Notes
Article history
The research reported in this issue of the journal was funded by the HTA programme as award number 17/136/10. The contractual start date was in July 2019. The draft report began editorial review in April 2023 and was accepted for publication in August 2023. The authors have been wholly responsible for all data collection, analysis and interpretation, and for writing up their work. The HTA editors and publisher have tried to ensure the accuracy of the authors’ report and would like to thank the reviewers for their constructive comments on the draft document. However, they do not accept liability for damages or losses arising from material published in this report.
Permissions
Copyright statement
Copyright © 2024 Goodacre et al. This work was produced by Goodacre et al. under the terms of a commissioning contract issued by the Secretary of State for Health and Social Care. This is an Open Access publication distributed under the terms of the Creative Commons Attribution CC BY 4.0 licence, which permits unrestricted use, distribution, reproduction and adaptation in any medium and for any purpose provided that it is properly attributed. See: https://creativecommons.org/licenses/by/4.0/. For attribution the title, original author(s), the publication source – NIHR Journals Library, and the DOI of the publication must be cited.
2024 Goodacre et al.
Chapter 1 Introduction
Background
The problem
Sepsis is a life-threatening reaction to an infection in which the immune system overreacts to infection and causes organ damage. The Third International Consensus Definitions for Sepsis and Septic Shock (sepsis-3) defines sepsis as life-threatening organ dysfunction caused by a dysregulated host response to infection, in which organ dysfunction can be represented by an increase in the Sequential (Sepsis-related) Organ Failure Assessment (SOFA) score of two points or more, and is associated with an in-hospital mortality > 10%. 1
This definition has recognised limitations. Sepsis is a broad term applied to an incompletely understood process with, as yet, no criteria that uniquely identify a septic patient. In adults, the clinical presentation of infection and new organ damage is often seen in people with long-term conditions where a self-limiting or easily treatable infection is exacerbating underlying comorbidity, rather than causing organ damage through a dysregulated response. 2 In such circumstances, comorbidities or frailty may be the main determinants of mortality. 3,4
Early recognition and treatment of sepsis has the potential to reduce mortality, depending upon the extent to which mortality risk is due to a dysregulated response to infection. Guidelines for sepsis highlight the importance of early recognition and treatment, with treatment recommended within 1 hour of presentation for those at highest risk. 5–9 This can only be achieved if sepsis is recognised and prioritised in the emergency care system.
Sepsis can be recognised by identifying clinical features of the host response or organ dysfunction, such as altered mental state, low blood pressure or rapid respiratory rate. Early warning scores use simple measurements to calculate a score, with a higher score indicating a higher risk of serious illness and adverse outcomes. They can be used by prehospital providers, such as ambulance paramedics, to identify people with suspected sepsis who need to be prioritised for treatment. 10 Prioritisation can involve a range of options based around pre-alerting the emergency department (ED), allowing the patient with sepsis to be seen before other patients. This could lead to the patient with sepsis being seen immediately on arrival by a clinician who is able to provide time-critical treatment for sepsis and refer for urgent specialist care. Early warning scores could also be used to select people for prehospital treatment for sepsis, such as intravenous fluids or antibiotic therapy, to further reduce treatment delays.
The recognised limitations of the definition of sepsis and uncertainty around the benefits of early treatment can lead to lack of clarity in determining what an early warning score for sepsis is intended to diagnose or predict. An early warning score could be used to identify patients with a diagnosis of sepsis according to the sepsis-3 definition, to predict patients with the highest risk of adverse outcome from sepsis, or to identify patients most likely to benefit from early treatment or a specific intervention for sepsis.
An effective early warning score needs to be accurate and implemented with an appropriate balance between sensitivity and specificity. High sensitivity is needed to ensure that people with the potential to benefit from urgent treatment are not missed, with consequent delayed treatment and avoidable mortality and morbidity. However, sacrificing specificity to maximise sensitivity can result in overtriage, in which people without sepsis or the potential to benefit from urgent treatment are inappropriately prioritised. This increases the pressure on EDs and impairs their ability to provide rapid treatment to those who require it. It may also result in inappropriate prehospital treatment with associated consequences, especially if prehospital scope of practice for sepsis includes antibiotic therapy.
The problem of overtriage is compounded if early warning scores are applied to an unselected population with a low prevalence of sepsis or a high prevalence of conditions that increase early warning scores in the absence of sepsis. National Institute for Health and Care Excellence (NICE) guidance advises thinking ‘could this be sepsis?’5 if a person presents with signs or symptoms that indicate possible infection and highlights that people with sepsis may have non-specific, non-localised presentations, and may not have a high temperature. This could result in sepsis being suspected in any patient attended by emergency ambulance for a medical complaint that is not attributable to a clear alternative cause. An early warning score with poor specificity could therefore result in overtriage of a huge number of patients, placing severe pressure on the emergency care system.
Uncertainty and evidence deficit
The NICE Guideline Development Group (GDG) identified a number of early warning scores that are easy to use, only require simple measurements and could therefore be used in the prehospital setting. 5 These are the Simple Triage Scoring System (STSS), Rapid Emergency Medicine Score (REMS) or modified-REMS, the Modified Early Warning score (MEWS) and National Early Warning score (NEWS). 11–14 They were developed through expert consensus or analysis of routine data from hospitalised patients and contain similar measures (heart rate, respiratory rate, oxygen saturation, blood pressure and conscious level) but differ in their calculation. REMS, MEWS and NEWS have been shown to predict adverse outcome in acute medical admissions, while STSS has been shown to predict mortality in inpatients with suspected infection.
The NICE GDG identified other early warning scores for use in hospital, such as Mortality in the Emergency Department, Sepsis and Predisposition, Infection, Response and Organ dysfunction, but did not recommend them for prehospital use on the basis that they include blood tests that are not currently available in the prehospital setting. 5
A meta-analysis and systematic review of in-hospital studies suggested that early warning scores predicted mortality in sepsis with limited accuracy, based on poor-quality data. 15 A systematic review of early warning scores undertaken for NICE guidance identified 47 relevant studies (including studies of in-hospital scores). All were judged as being of very low quality. There was significant variability in population, outcomes and analysis, so meta-analysis was not possible. No relevant economic evaluations were identified. The guideline recommended that clinicians consider using an early warning score to assess people with suspected sepsis in acute hospital settings and recommended research to determine whether early warning scores can be used to improve the detection of sepsis.
Two systematic reviews of prehospital identification of sepsis also reported limited existing evidence and a need for further research. Smyth et al. reported three studies developing prehospital sepsis screening tools for adults and six studies of paramedic diagnosis of sepsis. 16 Lane et al. reported nine studies of prehospital identification of sepsis. 17 Three of the studies from these systematic reviews evaluated sepsis-specific prehospital scores [Prehospital Early Sepsis Detection (PRESEP), Prehospital Severe Sepsis (PRESS) and the CIS], and the other studies evaluated MEWS, the Systemic Inflammatory Response Syndrome (SIRS) criteria and the Robson tool. 18 Study quality was poor, sensitivity (0.43–0.86) and specificity (0.47–0.87) varied markedly, and none of the screening tools had been validated.
More recently, the qSOFA score has been derived and validated to predict death in hospitalised patients with suspected sepsis and NEWS has been updated to become the National Early Warning Score, version 2 (NEWS2). 19–21 A meta-analysis of 38 recent studies of qSOFA reported pooled sensitivity of 0.61 and pooled specificity of 0.72 for mortality. 22 A systematic review comparing qSOFA and hospital early warning scores for prognosis in suspected sepsis in ED patients suggested that qSOFA has better specificity for predicting adverse outcome at its recommended threshold but NEWS has better sensitivity. 23
Other recent studies have developed scores in the prehospital setting. Smyth et al. derived and validated the Screening to Enhance PrehoSpital Identification of Sepsis (SEPSIS) tool to identify patients with high risk of sepsis in medical cases attended by emergency ambulance with an area under the receiver operating characteristic (ROC) curve of 0.86, and sensitivity 0.80 and specificity 0.78 at the recommended threshold. 24 Bayer et al. developed the PRESEP score to identify sepsis in prehospital patients with suspected sepsis with area under the ROC curve 0.93, sensitivity 0.85 and specificity 0.86. 25 Polito et al. developed the PRESS score to identify severe sepsis in physiologically abnormal prehospital patients with suspected sepsis with sensitivity 0.86 and specificity 0.47. 26
In developing our research proposal, we searched for studies evaluating the sensitivity and specificity or the effect of implementation of early warning scores for suspected sepsis in a prehospital population. 10 We identified 13 studies evaluating 20 scores. All but one were retrospective studies. The study populations varied from including all medical cases to including only those with presumed or diagnosed sepsis. Definitions of the reference standard included diagnosis (sepsis), prognosis (mortality) or health service use (admission to intensive care). Sensitivity and specificity varied substantially across the scores and studies. The most extensively studied score, qSOFA (studied in 9 of the 13 studies), had sensitivity ranging from 0.16 to 0.86 and specificity ranging from 0.16 to 0.97.
Two single-centre uncontrolled before versus after implementation studies have evaluated the potential effect of a score on practice. Polito et al. showed that implementation of the PRESS score was associated with improved sepsis recognition by prehospital personnel. 26 Borelli et al. showed that implementation of a prehospital sepsis screening tool was associated with improved clinician compliance with Surviving Sepsis Campaign 3-hour sepsis bundle recommendations. 27
Previous studies have important limitations other than the low quality identified in the NICE review:
-
Early warning scores should ideally identify patients who have the greatest potential to benefit from prioritisation and urgent treatment. Studies using mortality as the outcome or reference standard may not achieve this aim. 28 Firstly, patients whose lives are saved by urgent treatment will be categorised as reference standard negative despite having clearly benefited. Secondly, patients with severe pre-existing life-limiting conditions that make life-saving treatment futile or inappropriate will be classified as reference standard positive despite having little potential to benefit. Early warning scores developed to predict adverse outcomes such as mortality may therefore identify those with irreversible mortality while missing those with greatest potential to benefit from urgent treatment.
-
Early warning scores need to be operationalised by using a threshold for decision-making that optimises the trade-off between sensitivity and specificity in terms of the benefits, harms and costs of prioritisation. Existing studies have not explicitly examined the trade-off in these terms and the NICE review identified no relevant economic evaluations. Although the cost of applying an early warning score is small, the potential knock-on costs of overtriage are substantial.
-
Early warning scores should be evaluated in the population in whom the score will be used. REMS, MEWS and NEWS were developed and validated in acute medical inpatients with a range of medical complaints, while STSS and qSOFA were developed in inpatients with suspected sepsis. Inpatient populations, especially those identified as having suspected sepsis by hospital clinicians, are likely to have a higher prevalence of severe sepsis than prehospital populations. Using an early warning score developed for an inpatient population in the prehospital setting could lead to substantial overtriage.
Research therefore needs to use a reference standard or outcome that reflects potential to benefit from urgent treatment, examine the trade-off between sensitivity and specificity in terms of the benefit, harms and costs of using an early warning score to prioritise patients, and evaluate early warning scores in the prehospital population.
Research objectives
We aimed to determine the accuracy, impact and cost-effectiveness of prehospital early warning scores for adults with suspected sepsis. Our specific objectives were:
-
to estimate the accuracy of prehospital early warning scores for identifying sepsis requiring time-critical treatment in adults with possible sepsis who are attended by emergency ambulance
-
to estimate the impact of using prehospital early warning scores to guide key prehospital decisions, in terms of the operational consequences, and the cost-effectiveness of alternative strategies.
We planned two concurrent streams of work to address these objectives:
-
A retrospective cohort study using routine data sources to estimate the accuracy of prehospital early warning scores alongside paramedic diagnostic impression (index test) for identifying sepsis requiring time-critical treatment (reference standard) in adults with possible sepsis who are attended by emergency ambulance (population).
-
Decision-analytic modelling to determine the impact of using prehospital early warning scores to guide two key decisions: (1) alerting the receiving hospital so that the patient is seen immediately on arrival; (2) providing prehospital treatment for sepsis, such as intravenous antibiotics.
We focused on evaluating existing scores rather than developing a new score, because the existing evidence showed that numerous scores have been developed, but with very limited validation.
We limited our study to adults because the presentation and management of sepsis differ markedly between adults and children. The physiological constituents of early warning scores have different normal ranges and associations with outcome in adults and children, the confounding role of comorbidities is more significant in adults, and adults have higher rates of adverse outcome than children, when sepsis is suspected. For these reasons adults and children have separate guidelines for suspected sepsis and are studied in separate research projects.
Chapter 2 Retrospective cohort study
Methods
We used routine sources to collect data from a cohort of patients with possible sepsis who were transported by two ambulance services (Yorkshire and West Midlands) to four acute hospitals (Sheffield Northern General Hospital, Rotherham General Hospital, Barnsley Hospital, and University Hospitals Coventry and Warwickshire). We calculated early warning scores from routine data recorded on the ambulance service electronic patient-report form (ePRF) and determined the number of cases that would be prioritised using early warning scores alongside paramedic diagnostic impression.
We planned to determine the accuracy of early warning scores and paramedic diagnostic impression against a reference standard of sepsis needing urgent treatment, based on retrospective hospital record review. We planned to use the Data Access Request Service (DARS) from NHS Digital to link ambulance service data to Emergency Care Data Set, Hospital Episodes Statistics and Office for National Statistics mortality data. Hospitals would then be notified of cases with a diagnostic coding for sepsis that would undergo reference standard assessment. However, we were unable to implement this plan (see details below) and had to use an alternative approach, linking data from one ambulance service (Yorkshire) to one hospital (Sheffield Northern General Hospital).
Study population
We used routine ambulance service data to identify all episodes in which Yorkshire or West Midlands Ambulance Service transported adults as an emergency to the participating hospitals over the course of 2019 and a diagnosis of sepsis could have been suspected. We excluded episodes with injury, mental health problems, cardiac arrest or direct transfer to specialist services (including maternity, cardiac or stroke services). We also excluded episodes with no vital signs recorded since vital signs are essential to calculating early warning scores.
The participating ambulance services used an item on the ePRF to record the ambulance clinician diagnostic impression from a range of standardised options. These options differed between the two ambulance services, so clinical experts in the research team grouped the diagnostic impressions into five categories:
-
sepsis
-
infection (excluding sepsis)
-
non-specific diagnostic impression in which sepsis could be suspected
-
other diagnostic impression in which sepsis would not usually be suspected
-
diagnostic impression meets exclusion criteria.
Appendix 1 shows the categorisation of diagnostic impressions in the two ambulance services. We excluded episodes with a diagnostic impression in category 5 or no diagnostic impression recorded. Categories 1–4 were used to evaluate how the accuracy of early warning scores varied according to their application on the basis of diagnostic impression. NICE guidance suggests that sepsis could be suspected in any non-specific presentation but only applying early warning scores to those with suspected sepsis or suspected infection could provide a better balance of sensitivity and specificity.
The ambulance services differed in the way that diagnostic impression was recorded, with Yorkshire Ambulance Service only allowing one diagnosis to be recorded, while West Midlands Ambulance Service allowed multiple diagnoses, with no ranking in order of likelihood or importance. We categorised the West Midlands episodes according to whichever impression was nearest the top of our ranked order. For example, a patient with diagnostic impressions of atrial fibrillation, urinary tract infection and sepsis would be categorised in group 1. The exception was category 5, where any diagnosis meeting the exclusion criteria resulted in exclusion.
This represented a fundamental difference in the way that diagnostic category would be used in principle and in practice alongside an early warning scores. In Yorkshire, patients in category 1 would be those in whom the ambulance clinician considered sepsis to be the most likely diagnosis. In the West Midlands, patients in category 1 would be those in whom sepsis was one of the main differential diagnoses.
We identified patients with multiple episodes recorded so that we could use episode or patient as the unit of analysis. We only used data from the first episodes in analyses involving patient as the unit of analysis.
Prehospital early warning scores for sepsis
We evaluated any early warning scores that could be used by prehospital professionals and calculated from routinely available prehospital data. We included dichotomous scores (i.e. rules) that simply categorise into high- and low-risk groups, but refer collectively to the index test as constituting early warning scores for simplicity.
We searched EMBASE, CINHAL, PubMed, Clinicaltrials.gov, the ISRCTN registry and Research Registry for completed and ongoing studies of early warning scores for suspected sepsis in a prehospital population using the search strategy outlined in Appendix 2.
We planned to convene a group of 10–15 experts from prehospital care, emergency medicine and critical care to review the early warning scores identified by the literature search and select those that could be used in prehospital care and calculated from routinely available prehospital data. However, the COVID-19 pandemic substantially reduced the ability of relevant clinicians to participate in this activity, so we amended the study protocol to allow clinical experts from the research team to review early warning scores for evaluation and reduce the need for the project to draw upon overstretched clinical expertise.
The clinical experts convened over two online meetings and selected early warning scores that fulfilled the following criteria: (1) the variables constituting the score are likely to be measured and recorded in a standard manner; (2) categorisation and allocation of points to form a score follows a logical and intuitive process; and (3) the process of calculating a score is simple and reproducible, with a low risk of error. They also considered whether thresholds used to categorise a continuous measure were based on accepted cut-points, such as accepted normal ranges, but did not exclude scores that failed to meet these criteria.
The clinical experts selected 21 early warning scores that could potentially be used by prehospital professionals and calculated from routine ambulance service data. They determined whether any modification to the score was required to allow it to be calculated from routine data and then specified the modification required. They also specified how the score should be calculated if any elements were missing in routine data. This usually involved assuming that any missing variables were normal or negative. Finally, they identified a decision-making threshold for each score using existing literature or their knowledge of use of the scores in practice.
Public representatives reviewed the early warning scores to determine whether their use was likely to be acceptable to the patient and the public, taking into account whether measuring or recording variables for the score could be intrusive for the patient, and whether the score raises concerns about equity, such as in relation to age, gender, ethnic group or socioeconomic status. Several scores used age as a variable, which was felt to be acceptable given the association between age and risk of adverse outcome, provided use did not result in any age discrimination. None of the scores used gender, ethnic groups or socioeconomic variables. Some concerns were raised about use of residence in a care home as a variable in the PRESS score. This was dropped from the score on the basis of these concerns and lack of ambulance service data to confirm residence.
Table 1 outlines the scores and compares their constituent variables. Appendix 3 provides details of how each score is calculated, any modifications or assumptions in calculating the score from routine data, and the threshold for decision-making. The scores used combinations of age and six physiological measurements (temperature, heart rate, respiratory rate, peripheral oxygen saturation, conscious level, and blood pressure), along with a small number of other variables. Conscious level was assessed using either the Glasgow Coma Scale (GCS) or the alert, confusion, voice, pain, unresponsive (ACVPU) scale. Blood pressure was assessed using either the systolic blood pressure or the mean arterial pressure. Modification of the UK Sepsis Trust (UKST) red-flag criteria involved removing lactate, oliguria and recent chemotherapy from the criteria because these were not routinely recorded and lactate is not routinely measured in the prehospital setting.
Early warning score | Age | Temperature | Heart rate | Respiratory rate | Oxygen saturation | Conscious level | Blood pressure | Other |
---|---|---|---|---|---|---|---|---|
90-30-9029 | X | X | X | |||||
Borelli27 | X | X | X | X | X | X | Suspected infection | |
CIS30 | X | X | X | X | X | X | ||
HEWS31 | X | X | X | X | X | X | Inspired oxygen | |
MEWS13 | X | X | X | X | X | |||
NEWS214 | X | X | X | X | X | X | Inspired oxygen | |
NHS pre-alert32 | X | X | X | X | X | |||
PHANTASi33 | X | X | X | |||||
PITSTOP34 | X | X | Paramedic suspicion of infection | |||||
PreSAT35 | X | X | X | X | ||||
PRESEP25 | X | X | X | X | X | |||
PRESS26 | X | X | X | X | Dispatch chief complaint of sick person; nursing home resident | |||
PSP36 | X | X | X | X | ||||
REMS12 | X | X | X | X | X | X | ||
qSOFA19 | X | X | X | |||||
RST29 | X | X | X | X | Blood glucose | |||
SEPSIS24 | X | X | X | X | X | X | X | Skin appearance |
Sepsis Alert37 | X | X | X | X | Suspected or documented infection, hypoperfusion | |||
STSS11 | X | X | X | X | X | X | ||
Suffoletto strategy38 | X | X | ||||||
UKST red flag39,a | X | X | X | X | X | Skin appearance |
We planned, as part of the expert group work, to use consensus methods to create additional scores for evaluation. However, having reviewed the existing scores, the clinical experts determined that there was little potential to develop an additional score from routinely recorded data that would provide a clinically important improvement on the extensive range of existing scores.
Calculation of the early warning score from electronic patient-report form data
Age, heart rate, respiratory rate, systolic blood pressure, peripheral oxygen saturation, temperature and GCS were recorded on the ePRF. Mean arterial blood pressure was calculated using a formula in which the diastolic blood pressure is doubled and added to systolic blood pressure, and then divided by three. Conscious level and ACVPU were inferred from GCS. We used the first recorded measurement for each variable, if it was recorded multiple times. If the variable was not recorded in the first set of observations, then the first recorded measurement was used from a subsequent set of observations.
Appendix 3 outlines how other variables used in the early warning scores were calculated from the ePRF. Reference standard results were unknown at the time of early warning score calculation.
Use of the early warning score alongside diagnostic impression
The index tests for our evaluation were early warning scores and paramedic diagnostic impression, either used alone or in combination. We evaluated how early warning scores could be used alongside the prehospital diagnostic impression by applying each early warning scores in the following ways:
-
Score applied only to cases in category 1 (sepsis), with cases in categories 2–4 considered index test negative.
-
Score applied only to cases in categories 1 and 2 (sepsis or infection), with cases in categories 3 and 4 considered index test negative.
-
Score applied only to cases in categories 1–3 (sepsis, infection or non-specific), with cases in category 4 considered index test negative.
-
Score applied to all eligible cases, regardless of diagnostic category (i.e. evaluation of the early warning scores alone).
We also evaluated diagnostic category alone as an index test (with no early warning scores) using three different thresholds: (1) category 1 positive, categories 2 to 4 negative; (2) categories 1 and 2 positive, categories 3 and 4 negative; and (3) categories 1 to 3 positive, category 4 negative.
The reference standard: sepsis requiring urgent treatment
The purpose of prehospital early warning scores is to prioritise patients who have potential to benefit from urgent treatment for sepsis. We therefore defined the reference standard as being positive if the patient met the sepsis-3 definition of sepsis and received treatment for sepsis within 4 hours of initial assessment at hospital. This definition recognised that some patients with sepsis have life-limiting conditions (such as metastatic malignancy or end-stage chronic disease) that would make urgent treatment for sepsis futile or run counter their wishes. The reference standard for the primary analysis (treated sepsis) required both diagnosis and treatment. We undertook a secondary analysis using a reference standard that only required the sepsis-3 definition to be met (diagnosed sepsis). The rationale for this approach was that the primary analysis would determine the accuracy for identifying cases most likely to benefit from urgent treatment. The secondary analysis would determine whether accuracy differed when the reference standard included cases that could have benefited from early diagnosis (e.g. to allow prognostication or consideration of treatment options), even though they did not receive urgent treatment for sepsis.
Determining the reference standard involved hospital record review and expert judgement, but we needed a large sample size to detect a sufficient number of cases with a positive reference standard. We therefore used a screening process to select cases for reference standard review that were likely to meet the reference standard definition and avoid review for those unlikely to meet the definition. We used routine hospital data to select those with a primary or secondary International Classification of Disease (ICD) 10 admission code or cause of death compatible with sepsis, or an ED code for sepsis. Research nurses briefly reviewed the ED records of these cases and selected patients for expert review if they had a diagnosis of sepsis or any treatment for sepsis recorded in the ED notes or if sepsis was recorded as an admission diagnosis on the hospital discharge summary. Cases were excluded if there was no evidence of sepsis diagnosis or treatment, or if sepsis developed after hospital admission.
Two experts independently reviewed hospital records for all patients selected by the research nurses and determined whether the following criteria were met: (1) evidence of infection and life-threatening organ dysfunction (as defined by sepsis-3, the Third International Consensus Definitions for Sepsis and Septic Shock) within 4 hours of initial assessment; (2) treatment for sepsis was given within 4 hours. Evidence of infection could include microbiology reports identifying organisms, radiology reports identifying infective changes, or consistent markers of infection (elevated white cell count, C-reactive protein and temperature). Organ dysfunction, in accordance with the sepsis-3 definition, was defined as a SOFA score of 2 or more points worse than normal. The SOFA score was estimated using the ED observations chart and first blood results after admission. If arterial blood gas sampling had not been recorded, the arterial partial pressure of oxygen was estimated from the peripheral oxygen saturation. The normal SOFA score was estimated using previous hospital records and blood tests. Elements of the normal SOFA score were assumed to be zero unless there was clear evidence that they would not be normal, such as evidence of pre-existing confusion in patients with dementia or evidence of hypoxia or long-term oxygen therapy in patients with chronic lung disease. Treatment for sepsis usually involved evidence of administration of any antimicrobial that could be used to treat a sepsis-causing organism. Oral antimicrobial therapy was not considered to be treatment for sepsis unless there was a specific reason why intravenous antibiotics were not used (such as delays in gaining intravenous access). The expert reviewers were encouraged to make judgements in all cases, even when information was incomplete, since the potential bias from excluding cases with incomplete information was considered to be greater than the potential bias from misclassification. Index test results were not available to assessors during reference standard review, although assessors were aware of constituent elements of early warning scores recorded in ED notes.
If the two reviewers disagreed on the overall sepsis-3 judgement or whether treatment for sepsis was given, then a consensus decision was reached through discussion, with a third expert available to resolve any cases where consensus could not be reached. Disagreements over an element of the sepsis-3 definition (evidence of infection or change in SOFA score) were left unresolved if they did not affect the overall judgement, that is, if the reviewers agreed that the sepsis-3 definition was not met but disagreed on which criterion was not met.
Data linkage and management
Each ambulance service provided data from all eligible cases transported to one of the participating hospitals over 2019. The ambulance service created a unique study identification number for each case. Two linked databases were created: (1) containing the unique identification, time and date of call, and personal details, which was sent to NHS Digital; (2) containing the unique identification, time and date of call, and all non-personal details, which was sent to the Sheffield Clinical Trials Research Unit (CTRU).
We planned that NHS Digital would use the personal details from the ambulance data to link each case to the Emergency Care Data Set data for the related ED attendance and any related Hospital Episodes Statistics or Office for National Statistics data from subsequent hospital admissions. They would then send selected de-identified data from the Emergency Care Data Set, Hospital Episodes Statistics and Office for National Statistics data alongside the unique study identification number to the Sheffield CTRU, and would send a data set to a research nurse at each participating hospital consisting of the unique study identification number, personal data and the selected Emergency Care Data Set, Hospital Episodes Statistics and Office for National Statistics data. The research nurses would then undertake the process outlined above to determine the reference standard.
Our initial application to the NHS Digital DARS was rejected on the basis that the data flow process, involving NHS Digital sending personal data to multiple recipients (Sheffield CTRU and the four hospitals), incurred an unacceptable risk to NHS Digital. We therefore amended the data flow process so that NHS Digital would only send data to Sheffield CTRU, who would then send NHS numbers for patients requiring reference standard adjudication to the four hospitals. The regulatory authorities and NHS Digital approved the amended process.
After NHS Digital approved the application and signed a Data-Sharing Agreement with the University of Sheffield there was a prolonged delay in receiving data during which a scheduled date for data release (19 May 2022) passed without receiving any data. In September 2022, after 9 months of waiting for NHS Digital to provide data and no indication of when it would be provided, and with 4 months until the funder had stated that the project had to be completed, we still had not received linked data. At this point, after consultation with the study steering committee and public representatives, and approval from regulatory authorities, we implemented a rescue plan to allow us to collect reference standard data at one of the participating sites. Details of the timeline for seeking linked data from NHS Digital are outlined in Appendix 4.
The rescue plan involved Yorkshire Ambulance Service identifying all eligible cases transported to the Sheffield Northern General Hospital that had an NHS number recorded, excluding cases where the patient had opted out from access to their personal details for research purposes, and then adding NHS numbers to the data sent to Sheffield CTRU. The data were then sent to the Chief Investigator, who works as a clinician at the Sheffield Northern General Hospital. The Chief Investigator shared the data with the Scientific Computing team at Sheffield Teaching Hospitals NHS Trust, who searched the hospital information system for any ED attendances or hospital admissions with the same NHS number, occurring within 48 hours of ambulance transport to hospital. The Scientific Computing team informed Sheffield CTRU of any cases that could not be linked or had no coded attendance or admission within 48 hours. These were excluded from the analysis. The Scientific Computing team then provided the Chief Investigator with ED codes and admission ICD10 codes or causes of death for all cases identified as having a coded attendance or admission within 48 hours. The Chief Investigator then identified all cases with an ED code of septicaemia or neutropenic sepsis, or a primary or secondary ICD10 code for sepsis.
As outlined above, the research nurses then screened the ED records and hospital discharge summaries of cases with a code for sepsis and selected for expert review those with a diagnosis of sepsis or treatment for sepsis recorded. Four consultants in emergency medicine who work in the Northern General Hospital ED (SG, KI, SC and GF) undertook expert review, with two independently reviewing each case.
Data analysis
We originally planned to undertake analysis across both ambulance services and all four hospitals, but revised this plan to undertake a separate analysis for each hospital because: (1) inability to use NHS Digital linkage meant that we could only measure the reference standard at Sheffield Northern General Hospital; (2) the two ambulance services used diagnostic impressions in fundamentally different ways, and evaluation of early warning scores alongside diagnostic impression was considered to be crucial.
Descriptive analysis of the ambulance service data for each participating hospital used episode as the unit of analysis to compare the index tests (early warning scores and paramedic diagnostic impressions) in terms of the mean [standard deviation (SD)] number of episodes per day that would be positive (i.e. prioritised or selected for treatment). We also reported the mean total eligible attendances per day and the mean number per day that received a pre-alert from the ambulance service. We then calculated the proportion of eligible attendances that would be prioritised using each index test strategy. This indicated whether each strategy is likely to be manageable and result in meaningful prioritisation, on the basis that prioritisation requires sufficient staff resources, sepsis is one of a number of conditions that might benefit from prioritisation, and prioritisation will not be meaningful if too many attendances are prioritised. We used three categories to indicate whether each strategy was likely to be manageable, based on the opinions of clinicians in the research team:
-
Green: < 5% of the mean daily attendances would be prioritised – likely to be manageable.
-
Amber: 5–10% would be prioritised – uncertain.
-
Red: more than 10% would be prioritised – unlikely to be manageable.
We evaluated each index test based upon using the recommended threshold (see Appendix 3) with the exception of NEWS2 and qSOFA, where we evaluated all potential thresholds. NEWS2 is routinely used in NHS ambulance services and can be implemented at any threshold with minimal additional training, while qSOFA is simple to calculate and recommended in international guidelines. 1 Implementing other scores would require additional training and possibly decision-support tools. We therefore wanted to compare these scores to all NEWS2 thresholds to ensure that superior performance did not just reflect the chosen NEWS2 threshold.
When calculating early warning scores, missing parameters were generally assumed normal (scored zero): details for each score are outlined in Appendix 3. Cases were excluded from analysis of a specific early warning scores if more than half of the variables used to calculate the score were missing.
We used data from a subset of the cohort (patients with NHS numbers who were transported to the Sheffield Northern General Hospital) to estimate the accuracy of each index test compared to the reference standard. We used the patient as the unit of analysis and only included the first eligible episode per patient. Primary analysis used treated sepsis as the reference standard and secondary analysis used diagnosed sepsis (see The reference standard: sepsis requiring urgent treatment). Kappa scores were calculated to determine the agreement between adjudicators for each element of the reference standard, the overall reference standard judgement and the judgement of whether treatment for sepsis was provided.
We constructed ROC curves to evaluate sensitivity and specificity over the range of each score. We calculated the area under the ROC curve and sensitivities and specificities at key cut-points, each with a 95% confidence interval (CI).
In our proposal, we planned to explore whether it is possible to statistically derive a clinically credible new score using multivariable logistic regression. We ultimately did not include this in our statistical analysis plan for the following reasons: (1) the large number of existing scores using the same limited number of available variables suggested that there was little potential to derive a new score with superior accuracy (other than due to overfitting); (2) the limited time available after delays incurred waiting for NHS Digital compelled us to prioritise the core analysis; (3) limitations in the population sample with reference standard adjudication (single site, only those with NHS numbers) undermined the potential applicability of a derived model to other settings.
Selection of strategies for the decision-analytic modelling
We used the results of the primary (treatment) analysis to compare the sensitivity and specificity of each strategy (combination of early warning score and diagnostic impression) to all other strategies and exclude any strategy that had inferior sensitivity and specificity to another strategy (i.e. both parameters inferior or one parameter equivalent and the other inferior). We used the point estimate to three decimal places for this assessment. Clinical experts in the group then reviewed these strategies for inclusion in the decision-analytic modelling. They excluded the strategies that were considered unmanageable due to prioritising > 10% of eligible cases in the analysis of mean daily rates of attendances prioritised. They then excluded strategies that would require additional training for ambulance and ED staff if they did not offer clearly superior accuracy to points on the NEWS2 ROC curve or an alternative rationale for use instead of NEWS2. The rationale for this decision was that NEWS2 is widely used by NHS ambulance services and hospitals, so any alternative strategy requiring additional training would need to offer clearly superior accuracy. We included the accuracy of pre-alert practice in 2019 alongside diagnostic impression as a strategy in this assessment in case it could represent a worthwhile comparator strategy in the modelling.
Sample size
We based our planned sample size estimate on data from Smyth et al. reporting 3.7% prevalence of high-risk sepsis in adults transported to hospital with a non-specific emergency presentation,24 and data from reviews of sepsis by the National Confidential Enquiry into Patient Outcome and Death (NCEPOD) and the Intensive Care National Audit and Research Centre (ICNARC). 40,41 NCEPOD identified 3363 adults (≥ 16 years) seen by the Critical Care Outreach Team or admitted directly to critical care with a diagnosis of sepsis across 305 hospitals over a 2-week period. ICNARC reported 22,081 admissions to critical care with septic shock across 205 hospitals over 2012. These studies suggest that the key determinant of sample size is the number of reference standard positive cases (to estimate sensitivity with acceptable precision) and an incidence of around two reference standard positive cases per week at a typical hospital.
Our plan to collect data across four hospitals over 1 year was based on attempting to ensure generalisability across multiple sites and avoid seasonality. Data from Smyth suggested around 23,000 eligible episodes over 1 year at each participating hospital, including around 847 with the reference standard, providing a total sample size of 92,000 across four hospitals with 3388 reference standard positive cases. This would result in an overpowered study with unnecessary and excessive work delivering reference standard validation. We therefore planned to randomly sample cases at each site to achieve a sufficient number of reference standard positive cases.
We estimated that around 2000 episodes would be selected for research nurse review (500 at each site), with around 1000 for expert hospital record review, to identify 200 reference standard positive episodes. This sample size would allow us to estimate the sensitivity of an early warning score with a standard error of 2.1% assuming sensitivity of 90%, and the area under the ROC curve with a standard error of 2% assuming an area under the ROC curve of at least 0.75. 42 The sample size also satisfies the recommendation by Collins et al. that external validation studies be based on a minimum of 100–200 events. 43
Following the failure of NHS Digital to provide linked data and the activation of our rescue plan, we attempted to pragmatically deliver an appropriate sample size within the time and resources available. We decided that random sampling of cases would not be practical and instead used consecutive sampling of cases in time order. In the event we were able to review all available cases across 2019 at the Sheffield site and deliver a sample of reference standard positive cases that exceeded our planned sample size.
