Notes
Article history
The research reported in this issue of the journal was funded by the HTA programme as project number 17/93/06. The contractual start date was in September 2018. The draft report began editorial review in June 2020 and was accepted for publication in January 2021. The authors have been wholly responsible for all data collection, analysis and interpretation, and for writing up their work. The HTA editors and publisher have tried to ensure the accuracy of the authors’ report and would like to thank the reviewers for their constructive comments on the draft document. However, they do not accept liability for damages or losses arising from material published in this report.
Permissions
Copyright statement
Copyright © 2022 Gega et al. This work was produced by Gega et al. under the terms of a commissioning contract issued by the Secretary of State for Health and Social Care. This is an Open Access publication distributed under the terms of the Creative Commons Attribution CC BY 4.0 licence, which permits unrestricted use, distribution, reproduction and adaption in any medium and for any purpose provided that it is properly attributed. See: https://creativecommons.org/licenses/by/4.0/. For attribution the title, original author(s), the publication source – NIHR Journals Library, and the DOI of the publication must be cited.
2022 Gega et al.
Chapter 1 Background
Mental health
We can begin to understand mental health with two related, yet independent, concepts. Mental well-being (Figure 1, blue circle) refers to our sense of self, our ability to meet our potential and develop relationships, and our ability to do things that we consider important and worthwhile. Mental health problems (see Figure 1, purple circle) refer to the presence of specific signs and symptoms that indicate a diagnosable condition that affects our emotional state, physical function, behaviour and thinking.
FIGURE 1.
Mental well-being vs. mental health problems.
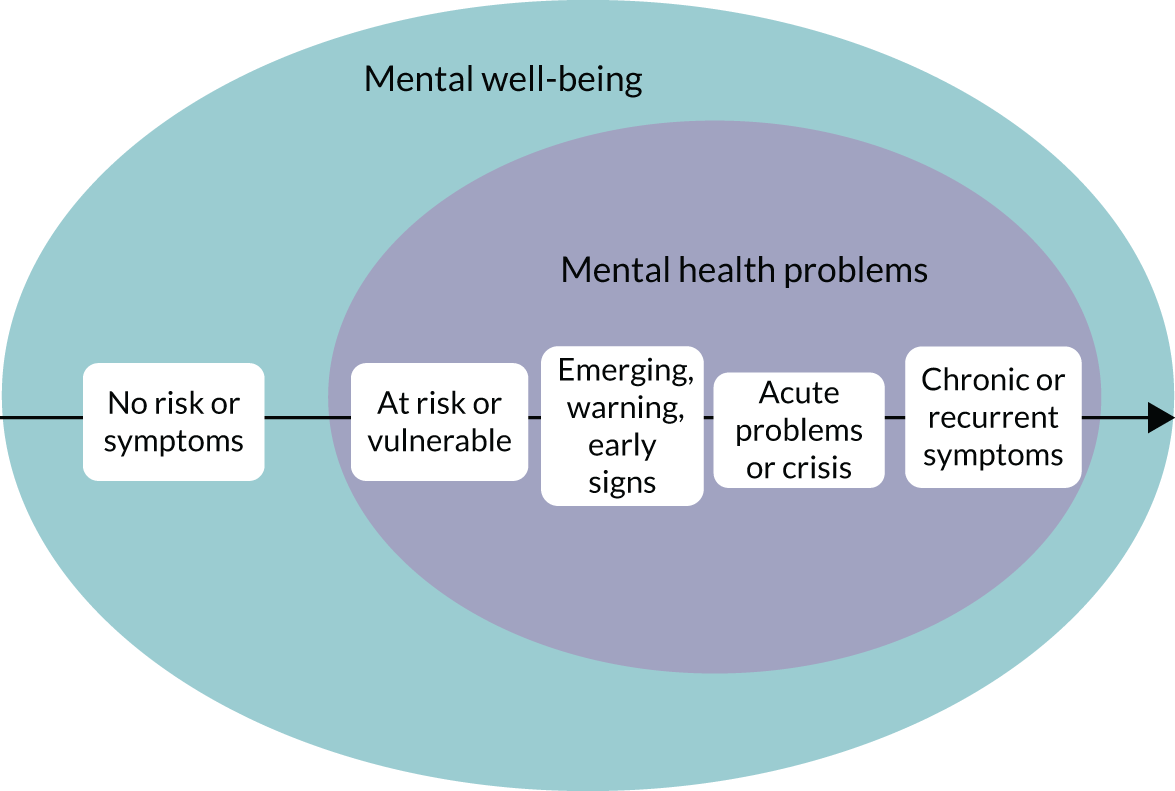
The diagnosis of mental health problems is based on specific signs and symptoms that follow the World Health Organization (WHO)’s classification criteria,1 which help clinicians group different types of mental health problems into specific diagnostic categories (Table 1). Clinical guidelines are also helpful to group different problems when they follow similar care pathways, for example the National Institute for Health and Care Excellence (NICE)’s clinical guidelines on common mental health problems. 2 Different mental health problems often coexist as comorbidities. In addition, the severity of mental health problems varies along a continuum not only between individuals, but also at different times in the same person; some people are more vulnerable than others, and the same person is more vulnerable at certain times in their lives, because of an accumulation of risk factors (e.g. family history of mental illness, abuse or bullying, and poverty). At any particular time, some susceptible individuals will be at one end of the continuum, with no symptoms and no risk of any mental health problems, while others will be further along the continuum, experiencing some degree of vulnerability to a specific mental health problem or exhibiting emerging or warning symptoms to some degree (see Figure 1, black arrow). Some people will move along the continuum to experience acute symptoms or a crisis, and, of those, some will recover and others will experience life-long and recurrent problems. This continuum gives a useful context to our work, as we will explore research studies in mental health, not only across different clinical populations, but also at different stages in the continuum for a specific clinical population represented by mild, moderate, severe or subthreshold states.
Classification group | Examples of conditions |
---|---|
Schizophrenia/psychosis | Schizophrenia, schizoaffective disorder, delusional disorder |
Mood disorders | Bipolar affective disorder, depressive episodes |
Anxiety or fear-related disorders | Generalised anxiety disorder, panic disorder, agoraphobia, specific phobia, social anxiety, separation anxiety, selective mutism |
Obsessive–compulsive or related disorders | Obsessive–compulsive disorder, body dysmorphic disorder, health anxiety, body-focused repetitive behaviour |
Disorders associated with stress | Post-traumatic stress disorder, prolonged grief disorder, adjustment disorder |
Feeding or eating disorders | Anorexia, bulimia, binge eating disorder, avoidant–restrictive food intake, pica |
Disorders of bodily distress or bodily experience | Bodily distress disorder, body integrity dysphoria |
Disorders due to substance use or addictive behaviours |
Disorders due to substance use: alcohol drugs, sedatives, hypnotics or anxiolytics, caffeine, nicotine, other psychoactive and non-psychoactive substances Disorders due to addictive behaviours: gambling disorder, gaming disorder |
Impulse control disorders | Pyromania, kleptomania, compulsive sexual behaviour |
Personality disorders and related traits | Personality disorder, prominent personality traits or patterns |
Mental or behavioural disorders associated with pregnancy, childbirth and the puerperium | Post-natal depression, post-natal psychosis |
So, what is the difference, and the relationship, between mental well-being and mental health problems? Although diagnosable mental health problems are a risk factor for poor mental well-being, a diagnosis does not necessarily lead to poor mental well-being; many people with diagnosable mental health problems flourish and maintain strong mental well-being. In contrast, the absence of diagnosable mental health problems does not guarantee strong mental well-being, as people have reported poor mental well-being without a diagnosis. This is an important distinction to delineate the scope of this work, which relates to emerging or existing diagnosable mental health problems and is not about general mental well-being.
Interventions in mental health
Interventions to promote or improve mental health can be mapped onto the two concepts of mental health – mental well-being and mental health problems – and across the four key points of the continuum in Figure 1 (at risk, emerging, acute problems, chronic problems). Interventions to support and improve mental well-being (Figure 2, the purple circle that sits in the background) are the foundation of good mental health for any population. This includes having an active lifestyle, eating and sleeping well, safeguarding people from abuse and bullying, providing good education, reducing poverty, ensuring equality and justice, and having meaningful relationships. Such activities are the foundation of mental well-being for any population, but they are beyond the scope of this work, unless they are specifically developed, implemented and evaluated in the context of preventing or improving emerging or existing mental health problems.
FIGURE 2.
Interventions to prevent or improve mental health problems.
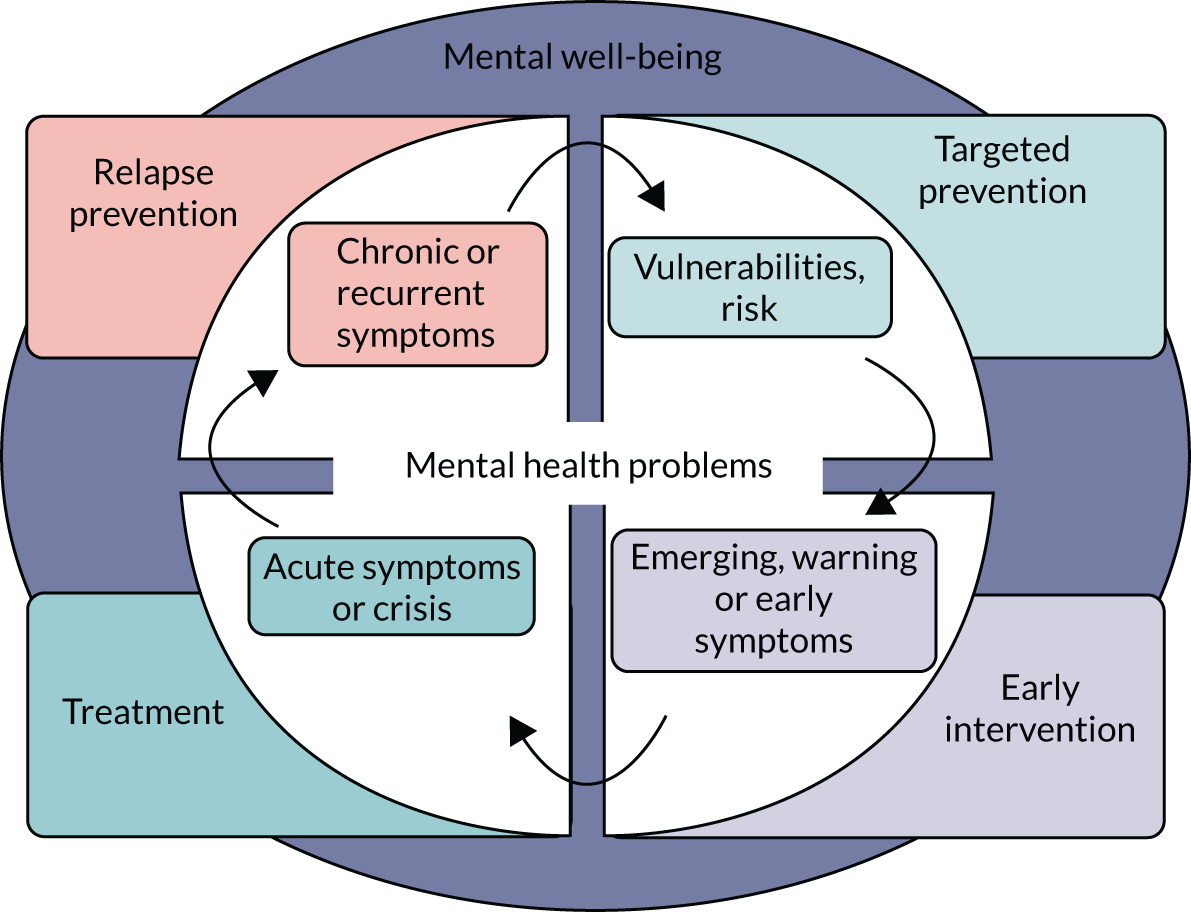
Typically, interventions within the scope of our work, that is interventions that aim to prevent or improve emerging or existing mental health problems, are either pharmacological (i.e. prescribed psychiatric medication indicated for a specific diagnosis) or non-pharmacological (i.e. psychological therapies, social interventions, physical and occupational therapies, behavioural interventions). The aims of these interventions, as shown in Figure 1, are to:
-
reduce the likelihood of occurrence of future mental illness among those at risk (targeted prevention)
-
reduce emerging and early symptoms before these manifest as a diagnosable illness (early intervention)
-
improve acute symptoms and manage crisis (treatment)
-
improve and manage chronic symptoms to minimise the likelihood of recurrence (relapse prevention).
Our project focused on mental health outcomes associated with an intervention, in the form of reducing the incidence/occurrence of mental health problems and the severity of clinical symptoms associated with those. Mental health interventions could instigate behaviour change, improve physical health outcomes and have a positive impact on proxy indicators and factors associated with mental well-being (e.g. employment and poverty). These are important outcomes but beyond the scope of this project.
Digital interventions
Digital interventions (DIs) use software programs that are accessed via computers, tablets, smartphones, audio-visual and virtual reality (VR) equipment, gaming consoles, robots and other devices to deliver interventions that aim to prevent or improve mental health problems, including depression, anxiety disorders, addictive behaviours and eating disorders. 3 DIs collect, store and retrieve clinical information: deliver standardised instructions via text, voice files or video clips: and guide users in the application of therapeutic activities. DIs can include varying levels of standardisation, self-help and clinician involvement; some are entirely self-administered by service users, whereas others are completely reliant on a clinician/therapist.
Digital interventions are often standardised, automated, user-directed, psychological therapies that use technology to help users work through a therapeutic activity either independently of, or alongside, a clinician or therapist. One common mental health therapy that features heavily in DIs, because of its structured approach, is cognitive–behavioural therapy (CBT). CBT treats a physical or mental health problem by identifying and changing certain beliefs and behaviours that maintain the problem. CBT places emphasis on activities completed by users outside therapy sessions; this is commonly referred to as ‘homework’, which fits in well with the self-directed nature of DIs.
An example of a DI is an internet-based self-help programme evaluated by Christensen et al. 4 The intervention was a 10-week structured therapy consisting of psychoeducation (weeks 1 and 2), CBT (weeks 3–7), relaxation (weeks 8 and 9) and physical activity (week 10). The psychoeducation section provided information on worry, stress, fear and anxiety; how to differentiate between types of anxiety disorders; risk factors for anxiety; comorbidity; consequences of anxiety; and available treatments. The CBT toolkit addressed typical anxious thoughts and included sections on dealing with the purpose and meaning of worry, the act of worrying and the content of worry. The relaxation modules guided participants on how to progressively tense and relax different muscle groups to induce relaxation and how to become aware of their breathing and body, acknowledging thoughts and external distractions but remaining focused on the present. The physical activity gave tailored advice depending on the level of the participant’s motivation and ability.
Another example of a DI is a mobile app (application) in a study by Pham et al. ,5 which engaged users in a series of minigames to learn and practise diaphragmatic breathing to alleviate symptoms of anxiety, in line with NHS protocols and evidence-based literature. The minigames had various themes, from sailing a boat down a river to flying balloons into the sky. Users touched the screen with their finger as they inhaled and removed their finger from the screen as they exhaled to control the gaming mechanics. A breathing indicator visually represented a full breath; users saw a circle expanding as they inhaled and contracting as they exhaled. This indicator provided a visual guide of a breathing retraining exercise. The goal of each minigame was to correctly follow the breathing indicator to progress in the game narrative; users progressed through levels and achieved goals by breathing correctly and staying calm.
Economic evaluations
Digital interventions are particularly important for mental health care in locations where access to services is limited and face-to-face contact with psychiatrists and psychologists is at a premium. The decision to adopt DIs into a health-care system is, at least in part, informed by an assessment of value for money. There is an assumption that DIs offer ‘good value for money’ because they have the potential to save clinician time and make clinical work more efficient by encouraging patient self-management, allowing remote delivery of interventions, enabling a less specialised workforce to deliver complex interventions, enhancing outcomes for the same level of therapeutic input and reducing waiting lists (WLs).
Economic evaluations can provide evidence to support or refute the assumption that DIs are good value for money, by comparing the costs and outcomes of DIs relative to the costs and outcomes of relevant alternatives. Economic evaluations are often built within clinical evaluations or trials, which compare the outcomes of a new intervention/service with the outcomes of a control (e.g. usual or standard care) over a specific period of time. Randomised controlled trials (RCTs) are considered the ‘gold standard’ of clinical evaluations because changes in the selected outcome measures are likely to be due to the effect of the intervention itself, rather than be due to chance or other confounding variables (e.g. spontaneous remission of symptoms over time, attention or measurement effects).
Outcomes in economic evaluations are often expressed in terms of quality-adjusted life-years (QALYs), which are generated by multiplying years of life by the utility scores associated with the specific health states experienced by the person. Costs are calculated by multiplying resources used (resource utilisation) over an appropriate time horizon by the price attached to each unit of that resource (unit cost). The costs can include direct costs (e.g. for mediation, therapies, social services and transportation), indirect costs (e.g. productivity loss due to time off work and criminal justice expenditure) and intangible costs (e.g. impaired quality of life and distress of living with pain).
The type of resources included in the final cost calculation depends on the perspective of the economic evaluation, that is who pays for or saves from the resources used that we are interested in, such as the society in general or the health service in particular. This is important when the intervention is expected to have different impacts on different sectors and stakeholders (e.g. one sector incurred the majority of the costs and another yields the benefits of an intervention). The perspective of an economic evaluation can be as narrow as a particular agency or government department (e.g. ministry of health) or can be broader to include the statutory/public sector as a whole (e.g. all health and social care services).
There are five common types of economic evaluations that compare costs and outcomes between different interventions: cost minimisation analysis (CMA), cost–consequences analysis (CCA), cost-effectiveness analysis (CEA), cost–utility analysis (CUA) and cost–benefit analysis (CBA). All five types are similar in the way they measure costs, but they differ in the ways they measure health outcomes and combine these with costs to reach decisions about value for money.
Cost-minimisation analysis starts from the basis that two interventions have similar outcomes (in terms of effectiveness and safety) but different costs; however, the lack of a statistically significant difference in outcomes does not mean that the interventions are equivalent. 6 A CCA considers all the health and non-health impacts and costs of different interventions across different sectors; it then lists or tabulates these in a disaggregated form for each intervention and does not attempt to synthesise the costs and outcomes within and between interventions.
Cost-effectiveness analysis, CBA and CUA are three types of economic evaluations that compare the costs and outcomes of an intervention with the costs and outcomes of its alternatives. Outcomes are measured in their natural units (e.g. symptom-free days, depression score) in a CEA; in units of utility or preference, often as a QALY, in a CUA; and in monetary units in a CBA. The relative costs and outcomes of an intervention and an alternative are then summarised into one number, known as an incremental cost-effectiveness ratio (ICER), by dividing the difference in costs (incremental cost) by the difference in outcomes (incremental effect).
Table 2 provides an overview of how the five types of economic evaluations differ in terms of outcomes and their synthesis with costs.
Analysis | Expression of outcomes | Synthesis of costs and outcomes |
---|---|---|
CMA | Outcomes are shown to be similar | Only costs are compared |
CCA | A group of different outcomes expressed in their natural units | Not applicable – costs and outcomes are not combined but presented in separate tables for qualitative comparison |
CEA | A single condition-specific outcome expressed in its natural units (e.g. points on a depression scale) | ICER: cost per natural unit |
CUA | Utilities: QALYs or disability-adjusted life-years | ICER: cost per utility |
CBA | Money | Net monetary benefit or cost |
These five types of economic evaluations are informed by short- or medium-term clinical outcomes (in mental health usually up to 2 years) when they are based on a within-trial analysis, depending on the length of time during which participants in a RCT are followed up. Within-trial CEAs, or ‘piggy-back’ economic evaluations as they are known, have limitations, for example the atypical nature of trial setting, inappropriate clinical alternatives, inadequate length of follow-up, inadequate sample size for economic analysis, protocol-driven costs and benefits, and inappropriate range of end points (for both costs and outcomes). Health economic decision models are used to guide the choice of interventions for a clinical population on the basis of expected benefits and costs, commonly over a lifetime. 7 Decision models are often implemented by using either decision trees or Markov models. In Markov models, patients move between clinical states of interest in discrete time periods. Each state is associated with certain costs and outcomes. Decision models are defined by parameters that include probabilities of transition between clinical states, costs and outcomes associated with each state, treatment effects and other covariates (e.g. comorbidities and age). All available relevant evidence should be used to inform these parameters, which may include RCTs and population observational studies.
Once the costs and outcomes of competing alternatives have been estimated, either through a within-trial economic evaluation or through modelling, standard decision rules can be used to conclude whether or not a DI should be adopted. 8 If CEAs demonstrate that DIs are likely to be both more effective and less costly than the alternatives, then DIs are the preferred option in terms of ‘value for money’. Decision-making is more complex if DIs yield better outcomes for a greater cost than their alternatives. Where costs are higher and outcomes better, or costs lower and outcomes poorer, the incremental gain for a DI (costs saved or QALYs gained) must be assessed according to the marginal productivity of the health-care system (i.e. how much health is gained with an increase in expenditure at the margin or how much health is lost with a decrease in expenditure at the margin). An acceptable cost per QALY is health system specific, and is estimated at £15,000 in the UK,9 although alternative figures of £50,00010 and £20,000–30,000 have been used in decision-making. 11
Making a choice in favour of DIs (even when they are likely to be cost-effective) may imply the sacrifice of alternative options, which may not always be possible for ethical, clinical or feasibility reasons (e.g. we cannot replace the family doctor with digital self-management, but we can use the latter in addition to visits to the family doctor). Moreover, the cost of software and hardware for DIs is often frontloaded, whereas savings (or improved outcomes) are accrued in the long run, so those paying for DIs need to have the money to invest up front. Finally, costs incurred for DIs and benefits accrued from DIs may relate to different budgets (e.g. DIs are paid for by the health service that looks after the employees of a company but savings are accrued in the employment sector by reducing absenteeism of these employees). 12 Health-care providers and users may not adopt DIs even when they are proven to be cost-effective, because this will require either disinvesting from existing care options that cannot be forgone, or generating ‘new monies’ to add DIs to existing care options.
An additional consideration for decision-making utilising cost-effectiveness evidence is uncertainty. This uncertainty pertains to the evidence base used to generate estimates of cost-effectiveness as well as assumptions that are required in compiling this evidence. To inform decision-making, we need to characterise this uncertainty appropriately, for example using probabilistic sensitivity analysis and/or scenario analyses, and we need to explore the implications of this uncertainty in terms of adoption decisions and recommendations for further research. 13 Although decisions are binary (yes/no) in terms of cost-effectiveness, the evidence underpinning decisions may be uncertain, and so there is a probability of making the ‘wrong decision’. The evidence base to support assessments of cost-effectiveness for DIs is likely to be less developed than, for example, pharmaceuticals because of different regulatory requirements associated with the adoption of digital health interventions compared with pharmaceuticals. This implies that an assessment of cost-effectiveness for DIs should reflect this uncertainty and communicate it appropriately to decision-makers.
Gaps and limitations
The first systematic review of economic evidence for DIs was published by NICE more than 10 years ago14 and included only one CEA available at the time, which was on computerised CBT (cCBT). 15 Recent syntheses of economic evidence relating to DIs have focused on a specific technology (e.g. the internet)16,17 or a specific intervention (e.g. CBT)18 or a combination of both (e.g. internet CBT). 18–21 Some reviews22,23 include a wider range of interventions, such as online problem-solving therapy and positive psychology interventions. Most reviews are of studies of the most common mental health problems, namely depression and anxiety, but, increasingly, more reviews of economic evidence relevant to psychological and behavioural interventions include addictive behaviours (e.g. smoking24) and physical health/somatic problems. 25,26
The number of economic evaluations is a fraction of the number of clinical trials of DIs. Reviews of economic evidence for the use digital technologies to support mental health care (irrespective of the targeted population or type of technologies and interventions used) are useful, not least because the potential investment in digital technologies is large and irreversible. The economic evidence base for DIs is uncertain, so we need to understand under what circumstances these technologies are conducive to efficient delivery of care and the degree of certainty in the conclusions regarding cost-effectiveness. There may also be particular core assumptions that are key to determining the cost-effectiveness of DIs, such as engagement with DIs by patients (which can considerably change outcomes) and variable provision of personal support as an adjunct to DIs (which can considerably change the cost, e.g. if support is given by specialist clinicians or laypeople).
Previous work27 has concluded that economic evaluations for DIs (not specific to, but including, mental health) may require more flexible approaches to reflect the complexity of the intervention and its outcomes. Data to inform CEAs may not capture all the information required to assess cost-effectiveness. In most CEAs for DIs, time horizons are short, and the full opportunity costs of DIs, such as development costs, are not usually captured. Wider social costs, including productivity losses, presenteeism and other intangible costs, which carry weight in mental health, are also inconsistently measured. In addition, CEAs rarely estimate the investment sum needed for implementing DIs or the budgetary impact of their implementation against existing alternatives.
To our knowledge, there is no consideration of the appropriateness of existing methods of CEA to assess the value of DIs. To do so requires a comprehensive overview and critique of the cost-effectiveness evidence relating to the use of digital technologies to promote or improve mental health outcomes. Such a review will help to highlight the key conditions that make DIs cost-effective based on current evidence, as well as to identify key issues for consideration in establishing their cost-effectiveness. The results of a review and critique can be used to generate guidance and a checklist for future CEAs of DIs.
Aims and objectives
Our main aim was to make best use of existing evidence so that we could (1) inform practice and future research about which DIs are likely to represent a good use of health-care resources, (2) evaluate how uncertain the evidence regarding their cost-effectiveness is and (3) determine what drives variation in their value for money. Our secondary aim was to explore how current economic and clinical evidence is understood and used by key stakeholders in making decisions about the future development, evaluation and adoption of DIs.
Our objectives were to:
-
identify and summarise all published and unpublished CEAs comparing the costs and outcomes of DIs for the prevention and treatment of any mental health condition to the costs and outcomes of relevant alternatives [e.g. interventions that do not involve digital technologies or no intervention (NI)]
-
identify key drivers of variation in the effects and costs of DIs (e.g. for different population subgroups, delivery methods, economic perspectives or outcome measures)
-
develop classification criteria to inform the categorisation of DIs and their comparators
-
critically evaluate the quality and appropriateness of the methods used by existing CEAs to establish the cost-effectiveness of DIs
-
determine what cost-effectiveness judgements can be made for DIs given current evidence from economic evaluations
-
conduct an exploratory analysis to quantify the short- and long-term cost-effectiveness of DIs using a de novo decision-analytic model informed by a systematic review and quantitative data synthesis of clinical trials on common mental health problems
-
conduct a value-of-information (VOI) analysis based on the decision model findings and make recommendations as to what further research is necessary to inform future decisions
-
suggest how the methods of future CEAs for DIs can be improved by producing a step-by-step guide and a quality assessment checklist
-
investigate how the results on CEAs of DIs can be most effectively communicated to and inform decision-making by:
-
commissioners to fund services that use DIs
-
practitioners and service managers to provide DIs in routine care
-
service users to engage with DIs to improve or promote their mental health
-
technologists and researchers to further develop and optimise DIs.
-
Project design
The project had four work packages (WPs):
-
WP1 was a systematic review, critical appraisal and narrative synthesis of economic evaluations of DIs across all mental health conditions.
-
WP2 was a systematic review and network meta-analysis (NMA) of RCTs on DIs for a selected mental health condition.
-
WP3 was the economic modelling and VOI analysis of DIs for the selected mental health condition.
-
WP4 was a series of knowledge exchange seminars with stakeholders focusing on costs and outcomes of DIs.
We have reported our methods and findings of the systematic reviews in accordance with the Preferred Reporting Items for Systematic Reviews and Meta-Analyses (PRISMA) statements. 28,29 We have reported our economic modelling in accordance with the Consolidated Health Economic Evaluation Reporting Standards (CHEERS) statement. 30
Changes to protocol
The protocol initially stated that the systematic review in WP2 and the model in WP3 would focus on depression and anxiety under the umbrella ‘common mental health problems’. These conditions were chosen because most of the available and best-quality clinical evidence relates to common mental health problems, as opposed to psychosis, eating disorders or autism. 31–35 During the course of the project, we concluded that the disease and treatment pathways across the group of conditions included under the original umbrella term of common mental health problems were too disparate to analyse in a single model. Related to this point, the international classification manuals removed some of the conditions that were previously considered under ‘anxiety disorders’, such as obsessive–compulsive disorder (OCD) and post-traumatic stress disorder, to their own diagnostic groups, making them even more disparate. Consequently, we refined the scope of the review in WP2 and the model in WP3 to an exemplar mental health condition, and the changes were approved by the project steering group’s independent members. The decision to focus on one exemplar condition, and that this condition should be generalised anxiety disorder (GAD) as opposed to a group of common mental health problems or a single condition other than GAD (e.g. depression), was based on the following factors:
-
Different common mental health problems have different illness trajectories and different treatment pathways, so they cannot be reasonably analysed in one model.
-
GAD is the most prevalent and least studied condition among other common mental health problems; its point prevalence is nearly double that of depression and it is often confused with panic disorder or depression when self-reported by survey participants. It is also commonly comorbid with other physical and mental health problems.
-
A substantial proportion of the papers identified in WP1 targeted GAD and related conditions, such as worry and stress, and so there was value in synthesising the findings from multiple studies and using this synthesis to inform the economic model.
-
We have not identified any anxiety models with an analysis time horizon > 18 months, and so a long-term model would provide a useful contribution to the body of evidence. In contrast, several long-term economic models for depression already exist, some of which have been used as a basis to assess the cost-effectiveness of DIs.
In the original protocol we thought that WP4 would require ethics approval by the University of York and the Health Research Authority. In the end, ethics approval was not necessary because our WP4 seminars were conducted as consultations and educational seminars, and we did not collect or report any information from individual participants. We did not record the sessions and we did not use any quotations or individual contributions, but reported only on general discussion themes across seminar groups. This was because our engagement with the stakeholders was an iterative process and it became apparent that it had to be embedded within the routine professional development activities of the stakeholders, such as clinical seminars and advisory group meetings, rather than as separate ‘research focus groups’.
Patient and public involvement
The patient and public involvement/service user member of our steering group attended all steering groups meetings and gave feedback during and after the meetings about how the project can lead to clear messages about the value for money of digital mental health interventions, especially ‘long-term’ value. He suggested that 6 months is a meaningful period of time to measure benefits and costs from a service user’s perspective as a way of distinguishing short-term and medium-term outcomes. We liaised regularly with him outside the steering group meetings to discuss decisions about literature search terms, inclusion/exclusion criteria and ways of organising information to carry out dissemination activities for our findings as part of WP4.
We have also had patient and public representation through our partners at the Mental Health Foundation [(MHF); London, UK], whom we met on a regular basis. Josefien Breedvelt, the Research Manager at the MHF at the time, acted as a conduit between the MHF’s regular patient and public consultations and this project. MHF is a public champion of mental health promotion and illness prevention, which has been a ‘grey area’ in our literature review for which we sought the MHF’s steer. DIs for mental health promotion and prevention were represented in a large and often overinclusive body of literature that could mean anything from universal emotional well-being initiatives to targeted or indicated interventions for populations with established symptoms or risk factors. The MHF participated in discussions about inclusion/exclusion criteria for our review in terms of appropriate interventions and outcomes around mental health promotion and prevention. One of the conclusions was that ‘prevention of mental health problems’ rather than ‘mental health promotion’ better described the focus of our review.
We have also had public involvement activities through the Closing the Gap network (University of York); one of the network’s themes, which was led by this project’s chief investigator, focused on improving physical outcomes in people with mental illness through digital technologies. Our project included only mental health outcomes and not physical health outcomes, but this was a point that we had to decide on early in the project so that we could agree on inclusion/exclusion criteria for the retrieved literature. The patient and public involvement members of the Closing the Gap network suggested that certain outcomes are directly related to physical health but can also be considered as mental health outcomes, such as smoking cessation and alcohol detoxification (‘addiction outcomes’). This was suggested as a good base for our review, which in the future can be extended to include physical health outcomes separate to mental health outcomes.
Conclusion
Digital interventions use software programs, accessed via computers, smartphones, audio-visual equipment and other devices, to deliver therapeutic activities that aim to make a difference to the symptoms and functioning of people with mental health and addiction problems. Economic evaluations can provide evidence as to whether or not DIs offer value for money, based on their costs and outcomes relative to the costs and outcomes of alternative care options. Our first aim was to review all published economic studies on DIs for mental health and addiction problems. Our second aim was to use an exemplar clinical condition to conduct a synthesis of clinical evidence, which would then inform our third aim of constructing an economic model that demonstrates how we can bring together evidence from different sources to assess the cost-effectiveness of DIs compared with all possible alternatives. To this end, we also aimed to develop classification criteria for categorising DIs and their alternatives so that they could be reasonably pooled together. Finally, we aimed to explore how evidence on costs and outcomes, as well as other factors, may influence stakeholder decisions to adopt DIs in mental health.
Chapter 2 Classification of digital interventions in mental health
Introduction
Systematic reviews can provide a comprehensive picture of the currently available evidence on DIs, but the way they often lump or split this evidence does not always lead to meaningful or useful conclusions. For example, technology-mediated interventions in mental health can include internet consultations [clinician–patient telecommunication by e-mail or via SkypeTM (Microsoft Corporation, Redmond, WA, USA)] and internet therapy (self-help with no support or some brief support from a clinician or lay person. These two types of interventions are ‘apples and oranges’ when it comes to funding and delivery of services, because internet consultations need a clinician to deliver them but do not require sophisticated software (so costs are mostly for human resources), whereas internet therapy needs software but can be delivered without clinician input (so costs are mostly for technology development and maintenance).
Using a classification system can help an evidence synthesis make best use of the currently available evidence by grouping together DIs that share key characteristics. Different stakeholders may have different views about how DIs should be lumped or split based on their key characteristics. For developers, the type of technology used [e.g. web based, mobile apps (applications) or artificial intelligence] may be the most important characteristic, whereas for clinicians the type of therapeutic approach may have an over-riding significance. For managers, DIs that increase service capacity are different from DIs that enhance usual care, whereas service users may consider it important to differentiate between DIs that enable them to stay in contact with clinicians and peers and DIs that are entirely automated self-help.
The WHO1 has produced a classification system for digital health interventions (not specific to mental health) according to four stakeholder groups (i.e. clients, health-care providers, health system managers and data services). The WHO classification groups reflect the different functions of DIs for each stakeholder group (e.g. self-monitoring for clients, training for health-care providers, management of budget and expenditures for managers, and data storage and aggregation for data services). The WHO system uses the term ‘intervention’ in a broad sense to include administrative activities and training that are important for health care but are not designed as patient-facing therapeutic activities to directly prevent or improve clinical symptoms.
For this project, we developed classification criteria that we could apply to the comparators used across the economic evaluations reviewed in WP1 and the RCTs reviewed in WP2. Using these criteria, we aimed to allocate each comparator (e.g. internet therapy, face-to-face therapy, control website and usual care) to a classification group. This would enable us to aggregate many complex and diverse comparators to a contained number of classification groups. Our evidence synthesis pooled together the costs and outcomes of comparators within the same classification group and compared the costs and outcomes of comparators in different classification groups. The granularity and number of classification groups aimed to strike a balance between the number of studies that could inform each classification group and the number of distinctive comparisons between groups; too many classification groups would have limited the number of combined studies within each group, whereas too few classification groups would have diluted the distinctiveness of comparisons between groups.
Classification of digital interventions and their comparators
We followed a five-step process for the allocation of comparators into classification groups. First, we extracted the key characteristics of comparison groups as reported in the reviewed studies. These comparison groups included at least one DI. We used existing frameworks of reporting complex interventions36 to ensure that we captured all the necessary components for each comparison group, as reported by the studies. Second, we identified common and differentiating features of the comparison groups between and within studies. Third, we consulted the literature and an advisory group of researchers, clinicians and service users about features within the available comparison groups that could be important for the relative costs and outcomes of DIs and their comparators. Fourth, we classified each comparison group in the reviewed studies based on specific criteria. Finally, we used combinations of these criteria to arrive at a list of classification groups.
We used three criteria, as shown in Figure 3, to classify each comparison group: (1) whether the group was an intervention or a control – the intervention could be either psychosocial/behavioural (I) or medication (M), and the control could be either a non-therapeutic control (C) or NI; (2) whether the intervention/control was digital (D) or non-digital (NoD); and (3) whether the intervention/control was supported (S) or unsupported (U). The criteria are defined in Table 3.
FIGURE 3.
Classification of DIs and controls.
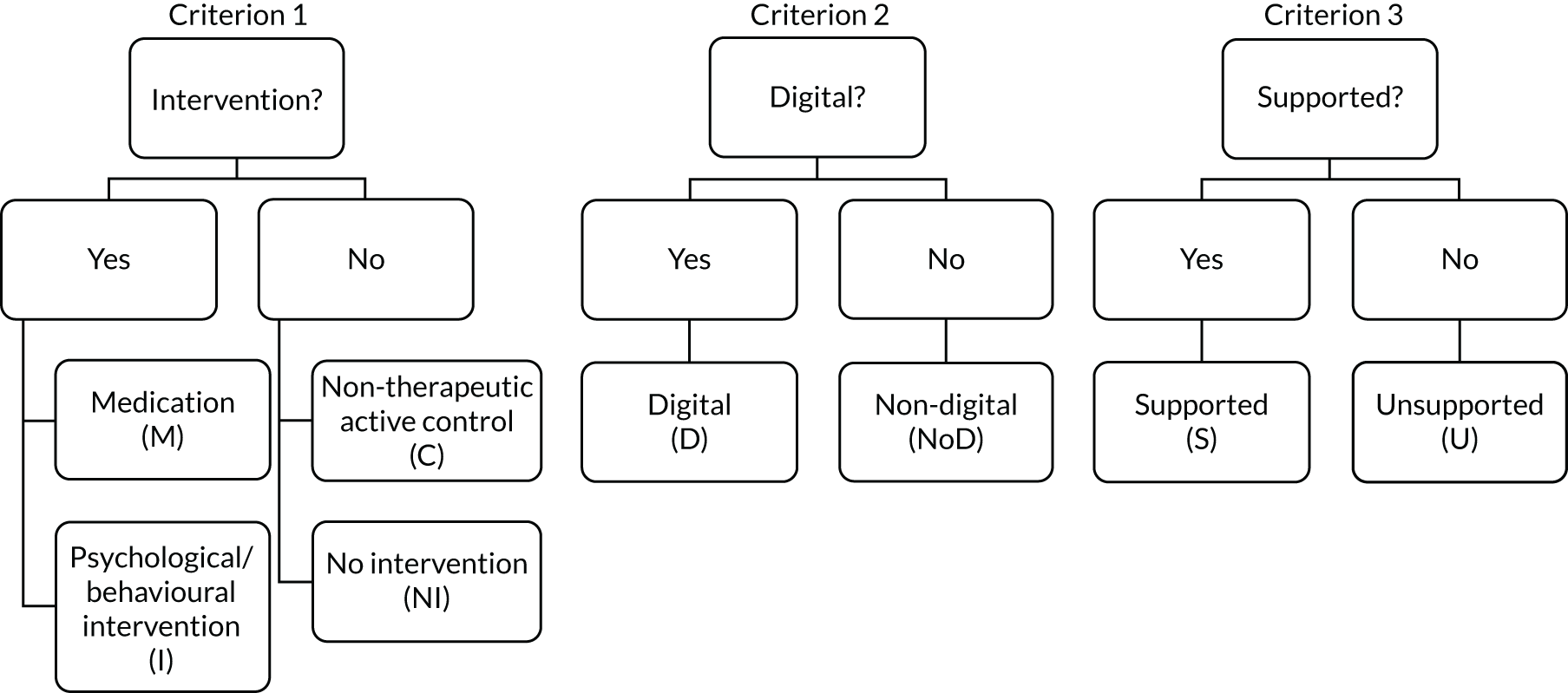
Group classification | Criteria |
---|---|
Psychosocial/behavioural intervention (I) | An activity offered as part of a research protocol for therapeutic purposes (i.e. we expect it to make a difference in a mental health problem by improving clinical symptoms and functioning, based on psychological, behavioural, social or educational theories, evidence and/or experience) |
Medication (M) | A pharmacological agent (pills, injections, etc.) offered as part of a research protocol |
Non-therapeutic control (C) | An activity offered as part of a research protocol that we do not expect to make a clinically important difference to a mental health problem. This may be a psychological placebo, an attention control, or a change in usual care introduced by the research team to keep participants safe and minimise attrition |
Digital (D) | Interventions/controls that include software processing of patient information to guide an activity |
Non-digital (NoD) | Interventions/controls that do not involve any technology; for example, they are delivered by printed materials or during face-to-face meetings, or involve telecommunications technology without software-led activities (e.g. consultations by e-mail, Skype or telephone) |
No intervention (NI) | No protocolled research activity and no changes in usual care introduced by the research team; this is typically when participants are placed on a WL or receive usual care. We used NI rather than ‘WL/usual care’ to differentiate from a ‘non-therapeutic control’ in which WL/usual care is enhanced by research activities (e.g. weekly contact for assessment) |
Supported (S) | Interventions/controls that include scheduled or regular reciprocal/two-way person-to-person interactions (e.g. between service user and clinician or researcher, or peer to peer) |
Unsupported (U) | Interventions/controls with no person-to-person interaction or ad hoc interaction (e.g. telephoning a helpline if any problems as a one-off) or non-reciprocal communication (e.g. reminders, posted or telephone messages without the expectation that there will be a conversation with the patient) |
Using a combination of two criteria (intervention vs. control and digital vs. non-digital), we arrived at six comparison groups: DI, digital control (DC), non-digital intervention (NoDI), non-digital control (NoDC), NI and medication (Figure 4). In the context of this report, NoDI infers that the intervention is psychosocial/behavioural in nature. It is worth reiterating that we merged WL and usual care into ‘no intervention’ to mean that no additional activities or input were offered as part of a research study over and above interventions and resources that were routinely accessible to all participants irrespective of group allocation. We have further addressed this by grouping usual care or WL interventions into active controls when they included activities over and above what would be expected in routine care, or when usual-care activities were not accessible to the intervention group.
FIGURE 4.
Combination of interventions vs. controls and digital vs. non-digital. M, medication.
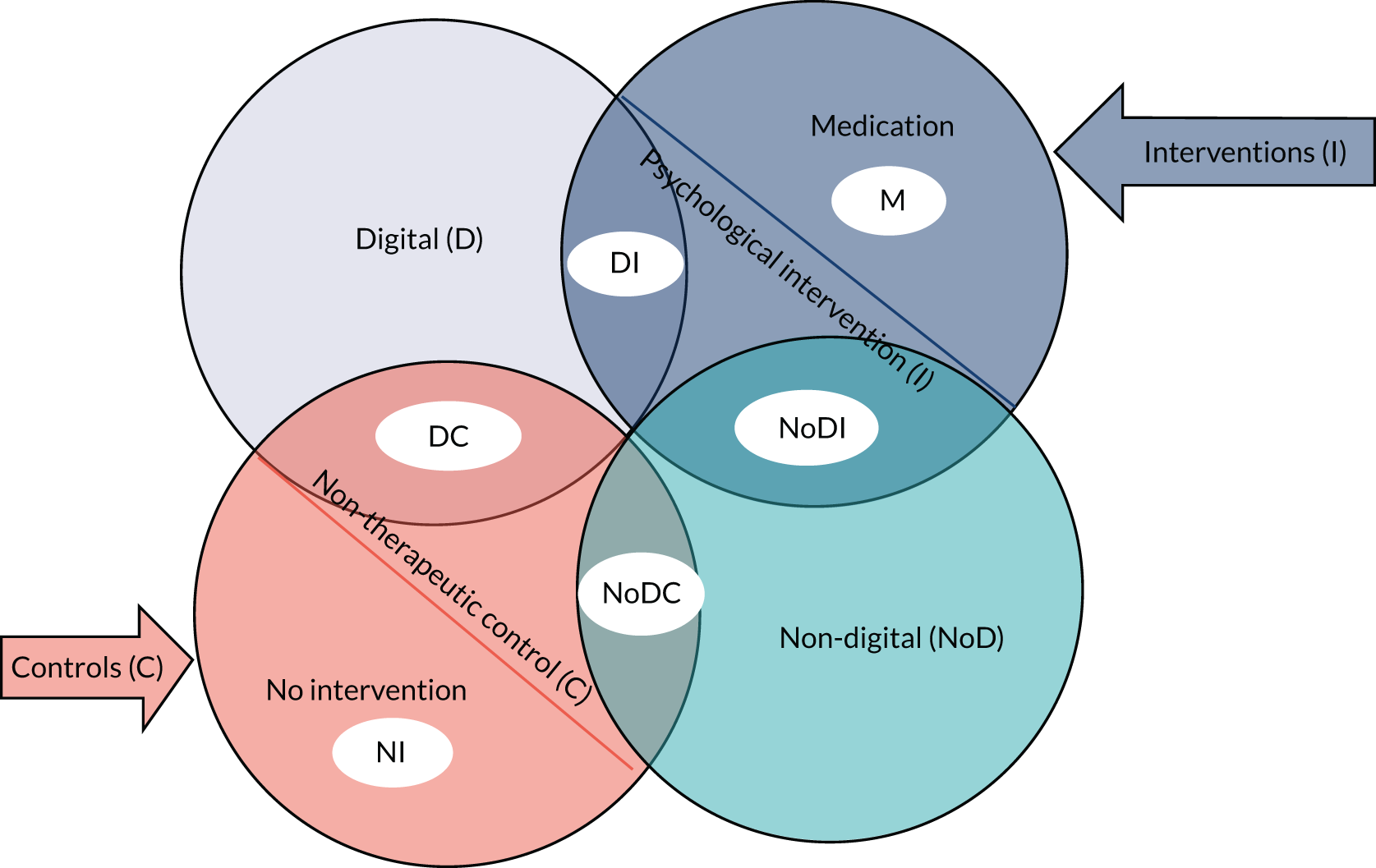
Using a combination of the three criteria (i.e. intervention vs. control, digital vs. non-digital and supported vs. unsupported), we arrived at 10 classification groups: medication (M), supported non-digital intervention (SNoDI), supported digital intervention (SDI), unsupported digital intervention (UDI), supported digital control (SDC), unsupported digital control (UDC), NI, unsupported non-digital intervention (UNoDI), unsupported non-digital control (UNoDC) and supported non-digital control (SNoDC) (Figure 5).
FIGURE 5.
Taxonomy groups based on combinations of intervention vs. control, digital vs. non-digital and supported vs. unsupported. M, medication.
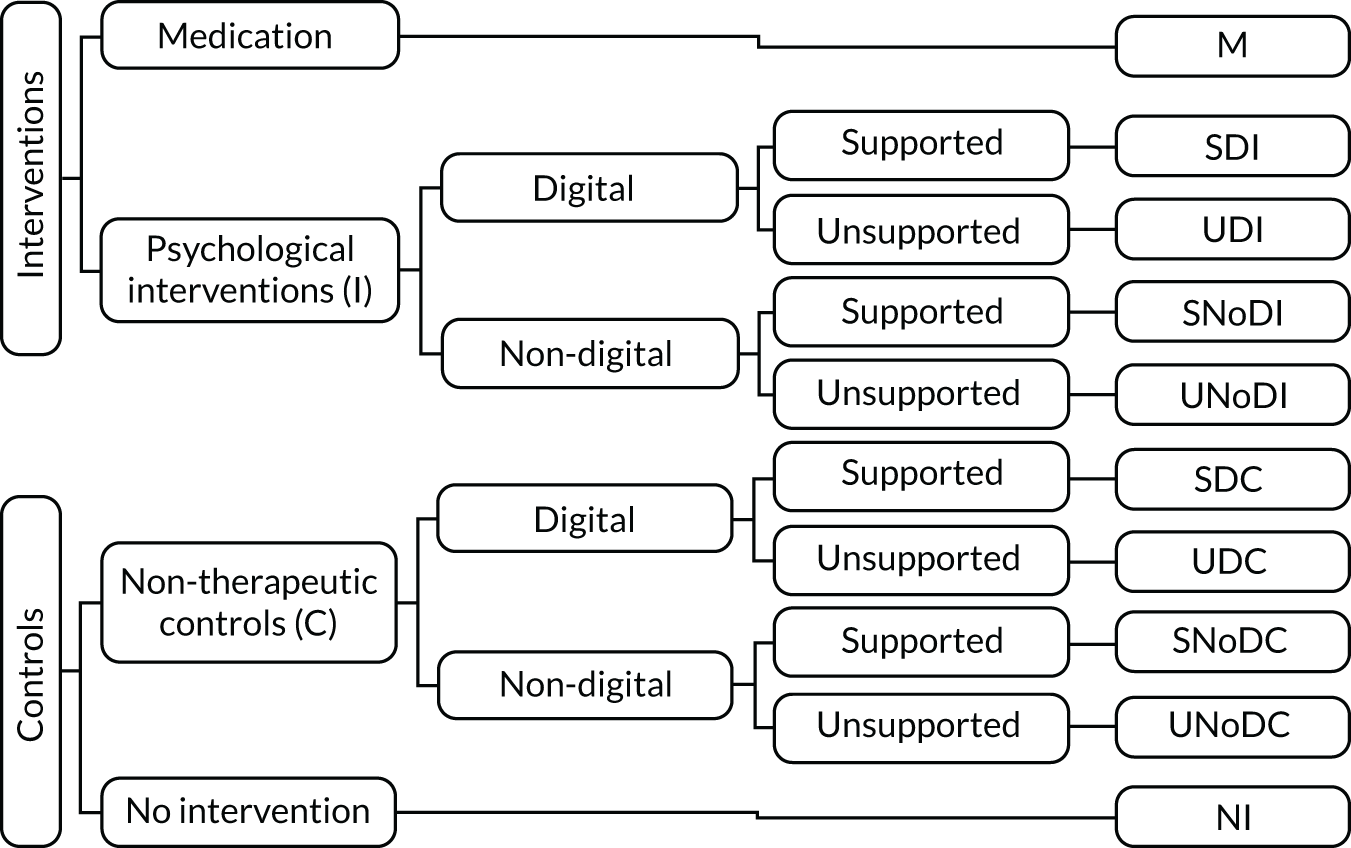
Table 4 provides examples for each classification group.
Acronym | Classification of intervention/control | Examples |
---|---|---|
M | Medication | Antidepressants, anxiolytics |
SDI | Supported digital intervention | Computerised CBT with weekly telephone support, clinician-delivered therapy with VR, mobile app with SMS communication with a facilitator |
UDI | Unsupported digital intervention | Internet self-help without any clinician contact, mobile app with automated reminders but without any two-way interaction with a facilitator |
SNoDI | Supported non-digital intervention | Individual or group therapy, telephone brief therapy |
UNoDI | Unsupported non-digital intervention | Stand-alone self-help using a treatment manual, bibliotherapy |
SDC | Supported digital control | Access to a general health education website with weekly check-in calls, computer-delivered ‘sham’ experiment |
UDC | Unsupported digital control | Access to an educational website with reminder e-mails |
SNoDC | Supported non-digital control | WL with weekly check-in communication with a person, usual care with weekly researcher assessments |
UNoDC | Unsupported non-digital control | General self-help (e.g. leaflets with health advice not specific to the mental health problem targeted) |
NI | No intervention | WL, usual care |
Challenges with classification of comparators within studies of digital interventions
We resolved discrepancies between reviewers who attributed the same comparators to different classification groups by refining and expanding the definitions of our classification criteria until two reviewers could independently arrive at the same classification for every comparator within the selected studies. The discrepancies between reviewers highlighted some of the difficulties in classifying complex interventions and some of the gaps in reporting comparators to a sufficient detail to enable us to classify them appropriately. Below, we discuss some of the challenges in the classification of comparators across the economic evaluations reviewed in WP1 and in the RCTs reviewed in WP2.
When a waiting list goes beyond ‘no intervention’
By default, we have classified WL as ‘no intervention’, assuming no regular input from the research team and no changes in usual care because of the research study. If a WL (or usual care) was enhanced through regular contact or therapeutic materials from the research team then we classified it as ‘non-therapeutic active control’. For example, in Johansson et al. ,37 participants on the WL received weekly assessments and substantial non-directive support via an online system by therapists, so this was classified as SDC. Teng et al. 38 invited all WL participants to have a weekly face-to-face assessment with a research assistant in a laboratory, which was classified as SNoDC.
In Pham et al. ,5 patients on the WL also received a weekly newsletter with curated content on breathing retraining exercises, matching content to the intervention they were to receive after coming off the WL, and e-mail reminders to complete assessments, albeit without any personal interaction; this was classified as UDC, although we could argue that the extent and therapeutic content of the information was on a par with an intervention. As the authors called this a ‘waiting list’, and the participants knew that they were being given information while waiting to receive an intervention, irrespective of the potential therapeutic effect of the WL, it could be classified only as a control.
In a study by Lovell et al. ,39 WL was a period of NI followed by individual high-intensity CBT. As the majority of the participants randomised to WL/usual care received treatment within the duration of the study, the comparator for internet CBT was more akin to a standard therapy than to NI. This raises the importance of monitoring and reporting what usual care is within RCTs so that sensitivity analyses can take into account the actual interventions that were received by the control group, rather than making assumptions about what the control group received.
There are occasions40,41 when participants on the WL engage in a research activity that would not be available to those receiving usual care (e.g. the provision of weekly online ratings or the option to telephone the research team if they encounter problems). In this case, ‘no intervention’ is still the appropriate classification because there participants in such a group receive no regular input from the research team (e.g. measurement of outcomes or scheduled ‘check-ins’ with participants).
When a comparator labelled as ‘therapy’ is better classified as a ‘control’
It can be difficult to classify an activity as ‘therapy’ or ‘control’ when the same activity could be therapeutic in some contexts but not in others. For example, in a study reported by McCrone et al. ,42 one of the randomisation arms was a computerised relaxation programme that was delivered in the same way as the intended intervention (i.e. cCBT for OCD) in a clinic using a self-administered software-based programme. Although applied relaxation can be used as a treatment for some conditions, such as GAD, there is no evidence or indication that it is a bona fide treatment for OCD, so the study used this as a psychological placebo.
In another study by Andersson et al. ,43 internet CBT was compared with ‘internet support therapy’, which was intended as a ‘control over attention effects and possible alleviating effects in having contact with a professional therapist’. The internet CBT was a self-help intervention that included 100 pages of materials and worksheets with established therapy components as well as weekly homework and written feedback from therapists through an integrated treatment platform. Internet support therapy included supportive communication with a therapist via the same integrated treatment platform but without the CBT content or homework. Interacting with a therapist via an online platform in a supportive way could have been classified as a therapeutic activity for a condition such as depression but not for OCD; therefore, the internet support therapy on this occasion was a control rather than an intervention.
When a technology-enabled intervention is classified as ‘non-digital’
We have made the distinction between DIs and telecommunication media that may use a digital interface (e.g. a computer or a mobile phone) without information processing. For example, short message service (SMS) texts or e-mails between a therapist and a user are not classified as ‘DIs’, whereas an online or telephone-based messaging system, as used in Johansson et al. ,37 that includes specially constructed backend software to provide a bespoke therapy environment with message encryption and centralised monitoring is classified as a DI. In another scenario, videoconferencing is not a DI unless it is part of a platform with software processing that guides some elements of the intervention.
When unsupported interventions/controls include some type of ‘contact’
In their most basic form, unsupported interventions do not involve any interpersonal contact but are entirely self-administered. Some unsupported interventions may include one-way reminders, postcards or telephone messages, or a helpline to call if there are any problems. What differentiates these from supported interventions is that, in the case of supported interventions, there is a regular and expected two-way interaction between the user and a facilitator or between peers.
Mixed interventions
Interventions could be a mix of medication and psychosocial/behavioural interventions (e.g. when a DI is used to assist a participant in taking their medication and to monitor adherence). Medication that is a usual/routine care intervention may still be part of a ‘psychosocial intervention’ group, a ‘no intervention’ group or an ‘active control’ group. If participants are offered psychosocial support as part of a controlled medication trial, then the intervention is classified as ‘medication’. Participants may still be offered medication as part of usual care in addition to the psychosocial intervention (the medication is not a ‘research intervention’).
Granularity of taxonomy
Digital interventions are complex and may include different therapeutic components (e.g. psychoeducation, cognitive techniques, behavioural techniques and motivational interviewing) and different layers of intensity (e.g. weekly 1-hour sessions or brief interventions). In addition, DIs increasingly follow a mixed model in which one intervention may include different types of technologies (e.g. telephone, website, biofeedback) and different types of personal support (e.g. face-to-face sessions with a therapist, telephone calls for technical support or standardised texts and e-mails from an assistant).
Conclusions
We propose a classification system for DIs and their alternatives based on three criteria: therapeutic intent (intervention vs. control), software processing (digital vs. non-digital) and interpersonal communication (supported vs. unsupported). Such classification requires a judgement based not only on predefined criteria, but also on understanding the nuances of technology-enabled interventions in the context of specific clinical applications. Distinguishing between digital and non-digital interventions is not always straightforward, especially as technologies can be used for patient–clinician telecommunication or for patient-directed activities, or for a blend of both. Classifying a DI as an intervention or control could also be complicated when interventions for one clinical condition may not be considered therapeutic for another. WLs and usual care are classified by default as ‘no intervention’; however, they may be more accurately described as ‘active controls’ (when the research design introduces additional processes such as monitoring) or as ‘interventions’ (when participants receive routine treatments such as medication or face-to-face therapy). The relative effects of DIs depend on how tough their comparators are (e.g. DIs may perform ‘better’ than ‘no intervention’ but perform less well against ‘gold standard’ treatments). To enable the appropriate analysis and meaningful interpretation of evidence syntheses, research studies need to describe in detail the comparators of DIs in accordance with existing frameworks for reporting complex interventions, including any support that participants have received in a WL or usual care.
Chapter 3 Review of economic studies
Introduction
To inform decision-makers about which DIs (under what circumstances) may offer good value for money, we needed to review the relevant body of economic evidence that currently exists in the form of economic evaluations. In addition, we needed to critically appraise whether or not the economic evidence takes into account all relevant costs and outcomes, for the full range of possible alternative interventions and care options, and over an appropriate time horizon, for the mental health conditions of interest. 44
This systematic review aimed to identify all economic evaluations of DIs and extract common themes, focusing on the methods used in the economic analyses and their appropriateness for decision-making. The review also considered whether methods have yet to be developed or utilised, and what further research is needed to inform economic analysis in the context of DIs.
Methods
Searches
Material in this section has been reproduced with permission from Jankovic et al. , Systematic review and critique of methods for economic evaluation of digital mental health interventions, Applied Health Economics and Health Policy, published 2020. 45 Copyright © 2020, Springer Nature Switzerland AG.
In December 2018, the following databases were searched to identify published and unpublished studies: MEDLINE, PsycInfo® (American Psychological Association, Washington, DC, USA), Cochrane Central Register of Controlled Trials (CENTRAL), Cochrane Database of Systematic Reviews (CDSR), Cumulative Index to Nursing and Allied Health Literature (CINAHL) Plus, Database of Abstracts of Reviews of Effects (DARE), EMBASE™ (Elsevier, Amsterdam, the Netherlands), Web of Science™ (Clarivate Analytics, Philadelphia, PA, USA) Core Collection, NHS Economic Evaluation Database (NHS EED), the Health Technology Assessment database and the National Institute for Health Research (NIHR) Journals Library and the Database of Promoting Health Effectiveness Reviews (DoPHER). The full search strategy is presented in Report Supplementary Material 1.
We also searched two clinical trial registries for ongoing studies (ClinicalTrials.gov and the WHO’s International Clinical Trials Registry Platform portal), searched the NIHR portfolio and conducted web searches using Google (Google Inc., Mountain View, CA, USA) and Google Scholar (Google Inc.) using simplified search terms. After searches were completed, we searched the studies included in the relevant systematic reviews identified as well as the references cited in the included studies and conducted forward citation chasing on all identified protocols and conference abstracts. We also contacted researchers in the field and searched the reference lists of the selected studies.
The searches were conducted from 1997, as no relevant studies of DIs could have been published before this date. Searches were restricted to studies written in English, as we anticipated that most economic studies written in other languages would also have a version published in English (e.g. the South Asia Cochrane Group). 46
Selection criteria
Material in this section has been reproduced with permission from Jankovic et al. , Systematic review and critique of methods for economic evaluation of digital mental health interventions, Applied Health Economics and Health Policy, published 2020. 45 Copyright © 2020, Springer Nature Switzerland AG.
Eligible studies included participants with symptoms or at risk of mental health problems as defined by the International Classification of Diseases, Eleventh Revision,1 criteria for mental, behavioural or neurodevelopmental disorders, with the exception of the conditions listed under the categories of neurodevelopmental, neurocognitive and disruptive behaviour or dissocial disorders. Studies were also excluded if the primary diagnosis of the participants was a physical or other condition other than those listed (e.g. cancer or insomnia). All DIs that expressly targeted mental health outcomes and patient-facing therapeutic activities were included, with the exception of those that were simply a communication medium (e.g. telephones or videoconferencing). A broad range of studies was considered in the review, including economic evaluations conducted alongside trials, modelling studies and analyses of administrative databases. Only full economic evaluations that compared two or more options and considered both costs and consequences (i.e. CMA, CEA, CUA and CBA) were included in the review. No studies were excluded on the basis of their comparator group. Study protocols, abstracts and reviews were marked to facilitate follow-up as described in Searches.
Study selection
Two reviewers, one of whom had expertise in health economics, independently assessed all titles and abstracts for eligibility. If either reviewer indicated that a study could be relevant, the full text for that study was sought and again assessed independently by two reviewers, with disagreements resolved through discussion or with a third reviewer.
Data extraction
The purpose of data extraction was to summarise methodology and identify challenges common in the evaluation of DIs. To do so, we extracted information reported in the studies on the population, intervention (underpinning principles, delivery mode, level of support, treatment duration) comparators, outcomes (clinical and economic outcomes, and the economic end point), study design, analytical approach (within-trial analysis, decision model, statistical model, epidemiological study), analysis time horizon, setting (country and analytical perspective), analytical framework (CMA, CEA, CUA, CCA or CBA) and methods employed to characterise uncertainty.
Critical analysis
Material in this section has been reproduced with permission from Jankovic et al. , Systematic review and critique of methods for economic evaluation of digital mental health interventions, Applied Health Economics and Health Policy, published 2020. 45 Copyright © 2020, Springer Nature Switzerland AG.
The identified studies were critically reviewed, to assess whether or not the existing evidence meets the requirements for decision-making in health care44 and to assess the challenges in generating cost-effectiveness evidence in this context. The following questions were asked of the methods used in the included studies:
-
Does the economic analysis estimate both costs and effects?
-
Does the analysis appropriately synthesise all of the available evidence?
-
Are the full ranges of possible alternative interventions and clinical strategies included?
-
Are costs and outcomes considered over an appropriate time horizon?
Quality assessment
Checklists are useful tools to assess the quality and applicability of economic evaluations. They also provide a framework for summarising the methods and results of economic evaluations in a consistent manner. Having an appropriate quality assessment checklist for economic evaluations of DIs in mental health can improve reporting standards and encourage harmonisation of key methods likely to drive heterogeneity in results from different economic evaluations. Following a checklist can help structure the narrative synthesis of results and critique of the methods used. This process helps the comparison of results across different studies, which are likely to be driven by methods employed to determine cost-effectiveness.
There are several checklists used in reviews of health economic evaluations, as summarised by Watts and Li. 47 Their paper notes that the Drummond48 checklist [or BMJ (British Medical Journal) checklist, as Watts and Li call it] and the Philips49 checklist are the most commonly used and are well regarded by the health economics community. Using the Drummond48 checklist, we assessed the included studies according to the clarity of their research questions, the quality and completeness of data used in the economic evaluation, the methods used to characterise uncertainty in the evaluation model, and the interpretation of their results. We have also used the Philips49 checklist, which is specific to model-based economic evaluations and describes attributes of good practice and questions for critical appraisal according to the model’s structure, data and consistency.
Results
Summary of available economic evaluations of digital interventions
Material in this section has been reproduced with permission from Jankovic et al. , Systematic review and critique of methods for economic evaluation of digital mental health interventions, Applied Health Economics and Health Policy, published 2020. 45 Copyright © 2020, Springer Nature Switzerland AG.
Our systematic literature search and study selection identified 63 primary studies,15,39,42,43,50–108 whose results were reported in 67 papers,15,39,42,43,50–112 as shown in Figure 6. After the removal of duplicates, 6931 of the 10,764 records originally identified remained and were screened, of which 6645 were excluded by title and abstract and 286 were assessed for eligibility by reviewing their full text. A total of 219 papers were excluded because the primary diagnosis was not a mental health problem (n = 27); the intervention was not a mental health intervention (n = 13); the study did not include health economic outcomes (n = 36); it was not an economic evaluation, or it was a review rather than a primary study (n = 10); it was a conference abstract rather than a peer-reviewed paper (n = 26); or it was a duplicate reference (n = 4) or a protocol (n = 103). Report Supplementary Material 2 gives the references of the excluded studies and the reasons for exclusion.
FIGURE 6.
The PRISMA flow diagram for the identification and selection of cost-effectiveness studies relating to DIs in mental health (search 1: up to November 2018). HE, health economic. Reproduced with permission from Jankovic et al. , Systematic review and critique of methods for economic evaluation of digital mental health interventions, Applied Health Economics and Health Policy, published 2020. 45 Copyright © 2020, Springer Nature Switzerland AG.
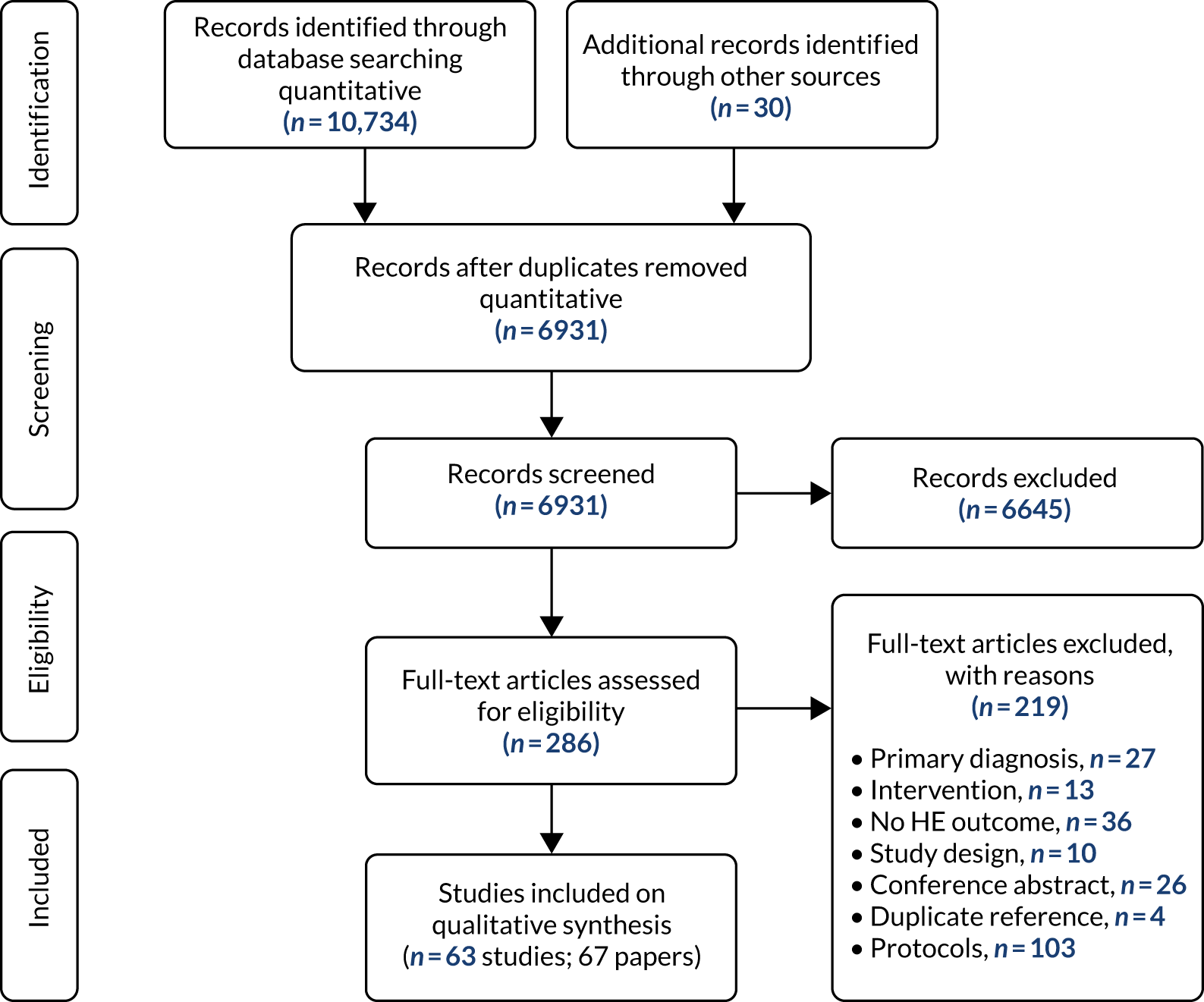
Two papers89,109 reported the results of identical analyses from the same study; therefore, the paper by Duarte et al. 109 was incorporated in the summary with Littlewood et al. 89 to avoid duplicate reporting. Two papers62,112 reported results from the same economic evaluation using different perspectives: the employer’s62 and the societal. 112 Three papers69,110,111 used the same sample and interventions but different economic evaluation methods: El Alaoui et al. ’s110 paper was based on the provider’s perspective and a 4-year time horizon, Hedman et al. 69 adopted a societal perspective and a 6-month time horizon, and Hedman et al. ’s111 paper was based on a societal perspective and a 4-year time horizon. It is worth highlighting that two papers43,51 were considered as reporting separate studies, although they used the same sample, as the study reported in the second paper51 was an extension of that reported in the first. 43 In conclusion, there were 66 papers with unique economic analyses and 63 studies with separate samples and interventions (hereafter referred to as 66 economic evaluations and 63 studies).
Approximately two-thirds of the studies (45/63) evaluated interventions that target anxiety and/or depression. Other conditions included suicidal ideation (n = 1), child disruptive behaviour (n = 1), eating disorders (n = 3), schizophrenia (n = 3) and addiction, including drug and alcohol addiction (n = 3 and n = 2, respectively) and smoking cessation (n = 8) (Table 5).
