Notes
Article history
The research reported in this issue of the journal was commissioned and funded by the Evidence Synthesis Programme on behalf of NICE as award number NIHR133836. The protocol was agreed in July 2021. The assessment report began editorial review in January 2022 and was accepted for publication in December 2022. The authors have been wholly responsible for all data collection, analysis and interpretation, and for writing up their work. The HTA editors and publisher have tried to ensure the accuracy of the authors’ manuscript and would like to thank the reviewers for their constructive comments on the draft document. However, they do not accept liability for damages or losses arising from material published in this manuscript.
Permissions
Copyright statement
Copyright © 2024 Westwood et al. This work was produced by Westwood et al. under the terms of a commissioning contract issued by the Secretary of State for Health and Social Care. This is an Open Access publication distributed under the terms of the Creative Commons Attribution CC BY 4.0 licence, which permits unrestricted use, distribution, reproduction and adaptation in any medium and for any purpose provided that it is properly attributed. See: https://creativecommons.org/licenses/by/4.0/. For attribution the title, original author(s), the publication source – NIHR Journals Library, and the DOI of the publication must be cited.
2024 Westwood et al.
Chapter 1 Objective
The overall objective of this assessment was to evaluate the clinical and cost-effectiveness of using artificial intelligence (AI)-derived software to support the review of computed tomography (CT) brain scans in acute stroke, in the NHS setting. The following research questions were defined to address the stated objective:
-
(1) Does AI-derived software-assisted review of non-enhanced CT brain scans for guiding thrombolysis treatment decisions for people with suspected acute stroke represent a clinically and cost-effective use of NHS resources?
-
(2a) Does AI-derived software-assisted review of CT angiography (CTA) brain scans for guiding mechanical thrombectomy treatment decisions for people with an ischaemic stroke represent a clinically and cost-effective use of NHS resources?
-
(2b) Does AI-derived software-assisted review of CT perfusion (CTP) brain scans for guiding mechanical thrombectomy treatment decisions for people with an ischaemic stroke after a CTA brain scan represent a clinically and cost-effective use of NHS resources?
Chapter 2 Background and definition of the decision problem(s)
Population
The primary population for this assessment was people presenting or attending secondary care with a suspected acute stroke, who were last known to be well within the previous 24 hours. Within this population, separate groups were considered for each research question (see Assessment of clinical effectiveness).
Depending on the availability of evidence, the following subpopulation could be considered: people over the age of 80 years with small-vessel disease and calcification of the cerebrovasculature.
The condition
Stroke is a serious life-threatening medical condition defined by the World Health Organization (WHO) as a clinical syndrome consisting of ‘rapidly developing clinical signs of focal (at times global) disturbance of cerebral function, lasting more than 24 hours or leading to death with no apparent cause other than that of vascular origin’. 1 Stroke can occur without any warning and leads to interruption or restriction of the blood flow to the brain causing reduction of the flow of oxygen and nutrients to the brain and subsequently brain cell death. The effects of a stroke depend on which area of the brain is affected, the extent of damage and the time to treatment. 2
There are two main types of stroke:
-
Ischaemic stroke – the most frequently occurring type of stroke resulting from reduced blood flow due to arterial occlusion. Approximately 87.1% of patients in the United Kingdom (UK) will suffer from this type of stroke. Arterial blockage can be caused by the formation of atherosclerotic plaques (fatty deposits building up in the walls of arteries). As well as narrowing the artery, making it harder for blood to pass through it, the fatty deposits can break down or become inflamed. When this happens, a blood clot forms, which can block the artery, or a clot can travel from a distant location, such as from the heart or blood vessels in the neck and block the blood vessel in the brain (embolisation); the majority of the ischaemic strokes are caused by this mechanism rather than in situ thrombosis. Other causes of ischaemic stroke are small-vessel disease leading to vessel damage, heart conditions such as atrial fibrillation (AF), patent foramen ovale, endocarditis or arterial dissection. 2,3
-
Haemorrhagic stroke, also referred to as intracranial haemorrhage (ICH) or cerebral haemorrhage, accounts for approximately 12.5% of all strokes in the UK and is caused by bleeding from blood vessels in or around the brain. This type of stroke can be intracerebral (bleed within the brain) or subarachnoid (bleed on the surface of the brain in the subarachnoid space). Intracerebral haemorrhagic stroke is most associated with high blood pressure, resulting in the bursting of an artery, whereas subarachnoid haemorrhagic stroke is most frequently caused by a burst aneurysm. 2,3
A transient ischaemic attack (TIA), sometimes known as a mini stroke, is differentiated from ischaemic stroke in that symptoms are time limited/self-resolving. Patients who have experienced one or more TIAs are at increased risk for ischaemic stroke. 2 The diagnosis of TIA is not considered in this assessment.
In 2018–19, there were 224,172 hospital admissions for stroke (including stroke mimics) in the UK and the in-hospital crude mortality rate for 2017–19 was reported to be 13.4%. 4 In the same year, there were over 1.2 million stroke survivors in the UK with stroke prevalence (defined as patients who have had a stroke or TIA on a general practice register) ranging from 1.77% in England to 2.28% in Scotland. 5
Symptoms and risk factors
Common symptoms include drooping of one side of the face, problems with speaking and vision, loss of sensation in an arm or leg and slurred or garbled speech. Other symptoms can include nausea, vomiting, vertigo and decreased level of consciousness. 2
The Sentinel Stroke National Audit Programme (SSNAP), the UK national healthcare quality improvement programme, collects patient data from England, Wales and Northern Ireland and provides information on patient characteristics and outcomes, and the infrastructure of stroke services. Among 89,280 stroke patients for whom data were collected between April 2019 and March 2020, the median age of patients with acute stroke in the UK was 77 years. 3 The risk of stroke increases with age due to continuous changes in brain arteries. 2 Females accounted for 48% of all acute stroke patients in the UK. 3
It is estimated that approximately 90% of strokes are attributable to risk factors that can be modified during a patient’s lifetime (e.g. management of high blood pressure, diabetes, changes in smoking habits and addressing physical inactivity). 2 According to SSNAP, 55.1% and 22.5% of acute stroke patients in the UK, respectively, suffered from hypertension and diabetes before their stroke. 3
Diagnosis and treatment
Timely and effective management of patients with suspected stroke substantially impacts their outcomes. As stroke mimics account for approximately 20–25% of all acute presentations, the patient’s history is crucial to establish the potential cause of their symptoms and to avoid misdiagnosis. 6
Outside the hospital setting, patients with suspected stroke should be assessed using the ‘face, arm, speech’ test (FAST) and they must be transported to the hospital as quickly as possible, preferably to a stroke unit. 7 Specialised stroke units are trained in the management of stroke patients and have access to specialist medical staff, diagnostic imaging equipment, time-sensitive procedures such as thrombectomy and thrombolysis and other services. In the UK, these units are known as comprehensive stroke centres (CSCs), defined as centres providing hyperacute, acute and inpatient rehabilitation including thrombectomy and neurosurgery services. Non-specialist units may be unable to provide access to specialist medical staff or some crucial medical procedures, which can affect the timely and effective selection and treatment of patients suffering from a stroke. In the UK, these units are known as acute stroke centres, defined as centres which provide hyperacute, acute and inpatient rehabilitation, but excluding thrombectomy and neurosurgery; all acute stroke centres are expected to have an intrahospital thrombectomy transfer pathway to transfer patients from acute stroke centres to CSCs.
In the emergency department, patients should be assessed with the Recognition of Stroke in the Emergency Room (ROSIER) scale. 7,8 After admission, a CT or a magnetic resonance imaging (MRI) brain scan should be performed at the next available imaging slot, within an hour from arrival, to rule out other causes of symptoms, to provide information on the potential cause and to show the extent of damage and decide on the best treatment option. 2 A CT scan is quick and effective method ruling out ICH, which is often sufficient to make thrombolysis decisions for patients with ischaemic stroke. However, the specificity of a CT scan might be compromised in patients with acute ischaemia because of continuing changes in the brain since the onset of symptoms. 6 Other tests may be needed, especially for patients with haemorrhagic stroke, to provide more information on the cause of stroke. In the UK, only 55.2% of patients with acute stroke are scanned within 1 hour from admission, with the numbers rising to 95.5% for a scan within 12 hours from patient admission. 3 Admission directly to a stroke unit and assessment by a stroke specialist can lead to improved patient outcomes and reduction in complications. Patients who are seen in a specialist stroke unit are also more likely to receive more targeted secondary care. 2 Based on the SSNAP, between April 2019 and March 2020, the stroke unit was the first ward of admission for 79.9% of acute stroke patients in the UK. 3 Some patients, however, may be initially transported to other units where direct specialist care is not available.
Patients with an ischaemic stroke can be treated with thrombolysis, which uses alteplase to dissolve the clot blocking the artery in the brain. 2 The shorter the time between symptom onset and thrombolysis, the higher a patient’s chance of better recovery; however, only a limited number of patients can benefit from this treatment due to the number of contraindications and potential complications that need to be considered. For stroke patients with unknown time of symptom onset, a 2021 systematic review showed that patients treated with alteplase thrombolysis had over three-times greater risk of symptomatic intracranial haemorrhage (sICH; an adverse effect of thrombolysis) when compared with patients receiving conservative medical treatment. There was no increase in the risk of death at 3 months, and patients had a similar likelihood of functional independence. 9 Treatment with alteplase is also associated with an increased risk of ICH, compared with conservative treatment, in patients with a clearly defined time of stroke onset. 10
Some patients with ischaemic stroke may benefit from thrombectomy (i.e. extraction of arterial obstruction with a device). Thrombectomy is considered if the obstruction is present in a large artery11 and has been shown to be superior to best medical therapy alone (e.g. thrombolysis alone) for patients with anterior circulation large artery occlusion. 6,12 In patients with an ischaemic stroke, thrombolysis can be administered before mechanical thrombectomy without an increase in the incidence of sICH or mortality at 90 days when compared with thrombectomy alone. Similarly, there is no difference between treatments (thrombolysis plus thrombectomy vs. thrombectomy alone) in the rates of successful recanalisation or the level of patients’ functional independence at 90 days. 13
Patients with haemorrhagic stroke require intensive blood pressure-lowering medications or reversal of antithrombotic medications at the early stages of their treatment. Patients may undergo surgery to seal a burst aneurysm or relieve the pressure on the brain. Severe headaches can be addressed with pain relief medication. 2
More information regarding the patient pathway, available treatments and patient eligibility for treatment in the NHS setting is provided in the Care pathway section.
Intervention technologies
Over recent years, a number of software products with AI-derived algorithms have been developed, which are intended to facilitate the review of CT images of the brain in conditions such as stroke. These products are not intended to provide a diagnosis but rather to support the review of scans, reporting by a radiologist and prioritisation of critical cases.
For patients with suspected stroke, software using AI-derived algorithms may be a useful tool in the early stages of the treatment pathway, particularly where neuroradiologist assessment of the CT images is not directly available. The use of AI-derived algorithms may potentially speed up the process of reviewing CT scans by identifying, quantifying and notifying about clinically relevant brain structures related to acute stroke. Highlighting stroke-related changes in the patient’s brain may assist in confirming a stroke and, together with other patient information, expedite the patient transfer and support assessments of the suitability of time-sensitive treatments such as thrombolysis and thrombectomy, leading to improvement of patient outcomes. Other potential benefits include improved report turnaround time and enabling rapid review of scans by a multisite clinical team.
These software products are typically designed to be incorporated into standard radiology CT workstations. This means that they can work with existing forms of brain imaging, including non-contrast CT (NCCT), CTA and CTP imaging, radiology information systems (RIS) and picture archiving and communication systems (PACS). They are typically hosted on a web cloud which is separate from image exchange portals used to transfer images between care providers.
The Royal College of Radiologists published a position statement in AI in medical imaging in 201814 and subsequently published guidance in 2021 on integrating AI with the radiology reporting workflows (RIS and PACS). 15 The guidance recommends that:
-
‘AI must be integrated in reporting (radiology information system [RIS] and picture archiving and communication system [PACS]) workflows seamlessly and in a way that does not add extra burden to radiologists.
-
The accuracy of the AI algorithms must be clearly declared for radiologists and others making decisions on patient management.
-
AI findings must be communicated to the RIS via existing, widely used global technical standards (HL7).
-
AI findings must be communicated to the PACS using existing, widely used global technical standards (Digital Imaging Communications in Medicine [DICOM]).
-
The workflow must be robust enough to ensure AI analysis is complete and available on PACS before a human reporter starts image interpretation’. 15
In March 2020, National Institute for Health and Care Excellence (NICE) published Medtech innovation briefing 207 (Artificial Intelligence for Analysing CT Brain Scans)16 describing AI-derived software for CT brain scans. Based on this briefing, ‘the intended place in therapy would be to support radiologists in secondary care when they are reviewing CT brain scans of people with suspected brain abnormalities. The technology may be of most benefit when images are not first reviewed by neuroradiologists’. 16
Several companies offer software with AI-derived algorithms for analysing CT brain scans in people with a suspected acute stroke. Some companies offer software that can be used to analyse NCCT, CTA and CTP scans (or have agreements between companies to offer their algorithms as a package), whereas others have software that can only analyse one of these types of scans. Some software packages do not have a dedicated platform through which they are delivered but may be housed on multivendor platforms (e.g. Blackford Analysis, Edinburgh, UK).
These technologies are classed as medical devices and require a CE mark. Details of the technologies considered in this assessment are provided in Table 1 and the following sections. Where less detail is given, this is because only information available in the public domain was able to be used.
Platform | Available to the NHS | Type of CT scan analysed | ||
---|---|---|---|---|
NCCT | CTA | CTP | ||
icobrain CTa | ✓ | ✓ | ||
Aidoc | ✓ | ✓ | ✓ | |
Aidoc + icobrain | NYD | ✓ | ✓ | ✓ |
RapidAI | ✓ | ✓b | ✓ | ✓ |
e-stroke | ✓ | ✓b | ✓ | ✓ |
Viz | ✓ | ✓ | ✓ | ✓ |
qERa | NYD | ✓ | ||
Zebra-Med | TBC | ✓ | ||
CTP 4D | TBC | ✓ | ||
BrainScan | TBC | ✓ | ||
Cercare Strokea | NYD | ✓ | ||
CINA Heada | ✓ | ✓b | ✓ | |
Accipioa | ✓ | ✓ | ||
BioMind | TBC | ✓ |
icobrain CT
The neuroimaging platform icobrain® CT (icometrix, Leuven, Belgium) is a CE marked class 1 medical device that uses AI-derived algorithms to detect abnormalities in brain CT scans; icobrain CT can generate two output reports related to stroke diagnosis:
-
Report 1, from icobrain CTP, details a quantitative assessment of perfusion in the brain based on a CT scan with contrast. It analyses the flow of blood in areas of the brain to determine the presence of potentially salvageable tissues in ischaemic stroke. The analysis includes a calculation of abnormality in parameters such as mean transit time, cerebral blood flow, cerebral blood volume and time to maximum of residue function.
-
Report 2, from icobrain TBI, can give a quantitative assessment of ICH based on a non-enhanced CT scan. This report also has application in traumatic brain injury. Some of the NCCT parameters measured include midline shift and asymmetry index between the left and right lateral ventricle.
The company notes that its AI-derived neuroimaging platform integrates with existing RIS and PACS. The software is intended for automatic labelling, visualisation and volumetric quantification of segmentable brain structures from a set of CT images. It receives digital images as input and generates an electronic report on quantitative parameters and annotated images. Results can be viewed as visual reports through digital imaging and communication in medicine (DICOM) output images, e-mail notifications and on a web browser. The report highlights stroke-related changes that guide clinician diagnosis. Data from and into the PACS are transferred securely over a software icobridge, installed on site. icobrain CT has had two major releases, versions 4.0 and 5.0. The company notes that performance of icobrain in detecting ICH and for CTP analysis has been tested on a series of scenarios that cover specific aspects of the software performance. icobrain CT algorithms send and receive information over a secure cloud ‘icometrix’. Icometrix is ISO13485 and ISO27001 certified and UK General Data Protection Regulation (GDPR) and United States Health Insurance Portability and Accountability Act (HIPAA) compliant for privacy and security.
The company provides a training manual for health professionals, which gives guidance on how to use the software and interpret reports. Customer support is also available from the company. Prior to deployment in clinical practice the company carries out a clinical and technical test phase. icobrain CT is currently a self-certified class 1 medical device under the Medical Device Directive. The company notes that it will be up-classified to a class 2a medical device under the Medical Device Regulation, in line with the transition from the Medical Device Directive to the Medical Device Regulation.
Aidoc intracranial haemorrhage, Aidoc large-vessel occlusion, Aidoc mobile
The Aidoc® software, also called ‘BriefCase’ (Aidoc, Tel Aviv, Israel), is a CE marked class 1 medical device AI triage and notification platform. This neuroimaging platform uses AI-derived algorithms to detect abnormalities in brain CT scans. Algorithms related to stroke diagnosis include:
-
Aidoc ICH for detecting suspected ICH on non-contrast head CT
-
Aidoc LVO for detecting suspected LVOs on CTA.
The third component of the platform relevant to stroke diagnosis is Aidoc mobile, which is for communication between clinical stakeholders in the stroke pathway to facilitate peer review.
The company notes that its software can integrate with existing radiology workstations, including PACS, reporting system and radiology workflow solutions. The platform can prioritise worklist, triage and generate notification on suspected stroke cases. Analysis done by the AI-derived software is intended to supplement CT scan review by a neuroradiologist or stroke specialist.
The company provides an initial product training, which lasts around 30 minutes, and where necessary, additional training on specific workflows can be provided. Recurring annual training is also available to review new features, enhancements and algorithms. Prior to deployment of the software on a site, the company carries out an automated performance assessment through its AI operations centre. Aidoc is ISO13485 and ISO27001 certified. The Aidoc software is currently a self-certified class 1 medical device under the Medical Device Directive; the company notes that it will be up-classified to a class 2a medical device under the Medical Device Regulation, in line with the transition from the Medical Device Directive to the Medical Device Regulation.
icometrix and Aidoc ‘comprehensive stroke solution’
Aidoc and icometrix have partnered to provide a stroke solution in which the Aidoc software detects ICH and large-vessel occlusion (LVO) and the icobrain software is used for CTP analysis to detect ischaemic stroke. Figure 1 shows how the technologies are intended to be implemented in clinical practice.
FIGURE 1.
The icometrix and Aidoc ‘comprehensive stroke solution’ pathways.

Rapid Alberta Stroke Program Early Computed Tomography Score, Rapid ICH, Rapid CTA, Rapid LVO, Rapid CTP algorithms
RapidAI® (iSchemaView, Menlo Park CA, USA) is a CE marked class 2a medical device neuroimaging platform that uses AI-derived software for detecting abnormalities in brain CT scans. The CT algorithms relevant to stroke diagnosis are:
-
Rapid ICH is an image processing software that analyses non-enhanced CT head scans to detect, and flag suspected ICH. Cases with suspected findings can be notified through e-mail and the mobile application. The notification includes compressed images that are for informational purposes only and not intended to be diagnostic. The notified clinician is responsible for viewing non-compressed images on a diagnostic viewer and carrying out necessary patient evaluation.
-
Rapid CTA is an image processing software that analyses head CT angiograms scans to provide neurological vasculature maps with indications of hemispheric differences in the intracranial internal carotid artery (ICA)/middle cerebral artery (MCA) region, which may indicate a LVO.
-
Rapid LVO is an image processing software that analyses head CT angiogram scans to highlight and notify cases with suspected LVO.
-
Rapid CTP enables the assessment of salvageable brain tissue through the delivery of quantified and colour-coded CTP maps that identify brain regions with reduced cerebral blood flow, volume and transit time that exceed prespecified thresholds. Imaging data sets acquired from CT or cone beam CT or magnetic resonance (MR) perfusion and mismatch, MR diffusion, and CT/MR angiography are analysed to measure parameters that determine suitability for thrombectomy.
-
RAPID Alberta Stroke Program Early CT Score (ASPECTS) is not intended for the primary interpretation of CT images. It assists the clinician in evaluating patients presenting for diagnostic imaging with known MCA or ICA occlusion, to assess the extent of disease on NCCT scans. Extent of disease refers to the number of ASPECTS regions affected. Image data and AI analysis of morphological features is used to generate a single ASPECTS. This score is useful in characterising early signs of brain ischaemia, areas of irreversible tissue injury and to help the clinician assess patient eligibility for thrombectomy or thrombolysis.
The RapidAI platform runs on a standard computer or a virtual platform, such as VMware® (VMware Inc., Palo Alto, CA, USA), and can be used to perform image viewing, processing and analysis. The software receives DICOM compliant images as input primarily CT, CTA, cone beam CT and MR. Results from on the Rapid platform can be viewed as visual reports through PACS, e-mail notifications and the Rapid mobile application. Notifications have a sound option for positive cases and can be set to user defined thresholds to enable prioritisation. Results from multiple sites can be viewed and organised in one location. RapidAI is ISO certified and complies with GDPR and data security requirements.
The company provides training, which includes online role-based product training, virtual instructor-led sessions led by clinical experts and performance support content.
e-Alberta Stroke Program Early Computed Tomography Score, e-computed tomography perfusion, e-computed tomography angiography
The e-Stroke platform (Brainomix Ltd, Oxford, UK) is a CE marked class 2a medical device neuroimaging platform that uses AI-derived software for detecting anomalies in brain CT scans. The platform includes the following algorithms relevant to stroke diagnosis:
-
e-ASPECTS analyses NCCT scans for clot detection, signs of hypodensity and generates a heat map of regional ischaemic change, volume of the change and an automatic ASPECTS score.
-
e-CTP analyses CTP scans to generate perfusion summary maps, report parameters, such as mismatch volume and ratio, hypoperfusion intensity ratio and assesses eligibility for mechanical thrombectomy.
-
e-CTA analyses CTA scans to detect the location of LVOs and to generate a CT collateral score, which is used to assesses eligibility for mechanical thrombectomy.
The software integrates with current imaging systems and results can be viewed as visual reports through DICOM output images, e-mail notifications and a web browser.
Viz
The Viz platform (Viz.ai, Viz.ai Inc., San Francisco, CA, USA) is a CE marked class 1 medical device software that uses static AI-derived algorithms to detect abnormalities in brain scans in clinical practice. The algorithms relevant to stroke detection include:
-
Viz LVO analyses CTA images of the brain and sends notification to the clinician if a suspected LVO has been detected. Notifications include compressed images that can be previewed for information purposes only. They are not intended to be diagnostic. The notified clinician is responsible for viewing non-compressed images on a diagnostic viewer and carrying out necessary patient evaluation.
-
Viz ICH analyses NCCT images of the brain and sends notification to the clinician if a suspected ICH has been detected.
-
Viz CTP has communication and analysis capabilities for CTP scans. The analysis includes the calculation of parameters related to tissue perfusion and tissue blood volume.
The company notes that the Viz platform integrates with currently available CT scanners and is designed to receive DICOM images, which can be transferred securely to Viz.ai’s GDPR-compliant Amazon Web Services cloud. Within the cloud, Viz.ai will analyse the imaging data for specific neurovascular disease. The platform can be used by hospital networks and trained clinicians.
The Viz platform is GDPR/HIPAA compliant and has ISO and SOC-2 certifications. Viz is currently a self-certified class 1 medical device under the Medical Device Directive, the company notes that it will be up-classified to a class 2a medical device under the Medical Device Regulation, in line with the transition from the Medical Device Directive to the Medical Device Regulation.
qER
qER (Qure.ai Technologies, Mumbai, India) is a CE marked triage and notification tool that detects and quantifies a range of brain abnormalities intracerebral bleeds and their subtypes, infarcts, mass effect, midline shift and cranial fractures following NCCT imaging. Based on information from AI for Radiology (https://grand-challenge.org/aiforradiology), qER currently has class 2a CE mark. The software populates a radiology reporting template with preliminary findings, patient prioritisation and alert systems including mobile notifications. It integrates with current imaging systems.
Zebra triage
Zebra-Med (Zebra Medical Vision, Shefayim, Israel) is a CE marked software that detects and annotates ICH after NCCT imaging and automates patient prioritisation and a real-time alert system. Based on information from AI for Radiology (https://grand-challenge.org/aiforradiology), Zebra-Med currently has class 2a CE mark. It integrates with the current imaging worklist and viewer with an accompanying alert widget.
Zebra Medical Vision was acquired by Nano-X Imaging in November 2021 and now operates as Nanox. The product is now called Neuro Solution.
CT Perfusion 4D neuro
CT Perfusion 4D Neuro® (GE HealthCare, Chalfont St Giles, UK) is a CE marked medical device for CTP image analysis of images obtained by cine imaging (in the head and body) after the intravenous (IV) injection of contrast. It produces image data and generates information regarding changes in image intensity over time and in calculation of the various perfusion-related parameters (including regional blood flow, regional blood volume, mean transit time and capillary permeability).
BrainScan
BrainScan® CT (BrainScan SA, Gdansk, Poland) is a CE marked AI-derived platform that enables automatic detection and classification of pathological changes occurring in CT examinations of the brain. Based on information from AI for Radiology (https://grand-challenge.org/aiforradiology), BrainScan CT currently has class 2a CE mark.
Cercare Stroke
Cercare® Stroke (Cercare Medical, Aarhus, Denmark) is a CE marked AI-enabled stroke CT and MRI software. The technology uses inputs from perfusion maps and additional maps of oxygen extraction and metabolism to provide an overview of brain tissues status in stroke. Based on information from AI for Radiology (https://grand-challenge.org/aiforradiology), Ceracare Stroke currently has class 2a CE mark.
CINA Head
CINA Head (Avicenna.ai, La Ciotat, France) uses CE marked class 1 medical device AI software for detecting abnormalities in brain CT scans. The algorithms in CINA head include:
-
CINA ICH identifies suspected ICH on NCCT scans and prioritises them on the radiologist’s worklist.
-
CINA LVO detects and prioritises the review of suspected LVOs on CTA.
-
CINA ASPECTS analyses NCCT and creates heat maps that indicate signs of hypodensity which help characterise early ischaemic brain tissue injury.
Accipio
Accipio® (MaxQ AI, Tel Aviv, Israel) is a CE marked AI-derived software that analyses NCCT scan to identify and prioritise suspected ICH. Based on information from AI for Radiology (https://grand-challenge.org/aiforradiology), Accipio has class 2b CE mark. It was discontinued in 2022.
BioMind
BioMind® [Hanalytics (BioMind), Singapore, Singapore] is a CE marked (class not available publicly) AI-derived software used for detecting the location of intracerebral haemorrhage on CT scans and assessing its severity.
Comparator
The comparator for this technology appraisal is review of CT brain scans, by a neuroradiologist or other healthcare professional unassisted by AI-derived software.
Care pathway
Stroke care service provision
The NHS Long Term Plan17 identifies stroke as a clinical priority and sets out in section 3.78 of the Plan the NHS’s ambition to support the national scaling of technology that will assist the expansion of life-changing treatments to more patients, which includes CTP scans to assess the reversibility of brain damage, improved access to MRI scanning and the potential use of AI in the interpretation of CT and MRI scans to support clinical decisions regarding suitability for thrombolysis and thrombectomy.
The National Stroke Service Model: Integrated Stroke Delivery Networks18 outlines best practices for stroke care, people with a suspected stroke should typically receive care within 4 hours in:
-
a hospital with a comprehensive stroke centre that provides hyperacute, acute and inpatient rehabilitation including thrombectomy and neurosurgery services, or
-
an acute stroke centre that provides hyperacute, acute and inpatient rehabilitation, but excluding thrombectomy and neurosurgery. All acute stroke centres are expected to have an intrahospital thrombectomy transfer pathway to transfer patients from acute stroke centres to CSCs.
Hyperacute stroke care usually covers the first 72 hours after a person is admitted. Services provided in the hyperacute phase include specialist clinical assessment, urgent imaging and skilled clinical interpretation of images, delivery of IV thrombolysis 24 hours a day, 7 days a week and transfer or treatment for thrombectomy. Imaging ensures that appropriate diagnosis is made, and time-dependent interventions are delivered. The guidance describes an optimal stroke imaging pathway.
Initial assessment
The diagnosis and initial management of suspected stroke are discussed in NICE guideline NG128. 7 For a diagnosis of stroke or TIA, patients with sudden onset of neurological symptoms outside hospital should be assessed using, for example, FAST and checked for a potential episode of hypoglycaemia. For patients admitted to the emergency department, the early diagnosis should be established using, for example, a ROSIER tool. 7
The guideline NG128 recommends: ‘Admit everyone with suspected stroke directly to a specialist acute stroke unit after initial assessment, from either the community, the emergency department, or outpatient clinics. (An acute stroke unit is a discrete area in the hospital that is staffed by a specialist stroke multidisciplinary team. It has access to equipment for monitoring and rehabilitating patients. Regular multidisciplinary team meetings occur for goal setting.). 7 Similarly, NICE Quality Standard QS21 states ‘Adults presenting at an accident and emergency (A&E) department with suspected stroke are admitted to a specialist acute stroke unit within 4 hours of arrival’.
For patients with an initial diagnosis of acute stroke and an indication of prompt brain imaging, NG1287 recommends immediate (i.e. ‘ideally the next slot and definitely within 1 hour, whichever is sooner’) brain imaging with a non-enhanced CT to rule out or confirm ICH, if any of the following apply:
-
indications for thrombolysis or thrombectomy
-
on anticoagulant treatment
-
a known bleeding tendency
-
a depressed level of consciousness [Glasgow Coma Scale (GCS) score below 13]
-
unexplained progressive or fluctuating symptoms
-
papilloedema, neck stiffness or fever
-
severe headache at onset of stroke symptoms.
For patients with ischaemic stroke, CT with contrast angiography should be performed following an initial non-enhanced CT scan to confirm the presence of occlusion and/or clot. Addition of CTP imaging, or MR equivalent, is recommended if thrombectomy is indicated beyond 6 hours of symptom onset to assess potential salvage of brain tissue. 7
Patients with suspected acute stroke without indication for immediate brain imaging should be scanned as soon as possible and within 24 hours of symptom onset. 7
The National Stroke Service Model guidance18 describes an optimal stroke imaging pathway and recommends that stroke imaging, interpretation and transfer decisions are made within 20 minutes of patient’s arrival.
Treatment
Initially, patients with acute stroke must have their blood glucose concentration maintained and can be offered supplemental oxygen therapy if oxygen saturation drops below 95%. 7 The treatment options for patients with suspected or confirmed ischaemic or haemorrhagic stroke are summarised below.
Ischaemic stroke
For patients with suspected or clinically confirmed ischaemic stroke, NICE NG1287 and Technology Appraisal Guidance 26419 recommend thrombolysis with alteplase (within its marketing authorisation) if:
-
treatment is started as early as possible within 4.5 hours of onset of stroke symptoms
-
and ICH has been excluded by appropriate imaging techniques.
Alteplase should be administered in a well-organised stroke service with appropriately trained staff to deliver thrombolysis and monitor for any complications, nursing staff trained in acute stroke care and immediate access to brain imaging with professionals trained to interpret images. The procedure can also be carried out in the emergency department if staff are appropriately trained and supported, and patients can be cared for after the procedure in an acute stroke service. 7
Thrombectomy for ischaemic stroke is recommended by NICE, with more information available in Interventional Procedures Guidance 548. 20
For patients with acute ischaemic stroke (AIS) and confirmed occlusion of the proximal anterior circulation demonstrated by CT or MR angiography, thrombectomy should be offered as soon as possible (if not contraindicated and within 6 hours of symptom onset), together with IV thrombolysis (within 4.5 hours). 7 Thrombectomy alone should be offered for the same patient population (AIS and confirmed occlusion of the proximal anterior circulation demonstrated by CT or MR angiography) last known to be well between 6 and 24 hours earlier (including wake-up strokes), with the potential to salvage brain tissue as shown by CTP or diffusion-weighted MRI sequence. 7
For patients last known to be well in the preceding 24 hours (including wake-up strokes) with AIS and who have confirmed occlusion of the proximal posterior circulation demonstrated by CT or MR angiography and the potential salvage brain tissue (as shown by CTP or diffusion-weighted MRI sequence), thrombectomy is recommended together with IV thrombolysis. 7,21
Patients with ischaemic stroke are recommended to receive pharmacological treatment (i.e. aspirin or an alternative antiplatelet agent if there is intolerance to aspirin) within 24 hours. Anticoagulant therapy with heparin and then warfarin is recommended for people diagnosed with cerebral venous sinus thrombosis (including those with secondary cerebral haemorrhage). 7
Haemorrhagic stroke
Surgical intervention following primary intracerebral haemorrhage can be considered for previously fit people. Initial medical treatment, instead of surgical intervention, should be offered for patients with:
-
small deep haemorrhages
-
lobar haemorrhage without either hydrocephalus or rapid neurological deterioration
-
a large haemorrhage and significant comorbidities before the stroke
-
a score on the GCS score below 8 unless this is because of hydrocephalus
-
posterior fossa haemorrhage. 7
The NICE guideline NG128 recommends a reversal of anticoagulation treatment using a combination of prothrombin complex concentrate and IV vitamin K, in people with a primary intracerebral haemorrhage who were receiving warfarin before their stroke. 7
A list of NICE guidance relevant to the management of stroke is provided in Appendix 5.
Chapter 3 Assessment of clinical effectiveness
Systematic review methods followed the principles outlined in the Centre for Reviews and Dissemination (CRD) guidance for undertaking reviews in health care,22 NICE Diagnostics Assessment Programme Manual23 and the Cochrane Handbook for Diagnostic Test Accuracy Reviews. 24
Systematic review methods
Search strategy
Searches were undertaken to identify interventions using AI to diagnose acute stroke, as recommended in the CRD guidance for undertaking reviews in health care and the Cochrane Handbook for Diagnostic Test Accuracy Reviews. 22,24
Candidate search terms were identified from target references, browsing database thesauri (e.g. MEDLINE MeSH and Embase). Strategy development involved an iterative approach testing candidate text and indexing terms across a sample of bibliographic databases, so as to reach a satisfactory balance of sensitivity and specificity. Search strategies were developed specifically for each database and the keywords and thesaurus terms were adapted according to the configuration of each database. No restrictions on language, publication status or date were applied.
-
MEDLINE (Ovid) 1946 to 7 July 2021
-
MEDLINE In-Process Citations (Ovid) to 7 July 2021
-
MEDLINE Daily Update (Ovid) to 7 July 2021
-
MEDLINE Epub Ahead of Print (Ovid) to 7 July 2021
-
Embase (Ovid) 1974 to 7 July 2021
-
Cochrane Database of Systematic Reviews (CDSR; Wiley) to July 2021/Iss7
-
Cochrane Central Register of Controlled Trials (CENTRAL; Wiley) to July 2021/Iss7
-
Science Citation Index (SCI; Web of Science) 1988 to 6 July 2021
-
Database of Abstracts of Reviews of Effects (DARE; www.crd.york.ac.uk/CRDWeb) to 31 March 2015
-
Health Technology Assessment Database (HTA; www.crd.york.ac.uk/CRDWeb) 31 March 2018
-
KSR Evidence (KSR Ltd) to 7 July 2021
-
Epistemonikos (www.epistemonikos.org) to 7 July 2021
-
International Network of Agencies for Health Technology Assessment (INAHTA) Publication (www.inahta.org) to 6 July 2021
-
National Institute for Health and Care Research (NIHR) HTA programme (www.nihr.ac.uk) to 2 July 2021
-
Aggressive Research Intelligence Facility (ARIF) database (www.birmingham.ac.uk/research/activity/mds/projects/HaPS/PHEB/ARIF/index.aspx) searched 2 July 2021
-
PROSPERO (CRD; www.crd.york.ac.uk/prospero) to 7 July 2021
-
International Platform of Registered Systematic Review and Meta-analysis Protocols (INPLASY, https://inplasy.com) to 2 July 2021
-
Latin American and Caribbean Health Sciences Literature (LILACS; http://regional.bvsalud.org/php/index.php?lang=en) to 2 July 2021.
The main Embase search strategy was independently peer reviewed by a second information specialist, using the Peer Review of Electronic Search Strategies checklist. 25
Completed and continuing trials were identified by searches of the following resources:
-
ClinicalTrials.gov (US National Institutes of Health; www.clinicaltrials.gov) to 2 July 2021
-
European Union Clinical Trials Register (www.clinicaltrialsregister.eu/ctr-search/search) to 28 July 2021
-
WHO International Clinical Trials Registry Platform (ICTRP; www.who.int/ictrp/en) to 2 July 2021
-
ScanMedicine (https://scanmedicine.com) to 2 July 2021.
Conference proceedings
To identify conference proceedings, searches in Embase were not restricted to exclude conference abstracts. Additional searches were also undertaken of the following specific conference proceedings resources:
-
Northern Light Life Sciences Conference Abstracts (Ovid) 2010 to 2021/Wk25
-
Conference Proceedings Citation Index (Web of Science) 1988 to 6 July 2021.
Named technologies
An additional search was undertaken combining named AI technologies and terms for stroke in order to ensure no relevant studies were missed. These supplementary searches were restricted from 2017 to the present and were undertaken in the following resources:
-
MEDLINE (Ovid) 1946 to 3 September 2021
-
MEDLINE In-Process Citations (Ovid) to 3 September 2021
-
MEDLINE Daily Update (Ovid) to 3 September 2021
-
MEDLINE Epub Ahead of Print (Ovid) to 3 September 2021
-
Embase (Ovid) 1974 to 3 September 2021
-
Northern Light Life Sciences Conference Abstracts (Ovid) 2010 to 2021/Wk34.
Preprints search
Given the fast-moving nature of this topic, the decision was made to conduct a further search of the medRxiv preprint server. All results retrieved from this resource were treated with due caution given the warning from the website’s homepage that ‘Preprints are preliminary reports of work that have not been certified by peer review. They should not be relied on to guide clinical practice or health-related behaviour and should not be reported in news media as established information’. 26
-
MedRxiv (www.medrxiv.org) to 29 September 2021.
Guidelines
A search of the following resources from 2017 to present was conducted to identify the latest guidelines for stroke:
-
Turning Research into Practice (TRIP) database (www.tripdatabase.com) to 26 October 2021
-
Guidelines International Network (GIN; https://g-i-n.net/international-guidelines-library) to 20 October 2021
-
HTA Database (CRD) to March 2018
-
NICE (www.nice.org.uk/guidance) to 20 October 2021
-
NIHR HTA (www.nihr.ac.uk) to 20 October 2021
-
ECRI Guidelines Trust (https://guidelines.ecri.org) to 20 October 2021
-
NHS Evidence (www.evidence.nhs.uk) to 20 October 2021
-
INAHTA (https://database.inahta.org) to 20 October 2021.
Update searches
To ensure that no new relevant papers had been published since the original core strategies were run in July 2021, the main Embase and MEDLINE searches were rerun in their entirety in October 2021 before submission of the draft report. Results were deduplicated against the original search results and for completeness the MedRxiv preprints search was also updated:
-
MEDLINE 1946 to 15 October 2021
-
MEDLINE In-Process Citations to 15 October 2021
-
MEDLINE Daily Update to 15 October 2021
-
MEDLINE Epub Ahead of Print to 15 October 2021
-
Embase 1974 to 18 October 2021
-
MedRxiv to 20 October 2021.
Search strategies for all the resources listed above are presented in Appendix 1.
Hand searching
The bibliographies of included articles and relevant systematic reviews were checked for additional studies.
All identified references were downloaded in EndNote™ (Clarivate Analytics, Philadelphia, PA, USA) software for further assessment and handling. Results for the searches described above were imported into a single project library and deduplicated against each other. All search results (both clinical and economics) were screened for all areas of interest. Rigorous records were maintained as part of the searching process. Individual records within the EndNote reference library were tagged with search information, including the name of the searcher, date searched, database name and host, strategy name and iteration.
Inclusion and exclusion criteria
Separate inclusion criteria were developed for each of the three research questions, and these are summarised in Table 2.
Decision question 1 | Is the use of AI-derived software to assist review of non-enhanced CT brain scans to guide thrombolysis treatment decisions for people with suspected acute stroke a clinically effective intervention? | |
---|---|---|
Decision question 2a | Is the use of AI-derived software to assist review of CTA brain scans for guiding mechanical thrombectomy treatment decisions for people with an ischaemic stroke a clinically effective intervention? | |
Decision question 2b | Is the use of AI-derived software-assisted review of CTP brain scans to guide mechanical thrombectomy treatment decisions for people with an ischaemic stroke, after a CTA brain scan, a clinically effective intervention? | |
Research question | What is the diagnostic performance of AI-derived software-assisted review of plain CT brain scans to rule out ICH and to rule in ischaemic stroke in people with suspected acute stroke? | What are the clinical effects of using AI-derived software-assisted review of plain CT brain scans to guide thrombolysis treatment decisions in people with suspected acute stroke? |
Participants | Adults (≥ 18 years) attending a secondary care stroke centre with suspected acute stroke and who were last known to be well within the past 24 hours | |
Interventions (index test) | AI-derived software-assisted review of plain CT brain scan by a healthcare professional other than a neuroradiologist | AI-derived software-assisted plain CT brain scan review by a neuroradiologist or other healthcare professional |
Comparators | AI-derived software-assisted plain CT brain scan review by a healthcare professional other than a neuroradiologist, using a different AI-derived technology, or unassisted plain CT brain scan review by a healthcare professional other than a neuroradiologist | Unassisted plain CT brain scan review by a neuroradiologist or other healthcare professional |
Reference standard | Unassisted plain CT brain scan review by a neuroradiologist, or by a consensus panel | Not applicable |
Outcomes | Test accuracy (the numbers of TP, FN, FP and TN test results), for the target conditions ICH and ischaemic stroke. Where reported, information will also be extracted on technical failure rates, time to intervention and ease of use/acceptability to cliniciansa |
Clinical/patient-perceived outcomes: mortality, function (e.g. mRS), health-related quality of life, adverse events (e.g. bleed subsequent to thrombolysis), length of hospital stay. Where reported, information will be extracted on technical failure rates, time to thrombolysis/rate of thrombolysis within the clinically appropriate time window, time in emergency department prior to admission or discharge and ease of use/acceptability to cliniciansa |
Study design | Diagnostic accuracy studies | All comparative study designs: study designs will be included in a hierarchical manner (RCTs, controlled clinical trials, observational studies); i.e. controlled clinical trials and observational studies will only be considered for inclusion where no RCTs are identified, or where there are concerns about the applicability (e.g. non-UK settings) or risk of bias for identified RCTs |
Research question | What is the diagnostic performance of AI-derived software-assisted review of CTA brain scans to guide thrombolysis treatment decisions in people with confirmed ischaemic acute stroke? | What are the clinical effects of using AI-derived software-assisted review of CTA to guide mechanical thrombectomy treatment decisions in people with confirmed ischaemic stroke? |
Participants | Adults (≥ 18 years) attending a secondary care stroke centre with AIS, who were last known to be well within the past 6 hours | |
Interventions (index test) | AI-derived software-assisted CTA brain scan review by a healthcare professional other than a neuroradiologist | AI-derived software-assisted CTA brain scan review by a neuroradiologist or other healthcare professional |
Comparators | AI-derived software-assisted CTA brain scan review by a healthcare professional other than a neuroradiologist, using a different AI-derived technology, or unassisted CTA brain scan review by a healthcare professional other than a neuroradiologist | Unassisted CTA brain scan review by a neuroradiologist or other healthcare professional |
Reference standard | Unassisted CTA scan review by a neuroradiologist or by a consensus panel | Not applicable |
Outcomes | Test accuracy (the numbers of TP, FN, FP and TN test results) for the target condition (LVO/occlusion of the proximal anterior circulation) | Clinical/patient-perceived outcomes: mortality, function (e.g. mRS), HRQoL, procedure-related adverse events (e.g. bleed subsequent to thrombolysis), length of hospital stay |
Where reported, information will also be extracted on technical failure rates, time to start of interventional procedure (insertion of catheter) and ease of use/acceptability to cliniciansa | Where reported, information will be extracted on technical failure rates, time to start of interventional procedure (insertion of catheter), reperfusion rates and ease of use/acceptability to cliniciansa | |
Study design | Diagnostic accuracy studies | All comparative study designs: study designs will be included in a hierarchical manner (RCTs, controlled clinical trials, observational studies); i.e. controlled clinical trials and observational studies will only be considered for inclusion where no RCTs are identified, or where there are concerns about the applicability (e.g. non-UK settings) or risk of bias for identified RCTs |
Research question | What is the diagnostic performance of AI-derived software-assisted review of CTA and CTP brain scans to guide thrombolysis treatment decisions in people with confirmed ischaemic acute stroke? | What are the clinical effects of using AI-derived software-assisted review of CTA and CTP brain scans to guide mechanical thrombectomy treatment decisions in people with confirmed ischaemic stroke? |
Participants | Adults (≥ 18 years) attending a secondary care stroke centre with suspected acute stroke, who were last known to be well more than 6 hours previously, but within 24 hours, and in whom ischaemic stroke has been confirmed on plain CT | |
Interventions (index test) | AI-derived software-assisted CTA and CTP brain scan review by a healthcare professional other than a neuroradiologist |
|
Comparators | AI-derived software-assisted CTA and CTP brain scan review by a healthcare professional other than a neuroradiologist, using a different AI-derived technology, or unassisted CTA and CTP brain scan review by a healthcare professional other than a neuroradiologist | Unassisted CTA brain scan review by a neuroradiologist or other healthcare professional and unassisted CTP brain scan review by a neuroradiologist |
Reference standard | Unassisted CTA and CTP scan review by a neuroradiologist or by a consensus panel | Not applicable |
Outcomes | Test accuracy (the numbers of TP, FN, FP and TN test results) for the target conditions (LVO/occlusion of the proximal anterior circulation for CTA and presence of salvageable tissue for CTP) | Clinical/patient-perceived outcomes: mortality, function (e.g. mRS), HRQoL, procedure-related adverse events (e.g. bleed subsequent to thrombolysis), length of hospital stay |
Where reported, information will also be extracted on technical failure rates, time to start of interventional procedure (insertion of catheter) and ease of use/acceptability to cliniciansa | Where reported, information will be extracted on technical failure rates, time to start of interventional procedure (insertion of catheter), reperfusion rates and ease of use/acceptability to cliniciansa | |
Study design | Diagnostic accuracy studies | All comparative study designs: study designs will be included in a hierarchical manner (RCTs, controlled clinical trials, observational studies); i.e. controlled clinical trials and observational studies will only be considered for inclusion where no RCTs are identified, or where there are concerns about the applicability (e.g. non-UK settings) or risk of bias for identified RCTs |
Comparative studies, which reported secondary outcomes only (time to intervention and acceptability to clinicians), were included to maximise the available information for these outcomes. However, it should be noted that these outcomes alone are not sufficient to inform meaningful estimates of the clinical and cost-effectiveness of software using AI-derived algorithms for analysing CT brain scans in people with a suspected acute stroke. Because it is possible, for example, for the use of such software to reduce time to intervention while also being associated with poorer clinical outcomes, secondary outcome data are only useful for decision-making when combined with data on higher-level outcomes (clinical outcomes or measures of diagnostic performance).
Inclusion screening and data extraction
Two reviewers independently screened the titles and abstracts of all reports identified by searches and any discrepancies were discussed and resolved by consensus. Full copies of all studies deemed potentially relevant were obtained and the same two reviewers independently assessed these for inclusion; any disagreements were resolved by consensus. Details of studies excluded at the full paper screening stage are presented in Appendix 4, together with reasons for exclusion.
Studies cited in materials provided by the manufacturers of software with AI-derived algorithms for analysing CT brain scans in people with suspected stroke were first checked against the project reference database, in EndNote X20; any studies not already identified by our searches were screened for inclusion following the process described above.
Where available, data were extracted on the following: study design/details, participant characteristics, details of the AI-derived software (e.g. manufacturer, version used, mode of implementation), details of the CT scanner and imaging protocol(s), details of comparator (i.e. who reviewed the scans), clinical outcomes such as the Modified Rankin Scale (mRS), 2 × 2 data to calculate test performance outcome measures [sensitivity, specificity, positive predictive value (PPV) and negative predictive value (NPV)], technical failure rates and time to intervention (time from imaging to IV thrombolysis or to groin puncture for mechanical thrombectomy). Data were extracted by one reviewer using standard data extraction forms. A second reviewer checked data extraction and any disagreements were resolved by consensus or discussion with a third reviewer.
Quality assessment
The methodological quality of studies reporting diagnostic accuracy data was assessed using QUADAS-2. 27 To provide optimal relevance to the current topic, the methodological quality of observational ‘before and after’ studies was assessed using a checklist devised by the authors for this review; this checklist included items relating to both risk of bias and reporting quality that were considered important for the interpretation of studies assessing the implementation of AI-derived software-assisted review of CT images in stroke patients. Quality assessment was undertaken by one reviewer and checked by a second reviewer, and any disagreements were resolved by consensus or discussion with a third reviewer.
The results of the quality assessments are summarised and presented in Tables 5 and 6 (see Study quality) and are provided in full, by study, in Appendix 3.
Methods of analysis/synthesis
Where multiple studies evaluated the accuracy of the same AI-derived software for the same target condition, the hierarchical summary receiver operating characteristic (HSROC) model was used to estimate summary sensitivity and specificity with 95% confidence intervals (CIs) and prediction regions around the summary points, and to plot HSROC curves. 28–30 This approach allows for between-study heterogeneity in sensitivity and specificity, and for the trade-off (negative correlation) between sensitivity and specificity commonly seen in diagnostic meta-analyses. Analyses were performed in Stata® 13 (StataCorp LP, College Station, TX, USA), mainly using the metandi command.
All other results, including those from ‘before and after’ studies of the implementation of AI-derived software technologies, were summarised in a narrative synthesis.
The results of included studies are grouped by research question addressed, AI-derived software evaluated and study type.
Results of the assessment of clinical effectiveness assessment
The literature searches of bibliographic databases conducted for this assessment identified 6145 unique references after deduplication. Following initial screening of titles and abstracts, 193 were considered to be potentially relevant and ordered for full paper screening; of these, 2 publications31,32 could not be obtained and 27 were included in the review. 33–59 An additional two publications,60,61 cited in documents supplied by the technology manufacturers, met the inclusion criteria for this assessment and were included in the review; one of these60 was an additional conference abstract relating to a study for which our searches had already identified two publications,37,38 and the other61 was published in a journal not indexed in the databases searched. One further study was provided, pre-publication, by a specialist committee member. 62 All remaining potentially relevant studies cited in documents supplied by the technology manufacturers had already been identified by bibliographic database searches. Figure 2 shows the flow of studies through the review process, Tables 3 and 4 provide an overview of the included studies and Appendix 4, Table 34 provides details, with reasons for exclusion, of all publications excluded at the full paper screening stage.
FIGURE 2.
Flow of studies through the review process.

