Notes
Article history
The research reported in this issue of the journal was commissioned and funded by the Evidence Synthesis Programme on behalf of NICE as award number NIHR135437. The protocol was agreed in March 2022. The draft manuscript began editorial review in August 2022 and was accepted for publication in March 2023. The authors have been wholly responsible for all data collection, analysis and interpretation, and for writing up their work. The HTA editors and publisher have tried to ensure the accuracy of the authors’ manuscript and would like to thank the reviewers for their constructive comments on the draft document. However, they do not accept liability for damages or losses arising from material published in this article.
Permissions
Copyright statement
Copyright © 2024 Cox et al. This work was produced by Cox et al. under the terms of a commissioning contract issued by the Secretary of State for Health and Social Care. This is an Open Access publication distributed under the terms of the Creative Commons Attribution CC BY 4.0 licence, which permits unrestricted use, distribution, reproduction and adaptation in any medium and for any purpose provided that it is properly attributed. See: https://creativecommons.org/licenses/by/4.0/. For attribution the title, original author(s), the publication source – NIHR Journals Library, and the DOI of the publication must be cited.
2024 Cox et al.
Chapter 1 Background and definition of the decision problem
Parkinson’s disease
Parkinson’s disease (PD) is a condition that affects the brain, resulting in a progressive loss of co-ordination as well as movement problems. It is caused by a loss of cells in the brain that are responsible for producing dopamine, which helps to control and co-ordinate body movements. In the early stages of PD, the three main symptoms are shaking (tremor), slowness of movement (bradykinesia) and muscle stiffness (rigidity). These develop gradually, in no particular order. 1 Other physical symptoms that can occur early on include balance problems, nerve pain, loss of sense of smell (hyposmia) and sleep disturbances. It can also impact mental health, with higher rates of anxiety and depression. There is no consistently reliable test that can distinguish PD from other conditions that have similar clinical presentations; diagnosis is primarily based on history and clinical examination. 2
Healthcare professionals often refer to different ‘stages’ of PD. 3 The early or diagnosis stage describes the period when someone is first experiencing symptoms, being diagnosed and coming to terms with this. The maintenance stage is when symptoms are controlled, perhaps by medication. Advanced PD is defined by the presence of more complex symptoms that significantly impact daily living, including anxiety, depression and dementia. Advanced PD has a severe negative impact on the quality of life of patients, their families and carers. The palliative stage involves providing relief from the symptoms, stress and pain of the condition. 3
The Parkinson’s UK report on the incidence and prevalence of PD states that there are around 145,500 people living with PD in the UK. 4 Men are more likely to develop PD than women, and the risk of developing the disease increases sharply with age. It is estimated that around 10% of patients have advanced disease. 5 In 2018, there were 6505 deaths due to PD in England and Wales. All deaths occurred in people aged 50 years or above, with 87% occurring in people aged 75 years or above. 5
Treatment for Parkinson’s disease
Recommendations for the treatment of PD are given in the National Institute for Health and Care Excellence (NICE) guideline for PD in adults (NG71). 2 Patients should be offered both non-pharmacological and pharmacological management for motor symptoms. This includes referral to a physiotherapist for physical activity regimes. This can also include referral to an occupational therapist for people with difficulties doing day-to-day activities.
Pharmacological treatment
Levodopa is the most commonly prescribed treatment for managing the motor symptoms of PD in the early stages. 2 However, it may be associated with significant motor complications, including response fluctuations and dyskinesias (involuntary movements), particularly after long-term use. Response fluctuations are characterised by large variations in motor performance, with normal function during the ‘on’ period, and weakness and restricted mobility during the ‘off’ period. ‘Wearing off’ of the drug or ‘End-of-dose’ deterioration with progressively shorter duration of benefit can also occur over time. Sleep disturbances such as insomnia, nocturia (night time urination) and restless leg syndrome (‘jumping’ of the legs and/or arms) can be caused by ‘wearing-off’ periods during the night. Dopaminergic therapies can also cause non-motor adverse effects, such as impulse control disorders, excessive sleepiness or sudden onset of sleep and psychotic symptoms such as hallucinations and delusions. Patient preferences are key to treatment decisions; the benefits of treatment must be balanced against the potential side effects.
Dopamine agonists, monoamine oxidase type B (MAO-B) inhibitors or catechol-O-methyltransferase (COMT) inhibitors are offered as additional treatment for people who have developed dyskinesia or motor fluctuations despite optimal levodopa therapy. If the dyskinesia remains uncontrolled, amantadine can be considered.
The NICE guideline for PD in adults recommends adjusting medicines to reduce the occurrence of daytime sleepiness or sudden onset of sleep, having first sought advice from a healthcare professional with specialist expertise in PD. Modafinil should be considered to treat excessive daytime sleepiness if a detailed sleep history has excluded reversible pharmacological and physical causes. Clonazepam or melatonin may be considered to treat rapid eye movement sleep behaviour disorder if a medicine’s review has addressed possible pharmacological causes. 2
Advanced Parkinson’s disease
The symptoms of advanced PD may still be responsive to adjustments in the dose and combination of levodopa with adjuvant MAO-B and/or COMT therapies. 6 Intermittent apomorphine injection and/or continuous apomorphine infusion may also be considered for people with advanced PD. Deep brain stimulation (DBS) can be considered in people with late-stage PD whose symptoms do not respond adequately to best medical therapy. Clinical experts highlighted that this procedure is only normally considered for people who have been taking medication for PD for over 5 years.
Levodopa–carbidopa intestinal gel (LCIG) is currently available through an NHS England clinical commissioning policy. It can be considered in certain people with advanced levodopa-responsive PD with severe motor fluctuations that have not responded to available medications. NICE recommends that this policy is reviewed in light of NG71 (NICE guidelines for PD in adults, section 1.8.4). 2
Description of the technologies under assessment
People with Parkinson’s disease (PwP) experience a range of motor symptoms, which can fluctuate in severity during the day and between days. Remote monitoring devices are intended to be used alongside clinical judgement to assess disease severity and help manage PD symptoms and the adverse effects of treatment. They can be used in any setting and are most likely to be used in people’s homes.
The results of the monitoring are analysed remotely, and a summary provided to the specialist physician and/or to the patient. The specialist should use this summary to assess motor symptoms (bradykinesia and dyskinesia) and other symptoms, including sleep disturbance and tremors, and how these are influenced by the use and timing of treatment. The data should be used to determine whether any changes in the treatment regimen are desirable, in consultation with the patient. Results of the monitoring devices are intended to complement existing methods of assessment, such as patient-reported symptoms and clinical assessment, and are not intended to replace them.
Results from the monitoring devices may also have more general benefits, alongside treatment modification. These include providing a clear and objective measurement of symptoms, which may enable both patient and clinician to better understand the patient’s condition, and provide clearer justification of the value of treatment and the need for modification. These devices may also be of particular use for patients who may have difficulty communicating, recalling or recording their symptoms, for example, due to learning difficulties or language barriers.
This assessment considers only wearable remote monitoring devices that produce results with no input, or limited input, from the user. All technologies assess, at minimum, bradykinesia and dyskinesia. Five relevant remote monitoring devices with CE marks (or in the process of seeking CE-marking) were identified for consideration:
-
Personal KinetiGraph (PKG) Movement Recording System (Global Kinetics);
-
Kinesia 360 motor assessment system (Great Lakes NeuroTechnologies);
-
KinesiaU motor assessment system (Great Lakes NeuroTechnologies);
-
PDMonitor (PD Neurotechnology); and
-
STAT-ON (Sense4Care).
Personal KinetiGraph Movement Recording System (Global Kinetics)
The PKG Movement Recording System (Global Kinetics) is a Class IIa CE marked system that uses a wrist-worn PKG watch/logger that continuously measures movement over a period of at least 6 days. It is intended to quantify kinematics of movement disorder symptoms in conditions such as PD, including tremor, bradykinesia (slowness) and dyskinesia (involuntary movements). It has event markers for medication reminders and patient acknowledgement. It is also intended to be used to monitor activity associated with movement during sleep. The company states that PKG is an adjunct to clinical practice and should be used in combination with patient and healthcare consultation. They envisage that the PKG is used twice a year, although there is some uncertainty about the best time to use the PKG; varying between every 6 months, regardless of current symptoms, to only when there is a suspicion that medication is not adequately controlling symptoms.
Healthcare professionals can order the PKG online. The company sends the watch directly to the person who will wear it (for a period of at least 6 days), also providing a paid and addressed envelope for the watch to be returned to the company. Data are then extracted and processed by cloud-based algorithms and a report is generated for the healthcare professional to view online.
The PKG measures bradykinesia, dyskinesia, tremors, motor fluctuations, immobility and when the watch is not being worn. It can also prompt the user to take their medication at prescribed times and the user can register when they have taken their medication. As well as providing the raw data, it can generate a report based on movement over a 6-day period using validated proprietary algorithms. The report includes summary graphs showing measurements over time and a summary following results, along with a suggested target range for interpretation:
-
a bradykinesia score;
-
a dyskinesia score;
-
a fluctuation dyskinesia score;
-
percentage of time with tremor; and
-
percentage of time immobile (indicative of daytime sleepiness).
The company has stated that new versions of the technology will include 24-hour measurements of sleep-related functions. The device is intended to be interpreted only by trained technicians or clinicians, and as an aid to existing clinical methods. It is not intended to be the sole or primary means of clinical assessment. The company states that the PKG is suitable for 70–80% of PwP, particularly when managing patients remotely, managing complex patients and those being considered for (or already on) advanced therapy. The company does not recommend use of the technology for patients who have restricted movement (e.g. patients confined to bed or wheelchair users) or for patients who operate heavy machinery for prolonged periods.
The company provides healthcare professionals with education and training, and state that healthcare professionals should complete an average of 15–20 PKGs to be proficient, supported by an eLearning module, which takes approximately 1–2 hours.
Kinesia 360 motor assessment system (Great Lakes NeuroTechnologies)
The Kinesia 360 motor assessment system (Great Lakes NeuroTechnologies) is a Class I CE-marked system that monitors physical motion and muscle activity to quantify movement disorder symptoms and assess activity. The Kinesia 360 system consists of a tablet, sensors and charge pad, USB cable and charge pad power cable. Sensors worn on the wrist and ankle combined with a mobile application continuously record data, including bradykinesia, dyskinesia and tremor. While the device can be worn at night, the motor sensors can record up to 16 hours of motion data continuously before they need to be recharged. Typical use involves wearing the sensors during the day and recharging/data upload overnight. The mobile application also includes electronic diaries for capturing patient-reported outcomes and customisable medication diaries.
When the Kinesia Sensor bands are returned to the charging pad, data from the motion sensors are automatically downloaded and then uploaded to the Kinesia Web Portal and algorithms are used to detect symptoms and calculate severity scores. Clinicians can view web-based reports that include:
-
a dyskinesia score;
-
total and percentage of time with tremor;
-
total and percentage of time at rest;
-
total and percentage of active time (but not walking);
-
number of steps;
-
a symptom summary report that displays how tremor, slowness, dyskinesia and walking change over time; and
-
a dose report that shows how tremor, slowness, dyskinesia and walking change as a function of different medication or therapy doses.
Healthcare staff can be trained in Kinesia 360 in approximately 30 minutes.
KinesiaU motor assessment system (Great Lakes NeuroTechnologies)
The KinesiaU motor assessment system (Great Lakes NeuroTechnologies) measures tremor, slowness and dyskinesia using a smartwatch and smartphone application. Patient symptoms can be monitored continuously during activities of daily living (iOS only as of February 2022) and discretely during standardised tasks (iOS and Android). Patients can view reports in real-time and healthcare professionals can view their patients’ data remotely through the KinesiaU provider portal. The product is to be used only under the direction of a qualified clinician and all changes to therapy regimens are to be based solely on the clinical judgement of the clinician. The company is seeking CE-marking. A number of new features are planned to be added to the KinesiaU system, including additional data reports, enhanced patient diaries, electronic health record integration, patient medication reminders and continuous monitoring for Android smartwatches.
The reports rate the severity of tremor, slowness and dyskinesia symptoms according to good, mild, moderate and severe categories. This can be measured through specific active tasks or through continuous recording. To start a continuous (all day) recording, the user must tap the ‘Continuous’ button on the home screen. The smartwatch application must be kept open during the recording. Active tasks may be performed during the continuous recording.
Reports can be produced throughout the day and over the course of days, weeks and months in response to therapy and activities. The report page on the smartwatch application displays the severity of the selected symptom (tremor, slowness and dyskinesia) averaged for the selected time range. The symptoms can be displayed individually or averaged together and shown as ‘All symptoms’. The mobile application also includes customisable medication and exercise diaries, which can be added to the report. Patients can view reports in real-time and share reports (PDF format) with their healthcare professionals.
Healthcare staff can be trained in KinesiaU in approximately 30 minutes.
PDMonitor (Parkinson’s disease Neurotechnology)
The PDMonitor system (PD Neurotechnology) is a Class IIa CE-marked system that measures activity/posture, bradykinesia, freezing of gait, gait disturbances, wrist tremor, leg tremor, dyskinesia and ‘on’ and ‘off’ periods. The duration and frequency of use are decided by the physician. The device should be removed when performing intense fitness activities.
The PDMonitor system consists of the SmartBox, five monitoring devices and a PDMonitor mobile application. The devices are worn on both wrists, both ankles and one is worn on the waist, and acquire movement data for assessing motor symptoms. The PDMonitor SmartBox is a docking station for charging the monitoring devices, collecting, storing and processing data and uploading it to the PD Neurotechnology storage service. The SmartBox must be connected to the PD Neurotechnology storage service to be properly configured, either via an ethernet cable or an available Wi-Fi network; this requires an internet connection. A web-based application can be used by healthcare professionals to view and download patient reports. The PDMonitor mobile application is an electronic diary for medications, diet and symptoms related to PD. It also provides a summary of daily activity as recorded by the PDMonitor system.
An ‘Induction and Usage Training’ is offered to healthcare professionals, either in groups or in person, to help them understand the PDMonitor system. There is also a physician user manual for the physician tool.
STAT-ON (Sense4Care)
The STAT-ON (Sense4Care) is a Class IIa CE-marked, waist-worn inertial recorder, configured by a doctor and used by the patient in clinical, ambulatory or home environments. It measures motor disorders and events when worn by someone with PD, but does not measure tremor. The device measures dyskinesia, ‘on’ and ‘off’ periods, gait parameters (including bradykinesia and freezing of gait), falls, energy expenditure and posture. It can also register when medication has been taken and up to 10 alarms per day can be set.
Health professionals should manage the use of the device; they should provide the sensor to the user correctly configured and charged. Results can be used to adjust or evaluate a therapy or to adjust a person’s diet.
The STAT-ON system consists of a monitoring device, its base charger, a belt and a mobile application. The device collects data and uses artificial intelligence algorithms to process it. Results are stored in its internal memory. The smartphone application connects to the STAT-ON device via Bluetooth. The mobile application is used for configuring the system and for downloading the data. It also sends the data as a report via e-mail.
The company has advised that the STAT-ON could be worn during the night to monitor movement. The user should wear the device for a minimum of 5 days (ideally for 7 days), totalling a minimum of 24 hours over the 5 days to generate sufficient data. After this, a report can be generated at any time. A health professional can download the report to their phone using the STAT-ON application, which automatically generates a report of the motor state and symptoms during time of use. Reports include a summary of activity and prevalence of symptoms during the monitored period, including:
-
total freezing of gait episodes and average number of episodes per day;
-
average minutes walking and number of steps per day;
-
number of falls;
-
time in ‘Off’/Intermediate/‘On’; and
-
time with dyskinesia.
As well as numerically, data are also presented in graphs. In addition to a summary report, a more detailed report with further data analysis can also be produced.
The STAT-ON device is not indicated for children or for PwP with Hoehn and Yahr (H&Y) Scale 5. The device should not be worn by a person in a wheelchair or using crutches as the results will not be valid.
Training sessions last an hour and a half. Quick guides are provided for healthcare professionals and quick videos to understand how the system is configured. A complete graphical document is also provided with user cases, examples and how to interpret the report.
Populations and relevant subgroups
The population of interest is PwP. The subgroups relevant to this appraisal are:
-
patients grouped according to disease stage (e.g. early, maintenance or late-stage), current treatment and treatment options;
-
people with advanced PD (however defined, but including patients receiving DBS, LCIG or apomorphine];
-
people with communication barriers, which limit ability to describe their symptoms; and
-
according to ethnicity.
Global Kinetics Corporation informed NICE that there are 34 hospitals across the UK using the PKG. However, use is limited by funding constraints within the care pathway (personal communication). 7 The PDMonitor is available in the UK and is currently in demo use at King’s College, St George’s and Belfast Trusts. 8 Kinesia 360 and STAT-ON are available in the UK,9,10 although there is no indication that they are currently being used in NHS practice. KinesiaU is not yet available in the UK. 11
Comparators
The comparator is clinical judgement of symptoms and need for treatment modification, without the use of remote monitoring devices.
The assessment of disease symptoms, including motor symptoms, in current clinical practice varies. It includes patient or carer-reported history taking, for example diaries, and use of rating scales; in NHS practice the Movement Disorders Society (MDS) Unified Parkinson’s Disease Rating Scale (UPDRS) – part 2, the Modified Bradykinesia Rating Scale (MBRS) and the H&Y scale are the most frequently used. Exact methodology and choice of rating scales may vary substantially between centres. Problems may arise when using clinical judgement to assess symptoms because of limited time to assess symptoms, long periods between clinical assessments, and difficulties in communicating symptoms between patients, carers and clinicians. Technologies such as mobile activity trackers and mobile applications may also be used to support information recorded in personal diaries, but these technologies do not appear to be in widespread use. Sleep diaries are also used.
Care pathways
Management of PD depends on the stage of the disease. In the early or diagnosis stage, patients may not require any medical treatment or will be managed with non-pharmacological treatment, such as physiotherapy. The maintenance stage is when symptoms are controlled, perhaps by medication. Levodopa is the most prescribed treatment for managing the motor symptoms of PD, but dopamine agonists, MAO-B inhibitors or COMT inhibitors may also be used. Advanced PD is defined by the presence of more complex symptoms that significantly impact daily living, including anxiety, depression and dementia. In this stage levodopa may still be beneficial, but patients might be given DBS or levodopoa–carbodopa intestinal gel.
It is important to regularly monitor PwP to assess disease progression and adverse effects of treatment. NICE recommends that PwP should be seen by a specialist every 6–12 months initially, then more often with increasing disease complexity (every 2–3 months), although this is often difficult because of the increasingly ageing population and demands on PD services. 12 The remote monitoring technologies considered in this assessment (see Description of the technologies under assessment) have all been proposed as a means of supporting clinical and patient evaluation of symptoms.
This assessment evaluates whether remote continuous monitoring devices are effective and reliable for monitoring motor symptoms, tremors and sleep disturbance in PwP. They could potentially be used alongside clinical judgement to help manage symptoms:
-
at all review appointments;
-
at a subset of review appointments (e.g. if motor fluctuations are not being adequately managed);
-
between review appointments (to allow for more frequent monitoring of symptoms, or where there is substantial time between appointments); and
-
in place of some in-person reviews (including remote management, remote appointments and where a patient might be unable to attend in person).
Outcomes
Outcomes considered fall into four key areas: (1) the association between monitoring results and clinical measures (such as bradykinesia and dyskinesia), (2) the intermediate impact of monitoring on treatment decisions and management, (3) the impact on clinical symptoms and disease severity and (4) benefits and value to patients, carers and health professionals.
Costs considered include those associated with the use of the remote monitoring devices (e.g. acquisition and operational costs), costs of clinical management of PD (including treatment costs and healthcare utilisation, e.g. review appointments), costs of hospitalisation, further tests and treatment-related adverse events. Costs were considered from an NHS and Personal Social Services (PSS) perspective.
Association outcomes
Association between outputs of remote monitoring (such as bradykinesia score, dyskinesia score, sleep disturbance and tremor measures) and clinical measures, including:
-
Rating scales such as the UPDRS, MBRS and the H&Y scales.
-
Other measures of bradykinesia and dyskinesia, sleep disturbance or tremor.
-
Clinical assessment.
-
Patient-reported symptoms.
-
Any measure of association, such as sensitivity and specificity, measure of correlation or results of regression models.
Intermediate impact of monitoring
All impacts on clinical decision-making:
-
Changes in therapy (e.g. starting levodopa).
-
Modification of current therapy dose or timing (primarily levodopa, and including potential changes to therapy identified, which were contraindicated or declined by the patient).
-
The use of additional interventions (including pharmacological and non-pharmacological interventions for management of motor and non-motor symptoms associated with PD).
-
Adherence to medication.
-
Number and length of clinical appointments.
-
Incidence of remote appointments.
-
Ease of use/acceptability by clinicians.
Clinical outcomes
Measurable clinical impact of using the technologies:
-
Change in clinical symptoms.
-
On–off periods.
-
UPDRS, MBRS, H&Y scores.
-
Dyskinesia and bradykinesia scores
-
Sleep disturbance.
-
Tremors.
-
Number and length of hospital admissions.
-
Other morbidities (including falls, hip fracture, cognitive functioning, other non-motor outcomes, adverse effects of treatment).
-
Mortality.
Patient- and carer-reported outcomes
-
Health-related quality of life (HRQoL).
-
Ease of use and acceptability for patients and carers.
-
Patient and carer experience (including quality of care, patient and carer satisfaction and engagement, e.g. impact on discussions about symptom management, communication and relationship between patients and clinicians).
It was expected that data would be unavailable for many of these outcomes. They are listed here to present a complete list of outcomes of interest.
Costs
Costs for consideration may include:
-
Costs related to using the intervention (including any time analysing and storing data, communicating results and arranging for use of the technology).
-
Cost of staff training.
-
Cost of review appointments.
-
Cost of further tests.
-
Cost of treatment (including costs of any adverse events).
Aims and objectives
The aim of the project is to determine the clinical and cost-effectiveness of remote monitoring devices that continuously monitor motor symptoms, tremors and sleep disturbance, alongside clinical judgement in PwP, specifically the five technologies described (see Description of the technologies under assessment).
To achieve this, the following objectives were set.
Clinical effectiveness
-
To perform a systematic review and, if feasible, a meta-analysis of the association between the output of the five remote monitoring devices and key indicators of disease symptoms and severity.
-
To perform a systematic review, narrative synthesis and, if feasible, a meta-analysis of the clinical impact of the remote monitoring devices and, in particular, consider their impact on change in treatment strategy and disease severity.
-
To perform a systematic review and narrative synthesis of patient and physician opinions on the value and ease of use of the remote monitoring devices.
Cost-effectiveness
-
To perform a systematic review of published cost-effectiveness studies of the use of the five remote monitoring devices in the management of PwP.
-
To develop a decision-analytic model to estimate the cost-effectiveness of the five remote monitoring devices as an adjunct to clinical judgement for the assessment of motor and non-motor symptoms in PwP compared to clinical judgement alone. If it is not feasible to estimate the cost-effectiveness for some of the devices due to a lack of comparative effectiveness evidence, the range of costs, resource consequences and potential clinical benefits associated with these devices will be described based on available information.
-
It is anticipated that the decision-analytic model will link the intermediate outcomes derived from the remote monitoring devices to short-term costs and consequences (e.g. the impact of a change in treatment). If feasible and appropriate, it will then aim to link the short-term consequences to potential longer-term costs and consequences (e.g. impact of a change in disease severity to incidence of motor symptoms such as falls and hip fractures) using the best available evidence.
-
The cost-effectiveness of the remote monitoring devices, if feasible, will be expressed in terms of incremental cost per quality-adjusted life-year (QALY) and/or net health (or monetary) benefits.
Chapter 2 Assessment of clinical effectiveness
Methods for reviewing clinical effectiveness
The systematic review was conducted following the general principles recommended in the Centre for Reviews and Dissemination (CRD) guidance and reported in accordance with the Preferred Reporting Items for Systematic Reviews and Meta-Analyses (PRISMA) statement. 13
Search strategies
Comprehensive searches of the literature were conducted to identify all studies relating to the use of the remote continuous monitoring devices PKG, Kinesia 360, KinesiaU, PDMonitor and STAT-ON for monitoring motor symptoms in PwP. An Information Specialist (HF) designed the search strategy in Ovid MEDLINE in consultation with the research team. The strategy consisted of terms for the population, which were then combined with specific interventions of interest, or broader terms that reflect remote monitoring technologies. Text word searches for terms appearing in the title, abstract or keyword fields of database records were included in the strategy alongside searches of relevant subject headings. Date, language and study design limits were not applied. The final MEDLINE strategy was adapted for use in all resources searched.
The searches were carried out on 1 February 2022. The following databases were searched: MEDLINE(R) ALL; EMBASE; EconLit; APA PsycInfo; Cochrane Central Register of Controlled Trials (CENTRAL); Cochrane Database of Systematic Reviews (CDSR); Database of Abstracts of Reviews of Effects (DARE); Health Technology Assessment (HTA) Database; NHS Economic Evaluation Database (NHS EED); and the International HTA Database.
In addition, the following resources were searched for ongoing, unpublished, or grey literature: ClinicalTrials.gov; EU Clinical Trials Register; and the World Health Organization (WHO) International Clinical Trials Registry Platform. All search strategies are presented in full in Appendix 1.
Search results were imported into EndNote 20 (Clarivate Analytics) and deduplicated. As a supplementary search method, reference lists of relevant reviews were scanned to identify additional potentially relevant studies. Company websites were also searched for additional relevant studies.
The companies who manufacture or control the devices of interest were contacted (via NICE) and invited to supply any material they considered relevant. This could include journal articles (published or unpublished), conference abstracts, lists of papers, study data and details of unpublished, ongoing or planned studies. These company submissions were examined for relevant eligible material.
Selection criteria
Two reviewers (RW and NM) independently screened all titles and abstracts using Covidence systematic review management software. Full papers of any titles and abstracts that were thought to be relevant were obtained where possible and independently screened by the two reviewers according to the criteria below. Any disagreements were resolved by consensus or by consulting a third reviewer (MS). Conference abstracts were included where sufficient data were reported to confirm eligibility.
Population
People with PD.
Interventions
Five remote monitoring devices with CE marks (or in the process of seeking CE-marking) for monitoring motor and non-motor symptoms in PwP:
-
PKG Movement Recording System (Global Kinetics);
-
Kinesia 360 motor assessment system (Great Lakes NeuroTechnologies);
-
KinesiaU motor assessment system (Great Lakes NeuroTechnologies);
-
PDMonitor (PD Neurotechnology); and
-
STAT-ON (Sense4Care).
Comparators
Clinical judgement of disease symptoms without the use of remote monitoring devices, which may include the use of rating scales. Single-arm studies without use of a comparator were eligible for inclusion.
Outcomes
See Outcomes for a full list of relevant outcomes.
Study designs
All study designs were eligible for inclusion, provided they reported evidence on the outcomes listed.
Scoping eligible studies
Studies that met the inclusion criteria were scoped in order to prioritise studies reporting the most relevant outcomes for full data extraction. Studies reported only as abstracts were not subject to full data extraction, but are tabulated in appendices.
Data extraction
A data extraction form was developed, piloted and finalised to extract study and patient characteristics and eligible outcomes. Data were extracted by one reviewer (RW or MS) and independently checked by a second reviewer (MS or RW), with discrepancies resolved through discussion. Data from relevant studies with multiple publications were extracted and reported as a single study, where it was possible to determine that the publications included the same patients. The most recent or most complete publication was used in situations where we could not exclude the possibility of overlapping populations across separate study reports.
Quality assessment
The quality of the diagnostic accuracy studies was assessed using the Quality Assessment of Diagnostic Accuracy Studies-2 (QUADAS-2) tool. 14 QUADAS-2 evaluates both risk of bias (associated with the population selection, index test, reference standard and patient flow) and study applicability (population selection, index test and reference standard) to the review question.
Risk of bias in randomised controlled trials (RCTs) was assessed using the latest version of the Cochrane risk of bias tool. 15 A tool for assessing the risk of bias of non-randomised studies was developed using relevant criteria as outlined in CRD’s guidance on undertaking systematic reviews. 16
Quality assessment was performed by one reviewer (RW or MS) and independently checked by a second reviewer (MS or RW). Disagreements were resolved through discussion. Quality assessment was performed only for included studies with full publications. Conference abstracts were not quality assessed due to the lack of information to merit a full assessment. Quality assessment was not performed for studies reporting association outcomes without reporting diagnostic accuracy.
Methods of data synthesis
The results of data extraction were presented in structured tables and as a narrative summary. A broad thematic synthesis was used to identify key issues arising from the extracted evidence, including key areas of agreement or disagreement across the included literature.
A statistical synthesis using meta-analysis was proposed in the protocol. However, due to the substantial diversity in study populations, conduct and outcomes reported, it was not possible to combine any studies in meta-analyses. Therefore, a narrative and thematic synthesis approach was used throughout.
Analysis of individual participant data
One clinical trial of PKG has deposited its original trial data on a repository for reanalysis. 17 The authors of the study gave permission to the External Assessment Group (EAG) to reanalyse the trial data, and have supplied it to the EAG.
The supplied data were checked, compared to the published results and reanalysed. Linear regression was used to analyse continuously distributed outcomes (e.g. UPDRS score) by considering the change from baseline to follow-up time for each outcome, and analysing the mean difference in change from baseline between PKG and non-PKG patients. Logistic regression was used for dichotomous outcomes (e.g. change in medication), analysing the odds ratio between PKG and non-PKG patients. Analyses were adjusted for potential confounding factors, chiefly the number of clinical visits and duration of PD (see Woodrow individual participant data for further details).
Methods for estimating quality of life
Health-related quality of life associated with disease severity was estimated. It was expected that measures of disease severity would be expressed in terms of different instruments of disease activity (e.g. UPDRS, Modified-UPDRS, MBRS, H&Y). In accordance with the NICE reference case, HRQoL utility values should be based on the EuroQoL – EuroQol-5 Dimensions (EQ-5D) instrument. Therefore, a pragmatic review of utility studies was carried out to identify relevant studies that (1) directly estimate EQ-5D utility values, and (2) establish the relationship between EQ-5D utility and measures of disease severity (including mapping studies).
Chapter 3 Results of the review of clinical effectiveness
General summary of evidence
The literature searches of bibliographic databases identified 1716 references. After the initial screening of titles and abstracts, 194 were considered to be potentially relevant and ordered for full paper screening. Sixty-three studies were eligible for inclusion in the review and 131 studies were excluded. Two additional studies were identified from scanning systematic review reference lists and 19 additional studies were identified from company submissions and websites. The full study selection process is illustrated in the PRISMA diagram in Figure 1. The 131 studies excluded at full paper stage are listed in Appendix 2, Table 49, along with the reasons for their exclusion.
FIGURE 1.
Flow diagram of the study selection process.

A total of 84 studies met the eligibility criteria; there were 7 ongoing studies with no results available (summarised in Appendix 3, Table 50) and 77 studies were included in the systematic review. Complete details of all included studies are given in Appendix 4 (see Tables 51–66). Where stated, most studies of PKG were conducted in Australia or the USA, most studies of STAT-ON were conducted in Spain, studies of Kinesia 360 and KinesiaU were conducted in USA or Canada and the study of PDMonitor was conducted in Greece and Italy. Few studies were conducted in the UK.
Fifty-seven studies evaluated PKG;17–73 15 studies evaluated STAT-ON;18–32 3 studies evaluated Kinesia 360;33–35 1 study evaluated KinesiaU;36 and 1 study evaluated PDMonitor. 37 There were no studies that directly compared one remote continuous monitoring device with another.
Additional ongoing studies and planned studies are reported in the company submissions. 8–11,38
Classification of studies by outcome reported
The included studies varied substantially, both within and across technologies, concerning the outcomes reported. To simplify the assessment of the studies they have been arranged into six categories by type of outcome reported as follows.
Diagnostic accuracy studies
Studies reporting whether the devices can predict symptoms and outcomes (such as bradykinesia, dyskinesia, sleep disturbance or tremor), or predict the need for medication change or similar. Studies must report sensitivity and specificity, or other diagnostic accuracy statistics.
Association studies
Studies reporting whether device output is associated with symptoms and outcomes, that report correlations, model fit, or other measures of association, without reporting diagnostic accuracy.
Intermediate impact of monitoring studies
Studies reporting how devices impact changes in treatment, treatment adherence and adherence to appointments.
Clinical outcome studies
Studies reporting how devices impact outcomes for patients, including changes in UPDRS and quality of life.
Patient and carer opinion studies
Studies reporting how patients or carers viewed the device, such as whether it was easy to use and useful.
Clinician opinion studies
Studies reporting opinions of clinicians on the devices, such as whether they provide useful information to inform treatment and management.
Table 1 illustrates the number of studies reporting the different types of outcomes according to technology for all studies reported in full journal articles. The numbers in this table exceed the total number of papers because some papers reported on multiple classes of outcome.
PKG | STAT-ON | Kinesia 360 | KinesiaU | PDMonitor | |
---|---|---|---|---|---|
Diagnostic accuracy | 7 | 8 | 1 | 0 | 0 |
Association study | 11 | 3 | 0 | 0 | 0 |
Intermediate impact | 8 | 0 | 1 | 1 | 0 |
Clinical outcomes | 6 | 0 | 2 | 1 | 0 |
Patient and carer opinions | 4 | 1 | 1 | 1 | 0 |
Clinician opinions | 4 | 1 | 0 | 0 | 0 |
Table 2 summarises the same data for studies reported only as conference abstracts.
PKG | STAT-ON | Kinesia 360 | KinesiaU | PDMonitor | |
---|---|---|---|---|---|
Diagnostic accuracy | 1 | 1 | 0 | 0 | 0 |
Association study | 10 | 1 | 0 | 0 | 1 |
Intermediate impact | 17 | 0 | 0 | 0 | 0 |
Clinical outcomes | 4 | 0 | 0 | 0 | 0 |
Patient and carer opinions | 4 | 1 | 0 | 0 | 0 |
Clinician opinions | 2 | 2 | 0 | 0 | 0 |
It can be seen from Tables 1 and 2 that most of the published evidence is for the PKG device; there is a modest amount of primarily diagnostic accuracy evidence for STAT-ON, and almost no evidence for Kinesia 360, KinesiaU or PDMonitor. Much of the evidence is categorised as either diagnostic accuracy or association studies. These were generally proof-of-concept studies to demonstrate that the devices could provide clinically viable measurements. Evidence on the intermediate impact of the devices, such as whether their use led to changes in treatment, was generally only available for PKG. Studies reporting clinical outcomes were few, and only three (one for PKG17 and two for Kinesia 36033,34) were comparative studies, comparing device use to standard clinical practice. There was limited evidence on patient, carer or clinical opinions, mostly for PKG.
In the sections below, the included studies are summarised for each of the five monitoring technologies and for each outcome class described above. The following sections provide a general summary of the evidence. Where additional data were available in publications, the complete data extraction is presented in Appendix 4 (see Tables 51–66).
Personal KinetiGraph
This section considers the results of all the studies that assessed the PKG device. Studies are summarised narratively, according to the type of outcomes reported. This section also presents the analysis of the IPD (individual participant data) supplied for one trial (Woodrow et al. ). 17
Diagnostic accuracy
Seven papers reporting diagnostic accuracy data [sensitivity and specificity, or area under the curve (AUC)] for PKG were identified. 39–45 One further conference abstract was found,46 which is not discussed here due to limited reporting of data.
The QUADAS-2 risk-of-bias assessment of these studies is summarised in Table 3.
Study | Risk of bias | Applicability concern | |||||
---|---|---|---|---|---|---|---|
Patient selection | Index test | Reference standard | Flow and timing | Patient selection | Index test | Reference standard | |
Braybrook (2016)39 | High | Unclear | High | Unclear | Low | Unclear | Low |
Horne (2015)41 | High | High | High | Unclear | Low | Unclear | Low |
Horne (2016)40 | High | High | Unclear | Low | Low | High | Low |
Khodakarami (2019) A42 | High | High | High | Unclear | Unclear | Unclear | Unclear |
Khodakarami (2019) B43 | High | High | Unclear | Unclear | Low | Unclear | Low |
McGregor (2018)44 | High | High | Low | Low | Low | Unclear | Low |
Watts (2021)45 | Unclear | Unclear | Unclear | Unclear | Low | Unclear | Low |
The risk-of-bias assessment identified substantial concerns with the included diagnostic accuracy studies. Reporting was frequently poor, leading to an ‘Unclear’ assessment and, where risk could be assessed, studies were often at high risk of bias. Four of the studies were case-control studies,40,41,43,44 which are generally accepted as having high risk of bias, as the patient’s condition is known before the PKG assessment is performed. In most studies the reference standard was not described in detail, often limited to just stating that it was clinical opinion. Similarly, the exact test being assessed was rarely described. The EAG have assumed that it was the output of the PKG device in some form, but it is unclear whether the output or algorithm used is the same as for the current device in actual use, hence our ‘Unclear’ classification for the applicability of the index test in these studies. There were also concerns with the flow and timing component of risk-of-bias assessment, because it was generally not clear when the reference standard and index tests were performed, and whether each was assessed blinded to the results of the other.
It should be noted, however, that some of these risk of bias issues may be due to the nature of the studies and the condition. There is no clearly established reference standard for measuring PD symptoms beyond clinician and patient assessment (e.g. by using UPDRS). This is unlikely to be a perfect reference standard. Indeed, a possible benefit of PKG (and the other technologies) is that they may provide a more accurate evaluation of symptoms than patient recall or clinical opinion; this cannot be easily determined from a diagnostic accuracy study. Also, the studies do not appear to have been designed as formal diagnostic accuracy studies. Most were proof-of-concept studies where diagnostic accuracy data were reported alongside other information. This may explain why some aspects of bias risk were not clearly reported.
A summary of the diagnostic accuracy data reported in the included studies is shown in Table 4. None of the studies were from the UK, but most were from Australia or the USA, and so are likely to have results that generalise to the UK population. Studies varied substantially in size: from 26 to 373.
Study | Study type | n | Reference standard | Outcome | Sensitivity | Specificity | AUC (%) |
---|---|---|---|---|---|---|---|
Braybrook (2016)39 Australia |
Prospective cohort | 85 (cohort 1) 87 (cohort 2) |
Clinical judgement | Tremor | 92.5 90.3 |
92.9a 92.7 |
92 |
Horne (2015)41 Australia |
Case-control | 36 cases, 16 controls | AIMS and UPDRS | Fluctuation (wearing off of DK) | 97.1 | 87.5a | 98 |
Horne (2016)40 Australia |
Case-control | 18 cases, 35 controls | AIMS and UPDRS | BKS | 100 | 83 | 96 |
Khodakarami (2019) A42 Australia |
Cohort | 172 | Clinical opinion | Suitability for device-assisted therapy | 89 | 86.6 | 93 |
Khodakarami (2019) B43 Holland, USA and Australia |
Case-control | 199 cases, 174 controls | Levodopa challenge test (clinic assessed) | Levodopa response | 92 | ||
McGregor (2018)44 Australia |
Case-control | 72 cases, 46 controls | Polysomnography | Sleep disturbance and quality | 80 | 86a | |
Watts (2021)45 USA |
Cohort | 26 | UPDRS | Treatment classification accuracy | 84.5 (± 0.7) | 81.7 (± 2.2) | 83.1 (± 1.1) |
Diagnostic accuracy results were generally poorly reported. None reported actual numbers of true positives, etc., and most did not report confidence intervals (CIs) or standard errors for the reported estimates. Each study examined a different outcome, with no replication of outcome across different studies. Most studies used some form of clinical judgement as the reference standard, generally using UPDRS to measure symptoms.
The three studies that reported bradykinesia, dyskinesia and tremor39–41 showed high diagnostic accuracy of PKG to detect these, with sensitivities above 90% and specificities ranging from 83% to 92.9%. This suggests that PKG is able to measure key PD motor outcomes. The one study of sleep disturbance showed slightly poorer diagnostic accuracy (80% sensitivity, 86% specificity),44 suggesting that PKG may not be as effective at identifying people with sleep disturbance.
Three studies examined the diagnostic accuracy of PKG for making treatment decisions. 42,43,45 The two studies by Khodakarami showed that PKG had a reasonably good ability to identify patients’ levodopa response (92% AUC) or need for device-assisted therapy (AUC 93%). One small study showed slightly poorer performance for accuracy of treatment classification (AUC 83.1%),45 but the clinical relevance of this classification was unclear.
Association outcomes
Eleven papers reporting association outcomes for PKG were identified. 47–57 Ten further conference abstracts were found, but are not discussed here due to limited reporting (see Appendix 4, Table 54). 58–67 A summary of the results of studies reporting association data for PKG is reported in Tables 5 and 7. One study was from the UK; others were mostly from Europe, Australia or the USA. Studies varied in size; from 18 to 228 patients.
Study | Study type | n | Reference standard | Outcome | Correlation/result | p-value |
---|---|---|---|---|---|---|
Chen (2020)47 China |
Prospective cohort | 100 | UPDRS III total | BKS | 0.546 | < 0.001 |
UPDRS III tremor | % time tremor | 0.434 | < 0.05 | |||
WOQ-9 | DKS | Very weak | > 0.05 | |||
WOQ-9 | FDS | Very weak | > 0.05 | |||
Evans (2014)48 Australia |
Prospective cohort | 25 | QUIP | Impulse control behaviour | 0.79 in 19 patients (6 patients were clear outliers) | Not significant |
Griffiths (2012)49 Australia |
Prospective cohort | 44 | Modified AIMS | DKS | 0.8 | < 0.0001 |
UPDRS IV (n = 25 with bilateral PD) | Global median DKS | Not stated | < 0.05 | |||
UPDRS III (n = 25 with bilateral PD) | Global median BKS | 0.64 | < 0.0005 | |||
‘Dot slide’ test | BKS | 0.63 | < 0.001 | |||
Guan (2021)50 USA |
Prospective cohort | 18 | On–off (using UPDRS III) | BKS | −0.547 (6 months) | 0.019 |
DKS | 0.133 (6 months) | 0.598 | ||||
PTT | −0.523 (6 months) | 0.1 | ||||
PDQ39 (ADL domain) | BKS | 0.381 | 0.119 | |||
DKS | −0.057 | 0.824 | ||||
PTT | 0.16 | 0.526 | ||||
Hoglund (2021)51 Sweden |
Prospective cohort | 53 | Motor and non-motor (mood and anxiety) fluctuations | Daytime sleep | Daytime sleepiness correlated with motor symptoms, mood and anxiety amongst motor fluctuators (n = 28) | Significant |
Sleepiness diary | Daytime sleep | Weak | Not significant | |||
Khodakarami (2021)52 Australia |
Retrospective cohort | 228 | UPDRS III | % time bradykinesia | 0.4 | < 0.0001 |
UPDRS Total | % time bradykinesia | 0.34 | < 0.0001 | |||
PDQ39 | % time bradykinesia | 0.35 | < 0.0001 | |||
Klingelhoefer (2016)53 UK |
Prospective cohort | 63 | NMSQuest (n = 30 with excessive daytime sleepiness) | PKG sleep assessment | Significant correlation (no significant correlation in ‘non-sleepy’ patients) |
Significant |
PDQ8 (n = 30 with excessive daytime sleepiness) | PKG sleep assessment | 0.46–0.6 | Not significant | |||
Knudson (2020)54 Denmark |
Prospective cohort | 34 | UPDRS II | BK change score | Not stated | 0.006 |
DKS | Not stated | 0.007 | ||||
Kotschet (2014)55 Australia |
Case control | 98 | Polysomnography (n = 7 with > 30 minutes immobile/day) | Sleep disturbance (immobility) | 85.2% concordance | < 0.0001 |
Epworth sleepiness score | % time immobile | Not stated | 0.01 | |||
Ossig (2016)56 Germany |
Prospective cohort | 24 | Patient diary | BKS, DKS, on–off periods (calibrated) | 0.404–0.658 | < 0.05 |
Tan (2019)57 USA |
Prospective cohort | 54 | Patient diary | Fluctuation score | PKG fluctuator scores significantly differentiated early and troublesome fluctuators, as well as dyskinetic and non-dyskinetic patients, but not subtler motor fluctuations | – |
Study | Dominey (2020)68 | Evans (2020)69 | Farzanehfar (2018)70 | Joshi (2019)71 | Krause (2021)72 | Nahab (2019)73 | Santiago (2019)74 | Sundgren (2021)75 |
---|---|---|---|---|---|---|---|---|
Inclusion criteria clearly defined | Yes | No | Yes | Yes | Yes | Yes | No | Yes |
Representative sample from relevant population | Yes | Unclear | Yes | Yes | Yes | Yes | Unclear | Yes |
Clearly described and consistently delivered intervention | Yes | Yes | Yes | Yes | Yes | Yes | Yes | Yes |
Clearly described and consistently delivered comparator (if applicable)a | N/A | N/A | Yes | Yes | Yes | Yes | Yes | Yes |
Outcome measures pre-specified, reliable and consistently assessed | Unclear | Unclear | Yes | Yes | Yes | Yes | Yes | Yes |
Outcome assessors blinded | N/A | N/A | Yes | Yes | Yes | Yes | Unclear | Yes |
Attrition low and accounted for in analysis | No | Yes | Yes | Yes | Yes | Yes | No | Yes |
Free from suggestion of selective reporting | Yes | Yes | Yes | Yes | Yes | Yes | Yes | Yes |
Overall judgement of risk of bias | High | High | Low | Low | Low | Low | High | Low |
Study | Study type | n | PKG use | Comparator | Intermediate impact of monitoring |
---|---|---|---|---|---|
Dominey (2020)68 UK |
Retrospective cohort | 166 (78 new patients and 88 follow-up) | PKG for 6 days | None | Treatment recommendations were made for 92% (152/166) of patients; most commonly relating to dopamine replacement and advice on sleep hygiene and bowel management. Treatment recommendations were implemented for 73% (83/114) of patients (available data); including advanced therapy (n = 6), additional motor agent (n = 34) and additional non-motor agent (n = 16). |
Information from the PKG confirmed initial judgement in 54.5% of cases and provided additional information in 45.5% of cases. | |||||
Evans (2020)69 UK |
Pilot cohort | 61 | PKG in a virtual clinical appointment | None | 79% (48/61) of appointments were deemed successful (the clinician felt the outcome of the consultation was likely to have been the same as a face to face). Reasons for unsuccessful consultations included complex phase of disease (n = 5), problems with the PKG (n = 5), needing a blood pressure reading (n = 2) and speech problems (n = 1). |
Farzanehfar (2018)70 Australia |
Prospective cohort | 103 | PKG for 6–7 days | Clinical assessment by a neurologist | The neurologist agreed with the PKG in 90% (93/103) of cases. In 61% (63/103) of cases the PKG added to the clinical findings to the extent that the therapeutic decision was influenced. Adjustment of oral therapy was attempted in 40/80 patients with uncontrolled motor function, 9/80 were referred for advanced therapy, no change was made in 5 cases because of risk of contraindications and 26/80 did not complete the study (protocol violations). |
Joshi (2019)71 USA |
Prospective cohort | 63 (85 routine care visits) | PKG for 6 days | Clinical assessment | In 48% of patients the PKG reported a symptom not reported by the patient (24% bradykinesia, 16% dyskinesia, 8% tremor). 24% of patients reported a symptom that didn’t appear in the PKG report. |
PKG data were used to make changes in treatment plans in 79% (50/63) of patients; most commonly addition of at least one medication or changed dosage and timing of medications. | |||||
Krause (2021)72 USA |
Retrospective cohort | 104 (170 PKG reports) |
PKG for 7 days | Clinical assessment by a movement disorder specialist | PKG complemented patient input in 82.9% (141/170) of PKG reports led to medication changes in 71% (100/141) of the complimented inputs; 79 led to increase in medications, 6 led to decrease in medications and 23 led to introduction of a new drug (some encounters led to more than one medication change). |
Nahab (2019)73 USA |
Prospective cohort | 28 (clinically stable patients using levodopa) | PKG for 6 days at 2 routine visits | Clinical assessment by a movement disorder specialist | PKG revealed a higher degree of symptom severity than was noted by clinical history alone in 18 patients (64%) at visit 1 and 8 patients (29%) at visit 2, resulting in clinical management plan changes. Medication changes included adding a new medication (6 instances), stopping a medication (2), increasing (14) or decreasing (1) medication dose or adjusting dose timing (5). |
64% of patients had an increase in levodopa dose; 11% had a dose reduction. | |||||
Santiago (2019)74 USA |
Physician survey | 89 (patients considered to benefit from continuous objective measurement; 112 assessments) | PKG for 6 days | Clinical assessment by a movement disorder specialist | 32% (36/112) had an alteration to patient care as a result of PKG. The PKG most commonly yielded new information on daily off time [50% (18/36)]. |
Sundgren (2021)75 Sweden |
Prospective cohort | 66 | PKG for 6 days | Clinical assessment by a neurologist | After clinical assessment, a treatment change was recommended for 52/66 people with PD; for the remaining 14 patients the current treatment was planned to be left unchanged. After PKG review, the treatment plan proposed after the clinical assessment was changed in 31.8% (21/66) PwP. |
The clinical assessment and the PKG review differed frequently, mainly regarding overall presence of motor problems (67%), characteristics of bradykinesia/wearing off (79%), dyskinesia (35%) and sleep (55%). | |||||
Almost all patients reported good compliance and no tendency to impulse control disorder. For these items there were few disagreements between the clinical and PKG assessments (3% for impulse control disorder and 5% for compliance). |
In general, PKG bradykinesia score, per cent time in bradykinesia (PTB) and per cent time in tremor (PTT) scores were moderately correlated with UPDRS III scores (across three studies). Bradykinesia score and PTB was also moderately correlated with Parkinson’s Disease Quality of Life 39 Questions (PDQ39) scores (two studies). There was a statistically significant correlation between PKG dyskinesia score and UPDRS II in one study. PKG dyskinesia score was also significantly correlated with modified abnormal involuntary movements scale (AIMS) and PKG bradykinesia score was significantly correlated with bradykinesia measured by the ‘dot slide’ test (one study). In the same study, a subgroup of patients with bilateral PD, there was significant correlation between ‘global median dyskinesia score’ and UPDRS IV, and ‘global median bradykinesia score’ and UPDRS III.
However, in one study the Wearing-off Questionnaire-9 (WOQ-9) had a very weak, non-significant correlation with the PKG fluctuation and dyskinesia score (FDS) and dyskinesia score. Another study found that PKG fluctuator scores significantly differentiated early fluctuators and troublesome fluctuators, as well as dyskinetic and non-dyskinetic patients, but could not discriminate subtler motor fluctuations.
Results relating to sleep outcomes were more mixed. In one study, high Epworth Sleepiness Score was correlated with PKG proportion of time immobile (PTI). In the two studies that reported them, correlations between PKG variables and 3-day daytime sleepiness diaries were generally weak and non-significant. In a subgroup of patients with excessive daytime sleepiness, the PKG’s parameters for quantity and quality of night-time sleep correlated significantly with the total burden of non-motor symptoms of PD as measured by Non-Motor Symptoms Questionnaire (NMSQuest) (one study). In non-sleepy patients there was no significant correlation. There was also a moderate to high (though non-statistically significant) correlation between PKG night-time sleep markers and the Parkinson’s Disease Questionnaire-8 (PDQ8) in the excessive daytime sleepiness group. In a subgroup of patients who were immobile for > 30 minutes/day and underwent ambulatory daytime polysomnography (n = 7), periods of immobility on PKG were highly correlated with detection of sleep by polysomnography.
The ratio of medication acknowledgements/number of doses was strongly correlated with ratings of Impulsive-Compulsive Behaviours in 19/25 patients; however, 6 patients were clear outliers and fell into the false negative group; these patients had normal response ratios, but high Impulsive-Compulsive Behaviour scores.
The Bergquist (2018)59 conference abstract is worthy of note; it describes preliminary data from the ongoing WestPORTS registry study, which compares randomly selected PwP in West Sweden (n = 154) with retrospective data from clinically motivated recordings in PwP suspected to have motor fluctuations (n = 248). The PKG scores were significantly different between the two populations: median bradykinesia score 30.4 versus 23.0 (p = 0.014) and dyskinesia score 1.0 versus 3.0 (p < 0.0001) in the randomly selected population and clinically motivated recordings, respectively.
Intermediate impact of monitoring
Twenty-five studies reporting on the intermediate impact of monitoring were identified; 8 reported in full publications68–75 and 17 as conference abstracts. 76–92 The conference abstracts are not discussed here due to limited reporting (see Appendix 4, Table 57); however, results were largely consistent with those of the full papers.
The eight studies reported in full were assessed for quality using a tool developed for the review using relevant criteria. The results are summarised in Table 6.
Five of the six comparative studies (where PKG was compared against clinical assessment before reviewing the PKG data) had a low overall risk of bias. 70–73,75 However, one of the comparative studies did not clearly define the study inclusion criteria, attrition was high and it was unclear whether the clinician was blinded to PKG results at the time of outcome assessment. 74 The two uncontrolled studies had a high risk of bias. 68,69
A summary of the results of studies reporting the intermediate impact of monitoring is reported in Table 7. Two studies were from the UK; others were from Australia, the USA and Sweden.
Three studies reported the level of agreement between the PKG and clinical assessment/initial judgement; there was agreement in 54.5–90% of cases. 68,70,72 Hence, there appears to be considerable cross-study uncertainty in the consistency between PKG assessments and standard clinical assessments.
Six studies reported the proportion of patients for whom the PKG provided additional information leading to a change in the clinical management plan; this was the case in 31.8–79% of patients. 68,70,71,73–75 The most common treatment changes were the addition of at least one medication or a change in dosage while a small proportion of patients were referred for advanced therapy. This suggests that there will be a proportion of patients for whom PKG will lead to changes in management, but also a substantial proportion where management will be unchanged. There is considerable uncertainty concerning exactly how many patients will have changes to management if PKG is used. It was unclear from the publications how a decision to change, or not change, management related to patient symptoms, nor exactly what the changes were (such as how much levodopa dosage was adjusted).
One study assessed PKG use in virtual clinical appointments; 79% of virtual appointments were deemed successful (the outcome of the consultation was likely to have been the same as a face-to-face appointment). 69 Reasons for unsuccessful consultations included complex phase of disease, problems with the PKG, needing a blood pressure reading and speech problems.
Clinical outcomes
We identified 10 studies that reported on clinical outcomes related to PKG. As the original trial data were supplied for the Woodrow trial it is analysed in Woodrow individual participant data. The remaining studies are summarised in Other studies reporting results for clinical outcomes.
Woodrow individual participant data
Full data were made available for the Australian trial of PKG by Woodrow et al. 17 This was not randomised; rather, 12 centres were selected to either use PKG for the management of patients or use standard clinical practice. PKG clinics were generally those with existing experience of using PKG in practice. Patients were assigned to clinics based on location and convenience. Hence, the trial can be thought of as a quasi-randomised cluster trial. All patients wore the PKG smartwatch, and so were blind to which arm they were in. In total, 200 patients were recruited to the study. After withdrawals and patients with incomplete data were excluded, the EAG analysed 162 patients; 77 patients were managed using PKG, and 85 using standard care.
PKG measurements were taken for all patients, but only given to clinicians in the PKG arm. Patients were seen every 5 weeks, with PKG measurements taken before each visit until their PKG measurements were judged to be ‘in target’ (defined as bradykinesia score < 26 and dyskinesia score < 7 from PKG assessment), with a maximum of five consultations.
The risk of bias of the trial was assessed using the Cochrane risk of bias tool, and is reported in Table 8. Although Cochrane risk of bias is intended for RCTs, it was considered the most suitable tool for assessing the Woodrow trial. The main risk of bias in the trial was because it was not strictly randomised. Other aspects of the trial were judged to be at low risk of bias.
Study | Woodrow17 |
Risk of bias arising from the randomisation process | High |
Risk of bias due to deviations from the intended interventions | Low |
Missing outcome data | Low |
Risk of bias in measurement of the outcome | Low |
Risk of bias in selection of the reported result | Low |
Overall judgement of risk of bias | Some concerns |
The supplied IPD was checked for potential bias problems, including imbalances across trial arms. When examining patient characteristics at recruitment, the EAG could not exactly match results presented in the trial publication,17 but inconsistences were small and most likely due to differences in how excluded patients were evaluated. The EAG found no substantial imbalance in patient characteristics between PKG and non-PKG patients, so, although the trial was not randomised, there does not appear to be any bias due to imbalance between arms. Missing data were largely confined to patients who were excluded or withdrew from the trial. There was no evidence of imbalance in missing data between arms.
The IPD included the following outcomes, which are reanalysed here:
-
UPDRS (I, II, III, IV and Total):
-
part I covers non-motor aspects of daily living (e.g. depression and anxiety);
-
part II covers motor aspects of daily living (e.g. walking and eating);
-
part III is the full motor assessment; and
-
part IV covers motor complications (dyskinesia and ‘on–off’ times).