Ethical approvals
The study gained initial approval from the London – Stanmore Research Ethics Committee (REC) (reference number 19/LO/1443) on 23 September 2019 and the Health Research Authority (HRA) on 30 January 2020. Due to the use of personal data for record linkage Confidentiality Advisory Group (CAG) approval was sought and granted on 13 January 2020. Over the course of the study, three substantial amendments were submitted to the REC, HRA and CAG. Dates of approval are provided in Table 2 and the resulting changes are outlined in the text below.
Amendment date | REC approval | HRA approval | CAG approval | |
---|---|---|---|---|
Substantial amendment 1 | 16 December 2019 | 20 December 2019 | 30 January 2020 | Not required |
Substantial amendment 2 | 24 May 2021 | 7 June 2021 | 15 June 2021 | 17 June 2021 |
Substantial amendment 3 | 6 July 2022 | 20 July 2022 | 26 July 2022 | 26 July 2022 |
Substantial amendment 1
A change to the inclusion criteria for the study on the Integrated Research Application System form, lowering the age of inclusion from 18+ to 16+ in order to bring the study inclusion criteria in line with the age the ambulance services consider to be an adult.
Substantial amendment 2
A change to the data flow in order to secure approval from NHS Digital. The initial data flow was rejected by NHS Digital as it involved the provision of data to more than one data processor. The new approach was for NHS Digital to send all data to Sheffield CTRU, who would act as the data processor and controller. Sheffield CTRU would then securely transfer NHS numbers to the appropriate participating hospitals for assessment of the patient for reference standard inclusion.
Substantial amendment 3
The introduction of an alternative data flow to be used in the case that NHS Digital data linkage was not delivered. This involved staff at one participating hospital (Northern General Hospital, Sheffield) using NHS numbers to link data form one ambulance service (Yorkshire) to the hospital data.
Results
Overview of the ambulance service data
There were 178,333 ambulance episodes provided from 2019: 27,564 from West Midlands Ambulance Service and 150,769 from Yorkshire Ambulance Service. The Yorkshire Ambulance Service data set (Doncaster, Sheffield and Rotherham sites) was missing a period of 9 days in February. The West Midlands Ambulance Service data set (Coventry site) was missing data for the second half of each of the last 6 months of the year.
We excluded 83,311 episodes that met study exclusion criteria, had no unique patient identifier or had no data to calculate early warning scores. The analysis set included 95,022 episodes from 71,204 unique patients across the four sites. Table 3 compares the characteristics of the 71,204 first episodes to those of the 23,818 repeat episodes. The repeat episodes involved patients who tended to be older (median age 75 vs. 66 years) and had slightly more abnormal physiological measurements. Diagnostic impressions of sepsis (4.4% vs. 3.8%), infection (9.7% vs. 8.8%) and non-specific presentations (41.8% vs. 37.1%) were slightly more common in the repeat attendances.
First (N = 71,204) | Repeat (N = 23,818) | Total (N = 95,022) | |
---|---|---|---|
Age (years) | |||
Mean (SD) | 61.7 (22.3) | 68.8 (19.8) | 63.5 (21.9) |
Median (IQR) | 66.0 (44.0–81.0) | 75.0 (56.0–84.0) | 69.0 (47.0–82.0) |
Range | 16.0–106.0 | 16.0–107.0 | 16.0–107.0 |
Sex | |||
Missing | 220 | 49 | 269 |
Female (%) | 37,588 (53.0) | 12,539 (52.8) | 50,127 (52.9) |
Male (%) | 33,396 (47.0) | 11,230 (47.2) | 44,626 (47.1) |
Ethnicity | |||
Missing | 29,005 | 9381 | 38,386 |
White (%) | 40,045 (94.9) | 13,979 (96.8) | 54,024 (95.4) |
Asian (%) | 968 (2.3) | 253 (1.8) | 1221 (2.2) |
Black (%) | 397 (0.9) | 69 (0.5) | 466 (0.8) |
Mixed (%) | 208 (0.5) | 41 (0.3) | 249 (0.4) |
Other (%) | 581 (1.4) | 95 (0.7) | 676 (1.2) |
ACVPU | |||
Missing | 1944 | 688 | 2632 |
Alert (%) | 63,059 (91.0) | 20,805 (89.9) | 83,864 (90.8) |
Confusion (%) | 2371 (3.4) | 991 (4.3) | 3362 (3.6) |
Pain (%) | 890 (1.3) | 282 (1.2) | 1172 (1.3) |
Unresponsive (%) | 1029 (1.5) | 282 (1.2) | 1311 (1.4) |
Voice (%) | 1911 (2.8) | 770 (3.3) | 2681 (2.9) |
GCS | |||
Mean (SD) | 14.5 (1.7) | 14.4 (1.7) | 14.5 (1.7) |
Median (IQR) | 15.0 (15.0–15.0) | 15.0 (15.0–15.0) | 15.0 (15.0–15.0) |
Range | 3.0–15.0 | 3.0–15.0 | 3.0–15.0 |
Diastolic BP (mmHg) | |||
Mean (SD) | 82.1 (17.4) | 79.9 (17.3) | 81.6 (17.4) |
Median (IQR) | 82.0 (71.0–93.0) | 79.0 (68.0–90.0) | 81.0 (70.0–92.0) |
Range | 0.0–197.0 | 0.0–191.0 | 0.0–197.0 |
Systolic BP (mmHg) | |||
Mean (SD) | 140.9 (28.1) | 138.7 (27.8) | 140.3 (28.1) |
Median (IQR) | 139.0 (122.0–157.0) | 137.0 (120.0–155.0) | 138.0 (122.0–157.0) |
Range | 32.0–285.0 | 36.0–288.0 | 32.0–288.0 |
Heart rate (beats/minute) | |||
Mean (SD) | 90.1 (23.2) | 90.3 (22.5) | 90.2 (23.0) |
Median (IQR) | 88.0 (74.0–104.0) | 88.0 (74.0–104.0) | 88.0 (74.0–104.0) |
Range | 0.0–220.0 | 0.0–220.0 | 0.0–220.0 |
Oxygen saturation (%) | |||
Mean (SD) | 95.7 (5.2) | 94.6 (6.0) | 95.4 (5.4) |
Median (IQR) | 97.0 (95.0–98.0) | 96.0 (94.0–98.0) | 97.0 (95.0–98.0) |
Range | 10.0–100.0 | 15.0–100.0 | 10.0–100.0 |
Supplemental oxygen | |||
Missing | 7433 | 2005 | 9438 |
No (%) | 60,228 (94.4) | 20,100 (92.1) | 80,328 (93.9) |
Yes (%) | 3543 (5.6) | 1713 (7.9) | 5256 (6.1) |
Respiration (breath/minute) | |||
Mean (SD) | 20.5 (6.4) | 21.4 (6.9) | 20.7 (6.6) |
Median (IQR) | 18.0 (16.0–22.0) | 20.0 (18.0–24.0) | 18.0 (16.0–22.0) |
Range | 0.0–98.0 | 0.0–98.0 | 0.0–98.0 |
Temp (°C) | |||
Mean (SD) | 37.0 (1.0) | 37.0 (0.9) | 37.0 (1.0) |
Median (IQR) | 36.9 (36.4–37.4) | 36.9 (36.4–37.4) | 36.9 (36.4–37.4) |
Range | 26.0–42.0 | 27.0–41.8 | 26.0–42.0 |
Glucose (mmol/l) | |||
Mean (SD) | 7.3 (3.4) | 7.5 (3.7) | 7.4 (3.5) |
Median (IQR) | 6.4 (5.5–7.9) | 6.5 (5.5–8.1) | 6.4 (5.5–7.9) |
Range | 0.0–49.0 | 0.6–50.0 | 0.0–50.0 |
Pre-alerted | |||
No (%) | 65,736 (92.3) | 22,011 (92.4) | 87,747 (92.3) |
Yes (%) | 5468 (7.7) | 1807 (7.6) | 7275 (7.7) |
Impression | |||
1 – sepsis (%) | 2700 (3.8) | 1045 (4.4) | 3745 (3.9) |
2 – infection (%) | 6293 (8.8) | 2321 (9.7) | 8614 (9.1) |
3 – non-specific (%) | 26,393 (37.1) | 9962 (41.8) | 36,355 (38.3) |
4 – others (%) | 35,818 (50.3) | 10,490 (44.0) | 46,308 (48.7) |
Table 4 shows the characteristics of the 71,204 patients by hospital site. Patients at the larger hospitals (Sheffield and Coventry) tended to be older and more ethnically diverse. Patients transported to Coventry by West Midlands Ambulance Service were more likely to have a diagnostic impression of sepsis (6.5% vs. 2.5–3.6%), infection (21.4% vs. 3.7–5.5%) or non-specific presentations (40.8% vs. 33.9–38.3%), reflecting the potential for paramedics in West Midlands Ambulance Service to record multiple diagnostic impressions.
DRI (N = 15,825) | NGH (N = 24,815) | RGH (N = 13,222) | UHCW (N = 17,342) | Total (N = 71,204) | |
---|---|---|---|---|---|
Age (years) | |||||
Mean (SD) | 63.1 (21.7) | 60.9 (22.7) | 62.2 (22.0) | 61.2 (22.2) | 61.7 (22.3) |
Median (IQR) | 68.0 (47.0–81.0) | 65.0 (42.0–80.0) | 67.0 (45.0–81.0) | 65.0 (44.0–80.0) | 66.0 (44.0–81.0) |
Range | 16.0–105.0 | 16.0–105.0 | 16.0–106.0 | 16.0–105.0 | 16.0–106.0 |
Sex | |||||
Missing | 7 | 22 | 6 | 185 | 220 |
Female (%) | 8564 (54.1) | 13,087 (52.8) | 7233 (54.7) | 8704 (50.7) | 37,588 (53.0) |
Male (%) | 7254 (45.9) | 11,706 (47.2) | 5983 (45.3) | 8453 (49.3) | 33,396 (47.0) |
Ethnicity | |||||
Missing | 3095 | 12,115 | 4461 | 9334 | 29,005 |
White (%) | 12,412 (97.5) | 11,931 (93.9) | 8493 (96.9) | 7209 (90.0) | 40,045 (94.9) |
Asian (%) | 90 (0.7) | 256 (2.0) | 137 (1.6) | 485 (6.1) | 968 (2.3) |
Black (%) | 43 (0.3) | 127 (1.0) | 16 (0.2) | 211 (2.6) | 397 (0.9) |
Mixed (%) | 53 (0.4) | 81 (0.6) | 12 (0.1) | 62 (0.8) | 208 (0.5) |
Other (%) | 132 (1.0) | 305 (2.4) | 103 (1.2) | 41 (0.5) | 581 (1.4) |
ACVPU | |||||
Missing | 2 | 0 | 1 | 1941 | 1944 |
Alert (%) | 14,497 (91.6) | 22,853 (92.1) | 12,166 (92.0) | 13,543 (87.9) | 63,059 (91.0) |
Confusion (%) | 456 (2.9) | 724 (2.9) | 417 (3.2) | 774 (5.0) | 2371 (3.4) |
Pain (%) | 189 (1.2) | 298 (1.2) | 124 (0.9) | 279 (1.8) | 890 (1.3) |
Unresponsive (%) | 196 (1.2) | 298 (1.2) | 164 (1.2) | 371 (2.4) | 1029 (1.5) |
Voice (%) | 485 (3.1) | 642 (2.6) | 350 (2.6) | 434 (2.8) | 1911 (2.8) |
GCS | |||||
Mean (SD) | 14.5 (1.7) | 14.5 (1.6) | 14.5 (1.6) | 14.4 (1.9) | 14.5 (1.7) |
Median (IQR) | 15.0 (15.0–15.0) | 15.0 (15.0–15.0) | 15.0 (15.0–15.0) | 15.0 (15.0–15.0) | 15.0 (15.0–15.0) |
Range | 3.0–15.0 | 3.0–15.0 | 3.0–15.0 | 3.0–15.0 | 3.0–15.0 |
Diastolic BP (mmHg) | |||||
Mean (SD) | 83.3 (18.1) | 82.6 (17.4) | 82.7 (17.3) | 80.0 (16.6) | 82.1 (17.4) |
Median (IQR) | 83.0 (71.0–94.0) | 82.0 (71.0–93.0) | 83.0 (72.0–93.0) | 79.0 (70.0–89.0) | 82.0 (71.0–93.0) |
Range | 0.0–197.0 | 0.0–195.0 | 0.0–192.0 | 0.0–196.0 | 0.0–197.0 |
Systolic BP (mmHg) | |||||
Mean (SD) | 142.7 (28.4) | 140.8 (27.1) | 141.3 (27.2) | 139.0 (30.0) | 140.9 (28.1) |
Median (IQR) | 141.0 (124.0–159.0) | 139.0 (123.0–156.0) | 140.0 (123.0–156.0) | 137.0 (118.0–158.0) | 139.0 (122.0–157.0) |
Range | 42.0–260.0 | 43.0–285.0 | 48.0–281.0 | 32.0–271.0 | 32.0–285.0 |
Heart rate (beats/minute) | |||||
Mean (SD) | 90.1 (23.4) | 89.1 (22.3) | 89.4 (22.4) | 92.2 (24.8) | 90.1 (23.2) |
Median (IQR) | 88.0 (74.0–103.0) | 86.0 (74.0–102.0) | 87.0 (74.0–102.0) | 90.0 (75.0–106.0) | 88.0 (74.0–104.0) |
Range | 0.0–220.0 | 0.0–218.0 | 0.0–220.0 | 0.0–220.0 | 0.0–220.0 |
Oxygen saturation (%) | |||||
Mean (SD) | 95.5 (5.5) | 95.8 (4.9) | 95.6 (5.1) | 95.9 (5.3) | 95.7 (5.2) |
Median (IQR) | 97.0 (95.0–98.0) | 97.0 (95.0–98.0) | 97.0 (95.0–98.0) | 97.0 (95.0–99.0) | 97.0 (95.0–98.0) |
Range | 13.0–100.0 | 10.0–100.0 | 16.0–100.0 | 17.0–100.0 | 10.0–100.0 |
Supplemental oxygen | |||||
Missing | 53 | 45 | 29 | 7306 | 7433 |
No (%) | 14,756 (93.6) | 23,466 (94.7) | 12,473 (94.5) | 9533 (95.0) | 60,228 (94.4) |
Yes (%) | 1016 (6.4) | 1304 (5.3) | 720 (5.5) | 503 (5.0) | 3543 (5.6) |
Respiration (breath/minute) | |||||
Mean (SD) | 20.7 (6.4) | 20.1 (6.0) | 20.6 (6.2) | 20.6 (7.1) | 20.5 (6.4) |
Median (IQR) | 18.0 (18.0–22.0) | 18.0 (16.0–22.0) | 18.0 (18.0–22.0) | 18.0 (16.0–22.0) | 18.0 (16.0–22.0) |
Range | 0.0–98.0 | 0.0–93.0 | 0.0–96.0 | 0.0–98.0 | 0.0–98.0 |
Temp (°C) | |||||
Mean (SD) | 37.0 (1.0) | 36.9 (1.0) | 37.0 (1.0) | 37.0 (1.0) | 37.0 (1.0) |
Median (IQR) | 36.9 (36.4–37.4) | 36.8 (36.4–37.4) | 36.9 (36.4–37.4) | 36.9 (36.4–37.5) | 36.9 (36.4–37.4) |
Range | 26.4–41.7 | 26.0–41.8 | 32.0–41.8 | 30.1–42.0 | 26.0–42.0 |
Glucose (mmol/l) | |||||
Mean (SD) | 7.3 (3.4) | 7.2 (3.3) | 7.3 (3.4) | 7.4 (3.6) | 7.3 (3.4) |
Median (IQR) | 6.4 (5.5–7.9) | 6.3 (5.5–7.8) | 6.4 (5.5–7.9) | 6.4 (5.6–7.9) | 6.4 (5.5–7.9) |
Range | 0.1–44.0 | 0.5–49.0 | 0.4–46.0 | 0.0–39.5 | 0.0–49.0 |
Pre-alert | |||||
No (%) | 14,584 (92.2) | 23,601 (95.1) | 12,036 (91.0) | 15,515 (89.5) | 65,736 (92.3) |
Yes (%) | 1241 (7.8) | 1214 (4.9) | 1186 (9.0) | 1827 (10.5) | 5468 (7.7) |
Impression | |||||
1 – sepsis (%) | 477 (3.0) | 623 (2.5) | 472 (3.6) | 1128 (6.5) | 2700 (3.8) |
2 – infection (%) | 585 (3.7) | 1377 (5.5) | 613 (4.6) | 3717 (21.4) | 6292 (8.8) |
3 – non-specific (%) | 6063 (38.3) | 8413 (33.9) | 4836 (36.6) | 7082 (40.8) | 26,394 (37.1) |
4 – other (%) | 8700 (55.0) | 14,402 (58.0) | 7301 (55.2) | 5415 (31.2) | 35,818 (50.3) |
Daily rates of prioritised attendances
Tables 5–8 show, for each hospital, the mean (SD) number of attendances per day that would be prioritised according to each strategy using the threshold in the second column and when applied to the paramedic diagnostic impression(s) in the remaining columns. The first row shows the number of attendances prioritised using paramedic diagnostic impression alone. The second row shows the number of attendances that were recorded as being pre-alerted to the ED in 2019.
Early warning score | Threshold | Impression | |||
---|---|---|---|---|---|
Sepsis | Sepsis/infection | Sepsis/infection/non-specific | All | ||
Impression alone | 1.9 (1.3) | 4.2 (2.2) | 28 (6.8) | 59.5 (10.8) | |
Pre-alerted | 0.9 (1) | 1 (1) | 2.6 (1.7) | 4.7 (2.4) | |
NEWS2 | 4 | 1.7 (1.2) | 2.9 (1.8) | 10.9 (4) | 15.1 (4.7) |
NEWS2 | 6 | 1.5 (1.2) | 2.1 (1.5) | 6.7 (2.9) | 8.4 (3.3) |
qSOFA | 0 | 1.7 (1.3) | 3.2 (1.9) | 15.6 (4.8) | 25.6 (6.4) |
qSOFA | 1 | 0.8 (0.9) | 1.1 (1.1) | 3.3 (2) | 4.6 (2.3) |
90-30-90 | 0 | 1.3 (1.1) | 2 (1.5) | 8.2 (3.2) | 10.8 (3.6) |
Borelli | 0 | 1.4 (1.1) | 2.1 (1.5) | 6.6 (3) | 8.4 (3.4) |
CIS | 0 | 1.8 (1.3) | 4 (2.2) | 25.9 (6.5) | 52.2 (9.9) |
CIS | 4 | 0.4 (0.6) | 0.5 (0.7) | 1.1 (1.1) | 1.4 (1.2) |
HEWS | 4 | 1.5 (1.2) | 2.3 (1.5) | 7.9 (3.3) | 11.1 (3.9) |
MEWS | 4 | 1.4 (1.1) | 1.9 (1.4) | 5.2 (2.5) | 7.1 (3) |
NHS | 0 | 1.5 (1.1) | 2.3 (1.6) | 9.3 (3.5) | 13.5 (4.1) |
PHANTASi | 0 | 1.4 (1.1) | 2.2 (1.5) | 5.9 (2.8) | 8.1 (3.4) |
PITSTOP | 0 | 0.2 (0.4) | 0.2 (0.5) | 0.4 (0.6) | 0.4 (0.7) |
PreSAT | 0 | 1.6 (1.2) | 2.8 (1.7) | 10.6 (3.8) | 15.4 (4.8) |
PRESEP | 3 | 1.6 (1.2) | 2.8 (1.7) | 8.8 (3.6) | 11.3 (4.2) |
PRESS | 1 | 0.3 (0.5) | 0.3 (0.6) | 0.9 (1.1) | 1.1 (1.2) |
PSP | 1 | 1.7 (1.2) | 2.9 (1.8) | 12.6 (4.2) | 22.3 (5.6) |
REMS | 2 | 1.8 (1.3) | 3.9 (2.1) | 24.9 (6.4) | 49.1 (9.5) |
RST | 0 | 1.8 (1.3) | 3.4 (1.9) | 15.2 (4.6) | 25.7 (6.3) |
SAS | 0 | 0.1 (0.3) | 0.1 (0.4) | 0.4 (0.6) | 0.5 (0.7) |
SEPSIS | 4 | 0.9 (0.9) | 1.1 (1) | 2.4 (1.7) | 2.7 (1.7) |
STSS | 1 | 1.4 (1.2) | 2.6 (1.7) | 11.7 (4.1) | 17 (4.9) |
Suffoletto | 0 | 1.4 (1.2) | 2.4 (1.6) | 7 (3.1) | 9.2 (3.6) |
UK | 0 | 1.7 (1.3) | 3.1 (1.9) | 14.2 (4.6) | 22.6 (5.7) |
Early warning score | Threshold | Impression | |||
---|---|---|---|---|---|
Sepsis | Sepsis/infection | Sepsis/infection/non-specific | All | ||
Impression alone | 2.5 (1.6) | 8 (3.2) | 41 (8.6) | 93.5 (14.7) | |
Pre-alerted | 1 (1) | 1.3 (1.1) | 2.9 (1.8) | 4.8 (2.3) | |
NEWS2 | 4 | 2.2 (1.5) | 5.3 (2.6) | 16.1 (5.1) | 22.7 (6.2) |
NEWS2 | 6 | 1.9 (1.4) | 3.6 (2.1) | 9.6 (3.5) | 12.3 (3.9) |
qSOFA | 0 | 2.2 (1.5) | 5.9 (2.7) | 23.1 (6.1) | 39.3 (8.2) |
qSOFA | 1 | 1.2 (1.1) | 2 (1.5) | 5.1 (2.5) | 7.4 (2.9) |
90-30-90 | 0 | 1.6 (1.2) | 3.3 (2) | 11.6 (4) | 15.4 (4.7) |
Borelli | 0 | 1.9 (1.4) | 3.9 (2.2) | 9.5 (3.7) | 12.4 (4.2) |
CIS | 0 | 2.5 (1.6) | 7.7 (3.1) | 37.3 (8) | 79 (12.9) |
CIS | 4 | 0.5 (0.8) | 0.6 (0.8) | 1.5 (1.3) | 1.9 (1.4) |
HEWS | 4 | 1.9 (1.4) | 4 (2.2) | 11.2 (3.9) | 15.7 (4.7) |
MEWS | 4 | 1.7 (1.3) | 3.3 (2) | 7.3 (3.1) | 10.1 (3.6) |
NHS | 0 | 1.8 (1.3) | 3.9 (2.2) | 13.3 (4.4) | 19.7 (5.4) |
PHANTASi | 0 | 1.8 (1.4) | 4.4 (2.3) | 8.9 (3.6) | 12.7 (4.4) |
PITSTOP | 0 | 0.2 (0.5) | 0.4 (0.6) | 0.5 (0.7) | 0.6 (0.8) |
PreSAT | 0 | 2.2 (1.5) | 5.5 (2.7) | 15.2 (5.1) | 23.1 (6.1) |
PRESEP | 3 | 2.2 (1.5) | 5.6 (2.7) | 12.9 (4.7) | 16.7 (5.3) |
PRESS | 1 | 0.4 (0.7) | 0.6 (0.8) | 1.5 (1.2) | 1.8 (1.3) |
PSP | 1 | 2.3 (1.5) | 5.6 (2.7) | 18.5 (5.1) | 35.1 (7.2) |
REMS | 2 | 2.4 (1.5) | 7.3 (3) | 35.4 (7.8) | 73.3 (12.3) |
RST | 0 | 2.4 (1.5) | 6.4 (2.8) | 22.6 (6.1) | 40.2 (8) |
SAS | 0 | 0.2 (0.5) | 0.3 (0.5) | 0.6 (0.8) | 0.8 (0.9) |
SEPSIS | 4 | 1.3 (1.2) | 2 (1.5) | 3.6 (2.1) | 4.1 (2.2) |
STSS | 1 | 2 (1.4) | 4.8 (2.4) | 17 (5.1) | 25.4 (6.4) |
Suffoletto | 0 | 2 (1.4) | 5 (2.5) | 10.6 (4) | 14.3 (4.6) |
UK | 0 | 2.2 (1.5) | 5.5 (2.7) | 21.2 (5.8) | 34.7 (7.8) |
Early warning score | Threshold | Impression | |||
---|---|---|---|---|---|
Sepsis | Sepsis/infection | Sepsis/infection/non-specific | All | ||
Impression alone | 1.9 (1.4) | 4.4 (2.3) | 23.8 (5.3) | 51.3 (8.9) | |
Pre-alerted | 1 (1.1) | 1.2 (1.1) | 2.8 (1.7) | 4.5 (2.2) | |
NEWS2 | 4 | 1.8 (1.4) | 3.1 (1.9) | 9.2 (3.5) | 12.5 (3.9) |
NEWS2 | 6 | 1.5 (1.3) | 2.2 (1.6) | 5.7 (2.7) | 6.9 (2.8) |
qSOFA | 0 | 1.8 (1.4) | 3.4 (2) | 13.7 (4) | 22.5 (5.2) |
qSOFA | 1 | 0.9 (1) | 1.3 (1.1) | 3.2 (1.9) | 4.3 (2) |
90-30-90 | 0 | 1.2 (1.1) | 2 (1.5) | 6.6 (2.9) | 8.6 (3.2) |
Borelli | 0 | 1.5 (1.2) | 2.3 (1.6) | 5.6 (2.5) | 7.1 (2.7) |
CIS | 0 | 1.9 (1.4) | 4.3 (2.2) | 21.7 (5.1) | 43.9 (8.1) |
CIS | 4 | 0.4 (0.6) | 0.4 (0.6) | 0.8 (1) | 1 (1) |
HEWS | 4 | 1.5 (1.3) | 2.3 (1.6) | 6.5 (2.8) | 8.8 (3.1) |
MEWS | 4 | 1.4 (1.2) | 2 (1.5) | 4.3 (2.2) | 5.8 (2.3) |
NHS | 0 | 1.5 (1.3) | 2.4 (1.7) | 7.7 (3.1) | 11.1 (3.7) |
PHANTASi | 0 | 1.5 (1.2) | 2.4 (1.6) | 5.1 (2.5) | 6.9 (2.8) |
PITSTOP | 0 | 0.2 (0.4) | 0.2 (0.4) | 0.4 (0.6) | 0.4 (0.6) |
PreSAT | 0 | 1.7 (1.3) | 3.1 (1.9) | 8.8 (3.3) | 12.9 (3.9) |
PRESEP | 3 | 1.7 (1.3) | 3 (1.8) | 7.5 (3.1) | 9.5 (3.5) |
PRESS | 1 | 0.3 (0.6) | 0.4 (0.6) | 0.8 (0.9) | 1 (1) |
PSP | 1 | 1.7 (1.3) | 3.1 (1.9) | 11.1 (3.6) | 19.7 (4.8) |
REMS | 2 | 1.9 (1.4) | 4.1 (2.2) | 20.7 (4.9) | 41 (7.9) |
RST | 0 | 1.8 (1.4) | 3.6 (2) | 13.1 (3.8) | 22.2 (5.2) |
SAS | 0 | 0.2 (0.4) | 0.2 (0.4) | 0.4 (0.6) | 0.5 (0.6) |
SEPSIS | 4 | 0.9 (1) | 1.2 (1.1) | 2.1 (1.6) | 2.3 (1.6) |
STSS | 1 | 1.5 (1.3) | 2.8 (1.8) | 10 (3.5) | 14.6 (4.2) |
Suffoletto | 0 | 1.6 (1.3) | 2.7 (1.7) | 6.1 (2.7) | 7.9 (3.1) |
UK | 0 | 1.7 (1.4) | 3.2 (1.9) | 12.3 (3.8) | 19.5 (4.9) |
Early warning score | Threshold | Impression | |||
---|---|---|---|---|---|
Sepsis | Sepsis/infection | Sepsis/infection/non-specific | All | ||
Impression alone | 5 (2.4) | 21.4 (6) | 52.1 (9.5) | 74 (11) | |
Pre-alerted | 2.5 (1.7) | 3.3 (1.9) | 4.2 (2.2) | 7.6 (2.8) | |
NEWS2 | 4 | 4.4 (2.3) | 11.6 (4.2) | 18.1 (5.1) | 23.7 (5.8) |
NEWS2 | 6 | 3.7 (2) | 7.6 (3.1) | 10.3 (3.7) | 12.9 (4) |
qSOFA | 0 | 4.5 (2.3) | 14.1 (4.7) | 25.9 (6.2) | 34.9 (7) |
qSOFA | 1 | 2.2 (1.5) | 4 (2.1) | 6 (2.5) | 7.5 (2.8) |
90-30-90 | 0 | 3.1 (1.9) | 6.9 (3.1) | 10.6 (3.8) | 13.2 (4.1) |
Borelli | 0 | 3.4 (2) | 7.1 (3.1) | 9.3 (3.5) | 11.3 (3.8) |
CIS | 0 | 4.9 (2.4) | 19.7 (5.7) | 44 (8.6) | 63.1 (10.1) |
CIS | 4 | 0.8 (0.9) | 1.1 (1.1) | 1.4 (1.2) | 1.8 (1.4) |
HEWS | 4 | 3.7 (2.1) | 8 (3.3) | 11.7 (4) | 15.4 (4.4) |
MEWS | 4 | 3.4 (1.9) | 6.7 (3) | 9.2 (3.4) | 11.7 (3.6) |
NHS | 0 | 3.8 (2) | 8.9 (3.5) | 14.6 (4.4) | 19.2 (4.8) |
PHANTASi | 0 | 3.1 (1.8) | 7.7 (3.2) | 9.8 (3.6) | 11.5 (3.9) |
PITSTOP | 0 | 0.5 (0.7) | 0.8 (1) | 0.9 (1) | 0.9 (1) |
PreSAT | 0 | 4.3 (2.1) | 11.8 (4.3) | 17.9 (5.1) | 22 (5.4) |
PRESEP | 3 | 3.8 (2.1) | 10.2 (4) | 13.2 (4.4) | 14.8 (4.7) |
PRESS | 1 | 1 (1) | 1.6 (1.4) | 2.1 (1.6) | 2.4 (1.6) |
PSP | 1 | 4.2 (2.2) | 13 (4.4) | 26.1 (5.8) | 33.7 (6.5) |
REMS | 2 | 4.8 (2.4) | 18.6 (5.5) | 40.3 (8.2) | 57.4 (9.4) |
RST | 0 | 4.6 (2.3) | 15 (4.9) | 27.5 (6.4) | 37 (7.2) |
SAS | 0 | 0.5 (0.7) | 0.7 (0.8) | 1 (1) | 1.1 (1) |
SEPSIS | 4 | 1.8 (1.4) | 3.1 (1.9) | 3.5 (2.1) | 3.7 (2.1) |
STSS | 1 | 3.7 (2.1) | 10.5 (3.8) | 16.5 (4.8) | 21.3 (5.4) |
Suffoletto | 0 | 3.5 (1.9) | 9 (3.6) | 12.5 (4.2) | 13.9 (4.4) |
UK | 0 | 4.4 (2.3) | 12.7 (4.3) | 22.2 (5.5) | 30.5 (6.3) |
The colours in Tables 5–8 indicate the proportion of eligible attendances that would be prioritised and thus whether the number of prioritised cases would be manageable. Green indicates that < 5% of the mean daily attendances would be prioritised (likely to be manageable), amber indicates that 5–10% would be prioritised (uncertain) and red indicates that more than 10% would be prioritised (unlikely to be manageable).
The mean (SD) number of daily attendances meeting the study inclusion criteria were 93.5 (14.7) at Sheffield Northern General Hospital, 59.5 (10.8) at Doncaster Royal Infirmary, 51.3 (8.9) at Rotherham General Hospital, and 74 (11) at University Hospitals Coventry and Warwickshire. The proportion of attendances that the ambulance service pre-alerted to the hospital varied from 5.1% (4.8/93.5) at Sheffield Northern General Hospital to 10.3% (7.6/74) at University Hospitals Coventry and Warwickshire, with around 1 in 5 of the pre-alerted attendances at the Yorkshire hospitals having a diagnostic impression of sepsis, compared to 1 in 3 in the West Midlands.
The proportions of cases in categories 1 and 2 were higher at University Hospitals Coventry and Warwickshire because paramedics at West Midlands Ambulance Service can select multiple diagnostic impressions whereas paramedics at Yorkshire Ambulance Service can select only one. As a consequence, the index tests would generally result in more attendances being prioritised at University Hospitals Coventry and Warwickshire than at the Yorkshire hospitals. The combination of an early warning score with a diagnostic impression of sepsis in the West Midlands would result in a similar proportion of attendances being prioritised as the combination of an early warning score with a diagnostic impression of sepsis or infection in Yorkshire.
Most early warning scores operating at their pre-specified threshold would prioritise a substantial proportion of attendances if they were applied to attendances other than just those with a diagnostic impression of sepsis or infection. The exceptions are qSOFA (threshold > 1), the SEPSIS score, the CIS (threshold > 4), the Paramedic Initiated Treatment of Sepsis Targeting Out-of-hospital Patients clinical trial (PITSTOP) rule, the PRESS score and the sepsis alert criteria. It should be noted that the PITSTOP rule and sepsis alert criteria, along with the Prehospital Sepsis Assessment Tool (PreSAT), Robson and Suffoletto criteria, all specify paramedic suspicion of sepsis or infection as a qualifying criterion, so should only be applied to diagnostic categories 1 and 2.
In summary, to avoid prioritising a substantial proportion of cases, most early warning scores would need to be applied only to attendances with a primary diagnostic impression of sepsis or infection, or with sepsis as one of a number of diagnostic impressions, depending upon how the ambulance service records diagnostic impression.
Data linkage to Sheffield Teaching Hospitals National Health Service Trust
National Health Service numbers were available to link ambulance service to hospital data for 14,050/24,955 (56.3%) patients transported to Sheffield Teaching Hospitals. Table 9 compares the characteristics of the patients with NHS numbers available to those without. The patients with NHS numbers were markedly older (median age 71 vs. 55 years). They were more likely to be female (54.7% vs. 53.0%) and white ethnicity (95.7% vs. 91.8%), although these characteristics may reflect their older age. They were more likely to have a diagnostic impression of sepsis (2.9% vs. 2.0%), infection (6.5% vs. 4.3%) or non-specific presentation (35.3% vs. 32.0%). The difference in median age between the linked and unlinked populations was greatest for those in the ‘other’ diagnostic impression category and was not seen in those with a diagnostic impression of sepsis.
