Notes
Article history
This article presents independent research funded by the National Institute for Health and Care Research (NIHR). The views and opinions expressed by authors in this publication are those of the authors and do not necessarily reflect those of the NHS, the NIHR, the HTA programme or the Department of Health and Social Care. If there are verbatim quotations included in this publication the views and opinions expressed by the interviewees are those of the interviewees and do not necessarily reflect those of the authors, those of the NHS, the NIHR, the HTA programme or the Department of Health and Social Care.
Permissions
Copyright statement
Copyright © 2024 Woods et al. This work was produced by Woods et al. under the terms of a commissioning contract issued by the Secretary of State for Health and Social Care. This is an Open Access publication distributed under the terms of the Creative Commons Attribution CC BY 4.0 licence, which permits unrestricted use, distribution, reproduction and adaptation in any medium and for any purpose provided that it is properly attributed. See: https://creativecommons.org/licenses/by/4.0/. For attribution the title, original author(s), the publication source – NIHR Journals Library, and the DOI of the publication must be cited.
2024 Woods et al.
Chapter 1 Introduction
This section reproduces and adapts, with permission, content previously published as part of the study protocol. 1
Antimicrobial resistance
Antimicrobial resistance (AMR) develops when bacteria with mutations that prevent the activity of antimicrobials (AMs) emerge through selection pressure exerted by the use of AM agents. There are two major genetic processes involved: mutations in the genes native to the organism usually associated with the mechanism of action of the compound; and acquisition of foreign DNA coding for resistance determinants through horizontal gene transfer (HGT) of plasmids/genes (e.g. transposons). 2,3 The majority of pathogenic microorganisms appear to have the capability to develop resistance to at least some AM agents. Mechanisms of resistance include limiting uptake of a drug by the microbe, modification of a drug target, inactivation of a drug and active efflux of a drug. Resistance to multiple agents can develop via successive mutations, through the dissemination of genes or through a combination of both processes.
The increased mobility of the global population has had the effect of promoting the evolution and movement of antibiotic resistance genes. For example, very high rates of extended-spectrum β-lactamase (ESBL) production among Enterobacterales strains in Asian countries has resulted in substantial use of carbapenem antibiotics worldwide, leading to the emergence of plasmid-mediated resistance to carbapenems. 4 These have spread across the globe and between species. Multidrug-resistant bacteria can also spread rapidly within both hospitals and community settings, further contributing to increased AM use and heightened resistance,5 and narrowing the choices available for antibiotic treatment.
Gram-negative bacteria (GNB) pose a significant public health problem due to their increasing levels of resistance to antibiotics. This can lead to severe consequences where infections cannot be treated effectively, or where the increased risk of mortality and morbidity from infection can prevent life-saving procedures such as transplants or other invasive procedures. Enterobacterales account for many Gram-negative infections in humans, including urinary tract infections (UTIs), pneumonia, diarrhoea, meningitis and sepsis, while the non-fermenter Gram-negative bacilli account for the largest share of infections caused by carbapenem-resistant GNB. 6
Carbapenem resistance is a particular problem in GNB, since it constitutes the most reliable drug class for treating bacterial infections. There are two main types of carbapenem resistance, and these can be expressed in multiple pathogens:
-
Carbapenemase-mediated carbapenem resistance occurs when the microorganism produces an enzyme (carbapenemase) that hydrolyses carbapenem antibiotics (such as penicillins, cephalosporins, monobactams and carbapenems) and renders them ineffective. There are multiple carbapenemase enzymes, and these are grouped based upon the similarity of their amino acid sequences according to the Ambler classification system as class A, B, C or D. Class A, C and D enzymes have a serine-based hydrolytic mechanism, while class B enzymes are metallo-beta-lactamases (MBL) that contain zinc in the active site. Each class comprises a number of variants, which include:
-
Class A: Klebsiella pneumoniae carbapenemase (KPC), Guiana extended-spectrum β-lactamase (GES), Imipenemase/non-metallo carbapenemase-A (IMI/NMC), and Serratia marcescens enzyme (SME).
-
Class B (MBLs): New Delhi metallo-beta-lactamases (NDM), Verona integrated-encoded metallo-beta-lactamases (VIM), Imipenemase (IMP), Sao Paulo metallo-beta-lactamases (SPM) and Germany imipenemase (GIM).
-
Class C: Ampicillinase C (AmpC), cephamycinases (CMY).
-
Class D: Oxacillinase (OXA)-23, OXA-24, OXA-48, OXA-58 and related enzymes.
-
Carbapenemases are produced by a small but growing number of Enterobacterales strains, especially Escherichia coli and Klebsiella pneumoniae, and some non-fermenter organisms such as Pseudomonas aeruginosa and Acinetobacter baumannii. Bacteria-producing carbapenemases may cause serious drug-resistant infections, though the profile of resistance is different for each specific variant and is influenced by the pathogen expressing the resistance, and other resistance genes the organism may have. Of the Ambler Class A carbapenemases, the KPC carbapenemases are the most prevalent, found mostly on plasmids in K. pneumoniae. The class D carbapenemases are frequently detected in A. baumannii. The class B (MBLs) have been detected primarily in P. aeruginosa; however, there are increasing numbers of reports worldwide of this group of β-lactamases in the Enterobacterales. The main serine carbapenemases among carbapenemases-producing Enterobacterales (CPE) (Enterobacterales) in the UK are OXA-48 and KPC. The main MBLs in the UK are NDM, VIM and IMP. 7 Specifically, 12.5% of Enterobacterales are KPC, 36.5% are OXA-48-like and 43.2% MBL (mostly NDM) in the UK. 6
-
Non-carbapenemase carbapenem resistance occurs through a variety of non-enzymatic mechanisms which include reduced cell membrane permeability to carbapenems through downregulation of porins (membrane proteins that allow carbapenems into the cell), or overexpression of efflux pumps which remove carbapenems from the periplasmic space. Such mechanisms are often considered to produce low-level resistance, and generally more treatment options are available that maintain activity against these mechanisms.
The World Health Organization (WHO) maintains a list of priority pathogens where, due to the development of resistance, new AMs are urgently needed. The pathogens that the WHO deems ‘critical’ priorities are: carbapenem-resistant A. baumannii; carbapenem-resistant P. aeruginosa; carbapenem-resistant Enterobacterales (CRE) (where Klebsiella pneumonia and E. coli account for the large majority of Enterobacterales). These pathogens are typically multidrug-resistant GNB that can cause severe infections in secondary care settings, such as pneumonia and bloodstream infections (BSIs) (bacteraemia), which can often be fatal. 8,9
Early, targeted, effective and safe AM treatment is key for the management of patients infected with carbapenemase-producing carbapenem-resistant bacteria; however, reliable AM treatment options remain scarce. Therefore, individual treatment options tailored to susceptibilities of pathogens and severity of infection are the mainstay of clinical practice. 7 Carbapenems are a class of β-lactams that are often reserved as a last-line treatment option for infections that are resistant to other β-lactams with a narrower spectrum of action. 10 Carbapenems are considered one of the most reliable drugs for treating bacterial infections;2 therefore, the emergence and spread of resistance to these antibiotics is particularly concerning, especially resistance mediated via carbapenemase which renders other treatment options ineffective. This constitutes a major public health problem due to the morbidity and mortality associated with ineffectively treated infections by these bacteria.
New antimicrobials
There is widespread recognition that the pipeline for new AMs is poor with few AM agents currently in clinical development. A range of policies have been implemented to address this lack of investment; however, these have focused on ‘push incentives’ that lower the costs of research and development (R&D). In 2015, a joint government and industry AMR working group was established that highlighted the need for the development of ‘pull mechanisms’ and in particular a more appropriate payment model for new AMs. The payment model should align payment with value, support stewardship goals by delinking payment from drug sales volumes and provide smooth revenue from the point of approval even for AMs which are expected to be subject to strict stewardship and only used as drug-resistance increases.
Cefiderocol received a marketing authorisation in April 2020 for treating infections due to aerobic Gram-negative organisms (GNO) in adults with limited treatment options. It is an injectable siderophore cephalosporin. It has a cephalosporin backbone with a catechol moiety at the three-position side chain. The catechol moiety chelates ferric iron, which allows cefiderocol to cross the outer membrane via the bacteria’s own active receptor-mediated iron transport system. 11,12 The cephalosporin core then binds primarily to penicillin-binding proteins, killing bacterial cells by inhibition of peptidoglycan cell wall biosynthesis.
Cefiderocol has been tested in three randomised controlled studies13–15 (one described as a descriptive study) which recruited patients with complicated urinary tract infection (cUTI), bacteraemia, hospital-acquired pneumonia (HAP), ventilator-associated pneumonia (VAP) and healthcare-associated pneumonia; and in two in vitro susceptibility studies16,17 against carbapenem non-sensitive isolates from global sources. Cefiderocol is active against all four Ambler classes of carbapenemase in GNB, including the MBLs (class B) most prevalent in the UK (NDM, VIM, IMP), and the serine carbapenemases most prevalent in the UK (KPC in class A and OXA-48 in class D)17,18 as well as the non-mutational causes of carbapenem resistance (Porin OprD and efflux pump) in P. aeruginosa. It is also active against other WHO priority pathogens on the critical list, including ESBL-producing E. coli, K. pneumoniae, Enterobacter spp., Serratia spp., Proteus spp., Providencia spp., and Morganella and as well as carbapenem-resistant P. aeruginosa and A. baumannii. 19
Chapter 2 Aims and objectives
This section reproduces and adapts, with permission, content previously published as part of the study protocol. 1
The aim of this evaluation is to assess the value of cefiderocol to the NHS in England for the treatment of severe aerobic GNB infections when used within its licensed indications.
Specific objectives are:
-
to identify two high-value clinical scenarios (HVCSs), within its broad licensed indications, for which cefiderocol is expected to have a significant impact on patients’ outcomes in terms of reducing mortality risks and improving health-related quality of life (HRQoL)
-
to undertake an ‘evidence mapping’ exercise and relevant systematic literature reviews to characterise the available clinical effectiveness evidence for the use of cefiderocol in the HVCSs
-
to establish an appropriate decision-analytic model to quantify the costs and health benefits of the use of cefiderocol under various usage scenarios compared with alternative treatments and management strategies (usage scenarios of other available AMs) in the HVCSs. The decision-analytic model was required to estimate costs and health effects at both the individual level and the aggregate population level, providing population-level incremental net health effects (INHEs)
-
drawing on the systematic reviews and evidence synthesis, national-level data on healthcare-associated infections and other sources as needed, to identify evidence to populate the decision-analytic models
-
to use structured expert elicitation as necessary to supplement the available evidence to populate the decision-analytic models at the levels of the individual patients and populations
-
to use available evidence and, where necessary, expert opinion to quantitatively extrapolate estimated population-level INHEs associated with cefiderocol in the HVCSs to other expected uses for the product beyond the HVCSs and within the product’s licensed indications.
A protocol for the study was developed and is available from the National Institute for Health and Care Excellence (NICE) website. 20
Chapter 3 Decision problem
This section reproduces and adapts, with permission, content previously published as part of the study protocol. 1
Decision-making context
The overarching purpose of the evaluation is to inform funding arrangements for cefiderocol in England. The drug’s funding will differ from that of drugs evaluated under NICE Technology Appraisals in two important ways. Firstly, the payment for cefiderocol will be delinked from usage volumes and, instead, represent a fixed annual payment over the term of the agreement (3 years in the first instance, followed by a potential extension to 10 years). Second, in a NICE Health Technology Assessment (HTA), the price is proposed by the manufacturer, whereas here the payment will be agreed via commercial discussions between the manufacturer (Shionogi) and NHS England, as informed by the evaluation. The role of the evaluation and subsequent NICE Committee deliberations will be to provide guidance on the value of cefiderocol to the NHS in England to inform these commercial discussions. This will include providing advice on the preferred usage of cefiderocol including the role of stewardship strategies (i.e. policies to ensure appropriate prescribing).
In previous work, the Policy Research Unit in Economic Methods of Evaluation in Health and Social Care Interventions (EEPRU) set out principles for quantitively evaluating the value of a new AM. 21 The starting point for this is to identify the range of ways in which cefiderocol can be used and to compare these scenarios to the range of ways in which other comparator AMs can be used (usage scenarios).
Value is defined as the expected impact of each usage scenario on population-level INHEs. Value is defined at the population rather than individual-patient level as payments to the manufacturer will reflect overall value to the English NHS. Population-level INHEs reflect expected population-level health benefits to patients and the wider population, expected population-level costs borne by (or savings accruing to) the NHS and a measure of the health opportunity cost of healthcare funds which allows NHS costs to be converted to health foregone. As the purpose of the evaluation work is to inform a value-based payment for cefiderocol, the drug acquisition cost for cefiderocol is excluded from the calculation of population-level INHE. The incremental value of cefiderocol is the difference between the population net health effects (NHE) associated with a given cefiderocol usage scenario and the highest-population NHE for clinically relevant usage scenarios that include only comparator AMs. This is shown in Box 1.
Assume a number of strategies are being compared for a given indication. AM(N)i represent strategies using the new AM, and AM(E)i are strategies for existing treatments. Table 1 provides illustrative estimates of the expected per patient treated costs (Column B) and health effects in terms of QALYs per patient (Column C), over the relevant time horizon. The costs of the new AM strategies assume zero acquisition cost for the new product. Any indirect effects on others through changes in resistance are assumed to be reflected in the QALYs per patient treated.
Column D shows the expected per patient NHEs in terms of QALYs. This is calculated as QALYs−Costk, where k is the estimate of health opportunity cost which in this illustration is £15,000 per QALY. Column E details the expected population NHEs in QALYs assuming the potential to benefit 6000 patients over the time horizon of the analysis. AM(N)3 represents the best of the strategies involving the new AM, with an expected population NHE of 54,104 QALYs for the new AM. To calculate the value of the new drug in NHEs, the difference in population NHE between AM(N)3 and the best of the strategies using existing treatments is calculated (54,104–50,400 = 3704 QALYs). This is the population INHE that is the focus of the current assessment as it will inform the value-based payment for the new treatment.
A | B | C | D | E |
---|---|---|---|---|
Strategy | Expected costs, per patient treated | Expected QALYs, per patient treated | Expected net health benefit (QALYs), per patient treated | Expected population net health benefit (QALYs) |
AM(N)1 | 6800 | 9.0 | 8.547 | 51,280 |
AM(N)2 | 7000 | 9.3 | 8.833 | 53,000 |
AM(N)3 | 7240 | 9.5 | 9.017 | 54,104 |
AM(E)1 | 7500 | 8.9 | 8.400 | 50,400 |
AM(E)2 | 7800 | 8.5 | 7.980 | 47,880 |
AM(E)3 | 7600 | 8.4 | 7.893 | 47,360 |
As the population-level INHEs will inform the value-based payment to the manufacturer, they should reflect the overall value resulting from expected NHS usage. Expected NHS usage, in principle, reflects both the preferred usage specified in NICE guidance and the implications of clinical decisions taken locally.
As documented in New antimicrobials, the licensed indication for cefiderocol is fairly broad, being available to any patient with limited treatment options, regardless of the site of the infection. In practice, to control the spread of resistance to cefiderocol and to preserve its long-term viability as an effective treatment option, cefiderocol is expected to be used in a more restricted group of patients than permitted by its license. Quantifying the health and cost implications of using cefiderocol across anticipated NHS usage, even within this restricted population, remains challenging as use is expected across infections which differ in causative organism (pathogen, susceptibility and resistance mechanism), site of the infection, healthcare setting and other underlying features of the health status of the patient.
This evaluation will seek to characterise the value of cefiderocol across its range of expected uses using two approaches. Firstly, decision modelling will be used to evaluate the value of cefiderocol quantitatively in two scenarios defined by features of the pathogen, site of infection, healthcare setting and other patient characteristics, considered to represent important uses of cefiderocol (referred to as the HVCSs). Secondly, we will provide additional information and quantitative estimates to support the NICE Committee in assessing value in the overall population expected to receive cefiderocol in the English NHS including patients who fall outside the HVCSs.
The literature on the economic evaluation of AMs has described a range of elements of value associated with AMs that are not relevant to other interventions and previous work by EEPRU has sought to explain how these elements of value can be quantified in terms of population-level INHEs. 21 As part of the current evaluation, we assess the extent to which these additional elements of value are likely to apply in the context of cefiderocol and quantify them where this is feasible and they are expected to be quantitatively important.
The resourcing for this project was equivalent to that of a diagnostic assessment review or multiple technology assessment for NICE, but the levels of analysis extend from the typical focus of those evaluations on a single type of patient for one indication and setting. In this evaluation, we also include population-level health effects now and over time, and across several indications and settings. The objective is to use appropriate analyses of the available evidence at every level, but the detail in those analyses is inevitably constrained by the time and resources available for the project.
High-value clinical scenarios
Pathogen and resistance mechanisms
An important determinant of the efficacy of existing treatment and, therefore, to defining those patients most likely to benefit from cefiderocol, is the pathogen causing the infection and its mechanism of resistance.
Feedback during the NICE scoping consultation for cefiderocol, and subsequent consultation with clinical experts, has emphasised that cefiderocol should be prioritised for the treatment of patients with infections with confirmed or suspected carbapenem-resistant GNB in secondary/tertiary care. Carbapenem-resistant pathogens can be categorised according to two main classes of resistance mechanisms as discussed in detail in Antimicrobial resistance. For infections caused by carbapenem-resistant organisms with non-carbapenemase resistance mechanisms, a range of treatment options remains available. Infections caused by carbapenemase-producing pathogens have fewer treatment options. There are two main classes of carbapenemase-producers: serine carbapenemases and MBLs. The main serine carbapenemases among CRE in the UK are OXA-48 and KPC. The main MBLs in the UK are NDM, VIM and IMP.
Cefiderocol is active against MBLs and serine carbapenemases. It is also effective against a wide range of pathogens, including Enterobacterales and the non-fermenters P. aeruginosa and A. baumannii. Based on the rates reported in the latest available data from the English Surveillance Programme for Antimicrobial Utilisation and Resistance (ESPAUR) (based on BSIs),6 the most common carbapenem-resistant organisms are P. aeruginosa and Enterobacterales. Infections by A. baumannii seem to represent a lower share of carbapenem-resistant GNB infections, but where the pathogen has OXA mechanisms of resistance (OXA-40/24, OXA-51, OXA-58, OXA-143), there are very limited treatment options. For MBLs, there are often even more limited treatment options, and in some cases only cefiderocol is expected to be active. There may also be a use for cefiderocol in serine Enterobacterales which have more treatment options than MBLs; for example, in a stewardship/diversity treatment strategy. For this evaluation, the HVCSs focus on the treatment of infections caused by MBL Enterobacterales and P. aeruginosa based on the limited available treatment options for these patients. Clinicians and stakeholders to the project emphasised that cefiderocol may also be a relevant treatment option for patients with infections caused by Stenotrophomonas which is innately MBL; however, this was only discussed subsequent to the development of the HVCSs.
Availability of susceptibility data during the course of an infection
Infections in secondary/tertiary care are typically initially treated with empirically chosen antibiotics. At this stage of treatment, there is limited information available to inform treatment choice. Indicators of an elevated risk of carbapenem resistance at this stage include a range of patient- and setting-specific risk factors. Patient-level factors include prior microbiology history, recent history of hospital or long-term care admissions or regular hospital-based treatments, epidemiological links to other carriers, international travel, immunosuppression and recent broad-spectrum antibiotic exposure. Setting-specific factors include being admitted to augmented care or high-risk units and local epidemiology (e.g. previous history of outbreaks). 22
In some hospitals and tertiary care centres, screening for carriage of carbapenem-resistant pathogens is carried out. Routine screening for colonisation with Enterobacterales at the point of admission has recently been recommended by Public Health England (PHE) for specific high-risk patients and healthcare settings. 22 The objective of this screening is primarily to support enhanced infection control measures, surveillance and outbreak management efforts. However, information obtained via screening may also support treatment choice as colonisation with Enterobacterales is a risk factor for an Enterobacterales infection. Currently, implementation of screening for Enterobacterales is variable in the UK despite the PHE guideline,22 and the level and timing of information provided via screening also varies.
At the point an invasive bacterial infection is suspected, where possible, specimens are obtained to support further diagnostic work. Various diagnostic technologies can be used to better understand the causative pathogen and how it may respond to treatment. There are broadly three layers to this:
-
A culture is undertaken to understand the type of pathogen causing the infection.
-
Antimicrobial-susceptibility testing is conducted to assess the in vitro activity of a range of AMs against the pathogen in question.
-
Gene testing may also be conducted to establish the presence of specific resistance mechanisms.
Cultures are typically available relatively quickly with AM-susceptibility testing and gene testing taking longer [typically more than 48 hours, although this depends on local availability of testing technology and laboratory capacity; e.g. centres with access to polymerase chain reaction (PCR) testing may have information much more quickly]. The availability of gene testing also varies geographically. There may be an increase in the use of gene testing in the UK in the future as PHE has recently recommended routine use of molecular or immunochromatographic assays to detect the main carbapenemase producers. 23
Overall, variability in local practice, laboratory capacity and availability of diagnostic technologies means that there is likely to be significant variation in the nature and timing of the information available to inform treatment decisions.
Overview of high-value clinical scenarios
Based on feedback from stakeholders via the NICE scoping consultation and further discussion with clinical experts, EEPRU has identified two HVCSs for the use of cefiderocol: microbiology-directed treatment and risk-based empiric treatment. We explain these separately here but, in practice, they are often linked in a single-patient pathway.
Microbiology-directed treatment refers to the use of cefiderocol in individuals with infections caused by a pathogen confirmed to have a specific pathogen and resistance mechanism. This group of patients has undergone susceptibility testing and gene testing to understand specific resistance mechanisms. As this usage of cefiderocol will require susceptibility/gene testing to have been undertaken prior to receipt of cefiderocol, this clinical scenario will focus on individuals with non-critical infections. Population, intervention, comparison, outcomes, study designs for high-value clinical scenarios describes in more detail the specific Population, Intervention, Comparison, Outcomes, Study designs (PICOS) considered for this scenario.
Risk-based empiric treatment refers to the use of cefiderocol in the empiric setting (ES) for clinically urgent patients with high suspicion (i.e. a high risk) of specific carbapenem resistance based on patient phenotype but for whom information about the pathogen is currently very limited (susceptibility data and gene testing not yet available). Use within this HVCS should be restricted only to those patients in whom microbiology-directed treatment is likely to be considered inappropriate due to the potential delay in time to appropriate therapy. The risk-based empiric treatment HVCS is, therefore, focused on patients who meet two criteria: (1) the infection is considered clinically urgent based on a range of information including infection site and severity, and broader information relating to the health status of the patient; and (2) the patient is considered at elevated risk of a specific type of carbapenem-resistant infection using the type of risk markers described in Availability of susceptibility data during the course of an infection. Population, intervention, comparison, outcomes, study designs for high-value clinical scenarios describes in more detail the PICOS for this scenario.
Population, intervention, comparison, outcomes, study designs for high-value clinical scenarios
Based on feedback from stakeholders via the NICE scoping consultation and further discussion with clinical experts, EEPRU has defined the PICOS for HVCS for the microbiology-directed and risk-based empiric treatment pathways (see Table 2). The PICOS refine the NICE scope, which is broad and reflects the license of cefiderocol, to reflect the HVCS.
Element | MDS | Risk-based ES |
---|---|---|
Population – patients | Where microbiological susceptibility testing with gene testing has been performed | With clinically urgent disease with high risk of an infection caused by a resistant pathogen. Suspicion of infection may be based on knowledge of the local epidemiology where a patient was previously hospitalised, outbreak in the ward where the patient is currently admitted or previous cultures (taken during the current or previous hospital stays) showing the patient was colonised by MBL-producing Enterobacterales or P. aeruginosa |
Population – pathogen mechanism | Infections (Enterobacterales and P. aeruginosa) confirmed to be caused by MBL of the following subtypes:
|
Infections (Enterobacterales or P. aeruginosa) suspected to be caused by MBLs of the following subtypes:
|
Population – site of infection |
|
HAP/VAP |
Intervention | Cefiderocol alone or in combination | Cefiderocol alone or in combination |
Comparators Please note: These comparators reflect NHS practice based on clinical advice. The available evidence determines which of those listed (and possible additional products including combinations) can be formally incorporated into the modelling |
Comparators used in clinical practice in England, as defined by susceptibility testing and/or gene testing and considering infection site and infiltration data. Potential comparators include: | Comparators used in clinical practice in England, based on high risk of an infection, which include: |
Enterobacterales
:
|
Enterobacterales:
|
|
P. aeruginosa:
|
P. aeruginosa:
|
|
Outcomes | The outcome measures to be considered include:
|
Same as MDS |
|
||
Study designs | The types of studies and data to be considered include:
|
Same as MDS |
Microbiology-directed treatment: In the microbiology-directed usage scenario, feedback from stakeholders and clinical experts indicated that cUTIs have high prevalence and a slower clinical course than, for example, HAP and VAP. They are also responsible for a high proportion of BSI, the reduction of which is a key priority for NHS England (NHSE). cUTIs were therefore selected as the infection site for the microbiology-directed HVCS, with additional analysis also provided for HAP/VAP in the microbiology-directed setting (MDS).