-
-
Levodopa equivalent dose (LED).
-
H&Y.
-
Median bradykinesia score, active bradykinesia score and dyskinesia score.
-
Montreal Cognitive Assessment (MOCA).
-
NMSQuest.
-
PDQ-39.
-
The severity of predominantly non-dopaminergic symptoms in Parkinson’s disease (SENS PD).
-
Percentage time with bradykinesia, dyskinesia or tremor.
-
Percentage time inactive or immobile.
These are broadly the same outcomes reported in the trial publication, except time inactive was not reported in the paper so there is no clear evidence of reporting bias. Figure 2 shows the difference between PKG and non-PKG patients in terms of change in outcome from baseline, for all the outcomes reported above. The circles show the estimated difference between PKG and non-PKG arms, and the horizontal lines are 95% CIs. Results to the left of the red line indicate those favouring PKG. Results for LED are divided by 100, to fit on this plot. Full numerical results for this analysis are given in Appendix 5, Table 67.
FIGURE 2.
Results from the Woodrow trial: difference between PKG and standard care. BKS, bradykinesia score; DKS, dyskinesia score; SENS-PD, severity of predominantly nondopaminergic symptoms in Parkinson’s disease.

The results show that the use of PKG appears to improve UPDRS scores, particularly UPDRS III (by around 3.1 points) and IV (by around 1.2 points) and hence, total score. This is likely to be because PKG use is reducing time with bradykinesia (by 2.1 percentage points) dyskinesia (by 1.5 percentage points) and tremor (by 0.6 percentage points), although none of these reductions achieved statistical significance. Results for other outcomes are mostly in the direction of favouring PKG, but effect sizes are mostly small and CIs wide. Use of PKG appears to improve symptoms, without requiring substantial increases in levodopa dose.
The main exception to the general trend favouring PKG was for time inactive, which was higher in PKG patients than in non-PKG patients, by about 2.7% points. It is not clear why this discrepancy might occur. It was notably not reported in the original trial publication.
We examined models that adjusted for potential confounding factors, namely: age, sex, PD duration, UPDRS III at baseline and number of clinic visits during follow-up. This found that sex and age had no impact on results, but the other factors could alter outcomes. We reanalysed the data for all outcomes adjusting for PD duration, UPDRS III at baseline and number of clinic visits during follow-up. Results of this analysis are given in Appendix 5, Figure 11 and Table 67.
Overall, the results for the adjusted analyses were similar to the original analyses (Figure 2). The benefit of PKG was marginally reduced for some outcomes. For example, the benefit of PKG on UPDRS IV declined from 1.2 to 0.7 points. This might suggest that some of the observed benefit of PKG is because people in the PKG arm had more clinic visits.
Further analysis of the impact of both number of visits and baseline UPDRS III on outcomes was performed, by analysing outcomes separately for each number of visits and by quartiles of UPDRS III score at recruitment. These analyses are summarised in Appendix 5, Figures 12 and 13. These show that the benefits of PKG were mostly in patients who had poor UPDRS III scores initially, and those who required more visits before symptoms became in target. This suggests that PKG may be of most benefit to patients with more severe, and less manageable, symptoms. However, numbers of patients in each group were small (e.g. 48 patients had only one visit; 29 required four visits).
To match analyses performed in the trial publication, we also performed a subgroup analysis examining the impact of PKG on all outcomes according to whether patients were judged to be ‘in target’ or ‘out of target’ at baseline, using their PKG results (28 patients were ‘in target’ and 134 were ‘out of target’). The results of this analysis are summarised in Figure 3, and given in full in Appendix 5, Table 68.
FIGURE 3.
Impact of PKG in the Woodrow trial, by ‘in target’ status at baseline. BKS, bradykinesia score; DKS, dyskinesia score; SENS-PD, severity of predominantly nondopaminergic symptoms in Parkinson’s disease.

These results suggest PKG use predominately improves symptoms (particularly bradykinesia and UPDRS scores) in people who were not ‘in target’ and whose condition was not adequately controlled. For people whose condition was ‘in target’, there are no improvements in UPDRS or time in bradykinesia. However, for ‘in target’ patients on PKG, the percentage time in dyskinesia and LED were lower compared to non-PKG patients, although neither result was statistically significant because patient numbers were limited in this group.
This suggests that using PKG can be useful in improving UPDRS, by reducing bradykinesia in patients whose disease is not being adequately controlled, while possibly allowing for levodopa dose reduction and consequent reduction in dyskinesia in patients whose condition was already well-controlled.
The supplied IPD permitted analysis for three further dichotomous outcomes: change in medication, referral for device-assisted therapy (exact therapies were not reported) and ‘in target’ status. The first two were not included in the trial publication, but of relevance to this assessment. Odds ratios for these three outcomes are given in Table 9. Models adjusted for PD duration, UPDRS III at baseline and number of clinic visits during follow-up gave broadly similar results. The data were insufficient for analyses by target status at baseline.
Outcome | Number with PKG | Number with standard care | Odds ratio | 95% CI | |
---|---|---|---|---|---|
Change in medication | 49 | 39 | 1.18 | 0.52 | 2.68 |
Referral for device-assisted technology | 36 | 13 | 4.01 | 1.82 | 8.85 |
In target at follow-up | 34 | 14 | 3.43 | 1.67 | 7.03 |
The results suggest that patients using PKG were substantially more likely to be ‘in target’ at follow-up and to be referred for device-assisted technologies. There was no clear evidence that patients using PKG were more likely to have a change in medication.
Other studies reporting results for clinical outcomes
Nine cohort studies reported on clinical outcomes related to PKG; five were reported in full publications70–73,75 and four were reported as conference abstracts. 65,82,84,88 The quality assessment results for the five studies that were reported in full are presented in Table 6, as these studies also reported intermediate outcomes. All five had a low overall risk of bias. 70–73,75 Quality assessment was not undertaken for the conference abstracts, owing to limited reporting.
A summary of the results of the studies reporting clinical outcomes related to PKG are reported in Table 10, including results for the conference abstracts, given the importance of clinical outcomes to the assessment of PKG.
Study | Study type | n | PKG use | Comparator | Clinical outcomes |
---|---|---|---|---|---|
Studies reported in full publications | |||||
Farzanehfar (2018)70 Australia |
Prospective cohort | 103 | PKG for 6–7 days | Clinical assessment by a neurologist | 33/80 uncontrolled PwP were treated with oral therapy; motor scores and function were brought under control in 14 cases. In 19/33 cases it was not possible to reach therapeutic targets by the end of the study; 7 were reclassified, 3 were referred to advanced therapy and 4 were classed as ‘treatment contraindicated’. |
Significant improvements from baseline to final visit were observed in the 33 treated patients: | |||||
|
|||||
Improvements in quality of life (PDQ39) were significant in the subgroup of 14 patients whose symptoms were brought under control (effect size = 8.5, p = 0.03), but not the full population of 33 treated patients (effect size = 10, p = 0.08). | |||||
Joshi (2019)71 USA |
Prospective cohort | 63 (85 routine care visits) | PKG for 6 days | Clinical assessment | No serious adverse events or adverse device effects were reported. |
Krause (2021)72 USA |
Retrospective cohort | 104 (170 PKG reports) |
PKG for 7 days | Clinical assessment by a movement disorder specialist | Out of 104 patients, 49 had more than 1 PKG encounter; 37 had 2 encounters (mean interval 6.3 months between encounters), 7 had 3 encounters (mean interval 11.4 months between first and last encounter) and 5 had 4 encounters (mean interval 15.8 months between first and last encounter). Most patients undergoing 3 or 4 PKG encounters did not reach a controlled state as defined by PKG until the 3rd or 4th encounter. |
Nahab (2019)73 USA |
Prospective cohort | 28 (clinically stable patients using levodopa) | PKG for 6 days at 2 routine visits | Clinical assessment by a movement disorder specialist | Mean MDS-UPDRS III summary score significantly reduced (improved) from 28.9 at visit 1 to 24.1 at visit 2 (p < 0.028). Mean MDS-UPDRS IV summary score reduced from 4.1 at visit 1 to 3.0 at visit 2 (p = 0.07). |
BKS, DKS, percentage time immobile and percentage time in tremor showed no clear evidence of change from visit 1 to visit 2. H&Y ratings were similar between visit 1 and visit 2; at visit 2, 5 patients (18%) were rated as having improved one H&Y stage and 6 had worsened one stage. |
|||||
On the CGI-I scale, 17/28 patients (61%) showed improvement, 9 (32%) no change and 2 (7%) minimally worse. On the PGI-I scale, 13/24 patients (54%) indicated improvement, 9 (38%) no change, 2 (8%) minimally worse and 4 patients did not respond. |
|||||
Sundgren (2021)75 Sweden |
Prospective cohort | 66 | PKG for 6 days | Clinical assessment by a neurologist | There were no significant differences in clinical variables when repeated after 3–6 months (mean score at baseline and follow-up) in Parkinson’s Disease Composite Scale (18.5, 18.8), NMSQ (9.0, 8.8), PDQ-8 (22.7, 21.5), EQ VAS (66.0, 66.7), BKS (27.9, 27.4), DKS (3.5, 3.2), FDS (8.5, 8.6). Overall change at follow-up (assessed using CGI-I Scale) was 3.6; a score of 4 represents no change, a lower score represents improvement. |
Studies reported as conference abstracts | |||||
Farzanehfar (2017)82 Location not stated |
Prospective feasibility study | 28 (with controlled symptoms) | PKG | Clinical assessment | Patients with uncontrolled bradykinesia (n = 21) showed statistically significant improvements in UPDRS III and total UPDRS following intervention. In the 8 patients recognised as poorly controlled by PKG alone, there were statistically significant improvements in UPDRS III and total UPDRS following intervention (median improvement = 13; p = 0.01). |
Horne (2016)65 Location not stated |
Interim findings of prospective cohort study | 19 (considered well controlled by general neurologists) | PKG | Assessment by a movement disorder specialist | Clinically significant changes in patient outcomes were noted in the UPDRS, PKG scores and PDQ-8. |
Horne (2018)84 Australia |
Pilot prospective cohort study | 103 | PKG | Clinical assessment | At the end of the study, 48% of patients were in target (22% at outset and 26% by treatment change, not including those referred for advanced therapy). In those in whom oral therapy was changed, total UPDRS and PDQ-39 improved (effect size 8 and 10, respectively). MOCA scores also improved significantly. |
Lynch (2018)88 Australia |
Cohort study | 80 (uncontrolled patients) | PKG | None | Among 33 patients treated with oral therapy, decreases were observed in: mean UPDRS II (−4; 9–13), mean UPDRS III (−3; 36–39), per cent over target (−8; 64–73) and PDQ-39 (10; 19–29). |
Two full papers reported results for UPDRS changes. 70,73 One study, which reported that PKG resulted in clinical management plan changes in 64% of patients at visit 1 and 29% of patients at visit 2, reported a significant reduction (improvement) in mean MDS-UPDRS III summary score between visit 1 and visit 2 (from 28.9 to 24.1, p = 0.028). Mean MDS-UPDRS IV summary score was also reduced (from 4.1 to 3.0, p = 0.07). 73
Another study reported the proportion of ‘uncontrolled’ patients whose symptoms were brought under control after changes in oral therapy following assessment using PKG; 14/33 patients were brought under control (within 2–4 visits), while it was not possible to reach therapeutic targets by the end of the study in 19/33 patients. Significant improvements were observed in UPDRS I, UPDRS II, UPDRS III, UPDRS IV and total UPDRS. 70
Four conference abstracts reported limited data on UPDRS and other outcomes. 65,82,84,88 One study randomised patients to clinical examination followed by PKG or PKG followed by clinical examination. 82 Twenty-four patients were identified as uncontrolled; 16 by both clinical history and PKG, 8 by PKG alone. Following intervention there were statistically significant improvements in UPDRS III and total UPDRS amongst all patients with uncontrolled bradykinesia and amongst the eight patients identified as uncontrolled by PKG alone. 82
Another study that randomised patients to clinical examination followed by PKG or PKG followed by clinical examination also reported clinically significant changes in PKG scores, UPDRS and PDQ-8 after treatment for bradykinesia or fluctuations; the movement disorder specialist recognised the same symptoms as PKG in 10 patients, but would not have recognised 6 patients at poorly controlled without the PKG. 65
In one study, oral therapy was recommended in 74% of patients due to PKG scores being outside the target range; there were significant improvements in total UPDRS, PDQ-39 and MOCA scores in patients whose oral therapy was changed. 84 The final conference abstract reported improvements in UPDRS II, UPDRS III, per cent over target and PDQ-39 amongst 33 patients treated with oral therapy, who were found to have uncontrolled disease with the assistance of PKG. 88 While these results appear promising, two of the studies were very small and it was not possible to assess their quality owing to limited reporting.
Other clinical outcomes were less thoroughly reported and outcomes reported varied amongst the studies reported as full publications. In the study that reported that PKG resulted in clinical management plan changes in 64% of patients at visit 1 and 29% of patients at visit 2, PKG scores and H&Y ratings were similar between visit 1 and visit 2. 73 On the Clinician Global Impression of Improvement (CGI-I) scale, 61% of patients showed improvement, 32% no change and 7% minimally worse. On the Patient Global Impression of Improvement (PGI-I) scale, 54% of respondents indicated improvement, 38% no change and 8% minimally worse (four patients did not respond). 73 However, it is not clear how many patients would have had a change in treatment anyway, regardless of PKG; reporting was unclear.
In the study that reported the proportion of ‘uncontrolled’ patients whose symptoms were brought under control after changes in oral therapy following PKG assessment, there were significant improvements in NMSQ and MOCA between baseline and the final visit amongst the 33 patients who had a change in therapy. Improvement in quality of life, measured using PDQ-39, was not statistically significant amongst the full population who had a change in therapy; however, there was a statistically significant improvement in the subgroup of 14 patients whose symptoms were brought under control. 70
Another study, which reported that PKG resulted in treatment plan changes in 31.8% of patients, reported that there were no significant differences in clinical variables (PKG bradykinesia score, PKG dyskinesia score, PKG Fluctuations dyskinesia score, PD Composite Scale, NMSQ, PDQ-8 and EuroQoL Visual Analogue Scale) when repeated after 3–6 months. 75 However, the CGI-I scale showed a slight overall improvement at follow-up (mean score = 3.6). 75
A retrospective cohort study reported that 49/104 patients required more than one PKG encounter to reach a controlled state; 37 patients had two encounters, 7 patients had three encounters and 5 patients had four encounters. Most patients undergoing three or four PKG encounters did not reach a controlled state as defined by PKG until the third or fourth encounter. 72
One study merely reported that there were no serious adverse events or adverse device effects reported. 71
Patient and carer opinions
A total of eight studies reported patient or carer opinions on PKG. 68,69,71,73,78,91,93,94 The four studies reported in full also reported intermediate or clinical outcomes, so the quality assessment of these studies is reported in Table 6. 68,69,71,73 Four conference abstracts only reported survey results. 78,91,93,94 These have not been assessed for quality due to lack of information in abstracts.
The results are summarised in Table 11, with further data in Appendix 4, Table 63.
Study | n | Topic/question asked | Agreed (%) | Disagreed (%) | Neutral (%) | Don’t know (%) |
---|---|---|---|---|---|---|
Full papers | ||||||
Dominey (2020)68 UK |
62 | Introductory information and instructions were helpful | 79.0 | |||
Process of returning the device simple | 98.0 | |||||
Valued the medication reminders | 80.0 | |||||
Perceived the results as reflective of their lived experience | 60.0 | |||||
Valuable in providing additional information to their clinical team | 59.0 | |||||
Willing to continue using it as part of their management | 97.0 | |||||
Satisfaction at not having to travel to clinic | 40.0 | 44.0 | ||||
Evans (2020)69 UK |
46 | Satisfied with virtual clinic | 89.0 | 7.0 | 7.0 | |
Joshi (2019)71 USA |
63 | PKG medication reminders assisted me with taking my medication on time | 75.3 | 2.4 | 11.8 | 10.6 |
PKG data assisted me with explaining my symptoms to my doctor | 61.4 | 4.8 | 24.1 | 9.6 | ||
The PKG provided additional data that assisted my doctor with making decisions about my care | 78.6 | 1.2 | 10.7 | 9.5 | ||
The PKG was easy to use | 85.7 | 3.6 | 0.0 | 10.7 | ||
I was able to wear the PKG and complete medication use confirmations as instructed by my doctor | 84.3 | 4.8 | 0.0 | 10.8 | ||
I would be willing to use the PKG again to assist in the management of my Parkinson’s disease in the future | 86.9 | 1.2 | 1.2 | 10.7 | ||
Other questions omitted due to similarity. Results presented as proportion of visits (n = 85) | ||||||
Nahab (2019)73 USA |
28 | PKG was easy to use | 93.0 | |||
Performed as expected | 96.0 | |||||
Would use it again (if not charged) | 100.0 | |||||
Would use it again (if payment required) | 32.0 | |||||
Device assisted with explaining symptoms to the physician | 79.0 | |||||
Assisted with taking medication on time | 100.0 | |||||
Valuable in providing data to the physician they could not provide | 89.0 | |||||
Abstracts | ||||||
Chhabria (2018)78 Location not stated |
50 | Overall, patients reported high satisfaction with wearing the device | ||||
Rasul (2017)93 USA |
28 | PKG was very easy to use (strongly agreed) | 68.0 | |||
Able to wear PKG and complete medication use confirmations as instructed by doctor | 96.0 | |||||
The feature of PKG for reminder was very helpful for medication compliance | 97.0 | |||||
Spengler (2016)94 USA |
5 | Patients felt that PKG was helpful with medication reminders and helped explain the symptoms better | ||||
Thomas (2019)91 UK |
NR | 98% of patients reported a positive/neutral user experience |
In surveys of patients and carers most agreed that PKG was generally easy to use. PKG appeared most useful to patients as a reminder to take medication, with between 75.3% (Joshi et al. )71 and 100% (Nahab et al. )73 agreeing that PKG was useful for that purpose. Results were more equivocal for whether PKG provided useful information on symptoms that the patients or carers could not themselves provide; between 59% (Dominey et al. )68 and 79% (Nahab et al. )73 of patients agreed this was the case. It is unclear whether this was because people felt the device was not providing useful information, or because their symptoms were such that the device did not provide extra information.
Patients were generally willing to use the PKG device again, although less so if required to cover the costs themselves (Nahab et al. ). 73 In the study that used PKG for the remote management of patients (Evans et al. ),69 patients were generally happy with the virtual clinic approach, suggesting that PKG could reasonably be used for fully remote monitoring and assessment.
Clinical opinions
A total of six studies reported clinicians’ opinions on the value of PKG. 71,73–75,87,90 These are summarised in Table 12. The studies reported in full also reported intermediate or clinical outcomes, so the quality assessment of these studies is reported in Table 6. Two conference abstracts only reported survey results. 87,90 These have not been assessed for quality due to the lack of information in the abstracts.
Study | n | Question asked | Agreed (%) | Disagreed (%) |
---|---|---|---|---|
Full papers | ||||
Joshi (2019)71 USA |
63 | Improved dialogue with patient | 59 | |
Improved ability to assess impact of a therapy | 38 | |||
Improved ability to assess need for additional tests or treatments | 7 | |||
Improved ability to assess patient PD symptoms | 33 | |||
Some questions omitted due to similarity | ||||
Nahab (2019)73 USA |
28 | Improved dialogue with patient | 89 (visit 2) | |
Improved ability to assess impact of a therapy | 89 (visit 2) | |||
Improved ability to assess need for additional tests or treatments | 4 (visit 2) | |||
Santiago (2019)74 USA |
112 | Did PKG provide additional information? | 41 | 59 |
Was a clinical management plan change made? | 32 | 9 | ||
Sundgren (2021)75 Sweden |
66 | PKG improved the dialogue with the participant | 88 | |
Abstracts | ||||
Langston (2017)87 USA |
89 | PKG provided additional information not available from clinical consultation alone | 38 | |
Thakur (2017)90 USA |
51 | PKG provided information not available from the clinical consultation that drove a clinical management plan change | 47 |
Clinicians’ opinions of PKG were generally less favourable than patient opinions. Most clinicians agreed that use of PKG improved dialogue with patients. 71,73,75 They were generally less convinced that PKG improved their assessment of symptoms or need for changes in therapy. Four of the studies found that PKG provided additional useful information in only 32–47% of patients. 71,74,87,90 Nahab73 was an outlier, where 89% of clinicians felt that PKG improved their ability to assess impact of therapy.
It should be noted that these clinical opinions somewhat contradict the actual evidence on changes in treatment (see Intermediate impact of monitoring and Table 7), where many patients did have a change in treatment as a result of PKG use.
Summary of Personal KinetiGraph evidence
Much of the published evidence for PKG consists of either diagnostic accuracy or association studies, mostly designed as proof-of-concept studies to show that PKG can usefully measure symptoms associated with PD. This evidence broadly suggests that PKG can accurately measure dyskinesia, bradykinesia and tremor. Sensitivity estimates (for bradykinesia and fluctuations in dyskinesia) were high (near 100%), but specificity estimates were generally lower (83–87.5%), suggesting a possibility that PKG may slightly overdiagnose symptoms. PKG appears to have lower accuracy for measuring sleep disturbance. Diagnostic accuracy studies also suggest that PKG has reasonable accuracy when diagnosing clinical factors, such as levodopa response and need for device-assisted therapy. It should be noted that the quality of diagnostic evidence is low and lacks replication, with most outcomes studied in only one study. This is partly due to the limitations of performing formal diagnostic accuracy studies in this field, as all outcomes are usually assessed by clinicians and patients, with no clear objective reference standards.
The results of the association studies generally supported those of the diagnostic accuracy studies; PKG bradykinesia, dyskinesia and tremor scores were generally moderately correlated with UPDRS scores, while the evidence for sleep and impulse control behaviours was less promising. The evidence on the impact of PKG on sleep disturbance, and other non-motor aspect PD, was extremely limited.
The studies investigating the intermediate impact of PKG were generally of good quality (5/8 studies had a low risk of bias). The evidence from those studies indicates that PKG is being used by clinicians to guide treatment decisions, primarily the addition of a new therapy or increase in treatment dose. PKG use is likely to lead to a change in management for a sizeable proportion of patients (plausibly 31.8–79% of patients). However, this also means that many patients will have no change in management after PKG use, although its use may still be helpful for confirming that no change is required. As these studies were not formal comparative studies there is a possibility that some medication changes made because of PKG results were unnecessary, or helpful medication changes may have been missed. The population of these studies was newly diagnosed PwP, those attending routine follow-up, clinically stable patients and those considered to benefit from continuous objective measurement, where stated. There was no comparison of different patient populations; therefore, no indication of which patients are more likely to have management changes as a result of PKG use.
The majority of the evidence on the clinical value of PKG came from a single trial of 162 patients (Woodrow et al. ). 17 This trial compared centres using PKG to those using standard assessment methods. It was not randomised but was otherwise at low risk of bias. The Woodrow trial demonstrated that patients managed with PKG appear to benefit more than those on clinical management alone, with improvements in UPDRS III and IV scores (by around 3.1 and 1.2 points, respectively), and plausibly reductions in bradykinesia and dyskinesia. This benefit seems to depend on whether patients were ‘in target’ (i.e. condition was under control with current treatment) before PKG use. Patients not ‘in target’ saw improved UPDRS scores, but those ‘in target’ did not. Patients ‘in target’ may, however, benefit from reduced levodopa dose and consequent reduction in dyskinesia, but this was inconclusive, as only 28 patients were ‘in target’. It should be noted that the Woodrow trial included multiple uses of the PKG device over a short period of time (once every 5 weeks); hence the clinical benefits that might occur if PKG is used less frequently might be different.
Other clinical evidence was more limited and drawn from non-comparative studies, although these studies generally had low risk of bias. This additional evidence supports PKG giving improvements in UPDRS scores; study populations varied, with some studies including clinically stable patients with well controlled symptoms, while others included uncontrolled patients. It also suggested improvements in outcomes in the majority of patients as assessed by patients and clinicians using PGI-I and CGI-I scales. As these studies were not comparative it is unclear how much of the benefit can be ascribed to PKG use specifically.
There was limited evidence on patient, carer or clinician opinions of PKG. Patients had generally favourable opinions as to the value of PKG, particularly valuing its ability to remind them to take medication. Patients often found that PKG helped with discussing or reporting symptoms with their clinician. Clinicians were more equivocal about the value of PKG, with many thinking that PKG provided no additional clinical information over their own judgement, although the majority agreed that PKG improved dialogue with the patient.
There was some evidence, from one study, that PKG can be used for remote management of PD. 69 In that study, clinicians judged that most remote assessments were successful, and patients were generally satisfied with a remote assessment of their condition.
Overall, the EAG concludes that there is a reasonable body of evidence to support the use of PKG in practice. PKG appears to be able to reliably measure bradykinesia and dyskinesia, and provides useful information that leads to changes in clinical management for at least some patients. There is some evidence that using PKG leads to genuine clinical improvements for patients when compared to standard management, in terms of reductions in UPDRS scores, but this is based on a single trial. However, multiple clinic visits and PKG assessments may be required before patients reach a controlled state.
STAT-ON
This section considers the results of the 15 publications that reported evidence on the STAT-ON device. It should be noted that several included publications, particularly those reporting diagnostic accuracy taken from the manufacturer website, did not explicitly name the device used as STAT-ON. However, as the papers were listed as relating to STAT-ON on the manufacturer website, they are included in this assessment.
Diagnostic accuracy
A total of eight papers reporting on diagnostic accuracy for STAT-ON were identified. 18,21,22,24,26,28,30,31 None of these explicitly identified the device used as STAT-ON; however, all were listed on the device website, so it is assumed that the device is the same as STAT-ON. Many of the papers overlap in authorship, and most report data from the REMPARK study, or related studies, supported by the manufacturer. All studies are reported here for completeness, but it should be noted that they are not independent and may have overlapping populations. One further conference abstract was found, which is not discussed here due to limited reporting of data (this study reported that no device-related adverse events were reported). 19
The quality assessment of the papers is given in Table 13.
Study | Risk of bias | Applicability concerns | |||||
---|---|---|---|---|---|---|---|
Patient selection | Index test | Reference standard | Flow and timing | Patient selection | Index test | Reference standard | |
Bayes (2018)18 | Unclear | Unclear | Unclear | Unclear | Low | Unclear | Unclear |
Perez-Lopez (2016)22 | Unclear | Unclear | Unclear | Unclear | Low | Unclear | Unclear |
Perez-Lopez (2016)21 | Unclear | Unclear | Unclear | High | Low | High | Unclear |
Rodriguez-Martin (2017)24 | Unclear | Unclear | Unclear | Unclear | Low | Unclear | Low |
Rodriguez-Molinero (2015)26 | Unclear | Unclear | Unclear | High | Low | High | Low |
Rodriguez-Molinero (2018)28 | Unclear | Unclear | Unclear | Low | Low | Unclear | Low |
Sama (2017) A30 | Unclear | Unclear | Unclear | Low | Low | High | Unclear |
Sama (2018) B31 | Unclear | Unclear | Unclear | Low | Low | High | Unclear |
As the STAT-ON diagnostic accuracy papers were mostly reporting the REMPARK study, and/or had overlapping authorship, the QUADAS-2 assessment was very similar across publications. Risk of bias for the index test was considered unclear as papers did not generally describe the index test directly, and in some it appeared to be derived as part of the data analysis itself. Hence, it was also unclear whether these index tests are part of the current device in commercial use. Although effort was made to have robust reference standards, such as by video-recording patients for clinical assessment, or adjusting patient diaries for possible errors, it remains unclear whether clinical or patient assessment represents a robust reference standard for the outcomes considered. Flow and timing risk was often rated as high or unclear due to lack of reporting as to exactly when index tests and reference standard assessments were performed, and whether all included patients were analysed.
Table 14 presents a summary of the diagnostic accuracy data from the STAT-ON publications. No studies were from the UK; studies were mostly conducted in Spain, or in various European countries as part of the REMPARK study. Sample sizes varied from 12 to 102.
Study | Study type | n | Reference standard | Outcome | Sensitivity | Specificity |
---|---|---|---|---|---|---|
Bayes (2018)18 Spain, Italy, Israel and Ireland |
Cohort | 41 | Patient diaries + UPDRS correction | On–off times | 97 | 88 |
Perez-Lopez (2016) (AIM)22 Spain, Ireland, Italy and Israel |
Cohort | 102 | Clinical assessment based on video | DK (all n = 35) | 57 | 81 |
DK (strong trunk n = 4) | 100 | 98 | ||||
Perez-Lopez (2016) (Sensors)21 Spain |
Cohort | 35 | Clinical assessment and patient diaries | On–off times | 90.28 (by outputs) | 92.11 |
Rodriguez-Martin (2017)24 Spain, Ireland, Italy and Israel |
Cohort | 21 | Clinical assessment based on video | Freezing of gait (SVM generic) | 74.7 | 79 |
(SVM personalised) | 88.1 | 80.1 | ||||
Rodriguez-Molinero (2015)26 Spain |
Cohort | 20 (total 35 in study, exact number unclear) | Trained observer | On–off times | 96 (IQR: 93–100) | 94 (IQR: 90–100) |
Rodriguez-Molinero (2018)28 Spain |
Cohort | 23 (with advanced PD) |
Patient diaries | On–off times | PPV: 92 95% CI: 87.33 to 97.3 |
NPV: 94 95% CI: 90.71 to 97.1 |
Sama (2017) (CBM)30 Spain |
Cohort | 12 | Clinical assessment based on video | BK | 92.52 | 89.07 |
Sama (2018) (PRL)31 Spain |
Cohort | 15 | Clinical assessment based on video | Freezing of gait | 91.7 | 87.4 |
Reporting of diagnostic accuracy data was limited, with only two studies reporting numbers of true positives, etc.,21,22 and most studies not reporting CIs. Four studies evaluated whether STAT-ON can diagnose ‘on–off’ times. 18,21,26,28 These all found that STAT-ON had high diagnostic sensitivity (between 90.3% and 97%) and high specificity (88–94%), and also high positive predictive value (PPV) and negative predictive value (NPV) (92% and 94%, respectively28). Two studies evaluated freezing of gait and found that STAT-ON had high sensitivity (88.1% and 91.7%), but lower specificity (80.1% and 87.4%). 24,31
One study found that STAT-ON had high accuracy for predicting bradykinesia,30 and one study found that STAT-ON had high accuracy for predicting strong trunk dyskinesia, but poor diagnostic accuracy for predicting dyskinesia in general. 22
Overall, these results suggest that STAT-ON is good at determining ‘on–off’ times, and symptoms in the lower limbs and trunk, such as freezing of gait and trunk dyskinesia.
Association outcomes
Three small cohort studies (n = 11–75) reported only association outcomes. 23,27,29 One conference abstract that reported patient satisfaction/usability also reported limited association outcome data, but is not considered further here (see Appendix 4, Table 55 for data). 20 A summary of the association data reported in the included studies is shown in Table 15.
Study | Study type | n | Reference standard | Outcome | Correlation/result | p-value |
---|---|---|---|---|---|---|
Perrote (2021)23 Argentina |
Cohort | 11 | Patient diary | Mean hours monitored | 60 ± 9.89 vs. 40 ± 16.4 | < 0.001 |
Freezing of gait | Higher with STAT-ON | 0.003 | ||||
Hours in ON state | Higher with STAT-ON | 0.002 | ||||
Rodriguez-Molinero (2017)27 Spain, Italy, Israel and Ireland |
Cohort | 75 | UPDRS III | STAT-ON output | –0.56 | < 0.001 |
Gait (UPDRS III) | STAT-ON output | –0.73 | < 0.001 | |||
Rodriguez-Molinero (2019)29 Spain, Italy, Israel and Ireland |
Cohort | 13 | UDysRS (based on video) | DKS | 0.7 | 0.01 |
Trunk and leg DKS | 0.91 | < 0.001 |
We note that the study by Perrote did not strictly report correlation between STAT-ON and patient diaries; however, the study meets inclusion criteria for the review and fits better within ‘association outcomes’ than any of the other outcomes of interest. 23
In one study, there was a significant correlation between STAT-ON and clinical assessment using the Unified Dyskinesia Rating Scale (UDysRS) (0.70, 95% CI 0.33 to 0.88), which was higher for trunk and legs scale subitems (0.91, 95% CI 0.76 to 0.97), since the sensor is located on the waist. 29 In the largest study (n = 75) there was moderate correlation between UPDRS III and STAT-ON outputs (rho −0.56); correlation between STAT-ON outputs and the gait item in the UPDRS III was good (rho −0.73). 27 The other study reporting association data on STAT-ON simply reported that mean hours monitored was greater by STAT-ON than that recorded by the movement diary (60 ± 9.89 vs. 40 ± 16.4, p < 0.001) and that reporting of freezing of gait episodes and hours in the ON state were higher with STAT-ON compared to the patient movement diary. 23
Clinical outcomes and intermediate impact of monitoring
No studies reporting either intermediate impact or clinical outcomes were identified for STAT-ON.
Patient-, carer- and clinician-reported opinions
One paper18 and one conference abstract25 reported patient or carer opinions on STAT-ON and one paper32 and two conference abstracts20,25 reported clinicians’ opinions.
Due to the limited reporting of these studies, quality assessment was not performed. Results of the studies are summarised in Table 16, and are given in full in Appendix 4, Table 64.
Study | n | Topic/question asked | Agreed (%) | Disagreed (%) | Neutral (%) |
---|---|---|---|---|---|
Full papers | |||||
Bayes (2018)18 Spain, Italy, Israel and Ireland |
33 | Satisfaction with device (QUEST results) | 76.0 | 5.0 | 20.0 |
Santos-Garcia (2020)32 Spain (Clinician survey) |
27 | STAT-ON was considered better than the diaries | 70.3 | ||
A useful device for identifying advanced Parkinson’s disease patients | 81.5 | ||||
STAT-ON was considered from quite to very useful | 74 | ||||
STAT-ON was considered very useful | 11 | ||||
Conference abstracts | |||||
Rodriguez-Martin (2021)25 Location not stated |
30 | Caregivers find it a good or very good solution | 80 | ||
Caregivers found it easy to use | 76 | ||||
41 | Patients found it very easy to use | 77.5 | |||
19 | Neurologists think it can detect advanced PD symptoms | 88 | |||
Neurologists find the information useful or very useful | 100 | ||||
Caballol (2020)20 Spain |
39 | Patient satisfaction was high (most items scored ‘quite satisfied’ to ‘very satisfied’) |
Based on the limited evidence available, patients and caregivers generally found STAT-ON easy to use and were satisfied with the device (over 75% in two studies). 18,25 The majority of neurologists considered the device/information ‘quite’ to ‘very useful’ and considered it a useful tool for identifying patients with advanced PD symptoms. 32
One small cohort study (n = 39) reported that patient satisfaction with STAT-ON was high (assessed using Quebec User Evaluation of Satisfaction with Assistive Technology) and the system was found to be easy to use (assessed using System Usability Scale). 20
Summary of STAT-ON evidence
While a substantial body of evidence on STAT-ON has been published, this evidence is largely restricted to diagnostic accuracy studies. The diagnostic accuracy studies were of uncertain risk of bias, largely because of lack of clarity in the exact index tests being used, and difficulties in establishing a robust reference standard. In general, the studies suggest that STAT-ON can accurately determine ‘on–off’ times and bradykinesia. STAT-ON may have particular benefits in identifying trunk and lower limb symptoms. It appears to have reasonably good accuracy for diagnosing freezing of gait, and possibly trunk dyskinesia, but not dyskinesia elsewhere. However, most of these results are based on very small numbers of patients. A larger study reporting association outcomes suggests that there is a moderate correlation between STAT-ON outputs and the UPDRS III; there was good correlation between STAT-ON outputs and the gait item in the UPDRS III. 27
A key limitation in the STAT-ON evidence base is that there is currently no evidence on the intermediate impact of STAT-ON (i.e. whether its use changes clinical management of patients) or on the clinical impact of STAT-ON (such as whether it leads to improved UPDRS scores). It is therefore unclear whether STAT-ON use will lead to treatment modification, and whether patients will see a benefit in terms of reduction in bradykinesia and dyskinesia, and improvements in UPDRS scores and quality of life.
There was limited evidence on patient, carer or clinical opinions of STAT-ON; most was generally favourable.
Overall, the EAG considers that the diagnostic accuracy evidence suggests that STAT-ON is a promising technology, that is likely to be able to identify ‘on–off’ periods, bradykinesia and freezing of gait. As it is worn on the waist it may be particularly useful for detecting lower limb and trunk symptoms. However, the lack of clinical evidence for STAT-ON means that it is not currently possible to determine if its diagnostic value will translate into a real clinical value for patients. The EAG considers that, because STAT-ON is a waist-worn device and because diagnostic accuracy data relates mostly to ‘on–off’ times and freezing of gait, it cannot be safely assumed that the possible clinical benefits observed when using PKG will also apply when using STAT-ON.
Kinesia 360 and KinesiaU motor assessment systems
This section considers the Kinesia 360 and KinesiaU technologies together, as they are produced by the same company. Overall, there were three eligible studies for Kinesia 360 and one for KinesiaU. All were conducted in the USA or Canada.
Diagnostic accuracy
One study reported diagnostic accuracy for a technology judged to be equivalent to Kinesia 360. 35 It should be noted that the device used was not explicitly named as Kinesia 360, and so may not be exactly the same as the true Kinesia 360 motor assessment system. The QUADAS-2 assessment is presented in Table 17, and the results are summarised in Table 18.
Study | Risk of bias | Applicability concern | |||||
---|---|---|---|---|---|---|---|
Patient selection | Index test | Reference standard | Flow and timing | Patient selection | Index test | Reference standard | |
Pulliam (2018)35 | Unclear | Low | Low | Low | Low | High | Low |
Study | Study type | n | Reference standard | Outcome | Sensitivity | Specificity | AUC (%) |
---|---|---|---|---|---|---|---|
Pulliam, (2018)35 USA |
Prospective cohort | 13 | Clinical assessment based on video | DK | 74 | 85 | 86 |
BK | 80 | 66 | 82 | ||||
Tremor | 90 | 80 | 89 |
No diagnostic accuracy studies for KinesiaU were identified.
The risk of bias for the study was generally rated as low because the index test was clearly described, although it was not explicitly named as Kinesia 360. The index test and reference standard were assessed in a blinded fashion.
Kinesia 360 was found to have moderate to good diagnostic accuracy to detect bradykinesia, dyskinesia and tremor, with AUCs ranging from 82% for bradykinesia to 89% for tremor.
Association outcomes
There were no studies reporting association outcomes for Kinesia 360 or KinesiaU.
Clinical outcomes and intermediate impact of monitoring
Two studies of Kinesia 36033,34 and one study of KinesiaU36 were eligible for inclusion. The studies were assessed for quality using tools appropriate to their study design; the two RCTs were assessed using the Cochrane risk of bias tool and the cohort study was assessed using a tool developed for the review using relevant criteria. The quality assessment results are summarised in Tables 19 and 20.
Study | Isaacson (2019)33 | Peacock (2021)34 |
---|---|---|
Risk of bias arising from the randomisation process | Some concerns | High |
Risk of bias due to deviations from the intended interventions | Low | Low |
Missing outcome data | Low | Low |
Risk of bias in measurement of the outcome | Low | Low |
Risk of bias in selection of the reported result | Low | Some concerns |
Overall judgement of risk of bias | Some concerns | High |
Study | Hadley (2021)36 |
---|---|
Inclusion criteria clearly defined | Yes |
Representative sample from relevant population | Unclear |
Clearly described and consistently delivered intervention | Unclear |
Outcome measures prespecified, reliable and consistently assessed | Unclear |
Outcome assessors blinded | No |
Attrition low and accounted for in analysis | No |
Free from suggestion of selective reporting | Yes |
Overall judgement of risk of bias | High |
Each of the included studies had a high risk of bias or some concerns regarding bias, limiting the reliability of their results.
A summary of the results of studies reporting clinical outcomes related to Kinesia 360 and KinesiaU is reported in Table 21.
Study | Study type | n | Intervention | Comparator | Intermediate impact of monitoring | Clinical outcomes |
---|---|---|---|---|---|---|
Isaacson (2019)33 USA |
Pilot RCT | PwP (n = 39) with insufficiently controlled motor symptoms, prescribed transdermal rotigotine. | Kinesia 360 data used to supplement standard care in adjusting rotigotine dosage (n = 19). Kinesia 360 was worn throughout the day on at least 2 consecutive days in weeks 1, 2, 3, 4 and 11. | Standard care to titrate the optimal rotigotine dosage (n = 20). | Mean rotigotine dose (Kinesia 360 vs. standard care): 4.8 vs.. 3.9 mg/24 hours. Mean rotigotine dosage increase (Kinesia 360 vs. standard care): + 2.8 vs. + 1.9 mg/24 hours. Mean number of dosage changes (Kinesia 360 vs. standard care): 2.8 vs. 1.8 changes. |
Change in UPDRS II at week 12 (Kinesia 360 vs. standard care): −2.1 vs. 0.5; p = 0.004. Change in UPDRS III at week 12 (Kinesia 360 vs. standard care): −5.3 vs. −1.0; p = 0.134 (no significant difference between groups). Change in PAM-13 score at week 12 (Kinesia 360 vs. standard care): −4.6 vs. −0.2; p = 0.164 (no significant difference between groups). |
There was no significant change in PDQ-39 in either group. Mean rotigotine dosage increase from baseline to week 12 (Kinesia 360 vs. standard care): + 2.8 vs. + 1.9 mg/24 hours. Mean number of dosage changes: 2.8 vs. 1.8. Three patients in the Kinesia 360 arm and 2 patients in the standard care arm discontinued rotigotine due to treatment-emergent adverse events. |
||||||
Peacock (2021)34 Canada |
RCT | PwP (n = 25) with bothersome tremor or dyskinesia identified as a treatment target. | Telehealth follow-up care with data from in-home Kinesia 360 (n = 13). Kinesia 360 was worn for 3 days. | In-person follow-up care with clinical examination and 3-day symptom diary (n = 12). | None | Average change in PDQ-39 summary index score from baseline to completion (telehealth follow-up vs. usual care): 4.7 points (95% CI −10.2 to 0.7) vs. + 0.9 (95% CI −3.6 to 5.5). |
LEDD change from baseline (mean 32 vs. 52 mg) and appointments per participant (mean 2.2 vs. 1.8) were not significantly different between groups. | ||||||
Hadley (2021)36 USA |
Prospective cohort study | PwP (n = 16) undergoing therapy changes. | KinesiaU alongside clinical judgement. KinesiaU was worn for 3 days. | None | Therapy recommendation made based on KinesiaU and clinical judgement. | 8/13 patients who successfully used KinesiaU and returned for follow-up demonstrated improvements from their new therapy and were instructed to continue with it, while 5 were instructed to discontinue (due to lack of benefit or side effect) and return to previous therapy/dose. |
Three patients were prescribed levodopa inhalation powder (2 improved, 1 discontinued), 1 patient was prescribed trihexyphenidyl (discontinued), 3 patients were prescribed istradefylline (1 improved, 2 discontinued), 1 was prescribed increased melatonin dose (improved), 2 were prescribed exercise (improved), 2 were prescribed an increase in carbidopa-levodopa dose (1 improved, 1 discontinued) and 1 patient was prescribed increased doses of carbidopa-levodopa and trihexyphenidyl (improved). |
A small pilot RCT (n = 39) compared Kinesia 360 to supplemented standard care versus standard care alone to titrate the optimal rotigotine dosage in PwP with insufficiently controlled motor symptoms, prescribed transdermal rotigotine. 33 Mean rotigotine dose, mean rotigotine dosage increase and mean number of dosage changes were higher in the Kinesia 360 arm than the standard care arm. At week 12 there was a statistically significant improvement in UPDRS II in the Kinesia 360 arm compared with a slight worsening in the standard care arm (−2.1 vs. 0.5, p = 0.004). The difference in improvement in UPDRS III was not statistically significant between groups (−5.3 vs. −1.0; p = 0.134). There was no significant change in PDQ-39 or PAM-13 score at week 12 in either study group.
A small RCT that was suspended due to COVID-19 (n = 25) compared telehealth follow-up care using data from Kinesia 360 with usual in-person follow-up care in PwP with bothersome tremor or dyskinesia identified as a treatment target at their most recent visit. 34 The average change in PDQ-39 summary index score from baseline to completion (primary outcome) was −4.7 points in the telehealth group (95% CI −10.2 to +0.7) and +0.9 (95% CI −3.6 to +5.5) in the usual care group (mean baseline PDQ-39 score was 29 in the telemedicine group and 25 in the usual care group). Secondary outcomes were not significantly different between groups. Repeat measurement of MDS-UPDRS Part III was not completed due to suspension of face-to-face clinical and research visits, due to COVID-19.
A small cohort study (n = 16) assessed KinesiaU in PwP undergoing therapy changes. 36 Fourteen patients successfully used the KinesiaU system, while two did not complete the recordings due to user difficulty or technical issues. Thirteen of the patients who successfully used the KinesiaU system returned for a follow-up visit; the clinician reviewed the KinesiaU report with each patient and made a therapy recommendation based on the report and clinical judgement. Eight patients demonstrated improvements with their new therapy and were instructed to continue with it while five were instructed to return to their previous therapy.
Patient-, carer- and clinician-reported outcomes
One paper reported patient opinions of Kinesia 360, and one paper reported patient opinions of KinesiaU. The quality assessment results of these studies are presented in Tables 19 and 20. Table 22 summarises the patient opinion data from the two studies.
Study | Study type | N | Topic/question asked | Agreed (%) | Disagreed (%) | Neutral (%) |
---|---|---|---|---|---|---|
Peacock (2021)34 Kinesia 360 Canada |
RCT | 13 | Comfortable or very comfortable using motion sensors | 54.0 | 8.0 | 39.0 |
Telehealth patients would have preferred to be in the usual care group | 46.0 | 8.0 | 46.0 | |||
Hadley (2021)36 KinesiaU USA |
Prospective cohort | 16 | The KinesiaU system was easy to understand and use | 88.0 | 12.0 | |
I looked at the KinesiaU reports often | 32.0 | 37.0 | 31.0 | |||
The KinesiaU reports were useful to look at | 38.0 | 19.0 | 44.0 | |||
The periodic tasks were easy to perform | 88.0 | 6.0 | 6.0 | |||
I would continue to use the system on my own if it was available to me | 44.0 | 31.0 | 25.0 | |||
I would recommend this device to a friend | 53.0 | 14.0 | 33.0 | |||
The KinesiaU reports made me more aware of changes in my symptoms | 44.0 | 13.0 | 44.0 |
In the RCT of telehealth follow-up care with data from in-home Kinesia 360,34 54% of telehealth patients reported feeling comfortable or very comfortable using motion sensors and 8% were uncomfortable or very uncomfortable using motion sensors. Forty-six per cent of telehealth patients would have preferred to be in the usual care group, 8% would not and 46% were undecided. Eight per cent of usual care patients would have preferred to be in the telehealth group, 67% would not and 25% were undecided.
In the cohort study of KinesiaU,36 88% of patients agreed or strongly agreed that the KinesiaU system was easy to understand and use, while 12% disagreed or strongly disagreed. Only 32% of patients agreed or strongly agreed that they looked at the KinesiaU reports often and only 38% agreed or strongly agreed that they were useful to look at. Forty-four per cent of patients agreed or strongly agreed that they would continue to use the system if it was available to them, 25% were neutral and 31% disagreed.
Summary of Kinesia 360 and KinesiaU evidence
The evidence on Kinesia 360 was mostly from two small RCTs (64 patients in total). These suggested favourable results when using Kinesia 360, with reductions in UPDRS scores, and improvements in quality of life comparable to those seen for PKG. However, neither study used the device during routine clinical visits for PwP. In one study patients with insufficiently controlled motor symptoms were being assessed to titrate the optimal rotigotine dosage,33 and in the other study Kinesia 360 was used in remote telehealth assessments. 34
One diagnostic accuracy study found that Kinesia 360 had moderate to good accuracy for diagnosing dyskinesia, bradykinesia and tremor. 35
Given the limited evidence base, the EAG considers that Kinesia 360 is a promising technology, but there is too little evidence at present to be confident about its clinical value.
Evidence on KinesiaU was limited to one small study (16 patients). 36 The EAG considers this to be too little evidence to draw any conclusions on the clinical value of KinesiaU. Patient opinion of the KinesiaU system was not particularly favourable.
PDMonitor
One paper95 and one conference abstract37 discussing the PDMonitor technology were identified.
Diagnostic accuracy and association outcomes
One small cohort study (n = 30), reported only as an abstract, reported that PDMonitor accurately detected and estimated the severity of arm bradykinesia, dyskinesia, gait impairment, wrist tremor, leg tremor and freezing of gait compared with a clinical assessment by a PD expert physician using UPDRS III and AIMS. 37
Clinical outcomes and intermediate impact of monitoring
One case series described two cases where PDMonitor revealed information leading to medication changes and improvement in symptoms. 95 As this study only included two patients, the EAG do not think it represents useful evidence for PDMonitor and do not consider it further.
Patient-, carer- and clinician-reported outcomes
No studies reporting patient, carer or clinician opinion for PDMonitor were identified.
Summary of PDMonitor evidence
As there are currently no fully published studies of PDMonitor, the EAG considers that no conclusion as to the clinical value of PDMonitor can be drawn. It cannot be safely assumed that any possible benefits of PKG (or other technologies) will apply if PDMonitor is used.
Discussion
Summary of key results
A full summary of the EAG’s conclusions for each technology is reported in the sections of the report for each technology (see Personal KinetiGraph, STAT-ON, Kinesia 360 and KinesiaU motor assessment systems and PDMonitor). Here we present a broad summary of all evidence.
Overall, the EAG considers that only PKG has a body of research evidence sufficient to properly assess its clinical potential. PKG appears to accurately measure dyskinesia and bradykinesia, with very high sensitivity and reasonably high specificity. Diagnostic accuracy was also reasonably high for measuring tremor and treatment-related outcomes. However, its accuracy for measuring sleep disturbance was lower.
PKG is being used by clinicians to guide treatment decisions, primarily the addition of a new therapy or increase in treatment dose; PKG use led to a change in management for 32–79% of patients. The majority of the evidence on the clinical value of PKG came from a single non-randomised trial of 162 patients by Woodrow et al. 17 The trial demonstrated that patients managed with PKG appear to benefit more than those on clinical management alone, with improvements in UPDRS III and IV scores. This benefit seems to depend on whether patients were ‘in target’ (i.e. condition was under control with current treatment) before PKG use. Patients not ‘in target’ saw improved UPDRS scores, but those ‘in target’ did not (but only 28 patients were ‘in target’). Also, PKG was used frequently (every 5 weeks) in the trial, and benefits may be different if PKG is used less frequently.
Therefore, there is some evidence that using PKG leads to genuine clinical improvements for some patients. However, multiple clinic visits and PKG assessments may be required before patients reach a controlled state.
For STAT-ON, evidence is almost entirely limited to diagnostic accuracy studies. These suggest that STAT-ON can accurately diagnose ‘on–off’ times and bradykinesia. STAT-ON also seems to have reasonably good accuracy for diagnosing freezing of gait and possibly trunk dyskinesia (as a waist-worn device), but not dyskinesia elsewhere.
There is currently no evidence on the intermediate impact of STAT-ON or on the clinical impact of STAT-ON; therefore, it is unclear whether STAT-ON use will lead to treatment modification and subsequent improvements in symptoms and quality of life. Overall, the EAG concludes that while STAT-ON is a promising technology, the lack of clinical evidence means that it is not currently possible to determine if its diagnostic value will translate into real clinical value for patients.
Two small RCTs suggest favourable clinical outcomes with Kinesia 360 use in populations receiving rotigotine or when Kinesia 360 was used for remote telehealth assessments. In view of the extremely limited evidence base, the EAG considers that Kinesia 360 is a promising technology in certain situations, but that there is currently too little evidence to be confident about its clinical value.
Evidence on KinesiaU and PDMonitor are too limited to draw any conclusions on their clinical value.
Almost all the available evidence for all devices focused on motor symptoms (bradykinesia, dyskinesia and tremor). Evidence on sleep disturbance and other non-motor aspect PD was extremely limited.
The EAG considers that, because of the very different natures of the technologies assessed, such as the specific symptoms they measure, the position of the sensors on the body and the characteristics of PwP the devices have been assessed in, it should not be assumed that any clinical benefits observed for PKG would also be found with the other technologies.
There were no studies that directly compared one remote continuous monitoring device against another. In addition, there was limited evidence on the use of remote monitoring devices in different patient subgroups. Therefore, it is unclear which patients are more likely to have management changes and subsequent improvements in clinical outcomes as a result of their use. The only evidence relating to adverse events was that there were no device-related adverse events reported (PKG and STAT-ON).
Generalisability of results
The EAG considers that the results observed in the included studies are likely to be broadly generalisable to PwP in the UK. Although few studies were conducted in the UK itself, most were conducted in Europe, North America or Australia, and so their results are likely to be broadly applicable to the UK. Most studies recruited PwP without any further limitations on inclusion criteria, and without focus on specific subgroups. This would suggest that the results will be generally applicable to other PwP.
A key generalisability concern is that almost all studies were conducted in patients receiving pharmacological therapy, primarily levodopa. The clinical evidence for PKG is largely focused on how PKG use can modify levodopa therapy, and the clinical impacts of those therapy changes. Consequently, there is little to no evidence on the possible benefits of the technologies in other types of patients, such as those receiving non-pharmacological therapy, or on more advanced therapies such as DBS. The EAG does not think it safe to assume that any benefits of PKG, or other technologies, will necessarily apply to these other patient groups.
There was no evidence relating to most patient subgroups of interest. In particular, there was no evidence specifically related to people with communication barriers or difficulties, and no evidence for specific ethnicities, or by socioeconomic status. It is unclear how PKG, or other technologies, might work in those key populations.
As noted elsewhere, because of the very different natures of the technologies assessed, the EAG does not consider that any clinical benefits observed for PKG would also be found with the other technologies.
Strengths and limitations of analysis
This is the first complete systematic review of all available diagnostic and clinical evidence for PKG, Kinesia 360, KinesiaU and PDMonitor (a review of STAT-ON was published while this project was underway). This review used extensive database searches to identify all published evidence on the included technologies and followed rigorous recommended review methods to identify relevant publications, assess their risk of bias and undertake a narrative synthesis of the results. As such, this is the first fully rigorous review of these technologies, and also the first to compare the technologies in one review.
The review was strengthened by the provision of individual-level data for the key clinical trial of PKG, which permitted a more thorough examination of the clinical impact of PKG than would otherwise have been possible.
A key limitation of the analyses is due to the lack of replication across studies. In general, most outcomes were reported in only one or two studies, or outcomes were reported in inconsistent ways across studies. This meant that no meta-analysis was possible for any included studies, and the narrative synthesis was severely limited by the consequent difficulties in comparing different studies. This lack of replicability raises some concerns as to how robust the findings of the review are. It should be noted that many of the review conclusions are based on individual studies.
A further limitation is the low quality of much of the evidence, particularly for diagnostic accuracy. This casts some doubt on the validity of the diagnostic accuracy evidence. It should be noted that few studies were formal diagnostic accuracy studies, and there are innate difficulties in this field in robustly assessing diagnostic accuracy, given the lack of clear reference standards, and lack of clarity over the exact algorithms used to convert device output into diagnostic assessments.
Chapter 4 Assessment of existing cost-effectiveness evidence
This section provides an overview of existing cost-effectiveness evidence relating to the use of remote continuous monitoring devices (PKG, Kinesia 360, KinesiaU, PDMonitor and STAT-ON) as an adjunct to clinical judgement for the assessment of motor and non-motor symptoms in PwP. Given that all technologies under assessment have only recently been commercialised, it was anticipated that there would be a dearth of relevant economic evidence for the remote monitoring devices. Therefore, to inform the development of a new decision-analytic model in PD, a broader review of published cost-effectiveness studies evaluating pharmacological (e.g. levodopa, dopamine agonists) and non-pharmacological (e.g. DBS) interventions for the management of symptoms in PwP was undertaken.
Methodology of the cost-effectiveness review of remote continuous monitoring devices for people with Parkinson’s disease
Searches
The bibliographic search detailed in the Methods for reviewing clinical effectiveness was used to identify studies reporting on the cost-effectiveness of PKG, Kinesia 360, KinesiaU, PDMonitor and STAT-ON devices.
Selection process
The review considered a broad range of economic studies including trial-based economic evaluations, modelling studies and analyses of administrative databases. The inclusion criteria considered were full economic evaluations comparing two or more alternative interventions in terms of both costs and consequences (i.e. cost-minimisation, cost-effectiveness, cost–utility and cost–benefit analyses).