Studya | Country | Patients (N) | Target condition(s) reported | Subgroups reported |
---|---|---|---|---|
(Q1) Is AI-derived software-assisted review of non-enhanced CT brain scans for guiding thrombolysis treatment decisions for people with suspected acute stroke a clinically effective intervention? | ||||
Viz ICH | ||||
Barriera 2018 39 | USA | 284 | ICH | None |
Brainomix (unspecified) | ||||
Herweh 2020 48 | NR | 160 | ICH | None |
Brainomix e-ASPECTS | ||||
Mair 2021 62 | UK and Germany | 4100 | ICH and AIS | None |
(Q2a) Is AI-derived software-assisted review of CTA brain scans for guiding mechanical thrombectomy treatment decisions for people with an ischaemic stroke a clinically effective use intervention? | ||||
iSchemaView Rapid CTA | ||||
b Amukotuwa 2019 35 | NR | 926 | Intracranial anterior circulation LVO (ICA, carotid terminus or M1-segment of the MCA) ICA occlusion M1-segment MCA occlusion M2-segment MCA occlusion Intracranial anterior LVO (ICA, carotid terminus or M1-segment of the MCA) or M2-segment of the MCA occlusion |
None |
b Amukotuwa 2019 36 | Australia | 477 | Intracranial anterior circulation LVO (ICA, carotid terminus or M1-segment of the MCA) M2-segment MCA occlusion Intracranial anterior LVO (ICA, carotid terminus or M1-segment of the MCA) or M2-segment of the MCA occlusion |
None |
iSchemaView Rapid LVO | ||||
Dehkharghani 2021 41 | USA; Switzerland; Brazil | 217 | Intracranial anterior circulation LVO (ICA, carotid terminus or M1-segment of the MCA) | Age: 20–39 years; 40–59 years; ≥ 60 years CT scanner: GE Medical Systems; Siemens; Toshiba |
Dehkharghani 202142 | ||||
Paz 2021 55 | Canada | 151 | LVO (ICA, carotid terminus or M1-segment of the MCA) or M2/3-segment of the MCA occlusion | None |
Viz LVO | ||||
Barreira 2018 60 | USA | 875 | Intracranial anterior circulation LVO (ICA, carotid terminus or M1-segment of the MCA) | None |
Barreira 201837 | ||||
Rodrigues 201938 | ||||
Chatterjee 2018 40 | USA | 54 | Intracranial anterior LVO (ICA, carotid terminus or M1-segment of the MCA) or M2-segment of the MCA occlusion | None |
Dornbos 2020 43 | USA | 680 | Intracranial anterior LVO (ICA, carotid terminus or M1-segment of the MCA), distal M2-segment of the MCA or posterior circulation occlusion | None |
Shalitin 2020 61 | USA | 2544 | LVO (not defined) | None |
Yahav-Dovrat 2021 59 | USA | 1167 | LVO (not defined) | ‘Stroke protocol’ patients |
Brainomix e-CTA | ||||
Seker 2021 56 | Germany | 301 | Proximal (ICA or proximal M1 segment of the MCA) or distal (distal M1 segment or proximal M2 segment of the MCA) LVO. Proximal LVO (terminal ICA and proximal M1 segment of the MCA) | None |
Seker 201957 | ||||
Seker 201958 | ||||
Avicenna CINA LVO | ||||
McLouth 2021 51 | USA | 378 | Intracranial anterior LVO (ICA, carotid terminus or M1-segment of the MCA) or M2-segment of the MCA occlusion | Age: 18–39 years; 40–70 years; > 70 years Male/Female CT scanner: GE Medical Systems; Philips; Siemens; Canon (formerly Toshiba) |
(Q2b) Is AI-derived software-assisted review of CTP brain scans for guiding mechanical thrombectomy treatment decisions for people with an ischaemic stroke after a CTA brain scan a clinically effective intervention? | ||||
iSchemaView Rapid CTP | ||||
Kauw 2020 50 | Netherlands; USA | 176 | Suitability for thrombectomy | None |
Studya | Country | N | Time to intervention outcome reported | Clinical outcome(s) reported |
---|---|---|---|---|
(Q1) Is AI-derived software-assisted review of non-enhanced CT brain scans for guiding thrombolysis treatment decisions for people with suspected acute stroke a clinically effective intervention? and (Q2a) Is AI-derived software-assisted review of CTA brain scans for guiding mechanical thrombectomy treatment decisions for people with an ischaemic stroke a clinically effective intervention? |
||||
Brainomix e-ASPECTS and e-CTA | ||||
Gunda 2020 44 | Hungary | 797 | Time from CTA to groin puncture (thrombectomy); time from door to needle (thrombolysis) | None |
(Q2a) Is AI-derived software-assisted review of CTA brain scans for guiding mechanical thrombectomy treatment decisions for people with an ischaemic stroke a clinically effective intervention? | ||||
iSchemaView Rapid CTA | ||||
Adhya 2021 33 | USA | 310 | Time from CTA to groin puncture (thrombectomy) | 90-day mRS |
Viz LVO | ||||
Hassan 2021 45 | USA | 188 | Time from door to groin puncture (thrombectomy), within CSC | mRS at discharge; in-hospital mortality; in-hospital complications; length of hospital stay |
Hassan 2020 46 | USA | 43 | Time from CTA at PSC to groin puncture at CSC in patients transferred for thrombectomy | mRS at discharge; in-hospital mortality; in-hospital complications; length of hospital stay |
Hassan 202147 | ||||
Morey 2021 52 | USA | 55 | Time from CTA to skin puncture (thrombectomy) | 90-day mRS |
Morey 202053 | ||||
Morey 202154 | ||||
(Q2a) Is AI-derived software-assisted review of CTA brain scans for guiding mechanical thrombectomy treatment decisions for people with an ischaemic stroke a clinically effective intervention? and (Q2b) Is AI-derived software-assisted review of CTP brain scans for guiding mechanical thrombectomy treatment decisions for people with an ischaemic stroke after a CTA brain scan a clinically effective intervention? |
||||
iSchemaView RapidAI mobile application | ||||
Al-Kawaz 2021 34 | USA | 64 | Time from door to groin puncture (thrombectomy) | None |
iSchemaView Rapid (unspecified) | ||||
Kamal 2017 49 | NR | 168 | Time from door to groin puncture (thrombectomy) | None |
Overview of included studies
Based on the searches and inclusion criteria described above, a total of 30 publications33–61 relating to 22 studies33–36,39–41,43–46,48–52,55,56,59–62 were included in the review; the results section of this report cites studies using the primary publication and, where this is different, the publication in which the referenced data were reported.
The studies included in this review evaluated AI-derived software technologies produced by iSchemaView, Viz, Brainomix and Avicenna. For iSchemaView, three studies evaluated Rapid CTA,33,35,36 two studies evaluated Rapid LVO,41,55 one study evaluated Rapid CTP50 and two studies assessed the effects of implementing RapidAI (comprising Rapid CTA and Rapid CTP). 34,49 Eight studies evaluated Viz LVO40,43,45,46,52,59–61 and one study evaluated Viz ICH. 39 For Brainomix, one study evaluated e-CTA,56 one study evaluated e-ASPECTS,62 one study assessed the effects of implementing the e-ASPECTS and e-CTA components of the e-Stroke Suite44 and one study evaluated an un specified ‘AI-based algorithm developed by Brainomix’. 48 The remaining study evaluated CINA LVO, produced by Avicenna. 51 We did not identify any studies that evaluated the remaining AI-derived software technologies described in the Intervention technologies section of this report.
We did not identify any studies conducted in the UK that met the inclusion criteria for this assessment. However, one study reported that, to assess whether the sample was clinically representative of patients admitted to hospital with stroke, it was prespecified that age, sex, stroke severity, time since symptom onset and final diagnosis of included participants would be similar to data from the UK SSNAP (April 2018 to March 2019; www.strokeaudit.org), pooled randomised controlled trials (RCTs) and registries. 62 A total of 12 of the 22 included studies were conducted in USA,33,34,39,40,43,45,46,51,52,59–61 1 study each was conducted in Australia,36 Canada,55 Germany56 and Hungary,44 3 studies were multicentre studies conducted in USA, Brazil and Switzerland,41 in USA and the Netherlands,50 and in the UK and Germany (population validated for applicability to the UK setting using UK SSNAP data);62 the remaining three studies did not report information on geographical location. 35,48,49
Of the 22 included studies, 8 reported receiving some support from the manufacturers of AI-derived software technologies (including shareholdings, consulting fees and employment in relation to individual study authors),35,36,41,46,51,56,59,61 3 studies reported receiving no funding,33,34,52 2 studies were publicly funded50,62 and 9 studies reported no information about funding. 39,40,43–45,48,49,55,60
Full details of the characteristics of study participants, study inclusion and exclusion criteria, AI-derived software technologies evaluated and reference standard (for diagnostic test accuracy studies) or comparator (for before and after studies) are reported in the data extraction tables presented in Appendix 2, Tables 32 and 33.
Study quality
The methodological quality of the 15 studies36,39–41,43,48,50,51,55,56,59–62 that reported diagnostic test accuracy data was assessed using QUADAS-2. 27 No study reported accuracy data for more than one AI-derived software technology. Studies were generally poorly reported and information about how the AI-derived software technology (index text) was implemented; for example threshold or criteria used to determine the presence or absence of the target condition, was lacking. Five studies were published as conference abstracts only,39,40,43,48,60 and two studies were prepublication (not yet peer reviewed) texts. 55,62 All but one61 of the included studies were retrospective analyses and the remaining study61 did not report sufficient information to determine whether participants were recruited prospectively or retrospectively. The main potential sources of bias in the included diagnostic test accuracy studies relate to patient spectrum. There were also concerns regarding the applicability of the patient population and the index test to the research questions specified for this assessment (see Objective and Inclusion and exclusion criteria, Table 2). The results of QUADAS-2 assessments are summarised in Table 5; full QUADAS-2 assessments for each study are provided in Appendix 3. A summary of the risks of bias and applicability concerns within each QUADAS-2 domain is provided below.
Study | Risk of bias | Risk of bias | |||||
---|---|---|---|---|---|---|---|
Patient selection | Index test | Reference standard | Flow and timing | Patient selection | Index test | Reference standard | |
Amukotuwa 201935 | ? | ✓ | ✓ | ✓ | ? | ✗ | ✓ |
Amukotuwa 201936 | ✓ | ✓ | ✓ | ✓ | ✓ | ✗ | ✓ |
Barreira 201860 | ? | ? | ? | ✓ | ? | ✗ | ✓ |
Barreira 201839 | ✗ | ? | ? | ✓ | ✗ | ✗ | ✓ |
Chatterjee 201840 | ? | ? | ? | ✓ | ? | ✗ | ? |
Dehkharghani 202141 | ✗ | ✓ | ✓ | ✓ | ✗ | ✗ | ✓ |
Dornbos 202043 | ? | ? | ? | ✓ | ? | ✗ | ? |
Herweh 202048 | ✗ | ? | ? | ✓ | ? | ✗ | ✓ |
Kauw 202050 | ? | ? | ✗ | ✓ | ? | ✗ | ✗ |
Mair 202162 | ? | ✓ | ✓ | ✓ | ✓ | ✗ | ✗ |
McLouth 202151 | ✗ | ? | ? | ✓ | ? | ✗ | ✓ |
Paz 202155 | ? | ? | ? | ? | ? | ✗ | ? |
Seker 202156 | ✗ | ? | ✓ | ✓ | ✗ | ✗ | ✓ |
Shalitin 202061 | ? | ? | ? | ✓ | ? | ✗ | ? |
Yahav-Dovrat 202159 | ✓ | ? | ✓ | ✓ | ? | ✗ | ✓ |
Patient spectrum
Five studies were rated as high risk of bias for patient selection. 39,41,48,51,56 Three of these studies were diagnostic case–control studies. 39,41,56 Diagnostic case–control studies enrol patients known to have the target condition (cases) and controls without the target condition, that is they do not include a representative sample of the patients in whom the test would be used in clinical practice (e.g. all patients presenting with symptoms suggestive of AIS); because they exclude patients with unclear diagnoses or alternative explanations for the presenting symptoms (differential diagnoses), these studies may produce exaggerated estimates of test accuracy. 63,64 One study was rated high risk of bias for patient selection because patients were excluded for reasons that were not specified in the reported methods. 48 The remaining study51 was rated high risk of bias for patient selection because it included patients identified using a keyword search of a database; it was considered that potential inconsistencies in database indexing could result in inclusion of a different spectrum of patients than if a consecutive or random sample had been enrolled. A further eight studies were rated as unclear risk of bias because they did not provide sufficient details to make a judgement on whether appropriate steps were taken to minimise bias when enrolling patients. 35,40,43,50,55,60–62
Only two of the included studies were considered to have low concerns regarding the applicability of the included patients to the research questions specified for this assessment. 36,62 The three diagnostic case–control studies were rated as having high concerns regarding applicability because the inclusion of patients known to have the target condition and controls without the target condition was not considered to be representative of the spectrum of patients in who the AI-derived software technologies (index tests) would be used in clinical practice. 39,41,56 The remaining 10 studies were considered to have unclear applicability, because they did not report any information about the time from symptom onset or ‘last known well’ for included participants. 36,40,43,48,50,51,55,59–61
Index test
A total of 11 studies were rated as unclear risk of bias for the index test because no information was reported about how the AI-derived software technology (index text) was implemented, for example threshold or criteria used to determine the presence or absence of the target condition. 39,40,43,48,50,51,55,56,59–61 Eight of these studies also reported no information about the version of the software assessed and, hence, it was unclear whether the results of these studies would be applicable to currently available versions. 40,43,48,50,55,56,59,61
All studies were considered to have high concern regarding the applicability of the index test to the research questions specified for this assessment; this was because, in all cases, the AI-derived software technology was evaluated as a stand-alone intervention, rather than as an adjunct or aid to human interpretation (i.e. not as it would be used in clinical practice, as its use is recommended by the manufacturers and as specified in the inclusion criteria for this assessment).
Reference standard
One study was rated as high risk of bias and high concerns regarding applicability, with respect to the refence standard and its application. 50 In this study, images were processed by Rapid CTP then reviewed for potential causes of post-processing failure, by two clinicians in consensus, who were blinded to clinical data but had access to all imaging data available at the time of patient evaluation (i.e. not blinded to the index test results). 50 The 2 × 2 data needed to calculate measures of test accuracy could only be derived by using treatment received (thrombectomy or no thrombectomy) as the reference standard and hence the reference standard was not considered to be applicable to the research questions specified for this assessment, as defined by the inclusion criteria (Table 2). 50 One further study was rated as having high concerns with respect to the applicability of the reference standard. 62 In this study, the reference standard diagnosis was determined at follow-up based on all clinical and imaging data, rather than being based on the determination of a clinical expert reader without AI-derived software and with information that would be available at the point of assessment in clinical practice. 62 Using the follow-up reference standard, this study reported data on the comparative performance of the AI-derived software and a panel of human expert readers, who were masked to all other clinical and imaging data. 62 Eight further studies were rated as unclear risk of bias with respect to the reference standard and its implementation,39,40,43,48,51,55,60,61 because insufficient information was reported to determine whether the human readers providing the reference standard imaging interpretation were blinded to the output from the AI-derived software technology (index test); four of these studies were also considered to have provided insufficient information to determine whether the reference standard likely to correctly classify the target condition and were rated unclear with respect to reference standard applicability. 40,43,55,61
Patient flow
All but one55 of the studies reporting test accuracy data were rated low risk of bias with respect to patient flow. The remaining study55 was rated unclear risk of bias because no information was reported about the reference standard for interpretation of images, and hence it was not clear that all participants had received the same reference standard.
The methodological quality of the seven33,34,44–46,49,52 observational ‘before and after’ studies was assessed using a checklist devised by the authors for this review. The results of this assessment are summarised in Table 6 and reported, in full, for each study, in Appendix 3.
Study details | Q1 | Q2 | Q3 | Q4 | Q5 | Q6 | Q7 | Q8 | Q9 |
---|---|---|---|---|---|---|---|---|---|
Adhya 202133 | N | U | Y | U | Y | N | N | N | Y |
Al-Kawaz 202134 | N | U | U | Y | U | Y | N | NA | N |
Gunda 202044 | N | U | Y | U | Y | N | N | Y | N |
Hassan 202046 | N | Y | Y | N | Y | Y | N | Y | Y |
Hassan 202145 | N | Y | Y | Y | U | N | N | NA | Y |
Kamal 201749 | N | U | U | Y | U | N | N | NA | U |
Morey 202052 | N | Y | U | N | U | N | N | NA | Y |
Questions (Q):
|
All of these studies were retrospective studies, which assessed the effects of implementing an AI-derived software technology in real-world settings. In all studies, the primary outcome was a measure of time to intervention (thrombectomy and, in one study,44 thrombectomy or thrombolysis). As noted in Inclusion and exclusion criteria, time to intervention outcomes alone are not sufficient to inform meaningful estimates of the clinical and cost-effectiveness of AI-derived software technologies. It is important to measure clinical outcomes alongside time to intervention outcomes because it is possible, for example, for the implementation of AI-derived software technologies to reduce time to intervention while also being associated with poorer clinical outcomes. Only four of the studies in this section reported a clear clinical outcome measure, together with time to intervention. 33,45,46,52 In addition, with respect to the applicability of these studies to the current decision problem. Four33,46,49,52 of the seven studies evaluated the implementation of an AI-derived software technology in the context of providing an automated alert system (i.e. not as specified in the scope for this assessment) and two further studies were reported as conference abstracts that did not provide sufficient detail to determine how the AI-derived software technology had been implemented. 44,45
Observational comparative studies provide a lower level of evidence with respect to the effects of an intervention than RCTs. Where observational study designs are used to provide estimates of effect, it is important to control, as far as possible, for potential confounding factors (factors other than the intervention that may affect the outcome or outcomes being assessed); for example, by matching participants in the intervention and comparator groups on key risk factors. Two of the studies in this section did not report sufficient information to assess whether participants were comparable before and after the implementation of the AI-derived software technology, with respect to baseline demographic characteristics, comorbid conditions and risk factors. 33,44 Two further studies reported information indicating that the before and after implementation populations differed with respect to one or more key characteristics. 45,52 In addition, only three studies33,44,46 reported that there were no changes in the care pathway, other than the implementation of then AI-derived software technology, between the two time periods assessed; the remaining studies did not report sufficient information to determine whether any other changes had occurred.
Studies in this section were generally poorly reported, with no study providing a clear description of the imaging criteria used to select patients for treatment (thrombectomy or thrombolysis), and only two34,46 studies reporting information about how the AI-derived software technology was implemented (e.g. at what point in the care pathway was the AI-derived software technology and by whom were the results used/interpreted). Information about participant selection was also poorly reported; four studies33,34,44,49 did not report sufficient information to determine whether the spectrum of included participants was applicable to the research questions specified for this assessment (see Q2 in Table 6), and three studies34,49,52 did not report sufficient information to assess whether study inclusion criteria were similar before and after implementation of the AI-derived software technology.
No study in this section compared clinical outcomes with time to intervention in populations that were comparable (with respect to key baseline characteristics) before and after the implementation of the AI-derived software technology, and where the AI-derived software technology was the only change to the care pathway.
Research question 1
-
Is the use of AI-derived software to assist review of non-enhanced CT brain scans to guide thrombolysis treatment decisions for people with suspected acute stroke a clinically effective intervention?
Four studies39,44,48,62 reported some limited information relevant to research question 1 and three of these four studies were reported as conference abstracts only. 39,44,48 The results of these studies are summarised below and detailed study characteristics are provided in Appendix 2. Three studies provided data on the diagnostic performance of AI-derived software technologies for the detection of ICH in patients with suspected AIS (Table 7); one study evaluated Viz ICH in a random sample taken from a cohort of stroke patients with and without ICH,39 the second study evaluated an un-specified Brainomix AI-derived software technology in patients with suspected AIS48 and the final study evaluated Brainomix e-ASPECTS in a clinically representative of patients admitted to hospital with stroke. 62 The sensitivity and specificity estimates were 90.2% (95% CI 83.9% to 94.2%) and 100% (95% CI 97.5% to 100%) for Viz ICH,39 91.1% (95% CI 82.8% to 95.6%) and 88.9 (95% CI 80.2% to 94.0%) for the unspecified Brainomix AI-derived software technology48 and 93.8% (95% CI 91.6% to 95.4%) and 82.8% (95% CI 81.4% to 84.1%) for Brainomix e-ASPECTS. 62 The study that evaluated Brainomix e-ASPECTS also provided sensitivity and specificity estimates for the detection of AIS; these were 68.5% (95% CI 66.4% to 70.5%) and 74.1% (95% CI 71.95% to 76.1%), respectively. 62 It should be noted that all these studies were retrospective analyses of previously acquired images that assessed the performance of the AI-derived software technology alone; no study provided information about the performance of an AI-derived software technology as an adjunct or aid to human interpretation (as it would be used in clinical practice, as its use is recommended by the manufacturer and as specified in the inclusion criteria for this assessment).
Study details | AI-derived software technology | Target condition | TP | FP | FN | TN | Sensitivity (95% CI) | Specificity (95% CI) | PPV (95% CI) | NPV (95% CI) |
---|---|---|---|---|---|---|---|---|---|---|
Barreira 201839 | Viz ICH | ICH | 119 | 0 | 13 | 152 | 90.2 (83.9 to 94.2) | 100 (97.5 to 100) | NAa | NAa |
Herweh 202048 | (un-specified) Brainomix | 72 | 9 | 7 | 72 | 91.1 (82.8 to 95.6) | 88.9 (80.2 to 94.0) | 88.9 (80.2 to 94.0) | 91.1 (82.8 to 95.6) | |
Mair 202162 | Brainomix e-ASPECTS | 588 | 531 | 39 | 2550 | 93.8 (91.6 to 95.4) | 82.8 (81.4 to 84.1) | 52.5 (49.6 to 55.5) | 98.5 (97.9 to 98.9) | |
AIS | 1382 | 438 | 636 | 1252 | 68.5 (66.4 to 70.5) | 74.1 (71.9 to 76.1) | 75.9 (73.9 to 77.8) | 66.3 (64.2 to 68.4) |
The remaining publication44 reported an observational ‘before and after’ study, evaluating the effects on time to treatment of implementing the e-ASPECTS and e-CTA modules of Brainomix e-Stroke in a centre which did not offer thrombectomy (patients requiring thrombectomy were transferred to another unit); the results of this study are summarised in Table 8. The publication stated that ‘delivery of stroke care was otherwise unchanged’. e-ASPECTS analyses NCCT scans for clot detection, signs of hypodensity and generates a heat map of regional ischaemic change, volume of the change and an automatic ASPECTS score, and e-CTA analyses CTP scans to generate perfusion summary maps, report parameters such as mismatch volume and ratio, hypoperfusion intensity ratio, and assesses eligibility for mechanical thrombectomy, hence, only the implementation of e-ASPECTS is relevant to research question 1. However, the effects of implementation were not reported separately for e-ASPECTS and e-CTA. 44 The proportion of patients receiving thrombolysis was 11.5% before implementation and 18.1% after implementation (absolute numbers not reported), and the proportion of patients transferred for thrombectomy was 2.8% before implementation and 4.8% after implementation (absolute numbers not reported). 44 For patients receiving thrombolysis, the mean time from door to treatment was 44 minutes before implementation and 41 minutes after implementation (no estimates of variance reported). 44 For patients transferred for thrombectomy, the mean time from first CT to groin puncture was 174 minutes before implementation and 145 minutes after implementation (no estimates of variance reported). 44 It should also be noted that this study did not report any information comparing clinical outcomes before and after implementation, such as would be needed to inform decision-making.
Study details | AI-derived software technology | Time to treatment outcome | Pre implementation | Post implementation | Clinical outcome | Pre implementation | Post implementation |
---|---|---|---|---|---|---|---|
Gunda 202044 | Brainomix e-ASPECTS and e-CTA | Mean (SD) minutes from door to needle (IV thrombolysis) | 44 (NR), (n = 46) | 41 (NR), (n = 72) | None reported | NA | NA |
Mean (SD) minutes from door to groin puncture (thrombectomy) | 174 (NR), (n = 11) | 145 (NR), (n = 19) | None reported | NA | NA |
We did not identify any studies, conducted in patients with suspected AIS, evaluating Aidoc ICH, Rapid ICH, Rapid ASPECTS, qER, Zebra-Med, Brainscan, Avicenna CINA ICH, Avicenna CINA ASPECTS, MaxQ AI Accipio, or Biomind, the remaining AI-derived software technologies used in the analysis of NCCT images, as indicated in Table 1 and described in the Intervention technologies section of this report.
Research question 2a
-
(2a) Is the use of AI-derived software to assist review of CTA brain scans for guiding mechanical thrombectomy treatment decisions for people with an ischaemic stroke a clinically effective intervention?
Eighteen studies reported information relevant to research question 2a. 33–36,40,41,43–46,49,51,52,55,56,59–61 Eleven studies reported sufficient information to allow calculation of measures of the diagnostic performance of AI-derived software technologies for the detection of LVO;35,36,40,41,43,51,55,56,59–61 2 studies evaluated Rapid CTA35,36 and 2 studies evaluated Rapid LVO,41,55 5 studies evaluated Viz LVO,40,43,59–61 1 study evaluated Brainomix e-CTA56 and 1 study evaluated Avicenna CINA LVO. 51 The remaining seven studies in this section were observational ‘before and after’ studies, which evaluated the effects of implementing AI-derived software technologies in clinical practice. 33,34,44–46,49,52 Four studies reported, specifically, on the implementation of AI-derived software technologies for the analysis of CTA images, one on the implementation of Rapid CTA33 and three on the implementation of Viz LVO. 45,46,52 The remaining three studies assessed the effects of implementation of AI-derived software technologies which were unclearly reported or included multiple components;34,44,49 one study44 reported on the implementation of e-ASPECTS and e-CTA and is described in Research question 1 and Table 8, and two studies34,49 reported on the implementation of Rapid technologies and are described in Research question 2b and Table 17. One study, which provided diagnostic performance data for Viz LVO, also reported the effect of implementing Viz LVO on time from door to groin puncture, in patients who were transferred for thrombectomy. 43
The results of studies in this section are grouped by AI-derived software technology. Detailed study characteristics are provided in Appendix 2.
We did not identify any studies conducted in patients with AIS that evaluated Aidoc LVO, the remaining AI-derived software technology used in the analysis of CTA images, as indicated in Table 1 and described in the Intervention technologies section of this report.
Rapid CTA and Rapid LVO
Two studies reported sufficient data to calculate the sensitivity and specificty of Rapid CTA for the detection of intracranial anterior circulation LVO, at the relative vessel density < 75–60% (green) threshold. 35,36
The RAPID CTA algorithm performs the following operations: (1) imports the CTA raw data in DICOM format; (2) motion and tilt corrects the images; (3) trims the CTA data to restrict coverage from the C1 vertebra to the vertex; (4) elastically aligns a human head template with the CTA data; (5) warps template of anatomic structures (e.g. bones and blood vessels) on to the CTA to create masks; (6) removes the skull base and calvarium using the bone mask; (7) identifies and dichotomises (into small and large diameter) intracranial vessels; (8) determines vessel density by assessing the length of large calibre vessels in the suprasellar cistern (supraclinoid IVA) and proximal Sylvian cistern (M1 segment of the MCA) as well as the sum of density values (Hounsfield units) of the voxels constituting these vessels; (9) determines vessel density for small-calibre vessels (distal M1, M2 and M3 segments) further distally in and adjacent to the Sylvian cistern; (10) performs left–right comparison to determine the relative vessel density ratio, within the suprasellar and proximal Sylvian cistern and progressing distally; (11) creates axial, coronal and sagittal maximum intensity projection (MIP) of the intracranial vasculature from the bone-masked CTA; (12) highlights the areas of reduced relative interhemispheric vessel density on these MIPs using colour thresholds 75–80% (blue), 60–74% (green), 45–59% (yellow) and < 45% (red); (13) sends these MIPs as deidentified outputs to the PACS.
Data from these studies were not pooled, as the study populations overlapped (Table 9). The sensitivity and specificity estimates from the larger study study35 were 96.9% (95% CI 94.3% to 98.3%) and 74.3% (95% CI 70.6% to 77.7%), respectively. Further analysis from this study indicated that sensitivity and specificity estimates did not change substantially when M2 segment occlusions were included in the target condition; the estimated sensitivity was 95.4% (95% CI 92.7% to 97.1%) and the estimated specificity was 79.4% (95% CI 75.8% to 82.6%). 35 This study also provided separate sensitivity and specificity estimates for Rapid CTA for detection of occlusions of the ICA, and M1 and M2 -segments of the MCA, using varying optimised thresholds (see Table 9). 35
Study details | Population | Target condition | Threshold | TP | FP | FN | TN | Sensitivity (95% CI) | Specificity (95% CI) | PPV (95% CI) | NPV (95% CI) |
---|---|---|---|---|---|---|---|---|---|---|---|
Rapid CTA | |||||||||||
Amukotuwa 201935 | All | Intracranial anterior circulation LVO (ICA, carotid terminus or M1 segment of the MCA) | < 75–60% relative vessel density (green) | 310 | 151 | 10 | 437 | 96.9 (94.3 to 98.3) | 74.3 (70.6 to 77.7) | 67.2 (62.8 to 71.4) | 97.8 (95.9 to 98.8) |
aAmukotuwa 201936 | < 75% relative vessel densityb | 73 | 93 | 5 | 303 | 93.6 (85.9 to 97.2) | 76.5 (72.1 to 80.4) | 44.0 (36.6 to 51.6) | 98.4 (96.3 to 99.3) | ||
Amukotuwa 201935 | Intracranial anterior LVO (ICA, carotid terminus or M1 segment of the MCA) or M2 segment of the MCA occlusion | < 75–60% relative vessel density (green) | 351 | 112 | 17 | 431 | 95.4 (92.7 to 97.1) | 79.4 (75.8 to 82.6) | 75.8 (71.7 to 79.5) | 96.2 (94.0 to 97.6) | |
aAmukotuwa 201936 | < 75% relative vessel densityb | 97 | 70 | 9 | 301 | 91.5 (84.6 to 95.5) | 81.1 (76.8 to 84.8) | 58.1 (50.5 to 65.3) | 97.1 (94.6 to 98.5) | ||
Amukotuwa 201935 | ICA occlusion | < 60–45% relative vessel density (yellow) | 129 | 7 | 4 | 459 | 97.0 (92.5 to 98.8) | 86.4 (83.3 to 89.1) | 64.2 (57.3 to 70.5) | 99.1 (97.8 to 99.7) | |
Amukotuwa 201935 | M1-segment MCA occlusion | < 75–60% relative vessel density (green) | 281 | 108 | 9 | 423 | 96.9 (94.2 to 98.4) | 79.7 (76.0 to 82.9) | 72.2 (67.6 to 76.5) | 97.9 (96.1 to 98.9) | |
Amukotuwa 201935 | M2-segment MCA occlusion | < 80–75% relative vessel density (blue) | 54 | 133 | 6 | 398 | 90.0 (79.9 to 95.3) | 75.0 (71.1 to 78.5) | 28.9 (22.9 to 35.7) | 98.5 (96.8 to 99.3) | |
aAmukotuwa 201936 | < 75% relative vessel densityb | 24 | 144 | 4 | 305 | 85.7 (68.5 to 94.3) | 67.9 (63.5 to 72.1) | 14.3 (9.8 to 20.4) | 98.7 (96.7 to 99.5) | ||
Rapid LVO | |||||||||||
Dehkharghani 202141 | All | Intracranial anterior circulation LVO (ICA, carotid terminus or M1-segment of the MCA) | < 60% relative vessel density | 105 | 2 | 4 | 106 | 96.3 (90.9 to 98.6) | 98.1 (93.5 to 99.5) | NAc | NAc |
Paz 202155 | LVO (ICA, carotid terminus or M1-segment of the MCA) or M2/3-segment of the MCA occlusion | NR | 42 | 12 | 24 | 73 | 63.6 (51.6 to 74.2) | 85.9 (76.9 to 91.7) | 77.8 (65.1 to 86.8) | 75.3 (65.8 to 82.8) | |
Dehkharghani 202141 | Subgroup, age 20–39 years | Intracranial anterior circulation LVO (ICA, carotid terminus or M1 segment of the MCA) | < 60% relative vessel density | 7 | 0 | 0 | 10 | 100 (64.6 to 100) | 100 (72.2 to 100) | NAc | NAc |
Subgroup, age 20–39 years | 29 | 1 | 0 | 38 | 100 (88.3 to 100) | 97.4 (86.8 to 99.5) | NAc | NAc | |||
Subgroup, age ≥ 60 years | 69 | 1 | 4 | 57 | 94.5 (86.7 to 97.8) | 98.3 (90.9 to 99.7) | NAc | NAc | |||
Subgroup, GE Medical Systems scanner | 62 | 1 | 2 | 32 | 96.9 (89.3 to 99.1) | 97.0 (84.7 to 99.5) | NAc | NAc | |||
Subgroup, Siemens scanner | 14 | 1 | 0 | 45 | 100 (78.5 to 100) | 97.8 (88.7 to 99.6) | NAc | NAc | |||
Subgroup, Toshiba scanner | 26 | 0 | 2 | 28 | 92.9 (77.4 to 98.0) | 100 (87.9 to 100) | NAc | NAc |
Two studies reported sufficient data to allow calculation of sensitivity and specificity estimates for Rapid LVO. 41,55 One study provided data to calculate the sensitivity and specificity of Rapid LVO for the detection of intracranial anterior circulation LVO, at the relative vessel density < 60% (green)* threshold; the sensitivity and specificity estimates were 96.3% (95% CI 90.9% to 98.6%) and 98.1% (95% CI 93.5% to 99.5%), respectively. 41 The results of subgroup analyses from this study41 indicated that the sensitivity and specificity of Rapid LVO for the detection of intracranial anterior circulation LVO did not vary substantially with patient age or between the different CT scanners used to acquire images (see Table 9). The sensitivity and specificity estimates for Rapid LVO, calculated from the second study, were substantially lower; the sensitivity estimate was 63.6% (95% CI 51.6% to 74.2%) and the specificity estimate was 85.9% (95% CI 76.9% to 91.7). 55 However, this study included a wider range of anatomical locations in its definition of LVO (see Table 9). 55
It should be noted that all the studies that provided data on the diagnostic performance of Rapid CTA or Rapid LVO were retrospective analyses of previously acquired images, which assessed the performance of the AI-derived software technology alone; no study provided information about the performance of an AI-derived software technology as an adjunct or aid to human interpretation (as it would be used in clinical practice, as its use is recommended by the manufacturer and as specified in the inclusion criteria for this assessment).
Full diagnostic performance data for Rapid CTA and Rapid LVO are provided in Table 9.
The remaining study of Rapid CTA was an observational ‘before and after’ study, which reported some limited information about the effects of implementing Rapid CTA in a ‘real-world’ clinical setting (Table 10). 33 The article reporting this study stated that: ‘All interventional equipment, endovascular therapists, neuroradiology staff, and hospitals serviced were identical during the study period, and the only significant change was the installation of Rapid CTA’. Data from this study appear to indicate that the implementation of Rapid CTA was associated with a reduction in the mean time from CTA to groin puncture, for patients undergoing thrombectomy, from 92 minutes before implementation to 68 minutes after implementation, however, no estimates of variance were reported. 33 There was no significant difference in the proportion of patients who were functionally independent (mRS ≤ 2) following implementation of Rapid CTA (odds ratio 1.75, 95% CI 0.84 to 3.67). It should also be noted that this study evaluated the implementation of an Rapid CTA in the context of providing an automated alert system (i.e. not as specified in the scope for this assessment). 33 Two further studies reported information about the effects on time to treatment of implementing an unspecified Rapid product49 and the RapidAI Mobile Application (iSchemaView, Menlo Park, CA, USA). 34 Neither study provided separate results for the effects of the CTA and CTP analysis algorithms in Rapid; the results of these studies are described in Research question 2b and Table 17.
Study details | Time to treatment outcome | Pre implementation | Post implementation | Clinical outcome | Pre implementation | Post implementation |
---|---|---|---|---|---|---|
Adhya 202133 | Mean (SD) minutes from CTA to groin puncture (thrombectomy), setting unclear | 92 (NR), (n = 74) | 68 (NR), (n = 72) | Mean (SD): 90-day mRS | 4.47 (NR), (n = 74) | 3.9 (NR), (n = 67) |
Proportion with 90-day mRS ≤ 2 | 17/74 (23%) | 23/67 (34%) |
It should be noted that, although studies of this type provide some information about the effects of implementing Rapid AI-derived software technologies in ‘real-world’ clinical settings, the information provided is limited to those patients who underwent thrombectomy; that is, there is no information about the effects of implementation of these technologies, with respect to identification of patients who are candidates for thrombectomy.
Viz LVO
Five studies reported sufficient information to calculate measures of the diagnostic performance of Viz LVO. 40,43,59–61 The target condition varied across studies, with respect to the anatomical location of occlusions,40,43,60 and two studies did not provide any definition of LVO. 59,61 The summary estimates of sensitivity and specificity, derived from all five studies, were 88.0% (95% CI 76.9% to 94.2%) and 89.9% (95% CI 85.5% to 93.0%), respectively (Figure 3). A sensitivity analysis, excluding one study where the reported target condition included posterior circulation occlusions,43 resulted in a higher summary estimate of sensitivity [91.3% (95% CI 84.9% to 95.1%)] and a similar summary estimate of specificity (89.3, 95% CI 83.5% to 93.2%; Figure 4). One study also reported that, for those patients who were transferred between centres for thrombectomy (number not reported), the median time from door to groin puncture was significantly shorter after implementation of Viz LVO, 141 (95% CI 128.5 to 168) minutes, compared with before implementation, 185 minutes (95% CI 151 to 241 minutes, p = 0.027). 43 This study did not report any comparison of clinical outcomes for the periods before and after implementation of Viz LVO. 43
FIGURE 3.
Summary receiver operating characteristic – all studies Viz LVO.The summary point, illustrated, should be interpreted with caution, given the absence of a clear definition of threshold in any of the included studies and the potential for variation in the anatomical area included in the target condition

FIGURE 4.
Summary receiver operating characteristic – sensitivity analysis Viz LVO.The summary point, illustrated, should be interpreted with caution, given the absence of a clear definition of threshold in any of the included studies and the potential for variation in the anatomical area included in the target condition

It should be noted that all five studies that provided data on the diagnostic performance of Viz LVO were retrospective analyses, of previously acquired images, which assessed the performance of the AI-derived software technology alone; no study provided information about the performance of Viz LVO as an adjunct or aid to human interpretation (as it would be used in clinical practice, as its use is recommended by the manufacturer and as specified in the inclusion criteria for this assessment). Full diagnostic performance data for Viz LVO are provided in Table 11.
Study details | Population | Target condition | Threshold | TP | FP | FN | TN | Sensitivity (95% CI) | Specificity (95% CI) | PPV (95% CI) | NPV (95% CI) |
---|---|---|---|---|---|---|---|---|---|---|---|
Barreira 2018a60 | All | Intracranial anterior circulation LVO (ICA, carotid terminus or M1 segment of the MCA) | NR | 362 | 83 | 40 | 390 | 90.0 (86.7 to 92.6) | 82.5 (78.8 to 85.6) | 81.3 (77.5 to 84.7) | 90.7 (87.6 to 93.1) |
Chatterjee 201840 | Intracranial anterior LVO (ICA, carotid terminus or M1 segment of the MCA) or M2 segment of the MCA occlusion | 31 | 3 | 3 | 17 | 91.2 (77.0 to 97.0) | 85.0 (64.0 to 94.8) | 91.2 (77.0 to 97.0) | 85.0 (64.0 to 94.8) | ||
Dornbos 202043 | Intracranial anterior LVO (ICA, carotid terminus or M1 segment of the MCA), distal M2 segment of the MCA or posterior circulation occlusion | 45 | 55 | 23 | 557 | 66.2 (54.3 to 76.3) | 91.0 (88.5 to 93.0) | 45.0 (35.6 to 54.8) | 96.0 (94.1 to 97.3) | ||
Shalitin 202061 | LVO (not defined) | 157 | 147 | 6 | 2234 | 96.3 (92.2 to 98.3) | 93.8 (92.8 to 94.7) | 51.6 (46.0 to 57.2) | 99.7 (99.4 to 99.9) | ||
Yahav-Dovrat 202159 | All ‘stroke protocol’ | LVO (not defined) | 59 | 33 | 13 | 299 | 81.9 (71.5 to 89.1) | 90.1(86.4 to 92.8) | 64.1 (53.9 to 73.2) | 95.8 (93.0 to 97.5) | |
Summary estimatea (five studies)40,43,59–61 | 88.0 (76.9 to 94.2) | 89.9 (85.5 to 93.0) | |||||||||
Sensitivity analysis,a excluding Dornbos 202043 | 91.3 (84.9 to 95.1) | 89.3 (83.5 to 93.2) |
Three further observational ‘before and after’ studies, reported information about the effects of implementing Viz LVO in clinical settings (Table 12). 45,46,52 One study reported that, for patients transferred between centres for thrombectomy, the median time from CTA to groin puncture was significantly shorter after implementation of Viz LVO, 127 minutes (range 39–622 minutes), compared with before implementation, 216 minutes (range 109–608 minutes, p = 0.026). 46 This study also reported a small reduction in the length of hospital stay after implementation of Viz LVO [mean difference (MD) −2.5 days, 95% CI −4.7 to −0.3 days)] and no significant change in the proportion of patients who were functionally independent at 90 days post-procedure (mRS ≤ 2, OR 1.67, 95% CI 0.45 to 6.23) or rates of in-hospital complications (OR 0.60, 95% CI 0.06 to 6.28) or in-hospital mortality (OR 1.33, 95% CI 0.31 to 5.73). 46 A second study from the same research group reported that the mean time from door to groin puncture was also reduced following implementation of Viz LVO for patients who were treated with thrombectomy within centre (MD −86.7 minutes, 95% CI −125.9 to −47.5 minutes). 45 Again, this study found no significant change in the proportion of patients who were functionally independent at 90 days post procedure (mRS ≤ 2, OR 0.88, 95% CI 0.46 to 1.69) or rates of in-hospital complications (OR 0.87, 95% CI 0.46 to 1.62) or in-hospital mortality (OR 1.10, 95% CI 0.55 to 2.21) and, additionally, reported no significant change (p = 0.103) in the median length of hospital stay. 45 The final study reported a significant reduction in the mean time from CTA to groin puncture (MD −44.6 minutes, 95% CI −68.6 to −20.6 minutes) after implementation of Viz LVO for patients transferred between centres for thrombectomy. 52 This study also reported no significant change in the mean 90-day mRS after implementation of Viz LVO (MD −1.0, 95% CI −2.1 to 0.1). 52
Study details | Time to treatment outcome | Pre implementation | Post implementation | Mean difference (95% CI) | Clinical outcome | Pre implementation | Post implementation | Mean difference (95% CI) or OR (95% CI) |
---|---|---|---|---|---|---|---|---|
Dornbos 202043 | Median (IQR) minutes from door to groin puncture, for transferred patients | 185 (151–241), (n = NR) | 141 (128.5–168), (n = NR) | NC | None reported | NA | NA | NA |
Hassan 202046 | Median (min, max) minutes from CTA to groin puncture, for transferred patients | 216 (109–608), (n = 28) | 127 (39–622), (n = 11) | NC | 90-day mRS ≤ 2 | 8/28 | 6/15 | 1.67 (0.45 to 6.23)a |
In-hospital complications | 3/28 | 1/15 | 0.60 (0.06 to 6.28)a | |||||
In-hospital mortality | 6/28 | 4/15 | 1.33 (0.31 to 5.73)a | |||||
Mean (SD) hospital stay (days) | 9.7 (4.9), (n = 28) | 7.2 (2.5), (n = 15) | −2.5 (−4.7 to −0.3)a | |||||
Hassan 202145 | Mean (SD) minutes from door to groin puncture, within centre | 206.6 (169.1), (n = 86) | 119.9 (83.0), (n = 102) | −86.7 (−125.9 to −47.5)a | 90-day mRS ≤ 2 | 24/86 | 26/102 | 0.88 (0.46 to 1.69)a |
In-hospital complications | 27/86 | 29/102 | 0.87 (0.46 to 1.62)a | |||||
In-hospital mortality | 18/86 | 23/102 | 1.10 (0.55 to 2.21)a | |||||
Median (IQR) hospital stay (days) | 7.0 (4.0–11.0) | 7.5 (4.0–12.0) | NC | |||||
Morey 202052 | Mean (SD) minutes from CTA to groin puncture, for transferred patients | 161.3 (51.1), (n = 29) | 146.7 (39.4), (n = 26) | −44.6 (−68.6 to −20.6)a | Mean (SD) 90-day mRS | 4.3 (2.1), (n = 29) | 3.3 (1.9), (n = 26) | −1.0 (−2.1 to 0.1)a |
All four studies43,45,46,52 that provided information about the effects of implementing Viz LVO in clinical settings reported that implementation was associated with reductions in time to treatment for thrombectomy patients and, where reported, with no significant change in clinical outcomes. 45,46,52 However, it should be noted that two of these studies46,52 evaluated the implementation of Viz LVO in the context of providing an automated alert system (i.e. not as specified in the scope for this assessment) and the remaining two studies43,45 were reported as conference abstracts, which did not provide sufficient information to determine how Viz LVO had been implemented. It should also be noted that, although these studies provide some information about the effects of implementing Viz LVO in ‘real-world’ clinical settings, the information provided is limited to those patients who underwent thrombectomy; that is, there is no information about the performance of Viz LVO on the identification of patients who are candidates for thrombectomy.
Brainomix e-CTA
One study reported sufficient information to calculate the sensitivity and specificity of Brainomix e-CTA for the detection of proximal (ICA or proximal M1 segment of the MCA) or distal (distal M1 segment or proximal M2 segment of the MCA) LVO (Table 13). 56 The sensitivity and specificity estimates were 83.8% (95% CI 77.3% to 88.7%) and 95.7% (95% CI 91.0% to 98.0%), respectively. When patients with distal LVOs were excluded for the analysis, the estimated sensitivity and specificity values for the detection of proximal LVOs were 91.6% (95% CI 84.3% to 95.7%) and 97.9% (95% CI 93.9% to 99.3%), respectively. The reference standard for this study was provided by a board-certified neuroradiologist with more than 10 years of experience and unrestricted access to all clinical and imaging data, including data on interventional therapy and follow-up. 56 Using a subset of 144 patients, this study also provided comparative accuracy data for e-CTA compared with human readers (a board-certified neuroradiologist, a radiology resident and two neurology residents) for the detection of proximal (ICA or proximal M1 segment of the MCA) or distal (distal M1 segment or proximal M2 segment of the MCA) LVO; these data are summarised in Table 14. 56 It should be noted that, while this study provides a comparison of the diagnostic performance of e-CTA alone compared with human readers with varying levels of expertise, it does not provide any information about the performance of e-CTA when implemented as an adjunct to a human reader (i.e. as it would be implemented in clinical practice as its use is recommended by the manufacturer and as specified in the inclusion criteria for this assessment).
Study details | Population | Target condition | Threshold | TP | FP | FN | TN | Sensitivity (95% CI) | Specificity (95% CI) | PPV (95% CI) | NPV (95% CI) |
---|---|---|---|---|---|---|---|---|---|---|---|
Seker 202156 | All | Proximal (ICA or proximal M1 segment of the MCA) or distal (distal M1 segment or proximal M2 segment of the MCA) LVO | NR | 134 | 6 | 26 | 135 | 83.8 (77.3 to 88.7) | 95.7 (91.0 to 98.0) | NAa | NAa |
Subgroup, excluding distal LVO | Proximal LVO | 87 | 3 | 8 | 138 | 91.6 (84.3 to 95.7) | 97.9 (93.9 to 99.3) | NAa | NAa |
Study details | Population | Reader | Threshold | TP | FP | FN | TN | Sensitivity (95% CI) | Specificity (95% CI) | PPV (95% CI) | NPV (95% CI) |
---|---|---|---|---|---|---|---|---|---|---|---|
Seker 202156 | All | e-CTA | NR | 59 | 3 | 11 | 71 | 84.3 (74.0 to 91.0) | 95.9 (88.7 to 98.6) | NAa | NAa |
Neuroradiologist | 68 | 1 | 2 | 73 | 97.1 (90.2 to 99.2) | 98.6 (92.7 to 99.8) | NAa | NAa | |||
Radiology resident | 67 | 6 | 3 | 68 | 95.7 (88.1 to 98.5) | 91.9 (83.4 to 96.2) | NAa | NAa | |||
Neurology resident 1 | 60 | 7 | 10 | 67 | 85.7 (75.7 to 92.1) | 90.5 (81.7 to 95.3) | NAa | NAa | |||
Neurology resident 2 | 64 | 0 | 6 | 74 | 91.4 (82.5 to 96.0) | 100 (95.1 to 100) | NAa | NAa |
One additional study44 reported information about the effects of implementing the e-ASPECTS and e-CTA modules of Brainomix e-Stroke in a centre that did not offer thrombectomy (patients requiring thrombectomy were transferred to another unit). 44 The results of this study, summarised in Research question 1 and Table 8, appeared to indicate that implementation was associated with a reduction in mean time from first CT to groin puncture for patients treated with thrombectomy. It should also be noted that this study did not report any information comparing clinical outcomes before and after implementation, such as would be needed to inform decision-making.
Avicenna CINA LVO
One study reported sufficient data to calculate the sensitivity and specificity of CINA LVO for the detection intracranial anterior LVO or M2-segment occlusion of the MCA (Table 15). 51 The sensitivity and specificity estimates were 98.1% (95% CI 94.5% to 99.3%) and 98.2% (95% CI 95.5% to 99.3%), respectively. 51 The results of subgroup analyses indicated that the sensitivity and specificity of CINA LVO for the detection of intracranial anterior LVO or M2 segment occlusion of the MCA did not vary substantially with patient age or between the different CT scanners used to acquire images (sees Table 15). It should be noted that this study was a retrospective analysis of previously acquired images, which assessed the performance of the CINA LVO technology alone; it does not provide information about the performance of the AI-derived software technology as an adjunct or aid to human interpretation (i.e. as it would be used in clinical practice, as its use is recommended by the manufacturer and as specified in the inclusion criteria for this assessment).
Study details | Population | Target condition | Threshold | TP | FP | FN | TN | Sensitivity (95% CI) |
Specificity (95% CI) |
PPV (95% CI) | NPV (95% CI) |
---|---|---|---|---|---|---|---|---|---|---|---|
McLouth 202151 | All | Intracranial anterior LVO (ICA, carotid terminus or M1 segment of the MCA) or M2 segment of the MCA occlusion | NR | 153 | 4 | 3 | 218 | 98.1 (94.5 to 99.3) | 98.2 (95.5 to 99.3) | 97.5 (93.6 to 99.0) | 98.6 (96.1 to 99.5) |
Subgroup, age 18–39 years | 4 | 0 | 1 | 21 | 80.0 (37.6 to 96.4) | 100 (84.5 to 100) | 100 (51.0 to 100) | 95.5 (78.2 to 99.2) | |||
Subgroup, age 40–70 years | 65 | 3 | 0 | 108 | 100 (94.4 to 100) | 97.3 (92.4 to 99.1) | 95.6 (87.8 to 98.5) | 100 (96.6 to 100) | |||
Subgroup, age > 70 years | 83 | 1 | 2 | 90 | 97.6 (91.8 to 99.4) | 98.9 (94.0 to 99.8) | 98.8 (93.6 to 99.8) | 97.8 (92.4 to 99.4) | |||
Subgroup, male | 73 | 2 | 1 | 109 | 98.6 (92.7 to 99.8) | 98.2 (93.7 to 99.5) | 97.3 (90.8 to 99.3) | 99.1 (95.0 to 99.8) | |||
Subgroup, female | 78 | 2 | 2 | 104 | 97.5 (91.3 to 99.3) | 98.1 (93.4 to 99.5) | 97.5 (91.3 to 99.3) | 98.1 (93.4 to 99.5) | |||
Subgroup, GE Medical Systems scanner | 46 | 4 | 4 | 75 | 92.0 (81.2 to 96.8) | 94.9 (87.7 to 98.0) | 92.0 (81.2 to 96.8) | 94.9 (87.7 to 98.0) | |||
Subgroup, Philips scanner | 52 | 2 | 10 | 73 | 83.9 (72.8 to 91.0) | 97.3 (90.8 to 99.3) | 96.3 (87.5 to 99.0) | 88.0 (79.2 to 93.3) | |||
Subgroup, Siemens scanner | 29 | 4 | 1 | 39 | 96.7 (83.3 to 99.4) | 90.7 (78.4 to 96.3) | 87.9 (72.7 to 95.2) | 97.5 (87.1 to 99.6) | |||
Subgroup, Canon (formerly Toshiba) scanner | 13 | 0 | 1 | 23 | 92.9 (68.5 to 98.7) | 100 (85.7 to 100) | 100 (77.2 to 100) | 95.8 (79.8 to 99.3) |
No studies were identified which evaluated the effects of implementing CINA LVO in clinical practice.
Research question 2b
-
(2b) Is the use of AI-derived software-assisted review of CT perfusion brain scans to guide mechanical thrombectomy treatment decisions for people with an ischaemic stroke, after a CTA brain scan, a clinically effective intervention?
Three studies, two reported as journal articles34,50 and one as a conference abstract,49 provided some limited information relevant to research question 2b. All three studies evaluated iSchemaView Rapid products. The results of these studies are summarised below; detailed study characteristics are provided in Appendix 2, Tables 32 and 33.
One article reported sufficient information to allow the calculation of measures of the diagnostic performance of Rapid CTP for identifying patients who are suitable candidates for thrombectomy (Table 16). 50 The objectives of the study concerned the quantification and characterisation of failures occurring during the automated post-processing of imaging data with Rapid CTP. The study was a retrospective analysis of AIS patients, from a database, who had undergone CTP for thrombectomy; potential causes of Rapid CTP post-processing failures were evaluated by two clinicians (experience not specified) in consensus, who had access to all imaging data available at the time of patient evaluation and failures were reprocessed manually using IntelliSpace software (Philips, Best, Netherlands). A total of 176 AIS patients were included in the analysis and Rapid CTP post-processing failures accrued in 20 (11%) patients. Causes for failures were severe motion (n = 14, 70%), streak artefact (n = 3, 15%) and poor arrival of contrast (n = 3, 15%). Of the 176 patients, 126 (72%) received thrombectomy, based on clinical information and interpretation of CTP imaging, which included correction for failures. Based on information about the results of Rapid CTP image analysis provided in the paper and using treatment received as the reference standard, it was possible to calculate measures of the diagnostic performance of Rapid CTP alone (without correction) in identifying patients who are suitable candidates for thrombectomy; the estimated sensitivity was 95.2% (95% CI 90.0% to 97.8%) and the estimated specificity was 80.0% (95% CI 67.0% to 88.8%), and the estimates of PPV and NPV were 92.3% (95% CI 86.4% to 95.8%) and 87.0% (95% CI 74.3% to 93.9%), respectively.
Study details | AI-derived software technology | Target condition | TP | FP | FN | TN | Sensitivity (95% CI) | Specificity (95% CI) | PPV (95% CI) | NPV (95% CI) |
---|---|---|---|---|---|---|---|---|---|---|
Kauw 202050 | Rapid CTP | Candidate for thrombectomy | 120 | 10 | 6 | 40 | 95.2 (90.0 to 97.8) | 80.0 (67.0 to 88.8) | 92.3 (86.4 to 95.8) | 87.0 (74.3 to 93.9) |
The remaining two publications reported the results of observational ‘before and after’ studies, evaluating the effects on time to treatment and clinical outcome of implementing Rapid (details not specified) in the context of providing an automated e-mail alert system49 (i.e. not as specified in the scope for this assessment) and the RapidAI Mobile Application. 34 Neither study provided separate results for the effects of the CTA and CTP analysis algorithms in Rapid. The results of these studies are summarised in Table 17. One study reported no significant change in the mean time from door to groin puncture, in thrombectomy patients, following the implementation of RapidAI (MD 2.0 minutes, 95% CI −12.9 to 16.9 minutes). 49 Clinical outcome, as indicated by the proportion of patients (for whom data were available) with a mRS ≤ 3 (time point not specified), was also similar before (58/119, 48.7%) and after (23/41, 56.1%), implementation (calculated OR 1.34, 95% CI 0.66 to 2.74). 49 By contrast, the study that assessed the effects of implementing the RapidAI Mobile Application reported a reduction in the mean time from door to groin puncture after implementation (MD −33.2 minutes, 95% CI −60.2 to −6.2 minutes); this study also reported that implementation of the RapidAI Mobile Application had no effect on mean 90-day mRS (2.9, no estimate of variance reported) both before (n = 29) and after (n = 26) implementation. 34
Study details | AI-derived software technology | Time to treatment outcome | Pre implementation | Post implementation | Mean difference (95% CI) | Clinical outcome | Pre implementation | Post implementation |
---|---|---|---|---|---|---|---|---|
Al-Kawaz 202134 | RapidAI Mobile Application | Mean (SD) minutes from door to groin puncture, thrombectomy, within centre | 104.3 (57.9), (n = 31) | 71.1 (51.7), (n = 33) | −33.2 (–60.2 to –6.2)a | Mean (SD) 90-day mRS | 2.9 (NR), (n = 29) | 2.9 (NR), (n = 26) |
Kamal 201749 | Rapid (un specified) | Mean (SD) minutes from door to groin puncture, thrombectomy, setting unclear | 116 (61), (n = 136) | 118 (39), (n = 50) | 2 (−12.9 to 16.9)a | mRS ≤ 3 (time point not reported) | 58/119 | 23/41 |
We did not identify any studies conducted in patients with LVO that evaluated icobrain CT, Brainomix e-CTP, Viz CTP, CTP 4D (CEC Healthcare) or Ceracare Stroke, the remaining AI-derived software technologies used in the analysis of CTP images, as indicated in Table 1 and described in the Intervention technologies section of this report.
Selection of diagnostic accuracy estimates for inclusion in cost-effectiveness modelling
There is no evidence, in any population, about the accuracy of AI-derived software technologies in combination with clinicians. The available diagnostic accuracy studies were retrospective analyses of previously acquired images, which assessed the performance of the AI-derived software technology alone; no study provided information about the performance of an AI-derived software technology as an adjunct or aid to clinician interpretation (as it would be used in clinical practice and as specified in the decision problem for this assessment). This might imply that a cost-effectiveness analysis (CEA) is not feasible for any of the three research questions (1, 2a or 2b). However, we have chosen to conduct a CEA in relation to the research question (2a), where there is most evidence about the performance of AI-derived software technologies alone and one study comparing an AI-derived software technology alone with clinicians alone. 56 These studies were not considered appropriate to inform cost-effectiveness modelling, but formed the basis by which the accuracy of AI plus human reader could be elicited by expert opinion. The expert elicitation process, undertaken to inform cost-effectiveness modelling, is described in detail in the Model parameters section.
Diagnostic accuracy data sets were selected for use in the background information provided with the expert elicitation tool, based on comparability of the target condition across the different AI-derived software technologies assessed by included studies, comparability with the target condition in the study used to inform estimates of the effectiveness of thrombectomy in cost-effectiveness modelling,65 availability of comparator data56 and match to the target condition specified during the scoping phase of this assessment (see Table 2). The common target condition was intracranial anterior circulation LVO (ICA, carotid terminus or M1 segment of the MCA) or M2 segment of the MCA occlusion and the corresponding diagnostic performance estimates, for AI-derived software technologies and the comparator (human readers alone), provided with the expert elicitation tool are given in Table 18. These estimates were presented to the clinical experts for elicitation of sensitivity and specificity of the intervention (clinician plus AI) and the comparator (clinician only). It should be noted that the estimates for clinician alone could have been used directly in the model to inform the effectiveness of the comparator. However, given that so few data were available, from only one study,56 it was considered more appropriated to use these estimates to inform expert elicitation.
Study details | Intervention/Comparator | Target condition | TP | FP | FN | TN | Sensitivity (95% CI) | Specificity (95% CI) | PPV (95% CI) | NPV (95% CI) |
---|---|---|---|---|---|---|---|---|---|---|
Amukotuwa 201935 | Rapid CTA | Intracranial anterior LVO (ICA, carotid terminus or M1 segment of the MCA) or M2 segment of the MCA occlusion | 351 | 112 | 17 | 431 | 95.4 (92.7 to 97.1) | 79.4 (75.8 to 82.6) | 75.8 (71.7 to 79.5) | 96.2 (94.0 to 97.6) |
Chatterjee 201840 | Viz LVO | Intracranial anterior LVO (ICA, carotid terminus or M1 segment of the MCA) or M2 segment of the MCA occlusion | 31 | 3 | 3 | 17 | 91.2 (77.0 to 97.0) | 85.0 (64.0 to 94.8) | 91.2 (77.0 to 97.0) | 85.0 (64.0 to 94.8) |
Seker 202156 | Brainomix e-CTA | Proximal (ICA or proximal M1 segment of the MCA) or distal (distal M1 segment or proximal M2 segment of the MCA) LVO | 134 | 6 | 26 | 135 | 83.8 (77.3 to 88.7) | 95.7 (91.0 to 98.0) | 95.7 (91.0 to 98.0) | 83.9 (77.4 to 88.7) |
McLouth 202151 | Avicenna CINA LVO | Intracranial anterior LVO (ICA, carotid terminus or M1 segment of the MCA) or M2 segment of the MCA occlusion | 153 | 4 | 3 | 218 | 98.1 (94.5 to 99.3) | 98.2 (95.5 to 99.3) | 97.5 (93.6 to 99.0) | 98.6 (96.1 to 99.5) |
Seker 202156 | Neuroradiologist | Proximal (ICA or proximal M1 segment of the MCA) or distal (distal M1 segment or proximal M2 segment of the MCA) LVO | 68 | 1 | 2 | 73 | 97.1 (90.2 to 99.2) | 98.6 (92.7 to 99.8) | 98.6 (92.2 to 99.7) | 97.3 (90.8 to 99.3) |
Radiology resident | 67 | 6 | 3 | 68 | 95.7 (88.1 to 98.5) | 91.9 (83.4 to 96.2) | 91.8 (83.2 to 96.2) | 95.8 (88.3 to 98.6) | ||
Neurology resident 1 | 60 | 7 | 10 | 67 | 85.7 (75.7 to 92.1) | 90.5 (81.7 to 95.3) | 89.6 (80.0 to 94.8) | 87.0 (77.7 to 92.8) | ||
Neurology resident 2 | 64 | 0 | 6 | 74 | 91.4 (82.5 to 96.0) | 100 (95.1 to 100) | 100 (94.3 to 100) | 92.5 (84.6 to 96.5) |
The decision to undertake an expert elicitation process was made, given the complete absence of applicable evidence in the literature, with a view to providing the diagnostic appraisal committee with a framework to consider the potential cost-effectiveness of AI as it would be used in practice and in order to facilitate the development of research recommendations. Nevertheless, no comparison of different AI-derived software technologies was feasible, and the results of this CEA (reported in the Assessment of cost-effectiveness section) need to be regarded with caution.
Chapter 4 Assessment of cost-effectiveness
Review of economic analyses of software with artificial intelligence-derived algorithms for analysing computed tomography brain scans in people with a suspected acute stroke
Search strategy
A series of literature searches were performed to identify published economic evaluations and cost-effectiveness data and utility studies for diagnostic techniques and procedures used in the investigation of patients with stroke that were not included within the scope of the clinical effectiveness searches. The searches aimed to identify studies that could be used to support the development of a health economic model, to estimate the model input parameters and to answer the research questions of the assessment but not to perform a systematic review. Searches were therefore pragmatic in design and date limits applied where appropriate.
Methodological study design filters were included in the search strategies where relevant. No restrictions on language or publication status were applied. Limits were applied to remove animal studies. The main Embase strategy for each search was independently peer reviewed by a second information specialist, using the Canadian Agency for Drugs and Technologies in Health peer review checklist. 25 Identified references were downloaded in EndNote software for further assessment and handling. References in retrieved articles were checked for additional studies. In addition, the EndNote library created for the clinical effectiveness section (Search strategy) was also screened to identify potentially relevant economic studies.
The following databases were searched for relevant studies with from 2005 to September 2021:
-
NHS Economic Evaluation Database (NHS EED; www.crd.york.ac.uk/CRDWeb) to March 2015
-
MEDLINE 1946 to 15 September 2021
-
MEDLINE In-Process Citations to 15 September 2021
-
MEDLINE Daily Update to 15 September 2021
-
MEDLINE Epub Ahead of Print to 15 September 2021
-
Embase 1974 to 15 September 2021
-
EconLit (EBSCO) to 21 September 2021
-
SCI 1988 to 21 September 2021
-
Research Papers in Economics (http://repec.org) to 21 September 2021.
Supplementary searches
As described by the NICE methods guide, the information process that supports the development of a model is ‘a process of assembling evidence and this reflects an iterative, emergent process of information gathering’. 66 The following additional searches were requested by the health economists as part of this process:
Health-related quality of life and utilities
Searches for utility weights and health-related quality-of-life (HRQoL) papers for stroke were conducted on the following resources:
-
Embase 1974 to 1 November 2021
-
CEA Registry (www.cearegistry.org) to 14 July 2021.
Review of reviews
To locate papers evaluating the effectiveness of diagnostic imaging techniques without the use of AI an additional focused search aimed at identifying existing systematic reviews was run without date limits on the following resources:
-
CDSR to October 2021/Iss10
-
KSR Evidence to 14 October 2021.
Accuracy of human readers
Estimates of the performance of human readers alone (without AI) in interpreting diagnostic images in stroke were required to provide comparator data for cost-effectiveness modelling. Previous searches had found insufficient data supporting this topic; therefore, a single targeted search was undertaken on MEDLINE:
-
MEDLINE 2017 to 15 October 2021
-
MEDLINE In-Process Citations to October 2021
-
MEDLINE Daily Update to October 2021
-
MEDLINE Epub Ahead of Print to October 2021.
Review of reviews: alteplase
To locate papers evaluating the effectiveness of IV thrombolysis (alteplase) in AIS, an additional focused search aimed at identifying existing systematic reviews was run without date limits on the following resources:
-
CDSR to November 2021/Iss11
-
KSR Evidence to 11 November 2021.
Full search strategies for all of the above are reported in Appendix 1.
Inclusion criteria
Studies reporting outcomes of a full CEA, examining (quality-adjusted) life-years, with (at least) one AI-derived software-assisted review strategy, were eligible for inclusion.
Results
The literature search identified 2990 records from bibliographic database searches and supplementary searching (e.g. reference/citation checking, additional database searches including the database search for the assessment of clinical effectiveness). After title and abstract screening, 28 records are considered to be potentially relevant; after full-text screening one cost-effectiveness study (identified by hand-searching as it was published after conducting the literature search), was considered eligible for inclusion. This study is described in more detail below. Figure 5 shows the flow of studies through the review process.
FIGURE 5.
Flow of studies through the review process (review of economic analyses).
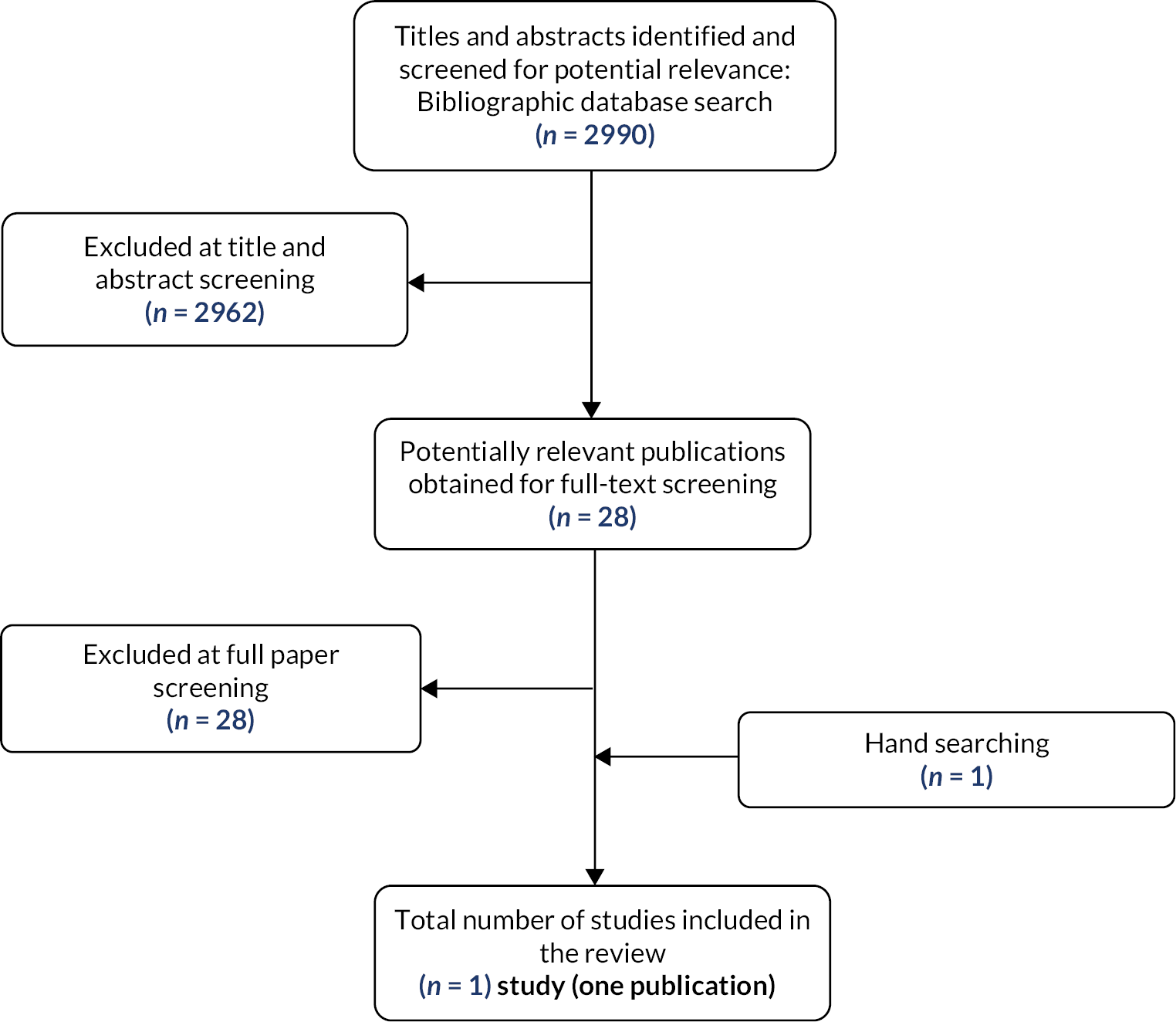
An additional economic model was submitted by Brainomix to NICE. This submission was not considered in this review as it was not specifically focused on one of the research questions nor does it adopt an approach (e.g. decision tree combined with a state transition model) typically adopted for diagnostic assessments.
van Leeuwen et al. 2021
van Leeuwen et al. 67 used a decision tree for the acute phase (90 days) combined with a state transition model (health states defined based on the mRS) with a life time time horizon (the economic model is available online https://grand-challenge.org/aiforradiology). The analyses were performed from a societal UK perspective (discount rates of 1.5% and 4.0% for effects and costs, respectively), while reporting the costs in 2018, US dollars for ease of interpretation (£1 = $1.283). The authors estimated the potential cost-effectiveness of using AI software in ischaemic stroke to aid intracranial LVO detection on CTA (with or without CTP) compared with standard care without the AI software. The population focused on vessel occlusions in the proximal anterior circulation (ICA, A1, M1, M2) as these were considered appropriate for the selection of patients to receive mechanical thrombectomy.
For the analysis it was assumed that AI software is capable of increasing the diagnostic sensitivity, especially for the detection of M2 occlusions, without a decrease in specificity. False positives generated by the AI software were assumed to be neutralised by the judgement of the reader, preventing overtreatment. It was noted that, besides providing a more accurate diagnosis, the use of AI may lead to shorter time to treatment, especially if it reduced the need for specialist review. However, as most currently available commercial products focus on triage and interactive decision support, the analyses only considered the claim that the use of AI could provide a more accurate diagnosis, that is reduce the number of missed LVOs.
The early HTA assessment considered the potential value of AI software in general without focus on a specific manufacturer. The main assumption (varied in uncertainty analyses) was that in standard care (without AI software) 6% of LVOs are missed and that with the addition of AI software this can be reduced by 50% (i.e. only 3% of LVOs are missed). It was acknowledged that although published accuracy data are available for AI software in isolation, there is no evidence of the performance of AI software combined with standard practice, that is it is unclear to what degree AI software can reduce the LVOs missed in standard practice. The price per patient for using the AI software was assumed to be $40. Additionally included costs were treatment related costs, acute stroke costs (90 days, depending on mRS) and long-term stroke costs (annual, depending on mRS). Scenario and deterministic multiway sensitivity analyses were performed (no probabilistic analysis).
The base-case analysis indicated that if the addition of AI software detected additional LVOs, this could potentially result in cost savings (of $156) while yielding additional quality-adjusted life-years (QALYs; 0.0095 QALY gained) compared with standard care without AI software. Sensitivity analyses seem to indicate that these results are sensitive to the percentage of LVOs missed by usual care, the percentage of missed LVOs detected by the AI software and the AI software costs per patient. Additional false-positive cases due to the addition of AI software only had very minor cost consequences ($0.07 per percentage point of false positives).
The authors noted that evidence is lacking regarding the percentage of missed LVOs (with standard care) that can be detected by AI software. Notably, this percentage cannot directly be derived from the sensitivity of an AI algorithm applied stand alone, as it is likely that the cases that were missed by a physician are also more likely to be missed by an algorithm (e.g. M2 occlusions). The authors specifically advised against using these sensitivity measures directly as model inputs.
Quality assessment (Drummond checklist68) of the study by van Leeuwen et al. 67 only indicated suboptimal score for reporting to (disaggregated/absolute) results as well as related to the uncertainty analyses (lack of CIs for stochastic data and justification for ranges over which the variables are varied).
Model structure and methodology
Intervention and comparators
The health economic analysis focused on research question 2a:
-
(2a) Does AI-derived software-assisted review of CT angiography brain scans for guiding mechanical thrombectomy treatment decisions for people with an ischaemic stroke represent a clinically and cost-effective use of NHS resources?
All diagnostic accuracy studies, identified by the systematic review conducted for this assessment (Assessment of clinical effectiveness section) assessed the accuracy of AI-derived software technologies as stand-alone interventions. As a result, information about how AI-derived software technologies would perform when used as an adjunct/aid to human readers (i.e. as recommended by the manufacturers, as specified for this assessment and as they would be used in clinical practice) is lacking. This is because the accuracy of the device by itself tells us nothing about how, or indeed if, it might improve the accuracy of a human reader. It would not make sense to infer that any of the variation in sensitivity observed between stand-alone AIs can tell us something about precisely the variation in a hypothetical, small improvement in sensitivity of the human reader. To still be able to perform a CEA, we elicited expert opinion to estimate the diagnostic accuracy of AI as adjunct to human reader. Experts were provided with the evidence on AI alone and human reader alone. Because it was considered too difficult for experts to differentiate between different AI-derived software-assisted review technologies, AI-derived software-assisted review in general (not specified by manufacturer or specific technology) is considered.
Model structure
This assessment uses the CEA by van Leeuwen et al. 67 (identified in the literature review as the only assessment focusing on a similar decision problem) as a starting point. In addition, recent cost-effectiveness assessments (mainly on the cost-effectiveness of thrombectomy) that have been identified informally (through the cost-effectiveness review, checking references) have also been used to support the development of the model. Consistent with the focus of AI-derived software-assisted review on triage and supporting the thrombectomy decision, the current assessment primarily considers the question of whether AI-derived software-assisted review could provide a more accurate diagnosis of LVO than usual care.
The de novo developed model consisted of a decision tree (short-term) and a state transition model (long-term) to calculate the mean expected costs and QALYs for people with ischaemic stroke and suspected LVO.
The decision tree was used to estimate short-term costs and consequences (first 90 days). For this purpose, a distinction is made between patients who have a LVO and those who do not. The definition of LVO was LVOs in the proximal anterior circulation (ICA, A1, M1, M2). This definition was chosen for two main reasons: consistency with the recommendations of NICE guidelines and with the meta-analysis by Román et al.,65 the source of the effectiveness of thrombectomy used in the model (Model parameters section). Subsequently, patients with LVO are classified as either eligible for thrombectomy or not eligible. Eligibility for thrombectomy is determined by a number of factors beyond the location of the occlusion, including timing and salvageability of brain tissue as determined by CTP scanning (Initial assessment section). Those with both LVO and eligibility for thrombectomy are further classified, based on the sensitivity of the diagnostic strategy, into whether a LVO was detected (and thus thrombectomy received) or not. Based on the classification in the decision tree, patients were subdivided into the health states according to the mRS. mRS is a commonly used scale for measuring the degree of disability or dependence in daily activities of people who have suffered a stroke and was the predominant outcome to define health states in published cost-effectiveness models in this disease area. Notably, patients without LVO were subdivided, based on the specificity of the diagnostic strategy, into whether a LVO was incorrectly detected or not. If a LVO was incorrectly detected (i.e. a false positive), this had cost consequences only (e.g. due to potential unnecessary transfer to experienced stroke centre qualified to perform thrombectomy) as, based on clinical opinion and consistent with the assessment by van Leeuwen et al. ,67 it was assumed that the LVO would be classified as a false positive (i.e. in fact no LVO) before proceeding to thrombectomy. The rationale for this was that specialists (e.g. neuroradiologists or neurointerventionalists) would review the imaging before agreeing to take patients for thrombectomy and then detect the false positive. The decision tree is shown in Figure 6.
FIGURE 6.
Decision tree structure (90 days).

The long-term consequences in terms of costs and QALYs were estimated using a state transition cohort model (Figure 7) with a lifetime horizon. The cycle time was 1 year. The following health states were included:
-
1) mRS 0
-
2) mRS 1
-
3) mRS 2
-
4) mRS 3
-
5) mRS 4
-
6) mRS 5
-
7) mRS 6 (death).
FIGURE 7.
State transition model structure.