Clinical and stakeholder advice also indicated that cefiderocol would be reserved for infections with limited treatment options, where susceptibility is demonstrated. This suggests that cefiderocol should be reserved to treat infections caused by carbapenemase-producing pathogens. As discussed in High-value clinical scenarios, cefiderocol is active against MBL and serine mechanisms, and against a wide range of pathogens. Based on clinical feedback, the patient group for the HVCS will be limited to patients with infections caused by MBL Enterobacterales and P. aeruginosa, since there are extremely limited treatment options for patients with these infections, and feedback from clinicians suggested that they would like to preserve cefiderocol’s effectiveness by restricting its use.
Cefiderocol can be used as a monotherapy but may also be used in combination with other treatments, as indicated by microbiology and gene testing. In clinical practice, alternative treatment options (comparators) would be defined by the results of susceptibility and gene testing.
Risk-based empiric treatment: In the risk-based empiric usage scenario, feedback from stakeholders and clinicians indicated that the most frequent clinically urgent infections are HAP/VAP and BSI. cUTIs were not considered relevant in this setting since they have a slower clinical course, giving time for susceptibility testing and genetic testing to be performed. Given the time and resources available for this project, the focus will be on the HAP/VAP sites as this was considered the most common indication for empirical antibiotics in high-risk patients such as those in the intensive care unit (ICU) or high-dependency unit (HDU) (whereas patients with BSI are more likely to have had microbiology). Patients will be those who have a high risk of a MBL Enterobacterales or P. aeruginosa infection. Focusing on this high-risk group was highlighted by the clinical advisors to this project as preferable to considering a broader group of patients with suspected carbapenem resistance, even if deteriorating rapidly on current therapy, as the latter group would be difficult to define and may lead to high levels of prescribing with associated risks of resistance emergence. Three patient characteristics were considered as relevant by our clinical advisors in identifying patients at high risk of a MBL Enterobacterales or P. aeruginosa infection: a high rate of MBL Enterobacterales or P. aeruginosa in a healthcare setting where the patient was previously admitted, an outbreak of MBL Enterobacterales or P. aeruginosa in the ward where the patient is currently admitted, or previous cultures (taken during the current or previous hospital stays) showing the patient was colonised by a MBL Enterobacterales or P. aeruginosa. Cefiderocol may be used as a monotherapy in this usage scenario or may be used in combination with other treatments to provide a broader spectrum of coverage. A range of comparators are relevant in this setting. Once microbiology has confirmed the susceptibility profile and mechanisms of resistance of the pathogen, treatment may be continued or stopped, dosage may be altered or different AMs may be initiated.
Chapter 4 Clinical evidence
This section reproduces and adapts, with permission, content previously published as part of the study protocol. 1
The evidence reviews reported within this section focus on the clinical evidence required to inform the patient-level component of the decision-analytic modelling. This includes estimating the comparative effectiveness of treatments, including both efficacy and safety, and the consequences of treatments in terms of long-term clinical outcomes, for both efficacy and safety. Clinical evidence that informs the population-level components of the analysis is described in Modelling direct population net health effects in high-value clinical scenario and Extrapolation from high-value clinical scenario to expected usage.
Approaches to estimating comparative effectiveness
Sources of evidence
In comparison to a standard HTA, the data available for evaluating new AMs are less straightforward. This has been discussed in detail in EEPRU’s framework. 21 This is largely because the randomised controlled trial (RCT) evidence is primarily generated for regulatory purposes, to demonstrate safety and efficacy against a range of pathogens. Trials are usually non-inferiority in design (usually with a −10% margin), and the comparators tend to be best available therapy (BAT). Patients with extensively drug-resistant infections, such as those with MBL infections, are usually excluded from these trials because it would be unethical to randomise patients to an ineffective comparator treatment, and testing patients to find out which treatments they are susceptible to could introduce critical time delays in treatment of very ill patients. Therefore, trials tend to recruit patients who are expected to be susceptible to the intervention and the comparator, that is, not extensively drug resistant. The relative treatment effect generated by such trials cannot be generalised to resistant populations, since this would overestimate the efficacy of the comparators, as resistant patients are unlikely to respond as well to BAT. In addition, BAT within the trials may not match clinical practice in England since best practice is highly variable due to local protocols reflecting testing capacities and the microbiological epidemiology in a given area. Regulatory trials also do not tend to address differences in treatment pathways, such as are found between the MDS and risk-based ES, or differences in stewardship protocols, such as rotation of AMs, mixing treatments or combination therapies. For the evaluation within the MDS, RCTs and observational studies are required that report outcomes in patients with the confirmed pathogen-mechanism combination of interest, while in the ES, patients will only be suspected of having an infection with the pathogen-mechanism combination of interest.
As such, from the outset, EEPRU were aware that additional sources of evidence may be required to fulfil the comparative effectiveness component, since it was unlikely that the RCTs would have been performed in patients with infections caused by the specific pathogen mechanisms of interest. The next levels of evidence in the evidence hierarchy are non-randomised studies and observational studies. EEPRU’s earlier work21 also highlighted the potential for using susceptibility studies to supplement clinical data. We therefore aimed to identify all these possible sources of evidence in our review (see Review questions). In the next section, a brief description of susceptibility studies is provided, since this study design is one that is not commonly encountered. Following this, a discussion of how the different study designs might be used to produce effectiveness estimates is provided (see Producing comparative efficacy estimates).
Susceptibility studies, pharmacokinetic/pharmacodynamic studies and breakpoints
Susceptibility studies are in vitro studies that report the results of AM-susceptibility testing (AST). AST is a laboratory method where isolates taken from patients (from infections or during screening) are grown in vitro (cultured) and tested for their susceptibility to various AM treatments. The AM being tested is applied at increasing concentrations to separate cultures of the sampled isolate, and the degree to which microbial growth is inhibited at each concentration is assessed. The lowest concentration at which microbial growth is inhibited is known as the minimum inhibitory concentration (MIC).
Clinical breakpoints distinguish between isolates where there is a likelihood of treatment success from those where treatment is more likely to fail. 24 If the MIC of a given isolate is at or below the breakpoint, the isolate is judged to be ‘susceptible’ (S). If it is above the breakpoint, the isolate is judged to be ‘resistant’ (R). For some AMs, there is also an intermediate category (I), which more recently has become ‘susceptible – increased exposure’ indicating that a higher dose of the drug should be used to elicit a response. They may also report the concentration at which 50% of isolates were inhibited (MIC 50), and the concentration at which 90% were inhibited (MIC 90).
The methods for setting breakpoints are not standardised. Currently, they are generally set by considering:24
-
the pharmacokinetic (PK) data: how the body affects the drug with respect to absorption, distribution, metabolism and excretion, usually obtained from studies in healthy volunteers
-
the pharmacodynamic (PD) data: how the drug affects the body (efficacy and toxicity) at its site(s) of action, usually obtained from in vitro studies, hollow fibre studies, animal studies and human studies. These data are used to set PD targets, for example for time above MIC
-
mathematical models (e.g. Monte Carlo simulation) to assess the likelihood of achieving the targets suggested by the PD data
-
any available clinical data linking treatment to clinical outcomes (e.g. from RCTs or observational studies).
Pharmacokinetic/pharmacodynamic studies are conducted to estimate how much drug will be available at the site of interest, and for what period of time at a given dose. One of its primary uses is by manufacturers and regulatory bodies to decide on the appropriate dose and dose frequency of the drug, such that it is likely to be available at concentrations that are likely to have an effect at the sites of interest.
There are two main organisations that set breakpoints, the Clinical Laboratory Standards Institute (CLSI) in the USA and the European Committee on Antimicrobial Susceptibility Testing (EUCAST) in Europe. These two organisations use different methodologies to set breakpoints, leading to differences in the breakpoints set both in absolute and relative terms, between treatments. They also describe different laboratory methods to assess MICs. In addition, many labs may use commercial assays, conducted according to manufacturer’s instructions. Clinical advisors to EEPRU indicated that it was unclear to what extent CLSI, EUCAST and commercial methods would produce the same absolute values, and in the event that values were different, whether relative values between treatments would also be different (i.e. the difference in absolute values was not consistent across treatments). In the UK, the British Society for Antimicrobial Chemotherapy (BSAC) now recommends use of EUCAST methods and breakpoints.
Susceptibility studies tend to report the proportion S, I and R, or list the number of isolates at each MIC. An example is given in Table 3. Here, for cefepime, the breakpoint is 1 mg/l, and since all isolates had MICs higher than the breakpoint, none were susceptible. For cefiderocol, with a breakpoint of 8 mg/l, 90.9% were susceptible, since only one isolate had a MIC above this point.
Treatment; breakpoint | Number susceptible, cumulative % susceptible | |||||||||||
---|---|---|---|---|---|---|---|---|---|---|---|---|
Drug concentration (mg/l) | ≤ 0.06 | 0.12 | 0.25 | 0.5 | 1 | 2 | 4 | 8 | 16 | 32 | > 32 | Susceptible (%) |
Cefepime (n = 11) BP: 1 mg/l |
4 36 |
3 64 |
1 73 |
3 100 |
0 | |||||||
Meropenem (n = 11) BP: 2 mg/l |
4 36 |
3 64 |
0 64 |
1 73 |
3 100 |
100 | ||||||
Cefiderocol (n = 11) BP: 8 mg/l |
1 9 |
0 9 |
0 9 |
1 18 |
0 18 |
3 45 |
5 91 |
0 91 |
0 91 |
0 91 |
1 100 |
90.9 |
Producing comparative efficacy estimates
Three main approaches, relating to the three main types of evidence available were developed:
-
Approach 1: review RCTs for any subgroup data relating to the pathogen-mechanism sites defined in the HVCSs and use these estimates to inform the model. A network meta-analysis (NMA) would likely be needed to provide estimates for the intervention and comparators, and all these studies would also need to be in the pathogen-mechanism sites defined in the HVCSs.
-
Approach 2: construct a network of observational studies relating to the pathogen-mechanism sites defined in the HVCSs, treated with cefiderocol and comparators. Individual patient data (IPD) would be required for at least one study to adjust for confounders.
-
Approach 3: use susceptibility studies (see Susceptibility studies, pharmacokinetic/pharmacodynamic studies and breakpoints above), that is, those that have tested relevant treatments in MBL Enterobacterales and P. aeruginosa isolates in vitro, to provide estimates of relative treatment effects. Conduct a NMA of susceptibility evidence, if necessary, to link the intervention and its comparators. Link in vitro susceptibility to clinical outcomes. Two approaches to linking susceptibility to clinical outcomes were considered:
-
Assume that, for patients who are susceptible to the treatment they are given, clinical outcomes would be similar regardless of the treatment received.
-
Assume that different treatments may result in different outcomes even among those susceptible to their treatment. Use evidence from a NMA of RCTs (in any susceptible pathogen mechanism, not just those considered within our HVCS) to estimate differences in treatment outcomes among susceptible patients. These relative treatment effects would then be applied to the proportion susceptible to the intervention and comparators, taken from the susceptibility NMA or epidemiological data.
-
Each of these approaches has its own merits and challenges.
In Approach 1, the difficulties with recruiting resistant patients mean subgroup data from RCTs may be underpowered and under-representative of the full spectrum of MBL Enterobacterales and P. aeruginosa infections. Where available, however, they could provide estimates with high internal validity (low risk of bias). Equivalent data for comparators from RCTs may be missing in the pathogen-mechanism sites of interest.
In Approach 2, comparative observational studies are often at high risk of confounding due to imbalances between prognostic and/or predictive factors at baseline, while comparisons across single-arm studies would require advanced synthesis techniques to mitigate against any apparent imbalances. Results from such analyses can be prone to a high degree of uncertainty and there may be residual confounding, for example, from imbalances in unknown or unobserved confounders. However, such studies may be able to include higher numbers of patients, since the barriers to recruitment described for RCTs are reduced.
In Approach 3, susceptibility studies have the advantage of testing all the treatments in the same sample of isolates, thereby reducing the chance of heterogeneity in patient samples between arms introducing confounding. They also tend to include higher numbers of patients/isolates. However, any given susceptibility study will have its own distribution of susceptibilities for each treatment, which give rise to the comparative treatment effects as expressed by % susceptibility, and this may not match the susceptibility profile of pathogens circulating in the UK, or that are likely to circulate in the future. In addition, susceptibility studies are in vitro, and no clinical outcomes are reported. In order to use this approach in the model, additional evidence requirements would be created since susceptibility can be considered a surrogate end point. It would be necessary to link susceptibility to clinical outcomes such as clinical cure, 30-day mortality, 90-day mortality, hospital length of stay (LoS), long-term mortality and recurrence of infections (see questions 4–6 below). As noted above, this approach would assume that, conditional upon susceptibility, clinical outcomes are similar across different AMs. An extension to this approach would be to use evidence from a NMA of RCTs (in broader populations than those considered within our HVCS) to estimate differences in treatment outcomes among susceptible patients regardless of the pathogen mechanism they are infected by, but dependent on the AM they were treated with. This would assume that relative treatment effects between AMs are generalisable across pathogen mechanisms, so long as patients were susceptible to the treatment they were given. For both approaches, these assumptions would need to be supported by empirical evidence and/or expert opinion.
Review questions
For each approach, a corresponding review question was developed. This section briefly states each review question, while sections Review methods, Review results and Statistical synthesis describe the PICOS, methods of evidence retrieval and results for each question. Subsequently, Additional review questions for Approach 3 describes three additional reviews (reviews 4–6) relating to Approach 3.
Review 1
Review question 1: Based on RCT evidence, what is the comparative effectiveness of the intervention and comparators in patients with cUTI or HAP/VAP caused by a MBL Enterobacterales or P. aeruginosa infection?
In addition to recruiting patients infected with the relevant pathogen-mechanism combination, the ideal study would be based on treatment in the UK or a country with a similar demographic and healthcare system, to reduce the impact of other factors on patient outcomes. Only evidence relating to the sites of interest would be relevant, since the risk of mortality and morbidity from infections at other sites is likely to be different.
Review 2
Review question 2: Based on observational studies, what is the comparative effectiveness of the intervention and comparators in patients with cUTI or HAP/VAP caused by a MBL Enterobacterales or P. aeruginosa infection?
Again, as well as recruiting patients infected with the relevant pathogen-mechanism combination, the ideal study would include patients in the UK or a country with a similar demographic and healthcare system, and would be in the sites of interest.
Review 3
Review question 3: What is the comparative effectiveness of the treatment and comparators based on in vitro susceptibility studies?
Because of their in vitro nature, and since clinical experts to EEPRU indicated that the site of the infection the isolate was obtained from was unlikely to affect the susceptibility profile of the infecting pathogen, isolates could be collected from any site.
Table 4 provides a summary of the alternative approaches to estimating comparative efficacy and safety.
Approach number | Studies design | Review question and number | Analytical approach | Taken forward (with reasons)? | Further detail |
---|---|---|---|---|---|
1 | RCTs |
|
NMA to estimate comparative efficacy | No, insufficient evidence in patients with MBL Enterobacterales or P. aeruginosa infections | See Reviews 1 and 2 |
2 | Observational studies |
|
Matched analysis | No, small studies, data not reported specific to the sites of interest; IPD not available | See Reviews 1 and 2 |
3 | Susceptibility studies |
|
NMA to estimate comparative efficacy from susceptibility studies; link susceptibility to clinical outcomes | Yes | See Review 3 |
Any clinical study |
|
See Review questions 4 and 5 | |||
Any clinical study |
|
To supplement approaches 1–3 | See Review questions 4 and 5 | ||
RCTs |
|
To supplement approaches 1–3 | See Review question 6 |
Review methods
Since review questions 1–3 were of central importance to estimating the comparative efficacy of treatments, a de novo search from database inception was undertaken to address all three questions. The nature and suitability of the evidence base were unknown but as already discussed, there was a strong expectation that RCT evidence would not be of high relevance, that is to say, would not have recruited patients with MBL Enterobacterales or P. aeruginosa infections. It was also unclear at this stage to what extent multiple HVCSs (e.g. including BSI) might be addressed in the evaluation (see Table 5 below). Therefore, a map of the available evidence was first constructed to maintain flexibility, and to aid an informed focusing of the inclusion criteria as the project proceeded (see Table 6). This methodology has been used elsewhere and is especially suited to topics such as this where the initial scope is broad. 25,26 The map comprised data extraction of key study characteristics. It was based on systematic literature searches of key bibliographic databases (see Search strategy below) supplemented by evidence submitted by experts and stakeholders, including the submission received from Shionogi and data requests to PHE, Shionogi and Pfizer [(who were participating in a concurrent EEPRU evaluation of ceftazidime-avibactam (CAZ-AVI)]. Evidence was then selected for further consideration according to a balance of relevance with study quality, as recommended in the Decision Support Unit Technical Support Document (TSD) 13. 27 Where preferred sources did not yield data, additional focused searches were employed to ensure that studies had not been missed or to fill evidence gaps. Where additional searches still did not yield data, elicitation was performed to fulfil the evidence requirement (see Structured expert elicitation).
Characteristic | Keyword mappinga | Key characteristics tabulationa | Selection for synthesis |
---|---|---|---|
Population | |||
Patients | Adults or children | Adults | Isolates from adults or children recruited consecutively, purposively, by convenience or as part of another study, for example, RCT Screening or invasive samples |
Pathogen mechanism | MDS: MBL Enterobacterales or P. aeruginosa; KPC Enterobacteralesb ES: suspected carbapenem-resistant Enterobacterales or P. aeruginosa treated empirically |
MDS: MBL Enterobacterales or P. aeruginosa; KPC Enterobacteralesb ES: suspected carbapenem-resistant Enterobacterales or P. aeruginosa treated empirically |
MBL Enterobacterales or P. aeruginosa |
Site of infection | RCTs: any site Observational studies and case series: cUTI, HAP/VAP or BSIb Susceptibility studies: any site |
RCTs, observational studies and case series: cUTI, HAP/VAP Susceptibility studies: any site |
Susceptibility studies: any site |
Setting | MDS or ES | MDS or ES | Any country; UK, Europe, USA, Canada, Australia, Asia and Middle East have highest relevance |
Intervention | |||
Cefiderocol | Cefiderocol | Cefiderocol | |
Comparators | |||
Any | Any | At least one of: colistin, meropenem, tigecycline, aztreonam, fosfomycin, gentamicin, amikacin, tobramycin | |
Outcomes | |||
As listed in section Population, intervention, comparison, outcomes, study designs for high-value clinical scenarios | As listed in section Population, intervention, comparison, outcomes, study designs for high-value clinical scenarios | In vitro susceptibility reported as proportion susceptible (not including intermediate) according to EUCAST or CLSI criteria Studies only reporting MIC50 and/or MIC90 with range were excluded |
|
Study designs | |||
RCT, observational studies, case series, susceptibility, PK/PD | RCT, observational studies, case series, susceptibility, PK/PD | Susceptibility studies where isolates were collected and tested retrospectively or prospectively |
Topic | Summary of clinical response |
---|---|
Location | Europe, USA, Canada, Australia, the Middle East and Asia have the most relevance since pathogens tend to arrive in the UK from these countries. South America to a lesser extent |
Date of recruitment | Studies from 2012 onwards have highest relevance. Likely to observe increases in resistance over time |
Sampling strategy and outbreaks | Consecutive sampling (which is often associated with studies of outbreaks) not necessarily more generalisable, since outbreaks will reflect a narrow spectrum of pathogens and may therefore underestimate diversity of susceptibility; multicentre studies should be more reflective of the diversity of isolates and should include outbreaks proportionate to their occurrence |
Isolates from screening | These are relevant since they will reflect the diversity of susceptibility found. Development of an infection is not dependent on the pathogen or mechanism per se, and so screening samples should be generalisable to infected patients |
AM-susceptibility testing laboratory methodologies | There are differences between EUCAST and CLSI methodologies (see Susceptibility studies, pharmacokinetic/pharmacodynamic studies and breakpoints), and it is unclear whether the two methodologies result in the same distribution of MICs at the same values for a given set of isolates. If the distribution or absolute values differ, the methodologies cannot be considered interchangeable. EEPRU were unable to identify any literature directly comparing the two methodologies for the treatments in the HVCSs and concluded that methodologies could not be assumed to be interchangeable |
Breakpoints | Expert advice indicated that CLSI and EUCAST breakpoints differ and cannot be assumed to be interchangeable (see Susceptibility studies, pharmacokinetic/pharmacodynamic studies and breakpoints). It is unclear whether studies using EUCAST laboratory methods and breakpoints would return the same percentage susceptible as studies using CLSI laboratory methods and breakpoints. It cannot be assumed that breakpoints from one guideline can be applied where laboratory methods from the other guideline have been used |
PK/PD data | Clinical advisors stated that the methodologies for conducting PK/PD data are not standardised and it is difficult to ascertain whether a study has been conducted well. Since the breakpoints set by EUCAST and CLSI are based on an assessment of the available PK/PD data, and as long as the treatment is known to infiltrate the appropriate site, it is reasonable to assume that susceptibility can be linked directly to clinical outcomes without further explicit consideration of PK/PD evidence. The advisors stated that cefiderocol and the comparators for each site penetrate to the sites of interest and it was therefore considered unnecessary to review these data |
Search strategy
The search strategy comprised terms relating to the treatment (cefiderocol). Terms relating to case reports, letters and (animals NOT humans) were used to narrow the search, but no other study design filters were applied.
The following electronic databases were searched from database inception:
-
MEDLINE and Epub Ahead of Print, In-Process, In-Data-Review and Other Non-Indexed Citations, Daily and Versions: Ovid, 1946 to present
-
EMBASE: Ovid, 1980 to present
-
The University of York Centre for Reviews and Dissemination (CRD) platform:
-
Database of Abstracts of Reviews of Effects (DARE): CRD, 1994–2015
-
HTA Database: CRD, 1989–2018
-
NHS Economic Evaluation Database (NHS EED): CRD, 1972–2015.
-
The search strategies are provided in Appendix 1.
In addition to the database searches, the following unpublished data were requested:
-
PHE.
Evidence on susceptibility to MBL Enterobacterales and P. aeruginosa for cefiderocol and the comparators defined by the HVCS were requested from PHE. This is detailed in Report Supplementary Material 1.
-
Data request to Shionogi (see Report Supplementary Material 1):
-
Submitted to NICE on 11 August 2021: any MBL Enterobacterales or P. aeruginosa susceptibility data they had access to, for cefiderocol and the HVCS comparators, specifically relating to SIDERO studies and studies reported in two other publications, at both CLSI and EUCAST breakpoints (see Susceptibility studies, pharmacokinetic/pharmacodynamic studies and breakpoints for description of breakpoints).
-
As will become apparent in Limitations of the data available from the published study reports, insufficient evidence was identified relating to fosfomycin, one of the comparators. An additional focused search for fosfomycin studies was conducted. Due to time constraints, the search was conducted in PubMed only (see Appendix 1, Fosfomycin search strategy for the search strategy) on 26 August 2021.
Two surveillance databases were also identified and queried for data that could be included in the review [Antimicrobial Testing Leadership And Surveillance (ATLAS) (https://atlas-surveillance.com/#/login) and SENTRY] (https://sentry-mvp.jmilabs.com/), but neither currently lists cefiderocol in the open access portal.
Keyword mapping, study selection, data extraction and quality assessment
Citations retrieved by the search were uploaded to EndNote (Clarivate Analytics, Philadelphia, PA, USA), deduplicated and considered for inclusion in the review.
Keyword mapping: citations that met the inclusion criteria listed in Table 5 were tagged in EndNote (Clarivate Analytics) by one reviewer, according to key study characteristics: treatment (cefiderocol); study design (RCT, observational, susceptibility, PK/PD); mechanism (MBL, other); pathogen (Enterobacterales, P. aeruginosa, other); and site (cUTI, HAP/VAP, BSI, other). All potential sources of evidence, including RCTs, observational studies, in vitro studies and national, local or international datasets identified in the grey literature were included in this stage of mapping.
Key characteristics mapping: a subset of studies that met the inclusion criteria listed in Table 5 were selected for key characteristics tabulation by one reviewer. The full text of RCT and observational studies identified as being potentially relevant based on their title and abstract were consulted in the first instance, and studies were tabulated and assessed for relevance against the key characteristics mapping criteria, as well as for relevance to the model. Since an assessment of this map concluded that insufficient relevant in vivo evidence was identified (see Reviews 1 and 2), the next level of evidence (susceptibility studies) was also tabulated.
Key study characteristics tailored to the study designs of interest (e.g. sample size, population, pathogen, mechanism, site, outcomes reported, susceptibility methodology, see Report Supplementary Material 1) were tabulated by one reviewer. Data relating to numeric outcomes were not extracted and quality assessment was not performed at this stage.
Study selection: the inclusion criteria for the mapping are listed in Table 5. At the final stage of study selection, only susceptibility studies were considered since other sources did not meet the requirements of the project. The reasons for this decision are detailed in Study selection results (reviews 1–3). Advice was sought from clinical advisors to aid the assessment of the relevance of susceptibility studies to the HVCSs, and to inform the final selection of evidence. Factors including location, date of recruitment, sampling strategy, screening and outbreak populations and susceptibility testing methodologies were considered, and decisions made (see Table 6). At this point, a decision was made not to review the PK/PD data, since these data are reviewed when setting breakpoints, and since clinical advisors to EEPRU stated that since the treatment and comparators penetrate to the sites of interest it was therefore reasonable to link directly between susceptibility and clinical outcomes (see Table 5).
