survival, it is not possible to make a straightforward comparison between the accuracy of the FPN prediction and the accuracy of AMPESs.
Palliative Prognostic Index
The PPI is calculated using five clinical variables (PPS, oral intake and the presence or absence of dyspnoea, oedema and delirium). The model stratifies into three groups: survival of < 3 weeks (score of > 6), survival of < 6 weeks (score of > 4) and survival of > 6 weeks (score of ≤ 4). In our patient population, 501 (49.2%) participants had a PPI score of > 6, 428 (23.4%) had a score of > 4 and 900 (49.2%) had a score of ≤ 4.
The discrimination of the PPI was investigated by plotting Kaplan–Meier survival curves for each risk group, which are shown in Figure 11.
FIGURE 11.
Kaplan–Meier survival curves for PPI predictions by risk group. This figure has been reproduced with permission from Stone et al. 59 This is an Open Access article distributed in accordance with the terms of the Creative Commons Attribution (CC BY 4.0) license, which permits others to distribute, remix, adapt and build upon this work, for commercial use, provided the original work is properly cited. See: http://creativecommons.org/licenses/by/4.0/. The figure includes minor additions and formatting changes to the original figure.
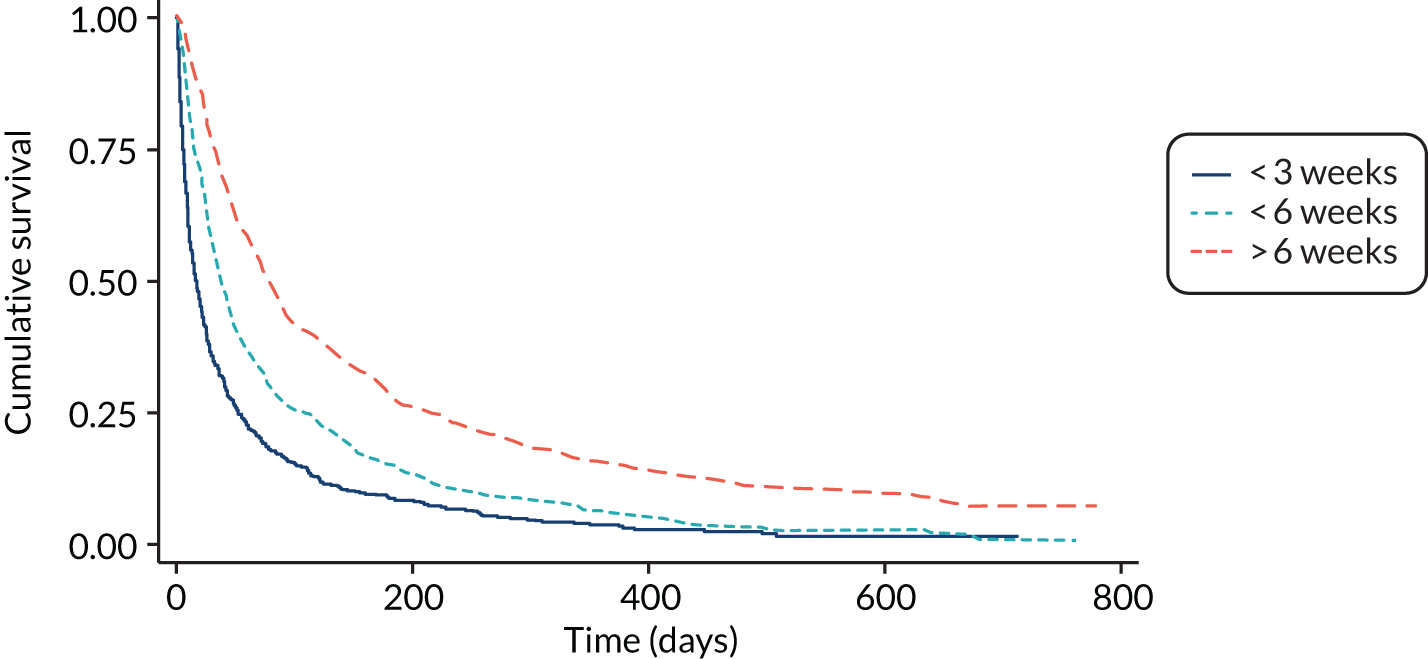
The median survival of patients in the PPI risk group that predicts survival of less than 3 weeks was 16 days (IQR 2–52 days). The median survival of patients in the PPI risk group that predicts survival of less than 6 weeks was 38 days (IQR 15–106 days). The median survival of patients in the PPI risk group that predicts survival of more than 6 weeks was 79 days (IQR 32–219 days).
The median survival of the patients predicted to die within 3 weeks, within 6 weeks or after 6 weeks was in the required range.
The discriminatory ability of the models has been assessed using the c-statistic. Separate c-statistic have been calculated using predicted survival for 3 weeks and 6 weeks to validate the PPI model. For the PPI risk category predicting survival of less than 3 weeks (n = 1829), the c-statistic was 0.675 (95% CI 0.652 to 0.699). For the PPI risk category predicting survival of more than 6 weeks (n = 1829), the c-statistic was 0.655 (95% CI 0.633 to 0.676).
Table 29 provides a comparison of the clinicians’ predictions and the model predictions with observed survival.
Variable | Observed survival (n) | Total (n) | ||
---|---|---|---|---|
< 3 weeks | < 6 weeks | > 6 weeks | ||
PPI predictions | ||||
< 3 weeks | 280 | 75 | 146 | 501 |
< 6 weeks | 130 | 99 | 199 | 428 |
> 6 weeks | 127 | 161 | 612 | 900 |
Total | 537 | 335 | 957 | 1829 |
Clinicians’ predictions | ||||
< 3 weeks | 196 | 25 | 15 | 236 |
< 6 weeks | 140 | 72 | 69 | 281 |
> 6 weeks | 186 | 237 | 869 | 1292 |
Total | 522 | 334 | 953 | 1809 |
Overall, the PPI correctly predicted the outcome on 991 out of 1829 (54.2%) occasions and the doctor’s estimate was correct on 1137 out of 1809 (62.9%) occasions. Table 30 shows a direct comparison between the number of correct predictions made by PPI and by the clinicians.
PPI predictions compared with observed deaths | Clinicians’ predictions compared with observed deaths | Total (n) | |
---|---|---|---|
Clinician was correct, n (%) | Clinician was incorrect, n (%) | ||
PPI was correct (%) | 738 (40.8) | 239 (13.2) | 977 |
PPI was incorrect (%) | 398 (22.0) | 432 (23.9) | 830 |
Total | 1136 | 671 | 1807 |
There was a statistically significant (p < 0.001) difference between the proportion of overall patient deaths predicted correctly by PPI and the corresponding proportion predicted correctly by the clinicians. The clinicians had a higher percentage of correct predictions than the PPI model.
Palliative Performance Scale
The PPS is a measure of functional status and is one of the variables included in the PPI score. The PPS is scored by health-care professionals and consists of 10 categories. A score of 10% represents the poorest functional status (totally bed-bound, unable to do any activity, mouth care only and drowsy or in a coma). A score of 100% represents the best functional status (full ambulation, normal activity and work with no evidence of disease, able to take care of themselves, normal intake of food and full conscious level).
The PPS scores for the participants are shown in Table 31. Just under half of the participants (n = 873; 27.6%) scored 50–60%. At 60%, the PPS score represents reduced ambulation, significant disease, occasionally needs assistance for self-care, normal or reduced food intake and full or confused conscious level. At 50%, the PPS score represents mainly sitting and lying, extensive disease (meaning that they are unable to do any work), considerable assistance required for self-care, normal or reduced food intake and full or confused conscious level.
PPS score (%) | n (%) |
---|---|
10 | 60 (3.3) |
20 | 78 (4.3) |
30 | 138 (7.6) |
40 | 273 (14.9) |
50 | 493 (27.0) |
60 | 376 (20.6) |
70 | 265 (14.5) |
80 | 109 (6) |
90 | 33 (1.8) |
100 | 2 (0.1) |
The discriminatory ability of the PPS as a prognostic tool was assessed by plotting the Kaplan–Meier survival curve for each of the PPS levels (Figure 12).
FIGURE 12.
Kaplan–Meier curve by PPS level. This figure has been reproduced with permission from Stone et al. 59 This is an Open Access article distributed in accordance with the terms of the Creative Commons Attribution (CC BY 4.0) license, which permits others to distribute, remix, adapt and build upon this work, for commercial use, provided the original work is properly cited. See: http://creativecommons.org/licenses/by/4.0/. The figure includes minor additions and formatting changes to the original figure.
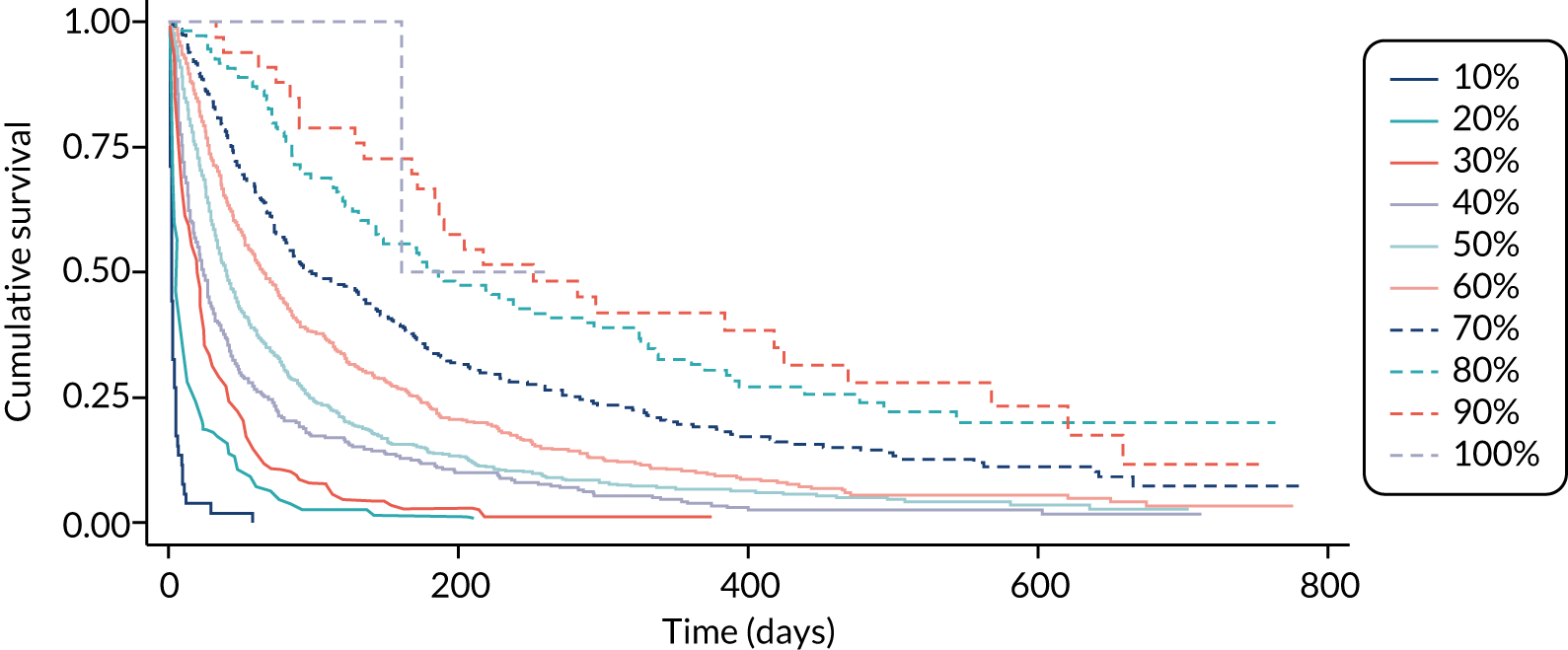
The median survival for each PPS level is shown in Table 32. With the exception of PPS level 100% (for which there were too few participants to judge), the median survival of each group increased in the expected direction as the performance status improved.
PPS score (%) | n | Survival time (days) | ||
---|---|---|---|---|
25% | 50% | 75% | ||
10 | 60 | 1 | 2 | 4 |
20 | 78 | 3 | 6 | 16 |
30 | 138 | 7 | 20 | 42 |
40 | 273 | 10 | 24 | 69 |
50 | 493 | 19 | 40 | 97 |
60 | 376 | 28 | 65 | 172 |
70 | 265 | 44 | 99 | 284 |
80 | 109 | 85 | 186 | 477 |
90 | 33 | 135 | 252 | 568 |
100 | 2a | 161 | 161 | – |
The PPS was not specifically developed as a prognostic tool, although it has previously been used to categorise patients into prognostic groups. 20 It is therefore not possible to make a straightforward comparison between the accuracy of the PPS prediction and the accuracy of clinicians’ predictions.
Results of nested qualitative substudy
Parts of this section have been reproduced with permission from Stone et al. 56 This is an Open Access article distributed in accordance with the terms of the Creative Commons Attribution (CC BY 4.0) license, which permits others to distribute, remix, adapt and build upon this work, for commercial use, provided the original work is properly cited. See: http://creativecommons.org/licenses/by/4.0/. The text below includes minor additions and formatting changes to the original text.
Data were collected between April 2017 and July 2018. Semistructured interviews were conducted with a purposive sample of patients, carers and clinicians to determine the acceptability of using prognostic indicators and barriers to and facilitators of clinical use.
Description of participants
Patients
A total of 29 patients were recruited to the qualitative substudy. Patients’ demographic details are shown in Table 33. The majority of patients (25/29; 86%) were recruited from two hospices in the Greater Manchester area. Patients had received a cancer diagnosis and were eligible for enrolment into the PiPS2 clinical study (i.e. estimate of survival for > 1 year). Five of the patients interviewed had declined to take part in the clinical study and their reasons for refusal were explored during the interview. Four patients were recruited from hospital day care and were receiving palliative care in a rural community trust.