The protocol for the selection of relevant studies defined two selection stages: (1) assessment and screening for possible inclusion of titles and abstracts identified by the search strategy, and (2) acquisition and screening for inclusion of the full texts of potentially relevant studies. Two researchers (RW and NM) independently screened the titles and abstracts of all reports identified by the bibliographic searches. Full-text papers were subsequently obtained for assessment and screened by at least two researchers, with any disagreement resolved by consensus.
Results of the cost-effectiveness review for remote continuous monitoring devices
A PRISMA diagram of the review of studies identified in the main systematic review is presented in Figure 4. The initial search identified a total of 1716 (after deduplication). A total of eight studies were identified as potentially relevant from their titles and/or abstracts. Following full-text assessment, none were considered to meet the full inclusion criteria. Two excluded studies, Lynch et al. and Rao et al. , were retrospectively included in the review because both aimed to assess the cost savings and QALY gains associated with PKG but were non-comparative assessments of cost-effectiveness (i.e. no comparator was considered in the analysis). 88,89
FIGURE 4.
Flow diagram of the study selection process for the cost-effectiveness review.

Following completion of the systematic review of previous cost-effectiveness studies for remote continuous monitoring devices, the company for PKG (Global Kinetics) made the EAG aware of a company-sponsored study examining the cost-effectiveness of PKG and clinical assessment in the management of PD. This study by Chaudhuri et al. was published online on 14 June 2022. 96 The study is directly relevant to the decision problem addressed in this report as it represents the first cost-effectiveness analysis performed for a continuous objective measurement system in PD in the context of the UK NHS. A detailed description and critique of Chaudhuri et al. is presented below.
Lynch et al.
Lynch et al. was a cost-consequence analysis considering the cost savings and QALY gains in people whose oral therapy was adjusted following assessment with the PKG and did not consider any comparator. 88 The analysis was based on a before-and-after study, enrolling 103 PwP in Australia, all of which were evaluated using the PKG. The economic evaluation focused specifically on a subgroup of 33 of these patients who had their oral therapy adjusted. In this subgroup, patients were observed to have experienced reductions in UPDRS II and UPDRS III scores and improvements in the PDQ-39 quality-of-life instrument.
No formal modelling was implemented as part of the economic analysis. Instead, the UPDRS domain scores were used to directly estimate reductions in costs and QALYs using evidence from published sources considering a 1-year time horizon. Cost savings were estimated using an algorithm reported in McCrone et al. , which suggested that a one-point reduction in UPDR II is associated with a $430 cost saving. 97 Based on reported changes in UPDRS II, this resulted in cost savings of $1719.42 per patient (exclusive of the cost of the PKG monitoring device). Benefits were estimated using regression models reported in the NICE PD guideline, which linked EQ-5D with several clinical measures. 98 Using reported improvements in UPDRS II, UPDRS III, per cent over target and PDQ-39, QALY gains of between 0.10 and 0.12 were estimated.
Rao et al.
Rao et al. sought to assess the impact of PKG on pharmacological treatment and decisions to initiate advanced treatment. 89 The study reports on 37 PwP attending a movement disorder clinic and who were clinically assessed as requiring advanced treatment. Following PKG, five of these were considered to need advanced treatment with the remaining five patients managed via dose adjustments to pharmacological care.
On the basis of this finding, the study estimated potential cost savings associated with postponing advanced treatment. This assumed transition to apomorphine either administered by pump or by pen. Cost savings associated with APO-go pump were estimated as £5400 per pump per year leading to a saving of £27,000 per year for five patients. For APO-go Pen treatment, equivalent cost savings were estimated as £3200 per year leading to a saving of £16,000 per year. Sources used to inform the costs were not stated.
Chaudhuri et al.
Chaudhuri et al. explored the cost-effectiveness of the PKG and clinical assessment in the management of PD compared to standard of care (SoC) in the context of the UK NHS. 96 A cost–utility model was developed using a Markov model structure. The model comprised three health states: (1) uncontrolled, (2) controlled and (3) death, with the ability to transition bidirectionally between the uncontrolled and controlled health states. The uncontrolled and controlled health states were based on the MDS-UPDRS domain scales of II (motor experiences of daily living) and III (motor examination). All individuals entering the model start in an uncontrolled state. The transitions between the uncontrolled and controlled health states are dependent on the improvement in MDS-UPDRS II and III with either the PKG, in addition to clinical assessment, or with clinical assessment alone (considered to represent SoC). The transition to the death state was based on UK all-cause mortality rates, with an elevated mortality risk of 2.22 associated with PD-specific relative mortality risk (based on Xu et al. , 2014). 99 The Markov model had a cycle length of 1 year and a lifetime horizon of 22 years in the base-case analysis. A discount rate of 3.5% was used for both costs and effects, in line with NICE recommendations. 100
The clinical efficacy of the PKG and clinical assessment compared with SoC was based on the study by Woodrow et al. 17 Full details of this study are described in the section Clinical outcomes of this report. In summary, the study is based on a population in Australia and evaluated comparative outcomes in a PKG+ arm (use of the PKG and clinical assessment) compared to a PKG− arm (clinical assessment without the use of the PKG). At the first screening visit, individuals were assessed to decide whether their PD motor features were ‘in target’ (no further treatment required) or ‘out of target’. In the latter case, a plan for changing treatment was provided until the next consultation 5 weeks later. The same assessment protocol was followed until the PD motor features were ‘in target’. A maximum of five visits were permitted, inclusive of the first screening visit. The primary study outcome was the difference in MDS-UPDRS total score from baseline to the end of the study. Woodrow et al. provide the outcomes of Total MDS-UPDRS score, as well as the subscores for domains I–IV, at the first and last study visit in the PKG+ and PKG− arms. 17 In the PKG+ arm, MDS-UPDRS total score and MDS-UPDRS domain scale III significantly improved by 8.5 points and 6.4 points, respectively, between the first and last study visit, while in the PKG− arm the change in MDS-UPDRS total score and MDS-UPDRS III failed to reach statistical significance.
The patient population used in the model by Chaudhuri et al. is based on the Woodrow et al. study. 17,96 The baseline patient characteristics for age, gender, levodopa equivalent daily dose (LEDD), MDS-UPDRS domain scales II and III and H&Y stage are the same as those reported in Table 1 of Woodrow et al. 17 For each intervention strategy in the model (i.e. PKG and clinical assessment, represented by PKG+ in Woodrow et al. , or SoC, represented by PKG− in Woodrow et al. ), individuals in the uncontrolled health state were assumed to have a MDS-UPDRS score in line with the MDS-UPDRS score obtained at the first screening visit in the corresponding arms of Woodrow et al. , while individuals in the controlled health state were assumed to have a MDS-UPDRS score in line with the final study visit in Woodrow et al. The probabilities of transitions between the uncontrolled and controlled health states in the first 6 months of the model were derived from the proportion of patients who were identified as controlled after the initial use of the PKG in Woodrow et al. After 6 months, transition probabilities were estimated using a bootstrap approach, but no details on the methods used are provided in Chaudhuri et al. The model did not include adverse events.
The treatment effectiveness was assumed to be maintained for a period of 5 years. This was based on a systematic literature review on the impact of LCIG in patients with advanced PD, where the results of the review suggest that LCIG extends the benefits of levodopa (in terms of reduced ‘off-time’) for at least 2–5 years. 101 From 6 years onwards, a long-term gradual waning of effectiveness was assumed in line with the natural disease progression of PD. The authors acknowledge that long-term progression rates are heterogeneous as they vary from person to person. Therefore, the authors used two alternative estimates for rates of progression: (1) a rate of progression based on a bootstrap analysis of published UPDRS III progression data based on a study by Holden et al. ,102 which equates to an average annual rate of progression of 10.9%, and (2) a published annual rate of progression of 2–7% based on a prospective study by Schrag et al. 103
The base-case analysis was modelled to mitigate the benefit observed in the Woodrow et al. study to 75%. This is because participating clinicians assigned to the PKG+ arm in Woodrow et al. received an additional 1-day training in interpreting the PKG for the assessment of PD compared to participating clinicians who were not assigned a PKG. The base case also assumed that PKG+ patients who are controlled will use two PKGs per year, while of the uncontrolled patients, 50% will use three PKGs per year and the other 50% will use four PKGs per year.
To derive HRQoL, the MDS-UPDRS domain scores for II and III obtained from the Woodrow et al. study were used in a published mapping algorithm to predict EQ-5D index values. The mapping algorithm was derived from a study population of 121 patients with idiopathic PD from study centres in Hessia, Germany. 104 The EQ-5D values were derived based on weights from a European population, valued by a visual analogue technique (European index) for the base-case analysis. Alternative values for a scenario analysis were based on weights derived from a German population, valued with the time trade-off approach (German index). The authors also conducted a scenario analysis using utility values derived from a model developed for the NICE PD guidelines, which estimated that HRQoL increases by 0.04 for every point reduction in UPDRS II and 0.02 for every point reduction in UPDRS III. 98 The resulting health state utility values from the alternative sources and approaches differed significantly.
PKG costs were based on the manufacturer’s list price of £225, equating to a total cost of £450 per year for controlled patients (two PKGs per year), and £787.50 per year for uncontrolled patients (50% with three PKGs per year and 50% with four PKGs per year). An outpatient and telephone visit with a movement disorder specialist was costed at £384 and £60 per year, respectively. Health state costs associated with PD progression were based on direct medical and non-medical costs associated with the H&Y scale from the NICE PD guidelines. 98 The authors state that the MDS-UPDRS scores in the model were applied to derive average annual costs by H&Y stage (see Table 23), but no details are provided. 96
H&Y stage | Annual costs (£) | Intervention (PKG+) % of patients | SoC (PKG−) % of patients |
---|---|---|---|
1 | 3918 | 13.85 | 11.65 |
2 | 7417 | 49.30 | 40.35 |
3 | 14,150 | 33.55 | 38.85 |
4 | 28,660 | 3.30 | 8.85 |
5 | 53,335 | 0 | 0.30 |
The cost-effectiveness results from Chaudhuri et al. showed that the intervention of PKG and clinical assessment is associated with lower total costs compared to SoC (£141,950 vs. £159,312) and improved total QALYs (7.88 vs. 7.61) over a lifetime horizon, which resulted in an incremental difference of £17,362 and 0.267 QALYs per patient and an ICER of −£64,979 per QALY. Sensitivity analysis by the authors indicated that the ICER was most sensitive to the annual cost of H&Y stage 4.
Critique of Chaudhuri et al.
Chaudhuri et al. 96 represents the first cost-effectiveness analysis of the PKG remote continuous monitoring system in PD. The study appears to be well conducted and has been performed from the perspective of the UK NHS, which makes it directly relevant to the decision problem addressed in this report. The study accounts for direct medical costs only and QALYs are accumulated over the lifetime of an individual with PD. The population is with moderate disease based on the inclusion criteria of Woodrow et al. (idiopathic PD of ≥ 4 years or taking ≥ 4 doses of levodopa per day because of a much greater likelihood of being out-of-target at baseline; aged 59–75 years because they are less likely to be candidates for DBS and less likely to have a high incidence of contraindications to increasing dopaminergic therapy; not receiving treatment with, or under consideration for, device-assisted therapy), while the intervention’s effectiveness is based on outcomes from the only comparative study for the PKG, where the PKG, in addition to clinical assessment, is compared to clinical assessment alone in Woodrow et al. In the absence of alternative sources of data for clinical efficacy, the data source, population and intervention strategies used by the authors are considered appropriate; however, it is not clear that individuals suitable for PKG in the UK NHS would use as many PKGs as assumed in Chaudhuri et al. (i.e. PwP classified as controlled and in-target are assumed to use two PKGs and have two movement disorder specialist visits per annum, while PwP classified as uncontrolled and out-of-target are assumed to use 3–4 PKGs with 3–4 visits per annum).
The clinical effectiveness of the PKG is based on the MDS-UPDRS domain scores II and III, while the primary end point of the Woodrow et al. study was total MDS-UPDRS. 17 Chaudhuri et al. justified the choice of domains II and III based on the availability of published mapping algorithms that use domains II and III to predict EQ-5D utility values for the model and on the basis that these domains constitute the largest components of the total MDS-UPDRS score. The EAG notes that this means that the results of Chaudhuri et al. do not reflect the impact of the PKG on non-motor experiences of daily living (MDS-UPDRS I) or on the most severe motor complications (MDS-UPDRS IV) associated with PD.
The model considered a lifetime horizon of 22 years in the base-case analysis. The study justified the choice of 22 years to approximate lifetime treatment and to capture the long-term costs and health effects of treatment. While the EAG acknowledges that a lifetime horizon is an appropriate time horizon to capture the effect of treatment on the long-term progression of PD, the EAG also has a major concern about the use of a lifetime horizon to capture the difference in costs and health effects of PKG compared to SoC when there is an absence of evidence to suggest that the benefits of PKG equates to changes in treatment that are sustained over the long-term, into a future of 22 years, particularly in recognition of the fact that there are no disease-modifying treatments available for PwP. The model by Chaudhuri et al. makes a number of strong assumptions about sustaining the 6–12 months of benefits observed in Woodrow et al. over the long-term:
-
In the model by Chaudhuri et al. the change in the MDS-UPDRS II and III scores between the first (uncontrolled) and last study visit (controlled) for the PKG+ arm compared with the PKG− arm in Woodrow et al. is used to capture a 6–12 months treatment effect. Although the authors mitigate this benefit to 75% to account for additional support provided to clinicians in the PKG+ arm and the effect of participating in a clinical trial, the benefits are assumed to be sustained in full (i.e. without any waning effect) for a period of 5 years. This assumption is not supported by any treatment data in patients with moderate PD and only justified by the authors as a proxy on the basis of data for the use of LCIG in patients with advanced PD.
-
It is only from year 6 onwards in the model that a long-term waning of treatment effect is included. The waning effect is assumed to gradually decline in line with the natural history of disease progression for PD; however, the authors acknowledge that the long-term progression rate of PD is heterogeneous and highly uncertain. An average progression rate of 10.9% per annum was used by the authors based on the progression of MDS-UPDRS III scores over 5 years from the Parkinson’s Progression Markers Initiative, which is an international, multicentre study in patients with de novo PD (early, initially untreated PD).
-
The transition probabilities representing the bidirectional movement between the uncontrolled and controlled health states in the model over time were based on the proportion of patients who were identified as controlled at the first visit in the Woodrow et al. study. The exact proportion used in the model at 6 months does not appear to be reported in Woodrow et al. but, more importantly, this proportion at 6 months, with an estimate of uncertainty, is used to establish the transition probabilities between the health states over a lifetime horizon.
The authors state that their modelling approach is conservative because they have mitigated the benefit from Woodrow et al. to 75% and have included the benefit of using the PKG for 1 year, whereas the cost of provision of the PKG for the following years is included in the model. However, the EAG notes that the benefits of using the PKG in the first year are sustained in full for a period of 5 years; it is simply that no additional ‘top-up’ benefit from using PKGs in the following years is included in the model. Furthermore, the changes in MDS-UPDRS scores from first to last visit in Woodrow et al. are based on up to five consultation visits, where a PKG was worn prior to each visit in the PKG+ arm. At each visit (5 weeks apart), a plan for changing treatment was provided until the motor features of the disease were considered to be in-target and no further treatment required. The EAG considers it unlikely that UK clinical practice would follow a similar protocol.
The movement between the uncontrolled and controlled health states over time, based on the MDS-UPDRS II and III scores from the Woodrow et al. study, affects the costs and utility values at each model cycle over the lifetime horizon of the model. The MDS-UPDRS II and III scores from Woodrow et al. are used in a mapping algorithm to predict EQ-5D index values for the uncontrolled and controlled health states in the model. The mapping algorithm by Dams et al. is based on a small study population of 121 PwP in Germany, where the health states identified by the EQ-5D were converted into EQ-5D indices employing weights from a pooled European population valued by a visual analogue technique. 104 The EAG notes that this method does not align with the NICE Reference case, where the valuation of HRQoL measured by patients (or their carers) should be based on a valuation of public preferences from a representative sample of the UK population using a choice-based method. 100
The mapping algorithm in Dams et al. is also based on UPDRS scores, rather than MDS-UPDRS scores. Chaudhuri et al. do not provide details on whether any conversion was made between UPDRS and MDS-UPDRS scales to derive the EQ-5D values. Furthermore, the resulting estimates of health state utility values differed substantially from other alternative sources and approaches considered by the authors. Therefore, even despite the concerns about the long-term extrapolation of benefits in the model, the health state utility values themselves are uncertain. This means that the accumulated total QALYs over a lifetime horizon are subject to considerable uncertainty.
The health state-related costs used in the model were based on MDS-UPDRS scores from Woodrow et al. and costs associated with the H&Y scale. The authors refer to the costs reported in the NICE PD guidelines Appendix F for the H&Y scale; however, despite a thorough review of the NICE PD guidelines,98 the EAG was unable to identify or validate the costs reported in Chaudhuri et al. No details are provided on how the annual costs by H&Y stage were derived in the model. Importantly, it is unclear how the MDS-UPDRS scores were converted onto the H&Y scale. The costs associated with the H&Y stages and the proportion of individuals in each stage by intervention strategy is a key driver of the total costs over a lifetime horizon. This is evident from Table 23, where the distribution of patients by intervention (PKG+) and SoC (PKG−) shows a greater proportion of patients in the less severe H&Y stages 1 and 2 and a smaller proportion in the higher stages of 3–5 for PKG+, whereas a smaller proportion are in stages 1 and 2 and a higher proportion in stages 3–5 for SoC. The annual cost associated with H&Y stages 1 and 2 is £3918 and £7417, respectively, whereas the annual cost associated with H&Y stages 3–5 varies from £14,150 to £53,335. Given that the annual health state costs are considerably larger than the annual cost of PKG and consultation visits (by an approximate order of magnitude of 10 for the lower H&Y stages and 100 for the higher stages), it is expected that the key driver of total costs in the model is the difference in the proportion of individuals in the H&Y stages by intervention over a lifetime horizon.
The base-case results suggest that PKG and clinical assessment compared to clinical assessment alone reduces the total costs to the NHS by £17,362 per patient. The cost savings for PKG+ are driven by a lower likelihood, on average, that a patient will end up in one of the more severe H&Y stages 3–5 (36.9%) compared to SoC (48%) over a lifetime horizon. The base-case results also suggest that PKG+ increases the total QALYs by 0.267 QALYs per patient compared to SoC. This is driven by a higher increment in utility for the controlled versus uncontrolled health states for PKG+ (0.036) compared to the increment in utility for the controlled versus uncontrolled health states for SoC (0.021), and a greater likelihood of being in the controlled health state versus uncontrolled health state for PKG+ compared to SoC over a lifetime horizon. Results of sensitivity and scenario analyses by the authors showed that the cost-effectiveness results were robust to the base-case conclusions; however, the EAG notes that the majority of these analyses did not address the key structural assumptions affecting the long-term efficacy, HRQoL and costs over a lifetime horizon in the model.
Methodology of the review of decision models evaluating interventions used in Parkinson’s disease
Given the very limited number of cost-effectiveness studies evaluating remote continuous monitoring devices in PD, a review of published cost-effectiveness studies evaluating broader interventions (both pharmacological and non-pharmacological) for the management of symptoms in people living with PD was conducted. The review targeted cost-effectiveness studies that included a decision-analytic model rather than economic evaluations conducted alongside clinical trials with no consideration of longer-term disease progression. The specific aims of the review were:
-
to help inform the conceptualisation of the decision problem for PwP and long-term progression of the disease;
-
to identify important structural assumptions used in previous models in PD, highlighting key areas of uncertainty and outlining the potential issues of generalising from the results of existing models; and
-
to identify any relevant sources of evidence (in particular, attention was given to identifying important parameter estimates and sources of data inputs for linking evidence on short- and long-term outcomes for PwP).
The studies identified from the review were not subject to a formal assessment using checklists to assess the quality of the included studies. Instead, a narrative review of key model features and modelling approach was used, key assumptions and data sources underpinning the link between short-term clinical outcomes (e.g. changes in symptom severity using different rating scales, time spent in ‘on-off’ periods) and long-term morbidity or disease progression and mortality in these studies was assessed.
Searches
The bibliographic search detailed in Methods for reviewing clinical effectiveness was used to identify relevant studies.
Study selection
Cost-effectiveness studies using decision-analytic modelling published after the year 2000 were considered for inclusion. Only cost-effectiveness, cost–utility and cost–benefit analyses were considered eligible. The population of this review was defined as people with diagnosed PD. Studies in people with other neurological disorders were excluded. The inclusion criteria further specified that only titles in English would be considered eligible. Titles that were books, editorials, letters to the editor and reviews that did not include a de novo model were excluded from the review.
Two researchers (EC, THP) conducted the two-step selection process consisting of first screening studies for inclusion based on the titles and abstracts of studies identified by the bibliographic searches, and then reviewing the full-text articles identified at the previous step as potentially relevant.
Results of the systematic review of decision models evaluating interventions in Parkinson’s disease
A total of 1285 records were identified during the initial search of the economic databases of which 744 remained after deduplication. The initial screening identified 41 titles as potentially relevant based on their titles and/or abstracts. The full-text articles of 32 of these records were obtained and assessed for eligibility. All were considered to meet the selection criteria and included in the review.
The majority of studies used a Markov cohort model with H&Y stages 1–5,105–115 off-time (e.g. ≥ 25% ‘off’ time/day),116–122 dyskinesia123,124 and motor fluctuation125,126 being the most popular discrete health states used to characterise disease progression in PD. In 11 studies, composite health states (i.e. health states encompassing multiple disease measures) were adopted; 10 combined H&Y stages and off-time,127–135 and one study combined off-time and cognitive function. 136 Two studies modelled disease progression according to treatment-specific health states within a Markov model framework, where interventions confer changes in UPDRS domain scores,137 and risk of developing dyskinesia. 124 Only one study used a decision tree, which was used to model operative complications associated with DBS and MR-guided focused ultrasound thalamotomy for tremor-dominant PD relative to medical therapy. 138 Two studies conducted individual-level simulation approaches, one implementing the total time spent without off-episode symptoms as the mechanism of treatment benefit139 and the other changes in MDS-UPDRS domain scores (among other measures). 140
In summary, most models in PD have adopted a Markov structure where the evaluation of treatment effectiveness and costs of different pharmacological or surgical interventions is modelled by transitions between health states. In most studies to date, these transitions between health states are used to track disease progression, which is predominantly measured by the H&Y scale (stages 1–5) and/or off-time per day. In most of the earliest studies, the mechanism of benefit from the interventions is derived by providing a difference in disease progression via treatment-specific transition probabilities between H&Y stages. In some studies, these are applied as ‘one-time’ shifts in the H&Y stage of disease (i.e. the treatment effect produces a one point in time shift in the H&Y stage of disease), while in other studies progression through the H&Y stages may occur in each model cycle over the course of the disease. In the latter case, the longer-term transition probabilities for each stage are typically derived from longitudinal studies of PwP. The use of discrete health states (e.g. ≥ 25% or < 25% off times per day) is considered to be quite a crude measure in the context of modelling complex diseases such as PD.
In the most recent studies, there has been a move away from the use of the H&Y stages to measure the severity of disease progression because H&Y only measures the motor symptoms of PD. More recently, the UPDRS or MDS-UPDRS domain scales (where the MDS-UPDRS is the revised version of the original Unified Parkinson’s Disease Rating Scale by the Movement Disorder Society) have been used in models to track disease progression over time because the UPDRS/MDS-UPDRS measures both motor and non-motor symptoms and is composed of four distinct parts: MDS-UPDRS I (non-motor aspects of experiences of daily living), MDS-UPDRS II (motor aspects of experiences of daily living), MDS-UPDRS III (motor examinations) and MDS-UPDRS IV (motor complications), where higher scores indicate increased disease severity. The UPDRS has been shown to have excellent internal consistency across multiple studies and stages of disease severity as measured by the H&Y staging system. The use of the UPDRS or MDS-UPDRS domain scales to track disease progression over time is also aligned with the outcomes reported in short-term clinical trial data of symptomatic therapies and devices used for PwP, where the primary or secondary efficacy outcome measures include the UPDRS or MDS-UPDRS domain scale.
Of the 32 studies reviewed, two analyses were considered good examples of contemporary modelling approaches that considered outcomes available from the clinical review (see Clinical outcomes). The first study by Fundament et al. was a Markov model where health states were based around treatment interventions and, for each treatment, disease progression was modelled according to changes in the UPDRS domain scores (parts I–IV). 137 The changes in UPDRS domain scores were recorded over time within the model, but they were not explicitly used to derive the health states. In the second study by Chandler et al. , disease progression was characterised in terms of changes in MDS-UPDRS, UPDRS and H&Y stages over a lifetime horizon. 140 Unlike Fundament et al. , the model was a discrete event simulation constructed to represent the individual-level heterogeneity observed in disease progression rates and capture the potential benefits of novel disease-modifying drugs when they become available for PD. A summary of both models is presented below.
Fundament et al.
Fundament et al. developed a Markov model to represent the progression of PD as rated using the UPDRS over time in patients with an early onset of motor complications. 137 The model was developed to evaluate the cost-effectiveness of DBS, compared to best medical treatment (BMT), for PD with early motor complications from a UK payer perspective. The study population was based on baseline characteristics for patients included in the Controlled Trial with DBS in Patients with Early Parkinson’s Disease (EARLYSTIM) trial, who all were assumed to have motor complications at model entry. The base-case analysis used a 15-year time horizon to capture the long-term progression to more advanced stages of PD.
Health states in the Markov model were based only on the treatment interventions (DBS and BMT) and death, but disease progression over time was modelled according to changes in UPDRS domain scores I–IV by treatment. Patients with DBS were assumed to continue therapy until withdrawal, after which they would continue with BMT until the end of the modelled time horizon or death. For the first 2 years of the model, clinical efficacy estimates in terms of changes in UPDRS scores for each intervention were derived from the EARLYSTIM trial, which collected UPDRS domain scores at treatment initiation and at 5, 12 and 24 months follow-up. These were used to calculate the percentage change in UPDRS from baseline in each domain for each intervention. Rates of disease progression beyond 2 years were modelled to be the same for both interventions (with the exception of UPDRS domain score IV) due to a lack of consistent long-term data on UPDRS outcomes by treatment. Annual rates of progression for each UPDRS domain were based on data pooled from various studies because the authors did not identify a single study that reported all domains of the UPDRS consistently. Supplemental appendix, table S1 in the paper provides the annual rates of progression in UPDRS scores by treatment group.
Mortality was incorporated into the model using a two-step approach. Firstly, age- and gender-specific baseline mortality was applied using UK all-cause mortality rates based on the baseline characteristics of patients in the EARLYSTIM trial. In the second step, a hazard ratio for mortality risk of 1.31 was applied to the baseline mortality for patients with more advanced disease. Using data from studies reporting on the relationship between UPDRS III and mortality, a per 10-point increase in UPDRS III score was associated with an increased risk of mortality, which was applied only in patients with a UPDRS III score > 15 to reflect the increased mortality associated with more advanced disease.
The model accounted for both treatment-specific and disease-related adverse events. The incidence of adverse events reported in the EARLYSTIM trial was used to inform the treatment-specific rates. For the disease-related adverse events, the authors highlighted that PD progression is associated with increasing postural instability, which may lead to falls and serious injury in some patients. To reflect the incidence of falls and associated hospitalisation rates, the authors pooled data from a series of studies to define a baseline proportion of patients falling per year of 42.8% based on patients with a UPDRS III score of 12 points. An odds ratio of 1.07 for each point increase in UPDRS III score was then applied to the baseline risk based on three studies reporting incidence of falls. 141 Of these falls, 50.9% were assumed to require hospitalisation.
Health-related quality of life was accounted for, in the short term, using a published mapping algorithm to map the PDQ-39 data from the EARLYSTIM trial to the EQ-5D, since EQ-5D was not collected in the trial. The corresponding utility weights by intervention were applied in the first 2 years of the model. For the longer term, after 2 years, the authors developed a new algorithm to link UPDRS scores to EQ-5D. The authors used an iterative approach to identify a statistical model that could accurately predict the EQ-5D index from the explanatory variables available from the EARLYSTIM trial (including UPDRS domain scores). The resulting EQ-5D function was given by Fundament et al. :
This algorithm was applied to changes in UPDRS scores over time in the model (beyond 2 years) to estimate the HRQoL by treatment group.
For resource use and costs used in the model, data were sourced from price lists, national drug prices, hospital payment tariffs and social care cost data. Due to a lack of standardised drug protocols for the management of PD, the authors analysed data from the UK Clinical Practice Research Datalink (CPRD) of 297 patients with PD for the period April 2003–March 2012 to derive information on drug formulations administered. Dosing information and the number of patients receiving each drug was combined with unit costs from the British National Formulary to calculate a drug cost per day for each treatment option of £4.16 for BMT and £2.28 for DBS. The costs of regular neurology outpatient appointments were accounted for by assuming four visits per year in the first year of treatment with DBS and two visits per year thereafter, while patients on BMT were assumed to have two visits per year. Home visits by a PD nurse were applied in both treatment groups with the same frequency as the neurology outpatient appointments. The unit costs associated with hospitalisation for falls and follow-up appointments were based on hospital payment tariffs and social care cost data. Supplemental appendix, table S1 in the paper provides a list of the unit costs used in the model.
The results of the cost-effectiveness analysis indicate that DBS leads to improved QALY outcomes and increased costs compared with BMT in patients with early onset of motor complications from a UK NHS perspective, leading to an ICER of £19,887 per QALY gained. Device costs made up the largest percentage of costs in the DBS treatment group, while in the BMT group, drug therapy and management of comorbidities were the main cost drivers. The model results were most sensitive to the time horizon used to model long-term costs and outcomes; when this was limited to 5 years, the ICER increased beyond £30,000 per QALY gained for DBS. The key uncertainty in the model was the lack of long-term follow-up data beyond 5 years for the interventions.
Chandler et al.
Chandler et al. developed a modelling framework to facilitate the estimation of long-term health and economic outcomes in PD. 140 The model was structured to simulate the long-term progression of PD from diagnosis through to a lifetime horizon, capturing both motor and non-motor symptoms and associated outcomes. The model was developed to support the cost-effectiveness evaluation of new disease-modifying drugs in PD when they become available. The authors recognise that there are currently no disease-modifying therapies available for the treatment of PwP, but new therapies are being studied and results from clinical trials are expected in the future.
The objective of the study by Chandler et al. was to develop and validate a novel model that addresses the unmet need to simulate the disease progression from diagnosis over a lifetime horizon. The authors also recognised the limitations of previous models in PD, where most have typically modelled transitions between health states defined based on the H&Y staging system and very few have tracked disease progression using UPDRS domain scores. The authors emphasised the need to reflect non-motor aspects of PD and therefore the need to develop models using the revised version of the original UPDRS (MDS-UPDRS). The model by Chandler et al. represents the first novel model in PD to track disease progression over a lifetime horizon using MDS-UPDRS domain scores (amongst others).
The model by Chandler et al. provides a conceptual modelling framework of long-term disease progression in PD, but it also simulates the progression of disease along the MDS-UPDRS and UPDRS subscales over time using predictive equations that capture the intercorrelation between MDS-UPDRS/UPDRS subscales and baseline population characteristics. The predictive equations for the simulation of disease progression for newly diagnosed PwP were developed from an analysis of longitudinal, observational data from the Parkinson’s Progression Markers Initiative, which is an international multicentre study that follows individuals from diagnosis (treatment naïve, age > 30 years) up to 6 years of follow-up. The data were analysed using a mixed-effect repeated measures (MMRM) model to predict change from previous values of MDS-UPDRS I (non-motor experiences of daily living), MDS-UPDRS II (motor experiences of daily living) and MDS-UPDRS III (motor examinations). In the MMRM model, the initiation of treatments for PD symptoms was identified as a predictor of MDS-UPDRS II and MDS-UPDRS III scores. For people treated with levodopa and/or dopamine agonists, MDS-UPDRS III was assessed both pre- and post-dose in the Parkinson’s Progression Markers Initiative data set, while for other medications (e.g. monoamine oxidase-B inhibitors) MDS-UPDRS III was assessed post dose. Additional predictors in the model included age, gender, disease duration, time, baseline MDS-UPDRS scores and prior MDS-UPDRS scores.
The form of the predictive equations was a general linear model. Supplementary Table 2 of Chandler et al. provides the mean predictor coefficients (with standard error) for change within each MDS-UPDRS subscale (I–III) from a prior visit for people with newly diagnosed PD based on baseline values for age, gender, disease duration and MDS-UPDRS scores, time from previous visit in days, MDS-UPDRS scores at a prior visit and PD medication. The mean scores within each MDS-UPDRS subscale increased year on year, which was indicative of greater levels of severity in both motor (subscales II and III) and non-motor (subscale I) symptoms in newly diagnosed PD. The authors also noted a significant difference in MDS-UPDRS II and III scores between people on no PD medication, people treated with levodopa and/or dopamine agonists, and other PD medications alone (e.g. monoamine oxidase-B inhibitors).
In addition to the predictive equations for newly diagnosed PD, the model by Chandler et al. also simulates disease trajectories when people initiate treatments for PD symptoms via a separate set of predictive equations for changes in UPDRS subscales. This second set of predictive equations was developed from an analysis of the National Institute of Neurological Disorders and Stroke Exploratory Trials of Parkinson Disease Long-term Study 1 (NET-PD LS-1), which was a large multicentre, randomised placebo-controlled efficacy trial of creatine, where eligible participants were within 5 years of PD diagnosis and treated with levodopa and/or dopamine agonists. The data from this study (1720 PwP with 5 years of follow-up) were used to develop equations to predict changes over time in UPDRS subscales I–IV, LED, and per cent off-time. Supplementary Table 3 of Chandler et al. provides the mean predictor coefficients (with standard error) for change within each UPDRS subscale (I–IV) from a prior visit based on baseline values for age, gender, disease duration and UPDRS scores, LED, time from the previous visit in days, off-time, UPDRS scores at prior visit, rate of change in prior UPDRS scores and interactions between variables. In the predictive model, LED is predicted to increase over time and impact on the UPDRS subscores I–IV.
Using the predictive equations for disease progression, Chandler et al. developed an individual patient-level simulation model to represent the heterogeneity observed in progression rates and capture the potential long-term benefits of new disease-modifying drugs when they become available, as distinct from symptomatic improvements. Disease progression was characterised in terms of changes in MDS-UPDRS, UPDRS and H&Y stages from diagnosis through to a lifetime horizon. Through the prediction equations, the simulation captures the benefits of starting symptomatic treatments, dose adjustments, increases in off-time and the associated complications of dopaminergic therapies.
A target population of newly diagnosed PwP was analysed by the authors to understand the potential value of treatment with new disease-modifying treatments. A profile of risk factors to predict disease progression, treatment changes and mortality was generated for each simulated individual in the model using the Parkinson’s Progression Markers Initiative data set. When individuals progressed to MDS-UPDRS or UPDRS subscale III, a UPDRS III threshold was used to predict the time to H&Y stage 3. H&Y was not collected in NET-PD LS-1; therefore, the longitudinal PRECEPT and PostCEPT studies in early-stage PD were used to predict time to H&Y stage 3. Conversions between the UPDRS and MDS-UPDRS subscales were based on the linear relationship published in Goetz et al. 142 The progression between H&Y stages 3 and 5 was based on published transition probabilities from a previous economic model in PD. 108 General population mortality estimates were adjusted to capture the impact of PD on mortality by applying hazard ratios by H&Y stage.
The model was validated in two ways: (1) the predictive equations developed for the MDS-UPDRS and UPDRS subscales were used to compare the predicted and observed scores each year post-baseline in the corresponding Parkinson’s Progression Markers Initiative and NET-PD LS-1 data sets, and (2) the simulated outcomes from the model with the predictive equations implemented within the model were compared to the observed data in the data sets. Supplemental Figures 1 and 2 demonstrated good validity between the simulated disease progression of UPDRS scores predicted over time and observed outcomes.
Chandler et al. also used their model to conduct an economic analysis for a new hypothetical Disease-modifying therapy (DMT), in addition to the current SoC compared to SoC alone. The analysis was conducted from a UK NHS perspective. The model was used to simulate newly diagnosed patients starting a DMT in addition to SoC. For the DMT, a 50% change in MDS-UPDRS progression compared to the natural history without the DMT was assumed, while a 5% discontinuation rate was included in the first year and a 2.5% in subsequent years. Patients who discontinued DMT were assumed to experience a gradual loss of treatment effect over 2 years, reverting to the natural history of progression on SoC.
For HRQoL, a MMRM model was developed by the authors to predict EuroQol-5 Dimensions, three-level version (EQ-5D-3L) utility values based on an analysis of data collected in the NET-PD LS-1 study and using the UK preference weights. This study had up to 6 years of follow-up with EQ-5D-3L index scores of 1741 observations at baseline and 330 at year 6. The statistical model was used to identify predictors of the association between EQ-5D utility values and PD severity; it found that gender, non-motor and motor aspects of PD (i.e. all four UPDRS subscales) were all significant predictors of EQ-5D-3L utility value. Supplemental appendix, table 4 in the paper provides the mean values (with standard error) for the coefficients of gender and each of UPDRS I–IV subscales to predict EQ-5D-3L index scores, while Supplemental figure 3 demonstrates that the utility equation performs well when validated with the observed data. In the authors’ hypothetical economic analysis for a new DMT, the predictive equation was used to derive utility values up to H&Y stage 3. Once individuals transition to H&Y stage 3, utility values are assigned conditional on H&Y stage and off-time using an approach adopted in a previous economic model in PD. 129 Caregiver disutilities were also assigned conditional on H&Y stage from a study reporting caregiver decrements by H&Y while controlling for age and gender. 130
For resource use and costs, the initiation of medications (levodopa and/or dopamine agonists or other PD medications such as monoamine oxidase-B inhibitors) for newly diagnosed PD was based on data observed in Parkinson’s Progression Markers Initiative for the likelihood of starting PD treatments over time. This was supplemented by symptomatic treatment costs from a study by Kalilani et al. who identified treatment patterns in the UK CPRD database for the period 2004–15 based on 7775 PD patients. 143 The most common PD medications included in the CPRD database (levodopa, 43%; pramipexole, 30.34%; ropinirole, 21.52%; and bromocriptine, 4.78%), the average cost per milligram and LED conversion factors for each treatment were used to derive a weighted average annual cost per milligram LED of £5.01. The percentage of patients receiving advanced therapies and associated treatment durations were based on the OBSERVE-PD (observational, cross-sectional Parkinson’s disease) study,144 while direct medical and non-medical costs per annum by H&Y stage were derived from a UK healthcare survey published by Findley et al. 145
The results of the cost-effectiveness analysis for the hypothetical DMT indicate that over a lifetime horizon, DMT in addition to SoC leads to improved discounted (at 3.5% per annum) QALY outcomes of 1.1 QALYs compared to SoC alone. Patients treated with SoC were projected to incur discounted costs of £232,619 over a lifetime (26% related to treatment costs and 55% from non-medical costs including respite care and nursing home costs). For the hypothetical DMT, without including acquisition and administration costs of the DMT, the total discounted costs were predicted to be lower than SoC by 16% due to reductions in hospitalisations, nursing home admissions and at-home care.
Conclusions of the assessment of existing cost-effectiveness evidence
The review did not identify any studies that evaluated the cost-effectiveness of PKG, Kinesia 360, KinesiaU, PDMonitor and STAT-ON devices. However, one study for PKG, which was published online after the cut-off date of our searches, was identified. 96
A supplementary review of decision models evaluating interventions in PD identified 32 studies with model-based economic evaluations in PD. Most studies adopted a Markov model that typically modelled the progression of PD according to transitions between health states defined by the H&Y staging system, off-time per day or a combination of both these measures. Only two models directly tracked disease progression using the UPDRS domain scores and considered both motor and non-motor aspects of PD;137,140 one of these models used the MDS-UPDRS domain scores. 140
The most relevant model to support the cost-effectiveness assessment of remote continuous monitoring devices in PD is the model by Chandler et al. 140 This model provides a new foundation for evaluating interventions in PD by taking account of motor and non-motor aspects of the disease and provides progression rates over time, which capture the benefits of starting symptomatic treatments, dose adjustments and longer-term implications of increasing off-time per day and the associated complications of dopaminergic therapies. The model is constructed as an individual patient level simulation for a target population of newly diagnosed, treatment naïve patients at baseline and is developed to aid the evaluation of new disease-modifying drugs in the future.
Despite the fact that the population likely to use remote continuous monitoring devices in PD does not match that of Chandler et al. , the model developed in this study provides a foundation for the underlying disease progression risk over time and associated complications of symptomatic therapies. In particular, the risk prediction equations from this study provide an invaluable source of the rate of progression of motor and non-motor symptoms that capture multiple factors and dependencies, including age, gender, duration of disease, PD medications and LED, as well as leveraging prior values and rates of change of the UPDRS domain subscales.
Chapter 5 Independent economic assessment: York model
Overview
At the time of the systematic literature review of cost-effectiveness studies (see Results of the cost-effectiveness review for remote continuous monitoring devices), no studies evaluating the cost-effectiveness of remote continuous monitoring devices (PKG, Kinesia 360, KinesiaU, PDMonitor and STAT-ON) were identified. Therefore, a de novo decision-analytic model was developed to formally estimate the cost-effectiveness of remote continuous monitoring devices, as an adjunct to clinical judgement, for the assessment of motor and non-motor symptoms in people with maintenance-phase PD, relative to clinical judgement alone (SoC) in the NHS. Due to not having identified any comparative evidence of clinical effectiveness for STAT-ON, KinesiaU, PDMonitor or for any device within an advanced disease setting (see Clinical outcomes), the full economic evaluation was limited to establishing the cost-effectiveness of PKG and Kinesia 360 compared to SoC in the maintenance stage of PD.
However, the costs associated with STAT-ON, KinesiaU and PDMonitor are descriptively assessed, alongside a number of threshold analyses based on uninformed assumptions about clinical efficacy. The conceptualisation, development and parameterisation of the economic model were informed by existing economic modelling studies described in Results of the systematic review of decision models evaluating interventions in Parkinson’s disease, and clinical findings reported in Results of the review of clinical effectiveness. The model provides a framework for combining and evaluating the impacts alternative remote monitoring schedules have across a range of parameters relevant in establishing patient outcomes, NHS costs and overall cost-effectiveness.
Three issues were considered central in developing and populating the decision-analytic model:
-
The need to link data from the use of remote continuous monitoring devices to meaningful changes in patient outcomes.
-
The need to model the underlying disease progression over time, while incorporating and extrapolating the potential benefits observed from the remote monitoring devices relative to SoC.
-
The need to consider and assess the impacts of alternative monitoring schedules (e.g. one-time use or repeated use of the devices over time).
The decision-analytic model provides a link between intermediate outcomes from remote continuous monitoring devices and final health outcomes expressed in terms of QALYs. The model provides a comparison between the potential health gains achieved by a remote monitoring intervention schedule, relative to their additional costs to NHS providers. Costs are expressed in Great British pounds (2019–20) and evaluated from the perspective of the NHS and PSS. Both costs and outcomes are discounted at a 3.5% annual discount rate, in line with current NICE guidelines. 100 The model was evaluated over a 5-year time-horizon to reflect maintenance-stage PD. The model is developed using Microsoft Excel.
The model is probabilistic, meaning that the uncertainty in the input parameters is reflected through the use of appropriate probability distributions, rather than using fixed mean parameter estimates. 146 Monte Carlo simulation is used to propagate uncertainty in input parameters through the model in order to capture the uncertainty in overall results. Scenario analyses are undertaken to explore the robustness of the cost-effectiveness results to changes in the parameter inputs and assumptions of the model.
The following sections outline the decision problem, the structure of the model and provide an overview of the key assumptions and data sources used to populate the model.
Decision problem and population
The decision problem the economic model seeks to address is whether devices for remote continuous monitoring of PwP (PKG, Kinesia 360, KinesiaU, STAT-ON or PDMonitor) represent a cost-effective use of NHS resources. 147
PD is a heterogeneous disease with many different patterns of progression, outcomes and associated cognitive decline. This complexity, combined with individual patient and service-specific circumstances, has led to considerable variation in the methods used in current clinical practice to assess PD symptoms. Monitoring methods include history taking, patient-reported diaries, activity data (e.g. mobile activity trackers, pedometers, etc.) and use of the UPDRS, H&Y and MBRS scales. 147 The NICE guideline for PD (NG71) recommends that people diagnosed with PD should be seen at regular intervals of 6–12 months to review their diagnosis. 2
The position of remote continuous monitoring device technologies within this clinical pathway remains uncertain. Remote technologies lend themselves to a variety of settings and configurations and can be applied at different stages of PD to routinely assist review appointments, as a means of substituting or screening the need for patient consultation, or as once-off applications in circumstances considered particularly beneficial for helping decisions about care. Specific circumstances may include patients having difficulties communicating symptoms, when motor fluctuations are not being adequately managed, where response to treatment is unclear or to inform the calibration of device-assisted therapies (e.g. DBS).
The target population of the model consists of PwP in the maintenance phase of disease, where symptoms have increased significantly since diagnosis and medication is routinely required and regularly reviewed. Although remote continuous monitoring devices may be used in advanced stages of PD (e.g. to aid programming DBS and to monitor the impact of advanced treatments), no evidence on the comparative effectiveness of the devices beyond the maintenance stage of PD was available.
The clinical subpopulation of patients experiencing uncontrolled motor symptoms (in-target and out-of-target – see Clinical outcomes) were considered potentially relevant subpopulations but were not incorporated into the design of the analysis due to the ex-post nature of the population definition (i.e. patients can only be identified as out-of-target after the use of a remote continuous monitoring device). It may be plausible that people with uncontrolled (out-of-target) motor symptoms are identified without the use of a continuous monitoring device, for example, following an assessment that is triggered due to self-referral; however, it is unclear how this population would be defined in practice and to what extent it would overlap with one identified as out-of-target using a remote continuous monitoring device. No other subpopulations were considered.
Strategies/comparators
The principal aim of remote continuous monitoring devices is to provide ‘objective’ ambulatory measurement and identify uncontrolled PD symptoms in order to inform necessary changes in treatment, thereby leading to improvements in patient outcomes. Other objectives include enhancing medication adherence, improving patient–physician dialogue, reducing unnecessary clinic visits, aiding patient autonomy and improving on broader elements of patient health and well-being (e.g. educational materials, strength and fitness training).
Given the complex and multifaceted nature of PD, as reflected by the broad range of information provided by remote monitoring devices, symptom status does not lend itself to a singular dichotomous primary end point (e.g. positive or negative status) or conventional assessments of diagnostic accuracy (e.g. sensitivity and specificity, predictive values, likelihood ratios). Instead, diagnostic evaluation studies have examined correlations between specific recorded variables (e.g. bradykinesia score) and broader symptom measures (e.g. disease-specific rating scales), the impact of diagnostic information on clinician decision-making, patient and clinician feedback, and the direct clinical benefits associated with remote monitoring on patient outcomes (see Results of the review of clinical effectiveness). Due to the inability to reliably link diagnostic accuracy, changes in treatment or clinician/patient feedback to patient outcomes, the EAG considered strategies on the basis of relevant models of care delivery and the extant evidence on comparative clinical impact.
Comprehensive searches of the literature identified three studies reporting comparative clinical outcomes for the remote monitoring devices under consideration (see Clinical outcomes):
-
Woodrow et al. : A quasi-randomised cluster trial (n = 154) assessing PKG’s ability to guide therapy and improve outcomes in PwP compared to SoC practice (see Personal Kinetigraph). 17
-
Isaacson et al. : A small pilot RCT (n = 39) evaluating Kinesia 360’s capability to inform optimal rotigotine dosage and improve outcomes in PD for people with insufficiently controlled motor symptoms, versus SoC alone (see Kinesia 360 and KinesiaU motor assessment systems). 33
-
Peacock et al. : A suspended small RCT (n = 25) comparing telehealth follow-up care using Kinesia 360 to SoC follow-up in PD for patients with bothersome tremor or dyskinesia (identified at a prior visit). 34
Due to the early termination of the trial, Peacock et al. did not provide any comparative outcome measures relevant to the analysis. 34 No comparative clinical effectiveness evidence could be identified for STAT-ON, KinesiaU or PDMonitor. Consequently, the full economic evaluation only considered intervention strategies using either PKG or Kinesia 360. To assess the potential cost-effectiveness of STAT-ON, KinesiaU and PDMonitor, an exploratory scenario analysis was presented using evidence from Woodrow et al. to model treatment effects across all interventions (including Kinesia 360). This scenario should be interpreted with caution as it is purely exploratory in nature due to the differences between the alternative remote continuous monitoring devices and the lack of evidence to suggest equivalence in outcomes.
In both Woodrow et al. and Isaacson et al. , the remote continuous monitoring devices were used to optimise current treatment as part of initial assessments and did not consider longer-term repeated use of remote continuous monitoring devices. However, based on company information, real-world applications of PKG and expert clinical advice, the EAG assessed two alternative monitoring strategies for PKG and Kinesia 360 within the decision-analytic model:
-
One-time use: Remote monitoring (PKG or Kinesia 360) implemented at model baseline as a one-time aid to clinical assessment.
-
Routine use: Remote monitoring (PKG or Kinesia 360) as an adjunct to SoC applied at every follow-up period to routinely assist regular clinical assessments.
One-time use attempts to approximate the use of the remote monitoring devices as used in Woodrow et al. and Isaacson et al. Consequently, it is expected to most closely reflect the available clinical evidence. Routine use assumes repeated use of remote monitoring devices as an adjunct to SoC. This strategy broadly reflects the use of remote monitoring devices as anticipated in the relevant company submissions for PKG and Kinesia 360.
A third strategy of recurrent use was considered in a scenario analysis and is presented as a variation to the routine use strategy. It explores the potential for remote monitoring assessments to replace SoC appointments. In this scenario, remote monitoring (PKG or Kinesia 360) was implemented at model baseline as an adjunct to SoC and, in the follow-up pathway, conducted 6 months between annual consultations to reduce the number of consultations and facilitate treatment titration prior to the next annual review. This strategy attempts to capture an approach to using remote monitoring devices reported in Dominey et al. 68
In the full economic evaluation, PKG and Kinesia 360 were compared to SoC within each alternative monitoring strategy delivery schedule. Further details of the alternative monitoring strategies are provided in Monitoring schedules and settings.
Model structure
A de novo economic analysis was developed to estimate the costs and health outcomes (in terms of QALYs) associated with alternative remote monitoring strategies and SoC. The economic analysis was designed to capture experiences of PwP during the maintenance phase of disease and is predicated on the assumption that remote monitoring provides a degree of symptomatic relief via improvements in clinical assessment and therapeutic decisions. The economic analysis does not attempt to explicitly model this link and instead directly captures improvements in symptom relief. Specifically, the model uses changes in MDS-UPDRS observed in Woodrow et al. and Isaacson et al. as an indicator of the degree of symptom control and to capture any health benefits resulting from remote continuous monitoring. 17,33
The economic analysis uses a Markov cohort structure consisting of three health states: enhanced maintenance, standard maintenance and death. Enhanced maintenance and standard maintenance are associated with a specific set of MDS-UPDRS domain scores (I–IV) representing the degree of symptom control associated with remote monitoring (enhanced monitoring health state) and SoC (standard monitoring health state). The difference between enhanced and standard maintenance is informed via evidence on comparative clinical efficacy and also linked to the changes in levodopa-equivalent titration associated with remote monitoring (see sections Model input parameters for further details of efficacy assumptions and Resource use and costs for resource assumptions).
For any remote monitoring strategy, the cohort enters the model in the enhanced maintenance health state. During the first cycle of the model (6 months) patients are assumed to either: (1) remain in the enhanced maintenance state, (2) transition to the standard maintenance health state or (3) transition to an absorbing death state. Transitions between the enhanced and standard maintenance health states are used to capture the effect of waning treatment, with the proportions in each health state determined by the proportion of treatment effect remaining (see Efficacy for further details on assumptions associated with the waning of treatment effect). Consequently, the monitoring schedule is determined by the monitoring strategy, rather than state membership (i.e. patients within the standard maintenance health state can still be undertaking remote monitoring).
Depending on the monitoring strategy and extrapolation assumptions, patients within the standard maintenance state can also transition back into the enhanced maintenance health state. This is assumed to only occur in strategies where repeated use of remote monitoring devices is permitted and represents a return to enhanced disease management for these patients. At base-case model settings, such back-transitions do not occur. For SoC, patients enter the model in the standard maintenance health state and can either: (1) remain in the standard maintenance health state, or (2) transition to an absorbing death state. The Markov model structure is presented in Figure 5.
FIGURE 5.
Markov model structure. a, Only applicable to patients receiving remote monitoring.

Since PD is a degenerative condition with no available cure or disease-modifying treatments, the model considers disease progression exogenous, meaning each monitoring strategy has no influence on disease progression. Disease progression is modelled according to changes in UPDRS domain scores (I–IV), where changes in UPDRS domain scores associated with remote monitoring differentiate the health states. Patients within the enhanced maintenance health state progress along the same disease trajectory as those within the standard maintenance health state, but experience an intermediate shift in UPDRS scores from alternative maintenance therapy representing the symptomatic relief associated with enhanced management.
The Markov cycle length is 6 months to align with the frequency of consultations made during the maintenance phase of PD. As indicated above, the base-case analysis uses a time horizon of 5 years. The NICE reference guide indicates that the time horizon used for estimating clinical and cost-effectiveness should be sufficiently long to reflect any differences in costs and benefits between the technologies being compared. 100 In considering the appropriate time horizon, the EAG considered the following points:
-
The primary benefits of monitoring result from the optimisation of treatment. The impact of monitoring devices on benefits and costs is therefore contingent upon the availability of alternative treatment strategies. As discussed previously, this is likely to be predominantly confined to the early and maintenance stages of the disease where alternative treatment strategies can offer improved symptom control.
-
Comparative clinical evidence on the use of remote continuous monitoring devices is confined to the maintenance stage of the disease, with only limited/no evidence in early and advanced populations.
-
The symptomatic benefits associated with improved monitoring relative to SoC are likely to be brief as a consequence of further disease progression and catch-up amongst patients receiving current SoC.
-
The lack of disease-modifying treatments (i.e. treatments that change how PD develops over time) means that improved monitoring cannot impact the long-term trajectory of patients.
-
The time horizon of the data used in the literature to establish key relationships (i.e. progression and HRQoL models use data with up to 6 years follow-up).
Reflecting on these points, the EAG considered a short 5-year horizon most appropriate. A lifetime horizon (more typically modelled in NICE appraisals) would require the model to extrapolate relationships beyond the time horizon of the data and would need to account for transitions to more advanced stages of disease, including the modelling of advanced treatments such as DBS, apomorphine injections and LCIG. While it is plausible that monitoring devices may impact on transitions to advanced therapies, the available clinical evidence to inform this is limited (see Association outcomes). Moreover, the lack of disease-modifying drugs implies convergence between patients on SoC with and without remote monitoring. Any impacts on costs and benefits would therefore be transitory, and most likely only impact the timing of when advanced treatments are initiated. The economic analysis also implicitly assumes that remote monitoring will not continue beyond the maintenance disease stage, reflecting the available clinical evidence. The 5-year time horizon therefore assumes that remote monitoring devices will be used for a maximum of 5 years (reflecting the approximate duration of the maintenance phase) with no lasting differences to costs and benefits after this time.
Model input parameters
Patient population
The target population in the model consists of patients in the maintenance phase of PD, where the management of symptomatic motor and non-motor features of the disease is routinely required. Patients in the model consist of the average characteristics of participants enrolled in the Woodrow et al. study, given that this study represents the largest comparative assessment of clinical effectiveness for remote continuous monitoring devices. Table 24 summarises the values used for the baseline patient characteristics in the model. Disease duration, levodopa daily dose, H&Y stage and baseline MDS-UPDRS scores all constitute key characteristics in determining baseline disease progression in the model. Sex and age characteristics establish disease progression, patient’s HRQoL and mortality risk.
Characteristics | Value |
---|---|
Female | 47% |
Disease duration | 6.35 years |
Age | 67.8 years |
LED | 718.6 mg |
H&Y stage | 2 |
Baseline MDS-UPDRS I | 10.86 |
Baseline MDS-UPDRS II | 10.12 |
Baseline MDS-UPDRS III | 35.46 |
Baseline MDS-UPDRS IV | 4.79 |
Expert clinical advice obtained by the EAG indicates the Australian study setting does not preclude its relevance to the UK context. Expert clinical advice sought by the EAG did not preclude the relevance of this population to the UK context. However, compared to the patient population in the UK, the trial population may represent a younger and more sex-balanced cohort than seen in UK clinical practice with the average age of Parkinson’s diagnosis in the UK occurring between 70 and 79 years of age and prevalence is notably higher amongst men. 4,148 The model population aims to reflect the average characteristics of patients with maintenance stage PD; however, the study by Isaacson et al. represents a more severe subpopulation of those experiencing clinically significant motor symptoms insufficiently controlled by current therapy. The EAG acknowledges these limitations.