Due to time restrictions on the project, only studies reporting susceptibility to both cefiderocol and also to any one of the comparators listed in Table 2 were included. This is a pragmatic approach to evidence retrieval, since ideally all susceptibility data relating to all comparators would have been included in the evidence synthesis but searches to identify this evidence would have been large. No studies reported combinations of AMs, the process for estimating efficacy for combination treatments using the results of the evidence synthesis are described in Clinical parameters – susceptibility evidence. Consequently, studies reporting susceptibility to both cefiderocol and also to any one of the comparators listed in Table 2 were included.
As mentioned previously (see Search strategy), no susceptibility data for fosfomycin were identified in the initial search and data requests, necessitating a separate search for studies relating to fosfomycin. This means a different approach has been taken for this comparator which may introduce bias if studies reporting data for fosfomycin are systematically different to those reporting cefiderocol. Studies were included if they met the inclusion criteria in Table 5, but reported data for fosfomycin and at least one comparator within the HVCSs.
Data extraction: data sources selected for inclusion in the review were data extracted by one reviewer and extractions were checked by a second. The initial key characteristics tabulation was expanded to include numerical outcome data for the susceptibility studies, and data were checked by a second reviewer. Data sources not selected for use in the model or clinical review were tabulated and reasons for their exclusion provided but were not assessed further.
Quality assessment: since there is no published quality assessment tool for susceptibility studies, a bespoke set of questions were developed and applied, relating to internal bias and relevance. This tool was developed by consulting two tools28,29 developed for the assessment of prevalence studies (since studies report the prevalence of susceptibility), the ROBINS-1 checklist30 for non-randomised studies (since the studies are comparative, but non-randomised), Cochrane’s RoB231 tool (since the NMA will assume the study arms are equivalent to randomised arms of a RCT) and the Newcastle–Ottawa Scale32 (since these are observational studies). Questions from all tools were considered for inclusion and adapted to the specifics of this review. The tool was reviewed by other members of the reviewing team, but no further validation work was undertaken. The final tool is reported in Appendix 2, Table 40, which shows the bespoke risk of bias assessment tool developed as part of this project to assess the in vitro susceptibility studies. Risk of bias was assessed using this tool by one reviewer.
Review results
Study selection results (reviews 1–3)
The electronic database searches, following the removal of duplicates, identified 261 records relating to cefiderocol. One additional record33 was identified from the company submission and two sets of data34,35 were obtained from the company through a data request (see Report Supplementary Material 1). After examination of the title and abstracts, 211 records were excluded because they did not meet the inclusion criteria for the key characteristics mapping stage (see Table 5), while 53 records were included in the key characteristics map. At this point, the decision was made to focus on MBL Enterobacterales or P. aeruginosa infections in cUTI and HAP/VAP in the first instance and not to review PK/PD data (see Table 6). This resulted in the exclusion of 3333,36–66 susceptibility and PK/PD studies (see Report Supplementary Material 1). A further five susceptibility and PK/PD studies (see Report Supplementary Material 1) were excluded upon examination of their full text because they did not report the percent susceptible (n = 1);67 there were insufficient data relating to comparators (n = 2)68,69 and there were fewer than 10 isolates included (n = 2). 70,71 The remaining citations related to three RCTs,13–15 six observational studies72–77 and four susceptibility studies reported across six sources,34,35,78–81 which were considered for use in the economic modelling. Ultimately, the four susceptibility studies reported across six sources34,36,78–81 entered the evidence synthesis. The reasons for exclusion of the RCT and observational studies from the synthesis are given in Reviews 1 and 2 and Review 3, and in Appendix 3, Review 1: randomised controlled trials and Review 2: Observational studies, Table 42.
A Preferred Reporting Items for Systematic Reviews and Meta-Analyses (PRISMA) flow diagram outlining the process of identifying relevant literature and the four included studies is provided in Figure 1.
FIGURE 1.
Preferred reporting items for systematic reviews and meta-analyses flow diagram for the cefiderocol clinical effectiveness review.

Reviews 1 and 2
The results of review questions 1 and 2 are reported in full in Appendix 3. A brief summary of the findings for each is provided here.
Review 1: three RCTs in cUTI and HAP/VAP were identified (APEKS cUTI,13 APEKS NP,14 CREDIBLE CR,15 for further details see Appendix 3, Review 1: randomised controlled trials, Table 41), but two excluded patients with carbapenem-resistant infections and therefore had low relevance to the HVCSs. No data were reported for patients with MBL infections in these two trials. CREDIBLE-CR15 recruited patients with carbapenem-resistant infections, and reported subgroup data for patients with MBL infections. However, the subgroup was small (n = 16 in the cefiderocol arm, n = 7 in the BAT arm) and was therefore not used due to the chance of baseline imbalances introducing bias. The RCTs indicated that cefiderocol was an effective treatment in the sites of interest.
Review 2: three observational studies were excluded since they did not report which pathogen mechanisms were recruited (n = 2)75,76 or did not include any MBL pathogens (n = 1). 72 Three73,74,77 observational studies reporting outcomes for patients with MBL infections treated with cefiderocol were identified. However, all reported infections across a range of sites, and in none of these was it possible to separate out patients with cUTI or HAP/VAP; there was insufficient time to obtain IPD. All studies were of a small sample size (range from n = 2 to 17 patients) and were highly heterogeneous in terms of key characteristics that are prognostic and expected to modify treatment response (e.g. site, pathogen, treatment line), limiting the conclusions that could be drawn from them and increasing the likely uncertainty associated with any synthesis performed.
Approaches 1 and 2 could therefore not be pursued since there was a lack of evidence relating to cUTI and HAP/VAP infections caused by MBLs to inform an assessment of comparative effectiveness. Approach 3 was considered the most viable option, and reviews relating to this approach are described in the remainder of this chapter.
Review 3
Studies reporting the susceptibility of MBL Enterobacterales or P. aeruginosa isolates to cefiderocol and at least one comparator
Since no RCTs or observational evidence were identified that met the requirements of the HVCSs, EEPRU considered the evidence relating to in vitro susceptibility. EEPRU’s approach is supported by the company submission,82 which states that ‘the in vitro data for cefiderocol should be considered the primary source of evidence to support the effectiveness and positioning of cefiderocol and is actually the only source of evidence for the risk-based empiric setting’.
Four susceptibility studies met the inclusion criteria of the review. 34,35,78–81 The characteristics of these studies are presented in Table 7. The three RCTs13–15 were also examined for relevant susceptibility data, but none were relevant to the susceptibility synthesis as they did not present data by mechanism. It is noted that the Shionogi company submission82 recommends PK/PD evidence should be used alongside clinical and susceptibility data to extrapolate to other infection sites. However, the clinical advisors to EEPRU advised that as cefiderocol and comparators penetrate to the sites of interest, it was reasonable to link directly between susceptibility and clinical outcomes (see Table 6). Furthermore, neither the Shionogi company submission nor discussion with the clinical advisors to EEPRU identified a quantitative approach to linking PK/PD evidence to clinical outcomes. Consequently, as part of the mapping exercise, PK/PD studies were excluded.
Study ID | Country Multisite? Year(s) of recruitment |
N | Inclusion criteria/β-lactamase testing selection criteria | % Mero non-susceptible | Laboratory methods Breakpoints |
Included in NMAs? |
---|---|---|---|---|---|---|
Cefiderocol studies | ||||||
SIDERO CR (data request data) [some detail from Longshaw et al. (2020)81 SIDERO CR 2014–6] |
Global Multisite 2014–6 |
305 (Enterobacterales 190; P. aeruginosa 115) | Enterobacterales and P. aeruginosa isolates from a surveillance collection with known AM susceptibility phenotypes and/or their species identification | EUCAST: Enterobacterales 96.8%; P. aeruginosa 99.1% CLSI: Enterobacterales 100%; P. aeruginosa 100% |
CLSI Data reported for both EUCAST and CLSI breakpoints |
Y: CLSI Enterobacterales CLSI P. aeruginosa EUCAST Enterobacterales EUCAST P. aeruginosa |
SIDERO WT (data Request data) [some detail from Kazmierczak et al. (2019)79 SIDERO-WT 2014] |
Global Multisite 2014–6 |
297 (Enterobacterales 131; P. aeruginosa 166) | Non-duplicate, Non-consecutive isolates of Gram-negative bacilli | EUCAST: Enterobacterales 96.2%; P. aeruginosa 100% CLSI: Enterobacterales 99.3%; P. aeruginosa 100% |
CLSI Data reported for both ECUAST and CLSI breakpoints |
Y: CLSI Enterobacterales CLSI P. aeruginosa EUCAST Enterobacterales EUCAST P. aeruginosa |
Johnston et al. (2020)78 | Europe and North America 2002–17 |
343 (all Enterobacterales) | CR clinical E. coli isolates | 100% | CLSI CLSI; FDA for cefiderocol |
Y: CLSI Enterobacterales |
Kohira (2016)80 | International Multisite 2000–11 |
NDM n = 49 VIM n = 12 IMP n = 8 |
Unclear how selected for inclusion or for β-lactamase testing | 17.4% | CLSI CLSI |
Y, CLSI CPE |
Fosfomycin studies | ||||||
EUCAST Enterobacterales | ||||||
Chakraborti et al. (2021)83 | Switzerland and Germany Multisite 2018–9 |
NDM n = 30 (excluded n = 3 from sewer, dog, river) | Unclear how selected from the surveillance study for inclusion in analysis | N/R | N/R EUCAST |
Y: EUCAST Enterobacterales |
Kaase et al. (2015)84 | Germany Multisite 2009–14 |
VIM n = 36 | Voluntary submission of isolates from German laboratories, all tested for VIM, IMP and NDM by PCR | 86.1% | EUCAST EUCAST |
Y: EUCAST Enterobacterales |
Livermore et al. (2011)85 | UK Unclear Unclear (pre 2011) |
IMP n = 13 NDM n = 17 VIM n = 5 |
Unclear how selected for inclusion, ‘diversity of carbapenem-resistance types’ | N/R (but all CR) | CLSI EUCAST |
Y: EUCAST Enterobacterales |
Ojdana et al. (2019)86 | Poland Single site 2009–14 |
NDM n = 10 | Isolates selected for testing according to EUCAST carbapenemase screening protocol | N/R (but all C non-susceptible) | EUCAST EUCAST |
Y: EUCAST Enterobacterales |
Perry et al. (2011)87 | Pakistan Multisite 2010 |
NDM1 n = 64 | Unclear how all isolates selected, some by random selection. All Gram-negative tested for VIM, IMP and NDM | 30% | EUCAST EUCAST, or if not available for a treatment, CLSI |
Y: EUCAST Enterobacterales |
EUCAST P. aeruginosa | ||||||
Cuba et al. (2020)88 | Unclear Unclear Unclear |
IMP n = 4 VIM n = 3 |
Unclear how selected for inclusion | 100% | EUCAST EUCAST |
Y: EUCAST P. aeruginosa |
CLSI Enterobacterales | ||||||
Aires et al. (2017)89 | Brazil Multisite 2013–4 |
NDM n = 16 | Unclear how selected for inclusion, selected for β-lactamase testing using EDTA, phenyl boronic acid and in vitro analysis of imipenem hydrolysis | 100% | eTest for mero, tig; agar dilution for others CLSI (except TIG-EUCAST) |
Y: CLSI Enterobacterales |
Sonnevend et al. (2020)90 | Arabian Peninsula Multisite 2009–17 |
MBL (NDM, VIM, IMP) n = 552 | All isolates received at laboratory were eligible, but unclear how selected for β-lactamase testing | 100% | N/R CLSI |
Y: CLSI Enterobacterales |
Vasso et al. (2015)91 | Singapore Multisite N/R |
Enterobacterales NDM n = 32 IMP n = 11 | Unclear how selected for inclusion | 90.7% | CLSI CLSI |
Y: CLSI Enterobacterales |
CLSI P. aeruginosa | ||||||
Jahan et al. (2021)92 |
USA Multisite 2016 |
P. aeruginosa n = 20 | 20 genetically unique MBLs selected from CDC and FDA AM resistance bank, unclear how selected for inclusion in the bank | N/R | Etest CLSI |
Y: CLSI P. aeruginosa |
PHE data | ||||||
PHE data | UK Multisite 2014–21* |
N = 159 | Enterobacterales isolates submitted to PHE AMRHAI with suspected carbapenem resistance and tested for all comparators | 5.0% | Unclear Unclear |
Y: EUCAST Enterobacterales EUCAST P. aeruginosa |
Policy Research Unit in Economic Methods of Evaluation in Health and Social Care Interventions first considered whether any one of the studies met all the requirements of the evaluation (ideally consecutive English data from a multisite study reporting outcomes for all relevant comparators, using BSAC/EUCAST breakpoints and laboratory methods), and could fulfil the evidence needs of the project without the need of a meta-analysis. Since the data received from PHE in response to EEPRU’s request did not report any evidence for cefiderocol, these data were not able to fulfil the requirements of the project, and a meta-analysis of susceptibility studies was planned. There were also a number of other limitations to the PHE data. Isolates have not historically been routinely submitted by testing centres which may limit how representative these data are of the true distribution of MBL susceptibilities in England. In addition, there is inconsistency in the testing methodologies used by local laboratories (albeit the majority use EUCAST). 93 Finally, not all isolates were tested for each comparator, and a compromise had to be made in conducting the analysis whereby to preserve internal validity only isolates tested among all comparators were included (see Report Supplementary Material 1) which may have introduced selection bias. Given this approach, we excluded PHE evidence for fosfomycin as this would have resulted in only 18 isolates being available for analysis across both MBL Enterobacterales and P. aeruginosa populations.
There was some uncertainty during the development of the PICOS about whether meropenem should be considered a relevant comparator. This led to inconsistencies in the inclusion of evidence relating to meropenem. Data from one study (Kohira et al. 201680) and the meropenem data from PHE were excluded erroneously from the synthesis. The sensitivity analyses performed to address this are described in Statistical synthesis plan.
Limitations of the data available from the published study reports
Two of the studies, Longshaw et al. (2020)81 and Kazmierczak et al. (2019),79 drew isolates from SIDERO CR and SIDERO WT (wild type), respectively. These were surveillance studies conducted by Shionogi. The two further studies78,94 identified by the review were also funded by Shionogi.
Policy Research Unit in Economic Methods of Evaluation in Health and Social Care Interventions identified limitations with all four studies with respect to their relevance to the synthesis being conducted to inform estimates of relative effectiveness in the HVCS:
-
Kazmierczak et al. (SIDERO WT)79 only reported MIC50, MIC90 and range, not % susceptibility. While these metrics could have been used to reconstruct the distribution curves and apply a breakpoint to generate an estimated % susceptibility, this was thought to introduce too much uncertainty to the estimates. In addition, not all three metrics were reported for all relevant subgroups in Kazmierczak et al. (SIDERO WT). 79
-
Data from SIDERO CR [Longshaw et al. (2020)]81 covered only Europe, whereas the full data set used in Shionogi’s response to a data request from EEPRU dated 14 June 2021 (see Report Supplementary Material 1) used worldwide data. After consultation with our clinical advisers, it was decided that, ideally, we would include worldwide data in the synthesis since resistance mechanisms frequently arrive in the UK from elsewhere (see Table 6).
-
Neither Johnston et al. (2020)78 nor Kohira et al. (2016)80 reported susceptibility using EUCAST breakpoints, only CLSI breakpoints.
-
All four sources of data used CLSI laboratory methods, which may not be equivalent to EUCAST laboratory methods.
-
None of the studies included data for fosfomycin.
Shionogi was approached to provide additional data to fulfil these requirements (data request dated 11 August 2021, see Report Supplementary Material 1) for all four studies. The request was for worldwide data, reporting percentage susceptibility using EUCAST and separately using CLSI breakpoints for cefiderocol and all relevant available comparators (in case data for fosfomycin was unpublished, but held by Shionogi). Shionogi supplied the requested analyses for SIDERO CR35 and SIDERO WT34 (see Table 7 below). The data were reported for Enterobacterales and P. aeruginosa separately, and included isolates with carriage or co-carriage of MBLs. The data were not restricted by carbapenem sensitivity, or any other sensitivity or phenotype, and counted intermediate susceptibility as resistant, in accordance with EEPRU’s requirements. Data were reported using CLSI and EUCAST breakpoints, but using CLSI laboratory methods. No data for fosfomycin were available. The company was unable to provide additional data for Johnston et al. (2020)78 or Dobias et al. (2017)67 as they did not have access to the raw data. Therefore, Dobias et al. (2017)67 was excluded from the syntheses, while Johnston et al. (2020)78 could only be included in a synthesis of data using CLSI breakpoints. Consequently, three studies (SIDERO CR, SIDERO WT and Johnston 2020)78 of Cefiderocol were eligible for inclusion in the statistical synthesis. Due to the uncertainty around comparators noted above, data for Kohira et al. (2016)80 were not requested, but the data reported in the journal publication were included in the review in the sensitivity analyses conducted to address this issue (see Statistical synthesis plan).
Characteristics of studies entering the network meta-analysis
Across the four cefiderocol studies, the sample size ranged from n = 6980 isolates to n = 34378 isolates. The cefiderocol studies collected isolates internationally, from multisite locations. Expert advice indicated that resistant infections tend to arrive in the UK from around the world, and consequently isolates collected from any location were of relevance to the assessment. All studies were therefore retained in the analysis.
The isolates were collected since 2014 for the SIDERO studies, Johnston et al. (2020)78 included isolates collected over the period 2002–17 and Kohira et al. (2016)80 included isolates from 2000 to 2011. Expert advice indicated that isolates collected since 2012 were of highest (but not exclusive) relevance, so all studies were retained in the analysis.
The PHE data did not meet the inclusion criteria for either the cefiderocol or fosfomycin review since it did not report data for cefiderocol or fosfomycin. However, it was considered the best source of UK data for comparators, which were not well covered by the Cefiderocol review, so the PHE data were included for this reason.
Fosfomycin studies
The supplementary search for fosfomycin studies yielded 113 citations, 45 studies were considered at full-text stage and 1083–92 studies were data extracted (see Table 7), and were eligible for inclusion in the statistical synthesis. The sample size ranged from n = 7 to n = 552 across the 10 fosfomycin studies. Studies were largely multisite studies in a single country (Germany,84 Pakistan,87 Brazil,89 Arabian Peninsula,90 Singapore,91 USA92), though one study drew from both Germany and Switzerland,83 one was a single-centre study from Poland,86 and two were unclear. 88,85 It should be noted that there are some limitations with these data, including:
-
Neither EUCAST nor CLSI have set a breakpoint for fosfomycin in P. aeruginosa. Both studies88,92 that reported susceptibility of fosfomycin in P. aeruginosa isolates used epidemiological breakpoints (breakpoints that distinguish between wild-type pathogens, and those with acquired resistance)95 that do not, according to EUCAST, predict clinical susceptibility.
-
Two of the studies89,92 did not use EUCAST or CLSI laboratory methods, but instead used a commercial assay (Etest).
-
Criteria used to select isolates for inclusion in the study, and then for β-lactamase testing, were often not well described, meaning it is unclear to what extent the studies reflect the true distribution of susceptibility for the population they drew from.
Despite these limitations, all studies were included since evidence was generally sparse across the network. These limitations should be noted when interpreting the results of the meta-analyses, especially with respect to the estimates for fosfomycin in P. aeruginosa.
Quality assessment of studies entering the meta-analysis
A summary of the quality assessment is presented in Table 8. No study scored low risk for all items.
Study ID | 1. Target population | 2. Sampling strategy | 3. Outcome measurement | 4. Missing data | ||||||||||
---|---|---|---|---|---|---|---|---|---|---|---|---|---|---|
Is the target population of the study broadly appropriate to the HVCS? | Were isolates selected based on resistance to comparators? | Were all isolates tested for the pathogen mechanism of interest in a standard way, and does this match the HVCS? | Was the beta-lactamase test appropriate? | Were data collected over an appropriate time period? | Overall judgement | Were isolates sampled from the target population in an appropriate way? | Overall judgement | Was susceptibility measured in an appropriate, standard way? | Does the study demonstrate selective analysis reporting, with respect to S, I and R? | Were S, I and R reported consistently for all treatments? | Overall judgement | Is there a risk of bias from missing data? | Overall judgement | |
Cefiderocol studies | ||||||||||||||
SIDERO CR (data request)35 | L | U | U | L | L | U | U | U | H | L | L | H | L | L |
SIDERO WT (data request)34 | L | U | U | L | L | U | U | U | H | L | L | H | L | L |
Johnston et al. (2020)78 | L | U | L | L | U | U | U | U | H | L | L | H | L | L |
Kohira (2016)80 | L | U | U | U | U | U | U | U | U | L | L | U | L | L |
Fosfomycin studies | ||||||||||||||
EUCAST CPE | ||||||||||||||
Chakraborti et al. (2021)83 | L | L | L | L | L | L | U | U | L | L | L | L | L | L |
Kaase et al. (2015)84 | L | L | U | L | U | U | U | U | L | L | L | L | L | L |
Livermore et al. (2011)85 | U | L | L | L | U | U | U | U | U | L | L | U | L | L |
Ojdana et al. (2019)86 | L | L | U | L | L | U | U | U | L | L | L | L | L | L |
Perry et al. (2011)87 | L | L | L | L | U | U | U | U | U | L | L | U | L | L |
EUCAST P. aeruginosa | ||||||||||||||
Cuba et al. (2020)88 | L | L | L | U | U | U | U | H | L | L | L | L | L | L |
CLSI CPE | ||||||||||||||
Aires et al. (2017)89 | L | L | U | L | L | L | U | U | U | L | L | U | L | L |
Sonnevend et al. (2020)90 | L | L | L | L | U | U | U | U | U | L | L | U | L | L |
Vasso et al. (2015)91 | L | L | L | U | U | U | U | U | U | L | L | L | L | L |
CLSI P. aeruginosa | ||||||||||||||
Jahan et al. (2021)92 | L | L | L | L | U | U | U | U | U | L | L | L | L | L |
PHE data | ||||||||||||||
PHE data | U | U | L | U | L | U | U | U | U | L | L | U | U | U |
Among the cefiderocol studies, CLSI laboratory methods were used but EUCAST breakpoints applied in three of four studies, and CLSI methods and breakpoints were used in the fourth. These issues led to a high risk of bias in ‘Outcome measurement’ compared to practice in England. All four scored unclear risk for ‘Target population’ and ‘Sampling strategy’, largely due to a lack of clarity about how isolates were selected for inclusion in the data request response, or how they were selected for β-lactamase testing from the wider population. All scored low risk for missing data, since all isolates were tested for all comparators.
Among the fosfomycin studies, only one scored low risk for ‘Target population’, while the remainder scored unclear high risk, largely due to a lack of clarity around how isolates were selected for β-lactamase testing, and whether isolates collected before 2012 would impact on susceptibility estimates. All studies scored unclear risk for ‘sampling strategy’, usually because it was not stated how isolates were selected, or where it was reported, it was not clear whether the strategy was appropriate. Outcome measurement was either low or unclear risk, due to a lack of clarity around laboratory methods. All scored low risk for missing data, since all isolates were tested for all comparators.
The PHE data were unclear on all domains, since PHE were unable to ascertain how isolates were tested, it was unclear what criteria led to submission of isolates, and it was unclear whether isolates were selected for testing of some interventions on the basis of suspected resistance or susceptibility, which may introduce selection bias.
Statistical synthesis
Statistical synthesis plan
Α NMA was planned to synthesise susceptibility studies identified by the review. Several sources of clinical heterogeneity were identified through the quality assessment and consideration of the study characteristics. As detailed in Table 6, location, and whether the sample included screened isolates were not considered to be important sources of heterogeneity by clinical advisors. This section details the source of heterogeneity that was considered potentially important, the reasons why it was considered important and the sensitivity analyses planned relating to these. A summary of the planned analyses is provided in bullet points at the end of this section. Statistical synthesis plan and Statistical synthesis methods for review question 3 detail the statistical methods used to conduct the NMA, while Susceptibility data entering the network meta-analysis and Results of the network meta-analysis report which studies entered each analysis, the results of these analyses and which were used in the decision-analytic model.
Susceptibility testing methodology: there were two main issues with respect to the susceptibility testing methodology used in the available evidence. The first relates to which breakpoints are used in England and the second relates to the breakpoint used for cefiderocol.
Breakpoints used in England: as detailed in Table 6, it cannot be assumed that all laboratory methods and breakpoints are interchangeable. In England, BSAC guidelines have recommended since 201696 that laboratories should use EUCAST laboratory methods and breakpoints. Therefore, currently in England, studies using EUCAST methods and breakpoints should have the highest clinical relevance. However, in their response to EEPRU’s data request, PHE noted that not all English laboratories comply with BSAC guidelines, and it is unclear to what extent CLSI and potentially other methods, implemented by commercial assays, may have been included in the PHE data.