ID | Gender | Age (years) | Primary tumour | Former occupation | Setting | In validation study |
---|---|---|---|---|---|---|
P1 | Male | 70 | Liver | Salesman | Hospice | Yes |
P2 | Female | 85 | Stomach | Carer | Hospice | Yes |
P3 | Male | 76 | Prostate | Joiner | Hospice | Yes |
P4 | Female | 61 | Breast | Chef | Hospice | Yes |
P5 | Male | 61 | Rectum | Manager | Hospice | Yes |
P6 | Female | 63 | Ovary | Teacher | Hospice | Yes |
P7 | Female | 71 | Skin | Teacher | Hospice | Yes |
P8 | Male | 63 | Pancreas | Accountant | Hospice | Yes |
P9 | Male | 80 | Lung | Printer | Hospice | Yes |
P10 | Male | 81 | Prostate | Manager | Hospice | Yes |
P11 | Male | 70 | Bile duct | Engineer | Hospice | Yes |
P12 | Male | 63 | Throat | Researcher | Hospice | Yes |
P13 | Female | 80 | Lymphoma | Tailor | Hospice | Yes |
P14 | Female | 67 | Pancreas | HSE inspector | Hospice | Yes |
P15 | Female | 61 | Bladder | Receptionist | Hospice | Yes |
P16 | Female | 84 | Colon | IT worker | Hospice | Yes |
P17 | Male | 64 | Myeloma | Police officer | Hospice | Yes |
P18 | Male | 65 | Oesophagus | Mill worker | Hospice | Yes |
P19 | Male | 79 | Lung | Boiler worker | Hospice | Yes |
P20 | Male | 88 | Prostate | Manager | Hospice | Yes |
P21a | Female | 92 | Lymphoma | Housewife | Hospice | No |
P22a | Male | 73 | Colon | Carer | Hospice | No |
P23a | Male | 58 | Oesophagus | Accountant | Hospice | No |
P24a | Female | 60 | Uterus | Housewife | Hospice | No |
P25a | Female | 69 | Breast | Housewife | Hospice | No |
P26 | Female | 85 | Neck | Researcher | Day care | Yes |
P27 | Female | 72 | Lung | Secretary | Day care | Yes |
P28 | Male | 62 | Bowel | Engineer | Day care | Yes |
P29 | Male | 69 | Prostate | Financial advisor | Day care | Yes |
Carers
A total of 20 carers were interviewed in the qualitative substudy. The majority of carers (17/20) were recruited from the two hospices in the Greater Manchester area. The remaining three carers were recruited from the rural community trust. All were relatives of patients recruited to the study. Most participants cared for patients who had consented to participate in the PiPS validation study and four carers were recruited from patients who had declined to participate. Carers’ demographic details are shown in Table 34.
ID | Gender | Age (years) | Relationship to patient | Occupation/former occupation | Setting | Patient in validation study |
---|---|---|---|---|---|---|
C1 | Female | 42 | Daughter | Unemployed | Hospice | Yes |
C2 | Female | 67 | Daughter | Company director | Hospice | Yes |
C3 | Female | 32 | Daughter | Administrative clerk | Hospice | Yes |
C4 | Female | 48 | Daughter-in-law | Carer | Hospice | Yes |
C5 | Female | 63 | Daughter | Personal assistant | Hospice | Yes |
C6 | Female | 78 | Wife | Teacher | Hospice | Yes |
C7 | Female | 35 | Wife | Housewife | Hospice | Yes |
C8 | Female | 86 | Wife | Shop owner | Hospice | Yes |
C9 | Male | 59 | Son | Entrepreneur | Hospice | Yes |
C10 | Female | 46 | Daughter | Carer | Hospice | Yes |
C11 | Female | 60 | Daughter | Nurse | Hospice | Yes |
C12 | Female | 65 | Daughter | NHS administrative clerk | Hospice | Yes |
C13 | Female | 53 | Daughter | Secretary | Hospice | Yes |
C14 | Female | 75 | Wife | Seamstress | Hospice | No |
C15 | Male | 50 | Son | Builder | Hospice | No |
C16 | Male | 65 | Brother | Engineer | Hospice | No |
C17 | Male | 64 | Son | Engineer | Hospice | No |
C18 | Female | 56 | Wife | Secretary | Day care | Yes |
C19 | Male | 63 | Husband | Roofer | Day care | Yes |
C20 | Female | 62 | Wife | Book-keeper | Day care | Yes |
Clinicians
A total of 32 clinicians were recruited into the qualitative substudy. Clinicians’ demographic details are shown in Table 35.
ID number | Gender | Age (years) | Professional group/grade |
---|---|---|---|
H1a | Female | 28 | Staff nurse (band 5) |
H2a | Female | 23 | Staff nurse (band 5) |
H3a | Female | 25 | Staff nurse (band 5) |
H4 | Male | 38 | GP |
H5 | Male | 29 | Trainee doctor (F2) |
H6 | Female | 34 | Trainee doctor (F1) |
H7 | Male | 42 | Consultant (palliative care) |
H8 | Female | 50 | Associate specialist (palliative care) |
H9 | Male | 39 | GP |
H10 | Male | 32 | GP |
H11 | Female | 47 | GP |
H12 | Female | 50 | GP |
H13 | Male | 45 | Consultant (palliative care) |
H14 | Male | 57 | Consultant (palliative care) |
H15 | Male | 28 | Trainee doctor (F2) |
H16 | Female | 30 | Specialist trainee doctor (palliative care) |
H17 | Female | 30 | Specialist trainee doctor (palliative care) |
H18 | Female | 30 | GP |
H19 | Female | 34 | GP |
H20 | Male | 48 | Consultant oncologist |
H21 | Female | 36 | Specialist palliative care nurse (band 7) |
H22 | Female | 45 | Specialist palliative care nurse (band 7) |
H23 | Female | 42 | Consultant (palliative care) |
H24 | Female | 44 | Consultant (palliative care) |
H25 | Female | 31 | Trainee doctor (F2) |
H26 | Female | 54 | Associate specialist (palliative care) |
H27 | Female | 45 | Consultant (palliative care) |
H28 | Female | 46 | Consultant oncologist |
H29 | Female | 39 | Consultant (palliative care) |
H30 | Female | 45 | Consultant (palliative care) |
H31 | Male | 44 | Consultant (palliative care) |
H32 | Female | 50 | Specialist community practitioner (district nurse) |
Findings
The patient and carer results are presented together, followed by the results of the interviews with the health-care professionals.
Patient and carer interviews
The primary aim of the nested qualitative research study was to assess the acceptability of the prognostic models to patients, carers and clinicians. The secondary objectives were to understand how clinicians discuss prognostic information with patients and relatives/carers from both the patients’ and clinicians’ perspectives. The main themes identified from analysis of the patient and carer interviews were desire for detailed prognostic information, acceptability of PiPS predictor models and preferred presentation of sensitive information.
Desire for detailed patient prognostic information
The majority of patients and carers expressed a desire for detailed information from doctors about life expectancy. Few reported that they had received this information, however, and reported that what information was given was not expressed clearly. A number of patients who said that they had asked about their life expectancy commented that doctors tended to be vague, overoptimistic and unwilling to deliver news that was considered bad or uncertain:
Whenever I have asked doctors about my prognosis, nearly everybody’s been vague, to be honest. I know that I’m going, but I want to know, even now, have I got a couple of weeks, a couple of months, the end of the year? I certainly know it isn’t anything further than that, but nobody dare tell me. I think doctors tend to be optimistic, I’d rather they would be more realistic.
P8: male patient aged 63 years (hospice)
Doctors are reluctant to give estimates of survival, their approach is very uncommunicative. When the prognosis is negative, you have to really probe and ask lots of questions, doctors would rather get out of giving you negative information altogether. I think it’s because they don’t want to give people bad news.
P5: male patient aged 61 years (hospice)
The specialist said to me it won’t be that long. Now, what does long mean, it’s a piece of string, isn’t it? I think they’re [doctors] very reluctant to say, ‘oh you’ve got 6 months’, and then you drop dead the following day. I would say the specialist was being extremely vague. He said, ‘it will kill you’, but he didn’t say when.
P3: male patient aged 76 years (hospice)
It is noteworthy that the first quotation seems to confirm our choice of presenting information in terms of days, weeks or months. A number of carers commented that, even if it was not shared with the patient, they would have preferred more detailed prognostic information. They described that they found clinicians reluctant to give a definite answer:
Nobody told him whether he’d got a week, a month or a year to live. They [the oncologist] just said the cancer was bad and we were just in shock because nobody could tell us what time my husband had left, the oncologists are reluctant to say, but I think as individuals you need some idea.
C18: female carer aged 56 years (community)
I was told that Mum had less than a year to live and that was 12 months ago . . . Mum wouldn’t want to know how long she has, plus she gets a bit confused, but the family would like more detailed information about her prognosis.
C2: female carer aged 67 years (hospice)
The main reason that both patients and carers gave for wanting more detailed prognostic information was to make plans and sort out family affairs. This related to sorting out individual and family finances and making funeral arrangements:
I said right from the start, if it’s terminal, tell me. I’d rather know so plans can be made and things can be put into place. I mean, I’m going to leave a wife behind so I wanted to get myself and my finances sorted out.
P29: male patient aged 69 years (community)
My husband has just turned 40 and is now on his seventh month without any treatment. The hardest part is the not knowing how long he has got. I think if I had a rough idea I think that would make things a little bit easier for me, with regards to organising his affairs and planning his funeral. But I am still in limbo, it could be next week or it could be 6 months.
C7: female carer aged 35 years (hospice)
A minority of patients explained that they did not want to discuss their life expectancy with clinicians. The main reason was that they preferred not to feel anxious or upset during the time they had left; in the following example, the patient was very elderly and did not have much time left irrespective of her advanced disease:
I would rather not know when I am going to go . . . I’ve lived a long life and I think I’d probably be more anxious, if I knew exactly when.
P21: female patient aged 92 years (hospice)
However, where patients did not want detailed prognostication, there was evidence in the carers’ data that patients were happy for relatives to receive it:
My wife doesn’t want to know what time she has left, she doesn’t, but we decided that I should be given detailed prognostic information.
C19: male carer aged 63 years (community)
One community care patient explained that his reluctance to discuss his prognosis was because he considered that he was still fighting his cancer, although the quotation perhaps also reveals his insight into his condition and the tension this represents for many patients between knowing and not knowing:
My doctor asked if I wanted to know my prognosis, but I said I didn’t. I know I have a life-limiting condition and I am still fighting the cancer, I am not ready to give up and think about my prognosis just yet. It is not the right time for me to be discussing my life expectancy.
P28: male patient aged 62 years (community)
The quotations on this theme clearly indicate that, for most patients, prognostic information is considered helpful, but there is likely to be a minority of patients who, for a number of reasons, do not wish to know.
Acceptability of PiPS predictor models
All participants considered that the PiPS models were acceptable for use in clinical practice and a useful aid in helping clinicians to more accurately predict life expectancy:
I think the tool is useful as it gives an indication of time left for people, however accurate it might be, that doctors can then pass on. At least it’s not this big open space that I have at the moment, the tool would help narrow the time frame, at least to give patients like me some idea.
P7: female patient aged 71 years (hospice)
I think developing this tool is massively needed. I’ve found the hardest part of going through all of this is the not knowing. I’m always asking, ‘can you tell me how long?’, and everybody says the same thing: ‘sadly, we can’t’. We were given a rough estimation, like, obviously 12 months being the longest survival rate from the oncologist, and we are actually past that now.
C7: female carer aged 35 years (hospice)
Patients and carers also considered that the PiPS model could help doctors initiate sensitive conversations with patients and carers about prognostication, potentially helping them to overcome their reported reluctance to do so (as cited in the context of the previous theme):
At the end of the day, doctors leave patients to ask about prognostic information because they [doctors] don’t want to volunteer the uncertainty. Any tool that helps must be useful for all concerned, especially in helping doctors explain this sort of information to patients.
P22: male patient aged 73 years (hospice)
I think the tool would be useful to help doctors start that sort of conversation about time left for people. I have felt that it is treated as a big secret, as if they [doctors] feel embarrassed to tell you or don’t know what to say. But I think if you’ve got something saying, look, we’ve had a look at this tool and it says maybe it’s months not years, you know, a bit of power to your elbow is always useful, isn’t it?
P15: female aged 61 years (hospice)
These are insightful comments from the patients and a theme that resonates with doctors’ concerns. We return to this theme when considering the views of the health-care professionals.
Presentation of sensitive information
Patients and carers agreed that the most appropriate way of receiving prognostic information was during face-to-face discussions and that this should be verbal rather than written:
I’ve had a number of face-to-face discussions with my doctors about my cancer and prognosis. I know talking about dying is a sensitive topic, it is part of life, I’ve accepted it.
P27: female patient aged 72 years (community)
I don’t think it is a good idea to show patients any written information about their prognosis, I think it could make people feel very anxious. I also think showing patients’ information about their probability of survival is hard for people to grasp. It could be very stressful for some, especially if they have a more negative outlook on things.
C1: female carer aged 42 years (hospice)
When having these conversations, patients and carers preferred doctors to convey prognostic information truthfully, honestly and sensitively. Participants confirmed that life expectancy presented as days, weeks or months was most meaningful to them:
I’d just like to know truthfully how quickly this is going to affect me . . . how soon am I going to get to the stage where I can’t swallow at all; what’s going to happen, when I’m going to die? I want to be well informed about the different stages.
P26: female aged 85 years (community)
Rather than being given statistical information about my husband’s time left, I’d rather doctors say to me, it’s days or weeks, rather than there’s a 50% chance he will still be here in 2 months. It’s just clearer to understand that way for me.
C7: female carer aged 35 years (hospice)
I’d personally go for the actual weeks, days, years, sort of number rather than percentages or a significant event, it’s more informative.
P27: female patient aged 72 years (community)
These data lend support to our presentation of PiPS results as days, weeks or months, although, overall, there appears to be misunderstanding among patients and carers about the ability of PiPS to predict longer survival. The scope and limits of PiPS would need to be clearly explained by clinicians.
Clinician interviews
The main themes identified from analysis of the clinicians’ interviews were (1) challenges and difficulties with predicting length of survival, (2) language used when discussing prognosis, (3) reasons for overestimating prognosis, (4) clinicians’ acceptability of PiPS models, (5) facilitators of use in clinical practice, (6) barriers to use in clinical practice and (7) clinicians’ views on existing prognostic models.
Challenges and difficulties with predicting length of survival
All clinicians commented on the complex nature of estimating length of survival for patients with advanced cancer and many found it difficult. A number of clinicians described how difficult it was to predict survival in ‘well’ patients who had not started to deteriorate. The following quotes are from two trainee doctors:
In the past I’ve found it extremely difficult to give prognostic information, especially when working in a hospital, as it seems more unpredictable and you are not really working in a specific palliative care environment, you do sometimes feel a bit lost with these kinds of conversations.
H15: trainee doctor (F2)
I think it’s difficult to predict exactly when someone is going to die and I think you do have to sort of explain about uncertainties to people because I know from talking to patients, in the past the oncologist may have said ‘oh, you’ve got 6 months’, and then 6 weeks later patients find themselves in a hospice and they’re dying.
H6: trainee doctor (F1)
Interestingly, this view was supported by more senior clinicians:
I find predicting length of survival extremely difficult. I think once there’s a change in somebody’s health condition it’s certainly a lot easier, because then you’ve got a reason to suspect that they may well be deteriorating. If somebody continues in a stable disease phase without the presence of imaging, I think it’s difficult to prognosticate.