Study (first author and year) | Design | Condition | Country | Target age group | Sample age: meana (SD), range (years) | Number randomised (number of men) | Number lost to follow-up/number completed | Perspective | Time horizon |
---|---|---|---|---|---|---|---|---|---|
Aardoom 201650 | 4-arm RCT | Eating disorder | The Netherlands | ≥ 16 years | None reported | 354 (4) | 152/202 | Societal | 3 months |
Andersson 201543 | 2-arm RCT | OCD | Sweden | Adults | (Mean, SD not reported), 18–67 | 101 (34) | 2/99 | Societal | 10 weeks (for economic outcomes, 4 months for clinical outcome) |
Andersson 201551 | 2-arm RCT | OCD | Sweden | Adults | (Mean, SD not reported), 20–70 | 93 (32) | 11/82 | Societal | 24 months |
Axelsson 201852 | 4-arm RCT | Health anxiety | Sweden | Adults | 38 (13), 20–72 | 132 (34) | 5/127 | Health system, societal | 12 weeks (post treatment) |
Bergman Nordgren 201453 | 2-arm RCT | Anxiety (not specified) | Sweden | Adults | 35 (SD not reported), 19–68 | 100 (37) | 21/79 | Societal | 1 year |
Bergström 201054 | 2-arm RCT | Panic disorder | Sweden | Adults | Not reported | 113 (40) | 26/87 | Health system | 6 months |
Bolier 201455 | 2-arm RCT | Depression | The Netherlands | ≥ 21 years | 43 (12), (range not reported) | 264 (58) | 86/198 | Societal | 6 months |
Brabyn 201656 | 2-arm RCT | Depression | UK | Adults | 41 (14), 8–77 | 369 (131) | 95/274 | Health system | 12 months |
Budney 201557 | 3-arm RCT | Cannabis addiction | USA | Adults | None reported | 75 (gender not reported) | 30/45 | Provider | 9 months |
Buntrock 201758 | 2-arm RCT | Depression | Germany | Adults | 45 (12) (range not reported) | 406 (106) | 118/288 | Health system, societal | 12 months |
Burford 201359 | 2-arm RCT | Smoking | Australia | Adults | (Mean, SD not reported), 18–30 | 160 (60) | 38/122 | Health system | 6 months |
Calhoun 201660 | 2-arm RCT | Smoking | USA | Not specified | 43 (14), (range not reported) | 413 (343) | 105/308 | Health-care provider | 12 months follow-up (lifelong QALY gain modelled) |
Dear 201561 | 2-arm RCT | Stress, anxiety, worry | Australia | ≥ 60 years | (Mean, SD not reported), 60–81 | 72 (28) | 10/62 | Health system | 12 weeks |
Ebert 201862 (linked with Kählke 2019112) | 2-arm RCT | Stress | Germany | Adults | 43 (10), (range not reported) | 264 (71) | 26/87 | Employer | 6 months |
El Alaoui 2017110 | 2-arm RCT | Social anxiety | Sweden | Adults | (Mean, SD not reported), 18–64 | 126 (81) | 25/101 | Provider | 4 years |
Garrido 201763 | 2-arm RCT | Schizophrenia | Spain | Adults | 33 (SD not reported), 18–55 | 67 (49) | 34/33 | Health system | 36 months |
Geraedts 201564 | 2-arm RCT | Depression | The Netherlands | Adults | None reported | 231 (87) | 106/125 | Societal, employer | 12 months |
Gerhards 201065 | 3-arm RCT | Depression | The Netherlands | 18- to 65-year olds | None reported | 303 (131) | 28/275 | Societal | 12 months |
Graham 201366 | 3-arm RCT | Smoking | USA | Adults | 36 (11), (range not reported) | 2005 (981) | 637/1368 | Payer | 18 months |
Guerriero 201367 | Modelling study based on pilot RCT | Smoking | UK | Not specified | Not applicable | 200 (gender not reported) | 16/184 | Health system | 31 weeks |
Hedman 201169 | 2-arm RCT | Social anxiety | Sweden | Adults | (Mean, SD not reported), 18–64 | 126 (81) | 25/101 | Societal | 6 months |
Hedman 201368 | 2-arm RCT | Health anxiety | Sweden | Adults | (Mean, SD not reported), 25–69 | 81 (21) | 6/75 | Societal | 12 weeks (post treatment) |
Hedman 2014111 | 2-arm RCT | Social anxiety | Sweden | Adults | (Mean, SD not reported), 18–64 | 126 (81) | Not reported | Societal | 4 years |
Hedman 201670 | 2-arm RCT | Health anxiety | Sweden | Adults | (Mean, SD not reported), 21–75 | 158 (33) | 16/142 | Societal | 12 weeks (post treatment) |
Hollinghurst 201071 | 2-arm RCT | Depression | UK | 18- to 75-year-olds | 35 (12), (range not reported) | 297 (95) | 87/210 | Health system | 8 months |
Holst 201872 | 2-arm RCT | Depression | Sweden | Adults | 37 (11), (range not reported) | 90 (38) | 25/65 | Health system, societal | 9 months |
Hunter 201773 | 2-arm RCT | Alcohol addiction | Italy | Adults | None reported | 763 (469) | 143/620 | Health system | 12 months |
Joesch 201274 | 2-arm RCT | Anxiety (mixed) | USA | Adults | None reported | 690 (195) | Not reported | Health system | 18 months (including treatment) |
Jolstedt 201875 | 2-arm RCT | Anxiety (mixed) | Sweden | 8- to 12-year-olds | 10 (1), (range not reported) | 131 (61) | 18/113 | Societal | 12 weeks (3 month follow-up but with crossover) |
Jones 200177 | 3-arm RCT | Schizophrenia | UK | Adults aged < 65 years | (Mean, SD not reported), 18–65 | 112 (gender not reported) | 56/66 | Societal | 3 months |
Jones 201476 | 2-arm RCT | Child disruptive disorder | USA | Children aged 3–8 years | 6 (SD, range not reported) | 22 (gender not reported) | 7/15 | Provider | Not reported |
Kählke 2019112 | 2-arm RCT | Stress | Germany | Adults | 43 (10), (range not reported) | 264 (71) | 26/87 | Employer | 6 months |
Kass 201778 | Modelling study | Eating disorder | USA | Not specified | Not applicable | Not applicable | Not applicable | Payer | Unclear (lifetime?) |
Kenter 201579 | 2-arm observational study | Depression and anxiety | The Netherlands | Adults | None reported | 4448 (2006) | Not reported | Provider | Varied (observational data) |
Kiluk 201680 | 3-arm RCT | Alcohol addiction | USA | Adults | 43 (12), (range not reported) | 68 (44) | 4/62 | Not reported | 6 months |
Koeser 201381 | Modelling study based on multiple studies | Depression | UK | Not specified | Not applicable | Not applicable | Not applicable | Health system | 8 months |
Kolovos 201682 | 2-arm RCT | Depression | The Netherlands | Adults | 38 (11), (range not reported) | 269 (124) | 158/111 | Health system, provider, societal | 12 months |
König 201883 | 2-arm RCT | Binge eating disorder | Germany and Switzerland | Adults | None reported | 178 (gender not reported) | 31/147 | Societal | 18 months |
Kraepelien 201884 | 3-arm RCT | Depression | Sweden | Adults | (Mean, SD not reported), 18–67 | 945 (250) | 717/228 | Health system, societal | Health-care perspective: 3 months. Societal perspective: 12 months |
Kumar 201885 | Modelling study based on multiple studies | GAD | USA | Adults | Not applicable | Not applicable | Not applicable | Payer, societal | Not reported |
Lee 201787 | Modelling study from multiple sources | Depression | Australia | Adolescents aged 11–17 years | Not applicable | Not applicable | Not applicable | Health system | 10 years |
Lee 201786 | Modelling study from multiple sources | Depression and anxiety | Australia | Adults aged < 60 years | None reported | Not applicable | Not applicable | Health system | 12 months |
Lenhard 201788 | 2-arm RCT | OCD | Sweden | Adolescents aged 12–17 years | 15 (2), (range not reported) | 67 (36) | 7/60 | Societal | 12 weeks |
Littlewood 201589 and Duarte 2017109 | 3-arm RCT | Depression | UK | Adults | 40 (13), (range not reported) | 691 (229) | 230/461 | Health system | 24 months |
Lovell 201739 | 3-arm RCT | OCD | UK | Adults | 33 (SD not reported), 18–77 | 475 (178) | 141/334 | Health system, societal | 12 months |
McCrone 200415 | 2-arm RCT | Depression and anxiety | UK | Adults | (Mean, SD not reported), 18–75 | 274 (72) | 12/262 | Health system, societal | 6 months |
McCrone 200742 | 3-arm RCT | OCD | USA and Canada | Adults | None reported | 218 (gender not reported) | 42/176 | Provider | Not reported |
McCrone 200990 | 3-arm RCT | Panic disorder | UK | Adults | 38 (13), (range not reported) | 90 (28) | 30/60 | Health system | 1 month |
Mihalopoulos 200591 | Modelling study from multiple sources | Panic disorder | Australia | Not specified | None reported | Not applicable | Not applicable | Health system | 12 months (reported in original model paper) |
Murphy 201692 | 2-arm RCT | Addiction to substances (mixed) | USA | Adults | 35 (11), (range not reported) | 507 (315) | Not reported | Payer, provider | 36 weeks |
Naughton 201793 | 2-arm RCT | Smoking | UK | Not specified | 27 (6), (range not reported) | 407 (gender not reported) | 146/261 | Payer | 11–36 weeks (final follow-up at 36 weeks of gestation) |
Naveršnik 201394 | Modelling study based on pilot trial | Depression | Slovenia | Adults | Not applicable | 46 (gender not reported) | 24/22 | Health system | 6 months |
Olmstead 201095 | 2-arm RCT | Addiction (mixed) | USA | Adults | 42 (10) (range not reported) | 77 (46) | 23/54 | Payer | Unclear (appears to be 8 weeks) |
Phillips 201496 | 2-arm RCT | Depression and/or anxiety | UK | Adults | None reported | 637 (296) | 406/231 | Not reported | 12 weeks (total) |
Romero-Sanchiz 201797 | 3-arm RCT | Depression | Spain | 18- to 65-year-olds | 43 (SD, range not reported) | 296 (70) | 83/203 | Societal | 12 months |
Smit 201398 | 3-arm RCT | Depression | Australia | Adults | None reported | 414 (165) | 183/231 | Health system | 6 months |
Solomon 201599 | Modelling study from multiple sources | Smoking | Australia | Adults | Not applicable | Not applicable | Not applicable | Societal | 12 months |
Španiel 2012100 | 2-arm RCT | Schizophrenia | Czech Republic and Slovakia | 18- to 60-year-olds | None reported | 158 (90) | 63/95 | Not reported | 12 months |
Stanczyk 2014101 | 3-arm RCT | Smoking | The Netherlands | Adults | None reported | 2551 (1273) | 1342/1209 | Health system, societal | 12 months |
Titov 2009102 | 2-arm RCT | Social anxiety | Australia | Adults | None reported | 193 (gender not reported) | 22/171 | Other | 10 weeks |
Titov 2015103 | 2-arm RCT | Depression | Australia | Elderly (no age specified) | (Mean, SD not reported), 61–78 | 54 (14) | 2/52 | Health system | 8 weeks |
van Spijker 2012104 | 2-arm RCT | Suicidal ideation | The Netherlands | Adults | 41 (14), (range not reported) | 236 (80) | 25/111 | Societal | 6 weeks |
Warmerdam 2010105 | 3-arm RCT | Depression | The Netherlands | Adults | 45 (12), (range not reported) | 263 (76) | 112/151 | Societal | 12 weeks |
Wijnen 2018106 | 2-arm RCT | Depression | The Netherlands | Adults | (Mean, SD not reported), 18–81 | 329 (80) | 92/237 | Health system, societal | 3 months |
Wright 2017107 | 2-arm RCT | Depression | UK | 12- to 18-year-olds | None reported | 91 (gender not reported) | 36/55 | Provider, societal | 4 months |
Wu 2014108 | Modelling study based on RCT | Smoking | UK | Adults | 45 (12), (range not reported) | 6911 (3163) | 1737/5174 | Health system | Lifetime |
The interventions varied both between and within individual conditions, in terms of their underlying principles (e.g. CBT, guided relaxation, self-help, exposure therapy, motivational support for smoking cessation), content (e.g. the number of modules), mode of delivery (e.g. mobile, computer or text based, or completed at home or at clinic), type of support (e.g. online chat, telephone, face to face), frequency of support (e.g. weekly, ad hoc), person delivering support (e.g. clinician, assistant, lay person) and extent of support (e.g. administrative support only, additional counselling) (see Table 10).
Comparators can be broadly categorised as NI, which could be WL or usual care, active non-therapeutic controls or standard therapy. There is limited reporting of what interventions patients had access to, or received, when allocated to a WL or to usual care. Non-therapeutic controls were designed as ‘psychological placebos’ or attention controls; they encourage patients to spend the same amount of time on treatment as active interventions, but without the ‘active’ component, such as CBT or problem-solving therapy. Non-therapeutic comparators included websites, printed reading and online relaxation not indicated as a ‘treatment’ for the condition under study (see Table 10).
Methods for costing interventions varied. The majority of the evaluations (50/66)15,39,42,43,50–75,77,80,82–84,88–90,92,95–98,100–106,110–112 included the cost of staff time required to deliver the intervention. Some studies included variable equipment costs and website maintenance and hosting, whereas very few considered the cost of development, capital costs or patient recruitment (or technology dissemination).
Most evaluations (52/66)15,39,42,43,50–75,77,80,82–84,88–90,92,95–98,100–106,110–112 were within-trial analyses, and one59 included a temporal extrapolation. A further three studies76,93,107 were within-pilot evaluations76,93 and feasibility trials,107 although one study79 used observational data. Ten studies67,78,81,85–87,91,94,99,108 used decision models to evaluate interventions. Eight78,81,85–87,91,94,99 of the 10 studies67,78,81,85–87,91,94,99,108 that used modelling to evaluate DIs did so because of the absence of head-to-head trial data, and used individual trials or non-comparative data sources to inform the treatment effect of DIs. The model for eating disorders used synthesised evidence of the treatment effect to derive the cost of different treatment options.
The vast majority of the papers reported some form of sensitivity analysis, with only 11 studies57,63,74,77,79,80,85,97,100,102,107 not reporting having conducted any. In total, 50 studies15,39,42,43,50–75,77,80,82–84,88–90,92,95–98,100–106,110–112 used both probabilistic and deterministic sensitivity analyses. Deterministic sensitivity analysis involved exploring alternative scenarios and assumptions; the most common parameter value that was varied was the cost of intervention. Other common scenarios included alternative methods for dealing with missing data or for estimating costs and effect.
Quality of the included studies under each item of the Drummond checklist
Table 6 reports the outcomes of the quality assessment for each economic study based on the Drummond et al. 48 checklist, which comprises 10 items that assess the following domains: (1) question definition, (2) description of competing alternatives, (3) established effectiveness, (4) inclusion of costs and consequences, (5) measurement of costs and consequences, (6) valuation of cost and consequences, (7) adjustment of costs and consequences for differential timing, (8) incremental analysis, (9) allowance for uncertainty and (10) presentation and discussion of results.
Study (first author and year) | Drummond checklist item | |||||||||
---|---|---|---|---|---|---|---|---|---|---|
1 | 2 | 3 | 4 | 5 | 6 | 7 | 8 | 9 | 10 | |
Aardoom 201650 | Yes | Unclear | Yes | Yes | Yes | Yes | NA | Yes | Yes | Yes |
Andersson 201543 | Yes | Unclear | Yes | Yes | Yes | Yes | No | Yes | Yes | Partial |
Andersson 201551 | Yes | Unclear | Yes | Yes | Yes | Yes | NA | Partial | Yes | Partial |
Axelsson 201852 | Yes | Yes | Yes | Yes | Yes | Yes | NA | Partial | Yes | Partial |
Bergman Nordgren 201453 | Yes | Unclear | Yes | Yes | Yes | Yes | NA | Yes | Yes | Partial |
Bergström 201054 | Partial | Yes | Yes | Partial | Yes | Yes | NA | Partial | Partial | Partial |
Bolier 201455 | Yes | Yes | Yes | Yes | Yes | Yes | NA | Yes | Yes | Partial |
Brabyn 201656 | Yes | Unclear | Yes | Yes | Yes | Yes | NA | Yes | Yes | Yes |
Budney 201557 | Yes | Yes | Yes | Yes | Yes | Yes | NA | No | No | Yes |
Buntrock 201758 | Yes | Yes | Yes | Yes | Yes | Yes | NA | Yes | Yes | Yes |
Burford 201359 | Yes | Partial | Yes | Yes | Yes | Partial | Yes | Partial | Partial | Yes |
Calhoun 201660 | Yes | Yes | Yes | Partial | Yes | Yes | Partial | No | No | Partial |
Dear 201561 | Yes | Unclear | Yes | Yes | Yes | Yes | NA | Yes | Partial | Partial |
Ebert 201862 (linked with Kählke 2019112) | Yes | Unclear | Yes | Yes | Yes | Yes | NA | Yes | Yes | Partial |
El Alaoui 2017110 | Yes | Yes | Yes | Yes | Yes | Yes | Yes | Yes | Yes | Yes |
Garrido 201763 | Partial | Unclear | Yes | Partial | Yes | Yes | No | No | No | Yes |
Geraedts 201564 | Yes | Yes | Yes | Yes | Yes | Yes | NA | Yes | Yes | Partial |
Gerhards 201065 | Yes | Yes | Yes | Yes | Yes | Yes | NA | Yes | Yes | Partial |
Graham 201366 | Yes | Unclear | Yes | Yes | Yes | Yes | No | Partial | No | Partial |
Guerriero 201367 | Partial | Yes | Partial | Yes | Yes | Yes | Partial | Yes | Yes | Yes |
Hedman 201169 | Yes | Yes | Yes | Yes | Yes | Yes | NA | Yes | Yes | Partial |
Hedman 201368 | Yes | Unclear | Yes | Yes | Yes | Yes | NA | Yes | Yes | Partial |
Hedman 2014111 | Yes | Yes | Yes | Yes | Yes | Yes | No | Yes | Yes | Partial |
Hedman 201670 | Yes | Unclear | Yes | Yes | Yes | Yes | NA | Yes | Yes | Partial |
Hollinghurst 201071 | Yes | Unclear | Yes | Yes | Yes | Yes | NA | Yes | Yes | Yes |
Holst 201872 | Yes | Yes | Yes | Yes | Yes | Yes | NA | Yes | Yes | Partial |
Hunter 201773 | Yes | Yes | Yes | Partial | Unclear | Yes | NA | Yes | Yes | Yes |
Joesch 201274 | Partial | Yes | Yes | Partial | Partial | Yes | No | Yes | No | Partial |
Jolstedt 201875 | Yes | Unclear | Yes | Yes | Yes | Yes | NA | Yes | Partial | Partial |
Jones 200177 | Partial | Yes | Yes | Unclear | Unclear | Unclear | Partial | No | Partial | Partial |
Jones 201476 | Partial | Yes | Partial | Partial | Yes | Yes | NA | No | No | Yes |
Kählke 2019112 | Yes | Unclear | Yes | Yes | Yes | Yes | NA | Yes | Yes | Yes |
Kass 201778 | Partial | No | No | No | Unclear | Partial | Unclear | No | No | Partial |
Kenter 201579 | Partial | Yes | Partial | Yes | Yes | Partial | Unclear | No | No | Yes |
Kiluk 201680 | No | Yes | Yes | Unclear | Yes | Unclear | NA | No | No | Yes |
Koeser 201381 | Yes | Yes | Partial | Yes | Yes | Yes | NA | Yes | Yes | Yes |
Kolovos 201682 | Yes | Yes | Yes | Yes | Yes | Yes | NA | Yes | Yes | Yes |
König 201883 | Yes | Yes | Yes | Yes | Yes | Yes | No | Yes | Yes | Yes |
Kraepelien 201884 | Yes | Yes | Yes | Yes | Yes | Yes | NA | Yes | Yes | Partial |
Kumar 201885 | Yes | Yes | No | Partial | Partial | Partial | Yes | Partial | Partial | Partial |
Lee 201787 | Yes | Yes | No | Yes | Yes | Yes | Yes | No | Yes | Partial |
Lee 201786 | Yes | Yes | No | Partial | Yes | Unclear | NA | Yes | Partial | Yes |
Lenhard 201788 | Yes | Unclear | Yes | Yes | Yes | Yes | NA | Partial | Yes | Partial |
Littlewood 201589 and Duarte 2017109 | Yes | Yes | Yes | Yes | Yes | Yes | Yes | Yes | Yes | Yes |
Lovell 201739 | Yes | Unclear | Yes | Yes | Yes | Yes | NA | Yes | Yes | Yes |
McCrone 200415 | Partial | Yes | Yes | Yes | Yes | Yes | NA | Partial | Partial | Yes |
McCrone 200742 | Partial | Yes | Yes | Yes | Unclear | Yes | Unclear | Yes | Yes | Yes |
McCrone 200990 | Partial | Yes | Yes | Partial | Yes | Yes | NA | Yes | Partial | Partial |
Mihalopoulos 200591 | Yes | Partial | No | Unclear | Yes | Unclear | NA | No | Partial | Partial |
Murphy 201692 | Yes | Yes | Yes | Partial | Yes | Yes | NA | Yes | Yes | Yes |
Naughton 201793 | Yes | Yes | Partial | No | Yes | Yes | NA | Yes | Yes | Yes |
Naveršnik 201394 | Yes | Yes | Partial | Unclear | Unclear | Yes | NA | Yes | Yes | Partial |
Olmstead 201095 | Yes | Yes | Yes | Partial | Yes | Yes | Unclear | Yes | Yes | Yes |
Phillips 201496 | No | Yes | Yes | Unclear | Yes | Yes | NA | No | Yes | Yes |
Romero-Sanchiz 201797 | Yes | Yes | Yes | Yes | Yes | Yes | NA | Yes | No | Yes |
Smit 201398 | Yes | Yes | Yes | Partial | Partial | Yes | NA | Yes | Yes | Yes |
Solomon 201599 | Partial | Yes | Yes | Yes | Yes | Yes | NA | Yes | Yes | Yes |
Španiel 2012100 | Partial | Yes | No | Partial | Yes | Yes | NA | No | Partial | Yes |
Stanczyk 2014101 | Yes | Unclear | Yes | Partial | Partial | Yes | NA | Yes | Yes | Partial |
Titov 2009102 | Partial | Unclear | Yes | No | Unclear | Partial | NA | Yes | No | No |
Titov 2015103 | Yes | Unclear | Yes | Yes | Yes | Yes | NA | Yes | Partial | Yes |
van Spijker 2012104 | Partial | Yes | Yes | Unclear | Yes | Yes | NA | Yes | Yes | Partial |
Warmerdam 2010105 | Yes | Yes | Yes | Yes | Yes | Yes | NA | Yes | Yes | Yes |
Wijnen 2018106 | Yes | Unclear | Yes | Yes | Yes | Yes | NA | Yes | Yes | Yes |
Wright 2017107 | Partial | Unclear | Partial | Yes | Yes | Yes | NA | No | No | Yes |
Wu 2014108 | Yes | Unclear | Yes | Partial | Yes | Yes | Yes | Yes | Yes | Yes |
Total | ||||||||||
N/A | 0 | 0 | 0 | 0 | 0 | 0 | 47 | 0 | 0 | 0 |
Yes | 48 | 41 | 53 | 42 | 56 | 57 | 6 | 45 | 42 | 35 |
Partial | 16 | 3 | 7 | 15 | 4 | 5 | 3 | 8 | 12 | 30 |
No | 2 | 1 | 6 | 3 | 0 | 0 | 6 | 13 | 12 | 1 |
Unclear | 0 | 21 | 0 | 6 | 6 | 4 | 4 | 0 | 0 | 0 |
Item 1: clarity of research question
The majority of the economic evaluations were conducted alongside trials and had the primary aim of establishing clinical effectiveness, with determination of cost-effectiveness being a secondary objective. Most studies (48/66)39,43,50–53,55–62,64–66,68–73,75,81–89,91–95,97,98,101,103,105,106,108,110–112 had a clearly defined objective (to compare costs and outcomes of DIs with the costs and outcomes of other treatment options), and the majority stated or implied their perspective (64/66). 15,39,42,43,50–79,81–95,97–108,110–112 The decision-making context was not always clear in the included economic evaluations. Each trial implied a different role for DIs, reflected in their approach to recruiting patients. Recruitment methods included self-referral; referral by clinician, whereby patients are identified ‘on the job’ and recruitment requires no additional resources; recruitment by screening medical records; and proactively inviting patients to participate. Target populations and recruitment methods were appropriate for the purpose of using DIs in the mental health care pathway. For example, recruitment of self-referred patients came with a role for DIs in the diagnosis and treatment of patients who may not have otherwise sought treatment, whereas targeting diagnosed patients implied that DIs were administered instead of or alongside existing treatment. Patients to whom DIs were offered for suicidal ideation and eating disorders were predominantly recruited by self-referral; all remaining conditions employed multiple methods, suggesting a varied role for DIs.
Item 2: description of competing alternatives
Digital interventions and their competing NoDIs were by and large described in sufficient detail to understand the underlying principles and modus operandi of the interventions. WL controls and treatment as usual (TAU) were very broad, and the difference between them was often not clear, particularly when participants had self-referred (recruited via adverts), as it is not possible to determine if TAU would have involved any care had the participants not signed up to the trials. We also assessed whether or not any important alternatives were omitted, or if treatment should have been considered, based on the proposed role of the intervention, and the role of the chosen comparator. In 41 of the trials15,42,52,54,55,57,58,60,64,65,67,69,72–74,76,77,79–87,89,90,92–100,104,105,110,111 the comparator was judged to be appropriate, being TAU or any other comparator justified in the study. In 21 trials39,61–63,66,68,70,71,75,88,101–103,106–108 it was not clear whether or not the comparator of choice was appropriate. This occurred when the study used a specific comparator (e.g. no treatment, attention control as opposed to TAU) without providing justification of whether or not this was a plausible treatment option.
Item 3: establishing effectiveness of the programme or services
The majority of the economic evaluations (53/66)15,39,42,43,50–66,68–75,77,80,82–84,88–90,92,95–99,101,103–106,108,110–112 established the effectiveness through a single trial; of these, 4715,39,42,43,50–66,68–75,77,80,82–84,88–90,92,95–99,101,103–106,108,110–112 included only within-trial analyses, two59,69 extrapolated the results over time and four59,77,86,100 used the treatment effect observed in a trial to populate a decision model. All these studies were assessed to have established the effectiveness of the programme in the appraisal. Seven papers67,76,79,81,93,94,107 used feasibility, pilot and observational studies, whereas another five85–87,91,100 used non-comparative data (single-arm studies or registry data) to inform the treatment effect in decision models; these were judged in the appraisal as having partially established and having not established the effectiveness of the programme, respectively. Finally, one study78 established the effectiveness of the programme through a systematic review and evidence synthesis, but the method of incorporating the findings into the decision model was not clear.
Item 4: identifying relevant costs and consequences
This item explored whether or not the range of costs and effects measured and included in the analysis was wide enough, whether or not they covered all relevant viewpoints, and whether or not they included capital costs as well as operating costs. The outcome measures and the included costs varied greatly between the studies. Admittedly, it was difficult to judge what was relevant. The majority of studies included some operating costs, such as staff time and website maintenance. Many recruited patients through public advertising, yet they did not include recruitment costs. If the interventions were to be rolled out, reaching out to the same patient population would incur costs, and so the cost of recruitment would potentially be relevant. In terms of effects, most trials included relevant effects for that trial. Effectiveness measured in terms of disease-specific outcome measures makes sense, but on a wider scale (e.g. if societal and health-care perspective are used) it is not clear how to judge whether or not an intervention is cost-effective, unless it is dominant or dominated.
Item 5: accuracy of measurement of costs and consequences
The majority of the studies (56/66)15,39,43,50–72,75,76,79–84,86–93,95–97,99,100,103–108,110–112 accurately measured costs and consequences. Six studies42,73,77,78,94,102 did not report sufficient detail about the costs and consequences included and, therefore, were assessed as ‘unclear’ on this item, and a further four studies74,85,98,101 omitted potentially relevant costs or outcomes, leading this item to be assessed as ‘partial’.
Item 6: were costs and consequences valued credibly?
This item explored the extent to which the sources of all values were clearly defined, market values were employed and the valuation of consequences was appropriate for the question posed. Overall, the majority of the studies included sufficient detail, with five studies59,78,79,85,102 providing partial information and four studies77,80,87,91 not stating clearly whether or not market values were employed.
Item 7: adjusting costs and consequences for differential timing
A total of 47 studies15,39,50–58,61,62,64,65,68–73,75,76,80–82,84,87,88,90–94,96–107,112 had an analysis time horizon shorter than 1 year, and so discounting was not required. Ten studies, reported in 11 papers,51,63,66,74,83,87,89,108,111 reported a time horizon longer than 12 months. Of those, six studies59,85,86,89,108,110 discounted both costs and effects, and two provided justification for the discount rate they used. 89,108 Six studies51,63,66,68,74,83 were assessed as ‘not discounted for differential timing’: one study reported a relatively short time horizon (18 months) as justification,74 whereas a further five studies51,63,66,68,83 did not report any discounting. Three studies60,67,77 were assessed as ‘partial’, one of which discounted only effects, because it did not model long-term costs,60 and the other two discounted only costs because it did not model long-term effects. 67,77 Finally, four studies42,78,79,95 had an unclear analysis time horizon. Of those, one discounted costs but not effects,78 and the other three did not report discounting. 42,79,95 These last three studies were assessed as ‘unclear’ as we could not tell whether or not discounting was required.
Item 8: incremental analysis of costs and outcomes
The majority of the studies (45/66)39,42,43,53,55,56,58,61,62,64,65,67–75,78,81–84,87,89,90,92–95,97–99,101–106,108,110–112 compared incremental costs with incremental outcomes. Twelve studies57,60,63,76,77,79,80,86,91,96,100,107 reported costs and outcomes separately, although the reported outcomes can be used to derive incremental costs and outcomes. Eight studies15,51,52,54,59,66,85,88 were assessed as partially reporting incremental analysis of costs and outcomes. Of these, three reported ICERs but for a limited range of scenarios (e.g. analysis assumptions, comparators or perspectives),52,59,66 whereas the remaining five estimated the incremental costs and effects but either did not report them (because they were not statistically significant51,88 or because one of the comparators was dominant85), reported non-incremental cost-effectiveness ratio and the incremental cost-effectiveness plane graphically54 or reported outcomes in terms of the probability that an intervention would be cost-effective. 15
Item 9: evaluation of uncertainty
The assessment of sensitivity analyses was based on whether or not the authors conducted any sensitivity analysis and provided the rationale for the types of sensitive analyses they conducted. The most common scenarios were alternative intervention costs and methods for dealing with missing data.
Item 10: discussion
The assessment of discussions was based on the extent to which the conclusions of the analysis were correctly drawn and interpreted intelligently and were compared with broader literature, and whether or not the discussion included generalisability of the findings, and implementation. In total, 35 studies15,39,42,50,56–59,63,67,71,73,76,79–83,87,89,92,93,95–100,103,105–108,110,112 included a satisfactory discussion, whereas a further 30 studies included a partial discussion. 43,51–55,60–62,64–66,68–70,72,74,75,77,78,84–86,88,90,91,94,101,104,111 Discussions were judged to be partial when they excluded at least one of the above criteria. Only one study102 was considered not to have critiqued methods for evaluation or discussed the generalisability and implications of the findings.
Quality of the included studies under each item of the Philips checklist
Tables 7–9 summarise the quality assessment for each model-based study based on the Philips et al. 49 checklist. The analysis methods are generally good; for example, in the majority of the studies the objective was clear (item S1), the choice of model was appropriate (item S6), structural assumptions were transparent and appropriate (items S3 and S4) and the sources of data for baseline outcomes were clear and justified (item D2a). The most common limitations were associated with the comparators (item S5), the time horizon (item S7) and the treatment effect (D2b). Specifically, none of the studies provided a systematic comparison of all possible comparators; the time horizon was either incomplete (potentially failing to capture the long-term effect of DIs) or unclear in 878,81,85–87,91,94,99 out of the 10 studies,67,78,81,85–87,91,94,99,108 and the treatment effect was synthesised from multiple trials in only one study78 (although the methods were not explicitly reported). In the remaining models, four studies87,91,99,108 informed the treatment effect using a single RCT, three studies81,85,86 used indirect evidence and two studies67,94 used a hypothetical treatment effect.
Checklist item | Study (first author and year) | |||||||||
---|---|---|---|---|---|---|---|---|---|---|
Guerriero 201367 | Kass 201778 | Koeser 201381 | Kumar 201885 | Lee 201787 | Lee 201786 | Mihalopoulos 200591 | Naveršnik 201394 | Solomon 201599 | Wu 2014108 | |
S1 statement of decision problem/objective | ||||||||||
Is there a clear statement of the decision problem? | Yes | Yes | Yes | Yes | Yes | Yes | Yes | Yes | Yes | Yes |
Is the objective of the evaluation and model specified and consistent with the stated decision problem? | Yes | Yes | Yes | Yes | Yes | Yes | Yes | Yes | Yes | Yes |
Is the primary decision-maker specified? | Yes | Yes | Yes | Yes | Yes | Yes | Yes | Yes | Yes | Yes |
S2 statement of scope/perspective | ||||||||||
Is the perspective of the model stated clearly? | Yes | Yes | Yes | Yes | Yes | Yes | Yes | Yes | Yes | Yes |
Are the model inputs consistent with the stated perspective? | Yes | Yes | Yes | Yes | Yes | Yes | Yes | Yes | Yes | Yes |
Has the scope of the model been stated and justified? | Yes | Partial | Yes | Partial | Yes | Yes | Yes | Yes | Yes | Yes |
Are the outcomes of the model consistent with the perspective, scope and overall objective of the model? | Yes | Yes | Yes | Yes | Yes | Yes | Yes | Yes | Yes | Yes |
S3 rationale for structure | ||||||||||
Is the structure of the model consistent with a coherent theory of the health condition under evaluation? | Yes | Partial | Yes | Yes | Yes | Yes | Yes | Yes | Yes | Yes |
Are the sources of data used to develop the structure of the model specified? | Yes | Yes | Yes | Yes | Yes | Yes | Yes | Yes | Yes | Yes |
Are the causal relationships described by the model structure justified appropriately? | Yes | Partial | Yes | Yes | Yes | Yes | Yes | Yes | Yes | Yes |
S4 structural assumptions | ||||||||||
Are the structural assumptions transparent and justified? | Yes | Yes | Yes | Yes | Yes | Yes | Yes | Yes | Yes | Yes |
Are the structural assumptions reasonable given the overall objective, perspective and scope of the model? | Yes | Yes | Yes | Yes | Yes | Yes | Yes | Yes | Yes | Yes |
S5 strategies/comparators | ||||||||||
Is there a clear definition of the options under evaluation? | Yes | Partial | Yes | Yes | Yes | Yes | Yes | Yes | Yes | Yes |
Have all feasible and practical options been evaluated? | No | Unclear | Yes | Unclear | Unclear | Unclear | Unclear | Unclear | Unclear | Unclear |
Is there justification for the exclusion of feasible options? | No | No | NA | No | No | Yes | No | No | No | No |
S6 model type | ||||||||||
Is the chosen model type appropriate given the decision problem and specified causal relationships within the model? | Yes | Yes | Yes | Yes | Yes | Yes | Yes | Yes | Yes | Yes |
S7 time horizon | ||||||||||
Is the time horizon of the model sufficient to reflect all important differences between options? | Yes | Unclear | Partial | Unclear | Unclear | Partial | Unclear | Unclear | Partial | Yes |
Are the time horizon of the model, the duration of treatment and the duration of treatment effect described and justified? | Yes | No | Partial | No | Partial | Partial | Partial | Partial | Partial | Yes |
S8 disease states/pathway | ||||||||||
Do the disease states (state transition model) or the pathways (decision tree model) reflect the underlying biological process of the disease in question and the impact of interventions? | Unclear | No | Yes | Yes | Yes | Yes | Yes | Yes | Unclear | Yes |
S9 cycle length | ||||||||||
Is the cycle length defined and justified in terms of the natural history of disease? | Yes | NA | NA | Yes | No | NA | Unclear | NA | NA | Yes |
Checklist item | Study (first author and year) | |||||||||
---|---|---|---|---|---|---|---|---|---|---|
Guerriero 201367 | Kass 201778 | Koeser 201381 | Kumar 201885 | Lee 201787 | Lee 201786 | Mihalopoulos 200591 | Naveršnik 201394 | Solomon 201599 | Wu 2014108 | |
D1 data identification | ||||||||||
Are the data identification methods transparent and appropriate given the objectives of the model? | Yes | Partial | Partial | Yes | Yes | Partial | Yes | Yes | Yes | Yes |
Where choices have been made between data sources, are these justified appropriately? | No | Yes | Yes | Yes | Yes | Partial | Yes | Yes | No | Yes |
Has particular attention been paid to identifying data for the important parameters in the model? | Unclear | Partial | Partial | No | Partial | Partial | Partial | Yes | Yes | Yes |
Has the quality of the data been assessed appropriately? | Unclear | Partial | Yes | Partial | Yes | Partial | Partial | Yes | Unclear | Yes |
Where expert opinion has been used, are the methods described and justified? | NA | NA | NA | Yes | NA | NA | NA | NA | NA | Yes |
D2 data modelling | ||||||||||
Is the data modelling methodology based on justifiable statistical and epidemiological techniques? | Implied | Yes | Yes | Yes | Yes | Yes | Yes | Yes | Implied | Yes |
D2a baseline data | ||||||||||
Is the choice of baseline data described and justified? | Yes | Yes | Yes | Yes | Yes | Yes | Yes | Yes | Yes | Yes |
Are transition probabilities calculated appropriately? | Yes | NA | NA | Yes | Yes | NA | NA | NA | Unclear | Yes |
Has a half-cycle correction been applied to both cost and outcome? | Unclear | NA | NA | No | No | NA | NA | NA | Unclear | No |
D2b treatment effect | ||||||||||
If relative treatment effects have been derived from trial data, have they been synthesised using appropriate techniques? | NA | Unclear | NA | NA | NA | NA | NA | NA | NA | NA |
Have the methods and assumptions used to extrapolate short-term results to final outcomes been documented and justified? | Yes | NA | NA | Yes | Yes | NA | NA | Yes | NA | Yes |
Have alternative assumptions been explored through sensitivity analysis? | No | Partial | No | Partial | Yes | Yes | No | Yes | Yes | No |
Have assumptions regarding the continuing effect of treatment once treatment is complete been documented and justified? Have alternative assumptions been explored through sensitivity analysis? | No | No | No | Partial | Yes | NA | No | Yes | Unclear | No |
D2c costs | ||||||||||
Are the costs incorporated into the model justified? | Yes | Partial | Yes | Yes | Yes | Yes | Yes | Yes | Yes | Partial |
Has the source for all costs been described? | Yes | Yes | Yes | Yes | Yes | No | Yes | Partial | Yes | Yes |
Have discount rates been described and justified given the target decision-maker? | Yes | No | Yes | Yes | Yes | No | Yes | No | NA | Yes |
D2d quality-of-life weights (utilities) | ||||||||||
Are the utilities incorporated into the model appropriate? | Implied | NA | Yes | Unclear | Yes | Yes | Yes | Yes | Implied | Yes |
Is the source for the utility weights referenced? | Yes | NA | Yes | Partial | Yes | Yes | Yes | Yes | Yes | Yes |
Are the methods of derivation for the utility weights justified? | Unclear | NA | Yes | Unclear | Yes | Yes | Yes | Yes | Unclear | Yes |
D3 data incorporation | ||||||||||
Have all data incorporated into the model been described and referenced in sufficient detail? | Unclear | Partial | Yes | Yes | Yes | Yes | Yes | Yes | Implied | Yes |
Has the use of mutually inconsistent data been justified (i.e. are assumptions and choices appropriate)? | NA | NA | NA | NA | NA | Partial | NA | NA | Unclear | NA |
Is the process of data incorporation transparent? | Yes | Partial | Yes | Partial | Yes | Partial | Yes | Yes | Partial | Yes |
If data have been incorporated as distributions, has the choice of distribution for each parameter been described and justified? | Yes | NA | Yes | NA | Yes | NA | Yes | Yes | Yes | Yes |
If data have been incorporated as distributions, is it clear that second order uncertainty is reflected? | Yes | NA | Yes | NA | Yes | NA | Yes | Yes | Yes | Yes |
D4 assessment of uncertainty | ||||||||||
Have the four principal types of uncertainty been addressed? | No | No | Partial | No | Partial | No | Partial | Yes | No | Partial |
If not, has the omission of particular forms of uncertainty been justified? | Partial | No | No | No | No | No | No | NA | Partial | |
D4a methodological | ||||||||||
Have methodological uncertainties been addressed by running alternative versions of the model with different methodological assumptions? | No | No | No | No | No | No | No | Yes | No | Yes |
D4b structural | ||||||||||
Is there evidence that structural uncertainties have been addressed via sensitivity analysis? | No | Partial | Partial | Partial | Yes | Yes | No | Yes | No | No |
D4c heterogeneity | ||||||||||
Has heterogeneity been dealt with by running the model separately for different subgroups? | No | Partial | No | Partial | Yes | No | Partial | No | No | No |
D4d parameter | ||||||||||
Are the methods of assessment of parameter uncertainty appropriate? | Yes | NA | Partial | NA | Yes | NA | Yes | Yes | Yes | Yes |
If data are incorporated as point estimates, are the ranges used for sensitivity analysis stated clearly and justified? | Yes | NA | NA | NA | NA | Partial | NA | NA | Yes | NA |
Checklist item | Study (first author and year) | |||||||||
---|---|---|---|---|---|---|---|---|---|---|
Guerriero 201367 | Kass (2017)78 | Koeser 201381 | Kumar 201885 | Lee 201787 | Lee 201786 | Mihalopoulos 200591 | Naveršnik 201394 | Solomon 201599 | Wu 2014108 | |
C1 internal consistency | ||||||||||
Is there evidence that the mathematical logic of the model has been tested thoroughly before use? | No | No | No | No | Yes | No | Yes | No | No | No |
C2 external consistency | ||||||||||
Are any counterintuitive results from the model explained and justified? | NA | NA | NA | NA | NA | NA | NA | NA | NA | NA |
If the model has been calibrated against independent data, have any differences been explained and justified? | NA | NA | NA | NA | NA | NA | NA | NA | NA | NA |
Have the results of the model been compared with those of previous models and any differences in results explained? | Yes | No | Yes | Yes | Yes | No | Yes | Yes | No | Yes |
Key challenges and limitations in economic evaluation of digital interventions
Material in this section has been reproduced with permission from Jankovic et al. , Systematic review and critique of methods for economic evaluation of digital mental health interventions, Applied Health Economics and Health Policy, published 2020. 45 Copyright © 2020, Springer Nature Switzerland AG.
The critical review of the studies identified a range of challenges arising from the complexity of DI interventions and the heterogeneity of evidence; we describe each in turn. 45
Estimation of costs and outcomes
The included studies use a variety of methods to measure costs and outcomes of DIs. Benefits were measured in terms of QALYs, disability-adjusted life-years, life-years, disease-free days, disease-specific outcome measures, response or clinical improvement, inpatient days avoided or days of abstinence in interventions that target addiction. Costs attributed to DIs included the cost of staff time required to deliver the intervention, a range of equipment costs, website maintenance and hosting, the cost of development, capital costs and the costs of patient recruitment (or technology dissemination).
The optimal method for measuring outcomes ultimately depends on the analysis perspective. An employer may be interested in measuring the effect of the intervention on productivity, a mental health-care provider may include a narrow range of benefits specific to the mental health condition targeted by the intervention and costs that fall on that provider, whereas a health system may aim to improve overall health, and so requires a broader health measure such as health-related quality of life (HRQoL) to allow comparison across different fields of medicine.
Although the majority of the studies that were identified in this review reported a range of different costs and outcomes, seven studies,52,54,59,66,74,86,90 evaluated interventions from a health-care system or payer perspective, but measured outcomes in terms of changes in clinical scores,59,66,86,90 clinical improvement/response/remission52,54 or disease-free days. 74 It is not clear how health gains in such disease-specific outcome measures can be used by decision-makers to allocate resources across different disease areas.
Similarly, the appropriate methods for measuring costs depend on the analysis perspective (e.g. whether to include the cost to employer, service provider, broader health system or the society), as well as the role of the intervention. When interventions target undiagnosed patients who would not have sought care otherwise, dissemination (e.g. advertising or public health campaigns) is an integral part of the intervention that is likely to affect its outcomes, and so the cost of dissemination should be included in the cost of the intervention. Conversely, when an intervention targets diagnosed patients, and is prescribed by clinicians, the dissemination costs are likely to be negligible. Studies that recruit self-referred patients through advertising rarely include recruitment costs in their analysis. Furthermore, the costs of developing and maintaining DIs are highly uncertain because very few studies included capital costs (e.g. computers, staff training or one-off software purchases), or costs for website maintenance and hosting. The subsequent cost per patient depends on the scale of roll-out, where wider delivery (e.g. providing an intervention nationally) is likely to dilute such fixed costs.
Use of all available evidence
Evidence synthesis is more complex for DIs than for interventions such as medication, as DIs are multilayered and subject to external factors, and, therefore, it is difficult to ensure uniform delivery or to disentangle the impact of each layer on outcomes. There is significant heterogeneity likely between interventions, the populations they target and the settings in which they are delivered; even when interventions target the same condition, they tend to vary in their underlying principles, content, and the type and extent of support. It is not clear whether each of the characteristics affect the treatment effect, or whether evidence from similar interventions can be reasonably pooled to make an overall recommendation about their cost-effectiveness.
Furthermore, interventions for the same mental health disorder can target different patient populations (e.g. according to disease severity). The population in which an intervention is evaluated can affect its comparability with other trials (i.e. it may not be appropriate to generalise costs and treatment effect observed in one target population to another, or to attempt to synthesise effectiveness of interventions observed in different patients populations).
Finally, DIs as well as comparators that involve behavioural therapy are likely to vary between settings (clinics and countries) in the referral system, capacity, waiting times and frequency of contact, and so synthesising evidence on resource use may not be appropriate.
Specification of comparators
Comparators included supported and unsupported DIs, medication, different types of face-to-face therapy, WL and usual care. The majority of economic evaluations were based on two-arm RCTs. WLs and usual care were the most common comparators. Their description was often limited and the distinction between them was not always clear. Treatment of mental health conditions tends to vary between health providers, and between different patient populations (e.g. diagnosed vs. undiagnosed), so a lack of understanding of a comparator in a trial can limit generalisability of the findings, as well as comparability of results across trials.
Time horizon for analysis
The majority of the evaluations were conducted alongside a trial or used retrospective data from a single study. Most economic evaluations did not explore the results beyond the trial end point, potentially failing to capture long-term costs and effects of DIs. This is considered inadequate for decision-making owing to the truncated time horizon. Mental illness is a lifetime condition for many patients, with periods of respite and relapse during which costs and outcomes can be influenced by any potential treatment received. Limiting the time horizon of an analysis can generate inaccurate estimates of cost-effectiveness. The lack of long-term modelling is likely to be due, in part, to the lack of reliable data about the long-term performance of DIs. In many cases, there are no empirical data on the duration of treatment effects and how these relate to a changing baseline (i.e. how illness progresses in the population that does not receive the treatment). This issue is likely to be confounded by comorbidities and future events, making long-term extrapolation challenging.
Cost-effectiveness conclusions
Cost-effectiveness results, which comprise estimates such as ICERs and net benefits, as reported in the reviewed studies, are summarised in Table 10. This table also shows which comparisons were between DIs (UDI, SDI) and non-digital psychological interventions (SNoDI, UNoDI), non-therapeutic controls (SDC, UDC, SNoDC, UNoDC) and NI. Only two studies86,99 reported cost-effectiveness comparisons with medication.
Study (first author and year) | Classification | Description of DIs and comparators | Results of economic evaluations |
---|---|---|---|
Aardoom 201650 | UDI |
|
Probability cost-effective when cost-effectiveness threshold = £0 per QALY: FB, > 20%; FBL, > 30%; FBH, ≈ 30%; WL, ≈ 0% Probability cost-effective when cost-effectiveness threshold > £20,000 per QALY: FB, 42–54%; FBL, 1–17%; FBH, 30–38%; WL, 5–15% |
SDI-1 |
|
||
SDI-2 |
|
||
NI |
|
||
Andersson 201543 | SDI |
|
ICER = US$931 per remission |
SDC |
|
||
Andersson 201551 | SDI |
|
Societal ICER = US$1489/relapse avoided. Provider ICER = US$1066/relapse avoided |
NI |
|
||
Axelsson 201852 | SDI |
|
ICERs from societal perspective (per responder): G-ICBT vs. U-CBT, £12,671; G-ICBT vs. BIB-CBT, £542,471; G-ICBT vs. WL, £416; U-ICBT vs. BIB-CBT, dominated; U-ICBT vs. WL, dominant; BIB-CBT vs. WL, £273 ICERs from health-care perspective (per responder): G-ICBT vs. U-CBT, £2902; G-ICBT vs. BIB-CBT, £290,670; G-ICBT vs. WL, £640; U-ICBT vs. BIB-CBT, dominated U-ICBT vs. WL, £80; BIB-CBT vs. WL, dominant |
UDI |
|
||
UNoDI |
|
||
NI |
|
||
Bergman Nordgren 201453 | SDI |
|
Dominant (when outcome is CORE-OM score or QALY gain) |
SNoDC |
|
||
Bergström 201054 | SDI |
|
Dominant |
SNoDI |
|
||
Bolier 201455 | UDI |
|
ICER = €21,319 per response |
NI |
|
||
Brabyn 201656 | UDI |
|
Telephone-facilitated MoodGym dominates minimally supported MoodGym |
SDI |
|
||
Budney 201557 | SDI |
|
Cost of computer and brief counselling significantly lower than therapist. Abstinence significantly higher after computer and therapist than brief counselling. ICERs not reported |
SNoDI |
|
||
SNoDI |
|
||
Buntrock 201758 | SDI |
|
Health system ICER = £13,500 per QALY, £1125/depression-free day Societal ICER = £13,400 per QALY, £1117/depression-free days |
UDC |
|
||
Burford 201359 | UDI |
|
ICER = AU$46 per additional quitter |
NI |
|
||
Calhoun 201660 | SDI |
|
Mean cost in intervention arm: US$178. Mean cost in control arm: US$26 Outcomes in intervention arm: 28 quits, 0.51 life-years and 0.27 QALYs gained Outcomes in control arm: 32 quits, 0.48 life-years and 0.27 QALYs gained ICERs not reported |
SNoDI |
|
||
Dear 201561 | SDI |
|
ICER = £8806 per QALY |
NI |
|
||
Ebert 201862 (linked with Kählke 2019)112 | SDI |
|
Dominant |
NI |
|
||
El Alaoui 2017110 | SDI |
|
Dominant |
SNoDI |
|
||
Garrido 201763 | SDI |
|
Cost significantly higher in the active control group. Observed improvement in four cognitive domains with treatment (ICERs not reported) |
SNoDC |
|
||
Geraedts 201564 | SDI |
|
Societal ICER = £532,959 per QALY, £314 per 1-point reduction in CES-D. Employer ICER = £382,354 per QALY, £223/CES-D point reduction |
NI |
|
||
Gerhards 201065 | UDI |
|
Cost of cCBT compared with TAU = –€711 (–€3111 to €1780). Cost of cCBT + TAU compared with TAU = €738 (€71871 to €3477). Effect not significant for either intervention |
SDI |
|
||
SNoDI |
|
||
Graham 201366 | UDI |
|
3 months: enhanced vs. basic internet ICER = US$4227/quitter; enhanced internet + telephone vs. enhanced internet ICER = US$1197/quitter; 6 months: enhanced vs. basic internet ICER = US$2305/quitter; enhanced internet + telephone vs. enhanced internet ICER = US$1841/quitter; 12 months: enhanced vs. basic internet ICER = dominated; enhanced internet + telephone vs. enhanced internet ICER = US$1528/quitter; 18 months: enhanced vs. basic internet ICER = dominated; enhanced internet + telephone vs. enhanced internet ICER = US$3781/quitter |
SDI |
|
||
UDC |
|
||
Guerriero 201367 | UDI |
|
Service with text-based support dominant |
NI |
|
||
Hedman 201169 | SDI |
|
ICBT dominant |
SNoDI |
|
||
Hedman 201368 | SDI |
|
ICBT dominant (per response and QALY gain) |
SNoDC |
|
||
Hedman 2014111 | SDI |
|
ICBT dominant |
SNoDI |
|
||
Hedman 201670 | SDI-1 |
|
ICER = US$10,000 per QALY, US$2124 per case improved |
SDI-2 |
|
||
Hollinghurst 201071 | SDI |
|
ICER = £17,173 per QALY, £3528 per recovery |
NI |
|
||
Holst 201872 | SDI |
|
Health system ICER = €537 per QALY, €41/BDI-II point reduction. Societal ICER = €5387 per QALY, €411/BDI-II point reduction |
NI |
|
||
Hunter 201773 | SDI |
|
Probability that INHS is cost-effective: 70% if costs include intervention delivery, training and website development; 84% if only the cost of training (excluding website development costs) is included; 75% if English NHS costs are used and intervention costs only are included |
SNoDI |
|
||
Joesch 201274 | SDI |
|
Incremental net benefit positive when cost-effectiveness threshold > US$5000 per QALY Incremental net benefit positive when cost-effectiveness threshold > US$4/anxiety-free day |
NI |
|
||
Jolstedt 201875 | SDI |
|
ICBT dominant (when outcome is response) |
SDC |
|
||
Jones 200177 | SDI-1 |
|
No significant difference in effect for a range of outcomes No cost-saving with DI |
SDI-2 |
|
||
SNoDI |
|
||
Jones 201476 | SDI |
|
TE-HNC had no significant effect, led to higher cost |
SNoDI |
|
||
Kass 201778 | SDI |
|
Cost of prevention: US$505.86. Cost of ‘wait and treat’: US$508.76. In-person therapy with prevention: 241 individuals per 1000 at risk. In-person therapy with wait and treat: 310 individuals per 1000 at risk. Clinical outcomes not considered |
NI |
|
||
Kenter 201579 | SDI |
|
Incremental cost = €585. Effect not significant |
SNoDI |
|
||
Kiluk 201680 | SDI-1 |
|
Costs CBT4CBT + TAU: US$410.83 CBT4CBT + monitoring: US$273.12 TAU: US$318.85 Significant reductions in alcohol found across all comparators. CBT4CBT + TAU demonstrated greater abstinence than TAU (numbers not reported, only p-values) |
SDI-2 |
|
||
SNoDI |
|
||
Koeser 201381 | UDI |
|
Beating the Blues: £2430 in moderate depression; £3016 in severe depression Guided self-help: £2488 in moderate depression; £4739 in severe depression TAU: £1510 in moderate depression; £2575 in severe depression |
SNoDI |
|
||
NI |
|
||
Kolovos 201682 | SDI |
|
ICER = £3222/unit reduction in CES-D score. Intervention dominated by usual care when health effect measured in terms of QALYs and remission |
UNoDI |
|
||
König 201883 | SDI |
|
ICER = €63 per binge-free day; intervention dominated by guided self-help when health effect measured in terms of QALYs |
SNoDI |
|
||
Kraepelien 201884 | SDI |
|
Health system ICERs: ICBT vs. TAU: €8817 per QALY; €3666/responder. Exercise vs. TAU: €14,571 per QALY; €7157/responder Societal ICERs: ICBT vs. TAU: €31,471 per QALY; €13,084/responder. Exercise vs. TAU: €37,974 per QALY; €18,652/responder |
SNoDI |
|
||
NI |
|
||
Kumar 201885 | SDI |
|
ICBT dominant relative to face-to-face CBT and status quo |
SNoDI |
|
||
NI |
|
||
Lee 201787 | SDI |
|
Not available (effectiveness of DIs not measured, but is assumed to be 50% and 100% of face-to-face therapy) |
SNoDI |
|
||
Lee 201786 | UDI |
|
Intervention dominant |
SNoDI |
|
||
M |
|
||
Lenhard 201788 | SDI |
|
ICBT dominant |
NI |
|
||
Littlewood 201589 Duarte 2017109 | SDI-1 |
|
Beating the Blues vs. TAU: dominated MoodGym vs. TAU: £6933 per QALY |
SDI-2 |
|
||
NI |
|
||
Lovell 201739 | SDI |
|
Health system ICERs supported cCBT vs. WL, £32,857 per QALY at 3 months, dominant after 12 months; supported cCBT vs. guided self-help, £94,167 per QALY at 3 months, dominant after 12 months; guided self-help vs. WL, £55,152 per QALY at 3 months, £3934 per QALY at 12 months Societal perspective ICERs supported cCBT vs. WL, £48,095 per QALY at 3 months, dominant after 12 months; supported cCBT vs. guided self-help, £45,417 per QALY at 3 months, £21,778 per QALY at 12 months; guided self-help vs. WL, £46,970 per QALY at 3 months, dominant after 12 months |
SNoDI |
|
||
NI |
|
||
McCrone 200415 | UDI |
|
Probability of cost-effectiveness: 85% if cost-effectiveness threshold = £0 per QALY 99% if cost-effectiveness threshold = £15,000 per QALY 14.5% if cost-effectiveness threshold = £0/depression-free day > 80% if cost-effectiveness threshold = £5/depression-free day 14% if cost-effectiveness threshold = £0/point reduction in the Beck Depression Inventory > 80% if cost-effectiveness threshold = £0/point reduction in the Beck Depression Inventory |
NI |
|
||
McCrone 200742 | SDI |
|
BTSteps vs. relaxation: £64/point reduction. Clinician-guided ERP vs. relaxation: £90/point reduction. Clinician-guided ERP vs. BTSteps: £133/point reduction |
SNoDI |
|
||
UNoDC |
|
||
McCrone200990 | SDI |
|
FF vs. relaxation: £64/point decrease in main problem. FF vs. clinician-led therapy: dominant |
SNoDI |
|
||
SDC |
|
||
Mihalopoulos 200591 | SDI-1 |
|
Psychologist delivered vs. TAU: AU$4300 per QALY. GP delivered vs. TAU: AU$3200 per QALY |
SDI-2 |
|
||
SNoDI |
|
||
NI |
|
||
Murphy 201692 | SDI |
|
ICER = £9073/abstinent year. Intervention dominated by usual care when effect measured in QALYs |
NI |
|
||
Naughton 201793 | UDI |
|
ICER = £133.53 per additional quitter |
NI |
|
||
Naveršnik 201394 | SDI |
|
€1400 per QALY |
NI |
|
||
Olmstead 201095 | SDI |
|
Clinic perspective: US$21 per drug-free specimen. Patient perspective: US$15 per drug-free specimen |
SNoDI |
|
||
Phillips 201496 | SDI |
|
Comparable QALY gain in two interventions; lower loss of employment and absence from work with intervention |
SDC |
|
||
Romero-Sanchiz 201797 | SDI |
|
TSG vs. TAU: dominant (for all outcomes). LITG vs. TAU: dominant (for all outcomes). TSG vs. LITG: not reported |
UDI |
|
||
NI |
|
||
Smit 201398 | SDI |
|
MT vs. UC: €5100/quitter (MTC dominated by UC). MTC vs. UC: €40,300 per QALY (MT dominated by UC) |
UDI |
|
||
NI |
|
||
Solomon 201599 | UDI |
|
MyCompass vs. CBT: AU$2966.37 per QALY. MyCompass vs. M: dominant |
M |
|
||
SNoDI |
|
||
Španiel 2012100 | SDI |
|
Intervention ineffective, cost not reported |
SDC |
|
||
Stanczyk 2014101 | UDI-1 |
|
Video vs. control: €1500/abstinence; €60,000 per QALY. Text vs. control: €50,400/abstinence; text dominated by control (when outcome measured in QALYs). Video dominant over text (for both outcomes) |
UDI-2 |
|
||
UDC |
|
||
Titov 2009102 | SDI |
|
AU$5686 per year lived with disability gained |
NI |
|
||
Titov 2015103 | SDI |
|
AU$4392 per QALY |
NI |
|
||
van Spijker 2012104 | UDI |
|
Intervention dominant |
UDC |
|
||
Warmerdam 2010105 | SDI-1 |
|
CBT vs. WL: €22,609 per QALY. PST vs. WL: €11,523 per QALY. CBT dominated by PST (when using QALYs). CBT vs. WL: €1817 per additional reliably improved patient. PST vs. WL: €1248/change in depressive symptoms. CBT dominated by PST (when effect measured in change in depressive symptoms) |
SDI-2 |
|
||
NI |
|
||
Wijnen 2018106 | UDI |
|
Web-based self-help dominant for all outcomes |
NI |
|
||
Wright 2017107 | SDI |
|
Stressbusters had no effect, led to reduction in costs |
UDC |
|
||
Wu 2014108 | SDI |
|
Short-term: £14,527 per QALY. Long term: £9700 per QALY |
UNoDI |
|
Comparisons of summary estimates, such as ICERs and their constituent parts (i.e. costs and outcomes), across economic evaluations of DIs would be misleading given their methodological differences, as described in Key challenges and limitations in economic evaluation of digital interventions. Our review focused on the appropriateness of the methods used to establish the cost-effectiveness of DIs to inform health-care decision-making, and identified areas for improving consistency in future studies on key methods, including time horizon, included costs, expression of outcomes and description of comparators.
We give an overview of the results of the studies into three groups according to whether or not the studies found that: (1) DIs dominated their alternatives (i.e. DIs had a lower cost and a better outcome), (2) DIs were dominated by their alternatives (i.e. DIs had a higher cost and a worse outcome) or (3) DIs achieved better outcomes with higher costs and, therefore, their cost-effectiveness depended on willingness-to-pay thresholds and the level of uncertainty associated with the results. As stated in Key challenges and limitations in economic evaluation of digital interventions, we do not attempt to directly compare summary estimates, such as ICERs, across different economic evaluations, but instead aim to provide a panoramic overview of the landscape of economic evaluations on DIs.
Digital interventions were dominant against alternatives
-
DIs were dominant against NI for:
-
DIs were dominant against non-therapeutic controls for:
-
DIs were dominant against NoDIs for:
-
panic disorder (Bergström et al. 54 against group CBT, McCrone et al. 90 against individual CBT)
-
social anxiety69,110,111 (against face-to-face CBT; note, the three economic evaluations were based on the same clinical trial)
-
GAD85 (against face-to-face CBT or medication)
-
mixed depression and anxiety86 (against medication and face-to-face CBT)
-
binge eating disorder83 (against face-to-face CBT when outcomes were expressed in QALYs rather than number of binge-free days)
-
OCD39 (against manual-based self-help at the 12-month but not 3-month follow-up).
-
Digital interventions achieved better outcomes with higher costs against alternatives
-
DIs achieved better outcomes with higher costs against NI for:
-
DIs achieved better outcomes with higher costs against non-therapeutic controls for:
-
DIs achieved better outcomes with higher costs against NoDIs for:
-
health anxiety52 (against manualised self-help).
-
Digital interventions were dominated by their alternatives
-
DIs were dominated by NI for:
-
DIs were dominated by NoDIs for:
It is worth noting that the following studies found that DIs did not confer any added value in terms of outcomes against their alternatives:
-
DIs compared with NI for:
-
OCD39 (at 3-month follow-up).
-
-
DIs compared with non-therapeutic controls for:
-
DIs compared with NoDIs for:
-
child disruptive disorder76 (against clinic-based parenting programme)
-
depression and anxiety79 (against individual CBT)
-
eating disorder83 (against individual CBT when outcome was number of binge-free days)
-
OCD39 (against manualised self-help at 3-month follow-up)
-
schizophrenia77 (against clinician sessions)
-
severe depression81 (against audio-based self-help)
-
smoking60 (against specialist clinic smoking cessation programme).
-
This panoramic overview of the results of individual studies reiterates the complexity in interpreting the results of economic evaluations; even within the same study, results were different depending on how outcomes were expressed (e.g. QALYs rather than abstinent years92 or binge-free days83), the different time horizons (e.g. 12 months rather than 3 months39) or the different perspectives (e.g. societal vs. health care52). When DIs were compared with NI or non-therapeutic controls, individual studies suggested that the DIs either were dominant or achieved better outcomes with higher costs. When DIs were compared with NoDIs, such as individual or group CBT, several studies indicated dominance of the DIs studied, but equally as many studies found that DIs did not confer any added value.
Updated literature searches and additional studies retrieved
As the systematic literature searches were conducted > 1 year before the completion of all WPs and submission of the report, we conducted an updated literature search to capture new economic evaluations published after November 2018 (when our first literature search was carried out) up to October 2020. We searched the same databases using the same search terms as in our first literature search (see Searches), and we applied the same inclusion/exclusion criteria (see Selection criteria) and the same identification and selection process with the same reviewers (see Study selection). We have included the details of our updated literature search in Report Supplementary Material 1.
After duplicates were removed, 2422 of the 4740 records identified remained and were screened, of which 2292 were excluded by title and abstract and 130 were assessed for eligibility by full text. A total of 120 papers were excluded because the primary diagnosis was not a mental health problem (n = 5), the intervention was not a mental health intervention (n = 10), the study did not include health economic outcomes (n = 48), it was not an economic evaluation (n = 5), it was a review rather than a primary study (n = 4), it was a conference abstract rather than a peer-reviewed paper (n = 12), or it was duplicate reference (n = 3) or a protocol (n = 33). Report Supplementary Material 2 gives the references of the excluded studies and the reasons for exclusion.
As a result of the updated literature search, we identified 10 additional studies that met the inclusion criteria of our review and had been published over the preceding 2 years,113–122 as shown in Figure 7. All 10 additional studies used DIs based on CBT delivered via the web/internet113,114,116–119,121,122 or via VR120 or a mobile app. 115 One study used a non-CBT DI (progressive muscle relaxation). 119 The comparators against which the DIs were evaluated included NI,114,115,117,120–122 non-therapeutic controls118 and NoDIs including face-to-face CBT. 113,116,119
FIGURE 7.
The PRISMA flow diagram for the identification and selection of cost-effectiveness studies relating to DIs in mental health (search 2: from November 2018 to October 2020).
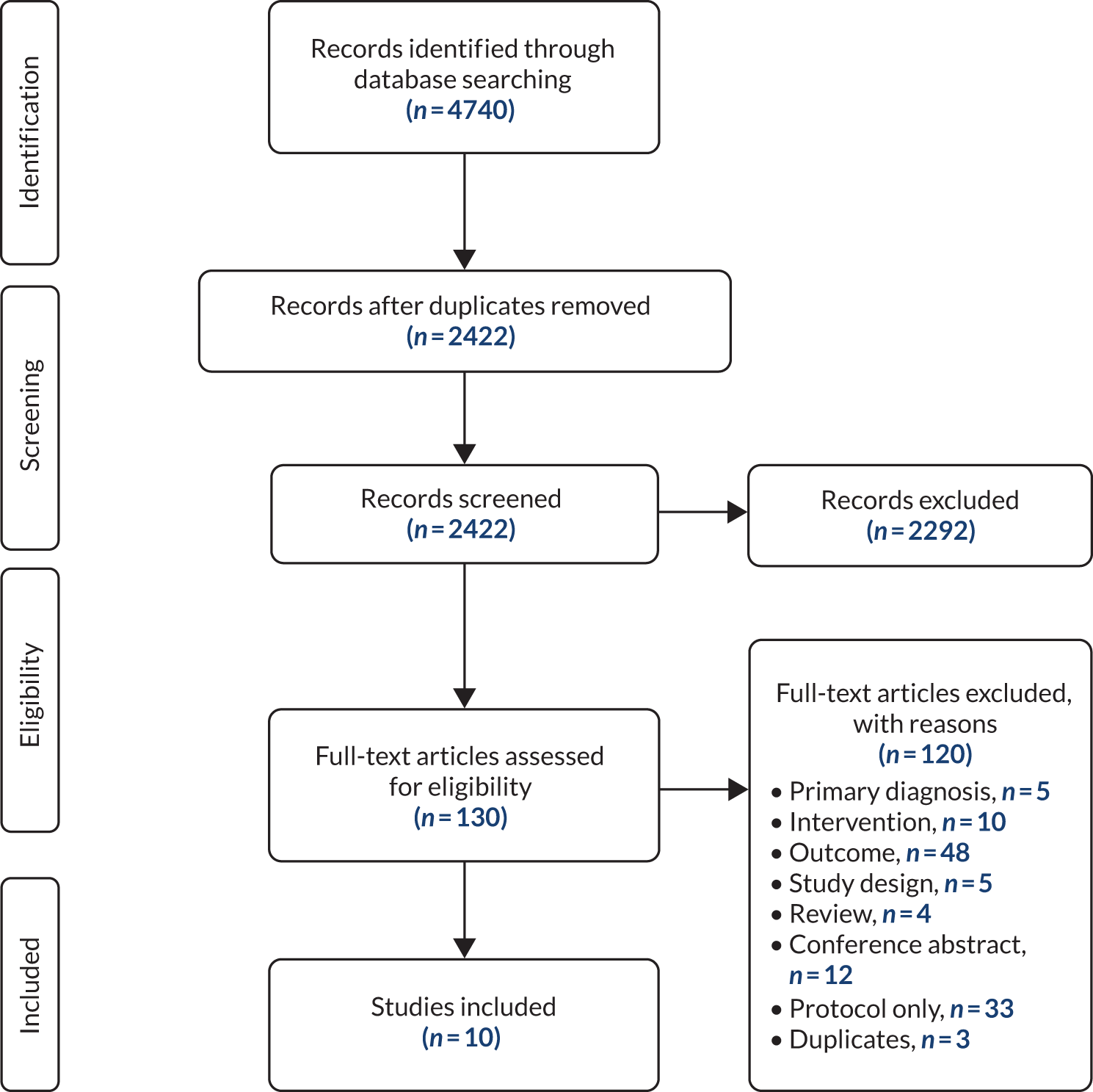
With the exception of one study that recruited adolescents with anxiety,118 the rest related to adult populations with depression,113–116 mixed depression and anxiety,122 social anxiety,121 OCD,119 paranoia in psychosis120 and stress-related disorders (i.e. adjustment disorder and exhaustion disorder). 117 The evaluations were conducted across six high-income countries: two in Germany,113,114 three in the Netherlands,115,116,120 one in Sweden,117 one in Canada,118 one in Australia119 and two in the UK. 121,122
One study was a model-based economic evaluation113 and the other nine used within-trial analyses. 114–122 It is worth noting that one within-trial analysis119 used a direct comparison between two DIs (CBT and relaxation) from a two-arm RCT benchmarked against a third intervention (face-to-face CBT) in an indirect comparison with data from previous meta-analyses. The time horizon across the 10 studies ranged from 8 weeks118 to 3 years. 113
The analytical framework used in the studies included CMA,114 CCA,118 CEA,113,115–117,119 CUA115–117,120–122 and CBA. 119 The perspectives adopted were either societal113,115–117,119,120 or that of the health-care provider. 114,116,117,119,121,122 One study118 did not report the relevant perspectives.
The model-based evaluation113 synthesised evidence from multiple sources (including 17 clinical trials meta-analysed to inform the treatment effect) to evaluate the cost-effectiveness of a DI (internet CBT) for the treatment of depression, compared with a NoDI (face-to-face CBT) from the societal perspective in Germany. It found that the internet CBT dominated face-to-face CBT, on the assumption that internet CBT had reduced waiting times compared with face-to-face CBT. This assumption was based on indirect evidence: the waiting time for face-to-face CBT was informed by average waiting times in Germany, whereas waiting time for internet CBT was based on a clinical trial among patients with OCD in Sweden. Sensitivity analysis suggested that internet CBT was less effective than face-to-face CBT when waiting times for the two treatments were equal.
Of the nine trial-based evaluations, the only one118 that compared a DI against a non-therapeutic control did not report any economic results because of low response rates and loss of data. Of the six studies that used NI as the comparator for DIs,114,115,117,120–122 one121 found a DI for social anxiety dominant at 12 months’ follow-up; three117,120,122 demonstrated that DIs for stress-related disorders, psychosis and depression/anxiety achieved better outcomes with higher cost; one114 found reduced costs for similar outcomes between a DI and usual care for depression; and one115 did not find a DI for depression cost-effective. Two studies compared DIs with face-to-face CBT and suggested that DIs are likely to be cost-effective for OCD119 and potentially for depression116 from a health-care perspective but not from a societal perspective.
The nature and results of these additional 10 economic evaluations are consistent with our findings from our earlier review of the 66 economic evaluations. Studies reported limited detail on comparators, making it difficult to distinguish between TAU and WL, or to determine similarity between comparators (e.g. TAU) in different settings. The use of trial-based analyses meant that the studies included a limited number of comparators, and a finite analysis time horizon. The findings could not be directly compared, as studies showed heterogeneity in terms of the target condition, population, analytical perspective, outcome measures and analysis time horizons. A panoramic overview of individual study results is consistent with the previous observation that DIs are dominant or achieve better outcomes with higher cost than NI, whereas the results of face-to-face comparisons of DIs and CBT are not consistent. The methods used in the model-based evaluation113 are comparable to those we used in our model in WPs 2 and 3 to analyse the cost-effectiveness of DIs for GAD, compared with all other treatment options.
Discussion
Material in this section has been reproduced with permission from Jankovic et al. , Systematic review and critique of methods for economic evaluation of digital mental health interventions, Applied Health Economics and Health Policy, published 2020. 45 Copyright © 2020, Springer Nature Switzerland AG.
Despite a growing literature on economic evaluation of DIs, including several systematic reviews, there is no conclusive evidence regarding their cost-effectiveness. The lack of consensus is often attributed to the heterogeneity in the interventions, the conditions they target and the methods used to evaluate them. This chapter aimed to assess the appropriateness of the methodology used to determine the cost-effectiveness of DIs and the challenges associated with estimating cost-effectiveness.
The review identified 66 economic evaluations from searches up to November 2018 and 10 additional economic evaluations up to October 2020. Our findings support conclusions from previous reviews that the methods used to evaluate DIs are heterogeneous. 14,18,19,21 The majority of trial-based economic evaluations are limited for decision-making purposes because of their truncated time horizon and inability to include the full range of possible alternative interventions and to incorporate all available evidence into the analysis. 44 The majority of the studies were conducted in high-income countries, so the results may not be readily generalisable to low- and middle-income countries. Differences in health systems (e.g. the US health system being primarily driven by insurance/private policies and the UK being supported by the NHS) is a contextual factor that affects the transferability of results across countries.