The de novo model was developed in R Shiny (R Foundation for Statistical Computing, Vienna, Austria)69 to leverage the benefits of using modern programming languages such as R (R Foundation for Statistical Computing, Vienna, Austria)70 while providing an accessible interface through the Shiny package. To improve model transparency as well as model credibility and for consistency with suggested good practices and conventions, the technical implementation of the computational model was inspired by recent work of the Data Analytics Research and Technology in Healthcare Group71,72 and others. 73
Model parameters
Decision tree probabilities
Proportion of ischaemic strokes that are large-vessel occlusions
The proportion of ischaemic strokes correctly suspected to be caused by LVOs was estimated by pooling the prevalence of LVOs in the diagnostic accuracy studies35,40,51 (random-effects model using logit transformation), resulting in an estimated prevalence of 46.1% (95% CI 43.0% to 49.1%).
Eligibility for medical thrombectomy
Not all patients with LVO are eligible for thrombectomy. Based on the UK study by McMeekin et al. in 2017,74 including early presenters (within 4 hours of onset) as well as late presenters and those for which the timing was unknown, the proportion of patients with LVO eligible for thrombectomy was 41.2% (95% CI 40.6% to 41.8%).
Accuracy of clinician and artificial intelligence-derived software-assisted review of computed tomography angiography brain scans
As was outlined in Selection of diagnostic accuracy estimates for inclusion in cost-effectiveness modelling, the available accuracy estimates were not appropriate for the decision problem. We therefore elicited expert opinion to inform sensitivity and specificity of clinician review of CTA brain scans and of AI-assisted review of CTA brain scans. In addition, we also elicited the throughput of patients with ischaemic stroke and suspected LVO per an average centre (this was not used in the end). This translated into five elicitation questions. The sensitivity question was phrased in terms of proportion of LVOs missed (= 1 – sensitivity). The specificity question was phrased in terms of proportion of non-LVOs falsely classed as LVOs (= 1 – specificity; screenshots of the questions and background information provided to clinical experts are presented in Appendix 6, Figures 22, 23 and 24).
We used the EXPLICIT tool (EXPert eLICItation Tool) developed by Grigore et al. 75 to facilitate remote expert elicitation. This tool has been validated, follows established methodological guidance for expert elicitation,76–78 and has the advantage that it is relatively easy to use. The tool includes an informed consent form, training exercises and explanations of some important heuristics. We also included background information on the evidence on accuracy of AI standalone and human reader alone, identified in Selection of diagnostic accuracy estimates for inclusion in cost-effectiveness modelling. Experts were asked for the mode and the upper and lower bounds to each estimate. A beta-PERT (program evaluation and review technique) distribution was then fitted. Mathematical aggregation of elicited expert estimates was performed using linear pooling, that is by taking the arithmetic average over all experts for each elicited quantity.
Five UK clinical experts sent complete responses (a consultant in emergency medicine, a clinical associate professor and honorary consultant stroke physician, a senior lecturer and honorary consultant neurosurgeon, a senior clinical lecturer and honorary consultant neuroradiologist and an honorary consultant neuroradiologist). The elicited mean sensitivity and specificity, as well as parameters for the beta-PERT distribution are presented in Table 19. Probability distributions are shown for the pooled experts’ estimates of sensitivity (Figure 8) and specificity (Figure 9) as well as for individual experts (Figure 10).
Mean | Lower bound | Mode | Upper bound | |
---|---|---|---|---|
Cliniciana only sensitivity | 93.00 | 83.60 | 94.20 | 97.60 |
Cliniciana only specificity | 94.09 | 88.00 | 94.58 | 98.20 |
AI + cliniciana sensitivity | 94.13 | 87.80 | 94.80 | 97.80 |
AI + cliniciana specificity | 93.77 | 84.80 | 94.80 | 98.60 |
FIGURE 8.
Elicited sensitivity estimates (pooled).
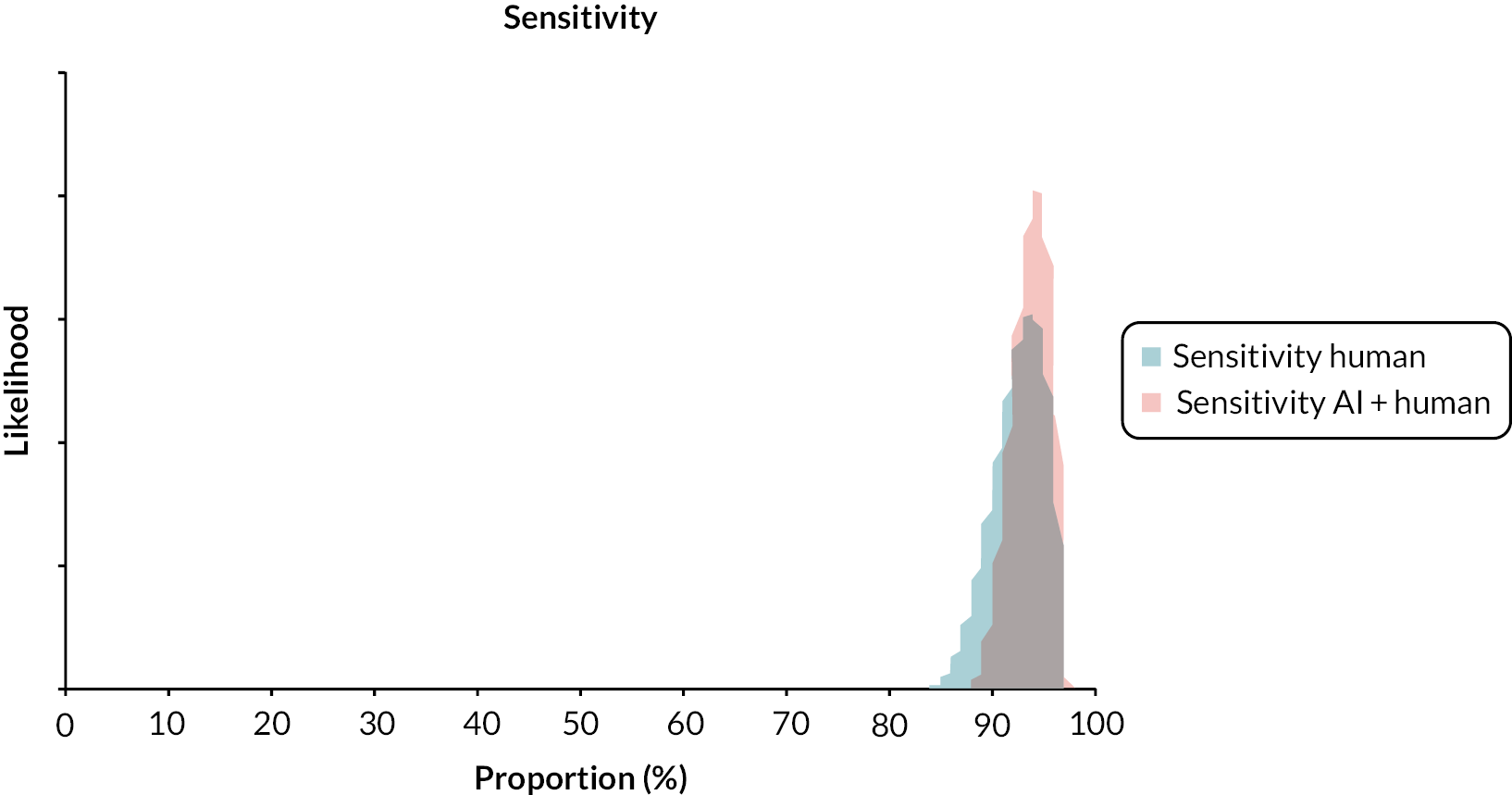
FIGURE 9.
Elicited specificity estimates (pooled).

FIGURE 10.
Probability distributions of individual experts.

Initial distribution over modified Rankin Score states for patients with large-vessel occlusion
We performed a pragmatic review to inform the distribution over the disability post stroke health states at 90 days after thrombectomy or standard medical therapy (i.e. for those ineligible for thrombectomy) at the end of the decision tree. A study by Román et al. 65 of the effectiveness of thrombectomy was identified in which mRS outcomes at 90 days were estimated based on an individual patient-level data meta-analysis, combining from seven randomised trials: Multicenter Randomized Clinical Trial of Endovascular Treatment for Acute Ischaemic Stroke in the Netherlands (MR CLEAN),79 Endovascular Treatment for Small Core and Anterior Circulation Proximal Occlusion with Emphasis on Minimizing CT to Recanalization Times (ESCAPE),80 Extending the Time for Thrombolysis in Emergency Neurological Deficits – Intra-Arterial (EXTEND-IA),81 Solitaire with the Intention for Thrombectomy as Primary Endovascular Treatment (SWIFT PRIME),82 Randomized Trial of Revascularization with Solitaire FR Device versus Best Medical Therapy in the Treatment of Acute Stroke Due to Anterior Circulation Large-vessel Occlusion Presenting within Eight Hours of Symptom Onset (REVASCAT),83 Mechanical Thrombectomy After Intravenous Alteplase Versus Alteplase Alone After Stroke (THRACE)84 and Pragmatic Ischaemic Stroke Thrombectomy Evaluation (PISTE). 85 This study was deemed to be the most recent meta-analysis on this topic and included all relevant, high-quality, randomised trials. Eligibility for thrombectomy in those trials was also consistent with the vessel occlusions in the proximal anterior circulation (ICA, A1, M1, M2). 65 Given that Román et al. 65 presented only stratified estimates of the distribution of mRS outcomes (i.e. stratified for ASPECTS categories), results were pooled to obtain an estimate for the full population (Table 20).
Treatment | mRS0 | mRS1 | mRS2 | mRS3 | mRS4 | mRS5 | mRS6 |
---|---|---|---|---|---|---|---|
mRS after LVO treated with IAT (n = 856) | 96 (11.1%) | 154 (18.1%) | 159 (18.6%) | 137 (16.1%) | 136 (15.9%) | 47 (5.5%) | 125 (14.7%) |
mRS after LVO treated without IAT (n = 862) | 54 (6.3%) | 84 (9.8%) | 122 (14.2%) | 139 (16.2%) | 216 (25.1%) | 94 (10.9%) | 150 (17.5%) |
It is unclear to what extent these distributions are generalisable to the current UK NHS setting, given that there is no information on the proportion of early compared with late presenters or proportion of patients receiving alteplase. The impact of potential problems with generalisability here is considered to be small as all patients will receive standard medical therapy, regardless of their true-negative or false-positive status. Likewise, the proportion of patients who are early presenters is the same irrespective of test outcome, which would mean that the distribution over mRS states would also be the same irrespective of test outcome.
Initial distribution over modified Rankin Score states for patients without large-vessel occlusion
To inform the distribution of patients with small-vessel occlusion (i.e. the true negatives and false positives) over mRS states, we performed a pragmatic review of five systematic reviews and meta-analyses9,86–89 of studies assessing the effectiveness of thrombolysis. None of these studies reported the distribution over mRS states, but individual studies included did; hence, we reviewed all studies informing these meta-analyses and ruled out those that did not report the distribution over mRS states at least 3 months after stroke that did not focus on small-vessel occlusion, and were based on small sample sizes in the thrombolysis group (n < 150). Only two studies were included: Choi et al. 90 and Paek et al. 91 Both were based on South Korean registries and had similar sample sizes. Choi et al. 90 used a retrospective analysis of the Clinical Research Center for Stroke – Fifth Division Registry database with n = 194 in the unmatched sample; Paek et al. 91 used a prospective registry of 15 South Korean stroke centres with n = 193 in the thrombolysis group (Table 21). Owing to limitations to data availability with Choi et al. (see NA in Table 21),90 we used the distribution reported by Paek et al. 91 in the evidence review group base case. While it is unclear whether this is representative of UK patients, the proportion of small-vessel occlusion and the accompanying mRS distribution is the same regardless of test outcome and will therefore not be influential in terms of incremental results.
Study | mRS0 (%) | mRS1 (%) | mRS2 (%) | mRS3 (%) | mRS4 (%) | mRS5 (%) | mRS6 (%) |
---|---|---|---|---|---|---|---|
Choi et al. 201590 (n = 194) | NA (39.2) | NA (31.4) | NA (11.3) | NA (10.3) | NA (NA) | NA (NA) | NA (NA) |
Paek et al. 201991 (n = 192) | 42 (21.8) | 68 (35.2) | 46 (23.8) | 24 (12.4) | 9 (4.7) | 4 (2.1) | 0 (0.0) |
We performed a pragmatic review to inform the transition probabilities for the state transition model using the identified CEA for thrombectomy studies as a starting point. Consistent with most CEA studies, we assumed that no transitions were possible between mRS states unless a recurrent stroke occurred (only in Lobotesis et al. 201692 patients could improve or deteriorate by one mRS state at the end of year 1). Other relevant transition probabilities included the probability of having a recurrent stroke and the mRS distribution after a recurrent stroke. After a recurrent stroke, we assumed that patients could either stay in their mRS state or move to a more severe mRS state. The distribution over the mRS states, after recurrent stroke, was based on that for patient’s ineligible for mechanical thrombectomy (see Table 20) to reflect a worse outlook after recurrent stroke compared with first stroke.
Recurrent stroke with transitions to the same or worse modified Rankin Score states
In the CEA model by van Leeuwen et al. ,67 the annual rate for recurrent stroke was 2.8%, based on a study by Pennlert et al. 93 In this study sex- and age-adjusted annual risk of stroke recurrence was estimated for patients at 28 days after an ischaemic stroke in the Swedish population-based Monitoring Trends and Determinants of Cardiovascular Disease stroke incidence registry (n = 5885 with ischaemic stroke, mean age = 64.2 years, range 24–74 years and proportion male = 60.6%). The index stroke occurred between 1995 and 1998. The average rate for recurrent stroke over the use calculated based on data provided in online supplement table 1 of Pennlert et al. 93 was 2.8%. Pennlert et al. 93 also observed that there was a decline in recurrent stroke rates over time, but we did not include this in the model.
An alternative source is the study by Mohan et al. ,94 who performed a systematic review and meta-analysis of 13 included studies reporting cumulative risk of recurrence after first-ever stroke (both ischaemic and haemorrhagic stroke). Only 3 of the 13 studies were from the UK. Some of these studies dated back a long time; for example, the oldest started data collection in 1961, whereas the newest started in 2003. The pooled cumulative risk of stroke recurrence was 3.1% (95% CI 1.7% to 4.4%) at 30 days; 11.1% (95% CI 9.0% to 13.3%) at 1 year; 26.4% (95% CI 20.1% to 32.8%) at 5 years; and 39.2% (95% CI 27.2% to 51.2%) at 10 years after initial stroke. 94 A Weibull model was fitted but model parameters were not provided. Lobotesis et al. 92 used the cumulative risk at 5 years reported by Mohan et al. 94 and estimated the annual risk of recurrence for the rest of patients’ lives at 2.0%.
We considered that Pennlert et al. 93 provided estimates specifically for ischaemic stroke while these were not available from Mohan et al. 94 We therefore used estimates by Pennlert et al. 93 in the base case and explored the use of Mohan et al. 94 in a scenario.
In the model, risk of recurrent stroke was the same across all mRS health states (consistent with assumptions in other CEA studies). 67,92
Death conditional on functional status after stroke
The study by Slot et al. 95 estimated the risk of stroke-related mortality conditional on functional dependency using two cohorts: the Oxfordshire Community Stroke Project (n = 320), the Lothian Stroke Register (n = 448) and the First International Stroke Trial (n = 1563), all UK studies. The authors found a significant impact of functional status on the cause of death. In particular, functionally dependent patients (i.e. those with mRS scores of 3–5) were more likely to die of recurrent stroke [relative risk (RR) 1.68 (95% CI 1.49 to 1.91)] than functionally independent patients. Stroke-related causes of death were present in 794 (49%) of the functionally dependent patients compared with 207 (29%) of the independent patients in all three cohorts combined and included International Classification of Diseases (ICD) codes for cerebrovascular diseases (ICD-9 430–438; ICD-10 I60–I69), either mentioned in the death certificate as a primary cause of death or a contributing factor (i.e. secondary, tertiary or quaternary cause of death). The risk of stroke-related cause of death increased by mRS score.
We estimated (recurrent stroke-related) mortality by: (1) multiplying recurrent stroke probability with the mRS 6 probability (see Table 20), and (2) applying the RR of dying per mRS state reported by Slot et al. 95 (Table 22) to the general population mortality. 96 The maximum probability of these two approaches was used in the economic model. This prevented underestimating mortality in the more severe mRS health states; ensured that mortality was consistent with the age-adjusted general population mortality while including mRS health state dependent mortality; and prevented double-counting.
mRS | RR (95% CI) |
---|---|
0–1 | 1.00 (baseline) |
2 | 1.12 (0.82 to 1.56) |
3 | 1.66 (1.24 to 2.23) |
4 | 1.92 (1.41 to 2.61) |
5 | 2.57 (1.92 to 3.43) |
To identify studies reporting utility values associated with the model health states (i.e. the mRS states), we performed a pragmatic search and review (see Search strategy). In addition, we also reviewed the identified CEA studies and searched their references. If necessary, references of articles identified in that way were also searched. This pragmatic review resulted in seven studies reporting utility values for the mRS states. 97–104 The studies reporting EuroQol Five Dimensions (EQ-5D) time-trade-off values using the UK value set were: Ali et al. ,101 Rivero-Arias et al. ,98 Rebchuk et al. 103 and Wang et al. 104 Ali et al. 101 was a multicountry study and the sample size of UK patients and utilities valued with the UK value set was small (n = 70). Rebchuk et al. 103 presented utility values averaged over nine studies that collected EQ-5D data, but most of these studies did not use the UK value set. Similarly, Wang et al. 104 presented utility values averaged over six studies, but not all of them using the UK value set. Therefore, we used total utility values from the study by Rivero-Arias et al. 98 in the base case (sample size of at least n = 365 at 1 month and more in subsequent months). Utilities by Rebchuk et al. 103 and Wang et al. 104 were applied in scenarios. Utility values are summarised in Table 23.
mRS | Rivero-Arias et al.98 utility values (SD) | Rebchuk et al.103 utility values (CI) (n) | Wang et al.104 utility values (SD) (n) |
---|---|---|---|
0 | 0.936 (0.127) | 0.93 (0.96 to 0.9) (3624) | 0.97 (0.1) (3148) |
1 | 0.817 (0.183) | 0.86 (0.89 to 0.83) (2376) | 0.88 (0.16) (4968) |
2 | 0.681 (0.211) | 0.68 (0.72 to 0.64) (1149) | 0.72 (0.21) (1950) |
3 | 0.558 (0.284) | 0.57 (0.61 to 0.53) (957) | 0.54 (0.25) (2327) |
4 | 0.265 (0.294) | 0.31 (0.35 to 0.26) (1101) | 0.23 (0.33) (1618) |
5 | −0.054 (0.264) | 0.06 (0.12 to 0.00) (400) | −0.17 (0.21) (858) |
Rivero-Arias et al. 98 derived mRS and EQ-5D three-level version (EQ-5D-3L) information from stroke or TIA patients identified as part of the Oxford Vascular (OXVASC) study. Ordinary least squares regression was used to predict UK EQ-5D-3L tariffs from mRS scores. Data were available at months 1, 6, 12 and 24 with sample sizes for the EQ-5D-3L varying by measurement point (n = 365, n = 478, n = 346 and n = 539, respectively).
Utility values used in the model were age-adjusted using the UK population norms for the EQ-5D-3L reported by Janssen et al. 105
Based on information provided by each company, mean costs per patient were calculated for using AI-derived software technologies. In the base-case analysis, a mean estimate was used based on all four technologies.
To calculate an overall mean price of the AI-derived software technologies, annual license fees for each device were applied to the UK situation in terms of number of CSCs, primary stroke centres (PSCs) and total number of stroke patients in the UK based on the SSNAP. 106 The mean cost price of AI-derived software technologies in the base-case analysis was assumed to be £49.24 (£12.31).
Table 24 presents all relevant inputs as well as intervention-specific cost estimates.
Fixed estimates for each AI technology | Source | ||
---|---|---|---|
Number of CSCs | 25 | Sentinel Stroke National Audit Programme | |
Number of PSCs | 177 | Sentinel Stroke National Audit Programme | |
Number of stroke patients in UK | 87,635 | Sentinel Stroke National Audit Programme | |
Intervention-specific inputs | Lowest price | Highest price | Source |
Rapid CTA | |||
AI licence annual fee for CSC: | £20,000 | N/A | Provided by company |
AI licence annual fee for PSC: | £20,000 | N/A | Provided by company |
Training costs: | £5000 | N/A | Assumption |
Total costs: | £5,050,000 | N/A | |
Cost per patient (Se*) | £57.63 (£14.41) | ||
Viz.ai | |||
AI licence annual fee for CSC: | £40,000 | £55,000 | Provided by company |
AI licence annual fee for PSC: | £20,000 | £30,000 | Provided by company |
Training costs: | £7241 | £7241 | Provided by company |
Total costs: | £6,002,682 | £8,147,682 | |
Cost per patient | £68.50 | £92.97 | |
Mean cost per patient (SE@)a | £80.73 (£20.18) | ||
Avicenna | |||
AI license Annual fee for CSC: | N/A | N/A | Avicenna only works with price per patient |
AI license Annual fee for PSC: | N/A | N/A | Avicenna only works with price per patient |
Training costs: | N/A | N/A | The company stated that no training was required to work with the software |
Mean cost per patient (SE@a) | £7.08 (£1.77) | Avicenna only works with price per patient (this price is for centres up to 5000 scans per year) | |
Brainomix | |||
AI license Annual fee for CSC: | £30,000 | £30,000 | Provided by company |
AI license Annual fee for PSC: | £15,000 | £15,000 | Provided by company |
Training costs: | £3000 | £8000 | Provided by company |
Total costs: | £4,011,000 | £5,021,000 | Provided by company |
Cost per patient | £45.77 | £57.29 | |
Mean cost per patient (Se*) | £51.53 (£12.88) | ||
Mean cost price of AI-derived software technologies in base-case analysis | |||
Mean cost per patient (Se*) | £49.24 (£12.31) |
We performed a pragmatic review to inform resource use and costs parameters in the model. A study by Lobotesis et al. 92 was identified, which served as the main source of input parameters related to resource use and costs. In that study, a UK healthcare provider perspective was assumed, and all (treatment) cost estimates were broken down into units and unit prices, enabling us to calculate treatment costs using a bottom-up approach. In line with that study, short-term costs (< 90 days) consisted of costs for treatment, hospitalisation and management of adverse events. In Lobotesis et al. ,92 to estimate treatment costs, unit costs for each cost item were presented in combination with the corresponding number of units that each cost item was used in which unit costs were sourced from Personal Social Services Research Unit (PSSRU), Unit Costs of Health and Social Care,107 and treatment and device costs for the stent retriever were provided by Medtronic. Costs and resource use associated with IV tissue plasminogen activator were derived from the NICE Technology Appraisal for alteplase. 19 Using these numbers, treatment costs were calculated using a bottom-up approach (Table 25).
Cost items | Unit price (£) | Source | Units | Total price (indexed to 2020) |
---|---|---|---|---|
Mechanical thrombectomy | ||||
Stent retriever | 3190 | Covidien internal pricing | 1.2 | 4161 |
Catheter/support kit | 920 | Covidien internal pricing | 1 | 1.000 |
Procedure pack | 35 | Covidien internal pricing | 1 | 38 |
Drapes/gowns/gloves | 80 | Covidien internal pricing | 1 | 87 |
Sheath | 15 | Expert clinical opinion | 1 | 16 |
Interventional suite | 150 | Expert clinical opinion | 3 | 489 |
Anaesthetist | 157 | Expert clinical opinion (cost not available in PSSRU) | 4 | 683 |
Anaesthetist assistant | 58 | PSSRU (nurse team manager) | 4 | 252 |
Radiographer | 58 | PSSRU (nurse team manager) | 3 | 189 |
Consultant interventional neuroradiologist | 140 | PSSRU (medical consultant) | 3 | 457 |
Registrar | 60 | PSSRU (registrar) | 3 | 196 |
Nurse (band 7) | 58 | PSSRU (nurse team manager) | 3 | 189 |
Scrub nurse (band 5) | 49 | PSSRU (nurse team leader) | 3 | 160 |
Subtotal | 7916 | |||
IV thrombolysis | ||||
Nurse activates stroke team | 49 | PSSRU (nurse team leader) | 0.08 | 4 |
Stroke team assessment (registrar grade) | 60 | PSSRU (registrar) | 0.5 | 33 |
Blood test | 5 | ISD Scotland24 | 1 | 6 |
Registrar accompanies patient to CT scan | 60 | PSSRU (registrar) | 1 | 65 |
Consultant reviews CT results and discusses with relatives | 140 | PSSRU (medical consultant) | 0.5 | 76 |
Nurse assessment | 58 | PSSRU (nurse team manager) | 0.08 | 5 |
IV t-PA infusion (registrar time) | 60 | PSSRU (registrar) | 1.25 | 82 |
Additional 12 routine observations | 49 | PSSRU (nurse team leader) | 1 | 53 |
1 : 1 care for 5 hours with senior nurse | 58 | PSSRU (nurse team manager) | 5 | 315 |
Junior staff review | 60 | PSSRU (registrar) | 0.42 | 27 |
Overnight junior staff review | 60 | PSSRU (registrar) | 0.17 | 11 |
Consultant review after infusion | 140 | PSSRU (medical consultant) | 0.33 | 50 |
Alteplase drug costs | 576 | BNF 25 | 1 | 626 |
Subtotal | 1354 | |||
Non-thrombolytic treatment | ||||
Emergency department doctor assessment | 140 | PSSRU (medical consultant) | 0.25 | 38 |
Blood test | 5 | ISD Scotland 24 | 1 | 6 |
CT scan (brain imaging) | 91 | NHS Reference Costs 26 | 1 | 98 |
Nurse to accompany to CT scan | 49 | PSSRU (nurse team leader) | 1 | 53 |
Nurse assessment | 49 | PSSRU (nurse team leader) | 0.08 | 4 |
Routine nurse observation (4 in 24 hours) | 49 | PSSRU (nurse team leader) | 0.33 | 18 |
Junior staff review | 60 | PSSRU (registrar) | 0.21 | 14 |
Consultant review at 24 hours | 140 | PSSRU (medical consultant) | 0.25 | 38 |
Subtotal | 269 |
In line with van Leeuwen et al. ,67 for patients with LVO receiving mechanical thrombectomy, it was assumed that 85% would receive both mechanical thrombectomy and IV thrombolysis, 10% to receive IV thrombolysis only and 5% to receive IV thrombolysis and going for mechanical thrombectomy but who appeared revascularised during angiography. Moreover, for patients with LVO not receiving mechanical thrombectomy, it was assumed that 40% would receive IV thrombolysis and 60% would receive non-thrombolytic treatment. 67 Treatment costs for non-LVO patients were assumed to be equal to the costs of one day in the acute stroke unit based on Patel et al. 108 Lastly, the additional costs of patients without LVO incorrectly classified as having LVO were assumed to be equal to the costs of an ambulance ride and a stroke unit day, using cost estimates from Patel et al. 108 An overview of the resulting short-term costs (< 90 days) for each branch of the decision tree is presented in Table 26.
Branch in decision tree | Costs (SE)a | Source |
---|---|---|
LVO receiving mechanical thrombectomy | 8794 (2198) | Lobotesis et al.,92 van Leeuwen et al.67 |
LVO not receiving mechanical thrombectomy | 702 (176) | Lobotesis et al.,92 van Leeuwen et al.67 |
LVO | 745 (186) | Patel et al.108 |
Non-LVO incorrectly classified as LVO | 559 (140) | Patel et al.108 |
Acute stroke costs (< 90 days) and long-term costs (annually) were attributed to the different mRS states and included costs of personal social services, such as nursing and residential care costs (i.e. for long-term costs). To this extent, Lobotesis et al. 92 used data from the OXVASC study. 109 As data were only available for three levels of post-stroke disability (i.e. mRS 0–2, mRS 3–4 and mRS 5), the authors employed a consensus-based approach by using three clinical experts from whom weights were elicited. By applying a weighting on the three levels of post-stroke disability, individual costs by mRS were calculated for mRS levels/states. Acute and long-term costs of acute ischaemic stroke by mRS are presented in Table 27.
mRS state | Mean acute costs (£) (SD) | Mean annual long-term costs (£) (SD) |
---|---|---|
0 | 3145 (8333) | 2846 (3998) |
1 | 3700 (8333) | 3348 (3998) |
2 | 4255 (8333) | 3850 (3998) |
3 | 16,409 (20,657) | 13,697 (8343) |
4 | 22,200 (20,657) | 18,532 (8343) |
5 | 26,367 (17,704) | 30,093 (16,209) |
6 (cost of death) | 3328 (3055) | – |
Overview of main model assumptions and input parameters
The main assumptions in the health economic analyses were:
-
Consistent with the focus of the AI-derived software-assisted review on triage and supporting the thrombectomy decision, the current assessment primarily considers the claim that AI-derived software-assisted review could provide a more accurate diagnosis of LVO.
-
Thrombectomy eligibility is independent of the diagnostic strategy.
-
For recurrent strokes, the mRS distribution of patients without thrombectomy is used.
-
Consistent with most cost-effectiveness studies in this disease area, it was assumed that transitions between health states mRS 0–5 were only possible in case a (recurrent) stroke occurred. After a recurrent stroke, patients could either stay in their mRS health state or move to a more severe mRS health state.
-
The risk of recurrent stroke was assumed the same across all mRS health states.
-
False positives have cost consequences only.
A summary of model input parameters is provided in Table 28.
Parameter | Description | Deterministic value | Probabilistic mean (95% CI) | Distribution |
---|---|---|---|---|
d_c | discount rate for costs | 0.035 | – | Fixed |
d_e | discount rate for effects | 0.035 | – | Fixed |
cycles | number of model cycles | 40 | – | Fixed |
age_init | starting age | 66 | – | Fixed |
p_male | proportion of patients that are male | 0.584 | – | Fixed |
p_prev | prevalence of LVO | 0.461 | 0.461 (0.430 to 0.491) | Logit normal |
p_mt_eligible_t1 | proportion of patients eligible for thrombectomy for t1 | 0.412 | 0.412 (0.406 to 0.418) | Beta |
p_mt_eligible_t2 | proportion of patients eligible for thrombectomy for t2 | 0.412 | 0.412 (0.406 to 0.418) | Beta |
p_se_t1 | sensitivity for t1 (clinician only) | 0.930 | 0.930 (0.876 to 0.969) | PERT |
p_sp_t1 | specificity for t1 (clinician only) | 0.941 | 0.941 (0.902 to 0.973) | PERT |
p_se_t2 | sensitivity for t2 (AI + clinician) | 0.941 | 0.941 (0.904 to 0.971) | PERT |
p_sp_t2 | specificity for t2 (AI + clinician) | 0.938 | 0.937 (0.885 to 0.978) | PERT |
p_mRS0_lvo_mt | proportion of patients with mRS0 after thrombectomy | 0.111 | 0.111 (0.091 to 0.133) | Dirichlet |
p_mRS1_lvo_mt | proportion of patients with mRS1 after thrombectomy | 0.181 | 0.181 (0.156 to 0.208) | Dirichlet |
p_mRS2_lvo_mt | proportion of patients with mRS2 after thrombectomy | 0.186 | 0.186 (0.161 to 0.212) | Dirichlet |
p_mRS3_lvo_mt | proportion of patients with mRS3 after thrombectomy | 0.161 | 0.161 (0.137 to 0.186) | Dirichlet |
p_mRS4_lvo_mt | proportion of patients with mRS4 after thrombectomy | 0.159 | 0.159 (0.135 to 0.185) | Dirichlet |
p_mRS5_lvo_mt | proportion of patients with mRS5 after thrombectomy | 0.055 | 0.055 (0.041 to 0.072) | Dirichlet |
p_mRS6_lvo_mt | proportion of patients with mRS6 after thrombectomy | 0.147 | 0.147 (0.124 to 0.172) | Dirichlet |
p_mRS0_lvo_no_mt | proportion of patients with mRS0 with LVO but without thrombectomy | 0.063 | 0.063 (0.048 to 0.080) | Dirichlet |
p_mRS1_lvo_no_mt | proportion of patients with mRS1 with LVO but without thrombectomy | 0.098 | 0.098 (0.079 to 0.119) | Dirichlet |
p_mRS2_lvo_no_mt | proportion of patients with mRS2 with LVO but without thrombectomy | 0.142 | 0.142 (0.119 to 0.167) | Dirichlet |
p_mRS3_lvo_no_mt | proportion of patients with mRS3 with LVO but without thrombectomy | 0.162 | 0.162 (0.138 to 0.188) | Dirichlet |
p_mRS4_lvo_no_mt | proportion of patients with mRS4 with LVO but without thrombectomy | 0.251 | 0.251 (0.222 to 0.280) | Dirichlet |
p_mRS5_lvo_no_mt | proportion of patients with mRS5 with LVO but without thrombectomy | 0.109 | 0.109 (0.089 to 0.130) | Dirichlet |
p_mRS6_lvo_no_mt | proportion of patients with mRS6 with LVO but without thrombectomy | 0.175 | 0.175 (0.150 to 0.200) | Dirichlet |
p_mRS0_no_lvo | proportion of non-LVO patients with mRS0 | 0.218 | 0.217 (0.161 to 0.278) | Dirichlet |
p_mRS1_no_lvo | proportion of non-LVO patients with mRS1 | 0.352 | 0.352 (0.287 to 0.421) | Dirichlet |
p_mRS2_no_lvo | proportion of non-LVO patients with mRS2 | 0.238 | 0.239 (0.181 to 0.301) | Dirichlet |
p_mRS3_no_lvo | proportion of non-LVO patients with mRS3 | 0.124 | 0.124 (0.082 to 0.174) | Dirichlet |
p_mRS4_no_lvo | proportion of non-LVO patients with mRS4 | 0.047 | 0.047 (0.022 to 0.080) | Dirichlet |
p_mRS5_no_lvo | proportion of non-LVO patients with mRS5 | 0.021 | 0.021 (0.006 to 0.044) | Dirichlet |
p_mRS6_no_lvo | proportion of non-LVO patients with mRS6 | 0.000 | – | Fixed |
p_mRS0_rec | proportion of patients with mRS0 after recurrent stroke | 0.063 | 0.063 (0.048 to 0.08) | Dirichlet |
p_mRS1_rec | proportion of patients with mRS1 after recurrent stroke | 0.098 | 0.098 (0.079 to 0.119) | Dirichlet |
p_mRS2_rec | proportion of patients with mRS2 after recurrent stroke | 0.142 | 0.142 (0.119 to 0.167) | Dirichlet |
p_mRS3_rec | proportion of patients with mRS3 after recurrent stroke | 0.162 | 0.162 (0.138 to 0.188) | Dirichlet |
p_mRS4_rec | proportion of patients with mRS4 after recurrent stroke | 0.251 | 0.251 (0.222 to 0.28) | Dirichlet |
p_mRS5_rec | proportion of patients with mRS5 after recurrent stroke | 0.109 | 0.109 (0.089 to 0.13) | Dirichlet |
p_mRS6_rec | proportion of patients with mRS6 after recurrent stroke | 0.175 | 0.175 (0.15 to 0.2) | Dirichlet |
p_rec_stroke | probability of recurrent stroke | 0.030 | 0.028 (0.016 to 0.043) | Beta |
rr_mRS0 | RR for mortality for patients with mRS0 | 1.000 | – | Fixed |
rr_mRS1 | RR for mortality for patients with mRS1 | 1.000 | – | Fixed |
rr_mRS2 | RR for mortality for patients with mRS2 | 1.120 | 1.137 (0.815 to 1.543) | Log-normal |
rr_mRS3 | RR for mortality for patients with mRS3 | 1.660 | 1.68 (1.237 to 2.227) | Log-normal |
rr_mRS4 | RR for mortality for patients with mRS4 | 1.920 | 1.948 (1.417 to 2.628) | Log-normal |
rr_mRS5 | RR for mortality for patients with mRS5 | 2.570 | 2.596 (1.926 to 3.439) | Log-normal |
u_mRS0 | utility for patients with mRS0 | 0.936 | 0.817 (0.507 to 0.993) | Truncated normal |
u_mRS1 | utility for patients with mRS1 | 0.817 | 0.752 (0.41 to 0.985) | Truncated normal |
u_mRS2 | utility for patients with mRS2 | 0.681 | 0.656 (0.28 to 0.964) | Truncated normal |
u_mRS3 | utility for patients with mRS3 | 0.558 | 0.552 (0.165 to 0.909) | Truncated normal |
u_mRS4 | utility for patients with mRS4 | 0.265 | 0.262 (-0.132 to 0.658) | Truncated normal |
u_mRS5 | utility for patients with mRS5 | -0.054 | -0.054 (-0.094 to -0.015) | Truncated normal |
u_mRS6 | utility for patients with mRS6 | 0.000 | – | Fixed |
c_mRS0_dt | decision tree costs for patients with mRS0 | £3419 | £3405 (£0 to £29,384) | Gamma |
c_mRS1_dt | decision tree costs for patients with mRS1 | £4022 | £4061 (£0 to £31,253) | Gamma |
c_mRS2_dt | decision tree costs for patients with mRS2 | £4625 | £4558 (£0 to £30,417) | Gamma |
c_mRS3_dt | decision tree costs for patients with mRS3 | £17,837 | £18,190 (£67 to £81,983) | Gamma |
c_mRS4_dt | decision tree costs for patients with mRS4 | £24,131 | £23,895 (£877 to £81,859) | Gamma |
c_mRS5_dt | decision tree costs for patients with mRS5 | £28,661 | £28,483 (£4045 to £77,477) | Gamma |
c_mRS6_dt | decision tree costs for patients with mRS6 | £3618 | £3565 (£133 to £12,152) | Gamma |
c_mRS0 | annual costs for patients with mRS0 | £3094 | £3118 (£4 to £16,017) | Gamma |
c_mRS1 | annual costs for patients with mRS1 | £3639 | £3612 (£24 to £15,874) | Gamma |
c_mRS2 | annual costs for patients with mRS2 | £4185 | £4176 (£82 to £15,882) | Gamma |
c_mRS3 | annual costs for patients with mRS3 | £14,889 | £14,913 (£2694 to £36,909) | Gamma |
c_mRS4 | annual costs for patients with mRS4 | £20,144 | £20,112 (£6488 to £42,020) | Gamma |
c_mRS5 | annual costs for patients with mRS5 | £32,711 | £32,727 (£7722 to £74,336) | Gamma |
c_mRS6 | annual costs for patients with mRS6 | £0 | – | Fixed |
c_t1 | technology costs for t1 | £0 | – | Fixed |
c_t2 | technology costs for t2 | £49 | £49 (£28 to £77) | Gamma |
c_treat_mt | initial treatment costs for patients with thrombectomy | £8794 | £8788 (£5386 to £13,130) | Beta and gamma |
c_treat_no_mt | initial treatment costs for LVO patients without thrombectomy | £702 | £705 (£411 to £1131) | Beta and gamma |
c_treat_non_LVO | initial treatment costs for non-LVO patients | £745 | £746 (£385 to £1220) | Beta and gamma |
c_FP | initial additional costs for non-LVO patients incorrectly classified as LVO | £559 | £558 (£322 to £864) | Gamma |
Model analyses
Discount rates of 3.5% and a half-cycle correction were applied for both costs and effects. Expected costs, life-years and QALYs were estimated from the perspective of the NHS. The incremental cost-effectiveness ratio was calculated by dividing the incremental costs by the incremental QALYs. Probabilistic sensitivity analyses (10,000 simulations) were performed, and cost-effectiveness acceptability curves and expected loss curves were constructed.
Sensitivity analysis
Deterministic one-way sensitivity analyses were performed, using all stochastic input parameters, to assess the impact of input parameters on the estimated outcomes. The results of these analyses are presented using optimal strategy plots and plotting the input parameters versus outcomes. Info-rank plots, based on the probabilistic sensitivity analyses, are presented to explore the relative ‘importance’ of each parameter in terms of the expected value of information. Finally, two-way sensitivity analyses were performed, including the most influential AI-specific parameters.
Scenario analyses
Various deterministic scenario analyses were performed to assess the impact of assumptions on the estimated outcomes:
-
assuming that the AI technology costs are increased to £100 per patient
-
assuming that the proportion of LVO patients eligible for mechanical thrombectomy with AI is increased to 50%
-
assuming that the AI technology plus clinician sensitivity is increased to 96%
-
assuming the AI technology plus clinician sensitivity is decreased to 90%
-
assuming that the LVO prevalence is increased to 50%
-
assuming that the LVO prevalence is decreased to 40%
-
assuming that recurrent strokes are LVOs eligible for thrombectomy with appropriate mRS distribution
-
assuming that recurrent strokes are non-LVOs
-
assuming that additional false positive costs are increased to £2000
-
assuming that the annual recurrent stroke probability is decreased to 2%
-
assuming that the annual recurrent stroke probability is increased to 4%
-
assuming that the proportion of patients eligible for thrombectomy is increased to 50% (both strategies)
-
assuming that the proportion of patients eligible for thrombectomy is decreased to 35% (both strategies)
-
utility values based on Rebchuk et al. (0.93, 0.86, 0.68, 0.57, 0.31 and 0.06 for mRS 0–5)
-
utility values based on Wang et al. (0.97, 0.88, 0.72, 0.54, 0.23 and –0.17 for mRS 0–5)
-
assuming no mortality cap (allowing mortality to be potentially lower than general population mortality)
-
assuming no utility cap (allowing utility values to be potentially higher than general population utility values)
-
assuming neither mortality cap nor utility cap
-
assuming accuracy for current practice without AI is based on Seker et al. 56 (neuroradiologist grader)
-
assuming that accuracy for current practice without AI is based on Seker et al. 56 (resident graders).
Results of cost-effectiveness analyses
The probabilistic base-case analyses were performed using 10,000 simulations. Although fewer simulations were deemed sufficient based on the convergence plots of the incremental results (Figure 11), the number of simulations was increased to increase the stability of the estimated results (given the small incremental differences) when rerunning the probabilistic sensitivity analysis with a different random seed.
Base-case analysis
The probabilistic results indicated that the addition of AI to detect LVO is potentially more effective, (QALY gain of 0.003), more costly (increased costs of £8.61) and cost-effective for willingness-to-pay thresholds of £3380 per QALY and higher (Table 29). The cost-effectiveness plane (see Figure 11) illustrates the negative correlation between incremental costs and incremental QALYs (i.e. if a technology is more effective it also tends to be less costly). The cost-effectiveness acceptability curves (Figure 12) indicates that at willingness-to-pay thresholds of £20,000 and £30,000 per QALY gained the probabilities of current practice with AI being cost-effective are 53.6% and 56.2%, respectively. The expected risks per patient associated with adding AI, at willingness-to-pay thresholds of £20,000 and £30,000 per QALY gained, were £80 and £95, respectively (these were £122 and £163, respectively, without adding AI; see expected loss curves; see Figure 12). On a population level (assuming 87,635 patients per annum in the UK) the estimated annual risks associated with adding AI were £7.0 million and £8.4 million, at willingness-to-pay thresholds of £20,000 and £30,000 per QALY gained, respectively. The deterministic results (Table 30) were similar to the probabilistic results.
Technology | Costs (£) | QALYs | Δ Costs (£) | Δ QALYs | Δ Costs (£)/Δ QALYs |
---|---|---|---|---|---|
Current practice without AI | 116,273 | 5.9000 | NA | NA | NA |
Current practice with AI | 116,281 | 5.9026 | 9 | 0.0025 | 3380 |
Technology | Costs (£) | QALYs | Δ Costs (£) | Δ QALYs | Δ Costs (£)/Δ QALYs |
---|---|---|---|---|---|
Current practice without AI | 117,267 | 6.2778 | NA | NA | NA |
Current practice with AI | 117,276 | 6.2806 | 10 | 0.0027 | 3490 |
FIGURE 11.
Convergence plot (incremental cost-effectiveness ratio), cost-effectiveness plane and expected incremental benefit.

FIGURE 12.
Cost-effectiveness acceptability and expected loss curves.
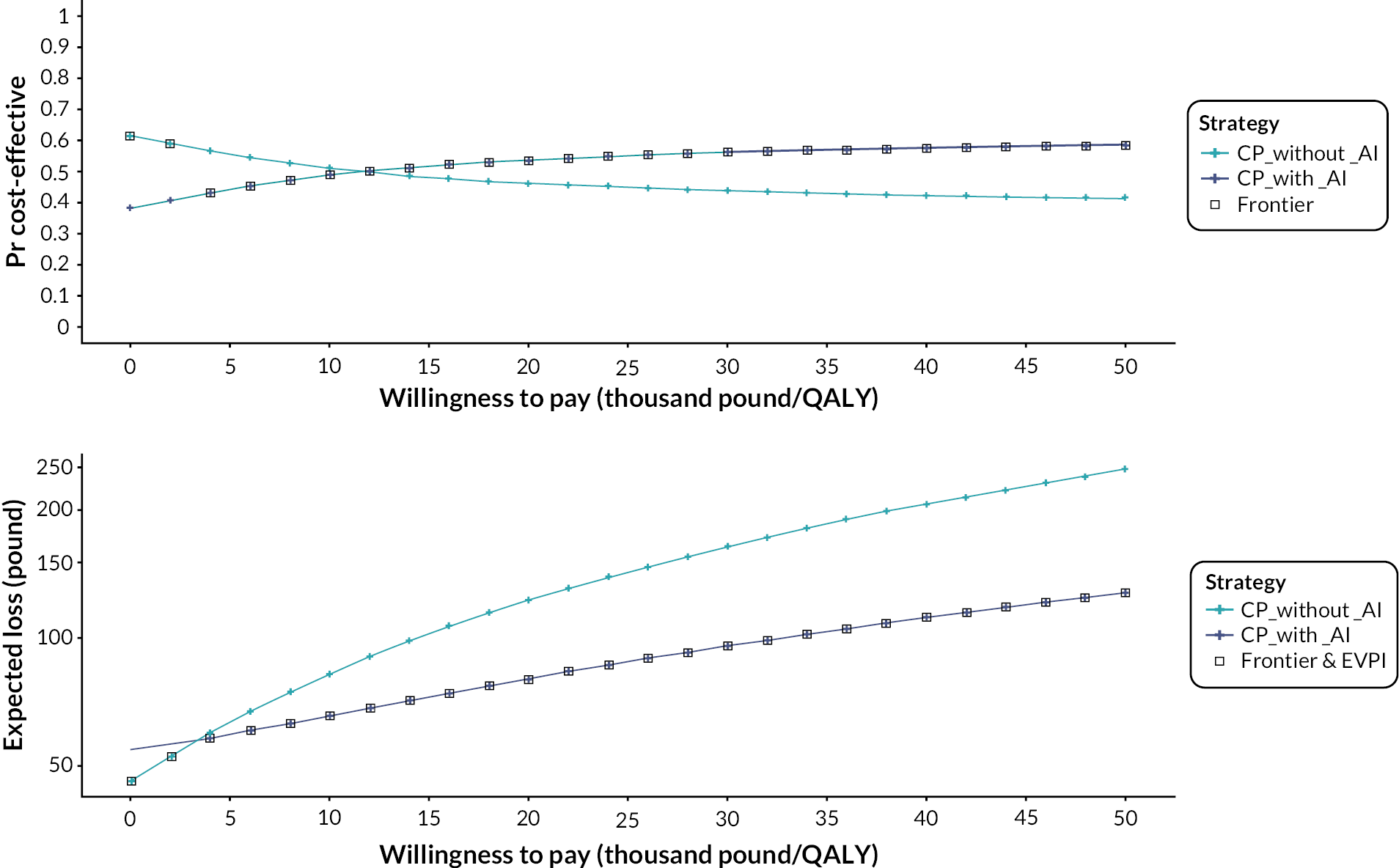
Intermediate results (probabilistic base case)
The diagnostic pathway (after the 90-day decision tree) results were similar for both strategies (Figure 13). The proportion of detected LVOs (and thus patients receiving thrombectomy) was increased from 17.6% to 17.9% when AI is added to current practice. As a result, the mRS 0–3 proportions were slightly higher while mRS 4–6 proportions were slightly lower when AI is added (differences < 0.1%). Moreover, the average traces (across all probabilistic sensitivity simulations) were very similar for both technologies (Figure 14). Similar to the 90-day decision tree results, the average trace differences per cycle were less than 0.1% with the addition of AI, resulting in slightly higher proportions in the lower mRS health states. When considering the cumulative costs and QALYs over time (Figure 15), the cost difference is largest in cycle 1 (the addition of AI resulting in a cost increase of £58) decreasing over time to £9 at the end of the time horizon. In contrast, the QALY difference (Figure 16) is smallest in cycle 1 (the addition of AI resulting in a QALY increase of 0.0002) increasing over time to 0.0025 QALY (at the end of the time horizon).
FIGURE 13.
Diagnostic pathway results for current practice with AI (t2) and without AI (t1).

FIGURE 14.
Average state transition trace for current practice with AI and without AI.

FIGURE 15.
Cumulative costs for current practice with AI and without AI.
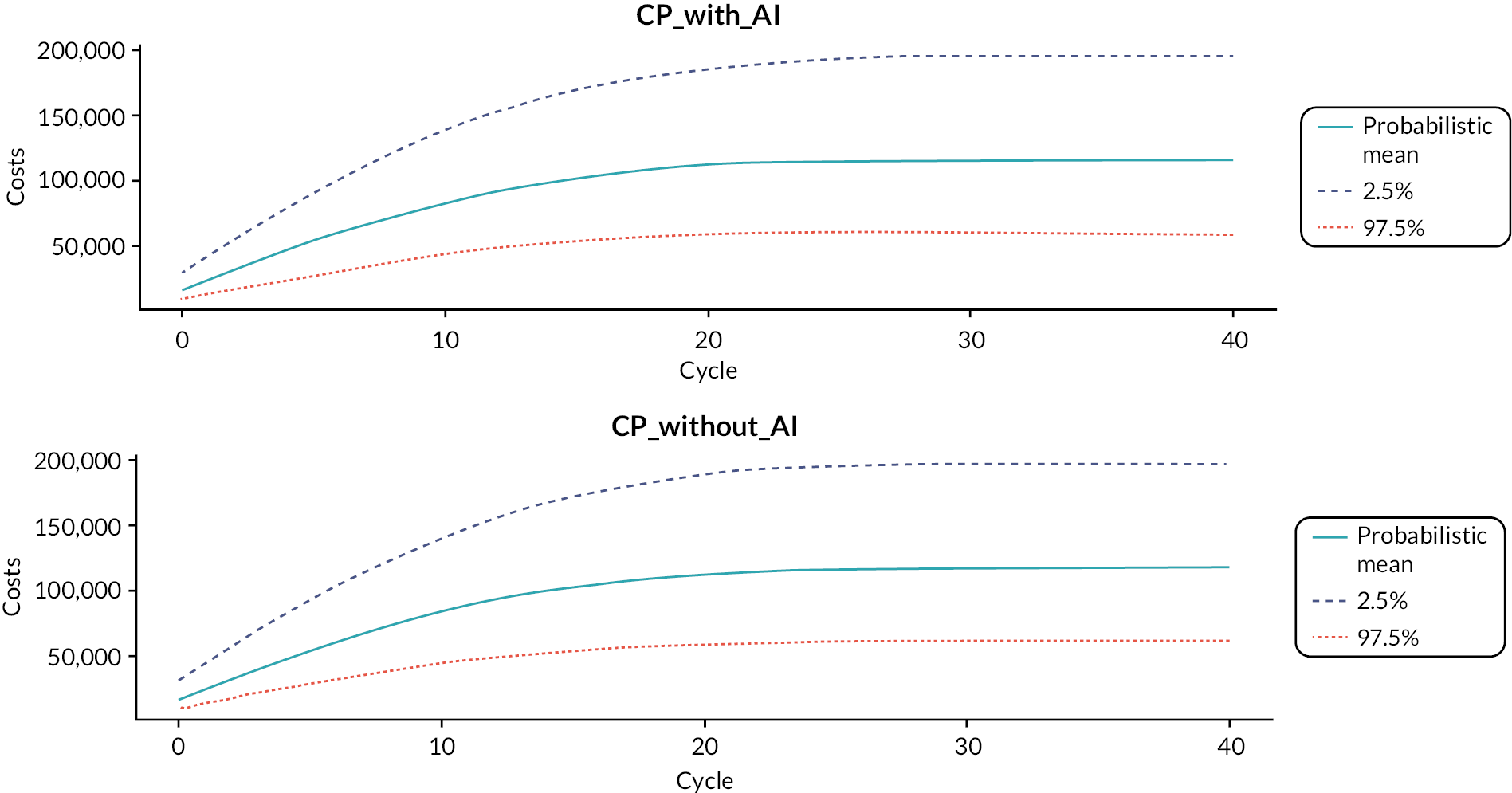
FIGURE 16.
Cumulative QALYs for current practice with AI and without AI.
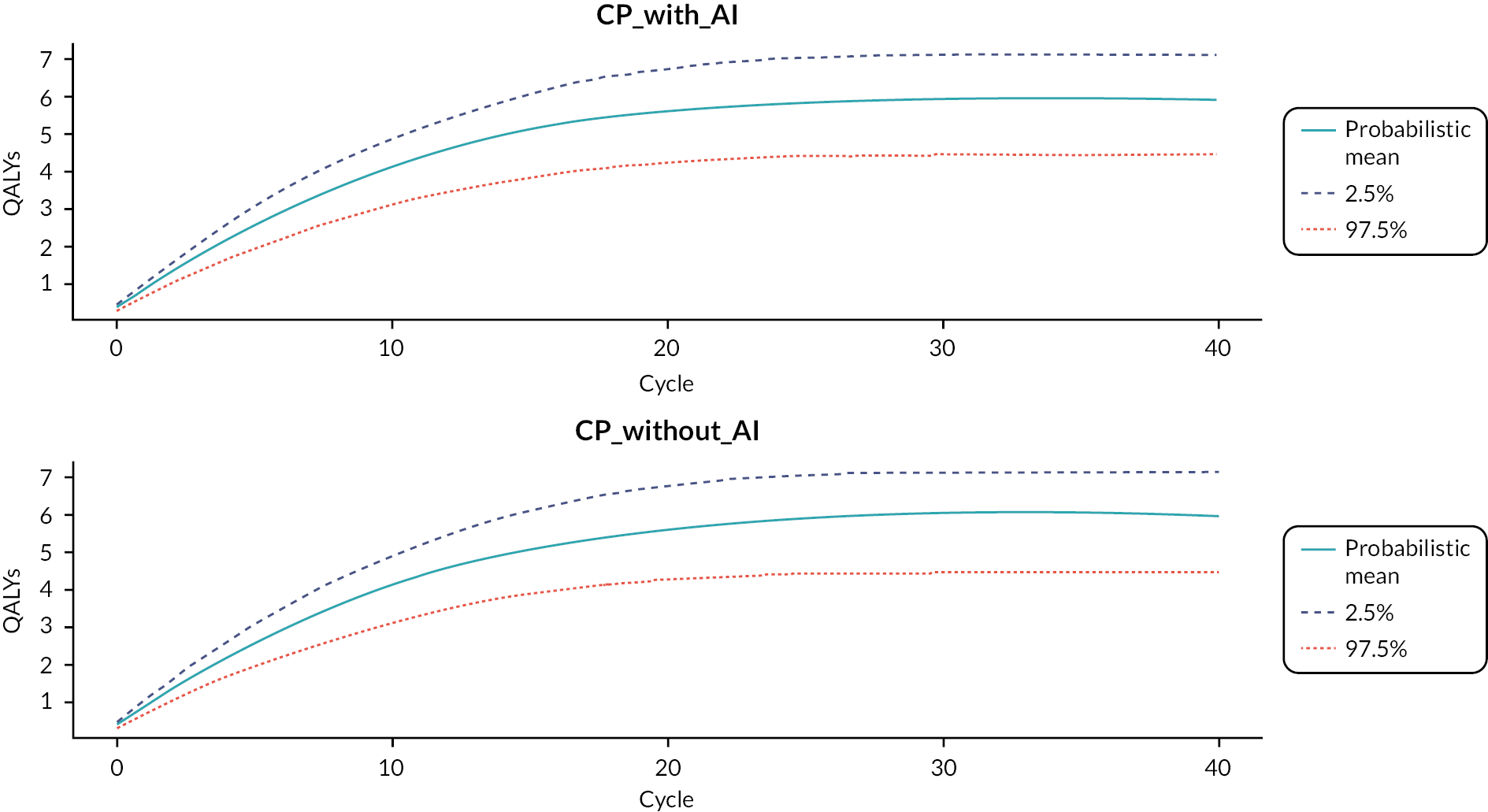
Considering the disaggregated costs, the cost increase for AI was mainly driven by the short-term costs (including the AI technology costs), while overall costs related to the mRS4 and mRS5 health states are lower (due to lower occupancy for these health states) when AI is added. Although incremental QALYs are very low and similar across health states, the increased QALYs for AI are driven by QALY differences in the mRS0 and mRS1 health states (due to higher occupancy for these health states). Finally, the estimated LYs were very similar for both strategies (10.847 vs. 10.848).
Sensitivity analyses
The info-rank plot indicated that the sensitivity of both technologies was the most important input parameter (Figure 17). In addition, the optimal strategy plots (Figure 18) indicated that the proportion of patients with LVO who are eligible for mechanical thrombectomy is important to determine the most optimal strategy in terms of costs and QALYs. For the estimated costs, specificity, the additional costs of the AI technology, costs related to mRS4 and mRS5 were input parameters (in addition to those mentioned above) that can change the strategy that is most optimal. Deterministic one-way sensitivity analyses for all stochastic parameters are presented in Figure 19 (costs) and Figure 20 (QALYs).
FIGURE 17.
Info-rank plots.

FIGURE 18.
Optimal strategy plots.

FIGURE 19.
One-way sensitivity analyses (costs).
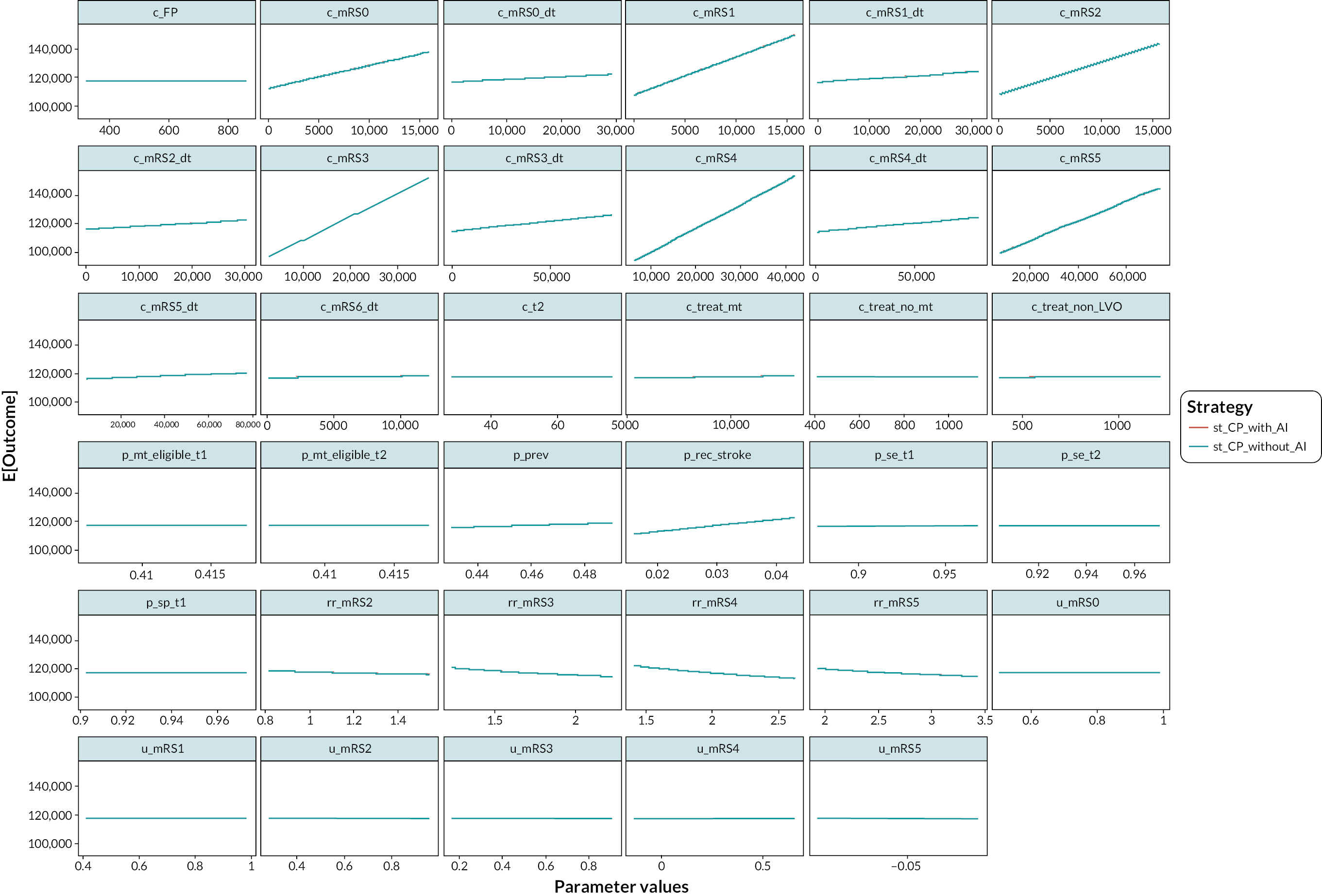
FIGURE 20.
One-way sensitivity analyses (QALYs).

Two-way sensitivity analyses were performed (Figure 21) between: (1) AI technology sensitivity; (2) AI technology costs; and (3) the proportion of LVO patients eligible for mechanical thrombectomy with AI. These analyses indicated that (given the 95% CI of these inputs), although the AI technology sensitivity is a main driver of the results, the AI technology costs and the proportion of LVO patients eligible for mechanical thrombectomy with AI can have an impact on the minimal AI technology sensitivity required for the AI technology to be cost-effective.
FIGURE 21.
Two-way sensitivity analyses (net monetary benefit with willingness to pay of £30,000 per QALY).