Consultant (palliative care), ID number H24
If somebody has other aspects of their general condition that shows they’re in decline, then it’s easy to give an estimation of time left because you’ve got some benchmarks to say, this person is deteriorating. Other than that, I think it’s just . . . we don’t have any more discernible information, really, that would allow us to give an accurate prediction.
Consultant (palliative care), ID number H27
If there’s nothing in the patient’s disease trajectory which would suggest a change in their condition, then I think it’s very difficult to prognosticate, so I think you’d struggle to give an accurate prognosis if somebody’s condition doesn’t change. If somebody’s condition deteriorates, then it’s the rapidity of that decline that gives you the best guide. And then it is just a guide.
Consultant oncologist, ID number H20
A number of senior clinicians said that they would generally explain to patients that accurately predicting length of survival was, in many cases, an impossible task owing to the amount of uncertainties involved:
I’ll often say it’s not like on TV, we’re not good enough to estimate accurately how long an individual is going to live, and then we talk about months or weeks, or . . . I’ll often tag onto the end of . . . so let’s say I think it’s likely to be weeks, because they’re going down quite quickly; I say how many weeks that is I’ve honestly no idea, things could flatten off and it might be quite a lot of weeks and that might turn into a few months, or things could get worse and we might be talking about a very small number of weeks.
Consultant oncologist, ID number H28
I say that, even with all of our experience, we’re often no better at predicting the future than relatives and patients are themselves. But patients and relatives don’t often get that. I’ve had one patient’s relative think I was withholding information from him, and that of course I must know, I’m the consultant, I must know how long the person has, and got really very angry with me. But I genuinely wasn’t certain with that patient.
Consultant (palliative care), ID number H13
These quotations are from interviews with senior clinicians with considerable experience of observing deterioration in patients with advanced cancer. It is interesting, therefore, that in the validation study, clinicians’ estimates were found to be quite good, with around 60% accuracy. There appears to be a mismatch between perceptions and reality. Clinicians’ lack of confidence in their ability to predict survival using clinically observed parameters might explain the next theme, which is the language they used with patients and families when discussing prognosis.
Language used when discussing prognosis
During the interviews, clinicians were asked about the type of language they used when discussing prognostication with patients and carers. The majority of clinicians, irrespective of experience or seniority, explained that they tended to avoid giving detailed prognostic information with specific time frames. Clinicians said that this was usually because they actually did not know, as these quotations illustrate:
Patients do ask but I keep it quite vague, especially just trying to sort of judge how much a patient wants to sort of talk to you about it. Yes, I wouldn’t just, answer just says weeks, or whatever, I think you just have to be quite kind and gentle. Also some patients just don’t want to know about prognosis. We just talk more in terms of sort of the rates of deterioration, that’s how I talk about it a bit more, you know, things are progressing over days, weeks, months, that kind of time frame.
H25: trainee doctor (F2)
Rather than giving an approximate time frame, I might say, as you’ve seen, things have been going downhill and if there are things that needs to be done, such as, you know, getting your financial, social affairs in order, I wouldn’t delay them any longer. I refrain from giving any specific time, like you’ve got 2 weeks or you’ve got – I don’t know – exactly 2 months, because I don’t think you’re going to know exactly how long it’s going to be.
Consultant (palliative care), ID number H31
Generally I try and keep the answer vague, I mean, you’re obviously able to give an indication as to when someone’s obviously breathing their last or when someone is very unwell, but when someone is in that stage where they’re kind of on a knife edge, I always say, well, it could be the next few weeks, but at the same time we’ve all been surprised and seen people last for months and months. So it’s about discussing the uncertainness involved in predicting survival.
Consultant (palliative care), ID number H30
In the third quotation, when predicting survival the clinician balances bad news with hope that the patient could live much longer. Referring back to the patient and carer data, this may be perceived as ‘vague’ rather than honest and kind. In the following quotation, the clinician describes how he explains to patients the process by which predictions are made and how observed changes support estimates or ‘guesses’. The process is shared with the patient and carer to some extent:
I’m careful to, I think, really try to avoid any certainty. And I usually, before I start talking about what my guess might be, I often talk about the fact that we don’t know, and that we guess, and how we guess. And I, sort of, try to give them a little bit of an understanding of how we’re prognosticating; that we’re using what we know about their illness, yes, and their scans, but actually, we’re using how they are, how things are changing, how they’ve been changing, and we use that to see how we’re going to predict.
Consultant (palliative care), ID number H23
Another clinician discussed how he avoids giving a number to patients because it will inevitably be wrong. He also considered that a vague prediction is probably more helpful:
I tend not to put numbers on it, I tend to think about things in terms of, you know, days, weeks, months, years. But I don’t usually put a number on it because that immediately means that I’m going to be wrong, if I put a number on it. And I tend to use that, sort of, language. Also, I think that actually, there’s no way of being sure, and often that, sort of, vague idea of a guess is often, for a lot of people, more helpful than adding numbers to the guess, is what I tend to say.
Consultant (palliative care), ID number H7
Junior clinicians also described how they avoided giving numbers to patients and preferred to convey vaguer estimations of prognosis:
I’ve always avoided numbers and will talk generally; well, it may be months. I talk about things will then change and then we might find that you’re changing and we’re looking at weeks, but nobody can say when that change is occurring, whether it’s going to occur tomorrow, next week, or in several months’ time. So, trying to get across that it’s a very inexact science.
H5: trainee doctor (F2)
I acknowledge that the prognostic information that I’m giving to patients is relatively vague and I apologise for that, but generally patients understand, and I don’t use numbers because I think patients and relatives pick up on the number and they focus on that number. Whereas actually, you’ve invariably got a scale of a very short time to a very long time and you’ve no idea where the patient is on that scale.
Specialty trainee doctor, ID number H16
A number of senior clinicians further considered that giving specific time frames could cause psychological harm to patients and carers, especially once patients had survived beyond their maximum given prediction. The following quotations illustrate this:
So, one of the questions that almost everyone wants to know is how long they’ve got, and I think it probably is the hardest question to answer because none of us know. I tend to be quite guarded and careful about how I answer that question, and usually probably far too vague for their liking, mainly because I’ve seen the negative effects of people having been given a very clear time scale, often from a hospital clinic they’re given x number of months, and more often than not they might exceed that and then they feel like they’re living on borrowed time, and actually, psychologically, for them and their family that’s often more damaging.
H9: GP
I’ll really try and avoid anything that might give them [patients] a number. And that’s, I suppose, because I’ve had many patients who feel like they’ve been given a number and then it causes them problems, because they’re counting on that. Others aren’t bothered so much by it, because then they get to the point, and once, when they outlive it, it can be a positive thing, but for some of them, they’re just waiting to die.
Consultant (palliative care), ID number H29
I don’t ever use numbers, because it fixes people’s focus onto a particular time scale, and rather than making what they can and enjoying each day for its own right and, you know, having an eye to the things that they need to be sorting out. I think that’s the sense of a much more vague time line from that point of view is far more helpful for most people.
Consultant (palliative care), ID number H31
Insights from this theme suggest that the ‘vague’ language that some patients and carers dislike is used by clinicians because they consider it to be a more honest response when so many factors are at play. There is also some reticence about giving a number in case a patient becomes fixated on it, which is a theme we pick up later when we discuss barriers to using PiPS.
Reasons for overestimating prognosis with patients
During the interviews, clinicians were asked about the accuracy of estimating length of survival for patients with advanced cancer and about existing studies suggesting that clinicians tend to give inaccurate and overoptimistic prognostic information. All agreed that the main reason they felt clinicians may give an overestimate of length of survival was that colleagues prefer to convey kinder, optimistic information that was perceived as less harmful. The following two quotes are from GPs:
I suppose you don’t want to cause harm if patients are in that good place, but also you’re then thinking, well, am I going to cause more harm by not addressing this now if patients are in a state of denial, and when it does hit them . . . those kinds of things. I suppose breaking any bad news, you do it at a rate the patient can cope with and there’s . . . I suppose there’s no rush, it’s not going to affect any change in the management, more how you’re handling the circumstances, in a way.
H12: GP
I don’t often get any negative criticism off patients, but when I have maybe a couple of times due to this factor where I’ve tried to sensitively discuss end of life and maybe the patient’s not been ready for it. Once I was on a home visit and the next time I saw the patient he was like, are you going to tell me I’m going to die again? And obviously that’s how he perceived it and I wasn’t aware . . . maybe I could have done it better or more sensitively. But he wasn’t ready for that discussion and it was me that was leading it instead of the patient. You can cause a lot of harm if you’re not careful, just by your manner and how you communicate with patients.
H11: GP
The following quotation further illustrates that this kinder, optimistic approach is also adopted by more junior clinicians:
It’s about all sorts of complex reasons why doctors may over estimate prognosis, really. It’s trying not to upset patients, not be a failing doctor, trying to understand what death and prognosis means to patients and what else is going on in people’s lives.
Specialty trainee doctor, ID number H17
Other clinicians also considered that it was better not to challenge patients’ or carers’ perceptions of the patient’s disease trajectory and described not wanting to reduce people’s hope if they were using denial as an effective coping mechanism:
It’s not that clinicians are overestimating prognosis it may be that sometimes, we can see that a patient is operating at a high level of denial, we wouldn’t necessarily challenge if we thought that was a useful coping mechanism for them, any challenge may cause stress and harm to the patient, but it might be that we then have to subtly bring along the patient and family’s understanding over time to try and prepare them for what’s going to happen.
Consultant (palliative care), ID number H14
In the next quotation, the clinician describes his experience of rapid deterioration in patients when such coping mechanisms are removed:
If they’re [patients] coping quite well with their perception of actually time left and we as clinicians change that quite drastically, that’s when you can do harm, and it can seem that people deteriorate very quickly then. So there’s that difficult line to tread between giving patients more optimistic information which may give patients time to still function, so they can plan things that are important, compared with taking away hope and doing harm.
Consultant (palliative care), ID number H24
I think when they [oncologists] have given prognostic indicators, I think they generally err on the side of saying they’ve got slightly longer than maybe they have to patients. They are trying not to destroy people’s hope. I think everybody wants to give people good news, don’t they, and in this scenario there isn’t much good news.
H19: GP
This theme therefore presents a tension. Clinicians withhold detailed estimations of survival because experience suggests they can get it wrong and that it can erode hope, and some consider denial to be an effective coping strategy. Yet the majority of patients want to know how long they have left. There appears to be a mismatch between what patients want and clinicians’ perception of this. This leads to the next theme, which presents their views on whether or not PiPS would help.
Clinicians’ acceptability of PiPS models
When asked about the acceptability of the PiPS models, clinicians gave a number of positive responses. The majority considered the model to be a useful algorithm that could offer a more scientific approach to estimating patients’ prognoses than a clinical judgement or hunch:
I think it’s useful to have it [PiPS] there and offer it to patients and say there is a scientific tool that can give you a better idea about what time you have left, which gives you closer numbers than I can, which might help your planning.
H4: GP
I think where PiPS would sit for me as a tool is if a patient asked me what I thought their prognosis would be, because currently I’ve got only my experience to use; whereas I wonder how it would work in my clinic. So, if, for example, a patient asked me, what do you think my prognosis is, we would have our usual probable discussion exploring what he thought, what they thought; and then I think I would probably say, there is an online tool that actually is more accurate than me, if you want us to use that tool we can do it now if you like.
Consultant oncologist, ID number H28
It would appear from the validation study that PiPS-B risk categories are as accurate as AMPESs, if not more so. Anticipating this as a possible outcome, we asked clinicians whether or not PiPS would still be beneficial. Clinicians stated that PiPS could help improve their confidence in making accurate predictions of life expectancy:
Even if PiPS is no more accurate than a clinician’s estimate, it would be useful for use in clinical practice, especially with less experienced staff, as when you’re a junior doctor you don’t necessarily have the experience to be confident in identifying a dying patient, so often you’re very hopeful. I think sometimes when you’re a junior doctor, you could err on the side of false reassurance. So I think because of the lack of experience, I think it’s more difficult for junior staff to prognosticate accurately.
Consultant (palliative care), ID number H7
I think we’re a little bit uncomfortable when we are asked the question about what time is left, because we know that it’s an estimate. We don’t want to be completely wrong, I suppose, and yet we understand that something sudden can happen at any point, can’t it? So, I think the PiPS tool perhaps gives you more confidence in making a prediction.
Consultant oncologist, ID number H20
I think for a lot of doctors having something more solid, where we can say actually it’s looked at blood tests, it’s looked at different clinical markers and it gives you something more firm to back you up and give you some numbers. We know that we can never be 100% sure, but what this tool does is gives you a bit more confidence. Maybe more doctors would have the conversation if they felt more confident about what they were saying, and actually that would be much better if doctors started talking about it more, that would be a really good thing.
Consultant (palliative care), ID number H14
I think for you to be confident with a consistent approximation, so a consistent level of knowledge that you can apply to how long, PiPS would be very useful, because it would give you some assurance that your approach every time is the same to the information that you’re being given. So you can use the tool to measure how accurate your own predictions are.
Specialist palliative care nurse, ID number H21
Importantly, and echoing findings from the patient and carer interviews, clinicians agreed that PiPS would be a useful communication prompt that could be used when conveying prognostic information. It could make starting, and holding, difficult conversations easier:
When communicating prognostic information it would be nice to wrap it around something, and I’m presuming with something like PiPS you can almost wrap it round, you know, we’ve filled in a tool, what we do is we look at you, we look at lots of things, such as your symptoms, we take them into consideration, and what the tool shows us that you’re very poorly and then I would maybe wait for the ‘how long?’ question.
Specialist district nurse, ID number H22
I mean PiPS is another way of starting this sort of difficult conversation which I do think a lot of clinicians have the gut reaction to shy away from. It is important to talk to people and to give them an idea, because I think the worst possible case scenario is these people who lose their relatives and it’s a terrible shock because nobody has had the balls to actually front it with them, so I think it could have a place.
H12: GP
A number of participants further discussed that PiPS would be helpful for encouraging clinicians to give a more realistic prediction by using it in a way that takes the responsibility for making that prediction away from clinicians themselves:
Sometimes oncologists might overestimate because they’re trying to be nice and maybe we [GPs] do that as well. So it might actually help a doctor who has that tendency to be more optimistic to use PiPS. I suppose then it’s not the doctor that’s giving the bad news, it’s like the tool, it’s the computer results that are saying this, which a doctor may find useful.
H10: GP
These points are particularly important when considering the use of PiPS in clinical practice, because, as the interviewees indicate, even if PiPS were to be no more accurate that clinician estimates, it might provide clinicians with more confidence and a framework around which to initiate a discussion of prognosis. For the clinician, using a prognostic tool like PiPS would be more akin to giving a patient the results of a test. This would remove some of the direct responsibility for the ‘bad news’ from the clinician and may make it easier for them to broach the subject. Nonetheless, the clinician will still need excellent communication skills to convey this information in a sensitive way and, therefore, if PiPS is to be used in this way it is clear that clinicians will need to develop good communication skills for this approach to be successful.