To draw conclusions regarding the cost-effectiveness of DIs, a synthesis of all available evidence is required, and in a way that models the long-term trajectory of mental health problems and includes all relevant comparators. Previous reviews have focused on specific types of DIs (e.g. internet-delivered CBT14,18,19,21 or guided internet interventions123) or on DIs for specific conditions (e.g. depression19,23,123 or anxiety disorders16). Given the complexity of DIs, their evaluation needs to be preceded by a taxonomy to inform what interventions can reasonably be pooled together and compared with groups of alternatives.
Decisions made about the appropriate method of CEA are, at least in part, driven by the intended role of the analysis. The role of some DIs reviewed in this study was unclear; studies recruited patients through self-referral or referral by clinician in which patients are identified ‘on the job’, by screening medical records and by proactively inviting patients to participate. Different target populations suggest different aims of interventions. Interventions that target self-referred patients have a role in the diagnosis and treatment of patients who may not have otherwise sought treatment, whereas those that target diagnosed patients imply that DIs are administered in addition to, or alongside, existing treatment. The role of therapy can affect whether or not evidence from different studies can be pooled and how we measure costs and effects, as well as what the appropriate comparators are.
The appropriate perspective for the evaluation of DIs can also vary according to the decision-making context. Evaluations can be commissioned at a local level (e.g. clinics, regional decision-makers), at a national level (e.g. NHS) or by employers and individuals themselves. Therefore, the costs and outcomes included in the analysis and the ‘decision rule’ used to interpret whether or not an intervention is cost-effective can also vary. An employer may be interested in measuring the effect of the intervention on productivity and a mental health care provider may include a narrow range of benefits specific to the mental health condition targeted by the intervention and the costs that fall on that provider, whereas a health system may aim to improve overall health, and therefore requires a broader health measure, such as HRQoL, to allow comparison across different fields of medicine. Furthermore, although NICE has an explicit decision rule (£20,000–30,000 per QALY), in other perspectives it is not clear how to interpret health gains that result in an additional cost, particularly when health benefits are measured using disease-specific outcomes; for example, how much should a provider spend on a 1-point increase in Generalised Anxiety Disorder-7 (GAD-7) score?
Conclusions
In this systematic review, we identified 76 economic evaluations of DIs, mostly conducted alongside trials with short-term follow-ups. Given that DIs are complex and heterogeneous, there are challenges specific to their economic evaluation and the synthesis of economic evidence, including estimation of all relevant costs and outcomes, analysis from different viewpoints and identification of relevant comparators. A classification system informed how DIs and comparators could be reasonably pooled together and compared in terms of cost-effectiveness, with the caveat of the different economic evaluation methods used and the diverse clinical problems addressed. The majority of studies that compared DIs with NI or with non-therapeutic controls found that DIs either achieved better outcomes at lower costs, and therefore were cost-effective, or achieved better outcomes with higher costs, in which case their cost-effectiveness would depend on how much we are willing to pay for them. When DIs were compared with NoDIs (e.g. individual or group CBT), several studies found DIs to be better and less costly, but an equal number found that DIs did not confer any added value in terms of outcomes and had no significant differences in costs.
Chapter 4 Review of clinical studies for generalised anxiety disorder
Parts of this chapter have been reproduced with permission from Saramago et al. 124 This is an Open Access article distributed in accordance with the terms of the Creative Commons Attribution (CC BY 4.0) license, which permits others to distribute, remix, adapt and build upon this work, for commercial use, provided the original work is properly cited. See: https://creativecommons.org/licenses/by/4.0/. The text below includes minor additions and formatting changes to the original text.
Introduction
Generalised anxiety disorder is the most common mental health condition in the UK, with 6% point-prevalence (measured over the preceding week), nearly double that of depression (3.3.%). 125 It is often confused with panic disorder or depression when self-reported by survey participants. 125 GAD is characterised by excessive worry that persists for several months and leads to significant distress or impairment in everyday life and functioning. 1 Other typical characteristics include free-floating anxiety and physical symptoms, such as muscle tension, headaches, restlessness, difficulty concentrating, irritability or sleep problems. GAD is associated with a low quality of life and high health-care costs. 126
Psychological interventions can be effective, especially CBT127 and applied relaxation. 128 Antidepressant medication can also be effective129 and is often the first choice for treatment by clinicians in view of limited access to psychological interventions. DIs, defined as software-based therapeutic activities accessed via technology platforms, such as the internet, VR and mobile phones, have been used as alternatives, or as add-ons, to conventional psychological therapies to improve access and increase patient choice. 3,130 DIs are included in the UK’s clinical guidelines for GAD2 in the context of a stepped-care model, in which patients are offered ‘self-help’ before medication or therapist-delivered CBT/applied relaxation.
Previous reviews of the effectiveness of DIs for GAD131,132 have included mixed populations of anxiety disorders and depression without reporting outcomes separately for GAD subgroups within these mixed samples. Reporting a disorder-specific outcome for mixed samples can be misleading because it implies that if an intervention works for the mixed sample it will also work for each of its constituent populations. Reviews and primary studies that include mixed samples do not answer the question of whether or not DIs are effective for GAD to inform disorder-specific clinical guidelines. To achieve this, we need to analyse GAD outcomes reported separately for GAD populations and for GAD subsamples within mixed populations.
Over the last two decades, NMA methods133 (also known as mixed treatment comparisons134,135) have enabled researchers to extend standard (pairwise) meta-analysis so that they can simultaneously compare interventions of interest and their alternatives within a single coherent analysis, even in the absence of direct comparisons from primary studies. Such an approach is being increasingly used in health technology assessments to inform the optimal intervention strategy for a given medical condition. 136 NMAs have been often used to inform estimates of cost-effectiveness and commissioning decisions.
This is a systematic review and quantitative synthesis of RCTs that compared DIs with other interventions and controls for GAD populations of varying severity (i.e. subthreshold, mild, moderate and severe). The review has five objectives:
-
categorise the DIs and its comparators into groups that can be pooled together
-
compare the pooled outcomes of DIs for GAD symptoms with the pooled outcomes of NoDIs, medication, non-therapeutic controls and NI
-
compare the pooled outcomes of different types of DIs against each other
-
identify limitations and gaps in the existing research on DIs for GAD
-
inform an economic model by using the pooled clinical evidence on effectiveness to assess the cost-effectiveness of DIs and alternative care options.
Methods
Search strategy
In December 2018, the following databases were searched to identify published and unpublished studies: MEDLINE, PsycInfo, CENTRAL, CDSR, CINAHL Plus, DARE, EMBASE, Web of Science Core Collection, DoPHER and ProQuest® (ProQuest LLC, Ann Arbor, MI, USA).
We searched two clinical trial registries for ongoing studies: ClinicalTrials.gov and the WHO’s International Clinical Trials Registry Platform portal. We also searched the NIHR portfolio, and conducted web searches using Google and Google Scholar using simplified search terms. After these searches were complete, we scanned the lists of included studies of relevant systematic reviews identified by the searches and the reference lists of all included studies; conducted forward citation chasing on all identified protocols, conference abstracts and the included studies using Google Scholar for any relevant publications; and contacted the authors of included studies for information on any other work in the field they were aware of.
The searches were conducted from 1997, as we did not anticipate finding relevant DIs from before that date, and were restricted to those written in English, as we anticipated that most studies written in other languages would also have a version published in English (e.g. the South Asia Cochrane Group46).
In June 2019, the searches were updated and widened to included terms based on unspecified anxiety disorders. At this time, we also conducted an additional pilot search using terms based on ‘worry’ and ‘anxiety prevention’ using only the Cochrane Library and PsycInfo databases to ensure that no articles were being missed. As no new included articles emerged from this pilot search, it was not deemed necessary to expand this to all the databases. The full search terms and outputs of the database searches are provided in Report Supplementary Material 3 and 4.
Study identification and selection
Two reviewers independently screened all titles and abstracts of the identified studies against our inclusion/exclusion criteria. If either reviewer thought that a study could be relevant, we retrieved the full text. The same two reviewers independently assessed the full texts against our inclusion/exclusion criteria. A third reviewer resolved any disagreements through discussion and arrived at the final list of included and excluded studies.
Eligible studies included those that featured the following:
-
Participants with symptoms or who were at risk of GAD within mental health populations or within the general population; we defined this as a certified diagnosis using a standardised diagnostic interview or a score above an accepted cut-off point for diagnosable GAD in standardised questionnaires.
-
Software-based systems and technology platforms designed for patient-facing delivery of a mental health intervention (i.e. an intervention to improve mental health outcomes).
-
All comparisons relevant to DIs, even when two or more DIs were compared with each other without other comparators.
-
GAD-specific measures of anxiety or worry, reported for GAD populations or GAD subsamples within mixed populations.
-
Randomised controlled trials to minimise risk of bias and confounding variables.
We excluded studies that featured the following:
-
Mixed populations of GAD with other conditions, when the outcomes were not reported separately for GAD subgroups.
-
Technology used as a means for telecommunication (e.g. e-mail, telephone or video) without any software-based processing.
-
Software-based systems designed for the training of health professionals or for administration without any patient-facing intervention components.
-
Outcomes that were not mental health related.
-
Study protocols, abstracts and reviews; these were marked so that we could check for RCTs that we may have missed in the database searches.
Data extraction and risk-of-bias assessment
Two researchers independently extracted data from published and unpublished study reports. Data were extracted on the sample, study design, intervention and comparator characteristics, baseline characteristics and results. Any discrepancies were resolved by a third reviewer. The same two reviewers who completed the data extraction (DM and HM) independently assessed the risk of bias of each study using the Cochrane risk-of-bias tool (RoB 2)137 and resolved disagreements by discussion with a third reviewer (RC). We assessed the risk of bias for each outcome measure reported in a study in the following six domains: randomisation process, deviations from intended interventions, missing outcome data, measurement of the outcome, selection of the reported results and overall bias. The risk-of-bias tool137 classifies each study as high risk, posing some concerns, or low risk based on responses to each domain.
Data synthesis and statistical analysis
An analysis of covariance (ANCOVA) modelling framework was used, in which the final measurement is synthesised, adjusting for baseline outcome measurements. With the ‘change from baseline’ approach, the ANCOVA avoids guessing within-patient correlation to enable the calculation of the standard error of change and subsequent sensitivity analyses for different correlation values. Treatment effect estimates based on ANCOVA methods have been shown to be more efficient, less biased and robust to chance baseline imbalance. 138–143 Hence, ANCOVA is the preferred method for estimating treatment effects from continuous outcomes. 144–147
A modelling approach in line with parameterisation for continuous data with normal likelihood and identity link used by Dias et al. 138,139 is taken throughout. Fixed-effects (FE) and random-effects (RE) models (accounting for potential correlation within multiarm trials) were fitted to the data, with each outcome of interest being modelled independently. In the model, patients who did not receive any treatment are expected to neither improve nor worsen during treatment (i.e. null placebo effect). In addition, it was assumed that the effect of the baseline measurement is common across all treatments, in other words that, when two active treatments are compared in a trial, the baseline effects are cancelled out.
All analyses were conducted within a Bayesian Markov chain Monte Carlo (MCMC) approach and fitted using WinBUGS software version 1.4.3, 2007 [Medical Research Council (UK) and Imperial College (UK)]148 and linked to the freely available software R (version 3.6.0; The R Foundation for Statistical Computing, Vienna, Austria) through the package R2WinBUGS. 149 In all models the MCMC Gibbs sampler was initially run for 10,000 iterations, and these were discarded as ‘burn-in’. Models were run for at least a further 5000 iterations, on which inferences were based. Chain convergence was checked using autocorrelation and Brooks–Gelman–Rubin diagram diagnostics. 150–152 Goodness of fit was assessed using the DIC (deviance information criterion) (with differences of ≥ 3 assumed to be important) and posterior mean residual deviance. 153,154
We presented the estimated results as relative treatment effect scores [and associated 95% credible intervals (CrIs)] in the selected outcome measures. We have estimated the probability of a treatment being the ‘best’ (i.e. being the most clinically effective),155 and presented rankograms for all interventions, which provide the probabilities of an intervention being ranked 1 (the highest) to 7 (the lowest). Finally, we reported the surface under the cumulative ranking curve (SUCRA), which is a numerical presentation of the overall ranking of each intervention. SUCRA values range from 0% to 100%: interventions are more likely to be placed towards the top ranks the closer their SUCRA value is to 100% and towards the bottom ranks the closer their SUCRA value is to 0%. 138,156
Appendix 1 gives further details on the analysis, including annotated synthesis WinBUGS code, sample data and initial values for the main synthesis model used (see Table 19).
Assessment of heterogeneity and consistency
For both end points, the model was extended to include study-level covariates as potential treatment effect modifiers. Clinical expectations were that potential sources of heterogeneity could include disease severity, concomitant medication and comorbidities. Meta-regression is the most commonly employed method to explore the influence of particular study-level covariates on the relative effect. However, this method requires that all studies report data on the covariate(s) in question. For the trials informing the NMA, complete data for disease severity (as a binary covariate mild to moderate/moderate to severe) were obtained, but not for the other two potential effect modifiers. To preserve all studies (and treatments), when a covariate was not reported by some studies, we allowed the model to impute missing covariate information (multiple imputation procedure assuming ‘missingness’ mechanism of ‘missing at random’).
As per guidance by Dias et al. ,156,157 inconsistency was assessed by comparing the DIC of our primary analyses (based on NMA models that assume consistency between direct and indirect evidence) and the DICs yielded by inconsistency models (which provide effect estimates based on direct evidence only). Results were assessed for coherence by qualitatively comparing estimates of pairwise ANCOVA meta-analysis (direct) and ANCOVA RE NMA (direct and indirect).
Sensitivity analysis
We conducted two types of sensitivity analysis. First, we evaluated how sensitive the networks were to individual trials. When network links were informed by more than one trial, we removed each trial one at a time (giving n – 1 for each analysis) and investigated the impact on the probability of each intervention being ‘best’. Second, we assessed the robustness of the synthesis results by repeating the analysis while excluding studies of fewer than 30 patients.
Results
Included and excluded studies
Initial systematic searches of bibliographic databases identified 16,272 records; in addition, 32 records were identified through secondary searches (e.g. citation searching of protocols and abstracts). After duplicates were removed, a total of 8920 records were screened by title and abstract, and 8543 records were excluded. We retrieved the full-text papers for the remaining 377 records and, as a result of further screening, 352 articles were excluded. In total, 21 studies (reported in 25 papers) were included in the review. The PRISMA flow diagram (Figure 8) summarises the number of records retrieved and selected at different stages of identification and screening.
FIGURE 8.
The PRISMA flow diagram for the identification and selection of clinical trials relating to DIs for GAD. Reproduced with permission from Saramago et al. 124 This is an Open Access article distributed in accordance with the terms of the Creative Commons Attribution (CC BY 4.0) license, which permits others to distribute, remix, adapt and build upon this work, for commercial use, provided the original work is properly cited. See: https://creativecommons.org/licenses/by/4.0/. The figure includes minor additions and formatting changes to the original figure.
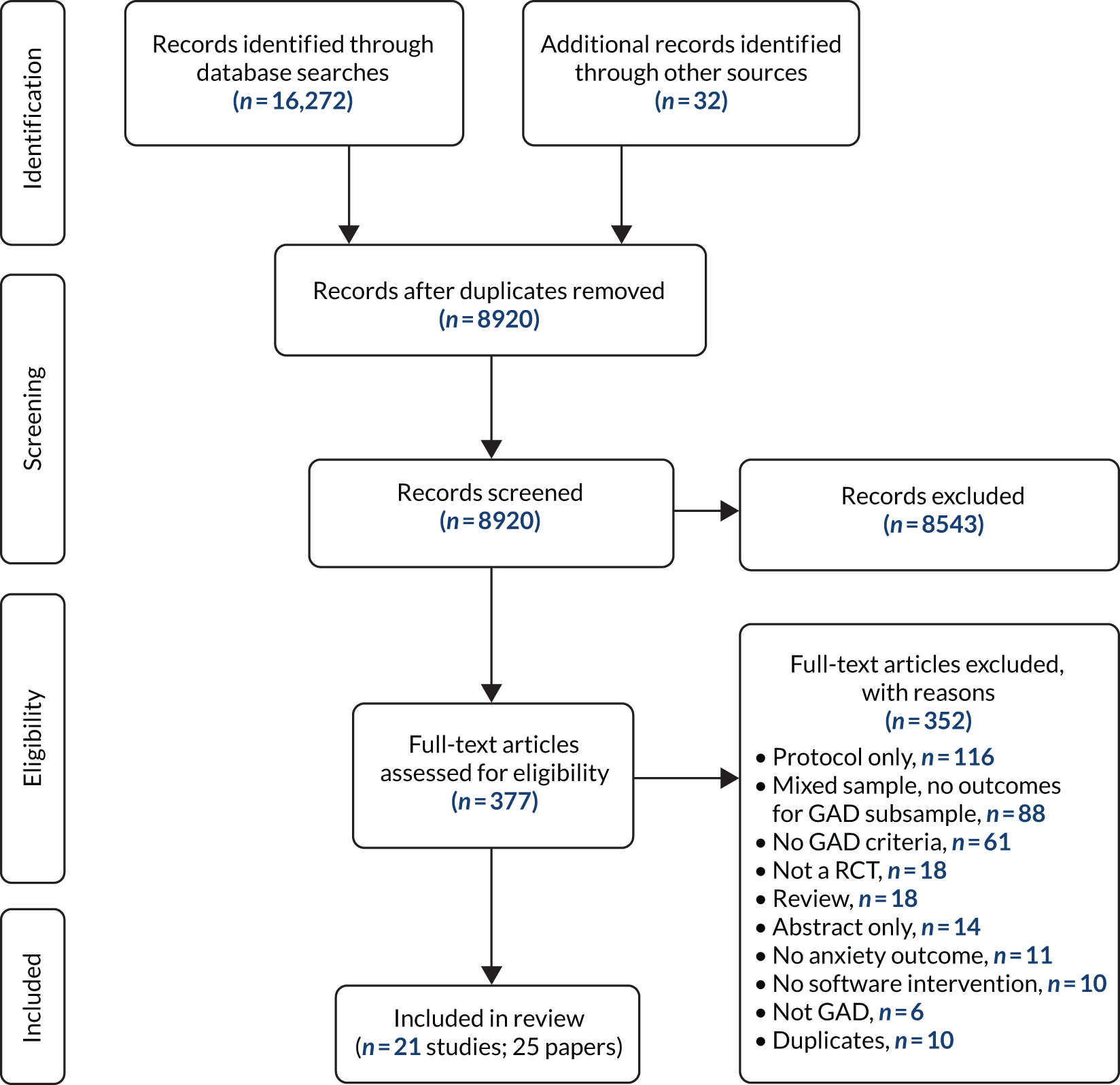
At the stage of full-text screening, we excluded 18 records because they were reviews, 14 that were abstract only and 116 that were protocols, and 10 duplicates that had not been picked up in the previous screening. We also excluded six studies that did not recruit GAD populations and 88 studies that recruited mixed populations, which may have included GAD among other conditions (e.g. depression) but did not report separate outcomes for the GAD sample. We excluded a further 61 studies in which the participants did not meet GAD criteria, 10 studies because the intervention was not digital (software based), 11 studies that reported no anxiety outcomes and 18 studies that were not RCTs. Report Supplementary Material 5 provides a full reference list of the excluded studies grouped according to reasons for exclusion.
Sample characteristics in randomised controlled trials of digital interventions for generalised anxiety disorder
The 21 RCTs included in the review,4,5,37,38,40,41,158–172 as detailed in Table 11, were conducted over 10 years, between 2009 and 2019, in 10 countries [Sweden, Australia, USA, UK, Canada, Spain, Italy, Ireland, Taiwan (Province of China) and the Netherlands] and involved 2547 randomised participants. Most participants were recruited from the general adult population, except in four studies161,163,168,172 that recruited students/young adults and one study164 involving the over-60s. GAD populations were defined as either meeting the criteria of an established diagnostic tool, such as the Mini-International Neuropsychiatric Interview (MINI),177 or scoring above an accepted cut-off value for diagnosable GAD in standardised questionnaires, such as the GAD-7 questionnaire178 or the Penn State Worry Questionnaire (PSWQ). 179
Study (first author and year) | RCT design | Comparison groups | Country | Population | Entry criteria | Number randomised | Number analysed | Treatment duration | Follow-up time points | Outcomes of interest | Other reported outcomes |
---|---|---|---|---|---|---|---|---|---|---|---|
Andersson 2012158 | 3-arm | SDI vs. SDI vs. NI | Sweden | General – adults | GAD diagnosis on SCID-I | 81 | 59 | 8 weeks |
8 weeks 3 months 18 months |
PSWQ | BAI, BDI-II, GAD-Q-IV, MADRS-S, QOLI, SCID-I, STAI-S, STAI-T |
Andersson 201740 | 2-arm | SDI vs. NI | Sweden | General – adults | PSWQ score > 56 | 140 | 132 | 10 weeks |
10 weeks 4 months 12 months |
PSWQ | BBQ, CAQ, HADS-A, IOU, MADRS-S, MCQ-30 |
Christensen 20144 | 5-arm | UDI vs. SDI vs. UDI vs. UDC vs. SDC | Australia | General – adults | GAD-7 score > 5 | 558 | 264 | 10 weeks |
10 weeks 6 months 12 months |
GAD-7 PSWQ | ASI, CES-D, days out of role, MINI |
Christensen 2014159 | 3-arm | SDI vs. SDC vs. M | Australia | General – adults | GAD diagnosis on ADIS-IV | 21 | 11 | 10 weeks |
10 weeks 6 months 12 months |
GAD-7 | CES-D, CGI |
Dahlin 201641 | 2-arm | SDI vs. NI | Sweden | General – adults | GAD diagnosis on SCID-I; PSWQ score > 45 | 103 | 85 | 9 weeks |
9 weeks 6 months |
GAD-7 PSWQ | BAI, GAD-Q-IV, MADRS-S, PHQ-9, QOLI |
aDear 2015160 | 2 × 2 factorial | SDI vs. SDI vs. UDI vs. UDI | Australia | General – adults |
GAD diagnosis on MINI GAD-7 score > 5 |
338 | 260 | 8 weeks |
9 weeks 3 months 12 months 24 months |
GAD-7 | K-10, MINI, Mini-SPIN, NEO-FFI-3, PDSS-SR, PHQ-9, SDS |
Hazen 2009161 | 2-arm | SDI vs. SDC | USA | University students | PSWQ score > 60 | 24 | 23 | 3–6 weeks | 3–6 weeks | PSWQ | BDI, STAI-T |
bHirsch 2018162 | 3-arm (analysed as 2-arm) | SDI vs. SDI vs. SDC | UK | General – adults |
Mixed sample anxiety/depressionc GAD diagnosis on SCID-I; GAD-7 score > 10 |
64 | 64 | 3–4 weeks |
3–4 weeks 1 month |
GAD-7 PSWQ | PHQ-9, RRS |
dHowell 2018163 | 2-arm | UDI vs. UDC | USA | University students | Mixed sample non-clinical (GAD < 4) and clinical mild GAD (4 < GAD-7 score < 10)e | 197 | NR | 4 weeks | 3 months | GAD-7d | None |
Johansson 201337 | 2-arm | SDI vs. SDC | Sweden | General – adults |
Mixed sample anxiety/depressionc GAD diagnosis on MINI GAD-7 score > 10 |
43 | NR | 10 weeks |
10 weeks 3 months |
GAD-7 | PHQ-9 |
Jones 2016164 | 2-arm | SDI vs. NI | Canada | Over 60s | GAD diagnosis or threshold subclinical on MINI; GAD-7 score > 10 | 46 | 41 | 7–10 weeks |
7–10 weeks 1 month |
GAD-7 | ACES, GAI, GDS, PHQ-9, PSWQ-A, WHOQOL |
Navarro-Haro 2019165 | 2-arm | SNoDI vs. SDI | Spain | Primary care – adults | GAD diagnosis on MINI | 42 | 30 | 12 weeks | 7–12 weeks | GAD-7 | DERS, FFMQ, HADS, MAIA |
Paxling 2011166 | 2-arm | SDI vs. NI | Sweden | General – adults |
GAD diagnosis on SCID-I; PSWQ score > 53 GAD-Q-IV > 5 |
89 | 72 | 8 weeks |
8 weeks 1 year 3 years |
PSWQ | BAI, BDI-II, GAD-Q-IV, MADRS-S, QOLI, STAI-S, STAI-T |
Pham 20165 | 2-arm | UDI vs. UDC | UK | General – adults |
Mixed sample common mental health problemsf GAD-7 score > 6, OASIS > 8, ASI > 16 |
63 | 42 | 4 weeks | 4 weeks | GAD-7 | Acceptability, ASI-3, OASIS, PDSS-SR, Q-LES-Q-SF |
Repetto 2013167 (linked with Gorini 2010,173 Gorini 2010174 and Pallavicini 2009175) | 3-arm | SDI vs. SDI vs. NI | Italy | Primary care – adults | GAD diagnosis (unspecified tool used) | 25 | 24 | NR | NR | GAD-7 PSWQ | BAI, HAM-A, STAI |
Richards 2016168 | 2-arm | SDI vs. NI | Ireland | University students | GAD-7 score > 10 | 137 | 112 | 6 weeks | 6 weeks | GAD-7 PSWQ | BDI-II, WASAS |
Robinson 2010169 | 3-arm | SDI vs. SDI vs. NI | Australia | General – adults | GAD diagnosis on MINI | 150 | 138 | 10 weeks |
11 weeks 3 months |
GAD-7 PSWQ | K-10, PHQ-9, SDS |
Teng 201938 | 3-arm | SDI vs. SDC vs. SNoDC | Taiwan (Province of China) | General – adults |
GAD diagnosis on DIS-IV PSWQ score > 60 |
93 | 82 | 4 weeks |
4 weeks 1 month |
PSWQ | BAI, BDI, STAI-S, STAI-T |
Titov 2009171 (linked with Lorian 2012176) | 2-arm | SDI vs. NI | Australia | General – adults | GAD diagnosis on MINI | 34 | NR | 8 weeks | 9 weeks | GAD-7 PSWQ | K-10, PHQ-9, SDS |
Titov 2010170 | 2-arm | SDI vs. NI | Australia | General – adults |
Mixed sample anxiety/depressionc GAD diagnosis on MINI |
48 | 19 | 9 weeks |
9 weeks 3 months |
PSWQ | DASS-21, K-10, NEO-FFI-3, PDSS-SR, PHQ-9, SDS, SPSQ |
Topper 2017172 | 3-arm | SNoDI vs. SDI vs. NI | The Netherlands | 15- to 22-year-olds | PSWQ score above 66th percentile (score 38) | 251 | 218 | 8–10 weeks |
8–10 weeks 3 months 12 months |
PSWQ | BDI-II, EDI-2-BU, GAD-Q-IV, MASQ-D30, PTQ, QDS, RRS |
Selection of studies and outcomes for the network meta-analyses
A total of 45 different outcome measures were reported in the included RCTs, as shown in Appendix 2. All 21 RCTs4,5,37,38,40,41,158–172 used either the GAD-7 (14 studies)4,5,37,41,159,160,162–165,167–169,171 or the PSWQ (14 studies),4,38,40,41,158,161,162,166–172 with seven studies4,41,162,167–169,171 using both to measure symptoms at baseline and outcomes at follow-up. Table 11 shows which studies reported GAD-7 and/or PSWQ scores. Apart from GAD-7 and PSWQ, the two most frequently reported outcomes were for depression, the Patient Health Questionnaire – 9 items (PHQ-9)180 and the Beck Depression Inventory, version 2 (BDI-II),181 which were reported in eight and six RCTs, respectively (see Appendix 2, Table 20). We focused on GAD-7 and PSWQ as our outcomes of choice for the NMAs because other commonly used GAD outcomes, such as the Beck Anxiety Inventory (BAI) and State–Trait Anxiety Inventory (STAI), were used in only five RCTs. An additional 25 outcome measures, including the Hamilton Anxiety Rating Scale (HAM-A)182 used in a recent NMA on medication for GAD,129 appeared only once in the included RCTs (see Appendix 2, Table 21).
Our first NMA was based on the GAD-7, a seven-item anxiety scale described in the literature as a valid and efficient tool to screen for GAD and assess symptom severity in clinical practice and research. 178 Our second NMA was based on the PSWQ, a measure that focuses on worry, which is one of the central features of GAD. 183 The measure is designed to capture the generality, excessiveness, and uncontrollability dimensions of pathological worry.
Our NMA for GAD-7 included 13 studies. 4,5,37,41,159,160,162,164,165,167–169,171 One study163 used GAD-7 but it was not included in the meta-analysis because it reported only categorical outcomes (i.e. mild, moderate, severe) rather than continuous scores. Another study164 reported the Penn State Worry Questionnaire – abbreviated (PSWQ-A), the abbreviated version of the PSWQ, so it was included only in the GAD-7 model. The measurement period ranged from 3 to 12 weeks because longer follow-ups were available for only a very few studies (e.g. for GAD-7, only four studies4,35,159,160 reported outcomes at 6 and/or 12 months, and one study160 reported outcomes at 24 months). Even when longer follow-ups were available, the control group had already crossed over to the intervention, so the randomisation was lost.
Risk-of-bias assessment
All but one161 of the 21 included studies were judged to have a high risk of bias in at least one domain of assessment for at least one outcome measure. This was largely because of outcome measurement, as all studies used self-reported (albeit standardised) questionnaires. The reason why self-reported outcomes are considered to have a high risk of bias in these studies is that participants are not masked to their allocation group, namely whether they have been allocated to a psychological intervention or to a WL or medication or a psychological placebo that is self-evidently non-therapeutic (e.g. a website with advice on general health rather than a sophisticated web-based programme specific to GAD).
The one study using self-reported measures that was assessed to be at low risk of bias involved the inclusion of a ‘sham arm’ very similar to the experimental arm,161 which made it more difficult for the participants to guess whether the arm to which they were allocated was an intervention or a control. One other study37 was assessed to be at low risk of bias but only for its researcher-administered diagnostic measure, the MINI. 177 Although researcher-administered outcomes are less open to inter-rater bias because they are completed by one person, or a few researchers who receive standardised training, a high risk of bias for the study remains if the researchers who administer the interviews are not masked to the participant’s allocation.
With regard to risk of bias, most studies were considered to be at a low risk for the randomisation process in general, although over half did not provide enough detail of allocation concealment to make an assessment. Similarly, most studies were rated as being at low risk of bias as a result of deviations from the intended intervention. Missing outcome data were of considerable concern with respect to bias, with over half the studies being rated as having a high risk of bias in this domain. Concerns about missing outcome data included high rates of withdrawals or exclusions at follow-up, differential attrition between groups and limited use of appropriate statistical procedures to mitigate these issues. The risk-of-bias outcome for each study under each domain is shown in Table 12 and a visual description of the risk-of-bias assessment across all studies is shown in Figure 9.
Study (first author and year) | Outcomes assessed individually for risk of bias | Randomisation process | Deviations from intended interventions | Missing outcome data | Measurement of outcome | Selection of the reported result | Overall bias |
---|---|---|---|---|---|---|---|
Andersson 2012158 |
Anxiety (GAD-Q-IV, STAI), worry (PSWQ), QoL (QOLI) Depression (MADRS-S, BDI-II), diagnosis (SCID-I) |
Low | Low | High | High | High | High |
Andersson 201740 | Worry (PSWQ), depression (MADRS-S, HADS-D), diagnosis (MINI) | Low | Low | Low | High | Concerns | High |
QoL (BBQ) | High | ||||||
Christensen 20144 | Anxiety (GAD-7), worry (PSWQ), depression (CES-D) | Low | Low | High | High | High | High |
Christensen 2014159 | Anxiety (GAD-7), worry (PSWQ), depression (CES-D) | Low | Concerns | High | High | Low | High |
Dahlin 201641 | Anxiety (GAD-7, GAD-Q-IV, BAI), worry (PSWQ), QoL (QOLI), depression (MADRS-S, PHQ-9) | Concerns | Low | Low | Low | Concerns | Concerns |
Dear 2015160 | Anxiety (GAD-7, PDSS) depression (PHQ-9), distress (K-10), diagnosis (MINI) | Low | High | High | High | Concerns | High |
Hazen 2009161 | Anxiety (STAI), worry (PSWQ), depression (BDI) | Concerns | Low | Low | High | Concerns | High |
Hirsch 2018162 | Anxiety (GAD-7), worry (PSWQ, RRS), depression (PHQ-9) | Low | High | High | High | Concerns | High |
Howell 2018163 | Anxiety (GAD-7) | Concerns | High | High | High | High | High |
Johansson 201337 | Anxiety (GAD-7), depression (PHQ-9), diagnosis (MINI) | Concerns | Low | Low | High | Concerns | High |
Jones 2016164 | Anxiety (GAD-7, GAI, ACES), depression (GDS, PHQ-9), worry (PSWQ-A), QoL (WHOQOL) | Low | Low | Low | High | Concerns | High |
Navarro-Haro 2019165 | Anxiety (GAD-7, HADS-A), depression (HADS-D) | Concerns | Concerns | High | High | Concerns | High |
Paxling 2011165 | Anxiety (GAD-Q-IV, STAI, BAI), worry (PSWQ), QoL (QOLI), depression (MADRS-S, BDI) | Concerns | Low | High | High | Low | High |
Pham 20165 | Anxiety (GAD-7, PDSS), QoL (Q-LES-Q-SF) | Concerns | Low | High | High | Concerns | High |
Repetto 2013167 | Anxiety (GAD-7, HAM-A, STAI, BAI), worry (PSWQ) | Concerns | Concerns | High | High | High | High |
Richards 2016168 | Anxiety (GAD-7), worry (PSWQ), depression (BDI-II) | Concerns | Low | Concerns | High | Low | High |
Robinson 2010169 | Anxiety (GAD-7), worry (PSWQ), depression (PHQ-9), general distress (K-10), disability (SDS) | Concerns | Low | Concerns | Low | Low | High |
Teng 201938 | Anxiety (STAI, BAI), worry (PSWQ), depression (BDI) | Concerns | Concerns | High | High | High | High |
Titov 2009171 | Anxiety (GAD-7), worry (PSWQ) | Low | Low | Concerns | Low | High | High |
Titov 2010170 | Anxiety (PDSS, SPSQ), stress (PSWQ), depression (PHQ-9) | Low | Low | Concerns | Low | High | High |
General distress (K-10), disability (SDS) | Low | Low | Concerns | Low | Concerns | High | |
Topper 2017172 | Anxiety (GAD-7, MASQ-A), worry (PSWQ, RRS, PTQ), general distress (MASQ-GD), depression (BDI-II) | Low | Concerns | High | Low | High | High |
FIGURE 9.
Proportion (%) of RCTs of DIs for GAD with ‘low risk’, ‘high risk’ or ‘some concerns’ in the six domains of the risk-of-bias tool. Reproduced with permission from Saramago et al. 124 This is an Open Access article distributed in accordance with the terms of the Creative Commons Attribution (CC BY 4.0) license, which permits others to distribute, remix, adapt and build upon this work, for commercial use, provided the original work is properly cited. See: https://creativecommons.org/licenses/by/4.0/. The figure includes minor additions and formatting changes to the original figure.
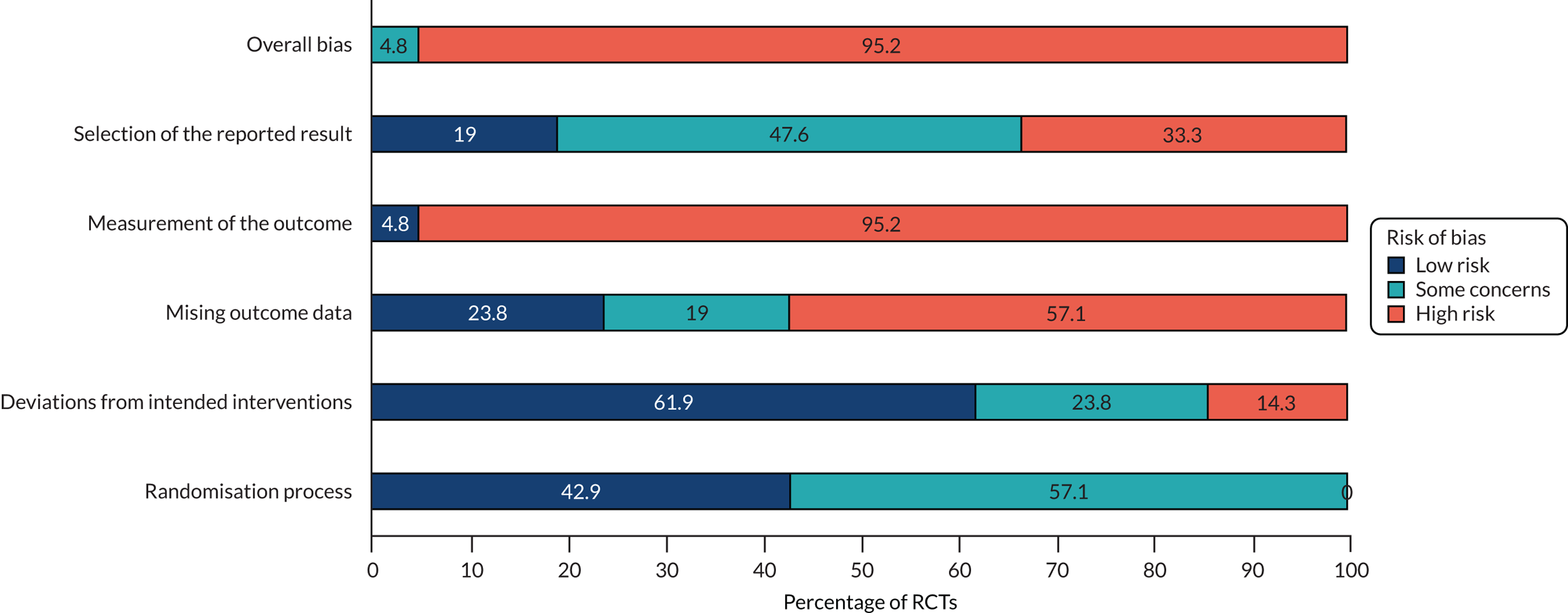
Classification of digital interventions and comparators
We classified DIs and their alternatives according to three criteria: (1) whether they were a psychological/behavioural intervention (I) or a non-therapeutic psychological/behavioural control (C); (2) whether they were digital (D) or non-digital (NoD); and (3) whether they were supported (S) or unsupported (U). WLs and usual care were classified under NI unless an active component (e.g. monitoring, sham activity) was introduced, in which case the WL/usual care was classified as non-therapeutic psychological/behavioural control. An additional classification group was included for pharmacological interventions, called medication (M).
The interventions and controls of the 21 included RCTs were allocated to one of the following eight classification groups: medication (M), NI, SDC, SDI, SNoDC, SNoDI, UDC and UDI. There were no available clinical studies that included UNoDIs or UNoDCs.
Table 13 describes all the interventions and controls included in each classification group for each study. The majority of DIs were supported (in 19 RCTs)4,37,38,40,41,158–162,164–172 and were compared against NI (in 12 RCTs). 5,40,41,158,164,166–172 Only three RCTs4,5,160 included UDIs; two were web-based CBT4,160 and one5 was a mobile game to practise breathing retraining. The only NoDI represented in two RCTs165,172 was group therapy [one CBT and one mindfulness-based intervention (MBI) and there was only one RCT that included medication (an antidepressant, sertraline)]. 159 Most of the non-therapeutic active controls reported in eight RCTs4,5,37,38,159,161–163 included a digital element, whereas only one RCT38 had a non-digital control in the form of a weekly face-to-face assessment with a research assistant in a laboratory.
Study (first author and year) | Intervention or control description (mode of delivery, therapy/control method, type of interpersonal contact/support) | Classification |
---|---|---|
Andersson 2012158 | Web-based psychodynamic therapy + weekly online support by psychology students/qualified psychologist | SDI |
Web-based CBT + weekly online support by psychology students/qualified psychologists | SDI | |
WL (crossover to web-based CBT at 3 months) | NI | |
Andersson 201740 | Web-based extinction therapy + daily online support by psychology students | SDI |
WL (+ weekly onlinea PSWQ ratings and option to telephone if symptoms worsen – crossover to web-based extinction therapy at 10 weeks) | NIb | |
Christensen 20144 | Web-based CBT – no interpersonal communication | UDI |
Web-based CBT + weekly telephone calls by ‘casual interviewers’ | SDI | |
Web-based CBT + weekly reminder e-mail similar in content to telephone calls by ‘casual interviewers’ but no two-way communication | UDI | |
Control website (information about general health) – no interpersonal communication | UDC | |
Control website (information general health) + weekly telephone calls by ‘casual interviewers’ | SDC | |
Christensen 2014159 | Web-based CBT + scheduled on-site meetings with psychologists/GPs | SDI |
Control website (information about general health) + scheduled meetings with psychologists/GPs | SDC | |
Medication (SSRI – 25 mg of sertraline up to 100 mg per day) + scheduled meetings with psychologists/GPs | M | |
Dahlin 201641 | Web-based MBT and ACT + weekly messages via a secure messaging system by psychology students | SDI |
WL (+ weekly onlinea GAD-7 and PSWQ ratings – contact with administrator implied for weekly measure completion but unclear – crossover to modified web-based MBT and ACT at 9 weeks) | NIb | |
Dear 2015160 | Web-based CBT (transdiagnostic model focusing on mental well-being) + weekly telephone/e-mail contact with qualified psychologists | SDI |
Web-based CBT (GAD-specific focusing on worry control) + weekly telephone/e-mail contact with qualified psychologists | SDI | |
Web-based CBT (transdiagnostic model focusing on mental well-being) + standardised weekly e-mail reminders and option to telephone/e-mail for technical support or other problems – no scheduled or regular interpersonal contact | UDI | |
Web-based CBT (GAD-specific focusing on worry control) + standardised weekly e-mail reminders and option to telephone/e-mail if needed technical support or had other problems – no scheduled or regular interpersonal contact | UDI | |
Hazen 2009161 | Computer-delivered attentional retraining + ‘non-therapy’ meetings with ‘experimenters’ every 6 days | SDI |
Sham training + ‘non-therapy’ meetings with ‘experimenters’ every 6 days | SDC | |
Hirsch 2018162 | Web-based CBM + one initial on-site meeting + regular (unspecified) contact by telephone/e-mail/SMS with researchers (unspecified qualifications) + RNT priming | SDI |
Web-based CBM + one initial on-site meeting + regular (unspecified) contact by telephone/e-mail/SMS with researchers (unspecified qualifications) – no RNT priming | SDI | |
Control website (neutral scenarios) + one initial on-site meeting + regular (unspecified) contact by telephone/e-mail/SMS with researchers (unspecified qualifications) | SDC | |
Howell 2018163 | Web-based CBT + standardised weekly e-mail reminders with information – no interpersonal contact | UDI |
Control website (online assessment and resources) + standardised weekly e-mail reminders with substantial information – no interpersonal contact | UDC | |
Johansson 201337 | Web-based psychodynamic therapy + weekly written messages via online messaging system by therapists (unspecified qualifications) | SDI |
WL + weekly assessment and non-directive support via online messaging system with therapists (unspecified qualifications) matching therapist support in the intervention | SDCc | |
Jones 2016164 | Web-based CBT + weekly messages via online messaging system by therapists (unspecified qualification) | SDI |
WL (crossover after 7–10 weeks) – no monitoring or other input specified | NI | |
Navarro-Haro 2019165 | Group MBI in weekly on-site meetings with a therapist | SNoDI |
VR mindfulness skills + group MBI in weekly on-site meetings with a therapist | SDI | |
Paxling 2011166 | Web-based CBT (like an online book) + weekly online/e-mail contact with therapist | SDI |
WL (crossover after 8 weeks) – no monitoring or other input specified | NI | |
Pham 20165 | Mobile game of breathing retraining – no interpersonal contact | UDI |
WL + weekly newsletter with curated content on breathing retraining exercises, matching content to mobile game, mindfulness meditation (assumed via mobile but not clear) + e-mail reminders to complete assessments (crossover to access the mobile game after 4 weeks) | UDCd | |
Repetto 2013167 | VR relaxation during weekly meetings with therapist + mobile phone home access of VR environments | SDI |
VR relaxation during weekly meetings with therapist + mobile phone home access of VR environments + biofeedback machine for therapist to adapt VR environments according to participant’s heart rate | SDI | |
WL (no monitoring or any other input specified) | NI | |
Richards 2016168 | Web-based CBT + weekly online messages by psychologists | SDI |
WL (crossover at week 7 – no monitoring or other input) | NI | |
Robinson 2010169 | Web-based CBT + weekly telephone/e-mail contact by a ‘clinician’ (clinical psychologist) | SDI |
Web-based CBT + weekly telephone/e-mail contact by a ‘technician’ (administrative clinic manager) | SDI | |
WL (crossover at week 11 – no monitoring or other input) | NI | |
Teng 201938 | Mobile app – home-delivered ABM + weekly ‘lab’ meeting with assistant + telephone call if missed sessions | SDI |
Mobile app – attention training + weekly ‘lab’ meeting with assistant + telephone call from the assistant if missed sessions | SDC | |
WL + weekly meetings with research assistant for matching assessment in a ‘lab’ | SNoDCe | |
Titov 2009171 | Web-based CBT + moderated online discussion forum + instant online messaging + one initial telephone contact + subsequent e-mail/telephone weekly contact with clinical psychologist | SDI |
WL (crossover at week 11 – no monitoring or other input) | NI | |
Titov 2010170 | Web-based CBT + moderated online discussion forum + instant online messaging + one initial telephone contact + subsequent e-mail/telephone weekly contact with clinical psychologist | SDI |
WL (+ unclear if contact with psychologist – crossover at week 9) | NI | |
Topper 2017172 | Group CBT in weekly meetings with psychologists | SNoDI |
Web-based CBT + weekly online personalised feedback from psychologists (unclear whether or not there was two-way communication between participant and psychologist in response to feedback) | SDI | |
WL (crossover at 12 months – no monitoring or other input from the research team) | NI |
Network meta-analysis results: Generalised Anxiety Disorder-7 scores at follow-up
Generalised Anxiety Disorder-7 scores were reported in 13 out of 20 studies (n = 1613 patients). 4,5,37,38,40,41,158–162,164–172 Each of these studies reported short-term outcomes (up to 12 weeks), with very few studies reporting outcomes beyond 12 weeks post treatment initiation. In those that did, crossovers were allowed at follow-up, biasing any long-term treatment effect. 184 Ten direct treatment comparisons were made in the 13 trials included in the GAD-7-based NMA; four of the 13 trials were multiarm trials (three three-arm trials159,167,169 and one five-arm trial4); five comparisons were informed by more than one trial when a pairwise ANCOVA meta-analysis was conducted (ANCOVA FE and ANCOVA RE models when n > 3).
We constructed a network plot to illustrate which interventions had been compared head to head (direct pairwise comparisons) for GAD-7 in the 13 included RCTs. An overview of these pairwise comparisons is shown in Appendix 3, Table 22. The structure of the network for GAD-7 is shown in Figure 10.
FIGURE 10.
Network plot for direct pairwise comparisons between all interventions and controls for GAD populations in RCTs with GAD-7 scores as an outcome. Line thickness around the node is proportional to the number of patients contributing to each intervention/control group. Line thickness connecting nodes is proportional to the number of patients contributing to each pairwise comparison between interventions/controls. n = number of trials informing each comparison. M, medication. Reproduced with permission from Saramago et al. 124 This is an Open Access article distributed in accordance with the terms of the Creative Commons Attribution (CC BY 4.0) license, which permits others to distribute, remix, adapt and build upon this work, for commercial use, provided the original work is properly cited. See: https://creativecommons.org/licenses/by/4.0/. The figure includes minor additions and formatting changes to the original figure.
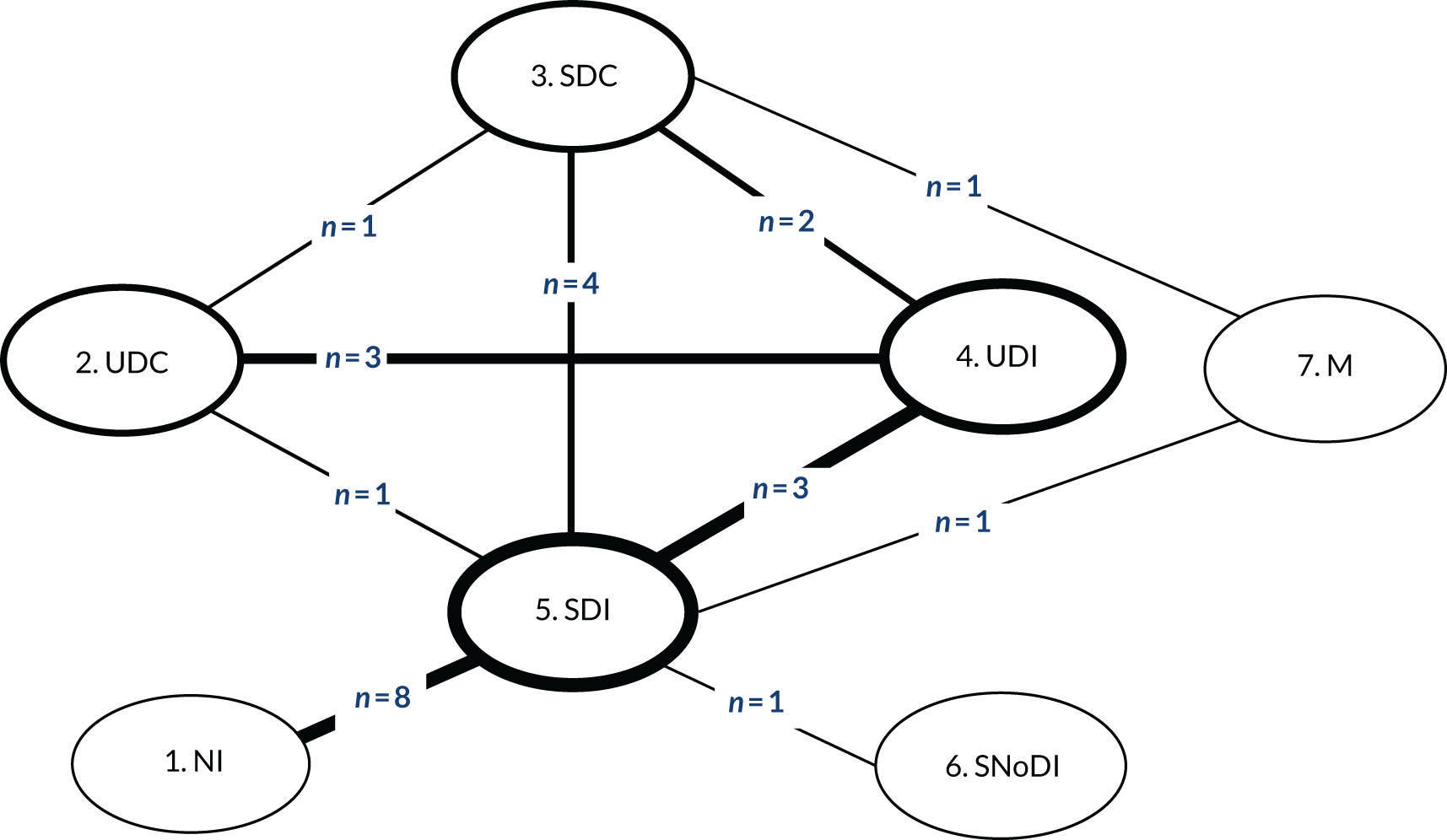
Fixed-effects and RE models were employed with minimal difference in mean residual deviances and DIC between the models tested. However, posterior estimates of between-study heterogeneity suggested considerable variability across studies, which was in line with the assessment made of the studies within the evidence base. Hence, a RE approach was preferred. There was a high degree of uncertainty in the network, especially links that were not informed by direct comparisons. Table 14 presents the full results of the NMA based on GAD-7 scores. Negative values suggest a positive effect for the first intervention in the direct pairwise comparisons, and that the intervention on the left column is ‘better’ in the NMA. GAD-7 score differences should be assessed in the light of the GAD-7 score range 0–21, from mild to severe.
GAD-7 network | Comparator | |||||||
---|---|---|---|---|---|---|---|---|
GAD-7 direct pairwise,a,b median (95% CrI) | ||||||||
NI | UDC | SDC | UDI | SDI | SNoDI | M | ||
FE | RE | |||||||
NI | Not available | Not available | Not available | –3.65 (–8.19, 0.9) | –1.26 b (–43.8, 40.93) | Not available | Not available | |
UDC | –0.26 (–16.82, 16.42) | –0.80a (–12.30, 10.70) | –0.77 (–7.81, 6.26) | –1.50 a (–11.34, 8.34) | Not available | Not available | ||
SDC | –0.14 (–15.52, 15.30) | 0.12 (–9.84, 10.05) | –0.51 (–31.75, 28.95) | –8.16 (–26.57, 13.04) | –1.07 b (–30.2, 27.19) | Not available | –8.20a (–22.07, 5.67) | |
UDI | –0.96 (–16.43, 14.54) | –0.7 (–9.10, 7.58) | –0.83 (–8.82, 7.29) | – | –0.14 (–8.85, 8.89) | –1.38 b (–26.23, 23.62) | Not available | Not available |
SDI | –1.75 (–15.72, 12.26) | –1.49 (–10.51, 7.47) | –1.61 (–8.03, 4.82) | –0.79 (–7.39, 5.80) | –0.71 a (–14.03, 12.61) | –2.70 a (–9.80, 4.40) | ||
SNoDI | –2.41 (–22.34, 17.46) | –2.21 (–18.69, 14.5) | –2.28 (–17.66, 13.16) | –1.45 (–16.92, 14.03) | — | –0.65 (–14.67, 13.23) | Not available | |
M | –4.95 (–21.09, 11.26) | –4.69 (–16.62, 7.11) | –4.81 (–14.59, 4.83) | –3.97 (–14.3, 6.20) | — | –3.18 (–11.32, 4.76) | –2.56 (–18.71, 13.54) |
Medication was the intervention associated with the largest decrease in GAD-7 median scores, although uncertainty was high in the NMA estimates, with all 95% CrIs including zero. These results were driven by the outcomes of a small (n = 21), three-arm trial159 that compared medication supplemented with scheduled face-to-face meetings with psychologists and general practitioners (GPs) with SDC (a general health website with scheduled meetings with psychologists and GPs) and SDI (a web-based CBT self-help programme with scheduled meetings with psychologists and GPs).
Based on SUCRA rankings and rankograms for each intervention (as detailed in Appendix 4), SDIs were estimated to be more effective than UDIs, which included unsupported web-based CBT4,160 and an unsupported mobile breathing retraining game;5 however, SDIs were less effective than SNoDI, which was a weekly group MBI with a therapist. 165 The adjustment for baseline scores indicated that the baseline effect on the final outcome was small with a 95% CrI including zero (median –0.14, 95% CrI –1.10 to 0.82).
The results of independently pooling direct evidence for each contrast (but not pooling when n = 1), were found to be generally consistent with the NMA results in terms of both direction and magnitude of the estimates (see Table 14; upper-right triangle, shaded). Of note are the differences in the estimates found when applying a FE and a RE ANCOVA meta-analysis model to direct evidence for the comparisons of SDIs and SDCs (n = 4) and of SDIs and NI (n = 8), evidencing non-negligible variability across the studies and the importance of accounting for between-study heterogeneity.
Network meta-analysis results: Penn State Worry Questionnaire scores at follow-up
The PSWQ follow-up scores were reported in 14 out of 20 studies4,31,40,41,158,161,162,166–172 (n = 1776 patients). Using these studies, we constructed a network plot to illustrate which interventions have been compared head to head (direct pairwise comparisons) for PSWQ score. An overview of these pairwise comparisons is shown in Appendix 3, Table 23. The structure of the network is shown in Figure 11.
FIGURE 11.
Network plot for direct pairwise comparisons between all interventions and controls for GAD populations in RCTs with PSWQ scores as an outcome (n = number of comparisons available across all RCTs). Line thickness around the node: proportional to the number of patients contributing to each intervention/control group. Line thickness connecting nodes: proportional to the number of patients contributing to each pairwise comparison between interventions/controls. n = number of trials informing each comparison. Reproduced with permission from Saramago et al. 124 This is an Open Access article distributed in accordance with the terms of the Creative Commons Attribution (CC BY 4.0) license, which permits others to distribute, remix, adapt and build upon this work, for commercial use, provided the original work is properly cited. See: https://creativecommons.org/licenses/by/4.0/. The figure includes minor additions and formatting changes to the original figure.
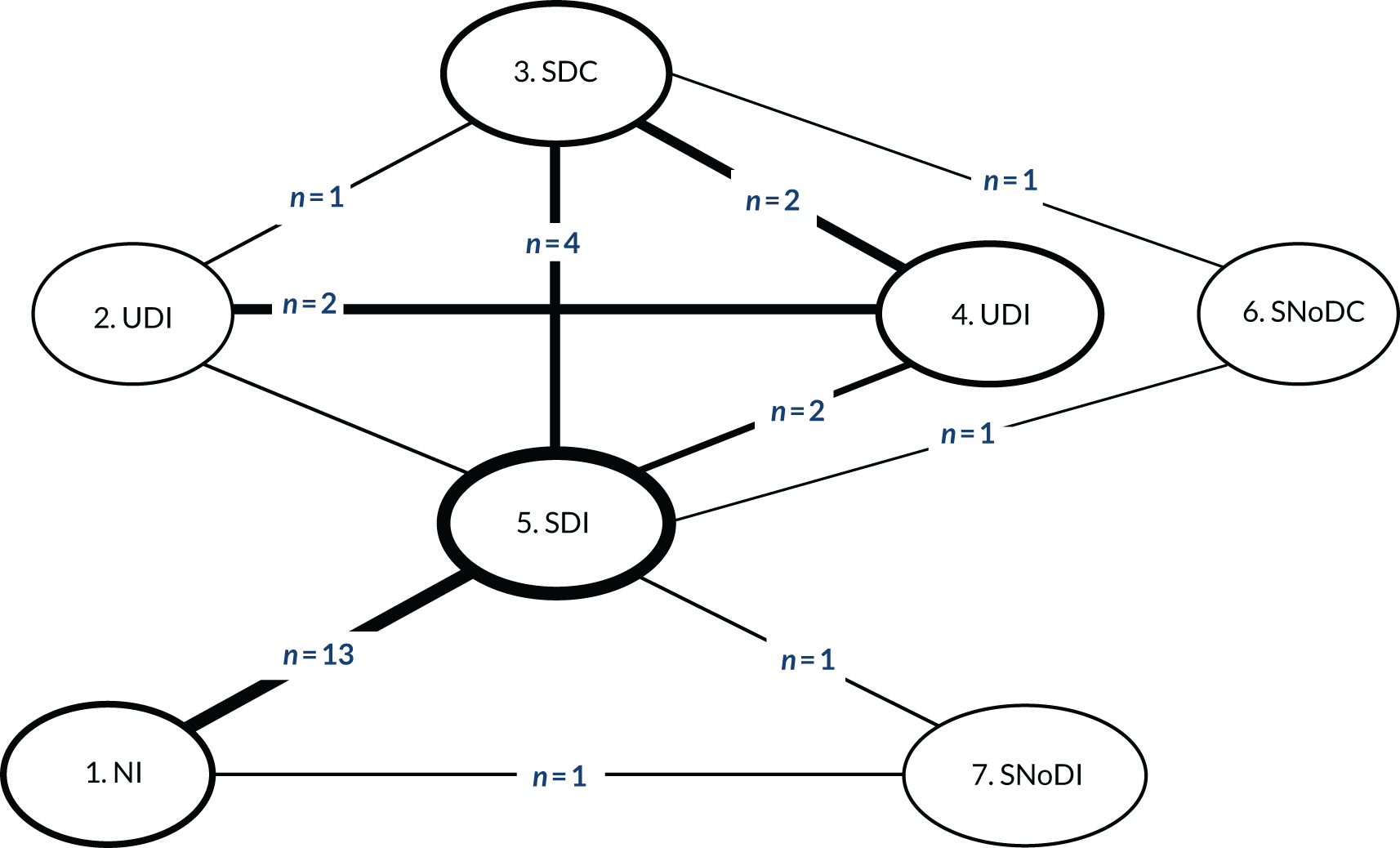
Eleven direct treatment comparisons were made in the 14 trials4,31,40,41,158,161,162,166–172 included in the NMA; 6 of the 14 trials were multiarm trials (five three-arm trials38,158,167,169,172 and one five-arm trial),4 five comparisons were informed by more than one trial in which pairwise ANCOVA meta-analysis was conducted (ANCOVA FE models and ANCOVA RE when n > 3).
Fixed-effects and RE models were employed with minimal difference in mean residual deviances and DIC between them. However, posterior estimates of between-study heterogeneity suggested considerable variability across studies, which was in line with the assessment made of the studies within the evidence base. Hence, a RE approach was preferred. Table 15 presents the full results of the NMA based on PSWQ scores. Negative values suggest a positive effect for the first intervention in the direct pairwise comparison, and that the intervention on the left column achieves better outcomes in the NMA. The PSWQ score differences should be assessed in the light of the PSWQ score ranging from 16 to 80, that is from mild to severe.
PSWQ network | Comparator | |||||||
---|---|---|---|---|---|---|---|---|
PSWQ direct pairwise,a,b median (95% CrI) | ||||||||
NI | UDC | SDC | UDI | SDI | SNoDC | SNoDI | ||
FE | RE | |||||||
NI | Not available | Not available | Not available | –5.23 (–12.91, 2.39) | 4.76 b (–51.7, 59.53) | Not available | –6.51a (–30.24, 17.22) | |
UDC | –2.13 (–45.14, 41.05) | –2.60a (–37.39, 32.19) | –6.73 (–33.99, 21.37) | –3.60 a (–35.42, 28.22) | Not available | Not available | ||
SDC | –3.76 (–46.77, 32.38) | –1.76 (–32.27, 28.68) | –2.52 (–26.55, 22.31) | –2.93 (–20.90, 15.00) | 0.95 b (–77.24, 80.71) | 3.15a (–20.58, 26.88) | Not available | |
UDI | –7.13 (–37.55, 24.61) | –4.96 (–35.07, 24.94) | –3.36 (–28.72, 21.82) | 1.53 (–21.48, 24.93) | — | Not available | Not available | |
SDI | –6.43 (–39.63, 39.15) | –4.35 (–34.27, 25.6) | –2.65 (–15.95, 10.74) | 0.67 (–23.71, 25.41) | 6.75 a (–20.97, 34.47) | –0.58 a (–24.74, 23.58) | ||
SNoDC | –0.39 (–53.64, 39.29) | 1.87 (–35.41, 38.99) | 3.51 (–19.87, 26.64) | 6.81 (–26.18, 39.67) | – | 6.15 (–18.36, 30.39) | Not available | |
SNoDI | –7.14 (–32.27, 28.68) | –4.98 (–44.2, 33.81) | –3.42 (–31.40, 24.76) | –0.06 (–34.59, 34.83) | – | –0.710 (–25.34, 23.9) | –6.91 (–41.53, 27.92) |
Uncertainty was high for all comparisons, with all 95% CrIs including zero. We observed no difference in median PSWQ scores between the SNoDI, which was a weekly group CBT with a therapist,172 and the UDI, an internet CBT self-help programme without therapist support. 4 Based on SUCRA rankings and rankograms for each intervention, as detailed in Appendix 4, SNoDI and UDI were estimated to be more effective than the remaining interventions and controls, including SDIs; however, SDIs were associated with larger median score reduction on the PSWQ than NI and UDC, which was a general health website without any personal communication. 4
Unexpectedly, SNoDC, a WL control supplemented by weekly face-to-face assessment with research assistant,38 was ‘worse’ than all other comparators, with similar effectiveness to NI. One explanation may be that repeated assessment without any supportive elements during interactions with the researcher may have accentuated participants’ awareness of their anxiety symptoms.
Adjustment for baseline in the PSWQ indicated that baseline effect on final outcome is negligible, with a 95% CrI including zero (median 0.01, 95% CrI –0.44 to 0.45). The results of independently pooling direct evidence on the PSWQ for each contrast (but not pooling when n = 1) were generally consistent with the NMA results (see Table 15; upper-right triangle, shaded).
Results of between-study heterogeneity and inconsistencies
Three sources of heterogeneity were considered relevant: disease severity, concomitant medication and comorbidities. Using data relating to disease severity and comorbidities was not feasible (see Appendix 1 for further details); therefore, only data on concomitant medication were included as a covariate in the synthesis modelling. For both outcomes, when this covariate was included, the between-study heterogeneity parameter was not reduced, suggesting that heterogeneity is not explained by this covariate. Crucially, even if the proportion receiving concomitant medication was found to be an important effect modifier, the meta-regression model is not necessarily suited to detect this intervention–covariate interaction, as patients were receiving medication before trial entry. Therefore, medication may have already exerted an effect on patients, being captured by the ANCOVA baseline adjustment component.
Several data loops existed in the networks for GAD-7 and PSWQ, where both direct and indirect data informed intervention effectiveness estimates; the possibility of inconsistencies was investigated. Tables 14 and 15 show no evidence of substantial discrepancies between the direct and the NMA results for both outcomes; given the uncertainty in the data, only very large differences were likely to result in statistical significance. The results of the consistency and inconsistency models for both outcomes indicated the existence of overall model consistency, as detailed in Appendix 5.
Sensitivity analysis results
The sensitivity of networks to specific studies was investigated. In total, 10 analyses with 12 (rather than the total 13) included studies for GAD-7, and 11 analyses with 13 (rather than the total 14) included studies for PSWQ were performed, and the probability of each intervention being the best was assessed. For GAD-7, the selective serotonin reuptake inhibitor (SSRI) and group CBT continued to have the highest chances of being ‘best’, with probabilities of around 43% and 30%, respectively. Similar results to the primary analysis were obtained for PSWQ. The exception was when a three-arm study comparing SDIs with NI was removed,158 which resulted in increased uncertainty around PSWQ scores for all active interventions.
Two studies159,167 in the evidence base informing the network for the GAD-7 outcome had included fewer than 30 patients. Not considering these studies from the network implied the exclusion of medication from the comparator set, altering the network structure. As expected, with the reduction in the number of studies informing the network, the uncertainty in the posterior effect distributions increased further. However, no significant changes were observed compared with the main model results. For PSWQ, two studies161,167 were also excluded from the network on the basis of lower patient numbers, with the comparator set prevailing. The ranking of active interventions in terms of median PSWQ score decrease compared with NI was unaltered, although higher score decreases were estimated.
Comparison of network meta-analysis results on Generalised Anxiety Disorder-7 and Penn State Worry Questionnaire for digital interventions
To compare the results of the NMAs across both outcome measures (i.e. GAD-7 and PSWQ), and only for comparisons between DIs and their alternatives (rather than between alternatives, e.g. medication vs. group therapy), we present an abridged version of the NMA results in Table 16. The table shows the network comparisons and the direct pairwise comparisons between DIs and alternatives for post treatment (3–12 weeks) scores on GAD-7 and PSWQ median scores (adjusted for baseline).
Comparators | GAD-7a score (95% confidence interval) | PSWQa score (95% confidence interval) | ||
---|---|---|---|---|
Network | Direct pairwise | Network | Direct pairwise | |
Medication | ||||
M vs. SDI | –3.18 (–11.32 to 4.76) | –2.70b (–9.80 to 4.40) | Not available | Not available |
M vs. UDI | –3.97 (–14.30 to 6.20) | Not available | Not available | Not available |
NI | ||||
SDI vs. NI | –1.75 (–15.72 to 12.26) | –3.66 (–8.19 to 0.90) FE; –1.26c (–43.8 to 40.93) RE | –6.43 (–39.63 to 39.15) | –5.23 (–12.91 to 2.39) FE; 4.76c (–51.7 to 59.53) RE |
UDI vs. NI | –0.96 (–16.43 to 14.54) | Not available | –7.13 (–37.55 to 24.61) | Not available |
Group therapy | ||||
SNoDI vs. SDI | –0.65 (–14.67 to 13.23) | –0.71b (–14.03 to 12.61) | –0.71 (–25.34 to 23.90) | –0.58b (–24.74 to 23.58) |
SNoDI vs. UDI | –1.45 (–16.92 to 14.03) | Not available | –0.06 (–34.59 to 34.83) | Not available |
Non-therapeutic controls | ||||
Digital | ||||
SDI vs. SDC | –1.61 (–8.03 to 4.82) | –8.16 (–26.57 to 13.04) FE; –1.07c (–30.2 to 27.19) RE | –2.65 (–15.95 to 10.74) | –2.93 (–20.90 to 15.00) FE; 0.95c (–77.24 to 80.71) RE |
SDI vs. UDC | –1.49 (–10.51 to 7.47) | –1.50b (–11.34 to 8.34) | –4.35 (–34.27 to 25.60) | –3.60b (–35.42 to 28.22) |
UDI vs. SDC | –0.83 (–8.82 to 7.29) | –0.51 (–31.75 to 28.95) | –3.36 (–28.72 to 21.82) | –2.52 (–26.55 to 22.31) |
UDI vs. UDC | –0.70 (–9.10 to 7.58) | –0.77 (–7.81 to 6.26) | –4.96 (–35.07 to 24.94) | –6.73 (–33.99 to 21.37) |
Non-digital | ||||
SNoDC vs. SDI | Not available | Not available | 6.15 (–18.36 to 30.39) | 6.75b (–20.97 to 34.47) |
SNoDC vs. UDI | Not available | Not available | 6.81 (–26.18 to 39.67) | Not available |
Variants of DIs | ||||
SDI vs. UDI | –0.79 (–7.39 to 5.80) | –0.14 (–8.85 to 8.89) FE; –1.38 (–26.23 to 23.62) RE | 0.67 (–23.71 to 25.41) | 1.53 (–21.48 to 24.93) FE |
Comparing the direction and magnitude of differences in median scores at follow-up between the GAD-7 and PSWQ results (where available for both) in Table 16, we make three observations. First, the difference in GAD-7 median scores at follow-up between medication and DIs is the largest across all comparators, and favours medication. Second, no data were available for comparisons between DIs and individual therapy, either face to face or by telephone, or between DIs and manualised guided self-help (which is the non-digital counterpart of most DIs). Third, the direction of effect favoured SDIs for GAD-7 and UDIs for PSWQ.
To compare the ranking of DIs relative to their comparators across both GAD-7 and PSWQ we produced two figures. Figure 12 shows the ranking of DIs (SDI and UDI) for GAD relative to NoDIs and controls based on their likelihood of being ‘best’ for outcomes up to 12 weeks’ follow-up (adjusted for baseline). Figure 12 shows the average ranking of each intervention based on SUCRAs. Appendix 4 shows in detail the SUCRA graphs and rankograms for each intervention and control for GAD-7 and PSWQ.
FIGURE 12.
Ranking of DIs (SDI and UDI) for GAD relative to NoDIs and controls based on their likelihood of being ‘best’ for outcomes up to 12 weeks’ follow-up (adjusted for baseline). Dotted line, top rank; bold line, middle rank; and dashed line, bottom rank. M, medication. Reproduced with permission from Saramago et al. 124 This is an Open Access article distributed in accordance with the terms of the Creative Commons Attribution (CC BY 4.0) license, which permits others to distribute, remix, adapt and build upon this work, for commercial use, provided the original work is properly cited. See: https://creativecommons.org/licenses/by/4.0/. The figure includes minor additions and formatting changes to the original figure.