Not linked (N = 10,905) | Linked (N = 14,050) | Total (N = 24,955) | |
---|---|---|---|
Age (years) | |||
Mean (SD) | 55.2 (23.3) | 65.3 (21.2) | 60.9 (22.7) |
Median (IQR) | 55.0 (34.0–76.0) | 71.0 (51.0–82.0) | 65.0 (42.0–80.0) |
Range | 16.0–102.0 | 16.0–105.0 | 16.0–105.0 |
Sex | |||
Missing | 0 | 22 | 22 |
Female (%) | 5484 (50.3) | 7672 (54.7) | 13,156 (52.8) |
Male (%) | 5421 (49.7) | 6356 (45.3) | 11,777 (47.2) |
Ethnicity | |||
Missing | 5290 | 6880 | 12,170 |
White (%) | 5153 (91.8) | 6860 (95.7) | 12,013 (94.0) |
Asian (%) | 136 (2.4) | 122 (1.7) | 258 (2.0) |
Black (%) | 73 (1.3) | 55 (0.8) | 128 (1.0) |
Mixed (%) | 49 (0.9) | 32 (0.4) | 81 (0.6) |
Other (%) | 204 (3.6) | 101 (1.4) | 305 (2.4) |
ACVPU | |||
Missing | 0 | 0 | 0 |
Alert (%) | 9754 (89.4) | 13,232 (94.2) | 22,986 (92.1) |
Confusion (%) | 341 (3.1) | 387 (2.8) | 728 (2.9) |
Pain (%) | 192 (1.8) | 107 (0.8) | 299 (1.2) |
Unresponsive (%) | 232 (2.1) | 67 (0.5) | 299 (1.2) |
Voice (%) | 386 (3.5) | 257 (1.8) | 643 (2.6) |
GCS | |||
Mean (SD) | 14.4 (2.0) | 14.7 (1.2) | 14.5 (1.6) |
Median (IQR) | 15.0 (15.0–15.0) | 15.0 (15.0–15.0) | 15.0 (15.0–15.0) |
Range | 3.0–15.0 | 3.0–15.0 | 3.0–15.0 |
Diastolic BP (mmHg) | |||
Mean (SD) | 83.1 (17.5) | 82.1 (17.2) | 82.6 (17.4) |
Median (IQR) | 83.0 (72.0–94.0) | 82.0 (71.0–93.0) | 82.0 (71.0–93.0) |
Range | 0.0–190.0 | 5.0–195.0 | 0.0–195.0 |
Systolic BP (mmHg) | |||
Mean (SD) | 139.0 (26.5) | 142.1 (27.4) | 140.8 (27.1) |
Median (IQR) | 138.0 (122.0–153.0) | 140.0 (124.0–158.0) | 139.0 (123.0–156.0) |
Range | 53.0–257.0 | 43.0–285.0 | 43.0–285.0 |
Heart rate (beats/minute) | |||
Mean (SD) | 89.5 (22.8) | 88.7 (21.9) | 89.1 (22.3) |
Median (IQR) | 87.0 (74.0–103.0) | 86.0 (73.0–102.0) | 86.0 (74.0–102.0) |
Range | 0.0–218.0 | 0.0–216.0 | 0.0–218.0 |
Oxygen saturation (%) | |||
Mean (SD) | 96.0 (4.9) | 95.6 (4.9) | 95.8 (4.9) |
Median (IQR) | 97.0 (95.0–98.0) | 97.0 (95.0–98.0) | 97.0 (95.0–98.0) |
Range | 18.0–100.0 | 10.0–100.0 | 10.0–100.0 |
Supplemental oxygen | |||
Missing | 18 | 27 | 45 |
No (%) | 10,345 (95.0) | 13,252 (94.5) | 23,597 (94.7) |
Yes (%) | 542 (5.0) | 771 (5.5) | 1313 (5.3) |
Respiration (breath/minute) | |||
Mean (SD) | 19.7 (6.0) | 20.5 (6.1) | 20.1 (6.0) |
Median (IQR) | 18.0 (16.0–20.0) | 18.0 (16.0–22.0) | 18.0 (16.0–22.0) |
Range | 0.0–93.0 | 0.0–91.0 | 0.0–93.0 |
Temp (°C) | |||
Mean (SD) | 36.8 (1.0) | 37.0 (1.0) | 36.9 (1.0) |
Median (IQR) | 36.8 (36.2–37.3) | 36.9 (36.4–37.4) | 36.8 (36.4–37.4) |
Range | 26.0–41.3 | 27.1–41.8 | 26.0–41.8 |
Glucose (mmol/l) | |||
Mean (SD) | 7.1 (3.2) | 7.4 (3.4) | 7.2 (3.3) |
Median (IQR) | 6.2 (5.4–7.6) | 6.4 (5.5–8.0) | 6.3 (5.5–7.8) |
Range | 0.5–36.6 | 0.9–49.0 | 0.5–49.0 |
Pre-alerted | |||
No (%) | 10,307 (94.5) | 13,419 (95.5) | 23,726 (95.1) |
Yes (%) | 598 (5.5) | 631 (4.5) | 1229 (4.9) |
Impression | |||
1 – sepsis (%) | 222 (2.0) | 407 (2.9) | 629 (2.5) |
2 – infection (%) | 471 (4.3) | 912 (6.5) | 1383 (5.5) |
3 – non-specific (%) | 3494 (32.0) | 4962 (35.3) | 8456 (33.9) |
4 – other (%) | 6718 (61.6) | 7769 (55.3) | 14,487 (58.1) |
Median (IQR) age by impression | |||
1 – sepsis | 76.5 (60.2–84.0) | 75.0 (63.0–83.0) | 75.0 (62.0–83.0) |
2 – infection | 71.0 (47.0–82.0) | 76.0 (62.0–84.0) | 74.0 (57.0–83.0) |
3 – non-specific | 63.0 (41.0–79.0) | 74.0 (57.0–83.0) | 71.0 (50.0–82.0) |
4 – other | 50.0 (31.0–72.8) | 67.0 (45.0–81.0) | 58.0 (37.0–78.0) |
The Scientific Computing department of Sheffield Teaching Hospitals NHS Trust identified a hospital admission or ED attendance within 48 hours for 12,870 of the 14,050 first episodes with an NHS number transported to the Northern General Hospital (91.6%). The remaining episodes comprised 431 (3.1%) with no data returned for the NHS number and 749 (5.3%) with no admission or attendance within 48 hours. Some 8260 (64.1%) had a hospital admission, of whom 275 (3.3%) had no primary diagnosis code and 347 (4.2%) had no secondary code. Some 10,964 (85.2%) had an ED attendance, of whom 14 (0.1%) had no ED code.
Overall, 684 episodes had a primary or secondary admission diagnosis of sepsis or an ED diagnosis of septicaemia or neutropenic sepsis, and were screened by the research nurses. Of these, 594 had a primary or secondary admission diagnosis of sepsis (315 primary, 322 secondary, 43 both) and 160 had an ED diagnosis of septicaemia or neutropenic sepsis (70 also has an admission primary or secondary diagnosis of sepsis, 90 did not).
The research nurses referred 655/684 (95.8%) for expert review. The experts judged that 368/655 (56.2%) episodes met the sepsis-3 definition and 348/368 (94.6%) of these received treatment for sepsis. Therefore, out of the 12,870 episodes that were linked with hospital attendance or admission, 684 (5.3%) had a diagnostic code for sepsis, 655 (5.1%) had evidence of sepsis diagnosis or treatment in their ED or admission records, 368 (2.9%) met the secondary (diagnosis) reference standard and 348 (2.7%) met the primary (treatment) reference standard. Figure 1 summarises the flow of cases to create the diagnostic accuracy cohort.
FIGURE 1.
Participant flow to create the Sheffield diagnostic accuracy cohort. NGH, Northern General Hospital.
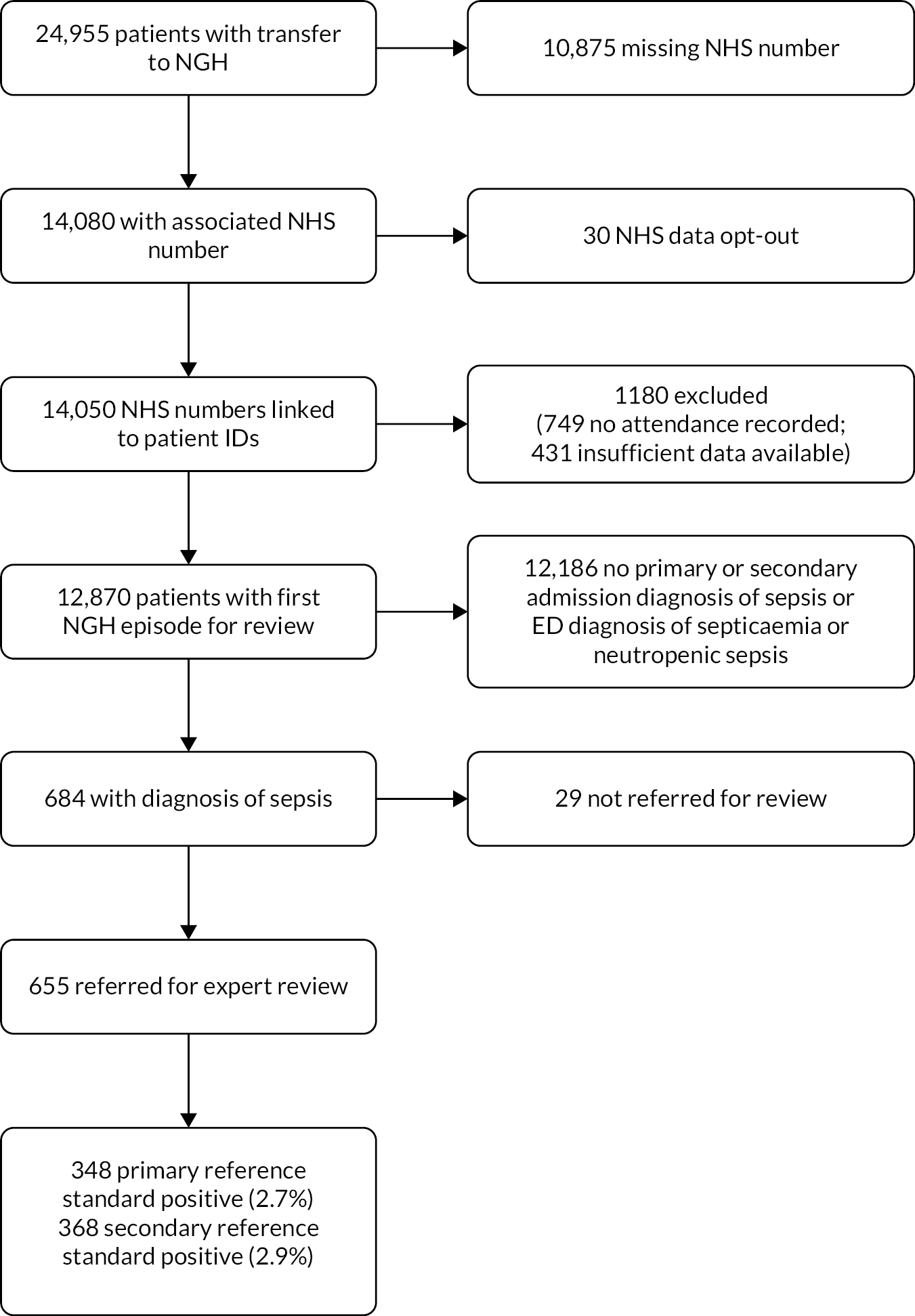
Adjudication of the reference standard
Table 10 shows the agreement between the two doctors adjudicating the reference standard. Agreement was very good on the two key judgements of whether the case met the sepsis-3 criteria and whether treatment for sepsis was given. Agreement was not as good on whether there was evidence of infection, but the cases in which there was disagreement tended to be those in which the doctors agreed that the SOFA score criterion was not met, so they did not affect overall judgement on the sepsis-3 definition.
Assessment | Doctor 1 (%) | Doctor 2 (%) | Consensus (%) | Kappa (95% CI) |
---|---|---|---|---|
Evidence of infection | 86.0 | 87.6 | 84.7 | 0.62 (0.53 to 0.71) |
SOFA score 2 + worse than normal | 60.2 | 61.1 | 60.0 | 0.87 (0.83 to 0.91) |
Patient meets sepsis-3 criteria | 56.0 | 55.0 | 56.2 | 0.89 (0.85 to 0.92) |
Treatment for sepsis given | 52.5 | 51.5 | 53.3 | 0.87 (0.83 to 0.91) |
There was radiological evidence of infection in 175/348 (50.1%) cases with the primary reference standard, microbiological evidence of infection 171 (49.0%) and other evidence of infection in 328 (94.0%). The site of suspected infection was the chest in 155 (44.4%) urine in 78 (22.3%), biliary in 43 (12.3%), abdominal in 16 (4.6%), skin in 25 (7.2%), other in 6 (1.7%) and unknown in 26 (7.4%). They had a mean clinical frailty score of 5.6 (median 6.0, range 2.0–9.0; 3 missing) and a mean SOFA score of 3.9 (median 3.0, range 2.0 to 14.0; 13 missing). They included 138 (39.5%) who had a Do Not Attempt Cardiopulmonary Resuscitation decision recorded, of whom 102 (29.2%) were pre-existing and 36 (10.3%) were made on attendance. Some 28 (8.0%) were admitted to critical care and 261 (74.8%) survived to hospital discharge or 30 days after attendance, whichever was sooner.
Accuracy for the primary (treatment) reference standard
Table 11 shows the area under the ROC curve for each early warning scores for the primary reference standard, when used alongside diagnostic impression. The first column indicates the early warning score and the remaining columns report the area under the ROC curve when the score is only applied to patients with a diagnostic impression of sepsis, only to patients with a diagnostic impression of sepsis or infection, only to patients with a diagnostic impression of sepsis, infection or a non-specific diagnosis, or when applied to all patients.
Early warning score | Sepsis | Sepsis or infection | Sepsis, infection or non-specific impression | All diagnostic impressions |
---|---|---|---|---|
NEWS2 | 0.655 (0.63, 0.68) | 0.756 (0.729, 0.783) | 0.858 (0.836, 0.88) | 0.877 (0.86, 0.895) |
qSOFA | 0.645 (0.62, 0.669) | 0.734 (0.707, 0.761) | 0.809 (0.785, 0.834) | 0.801 (0.778, 0.824) |
90-30-90 | 0.624 (0.601, 0.648) | 0.686 (0.66, 0.712) | 0.743 (0.717, 0.769) | 0.742 (0.717, 0.768) |
Borelli | 0.639 (0.615, 0.663) | 0.712 (0.686, 0.738) | 0.781 (0.755, 0.806) | 0.788 (0.764, 0.813) |
CIS | 0.654 (0.629, 0.679) | 0.755 (0.728, 0.782) | 0.845 (0.822, 0.867) | 0.838 (0.817, 0.859) |
HEWS | 0.654 (0.629, 0.679) | 0.751 (0.724, 0.778) | 0.841 (0.818, 0.863) | 0.837 (0.816, 0.858) |
MEWS | 0.654 (0.629, 0.679) | 0.753 (0.726, 0.78) | 0.851 (0.828, 0.873) | 0.857 (0.837, 0.876) |
NHS | 0.624 (0.601, 0.648) | 0.696 (0.67, 0.722) | 0.751 (0.725, 0.776) | 0.747 (0.723, 0.772) |
PHANTASi | 0.626 (0.602, 0.649) | 0.708 (0.682, 0.735) | 0.745 (0.719, 0.771) | 0.741 (0.716, 0.767) |
PITSTOP | 0.534 (0.52, 0.547) | 0.545 (0.53, 0.56) | 0.549 (0.533, 0.564) | 0.554 (0.537, 0.57) |
PreSAT | 0.648 (0.624, 0.673) | 0.734 (0.708, 0.761) | 0.789 (0.766, 0.813) | 0.775 (0.754, 0.797) |
PRESEP | 0.653 (0.628, 0.678) | 0.75 (0.723, 0.777) | 0.847 (0.824, 0.87) | 0.856 (0.834, 0.878) |
PRESS | 0.551 (0.534, 0.567) | 0.557 (0.54, 0.574) | 0.579 (0.559, 0.599) | 0.587 (0.566, 0.608) |
PSP | 0.654 (0.629, 0.679) | 0.754 (0.728, 0.781) | 0.84 (0.818, 0.862) | 0.832 (0.811, 0.852) |
REMS | 0.654 (0.629, 0.679) | 0.752 (0.725, 0.779) | 0.814 (0.792, 0.836) | 0.757 (0.732, 0.781) |
RST | 0.648 (0.623, 0.672) | 0.737 (0.711, 0.764) | 0.782 (0.761, 0.803) | 0.73 (0.712, 0.747) |
SAS | 0.537 (0.523, 0.551) | 0.542 (0.528, 0.557) | 0.558 (0.541, 0.576) | 0.563 (0.545, 0.581) |
SEPSIS | 0.654 (0.629, 0.679) | 0.755 (0.727, 0.782) | 0.862 (0.84, 0.884) | 0.882 (0.865, 0.899) |
STSS | 0.652 (0.627, 0.677) | 0.749 (0.722, 0.776) | 0.837 (0.814, 0.861) | 0.831 (0.809, 0.854) |
Suffoletto | 0.64 (0.616, 0.664) | 0.728 (0.701, 0.754) | 0.799 (0.775, 0.823) | 0.801 (0.778, 0.824) |
UK | 0.648 (0.623, 0.672) | 0.733 (0.707, 0.76) | 0.788 (0.766, 0.809) | 0.756 (0.737, 0.775) |
The area under the ROC curve for diagnostic impression alone was 0.822 (95% CI 0.799 to 0.845). The area under the ROC curve decreases as each early warning score is used more selectively on the basis of paramedic diagnostic impression. This could be interpreted as suggesting that early warning scores should not be used selectively. However, Figure 2 shows the ROC curves for NEWS2 alongside the categorisations of diagnostic impression. The area under the ROC curve is clearly smaller when NEWS2 is used selectively but the low prevalence of sepsis in the cohort and need to achieve high specificity means that the optimal threshold on the ROC curve is likely to lie where specificity exceeds 0.95. At this point, the ROC curves are very close together and the more selective strategies operating at lower NEWS2 thresholds offer slightly better accuracy.
FIGURE 2.
ROC curves for NEWS2 used alongside paramedic diagnostic impression.
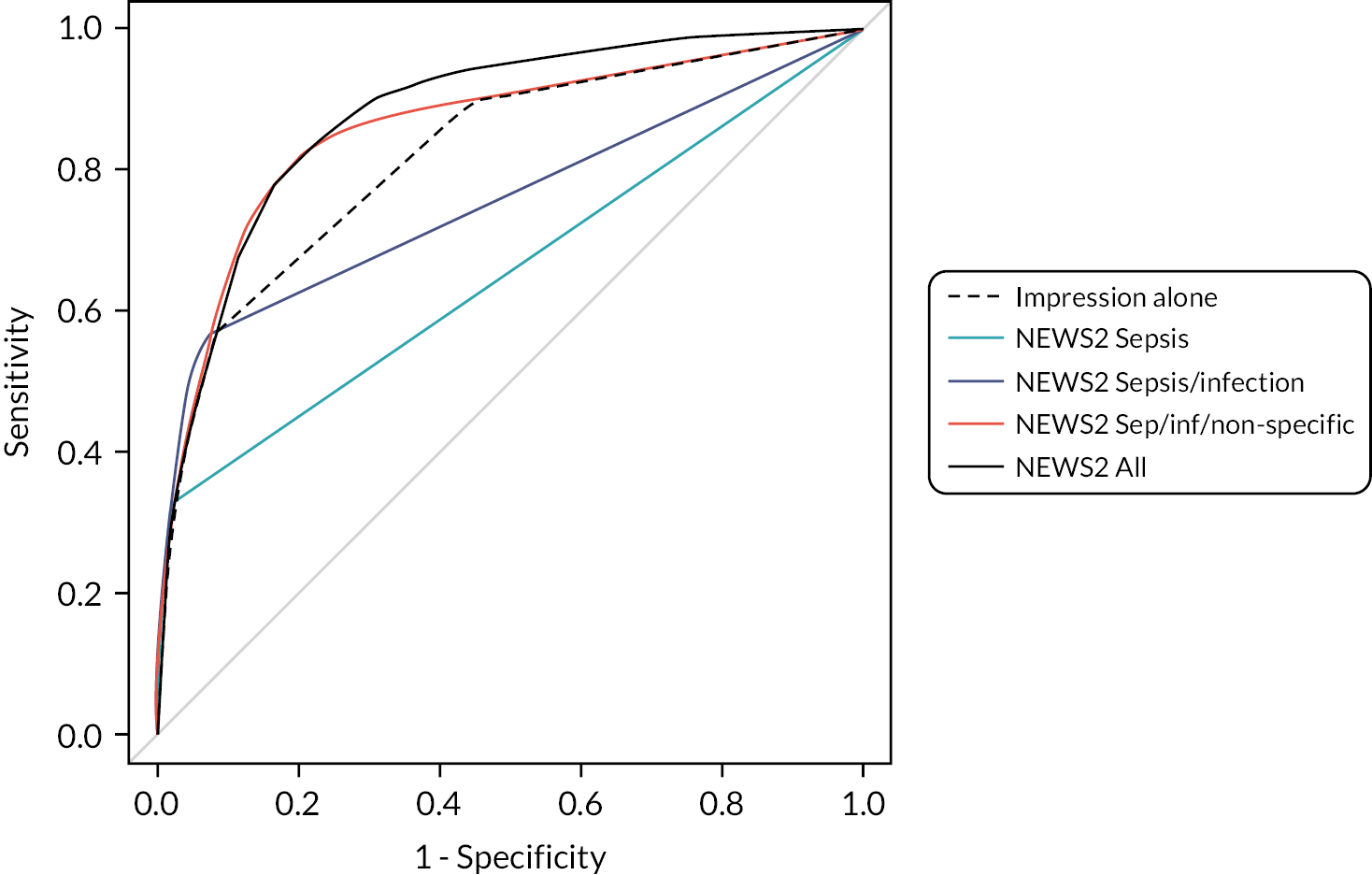
Table 11 also shows that the area under the ROC curve for NEWS2 is generally equivalent to or greater than the other early warning scores. The exceptions are the SEPSIS score when applied to those with non-specific presentations (including sepsis and infection) or all presentations. However, the SEPSIS score does not provide better accuracy than NEWS2 at thresholds providing a positive predictive value exceeding 0.2.
Figures 3–6 show the ROC curves for each score, or a plot of sensitivity and 1−specificity for dichotomised scores, when applied only to attendances with a diagnostic impression of sepsis (see Figure 3), only to attendances with a diagnostic impression of sepsis or infection (Figure 4), only to attendances with a diagnostic impression of sepsis, infection or a non-specific impression (Figure 5), and applied to all attendances (Figure 6). Each figure has two plots to avoid presenting too many overlapping curves on the same figure. The curves in Figures 3–5 have a straight line from the point on the curve at which the sensitivity of the combination of the score and paramedic diagnostic impression reaches the sensitivity of the paramedic diagnostic impression alone. This is because these strategies all involve applying the scores selectively on the basis of paramedic diagnostic impression. The sensitivity of the combination of the score and diagnostic impression cannot therefore exceed the sensitivity of the diagnostic impression alone.
FIGURE 3.
ROC curves for early warning scores applied to diagnostic impression of sepsis.

FIGURE 4.
ROC curves for early warning scores applied to diagnostic impression of sepsis or infection.

FIGURE 5.
ROC curves for early warning scores applied to diagnostic impression of sepsis, infection or non-specific presentation.
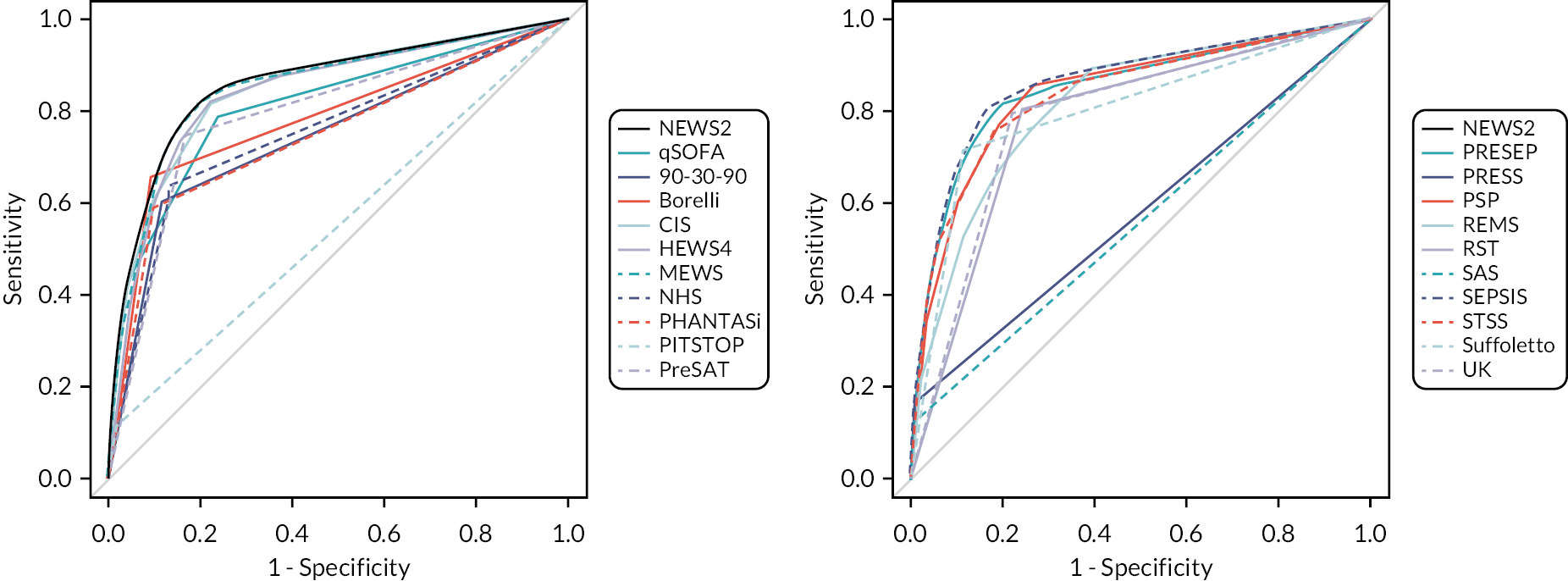
FIGURE 6.
ROC curves for early warning scores applied to all diagnostic impressions.

The figures show that NEWS2 has superior or similar accuracy to the other scores, with the possible exception of the SEPSIS score when applied to non-specific presentations or all cases at a threshold that gives sensitivity and specificity both around 0.8–0.9.
Table 12 shows the accuracy of the categorised paramedic diagnostic impression for the primary reference standard. Paramedic diagnostic impression of sepsis identified around 33% of treated sepsis cases, diagnostic impression of infection (including sepsis) identified around 57%, non-specific presentation (including sepsis and infection) identified around 90%, leaving around 10% with diagnostic impressions that would not suggest sepsis. Along with the limited positive predictive value (28.5% of those with a paramedic diagnostic impression of sepsis had the primary reference standard), this illustrates the diagnostic challenge of identifying sepsis in the prehospital setting.
Threshold | N | TP | FP | FN | TN | Sensitivity | Specificity | PPV | NPV |
---|---|---|---|---|---|---|---|---|---|
Sepsis | 12,870 | 114 | 286 | 234 | 12,236 | 0.328 (0.28, 0.379) | 0.977 (0.974, 0.98) | 0.285 (0.243, 0.331) | 0.981 (0.979, 0.983) |
Sepsis or infection | 12,870 | 199 | 1080 | 149 | 11,442 | 0.572 (0.519, 0.623) | 0.914 (0.909, 0.919) | 0.156 (0.137, 0.176) | 0.987 (0.985, 0.989) |
Sepsis, infection or non-specific impression | 12,870 | 312 | 5576 | 36 | 6946 | 0.897 (0.86, 0.924) | 0.555 (0.546, 0.563) | 0.053 (0.048, 0.059) | 0.995 (0.993, 0.996) |
Tables 13–16 show the diagnostic accuracy parameters for each threshold of the NEWS2 score when applied only to attendances with a diagnostic impression of sepsis (see Table 13), only to attendances with a diagnostic impression of sepsis or infection (see Table 14), only to attendances with a diagnostic impression of sepsis, infection or a non-specific impression (see Table 15), and applied to all attendances (see Table 16). The low prevalence of the reference standard (347/12,859, 2.7%) means that the PPV is relatively low even when specificity is high, and the NPV is relatively high even when sensitivity is low. Tables 17–20 report the same parameters for all thresholds of qSOFA and the pre-specified thresholds of the other early warning scores. The first row, marked ‘pre-alert’, shows the sensitivity and specificity of pre-alert practice in 2019, as recorded on the PRF.