Monitoring schedules and settings
Patients with PD require regular follow-up care. Appointments serve as an opportunity for healthcare professionals to review, test and treat a wide range of Parkinson’s-specific and age-related symptoms, events and complications (e.g. motor issues, behavioural abnormalities, falls, dementia, blood pressure, constipation, etc.). In UK clinical practice, appointments are conducted with a consultant or community PD nurse specialist, either via face-to-face consultations or remotely using phone or video appointments. At present, no standard template exists for monitoring within current care pathways with or without the introduction of remote monitoring devices. Remote monitoring devices have the potential to be introduced in a variety of ways, including on a targeted basis, where clinicians believe specific circumstances warrant its application; as a clinical aid used across all follow-up periods or as an intermediary tool to improve clinical understanding while facilitating targeted follow-up (only assessing those in need). After consultation with experts, reviewing real-world applications of remote monitoring devices and considering company responses, the EAG modelled one-time and routine applications of remote monitoring devices, with an alternative recurrent use assessed in a scenario analysis. The assumed schedules and settings for SoC and for the alternative remote monitoring strategies are detailed below.
Standard of care
Patients receiving SoC are assumed to undertake review appointments every 6 months, representing the lower bound of the recommended schedule in NICE clinical guidelines (every 6–12 months). 2 It is assumed 55% of consultations are conducted face to face and 45% remotely, in line with activities reported in the 2019–20 NHS reference costing schedule (from 158,768 recorded specialist Parkinson’s and Alzheimer’s nursing/liaison activities). 149
One-time use
Patients receiving a one-time remote monitoring assessment using PKG or Kinesia 360 are assumed to undertake remote assessment in conjunction with baseline consultation. Thereafter, patients’ follow-up schedule and setting align with SoC (see Table 25).
Base-case schedules | Baseline | 6 months | 12 months | 18 months | ... | Time horizon | |||||
---|---|---|---|---|---|---|---|---|---|---|---|
RMD FU | SoC FU | RMD FU | SoC FU | RMD FU | SoC FU | RMD FU | SoC FU | RMD FU | SoC FU | ||
SoC | ✕ | ✔ | ✕ | ✔ | ✕ | ✔ | ✕ | ✔ | … | ✕ | ✔ |
One-time use | |||||||||||
PKG | ✔ | ✔ | ✕ | ✔ | ✕ | ✔ | ✕ | ✔ | … | ✕ | ✔ |
Kinesia 360 | ✔ | ✔ | ✕ | ✔ | ✕ | ✔ | ✕ | ✔ | … | ✕ | ✔ |
Routine use | |||||||||||
PKG | ✔ | ✔ | ✔ | ✔ | ✔ | ✔ | ✔ | ✔ | … | ✔ | ✔ |
Kinesia 360 | ✔ | ✔ | ✔ | ✔ | ✔ | ✔ | ✔ | ✔ | … | ✔ | ✔ |
Recurrent use (scenario) | |||||||||||
PKG | ✔ | ✔ | ✔ | ✕a | ✕ | ✔ | ✔ | ✕a | … | ✕ | ✔ |
Kinesia 360 | ✔ | ✔ | ✔ | ✕a | ✕ | ✔ | ✔ | ✕a | … | ✕ | ✔ |
Routine use
Aligned with the companies’ positioning of the technologies, routine use strategies include remote monitoring (PKG or Kinesia 360) as an adjunct to SoC applied at every follow-up period to routinely assist clinical assessments. The model assumes that routine use of remote monitoring devices increases the rate of remote consultations up to 79% (vs. 45% in SoC). 69
Recurrent use (scenario)
In line with the management approach outlined in Dominey et al. ,68 the recurrent use scenario analysis introduces remote management as an intermediary assessment solution, replacing consultations between annual appointments where possible. In this scenario it was assumed that a proportion of intermediate consultations would not be required and that only 21% of patients would require interim (those between annual consultations) face-to-face consultations. This was based on the proportion of virtual clinic appointments deemed unsuccessful in Evans et al. (issues include the PKG, speech or hearing problems, complex care needs). 69 This strategy was only considered as a scenario due to its stylised nature. For simplicity, this scenario assumes equivalent effectiveness to the routine use strategy (see Efficacy for discussion).
Efficacy
The clinical efficacy of remote monitoring devices is modelled according to changes in UPDRS domain scores relative to SoC. The EAG considered changes in UPDRS to offer a broader and more appropriate measure of symptomatic benefit compared to changes in H&Y scales or other measures used in previous Parkinson’s-related economic evaluations (e.g. < 25% off-times per day, see Results of the systematic review of decision models evaluating interventions in Parkinson’s disease). The model considers the efficacy of remote monitoring devices as the health benefits attained via improved symptom control and management of the disease relative to SoC. These health benefits are confined to the enhanced maintenance health state of the model and are conferred via a one-time shift in the disease progression curve (independent of the rate of progression over time). Given the time horizon of the model, the benefits are considered to only occur within the management phase of PD. The magnitude of the changes in UPDRS associated with PKG and Kinesia 360 was informed by Woodrow et al. and Isaacson et al. , respectively. 17,33
To consider a common outcome measure across interventions, and to align with the progression risk and utility equations from Chandler et al. (see Progression of disease Section 0),140 MDS-UPDRS scores from Woodrow et al. were converted into the UPDRS scale using the linear relationships published by Goetz et al. 142 The EAG notes that Goetz et al. maps from UPDRS to MDS-UPDRS, and it is unclear how appropriate it is to apply this conversion in the reverse order; however, the EAG considers it preferable to use the conversion rather than assume that MDS-UPDRS and UPDRS are equivalent. As undertaken in Woodrow individual participant data, changes in UPDRS associated with PKG remote monitoring were adjusted for potential confounding factors (age, sex, PD duration, UPDRS III at baseline and number of clinical visits during follow-up).
Changes in UPDRS associated with Kinesia 360 were derived from the reported difference in UPDRS II and UPDRS III scores between baseline and 12-week follow-up. Due to an absence in reporting UPDRS I and UPDRS IV outcomes in Isaacson et al. , the EAG assume Kinesia 360 has no impact beyond the daily living (UPDRS II) and motor-symptom (UPDRS III) domains of the UPDRS. Without access to individual patient level data, the EAG were not able to adjust for potential confounding factors in Isaacson et al. It is also important to note that the study population in Isaacson et al. represents an enriched population compared to Woodrow et al. by recruiting patients with more severe disease, all of whom presented with clinically significant motor symptoms. Given these differences in the study populations, comparisons between PKG and Kinesia 360 should be interpreted with caution.
Estimated changes in UPDRS associated with PKG and Kinesia 360 are reported in Table 26. In line with findings on the MDS-UPDRS (see Woodrow individual participant data), PKG is also associated with a negligible and unfavourable impact on the UPDRS I and UPDRS II domains. In consideration of these highly uncertain results, the base-case analysis considered two efficacy configurations for PKG: (1) an unrestricted analysis that considers changes across all domains of the UPDRS, and (2) a restricted analysis that considers only UPDRS domains III and IV, with the average efficacy on UPDRS domains I and II set to zero (i.e. PKG having no detrimental impact on average) (see Table 26).
Intervention | Method | Source | UPDRS I | UPDRS II | UPDRS III | UPDRS IV |
---|---|---|---|---|---|---|
Base case | ||||||
PKG | Unrestricted and adjusted UPDRS changes (Section X) | Woodrow et al. (2020)17 | 0.02 | 0.33 | −2.65 | −1.16 |
PKG | Restricted and adjusted UPDRS changes (Section X) | Woodrow et al. (2020)17 | 0 | 0 | −2.65 | −1.16 |
Kinesia 360 | Observed UPDRS difference in differences (Table X) | Isaacson et al. (2019)33 | 0 | −2.6 | −4.3 | 0 |
Scenarios | ||||||
PKG | Unadjusted UPDRS changes | Woodrow et al. (2020)17 | −0.83 | 0.22 | −3.35 | −1.18 |
Kinesia 360 | Scaled (observed changes in UPDRS) | Woodrow et al. (2020)17 | −1.23 | 0.12 | −3.42 | −1.26 |
Woodrow individual participant data reports the significance of ‘target’ status in determining outcomes associated with remote monitoring devices. On the basis that ‘target’ status, as defined in Woodrow et al. , cannot be established a priori (i.e. in the absence of a remote monitoring device), the EAG apply average treatment-effects from the trial (i.e. considering all participants, with and without controlled disease). In practice however, healthcare professionals may, to an extent, be able to identify and focus remote monitoring on out-of-target patients (e.g. patients with insufficiently controlled symptoms). The EAG does not present cost-effectiveness results by, or averaged across, ‘target’ status on the basis that patients’ ‘target’ status, as defined in Woodrow et al. , does not represent, at the point of care, a valid ex-ante subgroup.
Low-quality diagnostic accuracy studies suggest that PKG and Kinesia 360 offer moderate short-term systematic benefits from remote monitoring, as observed through improvements in UPDRS II (Kinesia 360), III (PKG and Kinesia 360) and IV (PKG) scores. However, in the absence of any evidence on the efficacy of devices beyond the immediate term, the EAG explores alternative extrapolations in treatment effect that directly informs transitions between enhanced maintenance and standard maintenance health states in the model. In the base-case analysis, the EAG assume equal rates of treatment waning for PKG and Kinesia 360 with routine remote monitoring strategies and a recurrent scenario strategy incurring no waning in benefit over the maximum 5-year model time horizon (i.e. all patients remain within the enhanced maintenance health state) and one-time strategies with a 50% waning rate per cycle (i.e. every 6 months 50% of patients within the enhanced maintenance health state transition to the standard maintenance health state). Modelled strategy-specific efficacy on the UPDRS III domain is displayed in Figures 7 and 8. A wide range of potential values for the exponential waning rates were explored in sensitivity analysis (0–90%).
FIGURE 6.
Predicted disease progression in standard of care.

FIGURE 7.
Estimated one-time use monitoring-specific UPDRS III scores (50% decay rate).
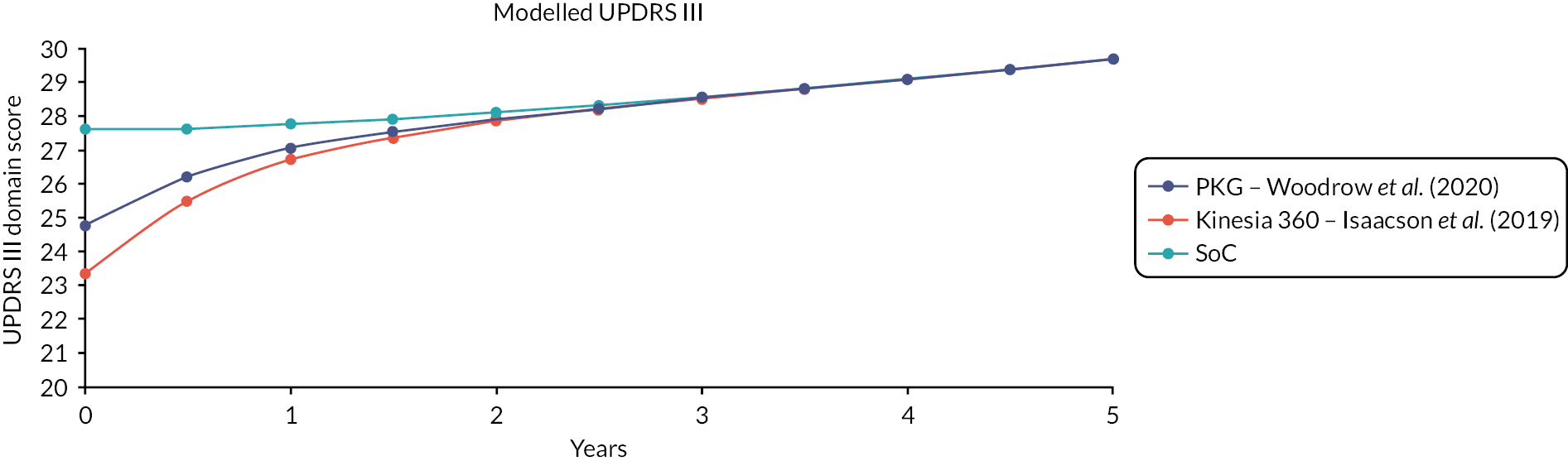
FIGURE 8.
Estimated routine monitoring-specific UPDRS III scores (0% decay rate).

Progression of disease
The model uses UPDRS domain scale scores to measure disease severity (behavioural problems, non-motor and motor symptoms and therapy complications) and progression over the analysis time horizon. Patients are assumed to progress across all four UPDRS domains according to the prediction equations published in Chandler et al. , which incorporate patient characteristics (age, gender), treatment factors (PD medications, LED, time since previous follow-up), condition (baseline severity, disease duration) and structural dependencies (rates of change and interactions). Patients in the enhanced maintenance health state progress along the SoC defined curve but with a decrement associated with their monitoring device (see Efficacy). Details regarding the data informing these equations are provided in Results of the systematic review of decision models evaluating interventions in Parkinson’s disease. See Table 27 for model coefficients and Figures 6–8 for progression predictions applicable to the model population.
UPDRS I | UPDRS II | UPDRS III | UPDRS IV | |||||
---|---|---|---|---|---|---|---|---|
β | SE | β | SE | β | SE | β | SE | |
Intercept | 0.19780 | 0.17220 | 0.65960 | 0.50540 | −2.78020 | 0.63200 | 2.50260 | 0.16600 |
Female | – | – | −0.19450 | 0.08112 | −0.75140 | 0.17200 | 0.21230 | 0.03852 |
Current disease duration (years) | −0.00350 | 0.01669 | 0.02299 | 0.03824 | 0.05820 | 0.07809 | −0.00107 | 0.01563 |
Current age (years) | −0.00048 | 0.00260 | −0.00924 | 0.00763 | 0.06763 | 0.00896 | −0.00522 | 0.00249 |
LEDD | −0.00001 | −0.00010 | 0.00029 | 0.00024 | −0.00109 | 0.00047 | 0.00036 | 0.00010 |
Time since last visit (days) | 0.00101 | 0.00016 | 0.00476 | 0.00039 | 0.00868 | 0.00079 | 0.00078 | 0.00015 |
No off-time | −0.17240 | 0.03450 | −0.57840 | 0.07832 | −0.48480 | 0.16470 | −2.34110 | 0.03305 |
Baseline score: UPDRS I | 0.25560 | 0.01893 | 0.00000 | 0.00000 | −0.07360 | 0.07479 | 0.08418 | 0.01601 |
Baseline score: UPDRS II | – | – | 0.06945 | 0.01612 | 0.05421 | 0.02600 | 0.00000 | 0.00000 |
Baseline score: UPDRS III | −0.00624 | 0.00311 | 0.02960 | 0.00563 | 0.17110 | 0.01695 | 0.00000 | 0.00000 |
Baseline score: UPDRS IV | – | – | – | – | – | – | 0.08793 | 0.01472 |
Prior visit score: UPDRS I | −1.08650 | 0.07079 | 0.20450 | 0.02509 | 0.33830 | 0.05784 | 0.05258 | 0.01142 |
Prior visit score: UPDRS II | – | – | −0.55090 | 0.05427 | – | – | – | – |
Prior visit score: UPDRS III | 0.01518 | 0.00245 | – | – | −0.30470 | 0.01428 | – | – |
Prior visit score: UPDRS IV | 0.03870 | 0.00927 | – | – | −0.09407 | 0.04415 | −0.62510 | 0.05252 |
Prior slope: UPDRS I | −37.06080 | 3.49530 | – | – | – | – | – | – |
Prior slope: UPDRS II | – | – | −48.64770 | 5.25890 | – | – | – | – |
Prior slope: UPDRS III | – | – | – | – | −89.65470 | 15.97280 | – | – |
Prior slope: UPDRS IV | – | – | – | – | – | – | −12.06010 | 1.98680 |
Current disease duration (years) * LEDD | 0.00005 | 0.00002 | 0.00000 | 0.00005 | 0.00006 | 0.00009 | 0.00005 | 0.00002 |
Baseline score: UPDRS I * Prior slope: UPDRS 1 | 4.70130 | 1.00190 | – | – | – | – | – | – |
Baseline score: UPDRS II * Prior slope: UPDRS II | – | – | 1.61260 | 0.49860 | – | – | – | – |
Prior visit score: UPDRS I * Current age (years) | 0.00809 | 0.00105 | – | – | – | – | −0.00193 | 0.00081 |
Prior visit score: UPDRS II * Current age (years) | – | – | 0.00494 | 0.00078 | – | – | – | – |
Prior visit score: UPDRS III * Prior slope: UPDRS III | – | – | – | – | −1.44400 | 0.23940 | – | – |
Adverse event costs
None of the studies identified in the systematic review reported any adverse events directly linked to the use of remote monitoring devices. It was therefore not possible to include adverse effects in the economic analysis.
Mortality
Mortality in the model is based on age- and sex-adjusted all-cause mortality probabilities from the UK Office of National Statistics Interim Life Tables 2018–20. 150 Parkinson’s disease is generally associated with increased mortality; however, survival is highly dependent on the stage and characteristics of the disorder. 151 Patients with Parkinson’s disease presenting with normal cognitive function seem to have a largely normal life expectancy. 151 A recent large, UK population-based study of more than 10,000 PwP and more than 50,000 in the control group without PD, showed a modest elevation in mortality risks for PwP. 148 Informed by this analysis, the base-case analysis adopts an elevated 1.14 hazard ratio for management-stage Parkinson’s patients, representing a modest overall increase in mortality associated with the condition compared to the general population. The model applies common mortality rates within patient traces, meaning no differential mortality impact is associated with SoC or the alternative remote monitoring strategies compared.
Health-related quality of life
Patient health-related quality of life
As previously discussed, health benefits from monitoring devices are assumed to be as a consequence of better symptom control and improved management of the disease. This is captured in the model via changes in UPDRS domain scores. To estimate HRQoL benefits associated with the monitoring devices, changes in UPDRS domain score were linked to HRQoL improvements using an algorithm reported in Chandler et al. 140 The Chandler et al. algorithm was based on a regression analysis using EQ-5D-3L data from the NET-PD LS-1 database, which includes 1741 PwP from the USA and Canada followed-up for maximum of 6 years. Data were analysed using UK preference weights for the EQ-5D-3L responses and a MMRM model to account for repeat measurement and included gender and UPDRS domain covariates (having tested for the impact of other patient covariates).
The EAG also considered two alternative sources of HRQoL data: NICE CG71 and Fundament et al. 98,137 Both alternative value sets were, however, based on data from patients with advanced disease and therefore did not reflect the modelled population. In the case of Fundament et al. , there were also specific methodological concerns with how the value set was generated (see Results of the systematic review of decision models evaluating interventions in Parkinson’s disease). The EAG, therefore, deemed Chandler et al. ’s algorithm the most appropriate source for estimating HRQoL within the UK decision-making context for management-phase PD. Table 28 reports the modelled coefficients.
Parameter | Coefficient | Standard error |
---|---|---|
Constant | 0.9434 | 0.006414 |
Male | 0.03955 | 0.006045 |
UPDRS I | −0.01913 | 0.001267 |
UPDRS II | −0.0133 | 0.000537 |
UPDRS III | −0.00161 | 0.00026 |
UPDRS IV | −0.00813 | 0.000966 |
The total incremental QALY gains for the remote continuous monitoring devices compared to SoC are derived from the magnitude of treatment effect (see Table 26), persistence of effect over time (see Efficacy) and HRQoL gains (see Table 28) associated with the UPDRS differentials between the alternate monitoring strategies. Differentials in UPDRS and associated HRQoL are estimated on a per cycle basis in the model (see Progression of disease). The average HRQoL utility values in the enhanced maintenance health state (using the restricted approach for estimating the efficacy of PKG) is displayed in Figure 9.
FIGURE 9.
Modelled HRQoL in the standard maintenance and in the treatment-specific enhanced maintenance health states for the remote monitoring devices.

Although PDQ-39 summary scores were available in Woodrow et al. , the omission of dimension-level individual participant data precluded the EAG from generating treatment-specific utility values by mapping the PDQ-39 onto EQ-5D. 152
Carer quality of life
Caring for PwP can place a burden on informal caregivers, negatively impacting their (health-related) quality of life. 153 Studies have shown that functional status is an important determinant of carer quality of life, with several studies emphasising mobility and cognitive impairment as drivers of carer quality of life. There is, however, no direct evidence linking the use of remote monitoring devices to carer quality of life. Moreover, the broader literature does not show any consistent relationship between relevant clinical outcomes such as UPDRS. 154 It was therefore not possible to account for any impacts on carer quality of life within the economic analysis. This may represent an uncaptured benefit given the observed improvements in UPDRS and other clinical outcomes.
Resource use and costs
This section details the resource use and costs applied in the model. The EAG did not establish a relationship between disease severity and costs. The model considers the costs of the remote continuous monitoring devices, implementation costs, follow-up consultations and medication costs. Details of each resource use and cost in the model are presented in the sections below.
Remote monitoring device costs
The costs of PKG, Kinesia 360, STAT-ON, KinesiaU and PDMonitor devices were based on company responses to the NICE request for information. The alternative devices had three types of payment mechanism: (1) pay per use, (2) subscription model or (3) outright purchase of the device. VAT was not applied to device costs as outlined in the NICE Diagnostics Assessment Programme manual. 100 The costs of the devices are reported in Table 29.
Cost (exc. VAT) (£) | Unit | Modelled cost per year (£) | Modelled cost per 5 years (base-case time horizon) (£) | |
---|---|---|---|---|
PKG | 225 | Per use per patient | 450a | 2250a |
Kinesia 360 | 224 | Monthly device subscription | 2688b | 13,440b |
STAT-ON | 1600 | Annual device subscription | 1920b | 9600b |
KinesiaU | 64 | Monthly subscription per patient | 768b | 3840b |
PDMonitor | 12,000 | Outright device purchase | 12,000b | 12,000b† |
The PKG device requires a payment of £225 per application. The cost is inclusive of the postage of the data logger to the patient, postage back to Global Kinetics Corporation (GKC) and the PKG report made available via the online portal. Kinesia devices use a subscription service cost model with monthly fees of £224 and £64 for Kinesia 360 and KinesiaU products, respectively. KinesiaU comprises patient-level costs for access to the company’s smartphone app (£5 per month) and clinician-specific costs for access to the KinesiaU portal (£59 per month). STAT-ON uses a subscription model with an annual licence fee (£1600). This grants the user(s) a device, charger kit and adjustable belt with clinical and technical support as well as a 2-year warranty. Kinesia 360, STAT-ON and PDMonitor allow multiple users to access the subscribed or purchased devices (albeit with new straps required). Besides PKG, it is unclear whether broader costs for the delivery and management of devices are included in the company costs (e.g. device delivery, administration, relevant support, etc.). The costs of potential loss or damage to devices is not stated by the companies and for simplicity is not included in the model.
The EAG assumes subscription models (Kinesia 360, KinesiaU and STAT-ON) continuously run over the course of the model time horizon for routine strategies and for the recurrent monitoring scenario. PDMonitor is treated as a one-time up-front cost, with no additional intervention costs for repeated use. The EAG believe this to be reasonable on the basis that the model time horizon falls within the company stated lifetime of the device (approximately 7 years). For one-time use remote monitoring strategies, it was assumed that a 3-month subscription was required for Kinesia products (in line with the 12-week follow-up in Isaacson et al. 33), and a 1-year subscription for STAT-ON. The EAG acknowledges that the one-time monitoring strategy does not align with the company’s positioning of purchased (PDMonitor) or subscription-based services (Kinesia 360, KinesiaU and STAT-ON) and may incur further administrative burden and implementation costs relative to one-time PKG use.
Implementation costs
The costs required to successfully implement remote monitoring strategies into service pathways were divided into fixed implementation costs (those irrespective of device use) and variable costs (those specifically incurred from using a remote monitoring device in clinical practice).
Fixed implementation costs were calculated in accordance with staff training times noted in company responses, with the mid-point selected within ranges (PKG and STAT-ON: 90 minutes; Kinesia 360, KinesiaU and PDMonitor: 30 minutes). This equated to a £56 cost per patient, assuming clinician-level fixed costs are distributed over eight patients. 69,155 Implementation costs may include clinician training (participating clinicians in the Woodrow study received a day of training in interpreting the PKG) and a variety of process factors (e.g. administration, procurement, etc.).
Variable implementation costs may exist given the potential for remote monitoring devices to require additional patient support and healthcare professional time required to arrange its application and review findings. The base-case analysis applies zero variable implementation costs, assuming consultation costs sufficiently cover potential broader service factor costs. Scenario analyses considered the removal of implementation costs and the addition of variable costs (an additional £39 cost per consultations using a remote monitoring device, equivalent to 15 minutes of general practitioner time).
Consultation costs
In line with Woodrow et al. , it was assumed that several initial face-to-face consultations would be required. For all remote monitoring strategies patients were assumed to undertake 2.57 initial visits, while patient receiving SoC were assumed to receive 2.17 visits.
For subsequent visits, the consultation setting and their associated costs are dependent on the monitoring strategy (i.e. one-time use or routine monitoring). As described previously (see Monitoring schedules and settings), patients receiving SoC are assumed to undertake review appointments every 6 months, with 55% of consultations conducted face to face and 45% remotely. This split was informed by activities reported in the 2019–20 NHS reference costing schedule (from 158,768 recorded specialist Parkinson’s and Alzheimer’s nursing/liaison activities). 149 Face-to-face and remote consultations were assumed to cost the NHS £81.41 and £56.41 respectively, in line with NHS reference costs 2019/20. 149
The one-time use remote monitoring strategies were assumed to align to the setting and follow-up schedule received with SoC. Relative to SoC and one-time use strategies, the routine and recurrent remote monitoring strategies are assumed to increase the proportion of consultations that can be conducted remotely based on evidence from Evans et al. 69 To incorporate this cost-saving, the routine and recurrent (scenario analysis) strategies assume 79% of consultations are conducted remotely (compared to 45% in SoC). The recurrent scenario strategy also allows 79% of patients to avoid an interim review between annual appointments (with the remaining 21% requiring face-to-face consultation). To consider the potential for remote monitoring strategies to mitigate the need for consultation, alternative consultation savings were explored in sensitivity analysis. Table 30 provides an overview of the costs and patient consultation settings for SoC and each monitoring strategy.
Face-to-face consultations | Cost source | Remote consultation | Cost source | Schedule | |||
---|---|---|---|---|---|---|---|
Proportion of patients (%) | Cost per consult (£) | Proportion of patients (%) | Cost per consult (£) | ||||
SoC | 55 | 81.41 | Tariff: N22AF | 45 | 56.41 | Tariff: N22AN | Every 6 months |
One-time use | 55 | 81.41 | Tariff: N22AF | 45 | 56.41 | Tariff: N22AN | Every 6 months |
Routine use | 21 | 81.41 | Tariff: N22AF | 79 | 56.41 | Tariff: N22AN | Every 6 months |
Scenario | |||||||
Recurrent use | 21 | 81.41 | Tariff: N22AF | 79 | 56.41 | Tariff: N22AN | Annuala |
Medication costs
Medication costs in the model were based on the average cost per milligram of LEDD regimens reported in Chandler et al. 140 The study calculates the average cost per milligram of LED in the UK to be £5.01 per year, from the medication composition published in an analysis of the UK Clinical Practice Research Datalink database between 2004 and 2015 (levodopa 43%, pramipexole 30.34%, ropinirole 21.52% and bromocriptine 4.78%) and drug costs from the Monthly Index of Medical Specialties database. 143
Across all remote monitoring strategies, patients within the enhanced maintenance health state in the model were prescribed an additional 22 mg LED, in line with the EAG adjusted estimates of the differences in LEDD consumption from the Woodrow et al. study (see Woodrow individual participant data). Based on previous economic evaluations, it was assumed LED doses increase at a rate of 10% per annum. 156–158 Differentials in LED between remote monitoring strategies diminish at the assumed rate of decay in treatment benefit, whereby differences are maintained over the model time horizon in the base-case routine and recurrent remote monitoring strategies (assuming no efficacy decay), and diminish at an assumed positive rate in the one-time remote monitoring strategies (50% base case).
Analytical methods
Base-case analysis
The base-case analyses present deterministic and probabilistic pairwise comparisons between PKG and SoC and Kinesia 360 and SoC for one-time use and routine remote monitoring strategies. Cost-effectiveness analyses of PKG were presented both with restricted and unrestricted efficacy (i.e. restricted to only assessing UPDRS domains III and IV). A base-case analysis with an incremental comparison of SoC, PKG and Kinesia 360 was also presented. The base-case parameters and their associated assumptions and sources are detailed in Table 31.
Parameter | Values | Source/assumptions | Probabilistic model setup | |
---|---|---|---|---|
Patient characteristics | ||||
Age | 67.8 | Woodrow et al. (2020)17 | N/A | |
Proportion of male individuals | 53% | Woodrow et al. (2020)17 | N/A | |
Disease duration | 6.35 years | Woodrow et al. (2020)17 | N/A | |
LEDD | 718.60 mg | Woodrow et al. (2020)17 | N/A | |
Proportion of patients experiencing no off-time | 0% | Woodrow et al. (2020)17 | N/A | |
Baseline MDS-UPDRS I | 10.86 | Woodrow et al. (2020)17 | N/A | |
Baseline MDS-UPDRS II | 10.12 | Woodrow et al. (2020)17 | N/A | |
Baseline MDS-UPDRS III | 35.46 | Woodrow et al. (2020)17 | N/A | |
Baseline MDS-UPDRS IV | 4.79 | Woodrow et al. (2020)17 | N/A | |
Efficacy | ||||
PKG: unrestricted adjusted estimates | ||||
UPDRS I | 0.00469 | Woodrow et al. (2020)17 | Gaussian distribution: Mean: 0.00469 SE: 0.24960 |
|
UPDRS II | 0.51770 | Woodrow et al. (2020)17 | Gaussian distribution: Mean: 0.51770 SE: 0.52475 |
|
UPDRS III | −2.84362 | Woodrow et al. (2020)17 | Gaussian distribution: Mean: −2.84362 SE: 1.00518 |
|
UPDRS IV | −0.72765 | Woodrow et al. (2020)17 | Gaussian distribution: Mean: −0.72765 SE: 0.58011 |
|
PKG: restricted adjusted estimates | ||||
UPDRS I | 0 | Woodrow et al. (2020)17 | Gaussian distribution: Mean: 0 SE: 0.24960 |
|
UPDRS II | 0 | Woodrow et al. (2020)17 | Gaussian distribution: Mean: 0 SE: 0.52475 |
|
UPDRS III | −2.84362 | Woodrow et al. (2020)17 | Gaussian distribution: Mean: −2.84362 SE: 1.00518 |
|
UPDRS IV | −0.72765 | Woodrow et al. (2020)17 | Gaussian distribution: Mean: −0.72765 SE: 0.58011 |
|
PKG: unrestricted unadjusted estimates | ||||
UPDRS I | −0.01318 | Woodrow et al. (2020)17 | Gaussian distribution: Mean: −0.01318 SE: 0.26946 |
|
UPDRS II | 0.23822 | Woodrow et al. (2020)17 | Gaussian distribution: Mean: 0.23822 SE: 0.52958 |
|
UPDRS III | −3.33473 | Woodrow et al. (2020)17 | Gaussian distribution: Mean: −3.33473 SE: 1.27556 |
|
UPDRS IV | −1.72282 | Woodrow et al. (2020)17 | Gaussian distribution: Mean: −1.72282 SE: 0.60529 |
|
PKG: restricted unadjusted estimates | ||||
UPDRS I | −0.01318 | Woodrow et al. (2020)17 | Gaussian distribution: Mean: −0.01318 SE: 0.26946 |
|
UPDRS II | 0 | Woodrow et al. (2020)17 | Gaussian distribution: Mean: 0 SE: 0.52958 |
|
UPDRS III | −3.33473 | Woodrow et al. (2020)17 | Gaussian distribution: Mean: −3.33473 SE: 1.27556 |
|
UPDRS IV | −1.72282 | Woodrow et al. (2020)17 | Gaussian distribution: Mean: −1.72282 SE: 0.60529 |
|
Kinesia 360 | ||||
UPDRS I | 0 | Isaacson et al. (2019)33 | Gaussian distribution: Mean: 0 SE: 0 |
|
UPDRS II | −2.60 | Isaacson et al. (2019)33 | Gaussian distribution: Mean: −2.60 SE: 0.6 |
|
UPDRS III | −4.30 | Isaacson et al. (2019)33 | Gaussian distribution: Mean: −4.30 SE: 2.0 |
|
UPDRS IV | 0 | Isaacson et al. (2019)33 | Gaussian distribution: Mean: 0 SE: 0 |
|
Long-term decay rate in efficacy (i.e. transitions between enhanced maintenance and standard maintenance health state) | ||||
One-time use remote monitoring | 50% | Assumption | N/A | |
Routine remote monitoring | 0% | Assumption | N/A | |
Recurrent remote monitoring (scenario) | 0% | Assumption | N/A | |
Disease progression | ||||
Disease progression model | Table 27 | Chandler et al. (2021)140 | Table 27 | |
HRQoL | ||||
UPDRS I | −0.01913 | Chandler et al. (2021)140 (see Health-related quality of life for full model) |
Gaussian distribution: Mean: −0.01913 SE: 0.001267 |
|
UPDRS II | −0.01330 | Chandler et al. (2021)140 (see Health-related quality of life for full model) |
Gaussian distribution: Mean: −0.01330 SE: 0.000537 |
|
UPDRS III | −0.00161 | Chandler et al. (2021)140 (see Health-related quality of life for full model) |
Gaussian distribution: Mean: −0.00161 SE: 0.00026 |
|
UPDRS IV | −0.00813 | Chandler et al. (2021)140 (see Health-related quality of life for full model) |
Gaussian distribution: Mean: −0.00813 SE: 0.000966 |
|
Costs | ||||
Intervention costs (£) | ||||
PKG | 225 (per use) | Company response | N/A | |
STAT-ON | 1600 (per year) | Company response | N/A | |
Kinesia 360 | 224 (per month) | Company response | N/A | |
KinesiaU | 64 (per month) | Company response | N/A | |
PDMonitor | 12,000 (one-time) | Company response | N/A | |
Fixed implementation cost per patient (£) | ||||
PKG | 29.25 | Calculated based on company-reported clinician training time and general practitioner time costs. It was assumed costs were distributed over an eight-patient cohort [in line with the eight-patient template in Evans et al. (2020)]. | N/A | |
STAT-ON | 29.25 | N/A | ||
Kinesia 360 | 9.75 | N/A | ||
KinesiaU | 9.75 | N/A | ||
PDMonitor | 9.75 | N/A | ||
Initial baseline consultations | ||||
SoC | 2.17 | Woodrow et al. (2020)17 | Gamma distribution: α: 4.15630 β: 0.52138 |
|
PKG | 2.57 | Woodrow et al. (2020)17 | Gamma distribution: α: 3.84598 β: 0.66797 |
|
STAT-ON | 2.57 | Woodrow et al. (2020)17 | Gamma distribution: α: 3.84598 β: 0.66797 |
|
Kinesia 360 | 2.57 | Woodrow et al. (2020)17 | Gamma distribution: α: 3.84598 β: 0.66797 |
|
KinesiaU | 2.57 | Woodrow et al. (2020)17 | Gamma distribution: α: 3.84598 β: 0.66797 |
|
PDMonitor | 2.57 | Woodrow et al. (2020)17 | Gamma distribution: α: 3.84598 β: 0.66797 |
|
Consultation costs (£) | ||||
Office appointment | 81.41 | NHS Reference costs (19/20): specialist nursing, Parkinson’s and Alzheimer’s nursing/liaison, adult, face-to-face (N22AF) | N/A | |
Remote appointment | 56.41 | NHS Reference costs (19/20): specialist nursing, Parkinson’s and Alzheimer’s nursing/liaison, adult, non-face-to-face (N22AN) | N/A | |
Medication costs | ||||
LED dosage change associated with remote monitoring | 21.62 mg | Woodrow et al. (2020)17 | Gaussian distribution: Mean: 21.62 mg SE: 43.95 mg |
|
Dopaminergic medication costs (per LED mg) | £5.01 | Chandler et al. (2021)140 | Gaussian distribution: Mean: 5.01 mg SE: 1.00 mg (20% mean) |
|
Non-dopaminergic medication costs (per annum) | £192.10 | Chandler et al. (2021)140 | Gaussian distribution: Mean: 192.10 SE: 38.42 (20% mean) |
|
Mortality | ||||
Disease-specific all-cause mortality hazard ratio | 1.14 | Okunoye et al. (2021)148 | Log normal distribution: Mean: 1.14 SE: 0.11 |
The core structural assumptions underlying the economic analysis are detailed in Table 32.
Assumption | Element | Description |
---|---|---|
1 | Costing | Cost differentials between SoC and alternative remote monitoring strategies only result from: (1) costs associated with using each remote monitoring device (PKG, Kinesia 360, etc.), (2) changes in LED medication use, (3) implementation costs and (4) follow-up care setting (face-to-face or remote consultation). The model considers the costs of the remote monitoring devices, implementation costs, follow-up consultations and medication costs. Costs were independent of disease progression. One-time applications of Kinesia devices and STAT-ON require a 3-month and annual subscription, respectively. |
2 | Efficacy | Treatment efficacy is represented via a one-time shift in severity (defined by UPDRS and used to differentiate the enhanced and standard maintenance health states) and linked in the model via changes in medication alone. The base-case analysis assumes a 0% and 50% per cycle waning in treatment benefit (i.e. transition from enhanced maintenance to standard maintenance health state and associated outcomes) for one-time use and routine remote monitoring strategies, respectively. |
3 | HRQoL | Health-related quality of life is only dependent on the UPDRS score and gender over the time horizon of the analysis. |
4 | Disease progression | Disease progression is defined on the UPDRS scale, with rates of progression deemed independent to remote monitoring. |
5 | Time horizon | A 5-year time horizon is sufficient for assessing the application of remote monitoring devices within the management phase of PD. |
6 | Adverse events | Patients do not experience adverse events. |
7 | Mortality | Patients experience an elevated 1.14 times greater all-cause mortality risk compared to the UK age- and sex-adjusted general population. On death all remote monitoring device subscriptions are assumed cancelled. |
Scenario analyses
A number of scenario analyses are considered in which the alternative strategies and assumptions are input into the economic model and results compared to base-case findings. These analyses are undertaken to assess the robustness of the base-case results to key uncertainties. Details of each scenario, including applicable model element, the relevant position taken in the base-case analysis, which strategies the scenario is applicable to and the alternative assumption applied is presented in Table 33.
Scenario | Element | Position in base-case analysis | Strategies | Variation in scenario analysis |
---|---|---|---|---|
1 | Remote monitoring strategies | One-time use and routine applications of remote monitoring devices considered (see Monitoring schedules and settings) | PKG, Kinesia 360 (recurrent strategies using PKG or the Kinesia 360 remote monitoring device) | An alternative remote monitoring strategy (recurrent) that places remote monitoring between annual clinic appointments in line with the schedule reported in Dominey et al. (2020) |
2 | PKG efficacy | Adjusted analysis of UPDRS data from Woodrow et al. (2020) | PKG (one-time use and routine remote monitoring strategies) | Unadjusted analysis of UPDRS data from Woodrow et al. (2020) |
3 | Implementation costs |
|
PKG, Kinesia 360 (one-time use and routine remote monitoring strategies) | Removing all implementation costs |
|
£39 variable cost (15 minutes of general practitioner time applied at each consultation with a corresponding remote monitoring device) | |||
4 | Consultation setting | SoC and one-time use remote monitoring strategies: 55% face to face, 45% remote appointment Routine remote monitoring strategies: 79% online, 21% remote |
PKG, Kinesia 360 (one-time use and routine remote monitoring strategies) | Equal consultation setting across all alternatives under investigation: 55% face to face, 45% remote appointment |
5 | Routine follow-up schedule | Follow-up schedule for one-time and routine monitoring strategies set to every 6 months (representing the lower bound of NICE guideline recommendations NG61) | PKG, Kinesia 360 (one-time use and routine remote monitoring strategies) | Follow-up schedule set to 12 months (representing the upper bound of NICE guideline recommendations).2 PKG routine remote monitoring strategy assumed to align with annual follow-up with other subscription and purchase models unaffected. |
6 | Incremental approach | Pairwise comparisons using base-case model settings | PKG, Kinesia 360, KinesiaU, STAT-ON, PDMonitor (routine remote monitoring strategies only) | A fully incremental comparison of routine remote strategies for PKG, Kinesia 360, KinesiaU, STAT-ON and PDMonitor assuming equal efficacy as estimated from Woodrow et al. (2020) |
Model validation
The model was developed in Excel by EC and validated by a second analyst (RH). As part of an overall quality assurance process, the internal validity of the model was assessed by extensively exploring logical consistency in the model results.
Chapter 6 Results of the independent economic assessment
Economic assessments comprised: (1) pairwise comparisons of PKG remote monitoring strategies with SoC, (2) pairwise comparisons between Kinesia 360 remote monitoring strategies and SoC, (3) incremental comparison with PKG, Kinesia 360 and SoC, (4) a cost comparison of all alternative monitoring derives including STAT-ON, KinesiaU and PDMonitor, and (5) a fully incremental exploratory scenario analysis that assumes equal efficacy across all the remote monitoring devices, but differences in costs of the devices. Scenario analyses applicable to each economic assessment are described in Table 33. Additional sensitivity analyses considered further uncertainties.
Personal KinetiGraph base-case scenario
Deterministic and probabilistic base-case findings for one-time use and routine PKG remote monitoring strategies are reported in Tables 34 and 35, respectively. Base-case ICERs for the PKG relative to SoC were dependent on monitoring strategy and whether efficacy estimates were restricted (those only considering UPDRS domains scales that demonstrated a beneficial impact of the PKG, see Table 26). The deterministic base-case ICERs for PKG one-time use and routine remote monitoring strategies were £67,856 (£202,363) per QALY and £57,877 (£172,602) per QALY when using a restricted (unrestricted) analysis, respectively. Incremental costs were markedly higher for the routine remote monitoring strategy (£2640) versus one-time use (£339). In both the restricted and unrestricted analyses, QALY gains were approximately 9.15 times greater for the routine remote monitoring strategy relative to one-time use. Restricted analyses significantly increased the HRQoL and consequent QALY-gain associated with PKG, increasing HRQoL gains in the enhanced maintenance health state from approximately 0.0036 to 0.0105 relative to SoC. In the restricted analysis, 44% of the HRQoL benefits associated with PKG were conferred via changes on the UPDRS III domain and the remainder via the UPDRS IV domain (56%). Mean and incremental probabilistic results were broadly comparable to deterministic values, albeit with minor reductions in estimated mean QALYs.
One-time use remote monitoring strategy | Incremental | ICER | ||||
---|---|---|---|---|---|---|
Costs (£) | QALY | Costs (£) | QALYs | (£/QALY) | ||
Deterministic analysis | ||||||
Restricted analysis | ||||||
SoC | 21,939 | 2.788 | ||||
PKG | 22,278 | 2.793 | 339 | 0.00499 | 67,856 | |
Unrestricted analysis | ||||||
SoC | 21,939 | 2.788 | ||||
PKG | 22,278 | 2.790 | 339 | 0.00167 | 202,363 | |
Probabilistic analysis | ||||||
Restricted analysis | ||||||
SoC | 21,886 | 2.760 | ||||
PKG | 22,225 | 2.765 | 339 | 0.00504 | 67,260 | |
Unrestricted analysis | ||||||
SoC | 21,953 | 2.755 | ||||
PKG | 22,291 | 2.757 | 338 | 0.00171 | 197,475 |
Routine remote monitoring strategy | Incremental | ICER | ||||
---|---|---|---|---|---|---|
Costs (£) | QALY | Costs (£) | QALYs | (£/QALY) | ||
Deterministic analysis | ||||||
Restricted analysis | ||||||
SoC | 21,939 | 2.788 | ||||
PKG | 24,580 | 2.834 | 2640 | 0.04562 | 57,877 | |
Unrestricted analysis | ||||||
SoC | 21,939 | 2.788 | ||||
PKG | 24,580 | 2.804 | 2640 | 0.01530 | 172,602 | |
Probabilistic analysis | ||||||
Restricted analysis | ||||||
SoC | 21,951 | 2.756 | ||||
PKG | 24,578 | 2.801 | 2627 | 0.04553 | 57,702 | |
Unrestricted analysis | ||||||
SoC | 21,875 | 2.756 | ||||
PKG | 24,527 | 2.771 | 2652 | 0.01509 | 175,711 |
In order to help understand the incremental results, the disaggregated incremental results for total expected costs for the PKG one-time use and routine remote monitoring strategies compared to SoC are presented in Table 36. The total incremental cost per strategy (relative to SoC) is £338.47 and £2640.34, respectively. Cost differentials were predominantly as a result of device-related costs, which made up 66% (one-time) and 83% (routine) of the cost differentials. PKG one-time remote monitoring incurred device and implementation costs while incurring additional consultation and medication costs relative to SoC. PKG routine remote monitoring found consultation cost savings via a higher proportion of remote consultations compared to face-to-face consultations and incurred additional costs relative to SoC via device-, implementation- and medication-related costs.
Strategy | PKG incremental costs vs. SoC | ||||
---|---|---|---|---|---|
Device costs (£) | Consultation costs (£) | Implementation costs (£) | Medication costs (£) | Total (£) | |
One-time use | 225.00 | 32.73 | 29.25 | 51.47 | 338.47 |
Routine monitoring | 2181.25 | −41.13 | 29.25 | 470.97 | 2640.34 |
Personal KinetiGraph scenario results
Table 37 shows the restricted (considering only UPDRS III and IV domains) and unrestricted (considering all UPDRS domains) results of each EAG scenario analysis. As for the base-case analysis, both one-time and routine use strategies where considered.
PKG: restricted analysis | PKG: unrestricted analysis | |||||||||
---|---|---|---|---|---|---|---|---|---|---|
Costs (£) | QALY | Incremental | ICER | Costs (£) | QALY | Incremental | ICER | |||
Costs (£) | QALYs | (£/QALY) | Costs (£) | QALYs | (£/QALY) | |||||
Scenario 1: recurrent monitoring strategy | ||||||||||
Routine use | ||||||||||
SoC | 21,939 | 2.788 | 21,939 | 2.788 | ||||||
PKG | 23,418 | 2.834 | 1479 | 0.04562 | 32,417 | 23,418 | 2.804 | 1479 | 0.01530 | 96,675 |
Scenario 2: unadjusted efficacy estimates Woodrow et al. (2020) | ||||||||||
One-time use | ||||||||||
SoC | 21,939 | 2.788 | 21,939 | 2.788 | ||||||
PKG | 22,278 | 2.795 | 338 | 0.00650 | 52,071 | 22,278 | 2.792 | 338 | 0.00422 | 80,239 |
Routine use | ||||||||||
SoC | 21,939 | 2.788 | 21,939 | 2.788 | ||||||
PKG | 24,580 | 2.848 | 2640 | 0.05945 | 44,413 | 24,580 | 2.827 | 2640 | 0.03858 | 68,438 |
Scenario 3(a): removal of implementation costs | ||||||||||
One-time use | ||||||||||
SoC | 21,939 | 2.788 | 21,939 | 2.788 | ||||||
PKG | 22,248 | 2.793 | 309.22 | 0.00499 | 61,992 | 22,248 | 2.790 | 309.22 | 0.00167 | 184,875 |
Routine use | ||||||||||
SoC | 21,939 | 2.788 | 21,939 | 2.788 | ||||||
PKG | 24,550 | 2.834 | 2611 | 0.04562 | 57,236 | 24,550 | 2.804 | 2611 | 0.01530 | 170,690 |
Scenario 3(b): inclusion of variable implementation costs (£39 per PKG) | ||||||||||
One-time use | ||||||||||
SoC | 21,939 | 2.788 | 21,939 | 2.788 | ||||||
PKG | 22,317 | 2.793 | 377.47 | 0.00499 | 75,675 | 22,317 | 2.790 | 377.47 | 0.00167 | 225,680 |
Routine use | ||||||||||
SoC | 21,939 | 2.788 | 21,939 | 2.788 | ||||||
PKG | 24,958 | 2.834 | 3018 | 0.04562 | 66,164 | 24,958 | 2.804 | 3018 | 0.01530 | 197,317 |
Scenario 4: consultation settings aligned across all strategies | ||||||||||
One-time use | ||||||||||
SoC | 21,939 | 2.788 | 21,939 | 2.788 | ||||||
PKG | 22,278 | 2.793 | 338.47 | 0.00499 | 67,856 | 22,278 | 2.790 | 338.47 | 0.00167 | 202,363 |
Routine use | ||||||||||
SoC | 21,939 | 2.788 | 21,939 | 2.788 | ||||||
PKG | 24,653 | 2.834 | 2714 | 0.04562 | 59,496 | 24,653 | 2.804 | 2714 | 0.01530 | 177,430 |
Scenario 5: annual routine follow-up (and annual PKG remote monitoring) | ||||||||||
Routine use | ||||||||||
SoC | 21,630 | 2.672 | 21,630 | 2.672 | ||||||
PKG | 23,317 | 2.718 | 1687 | 0.04562 | 36,973 | 23,317 | 2.687 | 1687 | 0.01530 | 110,260 |
Scenario 1 considers the recurrent monitoring strategy, a variation on the routine strategy in which interim consultations are avoided in a proportion of patients (see Monitoring schedules and settings for details). The recurrent remote monitoring strategy scenario generated ICERs of £32,417 and £96,675 per QALY when using the restricted and unrestricted analyses, respectively. This is substantially lower than the base-case routine strategy. This is driven by reductions in both the number of PKGs administered (approximately 50% reduction compared to routine use), as well as reductions in consultation costs, which are averted under this strategy.
Scenario 2 presents results using the naive unadjusted estimates from Woodrow et al. This scenario increases the QALY gains associated with remote monitoring. These are a result of greater efficacy on the UPDRS IV domain (adjusted: Δ−0.73; unadjusted Δ−1.16). The ICERs for the one-time use and routine remote monitoring strategies were £52,071 (£80,239) and £44,413 (£68,438) per QALY, respectively, for the restricted (unrestricted) analyses.
Scenario 3(a) and 3(b) consider alternative assumptions regarding model implementation costs. Scenario 3(a) removes the initial (fixed) implementation costs (£29.25), while scenario 3(b) adds variable implementation costs (£39 per PKG). Reflecting the removal of fixed implementation costs associated with PKG, scenario 3(a) results in a modest reduction in the ICER compared to the base-case analysis. The ICERs for the one-time use and routine remote monitoring strategies were £61,992 (£184,875) and £57,263 (£170,690) per QALY, respectively, for the restricted (unrestricted) analyses. These contrast with the results from scenario 3(b) where the respective ICERs increase. The ICERs for the one-time use and routine remote monitoring strategies were £75,675 (£225,680) and £66,164 (£197,317) per QALY, respectively, for the restricted (unrestricted) analyses.
Scenario 4, and the alignment of consultation settings (55% face to face, 45% remote), had a relatively minor impact on cost-effectiveness findings.
Scenario 5 explores annual (as opposed to six-monthly) routine follow-up and PKG applications. This scenario results in significantly reduced incremental costs compared to base-case findings, primarily driven by reduced device costs compared to the base case. Consequently, this scenario resulted in lower ICERs compared to the base-case analysis; £36,973 and £110,260 per QALY when using the restricted and unrestricted analyses, respectively. Note this scenario assumes equivalent outcomes to the base-case routine remote monitoring strategy configuration (i.e. biannual consultations with no waning in treatment efficacy).
Kinesia 360 base-case results
Deterministic and probabilistic base-case results for one-time use and routine Kinesia 360 remote monitoring strategies are reported in Tables 38 and 39, respectively. The deterministic base-case ICERs for one-time use (3-month subscription) and routine remote monitoring strategies were £38,828 and £67,203 per QALY, respectively. Incremental costs were markedly higher for the routine remote monitoring strategy (£12,125) versus one-time use (£766). QALY gains were approximately 9.15 times greater for the routine remote monitoring strategy (0.18) relative to one-time use (0.02). HRQoL benefits associated with Kinesia 360 remote monitoring were accrued via changes in the UPDRS II (83% of HRQoL gain) and III (17% of HRQoL gain) domains. Mean and incremental probabilistic results were broadly comparable to deterministic values, albeit with minor reductions in estimated mean QALYs.
One-time use remote monitoring strategy | Costs (£) | QALY | Incremental | ICER | |
---|---|---|---|---|---|
Costs (£) | QALYs | (£/QALY) | |||
Deterministic | |||||
SoC | 21,939 | 2.788 | |||
Kinesia 360 | 22,705 | 2.808 | 766 | 0.01973 | 38,828 |
Probabilistic | |||||
SoC | 21,886 | 2.760 | |||
Kinesia 360 | 22,651 | 2.780 | 765 | 0.01977 | 38,722 |
Routine remote monitoring strategy | Costs (£) | QALY | Incremental | ICER | |
---|---|---|---|---|---|
Costs (£) | QALYs | (£/QALY) | |||
Deterministic | |||||
SoC | 21,939 | 2.788 | |||
Kinesia 360 | 34,064 | 2.969 | 12,125 | 0.18042 | 67,203 |
Probabilistic | |||||
SoC | 21,951 | 2.756 | |||
Kinesia 360 | 34,061 | 2.936 | 12,110 | 0.17973 | 67,376 |
The disaggregated total expected costs for the Kinesia 360 one-time use and routine remote monitoring strategies versus SoC are presented in Table 40. The total incremental cost of Kinesia 360 one-time use relative to SoC is £765.97, and for routine remote monitoring is £12,124.90. Total incremental costs were almost entirely driven by device-related subscription costs, which made up 88% (one-time) and 96% (routine) of the cost differentials. As observed in the PKG analysis, small incremental cost savings are accrued in the consultation cost category when using a routine use strategy.
Strategy | PKG incremental costs vs. SoC | ||||
---|---|---|---|---|---|
Device costs (£) | Consultation costs (£) | Implementation costs (£) | Medication costs (£) | Total (£) | |
One-time use | 672.00 | 32.73 | 9.75 | 51.49 | 765.97 |
Routine monitoring | 11,685.31 | −41.13 | 9.75 | 470.97 | 12,124.90 |
Kinesia 360 scenario results
Table 41 reports the results of each EAG scenario analysis applicable to Kinesia 360 one-time use and routine remote monitoring strategies. Note that scenario 2 is not relevant to this comparison as only unadjusted effectiveness inputs are available for Kinesia 360. In all other respects the scenario analysis reflects those conducted for PKG.
Kinesia 360 | |||||
---|---|---|---|---|---|
Costs (£) | Incremental | ICER | |||
QALY | Costs (£) | QALYs | (£/QALY) | ||
Scenario 1: recurrent monitoring strategy | |||||
Routine use | |||||
SoC | 21,939 | 2.788 | |||
Kinesia 360 | 33,868 | 2.969 | 11,929 | 0.18042 | 66,115 |
Scenario 3(a): removal of implementation costs | |||||
One-time use | |||||
SoC | 21,939 | 2.788 | |||
Kinesia 360 | 22,695 | 2.808 | 756 | 0.01973 | 38,334 |
Routine use | |||||
SoC | 21,939 | 2.788 | |||
Kinesia 360 | 34,054 | 2.969 | 12,115 | 0.18042 | 67,149 |
Scenario 3(b): inclusion of variable implementation costs (£39 per consultation using remote monitoring) | |||||
One-time use | |||||
SoC | 21,630 | 2.672 | |||
Kinesia 360 | 22,744 | 2.808 | 805 | 0.01973 | 40,805 |
Routine use | |||||
SoC | 21,939 | 2.788 | |||
Kinesia 360 | 34,442 | 2.969 | 12,503 | 0.18042 | 69,298 |
Scenario 4: consultation settings aligned across all strategies (55% face to face, 45% remote) | |||||
One-time use | |||||
SoC | 21,939 | 2.788 | |||
Kinesia 360 | 22,705 | 2.808 | 766 | 0.01973 | 38,828 |
Routine use | |||||
SoC | 21,939 | 2.788 | |||
Kinesia 360 | 34,138 | 2.969 | 12,199 | 0.18042 | 67,612 |
Scenario 5: annual routine follow-up | |||||
Routine use | |||||
SoC | 21,630 | 2.672 | |||
Kinesia 360 | 33,793 | 2.852 | 12,162 | 0.18042 | 67,410 |
Results for scenarios 3(a), 3(b) and 4 demonstrate a similar pattern to those observed for PKG in all cases resulting in only minor variations in the ICER. Results for scenarios 1 and 5, however, contrast sharply with those reported for PKG. In the PKG comparisons, both scenarios 1 and 5 resulted in significant reductions in the ICER relative to the base case, primarily as a consequence of reductions in device acquisition costs. Similar reductions in device costs are, however, not generated for the respective Kinesia 360 scenarios and consequently only have minor impacts on incremental costs and overall cost-effectiveness. This is largely due to the EAG’s assumption that subscription services could not be repeatedly cancelled and reinitiated (i.e. continual subscription assumed), meaning device costs only varied across the predefined remote monitoring strategies considered (i.e. one-time use and routine use). ICERs varied between £38,334 and £40,805 per QALY for one-time use. When considering the routine remote monitoring strategy, ICERs ranged between £66,115 and £69,298 per QALY gained.