Breakpoint used for cefiderocol: Shionogi noted that the EUCAST breakpoint for cefiderocol is 2 mg/l, while the CLSI breakpoint is 4 mg/l. The company also stated that negotiations were underway with EUCAST to change the breakpoint to 2 mg/l. However, at the time of writing, no change had been made. Since it was also unclear whether CLSI and EUCAST breakpoints are (and have been) the same for all comparators, it would have been inappropriate to mix CLSI breakpoints and EUCAST breakpoints within one data set as this may affect the relative efficacy reported, especially given the difference in the cefiderocol breakpoint between EUCAST and CLSI.
Policy Research Unit in Economic Methods of Evaluation in Health and Social Care Interventions therefore planned to conduct NMAs for EUCAST and CLSI breakpoints separately. Since PHE data were of high relevance and included data for comparators, and since guidelines in England recommend EUCAST breakpoints, the base-case analysis was to include EUCAST breakpoints, and data from PHE. A sensitivity analysis was planned including only studies using CLSI breakpoints, including the higher 4 mg/l breakpoint for cefiderocol.
Pseudomonas aeruginosa and Enterobacterales isolates: since Enterobacterales and P. aeruginosa have different resistance profiles due to innate resistance or acquired resistance other than that due to MBLs, EEPRU planned to conduct NMAs for each separately.
Sources of data: three sources of data contributed to the networks: studies identified by the review of cefiderocol and related data requests; studies identified by the review of fosfomycin studies; and the PHE data. Since ideally the systematic review would have included systematic searches for all studies of all comparators in one network, there was some disparity in how the studies of fosfomycin had been identified compared to the other comparators, which may have introduced bias. Equally, the PHE data and fosfomycin studies did not, strictly speaking, meet the inclusion criteria for the review, since they did not report estimates for cefiderocol. Therefore, EEPRU planned analyses to test the impact of the inclusion of data not meeting the inclusion criteria in the economic model. Since PHE data were of high relevance, these data alone would be used wherever possible to inform the susceptibility for the comparators in a scenario analysis. The relative effect estimates for cefiderocol and fosfomycin (which are missing from the PHE data) could then come from one of two sources: a network including all three sources of data; or a network including only cefiderocol or only fosfomycin studies, respectively. Therefore, an analysis of studies reporting cefiderocol susceptibility and a separate analysis of studies reporting fosfomycin data were planned to provide the relative effects to apply to the PHE data.
Comparators included in the network: initially, when developing the HVCS PICOS, it was anticipated that in the ES, it would be unclear which pathogen was responsible for the infection. EEPRU therefore conducted the NMA including all comparators indicated for either MBL Enterobacterales or P. aeruginosa. However, during the course of the project, the ES HVCS was focused on patients where there was a high suspicion of the causative pathogen mechanism, leading to a change in the PICOS. This led to two analyses being conducted. One with all comparators included, and a sensitivity analysis with only the comparators relevant to the pathogen mechanism included. For the Enterobacterales networks, this sensitivity analysis meant removing meropenem, and for the P. aeruginosa networks, this meant removing aztreonam, tigecycline and gentamicin (since data were not available for amikacin and tobramycin). However, due to inconsistencies in the application of this decision, data from one study (Kohira et al. 201680) and the meropenem data from PHE were excluded erroneously from analyses conducted including all comparators. This affected the CLSI and EUCAST Enterobacterales networks, respectively (see bullet points below). Therefore, a sensitivity analysis was conducted to reinclude the missing data.
In summary, the following analyses were planned:
Main analysis:
-
Enterobacterales network including all studies using EUCAST breakpoints:
-
additional analysis including the missing meropenem data from PHE
-
additional analysis using only comparators specific to the pathogen.
-
-
Pseudomonas aeruginosa network including all studies using EUCAST breakpoints:
-
additional analysis using only comparators specific to the pathogen.
-
Sensitivity analyses:
-
Enterobacterales network including all studies using CLSI breakpoints:
-
additional analysis including the missing data from Kohira et al. (2016) 80
-
additional analysis using only comparators specific to the pathogen.
-
-
Pseudomonas aeruginosa network including all studies using CLSI breakpoints:
-
additional analysis using only comparators specific to the pathogen.
-
Additional analyses for use in the economic model:
-
Cefiderocol studies using EUCAST breakpoints and separately using CLSI breakpoints:
-
additional analysis using only comparators specific to the pathogen.
-
-
Fosfomycin studies using EUCAST breakpoints and separately using CLSI breakpoints:
-
additional analysis using only comparators specific to the pathogen.
-
Statistical synthesis methods for review question 3
A NMA was conducted to determine the relative susceptibility of cefiderocol and listed comparators. The data generation process was assumed to follow a binomial likelihood with probabilities modelled using a logit link function. Random-effect (RE) models were assumed to allow for expected between-study heterogeneity in relative effects. Further details of the statistical model are given in Appendix 4, Statistical model for the network meta-analysis.
All analyses were conducted using the freely available software package WinBUGS and R using the R2Winbugs interface package. Code was modified from NICE TSD 2 example 1c (RE models). 97
Convergence to the target posterior distributions was assessed using the Gelman–Rubin statistic, as modified by Brooks and Gelman, for two chains with different initial values. For all outcomes, a burn-in of 80,000 iterations of the Markov chain was used with a further 20,000 iterations retained to estimate parameters using one chain and thinning every 5 iterations.
The absolute goodness of fit was checked by comparing the total residual deviance to the total number of data points included in an analysis. The deviance information criterion (DIC) provides a relative measure of goodness of fit that penalises complexity and was used to compare different models for the same likelihood and data. Lower values of DIC are favourable, suggesting a more parsimonious model.
Inconsistency between direct and indirect evidence can arise because of an imbalance in treatment effect modifiers across studies comparing different pairs of treatments. 98,99 Consistency between direct and indirect evidence can be assessed where there are ‘loops’ of evidence in the network informed by separate, independent trials, so that both direct and indirect estimates are available.
Inconsistency was assessed by fitting unrelated mean effects (UME) models, based on a code from NICE TSD4. 98 In the UME model, the direct and indirect relative treatment effects are not constrained to be consistent with each other. This is equivalent to having separate, unrelated, meta-analyses for every pairwise contrast and with a common variance parameter in RE models. To explore whether the direct and indirect evidence for particular treatment comparisons is inconsistent, the contribution to the posterior mean residual deviance was plotted for the UME model against the NMA model in a deviance contribution plot. 98,99
Results are presented using the posterior median treatment effects, 95% credible intervals (CrI) and 95% prediction intervals (PrI). The 95% PrI indicates the extent of between-study heterogeneity by illustrating the range of odds ratios (ORs) that might be expected in a future study. Probabilities of treatment rankings were computed by counting the proportion of iterations of the Markov chain in which each intervention had each rank. Median treatment rankings and the probabilities of each treatment being the best treatment (i.e. ranks the first) are presented.
The estimated between-study standard deviation (SD) for each analysis is also presented. Values below 0.05 are considered to indicate low heterogeneity. Values between 0.05 and 0.5 are considered to indicate moderate heterogeneity. Values between 0.5 and 1.0 are considered to indicate high heterogeneity. Values above 1.0 are considered to indicate extremely high heterogeneity.
In the case of zero events, a continuity correction was applied by adding 1 to the denominator and 0.5 to the numerator as suggested as a solution by NICE Decision Support Unit TSD2 to stabilise the results. 97
Susceptibility data entering the network meta-analysis
Table 9 (A–D) presents the susceptibility data from the four cefiderocol studies (SIDERO CR, SIDERO WT, Johnston 2020, Kohira 201678,94), the 1083–92 fosfomycin studies and the PHE data included in the NMA. Considering the absolute data, susceptibility to cefiderocol was highest in the P. aeruginosa subgroups (96.9–99.1% in the P. aeruginosa EUCAST network and 100% in both studies for the P. aeruginosa CLSI subgroup). It was lowest in the Enterobacterales EUCAST subgroup (64.7% and 65.6%), but higher in the Enterobacterales CLSI subgroup (70.1 to 97.7%), where the breakpoint for cefiderocol is 4 mg/l (compared to 2 mg/l set by EUCAST).
The separate search for fosfomycin studies provided five data points for the Enterobacterales EUCAST network and three studies for the Enterobacterales CLSI network, but only one study for each of the P. aeruginosa EUCAST and CLSI networks, with relatively small numbers (n = 7 and n = 20, respectively).
In the Enterobacterales subgroups, colistin generally had high susceptibility in both the EUCAST (80.9% in the SIDERO WT study to 100%)83,84 and CLSI (78.6% in the SIDERO WT study to 90.7%)91 subgroups. However, in comparison to cefiderocol colistin had lower susceptibility in the Enterobacterales CLSI subgroup, whereas in the Enterobacterales EUCAST subgroup it had higher susceptibility. Fosfomycin also had generally good susceptibility in both subgroups [EUCAST 40%86 to 100%83,84 (four out of five studies > 74.3%);85 CLSI 76.7%91 to 93.8%]. 89
In the P. aeruginosa subgroups, susceptibility estimates for colistin (98.1% in the SIDERO WT34 data to 100% in the PHE data and SIDERO CR data)35 were comparable to cefiderocol (96.9% in SIDERO WT34 to 99.1% in SIDERO CR)35 in the EUCAST subgroup but lower than cefiderocol in the CLSI subgroup (colistin 80.7% in the SIDERO WT34 study to 100%,92 cefiderocol 100% in both studies). Fosfomycin had very different susceptibility in the EUCAST subgroup (14.3%) compared to the CLSI subgroup (80.0%), which may be due to chance since there was only one small study in each subgroup.
Results of the network meta-analysis
Summaries of the results of the base-case and sensitivity NMAs performed are presented in Tables 10 and 11. See Base-case network meta-analysis: metallo-beta-lactamasesEnterobacteralesinfections with European Committee on Antimicrobial Susceptibility Testing breakpoint, Base-case network meta-analysis: metallo-beta-lactamasesP. aeruginosainfections with European Committee on Antimicrobial Susceptibility Testing breakpoint and Sensitivity analyses for more detail about these analyses. The results are presented as ORs. Please note that they should not be assumed to be equivalent to risk ratios (RRs).
Analysis | Scenario | Cefiderocol OR, 95% CrI | Comparators | Section in report | Section in appendices |
---|---|---|---|---|---|
EUCAST Enterobacterales | All comparators, missing PHE meropenem data | 0.32, 0.04 to 2.47 | See Figure 3 | Base-case network meta-analysis: metallo-beta-lactamases | N/A |
All comparators, including PHE meropenem data | 0.31, 0.04 to 2.18 | Similar OR for comparators as for ‘all comparators’ analysis | |||
Only comparators specific to the pathogena | 0.34, 0.05 to 1.97 | ||||
Using only studies that report cefiderocol data (SIDERO) | 0.33, 0.06 to 1.65 | N/A – only cefiderocol OR used | Sensitivity analyses | Appendix 4, section Metallo-beta-lactamases, network diagram in Figure 20; forest plot in Figure 21 | |
Using only studies that report cefiderocol data (SIDERO), and only including in the network comparators specific to the pathogena | 0.33, 0.039 to 2.916 | N/A – only cefiderocol OR used | Sensitivity analyses | Appendix 4, section Metallo-beta-lactamases, no plot generated | |
EUCAST P. aeruginosa | All comparators | 0.44, 0.03 to 3.94 | See Figure 5 | Base-case network meta-analysis: metallo-beta-lactamases | N/A |
Only comparators specific to the pathogena | 0.44, 0.03 to 4.08 | Similar OR for comparators as for ‘all comparators’ analysis | Not presented | ||
Using only studies that report cefiderocol data (SIDERO) | 0.49, 0.03 to 5.29 | N/A – only cefiderocol OR used | Sensitivity analyses | Appendix 4, section Metallo-beta-lactamases, network diagram in Figure 22; forest plot in Figure 23 | |
Using only studies that report cefiderocol data (SIDERO), and only including in the network comparators specific to the pathogen | This network is the same as the ‘Using only SIDERO studies’ network | ||||
CLSI Enterobacterales | All comparators, missing Kohira et al. data | 1.38, 0.16 to 12.05 | See Appendix 4, section Metallo-beta-lactamases Enterobacterales network including all studies using Clinical Laboratory Standards Institute breakpoints, Figure 29a | Sensitivity analyses | Appendix 4, section Metallo-beta-lactamases Enterobacterales network including all studies using Clinical Laboratory Standards Institute breakpoints, network diagram in Figure 28, forest plot in Figure 29ab |
All comparators, including Kohira et al. data | 0.86, 0.11 to 7.05 | Small differences in comparator ORs | Sensitivity analyses | Appendix 4, section Metallo-beta-lactamases Enterobacterales network including all studies using Clinical Laboratory Standards Institute breakpoints, network diagram in Figure 28, forest plot in Figure 29b | |
Only comparators specific to the pathogen a | 1.30, 0.16 to 10.40 | Fosfomycin’s OR indicated susceptibility higher relative to colistin, rather than lower | Sensitivity analyses | Appendix 4, section Metallo-beta-lactamases, network diagram in Figure 28, forest plot in Figure 29c | |
Using only studies that report cefiderocol data (SIDERO, Johnston et al., Kohira et al.) | 15.70, 0.83 to 320.72 | N/A – only cefiderocol OR used | Sensitivity analyses | Appendix 4, section Metallo-beta-lactamases, network diagram in Figure 32, forest plot in Figure 33a | |
Using only studies that report cefiderocol data (SIDERO), and only including in the network comparators specific to the pathogena | 16.76, 1.19 to 285.30 | N/A – only cefiderocol OR used | Sensitivity analyses | Appendix 4, section Metallo-beta-lactamases, network diagram in Figure 32, forest plot in Figure 33b | |
CLSI P. aeruginosa | All comparators | 71.34, 4.33 to 5934.35 | See Appendix 4, section Metallo-beta-lactamases, Figure 31a | Sensitivity analyses | Appendix 4, section Metallo-beta-lactamases, network diagram in Figure 30, forest plot in Figure 31a |
Only comparators specific to the pathogen a | 64.19, 1.13 to 7672.55 | Similar OR for comparators as for ‘all comparators’ analysis | Sensitivity analyses | Appendix 4, section Metallo-beta-lactamases, network diagram in Figure 30, forest plot in Figure 31b | |
Using only studies that report cefiderocol data (SIDERO) | 66.73, 3.61 to 3284.37 | N/A – only cefiderocol OR used | Sensitivity analyses | Appendix 4, section Metallo-betalactamases P. aeruginosa, Clinical Laboratory Standards Institute breakpoints (SIDERO studies only), network diagram in Figure 34, forest plot in Figure 35 | |
Using only studies that report cefiderocol data (SIDERO), and only including in the network comparators specific to the pathogena | This network is the same as the ‘Using only SIDERO studies’ network |
FIGURE 2.
Network diagram of all studies contributing to the NMA (MBL Enterobacterales with EUCAST breakpoint for SIDERO, fosfomycin and PHE studies).

Analysis | Scenario | Fosfomycin OR, 95% CrI | Section in report | Section in appendices |
---|---|---|---|---|
EUCAST Enterobacterales | All comparators, missing PHE meropenem data | 0.34, 0.06 to 1.96 | Base-case network meta-analysis: metallo-beta-lactamases | N/A |
Using only fosfomycin studies | 0.24, 0.02 to 3.09 | Sensitivity analyses | Appendix 4, section European Committee on Antimicrobial Susceptibility Testing breakpoint with fosfomycin studies only, network diagram in Figure 24; forest plot Figure 25 | |
Using only fosfomycin studies and only including in the network comparators specific to the pathogena | 0.23, 0.02 to 2.36 | Sensitivity analyses | Appendix 4, section European Committee on Antimicrobial Susceptibility Testing breakpoint with fosfomycin studies only, forest plot Figure 25b | |
EUCAST P. aeruginosa | All comparators | 0.00, 0.00 to 0.19 | Base-case network meta-analysis: metallo-beta-lactamases | N/A |
Using only fosfomycin studies | 2.50, 0.04 to 52.37. Relative to aztreonam after applying a continuity correction. | Sensitivity analyses | Appendix 4, section European Committee on Antimicrobial Susceptibility Testing breakpoint with fosfomycin studies only, no forest plot | |
Using only fosfomycin studies and only including in the network comparators specific to the pathogena | 2.50, 0.04 to 52.37. Relative to aztreonam after applying a continuity correction | Sensitivity analyses | Appendix 4, section European Committee on Antimicrobial Susceptibility Testing breakpoint with fosfomycin studies only, no forest plot | |
CLSI Enterobacterales | All comparators, missing Kohira data | 0.69, 0.07 to 6.70 | Sensitivity analyses | Appendix 4, section Clinical Laboratory Standards Institute breakpoints sensitivity analysis, network diagram in Figure 28, forest plot Figure 29a |
Using only fosfomycin studies | 0.52, 0.06 to 4.01 | Sensitivity analyses | Appendix 4, section Clinical Laboratory Standards Institute breakpoint with fosfomycin studies only, network diagram in Figure 36, forest plot Figure 37a | |
Using only fosfomycin studies and only including in the network comparators specific to the pathogena | 0.59, 0.12 to 2.64 | Sensitivity analyses | Appendix 4, section Clinical Laboratory Standards Institute breakpoint with fosfomycin studies only, network diagram in Figure 36, forest plot Figure 37b | |
CLSI P. aeruginosa | All comparators | 0.06, 0.00 to 1.92 | Sensitivity analyses | Appendix 4, section Clinical Laboratory Standards Institute breakpoints sensitivity analysis, forest plot Figure 31a |
Using only fosfomycin studies | 0.10, 0.02 to 7.40. Relative to colistin after applying a continuity correction | Sensitivity analyses | Appendix 4, section Clinical Laboratory Standards Institute breakpoint with fosfomycin studies only, no forest plot | |
Using only fosfomycin studies and only including in the network comparators specific to the pathogena | 0.10, 0.02 to 7.40. Relative to colistin after applying a continuity correction | Sensitivity analyses | Appendix 4, section Clinical Laboratory Standards Institute breakpoint with fosfomycin studies only, no forest plot |
Base-case network meta-analysis: metallo-beta-lactamases Enterobacterales infections with European Committee on Antimicrobial Susceptibility Testing breakpoint
Eight studies contributed to the NMA of MBL Enterobacterales infections with EUCAST breakpoints, considering a total of eight comparators, and the full network diagram is shown in Figure 2. Three studies83,84,86 contained 100% susceptibility counts for one or more of the included comparators and therefore had a continuity correction applied prior to synthesis.
The relative susceptibility for each comparator relative to colistin is shown in Figure 3a. The model fitted the data well, with a total residual deviance of 33.50, which was close to the number of data points included in the analysis of 35. The between-study SD was 1.45 (95% CrI 0.93 to 2.35), which indicates extremely high heterogeneity. Cefiderocol was associated with a lower susceptibility relative to colistin (OR 0.32, 95% CrI 0.04 to 2.47), but the result was not statistically significant. Cefiderocol was also associated with an 11% probability of being the most effective treatment; median rank 3. The remainder of the treatments were also associated with lower susceptibility than colistin, but the results were not statistically significant. For all comparators, the high between-study SD results in wide 95% PrI. The updated analysis including the missing PHE meropenem data were very similar for cefiderocol (OR 0.31, CrI 0.04 to 2.18) and comparators.
Inconsistency checking was performed using the UME model. The model fitted the data well and the DIC was similar to the base-case NMA model (Appendix 4, Summary of network meta-analyses, Table 43). The estimated between-study SD is slightly smaller from the UME model compared to the base-case NMA model, but it still indicates extremely high heterogeneity (1.08, 95% CrI 0.67 to 1.82). The deviance plot (see Appendix 4, Inconsistency checks, Figures 38 and 39 show the deviance plots used to assess inconsistency) shows no obvious improvement in fit when using the UME model. As there is no evidence of inconsistency, no further steps were taken.
FIGURE 3.
Forest plot of OR vs. colistin for MBL Enterobacterales with EUCAST breakpoint (SIDERO, fosfomycin and PHE studies). (a) all comparators, missing PHE data; (b) All comparators, including missing PHE data; and (c) only comparators specific to the pathogen. PB, probability of being the best treatment.


Enterobacterales network including missed PHE meropenem data, using EUCAST breakpoints
When the meropenem data that were missed were included in the network, the OF for cefiderocol was very similar (OR 0.31, 95% CrI 0.04 to 2.18), and the same was true for the comparators.
Enterobacterales network restricted to comparators specific to the pathogen, using EUCAST breakpoints
When the network was restricted to comparators specific to the pathogen (see Figure 3b), the OR for cefiderocol was very similar (OR 0.34, CrI 0.05 to 1.97), and the same was true for all other comparators.
Base-case network meta-analysis: metallo-beta-lactamases P. aeruginosa infections with European Committee on Antimicrobial Susceptibility Testing breakpoint
Four studies contributed to the NMA of MBL P. aeruginosa infections with EUCAST breakpoints for SIDERO, fosfomycin and PHE studies, considering a total of four comparators, and the full network diagram is shown in Figure 4. All four studies (SIDERO WT data request,34 SIDERO CR data request,35 PHE data request and Cuba et al. )88 contained either zero or 100% susceptibility counts for one or more of the included comparators and therefore a numerical adjustment was applied prior to synthesis.
FIGURE 4.
Network diagram of all studies contributing to the NMA (MBL P. aeruginosa with EUCAST breakpoint for SIDERO, fosfomycin and PHE studies).

The relative susceptibility for each comparator relative to colistin is shown in Figure 5. The model fitted the data well, with a total residual deviance of 9.3, which was considered close to the number of data points included in the analysis of 11. The between-study SD was 0.87 (95% CrI 0.04 to 2.76), which indicates high heterogeneity. Cefiderocol was associated with a lower susceptibility relative to colistin (OR 0.44, 95% CrI 0.03, 3.94), but the result was not statistically significant. Cefiderocol was also associated with a 20% probability of being the most effective treatment; median rank 2. The remainder of the treatments were associated with no susceptibility. For all comparators, the high between-study SD results in wide 95% PrI. No inconsistency checking could be performed because the network does not include a feedback loop.
FIGURE 5.
Forest plot of OR vs. colistin for MBL P. aeruginosa with EUCAST breakpoint (SIDERO, fosfomycin and PHE studies). PB, probability of being the best treatment.

Pseudomonas aeruginosa network restricted to comparators specific to the pathogen, using EUCAST breakpoints
When the network was restricted to comparators specific to the pathogen, the OR for cefiderocol was very similar (OR 0.44, CrI 0.03 to 4.08), and the same was true for all other comparators.
Sensitivity analyses
A summary of the results of the sensitivity analyses is presented in this section. Details of the results are presented in Appendix 4, section Sensitivity analysis network meta-analysis results.
MBL Enterobacterales network including all studies using CLSI breakpoints
Further details can be found in Appendix 4, Metallo-beta-lactamases Enterobacterales network including all studies using Clinical Laboratory Standards Institute breakpoints, forest plot Figure 29a. When using CLSI breakpoints, cefiderocol was associated with a higher susceptibility relative to colistin (OR 1.38, 95% CrI 0.16 to 12.05), but the result was not statistically significant. The remainder of the treatments were associated with a lower susceptibility relative to colistin, and between-study SD was high.
When the missing study, Kohira (2016)94 was included in the analysis, the OR for cefiderocol was 0.86 (0.11–7.05) (see Appendix 4, Metallo-beta-lactamasesEnterobacteralesnetwork including all studies using Clinical Laboratory Standards Institute breakpoints, Figure 29b).
When the network was restricted to comparators specific to the pathogen, the OR for cefiderocol was very similar (OR 1.30, CrI 0.16 to 10.40), but fosfomycin’s OR indicated susceptibility higher relative to colistin, rather than lower) (see Appendix 4, Metallo-beta-lactamasesEnterobacteralesnetwork including all studies using Clinical Laboratory Standards Institute breakpoints, Figure 29c).
MBL Pseudomonas aeruginosa network including all studies using CLSI breakpoints
When using CLSI breakpoints, cefiderocol was associated with a statistically significantly higher susceptibility relative to colistin (OR 71.34, 95% CrI 4.33 to 5934.35). All other treatments (fosfomycin, aztreonam, meropenem, gentamicin) were associated with OR at or near 0, and none were statistically significant (see Appendix 4, Metallo-beta-lactamasesP. aeruginosanetwork including all studies using Clinical Laboratory Standards Institute breakpoints, Figure 31a). Between-study SD was high.
When the network was restricted to comparators specific to the pathogen, the OR for cefiderocol was a little lower (OR 64.19, CrI 1.13 to 7672.55). Other comparators (meropenem, fosfomycin) continued to be associated with OR at or near 0 (see Appendix 4, Metallo-beta-lactamasesP. aeruginosanetwork including all studies using Clinical Laboratory Standards Institute breakpoints, Figure 31b).
Networks using cefiderocol studies only
European Committee on Antimicrobial Susceptibility Testing breakpoints: when the NMAs were based on cefiderocol studies only (see Appendix 4, European Committee on Antimicrobial Susceptibility Testing breakpoint, only studies that report cefiderocol data [SIDERO]), the results were similar to the base-case NMAs for both MBL Enterobacterales and MBL P. aeruginosa isolates. The OR for cefiderocol versus colistin was 0.33 (95% CrI 0.06 to 1.65 compared to 0.32, 95% CrI 0.04 to 2.47) for MBL Enterobacterales infections and 0.49 (95% CrI 0.03 to 5.29) compared to 0.44 95% CrI 0.03, 3.94 for MBL P. aeruginosa infections. When the network also only included comparators specific to the pathogen, the Enterobacterales estimate was similar (0.33, 95% CrI 0.039 to 2.916) and the P. aeruginosa network did not change.