Threshold | N | TP | FP | FN | TN | Sensitivity | Specificity | PPV | NPV |
---|---|---|---|---|---|---|---|---|---|
0 | 12,859 | 114 | 282 | 233 | 12,230 | 0.329 (0.281, 0.38) | 0.977 (0.975, 0.98) | 0.288 (0.245, 0.334) | 0.981 (0.979, 0.984) |
1 | 12,859 | 114 | 278 | 233 | 12,234 | 0.329 (0.281, 0.38) | 0.978 (0.975, 0.98) | 0.291 (0.248, 0.338) | 0.981 (0.979, 0.984) |
2 | 12,859 | 114 | 273 | 233 | 12,239 | 0.329 (0.281, 0.38) | 0.978 (0.975, 0.981) | 0.295 (0.251, 0.342) | 0.981 (0.979, 0.984) |
3 | 12,859 | 113 | 262 | 234 | 12,250 | 0.326 (0.278, 0.377) | 0.979 (0.976, 0.981) | 0.301 (0.257, 0.35) | 0.981 (0.979, 0.983) |
4 | 12,859 | 111 | 245 | 236 | 12,267 | 0.32 (0.273, 0.371) | 0.98 (0.978, 0.983) | 0.312 (0.266, 0.362) | 0.981 (0.979, 0.983) |
5 | 12,859 | 108 | 228 | 239 | 12,284 | 0.311 (0.265, 0.362) | 0.982 (0.979, 0.984) | 0.321 (0.274, 0.373) | 0.981 (0.978, 0.983) |
6 | 12,859 | 105 | 190 | 242 | 12,322 | 0.303 (0.257, 0.353) | 0.985 (0.983, 0.987) | 0.356 (0.303, 0.412) | 0.981 (0.978, 0.983) |
7 | 12,859 | 95 | 157 | 252 | 12,355 | 0.274 (0.23, 0.323) | 0.987 (0.985, 0.989) | 0.377 (0.319, 0.438) | 0.98 (0.977, 0.982) |
8 | 12,859 | 86 | 129 | 261 | 12,383 | 0.248 (0.205, 0.296) | 0.99 (0.988, 0.991) | 0.4 (0.337, 0.467) | 0.979 (0.977, 0.982) |
9 | 12,859 | 73 | 94 | 274 | 12,418 | 0.21 (0.171, 0.256) | 0.992 (0.991, 0.994) | 0.437 (0.364, 0.513) | 0.978 (0.976, 0.981) |
10 | 12,859 | 59 | 65 | 288 | 12,447 | 0.17 (0.134, 0.213) | 0.995 (0.993, 0.996) | 0.476 (0.39, 0.563) | 0.977 (0.975, 0.98) |
11 | 12,859 | 40 | 36 | 307 | 12,476 | 0.115 (0.086, 0.153) | 0.997 (0.996, 0.998) | 0.526 (0.416, 0.635) | 0.976 (0.973, 0.978) |
12 | 12,859 | 25 | 21 | 322 | 12,491 | 0.072 (0.049, 0.104) | 0.998 (0.997, 0.999) | 0.543 (0.402, 0.678) | 0.975 (0.972, 0.977) |
13 | 12,859 | 8 | 12 | 339 | 12,500 | 0.023 (0.012, 0.045) | 0.999 (0.998, 0.999) | 0.4 (0.219, 0.613) | 0.974 (0.971, 0.976) |
14 | 12,859 | 4 | 7 | 343 | 12,505 | 0.012 (0.004, 0.029) | 0.999 (0.999, 1) | 0.364 (0.152, 0.646) | 0.973 (0.97, 0.976) |
15 | 12,859 | 0 | 3 | 347 | 12,509 | 0 (0, 0.011) | 1 (0.999, 1) | 0 (0, 0.561) | 0.973 (0.97, 0.976) |
16 | 12,859 | 0 | 0 | 347 | 12,512 | 0 (0, 0.011) | 1 (1, 1) | - | 0.973 (0.97, 0.976) |
Threshold | N | TP | FP | FN | TN | Sensitivity | Specificity | PPV | NPV |
---|---|---|---|---|---|---|---|---|---|
0 | 12,859 | 198 | 1032 | 149 | 11,480 | 0.571 (0.518, 0.622) | 0.918 (0.913, 0.922) | 0.161 (0.141, 0.183) | 0.987 (0.985, 0.989) |
1 | 12,859 | 197 | 967 | 150 | 11,545 | 0.568 (0.515, 0.619) | 0.923 (0.918, 0.927) | 0.169 (0.149, 0.192) | 0.987 (0.985, 0.989) |
2 | 12,859 | 197 | 889 | 150 | 11,623 | 0.568 (0.515, 0.619) | 0.929 (0.924, 0.933) | 0.181 (0.16, 0.205) | 0.987 (0.985, 0.989) |
3 | 12,859 | 191 | 776 | 156 | 11,736 | 0.55 (0.498, 0.602) | 0.938 (0.934, 0.942) | 0.198 (0.174, 0.224) | 0.987 (0.985, 0.989) |
4 | 12,859 | 181 | 658 | 166 | 11,854 | 0.522 (0.469, 0.574) | 0.947 (0.943, 0.951) | 0.216 (0.189, 0.245) | 0.986 (0.984, 0.988) |
5 | 12,859 | 171 | 540 | 176 | 11,972 | 0.493 (0.441, 0.545) | 0.957 (0.953, 0.96) | 0.241 (0.211, 0.273) | 0.986 (0.983, 0.987) |
6 | 12,859 | 155 | 410 | 192 | 12,102 | 0.447 (0.395, 0.499) | 0.967 (0.964, 0.97) | 0.274 (0.239, 0.313) | 0.984 (0.982, 0.986) |
7 | 12,859 | 133 | 314 | 214 | 12,198 | 0.383 (0.334, 0.435) | 0.975 (0.972, 0.978) | 0.298 (0.257, 0.342) | 0.983 (0.98, 0.985) |
8 | 12,859 | 109 | 218 | 238 | 12,294 | 0.314 (0.268, 0.365) | 0.983 (0.98, 0.985) | 0.333 (0.284, 0.386) | 0.981 (0.978, 0.983) |
9 | 12,859 | 91 | 146 | 256 | 12,366 | 0.262 (0.219, 0.311) | 0.988 (0.986, 0.99) | 0.384 (0.324, 0.447) | 0.98 (0.977, 0.982) |
10 | 12,859 | 68 | 91 | 279 | 12,421 | 0.196 (0.158, 0.241) | 0.993 (0.991, 0.994) | 0.428 (0.353, 0.505) | 0.978 (0.975, 0.98) |
11 | 12,859 | 47 | 48 | 300 | 12,464 | 0.135 (0.103, 0.175) | 0.996 (0.995, 0.997) | 0.495 (0.396, 0.594) | 0.976 (0.974, 0.979) |
12 | 12,859 | 29 | 28 | 318 | 12,484 | 0.084 (0.059, 0.117) | 0.998 (0.997, 0.998) | 0.509 (0.383, 0.634) | 0.975 (0.972, 0.978) |
13 | 12,859 | 11 | 17 | 336 | 12,495 | 0.032 (0.018, 0.056) | 0.999 (0.998, 0.999) | 0.393 (0.236, 0.576) | 0.974 (0.971, 0.976) |
14 | 12,859 | 5 | 8 | 342 | 12,504 | 0.014 (0.006, 0.033) | 0.999 (0.999, 1) | 0.385 (0.177, 0.645) | 0.973 (0.97, 0.976) |
15 | 12,859 | 0 | 3 | 347 | 12,509 | 0 (0, 0.011) | 1 (0.999, 1) | 0 (0, 0.561) | 0.973 (0.97, 0.976) |
16 | 12,859 | 0 | 0 | 347 | 12,512 | 0 (0, 0.011) | 1 (1, 1) | - | 0.973 (0.97, 0.976) |
Threshold | N | TP | FP | FN | TN | Sensitivity | Specificity | PPV | NPV |
---|---|---|---|---|---|---|---|---|---|
0 | 12,859 | 307 | 4638 | 40 | 7874 | 0.885 (0.847, 0.914) | 0.629 (0.621, 0.638) | 0.062 (0.056, 0.069) | 0.995 (0.993, 0.996) |
1 | 12,859 | 302 | 3833 | 45 | 8679 | 0.87 (0.831, 0.902) | 0.694 (0.686, 0.702) | 0.073 (0.065, 0.081) | 0.995 (0.993, 0.996) |
2 | 12,859 | 297 | 3248 | 50 | 9264 | 0.856 (0.815, 0.889) | 0.74 (0.733, 0.748) | 0.084 (0.075, 0.093) | 0.995 (0.993, 0.996) |
3 | 12,859 | 287 | 2598 | 60 | 9914 | 0.827 (0.784, 0.863) | 0.792 (0.785, 0.799) | 0.099 (0.089, 0.111) | 0.994 (0.992, 0.995) |
4 | 12,859 | 270 | 2048 | 77 | 10,464 | 0.778 (0.731, 0.819) | 0.836 (0.83, 0.843) | 0.116 (0.104, 0.13) | 0.993 (0.991, 0.994) |
5 | 12,859 | 252 | 1612 | 95 | 10,900 | 0.726 (0.677, 0.77) | 0.871 (0.865, 0.877) | 0.135 (0.12, 0.151) | 0.991 (0.989, 0.993) |
6 | 12,859 | 220 | 1152 | 127 | 11,360 | 0.634 (0.582, 0.683) | 0.908 (0.903, 0.913) | 0.16 (0.142, 0.181) | 0.989 (0.987, 0.991) |
7 | 12,859 | 181 | 823 | 166 | 11,689 | 0.522 (0.469, 0.574) | 0.934 (0.93, 0.938) | 0.18 (0.158, 0.205) | 0.986 (0.984, 0.988) |
8 | 12,859 | 147 | 513 | 200 | 11,999 | 0.424 (0.373, 0.476) | 0.959 (0.955, 0.962) | 0.223 (0.193, 0.256) | 0.984 (0.981, 0.986) |
9 | 12,859 | 111 | 311 | 236 | 12,201 | 0.32 (0.273, 0.371) | 0.975 (0.972, 0.978) | 0.263 (0.223, 0.307) | 0.981 (0.978, 0.983) |
10 | 12,859 | 82 | 181 | 265 | 12,331 | 0.236 (0.195, 0.284) | 0.986 (0.983, 0.987) | 0.312 (0.259, 0.37) | 0.979 (0.976, 0.981) |
11 | 12,859 | 55 | 90 | 292 | 12,422 | 0.159 (0.124, 0.201) | 0.993 (0.991, 0.994) | 0.379 (0.304, 0.46) | 0.977 (0.974, 0.979) |
12 | 12,859 | 34 | 47 | 313 | 12,465 | 0.098 (0.071, 0.134) | 0.996 (0.995, 0.997) | 0.42 (0.318, 0.528) | 0.976 (0.973, 0.978) |
13 | 12,859 | 12 | 28 | 335 | 12,484 | 0.035 (0.02, 0.059) | 0.998 (0.997, 0.998) | 0.3 (0.181, 0.454) | 0.974 (0.971, 0.976) |
14 | 12,859 | 6 | 12 | 341 | 12,500 | 0.017 (0.008, 0.037) | 0.999 (0.998, 0.999) | 0.333 (0.163, 0.563) | 0.973 (0.971, 0.976) |
15 | 12,859 | 0 | 3 | 347 | 12,509 | 0 (0, 0.011) | 1 (0.999, 1) | 0 (0, 0.561) | 0.973 (0.97, 0.976) |
16 | 12,859 | 0 | 0 | 347 | 12,512 | 0 (0, 0.011) | 1 (1, 1) | – | 0.973 (0.97, 0.976) |
Threshold | N | TP | FP | FN | TN | Sensitivity | Specificity | PPV | NPV |
---|---|---|---|---|---|---|---|---|---|
0 | 12,859 | 342 | 9189 | 5 | 3323 | 0.986 (0.967, 0.994) | 0.266 (0.258, 0.273) | 0.036 (0.032, 0.04) | 0.998 (0.996, 0.999) |
1 | 12,859 | 332 | 6749 | 15 | 5763 | 0.957 (0.93, 0.974) | 0.461 (0.452, 0.469) | 0.047 (0.042, 0.052) | 0.997 (0.996, 0.998) |
2 | 12,859 | 326 | 5210 | 21 | 7302 | 0.939 (0.909, 0.96) | 0.584 (0.575, 0.592) | 0.059 (0.053, 0.065) | 0.997 (0.996, 0.998) |
3 | 12,859 | 312 | 3801 | 35 | 8711 | 0.899 (0.863, 0.927) | 0.696 (0.688, 0.704) | 0.076 (0.068, 0.084) | 0.996 (0.994, 0.997) |
4 | 12,859 | 290 | 2792 | 57 | 9720 | 0.836 (0.793, 0.871) | 0.777 (0.769, 0.784) | 0.094 (0.084, 0.105) | 0.994 (0.992, 0.995) |
5 | 12,859 | 271 | 2088 | 76 | 10,424 | 0.781 (0.735, 0.821) | 0.833 (0.826, 0.84) | 0.115 (0.103, 0.128) | 0.993 (0.991, 0.994) |
6 | 12,859 | 235 | 1460 | 112 | 11,052 | 0.677 (0.626, 0.724) | 0.883 (0.878, 0.889) | 0.139 (0.123, 0.156) | 0.99 (0.988, 0.992) |
7 | 12,859 | 196 | 1018 | 151 | 11,494 | 0.565 (0.512, 0.616) | 0.919 (0.914, 0.923) | 0.161 (0.142, 0.183) | 0.987 (0.985, 0.989) |
8 | 12,859 | 155 | 616 | 192 | 11,896 | 0.447 (0.395, 0.499) | 0.951 (0.947, 0.954) | 0.201 (0.174, 0.231) | 0.984 (0.982, 0.986) |
9 | 12,859 | 117 | 364 | 230 | 12,148 | 0.337 (0.289, 0.388) | 0.971 (0.968, 0.974) | 0.243 (0.207, 0.284) | 0.981 (0.979, 0.984) |
10 | 12,859 | 86 | 214 | 261 | 12,298 | 0.248 (0.205, 0.296) | 0.983 (0.98, 0.985) | 0.287 (0.238, 0.34) | 0.979 (0.977, 0.982) |
11 | 12,859 | 57 | 110 | 290 | 12,402 | 0.164 (0.129, 0.207) | 0.991 (0.989, 0.993) | 0.341 (0.274, 0.416) | 0.977 (0.974, 0.98) |
12 | 12,859 | 36 | 57 | 311 | 12,455 | 0.104 (0.076, 0.14) | 0.995 (0.994, 0.996) | 0.387 (0.294, 0.489) | 0.976 (0.973, 0.978) |
13 | 12,859 | 13 | 35 | 334 | 12,477 | 0.037 (0.022, 0.063) | 0.997 (0.996, 0.998) | 0.271 (0.166, 0.41) | 0.974 (0.971, 0.977) |
14 | 12,859 | 6 | 15 | 341 | 12,497 | 0.017 (0.008, 0.037) | 0.999 (0.998, 0.999) | 0.286 (0.138, 0.5) | 0.973 (0.971, 0.976) |
15 | 12,859 | 0 | 4 | 347 | 12,508 | 0 (0, 0.011) | 1 (0.999, 1) | 0 (0, 0.49) | 0.973 (0.97, 0.976) |
16 | 12,859 | 0 | 1 | 347 | 12,511 | 0 (0, 0.011) | 1 (1, 1) | 0 (0, 0.793) | 0.973 (0.97, 0.976) |
EWS | Threshold | N | TP | FP | FN | TN | Sensitivity | Specificity | PPV | NPV |
---|---|---|---|---|---|---|---|---|---|---|
Pre-alert | 0 | 12,870 | 45 | 110 | 303 | 12,412 | 0.129 (0.098, 0.169) | 0.991 (0.989, 0.993) | 0.29 (0.225, 0.366) | 0.976 (0.973, 0.979) |
qSOFA | 0 | 12,869 | 107 | 249 | 241 | 12,272 | 0.307 (0.261, 0.358) | 0.98 (0.978, 0.982) | 0.301 (0.255, 0.35) | 0.981 (0.978, 0.983) |
qSOFA | 1 | 12,869 | 72 | 103 | 276 | 12,418 | 0.207 (0.168, 0.253) | 0.992 (0.99, 0.993) | 0.411 (0.341, 0.485) | 0.978 (0.976, 0.981) |
qSOFA | 2 | 12,869 | 19 | 21 | 329 | 12,500 | 0.055 (0.035, 0.084) | 0.998 (0.997, 0.999) | 0.475 (0.329, 0.625) | 0.974 (0.971, 0.977) |
90-30-90 | 0 | 12,857 | 91 | 169 | 256 | 12,341 | 0.262 (0.219, 0.311) | 0.986 (0.984, 0.988) | 0.35 (0.295, 0.41) | 0.98 (0.977, 0.982) |
Borelli | 0 | 12,835 | 102 | 203 | 245 | 12,285 | 0.294 (0.248, 0.344) | 0.984 (0.981, 0.986) | 0.334 (0.284, 0.389) | 0.98 (0.978, 0.983) |
CIS | 0 | 12,855 | 114 | 283 | 233 | 12,225 | 0.329 (0.281, 0.38) | 0.977 (0.975, 0.98) | 0.287 (0.245, 0.334) | 0.981 (0.979, 0.984) |
CIS | 4 | 12,855 | 32 | 42 | 315 | 12,466 | 0.092 (0.066, 0.127) | 0.997 (0.995, 0.998) | 0.432 (0.326, 0.546) | 0.975 (0.973, 0.978) |
HEWS | 4 | 12,835 | 101 | 209 | 246 | 12,279 | 0.291 (0.246, 0.341) | 0.983 (0.981, 0.985) | 0.326 (0.276, 0.38) | 0.98 (0.978, 0.983) |
MEWS | 4 | 12,859 | 88 | 189 | 259 | 12,323 | 0.254 (0.211, 0.302) | 0.985 (0.983, 0.987) | 0.318 (0.266, 0.375) | 0.979 (0.977, 0.982) |
NHS | 0 | 12,855 | 92 | 204 | 255 | 12,304 | 0.265 (0.221, 0.314) | 0.984 (0.981, 0.986) | 0.311 (0.261, 0.366) | 0.98 (0.977, 0.982) |
PHANTASi | 0 | 12,858 | 93 | 210 | 254 | 12,301 | 0.268 (0.224, 0.317) | 0.983 (0.981, 0.985) | 0.307 (0.258, 0.361) | 0.98 (0.977, 0.982) |
PITSTOP | 0 | 12,813 | 24 | 20 | 322 | 12,447 | 0.069 (0.047, 0.101) | 0.998 (0.998, 0.999) | 0.545 (0.401, 0.683) | 0.975 (0.972, 0.977) |
PreSAT | 0 | 12,835 | 110 | 250 | 237 | 12,238 | 0.317 (0.27, 0.368) | 0.98 (0.977, 0.982) | 0.306 (0.26, 0.355) | 0.981 (0.978, 0.983) |
PRESEP | 3 | 12,835 | 111 | 242 | 236 | 12,246 | 0.32 (0.273, 0.371) | 0.981 (0.978, 0.983) | 0.314 (0.268, 0.365) | 0.981 (0.979, 0.983) |
PRESS | 1 | 12,835 | 35 | 33 | 312 | 12,455 | 0.101 (0.073, 0.137) | 0.997 (0.996, 0.998) | 0.515 (0.398, 0.629) | 0.976 (0.973, 0.978) |
PSP | 1 | 12,835 | 111 | 258 | 236 | 12,230 | 0.32 (0.273, 0.371) | 0.979 (0.977, 0.982) | 0.301 (0.256, 0.349) | 0.981 (0.979, 0.983) |
REMS | 2 | 12,855 | 114 | 269 | 233 | 12,239 | 0.329 (0.281, 0.38) | 0.978 (0.976, 0.981) | 0.298 (0.254, 0.345) | 0.981 (0.979, 0.984) |
RST | 0 | 12,857 | 110 | 264 | 237 | 12,246 | 0.317 (0.27, 0.368) | 0.979 (0.976, 0.981) | 0.294 (0.25, 0.342) | 0.981 (0.978, 0.983) |
SAS | 0 | 12,836 | 26 | 14 | 321 | 12,475 | 0.075 (0.052, 0.108) | 0.999 (0.998, 0.999) | 0.65 (0.495, 0.779) | 0.975 (0.972, 0.977) |
SEPSIS | 4 | 12,856 | 78 | 123 | 269 | 12,386 | 0.225 (0.184, 0.272) | 0.99 (0.988, 0.992) | 0.388 (0.323, 0.457) | 0.979 (0.976, 0.981) |
STSS | 1 | 12,855 | 104 | 211 | 243 | 12,297 | 0.3 (0.254, 0.35) | 0.983 (0.981, 0.985) | 0.33 (0.281, 0.384) | 0.981 (0.978, 0.983) |
Suffoletto | 0 | 12,813 | 103 | 219 | 243 | 12,248 | 0.298 (0.252, 0.348) | 0.982 (0.98, 0.985) | 0.32 (0.271, 0.373) | 0.981 (0.978, 0.983) |
UK | 0 | 12,855 | 109 | 237 | 238 | 12,271 | 0.314 (0.268, 0.365) | 0.981 (0.979, 0.983) | 0.315 (0.268, 0.366) | 0.981 (0.978, 0.983) |
EWS | Threshold | N | TP | FP | FN | TN | Sensitivity | Specificity | PPV | NPV |
---|---|---|---|---|---|---|---|---|---|---|
Pre-alert | 0 | 12,870 | 52 | 133 | 296 | 12,389 | 0.149 (0.116, 0.191) | 0.989 (0.987, 0.991) | 0.281 (0.221, 0.35) | 0.977 (0.974, 0.979) |
qSOFA | 0 | 12,869 | 180 | 758 | 168 | 11,763 | 0.517 (0.465, 0.569) | 0.939 (0.935, 0.944) | 0.192 (0.168, 0.218) | 0.986 (0.984, 0.988) |
qSOFA | 1 | 12,869 | 106 | 192 | 242 | 12,329 | 0.305 (0.259, 0.355) | 0.985 (0.982, 0.987) | 0.356 (0.304, 0.412) | 0.981 (0.978, 0.983) |
qSOFA | 2 | 12,869 | 23 | 22 | 325 | 12,499 | 0.066 (0.044, 0.097) | 0.998 (0.997, 0.999) | 0.511 (0.37, 0.65) | 0.975 (0.972, 0.977) |
90-30-90 | 0 | 12,857 | 140 | 387 | 207 | 12,123 | 0.403 (0.353, 0.456) | 0.969 (0.966, 0.972) | 0.266 (0.23, 0.305) | 0.983 (0.981, 0.985) |
Borelli | 0 | 12,835 | 160 | 463 | 187 | 12,025 | 0.461 (0.409, 0.514) | 0.963 (0.959, 0.966) | 0.257 (0.224, 0.293) | 0.985 (0.982, 0.987) |
CIS | 0 | 12,855 | 198 | 1039 | 149 | 11,469 | 0.571 (0.518, 0.622) | 0.917 (0.912, 0.922) | 0.16 (0.141, 0.182) | 0.987 (0.985, 0.989) |
CIS | 4 | 12,855 | 38 | 54 | 309 | 12,454 | 0.11 (0.081, 0.147) | 0.996 (0.994, 0.997) | 0.413 (0.318, 0.515) | 0.976 (0.973, 0.978) |
HEWS | 4 | 12,835 | 154 | 477 | 193 | 12,011 | 0.444 (0.392, 0.496) | 0.962 (0.958, 0.965) | 0.244 (0.212, 0.279) | 0.984 (0.982, 0.986) |
MEWS | 4 | 12,859 | 135 | 412 | 212 | 12,100 | 0.389 (0.339, 0.441) | 0.967 (0.964, 0.97) | 0.247 (0.213, 0.285) | 0.983 (0.98, 0.985) |
NHS | 0 | 12,855 | 149 | 471 | 198 | 12,037 | 0.429 (0.378, 0.482) | 0.962 (0.959, 0.966) | 0.24 (0.208, 0.275) | 0.984 (0.981, 0.986) |
PHANTASi | 0 | 12,858 | 161 | 588 | 186 | 11,923 | 0.464 (0.412, 0.517) | 0.953 (0.949, 0.957) | 0.215 (0.187, 0.246) | 0.985 (0.982, 0.987) |
PITSTOP | 0 | 12,813 | 32 | 28 | 314 | 12,439 | 0.092 (0.066, 0.128) | 0.998 (0.997, 0.998) | 0.533 (0.409, 0.654) | 0.975 (0.973, 0.978) |
PreSAT | 0 | 12,835 | 183 | 732 | 164 | 11,756 | 0.527 (0.475, 0.579) | 0.941 (0.937, 0.945) | 0.2 (0.175, 0.227) | 0.986 (0.984, 0.988) |
PRESEP | 3 | 12,835 | 183 | 738 | 164 | 11,750 | 0.527 (0.475, 0.579) | 0.941 (0.937, 0.945) | 0.199 (0.174, 0.226) | 0.986 (0.984, 0.988) |
PRESS | 1 | 12,835 | 40 | 61 | 307 | 12,427 | 0.115 (0.086, 0.153) | 0.995 (0.994, 0.996) | 0.396 (0.306, 0.494) | 0.976 (0.973, 0.978) |
PSP | 1 | 12,835 | 188 | 723 | 159 | 11,765 | 0.542 (0.489, 0.593) | 0.942 (0.938, 0.946) | 0.206 (0.181, 0.234) | 0.987 (0.984, 0.989) |
REMS | 2 | 12,855 | 197 | 987 | 150 | 11,521 | 0.568 (0.515, 0.619) | 0.921 (0.916, 0.926) | 0.166 (0.146, 0.189) | 0.987 (0.985, 0.989) |
RST | 0 | 12,857 | 188 | 836 | 159 | 11,674 | 0.542 (0.489, 0.593) | 0.933 (0.929, 0.937) | 0.184 (0.161, 0.208) | 0.987 (0.984, 0.988) |
SAS | 0 | 12,836 | 30 | 22 | 317 | 12,467 | 0.086 (0.061, 0.121) | 0.998 (0.997, 0.999) | 0.577 (0.442, 0.701) | 0.975 (0.972, 0.978) |
SEPSIS | 4 | 12,856 | 107 | 216 | 240 | 12,293 | 0.308 (0.262, 0.359) | 0.983 (0.98, 0.985) | 0.331 (0.282, 0.384) | 0.981 (0.978, 0.983) |
STSS | 1 | 12,855 | 174 | 607 | 173 | 11,901 | 0.501 (0.449, 0.554) | 0.951 (0.948, 0.955) | 0.223 (0.195, 0.253) | 0.986 (0.983, 0.988) |
Suffoletto | 0 | 12,813 | 176 | 669 | 170 | 11,798 | 0.509 (0.456, 0.561) | 0.946 (0.942, 0.95) | 0.208 (0.182, 0.237) | 0.986 (0.984, 0.988) |
UK | 0 | 12,855 | 181 | 686 | 166 | 11,822 | 0.522 (0.469, 0.574) | 0.945 (0.941, 0.949) | 0.209 (0.183, 0.237) | 0.986 (0.984, 0.988) |
EWS | Threshold | N | TP | FP | FN | TN | Sensitivity | Specificity | PPV | NPV |
---|---|---|---|---|---|---|---|---|---|---|
Pre-alert | 0 | 12,870 | 71 | 313 | 277 | 12,209 | 0.204 (0.165, 0.249) | 0.975 (0.972, 0.978) | 0.185 (0.149, 0.227) | 0.978 (0.975, 0.98) |
qSOFA | 0 | 12,869 | 274 | 2984 | 74 | 9537 | 0.787 (0.741, 0.827) | 0.762 (0.754, 0.769) | 0.084 (0.075, 0.094) | 0.992 (0.99, 0.994) |
qSOFA | 1 | 12,869 | 149 | 551 | 199 | 11,970 | 0.428 (0.377, 0.481) | 0.956 (0.952, 0.959) | 0.213 (0.184, 0.245) | 0.984 (0.981, 0.986) |
qSOFA | 2 | 12,869 | 32 | 45 | 316 | 12,476 | 0.092 (0.066, 0.127) | 0.996 (0.995, 0.997) | 0.416 (0.312, 0.527) | 0.975 (0.972, 0.978) |
90-30-90 | 0 | 12,857 | 209 | 1463 | 138 | 11,047 | 0.602 (0.55, 0.652) | 0.883 (0.877, 0.889) | 0.125 (0.11, 0.142) | 0.988 (0.985, 0.99) |
Borelli | 0 | 12,835 | 227 | 1160 | 120 | 11,328 | 0.654 (0.603, 0.702) | 0.907 (0.902, 0.912) | 0.164 (0.145, 0.184) | 0.99 (0.987, 0.991) |
CIS | 0 | 12,855 | 310 | 5150 | 37 | 7358 | 0.893 (0.856, 0.922) | 0.588 (0.58, 0.597) | 0.057 (0.051, 0.063) | 0.995 (0.993, 0.996) |
CIS | 4 | 12,855 | 51 | 136 | 296 | 12,372 | 0.147 (0.114, 0.188) | 0.989 (0.987, 0.991) | 0.273 (0.214, 0.341) | 0.977 (0.974, 0.979) |
HEWS | 4 | 12,835 | 219 | 1381 | 128 | 11,107 | 0.631 (0.579, 0.68) | 0.889 (0.884, 0.895) | 0.137 (0.121, 0.155) | 0.989 (0.986, 0.99) |
MEWS | 4 | 12,859 | 177 | 909 | 170 | 11,603 | 0.51 (0.458, 0.562) | 0.927 (0.923, 0.932) | 0.163 (0.142, 0.186) | 0.986 (0.983, 0.988) |
NHS | 0 | 12,855 | 221 | 1696 | 126 | 10,812 | 0.637 (0.585, 0.686) | 0.864 (0.858, 0.87) | 0.115 (0.102, 0.13) | 0.988 (0.986, 0.99) |
PHANTASi | 0 | 12,858 | 204 | 1221 | 143 | 11,290 | 0.588 (0.535, 0.638) | 0.902 (0.897, 0.907) | 0.143 (0.126, 0.162) | 0.987 (0.985, 0.989) |
PITSTOP | 0 | 12,813 | 35 | 51 | 311 | 12,416 | 0.101 (0.074, 0.137) | 0.996 (0.995, 0.997) | 0.407 (0.309, 0.513) | 0.976 (0.973, 0.978) |
PreSAT | 0 | 12,835 | 258 | 2060 | 89 | 10,428 | 0.744 (0.695, 0.787) | 0.835 (0.828, 0.841) | 0.111 (0.099, 0.125) | 0.992 (0.99, 0.993) |
PRESEP | 3 | 12,835 | 255 | 1736 | 92 | 10,752 | 0.735 (0.686, 0.779) | 0.861 (0.855, 0.867) | 0.128 (0.114, 0.143) | 0.992 (0.99, 0.993) |
PRESS | 1 | 12,835 | 58 | 163 | 289 | 12,325 | 0.167 (0.132, 0.21) | 0.987 (0.985, 0.989) | 0.262 (0.209, 0.324) | 0.977 (0.974, 0.98) |
PSP | 1 | 12,835 | 268 | 2429 | 79 | 10,059 | 0.772 (0.725, 0.813) | 0.805 (0.798, 0.812) | 0.099 (0.089, 0.111) | 0.992 (0.99, 0.994) |
REMS | 2 | 12,855 | 309 | 4919 | 38 | 7589 | 0.89 (0.853, 0.919) | 0.607 (0.598, 0.615) | 0.059 (0.053, 0.066) | 0.995 (0.993, 0.996) |
RST | 0 | 12,857 | 279 | 3008 | 68 | 9502 | 0.804 (0.759, 0.842) | 0.76 (0.752, 0.767) | 0.085 (0.076, 0.095) | 0.993 (0.991, 0.994) |
SAS | 0 | 12,836 | 42 | 52 | 305 | 12,437 | 0.121 (0.091, 0.16) | 0.996 (0.995, 0.997) | 0.447 (0.35, 0.547) | 0.976 (0.973, 0.979) |
SEPSIS | 4 | 12,856 | 137 | 427 | 210 | 12,082 | 0.395 (0.345, 0.447) | 0.966 (0.963, 0.969) | 0.243 (0.209, 0.28) | 0.983 (0.98, 0.985) |
STSS | 1 | 12,855 | 262 | 2226 | 85 | 10,282 | 0.755 (0.707, 0.797) | 0.822 (0.815, 0.829) | 0.105 (0.094, 0.118) | 0.992 (0.99, 0.993) |
Suffoletto | 0 | 12,813 | 247 | 1451 | 99 | 11,016 | 0.714 (0.664, 0.759) | 0.884 (0.878, 0.889) | 0.145 (0.129, 0.163) | 0.991 (0.989, 0.993) |
UK | 0 | 12,855 | 275 | 2720 | 72 | 9788 | 0.793 (0.747, 0.832) | 0.783 (0.775, 0.79) | 0.092 (0.082, 0.103) | 0.993 (0.991, 0.994) |
EWS | Threshold | N | TP | FP | FN | TN | Sensitivity | Specificity | PPV | NPV |
---|---|---|---|---|---|---|---|---|---|---|
Pre-alert | 0 | 12,870 | 80 | 531 | 268 | 11,991 | 0.23 (0.189, 0.277) | 0.958 (0.954, 0.961) | 0.131 (0.106, 0.16) | 0.978 (0.975, 0.981) |
qSOFA | 0 | 12,869 | 301 | 4908 | 47 | 7613 | 0.865 (0.825, 0.897) | 0.608 (0.599, 0.617) | 0.058 (0.052, 0.064) | 0.994 (0.992, 0.995) |
qSOFA | 1 | 12,869 | 160 | 790 | 188 | 11,731 | 0.46 (0.408, 0.512) | 0.937 (0.933, 0.941) | 0.168 (0.146, 0.194) | 0.984 (0.982, 0.986) |
qSOFA | 2 | 12,869 | 33 | 65 | 315 | 12,456 | 0.095 (0.068, 0.13) | 0.995 (0.993, 0.996) | 0.337 (0.251, 0.435) | 0.975 (0.972, 0.978) |
90-30-90 | 0 | 12,857 | 222 | 1937 | 125 | 10,573 | 0.64 (0.588, 0.688) | 0.845 (0.839, 0.851) | 0.103 (0.091, 0.116) | 0.988 (0.986, 0.99) |
Borelli | 0 | 12,835 | 242 | 1505 | 105 | 10,983 | 0.697 (0.647, 0.743) | 0.879 (0.874, 0.885) | 0.139 (0.123, 0.156) | 0.991 (0.989, 0.992) |
CIS | 0 | 12,855 | 344 | 10,864 | 3 | 1644 | 0.991 (0.975, 0.997) | 0.131 (0.126, 0.137) | 0.031 (0.028, 0.034) | 0.998 (0.995, 0.999) |
CIS | 4 | 12,855 | 57 | 171 | 290 | 12,337 | 0.164 (0.129, 0.207) | 0.986 (0.984, 0.988) | 0.25 (0.198, 0.31) | 0.977 (0.974, 0.98) |
HEWS | 4 | 12,835 | 235 | 1923 | 112 | 10,565 | 0.677 (0.626, 0.724) | 0.846 (0.84, 0.852) | 0.109 (0.096, 0.123) | 0.99 (0.987, 0.991) |
MEWS | 4 | 12,859 | 190 | 1232 | 157 | 11,280 | 0.548 (0.495, 0.599) | 0.902 (0.896, 0.907) | 0.134 (0.117, 0.152) | 0.986 (0.984, 0.988) |
NHS | 0 | 12,855 | 241 | 2500 | 106 | 10,008 | 0.695 (0.644, 0.741) | 0.8 (0.793, 0.807) | 0.088 (0.078, 0.099) | 0.99 (0.987, 0.991) |
PHANTASi | 0 | 12,858 | 215 | 1710 | 132 | 10,801 | 0.62 (0.567, 0.669) | 0.863 (0.857, 0.869) | 0.112 (0.098, 0.127) | 0.988 (0.986, 0.99) |
PITSTOP | 0 | 12,813 | 39 | 67 | 307 | 12,400 | 0.113 (0.084, 0.15) | 0.995 (0.993, 0.996) | 0.368 (0.282, 0.463) | 0.976 (0.973, 0.978) |
PreSAT | 0 | 12,835 | 277 | 3099 | 70 | 9389 | 0.798 (0.753, 0.837) | 0.752 (0.744, 0.759) | 0.082 (0.073, 0.092) | 0.993 (0.991, 0.994) |
PRESEP | 3 | 12,835 | 270 | 2272 | 77 | 10,216 | 0.778 (0.731, 0.819) | 0.818 (0.811, 0.825) | 0.106 (0.095, 0.119) | 0.993 (0.991, 0.994) |
PRESS | 1 | 12,835 | 65 | 212 | 282 | 12,276 | 0.187 (0.15, 0.232) | 0.983 (0.981, 0.985) | 0.235 (0.189, 0.288) | 0.978 (0.975, 0.98) |
PSP | 1 | 12,835 | 292 | 4418 | 55 | 8070 | 0.841 (0.799, 0.876) | 0.646 (0.638, 0.655) | 0.062 (0.055, 0.069) | 0.993 (0.991, 0.995) |
REMS | 2 | 12,855 | 343 | 10,288 | 4 | 2220 | 0.988 (0.971, 0.996) | 0.177 (0.171, 0.184) | 0.032 (0.029, 0.036) | 0.998 (0.995, 0.999) |
RST | 0 | 12,857 | 304 | 5217 | 43 | 7293 | 0.876 (0.837, 0.907) | 0.583 (0.574, 0.592) | 0.055 (0.049, 0.061) | 0.994 (0.992, 0.996) |
SAS | 0 | 12,836 | 46 | 78 | 301 | 12,411 | 0.133 (0.101, 0.172) | 0.994 (0.992, 0.995) | 0.371 (0.291, 0.459) | 0.976 (0.974, 0.979) |
SEPSIS | 4 | 12,856 | 143 | 490 | 204 | 12,019 | 0.412 (0.362, 0.465) | 0.961 (0.957, 0.964) | 0.226 (0.195, 0.26) | 0.983 (0.981, 0.985) |
STSS | 1 | 12,855 | 282 | 3326 | 65 | 9182 | 0.813 (0.768, 0.85) | 0.734 (0.726, 0.742) | 0.078 (0.07, 0.087) | 0.993 (0.991, 0.994) |
Suffoletto | 0 | 12,813 | 263 | 1969 | 83 | 10,498 | 0.76 (0.712, 0.802) | 0.842 (0.836, 0.848) | 0.118 (0.105, 0.132) | 0.992 (0.99, 0.994) |
UK | 0 | 12,855 | 297 | 4291 | 50 | 8217 | 0.856 (0.815, 0.889) | 0.657 (0.649, 0.665) | 0.065 (0.058, 0.072) | 0.994 (0.992, 0.995) |
The low prevalence of the primary reference standard (2.7%) means that high specificity is required to achieve acceptable positive predictive value. The judgements made in the previous section around the feasibility of strategies that prioritised a large number of presentations, and the opinion of clinical experts in the research team, suggest that strategies with a positive predictive value below 0.2 are unlikely to be considered feasible (i.e. strategies resulting in fewer than 1 in 5 prioritised presentations actually having sepsis requiring urgent treatment). We would obviously like to prioritise as many cases of sepsis as possible (maximise sensitivity). The results are probably best interpreted by comparing the trade-off between sensitivity and positive predictive value, rather than sensitivity and specificity, because positive predictive value is more meaningful to clinicians and patients, and high specificity could be misinterpreted as being acceptable even though it is associated with poor positive predictive value.
Tables 13–16 show how sensitivity decreases and positive predictive value increases as the NEWS2 thresholds increases, and how selective application of NEWS2 on the basis of paramedic diagnostic impression improves positive predictive value at the expense of sensitivity. Determination of an optimal threshold depends upon a number of factors that may vary over time and between settings, but the results suggest that the best sensitivity that can be achieved with positive predictive value exceeding 0.2 is the sensitivity of 0.522 achieved by using NEWS2 with a threshold of > 4 in presentations with a diagnostic impression of sepsis or infection. The Academy of Medical Royal Colleges clinical decision support framework recommends that this threshold is used to identify presentations needing treatment within 3 hours of arrival. A threshold of > 6 is recommended to identify presentations needing treatment within 1 hour of arrival, which our data suggest has sensitivity of 0.447 and positive predictive value of 0.274.
Tables 17–20 show substantial variation in sensitivity and positive predictive value between the early warning scores at their recommended thresholds. However, none of the early warning scores appear to be clearly more accurate than NEWS2 if an appropriate threshold for NEWS2 is used. The widespread use of NEWS2 in the NHS suggests that this should be the default option unless an alternative score offers superior accuracy. One possible exception to this judgement is the use of qSOFA at a threshold > 1 in presentations with an impression of sepsis or infection. The sepsis-3 consensus group recommended using qSOFA > 1 in presentations with evidence of infection to allow early identification of possible sepsis and could offer an internationally recognised alternative to NEWS2. Our results suggest that this strategy would have sensitivity of 0.305 and positive predictive value of 0.356, which is similar to the sensitivity (0.314) and positive predictive value (0.333) of using NEWS2 > 8 in presentations with an impression of sepsis or infection. These tables also show that the sensitivity and positive predictive value of pre-alert practice in 2019, as recorded on the PRF, was relatively poor, with sensitivity and positive predictive value ranging from 0.129 (95% CI 0.098 to 0.169) and 0.29 (95% CI 0.225 to 0.366) when applied only to those with an impression of sepsis, to 0.23 (95% CI 0.189 to 0.277) and 0.131 (95% CI 0.106 to 0.16) when applied to all eligible cases. It was not mandatory to record pre-alerts in Yorkshire Ambulance Service in 2019, so these results may be affected by under-reporting.