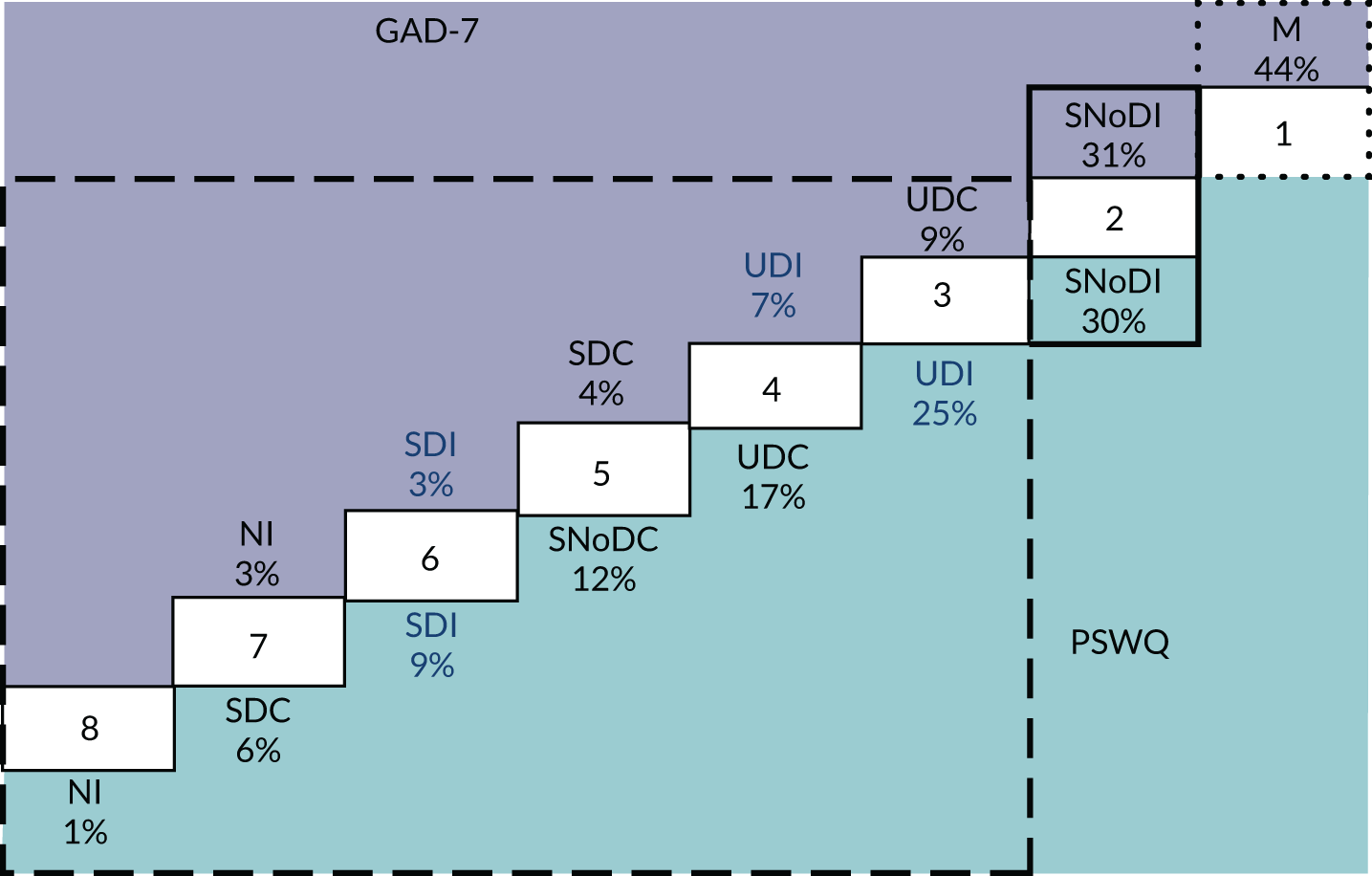
From Figure 13 we make three observations. First, medication and group therapy have a higher probability than DIs of being ‘best’ (i.e. they are associated with lower GAD-7 and PSWQ scores at 3–12 weeks’ follow-up). Second, UDIs are more likely than SDIs to be ‘best’. Third, several non-therapeutic controls (SDC, UDC and SNoDC) rank above SDIs for both GAD-7 and PSWQ.
FIGURE 13.
Ranking of DIs (SDI and UDI) for GAD relative to NoDIs and controls based on SUCRAs for outcomes up to 12 weeks’ follow-up (adjusted for baseline). Dotted line, top rank; bold line, upper-middle rank; double line, lower-middle rank. M, medication. Reproduced with permission from Saramago et al. 124 This is an Open Access article distributed in accordance with the terms of the Creative Commons Attribution (CC BY 4.0) license, which permits others to distribute, remix, adapt and build upon this work, for commercial use, provided the original work is properly cited. See: https://creativecommons.org/licenses/by/4.0/. The figure includes minor additions and formatting changes to the original figure.
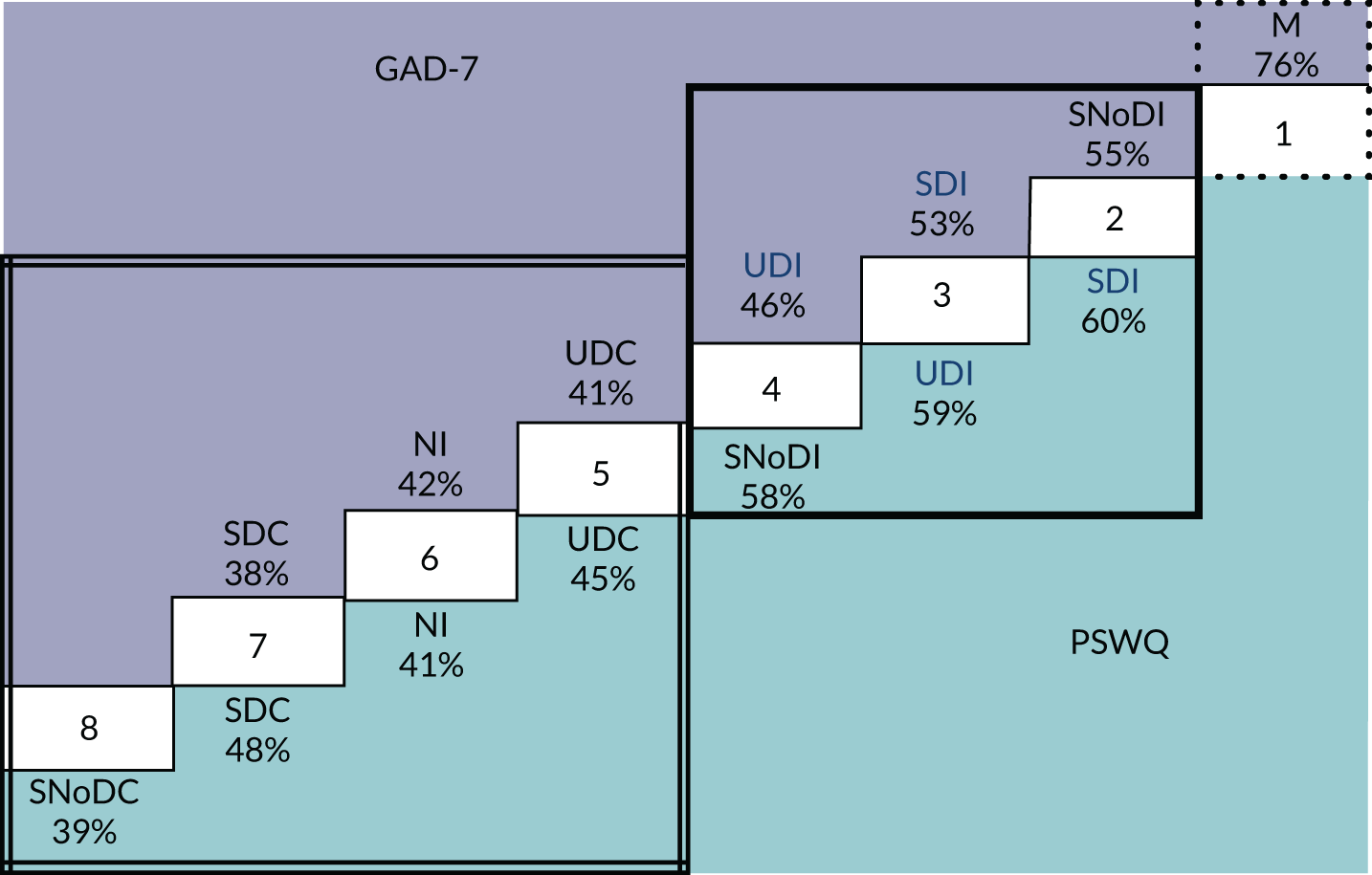
Discussion
Summary and interpretation
Our systematic review retrieved 21 studies4,5,37,38,40,41,158–172 and our analysis was based on 20 RCTs,4,5,37,38,40,41,158–162,164–172 which included 2325 adults with emerging or diagnosable GAD allocated to DIs or an alternative pathway of care, including NI. Comparisons varied depending on whether the intervention or control was digital or non-digital and supported or unsupported by clinicians or laypeople; the majority of comparisons were between SDIs and NI. Using an ANCOVA framework, our two NMAs pooled together post-treatment scores (adjusted for baseline) for two outcome measures: GAD-7 and PSWQ. In addition, the existence of treatment effect modifiers was assessed, several sensitivity analyses were carried out and network consistency was evaluated.
Our NMA results suggest that medication is associated with lower anxiety scores at follow-up relative to all other interventions and controls. Medication also ranks first in terms of its likelihood of being most effective, which considers the uncertainty in relative effect estimates. Medication results are supported by data from one study reporting GAD-7 and not PSWQ. Antidepressant medication as a treatment for GAD is supported by clinical guidelines2 and previous evidence syntheses. A large NMA129 of six trials comparing setraline with placebo for the treatment of GAD found that sertraline (the same antidepressant used in the study by Christensen et al. 159 included in our NMA) resulted in a greater improvement in HAM-A scores compared with baseline than did placebo (mean difference –2.88, 95% CrI –4.17 to –1.59). Another meta-analysis131 favoured a combined treatment of psychological therapy and medication for all anxiety disorders and depression, except GAD, where the direction of effect favoured antidepressant medication (venlafaxine) alone.
Our NMA makes best use of all currently available RCT-based evidence on DIs for GAD. Despite the sparsity and low quality of the data, a statistical synthesis can still be useful for mental health professionals who use DIs for GAD anyway, so that they can be informed about the current evidence base, understand which DIs have been shown to be more effective in reducing GAD and prioritise future research. Previous reviews of DIs that reported GAD-related outcomes131,132 used mixed samples of anxiety disorders and depression without reporting separate outcomes for GAD subgroups. There are no RCTs carried out in GAD populations comparing DIs with non-digital self-help interventions based on a manual rather that a web-based program. Nor are there any RCTs comparing DIs with individual therapy, either face to face or by telephone, as the only available comparisons within the literature are between DIs and group therapy.
Owing to very wide confidence intervals, our NMA results were inconclusive as to whether DIs for GAD are better than NI and non-therapeutic active controls, or whether they confer an additional benefit to standard therapy. Previous meta-analyses suggested that supported DIs could be as good as face-to-face therapy across depression, anxiety and somatic disorders. 185 Yet the mixed samples in these meta-analyses without separate analysis or reporting for GAD subsamples do not allow any conclusions to be drawn about the relative efficacy of DIs specific to the treatment and prevention of GAD.
Our NMA results about the comparative effectiveness of supported and unsupported DIs for GAD were counterintuitive, as we would expect SDIs to rank higher in terms of likelihood of being ‘best’, based on a previous meta-analysis in which SDIs were found to be four times more effective than DIs without any therapist contact. 186 We found that UDIs rank higher than SDIs in terms of being best, but the converse is true based on all rankings (SUCRAs). This is consistent with a recent review187 that reported mixed findings regarding guided versus unguided DIs and human versus automated support for DIs. This suggests that the design, content, technology platform and type of reinforcement offered in lieu of personal support in UDIs may bear more weight and account for greater variability in their outcomes.
The evidence base available in this setting is complex. In particular, the sheer volume of anxiety metrics (45 in total) being reported across the available studies suggests a lack of consensus on which measures to use in evaluating GAD outcomes. GAD-7 and PSWQ are commonly used and well-validated outcomes to capture change in GAD symptoms. The use of GAD-7 or PSWQ or both as outcome measures across all the included studies further strengthens our review, as we did not need to exclude any of the retrieved studies from the meta-analysis on the basis that it did not use GAD-7 or PSWQ. The only excluded study163 used GAD-7, but reported only categorical outcomes. It is worth noting that the HAM-A used in the large meta-analysis on medication for GAD129 featured in only one167 of our included studies, showing a discrepancy in the choice of outcomes for psychological and pharmacological interventions.
Limitations
There was substantial uncertainty around effect estimates of DIs against alternatives for GAD-7 and PSWQ. This was driven by the small number of studies informing most comparisons, as well as the small samples in some of these studies and their high risk of bias, limiting our confidence in any observed differences in anxiety scores between interventions and controls. These observed differences may simply be due to chance; therefore, we cannot make clear recommendations about the relative value of DIs compared with their comparators, but we can make recommendations for future research (both for primary studies and evidence synthesis) in view of the current evidence.
Caution is needed when interpreting the results of our NMA across all different interventions for GAD. Our review has been completed in the context of DIs; it included only RCTs in which at least one of the randomisation arms was a DI. Therefore, we cannot draw any conclusions about the comparative merit of NoDIs (psychological or pharmacological) for GAD when these are considered separately from DIs (e.g. group CBT vs. medication). To be able to do this, we would need to include RCTs in a NMA that would enable second or third order contrasts (e.g. RCTs comparing NI and medication), which was beyond the scope of this review. In addition, ranking based on likelihood of being best and on SUCRAs does not reflect differences in effectiveness estimates between interventions and controls and CrIs, that is, we cannot tell whether or not the differences between ranking position (e.g. between first, second, third and fourth) are clinically meaningful.
Another point of caution, as it is with all evidence synthesis, relates to pooling DIs and their alternatives into groups for analysis based on our classification criteria. Any classification implies interpretation and judgement, which is conditional on attained information from studies. We note the insufficient reporting of details about ‘non-therapeutic controls’ and WLs in some studies. Furthermore, we could have split DIs into further categories according to the technology used (e.g. VR, internet, mobile app), the function of the technology (e.g. adjunct to clinician-delivered therapy vs. patient self-help) or the type of support (e.g. telephone calls vs. meetings). This would have created more ‘nodes’ in the NMA models but also more uncertainty because comparisons between DIs and their alternatives within each subgroup would have been informed by fewer studies.
Many of our included RCTs had small samples and multiple arms, comparing different versions of the same intervention, thereby reducing the power of the study. Our evidence synthesis also shows that in the majority of RCTs either the time frame for follow-up is short (up to 12 weeks) or the control group has already crossed over to the intervention at the point of a longer follow-up (up to 2 years), which cancels the randomised comparison. Consequently, we did not include observations for further follow-up time points, when these were available, nor did we account for time differences in the short-term reporting (the timing of assessments post treatment varied from 3 to 12 weeks). Our NMA results reflect the short-term impact of DIs as there is scant evidence to inform randomised comparisons about effects beyond 12 weeks.
Recommendations
As GAD is the most prevalent and least studied condition among other common mental health problems, future evidence syntheses will be helpful to focus on GAD populations and GAD subgroups within mixed populations as a means of informing GAD-specific future research and clinical guidelines. 2 Feasibility and pilot studies, as well as user involvement in the development of the intervention and delivery protocols, could ensure that the final RCT tests the best possible intervention for GAD. Adaptive designs with improved intervention features and boosted recruitment numbers to a fully powered RCT are preferable to underpowered studies with multiple arms testing increments of the same DI.
Our NMAs and previous literature suggest that antidepressants are an important factor to consider in future studies on DIs for GAD. Studies of psychological interventions (whether digital or non-digital) often include participants who are taking medication as part of their routine care. It is difficult to disentangle the effects of medication and psychological support for GAD, and future RCTs need to report medication details (name, dose and duration) and include it as a covariate in their analysis to establish how outcomes with DIs and controls are influenced by concurrent medication use.
Having a consensus about GAD-specific outcome measures can prevent participant fatigue from completing batteries of different questionnaires and enable comparisons across studies and data syntheses. GAD-7 is more sensitive to changes associated with treatment and therefore may be more suitable for longitudinal clinical research. 188 Reporting continuous data on GAD-7 as a common measure in RCTs with GAD populations will make more studies available for a future statistical synthesis. Including HAM-A in studies of psychological therapies will enable us to compare results with those of pharmacological studies. Future analyses using multivariate models may be in a position to make better use of the available evidence by borrowing strength across different outcomes.
Many studies that include long-term follow up of participants offer the intervention to those randomised to the control group at a crossover point. Participants are also likely to receive some treatment as part of usual care the longer they remain on WLs or non-therapeutic controls, so studies cannot withhold interventions to enable long-term follow-up. As the typical duration of DIs is 3–12 weeks, the follow-up period of future RCTs needs to be longer than this, for example at least 6 months, to get a sense of the ‘stickiness’ of DIs beyond their delivery period. Usual care and WLs are poorly reported in RCTs, and the RCTs do not include data on concurrent interventions accessed by participants, including openly available self-help, which can influence the difference in outcomes between DIs and NIs.
Conclusions
To our knowledge, this study is the first to evaluate the effectiveness of DIs specifically in a GAD population. Using two outcome measures (GAD-7 and PSWQ), it is also the first to combine all the RCT-based effectiveness evidence from DIs and key comparators in a single modelling framework, allowing the estimation of relative treatment effects for all relevant comparisons. Our results suggest that medication is associated with lower anxiety scores at follow-up relative to all other interventions and controls. Results were inconclusive as to whether DIs are better than NI and non-therapeutic active controls for GAD, or whether they confer an additional benefit to standard therapy.
Future primary studies and meta-analyses need to focus on GAD populations rather than use mixed samples, or report outcomes specifically for GAD subsamples if they intend to answer questions about the comparative effectiveness of DIs for GAD. Comparing DIs with manualised (non-digital) self-help, for which there are no current RCTs for GAD populations, will be useful in the context of stepped care. Antidepressant medication as a first-line treatment for GAD compared with DIs deserves further research and economic modelling. As many decisions to use interventions are driven by ‘value for money’, and in the light of the scarcity of data on the costs and outcomes of DIs compared with non-digital alternatives for the treatment of GAD, it is necessary to assess the VOI to guide decisions of further research. To inform commissioning and potential disinvestment from non-digital alternatives, we need to put the findings of this evidence synthesis into context together with an assessment of the costs of developing and implementing DIs in clinical practice.
Chapter 5 Economic modelling for generalised anxiety disorder
Parts of this chapter have been reproduced with permission from Jankovic et al. 189 This is an Open Access article distributed in accordance with the terms of the Creative Commons Attribution-NonCommercial (CC BY-NC 4.0) license, which permits others to distribute, remix, adapt and build upon this work, for non-commercial use, provided the original work is properly cited. See: https://creativecommons.org/licenses/by/4.0/. The text below includes minor additions and formatting changes to the original text.
Introduction
Previous studies that evaluated the cost-effectiveness of DIs with GAD populations61,85 compared a specific DI against usual care or individual therapy. To our knowledge, no studies synthesised evidence on all DIs for GAD to evaluate their cost-effectiveness across different technologies and therapeutic modalities. Reviews of cost-effectiveness of DIs were for mixed mood and anxiety disorders, so they were dominated by DIs for depression and did not report outcomes for GAD populations separately. 16,20
This WP aims to evaluate the cost-effectiveness of DIs, across different types of technologies and therapeutic modalities, compared with (1) conventional therapy (without any digital components), (2) medication, (3) non-therapeutic controls and (4) NI, from the perspective of the UK’s health-care system. The analysis constitutes a cost-effectiveness model, specifically a Markov model, with 3-month cycles over the lifetime of an individual with GAD.
Methods
Classification of digital interventions and their alternatives
We compared seven types of interventions and controls for GAD based on our classification criteria: medication (M), SNoDI, SDI, UDI, SDC, UDC and NI. There were no available clinical studies that included UNoDIs or UNoDCs, and no studies of SNoDCs that used GAD-7 as an outcome. DCs identified in WP2 (see Classification of digital interventions and comparators) were included in the analysis, as patients are occasionally signposted to information sources in reputable websites when waiting times are long. Their exclusion from the analysis was explored in Scenario analysis.
Model structure
The CEA methods followed the NICE reference case. 11 An NHS and Personal Social Services perspective was used for the analysis. A full incremental analysis was undertaken comparing all seven interventions/controls simultaneously over a patient’s lifetime.
The model structure was based on GAD severity determined by the GAD-7 questionnaire, in which the scores denote no (scores 0–4), mild (5–9), moderate (10–14) or severe (15–21) anxiety. 178 A Markov cohort model was used, following the structure adapted from Kumar et al. 85 The health states included are shown in Figure 14.
FIGURE 14.
Decision model for GAD health states based on GAD-7 scores. Reproduced with permission from Jankovic et al. 189 This is an Open Access article distributed in accordance with the terms of the Creative Commons Attribution-NonCommercial (CC BY-NC 4.0) license, which permits others to distribute, remix, adapt and build upon this work, for non-commercial use, provided the original work is properly cited. See: https://creativecommons.org/licenses/by-nc/4.0/. The figure includes minor additions and formatting changes to the original figure.
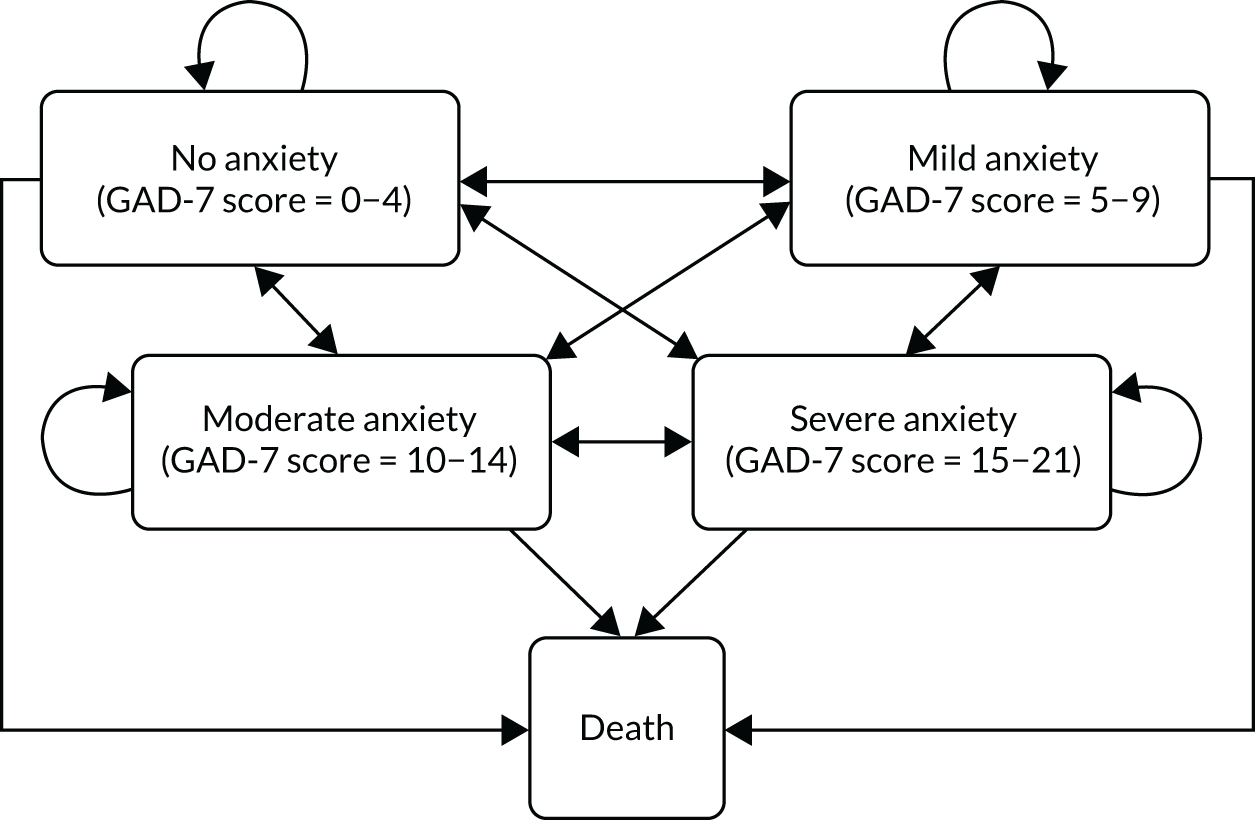
At the start of the model, patients are in one of four health states. At each subsequent cycle of the model, they can remain in this health state or transition to another, better or worse, health state. Health-care resource use and HRQoL are driven by GAD severity, both directly and through comorbidities. The intended effect of DIs was to reduce the severity of GAD, thereby reducing the number of patients in moderate and severe GAD states, with an associated impact on costs and HRQoL.
Model parameters
Intervention effectiveness
Changes in GAD-7 scores as a result of the seven interventions were informed by our NMA reported in Chapter 4. Using an ANCOVA framework,138 the meta-analysis reported median GAD-7 scores after treatment (3–12 weeks), adjusted by baseline scores. GAD-7 scores were modelled as a continuous variable. When scores generated in the ANCOVA model were between severity states (e.g. GAD-7 score 4.5), the score was rounded up.
Baseline and post-treatment GAD-7 scores were used to inform the model baseline GAD-7 scores and transitions after the first model cycle, respectively (see Appendix 7, Table 27). Changes in GAD-7 scores in the remaining cycles were estimated based on evidence from the literature. It was assumed that, without treatment, patients’ GAD symptoms would, on averge, improve over time, as reported by Yonkers et al. 190 Specifically, 15% of patients recovered in the first year, a further 10% recovered in the second year and a further 5% recovered in the third year. In the model, recovery was defined as a 5-point reduction in GAD-7 scores in the corresponding proportion of iterations with mild, moderate or severe anxiety, consistent with a move to a lower anxiety state. In the base case, the treatment effect was assumed to remain constant relative to no treatment indefinitely. Thus, the shift to lower GAD scores remained over a patient’s lifetime, although some patients were assumed to improve over time at the same rate as those in the base case.
State-specific utilities and costs
Targeted searches were conducted to inform state-specific health-care costs and utilities. To identify published economic evidence, we searched MEDLINE [via Ovid®; (Wolters Kluwer, Alphen aan den Rijn, the Netherlands)], EMBASE and PsycInfo. (Details of the searches, including the number of studies retrieved, are provided Report Supplementary Material 6.) The searches identified one study126 that reported state-specific utilities (no, mild, moderate and severe anxiety) with a measure of uncertainty (standard deviation). The utilities were derived from the Short Form questionnaire-6 Dimensions (SF-6D) scores using the UK scoring algorithm. The states were defined by the HAM-A182 score, an assessment tool highly correlated with the GAD-7 questionnaire (r = 0.852),191 which we used to inform the utilities in the model. Uncertainty in utilities was derived by fitting a beta distribution to the reported mean and standard error, using the method of moments. 192 The HRQoL/utility scores associated with the GAD-7 states were assumed to follow an underlying age depreciation over time, in accordance with UK population utility score norms (per year of age). 193
The targeted searches identified two studies that reported state-specific health-care resource use,85,194 and one further study reported state-specific costs in patients with anxiety and/or depression. 14 Study details are provided in Appendix 9, Table 30. Given the variation in the delivery of mental health services across settings, the UK-based study14 was used to inform health-care resource use in the base case, whereas the remaining two studies were used in scenario analyses. The costs from Kaltenthaler et al. 14 were adjusted to 2019 Great British pounds using the overall Consumer Price Index. 195,196 Costs and benefits were discounted at 3.5% over the time horizon of the model. 11
Mortality
Age- and sex-dependent mortality risk in patients with no anxiety was obtained from the Office for National Statistics. 197 Excess mortality in mild, moderate and severe anxiety states was derived from Michal et al. ,198 who reported the impact of anxiety or depression on all-cause mortality in cardiovascular outpatients on long-term oral anticoagulation, adjusted for age, sex, high school graduation, partnership, smoking, obesity and frailty in patients with mild, moderate and severe anxiety or depression, defined by Patient Health Questionnaire – 4 items (PHQ-4) scores. The excess mortality was assumed to capture deaths by suicide and due to GAD-related comorbidities. The reported risk ratios were applied to mortality without GAD.
Intervention cost
The intervention costs were derived from published literature. The cost of NI/WL was assumed to be £0. The only medication for which data were available was a SSRI. The cost of pharmacotherapy was assumed to represent the cost of medication (£16.42, representing the mean cost of all SSRIs199 weighted by the volume dispensed, as reported by OpenPrescribing.net200 in January 2020), the dispensing fees for SSRIs (12 prescriptions dispensed annually, at £1.26 each199) and GP appointments (7.5 in the first year of treatment and 4 thereafter, as per guidance for prescribing SSRIs from NICE,2 at £42.60 each). Medication was assumed to be prescribed for 5 years as a conservative estimate to prevent underestimating its cost. Antidepressant medication may be given for up to 2 years in the first instance, but relapse is common within the first year of stopping the medication, which may lead to another 2 years of prescription.
The cost of non-digital psychological interventions (SNoDI) was based on the time spent with a therapist multiplied by the cost of the therapist’s time (£53 per hour201). The SNoDI interventions in this evaluation used group therapy (group sizes of five to nine people) lasting 9–10.5 hours. 165,172 The intervention was therefore costed at 1.5 hours of therapist time per patient (based on 10.5 hours for seven patients). Non-attendance was assumed not to result in cancellation of the group session, and so this did not affect the average cost of therapy per patient.
The digital component of interventions/controls was assumed to carry no cost, on the basis that, if the intervention were rolled out nationally, the marginal cost per patient would be negligible. Alternative assumptions were explored in the sensitivity analysis. UDIs and UDCs were assumed to incur no additional costs. The cost of support for DCs and interventions was calculated separately, based on the level of support typically required to deliver them, as detailed in Appendix 6.
Cost-effectiveness analysis
Patients’ costs and HRQoL were tracked over time, until all patients in the cohort died. The cumulative costs and QALYs were then used to derive the net monetary benefit (NMB) conditional on the marginal productivity of the health system. NMB represents the difference between the benefit incurred by the intervention and the benefit forgone by displacing the resources elsewhere in the health system to fund the intervention. 48 The intervention with the highest NMB was the most cost-effective one.
Sensitivity analysis
Probabilistic analysis
Probabilistic uncertainty analysis was conducted to characterise the uncertainty associated with input parameters to the model and their impact on cost-effectiveness. Each parameter was sampled 25,000 times from its probability distribution. The number of iterations was chosen to match the number of random samples of the treatment effect generated in the meta-analysis. The model parameters and the probability distribution parameters are shown in Appendix 7 (see Tables 28 and 29).
Deterministic scenario analysis
A one-way scenario analysis was performed to evaluate the sensitivity of the model results to our assumptions. The following scenarios were explored regarding the GAD score trajectory, health-care resource use and intervention costs:
-
Five additional scenarios regarding the GAD score trajectory (see Appendix 8).
-
Two alternative scenarios regarding health-care resource use, in which state-specific health-care costs were informed using alternative studies (i.e. Vera-Llonch et al194 and Kumar et al. 85). The cost of health care was derived by multiplying the reported resource use by the unit costs available from the NHS England tariff for the year 2018/19201 (see Appendix 9, Table 31).
-
Alternative DI costs, where a threshold analysis was performed to identify the maximum cost of DIs that would make them good value for money.
-
Alternative levels of support in SDCs (5 minutes per patient, delivered by non-clinical staff or by clinical psychologists).
-
Exclusion of DCs (UDCs and SDCs) from the analysis.
Value-of-information analysis
The results of the probabilistic uncertainty analysis were used to estimate the VOI. The maximum value of further research per patient treated [expected value of perfect information (EVPI)] was derived from the difference between the NMB under perfect information (i.e. where the optimum treatment is known with certainty) and existing information (i.e. the expected net benefit from the treatment that is the most cost-effective under current knowledge). 202
The value of further research at population level [expected value of perfect information at the population level (EVPIP)] was then estimated by multiplying EVPI by the effective population size (Equation 1):202
where It represents GAD incidence in year t, T is the total number of years for which information from the research would be useful (usually representing the technology lifetime) and dr is the discount rate, set at 3.5%.
The incidence of GAD was 4.9% of the population in the UK in 2008203 and 4.3% in Germany in 2008. 204 Therefore, in the VOI analysis, the incidence was set at 250,000, representing a conservative estimate in which < 10% of patients in England (population of ≈ 55.9 million) who acquire GAD would receive the intervention. The relevant technology lifetime was assumed to be 5 years.
Finally, the expected value of partial perfect information at the population level (EVPPIP) was derived to understand the parameters in the model driving uncertainty relevant to the adoption decision. The non-parametric method developed by Strong et al. 205 was used. The method, conducted using the Sheffield Accelerated Value of Information (SAVI) interface (URL: http://savi.shef.ac.uk/SAVI/; accessed 7 September 2021), regresses random samples of all uncertain parameters against the net benefit of each intervention to derive the impact of uncertainty about individual parameters on the model results. All uncertain parameters in the model were used to derive EVPPIP for the following five groups of parameters: treatment effect (GAD-7 scores after the first cycle, seven parameters), state-specific costs (four parameters), state-specific utilities (four parameters), excess death [three parameters – relative risk (RR) of death in mild, moderate and severe anxiety] and age-related utility decrements (54 parameters, utility decrements every year until all patients in the model die).
The opportunity cost was assumed to be £15,000 per QALY, in line with the empirical estimate by Claxton et al. 206
Results
Generalised anxiety disorder score trajectory
Figure 15 shows the proportion of patients in each GAD-7 health state for the initial 5 years, without treatment. At the start of treatment almost 80% of patients had moderate GAD, a further 20% had mild GAD and the proportion with no and severe GAD was < 0.05. In the base case, for the first 3 years of the model, patients’ GAD was assumed to improve spontaneously, resulting in a decrease in the proportion who had moderate and severe GAD, and an increase in the proportion with no or mild GAD. After 3 years the proportions remained constant (in the living population). The proportion in each anxiety state after treatment is shown in Appendix 8.
FIGURE 15.
Movement through Markov states for the initial 5 years without treatment. Reproduced with permission from Jankovic et al. 189 This is an Open Access article distributed in accordance with the terms of the Creative Commons Attribution-NonCommercial (CC BY-NC 4.0) license, which permits others to distribute, remix, adapt and build upon this work, for non-commercial use, provided the original work is properly cited. See: https://creativecommons.org/licenses/by-nc/4.0/. The figure includes minor additions and formatting changes to the original figure.
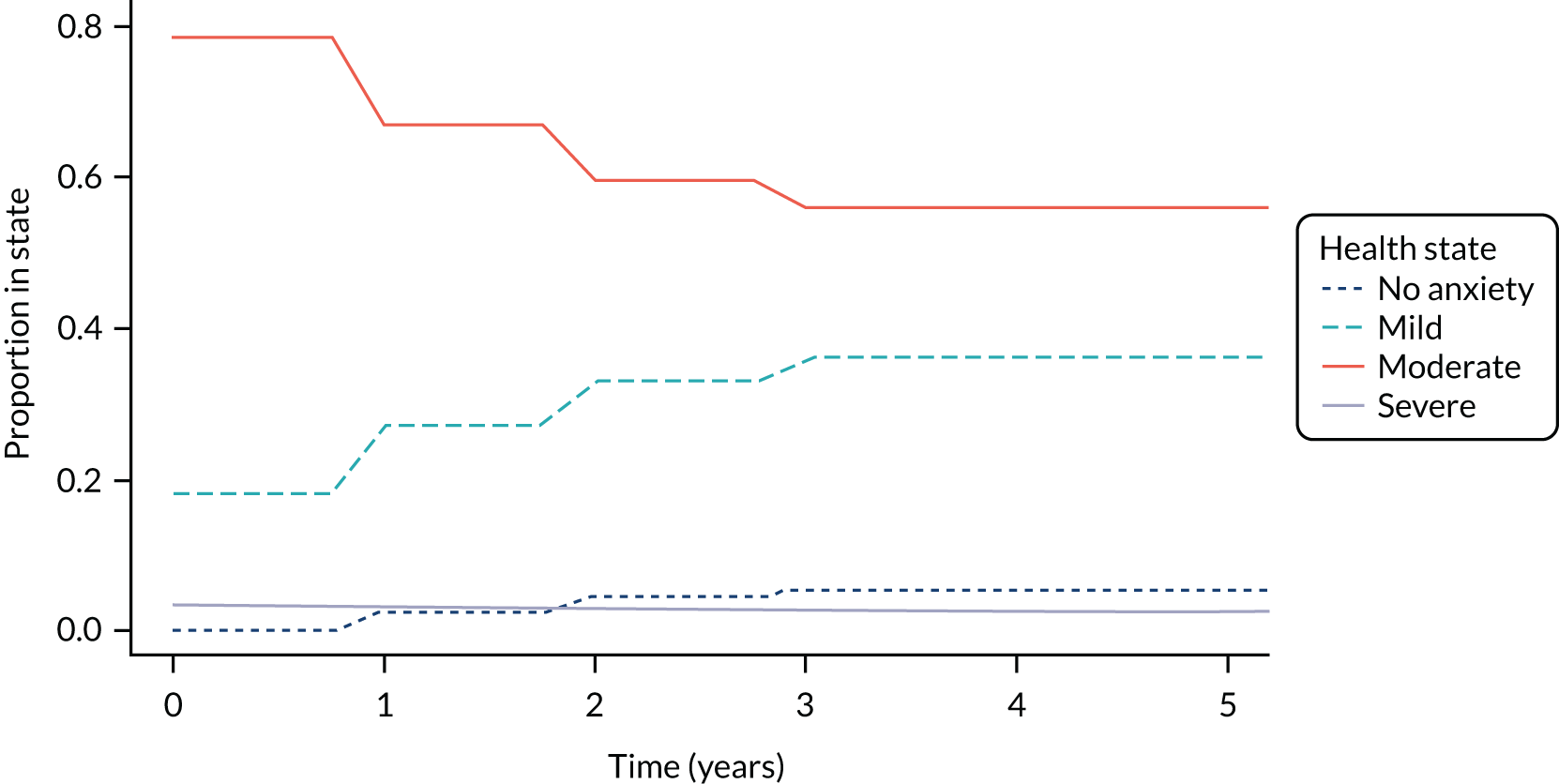
The reduction in GAD-7 scores after receiving treatment with the seven treatment options compared in the model is shown in Figure 16. The initial reduction in GAD-7 reflects the effect of treatment, as determined by our meta-analysis. The treatment effect was assumed to remain constant indefinitely, and after 1 year GAD-7 scores decreased further as patients’ symptoms continued to improve at the same rate as without treatment. However, the GAD-7 reduction decreased, as, following treatment, the number of patients who could recover spontaneously was lower than if no treatment had been received. The rate of decrease in GAD-7 score varied between interventions, depending on the magnitude and uncertainty of the treatment effect. More effective treatments were associated with fewer patients in mild, moderate and severe anxiety states, and so the spontaneous symptoms improvement affected fewer patients. Wider confidence intervals led to a higher probability of patients being in the no anxiety state, and so the spontaneous symptoms improvement affected fewer patients; this is why the reduction in GAD-7 scores after 1 year was higher in SDIs than in UDIs, despite SDIs being more effective. After 3 years, the reduction in GAD-7 remained constant.
FIGURE 16.
Change in mean GAD-7 score for the initial 5-year period after starting treatment. M, medication. Reproduced with permission from Jankovic et al. 189 This is an Open Access article distributed in accordance with the terms of the Creative Commons Attribution-NonCommercial (CC BY-NC 4.0) license, which permits others to distribute, remix, adapt and build upon this work, for non-commercial use, provided the original work is properly cited. See: https://creativecommons.org/licenses/by-nc/4.0/. The figure includes minor additions and formatting changes to the original figure.
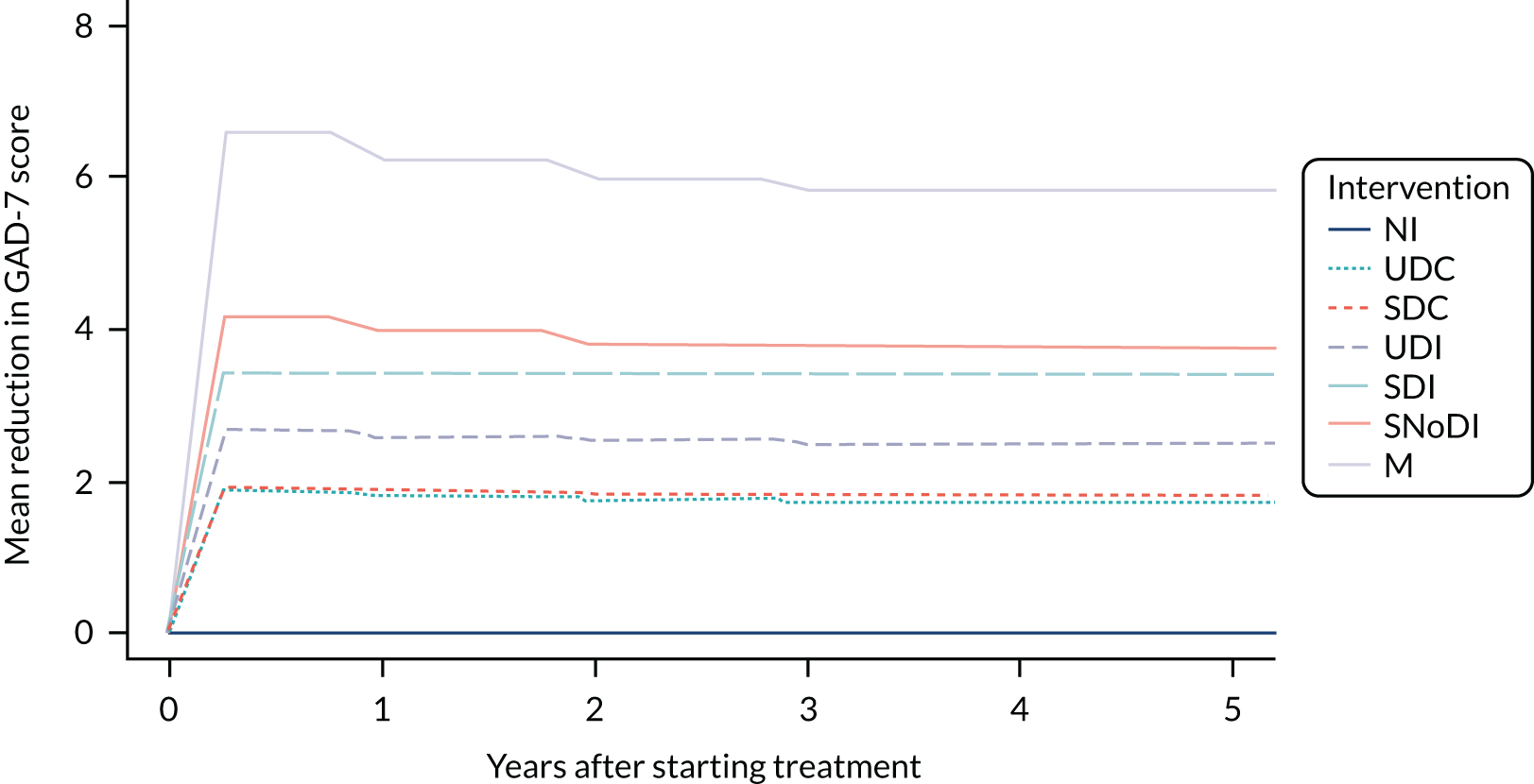
Cost and outcomes of digital interventions compared with alternatives
Table 17 shows the costs and outcomes associated with each of the seven comparators. The differences in QALY and life-years follow the same pattern; the differences between comparators were small and uncertain, reflected in wide and overlapping confidence intervals. The QALY and life-year gains reflect the results from the meta-analysis: on average, the greatest reduction in post-treatment anxiety scores was associated with medication, followed by SNoDIs, then by SDIs and then by UDIs. Both SDIs and UDIs were associated with lower scores post treatment than SDCs, UDCs and no treatment.
Outcome | Options | ||||||
---|---|---|---|---|---|---|---|
M | SNoDI | UDI | SDI | SDC | UDC | NI | |
QALYs | 12.9 (11.5 to 14.3) | 12.2 (10.4 to 14.2) | 11.8 (9.7 to 14.1) | 11.9 (10.5 to 13.7) | 11.6 (9.2 to 14.0) | 11.5 (8.9 to 14.1) | 11.1 (9.4 to 12.4) |
Life-years | 37.2 (34.1 to 38.6) | 36.4 (32.09 to 38.6) | 36.0 (31.4 to 38.8) | 36.4 (32.5 to 39.0) | 35.7 (31.0 to 38.7) | 35.6 (30.5 to 38.6) | 34.9 (30.9 to 38.6) |
Health-care cost (£) | 10,640 (725 to 45,948) | 12,362 (49 to 59,092) | 14,114 (25 to 68,008) | 14,218 (139 to 63,152) | 14,605 (13 to 74,297) | 14,822 (18 to 75,923) | 16,069 (1 to 87,123) |
Intervention cost (£) | 1115 (1113 to 1115) | 80 | 0 | 80 | 18 | 0 | 0 |
Total cost (£) | 11,754 (1839 to 47,063) | 12,442 (128 to 59,171) | 14,114 (25 to 68,008) | 14,298 (218 to 63,232) | 14,623 (31 to 74,315) | 14,822 (18 to 75,923) | 16,069 (1 to 87,123) |
Incremental cost compared with M (£) | – | 688 (–38,229 to 49,867) | 2360 (–37,391 to 57,798) | 25,43 (–36,862 to 51,940) | 2868 (–37,785 to 63,454) | 3067 (–38,072 to 65,451) | 4315 (–38,184 to 75,859) |
Incremental QALYs compared with M | – | –0.7 (–3.4 to 2.2) | –1.2 (–3.6 to 1.8) | –1.0 (–3.0 to 0.8) | –1.4 (–3.8 to 1.4) | –1.4 (–4.5 to 1.8) | –1.9 (–3.9 to 0.3) |
Mean ICER (£ per QALY) | Dominant | Dominated | Dominated | Dominated | Dominated | Dominated | Dominated |
k = £0 per QALY | |||||||
NMB (£) | –11,754 (–47,063 to –1839) | –12,442 (–59,171 to –128) | –14,114 (–68,008 to –25) | –14,298 (–63,232 to –218) | –14,623 (–74,315 to –31) | –14,822 (–75,923 to –18) | –16,069 (–87,123 to –1) |
PCE | 0.051 | 0.129 | 0.151 | 0.107 | 0.159 | 0.165 | 0.238 |
k = £15,000 per QALY | |||||||
NMB (£) | 181,975 (131,817 to 209,180) | 171,033 (110,947 to 208,548) | 162,334 (97,837 to 205,226) | 164,551 (111,833 to 197,813) | 158,586 (88,232 to 203,339) | 158,335 (81,276 to 205,462) | 149,671 (74,016 to 180,051) |
PCE | 0.342 | 0.232 | 0.111 | 0.089 | 0.085 | 0.105 | 0.035 |
Health-care costs were highly uncertain, with overlapping confidence intervals. Differences in health-care costs largely followed the reverse order of QALY gains; health-care costs were lowest for patients taking medication and highest for patients who received NI. This was due to the correlation between health-care costs and GAD severity (see Appendix 7).
Supported digital interventions and SNoDIs were associated with the same intervention costs, as they include the same level of human resources. Medication was more expensive, as it is administered over 5 years (and so requires contact with health-care professionals for 5 years), and, unlike other interventions, the costs were uncertain because the proportion of patients who were alive and taking medication was uncertain. The total costs of different comparators follow the same order as health-care costs, except SDIs that incur a higher total cost than UDIs owing to the higher intervention cost.
Table 17 shows the incremental costs and effects and the net benefit of all seven comparators, whereas Figure 17 shows the cost-effectiveness acceptability curves for each intervention and control, and how their probability of cost-effectiveness changes for different opportunity costs. In Table 17, net benefit and the probability of cost-effectiveness are shown for two opportunity costs: £0 per QALY, representing a decision-maker who will implement only cost-saving interventions, and £15,000 per QALY, close to the empirical estimate of the opportunity cost in England. 9 The model results did not change significantly at opportunity costs higher than £15,000 per QALY (as seen in Figure 17). The intervention most likely to be cost-effective depends on the opportunity cost. The most cost-effective intervention is NI for an opportunity cost of £0 per QALY, SNoDIs for an opportunity cost of £1000 per QALY and medication for an opportunity cost of £2000 per QALY or higher.
FIGURE 17.
Cost-effectiveness acceptability curves for each intervention and control. M, medication. Reproduced with permission from Jankovic et al. 189 This is an Open Access article distributed in accordance with the terms of the Creative Commons Attribution-NonCommercial (CC BY-NC 4.0) license, which permits others to distribute, remix, adapt and build upon this work, for non-commercial use, provided the original work is properly cited. See: https://creativecommons.org/licenses/by-nc/4.0/. The figure includes minor additions and formatting changes to the original figure.
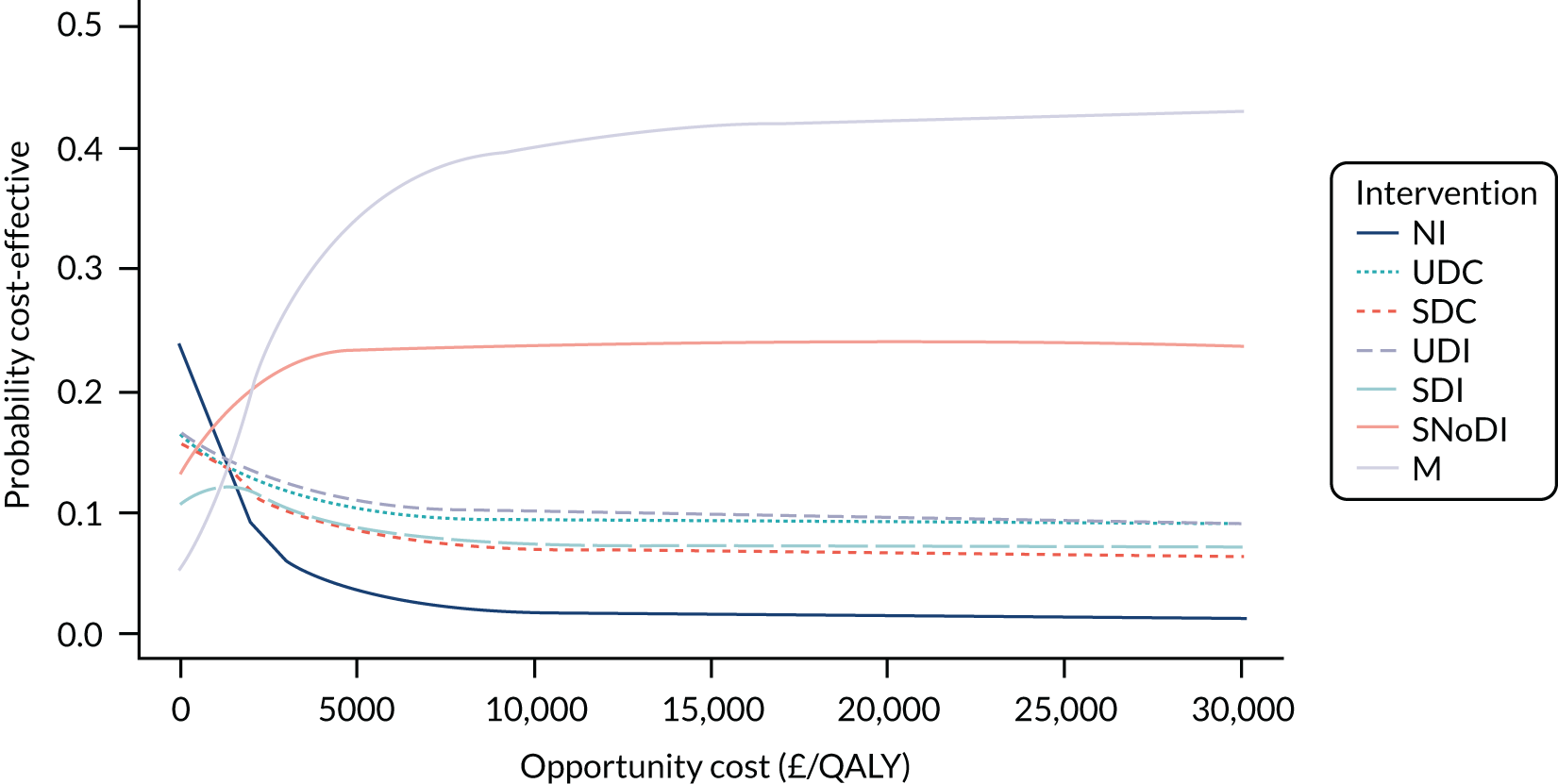
Medication was dominant, resulting in the highest NMB at all opportunity costs, followed by group therapy, as both led to lower total costs and greater QALY gains than DIs. NI had the lowest NMB because of the lower QALY gains and higher health-care costs. DIs (UDIs and SDIs) had a higher NMB than DCs (UDC and SDC) as they were associated with better outcomes and lower health-care costs. SDIs had greater QALY gains but also higher costs than UDIs, so their NMB depended on the opportunity cost of the health system. When the opportunity cost is £0 per QALY, UDIs are ranked above SDIs, whereas the opposite is the case when the opportunity cost increases to £15,000 per QALY.
These results are uncertain, which is reflected in the wide confidence intervals associated with NMB and the probability of each intervention being the most cost-effective (see Table 17 and Figure 18). For example, although medication has the highest NMB, there is a high probability that it is not the most cost-effective comparator: 0.949 probability at £0 per QALY opportunity cost and 0.658 at £15,000 per QALY.
FIGURE 18.
Cost-effectiveness ranking based on NMB for DIs and controls. Values in brackets are 95% CIs. k, opportunity cost; M, medication.
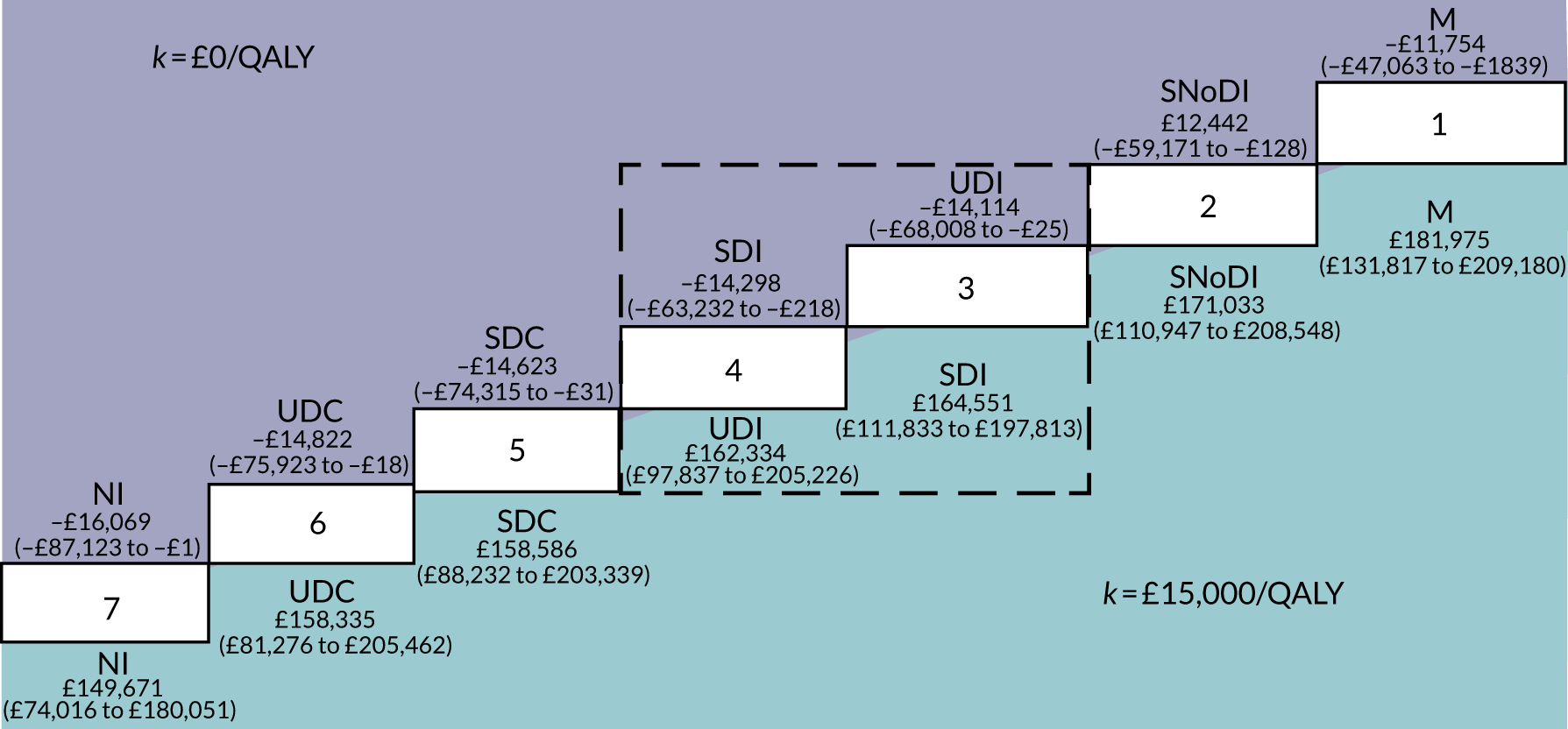
Accumulation of costs and outcomes over time is shown in Appendix 10. The longer the analysis time horizon, the greater the differences in the QALY gain (see Appendix 10, Figure 29), as the benefits accrue over time. Differences in health-care cost are driven by the clinical effectiveness of interventions, and so, as for QALY gains, the longer the time horizon the greater the cost differences (see Appendix 10, Figure 30), but the rate of change is diminishing. Medication is the most expensive intervention in the short term, but, as health-care cost savings accrue over time, and treatment cost reduces after 5 years, the total cost for medication increases at a lower rate than for other comparators, eventually becoming the second-cheapest treatment option, after SNoDI.
Value-of-information analysis
Figure 19 shows EVPIP over a range of opportunity costs. Even at its lowest, when opportunity cost is £4000 per QALY, the value of uncertainty is high (£11.4B), increasing to £16.2B and £19B when opportunity costs are £15,000 and £20,000 per QALY, respectively. The EVPPIP analysis suggested that uncertainty in the treatment effect had the greatest value, £12.9B. Parameters defining the effect of GAD on costs and HRQoL (state-related costs and utilities, and excess mortality) had low or negligible value, whereas age-related utility decrements were uncertain, valued at £6.8B.
FIGURE 19.
The EVPIP for a range of opportunity costs. Reproduced with permission from Jankovic et al. 189 This is an Open Access article distributed in accordance with the terms of the Creative Commons Attribution-NonCommercial (CC BY-NC 4.0) license, which permits others to distribute, remix, adapt and build upon this work, for non-commercial use, provided the original work is properly cited. See: https://creativecommons.org/licenses/by-nc/4.0/. The figure includes minor additions and formatting changes to the original figure.
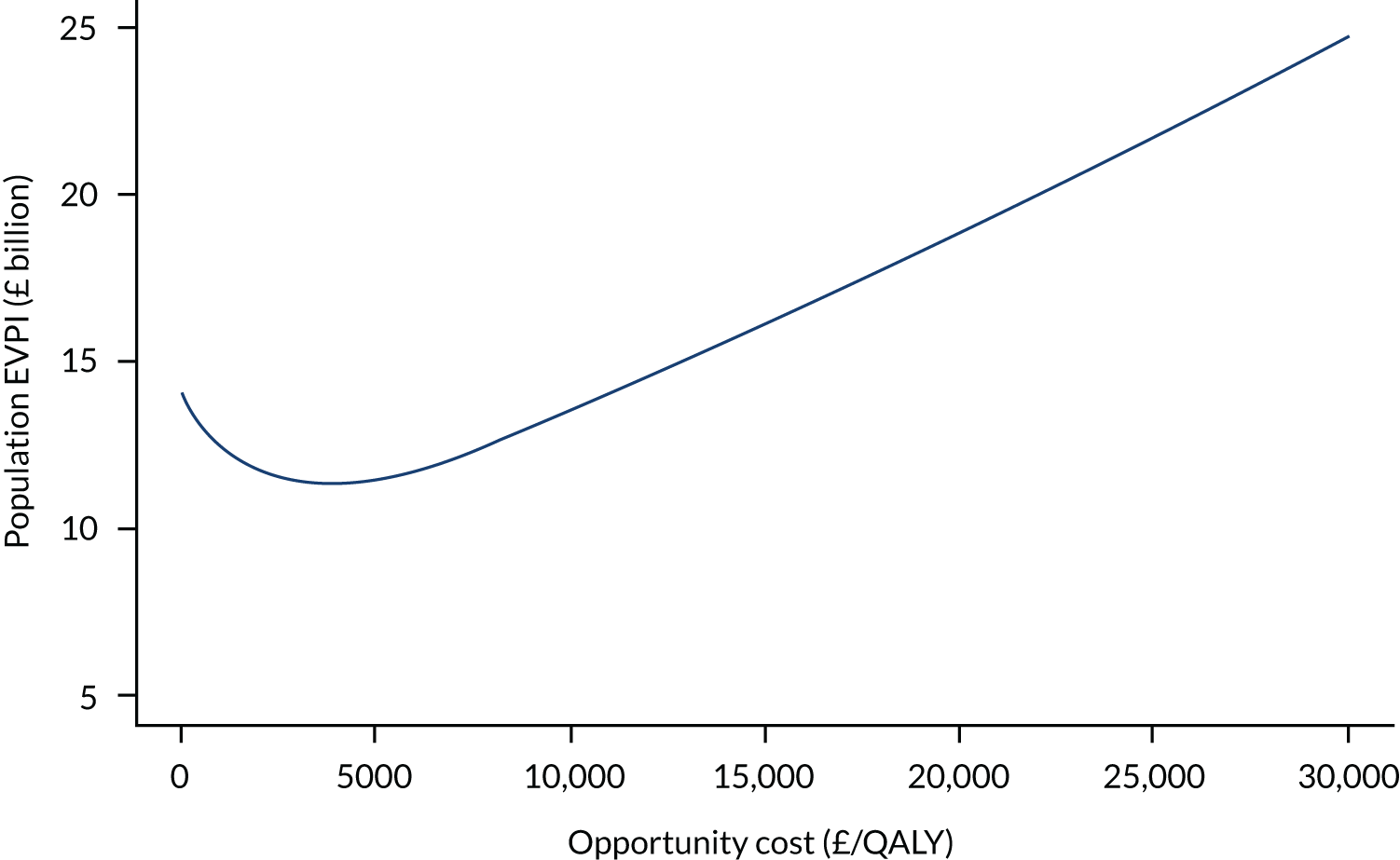
Scenario analysis
Scenario analyses were performed to explore the sensitivity of the findings to assumptions made regarding the GAD score trajectory, health-care costs and interventions costs. Appendix 11 shows the movement through Markov states, and the changes in GAD-7 scores in the initial 10-year period is shown in Appendix 11, Figures 31–33. The results were not sensitive to any of the alternative scenarios; the order of cost-effectiveness did not change, only the magnitude of the difference. Pharmacotherapy dominates all other interventions, and SDIs are more effective and costlier than UDIs (see Appendix 11). As DIs were costlier than face-to-face therapy and medication, the effect of increasing their cost further was not explored.
Discussion
To understand whether or not DIs represent value for money in the treatment of GAD, we constructed a decision-analytic model to evaluate the cost-effectiveness of supported and unsupported DIs, from the perspective of the UK health-care system, in comparison with group therapy, antidepressant medication, non-therapeutic controls and NI. The expected net benefit was the highest for medication followed by group therapy. All DIs and non-therapeutic controls led to higher net benefit than NI. SDIs led to higher costs than UDIs, but NMB was higher when the opportunity cost was ≥ £5000 per QALY.
These results are highly uncertain, with the VOI estimated to be > £11B. The VOI represents not only the value of further research in resolving model uncertainty, but also the scale of the loss of QALYs and incurred costs if the decision about whether to fund DIs, based on existing evidence, is incorrect. The EVPPIP analysis found that the effectiveness of DIs is highly uncertain. It is also a parameter fundamental to establishing the cost-effectiveness of DIs for GAD, given that costs are driven by clinical outcomes (better GAD outcomes lead to lower total health-care costs, compensating for higher DI costs). Therefore, the value of further research to establish the effectiveness of DIs for GAD is substantial: at least £12.9B.
We synthesised evidence on all DIs for GAD populations to evaluate the cost-effectiveness of these, whereas previous economic studies evaluated one specific DI for GAD using a single source for clinical data. Kumar et al. 85 evaluated the cost-effectiveness of a SDI (mobile self-directed cognitive–behavioural therapy: CBT) against individual CBT and NI, and found the SDI to be cost-saving against both comparators; however, clinical data came from a single-arm pilot study for the SDI and from a previous systematic review for the individual CBT, rather than from a RCT comparing SDI with individual CBT. In addition, Kumar et al. ’s85 CEA did not explore probabilistic uncertainty. Dear et al. 61 evaluated the cost-effectiveness of a SDI (internet-based self-directed CBT) compared with NI, using outcomes from a RCT. The authors found that the SDI was more effective but also costlier than NI, with a high probability of being cost-effective when the opportunity cost was AU$40,000 per QALY (≈ £20,000 per QALY). Our analysis supports the findings by Kumar et al. 85 and by Dear et al. 61 that SDIs may be more cost-effective than NI, albeit with great uncertainty. No clinical studies included head-to-head comparisons between DIs and individual CBT for GAD populations (only group CBT), so we could not confirm or refute the finding by Kumar et al. 85 that DIs are more cost-effective than individual CBT.
Limitations in data availability led to several assumptions regarding model parameters. Utilities and excess mortality in different severity states were informed by studies that used measures other than GAD-7 (i.e. HAM-A126 and PHQ-4198), but these measures have been found to be highly correlated with GAD-7. 191 Furthermore, excess mortality was estimated from the impact of anxiety and/or depression in cardiovascular outpatients with long-term oral anticoagulation; it is not clear how closely this relates to excess mortality from GAD in the general population, but the relatively small effect means that it is unlikely to have a large impact on the results. The cost of health care for different levels of GAD severity was based on data collected in 2005 (adjusted for inflation) in patients with anxiety and/or depression14 and on non-UK health-care resource use in the scenario analysis. 85,194 All three studies led to similar conclusions in terms of ranking and uncertainty regarding the cost-effectiveness of DIs and their alternatives.
Finally, the meta-analysis in WP2 did not provide information about relapse rates for each intervention and therefore it was not possible to include this potential impact of interventions. The relapse was assumed to be comparable across all comparators, and the rate of relapse was assumed to be lower than the rate of spontaneous improvement (as described in Model parameters), leading to a positive net change in symptoms. In the sensitivity analysis (see Appendix 11, Table 34), medication had a higher net benefit (£171,866, 95% CI £112,948 to £204,345) than other interventions in the base case when a constant treatment effect was assumed and, thus, we conclude that the results are not sensitive to assumptions about the rate of relapse.
Our model compared the lifetime effect of single treatments for GAD, whereas, in practice, patients can receive multiple cycles of the same therapy or a combination of therapies concurrently or in sequence. We did not model the cost-effectiveness of sequential or combined treatments owing to lack of data; therefore, we do not know whether or not DIs may be cost-effective as a first-line treatment in a stepped-care model before medication and individual or group therapy is offered to those who do not respond to DIs. The lack of GAD-specific cost data is another limitation, so having more up-to-date estimates of resource use for GAD patients as they move through a stepped-care pathway would enable more accurate estimates of cost-effectiveness.
Conclusions
As far as we are aware, this is the first study to evaluate the cost-effectiveness of DIs for GAD, across all types of DIs and in comparison to all types of alternatives, using a decision-analytic model. DIs are associated with a lower NMB than medication and group therapy, but with a higher NMB than non-therapeutic controls and NI. SDIs may be of better value than UDIs, but only for higher investment; if investment is zero, UDIs may be a better alternative. The high uncertainty of these results does not allow for any conclusions about the cost-effectiveness of DIs. NMB is driven by health-care resource use and HRQoL, which in turn are driven by GAD severity, both directly and through morbidity. This means that value for money is driven by clinical outcomes rather than intervention costs; therefore, focusing on treatment effects of DIs for GAD populations in future clinical studies will enable more certain results from cost-effectiveness evaluations.
Chapter 6 Knowledge transfer and stakeholder involvement
Introduction
We do not know how stakeholders, such as commissioners, practitioners, managers, patients, technologists and researchers, make sense of economic evidence about DIs and how they may use this evidence to inform their decisions to adopt, or not, certain DIs. Many concepts in economic evaluations (e.g. QALYs, uncertainty and ICERs) cannot be easily understood (or can be easily misunderstood) by audiences who are not health economists. For example, even the most basic message of an intervention being ‘cost-effective’ is often (mis)taken as the equivalent of being ‘cheap’ or ‘cost saving’. In a series of stakeholder seminars, we explored how individuals and organisations may understand, interpret and potentially use economic information to inform their decision-making about DIs, and how researchers can tailor knowledge transfer activities of economic evidence synthesis to fit the interests and priorities of each stakeholder group.
Stakeholder seminars
We have held seven seminars with groups of stakeholders (Figure 20), which included:
-
commissioners who may fund services that use DIs
-
practitioners and service managers who may provide DIs in routine care
-
service users who may engage with DIs to improve or promote their mental health
-
technologists and researchers who may further develop and optimise DIs.
FIGURE 20.
Stakeholder groups.
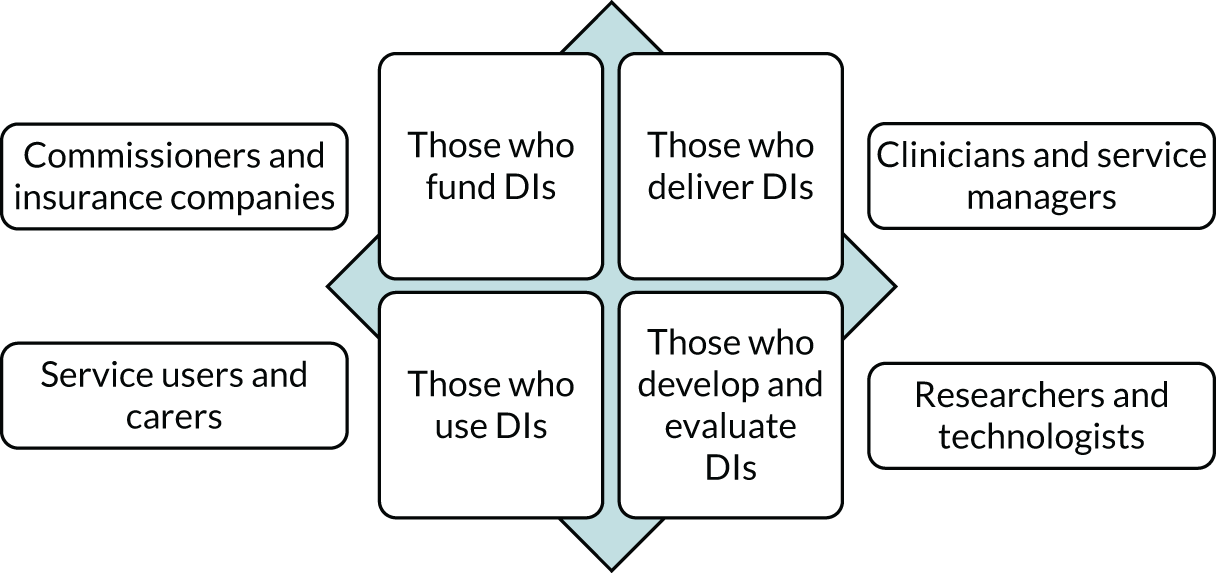
The groups were mixed to reflect the multidisciplinary nature of teams within health and community settings where DIs may be implemented. The seminars involved 2–18 participants and were delivered both via telecommunication [Skype, Zoom (Zoom Video Communications, San Jose, CA, USA) and Microsoft Teams (Microsoft Corporation, Redmond, WA, USA)] and in person by visiting NHS premises and holding classes at the University of York. We did not record the sessions but we took detailed notes. Most of the seminars were part of education and continuous professional development for health-care staff and students. The occurrence of the COVID-19 pandemic in the middle of our knowledge transfer activities meant that we had to be flexible about the way in which we carried out the seminars; we included as many representative stakeholders as possible within a naturalistic setting and with the changing priorities of the NHS. We did not cite any quotations from individual stakeholders, we summarised and reflected on only the key discussion points that arose from the seminars.
The seminars took into consideration a science communication framework207,208 and involved the following steps:
-
We presented a summary of our methods and findings in an appropriate way so that the audience could make sense of the presented information. To enable this, we used colour-coded diagrams and figures as much as possible, rather than tables with numbers or long narratives.
-
We discussed how the summary of evidence may speak to the values and intentions of stakeholders and how their decisions to invest time and resources in DIs may be supported or challenged in view of this evidence.