Scenario analyses
The results of the deterministic scenario analyses are provided in Table 31. The most influential scenario analyses improving the cost-effectiveness of the addition of AI were increasing the AI technology sensitivity to 96%, increasing the proportion of LVO patients eligible for mechanical thrombectomy with AI to 50%, removing the mortality cap and using Seker et al. 56 to inform accuracy for current practice without AI (resident graders); in these scenarios, the addition of AI was dominant. Decreasing the AI technology sensitivity to 90% and using Seker et al. 56 to inform accuracy for current practice without AI (neuroradiologist grader) resulted in current practice without AI being dominant while increasing the AI technology costs to £100 per patient would increase the incremental cost-effectiveness ratio to £22,072 per QALY gained.
Technology | Costs (£) | QALYs | Δ Costs (£) | Δ QALYs | Δ Costs (£)/Δ QALYs |
---|---|---|---|---|---|
Deterministic base case | |||||
Current practice without AI | 117,267 | 6.2778 | NA | NA | NA |
Current practice with AI | 117,276 | 6.2806 | 10 | 0.0027 | 3490 |
1 Assuming AI technology costs increased to £100 per patient | |||||
Current practice without AI | 117,267 | 6.2778 | NA | NA | NA |
Current practice with AI | 117,327 | 6.2806 | 60 | 0.0027 | 22,072 |
2 Assuming proportion of patients with LVO eligible for mechanical thrombectomy with AI increased to 50% | |||||
Current practice without AI | 117,267 | 6.2778 | NA | NA | NA |
Current practice with AI | 116,551 | 6.3293 | NA | NA | Dominance |
3 Assuming AI technology sensitivity increased to 96% | |||||
Current practice without AI | 117,267 | 6.2778 | NA | NA | NA |
Current practice with AI | 117,209 | 6.2851 | NA | NA | Dominance |
4 Assuming AI technology sensitivity decreased to 90% | |||||
Current practice without AI | 117,267 | 6.2778 | NA | NA | NA |
Current practice with AI | 117,425 | 6.2706 | NA | NA | Dominance |
5 Assuming LVO prevalence increased to 50% | |||||
Current practice without AI | 118,899 | 6.1535 | NA | NA | NA |
Current practice with AI | 118,905 | 6.1564 | 6 | 0.0030 | 2016 |
6 Assuming LVO prevalence decreased to 40% | |||||
Current practice without AI | 114,760 | 6.4688 | NA | NA | NA |
Current practice with AI | 114,775 | 6.4712 | 15 | 0.0024 | 6318 |
7 Assuming recurrent strokes are LVOs eligible for thrombectomy (with appropriate mRS distribution) | |||||
Current practice without AI | 112,941 | 6.4604 | NA | NA | NA |
Current practice with AI | 112,948 | 6.4632 | 7 | 0.0028 | 2612 |
8 Assuming recurrent strokes are non-LVOs | |||||
Current practice without AI | 108,203 | 6.6555 | NA | NA | NA |
Current practice with AI | 108,208 | 6.6585 | 5 | 0.0029 | 1649 |
9 Assuming additional FP costs increased to £2000 | |||||
Current practice without AI | 117,313 | 6.2778 | NA | NA | NA |
Current practice with AI | 117,324 | 6.2806 | 12 | 0.0027 | 4400 |
10 Assuming annual recurrent stroke probability decreased to 2% | |||||
Current practice without AI | 112,968 | 6.4541 | NA | NA | NA |
Current practice with AI | 112,976 | 6.4569 | 7 | 0.0028 | 2559 |
11 Assuming annual recurrent stroke probability increased to 4% | |||||
Current practice without AI | 121,340 | 6.1111 | NA | NA | NA |
Current practice with AI | 121,352 | 6.1138 | 12 | 0.0026 | 4426 |
12 Assuming proportion of patients eligible for thrombectomy increased to 50% (both strategies) | |||||
Current practice without AI | 116,550 | 6.3260 | NA | NA | NA |
Current practice with AI | 116,551 | 6.3293 | 1 | 0.0033 | 248 |
13 Assuming proportion of patients eligible for thrombectomy decreased to 35% (both strategies) | |||||
Current practice without AI | 117,769 | 6.2441 | NA | NA | NA |
Current practice with AI | 117,785 | 6.2464 | 16 | 0.0023 | 6735 |
14 Utility values based on Rebchuk et al.103 (0.93, 0.86, 0.68, 0.57, 0.31 and 0.06 for mRS 0–5) | |||||
Current practice without AI | 117,267 | 6.4529 | NA | NA | NA |
Current practice with AI | 117,276 | 6.4555 | 10 | 0.0025 | 3763 |
15 Utility values based on Wang et al.104 (0.97, 0.88, 0.72, 0.54, 0.23 and −0.17 for mRS 0–5) | |||||
Current practice without AI | 117,267 | 6.1980 | NA | NA | NA |
Current practice with AI | 117,276 | 6.2010 | 10 | 0.0030 | 3224 |
16 Assuming no mortality cap (allowing mortality to be potentially lower than general population mortality) | |||||
Current practice without AI | 230,687 | 9.7510 | NA | NA | NA |
Current practice with AI | 230,645 | 9.7550 | NA | NA | Dominance |
17 Assuming no utility cap (allowing utility values to be potentially higher than general population utility values) | |||||
Current practice without AI | 117,267 | 6.7299 | NA | NA | NA |
Current practice with AI | 117,276 | 6.7330 | 10 | 0.0030 | 3136 |
18 Assuming neither mortality cap nor utility cap | |||||
Current practice without AI | 230,687 | 10.4390 | NA | NA | NA |
Current practice with AI | 230,645 | 10.4434 | NA | NA | Dominance |
19 Assuming accuracy for current practice without AI based on Seker et al. 202156 (neuroradiologist grader) | |||||
Current practice without AI | 117,104 | 6.2879 | NA | NA | NA |
Current practice with AI | 117,276 | 6.2806 | NA | NA | Dominance |
20 Assuming accuracy for current practice without AI based on Seker et al. 202156 (resident graders) | |||||
Current practice without AI | 117,341 | 6.2729 | NA | NA | NA |
Current practice with AI | 117,276 | 6.2806 | NA | NA | Dominance |
Chapter 5 Discussion
Statement of principal findings
Clinical effectiveness
The evidence base, to inform assessment of the clinical effectiveness of AI-derived software technologies for analysing CT brain scans in people with suspected stroke, was limited. This assessment focused on evaluating the effectiveness of AI-derived software technologies as adjuncts or aid to human interpretation (i.e. as they would be used in clinical practice and as recommended by the manufacturers). Our assessment included a systematic review to identify evidence to address three specific research questions:
-
(1) Does AI-derived software-assisted review of non-enhanced CT brain scans for guiding thrombolysis treatment decisions for people with suspected acute stroke represent a clinically and cost-effective use of NHS resources?
-
(2a) Does AI-derived software-assisted review of CTA brain scans for guiding mechanical thrombectomy treatment decisions for people with an ischaemic stroke represent a clinically and cost-effective use of NHS resources?
-
(2b) Does AI-derived software-assisted review of CTP brain scans for guiding mechanical thrombectomy treatment decisions for people with an ischaemic stroke after a CTA brain scan represent a clinically and cost-effective use of NHS resources?
The scope included multiple software products, from 13 manufacturers (described in the Intervention technologies section). For 9 of the 13 manufacturers, no studies were identified that met the inclusion criteria (see Table 2) for our systematic review. All studies identified concerned AI-derived software technologies from four manufacturers, Avicenna, Brainomix, iSchemaView and Viz, and the majority 1833–36,40,41,43–46,49,51,52,55,56,59–61 of 22 studies33–36,39–41,43–46,48–52,55,56,59–62 reported data to inform research question 2a (i.e. evaluated AI-derived software for the interpretation of CTA). All the studies identified by our systematic review were either studies assessing the diagnostic accuracy of AI-derived software alone (i.e. not as it would be used in clinical practice, as recommended by the manufacturers and as specified in the inclusion criteria for this assessment),35,36,39–41,43,48,50,51,55,56,59–62 or ‘before and after’ observational studies reporting information about the effects of implementing AI-derived software technologies for treated patients only. 33,34,44–46,49,52
Is the use of artificial intelligence-derived software to assist review of non-enhanced computed tomography brain scans to guide thrombolysis treatment decisions for people with suspected acute stroke a clinically effective intervention?
Three studies39,48,62 provided information about the accuracy of AI-derived software technologies for the detection of ICH, in patients with suspected AIS. The sensitivity and specificity estimates were 90.2% (95% CI 83.9% to 94.2%) and 100% (95% CI 97.5% to 100%) for Viz ICH,39 91.1% (95% CI 82.8% to 95.6%) and 88.9 (95% CI 80.2% to 94.0%) for the unspecified Brainomix AI-derived software technology48 and 93.8% (95% CI 91.6% to 95.4%) and 82.8% (95% CI 81.4% to 84.1%) for Brainomix e-APECTS. 62 The study which evaluated Brainomix e-ASPECTS also provided sensitivity and specificity estimates for the detection of AIS; these were 68.5% (95% CI 66.4% to 70.5%) and 74.1% (95% CI 71.95% to 76.1%). 62 One additional study provided information about the effects on time to treatment of implementing the e-ASPECTS and e-CTA modules of Brainomix e-Stroke in a centre which did not offer thrombectomy (patients requiring thrombectomy were transferred to another unit). 44 This study reported increases in the proportions of patients receiving both IV thrombolysis and thrombectomy, following implementation, as well as a reduction in the mean time from first CT to groin puncture (174–145 minutes) for transferred thrombectomy patients. 44
Is the use of artificial intelligence-derived software to assist review of computed tomography angiography brain scans for guiding mechanical thrombectomy treatment decisions for people with an ischaemic stroke a clinically effective intervention?
Eleven studies provided information about the accuracy of various AI-derived software technologies for the detection of LVO on CTA scans in patients with AIS. 35,36,40,41,43,51,55,56,59–61 The anatomical locations of occlusions included in the definition of the target condition varied across studies. Where the target condition included occlusions of ICA, carotid terminus, or the M1 or M2 segments of the MCA, the sensitivity and specificity estimates were 95.4% (95% CI 92.7% to 97.1%) and 79.4% (95% CI 75.8% to 82.6%) for Rapid CTA,35 91.2% (95% CI 77.0% to 97.0%) and 85.0 (95% CI 64.0% to 94.8%) for Viz LVO,40 83.8% (95% CI 77.3% to 88.7%) and 95.7% (95% CI 91.0% to 98.0%) for Brainomix e-CTA,56 and 98.1% (95% CI 94.5% to 99.3%) and 98.2% (95% CI 95.5% to 99.3%) for Avicenna CINA LVO. 51 There was some evidence to indicate that where studies included more distal (e.g. M3 segment of the MCA) elements of the anterior circulation or included posterior circulation in their definition of the target condition, this was associated with markedly reduced estimates of sensitivity. One study provided an estimate of the sensitivity of Rapid LVO for detection of occlusions of the ICA, carotid terminus, or M1 or M2/3 segments of the MCA of 63.6% (95% CI 51.6% to 74.2%),55 and a further study provided an estimate of the sensitivity of Viz LVO for the detection of occlusions the ICA, carotid terminus, the M1 or M2 segments of the MCA, or posterior circulation occlusions of 66.2% (95% CI 54.3% to 76.3%). 43 All four studies that provided information about the effects of implementing Viz LVO43,45,46,52 and one study that provided information about the effects of implementing Rapid CTA33 in clinical settings reported that implementation was associated with reductions in time to treatment for thrombectomy patients and where reported, with no significant change in clinical outcomes, as indicated by mRS. 33,45,46,52 Three of these studies concerned the effects of implementing Viz LVO in patients who were transferred between centres for thrombectomy43,46,52 and one concerned the effects of implementing Viz LVO in patients who received thrombectomy within centre (no transfer);45 the study concerning the implementation of Rapid CTA was conducted in ‘a large multi-hospital network with CSCs and 24-hour neurointerventional coverage’, but did not state whether data were for patients who received thrombectomy following transfer, patients who received thrombectomy within centre, or a mixture of both. 33 It should be noted that two of these studies46,52 evaluated the implementation of Viz LVO in the context of providing an automated alert system (i.e. not as specified in the scope for this assessment) and the remaining two studies43,45 were reported as conference abstracts that did not provide sufficient information to determine how Viz LVO had been implemented; where studies have evaluated implementation of an AI-derived software technology in the context of provision of an automated alert system, it is plausible that any observed reductions in time to intervention may be driven by this ‘early alert’ step.
Is the use of artificial intelligence-derived software-assisted review of computed tomography perfusion brain scans to guide mechanical thrombectomy treatment decisions for people with an ischaemic stroke, after a computed tomography angiography brain scan, a clinically effective intervention?
One study provided information to allow the calculation of measures of the diagnostic performance of Rapid CTP for identifying patients who are suitable candidates for thrombectomy. 50 Based on information about the results of Rapid CTP image analysis provided in the paper and using treatment received as the reference standard, the estimated sensitivity was 95.2% (95% CI 90.0% to 97.8%) and the estimated specificity was 80.0% (95% CI 67.0% to 88.8%). Two further studies provided information about the effects of implementing Rapid AI (including Rapid CTA and Rapid CTP). 34,49 These studies reported inconsistent findings. One study reported no significant change in the mean time from door to groin puncture (MD 2.0 minutes, 95% CI −12.9 to 16.9 minutes) or the proportion of patients with a mRS ≤ 3 (calculated OR 1.34, 95% CI 0.66 to 2.74) for thrombectomy patients, following the implementation of RapidAI;49 it was not clear whether this study concerned patients who were transferred for thrombectomy or patients who were treated within centre. By contrast, the second study reported a reduction in the meantime from door to groin puncture after implementation (MD −33.2 minutes, 95% CI −60.2 to −6.2 minutes) and no change in mean 90-day mRS (2.9 before and after), for thrombectomy patients treated within a CSC (no transfer), following implementation of the RapidAI Mobile Application. 34
Cost-effectiveness
Does artificial intelligence-derived software-assisted review of computed tomography angiography brain scans for guiding mechanical thrombectomy treatment decisions for people with an ischaemic stroke represent a clinically and cost-effective use of NHS resources?
Our cost-effectiveness results are estimated based on expert elicitation to inform accuracy estimates for both AI-derived software technologies as an adjunct/aid to human readers and human readers without AI-derived software technologies. The probabilistic results indicated that the addition of AI to detect LVO is potentially more effective (QALY gain of 0.003), more costly (increased costs of £8.61) and cost-effective for willingness-to-pay thresholds of £3380 per QALY and higher. The cost-effectiveness analyses indicated that there is a negative correlation between incremental costs and incremental QALYs (i.e. if a technology is more effective it also tends to be associated with fewer costs). Differences between AI-derived software technologies as an adjunct/aid to human readers and human readers without AI-derived software technologies were in general very small. The cost increase for AI was mainly driven by the short-term costs (including the AI technology costs), while overall costs related to the mRS4 and mRS5 health states decrease when AI is added. The increased QALYs for AI were driven by QALY differences in the mRS0 and mRS1 health states. Finally, the estimated LYs were very similar.
Strengths and limitations of assessment
Clinical effectiveness
Extensive literature searches were conducted to maximise retrieval of relevant studies. These included electronic searches of a variety of bibliographic databases, as well as screening of clinical trials registers and conference abstracts to identify unpublished studies. Because of the known difficulties in identifying test accuracy studies using study design-related search terms,110 search strategies were developed to maximise sensitivity at the expense of reduced specificity. Thus, large numbers of citations were identified and screened, relatively few of which met the inclusion criteria of the review.
The possibility of publication bias remains a potential problem for all systematic reviews. Considerations may differ for systematic reviews of test accuracy studies. It is relatively simple to define a positive result for studies of treatment; for example, a significant difference between the treatment and control groups, which favours treatment. This is not the case for test accuracy studies, which measure agreement between index test and reference standard. It would seem likely that studies finding greater agreement (high estimates of sensitivity and specificity) will be published more often. In addition, test accuracy data are often collected as part of routine clinical practice or by retrospective review of records; test accuracy studies are not subject to the formal registration procedures applied to RCTs and are therefore more easily discarded when results appear unfavourable. The extent to which publication bias occurs in studies of test accuracy remains unclear; however, simulation studies have indicated that the effect of publication bias on meta-analytic estimates of test accuracy is minimal. 111 Formal assessment of publication bias in systematic reviews of test accuracy studies remains problematic and reliability is limited. 24 We did not undertake a statistical assessment of publication bias in this review. However, our search strategy included a variety of routes to identify unpublished studies and resulted in the inclusion of a number of conference abstracts.
The rapidly evolving nature of research in this topic area presented a particular challenge. To be as inclusive as possible, we conducted a search of the medRvix the preprint server and asked clinical experts (specialist committee members for this topic) to provide details of any potentially relevant ongoing or unpublished studies, of which they were aware. One included study was identified from the medRvix search55 and a further study was provided, pre-publication, by a specialist committee member. 62 Results from these studies should be treated with appropriate caution, as they have not yet undergone peer review. To minimise the chances of omitting relevant new articles published since the original core strategies were run in July 2021, the main Embase and MEDLINE searches and the medRvix search were rerun in their entirety in October 2021 before submission of our draft report.
Clear inclusion criteria were specified in the protocol for this review, the review has been registered on PROSPERO (CRD42021269609) and the protocol is available from www.nice.org.uk/guidance/gid-dg10044/documents/final-protocol. The eligibility of studies for inclusion is therefore transparent. In addition, we have provided specific reasons for exclusion for all of the studies that were considered potentially relevant at initial citation screening and were subsequently excluded on assessment of the full publication (see Appendix 4). The review process followed recommended methods to minimise the potential for error and/or bias;22 studies were independently screened for inclusion by two reviewers and data extraction and quality assessment were done by one reviewer and checked by a second. Any disagreements were resolved by consensus.
The main limitations for this assessment were the paucity of evidence, particularly in relation to research questions 1 and 2b and, where evidence was identified, the applicability of that evidence to the specified questions.
The concerns regarding the applicability of the included studies were common across all three research questions.
The primary applicability concern, for studies that provided test accuracy data, was in relation to the implementation of the index test (AI-derived software technology). In all of these studies,35,36,39–41,43,48,50,51,55,56,59–62 the AI-derived software technology was evaluated as a stand-alone intervention, rather than as an adjunct or aid to human interpretation [as it would be used in clinical practice, as recommended by the manufacturers and as specified in the inclusion criteria for this assessment (see Table 2)].
In addition to diagnostic test accuracy studies, this assessment included some observational ‘before and after’ studies33,34,44–46,49,52 that assessed the effects of implementing AI-derived software technologies in ‘real-world’ clinical settings on time to intervention and in some cases,33,45,46,52 on clinical outcome. The information provided by studies of this type is limited in that it concerns only treated (i.e. test positive) patients; no information is provided about test-negative patients, hence there is no information about the extent to which AI-derived software technologies, as implemented, may miss patients with the target condition(s). In addition, no ‘real-world’ implementation study, included in this assessment, compared clinical outcomes with time to intervention in populations that were comparable (with respect to key baseline characteristics) before and after the implementation of the AI-derived software technology and where the AI-derived software technology was the only change to the care pathway. Differences in the study population (before and after implementation) and/or additional changes in the care pathway (other than implementation of the AI-derived software technology) mean that the extent to which any observed changes in time to intervention or clinical outcome are attributable to the implementation of the AI-derived software technology is highly uncertain. Studies that report only the effects of implementation of AI-derived software technologies on time to intervention are deficient in that they do not provide the information about clinical outcomes needed to inform decision-making. A reduction in time to intervention may not always be advantageous; for example, if the time saving is associated with a detrimental effect on clinical outcomes.
With respect to research question 1, ‘Is the use of AI-derived software to assist review of non-enhanced CT brain scans to guide thrombolysis treatment decisions for people with suspected acute stroke a clinically effective intervention?’ we were only able to identify three studies that reported information about the accuracy of AI-derived software technologies for the interpretation of NCCT in people with suspected acute stroke39,48,62 and one further observational ‘before and after’ study44 that assessed the combined effects of implementing Brainomix e-ASPECTS and e-CTA. Studies that evaluated included AI-derived software technologies frequently did not meet the population inclusion criteria for this assessment; for example, studies that evaluated the accuracy of AI-derived software technologies for the detection of ICH in all head CTs (i.e. including trauma patients and other suspected pathologies), with no separate data for patients with suspected stroke.
During the inclusion screening phase of our systematic review, we noted a number of articles reporting multivariable regression analyses, where good clinical outcome/functional independence (90-day mRS 0–2) was the dependent variable and baseline Brainomix e-ASPECTS score or e-Stroke-derived ischaemic core volume, on NCCT, was evaluated as a potential predictor of clinical outcome following thrombectomy. 112–115 These studies do not meet the inclusion criteria for our assessment because they do not provide a comparison of image interpretation with versus without the assistance of AI-derived software technologies to guide treatment decisions (e.g. whether or not to perform mechanical thrombectomy). In the examples cited, all participants had anterior circulation LVO strokes and underwent mechanical thrombectomy. 112–115 One study reported that in a multivariable regression analysis, adjusting for potential confounders including age, sex, hypertension, diabetes mellitus, AF, smoking status, baseline blood glucose, baseline National Institute of Health Stroke Scale (NIHSS) score, receipt of IV thrombolysis (tissue-type plasminogen activator) and time from last-known-well to imaging low ischaemic core volume, based on Brainomix e-Stroke software interpretation of baseline NCCT, was independently predictive of good outcome (adjusted OR 0.98, 95% CI 0.97 to 0.99). 112 Two further studies113,115 reported that the results of multivariable regression analyses indicated that e-ASPECTS score, on baseline NCCT, was an independent predictive of good outcome; adjusted OR 1.30 (95% CI 1.06 to 1.60; adjusted for age, premorbid mRS, baseline NIHSS, hypertension, hypercholesterolaemia, diabetes mellitus and prior stroke)113 and OR 1.37 (95% CI 1.01 to 1.84), co-variables not reported. 115 The final study of this type included variables for age, premorbid mRS, AF, previous stroke, baseline blood glucose, and haemoglobin A1c, baseline NIHSS, hyperdense vessel sign, e-ASPECTS, general anaesthesia, recanalisation and secondary ICH following IV thrombolysis and reported that e-ASPECTS was not independently predictive of good outcome. 114 Although they do not directly inform the research questions specified for this assessment, studies of this type may be of clinical interest in that they describe the potential of AI-derived parameters, taken from initial NCCT imaging, to predict clinical outcome following thrombectomy.
With respect to research question 2b, ‘Is the use of AI-derived software-assisted review of CT perfusion brain scans to guide mechanical thrombectomy treatment decisions for people with an ischaemic stroke, after a CTA brain scan, a clinically effective intervention?’ we were only able to identify one study that reported sufficient information to allow the calculation of measures of the diagnostic accuracy of Rapid CTP for identifying patients who are suitable candidates for thrombectomy, using treatment received as the reference standard50 and two further observational ‘before and after’ studies34,49 that assessed the effects of implementing RapidAI.
Of further note, two of the multivariable regression analyses described above also reported that low ischaemic core volume, assessed using iSchemaview Rapid CTP, was independently predictive of good clinical outcome (90-day mRS 0–2), adjusted OR 0.98 (95% CI 0.96 to 1.00)113 and adjusted OR 0.98 (95% CI 0.97 to 0.99). 112
This assessment did not identify sufficient evidence to support modelling of the cost-effectiveness of AI-derived software-assisted review of CTP brain scans to guide mechanical thrombectomy treatment decisions for people with an ischaemic stroke, after a CTA brain scan (research question 2b). However, although a systematic review of the effectiveness of treatments outside the scope of this assessment, it is notable that a number of key RCTs conducted in USA82,116,117 and Australia,81 supporting the effectiveness of thrombectomy in addition to IV thrombolysis, for patients with anterior circulation intracranial LVOs, used ischaemic core volume as a component of the participant selection criteria; in all instances, ischaemic core volume on CT was assessed using iSchemaview Rapid CTP, which may perhaps indicate that iSchemaView Rapid software is already widely used for the interpretation of CTP images.
Cost-effectiveness
Our CEA is the most comprehensive analysis to date focusing on AI-derived software-assisted review of CTA brain scans for guiding mechanical thrombectomy treatment decisions for people with an ischaemic stroke. The de novo probabilistic model was based on a previously developed CEA by van Leeuwen et al. 67 For the present analysis, a number of adjustments were made to the model, but most of the assumptions were maintained. The adjustments included adding probabilistic analyses, discount rates in line with NICE reference and the choice of alternative input parameters where this was considered appropriate (e.g. for implementing mortality and health state utility values).
Our initial intention was to inform accuracy estimates in the economic model through a comprehensive, high-quality systematic review of diagnostic accuracy studies. However, the available evidence was not appropriate to inform of CEA, as the accuracy estimates available were for AI-derived software technologies as stand-alone interventions rather than as an adjunct or aid to human interpretation (as defined in the scope for this assessment). To be able to perform a CEA, we obtained accuracy estimates by means of elicitation of expert beliefs. For this, we used an established tool75 that has been validated and that follows established methodological guidance for expert elicitation. 76–78 We obtained responses from five clinical experts representing a range of relevant specialties. Additional parameters were, where necessary, based on a pragmatic literature review. Such a review is standard practice in economic modelling given the large number of parameters required.
As in any economic model, a number of major and minor assumptions had to be made. It is important to understand the impact of these assumptions, to correctly interpret the results of the model. The impact of most assumptions has been explored in sensitivity and scenario analyses. One assumption that might be a matter for discussion is the focus of the AI-derived software-assisted review on triage and supporting the thrombectomy decision, considering the claim that AI-derived software-assisted review could provide a more accurate diagnosis of LVO. Other potential benefits, such as potential reduced time to treatment through the addition of AI-derived software-assisted review were not considered in the base-case analyses. However, in scenario and sensitivity analyses the impact of additional benefits of AI-derived software-assisted review were considered, indicating that this might potentially be an influential assumption warranting further studies (see also discussion of uncertainties in the cost-effectiveness; see Cost-effectiveness).
Finally, another strength of this assessment was the use of R (instead of the commonly used Microsoft Excel®, Microsoft Corporation, Redmond, WA, USA) to estimate the cost-effectiveness. The use of R allows leveraging the benefits of using modern programming languages,70 including improved transparency, reproducibility, modifiability and computational efficiency. The accessibility of R models might be perceived as a barrier for users unfamiliar with programming languages. Therefore, an accessible user interface was provided to the R model through the R Shiny package. Through the R Shiny user interface, users can specify different assumptions, change input parameters values, run underlying R code and visualise results. With the simple instructions provided in the readme file (included with the submission), this model is accessible to those with no programming knowledge allowing critical inquiry from decision-makers and other stakeholders. Moreover, to aid model transparency, as well as model credibility and for consistency with suggested good practices and conventions, the technical implementation of the computational model was inspired by recent work by the Data Analytics Research and Technology in Healthcare group71,72 and others. 73
Uncertainties
Clinical effectiveness
The key question, that is whether or not the addition of AI-derived software technologies can improve the performance of human readers at the decision points specified in the three research questions, and hence improve clinical outcomes for stroke patients, is not adequately addressed by either the diagnostic test accuracy studies or the observational ‘before and after’ studies included in this assessment.
We did not identify any studies that evaluated the diagnostic accuracy of an AI-derived software technology when used as an adjunct to human readers. One study included in this assessment, provided a direct comparison of the accuracy of an AI-derived software technology (Brainomix e-CTA) alone compared with individual human readers with different training and experience, for the detection of LVO (see Table 14). 56 This study found that the sensitivity of e-CTA alone (84.3%, 95% CI 74.0% to 91.0%) was similar to neurology resident 1 (85.7%, 95% CI 75.7% to 92.1%) or lower than neurology resident 2 (91.4%, 95% CI 82.5% to 96.0%), radiology resident (95.7%, 95% CI 88.1% to 98.5%), neuroradiologist (97.1%, 95% CI 90.2% to 99.2%) and that of unassisted human readers. 56 Based on these results, for it to be possible for the AI-derived software technology to improve the performance of human readers there would need to be a systematic difference in the reasons for a false negative (missed LVO) between the AI-derived software technology and human readers such that some or all of the small proportion of LVOs missed by human readers would be detected by the AI-derived software technology. However, it should be noted that it is unclear whether these unfavourable comparative accuracy results are reproducible or generalisable across different AI-derived software technologies and human readers in UK clinical settings; higher sensitivity estimates have been reported, using other AI-derived software technologies alone (Rapid CTA, Viz LVO and Avicennna CINNA LVO), for the detection of LVO (see Research question 2a) and we did not identify any UK studies comparing the accuracy of AI-derived software technologies alone to that of human readers.
The 2018 position statement on AI from the Royal College of Radiologists includes the following text, under the heading of Regulation: ‘A robust regulatory framework for the integration of AI into medical practice needs to be drawn up. Many companies of varying sizes are developing AI tools for use in radiology and clinical oncology. These companies are making claims about the power of these tools - some of which are unsubstantiated’. 14 The position statement goes on to specify, under the heading of Quality Assurance/Governance/Veracity, that: ‘Published results for sensitivity and specificity of AI tools will be necessary prior to the introduction of any technology in the radiology/clinical workflow’. 14 None of the AI-derived software technologies included in this assessment meet this requirement, in that we have not identified any estimates of the sensitivity and specificity (published or unpublished) of these interventions as they would be used in clinical practice (as an adjunct/aid to human interpretation of CT images). Some sensitivity and specificity estimates have been reported for the following AI-derived software technologies, evaluated as stand-alone interventions: Viz ICH and Viz LVO; iSchemaview Rapid CTA, Rapid LVO and Rapid CTP; Brainomix e-ASPECTS and e-CTA; Avicenna CINA LVO. These data are provided in the following sections: Research question 1, Research question 2a and Research question 2b of this report. For the remaining AI-derived software technologies included in the scope for this assessment and described in the section on Intervention technologies in this report, we did not identify any studies that met the inclusion criteria for this assessment.
Seven33,34,43–46,52 of the eight33,34,43–46,49,52 observational ‘before and after’ studies that assessed the effects of implementing AI-derived software technologies, in patients undergoing thrombectomy, reported results indicating that implementation was associated with a reduction in time to intervention. However, no study reported information to suggest that these reductions in time to intervention were associated with improvements in clinical outcome; all six studies that assessed clinical outcome reported results suggesting that the implementation of an AI-derived software technology had no effect on functional outcome, as indicated by mRS. 33,34,45,46,49,52 There is evidence from a meta-analysis of individual patient data118 and a multicentre RCT (the MR CLEAN study)119 to indicate a negative correlation between time to intervention and functional outcome in patients with LVO who undergo thrombectomy. The results of the meta-analysis of individual patient data indicated that earlier treatment with thrombectomy in addition to pharmacological thrombolysis was associated with lower degrees of disability, as indicated by 90-day mRS, than pharmacological thrombolysis alone and that this benefit remained statistically significant up to 7 hours and 18 minutes from onset of symptoms to arterial puncture; each hour of reperfusion delay was associated with a reduction in the proportion of patients achieving function independence (mRS 0–2), absolute risk difference −5.2% (95% CI −8.3% to −2.1%). 118 Similarly, the MR CLEAN study reported that thrombectomy remained an effective intervention, with respect to the proportion of patients achieving functional independence, up to 6 hours and 18 minutes from onset of symptoms to arterial puncture and that the absolute risk difference for achieving a good functional outcome was reduced by 6% for every hour of delay to reperfusion. 119 However, it remains unclear whether the potential reductions in time to intervention that might be achieved as a result of implementing of AI-derived software technologies would translate into improved clinical outcomes in ‘real-world’ settings. In addition, it should be remembered that the implementation of an AI-derived software technology has the potential to change not only the outcomes of patients who undergo thrombectomy but also which patients are selected for thrombectomy. Hence, evidence of a beneficial effect of implementation for patients undergoing thrombectomy is insufficient to show clinical effectiveness. This is because it would remain possible for there to be no effect or a detrimental effect on overall clinical outcomes in the scenario where implementation resulted in more patients who were suitable candidates for thrombectomy being missed (e.g. where an AI-derived software technology misses LVO in the same types of patients as a less experienced human reader and hence provides false reassurance).
The scope for this assessment specified one clinically relevant subgroup: ‘People over the age of 80 years with small-vessel disease and calcification of the cerebrovasculature’. 120 We did not identify any evidence to inform an assessment of the clinical effectiveness of any of the specified AI-derived software technologies in this population.
The inclusion criteria for this assessment (see Table 2) specified an early (last known well within 6 hours) window for research question 2a, on the clinical and cost-effectiveness of AI-derived software technologies for the interpretation of CTA, and a later (last known well more than 6 hours previously, but within 24 hours) window for research question 2b, on the clinical and cost-effectiveness of AI-derived software technologies for the interpretation of CTP following CTA. However, it remains unclear to what extent patients in the early window may benefit from additional imaging (CTP). Randomised controlled trials conducted in the UK85 and in the Netherlands79 in patients with LVO (detected on CTA, MRA or DSA) who were treated within 6 hours of symptom onset (i.e. the population specified for research question 2a; see Table 2), reported absolute differences the proportion of patients who were functionally independent (mRS 0–2) at 90 days of 11%85 and 13.5%79 in favour of thrombectomy. Of note, trials that additionally used utilised ischaemic core volume, assessed using Rapid CTP, as an imaging criterion to select patients for inclusion, within the 6-hour time window specified for research question 2a reported larger absolute differences the proportion of patients who were functionally independent (mRS 0–2) at 90 days of 31%81 and 25%82 in favour of thrombectomy.
It is unclear to what extent the diagnostic accuracy of AI-derived software technologies may vary according to the precise way in which the target condition is defined (e.g. the extent of the arterial anatomy included in the definition of a LVO). In addition, what constitutes a clinically appropriate definition of the target condition LVO may change over time as thrombectomy techniques improve and the evidence base on the efficacy of thrombectomy evolves.
Cost-effectiveness
The cost-effectiveness acceptability curves indicated that, at willingness-to-pay thresholds of £20,000 and £30,000 per QALY gained, the probabilities of current practice with AI being cost-effective were 54% and 56%, respectively. Moreover, the estimated annual risks associated with the addition of AI were estimated to be £7.0 million and £8.4 million, at willingness-to-pay thresholds of £20,000 and £30,000 per QALY gained, respectively. To reduce these risks, further evidence on the sensitivity of both technologies was considered as most important. This is particularly relevant given that the current accuracy estimates were based on expert elicitation (since empirical evidence was lacking for AI-derived software technologies as an adjunct/aid to human readers) that would require confirmation. In addition, sensitivity analyses indicated that in case the addition of AI resulted in a reduced time to treatment thereby increasing the proportion of patients with LVO who are eligible for mechanical thrombectomy, this would be an important outcome to consider in future studies. In that case, the clinical consequences (e.g. in terms of distribution over mRS states) of the reduced time to treatment through the addition of AI are an important consideration. The current base-case assessment did not consider any consequences of potentially reduced time to treatment through AI as this claim was not supported by available evidence. First, it is unclear whether the addition of AI would indeed reduce time to treatment: in the only studies where a reduction in time to treatment was observed, it was unclear whether this was potentially caused by redesign/optimisation of the logistic process. Caution is needed in interpreting these studies, as such findings are likely heavily context dependent and rely on the exact implementation of the addition of AI (e.g. implementation with automated alert system). Hence, the optimal implementation and place of AI is a potentially relevant topic for research. Notably, scenario analyses using alternative accuracy estimates for care as usual without AI, indicated that AI might be especially useful for non-expert graders, but this requires confirmation in future studies. Second, it is unclear whether the addition of AI would reduce the time to treatment and what the consequences would be in terms of impact on clinical outcomes such as distribution between mRS states. Moreover, from a cost perspective more evidence regarding the additional costs of the AI technology, and costs related to mRS4 and mRS5 would be informative.
Chapter 6 Conclusions
Implications for service provision
The available evidence is not suitable to determine the clinical effectiveness of using AI-derived software to support the review of CT brain scans in acute stroke in the NHS setting.
All studies that assessed the diagnostic accuracy of AI-derived software technologies evaluated these technologies as stand-alone interventions, rather than as an adjunct or aid to image interpretation by a healthcare professional (i.e. not as AI-derived software technologies would be used in clinical practice, as their use is recommended by the manufacturers and as specified in the inclusion criteria for this assessment).
In addition to diagnostic test accuracy studies, this assessment included some observational ‘before and after’ studies that assessed the effects of implementing AI-derived software technologies in ‘real-world’ clinical settings. The information provided by studies of this type was limited in that it concerned only treated (i.e. test positive) patients; no information was provided about test-negative patients and hence there was no information about the extent to which AI-derived software technologies, as implemented, may miss patients with the target condition(s).
The economic analyses did not provide evidence to prefer the AI-derived software strategy over current clinical practice. However, results indicated that if the addition of AI-derived software-assisted review for guiding mechanical thrombectomy treatment decisions increased the sensitivity of the diagnostic pathway (i.e. reduced the proportion of undetected LVOs), then this may be considered cost-effective. Nevertheless, the sensitivity of AI-derived software-assisted review when added to current clinical practice is largely uncertain and likely depends on the implementation of AI-derived software-assisted review.
Suggested research priorities
Given the deficiencies in the evidence base, outlined in the section on Implications for service provision, large, preferably multicentre, studies are needed (for all AI-derived software technologies) that evaluate these technologies as they would be implemented in clinical practice.
Cross-sectional test accuracy studies should evaluate the performance of AI-derived software technologies, when used as an adjunct/aid to human readers. Ideally, such studies should compare the performance of the AI-derived software technology in combination with a human reader with that of the human reader alone, where interpretation by an experienced expert or panel of experts provides the reference standard. Studies should be conducted in the population and setting in which the AI-derived software technology would be applied in practice (e.g. for the interpretation of CTA to select patients for thrombectomy, studies should be conducted in adults with confirmed AIS who were last known to be well within the past 6 hours). Studies of this type would allow assessment of whether and to what extent the addition of AI-derived software technologies changes the performance of human readers in the relevant clinical context.
Observational studies evaluating the effects of implementing AI-derived software technologies in UK clinical settings may also be of interest. Again, the precise way in which the technologies are implemented is critical to the utility of such studies for UK decision-making. Based on the scope defined for this assessment, AI-derived software technologies would need to be implemented as a real-time adjunct/aid to human readers and not as, for example, an automated early alert system. Observational comparative studies provide a lower level of evidence with respect to the effects of an intervention than RCTs. Where observational study designs are used to provide estimates of effect, it is therefore important to control, as far as possible, for potential confounding factors (factors other than the AI-derived software technology that may affect the outcome or outcomes being assessed), for example, by matching participants in the intervention and comparator groups on key risk factors. It is also important that the care pathway remains unchanged, other than with respect to the implementation of the AI-derived software technology. Studies of the effects of implementation of AI-derived software technologies should measure clinical outcomes alongside intermediate outcomes such as time to intervention and should report outcomes for test negative as well as test positive patients (e.g. for the interpretation of CTA to select patients for thrombectomy, outcomes should be reported for both patients who received thrombectomy and those who did not).
Cluster RCTs, where stroke centres are randomised to implement AI-derived software technologies or to continue with current practice, would offer a more methodologically robust approach to evaluating the effects of implementation.
Finally, implementations of AI-derived software technologies other than as specified in the scope for this assessment (e.g. AI-derived software technologies used as stand-alone early alert systems used to select images/patients for further consideration by a human reader, or the potential of AI-derived parameters taken from initial NCCT imaging to predict clinical outcome following thrombectomy and hence the potential utility of these parameters to select patients for thrombectomy) may warrant consideration and further research.
Acknowledgements
The authors would like to thank Pawel Posadzki, Edyta Ryczek and Steve Ryder for their contributions to the inclusion screening stage of the systematic review. We would also like to thank all of the clinical specialist members of the NICE diagnostic appraisal committee for this topic for their assistance and expert input throughout this project, and Dr Carole Gavin (Consultant in Emergency Medicine), Mr Jonathan Shapey (Senior Clinical Lecturer and Honorary Consultant Neurosurgeon), Dr Grant Mair (Stroke Association Senior Clinical Lecturer and Honorary Consultant Neuroradiologist), Dr Tim England (Clinical Associate Professor/Honorary Consultant Stroke Physician) and Prof David Werring (Honorary Consultant Neurologist) for their participation in our expert elicitation process. Finally, the authors would like to thank the lay members of the NICE diagnostics advisory committee and assessment subgroup for providing input on the patients’ perspective at key stages of the assessment process.
The views expressed in this report are those of the authors and not necessarily those of the NIHR HTA Programme. Any errors are the responsibility of the authors.
Contributions of authors
Marie Westwood (https://orcid.org/0000-0002-6257-0653) planned and performed the systematic review and interpretation of evidence, and provided senior advice and support to the systematic review.
Bram Ramaekers (https://orcid.org/0000-0001-5785-9228) planned and performed the cost-effectiveness analyses and interpreted the results.
Sabine Grimm (https://orcid.org/0000-0002-2175-7999) planned and performed the cost-effectiveness analyses and interpreted the results.
Nigel Armstrong (https://orcid.org/0000-0002-7443-4798) planned and performed the systematic review and interpretation of evidence, contributed to the planning and interpretation of the cost-effectiveness analyses, acquisition of input data and conducted the model peer review.
Ben Wijnen (https://orcid.org/0000-0001-7993-1905) planned and performed the cost-effectiveness analyses and interpreted the results.
Charlotte Ahmadu (https://orcid.org/0000-0001-6420-9071) planned and performed the systematic review and interpretation of evidence, contributed to the planning and interpretation of the cost-effectiveness analyses, acquisition of input data and conducted the model peer review.
Shelley de Kock (https://orcid.org/0000-0002-3143-806X) devised and performed the literature searches and provided information support to the project.
Caro Noake (https://orcid.org/0000-0003-0329-4772) devised and performed the literature searches and provided information support to the project.
Manuela Joore (https://orcid.org/0000-0002-5649-6768) provided senior advice and support to the cost-effectiveness analyses.
All parties were involved in drafting and/or commenting on the report.
Ethics statement
This report concerns secondary research, for which ethics approval is not required.
Data-sharing statement
Requests for access to data should be addressed to the corresponding author.
Disclaimers
This manuscript presents independent research funded by the National Institute for Health and Care Research (NIHR). The views and opinions expressed by authors in this publication are those of the authors and do not necessarily reflect those of the NHS, the NIHR, the HTA programme or the Department of Health and Social Care. If there are verbatim quotations included in this publication the views and opinions expressed by the interviewees are those of the interviewees and do not necessarily reflect those of the authors, those of the NHS, the NIHR, the HTA programme or the Department of Health and Social Care.
References
- National Institute for Health and Care Excellence . Stroke in Adults. NICE Quality Standard QS2 2016. www.nice.org.uk/guidance/qs2 (accessed 25 May 2021).
- Campbell BCV, Khatri PS. Stroke. Lancet 2020;396:129-42.
- Sentinel Stroke National Audit Programme . SSNAP Annual Portfolio for April 2019–March 2020 Admissions and Discharges: National Results 2020. www.strokeaudit.org/Results2/Clinical-audit/National-Results.aspx (accessed 25 May 2021).
- Sentinel Stroke National Audit Programme . Springboard for Progress: The Seventh SSNAP Annual Report. Stroke Care Received for Patients Admitted to Hospital Between April 2019 to March 2020 2020. www.strokeaudit.org/Results2/Clinical-audit/National-Results.aspx (accessed 25 May 2021).
- Stroke Association . Stroke Statistics n.d. www.stroke.org.uk/what-is-stroke/stroke-statistics#Stroke%20prevalence%20in%20England (accessed 25 May 2021).
- Hurford R, Sekhar A, Hughes TAT, Muir KW. Diagnosis and management of acute ischaemic stroke. Pract Neurol 2020;20:304-16.
- National Institute for Health and Care Excellence . Stroke and Transient Ischaemic Attack in Over 16s: Diagnosis and Initial Management 2019. www.nice.org.uk/guidance/ng128 (accessed 25 May 2021).
- Nor AM, Davis J, Sen B, Shipsey D, Louw SJ, Dyker AG, et al. The Recognition of Stroke in the Emergency Room (ROSIER) scale: development and validation of a stroke recognition instrument. Lancet Neurol 2005;4:727-34.
- Wang C, Wang W, Ji J, Wang J, Zhang R, Wang Y. Safety of intravenous thrombolysis in stroke of unknown time of onset: a systematic review and meta-analysis. J Thromb Thrombolysis 2021;52:1173-81.
- National Institute of Neurological Disorders and Stroke rt-PA Stroke Study Group . Tissue plasminogen activator for acute ischaemic stroke. N Engl J Med 1995;333:1581-7.
- Tawil SE, Muir KW. Thrombolysis and thrombectomy for acute ischaemic stroke. Clin Med (Lond) 2017;17:161-5.
- Goyal M, Menon BK, van Zwam WH, Dippel DW, Mitchell PJ, Demchuk AM, et al. HERMES collaborators . Endovascular thrombectomy after large-vessel ischaemic stroke: a meta-analysis of individual patient data from five randomised trials. Lancet 2016;387:1723-31.
- Vidale S, Romoli M, Clemente Agostoni E. Mechanical thrombectomy with or without thrombolysis: a meta-analysis of RCTs. Acta Neurol Scand 2021;143:554-7.
- Royal College of Radiologists . RCR Position Statement on Artificial Intelligence 2018. www.rcr.ac.uk/posts/rcr-position-statement-artificial-intelligence#:~:text=The%20RCR%20believes%20that%20AI,technologies%20to%20enhance%20clinical%20practice (accessed 25 May 2021).
- Royal College of Radiologists . Integrating Artificial Intelligence With the Radiology Reporting Workflows (RIS and PACS) 2021. www.rcr.ac.uk/publication/integrating-artificial-intelligence-radiology-reporting-workflows-ris-and-pacs (accessed 21 May 2021).
- National Institute for Health and Care Excellence . NICE Medtech Innovation Briefing MIB207. Artificial Intelligence for Analysing CT Brain Scans 2020. www.nice.org.uk/guidance/mib207 (accessed 25 May 2021).
- NHS England . The NHS Long Term Plan 2019. www.longtermplan.nhs.uk (accessed 22 June 2021).
- NHS England . National Stroke Service Model: Integrated Stroke Delivery Networks 2021. www.england.nhs.uk/publication/national-stroke-service-model-integrated-stroke-delivery-networks (accessed 22 June 2021).
- National Institute for Health and Care Excellence . Technology Appraisal Guidance TA264. Alteplase for Treating Acute Ischaemic Stroke 2012. www.nice.org.uk/guidance/ta264 (accessed 24 May 2021).
- National Institute for Health and Care Excellence . Interventional Procedures Guidance IPG548. Mechanical Clot Retrieval for Treating Acute Ischaemic Stroke 2016. www.nice.org.uk/guidance/ipg548 (accessed 25 May 2021).
- Mechanical Thrombectomy for Acute Ischaemic Stroke: An Implementation Guide for the UK. Oxford: Oxford Academic Health Science Network; 2019.
- Centre for Reviews and Dissemination . Systematic Reviews: CRD’s Guidance for Undertaking Reviews in Health Care 2009. www.york.ac.uk/inst/crd/SysRev/!SSL!/WebHelp/SysRev3.htm (accessed 23 March 2021).
- National Institute for Health and Care Excellence . Diagnostics Assessment Programme Manual 2011. www.nice.org.uk/about/what-we-do/our-programmes/nice-guidance/nice-diagnostics-guidance (accessed 25 May 2021).
- Cochrane Diagnostic Test Accuracy Working Group . Cochrane Handbook for Systematic Reviews of Diagnostic Test Accuracy 2009. https://methods.cochrane.org/sdt/handbook-dta-reviews (accessed 25 March 2021).
- McGowan J, Sampson M, Lefebvre C. An evidence based checklist for the peer review of electronic search strategies (PRESS EBC). Evid Based Libr Inf Pract 2010;5:1-6.
- Cold Spring Harbor Laboratory, BMJ, Yale University . MedRxiv n.d. www.medrxiv.org (accessed 29 September 2021).
- Whiting PF, Rutjes AWS, Westwood ME, Mallett S, Deeks JJ, Reitsma JB, et al. QUADAS-2 Group . QUADAS-2: a revised tool for the quality assessment of diagnostic accuracy studies. Ann Intern Med 2011;155:529-36.
- Reitsma JB, Glas AS, Rutjes AWS, Scholten RJPM, Bossuyt PMM, Zwinderman AH. Bivariate analysis of sensitivity and specificity produces informative summary measures in diagnostic reviews. J Clin Epidemiol 2005;58:982-90.
- Harbord RM, Whiting P, Sterne JA, Egger M, Deeks JJ, Shang A, et al. An empirical comparison of methods for meta-analysis of diagnostic accuracy showed hierarchical models are necessary. J Clin Epidemiol 2008;61:1095-103.
- Harbord RM, Deeks JJ, Egger M, Whiting P, Sterne JA. A unification of models for meta-analysis of diagnostic accuracy studies. Biostatistics 2007;8:239-51.
- Acharya UR, Sree SV, Mookiah MR, Saba L, Gao H, Mallarini G, et al. Computed tomography carotid wall plaque characterization using a combination of discrete wavelet transform and texture features: a pilot study. Proc Inst Mech Eng H 2013;227:643-54.
- Cirio JJ, Ciardi C, Buezas M, Diluca P, Caballero ML, Lopez M, et al. Implementation of artificial intelligence in hyperacute arterial reperfusion treatment in a comprehensive stroke center. Neurol Argent 2021;13:212-20. https://doi.org/10.1016/j.neuarg.2021.07.003.
- Adhya J, Li C, Eisenmenger L, Cerejo R, Tayal A, Goldberg M, et al. Positive predictive value and stroke workflow outcomes using automated vessel density (RAPID-CTA) in stroke patients: one year experience. Neuroradiol J 2021;34:476-81. https://dx.doi.org/10.1177/19714009211012353.
- Al-Kawaz M, Primiani C, Urrutia V, Hui F. Impact of RapidAI mobile application on treatment times in patients with large-vessel occlusion. J Neurointerv Surg 2022;14:233-6.
- Amukotuwa SA, Straka M, Dehkharghani S, Bammer R. Fast automatic detection of large-vessel occlusions on CT angiography. Stroke 2019;50:3431-8.
- Amukotuwa SA, Straka M, Smith H, Chandra RV, Dehkharghani S, Fischbein NJ, et al. Automated detection of intracranial large-vessel occlusions on computed tomography angiography: a single center experience. Stroke 2019;50:2790-8.
- Barreira CM, Bouslama M, Haussen DC, Grossberg JA, Baxter B, Devlin T, et al. Automated large artery occlusion detection in stroke imaging-aladin study. Presented at American Heart Association/American Stroke Association 2018 International Stroke Conference and State-of-the-Science Stroke Nursing Symposium; 24–26 Jan 2018; Los Angeles. Stroke 2018;49.
- Rodrigues GM, Barreira C, Froehler M, Baxter B, Devlin T, Lim J, et al. Multicenter ALADIN: automated large artery occlusion detection in stroke imaging using artificial intelligence. Presented at the International Stroke Conference; 6–8 February 2019; Honolulu (HI). Stroke 2019;50.
- Barreira CM, Rahman HA, Bouslama M, Al-Bayati AR, Haussen DC, Grossberg JA, et al. Advance study: automated detection and volumetric assessment of intracerebral hemorrhage. Eur Stroke J 2018;3.
- Chatterjee A, Johnson C, Harvin A, Mullin P. Artificial intelligence detection of cerebrovascular large-vessel occlusion - VIZ algorithm diagnostic accuracy and clinical notification times in a retrospective evaluation n.d.
- Dehkharghani S, Lansberg M, Venkatsubramanian C, Cereda C, Lima F, Coelho H, et al. High-performance automated anterior circulation CT angiographic clot detection in acute stroke: a multireader comparison. Radiology 2021;298:665-70.
- Dehkharghani S, Lansberg MG, Venkatsubramanian C, Cereda CW, Lima FO, Coelho H, et al. Rapid-LVO for automated detection of intracranial large-vessel occlusion in CT angiography of the brain. Presented at International Stroke Conference Virtual; 17–19 March 2021. Stroke 2021;52.
- Dornbos D, Hoit D, Inoa-Acosta V, Nickele C, Arthur A, Elijovich L. Automated large-vessel occlusion by artificial intelligence improves stroke workflow metrics: 1st 100 patient experience in a hub and spoke stroke system. J Neurointerv Surg 2020;12.
- Gunda B, Sipos I, Stang R, Bojti P, Kis B, Harston G. Improved stroke care in a primary stroke centre using aidecision support. Presented at 12th World Stroke Congress; 12–15 May 2020; Vienna (Austria). Int J Stroke 2020;15.
- Hassan AE, Ringheanu VM, Preston L, Tekle W. CSC implementation of artificial intelligence software significantly improves door-in to groin puncture time interval and recanalization rates. Poster presented at International Stroke Conference Virtual; 17–19 March 2021. Stroke 2021;52.
- Hassan AE, Ringheanu VM, Rabah RR, Preston L, Tekle WG, Qureshi AI. Early experience utilizing artificial intelligence shows significant reduction in transfer times and length of stay in a hub and spoke model. Interv Neuroradiol 2020;26:615-22.
- Hassan AE, Ringheanu VM, Preston L, Tekle W. The implementation of artificial intelligence significantly reduces door-in door-out times in primary care center prior to transfer. Presented at the International Stroke Conference Virtual; 17–19 March 2021. Stroke 2021;52.
- Herweh C, Mokli Y, Bellot P, Schmitt N, Joly O, Weyland C, et al. AI-based automated detection of intracranial hemorrhage on nonenhanced CT scans. Presented at 12th World Stroke Congress; 12–15 May 2020; Vienna (Austria). Int J Stroke 2020;15.
- Kamal H, Abdelhamid N, Zhu L, Sarraj A. Does RAPID reduce groin puncture times in acute ischaemic stroke? Presented at International Stroke Conference; 21–24 February 2017; Houston (TX). Stroke 2017;48.
- Kauw F, Heit JJ, Martin BW, van Ommen F, Kappelle LJ, Velthuis BK, et al. Computed tomography perfusion data for acute ischaemic stroke evaluation using rapid software: pitfalls of automated postprocessing. J Comput Assist Tomogr 2020;44:75-7.
- McLouth J, Elstrott S, Chaibi Y, Quenet S, Chang PD, Chow DS, et al. Validation of a deep learning tool in the detection of intracranial hemorrhage and large-vessel occlusion. Front Neurol 2021;12.
- Morey JR, Zhang X, Yaeger KA, Fiano E, Marayati NF, Kellner CP, et al. Real-world experience with artificial intelligence-based triage in transferred large-vessel occlusion stroke patients. Cerebrovasc Dis 2021;50:450-5. https://doi.org/10.1159/000515320.
- Morey JR, Fiano E, Yaeger KA, Zhang X, Fifi JT. Impact of Viz LVO on time-to-treatment and clinical outcomes in large-vessel occlusion stroke patients presenting to primary stroke centers. MedRxiv 2020. https://doi.org/10.1101/2020.07.02.20143834.
- Morey J, Zhang X, Yaeger K, Fiano E, Marayati NF, Kellner CP, et al. Initial real-world experience with VIZ LVO in transferred large-vessel occlusion stroke patients. Poster presented at International Stroke Conference Virtual; 17–19 March 2021. Stroke 2021;52.
- Paz D, Yagoda D, Wein T. Single site performance of AI software for stroke detection and triage. MedRxiv 2021. https://doi.org/10.1101/2021.04.02.21253083.
- Seker F, Pfaff JAR, Mokli Y, Berberich A, Namias R, Gerry S, et al. Diagnostic accuracy of automated occlusion detection in CT angiography using e-CTA [published online ahead of print]. Int J Stroke 2021. https://doi.org/10.1177%2F1747493021992592 (accessed 30 July 2021).
- Seker F, Pfaff J, Herweh C, Berberich A, Mokli Y, Mohlenbruch M, et al. Automatic detection of large-vessel occlusion on CTA in acute ischaemic stroke using AI. Frankfurt Am. Clin Neuroradiol 2019;29.
- Seker F, Pfaff J, Moehlenbruch M, Gerry S, Ringleb P, Nagel S, et al. Automatic detection of large-vessel occlusion on CTA in acute ischaemic stroke. Presented at the 5th European Stroke Organisation Conference (ESOC); 22–24 May 2019; Milan (Italy). Eur Stroke J 2019;4:413-4.
- Yahav-Dovrat A, Saban M, Merhav G, Lankri I, Abergel E, Eran A, et al. Evaluation of artificial intelligence-powered identification of large-vessel occlusions in a comprehensive stroke center. AJNR Am J Neuroradiol 2021;42:247-54.
- Barreira C, Bouslama M, Lim J, Al-Bayati A, Saleem Y, Devlin T, et al. E-108 Aladin study: automated large artery occlusion detection in stroke imaging study – a multicenter analysis. J Neurointerv Surg 2018;10.
- Shalitin O, Sudry N, Mates J, Golan D. AI-powered stroke triage system performance in the wild. J Exp Stroke Transl Med 2020;12:1-4.
- Mair G, White P, Bath PM, Muir KW, Al-Shahi Salman R, Martin C, et al. External validation of Artificial Intelligence software to interpret brain CT in patients with acute stroke. The Real-world Independent Testing of e-ASPECTS Software Study (RITeS) 2021.
- Lijmer JG, Mol BW, Heisterkamp S, Bonsel GJ, Prins MH, van der Meulen JH, et al. Empirical evidence of design-related bias in studies of diagnostic tests. JAMA 1999;282:1061-6.
- Whiting PF, Smidt N, Sterne JA, Harbord R, Burton A, Burke M, et al. Systematic review: accuracy of anti-citrullinated peptide antibodies for diagnosing rheumatoid arthritis. Ann Intern Med 2010;152:456-64.
- Román LS, Menon BK, Blasco J, Hernández-Pérez M, Dávalos A, Majoie CBLM, et al. HERMES Collaborators . Imaging features and safety and efficacy of endovascular stroke treatment: a meta-analysis of individual patient-level data. Lancet Neurol 2018;17:895-904.
- Kaltenthaler E, Tappenden P, Paisley S, Squires H. Identifying and Reviewing Evidence to Inform the Conceptualisation and Population of Cost-effectiveness Models. Sheffield: NICE Decision Support Unit; 2011.
- van Leeuwen KG, Meijer FJA, Schalekamp S, Rutten MJCM, van Dijk EJ, van Ginneken B, et al. Cost-effectiveness of artificial intelligence aided vessel occlusion detection in acute stroke: an early health technology assessment. Insights Imaging 2021;12.
- Drummond MF, Jefferson TO. Guidelines for authors and peer reviewers of economic submissions to the BMJ. The BMJ economic evaluation working party. BMJ 1996;313:275-83.
- Hart R, Burns D, Ramaekers B, Ren S, Gladwell D, Sullivan W, et al. R and Shiny for cost-effectiveness analyses: why and when? A hypothetical case study. PharmacoEconomics 2020;38:765-76.
- Incerti D, Thom H, Baio G, Jansen JP. R you still using excel? The advantages of modern software tools for health technology assessment. Value Health 2019;22:575-9.
- Alarid-Escudero F, Krijkamp EM, Pechlivanoglou P, Jalal H, Kao SZ, Yang A, et al. A need for change! A coding framework for improving transparency in decision modeling. PharmacoEconomics 2019;37:1329-39.
- Alarid-Escudero F, Krijkamp E, Enns EA, Yang A, Hunink MGM, Pechlivanoglou P, et al. Med Decision-Making 2022;43:3-20.
- Smith R, Schneider P. Making health economic models Shiny: a tutorial. Wellcome Open Res 2020;5.
- McMeekin P, White P, James MA, Price CI, Flynn D, Ford GA. Estimating the number of UK stroke patients eligible for endovascular thrombectomy. Eur Stroke J 2017;2:319-26.
- Grigore B, Peters J, Hyde C, Stein K. EXPLICIT: a feasibility study of remote expert elicitation in health technology assessment. BMC Med Inform Decis Mak 2017;17.
- Bojke L, Claxton K, Bravo-Vergel Y, Sculpher M, Palmer S, Abrams K. Eliciting distributions to populate decision analytic models. Value Health 2010;13:557-64.
- O’Hagan A, Buck CE, Daneshkhah A, Eiser JR, Garthwaite PH, Jenkinson DJ, et al. Uncertain Judgements: Eliciting Experts’ Probabilities. Hoboken, NJ: Wiley; 2016.
- Bojke L, Grigore B, Jankovic D, Peters J, Soares M, Stein K. Informing reimbursement decisions using cost-effectiveness modelling: a guide to the process of generating elicited priors to capture model uncertainties. PharmacoEconomics 2017;35:867-77.
- Berkhemer OA, Fransen PS, Beumer D, van den Berg LA, Lingsma HF, Yoo AJ, et al. MR CLEAN Investigators . A randomized trial of intraarterial treatment for acute ischaemic stroke. N Engl J Med 2015;372:11-20.
- Goyal M, Demchuk AM, Menon BK, Eesa M, Rempel JL, Thornton J, et al. ESCAPE Trial Investigators . Randomized assessment of rapid endovascular treatment of ischaemic stroke. N Engl J Med 2015;372:1019-30.
- Campbell BC, Mitchell PJ, Kleinig TJ, Dewey HM, Churilov L, Yassi N, et al. EXTEND-IA Investigators . Endovascular therapy for ischaemic stroke with perfusion-imaging selection. N Engl J Med 2015;372:1009-18.
- Saver JL, Goyal M, Bonafe A, Diener HC, Levy EI, Pereira VM, et al. SWIFT PRIME Investigators . Stent-retriever thrombectomy after intravenous t-PA vs. t-PA alone in stroke. N Engl J Med 2015;372:2285-95.
- Jovin TG, Chamorro A, Cobo E, de Miquel MA, Molina CA, Rovira A, et al. REVASCAT Trial Investigators . Thrombectomy within 8 hours after symptom onset in ischaemic stroke. N Engl J Med 2015;372:2296-306.
- Bracard S, Ducrocq X, Mas JL, Soudant M, Oppenheim C, Moulin T, et al. THRACE Investigators . Mechanical thrombectomy after intravenous alteplase versus alteplase alone after stroke (THRACE): a randomised controlled trial. Lancet Neurol 2016;15:1138-47.
- Muir KW, Ford GA, Messow CM, Ford I, Murray A, Clifton A, et al. PISTE Investigators . Endovascular therapy for acute ischaemic stroke: the Pragmatic Ischaemic Stroke Thrombectomy Evaluation (PISTE) randomised, controlled trial. J Neurol Neurosurg Psychiatry 2017;88:38-44.
- Mac Grory B, Saldanha IJ, Mistry EA, Stretz C, Poli S, Sykora M, et al. Thrombolytic therapy for ‘wake-up stroke’: a systematic review and meta-analysis. Eur J Neurol 2021;28:2006-16.
- Karaszewski B, Wyszomirski A, Jablonski B, Werring DJ, Tomaka D. Efficacy and safety of intravenous rtPA in ischaemic strokes due to small-vessel occlusion: systematic review and meta-analysis. Transl Stroke Res 2021;12:406-15.
- Lan L, Rong X, Li X, Zhang X, Pan J, Wang H, et al. Reperfusion therapy for minor stroke: a systematic review and meta-analysis. Brain Behav 2019;9.
- Chen X, Shen Y, Huang C, Geng Y, Yu Y. Intravenous thrombolysis with 0.9 mg/kg alteplase for acute ischaemic stroke: a network meta-analysis of treatment delay. Postgrad Med J 2020;96:680-5.
- Choi JC, Jang MU, Kang K, Park JM, Ko Y, Lee SJ, et al. Comparative effectiveness of standard care with IV thrombolysis versus without IV thrombolysis for mild ischaemic stroke. J Am Heart Assoc 2015;4.
- Paek YM, Lee JS, Park HK, Cho YJ, Bae HJ, Kim BJ, et al. Intravenous thrombolysis with tissue-plasminogen activator in small vessel occlusion. J Clin Neurosci 2019;64:134-40.
- Lobotesis K, Veltkamp R, Carpenter IH, Claxton LM, Saver JL, Hodgson R. Cost-effectiveness of stent-retriever thrombectomy in combination with IV t-PA compared with IV t-PA alone for acute ischaemic stroke in the UK. J Med Econ 2016;19:785-94.
- Pennlert J, Eriksson M, Carlberg B, Wiklund PG. Long-term risk and predictors of recurrent stroke beyond the acute phase. Stroke 2014;45:1839-41.
- Mohan KM, Wolfe CD, Rudd AG, Heuschmann PU, Kolominsky-Rabas PL, Grieve AP. Risk and cumulative risk of stroke recurrence: a systematic review and meta-analysis. Stroke 2011;42:1489-94.
- Slot KB, Berge E, Sandercock P, Lewis SC, Dorman P, Dennis M. Oxfordshire Community Stroke Project . Causes of death by level of dependency at 6 months after ischaemic stroke in 3 large cohorts. Stroke 2009;40:1585-9.
- Office for National Statistics . National Life Tables: UK 2018–2020 2021. www.ons.gov.uk/peoplepopulationandcommunity/birthsdeathsandmarriages/lifeexpectancies/datasets/nationallifetablesunitedkingdomreferencetables (accessed 9 December 2021).
- Rethnam V, Bernhardt J, Dewey H, Moodie M, Johns H, Gao L, et al. AVERT Trial Collaboration Group . Utility-weighted modified Rankin Scale: still too crude to be a truly patient-centric primary outcome measure?. Int J Stroke 2020;15:268-77.
- Rivero-Arias O, Ouellet M, Gray A, Wolstenholme J, Rothwell PM, Luengo-Fernandez R. Mapping the modified Rankin scale (mRS) measurement into the generic EuroQol (EQ-5D) health outcome. Med Decis Making 2010;30:341-54.
- Dijkland SA, Voormolen DC, Venema E, Roozenbeek B, Polinder S, Haagsma JA, et al. MR CLEAN Investigators . Utility-weighted modified Rankin Scale as primary outcome in stroke trials: a simulation study. Stroke 2018;49:965-71.
- Chaisinanunkul N, Adeoye O, Lewis RJ, Grotta JC, Broderick J, Jovin TG, et al. DAWN Trial and MOST Trial Steering Committees . Adopting a patient-centered approach to primary outcome analysis of acute stroke trials using a utility-weighted modified Rankin scale. Stroke 2015;46:2238-43.
- Ali M, MacIsaac R, Quinn TJ, Bath PM, Veenstra DL, Xu Y, et al. Dependency and health utilities in stroke: data to inform cost-effectiveness analyses. Eur Stroke J 2017;2:70-6.
- Hong KS, Saver JL. Quantifying the value of stroke disability outcomes: WHO global burden of disease project disability weights for each level of the modified Rankin Scale. Stroke 2009;40:3828-33.
- Rebchuk AD, O’Neill ZR, Szefer EK, Hill MD, Field TS. Health utility weighting of the modified rankin scale: a systematic review and meta-analysis. JAMA Netw Open 2020;3.
- Wang X, Moullaali TJ, Li Q, Berge E, Robinson TG, Lindley R, et al. Utility-weighted modified rankin scale scores for the assessment of stroke outcome: pooled analysis of 20 000+ patients. Stroke 2020;51:2411-7.
- Janssen MF, Szende A, Cabases J, Ramos-Goñi JM, Vilagut G, König HH. Population norms for the EQ-5D-3L: a cross-country analysis of population surveys for 20 countries. Eur J Health Econ 2019;20:205-16.
- Bragg S, Paley L, Kavanagh M, McCurran V, Hoffman A, Rudd A. Sentinel Stroke National Audit Programme (SSNAP) Clinical Audit August 2017–November 2017 Public Report 2018. www.strokeaudit.org/Documents/National/Clinical/AugNov2017/AugNov2017-PublicReport.aspx (accessed 7 December 2021).
- Personal Social Services Research Unit . Unit Costs of Health and Social Care 2014 2014. www.pssru.ac.uk/project-pages/unitcosts/2014 (accessed 22 June 2023).
- Patel A, Berdunov V, Quayyum Z, King D, Knapp M, Wittenberg R. Estimated societal costs of stroke in the UK based on a discrete event simulation. Age Ageing 2020;49:270-6.
- Luengo-Fernandez R, Yiin GS, Gray AM, Rothwell PM. Population-based study of acute- and long-term care costs after stroke in patients with AF. Int J Stroke 2013;8:308-14.
- Whiting P, Westwood M, Beynon R, Burke M, Sterne JA, Glanville J. Inclusion of methodological filters in searches for diagnostic test accuracy studies misses relevant studies. J Clin Epidemiol 2011;64:602-7.
- Deeks JJ, Macaskill P, Irwig L. The performance of tests of publication bias and other sample size effects in systematic reviews of diagnostic test accuracy was assessed. J Clin Epidemiol 2005;58:882-93.
- Bouslama M, Ravindran K, Harston G, Rodrigues GM, Pisani L, Haussen DC, et al. Noncontrast computed tomography e-Stroke infarct volume is similar to RAPID computed tomography perfusion in estimating postreperfusion infarct volumes. Stroke 2021;52:634-41.
- Demeestere J, Scheldeman L, Cornelissen SA, Heye S, Wouters A, Dupont P, et al. Alberta stroke program early CT score versus computed tomographic perfusion to predict functional outcome after successful reperfusion in acute ischaemic stroke. Stroke 2018;49:2361-7.
- Pfaff J, Herweh C, Schieber S, Schonenberger S, Bosel J, Ringleb PA, et al. e-ASPECTS correlates with and is predictive of outcome after mechanical thrombectomy. AJNR Am J Neuroradiol 2017;38:1594-9.
- Olive-Gadea M, Martins N, Boned S, Carvajal J, Moreno MJ, Muchada M, et al. Baseline ASPECTS and e-ASPECTS correlation with infarct volume and functional outcome in patients undergoing mechanical thrombectomy. J Neuroimaging 2019;29:198-202.
- Albers GW, Marks MP, Kemp S, Christensen S, Tsai JP, Ortega-Gutierrez S, et al. DEFUSE 3 Investigators . Thrombectomy for stroke at 6 to 16 hours with selection by perfusion imaging. N Engl J Med 2018;378:708-18.
- Nogueira RG, Jadhav AP, Haussen DC, Bonafe A, Budzik RF, Bhuva P, et al. DAWN Trial Investigators . Thrombectomy 6 to 24 hours after stroke with a mismatch between deficit and infarct. N Engl J Med 2018;378:11-2.
- Saver JL, Goyal M, van der Lugt A, Menon BK, Majoie CB, Dippel DW, et al. HERMES Collaborators . Time to treatment with endovascular thrombectomy and outcomes from ischaemic stroke: a meta-analysis. JAMA 2016;316:1279-88.
- Fransen PS, Berkhemer OA, Lingsma HF, Beumer D, van den Berg LA, Yoo AJ, et al. Multicenter Randomized Clinical Trial of Endovascular Treatment of Acute Ischaemic Stroke in the Netherlands Investigators . Time to reperfusion and treatment effect for acute ischaemic stroke: a randomized clinical trial. JAMA Neurol 2016;73:190-6.
- National Institute for Health and Care Excellence . Software With Artificial Intelligence Derived Algorithms for Analysing CT Brain Scans in People With a Suspected Acute Stroke: Final Scope 2021. www.nice.org.uk/guidance/gid-dg10044/documents/final-scope (accessed 12 August 2021).