Clinical Laboratory Standards Institute breakpoints: when the NMAs were based on cefiderocol studies only (see Appendix 4, section Clinical Laboratory Standards Institute breakpoint including only studies reporting cefiderocol data), the results for Enterobacterales were much higher (OR 15.70, 95% CrI 0.83 to 320.72 compared with 1.38, 95% CrI 0.16 to 12.05) and for P. aeruginosa were similar (OR 66.73, 95% CrI 3.61 to 3284.37 compared to 71.34, 95% CrI 4.33 to 5934.35). When the network also only included comparators specific to the pathogen, the Enterobacterales estimate was similar (OR 16.76, 95% CrI 1.19 to 285.30) and the P. aeruginosa network did not change.
Networks using fosfomycin studies only
European Committee on Antimicrobial Susceptibility Testing breakpoints: when the NMAs were based on fosfomycin studies only (see Appendix 4, European Committee on Antimicrobial Susceptibility Testing breakpoint with fosfomycin studies only), fosfomycin was again associated with a lower susceptibility relative to colistin (OR 0.24, 95% CrI 0.02 to 3.09) for MBL Enterobacterales infection, but this effect was smaller compared to the base-case NMA. When the network also only included comparators specific to the pathogen, the Enterobacterales estimate was similar (OR 0.23, 95% CrI 0.02 to 2.36). No NMA was performed for MBL P. aeruginosa infection population using fosfomycin studies only because the network only consists of one study (see Table 9 for details).
Clinical Laboratory Standards Institute breakpoints: when the NMAs were based on fosfomycin studies only (see Appendix 4, section Clinical Laboratory Standards Institute breakpoint with fosfomycin studies only), fosfomycin was again associated with a lower susceptibility relative to colistin (OR 0.52, 95% CrI 0.06, 4.01) for MBL Enterobacterales infection, but this effect was smaller compared to the base-case NMA (OR 0.69, 95% CrI 0.07 to 6.70). When the network also only included comparators specific to the pathogen, the Enterobacterales estimate was similar (OR 0.59, 95% CrI 0.12 to 2.64). No NMA was performed for MBL P. aeruginosa infection population using fosfomycin studies only because the network only consists of one study (see Table 9 for details).
Additional review questions for Approach 3
Review questions 4–6 were defined in order to supply estimates to populate the decision-analytic model. This section describes the rationale for and requirements of each additional question, while Review questions 4 and 5 and Review question 6 describe the methods and results for each question. The approach to evidence identification and selection differed for each of these three questions, due to their perceived importance to the model, time constraints and the availability of existing reviews.
The additional review questions were:
Review question 4: What is the link between in vitro susceptibility and clinical outcomes from the published literature, in the sites of relevance, in patients according to their susceptibility to the treatment they were given?
As described above in Susceptibility studies, pharmacokinetic/pharmacodynamic studies and breakpoints, susceptibility studies do not report clinical outcomes; therefore, it was necessary to establish the link between susceptibility in vitro, and clinical outcomes. Two approaches to evidencing this link were proposed:
-
Assume that clinical outcomes do not differ according to the specific antibiotic used or the specific pathogen mechanism causing the infection, conditional upon susceptibility to that antibiotic. This assumption was validated by our clinical experts.
-
Assume that different treatments may result in different outcomes, conditional on susceptibility to the antibiotic given.
In both approaches, studies should have tested the susceptibility of a patient to the treatment they were given, and report clinical outcomes for those susceptible or not in cUTI and HAP/VAP separately. In Approach 2 data on effectiveness conditional upon susceptibility would be required for the intervention and comparators, and would need to comprise a viable NMA. Initial scoping work based on a previous systematic review [reported as part of Shionogi’s application to the European Network for Health Technology Assessment (EUNETHTA, Project PTJA11)]100 indicated that the RCTs in the HVCS sites provided poor coverage of the comparators of interest. Clinical advisors were also supportive of Approach 1, and consequently Approach 2 was not pursued further.
Review question 5: What is the long-term risk of mortality (and other outcomes) for patients with carbapenem-resistant cUTI or HAP/VAP?
This question became necessary since review question 4 did not identify any studies that reported long-term clinical outcomes. The question was widened to include any carbapenem-resistant infections.
Review question 6: What are the important safety implications of cefiderocol?
This question was required to inform the modelling of important adverse events.
Review questions 4 and 5
The methods and results for review questions 4 and 5 are presented in Appendix 5 (see Appendix 5, Tables 44 and 55 for additional inclusion criteria applied within these reviews). Review 4 (link between susceptibility and clinical outcomes) identified three studies in patients with HAP/VAP conducted solely in the ES. No useful evidence relating to the MDS was identified. Review 5 (link between susceptibility and long-term clinical outcomes) did not identify any relevant literature.
Review question 6
What are the important safety implications of cefiderocol?
Methods
A comprehensive review of the safety of comparators was not possible within the time frame of this evaluation. Adverse events included in the model for the intervention and comparators are described in Model structure for the risk-based empiric setting. Clinical advisors to EEPRU indicated that cefiderocol is predominantly a safe treatment, but that colistin and aminoglycosides have significant adverse events relating to acute kidney injury (AKI). Another key adverse event related to antibiotic use is the emergence of Clostridium difficile in a patient’s digestive tract, which can lead to diarrhoea and serious damage to the colon. EEPRU conducted a review of the RCT trial evidence for cefiderocol to establish whether it supported the clinical view that cefiderocol is a safe treatment. EEPRU were especially interested in establishing safety compared to toxic alternatives (colistin and aminoglycosides) and the other ‘safer’ treatments used in the HVCSs.
Rates of serious treatment-related adverse events, nephrotoxicity adverse events and C. difficile infections were extracted from the included RCT publications and/or their ClinicalTrials.gov national clinical trial record. Only RCTs relating to the sites of interest were reviewed, due to time and resource constraints. Only RCTs were considered as these give comparative data. In the absence of nephrotoxicity events, other kidney and renal adverse events were extracted. The data were then synthesised narratively for any important safety signals.
A pooled analysis of CREDIBLE-CR, APEKS-NP and APEKS-cUTI was presented by Shionogi in their submission to NICE,82 but since comparators in the studies differed, EEPRU preferred to consider the data from each RCT separately.
Results
The extracted adverse event data in the RCTs for cefiderocol are presented in Table 12. Across three RCTs, the proportion of patients with serious adverse events was lower with Cefiderocol compared to: imipenem/cilastatin (4.7% vs. 8.1%; APEKs-cUTI),13 meropenem (2.0% vs. 3.3%; APEKs-NP)14 and BAT [which comprised colistin-based regimens n = 30 (61%); non-colistin-based regimens (n = 19 (39%)] (1% vs. 10%, Bassetti et al. 2019/20 CREDIBLE). 15 A statistical significance for the between-group differences was not reported by any RCT.
Author (date) acronym | Intervention and comparator | Site | Serious treatment-related AE (total safety pop), n (%) | BAT therapies used | Nephrotoxicity, n (%) | C. difficile infection n (%) | Any other category that is important (name, n/N and %) | ||||
---|---|---|---|---|---|---|---|---|---|---|---|
Intervention | Comparator | Intervention | Comparator | Intervention | Comparator | Intervention | Comparator | ||||
Portsmouth et al. (2018) APEKs-cUTI13 | Cefiderocol vs. imipenem/cilastatin | cUTI | (n = 300) 14 (4.7%) (SAE) |
(n = 148) 12 (8.1%) (SAE) |
- | AKI 0 Renal disorder 0 |
AKI 0 Renal disorder 0 |
0 | 0 | Investigations 5 (1.7) |
Investigations 2 (1.4) |
Wunderink et al. (2021) APEKs-NP14 | Cefiderocol vs. meropenem | HAP/VAP/HCAP, cUTI or BSI/sepsis | (n = 148) 3 (2.0) |
(n = 150) 5 (3.3) |
- | AKI 0 Renal disorder 0 |
AKI 0 Renal disorder 0 |
1 (0.7) | 2 (1.3) | Respiratory, thoracic and mediastinal disorders 18 (12.2) Investigations 4 (2.7) |
Respiratory, thoracic and mediastinal disorders 14 (9.3) Investigations 4 (2.7) |
Bassetti et al. (2019, 2020) CREDIBLE-CR101,102 | Cefiderocol vs. BAT | HAP/VAP/HCAP, cUTI or BSI/sepsis | (n = 101) 1 (1%) |
(n = 49) 5 (10%) |
Colistin-based regimens n 30 (61%); non-colistin-based regimens n 19 (39%) | AKI 0 Renal disorder 0 |
AKI 4 (8.2) Renal disorder 1 (2.0) |
0 | 0 | Respiratory, thoracic and mediastinal disorders 7 (7) Infections and infestations 29 (29) Investigations 8 (7.9) |
Respiratory, thoracic and mediastinal disorders 2 (4%) Infections and infestations 11 (22) Investigations 2 (4.1) |
Cefi pooled data (from Shionogi submission) (n = 549 vs. n = 347)82 | N/R | N/R | AKI 0 Renal disorder 0 |
AKI 4 (1.2) Renal disorder 1 (0.3) |
1 (0.2) | 2 (0.6) | Investigations (alanine aminotransferase increased; gamma-glutamyltransferase increased; Aspartate aminotransferase increased) 17 (3.1) |
Investigations (alanine aminotransferase increased; gamma-glutamyltransferase increased; aspartate aminotransferase increased) 8 (2.3) |
None of the included studies reported specifically on nephrotoxicity. Related adverse events included AKI and renal disorder. All three RCTs reported both of these adverse events. The proportions of patients with either AKI or renal disorder was 0.0% for both cefiderocol and the comparator in the two RCTs that did not use colistin in the comparator arm. 13,14 The proportions of patients with AKI and renal disorder were greater with the comparator in the RCT by Bassetti et al. ,15 where colistin was used within the comparator arm (BAT 8.2% vs. cefiderocol 0.0% and 2.0% vs. 0.0%, respectively). A statistical significance for the between-group difference was not reported by any RCT.
One study reported C. difficile infections. The proportion of patients with C. difficile was greater with the comparator (meropenem) compared to cefiderocol (1.3% vs. 0.7%). 14 A statistical significance for the between-group differences was not reported.
Other adverse events were generally very low across the studies, with slightly greater proportions of patients experiencing adverse events with cefiderocol compared to the comparator in all studies. Statistical significance for the between-group differences was not reported.
Overview and critique of evidence in Shionogi submission to National Institute for Health and Care Excellence
In their submission,82 Shionogi include evidence on cefiderocol from RCTs, non-RCTs and susceptibility studies. EEPRU checked that the evidence submitted by Shionogi, which met EEPRU’s inclusion criteria, had been identified by EEPRU searches and included in our evidence review.
Shionogi presented clinical data from RCTs and observational studies, including CREDIBLE-CR15 and compassionate use data. In both cases, outcomes were not reported by site and by pathogen mechanism, so could not be used in EEPRU’s evaluation (see also Appendix 3, Review 1: randomised controlled trials for more details about CREDIBLE-CR). 15
Shionogi state that use of in vitro susceptibility studies has a number of advantages (see section 2.3.1.2 of the company submission). 82 EEPRU are largely in agreement that given the difficulties with conducting RCTs of new AMs, in vitro evidence may provide useful information that can contribute to estimates of comparative efficacy. However, EEPRU also identified a number of drawbacks to this approach, as noted in the Discussion and conclusions: cefiderocol clinical evidence review and in Producing comparative efficacy estimates.
Several in vitro susceptibility studies were listed by Shionogi in section 2.3.1 of the company submission,82 but it was unclear whether these were identified by a systematic review. The main studies cited were SIDERO WT79 and SIDERO CR,79 both of which were the subject of subsequent data requests made by EEPRU to Shionogi, and were then included in EEPRU’s synthesis [see section Study selection results (reviews 1–3)]. SENTRY, a surveillance study which publishes some data through an open access portal (https://sentry-mvp.jmilabs.com/), was noted in the submission, but Shionogi did not submit data from this source in response to EEPRU’s data request, and data relating to cefiderocol were not available to EEPRU via the open access portal. The UK susceptibility studies103,104 referred to by Shionogi were not eligible for inclusion in EEPRU’s review, since they did not report data for comparators and were only published as meeting or conference abstracts.
Shionogi also noted that they were at the time of the assessment applying to EUCAST for the breakpoint for cefiderocol to be changed. This has already been discussed in Statistical synthesis plan, and two analyses were planned relating to this: one using EUCAST breakpoints for cefiderocol and comparators, and one using CLSI breakpoints for cefiderocol and comparators.
Shionogi also presented PK and PD data. EEPRU did not review PK and PD data, as discussed in Table 6.
Shionogi included a pooled analysis of adverse event data. EEPRU did not use this analysis but did use the individual relevant studies as discussed in Review question 6.
Discussion and conclusions: cefiderocol clinical evidence review
There are evidential challenges when evaluating the use of new AMs to treat infections caused by multidrug resistant (MDR) pathogens. RCTs are of generally low relevance. They tend not to recruit patients with MDR pathogens, since it would be unethical to randomise patients to potentially ineffective existing treatments in the comparator arm. Therefore, relative treatment effects between the intervention and comparator cannot be generalised to MDR pathogens, as this would overestimate the efficacy of the comparator.
Since it was anticipated that RCTs were unlikely to be the primary source of evidence, three approaches to estimating comparative efficacy between the intervention and comparators were considered. In Approaches 1 and 2, RCTs and observational studies (respectively), with data for patients with HAP/VAP or cUTI infections caused by MBL Enterobacterales or P. aeruginosa, could be used to construct a NMA to compare the intervention and comparators. In Approach 3, in vitro susceptibility studies could be used to indicate the proportion of MBL Enterobacterales or P. aeruginosa isolates that are susceptible to treatment; additional evidence would be required to link susceptibility to clinical outcomes in cUTI and HAP/VAP.
Approaches 1 and 2 were not pursued since insufficient evidence from RCTs and observational studies was identified during the mapping review. The key limitations of the RCTs and observational studies that were identified included small numbers of MBL infections, and data not being reported for the sites of interest.
In Approach 3, relatively large samples of MBL Enterobacterales and P. aeruginosa isolates obtained from a range of clinical sites of infection were available from in vitro susceptibility studies, and susceptibility (unlike clinical outcomes) was expected to generalise across sites. Therefore, a NMA was conducted. Three studies (SIDERO CR, SIDERO WT and Johnston et al. ) reporting data for cefiderocol and at least one comparator met the inclusion criteria and were synthesised. Data from PHE were included alongside these studies in the synthesis as although these data did not include cefiderocol, it was considered highly relevant to estimating the comparative effectiveness of other AMs in the UK. No data were available for fosfomycin from the review, or from the PHE data. Therefore, a separate search was conducted for fosfomycin studies and 10 were included alongside the PHE data and the cefiderocol studies.
Separate networks were produced for MBL Enterobacterales and P. aeruginosa, and these were run using both EUCAST and CLSI breakpoints, since the CLSI breakpoint for cefiderocol is 4 mg/l, while for EUCAST it is 2 mg/l. Additionally, relative treatment effects for comparators may differ when using CLSI or EUCAST breakpoints.
The main NMAs used the EUCAST breakpoints. The between-study SDs indicated high heterogeneity. In the MBL Enterobacterales EUCAST analysis, cefiderocol was associated with a lower susceptibility relative to colistin (OR 0.32, 95% CrI 0.04 to 2.47), but the result was not statistically significant. Fosfomycin had a similar OR (OR 0.34, 95% CrI 0.06 to 1.96) compared to colistin as cefiderocol. The remainder of the treatments were also associated with lower susceptibility than colistin, but the results were not statistically significant. Four studies contributed to the NMA of MBL P. aeruginosa infections with EUCAST breakpoints. Cefiderocol was associated with a lower susceptibility relative to colistin (OR 0.44, 95% CrI 0.03 to 3.94), but the result was not statistically significant. The remainder of the treatments were associated with no susceptibility. The EUCAST network results were selected as the base-case source of evidence for the economic model as these are more commonly used in England.
Sensitivity analyses were performed using CLSI breakpoints. In these analyses, where the breakpoint for cefiderocol is higher, cefiderocol was associated with a higher susceptibility relative to colistin, rather than a lower susceptibility. However, the results were very uncertain in some of the NMAs due to sparse data, and large number of treatment arms were with either 0% or 100% susceptibility. These scenario analyses were run in the economic model.
Sensitivity analyses were also conducted to test assumptions about the inclusion of comparators in the network, and to assess the impact of missed data on the analysis. The estimates of comparative efficacy from the EUCAST networks were very similar in these sensitivity analyses, and it was not necessary to run these scenarios in the economic model. However, the ORs from the CLSI networks were noticeably lower for cefiderocol in two sensitivity analyses (when including missed data from Kohira et al. in the CLSI Enterobacterales network, and when only using the comparators specific to the pathogen in the CLSI P. aeruginosa network). Further, the OR for fosfomycin was higher in a third sensitivity analysis (when only using the comparators specific to the pathogen in the CLSI Enterobacterales network). These scenarios were also considered in the economic modelling – see section Clinical parameters – susceptibility evidence for further discussion.
Additional scenarios were performed, where the PHE data were used for comparators, but the relative treatment effect for fosfomycin was ascertained from a network including only the fosfomycin studies (since these were obtained from a separate review), and the cefiderocol relative treatment effect was ascertained from a network including only the cefiderocol studies (i.e. the SIDERO studies) rather than from the analysis including all studies. This was in case the separate review of fosfomycin studies introduced bias, should studies of fosfomycin be systematically different from studies of cefiderocol, for example, in the isolates selected for testing. Sensitivity analyses were conducted to test the effect of assumptions about the inclusion of comparators in the network. Results from these analyses were similar when using a single network for cefiderocol and fosfomycin, so were not used in the economic model.
Review 4 (link between susceptibility and clinical outcomes) identified two studies that reported mortality or hospital LoS conditional on susceptibility to empiric treatment and were selected for use in the model for the ES. No useful evidence relating to the MDS was identified. Review 5 did not identify any relevant literature, but an unpublished study (CARBAR) was submitted by Shionogi that contained useful data. Review 6 indicated that cefiderocol does not appear to increase the risk of AKI, C. difficile, or any other serious adverse event, compared to non-toxic comparators (i.e. comparators that were not colistin or an aminoglycoside). No study reported a comparison of cefiderocol exclusively to colistin or aminoglycosides. Event rates were generally very low or zero.
Strengths
The clinical review was conducted using a mapping approach based on robust systematic searches to capture relevant literature. This allowed EEPRU to focus resources from a relatively early stage on a viable approach to deriving clinical efficacy estimates, while still conducting a comprehensive search despite a paucity of high-quality evidence. Data extractions were checked by a second reviewer to ensure data integrity, and statistical analyses were performed using standard NMA approaches. At all stages of the clinical review, clinical advisors were consulted where there was uncertainty, and the resulting methods of synthesis have attempted to account for clinical sources of heterogeneity where feasible. Susceptibility studies, while not reporting clinical outcomes, have the advantage of testing all the treatments in the same sample of isolates, thereby reducing the chance of heterogeneity in patient samples between arms introducing confounding. They also tend to include higher numbers of patients/isolates compared to RCTs and observational studies.
Limitations
There are limitations to the clinical review, largely due to the availability of evidence and time available to conduct the evaluation.
A lack of availability of relevant RCT or observational evidence to inform clinical outcomes has resulted in the use of in vitro susceptibility data, which can be considered a surrogate outcome. This subsequently required a link to be made between susceptibility and clinical outcomes using published data and expert elicitation. No pre-specified criteria for judging the suitability of the surrogate or the linked evidence were applied. The data available to evidence the link between susceptibility and clinical outcomes were sparse and was not specifically for the pathogen mechanism of interest but rather a wider population of carbapenem-resistant patients. For the MDS, expert elicitation was used to derive the link between susceptibility and clinical outcomes (see Chapter 5).
Other limitations relate to the review methods applied in this evaluation. Because this was the first evaluation of this type commissioned by NICE, and because EEPRU could not foresee the evidence and synthesis requirements at the inception of the project, no registration with PROSPERO was performed. The statistical plan was made in response to the available data, rather than being formulated a priori, since the types of heterogeneity that would be encountered and their importance were largely unknown at the project outset. Due to time constraints, many stages of the review process were done by only one reviewer, which introduces a risk of inaccuracy. Data were checked by a second reviewer for the susceptibility review, but study selection and risk of bias assessment were conducted by only one. Since there was no suitable risk of bias tool available, EEPRU created a bespoke tool. This was done by consulting other available tools, but no face validity checks were performed by experts in susceptibility testing, and no other validation of the tool has been undertaken. This could be an area of future research. To allow for this, risk of bias scores were not used in the statistical synthesis to weight studies, subgroup studies or exclude studies, and instead aspects of clinical heterogeneity were considered in sensitivity analyses individually. Agreeing on the choice of comparators for pathogen mechanisms was a challenge throughout the project, and some inconsistencies were introduced into the review by this issue. Sensitivity analyses have been used in the NMA to explore how respecifying these affects the NMA outputs, and in most instances the impact was small. Given the resources available in the project, there were limits to the extent to which these could be implemented in the economic model. However, the most impactful scenarios have been incorporated into the modelling scenarios.
Due to the time constraints available for the evaluation, the review initially only included studies that reported data for cefiderocol and at least one comparator, whereas ideally all susceptibility evidence for all comparators would have been sought to construct the network, regardless of whether cefiderocol had also been tested. As there were no data for fosfomycin in the cefiderocol studies, and the numbers in the PHE data were too small to use, a separate search was conducted for fosfomycin studies, which may introduce bias if studies reporting data for fosfomycin are systematically different to those reporting data for cefiderocol. Equally, the PHE data did not strictly meet the inclusion criteria for the review, since they did not report estimates for cefiderocol. To minimise the impact of these potential concerns, sensitivity analyses were planned using each source separately in the economic model, as well as using the network including all studies. In terms of statistical techniques, while EEPRU undertook consistency checks, further investigations relating to the high heterogeneity in the network were not undertaken due to time constraints.
There were also some limitations introduced by susceptibility testing and implementation in clinical practice in England, and available susceptibility data. Setting clinical breakpoints is a subjective process conducted by relevant experts taking into account a range of evidence, which may have been generated differently for different comparators. Therefore, any given breakpoint may not reflect the true activity of a treatment in clinical practice, and the extent to which it does may vary between treatments. Breakpoints also change over time as pathogens increasingly acquire resistance. EEPRU were not able to resolve whether it is better to use breakpoints contemporaneous to the sample collection date, or apply current breakpoints to the available data (where data allowed), and for pragmatic reasons used data as reported in the published reports. Laboratory methods of susceptibility testing recommended by EUCAST and CLSI have also changed over time; before 2016, BSAC had its own set of methods, which may have affected the estimates of susceptibility derived before and since then, as practice, did not change immediately. As noted in Statistical synthesis plan, PHE data were a mixture of BSAC, CLSI and EUCAST methods and breakpoints, which could potentially affect the estimates of susceptibility derived. The data entering the synthesis did not match practice in England in that CLSI laboratory methods were used rather than EUCAST laboratory methods; it is unclear to what extent CLSI laboratory methods produce the same absolute values as EUCAST methods, and therefore applying EUCAST breakpoints to data derived using CLSI methods may affect relative estimates of efficacy. Clinical advisors also noted that in vitro susceptibility to meropenem, in particular, does not always indicate how well a patient will respond to this treatment in clinical practice. A similar issue arose for fosfomycin because there is no clinical breakpoint for P. aeruginosa isolates. In this case, the epidemiological cut-off, which differentiates between isolates with and without resistance mechanisms but does not link to expected clinical outcomes, was used by the MBL P. aeruginosa studies identified by the review. In addition, the evidence available for fosfomycin in P. aeruginosa included very small studies.
Conclusions
Susceptibility estimates have been used to estimate the clinical effectiveness of cefiderocol and its comparators in the HVCSs. In the EUCAST networks for MBL Enterobacterales and P. aeruginosa, cefiderocol had lower susceptibility relative to colistin, but the result was not statistically significant, and heterogeneity was high. In the CLSI network, where the breakpoint for cefiderocol is higher (4 mg/l compared to EUCAST’s 2 mg/l), cefiderocol was associated with a higher susceptibility relative to colistin, rather than a lower susceptibility. However, the results are highly uncertain. There are a number of limitations to the evidence available and the analyses done, which should be borne in mind when interpreting the evidence.
For the economic evaluation, the base-case analysis uses the full EUCAST networks to provide relative efficacy of cefiderocol and comparators. Scenario analyses are conducted using the CLSI networks, and using PHE data for the available comparators with separate networks including only cefiderocol studies and fosfomycin studies providing the relative efficacy for cefiderocol and fosfomycin, respectively.
Chapter 5 Structured expert elicitation
The review in Review questions 4 and 5 did not identify any studies that informed clinical outcomes in HAP/VAP and cUTI patients conditional upon susceptibility, following microbiology-directed treatment. In the absence of empirical evidence, outcomes were informed by eliciting judgements of individuals who have expertise on the subject matter.