-
We listened to the audience to understand the decisions they have to make about DIs and the values and intentions that underpin these decisions.
-
We discussed how we can improve the understanding and use of economic evidence by stakeholders.
Each seminar had two parts. The first part was the communication of our methods and findings through an interactive presentation. The second part was a question–answer and discussion session when we asked the audience to identify the highlights of our findings that were important to them, identify any aspects of the presentation that were not clear, and offer comments and feedback in general. We did not follow a structured discussion topic guide so that we could understand, in the first instance, what areas the stakeholders identified as important without being prompted or coached in a certain direction. Using a thematic analysis,209 we identified key themes and were able to draw comparisons about the understanding and potential use of our evidence within and between the different stakeholder groups. In our final expert oversight group, which included health economists, statisticians, researchers and a representative from Public Health England, we presented the findings of the stakeholder workshops and a proposal of how a series of future evidence briefings will be structured and communicated to a broader audience.
Summary of feedback
We produced a matrix (Table 18) to map out the areas of importance for conducting and communicating research on costs and outcomes of DIs in mental health that emerged from the stakeholder seminars within and between the four stakeholder groups.
Areas of importance for conducting and communicating research on DIs | Stakeholders | |||
---|---|---|---|---|
Those who fund DIs | Those who deliver DIs | Those who use DIs | Those who develop and evaluate DIs | |
Populations | ||||
Children and young people | ✗ | |||
Rural vs. urban areas | ✗ | |||
Older adults | ✗ | ✗ | ||
Interventions | ||||
Making a difference | ✗ | |||
Therapeutic relationship | ✗ | |||
Non-specific effect of technology | ✗ | |||
Safety and adverse effects | ✗ | ✗ | ✗ | |
Meaning, ubiquity | ✗ | ✗ | ||
Sustainability | ✗ | ✗ | ||
Tracking/monitoring | ✗ | ✗ | ||
Communication with peers | ✗ | |||
Communication with clinicians | ✗ | |||
Outcomes and service use | ||||
Relapse occurrence | ✗ | |||
Risk increase | ✗ | |||
Attendance/completion of sessions | ✗ | |||
Waiting time | ✗ | |||
Admission rates | ✗ | ✗ | ||
Remission and recovery rates | ✗ | |||
Re-admission rates | ✗ | ✗ | ||
Treatment duration and discharge rates | ✗ | |||
Transition experience | ✗ | ✗ | ||
Number of patients per clinician | ✗ | |||
Values | ||||
Choice | ✗ | ✗ | ||
Inevitability | ✗ | ✗ | ✗ | |
Access/reach | ✗ | ✗ | ||
Continuity of care | ✗ |
Some stakeholders questioned what we really mean by ‘digital interventions’. A lot of people confuse digital platforms (e.g. Skype and smartphones) with DIs (i.e. an activity underpinned by a clinical rationale). There is also the added complication of social media, which are sometimes used as platforms to increase awareness, reduce stigma and encourage help-seeking in mental health, but are not really DIs. Although an important function of digital media is to start a conversation about mental health and encourage help-seeking, they may also have the inadvertent effect of medicalising normal emotional responses and inflating the demand for mental health services.
When we talked about DIs in mental health, some of the stakeholders reflected that it is important to differentiate whether these are for specific diagnosable mental health problems or for factors that may contribute to mental health problems, such as isolation, loneliness, lack of well-being or lack of awareness. In our evidence synthesis, we included DIs only for specific mental health problems, because we wanted to make it relevant to health services that fund and deliver DIs to those with emerging or existing diagnosable conditions, rather than to the general population.
Stakeholders’ experiences of recovery with DIs indicated that their effects were much lower in real life and within health-care services than is reported in research and evaluation studies. This is not surprising given that many of the studies we reviewed had a high risk of bias due to the ways in which the results were reported. In the seminars, we discussed what we would want to see reported in research studies, which is not there now, to enable us to make better use of the available evidence. One of the areas for improvement is reporting rates of remission and recovery, for example the percentage of people who score below ‘caseness’, as well as reporting outcomes according to ‘health states’ (e.g. not only the mean scores at follow-up but also the percentage of people with no symptoms and with mild, moderate and severe symptoms).
With regard to the role of DIs for different age groups, a popular view is that DIs lend themselves better to the mental health care of children and young people (CYP). Are CYP more likely to engage with DIs because they are used to technology and because they enjoy it more? Stakeholders reported that this is not always the case as young people may prefer something different from technology, if they associate ‘digital’ with schoolwork or social peer pressure. Misconceptions of older generations not wishing or being able to use technology may also raise barriers and lead to patronising attitudes in the way DIs are designed for and offered to older generations.
Can technology alone be therapeutic for CYP who use it simply because it is enjoyable (e.g. children who enjoy playing computer games may find that their mood improves with a game-based DI because they find it enjoyable rather than because of the therapy techniques included in it)? We discussed the value of non-therapeutic DCs as a way of testing the differential effects of technology over therapy, by having a ‘control’ game without any therapeutic content. In our review, we found that some of the DCs ranked higher than interventions in terms of the probability of being effective, which may be explained by the non-specific effect of the technology itself (e.g. enjoyable, distracting and novel) rather than the content of the technology. Based on this question, future research will be helpful if it focuses on comparisons between DIs and their non-digital counterpart interventions, for example self-help using a digital medium and a non-digital one (a book or a manual). In this way, we can understand whether or not the digital element adds value over and above the content of the intervention.
Given the inconclusive evidence of our review that SDIs may not necessarily be better than UDIs, a member of the audience in one of our seminars asked whether or not the therapeutic relationship is still important. We discussed that the therapeutic relationship may contribute to clinical outcomes in SDIs, but that, equally, the therapeutic ingredients of UDIs may be independent mediators of outcomes. The assumption that the therapeutic relationship synergistically contributes to change in clinical outcomes alongside the therapeutic ingredients of an intervention is challenged by our findings that UDIs may yield similar outcomes as supported ones. This does not minimise the role of the therapeutic relationship but, rather, it emphasises the fact that various elements of DIs make independent contributions. Yet SDIs are advocated by service users, who value the experience of having a person to communicate with, and by clinicians, who suggest that fulfilling patients’ needs and preferences to have a person to communicate with at low-intensity care (when DIs are commonly offered) may lead to less need for high-intensity treatment.
In the seminars, we discussed what factors may influence adoption of DIs by decision-makers, other than costs and outcomes. The stakeholders identified two contexts that may drive investment in DIs: first, the belief that technology has the ability to provide a solution to complex mental health problems and, second, the desire to invest in technology to overcome severe NHS staff shortages and vacancies. Some seminar audiences raised concerns that investing in technology has happened on the basis of assumptions and wishful thinking (and also political agendas), rather than on clinical evidence that technology can indeed be a solution to complex problems, and without economic evidence that money is better spent on technology rather than, for example, workforce and care environment. Clinicians spoke of valuing patient choice and the fact that logistically DIs have proven a good way of making interventions available at all times, so that people can access them from their home, outside working hours (e.g. if they are young parents or work shifts). Service users can also use DIs to suit their pace, go over therapeutic activities again and again and, more importantly, have access to interventions well beyond their discharge from the service.
Relapse prevention following discharge from a hospital or a community care team was raised as a potential area in which DIs have a role to play. We discussed service users valuing continuing contact with clinicians after discharge, but also having access to entirely self-administered interventions. We also discussed that most DIs in the reviewed literature have been for common mental health problems in primary care, but for mental health professionals who care for people with severe mental illness, DIs have most value when incorporated within secondary care pathways as a means of relapse prevention. This is an important point given that very few studies have implemented and evaluated DIs for relapse prevention, and relapse is rarely used as a research outcome metric. Having longer-term follow-ups after the end of an intervention during the ‘relapse period’ has also been flagged by researchers as a way of improving data availability for the potential long-term modelling of intervention effectiveness and cost-effectiveness.
Although relapse prevention is an important area for DI use, and sleep disturbance is a warning of relapse, we did not include sleep-tracking devices in our current reviews, because their reported outcomes do not capture the occurrence or deterioration of symptoms of mental illness; they detect only changes in sleep patterns. There is a whole body of literature around behaviour change and physical health outcomes in psychiatric populations that is beyond the scope of this work but could be explored in future evidence synthesis. In addition, stakeholders mentioned the value of having an overview of mental health outcomes for people with physical problems (such as depression in people with sleep disorders); this was not within the remit of the current review but could be considered in a future evidence synthesis that focuses on mental health outcomes with DIs used by people with a primary medical/physical problem.
Future communication strategies
The communication of our findings involving stakeholders is ongoing. The next step is to carry out a webinar involving a panel of representative stakeholders in a facilitated discussion. This builds on a model we have already applied in Cochrane Common Mental Disorders and which is becoming the cornerstone for our knowledge mobilisation on selected reviews. The webinar will be widely advertised with an open invitation, but with some targeted approaches to key individuals as part of the audience, including members of the research team, researchers working in the field, commissioners, clinicians and service users. Following the panel discussion, the audience will be invited to ask questions, contribute information and offer ideas for next steps.
This webinar discussion will provide the foundation from which we will develop more tailored dissemination products for each of our target stakeholder audiences. When appropriate, the contents of the webinar will be used to shape the outputs targeting different audiences. The advantage of this approach is that it provides a neutral space, largely independent of the research team, in which the findings of our evidence synthesis can be discussed and interpreted by representatives of different stakeholder groups, and in which the positions of each can be explored both independently and together. This is especially important when the findings are not obviously conclusive, when different stakeholder groups hold different perspectives or assumptions and when the interpretation of findings is nuanced. The webinar will be recorded and made publicly available as part of the project outputs materials on an appropriate digital platform.
As a follow-on, we will produce bespoke outputs for each audience, which summarise the key findings of our review but, in addition, provide specific stakeholder commentary (preferably submitted by the panellist following the discussion) to better engage and enable decision-makers to consider and use the findings. We have a variety of ‘briefing’ templates for policy-makers and commissioners that we can build on, although the key issue is that these are brief (preferably one page), clearly laid out and not too dense. We will consider something different for service users and the public; brief plain-language summaries are useful and well-established methods, but blogs, infographics and short podcasts can be much more appealing for broad audiences, and can be easily shared via social media [Twitter (Twitter, Inc., San Francisco, CA, USA; www.twitter.com) is a key channel for both non-academic and academic audiences, and is also a place for the webinar recording link to be shared].
Conclusion
A series of seminars with stakeholders (i.e. individuals and organisations who would potentially fund, deliver, develop, evaluate and use DIs) helped inform the interpretation of our findings; raised questions for future research; highlighted limitations in our evidence syntheses; and helped us expand, clarify and add to the discussion of our results. An aspect of our findings that was not picked up by the seminar audiences was the role of medication as a comparator or a mediator of outcomes for research on DIs in mental health, or even the role of DIs to support medication use (e.g. using software-based activities to monitor benefits and side-effects or to ensure appropriate and consistent use of medication). DIs are seen as a platform to psychological therapies, and, as such, they may be perceived as separate to medication. This is reflected in the limited research and reporting of medication alongside DIs, despite medication being one of the most commonly offered interventions for mental health problems.
Chapter 7 Conclusions and recommendations
Future evaluations of costs and outcomes of digital interventions in mental health
Populations: reporting subgroup data by diagnosis, severity and comorbidity
Many studies and reviews on DIs that we retrieved through our literature searches included mixed populations (e.g. mixed anxiety disorders, or mixed anxiety and depression) without reporting outcomes separately for each diagnostic subgroup. Reporting outcomes for mixed samples, even if the outcome is measured by a disorder-specific tool, such as the GAD-7, can be misleading because it implies that if an intervention works for the mixed sample, then it will also work for each of its constituent populations. Mixed samples do not answer the question of whether or not DIs are effective with a view to informing condition-specific clinical guidelines. To achieve this, future studies with mixed samples need to report outcomes separately for condition-specific subgroups; even if the sample size is not sufficiently large to explore differences by condition within a study, reporting the data by the condition will facilitate data synthesis across studies.
Apart from reporting outcomes for condition-specific subgroups in mixed populations, symptom severity is another important variant for which outcomes need to be reported for ‘mild, moderate and severe’ subgroups in future studies using established cut-off scores for the chosen outcome measure. This is important for two reasons. First, policy decisions may be based on symptom severity in addition to mental health condition, for example recommending the use of DIs only for patients with mild to moderate depression or GAD. Second, any participant heterogeneity needs to be reflected in the analysis of effectiveness data, as we did in WP2, particularly when pooling data from multiple studies with different distributions of symptom severity. Reporting data on patient subgroups by symptom severity will enable more accurate synthesis of evidence for economic modelling in the future.
Related to the issue of heterogeneity in patient characteristics, many study participants are affected by comorbidities in the form of multiple mental health problems (e.g. substance misuse and depression), but also in the form of mixed physical and mental health problems (e.g. chronic pain and depression). Each of these comorbidities acts as a competing risk, and, as such, it should be accounted for when determining the effectiveness of DIs. Techniques are available to do this, including the use of meta-regression adjusting for differences in the proportions of patients with comorbidities that have been pooled together from individual studies, as we have done in WP2. To be able to achieve this, data on key comorbidities need to be reported in a systematic manner, for example clearly reporting the proportion of patients with chronic pain or with substance misuse within a group of participants with depression. Many studies included in WP2 did not include this detail, and future research needs to collect and report data on the mental and physical health conditions that participants experience in addition to the condition under investigation.
Digital interventions: granularity of classification
We developed a classification system in which interventions were grouped according to whether they were psychological or pharmacological (medication), and controls were grouped according to whether they were non-therapeutic activities (e.g. monitoring) or NI. By ‘no intervention’ we mean that no additional activities or input were offered as part of the research study over and above interventions and resources that were routinely accessible to all participants irrespective of group allocation. Psychological interventions and non-therapeutic controls were further classified as (1) either digital (software driven) or non-digital (lack of technology, or use of technology only for telecommunication, such as speaking on the telephone or via Skype) and (2) either supported (involving interpersonal communication) or unsupported (pure self-help).
This classification system helped us pool DIs and their comparators in the evidence synthesis for WPs 1 and 2. Our classification system did not differentiate between different types of technology platforms (e.g. computer or smartphone) or different types of therapies (e.g. CBT or psychodynamic). This would have made us lose sight of the bigger picture and diluted the evidence synthesis with too few studies in each group. Moreover, DIs using the same technology are not necessarily more alike than those using different technologies; for example, the same self-help programme may be delivered via a computer or a smartphone, whereas an internet platform for live teleconsultation with a therapist is entirely different from an internet self-help programme. The optimal level of granularity for future reviews needs to be driven by its research questions; reviews that focus on incremental iterations of DIs to inform their future development need greater granularity, whereas reviews that are interested in a panoramic overview of DIs to inform pathways of care need to group DIs and their alternatives using higher-order characteristics.
Comparators: determined by care pathways
The DIs evaluated in WP1 reflect the use of such treatments at different points in the clinical pathway for people experiencing mental health problems. DIs can be used to identify risk, using either a targeted or a universal population approach, and to prevent the onset, deterioration or relapse of mental health and addiction problems. Given the heterogeneity of DIs in their functions, delivery setting and targeted clinical conditions, decisions are often made in the context of the services and care pathways within which DIs are implemented. On this basis, future within-trial economic evaluations need to use appropriate alternatives, which would be displaced by a potentially cost-effective DI in a specific care pathway.
Figure 21 depicts the four levels of an inverse pyramid representing the care options within a stepped-care model. The care options that feature at each level should act as alternatives in future economic evaluations of DIs. Step 1, or ‘watchful waiting’, includes alternatives such as assessment, education and monitoring (typically delivered in the community) for populations with subthreshold or mild symptoms or who are at risk of developing a mental health problem. Step 2 includes ‘low-intensity’ interventions in the form of supported and unsupported self-help, as well as education groups. Step 3, or ‘high-intensity’ interventions, includes individual or group therapy and medication. Both step 2 and step 3 are typically delivered in primary care. Step 4, or ‘specialist’ interventions, includes crisis and hospital care as well as complex therapy and medication regimes, typically delivered in secondary care.
FIGURE 21.
Digital interventions and their alternatives in the context of the stepped-care model.
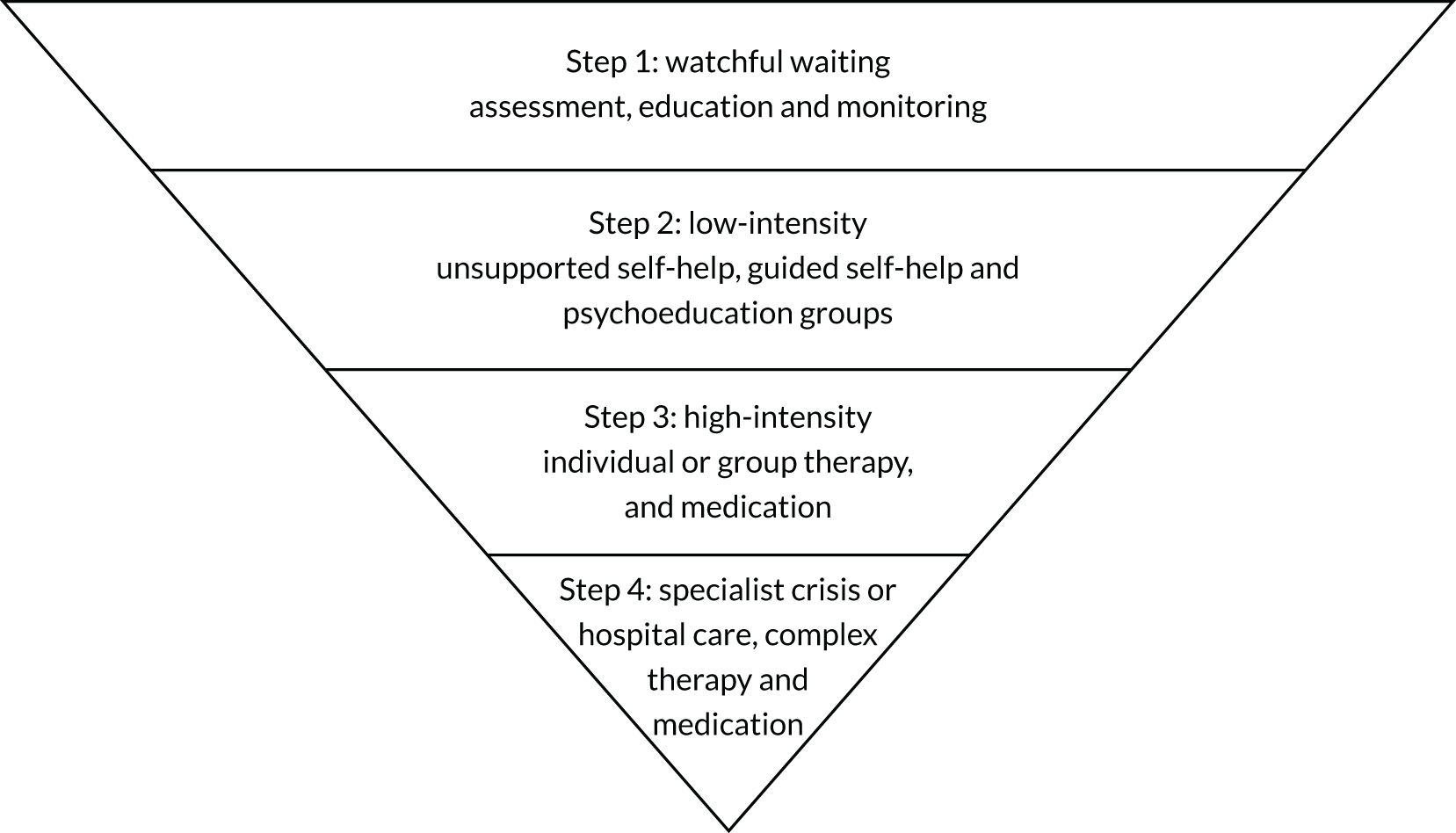
Future research needs to consider the role of DIs in clinician-led therapy, especially as this was discussed by our stakeholders, who suggested that DIs are underused in level 3 (high-intensity interventions) and level 4 (specialist interventions) of the stepped-care model when clinicians are at the forefront of treatment delivery. Supported DIs were valued by service users who participated in our stakeholder groups, reflecting their preference for communicating with a professional rather than doing pure self-help. Future economic studies need to address the evidence gap in comparing DIs with individual therapy and to evaluate how individual therapy and DIs may complement one another, especially at levels 3 and 4 of the stepped-care model. Given our conclusion that the effectiveness of DIs drives their value for money, future research should explore whether or not the synergy of DIs and individual therapy in a blended approach can improve clinical outcomes, and, in turn, the cost-effectiveness of DIs, in the context of clinician-led treatment or supported self-help.
Clinical outcomes: choice of standardised measures
In WP2 we used a NMA to combine multiple sources of evidence into a single estimate. This allows the body of evidence to be reflected in a way that accounts for any underlying trends in treatment effect, but also captures heterogeneity between studies. The challenge in such a synthesis of evidence is that clinical outcomes have to be measured by the same standardised tool. As we found in WP2, 45 different outcome measures were used across 20 studies, but only two of those (the GAD-7 and the PSWQ) were used in enough studies (14 in each case) to enable us to carry out a meta-analysis. In addition, standardised tools measure different constructs (e.g. clinical symptoms in GAD-7 and worry in PSWQ), so the conclusions of the two meta-analyses were not directly comparable. Future studies of DIs need to include outcome measures that will enable comparisons and synthesis of evidence with other studies for the same clinical population. As there is no consensus on recommended outcome measures for specific conditions, a literature review should inform the selection of at least one measure by individual studies on the basis that it will allow comparisons and integration of results with those of other studies.
Cost estimation: reporting all technology-related costs
In determining cost-effectiveness, we often need to consider multiple forms and sources of evidence to estimate the costs of the interventions and their comparators. DIs are difficult to establish a cost for, as discussed by McNamee et al. 27 For example, there may be significant costs of software development and regular maintenance of digital platforms, which are difficult to disentangle and allocate on a per-patient basis. There are two types of costs that have been under-reported in current economic studies of DIs, and which need to be included in future research studies, or at least reasons for exclusion reported: costs associated with the use of technology (e.g. cost of having Wi-Fi, cost of a computer/smartphone and cost of licences to access software, website maintenance and staff training) and costs associated with reaching potential users (e.g. the cost of advertising if recruiting among the general public and the cost of clinician time if recruitment requires assessment and signposting within clinical services).
Cost estimation: justifying differences according to perspectives
The perspectives of future economic evaluations on DIs will determine which costs should be included in their final cost estimation. For example, costs to develop the technology may be incurred by private companies and not by the health service that adopts the DI; therefore, such costs may be included only if the evaluation is conducted from a societal perspective rather than from a health-care perspective. Future studies need to explicitly discuss how the perspective they used influenced their cost estimation, especially for costs that are technology specific but under-reported, such as capital costs (computers, staff training, one-off software purchases) or costs for website maintenance. Furthermore, future economic studies need to explain and discuss the costs that account for differences in cost-effectiveness results driven by different perspectives, as we have seen in the results of many economic studies in WP1.
Time horizon: need for long-term data
Owing to the chronic nature of mental health problems, it is useful to model the impact of short-term interventions over a longer time horizon to understand how differences in treatments translate to differences in costs and outcomes over the lifetime of individuals. Unfortunately, there are few data to support assumptions regarding the ‘stickiness’ of DIs in mental health beyond the initial treatment period. In addition, there are sparse long-term data regarding the trajectory of mental health conditions, such as GAD, over the longer term. To establish cost-effectiveness over an appropriate time horizon, some extrapolation of shorter-term trial evidence over a longer time horizon is necessary. This leads to assumptions about longer-term treatment effect and the natural history of mental health conditions, which must be validated or tested using sensitivity analysis.
Available data on outcomes and resource use within RCTs are typically short term. This is because of the high costs of long-term follow-ups as part of a research trial, but also because the longer the follow-up, the more likely it is that the trial will lose participants. Our meta-analysis in WP2 showed that typical follow-up was 3–12 weeks before the control group crossed over to an active intervention; this limited the usefulness of longer-term data in estimating treatment effects because the groups were no longer randomised. Although crossover for the control group from ‘no intervention’ to an active intervention may seem reasonable, especially when studies recruit from the general public and use the crossover as enticement for randomisation, it limits the value of the research study for long-term outcomes. Our modelling study in WP3 relied on assumptions regarding long-term effectiveness in the absence of longer-term trial data. Determining how effective DIs are over the longer term, even with extrapolation, is possible only with longer follow-up in primary studies. Future RCTs on DIs should have at least a 6-month follow-up and avoid crossover designs, especially when participants in the usual-care control group are likely to have access to routine interventions anyway.
Economic analysis methods: trial based versus economic modelling
Future economic evaluations of DIs need to take into account the body of existing evidence and how the planned evaluation will contribute towards the literature. To inform decision-making, new evidence on DIs must be put into context with the existing relevant evidence. Trial-based evaluations are often insufficient in follow-up and cannot capture all the relevant differences in costs and outcomes between alternatives, for example between DIs and NoDIs, medication or usual care. The requirement to include all relevant comparators motivates the need for evidence syntheses that pool outcomes from different primary studies, in particular more complex forms of synthesis that reflect multiple comparisons within a single framework, such as the NMA we have conducted in WP2. The use of modelling, specifically extrapolation modelling, gives the opportunity to compare many different alternatives with DIs, which is not possible to do as part of a single within-trial evaluation. Yet the use of extrapolation modelling does not negate the need for robust primary studies that have large samples and long-term follow-ups.
Research questions: value beyond symptom reduction and cost per unit gained
Stakeholders asked whether economic studies can tell us if DIs can reduce relapse, risk and admission/re-admission rates; can improve attendance/engagement with health services; can reduce waiting time for treatment or improve remission and recovery rates; can help deliver treatment more quickly and speed up discharge; or can help clinicians treat more patients within a given time. Clinical and economic studies of DIs usually collect all the necessary data to answer these questions, so it will be useful to explicitly formulate questions that speak to the stakeholders’ interests and report results in a way that enable us to answer these questions beyond symptom reduction (e.g. GAD-7 scores) and costs per unit of outcome gained (e.g. depression-free days or QALYs).
When stakeholders were asked about what would influence their decisions to adopt DIs other than costs and outcomes, they cited four factors: increasing choice in the way patients and service users access help, improving access/reach for underserved or underengaged populations, enabling continuity of care and accepting the ‘inevitability of going digital’. These four factors can be used to steer research questions and discussion topics in future qualitative studies to inform the body of evidence on the impact of DIs on choice, access/reach and continuity of care, as well as the impact of the ‘inevitability of going digital’ on service users who are not digital natives. These questions may be beyond the scope of standard economic evaluations but are important for decision-making on adopting DIs alongside value for money.
The current economic evidence does not consider costs associated with implementation. Although issues of budget and capacity are methodologically separate to determining cost-effectiveness,11 decisions to adopt DIs are also driven by the need to deliver interventions in a resource-conscious system, such as the UK’s NHS. Beyond standard methods to establish the cost-effectiveness of DIs, future research needs to consider the actual gains that can be achieved by a system that recommends them, and the investments that may be required to achieve those gains in practice,210 for example where capital investment is required to build technological infrastructure or to train the workforce in technology literacy.
Digital therapeutic alliance: measure and relationship with outcomes
Future research needs to consider incorporating standardised tools that measure digital therapeutic alliance, like the one developed by Berry et al. ,211 to capture the ‘working relationship’ between users and the digital medium itself. This is grounded on our stakeholders’ interest in understanding whether or not using a digital medium mediates clinical outcomes. The value of digital technologies beyond their therapeutic content has been the objective of two groups of economic evaluations we identified in our systematic review. First, studies that compare DIs with their non-digital counterparts (e.g. Jones et al. 76) can inform us about the added value of the digital medium all things being equal in the intervention itself (same content with and without the digital medium). Second, studies that compare DIs with ‘digital dummies’, for example a technology-aided programme with and without a therapeutic element (e.g. in Španiel et al. 100) can inform us about the non-specific effect of the digital medium on outcomes other than the therapeutic intervention itself.
Recent literature reviews212,213 suggest a relationship between digital therapeutic alliance and outcomes with DIs, perhaps indirectly via improved engagement with therapy. Designing and delivering research studies that compare DIs with appropriate alternatives to control for differences in the content of the digital medium (therapeutic vs. non-therapeutic) or the delivery of the intervention (digital vs. non-digital) can answer questions about the mediating role of the digital medium on clinical outcomes; however, such studies are resource intensive. Alternatively, future studies that compare DIs with NI or with face-to-face therapy can incorporate a measure of digital therapeutic alliance to help us understand and improve the working relationship between users and DIs, and subsequently improve outcomes that can drive value for money.
Decision-making for digital interventions in mental health
Health service commissioners and providers are particularly concerned with delivering the best-quality service for the least possible cost. Economic evaluations, especially CEAs and CUAs, are important tools to help us make decisions about resource allocation to DIs based on their ‘value for money’. Figure 22 is a visual representation of how economic evaluations can inform decision-making based on the costs and outcomes of DIs against the costs and outcomes of their alternatives. The figure is a cost-effectiveness plane214 whose four quadrants represent four possible combinations of relative costs and outcomes. In the south-east quadrant DIs are more effective and less costly than their alternatives. The opposite is true in the north-west quadrant, where DIs are less effective and more costly. The north-east quadrant corresponds to more effective and more costly DIs, whereas in the south-west quadrant DIs are less effective and less costly than their alternatives.
FIGURE 22.
Decision-making based on costs and outcomes of DIs compared with alternatives.
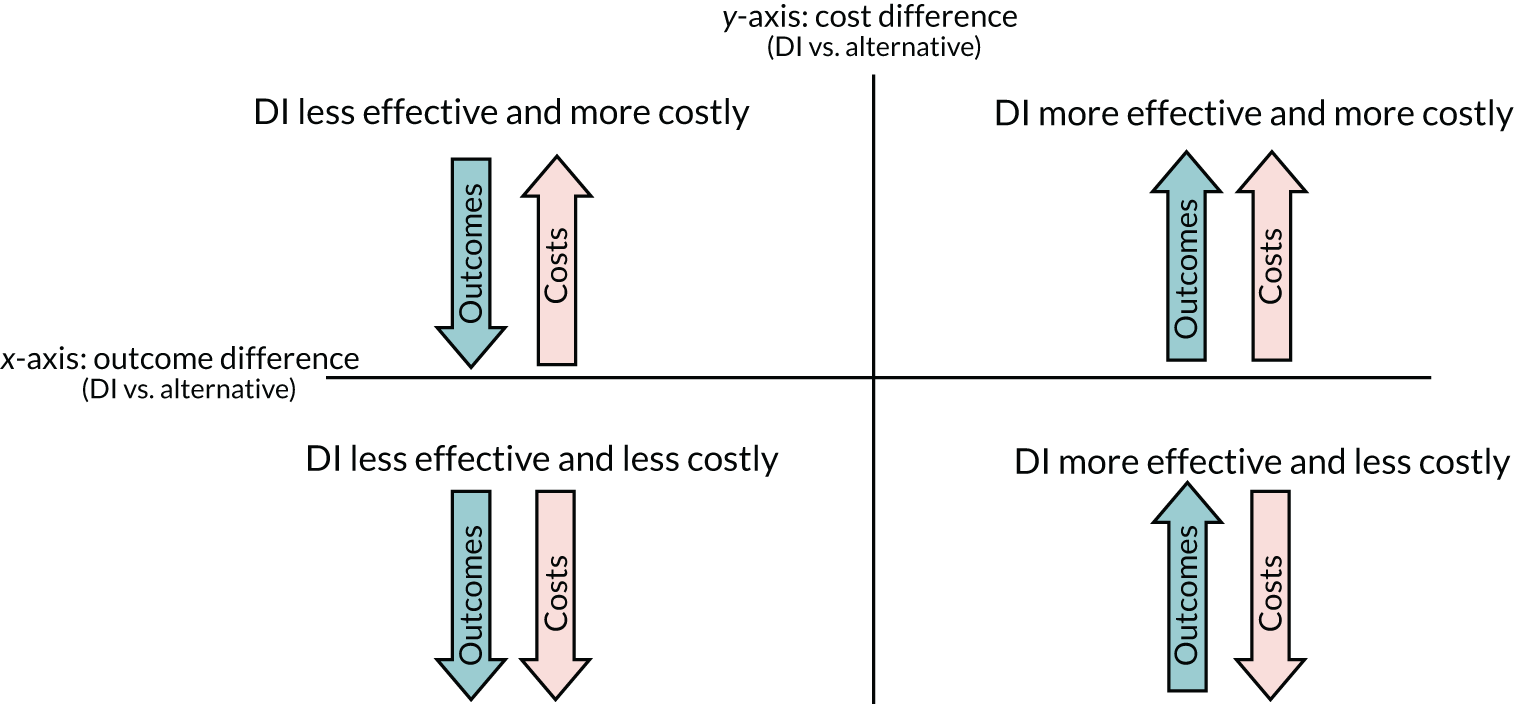
Decision-making is relatively straightforward when DIs are more effective and less costly, or so-called ‘dominant’ (south-east quadrant), as they can save money. Examples of such DIs from our review include an automated motivational text-messaging service and an internet CBT programme for smoking67,99 (which dominated ‘usual care’) and an online self-help intervention to reduce the frequency and intensity of suicidal ideation104 (which dominated the alternative of signposting participants to a website with general information about suicide). Yet, even in cases in which DIs achieve better outcomes at lower costs, their adoption may require disinvesting from alternatives that are valued and preferred by service users or professionals (e.g. face-to-face consultation with a clinician). In this case, decision-makers may opt for the status quo based on people’s personal values and preferences, rather than an intervention’s value for money.
When DIs are more effective and more costly than their alternatives (north-east quadrant), health-care commissioners and providers need to decide whether or not they get enough ‘bang for their buck’ from an intervention for it to be worth their investment, and to consider whether or not they can afford the extra cost to obtain the extra gain. This was the case in several economic evaluations of internet CBT, which was found to be more effective but also more costly than usual care for depression15 and panic,95 or than relaxation for OCD,42 or than WL for depression. 105 In these cases, the decision about whether or not DIs are a cost-effective option depends on how much money we are willing, or able, to invest in them.
On the flipside, when DIs are shown to be less effective and less costly (south-west quadrant), decision-makers have to weigh up the loss in benefits against the need to deliver services within budget in a system that does not have infinite capacity such as the UK’s NHS. An example from our economic modelling speaks to the relative value of UDIs for GAD, which ranked marginally lower than unsupported ones in terms of outcomes, yet their NMB was higher for no investment (£0) because of their lower intervention costs. For a health-care system that cannot afford or cannot recruit the human resources to support DIs, our economic model opens up the hypothesis (albeit with uncertainty) that, for no investment, UDIs may have better value than SDIs if the alternative is ‘doing nothing’.
Finally, in the scenario in which a DI is less effective but also more costly (north-west quadrant), so the DI is ‘dominated’ by an alternative, it does not automatically follow that this DI is rejected, because decision-making in health care involves social and moral considerations, alongside economic ones. An example from our review is a web-based CBT self-help programme for health anxiety52 that was dominated by a paper-based CBT self-help manual; the web-based CBT may still be considered for adoption if it reaches younger populations who are digital natives and may prefer to access help through a web-based programme rather than by using a printed manual.
It is important to recognise that decisions about DIs need to be made in a timely manner because delaying decisions until perfect cost-effectiveness evidence becomes available may result in HRQoL losses and increased health-care costs. As this report was completed during the COVID-19 pandemic, when mental health and addiction were areas of increasing concern, and digital technologies became the ‘default’ option for health care and other activities, a major determinant of the use of DIs in the future may be necessity and established practice owing to the pandemic circumstances.
Conclusion
The NHS investment in digital technologies is growing rapidly. 215 According to the National Information Board’s strategy for personalised health and care 2020,216 use of an online digital health service, rather visiting a health professional, can improve patient choices, access to services, clinical outcomes and self-care. In the treatment of patients with mental health conditions, this can offer the potential to elevate a system that can face challenges in providing face-to-face appointments across all geographical locations. Digitalisation of mental health care needs to demonstrate value for money as a way of improving access to and outcomes with services. DIs are complex interventions, which require complex methodology to evaluate cost-effectiveness appropriately. 217
Complexities, although not unique to DIs, do pose a challenge for the evaluation and the eventual usefulness of the evidence generated. It is difficult to conclude on the overall cost-effectiveness of DIs, given the heterogeneity of the populations, interventions, comparators and outcomes used across different studies that evaluate DIs. Moreover, a lack of data limits the extent to which conclusions can be drawn regarding particular conditions, even those as common as GAD. However, if we were to make best use of the available evidence, albeit with the uncertainty and limitations that come with it, we can, at the very least, observe trends in the costs and outcomes of DIs and identify research gaps in the context of stepped care for mental health.
With this in mind, DIs may have a place as first-line treatments instead of ‘doing nothing’ or doing something without an expected therapeutic benefit (e.g. monitoring or having a general discussion); however, DIs may not confer any clinical or financial value when they are used instead of or in addition to medication or individual therapy. Clinical outcomes rather than intervention costs drive ‘value for money’ when DIs are compared with alternative care options; to put it simply, it is better to make DIs more effective rather than cheaper than their alternatives. Future research needs to consider two ways of making DIs more effective: improving the technology and/or improving the interpersonal support offered alongside the technology. The value of integrating digital and interpersonal elements, as well as the choice of alternatives with which to compare DIs, depends on where in the stepped-care model we want DIs to fit.
Considerations other than costs and outcomes during decision-making relevant to DIs, such as geographical reach and limited workforce, are beyond the remit of standard economic evaluations. Complexity and heterogeneity are inherent features not only of DIs, but also of the methods used to evaluate them. We need to cut through this complexity and heterogeneity and make the best use of currently available evidence by classifying interventions and controls in a way that fits clinically meaningful research questions, pooling together costs and outcomes across different studies, and presenting the results in a way that speaks to what is important to decision-makers and end users. Ultimately, no individual interventions or evidence syntheses are of any value by themselves, unless they are considered in the context of a health and social care system that would invest in and use interventions (digital or non-digital) to promote and improve mental health.
Acknowledgements
We thank our NHS colleagues, and in particular Mrs Sarah Daniel, Head of Research at Tees, Esk and Wear Valleys NHS Foundation Trust for organising workshops with stakeholders within the health service. We thank the service user representatives and academic colleagues for their thoughts on this programme of work and our findings. We thank Professor Paul McNamee, Professor Nicola Cooper, Dr Alrinda Cerga-Pashoja and Mr Daniel Horne for their guidance and support as members of our advisory/steering group.
Contributions of authors
Lina Gega (https://orcid.org/0000-0003-2902-9256) (Reader in Mental Health and Honorary Nurse Consultant) conceived this programme of work; wrote the protocol; obtained the funding; co-ordinated and supervised all WPs; organised the steering group and incorporated its feedback; drafted the progress reports; and contributed to and checked the literature searches, screening of records, data extractions, analyses and write-up across all WPs. She developed and applied the classification system; produced the figures and tables; led the clinical and lay interpretation, presentation and discussion of the results; conducted and summarised the stakeholder consultation groups; and produced the final report.
Dina Jankovic (https://orcid.org/0000-0002-9311-1409) (Research Fellow in Health Economics) completed the data extraction, quality assessment and methodological discussion of economic studies for WP1; led the scoping, developing, coding, analysing and write-up for the modelling study for WP3; contributed to the NMA for WP2; contributed to the screening of literature for WPs 1 and 2; and led the paper submissions to peer-reviewed journals for WPs 1 and 3.
Pedro Saramago (https://orcid.org/0000-0001-9063-8590) (Research Fellow in Health Economics) led the NMA for WP2; contributed to the data extraction, quality assessment and methodological discussion of economic studies for WP1; contributed to the classification system; contributed to the scoping, developing, coding, analysing and write-up for the modelling study for WP3; contributed to the screening of literature for WPs 1 and 2; and led the submission of a paper to a peer-reviewed journal for WP2.
David Marshall (https://orcid.org/0000-0001-5969-9539) (Research Fellow in Evidence Synthesis) co-ordinated the literature searches for WPs 1–3, screened the retrieved records for WPs 1–3, contributed to the classification system for WPs 1 and 2, carried out the methodological assessment for WP2, conducted the clinical data extraction for WPs 1 and 2, and co-ordinated the referencing of the final report.
Sarah Dawson (https://orcid.org/0000-0002-6682-063X) (Senior Research Associate in Information Retrieval) conducted the literature searches for WPs 1 and 2 and updated the literature searches as needed. She contributed to the defining and refining of the inclusion/exclusion criteria for WPs 1 and 2.
Sally Brabyn (https://orcid.org/0000-0001-5381-003X) (Research Fellow in Mental Health) contributed to the data extraction for WP1 and the stakeholder seminars for WP4.
Georgios F Nikolaidis (https://orcid.org/0000-0001-9008-6896) (Research Fellow in Health Economics) conducted part of the NMA for WP2 and contributed to the write-up of the results for WP2.
Hollie Melton (https://orcid.org/0000-0003-3837-510X) (Research Fellow in Evidence Synthesis) contributed to the screening of records for WPs 1 and 2, and to the data extraction and quality assessment of the clinical studies for WP2.
Rachel Churchill (https://orcid.org/0000-0002-1751-0512) (Chair in Evidence Synthesis) supervised the work of colleagues from the Centre for Reviews and Dissemination and from the Cochrane Common Mental Disorders Group. She is leading the planned webinar as part of WP4.
Laura Bojke (https://orcid.org/0000-0001-7921-9109) (Reader in Health Economics) supervised and contributed to the economic review for WP1; the NMA for WP2; the scoping, developing, coding, analysing and write-up of the modelling study for WP3; and the write-up of recommendations and conclusions.
Publication
Jankovic D, Bojke L, Marshall D, Saramago Goncalves P, Churchill R, Melton H, et al. Systematic review and critique of methods for economic evaluation of digital mental health interventions. Appl Health Econ Health Pol 2021;19:17–27.
Saramago P, Gega L, Marshall D, Nikolaidis GF, Jankovic D, Melton H, et al. Digital interventions for generalised anxiety disorder (GAD): systematic review and network meta-analysis. Front Psychiatry 2021;12:726222.
Jankovic D, Saramago PG, Gega L, Marshall D, Wright K, Hafidh M, et al. Cost Effectiveness of Digital Interventions for Generalised Anxiety Disorder: A Model-Based Analysis [published online ahead of print 27 December 2021]. PharmacoEconomics Open 2021.
Data-sharing statement
Our data were from published literature. We will make extracted data available to the scientific community with as few restrictions as feasible, while retaining exclusive use until the publication of major outputs. Stakeholder consultation notes may not be suitable for sharing but these can be discussed with the corresponding author.
Disclaimers
This report presents independent research funded by the National Institute for Health Research (NIHR). The views and opinions expressed by authors in this publication are those of the authors and do not necessarily reflect those of the NHS, the NIHR, NETSCC, the HTA programme or the Department of Health and Social Care. If there are verbatim quotations included in this publication the views and opinions expressed by the interviewees are those of the interviewees and do not necessarily reflect those of the authors, those of the NHS, the NIHR, NETSCC, the HTA programme or the Department of Health and Social Care.
References
- World Health Organization . International Classification of Diseases 2018.
- National Institute for Health and Care Excellence (NICE) . Generalised Anxiety Disorder and Panic Disorder in Adults: Management 2011.
- Gega L, Gilbody S, Aboujaoude E, Starcevic V. Mental Health in the Digital Age: Grave Dangers, Great Promise. Oxford: Oxford University Press; 2015.
- Christensen H, Batterham P, Mackinnon A, Griffiths KM, Kalia Hehir K, Kenardy J, et al. Prevention of generalized anxiety disorder using a web intervention, iChill: randomized controlled trial. J Med Internet Res 2014;16. https://doi.org/10.2196/jmir.3507.
- Pham Q, Khatib Y, Stansfeld S, Fox S, Green T. Feasibility and efficacy of an mHealth game for managing anxiety: ‘Flowy’ randomized controlled pilot trial and design evaluation. Games Health J 2016;5:50-67. https://doi.org/10.1089/g4h.2015.0033.
- Briggs AH, O’Brien BJ. The death of cost-minimization analysis?. Health Econ 2001;10:179-84. https://doi.org/10.1002/hec.584.
- National Institute for Health and Care Excellence (NICE) . Guide to the Methods of Technology Appraisal 2008.
- Karlsson G, Johannesson M. The decision rules of cost-effectiveness analysis. PharmacoEconomics 1996;9:113-20. https://doi.org/10.2165/00019053-199609020-00003.
- Lomas J, Martin S, Claxton K. Estimating the marginal productivity of the English National Health Service from 2003 to 2012. Value Health 2019;22:995-1002. https://doi.org/10.1016/j.jval.2019.04.1926.
- Grosse SD. Assessing cost-effectiveness in healthcare: history of the $50,000 per QALY threshold. Expert Rev Pharmacoecon Outcomes Res 2008;8:165-78. https://doi.org/10.1586/14737167.8.2.165.
- National Institute for Health and Care Excellence (NICE) . Guide to the Methods of Technology Appraisal 2013.
- Aboujaoude E, Gega L. From digital mental health interventions to digital ‘addiction’: where the two fields converge. Front Psychiatry 2019;10. https://doi.org/10.3389/fpsyt.2019.01017.
- Claxton K. The irrelevance of inference: a decision-making approach to the stochastic evaluation of health care technologies. J Health Econ 1999;18:341-64. https://doi.org/10.1016/S0167-6296(98)00039-3.
- Kaltenthaler E, Brazier J, De Nigris E, Tumur I, Ferriter M, Beverly C, et al. Computerized cognitive behavior therapy for depression and anxiety update: a systematic review and economic evaluation. Health Technol Assess 2006;10. https://doi.org/10.3310/hta10330.
- McCrone P, Knapp M, Proudfoot J, Ryden C, Cavanagh K, Shapiro DA, et al. Cost-effectiveness of computerised cognitive-behavioural therapy for anxiety and depression in primary care: randomised controlled trial. Br J Psychiatry 2004;185:55-62. https://doi.org/10.1192/bjp.185.1.55.
- Arnberg FK, Linton SJ, Hultcrantz M, Heintz E, Jonsson U. Internet-delivered psychological treatments for mood and anxiety disorders: a systematic review of their efficacy, safety, and cost-effectiveness. PLOS ONE 2014;9. https://doi.org/10.1371/journal.pone.0098118.
- Donker T, Blankers M, Hedman E, Ljótsson B, Petrie K, Christensen H. Economic evaluations of internet interventions for mental health: a systematic review. Psychol Med 2015;45:3357-76. https://doi.org/10.1017/S0033291715001427.
- Hedman E, Ljótsson B, Lindefors N. Cognitive behavior therapy via the internet: a systematic review of applications, clinical efficacy and cost-effectiveness. Expert Rev Pharmacoecon Outcomes Res 2012;12:745-64. https://doi.org/10.1586/erp.12.67.
- Ahern E, Kinsella S, Semkovska M. Clinical efficacy and economic evaluation of online cognitive behavioral therapy for major depressive disorder: a systematic review and meta-analysis. Expert Rev Pharmacoecon Outcomes Res 2018;18:25-41. https://doi.org/10.1080/14737167.2018.1407245.
- Massoudi B, Holvast F, Bockting CLH, Burger H, Blanker MH. The effectiveness and cost-effectiveness of e-health interventions for depression and anxiety in primary care: a systematic review and meta-analysis. J Affect Disord 2019;245:728-43. https://doi.org/10.1016/j.jad.2018.11.050.
- Naidu VV, Giblin E, Burke KM, Madan I. Delivery of cognitive behavioural therapy to workers: a systematic review. Occup Med 2016;66:112-17. https://doi.org/10.1093/occmed/kqv141.
- Kolovos S, van Dongen JM, Riper H, Buntrock C, Cuijpers P, Ebert DD, et al. Cost effectiveness of guided internet-based interventions for depression in comparison with control conditions: an individual-participant data meta-analysis. Depress Anxiety 2018;35:209-19. https://doi.org/10.1002/da.22714.
- Paganini S, Teigelkötter W, Buntrock C, Baumeister H. Economic evaluations of internet- and mobile-based interventions for the treatment and prevention of depression: a systematic review. J Affect Disord 2018;225:733-55. https://doi.org/10.1016/j.jad.2017.07.018.
- Chen YF, Madan J, Welton N, Yahaya I, Aveyard P, Bauld L, et al. Effectiveness and cost-effectiveness of computer and other electronic aids for smoking cessation: a systematic review and network meta-analysis. Health Technol Assess 2012;16. https://doi.org/10.3310/hta16380.
- Badawy SM, Kuhns LM. Texting and mobile phone app interventions for improving adherence to preventive behavior in adolescents: a systematic review. JMIR Mhealth Uhealth 2017;5. https://doi.org/10.2196/mhealth.6837.
- de la Torre-Díez I, López-Coronado M, Vaca C, Aguado JS, de Castro C. Cost–utility and cost-effectiveness studies of telemedicine, electronic, and mobile health systems in the literature: a systematic review. Telemed J E Health 2015;21:81-5. https://doi.org/10.1089/tmj.2014.0053.
- McNamee P, Murray E, Kelly MP, Bojke L, Chilcott J, Fischer A, et al. Designing and undertaking a health economics study of digital health interventions. Am J Prev Med 2016;51:852-60. https://doi.org/10.1016/j.amepre.2016.05.007.
- Moher D, Liberati A, Tetzlaff J, Altman DG. PRISMA Group . Preferred reporting items for systematic reviews and meta-analyses: the PRISMA statement. Ann Intern Med 2009;151:264-9. https://doi.org/10.7326/0003-4819-151-4-200908180-00135.
- Moher D, Shamseer L, Clarke M, Ghersi D, Liberati A, Petticrew M, et al. Preferred reporting items for systematic review and meta-analysis protocols (PRISMA-P) 2015 statement. Syst Rev 2015;4. https://doi.org/10.1186/2046-4053-4-1.
- Husereau D, Drummond M, Petrou S, Carswell C, Moher D, Greenberg D, et al. Consolidated Health Economic Evaluation Reporting Standards (CHEERS) statement. Int J Technol Assess Health Care 2013;29:117-22. https://doi.org/10.1017/S0266462313000160.
- Barak A, Hen L, Boniel-Nissim M, Shapira N. A comprehensive review and a meta-analysis of the effectiveness of internet-based psychotherapeutic interventions. J Technol Hum Serv 2008;26:109-60. https://doi.org/10.1080/15228830802094429.
- Cuijpers P, van Straten A, Andersson G. Internet-administered cognitive behavior therapy for health problems: a systematic review. J Behav Med 2008;31:169-77. https://doi.org/10.1007/s10865-007-9144-1.
- Hollis C, Falconer CJ, Martin JL, Whittington C, Stockton S, Glazebrook C, et al. Annual research review: digital health interventions for children and young people with mental health problems – a systematic and meta-review. J Child Psychol Psychiatry 2017;58:474-503. https://doi.org/10.1111/jcpp.12663.
- Marks I, Cavanagh K, Gega L. Hands-on Help: Computer-aided Psychotherapy. London: Psychology Press; 2007.
- Mayo-Wilson E, Montgomery P. Media-delivered cognitive behavioural therapy and behavioural therapy (self-help) for anxiety disorders in adults. Cochrane Database Syst Rev 2013;9. https://doi.org/10.1002/14651858.CD005330.pub4.
- Schulz R, Czaja SJ, McKay JR, Ory MG, Belle SH. Intervention taxonomy (ITAX): describing essential features of interventions. Am J Health Behav 2010;34:811-21. https://doi.org/10.5993/AJHB.34.6.15.
- Johansson R, Björklund M, Hornborg C, Karlsson S, Hesser H, Ljótsson B, et al. Affect-focused psychodynamic psychotherapy for depression and anxiety through the internet: a randomized controlled trial. Peer J 2013;1. https://doi.org/10.7717/peerj.102.
- Teng MH, Hou YM, Chang SH, Cheng HJ. Home-delivered attention bias modification training via smartphone to improve attention control in sub-clinical generalized anxiety disorder: a randomized, controlled multi-session experiment. J Affect Disord 2019;246:444-51. https://doi.org/10.1016/j.jad.2018.12.118.
- Lovell K, Bower P, Gellatly J, Byford S, Bee P, McMillan D, et al. Clinical effectiveness, cost-effectiveness and acceptability of low-intensity interventions in the management of obsessive-compulsive disorder: the Obsessive-Compulsive Treatment Efficacy randomised controlled Trial (OCTET). Health Technol Assess 2017;21. https://doi.org/10.3310/hta21370.
- Andersson E, Hedman E, Wadström O, Boberg J, Andersson EY, Axelsson E, et al. Internet-based extinction therapy for worry: a randomized controlled trial. Behav Ther 2017;48:391-402. https://doi.org/10.1016/j.beth.2016.07.003.
- Dahlin M, Andersson G, Magnusson K, Johansson T, Sjögren J, Håkansson A, et al. Internet-delivered acceptance-based behaviour therapy for generalized anxiety disorder: a randomized controlled trial. Behav Res Ther 2016;77:86-95. https://doi.org/10.1016/j.brat.2015.12.007.
- McCrone P, Marks IM, Greist JH, Baer L, Kobak KA, Wenzel KW, et al. Cost-effectiveness of computer-aided behaviour therapy for obsessive-compulsive disorder. Psychother Psychosom 2007;76:249-50. https://doi.org/10.1159/000101504.
- Andersson E, Hedman E, Ljotsson B, Wikstrom M, Elveling E, Lindefors N, et al. Cost-effectiveness of internet-based cognitive behavior therapy for obsessive-compulsive disorder: results from a randomized controlled trial. J Obsessive Compuls Relat Disord 2015;4:47-53. https://doi.org/10.1016/j.jocrd.2014.12.004.
- Sculpher MJ, Claxton K, Drummond M, McCabe C. Whither trial based economic evaluation for health care decision making?. Health Econ 2006;15:677-87. https://doi.org/10.1002/hec.1093.
- Jankovic D, Bojke L, Marshall D, Saramago Goncalves P, Churchill R, Melton H, et al. Systematic review and critique of methods for economic evaluation of digital mental health interventions. Appl Health Econ Health Policy 2020:1-11. https://doi.org/10.1007/s40258-020-00607-3.
- Hodgins DC, Peden N. Cognitive-behavioral treatment for impulse control disorders. Braz J Psychiatry 2008;30:31-40.
- Watts RD, Li IW. Use of checklists in reviews of health economic evaluations, 2010 to 2018. Value Health 2019;22:377-82. https://doi.org/10.1016/j.jval.2018.10.006.
- Drummond MF, Sculpher MJ, Claxton K, Stoddart GL, Torrance GW. Methods for the Economic Evaluation of Health Care Programmes. Oxford: Oxford University Press; 2015.
- Philips Z, Bojke L, Sculpher M, Claxton K, Golder S. Good practice guidelines for decision-analytic modelling in health technology assessment. PharmacoEconomics 2006;24:355-71. https://doi.org/10.2165/00019053-200624040-00006.
- Aardoom JJ, Dingemans AE, van Ginkel JR, Spinhoven P, Van Furth EF, Van den Akker-van Marle ME. Cost-utility of an internet-based intervention with or without therapist support in comparison with a waiting list for individuals with eating disorder symptoms: a randomized controlled trial. Int J Eat Disord 2016;49:1068-76. https://doi.org/10.1002/eat.22587.
- Andersson E, Ljotsson B, Hedman E, Mattson S, Enander J, Andersson G, et al. Cost-effectiveness of an internet-based booster program for patients with obsessive-compulsive disorder: results from a randomized controlled trial. J Obsessive-Compuls Relat Disord 2015;4:14-9. https://doi.org/10.1016/j.jocrd.2014.10.002.
- Axelsson E, Andersson E, Ljótsson B, Hedman-Lagerlöf E. Cost-effectiveness and long-term follow-up of three forms of minimal-contact cognitive behaviour therapy for severe health anxiety: results from a randomised controlled trial. Behav Res Ther 2018;107:95-105. https://doi.org/10.1016/j.brat.2018.06.002.
- Bergman Nordgren L, Hedman E, Etienne J, Bodin J, Kadowaki A, Eriksson S, et al. Effectiveness and cost-effectiveness of individually tailored internet-delivered cognitive behavior therapy for anxiety disorders in a primary care population: a randomized controlled trial. Behav Res Ther 2014;59:1-11. https://doi.org/10.1016/j.brat.2014.05.007.
- Bergström J, Andersson G, Ljótsson B, Rück C, Andréewitch S, Karlsson A, et al. Internet-versus group-administered cognitive behaviour therapy for panic disorder in a psychiatric setting: a randomised trial. BMC Psychiatry 2010;10. https://doi.org/10.1186/1471-244X-10-54.
- Bolier L, Majo C, Smit F, Westerhof GJ, Haverman M, Walburg JA, et al. Cost-effectiveness of online positive psychology: randomized controlled trial. The J Posit Psychol 2014;9:460-71. https://doi.org/10.1080/17439760.2014.910829.
- Brabyn S, Araya R, Barkham M, Bower P, Coo per C, Duarte A, et al. The second Randomised Evaluation of the Effectiveness, cost-effectiveness and Acceptability of Computerised Therapy (REEACT-2) trial: does the provision of telephone support enhance the effectiveness of computer-delivered cognitive behaviour therapy? A randomised controlled trial. Health Technol Assess 2016;20. https://doi.org/10.3310/hta20890.
- Budney AJ, Stanger C, Tilford JM, Scherer EB, Brown PC, Li Z, et al. Computer-assisted behavioral therapy and contingency management for cannabis use disorder. Psychol Addict Behav 2015;29:501-11. https://doi.org/10.1037/adb0000078.
- Buntrock C, Berking M, Smit F, Lehr D, Nobis S, Ri per H, et al. Preventing depression in adults with subthreshold depression: health-economic evaluation alongside a pragmatic randomized controlled trial of a web-based intervention. J Med Internet Res 2017;19. https://doi.org/10.2196/jmir.6587.
- Burford O, Jiwa M, Carter O, Parsons R, Hendrie D. Internet-based photoaging within Australian pharmacies to promote smoking cessation: randomized controlled trial. J Med Internet Res 2013;15. https://doi.org/10.2196/jmir.2337.
- Calhoun PS, Datta S, Olsen M, Smith VA, Moore SD, Hair LP, et al. Comparative effectiveness of an internet-based smoking cessation intervention versus clinic-based specialty care for veterans. J Subst Abuse Treat 2016;69:19-27. https://doi.org/10.1016/j.jsat.2016.06.004.
- Dear BF, Zou JB, Ali S, Lorian CN, Johnston L, Sheehan J, et al. Clinical and cost-effectiveness of therapist-guided internet-delivered cognitive behavior therapy for older adults with symptoms of anxiety: a randomized controlled trial. Behav Ther 2015;46:206-17. https://doi.org/10.1016/j.beth.2014.09.007.
- Ebert DD, Kählke F, Buntrock C, Berking M, Smit F, Heber E, et al. A health economic outcome evaluation of an internet-based mobile-supported stress management intervention for employees. Scand J Work Environ Health 2018;44:171-82. https://doi.org/10.5271/sjweh.3691.
- Garrido G, Penadés R, Barrios M, Aragay N, Ramos I, Vallès V, et al. Computer-assisted cognitive remediation therapy in schizophrenia: durability of the effects and cost–utility analysis. Psychiatry Res 2017;254:198-204. https://doi.org/10.1016/j.psychres.2017.04.065.
- Geraedts AS, van Dongen JM, Kleiboer AM, Wiezer NM, van Mechelen W, Cuijpers P, et al. Economic evaluation of a web-based guided self-help intervention for employees with depressive symptoms: results of a randomized controlled trial. J Occup Environ Med 2015;57:666-75. https://doi.org/10.1097/JOM.0000000000000423.
- Gerhards SA, de Graaf LE, Jacobs LE, Severens JL, Huibers MJ, Arntz A, et al. Economic evaluation of online computerised cognitive–behavioural therapy without support for depression in primary care: randomised trial. Br J Psychiatry 2010;196:310-18. https://doi.org/10.1192/bjp.bp.109.065748.
- Graham AL, Chang Y, Fang Y, Cobb NK, Tinkelman DS, Niaura RS, et al. Cost-effectiveness of internet and telephone treatment for smoking cessation: an economic evaluation of the iQUITT Study. Tob Control 2013;22. https://doi.org/10.1136/tobaccocontrol-2012-050465.
- Guerriero C, Cairns J, Roberts I, Rodgers A, Whittaker R, Free C. The cost-effectiveness of smoking cessation support delivered by mobile phone text messaging: Txt2stop. Eur J Health Econ 2013;14:789-97. https://doi.org/10.1007/s10198-012-0424-5.
- Hedman E, Andersson E, Lindefors N, Andersson G, Rück C, Ljótsson B. Cost-effectiveness and long-term effectiveness of internet-based cognitive behaviour therapy for severe health anxiety. Psychol Med 2013;43:363-74. https://doi.org/10.1017/S0033291712001079.
- Hedman E, Andersson E, Ljótsson B, Andersson G, Rück C, Lindefors N. Cost-effectiveness of internet-based cognitive behavior therapy vs. cognitive behavioral group therapy for social anxiety disorder: results from a randomized controlled trial. Behav Res Ther 2011;49:729-36. https://doi.org/10.1016/j.brat.2011.07.009.
- Hedman E, Andersson E, Ljótsson B, Axelsson E, Lekander M. Cost effectiveness of internet-based cognitive behaviour therapy and behavioural stress management for severe health anxiety. BMJ Open 2016;6. https://doi.org/10.1136/bmjopen-2015-009327.
- Hollinghurst S, Peters TJ, Kaur S, Wiles N, Lewis G, Kessler D. Cost-effectiveness of therapist-delivered online cognitive-behavioural therapy for depression: randomised controlled trial. Br J Psychiatry 2010;197:297-304. https://doi.org/10.1192/bjp.bp.109.073080.
- Holst A, Björkelund C, Metsini A, Madsen JH, Hange D, Petersson EL, et al. Cost-effectiveness analysis of internet-mediated cognitive behavioural therapy for depression in the primary care setting: results based on a controlled trial. BMJ Open 2018;8. https://doi.org/10.1136/bmjopen-2017-019716.
- Hunter R, Wallace P, Struzzo P, Vedova RD, Scafuri F, Tersar C, et al. Randomised controlled non-inferiority trial of primary care-based facilitated access to an alcohol reduction website: cost-effectiveness analysis. BMJ Open 2017;7. https://doi.org/10.1136/bmjopen-2016-014577.
- Joesch JM, Sherbourne CD, Sullivan G, Stein MB, Craske MG, Roy-Byrne P. Incremental benefits and cost of coordinated anxiety learning and management for anxiety treatment in primary care. Psychol Med 2012;42:1937-48. https://doi.org/10.1017/S0033291711002893.
- Jolstedt M, Wahlund T, Lenhard F, Ljótsson B, Mataix-Cols D, Nord M, et al. Efficacy and cost-effectiveness of therapist-guided internet cognitive behavioural therapy for paediatric anxiety disorders: a single-centre, single-blind, randomised controlled trial. Lancet Child Adolesc Health 2018;2:792-801. https://doi.org/10.1016/S2352-4642(18)30275-X.
- Jones DJ, Forehand R, Cuellar J, Parent J, Honeycutt A, Khavjou O, et al. Technology-enhanced program for child disruptive behavior disorders: development and pilot randomized control trial. J Clin Child Adolesc Psychol 2014;43:88-101. https://doi.org/10.1080/15374416.2013.822308.
- Jones RB, Atkinson JM, Coia DA, Paterson L, Morton AR, McKenna K, et al. Randomised trial of personalised computer based information for patients with schizophrenia. BMJ 2001;322:835-40. https://doi.org/10.1136/bmj.322.7290.835.
- Kass AE, Balantekin KN, Fitzsimmons-Craft EE, Jacobi C, Wilfley DE, Taylor CB. The economic case for digital interventions for eating disorders among United States college students. Int J Eat Disord 2017;50:250-8. https://doi.org/10.1002/eat.22680.
- Kenter RMF, van de Ven PM, Cuijpers P, Koole G, Niamat S, Gerrits RS, et al. Costs and effects of internet cognitive behavioral treatment blended with face-to-face treatment: results from a naturalistic study. Internet Interventions 2015;2:77-83. https://doi.org/10.1016/j.invent.2015.01.001.
- Kiluk BD, Devore KA, Buck MB, Nich C, Frankforter TL, LaPaglia DM, et al. Randomized trial of computerized cognitive behavioral therapy for alcohol use disorders: efficacy as a virtual stand-alone and treatment add-on compared with standard outpatient treatment. Alcohol Clin Exp Res 2016;40:1991-2000. https://doi.org/10.1111/acer.13162.
- Koeser L, Dobbin A, Ross S, McCrone P. Economic evaluation of audio based resilience training for depression in primary care. J Affect Disord 2013;149:307-12. https://doi.org/10.1016/j.jad.2013.01.044.
- Kolovos S, Kenter RM, Bosmans JE, Beekman AT, Cuijpers P, Kok RN, et al. Economic evaluation of internet-based problem-solving guided self-help treatment in comparison with enhanced usual care for depressed outpatients waiting for face-to-face treatment: a randomized controlled trial. J Affect Disord 2016;200:284-92. https://doi.org/10.1016/j.jad.2016.04.025.
- König HH, Bleibler F, Friederich HC, Herpertz S, Lam T, Mayr A, et al. Economic evaluation of cognitive behavioral therapy and internet-based guided self-help for binge-eating disorder. Int J Eat Disord 2018;51:155-64. https://doi.org/10.1002/eat.22822.
- Kraepelien M, Mattsson S, Hedman-Lagerlöf E, Petersson IF, Forsell Y, Lindefors N, et al. Cost-effectiveness of internet-based cognitive-behavioural therapy and physical exercise for depression. BJPsych Open 2018;4:265-73. https://doi.org/10.1192/bjo.2018.38.
- Kumar S, Jones Bell M, Juusola JL. Mobile and traditional cognitive behavioral therapy programs for generalized anxiety disorder: a cost-effectiveness analysis. PLOS ONE 2018;13. https://doi.org/10.1371/journal.pone.0190554.
- Lee YC, Gao L, Dear BF, Titov N, Mihalopoulos C. The cost-effectiveness of the Online MindSpot Clinic for the treatment of depression and anxiety in Australia. J Ment Health Policy Econ 2017;20:155-66.
- Lee YY, Barendregt JJ, Stockings EA, Ferrari AJ, Whiteford HA, Patton GA, et al. The population cost-effectiveness of delivering universal and indicated school-based interventions to prevent the onset of major depression among youth in Australia. Epidemiol Psychiatr Sci 2017;26:545-64. https://doi.org/10.1017/S2045796016000469.
- Lenhard F, Ssegonja R, Andersson E, Feldman I, Rück C, Mataix-Cols D, et al. Cost-effectiveness of therapist-guided internet-delivered cognitive behaviour therapy for paediatric obsessive-compulsive disorder: results from a randomised controlled trial. BMJ Open 2017;7. https://doi.org/10.1136/bmjopen-2016-015246.
- Littlewood E, Duarte A, Hewitt C, Knowles S, Palmer S, Walker S, et al. A randomised controlled trial of computerised cognitive behaviour therapy for the treatment of depression in primary care: the Randomised Evaluation of the Effectiveness and Acceptability of Computerised Therapy (REEACT) trial. Health Technol Assess 2015;19. https://doi.org/10.3310/hta191010.
- McCrone P, Marks IM, Mataix-Cols D, Kenwright M, McDonough M. Computer-aided self-exposure therapy for phobia/panic disorder: a pilot economic evaluation. Cogn Behav Ther 2009;38:91-9. https://doi.org/10.1080/16506070802561074.
- Mihalopoulos C, Kiropoulos L, Shih ST, Gunn J, Blashki G, Meadows G. Exploratory economic analyses of two primary care mental health projects: implications for sustainability. Med J Aust 2005;183:S73-6. https://doi.org/10.5694/j.1326-5377.2005.tb07184.x.
- Murphy SM, Campbell AN, Ghitza UE, Kyle TL, Bailey GL, Nunes EV, et al. Cost-effectiveness of an internet-delivered treatment for substance abuse: data from a multisite randomized controlled trial. Drug Alcohol Depend 2016;161:119-26. https://doi.org/10.1016/j.drugalcdep.2016.01.021.
- Naughton F, Coo per S, Foster K, Emery J, Leonardi-Bee J, Sutton S, et al. Large multi-centre pilot randomized controlled trial testing a low-cost, tailored, self-help smoking cessation text message intervention for pregnant smokers (MiQuit). Addiction 2017;112:1238-49. https://doi.org/10.1111/add.13802.
- Naveršnik K, Mrhar A. Cost-effectiveness of a novel e-health depression service. Telemed J E Health 2013;19:110-16. https://doi.org/10.1089/tmj.2012.0081.
- Olmstead TA, Ostrow CD, Carroll KM. Cost-effectiveness of computer-assisted training in cognitive-behavioral therapy as an adjunct to standard care for addiction. Drug Alcohol Depend 2010;110:200-7. https://doi.org/10.1016/j.drugalcdep.2010.02.022.
- Phillips R, Schneider J, Molosankwe I, Leese M, Foroushani PS, Grime P, et al. Randomized controlled trial of computerized cognitive behavioural therapy for depressive symptoms: effectiveness and costs of a workplace intervention. Psychol Med 2014;44:741-52. https://doi.org/10.1017/S0033291713001323.
- Romero-Sanchiz P, Nogueira-Arjona R, García-Ruiz A, Luciano JV, García Campayo J, Gili M, et al. Economic evaluation of a guided and unguided internet-based CBT intervention for major depression: results from a multi-center, three-armed randomized controlled trial conducted in primary care. PLOS ONE 2017;12. https://doi.org/10.1371/journal.pone.0172741.
- Smit ES, Evers SM, de Vries H, Hoving C. Cost-effectiveness and cost-utility of internet-based computer tailoring for smoking cessation. J Med Internet Res 2013;15. https://doi.org/10.2196/jmir.2059.
- Solomon D, Proudfoot J, Clarke J, Christensen H. e-CBT (myCompass), antidepressant medication, and face-to-face psychological treatment for depression in Australia: a cost-effectiveness comparison. J Med Internet Res 2015;17. https://doi.org/10.2196/jmir.4207.
- Španiel F, Hrdlička J, Novák T, Kožený J, Höschl C, Mohr P, et al. Effectiveness of the information technology-aided program of relapse prevention in schizophrenia (ITAREPS): a randomized, controlled, double-blind study. J Psychiatr Pract 2012;18:269-80. https://doi.org/10.1097/01.pra.0000416017.45591.c1.
- Stanczyk NE, Smit ES, Schulz DN, de Vries H, Bolman C, Muris JW, et al. An economic evaluation of a video- and text-based computer-tailored intervention for smoking cessation: a cost-effectiveness and cost-utility analysis of a randomized controlled trial. PLOS ONE 2014;9. https://doi.org/10.1371/journal.pone.0110117.
- Titov N, Andrews G, Johnston L, Schwencke G, Choi I. Shyness programme: longer term benefits, cost-effectiveness, and acceptability. Aust N Z J Psychiatry 2009;43:36-44. https://doi.org/10.1080/00048670802534424.
- Titov N, Dear BF, Ali S, Zou JB, Lorian CN, Johnston L, et al. Clinical and cost-effectiveness of therapist-guided internet-delivered cognitive behavior therapy for older adults with symptoms of depression: a randomized controlled trial. Behav Ther 2015;46:193-205. https://doi.org/10.1016/j.beth.2014.09.008.
- van Spijker BA, Majo MC, Smit F, van Straten A, Kerkhof AJ. Reducing suicidal ideation: cost-effectiveness analysis of a randomized controlled trial of unguided web-based self-help. J Med Internet Res 2012;14. https://doi.org/10.2196/jmir.1966.
- Warmerdam L, Smit F, van Straten A, Ri per H, Cuijpers P. Cost-utility and cost-effectiveness of internet-based treatment for adults with depressive symptoms: randomized trial. J Med Internet Res 2010;12. https://doi.org/10.2196/jmir.1436.
- Wijnen BF, Lokman S, Leone S, Evers SM, Smit F. Complaint-directed mini-interventions for depressive symptoms: a health economic evaluation of unguided web-based self-help interventions based on a randomized controlled trial. J Med Internet Res 2018;20. https://doi.org/10.2196/10455.