Facilitators for use in clinical practice
All clinicians commented that the online PiPS prognosticator tool was user friendly and quick and easy to use:
The tool is quick and easy to use, I could put one of our junior doctors in front of PiPS, and they would complete it, without any training really.
Consultant (palliative care), ID number H14
I find the online tool quite straightforward to use, once everything is entered it is just a case of submit and the results appear.
Consultant (palliative care), ID number H27
I think PiPS is very straightforward. It’s available online, so you can put the data in. I have no difficulty at all with it.
Consultant oncologist, ID number H20
A number of clinicians considered that PiPS could be beneficially used as an educational training tool, especially for less experienced staff:
It would be interesting for junior staff like me to use PiPS that are new to palliative care. Say, OK, for your first month in your job, try and plug in the details of the patients that we’ve actually got the relevant details for, and then just get a feel yourself on how that’s matching up to your own reality and clinical judgement of what time patients have left.
H6: trainee doctor (F1)
Well, PiPS would be useful in clinical practice, if you found the tool gives the same prediction as an experienced clinician on more or less every occasion, then your junior staff should be trained to that standard; and that would be a way of saying, right, let’s look at a patient, OK, tell me what your gut feeling is, assess your patient. Then you could say, well, why do you feel that, and I feel this . . . OK, let’s have a look on the PiPS tool, let’s see if you are right or not . . . that would be something that would help with learning and development, wouldn’t it?
Specialist district nurse, ID number H22
Participants further commented on how the PiPS tool could help inform decision-making in relation to treatment options and/or discharge planning:
If it’s [PiPS] no more accurate than clinical judgement, it’s probably got a good basis for training, I guess. You may have a particular patient and you may think to yourself, I wonder what the numbers are re[garding] length of time. And then you could use the tool and see how close you get, to help build up your experience.
H10: GP
Even if it’s no more accurate than clinicians’ estimate, I would still use it, especially in the hospital setting. The reason being I think one of the things . . . or at least from a palliative care point of view, is that it could aid any MDT [multidisciplinary team] discussions as sometimes it is difficult to convince other clinicians about a patient’s prognosis. If we can use PiPS, then at least we could use this as an evidence base to say why we think the particular patient has got weeks or months.
Consultant (palliative care), ID number H30
Interestingly, a trainee doctor (F2) described that PiPS could support doctors attempting to convince more senior colleagues about a patient’s prognosis. The following quotation illustrates this point:
I think PiPS is a useful tool, especially for us junior palliative care staff working in a hospital setting, trying to convince more senior clinicians about a patient’s prognosis. It also aids the decision about stopping curative treatment or not and thinking what is best for the patient and the time they have left.
H5: trainee doctor (F2)
Clinicians further highlighted that PiPS would be helpful when commissioning care packages for patients:
PiPS is definitely helpful, with commissioning care packages, so commissioners like statistics, and certainty, because they don’t want to think that they’re going to be funding continuing health care for ages and ages, so being able to put some statistics in from a prediction, I think is helpful in the right people getting continuing health care, when we’re looking at arranging discharges and care packages.
Consultant (palliative care), ID number H27
A few participants considered that PiPS would be a helpful tool for use outside specialist palliative care, such as in the community. For example, the GP in the next quotation commented that PiPS would be useful in helping colleagues estimate length of survival for patients:
The value, I suppose, comes down to if we do have to diagnose somebody in primary care and they say, well, how long have they got, and the usual answer at GP level at the moment is, I don’t really know, why don’t you go and see the specialist and they will give you more information. At least then if we had PIPS in place we could run the patient’s details through it to generate an estimate.
H9: GP
Overall, therefore, there are a number of reasons why PiPS might be adopted in routine clinical practice.
Barriers to use in clinical practice
A minority of participants considered that it was not appropriate to use the PiPS tool, especially if blood tests were needed to improve the accuracy of the prediction. This related to both the practicalities of organising blood tests for patients with advanced cancer and the ethics implications of drawing blood from very unwell patients:
I tend not to run a lot of blood tests on patients that are palliative and cancer based. I am not sure how ethical it is to be taking bloods with patients that have advanced cancer, especially if I am seeing them at home. It is about making them comfortable, not sticking them with needles.
H4: GP
I think what does sit with me a little is to say to patients, well, we might be able to work out how long you are going to live but you’ve got to wait a couple of weeks to have a blood test done, because it’s a couple of weeks’ wait to get them back for their bloods.
Consultant oncologist, ID number H20
I don’t think PiPS is a waste of time. I think it’s potentially a waste of resources but wouldn’t say it’s a waste of time, but I’d say it’s a waste of blood tests and cost.
H5: trainee doctor (F2)
A few GPs considered that introducing PiPS into clinical practice could be time-consuming, both completing the tool and finding time to support patients emotionally:
We’re saturated at the moment in primary care, so to have to do something else like complete and run a PiPS estimate could be time-consuming. Also then you’ve got to find time to give patients the result. You’re grappling with time to deal with the results and to give patients time to talk and that kind of thing, like making time in supporting the patient emotionally.
H11: GP
Another, potentially more important, barrier relates to previous discussion about clinicians preferring to rely on their clinical judgement and avoiding prognostic discussions with patients and carers. The latter was due to difficulties with balancing a kinder, more optimistic stance with being realistic with patients considered not ready for receiving devastating news. If PiPS is perceived to potentially do more harm than good, it is unlikely to be used:
All the statistics doesn’t fit well with everyone and I just would always trust my own clinical judgement because that’s what I’ve always done. And if using a tool doesn’t benefit the patient in any way then it won’t be used. If you don’t actually feel that it’s going to actually help, it’s just another box-ticking exercise.
H9: GP
I think people do feel that if they’re told they’ve got 3 to 6 months they’ll come to my clinic and say, ‘I’m now 6 months and a week, what am I going to do now, what does that mean now?’. I’ve certainly looked after a few people who have been stuck because they’re either dying or just here waiting because somebody said they should have died. So, they’re left with, well what does that mean, where am I now, what do I do with life while I wait? It can feel much more that they’re waiting for something rather than just getting the most out of life, which is clearly what we’re aiming to help them do.
Consultant (palliative care), ID number H11
Clinicians’ attitudes to existing prognostic models
Clinicians were shown existing prognostic models (PPI, PPS, PaP and FPN) and asked to comment on their impressions of their usefulness. Clinicians considered these prognostics tools to be cumbersome and difficult to use compared with PiPS. They liked being able to complete PiPS online:
Compared with PiPS, the PPS tool scores are too wide-ranging and the PPI I suppose it’s not bad, it’s not as easy to do as running the PiPS on the computer one. I suppose the [FPN] is similar in a little way because it’s looking at some of the same parameters in the bloods and then it’s giving you the probability scores a bit like a percentage, 0.9–90%. I suppose it’s how user friendly it is in that format there, it doesn’t look that easy to use. Yeah, so it’s a lot easier to be able to press a button on the computer and get the results.
H12: GP
I think all these tools [PPI, PPS, PaP and FPN] are a bit cumbersome unless you’re of a certain mind and you like your stats and your figures . . . you’re going to have a low uptake of clinicians using them, whereas if it’s a click of a button we have the answer it’s a lot easier for people to use.
H11: GP
For some of these existing tools we’ve not got the influence of those recognised markers in the bloods, so I would have less confidence in it. They also just look more difficult to use than PiPS.
Consultant (palliative care), ID number H14
Others described the outcome from the tools other than PiPS as vague:
The PPS tool is rather vague and a bit wishy-washy. I probably wouldn’t find it useful. For the PPI tool the information is more detailed there, it makes a bit more sense in the sense it’s less vague in re[garding] the time frames.
H4: GP
The PPS results are a little wide-ranging to explain to the patient, therefore it is not very useful really. It wouldn’t be easy explaining to patients that they have a 30–70% chance of surviving 30 days. Patients prefer less statistical information.
Consultant (palliative care), ID number H24
Clinicians clearly found PiPS easier to use and were happier with the way the results are presented by PiPS than by other prognostic indicators that are available.
Summary of qualitative results
Patients’ and carers’ information needs and acceptability of PiPS
The majority of patients and carers clearly expressed a desire for detailed prognostic information but found clinicians vague, overoptimistic and unwilling to deliver accurate information about length of patient survival. The main reasons for wanting detailed information were to put finances in order and to make funeral plans. All patients and carers considered that the PiPS models/tools were acceptable for use in clinical practice and were a potentially useful aid in helping clinicians more accurately predict life expectancy and initiate sensitive conversations about prognostication with patients and carers. Participants confirmed that life expectancy presented as days, weeks or months was most meaningful to them.
Clinicians’ perspectives
Clinicians found estimating length of survival complex and often challenging and the process of conveying prognostic information to patients and carers a difficult and uncomfortable task. Clinicians, regardless of seniority, explained that they avoided giving specific time frames in discussions, either because they did not know or because they did not want the discussion to have a negative impact on the patient or carer. All clinicians described the challenges and difficulties they faced in predicting length of survival and admitted to being vague and avoiding the discussion of time frames with patients and carers. Clinicians considered that PiPS might have a place as an educational training tool, especially for training less experienced staff. Clinicians further commented on how PiPS could help inform decision-making in relation to treatment options, discharge planning and admission to hospices, or when commissioning care packages for patients. Clinicians said that even if PiPS was no more accurate than a clinician’s estimate they would still regard it as a potentially beneficial tool that could help improve their confidence in making predictions about survival. Clinicians considered that PiPS could be a useful communication aid when conveying prognostic information to patients and carers. Clinicians further discussed that PiPS might be a helpful way of encouraging clinicians to give more realistic predictions and could possibly be used in a way that took the responsibility of making predictions away from clinicians themselves.
We identified a few barriers to using PiPS in clinical practice. The first was the practical and ethics difficulties of organising blood tests for patients with advanced cancer. Two GPs considered that introducing PiPS into clinical practice could be time-consuming in relation to both completing the tool and finding time to communicate the results to patients and families. This barrier was not mentioned by their hospice colleagues. Other barriers related to clinicians preferring to rely on their own clinical judgement or wanting to avoid prognostic discussions with patients and carers.
Chapter 4 Discussion
Statement of principal findings
This study has demonstrated that the PiPS-B risk categories to predict whether a patient will survive for days, weeks or months+ are as accurate as an AMPES in palliative care patients with advanced cancer. The study also found that the PiPS-A risk categories were not as accurate as an AMPES. All four models (PiPS-A14, PiPS-A56, PiPS-B14 and PiPS-B56) performed well individually. The discriminatory ability (c-statistic) of each model varied from 0.776 (95% CI 0.755 to 0.797) for PiPS-A56 to 0.837 (95% CI 0.810 to 0.863) for PiPS-B14. The calibration of PiPS-A14 [CiL –0.037 (95% CI –0.168 to 0.095), CS 0.981 (95% CI 0.872 to 1.09)] and of PiPSA-56 [CiL 0.109 (95% CI 0.002 to 0.215), CS 0.946 (95% CI 0.842 to 1.05)] was good. The calibration of PiPS-B14 [CiL –0.202 (95% CI –0.364 to –0.039), CS 0.840 (95% CI 0.730 to 0.950)] and PiPS-B56 [CiL 0.152 (95% CI 0.030 to 0.273), CS 0.914 (95% CI 0.808 to 1.02)] was less good.
This study also provided validation data about other prognostic tools. The study was not powered to detect differences in the predictive accuracy of these other tools compared with clinicians’ predictions, and for methodological reasons a direct comparison of the performance of different methods of prognostication was not always possible. Where a direct comparison was possible, the discriminatory ability of the FPN [c-index 0.684 (95% CI 0.669 to 0.700)] and the PPI [c-statistic for > 6 weeks’ survival 0.655 (95% CI 0.633 to 0.676); c-statistic for < 3 weeks’ survival 0.675 (95% CI 0.652 to 0.699)] was lower than PiPS-A14 [c-statistic 0.776 (95% CI 0.755 to 0.797)], PiPS-A56 [c-statistic 0.825 (95% CI 0.803 to 0.848)], PiPS-B14 [0.810 (95% CI 0.788 to 0.832)] or PiPS-B56 [c-statistic 0.837 (95% CI 0.810 to 0.863)]. Moreover, the PPI predictions were statistically significantly (p < 0.001) worse than those of clinicians.
The nested qualitative substudy found that patients and their carers were generally in favour of having access to more accurate prognostic information and preferred the PiPS method of couching a prognostic estimate in terms of whether individuals were expected to survive for days, weeks or months+. Clinicians were generally in favour of using PiPS, even if it were no better than an AMPES, because it could have other benefits in terms of communication, training, supporting junior colleagues and providing objective information for the commissioning of care packages. Some clinicians expressed the view that they would be reluctant to use PiPS-B because they preferred to use their own clinical judgement or because they did not believe that taking a blood specimen was appropriate in these circumstances.
Strengths of the study
Validation study
This study – PiPS2 – has been one of the largest prospective palliative care studies undertaken in the UK. A total of 1833 patients across 27 sites in England and Wales were included in the study. PiPS2 has demonstrated that there is an enthusiasm to participate in research among palliative care service professionals, patients and their carers, even when the patient population concerned is gravely ill. The final sample size exceeded the minimum required for the primary analysis and was achieved within the originally estimated time frame.
One of the main strengths of PiPS2 is that it was powered to demonstrate a difference between the accuracy of PiPS-B risk categories compared with those of an AMPES. Previous prognostic studies have simply validated various prognostic tools statistically and have reported their discrimination, calibration and accuracy. However, the current default method for predicting survival in palliative care practice is to use clinician predictions. Therefore, it is important that any newly proposed prognostic method should be at least as accurate as this before being considered for adoption into clinical practice.
Another strength of PiPS2 has been the robustness of the data collection and validation procedures. Data were collected at 27 different sites, a number of which had never been involved in multicentre research projects. Data were entered onto a secure online database and the accuracy of data were checked remotely by the trial manager. In addition, each site was subject to at least one data-monitoring review visit at which CRFs and source documents were checked for accuracy and consistency and at which further data queries were raised. A final check of all data was independently conducted by the trial manager and statistician. Therefore, it is possible to have high confidence in the robustness, consistency and reliability of the study data. Furthermore, the PiPS database will be made available (open access) after the main results have been published, and this will provide a valuable resource for answering other research questions and/or for the development/refinement of prognostic models.