Incremental analysis of Personal KinetiGraph and Kinesia 360
Tables 42 and 43 display the fully incremental deterministic and probabilistic base-case cost-effectiveness results for PKG and Kinesia 360 for one-time use and routine remote monitoring strategies. In each analysis, PKG is extendedly dominated by Kinesia 360, suggesting Kinesia 360 offers better value relative to PKG. The extended dominance of PKG occurs due to Kinesia 360 generating health benefits at a lower incremental cost per QALY gained (i.e. has lower ICER for PKG vs. SoC). Note that caution must be taken when comparing the cost-effectiveness of PKG versus Kinesia 360 given the fundamental differences in the underlying evidence base that informs the expected improvements in patient outcomes.
One-time use remote monitoring strategies | Costs (£) | QALY | Incremental | ICER | ||
---|---|---|---|---|---|---|
Costs (£) | QALYs | (£/QALY) | ||||
Deterministic analysis | ||||||
Restricted analysis | ||||||
SoC | 21,939 | 2.788 | ||||
PKG | 22,278 | 2.793 | 338 | 0.00499 | Ext. dominated | |
Kinesia 360 | 22,705 | 2.808 | 766 | 0.01973 | 38,828 | |
Unrestricted analysis | ||||||
SoC | 21,939 | 2.788 | ||||
PKG | 22,278 | 2.790 | 338 | 0.00167 | Ext. dominated | |
Kinesia 360 | 22,705 | 2.808 | 766 | 0.01973 | 38,828 | |
Probabilistic analysis | ||||||
Restricted analysis | ||||||
SoC | 21,886 | 2.760 | ||||
PKG | 22,225 | 2.765 | 339 | 0.00504 | Ext. dominated | |
Kinesia 360 | 22,651 | 2.780 | 765 | 0.01977 | 38,722 | |
Unrestricted analysis | ||||||
SoC | 21,869 | 2.768 | ||||
PKG | 22,291 | 2.757 | 338 | 0.00171 | Ext. dominated | |
Kinesia 360 | 22,651 | 2.780 | 765 | 0.01977 | 38,722 |
Routine remote monitoring strategy | Incremental | ICER | ||||
---|---|---|---|---|---|---|
Costs (£) | QALY | Costs (£) | QALYs | (£/QALY) | ||
Deterministic analysis | ||||||
Restricted analysis | ||||||
SoC | 21,939 | 2.788 | ||||
PKG | 24,580 | 2.834 | 2640 | 0.04562 | Ext. dominated | |
Kinesia 360 | 34,064 | 2.969 | 9485 | 0.13480 | 67,203 | |
Unrestricted analysis | ||||||
SoC | 21,939 | 2.788 | ||||
PKG | 24,580 | 2.804 | 2640 | 0.01530 | Ext. dominated | |
Kinesia 360 | 34,064 | 2.969 | 12,125 | 0.18042 | 67,203 | |
Probabilistic analysis | ||||||
Restricted analysis | ||||||
SoC | 22,116 | 2.765 | ||||
PKG | 24,601 | 2.810 | 2485 | 0.04549 | Ext. dominated | |
KinesiaU | 34,087 | 2.947 | 9486 | 0.13643 | 65,800 | |
Unrestricted analysis | ||||||
SoC | 22,325 | 2.750 | ||||
PKG | 24,783 | 2.767 | 2458 | 0.01658 | Ext. dominated | |
Kinesia 360 | 34,087 | 2.947 | 9486 | 0.13643 | 65,800 |
KinesiaU, STAT-ON and PDMonitor base-case results
In the absence of any evidence of comparative effectiveness for KinesiaU, STAT-ON and PDMonitor, a cost comparison was conducted between all devices. Figure 10 displays the discounted device costs over a 5-year time horizon for all the remote monitoring devices under consideration. Costs were markedly different between the remote monitoring devices with a 5-year subscription to Kinesia 360 as the most expensive alternative (£12,136), followed by purchasing a PDMonitor (£12,000), then by 5-year subscriptions of STAT-ON (£7224) and KinesiaU (£3468). PKG had the lowest device costs provided being applied triannually or less (at four PKGs per annum KinesiaU has the cheapest 5-year device costs).
FIGURE 10.
Discounted remote monitoring device costs.

Table 44 reports modelled disaggregated pairwise incremental costs (relative to SoC) for each alternative device. Across all alternative devices and remote monitoring strategies device-related costs constituted the largest share of incremental costs. Note that the modelled device costs marginally differ from those aforementioned and reported in Figure 10 due to mortality effects. The EAG acknowledges that one-time use strategies may be illogical (e.g. PDMonitor) and potentially infeasible in some cases. Furthermore, PDMonitor and Kinesia 360 devices have the potential to be shared between patients, which would significantly reduce the average device costs per patient but may also incur transfer costs (e.g. strap changes) as well as other costs due to the administrative burden associated with a shared usage model. Given the uncertainties in how sharing models for remote monitoring strategies would occur in practice, the EAG only considered individual patient-level applications for devices.
Strategy | PKG incremental costs vs. SoC at base-case settings | ||||
---|---|---|---|---|---|
Device costs (£) | Consultation costs (£) | Implementation costs (£) | Medication costs (£) | Total (£) | |
One-time use | |||||
KinesiaU | 192.00 | 32.73 | 9.75 | 51.49 | 285.97 |
PKG | 225.00 | 32.73 | 9.75 | 51.49 | 337.47 |
Kinesia 360 | 672.00 | 32.73 | 9.75 | 51.49 | 765.97 |
STAT-ON | 1600.00 | 32.73 | 29.95 | 51.49 | 1713.47 |
PDMonitor | 12,000.00 | 32.73 | 9.75 | 51.49 | 12,093.97 |
Routine use | |||||
PKG | 2181.25 | −41.13 | 29.95 | 470.97 | 2640.34 |
KinesiaU | 3338.66 | −41.13 | 9.75 | 470.97 | 3778.25 |
STAT-ON | 6955.54 | −41.13 | 29.95 | 470.97 | 7414.63 |
Kinesia 360 | 11,685.31 | −41.13 | 9.75 | 470.97 | 12,124.90 |
PDMonitor | 12,000.00 | −41.13 | 9.75 | 470.97 | 12,439.59 |
Tables 45 and 46 show the results of scenario 6, a fully incremental comparison of the one-time and routine remote monitoring device strategies respectively. In this scenario, analysis of all monitoring derives are assumed to be equally effective, as calculated using adjusted efficacy estimates from Woodrow et al. The EAG presents this scenario for purely exploratory purposes and advises caution interpreting the results given the lack of evidence to suggest equivalence in outcomes across remote monitoring devices. Exploratory results were presented for both restricted and unrestricted adjusted estimates from Woodrow et al. Using a one-time use strategy, KinesiaU (assuming a 3-month subscription) had the lowest costs and an ICER of £53,331 per QALY when using the restricted analysis. This increased to £170,975 per QALY in the unrestricted analysis. As KinesiaU was the cheapest alternative, it dominated all other equally efficacious one-time use alternatives. For the routine remote monitoring strategy, PKG had the lowest costs and an ICER of £57,877 per QALY when using the restricted analysis. This increased to £172,602 in the unrestricted analysis. Again, as PKG was the cheapest alternative, all other equally efficacious alternatives were dominated.
One-time use remote monitoring strategies | Costs (£) | QALY | Incremental | ICER | |
---|---|---|---|---|---|
Costs (£) | QALYs | (£/QALY) | |||
Scenario 6: fully incremental comparison of all remote monitoring strategies assuming equal efficacy | |||||
Restricted analysis | |||||
SoC | 21,939 | 2.788 | |||
KinesiaU | 22,225 | 2.793 | 286 | 0.00499 | 57,331 |
PKG | 22,278 | 2.793 | 53 | 0.00000 | Dominated |
Kinesia 360 | 22,705 | 2.793 | 480 | 0.00000 | Dominated |
STAT-ON | 23,653 | 2.793 | 1428 | 0.00000 | Dominated |
PDMonitor | 34,033 | 2.793 | 11,808 | 0.00000 | Dominated |
Unrestricted analysis | |||||
SoC | 21,939 | 2.788 | |||
KinesiaU | 22,225 | 2.790 | 286 | 0.00167 | 170,975 |
PKG | 22,278 | 2.790 | 53 | 0.00000 | Dominated |
Kinesia 360 | 22,705 | 2.790 | 480 | 0.00000 | Dominated |
STAT-ON | 23,653 | 2.790 | 1428 | 0.00000 | Dominated |
PDMonitor | 34,033 | 2.790 | 11,808 | 0.00000 | Dominated |
Routine remote monitoring strategies | Costs (£) | QALY | Incremental | ICER | |
---|---|---|---|---|---|
Costs (£) | QALYs | (£/QALY) | |||
Scenario 6: fully incremental comparison of all remote monitoring strategies assuming equal efficacy | |||||
Restricted analysis | |||||
SoC | 21,939 | 2.788 | |||
PKG | 24,580 | 2.834 | 2640 | 0.04562 | 57,877 |
KinesiaU | 25,717 | 2.834 | 1138 | 0.00000 | Dominated |
STAT-ON | 29,354 | 2.834 | 4774 | 0.00000 | Dominated |
Kinesia 360 | 34,064 | 2.834 | 9485 | 0.00000 | Dominated |
PDMonitor | 34,379 | 2.834 | 9799 | 0.00000 | Dominated |
Unrestricted analysis | |||||
SoC | 21,939 | 2.788 | |||
PKG | 24,580 | 2.804 | 2640 | 0.01530 | 172,602 |
KinesiaU | 25,717 | 2.804 | 1138 | 0.00000 | Dominated |
STAT-ON | 29,354 | 2.804 | 4774 | 0.00000 | Dominated |
Kinesia 360 | 34,064 | 2.804 | 9485 | 0.00000 | Dominated |
PDMonitor | 34,379 | 2.804 | 9799 | 0.00000 | Dominated |
Sensitivity analyses
As discussed in Efficacy, there is limited evidence to evaluate the impacts of remote monitoring on patient outcomes beyond the immediate term. To explore this uncertainty, a sensitivity analysis assessed the impact of alternative waning rates (0–90%). Table 47 reports pairwise ICERs for PKG and Kinesia 360, considering both one-time use and routine remote monitoring strategies. The results of this analysis show that the ICER is highly sensitive to the assumed waning rate. The restricted analysis for one-time PKG found ICERs of below £20,000 per QALY when assuming a waning rate of 10% or less, while a 20% waning rate resulted in an ICER of less than £30,000 per QALY (£29,755). When using an unrestricted analysis, PKG’s one-time use ICER did not fall below £49,548. Kinesia 360’s ICERs fell below £20,000 per QALY for waning rates of 30% or less, while a 40% waning rate resulted in ICER below £30,000 per QALY (£28,080). Since the base-case analysis assumes routine remote monitoring strategies maintain treatment-specific reductions in UPDRS (i.e. no waning of the treatment effect), alternative waning rates only made PKG and Kinesia 360 remote monitoring strategies less favourable.
Pairwise ICERs (vs. SoC) | Efficacy decay rates | |||||||||
---|---|---|---|---|---|---|---|---|---|---|
One-time use (%) | 0 | 10 | 20 | 30 | 40 | 50a | 60 | 70 | 80 | 90 |
PKG: restricted analysis (£) | 16,614 | 20,816 | 27,174 | 36,352 | 49,310 | 67,856 | 95,853 | 142,573 | 236,032 | 516,422 |
PKG: unrestricted analysis (£) | 49,548 | 62,078 | 81,039 | 108,410 | 147,054 | 202,363 | 285,854 | 425,185 | 703,902 | 1,540,087 |
Kinesia 360 (£) | 6570 | 9215 | 13,218 | 18,996 | 27,153 | 38,828 | 56,452 | 85,863 | 144,697 | 321,206 |
Routine use (%) | 0a | 10 | 20 | 30 | 40 | 50 | 60 | 70 | 80 | 90 |
PKG: restricted analysis (£) | 57,877 | 90,786 | 140,284 | 211,507 | 311,919 | 455,559 | 672,355 | 1,034,136 | 1,757,838 | 3,929,031 |
PKG: unrestricted analysis (£) | 172,602 | 270,743 | 418,357 | 630,760 | 930,214 | 1,358,581 | 2,005,114 | 3,084,027 | 5,242,268 | 11,717,253 |
Kinesia 360 (£) | 67,203 | 110,636 | 176,285 | 270,991 | 404,667 | 595,972 | 884,745 | 1,366,650 | 2,330,650 | 5,222,771 |
As discussed in Consultation costs, remote monitoring may reduce the number of consultations required between patients and healthcare practitioners. To consider this uncertainty, the EAG have explored the impact of assuming fewer consultations are required when using remote monitoring. Table 48 displays the pairwise ICERs for PKG and Kinesia 360 across a 0–50% range of face-to-face and remote consultation cost savings. The EAG did not believe this sensitivity analysis was applicable for one-time use strategies and therefore this analysis only considers the routine remote monitoring strategy. The results of this analysis show that the ICER is broadly insensitive to this parameter. This was likely due to two reasons: (1) consultation costs represent a relatively small proportion of total overall costs, and (2) routine strategies were assumed to predominantly undertake cheaper remote appointments (79%), thus providing less scope for cost saving.
Pairwise ICER (vs. SoC) | Remote monitoring consultation savings | |||||
---|---|---|---|---|---|---|
Routine use (%) | 0a | 10 | 20 | 30 | 40 | 50 |
PKG: restricted analysis (£) | 57,877 | 56,702 | 55,527 | 54,351 | 53,176 | 52,001 |
PKG: unrestricted analysis (£) | 172,602 | 169,097 | 165,593 | 162,088 | 158,583 | 155,079 |
Kinesia 360 (£) | 67,203 | 66,906 | 66,609 | 66,311 | 66,014 | 65,717 |
Discussion of the independent economic assessment
The decision problem addressed by the model relates to the cost-effectiveness of remote monitoring devices in providing ‘objective’ ambulatory measurements to aid in the identification and treatment of uncontrolled PD symptoms. In the absence of any evidence to reliably establish the clinical value of remote monitoring within early- or advanced-stage PD, or use of STAT-ON, KinesiaU or PDMonitor, cost-effectiveness assessments were confined to PKG and Kinesia 360 technologies during management-stage PD. The cost-effectiveness analysis assessed two remote monitoring strategies for PKG and Kinesia 360: (1) one-time use (one PKG, 3-month Kinesia 360 subscription), and (2) routine use (biannual PKG assessment, continuous subscription to Kinesia 360).
The clinical efficacy for PKG was established using restricted (considering only the clinical benefit associated with UPDRS domains III and IV) and unrestricted analyses (considering the impact on all UPDRS domains I–IV) of trial data (see Woodrow individual participant data), and for Kinesia 360 via a difference-in-difference analysis of UPDRS outcomes reported in a small pilot study. 33 Base-case cost-effectiveness estimates considered the costs and consequences associated with PKG (restricted and unrestricted) and Kinesia 360 remote monitoring strategies. The analysis also considered the cost of each technology together with relevant consultation, medication and implementation costs over a 5-year time horizon.
The base-case ICERs for PKG one-time use and routine remote monitoring strategies were £67,856 (£202,363) per QALY and £57,877 (£172,602) per QALY gained, respectively, when using a restricted (unrestricted) analysis. The base-case ICERs for Kinesia 360 one-time use (3-month subscription) and routine remote monitoring strategies were £38,828 and £67,203 per QALY, respectively. Cost-effectiveness results were largely insensitive to the number and type of consultations and to the implementation costs considered. The main drivers of cost-effectiveness identified were: (1) the direction and magnitude of changes on the UPDRS associated with remote monitoring strategies, (2) the persistence in changes to UPDRS over time (treatment waning) and (3) the number of devices requested (PKG). Over a 5-year time horizon, PKG incurred the lowest device-related costs (provided that the number of devices ordered per annum was ≤ 3), followed by KinesiaU, STAT-ON, Kinesia 360 and PDMonitor. On a one-time use basis, KinesiaU incurred the lowest device costs (provided subscription ≤ 3 months) followed by PKG, Kinesia 360 (subscription ≤ 3 months), STAT-ON (1 year minimum subscription) and PDMonitor.
Despite evaluating the PKG remote monitoring system within the same context and utilising the same efficacy data, the EAG’s findings significantly differed from those reported in Chaudhuri et al. who found PKG to be highly cost-effective. This misalignment could be due to a number of reasons. First, the EAG model considered a 5-year time horizon, meaning costs and benefits were broadly limited to management-stage PD and ensured the model cohort was aligned to the populations and follow-up horizons used to inform key relationships within the model (i.e. efficacy, progression and HRQoL). In contrast, Chaudhuri et al. considered a lifetime horizon that allows treatment effects to accrue over the entire lifetime of a Parkinson’s patient (up to 22 years into the future). Second, this economic assessment only considered PKG-related cost savings via facilitating more remote consultations and potentially reducing the number of consultations overall (e.g. recurrent strategy). In contrast, Chaudhuri et al. incorporated PKG cost savings as those directly related to the associated shifts in disease severity (defined on the H&Y scale). Third, the utilities associated with changes on the UPDRS, and the sources and methods used to calculate them, are markedly different between the analyses. Fourth, different PKG schedules are assumed (EAG: one-time and routine biannual applications, whereas Chaudhuri et al. controlled patients: 2 PKGs, uncontrolled: 3–4 PKGs) and consider different UPDRS domains (Chaudhuri et al. only considers changes in MDS-UPDRS domains II and III). Lastly, differences in model structure and the estimation methods used to derive efficacy estimates may also contribute to different findings.
With respect to each of the differences above, the EAG states the following positions. Firstly, the EAG believes assessing costs and outcomes within a shorter-term horizon allows for a more internally consistent assessment of remote monitoring strategies in the context of the clinical evidence (confined to the maintenance stage of the disease), therapeutic options (no disease-modifying treatments to impact the long-term trajectory of patients) and disease progression (where treatment strategies may progressively converge between remote monitoring and SoC).
Secondly, it is not clear whether the moderate reductions in MDS-UPDRS associated with remote monitoring translate into meaningful reductions in NHS costs at the management-stage of PD. In line with Chandler et al. ’s conceptual model (for potential disease-modifying therapies), Chaudhuri et al. ’s analysis suggests remote monitoring can shift disease status on the H&Y scale and consequently delay patients’ progression through progressively costly H&Y states. The EAG appreciate the potential for symptomatic benefits from remote monitoring to reduce healthcare utilisation, but question whether such changes can reliably prompt changes on the H&Y scale (progression on the H&Y scale often takes years under normal circumstances)159 and whether such changes can be reliably extrapolated over a lifetime horizon. The EAG’s approach to omit any disease-related cost savings could be considered conservative; nevertheless the EAG has concerns regarding Chaudhuri et al. ’s approach to modelling cost savings given the uncertainties surrounding the validity of the methodological approach taken (i.e. conversion from MDS-UPDRS to H&Y, the derivation of differential H&Y distributions and their life-time extrapolation) and the H&Y health state costs used (note that the EAG could not validate the values reported due to a lack of details).
Thirdly, the utilities in this analysis align with NICE method guidelines by representing robust estimates of the association between UPDRS scores and UK EQ-5D-3L preference weights (see Patient health-related quality of life). 100,140 The base-case utilities from Chaudhuri et al. mapped UPDRS scores onto EQ-5D values using an algorithm derived using weights from a European population valued by a visual analogue technique, thereby not aligning with NICE method guidelines. The authors do not state whether efforts were made to convert Woodrow et al. MDS-UPDRS scores into the UPDRS scale. 104
The current findings suggest that one-time use and routine applications of PKG and Kinesia 360 remote monitoring devices are not cost-effective relative to SoC at a £30,000 per QALY threshold. This finding is, however, subject to significant uncertainty.
First, remote monitoring devices are not confined to management-stage PD or to any single monitoring strategy configuration. Applications in an advanced disease setting (e.g. DBS) to patients receiving non-pharmacological therapies, or delivered using alternative configurations than those modelled in the EAG analysis, may significantly alter study findings. In the absence of any comparative clinical evidence on the longer-term and/or repeated use of the technologies, the base-case treatment effectiveness for alternative remote monitoring strategies was assumed rather than based on empirical data. While explored in sensitivity analysis, this remains a key uncertainty.
Second, consultation costs associated with SoC and remote monitoring in the model may be underestimated. Parkinson’s patients interact with a variety of healthcare professionals. A UK survey analysis reports PwP engagement across 18 alternative healthcare professions. The authors calculate the average NHS consultation cost for Parkinson’s to be £443.04 per annum (2015), markedly larger than SoC consultation costs in this analysis (£140.31 per annum from face-to-face and remote specialist nurse consultations). 160 A broader consideration of the healthcare professions involved with patient consultation may provide more information on potential cost savings from facilitating remote appointments or averting consultations.
Third, several broader cost and benefit factors related to remote monitoring strategies were considered beyond the scope of this analysis. These include the potential risks and costs associated with the loss, damage or theft of devices (not outlined in company responses), carer quality of life, the alleviation of capacity constraints on service providers and indirect costs to Parkinson’s patients and their carers (e.g. travel, out-of-pocket payments for private services, informal care, etc.). The benefits of remote monitoring may be amplified for those with particular difficulties attending consultations or those accessing care from services at full capacity.
The cost-effectiveness of PKG and Kinesia 360 was largely contingent on uncertain estimates in the magnitude and persistence of the symptomatic benefits (as defined according to UPDRS domains I–IV) patients can achieve with each remote monitoring technology. The average costs associated with each technology are largely dependent on its configuration within a remote monitoring strategy (e.g. one-time use, routine use, recurrent use, etc.). From a resourcing perspective, the PKG technology appears most flexible to cost considerations, albeit with Kinesia 360 and PDMonitor offering avenues for potential cost sharing between patients. From an efficacy perspective, subscription and purchase models may be advantageous relative to PKG ordering, provided patients benefit from more regular remote self-assessments.
Chapter 7 Discussion
Statement of principal findings
Clinical effectiveness
Overall, the EAG considers that only PKG has a substantial body of research evidence. PKG appears to accurately measure dyskinesia and bradykinesia, with very high sensitivity and reasonably high specificity. Diagnostic accuracy was also reasonably high for measuring tremor and treatment-related outcomes. However, its accuracy for measuring sleep disturbance was lower.
PKG is being used by clinicians to guide treatment decisions, primarily the addition of a new therapy or increase in treatment dose; PKG use led to a change in management for 32–79% of patients. Patients managed with PKG appear to benefit more than those on clinical management alone, with improvements in UPDRS III and IV scores. This benefit seems to depend on whether patients were in-target (i.e. their condition was under control with current treatment) before PKG use. Patients not in-target saw improved UPDRS scores, but those in-target did not.
For STAT-ON, evidence is almost entirely limited to diagnostic accuracy studies. These suggest that STAT-ON can accurately diagnose on–off times and bradykinesia. STAT-ON also seems to have reasonably good accuracy for diagnosing freezing of gait and possibly trunk dyskinesia (as a waist-worn device), but not dyskinesia elsewhere.
There is currently no evidence on the intermediate impact of STAT-ON or on the clinical impact of STAT-ON. Therefore, it is unclear whether STAT-ON use will lead to treatment modification and subsequent improvements in symptoms and quality of life.
Two small RCTs suggest favourable clinical outcomes with Kinesia 360 use in populations receiving rotigotine or when Kinesia 360 was used for remote telehealth assessments. However, the EAG considers that there is currently too little evidence to be confident about its clinical value.
Evidence on KinesiaU and PDMonitor are too limited to draw any conclusions on their clinical value.
The only evidence relating to adverse events was that there were no device-related adverse events reported (PKG and STAT-ON). Almost all the available evidence for all devices focused on motor symptoms (bradykinesia, dyskinesia and tremor). Evidence on sleep disturbance, and other non-motor aspect PD was extremely limited.
Cost-effectiveness
The base-case cost-effectiveness results for one-time use and routine PKG and Kinesia 360 remote monitoring strategies found ICERs exceeding £30,000 per QALY gained. The EAG were not able to evaluate the cost-effectiveness of STAT-ON, KinesiaU or PDMonitor. Over a 5-year time horizon, modelled device costs for routine use were lowest for PKG, provided three devices or less were ordered per annum, followed by KinesiaU, STAT-ON, Kinesia 360 and PDMonitor. Base-case QALY gains from PKG remote monitoring strategies were highly sensitive to the inclusion of associated small, unfavourable and statistically insignificant changes on the UPDRS II domain.
Scenario analyses considered an additional monitoring strategy and alternative model assumptions from those used as part of the base-case analysis. Cost-effectiveness results were largely robust to changes in consultation setting (face to face vs. remote), fixed implementation costs and potential consultation savings. The scenarios were also used to identify the main drivers of cost-effectiveness. The key drivers identified were: (1) the direction and magnitude of changes on the UPDRS associated with remote monitoring strategies, (2) the persistence in changes to UPDRS (treatment waning) and (3) the number of devices requested (PKG).
The EAG was not able to evaluate the cost-effectiveness of STAT-ON, KinesiaU or PDMonitor due to a lack of comparative clinical effectiveness evidence. In a cost comparison (assuming a 5-year time horizon), modelled device costs were lowest for PKG, provided three devices or less were ordered per annum, followed by KinesiaU, STAT-ON, Kinesia 360 and PDMonitor.
Strengths and limitations of the assessment
Strengths
This is the first complete systematic review of all available diagnostic and clinical evidence for PKG, Kinesia 360, KinesiaU, STAT-ON and PDMonitor. This review used extensive database searches to identify all published evidence on the included technologies and followed rigorous recommended review methods to identify relevant publications, assess their risk of bias and undertake a narrative synthesis of the results. As such, this is the first fully rigorous review of these technologies, and also the first to compare the technologies in one review.
The review was strengthened by the provision of individual-level data for the key clinical trial of PKG, which permitted a more thorough examination of the clinical impact of PKG than would otherwise have been possible.
This is also the first study to review and estimate the cost and cost-effectiveness of PKG, Kinesia 360, KinesiaU, STAT-ON and PDMonitor technologies relative to SoC and each other (where possible). A de novo economic model was developed to assess the costs and consequences associated with one-time and routine applications of each technology within management-stage PD. The model made the best use of systematically identified evidence of clinical effectiveness, considered a broad and sensitive measure of PD severity, progression and symptomatic benefit, and utilised contemporary evidence of disease progression, HRQoL and NHS service utilisation associated with PD and remote monitoring.
Limitations
There was a lack of replication across studies. In general, most outcomes were reported in only one or two studies, or outcomes were reported in inconsistent ways across studies. This meant that no meta-analysis was possible for any included studies and the narrative synthesis was severely limited by the consequent difficulties in comparing different studies. This lack of replicability raises some concerns as to how robust the findings of the review are. It should be noted that many of the review conclusions are based on individual studies.
A further limitation is the low quality of much of the evidence, particularly for diagnostic accuracy. There were many low-quality case-control studies, and in most studies it was unclear whether the reference standard (usually clinical opinion) was robust. This casts some doubt on the validity of the diagnostic accuracy of evidence. It should be noted that few studies were formal diagnostic accuracy studies, and there are innate difficulties in this field in robustly assessing diagnostic accuracy given the lack of clear reference standards, and lack of clarity over the exact algorithms used to convert device output into diagnostic assessments. There were also quality concerns for the clinical evidence, as most studies were not comparative, and no randomised studies have been performed for any device.
Cost-effectiveness results were limited for a number of reasons and should be interpreted with caution. The EAG identified no evidence to reliably establish the clinical value of STAT-ON, KinesiaU or PDMonitor, thereby restricting any meaningful assessments of cost-effectiveness for these devices. The evidence used to inform the clinical effectiveness of Kinesia 360 is extremely limited and unlikely to be comparable with that used for PKG, making comparisons problematic. Conversions made between MDS-UPDRS scores and UPDRS are at risk of bias. Cost-effectiveness results were highly sensitive to the persistence in initial clinical improvements, a variable that could not be informed with current evidence. Furthermore, cost-effectiveness assessments were confined to management-phase PD assessments only. Remote monitoring devices are applicable to a wide variety of potential schedules, contexts and settings. The consequences, implementation costs (e.g. healthcare professional time and administration) and risks (e.g. loss, damage or theft of devices) associated with alternative delivery model configurations is unknown and likely to impact cost-effectiveness.
Uncertainties
The primary clinical uncertainty in this review is the clinical value of STAT-ON, KinesiaU and PDMonitor, as these technologies currently have no evidence, and particularly no formal comparison with standard care, to demonstrate that they produce clinical benefit for patients. The trial evidence for Kinesia 360 is currently too limited to be confident of its clinical value. Because of the very different natures of the technologies assessed, the EAG does not consider that any clinical benefits observed for PKG would also be found with the other technologies. Even for PKG the comparative evidence with standard care is limited to one trial that was not strictly randomised.
Almost all studies were conducted in patients receiving pharmacological therapy, primarily levodopa. The clinical evidence for PKG is largely focused on how PKG use can modify levodopa therapy, and the clinical impacts of those therapy changes. Consequently, there is little to no evidence on the possible benefits of the technologies in other types of patients, such as those receiving non-pharmacological therapy, or on more advanced therapies such as DBS. The EAG does not think it safe to assume that any clinical benefits observed will necessarily apply to these other patient groups.
There were no studies that directly compared one remote continuous monitoring device with another. In addition, there was limited evidence on the use of remote monitoring devices in different patient subgroups. Therefore, it is unclear which patients are more likely to have management changes and subsequent improvements in clinical outcomes as a result of device use.
There is currently no evidence on the long-term use or repeated use of the technologies. It is currently uncertain for how long the observed clinical benefits with PKG will persist, or how frequently PKG (or other technologies) should be used to maintain clinical benefit (e.g. every 6 months or every year).
Uncertainties in the economic analysis largely reflect the limitations of available clinical evidence. Additionally, uncertainties relate to uncaptured costs and benefits associated with remote monitoring strategies. These include additional administration and training costs, potential risks and costs associated with the loss, damage or theft of devices, carer quality-of-life benefits, capacity constraints on service providers and indirect costs to Parkinson’s patients and their carers.
Patient and public involvement
Patient and public involvement (PPI) representatives sat on the NICE committee that oversaw this project, and had roles in planning the project and discussing its findings. The EAG did not seek any further PPI representation.
Equality, diversity and inclusion
As this was a systematic review and economic analysis there was no scope to directly include or involve potentially under-represented groups. For discussion around generalisability of findings to the population with PD see Generalisability of results.
Responsibility for diversity and inclusion in the project as a whole, and its application to UK medical practice, lies with the NICE committee responsible for the project, and is outside the scope of the work of the EAG.
Chapter 8 Conclusions
Implications for service provision
The EAG considers that the evidence for PKG shows that it could be of use in clinical practice, provided it can be made cost-effective. It provides useful information on key symptoms of PD, including bradykinesia, dyskinesia and tremor. This leads to changes in treatment management for at least some patients, and consequent improvement in symptoms. There is some evidence that PKG provides the most clinical benefits in patients whose symptoms are inadequately controlled by their current treatment, and PKG use may be best used if targeted at such patients. The EAG notes, however, that PKG may be required to identify such patients.
Although there is some promising evidence to support the clinical value of STAT-ON and Kinesia 360, the EAG considers that the evidence is currently not sufficient to be confident that these technologies will produce clinical benefits for patients. The EAG considers that there is too little evidence for KinesiaU or PDMonitor to draw any conclusions as to their clinical value.
Almost all current evidence relates to patients receiving pharmacological therapy, mainly levodopa. The EAG notes that, at present, it is unclear whether PKG or other technologies offer any clinical benefit in other patients, such as those receiving advanced therapies.
Concerns about potential bias together with the other limitations in the available evidence mean that cost-effectiveness estimates are highly uncertain. Uncertainties regarding the magnitude and durability of treatment effects are a primary concern and are key drivers of cost-effectiveness. Moreover, concerns about uncaptured implementation costs and benefits further increase uncertainties. Taken at face value, the results of the economic analysis are largely unfavourable, with ICERs in excess of thresholds of £20–30K typically adopted by NICE. Given the current clinical evidence base, establishing the cost-effectiveness of remote monitoring devices is likely to require either a reduction in total device costs (the primary driver of total costs) or the identification of additional cost savings not accounted for in the EAG’s analysis.
Suggested research priorities
The primary research priority should be to conduct further studies into the clinical impact of remote monitoring devices and with this information an assessment of cost-effectiveness of the devices may be more fully understood. This should focus on expanding the evidence base for PKG and Kinesia 360, where there is currently limited evidence on clinical effects, as well as conducting studies of STAT-ON, KinesiaU and PDMonitor, where there is currently no evidence of clinical effects. This requires RCTs comparing the devices to standard clinical management without the use of remote continuous monitoring devices. Cluster RCTs (clustered by centre or clinic) and quasi-randomised trials would also be of value. Non-randomised comparative studies would also be useful, provided they can be shown to be free of selection biases.
These trials should, at least, record the following outcomes:
-
number of patients with changes in clinical management;
-
changes in treatment, such as LED;
-
UPDRS (all subscales), H&Y, bradykinesia and dyskinesia; and
-
patient opinions and patient-centred outcomes, including quality of life (e.g. PDQ-39).
All trials should examine whether clinical benefits vary according to key patient subgroups, such as by symptom severity at randomisation. Studies should be carefully designed to consider the most applicable remote monitoring schedules and settings, as there is significant potential for variation in how remote monitoring devices could be used in practice. Specific consideration should be given to longer-term routine use of remote monitoring devices; currently all evidence pertains to short-term applications. Future studies of remote monitoring devices for PD may also consider patients with early and advanced disease. There is currently no evidence in these populations for any device.
Implementing remote monitoring may have a range of resource consequences that are currently not fully understood and may impact significantly on cost-effectiveness. This may include impacts on healthcare professionals’ time and administration of the devices, as well as risks such as loss, damage or theft of devices. Where possible, future studies should seek to address these uncertainties by collecting appropriate data on the long-term resource implications. Understanding the link between intermediate outcomes derived from the remote monitoring devices on short-term costs and consequences (e.g. the impact of a change in treatment) to potential longer-term costs and consequences (e.g. incidence of motor symptoms, falls and hip fractures) using the best available evidence is a priority for the assessment of cost-effectiveness.
The EAG considers that collecting further diagnostic accuracy evidence is a lower priority, but would be useful for Kinesia 360, KinesiaU and PDMonitor. Diagnostic accuracy studies should evaluate the accuracy of these technologies for measuring bradykinesia and dyskinesia. Care will have to be taken to ensure the reference standard is robust and at low risk of bias. It may be helpful for such studies to compare the technologies to PKG.
If deemed clinically useful, observational studies to investigate the value of all technologies in patients receiving advanced therapies (such as DBS), or patients receiving non-pharmacological therapies, may be worthwhile.
Additional information
Contributions of authors
Edward Cox (https://orcid.org/0000-0001-8981-0699) (Research Fellow in Health Economics) contributed to the protocol, developed the economic model, contributed to the writing of the cost-effectiveness sections of the report and performance of the economic analysis.
Ros Wade (https://orcid.org/0000-0002-8666-8110) (Research Fellow in Systematic Reviews) contributed to the protocol, the systematic review and writing of the clinical effectiveness sections of the report.
Robert Hodgson (https://orcid.org/0000-0001-6962-2893) (Senior Research Fellow in Health Economics) contributed to the protocol, validated the economic model, contributed to the writing of the cost-effectiveness sections of the report and performance of the economic analysis.
Helen Fulbright (https://orcid.org/0000-0002-1073-1099) (Information Specialist) produced the search strategies and performed the searches.
Thai Han Phung (https://orcid.org/0000-0001-6193-4673) (Research Fellow in Health Economics) contributed to the systematic review.
Nicholas Meader (https://orcid.org/0000-0001-9332-6605) (Research Fellow in Systematic reviews) contributed to the protocol and the systematic review.
Simon Walker (https://orcid.org/0000-0002-5750-3691) contributed to the development of the economic model and the review of the cost-effectiveness sections of the report.
Claire Rothery (https://orcid.org/0000-0002-7759-4084) (Senior Research Fellow in Health Economics) contributed to the protocol, writing of the cost-effectiveness sections of the report, performance of the economic analysis, economic model development and had overall responsibility for the cost-effectiveness sections of the report and takes joint responsibility for the report as a whole.
Mark Simmonds (https://orcid.org/0000-0002-1999-8515) (Senior Research Fellow and Statistician) contributed to the protocol, undertook analyses using the individual patient data supplied by Global Kinetics Corporation and oversaw the conduct and writing of the clinical effectiveness sections and takes joint responsibility for the report as a whole.
Acknowledgements
We would like to thank Dr Biju Mohammad, Dr Lynn Osbourne and Dr Alistair Church for helpful clinical advice during the project. We thank Professor Malcolm K. Horne for provision of trial data and Mr Conor Chandler for providing clarification.
Data-sharing statement
This study did not generate any new data as it used existing sources. All data extracted from publications are contained within the report. Any queries or data requests should be submitted to the corresponding author for consideration.
Ethics statement
As no new data were created for this project no ethical approval was sought or required.
Information governance statement
This report did not generate or collect any personal data, as all data were drawn from existing published sources.
Disclosure of interests
Full disclosure of interests: Completed ICMJE forms for all authors, including all related interests, are available in the toolkit on the NIHR Journals Library report publication page at https://doi.org/10.3310/YDSL3294.
Primary conflicts of interest: Claire Rothery is a member of HTA Commissioning Committee (2021–2).
Disclaimers
This article presents independent research funded by the National Institute for Health and Care Research (NIHR). The views and opinions expressed by authors in this publication are those of the authors and do not necessarily reflect those of the NHS, the NIHR, the HTA programme or the Department of Health and Social Care. If there are verbatim quotations included in this publication the views and opinions expressed by the interviewees are those of the interviewees and do not necessarily reflect those of the authors, those of the NHS, the NIHR, the HTA programme or the Department of Health and Social Care.
References
- NHS England . Overview: Parkinson’s Disease 2019. www.nhs.uk/conditions/parkinsons-disease/ (accessed 30 January 2023).
- National Institute for Health and Care Excellence . Parkinson’s Disease in Adults 2017.
- Parkinson’s UK . Advanced Parkinson’s 2021. www.parkinsons.org.uk/information-and-support/advanced-parkinsons (accessed 30 January 2023).
- Parkinson’s UK . The Incidence and Prevalence of Parkinson’s in the UK: Results from the Clinical Practice Research Datalink Summary Report 2018.
- NHS England . Clinical Commissioning Policy: Levodopa–Carbidopa Intestinal Gel (LCIG) 2015.
- Ferreira JJ, Katzenschlager R, Bloem BR, Bonuccelli U, Burn D, Deuschl G, et al. Summary of the recommendations of the EFNS/MDS-ES review on therapeutic management of Parkinson’s disease. Eur J Neurol 2013;20:5-15.
- [Additional Information from Global Kinetics Corporation from Email Threads] n.d.
- National Institute for Health and Care Excellence . [Request for Information: PDMonitor]. Diagnostics Assessment Programme: Devices for Remote Continuous Monitoring of People With Parkinson’s Disease (Provisional Title) n.d.
- National Institute for Health and Care Excellence . [Request for Information: Kinesia 360]. Diagnostics Assessment Programme: Devices for Remote Continuous Monitoring of People With Parkinson’s Disease (Provisional Title) n.d.
- National Institute for Health and Care Excellence . [Request for Information: STAT-ON]. Devices for Remote Continuous Monitoring of People With Parkinson’s Disease (Provisional Title) n.d.
- National Institute for Health and Care Excellence . [Request for Information: KinesiaU]. Diagnostics Assessment Programme: Devices for Remote Continuous Monitoring of People With Parkinson’s Disease (Provisional Title) n.d.
- National Institute for Health and Care Excellence . Personal KinetiGraph for Remote Clinical Management of Parkinson’s Disease – Medtech Innovation Briefing 2021. www.nice.org.uk/advice/mib258/resources/personal-kinetigraph-for-remote-clinical-management-of-parkinsons-disease-pdf-2285965700131525 (accessed 30 January 2023).
- Moher D, Liberati A, Tetzlaff J, Altman DG, Group P. Preferred reporting items for systematic reviews and meta-analyses: the PRISMA statement. PLOS Med 2009;6.
- Whiting PF, Rutjes AWS, Westwood ME, Mallett S, Deeks JJ, Reitsma JB, et al. QUADAS-2 Group . QUADAS-2: a revised tool for the quality assessment of diagnostic accuracy studies. Ann Intern Med 2011;155:529-36.
- Sterne JAC, Savovic J, Page MJ, Elbers RG, Blencowe NS, Boutron I, et al. RoB 2: a revised tool for assessing risk of bias in randomised trials. BMJ 2019;366.
- Centre for Reviews and Dissemination . Systematic Reviews: CRD’s Guidance for Undertaking Reviews in Health Care 2009.
- Woodrow H, Horne MK, Fernando CV, Kotschet KE. Treat to Target Study Group . A blinded, controlled trial of objective measurement in Parkinson’s disease. NPJ Parkinsons Dis 2020;6.
- Bayés A, Samá A, Prats A, Pérez-López C, Crespo-Maraver M, Moreno JM, et al. A ‘holter’ for Parkinson’s disease: validation of the ability to detect on–off states using the rempark system. Gait Posture 2018;59:1-6.
- Bougea A, Palkopoulou M, Pantinaki S, Antonoglou A, Efthymiopoulou E. Validation of a real- time monitoring system to detect motor symptoms in patients with Parkinson’s disease treated with Levodopa Carbidopa Intestinal Gel. Mov Disord 2021;36.
- Caballol N, Prats A, Quispe P, Ranchal MA, Alcaine S, Fondevilla F, et al. Early detection of Parkinson’s disease motor fluctuations with a wearable inertial sensor. Mov Disord 2020;35.
- Pérez-López C, Samà A, Rodríguez-Martín D, Català A, Cabestany J, Moreno-Arostegui JM, et al. Assessing motor fluctuations in Parkinson’s disease patients based on a single inertial sensor. Sensors 2016;16.
- Pérez-López C, Samà A, Rodríguez-Martín D, Moreno-Aróstegui JM, Cabestany J, Bayes A, et al. Dopaminergic-induced dyskinesia assessment based on a single belt-worn accelerometer. Artif Intell Med 2016;67:47-56.
- Perrote F, Zeppa G, Coca H, Figueroa S, de Battista JC. Evaluación de un sistema de sensores inerciales externos tipo holter en pacientes con enfermedad de Parkinson en Argentina. Neurologia 2021;13:153-8.
- Rodríguez-Martín D, Samà A, Pérez-López C, Català A, Moreno Arostegui JM, Cabestany J, et al. Home detection of freezing of gait using support vector machines through a single waist-worn triaxial accelerometer. PLOS ONE 2017;12.
- Rodriguez-Martin DRM, Perez-Lopez CPL, Pie MP, Calvet JC, Catala ACM, Rodriguez-Molinero ARM, et al. Satisfaction survey on a Parkinson’s holter, a medical device for the monitoring of motor symptoms. Mov Disord 2021;36:S569-70.
- Rodríguez-Molinero A, Samà A, Pérez-Martínez DA, Pérez López C, Romagosa J, Bayés A, et al. Validation of a portable device for mapping motor and gait disturbances in Parkinson’s disease. JMIR Mhealth Uhealth 2015;3.
- Rodríguez-Molinero A, Samà A, Pérez-López C, Rodríguez-Martín D, Quinlan LR, Alcaine S, et al. Analysis of correlation between an accelerometer-based algorithm for detecting Parkinsonian gait and updrs subscales. Front Neurol 2017;8.
- Rodríguez-Molinero A, Pérez-López C, Samà A, de Mingo E, Rodríguez-Martín D, Hernández-Vara J, et al. A kinematic sensor and algorithm to detect motor fluctuations in Parkinson disease: validation study under real conditions of use. JMIR Rehabil Assist Technol 2018;5.
- Rodríguez-Molinero A, Pérez-López C, Samà A, Rodríguez-Martín D, Alcaine S, Mestre B, et al. Estimating dyskinesia severity in Parkinson’s disease by using a waist-worn sensor: concurrent validity study. Sci Rep 2019;9.
- Samà A, Pérez-López C, Rodríguez-Martín D, Català A, Moreno-Aróstegui JM, Cabestany J, et al. Estimating bradykinesia severity in Parkinson’s disease by analysing gait through a waist-worn sensor. Comput Biol Med 2017;84:114-23.
- Samà A, Rodríguez-Martín D, Pérez-López C, Català A, Alcaine S, Mestre B, et al. Determining the optimal features in freezing of gait detection through a single waist accelerometer in home environments. Pattern Recognit Lett 2018;105:135-43.
- Santos Garcia D, Lopez Ariztegui N, Cubo E, Vinagre Aragon A, Garcia-Ramos R, Borrue C, et al. Clinical utility of a personalized and long-term monitoring device for Parkinson’s disease in a real clinical practice setting: an expert opinion survey on stat-on TM. Neurologia (Engl Ed) 2020;24.
- Isaacson SH, Boroojerdi B, Waln O, McGraw M, Kreitzman DL, Klos K, et al. Effect of using a wearable device on clinical decision-making and motor symptoms in patients with Parkinson’s disease starting transdermal rotigotine patch: a pilot study. Parkinsonism Relat Disord 2019;64:132-7.
- Peacock D, Yoneda J, Thomson V, Wile D. Tailoring the use of wearable systems and telehealth for Parkinson’s disease. Parkinsonism Relat Disord 2021;89:111-2.
- Pulliam CL, Heldman DA, Brokaw EB, Mera TO, Mari ZK, Burack MA. Continuous assessment of levodopa response in Parkinson’s disease using wearable motion sensors. IEEE Trans Biomed Eng 2018;65:159-64.
- Hadley AJ, Riley DE, Heldman DA. Real-world evidence for a smartwatch-based Parkinson’s motor assessment app for patients undergoing therapy changes. Digit Biomark 2021;5:206-15.
- Kostikis N, Rigas G, Konitsiotis S, Fotiadis D. PDMonitor: a novel system for objective monitoring of Parkinson’s disease symptoms-efficacy and usability study. Mov Disord Clin Pract 2020;7:S21-2.
- National Institute for Health and Care Excellence . [Request for Information: Parkinsons KinetiGraph Movement Recording System]. Parkinson’s KinetiGraph Movement Recording System for Remote Clinical Management of Parkinson’s Disease (Provisional Title) n.d.
- Braybrook M, O’Connor S, Churchward P, Perera T, Farzanehfar P, Horne M. An ambulatory tremor score for Parkinson’s disease. J Parkinsons Dis 2016;6:723-31.
- Horne M, Kotschet K, McGregor S. The clinical validation of objective measurement of movement in Parkinson’s disease. Oruen 2016;2:16-23.
- Horne MK, McGregor S, Bergquist F. An objective fluctuation score for Parkinson’s disease. PLOS ONE 2015;10.
- Khodakarami H, Farzanehfar P, Horne M. The use of data from the Parkinson’s KinetiGraph to identify potential candidates for device assisted therapies. Sensors 2019;19.
- Khodakarami H, Ricciardi L, Contarino MF, Pahwa R, Lyons KE, Geraedts VJ, et al. Prediction of the levodopa challenge test in Parkinson’s disease using data from a wrist-worn sensor. Sensors 2019;19.
- McGregor S, Churchward P, Soja K, O’Driscoll D, Braybrook M, Khodakarami H, et al. The use of accelerometry as a tool to measure disturbed nocturnal sleep in Parkinson’s disease. NPJ Parkinsons Dis 2018;4.
- Watts J, Khojandi A, Vasudevan R, Nahab FB, Ramdhani RA. Improving medication regimen recommendation for Parkinson’s disease using sensor technology. Sensors 2021;21.
- Horne M, Volkmann J, Sannelli C, Luyet PP, Moro E. An evaluation of the Parkinson’s KinetiGraph (PKG) as a tool to support deep brain stimulation eligibility assessment in patients with Parkinson’s disease. Mov Disord 2017;32:458-9.
- Chen L, Cai G, Weng H, Yu J, Yang Y, Huang X, et al. More sensitive identification for bradykinesia compared to tremors in Parkinson’s disease based on Parkinson’s KinetiGraph (PKG). Front Aging Neurosci 2020;12.
- Evans AH, Kettlewell J, Osborn S, Kotschet K, Griffiths RI, Horne M. A conditioned response as a biomarker of impulsive–compulsive behaviours in Parkinson’s disease. Mov Disord 2014;1.
- Griffiths RI, Kotschet K, Arfon S, Xu ZM, Johnson W, Drago J, et al. Automated assessment of bradykinesia and dyskinesia in Parkinson’s disease. J Parkinsons Dis 2012;2:47-55.
- Guan I, Trabilsy M, Barkan S, Malhotra A, Hou Y, Wang F, et al. Comparison of the Parkinson’s KinetiGraph to off/on levodopa response testing: single center experience. Clin Neurol Neurosurg 2021;209.
- Hoglund A, Hagell P, Broman JE, Palhagen S, Sorjonen K, Fredrikson S, et al. Associations between fluctuations in daytime sleepiness and motor and non-motor symptoms in Parkinson’s disease. Mov Disord Clin Pract 2021;8:44-50.
- Khodakarami H, Shokouhi N, Horne M. A method for measuring time spent in bradykinesia and dyskinesia in people with Parkinson’s disease using an ambulatory monitor. J Neuroeng Rehabil 2021;18.
- Klingelhoefer L, Rizos A, Sauerbier A, McGregor S, Martinez-Martin P, Reichmann H, et al. Night-time sleep in Parkinson’s disease – the potential use of Parkinson’s KinetiGraph: a prospective comparative study. Eur J Neurol 2016;23:1275-88.
- Knudson M, Thomsen TH, Kjaer TW. Comparing objective and subjective measures of Parkinson’s disease using the Parkinson’s KinetiGraph. Front Neurol 2020;11.
- Kotschet K, Johnson W, McGregor S, Kettlewell J, Kyoong A, O’Driscoll DM, et al. Daytime sleep in Parkinson’s disease measured by episodes of immobility. Parkinsonism Relat Disord 2014;20:578-83.
- Ossig C, Gandor F, Fauser M, Bosredon C, Churilov L, Reichmann H, et al. Correlation of quantitative motor state assessment using a kinetograph and patient diaries in advanced PD: data from an observational study. PLOS ONE 2016;11.
- Tan EE, Hogg EJ, Tagliati M. The role of personal KinetiGraph TM fluctuator score in quantifying the progression of motor fluctuations in Parkinson’s disease. Funct Neurol 2019;34:21-8.
- Bergquist F, Ax A, Sjostrom A, Wallerstedt S. West Sweden Parkinson objective measurement registry study (westports). Mov Disord 2018;33:S356-7.
- Bergquist F, Gudmundsdottir T. Objective characterisation of Parkinson’s disease motor fluctuations with the Parkinson KinetiGraph – three years experience at a movement disorder clinic. J Parkinsons Dis 2016;6.
- Bogdanova-Mihaylova P, Kavanagh N, Walsh RA. Automated assessment of advanced motor Parkinson’s disease; a pilot study of the Parkinson’s KinetiGraph as an objective tool for measurement of motor fluctuations. Mov Disord 2016;31.
- Dahlen M, Eriksson B, Bergquist F. Poor correlation between patients’ assessments of medication state and clinician’s interpretation of Parkinson’s KinetiGraph (PKG) objective recordings. Mov Disord 2014;1.
- Dominey T, Carroll C. Using remotely collected data to identify Parkinson’s disease (PD) subtypes. Mov Disord 2018;33:S461-2.
- Fowler A, Lyons K, Sharma V, Pahwa R. Evaluating the clinical efficacy of the personal KinetiGraph movement recording system. Mov Disord 2017;32:449-50.
- Horne M, McGregor S, Hamilton G, O’Driscoll D, Blaze R, Churchward P. Measurement of night time sleep using an accelerometry based system. Mov Disord 2016;31:S120-1.
- Horne M, O’Connor S, Churchward P, Perera T, Braybrook M. Ambulatory assessment of tremor. Mov Disord 2016;31.
- Lina C, Guoen C, Huidan W, Yingqing W, Ying C, Xiaochun C, et al. Application of PKG system for objective measurement and early identification in Parkinson’s disease. Mov Disord 2020;35.
- Margolesky J, Luca C. Personal KinetiGraph devices assessing efficacy of continuous enteral carbidopa/levodopa infusion therapy. Mov Disord 2017;32.
- Dominey T, Kehagia AA, Gorst T, Pearson E, Murphy F, King E, et al. Introducing the Parkinson’s KinetiGraph into routine Parkinson’s disease care: a 3-year single centre experience. J Parkinsons Dis 2020;10:1827-32.
- Evans L, Mohamed B, Thomas EC. Using telemedicine and wearable technology to establish a virtual clinic for people with Parkinson’s disease. BMJ Open Qual 2020;9.
- Farzanehfar P, Woodrow H, Braybrook M, McGregor S, Evans A, Nicklason F, et al. Objective measurement in routine care of people with Parkinson’s disease improves outcomes. NPJ Parkinsons Dis 2018;4.
- Joshi R, Bronstein JM, Keener A, Alcazar J, Yang DD, Joshi M, et al. PKG movement recording system use shows promise in routine clinical care of patients with Parkinson’s disease. Front Neurol 2019;10.
- Krause E, Randhawa J, Mehanna R. Comparing subjective and objective response to medications in Parkinson’s disease patients using the personal KinetiGraph TM. Parkinsonism Relat Disord 2021;87:105-10.
- Nahab F, Abu-Hussain H, Moreno L. Evaluation of clinical utility of the personal KinetiGraph® in the management of Parkinson disease. Adv Parkinson’s Dis 2019;8:42-61.
- Santiago A, Langston JW, Gandhy R, Dhall R, Brillman S, Rees L, et al. Qualitative evaluation of the Personal KinetiGraphTM movement recording system in a Parkinson’s clinic. J Parkinsons Dis 2019;9:207-19.
- Sundgren M, Andreasson M, Svenningsson P, Noori RM, Johansson A. Does information from the Parkinson KinetiGraph TM (PKG) influence the neurologist’s treatment decisions? – an observational study in routine clinical care of people with Parkinson’s disease. J Pers Med 2021;11.
- Andriola M. Personal kineti graph (PKG) use in the identification of unknown, under-medicated Parkinson’s disease patients. Mov Disord 2017;32.
- Bergquist F, Cvejtkovic A, Sjostrom AC, Wallerstedt S. Parkinson KinetiGraph – does it change the management of PD patients?. Mov Disord 2019;34:S28-9.
- Chhabria N, Isaacson S. Clinical effect of the PKG watch in the management of Parkinson’s patients. Mov Disord 2018;33:S34-5.
- Duja S. PKG for the management of advance Parkinson’s disease. Mov Disord 2021;36.
- Duja S, Mujeeb Q. Role of Parkinson’s KinetiGraph in routine management of Parkinson’s disease. Eur J Neurol 2021;28.
- Evans LA, Mohamed B, Thomas EC. Feasibility of a Wearable Technology Based Virtual Clinic for People with Parkinson’s 2019;48.
- Farzanehfar P, Braybrook M, Kotschet K, Horne M. Objective measurement in clinical care of patients with Parkinson’s disease: an RCT using the PKG. Mov Disord 2017;32:445-6.
- Horne M, Kotschet K, Braybrook M. Objective measurement in clinical care of patients with Parkinson’s disease. Mov Disord 2016;31.
- Horne M, Farzanehfar P, Woodrow H, Braybrook M, McGregor S, Evans A, et al. Objective measurement in routine care of people with Parkinson’s disease improves outcomes. Mov Disord 2018;33:S470-1.