Accuracy for the secondary (diagnostic) reference standard
We repeated the accuracy analysis using the secondary (diagnostic) reference standard instead of the primary (treatment) reference standard, but there was no meaningful difference in the results. This is unsurprising, given that there were only 20 additional reference standard positive cases in the secondary analysis. The results are available in Report Supplementary Material 1.
Selection of strategies for the decision-analytic modelling
None of the alternative strategies were judged to offer clearly superior accuracy to NEWS2, so only NEWS2 strategies were included in the modelling. We also limited the NEWS2 strategies to those applied alongside a diagnostic impression of sepsis or a diagnostic impression of infection or sepsis. Although NEWS2 could prioritise a manageable number of cases when applied to non-specific presentations or all cases if a high threshold were used, we noted that equivalent or better accuracy could be achieved at a lower threshold when applied to patients with a diagnostic impression of infection or sepsis. The clinical experts decided to include one additional strategy in the modelling – a strategy of using a qSOFA score > 1 in patients with a diagnostic impression of infection or sepsis. This strategy is recommended in the sepsis-3 guidance and represents a relatively simple strategy with equivalent accuracy to a point on the NEWS2 ROC curve.
Chapter 3 Decision-analytic modelling
Introduction
Prehospital early warning scores can be used to prioritise people with suspected sepsis for urgent treatment. There will be a trade-off between the benefits that can be gained through early treatment for sepsis and the harms of delaying care for people with other urgent conditions who were seen later due to the prioritisation of patients with suspected sepsis. Further, there is the possibility that prioritising too many patients risks overstretching resources in hospitals.
There is potential for prehospital treatment for suspected sepsis. The decision to start treatment involves weighing the benefits for patients who have sepsis against the costs and risks of unnecessary treatment, especially antibiotic use.
We used decision-analytic modelling to determine the impact of using prehospital early warning scores to guide two key decisions: (1) alerting the receiving hospital that there is a patient who should be seen immediately on arrival; and (2) providing prehospital treatment for sepsis. No formal health economic analysis plan was developed, with the preference being to determine the methods used and the analyses on receipt of the data.
Literature reviews
A literature review was undertaken to identify previously published economic evaluations related to sepsis interventions with the aim (1) to identify any previous economic evaluations ‘relating to the improvement in patients prognoses due to earlier treatment’ and (2) to provide sources to populate the parameters used within the model.
The team were aware of a systematic review of health economic evaluations of sepsis interventions in critically ill adults published in 202044 which included studies published prior to 17 July 2018. This search was updated twice using the EMBASE, MEDLINE and Cochrane Library searches, and the inclusion criteria, provided in Higgins et al. ,44 initially on 11 May 2020 and then on 30 November 2022 (Figure 7). This search will henceforth be referred to as Search 1.
FIGURE 7.
PRISMA diagram for included studies of economic evaluations for sepsis interventions (Search 1). Adapted from Moher et al. 45
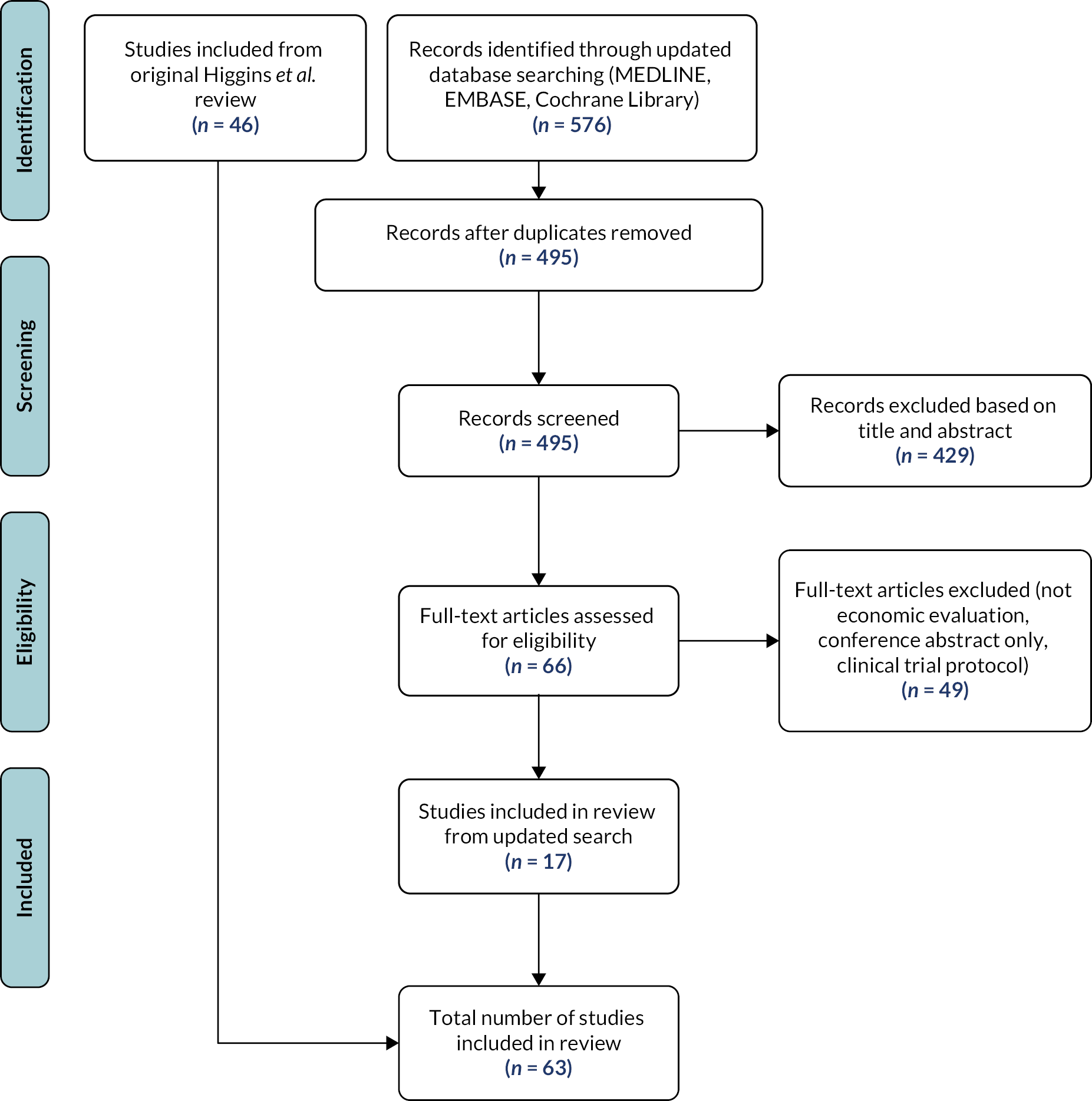
In total 63 economic evaluations were identified, 46 from the original systematic review and 17 from the updated searches. Four of the included studies in the original systematic review could not be accessed but this was not deemed to adversely impact on the conclusions as three studies were published in 1992/93; the remaining study was written in Russian.
None of the 63 economic evaluations identified in Search 1 evaluated the use of prehospital early warning scores to guide earlier treatment. Eight studies assessed the use of early goal-directed therapy (EGDT) or other integrated sepsis protocols, all of which consisted of different bundles of care, mainly focused around EGDT being administered in a timely manner. EGDT consists of achieving a set of physiological targets (mainly targeting central venous pressure, mean arterial pressure and central venous oxygen saturation) through providing therapeutic interventions with continuous monitoring. These studies varied in the package of care provided compared to usual care, with four of the studies being before and after studies of protocols now in place as standard care. The one included UK study of EGDT46 required all patients to have been identified as having sepsis and to have been received antibiotics prior to being randomised to usual UK resuscitation protocol or EGDT. As such, effectiveness data from this study on mortality and hospital length of stay (LoS) were not applicable to the current decision problem of using early warning scores to identify patients with sepsis prior to hospital admission and/or providing immediate treatment. However, as these studies may include data on other important parameters, such as health-related quality of life (HRQoL), they were included in the review for the relevant sections and are discussed in Model parameterisation.
In order to obtain estimates for additional parameters required in the mathematical model a second search was undertaken, henceforth named Search 2, updating a systematic review undertaken by Smyth et al. 47 in 2016 which identified studies assessing the impact of prehospital care on outcomes for sepsis. The Smyth et al. 47 review was identified through a targeted literature search for the effectiveness of prehospital antibiotic treatment. The search was twice updated, using MEDLINE, EMBASE, CINAHL and Cochrane Library, once on 11 October 2020, and once on 22 November 2022. Only papers estimating the impact on patient outcomes (related to either mortality or resource use) of prehospital antibiotic treatment or prehospital sepsis alerts leading to immediate antibiotic treatment were retained for use in the model. Studies comparing method of arrival at hospital or assessing the impact of non-antibiotic prehospital treatment were not included. Three studies were included from the Smyth et al. 47 review and a further nine studies were identified from the updated review (Figure 8). These studies will be discussed in Model parameterisation.
FIGURE 8.
PRISMA diagram of included studies for prehospital sepsis alerts or antibiotic treatment (Search 2). Adapted from Moher et al. 45
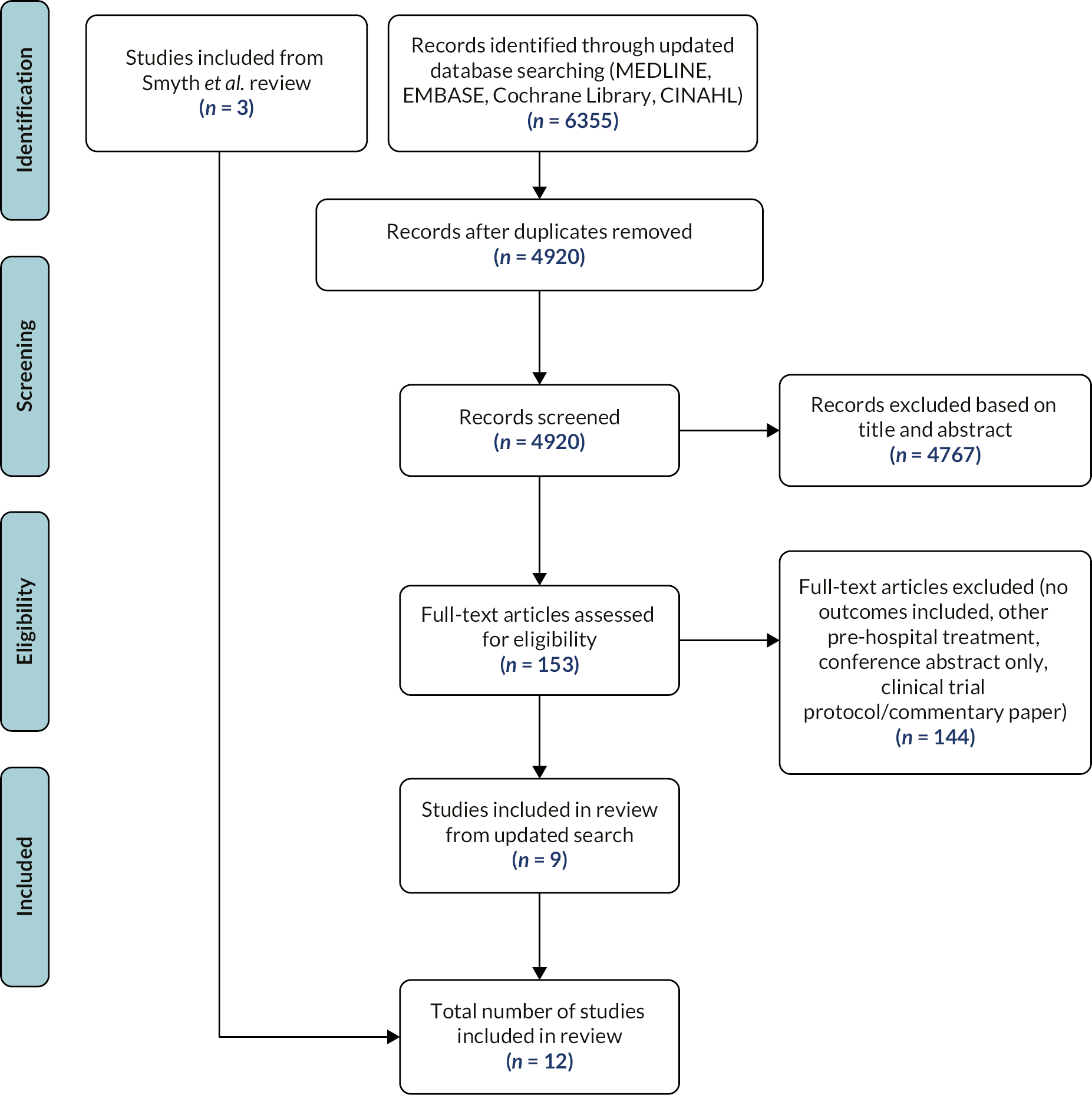
Model structure
A simple decision tree structure was chosen following discussions with clinicians, which incorporates an aggregated estimate of the increased delays to other patients in the ED. The model focuses on adult patients with possible sepsis who arrive at the ED by emergency ambulance. It evaluates a range of strategies that use a prehospital early warning score implemented in combination with prehospital diagnostic impression judged by paramedics to select which patients are prioritised. The prehospital diagnostic impression has the following categories: (1) sepsis; (2) infection (excluding sepsis); (3) (other diagnostic impression in which sepsis could be suspected); and (4) (other diagnostic impression in which sepsis would not be suspected). Within the modelling only categories 1 and 2 were considered for prioritisation for sepsis-related reasons.
All strategies were compared alongside a ‘zero option’ strategy of prioritisation for no patients. We did not include a strategy reflecting ‘routine practice’ since our best estimate of this, pre-alert recorded in the 2019 data, had inferior accuracy to the strategies we planned to include (see Selection of strategies for the decision-analytic modelling).
The strategies are based on early warning scores with a range of sensitivities and specificities (as determined by the retrospective cohort study) and the prehospital diagnostic impression categories for which the early warning score is applied.
-
Score applied only to cases in category 1, with cases in categories 2–4 considered index test negative.
-
Score applied only to cases in categories 1 and 2, with cases in categories 3 and 4 considered index test negative.
For example, a strategy may consist of applying an early warning score to patients with a diagnostic impression of 1 or 2 only, then prioritising those patients who reach the early warning score threshold. Patients who do not reach the threshold or who are given a diagnostic impression of 3 or 4 are not prioritised, as shown in Figure 9. This equates to 2 above.
FIGURE 9.
Model diagram.

Patients who have a strategy applied are categorised into four groups, depending upon whether the strategy (index test) is positive and whether they have sepsis or not (reference standard):
-
score and diagnostic impression category positive, sepsis (true positive)
-
score and diagnostic impression category negative, sepsis (false negative)
-
score and diagnostic impression category positive, not sepsis (false positive)
-
score and diagnostic impression category negative, not sepsis (true negative).
True positives are assumed to receive early treatment for sepsis and potential benefits. False positives are assumed to receive unnecessary antibiotic treatment and to incur additional costs associated with inappropriate use of a resuscitation bay. Both true negatives and false negatives receive care as normal. There are no changes in outcomes for those patients who are not prioritised (independent of whether they have sepsis or not).
The NICE Reference Case requires cost-effectiveness to be presented in terms of cost per quality-adjusted life-years (QALY) gained. 48 It is assumed that incremental costs are affected by false-positive patients through the cost of unnecessary antibiotic treatment and the inappropriate use of resuscitation bays as opposed to general ED bays. The impact on incremental costs due to reductions in LoS (both ICU and general bed-days) due to receiving timely treatment (true positives) is explored.
The perspective undertaken for costs was that of the NHS with costs in pounds sterling inflated to 2019–20 prices where appropriate. A lifetime horizon was approximated by the use of QALY gained due to the prevention of a sepsis mortality. This value used a discount rate of 3.5% per annum as recommended by NICE. 48 No costs were assumed to be incurred over more than 1 year and so discounting of costs was not undertaken.
Model parameterisation
Model parameters were sourced from a combination of retrospective cohort data, published literature identified in Search 1 or Search 2 or clinical opinion. These are discussed in further detail below.
Population
Members of the study team recently undertook a retrospective single-centre descriptive study based in Sheffield Northern General Hospital on the characteristics and outcomes of suspected sepsis patients in the ED. 2 The median age of the subset of patients with diagnosed sepsis meeting the sepsis-3 definition was 77 years [interquartile range (IQR) 65–85] and 39.8% (100/251) of the population were female. These figures are used in the model with the median assumed equal to the mean. The sepsis prevalence used in the model is 2.70% based on data in the retrospective cohort study presented in Chapter 2.
Two ambulance populations are modelled to represent large and small service sizes, with the difference being the number of daily ambulances arrivals (excluding children, trauma, maternity and mental health). Data on the average number of daily ambulance arrivals for a small hospital were taken from Rotherham General Hospital data (average of 51.3) and from Sheffield Teaching Hospital data for a large hospital (average of 93.5), both part of the retrospective cohort study.
Strategies implemented – early warning score sensitivity and specificity
Based on analyses of sensitivity and specificity in conjunction with clinical advice, 23 strategies were compared with no prioritisation (see Selection of strategies for the decision-analytic modelling) These were 10 strategies for people classified as category 1 (sepsis) and 13 strategies were analysed for patients classified as either category 1 or category 2 (sepsis or infection). These strategies are shown in Table 21. All early warning scores were compared to a strategy of no prioritisation of patients. Due to insufficient data, no formal comparisons of early warning scores were performed; decision-makers would need to assess this individually based on the total number of pre-alerts per day, and assessing the likelihood that there would be reductions in mortality, morbidity and LoS generated from using each early warning score.
Strategy | Category 1 (sepsis) |
Category 1 or 2 (sepsis and infection) |
---|---|---|
No prioritisation | ✓ | ✓ |
NEWS2 > 0 | ✓ | ✓ |
NEWS2 > 1 | ✓ | ✓ |
NEWS2 > 2 | ✓ | ✓ |
NEWS2 > 3 | ✓ | ✓ |
NEWS2 > 4 | ✓ | ✓ |
NEWS2 > 5 | ✕ | ✓ |
NEWS2 > 6 | ✕ | ✓ |
NEWS2 > 7 | ✓ | ✓ |
NEWS2 > 8 | ✓ | ✓ |
NEWS2 > 9 | ✓ | ✓ |
NEWS2 > 10 | ✓ | ✓ |
NEWS2 > 11 | ✓ | ✓ |
qSOFA > 1 | ✕ | ✓ |
Sensitivity and specificity data for each combination of early warning score and the diagnostic impression category to which it is applied were sourced from the retrospective cohort study. As NEWS2 was recently endorsed by NHS England for use in ambulance settings49,50 and the Academy of Medical Royal Colleges has recently produced guidance for prioritisation of sepsis based on varying thresholds of NEWS2,51 scenarios were included which evaluate the NEWS2 score across its varying thresholds when applied to patients in category 1 and in categories 1 or 2. As discussed in Methods, data on the reference standard needed to estimate the sensitivity and specificity could only be measured with data from Sheffield Northern General Hospital rather than the four ambulance services as initially planned. The specificity and sensitivity of each strategy (combination of early warning score and diagnostic impression to which it is applied) are shown in Accuracy for the primary (treatment) reference standard.
Baseline mortality risk due to sepsis
In order to estimate the benefit of prehospital treatment or prehospital early warning score leading to immediate treatment, an estimate of baseline sepsis mortality rate was required.
Many studies found in Search 1 used the control arms of studies or country-specific cohort data for baseline mortality; however. the majority of these were based in North America or Europe (France, Spain, Italy) and therefore not necessarily generalisable to our research. Nine studies used mortality data from the PROWESS trial, a worldwide randomised controlled trial (RCT) of Drotrecogin alfa (activated) for severe sepsis. A UK health technology assessment conducted by Westwood et al. 52 used a range of probabilities for baseline mortality taken from previous literature (Christ-Crain et al.;53,54 Bouadma et al. ;55 Qu et al. 56 Roh et al. 57). The probability of mortality for adults ranged from 6.2% to 7.2% in the ED and from 16.9% to 18.2% for adults in the ICU. Mouncey et al. 46 reported a 24% 28-day mortality and 29% 90-day mortality rate for the usual-care arm of their UK trial.
Stevenson et al. 58 used a baseline 30-day mortality rate of 13% and a hospital mortality rate of 21%, both taken from a previous health technology assessment report59 reporting on data from four NHS hospitals in the North-West of England. The 29% 90-day mortality rate reported by Mouncey et al. 46 was then used in sensitivity analyses. Both Soares et al. 60 and Green et al. 61,62 reported a baseline mortality rate relevant to the UK. They used figures from the ICNARC case-mix programme which identified admissions of severe sepsis in England, Wales and Northern Ireland. Soares et al. 60 used the time period of 2007–9, which was the most recent available at the time of their study. Overall hospital mortality was 40.6% (95% CI 40.0% to 41.2%) and baseline mortality for critical care units was 29.1% (95% CI 28.6% to 29.7%). Green et al. 18,19 did not report the time period used but reported a 28-day mortality rate of 41.5% (95% CI 40.8% to 42.3%) for patients with severe sepsis and 46.2% (95% CI 45.3% to 47.1%) for patients with severe sepsis and multiple organ dysfunctions.
Members of the team were aware from personal communication with Dr Lisa Sabir (Academic Clinical Fellow in Emergency Medicine) of a single-centre retrospective study of adult patients with suspected sepsis in ED. Other studies usually have a selected cohort from which sepsis mortality is estimated due to the primary aim being to study a treatment or diagnostic test. Dr Sabir’s study was specifically designed to describe the characteristics and outcomes of a randomly selected cohort of patients attending an ED with suspected sepsis and diagnosed with sepsis according to the sepsis-3 definition. 1 Due to this, this study was deemed most relevant to our current study. Sabir et al. (personal communication) communicated that there were 50 deaths within 30 days from 192 patients where data were fully known in the sepsis-3-defined cohort, resulting in a 30-day mortality rate of 26%. As this study was deemed the most relevant population this was chosen to be used in our model.
Health-related quality of life
Health-related quality of life after sepsis was required to estimate the QALY gains associated with reductions in mortality due to prehospital sepsis alerts/antibiotics. Only two of the identified economic evaluations in Search 1 provided utility values that were sepsis-specific, published as full papers and relevant to the UK. Therefore, the utility values provided in Stevenson et al. 58 (taken from Cuthbertson et al. 63) and Mouncey et al. ,46 as part of the PROmisE trial, were considered the most applicable to be used. The utility values for sepsis survivors used in the model are shown below:
-
Initial 90 days: average of intervention (0.609, SD 0.319) and comparator group (0.603, SD 0.312) in PROmisE trial at 90 days = 0.611.
-
91 days to 1 year: average of intervention (0.620, SD 0.307) and comparator group (0.653, 0.323 SD) in PROmisE trial at 1 year = 0.637.
-
After 1 year: 0.676 calculated from the value used between 91 days and 1 year (0.636) increased by a multiplier of 1.0625, which was calculated from dividing the value at 5 years post sepsis and 3 years post sepsis reported in Cuthbertson et al. (0.68/0.64). Utilities are switched to age–sex-matched general population utility values22 where these were below 0.676.
The quality-adjusted life-year gains associated with preventing 30-day mortality
Following the methods used in Stevenson et al.,58 it is assumed in the model that each 30-day mortality prevented due to correct prioritisation of sepsis patients is associated with a QALY gain of 5.94. This figure is based on the following:
-
The estimated number of discounted life-years for a typical patient. The remaining life expectancy of each patient with an assumed age of 77 years and 39.8% (female)/60.2% (male) gender split2 was calculated using National life tables for England and Wales (2018–20). 64 The model assumes that life expectancy following sepsis for those immediately treated is equal to that of the general population.
-
Quality of life post sepsis. The utility values used are those described in Health-related quality of life.
Costs associated with false positives
The only costs included in the model are in relation to patients being incorrectly prioritised (false positives). These include unnecessary antibiotic treatment and a penalty cost for taking up a resuscitation bay as opposed to a general ED bay.
Following advice from clinical experts, it was advised that Tazocin (piperacillin/tazobactam) was the most likely antibiotic to be used in a prehospital setting for sepsis and is also used in the ED in Sheffield Northern General Hospital; therefore, it is able to represent either prehospital treatment or immediate treatment on arrival. The cost of Tazocin 4 g/0.5 g powder solution for infusion vials (Pfizer Ltd) was sourced from the British National Formulary65 with a cost of £15.17 applied to all false-positive patients to represent the first dose of antibiotics.
Patients incorrectly prioritised will take up resources in ED as they are likely to be immediately sent to a resuscitation bay rather than a general ED bay. As NHS Reference Costs do not provide estimates of the costs of resuscitation and general ED bays, the costs per day of an ICU (critical care) bed and general ward bed were used to represent resuscitation bay and general ED bay, respectively, and assumed to reflect the differences in staffing requirements associated with each. After discussions with clinical experts, it was assumed that the penalty cost of incorrectly prioritising patients would equal the difference between 4 hours in an ICU bed and 4 hours in a general ward bed. The main limitation of this assumption is that it assumes there is capacity to increase resuscitation bays and the associated staffing to accommodate the prioritised patients. Sources and costs used to reflect the penalty cost are shown in Table 22. No further analyses were undertaken to evaluate the potential harm to non-prioritised patients who have care delayed due to prioritised patients as it is assumed that this would be incorporated within the additional costs associated with the additional resuscitation bed cost.
Parameter | Value | Source |
---|---|---|
Cost per ICU/day (per 4 hours) |
£1096.41 (£182.74) | NHS Reference Costs 2019–20.66 CCU01-non-specific, general adult critical care patients predominate, Organs supported 0 |
Cost per ward/day (per 4 hours) |
£351.15 (58.53) | NHS Reference Costs 2017–8.67 Non-elective inpatient excess bed-days inflated to 2019–20 prices using PSSRU NHSCII68 |
Penalty cost of incorrectly prioritising non-sepsis patients | £124.21 | Calculated. Cost difference between 4 hours ICU bed and 4 hours general ward bed |
Effectiveness of interventions (prehospital sepsis alerts or prehospital antibiotics)
Seven studies found in Search 2 assessed the impact of a prehospital alert/documentation by emergency medical staff (EMS) in the ambulance to warn arriving hospitals of a potential sepsis case (Table 23). Four of these studies were based in the USA (Borrelli et al. ;27 Hunter et al. ;69 Mixon et al. ;70 Guerra et al. 37), while the remaining three were based in Europe; UK (McClelland and Jones71), the Netherlands (Alam et al. 72) and Germany (Floer et al. 73). None of these studies were RCTs and all used retrospective data with conflicting results for the interventions in terms of impact on LoS and mortality. Two out of the seven studies found a significant difference in mortality (McClelland and Jones;71 Guerra et al. 37). Three studies assessed the impact of prehospital alerts on hospital LoS and only one of these (Borrelli et al. 27) found a significant difference in LoS with the intervention group (sepsis screening tool and prehospital alert) having a significantly lower median LoS of 5 days (IQR 3–6) versus 8 days (IQR 5–12), p = 0.01. McClelland and Jones was the only UK study looking at prehospital sepsis alerts and found a significant impact on 3-month mortality rates; however, this was a small pilot study with only 49 patients included in total. 71 As these studies were retrospective they could be subject to confounding bias, as patients’ characteristics such as age or SOFA score may have differed between the groups, as in Mixon et al. 69 and Hunter et al. 70 These studies did, however, show that pre-alerts have significant improvement in the time to antibiotics, reducing time to antibiotics to below 1 hour. However, it is unknown from these current studies if this has an impact on mortality and morbidity.
Author, year | Study details | Mortality impact | LOS impact | TTA |
---|---|---|---|---|
Prehospital alert | ||||
Alam et al., 201672 | Country: Netherlands Intervention: recognition and documentation of sepsis during handover by GPs and EMS personnel Comparator: patients not recognised by GPs/EMS Study type: prospective observational study Patient details: 301 patients with sepsis |
Patients who died during admission did not have a significantly longer TTA (mean: 111.46 minutes ± 115.92) compared to patients that did not die (mean: 118.21 minutes ± 90.76) | NR | TTA lower in group in which sepsis was documented by EMS staff vs. not: 65.6 minutes vs. 101.5 minutes (p = 0.024) |
Borrelli et al., 201927 | Country: USA Intervention: EMS sepsis screening tool implemented. EMS alert Emergency Communications Registered Nurse while on route to ED who direct fluid administration by EMS and inform attending physician of possible sepsis Comparator: retrospective historical control group of patients who presented to the ED with an initial presentation of severe sepsis or septic shock Study type: retrospective cohort Patient details: 43 control group, 20 EMS tool group |
Hospital mortality was lower in the post-EMS tool group (11.6% vs. 0%, p = 0.14) | Median hospital LOS was significantly shorter in the post-EMS tool group [8 days (IQR 5–12) vs. 5 days (IQR 3–6), p = 0.01]. Median ICU LOS significantly shorter in the post-EMS tool group [3 days (IQR 0–6) vs. 0 days (IQR 0), p = 0.001] Lower ICU admission rate (33% vs. 52%, p = 0.003) |
Median TTA was lower in the pre-EMS tool group: 63.5 minutes (IQR 44–92) vs. 72 minutes (IQR 59.5–112), p = 0.26 |
Hunter et al., 201969 | Country: USA Intervention: prehospital sepsis alert (pre-arrival alert) Comparator: no prehospital sepsis alert Study type: retrospective cohort Patient details: 162 pre-alert notification, 110 no notification. Prehospital sepsis alert group was significantly older (69 vs. 64 years old, p = 0.024) |
No change in mortality between groups: no alert: 15 (14%) vs. alert group: 16 (11%), p = 0.565 | NR | TTA lower in prehospital alert group: 33 minutes (95% CI 26 to 40 minutes) vs. 61 minutes (95% CI 44 to 78 minutes), p = 0.004 |
Mixon et al., 202070 | Country: USA Intervention: sepsis alert calls via EMS Comparator: sepsis alert calls in ED Study type: multicentre retrospective Patient details: 419 ED alert, 88 EMS alert |
No significant difference in 60-day in-hospital mortality: 16.28% vs. 9.64%, p = 0.07 | No difference between sepsis alerts initiated in the field and those initiated in the ED in regard to hospital LOS (3.94 days vs. 3.77 days, p = 0.72), ICU LOS (2.0 vs. 2.0, p = 0.62) No sig difference in ICU admission (18.18% vs. 15.27%, p = 0.62) |
TTA significantly lower in EMS alert group: 48.5 minutes vs. 65 minutes, p < 0.01 EMS alert group more likely to receive antibiotics within 60 minutes than ED alert: 59.1% vs. 44%, p < 0.01 |
Guerra et al., 201337 | Country: USA Intervention: training of EMS staff to recognise sepsis and pre-alert EDs (implementing a Sepsis Alert protocol). Comparator: no Sepsis Alert protocol implemented: patients unrecognised by EMS who arrived at the ED in severe sepsis Study type: retrospective case control study (pilot study) Patient details: 112 severe sepsis patients; 37 pre-alerts group, 75 no alert group |
If hospital was ‘pre-alerted’, survival to discharge improved OR 3.19 (95% CI 1.14 to 8.88, p = 0.040) | No significant reduction in LoS: mean 7.3 days (SD 6.8 days, pre-alert) vs. 8.4 days (SD 8.8 days, no pre-alert, p = 0.65) | No significant reduction in mean TTA: pre-alert 72.6 minutes (SD 59.3 minutes) vs. 98.5 minutes no pre-alert (SD 89.9 minutes, p = 0.07) |
McClelland and Jones., 201671 | Country: UK Intervention: patients recognised by EMS Comparator: patients not recognised as sepsis by EMS Study type: pilot study, non RCT Patient details: 49 patients |
3-month mortality higher in EMS-identified patients: 21% (5/24) vs. 16% (4/25) (not identified) | ICU admission lower in EMS-identified patients: 4% (1/23) (EMS-identified) vs. 13% (3/23) (not identified) | Does not separately report TTA but instead look at Time to Sepsis Six bundle (oxygen, cultures, antibiotics, fluids, lactate measurement and urine output monitoring): EMS identified mean 205 minutes (SD 271 minutesa) vs. 120 minutes (SD 110) (not identified) |
Floer et al., 202173 | Country: Germany Intervention: documentation of suspected sepsis by EMS Comparator: sepsis not documented by EMS Study type: retrospective single-centre cohort study Patient details: sepsis recognised (n = 25); sepsis not recognised (n = 238) |
In hospital mortality 8.0% if suspected sepsis identified by EMS staff vs. 22.8% if not (p = 0.0292) | NR | 136.50 minutes for sepsis diagnosed by EMS compared to non-diagnosed sepsis 206.98 minutes after the arrival of EMS on the scene (p = 0.0069) |
Prehospital antibiotics | ||||
Chamberlain et al., 200975 | Country: Australia Intervention: prehospital antibiotics and fluids Comparator: prehospital fluids only Study type: prospective RCT (abstract only) Patient details: 198 septic shock patients |
28-day mortality reduced: 42.4% (intervention) vs. 56.7% (control), OR 0.56 (95% CI 0.32 to 1.00; p = 0.049) | Mean ICU LOS: reduced 6.8 ± 2.1 days (intervention) vs. 11.2 ± 5.2 days (control, p = 0.001) |
TTA was reduced by 3.4 ± 2.6 hours (p = 0.02) |
Jouffroy et al., 202074 | Country: France Intervention: prehospital antibiotics provided by MICU Control: no prehospital antibiotics provided by MICU Study type: multicentre retrospective cohort Patient details: septic shock patients only. 308 patients: 98 received prehospital antibiotics. Mean age = 70 |
Significant association between prehospital antibiotic therapy and 30-day mortality: HR – 0.56, 95% CI (0.35 to 0.90), p = 0.01 | ICU LOS: prehospital treatment = 4 days [2–8] vs. no prehospital treatment = 5 days (3–10) (p = 0.478) In-hospital LOS: prehospital treatment = 7 days (2–13) vs. no TX = 17 days (10–29) (p < 0.001) |
|
Alam et al., 201833 | Country: the Netherlands Intervention: open-label intravenous ceftriaxone 2000 mg in the ambulance in addition to usual care (following training for EMS staff). Comparator: usual care in the ambulance of fluid resuscitation and supplementary oxygen Study type: prospective RCT Patient details: 2698 patients: 1535 intervention vs. 1137 control. All severity of sepsis included |
28-day mortality: intervention group 120 (8%), control 93 (8%); relative risk = 0.95 (95% CI 0.74 to 1.24); risk difference –0.37 (–2.5 to 1.7); p = 0.78 90-day mortality: intervention group 178 (12%), control group 134 (12%) (p = 0.87). Relative risk = 0.98 No significant difference in 28-day mortality between groups in any of the subgroups (< 65 vs. > 65; sepsis severity, prehospital qSOFA) |
No significant difference in length of hospital stays | Median TTA before arriving at the ED for patients in the intervention group was 26 minutes (IQR 19–34). Median TTA after arriving at the ED in the usual care group was 70 minutes (IQR 36–128) |
Cunningham et al., 202276 | Country: USA Intervention: paramedics trained for sepsis recognition and initiation of sepsis protocol which includes initiation of prehospital antibiotics and drawing of blood cultures (in order to assess contamination rates of blood cultures drawn in the prehospital setting) Comparator: retrospectively analysed group of patients who would have met protocol criteria based on PCR results. Study type: pilot study, non-RCT Patient details: patients with sepsis with hypotension and septic shock. Prospective intervention cohort (n = 29), historical cohort (n = 34) |
No significant difference | No significant difference | 911 call receipt to TTA significantly lower for the prospective cohort (intervention) than the historical cohort (mean = 36.04 minutes vs. 220.76 minutes; p < 0.005) |
Jones et al., 202277 | Country: UK Intervention: paramedics trained in sepsis recognition protocol. Intervention group (usual care and collection of blood cultures and IV administration of 2 g cefotaxime). Comparator: usual care (maintaining oxygen saturation and alerting the hospital if deemed serious condition) Study type: RCT feasibility study Patient details: 62 intervention vs. 52 control |
90-day mortality: intervention group: 21 (33.9%) vs. control group: 11 (21.2%) Odds ratio = 1.9 (0.82, 4.5) (p = 0.13) |
NR | NR |
Five studies in Search 2 assessed the impact of prehospital antibiotic treatment on sepsis outcomes. This also showed conflicting evidence on mortality and LoS, with two out of the five studies showing a significant difference in mortality (Jouffroy et al.;74 Chamberlain et al. 75). These same studies were the only ones to also show a significant difference in ICU LoS. The PHANTASi trial is the only large-scale multicentre RCT to look at the impact of prehospital antibiotic treatment. 33 However, the results of this study did not show a significant impact on either mortality or LoS. Only one of the five studies was based in the UK (Jones et al. 77). This RCT feasibility study (PhRASe study) was designed to assess the viability of paramedics recognising and screening patients for severe sepsis and collecting blood cultures and administering intravenous antibiotics. The results showed no significant difference in mortality and ICU admission between the control and intervention groups. However, this study was not powered to assess significant differences in these outcomes.