Methods
A structured elicitation process was used to improve accountability and transparency. Specifically, the reference methods for HTA developed at York105 were employed. The full elicitation protocol describes the process used and is presented in Report Supplementary Material 2.
Approach to elicitation
Clinical experts were recruited to take part in the elicitation exercise, and their beliefs were elicited individually and remotely using an application developed in R, SHINY package. 106
Experts were trained in the approach to elicitation prior to the task, using an online training webinar (slides are presented in Report Supplementary Material 2). Experts were asked to express their uncertainty about the outcomes of interest using a histogram (Chips and Bins approach). 107 This approach has been shown to work well for experts not trained in probabilities and statistics. 105 Once experts were individually asked to express their beliefs, these were then aggregated using linear opinion pooling. First, a probability distribution was fitted to each expert’s beliefs from the histogram and then these were pooled, assuming that each expert contributed equally to the group overall distribution. This overall distribution was then relayed back to experts, and they were given the opportunity to revise their own beliefs on the histograms they previously completed.
Expert recruitment
Experts recruited to take part in the elicitation exercise included medical consultants, ICU consultants, pulmonary consultants and microbiologists. The literature suggests that around 10 experts should be included in an individual elicitation, and that recruitment should strive for a representative sample. 105 To this end, we sought to recruit experts from across the UK using our clinical leads. We approached experts directly and asked for their participation. Experts that agreed to participate were invited to attend a training webinar. The majority of experts attended this session, with a few choosing to view the pre-recorded slides instead. Experts’ identities were known to the modelling team; however, in aggregating, feeding back and reporting, all experts’ identities were anonymised.
Parameters elicited
The elicitation was conducted to inform outcomes in HAP, VAP and cUTIs caused by carbapenem-resistant GNB of interest, following microbiology-directed treatment. The elicitation exercise was used to inform outcomes in two distinct reports where the pathogens of interest included Enterobacterales OXA-48, MBL Enterobacterales or MBL P. aeruginosa.
For each of the three sites of infection, we elicited outcomes depending on whether the infectious pathogen is susceptible to treatment. Therefore, outcomes only depend on whether a patient is susceptible to treatment or not, and not to the specific treatment given. The outcomes of interest were 30-day mortality, LoS in hospital and the type of ward these patients would stay on in hospital. As background information, we presented several related studies to experts (see Report Supplementary Material 2 for details).
Results
Completion rate
Eleven experts agreed to take part in the elicitation task and took part in the training. Only five provided usable data (see Appendix 6).
Group summaries and use in the modelling
Pooled summaries for each elicited quantity are shown in Appendix 6. The group summaries on 30-day mortality (see Appendix 6, Results, Figures 40, 42 and 44) indicate that survival is the lowest for VAP patients and highest for cUTI patients, and that susceptibility to treatment increases the probability of survival, for all three sites of infection. The group summaries on LoS (see Appendix 6, Results, Figures 41, 43 and 45) indicate that the LoS is the shortest in patients with cUTIs and the longest for patients with VAP. For all three sites of infection, susceptibility to treatment decreased the LoS. The group summaries for the proportion of time spent on different types of wards (see Appendix 6, Results, Tables 46–48) indicate that patients with VAP spend the most time in ICU and the least time on general medical wards, followed by HAP, then cUTIs. Furthermore, patients who are susceptible to treatment are expected to spend more time on the general medical ward and less on ICU and HDU, for all three sites of infection.
In the model, outcomes of HAP and VAP were modelled together, and so experts’ responses were pooled. When pooling, outcomes for HAP and VAP were weighted by their relative occurrence in Tumbarello et al. (2013) – 0.283 (28/99) for HAP and 0.717 (71/99) for VAP. Tumbarello was chosen as the study where participants were the most representative of patients in the HVCSs that reported the proportion of patients with HAP that was ventilator associated.
The pooled results for expert beliefs are shown in Figure 6 and summarised in Table 13.
FIGURE 6.
Survival and LoS by susceptibility. (a) 30-day survival with HAP/VAP combined; and (b) expected LoS with HAP/VAP combined.

ICU | HDU | General medical ward | |
---|---|---|---|
HAP/VAP, susceptible | 49.90 | 14.94 | 35.16 |
HAP/VAP, not susceptible | 58.92 | 17.21 | 23.86 |
cUTI, susceptible | 15.00 | 17.00 | 68.00 |
cUTI, not susceptible | 23.33 | 18.33 | 58.33 |
Validation of experts’ estimates
We explored alternative sources of evidence to inform LoS in the model, in order to validate the elicitation results. This suggested that the LoS elicited from the experts is broadly comparable to the estimates within the literature; for further details, see Appendix 6.
Chapter 6 Existing economic evidence
Assessment of existing cost-effectiveness evidence and modelling approaches
A series of reviews of existing cost-effectiveness evidence and modelling approaches was conducted:
-
A review of existing cost-effectiveness evidence for cefiderocol with a focus on studies that include decision-analytic models. The aims were to establish the existence of potentially policy-relevant models to guide NICE and NHS decisions; and to identify relevant analytical methods and data sources.
-
A review of existing approaches to modelling resistant pathogens in the target population, currently and over time. The aim of this review was to identify methods that could be adopted for this purpose in EEPRU’s modelling.
-
A review of existing cost-effectiveness models in HAP/VAP to understand modelling approaches and data sources.
-
A review of existing cost-effectiveness models in cUTI. Again, the purpose was to understand modelling approaches and data sources.
Each review involved searches of bibliographic databases using standardised search terms, selection of studies based on explicit inclusion criteria and data extraction using an agreed template. Details of each review are provided in Report Supplementary Material 3. Here, the key results of each review are outlined.
Review 1: existing cost-effectiveness evidence for cefiderocol
A total of 89 potentially relevant papers or abstracts were identified for the review from the searches. All the publications were screened using their titles and abstracts, and the only study included was in the form of a poster and provided limited detail regarding the sources of clinical evidence and how these were used in the modelling. 108 This, together with the study’s US focus, means it provides no basis to inform the current evaluation of cefiderocol.
Review 2: review of existing approaches for resistance modelling
Nine studies were included in this review. Five economic evaluations of ceftazidime/avibactam were included in this group. None of these assessed the implications of changes in resistance over time. Three analyses made assumptions (rather than drawing on evidence) about the proportions of patients with resistant infection in the relevant population, and the impact of resistance on clinical parameters. 109–111 The other two studies drew on evidence from observational studies to quantify the impact of resistance on relevant parameters in the modelling. 111,112
The other four studies in this review provided some indications of how resistance could be captured. One study assessed the appropriateness of alternative empiric therapies based on susceptibility data from a specific Taiwanese hospital. 113 Another looked at procalcitonin-guided antibiotic stewardship and estimated the correlation between the percentage reduction in days of antibiotic use resulting from the procalcitonin-guided test and antibiotic resistance. 114
The other two studies in this review attempted to deal with resistance through mechanistic infectious disease modelling. One used hypothetical data for illustrative purposes. 115 The other used the combination of a dynamic transmission model and a treatment pathway model as a generic framework to evaluate antibiotics for different indications and pathogens. 116 In principle, such a model could be capable of quantifying not just the direct health effects of a new antibiotic but also the indirect impacts via any reduction in transmission of relevant pathogens. It could also reflect changes in resistance over time in response to different stewardship strategies and the introduction of new AMs. However, whether the model can achieve this in practice will inevitably depend on the available evidence and the assumptions necessary to address the evidence gaps.
Review 3: existing cost-effectiveness models in hospital-acquired pneumonia/ventilator-associated pneumonia
This review used an earlier systematic review117 to extract information on the characteristics of three relevant studies including target population, modelling assumptions, model structure and key evidence. 118–120 All of these studies included standard cost-effectiveness models and did not consider the impact of alternative therapies on resistance patterns over time. One study attempted to include transmission rates in the modelling, but this was not extrapolated to estimate population-level health effects. 120 As a UK study, one study provided some potentially useful evidence sources for the current evaluation. 118
Review 4: existing cost-effectiveness models in complicated urinary tract infection
One study was identified121 in addition to the models in cUTI identified in Review 2. 108,110,111,113,116 As for Review 3, the UK-based studies provided some insights on evidence sources. The additional study121 was US based and used microsimulation to track patients allowing for treatment switching as microbiological information becomes available. A surveillance data set was used to sample isolates and to determine susceptibility to different treatments. This use of susceptibility data, rather than standard in vivo evidence from RCTs and other designs, is novel and has the potential to address modelling challenges.
Chapter 7 Methods for quantitative assessment of value
This section reproduces and adapts, with permission, content previously published as part of the study protocol. 1
Overview of methods
The quantitative economic analysis developed for this evaluation comprises three components: an assessment of the INHEs of introducing cefiderocol within the HVCSs at the patient level; an assessment of INHEs within the HVCSs at the population level; and an assessment of how population-level INHEs within the HVCSs might appropriately be rescaled to reflect expected usage across the NHS. An overview of each component is provided below, and the methods for each component are described in the following sections. In line with the NICE Reference Case, the model perspective is the NHS and Personal and Social Services, health benefits are expressed in terms of quality-adjusted life-years (QALYs) and both costs and QALYs are discounted at a rate of 3.5% per annum.
The patient-level component of the model is structured similarly to models developed as part of other NICE activities, and characterises the likely comparative effectiveness of cefiderocol and existing AM usage scenarios; also the impact of cefiderocol and existing AM usage scenarios on costs, HRQoL and mortality over the lifetime of the patient.
The population-level component aggregates the patient-level predictions to the population level accounting for the size of, and growth over time in, the eligible patient population in England within each HVCS. This component also reflects how resistance is likely to develop to cefiderocol and existing AMs over time. The previous EEPRU framework outlined two broad approaches to modelling this: mechanistic dynamic transmission modelling which attempts to explain the way in which susceptible and resistant pathogens spread through the population; and statistical forecasting models that predict the number of people with infections with specific resistance profiles without explicitly modelling the underlying mechanistic processes of pathogen transmission and resistance acquisition. 21 We considered both approaches, but ultimately used a forecast-based approach, for reasons detailed below.
The use of a transmission model was considered but not pursued on three grounds. Firstly, developing a mechanistic transmission model that characterises the spread of carbapenem-resistant organisms, with an adequate level of detail to model the introduction of cefiderocol, and that is appropriately calibrated to historical epidemiological data, was not considered feasible within the time and resources available for this 9-month project. Secondly, our clinical advisors considered that the direction and magnitude of the effects of the new treatments on transmission were uncertain and not well evidenced (see Additional elements of value relevant to antimicrobials). Thirdly, advice during our previous EEPRU work21 indicated that transmission modelling in AMR is an evolving science where the degree of parameter and structural uncertainty can lead to instability in model predictions and that, although there is no guarantee that a forecast-based approach will offer more certain or robust predictions, it should offer greater transparency.
The final quantitative assessment performed is to rescale the population-level INHEs observed in the HVCSs to reflect expected usage. This part of the quantitative assessment takes a very pragmatic approach seeking to identify the range of clinical scenarios in which cefiderocol is expected to be used, enumerate the corresponding population sizes using the best available evidence, and rescale the population-level INHEs estimated for the HVCS accordingly.
The literature on the economic evaluation of AMs has described a range of elements of value associated with these products that are not relevant to and, therefore, do not feature in evaluations of other drugs and health technologies. 21,122 Following presentation of the quantitative assessments of value, we therefore discuss whether these additional elements of value might be delivered via use of cefiderocol, the extent to which they are captured by our quantitative assessments and, where they are not captured, whether they are likely to substantively modify the estimates of value presented (see Additional elements of value relevant to antimicrobials).
Modelling direct patient net health effects in high-value clinical scenarios
Relationship with decision problem
Population
The patient populations modelled align with the decision problem outlined in Decision problem. These are summarised in Table 14.
Site | Pathogen | Mechanism | Setting |
---|---|---|---|
HAP/VAP | Enterobacterales | MBL | Microbiology-directed |
HAP/VAP | P. aeruginosa | MBL | Microbiology-directed |
HAP/VAP | Enterobacterales | MBL | Risk-based empiric treatment |
HAP/VAP | P. aeruginosa | MBL | Risk-based empiric treatment |
cUTI | Enterobacterales | MBL | Microbiology-directed |
cUTI | P. aeruginosa | MBL | Microbiology-directed |
Intervention
Cefiderocol is considered as monotherapy only due to a lack of in vivo or in vitro evidence about how it performs in combination with other agents. The clinical advisors confirmed that monotherapy was more likely to be used in practice but indicated that combination therapy was more likely for P. aeruginosa.
Comparators
A wide range of drugs is considered relevant in the HVCSs, and different drugs were considered relevant depending on the site, pathogen, mechanism and setting. The full list of comparators is provided in Population, intervention, comparison, outcomes, study designs for high-value clinical scenarios. Due to the paucity of data available to inform the comparative effectiveness assessment (see Clinical evidence), and our reliance on in vitro susceptibility data to inform comparative effectiveness, it was possible to take a simplified approach to modelling these comparators rather than conducting a fully incremental analysis of all available AM options as is typically recommended in economic evaluation. The approach taken is documented in the following section.
Model structure
The model structure differs according to the setting (MDS or ES) but not the site, pathogen or mechanism of resistance. We describe the structure for the MDS first as it is more straightforward and forms part of the ES model structure.
Due to the paucity of in vivo data relevant to the modelled HVCSs (see Review results), we have assumed that differences across treatments in in vitro susceptibility are predictive of in vivo clinical outcomes. This was considered reasonable by the clinical advisors to this project, and evidence relating to the treatment susceptibility as a surrogate for clinical outcomes is reviewed in Review questions 4 and 5. We link susceptibility to time in hospital and mortality. We do not model the development of infection sequelae such as sepsis. This would have required a range of additional evidence including the rate of development of sepsis, how this relates to susceptibility to the treatment administered and mortality and hospitalisation outcomes conditional upon whether a patient developed sepsis. Given the sparsity of evidence available, including these additional parameters was not considered appropriate. We would, however, expect 30-day mortality and hospitalisation outcomes to implicitly reflect the possibility that patients may develop additional complications including sepsis. Repeat infection following discharge was also not explicitly modelled (though will be implicitly reflected in the mortality data) as these were considered unlikely to be a significant driver of population-level INHEs in the HVCSs.
In addition to differences in effectiveness, we model differences in treatment safety. We focus on nephrotoxicity and, in particular, the occurrence of AKI as this was considered to have the most significant implications for the modelling in terms of driving treatment choices (with clinicians keen to avoid highly nephrotoxic comparator drugs) and influencing INHEs as cefiderocol is expected to be associated with lower rates of nephrotoxicity than some comparators.
Ototoxicity was raised by our clinical advisors as a safety concern associated with use of aminoglycosides. This was not modelled as it was expected that significant hearing impairment associated with aminoglycosides would be rare in this patient group. 123 Reduced rates of C. difficile infection were highlighted by a number of stakeholders as a potential benefit of the new drugs. This was not included in the modelling as rates of C. difficile are very low124 (see Review question 6).
Shionogi’s manufacturer submission did not include an economic model. However, it suggested modelling the benefits of improved empiric therapy choice using data linking time to appropriate therapy to key outcomes (mortality, hospitalisation). This approach was not pursued as it was not clear how patients who never receive appropriate therapy (e.g. because they are not susceptible to any available treatment options) should be reflected in this type of model structure. In particular, it was unclear how a model based around time to appropriate therapy could accommodate all comparisons of interest, that is, use of cefiderocol in the ES, use of cefiderocol in the MDS and no use of cefiderocol. The latter two treatment pathways would both involve a similar time taken to reach the MDS, but if cefiderocol were used in the MDS, a higher proportion of people would be expected to receive appropriate therapy at this point.
Model structure for microbiology-directed setting
In the MDS, each patient’s susceptibility to available treatment options is known, and treatment can be tailored accordingly. Based on feedback from our clinical advisors, the two main reasons for initiating treatment with cefiderocol (provided patients are susceptible to it) within the MDS HVCSs would be that patients are either: (1) not susceptible to any other available treatment options (i.e. patients are completely MDR to relevant existing treatment options); or (2) that the only other treatments to which they are susceptible carry an elevated risk of nephrotoxicity. We include colistin and aminoglycosides within the category of nephrotoxic drugs as our clinical advisors indicated that they are likely to be associated with elevated levels of nephrotoxicity. To reflect these considerations, patients within the MDS are divided into three categories based on their susceptibility to existing therapies and, within each category, further subdivided according to their susceptibility to cefiderocol. Table 15 shows these subgroups, how they determine treatment choice under existing care and how that would change if cefiderocol was to become available to this patient group. The groups for which a switch to cefiderocol is expected are highlighted in bold.
Susceptibility to existing drugs | Susceptibility to cefiderocol | Therapy under existing care | Therapy with new drug available |
---|---|---|---|
Susceptible to one or more non-colistin/aminoglycosides option | Susceptible | Non-colistin/amino | Non-colistin/amino |
Resistant | Non-colistin/amino | Non-colistin/amino | |
Susceptible only to colistin or aminoglycosides | Susceptible | Colistin/amino-based | Cefiderocol |
Resistant | Colistin/amino-based | Colistin/amino-based | |
Not susceptible to any available treatment options | Susceptible | Multidrug salvage | Cefiderocol |
Resistant | Multidrug salvage | Multidrug salvage |
In the group of patients who are susceptible only to colistin or an aminoglycoside, and susceptible to cefiderocol, cefiderocol offers a safety advantage. In the group of patients who are not susceptible to any available treatment options and, in the absence of the new treatment under evaluation, would receive multidrug salvage therapy, cefiderocol offers a safety and efficacy advantage. This is because, for many patients, multidrug salvage therapy would be expected to include a colistin or aminoglycoside component. Throughout the modelling, isolates classed as intermediate resistant are grouped with those which are resistant as patients infected with intermediate resistant and resistant pathogens are expected to experience similar outcomes in the HVCS based on feedback from EEPRU’s clinical advisors, and much of the data relating mortality and hospitalisation to susceptibility follow this grouping. Our clinical advisors noted that it may be possible to overcome intermediate resistance via higher dosing, but also considered that it would be difficult to evidence this within the model. Given the diverse range of data sources informing susceptibility and the link between susceptibility and outcomes, and the level of reporting within these studies, it was not feasible to explore the implications of differential outcomes between intermediate resistant and resistant patients.
In the MDS, the model is, therefore, driven by the proportion of individuals within each category of ‘susceptibility to existing drugs’ and the proportion of individuals susceptible to cefiderocol. This is based on susceptibility data as documented in section Clinical parameters – susceptibility evidence. The comparison made within the model is between the overall MDS cohort who receive tailored therapy with the new drug available (column four of Table 15) and the overall MDS cohort who receive tailored therapy under existing treatment options only (column 3 of Table 15).
Importantly, the fact that the susceptibility profile is known prior to initiation of treatment in the MDS, alongside the assumption that susceptibility is the sole predictor of treatment effectiveness, means that we do not need to model each individual treatment option within the MDS. For example, it is not relevant (to clinical outcomes) whether a patient is susceptible to fosfomycin or aztreonam as susceptibility to these treatments would be assumed to result in the same outcomes. Although there are differences in the costs of therapies, these are modest in relation to other costs such as that of hospitalisations which may include periods in the ICU/HDU. In practice, patients may receive a combination of agents, but this is not modelled explicitly due to a lack of evidence. EEPRU’s clinical advisors considered it reasonable to assume that, in the MDS, patients susceptible to a single AM within a multiagent regimen perform as well as those susceptible to all components of that regimen (i.e. it does not matter if you are susceptible to drug A, drug B or drug A and B, as long as you are susceptible to one of the agents received).
Following receipt of treatment in the MDS, patients are modelled to experience one of the four alternative 30-day outcomes which determine their long-term outcomes (see Figure 7). A decision tree is used to determine the distribution of patients across these categories at 30 days. This is as shown in Figure 8. Probabilities highlighted in bold differ by treatment in this figure. In the MDS, we only model one line of treatment explicitly, though hospitalisation and mortality evidence will reflect the fact that some patients entering the MDS receive multiple lines of therapy.
FIGURE 7.
Thirty-day outcomes in the MDS.

FIGURE 8.
Decision tree used to calculate impact of AKIs on 30-day outcomes in MDS.

All patients face a risk of death due to their infection and comorbidities (p_bgrdD30d_MDS). The risk differs according to whether patients have received a treatment to which they are susceptible or not. In the MDS, given that treatments are tailored according to patients’ known susceptibility profiles, only patients infected with a fully MDR infection (who receive multidrug salvage treatment) are expected to face the elevated risk of death of those non-susceptible to treatment. The efficacy advantage of cefiderocol is, therefore, driven by the proportion of people who, rather than having ‘multidrug salvage’, are given cefiderocol (see Table 15), as these patients switch from experiencing the mortality of non-susceptible patients to experiencing the mortality of susceptible patients.
In addition, patients face differing drug-related risks of experiencing an AKI. Patients who experience an AKI face an elevated risk of death compared to those who do not. When modelling the effect of AKI on mortality, we account for the fact that the available mortality data already reflect both the underlying risk of AKI associated with currently available non-colistin/aminoglycoside AMs, and the background risk of AKI associated with patients underlying comorbidities and infection (see Figure 8). Patients who experience an AKI and survive until 30 days face a risk of adverse long-term outcomes according to whether they have: (1) recovered their renal function; or (2) suffered irreversible renal failure, that is, developed chronic kidney disease (CKD).
At 30 days, patients who are discharged alive without renal dysfunction are assigned a comorbidity-adjusted QALY outcome estimated using an alive–dead area-under-the-curve approach. This is independent of the assigned treatment, as patients alive at 30 days without a history of AKI are assumed to experience similar outcomes regardless of the treatment they received for their infection.
Patients discharged with recovered renal function face the same HRQoL outcomes, but they face an additional risk of progressing to CKD and elevated mortality. Patients discharged with CKD or who develop CKD face further elevated mortality, reduced HRQoL and additional healthcare costs. The experience of the two groups of patients with a history of AKI is modelled as a semi-Markov process (with transition probabilities dependent on time in model) for all transitions as shown in Figure 9.
FIGURE 9.
Markov model used to calculate post-30-day outcomes in patients with recovered renal function and irreversible renal failure.

Model structure for the risk-based empiric setting
The approach taken in the ES is similar to that taken in the MDS in terms of the possible 30-day outcomes patients can experience and the long-term implications of these outcomes. However, the decision tree describing differences across comparators in the first 30 days is more complex for two reasons. Firstly, there is a need to model outcomes both in those correctly identified as having the pathogen-mechanism combination suspected, as well as those who were labelled as high risk but in fact have a different causative pathogen or mechanism. Secondly, there is a need to model both the ES phase of treatment, and progression of some patients to the MDS for further treatment.
Unlike in the MDS, in the ES the susceptibility of patients to treatments provided is unknown at the time of initiating empiric treatment. It is, therefore, necessary to model the probability of susceptibility to individual treatment combinations as this determines clinical outcomes and, in particular, the need for further treatment. Since, as documented in Population, intervention, comparison, outcomes, study designs for high-value clinical scenarios, there are a number of feasible treatment combinations for these patients, to simplify the modelling we compare empiric use of cefiderocol in the ES with two alternative treatment options:
-
The non-colistin or aminoglycoside-based treatment combination with the current highest estimated susceptibility in the UK population.
-
The colistin or aminoglycoside-based treatment combination with the current highest estimated susceptibility in the UK population.
When considering possible treatment pathways in the ES, the following possible pathways are relevant (shorthand labels: E1, E2nca, etc. are used in the results section):
-
E1: empiric use of cefiderocol followed by existing treatments in the MDS.
-
E2nca: empiric treatment using non-colistin or aminoglycoside-based existing therapies followed by existing therapies in the MDS.
-
E2ca: empiric treatment using colistin or aminoglycoside-based existing therapies followed by existing therapies in the MDS.
-
E3nca: empiric treatment using non-colistin or aminoglycoside-based existing therapies followed by cefiderocol use if indicated in the MDS.
-
E3ca: empiric treatment using colistin or aminoglycoside-based existing therapies followed by cefiderocol use if indicated in the MDS.
Repeated usage of cefiderocol in the MDS for patients who fail cefiderocol in the ES was not modelled as this was not considered to represent a priority use for cefiderocol.
Thirty-day outcomes in the ES are determined by a decision tree which comprises three subcomponents:
-
the risk of carrying the pathogen mechanism of concern
-
outcomes at the point at which patients are assessed for MDS treatment, that is at around 3–5 days when susceptibility results report and
-
30-day outcomes following MDS assessment.
Each of these is considered in more detail below.
-
Risks of carrying the pathogen mechanism of concern.
Patients may or may not have the suspected pathogen mechanism of concern. We assume that patients who do not have the pathogen mechanism of interest experience the same effectiveness outcomes regardless of the choice of empiric treatment (though safety differs), as our clinical advisors confirmed that these patients represented a broadly susceptible population (rather than a population enriched with pathogens carrying other resistance mechanisms), and that for this reason effectiveness is likely to be similar across all empiric treatment options considered. For simplicity, we assume that patients who have a different pathogen-mechanism experience the susceptibility associated with colistin/aminoglycoside-based therapy in people with the pathogen mechanism of interest regardless of the choice of treatment. Colistin/aminoglycoside-based therapy was chosen as more representative of outcomes across susceptible patients as this treatment class showed robust and high susceptibility across subgroups and scenarios. The structure of this element of the ES model is presented as Figure 10.