Qualitative substudy
As well as conducting a large quantitative validation of prognostic tools, PiPS2 included an embedded qualitative substudy designed to investigate patients’, carers’ and clinicians’ perspectives on the use of the predictor models of survival. This complementary work was a great strength of the study because it allowed a greater understanding of the perceived value of these tools to patients, their families and the health-care professionals looking after them. The qualitative benefits and disadvantages of routine use of prognostic algorithms is not something that has been adequately explored in research studies previously. A major strength of the qualitative substudy is that the patient and carer sample was comparatively large and varied and data saturation was reached. We are confident, therefore, that the views of patients and carers presented in this report are transferable to other settings in the UK. The clinician sample was similarly large and varied and included the views of practitioners across disciplines who would potentially use PiPS in routine clinical practice. Again, we are confident that the clinicians’ findings are transferable.
Another strength of the qualitative substudy was that data collection was carried out over a long period of time, allowing constant comparative analysis throughout the study, and iteration of the topic guides. We were able to constantly revise the topics to be discussed with prospective participants and feed their views into subsequent interviews. A wide range of views was therefore captured.
The study was greatly strengthened by the participation of service user representatives throughout, from generation of the research proposal to membership of the Study Management Group and Study Steering Committee. Our service user representatives fully engaged in the research endeavour and will continue to be involved in the dissemination of study results.
Weaknesses of the study
Validation study
One of the main limitations of this study was that it has not always been possible to make a straightforward comparison between the accuracy of the prognostic tools and the accuracy of clinicians’ predictions, nor to directly compare the prognostic accuracy of different tools. Where head-to-head comparisons have been possible, they have been undertaken and have shown that the PiPS tools (both PiPS-A and PiPS-B) show greater discrimination than FPN or PPI and that the PPI is less accurate than clinicians’ predictions. However, it has not been possible to directly compare the performance of the PaP or the PPS to either the PiPS models or AMPESs. It is therefore not possible to make a recommendation about which of these tools would be best to use in clinical practice.
Another potential limitation of this research is that a number of the assessments required for the calculation of prognostic scores were rather subjective in nature or were challenging to obtain. For instance, the calculation of the FPN requires an estimate of the TTD, which is defined as the time elapsing between diagnosis and the point at which the disease becomes ‘terminal’. This was not always easy or straightforward to obtain. As another example, both PiPS-A and PiPS-B require clinicians to score the patient’s general health on a 7-point scale and this requires an element of subjective judgement.
It is important for cohort studies to have a clear inception point. In PiPS2 the inception point was recent referral to palliative care services. However, the nature of palliative care practice is such that the type of patients referred to each service can vary from place to place according to local practice and admission guidelines. Patients in our study are therefore likely to have been referred to palliative care services at different stages in their disease trajectory. Moreover, ‘referral to palliative care services’ as an inception point may not be easily transferable to the population of patients seen in other countries. Nonetheless, all of the patients included in the study had advanced, incurable cancer and were thus clearly recognisable as a defined population.
It should be noted that PiPS2 was exclusively concerned with determining the prognosis of patients with advanced cancer. This is because the primary aim of the study was to validate the PiPS-B prognostic tool, which was developed solely as a cancer prognosis tool. There is an increasing recognition of the need to widen the access to palliative care services to more patients with non-malignant disease. Moreover, some authors have described cancer as presenting with a more predictable trajectory than other diseases, with ‘a short period of evident decline’. 60,61 This suggests that there may be a greater need to develop and validate prognostic tools in non-malignant palliative care populations. Nonetheless, it remains the case that cancer patients currently make up the majority of palliative care referrals.
Qualitative substudy
One potential limitation was that the Manchester-based research fellow (KS) recruited patients to both the quantitative and qualitative studies and conducted the qualitative interviews. It is possible that when patients were being asked about the PiPS tools there was risk of reporting overly positive experiences. However, methodologically (and ethically), it was appropriate for KS to recruit to the nested study because we were purposively sampling according to certain characteristics. In terms of ethics, the patients were very unwell and we needed to recruit as sensitively as possible. In addition, although KS was part of the research team for PiPS2, she was not involved in the development of PiPS and had no vested interest in a positive or negative response from patients.
Relatively few views were represented from patients who did not want to participate in the PiPS2 quantitative study. A small number of patients who took part in the interview study (n = 5) had declined to take part in the larger clinical study. The reason that they were not recruited to the validation study was because they did not want to give a blood sample. However, these patients were equally positive about the PiPS tool. Another small number (n = 4) of patients declined to take part in both the clinical study and the nested qualitative interview study. Reasons given were that they were in too much pain or discomfort to take part in either study.
The number of community patients interviewed was small (n = 4), so findings from these patients should be interpreted with caution. It appears that their views were slightly different from the other participants’ because they were generally at an earlier stage in the disease trajectory and not ready to think about how long they had left to live.
Relation to other research
Comparison with PiPS1
There were some differences between the patient populations included in PiPS111 and PiPS2. In PiPS1, the median survival of participants was 34 days, and in PiPS2 it was 45 days. There are several possible reasons why the population in PiPS2 had a longer survival than that in PiPS1. It is possible, for instance, that referral criteria to palliative care services have changed between the two studies and that patients are being referred earlier in their disease trajectories. Another explanation may be that the types of patients included in the two studies were different. In PiPS1, the proportion of patients recruited from IPCUs, HPCTs and community settings was 69%, 18%, and 12%, respectively. 62 The corresponding figures for PiPS2 were 68%, 7% and 25%, respectively. Thus, a greater proportion of participants in PiPS2 were recruited from community settings and a smaller proportion from HPCTs. As shown in Figure 3, the survival of patients in each setting was different, with those recruited from IPCUs (hospices) having the worst prognosis and those recruited from the community having the best prognosis. In PiPS1, none of the patients were receiving chemotherapy, whereas in PiPS2 10.4% were doing so. It is increasingly the case that patients under the care of palliative care services receive simultaneous cancer therapies (chemotherapy, radiotherapy, endocrine treatments, immunotherapies and biological therapies). For this reason, we did not exclude patients who were receiving palliative cancer treatments from PiPS2. In a subgroup analysis in a previous study, Baba et al. 63 reported that the PiPS prognostic models performed just as well in patients who were or were not receiving palliative cancer therapies.
It has been hypothesised that it is easier to predict survival in patients who are closer to death. By analogy with weather forecasting, this phenomenon is known as the ‘horizon effect’. 64 Just as it is easier to predict that it will rain in the afternoon when one can see storm clouds on the horizon in the morning, and more difficult to predict the weather 1 month in advance, so it is hypothesised that it is easier to predict survival when someone is going to die imminently than when they are expected to live longer. Therefore, one might have expected that the longer survival of patients in PiPS2 would have made them a more difficult population in which to prognosticate than that of PiPS1. In fact, in PiPS1, the AMPESs were accurate on 57.5% of occasions, whereas in PiPS2 their accuracy was 61.9%. The reasons for the superior prognostic accuracy in PiPS2 is not clear; it perhaps represents an improvement in clinical prognostic skills between the two studies. It is noteworthy that the overall accuracy of the PiPS-B risk categories was unchanged between the two studies, at 61.5% in PiPS1 and 61.9% in PiPS2.
The primary tool of interest in PiPS2 was PiPS-B, which consists of two prognostic models (PiPS-B14 and PiPS-B56) combined by a decision rule to create three prognostic risk categories (days, weeks and months+). In the original study by Gwilliam et al. ,11 the PiPS-B models and decision rule together were found to be slightly more accurate than a clinician’s or a nurse’s estimate of survival (but not better than an AMPES). In PiPS2, the PiPS-B14 and PiPS-B56 algorithms performed well individually but the PiPS-B risk categories (created using the decision rule) performed less well. It is possible that the performance of the PiPS-B may be improved by recalibration and/or adjustment of the decision rule.
Relation to other studies
PiPS
Since PiPS1 was published,11 Baba et al. 63 have undertaken an independent validation of the PiPS models in 2426 Japanese cancer patients in palliative care units, community services and hospitals, including patients receiving palliative chemotherapy. They had data for 2212 patients who completed PiPS-A and for 1257 patients who completed PiPS-B. They reported that the PiPS instruments performed as well as in PiPS1. 11 However, the Japanese study did not compare the accuracy of PiPS risk categories with the accuracy of CPS. In a subsequent subanalysis, the same group also reported that PiPS-A was feasible to use in palliative care populations because the variables required for its calculation were routinely available in 91–96% of patients, depending on setting,65 whereas the data required to calculate PiPS-B were only routinely available in 29–65% of patients dependent on setting, with the lowest availability being in the community, where fewer patients have blood tests. In a secondary analysis using the same data, the researchers developed an alternative prognostic tool that did not rely on the presence or absence of symptoms, which the authors regarded as being ‘too subjective’ to include in a prognostic score. 66 They termed their newly developed tool the Objective Prognostic Indicator for Advanced Cancer (OPI-AC) and reported that PiPS-B was better than the OPI-AC for predicting 14-day survival (AUC 0.86 vs. 0.81, respectively) but worse than the OPI-AC at predicting 56-day survival (AUC 0.83 vs. 0.90, respectively). Kim et al. 67 conducted a prospective study in 202 advanced cancer patients admitted to a Republic of Korea cancer centre and reported that the PiPS instruments performed approximately as well as in PiPS1, and confirmed that the PiPS risk categories were better than a clinician’s estimate of survival.
Palliative Prognostic score
The PaP score has previously been independently validated by numerous groups23–26 and the proportion of patients surviving in each of the three prognostic categories is usually found to fall within the specified boundaries (< 30% probability of surviving 30 days, 30–70% probability of surviving 30 days and > 70% probability of surviving 30 days). Some studies have intended to compare the accuracy of the PaP with the accuracy of clinicians’ estimates of survival. 24,27,68–73 However, there are methodological challenges to doing this because the PaP score does not make a specific prediction about whether a patient will or will not survive for 30 days. It is therefore not possible to compare directly the prediction made by PaP with that made by a clinician. To illustrate the problem, consider what it means for the PaP score to place someone in risk group B (30–70% probability of surviving 30 days). How could one judge whether or not such a prediction was correct or incorrect? It could be compatible with either outcome (died or survived for 30 days). How informative, therefore, would this information be to clinicians?
These methodological difficulties are illustrated in a study by Tavares et al. 73 These authors attempted to compare the accuracy of the PaP with the accuracy of clinician predictions. They did this by categorising predictions as ‘adequate’, ‘optimistic’ or ‘pessimistic’. For the CPS, this was relatively straightforward (CPS was ‘adequate’ if it was within 1 week of actual survival). However, for the PaP predictions, it was not straightforward to determine whether or not the ‘prediction’ made by PaP was accurate or inaccurate. The authors decided that, if patients in the best risk group survived > 21 days, then the PaP estimate was adequate; if the patients in the intermediate risk group survived 21–35 days, then the PaP estimate was adequate; and, if the patients in the worst risk group survived for 0 to 35 days, then the PaP estimate was adequate. On this basis, the authors concluded that PaP estimates were adequate on 47% of occasions compared with adequate predictions by junior doctors on 10.5% of occasions and by palliative care experts on 23.7% of occasions. However, the median survival of the patients in this study was 24 days and, according to the authors’ definitions of ‘acceptable’ predictions, the PaP would provide an adequate prediction for any patient surviving between 22 and 35 days whichever prognostic category they were placed in.
To get around this problem, most authors have evaluated the performance of the PaP score (which can range between 0 and 17.5) as a continuous prognostic variable and have compared it with the temporal survival predictions made by clinicians. 24,27,68,69 Using this scoring method, a higher PaP score is simply regarded as representing a worse prognosis and the three prognostic risk groups (< 30% probability of surviving 30 days, 30–70% probability of surviving 30 days and > 70% probability of surviving 30 days) as described in the original development study are ignored. 22 Using this approach, Hui et al. 27 studied 222 cancer patients and reported that the c-index for the PaP was 0.64 (95% CI 0.54 to 0.74) and that this was significantly better (p < 0.0001) than the c-index for the CPS [0.56 (95% CI 0.46 to 0.66)]. Ermacora68 studied 334 advanced cancer patients and reported that the AUC of the PaP was 0.82 (95% CI 0.77 to 0.86) and that this was higher than the AUC reported for two physicians [physician 1, AUC 0.77 (95% CI 0.72–0.81); physician 2, AUC 0.76 (95% CI 0.71–0.81)] and one nurse [AUC 0.78 (95% CI 0.72–0.82)].
Palliative Prognostic Index
Palliative Prognostic Index scores can vary between 0 and 15. If patients score ≤ 4 they are predicted to survive for > 6 weeks. If the PPI score is > 6 then the patient is predicted to survive < 3 weeks. Therefore, it ought to be relatively straightforward to compare the accuracy of PPI predictions with CPS at 3 or 6 weeks. In PiPS2, we found that there was a statistically significant (p < 0.001) difference between the proportion of overall patient deaths predicted correctly by PPI and the corresponding proportion predicted correctly by clinicians (see Table 30). The doctors were better than the PPI model at correctly identifying the prognosis. However, none of the other published studies has directly compared the accuracy of the PPI and clinicians in this manner. 68,69,72–74 Studies have reported on the performance of the PPI for predicting survival at 3 and 6 weeks (which the PPI is designed to predict)68 but also its performance at predicting 30-day,69,74 100-day74 or 90-day survival. 73 One study reported the accuracy of the PPI and the accuracy of CPS using the AUC,68 one used the c-index69 and two studies used a correlation coefficient. 72,73 Stiel et al. 72 reported that the Spearman’s rank-order correlation between PPI and actual survival was –0.678, whereas the correlation between the clinicians’ predictions and actual survival was −0.556.
Feliu Prognostic Nomogram
The FPN uses five variables (i.e. ECOG performance status, serum albumin concentration, LDH concentration, lymphocyte count and TTD) to predict probability of survival at 15, 30 and 60 days. 18 Since its publication, the FPN has not been validated by any independent groups. Because the FPN does not make a temporal prediction of survival, it is difficult to directly compare its accuracy with that of clinicians’ predictions. In Feliu et al. ’s18 original report, the c-index for the FPN was 0.70 and it was well calibrated. In PiPS2, we found that the discrimination of the FPN was similar [c-index 0.684, 95% CI 0.669 to 0.700] and confirmed that it was well calibrated.
Palliative Performance Scale
The PPS was not specifically designed to be used as a prognostic tool. However, in a large (n = 6066) retrospective analysis20 of referrals to a Canadian hospice service, the PPS was found to discriminate between groups with different survival prospects. Lau et al. 20 reported that the median survival of patients with a PPS of 10% was 1 day, with PPS 20% was 2 days, with PPS 30% was 5 days, with PPS 40% was 13 days, with PPS 50% was 28 days, with PPS 60% was 43 days and with PPS 70% was 63 days. In PiPS2 we similarly found that the PPS was able to discriminate between groups with different survival prospects, although the median survival for each PPS group was different in our population (see Table 46). Because the PPS does not make specific survival predictions, it was not possible to directly compare its performance with that of clinicians.