Due to the large variability in results found, there was deemed to be no single estimate that could be chosen to accurately represent the effectiveness of either prehospital sepsis alerts or prehospital antibiotics on mortality or LoS outcomes. The gains associated with earlier treatment (if any) therefore cannot be currently quantified. In order to provide information for decision-makers, threshold analyses were performed to provide, in isolation, the reduction in: mortality; general ward LoS; ICU LoS; and the gains in QALYs, in isolation, that would be required for a strategy to be cost-effective at a standard NICE cost per QALY threshold of £20,000 compared with no prioritisation. 47
Analyses undertaken
It was initially anticipated that the economic model would separately analyse the impact of prehospital early warning scores in providing earlier treatment to patients by (1) alerting the receiving hospital so that the patient is seen immediately on arrival and/or (2) providing prehospital treatment for sepsis. After reviewing the literature, however, it was deemed that there was inconclusive evidence on the benefits of prehospital sepsis alerts for immediate treatment (including prehospital antibiotic treatment). Therefore, the focus was changed to concentrate on patients being seen, and treated, immediately on arrival at the ED and assessing the operational consequences and cost-effectiveness of relevant strategies. Due to inconclusive benefits associated with prehospital alerts/treatment, threshold analyses were performed to provide, in isolation, the reduction in: mortality; general ward LoS; ICU LoS; and the gains in QALYs, in isolation, that would be required for a strategy to be cost-effective at a standard NICE cost per QALY threshold of £20,000 compared with no prioritisation.
Due to a lack of data, the impact of prehospital alerts on operational consequences was also simplified to focus only on the number of alerts per day received by the ED. This would allow an assessment of whether particular strategies were feasible.
The key outputs of the model are as follows:
-
number of patients prioritised (i.e. number of additional calls)
-
number of patients not prioritised
-
incremental costs (due to incorrectly prioritised patients taking up ICU beds and receiving unnecessary antibiotics)
-
the reduction in general ward LoS that would be required for each strategy to become cost-effective compared with no prioritisation
-
the reduction in ICU LoS that would be required for each strategy to become cost-effective with no prioritisation
-
the reduction in mortality that would be required for each strategy to become cost-effective with no prioritisation
-
the net gains in QALYs that would be needed for each strategy to become cost-effective with no prioritisation. These QALYs could come from less long-term morbidity associated with sepsis patients or with prioritisation of non-sepsis patients who benefited from earlier treatment, although QALYs could be lost due to the overuse of antibiotics.
The threshold analyses have been undertaken in isolation; the thresholds needed would be lower if more than one component were considered simultaneously, for example, if both mortality benefits and a reduced LoS was changed. There is considerable uncertainty in the relative results of each strategy but given the lack of data on key model parameters, such as the benefit of providing early treatment for patients with sepsis, no further quantification of uncertainty was undertaken beyond the threshold analyses.
Probabilistic analyses were undertaken in a nearly finalised model which showed that the model was linear, with the probabilistic results being very similar to the deterministic results. Given this, and that the primary analyses would be threshold-based, we have provided deterministic answers only and did not run probabilistic results in the final model.
Analyses were undertaken that compared all strategies simultaneously at selected relative risk of mortality associated with early treatment between 0.900 and 1.00. These analyses used incremental net monetary benefit (iNMB) for all strategies compared with no prioritisation. iNMB is defined as the cost per QALY gained threshold multiplied by the incremental QALY gain minus the incremental cost; under this framework the strategy with the largest estimated iNMB is deemed to be the most cost-effective, which could be zero if the benchmark intervention is most cost-effective. 78 The absolute loss (valued in terms of cost) of moving to a different strategy is calculated by comparing the estimated iNMBs.
The fully incremental analysis was undertaken again assuming that the costs of false positives had been underestimated, with the cost increased from £124 to £500.
Decision-analytic modelling results
The following sections provide the results of the modelling: to aid readability of the figures each strategy has been referenced by the early warning score, the threshold score and the description of the highest category number that is used. Thus, as examples, NEWS2 > 5_infection refers to using the NEWS2 score and prioritising all patients with sepsis or infection with a score > 5, whereas NEWS2 > 9_sepsis refers to using the NEWS2 score and prioritising all patients with sepsis with a score > 9.
The results are presented ranked in terms of sensitivity, with the most sensitive strategy [applying the NEWS2 score to people categorised as 1 or 2 (sepsis or infection) and prioritising people with a score > 1] on the left and the least sensitive strategy [applying the NEWS2 score to people categorised as 1 (sepsis only) and prioritising people with a score > 11] on the right.
The number of prehospital alerts by each strategy
The operational consequences of each strategy need to be considered. Figure 10 provides the estimated total number of alerts for each strategy for a large hospital which is assumed to have, on average, 93.5 patients arriving by ambulance each day and also the number of times of prioritisation when the patient had sepsis. Figure 11 provides the estimated total number of alerts for each strategy for a small hospital which is assumed to have, on average, 51.3 patients arriving by ambulance each day and also the number of times of prioritisation when the patient had sepsis. Table 24 shows the number prioritised, number with sepsis correctly prioritised and number with sepsis not prioritised at a large and a small hospital. It is seen in both Figures 10 and 11 that the ratio of correctly prioritised patients to the total number of prioritised patients varies widely by strategy.
FIGURE 10.
The number of cases prioritised each day in a large hospital using each strategy.

FIGURE 11.
The number of cases prioritised each day in a small hospital using each strategy.

Large hospital | Small hospital | |||||
---|---|---|---|---|---|---|
Number prioritised | Number correctly prioritised | Number sepsis not prioritised | Number prioritised | Number correctly prioritised | Number sepsis not prioritised | |
NEWS2 > 0_infection | 8.95 | 1.44 | 1.09 | 4.91 | 0.79 | 0.60 |
NEWS2 > 1_infection | 8.47 | 1.44 | 1.09 | 4.65 | 0.79 | 0.60 |
NEWS2 > 2_infection | 7.90 | 1.44 | 1.09 | 4.33 | 0.79 | 0.60 |
NEWS2 > 3_infection | 7.03 | 1.39 | 1.14 | 3.86 | 0.76 | 0.62 |
NEWS2 > 4_infection | 6.10 | 1.32 | 1.21 | 3.35 | 0.72 | 0.66 |
NEWS2 > 5_infection | 5.17 | 1.25 | 1.28 | 2.84 | 0.68 | 0.70 |
NEWS2 > 6_infection | 4.11 | 1.13 | 1.40 | 2.26 | 0.62 | 0.77 |
NEWS2 > 7_infection | 3.25 | 0.97 | 1.56 | 1.78 | 0.53 | 0.86 |
NEWS2 > 0_sepsis | 2.88 | 0.83 | 1.70 | 1.58 | 0.46 | 0.93 |
NEWS2 > 1_sepsis | 2.85 | 0.83 | 1.70 | 1.56 | 0.46 | 0.93 |
NEWS2 > 2_sepsis | 2.82 | 0.83 | 1.70 | 1.54 | 0.46 | 0.93 |
NEWS2 > 3_sepsis | 2.73 | 0.82 | 1.70 | 1.50 | 0.45 | 0.94 |
NEWS2 > 4_sepsis | 2.59 | 0.81 | 1.72 | 1.42 | 0.44 | 0.94 |
NEWS2 > 8_infection | 2.38 | 0.79 | 1.73 | 1.31 | 0.44 | 0.95 |
qSOFA > 1_infection | 2.17 | 0.77 | 1.76 | 1.19 | 0.42 | 0.96 |
NEWS2 > 7_sepsis | 1.83 | 0.69 | 1.84 | 1.01 | 0.38 | 1.01 |
NEWS2 > 9_infection | 1.72 | 0.66 | 1.87 | 0.95 | 0.36 | 1.02 |
NEWS2 > 8_sepsis | 1.56 | 0.63 | 1.90 | 0.86 | 0.34 | 1.04 |
NEWS2 > 9_sepsis | 1.22 | 0.53 | 2.00 | 0.67 | 0.29 | 1.10 |
NEWS2 > 10_infection | 1.16 | 0.50 | 2.03 | 0.63 | 0.27 | 1.12 |
NEWS2 > 10_sepsis | 0.90 | 0.43 | 2.10 | 0.50 | 0.24 | 1.15 |
NEWS2 > 11_infection | 0.69 | 0.34 | 2.19 | 0.38 | 0.19 | 1.20 |
NEWS2 > 11_sepsis | 0.55 | 0.29 | 2.24 | 0.30 | 0.16 | 1.23 |
Managers and clinicians can use Figures 10 and 11, and Table 24, to predict the consequences of using each strategy at their hospital. They can thus predict the consequences of implementing national recommendations or guidelines. For example, the Academy of Medical Royal Colleges clinical decision support framework recommends using NEWS2 > 6 to identify presentations with evidence of infection for urgent treatment within 1 hour. Table 24 suggests that this would result in 4.11 cases being prioritised per day at a large hospital, including 1.13 with sepsis, while 1.40 people with sepsis were not prioritised. At a small hospital 2.26 cases would be prioritised per day, including 0.62 with sepsis, while 0.77 people with sepsis were not prioritised.
Threshold analysis based on length of stay
Figure 12 shows the average reduction in LoS for patients correctly prioritised that would be required for each strategy to be estimated to be cost-effective assuming a willingness to pay of £20,000 per QALY compared to no prioritisation. These are provided in isolation for general ward stay and ICU stay. These values would be lower if there was a reduction in both general ward stay and ICU stay.
FIGURE 12.
The threshold levels for reduction in general ward LoS and reduction in ICU LoS at which each strategy becomes cost-effective assuming a willingness to pay £20,000 per QALY gained compared with no prioritisation.
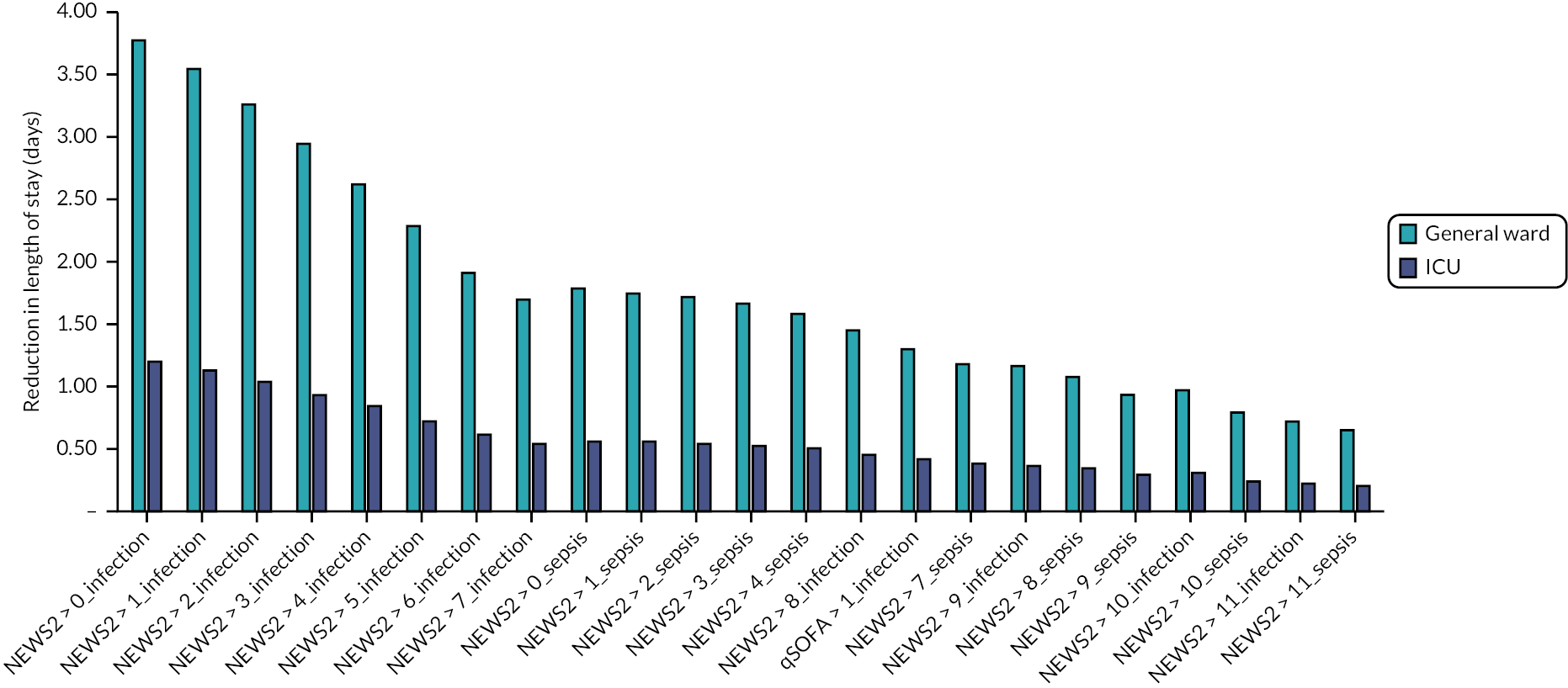
Threshold analysis based on relative risk of mortality
Figure 13 provides the relative risk of mortality for patients with sepsis who have been correctly prioritised at which each strategy would be cost-effective assuming a willingness to pay of £20,000 per QALY compared to no prioritisation. The y-axis starts at 0.975, indicating that only minor gains in mortality are required in order for even the most sensitive strategies to be cost-effective.
FIGURE 13.
The threshold levels for relative risk of mortality at which each strategy becomes cost-effective assuming a willingness to pay £20,000 per QALY gained compared with no prioritisation.

Threshold based on QALYs gained
Figure 14 provides the total number of QALYs gained per patient with sepsis correctly prioritised at which each strategy is estimated to become cost-effective assuming a willingness to pay of £20,000 per QALY compared to no prioritisation. It is seen that these numbers are very low, with all strategies having a value below 0.0006.
FIGURE 14.
The threshold levels for QALYs gained per patient at which each strategy becomes cost-effective assuming a willingness to pay £20,000 per QALY gained compared with no prioritisation.
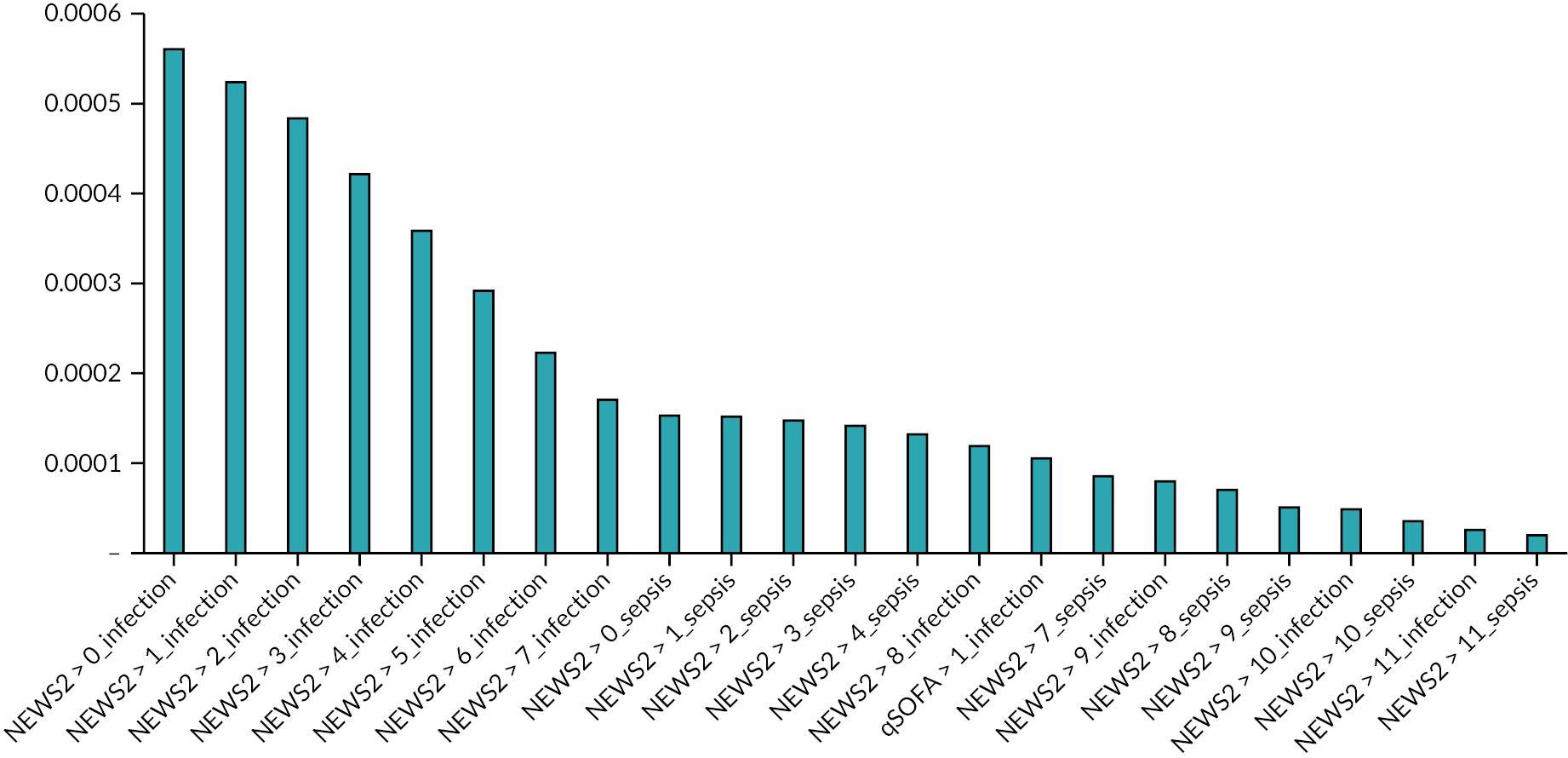
The threshold analyses only show the threshold of effectiveness required for each strategy to be cost-effective compared to no prioritisation. We used this approach because none of the alternative strategies represent routine practice. The strategies can be compared to each other by examining the difference in the threshold level between the strategies. Given that the threshold relative risk reduction for mortality required for even the most sensitive strategy to be cost-effective was very small, we can conclude that more sensitive (effective) strategies are likely to be cost-effective compared to less sensitive strategies, if we believe that prioritisation results in a meaningful reduction in mortality. It is also worth noting that we excluded the most sensitive strategies from the decision-analytic modelling on the basis that they would prioritise an unmanageable number of cases. These strategies would be cost-effective if prioritisation reduced mortality and hospitals could release resources to increase capacity for prioritisation.
Incremental analyses comparing all strategies while varying the benefit associated with prioritisation
Figure 15 provides the iNMB for all strategies assuming a willingness to pay of £20,000 per QALY. At all relative risks, there are multiple strategies with similar iNMB values.
FIGURE 15.
The iNMB compared with no prioritisation assuming a willingness to pay £20,000 per QALY gained at different benefits associated with prioritisation.

The strategy with the greatest iNMB associated with prioritisation between ranges of relative risks of mortality is shown in Table 25 assuming a willingness to pay of £20,000 per QALY gained. The strategy with the highest iNMB changes multiple times between a range of 0.900 and 1.000. The results suggest that strategies prioritising patients at relatively low thresholds of NEWS2 (> 2 or > 3) are the most cost-effective strategies when the relative risk of mortality is 0.9–0.946. These strategies would prioritise large numbers of patients that would potentially exceed the capacity of the ED.
Relative risk of mortality associated with prioritisation | Strategy with the highest iNMB |
---|---|
0.900–0.914 | NEWS2 > 2_infection |
0.916–0.946 | NEWS2 > 3_infection |
0.948–0.962 | NEWS2 > 5_infection |
0.964–0.980 | NEWS2 > 6_infection |
0.982–0.984 | qSOFA > 1_infection |
0.986 | NEWS2 > 7_sepsis |
0.988–0.990 | NEWS2 > 9_sepsis |
0.992 | NEWS2 > 10_sepsis |
0.994 | NEWS2 > 11_sepsis |
0.996–1.000 | No prioritisation |
Incremental analyses comparing all strategies whilst varying the benefit associated with prioritisation having increased the costs of false positives
Figure 16 provides the iNMB, assuming a willingness to pay of £20,000 per QALY, for all strategies compared with no prioritisation assuming that the costs of false positives was increased from £124 to £500. The iNMBs for all strategies were noticeably reduced, with many strategies having a negative iNMB even at a relative risk of 0.980.
FIGURE 16.
The iNMB compared with no prioritisation assuming a willingness to pay £20,000 per QALY gained at different benefits associated with prioritisation.

The strategy with the greatest iNMB associated with prioritisation between ranges of relative risks of mortality is shown in Table 26 assuming a willingness to pay of £20,000 per QALY gained. The strategy with the highest iNMB changes multiple times between a range of 0.900 and 1.000. This suggests that the findings are sensitive to our estimate of the cost of false-positive prioritisation. Strategies using relatively low thresholds of NEWS2 (> 2 or > 3) are not the most cost-effective at relative risk estimates from 0.900 to 0.946 if the cost of false positives is markedly higher than our baseline estimate.
Relative risk of mortality associated with prioritisation | Strategy with the highest iNMB |
---|---|
0.900–0.926 | NEWS2 > 6_infection |
0.928–0.944 | qSOFA > 1_infection |
0.946–0.948 | NEWS2 > 7_sepsis |
0.950–0.954 | NEWS2 > 8_sepsis |
0.956–0.964 | NEWS2 > 9_sepsis |
0.966–0.974 | NEWS2 > 10_sepsis |
0.976–0.984 | NEWS2 > 11_sepsis |
0.986–1.000 | No prioritisation |
Chapter 4 Discussion
Main findings
Our findings demonstrate the challenge of prehospital identification of sepsis. People with sepsis may have non-specific presentations,5 so sepsis could be considered as a possible diagnosis in any patient transported to hospital by ambulance with a medical complaint. We found that if prehospital early warning scores for sepsis are applied to those with non-specific presentations (using the recommended threshold) or all medical cases, then most would prioritise over 10% of medical cases. Prioritising a large proportion of cases is likely to overburden the ED and would not result in meaningful prioritisation.
Lower rates of prioritisation can be achieved if paramedics apply early warning scores selectively to those with a diagnostic impression of sepsis or infection. However, this approach will result in patients with sepsis not being prioritised. When we were able to link ambulance service data to hospital data, we found that only 33% of patients with the primary reference standard (sepsis-3 definition receiving treatment for sepsis) presented with a paramedic diagnostic impression of sepsis and only 57% presented with a diagnostic impression of sepsis or infection. We were therefore unable to identify a strategy that would prioritise a substantial majority of patients with sepsis without prioritising a potentially unmanageable number of patients for the ED.
While recognising that none of the strategies could be considered ideal, our findings provide useful comparisons between early warning scores. The NEWS2 score is widely used across NHS ambulance services and hospitals. 49,50 Paramedics are trained to record and interpret NEWS2, and NHS prehospital data-collection systems often automatically calculate a NEWS2 score. NEWS2 could therefore be considered the default option for a prehospital early warning score for sepsis, requiring an alternative score to demonstrate superior accuracy. We found that NEWS2 accuracy had similar or superior accuracy to other early warning scores. The only exception was the SEPSIS score,24 which had a slightly higher area under the ROC curve when applied to non-specific presentations or all medical cases. This may reflect the development of the SEPSIS score using prehospital data to identify sepsis among all medical cases. However, the SEPSIS score was not more accurate than NEWS2 at thresholds that provided acceptable specificity (i.e. high enough to avoid prioritising an unmanageable number of cases). We therefore found no evidence that an alternative early warning score can provide better prioritisation than NEWS2.
Our findings provide diagnostic accuracy estimates for strategies that have been recommended in recent guidelines. The Academy of Medical Royal Colleges clinical decision support framework recommends that patients with evidence of infection and NEWS2 > 4 or NEWS2 > 6 should respectively receive treatment for sepsis within 3 hours or 1 hour of arrival. 51 Our findings suggest that the sensitivity and positive predictive value would be 0.533 and 0.2 for the NEWS2 > 4 strategy and 0.447 and 0.274 for the NEWS2 > 6 strategy. The sepsis-3 guidelines recommend using evidence of infection and qSOFA > 1 to identify potential sepsis. Our findings suggest this strategy would have sensitivity of 0.305 and positive predictive value of 0.356. Similar sensitivity (0.314) and positive predictive value (0.333) could be achieved using NEWS2 > 8 in presentations with a diagnostic impression of sepsis or infection. These strategies therefore provide a range of practical options that ambulance services and hospitals could implement based upon local capacity to handle prioritised cases.
The modelling provides insights into how many cases (overall and with sepsis) would be prioritised in different-size hospitals using alternative strategies. A large hospital receiving a mean of 93.5 medical cases per day via emergency ambulance would prioritise 6.10, 4.11 and 2.38 cases per day if patients with a diagnostic impression of sepsis or infection were prioritised with a NEWS2 score > 4, > 6, > 8, respectively. Of these, 1.32, 1.13 and 0.79 per day would have sepsis, while 1.21, 1.40 and 1.73 cases with sepsis would not be prioritised. A small hospital receiving a mean of 51.3 medical cases per day via emergency ambulance would prioritise 3.35, 2.26 and 1.31 cases per day if patients with a diagnostic impression of sepsis or infection were prioritised with a NEWS2 score > 4, > 6 and > 8 respectively. Of these, 0.72, 0.62 and 0.44 per day would have sepsis, while 0.66, 0.77 and 0.95 cases with sepsis would not be prioritised.
Our efforts to determine the cost-effectiveness of alternative strategies were undermined by a lack of evidence or consensus regarding the effectiveness of early treatment for sepsis. There is a clear biological rationale for expecting early treatment to improve outcomes but existing evidence, summarised in Model parameterisation, was insufficient to provide a reliable estimate of effectiveness for the modelling. We therefore undertook threshold analyses to explore whether strategies prioritising patients for early treatment would be cost-effective compared to no prioritisation at a willingness-to-pay threshold of £20,000/QALY under various assumptions regarding the effectiveness of early treatment. The most striking finding was that the prioritisation strategies would generally be cost-effective even if the mortality risk reduction associated with early treatment was relatively small. All the strategies tested would be cost-effective, compared to no prioritisation, if the relative risk of mortality associated with early treatment was 0.975 (see Figure 13). Strategies with low NEWS2 thresholds (> 2 or > 3) would be the most cost-effective if the relative risk of mortality was 0.900–0.946. The largest randomised trial of prehospital antibiotics for sepsis, the PHANTASi trial, reported a point estimate of 0.95 for 28-day mortality, albeit with a 95% CI of 0.74 to 1.24. 33 If we believe that prioritisation results in a meaningful reduction in mortality, then sensitive strategies are potentially cost-effective, although they will prioritise large numbers of patients that are likely to exceed the capacity of the ED to provide meaningful prioritisation.
These findings suggest that prioritisation strategies, including those with poor positive predictive value, could be cost-effective even if the mortality benefit of prioritisation was relatively small. This may reflect other assumptions in our model and the difficulty of estimating the true cost of prioritising people without sepsis. Our model assumed that the negative impact of unnecessary prioritisation was measured by the cost of managing a patient in a resuscitation bay rather than a general ED bay. This assumption implies that resources are available to increase the number of resuscitation bays and thus avoid negative effects on other patients who might benefit from prioritisation. If this is not possible, then the negative effects on other patients could be substantial and difficult to predict. Our findings were sensitive to variation in the potential cost of false-positive prioritisation. Strategies with a low NEWS2 threshold (> 2 or > 3) were no longer the most cost-effective at relative risk estimates between 0.9 and 0.946 when we assumed the cost of false positives was markedly higher than the baseline estimate.
Comparison to other studies
We recently searched for studies validating the accuracy early warning scores for suspected sepsis in a prehospital population and identified 13 studies evaluating the scores included in this study. 10 There was substantial variation in the reported results, with no consistent evidence that any score was superior to the others. The variation in the results may be explained by differences in study populations and outcomes, rather than variation in the composition of the scores. Furthermore, variation in the thresholds used in different studies makes comparisons difficult. A systematic review23 of hospital studies found that at established thresholds NEWS tended to have higher sensitivity while qSOFA tended have to higher specificity. Our study suggests that this difference reflects the chosen threshold. The sensitivity and specificity of NEWS2 at a higher threshold than usually recommended (> 8) are similar to the sensitivity and specificity of qSOFA > 1.
Two previous studies have, similarly to ours, evaluated many scores in the same study. Lane et al. 17 evaluated 21 strategies, including 14 scores, in a retrospective cohort of 12,740 adult ambulance transfers to the ED who had infection subsequently diagnosed in the ED. No single strategy had high sensitivity and specificity for classifying sepsis, but the Critical Illness Prediction score, NEWS and qSOFA showed good prediction for sepsis. Smyth et al. 16 evaluated eight scores in a retrospective cohort of 6682 adult medical cases using a reference standard of high risk of severe illness or death from sepsis. Three strategies appeared to offer an acceptable balance between sensitivity and PPV: SEPSIS > 2 (sensitivity 0.80, PPV 0.12), SIRS > 1 (sensitivity 0.80, PPV 0.08) and NEWS > 4 (sensitivity 0.85, PPV 0.10). In common with our study, these studies did not identify any early warning score with clearly superior accuracy to NEWS2. More detailed comparison is limited by differences in the reference standard used in the different studies.
Strengths and limitations
We used a large sample of routinely recorded ambulance data from two ambulance services transporting patients to four NHS hospitals. The ambulance services used different approaches to recording paramedic diagnostic impression and the hospitals varied in size and specialist services. This allowed us to explore the number of presentations prioritised in different settings and using different systems. The linked data used to analyse early warning score accuracy involved just one hospital but was still a large sample with a substantial number of reference standard positive cases, allowing us to estimate the sensitivity of early warning scores with reasonable precision. Our sample size calculation was based on an assumption of 200 reference standard positive cases, whereas we actually identified 348 cases with the primary reference standard. Our definition of the reference standard was based on the most recent internationally recognised definition of sepsis1 and was adjudicated in a robust way by two independent clinicians identifying evidence of infection and a two-point change in the SOFA score with acceptable interobserver agreement.
This study has a number of limitations that need to be taken into account when interpreting the findings. Our rescue plan was the only viable option for completing the study within the time constraints after NHS Digital failed to deliver the linked data. The accuracy analysis used data from just over half the eligible cases transported to Sheffield, with exclusions mainly due to a lack of the patient’s NHS number. The eligible patients tended to be much older than those without NHS numbers, possibly reflecting more frequent contact with health services and hence more frequent opportunities to record the NHS number. Sepsis is associated with age and comorbidity, but our findings may not be generalisable to younger patients with no or little comorbidity. Delays caused by the COVID-19 pandemic and waiting for NHS Digital meant that the study data from 2019 may reflect historical practice, particularly in the use of pre-alerts as a way of identifying how ambulance services prioritise patients in usual practice. The differences between ambulance services in the way diagnostic impressions are recorded makes it difficult to draw generalisable conclusions across different NHS ambulance services. We were only able to analyse early warning score accuracy using data from one hospital, thus limiting the generalisability of the findings. We collected data over a year to mitigate the effects of seasonality and used data from 2019 as we felt that this was a typical year in terms of the prevalence of respiratory pathogens (if such a thing exists), but rates of presentations requiring prioritisation may show marked seasonality and variation according to the prevalence of respiratory pathogens. Furthermore, both ambulance services provided data with a number of days missing, which may have limited our ability to take seasonality into account.
Our reference standard had important strengths, noted above, but also some limitations. While adjudicating the reference standard we noticed that the change in SOFA score often reflected the local effects of infection (e.g. respiratory failure in pneumonia or raised bilirubin in biliary infection) or an exacerbation of underlying comorbidity, rather than organ failure likely to reflect a dysregulated host response to infection. This reflects limitations of the sepsis-3 definition and our current inability to measure a dysregulated host response in clinical practice. 1 The consequence of this issue is that our reference standard is likely to include many patients who do not have a dysregulated response to infection and are unlikely to benefit from early treatment. We tried to address this issue by defining the primary reference standard based on the sepsis-3 definition and early receipt of treatment for sepsis, with a secondary reference standard based on the sepsis-3 definition alone. However, 95% of presentations meeting the sepsis-3 definition received early treatment for sepsis, so there was little difference between our primary and secondary reference standard definitions in practice. This issue may relate to the uncertainty identified in the decision-analytic modelling around the effect of early treatment upon sepsis outcomes. If the sepsis-3 definition identifies patients at risk of mortality due to underlying comorbidities rather than acute infection, then the effect of early antibiotic treatment will be attenuated. 79
Widespread use of NEWS2 across the NHS may have influenced paramedic assessment of diagnostic impression, particularly in terms of differentiating sepsis from other infections. This may mean that paramedic diagnostic impression and NEWS2 scores are correlated to a degree. Use of NEWS2 in the ED may have prompted greater investigation for infection in patients with a higher NEWS2 scores. However, NEWS2 scores were not routinely recorded in the hospital records used in reference standard assessment, so the reference standard adjudicators were not aware of the patient’s NEWS2 (or any other) score.
We used decision-analytic modelling to explore the trade-off between the costs and benefits of different strategies for prioritising people with suspected sepsis and to determine the cost-effectiveness of alternative strategies. We modelled the operational consequences of using different strategies at small and large hospitals, but the cost-effectiveness analysis was limited by substantial uncertainty around the benefits of early treatment and the negative consequences of unnecessary prioritisation. We decided that it would not be possible to identify a credible estimate of the effectiveness of early treatment of sepsis that would be accepted across the spectrum of opinion on this issue, so we adopted an alternative approach of identifying the threshold of treatment effect at which each strategy would be cost-effective compared to no prioritisation. This at least allows readers with different opinions to interpret the findings in the light of their beliefs, for example, on the effectiveness of early treatment. The analysis suggested that sensitive strategies are likely to be cost-effective, if we believe that early treatment reduces mortality, which is seen in the strategies that produce the highest iNMB at lower relative risk of mortality associated with prioritisation. The cost associated with false positives was shown to be a key driver of results, with ‘no prioritisation’ having the highest iNMB at relative risks of 0.996 or greater when the costs were assumed to be £124 but having the highest iNMB at relative risks of 0.986 or greater when the costs were increased to £500.