FIGURE 10.
First component of 30-day outcomes model for ES: risk of carrying pathogen mechanism of concern.
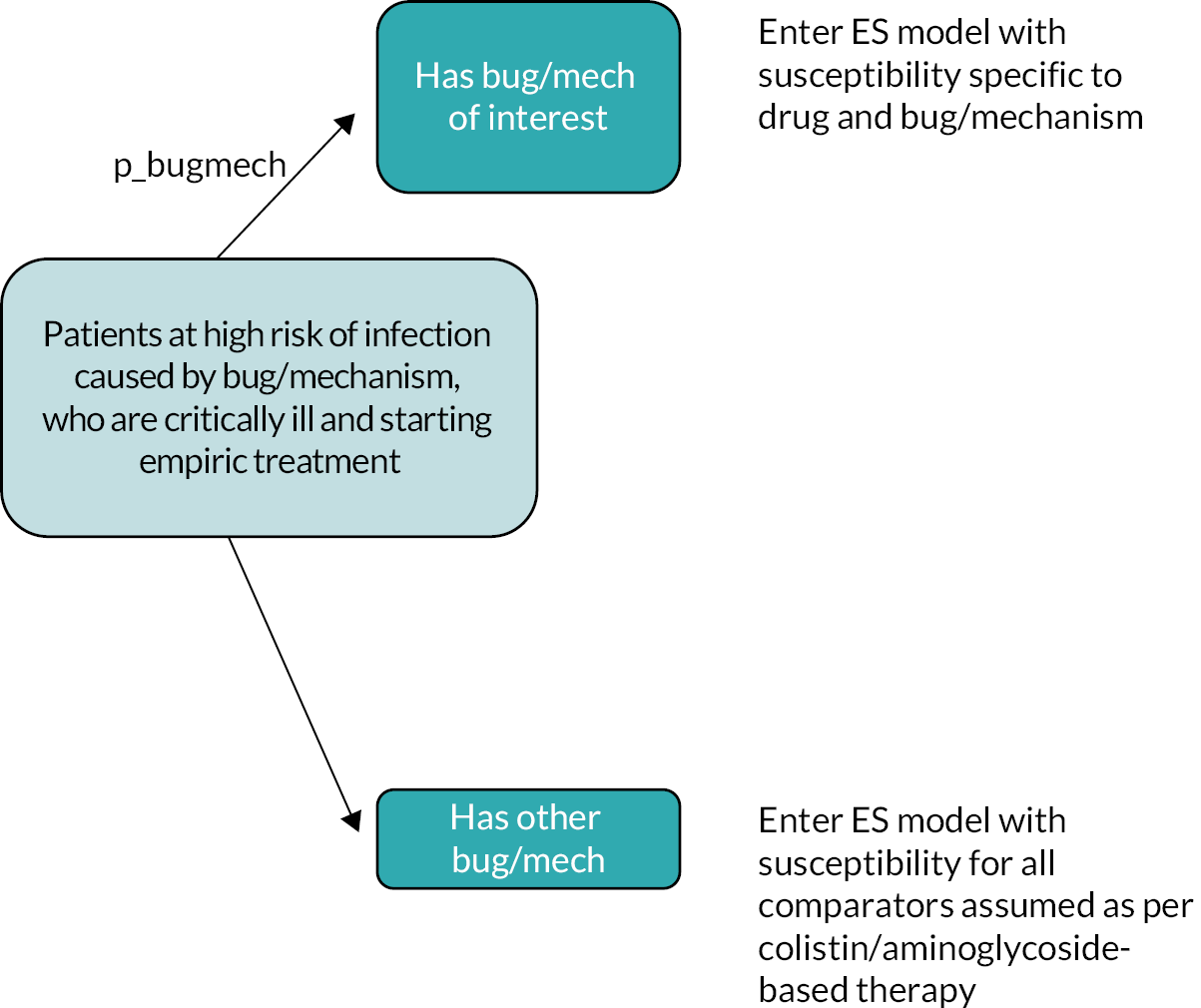
-
Outcomes at the point at which patients are assessed for MDS treatment.
At initiation of empiric treatment, patients are classified by the model as susceptible or non-susceptible to their empiric therapy. As in the MDS, susceptibility is the driver of differences in effectiveness across treatments. Note that we are able to model differences in susceptibility across treatments used in the ES dependent on whether a patient is susceptible or non-susceptible, even though clinicians will not observe this information until patients enter the MDS.
At the point at which patients’ microbiology results become available, patients may have died, may require initiation of a new AM treatment (e.g. due to lack of efficacy) or may complete their course of empiric treatment. The probability of these three outcomes depends on whether patients were susceptible to their empiric treatment or not, but not directly on the choice of specific treatment. Patients who have received empirically a treatment to which they are later found not to be susceptible are all assumed to require further treatment in the MDS, provided they survive until microbiology results are available. This assumption is based on evidence presented in Tumbarello et al. (2013), which found that all patients who received inappropriate empiric treatment and survived until their microbiology results were received switched to appropriate therapy (further details on Tumbarello et al. are provided in 8.2.3.4). 125 The structure of this element of the ES is shown in Figure 11.
FIGURE 11.
Second component of 30-day outcomes model for ES: outcomes at the point at which patients are assessed for MDS treatment. NB: Mortality (p_bgrd_Dst_S and p_bgrdDst_nonS) is also adjusted to reflected differences in mortality due to AKI, in the same way as shown in Figure 12, but this is not shown for parsimony.

-
30-day outcomes following assessment for MDS treatment.
People who survive until the time point of assessment for MDS treatment enter the third part of the decision tree which is shown in Figure 12. Those requiring no further treatment face a risk of dying between this point and 30 days which depends on whether they experienced an AKI. Those surviving to 30 days face the possibility of entering the (1) alive; (2) alive with recovered renal function or (3) alive with irreversible renal failure health states described in Figure 12. While patients may experience an AKI following empiric treatment, clinicians confirmed that in this patient group, where treatment options are limited, the AKI alone would not typically trigger a treatment switch, provided the treatment was effective.
FIGURE 12.
Third component of 30-day outcomes model for ES: 30-day outcomes following assessment for MDS treatment.

Patients who require further treatment enter the MDS component of the model. Their outcomes depend on whether they experienced an AKI following first-line treatment (i.e. this is ‘remembered’ within the model) as this determines both their outcomes (patients who experience an AKI experience elevated mortality and the implications of reversible or irreversible kidney damage) and their choice of treatment in the MDS. Our clinical advisors informed us that patients requiring further treatment in the MDS, who experienced an AKI following treatment in the ES are unlikely to receive colistin- or aminoglycoside-based treatment in the MDS. Patients who fit this profile, and are only susceptible to colistin or aminoglycoside-based treatment are, therefore, assumed to receive multidrug salvage therapy in the MDS, or the new drug if available. For these patients, multidrug salvage therapy is assumed not to include colistin or an aminoglycoside. Instead, they are assumed to receive the outcomes of multidrug salvage therapy without elevated risk of AKI.
In the absence of evidence to support more detailed modelling, we assume that a patient’s susceptibility to treatment is the same in the ES and MDS. In reality, patients entering the MDS who were already assessed as high risk of carrying a highly resistant pathogen in the ES are likely to receive aggressive treatments in the ES which may change their resistance profile in the MDS. The nature of the effects on individual resistance are hard to predict as they are influenced by the treatment received in the ES, the effectiveness of this treatment and the development of acquired resistance. These are not, therefore, considered within the model.
Sources of evidence
Identification of evidence
Susceptibility evidence and evidence linking susceptibility to mortality and hospitalisation were obtained via the systematic reviews and structured expert elicitation as described in Review results, Statistical synthesis and Review questions 4 and 5. Other key clinical parameters were obtained from existing systematic reviews where possible; otherwise, clinical parameters were obtained from existing UK cost-effectiveness models. Quality-of-life weights (utilities) were obtained from a systematic review (described below) and cost parameters via targeted searches.
Clinical parameters – susceptibility evidence
The susceptibility data used in the model base-case analysis are summarised in Table 16. These represent the mean values of the samples used in the probabilistic sensitivity analysis (PSA), along with 95% percentile-based confidence intervals. Five key susceptibility parameters inform the model:
Pathogen-mechanism subgroup | Description | Value (%) | 95% CI (%) |
---|---|---|---|
MBL Enterobacterales | Proportion of isolates susceptible to one or more non-colistin/aminoglycosides option | 91 | 87 to 95 |
MBL Enterobacterales | Proportion of isolates susceptible only to colistin or aminoglycosides | 7 | 4 to 12 |
MBL Enterobacterales | Proportion of isolates susceptible to cefiderocol | 67 | 22 to 95 |
MBL Enterobacterales | Proportion of isolates susceptible to the most effective non-colistin/aminoglycoside-based empiric treatment | Not applicable | Not applicable |
MBL Enterobacterales | Proportion of isolates susceptible to the most effective colistin/aminoglycoside-based empiric treatment | 96 | 90 to 99 |
MBL P. aeruginosa | Proportion of isolates susceptible to one or more non-colistin/aminoglycosides option | 28 | 0 to 100 |
MBL P. aeruginosa | Proportion of isolates susceptible only to colistin or aminoglycosides | 71 | 0 to 100 |
MBL P. aeruginosa | Proportion of isolates susceptible to cefiderocol | 98 | 85 to 100 |
MBL P. aeruginosa | Proportion of isolates susceptible to the most effective non-colistin/aminoglycoside-based empiric treatment | 28 | 0 to 100 |
MBL P. aeruginosa | Proportion of isolates susceptible to the most effective colistin/aminoglycoside-based empiric treatment | 100 | 97 to 100 |
-
One parameter describes susceptibility to cefiderocol in the MDS and ES.
-
Two parameters describe susceptibility to colistin/aminoglycoside-based therapy and to non-colistin/aminoglycoside-based therapy in the ES.
-
Two parameters describe the number of individuals in each category of susceptibility in the MDS as shown in Table 15 (namely, susceptible to a non-colistin/aminoglycoside AM, susceptible only to a colistin/aminoglycoside AM).
Susceptibility to existing drugs is obtained from both the analysis of PHE data and the NMA, as described in Statistical synthesis. These analyses can be combined to provide evidence on absolute rates of susceptibility to AMs for the HVCS. This evidence required further adjustment before it could be used in the economic model, with different adjustments for the ES and MDS. The methods employed to obtain estimates for these two settings are discussed in turn, with further details provided in Appendix 7. Of note, while evidence on susceptibility to meropenem was available, this was not used in the economic modelling. This is because clinical advice was that, for meropenem, susceptibility among carbapenem-producing pathogens was not a good surrogate predictor of clinical outcomes. This reflects advice in the literature. 126,127 Hence, while meropenem is included as a comparator in the PICOS, it is assumed to have zero efficacy in the economic modelling (and so not actively modelled).
Susceptibility is estimated to be specific to the pathogen-mechanism subgroup of interest but is assumed to generalise across sites and settings. This was considered a reasonable assumption by our clinical advisors and preferable to further subgrouping the susceptibility data given the small sample sizes available to inform these parameter estimates for the HVCS.
Susceptibility for AMs used in the ES
Clinical advice, as reflected in the PICO, was that combination treatment was frequently used in the ES. Hence, evidence on absolute susceptibilities for individual drugs needed to be converted to evidence on overall susceptibility to combination treatments, to identify the most effective combination treatments. This requires information on conditional susceptibility (e.g. for combination treatment of AM ‘X’ and AM ‘Y’, evidence is required on the susceptibility to AM ‘Y’ conditional on being resistant to AM ‘X’). For use in the model, the most effective ES treatment which did not include colistin or an aminoglycoside was considered, as well as the most effective ES which did. A discussion of the available evidence on conditional susceptibility is provided in Appendix 7.
For the base-case analysis, evidence on absolute susceptibilities for combination treatments and monotherapies was obtained from the NMA based on EUCAST studies (see Statistical synthesis) applied to the absolute colistin susceptibility from the PHE data (colistin was chosen as the reference AM as it appeared in the majority of studies, and susceptibility to this AM was relatively constant over time as illustrated by an analysis of PHE data; see Report Supplementary Material 3). Where the NMA provided evidence for multiple AMs within the same class (such as aminoglycosides), the most effective AM was used. The assumption of independence of absolute susceptibilities was relaxed in scenario analyses, as detailed in Appendix 7 (relaxing this assumption was not possible for the P. aeruginosa population due to an absence of evidence).
There are two main approaches for defining breakpoints for susceptibility evidence (which in turn affect relative and absolute rates of susceptibility): EUCAST and CLSI (see Susceptibility studies, pharmacokinetic/pharmacodynamic studies and breakpoints). The former was judged to be most relevant to the UK, hence evidence from studies using EUCAST breakpoints was used in the base case. A scenario analysis included only CLSI studies.
Another scenario analysis used only evidence from PHE, as this represents UK-specific evidence. In this scenario, evidence for cefiderocol comes from the EUCAST NMA as no susceptibility evidence was available for cefiderocol from PHE. As there was insufficient evidence for fosfomycin in the PHE data, this scenario assumes that fosfomycin is not used (as fosfomycin and colistin are the only comparators for the Pseudomonas population, this scenario was only run for the Enterobacterales-MBL population). Additional scenarios were conducted in which evidence for all other treatments came from the PHE data with cefiderocol and fosfomycin evidence coming from their own separate networks. For each population, there were two such scenarios: one which was restricted to EUCAST studies and one which was restricted to CLSI studies.
For the PSA (which was performed for the base-case analysis only), two sources of uncertainty were considered:
-
Uncertainty in the OR obtained from the NMA posterior distribution.
-
Uncertainty in the absolute susceptibility of colistin (to which OR are applied), obtained from PHE data and modelled using a beta distribution.
As discussed in Sensitivity analyses and Discussion and conclusions: cefiderocol clinical evidence review agreeing on the choice of comparators for pathogen mechanisms was a challenge throughout the project, and some inconsistencies were introduced into the review and NMA by this issue. These were explored through a series of sensitivity analyses (see Sensitivity analyses and Discussion and conclusions: cefiderocol clinical evidence review), which indicated that the results of the NMA of CLSI studies were somewhat sensitive to these choices. Results for the CLSI Enterobacterales network varied according to the choice of comparators and inclusion of the Kohira et al. study, while results of the CLSI P. aeruginosa network varied according to the choice of comparators. For the CLSI P. aeruginosa NMA, including only comparators specific to the pathogen resulted in changes to the ORs estimated from the NMA, but these did not translate to substantive differences in absolute susceptibilities and so this scenario was not explored in the modelling. For the CLSI Enterobacterales, network differences in absolute susceptibility were observed across both scenarios. Due to limitations on the number of scenarios that could feasibly be explored in this project, results are included for the CLSI scenario that contrasted most with the base case (restricting to pathogen-specific comparators, including Kohira et al. data). We note that of these CLSI Enterobacterales scenarios, the scenario included within the modelling has the most favourable susceptibility for cefiderocol.
Susceptibility for AMs used in the MDS
When microbiology test results are available, it is assumed that patients will receive an AM to which they are susceptible (if they are susceptible to an AM). It was further assumed that, given their toxicity, use of either colistin or an aminoglycoside would be reserved for when a patient was not susceptible to any other relevant AMs. Hence, for use in the economic model, it was necessary to convert absolute susceptibility evidence for each AM into the proportion of patients falling into each of the following mutually exclusive groups:
-
susceptible to an AM that is not colistin or an aminoglycoside
-
susceptible only to colistin or an aminoglycoside
-
not susceptible to any available treatment options.
The AMs contributing to the first susceptibility grouping are:
-
fosfomycin (both populations)
-
aztreonam (Enterobacterales-MBL only)
-
tigecycline (Enterobacterales-MBL only).
In the ES, susceptibility to a given AM was assumed independent of susceptibility to any other AM. This assumption could also be used to derive the proportion in each susceptibility group for the MDS. A discussion of the appropriateness of this assumption is provided in Appendix 7. This suggested that, in the MDS, the assumption of independence did not hold. Instead, evidence from PHE was used to estimate the bias arising when assuming independence to derive the proportion in each susceptibility group. This was quantified as a scaling factor which was then used to adjust estimates of the proportion in each susceptibility group obtained from the NMA using an assumption of independence of susceptibilities. Note that, for P. aeruginosa, there is only one non-toxic AM in the MDS, so no adjustment is required. A scenario analysis that only used isolate-level data from PHE was also considered. Due to insufficient evidence, this scenario assumes that fosfomycin is not used.
For the PSA, two primary sources of uncertainty were considered:
-
uncertainty in the ORs obtained from the NMA posterior distribution
-
uncertainty in the scaling factor used. This in turn had two components: variation in the true proportions in each susceptibility group from the PHE data (modelled using a Dirichlet distribution), and variation in the absolute susceptibility to each AM in the PHE data (modelled using a beta distribution).
Overview of options for including susceptibility data in the economic model
A base case and four scenarios were used when generating susceptibility probabilities for use in the economic model (for both cefiderocol and the comparators):
-
In the base-case analysis, the NMA of EUCAST studies was used.
-
In Scenario 1 (S1), the NMA of CLSI studies was used.
-
In Scenario 2 (S2), PHE data were used, with cefiderocol and fosfomycin effects obtained from separate cefiderocol and fosfomycin networks using EUCAST studies.
-
In Scenario 3 (S3), PHE data were used, with cefiderocol and fosfomycin effects obtained from separate cefiderocol and fosfomycin networks using CLSI studies.
-
In Scenario 4 (S4), for MBL Enterobacterales, PHE data were used, with cefiderocol effects obtained from the separate cefiderocol network of EUCAST studies and fosfomycin excluded. In Scenario 4 (S4), for MBL Pseudomonas, the NMA using EUCAST breakpoints was used and absolute colistin susceptibility values were obtained from SIDERO WT.
The susceptibility probabilities used in each analysis are shown in Table 17. In MBL Enterobacterales, cefiderocol susceptibility is sensitive to the breakpoints used. In the scenarios using the EUCAST breakpoints, cefiderocol susceptibility is 65–69%, whereas in the scenarios using CLSI breakpoints, this increases to 87–98%. Empiric use of colistin/aminoglycoside-based therapy is associated with similar susceptibility across scenarios of 94–97%. In the MDS, comparator outcomes are similar across Scenarios 1–3 with 91–97% of patients susceptible to a non-colistin/aminoglycoside-based treatment, and approximately 3–7% only to a colistin/aminoglycoside-based treatment. Use of the PHE data, which does not include fosfomycin, increases the proportion of patients susceptible only to a colistin/aminoglycoside-based treatment to 20%.
Base case | S1 | S2 | S3 | S4 | |
---|---|---|---|---|---|
Enterobacterales -MBL population | |||||
Empiric treatment setting | |||||
Susceptibility to the most effective non-colistin/aminoglycoside | Not applicable | ||||
Most effective non-colistin/aminoglycoside | None | ||||
Susceptibility to the most effective colistin/aminoglycoside (%) | 96 | 97 | 95 | 97 | 94 |
Most effective colistin/aminoglycoside | Colistin + fosfomycin | Colistin + fosfomycin | Colistin + fosfomycin | Colistin + fosfomycin | Colistin + tigecycline |
MDS (%) | |||||
Susceptibility to a non-colistin/aminoglycoside | 91 | 97 | 91 | 95 | 77 |
Susceptibility to colistin/aminoglycoside | 7 | 3 | 7 | 4 | 20 |
Susceptibility to cefiderocol | 67 | 87 | 69 | 98 | 65 |
P. aeruginosa population | |||||
Empiric treatment setting (%) | |||||
Susceptibility to the most effective non-colistin/aminoglycoside | 28 | 86 | 34 | 96 | 3 |
Most effective non-colistin/aminoglycoside | Fosfomycin | Fosfomycin | Fosfomycin | Fosfomycin | Fosfomycin |
Susceptibility to the most effective colistin/aminoglycoside based | 99.51 | 99.85 | 99.64 | 99.95 | 81.34 |
Most effective colistin/aminoglycoside based | Colistin + fosfomycin | Colistin + fosfomycin | Colistin + fosfomycin | Colistin + fosfomycin | Colistin + fosfomycin |
MDS (%) | |||||
Susceptibility to a non-colistin/aminoglycoside | 28 | 86 | 34 | 96 | 3 |
Susceptibility to colistin/aminoglycoside | 71 | 14 | 66 | 4 | 78 |
Susceptibility to cefiderocol | 97.83 | 99.98 | 97.46 | 99.97 | 62.37 |
In MBL P. aeruginosa, in most scenarios, cefiderocol is associated with a high susceptibility of 98–100%, which is comparable to the susceptibility for empiric use of colistin/aminoglycoside-based therapy (100% across scenarios). Scenario 4, which uses a lower baseline susceptibility for colistin, results in a lower susceptibility for cefiderocol (62%) than colistin/aminoglycoside-based empiric therapy (susceptibility 81%). Susceptibility to non-colistin/aminoglycoside-based empiric therapy varies markedly across scenarios (3–96%), with susceptibility to comparators much higher when evidence using the CLSI breakpoints is included. A similar pattern is observed in the MDS where the proportion of patients susceptible to a non-colistin/aminoglycoside-based treatment is much higher when evidence using the CLSI breakpoints is included.
As previously noted, to obtain absolute susceptibilities for each AM, ORs from the NMA were applied to the absolute PHE colistin susceptibility values. This was achieved by converting the probabilities of susceptibility to odds, applying the ORs and converting the resulting treatment-specific odds back to probabilities. For the P. aeruginosa population, colistin susceptibility was 100%, so a continuity correction was applied. As this baseline susceptibility was very high, resulting estimates for the other AMs could end up being large, even if the ORs were small. This effect was compounded by the skewed distributions for the ORs. Hence, even though fosfomycin was associated with very low ORs, it still had a resulting high susceptibility in some of the scenarios considered. Therefore, a scenario using a lower baseline colistin susceptibility level was run based on values from SIDERO WT.
Three other sensitivity analyses of the NMA results were found to modify the relative differences in susceptibility across comparators (see Sensitivity analyses).
Furthermore, NICE’s consultation and Committee deliberations indicated that, in terms of existing therapies (i.e. in a world without cefiderocol), there is a proportion of patients who would not receive colistin/aminoglycosides, even if they were susceptible, and no other effective therapy was available. This would be due to a patient’s high clinical risk of renal toxicity. For such patients, it can be assumed that they would only receive salvage therapy. The size of this subgroup of patients with an absolute contraindication to colistin/aminoglycosides was considered small by EEPRU’s clinical advisors. However, on request from NICE, a separate scenario was run where a proportion of patients who, without cefiderocol, were only susceptible to colistin, received multidrug salvage therapy. Considering the lack of empirical evidence, the NICE Committee suggested the plausible range for the proportion of patients to be 20–40%. Details of the methods are provided in Appendix 8, Additional scenario: contraindications to colistin and aminoglycosides and model parameters are presented in Appendix 8, Additional scenario: contraindications to colistin and aminoglycosides, Table 60.
Clinical parameters – linking susceptibility to short-term mortality in the MDS
The remaining clinical evidence predicting 30-day outcomes in the MDS is presented in Table 18. Thirty-day mortality differs across comparators via two mechanisms in the MDS. Thirty-day mortality does not differ in the MDS setting if infections are susceptible to existing treatments because patients will be treated with the correct AM, though it does differ if patients have infections resistant to existing options but susceptible to cefiderocol, as their recovery will be more likely if they take cefiderocol. In addition, patients’ mortality risk varies according to the AKI rate associated with the AM used.
Site | Parameter name | Description | Value | Uncertainty (measure) | Source |
---|---|---|---|---|---|
cUTI | p_bgrdS30d_MDS_S | 30-day survival in cUTI patients receiving a treatment to which they are susceptible | 0.854 | Beta (12.10, 2.07) 95% CI (0.636 to 0.979) |
Structured expert elicitation |
cUTI | p_bgrdS30d_MDS_nonS | 30-day survival in cUTI patients receiving a treatment to which they are resistant | 0.610 | Beta (3.55, 2.27) 95% CI (0.227 to 0.923) |
Structured expert elicitation |
HAP/VAP | p_bgrdS30d_MDS_S | 30-day survival in HAP/VAP patients receiving a treatment to which they are susceptible | 0.578 | Beta (3.99, 2.91) 95% CI (0.226 to 0.888) |
Structured expert elicitation |
HAP/VAP | p_bgrdS30d_MDS_nonS | 30-day survival in HAP/VAP patients receiving a treatment to which they are resistant | 0.376 | Beta (2.71, 4.51) 95% CI (0.090 to 0.726) |
Structured expert elicitation |
All | p_AKI_ca | Risk of AKI in patients receiving colistin or an aminoglycoside | 0.45 | 95% CI (0.41 to 0.49) | Sisay (2021)128 |
All | OR_AKI_ca | Elevation in risk of AKI associated with colistin or aminoglycosides compared to other less nephrotoxic therapies | 1.81 | 95% CI (1.13 to 2.92) | Chien (2020)129 |
All | OR_AKI_death | OR of mortality for AKI compared with no AKI | 5.11 | 95% CI (4.23 to 6.17) | Kerr (2014)130 |
HAP/VAP | p_AKIirrec | Proportion of individuals who experience an AKI who have ESRD | 0.003 | 0.002 | Sisay (2021)128 |
cUTI | p_AKIirrec | Proportion of individuals who experience an AKI who have ESRD | 0.001 | 0.002 |
As documented in Review questions 4 and 5, several studies have explored the link between whether patients have been administered a treatment to which they are susceptible and their 30-day mortality outcomes in the infection sites of interest for the HVCSs. However, these studies have focused on the ES and none was available relating specifically to the MDS where outcomes are expected to differ substantively. Patients in the MDS who receive an inappropriate drug are much more likely to be MDR than patients receiving inappropriate treatment in the ES and are more likely to be in a critical state that reduces the possibility for further treatment.