Chapter 5 Conclusions
Implications for health care
Our study has shown that, for patients with advanced cancer newly referred to palliative care services in the UK, the PiPS-B risk categories are as accurate as an AMPES. There are reasons to believe that the PiPS-B may be a valuable addition to clinical practice. Clinicians have said that they would value a prognostic tool even if it were no better than a CPS because it would be more objective and reproducible and could be used as an educational, training and communication aid. Clinicians find that prognosticating is an uncomfortable task and they are more likely to avoid discussing time scales, instead choosing to give only vague estimates or avoiding the issue altogether.
However, we believe that, on the basis of the evidence, it would be premature to recommend the routine use of PiPS-B in palliative care clinical practice. Further work is needed to assess the impact of introducing such a prognostic tool on the outcomes for patients and their families. Moreover, although PiPS-B shows promising characteristics (in terms of discrimination), it may be in need of recalibration and may benefit from a modification in the existing decision rule that is used to categorise patients into the three prognostic categories.
Our study provides good evidence that PPI is significantly worse than clinicians’ predictions of survival and should not be recommended for use in routine clinical practice. The PiPS-A risk categories (created using the existing decision rule) were also significantly worse than an AMPES and should not be used in clinical practice in their current form. Our study also shows that, in this population, the FPN has poorer discrimination than PiPS-A or PiPS-B.
Unanswered questions and further research
Further research is needed to determine whether or not the routine use of prognostic tools can improve outcomes for palliative care patients. This will probably require a large, multicentre randomised controlled trial comparing usual practice with the use of one or more validated prognostic tool. One of the difficulties with the design of such a study will be determining which clinical outcomes are the most likely to be affected by better prognostication and identifying suitable instruments with which to reliably measure the desired outcomes.
Further exploratory analyses are required to optimise the performance of the PiPS tools, by adjustment of the decision rules, recalibration or a combination of the two. It may also be possible to use the PiPS2 data set (possibly combined with the PiPS1 data and other existing data sets) to create a new prognostic model or to adjust the PiPS-B model to produce incremental improvements in performance.
The AMPESs in PiPS2 were more accurate than those in PiPS1. The predictions in both studies were made by specialist palliative care clinicians and nurses. It is possible that in other clinical settings (e.g. primary care or acute oncology) or among other practitioners (e.g. junior doctors or nurses) the CPS may be less accurate. In those circumstances, PiPS-B may have a greater role as an aid to prognostication. Our qualitative work also suggests that PiPS-B may have a role to play in educating less experienced staff in how to prognosticate and in improving prognostic communication between clinicians and patients.
Acknowledgements
We would like to thank NIHR for providing funding for this research through the HTA programme. We would also like to thank the UCL PRIMENT™ Clinical Trials Unit for its support, Karolina Christodoulides and Jane Harrington for their help with administrative tasks and data-monitoring and Florence Todd-Fordham for contributing to data quality control procedures. We would like to thank all the patients, carers and clinicians who participated in this study and our collaborators across participating sites. Thanks are also owed to our Study Steering Committee members: Professor Miriam Johnson (chairperson), Susan Charman (statistician) and Dr Angela McCullagh (public representative). This work was supported by Marie Curie and the NIHR British Research Council.
Contributions of authors
Patrick Stone (https://orcid.org/0000-0002-5765-9047) (Professor, Palliative Medicine) designed the study and obtained funding. He contributed to analysis and interpretation of the results and drafted, reviewed and approved the manuscript. He is the guarantor of the study.
Anastasia Kalpakidou (https://orcid.org/0000-0001-9453-9717) (Research Associate) was the study manager and was responsible for day-to-day running of the study and data quality control. She contributed to analysis and interpretation of the results and reviewed and approved the manuscript.
Chris Todd (https://orcid.org/0000-0001-6645-4505) (Professor, Primary Care and Community Health) designed the study and obtained funding. He contributed to analysis and interpretation of the results and reviewed and approved the manuscript.
Jane Griffiths (https://orcid.org/0000-0002-6674-6361) (Lecturer, Nursing) designed the study and obtained funding. She contributed to analysis and interpretation of the results and reviewed and approved the manuscript.
Vaughan Keeley (https://orcid.org/0000-0002-3004-2821) (Professor, Palliative Medicine) designed the study and obtained funding. He contributed to analysis and interpretation of the results and reviewed and approved the manuscript.
Karen Spencer (https://orcid.org/0000-0003-2038-2600) (Research Associate, Nursing) contributed to the design and analysis of the qualitative study. She contributed to analysis and interpretation of the results and reviewed and approved the manuscript.
Peter Buckle (https://orcid.org/0000-0002-3985-2441) (Public Representative, bereaved relative) provided a service users’ perspective and contributed to the design of PISs and oversight of the study. He contributed to analysis and interpretation of the results and reviewed and approved the manuscript.
Dori-Anne Finlay (https://orcid.org/0000-0001-7979-8251) (Public Representative, bereaved relative) provided a service user’s perspective and contributed to the design of PISs and oversight of the study. She contributed to analysis and interpretation of the results and reviewed and approved the manuscript.
Victoria Vickerstaff (https://orcid.org/0000-0002-3119-670X) (Research Associate, Statistician) performed the statistical analyses. She contributed to analysis and interpretation of the results and reviewed and approved the manuscript.
Rumana Z Omar (https://orcid.org/0000-0003-1483-1932) (Professor, Statistics) designed the study and obtained funding. She performed the statistical analyses, contributed to analysis and interpretation of the results and reviewed and approved the manuscript.
Publications
Kalpakidou AK, Todd C, Keeley V, Griffiths J, Spencer K, Vickerstaff V, et al. The Prognosis in Palliative care Study II (PiPS2): study protocol for a multi-centre, prospective, observational, cohort study. BMC Palliat Care 2018;17:101.
Stone PC, Kalpakidou A, Todd C, Griffiths J, Keeley V, Spencer K, et al. The Prognosis in Palliative care Study II (PiPS2): a prospective observational validation study of a prognostic tool with an embedded qualitative evaluation. PLOS ONE 2021;16:e0249297.
Stone P, Vickerstaff V, Kalpakidou A, Todd C, Griffiths J, Keeley V, et al. Prognostic tools or clinical predictions: Which are better in palliative care? PLOS ONE 2021;16:e0249763.
Kalpakidou A, Todd C, Omar RZ, Keeley V, Griffiths J, Spencer K, et al. Study recruitment factors in advanced cancer: the Prognosis in Palliative care Study II (PiPS2) – a multicentre, prospective, observational cohort project [published online ahead of print May 5 2021]. BMJ Support Palliat Care 2021.
Data-sharing statement
All available data from the validation study can be obtained by contacting the corresponding author. The data generated by the nested qualitative substudy are not suitable for sharing beyond that contained in the report owing to the qualitative nature of the research. Further information can be obtained from the corresponding author on request.
Patient data
This work uses data provided by patients and collected by the NHS as part of their care and support. Using patient data is vital to improve health and care for everyone. There is huge potential to make better use of information from people’s patient records, to understand more about disease, develop new treatments, monitor safety, and plan NHS services. Patient data should be kept safe and secure, to protect everyone’s privacy, and it’s important that there are safeguards to make sure that it is stored and used responsibly. Everyone should be able to find out about how patient data are used. #datasaveslives You can find out more about the background to this citation here: https://understandingpatientdata.org.uk/data-citation.
Disclaimers
This report presents independent research funded by the National Institute for Health Research (NIHR). The views and opinions expressed by authors in this publication are those of the authors and do not necessarily reflect those of the NHS, the NIHR, NETSCC, the HTA programme or the Department of Health and Social Care. If there are verbatim quotations included in this publication the views and opinions expressed by the interviewees are those of the interviewees and do not necessarily reflect those of the authors, those of the NHS, the NIHR, NETSCC, the HTA programme or the Department of Health and Social Care.
References
- Adams E, Boulton M, Watson E. The information needs of partners and family members of cancer patients: a systematic literature review. Patient Educ Couns 2009;77:179-86. https://doi.org/10.1016/j.pec.2009.03.027.
- Degner LF, Kristjanson LJ, Bowman D, Sloan JA, Carriere KC, O’Neil J, et al. Information needs and decisional preferences in women with breast cancer. JAMA 1997;277:1485-92. https://doi.org/10.1001/jama.1997.03540420081039.
- Steinhauser KE, Christakis NA, Clipp EC, McNeilly M, Grambow S, Parker J, et al. Preparing for the end of life: preferences of patients, families, physicians, and other care providers. J Pain Symptom Manage 2001;22:727-37. https://doi.org/10.1016/S0885-3924(01)00334-7.
- Kirk P, Kirk I, Kristjanson LJ. What do patients receiving palliative care for cancer and their families want to be told? A Canadian and Australian qualitative study. BMJ 2004;328. https://doi.org/10.1136/bmj.38103.423576.55.
- Kutner JS, Steiner JF, Corbett KK, Jahnigen DW, Barton PL. Information needs in terminal illness. Soc Sci Med 1999;48:1341-52. https://doi.org/10.1016/S0277-9536(98)00453-5.
- Glare P, Virik K, Jones M, Hudson M, Eychmuller S, Simes J, et al. A systematic review of physicians’ survival predictions in terminally ill cancer patients. BMJ 2003;327:195-8. https://doi.org/10.1136/bmj.327.7408.195.
- Neuberger J, Guthrie C, Aaronovitch D, Hameed K, Bonser T, Pentregarh H, et al. More Care, Less Pathway: A Review of the Liverpool Care Pathway. London: Department of Health and Social Care; 2013.
- Ellershaw J, Foster A, Murphy D, Shea T, Overill S. Developing an integrated care pathway for the dying patient. Eur J Palliati Care 1997;4:203-7.
- Department of Health and Social Care (DHSC) . End of Life Care Strategy – Promoting High Quality Care for All Adults at the End of Life 2008. https://assets.publishing.service.gov.uk/government/uploads/system/uploads/attachment_data/file/136431/End_of_life_strategy.pdf (accessed 28 July 2020).
- Thomas K. Gold Standards Framework Prognostic Indicator Guidance (PIG) n.d. www.goldstandardsframework.org.uk/PIG (accessed 28 January 2020).
- Gwilliam B, Keeley V, Todd C, Gittins M, Roberts C, Kelly L, et al. Development of prognosis in palliative care study (PiPS) predictor models to improve prognostication in advanced cancer: prospective cohort study. BMJ 2011;343. https://doi.org/10.1136/bmj.d4920.
- National Institute for Health Research (NIHR) . Prognostic Models for People With Advanced Cancer 2013. https://fundingawards.nihr.ac.uk/award/13/20/01? (accessed August 2020).
- Stone PC, Lund S. Predicting prognosis in patients with advanced cancer. Ann Oncol 2007;18:971-6. https://doi.org/10.1093/annonc/mdl343.
- Lund S, Stone P. Predicting survival in advanced cancer patients. Eur J Pall Care 2013;20:58-61.
- Morita T, Tsunoda J, Inoue S, Chihara S. The Palliative Prognostic Index: a scoring system for survival prediction of terminally ill cancer patients. Support Care Cancer 1999;7:128-33. https://doi.org/10.1007/s005200050242.
- Anderson F, Downing GM, Hill J, Casorso L, Lerch N. Palliative Performance Scale (PPS): a new tool. J Palliat Care 1996;12:5-11. https://doi.org/10.1177/082585979601200102.
- Pirovano M, Maltoni M, Nanni O, Marinari M, Indelli M, Zaninetta G, et al. A new palliative prognostic score: a first step for the staging of terminally ill cancer patients. Italian Multicenter and Study Group on Palliative Care. J Pain Symptom Manage 1999;17:231-9. https://doi.org/10.1016/S0885-3924(98)00145-6.
- Feliu J, Jiménez-Gordo AM, Madero R, Rodríguez-Aizcorbe JR, Espinosa E, Castro J, et al. Development and validation of a prognostic nomogram for terminally ill cancer patients. J Natl Cancer Inst 2011;103:1613-20. https://doi.org/10.1093/jnci/djr388.
- Stone CA, Tiernan E, Dooley BA. Prospective validation of the palliative prognostic index in patients with cancer. J Pain Symptom Manage 2008;35:617-22. https://doi.org/10.1016/j.jpainsymman.2007.07.006.
- Lau F, Downing M, Lesperance M, Karlson N, Kuziemsky C, Yang J. Using the Palliative Performance Scale to provide meaningful survival estimates. J Pain Symptom Manage 2009;38:134-44. https://doi.org/10.1016/j.jpainsymman.2008.05.017.
- Olajide O, Hanson L, Usher BM, Qaqish BF, Schwartz R, Bernard S. Validation of the palliative performance scale in the acute tertiary care hospital setting. J Palliat Med 2007;10:111-17. https://doi.org/10.1089/jpm.2006.0125.
- Maltoni M, Nanni O, Pirovano M, Scarpi E, Indelli M, Martini C, et al. Successful validation of the palliative prognostic score in terminally ill cancer patients. Italian Multicenter Study Group on Palliative Care. J Pain Symptom Manage 1999;17:240-7. https://doi.org/10.1016/S0885-3924(98)00146-8.
- Glare P, Virik K. Independent prospective validation of the PaP score in terminally ill patients referred to a hospital-based palliative medicine consultation service. J Pain Symptom Manage 2001;22:891-8. https://doi.org/10.1016/S0885-3924(01)00341-4.
- Glare PA, Eychmueller S, McMahon P. Diagnostic accuracy of the palliative prognostic score in hospitalized patients with advanced cancer. J Clin Oncol 2004;22:4823-8. https://doi.org/10.1200/JCO.2004.12.056.
- Naylor C, Cerqueira L, Costa-Paiva LH, Costa JV, Conde DM, Pinto-Neto AM. Survival of women with cancer in palliative care: use of the palliative prognostic score in a population of Brazilian women. J Pain Symptom Manage 2010;39:69-75. https://doi.org/10.1016/j.jpainsymman.2009.05.020.
- Tarumi Y, Watanabe SM, Lau F, Yang J, Quan H, Sawchuk L, et al. Evaluation of the Palliative Prognostic Score (PaP) and routinely collected clinical data in prognostication of survival for patients referred to a palliative care consultation service in an acute care hospital. J Pain Symptom Manage 2011;42:419-31. https://doi.org/10.1016/j.jpainsymman.2010.12.013.
- Hui D, Park M, Liu D, Paiva CE, Suh SY, Morita T, et al. Clinician prediction of survival versus the Palliative Prognostic Score: which approach is more accurate?. Eur J Cancer 2016;64:89-95. https://doi.org/10.1016/j.ejca.2016.05.009.