Our estimate of the cost of unnecessary prioritisation assumes that hospitals can find the necessary resources to increase capacity to handle additional prioritised cases, if additional prioritisation is cost-effective. This assumption is unlikely to hold in practice, given the limited availability of staff with the necessary expertise. The more likely consequence of unnecessary prioritisation would be delays to other urgent cases, with extremely uncertain and unpredictable impacts. We were also unable to identify a credible way of estimating the impact of unnecessary prioritisation on antimicrobial resistance. We considered developing a more complex model to explore the effects of unnecessary prioritisation but decided that a more complex model would not produce robust estimates of cost-effectiveness that would be accepted by decision-makers. We believe that the estimates of the operational consequence of different strategies that we were able to produce are likely to be far more useful to decision-makers.
In retrospect, our plans for economic analysis were probably overambitious and did not reflect limitations in conceptual understanding of sepsis or evidence around the effect of treatment delays. The definition of sepsis is contested and includes patients whose risk of adverse outcome is related to their comorbidities rather than infection. 80 As a consequence, and as we have outlined, the effect of urgent treatment is uncertain and likely to vary substantially across this heterogeneous patient group. We also underestimated the complexity of prioritisation and the impact of deteriorating performance in the emergency care system. The effect of prioritisation is likely to be context-dependent and related to the capacity of the emergency care system to respond. The effect of prioritisation on an over-stretched system in which patient safety is compromised is very difficult to predict.
Implications for decision-makers
In common with other similar studies, we were unable to identify an ideal strategy that prioritised a substantial majority of presentations with sepsis without prioritising a potentially unmanageable number of presentations. Even the most accurate strategy we examined (NEWS2 alongside diagnostic impression of sepsis or infection) would only prioritise just over half the presentations with sepsis, while prioritising four patients without sepsis for every one with confirmed sepsis, although the cases without sepsis may benefit from prioritisation for other reasons. It is unlikely that hospitals would be able to manage higher rates of prioritisation, so hospitals need to ensure that failure to prioritise suspected sepsis does not lead to harmful delays to treatment.
Our findings can assist decision-makers to determine the consequences of implementing strategies advocated in sepsis guidelines. As outlined above, our findings suggest that strategies based on evidence of infection and NEWS2 > 4 or NEWS2 > 6 incorporated in the Academy of Medical Royal Colleges decision support tool offer reasonable alternatives that could optimise sensitivity (NEWS2 > 4) or optimise positive predictive value (NEWS2 > 6) depending on ED capacity and need to prioritise sepsis. 51 The sepsis-3 recommendation1 to use qSOFA > 1 alongside evidence of infection offers better positive predictive value at the expense of sensitivity, although similar accuracy could be achieved using NEWS2 > 8 if additional training in using qSOFA were a barrier to implementation.
Other relevant guidelines can be compared to using NEWS2 at a specified threshold in presentations with a diagnostic impression of infection or sepsis. The NHS pre-alert criteria for ambulance services6 have sensitivity of 0.429 and positive predictive value of 0.24 when applied to presentations with a diagnostic impression of infection or sepsis, which is inferior to NEWS > 6 applied to the same presentations (sensitivity 0.447, positive predictive value 0.274). The UKST Red Flag criteria8 (excluding skin, lactate, recent chemotherapy and urine output criteria) had sensitivity of 0.522 and positive predictive value of 0.209 when applied to presentations with a diagnostic impression of infection or sepsis, almost equivalent to NEWS2 > 4 applied to the same presentations (sensitivity 0.522, positive predictive value 0.216). Including the skin, lactate, chemotherapy and urine output criteria would be expected to increase sensitivity at the expense of positive predictive value.
Decision-makers can therefore use our findings, particularly the estimates of accuracy and operational consequences of using NEWS2 at thresholds of > 4, > 6 or > 8 in patients with a diagnostic impression of infection or sepsis, to determine an appropriate strategy for local circumstances. National guideline developers, such as NICE, can use the estimates to inform national guidance. However, it is worth noting that our estimates are based on mean values across 2019. The incidence of sepsis, and other conditions that lead to a diagnostic impression of infection or sepsis and NEWS2 exceeding a specified threshold, is likely to be influenced by seasonality and the prevalence of respiratory pathogens. Hence, it may be appropriate for guideline developers and decision-makers to indicate a need for flexibility in the choice of threshold.
Finally, our decision-analytic modelling suggested that if we believe that prioritisation of cases with sepsis has a meaningful effect on mortality, then more sensitive strategies are likely to be cost-effective compared to less sensitive strategies. This suggests that strategies with high sensitivity could be cost-effective if capacity were available to manage increased numbers of prioritised cases. However, the potential benefit of prioritisation is probably inversely related to the capacity of the ED (the more limited ED is, the greater the need for prioritisation). The practical implications of this observation are probably that, if we believe that early treatment for sepsis has a meaningful effect on mortality, then it is worth seeking resources to increase ED capacity and reduce the risk of delayed assessment on arrival.
Recommendations for future research
The limitations of our study outlined above mean that it would be helpful to determine whether our findings are consistent across different hospitals and over time. Our experience suggests that future studies should not use NHS Digital to facilitate data linkage between ambulance services and hospitals. The most feasible alternative is likely to involve repeating the process we used at Sheffield Teaching Hospitals Trust across multiple sites, co-ordinated by a Clinical Trials Unit. However, it is worth noting that this process was facilitated by the presence of the Chief Investigator as a clinician with an honorary contract. Any recommendations for future research will need to take into account the barriers to using routine data to support research in the NHS. 80 Although multicentre research is clearly desirable to enhance generalisability, the substantial barriers to multicentre research involving routine data need to be taken into account. The increased availability of NHS numbers to ambulance services mean that our study could be relatively quickly repeated at a single hospital and ambulance service, with a much higher proportion of linked cases, but repeating this process across multiple sites could be challenging.
We did not identify any strategy that could prioritise a substantial proportion of patients with sepsis without prioritising a potentially unmanageable number of cases. We clearly require better early warning scores, but further research based upon existing variables is unlikely to be helpful. We examined multiple alternative scores and nearly all were equivalent or inferior to NEWS2. Even the SEPSIS score, which was specifically developed as a prehospital early warning score for sepsis for sepsis in the NHS, only had marginally superior accuracy to NEWS2 in selected analyses. Improving performance is therefore likely to involve identifying new predictors or developing new tests for use in the prehospital setting.
Recommendations for future research need to take into account the limitations of the current sepsis-3 definition. We noted that many cases fulfilled the sepsis-3 definition on the basis of organ failure that was likely to represent the direct effects of infection or exacerbation of comorbidity, rather than a dysregulated host response to infection. This may explain the lack of evidence we identified to estimate the benefit of early treatment for sepsis. If the definition of sepsis includes many patients who do not have a dysregulated host response to infection, then studies of early treatment for sepsis are likely to yield uncertain, conflicting or negative findings. Substantial research is currently developing and evaluating biomarkers that could clinically measure the dysregulated host response that characterises sepsis. This research could lead to better definition of sepsis and clinical markers that predict response to treatment rather than just adverse outcome (which may reflect severity of frailty and comorbidities rather than severity of infection or host response). In the meantime, it is important to recognise that research into early recognition of sepsis will be limited by the lack of a reference standard definition that appropriately reflects the characterisation of sepsis as a dysregulated host response to infection.
Equality, diversity and inclusion
The study design provided limited opportunities to address issues of equality, diversity or inclusion, which were compounded by the challenges we faced in obtaining and linking routine data. We planned to undertake the study across four diverse hospitals serving diverse populations but were only able to undertake the main analysis using linked data from one hospital. The ambulance services were able to provide data on ethnicity, and this raised some concerns. Ethnicity was missing from 29,005/71,204 (41%) of the population. Among those with ethnicity recorded, the population was overwhelmingly white (95%). This may reflect a high proportion of white population in the participating sites (especially Doncaster and Barnsley) or a higher rate of missing ethnicity data for non-white populations. Either way, the ethnicity data limit our ability to apply our findings to minority ethnic populations. This is an important limitation because early warning scores are based upon variables that may differ between populations and ethnicity may influence paramedic diagnostic impression. Measurement of peripheral oxygen saturation is included in a number of early warning scores, including NEWS2, but may overestimate oxygenation in people of black ethnicity. 81,82
Patient and public involvement
Patient and public involvement (PPI) was invaluable to the study in ensuring that the study was respectful of patient dignity, autonomy and confidentiality, particularly in its use of routine data. PPI representatives made the following contributions to the study:
-
Development of the proposal. PPI representatives helped to develop the proposal through meetings with the lead applicant and contributed to drafting the proposal, including the plain English summary. The lead applicant also presented the proposal for discussion at a meeting of the Sheffield Emergency Care Forum (SECF), an established group dedicated to providing PPI for research in emergency care.
-
Development and implementation of the study protocol. PPI representatives met regularly with the study manager and chief investigator and attended project management group meetings. PPI representatives shared regular updates on the project with the wider SECF group and on the SECF website.
-
Review of early warning scores for inclusion in the evaluation. As outlined in the methods, PPI representatives reviewed the early warning scores to determine whether their use was likely to be acceptable to the patient and the public, taking into account whether measuring or recording variables for the score could be intrusive for the patient, and whether the score raises concerns about equity, such as in relation to age, gender, ethnic group or socioeconomic status. This resulted in modification of one of the scores to remove a variable relating to residence in a care home.
-
Development of the decision-analytic model. The research team presented the developing model at PPI meetings and identified key assumptions in the model. Discussions focused on the role of prioritisation in emergency care and the use of early warning scores. The PPI representatives felt that prioritisation was appropriate provided it reflected need for urgent care and did not discriminate of the basis of personal characteristics, such as age, gender, ethnic group or socioeconomic status. The use of age in early warning scores was considered appropriate if it was related to need for urgent treatment. Other personal characteristics would not influence prioritisation as they were not included as (or clearly associated with) variables in the early warning scores. The PPI representatives contributed to consideration of the difficult issue of how to estimate the impact of unnecessary prioritisation upon the emergency care system. They generally supported the modelling approach of providing hospitals with estimates of the number of patients who would be prioritised using different strategies, which could then inform policies at each hospital, rather than trying to find a ‘one size fits all’ approach.
-
Review of study outputs. PPI representatives reviewed the study conclusions, implications for practice and research recommendations, and considered whether these reflected the needs, preferences and values of patients and the public.
-
Co-production of public-facing material. The PPI group co-produced the Public Awareness Poster which was displayed at prominent locations in the ED of the Northern General Hospital. This ensured that the design and content of the poster was accessible and clear and led to the inclusion of a QR code linked to the study website.
-
Study oversight. The study steering committee included two PPI representatives to provide study oversight.
-
UK Sepsis Trust. The study team contacted the UKST several times to seek PPI representatives to join the PPI group. Following an initially positive response, we were unable to identify representatives to join the PPI group.
-
NHS Digital. The PPI representatives expressed their concerns that the failure of NHS Digital to provide data linkage had substantially undermined the study. They reported similar experiences with other studies and raised concerns that difficulties using NHS data were creating substantial barriers to research and were not in patients’ or the public interest.
Chapter 5 Conclusions
We were unable to identify an ideal strategy for prehospital prioritisation of patients with possible sepsis that would prioritise a substantial majority of those with sepsis without prioritising a potentially unmanageable number of patients. Using NEWS2 in patients with a diagnostic impression of infection or sepsis could offer practical (albeit suboptimal) options for prioritisation, with sensitivity and positive predictive value ranging from 0.522 and 0.216 with NEWS2 > 4, to 0.447 and 0.274 with NEWS2 > 6, or 0.314 and 0.333 with NEWS2 > 8. NEWS2 has the advantage of being widely used and understood in the NHS and increasingly integrated into ambulance and hospital information systems. None of the other early warning scores, which would require additional measures to implement, offered any meaningful improvement on NEWS2.
Acknowledgements
We thank Anna Wilson and Sarah Bird (Research Nurses, Sheffield Teaching Hospitals NHS Foundation Trust) for screening cases for reference standard adjudication.
We thank Erica Wallis (Research Co-ordinator, Sheffield Teaching Hospitals NHS Foundation Trust) for her support and advice on ethical and governance issues.
We thank Linda Abouzeid, Lynn Simmons and Enid Hirst (public representatives, Sheffield Emergency Care Forum) and Peter Hewkin (independent public representative) for their insight and guidance.
We thank Katie Ridsdale and Jack Rose (Trial Support Officers, University of Sheffield CTRU) for providing central administrative support.
We thank Martin Bayley, Steven Wood and Thomas Butterfield (Healthcare Computer Scientists, Sheffield Teaching Hospitals NHS Foundation Trust) for linking ambulance service to hospital data.
We thank Caroline Leech (University Hospitals Coventry and Warwickshire), Sheriff Adewunmi (Doncaster Royal Infirmary) and Kate Rankin (Rotherham General Hospital) for their help with initial hospital site set-up and interpreting the ambulance service data for their respective hospitals.
We thank Janette Turner (Reader in Urgent and Emergency Care, University of Sheffield) and Catheryn James (Clinical Manager, Yorkshire Ambulance Service) for their help with developing the proposal and involvement in the project management group.
We thank Sarah Lay-Flurrie (Senior Statistician, University of Oxford) for her initial role in the Study Steering Committee.
We thank the NIHR Sheffield Clinical Research Facility for supporting the study.
We thank the members of our oversight committee, the Study Steering Committee: Tim Coats (Professor of Emergency Medicine, University of Leicester), Alice Riddell (Patient representative, Sheffield Emergency Care Forum), Shan Bennett (Patient representative, Sheffield Emergency Care Forum), Duncan Robertson (Consultant Paramedic and Regional Clinical Lead, Welsh Ambulance Service), James Fullerton (Specialist Registrar in Clinical Pharmacology and Therapeutics, University of Oxford), Graham McClelland (Paramedic Research Fellow, North East Ambulance Service), Fenella Wrigley (Chief Medical Officer, London Ambulance Service).
Ethical approval
The study was approved by London – Stanmore REC (reference number 19/LO/1443) on 23 September 2019, HRA on 30 January 2020, and CAG on 13 January 2020.
Information governance statement
The University of Sheffield is committed to handling all personal information in line with the UK Data Protection Act (2018) and the General Data Protection Regulation (EU GDPR) 2016/679.
Under the Data Protection legislation, The University of Sheffield is the Data Controller, and you can find out more about how we handle personal data, including how to exercise your individual rights and the contact details for our Data Protection Officer, here: www.sheffield.ac.uk/scharr/research/centres/ctru/phews.
Department of Health disclaimer
This report presents independent research commissioned by the National Institute for Health and Care Research (NIHR). The views and opinions expressed by authors in this publication are those of the authors and do not necessarily reflect those of the NHS, the NIHR, MRC, CCF, NETSCC, the HTA programme or the Department of Health.
Data-sharing statement
All data requests should be submitted to the corresponding author for consideration. Access to anonymised data may be granted following review.
Acknowledgement of patient data
This work uses data provided by patients and collected by the NHS as part of their care and support. Using patient data is vital to improve health and care for everyone. There is huge potential to make better use of information from people’s patient records, to understand more about disease, develop new treatments, monitor safety, and plan NHS services. Patient data should be kept safe and secure, to protect everyone’s privacy, and it is important that there are safeguards to make sure that they are stored and used responsibly. Everyone should be able to find out about how patient data are used. #datasaveslives. You can find out more about the background to this citation here: https://understandingpatientdata.org.uk/data-citation.
Contributions of authors
Steve Goodacre (https://orcid.org/0000-0003-0803-8444) (Professor of Emergency Medicine) produced the first draft of the report, conceived of or designed the work, was involved in the acquisition of data for the work and interpretation of data for the work, revised the work critically for important intellectual content and was involved in the final approval of the version to be published.
Laura Sutton (https://orcid.org/0000-0003-3327-5927) (Lecturer in Epidemiology and Statistics), produced the first draft of the report, was involved in the analysis of data and the interpretation of data for the work, revised the work critically for important intellectual content and was involved in the final approval of the version to be published.
Kate Ennis (https://orcid.org/0000-0003-4284-217X) (Research Associate, Health Economics and Decision Science) produced the first draft of the report, was involved in the acquisition of data for the work, the analysis of data and the interpretation of data for the work, revised the work critically for important intellectual content and was involved in the final approval of the version to be published.
Ben Thomas (https://orcid.org/0000-0002-6659-6930) (Study Manager) was involved in the acquisition of data for the work and the interpretation of data for the work, revised the work critically for important intellectual content and was involved in the final approval of the version to be published.
Olivia Hawksworth (https://orcid.org/0000-0001-6513-100X) (Study Manager) was involved in the interpretation of data for the work, revised the work critically for important intellectual content and was involved in the final approval of the version to be published.
Khurram Iftikhar (https://orcid.org/0000-0002-9721-6232) (Consultant in Emergency Medicine and Major Trauma) was involved in the acquisition of data for the work and the interpretation of data for the work, revised the work critically for important intellectual content and was involved in the final approval of the version to be published.
Susan J Croft (https://orcid.org/0000-0003-4190-2599) (Consultant in Emergency Medicine) was involved in the acquisition of data for the work and the interpretation of data for the work, revised the work critically for important intellectual content and was involved in the final approval of the version to be published.
Gordon Fuller (https://orcid.org/0000-0001-8532-3500) (Clinical Lecturer in Emergency Medicine) conceived of or designed the work, was involved in the acquisition of data for the work and the interpretation of data for the work, revised the work critically for important intellectual content and was involved in the final approval of the version to be published.
Simon Waterhouse (https://orcid.org/0000-0002-6303-9610) (Lead Data Specialist) was involved in the acquisition of data for the work and the interpretation of data for the work, revised the work critically for important intellectual content and was involved in the final approval of the version to be published.
Daniel Hind (https://orcid.org/0000-0002-6409-4793) (Professor of Evaluation) conceived of or designed the work, was involved in the interpretation of data for the work, revised the work critically for important intellectual content and was involved in the final approval of the version to be published.
Matt D Stevenson (https://orcid.org/0000-0002-3099-9877) (Professor of Health Technology Assessment) produced the first draft of the report, conceived of or designed the work, was involved in the analysis of data and the interpretation of data for the work, revised the work critically for important intellectual content and was involved in the final approval of the version to be published.
Mike J Bradburn (https://orcid.org/0000-0002-3783-9761) (Senior Medical Statistician) conceived of or designed the work, was involved in the interpretation of data for the work, revised the work critically for important intellectual content and was involved in the final approval of the version to be published.
Michael Smyth (https://orcid.org/0000-0003-0220-2223) (Research Fellow) conceived of or designed the work, was involved in the interpretation of data for the work, revised the work critically for important intellectual content and was involved in the final approval of the version to be published.
Gavin D Perkins (https://orcid.org/0000-0003-3027-7548) (Professor of Critical Care Medicine) conceived of or designed the work, was involved in the interpretation of data for the work, revised the work critically for important intellectual content and was involved in the final approval of the version to be published.
Mark Millins (https://orcid.org/0000-0003-3065-0330) (Associate Director for Paramedic Practice) conceived of or designed the work, was involved in the interpretation of data for the work, revised the work critically for important intellectual content and was involved in the final approval of the version to be published.
Andy Rosser (https://orcid.org/0000-0002-5477-4269) (Lead Research Paramedic) conceived of or designed the work, was involved in the interpretation of data for the work, revised the work critically for important intellectual content and was involved in the final approval of the version to be published.
Jon Dickson (https://orcid.org/0000-0002-1361-2714) (Senior Clinical Lecturer and GP) conceived of or designed the work, was involved in the interpretation of data for the work, revised the work critically for important intellectual content and was involved in the final approval of the version to be published.
Matthew Wilson (https://orcid.org/0000-0002-9704-5189) (Senior Lecturer in Anaesthesia) conceived of or designed the work, was involved in the interpretation of data for the work, revised the work critically for important intellectual content and was involved in the final approval of the version to be published.
All authors agree to be accountable for all aspects of the work in ensuring that questions related to the accuracy or integrity of any part of the work are appropriately investigated and resolved.
Disclaimers
This report presents independent research funded by the National Institute for Health and Care Research (NIHR). The views and opinions expressed by authors in this publication are those of the authors and do not necessarily reflect those of the NHS, the NIHR, the HTA programme or the Department of Health and Social Care. If there are verbatim quotations included in this publication the views and opinions expressed by the interviewees are those of the interviewees and do not necessarily reflect those of the authors, those of the NHS, the NIHR, the HTA programme or the Department of Health and Social Care.
References
- Singer M, Deutschman CS, Seymour CW, Shankar-Hari M, Annane D, Bauer M, et al. The third international consensus definitions for sepsis and septic shock (sepsis-3). JAMA 2016;315:801-10. https://doi.org/10.1001/jama.2016.0287.
- Sabir L, Wharton L, Goodacre S. Retrospective single-centre descriptive study of the characteristics, management and outcomes of adult patients with suspected sepsis in the emergency department. Emerg Med J 2022;39:272-8. https://doi.org/10.1136/emermed-2020-211111.
- Rhee C, Jones TM, Hamad Y, Pande A, Varon J, O’Brien C, et al. Centers for Disease Control and Prevention (CDC) Prevention Epicenters Program . Prevalence, underlying causes, and preventability of sepsis-associated mortality in US acute care hospitals. JAMA Netw Open 2019;2. https://doi.org/10.1001/jamanetworkopen.2018.7571.
- Kopczynska M, Sharif B, Cleaver S, Spencer N, Kurani A, Lee C, et al. Welsh Digital Data Collection Platform Collaborators . Sepsis-related deaths in the at-risk population on the wards: attributable fraction of mortality in a large point-prevalence study. BMC Res Notes 2018;11. https://doi.org/10.1186/s13104-018-3819-2.
- Sepsis: recognition, diagnosis and early management. NICE; n.d.
- JRCALC Clinical Guidelines 2019. Bridgwater: Class Professional Publishing; 2019.
- Evans L, Rhodes A, Alhazzani W, Antonelli M, Coopersmith CM, French C, et al. Surviving sepsis campaign: international guidelines for management of sepsis and septic shock 2021. Intensive Care Med 2021;47:1181-247. https://doi.org/10.1007/s00134-021-06506-y.
- Nicholls T, Wenman J, Nutbeam T, Daniels R. Toolkit: Prehospital Management of Sepsis in Adults and Young People over 12 Years – 2016 2016. https://sepsistrust.org/wp-content/uploads/2018/06/PH-toolkit-FINAL-2.pdf (accessed 16 September 2022).
- Academy of Medical Royal Colleges . Statement on the Initial Antimicrobial Treatment of Sepsis V2.0 2022.
- Goodacre S, Thomas B, Smyth M, Dickson JM. Should prehospital early warning scores be used to identify which patients need urgent treatment for sepsis?. BMJ 2021;375. https://doi.org/10.1136/bmj.n2432.
- Talmor D, Jones AE, Rubinson L, Howell MD, Shapiro NI. Simple Triage Scoring System predicting death and the need for critical care resources for use during epidemics. Crit Care Med 2007;35:1251-6. https://doi.org/10.1097/01.CCM.0000262385.95721.CC.
- Olsson T, Terent A, Lind L. Rapid Emergency Medicine score: a new prognostic tool for in-hospital mortality in nonsurgical emergency department patients. J Intern Med 2004;255:579-87. https://doi.org/10.1111/j.1365-2796.2004.01321.x.
- Jouffroy R, Saade A, Ellouze S, Carpentier A, Michaloux M, Carli P, et al. Prehospital triage of septic patients at the SAMU regulation: comparison of qSOFA, MRST, MEWS and PRESEP scores. Am J Emerg Med 2018;36:820-4. https://doi.org/10.1016/j.ajem.2017.10.030.
- Silcock DJ, Corfield AR, Gowens PA, Rooney KD. Validation of the National Early Warning Score in the prehospital setting. Resuscitation 2015;89:31-5. https://doi.org/10.1016/j.resuscitation.2014.12.029.
- Hamilton F, Arnold D, Baird A, Albur M, Whiting P. Early Warning Scores do not accurately predict mortality in sepsis: a meta-analysis and systematic review of the literature. J Infect 2018;76:241-8. https://doi.org/10.1016/j.jinf.2018.01.002.
- Smyth MA, Brace-McDonnell SJ, Perkins GD. Identification of adults with sepsis in the prehospital environment: a systematic review. BMJ Open 2016;6. https://doi.org/10.1136/bmjopen-2016-011218.
- Lane D, Ichelson RI, Drennan IR, Scales DC. Prehospital management and identification of sepsis by emergency medical services: a systematic review. Emerg Med J 2016;33:408-13. https://doi.org/10.1136/emermed-2015-205261.
- Robson W, Nutbeam T, Daniels RS. A need for prehospital intervention?. Emerg Med J 2009;26:535-8. https://doi.org/10.1136/emj.2008.064469.
- Seymour CW, Liu VX, Iwashyna TJ, Brunkhorst FM, Rea TD, Scherag A, et al. Assessment of clinical criteria for sepsis: for the third international consensus definitions for sepsis and septic shock (sepsis-3). JAMA 2016;315:762-74. https://doi.org/10.1001/jama.2016.0288.
- Freund Y, Lemachatti N, Krastinova E, Van Laer M, Claessens Y-E, Avondo A, et al. French Society of Emergency Medicine Collaborators Group . Prognostic accuracy of sepsis-3 criteria for in-hospital mortality among patients with suspected infection presenting to the emergency department. JAMA 2017;317:301-8. https://doi.org/10.1001/jama.2016.20329.
- National Early Warning Score (NEWS) 2. London: RCP; 2017.
- Fernando SM, Tran A, Taljaard M, Cheng W, Rochwerg B, Seely AJE, et al. Prognostic accuracy of the quick sequential organ failure assessment for mortality in patients with ssuspected infection. Ann Intern Med 2018;168:266-75. https://doi.org/10.7326/M17-2820.
- Sabir L, Ramlakhan S, Goodacre S. Comparison of qSOFA and Hospital Early Warning Scores for prognosis in suspected sepsis in emergency department patients: a systematic review. Emerg Med J 2022;39:284-94. https://doi.org/10.1136/emermed-2020-210416.
- Smyth MA, Gallacher D, Kimani PK, Ragoo M, Ward M, Perkins GD. Derivation and internal validation of the Screening to Enhance Prehospital Identification of Sepsis (SEPSIS) score in adults on arrival at the emergency department. Scand J Trauma Resusc Emerg Med 2019;27. https://doi.org/10.1186/s13049-019-0642-2.
- Bayer O, Schwarzkopf D, Stumme C, Stacke A, Hartog CS, Hohenstein C, et al. An early warning scoring system to identify eptic patients in the prehospital setting: the PRESEP score. Acad Emerg Med 2015;22:868-71. https://doi.org/10.1111/acem.12707.
- Polito CC, Isakov A, Yancey AH, Wilson DK, Anderson BA, Bloom I, et al. Prehospital recognition of severe sepsis: development and validation of a novel EMS screening tool. Am J Emerg Med 2015;33:1119-25. https://doi.org/10.1016/j.ajem.2015.04.024.
- Borrelli G, Koch E, Sterk E, Lovett S, Rech MA. Early recognition of sepsis through emergency medical services pre-hospital screening. Am J Emerg Med 2019;37:1428-32. https://doi.org/10.1016/j.ajem.2018.10.036.
- Challen K, Bradburn M, Goodacre SW. Development and validation of a score to identify in the Emergency Department patients who may benefit from a time-critical intervention: a cohort study. Scand J Trauma Resusc Emerg Med 2015;23. https://doi.org/10.1186/s13049-015-0150-y.
- Wallgren UM, Castrén M, Svensson AEV, Kurland L. Identification of adult septic patients in the prehospital setting: a comparison of two screening tools and clinical judgment. Eur J Emerg Med 2014;21:260-5. https://doi.org/10.1097/MEJ.0000000000000084.
- Seymour CW, Kahn JM, Cooke CR, Watkins TR, Heckbert SR, Rea TD. Prediction of critical illness during out-of-hospital emergency care. JAMA 2010;304:747-54. https://doi.org/10.1001/jama.2010.1140.
- Tam B, Xu M, Kwang M, Wardell C, Kwong A, Fox-Robichaud A. The admission Hamilton Early Warning Score (HEWS) predicts the risk of critical event during hospitalization. Can J Gen Intern Med 2017;11:24-7. https://doi.org/10.22374/cjgim.v11i4.190.
- Association of Ambulance Chief Executives, The Royal College of Emergency Medicine . UK NHS Ambulance Services Pre-Alert Guideline for the Deteriorating Adult Patient 2020. https://rcem.ac.uk/wp-content/uploads/2021/10/NHS_Ambulance_Services_pre_alert_criteria_guideline.pdf (accessed 27 July 2023).
- Alam NO, Oskam E, Stassen PM, van Exter P, van de Ven PM, Haak HR, et al. Prehospital antibiotics in the ambulance for sepsis: a multicentre, open label, randomised trial. Lancet Respir Med 2018;6:40-5.
- Scales DD. Paramedic Initiated Treatment of Sepsis Targeting Out-of-Hospital Patients (PITSTOP) 2023. clinicaltrials.gov (accessed 12 July 2023).
- Walchok JG, Pirrallo RG, Furmanek D, Lutz M, Shope C, Giles B, et al. Paramedic-initiated CMS sepsis core measure bundle prior to hospital arrival: a stepwise approach. Prehosp Emerg Care 2017;21:291-300. https://doi.org/10.1080/10903127.2016.1254694.
- Baez AA, Cochon L. Acute Care Diagnostics Collaboration: assessment of a Bayesian clinical decision model integrating the Prehospital Sepsis Score and point-of-care lactate. Am J Emerg Med 2016;34:193-6. https://doi.org/10.1016/j.ajem.2015.10.007.
- Guerra WF, Mayfield TR, Meyers MS, Clouatre AE, Riccio JC. Early detection and treatment of patients with severe sepsis by prehospital personnel. J Emerg Med 2013;44:1116-25. https://doi.org/10.1016/j.jemermed.2012.11.003.
- Suffoletto B, Frisch A, Prabhu A, Kristan J, Guyette FX, Callaway CW. Prediction of serious infection during prehospital emergency care. Prehosp Emerg Care 2011;15:325-30. https://doi.org/10.3109/10903127.2011.561411.
- UK Sepsis Trust . Sepsis Screening Tool Prehospital n.d. https://sepsistrust.org/wp-content/uploads/2020/08/Sepsis-Prehospital-12-231219.pdf (accessed 15 March 2023).
- Goodwin APL, Srivastava V, Shotton H, Protopapa K, Butt A, Mason M. Just Say Sepsis. A Review of the Process of Care Received by Patients With Sepsis 2015. www.ncepod.org.uk/2015report2/downloads/JustSaySepsis_FullReport.pdf (accessed 16 September 2022).
- Parrott F. Length of Stay, Survival and Organ Support of Admissions With Septic Shock to Adult, General Critical Care Units in England, Wales and Northern Ireland 2014. www.icnarc.org/DataServices/Attachments/Download/ac215f3c-0a4b-e411-a65b-d48564544b14.
- Hanley JA, McNeil BJ. The meaning and use of the area under a receiver operating characteristic (ROC) curve. Radiology 1982;143:29-36. https://doi.org/10.1148/radiology.143.1.7063747.
- Collins GS, Ogundimu EO, Altman DG. Sample size considerations for the external validation of a multivariable prognostic model: a resampling study. Stat Med 2016;35:214-26. https://doi.org/10.1002/sim.6787.
- Higgins AM, Brooker JE, Mackie M, Cooper DJ, Harris AH. Health economic evaluations of sepsis interventions in critically ill adult patients: a systematic review. J Intensive Care 2020;8. https://doi.org/10.1186/s40560-019-0412-2.
- Moher D, Liberati A, Tetzlaff J, Altman DG. The PRISMA Group . Preferred Reporting Items for Systematic Reviews and Meta-analyses: the PRISMA statement. PLOS Med 2009;6.
- Mouncey PR, Osborn TM, Power GS, Harrison DA, Sadique MZ, Grieve RD, et al. Protocolised Management In Sepsis (ProMISe): a multicentre, randomised controlled trial of the clinical and cost-effectiveness of early protocolised resuscitation for emerging septic shock. Health Technol Assess 2015;19:1-150. https://doi.org/10.3310/hta19970.
- Smyth MA, Brace-McDonnell SJ, Perkins GD. Impact of prehospital care on outcomes in sepsis: a systematic review. West J Emerg Med 2016;17:427-37. https://doi.org/10.5811/westjem.2016.5.30172.
- NICE Health Technology Evaluations: The Manual (PMG36). London, UK; 2022.
- National Early Warning Score (NEWS). London, UK; 2022.
- Royal College of Physicians . National Early Warning Score (NEWS) 2: Standardising the Assessment of Acute-Illness Severity in the NHS. Updated Report of a Working Party 2017.
- Bion J BG, Boyle A, Carrol E, Christian W, Crossland S, Faust S, et al. Academy of Medical Royal Colleges Statement on the Initial Antimicrobial Treatment of Sepsis. London, UK: Academy of Medical Royal Colleges; 2022.
- Westwood M, Ramaekers B, Whiting P, Tomini F, Joore M, Armstrong N, et al. Procalcitonin testing to guide antibiotic therapy for the treatment of sepsis in intensive care settings and for suspected bacterial infection in emergency department settings: a systematic review and cost-effectiveness analysis. Health Technol Assess 2015;19:v-xxv, 1. https://doi.org/10.3310/hta19960.
- Christ-Crain M, Jaccard-Stolz D, Bingisser R, Gencay MM, Huber PR, Tamm M, et al. Effect of procalcitonin-guided treatment on antibiotic use and outcome in lower respiratory tract infections: cluster-randomised, single-blinded intervention trial. Lancet 2004;363:600-7. https://doi.org/10.1016/S0140-6736(04)15591-8.
- Mirjam C-C, Daiana S, Roland B, Christian M, David M, Peter RH, et al. Procalcitonin guidance of antibiotic therapy in community-acquired pneumonia. Am J Respir Crit Care Med 2006;174:84-93. https://doi.org/10.1164/rccm.200512-1922OC.
- Bouadma L, Luyt C-E, Tubach F, Cracco C, Alvarez A, Schwebel C, et al. PRORATA trial group . Use of procalcitonin to reduce patients’ exposure to antibiotics in intensive care units (PRORATA trial): a multicentre randomised controlled trial. Lancet 2010;375:463-74. https://doi.org/10.1016/S0140-6736(09)61879-1.
- Qu R, Ji Y, Ling Y, Ye CY, Yang SM, Liu YY, et al. Procalcitonin is a good tool to guide duration of antibiotic therapy in patients with severe acute pancreatitis. A randomized prospective single-center controlled trial. Saudi Med J 2012;33:382-7.