- Jones S, Grose C, Mahon S, Williams T, Thomas C, Mohamed B. Does the Parkinson’s KinetiGraph change clinical practice?. Mov Disord 2018;33:S507-8.
- Klingelhoefer L, Rizos A, Sauerbier A, Trivedi D, Inniss R, Perkins L, et al. Usefulness of Parkinson’s KinetiGraph in a Parkinson’s disease clinic – survey of 82 patients. Parkinsonism Relat Disord 2016;2.
- Parkinson Study Group, Huntington Study Group, Dystonia Study Group,Tourette Syndrome Study Group, Cooperative Ataxia Group, and Tremor Research Group 2017;32:e1-19. https://doi.org/10.1002/mds.27134.
- Lynch P, Jackson D, Tilden D, Horne M. Costs and outcomes for Parkinson’s disease patients who have their management adjusted by personal KinetiGraph (PKG). Mov Disord 2018;33.
- Rao S, Ebenezer L, Raha S. Parkinson’s KinetiGraph (PKG) in clinical management of Parkinson’s disease. J Parkinsons Dis 2019;9:166-7.
- Thakur N, Ramatowski L. Personal kinetigraphtm (PKGTM) use in the routine clinical care of patients with Parkinson’s disease. Mov Disord 2017;32.
- Thomas C, Mohamed B, Silverdale M, Kobylecki C, Osborne L, Saha R, et al. Impact of quantitative assessment of Parkinsonian symptoms using wearable technology on treatment decisions. Mov Disord 2019;34:S455-6.
- Wilson N, Mappilakkandy R, Smith M, Nithi K. Does Parkinsons KinetiGraph (PKG) recording help in clinical decision making? A local experience. Mov Disord 2017;32.
- Rasul A, Farooqi A, Martinez J, Margolesky J, Luca C. Objective Assessment of Bradykinesia and Dyskinesia in Advanced Parkinson’s Disease 2017;32.
- Spengler D, Velez-Aldahondo V, Singer C, Luca C. Initial Deep Brain Stimulation Programming Optimization Using the Personal KinetiGraph (PKG) Movement Recording System 2016;86.
- Tsamis KI, Rigas G, Nikolaos K, Fotiadis DI, Konitsiotis S. Accurate monitoring of Parkinson’s disease symptoms with a wearable device during COVID-19 pandemic. In Vivo 2021;35:2327-30.
- Chaudhuri KR, Hand A, Obam F, Belsey J. Cost-effectiveness analysis of the Parkinson’s KinetiGraph and clinical assessment in the management of Parkinson’s disease. J Med Econ 2022;25:774-82.
- McCrone P, Allcock LM, Burn DJ. Predicting the cost of Parkinson’s disease. Mov Disord 2007;22:804-12.
- National Institute for Health and Care Excellence . Parkinson’s Disease. Appendix F: Full Health Economics Report 2016. www.nice.org.uk/guidance/ng71/evidence/appendix-f-he-report-pdf-4538466259 (accessed 30 January 2023).
- Xu J, Gong DD, Man CF, Fan Y. Parkinson’s disease and risk of mortality: meta-analysis and systematic review. Acta Neurol Scand 2014;129:71-9.
- National Institute for Health and Care Excellence . NICE Health Technology Evaluations: The Manual (PMG36) 2022. www.nice.org.uk/process/pmg36/resources/nice-health-technology-evaluations-the-manual-pdf-72286779244741 (accessed 30 January 2023).
- Antonini A, Odin P, Pahwa R, Aldred J, Alobaidi A, Jalundhwala YJ, et al. The long-term impact of levodopa/carbidopa intestinal gel on ‘off’-time in patients with advanced Parkinson’s disease: a systematic review. Adv Ther 2021;38:2854-90.
- Holden SK, Finseth T, Sillau SH, Berman BD. Progression of MDS-UPDRS scores over five years in de novo parkinson disease from the Parkinson’s progression markers initiative cohort. Mov Disord Clin Pract 2018;5:47-53.
- Schrag A, Dodel R, Spottke A, Bornschein B, Siebert U, Quinn NP. Rate of clinical progression in Parkinson’s disease: a prospective study. Mov Disord 2007;22:938-45.
- Dams J, Klotsche J, Bornschein B, Reese JP, Balzer-Geldsetzer M, Winter Y, et al. Mapping the EQ-5D index by UPDRS and PDQ-8 in patients with Parkinson’s disease. Health Qual Life Outcomes 2013;11.
- Davey P, Rajan N, Lees M, Aristides M. Cost-effectiveness of pergolide compared to bromocriptine in the treatment of Parkinson’s disease: a decision-analytic model. Value Health 2001;4:308-15.
- Fann JC, Chang KC, Yen AM, Chen SL, Chiu SY, Chen HH, et al. Cost-effectiveness analysis of deep brain stimulation for Parkinson disease in Taiwan. World Neurosurg 2020;138:e459-68.
- Hjelmgren J, Ghatnekar O, Reimer J, Grabowski M, Lindvall O, Persson U, et al. Estimating the value of novel interventions for Parkinson’s disease: an early decision-making model with application to dopamine cell replacement. Parkinsonism Relat Disord 2006;12:443-52.
- Johnson SJ, Diener MD, Kaltenboeck A, Birnbaum HG, Siderowf AD. An economic model of Parkinson’s disease: implications for slowing progression in the United States. Mov Disord 2013;28:319-26.
- Lindgren P, Jönsson B, Duchane J. The cost-effectiveness of early cabergoline treatment compared to levodopa in Sweden. Eur J Health Econ 2003;4:37-42.
- Nuijten MJ, Kosa J, Engelfriet P. Modeling the cost-effectiveness and budgetary impact for subpopulations. Eur J Health Econ 2003;4:70-8.
- Postma MJ, Boersma C. Flexibility of Markov modeling for clinical pharmacoeconomics: illustration for cost-effectiveness in early Parkinson’s disease. Expert Rev Clin Pharmacol 2012;5:1-4.
- Shimbo T, Hira K, Takemura M, Fukui T. Cost-effectiveness analysis of dopamine agonists in the treatment of Parkinson’s disease in Japan. PharmacoEcon 2001;19:875-86.
- Smala AM, Spottke EA, Machat O, Siebert U, Meyer D, Kohne-Volland R, et al. Cabergoline versus levodopa monotherapy: a decision analysis. Mov Disord 2003;18:898-905.
- Findley LJ, Lees A, Apajasalo M, Pitkanen A, Turunen H. Cost-effectiveness of levodopa/carbidopa/entacapone (Stalevo) compared to standard care in UK Parkinson’s disease patients with wearing-off. Curr Med Res Opin 2005;21:1005-14.
- Linna M, Taimela E, Apajasalo M, Marttila RJ. Probabilistic sensitivity analysis for evaluating cost–utility of entacapone for Parkinson’s disease. Expert Rev Pharmacoecon Outcomes Res 2002;2:91-7.
- Nuijten MJ, van Iperen P, Palmer C, van Hilten BJ, Snyder E. Cost-effectiveness analysis of entacapone in Parkinson’s disease: a Markov process analysis. Value Health 2001;4:316-28.
- Palmer CS, Nuijten MJ, Schmier JK, Subedi P, Snyder EH. Cost effectiveness of treatment of Parkinson’s disease with entacapone in the United States. PharmacoEcon 2002;20:617-28.
- Arnold RJG, Layton A, Rustay NR, Chen S. Cost-effectiveness of extended-release carbidopa-levodopa for advanced Parkinson’s disease. Am J Pharm Benefits 2017;9:23-9.
- Groenendaal H, Tarrants ML, Armand C. Treatment of advanced Parkinson’s disease in the United States: a cost–utility model. Clin Drug Investig 2010;30:789-98.
- Hansen RN, Suh K, Serbin M, Yonan C, Sullivan SD. Cost-effectiveness of opicapone and entacapone in reducing OFF-time in Parkinson’s disease patients treated with levodopa/carbidopa. J Med Econ 2021;24:563-9.
- Hudry J, Rinne JO, Keranen T, Eckert L, Cochran JM. Cost–utility model of rasagiline in the treatment of advanced Parkinson’s disease in Finland. Ann Pharmacother 2006;40:651-7.
- Rudakova AV, Levin OS. Pharmacoeconomic aspects of combined treatment of advanced stage of Parkinson’s disease. Zh Nevrol Psikhiatr Im S S Korsakova 2017;117:96-100.
- Haycox A, Armand C, Murteira S, Cochran J, Francois C. Cost effectiveness of rasagiline and pramipexole as treatment strategies in early Parkinson’s disease in the UK setting: an economic Markov model evaluation. Drugs Aging 2009;26:791-80.
- Farkouh RA, Wilson MR, Tarrants ML, Castelli-Haley J, Armand C. Cost-effectiveness of rasagiline compared with first-line early Parkinson disease therapies. Am J Pharm Benefits 2012;4:99-107.
- Nuijten MJ. Incorporation of statistical uncertainty in health economic modelling studies using second-order Monte Carlo simulations. PharmacoEcon 2004;22:759-69.
- Nuijten MJ, Rutten F. Combining a budgetary-impact analysis and a cost-effectiveness analysis using decision-analytic modelling techniques. PharmacoEcon 2002;20:855-67.
- Pietzsch JB, Garner AM, Marks WJ. Cost-effectiveness of deep brain stimulation for advanced Parkinson’s disease in the United States. Neuromodulation 2016;19:689-97.
- Eggington S, Valldeoriola F, Chaudhuri KR, Ashkan K, Annoni E, Deuschl G. The cost-effectiveness of deep brain stimulation in combination with best medical therapy, versus best medical therapy alone, in advanced Parkinson’s disease. J Neurol 2014;261:106-16.
- Lowin J, Bergman A, Chaudhuri KR, Findley LJ, Roeder C, Schifflers M, et al. A cost-effectiveness analysis of levodopa/carbidopa intestinal gel compared to standard care in late stage Parkinson’s disease in the UK. J Med Econ 2011;14:584-93.
- Kalabina S, Belsey J, Pivonka D, Mohamed B, Thomas C, Paterson B. Cost–utility analysis of levodopa carbidopa intestinal gel (Duodopa) in the treatment of advanced Parkinson’s disease in patients in Scotland and Wales. J Med Econ 2019;22:215-25.
- Walter E, Odin P. Cost-effectiveness of continuous subcutaneous apomorphine in the treatment of Parkinson’s disease in the UK and Germany. J Med Econ 2015;18:155-65.
- Dams J, Balzer-Geldsetzer M, Siebert U, Deuschl G, Schuepbach WM, Krack P, et al. EARLYSTIM-Investigators . Cost-effectiveness of neurostimulation in Parkinson’s disease with early motor complications. Mov Disord 2016;31:1183-91.
- Lowin J, Sail K, Baj R, Jalundhwala YJ, Marshall TS, Konwea H, et al. The cost-effectiveness of levodopa/carbidopa intestinal gel compared to standard care in advanced Parkinson’s disease. J Med Econ 2017;20:1207-15.
- van Boven JF, Novak A, Driessen MT, Boersma C, Boomsma MM, Postma MJ. Economic evaluation of ropinirole prolonged release for treatment of Parkinson’s disease in the Netherlands. Drugs Aging 2014;31:193-201.
- All Wales Medicines Strategy Group . Co-Careldopa Intestinal Gel (Duodopa®) 2007. https://awttc.nhs.wales/files/appraisals-asar-far/appraisal-report-levodopa-carbidopa-intestinal-gel-duodopa-3397/ (accessed 30 January 2023).
- Espay AJ, Vaughan JE, Marras C, Fowler R, Eckman MH. Early versus delayed bilateral subthalamic deep brain stimulation for Parkinson’s disease: a decision analysis. Mov Disord 2010;25:1456-63.
- Fundament T, Eldridge PR, Green AL, Whone AL, Taylor RS, Williams AC, et al. Deep brain stimulation for Parkinson’s disease with early motor complications: a UK cost-effectiveness analysis. PLOS ONE 2016;11.
- Meng Y, Pople CB, Kalia SK, Kalia LV, Davidson B, Bigioni L, et al. Cost-effectiveness analysis of MR-guided focused ultrasound thalamotomy for tremor-dominant Parkinson’s disease. J Neurosurg 2021;135:273-8.
- Thach A, Kirson N, Zichlin ML, Dieye I, Pappert E, Williams GR. Cost-effectiveness of apomorphine sublingual film as an ‘on-demand’ treatment for ‘off’ episodes in patients with Parkinson’s disease. J Health Econ Outcomes Res 2021;8:82-9.
- Chandler C, Folse H, Gal P, Chavan A, Proskorovsky I, Franco-Villalobos C, et al. Modeling long-term health and economic implications of new treatment strategies for Parkinson’s disease: an individual patient simulation study. J Mark Access Health Policy 2021;9.
- Ashburn A, Stack E, Pickering RM, Ward CD. A community-dwelling sample of people with Parkinson’s disease: characteristics of fallers and non-fallers. Age Ageing 2001;30:47-52.
- Goetz CG, Stebbins GT, Tilley BC. Calibration of unified Parkinson’s disease rating scale scores to movement disorder society-unified Parkinson’s disease rating scale scores. Mov Disord 2012;27:1239-42.
- Kalilani L, Friesen D, Boudiaf N, Asgharnejad M. The characteristics and treatment patterns of patients with Parkinson’s disease in the United States and United Kingdom: a retrospective cohort study. PLOS ONE 2019;14.
- Fasano A, Fung VSC, Lopiano L, Elibol B, Smolentseva IG, Seppi K, et al. Characterizing advanced Parkinson’s disease: OBSERVE-PD observational study results of 2615 patients. BMC Neurol 2019;19.
- Findley LJ, Wood E, Lowin J, Roeder C, Bergman A, Schifflers M. The economic burden of advanced Parkinson’s disease: an analysis of a UK patient dataset. J Med Econ 2011;14:130-9.
- Claxton K, Sculpher MJ, Briggs AH. Decision Modelling for Health Economic Evaluation. Oxford: Oxford University Press; 2006.
- National Institute for Health and Care Excellence . Diagnostics Assessment Programme. Devices for Remote Continuous Monitoring of People With Parkinson’s Disease: Final Scope 2022. www.nice.org.uk/guidance/gid-dg10047/documents/final-scope (accessed 30 January 2023).
- Okunoye O, Horsfall L, Marston L, Walters K, Schrag A. Mortality of people with Parkinson’s disease in a large UK-based cohort study: time trends and relationship to disease duration. Mov Disord 2021;36:2811-20.
- England N. 2019/20 National Cost Collection Data Version 2. London: NHS England; n.d.
- Office for National Statistics . National Life Tables – Life Expectancy in the UK: 2018 to 2020 2021. www.ons.gov.uk/peoplepopulationandcommunity/birthsdeathsandmarriages/lifeexpectancies/bulletins/nationallifetablesunitedkingdom/2018to2020 (accessed 30 January 2023).
- Bäckström D, Granåsen G, Domellöf ME, Linder J, Jakobson Mo S, Riklund K, et al. Early predictors of mortality in parkinsonism and Parkinson disease: a population-based study. Neurology 2018;91:e2045-56.
- Young MK, Ng S-K, Mellick G, Scuffham PA. Mapping of the PDQ-39 to EQ-5D scores in patients with Parkinson’s disease. Qual Life Res 2012;22:1065-72.
- Greenwell K, Gray WK, van Wersch A, van Schaik P, Walker R. Predictors of the psychosocial impact of being a carer of people living with Parkinson’s disease: a systematic review. Parkinsonism Relat Disord 2015;21:1-11.
- Gumber A, Ramaswamy B, Thongchundee O. Effects of Parkinson’s on employment, cost of care, and quality of life of people with condition and family caregivers in the UK: a systematic literature review. Patient Relat Outcome Meas 2019;10:321-33.
- Curtis LB. A Unit Costs of Health and Social Care 2020. Kent, Canterbury: Personal Social Services Research Unit, University of Kent; 2020.
- Ng JH, See AAQ, Xu Z, King NKK. Longitudinal medication profile and cost savings in Parkinson’s disease patients after bilateral subthalamic nucleus deep brain stimulation. J Neurol 2020;267:2443-54.
- David Charles P, Padaliya BB, Newman WJ, Gill CE, Covington CD, Fang JY, et al. Deep brain stimulation of the subthalamic nucleus reduces antiparkinsonian medication costs. Parkinsonism Relat Disord 2004;10:475-9.
- Hacker ML, Currie AD, Molinari AL, Turchan M, Millan SM, Heusinkveld LE, et al. Subthalamic nucleus deep brain stimulation may reduce medication costs in early stage Parkinson’s disease. J Parkinsons Dis 2016;6:125-31.
- Zhao YJ, Wee HL, Chan Y-H, Seah SH, Au WL, Lau PN, et al. Progression of Parkinson’s disease as evaluated by Hoehn and Yahr stage transition times. Mov Disord 2010;25:710-6.
- Gumber A, Ramaswamy B, Ibbotson R, Ismail M, Thongchundee O, Harrop D, et al. Economic, Social and Financial Cost of Parkinson’s on Individuals, Carers and Their Families in the UK. Project Report. Sheffield: Centre for Health and Social Care Research, Sheffield Hallam University; 2017.
- Abou L, Peters J, Wong E, Akers R, Dossou MS, Sosnoff JJ, et al. Gait and balance assessments using smartphone applications in Parkinson’s disease: a systematic review. J Med Syst 2021;45.
- Adams JL, Lizarraga KJ, Waddell EM, Myers TL, Jensen-Roberts S, Modica JS, et al. Digital technology in movement disorders: updates, applications, and challenges. Curr Neurol Neurosci Rep 2021;21.
- AlMahadin G, Lotfi A, Zysk E, Siena FL, Carthy MM, Breedon P. Parkinson’s disease: current assessment methods and wearable devices for evaluation of movement disorder motor symptoms – a patient and healthcare professional perspective. BMJ Neurol Open 2020;20.
- Ancona S, Faraci FD, Khatab E, Fiorillo L, Gnarra O, Nef T, et al. Wearables in the home-based assessment of abnormal movements in Parkinson’s disease: a systematic review of the literature. J Neurol 2022;269:100-10.
- Barrachina-Fernandez M, Maitin AM, Sanchez-Avila C, Romero JP. Wearable technology to detect motor fluctuations in Parkinson’s disease patients: current state and challenges. Sensors 2021;21.
- Battista L, Romaniello A. A wearable tool for selective and continuous monitoring of tremor and dyskinesia in Parkinsonian patients. Parkinsonism Relat Disord 2020;77:43-7.
- Battista L, Romaniello A. A wearable tool for continuous monitoring of movement disorders: clinical assessment and comparison with tremor scores. Neurol Sci 2021;42:4241-8.
- Bendig J, Prieto-Jarabo M, Koppitz A, Falkenburger B, Reichmann H, Lowenbruck K. Usability assessments in patients with Parkinson’s disease: the foundation for a holistic telemedical solution (telepark). Mov Disord 2020;35.
- Blaze RL, Tan J, Evans AH. Quantitative assessment of advanced therapies in Parkinson’s disease using the Parkinson KinetiGraph (PKG). Mov Disord 2016;31.
- Brillman S, Isaacson SH. Assessment of dose failure and delayed-on with the time-to-on questionaire (TOQ) and Parkinson’s KinetiGraph in PD patients with motor fluctuations. Mov Disord 2015;1.
- Canento T, Tan J, Cruse B, Wools C, Ghaly M, Beckley M, et al. Daytime sleepiness in Parkinson’s disease: an indicator of impaired activities of daily living. Mov Disord 2019;34:S244-5.
- Carroll C, Kobylecki C, Silverdale M, Thomas C, group PKG. Impact of quantitative assessment of Parkinson’s disease-associated symptoms using wearable technology on treatment decisions. J Parkinsons Dis 2019;9.
- Channa A, Popescu N, Ciobanu V. Wearable solutions for patients with Parkinson’s disease and neurocognitive disorder: a systematic review. Sensors 2020;20.
- David Prakash B, Seah ISH, Tan LCS, Au WL. Differential effects of age and gender on hand motion tasks in an Asian population. Mov Disord 2013;1.
- Del Prete E, Schmitt E, Meoni S, Fraix V, Castrioto A, Pelissier P, et al. Do non-motor fluctuations temporarily match on/off motor condition?. Mov Disord 2019;34.
- Del Prete E, Schmitt E, Meoni S, Fraix V, Castrioto A, Pelissier P, et al. Do neuropsychiatric fluctuations temporally match motor fluctuations in Parkinson’s disease?. Neurol Sci 2022;19.
- Dominey T, Hutchinson L, Pearson E, Murphy F, Bell L, Carroll C. Evaluating the clinical utility of the Parkinson’s KinetiGraph (PKGTM) in the remote management of Parkinson’s disease. Mov Disord 2018;33:S527-8.
- Edwards E, Partridge R, Ankeny U, Langley J, Whipps S, Whipps J, et al. Home based care: a care pathway innovation for Parkinson’s disease. Mov Disord 2020;35:S148-9.
- Edwards E, Partridge R, Ankeny U, Langley J, Whipps S, Whipps J, et al. Development of a home based care pathway for people with Parkinson’s. Mov Disord Clin Pract 2020;7.
- Evans L, Mohamed B, Thomas EC. ‘So much easier’; patient’s perceptions of virtual clinics for Parkinson’s disease. Mov Disord 2019;34:S248-50.
- Evans L, Mohamed B, Thomas C. Using telemedicine and wearable technology to establish a virtual clinic for people with Parkinson’s. Mov Disord 2020;35:S648-9.
- Evans L, Mohamed B, Thomas C. Is Parkinson’s Kinetigraph useful in frail patients with Parkinson’s disease?. Prog Neurol Psychiatry 2021;25:24-6.
- Farley B, Bullock A, Kaul I, Nguyen D, Belfort G, Kanes S, et al. Validation of a wearable device for continuous tremor measurement in Parkinson’s disease and essential tremor. Mov Disord 2018;33:S66-7.
- Farzanehfar P, Churchward P, Horne M. Using an objective measure of movement to quantify night time sleep. Mov Disord 2017;32.
- Farzanehfar P, Horne M. Evaluation of the Parkinson’s KinetiGraph in monitoring and managing Parkinson’s disease. Expert Rev Med Devices 2017;14:583-91.
- Flisar D, Piks B, Berlot R, Dreo J, Kramberger Gregoric M, Trost M, et al. Accelerometric assessment of movements in Parkinson’s disease fluctuators. Eur J Neurol 2016;2.
- Flisar D, Piks B, Meglic B, Pirtosek Z, Kramberger G. Objective movement recording in PD patient before and after stndbs. Mov Disord 2016;31:S182-3.
- Flisar D, Trost M, Zupancic Kriznar N, Kramberger Gregoric M, Meglic B, Georgiev D, et al. Accelerometric evaluation of motor performance in PD patients before and after stn-dbs treatment. Eur J Neurol 2018;25.
- Gao C, Smith S, Lones M, Jamieson S, Alty J, Cosgrove J, et al. Objective assessment of bradykinesia in Parkinson’s disease using evolutionary algorithms: clinical validation. Transl Neurodegener 2018;7.
- Gernon S, Fowler A, Lyons K, Pahwa R. Clinical Experience with Personal KinetiGraph Before and After Deep Brain Stimulation for Parkinson’s Disease 2018;90.
- Ghoraani B, Galvin JE, Jimenez-Shahed J. Point of view: wearable systems for at-home monitoring of motor complications in Parkinson’s disease should deliver clinically actionable information. Parkinsonism Relat Disord 2021;84:35-9.
- Giuffrida JP, Riley DE, Maddux BN, Heldman DA. Clinically deployable Kinesia technology for automated tremor assessment. Mov Disord 2009;24:723-30.
- Giuffrida JP, Riley DE, Maddux BN, Heldmann DA. Clinically deployable kinesiatm technology for automated tremor assessment. Mov Disord 2009;24:723-30.
- Griffiths R, Kotschet K, Johnson W, Drago J, Evans A, Kempster P, et al. Automated ambulatory measurement of dyskinesia and bradykinesia. Mov Disord 2012;1.
- Heldman DA, Espay AJ, LeWitt PA, Giuffrida JP. Clinician versus machine: reliability and responsiveness of motor endpoints in Parkinson’s disease. Parkinsonism Relat Disord 2014;20:590-5.
- Heldman DA, Giuffrida JP, Cubo E. Wearable sensors for advanced therapy referral in Parkinson’s disease. J Parkinsons Dis 2016;6:631-8.
- Horne M, Osborn S, Evans A, Kotschet K. The effect on fluctuations of PD of advanced therapies measured by an objective long term monitoring system. Mov Disord 2014;1:S185-6.
- Isaacson S, Boroojerdi B, Klos K, Carson S, Markowitz M, Heldman D, et al. The impact of a wearable device on Parkinson’s disease motor symptom management in patients starting rotigotine transdermal patch. Mov Disord 2018;33:S520-1.
- Jansa J, Piks B, Flisar D, Gregoric Kramberger M, Pirtosek Z. Self-perception of daily performance in relation to motor performance in advanced Parkinson’s disease-case studies. Mov Disord 2016;31.
- Johansson A, Lundgren M, Othman M, Nyholm D. Evaluation of device-assisted treatment using a wearable accelerometry wrist sensor. Mov Disord 2019;34.
- Joshi R, Bronstein JM, Alcazar J, Yang D, Joshi M, Hermanowicz N. An observational study of PKG movement recording system use in routine clinical care of patients with Parkinson’s disease. Mov Disord 2019;34:S16-7.
- Karl JA, Ouyang B, Goetz S, Metman LV. A novel dbs paradigm for axial features in Parkinson’s disease: a randomized crossover study. Mov Disord 2020;35:1369-78.
- Keogh A, Argent R, Anderson A, Caulfield B, Johnston W. Assessing the usability of wearable devices to measure gait and physical activity in chronic conditions: a systematic review. J Neuroeng Rehabil 2021;18.
- Kilincalp G, Sjostrom AC, Eriksson B, Holmberg B, Constantinescu R, Bergquist F. Predictive value of ambulatory objective movement measurement for outcomes of levodopa/carbidopa intestinal gel infusion. J Pers Med 2022;12.
- King E, Abraham J, Edwards E, Gorst T, Holley M, Inches J, et al. Evaluating a novel home-based care pathway for people with Parkinson’s disease. Mov Disord 2021;36.
- Klingelhoefer L, Horne M, Rizos A, Sauerbier A, McGregor S, Trivedi D, et al. Sleep assessment in Parkinson’s disease-the use of Parkinson’s Kineti Graph. Mov Disord 2015;1.
- Klingelhofer L, Rizos A, Horne M, Sauerbier A, McGregor S, De Micco R, et al. First comparison of continuous movement data (hauser-diary versus Parkinson’s KinetiGraph) in patients with Parkinson’s disease. Eur J Neurol 2016;2.
- Koivu M, Scheperjans F, Pekkonen E. Ambulatory movement measurement in evaluating deep brain stimulation effect in patients with advanced Parkinson’s disease. Mov Disord 2017;32:906-7.
- Kostikis N, Rigas G, Konitsiotis S, Fotiadis DI. Configurable offline sensor placement identification for a medical device monitoring Parkinson’s disease. Sensors 2021;21.
- Kotschet K, Johnson W, Griffiths R, Horne M. Quantifying daytime sleepiness in Parkinson’s disease. Mov Disord 2012;1:S218-9.
- Krause E, Randhawa J, Mehanna R. Is the personal KinetiGraph useful in the management of Parkinson’s disease patients? A retrospective study from a tertiary movement disorder center. Mov Disord 2019;34:S379-80.
- Leake A, De Angelis A, Horne M, Paviour D, Coebergh J, Edwards M, et al. Exploring the relationship between motor and non-motor fluctuations in Parkinson’s disease: patient’s perspective, clinician’ s assessment and objective measures from a wearable device. Mov Disord 2019;34:S647-8.
- Lynch P, Zoellner Y, McGregor S, Home M. Objective data in Parkinson’s disease therapy management-a retrospective analysis of the Parkinson’s KinetiGraph (PKG) database. Mov Disord 2016;31.
- Lynch P, Pahwa R, Bergquist F, Horne M. Objective data in Parkinson’s disease: a description of over 20,000 Parkinson’s symptom scores across the world using the personal KinetiGraph (PKG). Mov Disord 2018;33.
- Lynch P, Pahwa R, Bergquist F, Horne M. Objective Data in Parkinson’s Disease: A Description of Over 10,000 Parkinson’s Symptom Scores Across the World Using the Personal KinetiGraph (PKG) 2018;90.
- Lynch P, Pahwa R, Bergquist F, Horne M. Continuous Objective Monitoring in Parkinson’s Disease: A Description of Over 25,000 Parkinson’s Symptom Scores Across the World Using the Personal KinetiGraph (PKG) Wearable Monitoring Device 2019;92.
- Malhotra A, Barkan S, Lee A, Hellmers N, Sarva H, Henchcliffe C. Is the Parkinson’s KinetiGraph reflective of clinical off/on motor testing: single center experience. Mov Disord Clin Pract 2020;7.
- Metta V, Batzu L, Leta V, Trivedi D, Powdleska A, Mridula KR, et al. Parkinson’s disease: personalized pathway of care for device-aided therapies (DAT) and the role of continuous objective monitoring (COM) using wearable sensors. J Pers Med 2021;11.
- Mirelman A, Hillel I, Rochester L, Del Din S, Bloem BR, Avanzino L, et al. Tossing and turning in bed: nocturnal movements in Parkinson’s disease. Mov Disord 2020;35:959-68.
- Mohamed B, Evans L, Shukir M, Abdullah A, Thomas C. Evaluating the use of Parkinson’s kinetigraphs in patients with Parkinson’s disease and frailty. Mov Disord 2020;35.
- Morgan C, Rolinski M, McNaney R, Jones B, Rochester L, Maetzler W, et al. Systematic review looking at the use of technology to measure free-living symptom and activity outcomes in Parkinson’s disease in the home or a home-like environment. J Parkinsons Dis 2020;10:429-54.
- Morgante F, De Angelis A, Siri C, Horne M, Leake A, Paviour D, et al. Shedding light on the relationship between dyskinesia assessed by a wearable device and impulsive compulsive behaviour in Parkinson’s disease. Mov Disord 2019;34.
- Mostile G, Giuffrida JP, Adam OR, Davidson A, Jankovic J. Correlation between kinesia system assessments and clinical tremor scores in patients with essential tremor. Mov Disord 2010;25:1938-43.
- Nahab F, Abuhussain H, Moreno L. Personal KinetiGraphTM movement recording system: an assessment of utility in a movement disorder clinic. Mov Disord 2018;33.
- Pahwa R, Isaacson SH, Torres-Russotto D, Nahab FB, Lynch PM, Kotschet KE. Role of the personal KinetiGraph in the routine clinical assessment of Parkinson’s disease: recommendations from an expert panel. Expert Rev Neurother 2018;18:669-80.
- Pahwa R, Dorsey R, Isaacson S, Kandukuri P, Jalundhwala Y, Kukreja P, et al. Evaluating the real-world impact of levodopa/carbidopa intestinal gel (LCIG) on motor symptoms using wearable sensors: evidence from provide study. Mov Disord 2019;34:S70-2.
- Pahwa R, Bergquist F, Horne M, Minshall ME. Objective measurement in Parkinson’s disease: a descriptive analysis of Parkinson’s symptom scores from a large population of patients across the world using the personal KinetiGraph R. J Clin Mov Disord 2020;7.
- Pai H. Exploring the use of wearable sensors in Parkinson’s disease and the detection of early morning periods. Eur J Neurol 2020;27.
- Papapetropoulos S, Mitsi G. A 12-month, 2-arm, 2-period, randomized, controlled trial of a digital solution for the management of Parkinson’s disease (PD): rationale and study design. Mov Disord 2016;31.
- Phillips RS, Wilson KA, Walter BL, Ridgel AL. Bradykinesia and timed up and go are improved after dynamic cycling in Parkinson’s disease. J Parkinsons Dis 2013;1.
- Podlewska A, Van Wamelen D, Sauerbier A, Leta V, Trivedi D, Parry M, et al. Wearable sensor use and monitoring effect of dopamine replacement therapy on motor parameters in a real life clinical setting. Mov Disord 2019;34:S295-6.
- Potter A, Newsome G, Kern G, Parsons A, Page D, Dalati Y, et al. Personal KinetiGraph and deep brain stimulation with Parkinson’s disease. Mov Disord 2020;35.
- Powell A, Graham D, Portley R, Snowdon J, Hayes MW. Wearable technology to assess bradykinesia and immobility in patients with severe depression undergoing electroconvulsive therapy: a pilot study. J Psychiatr Res 2020;130:75-81.
- Pulliam CL, Heldman DA, Burack MA, Mera TO. Continuous motion sensor assessment of Parkinson’s disease during activities of daily living. Mov Disord 2015;1.
- Robertson EE, Hall DA, Pal G, Ouyang B, Liu Y, Joyce JM, et al. Tremorography in fragile x-associated tremor/ataxia syndrome, Parkinson’s disease and essential tremor. Clin Park Relat Disord 2020;3.
- Rodriguez-Martin D, Perez-Lopez C, Sama A, Pie M, Catala A, Cabestany J, et al. Stat-on: a wearable inertial system to objectively evaluate motor symptoms in Parkinson’s disease. Mov Disord 2019;34:S296-7.
- Rodriguez-Martin DRM, Perez-Lopez CPL, Pie MP, Calvet JC, Catala ACM, Cabestany JCM, et al. Feasibility to detect Parkinson’s motor symptoms with a waist-worn Parkinson’s holter. Mov Disord 2021;36.
- Rovini E, Maremmani C, Cavallo F. Automated systems based on wearable sensors for the management of Parkinson’s disease at home: a systematic review. Telemed J E Health 2019;25:167-83.
- Sachdev B, Buttery P, Morris R. Assessing the impact on bradykinesia and dyskinesia in patients with Parkinson’s disease undergoing deep brain stimulation using a novel and objective automated assessment tool. Stereotact Funct Neurosurg 2017;95.
- Santos García D, López Ariztegui N, Cubo E, Vinagre Aragón A, García Ramos R, Borrué C, et al. Use in clinical practice of a personalized and long-term monitoring device for Parkinson’s disease: STAT-ON. Mov Disord 2020;35:S663-4.
- Sasaki F, Oyama G, Sekimoto S, Nakamura R, Jo T, Iwamuro H, et al. Closed loop programming evaluation using external responses for deep brain stimulation (CLOVER-DBS). Mov Disord 2018;33.
- Sica M, Tedesco S, Crowe C, Kenny L, Moore K, Timmons S, et al. Continuous home monitoring of Parkinson’s disease using inertial sensors: a systematic review. PLOS ONE 2021;16.
- Sringean J, Taechalertpaisarn P, Jitkritsadakul O, Bhidayasiri R. Bilateral recording of Parkinson’s signs with the Parkinson’s KinetiGraph: assessing its utility to evaluate asymmetric features. Mov Disord 2014;2:S36-7.
- Stuijt C, Laar TV. Effect of pharmacist-led interventions on motor symptoms and quality of life in Parkinson’s patients: a pilot study. Mov Disord 2016;31.
- Stuijt C, Karapinar F, Van Den Bemt B, Van Laar T. Effect of pharmacist-led interventions on motor symptoms and quality of life in Parkinson’s patients: a pilot study. Int J Clin Pharm 2017;39.
- Stuijt C, Karapinar-Carkit F, van den Bemt B, van Laar T. Effect of pharmacist-led interventions on (non)motor symptoms, medication-related problems, and quality of life in Parkinson disease patients: a pilot study. Clin Neuropharmacol 2018;41:14-9.
- Sundgren M, Rousu P, Johansson A. Does information from the personal kinetigraphtm (PKG) influence neurologists’ treatment decisions?. Mov Disord 2019;34:S299-300.
- Sung C, Danoudis M, Iansek R. Liquid sinemet in the management of complex motor fluctuations in advanced Parkinson’s disease. Mov Disord 2018;33:S128-9.
- Suttrup I, Zentsch V, Schroeder J, Warnecke T. Effects of safinamide in patients with Parkinson’s disease measured by Parkinson’s KinetiGraphTM. Mov Disord 2016;31.
- Taddei RN, Leta V, Sauerbier A, Parry M, Podlewska A, Hall L, et al. Combined catechol-o-methyl-transferase inhibition and intrajejunal levodopa infusion: a real-life single-centre experience. Mov Disord 2018;33.
- Tan E, Tagliati M, Hogg E, Horne M. The personal KinetiGraph fluctuator score identifies motor fluctuations in Parkinson’s disease. Mov Disord 2017;32.
- Thomas C, Mohammed B, Abdelgadir E, Silverdale MA, Kobylecki C, Osborne L, et al. Can implementation of technology transform the management of Parkinson’s? Lessons learnt from the Parkinson’s KinetiGraph (TM) (PKG (TM)) service evaluation project. Mov Disord 2017;32.
- Thomas I, Bergquist F, Johansson D, Nyholm D, Memedi M, Westin J. Automated dosing schemes for administration of microtablets of levodopa for Parkinson’s disease, using wearable sensors. Mov Disord 2017;32:914-5.
- Thomas I, Alam M, Bergquist F, Johansson D, Memedi M, Nyholm D, et al. Sensor-based algorithmic dosing suggestions for oral administration of levodopa/carbidopa microtablets for Parkinson’s disease: a first experience. J Neurol 2019;266:651-8.
- Thomsen T, Kjaer T, Jorgensen L, Haahr A, Winge K. A characterization of the ADL-level in daily life with Parkinson’s disease based on objective measurements and subjective experiences. Mov Disord 2019;34.
- Titova N, Trivedi D, Bezdolny Y, Katunina E, Ray Chaudhuri K. Wearable sensor monitoring in denovo Parkinson’s disease using the Parkinson’s KinetiGraph: pilot data and correlations from the MoNS-PD cohort. Mov Disord 2020;35.
- van den Bergh R, Bloem BR, Meinders MJ, Evers LJW. The state of telemedicine for persons with Parkinson’s disease. Curr Opin Neurobiol 2021;34:589-97.
- van Uem JMT, Maier KS, Santos AT, Fagerbakke O, Larsen F, Ferreira JJ, et al. Twelve-week sensor assessment in Parkinson’s disease: impact on quality of life. Mov Disord 2016;31.
- van Uem JMT, Cerff B, Kampmeyer M, Prinzen J, Zuidema M, Hobert MA, et al. The association between objectively measured physical activity, depression, cognition, and health-related quality of life in Parkinson’s disease. Parkinsonism Relat Disord 2018;48:74-81.
- van Wamelen D, Leta V, Podlewska A, Trivedi D, Min Wan Y, Metta V, et al. Wearable Sensor (Parkinson’s KinetiGraph) and Dopamine Transporter Imaging as Potential Biosignature for Constipation in Parkinson’s 2019;92.
- van Wamelen D, Bendriss-Otiko T, Podlewska A, Leta V, Lazcano Ocampo C, Trivedi D, et al. Dyskinesia and bradykinesia severity patterns in Parkinson’s disease using a wearable sensor. Mov Disord 2020;35:S159-61.
- van Wamelen D, Batzu L, Podlewska A, Trivedi D, Ray Chaudhuri K. Wearable sensor motor outcomes across patients with Parkinson’s disease from Asian, Black, and Caucasian Ethnicity in the United Kingdom. Mov Disord 2021;36.
- van Wamelen DJ, Sringean J, Trivedi D, Carroll CB, Schrag AE, Odin P, et al. International Parkinson and Movement Disorder Society Non Motor Parkinson’s Disease Study Group . Digital health technology for non-motor symptoms in people with Parkinson’s disease: futile or future?. Parkinsonism Relat Disord 2021;89:186-94.
- Watts J, Khojandi A, Niethammer M, Ramdhani R. Predicting MDS-UPDRS ratings for deep brain stimulation patients using wearable sensor data. Mov Disord 2021;36:S552-3.
- Williamson JR, Telfer B, Mullany R, Friedl KE. Detecting Parkinson’s disease from wrist-worn accelerometry in the U.K. Biobank. Sensors 2021;21.
- Zampogna A, Manoni A, Asci F, Liguori C, Irrera F, Suppa A. Shedding light on nocturnal movements in Parkinson’s disease: evidence from wearable technologies. Sensors 2020;20.
- Zhang H, Li C, Liu W, Wang J, Zhou J, Wang S. A multi-sensor wearable system for the quantitative assessment of Parkinson’s disease. Sensors 2020;20.
- Zhang H, Song C, Rathore AS, Huang M-C, Zhang Y, Xu W. mHealth technologies towards Parkinson’s disease detection and monitoring in daily life: a comprehensive review. IEEE Rev Biomed Eng 2021;14:71-8.
- Price J, Martin H, Ebenezer L, Cotton P, Shuri J, Martin A, et al. A service evaluation by Parkinson’s disease nurse specialists, of Parkinson’s KinetiGraph (PKG) movement recording system use in routine clinical care of patients with Parkinson’s disease. J Parkinsons Dis 2016;6.
- Price J, Martin H, Ebenezer L. A service evaluation of the clinical use of Parkinson’s KinetiGraph (PKG) movement recording system in the assessment of Parkinson’s disease patients with tremor. Mov Disord 2017;32.
- Rodríguez-Molinero A, Hernández-Vara J, Parez D, Catala A, Bayes A, Martínez JC. Randomized multicenter single-blind parallel-group trial to compare the efficacy of a holter for Parkinson symptoms against other clinical follow-up methods. J Parkinsons Dis 2019;9.
- UK PKG Registry: Multi-Centre Real-World Registry of Personal KinetiGraph in 441 Patients With Parkinson’s Disease n.d. www.hra.nhs.uk/planning-and-improving-research/application-summaries/research-summaries/pkgreg/ (accessed 30 January 2023).
- ClinicalTrials.gov . Monitoring of Mobility of Parkinson’s Patients for Therapeutic Purposes – Clinical Trial 2019. https://clinicaltrials.gov/show/NCT04176302 (accessed 30 January 2023).
- ClinicalTrials.gov . Evaluation of the Personal KinetiGraph™ (PKG™) to Improve Insight into Parkinson’s Disease Status 2018. https://clinicaltrials.gov/show/NCT03741920 (accessed 30 January 2023).
Appendix 1 Literature search strategies
Search strategies for identification of clinical studies (February 2022)
Ovid MEDLINE(R) ALL
Via Ovid http://ovidsp.ovid.com/
Date range searched: 1946 to 31 January 2022
Date searched: 1 February 2022
Records retrieved: 687
-
Parkinson Disease/ (74617)
-
(parkinson* adj2 (disease* or syndrom* or disorder* or complex)).ti,ab,kw. (111790)
-
or/1-2 (124681)
-
Wearable Electronic Devices/ (5431)
-
Telemetry/ (10052)
-
Remote Sensing Technology/ (3551)
-
((continuous* or remote*) adj2 (measure* or monitor* or sensor*)).ti,ab,kw. (50230)
-
(((wear* or worn or wrist* or ankle* or body* or waist* or belt*) adj2 (tech* or device* or sensor*)) and (remote* or continuous*)).ti,ab,kw. (2412)
-
(((inertia* or kinetic* or motor or gait or bradykine* or dyskine* or tremor* or shaking or instability or stability or balance or sleep*) adj2 (tech* or device* or sensor*)) and (remote* or continuous*)).ti,ab,kw. (1523)
-
telemetr*.ti,ab,kw. (9720)
-
((smart watch* or smart-watch* or smartwatch*) and (remote* or continuous*)).ti,ab,kw. (171)
-
(((mobile adj (health* or app*)) or (e-health or eHealth or m-health or mHealth)) and (remote* or continuous*)).ti,ab,kw. (2230)
-
((remote* or continuous*) and ((ambulatory or outpatient* or patient* or physiologic*) adj2 (monitor* or manage*))).ti,ab,kw. (8954)
-
or/4-13 (81597)
-
3 and 14 (633)
-
KinetiGraph*.ti,ab,kw,rn. (33)
-
(PKG* not “protein kinase”).ti,ab,kw,rn. (1002)
-
(kineti* adj graph*).ti,ab,kw,rn. (76)
-
Kinesia*.ti,ab,kw,rn. (102)
-
(STAT ON* or STAT-ON*).ti,ab,kw,rn. (30)
-
(PDMonitor* or (PD adj monitor*)).ti,ab,kw,rn. (37)
-
or/16-21 (1251)
-
3 and 22 (101)
-
15 or 23 (713)
-
exp animals/ not humans/ (4951717)
-
24 not 25 (688)
-
remove duplicates from 26 (687)
Key
-
/ = indexing term (Medical Subject Heading: MeSH)
-
* = truncation
-
ti,ab,kw = terms in either title, abstract, or keyword fields
-
rn = registry number/name of substance field
-
adj3 = terms within three words of each other (any order)
EMBASE
Via Ovid http://ovidsp.ovid.com/
Date range searched: 1974 to 31 January 2022
Date searched: 1 February 2022
Records retrieved: 1108
-
Parkinson disease/ (170324)
-
(parkinson* adj2 (disease* or syndrom* or disorder* or complex)).ti,ab,kw. (159760)
-
or/1-2 (200059)
-
wearable sensor/ (966)
-
telemetry/ (19025)
-
remote sensing/ (11647)
-
((continuous* or remote*) adj2 (measure* or monitor* or sensor*)).ti,ab,kw. (71013)
-
(((wear* or worn or wrist* or ankle* or body* or waist* or belt*) adj2 (tech* or device* or sensor*)) and (remote* or continuous*)).ti,ab,kw. (3192)
-
(((inertia* or kinetic* or motor or gait or bradykine* or dyskine* or tremor* or shaking or instability or stability or balance or sleep*) adj2 (tech* or device* or sensor*)) and (remote* or continuous*)).ti,ab,kw. (2334)
-
telemetr*.ti,ab,kw. (15101)
-
((smart watch* or smart-watch* or smartwatch*) and (remote* or continuous*)).ti,ab,kw. (231)
-
(((mobile adj (health* or app*)) or (e-health or eHealth or m-health or mHealth)) and (remote* or continuous*)).ti,ab,kw. (2726)
-
((remote* or continuous*) and ((ambulatory or outpatient* or patient* or physiologic*) adj2 (monitor* or manage*))).ti,ab,kw. (15311)
-
or/4-13 (116848)
-
3 and 14 (962)
-
KinetiGraph*.ti,ab,kw,dv. (131)
-
(PKG* not “protein kinase”).ti,ab,kw,dv. (1714)
-
(kineti* adj graph*).ti,ab,kw,dv. (112)
-
Kinesia*.ti,ab,kw,dv. (213)
-
(STAT ON* or STAT-ON*).ti,ab,kw,dv. (65)
-
(PDMonitor* or (PD adj monitor*)).ti,ab,kw,dv. (73)
-
or/16-21 (2178)
-
3 and 22 (299)
-
15 or 23 (1203)
-
animal/ (1551022)
-
exp animal experiment/ (2794289)
-
nonhuman/ (6785311)
-
(rat or rats or mouse or mice or hamster or hamsters or animal or animals or dog or dogs or cat or cats or bovine or sheep).ti,ab,sh. (6074833)
-
or/25-28 (9600168)
-
exp human/ (23201428)
-
human experiment/ (563905)
-
30 or 31 (23203414)
-
29 not (29 and 32) (6877011)
-
24 not 33 (1132)
-
remove duplicates from 34 (1108)
Key
-
/ or.sh. = indexing term (Emtree Subject Heading)
-
* = truncation
-
ti,ab,kw = terms in either title, abstract, or keyword fields
-
dv = device trade name field
-
adj3 = terms within three words of each other (any order)
APA PsycInfo
Via Ovid http://ovidsp.ovid.com/
Date range searched: 1806 to January Week 4 2022
Date searched: 1 February 2022
Records retrieved: 134
-
Parkinson’s Disease/ (24607)
-
Parkinson Disease.mh. (13837)
-
(parkinson* adj2 (disease* or syndrom* or disorder* or complex)).ti,ab. (31456)
-
or/1-3 (33506)
-
wearable devices/ (444)
-
Wearable Electronic Devices.mh. (121)
-
Telemetry.sh,mh. (746)
-
Remote Sensing Technology.mh. (66)
-
((continuous* or remote*) adj2 (measure* or monitor* or sensor*)).ti,ab. (4631)
-
(((wear* or worn or wrist* or ankle* or body* or waist* or belt*) adj2 (tech* or device* or sensor*)) and (remote* or continuous*)).ti,ab. (172)
-
(((inertia* or kinetic* or motor or gait or bradykine* or dyskine* or tremor* or shaking or instability or stability or balance or sleep*) adj2 (tech* or device* or sensor*)) and (remote* or continuous*)).ti,ab. (351)
-
telemetr*.ti,ab. (1062)
-
((smart watch* or smart-watch* or smartwatch*) and (remote* or continuous*)).ti,ab. (21)
-
(((mobile adj (health* or app*)) or (e-health or eHealth or m-health or mHealth)) and (remote* or continuous*)).ti,ab. (269)
-
((remote* or continuous*) and ((ambulatory or outpatient* or patient* or physiologic*) adj2 (monitor* or manage*))).ti,ab. (494)
-
or/5-15 (7495)
-
4 and 16 (104)
-
KinetiGraph*.ti,ab. (8)
-
(PKG* not “protein kinase”).ti,ab. (70)
-
(kineti* adj graph*).ti,ab. (2)
-
Kinesia*.ti,ab. (33)
-
(STAT ON* or STAT-ON*).ti,ab. (0)
-
(PDMonitor* or (PD adj monitor*)).ti,ab. (2)
-
or/18-23 (106)
-
4 and 24 (36)
-
17 or 25 (135)
-
remove duplicates from 26 (134)
Key
-
/ = indexing term (Thesaurus of Psychological Index Terms)
-
mh = indexing term MeSH
-
* = truncation
-
ti,ab = terms in either title or abstract fields
-
adj3 = terms within three words of each other (any order)
Econlit
Via Ovid http://ovidsp.ovid.com/
Date range searched: 1886 to 27 January 2022
Date searched: 1 February 2022
Records retrieved: 0
-
(parkinson* adj2 (disease* or syndrom* or disorder* or complex)).ti,ab,kw. (36)
-
((continuous* or remote*) adj2 (measure* or monitor* or sensor*)).ti,ab,kw. (363)
-
(((wear* or worn or wrist* or ankle* or body* or waist* or belt*) adj2 (tech* or device* or sensor*)) and (remote* or continuous*)).ti,ab,kw. (3)
-
(((inertia* or kinetic* or motor or gait or bradykine* or dyskine* or tremor* or shaking or instability or stability or balance or sleep*) adj2 (tech* or device* or sensor*)) and (remote* or continuous*)).ti,ab,kw. (7)
-
telemetr*.ti,ab,kw. (15)
-
((smart watch* or smart-watch* or smartwatch*) and (remote* or continuous*)).ti,ab,kw. (0)
-
(((mobile adj (health* or app*)) or (e-health or eHealth or m-health or mHealth)) and (remote* or continuous*)).ti,ab,kw. (7)
-
((remote* or continuous*) and ((ambulatory or outpatient* or patient* or physiologic*) adj2 (monitor* or manage*))).ti,ab,kw. (10)
-
KinetiGraph*.ti,ab,kw. (0)
-
(PKG* not “protein kinase”).ti,ab,kw. (1)
-
(kineti* adj graph*).ti,ab,kw. (0)
-
Kinesia*.ti,ab,kw. (0)
-
(STAT ON* or STAT-ON*).ti,ab,kw. (1)
-
(PDMonitor* or (PD adj monitor*)).ti,ab,kw. (0)
-
or/2-14 (402)
-
1 and 15 (0)
Key
-
* = truncation
-
ti,ab,kw = terms in either title, abstract, or keyword fields
-
adj3 = terms within three words of each other (any order)
Cochrane Central Register of Controlled Trials
Via Wiley http://onlinelibrary.wiley.com/
Date range: Issue 2 of 12 February 2022
Date searched: 1 February 2022
Records retrieved: 125
-
[mh ^“Parkinson Disease”] 4556
-
(parkinson* NEAR/2 (disease* or syndrom* or disorder* or complex)):ti,ab,kw 10982
-
{OR #1-#2} 10982
-
[mh ^“Wearable Electronic Devices”] 115
-
[mh ^Telemetry] 257
-
[mh ^“Remote Sensing Technology”] 50
-
((continuous* or remote*) NEAR/2 (measure* or monitor* or sensor*)):ti,ab,kw 8741
-
(((wear* or worn or wrist* or ankle* or body* or waist* or belt*) NEAR/2 (tech* or device* or sensor*)) and (remote* or continuous*)):ti,ab,kw 396
-
(((inertia* or kinetic* or motor or gait or bradykine* or dyskine* or tremor* or shaking or instability or stability or balance or sleep*) NEAR/2 (tech* or device* or sensor*)) and (remote* or continuous*)):ti,ab,kw 466
-
telemetr*:ti,ab,kw 843
-
((smart NEXT watch* or smartwatch*) and (remote* or continuous*)):ti,ab,kw 37
-
(((mobile NEXT (health* or app*)) or (eHealth or mHealth)) and (remote* or continuous*)):ti,ab,kw 694
-
((remote* or continuous*) and ((ambulatory or outpatient* or patient* or physiologic*) NEAR/2 (monitor* or manage*))):ti,ab,kw 3248
-
{OR #4-#13} 12526
-
#3 and #14 83
-
KinetiGraph*:ti,ab,kw 13
-
(PKG* not “protein kinase”):ti,ab,kw 93
-
(kineti* NEXT graph*):ti,ab,kw 11
-
Kinesia*:ti,ab,kw 37
-
(“STAT ON”):ti,ab,kw 1265
-
(PDMonitor* or (PD NEXT monitor*)):ti,ab,kw 7
-
{OR #16-#21} 1406
-
#3 and #22 50
-
#15 or #23 in Trials 125
Key
-
mh = exploded indexing term (MeSH)
-
mh ^ = unexploded indexing term (MeSH)
-
* = truncation
-
ti,ab,kw = terms in either title or abstract or keyword fields
-
near/3 = terms within three words of each other (any order)
-
next = terms are next to each other
Cochrane Database of Systematic Reviews
via Wiley http://onlinelibrary.wiley.com/
Date range: Issue 2 of 12 February 2022
Date searched: 1 February 2022
Records retrieved: 4
-
[mh ^“Parkinson Disease”] 4556
-
(parkinson* NEAR/2 (disease* or syndrom* or disorder* or complex)):ti,ab,kw 10982
-
{OR #1-#2} 10982
-
[mh ^“Wearable Electronic Devices”] 115
-
[mh ^Telemetry] 257
-
[mh ^“Remote Sensing Technology”] 50
-
((continuous* or remote*) NEAR/2 (measure* or monitor* or sensor*)):ti,ab,kw 8741
-
(((wear* or worn or wrist* or ankle* or body* or waist* or belt*) NEAR/2 (tech* or device* or sensor*)) and (remote* or continuous*)):ti,ab,kw 396
-
(((inertia* or kinetic* or motor or gait or bradykine* or dyskine* or tremor* or shaking or instability or stability or balance or sleep*) NEAR/2 (tech* or device* or sensor*)) and (remote* or continuous*)):ti,ab,kw 466
-
telemetr*:ti,ab,kw 843
-
((smart NEXT watch* or smartwatch*) and (remote* or continuous*)):ti,ab,kw 37
-
(((mobile NEXT (health* or app*)) or (eHealth or mHealth)) and (remote* or continuous*)):ti,ab,kw 694
-
((remote* or continuous*) and ((ambulatory or outpatient* or patient* or physiologic*) NEAR/2 (monitor* or manage*))):ti,ab,kw 3248
-
{OR #4-#13} 12526
-
#3 and #14 83
-
KinetiGraph*:ti,ab,kw 13
-
(PKG* not “protein kinase”):ti,ab,kw 93
-
(kineti* NEXT graph*):ti,ab,kw 11
-
Kinesia*:ti,ab,kw 37
-
(“STAT ON”):ti,ab,kw 1265
-
(PDMonitor* or (PD NEXT monitor*)):ti,ab,kw 7
-
{OR #16-#21} 1406
-
#3 and #22 50
-
#15 or #23 in Cochrane Reviews 4
Key
-
mh = exploded indexing term (MeSH)
-
mh ^ = unexploded indexing term (MeSH)
-
* = truncation
-
ti,ab,kw = terms in either title or abstract or keyword fields
-
near/3 = terms within three words of each other (any order)
-
next = terms are next to each other
International Health Technology Assessment database
Via https://database.inahta.org/
Date range: Inception to 1 February 2022
Date searched: 1 February 2022
Records retrieved: 36
((((continuous* OR remote*) AND (measure* OR monitor* OR sensor*)))[Title] OR (((continuous* OR remote*) AND (measure* OR monitor* OR sensor*)))[abs] OR (((continuous* OR remote*) AND (measure* OR monitor* OR sensor*)))[Keywords] OR ((((wear* OR worn OR wrist* OR ankle* OR body* OR waist* OR belt*) AND (tech* OR device* OR sensor*)) AND (remote* OR continuous*)))[Title] OR ((((wear* OR worn OR wrist* OR ankle* OR body* OR waist* OR belt*) AND (tech* OR device* OR sensor*)) AND (remote* OR continuous*)))[abs] OR ((((wear* OR worn OR wrist* OR ankle* OR body* OR waist* OR belt*) AND (tech* OR device* OR sensor*)) AND (remote* OR continuous*)))[Keywords] OR ((((inertia* OR kinetic* OR motor OR gait OR bradykine* OR dyskine* OR tremor* OR shaking OR instability OR stability OR balance OR sleep*) AND (tech* OR device* OR sensor*)) AND (remote* OR continuous*)))[Title] OR ((((inertia* OR kinetic* OR motor OR gait OR bradykine* OR dyskine* OR tremor* OR shaking OR instability OR stability OR balance OR sleep*) AND (tech* OR device* OR sensor*)) AND (remote* OR continuous*)))[abs] OR ((((inertia* OR kinetic* OR motor OR gait OR bradykine* OR dyskine* OR tremor* OR shaking OR instability OR stability OR balance OR sleep*) AND (tech* OR device* OR sensor*)) AND (remote* OR continuous*)))[Keywords] OR ((telemetr* OR ((smart watch* OR smart-watch* OR smartwatch*) AND (remote* OR continuous*))))[Title] OR ((telemetr* OR ((smart watch* OR smart-watch* OR smartwatch*) AND (remote* OR continuous*))))[abs] OR ((telemetr* OR ((smart watch* OR smart-watch* OR smartwatch*) AND (remote* OR continuous*))))[Keywords] OR ((((mobile AND (health* OR app*)) OR (e-health OR eHealth OR m-health OR mHealth)) AND (remote* OR continuous*)))[Title] OR ((((mobile AND (health* OR app*)) OR (e-health OR eHealth OR m-health OR mHealth)) AND (remote* OR continuous*)))[abs] OR ((((mobile AND (health* OR app*)) OR (e-health OR eHealth OR m-health OR mHealth)) AND (remote* OR continuous*)))[Keywords] OR (((remote* OR continuous*) AND ((ambulatory OR outpatient* OR patient* OR physiologic*) AND (monitor* OR manage*))))[Title] OR (((remote* OR continuous*) AND ((ambulatory OR outpatient* OR patient* OR physiologic*) AND (monitor* OR manage*))))[abs] OR (((remote* OR continuous*) AND ((ambulatory OR outpatient* OR patient* OR physiologic*) AND (monitor* OR manage*))))[Keywords] OR ((KinetiGraph* OR PKG* OR Kinesia* OR STAT ON* OR STAT-ON* OR PDMonitor* OR (kineti* AND graph*) OR (PD AND monitor*)))[Title] OR ((KinetiGraph* OR PKG* OR Kinesia* OR STAT ON* OR STAT-ON* OR PDMonitor* OR (kineti* AND graph*) OR (PD AND monitor*)))[abs] OR ((KinetiGraph* OR PKG* OR Kinesia* OR STAT ON* OR STAT-ON* OR PDMonitor* OR (kineti* AND graph*) OR (PD AND monitor*)))[Keywords]) AND ((“Parkinson Disease”[mh]) OR ((parkinson* AND (disease* OR syndrom* OR disorder* OR complex)))[Title] OR ((parkinson* AND (disease* OR syndrom* OR disorder* OR complex)))[abs] OR ((parkinson* AND (disease* OR syndrom* OR disorder* OR complex)))[Keywords])
Key
-
[mh] = indexing term: Medical Subject Heading (MeSH)
-
[Keywords] = search of keywords field
-
[abs] = search of abstract field
-
[Title] = search of title field
-
* = truncation
Database of Abstracts of Reviews of Effects
Via https://www.crd.york.ac.uk/CRDWeb/
Date range searched: Inception to 31 March 2015
Date searched: 1 February 2022
Records retrieved: 2
-
MeSH DESCRIPTOR Parkinson Disease IN DARE 144
-
(parkinson* NEAR2 (disease* or syndrom* or disorder* or complex)) IN DARE 256
-
#1 OR #2 256
-
MeSH DESCRIPTOR Wearable Electronic Devices IN DARE 0
-
MeSH DESCRIPTOR Telemetry IN DARE 6
-
MeSH DESCRIPTOR Remote Sensing Technology IN DARE 1
-
((continuous* or remote*) NEAR2 (measure* or monitor* or sensor*)) IN DARE 190
-
(((wear* or worn or wrist* or ankle* or body* or waist* or belt*) NEAR2 (tech* or device* or sensor*)) and (remote* or continuous*)) IN DARE 2
-
(((inertia* or kinetic* or motor or gait or bradykine* or dyskine* or tremor* or shaking or instability or stability or balance or sleep*) NEAR2 (tech* or device* or sensor*)) and (remote* or continuous*)) IN DARE 3
-
telemetr* IN DARE 14
-
((smart watch* or smart-watch* or smartwatch*) and (remote* or continuous*)) IN DARE 0
-
(((mobile NEAR (health* or app*)) or (e-health or eHealth or m-health or mHealth)) and (remote* or continuous*)) IN DARE 2
-
((remote* or continuous*) and ((ambulatory or outpatient* or patient* or physiologic*) NEAR2 (monitor* or manage*))) IN DARE 35
-
#4 OR #5 OR #6 OR #7 OR #8 OR #9 OR #10 OR #11 OR #12 OR #13 233
-
#3 AND #14 2
-
KinetiGraph* IN DARE 0
-
(PKG* NOT protein kinase) IN DARE 0
-
(kineti* NEAR graph*) IN DARE 0
-
Kinesia* IN DARE 1
-
(STAT ON* or STAT-ON*) IN DARE 0
-
(PDMonitor* or (PD NEAR monitor*)) IN DARE 0
-
#16 OR #17 OR #18 OR #19 OR #20 OR #21 1
-
#3 AND #22 0
-
#15 OR #23 2
Key
-
MeSH DESCRIPTOR = indexing term: Medical Subject Heading (MeSH)
-
* = truncation
-
NEAR3 = terms within three words of each other (only in the order specified)
NHS Economic Evaluation Database
Via https://www.crd.york.ac.uk/CRDWeb/
Date range searched: Inception to 31 March 2015
Date searched: 1 February 2022
Records retrieved: 1
-
MeSH DESCRIPTOR Parkinson Disease IN EED 33
-
(parkinson* NEAR2 (disease* or syndrom* or disorder* or complex)) IN EED 46
-
#1 OR #2 46
-
MeSH DESCRIPTOR Wearable Electronic Devices IN EED 0
-
MeSH DESCRIPTOR Telemetry IN EED 14
-
MeSH DESCRIPTOR Remote Sensing Technology IN EED 2
-
((continuous* or remote*) NEAR2 (measure* or monitor* or sensor*)) IN EED 36
-
(((wear* or worn or wrist* or ankle* or body* or waist* or belt*) NEAR2 (tech* or device* or sensor*)) and (remote* or continuous*)) IN EED 0
-
(((inertia* or kinetic* or motor or gait or bradykine* or dyskine* or tremor* or shaking or instability or stability or balance or sleep*) NEAR2 (tech* or device* or sensor*)) and (remote* or continuous*)) IN EED 0
-
telemetr* IN EED 26
-
((smart watch* or smart-watch* or smartwatch*) and (remote* or continuous*)) IN EED 0
-
(((mobile NEAR (health* or app*)) or (e-health or eHealth or m-health or mHealth)) and (remote* or continuous*)) IN EED 8
-
((remote* or continuous*) and ((ambulatory or outpatient* or patient* or physiologic*) NEAR2 (monitor* or manage*))) IN EED 34
-
#4 OR #5 OR #6 OR #7 OR #8 OR #9 OR #10 OR #11 OR #12 OR #13 89
-
#3 AND #14 0
-
KinetiGraph* IN EED 0
-
(PKG* NOT protein kinase) IN EED 0
-
(kineti* NEAR graph*) IN EED 0
-
Kinesia* IN EED 0
-
(STAT ON* or STAT-ON*) IN EED 0
-
(PDMonitor* or (PD NEAR monitor*)) IN EED 1
-
#16 OR #17 OR #18 OR #19 OR #20 OR #21 1
-
#3 AND #22 1
-
#15 OR #23 1
Key
-
MeSH DESCRIPTOR = indexing term: Medical Subject Heading (MeSH)
-
* = truncation
-
NEAR3 = terms within three words of each other (only in the order specified)
Health Technology Assessment
Via https://www.crd.york.ac.uk/CRDWeb/
Date range searched: Inception to March 2018
Date searched: 1 February 2022
Records retrieved: 0
-
MeSH DESCRIPTOR Parkinson Disease IN HTA 66
-
(parkinson* NEAR2 (disease* or syndrom* or disorder* or complex)) IN HTA 86
-
#1 OR #2 86
-
MeSH DESCRIPTOR Wearable Electronic Devices IN HTA 0
-
MeSH DESCRIPTOR Telemetry IN HTA 17
-
MeSH DESCRIPTOR Remote Sensing Technology IN HTA 5
-
((continuous* or remote*) NEAR2 (measure* or monitor* or sensor*)) IN HTA 66
-
(((wear* or worn or wrist* or ankle* or body* or waist* or belt*) NEAR2 (tech* or device* or sensor*)) and (remote* or continuous*)) IN HTA 2
-
(((inertia* or kinetic* or motor or gait or bradykine* or dyskine* or tremor* or shaking or instability or stability or balance or sleep*) NEAR2 (tech* or device* or sensor*)) and (remote* or continuous*)) IN HTA 1
-
telemetr* IN HTA 23
-
((smart watch* or smart-watch* or smartwatch*) and (remote* or continuous*)) IN HTA 0
-
(((mobile NEAR (health* or app*)) or (e-health or eHealth or m-health or mHealth)) and (remote* or continuous*)) IN HTA 1
-
((remote* or continuous*) and ((ambulatory or outpatient* or patient* or physiologic*) NEAR2 (monitor* or manage*))) IN HTA 19
-
#4 OR #5 OR #6 OR #7 OR #8 OR #9 OR #10 OR #11 OR #12 OR #13 92
-
#3 AND #14 0
-
KinetiGraph* IN HTA 0
-
(PKG* NOT protein kinase) IN HTA 0
-
(kineti* NEAR graph*) IN HTA 0
-
Kinesia* IN HTA 0
-
(STAT ON* or STAT-ON*) IN HTA 0
-
(PDMonitor* or (PD NEAR monitor*)) IN HTA 1
-
#16 OR #17 OR #18 OR #19 OR #20 OR #21 1
-
#3 AND #22 0
-
#15 OR #23 0
Key
-
MeSH DESCRIPTOR = indexing term: Medical Subject Heading (MeSH)
-
* = truncation
-
NEAR3 = terms within three words of each other (only in the order specified)
ClinicalTrials.gov
Via https://clinicaltrials.gov/
Date searched: 1 February 2022
Records retrieved: 271
Advanced search screen used. Two separate searches were used, retrieving 271 records in total, which were imported into EndNote 20 and deduplicated.