- Abdelkhaleq R, Lopez-Rivera V, Salazar-Marioni S, Lee S, Kim Y, Giancardo L, et al. Optimizing predictions of infarct core using machine learning. Presented at International Stroke Conference Virtual; 17–19 March 2021. Stroke 2021;52.
- Aboutaleb P, Barman A, Lopez-Rivera V, Lee S, Vahidy F, Fan J, et al. Automated detection of hemorrhagic stroke from non-contrast computed tomography: a machine learning approach. Presented at International Stroke Conference; 19–21 February 2020; Los Angeles (CA). Stroke 2020;51.
- Aghaebrahim A, Desai S, Monteiro A, Granja M, Agnoletto G, Cortez G, et al. Outcomes of large-vessel occlusion thrombectomy in patients with CT perfusion defined large core stroke. J Neurointerv Surg 2020;12.
- Aktar M, Tampieri D, Rivaz H, Kersten-Oertel M, Xiao Y. Automatic collateral circulation scoring in ischaemic stroke using 4D CT angiography with low-rank and sparse matrix decomposition. Int J Comput Assist Radiol Surg 2020;15:1501-11.
- Albers GW, Wald MJ, Mlynash M, Endres J, Bammer R, Straka M, et al. Automated calculation of Alberta Stroke Program Early CT Score: validation in patients with large hemispheric infarct. Stroke 2019;50:3277-9.
- Alderson J, O’Cearbhaill R, Harston G, Greveson E, Joly O, Griffin E, et al. Large-vessel occlusion identification using non-contrast CT and CTA. Presented at 12th World Stroke Congress; 12–15 May 2020; Vienna (Austria). Int J Stroke 2020;15.
- Alderson J, O’Cearbhaill R, Joly O, Harston G, Greveson E, Griffin E, et al. Prediction of clinical outcome in a cohort of patients with LVO using automated artificial intelligence image analysis of multiphase CT angiography. Int J Stroke 2020;15.
- Apterbach W, Garra G, Gupta S. The impact of new advanced imaging requirements on tissue plasminogen activator administration. Acad Emerg Med 2021;28.
- Austein F, Fischer AC, Jurgensen N, Jansen O. Evaluation of conventional automated and volume weighted ASPECTS vs CT perfusion core volume to predict the final infarct volume after successful thrombectomy. Clin Neuroradiol 2018;28.
- Austein F, Langguth P, Jansen O. Evaluation of conventional automated and volume weighted automated aspects vs. CT perfusion core volume to predict the final infarct volume after successful endovascular therapy. Fortschr Rontgenstr 2019;191.
- Austein F, Wodarg F, Jurgensen N, Huhndorf M, Meyne J, Lindner T, et al. Automated versus manual imaging assessment of early ischaemic changes in acute stroke: comparison of two software packages and expert consensus. Eur Radiol 2019;29:6285-92.
- Austein F, Joly O, Harston G, Watkinson JI, Langguth P, Jansen O. Stability and equivalence of a newly developed fully-automated CT-perfusion post processing software for analysis in patients with acute anterior large-vessel occlusion. Presented at 12th World Stroke Congress 2020; 12–15 May 2020; Vienna Austria. Int J Stroke 2020;15:107-8.
- Bacchi S, Zerner T, Oakden-Rayner L, Kleinig T, Patel S, Jannes J. Deep learning in the prediction of ischaemic stroke thrombolysis functional outcomes: a pilot study. Acad Radiol 2020;27:e19-23.
- Bar M, Kral J, Cabal M, Havelka J, Kasickova L. The correlation of the final infarct volume measurement between NCCT and MRI DWI in acute stroke patients after mechanical thrombectomy. Presented at 5th Congress of the European Academy of Neurology (EAN 2019); 26 June–2 July 2019; Oslo Norway. Eur J Neurol 2019;26.
- Barman A, Inam ME, Lee S, Savitz S, Sheth S, Giancardo L. Determining ischaemic stroke from CT-angiography imaging using symmetry-sensitive convolutional networks 2019:1873-7. https://dx.doi.org/10.1109/ISBI.2019.8759475.
- Barros RS, Van Der Steen WE, Ponomareva E, Boers AM, Zijlstra IJ, Van Der Berg R, et al. Detection and segmentation of subarachnoid hemorrhages with deep learning. Presented at American Heart Association/American Stroke Association 2019 International Stroke Conference and State-of-the-Science Stroke Nursing Symposium; 6–8 Feb 2019; Honolulu, HI. Stroke 2019;50. https://doi.org/10.1161/str.50.suppl_1.WMP29.
- Beijing Tiantan Hospital . An AI-Based CDSS for Integrated Management of Patients With Acute Ischaemic Stroke (GOLDEN BRIDGE II). NCT04524624 2020. https://ClinicalTrials.gov/show/NCT04524624 (accessed 15 December 2021).
- Bentley P, Ganesalingam J, Dias A, Mehta A, Sharma P, Halse O, et al. Hyperacute fingerprinting: CT brain machine-learning predicts response to thrombolysis. Cerebrovasc Dis 2013;35.
- Bentley P, Ganesalingam J, Carlton Jones AL, Mahady K, Epton S, Rinne P, et al. Prediction of stroke thrombolysis outcome using CT brain machine learning. Neuroimage Clin 2014;4:635-40.
- Bhagat R, Madireddy K, Naik S, Kutty G, Liu W. A volumetric comparison of computed tomography perfusion rapid core volume in different time frames with diffusion-weighted imaging infarct volume in the post-thrombectomy patients after large-vessel occlusion. Presented at American Stroke Association International Stroke Conference (ISC 2021); 17–19 March 2021; Virtual. Stroke 2021;52.
- Biswas V, McVerry F, Macdougall N, Huang X, Muir K. Interaction of hypoperfusion intensity ratio and hyperglycaemia predicts functional outcome in ischaemic stroke. Presented at 12th World Stroke Congress 2020; 12–15 May 2020; Vienna, Austria. Int J Stroke 2020;15.
- Bouslama M, Rodrigues G, Ravindran K, Haussen D, Frankel M, Nogueira R. CT perfusion and e-aspects automated noncontract CT ischaemic core volumes: correlations and clinical outcome prediction. Presented at 5th European Stroke Organisation Conference (ESOC 2019); 22–24 May 2019; Milan Italy. Eur Stroke J 2019;4.
- Bouvy C, Maldonado Slootjes S, Ackermans N, Gille M, Paindeville P, Rutgers M. Full-automated A.I. CT perfusion seems highly reliable to exclude large-vessel occlusion. Presented at 12th World Stroke Congress 2020; 12–15 May 2020; Vienna Austria. Int J Stroke 2020;15.
- Brinjikji W, Benson J, Campeau N, Carr C, Cogswell P, Klaas J, et al. Brainomix easpects software improves interobserver agreement and accuracy of neurologist and neuroradiologists in interpretation of aspects score and outperforms human readers in prediction of final infarct. J Neurointerv Surg 2020;12:A112-13.
- Brinjikji W, Abbasi M, Arnold C, Benson JC, Braksick SA, Campeau N, et al. e-ASPECTS software improves interobserver agreement and accuracy of interpretation of aspects score. Interv Neuroradiol 2021;27:781-7. https://doi.org/10.15910199211011861.
- Brinjikji W, Rabinstein AA, Harston G, Joly O, Abbasi M, Kallmes D. Eloquence mapping in acute ischaemic stroke. Presented at American Stroke Association International Stroke Conference (ISC 2021); 17–19 March 2021. Stroke 2021;52.
- Bruggeman AA, Koopman M, Soomro J, Yoo AJ, Marquering HA, Emmer BJ, et al. Automated artificial intelligence based detection and location specification of large-vessel occlusion on CT angiography in stroke. Presented at Presented at American Stroke Association International Stroke Conference (ISC 2021); 17–9 March 2021; Virtual. Stroke 2021;52.
- Brugnara G, Neuberger U, Mahmutoglu MA, Foltyn M, Herweh C, Nagel S, et al. Multimodal predictive modeling of endovascular treatment outcome for acute ischaemic stroke using machine-learning. Stroke 2020;51:3541-51.
- Buls N, Watte N, Nieboer K, Ilsen B, de Mey J. Performance of an artificial intelligence tool with real-time clinical workflow integration - detection of intracranial hemorrhage and pulmonary embolism. Phys Med 2021;83:154-60.
- Bulwa Z, Dasenbrock H, Osteraas N, Cherian L, Crowley RW, Chen M. Incidence of unreliable automated computed tomography perfusion maps. J Stroke Cerebrovasc Dis 2019;28.
- Campbell BCV, Yassi N, Ma H, Sharma G, Salinas S, Churilov L, et al. Imaging selection in ischaemic stroke: feasibility of automated CT-perfusion analysis. Int J Stroke 2015;10:51-4.
- Capasso R, Vallone S, Serra N, Zelent G, Verganti L, Sacchetti F, et al. Qualitative versus automatic evaluation of CT perfusion parameters in acute posterior circulation ischaemic stroke. Neuroradiology 2021;63:317-30.
- Chatterjee A, Somayaji NR, Kabakis IM. Artificial intelligence detection of cerebrovascular large-vessel occlusion: nine month, 650 patient evaluation of the diagnostic accuracy and performance of the Viz.ai LVO algorithm. Presented at International Stroke Conference 2019; 6–8 Feb 2019; Honolulu, Hawaii. Stroke 2019;50.
- Chilamkurthy S, Ghosh R, Tanamala S, Biviji M, Campeau NG, Venugopal VK, et al. Deep learning algorithms for detection of critical findings in head CT scans: a retrospective study. Lancet 2018;392:2388-96.
- Chriashkova J, Goncalves C, Aslam M, Perera S, Walter S, Fisher J, et al. Can artificial intelligence improve physician sensitivity in detecting early ischaemic damage on computed tomography?. Insights Imaging 2019;10.
- Chriashkova J, Menon N, Chakrabarti A, Guyler P, Kelavkar S, Kuhn A, et al. E-ASPECTS improves sensitivity to early ischaemic injury on acute computed tomography scans. Presented at International Stroke Conference 2019; 6–8 Feb 2019; Honolulu, HI. Stroke 2019;50.
- Chung CY, Rodrigues GM, Haussen DC, Barreira CM, Grossberg J, Frankel MR, et al. Automated detection of hyperdense mca sign and automated notification of large-vessel occlusion using artificial intelligence. Presented at International Stroke Conference 2019; 6–8 Feb 2019; Honolulu, HI. Stroke 2019;50.
- Chung C, Pisani L, Peterson R, Mohammaden M, Harston G, Joly O, et al. Automated detection of hyperdense vessel sign on acute ischaemic stroke patients with large-vessel occlusion. Presented at 12th World Stroke Congress; 12–15 May 2020; Vienna (Austria). Int J Stroke 2020;15.
- Cimflova P, Volny O, Mikulik PR, Tyshchenko B, Belaskova S, Vinklarek J, et al. Detection of ischaemic changes on baseline multimodal computed tomography: expert reading vs. Brainomix and RAPID software. J Stroke Cerebrovasc Dis 2020;29.
- Cimflova P, Volny O, Mikulik R, Tyshchenko B, Belaskova S, Vinklarek J, et al. Detection of ischaemic changes on baseline multimodal computed tomography: expert reading vs. brainomix and rapid software. Presented at 12th World Stroke Congress; 12–15 May 2021; Vienna (Austria). Int J Stroke 2020;15.
- Copelan AZ, Smith ER, Drocton GT, Narsinh KH, Murph D, Khangura RS, et al. Recent administration of iodinated contrast renders core infarct estimation inaccurate using RAPID software. AJNR Am J Neuroradiol 2020;41:2235-42.
- D’Esterre CD, Qazi E, Patil S, Lee TY, Almekhlafi M, Demchuk AM, et al. CT Perfusion thresholds to separate acute infarct core from penumbra using optimized imaging and advanced post-processing. Presented at the 23rd European Stroke Conference; 6–9 May 2014; Nice (France). Cerebrovasc Dis 2014;37.
- Davidovic K, Stankovic A, Kostic J, Crnovrsanin F, Masulovic D, Maksimovic R. Diagnostic importance of brain CT perfusion 4D in the detection of acute suptratentorial infarctions. Presented at European Congress of Radiology 2017, Vienna, Austria, 1–5 March 2017. Abstract B-0097. Insights Imaging 2017;8.
- Davis MA, Rao B, Cedeno PA, Saha A, Zohrabian VM. Machine learning and improved quality metrics in acute intracranial hemorrhage by noncontrast computed tomography published online ahead of print. Curr Probl Diagn Radiol 2022;51:556-61.
- Dehkharghani S, Bammer R, Straka M, Albin LS, Kass-Hout O, Allen JW, et al. Performance and predictive value of a user-independent platform for CT perfusion analysis: threshold-derived automated systems outperform examiner-driven approaches in outcome prediction of acute ischaemic stroke. AJNR Am J Neuroradiol 2015;36:1419-25.
- Delio PR, Wong ML, Tsai JP, Hinson HE, McMenamy J, Le T, et al. Assistance from automated aspects software improves reader performance. Presented at International Stroke Conference Virtual; 17–19 March 2021. Stroke 2021;52.
- Delio PR, Wong ML, Tsai JP, Hinson HE, McMenamy J, Le TQ, et al. Assistance from automated ASPECTS software improves reader performance. J Stroke Cerebrovasc Dis 2021;30.
- Demeestere J, Scheldeman L, Cornelissen S, Heye S, Christensen S, Mlynash M, et al. Conventional and automated aspects vs. CT perfusion core volume to predict functional outcome in reperfused acute ischaemic stroke patients undergoing endovascular therapy. Presented at the the American Heart Association/American Stroke Association 2018 International Stroke Conference and State-of-the-Science Stroke Nursing Symposium; 23–26 January 2018; Los Angeles (CA). Stroke 2018;49.
- Desai S, Jadhav A, Rocha M, Jovin T, Molyneaux B. Association of automated aspects and ischaemic core volume of anterior circulation large-vessel occlusion stroke within 24-hours of onset. Presented at the 5th European Stroke Organisation Conference (ESOC); 22–24 May 2019; Milan (Italy). Eur Stroke J 2019;4.
- Devlin T, Shah R, Patterson J, Fleming J, Nichols J, Knowles B, et al. DISTINCTION: Automated Detection, Identification, Selection, And Triage Using Artificial Intelligence In Large-vessel Occlusions Requiring Critical And Timely InterventION. Presented at International Stroke Conference 2019; 6–8 Feb 2019; Honolulu, HI. Stroke 2019;50.
- Docema R, Cardoso EFR, Eduardo Chenedezi RF. Diagnostic accuracy of artificial intelligence algorithms to detect intracranial haemorrhage in head computed scans n.d. www.crd.york.ac.uk/prospero/display_record.php?ID=CRD42021233801.
- Elijovich L, Dornbos D, Nickele C, Alexandrov A, Inoa-Acosta V, Arthur AS, et al. Automated emergent large-vessel occlusion detection by artificial intelligence improves stroke workflow in a hub and spoke stroke system of care. J Neurointerv Surg 2021;14:704-8.
- Ferreti LA, Leitao CA, Teixeira BCA, Lopes Neto FDN, VF ZE, Lange MC. The use of e-ASPECTS in acute stroke care: validation of method performance compared to the performance of specialists. Arq Neuropsiquiatr 2020;78:757-61.
- Ferreti L, Leitao C, Teixeira B, Zetola V, Lange M. The E-ASPECTS improves the performance of emergencists in the evaluation of early signs of ischemia. Neurology 2020;94.
- Fischer J, Friedrich B, Monch S, Berndt M, Wunderlich S, Seifert C, et al. Software-based automatic ASPECTS calculation is superior in comparison to human readers. Clin Neuroradiol 2018;28.
- Ford LM, Commet ME, Ahluwalia JS, Cenzer CW, Aftab M. 110 Reliability of automated interpretation of computed tomography images in the management of acute stroke: a single-center analysis. Presented at American College of Emergency Physicians (ACEP) 2020 Research Forum Virtual; 26–29 October 2020. Ann Emerg Med 2020;76.
- Ginat DT. Analysis of head CT scans flagged by deep learning software for acute intracranial hemorrhage. Neuroradiology 2020;62:335-40.
- Ginat D. Implementation of machine learning software on the radiology worklist decreases scan view delay for the detection of intracranial hemorrhage on CT. Brain Sci 2021;11.
- Goebel J, Stenzel E, Guberina N, Wanke I, Koehrmann M, Kleinschnitz C, et al. Automated ASPECT rating: comparison between the Frontier ASPECT Score software and the Brainomix software. Neuroradiology 2018;60:1267-72.
- Goebel J, Stenzel E, Wanke I, Kohrmann M, Kleinschnitz C, Forsting M, et al. Computer aided diagnosis for ASPECT rating: Initial experiences with the Frontier ASPECT Score software. Clin Neuroradiol 2018;28.
- Goebel J, Stenzel E, Zulow S, Wanke I, Kohrmann M, Kleinschnitz C, et al. Automated ASPECT rating: comparison between the Frontier ASPECT Score software and the Brainomix software. Clin Neuroradiol 2018;28:S5-6.
- Goncalves C, Bowman S, Liyanage S, OrathPrabakaran R, Shah S, Gerry S, et al. Automated assessment of early ischaemic damage on CT scans: as good as an expert? n.d.
- Grunwald I, Sinha D, Day D, Reith W, Chapot R, Papanagiotou P, et al. Evaluation of the novel medical imaging software e-ASPECTS for patient selection in stroke. Presented at the UK Stroke Forum 2015 Conference; 1–3 December 2015; Liverpool (UK). Int J Stroke 2015;10.
- Grunwald I, Ragoschke-Schummbb A, Kettnerc M, Walterb S, Shah S, Fassbenderb K. e-ASPECTS in pre-hospital stroke treatment on a mobile stroke unit. Presented at UK Stroke Forum 2016 Conference; 28–30 November 2016; Liverpool (UK). Int J Stroke 2016;11.
- Grunwald IQ, Ragoschke-Schumm A, Kettner M, Schwindling L, Roumia S, Helwig S, et al. First automated stroke imaging evaluation via electronic Alberta stroke program early CT score in a mobile stroke unit. Cerebrovasc Dis 2016;42:332-8.
- Grunwald IQ, Sinha D, Roffe C, Walter S. Evaluation of the e-ASPECTS automated software for detection of acute ischaemic stroke. Presented at the International Stroke Conference; 17–19 February 2016; Los Angeles (CA). Stroke 2016;47.
- Grunwald IQ, Kulikovski J, Reith W, Gerry S, Namias R, Politi M, et al. Collateral automation for triage in stroke: evaluating automated scoring of collaterals in acute stroke on computed tomography scans. Cerebrovasc Dis 2019;47:217-22.
- Guberina N, Dietrich U, Radbruch A, Goebel J, Deuschl C, Ringelstein A, et al. Detection of early infarction signs with machine learning-based diagnosis by means of the Alberta Stroke Program Early CT score (ASPECTS) in the clinical routine. Neuroradiology 2018;60:889-901.
- Heit JJ, Coelho H, Lima FO, Granja M, Aghaebrahim A, Hanel R, et al. Automated cerebral hemorrhage detection using RAPID. AJNR Am J Neuroradiol 2021;42:273-8.
- Herweh C, Ringleb PA, Rauch G, Behrens L, Moehlenbruch M, Gottorf R, et al. Similar performance on aspect scoring between stroke experts and an automated algorithm (e-ASPECTS) on CT scans of acute ischaemic stroke patients. Int J Stroke 2014;9:52-3.
- Herweh C, Ringleb PA, Rauch G, Gerry S, Behrens L, Mohlenbruch M, et al. Performance of e-ASPECTS software in comparison to that of stroke physicians on assessing CT scans of acute ischaemic stroke patients. Int J Stroke 2016;11:438-45.
- Herweh C, Bellot P, Seker F, Joly O, Mokli Y, Bendszus M, et al. AI-based automated detection of large-vessel occlusion on nonenhanced CT scans in acute ischaemic stroke. Presented at 12th World Stroke Congress; 12–15 May 2020; Vienna (Austria). Int J Stroke 2020;15:292-3.
- Hoelter P, Muehlen I, Goelitz P, Beuscher V, Schwab S, Doerfler A. Automated ASPECT scoring in acute ischaemic stroke: comparison of three software tools. Neuroradiology 2020;62:1231-8.
- Hoffmann RS, Saban M, Abergel E, Eran A. n.d.
- Hokkinen L, Makela T, Savolainen S, Kangasniemi M. Evaluation of a CTA-based convolutional neural network for infarct volume prediction in anterior cerebral circulation ischaemic stroke. Eur Radiol Exp 2021;5.
- Hoving JW, Marquering HA, Majoie C, Yassi N, Sharma G, Liebeskind DS, et al. Volumetric and spatial accuracy of computed tomography perfusion estimated ischaemic core volume in patients with acute ischaemic stroke. Stroke 2018;49:2368-75.
- Hoyte LC, Al Sultan AS, Finkelstein S, Boyko M, Fok D, Pordeli P, et al. Reliability of automated software to assign e-ASPECTS to CT scans for acute ischaemic changes. Neurology 2017;88.
- Jankowitz BT, Stinson T, Begun D, Davies J. Large scale, CT evaluation can improve screening for multi-center stroke trials. Presented at International Stroke Conference Virtual; 17–19 March 2021. Stroke 2021;52.
- John S, Hussain SI, Piechowski B, Dogar MA. Discrepancy in core infarct between CT aspects and CT perfusion when selecting for mechanical thrombectomy. Presented at XXIV World Congress of Neurology; 27–31 October 2019; Dubai (UAE). J Neurol Sci 2019;405.
- John S, Hussain SI, Piechowski-Jozwiak B, Dogar MA. Discrepancy in core infarct between non-contrast CT and CT perfusion when selecting for mechanical thrombectomy. J Cerebrovasc Endovasc Neurosurg 2020;22:8-14.
- Katramados AM, Kole M, Marin H, Alsrouji O, Varun P, Miller D, et al. Real-word performance of two automated software platforms for large-vessel occlusion identification in acute ischaemic stroke patients: a single center experience. Presented at International Stroke Conference Virtual; 17–19 March 2021. Stroke 2021;52.
- Kelavkar S, Grunwald Q, Shah S. In how far can the CE-marked e-ASPECTS software (Brainomix, Oxford) assist clinicians?. Cerebrovasc Dis 2017;43.
- Kettenberger P, Jansen O, Riedel C. Automatic clot detection in NECT images of acute ischaemic stroke patients using a convolutional neural network. Clin Neuroradiol 2018;28.
- Kettenberger P, Jansen O, Larsen N, Riedel C. Automatic clot detection in NECT images of acute ischaemic stroke patients using a convolutional neural network. Presented at the 5th European Stroke Organisation Conference (ESOC); 22–24 May 2019; Milan (Italy). Eur Stroke J 2019;4:416-7.
- Kim CH, Hahm MH, Lee DE, Choe JY, Ahn JY, Park SY, et al. Clinical usefulness of deep learning-based automated segmentation in intracranial hemorrhage published online ahead of print. Technol Health Care 2021;29:881-95. https://dx.doi.org/10.3233/THC-202533.
- Kniep HC, Sporns PB, Broocks G, Kemmling A, Nawabi J, Rusche T, et al. Posterior circulation stroke: machine learning-based detection of early ischaemic changes in acute non-contrast CT scans. J Neurol 2020;267:2632-41.
- Knight-Greenfield A, Beecy A, Chang Q, Anchouche K, Baskaran L, Elmore K, et al. A novel deep learning approach for automated diagnosis of cerebral infarction on computed tomography. Presented at the American Heart Association/American Stroke Association 2018 International Stroke Conference and State-of-the-Science Stroke Nursing Symposium; 23–26 January 2018; Los Angeles (CA). Stroke 2018;49.
- Kral J, Cabal M, Kasickova L, Havelka J, Jonszta T, Volny O, et al. Machine learning volumetry of ischaemic brain lesions on CT after thrombectomy-prospective diagnostic accuracy study in ischaemic stroke patients. Neuroradiology 2020;62:1239-45.
- Kuang HL, Teleg E, Najm M, Wilson AT, Sohn SI, Goyal M, et al. Automated ASPECTS scoring of CT scans for acute ischaemic stroke patients using machine learning. Presented at the International Stroke Conference; 23–26 January 2018; Los Angeles (CA). Stroke 2018;49.
- Kuang H, Najm M, Chakraborty D, Maraj N, Sohn SI, Goyal M, et al. Automated ASPECTS on noncontrast CT scans in patients with acute ischaemic stroke using machine learning. AJNR Am J Neuroradiol 2019;40:33-8.
- Kuang HL, Qiu W, Najm M, Dowlatshahi D, Mikulik R, Poppe AY, et al. Validation of an automated ASPECTS method on non-contrast computed tomography scans of acute ischaemic stroke patients. Int J Stroke 2020;15:528-34.
- Kuo W, Hane C, Mukherjee P, Malik J, Yuh EL. Expert-level detection of acute intracranial hemorrhage on head computed tomography using deep learning. Proc Natl Acad Sci U S A 2019;116:22737-45.
- Lasocha B, Pulyk R, Brzegowy P, Latacz P, Slowik A, Popiela TJ. Real-world comparison of human and software image assessment in acute ischaemic stroke patients’ qualification for reperfusion treatment. J Clin Med 2020;9.
- Lee JY, Kim JS, Kim TY, Kim YS. Detection and classification of intracranial haemorrhage on CT images using a novel deep-learning algorithm. Sci Rep 2020;10.
- Liu QC, Jia ZY, Zhao LB, Cao YZ, Ma G, Shi HB, et al. Agreement and accuracy of ischaemic core volume evaluated by three CT perfusion software packages in acute ischaemic stroke. J Stroke Cerebrovasc Dis 2021;30.
- Lo CM, Hung PH, Lin DT. Rapid assessment of acute ischaemic stroke by computed tomography using deep convolutional neural networks. J Digit Imaging 2021;34:637-46. https://dx.doi.org/10.1007/s10278-021-00457-y.
- Loffler MT, Sollmann N, Monch S, Friedrich B, Zimmer C, Baum T, et al. Improved reliability of automated ASPECTS evaluation using iterative model reconstruction from head CT scans. J Neuroimaging 2021;31:341-7.
- Maegerlein C, Fischer J, Monch S, Berndt M, Wunderlich S, Seifert CL, et al. Automated calculation of the Alberta Stroke Program Early CT Score: feasibility and reliability. Radiology 2019;291:141-8.
- Mair G, Bath P, Muir K, Von Kummer R, Al-Shahi Salman R, Sandercock P, et al. Real-world independent testing of easpects software (RITES). Presented at 12th World Stroke Congress; 12–15 May 2020; Vienna (Austria). Int J Stroke 2020;15.
- Mansour OY, Ramadan I, Abdo A, Hamdi M, Eldeeb H, Marouf H, et al. Deciding thrombolysis in AIS based on automated versus on WhatsApp interpreted ASPECTS, a reliability and cost-effectiveness analysis in developing system of care. Front Neurol 2020;11.
- Meijs M, Patel A, van de Leemput SC, Prokop M, van Dijk EJ, de Leeuw FE, et al. Robust segmentation of the full cerebral vasculature in 4D CT of suspected stroke patients. Sci Rep 2017;7.
- Meijs M, Meijer FJA, Prokop M, Ginneken BV, Manniesing R. Image-level detection of arterial occlusions in 4D-CTA of acute stroke patients using deep learning. Med Image Anal 2020;66.
- Modak JM, Lee JW, Reeves C, Staff I, Ollenschleger MD. CT perfusion and radiation exposure in acute ischaemic stroke: a quality improvement study. Presented at International Stroke Conference; 6–8 February 2019; Honolulu (HI). Stroke 2019;50.
- Morey J, Zhang X, Yaeger K, Fiano E, Marayati NF, Kellner CP, et al. Initial real-world experience with Viz LVO in transferred large-vessel occlusion stroke patients. Stroke 2021;52.
- Murray N. Artificial intelligence in acute stroke diagnostics: application in large-vessel occlusions. Neurology 2019;92.
- Nagel S, Sinha D, Day D, Reith W, Chapot R, Papanagiotou P, et al. e-ASPECTS software is non-inferior to neuroradiologists in applying the ASPECT score to computed tomography scans of acute ischaemic stroke patients. Int J Stroke 2017;12:615-22.
- Nagel S, Wang X, Carcel C, Robinson T, Lindley RI, Chalmers J, et al. ENCHANTED Investigators . Clinical utility of electronic Alberta Stroke Program Early Computed Tomography Score software in the ENCHANTED trial database. Stroke 2018;49:1407-11.
- Nagel S, Joly O, Pfaff J, Papanagiotou P, Fassbender K, Reith W, et al. E-aspects derived acute ischaemic volumes on non-contrast enhanced computed tomography images correlate with diffusion weighted imaging lesion volumes and predict clinical outcome in acute ischaemic stroke patients. Presented at the International Stroke Conference; 6–8 February 2019; Honolulu (HI). Stroke 2019;50.
- Nagel S, Joly O, Pfaff J, Papanagiotou P, Fassbender K, Reith W, et al. e-ASPECTS derived acute ischaemic volumes on non-contrast-enhanced computed tomography images. Int J Stroke 2020;15:995-1001.
- Neuberger U, Pfaff J, Nagel S, Ringleb PA, Herweh C, Bendszus M, et al. Impact of slice thickness on robustness of electronic Alberta stroke program early computed tomography scores (e-ASPECTS). Clin Neuroradiol 2019;29.
- Neuberger U, Nagel S, Pfaff J, Ringleb PA, Herweh C, Bendszus M, et al. Impact of slice thickness on clinical utility of automated Alberta Stroke Program Early Computed Tomography Scores. Eur Radiol 2020;30:3137-45.
- Neuhaus A, Seyedsaadat SM, Mihal D, Benson J, Mark I, Kallmes DF, et al. Region-specific agreement in ASPECTS estimation between neuroradiologists and e-ASPECTS software. J Neurointerv Surg 2020;12:720-3.
- Nishio M, Koyasu S, Noguchi S, Kiguchi T, Nakatsu K, Akasaka T, et al. Automatic detection of acute ischaemic stroke using non-contrast computed tomography and two-stage deep learning model. Comput Methods Programs Biomed 2020;196.
- Ojeda P, Zawaideh M, Mossa-Basha M, Haynor D. Medical Imaging 2019: Image Processing. Proceedings of SPIE. Bellingham: Spie-Int Soc Optical Engineering; 2019.
- Olive-Gadea M, Martins N, Boned S, Carvajal J, Rios MA, Muchada M, et al. Aspects and easpects correlation with baseline and final infarct volume in acute ischaemic stroke thrombectomy patients. Presented at the American Heart Association/American Stroke Association 2018 International Stroke Conference and State-of-the-Science Stroke Nursing Symposium; 23–26 January 2018; Los Angeles (CA). Stroke 2018;49.
- Olive-Gadea M, Martins N, Boned S, Carvajal J, Rios MA, Muchada M, et al. Time dependency of aspects and e-ASPECTS correlation with infarct volume. Presented at the 4th European Stroke Organisation Conference (ESOC); 16–18 May 2018; Gothenburg (Sweden). Eur Stroke J 2018;3.
- Olive-Gadea M, Crespo C, Granes C, Hernandez-Perez M, Perez de la Ossa N, Laredo C, et al. Deep learning based software to identify large-vessel occlusion on noncontrast computed tomography. Stroke 2020;51:3133-7.
- Olive-Gadea M, Crespo C, Granes C, Hernandez-Perez M, Perez De La Ossa N, Laredo C, et al. Identification of large-vessel occlusion on non-contrast CT using a deep learning software. Presented at 12th World Stroke Congress; 12–15 May 2020; Vienna (Austria). Int J Stroke 2020;15:134-5.
- Pfaff J, Herweh C, Schieber S, Schonenberger S, Bosel J, Ringleb P, et al. The e-aspects correlates with and is predictive of outcome after mechanical thrombectomy. Presented at the 3rd European Stroke Organisation Conference (ESOC); 16–18 May 2017; Prague (Czech). Eur Stroke J 2017;2:255-6.
- Pisani L, Haussen D, Mohammaden M, Camara C, Rodrigues G, Liberato B, et al. Comparison of two automated CT perfusion packages on acute stroke assessment. Presented at 12th World Stroke Congress; 12–15 May 2020; Vienna (Austria). Int J Stroke 2020;15.
- Pisani L, Mohammaden M, Bouslama M, Al-bayati AR, Haussen DC, Frankel MR, et al. Comparison of three automated CT perfusion software packages for thrombectomy eligibility and final infarct volume prediction. Presented at the International Stroke Conference Virtual; 17–19 March 2021. Stroke 2021;52.
- Prokhorikhin A, Baystrukov V, Boykov A, Malaev D, Tarkova A, Shayakhmetova S, et al. Neural network-based system of acute stroke non-contrast computed tomography diagnostics: a comparative study. Russ Electron J Radiol 2020;10:36-45.
- Providence Little Company of Mary-Torrance . Automated detection and triage of large-vessel occlusions using artificial intelligence for early and rapid treatment (ALERT). NCT04142879 2019. https://ClinicalTrials.gov/show/NCT04142879 (accessed 30 July 2021).
- Psychogios MN, Sporns PB, Ospel J, Katsanos AH, Kabiri R, Flottmann FA, et al. Automated perfusion calculations vs. visual scoring of collaterals and CBV-ASPECTS has the machine surpassed the eye?. Clin Neuroradiol 2021;31:499-506.
- Purrucker J, Mattern N, Herweh C, Nagel S, Gumbinger C. Realworld hospital transfer times and loss of braintissue measured with e-ASPECTS underlines importance of improvement of stroke care delivery. Presented at the 4th European Stroke Organisation Conference; 16–18 May 2018; Gothenburg (Sweden). Eur Stroke J 2018;3.
- Purrucker JC, Mattern N, Herweh C, Mohlenbruch M, Ringleb PA, Nagel S, et al. Electronic Alberta Stroke Program Early CT score change and functional outcome in a drip-and-ship stroke service. J Neurointerv Surg 2020;12:252-5.
- Qiu W, Kuang H, Ospel JM, Hill MD, Demchuk AM, Goyal M, et al. Automated prediction of ischaemic brain tissue fate from multiphase computed tomographic angiography in patients with acute ischaemic stroke using machine learning. J Stroke 2021;23:234-43.
- Rao B, Zohrabian V, Cedeno P, Saha A, Pahade J, Davis MA. Utility of artificial intelligence tool as a prospective radiology peer reviewer: detection of unreported intracranial hemorrhage. Acad Radiol 2021;28:85-93.
- Rava RA, Podgorsak AR, Waqas M, Snyder KV, Mokin M, Levy EI, et al. Investigation of convolutional neural networks using multiple computed tomography perfusion maps to identify infarct core in acute ischaemic stroke patients. J Med Imaging (Bellingham) 2021;8.
- Reidler P, Stueckelschweiger L, Puhr-Westerheide D, Feil K, Kellert L, Dimitriadis K, et al. Performance of automated attenuation measurements at identifying large-vessel occlusion stroke on CT angiography. Clin Neuroradiol 2020;31:763-72.
- Sachdev H, Ong K, Paulson A, Emami M, Tolley U, Wang W, et al. Utilization of ‘RAPID’ CT perfusion in treatment of acute ischaemic stroke (AIS): a community hospital experience in California, United States. Interv Neuroradiol 2015;21.
- Seo K, Kim GS, Yun PH, Suh SH. An introduction of the rapid software increased the number of mechanical thrombectomy with favorable outcome in stroke patients. Neuroradiology 2019;61.
- Shah S, Kelavkar S, Johnson M, Vlahovic I, Guyler P, Kiihn AL, et al. What is the optimal way to integrate the e-ASPECTS software into a stroke pathway? Presented at 26th European Stroke Conference; 24–26 May 2017; Berlin, Germany. Cerebrovasc Dis 2017;43.
- Sheth SA, Inam ME, Barman A, Lee S, Savitz SI, Giancardo L. Automated accurate determinations of acute infarct core volumes from CT angiography using machine learning. Presented at the International Stroke Conference; 6–8 February 2019; Honolulu (HI). Stroke 2019;50.
- Sheth SA, Lopez-Rivera V, Barman A, Grotta JC, Yoo AJ, Lee S, et al. Machine learning-enabled automated determination of acute ischaemic core from computed tomography angiography. Stroke 2019;50:3093-100.
- Shinohara Y, Takahashi N, Lee Y, Ohmura T, Kinoshita T. Development of a deep learning model to identify hyperdense MCA sign in patients with acute ischaemic stroke. Jpn J Radiol 2020;38:112-7.
- Shinohara Y, Takahashi N, Lee Y, Ohmura T, Umetsu A, Kinoshita F, et al. Usefulness of deep learning-assisted identification of hyperdense MCA sign in acute ischaemic stroke: comparison with readers’ performance. Jpn J Radiol 2020;38:870-7.
- Siegler JE, Rosenberg J, Cristancho D, Olsen A, Pulst-Korenberg J, Raab L, et al. Computed tomography perfusion in stroke mimics. Int J Stroke 2020;15:299-307.
- Sundaram VK, Goldstein J, Wheelwright D, Aggarwal A, Pawha PS, Doshi A, et al. Automated aspects in acute ischaemic stroke: a comparative analysis with CT perfusion. AJNR Am J Neuroradiol 2019;40:2033-8.
- Suomalainen O, Curtze S, Abou Elseoud A. E-ASPECTS and rapid in the evaluation of ischaemic core in acute stroke patients (Helskinki Stroke Registry). Presented at the 5th European Stroke Organisation Conference (ESOC); 22–24 May 2019; Milan (Italy). Eur Stroke J 2019;4.
- Suomalainen O, Abou A, Martinez-Majander N, Forss N, Tiainen M, Curtze S. Evaluation of infarct core volume; comparison of e-aspects volume feature (NCCTCORE) with rapid perfusion imaging. Presented at 12th World Stroke Congress; 12–15 May 2020; Vienna (Austria). Int J Stroke 2020;15:294-5.
- Timaran D, Mateo-Camacho Y, Morales L, Fuentes D, Torres C, Punzo R, et al. Diagnostic performance of a semiautomated (syngo.via-Vb20) and automated (rapid-AI) workstations estimating favorability of patients with acute ischaemic stroke to undergo extended thrombolysis and/or endovascular treatment. Presented at European Stroke Organisation Conference (ESOC 2021); 1–3 Sept 2021; Virtual. Eur Stroke J 2021;6:354-5.
- Tolhuisen ML, Ponomareva E, Koopman MS, Jansen IG, Boers AM, Majoie CB, et al. Artificial intelligence based detection of large-vessel occlusion on non-contrast computed tomography in stroke. Presented at the International Stroke Conference; 6–8 February 2019; Honolulu (HI). Stroke 2019;50.
- Tsang ACO, Lenck S, Hilditch C, Nicholson P, Brinjikji W, Krings T, et al. Automated CT perfusion imaging versus non-contrast CT for ischaemic core assessment in large-vessel occlusion. Clin Neuroradiol 2020;30:109-14.
- Tyan YS, Wu MC, Chin CL, Kuo YL, Lee MS, Chang HY. Ischaemic stroke detection system with a computer-aided diagnostic ability using an unsupervised feature perception enhancement method. Int J Biomed Imaging 2014;2014.
- University of Guadalajara . Automated diagnosis of stroke in computed tomography with the use of artificial intelligence. NCT03874702 2019. https://ClinicalTrials.gov/show/NCT03874702 (accessed 30 July 2021).
- Vargas J, Moorhead S, Chaudry M, Turner R, Turk A. A comparison of two automated CTP algorithms for estimation of core infarct. J Neurointerv Surg 2021;13.
- Voter AF, Meram E, Garrett JW, Yu JJ. Diagnostic accuracy and failure mode analysis of a deep learning algorithm for the detection of intracranial hemorrhage. J Am Coll Radiol 2021;18:1143-52. https://dx.doi.org/10.1016/j.jacr.2021.03.005.
- Voter AF, Meram E, Garrett JW, Yu JJ. Diagnostic accuracy and failure mode analysis of a deep learning algorithm for the detection of intracranial hemorrhage. J Am Coll Radiol 2021;18:1143-52.
- Vyas D, Bohra V, Karan V, Huded V. Rapid processing of perfusion and diffusion for ischaemic strokes in the extended time window: an Indian experience. Ann Indian Acad Neurol 2019;22:96-9.
- Wang C, Shi Z, Yang M, Huang L, Fang W, Jiang L, et al. Deep learning-based identification of acute ischaemic core and deficit from non-contrast CT and CTA. J Cereb Blood Flow Metab 2021;41:3028-38. https://dx.doi.org/10.1177/0271678X211023660.
- Wang TG, Chen LG, Jin XL, Yuan Y, Zhang QW, Shao CW, et al. CT perfusion based ASPECTS improves the diagnostic performance of early ischaemic changes in large-vessel occlusion. BMC Med Imaging 2021;21.
- Weiss D, Chuang D, Fadhil A, Duncan K, Smith M, Weiss A, et al. E-Aspects predicts decompressive hemicraniectomy. Neurology 2020;94.
- Weiss DL, Chuang DY, Fadhil A, Duncan KR, Weiss A, Smith ML, et al. Use of the electronic Alberta stroke program early CT score software to guide treatment of patients with acute ischaemic stroke. Presented at the International Stroke Conference Virtual; 17–19 March 2021. Stroke 2021;52.
- Yang L, Liu Q, Zhao Q, Zhu X, Wang L. Machine learning is a valid method for predicting prehospital delay after acute ischaemic stroke. Brain Behav 2020;10.
- Yang W, Hong JY, Kim JY, Paik SH, Lee SH, Park JS, et al. A novel singular value decomposition-based denoising method in 4-dimensional computed tomography of the brain in stroke patients with statistical evaluation. Sensors 2020;20.
- Zamarro Parra J, Parrilla G, Espinosa de Rueda Ruiz M, Blanca GVN, Jose DP, Diego PG. Automated acute infarct volume and collateral assessment strongly predicts clinical outcome in patients undergoing mechanical thrombectomy. Presented at the 5th European Stroke Organisation Conference (ESOC); 22–24 May 2019; Milan (Italy). Eur Stroke J 2019;4.
Appendix 1 Literature search strategies
Main clinical effectiveness searches
Database | Dates covered | Hits |
---|---|---|
Embase | 1974–2021/07/07 | 1960 |
MEDLINE + PreMedline | 1946–2021/07/07 | 1110 |
CDSR | up to 2021/07/Iss7 | 135 |
CENTRAL | up to 2021/07/Iss7 | 406 |
DARE + HTA (CRD) | up to 2015/03 & 2018/03 | 361 |
Science Citation Index (SCI) + CPCI-S | 1988–2021/07/06 | 857 |
KSR Evidence | up to 2021/07/07 | 42 |
Epistemonikos | up to 2021/07/07 | 3 |
NIHR HTA | up to 2021/07/02 | 5 |
INAHTA | up to 2021/07/06 | 265 |
ARIF | up to 2021/07/02 | 0 |
PROSPERO | up to 2021/07/07 | 23 |
INPLASY | up to 2021/07/02 | 1 |
LILACs | up to 2021/07/02 | 374 |
ClinTrials.gov | up to 2021/07/02 | 39 |
EUCTR | up to 2021/07/28 | 16 |
WHO ICTRP | up to 2021/07/02 | 14 |
ScanMedicine | up to 2021/07/02 | 28 |
Northern Light | 2010–2021/Wk25 | 64 |
Total | 5703 |
Embase (Ovid): 1974–2021/07/07
Searched: 8.7.21
Stroke + Diagnostic/Scan + AI (NoA)
-
exp brain ischemia/ (199232)
-
exp brain hemorrhage/ (150355)
-
basal ganglion hemorrhage/ (654)
-
cerebrovascular accident/ (226798)
-
brain infarction/ (55721)
-
blood vessel occlusion/ (11569)
-
(Stroke$ or apople$ or cerebral vasc$ or cerebrovasc$ or cerebro vasc$ or poststroke$ or encephalorrhag$ or hematencephalon$ or large-vessel occlusion$).ti,ab,ot. (498658)
-
((brain or blood flow) adj2 disturb$).ti,ab,ot. (2899)
-
((sinus or sagittal) adj3 thromb$).ti,ab,ot. (7142)
-
(isch?emi$ adj3 (seizure$ or attack$ or thrombo$ or embolic or encephalopath$ or neural)).ti,ab,ot. (40502)
-
((Bleed$ or h?emorrhag$) adj2 corpus callosum).ti,ab,ot. (27)
-
((brain or cerebr$ or cerebell$ or cortical or Intraparenchymal or intracortical or vertebrobasil$ or hemispher$ or intracran$ or intra-cran$ or intracerebral or intratentorial or intra-tentorial or intraventricular or intra-ventricular or periventricular or peri-ventricular or supratentorial or supra-tentorial or anterior circulat$ or posterior circulat$ or basal gangli$ or global or focal or parenchymal or subarachnoid or sub-arachnoid or putaminal or putamen or posterior fossa or intra-axial or intraaxial or lacunar) adj3 (arrest$ or attack$ or isch?emi$ or infarct$ or insufficien$ or emboli$ or occlus$ or hypox$ or vasospasm or obstruction or vasculopath$ or failure$ or thromb$ or h?emorrhag$ or microh?emorrhag$ or accident$ or h?ematoma$ or bleed$ or microbleed$ or insult$)).ti,ab,ot. (292691)
-
(CVA or CVAS or MCA$ or ICH or ICHs or CVST or CVSTs or CVDST or CVT or CVDSTs or CVTs or LVO or LVOs).ti,ab. (81862)
-
or/1-13 (861830)
-
((diagnos$ or predict$ or specificity or sensitiv$) adj4 (criteria or criterion or guideline$ or pattern$ or trend$ or utili$ or management or prevalence or initiat$ or distribution$ or coverage or variety or selection or spread or alternative$ or frequen$)).ti,ab,ot. (493299)
-
diagnosis/ or early diagnosis/ (1442538)
-
exp brain scintiscanning/ (9831)
-
Neurologic examination/ (70389)
-
Computer assisted tomography/ (776896)
-
Brain radiography/ (7759)
-
((Brain or cerebral or neurologic$ or CT or head) adj2 (scan$ or scintigraph$ or examination$ or angiograph$ or image analys$ or perfusion$ or radiograph$)).ti,ab,ot,hw. (388831)
-
(CAT scan$ or CTA or CTP or neuroimag$ or neuro-imag$ or (comput$ adj2 tomograph$)).ti,ab,ot,hw. (1298946)
-
(Gamma encephalograph$ or Gammaencephalograph$ or Radio encephalograph$ or Radioencephalograph$).ti,ab,ot,hw. (48)
-
or/15-23 (3118232)
-
exp artificial intelligence/ (49699)
-
automated pattern recognition/ (16903)
-
decision support system/ (23908)
-
computer assisted diagnosis/ (40299)
-
Convolutional neural network/ (9836)
-
(Artificial intelligence or AI or machine intelligence or computer-aided triage$ or support vector machine$ or relevance vector machine$).ti,ab,ot. (75268)
-
((automat$ or computer) adj2 (analys$ or diagnos$ or detect$)).ti,ab,ot. (54307)
-
((deep or machine) adj learning).ti,ab,ot. (65494)
-
(decision support$ adj (software or tool$)).ti,ab,ot. (4658)
-
(CNN or CNNs or convNet or (convolut$ adj2 neural network$) or convolutional ANNs or convolutional ANN or convolutional NNs or convolutional NN).ti,ab. (15300)
-
automat$ hierarch$ evaluat$.ti,ab. (1)
-
(Aidoc or e-CTA or e-ASPECTS or e-stroke or brainomix or brainscan or "brainscan.ai" or icobrain or icometrix or qER or Qure or Zebra$ or e-CTP or briefcase or rapid CTA or rapid LVO or rapid core or rapid ASPECTS or rapid ICH or rapidai or blackford or "viz.ai" or viz or "ct perfusion 4d" or cercare or cina$ or Avicenna or accipio$ or maxQ AI or biomind or "biomind.ai" or ischemaview or rapid CTP or "qure.ai").ti,ab. (125734)
-
or/25-36 (395358)
-
14 and 24 and 37 (2069)
-
(letter or editorial or note).pt. (2732767)
-
38 not 39 (2006)
-
animal/ (1515289)
-
animal experiment/ (2691055)
-
(rat or rats or mouse or mice or murine or rodent or rodents or hamster or hamsters or pig or pigs or porcine or rabbit or rabbits or animal or animals or dogs or dog or cats or cow or bovine or sheep or ovine or monkey or monkeys).ti,ab,ot,hw. (7014819)
-
or/41-43 (7014819)
-
exp human/ (22461462)
-
human experiment/ (549308)
-
or/45-46 (22463344)
-
44 not (44 and 47) (5340409)
-
40 not 48 (1960)
MEDLINE and Epub Ahead of Print, In-Process, In-Data-Review & Other Non-Indexed Citations, Daily and Versions (Ovid): 1946-2021/07/07
Searched: 8.7.21
-
exp Brain Ischemia/ (114022)
-
exp Intracranial Hemorrhages/ (74140)
-
Stroke/ (110696)
-
(Stroke$ or apople$ or cerebral vasc$ or cerebrovasc$ or cerebro vasc$ or poststroke$ or encephalorrhag$ or hematencephalon$ or large-vessel occlusion$).ti,ab,ot. (321196)
-
((brain or blood flow) adj2 disturb$).ti,ab,ot. (2094)
-
((sinus or sagittal) adj3 thromb$).ti,ab,ot. (4982)
-
(isch?emi$ adj3 (seizure$ or attack$ or thrombo$ or embolic or encephalopath$ or neural)).ti,ab,ot. (26336)
-
((Bleed$ or h?emorrhag$) adj2 corpus callosum).ti,ab,ot. (23)
-
((brain or cerebr$ or cerebell$ or cortical or Intraparenchymal or intracortical or vertebrobasil$ or hemispher$ or intracran$ or intra-cran$ or intracerebral or intratentorial or intra-tentorial or intraventricular or intra-ventricular or periventricular or peri-ventricular or supratentorial or supra-tentorial or anterior circulat$ or posterior circulat$ or basal gangli$ or global or focal or parenchymal or subarachnoid or sub-arachnoid or putaminal or putamen or posterior fossa or intra-axial or intraaxial or lacunar) adj3 (arrest$ or attack$ or isch?emi$ or infarct$ or insufficien$ or emboli$ or occlus$ or hypox$ or vasospasm or obstruction or vasculopath$ or failure$ or thromb$ or h?emorrhag$ or microh?emorrhag$ or accident$ or h?ematoma$ or bleed$ or microbleed$ or insult$)).ti,ab,ot. (207796)
-
(CVA or CVAS or MCA$ or ICH or ICHs or CVST or CVSTs or CVDST or CVT or CVDSTs or CVTs or LVO or LVOs).ti,ab. (47842)
-
or/1-10 (547945)
-
((diagnos$ or predict$ or specificity or sensitiv$) adj4 (criteria or criterion or guideline$ or pattern$ or trend$ or utili$ or management or prevalence or initiat$ or distribution$ or coverage or variety or selection or spread or alternative$ or frequen$)).ti,ab,ot. (343809)
-
Diagnosis/ (17448)
-
Early Diagnosis/ (28246)
-
Brain/dg [Diagnostic Imaging] (49771)
-
Stroke/dg [Diagnostic Imaging] (7424)
-
Radiography/ (321804)
-
exp Radionuclide Imaging/ (221021)
-
Neurologic Examination/ (27644)
-
Tomography, X-Ray Computed/ (395500)
-
((Brain or cerebral or neurologic$ or CT or head) adj2 (scan$ or scintigraph$ or examination$ or angiograph$ or image analys$ or perfusion$ or radiograph$)).ti,ab,ot,hw. (226174)
-
(CAT scan$ or CTA or CTP or neuroimag$ or neuro-imag$ or (comput$ adj2 tomograph$)).ti,ab,ot,hw. (465619)
-
(Gamma encephalograph$ or Gammaencephalograph$ or Radio encephalograph$ or Radioencephalograph$).ti,ab,ot,hw. (155)
-
or/12-23 (1606067)
-
exp Artificial Intelligence/ (117654)
-
Pattern Recognition, Automated/ (25872)
-
Neural Networks, Computer/ (31087)
-
(Artificial intelligence or AI or machine intelligence or computer-aided triage$ or support vector machine$ or relevance vector machine$).ti,ab,ot. (58543)
-
((automat$ or computer) adj2 (analys$ or diagnos$ or detect$)).ti,ab,ot. (42174)
-
((deep or machine) adj learning).ti,ab,ot. (54334)
-
(decision support$ adj (software or tool$)).ti,ab,ot. (3372)
-
(CNN or CNNs or convNet or (convolut$ adj2 neural network$) or convolutional ANNs or convolutional ANN or convolutional NNs or convolutional NN).ti,ab. (12557)
-
automat$ hierarch$ evaluat$.ti,ab. (1)
-
(Aidoc or e-CTA or e-ASPECTS or e-stroke or brainomix or brainscan or "brainscan.ai" or icobrain or icometrix or qER or Qure or Zebra$ or e-CTP or briefcase or rapid CTA or rapid LVO or rapid core or rapid ASPECTS or rapid ICH or rapidai or blackford or "viz.ai" or viz or "ct perfusion 4d" or cercare or cina$ or Avicenna or accipio$ or maxQ AI or biomind or "biomind.ai" or ischemaview or rapid CTP or "qure.ai").ti,ab. (102411)
-
or/25-34 (341861)
-
11 and 24 and 35 (1151)
-
(letter or editorial or note).pt. (1715243)
-
exp animals/ not (exp animals/ and humans/) (4857607)
-
36 not (37 or 38) (1110)
Cochrane Database of Systematic Reviews (CDSR) (Wiley): up to 2021/07/Iss7
Cochrane Central Register of Controlled Trials (CENTRAL) (Wiley): up to 2021/07/Iss7
Searched: 8.7.21
-
MeSH descriptor: [Brain Ischemia] explode all trees 3746
-
MeSH descriptor: [Intracranial Hemorrhages] explode all trees 2038
-
(Stroke* or apople* or cerebral-vasc* or cerebrovasc* or cerebro-vasc* or poststroke* or encephalorrhag* or hematencephalon* or large-vessel-occlusion*):ti,ab,kw 67048
-
((brain or blood flow) near/2 disturb*):ti,ab,kw 164
-
((sinus or sagittal) near/3 thromb*):ti,ab,kw 207
-
((ischaemi* or ischemi*) near/3 (seizure* or attack* or thrombo* or embolic or encephalopath* or neural)):ti,ab,kw 4773
-
((Bleed* or hemorrhag* or haemorrhag*) near/2 corpus-callosum):ti,ab,kw 0
-
((brain or cerebr* or cerebell* or cortical or Intraparenchymal or intracortical or vertebrobasil* or hemispher* or intracran* or intra-cran* or intracerebral or intratentorial or intra-tentorial or intraventricular or intra-ventricular or periventricular or peri-ventricular or supratentorial or supra-tentorial or anterior-circulat* or posterior-circulat* or basal-gangli* or global or focal or parenchymal or subarachnoid or sub-arachnoid or putaminal or putamen or posterior-fossa or intra-axial or intraaxial or lacunar) near/3 (arrest* or attack* or ischaemi* or ischemi* or infarct* or insufficien* or emboli* or occlus* or hypox* or vasospasm or obstruction or vasculopath* or failure* or thromb* or hemorrhag* or haemorrhag* or microhemorrhag* or microhaemorrhad or haemorrhag* or accident* or hematoma* or haemotoma* or bleed* or microbleed* or insult*)):ti,ab,kw 34886
-
(CVA or CVAS or MCA* or ICH or ICHs or CVST or CVSTs or CVDST or CVT or CVDSTs or CVTs or LVO or LVOs):ti,ab,kw 4959
-
#1 or #2 or #3 or #4 or #5 or #6 or #7 or #8 or #9 82032
-
((diagnos* or predict* or specificity or sensitiv*) near/4 (criteria or criterion or guideline* or pattern* or trend* or utili* or management or prevalence or initiat* or distribution* or coverage or variety or selection or spread or alternative* or frequen*)):ti,ab,kw 30643
-
MeSH descriptor: [Diagnosis] explode all trees 342030
-
MeSH descriptor: [Early Diagnosis] explode all trees 1796
-
MeSH descriptor: [Brain] explode all trees and with qualifier(s): [diagnostic imaging - DG] 1679
-
MeSH descriptor: [Radiography] explode all trees 21097
-
MeSH descriptor: [Radionuclide Imaging] explode all trees 4662
-
MeSH descriptor: [Neurologic Examination] explode all trees 23937
-
MeSH descriptor: [Tomography, X-Ray Computed] explode all trees 5168
-
((Brain or cerebral or neurologic* or CT or head) near/2 (scan* or scintigraph* or examination* or angiograph* or image analys* or perfusion* or radiograph*)):ti,ab,kw 15653
-
(Gamma-encephalograph* or Gammaencephalograph* or Radio-encephalograph* or Radioencephalograph*):ti,ab,kw 0
-
(CAT scan* or CTA or CTP or neuroimag* or neuro-imag* or (comput* near/2 tomograph*)):ti,ab,kw 24102
-
#11 or #12 or #13 or #14 or #15 or #16 or #17 or #18 or #19 or #20 or #21 391257
-
MeSH descriptor: [Artificial Intelligence] explode all trees 1128
-
MeSH descriptor: [Pattern Recognition, Automated] explode all trees 184
-
MeSH descriptor: [Diagnosis, Computer-Assisted] explode all trees 1867
-
MeSH descriptor: [Neural Networks, Computer] explode all trees 129
-
(Artificial-intelligence or AI or machine-intelligence or computer-aided-triage* or support-vector-machine* or relevance-vector-machine*):ti,ab,kw 5045
-
((automat* or computer) near/2 (analys* or diagnos* or detect*)):ti,ab,kw 3064
-
((deep or machine) near/1 learning):ti,ab,kw 1791
-
(decision-support* near/1 (software or tool*)):ti,ab,kw 552
-
(CNN or CNNs or convNet or (convolut* near/2 neural-network*) or convolutional-ANNs or convolutional-ANN or convolutional-NNs or convolutional-NN):ti,ab,kw 326
-
"automat* hierarch* evaluat*":ti,ab,kw 0
-
(Aidoc or e-CTA or e-ASPECTS or e-stroke or brainomix or brainscan or "brainscan.ai" or icobrain or icometrix or qER or Qure or Zebra* or e-CTP or briefcase or rapid-CTA or rapid-LVO or rapid-core or rapidai or rapid-ASPECTS or rapid-LCH or blackford or "viz.ai" or viz or "ct perfusion 4d" or cercare or cina* or Avicenna or accipio* or maxQ-AI or biomind or "biomind.ai" or ischemaview or rapid-CTP or "qure.ai"):ti,ab,kw 4601
-
#23 or #24 #25 or #26 or #27 or #28 or #29 or #30 or #31 or #32 or #33 15237
-
#10 and #22 and #34 541
CDSR = 135
CENTRAL = 406
Science Citation Index Expanded (Web of Science): 1988–2021/07/06
Conference Proceedings Citation Index (Web of Science): 1988–2021/07/06
Searched: 6.7.21
-
#24 857 #22 NOT #23 Indexes=SCI-EXPANDED, CPCI-S Timespan=All years
-
#23 4,041,528 TS=(cat or cats or dog or dogs or animal or animals or rat or rats or hamster or hamster or feline or ovine or canine or bovine or sheep or mice)
-
#22 890 #21 AND #13 AND #8
-
#21 704,777 #20 OR #19 OR #18 OR #17 OR #16 OR #15 OR #14
-
#20 161,375 TS=(Aidoc OR e-CTA OR e-ASPECTS OR e-stroke OR brainomix OR brainscan OR "brainscan.ai" OR icobrain OR icometrix OR qER OR Qure OR Zebra* OR e-CTP OR briefcase OR “rapid CTA” OR “rapid LVO” OR “rapid core” OR “rapid ASPECTS” OR “rapid ICH” OR rapidai OR blackford OR "viz.ai" OR viz OR "ct perfusion 4d" OR cercare OR cina* OR Avicenna OR accipio* OR “maxQ AI” OR biomind OR "biomind.ai" OR ischemaview OR “rapid CTP” OR "qure.ai")
-
#19 2 TS=“automat* hierarch* evaluat*”
-
#18 71,652 TS=(CNN OR CNNs OR convNet OR (convolut* NEAR/2 “neural network*”) OR “convolutional ANNs” OR “convolutional ANN” OR “convolutional NNs” OR “convolutional NN”)
-
#17 9,599 TS=(“decision support*” NEAR/2 (software OR tool*) )
-
#16 242,068 TS=((deep OR machine) NEAR/2 learning)
-
#15 124,965 TS=((automat* OR computer) NEAR/2 (analys* OR diagnos* OR detect*) )
-
#14 192,179 TS=(“Artificial intelligence” OR AI OR “machine intelligence” OR “computer-aided triage*” OR “support vector machine*” OR “relevance vector machine*”)
-
#13 950,006 #12 OR #11 OR #10 OR #9
-
#12 1 TS=(“Gamma encephalograph*” OR Gammaencephalograph* OR “Radio encephalograph*” OR Radioencephalograph*)
-
#11 404,804 TS=(“CAT scan*” OR CTA OR CTP OR neuroimag* OR neuro-imag* OR (comput* NEAR/2 tomograph*) )
-
#10 161,458 TS=((Brain OR cerebral OR neurologic* OR CT OR head) NEAR/2 (scan* OR scintigraph* OR examination* OR angiograph* OR "image analys*" OR perfusion* OR radiograph*) )
-
#9 473,469 TS=((diagnos* OR predict* OR specificity OR sensitiv*) NEAR/4 (criteria OR criterion OR guideline* OR pattern* OR trend* OR utili* OR management OR prevalence OR initiat* OR distribution* OR coverage OR variety OR selection OR spread OR alternative* OR frequen*) )
-
#8 501,283 #7 OR #6 OR #5 OR #4 OR #3 OR #2 OR #1
-
#7 53,057 TI=(CVA OR CVAS OR MCA* OR ICH OR ICHs OR CVST OR CVSTs OR CVDST OR CVT OR CVDSTs OR CVTs OR LVO OR LVOs) OR AB=(CVA OR CVAS OR MCA* OR ICH OR ICHs OR CVST OR CVSTs OR CVDST OR CVT OR CVDSTs OR CVTs OR LVO OR LVOs)
-
#6 125,500 TS=((brain OR cerebr* OR cerebell* OR cortical OR Intraparenchymal OR intracortical OR vertebrobasil* OR hemispher* OR intracran* OR intra-cran* OR intracerebral OR intratentorial OR intra-tentorial OR intraventricular OR intra-ventricular OR periventricular OR peri-ventricular OR supratentrial OR supra-tentorial OR “anterior circulat*” OR “posterior circulat*” OR “basal gangli*” OR global OR focal OR parenchymal OR subarachnoid OR sub-arachnoid OR putaminal OR putamen OR “posterior fossa” OR intra-axial OR intraaxial OR lacunar) NEAR/3 (arrest* OR attack* OR isch?emi* OR infarct* OR insufficien* OR emboli* OR occlus* OR hypox* OR vasospasm OR obstruction OR vasculopath* OR failure* OR thromb* OR h?emorrhag* OR microh?emorrhag* OR accident* OR h?ematoma* OR bleed* OR microbleed* OR insult*) )
-
#5 9 TS=((Bleed* OR h?emorrhag*) NEAR/2 “corpus callosum”)
-
#4 4,227 TS=(isch?emi* NEAR/3 (seizure* OR attack* OR thrombo* OR embolic OR encephalopath* OR neural) )
-
#3 5,016 TS=((sinus OR sagittal) NEAR/3 thromb*)
-
#2 2,426 TS=((brain OR “blood flow”) NEAR/2 disturb*)
-
#1 395,490 TS=(Stroke* OR apople* OR “cerebral vasc*” OR cerebrovasc* OR “cerebro vasc*” OR poststroke* OR encephalorrhag* OR hematencephalon* OR “large-vessel occlusion*”)
Database of Abstracts of Reviews of Effects (DARE) (Internet) ( www.crd.york.ac.uk/CRDWeb/ ): up to 2015/03/31
Health Technology Assessment Database (HTA) (Internet) ( www.crd.york.ac.uk/CRDWeb/ ): up to 2018/03/31
Searched: 7.7.21
1 | MeSH DESCRIPTOR Brain Ischemia EXPLODE ALL TREES | 328 |
2 | MeSH DESCRIPTOR Intracranial Hemorrhages EXPLODE ALL TREES | 258 |
3 | MeSH DESCRIPTOR Stroke EXPLODE ALL TREES | 1356 |
4 | MeSH DESCRIPTOR Ischaemic Stroke EXPLODE ALL TREES | 0 |
5 | MeSH DESCRIPTOR Hemorrhagic Stroke EXPLODE ALL TREES | 0 |
6 | (Stroke* or apople* or "cerebral vasc*" or cerebrovasc* or "cerebro vasc*" or poststroke* or encephalorrhag* or hematencephalon* or "large-vessel occlusion*") | 3402 |
7 | (((brain or "blood flow") and disturb*)) OR (((sinus or sagittal) and thromb*)) OR (((ischemi* or ischaemi*) and (seizure* or attack* or thrombo* or embolic or encephalopath* or neural))) | 691 |
8 | (((Bleed* or hemorrhag* or haemorrhag*) and "corpus callosum")) OR (((brain or cerebr* or cerebell* or cortical or Intraparenchymal or intracortical or vertebrobasil* or hemispher* or intracran* or intra-cran* or intracerebral or intratentorial or intra-tentorial or intraventricular or intra-ventricular or periventricular or peri-ventricular or supratentorial or supra-tentorial or anterior circulat* or "posterior circulat*" or "basal gangli*" or global or focal or parenchymal or subarachnoid or sub-arachnoid or putaminal or putamen or "posterior fossa" or intra-axial or intraaxial or lacunar) and (arrest* or attack* or ischemi* or ischaemi* or infarct* or insufficien* or emboli* or occlus* or hypox* or vasospasm or obstruction or vasculopath* or failure* or thromb* or hemorrhag* or haemorrhag* or microhemorrhag* or microhaemorrhag* or accident* or hematoma* or haematoma* or bleed* or microbleed* or insult*))) OR (CVA or CVAS or MCA* or ICH or ICHs or CVST or CVSTs or CVDST or CVT or CVDSTs or CVTs or LVO or LVOs) | 2618 |
9 | #1 OR #2 OR #3 OR #4 OR #5 OR #6 OR #7 OR #8 | 5187 |
10 | MeSH DESCRIPTOR Diagnosis EXPLODE ALL TREES | 29251 |
11 | MeSH DESCRIPTOR Early Diagnosis EXPLODE ALL TREES | 413 |
12 | MeSH DESCRIPTOR brain EXPLODE ALL TREES WITH QUALIFIER DG IN DARE,HTA | 0 |
13 | MeSH DESCRIPTOR stroke EXPLODE ALL TREES WITH QUALIFIER DG IN DARE,HTA | 0 |
14 | MeSH DESCRIPTOR Radionuclide Imaging EXPLODE ALL TREES | 725 |
15 | MeSH DESCRIPTOR Neurologic Examination EXPLODE ALL TREES | 772 |
16 | (((Brain or cerebral or neurologic* or CT or head) and (scan* or scintigraph* or examination* or angiograph* or "image analys*" or perfusion* or radiograph*))) OR (((diagnos* or predict* or specificity or sensitiv*) and (criteria or criterion or guideline* or pattern* or trend* or utili* or management or prevalence or initiat* or distribution* or coverage or variety or selection or spread or alternative* or frequen*))) OR (("CAT scan*" or CTA or CTP or neuroimag* or neuro-imag* or (comput* and tomograph*))) | 25348 |
17 | ("Gamma encephalograph*" or Gammaencephalograph* or "Radio encephalograph*" or Radioencephalograph*) | 0 |
18 | #10 OR #11 OR #12 OR #13 OR #14 OR #15 OR #16 OR #17 | 40752 |
19 | MeSH DESCRIPTOR Artificial Intelligence EXPLODE ALL TREES | 290 |
20 | MeSH DESCRIPTOR Pattern Recognition, Automated EXPLODE ALL TREES | 3 |
21 | MeSH DESCRIPTOR Neural Networks, Computer EXPLODE ALL TREES | 0 |
22 | ("Artificial intelligence" or AI or "machine intelligence" or "computer-aided triage*" or "support vector machine*" or "relevance vector machine*") OR (((automat* or computer) and (analys* or diagnos* or detect*))) OR (((deep or machine) and learning)) | 2249 |
23 | (("decision support*" and (software or tool*))) OR (CNN or CNNs or convNet or (convolut* and "neural network*") or "convolutional ANNs" or "convolutional ANN" or "convolutional NNs" or "convolutional NN") OR ("automat* hierarch* evaluat*") | 176 |
24 | (Aidoc OR "e-CTA" OR "e-ASPECTS" OR "e-stroke" OR brainomix OR brainscan OR "brainscan.ai" OR icobrain OR icometrix OR qER OR Qure OR Zebra* OR "e-CTP" OR briefcase OR "rapid CTA" OR "rapid LVO" OR "rapid core" OR "rapid ASPECTS" OR "rapid ICH" OR rapidai OR blackford OR "viz.ai" OR viz OR "ct perfusion 4d" OR cercare OR cina* OR Avicenna OR accipio* OR maxQ AI OR biomind OR "biomind.ai" OR ischemaview OR "rapid CTP" OR "qure.ai") | 5365 |
25 | #19 OR #20 OR #21 OR #22 OR #23 OR #24 | 7756 |
26 | #9 AND #18 AND #25 | 497 |
27 | * IN DARE, HTA | 62769 |
28 | #26 AND #27 | 361 |
KSR Evidence (KSR Ltd) (https://ksrevidence.com/): up to 2021/07/07
Searched: 7.7.21
-
(Stroke* or apople* or "cerebral vasc*" or cerebrovasc* or "cerebro vasc*" or poststroke* or encephalorrhag* or hematencephalon* or "large-vessel occlusion*") in Title or Abstract 6910 results
-
((brain or "blood flow") adj2 disturb*) in Title or Abstract 14 results
-
((sinus or sagittal) adj3 thromb*) in Title or Abstract 34 results
-
((ischemi* or ischaemi*) adj3 (seizure* or attack* or thrombo* or embolic or encephalopath* or neural)) in Title or Abstract 601 results
-
((Bleed* or hemorrhag* or haemorrhag*) adj2 "corpus callosum") in Title or Abstract 1 result
-
CVA or CVAS or MCA* or ICH or ICHs or CVST or CVSTs or CVDST or CVT or CVDSTs or CVTs or LVO or LVOs in Title or Abstract 534 results
-
((brain or cerebr* or cerebell* or cortical or Intraparenchymal or intracortical or vertebrobasil* or hemispher* or intracran* or intra-cran* or intracerebral or intratentorial or intra-tentorial or intraventricular or intra-ventricular or periventricular or peri-ventricular or supratentorial or supra-tentorial or "anterior circulat*" or "posterior circulat*" or "basal gangli*" or global or focal or parenchymal or subarachnoid or sub-arachnoid or putaminal or putamen or "posterior fossa" or intra-axial or intraaxial or lacunar) adj3 (arrest* or attack* or ischemi* or ischaemi* or infarct* or insufficien* or emboli* or occlus* or hypox* or vasospasm or obstruction or vasculopath* or failure* or thromb* or hemorrhag* or haemorrhag* or microhemorrhag* or microhaemorrhag* or accident* or hematoma* or haematoma* or bleed* or microbleed* or insult*)) in Title or Abstract 2211 results
-
#1 or #2 or #3 or #4 or #5 or #6 or #7 in All text 8102 results
-
((diagnos* or predict* or specificity or sensitiv*) adj4 (criteria or criterion or guideline* or pattern* or trend* or utili* or management or prevalence or initiat* or distribution* or coverage or variety or selection or spread or alternative* or frequen*)) in Title or Abstract 5140 results
-
((Brain or cerebral or neurologic* or CT or head) adj2 (scan* or scintigraph* or examination* or angiograph* or "image analys*" or perfusion* or radiograph*)) in Title or Abstract 704 results
-
("CAT scan*" or CTA or CTP or neuroimag* or neuro-imag* or (comput* adj2 tomograph*)) in Title or Abstract 2625 results
-
"Gamma encephalograph*" or Gammaencephalograph* or "Radio encephalograph*" or Radioencephalograph* in Title or Abstract 0 results
-
#9 or #10 or #11 or #12 in Title or Abstract 7867 results
-
"Artificial intelligence" or AI or "machine intelligence" or "computer-aided triage*" or "support vector machine*" or "relevance vector machine*" in Title or Abstract 421 results
-
((automat* or computer) adj2 (analys* or diagnos* or detect*)) in Title or Abstract 181 results
-
((deep or machine) adj learning) in Title or Abstract 354 results
-
("decision support*" adj (software or tool*)) in Title or Abstract 56 results
-
CNN or CNNs or convNet or (convolut* adj2 "neural network*") or "convolutional ANNs" or "convolutional ANN" or "convolutional NNs" or "convolutional NN" in Title or Abstract 34 results
-
Aidoc OR "e-CTA" OR "e-ASPECTS" OR "e-stroke" OR brainomix OR brainscan OR "brainscan ai" OR icobrain OR icometrix OR qER OR Qure OR Zebra* OR "e-CTP" OR briefcase OR "rapid CTA" OR "rapid LVO" OR "rapid core" OR "rapid ASPECTS" OR "rapid ICH" OR rapidai OR blackford OR "viz ai" OR viz OR "ct perfusion 4d" OR cercare OR cina* OR Avicenna OR accipio* OR maxQ AI OR biomind OR "biomind ai" OR ischemaview OR "rapid CTP" OR "qure ai" in Title or Abstract 16149 results
-
#14 or #15 or #16 or #17 or #18 or #19 in All text 16916 results
-
#20 and #13 and #8 in All text 42 results
Epistemonikos ( www.epistemonikos.org/ ): up to 2021/07/07
Searched: 7.7.21
(title:((title:(stroke* OR "brain haemorrhag*" OR "brain hemorrhag*" OR "brain bleed*" OR "cerebr* bleed*") OR abstract:(stroke* OR "brain haemorrhag*" OR "brain hemorrhag*" OR "brain bleed*" OR "cerebr* bleed*")) AND (title:(diagnos* OR "brain scan*" OR "CT scan*" OR "CAT scan*" OR "comput* tomograph*") OR abstract:(diagnos* OR "brain scan*" OR "CT scan*" OR "CAT scan*" OR "comput* tomograph*")) AND (title:("artificial intelligence" OR AI OR "machine intelligence" OR "computer-aided triage*" OR "decision support software") OR abstract:("artificial intelligence" OR AI OR "machine intelligence" OR "computer-aided triage*" OR "decision support software"))) OR abstract:((title:(stroke* OR "brain haemorrhag*" OR "brain hemorrhag*" OR "brain bleed*" OR "cerebr* bleed*") OR abstract:(stroke* OR "brain haemorrhag*" OR "brain hemorrhag*" OR "brain bleed*" OR "cerebr* bleed*")) AND (title:(diagnos* OR "brain scan*" OR "CT scan*" OR "CAT scan*" OR "comput* tomograph*") OR abstract:(diagnos* OR "brain scan*" OR "CT scan*" OR "CAT scan*" OR "comput* tomograph*")) AND (title:("artificial intelligence" OR AI OR "machine intelligence" OR "computer-aided triage*" OR "decision support software") OR abstract:("artificial intelligence" OR AI OR "machine intelligence" OR "computer-aided triage*" OR "decision support software"))))
3 results filtered to systematic review
NIHR Health Technology Assessment (HTA) (Internet) (www.nihr.ac.uk/): up to 2021/07/02
Searched: 2.7.21
Search terms | Journal reports | Research Projects |
---|---|---|
“artificial intelligence” | 0 | 5 |
INAHTA (www.inahta.org/): up to 2021/07/06
Searched: 6.7.21
((((“Artificial intelligence” OR AI OR “machine intelligence” OR “computer-aided triage*” or "automat* analys*" or "computer analys*" or "decision support* software")[abs]) OR (((“Artificial intelligence” OR AI OR “machine intelligence” OR “computer-aided triage*” or "automat* analys*" or "computer analys*" or "decision support* software")[Title]) OR ("Artificial Intelligence"[mhe])) AND (((stroke* or "intracranial haemorrhag*" or "intracranial hemorrhag*" or "brain ischaemi*" or "brain ischemi*")[abs]) OR ((stroke* or "intracranial haemorrhag*" or "intracranial hemorrhag*" or "brain ischaemi*" or "brain ischemi*")[Title]) OR ("Stroke"[mh]) OR ("Intracranial Hemorrhages"[mhe]) OR ("Brain Ischemia"[mhe]))
265 results
Aggressive Research Intelligence Facility (ARIF) (Internet) ( www.birmingham.ac.uk/research/activity/mds/projects/HaPS/PHEB/ARIF/index.aspx )
Searched: 2.7.21
Unable to search as ARIF databases were unavailable due to ongoing server issues
PROSERO (CRD) ( www.crd.york.ac.uk/prospero/ ): up to 2021/07/07
Searched: 7.7.21
-
#1 MeSH DESCRIPTOR Stroke EXPLODE ALL TREES 1371
-
#2 MeSH DESCRIPTOR Ischaemic Stroke EXPLODE ALL TREES 36
-
#3 MeSH DESCRIPTOR Hemorrhagic Stroke EXPLODE ALL TREES 1
-
#4 stroke* OR "brain haemorrhag*" OR "brain hemorrhag*" OR "brain bleed*" OR "cerebr* bleed*" 7515
-
#5 #4 OR #3 OR #2 OR #1 7530
-
#6 MeSH DESCRIPTOR Diagnosis EXPLODE ALL TREES 16729
-
#7 MeSH DESCRIPTOR Early Diagnosis EXPLODE ALL TREES 389
-
#8 MeSH DESCRIPTOR Tomography, X-Ray Computed EXPLODE ALL TREES 386
-
#9 "brain scan*" OR "CT scan*" OR "CAT scan*" OR "comput* tomograph*" 2310
-
#10 #6 OR #7 OR #8 OR #9 18292
-
#11 MeSH DESCRIPTOR Artificial Intelligence EXPLODE ALL TREES 357
-
#12 MeSH DESCRIPTOR Pattern Recognition, Automated EXPLODE ALL TREES 1
-
#13 "artificial intelligence" OR AI OR "machine intelligence" OR "computer-aided triage*" OR "decision support software" 943
-
#14 #13 OR #12 OR #11 1170
-
#15 #5 AND #10 AND #14 23
INPLASY (Internet) (https://inplasy.com/): up to 2021/07/02
Searched: 2.7.21
MeSH / Keyword search | Hits |
---|---|
Artificial intelligence | 1 |
LILACS (Internet) (http://regional.bvsalud.org/php/index.php?lang=en): up to 2021/07/02
Searched: 2.7.21
(mh:(stroke or "brain ischaemia" or "brain ischemia" or "intracranial haemorrhage*" or "intracranial hemorrhage*" or "large-vessel occlusion*" )) AND (diagnosis or "cat scan" or "CT scan" or "brain scan" or "neuroimag*" or "neuro-imag*") AND ("artificial intelligence" or AI or "machine intelligence" or "computer aided triage" or "automat* diagnos*" or "computer diagnos*" or "decision support software")
374 results
ClinicalTrials.gov (Internet) ( www.who.int/clinical-trials-registry-platform ): up to 2021/07/02
Searched: 2.7.21
((stroke OR "brain ischemia" OR "brain ischaemia" or "blood vessel occlusion" OR "cerebral ischemia" or "cerebral ischaemia" or "large-vessel occlusion" OR "intracranial haemorrhage" OR "intracranial hemorrhage") AND ("artificial intelligence" OR "automated pattern recognition" OR "computer assisted diagnosis" OR "computer aided triage" OR "decision support software" OR "automated diagnosis"))
39 results
EU Clinical Trials Register (Internet) ( www.clinicaltrialsregister.eu/ctr-search/search ): up to 2021/07/28
Searched: 28.7.21
Search terms | Hits |
---|---|
“artificial intelligence” | 2 |
“machine intelligence” | 0 |
Aidoc | 0 |
e-cta | 0 |
e-aspects | 0 |
e-stroke | 0 |
Brainomix | 0 |
Brainscan* | 0 |
Icobrain | 0 |
Icometrix | 0 |
Qer | 0 |
qure | 1 |
Zebra* | 3 |
c-ctp | 0 |
Briefcase | 0 |
“rapid CTA” | 0 |
“rapid LVO” | 0 |
“rapid core” | 0 |
“rapid aspects” | 0 |
“rapid ICH” | 0 |
Rapidai | 0 |
Blackford | 0 |
Viz.ai | 0 |
Viz | 8 |
“ct perfusion 4d” | 0 |
Cercare | 0 |
Cina* AND stroke | 2 |
Avicenna | 0 |
Accipio* | 0 |
“maxq ai” | 0 |
Biomind* | 0 |
Ischemaview | 0 |
“rapid ctp” | 0 |
Qure.ai | 0 |
Total | 16 |
WHO ICTRP (Internet) (https://ictrptest.azurewebsites.net/Default.aspx): up to 2021/07/02
Searched: 2.7.21
Search terms | Hits |
---|---|
Artificial intelligence AND stroke | 14 |
ScanMedicine (Internet) ( https://scanmedicine.com /): up to 2021/07/02
Searched: 2.7.21
Search terms | Hits |
---|---|
"artificial intelligence" + stroke [only] | 28 |
Northern Light Life Sciences Conference Abstracts (Ovid): 2010–2021/Wk25
Searched: 7.7.21
-
exp Brain Ischemia/ (5706)
-
exp Intracranial Hemorrhages/ (12738)
-
Stroke/ (37884)
-
(Stroke$ or apople$ or cerebral vasc$ or cerebrovasc$ or cerebro vasc$ or poststroke$ or encephalorrhag$ or hematencephalon$ or large-vessel occlusion$).ti,ab. (50748)
-
(CVA or CVAS or MCA$ or ICH or ICHs or CVST or CVSTs or CVDST or CVT or CVDSTs or CVTs or LVO or LVOs).ti,ab. (7951)
-
((brain or cerebr$ or cerebell$ or cortical or Intraparenchymal or intracortical or vertebrobasil$ or hemispher$ or intracran$ or intra-cran$ or intracerebral or intratentorial or intra-tentorial or intraventricular or intra-ventricular or periventricular or peri-ventricular or supratentorial or supra-tentorial or anterior circulat$ or posterior circulat$ or basal gangli$ or global or focal or parenchymal or subarachnoid or sub-arachnoid or putaminal or putamen or posterior fossa or intra-axial or intraaxial or lacunar) adj3 (arrest$ or attack$ or isch?emi$ or infarct$ or insufficien$ or emboli$ or occlus$ or hypox$ or vasospasm or obstruction or vasculopath$ or failure$ or thromb$ or h?emorrhag$ or microh?emorrhag$ or accident$ or h?ematoma$ or bleed$ or microbleed$ or insult$)).ti,ab. (18023)
-
((brain or blood flow) adj2 disturb$).ti,ab. (104)
-
((sinus or sagittal) adj3 thromb$).ti,ab. (543)
-
(isch?emi$ adj3 (seizure$ or attack$ or thrombo$ or embolic or encephalopath$ or neural)).ti,ab. (2184)
-
((Bleed$ or h?emorrhag$) adj2 corpus callosum).ti,ab. (2)
-
or/1-10 (86665)
-
Diagnosis/ (0)
-
Early Diagnosis/ (21707)
-
Radiography/ (0)
-
exp Radionuclide Imaging/ (0)
-
Neurologic Examination/ (0)
-
Tomography, X-Ray Computed/ (0)
-
((Brain or cerebral or neurologic$ or CT or head) adj2 (scan$ or scintigraph$ or examination$ or angiograph$ or image analys$ or perfusion$ or radiograph$)).ti,ab. (19256)
-
(CAT scan$ or CTA or CTP or neuroimag$ or neuro-imag$ or (comput$ adj2 tomograph$)).ti,ab. (24365)
-
(Gamma encephalograph$ or Gammaencephalograph$ or Radio encephalograph$ or Radioencephalograph$).ti,ab. (0)
-
or/12-20 (61593)
-
exp Artificial Intelligence/ (0)
-
Pattern Recognition, Automated/ (0)
-
Neural Networks, Computer/ (0)
-
(CNN or CNNs or convNet or (convolut$ adj2 neural network$) or convolutional ANNs or convolutional ANN or convolutional NNs or convolutional NN).ti,ab. (1290)
-
(Artificial intelligence or AI or machine intelligence or computer-aided triage$ or support vector machine$ or relevance vector machine$).ti,ab. (6547)
-
((automat$ or computer) adj2 (analys$ or diagnos$ or detect$)).ti,ab. (4358)
-
((deep or machine) adj learning).ti,ab. (8611)
-
automat$ hierarch$ evaluat$.ti,ab. (0)
-
(decision support$ adj (software or tool$)).ti,ab. (775)
-
(Aidoc or e-CTA or e-ASPECTS or e-stroke or brainomix or brainscan or "brainscan.ai" or icobrain or icometrix or qER or Qure or Zebra$ or e-CTP or briefcase or rapid CTA or rapid LVO or rapid core or rapid ASPECTS or rapid ICH or rapidai or blackford or "viz.ai" or viz or "ct perfusion 4d" or cercare or cina$ or Avicenna or accipio$ or maxQ AI or biomind or "biomind.ai" or ischemaview or rapid CTP or "qure.ai").ti,ab. (7552)
-
or/22-31 (27321)
-
11 and 21 and 32 (64)
Named technologies
Database | Dates covered | Hits |
---|---|---|
Embase | 2017–2021/09/03 | 1361 |
MEDLINE + PreMedline | 2017–2021/09/03 | 915 |
Northern Light | 2017–2021/Wk34 | 46 |
Total | 2322 |
Embase (Ovid): 2017–2021/09/03
Date searched: 7.9.21
Stroke + named tech + (Limits: NoA/2017-C)
-
exp brain ischemia/ (200071)
-
exp brain hemorrhage/ (152081)
-
basal ganglion hemorrhage/ (662)
-
cerebrovascular accident/ (230100)
-
brain infarction/ (56277)
-
blood vessel occlusion/ (11766)
-
(Stroke$ or apople$ or cerebral vasc$ or cerebrovasc$ or cerebro vasc$ or poststroke$ or encephalorrhag$ or hematencephalon$ or large-vessel occlusion$).ti,ab,ot. (503730)
-
((brain or blood flow) adj2 disturb$).ti,ab,ot. (2924)
-
((sinus or sagittal) adj3 thromb$).ti,ab,ot. (7228)
-
(isch?emi$ adj3 (seizure$ or attack$ or thrombo$ or embolic or encephalopath$ or neural)).ti,ab,ot. (40907)
-
((Bleed$ or h?emorrhag$) adj2 corpus callosum).ti,ab,ot. (27)
-
((brain or cerebr$ or cerebell$ or cortical or Intraparenchymal or intracortical or vertebrobasil$ or hemispher$ or intracran$ or intra-cran$ or intracerebral or intratentorial or intra-tentorial or intraventricular or intra-ventricular or periventricular or peri-ventricular or supratentorial or supra-tentorial or anterior circulat$ or posterior circulat$ or basal gangli$ or global or focal or parenchymal or subarachnoid or sub-arachnoid or putaminal or putamen or posterior fossa or intra-axial or intraaxial or lacunar) adj3 (arrest$ or attack$ or isch?emi$ or infarct$ or insufficien$ or emboli$ or occlus$ or hypox$ or vasospasm or obstruction or vasculopath$ or failure$ or thromb$ or h?emorrhag$ or microh?emorrhag$ or accident$ or h?ematoma$ or bleed$ or microbleed$ or insult$)).ti,ab,ot. (295196)
-
(CVA or CVAS or MCA$ or ICH or ICHs or CVST or CVSTs or CVDST or CVT or CVDSTs or CVTs or LVO or LVOs).ti,ab. (82820)
-
or/1-13 (870499)
-
(Aidoc or e-CTA or e-ASPECTS or e-stroke or brainomix or brainscan or "brainscan.ai" or icobrain or icometrix or qER or Qure or Zebra$ or e-CTP or briefcase or rapid CTA or rapid LVO or rapid core or rapid ASPECTS or rapid ICH or rapidai or blackford or "viz.ai" or viz or "ct perfusion 4d" or cercare or cina$ or Avicenna or accipio$ or maxQ AI or biomind or "biomind.ai" or ischemaview or rapid CTP or "qure.ai").ti,ab. (127715)
-
14 and 15 (3409)
-
(letter or editorial or note).pt. (2753204)
-
16 not 17 (3392)
-
animal/ (1525609)
-
animal experiment/ (2713339)
-
(rat or rats or mouse or mice or murine or rodent or rodents or hamster or hamsters or pig or pigs or porcine or rabbit or rabbits or animal or animals or dogs or dog or cats or cow or bovine or sheep or ovine or monkey or monkeys).ti,ab,ot,hw. (7057425)
-
or/19-21 (7057425)
-
exp human/ (22670126)
-
human experiment/ (552250)
-
or/23-24 (22672045)
-
22 not (22 and 25) (5366393)
-
18 not 26 (3030)
-
limit 27 to yr="2017 -Current" (1361)
MEDLINE and Epub Ahead of Print, In-Process, In-Data-Review & Other Non-Indexed Citations, Daily and Versions (Ovid): 2017–2021/09/03
Searched: 7.9.21
-
exp Brain Ischemia/ (114939)
-
exp Intracranial Hemorrhages/ (74704)
-
Stroke/ (112246)
-
(Stroke$ or apople$ or cerebral vasc$ or cerebrovasc$ or cerebro vasc$ or poststroke$ or encephalorrhag$ or hematencephalon$ or large-vessel occlusion$).ti,ab,ot. (325118)
-
((brain or blood flow) adj2 disturb$).ti,ab,ot. (2108)
-
((sinus or sagittal) adj3 thromb$).ti,ab,ot. (5061)
-
(isch?emi$ adj3 (seizure$ or attack$ or thrombo$ or embolic or encephalopath$ or neural)).ti,ab,ot. (26633)
-
((Bleed$ or h?emorrhag$) adj2 corpus callosum).ti,ab,ot. (23)
-
((brain or cerebr$ or cerebell$ or cortical or Intraparenchymal or intracortical or vertebrobasil$ or hemispher$ or intracran$ or intra-cran$ or intracerebral or intratentorial or intra-tentorial or intraventricular or intra-ventricular or periventricular or peri-ventricular or supratentorial or supra-tentorial or anterior circulat$ or posterior circulat$ or basal gangli$ or global or focal or parenchymal or subarachnoid or sub-arachnoid or putaminal or putamen or posterior fossa or intra-axial or intraaxial or lacunar) adj3 (arrest$ or attack$ or isch?emi$ or infarct$ or insufficien$ or emboli$ or occlus$ or hypox$ or vasospasm or obstruction or vasculopath$ or failure$ or thromb$ or h?emorrhag$ or microh?emorrhag$ or accident$ or h?ematoma$ or bleed$ or microbleed$ or insult$)).ti,ab,ot. (209642)
-
(CVA or CVAS or MCA$ or ICH or ICHs or CVST or CVSTs or CVDST or CVT or CVDSTs or CVTs or LVO or LVOs).ti,ab. (48496)
-
or/1-10 (553497)
-
(Aidoc or e-CTA or e-ASPECTS or e-stroke or brainomix or brainscan or "brainscan.ai" or icobrain or icometrix or qER or Qure or Zebra$ or e-CTP or briefcase or rapid CTA or rapid LVO or rapid core or rapid ASPECTS or rapid ICH or rapidai or blackford or "viz.ai" or viz or "ct perfusion 4d" or cercare or cina$ or Avicenna or accipio$ or maxQ AI or biomind or "biomind.ai" or ischemaview or rapid CTP or "qure.ai").ti,ab. (104207)
-
11 and 12 (2182)
-
(letter or editorial or note).pt. (1729571)
-
exp animals/ not (exp animals/ and humans/) (4881960)
-
13 not (14 or 15) (1953)
-
limit 16 to yr="2017 -Current" (915)