- Gwilliam B, Keeley V, Todd C, Roberts C, Gittens M, Kelly L, et al. Prognosticating in patients with advanced cancer – observational study comparing the accuracy of clinicians’ and patients’ estimates of survival. Ann Oncol 2013;24:482-8. https://doi.org/10.1093/annonc/mds341.
- Kalpakidou AK, Todd C, Keeley V, Griffiths J, Spencer K, Vickerstaff V, et al. The Prognosis in Palliative care Study II (PiPS2): study protocol for a multi-centre, prospective, observational, cohort study. BMC Palliat Care 2018;17. https://doi.org/10.1186/s12904-018-0352-y.
- Craig P, Dieppe P, Macintyre S, Michie S, Nazareth I, Petticrew M. Medical Research Council Guidance . Developing and evaluating complex interventions: the new Medical Research Council guidance. BMJ 2008;337. https://doi.org/10.1136/bmj.a1655.
- Department of Health and Social Care (DHSC) . Mental Capacity Act 2005.
- Royal College of General Practitioners . Mental Capacity Act Toolkit for Adults in England and Wales 2011. www.rcgp.org.uk/-/media/Files/CIRC/Learning-disabilities/Learning-disabilities-resources/CIRC_Mental-Capacity-Act-Toolkit-2011.ashx?la=en (accessed 20 January 2020).
- Hodkinson HM. Evaluation of a mental test score for assessment of mental impairment in the elderly. Age Ageing 1972;1:233-8. https://doi.org/10.1093/ageing/1.4.233.
- Oken MM, Creech RH, Tormey DC, Horton J, Davis TE, McFadden ET, et al. Toxicity and response criteria of the Eastern Cooperative Oncology Group. Am J Clin Oncol 1982;5:649-55. https://doi.org/10.1097/00000421-198212000-00014.
- Karnofsky D, Burchenal J, MacLeod CM. Evaluation of Chemotherapeutic Agents. New York, NY: Columbia University Press; 1949.
- Machin D, Campbell MJ, Tan SB, Tan SH. Sample Size Tables for Clinical Studies. Oxford: Wiley-Blackwell; 2009.
- Harrell F. Regression Modelling Strategies with Applications to Linear Models, Logistic Regression and Survival Analysis. New York, NY: Springer Publishing; 2002.
- Adams G, Gulliford MC, Ukoumunne OC, Eldridge S, Chinn S, Campbell MJ. Patterns of intra-cluster correlation from primary care research to inform study design and analysis. J Clin Epidemiol 2004;57:785-94. https://doi.org/10.1016/j.jclinepi.2003.12.013.
- Alba AC, Agoritsas T, Walsh M, Hanna S, Iorio A, Devereaux PJ, et al. Discrimination and calibration of clinical prediction models: users’ guides to the medical literature. JAMA 2017;318:1377-84. https://doi.org/10.1001/jama.2017.12126.
- Miller ME, Langefeld CD, Tierney WM, Hui SL, McDonald CJ. Validation of probabilistic predictions. Med Decis Making 1993;13:49-58. https://doi.org/10.1177/0272989X9301300107.
- Steyerberg EW, Vickers AJ, Cook NR, Gerds T, Gonen M, Obuchowski N, et al. Assessing the performance of prediction models: a framework for traditional and novel measures. Epidemiology 2010;21:128-38. https://doi.org/10.1097/EDE.0b013e3181c30fb2.
- Steyerberg E. Clinical Prediction Models: A Practical Approach to Development, Validation and Updating. Rotterdam: Springer Publishing; 2009.
- Bland JM, Altman DG. Multiple significance tests: the Bonferroni method. BMJ 1995;310. https://doi.org/10.1136/bmj.310.6973.170.
- White IR, Royston P, Wood AM. Multiple imputation using chained equations: issues and guidance for practice. Stat Med 2011;30:377-99. https://doi.org/10.1002/sim.4067.
- Walshe C, Todd C, Caress AL, Chew-Graham C. Judgements about fellow professionals and the management of patients receiving palliative care in primary care: a qualitative study. Br J Gen Pract 2008;58:264-72. https://doi.org/10.3399/bjgp08X279652.
- McNamara B. Fragile Lives: Death, Dying and Care. Sydney, NSW: Allen & Unwin; 2001.
- Chapple A, Ziebland S. Prostate cancer: embodied experience and perceptions of masculinity. Sociol Health Illn 2002;24:820-41. https://doi.org/10.1111/1467-9566.00320.
- Sinding C, Wiernikowski J. Disruption foreclosed: older women’s cancer narratives. Health 2008;12:389-411. https://doi.org/10.1177/1363459308090055.
- Bury M. Chronic illness as biographical disruption. Sociol Health Illn 1982;4:167-82. https://doi.org/10.1111/1467-9566.ep11339939.
- Higginson IJ, Evans CJ, Grande G, Preston N, Morgan M, McCrone P, et al. Evaluating complex interventions in end of life care: the MORECare statement on good practice generated by a synthesis of transparent expert consultations and systematic reviews. BMC Med 2013;11. https://doi.org/10.1186/1741-7015-11-111.
- Ritchie J, Lewis J, Ritchie J, Lewis J, Elam G. Qualitative Research Practice: A Guide for Social Science Students and Researchers. Newbury Park, CA: SAGE Publications Ltd; 2003.
- Srivastava A, Thompson S. Framework analysis: a qualitative methodology for applied policy research. J Admin Govern 2009;4:72-9.
- Bernard H, Ryan G. Analysing Qualitative Data; Systematic Approaches. Newbury Park, CA: SAGE Publications Ltd; 2010.
- Ritchie J, Spencer L, Bryman A, Burgess R. Analysing Qualitative Data. Oxon: Routledge; 1994.
- Hosmer D, Lemeshow S, Sturdivant R. Applied Logistic Regression. New York, NY: John Wiley & Sons; 2013.
- Stone PC, Kalpakidou A, Todd C, Griffiths J, Keeley V, Spencer K, et al. The Prognosis in Palliative care Study II (PiPS2): a prospective observational validation study of a prognostic tool with an embedded qualitative evaluation. PLOS ONE 2021;16. https://doi.org/10.1371/journal.pone.0249297.
- Rubin DB. Multiple Imputation for Nonresponse in Surveys. New York, NY: John Wiley & Sons; 1987.
- Li K-H, Meng X-L, Raghunathan TE, Rubin DB. Significance levels from repeated p-values with multiply-imputed data. Statistica Sinica 1991;1:65-92.
- Stone P, Vickerstaff V, Kalpakidou A, Todd C, Griffiths J, Keeley V, et al. Prognostic tools or clinical predictions: Which are better in palliative care?. PLOS ONE 2021;16. https://doi.org/10.1371/journal.pone.0249763.
- Murray S, Kendall M, Boyd K, Sheikh A. Illness trajectories and palliative care. BMJ 2005;330:1007-11. https://doi.org/10.1136/bmj.330.7498.1007.
- Seow H, O’Leary E, Perez R, Tanuseputro P. Access to palliative care by disease trajectory: a population-based cohort of Ontario decedents. BMJ Open 2018;8. https://doi.org/10.1136/bmjopen-2017-021147.
- Stone P, Gwilliam B, Keeley V, Todd C, Kelly L, Barclay S. Factors affecting recruitment to an observational multicentre palliative care study. BMJ Support Palliat Care 2013;3:318-23. https://doi.org/10.1136/bmjspcare-2012-000396.
- Baba M, Maeda I, Morita T, Hisanaga T, Ishihara T, Iwashita T, et al. Independent validation of the modified prognosis palliative care study predictor models in three palliative care settings. J Pain Symptom Manage 2015;49:853-60. https://doi.org/10.1016/j.jpainsymman.2014.10.010.
- Chow E, Harth T, Hruby G, Finkelstein J, Wu J, Danjoux C. How accurate are physicians’ clinical predictions of survival and the available prognostic tools in estimating survival times in terminally ill cancer patients? A systematic review. Clin Oncol (R Coll Radiol) 2001;13:209-18. https://doi.org/10.1007/s001740170078.
- Baba M, Maeda I, Morita T, Inoue S, Ikenaga M, Matsumoto Y, et al. Survival prediction for advanced cancer patients in the real world: a comparison of the Palliative Prognostic Score, Delirium-Palliative Prognostic Score, Palliative Prognostic Index and modified Prognosis in Palliative Care Study predictor model. Eur J Cancer 2015;51:1618-29. https://doi.org/10.1016/j.ejca.2015.04.025.
- Hamano J, Takeuchi A, Yamaguchi T, Baba M, Imai K, Ikenaga M, et al. A combination of routine laboratory findings and vital signs can predict survival of advanced cancer patients without physician evaluation: a fractional polynomial model. Eur J Cancer 2018;105:50-6. https://doi.org/10.1016/j.ejca.2018.09.037.
- Kim ES, Lee JK, Kim MH, Noh HM, Jin YH. Validation of the prognosis in palliative care study predictor models in terminal cancer patients. Korean J Fam Med 2014;35:283-94. https://doi.org/10.4082/kjfm.2014.35.6.283.
- Ermacora P, Mazzer M, Isola M, Pascoletti G, Gregoraci G, Basile D, et al. Prognostic evaluation in palliative care: final results from a prospective cohort study. Support Care Cancer 2019;27:2095-102. https://doi.org/10.1007/s00520-018-4463-z.
- Maltoni M, Scarpi E, Pittureri C, Martini F, Montanari L, Amaducci E, et al. Prospective comparison of prognostic scores in palliative care cancer populations. Oncologist 2012;17:446-54. https://doi.org/10.1634/theoncologist.2011-0397.
- Mendis R, Soo WK, Zannino D, Michael N, Spruyt O. Multidisciplinary prognostication using the Palliative Prognostic Score in an Australian cancer center. Palliat Care 2015;9:7-14. https://doi.org/10.4137/PCRT.S24411.
- Numico G, Occelli M, Russi EG, Silvestris N, Pasero R, Fea E, et al. Survival prediction and frequency of anticancer treatment in cancer patients hospitalized due to acute conditions. Role of clinical parameters and PaP score. Support Care Cancer 2011;19:1823-30. https://doi.org/10.1007/s00520-010-1024-5.
- Stiel S, Bertram L, Neuhaus S, Nauck F, Ostgathe C, Elsner F, et al. Evaluation and comparison of two prognostic scores and the physicians’ estimate of survival in terminally ill patients. Support Care Cancer 2010;18:43-9. https://doi.org/10.1007/s00520-009-0628-0.
- Tavares T, Oliveira M, Gonçalves J, Trocado V, Perpétuo J, Azevedo A, et al. Predicting prognosis in patients with advanced cancer: a prospective study. Palliat Med 2018;32:413-16. https://doi.org/10.1177/0269216317705788.
- Farinholt P, Park M, Guo Y, Bruera E, Hui D. A comparison of the accuracy of clinician prediction of survival versus the Palliative Prognostic Index. J Pain Symptom Manage 2018;55:792-7. https://doi.org/10.1016/j.jpainsymman.2017.11.028.
- K Inouye SK, van Dyck CH, Alessi CA, Balkin S, Siegal AP, Horwitz RI. Clarifying confusion: the confusion assessment method. A new method for detection of delirium. Ann Intern Med 1990;113:941-8. https://doi.org/10.7326/0003-4819-113-12-941.
- Victoria Hospice Society . Palliative Performace Scale Version 2 n.d. www.victoriahospice.org/health-professionals/clinical-tools (accessed August 2020).
Appendix 1 The PiPS2 qualitative substudy interview topic guide: patients and carers/relatives
This appendix has been reproduced with permission from Stone et al. 56 This is an Open Access article distributed in accordance with the terms of the Creative Commons Attribution (CC BY 4.0) license, which permits others to distribute, remix, adapt and build upon this work, for commercial use, provided the original work is properly cited. See: http://creativecommons.org/licenses/by/4.0/. The text below includes minor additions and formatting changes to the original text.
At the start
We have approached you to ask you to share your experiences of being approached and/or involved with the Prognosis in Palliative Care Study. We would also like to ask your views about the development of a prognosis tool for palliative care patients and what you think about doctors and nurses using prognostic tools with patients.
Where necessary, prompts will be used to guide the discussion.
Sample questions
-
I wondered if I could start by asking you a little about your illness/the illness of your relative/the person you are caring for?
-
Can you tell me about some of the reasons why you or your relative or the person you care for decided to or not to take part in the prognostic study?
-
Was the decision made following discussion with others around you/them?
-
What opinions do you have about the development of a tool/indicator that can estimate the life expectancy of palliative care patients?
-
If we could develop a method/tool that is similar or better than a clinician’s prediction of life expectancy, would you want it?
-
Should health professionals check what prognostic information patients/carers desire before initiating prognostic conversations? (Respecting patients’/carers’ information wishes).
-
Do you believe that health professionals should always share prognostic information with patients to help patients/family carers make decisions and plans? Why/why not?
-
If it is no more accurate than clinicians’ predictions is it a useful way of starting the conversation with palliative patients/their carers about life expectancy?
-
What do you think is the best and most sensitive way to present prognostic information to patients and/or relatives/carers? For example: how do you think the tool/indicator should be introduced to patients/relatives/carers? Prompt: over time, in writing, via face-to-face conversations.
-
Do you think that patients/carers would prefer/find it easier to understand if the prognostic tool/indicator said: (1 – probability) the patient/person you care for has X% probability of surviving for X amount of time, (2 – length of survival – precise) the patient/person you care for is predicted to live for this long (number of days, weeks, months or years) or (3 – length of survival – vaguer) the outlook for patients like you/the person you care for is good, bad or average?
-
Do you think that patients would be more comfortable receiving prognostic information in relation to the probability of reaching a significant future event?
-
What are your opinions about the usefulness of such a tool/indicator?
-
Do you think that there are any disadvantages for patients or carers? If yes, can you tell me about what they are?
-
Do you think that most palliative care patients and their carers want to know this information?
Appendix 2 The PiPS2 qualitative substudy interview topic guide: clinicians (palliative care specialists, oncologists, general practitioners and specialist nurses)
This appendix has been reproduced with permission from Stone et al. 56 This is an Open Access article distributed in accordance with the terms of the Creative Commons Attribution (CC BY 4.0) license, which permits others to distribute, remix, adapt and build upon this work, for commercial use, provided the original work is properly cited. See: http://creativecommons.org/licenses/by/4.0/. The text below includes minor additions and formatting changes to the original text.
At the start
We have approached you to take part in this research to share your views and opinions about giving prognostic information to patients/carers as well as using prognostic tools to possibly improve the delivering of survival-length prognosis to patients/carers with advanced cancer.
-
Can you tell me about your clinical role and how long you have worked in palliative care?
-
What are your experiences of making predictions of survival length to patients, relatives or carers?