Search strategies:
-
Condition or disease: Parkinson
-
Other terms: (“Kineti Graph” OR KinetiGraph OR PKG OR Kinesia OR “STAT ON” OR PDMonitor OR “PD Monitor”) = 46 hits
-
-
Condition or disease: Parkinson
-
Other terms: ((continuous OR remote) AND (measure OR monitor OR sensor)) = 225 hits
-
European Union Clinical Trials Register
Via www.clinicaltrialsregister.eu/ctr-search/search
Date searched: 1 February 2022
Records retrieved: 11
Advanced search screen used. Two separate searches were used, retrieving 11 records in total, which were imported into EndNote 20 and deduplicated.
Search strategies:
-
(Parkinson AND (“Kineti Graph” OR KinetiGraph OR PKG OR Kinesia OR “STAT ON” OR PDMonitor OR “PD Monitor”)) = 6 hits
-
Parkinson AND ((continuous OR remote) AND (measure OR monitor OR sensor)) = 5 hits
World Health Organization International Clinical Trials Registry Platform
Via https://trialsearch.who.int/AdvSearch.aspx
Date searched: 1 February 2022
Records retrieved: 12
Advanced search screen used. Two separate searches were used, retrieving 12 records in total, which were imported into EndNote 20 and deduplicated.
Search strategies:
-
Condition: Parkinson
-
Intervention: (Kineti Graph OR KinetiGraph OR PKG OR Kinesia OR STAT ON OR STAT-ON OR PDMonitor OR PD Monitor)
-
Recruitment Status: ALL
-
= 11 records for 11 trials found
-
-
Condition: Parkinson
-
Intervention: ((continuous OR remote) AND (measure OR monitor OR sensor))
-
Recruitment Status: ALL
-
= 1 records for 1 trials found
-
Search strategies for identification of economic studies (March 2022)
Ovid MEDLINE(R) ALL
Via Ovid http://ovidsp.ovid.com/
Date range searched: 1946 to 1 March 2022
Date searched: 2 March 2022
Records retrieved: 529
-
Parkinson Disease/ (75106)
-
(parkinson* adj2 (disease* or syndrom* or disorder* or complex)).ti,ab,kw. (112396)
-
1 or 2 (125301)
-
*economics/ (10787)
-
exp *“costs and cost analysis”/ (78000)
-
(economic adj2 model*).mp. (14362)
-
(cost minimi* or cost-utilit* or health utilit* or economic evaluation* or economic review* or cost outcome* or cost analys?s or economic analys?s or budget* impact analys?s).ti,ab,kf,kw. (38225)
-
(cost-effective* or pharmacoeconomic* or pharmaco-economic* or cost-benefit or costs).ti,kf,kw. (80925)
-
(life year or life years or qaly* or cost-benefit analys?s or cost-effectiveness analys?s).ab,kf,kw. (35175)
-
(cost or economic*).ti,kf,kw. and (costs or cost-effectiveness or markov or monte carlo or model or modeling or modelling).ab. (75922)
-
or/4-10 (205434)
-
3 and 11 (546)
-
exp animals/ not humans/ (4965507)
-
12 not 13 (544)
-
letter.pt. (1170841)
-
editorial.pt. (596777)
-
historical article.pt. (367835)
-
or/15-17 (2114647)
-
14 not 18 (529)
Key
-
/ = indexing term (Medical Subject Heading: MeSH)
-
* before a MeSH term = focused subject heading
-
* = truncation
-
ti,ab,kw = terms in either title, abstract, or keyword fields
-
mp = multipurpose field
-
pt = publication type
-
? = optional wild card character – stands for zero or one letters
-
adj3 = terms within three words of each other (any order)
EMBASE
Via Ovid http://ovidsp.ovid.com/
Date range searched: 1974 to 1 March 2022
Date searched: 2 March 2022
Records retrieved: 548
-
Parkinson Disease/ (171312)
-
(parkinson* adj2 (disease* or syndrom* or disorder* or complex)).ti,ab,kw. (160723)
-
1 or 2 (201252)
-
*economics/ (27548)
-
*“cost benefit analysis”/ (12396)
-
*“cost effectiveness analysis”/ (34235)
-
*“cost utility analysis”/ (2741)
-
*“cost minimization analysis”/ (817)
-
(economic adj2 model*).mp. (8770)
-
(cost minimi* or cost-utilit* or health utilit* or economic evaluation* or economic review* or cost outcome or cost analys?s or economic analys?s or budget* impact analys?s).ti,ab,kw. (56649)
-
(cost-effective* or pharmacoeconomic* or pharmaco-economic* or cost-benefit or costs).ti,kw. (111273)
-
(life year or life years or qaly* or cost-benefit analys?s or cost-effectiveness analys?s).ab,kw. (54031)
-
(cost or economic*).ti,kw. and (costs or cost-effectiveness or markov).ab. (93389)
-
or/4-13 (232822)
-
3 and 14 (872)
-
animal/ (1558504)
-
exp animal experiment/ (2806299)
-
nonhuman/ (6815251)
-
(rat or rats or mouse or mice or hamster or hamsters or animal or animals or dog or dogs or cat or cats or bovine or sheep).ti,ab,sh. (6095552)
-
or/16-19 (9639196)
-
exp human/ (23327515)
-
human experiment/ (567633)
-
or/21-22 (23329520)
-
20 not (20 and 23) (6898393)
-
15 not 24 (864)
-
letter.pt. (1213039)
-
editorial.pt. (718624)
-
note.pt. (885196)
-
conference abstract.pt. (4333669)
-
or/26-29 (7150528)
-
25 not 30 (548)
Key
-
/ or.sh. = indexing term (Emtree Subject Heading)
-
* before an Emtree term = focused subject heading
-
* = truncation
-
ti,ab,kw = terms in either title, abstract, or keyword fields
-
mp = multipurpose field
-
pt = publication type
-
? = optional wild card character – stands for zero or one letters
-
adj3 = terms within three words of each other (any order)
Econlit
Via Ovid http://ovidsp.ovid.com/
Date range searched: 1886 to 24 February 2022
Date searched: 2 March 2022
Records retrieved: 36
1 (parkinson* adj2 (disease* or syndrom* or disorder* or complex)).ti,ab,kw. (36)
Key
-
* = truncation
-
ti,ab,kw = terms in either title, abstract or keyword fields
-
adj3 = terms within three words of each other (any order)
NHS Economic Evaluation Database
Via https://www.crd.york.ac.uk/CRDWeb/
Date range searched: Inception to 31 March 2015
Date searched: 2 March 2022
Records retrieved: 46
-
MeSH DESCRIPTOR Parkinson Disease IN EED 33
-
(parkinson* NEAR2 (disease* or syndrom* or disorder* or complex)) IN EED 46
-
#1 OR #2 46
Key
-
MeSH DESCRIPTOR = indexing term: Medical Subject Heading (MeSH)
-
* = truncation
-
NEAR2 = terms within three words of each other (only in the order specified)
Health Technology Assessment
Via https://www.crd.york.ac.uk/CRDWeb/
Date range searched: Inception to March 2018
Date searched: 2 March 2022
Records retrieved: 86
-
MeSH DESCRIPTOR Parkinson Disease IN HTA 66
-
(parkinson* NEAR2 (disease* or syndrom* or disorder* or complex)) IN HTA 86
-
#1 OR #2 86
Key
-
MeSH DESCRIPTOR = indexing term: Medical Subject Heading (MeSH)
-
* = truncation
-
NEAR2 = terms within three words of each other (only in the order specified)
International Health Technology Assessment database
Via https://database.inahta.org/
Date range: Inception to 2 March 2022
Date searched: 2 March 2022
Records retrieved: 95
((“Parkinson Disease”[mh]) OR ((parkinson* AND (disease* OR syndrom* OR disorder* OR complex)))[Title] OR ((parkinson* AND (disease* OR syndrom* OR disorder* OR complex)))[abs] OR ((parkinson* AND (disease* OR syndrom* OR disorder* OR complex)))[Keywords])
Key
-
[mh] = indexing term: Medical Subject Heading (MeSH)
-
[Keywords] = search of keywords field
-
[abs] = search of abstract field
-
[Title] = search of title field
-
* = truncation
Appendix 2 Excluded studies with rationale
Study | Reason for exclusion |
---|---|
Abou (2021)161 | Not an eligible device |
Adams (2021)162 | Not an eligible device |
AlMahadin (2020)163 | Not an eligible device |
Ancona (2022)164 | Review article |
Barrachina-Fernandez (2021)165 | Not an eligible device |
Battista (2020)166 | Not an eligible device |
Battista (2021)167 | Not an eligible device |
Bendig (2020)168 | No relevant outcome data |
Blaze (2016)169 | Monitoring device not the intervention under assessment |
Brillman (2015)170 | No relevant outcome data |
Canento (2019)171 | No relevant outcome data |
Carroll (2019)172 | Duplicate report |
Channa (2020)173 | Review article |
David Prakash (2013)174 | Not an eligible device |
DelPrete (2019)175 | No relevant outcome data |
DelPrete (2022)176 | Monitoring device not the intervention under assessment |
Dominey (2018)177 | Duplicate report |
Edwards (2020)178 | Monitoring device not the intervention under assessment |
Edwards (2020)179 | No relevant outcome data |
Evans (2014)48 | Monitoring device not the intervention under assessment |
Evans (2019)180 | Duplicate report |
Evans (2020)181 | Duplicate report |
Evans (2021)182 | No relevant outcome data |
Farley (2018)183 | Not an eligible device |
Farzanehfar (2017)184 | Duplicate report |
Farzanehfar (2017)185 | Review article |
Flisar (2016)186 | No relevant outcome data |
Flisar (2016)187 | No relevant outcome data |
Flisar (2018)188 | No relevant outcome data |
Gao (2018)189 | Not an eligible device |
Gernon (2018)190 | No relevant outcome data |
Ghoraani (2021)191 | Review article |
Giuffrida (2009)192 | Not an eligible device |
Giuffrida (2009)193 | Not an eligible device |
Griffiths (2012)194 | Duplicate report |
Heldman (2014)195 | Not an eligible device |
Heldman (2016)196 | Not an eligible device |
Horne (2014)197 | No relevant outcome data |
Isaacson (2018)198 | Duplicate report |
Jansa (2016)199 | No relevant outcome data |
Johansson (2019)200 | Monitoring device not the intervention under assessment |
Joshi (2019)201 | Duplicate report |
Karl (2020)202 | Monitoring device not the intervention under assessment |
Keogh (2021)203 | Review article |
Kilincalp (2022)204 | Monitoring device not the intervention under assessment |
King (2021)205 | Not an eligible device |
Klingelhoefer (2015)206 | Duplicate report |
Klingelhoefer (2016)207 | No relevant outcome data |
Koivu (2017)208 | No relevant outcome data |
Kostikis (2021)209 | No relevant outcome data |
Kotschet (2012)210 | No relevant outcome data |
Krause (2019)211 | Same patients as another study |
Leake (2019)212 | No relevant outcome data |
Lynch (2016)213 | No relevant outcome data |
Lynch (2018)214 | No relevant outcome data |
Lynch (2018)215 | No relevant outcome data |
Lynch (2019)216 | No relevant outcome data |
Malhotra (2020)217 | No relevant outcome data |
Metta (2021)218 | No relevant outcome data |
Mirelman (2020)219 | Not an eligible device |
Mohamed (2020)220 | No relevant outcome data |
Morgan (2020)221 | Review article |
Morgante (2019)222 | Monitoring device not the intervention under assessment |
Mostile (2010)223 | Not an eligible device |
Nahab (2018)224 | Duplicate report |
Pahwa (2018)225 | No relevant outcome data |
Pahwa (2019)226 | No relevant outcome data |
Pahwa (2020)227 | No relevant outcome data |
Pai (2020)228 | No relevant outcome data |
Papapetropoulos (2016)229 | Not an eligible device |
Phillips (2013)230 | Monitoring device not the intervention under assessment |
Podlewska (2019)231 | No relevant outcome data |
Potter (2020)232 | No relevant outcome data |
Powell (2020)233 | Not PD |
Pulliam (2015)234 | Not an eligible device |
Robertson (2020)235 | Not an eligible device |
Rodriguez-Martin (2019)236 | Review article |
Rodriguez-Martin (2021)237 | No relevant outcome data |
Rovini (2019)238 | Review article |
Sachdev (2017)239 | No relevant outcome data |
Santos Garcia (2020)240 | Duplicate report |
Sasaki (2018)241 | No relevant outcome data |
Sica (2021)242 | Review article |
Sringean (2014)243 | No relevant outcome data |
Stuijt (2016)244 | Monitoring device not the intervention under assessment |
Stuijt (2017)245 | Duplicate report |
Stuijt (2018)246 | Monitoring device not the intervention under assessment |
Sundgren (2019)247 | Duplicate report |
Sung (2018)248 | No relevant outcome data |
Suttrup (2016)249 | No relevant outcome data |
Taddei (2018)250 | Monitoring device not the intervention under assessment |
Tan (2017)251 | No relevant outcome data |
Thomas (2017)252 | Duplicate report |
Thomas (2017)253 | Monitoring device not the intervention under assessment |
Thomas (2019)254 | Monitoring device not the intervention under assessment |
Thomsen (2019)255 | No relevant outcome data |
Titova (2020)256 | No relevant outcome data |
van den Bergh (2021)257 | Review article |
van Uem (2016)258 | Not an eligible device |
van Uem (2018)259 | Not an eligible device |
van Wamelen (2019)260 | Monitoring device not the intervention under assessment |
van Wamelen (2020)261 | Monitoring device not the intervention under assessment |
van Wamelen (2021)262 | Monitoring device not the intervention under assessment |
van Wamelen (2021)263 | Review article |
Watts (2021)45 | Same patients as another study (although this paper was used to supplement data extraction of diagnostic accuracy outcomes) |
Watts (2021)264 | No relevant outcome data |
Williamson (2021)265 | Not an eligible device |
Zampogna (2020)266 | Not an eligible device |
Zhang (2020)267 | Not an eligible device |
Zhang (2021)268 | Review article |
#2178 (no author name or year or abstract){#314} | No relevant outcome data |
#2120 (no author name or year or abstract){#316} | No relevant outcome data |
#2075 (no author name or year or abstract){#315} | No relevant outcome data |
#1616 (no author name or year or abstract){#317} | No relevant outcome data |
#1971 (no author name or year or abstract){#313} | Not an eligible device |
#1581 (no author name or year or abstract){#310} | No relevant outcome data |
#1580 (no author name or year or abstract) #308} | No relevant outcome data |
#1434 (no author name or year or abstract){#318} | No relevant outcome data |
#552 (no author name or year or abstract){#312} | Not an eligible device |
#1319 (no author name or year or abstract){#309} | No relevant outcome data |
Euctr-000346-19-Se, 2018{Euctr-000346-19-Se, 2018 #301} | Monitoring device not the intervention under assessment |
Euctr-005170-19-Se, 2018{Euctr-005170-19-Se, 2018 #300} | Monitoring device not the intervention under assessment |
NCT04381065, 2020{Nct, 2020 #302} | Study withdrawn |
NCT03984305, 2019{Nct, 2019 #297} | Study terminated |
NCT03152721, 2017{Nct, 2017 #305} | Duplicate report |
NCT03103919, 2017{Nct, 2017 #296} | Duplicate report |
NCT04653688, 2020{NCT04653688, 2020 #307} | Monitoring device not the intervention under assessment |
NCT031305959 | Duplicate report |
NCT04719468, 2021{Nct, 2021 #299} | Monitoring device not the intervention under assessment |
NCT02152319, 2014{Nct, 2014 #306} | Not an eligible device |
NCT03531060, 2018{Nct, 2018 #303} | Monitoring device not the intervention under assessment |
Appendix 3 Ongoing studies
Study details | Study design and funding source | Population | Intervention | Comparator(s) | Outcomes |
---|---|---|---|---|---|
Price (2016)269 UK |
Prospective cohort study Funding: not stated |
PwP (n = 5 to date) | PKG | – | Patient satisfaction |
Price (2017)270 Location: not stated |
Prospective cohort study Funding: not stated |
PwP (n = 6 to date) | PKG to assess tremor | Patient report | Patient satisfaction |
Rodriguez-Molinero (2019)271 Spain |
RCT Funding: not stated (2 authors’ affiliations are Sense4Care) |
PwP | STAT-ON | Patient diary Clinical assessment in clinic |
Change in clinical management and number of visits to the doctor for medication adjustment Motor fluctuations diary, UPDRS, Freezing of Gait Questionnaire |
UK PKG Registry: Multi-centre real-world registry of PKG in 441 patients with PD. NIHR portfolio study ID: 35053272 UK |
Registry Funding: not stated |
PwP (n = 441) | PKG | – | |
#1692 (NCT05153356){, #311} USA |
Prospective cohort study Funding: not stated |
PwP (estimated enrolment n = 20) | PKG Kinesia 360 |
Patient questionnaires and clinician rating scales | Correlation, patient satisfaction |
NCT04176302 (2019)273 Spain |
RCT (MoMoPa-EC) Funding: not stated |
PwP | STAT-ON | Clinical assessment Patient diary |
Clinical outcomes, change in clinical management, physician and patient satisfaction |
NCT03741920 (2018)274 USA |
RCT (APPRISE) Funding: not stated |
PwP (n = 231) | PKG | Clinical assessment | Change in clinical management |
Appendix 4 Data extraction tables
Diagnostic accuracy
For diagnostic accuracy results (sensitivity/specificity etc.), see Table 4 of main report.
PKG (7 full papers, 1 abstract)
Study details | Study design and funding source | Population (incl. n) | Reference standard |
---|---|---|---|
Full papers | |||
Braybrook (2016)39 Australia |
Prospective cohort study Funding: GKC |
PwP (n = 172) | Examination UPDRS III History |
Horne (2015)41 Australia |
Case-control Funding: GKC |
PwP (n = 36) and healthy controls (n = 16) | UPDRS III AIMS |
Horne (2016)40 Australia |
Case-control Funding: not stated |
PwP (n = 18) and healthy controls (n = 35) | UPDRS III AIMS |
Khodakarami (2019)42 Australia |
Retrospective cohort study Funding: GKC (1 author is employed by GKC, 1 author has financial interests in GKC) |
PwP (construction set n = 112) and PwP (test set n = 60) | Clinical assessment for selecting patients for DAT referrals (including MDS-UPDRS) |
Khodakarami (2019)43 Holland, USA and Australia |
Case-control Funding: none (1 author is employed by GKC, 1 author has financial interests in GKC) |
PwP (n = 199), people without Parkinson’s disease (n = 174) and PwP who underwent DBS (n = 24) | LDCT as part of routine clinical care (including UPDRS III) |
McGregor (2018)44 Australia |
Case-control Funding: GKC |
PwP (n = 72), healthy controls (n = 155) and healthy people undergoing polysomnogram (n = 46) | PDSS-2 in PwP PSG in non-PD controls (n = 46) |
Watts (2021)45 USA |
Cohort Funding: partially supported by the Science Alliance, The University of Tennessee, The Parkinson’s Alliance and by the Laboratory Directed Research and Development Program of Oak Ridge National Laboratory managed by UT-Battelle, LLC, for the US Department of Energy (1 author is employed by GKC) |
PwP (n = 26) | MDS-UPDRS III |
Abstracts | |||
Horne (2017)46 Germany and France |
Cohort Funding: not stated |
PwP (n = 36) under consideration for DBS | Clinical assessment |
STAT-ON (8 full papers, 1 abstract)
Study details | Study design and funding source | Population (incl. n) | Reference standard | Additional results |
---|---|---|---|---|
Full papers | ||||
Bayes (2018)18 Spain, Italy, Israel and Ireland |
Prospective pilot cohort study Funding: European Community |
PwP (n = 41) | Patient diaries and clinical assessment | |
Perez-Lopez (2016) (AIM)22 Spain, Ireland, Italy and Israel |
Prospective cohort study Funding: European Community |
PwP (n = 92) and historical PwP patients (n = 10) | Clinical assessment (video-recording used for labelling the signal by a trained expert) | |
Perez-Lopez (2016) (Sensors)21 Spain |
Prospective cohort study Funding: Instituto de Salud Carlos III-Ministerio de Economia y Competividad and the European Regional Development Fund (6 authors are shareholders of Sense4Care) |
PwP (n = 15) and historical PwP patients (n = 20) | Clinical assessment using UPDRS Patient diaries |
|
Rodriguez-Martin (2017)24 Spain, Ireland, Italy and Israel |
Prospective cohort study Funding: La Fundacio La Marato de TV3 20140431 and European Community (6 authors are shareholders of Sense4Care) |
PwP (n = 21) Subgroup of patients from Perez-Lopez (2016) (AIM)22 who had Freezing of Gait score above 6 |
Clinical assessment (video-recording used for labelling the signal by an experienced clinician) | |
Rodriguez-Molinero (2015)26 Spain |
Prospective cohort study Funding: Instituto de Salud Carlos III – Ministerio de Economia y Competitividad and the European Regional Development Fund |
PwP (n = 20) and PwP (n = 15) | Clinical assessment (video-recording used for labelling the signal by experienced clinicians) and patient report | |
Rodriguez-Molinero (2018)28 Spain |
Prospective cohort study Funding: Instituto de Salud Carlos III (4 authors are shareholders of Sense4Care) |
PwP – advanced (n = 23) | Patient diaries | |
Sama (2017)30 Spain |
Prospective cohort study Funding: Instituto de Salud Carlos III-Ministerio de Economia y Competividad and the European Regional Development Fund (6 authors are shareholders of Sense4Care) |
PwP (n = 12) | Clinical assessment (video-recording used for labelling the signal by a trained expert) UPDRS |
|
Sama (2018)31 Spain |
Prospective cohort study Funding: La Fundacio La Marato de TV3 436/C/2014 (3 authors are shareholders of Sense4Care) |
PwP (n = 15) | Clinical assessment (video-recording used for labelling the signal by a trained expert) | |
Abstracts | ||||
Bougea (2021)19 Location: not stated |
Prospective cohort study Funding: not stated |
PwP with motor fluctuations and dyskinesia, treated with LCIG (n = 51) | Patient diary completed once every hour | STAT-ON demonstrated 93.4% sensitivity and 89% specificity in detecting off-state, 94% sensitivity and 87% specificity in dyskinesia and 94.2% sensitivity and 87.1% specificity in falls compared with patient-completed diaries (off: 71.6% and 80.3%, dyskinesia: 78.2% and 81.4%, falls: 78.2% and 81.4%). The overall classification accuracy was 92.2%. No device-related adverse events were reported. |
Kinesia 360 (1 full paper, 0 abstracts)
Study details | Study design and funding source | Population (incl. n) | Reference standard |
---|---|---|---|
Full papers | |||
Pulliam (2018)35 USA |
Prospective cohort study Funding: National Institutes of Health (4 authors are employees of Great Lakes Neuro-Technologies) |
PwP (n = 13) | Clinical assessment (clinician ratings based on video-recordings) UPDRS III |
KinesiaU (0 papers/abstracts)
PDMonitor (0 papers/abstracts)
Association outcomes
PKG (11 full papers, 10 abstracts)
Study details | Study design and funding source | Population | Intervention | Comparator(s) | Results |
---|---|---|---|---|---|
Full papers | |||||
Chen (2020)47 China |
Prospective cohort study Funding: Joint Funds for the innovation of Science and Technology, Fujian province and the Central Government Directs Special Funds for Local Science and Technology Development |
PwP (n = 100) | PKG for ≥ 6 days | UPDRS III WOQ-9 (n = 75 PwP receiving stable levodopa treatment 125 mg t.i.d.) |
The PKG BKS was moderately correlated with UPDRS III scores: UPDRS III total score (r = 0.546, p < 0.0001), UPDRS III-B (r = 0.588, p < 0.001) and UPDRS III-R (r = 0.479, p < 0.001), especially in the early stage (H&Y stage 1–2) group (r = 0.682, p < 0.001). The PKG PTT scores and UPDRS tremor scores were also significantly correlated: UPDRS III-T (r = 0.434, p < 0.05) and UPDRS II-T (r = 0.269, p < 0.05). However, the WOQ-9 had a very weak, non-significant correlation with the PKG DKS and FDS; p > 0.05. |
Evans (2014)48 Australia |
Prospective cohort study Funding: Medical Research Council Funds (4 authors have a financial interest in GKC) |
PwP (n = 25) | PKG worn for 10 days (acknowledgement of medication consumption) | A blinded examiner administered the Starkstein AS and the QUIP Patients also completed the BDI, BIS/BAS and the STAI |
A response ratio, representing the number of acknowledgements/number of doses (expressed as a percentage) was strongly correlated with ratings of impulsive-compulsive behaviours (r2 = 0.79) in 19/25 patients. However, 6 patients were clear outliers and fell into the false negative group; these patients had normal response ratios, but high impulsive-compulsive behaviour scores, as well as higher apathy scores and low levels of dyskinesia. |
Griffiths (2012)49 Australia |
Prospective cohort study Funding: Florey Neuroscience Institute, the Pfizer Neuroscience Research grant, Medical Research Council Research Funds and the Victorian Government’s Operational Infrastructure Support Program (4 authors have financial interest in Global Kinetics, Global Kinetics provided the PKG devices) |
PwP (n = 34) and age-matched controls (n = 10) | PKG worn for up to 10 days | Conventional clinical rating methods: rapid alternating movements, AIMS and UPDRS III and IV | There was a significant correlation between modified AIMS (assessed by three movement disorder specialists) and PKG DKS (r = 0.80, p < 0.0001). The margin of error in predicting the AIMS from DKS was 3.2 AIMS units compared with −3.4 to +4.3 AIMS units by the neurologists (with a maximum score of 20). The PKG ‘global median DKS’ was obtained from 25 patients with established bilateral PD over 10 days; there was a significant correlation between Global DKS and UPDRS IV (p < 0.05). The Global DKS predicted the UPDRS IV with a margin of error of 3.9 over a possible range of 0–8. PKG BKS correlated well with bradykinesia measured by the ‘dot slide’ test (r = 0.63, p < 0.001), with a specificity of 88% and sensitivity of 95%. The PKG ‘global median BKS’ was obtained from the 25 patients with established bilateral PD in the on-state; there was a significant correlation between Global BKS and UPDRS III (r = 0.64, p < 0.0005). The margin of error in predicting the UPDRS III from BKS was 18 UPDRS III units. |
Guan (2021)50 USA |
Prospective cohort study (substudy of ATLaS-Parkinson’s disease) Funding: National Institute of Neurological Disorders and Stroke and Neurodegenerative Disorders Development Trust |
PwP (n = 18) | PKG worn for 6 days | Traditional in-clinic off-on testing using MDS-UPDRS part III | There was a moderate inverse correlation between off-on improvement and BKS at baseline (r = −0.552, p = 0.017) and 6-month (r = −0.547, p = 0.019) visits. There were no significant associations between off-on improvement and the remaining PKG scores (DKS: r = 0.133, p = 0.598 and PTT: r = −0.523, p = 0.1 at 6 months). There was a moderate positive linear association between bradykinesia and the ADL domain of the PDQ-39 (coefficient of 0.38). However, this did not reach statistical significance. There were no other significant associations between the PKG scores and off-on improvement to the ADL and mobility domains of the PDQ-39. |
Hoglund (2021)51 Sweden |
Prospective cohort study Funding: The Swedish Parkinson Foundation (Global Kinetics provided the PKG devices) |
PwP (n = 53) | PKG worn for 6 days (during the daytime: 09:00–18:00) | Three-day home diary of daytime sleepiness, other non-motor (mood and anxiety) and motor fluctuations | Twenty-eight patients were classified as motor fluctuators and 24 as non-fluctuators. Daytime sleepiness correlated significantly with motor symptoms, mood and anxiety amongst motor fluctuators (n = 28). Motor fluctuators showed stronger correlations between the individual mean level of all diary variables (daytime sleepiness, anxiety, mood and motor symptoms) when compared to the non-fluctuators (n = 24). Stronger positive within-individual correlations were found among fluctuators in comparison to non-fluctuators. Correlations between diary variables and PKG variables were generally weak and non-significant. |
Khodakarami (2021)52 Australia |
Retrospective cohort study [patient overlap with Farzanehfar (2018) and Woodrow (2020)] Funding: none (2 authors are employed by Global Kinetics, 1 author has financial interests in Global Kinetics) |
PwP (n = 228) and people without PD (n = 157) | PKG worn for 6 days | MDS-UPDRS III, total UPDRS and PDQ-39 | A normal range of PTB and PTD based on control subjects was developed. The level of PTB and PTD experienced by PwP was compared with their levels of fluctuation. There was a correlation (Pearson’s ρ = 0.4, p < 0.0001) between UPDRS III scores and PTB. The correlation between PDQ-39 scores and UPDRS total scores and PTB was slightly lower (Pearson’s ρ = 0.35, p < 0.0001 and Pearson’s ρ = 0.34, p < 0.0001, respectively). PTB and PTD fell in response to treatment for bradykinesia or dyskinesia (respectively) with greater sensitivity than clinical scales. |
Klingelhoefer (2016)53 UK |
Prospective cohort study Funding: not stated |
PwP (n = 63) | PKG worn for 6 days and 5 nights | Hauser diaries and scales of motor state (including UPDRS IV and AIMS), non-motor state and sleep assessments (including NMSQuest, ESS, PDSS 1 and HADS) and HRQoL (PDQ-8) | In patients with excessive daytime sleepiness (n = 30) the PKG’s parameters for quantity and quality of night-time sleep correlated significantly with the total burden of non-motor symptoms of PD as measured by NMSQuest as well as overall sleep disturbances as measured by PDSS. In ‘non-sleepy’ PwP (n = 33) there was no significant correlation. There were no significant correlations of night-time sleep quantity parameters of the Hauser diary with subjective sleep perception (NMSQuest and PDSS) in either patient group. A moderate to high correlation of quantitative and qualitative night-time sleep markers of the PKG was noted with the PDQ-8 (rs = 0.46–0.60) in the excessive daytime sleepiness group. |
Knudson (2020)54 Denmark |
Prospective cohort study Funding: Carleton College, DIS Copenhagen, and the Department of Clinical Neurophysiology and Neurology at Zealand University Hospital |
PwP (n = 34) | PKG worn for 6 days and patient questionnaire | MDS-UPDRS II | There was a significant correlation between MDS-UPDRS II and PKG bradykinesia change score (p = 0.006), PKG DKS (p = 0.007) and subjective score (p = 0.0009). |
Kotschet (2014)55 Australia |
Prospective cohort study Funding: Medical Research Council Funds and GKC |
PwP (n = 68) and healthy controls (n = 30) | PKG | Daytime PSG (7 patients) ESS |
Seven PwP whose time immobile was > 30 minutes/day (measured on a previous PKG recording) had daytime PSG performed, periods of immobility on PKG had an 85.2% concordance with the detection of sleep by ambulatory daytime PSG (p < 0.0001). High ESS was associated with PTI (p = 0.01). PwP had a higher PTI than healthy controls (p < 0.0001). PwP with a high PTI had more bradykinesia, less dyskinesia and higher PDQ-39 scores than those with low PTI. There was no relationship between PTI and dose or type of PD medications, although in 53% of patients PTI increased in the 30–60 minutes after levodopa, confirming that in some patients levodopa results in increased sleepiness. |
Ossig (2016)56 Germany |
Prospective cohort study Funding: GKC |
PwP inpatients (n = 24) | PKG data recorded between 6 a.m. and 10 p.m. | Patient diaries completed every hour for 5 consecutive days | Distribution of total motor state hours per day measured by PKG showed a moderate-to-strong correlation to those assessed by diaries for the different motor states (Pearson’s correlation coefficients: 0.404–0.658). Inter-rating method agreements on the single-hour level were only low to-moderate (κ = 0.215–0.324). |
Tan (2019)57 USA |
Prospective cohort study Funding: not stated |
PwP (n = 54). Patients were split into different fluctuator groups [non-fluctuators (n = 14), early fluctuators (n = 15), moderate fluctuators (n = 15) and troublesome fluctuators (n = 10)] according to WOQ-9 and MDS-UPDRS IV scores | PKG fluctuator scores (worn for 6 days) | Patient 2-day motor symptom diary (39 patients returned valid 2-day motor diaries) | PKG fluctuator scores significantly differentiated early fluctuators and troublesome fluctuators, as well as dyskinetic and non-dyskinetic subjects, but could not discriminate subtler motor fluctuations. Patient diaries could not distinguish the four study groups on the basis of average off-time. The diaries distinguished non-fluctuators from moderate fluctuators for average dyskinesia time. |
Abstracts | |||||
Bergquist (2016)59 Sweden |
Retrospective cohort study Funding: not stated |
PwP (n = 258) | PKG | Visual assessment by a trained specialist | The agreement between visual assessment and assessments based on median PKG scores was low (ĸ = 0.11). In particular, the PKG FDS identified fewer off-fluctuators than visual assessment by a trained specialist as 25% of the population had significantly increased FDS, but visual assessment identified off-fluctuations in an additional 45% of the population. Patients tolerated the PKG well and only 8% had problems handling the reminder function or wearing the device. |
Bergquist (2018)58 Sweden |
Prospective cohort study (WestPORTS study) Funding: not stated |
PwP (n = 154) and historical PwP (n = 248) | PKG in a randomly selected population of PwP | Clinically motivated PKG recordings in a historical cohort of PwP suspected to have motor fluctuations | PKG BKS and DKS were significantly different between a randomly selected population of PwP and a historical PwP population with clinically motivated PKG recordings; median BKS 30.4 and 23.0 (p = 0.014), median DKS 1.0 and 3.0 (p < 0.0001), respectively. |
Bogdanova-Mihaylova (2016)60 Location: not stated |
Prospective cohort study Funding: not stated |
PwP (n = 20 with advanced disease) | PKG for 6 days | Patient diaries, patient derived dyskinesia severity data (UPDRS IV) and patient perception of motor disability (ranging from 1 to 5) | By history, the mean total daily duration of the off-time was 3 hours. There was no significant correlation between PKG derived BKS with subjectively reported off-time (r = 0.29, p = 0.25) and UPDRS IV part B fluctuations (r = 0.25, p = 0.28). Of the patients, 47% who completed the diary (n = 17) reported troublesome dyskinesia with a mean duration of 17.85% of the waking day. Correlation between PKG derived DKS and UPDRS IV part A dyskinesia was approaching significance (r = 0.38, p = 0.09). A statistically significant association was found between patients’ perception of disability due to motor fluctuations and FS derived from variations in DKS and BKS (r = 0.52, p = 0.018). |
Dahlen (2014)61 Location: not stated |
Prospective cohort study Funding: not stated |
PwP (n = 15 with suspected motor fluctuations) | PKG worn for 10 days | Hauser-style patient diaries for 3 days | A positive linear correlation (slope 0.73 ± 0.33) was found for sleep time (r2 = 50.275, p = 0.0447), but not for time spent in off, on or bad on. Seven patients reported on-time that correlated with PKG recordings, two patients reported off-time that correlated, and two reported bad on-time correlating with high DK. |
Dominey (2018)62 Location: not stated |
Prospective or retrospective cohort study Funding: not stated |
PwP (n = 62 newly diagnosed patients) | PKG | NMSQuest | Patients were allocated to one of 5 phenotypic subgroups based on PKG data and NMSQuest data. Of the patients, 19% met full criteria for inclusion in 1 of the 5 subgroups. Of the remaining patients, 51% failed to be allocated to a subgroup due to missing one of the required criteria for inclusion. |
Fowler (2017)63 Location: not stated |
Prospective cohort study Funding: not stated |
PwP (n = 26 patients being evaluated for advanced therapy) | PKG worn for 6 days | Clinical assessment by a movement disorder specialist | All patients met clinical criteria for advanced therapy. 16/26 patients (61.54%) met criteria by PKG for additional therapy. Of the 10 patients who did not meet the criteria, the BKS score ranged from 18.6 to 25.9, the DKS score ranged from 0.6 to 7.3 and the FDS score ranged from 7.3 to 11.5. |
Horne (2016)64 Location: not stated |
Prospective cohort study Funding: not stated |
PwP (n = 45), additional PwP (n = 35) and age-matched non-PD controls (n = 24) | PKG worn for 6 nights | PSG for sleep assessment and PDSS-2 questionnaire (n = 35 PwP) | The PKG score combining the percentage time asleep and the median time immobile predicted normal and abnormal sleep (according to the polysomnography) with 100% selectivity and sensitivity. 2/24 non-PD controls had abnormal sleep according to the PKG, 1 of which gave a history of restless legs. Amongst PwP, 28% had normal sleep according to the PKG criteria. In those interviewed, PKG values had a good correlation with the PDSS-2 scale (r = 250.49). |
Horne (2016)65 Location: not stated |
Retrospective cohort study Funding: not stated |
PwP (n = 200) | PKG worn for 6 days | Clinical assessment for the presence and nature of tremor | A percentage time that tremor was present (between 09:00 and 18:00) ≥ 0.8 provided a high sensitivity (92.5%) and selectivity (92.9%) in identifying tremor. False negatives were mainly low-amplitude kinetic or postural tremor, which were frequently not apparent to the patient or tremors that did not affect the upper limb. A percentage time that tremor was present ≥ 1 indicated a high likelihood of the presence of clinically meaningful tremor and a ‘grey zone’ was identified between 0.6 and 1.0. Tremor did not produce false increase in DKS in this sample of 200. |
Lina (2020)66 Location: not stated |
Prospective or retrospective cohort study Funding: not stated |
PwP (n = 100) | PKG worn for ≥ 6 days | UPDRS III | There was significant correlation between PKG BKS and UPDRS III, including UPDRS III total scores, UPDRS III-BKSs and UPDRS III-rigidity scores (Pearson’s correlation coefficients: 0.479–0.588, p < 0.05). There was also significant correlation between PKG PTT score and UPDRS III-tremor scores and UPDRS II-tremor scores (r = 0.223, r = 0.343, p < 0.05). Subgroup analysis showed that early stage (H&Y stage 1–2) or early disease course (< 3 years) PKG BKS and UPDRS III scores were better correlated (r2 = 0.465, r2 = 0.441, p < 0.05). |
Margolesky (2017)67 Location: not stated |
Prospective or retrospective cohort study Funding: not stated |
PwP on continuous enteral CD/LD infusion therapy (n = 2) | PKG worn for 6 days prior to initiation of CD/LD therapy and after a 3-month titration period of enteral CL/LD therapy | Patient report | Patients’ subjective results were not fully reflected in the objective PKG results. Patient 1 noted mild dyskinesia and the PKG noted an increase in dyskinesia. Patient 2 noted moderate dyskinesia and the PKG device noted no change in dyskinesia but an increase in per cent time with tremor. |
STAT-ON (3 full papers, 1 abstract)
Study details | Study design and funding source | Population (incl. N) | Intervention | Reference standard/comparator(s) | Brief results |
---|---|---|---|---|---|
Full papers | |||||
Perrote (2021)23 Argentina |
Retrospective cohort study Funding: none |
PwP (n = 11) | STAT-ON (not stated in paper, but in Sense4Care submission) | Self-administered movement diary | The mean hours monitored was greater by the Holter than recorded by the movement diary (60 ± 9.89 vs. 40 ± 16.4, p < 0.001). The report of freezing of gait episodes and hours in the on-state were higher with the Holter compared to the movement diary. |
Rodriguez-Molinero (2017)27 Spain, Italy, Israel and Ireland |
Prospective cohort study Funding: The European Commission and partially supported by the Instituto de Salud Carlos III-Ministerio de Economia y Competividad and the European Regional Development Fund (5 authors are shareholders of Sense4Care) |
PwP (n = 75) asked to walk both in the off- and the on-state in the patient’s home | STAT-ON (not stated in paper, but on Sense4Care website) | Motor section of the UPDRS III administered in both motor phases | Correlation between UPDRS III and algorithm outputs was moderate (rho −0.56, p < 0.001). Correlation between the algorithm outputs and the gait item in the UPDRS III was good (rho −0.73, p < 0.001). The factorial analysis of the UPDRS III has repeatedly shown that several of its items can be clustered under the so-called Factor 1: ‘axial function, balance, and gait.’ The correlation between the algorithm outputs and this factor of the UPDRS III was −0.67 (p < 0.01). |
Rodriguez-Molinero (2019)29 Spain, Italy, Israel and Ireland |
Prospective cohort study Funding: Instituto de Salud Carlos III-Ministerio de Economia y Competividad and the European Regional Development Fund (5 authors are shareholders of Sense4Care) |
PwP (n = 13) performing normal daily life activities (for an average period of 30 minutes) at home Possible patient overlap with other REMPARK studies |
STAT-ON (not stated in paper, but in Sense4Care submission) | Clinical assessment (video-recording used for labelling the signal by a trained expert) determining the severity of dyskinesia through the UDysRS | The correlation coefficient between the sensor output and UDysRS result was 0.70 (95% CI 0.33 to 0.88, p = 0.01). Since the sensor was located on the waist, the correlation between the sensor output and the results of the trunk and legs scale subitems was calculated: 0.91 (95% CI 0.76 to 0.97, p < 0.001). |
Abstracts | |||||
Caballol (2020)20 Spain |
Prospective cohort study Funding: not stated |
PwP (n = 39) Dates: not stated |
STAT-ON worn for 1 week (device not stated in abstract, but abstract in Sense4Care submission) | Patient report | Of the patients, 74% reported having motor fluctuations, 31% freezing of gait and 54% dyskinesia. According to the information provided by the sensor, 100% of patients had motor fluctuations, 61% freezing of gait and 79% dyskinesia. The proportion of patients reporting motor fluctuations increased to 79% after returning the device. According to PD specialist experience, all patients who still reported not having motor fluctuations actually presented with them, when analysing clinical symptoms and data provided by the sensor. |
Kinesia 360 (0 full papers, 0 abstracts)
KinesiaU (0 full papers, 0 abstracts)
PDMonitor (0 full papers, 1 abstract)
Study details | Study design and funding source | Population (incl. n) | Intervention | Reference standard/comparator(s) | Brief results |
---|---|---|---|---|---|
Abstracts | |||||
Kostikis (2020)37 Greece and Italy |
Prospective cohort study Funding: not stated |
PwP (n = 30) | PDMonitor in the clinic for 3 hours | Clinical assessment by a PD expert physician every half hour using UPDRS III and AIMS | The device accurately detected and estimated the severity of arm bradykinesia (r2 = 0.46 with UPDRS items 23, 24, 25), dyskinesia (accuracy 90% compared to AIMS score), gait impairment (r2 = 0.6 with UPDRS item 29), wrist tremor (accuracy 89% and r2 = 0.67 with UPDRS item 20), leg tremor (accuracy 93%) and freezing of gait (accuracy 93% compared to UPDRS item 14). A 900-hour wearability study was also conducted with PwP (n = 10) and healthy volunteers (n = 19) who wore PDMonitor at home. Users found it relatively easy to wear the device. |
Intermediate impact
PKG (8 full papers, 17 abstracts)
Study details | Study design and funding source | Population | Intervention | Comparator(s) | Results |
---|---|---|---|---|---|
Full papers | |||||
Dominey (2020)68 [some data extracted from Dominey (2018) abstract177 and Carroll (2019) letter172 have overlapping patients with this study] UK |
Retrospective cohort study Funding: not stated (PKG recordings were provided by GKC or funded by a licence arrangement with GKC) |
PwP (n = 166; 78 new patients and 88 follow-up) Dates: July 2015–January 2018 Reported in Dominey (2018b) abstract:177 PwP (n = 216; 104 new patients, 5 complex care and 107 follow-up). Dates: July 2015–January 2018 Reported in Carroll (2019) letter:172 PwP (n = 209). Dates: July 2015–July 2017 |
PKG worn for 6 days | – | Treatment recommendations were made by reporters for 152/166 patients (92%) with the most common changes relating to dopamine replacement and advice on sleep hygiene and bowel management, for example upon detection of dose failure. Final treatment outcomes obtained retrospectively from follow-up letters were available for 133/166 reports (80%); treatment recommendations were implemented for 83/114 patients (73%), with advanced therapy in 6, additional motor agent in 34 and additional non-motor agent in 16. Reported in Dominey177 (abstract): the most frequent purpose of the PKG was to investigate medication response (55%). There were remotely implemented treatment changes made in 93% of cases. |
Costs calculated by the NHS business advisory service: implementation of the PKG in routine PD care has the potential to reduce routine consultant follow-up appointments by 50%, leading to an estimated saving of £104,000 per 250 patients. Reported in Carroll172 (letter): information from the PKG confirmed initial judgement in 54.5% cases and provided additional information in 45.5% cases. |
|||||
Evans (2020)69 [some data extracted from Evans (2019) abstract,180 which has overlapping patients with this study] UK |
Pilot cohort study (PKG recordings taken prior to enrolment) Funding: Bevan Commission |
PwP (n = 61 who had a PKG within the last 2 months and face-to-face appointment due in 1–2 months) Dates: From September 2018 |
PKG (used in a virtual clinic appointment) | Face-to-face clinic appointment | A consultation was deemed successful if the clinician felt that the outcome of the consultation was likely to have been the same as a face-to-face clinic. This could include a decision to change medications, a referral to another multidisciplinary team member or a decision to follow-up only. If the face-to-face clinic appointment was required within 8 weeks after the virtual clinic, it was considered unsuccessful. By this definition 48/61 appointments (79%) were successful. Reasons that the consultation was unsuccessful included complex phase of disease (n = 5), problems with the PKG (n = 5), needing a blood pressure reading (n = 2) and speech problems (n = 1). |
Face-to-face clinic template has a combination of 40-minute new patient and 20-minute follow-up slots. Virtual clinic appointments had an average phone consultation length of 12 minutes, but an administration time (PKG to be reviewed and interpreted prior to the call, pro-forma filled in and letter typed) of 10 minutes per patient; total clinic time of 22 minutes, compared with a regular follow-up slot of 20 minutes (not including dictation and typing of the clinic letter). Colleague consensus is that face-to-face appointments often run late, longer than the 20 minute slot allocated and the extra administration time can vary from 30 minutes to 2 hours. Of the 61 consultations, 35 of the previously planned face-to-face contacts could be postponed a median time of 6 months. A face-to-face follow-up appointment in movement disorder clinic costs £116 including the premises, support staff and clinician time. Currently the cost of each PKG is £225, including the postage of the data logger to the patient, postage back to GKC and the PKG report made available via the online portal. Even without accounting for the cost of a clinician’s session, this makes virtual clinics using PKG appear more expensive than a normal clinic. However, this does not take into account the value-added features mentioned above, reduced use of ambulance transport and new patient slots created to reduce waiting lists. | |||||
Farzanehfar (2018)70 Australia |
Prospective cohort study Funding: GKC |
PwP (n = 103) Dates: not stated |
PKG worn for 6–7 days | Clinical assessment by neurologist | After reviewing the patient in conjunction with the PKG, the study neurologist agreed with the pre-reporting of the PKG in 93/103 cases (90%). In 63 (61%) of these cases the PKG added to the clinical findings to an extent that the therapeutic decision was influenced. There was artefactual elevation of the BKS or DKS plot in 10 cases, mostly due to exercise (3 cases) and increased somnolence (5 cases). In 9 cases, these artefacts were noted and reported and thus would not have affected therapeutic decisions. If the PKG had not been acted on without consideration of the interpretation, then it may have led to the changing of a dose at the time that sleep or exercise occurred. A low frequency tremor caused artefactual elevation of the DKS in one case but this was noted by the PKG reporter. The PKG did fail to detect truncal dyskinesia in one case but this did not alter the therapeutic decision. |
At the beginning of the study, 23/103 PwP had controlled motor function, according to the neurologist’s clinical judgement (based on history, examination and inspection of the PKG), and 80 PwP had uncontrolled motor function. Adjustment of oral therapy was attempted in 40 of these 80 PwP (uncontrolled), 9 were immediately referred for advanced therapy, no attempt was made in 5 cases because of risk of contraindications and 26 did not complete the study (protocol violations). |
|||||
Joshi (2019)71 USA |
Prospective cohort study Funding: GKC |
PwP (n = 63; 85 routine care visits) Dates: not stated |
PKG worn for 6 days | Clinical assessment (discussion of PD symptoms with patient and a motor examination) | In 48% of patients, the PKG reported a symptom not reported by the patient. Of the patients, 24% reported a symptom that did not appear in the PKG report. Data from the PKG were used to make changes in treatment plans in 50 patients (79%). The most common treatment changes included the addition of at least one medication or changed dosage and timing of medications. |
Krause (2021)72 USA |
Retrospective cohort study Funding: none (1 author serves as a consultant for Global Kinetic Corporation) |
PwP (n = 104; 170 PKG reports) Dates: 1 December 2016–30 October 2018 |
PKG worn for 7 days | Clinical assessment by a movement disorder specialist | PKG complemented patient input in 141/170 PKG reports (82.9%) and led to medication changes in 100/141 (71%) of the complimented inputs. Of these medication changes, 79 led to increase in medications and 23 led to the introduction of a new drug; 7 of which were amantadine immediate or extended release for dyskinesia. Six PKG encounters led to a decrease in medication; 4 because of levodopa-induced dyskinesia and 2 for lack of response. Some encounters led to more than one medication change (hence 108 medication changes resulting from 100 encounters resulting in medication change). |
Nahab (2019)73 USA |
Prospective cohort study Funding: not stated |
PwP (n = 28) clinically stable patients using levodopa who attended both routine visits Dates: 2 June 2016–16 March 2017 |
PKG worn for 6 days | Routine clinical assessment by a movement disorder specialist (including symptom review, medication review and routine clinical exams), undertaken twice, with UPDRS data reviewed by the movement disorder specialist after the second visit | PKG revealed a higher degree of symptom severity than was noted by clinical history alone in 18 patients (64%) at visit 1 and 8 patients (29%) at visit 2, resulting in clinical management plan changes. Medication changes included adding a new medication (6 instances), stopping a medication (2), increasing (14) or decreasing (1) medication dose or adjusting dose timing (5). Of the patients, 64% had an increase in levodopa dose and 11% had a dose reduction. |
Santiago (2019)74 USA |
Prospective physician survey Funding: GKC provided PKG system product for use in this research project, research service support and funds for manuscript submission |
Physicians of PwP (n = 4 movement disorder specialists who ordered 143 PKGs on 89 patients; 112 completed surveys on 81 patients were included in the analysis) Dates: December 2015–July 2016 |
PKG worn for 6 days | Clinical consultation alone (routine clinic visit before reviewing PKG data) | Physicians targeted PKG use in patient populations they believed continuous objective measurement would improve the value of clinical encounters. Patients generally fell into 1 of 4 categories: first patient visit in clinic, PwP disease symptom fluctuations, patients with indeterminate history, and patients considering or using DBS or Duopa. Of 112 completed physician surveys, 46 (41%) indicated the PKG provided relevant additional information sufficient to consider adjusting their therapeutic management plan; 66 (59%) indicated the PKG provided no further information to support a therapeutic decision differing from that made during a routine clinic visit. |
Upon further review of these 46 surveys, 36 surveys (78%) stated that the information provided by the PKG ultimately resulted in an alteration in patient care, whereas 10 surveys stated the PKG provided additional information but that no alteration in patient care occurred based on this information. Overall, 36/112 patients (32%) had an alteration to patient care as a result of PKG. The PKG most commonly yielded new information on daily off-time [50% (18/36)]. | |||||
Sundgren (2021)75 Sweden |
Prospective cohort study Funding: GKC |
PwP (n = 66) Dates: March 2018–February 2020 |
PKG worn for 6 days | Clinical assessment (before reviewing PKG data) | After clinical assessment, a treatment change was recommended for 52/66 PwP; for the remaining 14 patients the current treatment was planned to be left unchanged. After PKG review, the treatment plan proposed after the clinical assessment was changed in 21/66 PwP (31.8%). The clinical assessment and the PKG review differed frequently (defined as non-identical choices among the predefined options), mainly regarding overall presence of motor problems (67%), characteristics of bradykinesia/wearing off (79%), dyskinesia (35%) and sleep (55%). Almost all patients reported good compliance and no tendency to impulse control disorder. For these items there were few disagreements between the clinical and PKG assessments (3% for impulse control disorder and 5% for compliance). |