Northern Light Life Sciences Conference Abstracts (Ovid): 2017–2021/Wk34
Searched: 7.9.21
-
exp Brain Ischemia/ (6060)
-
exp Intracranial Hemorrhages/ (13588)
-
Stroke/ (40328)
-
(Stroke$ or apople$ or cerebral vasc$ or cerebrovasc$ or cerebro vasc$ or poststroke$ or encephalorrhag$ or hematencephalon$ or large-vessel occlusion$).ti,ab. (54065)
-
(CVA or CVAS or MCA$ or ICH or ICHs or CVST or CVSTs or CVDST or CVT or CVDSTs or CVTs or LVO or LVOs).ti,ab. (8472)
-
((brain or cerebr$ or cerebell$ or cortical or Intraparenchymal or intracortical or vertebrobasil$ or hemispher$ or intracran$ or intra-cran$ or intracerebral or intratentorial or intra-tentorial or intraventricular or intra-ventricular or periventricular or peri-ventricular or supratentorial or supra-tentorial or anterior circulat$ or posterior circulat$ or basal gangli$ or global or focal or parenchymal or subarachnoid or sub-arachnoid or putaminal or putamen or posterior fossa or intra-axial or intraaxial or lacunar) adj3 (arrest$ or attack$ or isch?emi$ or infarct$ or insufficien$ or emboli$ or occlus$ or hypox$ or vasospasm or obstruction or vasculopath$ or failure$ or thromb$ or h?emorrhag$ or microh?emorrhag$ or accident$ or h?ematoma$ or bleed$ or microbleed$ or insult$)).ti,ab. (19017)
-
((brain or blood flow) adj2 disturb$).ti,ab. (113)
-
((sinus or sagittal) adj3 thromb$).ti,ab. (576)
-
(isch?emi$ adj3 (seizure$ or attack$ or thrombo$ or embolic or encephalopath$ or neural)).ti,ab. (2281)
-
((Bleed$ or h?emorrhag$) adj2 corpus callosum).ti,ab. (2)
-
or/1-10 (92032)
-
(Aidoc or e-CTA or e-ASPECTS or e-stroke or brainomix or brainscan or "brainscan.ai" or icobrain or icometrix or qER or Qure or Zebra$ or e-CTP or briefcase or rapid CTA or rapid LVO or rapid core or rapid ASPECTS or rapid ICH or rapidai or blackford or "viz.ai" or viz or "ct perfusion 4d" or cercare or cina$ or Avicenna or accipio$ or maxQ AI or biomind or "biomind.ai" or ischemaview or rapid CTP or "qure.ai").ti,ab. (7830)
-
11 and 12 (86)
-
limit 13 to yr="2017 -Current" (46)
Preprints Search
Database | Dates covered | Hits |
---|---|---|
MedRxiv | up to 2021/09/29 | 538 |
Total | 538 |
MedRxiv: the preprint server for Health Sciences ( www.medrxiv.org/ ): up to 2021/09/29
Searched 29.9.21
Advanced search
Full text or abstract or title (match whole all) | Hits |
---|---|
stroke* Aidoc | 1 |
Stroke* e-CTA | 0 |
Stroke* e-ASPECTS | 0 |
e-stroke | 14 |
Stroke* brainomix | 0 |
Stroke* brainscan | 1 |
Stroke* brainscan.ai | 0 |
stroke icobrain | 1 |
Stroke* icometrix | 2 |
Stroke* qER | 0 |
Stroke* Qure | 3 |
Stroke* Zebra* | 1 |
Stroke* e-CTP | 0 |
Stroke* briefcase | 0 |
Stroke* rapid CTA | 14 |
Stroke* rapid LVO | 8 |
Stroke* rapid core | 247 |
Stroke* rapid ASPECTS | 331 |
Stroke* rapid ICH | 27 |
Stroke* rapidai | 1 |
Stroke* blackford | 1 |
Stroke* viz.ai | 2 |
Stroke* viz | 23 |
Stroke* ct perfusion 4d | 15 |
Stroke* cercare | 0 |
Stroke* cina* | 2 |
Stroke* Avicenna | 2 |
Stroke* accipio* | 0 |
Stroke* maxQ AI | 0 |
Stroke* biomind | 0 |
Stroke* biomind.ai | 0 |
Stroke* ischemaview | 1 |
Stroke* rapid CTP | 5 |
Stroke* qure.ai | 0 |
Total | 702 |
Total without dupes | 538 |
Guidelines
Database | Dates covered | Hits |
---|---|---|
TRIP | 2017–2021/10/26 | 59 |
GIN | 2017–2021/10/20 | 7 |
HTA | 2017–2018/03 | 17 |
NICE | 2017–2021/10/20 | 1 |
NIHR HTA | 2017–2021/10/20 | 8 |
ECRI | 2017–2021/10/20 | 39 |
NHS Evidence | 2017–2021/10/20 | 358 |
INAHTA | 2017–2021/10/20 | 64 |
Total | 553 |
TRIP database (www.tripdatabase.com/): 2017–2021/10/26
Date searched: 26.10.21
Limits: All of these words in Title
Publication year – 2017–2021
Search term (in Title) | Results |
---|---|
Stroke | 59 |
TIA | 59 |
transient ischaemic attack | 2 |
transient ischaemic attack | 2 |
brain ischaemia | 0 |
brain ischemia | 0 |
intracranial haemorrhage | 0 |
intracranial hemorrhage | 0 |
vessel occlusion | 1 |
Total | 123 |
Total (after deduplication) | 59 |
Guidelines International Network (GIN) ( https://g-i-n.net/international-guidelines-library/ ): 2017–2021/10/20
Searched: 20.10.21
Limits:
Publication year – 2017–2021
Guideline publication status – Published
Search term | Results |
---|---|
Stroke | 7 |
TIA | 0 |
Total | 7 |
Health Technology Assessment Database (HTA) (CRD): 2017–2018/03
Searched 20.10.21
-
MeSH DESCRIPTOR Brain Ischemia EXPLODE ALL TREES 328 Delete
-
MeSH DESCRIPTOR Intracranial Hemorrhages EXPLODE ALL TREES 258 Delete
-
((Stroke* or apople* or cerebral-vasc* or cerebrovasc* or cerebro-vasc* or poststroke* or encephalorrhag* or hematencephalon* or large-vessel-occlusion*)) 3402 Delete
-
(((brain or blood flow) NEAR2 disturb*)) 1 Delete
-
(((sinus or sagittal) NEAR3 thromb*)) 5 Delete
-
(((ischaemi* or ischemi*) NEAR3 (seizure* or attack* or thrombo* or embolic or encephalopath* or neural))) 342 Delete
-
(((Bleed* or hemorrhag* or haemorrhag*) NEAR2 corpus-callosum)) 0 Delete
-
(((brain or cerebr* or cerebell* or cortical or Intraparenchymal or intracortical or vertebrobasil* or hemispher* or intracran* or intra-cran* or intracerebral or intratentorial or intra-tentorial or intraventricular or intra-ventricular or periventricular or peri-ventricular or supratentorial or supra-tentorial or anterior-circulat* or posterior-circulat* or basal-gangli* or global or focal or parenchymal or subarachnoid or sub-arachnoid or putaminal or putamen or posterior-fossa or intra-axial or intraaxial or lacunar) NEAR3 (arrest* or attack* or ischaemi* or ischemi* or infarct* or insufficien* or emboli* or occlus* or hypox* or vasospasm or obstruction or vasculopath* or failure* or thromb* or hemorrhag* or haemorrhag* or microhemorrhag* or microhaemorrhad or haemorrhag* or accident* or hematoma* or haemotoma* or bleed* or microbleed* or insult*))) 1054 Delete
-
((CVA or CVAS or MCA* or ICH or ICHs or CVST or CVSTs or CVDST or CVT or CVDSTs or CVTs or LVO or LVOs)) 309 Delete
-
#1 OR #2 OR #3 OR #4 OR #5 OR #6 OR #7 OR #8 OR #9 4155 Delete
-
(#10) IN HTA 515 Delete
-
(#10) IN HTA FROM 2017 TO 2021 17 Delete
National Institute for Health and Care Excellence (NICE) ( www.nice.org.uk/guidance/ ): 2017–2021/10/20
Searched: 20.10.21
Browsed 'Stroke and transient ischaemic attack' section at: www.nice.org.uk/guidance/conditions-and-diseases/cardiovascular-conditions/stroke-and-transient-ischaemic-attack/products?Status=Published
Limited to publication date 2017–2021
Records found: 1
NIHR Health Technology Assessment (HTA) (www.nihr.ac.uk/): 2017–2021/10/20
Searched 20.10.21
Home/Researchers/Data and publications
2017-C: limited PDF
Search term | Results |
---|---|
Stroke | 8 |
TIA | 0/1 (dupe) |
'transient ischaemic attack' | 0 |
'transient ischaemic attack' | 0 |
'brain ischaemia' | 0 |
'brain ischemia' | 0 |
'intracranial haemorrhage' | 0/1 |
'intracranial hemorrhage' | 0 |
'vessel occlusion' | 0 |
Total | 10 |
Total (after deduplication) | 8 |
ECRI Guidelines Trust ( https://guidelines.ecri.org/ ): 2017–2021/10/20
Searched: 20.10.21
Limits:
Publication year – 2017–2021
Search term | Results |
---|---|
Stroke | 39 |
TIA | 1 |
'transient ischaemic attack' | 3 |
'transient ischaemic attack' | 2 |
'brain ischaemia' | 0 |
'brain ischemia' | 0 |
'intracranial haemorrhage' | 0 |
'intracranial hemorrhage' | 0 |
'vessel occlusion' | 0 |
Total (after deduplication) | 39 |
NHS Evidence (www.evidence.nhs.uk/): 2017–2021/10/20
Searched 20.10.21
Limited to Guidance and HTAs (2017-C)
Terms searched | Hits |
---|---|
(stroke or "brain ischemia" or "brain ischaemia" or "blood vessel occlusion" or "cerebral ischemia" or "cerebral ischaemia" or "large-vessel occlusion" or "intracranial haemorrhage" or "intracranial hemorrhage") AND (scan* or scintigraph* or examination* or angiograph* or image analys* or perfusion* or radiograph* or CTA or CTP or CTAs or CTPs or neuroimag* or neuro-imag*) | 358 |
Total | 358 |
International HTA Database (INAHTA)( https://database.inahta.org/): 2017–2021/10/20
Searched: 20.10.21
Records found: 64
-
14 #13 OR #12 OR #11 OR #10 OR #9 OR #8 OR #7 OR #6 OR #5 OR #4 OR #3 OR #2 OR #1 435
-
13 "blood vessel occlusion" 0
-
12 "intracranial hemorrhage" 6
-
11 "intracranial haemorrhage" 4
-
10 "large-vessel occlusion" 2
-
9 "cerebral ischaemia" 2
-
8 "cerebral ischemia" 1
-
7 "brain ischaemia" 0
-
6 "brain ischemia" 2
-
5 TIA 16
-
4 "transient ischaemic attack" 9
-
3 "transient ischaemic attack" 7
-
2 stroke* 409
-
1 "Stroke"[mhe] 225
Limits: Publication year – 2017–2021
Project status – Completed
October Update searches
Database | Dates covered | Hits |
---|---|---|
Embase | 1974–2021/10/18 | 2098 |
MEDLINE + PreMedline | 1946–2021/10/15 | 1192 |
medRxiv | Up to 2021/10/20 | 37 |
Total | 3327 |
Embase (Ovid): 1974–2021/10/18
Searched: 19.10.21
-
exp brain ischemia/ (200949)
-
exp brain hemorrhage/ (153883)
-
basal ganglion hemorrhage/ (672)
-
cerebrovascular accident/ (232943)
-
brain infarction/ (56774)
-
blood vessel occlusion/ (12030)
-
(Stroke$ or apople$ or cerebral vasc$ or cerebrovasc$ or cerebro vasc$ or poststroke$ or encephalorrhag$ or hematencephalon$ or large-vessel occlusion$).ti,ab,ot. (509763)
-
((brain or blood flow) adj2 disturb$).ti,ab,ot. (2932)
-
((sinus or sagittal) adj3 thromb$).ti,ab,ot. (7391)
-
(isch?emi$ adj3 (seizure$ or attack$ or thrombo$ or embolic or encephalopath$ or neural)).ti,ab,ot. (41377)
-
((Bleed$ or h?emorrhag$) adj2 corpus callosum).ti,ab,ot. (27)
-
((brain or cerebr$ or cerebell$ or cortical or Intraparenchymal or intracortical or vertebrobasil$ or hemispher$ or intracran$ or intra-cran$ or intracerebral or intratentorial or intra-tentorial or intraventricular or intra-ventricular or periventricular or peri-ventricular or supratentorial or supra-tentorial or anterior circulat$ or posterior circulat$ or basal gangli$ or global or focal or parenchymal or subarachnoid or sub-arachnoid or putaminal or putamen or posterior fossa or intra-axial or intraaxial or lacunar) adj3 (arrest$ or attack$ or isch?emi$ or infarct$ or insufficien$ or emboli$ or occlus$ or hypox$ or vasospasm or obstruction or vasculopath$ or failure$ or thromb$ or h?emorrhag$ or microh?emorrhag$ or accident$ or h?ematoma$ or bleed$ or microbleed$ or insult$)).ti,ab,ot. (298073)
-
(CVA or CVAS or MCA$ or ICH or ICHs or CVST or CVSTs or CVDST or CVT or CVDSTs or CVTs or LVO or LVOs).ti,ab. (83973)
-
or/1-13 (879622)
-
((diagnos$ or predict$ or specificity or sensitiv$) adj4 (criteria or criterion or guideline$ or pattern$ or trend$ or utili$ or management or prevalence or initiat$ or distribution$ or coverage or variety or selection or spread or alternative$ or frequen$)).ti,ab,ot. (504238)
-
diagnosis/ or early diagnosis/ (1452121)
-
exp brain scintiscanning/ (9890)
-
Neurologic examination/ (71955)
-
Computer assisted tomography/ (791392)
-
Brain radiography/ (7979)
-
((Brain or cerebral or neurologic$ or CT or head) adj2 (scan$ or scintigraph$ or examination$ or angiograph$ or image analys$ or perfusion$ or radiograph$)).ti,ab,ot,hw. (397707)
-
(CAT scan$ or CTA or CTP or CTAs or CTPs or neuroimag$ or neuro-imag$ or (comput$ adj2 tomograph$)).ti,ab,ot,hw. (1331476)
-
(Gamma encephalograph$ or Gammaencephalograph$ or Radio encephalograph$ or Radioencephalograph$).ti,ab,ot,hw. (48)
-
or/15-23 (3169335)
-
exp artificial intelligence/ (53172)
-
automated pattern recognition/ (16993)
-
decision support system/ (24298)
-
computer assisted diagnosis/ (40643)
-
Convolutional neural network/ (11478)
-
(Artificial intelligence or AI or machine intelligence or computer-aided triage$ or support vector machine$ or relevance vector machine$).ti,ab,ot. (79275)
-
((automat$ or computer) adj2 (analys$ or diagnos$ or detect$)).ti,ab,ot. (55546)
-
((deep or machine) adj learning).ti,ab,ot. (72859)
-
(decision support$ adj (software or tool$)).ti,ab,ot. (4842)
-
(CNN or CNNs or convNet or (convolut$ adj2 neural network$) or convolutional ANNs or convolutional ANN or convolutional NNs or convolutional NN).ti,ab. (17172)
-
automat$ hierarch$ evaluat$.ti,ab. (1)
-
(Aidoc or e-CTA or e-ASPECTS or e-stroke or brainomix or brainscan or "brainscan.ai" or icobrain or icometrix or qER or Qure or Zebra$ or e-CTP or briefcase or rapid CTA or rapid LVO or rapid core or rapid ASPECTS or rapid ICH or rapidai or blackford or "viz.ai" or viz or "ct perfusion 4d" or cercare or cina$ or Avicenna or accipio$ or maxQ AI or biomind or "biomind.ai" or ischemaview or rapid CTP or "qure.ai").ti,ab. (129176)
-
or/25-36 (411932)
-
14 and 24 and 37 (2210)
-
(letter or editorial or note).pt. (2769185)
-
38 not 39 (2145)
-
animal/ (1534498)
-
animal experiment/ (2730003)
-
(rat or rats or mouse or mice or murine or rodent or rodents or hamster or hamsters or pig or pigs or porcine or rabbit or rabbits or animal or animals or dogs or dog or cats or cow or bovine or sheep or ovine or monkey or monkeys).ti,ab,ot,hw. (7090039)
-
or/41-43 (7090039)
-
exp human/ (22842436)
-
human experiment/ (556748)
-
or/45-46 (22844369)
-
44 not (44 and 47) (5387198)
-
40 not 48 (2098)
MEDLINE and Epub Ahead of Print, In-Process, In-Data-Review & Other Non-Indexed Citations, Daily and Versions (Ovid): 1946–2021/10/15
Searched: 19.10.21
-
exp Brain Ischemia/ (115589)
-
exp Intracranial Hemorrhages/ (75053)
-
Stroke/ (113288)
-
(Stroke$ or apople$ or cerebral vasc$ or cerebrovasc$ or cerebro vasc$ or poststroke$ or encephalorrhag$ or hematencephalon$ or large-vessel occlusion$).ti,ab,ot. (327818)
-
((brain or blood flow) adj2 disturb$).ti,ab,ot. (2117)
-
((sinus or sagittal) adj3 thromb$).ti,ab,ot. (5117)
-
(isch?emi$ adj3 (seizure$ or attack$ or thrombo$ or embolic or encephalopath$ or neural)).ti,ab,ot. (26850)
-
((Bleed$ or h?emorrhag$) adj2 corpus callosum).ti,ab,ot. (23)
-
((brain or cerebr$ or cerebell$ or cortical or Intraparenchymal or intracortical or vertebrobasil$ or hemispher$ or intracran$ or intra-cran$ or intracerebral or intratentorial or intra-tentorial or intraventricular or intra-ventricular or periventricular or peri-ventricular or supratentorial or supra-tentorial or anterior circulat$ or posterior circulat$ or basal gangli$ or global or focal or parenchymal or subarachnoid or sub-arachnoid or putaminal or putamen or posterior fossa or intra-axial or intraaxial or lacunar) adj3 (arrest$ or attack$ or isch?emi$ or infarct$ or insufficien$ or emboli$ or occlus$ or hypox$ or vasospasm or obstruction or vasculopath$ or failure$ or thromb$ or h?emorrhag$ or microh?emorrhag$ or accident$ or h?ematoma$ or bleed$ or microbleed$ or insult$)).ti,ab,ot. (210934)
-
(CVA or CVAS or MCA$ or ICH or ICHs or CVST or CVSTs or CVDST or CVT or CVDSTs or CVTs or LVO or LVOs).ti,ab. (48905)
-
or/1-10 (557308)
-
((diagnos$ or predict$ or specificity or sensitiv$) adj4 (criteria or criterion or guideline$ or pattern$ or trend$ or utili$ or management or prevalence or initiat$ or distribution$ or coverage or variety or selection or spread or alternative$ or frequen$)).ti,ab,ot. (350992)
-
Diagnosis/ (17472)
-
Early Diagnosis/ (28758)
-
Brain/dg [Diagnostic Imaging] (51780)
-
Stroke/dg [Diagnostic Imaging] (7712)
-
Radiography/ (322703)
-
exp Radionuclide Imaging/ (223371)
-
Neurologic Examination/ (27754)
-
Tomography, X-Ray Computed/ (399785)
-
((Brain or cerebral or neurologic$ or CT or head) adj2 (scan$ or scintigraph$ or examination$ or angiograph$ or image analys$ or perfusion$ or radiograph$)).ti,ab,ot,hw. (229398)
-
(CAT scan$ or CTA or CTP or CTAs or CTPs or neuroimag$ or neuro-imag$ or (comput$ adj2 tomograph$)).ti,ab,ot,hw. (475578)
-
(Gamma encephalograph$ or Gammaencephalograph$ or Radio encephalograph$ or Radioencephalograph$).ti,ab,ot,hw. (155)
-
or/12-23 (1629565)
-
exp Artificial Intelligence/ (125230)
-
Pattern Recognition, Automated/ (25989)
-
Neural Networks, Computer/ (33266)
-
(Artificial intelligence or AI or machine intelligence or computer-aided triage$ or support vector machine$ or relevance vector machine$).ti,ab,ot. (61743)
-
((automat$ or computer) adj2 (analys$ or diagnos$ or detect$)).ti,ab,ot. (43089)
-
((deep or machine) adj learning).ti,ab,ot. (60757)
-
(decision support$ adj (software or tool$)).ti,ab,ot. (3515)
-
(CNN or CNNs or convNet or (convolut$ adj2 neural network$) or convolutional ANNs or convolutional ANN or convolutional NNs or convolutional NN).ti,ab. (14199)
-
automat$ hierarch$ evaluat$.ti,ab. (1)
-
(Aidoc or e-CTA or e-ASPECTS or e-stroke or brainomix or brainscan or "brainscan.ai" or icobrain or icometrix or qER or Qure or Zebra$ or e-CTP or briefcase or rapid CTA or rapid LVO or rapid core or rapid ASPECTS or rapid ICH or rapidai or blackford or "viz.ai" or viz or "ct perfusion 4d" or cercare or cina$ or Avicenna or accipio$ or maxQ AI or biomind or "biomind.ai" or ischemaview or rapid CTP or "qure.ai").ti,ab. (105422)
-
or/25-34 (356783)
-
11 and 24 and 35 (1237)
-
(letter or editorial or note).pt. (1738660)
-
exp animals/ not (exp animals/ and humans/) (4898472)
-
36 not (37 or 38) (1192)
medRxiv: the preprint server for Health Sciences ( www.medrxiv.org/ ): up to 2021/10/20
Searched 20.10.21
Advanced search
Full text or abstract or title (match whole all) | Update (20.10.21) Hits |
---|---|
stroke* Aidoc | 0 |
Stroke* e-CTA | 0 |
Stroke* e-ASPECTS | 0 |
e-stroke | 0 |
Stroke* brainomix | 0 |
Stroke* brainscan | 1 |
Stroke* brainscan.ai | 0 |
stroke icobrain | 0 |
Stroke* icometrix | 0 |
Stroke* qER | 0 |
Stroke* Qure | 0 |
Stroke* Zebra* | 0 |
Stroke* e-CTP | 0 |
Stroke* briefcase | 0 |
Stroke* rapid CTA | 0 |
Stroke* rapid LVO | 0 |
Stroke* rapid core | 22 |
Stroke* rapid ASPECTS | 23 |
Stroke* rapid ICH | 1 |
Stroke* rapidai | 0 |
Stroke* blackford | 0 |
Stroke* viz.ai | 0 |
Stroke* viz | 1 |
Stroke* ct perfusion 4d | 0 |
Stroke* cercare | 0 |
Stroke* cina* | 0 |
Stroke* Avicenna | 0 |
Stroke* accipio* | 0 |
Stroke* maxQ AI | 0 |
Stroke* biomind | 0 |
Stroke* biomind.ai | 0 |
Stroke* ischemaview | 1 |
Stroke* rapid CTP | 1 |
Stroke* qure.ai | 0 |
Total | 50 |
Total without dupes | 37 |
Cost-Effectiveness Searches
Database | Dates covered | Hits |
---|---|---|
Embase | 2005–2021/09/15 | 988 |
MEDLINE + PreMedline | 2005–2021/09/15 | 1233 |
NHS EED | 2005–2015/03 | 559 |
EconLit | 2005–2021/09/21 | 82 |
Science Citation Index (SCI) + CPCI-S | 2005–2021/09/21 | 1007 |
RePeC (Ideas) | 2005–2021/09/21 | 79 |
Total | 3948 |
Embase (Ovid): 2005-2021/09/15
Searched: 16.9.21
Stroke + (Cat Scan/diagnostics) + NHSEED SD filter (20015-C)
-
exp brain ischemia/ (200456)
-
exp brain hemorrhage/ (152656)
-
basal ganglion hemorrhage/ (669)
-
cerebrovascular accident/ (230904)
-
brain infarction/ (56442)
-
blood vessel occlusion/ (11828)
-
(Stroke$ or apople$ or cerebral vasc$ or cerebrovasc$ or cerebro vasc$ or poststroke$ or encephalorrhag$ or hematencephalon$ or large-vessel occlusion$).ti,ab,ot. (505578)
-
((brain or blood flow) adj2 disturb$).ti,ab,ot. (2928)
-
((sinus or sagittal) adj3 thromb$).ti,ab,ot. (7285)
-
(isch?emi$ adj3 (seizure$ or attack$ or thrombo$ or embolic or encephalopath$ or neural)).ti,ab,ot. (41063)
-
((Bleed$ or h?emorrhag$) adj2 corpus callosum).ti,ab,ot. (27)
-
((brain or cerebr$ or cerebell$ or cortical or Intraparenchymal or intracortical or vertebrobasil$ or hemispher$ or intracran$ or intra-cran$ or intracerebral or intratentorial or intra-tentorial or intraventricular or intra-ventricular or periventricular or peri-ventricular or supratentorial or supra-tentorial or anterior circulat$ or posterior circulat$ or basal gangli$ or global or focal or parenchymal or subarachnoid or sub-arachnoid or putaminal or putamen or posterior fossa or intra-axial or intraaxial or lacunar) adj3 (arrest$ or attack$ or isch?emi$ or infarct$ or insufficien$ or emboli$ or occlus$ or hypox$ or vasospasm or obstruction or vasculopath$ or failure$ or thromb$ or h?emorrhag$ or microh?emorrhag$ or accident$ or h?ematoma$ or bleed$ or microbleed$ or insult$)).ti,ab,ot. (296096)
-
(CVA or CVAS or MCA$ or ICH or ICHs or CVST or CVSTs or CVDST or CVT or CVDSTs or CVTs or LVO or LVOs).ti,ab. (83193)
-
or/1-13 (873429)
-
((diagnos$ or predict$ or specificity or sensitiv$) adj4 (criteria or criterion or guideline$ or pattern$ or trend$ or utili$ or management or prevalence or initiat$ or distribution$ or coverage or variety or selection or spread or alternative$ or frequen$)).ti,ab,ot. (500975)
-
diagnosis/ or early diagnosis/ (1447537)
-
exp brain scintiscanning/ (9877)
-
Neurologic examination/ (71424)
-
Computer assisted tomography/ (787646)
-
Brain radiography/ (7923)
-
((Brain or cerebral or neurologic$ or CT or head) adj2 (scan$ or scintigraph$ or examination$ or angiograph$ or image analys$ or perfusion$ or radiograph$)).ti,ab,ot,hw. (394865)
-
(CAT scan$ or CTA or CTP or CTAs or CTPs or neuroimag$ or neuro-imag$ or (comput$ adj2 tomograph$)).ti,ab,ot,hw. (1322884)
-
(Gamma encephalograph$ or Gammaencephalograph$ or Radio encephalograph$ or Radioencephalograph$).ti,ab,ot,hw. (48)
-
or/15-23 (3154111)
-
14 and 15 (18433)
-
health-economics/ (33663)
-
exp economic-evaluation/ (323525)
-
exp health care-cost/ (307833)
-
exp pharmacoeconomics/ (212823)
-
or/26-29 (684070)
-
(econom$ or cost or costs or costly or costing or price or prices or pricing or pharmacoeconomic$).ti,ab. (1186225)
-
(expenditure$ not energy).ti,ab. (44234)
-
(value adj2 money).ti,ab. (2638)
-
budget$.ti,ab. (41819)
-
or/31-34 (1225642)
-
30 or 35 (1565097)
-
letter.pt. (1190591)
-
editorial.pt. (702926)
-
note.pt. (865546)
-
or/37-39 (2759063)
-
36 not 40 (1440016)
-
(metabolic adj cost).ti,ab. (1642)
-
((energy or oxygen) adj cost).ti,ab. (4612)
-
((energy or oxygen) adj expenditure).ti,ab. (33824)
-
or/42-44 (38934)
-
41 not 45 (1432035)
-
exp animal/ (27569658)
-
exp animal-experiment/ (2743270)
-
nonhuman/ (6663210)
-
(rat or rats or mouse or mice or hamster or hamsters or animal or animals or dog or dogs or cat or cats or bovine or sheep).ti,ab,sh. (5992658)
-
or/47-50 (29634110)
-
exp human/ (22733515)
-
exp human-experiment/ (554891)
-
52 or 53 (22735496)
-
51 not (51 and 54) (6899644)
-
46 not 55 (1300585)
-
25 and 56 (1126)
-
limit 57 to yr="2005 -Current" (988)
Economics terms based on Costs filter:
Centre for Reviews and Dissemination. Search strategies: NHS EED Embase using OvidSP (economics filter) [Internet]. York: Centre for Reviews and Dissemination; 2014 [accessed 2.6.14]. Available from: www.crd.york.ac.uk/crdweb/searchstrategies.asp#nhseedembase
MEDLINE(Ovid) and Epub Ahead of Print, In-Process, In-Data-Review & Other Non-Indexed Citations and Daily: 2005–2021/09/15
Searched 16.9.21
-
exp Brain Ischemia/ (115093)
-
exp Intracranial Hemorrhages/ (74784)
-
Stroke/ (112477)
-
(Stroke$ or apople$ or cerebral vasc$ or cerebrovasc$ or cerebro vasc$ or poststroke$ or encephalorrhag$ or hematencephalon$ or large-vessel occlusion$).ti,ab,ot. (325891)
-
((brain or blood flow) adj2 disturb$).ti,ab,ot. (2108)
-
((sinus or sagittal) adj3 thromb$).ti,ab,ot. (5069)
-
(isch?emi$ adj3 (seizure$ or attack$ or thrombo$ or embolic or encephalopath$ or neural)).ti,ab,ot. (26691)
-
((Bleed$ or h?emorrhag$) adj2 corpus callosum).ti,ab,ot. (23)
-
((brain or cerebr$ or cerebell$ or cortical or Intraparenchymal or intracortical or vertebrobasil$ or hemispher$ or intracran$ or intra-cran$ or intracerebral or intratentorial or intra-tentorial or intraventricular or intra-ventricular or periventricular or peri-ventricular or supratentorial or supra-tentorial or anterior circulat$ or posterior circulat$ or basal gangli$ or global or focal or parenchymal or subarachnoid or sub-arachnoid or putaminal or putamen or posterior fossa or intra-axial or intraaxial or lacunar) adj3 (arrest$ or attack$ or isch?emi$ or infarct$ or insufficien$ or emboli$ or occlus$ or hypox$ or vasospasm or obstruction or vasculopath$ or failure$ or thromb$ or h?emorrhag$ or microh?emorrhag$ or accident$ or h?ematoma$ or bleed$ or microbleed$ or insult$)).ti,ab,ot. (209964)
-
(CVA or CVAS or MCA$ or ICH or ICHs or CVST or CVSTs or CVDST or CVT or CVDSTs or CVTs or LVO or LVOs).ti,ab. (48598)
-
or/1-10 (554564)
-
((diagnos$ or predict$ or specificity or sensitiv$) adj4 (criteria or criterion or guideline$ or pattern$ or trend$ or utili$ or management or prevalence or initiat$ or distribution$ or coverage or variety or selection or spread or alternative$ or frequen$)).ti,ab,ot. (348884)
-
Diagnosis/ (17470)
-
Early Diagnosis/ (28588)
-
Brain/dg [Diagnostic Imaging] (51013)
-
Stroke/dg [Diagnostic Imaging] (7589)
-
Radiography/ (322399)
-
exp Radionuclide Imaging/ (222597)
-
Neurologic Examination/ (27713)
-
Tomography, X-Ray Computed/ (398463)
-
((Brain or cerebral or neurologic$ or CT or head) adj2 (scan$ or scintigraph$ or examination$ or angiograph$ or image analys$ or perfusion$ or radiograph$)).ti,ab,ot,hw. (228460)
-
(CAT scan$ or CTA or CTP or CTAs or CTPs or neuroimag$ or neuro-imag$ or (comput$ adj2 tomograph$)).ti,ab,ot,hw. (472827)
-
(Gamma encephalograph$ or Gammaencephalograph$ or Radio encephalograph$ or Radioencephalograph$).ti,ab,ot,hw. (155)
-
or/12-23 (1622669)
-
11 and 24 (99863)
-
economics/ (27366)
-
exp "costs and cost analysis"/ (249120)
-
economics, dental/ (1919)
-
exp "economics, hospital"/ (25299)
-
economics, medical/ (9153)
-
economics, nursing/ (4006)
-
economics, pharmaceutical/ (3018)
-
(economic$ or cost or costs or costly or costing or price or prices or pricing or pharmacoeconomic$).ti,ab. (888235)
-
(expenditure$ not energy).ti,ab. (32593)
-
(value adj1 money).ti,ab. (36)
-
budget$.ti,ab. (31710)
-
or/26-36 (1045094)
-
((energy or oxygen) adj cost).ti,ab. (4365)
-
(metabolic adj cost).ti,ab. (1538)
-
((energy or oxygen) adj expenditure).ti,ab. (26701)
-
or/38-40 (31589)
-
37 not 41 (1037831)
-
letter.pt. (1151819)
-
editorial.pt. (580627)
-
historical article.pt. (365432)
-
or/43-45 (2077389)
-
42 not 46 (999755)
-
25 and 47 (1716)
-
exp animals/ not (exp animals/ and humans/) (4885879)
-
48 not 49 (1684)
-
limit 50 to yr="2005 -Current" (1233)
Costs filter:
Centre for Reviews and Dissemination. NHS EED Economics Filter: Medline (Ovid) monthly search [Internet]. York: Centre for Reviews and Dissemination; 2010 [cited 28.9.10]. Available from: www.york.ac.uk/inst/crd/intertasc/nhs_eed_strategies.html
NHS Economic Evaluation Database (NHS EED) (Internet) (www.crd.york.ac.uk/CRDWeb/): 2005–2015/03
Searched: 16.9.2021
-
MeSH DESCRIPTOR Brain Ischemia EXPLODE ALL TREES 328 Delete
-
MeSH DESCRIPTOR Intracranial Hemorrhages EXPLODE ALL TREES 258 Delete
-
MeSH DESCRIPTOR Stroke EXPLODE ALL TREES 1356 Delete
-
MeSH DESCRIPTOR Ischaemic Stroke EXPLODE ALL TREES 0 Delete
-
MeSH DESCRIPTOR Hemorrhagic Stroke EXPLODE ALL TREES 0 Delete
-
((Stroke* or apople* or "cerebral vasc*" or cerebrovasc* or "cerebro vasc*" or poststroke* or encephalorrhag* or hematencephalon* or "large-vessel occlusion*")) 3402 Delete
-
((((brain or "blood flow") and disturb*)) OR (((sinus or sagittal) and thromb*)) OR (((ischemi* or ischaemi*) and (seizure* or attack* or thrombo* or embolic or encephalopath* or neural)))) 691 Delete
-
((((Bleed* or hemorrhag* or haemorrhag*) and "corpus callosum")) OR (((brain or cerebr* or cerebell* or cortical or Intraparenchymal or intracortical or vertebrobasil* or hemispher* or intracran* or intra-cran* or intracerebral or intratentorial or intra-tentorial or intraventricular or intra-ventricular or periventricular or peri-ventricular or supratentorial or supra-tentorial or anterior circulat* or "posterior circulat*" or "basal gangli*" or global or focal or parenchymal or subarachnoid or sub-arachnoid or putaminal or putamen or "posterior fossa" or intra-axial or intraaxial or lacunar) and (arrest* or attack* or ischemi* or ischaemi* or infarct* or insufficien* or emboli* or occlus* or hypox* or vasospasm or obstruction or vasculopath* or failure* or thromb* or hemorrhag* or haemorrhag* or microhemorrhag* or microhaemorrhag* or accident* or hematoma* or haematoma* or bleed* or microbleed* or insult*))) OR (CVA or CVAS or MCA* or ICH or ICHs or CVST or CVSTs or CVDST or CVT or CVDSTs or CVTs or LVO or LVOs)) 2618 Delete
-
(#1 OR #2 OR #3 OR #4 OR #5 OR #6 OR #7 OR #8) 5187 Delete
-
MeSH DESCRIPTOR Diagnosis EXPLODE ALL TREES 29251 Delete
-
MeSH DESCRIPTOR Early Diagnosis EXPLODE ALL TREES 413 Delete
-
MeSH DESCRIPTOR brain EXPLODE ALL TREES WITH QUALIFIER DG IN NHSEED 0 Delete
-
MeSH DESCRIPTOR stroke EXPLODE ALL TREES WITH QUALIFIER DG IN NHSEED 0 Delete
-
MeSH DESCRIPTOR Radionuclide Imaging EXPLODE ALL TREES 725 Delete
-
MeSH DESCRIPTOR Neurologic Examination EXPLODE ALL TREES 772 Delete
-
((((Brain or cerebral or neurologic* or CT or head) and (scan* or scintigraph* or examination* or angiograph* or "image analys*" or perfusion* or radiograph*))) OR (((diagnos* or predict* or specificity or sensitiv*) and (criteria or criterion or guideline* or pattern* or trend* or utili* or management or prevalence or initiat* or distribution* or coverage or variety or selection or spread or alternative* or frequen*))) OR (("CAT scan*" or CTA or CTP or neuroimag* or neuro-imag* or (comput* and tomograph*)))) 25348 Delete
-
(("Gamma encephalograph*" or Gammaencephalograph* or "Radio encephalograph*" or Radioencephalograph*)) 0 Delete
-
(#10 OR #11 OR #12 OR #13 OR #14 OR #15 OR #16 OR #17) 40752 Delete
-
#9 AND #18 3280 Delete
-
(#19) IN NHSEED 1081 Delete
-
(#19) IN NHSEED FROM 2005 TO 2021 559 Delete
Econlit (EBSCO): 2005–2021/09/21
Searched: 21.9.21
-
S16 S13 AND S14 Limiters – Published Date: 20050101–20211231 82
-
S15 S13 AND S14 93
-
S14 S8 OR S9 OR S10 OR S11 OR S12 94,023
-
S13 S1 OR S2 OR S3 OR S4 OR S5 OR S6 430
-
S12 “Gamma encephalograph*” OR Gammaencephalograph* OR “Radio encephalograph*” OR Radioencephalograph* 0
-
S11 comput* N2 tomograph* 36
-
S10 “CAT scan*” OR CTA OR CTP OR neuroimag* OR neuro-imag* 174
-
S9 scan* OR scintigraph* OR examination* OR angiograph* OR "image analys*" OR perfusion* OR radiograph* 20,848
-
S8 diagnos* OR predict* 74,198
-
S7 TI ( CVA OR CVAS OR MCA* OR ICH OR ICHs OR CVST OR CVSTs OR CVDST OR CVT OR CVDSTs OR CVTs OR LVO OR LVOs ) OR AB ( CVA OR CVAS OR MCA* OR ICH OR ICHs OR CVST OR CVSTs OR CVDST OR CVT OR CVDSTs OR CVTs OR LVO OR LVOs ) 516
-
S6 TI (brain OR cerebr* OR cerebell* OR cortical OR Intraparenchymal OR intracortical OR vertebrobasil* OR hemispher* OR intracran* OR intra-cran* OR intracerebral OR intratentorial OR intra-tentorial OR intraventricular OR intra-ventricular OR periventricular OR peri-ventricular OR supratentrial OR supra-tentorial OR “anterior circulat*” OR “posterior circulat*” OR “basal gangli*” OR global OR focal OR parenchymal OR subarachnoid OR sub-arachnoid OR putaminal OR putamen OR “posterior fossa” OR intra-axial OR intraaxial OR lacunar ) AND TI( arrest* OR attack* OR isch?emi* OR infarct* OR insufficien* OR emboli* OR occlus* OR hypox* OR vasospasm OR obstruction OR vasculopath* OR failure* OR thromb* OR h?emorrhag* OR microh?emorrhag* OR accident* OR h?ematoma* OR bleed* OR microbleed* OR insult* ) 68
-
S5 (Bleed N4 “corpus callosum”) or (h?emorrhag* n4 “corpus callosum”) 0
-
S4 TX isch?emi* 14
-
S3 TX (sinus N3 thromb*) or (sagittal N3 thromb*) 0
-
S2 TX (brain N2 disturb*) or (“blood flow” N2 disturb*) 1
-
S1 TX Stroke* OR apople* OR “cerebral vasc*” OR cerebrovasc* OR “cerebro vasc*” OR poststroke* OR encephalorrhag* OR hematencephalon* OR “large-vessel occlusion*” 353
Science Citation Index Expanded (Web of Science): 2005–2021/09/21
Conference Proceedings Citation Index (Web of Science): 2005–2021/09/21
Searched: 21.9.21
-
27 #26 results from Science Citation Index Expanded (SCI-EXPANDED), Conference Proceedings Citation Index – Science (CPCI-S) 1,007
-
26 #14 AND 24 and 2005 or 2006 or 2007 or 2008 or 2009 or 2010 or 2011 or 2012 or 2013 or 2014 or 2015 or 2016 or 2017 or 2018 or 2019 or 2020 or 2021 (Publication Years) 1,106
-
25 #14 AND #24 1,350
-
24 #19 NOT #23 2,887,051
-
23 #20 OR #21 OR #22 319,156
-
22 TS=((energy or oxygen) SAME expenditure) 49,598
-
21 TS=(metabolic SAME cost) 17,108
-
20 TS=((energy or oxygen) SAME cost) 266,150
-
19 #15 OR #16 OR #17 OR #18 3,165,727
-
18 TS=(budget*) 146,577
-
17 TS=(value NEAR/1 money) 3,953
-
16 TS=(expenditure* not energy) 67,519
-
15 TS=(economic* or cost or costs or costly or costing or price or prices or pricing or pharmacoeconomic*) 3,030,437
-
14 #8 AND #13 48,914
-
13 #12 OR #11 OR #10 OR #9 1,063,103
-
12 TS=(“Gamma encephalograph*” OR Gammaencephalograph* OR “Radio encephalograph*” OR Radioencephalograph*) 1
-
11 TS=(“CAT scan*” OR CTA OR CTP OR CTAs OR CTPs OR neuroimag* OR neuro-imag* OR (comput* NEAR/2 tomograph*) ) 447,821
-
10 TS=((Brain OR cerebral OR neurologic* OR CT OR head) NEAR/2 (scan* OR scintigraph* OR examination* OR angiograph* OR "image analys*" OR perfusion* OR radiograph*) ) 177,922
-
9 TS=((diagnos* OR predict* OR specificity OR sensitiv*) NEAR/4 (criteria OR criterion OR guideline* OR pattern* OR trend* OR utili* OR management OR prevalence OR initiat* OR distribution* OR coverage OR variety OR selection OR spread OR alternative* OR frequen*) )
537,076
-
8 #7 OR #6 OR #5 OR #4 OR #3 OR #2 OR #1 541,925
-
7 TI=(CVA OR CVAS OR MCA* OR ICH OR ICHs OR CVST OR CVSTs OR CVDST OR CVT OR CVDSTs OR CVTs OR LVO OR LVOs) OR AB=(CVA OR CVAS OR MCA* OR ICH OR ICHs OR CVST OR CVSTs OR CVDST OR CVT OR CVDSTs OR CVTs OR LVO OR LVOs) 60,217
-
6 TS=((brain OR cerebr* OR cerebell* OR cortical OR Intraparenchymal OR intracortical OR vertebrobasil* OR hemispher* OR intracran* OR intra-cran* OR intracerebral OR intratentorial OR intra-tentorial OR intraventricular OR intra-ventricular OR periventricular OR peri-ventricular OR supratentrial OR supra-tentorial OR “anterior circulat*” OR “posterior circulat*” OR “basal gangli*” OR global OR focal OR parenchymal OR subarachnoid OR sub-arachnoid OR putaminal OR putamen OR “posterior fossa” OR intra-axial OR intraaxial OR lacunar) NEAR/3 (arrest* OR attack* OR isch?emi* OR infarct* OR insufficien* OR emboli* OR occlus* OR hypox* OR vasospasm OR obstruction OR vasculopath* OR failure* OR thromb* OR h?emorrhag* OR microh?emorrhag* OR accident* OR h?ematoma* OR bleed* OR microbleed* OR insult*) ) 133,540
-
5 TS=((Bleed* OR h?emorrhag*) NEAR/2 “corpus callosum”) 10
-
4 TS=(isch?emi* NEAR/3 (seizure* OR attack* OR thrombo* OR embolic OR encephalopath* OR neural) ) 4,529
-
3 TS=((sinus OR sagittal) NEAR/3 thromb*) 5,630
-
2 TS=((brain OR “blood flow”) NEAR/2 disturb*) 2,569
-
1 TS=((Stroke* OR apople* OR “cerebral vasc*” OR cerebrovasc* OR “cerebro vasc*” OR poststroke* OR encephalorrhag* OR hematencephalon* OR “large-vessel occlusion*”) ) 426,003
RePEc: Research Papers in Economics (http://repec.org/): 2005–2021/09/21
Searched 21.9.21
Keywords in whole record
((stroke | "brain ischemia" | "brain ischaemia" | "blood vessel occlusion" | "cerebral ischemia" | "cerebral ischaemia" | "large-vessel occlusion" | "intracranial haemorrhage" | "intracranial hemorrhage") + (diagnose | diagnostic | diagnostics | scan | scans | scintigraph | angiograph | radiograph | CTA | CTP | CTAs | CTPs | neuroimaging | neuro-imaging ))
Limit: 2005–2021
Found 79 records
HRQoL and Utilities
Database | Dates covered | Hits |
---|---|---|
Embase | 1974–2021/11/01 | 1254 |
CEA Registry | up to 2021/07/14 | 788 |
Total | 2042 |
Embase (Ovid): 1974-2021/11/01
Searched: 12.8.21
Stroke + EQ5D only
-
exp brain ischemia/ (201252)
-
exp brain hemorrhage/ (154320)
-
basal ganglion hemorrhage/ (674)
-
cerebrovascular accident/ (233847)
-
brain infarction/ (56899)
-
blood vessel occlusion/ (12067)
-
(Stroke$ or apople$ or cerebral vasc$ or cerebrovasc$ or cerebro vasc$ or poststroke$ or encephalorrhag$ or hematencephalon$ or large-vessel occlusion$).ti,ab,ot. (511132)
-
((brain or blood flow) adj2 disturb$).ti,ab,ot. (2937)
-
((sinus or sagittal) adj3 thromb$).ti,ab,ot. (7415)
-
(isch?emi$ adj3 (seizure$ or attack$ or thrombo$ or embolic or encephalopath$ or neural)).ti,ab,ot. (41482)
-
((Bleed$ or h?emorrhag$) adj2 corpus callosum).ti,ab,ot. (27)
-
((brain or cerebr$ or cerebell$ or cortical or Intraparenchymal or intracortical or vertebrobasil$ or hemispher$ or intracran$ or intra-cran$ or intracerebral or intratentorial or intra-tentorial or intraventricular or intra-ventricular or periventricular or peri-ventricular or supratentorial or supra-tentorial or anterior circulat$ or posterior circulat$ or basal gangli$ or global or focal or parenchymal or subarachnoid or sub-arachnoid or putaminal or putamen or posterior fossa or intra-axial or intraaxial or lacunar) adj3 (arrest$ or attack$ or isch?emi$ or infarct$ or insufficien$ or emboli$ or occlus$ or hypox$ or vasospasm or obstruction or vasculopath$ or failure$ or thromb$ or h?emorrhag$ or microh?emorrhag$ or accident$ or h?ematoma$ or bleed$ or microbleed$ or insult$)).ti,ab,ot. (298685)
-
(CVA or CVAS or MCA$ or ICH or ICHs or CVST or CVSTs or CVDST or CVT or CVDSTs or CVTs or LVO or LVOs).ti,ab. (84175)
-
or/1-13 (881919)
-
(eq-5d or eq5d or eq-5 or eq5 or euro qual or euroqual or euro qual5d or euroqual5d or euro qol or euroqol or euro qol5d or euroqol5d or euro quol or euroquol or euro quol5d or euroquol5d or eur qol or eurqol or eur qol5d or eur qol5d or eur?qul or eur?qul5d or euro$ quality of life or european qol).ti,ab. (25202)
-
(euro$ adj3 (5 d or 5d or 5 dimension$ or 5dimension$ or 5 domain$ or 5domain$)).ti,ab. (7370)
-
or/15-16 (25235)
-
14 and 17 (1510)
-
(letter or editorial or note).pt. (2774277)
-
conference.so. (589741)
-
18 not (19 or 20) (1254)
CEA Registry (www.cearegistry.org): up to 2021/07/14
Searched: 14.7.21
Keywords | Ratios | Utility weights |
---|---|---|
Ischaemic stroke | 44 | 100/130 |
Ischaemic stroke | 100/243 | 100/502 |
haemorrhagic stroke | 9 | 57 |
large-vessel occlusion | 9 | 13 |
hemorrhagic stroke | 31 | 100/136 |
intracranial haemorrhage | 8 | 98 |
intracranial hemorrhage | 49 | 100/228 |
Total | 220/250 (dupes removed) | 568/1,164 |
Review of reviews
Database | Dates covered | Hits |
---|---|---|
CDSR | up to 2021/10/Iss10 | 404 |
KSR Evidence | up to 2021/10/14 | 498 |
Total | 902 |
CDSR (Wiley): up to 2021/10/Iss10
Searched: 14.10.21
Stroke + CTscan/Diagnostics
-
MeSH descriptor: [Brain Ischemia] explode all trees 3805
-
MeSH descriptor: [Intracranial Hemorrhages] explode all trees 2064
-
(Stroke* or apople* or cerebral-vasc* or cerebrovasc* or cerebro-vasc* or poststroke* or encephalorrhag* or hematencephalon* or large-vessel-occlusion*):ti,ab,kw 68306
-
((brain or blood flow) near/2 disturb*):ti,ab,kw 168
-
((sinus or sagittal) near/3 thromb*):ti,ab,kw 216
-
((ischaemi* or ischemi*) near/3 (seizure* or attack* or thrombo* or embolic or encephalopath* or neural)):ti,ab,kw 4859
-
((Bleed* or hemorrhag* or haemorrhag*) near/2 corpus-callosum):ti,ab,kw 0
-
((brain or cerebr* or cerebell* or cortical or Intraparenchymal or intracortical or vertebrobasil* or hemispher* or intracran* or intra-cran* or intracerebral or intratentorial or intra-tentorial or intraventricular or intra-ventricular or periventricular or peri-ventricular or supratentorial or supra-tentorial or anterior-circulat* or posterior-circulat* or basal-gangli* or global or focal or parenchymal or subarachnoid or sub-arachnoid or putaminal or putamen or posterior-fossa or intra-axial or intraaxial or lacunar) near/3 (arrest* or attack* or ischaemi* or ischemi* or infarct* or insufficien* or emboli* or occlus* or hypox* or vasospasm or obstruction or vasculopath* or failure* or thromb* or hemorrhag* or haemorrhag* or microhemorrhag* or microhaemorrhad or haemorrhag* or accident* or hematoma* or haemotoma* or bleed* or microbleed* or insult*)):ti,ab,kw 35512
-
(CVA or CVAS or MCA* or ICH or ICHs or CVST or CVSTs or CVDST or CVT or CVDSTs or CVTs or LVO or LVOs):ti,ab,kw 5080
-
#1 or #2 or #3 or #4 or #5 or #6 or #7 or #8 or #9 83530
-
((diagnos* or predict* or specificity or sensitiv*) near/4 (criteria or criterion or guideline* or pattern* or trend* or utili* or management or prevalence or initiat* or distribution* or coverage or variety or selection or spread or alternative* or frequen*)):ti,ab,kw 31376
-
MeSH descriptor: [Diagnosis] explode all trees 347283
-
MeSH descriptor: [Early Diagnosis] explode all trees 1859
-
MeSH descriptor: [Brain] explode all trees and with qualifier(s): [diagnostic imaging - DG] 1750
-
MeSH descriptor: [Radiography] explode all trees 21297
-
MeSH descriptor: [Radionuclide Imaging] explode all trees 4690
-
MeSH descriptor: [Neurologic Examination] explode all trees 24248
-
MeSH descriptor: [Tomography, X-Ray Computed] explode all trees 5244
-
((Brain or cerebral or neurologic* or CT or head) near/2 (scan* or scintigraph* or examination* or angiograph* or image analys* or perfusion* or radiograph*)):ti,ab,kw 16002
-
(Gamma-encephalograph* or Gammaencephalograph* or Radio-encephalograph* or Radioencephalograph*):ti,ab,kw 0
-
(CAT scan* or CTA or CTP or neuroimag* or neuro-imag* or (comput* near/2 tomograph*)):ti,ab,kw 24745
-
#11 or #12 or #13 or #14 or #15 or #16 or #17 or #18 or #19 or #20 or #21 397794
-
#10 and #22 23138
CDSR retrieved = 404
KSR Evidence (KSR Ltd): up to 2021/10/14
Searched: 14.10.21
-
# QueryResults
-
1 (Stroke* or apople* or "cerebral vasc*" or cerebrovasc* or "cerebro vasc*" or poststroke* or encephalorrhag* or hematencephalon* or "large-vessel occlusion*") in Title or Abstract 7315 results
-
2 ((brain or "blood flow") adj2 disturb*) in Title or Abstract 14 results
-
3 ((sinus or sagittal) adj3 thromb*) in Title or Abstract 37 results
-
4 ((ischemi* or ischaemi*) adj3 (seizure* or attack* or thrombo* or embolic or encephalopath* or neural)) in Title or Abstract 639 results
-
5 ((Bleed* or hemorrhag* or haemorrhag*) adj2 "corpus callosum") in Title or Abstract 1 result
-
6 CVA or CVAS or MCA* or ICH or ICHs or CVST or CVSTs or CVDST or CVT or CVDSTs or CVTs or LVO or LVOs in Title or Abstract 582 results
-
7 ((brain or cerebr* or cerebell* or cortical or Intraparenchymal or intracortical or vertebrobasil* or hemispher* or intracran* or intra-cran* or intracerebral or intratentorial or intra-tentorial or intraventricular or intra-ventricular or periventricular or peri-ventricular or supratentorial or supra-tentorial or "anterior circulat*" or "posterior circulat*" or "basal gangli*" or global or focal or parenchymal or subarachnoid or sub-arachnoid or putaminal or putamen or "posterior fossa" or intra-axial or intraaxial or lacunar) adj3 (arrest* or attack* or ischemi* or ischaemi* or infarct* or insufficien* or emboli* or occlus* or hypox* or vasospasm or obstruction or vasculopath* or failure* or thromb* or hemorrhag* or haemorrhag* or microhemorrhag* or microhaemorrhag* or accident* or hematoma* or haematoma* or bleed* or microbleed* or insult*)) in Title or Abstract 2368 results
-
8 #1 or #2 or #3 or #4 or #5 or #6 or #7 in All text 8597 results
-
9 ((diagnos* or predict* or specificity or sensitiv*) adj4 (criteria or criterion or guideline* or pattern* or trend* or utili* or management or prevalence or initiat* or distribution* or coverage or variety or selection or spread or alternative* or frequen*)) in Title or Abstract 5493 results
-
10 ((Brain or cerebral or neurologic* or CT or head) adj2 (scan* or scintigraph* or examination* or angiograph* or "image analys*" or perfusion* or radiograph*)) in Title or Abstract 759 results
-
11 ("CAT scan*" or CTA or CTP or neuroimag* or neuro-imag* or (comput* adj2 tomograph*)) in Title or Abstract 2808 results
-
12 "Gamma encephalograph*" or Gammaencephalograph* or "Radio encephalograph*" or Radioencephalograph* in Title or Abstract 0 results
-
13 #9 or #10 or #11 or #12 in All text 8410 results
-
14 #8 and #13 in All text 498 results
Accuracy of human readers
Database | Dates covered | Hits |
---|---|---|
Medline + PreMedline | 2017–2021/10/15 | 2,726 |
Total | 2,726 |
MEDLINE and Epub Ahead of Print, In-Process, In-Data-Review & Other Non-Indexed Citations, Daily and Versions (Ovid): 2017-2021/10/15
Searched 19.10.21
Stroke + CTscan/Diagnostics + reader (Limits 2017-C, Not Covid)
-
exp Brain Ischemia/ (115589)
-
exp Intracranial Hemorrhages/ (75053)
-
Stroke/ (113288)
-
(Stroke$ or apople$ or cerebral vasc$ or cerebrovasc$ or cerebro vasc$ or poststroke$ or encephalorrhag$ or hematencephalon$ or large-vessel occlusion$).ti,ab,ot. (327818)
-
((brain or blood flow) adj2 disturb$).ti,ab,ot. (2117)
-
((sinus or sagittal) adj3 thromb$).ti,ab,ot. (5117)
-
(isch?emi$ adj3 (seizure$ or attack$ or thrombo$ or embolic or encephalopath$ or neural)).ti,ab,ot. (26850)
-
((Bleed$ or h?emorrhag$) adj2 corpus callosum).ti,ab,ot. (23)
-
((brain or cerebr$ or cerebell$ or cortical or Intraparenchymal or intracortical or vertebrobasil$ or hemispher$ or intracran$ or intra-cran$ or intracerebral or intratentorial or intra-tentorial or intraventricular or intra-ventricular or periventricular or peri-ventricular or supratentorial or supra-tentorial or anterior circulat$ or posterior circulat$ or basal gangli$ or global or focal or parenchymal or subarachnoid or sub-arachnoid or putaminal or putamen or posterior fossa or intra-axial or intraaxial or lacunar) adj3 (arrest$ or attack$ or isch?emi$ or infarct$ or insufficien$ or emboli$ or occlus$ or hypox$ or vasospasm or obstruction or vasculopath$ or failure$ or thromb$ or h?emorrhag$ or microh?emorrhag$ or accident$ or h?ematoma$ or bleed$ or microbleed$ or insult$)).ti,ab,ot. (210934)
-
(CVA or CVAS or MCA$ or ICH or ICHs or CVST or CVSTs or CVDST or CVT or CVDSTs or CVTs or LVO or LVOs).ti,ab. (48905)
-
or/1-10 (557308)
-
((diagnos$ or predict$ or specificity or sensitiv$) adj4 (criteria or criterion or guideline$ or pattern$ or trend$ or utili$ or management or prevalence or initiat$ or distribution$ or coverage or variety or selection or spread or alternative$ or frequen$)).ti,ab,ot. (350992)
-
Diagnosis/ (17472)
-
Early Diagnosis/ (28758)
-
Brain/dg [Diagnostic Imaging] (51780)
-
Stroke/dg [Diagnostic Imaging] (7712)
-
Radiography/ (322703)
-
exp Radionuclide Imaging/ (223371)
-
Neurologic Examination/ (27754)
-
Tomography, X-Ray Computed/ (399785)
-
((Brain or cerebral or neurologic$ or CT or head) adj2 (scan$ or scintigraph$ or examination$ or angiograph$ or image analys$ or perfusion$ or radiograph$)).ti,ab,ot,hw. (229398)
-
(CAT scan$ or CTA or CTP or CTAs or CTPs or neuroimag$ or neuro-imag$ or (comput$ adj2 tomograph$)).ti,ab,ot,hw. (475578)
-
(Gamma encephalograph$ or Gammaencephalograph$ or Radio encephalograph$ or Radioencephalograph$).ti,ab,ot,hw. (155)
-
or/12-23 (1629565)
-
11 and 24 (100335)
-
(rater$ or reader$ or inter-rater$ or inter-reader$ or radiologist$ or resident$ or consultant$ or expert$ or experience$).ti,ab,ot. (1674641)
-
25 and 26 (9582)
-
limit 27 to yr="2017 -Current" (2790)
-
coronavirus/ or betacoronavirus/ or coronavirus infections/ (46824)
-
(Betacoronavirus$ or Sars-cov-2 or sars-cov2 or sarscov-2 or SARSCOV2 or Coronavirus$ or corona virus$ or covid-19 or covid19$ or 2019-ncov or corona-virus$ or wuhan-2019-ncov or cov19 or cov-19 or coronavirinae or Coronaviridae or CV19 or 2019nCoV or 19nCoV or nCoV$ or COVID).ti,ab,ot,hw,kw. (203858)
-
((new or novel or "19" or "2019" or Wuhan or Hubei or China or Chinese) adj5 (virus$ or pneumonia$ or outbreak$ or epidemic$ or pandemic$ or influenza or flu or CoV or HCoV)).ti,ab,ot,hw,kw. (129317)
-
or/29-31 (243973)
-
28 not 32 (2726)
Review of reviews: Alteplase
Database | Dates covered | Hits |
---|---|---|
CDSR | up to 2021/11/Iss11 | 15 |
KSR Evidence | up to 2021/11/11 | 191 |
Total | 206 |
Cochrane Database of Systematic Reviews (CDSR)(Wiley): up to 2021/11/Iss11
Searched 11.11.21
-
ID SearchHits
-
#1 MeSH descriptor: [Tissue Plasminogen Activator] explode all trees 1729
-
#2 (Alteplase or Activase or Actilyse or activacin or atlepase or Cathflo Activase or g 11021 or g 11035 or g 11044 or g11021 or g11035 or g11044 or gmk 527 or gmk527 or grtpa or ly 210825 or ly210825 or mmr 701 or mmr701 or td 2061 or td2061 or tisokinase):ti,ab 1158
-
#3 (t-PA or rt-PA or rtpa or ttpa):ti,ab 2903
-
#4 (tissue* near/3 plasminogen near/3 activator):ti,ab 2485
-
#5 (tissue* near/3 activator near/3 plasminogen):ti,ab 2480
-
#6 (plasminogen near/3 activator near/3 tissue*):ti,ab 2486
-
#7 (plasminogen near/3 tissue* near/3 activator):ti,ab 2485
-
#8 #1 or #2 or #3 or #4 or #5 or #6 or #7 5068
CDSR retrieved 15 results
KSR evidence: up to 2021/11/11
Searched 11.11.21
-
(Alteplase or Activase or Actilyse or activacin or atlepase or Cathflo Activase or g 11021 or g 11035 or g 11044 or g11021 or g11035 or g11044 or gmk 527 or gmk527 or grtpa or ly 210825 or ly210825 or mmr 701 or mmr701 or td 2061 or td2061 or tisokinase) in All text 79 results
-
(t-PA or rt-PA or rtpa or ttpa) in Title or Abstract 50 results
-
(tissue* near/3 plasminogen near/3 activator) in Title or Abstract 118 results
-
(tissue* near/3 activator near/3 plasminogen) in Title or Abstract 118 results
-
(plasminogen near/3 activator near/3 tissue*) in Title or Abstract 118 results
-
(plasminogen near/3 tissue* near/3 activator) in Title or Abstract 118 results
-
#1 or #2 or #3 or #4 or #5 or #6 in All text 191 results
Appendix 2 Data extraction tables
Study details | Selection criteria | Participant details | AI intervention |
---|---|---|---|
Adhya et al.33 Publication type: full paper Setting: USA, multi-hospital network (number of sites unclear) |
Inclusion criteria: All patients who received CTA for the evaluation of AIS or neurological deficit that included RAPID-CTA with relative vessel density of 60% or less | Mean (SD) age, years: 70 (NR) Male (%): 145 (47) No further participant characteristics were reported |
Rapid CTA |
Funding: none: ‘The author(s) received no financial support for the research, authorship, and/or publication of this article’. Recruitment: November 2019–November 2020 (retrospective) Participants (n): 310 |
Exclusion criteria: NR Research question: (Q2a) Is AI-derived software-assisted review of CTA brain scans for guiding mechanical thrombectomy treatment decisions for people with an ischaemic stroke a clinically effective intervention? |
||
Al-Kawaz et al.34 Publication type: full paper |
Inclusion criteria: patients presenting with LVO Exclusion criteria: NR |
Intervention: Median (IQR) age, years: 67 (57–81) |
RapidAI |
Setting: USA, single centre (CSC) Funding: none: ‘The authors have not declared a specific grant for this research from any funding agency in the public, commercial or not-for-profit sectors’. |
Research questions: (Q2a) Is AI-derived software-assisted review of CTA brain scans for guiding mechanical thrombectomy treatment decisions for people with an ischaemic stroke a clinically effective intervention? |
Male (%): 17 (51.5) Diabetes (%): 11 (33.3) Hypertension (%): 27 (81.8) Baseline NIHSS, median (IQR): 15 (10–22) |
|
Recruitment: June 2019–October 2020 (retrospective) Participants (n): 64 |
(Q2b) Is AI-derived software-assisted review of CTP brain scans for guiding mechanical thrombectomy treatment decisions for people with an ischaemic stroke after a CTA brain scan a clinically effective intervention? | Comparator: Median (IQR) age, years: 69.5 (60–77) Male (%):16 (48.5) Diabetes (%): 11 (33.3) Hypertension (%): 25 (80.6) Baseline NIHSS, median (IQR): 11 (9–18) There were no significant differences, in baseline characteristics, between groups |
|
Amukotuwa et al.35 DEFUSE 2 and 3, plus 3 additional cohorts (1 of which was the Amukotwa et al.35 cohort) Publication type: full paper Setting: NR, multicentre Funding: public: ‘This study was funded by grants from the National Institutes of Health: 1R01EB002711, 1R01NS039325, and 1U10NS086487’. Individual study authors disclosed shareholdings in or fees from iScemaView Recruitment: July 2008–December 2018 (retrospective) Participants (n): 926 |
Inclusion criteria: NR Exclusion criteria: screen failure; CTA not included in the acute CT protocol; inadequate data format; CTA deemed, by an experienced neuroradiologist, to be technically inadequate to allow accurate interpretation by a human reader Research question: (Q2a) Is AI-derived software-assisted review of CTA brain scans for guiding mechanical thrombectomy treatment decisions for people with an ischaemic stroke a clinically effective intervention? |
Median (IQR) age, years: 70 (58–80) Male (%): 504 (54.4) Baseline NIHSS, median (IQR): 14 (9–19) No further participant characteristics were reported |
Rapid CTA |
Amukotuwa et al.36 Publication type: full paper Setting: Australia, multicentre Funding: none; individual study authors disclosed receipt of support and/or consulting fees from iScemaView Recruitment: January 2017–December 2018 (retrospective) Participants (n): 477 |
Inclusion criteria: Consecutive adult (≥ 18 years) patients who had undergone multimodal brain CT for suspected AIS within 24 hours of symptom onset or last seen well Exclusion criteria: Technically inadequate CTA (poor contrast bolus or substantial motion or metal artefact that precluded accurate assessment of the intracranial arteries to the level of the distal M2 segments of the middle cerebral arteries by an experienced neuroradiologist); thin slice CTA images unavailable |
Median (IQR) age, years: 70(60–80) Male (%): 271 (56.8) Baseline NIHSS, median (IQR): 6 (2–9) No further participant characteristics were reported |
Rapid CTA |
Research question: (Q2a) Is AI-derived software-assisted review of CTA brain scans for guiding mechanical thrombectomy treatment decisions for people with an ischaemic stroke a clinically effective intervention? |
|||
Barreira et al.60 ALADIN Barreira et al.37 Rodrigues et al.38 Publication type: conference abstract Setting: USA, multicentre Funding: NR Recruitment: NR 201–NR 2017 (retrospective) Participants (n): 875 |
Inclusion criteria: random sample from a retrospective cohort of AIS patients with and without anterior circulation LVOs Exclusion criteria: NR Research question: (Q2a) Is AI-derived software-assisted review of CTA brain scans for guiding mechanical thrombectomy treatment decisions for people with an ischaemic stroke a clinically effective intervention? |
Male (%): 433 (49.5) Baseline NIHSS, median (IQR): 15 (10–20) No further participant characteristics were reported |
Viz LVO |
Barreira et al.39 ADVANCE Publication type: conference abstract Setting: USA, single centre Funding: NR Recruitment: NR 201–NR 2017 (retrospective) Participants (n): 284 |
Inclusion criteria: random sample from a cohort of stroke patients with and without ICH Exclusion criteria: NR Research question: (Q1) Is AI-derived software-assisted review of non-enhanced CT brain scans for guiding thrombolysis treatment decisions for people with suspected acute stroke a clinically effective intervention? |
No participant characteristics were reported | Viz ICH |
Chatterjee et al.40 Publication type: conference abstract Setting: USA, single centre Funding: NR Recruitment: NR (retrospective) Participants (n): 54 |
Inclusion criteria: patients with acute stroke CTA studies Exclusion criteria: NR Research question: (Q2a) Is AI-derived software-assisted review of CTA brain scans for guiding mechanical thrombectomy treatment decisions for people with an ischaemic stroke a clinically effective intervention? |
No participant characteristics were reported | Viz LVO |
Dehkharghani et al.41 Dehkharghani et al.42 Publication type: full paper Setting: USA; Switzerland; Brazil, multi-centre Funding: industry: ‘Supported by iSchemaView’. Recruitment: NR (retrospective) Participants (n): 217 |
Inclusion criteria: Individuals undergoing cerebrovascular CTA, from the CRISP and DASH trials and from institutional registries of participating hospitals; technically adequate, thin section (≤ 2 mm) contiguous cerebrovascular CTA sources axial images, free of artefacts that would degrade interpretation by human readers (e.g. those related to severe metallic streak or beam hardening) | Mean (SD) age, years: 64 (16) Male (%): 116 (54) No further participant characteristics were reported |
Rapid CTA |
Exclusion criteria: Age < 18 years Research question: (Q2a) Is AI-derived software-assisted review of CTA brain scans for guiding mechanical thrombectomy treatment decisions for people with an ischaemic stroke a clinically effective intervention? |
|||
Dornbos et al.43 Publication type: conference abstract Setting: USA, multicentre (1 CSC and 2 spoke hospitals) Funding: NR Recruitment: May 2019–December 2019 (retrospective) Participants (n): 680 |
Inclusion criteria: consecutive stroke cases Exclusion criteria: NR Research question: (Q2a) Is AI-derived software-assisted review of CTA brain scans for guiding mechanical thrombectomy treatment decisions for people with an ischaemic stroke a clinically effective intervention? |
No participant characteristics were reported | Viz LVO |
Gunda et al.44 Publication type: conference abstract Setting: Hungary, single centre Funding: NR Recruitment: ‘Two identical 7-month periods in 2017 and 2108’ (retrospective) Participants (n): 797 |
Inclusion criteria: stroke patients (no further details reported) Exclusion criteria: NR Research question: (Q1) Is AI-derived software-assisted review of non-enhanced CT brain scans for guiding thrombolysis treatment decisions for people with suspected acute stroke a clinically effective intervention? |
No participant characteristics were reported | Brainomix eASPECTS and eCTA |
(Q2a) Is AI-derived software-assisted review of CTA brain scans for guiding mechanical thrombectomy treatment decisions for people with an ischaemic stroke a clinically effective intervention? | |||
Hassan et al.45 Publication type: conference abstract Setting: USA, single centre Funding: NR Recruitment: November 2016–November 2020 (retrospective) Participants (n): 188 |
Inclusion criteria: LVO transfer patients who arrived at a comprehensive care centre for 2 years before and after implementation of AI software in November 2018 Exclusion criteria: NR Research question: (Q2a) Is AI-derived software-assisted review of CTA brain scans for guiding mechanical thrombectomy treatment decisions for people with an ischaemic stroke a clinically effective intervention? |
Intervention: Mean (SD) age, years: 69.9 (15.8) Male (%): 58 (56.9) Ethnicity (%): white 26 (25.5); Hispanic 78 (76.5); African American 0 (0); Asian 0 (0) AF (%): 21 (20.6) Diabetes (%): 51 (50) Smoking (%): 9 (8.8) Hypertension (%): 81 (79.4) Previous TIA/stroke (%): 24 (23.5) |
Viz LVO |
Baseline NIHSS, mean (SD): 15.9 (7.1) Comparator: Mean (SD) age, years: 68.5 (13.1) Male (%): 51 (59.3) Ethnicity: white 16 (18.6); Hispanic 68 (79.1); African American 1 (1.2); Asian 1 (1.2) AF (%): 19 (22.1) Diabetes (%): 45 (52.3) Smoking (%): 7 (8.1) Hypertension (%): 69 (80.2) Previous TIA/stroke (%): 23 (26.7) Baseline NIHSS, mean (SD): 16.1 (8.3) There were no significant differences, in baseline characteristics, between groups |
|||
Hassan et al.46 Hassan et al.47 Publication type: full paper Setting: USA, single centre Funding: none: ‘The author(s) received no financial support for the research, authorship, and/or publication of this article’. One study author disclosed receipt of fees from Viz.ai. Recruitment: February 2017–May 2019 (retrospective) Participants (n): 43 |
Inclusion criteria: LVO transfer patients from a single primary care centre, transferred to a comprehensive care centre Exclusion criteria: NR Research question: (Q2a) Is AI-derived software-assisted review of CTA brain scans for guiding mechanical thrombectomy treatment decisions for people with an ischaemic stroke a clinically effective intervention? |
Intervention: Mean (SD) age, years: 69.1 (13.3) Male (%): 6 (40.0) Ethnicity: white 5 (30); Hispanic 10 (70); African American 0 (0); Asian 0 (0) AF (%): 1 (6.7) Diabetes (%): 7 (46.7) Smoking (%): 2 (13.3) Hypertension (%): 13 (86.7) Baseline NIHSS, mean (SD): 14.1 (6.8) Comparator: Mean (SD) age, years: 71.6 (12.3) Male (%): 15 (53.4) |
Viz LVO |
Ethnicity: white 5 (17.9); Hispanic 23 (82.1); African American 0 (0); Asian 0 (0) AF (%): 10 (35.7) Diabetes (%): 12 (42.9) Smoking (%): 2 (7.1) Hypertension (%): 25 (89.3) Baseline NIHSS, mean (SD): 18.3 (7.4) There were no significant differences, in baseline characteristics, between groups |
|||
Herweh et al.48 Publication type: conference abstract Setting: Germany, single centre Funding: NR Recruitment: NR (retrospective) Participants (n): 160 |
Inclusion criteria: patients with suspected AIS Exclusion criteria: NR Research question: (Q1) Is AI-derived software-assisted review of non-enhanced CT brain scans for guiding thrombolysis treatment decisions for people with suspected acute stroke a clinically effective intervention? |
No participant characteristics were reported | Brainomix |
Kamal et al.49 Publication type: conference abstract Setting: NR Funding: NR Recruitment: January 2014–July 2016 (retrospective) Participants (n): 186 |
Inclusion criteria: patients undergoing thrombectomy (no further details reported) Exclusion criteria: NR Research question: (Q2a) Is AI-derived software-assisted review of CTA brain scans for guiding mechanical thrombectomy treatment decisions for people with an ischaemic stroke a clinically effective intervention? (Q2b) Is AI-derived software-assisted review of CTP brain scans for guiding mechanical thrombectomy treatment decisions for people with an ischaemic stroke after a CTA brain scan a clinically effective intervention? |
Intervention: Mean (SD) age, years: 63.0 (16.0) Male (%): 24 (48.0) Diabetes (%): 12/43 (27.9) Smoking (%): 15/43 (24.9) Baseline NIHSS, mean (SD): 20.0 (7.0) Comparator: Mean (SD) age, years: 61.0 (15.0) Male (%): 89(65.4) Diabetes (%): 26/100 (26) Smoking (%): 21/101 (20.8) |
RapidAI |
Baseline NIHSS, mean (SD): 17.0 (6.0) There were no significant differences, in baseline characteristics, between groups |
|||
Kauw et al.50 Publication type: full paper Setting: Netherlands; USA, multicentre Funding: public: ‘Dutch Heart Foundation and the Netherlands Organization for Scientific Research, domain Applied and Engineering Sciences, as part of their joint strategic research program: Earlier Recognition of Cardiovascular Disease (grant number 14732)’. |
Inclusion criteria: Consecutive patients with AIS undergoing CTP for thrombectomy triage Exclusion criteria: NR Research question: (Q2b) Is AI-derived software-assisted review of CTP brain scans for guiding mechanical thrombectomy treatment decisions for people with an ischaemic stroke after a CTA brain scan a clinically effective intervention? |
Mean (SD) age, years: 72 (15) Male (%): 86 (49) No further participant characteristics were reported |
Rapid CTP |
Recruitment: NR 2012–NR 2018 (retrospective) Participants (n): 176 |
|||
Mair et al.62 RITeS Publication type: full paper Setting: UK, multicentre Funding: public: principal funder: Stroke Association (TSA_CR_2017/01). Supported software purchase: Medical Research Council (MC_PC_17188). Recruitment: June 2003–May 2018 (retrospective) Participants (n): 4100 |
Inclusion criteria: A clinically representative sample of CT brain scans performed soon after stroke onset from 7 national/international multicentre RCTs and 2 single-centre prospective observational studies. Studies recruited patients with acute stroke since May 2000 and one was still recruiting during RITeS. Of the RCTs, 6 included ischaemic stroke only, 2 haemorrhagic stroke only and 1 included ischaemic or haemorrhagic stroke or stroke mimics. 1 observational study studied haemorrhagic and the other ischaemic stroke. To assess whether the sample was clinically representative of patients admitted to hospital with stroke, it was prespecified that age, sex, stroke severity, time since symptom onset and final diagnosis in RITeS would be similar to data from the UK SSNAP (April 2018–March 2019, www.strokeaudit.org), pooled RCTs and registries. | Median (IQR) age, years: 78 (68, 85) Male (%): 2031 (49.5) Baseline NIHSS, median (IQR): 10 (6–16) Time from symptom onset, median (IQR), hours: 2.5 (1.8–3.8) |
Brainomix e-ASPECTS |
Exclusion criteria: NR Research question: (Q1) Is AI-derived software-assisted review of non-enhanced CT brain scans for guiding thrombolysis treatment decisions for people with suspected acute stroke a clinically effective intervention? |
|||
McLouth et al.51 Publication type: full paper Setting: USA, multicentre Funding: NR; individual study authors declared employment by or stockholding in Avicenna.ai Recruitment: NR 2017–NR 2019 (retrospective) Participants (n): 378 |
Inclusion criteria: patients with suspected LVO, on clinical grounds, in whom CTA studies had been performed, identified from University of California, Irvine and a teleradiology service, vRAD (Minneapolis, MN, USA) databases using key words such as ‘CTA’, ‘head’ and ‘LVO’. Exclusion criteria: NR Research question: (Q2a) Is AI-derived software-assisted review of CTA brain scans for guiding mechanical thrombectomy treatment decisions for people with an ischaemic stroke a clinically effective intervention? |
Male (%): 185 (40.9) No further participant characteristics were reported |
CINA LVO |
Morey et al.52 Morey et al.53 Morey et al.54 Publication type: full paper Setting: USA, single centre (PSC) Funding: none: ‘This research received no specific grant from any funding agency in the public, commercial, or not-for-profit sectors’. Recruitment: July 2018–March 2020 (retrospective) Participants (n): 55 |
Inclusion criteria: Consecutive patients who presented to a PSC that used Viz LVO and who were transferred to a thrombectomy capable stroke centre or CSC for LVO stroke and underwent thrombectomy Exclusion criteria: inpatient at the time of stroke; thrombectomy decision delayed due to fluctuating symptoms Research question: (Q2a) Is AI-derived software-assisted review of CTA brain scans for guiding mechanical thrombectomy treatment decisions for people with an ischaemic stroke a clinically effective intervention? |
Intervention: Mean (SD) age, years: 72.8 (15.4) Male (%): 13 (50) AF (%): 14 (53.8) Diabetes (%): 8 (30.8) Hypertension (%): 14 (53.8) Previous TIA/stroke (%): 2 (7.7) Baseline NIHSS, median (IQR): 14 (NR–NR) Comparator: Mean (SD) age, years: 76.2 (13.9) Male (%): 14 (48.3) AF (%): 15 (55.6) Diabetes (%): 12 (42.9) |
Viz LVO |
Hypertension (%): 23 (82.1) Previous TIA/stroke (%): 6 (20.7) Baseline NIHSS, median (IQR): 17 (NR–NR) Proportion of patients with hypertension significantly lower in the intervention than in the comparator group |
|||
Paz et al.55 Publication type: full paper Setting: Canada, single centre Funding: NR Recruitment: Retrospective (July 2020–December 2020) Participants (n): 151 |
Inclusion criteria: patients who presented with suspected acute stroke symptoms and whose imaging studies were processed by RAPID LVO. Exclusion criteria: NR Research question: (Q2a) Is AI-derived software-assisted review of CTA brain scans for guiding mechanical thrombectomy treatment decisions for people with an ischaemic stroke a clinically effective intervention? |
Mean (SD) age, years: 70.6 (15.9) Male (%): 69 (45.7) No further participant characteristics were reported |
Rapid LVO |
Seker et al.56 Seker et al.57 Seker et al.58 Publication type: full paper Setting: Germany, single centre Funding: none: ‘The author(s) received no financial support for the research, authorship, and/or publication of this article’. Individual study authors declared receipt of support and/or fees from Brainomix Recruitment: January 2014–December 2017 (retrospective) Participants (n): 301 |
Inclusion criteria: case–control validation study: Cases comprised patients with LVO of the terminal carotid artery or MCA up to the proximal M2 level who had CTA images of sufficient quality (CT scan primarily in the arterial phase without severe motion artefacts and with a slice thickness of ≤ 1 mm); controls comprised CTA examinations from 141 consecutive AIS patients without LVO Exclusion criteria: NR Research question: (Q2a) Is AI-derived software-assisted review of CTA brain scans for guiding mechanical thrombectomy treatment decisions for people with an ischaemic stroke a clinically effective intervention? |
No participant characteristics were reported | Brainomix eCTA |
Shalitin et al.61 Publication type: full paper Setting: USA, multicentre Funding: NR; individual study authors appear to have been employees of Viz.ai Recruitment: NR Participants (n): 2544 |
Inclusion criteria: NR Exclusion criteria: NR Research question: (Q2a) Is AI-derived software-assisted review of CTA brain scans for guiding mechanical thrombectomy treatment decisions for people with an ischaemic stroke a clinically effective intervention? |
Mean (SD) age, years: 66.0 (17.4) Male (%): 1186 (46.6) No further participant characteristics were reported |
Viz LVO |
Yahav-Dovrat et al.59 Publication type: full paper Setting: USA, single centre (CSC) Funding: NR; individual study authors disclosed receipt of support and/or consulting fees from Viz.ai Recruitment: January 2018–March 2019 (retrospective) Participants (n): 1167 |
Inclusion criteria: All CTA scans including non-acute ischaemic stroke cases (subgroup data for stroke protocol patients) Exclusion criteria: Examinations with metal artefact, severe motion, or incomplete skull scanning Research Question: (Q2a) Is AI-derived software-assisted review of CTA brain scans for guiding mechanical thrombectomy treatment decisions for people with an ischaemic stroke a clinically effective intervention? |
Mean (SD) age, years: 62.2 (19.6) Male (%): 689 (59) No further participant characteristics were reported |
Viz LVO |
Study details | Imaging details | AI-derived software technology | Reference standard/comparator |
---|---|---|---|
Adhya et al.33 Research question: (Q2a) Is AI-derived software-assisted review of CTA brain scans for guiding mechanical thrombectomy treatment decisions for people with an ischaemic stroke a clinically effective intervention? |
No details were reported | AI-derived software technology: Rapid CTA, version NR Analysis: Unclear (routine practice, post implementation of Rapid CTA) |
Comparator image interpretation: unclear (routine practice, pre implementation of Rapid CTA) |
Al-Kawaz et al.34 Research question: (Q2a) Is AI-derived software-assisted review of CTA brain scans for guiding mechanical thrombectomy treatment decisions for people with an ischaemic stroke a clinically effective intervention? (Q2b) Is AI-derived software-assisted review of CTP brain scans for guiding mechanical thrombectomy treatment decisions for people with an ischaemic stroke after a CTA brain scan a clinically effective intervention? |
No details were reported | AI-derived software technology: RapidAI Mobile Application Analysis: unclear (routine practice, post implementation of RapidAI Mobile App) |
Comparator image interpretation: unclear (routine practice, pre implementation of RapidAI Mobile App) |
Amukotuwa et al.35 DEFUSE 2 and 3, plus three additional cohorts (one of which was the Amukotwa 2019b36 cohort) Research question: (Q2a) Is AI-derived software-assisted review of CTA brain scans for guiding mechanical thrombectomy treatment decisions for people with an ischaemic stroke a clinically effective intervention? |
No details were reported The article stated that study sites used a ‘representative sample of scanner models from all major CT vendors’ |
AI-derived software technology: Rapid CTA, version 4.9.1 Analysis: AI alone |
Reference standard image interpretation: for patients from DEFUSE 2 and 3, the presence and location of occlusive lesion had already been determined by the study investigators and was verified by a neuroradiologist with 8 years post-fellowship experience. For the remaining cohorts, 2 neuroradiologists with 9 years post-fellowship experience determined the presence and site of occlusive lesions, in consensus, based on multimodal CT including CTA and with access to all clinical and imaging data (including perfusion); any disagreements were resolved by review of all available imaging, including perfusion |
Amukotuwa et al.36 Research question: (Q2a) Is AI-derived software-assisted review of CTA brain scans for guiding mechanical thrombectomy treatment decisions for people with an ischaemic stroke a clinically effective intervention? |
CT scanner: 256-slice multi-detector CT (iCT 256, Philips Health care, Cleveland, OH) CTA image acquisition: 80 ml of non-ionic contrast (Omnipaque 350, GE Health care, WI) IV at 5 ml/s followed by a 40 ml saline flush at 6 ml/s; helical acquisition; tube voltage 100 kV; slice collimation width 0.625 mm; image matrix 512 × 512; spiral pitch factor 0.518; slice thickness 4 mm |
AI-derived software technology: Rapid CTA, version 4.9 Analysis: AI alone |
Reference standard image interpretation: Two diagnostic neuroradiologists with 8- and 9-year post-fellowship experience and access to the complete multimodal CT (NCCT, CTP and CTA) and details of the clinical presentation. Consensus was recorded and verified by an interventional neuroradiologist with 7 years’ experience |
Barreira et al.60 Research question: (Q2a) Is AI-derived software-assisted review of CTA brain scans for guiding mechanical thrombectomy treatment decisions for people with an ischaemic stroke a clinically effective intervention? |
No details were reported | AI-derived software technology: Viz LVO, version 3.04 Analysis: AI alone |
Reference standard image interpretation: CTAs were analysed and graded by experienced stroke neuroradiologists (no further details were reported) |
Barreira et al.39 Research question: (Q1) Is AI-derived software-assisted review of non-enhanced CT brain scans for guiding thrombolysis treatment decisions for people with suspected acute stroke a clinically effective intervention? |
No details were reported | AI-derived software technology: Viz ICH, version 2.0 Analysis: AI alone |
Reference standard image interpretation: experienced stroke neurologists grading the same NCCTs with a semi-automated tool (OsiriX MD version 9.0.1) |
Chatterjee et al.40 Research question: (Q2a) Is AI-derived software-assisted review of CTA brain scans for guiding mechanical thrombectomy treatment decisions for people with an ischaemic stroke a clinically effective intervention? |
No details were reported | AI-derived software technology: Viz LVO, version NR Analysis: AI alone |
Reference standard image interpretation: No details were reported |
Dehkharghani et al.41 Research question: (Q2a) Is AI-derived software-assisted review of CTA brain scans for guiding mechanical thrombectomy treatment decisions for people with an ischaemic stroke a clinically effective intervention? |
CT scanner: GE Medical, Philips, Siemens or Toshiba (no further details reported) CTA image acquisition: no details were reported |
AI-derived software technology: Rapid LVO, version 1.0 Analysis: AI alone |
Reference standard image interpretation: 2 board-certified neuroradiologists, with 11- and 7-years’ experience, blinded to clinical history and imaging outcome, independently scored all examinations for LVO. A LVO was defined as occlusion or near occlusion by a focal stenosis > 80%. Discrepancies between the two readers were adjudicated by a third board-certified neuroradiologist with 7 years’ experience. For examinations classified as positive, readers were subsequently presented with the automated output and asked to assess it for presence of LVO, LVO side and inclusion of compromised vessel segment within the region of interest; All three criteria had to be met in order for an automated image to be classified as true positive |
Dornbos et al.43 Research question: (Q2a) Is AI-derived software-assisted review of CTA brain scans for guiding mechanical thrombectomy treatment decisions for people with an ischaemic stroke a clinically effective intervention? |
No details were reported | AI-derived software technology: Viz LVO, version NR Analysis: AI alone |
Reference standard image interpretation: blinded neuroradiologists (no further details were reported) |
Gunda et al.44 Research question: (Q1) Is AI-derived software-assisted review of non-enhanced CT brain scans for guiding thrombolysis treatment decisions for people with suspected acute stroke a clinically effective intervention? (Q2a) Is AI-derived software-assisted review of CTA brain scans for guiding mechanical thrombectomy treatment decisions for people with an ischaemic stroke a clinically effective intervention? |
No details were reported | AI-derived software technology: e-ASPECTS and e-CTA, version NR Analysis: Unclear, ‘AI decision support software was implemented in 2018 and delivery of stroke care was otherwise unchanged’ |
Comparator image interpretation: unclear (standard stroke care before implementation of AI decision support software) |
Hassan et al.45 Research question: (Q2a) Is AI-derived software-assisted review of CTA brain scans for guiding mechanical thrombectomy treatment decisions for people with an ischaemic stroke a clinically effective intervention? |
No details were reported | AI-derived software technology: Viz LVO, version NR Analysis: unclear (routine practice, post implementation of Viz LVO) |
Comparator image interpretation: unclear (routine practice, pre implementation of Viz LVO) |
Hassan et al.46 Research question: (Q2a) Is AI-derived software-assisted review of CTA brain scans for guiding mechanical thrombectomy treatment decisions for people with an ischaemic stroke a clinically effective intervention? |
No details were reported | AI-derived software technology: Viz LVO, version NR Analysis: unclear (routine practice, post implementation of Viz LVO) |
Comparator image interpretation: unclear (routine practice, pre implementation of Viz LVO) |
Herweh et al.48 Research question: (Q1) Is AI-derived software-assisted review of non-enhanced CT brain scans for guiding thrombolysis treatment decisions for people with suspected acute stroke a clinically effective intervention? |