- Roh YH, Lee BJ. Treatment of elderly patients with community-acquired pneumonia with the guidance of procalcitonin. Chest 2013;144. https://doi.org/10.1378/chest.1703693.
- Stevenson M, Pandor A, Martyn-St James M, Rafia R, Uttley L, Stevens J, et al. Sepsis: the LightCycler SeptiFast Test MGRADE®, SepsiTestTM and IRIDICA BAC BSI assay for rapidly identifying bloodstream bacteria and fungi – a systematic review and economic evaluation. Health Technol Assess 2016;20:1-246. https://doi.org/10.3310/hta20460.
- Warhurst G, Dunn G, Chadwick P, Blackwood B, McAuley D, Perkins GD, et al. Rapid detection of health-care-associated bloodstream infection in critical care using multipathogen real-time polymerase chain reaction technology: a diagnostic accuracy study and systematic review. Health Technol Assess 2015;19:1-142. https://doi.org/10.3310/hta19350.
- Soares MO, Welton NJ, Harrison DA, Peura P, Shankar-Hari M, Harvey SE, et al. An evaluation of the feasibility, cost and value of information of a multicentre randomised controlled trial of intravenous immunoglobulin for sepsis (severe sepsis and septic shock): Incorporating a systematic review, metaanalysis and value of information analysis. Health Technol Assess 2012;16.
- Green CDJ, Dinnes J, Takeda A, Shepherd J, Hartwell D. Clinical effectiveness and cost-effectiveness of drotrecogin alfa (activated) (Xigris) for the treatment of severe sepsis in adults: a systematic review and economic evaluation. Health Technol Assess 2005;9.
- Green C, Dinnes J, Takeda AL, Cuthbertson BH. Evaluation of the cost-effectiveness of drotrecogin alfa (activated) for the treatment of severe sepsis in the United Kingdom. Int J Technol Assess Health Care 2006;22:90-100. https://doi.org/10.1017/S0266462306050884.
- Cuthbertson BH, Elders A, Hall S, Taylor J, MacLennan G, Mackirdy F, et al. Scottish Critical Care Trials Group . Mortality and quality of life in the five years after severe sepsis. Crit Care 2013;17. https://doi.org/10.1186/cc12616.
- ONS . National Life Tables: England and Wales. 2018–2020 2021.
- BNF . British National Formulary 2022.
- NHS England . NHS Reference Costs 2019 20 2022.
- NHS England . NHS Reference Costs 2017 18 2018.
- PSSRU . Unit Costs of Health and Social Care 2020: NHS Cost Inflation Index 2019 20 2020.
- Hunter CL, Silvestri S, Stone A, Shaughnessy A, Miller S, Rodriguez A, et al. Prehospital sepsis alert notification decreases time to initiation of CMS sepsis core measures. Am J Emerg Med 2019;37:114-7.
- Mixon M, Dietrich S, Floren M, Rogoszewski R, Kane L, Nudell N, et al. Time to antibiotic administration: sepsis alerts called in emergency department versus in the field via emergency medical services. Am J Emerg Med 2020;11.
- McClelland G, Jones J. A pilot study exploring the accuracy of pre-hospital sepsis recognition in the North East Ambulance Service. J Paramed Pract 2015;7:459-65. https://doi.org/10.12968/jpar.2015.7.9.459.
- Alam N, Doerga KB, Hussain T, Hussain S, Holleman F, Kramer MH, et al. Epidemiology, recognition and documentation of sepsis in the pre-hospital setting and associated clinical outcomes: a prospective multicenter study. Acute Med 2016;15:168-75.
- Floer M, Ziegler M, Lenkewitz B, Auer A, Meister T. Out-of-hospital sepsis recognition by paramedics improves the course of disease and mortality: a single center retrospective study. Adv Clin Exp Med 2021;30:1115-25.
- Jouffroy R, Gilbert B, Tourtier JP, Bloch-Laine E, Ecollan P, Bounes V, et al. Impact of prehospital antibiotic therapy on septic shock mortality. Prehosp Emerg Care 2020;25:317-24.
- Chamberlain D. Prehospital administered intravenous antimicrobial protocol for septic shock: a prospective randomized clinical trial. Crit Care 2009;13. https://doi.org/10.1186/cc7481.
- Cunningham CT, Sanseverino A, Reznek M, Borges E, Beth Urhoy M, Gross K, et al. A pilot study of prehospital antibiotics for severe sepsis. Acad Emerg Med 2022;29:231-3. https://doi.org/10.1111/acem.14388.
- Jones J, Allen S, Davies J, Driscoll T, Ellis G, Fegan G, et al. Randomised feasibility study of prehospital recognition and antibiotics for emergency patients with sepsis (PhRASe). Sci Rep 2021;11. https://doi.org/10.1038/s41598-021-97979-w.
- Stinnett AA, Mullahy J. Net health benefits: a new framework for the analysis of uncertainty in cost-effectiveness analysis. Med Decis Making 1998;18:S68-80. https://doi.org/10.1177/0272989X98018002S09.
- Goodacre S, Fuller G, Conroy S, Hendrikse C. Diagnosis and management of sepsis in the older adult. BMJ 2023;382. https://doi.org/10.1136/bmj-2023-075585.
- Taylor JA, Crowe S, Pujol FE, Franklin RC, Feltbower RG, Norman LJ, et al. The road to hell is paved with good intentions: the experience of applying for national data for linkage and suggestions for improvement. BMJ Open 2021;11. https://doi.org/10.1136/bmjopen-2020-047575.
- Bangash MN, Hodson J, Evison F, Patel JM, Johnston AM, Gallier S, et al. Impact of ethnicity on the accuracy of measurements of oxygen saturations: a retrospective observational cohort study. EClinicalMedicine 2022;48. https://doi.org/10.1016/j.eclinm.2022.101428.
- Fawzy A, Wu TD, Wang K, Robinson ML, Farha J, Bradke A, et al. Racial and ethnic discrepancy in pulse oximetry and delayed identification of treatment eligibility among patients with COVID-19. JAMA Intern Med 2022;182:730-8.
- UK Sepsis Trust . The Yellow Manual 2022. https://sepsistrust.org/wp-content/uploads/2022/06/Yellow-Manual-6th-Edition.pdf (accessed 21 July 2023).
Appendix 1 Categorisation of diagnostic impressions in each ambulance service
Category | West Midlands Ambulance Service | Yorkshire Ambulance Service |
---|---|---|
1 | Sepsis, cold sepsis | Sepsis |
2 | Cellulitis, wound infection, acute otitis media, acute bacterial sinusitis, quinsy, tonsillitis, abscess, cellulitis, hepatitis, HIV, influenza, meningitis, pyrexia of unknown origin, TB, tonsillitis, UTI, viral, bronchiolitis, bronchitis, chest infection, pneumonia, pyelonephritis | Cold and flu, febrile illness, meningitis, pyrexia of unknown origin, chest infection-pneumonia, UTI |
3 | Hypotension, tachycardia, undetermined rash, dermatology (other), sore throat, abdominal pain, appendicitis, cholecystitis, diarrhoea, diverticulitis, pancreatitis, vomiting, Addison’s disease, coma, convulsion, headache, COPD, emphysema, pleuritic chest pain, respiratory (other), haematuria, renal colic, retention, urological (other), unable to determine, generalised weakness, faint (vaso vagal) | Catheter problems, COPD, convulsion/fitting, collapse-reason unknown, confused/distressed/upset, diarrhoea/constipation, dizzy/near faint/loss of co-ordination, shortness of breath, generally unwell, haematuria, headache, hypotension, other medical condition, urinary retention, shock (hypovolemic), transient loss of consciousness, unconscious, vomiting |
4 | Cancer (other), acute coronary syndrome, atrial fibrillation, bradycardia, cardiac arrest, dysrhythmia, heart failure, hypertension, STEMI, supraventricular tachycardia, cardiac (other), eczema, pressure sores, thrombophlebitis, ulcer, urticaria, epistaxis, vertigo, ENT, acid reflux, Crohn’s disease, GI bleed, haematemesis, malena, obstruction, PR bleed, ulcerative colitis, anaphylaxis, hyperglycaemia, hyperthyroidism, hypoglycaemia, hypothyroidism, lupus, endocrine, hearing impairment, dyslexia, dyspraxia, dyscalculia, dysgraphia, dysphasia/aphasia, auditory processing disorder, visual processing disorder, ADD/ADHD, autism, Asperger’s syndrome, arthritis, back pain, carpal tunnel, tendonitis, Alzheimer’s, epilepsy, migraine, motor neurone disease, multiple sclerosis, Parkinson’s disease, stroke, subarachnoid haemorrhage, TIA, neurological (other), conjunctivitis, corneal abrasion, foreign body in eye, loss of vision, eye pain, ophthalmology (other), overdose, intoxication, asthma, haemopneumothorax, haemothorax, pneumothorax, pulmonary embolism, aortic aneurysm, DVT, limb ischaemia, vascular (other), visual impairment, deceased | Abdominal pain, alcohol related, allergic reaction/rash, anaphylactic shock, AAA, asthma, bite/sting, bleeding PR, bleeding PV, cardiac arrest, cardiac STEMI, cardiac chest pain (ACS), cardiac NSTEMI, choking, carbon monoxide poisoning, dental, drug overdose, end of life care/palliative, epileptic fit, epistaxis, eye injury/eye problem, falls, gynaecological, haematemesis, haemoptysis, haemorrhage/lacerations, hyperglycaemia, hypertension, hypoglycaemia, neurological problems, pain – back non-traumatic, pain – other, panic attack, poisoning, pulmonary embolism, rape/sexual assault, pneumothorax (spontaneous), renal problems/colic, respiratory arrest, seizures (non-EP), smoke inhalation, solvent related, stroke – FAST positive, unable to cope, no injury or illness, cardiac arrhythmia, vascular emergency (non-AAA), Dead on EMS arrival – signs inconsistent with life, resuscitation unsuccessful |
5 | Eclampsia, ectopic, hyperemesis, labour, miscarriage, pregnancy, PV bleed, child birth, obstetric (other), schizophrenia, depression, bipolar disorder, anxiety disorder, OCD, eating disorder, personality disorder, dementia, brain injury, Alzheimer’s, schizoaffective disorder, chemical, biological, radiological, nuclear explosive, burns/scalds, drowning, electrocution, hyperthermia, hypothermia, immersion, inhalation, asphyxiation, environmental (other), bite, sting, gunshot, stabbing, inflicted (other), blunt trauma, crush injury, C-spine injury, dislocation, fracture closed, fracture open, head injury, multiple injuries, muscular, penetrating, sprain/strain, injury (other) | Burns, drowning, electrocution, fracture/possible fracture, hanging, head injury, major trauma, minor cuts and bruising, minor injuries – other, multiple injuries, obstetric – BBA, obstetric – birth imminent, obstetric – miscarriage, obstetric – normal labour, obstetric – premature labour, obstetric emergency (other), psychiatric problems, spinal injury, sprain/strain/dislocation, stabbed/shot/weapon wound, wound closure, non-accidental injury |
Appendix 2 Search strategy to identify early warning scores for evaluation
We used the search strategy from Goodacre et al. to identify early warning scores for evaluation. 10
-
Ambulances/
-
Air ambulances/
-
Paramedic*
-
“Emergency service*” [Title/Abstract]
-
Allied health personnel/
-
Emergency medical technicians/
-
“Out of hospital”
-
“Emergency medical service*”
-
EMS
-
Prehospital [Title/Abstract]
-
Emergency treatment/
-
“Transportation of patients”/
-
EMT
-
1 or 2 or 3 or 4 or 5 or 6 or 7 or 8 or 9 or 10 or 11 or 12 or 13
-
Sepsis/
-
Septicemia*
-
Septicaemia*
-
Sepsis
-
Septic
-
Systemic inflammatory response syndrome/
-
“Systemic inflammatory response syndrome” [Title/Abstract]
-
SIRS
-
“Serious infection*” [Title/Abstract]
-
15 or 16 or 17 or 18 or 19 or 20 or 21 or 22 or 23
-
Risk assessment/ classification
-
Risk assessment/ methods*
-
Point-of-care systems/
-
Severity of illness index/
-
EWS [Title/Abstract]
-
“Early warning scoring” [Title/Abstract]
-
“Early warning” [Title/Abstract]
-
“Warning system*” [Title/Abstract]
-
“Warning scoring*” [Title/Abstract]
-
“Early detection” [Title/Abstract]
-
Prediction [Title/Abstract]
-
“Screening tool*” [Title/Abstract]
-
24 or 25 or 26 or 27 or 28 or 29 or 30 or 31 or 32 or 33 or 34 or 35 or 36
-
14 and 24 and 37
Appendix 3 Details of each early warning score
90-30-9029
Dichotomous assessment, positive if any of the following criteria are met:
-
systolic BP < 90 mmHg;
-
respiratory rate > 30/minute;
-
oxygen saturation < 90%.
Modification:
If the oxygen saturation is measured on supplemental oxygen, it is assumed to be < 90% on air (i.e. the criterion is positive).*
Missing data:
Assume any missing criterion is negative/normal.
The Borrelli strategy:27
Dichotomous assessment, positive if three or more criteria are met:
-
respiratory rate > 20/minute;
-
heart rate > 90/minute;
-
systolic BP < 90 mmHg;
-
documented fever or temperature > 38.3°C or < 36°C;
-
new onset of mental status change;
-
O2 saturation < 90%;
-
suspected infection.
Modification:
Documented fever or temperature > 38.3°C or < 36°C, is effectively just temperature > 38.3°C or < 36°C.
New onset of mental status change assumed if the GCS verbal scale is < 5. If the GCS verbal scale is missing, then mental status change is assumed if GCS < 15 or AVPU <A.
If the oxygen saturation is measured on supplemental oxygen, it is assumed to be < 90% on air (i.e. the criterion is positive).
Missing data:
Assume any missing variable is negative.
CIS. 30
Score | 0 | 1 | 2 |
---|---|---|---|
Respiratory rate | 12–23 | < 12 or 24–35 | > 35 |
Heart rate | < 120 | ≥ 120 | |
Systolic BP | > 90 | ≤ 90 | |
Age | < 45 | ≥ 45 | |
SpO2 | ≥ 88 | < 88 | |
GCS | 15 | 8–14 | < 8 |
Thresholds of > 4 or > 0 are suggested, depending upon whether specificity or sensitivity is to be optimised.
Modification:
If the oxygen saturation is measured on supplemental oxygen, it is assumed to be < 88% on air (i.e. scores 1 point).
Missing data:
Assume any missing variable scores zero.
HEWS. 31
Score | 3 | 2 | 1 | 0 | 1 | 2 | 3 |
---|---|---|---|---|---|---|---|
Respiratory rate | < 8 | 8–13 | 14–20 | 21–30 | > 30 | ||
Oxygen saturation | < 85 | 85–91 | > 91 | ||||
Heart rate | < 40 | 40–50 | 51–100 | 101–110 | 111–130 | > 130 | |
Systolic BP | < 70 | 71–90 | 91–170 | 171–200 | > 200 | ||
Temperature | < 35.0 | 35.1–36.4 | 36.5–38.0 | 38.1–39.0 | > 39.0 | ||
Neurology | Alert | Voice | Pain | Unresponsive | |||
Air or oxygen | Air | ≤ 5 l/minute or ≤ 50% by mask | > 5 l/minute or > 50% by mask |
Threshold > 4
Modification:
If AVPU is missing, infer from GCS.
If on oxygen but amount unknown, score 2 points.
Missing data:
Assume any missing variable scores zero.
MEWS. 13
MEWS has five parameters, each of which is scored from 0 to 2 or 3, providing an overall score between 0 and 14.
Score | 3 | 2 | 1 | 0 | 1 | 2 | 3 |
---|---|---|---|---|---|---|---|
Respiratory rate | < 9 | 9–14 | 15–20 | 21–29 | ≥ 30 | ||
Heart rate | ≤ 40 | 41–50 | 51–100 | 101–110 | 111–129 | ≥ 130 | |
Systolic BP | ≤ 70 | 71–80 | 81–100 | 101–199 | ≥ 200 | ||
Temperature | < 35.0 | 35.0–38.4 | ≥ 38.5 | ||||
AVPU | Alert | Voice | Pain | Unresponsive |
A threshold of 5 or more has been shown to be associated with an increased risk of death.
Modification:
If AVPU missing, infer AVPU from GCS.
Missing data:
Assume any missing variable scores zero.
NEWS2. 14
The NEWS2 has seven parameters, each of which is scored from 0 to 3, providing an overall score between 0 and 20.
Score | 3 | 2 | 1 | 0 | 1 | 2 | 3 |
---|---|---|---|---|---|---|---|
Respiratory rate | ≤ 8 | 9–11 | 12–20 | 21–24 | ≥ 25 | ||
Oxygen saturation | ≤ 91 | 92–93 | 94–95 | ≥ 96 | |||
Heart rate | ≤ 40 | 41–50 | 51–90 | 91–110 | 111–130 | ≥ 131 | |
Systolic BP | ≤ 90 | 91–100 | 101–110 | 111–219 | ≥ 220 | ||
Temperature | ≤ 35.0 | 35.1–36.0 | 36.1–38.0 | 38.1–39.0 | ≥ 39.1 | ||
Neurology | Alert | Confusion, voice, pain, unresponsive | |||||
Air or oxygen | Oxygen (based on FiO2 > 21%, or FiO2 > 0 l/minute) | Air |
We will not use the scale for patients with confirmed hypercapnic respiratory failure.
Modification:
If AVPU is missing, infer AVPU from GCS.
Missing data:
Assume any missing variable scores zero.
National Health Service pre-alert. 32
Pre-alert if any of the following are present:
-
respiratory rate ≤ 8 or ≥ 25;
-
O2 saturations on oxygen < 92% (patients usually running normal oxygen saturations) or < 4% (patients with chronic hypercapnic respiratory failure);
-
systolic < 90 mmHg OR downward-trending systolic where symptomatic;
-
tachycardia ≥ 131;
-
GCS motor < 4.
Sepsis red-flag criteria evaluated as part of UKST criteria.
Modification:
Drop < 84% oxygen saturation threshold for patients with chronic hypercapnic respiratory failure.
Drop downward-trending systolic where symptomatic.
Missing data:
Assume any missing criterion is negative.
Prehospital ANTibiotics Against Sepsis (PHANTASi). 33
Dichotomous assessment, positive if both the following criteria are met:
-
Temperature > 38°C or < 36°C;
-
Heart rate > 90 beats per minute or respiratory rate > 20/minute.
Modification:
None required.
Missing data:
Assume any missing variable is negative/normal.
PITSTOP. 34
Dichotomous assessment, positive if all the following three criteria are met:
-
paramedic suspects possible infection
-
temperature ≥ 38.0°C
-
systolic BP < 100 mmHg.
Modification:
None required.
Missing data:
Assume any missing criterion is negative/normal.
PreSAT. 35
Dichotomous assessment, positive if both the following criteria are met:
-
presentation suggestive of infection
-
any two from (1) temperature > 38°C or < 36°C, (2) heart rate > 90/minute, (3) respiratory rate > 20/minute, (4) systolic BP < 90 mmHg.
Modification:
None required.
Missing data:
Assume any missing variable is negative/normal.
PRESEP. 25
Parameter | Score |
---|---|
Temperature > 38°C | 4 |
Temperature < 36°C | 1 |
SaO2 < 92% | 2 |
RR > 22 breaths/minute | 1 |
HR > 90 beats/minute | 2 |
BP < 90 mmHg | 2 |
Recommended threshold > 3
Modification:
If the oxygen saturation is measured on supplemental oxygen, it is assumed to be < 92% on air (i.e. score 2 points).
Missing data:
Assume any missing variable scores zero.
PRESS. 26
The score is only applied to patients meeting all three of the following criteria, so patients not meeting these criteria should score zero:
-
heart rate > 90/minute
-
respiratory rate > 20/minute
-
systolic blood pressure BP < 110 mmHg.
Score | 0 | 1 | 2 | 3 | 4 | 5 |
---|---|---|---|---|---|---|
Age | < 40 | ≥ 60 | 40–59 | |||
SpO2 | ≥ 90 | 80–89 | 70–79 | 60–69 | < 60 | |
Systolic BP | 100–109 | 90–99 | 80–89 | 70–79 | 60–69 | < 60 |
Hot tactile temperature | X | |||||
ED chief concern: sick person | X | |||||
Nursing home transport | X |
Threshold > 1.
Modification:
If the oxygen saturation is measured on supplemental oxygen, it is assumed to be < 90% on air and scored 2 points.
Infer hot tactile temperature from recorded temperature > 38°C.
Drop ED chief concern sick person – address this through diagnostic impression.
Drop nursing home transport.
Missing data:
Assume any missing variable scores zero.
Prehospital Sepsis Project (PSP). 36
Parameter | Score |
---|---|
Temperature > 38°C | 1 |
Heart rate/systolic BP ≥ 0.7 | 2 |
Respiratory rate > 22/minute | 1 |
Low risk = 0–1 point, moderate risk = 2 points, high risk = 3–4 points.
Modification:
None required.
Missing data:
Assume missing temperature or respiratory rate scores zero.
Assume heart rate/systolic BP scores zero unless either (1) heart rate is > 100 and systolic BP is missing, or (2) systolic BP is < 100 and heart rate is missing.
qSOFA. 19
Parameter | Score |
---|---|
GCS < 15 | 1 |
Respiratory rate ≥ 22 | 1 |
Systolic BP ≤ 100 | 1 |
Total score 0–3.
Low risk = 0 or 1.
High risk = 2 or 3.
Modification:
None required.
Missing data:
Assume any missing variable scores zero.
REMS. 12
Score | 0 | 1 | 2 | 3 | 4 | 5 | 6 |
---|---|---|---|---|---|---|---|
Age | < 45 | 45–54 | 55–64 | 65–74 | > 65 | ||
MAP | 70–109 | 50–69 or 110–129 | 130–159 | > 159 or < 50 | |||
Heart rate | 70–109 | 55–69 or 110–139 | 40–54 or 140–179 | < 179 or < 40 | |||
Respiratory rate | 12–24 | 10–11 or 25–34 | 6–9 | 35–49 | < 6 or > 49 | ||
SpO2 (%) | > 89 | 86–89 | 75–85 | < 75 | |||
GCS | > 13 | 11–13 | 8–10 | 5–7 | 3–4 |
High risk (REMS ≥ 3): patient may need aggressive treatment.
Low risk (REMS < 3): patient may be appropriate to triage for routine treatment.
Modification:
If the oxygen saturation is measured on supplemental oxygen, it is assumed to be < 89% on air and scored 2 points.
Missing data:
Assume any missing variable scores zero.
Robson Screening Tool (RST). 18
Dichotomous assessment, positive if presentation suggestive of infection and any two of:
-
temperature > 38.3°C or < 36°C
-
heart rate > 90 beats/minute
-
respiratory rate > 20 breaths/minute
-
acutely altered mental status
-
plasma glucose > 6.6 mmol/l (unless diabetic).
Modification:
Criterion 5 applies regardless of whether they are diabetic.
Acutely altered mental status change assumed if the GCS verbal scale is < 5. If the GCS verbal scale is missing, then acutely altered mental status is assumed if GCS < 15 or AVPU = A.
Missing data:
Assume any missing criterion is negative.
SEPSIS24
Parameter | −1 | 0 | 1 | 2 |
---|---|---|---|---|
Age | ≤ 60 | > 60 | ||
Respiratory rate | ≤ 20 or > 60 | 21–40 | 40–60 | |
SpO2 | ≥ 94 | < 94 | ||
Heart rate | ≤ 100 | 101–140 | 141–160 | |
Systolic BP | > 160 | < 60 or 100–160 | 60–99 | |
GCS | 13–15 | 3–12 | ||
Temperature | < 37.5 | 37.5 to 39.5 | > 39.5 | |
Skin | Jaundice, pallor, mottling |
High risk if score > 4.
Modification:
Skin features dropped from the score (not recorded on ePFR).
Missing data:
Assume any missing variable scores zero.
Give 1 point if SpO2 > 94% on oxygen.
Sepsis alert. 37
At least two SIRS criteria:
-
Temperature > 38°C or < 36°C
-
Pulse > 90 beats/minute
-
Respiratory rate > 20 breaths/minute or mechanically ventilated.
And
Suspected or documented infection
And
Hypoperfusion, as manifested by one of the following:
-
systolic BP < 90 mmHg
-
mean arterial pressure < 65 mmHg
-
lactate level ≥ 4 mmol/l.
Modification:
Drop lactate level ≥ 4 mmol/l.
Missing data:
Assume negative if missing.
Simple Triage Scoring System (STSS)11
Parameter | Score |
---|---|
Age > 65 years | 1 |
Altered mental status | 1 |
Respiratory rate of > 30 breaths/minute | 1 |
Low oxygen saturation | 1 |
Shock index of > 1 (heart rate > systolic BP) | 1 |
Modification:
Altered mental status change assumed if the GCS verbal scale is < 5. If the GCS verbal scale is missing, then altered mental status is assumed if GCS < 15 or AVPU < A.
Score 1 point for low oxygen saturation if oxygen saturation is < 94% or measured on supplemental oxygen.
Missing data:
Assume any missing variable scores zero.
Suffoletto strategy. 38
Dichotomous assessment, positive if any of the following criteria are met:
-
systolic BP < 100 mmHg
-
history or suspicion of fever
-
prehospital judgement of infection.
Modification:
Infer history or suspicion of fever from temperature > 38°C.
Missing data:
Assume any missing criterion is negative.
UK Sepsis Trust Red Flags. 39,83
Any of the following gives a positive score:
-
objective evidence of new or altered mental state
-
systolic BP ≤ 90 mmHg (or drop of > 40 from normal)
-
heart rate ≥ 130/minute
-
respiratory rate ≥ 25/minute
-
needs O2 to keep SpO2 ≥ 92% (88% in COPD)
-
non-blanching rash/mottled/ashen/cyanotic
-
lactate ≥ 2 mmol/l
-
recent chemotherapy
-
not passed urine in 18 hours.
Modification:
New or altered mental state assumed if the GCS verbal scale is < 5. If the GCS verbal scale is missing, then new or altered mental state is assumed if GCS < 15 or AVPU < A.
Drop BP change from normal and just use systolic BP ≤ 90 mmHg.
Simplify O2 criteria to SpO2 < 92% or measured on supplemental oxygen.
Drop non-blanching rash/mottled/ashen/cyanotic.
Drop lactate ≥ 2 mmol/l.
Drop recent chemotherapy.
Drop not passed urine.
Missing data:
Assume any missing criterion is negative.
Appendix 4 Timeline for seeking linked data from NHS Digital
The table below outlines the timeline for seeking linked data from NHS Digital. Our original plan involved securing HRA, CAG and REC approval during the first 6 months of the project, concurrently agreeing the NHS Digital specification in months 4–6, and receiving the linked data in months 7–9. Our submissions to HRA, CAG and the REC were slightly delayed and approvals were received in month 7. We started work on the NHS Digital specification alongside HRA submission and shared the initial DARS application with NHS Digital in month 8.
From month 7 to month 9 of the project, the Chief Investigator and Study Manager started work on a prioritised urgent public health study (PRIEST, the Pandemic Respiratory Infection Emergency System Triage study). In agreement with NIHR, we suspended the Prehospital Early Warning Scores for Sepsis (PHEWS) study from March to December 2020 to allow the study team to prioritise the PRIEST study. Allowing for time taken to wind down and then restart the study, the project timeline was effectively set back by 1 year, with January 2021 becoming month 7.
We submitted the DARS application to NHS Digital in January 2021 (effectively month 7). The study team responded to queries from NHS Digital over the next 3 months, but NHS Digital unexpectedly rejected the application in May 2021 after they raised new concerns about the risk associated with the planned flow of their data to multiple organisations. NHS Digital had not raised these concerns when we shared the initial draft of the application with them in February 2020 nor when we submitted the application in February 2021.
NHS Digital reported concerns that sending linked data to the four hospital sites involved an unacceptable risk to them. We therefore revised the proposed data flow so that NHS Digital would send all linked data to Sheffield CTRU, who would then send relevant NHS numbers to the participating sites for reference standard review. We rapidly developed this proposal, submitted a substantial amendment to the HRA, and received approval from the HRA (including CAG and REC review) on 17 June 2021. NHS Digital reviewed the revised DARS application, approved the application on 7 August 2021, and provided a Data-Sharing Agreement on 22 September 2021. The total time from DARS submission to provision of the Data-Sharing Agreement was therefore 8 months.
The University of Sheffield signed the Data-Sharing Agreement after a 3-week delay and there was a further delay until 13 December while the ambulance services submitted data to NHS Digital (total delay of 3 months).
On 7 January 2022 the study team identified a data breach, whereby Yorkshire Ambulance Service had sent data to NHS Digital from all patients transferred to hospital rather than just those transferred to the participating hospitals. NHS Digital reported that data linkage had not commenced and on 31 January confirmed that they had deleted the data from patients transported to non-participating sites.
From this point onwards, the study team were waiting for NHS Digital to deliver the linked data. In response to requests for updates, NHS Digital reports a backlog of requests to their Data Production Team. A scheduled release date of 19 May 2022 passed without data release.
In June 2022 the study team recognised that the lack of NHS Digital data had become a critical threat to the delivery of the project. NIHR had agreed an 18-month extension to the project to reflect the delays due to the pandemic and due to not receiving NHS Digital data but was unable to allow any further extension beyond the new end date of 31 December 2022. We therefore developed a rescue plan that would allow completion of the project at the lead NHS site (Sheffield) using direct data linkage between the ambulance service and hospital rather than NHS Digital. We developed substantial amendment 3 to include the rescue plan, which the HRA approved in July 2022. On 19 September 2022 we decided to formally activate the rescue plan and withdraw the NHS Digital application, after the 9-month delay waiting for the linked data.
In retrospect, our original timelines were extremely overoptimistic. Although we developed the DARS application concurrent with HRA submission, we could not submit it until we received HRA, CAG and REC approvals. The subsequent 8-month delay between DARS submission and provision of the Data-Sharing Agreement reflects the need for NHS Digital to have robust review processes and the potential for rejection and reapplication. Future projects should allow at least 12 months for regulatory approvals and NHS Digital review of the DARS application.
The subsequent delay and failure to deliver linked data are more difficult (and potentially impossible) to accommodate in a time-limited contract-funded research project. After 9 months we had received no data nor any indication of when the data would be delivered. The funder was understandably unwilling to extend the research contract under these circumstances, so our only option was to withdraw the DARS application and implement our rescue plan. It is possible that the delay and ultimate failure to deliver data reflects exceptional circumstances following the COVID-19 pandemic. However, our experience suggests that using NHS Digital data is not a viable option for future time-limited contract-funded research projects.
Date | Event |
---|---|
13 January 2020 | HRA, REC and CAG approvals secured |
February 2020 | Initial draft of DARS application shared with NHS Digital and feedback received |
March 2020 | Study suspended due to COVID-19 pandemic |
January 2021 | Study reactivated and DARS application submitted to NHS Digital |
4 February 2021 to 11 March 2021 | Study manager receives queries from NHS Digital about the application and responds to queries |
20 April 2021 | NHS Digital raise concerns about sending data to multiple recipients |
7 May 2021 | NHS Digital reject DARS application on the basis that it is too high risk for them to send data to multiple recipients |
24 May 2021 | Study team submit substantial amendment 2 to HRA outlining alternative data flow |
8 June 2021 | Study team submit amended DARS to NHS Digital with amended data flow so that all NHS Digital data go to Sheffield CTRU |
17 June 2021 | HRA approval received for substantial amendment 2 |
June–July 2021 | Study manager receives queries from NHS Digital about the application and responds to queries |
15 July 2021 | NHS Digital confirm that the DARS application has been passed to a Senior Case Officer for review |
9 August 2021 | NHS Digital approve DARS application subject to conditions |
13 August 2021 | Study manager responds to conditions |
24 August 2021 | NHS Digital approval of DARS application |
22 September 2021 | NHS Digital provide Data-Sharing Agreement for signing |
15 October 2021 | Sheffield University sign off Data-Sharing Agreement |
13 December 2021 | Ambulance services complete submission of data to NHS Digital |
7 January 2022 | Study manager contacts NHS Digital to advise of ambulance service data breach |
31 January 2022 | NHS Digital confirm that data for transfers to non-participating hospitals have been deleted |
1 April 2022 | Study manager asks NHS Digital to provide a date when linked data will be issued |
19 April 2022 | NHS Digital Data Production Team report a backlog of applications but state that the PHEWS data are scheduled for release on 19 May 2022 |
20 May 2022 | Study manager contacts NHS Digital as no data were received |
23 May 2022 | NHS Digital respond that the Data Production Team have a backlog and release of the PHEWS data will be delayed |
June–July 2022 | Study manager requests further updates on when the data will be released but receives no confirmed date |
6 July 2022 | Substantial amendment 3 submitted to HRA with the option of the rescue plan, if NHS Digital fails to deliver linked data |
26 July 2022 | HRA approve substantial amendment 3 |
August–September 2022 | Practicalities of implementing the rescue plan explored with Sheffield Teaching Hospitals NHS Trust |
19 September 2022 | Rescue plan implemented and NHS Digital informed that the DARS application has been withdrawn |
List of abbreviations
- ACVPU
- alert, confusion, voice, pain, unresponsive
- CAG
- Confidentiality Advisory Group
- CIS
- Critical Illness Score
- CTRU
- Clinical Trials Research Unit
- ED
- emergency department
- EGDT
- early goal-directed therapy
- ePRF
- electronic patient report form
- GCS
- Glasgow Coma Scale
- GDG
- Guideline Development Group
- HEWS
- Hamilton Early Warning Score
- HRA
- Health Research Authority
- HRQoL
- health-related quality of life
- ICD
- International Classification of Diseases
- ICNARC
- Intensive Care National Audit and Research Centre
- iNMB
- incremental net monetary benefit
- LoS
- length of stay
- MEWS
- Modified Early Warning Score
- NCEPOD
- National Confidential Enquiry into Patient Outcome and Death
- NEWS
- National Early Warning Score
- NEWS2
- National Early Warning Score, version 2
- NICE
- National Institute for Health and Care Excellence
- PHEWS
- prehospital early warning scores for sepsis
- PITSTOP
- Paramedic-Initiated Treatment of Sepsis Targeting Out-of-hospital Patients clinical trial
- PreSAT
- Prehospital Sepsis Assessment Tool
- PRESEP
- Prehospital Early Sepsis Detection
- PRESS
- Prehospital Severe Sepsis
- PSP
- Prehospital Sepsis Project
- QALY
- quality-adjusted life-year
- REC
- Research Ethics Committee
- REMS
- Rapid Emergency Medicine Score
- ROC
- receiver operating characteristic
- RST
- Robson Screening Tool
- SEPSIS
- Screening to Enhance PrehoSpital Identification of Sepsis
- SIRS
- systemic inflammatory response syndrome
- SOFA
- Sequential (sepsis-related) Organ Failure Assessment
- STSS
- Simple Triage Scoring System
- UKST
- UK Sepsis Trust
Notes
Supplementary material can be found on the NIHR Journals Library report page (https://doi.org/10.3310/NDTY2403).
Supplementary material has been provided by the authors to support the report and any files provided at submission will have been seen by peer reviewers, but not extensively reviewed. Any supplementary material provided at a later stage in the process may not have been peer reviewed.