Abstracts | |||||
Andriola (2017)76 USA |
Prospective cohort study Funding: not stated |
PwP (n = 49) Dates: May 2016–March 2017 |
PKG worn for 6 days | Clinical assessment conducted during a routine PD follow-up visit | Three patients were excluded from the analysis because the recording was not complete. Reason for PKG: confirm need for DBS (n = 11), optimisation of DBS (n = 12) and other routine therapy assessment (n = 23). PKG confirmed the need for DBS in 10/11 patients (91%). 8/12 post-DBS patients (67%) had clinical management adjustments post-PKG. 14/23 non-DBS patients (61%) subsequently had clinical management plan changes post-PKG and 3 (13%) were identified as DBS candidates. Overall, clinical management changes were made in 34/46 patients (74%) after PKG. |
Bergquist (2019)77 Sweden |
RCT (substudy of the WestPORTS study) Funding: not stated |
PwP [n = 121 (stated in abstract, however, 61 + 59 = 120)] Dates: not stated |
Managing physician provided with PKG report (n = 61) | Managing physician received results of patients’ self-rating using PDQ-8 and NMSQ self-rating (n = 59) | Patients were re-evaluated with PKG and self-ratings 3 months after the visit, changes in medication between the visit and follow-up were identified based on medical records and reported use. Over 2/3 patients could be classified as ‘uncontrolled’ based on the PKG. There was no difference in the frequency or type of management change between the 2 groups. |
Chhabria (2018)78 Location: not stated |
Retrospective cohort study Funding: not stated |
PwP (n = 50) Dates: not stated |
PKG | – | Reason for prescribing the PKG watch was most commonly difficulty distinguishing timing of tremor and dyskinesia, unclear off-periods, unclear response to doses of levodopa, and periods of somnolence. Over 50% of patients that wore the PKG watch had changes made to their medications. Compliance with individual doses of medications seemed improved. |
Duja (2021)79 Location: not stated |
Retrospective cohort study Funding: not stated |
PwP (n = 50), described as advanced disease Dates: not stated but it was ‘the last 4 years and during pandemic…’ |
PKG worn for 7 days | – | As a result of PKG 31.11% of patients had their levodopa increased, 22.22% had their medication timing adjusted, 4.44% had COMT inhibitor added, another 4.44% had MAO-B inhibitor added and 2.22% had dopamine agonist increased, 4.44% had their apomorphine dose adjusted and 17.78% were referred for advance treatment (half for apomorphine, rest for Duodopa and DBS), 22.22% had more than one adjustment. Patient satisfaction was high. |
Duja (2021)80 Location: not stated |
Retrospective cohort study Funding: not stated |
PwP (83 patients had PKG; currently data are available for 48 patients) Dates: not stated |
PKG worn for 7 days | – | Changes in medical management were made for 41 patients (85.42%). Eighteen (43.9%) had their medication increased, 16 (39%) had medication changed to a different group of medication, 4 (9.7%) were referred for advanced treatment and 3 (7.3%) had their treatment reduced in view of failure to respond and an alternate diagnosis was made. No changes were made in the management of 7 patients (14.58%). |
Evans (2019)81 UK |
Retrospective cohort study Funding: not stated |
PwP (n = 28) Dates: not stated |
PKG (used in a virtual clinic appointment) | – | Of the appointments, 82% were successful where a clinical decision could be made. This could be a medication change (n = 13), or no action required (n = 10). The reasons that clinical decisions could not be made included needing a blood pressure reading (n = 2) and complex stage of disease (n = 2). |
Farzanehfar (2017)82 Location: not stated |
Prospective feasibility study Funding: not stated |
PwP (n = 28 where patient and doctor perceived that PD symptoms were controlled) Dates: not stated |
PKG | Clinical assessment | There were 4/28 patients identified as optimally controlled by both the clinical history and PKG. Of the patients, 24 were uncontrolled (3 with dyskinesia and 21 with bradykinesia), 16 were identified as uncontrolled by both the clinical history and PKG, 8 were identified as uncontrolled only via the PKG. |
Horne (2016)83 Location: not stated |
Interim findings of a prospective cohort study Funding: not stated |
PwP (n = 19 considered to be well controlled by general neurologists) Dates: not stated |
PKG | Assessment by a movement disorder specialist | Three patients were considered well controlled and 16 patients were considered poorly controlled and consequently treatment was changed. The movement disorder specialist would have not recognised 6 patients as poorly controlled without the PKG and in 10 patients the movement disorder specialist recognised the same symptoms as the PKG. There were no examples in which the PKG failed to recognise treatment symptoms. The 15/16 poorly controlled patients (94%) were treated for bradykinesia or fluctuations (wearing-off). |
Horne (2018)84 Australia |
Pilot prospective cohort study Funding: not stated |
PwP (n = 103) Dates: not stated |
PKG | Clinical assessment | The PKG motor scores of 78% of participants were outside the target for optimum control and changes in oral therapy were recommended in 74%, advanced therapy in 12% and treatment was contraindicated in 9%. At the end of the study, 48% were in-target (22% at outset and a further 26% by treatment change and not including those referred for advanced therapy – 19%). Advanced therapy had not previously been discussed in these patients. Contraindications prevented therapy change in 17%. |
Jones (2018)85 Location: not stated |
Retrospective cohort study Funding: not stated |
PwP (n = 70) with either self-reported severe or worsening symptomatology or an uncertain response to a treatment change and a clinical query over the next best management course Dates: December 2015–February 2017 |
PKG | Clinical assessment | Increasing symptoms and wearing-off were the most common reasons for undertaking PKG. PKG was consistent with clinical impression in 53 patients (76%). It gave additional clinically relevant information (unidentified bradykinesia or dyskinesia) in 18 patients (25%). Clinical decision changed in 24 patients (34%) based on the results of PKG. Four patients were (6%) clinically considered to require an advanced treatment had current medication titrated instead. Five patients (7%) in whom advanced treatments were not being considered pre-PKG were deemed to require them and were subsequently referred. In 2 patients the PKG demonstrated a poor response to medication, which led to revision of the diagnosis. |
Klingelhoefer (2016)86 Location: not stated |
Prospective cohort study Funding: not stated |
PwP (n = 82) Dates: not stated |
PKG worn for 6 days | Routinely completed self-ratings (Hauser diary and other measures) | Of the patients, 68.3% were compliant with PKG and Hauser diary assessment, 15.9% were not compliant concerning both PKG and Hauser diary, 11% patients were compliant with PKG but not Hauser diary assessment, whereas 4.9% were compliant concerning Hauser diary but not PKG assessment. |
Langston (2017)87 USA |
Prospective or retrospective cohort study Funding: not stated |
PwP (n = 89; 123 movement disorder surveys completed, 44 patients had multiple PKGs) Dates: not stated |
PKG worn for 6 days | Clinical assessment by a movement disorder specialist | Physicians reported that the PKG provided additional information not available from clinical consultation alone in 38% of visits overall and in 53% of visits for patients noted as having fluctuations and/or unclear histories. Overall clinical management changes were made in 58% of visits. Physicians were more likely to make a change when the PKG provided additional information (78%) compared to only 44% of cases when PKG did not provide additional information. |
Lynch (2018)88 Australia |
Prospective or retrospective cohort study Funding: not stated |
PwP (n = 80 uncontrolled patients) Dates: not stated |
PKG | – | Of the 80 uncontrolled patients, 33 were treated with oral therapy, with the assistance of PKG. |
Rao (2019)89 UK |
Retrospective cohort study Funding: not stated |
PwP (n = 37; 45 PKG reports) Dates: February 2016–May 2018 |
PKG | Clinical assessment | There were multiple indications for PKG for most patients, including dose failure (14 patients), off-periods and wearing off (11 patients), possible off dystonia or dyskinesia (5 patients), freezing, falls and relationship with medication (6 patients), bradykinesia and pain (7 patients) and to quantify dyskinesia (3 patients). On clinical grounds, it was felt that 10/36 patients were likely to need complex treatment before PKG (one was already on apomorphine – APO-go pen). After PKG, 4 patients started complex treatment (APO-go pen), 1 is being assessed for DBS and 5 did not need complex treatment but changed their medication with increase in dosage of levodopa in 4 patients and a reduction in dosage in 1 patient. The authors envisage that 2 of these 5 patients are likely to need a form of complex treatment in the near future. |
Cost of postponing advanced treatment for 5 patients: APO-go pump (average cost of £5400/pump/year) led to a saving of £27,000/year. Postponement of apomorphine (APO-go) pen treatment (average cost of £3200/year) led to a saving of approx. £16,000/year. Thirty-five patients changed their PD medication after PKG. Furthermore, 13 were found to have mild to significant dyskinesia with 6 needing a reduction in drug doses, 26 patients were under-treated, mostly with off-periods, with 23 needing an increase in drug dosage. |
|||||
Thakur (2017)90 USA |
Retrospective cohort study Funding: not stated |
PwP (n = 51) Dates: November 2015–November 2016 |
PKG worn for 6 days | Clinical assessment during routine PD follow-up by movement disorder specialists | Two patients did not tolerate the PKG due to wristband irritation and 5 patients were lost to follow-up or their appointment was delayed. Physicians reported that the PKG provided information not available from the clinical consultation that drove a clinical management plan change in 21/44 patients (47%). Common clinical management changes were increase or reduction in medication dose and/or frequency in 19/21 patients (91%). The PKG provided supporting evidence in 2 patients for recommending advanced therapies with DBS. |
Thomas (2019)91 UK |
Retrospective cohort study Funding: not stated |
PwP (n = 256 evaluations) Dates: not stated |
PKG | Clinical assessment | The 209 completed evaluations provided information about the impact of the PKG test on clinical decision-making. The most frequent reasons for performing a PKG were ‘increased symptoms’ and ‘wearing-off’. Information from the PKG confirmed initial clinical judgement in 54.5% of cases and provided additional information to inform the clinical decision in 45.5% cases. Changes in decision-making included 10 patients where the PKG results prompted a treatment change when clinicians initially predicted no changes were necessary, and 15 patients who went on to receive advanced therapies where oral medication titration had initially been considered. Conversely, information from the PKG prompted clinicians to try other options in 18 patients originally considering advanced therapies. |
Wilson (2017)92 Location: not stated |
Prospective cohort study Funding: not stated |
PwP (n = 10 where follow-up data available) Dates: not stated |
PKG worn for 7 days | Patient 7 day symptom diary | In 2 patients with early-stage disease, bradykinesia was over-reported by PKG; on clinical assessment and with patient diaries there was no reported bradykinesia. One patient who felt under-treated was identified as having significant periods of dyskinesia, and the PKG report was used to explain that he was in fact over-treated. One patient who had reported freezing episodes was found to have significant episodes of bradykinesia; PKG helped guide apomorphine use. |
In 3 patients with advanced disease, PKG showed significant bradykinesia; 1 patient had previously declined increase in medication dose and subsequently was persuaded to comply, following PKG study. One of these patients had recurrent erroneous activation of the medication sensor, which may reflect impulse control disorder. One patient with cognitive impairment failed to report his bradykinesia, which was detected by PKG. |
STAT-ON (0 full papers, 0 abstracts)
Kinesia 360 (1 full paper, 0 abstracts)
Study details | Study design and funding source | Population | Intervention | Comparator(s) | Results |
---|---|---|---|---|---|
Full papers | |||||
Isaacson (2019)33 USA |
Pilot RCT Funding: UCB Pharma |
PwP with insufficiently controlled motor symptoms, prescribed transdermal rotigotine (n = 39) Dates: March 2017–January 2018 |
Kinesia 360 data used to supplement standard care in adjusting rotigotine dosage (n = 19). Kinesia 360 was used throughout the day on at least two consecutive days in weeks 1, 2, 3, 4 and 11 | Standard care to titrate the optimal rotigotine dosage (n = 20) | Mean rotigotine dose was higher in the Kinesia 360 arm than the usual care arm (4.8 vs. 3.9 mg/24 hours). Mean rotigotine dosage increase (+2.8 vs. +1.9 mg/24 hours) and mean number of dosage changes (2.8 vs. 1.8 changes) during the study were also higher in the Kinesia 360 arm. |
KinesiaU (1 full paper, 0 abstracts)
Study details | Study design and funding source | Population | Intervention | Comparator(s) | Results |
---|---|---|---|---|---|
Full papers | |||||
Hadley (2021)36 USA |
Prospective cohort study Funding: National Institutes of Health (2 authors are employees of and own stock in Great Lakes Neuro-Technologies) |
PwP (n = 16) undergoing therapy changes | KinesiaU (alongside clinical judgement) worn for at least 3 days in the week prior to instituting a therapy change and for at least 3 days during weeks 3 and 5 after the therapy change | – | Fourteen patients successfully used the KinesiaU system for the duration of the study; 2 did not complete the recordings due to user difficulty or technical issues. The 14 participants averaged 4.9 assessments per day for 3 days per week during the study. Of the patients, 13 successfully used the KinesiaU system and returned for the follow-up visit with the clinician; 1 patient could not return due to COVID-19 travel difficulties. The clinician reviewed the KinesiaU reports with each patient and made a therapy recommendation based on the reports and clinical judgement. Eight patients demonstrated improvements from their new therapy and were instructed to continue with it, while 5 were instructed to discontinue (due to lack of benefit or side effect) and return to their previous therapy/dose. Three patients were prescribed levodopa inhalation powder (2 improved, 1 discontinued), 1 patient was prescribed trihexyphenidyl (discontinued), 3 patients were prescribed istradefylline (1 improved, 2 discontinued), 1 was prescribed increased melatonin dose (improved), 2 were prescribed exercise (improved), 2 were prescribed an increase in carbidopa-levodopa dose (1 improved, 1 discontinued) and 1 patient was prescribed increased doses of carbidopa-levodopa and trihexyphenidyl (improved). |
PDMonitor (0 full papers, 0 abstracts)
Clinical outcomes
PKG (6 full papers, 4 abstracts)
Study details | Study design and funding source | Population | Intervention | Comparator(s) | Results |
---|---|---|---|---|---|
Full papers | |||||
Farzanehfar (2018)70 Australia |
Prospective cohort study Funding: GKC |
PwP (n = 103) Dates: not stated |
PKG worn for 6–7 days | Clinical assessment by neurologist | The 33/80 PwP (uncontrolled) were treated with oral therapy and it was possible to bring the motor scores and function under control in 14 cases. In 19/33 cases it was not possible to reach therapeutic targets by the end of the study. After attempting treatment, 7 of these cases were reclassified: 3 were referred for advanced therapy and 4 were reassigned to the ‘treatment contraindicated’ group because they could not tolerate any change in medications. |
Significant improvements from baseline to final visit were observed in the 33 treated patients: | |||||
|
|||||
Improvements in quality of life (PDQ-39) were significant in the subgroup of 14 patients whose symptoms were brought under control (effect size = 8.5, p = 0.03), but not the full population of 33 treated patients (effect size = 10, p = 0.08). | |||||
Joshi (2019)71 USA |
Prospective cohort study Funding: GKC |
PwP (n = 63; 85 routine care visits) Dates: not stated |
PKG worn for 6 days | Clinical assessment (discussion of PD symptoms with patient and a motor examination) | No serious adverse events or adverse device effects were reported. |
Krause (2021)72 USA |
Retrospective cohort study Funding: none (1 author serves as a consultant for Global Kinetic Corporation) |
PwP (n = 104; 170 PKG reports) Dates: 1 December 2016–30 October 2018 |
PKG worn for 7 days | Clinical assessment by a movement disorder specialist | Out of 104 patients, 49 had more than 1 PKG encounter; 37 had 2 encounters (mean interval 6.3 months between encounters), 7 had 3 encounters (mean interval 11.4 months between first and last encounter) and 5 had 4 encounters (mean interval 15.8 months between first and last encounter). Most patients undergoing 3 or 4 PKG encounters did not reach a controlled state as defined by PKG until the 3rd or 4th encounter, suggesting that repeated use of PKG might be needed to optimise motor control as therapy changes done after one encounter might not be enough. |
Nahab (2019)73 USA |
Prospective cohort study Funding: not stated |
PwP (n = 28) clinically stable patients using levodopa who attended both routine visits Dates: 2 June 2016–16 March 2017 |
PKG worn for 6 days | Routine clinical assessment by a movement disorder specialist (including symptom review, medication review and routine clinical exams), undertaken twice, with UPDRS data reviewed by the movement disorder specialist after the second visit | Mean MDS-UPDRS III summary score significantly reduced (improved) from 28.9 at visit 1 to 24.1 at visit 2 (p < 0.028). Mean MDS-UPDRS IV summary score reduced from 4.1 at visit 1 to 3.0 at visit 2 (p = 0.07). Overall, PKG scores were similar between visit 1 and 2; 16 patients (57%) had improvement and 12 patients (43%) had worsening median BKS scores. Overall H&Y ratings were similar between visit 1 and visit 2; at visit 2, 5 patients (18%) were rated as having improved one H&Y stage and 6 had worsened one stage. |
On the CGI-I scale, the movement disorder specialist ranked 17/28 patients (61%) as having improvement, 9 (32%) as no change and 2 (7%) as minimally worse. On the PGI-I scale, 13/24 patients (54%) indicated their PD was improved, 9 (38%) as no change, 2 (8%) as minimally worse and 4 patients did not respond. |
|||||
Sundgren (2021)75 Sweden |
Prospective cohort study Funding: GKC |
PwP (n = 66) Dates: March 2018–February 2020 |
PKG worn for 6 days | Clinical assessment (before reviewing PKG data) | There were no significant differences in clinical variables when repeated after 3–6 months (mean score at baseline and follow-up) in Parkinson’s Disease Composite Scale (18.5, 18.8), NMSQ (9.0, 8.8), PDQ-8 (22.7, 21.5), EQ-VAS (66.0, 66.7), BKS (27.9, 27.4), DKS (3.5, 3.2), FDS (8.5, 8.6). Overall change at follow-up (assessed using CGI-I Scale) was 3.6; a score of 4 represents no change, a lower score represents improvement. |
Woodrow (2020)17 Australia (12 clinics; 6 using PKG) |
Blinded controlled trial (allocated based on convenience of clinic location) Funding: Parkinson’s Victoria, Shake It Up Australia Foundation and the Michael J Fox Foundation (GKC provided the PKG and loggers as a grant in aid) |
PwP, ≥ 4-year duration or taking ≥ 4 doses of levodopa/day (n = 154) Patients referred for device-assisted therapies were excluded from the study |
Assessment using history, examination and PKG information (worn for 6 days) (PKG+; n = 75) | Assessment using history and examination alone (patients wore the PKG logger for blinding purposes) (PKG−; n = 79) As per the PKG+ arm, patients were assessed at the first consultation to decide whether treatment was adequate or whether further treatment was required |
The 54/75 patients (72%) in the PKG+ arm and 57/79 patients (72%) in the PKG− arm were out-of-target at the first consultation, therefore, had a treatment change and were re-assessed after 5 weeks. The median number of visits (2) was the same in both arms; however, there were significantly more visits in the PKG+ arm (p = 0.01). In the subgroup of patients who were out-of-target at the first consultation, the median number of visits in the PKG+ arm was 3 (IQR = 3) vs. 2 (IQR = 2) in the PKG− arm (p = 0.0009). |
One of the authors is the inventor of the PKG and a paid consultant to GKC. Two authors have a financial interest in GKC | Dates: March 2018–December 2019 | At the first consultation patients were assessed to decide whether their motor features were in-target (BKS target < 26, DKS target < 7) or out-of-target | Patients requiring a treatment change were assessed again 5 weeks later; this was repeated until patients were in-target (with a maximum of 5 visits permitted), with the same caveats to changing treatment that applied to the PKG+ arm (see paper for further information) | There was a statistically significant improvement in mean UPDRS total score (primary outcome) between the first and last visit in the PKG+ arm (59.6 vs. 51.1; p = 0.001), but the improvement in the PKG− arm (62.3 vs. 57.4; p = 0.10) did not reach statistical significance. A direct comparison of the final MDS-UPDRS total score between treatment groups (51.1 vs. 57.4; p = 0.02) was statistically significant. | |
If out-of-target, a plan for changing treatment was provided and patients were assessed again 5 weeks later; this was repeated until PKG data were in-target (with a maximum of 5 visits permitted) See paper for full out-of-target criteria and treatment change caveats |
There was also a statistically significant improvement in MDS-UPDRS IV score (5.0 vs. 3.5), MDS-UPDRS III score (35.1 vs. 28.6) and PTOT score (19.9 vs. 13.0) between the first and last visit in the PKG+ arm. However, there was no significant difference in PDQ-39, SENS PD and NMSQ (amongst others) between the first and last visit in the PKG+ arm. Scores in the PKG− arm improved between the first and last visit, although none of the differences were statistically significant. |
||||
The 12% of the PKG+ group who were out-of-target were not treated, compared with 30% of the PKG− group, suggesting that PKG had a larger influence on clinical decisions than history and examination alone. Similarly, in the PKG− arm, 52% of cases reported as in-target were treated, compared with 17% in the PKG+ arm. Clinical scores of in-target cases were similar between the two treatment groups and remained unchanged 3 months later (data not shown). In the subgroup of patients who were out-of-target at the first consultation in the PKG+ arm (n = 54), there was a statistically significant improvement in mean MDS-UPDRS total (62.8 vs. 51.1; p < 0.001), MDS-UPDRS IV (5.6 vs. 3.3; p < 0.001), MDS-UPDRS III (36.5 vs. 28.6; p < 0.001), PDQ-39 (28.1 vs. 22.1; p = 0.045) and PTOT (23.8 vs. 14.3; p = 0.001) scores between the first and final visit. |
|||||
None of the scores changed significantly from first to final visit in the subgroup of patients who were out-of-target at the first consultation in the PKG− arm (n = 57). There were statistically significant increases in LEDD between the first and last visit in the PKG+ arm (675 vs. 799; p = 0.04) and the out-of-target subgroup of the PKG+ arm (669 vs. 823; p = 0.02). The increases in LEDD between the first and last visit in the PKG− arm (760 vs. 870) did not reach statistical significance (p = 0.06) but the increase in LEDD was statistically significant in the out-of-target subgroup of the PKG− arm (760 vs. 933; p = 0.02). There was no significant difference in final LEDD score between treatment groups. However, there was a statistically significant difference in the change in D2 agonist use between treatment groups (change in 33% patients in the PKG+ arm and 18% patients in the PKG− arm; p = 0.0001). Subgroup results are also presented for the subgroup of patients who were out-of-target due to bradykinesia. |
|||||
Abstracts | |||||
Farzanehfar (2017)82 Location: not stated |
Prospective feasibility study Funding: not stated |
PwP (n = 28 where patient and doctor perceived that Parkinson’s disease symptoms were controlled) Dates: not stated |
PKG | Clinical assessment | Analysis of the 21 patients with uncontrolled bradykinesia showed statistically significant changes in the UPDRS III (p = 0.005; median improvement = 7) and total UPDRS (p = 0.002; median improvement = 15) following intervention. In 8 patients recognised as poorly controlled only via the PKG, there was a statistically significant improvement in the UPDRS III (p = 0.01; median improvement = 13) and total UPDRS (p = 0.01, median improvement = 13). |
Horne (2016)83 Location: not stated |
Interim findings of a prospective cohort study Funding: not stated |
PwP (n = 19 considered to be well controlled by general neurologists) Dates: not stated |
PKG | Assessment by a movement disorder specialist | Clinically significant changes in patient outcomes were noted in the UPDRS, PKG scores and PDQ-8. |
Horne (2018)84 Australia |
Pilot prospective cohort study Funding: not stated |
PwP (n = 103) Dates: not stated |
PKG | Clinical assessment | At the end of the study, 48% patients were in-target (22% at outset and 26% by treatment change, not including those referred for advanced therapy). For those in whom oral therapy was changed, total UPDRS and PDQ-39 improved (effect size 8 and 10 respectively). MOCA scores also improved significantly. |
Lynch (2018)88 Australia |
Prospective or retrospective cohort study Funding: not stated |
PwP [n = 80 uncontrolled patients from Farzanehfar (2018) – excluded as doesn’t report data on relevant outcomes] Dates: not stated |
PKG | – | Among the 33 patients treated with oral therapy after PKG, decreases were observed in: mean UPDRS II (−4; 9–13), mean UPDRS III (−3; 36–39), per cent over target (−8; 64–73) and PDQ-39 (10; 19–29). It was estimated that over the relevant range of UPDRS II scores, a one-point reduction in UPDRS II correlates with an average of $430 in cost savings from lower resource utilisation. Improved disease management contributed to total resource utilisation cost savings of $1719.42 per patient over a 12-month period. Using PKG efficacy for UPDRS III (−3), UPDRS II (−4), per cent over target (−8) and PDQ-39 (−10), a QALY gain of between 0.10 and 0.12 was estimated over a 12-month period. |
STAT-ON (0 full papers, 0 abstracts)
Kinesia 360 (2 full papers, 0 abstracts)
Study details | Study design and funding source | Population | Intervention | Comparator(s) | Results |
---|---|---|---|---|---|
Full papers | |||||
Isaacson (2019)33 USA |
Pilot RCT Funding: UCB Pharma |
PwP with insufficiently controlled motor symptoms, prescribed transdermal rotigotine (n = 39) Dates: March 2017–January 2018 |
Kinesia 360 data used to supplement standard care in adjusting rotigotine dosage (n = 19) Kinesia 360 was used throughout the day on at least two consecutive days in weeks 1, 2, 3, 4 and 11 |
Standard care to titrate the optimal rotigotine dosage (n = 20) | At week 12, there was a statistically significant improvement in UPDRS II in the Kinesia 360 arm compared with a slight worsening in the standard care arm (−2.1 vs. 0.5; p = 0.004). The difference in improvement in UPDRS III was not statistically significant between groups (−5.3 vs. −1.0; p = 0.134). There was no significant change in PDQ-39 score at week 12 in either study group (−5.1 vs. −3.5; p = 0.471). Change in PAM-13 score at week 12 (Kinesia 360 vs. standard care): −4.6 versus −0.2; p = 0.164 (no significant difference between groups). Mean rotigotine dosage increase from baseline to week 12 (Kinesia 360 vs. standard care): +2.8 verus +1.9 mg/24 hours. Mean number of dosage changes: 2.8 versus 1.8. 3 patients in the Kinesia 360 arm and 2 patients in the standard care arm discontinued rotigotine due to treatment-emergent adverse events. |
Peacock (2021)34 Canada |
RCT Funding: not reported |
PwP (n = 25 – study suspended due to COVID-19) with bothersome tremor or dyskinesia identified as a treatment target at their most recent clinic visit Dates: May 2019–March 2020 |
Telehealth (video-conference) follow-up care with data from in-home Kinesia 360 (worn for 3 days), by movement disorder neurologist, at baseline, 6 weeks, 3 months and 6 months (n = 13) | Usual in-person follow-up care with clinical examination and review of 3 days of symptom diaries, by movement disorder neurologist, at baseline, 6 weeks, 3 months and 6 months (n = 12) | The average change in PDQ-39 summary index score from baseline to completion (primary outcome) was −4.7 points in the telehealth group (95% CI −10.2 to +0.7) and +0.9 (95% CI −3.6 to +5.5) in the usual care group. Note: mean baseline PDQ-39 score was 29 in the telemedicine group and 25 in the usual care group. Secondary outcomes were not significantly different between groups [LEDD change from baseline (mean 32 vs. 52 mg) and appointments per participant with sensor or diary data available (mean 2.2 vs. 1.8)]. Repeat measurement of MDS-UPDRS III was not completed due to suspension of face-to-face clinical and research visits, due to COVID-19. |
KinesiaU (1 full paper, 0 abstracts)
Study details | Study design and funding source | Population | Intervention | Comparator(s) | Results |
---|---|---|---|---|---|
Full papers | |||||
Hadley (2021)36 USA |
Prospective cohort study Funding: National Institutes of Health (2 authors are employees of and own stock in Great Lakes Neuro-Technologies) |
PwP (n = 16) undergoing therapy changes | KinesiaU (alongside clinical judgement) worn for at least 3 days in the week prior to instituting a therapy change and for at least 3 days during weeks 3 and 5 after the therapy change | – | There were 14 patients who successfully used the KinesiaU system for the duration of the study; 2 did not complete the recordings due to user difficulty or technical issues. The 14 participants averaged 4.9 assessments per day for 3 days per week during the study. The 13 patients who successfully used the KinesiaU system returned for the follow-up visit with the clinician; 1 patient could not return due to COVID-19 travel difficulties. The clinician reviewed the KinesiaU reports with each patient and made a therapy recommendation based on the reports and clinical judgement. Eight patients demonstrated improvements from their new therapy and were instructed to continue with it, while 5 were instructed to discontinue (due to lack of benefit or side effect) and return to their previous therapy/dose. Three patients were prescribed levodopa inhalation powder (2 improved, 1 discontinued), 1 patient was prescribed trihexyphenidyl (discontinued), 3 patients were prescribed istradefylline (1 improved, 2 discontinued), 1 was prescribed increased melatonin dose (improved), 2 were prescribed exercise (improved), 2 were prescribed an increase in carbidopa-levodopa dose (1 improved, 1 discontinued) and 1 patient was prescribed increased doses of carbidopa-levodopa and trihexyphenidyl (improved). |
PDMonitor (0 full papers, 0 abstracts)
Patient, carer and clinician opinions
PKG (6 full papers, 6 abstracts)
Study details | Study design and funding source | Population | Intervention | Comparator(s) | Results |
---|---|---|---|---|---|
Full papers | |||||
Dominey (2020)68 UK |
Retrospective cohort study Funding: not stated (PKG recordings were provided by GKC or funded by a licence arrangement with GKC) |
PwP (n = 166; 78 new patients and 88 follow-up) Dates: July 2015–January 2018 |
PKG worn for 6 days | – | Patient opinion (n = 62): 41/51 respondents (80%) valued the medication reminders and 23/39 (59%) rated use of the PKG as valuable in providing additional information to their clinical team, 24/40 respondents (60%) perceived the PKG results as reflective of their lived experience, 57/59 respondents (97%) were willing to continue using the PKG as part of their management, while 19/48 (40%) reported satisfaction at not having to travel to a clinic. |
Evans (2020)69 UK |
Pilot cohort study (PKG recordings taken prior to enrolment) Funding: Bevan Commission |
PwP (n = 61 who had a PKG within the last 2 months and face-to-face appointment due in 1–2 months) Dates: from September 2018 Reported in Evans180 abstract: PwP (n = 30 patients who returned questionnaires) |
PKG (used in a virtual clinic appointment) | Face-to-face clinic appointment | Patient satisfaction questionnaire (n = 46): 41/46 respondents (89%) agreed or strongly agreed they were satisfied with the virtual clinic. Reported in Evans180 (abstract): 27/30 patients (90%) agreed or strongly agreed that they were satisfied with the virtual clinic and that their concerns were suitably addressed, 23/30 (77%) felt they could talk to the doctor as if it were a regular consultation and 19/30 (63%) would recommend a virtual clinic consultation to other PwP. |
Joshi (2019)71 USA |
Prospective cohort study Funding: GKC |
PwP (n = 63; 85 routine care visits) Dates: not stated |
PKG worn for 6 days | Clinical assessment (discussion of PD symptoms with patient and a motor examination) | Patient and caregiver satisfaction: 82% agreed or strongly agreed that the PKG was easy to learn, easy to use, enabled them to confirm medication administration, performed as expected and would use it again. Physician’s assessment of global impact on patient care: the PKG improved dialogue with the patient in 59% of visits, improved ability to assess impact of a therapy in 38% of visits, improved ability to assess need for additional tests or treatments in 7% of visits, improved ability to assess PD symptoms in 33% of visits and improved patient education about symptoms in 29% of visits. |
Nahab (2019)73 USA |
Prospective cohort study Funding: not stated |
PwP (n = 28) clinically stable patients using levodopa who attended both routine visits | PKG worn for 6 days | Routine clinical assessment by a movement disorder specialist (including symptom review, medication review and routine clinical exams), undertaken twice, with UPDRS data reviewed by the movement disorder specialist after the second visit | The movement disorder specialist assessed the PKG as having an overall positive impact on patient care with high responses (79–100%) across clinical visits in improved dialogue, improved patient education and improved ability to assess the impact of therapy. Patients had a positive response on the use of the PKG; they agreed it was easy to use (93%), performed as expected (96%) and they would use it again (100%). Patients assessed the PKG as having an overall positive impact on their care; the device assisted with explaining symptoms (79%), in providing data to the physician they could not provide (89%), in assessing their daily activity levels (96%) and in providing data that contributed to the overall management of their PD (93%). |
When asked if they would be willing to pay for the device if their insurance didn’t cover the cost, 32% of patients said they would, 25% said it would depend on cost and 43% said they would not pay for the device. | |||||
Santiago (2019)74 USA |
Prospective physician survey Funding: GKC provided PKG System product for use in this research project, research service support and funds for manuscript submission |
Physicians of PwP (n = 4 movement disorder specialists who ordered 143 PKGs on 89 patients; 112 completed surveys on 81 patients were included in the analysis) Dates: December 2015–July 2016 |
PKG worn for 6 days | Clinical consultation alone (routine clinic visit before reviewing PKG data) | Of the 112 completed physician surveys, 46 (41%) indicated the PKG provided relevant additional information sufficient to consider adjusting their therapeutic management plan, 66 (59%) indicated the PKG provided no further information to support a therapeutic decision differing from that made during a routine clinic visit. |
Sundgren (2021)75 Sweden |
Prospective cohort study Funding: GKC |
PwP (n = 66) Dates: March 2018–February 2020 |
PKG worn for 6 days | Clinical assessment (before reviewing PKG data) | Physicians considered that the PKG improved the dialogue with the patient in 58/66 visits (88%). |
Abstracts | |||||
Chhabria (2018)78 Location: not stated |
Retrospective cohort study Funding: not stated |
PwP (n = 50) Dates: not stated |
PKG | – | Overall, patients reported high satisfaction with wearing the device. |
Langston (2017)87 USA |
Prospective or retrospective cohort study Funding: not stated |
PwP (n = 89; 123 movement disorder surveys completed, 44 patients had multiple PKGs) Dates: not stated |
PKG worn for 6 days | Clinical assessment by a movement disorder specialist | Of the patients, 38% agreed that the PKG provided additional information not available from clinical consultation alone. |
Rasul (2017)93 USA |
Prospective cohort study Funding: not stated |
PwP (n = 51; 28 provided feedback regarding their experience of using PKG) Dates: not stated |
PKG worn for 6 days | – | Of the patients, 68% strongly agreed that PKG was very easy to use, 96% agreed or strongly agreed that they were able to wear PKG and complete medication use confirmations as instructed by the doctor and 97% found that the feature of PKG for reminder was very helpful for medication compliance. |
Spengler (2016)94 USA |
Pilot prospective cohort study Funding: not stated |
PwP with newly implanted STN leads for DBS therapy (n = 5) Dates: not stated |
PKG | – | Patients felt that PKG was helpful with medication reminders and helped explain the symptoms better. |
Thakur (2017)90 USA |
Retrospective cohort study Funding: not stated |
PwP (n = 51) Dates: November 2015–November 2016 |
PKG worn for 6 days | Clinical assessment during routine Parkinson’s disease follow-up by movement disorder specialists | Two patients did not tolerate the PKG due to wristband irritation; 47% of clinicians agreed that the PKG provided information not available from the clinical consultation that drove a clinical management plan change. |
Thomas (2019)91 UK |
Retrospective cohort study Funding: not stated |
PwP (n = 256 evaluations) Dates: not stated |
PKG | Clinical assessment | Patient feedback on the PKG was favourable with 98% patients reporting a positive or neutral user experience. |
STAT-ON (2 full papers, 2 abstracts)
Study details | Study design and funding source | Population | Intervention | Comparator(s) | Results |
---|---|---|---|---|---|
Full papers | |||||
Bayes (2018)18 Spain, Italy, Israel and Ireland |
Prospective pilot cohort study and patient survey Funding: European Community |
PwP (n = 41) | STAT-ON | Patient diaries and clinical assessment | Usability and user satisfaction: median SUS in this study was 70 (IQR 25). A SUS score > 50 is considered acceptable and above 68–70 is good. In the QUEST questionnaire no patient was ‘not satisfied at all’ with the system, 5% were ‘not very satisfied’, 20% ‘more or less satisfied’ and 76% ‘satisfied’ or ‘very satisfied’. Comfort is the element with the lowest score and security with the highest. |
Santos Garcia (2020)32 Spain |
Prospective physician survey Funding: not stated (STAT-ON was provided by Sense4Care) |
Physicians of PwP (n = 27 neurologists treating 114 patients) Dates: October–December 2019 (survey sent in February 2020) |
STAT-ON | – | 74% of neurologists rated STAT-ON ‘quite’ to ‘very useful’. Subjective general opinion about the device (from 0 to 10) was 6.9 ± 1.7. On a scale of 1 (unhelpful) to 7 (very helpful), ‘on–off daily distribution’ was the best scored item (5.5 ± 1.5), whereas ‘falls detection’ (3.6 ± 2.0) and ‘number of freezing of gait episodes and duration’ (3.7 ± 1.7) were the worst. |
70.3% of neurologists rated STAT-ON better than diaries and 81.5% considered it a useful tool for the identification of patients with advanced PD. Most frequently reported limitations of the information provided by the device were ‘problems with the interpretation of time inactive’ (n = 6 neurologists) and ‘proper identification of freezing of gait episodes and/or falls’ (n = 5 neurologists). 89% of neurologists would use the device in their daily clinical practice. |
|||||
Abstracts | |||||
Rodriguez-Martin (2021)25 Location: not stated |
Prospective survey Funding: not stated |
PwP (n = 41), caregivers (n = 30), neurologists (n = 17) and health professionals (n = 19) Dates: not stated |
STAT-ON | – | 88% of neurologists surveyed think that the medical device can detect advanced PD symptoms. The average score of the sensor is 7.9/10 and all neurologists find the information useful or very useful. Health professionals scored the sensor 8.6/10 and all of them see the sensor as a good or very good solution. Patients rate the sensor 8.5/10 and 77.5% think it is very easy to use. The belt is rated 8.1/10. |
80% of caregivers find the sensor a good or very good solution and no-one dislikes the sensor. 76% think it is easy to use and no caregiver thinks the belt is difficult to wear and adjust. | |||||
Caballol (2020)20 Spain |
Prospective cohort study Funding: not stated |
PwP (n = 39) Dates: not stated |
STAT-ON worn for 1 week (device not stated in abstract, but abstract in Sense4Care submission) | Patient report | Satisfaction with the device (assessed using QUEST) was high: all items scored between 4, ‘quite satisfied’, and 5, ‘very satisfied’, except for the item ‘easy in adjusting’ which had a lower score. The system was found to be easy to use (assessed using SUS). |
Kinesia 360 (1 full paper, 0 abstracts)
Study details | Study design and funding source | Population | Intervention | Comparator(s) | Results |
---|---|---|---|---|---|
Full papers | |||||
Peacock (2021)34 Canada |
RCT Funding: not reported |
PwP (n = 25 – study suspended due to COVID-19) with bothersome tremor or dyskinesia identified as a treatment target at their most recent clinic visit Dates: May 2019–March 2020 |
Telehealth (video-conference) follow-up care with data from in-home Kinesia 360 (worn for 3 days), by movement disorder neurologist, at baseline, 6 weeks, 3 months and 6 months (n = 13) | Usual in-person follow-up care with clinical examination and review of 3 days of symptom diaries, by movement disorder neurologist, at baseline, 6 weeks, 3 months and 6 months (n = 12) | 54% of telehealth patients reported feeling comfortable or very comfortable using motion sensors, 39% were neither comfortable nor uncomfortable and 8% were uncomfortable or very uncomfortable using motion sensors. 50% of usual care patients reported feeling comfortable or very comfortable using symptom diaries, 50% were neither comfortable nor uncomfortable. 85% of telehealth patients felt comfortable or very comfortable talking to their doctor through a computer screen, while 15% felt uncomfortable or very uncomfortable. 46% of telehealth patients would have preferred to be in the usual care group, 8% would not and 46% were undecided. 8% of usual care patients would have preferred to be in the telehealth group, 67% would not and 25% were undecided. |
KinesiaU (1 full paper, 0 abstracts)
Study details | Study design and funding source | Population | Intervention | Comparator(s) | Results |
---|---|---|---|---|---|
Full papers | |||||
Hadley (2021)36 USA |
Prospective cohort study Funding: National Institutes of Health (2 authors are employees of and own stock in Great Lakes Neuro-Technologies) |
PwP (n = 16) undergoing therapy changes | KinesiaU (alongside clinical judgement) worn for at least 3 days in the week prior to instituting a therapy change and for at least 3 days during weeks 3 and 5 after the therapy change | – | 88% of patients agreed or strongly agreed that the KinesiaU system was easy to understand and use, while 12% disagreed or strongly disagreed. 88% agreed or strongly agreed that the periodic tasks were easy to perform, while 6% were neutral and 6% disagreed. Only 32% of patients agreed or strongly agreed that they looked at the KinesiaU reports often and only 38% agreed or strongly agreed that they were useful to look at (44% were neutral and 19% disagreed or strongly disagreed). 44% of patients agreed or strongly agreed that they would continue to use the system if it was available to them, 25% were neutral and 31% disagreed. |
PDMonitor (0 full papers, 0 abstracts)
Appendix 5 Further results from Woodrow individual participant data analysis
Variable | Unadjusted analysis | Adjusted analysis | ||
---|---|---|---|---|
Mean difference | Standard error | Mean difference | Standard error | |
Median active BKS | −0.665 | 0.457 | −0.412 | 0.449 |
Median BKS | −0.028 | 0.544 | 0.289 | 0.527 |
Median DKS | −0.432 | 0.540 | −0.516 | 0.561 |
H&Y | −0.025 | 0.095 | −0.044 | 0.097 |
LED (/100) | 0.216 | 0.440 | −0.224 | 0.413 |
MOCA | −0.164 | 0.292 | −0.200 | 0.300 |
NMS | 0.717 | 0.522 | 0.829 | 0.540 |
PDQ-39 | −1.077 | 1.553 | −0.650 | 1.600 |
Time in bradykinesia (%) | −2.106 | 1.734 | −1.223 | 1.699 |
Time in dyskinesia (%) | −1.507 | 1.344 | −2.059 | 1.390 |
Time immobile (%) | −0.636 | 0.495 | −0.558 | 0.516 |
Time inactive (%) | 2.701 | 1.203 | 2.685 | 1.252 |
Time in tremor (%) | −0.589 | 0.615 | −0.325 | 0.616 |
SENS-PD | −0.514 | 0.631 | −0.391 | 0.655 |
UPDRS I | 0.012 | 0.555 | −0.015 | 0.577 |
UPDRS II | 0.381 | 0.541 | 0.589 | 0.557 |
UPDRS III | −3.166 | 1.300 | −3.300 | 1.172 |
UPDRS IV | −1.151 | 0.578 | −0.719 | 0.576 |
UPDRS total | −4.236 | 1.884 | −3.540 | 1.810 |
FIGURE 11.
Woodrow IPD analysis: continuous outcomes using unadjusted and adjusted models.

FIGURE 12.
Woodrow IPD analysis: continuous outcomes by number of visits required.

FIGURE 13.
Woodrow IPD analysis: continuous outcomes by UPDRS III score at baseline.

Variable | Target | Odds ratio | Standard error | 95% CI | |
---|---|---|---|---|---|
Median active BKS | In-target | −1.585 | 1.101 | −3.743 | 0.574 |
Median active BKS | Not in-target | −0.073 | 0.510 | −1.073 | 0.928 |
Median BKS | In-target | −0.845 | 1.172 | −3.142 | 1.452 |
Median BKS | Not in-target | 0.524 | 0.613 | −0.677 | 1.725 |
Median DKS | In-target | −0.426 | 0.590 | −1.581 | 0.730 |
Median DKS | Not in-target | −0.637 | 0.685 | −1.979 | 0.705 |
H&Y | In-target | −0.029 | 0.228 | −0.476 | 0.418 |
H&Y | Not in-target | −0.035 | 0.113 | −0.258 | 0.187 |
LED (/100) | In-target | −1.000 | 1.126 | −3.207 | 1.208 |
LED (/100) | Not in-target | −0.192 | 0.466 | −1.105 | 0.722 |
MOCA | In-target | −1.692 | 0.760 | −3.182 | −0.201 |
MOCA | Not in-target | −0.010 | 0.335 | −0.666 | 0.646 |
NMS | In-target | 1.374 | 1.415 | −1.399 | 4.147 |
NMS | Not in-target | 0.381 | 0.630 | −0.853 | 1.616 |
PDQ-39 | In-target | 0.893 | 5.475 | −9.838 | 11.624 |
PDQ-39 | Not in-target | −2.438 | 1.724 | −5.818 | 0.941 |
Time in bradykinesia (%) | In-target | 0.463 | 2.783 | −4.992 | 5.918 |
Time in bradykinesia (%) | Not in-target | −0.711 | 1.996 | −4.624 | 3.202 |
Time in dyskinesia (%) | In-target | −2.356 | 2.578 | −7.410 | 2.698 |
Time in dyskinesia (%) | Not in-target | −2.337 | 1.637 | −5.546 | 0.871 |
Time immobile (%) | In-target | −2.766 | 1.466 | −5.640 | 0.108 |
Time immobile (%) | Not in-target | −0.093 | 0.559 | −1.189 | 1.003 |
Time inactive (%) | In-target | 6.777 | 3.162 | 0.579 | 12.975 |
Time inactive (%) | Not in-target | 2.009 | 1.422 | −0.778 | 4.795 |
Time in tremor (%) | In-target | −1.409 | 1.068 | −3.502 | 0.685 |
Time in tremor (%) | Not in-target | 0.003 | 0.744 | −1.454 | 1.461 |
SENS-PD | In-target | 2.242 | 1.449 | −0.599 | 5.083 |
SENS-PD | Not in-target | −0.858 | 0.748 | −2.323 | 0.607 |
UPDRS I | In-target | 1.448 | 1.477 | −1.447 | 4.344 |
UPDRS I | Not in-target | −0.319 | 0.661 | −1.614 | 0.977 |
UPDRS II | In-target | 0.115 | 1.628 | −3.076 | 3.307 |
UPDRS II | Not in-target | 0.323 | 0.599 | −0.851 | 1.498 |
UPDRS III | In-target | −0.856 | 2.437 | −5.633 | 3.921 |
UPDRS III | Not in-target | −3.179 | 1.551 | −6.219 | −0.140 |
UPDRS IV | In-target | −0.379 | 1.645 | −3.603 | 2.845 |
UPDRS IV | Not in-target | −1.236 | 0.627 | −2.464 | −0.008 |
UPDRS total | In-target | −5.111 | 4.431 | −13.796 | 3.574 |
UPDRS total | Not in-target | −4.110 | 2.154 | −8.331 | 0.111 |
Glossary
- Area under the curve
- Area under a receiver operator characteristic curve (for assessing diagnostic accuracy).
- Bradykinesia
- Slowness of movement.
- Cost-effectiveness analysis
- An economic analysis that converts effects into health terms and describes the costs for additional health gain.
- Decision modelling
- A theoretical construct that allows the comparison of the relationship between costs and outcomes of alternative healthcare interventions.
- Dyskinesia
- Involuntary and uncontrollable movement.
- False negative
- Incorrect negative test result; number of diseased persons with a negative test result.
- False positive
- Incorrect positive test result; number of non-diseased persons with a positive test result.
- Incremental cost-effectiveness ratio
- The difference in the mean costs of two interventions in the population of interest divided by the difference in the mean outcomes in the population of interest.
- Index test
- The test whose performance is being evaluated.
- Markov model
- An analytic method particularly suited to modelling repeated events or the progression of a chronic disease over time.
- Sensitivity
- Proportion of people with the condition of interest who have a positive test result.
- Specificity
- Proportion of people without the condition of interest who have a negative test result.
- True negative
- Correct negative test result; number of non-diseased persons with a negative test result.
- True positive
- Correct positive test result; number of diseased persons with a positive test result.
List of abbreviations
- AIMS
- Abnormal Involuntary Movements Scale
- AUC
- area under the curve
- BMT
- best medical treatment
- CGI-I
- Clinician Global Impression of Improvement
- COMT
- catechol-O-methyltransferase
- CRD
- Centre for Reviews and Dissemination
- DBS
- deep brain stimulation
- EAG
- External Assessment Group
- EQ-5D
- EuroQol-5 Dimensions
- ESS
- Epworth sleepiness score
- FDS
- fluctuations dyskinesia score
- GKC
- Global Kinetics Corporation
- HRQoL
- health-related quality of life
- H&Y
- Hoehn and Yahr
- ICER
- incremental cost-effectiveness ratio
- IPD
- individual participant data
- LCIG
- levodopa–carbidopa intestinal gel
- LED
- levodopa equivalent dose
- LEDD
- levodopa equivalent daily dose
- MAO-B
- monoamine oxidase type B
- MDS-UPDRS
- Movement Disorder Society-Unified Parkinson’s Disease Rating Scale
- MOCA
- Montreal Cognitive Assessment
- NICE
- National Institute for Health and Care Excellence
- NMSQ
- non-motor symptom questionnaire
- NMSQuest
- non-motor symptom questionnaire
- NPV
- negative predictive value
- PDQ-8
- Parkinson’s disease questionnaire-8
- PDQ-39
- Parkinson’s disease quality of life 39 questions
- PGI-I
- Patient Global Impression of Improvement
- PKG
- Personal KinetiGraph
- PPI
- Patient and public involvement
- PPV
- positive predictive value
- PRISMA
- Preferred Reporting Items for Systematic Reviews and Meta-Analyses
- PSS
- Personal Social Services
- PTB
- per cent time in bradykinesia
- PTD
- per cent time in dyskinesia
- PTI
- proportion of time immobile
- PTT
- per cent time tremor
- PwP
- people with Parkinson’s disease
- QALY
- quality-adjusted life-year
- QUADAS-2
- Quality Assessment of Diagnostic Accuracy Studies-2
- QUIP
- Questionnaire for Impulsive-Compulsive Disorders in Parkinson’s Disease
- RCT
- randomised controlled trial
- SoC
- standard of care
- UDysRS
- Unified Dyskinesia Rating Scale
- UPDRS
- Unified Parkinson’s Disease Rating Scale
- WOQ-9
- 9-item wearing-off questionnaire