- Wright B, Tindall L, Littlewood E, Allgar V, Abeles P, Trépel D, et al. Computerised cognitive-behavioural therapy for depression in adolescents: feasibility results and 4-month outcomes of a UK randomised controlled trial. BMJ Open 2017;7. https://doi.org/10.1136/bmjopen-2016-012834.
- Wu Q, Parrott S, Godfrey C, Gilbert H, Nazareth I, Leurent B, et al. Cost-effectiveness of computer-tailored smoking cessation advice in primary care: a randomized trial (ESCAPE). Nicotine Tob Res 2014;16:270-8. https://doi.org/10.1093/ntr/ntt136.
- Duarte A, Walker S, Littlewood E, Brabyn S, Hewitt C, Gilbody S, et al. Cost-effectiveness of computerized cognitive-behavioural therapy for the treatment of depression in primary care: findings from the Randomised Evaluation of the Effectiveness and Acceptability of Computerised Therapy (REEACT) trial. Psychol Med 2017;47:1825-35. https://doi.org/10.1017/S0033291717000289.
- El Alaoui S, Hedman-Lagerlöf E, Ljótsson B, Lindefors N. Does internet-based cognitive behaviour therapy reduce healthcare costs and resource use in treatment of social anxiety disorder? A cost-minimisation analysis conducted alongside a randomised controlled trial. BMJ Open 2017;7. https://doi.org/10.1136/bmjopen-2017-017053.
- Hedman E, El Alaoui S, Lindefors N, Andersson E, Rück C, Ghaderi A, et al. Clinical effectiveness and cost-effectiveness of internet- vs. group-based cognitive behavior therapy for social anxiety disorder: 4-year follow-up of a randomized trial. Behav Res Ther 2014;59:20-9. https://doi.org/10.1016/j.brat.2014.05.010.
- Kählke F, Buntrock C, Smit F, Berking M, Lehr D, Heber E, et al. Economic evaluation of an internet-based stress management intervention alongside a randomized controlled trial. JMIR Ment Health 2019;6. https://doi.org/10.2196/10866.
- Baumann M, Stargardt T, Frey S. Cost-utility of internet-based cognitive behavioral therapy in unipolar depression: a Markov model simulation. Appl Health Econ Health Policy 2020;18:567-78. https://doi.org/10.1007/s40258-019-00551-x.
- Gräfe V, Berger T, Hautzinger M, Hohagen F, Lutz W, Meyer B, et al. Health economic evaluation of a web-based intervention for depression: the EVIDENT-trial, a randomized controlled study. Health Econ Rev 2019;9. https://doi.org/10.1186/s13561-019-0233-y.
- Klein NS, Bockting CL, Wijnen B, Kok GD, van Valen E, Ri per H, et al. Economic evaluation of an internet-based preventive cognitive therapy with minimal therapist support for recurrent depression: randomized controlled trial. J Med Internet Res 2018;20. https://doi.org/10.2196/10437.
- Kooistra LC, Wiersma JE, Ruwaard J, Neijenhuijs K, Lokkerbol J, van Oppen P, et al. Cost and effectiveness of blended versus standard cognitive behavioral therapy for outpatients with depression in routine specialized mental health care: pilot randomized controlled trial. J Med Internet Res 2019;21. https://doi.org/10.2196/14261.
- Lindsäter E, Axelsson E, Salomonsson S, Santoft F, Ljótsson B, Åkerstedt T, et al. Cost-effectiveness of therapist-guided internet-based cognitive behavioral therapy for stress-related disorders: secondary analysis of a randomized controlled trial. J Med Internet Res 2019;21. https://doi.org/10.2196/14675.
- O’Connor K, Bagnell A, McGrath P, Wozney L, Radomski A, Rosychuk RJ, et al. An internet-based cognitive behavioral program for adolescents with anxiety: pilot randomized controlled trial. JMIR Ment Health 2020;7. https://doi.org/10.2196/13356.
- Osborne D, Meyer D, Moulding R, Kyrios M, Bailey E, Nedeljkovic M. Cost-effectiveness of internet-based cognitive-behavioural therapy for obsessive-compulsive disorder. Internet Interv 2019;18. https://doi.org/10.1016/j.invent.2019.100277.
- Pot-Kolder R, Veling W, Geraets C, Lokkerbol J, Smit F, Jongeneel A, et al. Cost-effectiveness of virtual reality cognitive behavioral therapy for psychosis: health-economic evaluation within a randomized controlled trial. J Med Internet Res 2020;22. https://doi.org/10.2196/17098.
- Powell J, Williams V, Atherton H, Bennett K, Yang Y, Davoudianfar M, et al. Effectiveness and cost-effectiveness of a self-guided internet intervention for social anxiety symptoms in a general population sample: randomized controlled trial. J Med Internet Res 2020;22. https://doi.org/10.2196/16804.
- Richards D, Enrique A, Eilert N, Franklin M, Palacios J, Duffy D, et al. A pragmatic randomized waitlist-controlled effectiveness and cost-effectiveness trial of digital interventions for depression and anxiety. NPJ Digit Med 2020;3. https://doi.org/10.1038/s41746-020-0293-8.
- Kolovos S, Van Dongen JM, Ri per H, Van Tulder MW, Bosmans JE. Cost-effectiveness of guided internet-based treatments for depression in comparison with control conditions: an individual-participant data meta-analysis. Value Health 2017;20:PA714-A715. https://doi.org/10.1016/j.jval.2017.08.1898.
- Saramago P, Gega L, Marshall D, Nikolaidis GF, Jankovic D, Melton H, et al. Digital interventions for generalised anxiety disorder (GAD): systematic review and network meta-analysis. Front Psychiatry 2021;12. https://doi.org/10.3389/fpsyt.2021.726222.
- McManus S, Bebbington P, Jenkins R, Brugha T. Mental Health and Wellbeing in England: Adult Psychiatric Morbidity Survey 2014. A Survey Carried Out for NHS Digital by NatCen Social Research and the Department of Health Sciences. Leicester: University of Leicester; 2016.
- Revicki DA, Travers K, Wyrwich KW, Svedsäter H, Locklear J, Mattera MS, et al. Humanistic and economic burden of generalized anxiety disorder in North America and Europe. J Affect Disord 2012;140:103-12. https://doi.org/10.1016/j.jad.2011.11.014.
- Hunot V, Churchill R, Silva de Lima M, Teixeira V. Psychological therapies for generalised anxiety disorder. Cochrane Database Syst Rev 2007;1. https://doi.org/10.1002/14651858.CD001848.pub4.
- Hayes-Skelton SA, Roemer L, Orsillo SM, Borkovec TD. A contemporary view of applied relaxation for generalized anxiety disorder. Cogn Behav Ther 2013;42:292-30. https://doi.org/10.1080/16506073.2013.777106.
- Slee A, Nazareth I, Bondaronek P, Liu Y, Cheng Z, Freemantle N. Pharmacological treatments for generalised anxiety disorder: a systematic review and network meta-analysis. Lancet 2019;393:768-77. https://doi.org/10.1016/S0140-6736(18)31793-8.
- Aboujaoude E, Gega L, Parish MB, Hilty DM. Editorial: digital interventions in mental health: current status and future directions. Front Psychiatry 2020;11. https://doi.org/10.3389/fpsyt.2020.00111.
- Cuijpers P, Sijbrandij M, Koole SL, Andersson G, Beekman AT, Reynolds CF. Adding psychotherapy to antidepressant medication in depression and anxiety disorders: a meta-analysis. World Psychiatry 2014;13:56-67. https://doi.org/10.1002/wps.20089.
- Richards D, Richardson T, Timulak L, McElvaney J. The efficacy of internet-delivered treatment for generalized anxiety disorder: a systematic review and meta-analysis. Internet Interven 2015;2:272-82. https://doi.org/10.1016/j.invent.2015.07.003.
- Lumley T. Network meta-analysis for indirect treatment comparisons. Stat Med 2002;21:2313-24. https://doi.org/10.1002/sim.1201.
- Lu G, Ades AE. Combination of direct and indirect evidence in mixed treatment comparisons. Stat Med 2004;23:3105-24. https://doi.org/10.1002/sim.1875.
- Caldwell DM, Ades AE, Higgins JP. Simultaneous comparison of multiple treatments: combining direct and indirect evidence. BMJ 2005;331:897-900. https://doi.org/10.1136/bmj.331.7521.897.
- Cooper NJ, Peters J, Lai MC, Juni P, Wandel S, Palmer S, et al. How valuable are multiple treatment comparison methods in evidence-based health-care evaluation?. Value Health 2011;14:371-80. https://doi.org/10.1016/j.jval.2010.09.001.
- Sterne JAC, Savović J, Page MJ, Elbers RG, Blencowe NS, Boutron I, et al. RoB 2: a revised tool for assessing risk of bias in randomised trials. BMJ 2019;366. https://doi.org/10.1136/bmj.l4898.
- Dias S, Sutton AJ, Ades AE, Welton NJ. Evidence synthesis for decision making 2: a generalized linear modelling framework for pairwise and network meta-analysis of randomized controlled trials. Med Decis Making 2013;33:607-17. https://doi.org/10.1177/0272989X12458724.
- Dias S, Welton NJ, Sutton AJ, Ades AE. NICE DSU Technical Support Document 2: A Generalised Linear Modelling Framework for Pairwise and Network Meta-Analysis of Randomised Controlled Trials. London: National Institute for Health and Care Excellence; 2014.
- Higgins JPT. Cochrane Handbook for Systematic Reviews of Interventions. Hoboken, NJ: Wiley-Blackwell; 2020.
- Riley RD, Kauser I, Bland M, Thijs L, Staessen JA, Wang J, et al. Meta-analysis of randomised trials with a continuous outcome according to baseline imbalance and availability of individual participant data. Stat Med 2013;32:2747-66. https://doi.org/10.1002/sim.5726.
- van Breukelen GJ. ANCOVA versus CHANGE from baseline in nonrandomized studies: the difference. Multivariate Behav Res 2013;48:895-922. https://doi.org/10.1080/00273171.2013.831743.
- Winkens B, van Breukelen GJ, Schouten HJ, Berger MP. Randomized clinical trials with a pre- and a post-treatment measurement: repeated measures versus ANCOVA models. Contemp Clin Trials 2007;28:713-19. https://doi.org/10.1016/j.cct.2007.04.002.
- Fu R, Vandermeer BW, Shamliyan TA, O’Neil ME, Yazdi F, Fox SH, et al. Methods Guide for Effectiveness and Comparative Effectiveness Reviews. Rockville, MD: Agency for Healthcare Research and Quality; 2008.
- Vickers AJ. The use of percentage change from baseline as an outcome in a controlled trial is statistically inefficient: a simulation study. BMC Med Res Methodol 2001;1. https://doi.org/10.1186/1471-2288-1-6.
- Vickers AJ, Altman DG. Statistics notes: analysing controlled trials with baseline and follow up measurements. BMJ 2001;323:1123-4. https://doi.org/10.1136/bmj.323.7321.1123.
- Deeks JJ, Higgins JPT, Altman DG, Higgins JPT, Thomas J, Chandler J, et al. Cochrane Handbook for Systematic Reviews of Interventions. Version 5.0.1 (Updated September 2008). 2008.
- Lunn DJ, Thomas A, Best N, Spiegelhalter D. WinBUGS – a Bayesian modelling framework: concepts, structure, and extensibility. Stat Computing 2000;10:325-37. https://doi.org/10.1023/A:1008929526011.
- Sturtz S, Ligges U, Gelman A. R2WinBUGS: a package for running WinBUGS from R. J Stat Softw 2005;12:1-16. https://doi.org/10.18637/jss.v012.i03.
- Brooks S, Gelman A. Some issues in monitoring convergence of iterative simulations. Dimension Reduct Computat Complex Info 1998;30:30-6.
- Brooks S, Gelman A. General methods for monitoring convergence of iterative simulations. J Comput Graph Stat 1998;7:434-55. https://doi.org/10.2307/1390675.
- Gelman A, Rubin DB. Inference from iterative simulation using multiple sequences. Stat Sci 1992;7:457-72. https://doi.org/10.2307/2246093.
- Spiegelhalter DJ, Best NG, Carlin BR, van der Linde A. Bayesian measures of model complexity and fit. J R Stat Soc Series B Stat Methodol 2002;64:583-616. https://doi.org/10.1111/1467-9868.00353.
- Saramago P, Woods B, Weatherly H, Manca A, Sculpher M, Khan K, et al. Methods for network meta-analysis of continuous outcomes using individual patient data: a case study in acupuncture for chronic pain. BMC Med Res Methodol 2016;16. https://doi.org/10.1186/s12874-016-0224-1.
- Ades AE, Sculpher M, Sutton A, Abrams K, Coo per N, Welton N, et al. Bayesian methods for evidence synthesis in cost-effectiveness analysis. PharmacoEconomics 2006;24:1-19. https://doi.org/10.2165/00019053-200624010-00001.
- Dias S, Welton NJ, Sutton AJ, Caldwell DM, Lu G, Ades AE. Evidence synthesis for decision making 4: inconsistency in networks of evidence based on randomized controlled trials. Med Decis Making 2013;33:641-56. https://doi.org/10.1177/0272989X12455847.
- Dias S, Welton NJ, Sutton AJ, Caldwell DM, Lu G, Ades AE. NICE DSU Technical Support Document 4: Inconsistency in Networks of Evidence Based on Randomised Controlled Trials. London: National Institute for Health and Care Excellence; 2014.
- Andersson G, Paxling B, Roch-Norlund P, Östman G, Norgren A, Almlöv J, et al. Internet-based psychodynamic versus cognitive behavioral guided self-help for generalized anxiety disorder: a randomized controlled trial. Psychother Psychosom 2012;81:344-55. https://doi.org/10.1159/000339371.
- Christensen H, Mackinnon AJ, Batterham PJ, O’Dea B, Guastella AJ, Griffiths KM, et al. The effectiveness of an online e-health application compared to attention placebo or sertraline in the treatment of generalised anxiety disorder. Internet Interv 2014;1:169-74. https://doi.org/10.1016/j.invent.2014.08.002.
- Dear BF, Staples LG, Terides MD, Karin E, Zou J, Johnston L, et al. Transdiagnostic versus disorder-specific and clinician-guided versus self-guided internet-delivered treatment for generalized anxiety disorder and comorbid disorders: a randomized controlled trial. J Anxiety Disord 2015;36:63-77. https://doi.org/10.1016/j.janxdis.2015.09.003.
- Hazen RA, Vasey MW, Schmidt NB. Attentional retraining: a randomized clinical trial for pathological worry. J Psychiatr Res 2009;43:627-33. https://doi.org/10.1016/j.jpsychires.2008.07.004.
- Hirsch CR, Krahé C, Whyte J, Loizou S, Bridge L, Norton S, et al. Interpretation training to target repetitive negative thinking in generalized anxiety disorder and depression. J Consult Clin Psychol 2018;86:1017-30. https://doi.org/10.1037/ccp0000310.
- Howell AN, Rheingold AA, Uhde TW, Guille C. Web-based CBT for the prevention of anxiety symptoms among medical and health science graduate students. Cogn Behav Ther 2019;48:385-40. https://doi.org/10.1080/16506073.2018.1533575.
- Jones SL, Hadjistavropoulos HD, Soucy JN. A randomized controlled trial of guided internet-delivered cognitive behaviour therapy for older adults with generalized anxiety. J Anxiety Disord 2016;37:1-9. https://doi.org/10.1016/j.janxdis.2015.10.006.
- Navarro-Haro MV, Modrego-Alarcón M, Hoffman HG, López-Montoyo A, Navarro-Gil M, Montero-Marin J, et al. Evaluation of a mindfulness-based intervention with and without Virtual Reality Dialectical Behavior Therapy® mindfulness skills training for the treatment of generalized anxiety disorder in primary care: a pilot study. Front Psychol 2019;10. https://doi.org/10.3389/fpsyg.2019.00055.
- Paxling B, Almlöv J, Dahlin M, Carlbring P, Breitholtz E, Eriksson T, et al. Guided internet-delivered cognitive behavior therapy for generalized anxiety disorder: a randomized controlled trial. Cogn Behav Ther 2011;40:159-73. https://doi.org/10.1080/16506073.2011.576699.
- Repetto C, Gaggioli A, Pallavicini F, Cipresso P, Raspelli S, Riva G. Virtual reality and mobile phones in the treatment of generalized anxiety disorders: a phase-2 clinical trial. Pers Ubiquitous Comput 2013;17:253-60. https://doi.org/10.1007/s00779-011-0467-0.
- Richards D, Timulak L, Rashleigh C, McLoughlin O, Colla A, Joyce C, et al. Effectiveness of an internet-delivered intervention for generalized anxiety disorder in routine care: a randomised controlled trial in a student population. Internet Interv 2016;6:80-8. https://doi.org/10.1016/j.invent.2016.10.003.
- Robinson E, Titov N, Andrews G, McIntyre K, Schwencke G, Solley K. Internet treatment for generalized anxiety disorder: a randomized controlled trial comparing clinician vs. technician assistance. PLOS ONE 2010;5. https://doi.org/10.1371/journal.pone.0010942.
- Titov N, Andrews G, Johnston L, Robinson E, Spence J. Transdiagnostic internet treatment for anxiety disorders: a randomized controlled trial. Behav Res Ther 2010;48:890-9. https://doi.org/10.1016/j.brat.2010.05.014.
- Titov N, Andrews G, Robinson E, Schwencke G, Johnston L, Solley K, et al. Clinician-assisted internet-based treatment is effective for generalized anxiety disorder: randomized controlled trial. Aust N Z J Psychiatry 2009;43:905-12. https://doi.org/10.1080/00048670903179269.
- Topper M, Emmelkamp PMG, Watkins E, Ehring T. Prevention of anxiety disorders and depression by targeting excessive worry and rumination in adolescents and young adults: a randomized controlled trial. Behav Res Ther 2017;90:123-36. https://doi.org/10.1016/j.brat.2016.12.015.
- Gorini A, Pallavicini F, Algeri D, Repetto C, Gaggioli A, Riva G. Virtual reality in the treatment of generalized anxiety disorders. Stud Health Technol Inform 2010;154:39-43.
- Gorini A, Pallavicini F, Algeri D, Repetto C, Gaggioli A, Riva G. Virtual reality in the treatment of generalized anxiety disorders. Annu Rev CyberTherapy Telemed 2010;8:31-5.
- Pallavicini F, Algeri D, Repetto C, Gorini A, Riva G. Biofeedback, virtual reality and mobile phones in the treatment of generalized anxiety disorder (GAD): a phase-2 controlled clinical trial. J Cyber Ther Rehabil 2009;2:315-27.
- Lorian CN, Titov N, Grisham JR. Changes in risk-taking over the course of an internet-delivered cognitive behavioral therapy treatment for generalized anxiety disorder. J Anxiety Disord 2012;26:140-9. https://doi.org/10.1016/j.janxdis.2011.10.003.
- Sheehan DV, Lecrubier Y, Sheehan KH, Amorim P, Janavs J, Weiller E, et al. The Mini-International Neuropsychiatric Interview (M.I.N.I.): the development and validation of a structured diagnostic psychiatric interview for DSM-IV and ICD-10. J Clin Psychiatry 1998;59:22-33.
- Spitzer RL, Kroenke K, Williams JB, Löwe B. A brief measure for assessing generalized anxiety disorder: the GAD-7. Arch Intern Med 2006;166:1092-7. https://doi.org/10.1001/archinte.166.10.1092.
- Meyer TJ, Miller ML, Metzger RL, Borkovec TD. Development and validation of the Penn State Worry Questionnaire. Behav Res Ther 1990;28:487-95. https://doi.org/10.1016/0005-7967(90)90135-6.
- Kroenke K, Spitzer RL. The PHQ-9: A new depression diagnostic and severity measure. Psychiatr Ann 2002;32:509-15. https://doi.org/10.3928/0048-5713-20020901-06.
- Beck A, Steer R, Brown G. Manual for Beck Depression Inventory-II (BDI-II). San Antonio, TX: Psychology Corporation; 1996.
- Maier W, Buller R, Philipp M, Heuser I. The Hamilton Anxiety Scale: reliability, validity and sensitivity to change in anxiety and depressive disorders. J Affect Disord 1988;14:61-8. https://doi.org/10.1016/0165-0327(88)90072-9.
- Barlow DH. Anxiety and its Disorders: The Nature and Treatment of Anxiety and Panic. New York, NY: Guilford Press; 2002.
- Latimer NR, Abrams KR, Lambert PC, Crowther MJ, Wailoo AJ, Morden JP, et al. Adjusting survival time estimates to account for treatment switching in randomized controlled trials – an economic evaluation context: methods, limitations, and recommendations. Med Decis Making 2014;34:387-402. https://doi.org/10.1177/0272989X13520192.
- Cuij pers P, Donker T, van Straten A, Li J, Andersson G. Is guided self-help as effective as face-to-face psychotherapy for depression and anxiety disorders? A systematic review and meta-analysis of comparative outcome studies. Psychol Med 2010;40:1943-57. https://doi.org/10.1017/S0033291710000772.
- Spek V, Cuij pers P, Nyklícek I, Ri per H, Keyzer J, Pop V. Internet-based cognitive behaviour therapy for symptoms of depression and anxiety: a meta-analysis. Psychol Med 2007;37:319-28. https://doi.org/10.1017/S0033291706008944.
- Shim M, Mahaffey B, Bleidistel M, Gonzalez A. A scoping review of human-support factors in the context of internet-based psychological interventions (IPIs) for depression and anxiety disorders. Clin Psychol Rev 2017;57:129-40. https://doi.org/10.1016/j.cpr.2017.09.003.
- Dear BF, Titov N, Sunderland M, McMillan D, Anderson T, Lorian C, et al. Psychometric comparison of the Generalized Anxiety Disorder scale-7 and the Penn State Worry Questionnaire for measuring response during treatment of generalised anxiety disorder. Cogn Behav Ther 2011;40:216-27. https://doi.org/10.1080/16506073.2011.582138.
- Jankovic D, Saramago PG, Gega L, Marshall D, Wright K, Hafidh M, et al. Cost Effectiveness of Digital Interventions for Generalised Anxiety Disorder: A Model-Based Analysis. PharmacoEconomics Open 2021. https://doi.org/10.1007/s41669-021-00318-y.
- Yonkers KA, Warshaw MG, Massion AO, Keller MB. Phenomenology and course of generalised anxiety disorder. Br J Psychiatry 1996;168:308-13. https://doi.org/10.1192/bjp.168.3.308.
- Ruiz MA, Zamorano E, García-Campayo J, Pardo A, Freire O, Rejas J. Validity of the GAD-7 scale as an outcome measure of disability in patients with generalized anxiety disorders in primary care. J Affect Disord 2011;128:277-86. https://doi.org/10.1016/j.jad.2010.07.010.
- Willan AR, Briggs AH. Statistical Analysis of Cost-effectiveness Data. Hoboken, NY: John Wiley & Sons, Inc.; 2006.
- Kind P, Hardman G, Macran S. UK Population Norms for EQ-5D (Working Papers 172). York: Centre for Health Economics, University of York; 1999.
- Vera-Llonch M, Dukes E, Rejas J, Sofrygin O, Mychaskiw M, Oster G. Cost-effectiveness of pregabalin versus venlafaxine in the treatment of generalized anxiety disorder: findings from a Spanish perspective. Eur J Health Econ 2010;11:35-44. https://doi.org/10.1007/s10198-009-0160-7.
- Office for National Statistics . Consumer Price Inflation, UK: May 2015 2015. www.ons.gov.uk/economy/inflationandpriceindices/bulletins/consumerpriceinflation/2015-06-16 (accessed 3 April 2020).
- Office for National Statistics . Consumer Price Inflation, UK: May 2019 2019. www.ons.gov.uk/economy/inflationandpriceindices/bulletins/consumerpriceinflation/may2019 (accessed 3 April 2020).
- Office for National Statistics . National Life Tables: UK 2019. www.ons.gov.uk/peoplepopulationandcommunity/birthsdeathsandmarriages/lifeexpectancies/datasets/nationallifetablesunitedkingdomreferencetables (accessed 3 April 2020).
- Michal M, Prochaska JH, Keller K, Göbel S, Coldewey M, Ullmann A, et al. Symptoms of depression and anxiety predict mortality in patients undergoing oral anticoagulation: results from the thrombEVAL study program. Int J Cardiol 2015;187:614-19. https://doi.org/10.1016/j.ijcard.2015.03.374.
- Pharmaceutical Services Negotiating Committee . Professional Fees (Drug Tariff Part IIIA) 2019. https://psnc.org.uk/dispensing-supply/endorsement/fees-allowances/ (accessed 3 April 2020).
- OpenPrescribing.net, EDM DataLab, University of Oxford. OpenPrescribing.Net 2020. https://OpenPrescribing.net (accessed March 2020).
- NHS Improvement . National Cost Collection 2018 19 2019. https://improvement.nhs.uk/resources/national-cost-collection/ (accessed 3 April 2020).
- Claxton KP, Sculpher MJ. Using value of information analysis to prioritise health research: some lessons from recent UK experience. PharmacoEconomics 2006;24:1055-68. https://doi.org/10.2165/00019053-200624110-00003.
- Walters K, Rait G, Griffin M, Buszewicz M, Nazareth I. Recent trends in the incidence of anxiety diagnoses and symptoms in primary care. PLOS ONE 2012;7. https://doi.org/10.1371/journal.pone.0041670.
- Beesdo K, Pine DS, Lieb R, Wittchen HU. Incidence and risk patterns of anxiety and depressive disorders and categorization of generalized anxiety disorder. Arch Gen Psychiatry 2010;67:47-5. https://doi.org/10.1001/archgenpsychiatry.2009.177.
- Strong M, Oakley JE, Brennan A. Estimating multiparameter partial expected value of perfect information from a probabilistic sensitivity analysis sample: a nonparametric regression approach. Med Decis Making 2014;34:311-26. https://doi.org/10.1177/0272989X13505910.
- Claxton K, Martin S, Soares M, Rice N, Spackman E, Hinde S, et al. Methods for the estimation of the National Institute for Health and Care Excellence cost-effectiveness threshold. Health Technol Assess 2015;19. https://doi.org/10.3310/hta19140.
- von Winterfeldt D. Bridging the gap between science and decision making. Proc Natl Acad Sci USA 2013;110:14055-61. https://doi.org/10.1073/pnas.1213532110.
- Bruine de Bruin W, Bostrom A. Assessing what to address in science communication. Proc Natl Acad Sci USA 2013;110:14062-8. https://doi.org/10.1073/pnas.1212729110.
- Braun V, Clarke V. Using thematic analysis in psychology. Qual Res Psychol 2006;3:77-101. https://doi.org/10.1191/1478088706qp063oa.
- Whyte S, Dixon S, Faria R, Walker S, Palmer S, Sculpher M, et al. Estimating the cost-effectiveness of implementation: is sufficient evidence available?. Value Health 2016;19:138-44. https://doi.org/10.1016/j.jval.2015.12.009.
- Berry K, Salter A, Morris R, James S, Bucci S. Assessing therapeutic alliance in the context of mHealth interventions for mental health problems: development of the Mobile Agnew Relationship Measure (mARM) Questionnaire. J Med Internet Res 2018;20. https://doi.org/10.2196/jmir.8252.
- Henson P, Wisniewski H, Hollis C, Keshavan M, Torous J. Digital mental health apps and the therapeutic alliance: initial review. BJPsych Open 2019;5. https://doi.org/10.1192/bjo.2018.86.
- Tremain H, McEnery C, Fletcher K, Murray G. The therapeutic alliance in digital mental health interventions for serious mental illnesses: narrative review. JMIR Ment Health 2020;7. https://doi.org/10.2196/17204.
- Black WC. The CE plane: a graphic representation of cost-effectiveness. Med Decis Making 1990;10:212-14. https://doi.org/10.1177/0272989X9001000308.
- NHS England . Five Year Forward View 2014. www.england.nhs.uk/wp-content/uploads/2014/10/5yfv-web.pdf (accessed 31 January 2018).
- National Information Board . Personalised Health and Care 2020: Using Data and Technology to Transform Outcomes for Patients and Citizens: A Framework for Action 2014.
- Craig P, Dieppe P, Macintyre S, Michie S, Nazareth I, Petticrew M. Medical Research Council guidance . Developing and evaluating complex interventions: the new Medical Research Council guidance. BMJ 2008;337. https://doi.org/10.1136/bmj.a1655.
- Cooper NJ, Sutton AJ, Morris D, Ades AE, Welton NJ. Addressing between-study heterogeneity and inconsistency in mixed treatment comparisons: application to stroke prevention treatments in individuals with non-rheumatic atrial fibrillation. Stat Med 2009;28:1861-81. https://doi.org/10.1002/sim.3594.
Appendix 1 Methods for statistical analysis and synthesis model
Reproduced with permission from Saramago et al. 124 This is an Open Access article distributed in accordance with the terms of the Creative Commons Attribution (CC BY 4.0) license, which permits others to distribute, remix, adapt and build upon this work, for commercial use, provided the original work is properly cited. See: https://creativecommons.org/licenses/by/4.0/. The text below includes minor additions and formatting changes to the original text.
Statistical synthesis model
Using the RE approach, the NMA ANCOVA model used takes the following form:
The set of treatments included in these trials are labelled [A, B, C, . . .], where A is the reference treatment and y1i,k and σ1i,k2 are the study i- and arm k-specific post-treatment measurement (the assessment ranging from 3 to 12 weeks) and their associated standard errors. θi,k is the linear predictor that uses the identity link function, with µi being the study-specific baseline parameters for the reference treatment b in each study (which is not necessarily the reference treatment of the network, i.e. treatment A) and δi,b,k is the study-specific relative treatment effects between the treatment included in arm k and the treatment included in the baseline arm b of study i. βbk represent the treatment-specific coefficients that adjust for the pre-treatment (i.e. baseline) measurements y0i,k under the ANCOVA model. δi,k,l are assumed to follow a RE approach with mean dbk and a between-study heterogeneity τ2 that is assumed to be common across all treatment comparisons to assist identification. For trials that use an active control treatment (i.e. b ≠ A) the consistency assumption is imposed in the form of a set of functional relationships among basic parameters (e.g. dAk). Note that βAA is assumed to be zero, indicating that patients who did not receive any treatment are expected to neither improve nor worsen during the duration of treatment (i.e. null placebo effect). Finally, we assume that the effect of the baseline measurement is common across all treatments so that βAk = β, implying that, when two active treatments are compared in a trial, the baseline effects are offset. Vague prior distributions were assigned to all parameters [i.e. dAk, β ∼ N(0,10-6) and τ ∼ Unif(0,10)].
Meta-regression is the most commonly employed method to explore the influence of particular study-level covariates on the relative effect. A range of approaches can be used to model comparison-specific treatment–effect interactions. 218 In this analysis, we assumed a common effect interaction (i.e. a single interaction term was assumed to apply to all comparisons with NI), as this was deemed more clinically plausible and also less data demanding. However, this method requires that all studies report data on the covariate(s) in question. For the trials informing the NMA, complete data were obtained for disease severity (as a binary covariate mild to moderate/moderate to severe) but not for the other two potential effect modifiers. Under these circumstances, one option is to exclude studies for which data on the covariate are missing and perform a meta-regression on the subset of studies that provide covariate information; however, this approach may lead to a smaller (with fewer interventions being compared) and ‘weaker’ network (with less evidence informing it). Alternatively, to preserve all studies (and treatments), we may assume that the covariate is distributed across studies in accordance with a beta distribution, the hyperparameters of which are assigned non-informative priors and are estimated in the model through the MCMC simulation to impute missing covariate information (multiple imputation procedure assuming ‘missingness’ mechanism of ‘missing at random’). The meta-regression model extends the aforementioned NMA ANCOVA model so that the linear predictor is now:
BAk are again assumed independent of treatment comparison so that BAk = B, which represents the additional effect that is observed not because of the treatment, but because of the interaction of the treatment with the study-level covariate. Xi represent the study-level covariate values, and are assigned a beta distribution with hyperparameters a, b, which are estimated in the model and are assigned vague priors a, b ∼ Unif(0,1000). As Xi are proportions, a beta distribution is perhaps the most reasonable distributional assumption. The effect modification for the reference treatment is also assumed to be zero.
WinBUGS code for main synthesis model
The WinBUGS modelling code is provided followed by a summary table of all variables included in the data set and R code describing the specification of initial values for two chains.
WinBUGS model code
model {
for(i in 1:NS) {
w[i,1]<- 0
delta[i,1]<- 0
mu[i] ∼ dnorm(0,1.0E-6)
for (k in 1:na[i]) {
y1[i,k] ∼ dnorm(theta[i,k], prec[i,k]) #likelihood function
theta[i,k] <- mu[i] + delta[i,k]
var[i,k] <- pow(se1[i,k], 2)
prec[i,k] <- 1/var[i,k]
dev[i,k] <- (y1[i,k] - theta[i,k]) * (y1[i,k] – theta[i,k]) * prec[i,k] #residual deviance
}
resdev[i] <- sum(dev[i,1:na[i]])
for (k in 2:na[i]) {
#consistency model for treatment effects and baseline adjustment
delta[i,k] ∼ dnorm(md[i,k],precd[i,k])
md[i,k]<- d[t[i,k]] - d[t[i,1]] + (b_base[t[i,k]] – b_base[t[i,1]]) * y0[i,k] + sw[i,k]
precd[i,k] <- pre * 2 * (k – 1)/k
#correction for multi-arm trials
w[i,k]<- delta[i,k] – d[t[i,k]] + d[t[i,1]]
sw[i,k]<- sum(w[i,1:k-1])/(k – 1)
}
}
#total Residual Deviance
totresdev <- sum(resdev[])
d[1]<-0
for (k in 2:NT) {
#prior on treatment effects and baseline score effects
d[k] ∼ dnorm(0,1.0E-6)
b_base[k] <- b_basey
}
#prior on random treatment effect variance
tau ∼ dunif(0,10)
tau.sq<- tau * tau
pre<- 1/(tau.sq)
#prior on impact of baseline score on final outcome score
b_basey ∼ dnorm(0,1.0E-6)
b_base[1]<-0
# pairwise effects
for (c in 1:(NT – 1)) {
for (k in (c + 1):NT) {
ef[c,k] <- d[k] – d[c]
}
}
# Treatment A baseline, based on average of the trials including No intervention
for (i in 1:NS) {
mu1[i] <- mu[i] * equals(t[i,1],1)
}
mn.mu1<- sum(mu1[]) / 6
#Posterior distributions of absolute post-treatment scores
for (k in 1:NT) {
T[k]<- mn.mu1 + d[k] + b_base[k]*mn.mu1
}
# ranking and prob{treatment k is the best}
for (k in 1:NT) {
rk[k]<- NT + 1 – rank(T[],k)
best[k]<- equals(rk[k],7)
}
Object | Variable | Description |
---|---|---|
Data set descriptors/constants | na | Number of arms in studies in data set |
NS | Number of trials in data set | |
NT | Number of treatments in data set | |
Data | y1 | Data on final outcome mean score (arm level) |
y0 | Data on baseline outcome mean score (arm level) | |
t | Treatment code | |
se1 | Data on final outcome standard error of mean score (arm level) |
The R code used to generate initial values (only one set shown for didactic purposes) was:
list(d = c(NA,0,0,0,0,0,0), mu = c(0,0,0,0,0, 0,0,0,0,0,0,0,0), b_basey = c(0), tau = c(1))
Appendix 2 Outcome measures used in randomised controlled trials of digital interventions for generalised anxiety disorder
Reproduced with permission from Saramago et al. 124 This is an Open Access article distributed in accordance with the terms of the Creative Commons Attribution (CC BY 4.0) license, which permits others to distribute, remix, adapt and build upon this work, for commercial use, provided the original work is properly cited. See: https://creativecommons.org/licenses/by/4.0/. The text below includes minor additions and formatting changes to the original text.
Study (first author and year) | Outcome measure | |||||||||||||||||||
---|---|---|---|---|---|---|---|---|---|---|---|---|---|---|---|---|---|---|---|---|
GAD-7 | PSWQ | PHQ-9 | BDI/BDI-II | STAI-T | BAI | STAI-S | MADRS-S | PDSS-SR | GAD-Q-IV | QOLI | K-10 | SDS | NEO-FFI-3 | RRS | HADS/HADS-A | MINI | CGI | ASI/ASI-3 | CES-D | |
Andersson 2012158 | ✗ | ✗ | ✗ | ✗ | ✗ | ✗ | ✗ | ✗ | ✗ | |||||||||||
Andersson 201740 | ✗ | ✗ | ✗ | |||||||||||||||||
Christensen 20144 | ✗ | ✗ | ✗ | ✗ | ✗ | |||||||||||||||
Christensen 2014159 | ✗ | ✗ | ✗ | |||||||||||||||||
Dahlin 201641 | ✗ | ✗ | ✗ | ✗ | ✗ | ✗ | ✗ | |||||||||||||
Dear 2015160 | ✗ | ✗ | ✗ | ✗ | ✗ | |||||||||||||||
Hazen 2009161 | ✗ | ✗ | ✗ | |||||||||||||||||
Hirsch 2018162 | ✗ | ✗ | ✗ | ✗ | ||||||||||||||||
Howell 2018163 | ✗ | |||||||||||||||||||
Johansson 201337 | ✗ | ✗ | ||||||||||||||||||
Jones 2016164 | ✗ | ✗ | ||||||||||||||||||
Navarro-Haro 2019165 | ✗ | ✗ | ||||||||||||||||||
Paxling 2011166 | ✗ | ✗ | ✗ | ✗ | ✗ | ✗ | ✗ | ✗ | ||||||||||||
Pham 20165 | ✗ | ✗ | ✗ | |||||||||||||||||
Repetto 2013167 | ✗ | ✗ | ✗ | ✗ | ✗ | |||||||||||||||
Richards 2016168 | ✗ | ✗ | ✗ | |||||||||||||||||
Robinson 2010169 | ✗ | ✗ | ✗ | ✗ | ✗ | |||||||||||||||
Teng 201938 | ✗ | ✗ | ✗ | ✗ | ✗ | |||||||||||||||
Titov 2009171 | ✗ | ✗ | ✗ | ✗ | ✗ | |||||||||||||||
Titov 2010170 | ✗ | ✗ | ✗ | ✗ | ✗ | ✗ | ||||||||||||||
Topper 2017172 | ✗ | ✗ | ✗ | ✗ | ||||||||||||||||
Total number of comparisons | 14 | 14 | 8 | 6 | 5 | 5 | 4 | 4 | 3 | 4 | 3 | 3 | 3 | 2 | 2 | 2 | 2 | 2 | 2 | 2 |
Study (first author and year) | Outcome measure | DASS-21 | PTQ | MASQ-D30 | EDI-2-BU | QDS | |||||||||||||||||||
---|---|---|---|---|---|---|---|---|---|---|---|---|---|---|---|---|---|---|---|---|---|---|---|---|---|
PSWQ-A | SCID-I | CAQ | BBQ | MCQ-30 | IOU | Days out of role | Mini-SPIN | GAI | GDS | WHOQOL | ACES | FFMQ | DERS | MAIA | OASIS | Q-LES-Q-SF | HAM-A | WASAS | SPSQ | ||||||
Andersson 2012158 | ✗ | ||||||||||||||||||||||||
Andersson 201740 | ✗ | ✗ | ✗ | ✗ | |||||||||||||||||||||
Christensen 20144 | ✗ | ||||||||||||||||||||||||
Christensen 2014159 | |||||||||||||||||||||||||
Dahlin 201641 | |||||||||||||||||||||||||
Dear 2015160 | ✗ | ||||||||||||||||||||||||
Hazen 2009161 | |||||||||||||||||||||||||
Hirsch 2018162 | |||||||||||||||||||||||||
Howell 2018163 | |||||||||||||||||||||||||
Johansson 201337 | |||||||||||||||||||||||||
Jones 2016164 | ✗ | ✗ | ✗ | ✗ | ✗ | ||||||||||||||||||||
Navarro-Haro 2019165 | ✗ | ✗ | ✗ | ||||||||||||||||||||||
Paxling 2011166 | |||||||||||||||||||||||||
Pham 20165 | ✗ | ✗ | |||||||||||||||||||||||
Repetto 2013167 | ✗ | ||||||||||||||||||||||||
Richards 2016168 | ✗ | ||||||||||||||||||||||||
Robinson 2010169 | |||||||||||||||||||||||||
Teng 201938 | |||||||||||||||||||||||||
Titov 2009171 | |||||||||||||||||||||||||
Titov 2010170 | ✗ | ✗ | |||||||||||||||||||||||
Topper 2017172 | ✗ | ✗ | ✗ | ✗ | |||||||||||||||||||||
Total number of comparisons | 1 | 1 | 1 | 1 | 1 | 1 | 1 | 1 | 1 | 1 | 1 | 1 | 1 | 1 | 1 | 1 | 1 | 1 | 1 | 1 | 1 | 1 | 1 | 1 | 1 |
Appendix 3 Results of randomised controlled trials of digital interventions for generalised anxiety disorder synthesised in the network meta-analysis models
Reproduced with permission from Saramago et al. 124 This is an Open Access article distributed in accordance with the terms of the Creative Commons Attribution (CC BY 4.0) license, which permits others to distribute, remix, adapt and build upon this work, for commercial use, provided the original work is properly cited. See: https://creativecommons.org/licenses/by/4.0/. The text below includes minor additions and formatting changes to the original text.
Study (first author and year) | Intervention | Number of participants | Baseline GAD score | Post-treatment GAD score | ||
---|---|---|---|---|---|---|
y0, mean | se0, SE | y1, mean | se1, SE | |||
Christensen 20144 | UDC | 111 | 7.00 | 3.80 | 6.10 | 4.10 |
SDC | 113 | 6.60 | 3.70 | 5.30 | 4.20 | |
UDI | 111 | 6.80 | 3.90 | 6.10 | 4.70 | |
UDI | 110 | 6.80 | 3.60 | 4.70 | 3.60 | |
SDI | 113 | 6.20 | 3.90 | 4.60 | 2.90 | |
Christensen 2014159 | SDC | 7 | 11.70 | 4.80 | 12.00 | 6.50 |
SDI | 8 | 11.50 | 3.70 | 6.50 | 2.30 | |
M | 6 | 14.80 | 5.20 | 3.80 | 2.80 | |
Dahlin 201641 | NI | 51 | 13.51 | 4.14 | 10.72 | 4.20 |
SDI | 52 | 13.83 | 3.66 | 6.90 | 3.52 | |
Dear 2015160 | UDI | 170 | 12.42 | 4.34 | 6.23 | 4.05 |
SDI | 168 | 12.61 | 4.40 | 6.09 | 3.96 | |
aHirsch 2018162 | SDC | 20 | 14.55 | 3.46 | 11.15 | 4.33 |
SDI | 44 | 14.00 | 3.18 | 11.59 | 4.91 | |
Johansson 201337 | SDC | 21 | 12.67 | 2.80 | 8.90 | 4.70 |
SDI | 22 | 12.23 | 3.80 | 6.95 | 5.30 | |
Jones 2016164 | NI | 21 | 11.99 | 4.82 | 10.16 | 4.22 |
SDI | 24 | 11.78 | 4.87 | 6.50 | 4.55 | |
Navarro-Haro 2019165 | SDI | 19 | 14.05 | 4.61 | 9.79 | 5.60 |
SNoDI | 20 | 15.33 | 4.03 | 9.08 | 3.85 | |
Pham 20165 | UDC | 32 | 10.66 | 4.63 | 9.53 | 4.79 |
UDI | 31 | 11.55 | 5.05 | 9.39 | 5.21 | |
Repetto 2013167 | NI | 4 | 14.25 | 4.57 | 8.75 | 6.19 |
SDI | 4 | 10.25 | 5.56 | 8.25 | 3.95 | |
SDI | 4 | 16.00 | 8.37 | 6.50 | 4.51 | |
Richards 2016168 | NI | 67 | 13.19 | 2.78 | 9.13 | 4.13 |
SDI | 70 | 12.84 | 2.39 | 7.73 | 4.44 | |
Robinson 2010169 | NI | 48 | 12.94 | 4.07 | 11.25 | 4.70 |
SDI | 50 | 11.90 | 3.38 | 6.02 | 3.43 | |
SDI | 47 | 12.45 | 4.14 | 5.55 | 4.73 | |
Titov 2009171 | NI | 21 | 13.62 | 3.51 | 12.29 | 4.26 |
SDI | 24 | 14.33 | 4.50 | 6.92 | 4.40 |
Study (first author and year) | Intervention | Number of participants | Baseline PSWQ score | Post-treatment PSWQ score | ||
---|---|---|---|---|---|---|
y0, mean | se0, SE | y1, mean | se1, SE | |||
Andersson 2012158 | NI | 27 | 68.52 | 6.24 | 62.88 | 9.39 |
SDI | 27 | 67.89 | 6.19 | 60.78 | 9.83 | |
SDI | 27 | 69.74 | 5.56 | 61.88 | 7.73 | |
Andersson 201740 | NI | 70 | 66.59 | 6.84 | 66.31 | 7.84 |
SDI | 70 | 65.60 | 6.20 | 52.92 | 11.16 | |
Christensen 20144 | UDC | 111 | 40.30 | 12.00 | 41.00 | 12.30 |
SDC | 113 | 39.20 | 10.80 | 38.40 | 12.80 | |
UDI | 111 | 40.50 | 12.20 | 39.00 | 13.20 | |
UDI | 110 | 37.90 | 12.50 | 33.80 | 11.50 | |
SDI | 113 | 39.50 | 11.60 | 37.40 | 10.60 | |
Dahlin 201641 | NI | 51 | 67.45 | 6.77 | 63.35 | 8.4 |
SDI | 52 | 66.88 | 7.16 | 55.29 | 10.02 | |
Hazen 2009161 | SDC | 12 | 67.96 | 6.05 | 67.83 | 8.05 |
SDI | 12 | 71.09 | 4.70 | 62.82 | 8.75 | |
aHirsch 2018162 | SDC | 20 | 67.10 | 6.54 | 65.80 | 6.84 |
SDI | 44 | 69.48 | 6.22 | 65.32 | 9.39 | |
Paxling 2011166 | NI | 45 | 69.32 | 6.55 | 69.39 | 7.06 |
SDI | 44 | 68.74 | 5.94 | 57.82 | 13.01 | |
Repetto 2013167 | NI | 4 | 51.25 | 9.85 | 50.00 | 5.29 |
SDI | 4 | 48.50 | 12.66 | 47.25 | 8.73 | |
SDI | 4 | 41.25 | 13.24 | 48.50 | 12.40 | |
Richards 2016168 | NI | 67 | 63.48 | 6.95 | 60.33 | 8.79 |
SDI | 70 | 63.04 | 8.11 | 58.53 | 10.97 | |
Robinson 2010169 | NI | 48 | 65.81 | 10.24 | 64.22 | 11.81 |
SDI | 50 | 63.12 | 9.46 | 52.28 | 10.73 | |
SDI | 47 | 64.02 | 9.27 | 51.45 | 12.28 | |
Teng 201938 | SDC | 31 | 60.60 | 10.09 | 57.03 | 8.23 |
SDI | 31 | 59.80 | 8.86 | 53.43 | 11.01 | |
SNoDC | 31 | 62.27 | 8.99 | 60.18 | 8.88 | |
Titov 2009171 | NI | 21 | 66.33 | 12.70 | 66.14 | 8.70 |
SDI | 24 | 66.13 | 8.25 | 56.75 | 10.78 | |
Topper 2017172 | NI | 85 | 59.15 | 6.78 | 57.80 | 8.54 |
SDI | 84 | 58.73 | 6.96 | 51.87 | 8.85 | |
SNoDI | 82 | 58.20 | 6.59 | 51.29 | 8.58 |
Appendix 4 Surface under the cumulative ranking curve graphs and rankograms
Reproduced with permission from Saramago et al. 124 This is an Open Access article distributed in accordance with the terms of the Creative Commons Attribution (CC BY 4.0) license, which permits others to distribute, remix, adapt and build upon this work, for commercial use, provided the original work is properly cited. See: https://creativecommons.org/licenses/by/4.0/. The text below includes minor additions and formatting changes to the original text.
FIGURE 23.
Ranking of each intervention based on surface under the cumulative ranking curve values for GAD-7: (a) NI; (b) UDC; (c) SDC; (d) UDI; (e) SDI; (f) SNoDI; and (g) medication.
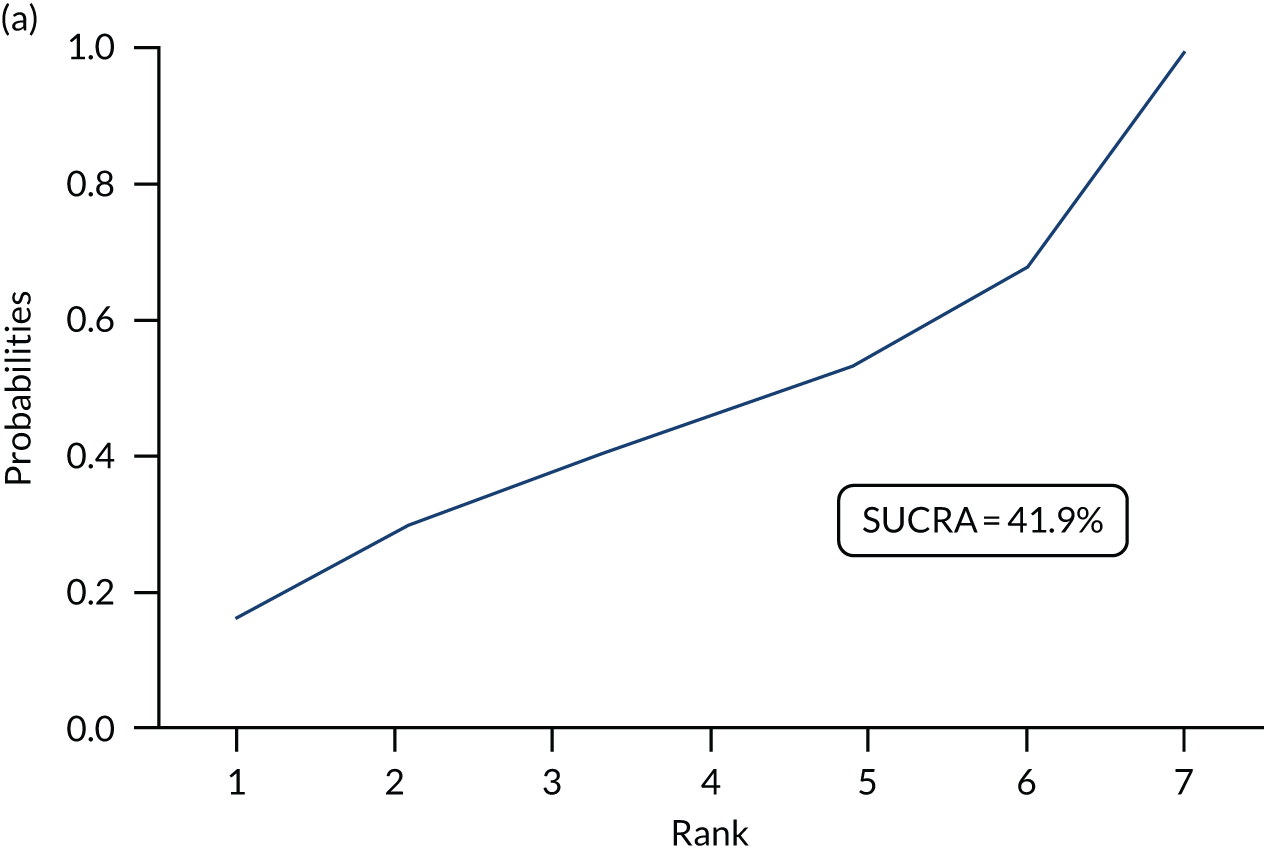
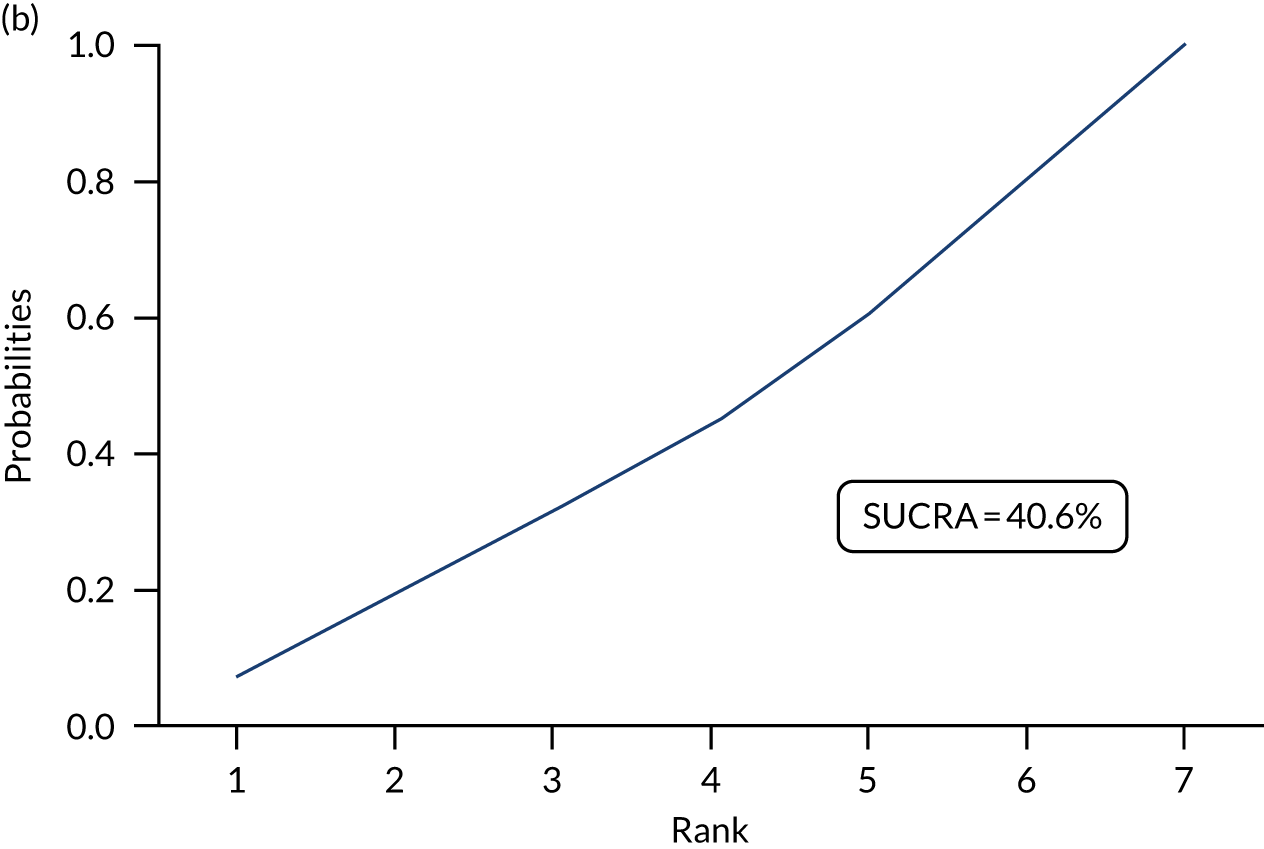
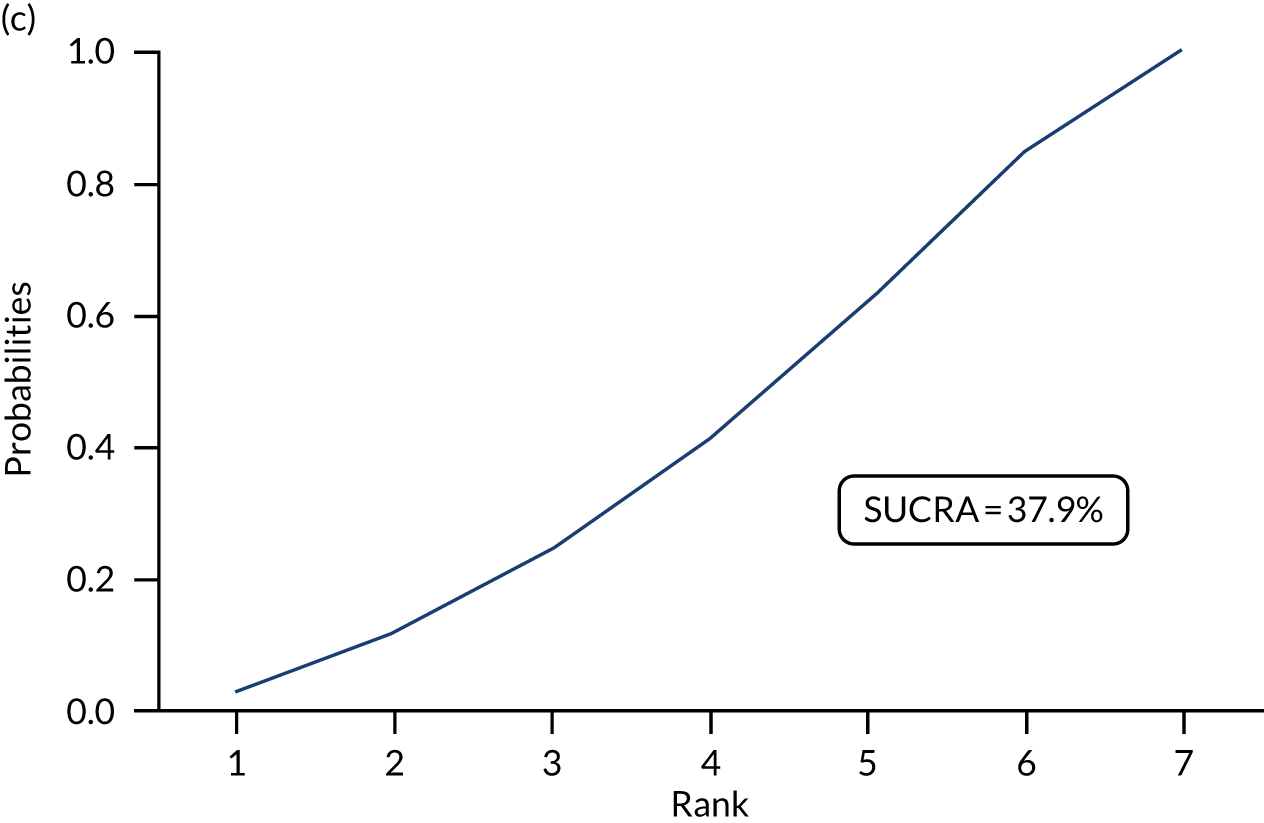
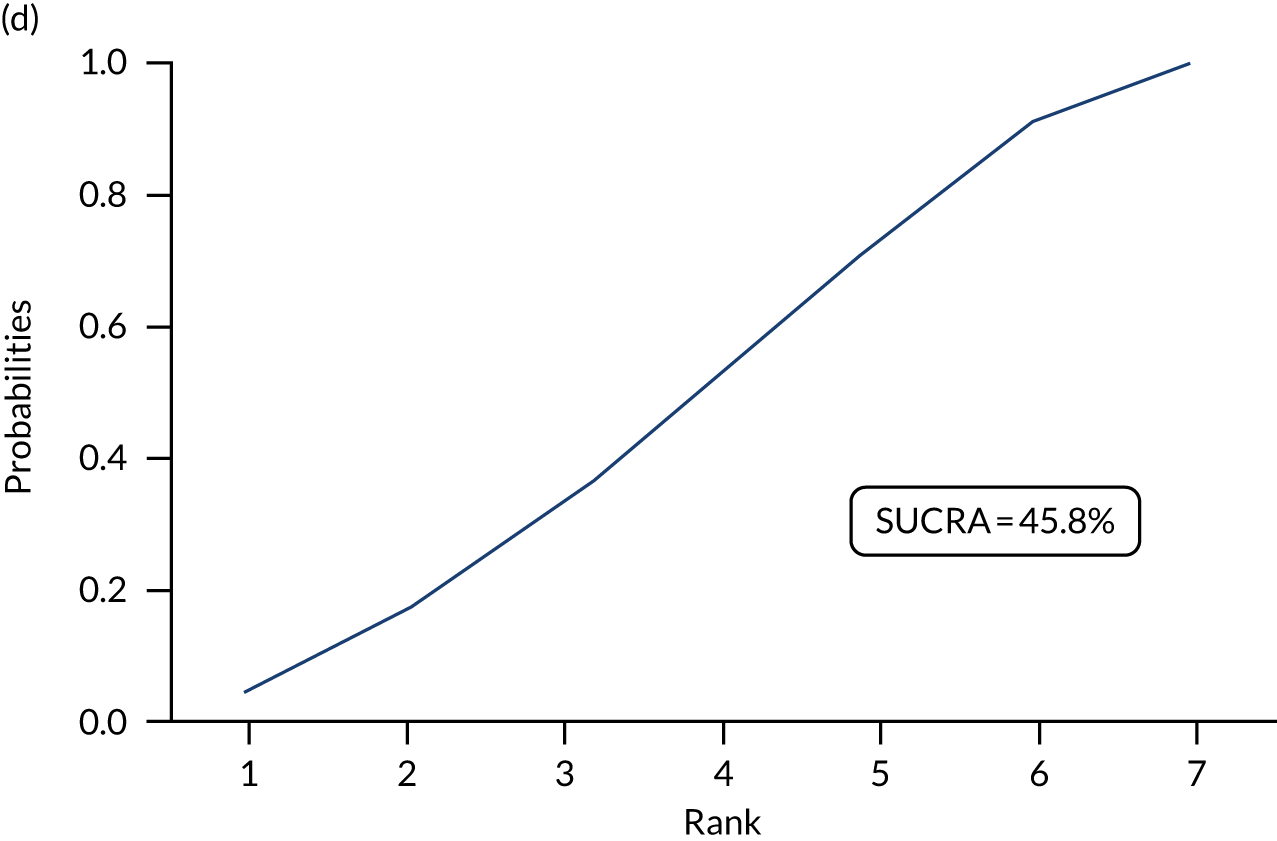
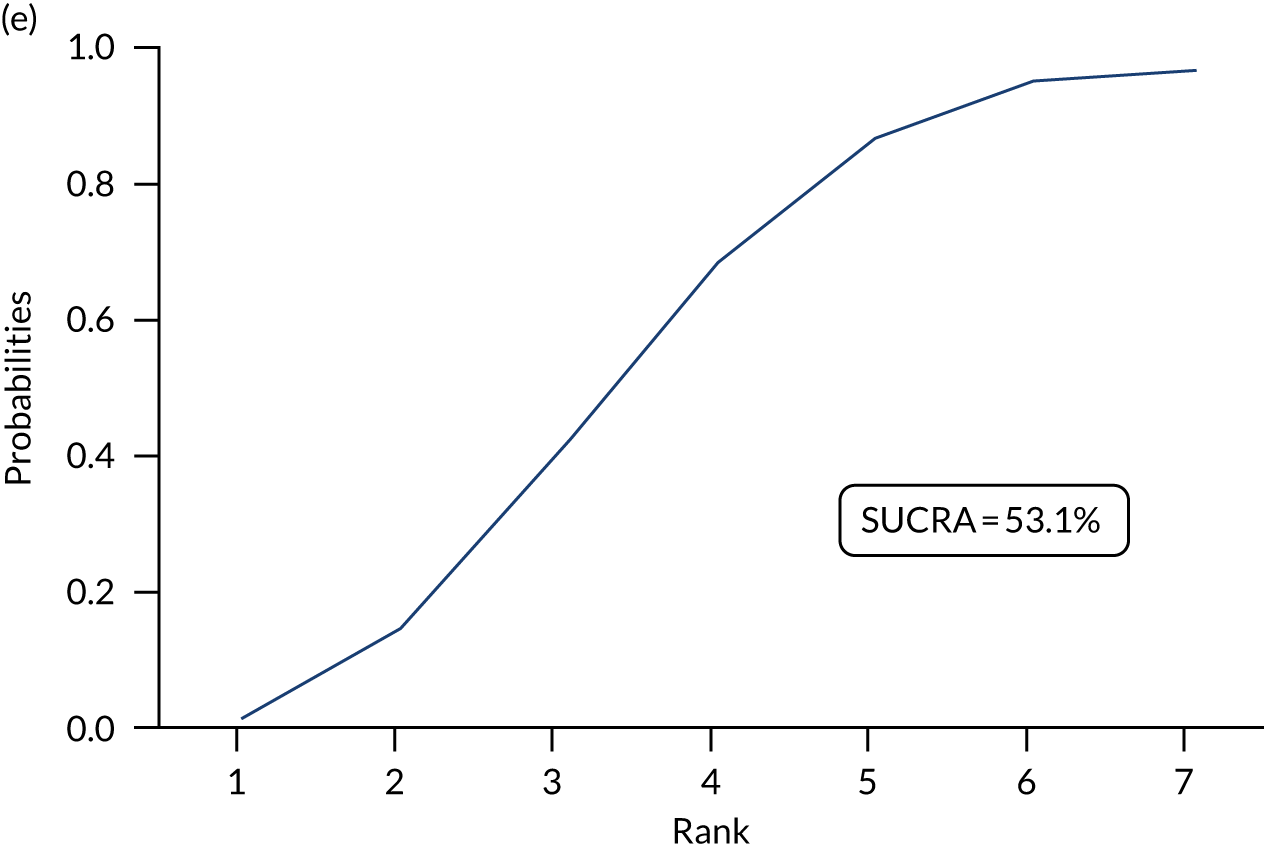
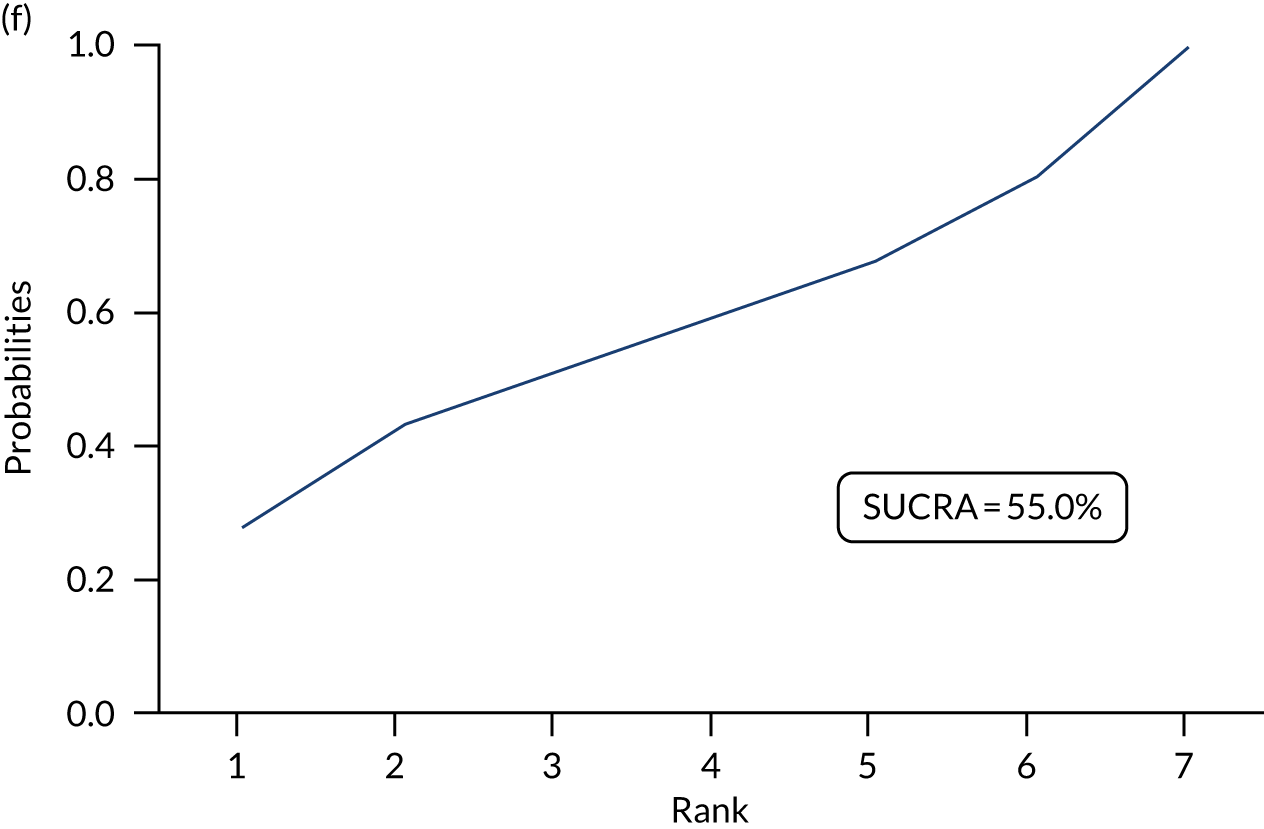
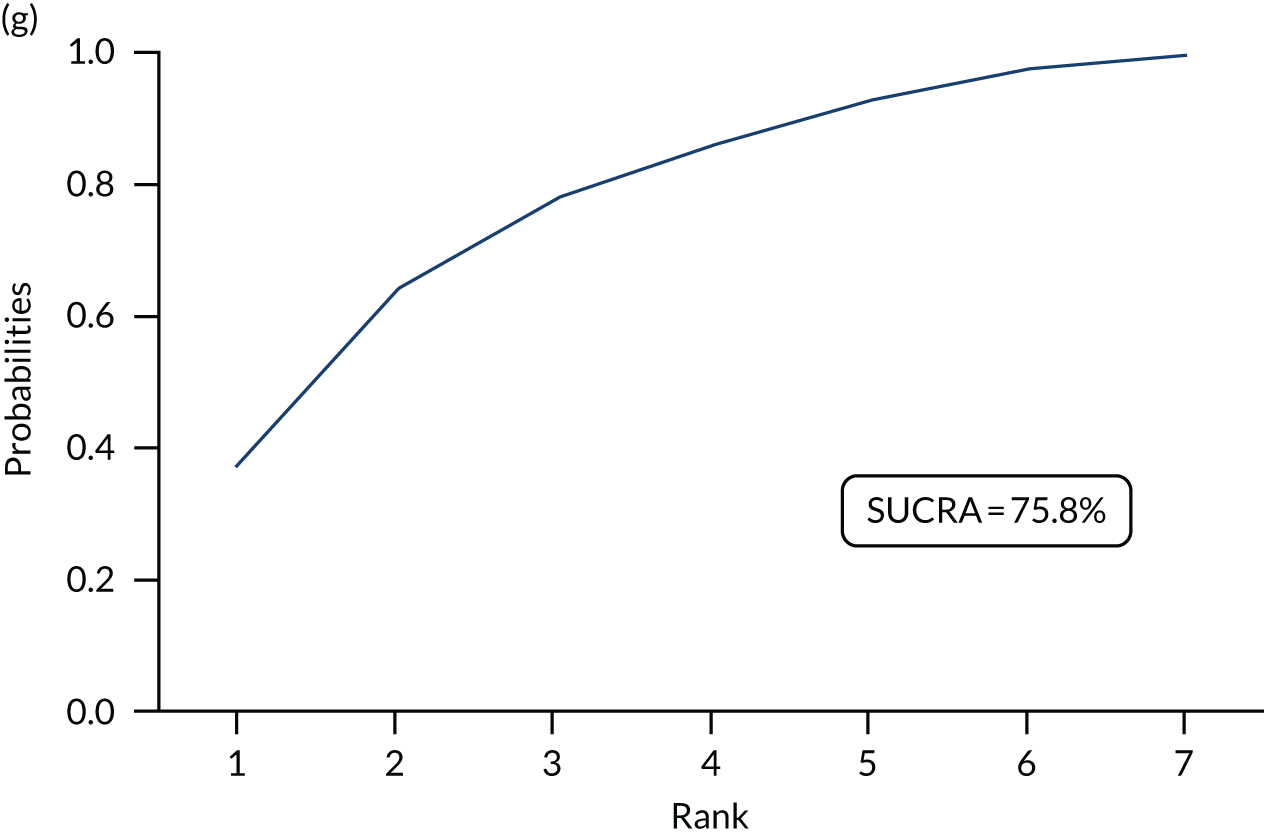
FIGURE 24.
Generalised Anxiety Disorder-7 rankograms: rankogram of each intervention for GAD-7: (a) NI; (b) UDC; (c) SDC; (d) UDI; (e) SDI; (f) SNoDI; and (g) medication.