-
Do you discuss with patients/carers how accurate/inaccurate survival predictions can be?
-
How easy or difficult do you find it to predict length of survival?
-
What information do you share with patients/carers about prognosis and how do you convey it?
-
How do you feel delivering such news to relatives or carers? Prompt: uncomfortable, stressful, etc. Is there anything that makes it easier? More difficult?
-
If patients/carers don’t specifically ask about the time that they/patients have left, do you share your estimate of survival time?
-
If patients do specifically ask about the time they have left, how do you respond?
-
How accurate do you find the estimates that you make?
-
Do you/health professionals document in patients’ notes the length of time they have left?
-
Is professional guidance (e.g. standards) available for clinicians on the best way of approaching prognostic discussions?
-
Do you check and discuss with patients/carers first if they want prognostic information and what type of information they would prefer, such as the probabilities of cure, survival rates, general expected outcome of the disease?
Next, I would like to start by showing you some prognostic models/tools:
www.pips.sgul.ac.uk/pipsforma.php (online link PiPS)
www.eoc.ch/dms/site-eoc/documenti/pallclick/strumenti/I-UCP-003—PaP/I-UCP-003%20-%20PaP.pdf (online link PaP)
http://jnci.oxfordjournals.org/content/suppl/2011/10/04/djr388.DC1/S1_JNCI-10–1736-s02.pdf (online link FPN)
www.sciencedirect.com/science/article/pii/S0885392407007981 (online link PPI)
www.collaborativecurriculum.ca/en/modules/PPS/PPS-thepalliativeperformancescale-01.jsp (online link PPS)
What are your first impressions of the models/tools?
-
Clinical usefulness
-
Ease of completion
-
Interpretability of outputs
Barriers to and facilitators of clinical use
-
If the tool is no more accurate than clinicians’ predictions, is it something that you would use? Prompt: could it be a useful way of having that conversation with patients/carers?
-
If such a tool was subject to the same clinical errors in judgement, would you use it or would you just rely on your own clinical judgement?
-
How would you explain to patients the probability/statistics of survival rates?
-
If such a tool absolved you of responsibility of delivering inaccurate prognostic information to patients/carers, would that make it more acceptable for you to use?
-
Would you consider using these types of prognostic tools in day-to-day clinical practice? Prompt: why?
-
What processes and structures in your work place would help or hinder you using such tools?
Appendix 3 List of participating units and the principal investigators at each site
This appendix has been reproduced with permission from Stone et al. 56 This is an Open Access article distributed in accordance with the terms of the Creative Commons Attribution (CC BY 4.0) license, which permits others to distribute, remix, adapt and build upon this work, for commercial use, provided the original work is properly cited. See: http://creativecommons.org/licenses/by/4.0/. The text below includes minor additions and formatting changes to the original text.
The list of participating sites along with the names of the PIs are shown in Table 36.
Site (n = 28) | PI |
---|---|
Birmingham St Mary’s Hospice | Dr Christina Radcliffe |
Bronglais General Hospital | Dr Gokulkrishnan Lingesan |
Coventry & Warwickshire Partnership NHS Trust | Ms Claire Plumb |
Derby Teaching Hospitals NHS Foundation Trust | Professor Vaughan Keeley |
Douglas Macmillan Hospice | Dr Claire Hookey |
ELiPSe community palliative care team | Dr Sarah Yardley |
John Eastwood Hospice | Dr Alpna Chauhan |
King’s College London | Dr Matthew Maddocks |
St Gemma’s Hospice, Leeds Community Healthcare NHS Trust | Professor Michael Bennett |
LOROS Hospice | Professor Christina Faull |
Marie Curie West Midlands Hospice | Dr Claire Ferguson |
Norfolk Community Health and Care NHS Trust | Dr Katie Carpenter (née Soden) |
Nottingham University Hospital | Dr Andrew Wilcock |
Phyllis Tuckwell Hospice Care | Dr Joanna Vriens |
Pilgrims Hospice | Dr Stephen Cox |
Princess Alice Hospice | Dr Jennifer Todd |
Royal Surrey County Hospital NHS Foundation Trust | Dr Andrew Davies |
St Andrew’s Hospice | Dr Jason Boland |
St Ann’s Hospice (Heald Green) | Dr Ashique Ahamed |
St Ann’s Hospice (Little Hulton) | Dr Ashique Ahamed |
St Catherine’s Hospice | Dr Amanda Gregory |
St George’s University Hospitals NHS Foundation Trust | Dr Ollie Minton |
St Giles Hospice (Walsall) | Ms Katie Burbridge (née Taroni) |
St Giles Hospice (Whittington) | Ms Katie Burbridge (née Taroni) |
St Richard’s Hospice | Dr Nicola Wilderspin |
Sue Ryder Leckhampton Court Hospice | Dr Paul Perkins |
University College London Hospitala | Dr Sarah Yardley |
Worcestershire Royal Hospital | Dr Nicola Heron |
Appendix 4 Case report form used for validation study
Parts of the CRF are reproduced with permission, as detailed below.
The Record of Capacity Assessment Under the Mental Capacity Act – CURB has been reproduced with permission from the Royal College of General Practitioners (RCGP). Copyright RCGP 2011. The Confusion Assessment Method (CAM) has been reproduced with permission from the American Geriatrics Society (AGS) CoCare: HELP™ program, (AGS, 2020, personal communication). 75 Confusion Assessment Method. Copyright 2003, Hospital Elder Life Program, LLC. Not to be reproduced without permission. No responsibility is assumed by the AGS or the Hospital Elder Life Program, LLC for any injury and/or damage to persons or property arising out of the application of any of the content at help.agscocare.org.
The Palliative Performance Scale version 2 (PPSv2) has been reproduced with permission from Victoria Hospice Society. 76 Copyright Victoria Hospice Society, BC, Canada (2001) www.victoriahospice.org.
The Victoria Hospice logo has been reproduced with permission from Victoria Hospice Society (Victoria Hospice Society, 2020, personal communication).
Appendix 5 Number of participants recruited at each site
Site | Type of service | Number of participants recruited at each service (excluding withdrawals)a | Number of participants recruited at each site (excluding withdrawals)a |
---|---|---|---|
Birmingham St Mary’s Hospice | CPCT | 51 | 51 |
Bronglais General Hospital |
IPCU CPCT |
21 8 |
29 |
Coventry and Warwickshire Partnership NHS Trust | CPCT | 21 | 21 |
Derby Teaching Hospitals NHS Foundation Trust |
CPCT HPCT IPCU |
60 3 125 |
188 |
Douglas Macmillan Hospice | CPCT | 68 | 68 |
ELiPSe community palliative care team | CPCT | 28 | 28 |
John Eastwood Hospice |
CPCT IPCU |
56 98 |
154 |
King’s College London | HPCT | 42 | 42 |
St Gemma’s Hospice, Leeds Community Healthcare NHS Trust |
CPCT IPCU |
5 182 |
187 |
LOROS Hospice | IPCU | 114 | 114 |
Marie Curie West Midlands Hospice | IPCU | 83 | 83 |
Norfolk Community Health and Care NHS Trust | IPCU | 133 | 133 |
Nottingham University Hospital |
CPCT IPCU |
11 138 |
149 |
Phyllis Tuckwell Hospice Care | CPCT | 41 | 41 |
Pilgrims Hospice |
CPCT IPCU |
39 49 |
88 |
Princess Alice Hospice | CPCT | 17 | 17 |
Royal Surrey County Hospital NHS Foundation Trust |
CPCT HPCT |
19 31 |
50 |
St Andrew’s Hospice | IPCU | 10 | 10 |
St Ann’s Hospice (Heald Green) | IPCU | 76 | 76 |
St Ann’s Hospice (Little Hulton) | IPCU | 40 | 40 |
St Catherine’s Hospice | IPCU | 101 | 101 |
St George’s University Hospitals NHS Foundation Trust | HPCT | 40 | 40 |
St Giles Hospice (Walsall) | IPCU | 26 | 26 |
St Giles Hospice (Whittington) | IPCU | 6 | 6 |
St Richard’s Hospice | IPCU | 47 | 47 |
Sue Ryder Leckhampton Court Hospice | IPCU | 36 | 36 |
University College London Hospitalb | HPCT | NA | NA |
Worcestershire Royal Hospital | HPCT | 8 | 8 |
Total | 1833 | 1833 |
Appendix 6 Comparison of variables included in PiPS-A and PiPS-B
The differences between the variables included in PiPS-A and PiPS-B are summarised in Table 38.
Variable | PiPS-A | PiPS-B |
---|---|---|
ECOG performance status | ✗ | ✗ |
General health status | ✗ | ✗ |
AMTS > 3 | ✗ | ✗ |
Primary breast cancer | ✗ | |
Primary prostate cancer | ✗ | ✗ |
Distant metastases (any) | ✗ | ✗ |
Liver metastases | ✗ | |
Bone metastases | ✗ | ✗ |
Anorexia | ✗ | ✗ |
Dysphagia | ✗ | |
Dyspnoea at rest | ✗ | |
Weight loss in last month | ✗ | |
Pulse rate | ✗ | ✗ |
Fatigue | ✗ | |
Albumin concentration | ✗ | |
Alkaline phosphatase concentration | ✗ | |
Alanine transaminase concentration | ✗ | |
CRP concentration | ✗ | |
Lymphocyte count | ✗ | |
Neutrophil count | ✗ | |
Platelet count | ✗ | |
Urea concentration | ✗ | |
White blood cell count | ✗ |
Appendix 7 The PiPS model regression equations
This appendix has been reproduced with permission from Stone et al. 56 This is an Open Access article distributed in accordance with the terms of the Creative Commons Attribution (CC BY 4.0) license, which permits others to distribute, remix, adapt and build upon this work, for commercial use, provided the original work is properly cited. See: http://creativecommons.org/licenses/by/4.0/. The text below includes minor additions and formatting changes to the original text.
For both PiPS-A and PiPS-B, two separate models have been developed to predict the 2-week (14-day) and 2-month (56-day) survival of patients (thus generating three prognostic categories: < 2 weeks, 2 weeks to 2 months and > 2 months). The week and month models include different sets of predictors. For both models (weeks and months), if the predicted probability of the event exceeded 50% for a patient then the patient was classified as having the event. Otherwise, it was assumed that the patient did not have the event. Thus, if, for example, the models predicted that a patient would survive 2 weeks and that the patient would die within 2 months then the PiPS model outcome would be that the patient was predicted to die in weeks.
PiPS-A14
The PiPS-A14 log-odds are:
where amts refers to AMTS (if ≤ 3 then = 0, if > 3 then = 1), pulse refers to pulse rate, distantmets to presence of distant metastases (no = 0, yes = 1), metsliver to presence of liver metastases (no = 0, yes = 1), ecog to ECOG score, overallhealth to Global Health Score, anorexia to anorexia (no = 0, yes = 1), metsbone to presence of bone metastases (no = 0, yes = 1), dyspnoea to dyspnoea (no = 0, yes = 1) and dysphagia to dysphagia (no = 0, yes = 1).
The corresponding probability of survival for PiPS-A14 is:
PiPS-A56
The PiPS-A56 log-odds are:
where amts refers to AMTS (if ≤ 3 then = 0, if > 3 then = 1), pulse refers to pulse rate, distantmets to presence of distant metastases (no = 0, yes = 1), metsliver to presence of liver metastases (no = 0, yes = 1), ecog to ECOG score, overallhealth to Global Health Score, primarybreast to primary cancer breast (no = 0, yes = 1), mgocancer to primary cancer MGO [male genital organ cancer (prostate)] (no = 0, yes = 1) and lostweight to lost weight (no = 0, yes = 1).
The corresponding probability of survival for PiPS-A56 is:
PiPS-B14
The PiPS-B14 log-odds are:
where amts refers to AMTS (if ≤ 3 then = 0, if > 3 then = 1), pulse refers to pulse rate, distantmets to presence of distant metastases (no = 0, yes = 1), metsbone to presence of bone metastases (no = 0, yes = 1), anorexia to anorexia (no = 0, yes = 1), ecog to ECOG score, overallhealth to General Health Score, wbc to WBC (× 109/l), platelet to platelet count (× 109/l), urea to urea concentration (mmol/l), alanine to alanine aminotransferase concentration (U/l) and creactive to CRP concentration (mg/l).
The corresponding probability of survival for PiPS-B14 model is:
PiPS-B56
The PiPS-B56 log-odds are:
where pulse refers to pulse rate, wbc to WBC (× 109/l), platelet to platelet count (× 109/l), neutrophil to neutrophil count (× 109/l), lymphocyte10exp9 to lymphocyte count (× 109/l), urea to urea concentration (mmol/l), alkaline to alkaline phosphatase concentration (U/l), creactive to CRP concentration (mg/l), mgocancer to primary cancer MGO (no = 0, yes = 1), fatigue to fatigue (no = 0, yes = 1) and overallhealth to General Health Score.
The corresponding probability of survival for PiPS-B56 is:
List of abbreviations
- AMPES
- agreed multiprofessional estimate of survival
- AMTS
- Abbreviated Mental Test Score
- AUC
- area under the curve
- CI
- confidence interval
- CiL
- calibration in the large
- CPCT
- community palliative care team
- CPS
- clinician prediction of survival
- CRF
- case report form
- CRP
- C-reactive protein
- CS
- calibration slope
- ECOG
- Eastern Co-operative Oncology Group
- eCRF
- electronic case report form
- FPN
- Feliu Prognostic Nomogram
- GP
- general practitioner
- HPCT
- hospital palliative care team
- HRA
- Health Research Authority
- HTA
- Health Technology Assessment
- IPCU
- inpatient palliative care unit
- IQR
- interquartile range
- KPS
- Karnofsky Performance Scale
- LDH
- lactate dehydrogenase
- NIHR
- National Institute for Health Research
- OPI-AC
- Objective Prognostic Indicator for Advanced Cancer
- PaP
- Palliative Prognostic
- PI
- principal investigator
- PiPS
- Prognosis in Palliative care Study
- PiPS1
- Prognosis in Palliative care Study I
- PiPS2
- Prognosis in Palliative care Study II
- PiPS-A
- Prognosis in Palliative care Study – All
- PiPS-A14
- Prognosis in Palliative care Study – All 14-day model
- PiPS-A56
- Prognosis in Palliative care Study – All 56-day model
- PiPS-B
- Prognosis in Palliative care Study – Blood
- PiPS-B14
- Prognosis in Palliative care Study – Blood 14-day model
- PiPS-B56
- Prognosis in Palliative care Study – Blood 56-day model
- PIS
- patient information sheet
- PPI
- Palliative Prognostic Index
- PPS
- Palliative Performance Scale
- REC
- Research Ethics Committee
- SD
- standard deviation
- SE
- standard error
- TTD
- time to terminal disease
- UCL
- University College London