No details were reported | AI-derived software technology: BrainomixVR (Brainomix, Oxford, UK) Analysis: AI alone |
Reference standard image interpretation: image interpretation by a board-certified neuroradiologist |
Kamal et al.49 Research question: (Q2a) Is AI-derived software-assisted review of CTA brain scans for guiding mechanical thrombectomy treatment decisions for people with an ischaemic stroke a clinically effective intervention? (Q2b) Is AI-derived software-assisted review of CTP brain scans for guiding mechanical thrombectomy treatment decisions for people with an ischaemic stroke after a CTA brain scan a clinically effective intervention? |
No details were reported | AI-derived software technology: RapidAI Analysis: unclear, ‘implementation of automated software analysis with instant e-mail distribution to treating clinicians’ |
Comparator image interpretation: unclear |
Kauw et al.50 Research question: (Q2b) Is AI-derived software-assisted review of CTP brain scans for guiding mechanical thrombectomy treatment decisions for people with an ischaemic stroke after a CTA brain scan a clinically effective intervention? |
CT scanner: no details were reported CTA and CTP image acquisition: CTP and CTA were performed as part of routine stroke work-up. The CTP was performed with cine mode on 80 kV and 100 mAs with 37 phases at 1 second interval, followed by 33 phases at 3 seconds interval, on a 128-slice scanner. The CTA was performed on 120 kV and 225 mAs and covered the aortic arch to the brain apex, with a slice thickness of 0.625 mm. |
AI-derived software technology: Rapid CTP, version NR Analysis: AI alone |
Reference standard image interpretation: images were reviewed, for potential causes of post-processing failure, by two clinicians (experience not specified) in consensus, who were blinded to clinical data but had access to all imaging data available at the time of patient evaluation. RAPID CTP post-processing failures were re-processed manually using IntelliSpace software (Philips, Best, The Netherlands). For this assessment, treatment received was used as the reference standard. |
Mair et al.62 Research question: (Q1) Is AI-derived software-assisted review of non-enhanced CT brain scans for guiding thrombolysis treatment decisions for people with suspected acute stroke a clinically effective intervention? |
CT scanner: no details were reported CTA image acquisition: no details were reported |
AI-derived software technology: Brainomix e-ASPECTS, versions 9–10 Analysis: AI alone |
Reference/comparator standard image interpretation: final diagnosis was treated as the reference standard: Final diagnosis (ischaemic stroke, brain haemorrhage, stroke mimic) was determined similarly in each study based on the local principal investigator’s diagnosis, and central adjudication of all available baseline and follow-up data including imaging expert scan interpretation. For the comparator (human expert reader) prior to RITeS, CT in the original nine studies had been rated by central expert panels, masked to all other clinical and imaging data. Five of seven RITeS studies that included primarily ischaemic stroke performed imaging assessment using the same validated online viewing platform (SIRS 1/2, https://sirs2.ccbs.ed.ac.uk/sirs2). CT was scored for: ASPECTS; ischaemia in all arterial territories; presence of hyperattenuated arteries; ICH location and size; structural mimics; and pre-stroke brain changes (atrophy, leukoaraiosis, old stroke lesions). CT image quality was recorded as good, moderate or poor. Two other ischaemic stroke studies in RITeS assessed CT for ischaemic brain lesions, ASPECTS and hyperattenuated arteries only. Two RITeS studies evaluating haemorrhagic stroke included assessment of haemorrhage location and size but not ASPECTS |
McLouth et al.51 Research question: (Q2a) Is AI-derived software-assisted review of CTA brain scans for guiding mechanical thrombectomy treatment decisions for people with an ischaemic stroke a clinically effective intervention? |
CT scanner: GE Medica Systems, Philips, Siemens, Canon (formerly Toshiba), or NMS. No further details reported. CTA image acquisition: inclusion criteria for CTA scans: strict axial acquisition; 512 × 512 matrix; slice thickness ≤ 1.25 mm; kVp range 80–140; arterial phase timing of contrast bolus confirmed by mini test bolus or automatic bolus tracking software; arterial (or other sharp) reconstruction kernel |
AI-derived software technology: CINA LVO, version 1.0 Analysis: AI alone |
Reference standard image interpretation: CTA interpreted by 2 US board-certified neuroradiologists, with consensus determined by a third board-certified neuroradiologist |
Morey et al.52 Research question: (Q2a) Is AI-derived software-assisted review of CTA brain scans for guiding mechanical thrombectomy treatment decisions for people with an ischaemic stroke a clinically effective intervention? |
No details were reported | AI-derived software technology: Viz LVO, version NR Analysis: unclear (routine practice, post implementation of Viz LVO) |
Comparator image interpretation: unclear (routine practice, pre implementation of Viz LVO) |
Paz et al.55 Research question: (Q2a) Is AI-derived software-assisted review of CTA brain scans for guiding mechanical thrombectomy treatment decisions for people with an ischaemic stroke a clinically effective intervention? |
CT scanner: no details were reported CTA image acquisition: institutional stroke protocol performed on all patients, comprising NCCT acquisition of the head followed by CTA (section thickness 0.8–1.0 mm) of the head; Toshiba Aquilion One 320 slices scanner; 80 kV, 310 mA for the mask, 150 mA for the pre-arterial phase, 300 mA for the arterial phase and 150 mA for the remainder of the acquisition; contrast ISOVUE 370; total scan time 60 seconds; axial thickness 1 mm, with interval of 0.8 mm; MIP on all 19 volumes coronal and sagittal 2 m, with 2 mm interval; DSA movie and perfusion maps |
AI-derived software technology: Rapid LVO, version NR Analysis: AI alone |
Reference standard image interpretation: no details were reported |
Seker et al.56 Research question: (Q2a) Is AI-derived software-assisted review of CTA brain scans for guiding mechanical thrombectomy treatment decisions for people with an ischaemic stroke a clinically effective intervention? |
CT scanner: article stated that: ‘CTA imaging was performed using a variety of multi-slice CT scanners at stroke centres participating in a regional network’. CTA image acquisition: CT acquisition protocols varied, reflecting real-world practice. In general, a single contrast bolus was given IV, followed by a saline flush. Aortic contrast opacification was monitored using bolus tracking. CT scans were from the aortic arch to the vertex. Only axial reformations with a slice thickness between 0.6 and 1 mm were included |
AI-derived software technology: e-CTA, version NR Analysis: AI alone |
Reference standard image interpretation: CTA interpreted by a board-certified neuroradiologist with >10 years’ experience and access to all clinical and imaging data, including data on interventional therapy and follow-up Comparator image interpretation: for a subgroup of the study population, diagnostic accuracy data were reported for 4 comparators (1 board-certified neuroradiologist, 1 radiology resident and 2 neurology residents) |
Shalitin et al.61 Research question: (Q2a) Is AI-derived software-assisted review of CTA brain scans for guiding mechanical thrombectomy treatment decisions for people with an ischaemic stroke a clinically effective intervention? |
CT scanner: GE Medical, Philips, Siemens, Toshiba or ‘other’ (no further details reported) CTA image acquisition: No details were reported |
AI-derived software technology: Viz LVO, version NR Analysis: AI alone |
Reference standard image interpretation: image interpretation by ‘a team of radiology trained annotators’ (no further details were reported) |
Yahav-Dovrat et al.59 Research question: (Q2a) Is AI-derived software-assisted review of CTA brain scans for guiding mechanical thrombectomy treatment decisions for people with an ischaemic stroke a clinically effective intervention? |
No details were reported | AI-derived software technology: Viz LVO, version NR Analysis: AI alone |
Reference standard image interpretation: interpretation of CTA by 1 of 4 senior neuroradiologists with 7–25 years of experience |
Appendix 3 Study quality
QUADAS-2 Assessments
Study: DEFUSE 2 and DEFUSE 335
Domain 1: patient selection
A. RISK OF BIAS | ||
The study population comprised five cohorts, DEFUSE 2 and 3, plus three additional cohorts (one of which was the Amukotwa et al.36 cohort), of patients who had undergone acute CTA. CTA deemed, by an experienced neuroradiologist, to be technically inadequate to allow accurate interpretation by a human reader, were excluded. | ||
Was a consecutive or random sample of patients enrolled? | Unclear | |
Was a case–control design avoided? | Yes | |
Did the study avoid inappropriate exclusions? | Yes | |
Could the selection of patients have introduced bias? | RISK: unclear | |
B. APPLICABILITY | ||
Retrospective analysis of five cohorts of patients from stroke studies, with no clear inclusion criteria reported for this study. | ||
Do the included patients match the question? | Concerns: unclear |
Domain 2: index test(s)
A. RISK OF BIAS | ||
RAPID CTA: The study reports the diagnostic performance of the AI technology alone (hence, blinding to reference standard results NA), for the target condition LVO (subgroups for various anatomical locations reported), using various thresholds. | ||
Were the index test results interpreted without knowledge of the results of the reference standard? | NA | |
If a threshold was used, was it prespecified? | Yes | |
Could the conduct or interpretation of the index test have introduced bias? | RISK: low | |
B. APPLICABILITY | ||
The AI technology was evaluated as a stand-alone intervention, rather than as an adjunct to human interpretation. | ||
Are there concerns that the index test, its conduct or interpretation differ from the review question? | Concerns: high |
Domain 3: reference standard
A. RISK OF BIAS | ||
For patients from DEFUSE 2 and 3, the presence and location of occlusive lesion had already been determined by the study investigators and was verified by a neuroradiologist with 8 years post-fellowship experience. For the remaining cohorts, two neuroradiologists with 9 years of post-fellowship experience determined the presence and site of occlusive lesions, in consensus, based on multimodal CT including CTA and with access to all clinical and imaging data (including perfusion); any disagreements were resolved by review of all available imaging, including perfusion. The reference standard determination was made before application of the AI intervention. | ||
Is the reference standard likely to correctly classify the target condition? | Yes | |
Were the reference standard results interpreted without knowledge of the results of the index test? | Yes | |
Could the reference standard, its conduct or its interpretation have introduced bias? | RISK: low | |
B. APPLICABILITY | ||
Is there concern that the target condition as defined by the reference standard does not match the review question? | Concerns: low |
Domain 4: flow and timing
A. RISK OF BIAS | ||
The study used CTA images from patients for whom a reference standard diagnosis had already been established. All patients appear to have been included in the analysis. | ||
Was there an appropriate time interval between index test and reference standard? | Yes | |
Did patients receive the same or a similar reference standard? | Yes | |
Were all patients included in the analysis? | Yes | |
Could the patient flow have introduced bias? | RISK: low |
Study: Amukotuwa et al.36
Domain 1: patient selection
A. RISK OF BIAS | ||
Retrospective analysis of consecutive adult (≥ 18 years) patients who had undergone multimodal brain CT for suspected AIS within 24 hours of symptom onset or last seen well. Technically inadequate CTAs (poor contrast bolus or substantial motion or metal artefact that precluded accurate assessment of the intracranial arteries to the level of the distal M2 segments of the MCAs by an experienced neuroradiologist) were excluded. | ||
Was a consecutive or random sample of patients enrolled? | Yes | |
Was a case–control design avoided? | Yes | |
Did the study avoid inappropriate exclusions? | Yes | |
Could the selection of patients have introduced bias? | RISK: low | |
B. APPLICABILITY | ||
Do the included patients match the question? | Concerns: low |
Domain 2: index test(s)
A. RISK OF BIAS | ||
RAPID CTA: The study reports the diagnostic performance of the AI technology alone (hence, blinding to reference standard results NA), for the target condition LVO (subgroups for various anatomical locations reported), using a prespecified threshold. | ||
Were the index test results interpreted without knowledge of the results of the reference standard? | NA | |
If a threshold was used, was it prespecified? | Yes | |
Could the conduct or interpretation of the index test have introduced bias? | RISK: low | |
B. APPLICABILITY | ||
The AI technology was evaluated as a stand-alone intervention, rather than as an adjunct to human interpretation. | ||
Are there concerns that the index test, its conduct, or interpretation differ from the review question? | Concerns: high |
Domain 3: reference standard
A. RISK OF BIAS | ||
Two diagnostic neuroradiologists with 8 and 9 years of post-fellowship experience who had access to the complete multimodal CT (NCCT, CTP and CTA) and details of the clinical presentation. Consensus was recorded and verified by an interventional neuroradiologist with 7 years of experience. The reference standard determination was made before application of the AI intervention. | ||
Is the reference standard likely to correctly classify the target condition? | Yes | |
Were the reference standard results interpreted without knowledge of the results of the index test? | Yes | |
Could the reference standard, its conduct or its interpretation have introduced bias? | RISK: low | |
B. APPLICABILITY | ||
Is there concern that the target condition as defined by the reference standard does not match the review question? | Concerns: low |
Domain 4: flow and timing
A. RISK OF BIAS | ||
The study used CTA images from patients for whom a reference standard diagnosis had already been established. All patients appear to have been included in the analysis. | ||
Was there an appropriate time interval between index test and reference standard? | Yes | |
Did patients receive the same or a similar reference standard? | Yes | |
Were all patients included in the analysis? | Yes | |
Could the patient flow have introduced bias? | RISK: low |
Study: ALADIN60
Domain 1: patient selection
A. RISK OF BIAS | ||
A random sample from a retrospective cohort of AIS patients with and without anterior circulation LVOs. No exclusion criteria were reported. | ||
Was a consecutive or random sample of patients enrolled? | Yes | |
Was a case–control design avoided? | Yes | |
Did the study avoid inappropriate exclusions? | Unclear | |
Could the selection of patients have introduced bias? | RISK: unclear | |
B. APPLICABILITY | ||
Time from symptom onset or ‘last seen well’ not reported | ||
Do the included patients match the question? | Concerns: unclear |
Domain 2: index test(s)
A. RISK OF BIAS | ||
Viz LVO: The study reports the diagnostic performance of the AI technology alone (hence, blinding to reference standard results NA), for the target condition LVO. No threshold was specified. | ||
Were the index test results interpreted without knowledge of the results of the reference standard? | NA | |
If a threshold was used, was it prespecified? | Unclear | |
Could the conduct or interpretation of the index test have introduced bias? | RISK: unclear | |
B. APPLICABILITY | ||
The AI technology was evaluated as a stand-alone intervention, rather than as an adjunct to human interpretation. | ||
Are there concerns that the index test, its conduct or interpretation differ from the review question? | Concerns: high |
Domain 3: reference standard
A. RISK OF BIAS | ||
CTAs analysed and graded by experienced stroke neuroradiologists (no further details reported). | ||
Is the reference standard likely to correctly classify the target condition? | Yes | |
Were the reference standard results interpreted without knowledge of the results of the index test? | Unclear | |
Could the reference standard, its conduct or its interpretation have introduced bias? | RISK: unclear | |
B. APPLICABILITY | ||
Is there concern that the target condition as defined by the reference standard does not match the review question? | Concerns: low |
Domain 4: flow and timing
A. RISK OF BIAS | ||
The index test and reference standard used the same CTA images. All patients appear to have been included in the analysis. | ||
Was there an appropriate time interval between index test and reference standard? | Yes | |
Did patients receive the same or a similar reference standard? | Yes | |
Were all patients included in the analysis? | Yes | |
Could the patient flow have introduced bias? | RISK: low |
Study: Barreira et al.39
Domain 1: patient selection
A. RISK OF BIAS | ||
Retrospective analysis of a random sample from a cohort of stroke patients with and without ICH (cases and controls). No exclusion criteria reported. | ||
Was a consecutive or random sample of patients enrolled? | No | |
Was a case–control design avoided? | No | |
Did the study avoid inappropriate exclusions? | Unclear | |
Could the selection of patients have introduced bias? | RISK: high | |
B. APPLICABILITY | ||
Not patients with suspected AIS (case–control design) | ||
Do the included patients match the question? | Concerns: high |
Domain 2: index test(s)
A. RISK OF BIAS | ||
Viz ICH: The study reports the diagnostic performance of the AI technology alone (hence, blinding to reference standard results NA), for the target condition ICH. No threshold was specified. | ||
Were the index test results interpreted without knowledge of the results of the reference standard? | NA | |
If a threshold was used, was it prespecified? | Unclear | |
Could the conduct or interpretation of the index test have introduced bias? | RISK: unclear | |
B. APPLICABILITY | ||
The AI technology was evaluated as a stand-alone intervention rather than as an adjunct to human interpretation. | ||
Are there concerns that the index test, its conduct or interpretation differ from the review question? | Concerns: high |
Domain 3: reference standard
A. RISK OF BIAS | ||
Experienced stroke neurologists grading the same NCCTs with a semi-automated tool (OsiriX MD v.9.0.1) | ||
Is the reference standard likely to correctly classify the target condition? | Yes | |
Were the reference standard results interpreted without knowledge of the results of the index test? | Unclear | |
Could the reference standard, its conduct, or its interpretation have introduced bias? | RISK: unclear | |
B. APPLICABILITY | ||
Is there concern that the target condition as defined by the reference standard does not match the review question? | Concerns: low |
Domain 4: flow and timing
A. RISK OF BIAS | ||
The index test and reference standard utilised the same CTA images. All patients appear to have been include in the analysis. | ||
Was there an appropriate time interval between index test and reference standard? | Yes | |
Did patients receive the same or a similar reference standard? | Yes | |
Were all patients included in the analysis? | Yes | |
Could the patient flow have introduced bias? | RISK: low |
Study: Chatterjee et al.40
Domain 1: patient selection
A. RISK OF BIAS | ||
Retrospective analysis of images from patients with stroke CTA studies. No exclusion criteria specified. | ||
Was a consecutive or random sample of patients enrolled? | Unclear | |
Was a case–control design avoided? | Yes | |
Did the study avoid inappropriate exclusions? | Unclear | |
Could the selection of patients have introduced bias? | RISK: unclear | |
B. APPLICABILITY | ||
Time from symptom onset or ‘last seen well’ not reported | ||
Do the included patients match the question? | Concerns: unclear |
Domain 2: index test(s)
A. RISK OF BIAS | ||
Viz LVO: The study reports the diagnostic performance of the AI technology alone (hence, blinding to reference standard results NA), for the target condition LVO. No threshold was specified. | ||
Were the index test results interpreted without knowledge of the results of the reference standard? | NA | |
If a threshold was used, was it prespecified? | Unclear | |
Could the conduct or interpretation of the index test have introduced bias? | RISK: unclear | |
B. APPLICABILITY | ||
The AI technology was evaluated as a stand-alone intervention rather than as an adjunct to human interpretation. | ||
Are there concerns that the index test, its conduct, or interpretation differ from the review question? | Concerns: high |
Domain 3: reference standard
A. RISK OF BIAS | ||
‘Conventional angiography’ (no further details reported). | ||
Is the reference standard likely to correctly classify the target condition? | Unclear | |
Were the reference standard results interpreted without knowledge of the results of the index test? | Unclear | |
Could the reference standard, its conduct or its interpretation have introduced bias? | RISK: unclear | |
B. APPLICABILITY | ||
Is there concern that the target condition as defined by the reference standard does not match the review question? | Concerns: unclear |
Domain 4: flow and timing
A. RISK OF BIAS | ||
The index test and reference standard used the same CTA images. All patients appear to have been include in the analysis. | ||
Was there an appropriate time interval between index test and reference standard? | Yes | |
Did patients receive the same or a similar reference standard? | Yes | |
Were all patients included in the analysis? | Yes | |
Could the patient flow have introduced bias? | RISK: low |
Study: CRISP and DASH41
Domain 1: patient selection
A. RISK OF BIAS | ||
Retrospective analysis of adult (≥ 18 years) individuals undergoing cerebrovascular CTA, from the CRISP and DASH trials and from institutional registries of participating hospitals; technically adequate, thin section (≤ 2 mm) contiguous cerebrovascular CTA sources axial images, free of artefacts that would degrade interpretation by human readers (e.g. those related to severe metallic streak or beam hardening). The study used a random selection of at least 100 patients who were LVO positive and 100 who were LVO negative, with enrichment to balance subgroup imbalances in age groupings and scanner manufacturer. | ||
Was a consecutive or random sample of patients enrolled? | No | |
Was a case–control design avoided? | No | |
Did the study avoid inappropriate exclusions? | Yes | |
Could the selection of patients have introduced bias? | RISK: high | |
B. APPLICABILITY | ||
Not patients with AIS and suspected LVO (case–control type design). | ||
Do the included patients match the question? | Concerns: high |
Domain 2: index test(s)
A. RISK OF BIAS | ||
RAPID CTA: The study reports the diagnostic performance of the AI technology alone (hence, blinding to reference standard results NA), for the target condition LVO (subgroups for age and scanner manufacturer reported), using a pre-specified threshold. | ||
Were the index test results interpreted without knowledge of the results of the reference standard? | NA | |
If a threshold was used, was it prespecified? | Yes | |
Could the conduct or interpretation of the index test have introduced bias? | RISK: low | |
B. APPLICABILITY | ||
The AI technology was evaluated as a stand-alone intervention, rather than as an adjunct to human interpretation. | ||
Are there concerns that the index test, its conduct or interpretation differ from the review question? | Concerns: high |
Domain 3: reference standard
A. RISK OF BIAS | ||
Two board-certified neuroradiologists, with 11 and 7 years of experience, blinded to clinical history and imaging outcome, independently scored all examinations for LVO. A LVO was defined as occlusion or near occlusion by a focal stenosis > 80%. Discrepancies between the two readers were adjudicated by a third board-certified neuroradiologist with 7 years of experience. For examinations classified as positive, readers were subsequently presented with the automated output and asked to assess it for presence of LVO, LVO side and inclusion of compromised vessel segment within the region of interest; All three criteria had to be met for an automated image to be classified as true positive. | ||
Is the reference standard likely to correctly classify the target condition? | Yes | |
Were the reference standard results interpreted without knowledge of the results of the index test? | Yes | |
Could the reference standard, its conduct or its interpretation have introduced bias? | RISK: low | |
B. APPLICABILITY | ||
Is there concern that the target condition as defined by the reference standard does not match the review question? | Concerns: low |
Domain 4: flow and timing
A. RISK OF BIAS | ||
The study used CTA images from patients for whom a reference standard diagnosis had already been established. All patients appear to have been include in the analysis. | ||
Was there an appropriate time interval between index test and reference standard? | Yes | |
Did patients receive the same or a similar reference standard? | Yes | |
Were all patients included in the analysis? | Yes | |
Could the patient flow have introduced bias? | RISK: low |
Study: Dornbos et al.43
Domain 1: patient selection
A. RISK OF BIAS | ||
A retrospective chart review of consecutive code stroke cases at a CSC and two spoke hospitals. No exclusion criteria were reported. | ||
Was a consecutive or random sample of patients enrolled? | Yes | |
Was a case–control design avoided? | Yes | |
Did the study avoid inappropriate exclusions? | Unclear | |
Could the selection of patients have introduced bias? | RISK: unclear | |
B. APPLICABILITY | ||
Time from symptom onset or ‘last seen well’ not reported | ||
Do the included patients match the question? | Concerns: unclear |
Domain 2: index test(s)
A. RISK OF BIAS | ||
Viz LVO: The study reports the diagnostic performance of the AI technology alone (hence, blinding to reference standard results NA), for the target condition LVO. No threshold was specified. | ||
Were the index test results interpreted without knowledge of the results of the reference standard? | NA | |
If a threshold was used, was it prespecified? | Unclear | |
Could the conduct or interpretation of the index test have introduced bias? | RISK: unclear | |
B. APPLICABILITY | ||
The AI technology was evaluated as a stand-alone intervention rather than as an adjunct to human interpretation. | ||
Are there concerns that the index test, its conduct, or interpretation differ from the review question? | Concerns: high |
Domain 3: reference standard
A. RISK OF BIAS | ||
CT/CTA interpretation by ‘blinded neuroradiologists’ (no further details reported). | ||
Is the reference standard likely to correctly classify the target condition? | Unclear | |
Were the reference standard results interpreted without knowledge of the results of the index test? | Unclear | |
Could the reference standard, its conduct or its interpretation have introduced bias? | RISK: unclear | |
B. APPLICABILITY | ||
Is there concern that the target condition as defined by the reference standard does not match the review question? | Concerns: unclear |
Domain 4: flow and timing
A. RISK OF BIAS | ||
The index test and reference standard used the same CTA images. All patients appear to have been included in the analysis. | ||
Was there an appropriate time interval between index test and reference standard? | Yes | |
Did patients receive the same or a similar reference standard? | Yes | |
Were all patients included in the analysis? | Yes | |
Could the patient flow have introduced bias? | RISK: low |
Study: Herweh et al.48
Domain 1: patient selection
A. RISK OF BIAS | ||
‘Selected’ NCCT scans (slice thickness 1 mm) from patients with suspected AIS. No exclusion criteria were reported. | ||
Was a consecutive or random sample of patients enrolled? | No | |
Was a case–control design avoided? | Yes | |
Did the study avoid inappropriate exclusions? | Unclear | |
Could the selection of patients have introduced bias? | RISK: high | |
B. APPLICABILITY | ||
Time from symptom onset or ‘last seen well’ not reported | ||
Do the included patients match the question? | Concerns: unclear |
Domain 2: index test(s)
A. RISK OF BIAS | ||
Brainomix: The study reports the diagnostic performance of the AI technology alone (hence, blinding to reference standard results NA), for the target condition ICH. No threshold was specified. | ||
Were the index test results interpreted without knowledge of the results of the reference standard? | NA | |
If a threshold was used, was it prespecified? | Unclear | |
Could the conduct or interpretation of the index test have introduced bias? | RISK: unclear | |
B. APPLICABILITY | ||
The AI technology was evaluated as a stand-alone intervention rather than as an adjunct to human interpretation. | ||
Are there concerns that the index test, its conduct or interpretation differ from the review question? | Concerns: high |
Domain 3: reference standard
A. RISK OF BIAS | ||
Image interpretation by a board-certified neuroradiologist (no further details reported). | ||
Is the reference standard likely to correctly classify the target condition? | Yes | |
Were the reference standard results interpreted without knowledge of the results of the index test? | Unclear | |
Could the reference standard, its conduct or its interpretation have introduced bias? | RISK: unclear | |
B. APPLICABILITY | ||
Is there concern that the target condition as defined by the reference standard does not match the review question? | Concerns: low |
Domain 4: flow and timing
A. RISK OF BIAS | ||
The index test and reference standard used the same CTA images. All patients appear to have been included in the analysis. | ||
Was there an appropriate time interval between index test and reference standard? | Yes | |
Did patients receive the same or a similar reference standard? | Yes | |
Were all patients included in the analysis? | Yes | |
Could the patient flow have introduced bias? | RISK: low |
Study: Kauw et al.50
Domain 1: patient selection
A. RISK OF BIAS | ||
Retrospective analysis of images from a database of consecutive patients with AIS undergoing CTP for thrombectomy triage. No exclusion criteria were reported. | ||
Was a consecutive or random sample of patients enrolled? | Yes | |
Was a case–control design avoided? | Yes | |
Did the study avoid inappropriate exclusions? | Unclear | |
Could the selection of patients have introduced bias? | RISK: unclear | |
B. APPLICABILITY | ||
Time from symptom onset or ‘last seen well’ not reported | ||
Do the included patients match the question? | Concerns: unclear |
Domain 2: index test(s)
A. RISK OF BIAS | ||
Rapid CTP: The study reports the diagnostic performance of the AI technology alone (hence, blinding to reference standard results NA) to determine the suitability of patients for thrombectomy. No threshold was specified. | ||
Were the index test results interpreted without knowledge of the results of the reference standard? | NA | |
If a threshold was used, was it prespecified? | Unclear | |
Could the conduct or interpretation of the index test have introduced bias? | RISK: unclear | |
B. APPLICABILITY | ||
The AI technology was evaluated as a stand-alone intervention rather than as an adjunct to human interpretation. | ||
Are there concerns that the index test, its conduct or interpretation differ from the review question? | Concerns: high |
Domain 3: reference standard
A. RISK OF BIAS | ||
Images were reviewed, for potential causes of post-processing failure, by two clinicians (experience not specified) in consensus who were blinded to clinical data but had access to all imaging data available at the time of patient evaluation. RAPID CTP post-processing failures were reprocessed manually using IntelliSpace software (Philips, Best, Netherlands). 2 × 2 data have could only be derived for the performance of the AI intervention by using treatment received as the reference standard. | ||
Is the reference standard likely to correctly classify the target condition? | Unclear | |
Were the reference standard results interpreted without knowledge of the results of the index test? | No | |
Could the reference standard, its conduct or its interpretation have introduced bias? | RISK: high | |
B. APPLICABILITY | ||
Is there concern that the target condition as defined by the reference standard does not match the review question? | Concerns: high |
Domain 4: flow and timing
A. RISK OF BIAS | ||
The index test and reference standard used the same CTA images. All patients appear to have been included in the analysis. | ||
Was there an appropriate time interval between index test and reference standard? | Yes | |
Did patients receive the same or a similar reference standard? | Yes | |
Were all patients included in the analysis? | Yes | |
Could the patient flow have introduced bias? | RISK: low |
Study: RITeS62
Domain 1: patient selection
A. RISK OF BIAS | ||
‘Representative population’: To reflect the hospital ‘front door’ for all patients with suspected stroke. Retrospective sample taken from 9 completed clinical trials, with the sample enriched to include realistic proportions of patients with a final diagnosis of ischaemic stroke, brain haemorrhage and stroke mimics. No exclusion criteria were reported. To assess whether the sample was clinically representative of patients admitted to hospital with stroke, it was prespecified that age, sex, stroke severity, time since symptom onset and final diagnosis in RITeS would be similar to data from the UK SSNAP (April 2018–March 2019, www.strokeaudit.org), pooled RCTs and registries. | ||
Was a consecutive or random sample of patients enrolled? | No | |
Was a case–control design avoided? | Yes | |
Did the study avoid inappropriate exclusions? | Unclear | |
Could the selection of patients have introduced bias? | RISK: unclear | |
B. APPLICABILITY | ||
To assess whether the sample was clinically representative of patients admitted to hospital with stroke, it was prespecified that age, sex, stroke severity, time since symptom onset and final diagnosis in RITeS would be similar to data from the UK SSNAP (April 2018–March 2019, www.strokeaudit.org), pooled RCTs and registries. The median time from symptom onset was 2.5 hours (IQR 1.8–3.8 hours). | ||
Do the included patients match the question? | Concerns: low |
Domain 2: index test(s)
A. RISK OF BIAS | ||
Brainomix e-ASPECTS: study reports the diagnostic performance of the AI technology alone (hence, blinding to reference standard results NA), for the target conditions ICH and AIS. Qualitative criteria for determining ICH and AIS were described. | ||
Were the index test results interpreted without knowledge of the results of the reference standard? | NA | |
If a threshold was used, was it prespecified? | Yes | |
Could the conduct or interpretation of the index test have introduced bias? | RISK: low | |
B. APPLICABILITY | ||
The AI technology was evaluated as a stand-alone intervention rather than as an adjunct to human interpretation. | ||
Are there concerns that the index test, its conduct or interpretation differ from the review question? | Concerns: high |
Domain 3: reference standard
A. RISK OF BIAS | ||
Final diagnosis was treated as the reference standard: final diagnosis (ischaemic stroke, brain haemorrhage, stroke mimic) was determined similarly in each study based on the local principal investigator’s diagnosis and central adjudication of all available baseline and follow-up data including imaging expert scan interpretation. Final diagnosis was established in each study prior to the start of RITeS. | ||
Is the reference standard likely to correctly classify the target condition? | Yes | |
Were the reference standard results interpreted without knowledge of the results of the index test? | Yes | |
Could the reference standard, its conduct, or its interpretation have introduced bias? | RISK: low | |
B. APPLICABILITY | ||
Is there concern that the target condition as defined by the reference standard does not match the review question? | Concerns: high |
Domain 4: flow and timing
A. RISK OF BIAS | ||
The index test and reference standard used the same CTA images. All patients appear to have been included in the analysis. | ||
Was there an appropriate time interval between index test and reference standard? | Yes | |
Did patients receive the same or a similar reference standard? | Yes | |
Were all patients included in the analysis? | Yes | |
Could the patient flow have introduced bias? | RISK: low |
Study: McLouth et al.51
Domain 1: patient selection
A. RISK OF BIAS | ||
Retrospective study using images from patients with suspected LVO, on clinical grounds, in whom CTA studies had been performed, identified from University of California, Irvine, and a teleradiology service, vRAD (Minneapolis, USA) databases using key words such as ‘CTA’, ‘head’ and ‘LVO’. No exclusion criteria were reported. | ||
Was a consecutive or random sample of patients enrolled? | No | |
Was a case–control design avoided? | Yes | |
Did the study avoid inappropriate exclusions? | Unclear | |
Could the selection of patients have introduced bias? | RISK: high | |
B. APPLICABILITY | ||
Time from symptom onset or ‘last seen well’ not reported | ||
Do the included patients match the question? | Concerns: unclear |
Domain 2: index test(s)
A. RISK OF BIAS | ||
CINA LVO: study reports the diagnostic performance of the AI technology alone (hence, blinding to reference standard results NA) for the target condition LVO (subgroups for age and scanner manufacturer). No threshold was specified. | ||
Were the index test results interpreted without knowledge of the results of the reference standard? | NA | |
If a threshold was used, was it prespecified? | Unclear | |
Could the conduct or interpretation of the index test have introduced bias? | RISK: unclear | |
B. APPLICABILITY | ||
The AI technology was evaluated as a stand-alone intervention rather than as an adjunct to human interpretation. | ||
Are there concerns that the index test, its conduct or interpretation differ from the review question? | Concerns: high |
Domain 3: reference standard
A. RISK OF BIAS | ||
CTA interpreted by two US board-certified neuroradiologists, with consensus determined by a third board-certified neuroradiologist. | ||
Is the reference standard likely to correctly classify the target condition? | Yes | |
Were the reference standard results interpreted without knowledge of the results of the index test? | Unclear | |
Could the reference standard, its conduct or its interpretation have introduced bias? | RISK: unclear | |
B. APPLICABILITY | ||
Is there concern that the target condition as defined by the reference standard does not match the review question? | Concerns: low |
Domain 4: flow and timing
A. RISK OF BIAS | ||
The index test and reference standard utilised the same CTA images. All patients appear to have been included in the analysis. | ||
Was there an appropriate time interval between index test and reference standard? | Yes | |
Did patients receive the same or a similar reference standard? | Yes | |
Were all patients included in the analysis? | Yes | |
Could the patient flow have introduced bias? | RISK: low |
Study: Paz et al.55
Domain 1: patient selection
A. RISK OF BIAS | ||
Retrospective study of all patients with suspected acute stroke symptoms whose images had been analysed using Rapid LVO. No exclusion criteria were reported. | ||
Was a consecutive or random sample of patients enrolled? | Unclear | |
Was a case–control design avoided? | Yes | |
Did the study avoid inappropriate exclusions? | Unclear | |
Could the selection of patients have introduced bias? | RISK: unclear | |
B. APPLICABILITY | ||
Time from symptom onset or ‘last seen well’ not reported | ||
Do the included patients match the question? | Concerns: unclear |
Domain 2: index test(s)
A. RISK OF BIAS | ||
Rapid LVO: The study reports the diagnostic performance of the AI technology alone (hence, blinding to reference standard results NA) for the target condition LVO. No threshold was specified. | ||
Were the index test results interpreted without knowledge of the results of the reference standard? | NA | |
If a threshold was used, was it prespecified? | Unclear | |
Could the conduct or interpretation of the index test have introduced bias? | RISK: unclear | |
B. APPLICABILITY | ||
The AI technology was evaluated as a stand-alone intervention rather than as an adjunct to human interpretation. | ||
Are there concerns that the index test, its conduct or interpretation differ from the review question? | Concerns: high |
Domain 3: reference standard
A. RISK OF BIAS | ||
No details were reported regarding how the reference standard diagnosis was determined. | ||
Is the reference standard likely to correctly classify the target condition? | Unclear | |
Were the reference standard results interpreted without knowledge of the results of the index test? | Unclear | |
Could the reference standard, its conduct or its interpretation have introduced bias? | RISK: unclear | |
B. APPLICABILITY | ||
Is there concern that the target condition as defined by the reference standard does not match the review question? | Concerns: unclear |
Domain 4: flow and timing
A. RISK OF BIAS | ||
The index test and reference standard used the same CTA images but no details of the reference standard for interpretation of images were reported. All patients appear to have been included in the analysis. | ||
Was there an appropriate time interval between index test and reference standard? | Yes | |
Did patients receive the same or a similar reference standard? | Unclear | |
Were all patients included in the analysis? | Yes | |
Could the patient flow have introduced bias? | RISK: unclear |
Study: Seker et al.56
Domain 1: patient selection
A. RISK OF BIAS | ||
Case–control validation study: cases comprised patients with LVO of the terminal carotid artery or MCA up to the proximal M2 level who had CTA images of sufficient quality (CT scan primarily in the arterial phase without severe motion artefacts and with a slice thickness of ≤ 1 mm); controls comprised CTA examinations from 141 consecutive AIS patients without LVO. No exclusion criteria were reported. | ||
Was a consecutive or random sample of patients enrolled? | No | |
Was a case–control design avoided? | No | |
Did the study avoid inappropriate exclusions? | Unclear | |
Could the selection of patients have introduced bias? | RISK: high | |
B. APPLICABILITY | ||
Case–control type study; not patients with AIS and suspected LVO. | ||
Do the included patients match the question? | Concerns: high |
Domain 2: index test(s)
A. RISK OF BIAS | ||
Brainomix eCTA: study reports the diagnostic performance of the AI technology alone (hence, blinding to reference standard results NA) for the target condition LVO (subgroups for anatomical location). No threshold was specified. | ||
Were the index test results interpreted without knowledge of the results of the reference standard? | NA | |
If a threshold was used, was it prespecified? | Unclear | |
Could the conduct or interpretation of the index test have introduced bias? | RISK: unclear | |
B. APPLICABILITY | ||
The AI technology was evaluated as a stand-alone intervention rather than as an adjunct to human interpretation. | ||
Are there concerns that the index test, its conduct or interpretation differ from the review question? | Concerns: high |
Domain 3: reference standard
A. RISK OF BIAS | ||
CTA interpreted by a board-certified neuroradiologist with > 10 years’ experience and access to all clinical and imaging data, including data on interventional therapy and follow-up. The study used CTA images from patients for whom a reference standard diagnosis had already been established (case–control). | ||
Is the reference standard likely to correctly classify the target condition? | Yes | |
Were the reference standard results interpreted without knowledge of the results of the index test? | Yes | |
Could the reference standard, its conduct or its interpretation have introduced bias? | RISK: low | |
B. APPLICABILITY | ||
Is there concern that the target condition as defined by the reference standard does not match the review question? | Concerns: low |
Domain 4: flow and timing
A. RISK OF BIAS | ||
The study used CTA images from patients for whom a reference standard diagnosis had already been established. All patients appear to have been included in the analysis. | ||
Was there an appropriate time interval between index test and reference standard? | Yes | |
Did patients receive the same or a similar reference standard? | Yes | |
Were all patients included in the analysis? | Yes | |
Could the patient flow have introduced bias? | RISK: low |
Study: Shalitin et al.61
Domain 1: patient selection
A. RISK OF BIAS | ||
Patients with CTA analysed using Viz LVO. No inclusion or exclusion criteria were reported. ‘All sequential scans within a defined date range were reviewed and analysed’. | ||
Was a consecutive or random sample of patients enrolled? | Yes | |
Was a case–control design avoided? | Yes | |
Did the study avoid inappropriate exclusions? | Unclear | |
Could the selection of patients have introduced bias? | RISK: unclear | |
B. APPLICABILITY | ||
No inclusion or exclusion criteria were reported. Time from symptom onset or ‘last seen well’ not reported | ||
Do the included patients match the question? | Concerns: unclear |
Domain 2: index test(s)
A. RISK OF BIAS | ||
Viz LVO: The study reports the diagnostic performance of the AI technology alone (hence, blinding to reference standard results NA), for the target condition LVO. No threshold was specified. | ||
Were the index test results interpreted without knowledge of the results of the reference standard? | NA | |
If a threshold was used, was it prespecified? | Unclear | |
Could the conduct or interpretation of the index test have introduced bias? | RISK: unclear | |
B. APPLICABILITY | ||
The AI technology was evaluated as a stand-alone intervention rather than as an adjunct to human interpretation. | ||
Are there concerns that the index test, its conduct or interpretation differ from the review question? | Concerns: high |
Domain 3: reference standard
A. RISK OF BIAS | ||
Image interpretation by ‘a team of radiology trained annotators’ (no further details reported). | ||
Is the reference standard likely to correctly classify the target condition? | Unclear | |
Were the reference standard results interpreted without knowledge of the results of the index test? | Unclear | |
Could the reference standard, its conduct or its interpretation have introduced bias? | RISK: unclear | |
B. APPLICABILITY | ||
Is there concern that the target condition as defined by the reference standard does not match the review question? | Concerns: unclear |
Domain 4: flow and timing
A. RISK OF BIAS | ||
The index test and reference standard used the same CTA images. All patients appear to have been included in the analysis. | ||
Was there an appropriate time interval between index test and reference standard? | Yes | |
Did patients receive the same or a similar reference standard? | Yes | |
Were all patients included in the analysis? | Yes | |
Could the patient flow have introduced bias? | RISK: low |
Study: Yahav-Dovrat et al.59
Domain 1: patient selection
A. RISK OF BIAS | ||
All consecutive head and neck CTA scans in a CSC. Examinations with metal artefact, severe motion or incomplete skull scanning were excluded from the analysis. | ||
Was a consecutive or random sample of patients enrolled? | Yes | |
Was a case–control design avoided? | Yes | |
Did the study avoid inappropriate exclusions? | Yes | |
Could the selection of patients have introduced bias? | RISK: low | |
B. APPLICABILITY | ||
Time from symptom onset or ‘last seen well’ not reported | ||
Do the included patients match the question? | Concerns: unclear |
Domain 2: index test(s)
A. RISK OF BIAS | ||
Viz LVO: study reports the diagnostic performance of the AI technology alone (hence, blinding to reference standard results NA) for the target condition LVO (subgroup data for ‘stroke protocol’ patients). No threshold was specified. | ||
Were the index test results interpreted without knowledge of the results of the reference standard? | NA | |
If a threshold was used, was it prespecified? | Unclear | |
Could the conduct or interpretation of the index test have introduced bias? | RISK: unclear | |
B. APPLICABILITY | ||
The AI technology was evaluated as a stand-alone intervention rather than as an adjunct to human interpretation. | ||
Are there concerns that the index test, its conduct or interpretation differ from the review question? | Concerns: high |
Domain 3: reference standard
A. RISK OF BIAS | ||
Interpretation of CTA by one of four senior neuroradiologists with 7–25 years of experience. The reference standard interpretation was taken from the patients’ files (i.e. determined before application of Viz LVO). | ||
Is the reference standard likely to correctly classify the target condition? | Yes | |
Were the reference standard results interpreted without knowledge of the results of the index test? | Yes | |
Could the reference standard, its conduct or its interpretation have introduced bias? | RISK: low | |
B. APPLICABILITY | ||
Is there concern that the target condition as defined by the reference standard does not match the review question? | Concerns: low |
Domain 4: flow and timing
A. RISK OF BIAS | ||
The index test and reference standard used the same CTA images. All patients appear to have been include in the analysis. | ||
Was there an appropriate time interval between index test and reference standard? | Yes | |
Did patients receive the same or a similar reference standard? | Yes | |
Were all patients included in the analysis? | Yes | |
Could the patient flow have introduced bias? | RISK: low |
Quality assessment of observational ‘before and after’ studies
Adhya et al.33
Q1. Did the study have a prospective design? | No |
Retrospective study reporting 1-year real-world experience of rapid CTA. | |
Q2. Did the study population include an appropriate spectrum of patients? | Unclear |
No information was reported about the time from symptom onset or ‘last known well’ for included participants. | |
Q3. Were the criteria used to select patients for CT imaging similar, before and after the introduction of the AI intervention? | Yes |
All patients at the emergency department for stroke or neurological deficit, during 2 periods, before and after implementation of Rapid CTA. | |
Q4. Were the study populations, before and after the introduction of the AI intervention, similar with respect to baseline demographic characteristics (e.g. age, male/female), comorbid conditions (e.g. hypertension, diabetes, AF) and risk factors (e.g. smoking status, previous history)? | Unclear |
Insufficient comparative baseline characteristics reported. | |
Q5. Other than the availability of AI software, was the care pathway similar before and after the introduction of the AI intervention? | Yes |
All interventional equipment, endovascular therapists, neuroradiology staff and hospitals serviced were identical during the study period; the only significant change was the installation of RAPID-CTA. | |
Q6. Was the implementation of the AI intervention clearly described (e.g. how and when it was used to assist human readers and what was the level of training and experience of the human readers)? | No |
No information reported. | |
Q7. Were the CT imaging criteria used to select patients for treatment (e.g. thrombectomy) clearly reported for both the periods before and after the introduction of the AI intervention? | No |
No information reported. | |
Q8. In addition to time to intervention outcomes, did the study report the proportion of patients who received treatment (e.g. thrombectomy) before and after the introduction of the AI intervention? | No |
Total number of patients evaluated in each time period not reported. | |
Q9. In addition to time to intervention outcomes, did the study report clinical outcomes (e.g. 90-day mRS) before and after the introduction of the AI intervention? | Yes |
Mean 90-day mRS and number of participants with mRS ≤ 2 reported. |
Al-Kawaz et al.34
Q1. Did the study have a prospective design? | No |
Retrospective analysis of prospectively collected data of patients presenting with LVOs between June 2019 and October 2020. | |
Q2. Did the study population include an appropriate spectrum of patients? | Unclear |
Patients with LVOs. No information was reported about the time from symptom onset or ‘last known well’ for included participants. | |
Q3. Were the criteria used to select patients for CT imaging similar, before and after the introduction of the AI intervention? | Unclear |
No information reported. | |
Q4. Were the study populations, before and after the introduction of the AI intervention, similar with respect to baseline demographic characteristics (e.g. age, male/female), comorbid conditions (e.g. hypertension, diabetes, AF) and risk factors (e.g. smoking status, previous history)? | Yes |
There were no significant differences in baseline demographic characteristics (age and proportion male), comorbid conditions (hypertension or diabetes mellitus) or NIHSS. | |
Q5. Other than the availability of AI software, was the care pathway similar before and after the introduction of the AI intervention? | Unclear |
No information reported. | |
Q6. Was the implementation of the AI intervention clearly described (e.g. how and when it was used to assist human readers and what was the level of training and experience of the human readers)? | Yes |
The interhospital treatment times analysis included patients presenting from a primary stroke centre affiliated with the CSC that used the RapidAI mobile application. Stroke neurologists provided tele-stroke services to the primary stroke centre and had remote access to imaging. All remaining patients in the analyses presented from the CSC ER. | |
Q7. Were the CT imaging criteria used to select patients for treatment (e.g. thrombectomy) clearly reported for both the periods before and after the introduction of the AI intervention? | No |
No information reported. | |
Q8. In addition to time to intervention outcomes, did the study report the proportion of patients who received treatment (e.g. thrombectomy) before and after the introduction of the AI intervention? | NA |
All included participants received thrombectomy. | |
Q9. In addition to time to intervention outcomes, did the study report clinical outcomes (e.g. 90-day mRS) before and after the introduction of the AI intervention? | No |
Study reports time from door to groin puncture only. |
Gunda et al.44
Q1. Did the study have a prospective design? | No |
Two identical 7-month periods, in 2017 and 2018, were retrospectively evaluated. | |
Q2. Did the study population include an appropriate spectrum of patients? | Unclear |
Insufficient information (study includes admitted stroke patients with no further details reported). | |
Q3. Were the criteria used to select patients for CT imaging similar, before and after the introduction of the AI intervention? | Yes |
The AI-derived software technology was implemented in 2018 and delivery of stroke care was otherwise unchanged over the 2 years. | |
Q4. Were the study populations, before and after the introduction of the AI intervention, similar with respect to baseline demographic characteristics (e.g. age, male/female), comorbid conditions (e.g. hypertension, diabetes, AF) and risk factors (e.g. smoking status, previous history)? | Unclear |
No information reported. | |
Q5. Other than the availability of AI software, was the care pathway similar before and after the introduction of the AI intervention? | Yes |
The AI-derived software technology was implemented in 2018 and delivery of stroke care was otherwise unchanged over the two years. | |
Q6. Was the implementation of the AI intervention clearly described (e.g. how and when it was used to assist human readers and what was the level of training and experience of the human readers)? | No |
No information reported. | |
Q7. Were the CT imaging criteria used to select patients for treatment (e.g. thrombectomy) clearly reported for both the periods before and after the introduction of the AI intervention? | No |
No information reported. | |
Q8. In addition to time to intervention outcomes, did the study report the proportion of patients who received treatment (e.g. thrombectomy) before and after the introduction of the AI intervention? | Yes |
The proportion of patients transferred for thrombectomy increased from 2.8% to 4.8% and the proportion receiving thrombolysis increased from 11.5% to 18.1% after implementation of the AI-derived software technology. | |
Q9. In addition to time to intervention outcomes, did the study report clinical outcomes (e.g. 90-day mRS) before and after the introduction of the AI intervention? | No |
No clinical outcomes were reported (treatment rates and time to treatment only). |
Hassan et al.46
Q1. Did the study have a prospective design? | No |
A retrospective study of LVO patients who presented to a primary stroke centre and were transferred to a CSC. | |
Q2. Did the study population include an appropriate spectrum of patients? | Yes |
Patients who presented at the primary stroke centre with a LVO on CTA and were transferred to the CSC with the intent of having endovascular treatment. | |
Q3. Were the criteria used to select patients for CT imaging similar, before and after the introduction of the AI intervention? | Yes |
The selection criteria were the same for both populations. | |
Q4. Were the study populations, before and after the introduction of the AI intervention, similar with respect to baseline demographic characteristics (e.g. age, male/female) comorbid conditions (e.g. hypertension, diabetes, AF) and risk factors (e.g. smoking status, previous history)? | No |
There were no significant differences in baseline demographic characteristics (age, proportion male or ethnicity), comorbid conditions (diabetes mellitus or hypertension) and risk factors (smoking status). The proportion of patients with AF and the mean baseline NIHSS were higher in the before implementation population. | |
Q5. Other than the availability of AI software, was the care pathway similar before and after the introduction of the AI intervention? | Yes |
The article includes a flowchart showing the care pathway before and after the introduction of the AI-derived software technology; only the imaging interpretation steps differ. | |
Q6. Was the implementation of the AI intervention clearly described (e.g. how and when it was used to assist human readers and what was the level of training and experience of the human readers)? | Yes |
After the implementation of the AI-derived technology, the physician at the CSC sees CTA results and confirms LVO on the app, before accepting the patient for transfer. | |
Q7. Were the CT imaging criteria used to select patients for treatment (e.g. thrombectomy) clearly reported for both the periods before and after the introduction of the AI intervention? | No |
Insufficient information reported. | |
Q8. In addition to time to intervention outcomes, did the study report the proportion of patients who received treatment (e.g. thrombectomy) before and after the introduction of the AI intervention? | Yes |
Before implementation of the AI-derived software technology, all 28 transferred patients received thrombectomy. After implementation, thrombectomy was withheld from 4 of the 15 transferred patients due to thrombolytic recanalisation following IV thrombolysis or extensive infarction. | |
Q9. In addition to time to intervention outcomes, did the study report clinical outcomes (e.g. 90-day mRS) before and after the introduction of the AI intervention? | Yes |
Number of patients with mRS at discharge ≤ 2, length of hospital stay, in-hospital complications and in-hospital mortality reported for all patients (including those who did not receive thrombectomy). |
Hassan et al.45
Q1. Did the study have a prospective design? | No |
The study used information from a ‘prospectively collected database’. | |
Q2. Did the study population include an appropriate spectrum of patients? | Yes |
All LVO transfer patients arriving at a CSC. | |
Q3. Were the criteria used to select patients for CT imaging similar, before and after the introduction of the AI intervention? | Yes |
All LVO transfer patients arriving at a CSC for approximately 2 years prior to and following implementation of the AI-derived software technology. | |
Q4. Were the study populations, before and after the introduction of the AI intervention, similar with respect to baseline demographic characteristics (e.g. age, male/female), comorbid conditions (e.g. hypertension, diabetes, AF) and risk factors (e.g. smoking status, previous history)? | Yes |
There were no significant differences in baseline demographic characteristics (age, proportion male or ethnicity), comorbid conditions (diabetes mellitus, hypertension, AF), risk factors (history of stroke/TIA or smoking status) or NIHSS. | |
Q5. Other than the availability of AI software, was the care pathway similar before and after the introduction of the AI intervention? | Unclear |
No information reported. | |
Q6. Was the implementation of the AI intervention clearly described (e.g. how and when it was used to assist human readers and what was the level of training and experience of the human readers)? | No |
No information reported. | |
Q7. Were the CT imaging criteria used to select patients for treatment (e.g. thrombectomy) clearly reported for both the periods before and after the introduction of the AI intervention? | No |
No information reported. | |
Q8. In addition to time to intervention outcomes, did the study report the proportion of patients who received treatment (e.g. thrombectomy) before and after the introduction of the AI intervention? | NA |
All included participants received thrombectomy. | |
Q9. In addition to time to intervention outcomes, did the study report clinical outcomes (e.g. 90-day mRS) before and after the introduction of the AI intervention? | Yes |
Number of patients with mRS at discharge ≤ 2, length of hospital stay and in-hospital mortality reported. |
Kamal et al.49
Q1. Did the study have a prospective design? | No |
A retrospective cohort study of AIS patients undergoing thrombectomy. | |
Q2. Did the study population include an appropriate spectrum of patients? | Unclear |
Insufficient information reported (AIS patients undergoing thrombectomy, no further details reported). | |
Q3. Were the criteria used to select patients for CT imaging similar, before and after the introduction of the AI intervention? | Unclear |
No information reported. | |
Q4. Were the study populations, before and after the introduction of the AI intervention, similar with respect to baseline demographic characteristics (e.g. age, male/female), comorbid conditions (e.g. hypertension, diabetes, AF) and risk factors (e.g. smoking status, previous history)? | Yes |
There were no significant differences in baseline demographic characteristics (age or proportion male), comorbid conditions (diabetes mellitus or hypertension), risk factors (smoking status), or NIHSS. | |
Q5. Other than the availability of AI software, was the care pathway similar before and after the introduction of the AI intervention? | Unclear |
No information reported. | |
Q6. Was the implementation of the AI intervention clearly described (e.g. how and when it was used to assist human readers and what was the level of training and experience of the human readers)? | No |
No information reported. | |
Q7. Were the CT imaging criteria used to select patients for treatment (e.g. thrombectomy) clearly reported for both the periods before and after the introduction of the AI intervention? | No |
No information reported. | |
Q8. In addition to time to intervention outcomes, did the study report the proportion of patients who received treatment (e.g. thrombectomy) before and after the introduction of the AI intervention? | NA |
All included patients received thrombectomy. | |
Q9. In addition to time to intervention outcomes, did the study report clinical outcomes (e.g. 90-day mRS) before and after the introduction of the AI intervention? | Unclear |
Proportion of patients with mRS ≤ 3 reported (no time point specified) |
Morey et al.52
Q1. Did the study have a prospective design? | No |
A retrospective analysis of a prospectively maintained database. | |
Q2. Did the study population include an appropriate spectrum of patients? | Yes |
Consecutive patients who were transferred to a TSC or CSC with LVO and who underwent thrombectomy. Inpatients and patients in whom the thrombectomy decision was delayed due to fluctuating symptoms were excluded. | |
Q3. Were the criteria used to select patients for CT imaging similar, before and after the introduction of the AI intervention? | Unclear |
No information reported. | |
Q4. Were the study populations, before and after the introduction of the AI intervention, similar with respect to baseline demographic characteristics (e.g. age, male/female), comorbid conditions (e.g. hypertension, diabetes, AF) and risk factors (e.g. smoking status, previous history)? | No |
There were no significant differences in baseline demographic characteristics (age or proportion male), comorbid conditions (diabetes mellitus or hypertension), risk factors (previous stroke/TIA) or NIHSS. The proportion or patients with hypertension was higher in the before implementation population. | |
Q5. Other than the availability of AI software, was the care pathway similar before and after the introduction of the AI intervention? | Unclear |
No information reported. | |
Q6. Was the implementation of the AI intervention clearly described (e.g. how and when it was used to assist human readers and what was the level of training and experience of the human readers)? | No |
No information reported. | |
Q7. Were the CT imaging criteria used to select patients for treatment (e.g. thrombectomy) clearly reported for both the periods before and after the introduction of the AI intervention? | No |
No information reported. | |
Q8. In addition to time to intervention outcomes, did the study report the proportion of patients who received treatment (e.g. thrombectomy) before and after the introduction of the AI intervention? | NA |
All included participants received thrombectomy. | |
Q9. In addition to time to intervention outcomes, did the study report clinical outcomes (e.g. 90-day mRS) before and after the introduction of the AI intervention? | Yes |
Median 90-day mRS and number of participants with 90-day mRS ≤ 2 were reported. |
Appendix 4 Details of excluded studies with rationale
To be included in the review, studies had to fulfil the following criteria:
-
Population: Adults (≥ 18 years) attending a secondary care stroke centre with: (Q1) suspected acute stroke and who were last known to be well within 24 hours; (Q2a) AIS, who were last known to be well within the past 6 hours; (Q2b) suspected acute stroke, who were last known to be well more than 6 hours previously, but within 24 hours, and in whom ischaemic stroke has been confirmed on plain CT.
-
Index Test: AI-derived software: Aidoc ICH, Aidoc LVO, Aidoc mobile (Aidoc); Accipio (MaxQ AI); e-ASPECTS, e-CTP, e-CTA (Brainomix); icobrain CT (Icometrix); Biomind (Biomind.ai); Brainscan; Cercare stroke (Cercare Medical); CINA ICH, CINA LVO, CINA ASPECTS (Avicenna); CTP 4D (GE Healthcare); qER (Qure.ai); Rapid ASPECTS, Rapid ICH, Rapid CTA, Rapid LVO, Rapid CTP, RapidAI (iSchemaView); Viv ICH, Viz LVO, Viz CTP (Viz.ai); Zebra-Med (Zebra Medical Vision). (Q1) AI-derived software-assisted review of plain CT by a healthcare professional other than a neuroradiologist. (Q2a) AI-derived software-assisted CTA by a healthcare professional other than a neuroradiologist. (Q2b) AI-derived software-assisted CTA and CTP review by a healthcare professional other than a neuroradiologist.
-
Reference Standard: Unassisted, (Q1) plain CT, (Q2a) CTA, (Q2b) CTP, review by a neuroradiologist, or by a consensus panel.
-
Comparator: (Q1) Unassisted plain CT review by a neuroradiologist or other healthcare professional. (Q2a) Unassisted CTA review by a neuroradiologist or other healthcare professional. (Q2b) AI-derived software-assisted CTA and AI-derived software-assisted CTP brain scan review by a neuroradiologist or other healthcare professional or Unassisted CTA and AI-derived software-assisted CTP brain scan review by a neuroradiologist or other healthcare professional.
-
Outcome: Test accuracy (the numbers of true-positive, false-negative, false-positive and true-negative test results), for the target condition: (Q1) ICH or ischaemic stroke; (Q2a) LVO/occlusion of the proximal anterior circulation; (Q2b) LVO/occlusion of the proximal anterior circulation for CTA and presence of salvageable tissue for CTP. Clinical/patient-perceived outcomes: mortality, function (e.g. mRs), HRQoL, procedure-related adverse events (e.g. bleed subsequent to thrombolysis), length of hospital stay.
Table 34 summarises studies that were screened for inclusion based on full-text publication but did not fulfil one or more of the above criteria. Studies were assessed sequentially against criteria; as soon as a study had failed based on one of the criteria it was not assessed against subsequent criteria. The table shows which of the criteria each study fulfilled (‘Y’) and on which item it failed (‘N’) or was unclear.
Study details | Study design | Population | Index test | Reference standard OR comparator | Outcome |
---|---|---|---|---|---|
Abdelkhaleq et al.121 | Y | N | Y | N | N |
Aboutaleb et al.122 | Y | Y | N | ||
Aghaebrahim et al.123 | N | N | |||
Aktar et al.124 | Y | N | Y | N | |
Albers et al.125 | Y | Y | Y | N | N |
Alderson et al.126 | Y | Y | Y | N | N |
Alderson et al.127 | N | ||||
Apterbach et al.128 | Y | Y | N | ||
Austein et al.129 | Y | Y | Y | Y | N |
Austein et al.130 | Y | Y | Y | Y | N |
Austein et al.131 | Y | N | |||
Austein et al.132 | Y | Y | Y | Y | N |
Bacchi et al.133 | Y | Y | N | ||
Bar et al.134 | Y | Y | Y | N | N |
Barman et al.135 | Y | Y | N | ||
Barros et al.136 | Y | Y | N | ||
Beijing Tiantan Hospital137 | Y | Y | N | ||
Bentley et al.138 | Y | N | |||
Bentley et al.139 | Y | N | |||
Bhagat et al.140 | Y | N | |||
Biswas et al.141 | N | ||||
Bouslama et al.142 | Y | Y | Y | N | |
Bouslama et al.112 | Y | Y | Y | N | |
Bouvy et al.143 | Y | N | |||
Brinjikji et al.144 | Y | Y | Y | Y | N |
Brinjikji et al.145 | Y | Y | Y | Y | N |
Brinjikji et al.146 | N | ||||
Bruggeman et al.147 | Y | Y | N | ||
Brugnara et al.148 | Y | Y | N | ||
Buls et al.149 | Y | N | |||
Bulwa et al.150 | Y | Y | Y | Y | N |
Campbell et al.151 | Y | Y | N | ||
Capasso et al.152 | Y | Y | Y | N | |
Chatterjee et al.153 | Y | Y | Y | Y | N |
Chilamkurthy et al.154 | Y | N | |||
Chriashkova et al.155 | Y | Y | Y | Y | N |
Chriashkova et al.156 | Y | Y | Y | Y | N |
Chung et al.157 | Y | Y | Y | Y | N |
Chung et al.158 | Y | N | |||
Cimflova et al.159 | Y | Y | Y | N | |
Cimflova et al.160 | Y | Y | Y | N | |
Copelan et al.161 | Y | Y | Y | Y | N |
D’Esterre et al.162 | Y | Y | N | ||
Davidovic et al.163 | Y | Y | N | ||
Davis et al.164 | Y | N | |||
Dehkharghani et al.165 | N | ||||
Delio et al.166 | Y | Y | Y | Y | N |
Delio et al.167 | Y | Y | Y | Y | N |
Demeestere et al.168 | N | ||||
Demeestere et al.113 | N | ||||
Desai et al.169 | Y | Y | Y | Y | N |
Devlin et al.170 | Y | Y | Y | Y | N |
Docema et al.171 | Y | Y | N | ||
Elijovich et al.172 | Y | Y | Y | N | N |
Ferreti et al.173 | Y | Y | Y | N | |
Ferreti et al.174 | Y | Y | Y | Y | N |
Fischer et al.175 | Y | Y | Y | Y | N |
Ford et al.176 | Y | Y | Y | Y | N |
Ginat et al.177 | Y | N | |||
Ginat et al.178 | Y | N | |||
Goebel et al.179 | Y | Y | Y | Y | N |
Goebel et al.180 | Y | Y | Y | Y | N |
Goebel et al.181 | Y | Y | Y | Y | N |
Goncalves et al.182 | Y | Unclear | Y | Y | N |
Grunwald et al.183 | Y | Y | Y | Y | N |
Grunwald et al.184 | N | ||||
Grunwald et al.185 | N | ||||
Grunwald et al.186 | N | ||||
Grunwald et al.187 | Y | Y | Y | Y | N |
Guberina et al.188 | Y | Y | Y | N | |
Heit et al.189 | Y | N | |||
Herweh et al.190 | Y | Y | Y | N | |
Herweh et al.191 | Y | Y | Y | N | |
Herweh et al.192 | Y | Y | N | ||
Hoelter et al.193 | Y | Y | Y | Y | N |
Hoffmann et al.194 | Y | N | |||
Hokkinen et al.195 | Y | Y | Y | Y | N |
Hoving et al.196 | Y | Y | Y | Y | N |
Hoyte et al.197 | Y | Y | Y | Y | N |
Jankowitz et al.198 | Y | N | |||
John et al.199 | Y | Y | Y | Y | N |
John et al.200 | Y | Y | Y | Y | N |
Katramados et al.201 | Y | Y | N | ||
Kelavkar et al.202 | Y | Y | N | ||
Kettenberger et al.203 | Y | Y | N | ||
Kettenberger et al.204 | Y | Y | N | ||
Kim et al.205 | Y | Y | N | ||
Kniep et al.206 | Y | Y | N | ||
Knight-Greenfield et al.207 | Y | Y | N | ||
Kral et al.208 | Y | Y | Y | N | |
Kuang et al.209 | Y | Y | Y | Y | N |
Kuang et al.210 | Y | Y | Y | N | N |
Kuang et al.211 | Y | Y | Y | Y | N |
Kuo et al.212 | Y | Y | N | ||
Lasocha et al.213 | Y | Y | Y | Y | N |
Lee et al.214 | Y | Y | N | ||
Liu et al.215 | Y | Y | Y | N | |
Lo et al.216 | Y | Y | N | ||
Loffler et al.217 | Y | Y | Y | Y | N |
Maegerlein et al.218 | Y | Y | Y | Y | N |
Mair et al.219 | Y | Y | Y | Y | N |
Mansour et al.220 | Y | Y | Y | Y | N |
Meijs et al.221 | Y | Y | Y | Y | N |
Meijs et al.222 | Y | Y | N | ||
Modak et al.223 | Y | Y | Y | Y | N |
Morey et al.224 | Y | Y | Y | Y | N |
Murray et al.225 | N | ||||
Nagel et al.226 | Y | Y | Y | Y | N |
Nagel et al.227 | N | ||||
Nagel et al.228 | Y | Y | Y | Y | N |
Nagel et al.229 | Y | Y | Y | Y | N |
Neuberger et al.230 | Y | Y | Y | Y | N |
Neuberger et al.231 | Y | Y | Y | Y | N |
Neuhaus et al.232 | Y | Y | Y | Y | N |
Nishio et al.233 | Y | Y | N | ||
Ojeda et al.234 | Y | N | |||
Olive-Gadea et al.235 | Y | Y | Y | Y | N |
Olive-Gadea et al.236 | Y | Y | Y | Y | N |
Olive-Gadea et al.113 | Y | Y | Y | Y | N |
Olive-Gadea et al.237 | Y | Y | N | ||
Olive-Gadea et al.238 | Y | Y | N | ||
Pfaff et al.239 | Y | Y | Y | Y | N |
Pfaff et al.114 | Y | Y | Y | Y | N |
Pisani et al.240 | Y | Y | Y | Y | N |
Pisani et al.241 | Y | Y | Y | Y | N |
Prokhorikhin et al.242 | Y | Y | N | ||
Providence Little Company243 | N | ||||
Psychogios et al.244 | Y | Y | Y | Y | N |
Purrucker et al.245 | Y | Y | Y | Y | N |
Purrucker et al.246 | Y | Y | Y | Y | N |
Qiu et al.247 | Y | Y | N | ||
Rao et al.248 | Y | Y | N | ||
Rava et al.249 | Y | Y | Y | Y | N |
Reidler et al.250 | Y | Y | N | ||
Sachdev et al.251 | Y | Y | Y | Y | N |
Seo et al.252 | Y | Y | Y | Y | N |
Shah et al.253 | Y | Y | Y | Y | N |
Sheth et al.254 | Y | Y | Y | Y | N |
Sheth et al.255 | Y | Y | N | ||
Shinohara et al.256 | Y | Y | N | ||
Shinohara et al.257 | Y | Y | N | ||
Siegler et al.258 | Y | Y | Y | Y | N |
Sundaram et al.259 | Y | Y | Y | Y | N |
Suomalainen et al.260 | Y | Y | Y | Y | N |
Suomalainen et al.261 | Y | Y | Y | Y | N |
Timaran et al.262 | Y | Y | Y | N | |
Tolhuisen et al.263 | Y | Y | N | ||
Tolhuisen et al.264 | Y | Y | N | ||
Tsang et al.264 | Y | Y | Y | Y | N |
Tyan 2014265 | Y | Y | N | ||
University of Guadalajara266 | Y | Y | N | ||
Vargas et al.267 | Y | Y | Y | N | N |
Voter et al.268 | Y | N | |||
Voter et al.269 | Y | N | |||
Vyas et al.270 | N | ||||
Wang C et al.271 | Y | Y | N | ||
Wang TG et al.272 | Y | Y | Y | N | |
Weiss et al.273 | Y | Y | Y | Y | N |
Weiss et al.274 | Y | Y | Y | Y | N |
Yang L et al.275 | Y | Y | N | ||
Yang W et al.276 | Y | Y | N | ||
Zamarro Parra et al.277 | Y | Y | Y | Y | N |
Appendix 5 National Institute for Health and Care Excellence guidance relevant to the management of suspected acute stroke
-
Stroke and Transient Ischaemic Attack in Over 16s: Diagnosis and Initial Management. NICE Guideline NG128; published 1 May 2019. URL: www.nice.org.uk/guidance/ng128
-
Alteplase for Treating Acute Ischaemic Stroke. Technology Appraisal Guidance TA264; published 26 September 2012. URL: www.nice.org.uk/guidance/ta264/chapter/1-Guidance
-
Mechanical Clot Retrieval for Treating Acute Ischaemic Stroke. Interventional Procedures Guidance IPG548; published 24 February 2016. URL: www.nice.org.uk/guidance/ipg548
-
Stroke in Adults. Quality Standard QS2; published 29 June 2010, last updated 12 April 2016. URL: www.nice.org.uk/guidance/qs2
-
Mechanical Thrombectomy Devices for Acute Ischaemic Stroke. Medtech Innovation Briefing MIB153; published 30 July 2018. URL: www.nice.org.uk/advice/mib153
-
RapidAI for Analysing CT/MRI Brain Scans in People with Suspected Acute Stroke. Medtech Innovation Briefing MIB262; published 1 June 2021. URL: www.nice.org.uk/advice/mib262
Appendix 6 Explicit tool screenshots
FIGURE 22.
Background information provided to experts.
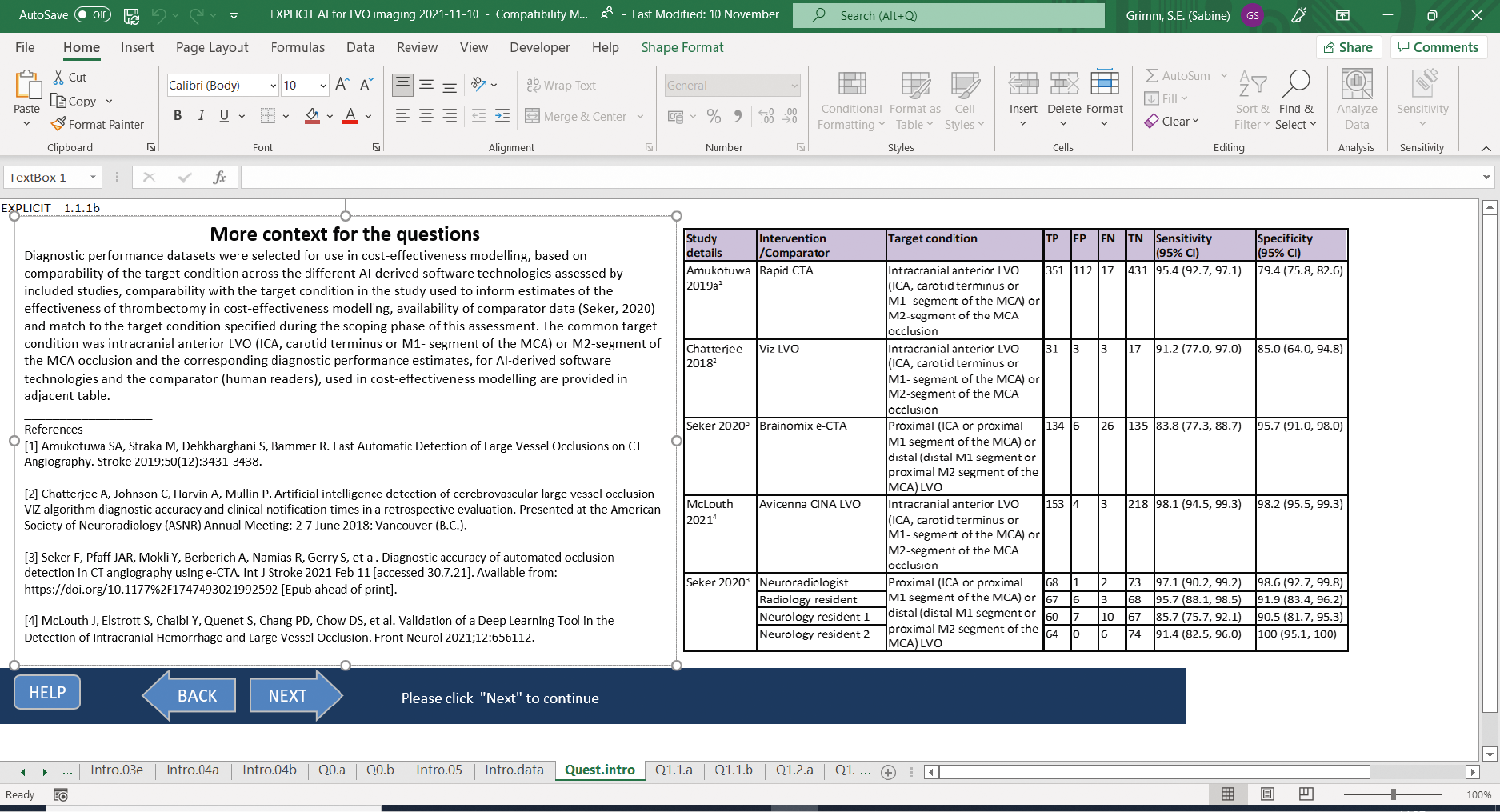
FIGURE 23.
Sensitivity question AI + human.