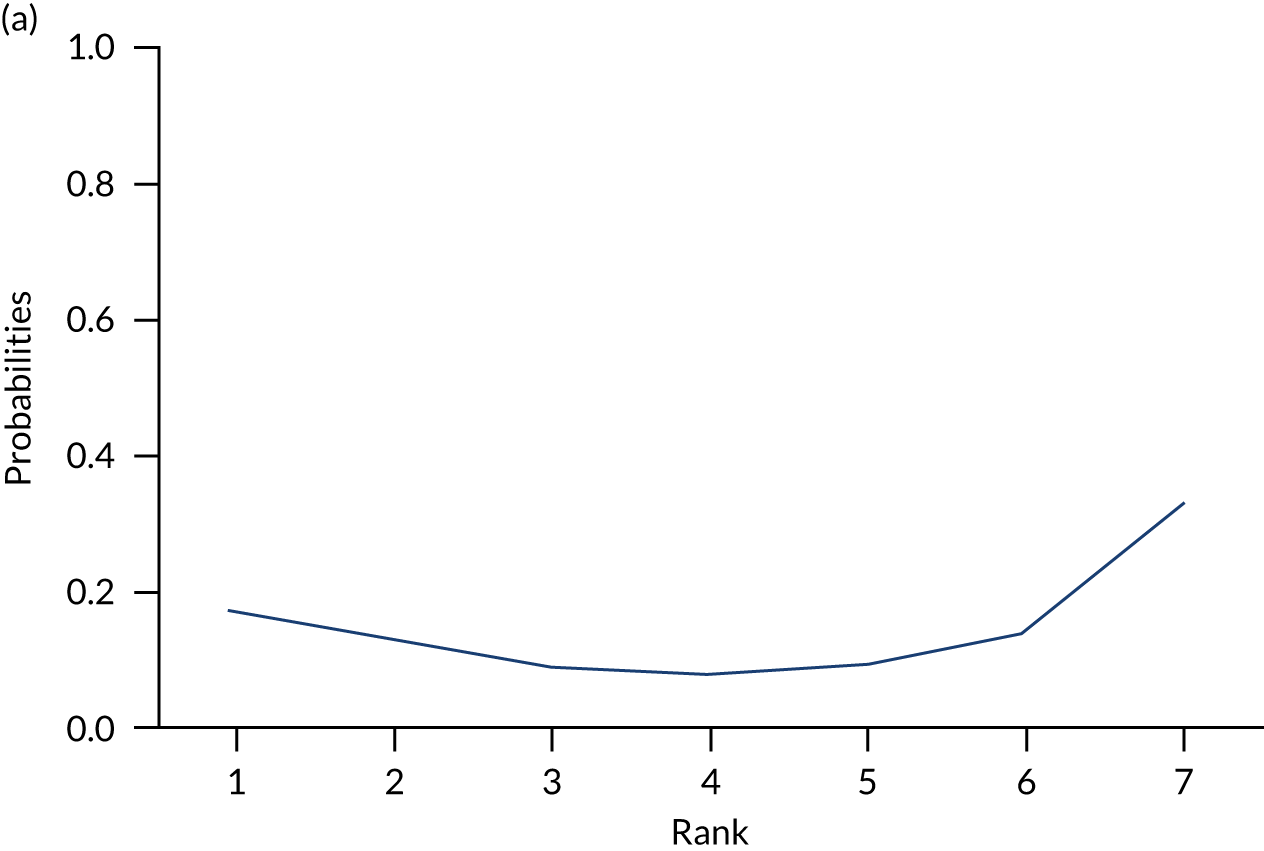
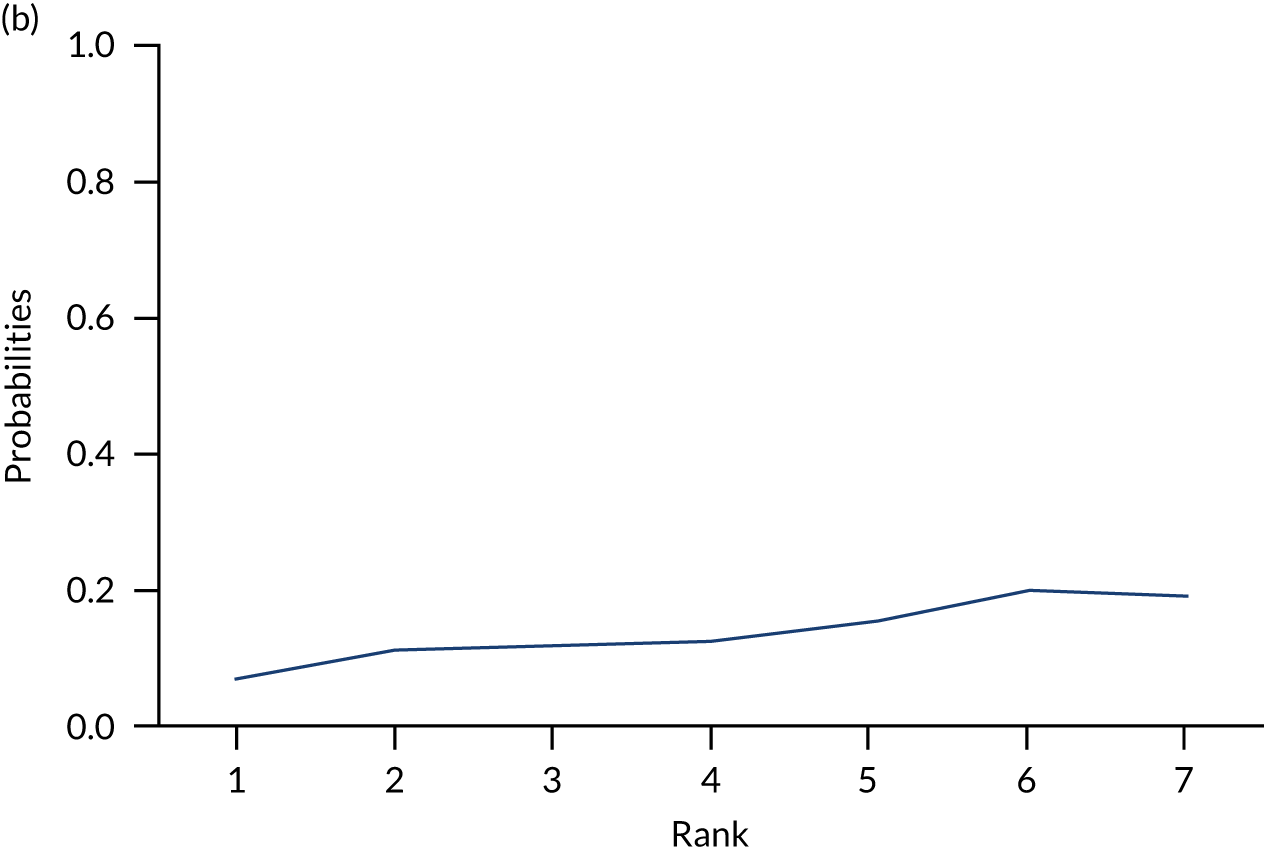
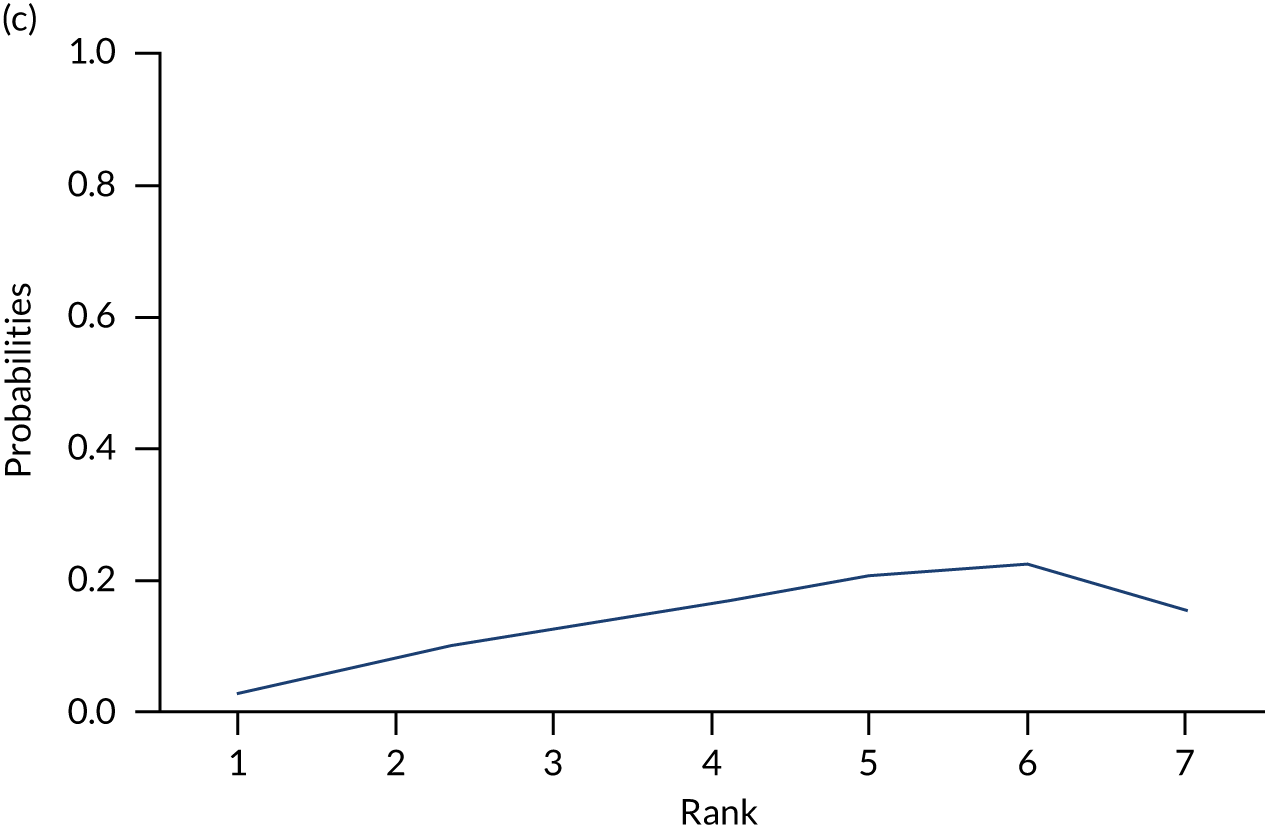
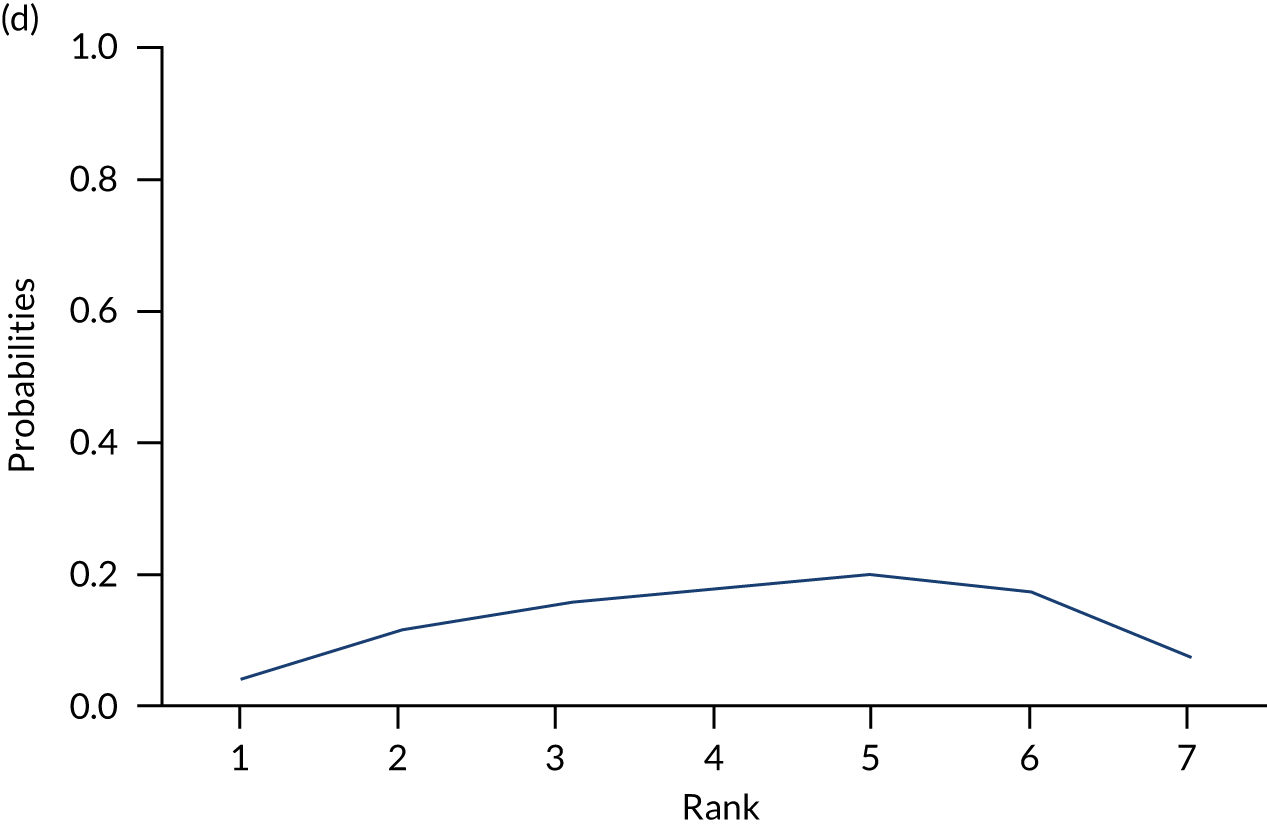
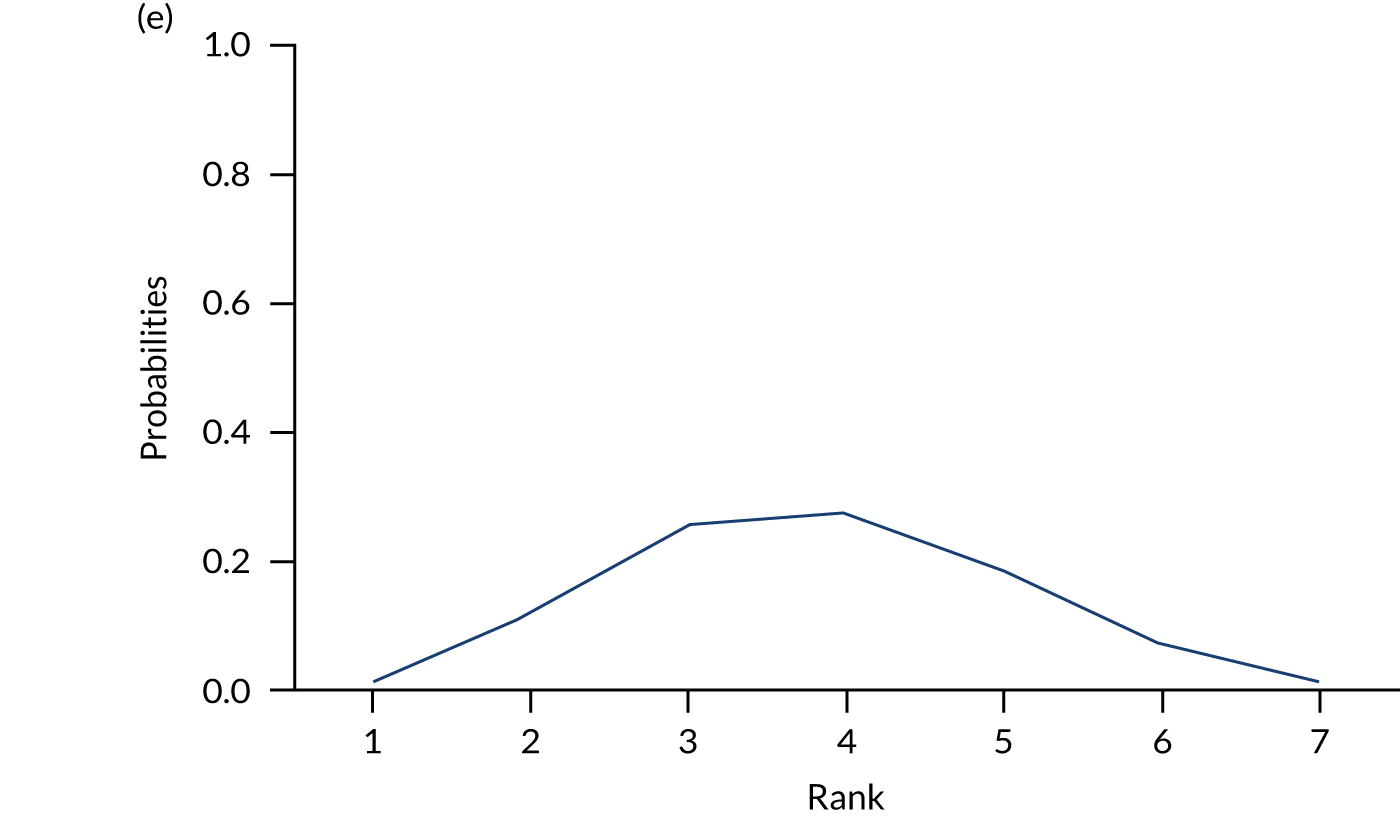
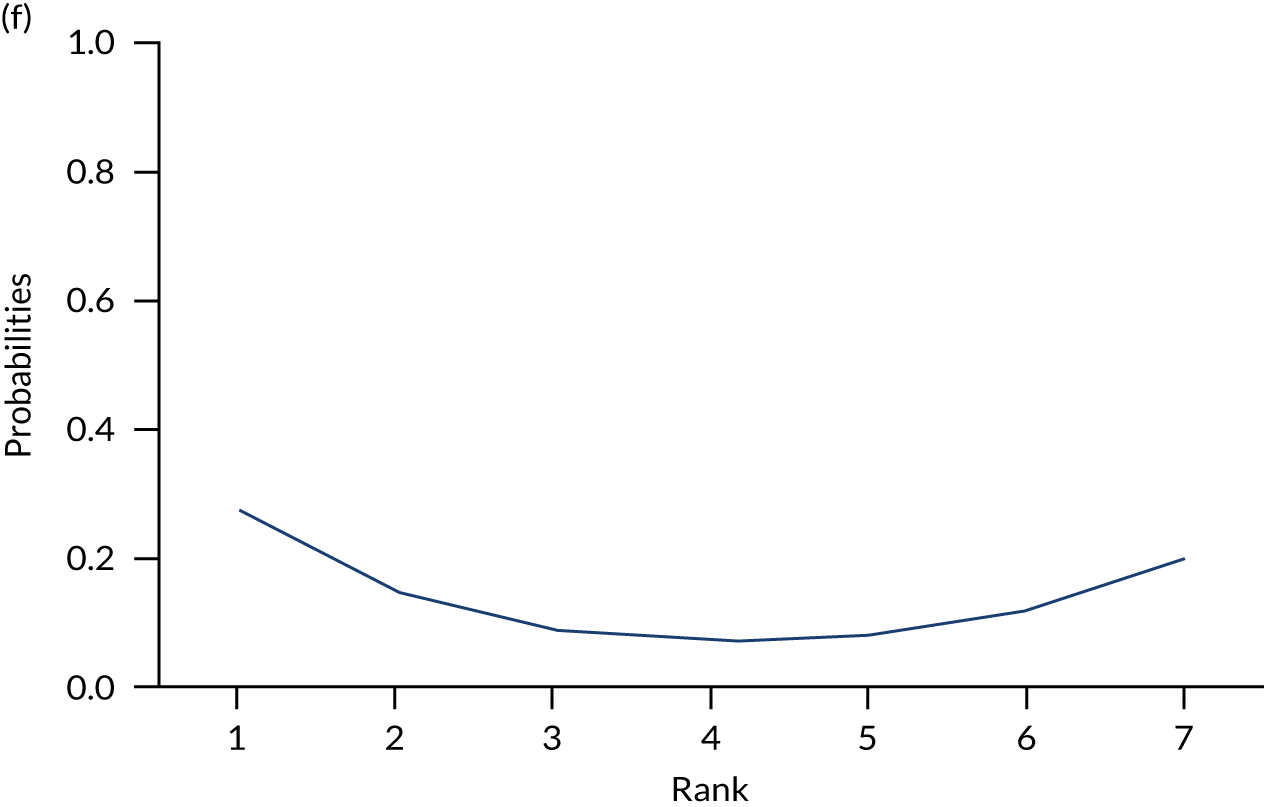
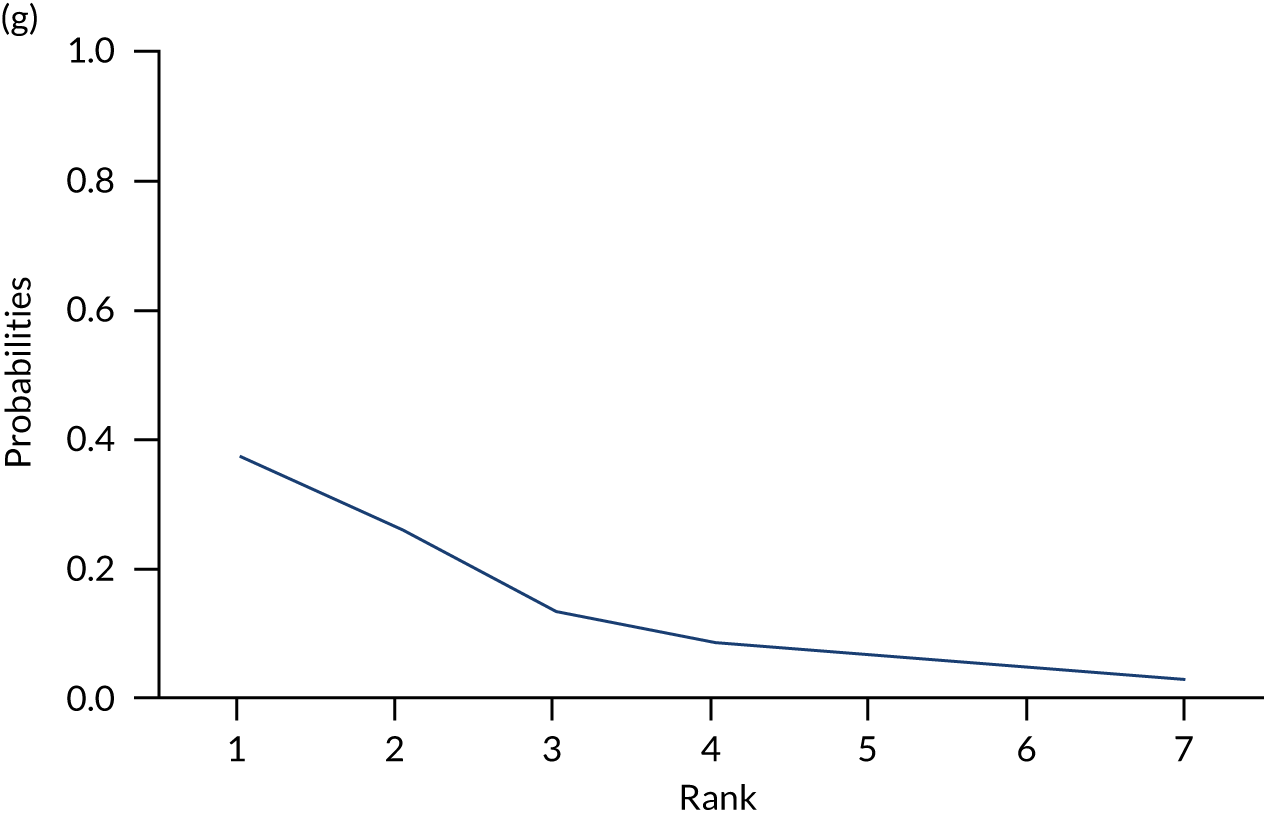
FIGURE 25.
Penn State Worry Questionnaire surface under the cumulative ranking curves: ranking of each intervention based on surface under the cumulative ranking curve values for the PSWQ: (a) NI; (b) UDC; (c) SDC; (d) UDI; (e) SDI; (f) SNoDC; and (g) SNoDI.
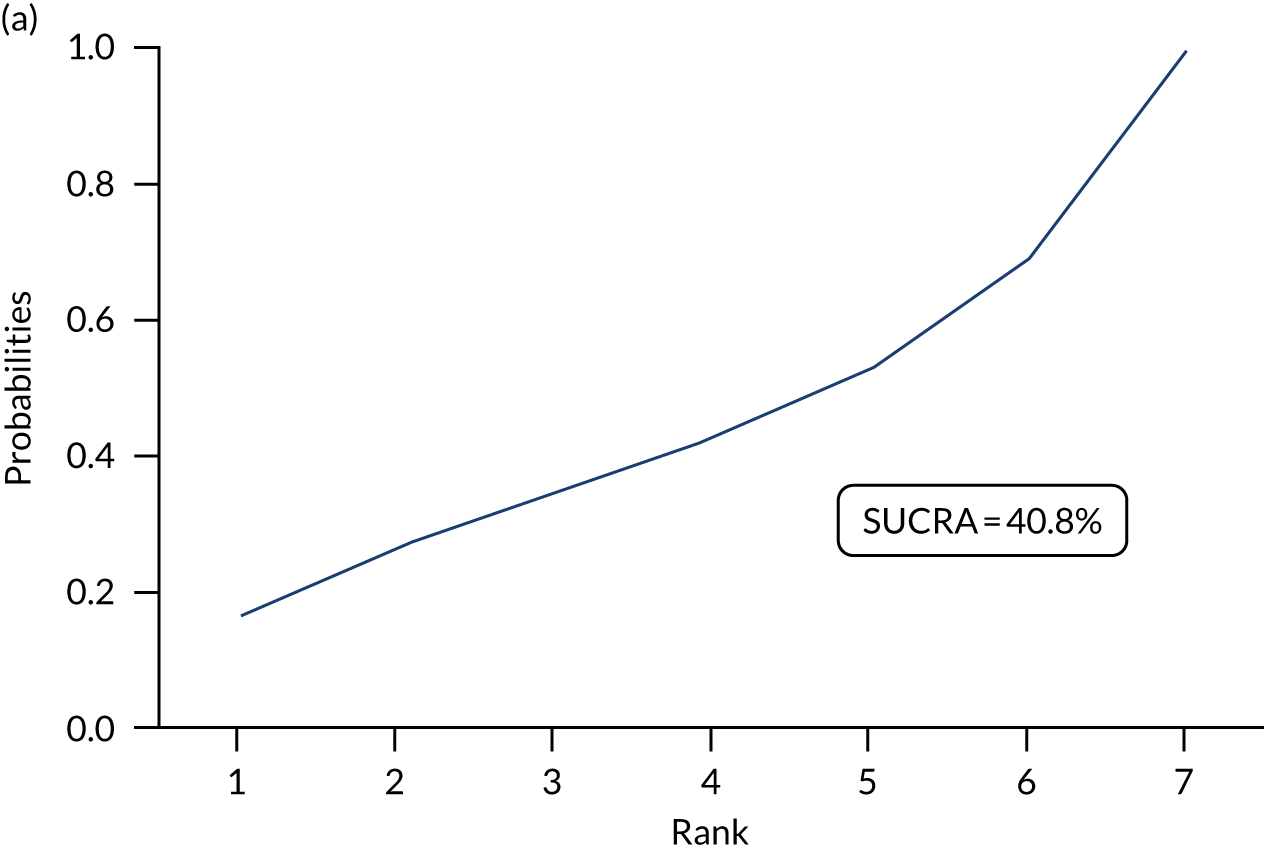
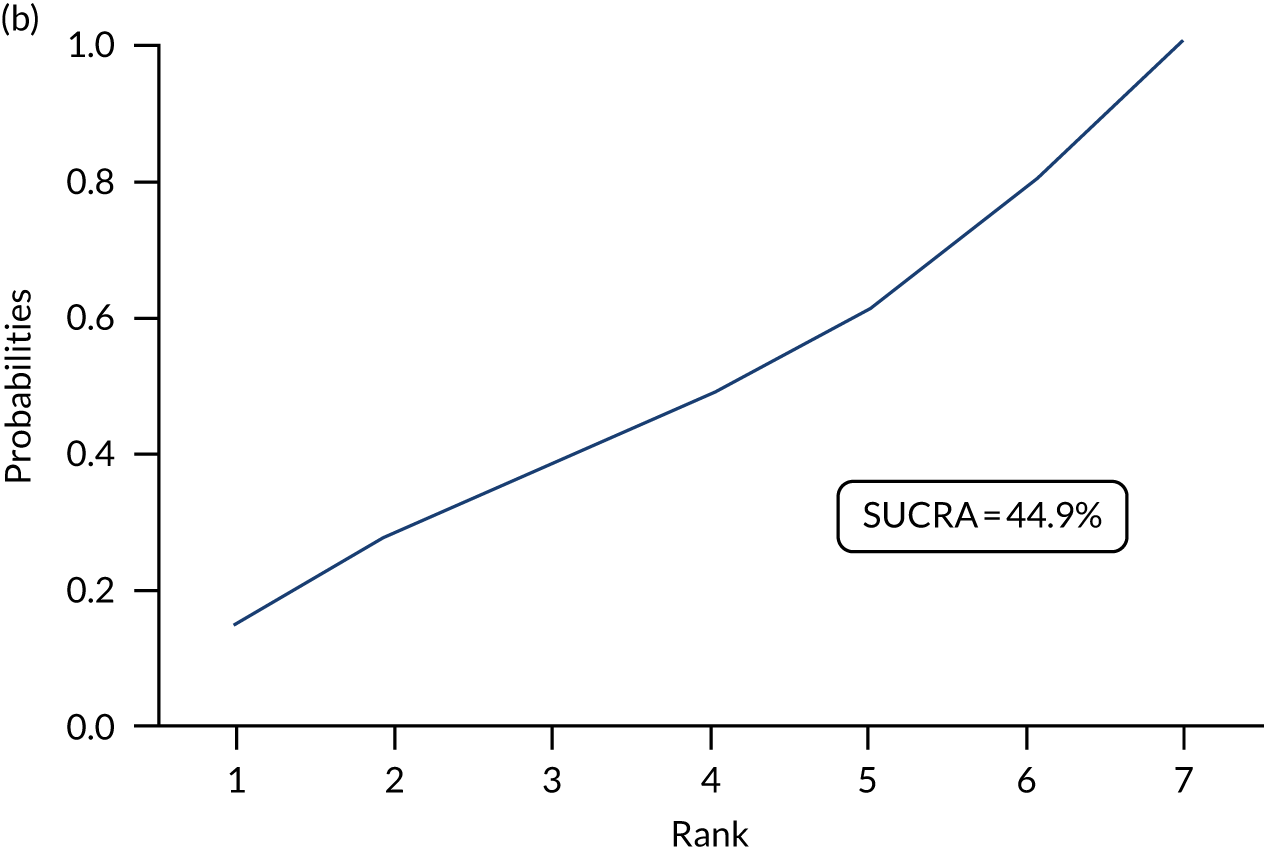
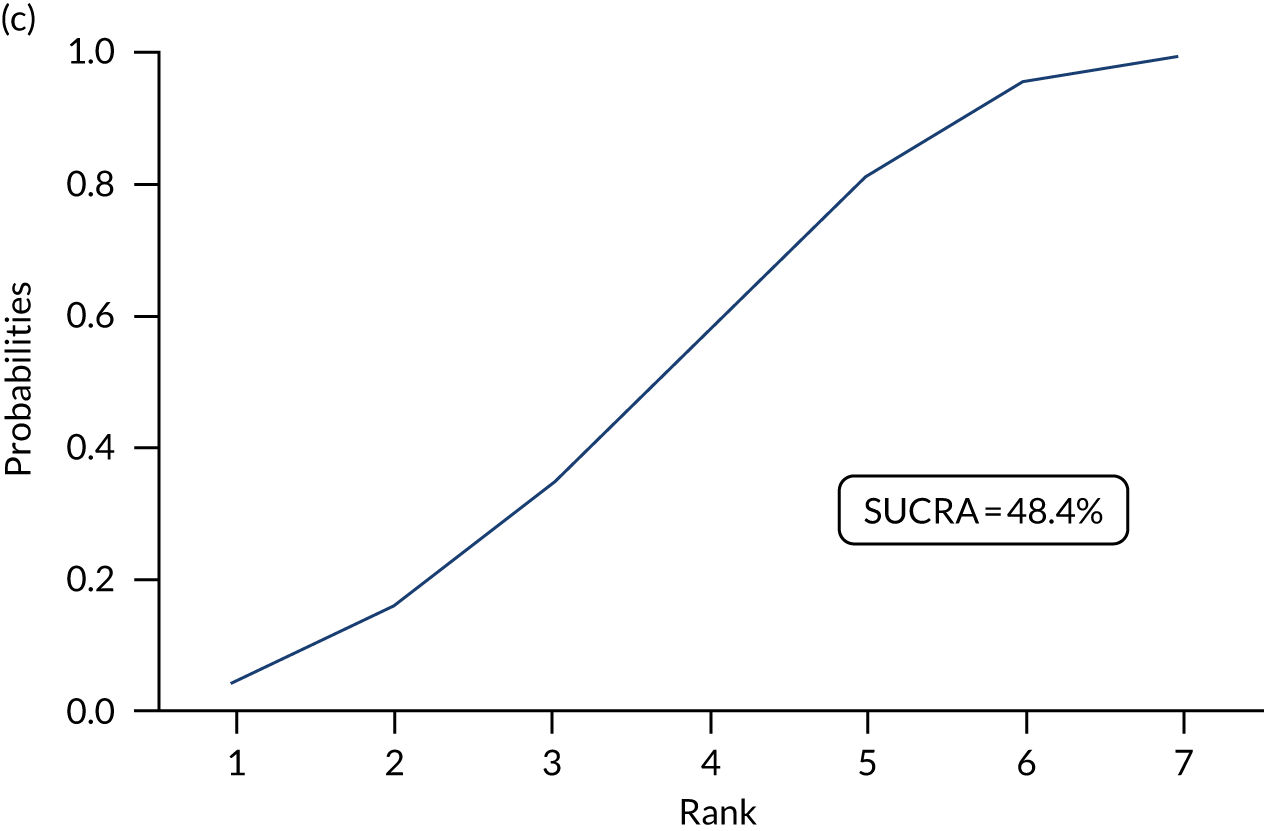
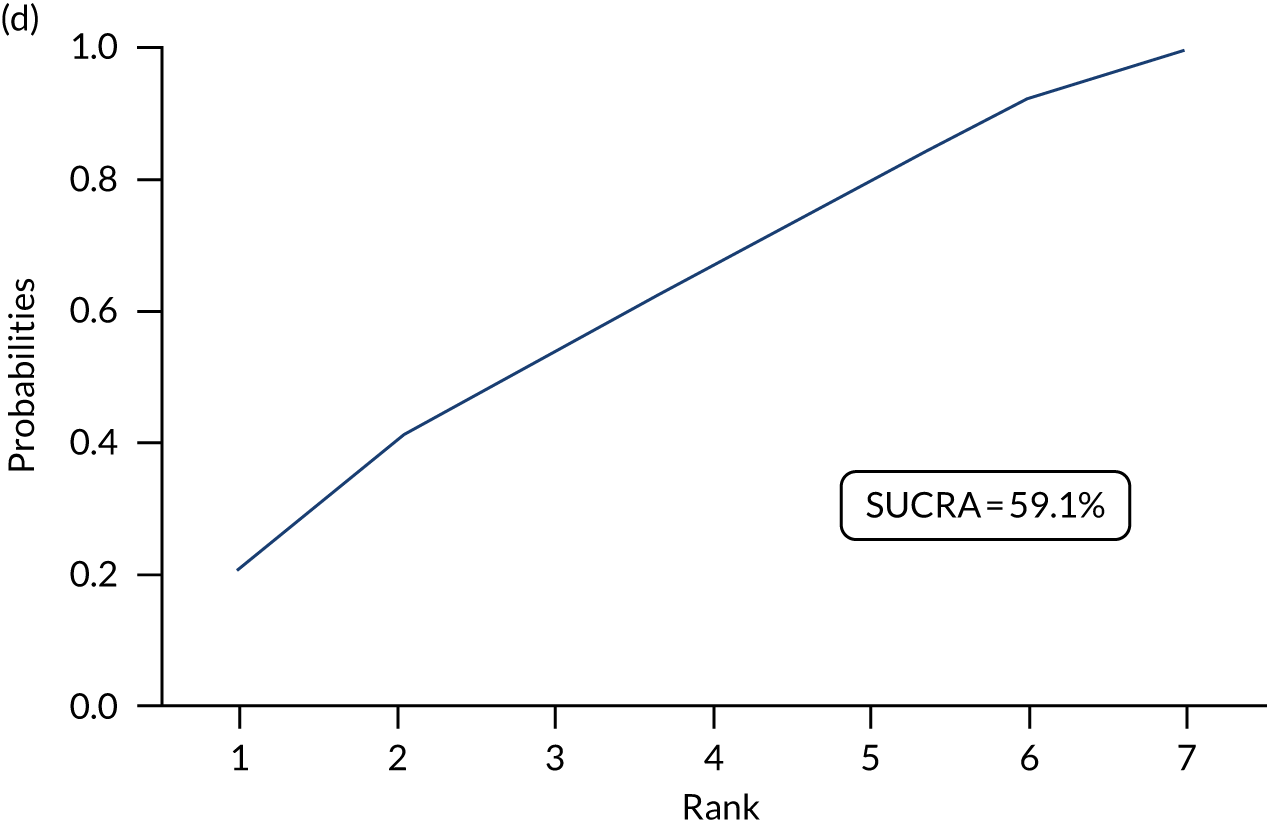
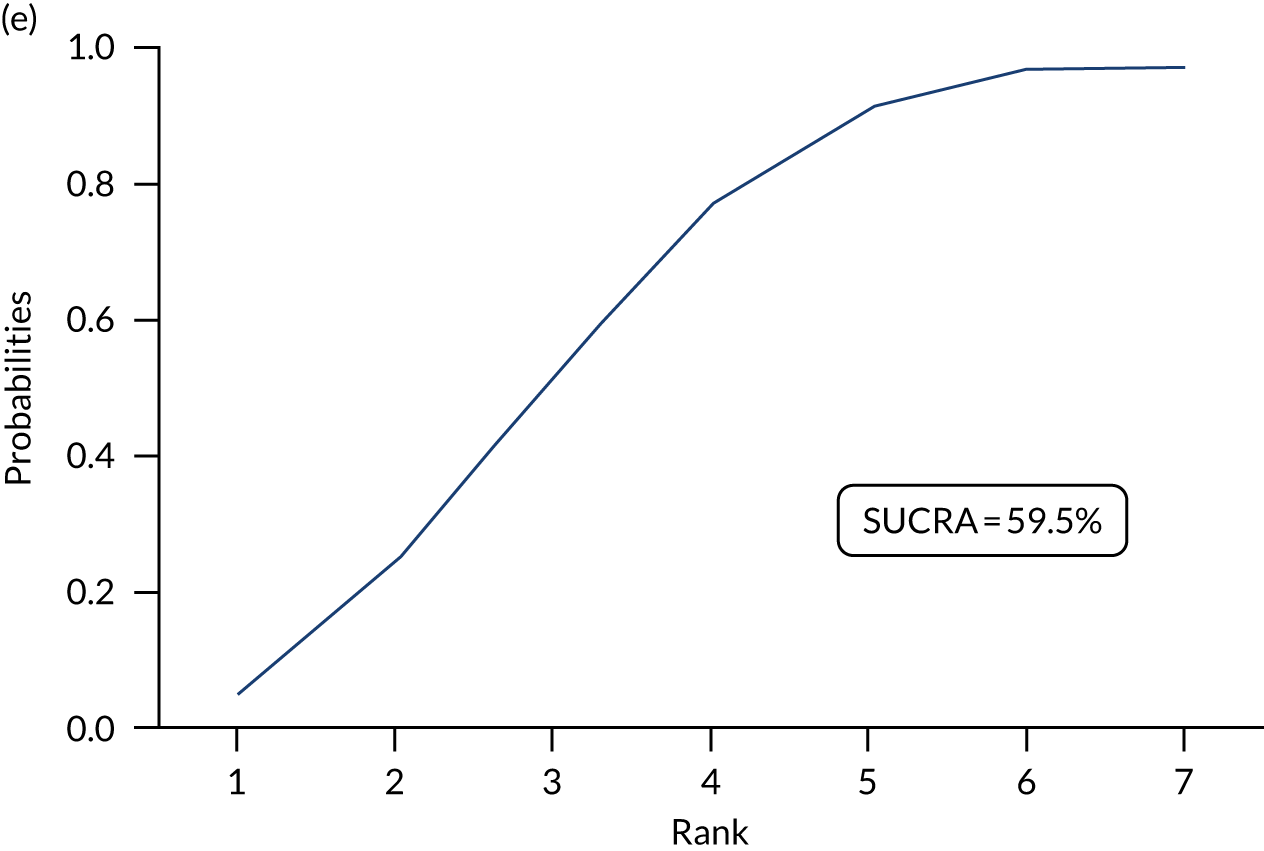
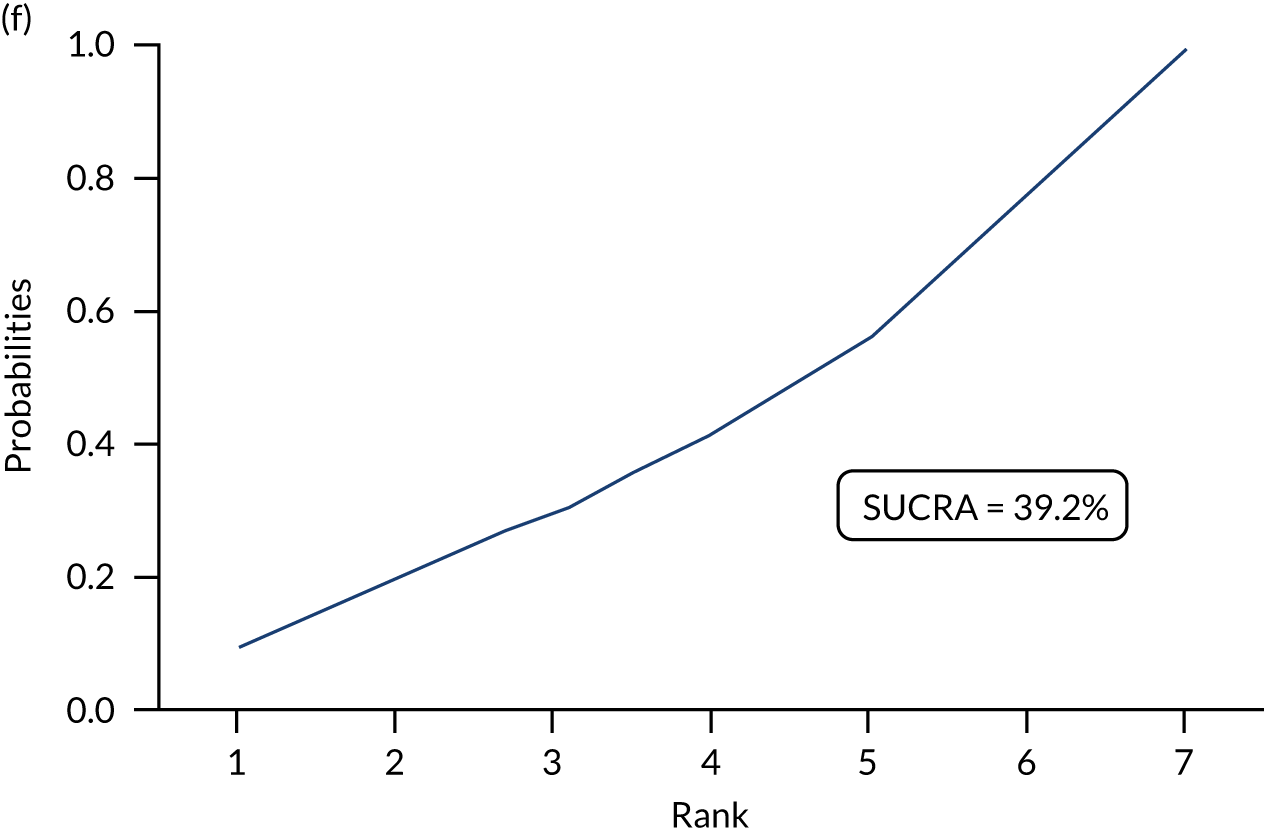
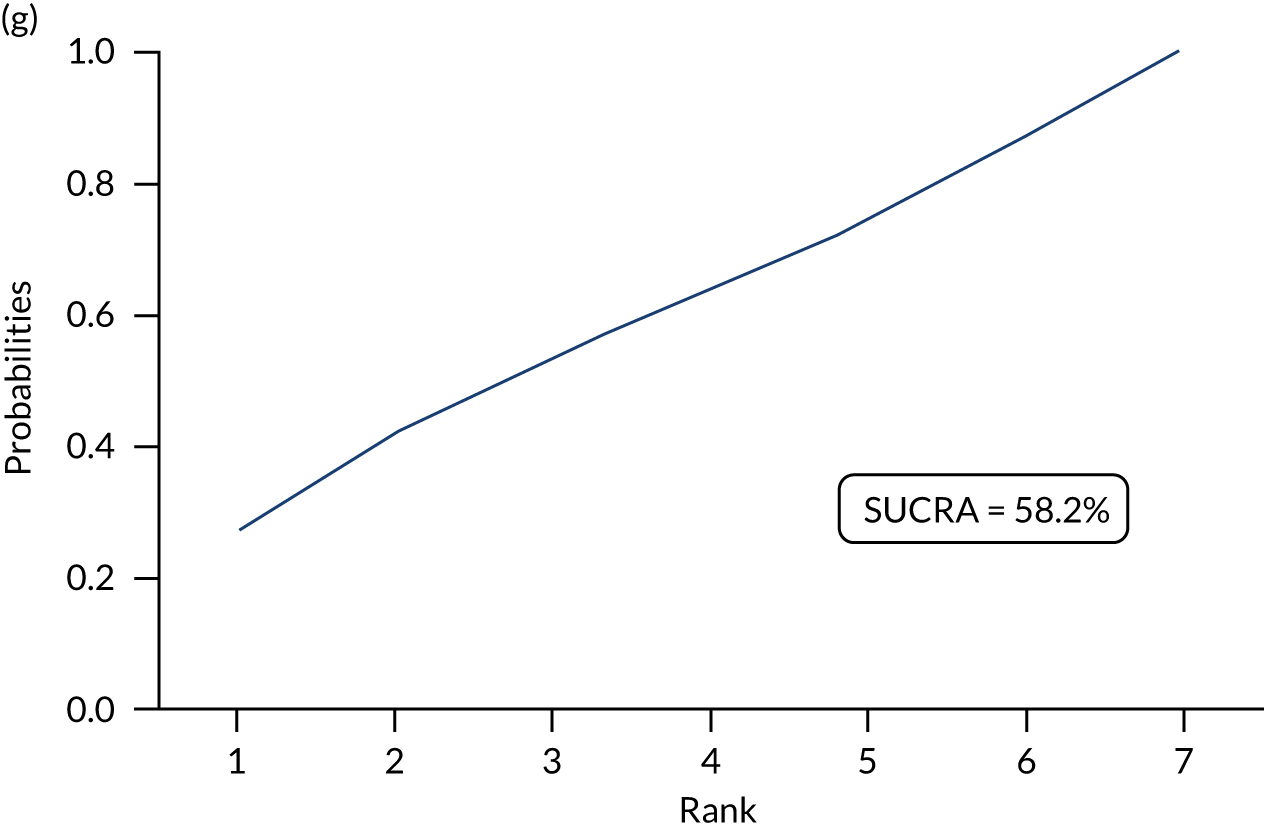
FIGURE 26.
Penn State Worry Questionnaire rankograms: rankogram of each intervention for PSWQ: (a) NI; (b) UDC; (c) SDC; (d) UDI; (e) SDI; (f) SNoDC; and (g) SNoDI.
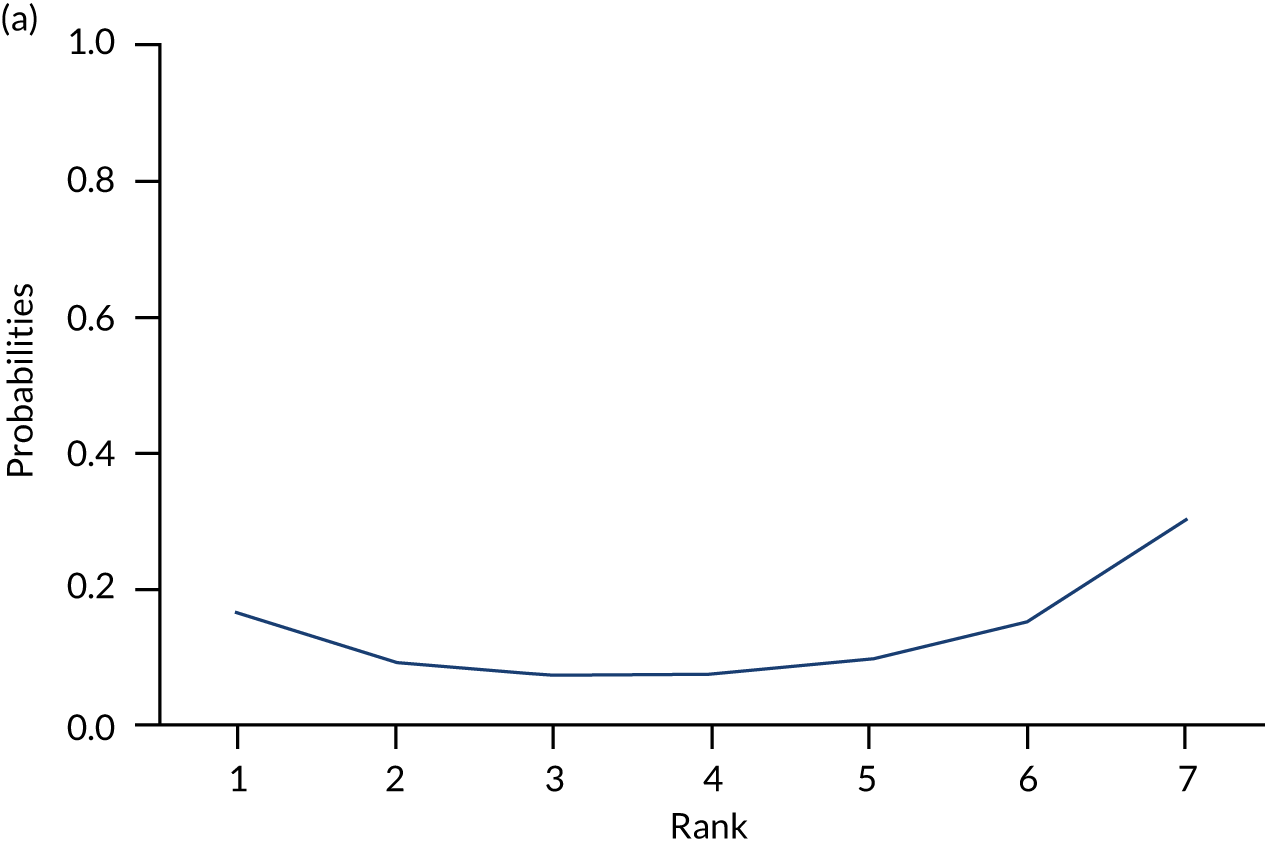
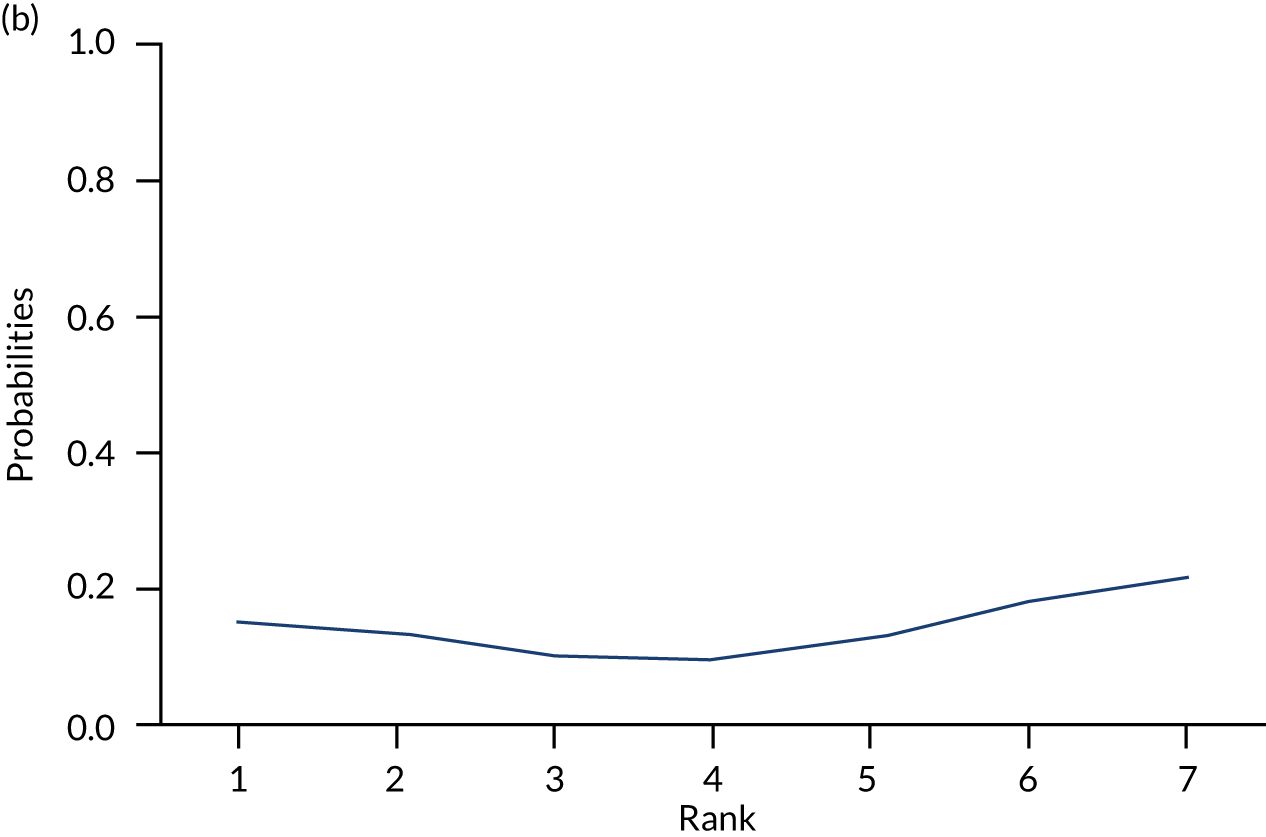
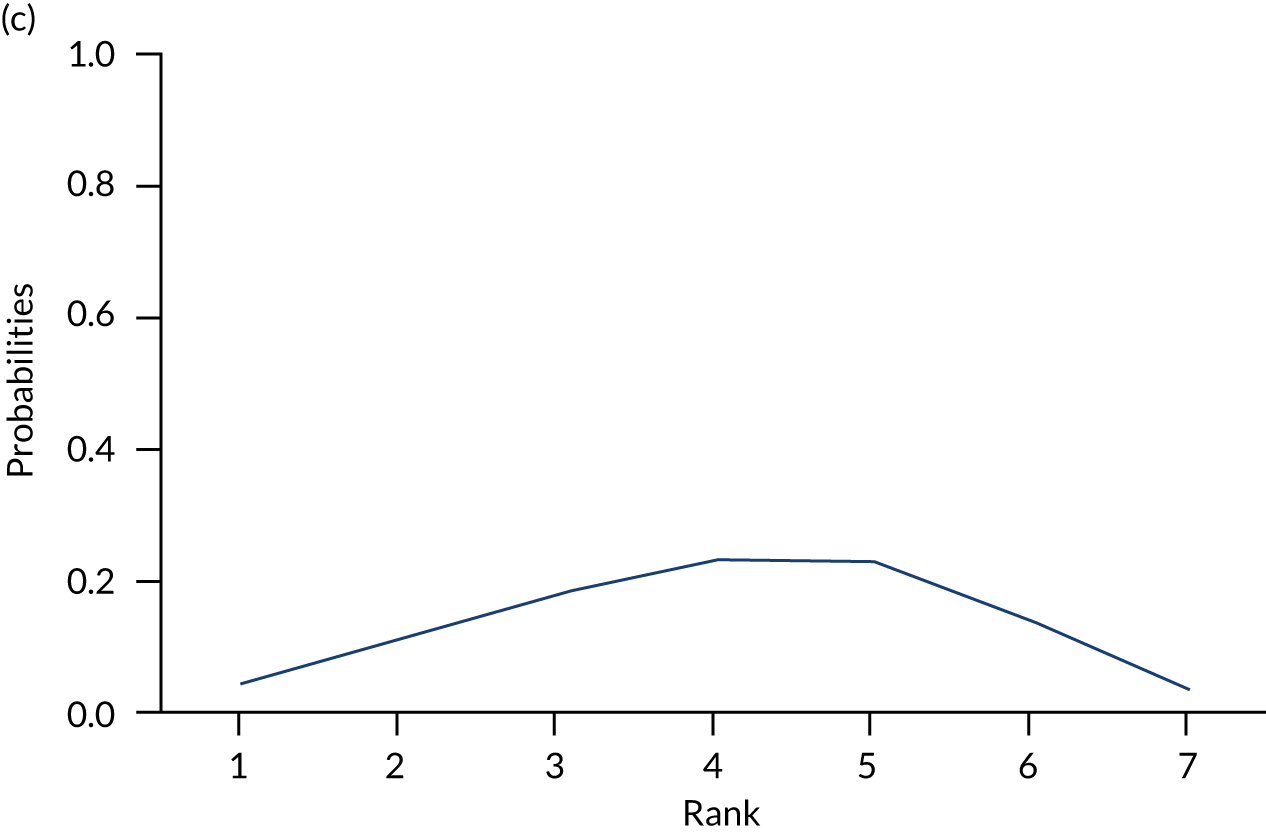
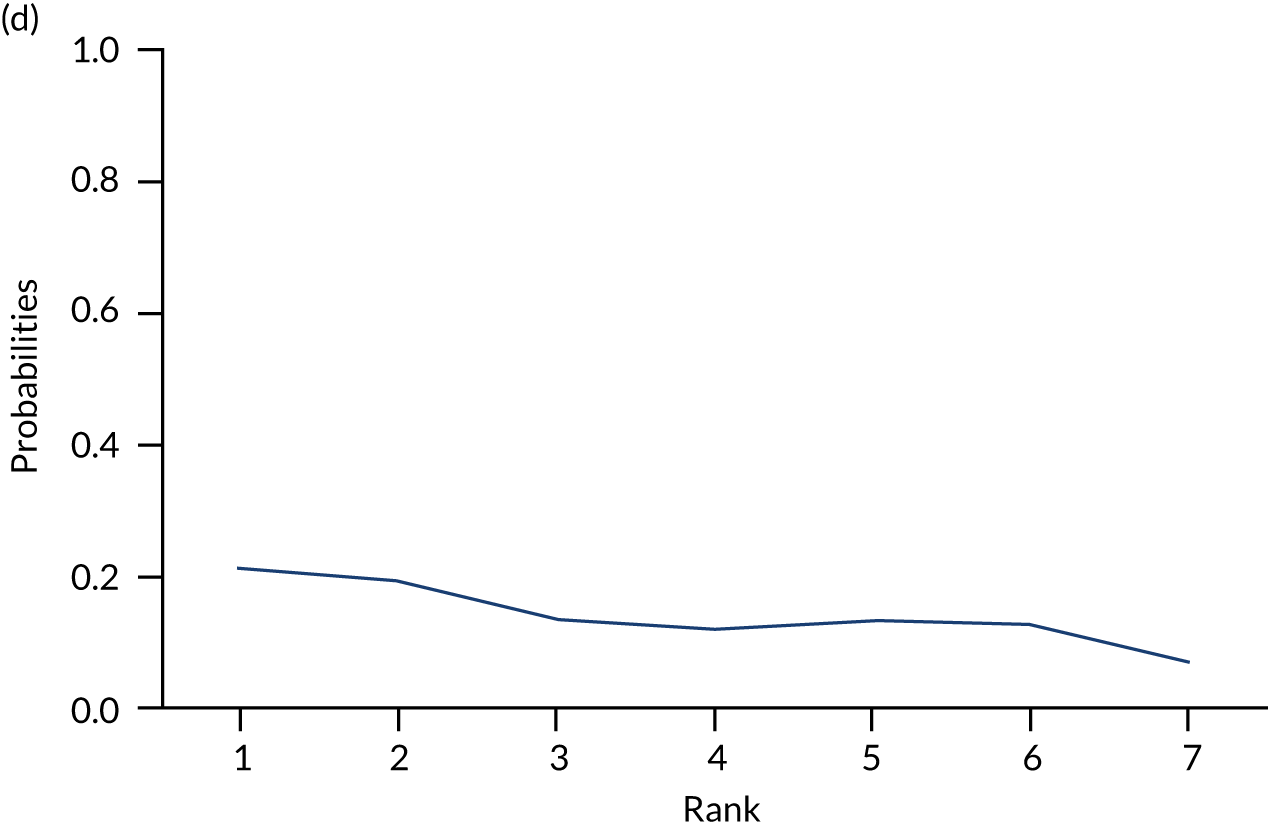
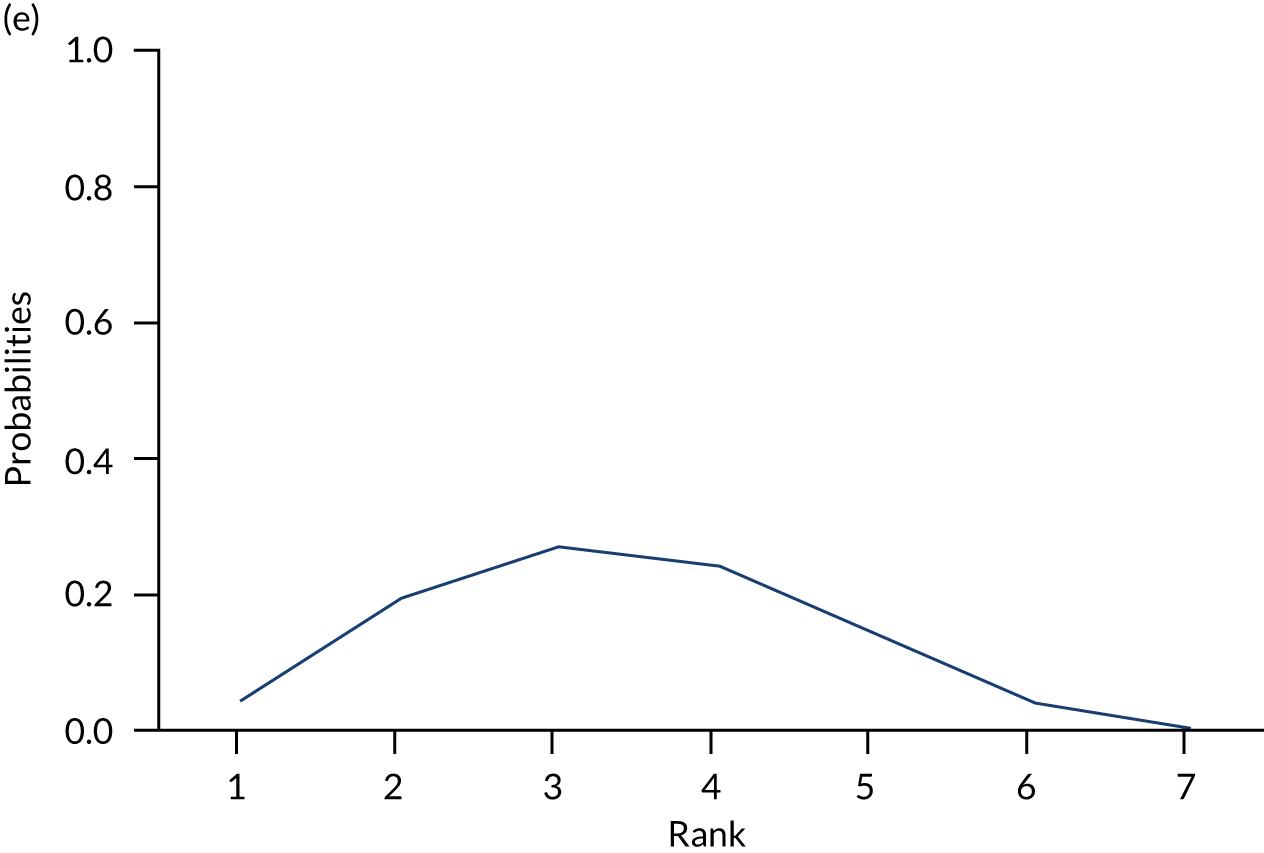
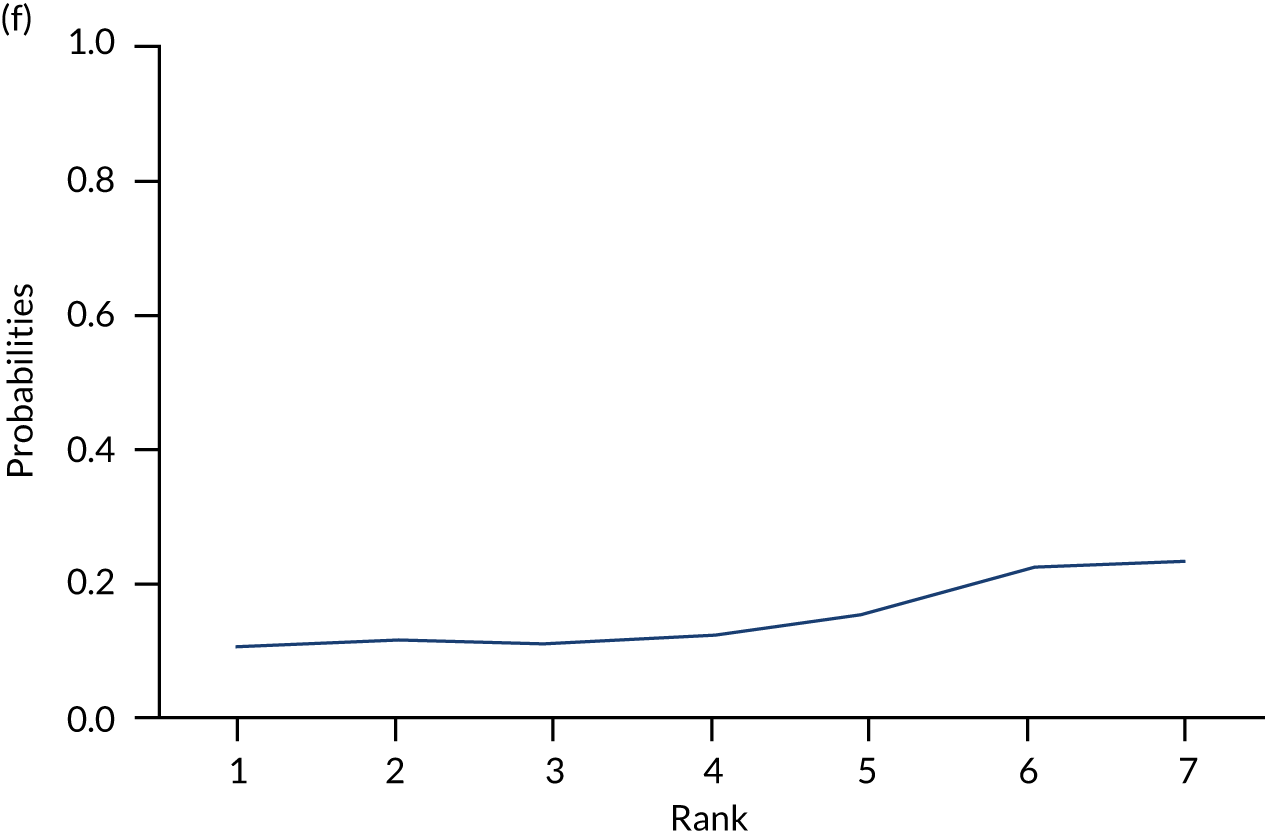
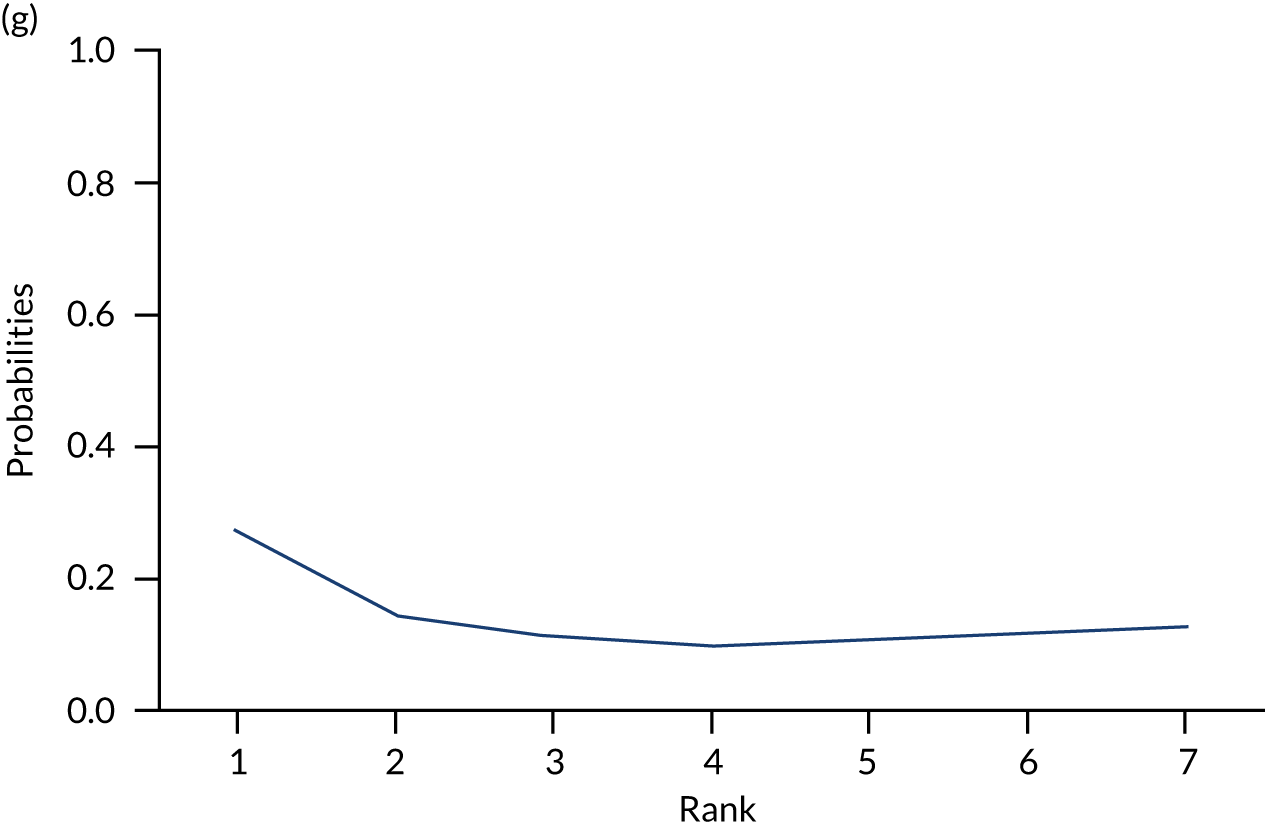
Appendix 5 Assessment of between-study heterogeneity and inconsistencies
Reproduced with permission from Saramago et al. 124 This is an Open Access article distributed in accordance with the terms of the Creative Commons Attribution (CC BY 4.0) license, which permits others to distribute, remix, adapt and build upon this work, for commercial use, provided the original work is properly cited. See: https://creativecommons.org/licenses/by/4.0/. The text below includes minor additions and formatting changes to the original text.
As only one study was performed in a population with milder disease, the NMA ANCOVA RE meta-regression model considering a binary covariate on severity (1, mild/moderate; 0, moderate/severe) did not converge for either of the end points. For comorbidities, the number of studies reporting information on the proportion of individuals within the trial with comorbidities was limited (GAD-7, 2 out of 13; PSWQ, 6 out of 14), impairing the use of multiple imputation. For this reason, we did not explore this variable further. Information on the proportion of individuals with concomitant medication was more prevalent across the evidence base (GAD-7, 9/13; PSWQ, 9/14); thus, data on concomitant medication were included as a covariate in the synthesis modelling.