FIGURE 24.
Specificity question AI + human.

Glossary
- Cost-effectiveness analysis
- An economic analysis that converts effects into health terms and describes the costs for additional health gain.
- Decision modelling
- A mathematical construct that allows the comparison of the relationship between costs and outcomes of alternative healthcare interventions.
- False negative
- Incorrect negative test result – number of diseased persons with a negative test result.
- False positive
- Incorrect positive test result – number of non-diseased persons with a positive test result.
- Incremental cost-effectiveness ratio
- The difference in the mean costs of two interventions in the population of interest divided by the difference in the mean outcomes in the population of interest.
- Index test
- The test whose performance is being evaluated.
- Meta-analysis
- Statistical techniques used to combine the results of two or more studies and obtain a combined estimate of effect.
- Meta-regression
- Statistical technique used to explore the relationship between study characteristics and study results.
- Opportunity costs
- The cost of foregone outcomes that could have been achieved through alternative investments.
- Publication bias
- Bias arising from the preferential publication of studies with statistically significant results.
- Quality of life
- An individual’s emotional, social and physical well-being and their ability to perform the ordinary tasks of living.
- Quality-adjusted life-year
- A measure of health gain, used in economic evaluations, in which survival duration is weighted or adjusted by the patient’s quality of life during the survival period.
- Receiver operating characteristic curve
- A graph which illustrates the trade-off between sensitivity and specificity that results from varying the diagnostic threshold.
- Reference standard
- The best currently available method for diagnosing the target condition. The index test is compared against this method to allow calculation of estimates of accuracy.
- Sensitivity
- Proportion of people with the target disorder who have a positive test result.
- Specificity
- Proportion of people without the target disorder who have a negative test result.
- State–transition model
- A model in which individuals move (transition) between disease states as their condition changes over time. Time spent in each disease state for a single model cycle (and transitions between states) is associated with a cost and a health outcome.
- True negative
- Correct negative test result – number of non-diseases persons with a negative test result.
- True positive
- Correct positive test result – number of diseased persons with a positive test result.
List of abbreviations
- AF
- atrial fibrillation
- AI
- artificial intelligence
- AIS
- acute ischaemic stroke
- ARIF
- Aggressive Research Intelligence Facility
- ASPECTS
- Alberta Stroke Program Early CT Score
- CDSR
- Cochrane Database of Systematic Reviews
- CEA
- cost-effectiveness analysis
- CENTRAL
- Cochrane Central Register of Controlled Trials
- CI
- confidence interval
- CRD
- Centre for Reviews and Dissemination
- CSC
- comprehensive stroke centre
- CT
- computed tomography
- CTA
- computed tomography angiography
- CTP
- computed tomography perfusion
- DARE
- Database of Abstracts of Reviews of Effects
- DICOM
- Digital Imaging Communications in Medicine
- ED
- emergency department
- EED
- Economic Evaluations Database
- FAST
- face arm speech test
- GDPR
- General Data Protection Regulation
- GIN
- Guidelines International Network
- HIPAA
- Health Insurance Portability and Accountability Act
- HRQoL
- health-related quality of life
- HSROC
- hierarchical summary receiver operating characteristic
- HTA
- Health Technology Assessment
- ICA
- internal carotid artery
- ICD
- International Classification of Diseases
- ICH
- intracranial haemorrhage
- ICTRP
- International Clinical Trials Registry Platform
- INAHTA
- International Network of Agencies for Health Technology Assessment
- INPLASY
- International Platform of Registered Systematic Review and Meta-analysis Protocols
- IV
- intravenous
- KSR
- Kleijnen Systematic Reviews
- LILACS
- Latin American and Caribbean Health Sciences Literature
- LVO
- large-vessel occlusion
- MCA
- middle cerebral artery
- MD
- mean difference
- MIP
- maximum intensity projection
- MR
- magnetic resonance
- MRI
- magnetic resonance imaging
- mRS
- Modified Rankin Score
- NA
- not applicable
- NCCT
- non-contrast computed tomography
- NHS
- National Health Service
- NICE
- National Institute for Health and Care Excellence
- NIHR
- National Institute for Health and Care Research
- NIHSS
- National Institute of Health Stroke Scale
- NPV
- negative predictive value
- OR
- odds ratio
- OXVASC
- Oxford Vascular
- PACS
- picture archiving and communications systems
- PPV
- positive predictive value
- PSSRU
- Personal Social Services Research Unit
- QALY
- quality-adjusted life-year
- RCT
- randomised controlled trial
- RIS
- radiology information systems
- ROSIER
- Recognition of Stroke In Emergency Rooms
- RR
- relative risk
- SCI
- Science Citation Index
- SD
- standard deviation
- sICH
- symptomatic intracranial haemorrhage
- SSNAP
- Sentinel Stroke National Audit Programme
- TIA
- transient ischaemic attack
- t-PA
- tissue-type plasminogen activator
- TRIP
- Turning Research into Practice
- UK
- United Kingdom
- WHO
- World Health Organization
Notes
-
Updated systematic review, completed July 2023 (NIHR Evidence Synthesis Programme project number NIHR135996)
Supplementary material can be found on the NIHR Journals Library report page (https://doi.org/10.3310/RDPA1487).
Supplementary material has been provided by the authors to support the report and any files provided at submission will have been seen by peer reviewers, but not extensively reviewed. Any supplementary material provided at a later stage in the process may not have been peer reviewed.