Network meta-analysis models for GAD-7 and PSWQ, which account for the proportion of patients receiving concomitant medication, fit comparably with those that did not, suggesting that no improvement in model fit was achieved. The effect modification coefficient is of the expected direction [GAD-7: βmed = –1.8 (95% CrI –28.6 to 24.2); PSWQ: βmed = –7.7 (95% CrI –81.2 to 64.9)], suggesting that, as the proportion of patients receiving concomitant medication increases, GAD-7 and PSWQ scores are reduced. However, for both outcomes, the covariate effect is not statistically significant and highly uncertain. For both outcomes, when this covariate is included, the between-study heterogeneity parameter, τ2, is not reduced, suggesting that heterogeneity is not explained by this covariate. Crucially, even if the proportion receiving concomitant medication is found to be an important effect modifier, the described meta-regression model is not necessarily suited to detect this intervention–covariate interaction as patients were receiving medication before trial entry. Therefore, medication may have already exerted an effect on patients, being captured by the ANCOVA baseline adjustment component, βk.
The consistency models produced lower DIC (difference in GAD-7 end point > 3 points) than the inconsistency models and, therefore, the additional model complexity that is due to the consistency assumptions is supported by the data (Table 24). The consistency plots (Figures 27 and 28) show that there are a few deviant data points for which the inconsistency models lead to higher residual deviance than the consistency models, further supporting the latter.
Model | Dres, median (95% CrI) | DIC | τ2, median (95% CrI) | B_base, median (95% CrI) |
---|---|---|---|---|
Outcome: GAD-7 | ||||
NMA ANCOVA RE: consistency model | 21.28 (11.23, 36.39) | 194.50 | 1.85 (0.004, 25.47) | –0.09 (–0.85, 0.68) |
NMA ANCOVA RE: inconsistency model | 23.11 (12.45, 38.73) | 198.17 | 1.95 (0.004, 29.37) | –0.08 (–1.77, 1.65) |
Outcome: PSWQ | ||||
NMA ANCOVA RE: consistency model | 21.33 (11.34, 36.33) | 253.82 | 8.03 (0.02, 77.54) | 0.01 (–0.45, 0.48) |
NMA ANCOVA RE: inconsistency model | 21.58 (11.61, 36.51) | 254.27 | 7.06 (0.01, 73.4) | –0.01 (–1.13, 1.13) |
FIGURE 27.
Consistency plot for GAD-7.
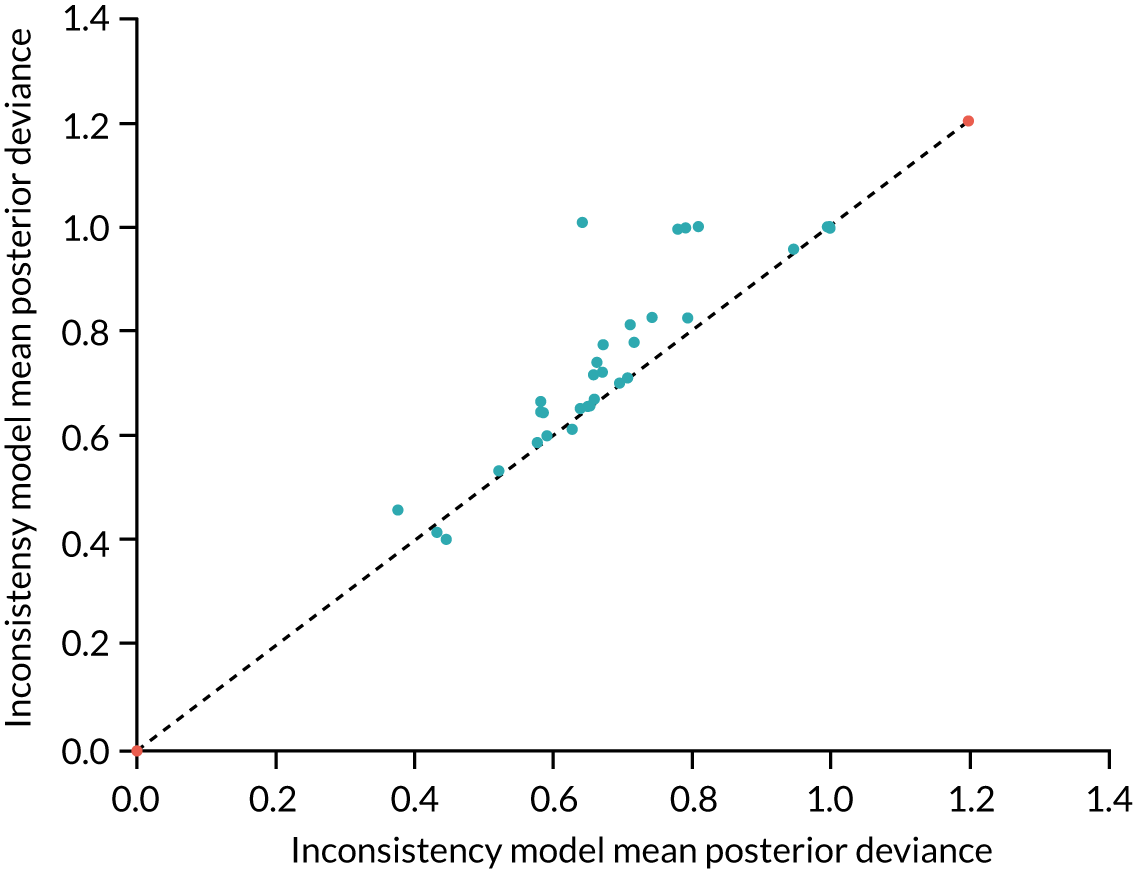
FIGURE 28.
Consistency plot for PSWQ.
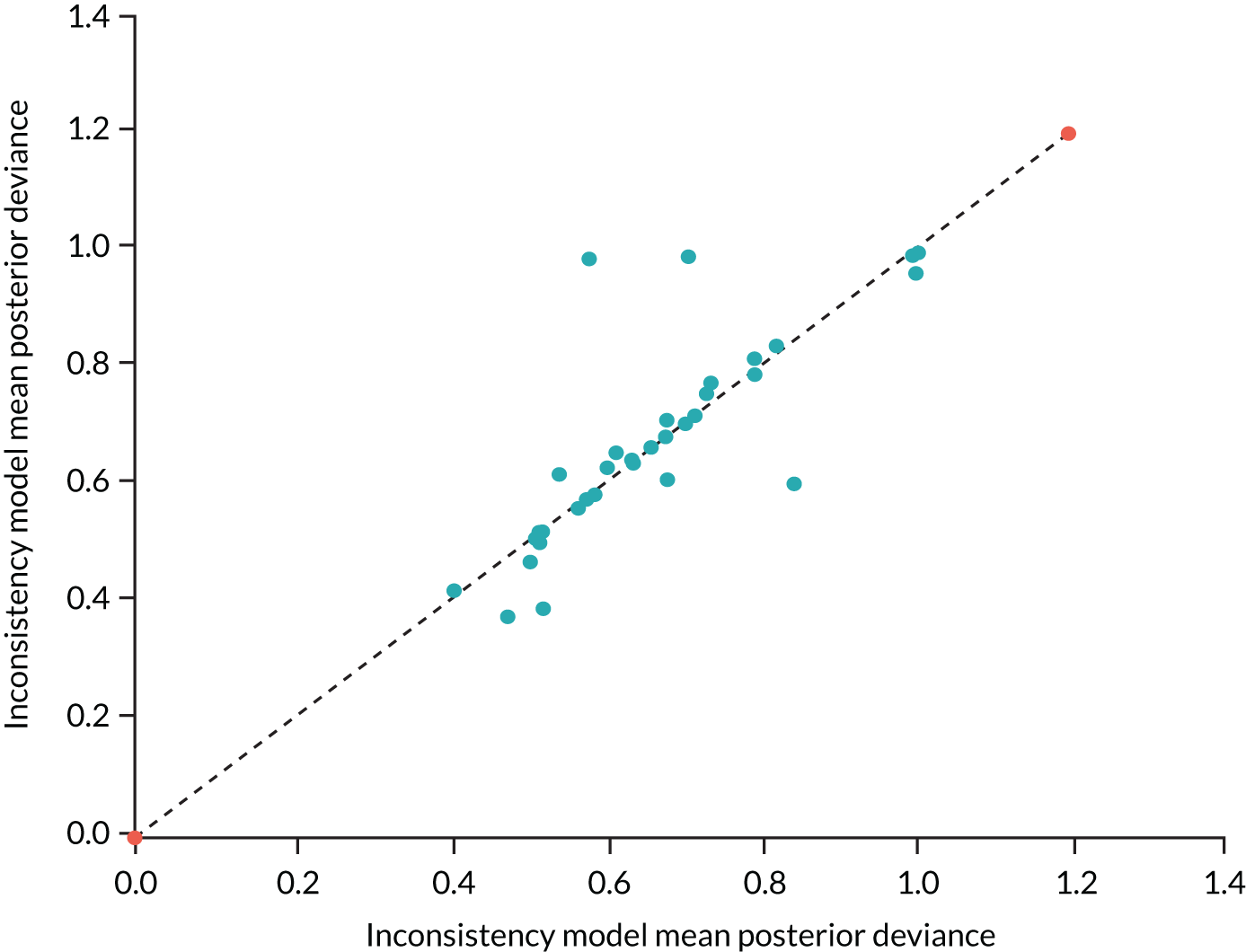
Appendix 6 Costing the support for digital interventions and controls
Parts of this appendix have been reproduced with permission from Jankovic et al. 189 This is an Open Access article distributed in accordance with the terms of the Creative Commons Attribution-NonCommercial (CC BY-NC 4.0) license, which permits others to distribute, remix, adapt and build upon this work, for non-commercial use, provided the original work is properly cited. See: https://creativecommons.org/licenses/by-nc/4.0/. The text below includes minor additions and formatting changes to the original text.
The cost of SDIs and SDCs was derived from (1) the cost of time spent by the facilitators of SDI/SDCs, as reported in the studies we included in our meta-analysis of RCTs on DIs for GAD, and (2) the cost of the person who provided the support. In SDCs (Table 25), the duration of contact varied between 5 minutes and 2.5 hours, and therapy was delivered by both clinical psychologists and non-clinical staff. In the base case, we assumed that interventions required 20 minutes of clinical psychologists’ time, and additional costs were explored in scenario analyses. In SDIs (Table 26), the duration of one-on-one contact varied from 33.54 to 130 minutes. The vast majority of studies that reported who delivered the intervention stated that the support was provided by clinical psychologists. Hence, in the base case, the interventions were assumed to last 90 minutes and to be delivered by a clinical psychologist, and additional costs were explored in scenario analyses.
Study (first author and year) | Contact time | Type of therapist |
---|---|---|
Christensen 20144 | 5–20 minutes | ‘Casual telephone interviewers’ |
Christensen 2014159 | Four appointments duration, NR; three appointments duration, NR | Clinical psychologist; GP |
Hazen 2009161 | 5 × 30 minutes | Not reported |
Hirsch 2018162 | NR | Researchers (unspecified) |
Johansson 201337 | 10 × 2.3 minutes (SD 0.86 minutes) | Master’s-level students in their last semester of a 5-year clinical psychologist programme |
Study (first author and year) | Contact time | Type of therapist |
---|---|---|
Andersson 2012158 | 113 minutes (SD 41 minutes) | Therapists in their final year of a 5-year clinical psychology programme and licensed psychologist |
Andersson 2012158 | 92 minutes (SD 61 minutes) | Psychologists with experience guiding internet treatment for GAD, and psychology students in their final year |
Andersson 201740 | 117 minutes (SD 96 minutes) | Clinical psychology students in their final year of the 5-year psychologist programme |
Christensen 20144 | 5–20 minutes | ‘Casual interviewers’ |
Christensen 2014159 | Four appointments duration, NR; three appointments duration, NR | Clinical psychologist; GP |
Dahlin 201641 | 78.78 minutes (range 1–226 minutes) | Clinical psychologist graduate students, supervised by clinical psychologist |
Dear 2015160 | NR, not significantly different from guided CBT (below) | Qualified psychologist |
Dear 2015160 | 33.54 minutes (SD 18.07 minutes) | Qualified psychologist |
Hazen 2009161 | 2.5 hours (5 × 30 minutes) | NR |
Hirsch 2018162 | NR | Researchers (unspecified) |
Hirsch 2018162 | NR | Researchers (unspecified) |
Johansson 201337 | 95 minutes (10 × 9.5 minutes; SD 4.0 minutes) | Master’s-level students in their last semester of a 5-year clinical psychologist programme |
Jones 2016164 | 105–210 minutes intended | Therapist, unspecified qualifications |
Navarro-Haro 2019165 | 9 hours, group; 90 minutes, individual | NR |
Paxling 2011166 | 9 minutes (SD 52 minutes) | Therapist in the final year of psychologist training |
Repetto 2013167 | NR | NR |
Repetto 2013167 | NR | NR |
Richards 2016168 | 60–90 minutes | Psychologists with a Master’s degree or higher |
Robinson 2010169 | 80.8 minutes (SD 22.6 minutes) | Qualified and registered clinical psychologist |
Robinson 2010169 | 74.5 minutes (SD 7.8 minutes) | Technician employed in an administrative role as a clinic manager |
Teng 201938 | 2.5 hours (5 × 30 minutes) | NR |
Titov 2009171 | 130 minutes with clinical psychologist; 30 minutes with administrator | Clinical psychologist; administrator |
Titov 2010170 | 46 minutes (SD 16 minutes) | Clinical psychologist |
Topper 2017172 | 3.96 sessions (SD 1.65 minutes) | Clinical psychologist |
Appendix 7 Model parameters
Parts of this appendix have been adapted with permission from Jankovic et al. 189 This is an Open Access article distributed in accordance with the terms of the Creative Commons Attribution-NonCommercial (CC BY-NC 4.0) license, which permits others to distribute, remix, adapt and build upon this work, for non-commercial use, provided the original work is properly cited. See: https://creativecommons.org/licenses/by-nc/4.0/. The text below includes minor additions and formatting changes to the original text.
Table 27 shows the distribution of patients across health states after treatment. At baseline (i.e. before treatment) the distribution was assumed to be identical to NI for all comparators.
Health state | Intervention | ||||||
---|---|---|---|---|---|---|---|
NI | UDC | SDC | UDI | SDI | SNoDIa | Mb | |
No anxiety | 0 | 0.114 | 0.073 | 0.115 | 0.038 | 0.290 | 0.492 |
Mild anxiety | 0.179 | 0.411 | 0.451 | 0.505 | 0.800 | 0.422 | 0.598 |
Moderate anxiety | 0.786 | 0.380 | 0.428 | 0.351 | 0.162 | 0.288 | 0 |
Severe anxiety | 0.035 | 0.094 | 0.047 | 0.029 | 0 | 0 | 0 |
Tables 28 and 29 show the model parameter values, with measures of uncertainty.
Parameter | Mean | Probability distribution | Source |
---|---|---|---|
Baseline GAD-7 score | 10.68 | ∼N(10.68, 1.83) | Chapter 4 |
GAD-7 score after treatment | |||
UDC | 8.77 | ∼N(8.77, 3.72) | Chapter 4 |
SDC | 8.76 | ∼N(8.76, 3.14) | |
UDI | 8.01 | ∼N(8.01, 3.16) | |
SDI | 7.22 | ∼N(7.22, 1.82) | |
SNoDI | 6.51 | ∼N(6.51, 3.59) | |
Medication | 4.08 | ∼N(4.08, 1.89) | |
Intervention cost | |||
NI, UDC, UDI | £0 | – | Assumed |
SDC | £18 | – | Derived |
SDI | £80 | – | Derived |
SNoDI | £80 | – | Derived |
Medication (per year) | £351.04 in year 1, £201.94 thereafter | – | Derived |
Health-care cost | Gamma (shape, scale) | Kaltenthaler et al.14 | |
No anxiety | £86 | (1.960, 0.016) | |
Mild anxiety | £200 | (0.848, 0.003) | |
Moderate anxiety | £210 | (0.295, 0.001) | |
Severe anxiety | £324 | (0.320, 0.0007) | |
Dead | £0 | – | |
Utilities | Mean (SD) used to derive beta parametersa | Revicki et al.126 | |
No anxiety | 0.72 | 0.72 (0.10) | |
Mild anxiety | 0.64 | 0.64 (0.10) | |
Moderate anxiety | 0.60 | 0.60 (0.10) | |
Severe anxiety | 0.53 | 0.60 (0.10) | |
Dead | 0 | – | |
Age-related utility decrements | Age specific (see Table 29) | ||
Mortality – general population | Sex and age specific (see Table 29) | ||
Excess mortality (RR) | Non-parametric CIb | Michal et al.198 | |
Mild anxiety | 1.20 | 0.82 to 1.67 | |
Moderate anxiety | 1.58 | 1.06 to 2.24 | |
Severe anxiety | 2.17 | 1.47 to 3.05 |
Age (years) | Mortality | Utility (SE) | |
---|---|---|---|
Male | Female | ||
0 | 0.004288 | 0.003592 | – |
1 | 0.000257 | 0.000228 | 0.94 (0.002) |
2 | 0.000129 | 0.000128 | 0.94 (0.002) |
3 | 0.000118 | 0.000096 | 0.94 (0.002) |
4 | 0.000095 | 0.000071 | 0.94 (0.002) |
5 | 0.000095 | 0.000072 | 0.94 (0.002) |
6 | 0.000067 | 0.00007 | 0.94 (0.002) |
7 | 0.000079 | 0.000063 | 0.94 (0.002) |
8 | 0.000066 | 0.000058 | 0.94 (0.002) |
9 | 0.000072 | 0.000063 | 0.94 (0.002) |
10 | 0.000072 | 0.000061 | 0.94 (0.002) |
11 | 0.000086 | 0.000074 | 0.94 (0.002) |
12 | 0.000099 | 0.000066 | 0.94 (0.002) |
13 | 0.000106 | 0.000074 | 0.94 (0.002) |
14 | 0.000131 | 0.000091 | 0.94 (0.002) |
15 | 0.000176 | 0.000107 | 0.94 (0.002) |
16 | 0.000225 | 0.000146 | 0.94 (0.002) |
17 | 0.000303 | 0.00015 | 0.94 (0.002) |
18 | 0.000391 | 0.000204 | 0.94 (0.002) |
19 | 0.000411 | 0.000187 | 0.94 (0.002) |
20 | 0.000485 | 0.00019 | 0.94 (0.002) |
21 | 0.000489 | 0.000213 | 0.94 (0.002) |
22 | 0.000483 | 0.000204 | 0.94 (0.002) |
23 | 0.000487 | 0.000196 | 0.94 (0.002) |
24 | 0.000515 | 0.00021 | 0.94 (0.002) |
25 | 0.00054 | 0.000252 | 0.94 (0.002) |
26 | 0.000548 | 0.000249 | 0.93 (0.003) |
27 | 0.00056 | 0.000269 | 0.93 (0.003) |
28 | 0.000628 | 0.000315 | 0.93 (0.003) |
29 | 0.000653 | 0.000304 | 0.93 (0.003) |
30 | 0.000692 | 0.000364 | 0.93 (0.003) |
31 | 0.00077 | 0.00037 | 0.93 (0.003) |
32 | 0.000776 | 0.000464 | 0.93 (0.003) |
33 | 0.000857 | 0.000466 | 0.93 (0.003) |
34 | 0.000904 | 0.000521 | 0.93 (0.003) |
35 | 0.000964 | 0.000552 | 0.93 (0.003) |
36 | 0.001083 | 0.000603 | 0.91 (0.003) |
37 | 0.001145 | 0.000714 | 0.91 (0.003) |
38 | 0.001142 | 0.0007 | 0.91 (0.003) |
39 | 0.001277 | 0.000772 | 0.91 (0.003) |
40 | 0.001413 | 0.000814 | 0.91 (0.003) |
41 | 0.001571 | 0.000929 | 0.91 (0.003) |
42 | 0.001693 | 0.001014 | 0.91 (0.003) |
43 | 0.001951 | 0.001122 | 0.91 (0.003) |
44 | 0.002024 | 0.001274 | 0.91 (0.003) |
45 | 0.002157 | 0.001375 | 0.91 (0.003) |
46 | 0.002296 | 0.00148 | 0.85 (0.004) |
47 | 0.002591 | 0.001651 | 0.85 (0.004) |
48 | 0.002747 | 0.001767 | 0.85 (0.004) |
49 | 0.003004 | 0.001895 | 0.85 (0.004) |
50 | 0.003224 | 0.002047 | 0.85 (0.004) |
51 | 0.003421 | 0.002281 | 0.85 (0.004) |
52 | 0.003744 | 0.002482 | 0.85 (0.004) |
53 | 0.003967 | 0.002657 | 0.85 (0.004) |
54 | 0.004248 | 0.002822 | 0.85 (0.004) |
55 | 0.004732 | 0.003163 | 0.85 (0.004) |
56 | 0.00514 | 0.003484 | 0.8 (0.004) |
57 | 0.005696 | 0.00377 | 0.8 (0.004) |
58 | 0.006252 | 0.00418 | 0.8 (0.004) |
59 | 0.006755 | 0.004536 | 0.8 (0.004) |
50 | 0.007549 | 0.004914 | 0.8 (0.004) |
61 | 0.008237 | 0.005432 | 0.8 (0.004) |
62 | 0.009032 | 0.006128 | 0.8 (0.004) |
63 | 0.010114 | 0.006559 | 0.8 (0.004) |
64 | 0.010879 | 0.007089 | 0.8 (0.004) |
65 | 0.011916 | 0.0077 | 0.8 (0.004) |
66 | 0.013085 | 0.008553 | 0.78 (0.004) |
67 | 0.014063 | 0.009173 | 0.78 (0.004) |
68 | 0.015525 | 0.010067 | 0.78 (0.004) |
69 | 0.016679 | 0.010969 | 0.78 (0.004) |
70 | 0.018153 | 0.012068 | 0.78 (0.004) |
71 | 0.020201 | 0.013331 | 0.78 (0.004) |
72 | 0.022407 | 0.015463 | 0.78 (0.004) |
73 | 0.025271 | 0.016993 | 0.78 (0.004) |
74 | 0.027758 | 0.01867 | 0.78 (0.004) |
75 | 0.031326 | 0.021376 | 0.78 (0.004) |
76 | 0.034938 | 0.024237 | 0.73 (0.005) |
77 | 0.039053 | 0.02692 | 0.73 (0.005) |
78 | 0.042973 | 0.030324 | 0.73 (0.005) |
79 | 0.047425 | 0.033515 | 0.73 (0.005) |
80 | 0.053347 | 0.037897 | 0.73 (0.005) |
81 | 0.059653 | 0.042929 | 0.73 (0.005) |
82 | 0.066297 | 0.048841 | 0.73 (0.005) |
83 | 0.075312 | 0.056501 | 0.73 (0.005) |
84 | 0.085068 | 0.063635 | 0.73 (0.005) |
85 | 0.094756 | 0.072676 | 0.73 (0.005) |
86 | 0.106853 | 0.082822 | 0.73 (0.005) |
87 | 0.119617 | 0.094077 | 0.73 (0.005) |
88 | 0.133736 | 0.106986 | 0.73 (0.005) |
89 | 0.149832 | 0.119952 | 0.73 (0.005) |
90 | 0.162588 | 0.13548 | 0.73 (0.005) |
91 | 0.178751 | 0.151395 | 0.73 (0.005) |
92 | 0.198581 | 0.167872 | 0.73 (0.005) |
93 | 0.218678 | 0.1851 | 0.73 (0.005) |
94 | 0.237316 | 0.204495 | 0.73 (0.005) |
95 | 0.262646 | 0.228609 | 0.73 (0.005) |
96 | 0.285623 | 0.247393 | 0.73 (0.005) |
97 | 0.307486 | 0.268697 | 0.73 (0.005) |
98 | 0.322356 | 0.288444 | 0.73 (0.005) |
99 | 0.363244 | 0.314599 | 0.73 (0.005) |
100 | 0.391216 | 0.336838 | 0.73 (0.005) |
101 | – | – | 0.73 (0.005) |
Appendix 8 Generalised anxiety disorder score trajectory in scenario analysis
Without treatment, patients’ GAD symptoms were assumed to improve over time, as reported by Yonkers et al. ,190 where 15% of patients recovered in the first year, a further 10% recovered in the second year and a further 5% recovered in the third year. The treatment effect was assumed to remain constant indefinitely (i.e. any change in GAD severity remained over a patient’s lifetime). In addition, in the base case, patients on treatment were assumed to improve over time at the same rate as those who had not received treatment. Five additional scenarios regarding the GAD score trajectory are summarised in Table 30.
Period for which treatment effect lasts | GAD score trajectory with treatment over time | GAD score trajectory with ‘no treatment’ over time | |
---|---|---|---|
Spontaneous recovery: GAD-7 scores decrease | No change: GAD-7 scores are constant | ||
Indefinitely | Constant: GAD-7 scores remain at post-treatment level indefinitely | Scenario 1 (base case) | Scenario 4 |
For 1 yeara then disappears | GAD-7 scores remain at post-treatment level for 1 year, then return to ‘no treatment’ level | Scenario 2 | Scenario 5 |
For 1 yeara then diminishes gradually for 10 years | GAD-7 scores decline over 10 years until they return to ‘no treatment’ level | Scenario 3 | Scenario 6 |
Appendix 9 Health-care costs in scenario analysis
Parts of this appendix have been reproduced with permission from Jankovic et al. 189 This is an Open Access article distributed in accordance with the terms of the Creative Commons Attribution-NonCommercial (CC BY-NC 4.0) license, which permits others to distribute, remix, adapt and build upon this work, for non-commercial use, provided the original work is properly cited. See: https://creativecommons.org/licenses/by-nc/4.0/. The text below includes minor additions and formatting changes to the original text.
Two alternative scenarios regarding health-care resource use informed state-specific health-care costs using alternative studies: Vera-Llonch et al. 194 and Kumar et al. 85 The cost of health care (Table 31) was derived by multiplying the reported resource use by the unit costs available from the NHS England tariff, year 2018/19. 201
It is important to note that Vera-Llonch et al. 194 evaluated medication for GAD, and so some of the health-care costs shown in Table 31 are likely to be associated with pharmacotherapy. We could not ascertain which resources were used for the pharmacotherapy from the available data, but we caution that the estimates derived using data from Vera-Llonch et al. 194 could overestimate health-care costs in the model.
Source (first author and year) | Health-care resource use | Unit cost of health services (£) | ||||
---|---|---|---|---|---|---|
Health-care service | No anxiety | Mild anxiety | Moderate anxiety | Severe anxiety | ||
Kumar 201885 | Primary care visits (n) | 1.2 | 1.7 | 2.2 | 2.4 | 31 (GP appointment) |
Emergency care visits (n) | 0.014 | 0.019 | 0.025 | 0.027 | 222 | |
Inpatient days (n) | 0.014 | 0.019 | 0.025 | 0.027 | 1603 | |
Total cost (£) per model cycle | 188 | 262 | 341 | 371 | – | |
Vera-Llonch 2010194 | Primary care visits (n) | 0.44 | 1.03 | 1.26 | 1.80 | 31 (GP appointment) |
Specialist visits (n) | ||||||
Psychiatrist | 0.42 | 0.48 | 0.48 | 0.49 | 109 (1 hour, consultant) | |
Psychologist | 0.48 | 0.52 | 1.03 | 1.37 | 53 (1 hour, grade 7) | |
Emergency room | 0.14 | 0.26 | 0.37 | 0.56 | 222 | |
Other | 0.33 | 0.37 | 0.58 | 0.52 | 0 | |
Blood counts (n) | 0.35 | 0.38 | 0.5 | 0.43 | 6.75 | |
Electrocardiography (n) | 0.33 | 0.35 | 0.33 | 0.18 | 58 | |
Thyroid function tests (n) | 0.33 | 0.33 | 0.36 | 0.35 | 1.84 | |
Inpatient days (mean) | 0.12 | 0.18 | 0.37 | 0.49 | 1603 | |
Total cost per model cycle (£) | 911 | 1444 | 2531 | 3316 | – |
Appendix 10 Cumulative costs and outcomes over time
FIGURE 29.
Mean cumulative QALY gain over time. M, medication. Reproduced with permission from Jankovic et al. 189 This is an Open Access article distributed in accordance with the terms of the Creative Commons Attribution-NonCommercial (CC BY-NC 4.0) license, which permits others to distribute, remix, adapt and build upon this work, for non-commercial use, provided the original work is properly cited. See: https://creativecommons.org/licenses/by-nc/4.0/. The figure includes minor additions and formatting changes to the original figure.
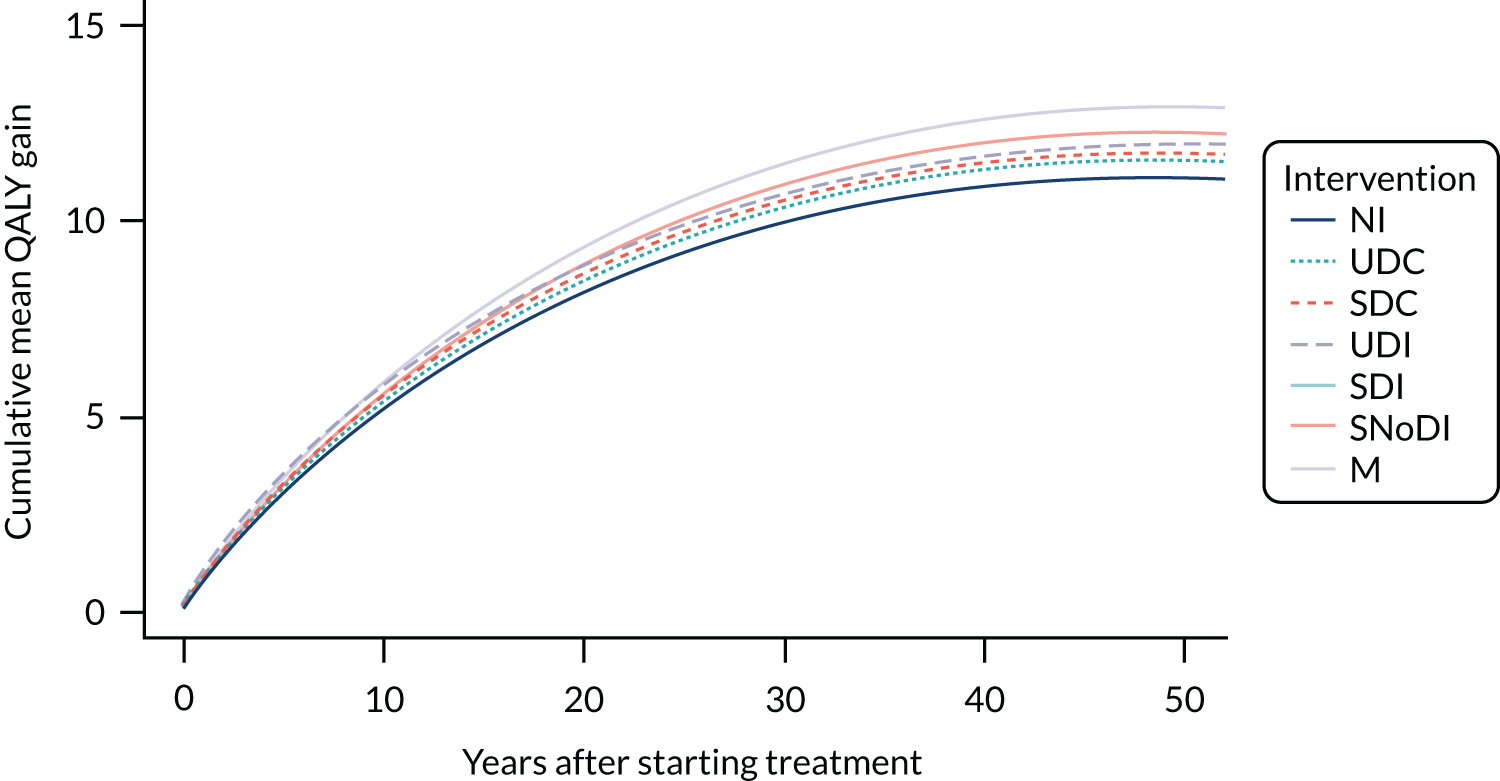
FIGURE 30.
Mean cumulative total cost over time. M, medication. Reproduced with permission from Jankovic et al. 189 This is an Open Access article distributed in accordance with the terms of the Creative Commons Attribution-NonCommercial (CC BY-NC 4.0) license, which permits others to distribute, remix, adapt and build upon this work, for non-commercial use, provided the original work is properly cited. See: https://creativecommons.org/licenses/by-nc/4.0/. The figure includes minor additions and formatting changes to the original figure.
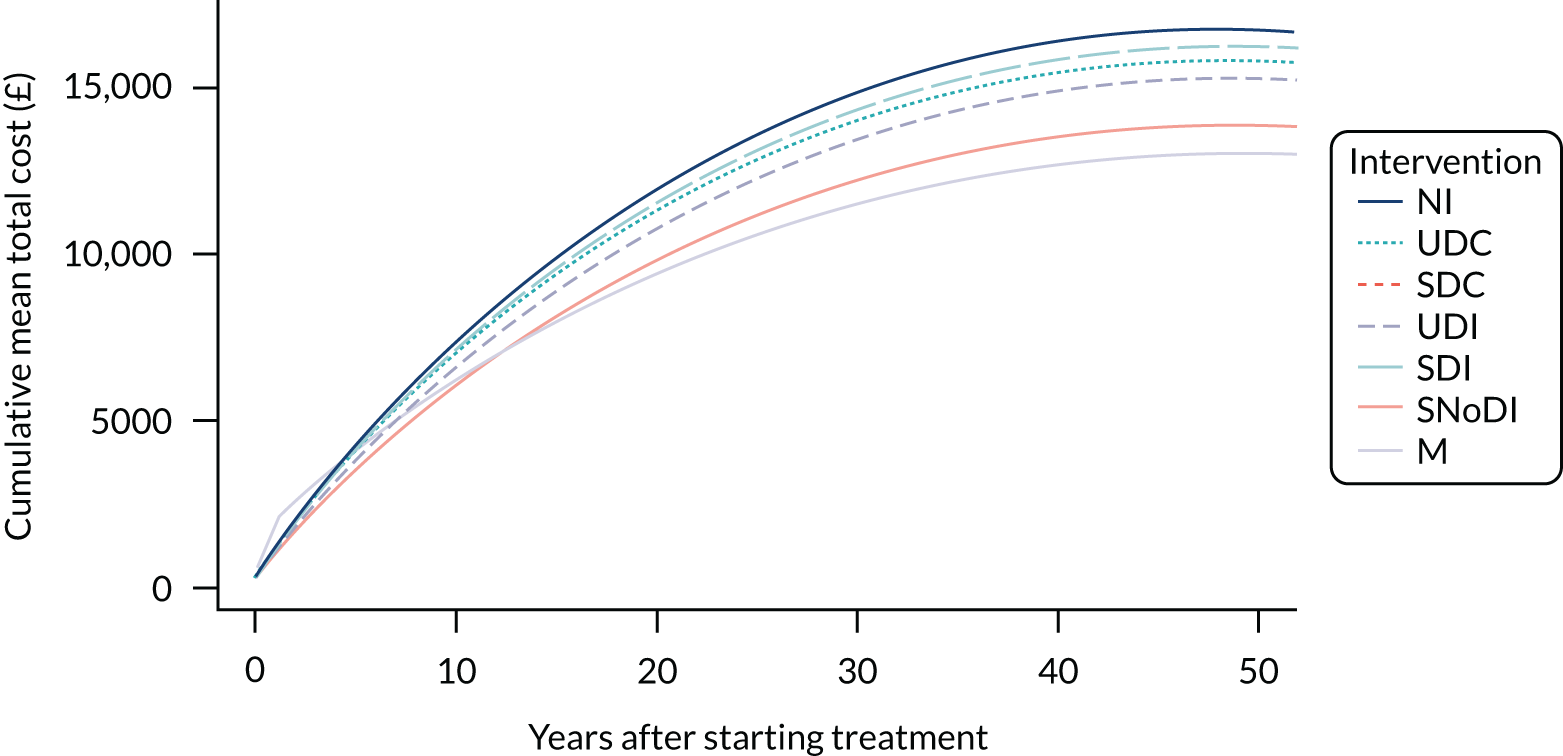
Appendix 11 Results from scenario analyses
Parts of this appendix have been adapted with permission from Jankovic et al. 189 This is an Open Access article distributed in accordance with the terms of the Creative Commons Attribution-NonCommercial (CC BY-NC 4.0) license, which permits others to distribute, remix, adapt and build upon this work, for non-commercial use, provided the original work is properly cited. See: https://creativecommons.org/licenses/by-nc/4.0/. The text below includes minor additions and formatting changes to the original text.
Alternative assumptions about the generalised anxiety disorder score trajectory with and without treatment
Six scenarios were explored for the GAD-7 score trajectory over time. The movement through the states and the changes in GAD-7 scores in the initial 10-year period are shown in Figures 31–33. After 10 years, outcomes were assumed to remain constant in all scenarios.
FIGURE 31.
Movement through Markov states without treatment in the initial 10-year period, where GAD-7 scores improve over time (scenarios 1–3). Adapted with permission from Jankovic et al. 189 This is an Open Access article distributed in accordance with the terms of the Creative Commons Attribution-NonCommercial (CC BY-NC 4.0) license, which permits others to distribute, remix, adapt and build upon this work, for non-commercial use, provided the original work is properly cited. See: https://creativecommons.org/licenses/by-nc/4.0/. The figure includes minor additions and formatting changes to the original figure.
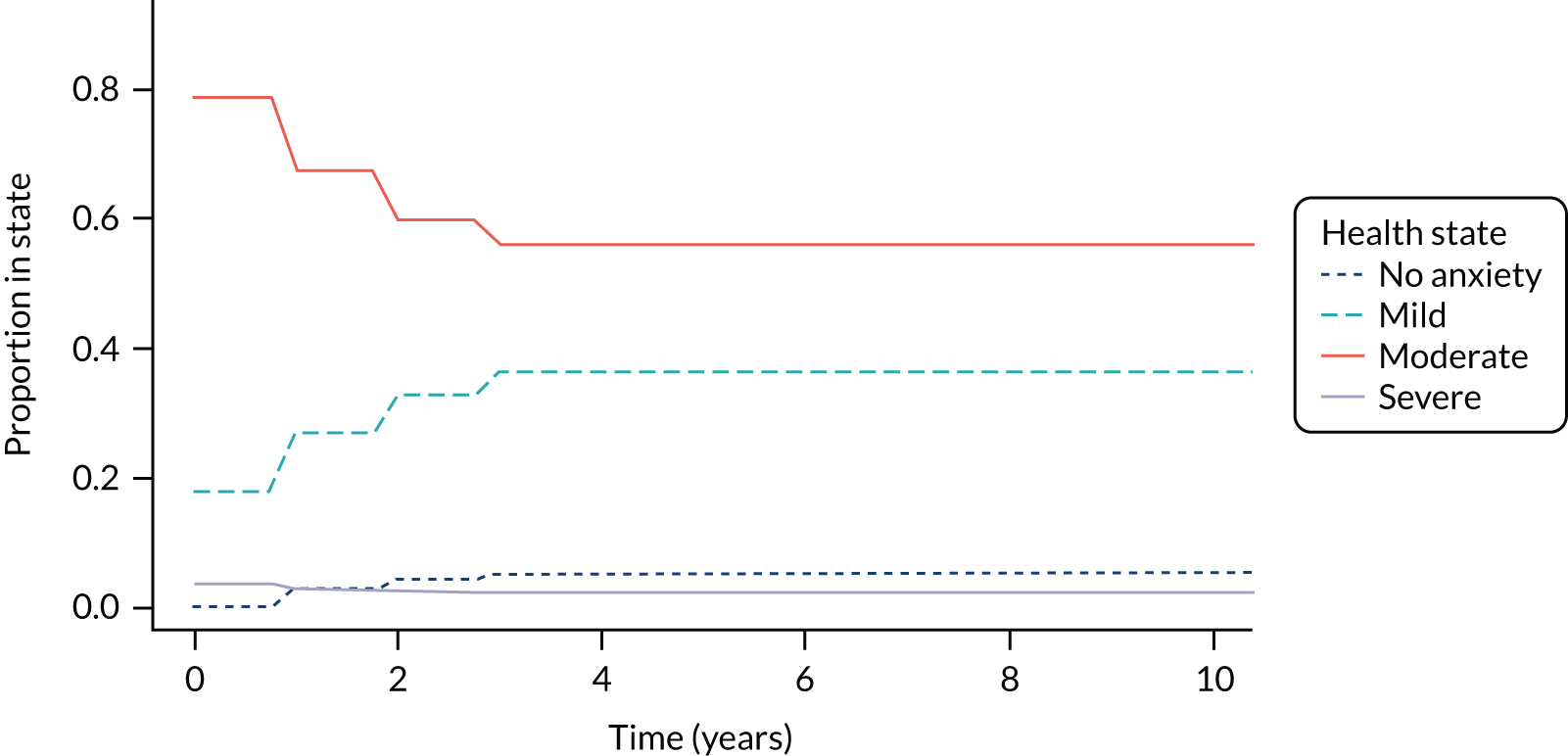
FIGURE 32.
Movement through Markov states without treatment in the initial 10-year period, where GAD-7 scores are constant (scenarios 4–6). Adapted with permission from Jankovic et al. 189 This is an Open Access article distributed in accordance with the terms of the Creative Commons Attribution-NonCommercial (CC BY-NC 4.0) license, which permits others to distribute, remix, adapt and build upon this work, for non-commercial use, provided the original work is properly cited. See: https://creativecommons.org/licenses/by-nc/4.0/. The figure includes minor additions and formatting changes to the original figure.
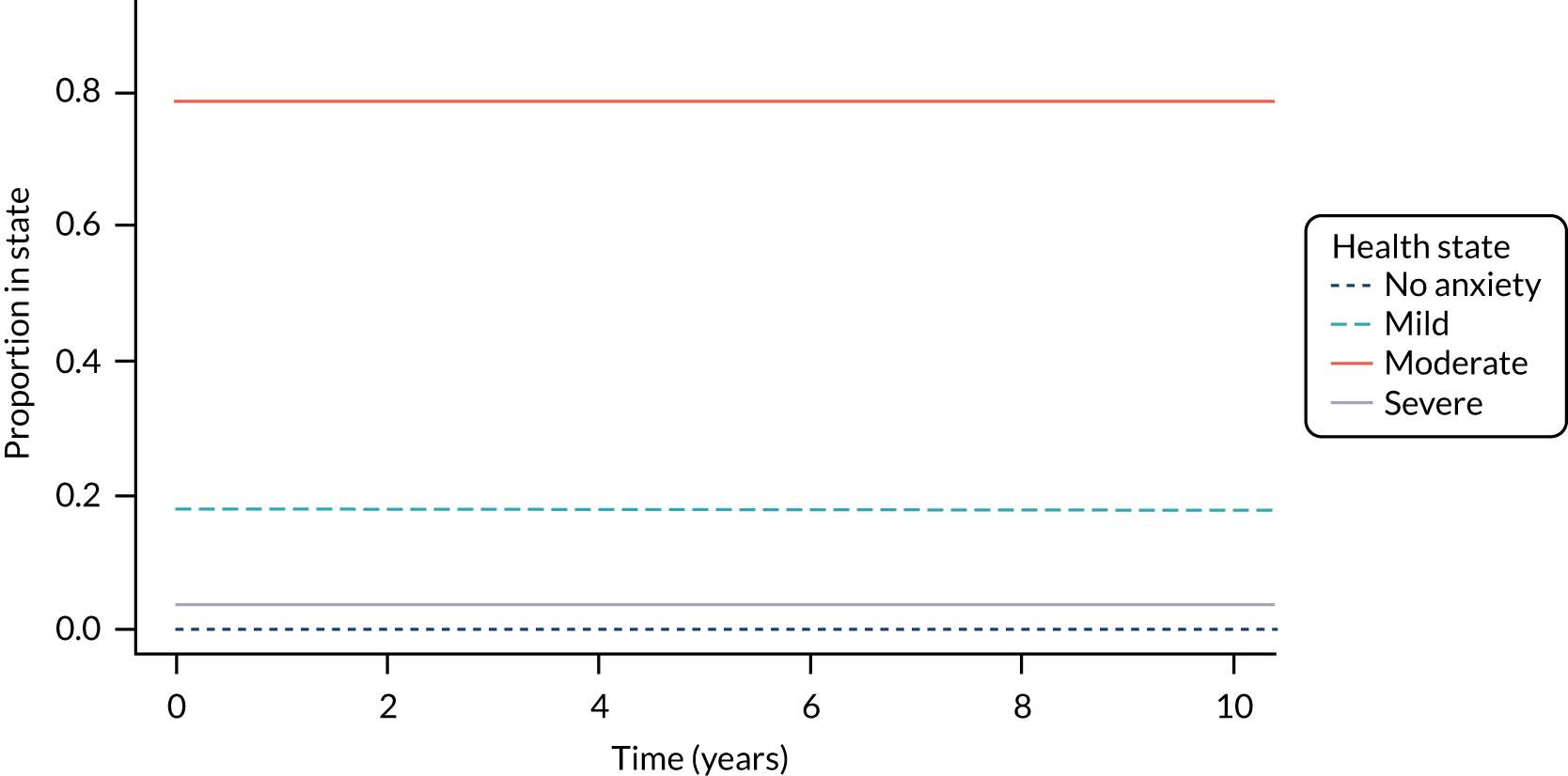
FIGURE 33.
Generalised Anxiety Disorder-7 scores over time, in six scenarios. Mean QALYs, costs and net benefit for each treatment in each scenario are shown in Tables 32–34, respectively. Adapted with permission from Jankovic et al. 189 This is an Open Access article distributed in accordance with the terms of the Creative Commons Attribution-NonCommercial (CC BY-NC 4.0) license, which permits others to distribute, remix, adapt and build upon this work, for non-commercial use, provided the original work is properly cited. See: https://creativecommons.org/licenses/by-nc/4.0/. The figure includes minor additions and formatting changes to the original figure.
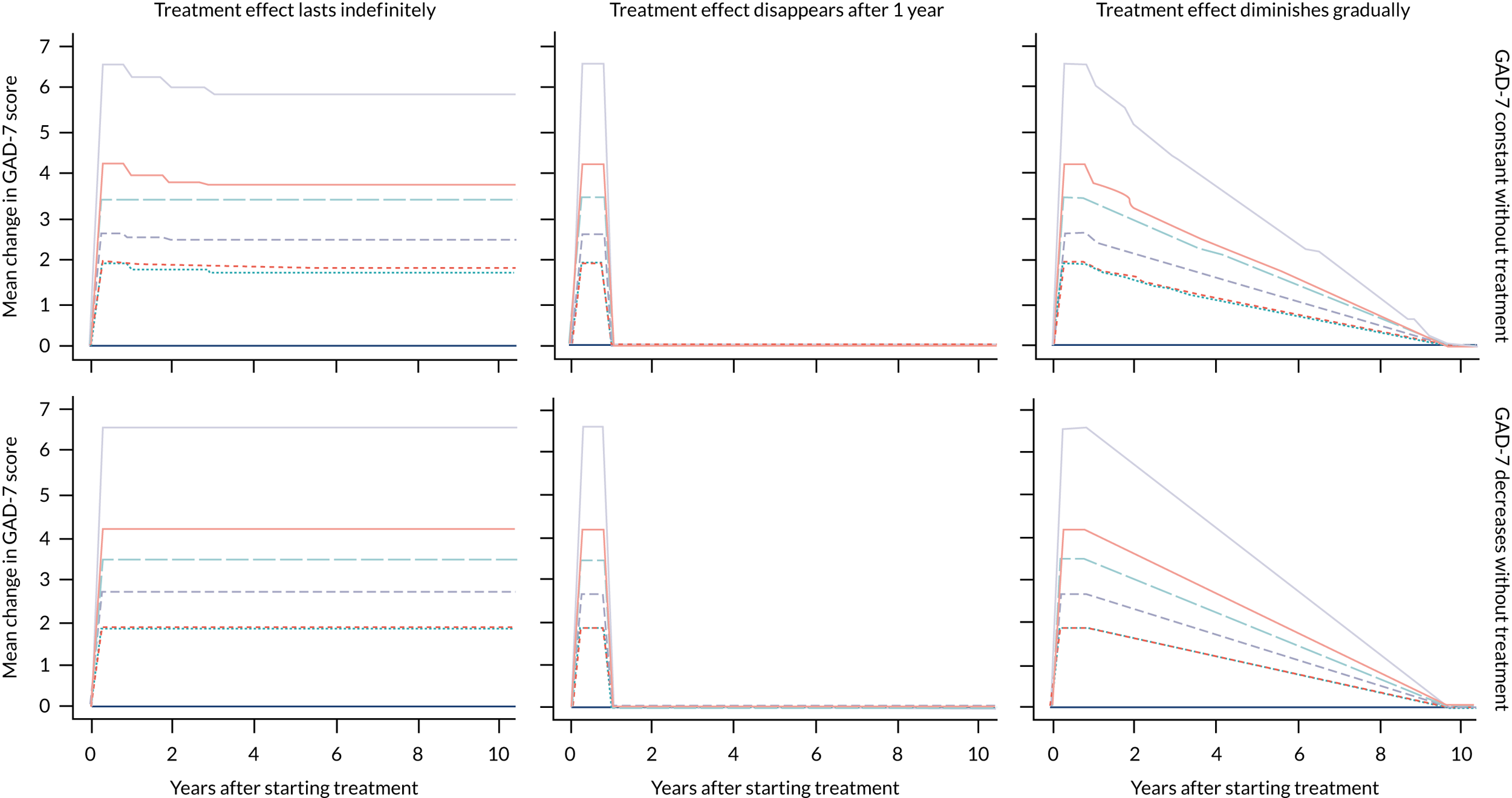
In Table 32, differences in costs and QALY gains were very small. An alternative assumption about the GAD score trajectory without treatment led to a 0.1 reduction in mean QALYs, whereas alternative assumptions about the treatment effect led to a maximum difference of 0.54 QALYs (medication when comparing no spontaneous improvement and a diminishing treatment effect, and spontaneous improvement with indefinite treatment effect).
Intervention | Treatment effect, mean QALY gain (95% confidence interval) | |||||
---|---|---|---|---|---|---|
No spontaneous improvement | Spontaneous improvement | |||||
Constant | Disappears after 1 year | Gradually diminishes | Constant | Disappears after 1 year | Gradually diminishes | |
NI | 10.95 (9.22 to 12.35) | 11.05 (9.37 to 12.40) | ||||
UDC | 11.46 (8.80 to 14.07) | 11.31 (9.24 to 13.54) | 11.31 (9.20 to 13.57) | 11.54 (8.89 to 14.07) | 11.41 (9.31 to 13.70) | 11.42 (9.28 to 13.7) |
SDC | 11.47 (9.09 to 13.96) | 11.30 (9.55 to 13.34) | 11.31 (9.52 to 13.38) | 11.55 (9.19 to 13.96) | 11.41 (9.65 to 13.51) | 11.42 (9.61 to 13.52) |
UDI | 11.69 (9.49 to 14.05) | 11.46 (9.85 to 13.55) | 11.48 (9.83 to 13.58) | 11.76 (9.65 to 14.05) | 11.57 (9.92 to 13.69) | 11.58 (9.92 to 13.72) |
SDI | 11.85 (10.45 to 13.71) | 11.56 (10.48 to 13.01) | 11.57 (10.49 to 13.07) | 11.92 (10.53 to 13.71) | 11.67 (10.56 to 13.14) | 11.68 (10.56 to 13.17) |
SNoDI | 12.17 (10.35 to 14.24) | 11.85 (10.34 to 13.82) | 11.87 (10.35 to 13.84) | 12.23 (10.40 to 14.24) | 11.96 (10.40 to 13.98) | 11.98 (10.42 to 13.99) |
M | 12.88 (11.41 to 14.33) | 12.38 (10.97 to 13.96) | 12.42 (11.03 to 13.97) | 12.92 (11.48 to 14.33) | 12.50 (11.03 to 14.10) | 12.53 (11.10 to 14.12) |
Differences in QALY gains between different scenarios follow a logical pattern. Without treatment, the QALY gain is slightly higher when spontaneous improvement is expected. The QALY impact of all treatments is lowest when the treatment effect is assumed to disappear after 1 year, and highest when it is assumed to remain constant indefinitely.
In Table 33, total costs follow a similar pattern; when patients were assumed to improve spontaneously, the total cost of treatment was slightly higher, probably because of lower mortality. However, differences between mean costs in different scenarios were small, with the maximum difference in mean costs of £557 (medication when comparing no spontaneous improvement and a diminishing treatment effect, and spontaneous improvement with indefinite treatment effect).
Intervention | Treatment effect, mean total cost (£) (95% confidence interval) | |||||
---|---|---|---|---|---|---|
No spontaneous improvement | Spontaneous improvement | |||||
Constant | Disappears after 1 year | Gradually diminishes | Constant | Disappears after 1 year | Gradually diminishes | |
NI | 16,530 (0 to 99,304) | 16,069 (1 to 87,123) | ||||
UDC | 16,059 (7 to 86,001) | 16,617 (7 to 97,025) | 16,590 (8 to 88,143) | 14,822 (18 to 75,923) | 16,133 (19 to 87,277) | 15,992 (20 to 78,709) |
SDC | 15,891 (23 to 82,488) | 16,542 (22 to 93,904) | 16,397 (23 to 86,704) | 14,623 (31 to 74,315) | 16,003 (32 to 84,115) | 15,881 (33 to 78,442) |
UDI | 15,377 (9 to 76,047) | 16,349 (9 to 96,453) | 16,224 (9 to 86,419) | 14,114 (25 to 68,008) | 15,769 (22 to 84,580) | 15,715 (30 to 77,420) |
SDI | 16,325 (158 to 69,394) | 16,608 (129 to 94,607) | 16,519 (157 to 85,324) | 14,298 (218 to 63,232) | 15,939 (160 to 83,243) | 15,675 (228 to 74,955) |
SNoDI | 13,500 (96 to 66,496) | 16,300 (96 to 94,607) | 15,995 (99 to 82,263) | 12,442 (128 to 59,171) | 15,867 (117 to 85,199) | 15,429 (131 to 73,077) |
M | 13,012 (1698 to 53,201) | 17,197 (1227 to 93,790) | 16,821 (1616 to 75,716) | 11,754 (1839 to 47,063) | 16,641 (1253 to 82,556) | 16,144 (1716 to 69,824) |
Finally, although the mean NMB varied across scenarios (Table 34), the ranking of interventions did not.
Intervention | Treatment effect, mean NMB (£) (95% confidence interval) | |||||
---|---|---|---|---|---|---|
No spontaneous improvement | Spontaneous improvement | |||||
Constant | Disappears after 1 year | Gradually diminishes | Constant | Disappears after 1 year | Gradually diminishes | |
NI | 147,661 (61,801 to 179,119) | 149,671 (74,016 to 180,051) | ||||
UDC | 155,905 (72,746 to 205,462) | 152,973 (69,172 to 197,625) | 153,114 (76,377 to 195,729) | 158,335 (81,276 to 205,462) | 155,082 (79,412 to 198,816) | 155,304 (85,579 to 197,580) |
SDC | 156,100 (79,038 to 203,339) | 152,984 (71,178 to 193,926) | 153,243 (77,863 to 192,218) | 158,586 (88,232 to 203,339) | 155,167 (83,412 to 194,860) | 155,361 (86,983 to 194,260) |
UDI | 159,919 (88,552 to 205,226) | 155,598 (72,214 to 198,097) | 155,912 (80,999 to 196,581) | 162,334 (97,837 to 205,226) | 157,821 (84,553 to 198,986) | 158,039 (91,794 to 198,295) |
SDI | 161,446 (104,622 to 197,813) | 156,745 (77,084 to 186,877) | 157,087 (85,918 to 185,869) | 164,551 (111,833 to 197,813) | 159,059 (89,223 to 188,411) | 159,529 (97,334 to 187,107) |
SNoDI | 169,043 (102,566 to 208,548) | 161,376 (79,787 to 202,462) | 162,027 (90,262 to 201,040) | 171,033 (110,947 to 208,548) | 163,517 (90,086 to 203,790) | 164,248 (99,994 to 203,032) |
M | 180,191 (125,503 to 209,180) | 168,526 (86,875 to 203,686) | 169,506 (104,381 to 202,389) | 181,975 (131,817 to 209,180) | 170,837 (100,380 to 204,893) | 171,866 (112,948 to 204,345) |
Alternative assumptions about the cost of health care
Two alternative costs of health care were considered, obtained from different sources. Comparison of state-specific costs are shown in Table 35.
Study (first author and year) | State-specific costs (£) | |||
---|---|---|---|---|
No anxiety | Mild anxiety | Moderate anxiety | Severe anxiety | |
Kaltenthaler 200614 (base case) | 86 | 200 | 210 | 324 |
Kumar 201885 | 188 | 262 | 341 | 371 |
Vera-Llonch 2010194 | 911 | 1444 | 2531 | 3316 |
The updated costs and NMB are shown in Table 36. The health-care costs did not affect the ranking of interventions and uncertainty (the NMB confidence intervals remain overlapping), only the magnitude of costs and the net benefit. Health-care resource use informed by Vera-Llonch et al. 194 led to the highest costs and, consequently, the lowest net benefit.
Intervention | Study (first author and year) | |||||
---|---|---|---|---|---|---|
Kaltenthaler 200614 (base case) | Kumar 201885 | Vera-Llonch 2010194 | ||||
Total cost (£) | NMB (£) | Total cost (£) | NMB (£) | Total cost (£) | NMB (£) | |
NI | 16,816 (0 to 99,741) | 149,671 (74,016 to 180,051) | 24,350 (15,907 to 31,631) | 141,346 (113,133 to 168,088) | 165,947 (84,946 to 237,267) | –209 (–79,666 to 98,286) |
UDC | 16,210 (7 to 86,259) | 158,335 (81,276 to 205,462) | 22,124 (14,004 to 31,217) | 151,015 (105,954 to 195,263) | 143,289 (75,350 to 257,370) | 29,838 (–121,839 to 130,949) |
SDC | 16,121 (23 to 83,355) | 158,586 (88,232 to 203,339) | 22,198 (14,169 to 31,021) | 151,119 (110,691 to 193,520) | 141,588 (76,197 to 237,970) | 31,622 (–91,709 to 127,800) |
UDI | 15,387 (9 to 76,428) | 162,334 (97,837 to 205,226) | 21,473 (13,908 to 30,711) | 154,969 (117,761 to 195,429) | 132,913 (75,209 to 232,125) | 43,549 (–73,383 to 130,796) |
SDI | 16,342 (149 to 71,053) | 164,551 (111,833 to 197,813) | 20,627 (14,199 to 29,183) | 158,224 (131,015 to 189,786) | 118,687 (76,055 to 217,748) | 60,181 (–55,378 to 120,708) |
SNoDI | 13,842 (93 to 69,453) | 171,033 (110,947 to 208,548) | 20,275 (13,505 to 30,176) | 163,102 (128,957 to 198,212) | 120,905 (73,304 to 224,888) | 62,564 (–62,082 to 135,299) |
M | 12,968 (1,656 to 53,015) | 181,975 (131,817 to 209,180) | 18,931 (14,297 to 25,775) | 174,836 (149,400 to 198,491) | 97,493 (73,262 to 135,514) | 96,277 (43,886 to 136,525) |
Alternative assumptions about the cost of therapy
Two alternative costs of support in SDCs. Comparisons of different assumptions are shown in Table 37. The cost difference is very small (smaller than the mean cost difference between SDCs and the next most expensive or next cheapest alternative), and therefore the results were concluded to be insensitive without running additional analyses.
Support required | Intervention cost (£) |
---|---|
30 minutes with psychologist (base case) | 26.50 |
5 minutes with psychologist | 4.42 |
5 minutes with administrative support | 1.50 |
Exclusion of digital controls (unsupported digital control and supported digital control)
In a constrained health system, patients may be referred to reputable online resources to access information about their condition. However, it is not clear to what extent this occurs in the NHS in England, and so we explored a scenario in which UDCs and SDCs are not considered as possible treatment options. Exclusion of DCs does not affect the costs, QALYs or net benefit of other treatments, only their probability of being cost-effective and the VOI. The results are shown in Figures 34 and 35. Figure 34 shows that the ranking of interventions is unchanged. The intervention most likely to be cost-effective depends on the opportunity cost; it is NI for opportunity cost £0 per QALY, SNoDIs for opportunity cost £1000 per QALY and medication for opportunity costs £2000 per QALY or greater.
FIGURE 34.
Cost-effectiveness acceptability curves without DCs. M, medication. Adapted with permission from Jankovic et al. 189 This is an Open Access article distributed in accordance with the terms of the Creative Commons Attribution-NonCommercial (CC BY-NC 4.0) license, which permits others to distribute, remix, adapt and build upon this work, for non-commercial use, provided the original work is properly cited. See: https://creativecommons.org/licenses/by-nc/4.0/. The figure includes minor additions and formatting changes to the original figure.
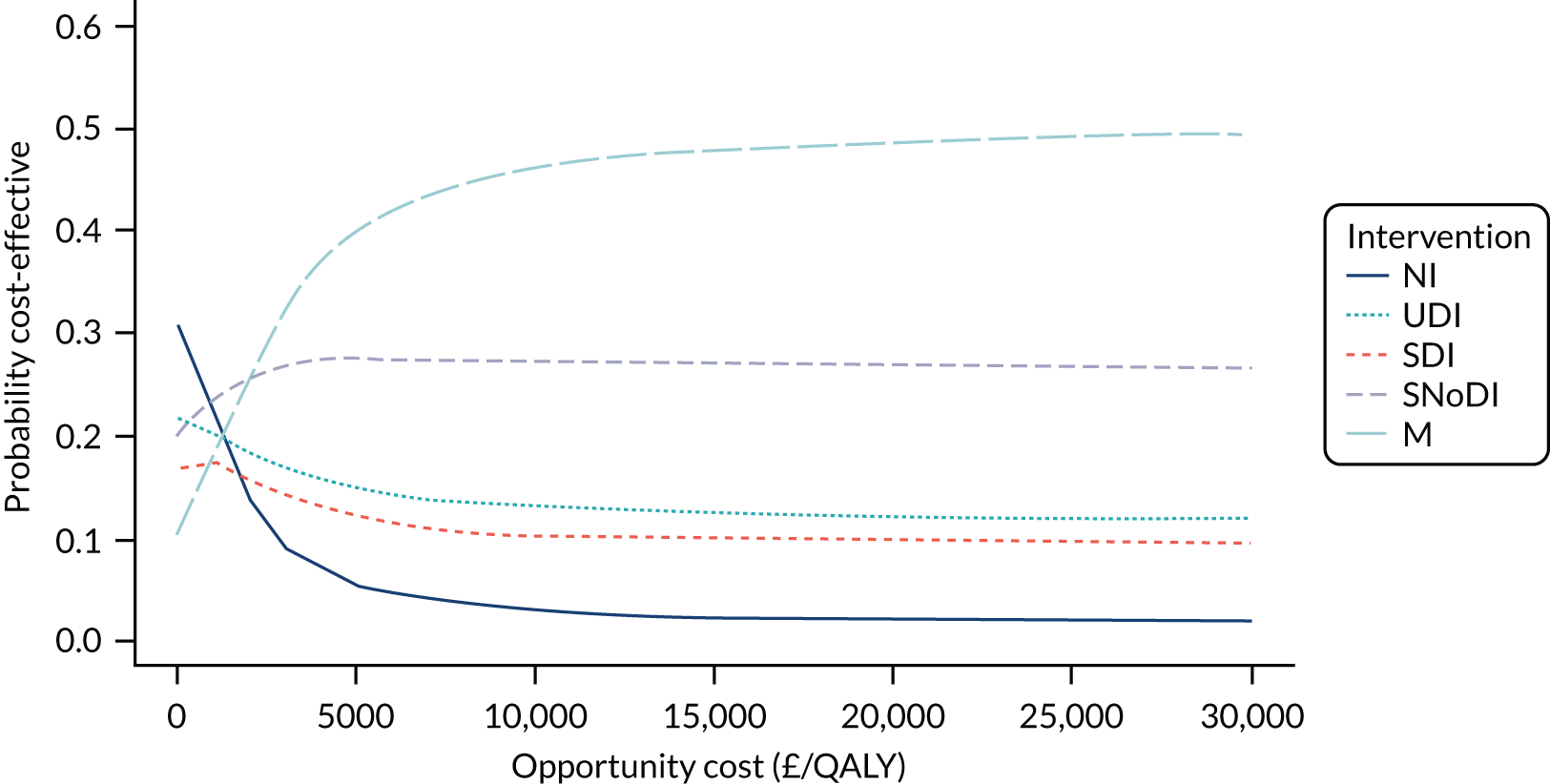
Figure 35 shows EVPIP over a range of opportunity costs. The relationship between EVPIP and the opportunity cost is comparable to that in the base case; VOI is lowest when opportunity cost is £4000 per QALY. The value of uncertainty is high, a minimum of £9.9B, increasing to £12.8B and £15.1B when the opportunity cost is £15,000 and £20,000 per QALY, respectively.
FIGURE 35.
The EVPIP for a range of opportunity costs. Adapted with permission from Jankovic et al. 189 This is an Open Access article distributed in accordance with the terms of the Creative Commons Attribution-NonCommercial (CC BY-NC 4.0) license, which permits others to distribute, remix, adapt and build upon this work, for non-commercial use, provided the original work is properly cited. See: https://creativecommons.org/licenses/by-nc/4.0/. The figure includes minor additions and formatting changes to the original figure.
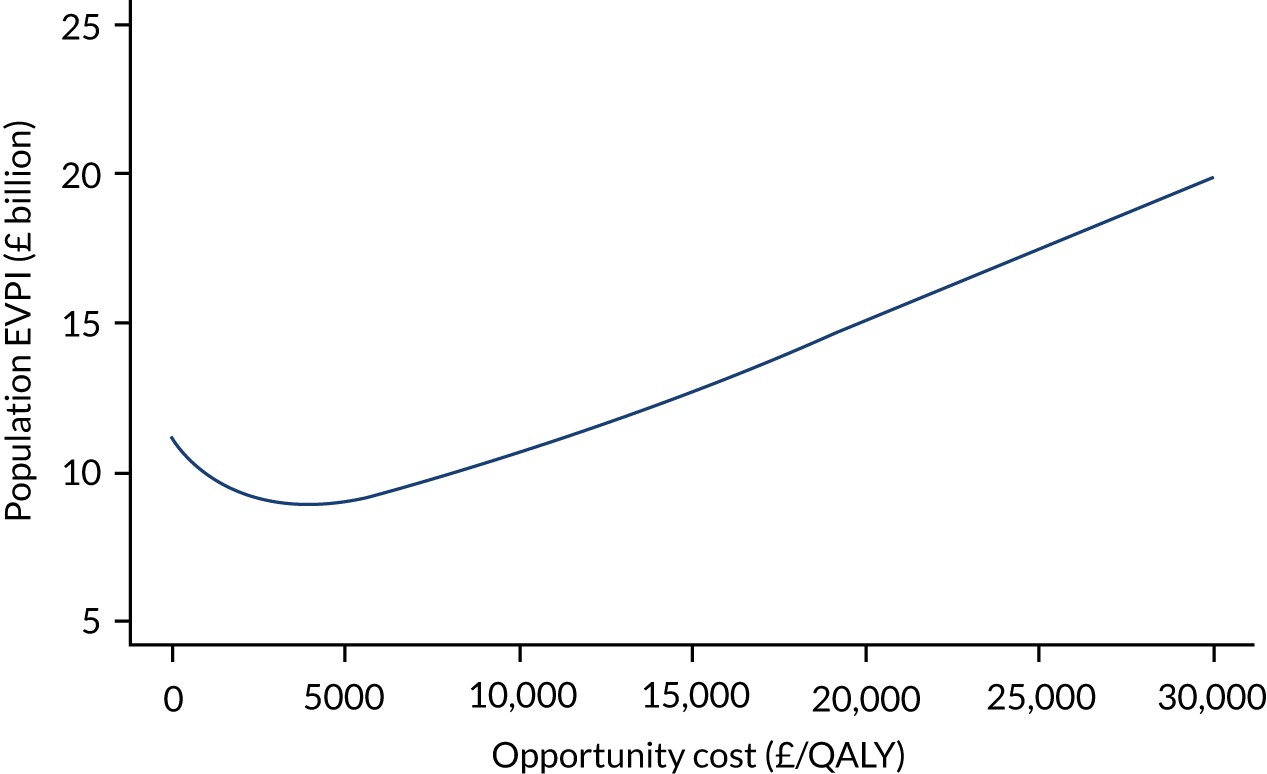
List of abbreviations
- ANCOVA
- analysis of covariance
- BAI
- Beck Anxiety Inventory
- BDI
- Beck Depression Inventory
- BDI-II
- Beck Depression Inventory, version 2
- CBA
- cost–benefit analysis
- CBT
- cognitive–behavioural therapy
- CCA
- cost–consequences analysis
- cCBT
- computerised cognitive–behavioural therapy
- CDSR
- Cochrane Database of Systematic Reviews
- CEA
- cost-effectiveness analysis
- CENTRAL
- Cochrane Central Register of Controlled Trials
- CINAHL
- Cumulative Index to Nursing and Allied Health Literature
- CMA
- cost minimisation analysis
- CrI
- credible interval
- CUA
- cost–utility analysis
- CYP
- children and young people
- DARE
- Database of Abstracts of Reviews of Effects
- DC
- digital control
- DI
- digital intervention
- DIC
- deviance information criterion
- DoPHER
- Database of Promoting Health Effectiveness Reviews
- EVPI
- expected value of perfect information
- EVPIP
- expected value of perfect information at the population level
- EVPPIP
- expected value of partial perfect information at the population level
- FE
- fixed effects
- GAD
- generalised anxiety disorder
- GAD-7
- Generalised Anxiety Disorder-7
- GAD-Q-IV
- Generalised Anxiety Disorder Questionnaire-IV
- GP
- general practitioner
- HAM-A
- Hamilton Anxiety Rating Scale
- HRQoL
- health-related quality of life
- ICER
- incremental cost-effectiveness ratio
- MBI
- mindfulness-based intervention
- MCMC
- Markov chain Monte Carlo
- MHF
- Mental Health Foundation
- MINI
- Mini-International Neuropsychiatric Interview
- NI
- no intervention
- NICE
- National Institute for Health and Care Excellence
- NIHR
- National Institute for Health Research
- NMA
- network meta-analysis
- NMB
- net monetary benefit
- NoDI
- non-digital intervention
- OCD
- obsessive–compulsive disorder
- PHQ-4
- Patient Health Questionnaire – 4 items
- PHQ-9
- Patient Health Questionnaire – 9 items
- PRISMA
- Preferred Reporting Items for Systematic Reviews and Meta-Analyses
- PSWQ
- Penn State Worry Questionnaire
- PSWQ-A
- Penn State Worry Questionnaire – abbreviated
- QALY
- quality-adjusted life-year
- RCT
- randomised controlled trial
- RE
- random effects
- RR
- relative risk
- SDC
- supported digital control
- SDI
- supported digital intervention
- SMS
- short message service
- SNoDC
- supported non-digital control
- SNoDI
- supported non-digital intervention
- SSRI
- selective serotonin reuptake inhibitor
- STAI
- State–Trait Anxiety Inventory
- SUCRA
- surface under the cumulative ranking curve
- TAU
- treatment as usual
- UDC
- unsupported digital control
- UDI
- unsupported digital intervention
- UNoDC
- unsupported non-digital control
- UNoDI
- unsupported non-digital intervention
- VOI
- value of information
- VR
- virtual reality
- WHO
- World Health Organization
- WL
- waiting list
- WP
- work package
Notes
-
Literature search for economic evaluations of DIs in mental health
-
Excluded studies by reason from review of economic evaluations of DIs in mental health
-
Literature search for RCTs of DIs for GAD, mixed anxiety and depression (up to December 2018)
-
Excluded studies by reason from review of RCTs of DIs for GAD
-
Targeted searches for informing state-specific costs and utilities for GAD model
Supplementary material can be found on the NIHR Journals Library report page (https://doi.org/10.3310/RCTI6942).
Supplementary material has been provided by the authors to support the report and any files provided at submission will have been seen by peer reviewers, but not extensively reviewed. Any supplementary material provided at a later stage in the process may not have been peer reviewed.