This data gap is perhaps unsurprising as multidrug resistance (including to colistin) remains rare and it may, therefore, be difficult to recruit or include sufficient patients in this setting. Given the absence of data to inform this important parameter, a structured expert elicitation exercise was conducted. The methods for the expert elicitation are described in Structured expert elicitation. These estimates were elicited separately for cUTI, HAP and VAP as these infection sites are expected to have quite different mortality rates. Separate estimates were not produced by causative pathogen mechanism. This is because, among those receiving a treatment to which they are susceptible, outcomes are expected to be similar across the pathogen-mechanism combinations relevant to cefiderocol HVCSs. Similarly, among patients receiving multidrug salvage therapy due to multidrug resistance, outcomes are expected to be similar across the pathogen-mechanism combinations relevant to the cefiderocol HVCSs.
Clinical parameters – acute kidney injury risk and subsequent outcomes
Rates of nephrotoxic drug-induced AKI and their implications are assumed to generalise across sites, pathogens and mechanisms in the absence of subgroup-specific data. The evidence from the cefiderocol RCTs provided limited evidence on the safety implications of aminoglycoside/colistin use (see Results) and these data were not considered by our advisors to be generalisable to the highly comorbid patients who tend to acquire carbapenem-resistant infections. These parameters were obtained from existing systematic reviews where possible or, if not available, from UK-specific sources.
Several recent systematic reviews and meta-analyses estimated the pooled cumulative incidence of AKI in patients treated with colistin or polymyxins B,128,129,131,132 and two reported differences in the rates of AKI between colistin or polymyxin B-based therapy and other agents. 131,129 The absolute risk of an AKI and the likelihood that an AKI resulted in irrecoverable kidney damage was derived from Sisay (2021),128 as this study had the most recent searches, included a broad range of study designs and was restricted to studies using the Risk Injury Failure Loss and End-Stage Renal Disease (RIFLE) criteria. The difference between colistin (or polymyxin B, a similar drug from the same class)–based therapy and other agents was obtained from Chien et al. (2020)129 as this review made some attempt to control for confounding. Chien et al. (2020)129 included both RCTs and comparative cohort studies, but excluded studies considered poor quality as assessed by the Newcastle–Ottawa scale (in particular, the authors state that only cohort studies of parallel design with patients with comparable clinical characteristics were included). Alternative sources for these parameters are explored as scenario analyses.
The excess death rate from AKI was derived by comparing in-hospital mortality rates in the UK for individuals who experienced an AKI, as defined by the Acute Kidney Injury Network (AKIN) criteria, and individuals without AKI using the East Kent Hospitals University NHS Foundation Trust (EKHUFT) data set from Kerr et al. (2014). 130 The latter gathers admission records from three inpatient hospitals in the South of England. The analysis of the EKHUFT data set was deemed more appropriate than that obtained using the Hospital Episode Statistics (HES) data set, as EKHUFT includes older and more comorbid patients that are, therefore, more similar to the patient population in this evaluation, and is more likely to include all AKIs than the HES data set. The impact of AKI on mortality was estimated by the authors adjusting for a range of covariates including history of hospital admission, comorbidities and primary diagnosis. We assumed the relative increase in mortality associated with AKI observed in the Kerr et al. analysis applied to the baseline risk of mortality in our HVCSs despite the patients within our HVCSs exhibiting a much higher baseline mortality risk. AKI is more prevalent in patients with poor prognosis and, although Kerr et al. attempted to adjust for these factors, the elevated mortality estimated was considered high by expert advisors. A scenario analysis was, therefore, run whereby the excess mortality associated with AKI was halved from the reported value.
Clinical evidence – linking susceptibility to 30-day outcomes in the empiric setting
The evidence informing the decision tree predicting 30-day outcomes in the ES is presented in Table 19. The mechanisms database described in section Appendix 1 was searched to identify papers providing quantitative estimates of the risk of carrying the pathogen mechanism of interest among patients with specific characteristics. This was supplemented by papers known to the study team. Two searches were conducted. The first was to identify UK-specific studies. This had two concepts; the first was to identify ‘risk’ studies (any field containing any of ‘risk’, ‘prevalence’, ‘incidence’, ‘character*’ or ‘outbreak’), returning 1696 studies. The second concept was for UK studies (abstract contains any of ‘United Kingdom’, ‘Great Britain’, ‘England’, ‘UK’, ‘NHS’, ‘Trust’, ‘London’, ‘*Shire’), returning 119 studies. Combining both concepts provided 61 studies for the first search. The second search was expanded to identify non-UK risk models and returned 51 studies based on their title containing ‘Risk’. No risk models were identified from either search. Indeed, even in the wider population of patients at risk of a carbapenem-resistant infection, there is a paucity of UK data available to estimate the risk of having a carbapenem-resistant infection among patients with relevant risk factors. 133
Pathogen/mechanism subgroup | Parameter name | Description | Value | Uncertainty (measure) | Source |
---|---|---|---|---|---|
MBL Enterobacterales | p_bug_mech_EMBL | Proportion of people in ES who have the suspected pathogen mechanism | 0.15 | 92 (n) | PHE SGSSa |
MBL P. aeruginosa | p_bug_mech_PMBL | 0.14 | 51 (n) | PHE SGSSa | |
All | p_bgrdDst_S | Proportion of patients who received a treatment to which they are susceptible who are dead at the point MDS results become available (assumed to be at 5 days based on CARBAR study) | 0.03 | 0.02 (SE) | Tumbarello (2013)125 |
All | p_bgrdDst_nonS | Proportion of patients who received a treatment to which they are not susceptible who are dead at the point MDS results become available (assumed to be at 5 days based on CARBAR study) | 0.11 | 0.04 (SE) | Tumbarello (2013)125 |
All | prtxF_S | Proportion of patients who received a treatment to which they are susceptible who require further treatment | 0.07 | 0.02 (SE) | APEKS-NP14 |
All | p_bgrdD30d_S | Proportion of patients who survive to MDS assessment, and do not require further treatment who die by 30 days | 0.32 | 0.06 (SE) | Tumbarello (2013)125 outcomes from susceptible cohort |
The probability that a patient entering the ES actually has the suspected pathogen mechanism was obtained from Second-Generation Surveillance System (SGSS) data supplied by PHE as shown in Table 19 (for further discussion see Quantitative extrapolation to expected usage). These data provide the number of tests for a given mechanism of resistance and the proportion of those tests that returned a positive result. For MBL mechanisms, separate tests are run for each specific type of mechanism (i.e. a separate test is run for IMP, VIM, NDM and in the case of P. aeruginosa Dutch imipenemase, DIM). We assume when calculating these figures that, if MBL is suspected, all MBL mechanism tests are run. These data are unlikely solely to reflect the ES HVCSs of focus in the current analysis, for example testing may be conducted due to a suspicion in the lab rather than at the level of the treating clinician (e.g. a lab finding of carbapenem non-susceptibility might trigger a test), some tests may be run following treatment failure or may be run in the ES but at a lower level of suspicion than considered in our HVCSs.
Given these uncertainties in the available data, we also conducted a survey of the mailing list of the BSAC. This survey asked microbiologists and infectious disease specialists how many times they saw patients who would fall into our ES HVCSs of interest, and the proportion of those who actually had the pathogen mechanism of interest. A survey was used in preference to the structured expert elicitation as this parameter was expected to vary according to local epidemiology and history of outbreaks of resistant infections, and it was not considered realistic that the expert elicitation exercise could include enough experts to adequately reflect this geographical heterogeneity. Unfortunately, the response to the survey was low with only nine experts providing usable responses. On average, these experts reported that, of the MBL Enterobacterales or P. aeruginosa HAP/VAP cases seen where there was a high suspicion of the mechanism of interest, 71% of patients would be confirmed as having the mechanism. These values are used in a sensitivity analysis. Given the high level of uncertainty around this parameter, sensitivity analysis results are shown for a wide range of alternative values.
Mortality at the time of assessment for entry to the MDS conditional upon susceptibility status, and 30-day mortality among patients not requiring further treatment, was obtained from Tumbarello (2013). 125 This study was conducted in 110 ICU patients with confirmed P. aeruginosa pneumonia in a hospital in Italy, and compared 30-day mortality in patients who were susceptible to initial empiric treatment and those who were not. Surviving patients who were not susceptible to empiric treatment were switched to definitive therapy, on average approximately 62 hours after symptom onset. Tumbarello (2013)125 was chosen as it reported a relatively high incidence of multidrug-resistant strains in infecting organisms (42/110) compared to the other studies identified in the review and was the only study reporting Kaplan–Meier curves (see Review questions 4 and 5 for details of review). No UK studies were identified.
The probability of requiring further treatment for susceptible patients was taken from the cefiderocol arm of the APEKS-NP study. Within the studies included in the cefiderocol evidence mappings, this was identified as the only study representing a predominantly empirically treated susceptible population of HAP/VAP patients that also reported subsequent treatment rates.
Clinical evidence – long-term mortality
All patients surviving to 30 days face an ongoing mortality risk based on the CARBAR134 and Merrick135 studies. Both studies included UK patients with infections caused by carbapenem-resistant organisms and were, therefore, considered relevant in terms of capturing the highly comorbid nature of patients who acquire these infections. Searches were conducted as described in Appendix 1 but did not identify any further evidence of relevance. A targeted search indicated a lack of data on long-term outcomes in both HAP/VAP and cUTI. It also seemed unlikely that outcomes in all-comer HAP/VAP and cUTI patients would reflect those of MDR patients who tend to have developed MDR infections as a result of multiple contacts with the health system, reflecting a wide range of comorbidities. We therefore chose to focus our review of long-term mortality on patients with resistant infections.
CARBAR134 was used to inform mortality in the base case as it included more geographically diverse patients, had a longer follow-up (2 years compared to 1 year in Merrick) and provided continuous survival estimates over time (i.e. Kaplan–Meier curves). Merrick135 reported all-cause mortality at 1 year of 31% which is similar to the 1-year mortality in CARBAR of 34%.
Kaplan–Meier curves from CARBAR were digitised and a published algorithm136 was used to recover pseudo IPD from the Kaplan–Meier curve for analysis. Parametric survival models were fitted to these data to facilitate extrapolation beyond the observed data. Data from 30 days onwards were used as these were of most relevance to the model. We followed guidance from the NICE Technical Support137 document and fitted a range of parametric survival models: exponential, Weibull, Gompertz, log-logistic, log-normal and generalised gamma. Model fit was assessed according to Akaike’s Information Criteria (AIC), log-cumulative hazard plots, hazard plots and visual assessment of the concordance between model predictions and Kaplan–Meier plots. No specific external data were identified to support validation of long-term predictions, so probabilities of death predicted by each model were compared to general population mortality over 20 years to assess plausibility. A summary of these assessments is provided in Appendix 7, Table 49. Overall, the Weibull, log-logistic and log-normal models were all considered plausible candidates and, in the absence of further evidence, log-normal was selected to offer a middle ground with the Weibull and log-logistic used in scenario analyses. Mortality is restricted so that it remains above that in the general population within the model.
In addition, patients alive with recovered renal function face an elevated risk of death and a risk of developing irreversible renal failure (CKD). 138 Patients alive with irreversible renal failure face the elevated risk of death of CKD patients.
A recent body of evidence, with which our group of experts agreed, suggests that AKI and CKD are closely linked and interconnected, whereby CKD is a risk factor for experiencing subsequent AKI and AKI is a promoter or instigator of CKD. It was, therefore, considered important to capture the fact that AKI is not a ‘self-limited process’ and that patients with recovered renal function post AKI are at risk of adverse renal outcomes and of developing CKD.
In our literature searches to identify evidence of the impact of AKI on the development of CKD and on long-term survival, we looked for studies that would control for the confounding impact of comorbidities as stringently as possible, as we aimed to estimate the causal effect of AKI on subsequent outcomes. The US study by Bucaloiu (2012)138 was selected as it compared outcomes of patients with hospital-associated AKI (with recovered renal function) against a non-AKI patient population matched for a wide range of relevant clinical and demographic characteristics. One thousand six hundred and ten patients with AKI and 3652 without were followed up from 90 days post discharge to approximately 6 years. A limitation of this study is that the propensity score matching process excluded the most comorbid patients due to a lack of sufficiently closely matching controls, and the study excluded patients with impaired kidney function prior to hospitalisation. This evidence was used to inform the increased risk of death and the increased risk of developing CKD in patients with recovered renal function after an AKI. Relevant parameters are shown in Table 20.
Site | Pathogen/mechanism subgroup | Parameter name | Description | Value | Uncertainty (measure) | Source |
---|---|---|---|---|---|---|
All | All | TPnoAKItoCKD | 1-year absolute probability of experiencing CKD in non-AKI patients. Approach to computation: baseline risk of CKD development in non-AKI: 1218 events over a median follow-up of 4.3 years in 3652 individuals [= 1218/(4.3 × 3652)] |
0.078 | SE for baseline risk assumed 10% of mean | Bucaloiu (2012)138 (note that these probabilities are assumed to apply from the second cycle onwards as Bucaloiu measured outcomes from 90 days post discharge) |
All | All | TPAKItoCKD | 1-year absolute probability of experiencing CKD in post-AKI patients with recovered renal function. Approach to computation: baseline risk of CKD development in non-AKI (0.078) multiplied by adjusted HR 1.91 (95% CI 1.75 to 2.09) |
0.143 | SE around HR of CKD development: 0.087, SE for baseline risk assumed 10% of mean | |
All | All | AKIodeath | 1-year probability of death in post->AKI patients with recovered renal function. Derived by multiplying the mortality from CARBAR by the HR of excess death adjusted for de novo CKD development from Bucaloiu (2012): 1.18 (95% CI 0.95 to 1.46) |
1.18 * mortality rate in non-AKI | SE 0.119 for HR | |
All | All | TPCKDtodeath | 1-year probability of death in CKD patients. Derived by multiplying AKI mortality by the HR of excess death in CKD patients compared with AKI patients in Bucaloiu (2012): 3.65 (95% CI 2.42 to 5.52) |
3.65 * mortality rate in AKI | SE 0.783 for HR |
There are a number of limitations to the approach taken to reflect the long-term implications of AKI within the model:
-
The CARBAR134 mortality data will have included patients who experienced AKI and, therefore, including additional mortality risk associated with AKI and CKD development is likely to exaggerate mortality risk in the model.
-
The risk of CKD development is likely to be higher than estimated from Bucaloiu138 in the highly comorbid patient group considered within the HVCSs.
-
The hazard ratios on mortality are applied multiplicatively despite the much higher baseline risk of death in the patient population considered within the HVCS.
Scenarios are explored to address each of these assumptions in turn:
-
CARBAR mortality rate is reduced by 10% reflecting an assumed AKI rate of 20% and an assumed excess mortality associated with AKI of 1.48 (95% CI 1.19 to 1.82) based on Bucaloiu (2012). 138
-
Patients in the HVCSs face double risk of CKD compared to patients in Bucaloiu (2012). 138
-
Patients in the HVCSs face an absolute increase in mortality risk observed in Bucaloiu (2012). 138
-
All of the above applied simultaneously.
We did not account for life years accrued within the first 30 days in the model as these were expected to have a marginal effect on the model results.
Health-related quality of life
The HRQoL implications of the infection are not modelled as these are expected to be short- lived and, therefore, are not expected to impact substantively on the model results. However, to quality adjust the life expectancy estimates accurately, we did consider it important to reflect the underlying comorbidities of the patients within the HVCSs. We did not identify any relevant utility data from existing models, most of which assumed that, post infection, patients would return to the HRQoL of the general population. Therefore, we conducted a review of utility studies that provide evidence according to the Charlson Comorbidity Index (CCI). The CCI is a summary score of comorbidity based on 17 included comorbidities. The comorbidities considered in the CCI have been selected and then weighted based on their ability to predict 1-year mortality among hospitalised patients. Importantly, the CCI is reported within the CARBAR study for patients with infections caused by carbapenem-resistant organisms, allowing the utility values presented by CCI score to be reweighted to reflect the CCI scores in a population similar to that included in our HVCSs.
The methods for this review are described in Appendix 1. This identified two studies reporting utilities by CCI in the general population. Both studies were based on large national surveys in France and Germany and estimated the SF-6D based on the SF-36 and SF-12, respectively. The French study was chosen in preference to the German study as the latter controlled for a number of variables likely to be associated with CCI (pain level, sociodemographic variables and health behaviours). Utility values by CCI score are reported in Appendix 7, Table 50; these reflect UK general population valuations. These are weighted by the distribution of CCI scores observed in CARBAR,134 also shown in Appendix 7, Table 50. This produced an overall weighted utility score of 0.66 for the CARBAR population based on their comorbidities; this is intended to reflect their long-term quality of life rather than the immediate impact of infection. This was used to compute a multiplicative reduction in HRQoL associated with comorbidities by comparing the CARBAR population to the general population (assumed to have a CCI score of 0). General population HRQoL was derived from a regression model estimated by Ara et al. (2010)139 using Health Survey for England (HSE) survey data for the years 2003 and 2006 (n = 26,679). This produced a baseline utility value of 0.73 for all patients. This resulted in a utility multiplier of 0.66/0.73 = 0.90. This was then applied to the age and gender-specific EuroQol-5 Dimensions (EQ-5D) utilities of the general UK population based on Ara et al. 139
Patients who have recovered their renal function post AKI are not expected to experience further disutility unless they develop CKD. The HRQoL decrement applied to the CKD patients is computed using pooled estimates from a systematic review and meta-analysis by Wyld et al. (2011). 141 The authors reported decrements of 0.02 (−0.04 to 0.09) for those in CKD pre treatment, and of 0.11 (0.08 to 0.15) for those with CKD in dialysis, where the latter was estimated to represent 2% of the diagnosed CKD population based on UK data. 142 These were applied to the baseline utility value of 0.73 such that the utility of those with CKD pre treatment was 0.71 and the utility of those with CKD in dialysis was 0.62.
Resource use and costs
The model includes costs relating to hospital stay, infection control during hospitalisation, AKI-related costs during hospitalisation, long-term costs associated with CKD and costs relating to use of existing AMs. The purchase price of cefiderocol is not included in the costings as the objective of the evaluation is to inform the payment for cefiderocol. Costs relating to testing (for pathogen, resistance mechanism or AM susceptibility) were not included as, in the HVCS populations, these tests were expected to be conducted to the same degree regardless of the introduction of cefiderocol.
An important cost driver in the model is time spent in hospital. Data on time in hospital for patients according to their treatment pathway and outcomes are presented in Table 21. As for 30-day mortality, we did not identify any studies in the MDS linking treatment susceptibility to duration or type of hospitalisation. This was, therefore, elicited as part of the structured expert elicitation exercise. LoS and the proportion of time spent in ICU or HDU was estimated conditional upon susceptibility for patients with cUTI and HAP/VAP separately. In the base case, all patients in the ES were assumed to spend 5 days in hospital prior to receipt of their microbiology results, the median wait reported in CARBAR. 134
Site | Parameter name | Description | Value | Uncertainty (measure) | Source (costing year) |
---|---|---|---|---|---|
cUTI | los_MDS_S | LoS following treatment in the MDS for cUTI patients who received a treatment to which they are susceptible (days) | 12.9 | Lnorm (2.507, 0.321) 95% CI (6.54 to 23.02) |
Structured expert elicitation |
cUTI | los_MDS_nonS | LoS following treatment in the MDS for cUTI patients who received a treatment to which they are not susceptible (days) | 17.7 | Lnorm (2.817, 0.334) 95% CI (8.68 to 32.2) |
Structured expert elicitation |
cUTI | p_ICU_MDS_S | Proportion of time in hospital in ICU for cUTI patients who received a treatment to which they are susceptible | 0.150 | N/Aa | Structured expert elicitation |
cUTI | P_ICU_MDS_nonS | Proportion of time in hospital in ICU for cUTI patients who received a treatment to which they are not susceptible | 0.233 | N/Aa | Structured expert elicitation |
cUTI | p_HDU_MDS_S | Proportion of time in hospital in HDU for cUTI patients who received a treatment to which they are susceptible | 0.170 | N/Aa | Structured expert elicitation |
cUTI | p_HDU_MDS_nonS | Proportion of time in hospital in HDU for cUTI patients who received a treatment to which they are not susceptible | 0.183 | N/Aa | Structured expert elicitation |
HAP/VAP | los_prior_ast | Time from empiric treatment initiation to receiving microbiology results (days) | 5b | N/A | CARBAR134 |
HAP/VAP | los_txsucc1 | Relative reduction in LoS for patients not requiring further treatment | 0.503 | N/A | Muscedere (2012)143 |
HAP/VAP | LoS_ES_success | LoS in ES for patients not requiring further treatment (days) | 12.8 | Assume uncertainty as for LoS HAPVAP_MDS_S, with fixed time to MDS (5 days) and relative reduction in LoS (0.503) | Derived from structured expert elicitation and Muscedere (2012)143 |
HAP/VAP | p_ICU_tx_succ1 | Proportion of time in ICU following receipt of empiric treatment for patients not requiring further treatment | 0.300c | N/Aa | Derived from Muscedere (2012)143 |
HAP/VAP | los_MDS_S | LoS following treatment in the MDS for HAP/VAP patients who received a treatment to which they are susceptible (days) | 20.4 | Lnorm (2.971, 0.298) 95% CI (10.88 to 34.97) |
Structured expert elicitation |
HAP/VAP | los_MDS_nonS | LoS following treatment in the MDS for HAP/VAP patients who received a treatment to which they are not susceptible (days) | 24.3 | Lnorm (3.118, 0.380) 95% CI (10.73 to 47.63) |
Structured expert elicitation |
HAP/VAP | p_ICU_MDS_S | Proportion of time in hospital in ICU for HAP/VAP patients who received a treatment to which they are susceptible | 0.499 | N/Aa | Structured expert elicitation |
HAP/VAP | P_ICU_MDS_nonS | Proportion of time in hospital in ICU for HAP/VAP patients who received a treatment to which they are not susceptible | 0.589 | N/Aa | Structured expert elicitation |
HAP/VAP | p_HDU_MDS_S | Proportion of time in hospital in HDU for HAP/VAP patients who received a treatment to which they are susceptible | 0.149 | N/Aa | Structured expert elicitation |
HAP/VAP | p_HDU_MDS_nonS | Proportion of time in hospital in HDU for HAP/VAP patients who received a treatment to which they are not susceptible | 0.172 | N/Aa | Structured expert elicitation |
All | c_AKI | Increase in in-hospital cost associated with experiencing an AKI | £5138 | (4724 to 5548) | Kolhe (2014)144 (2008 prices updated to 2019) |
cUTI | c_genward | Unit cost per day for cUTI patient on general ward | £687.08 | N/A | NHS reference costs |
HAP/VAP | c_genward | Unit cost per day for HAP/VAP patient on general ward | £870.51 | N/A | NHS reference costs |
All | c_ICU | Unit cost per day for person in ICU | £1689.09 | N/A | Derived from NHS reference costs and CARBAR |
All | c_HDU | Unit cost per day for HDU | £1299.67 | N/A | NHS reference costs |
All | c_Isolation | Daily cost of isolation | £21.96 | N/A | Knight (2018)145 |
The LoS for patients successfully treated in the ES was estimated from the LoS in patients who are susceptible to treatment in the MDS as estimated from the structured expert elicitation, the time to receiving MDS from CARBAR134 and the relative reduction in the LoS associated with receiving appropriate empiric treatment from Muscedere (2012). 143 The proportion of time spent in the ICU for patients who received a treatment to which they are susceptible and who did not require further treatment was derived from Muscedere (2012). 143 The study was conducted in 350 adult ICU patients with VAP (any pathogen and resistance profile) in Canada who received empiric treatment with meropenem or meropenem + ciprofloxacin. The study reported hospital and ICU LoS in patients who were susceptible to their empiric treatment and those who were not. Muscedere143 was chosen as it was the only study identified in the review in Review questions 4 and 5 that reported LoS conditional upon susceptibility in patients with HAP/VAP. The LoS reported by Muscedere (2012) was skewed. The mean LoS was derived by fitting a log-normal distribution to the reported median and interquartile range. The derived mean LoS in patients who received appropriate and inappropriate treatment (43.1 and 85.7 days, respectively) were used to derive the relative reduction in the LoS associated with receiving appropriate treatment. The derived mean LoS and stay in ICU were used to derive the proportion of hospital stay that was spent in ICU.
The additional hospitalisation costs associated with in-hospital AKI are informed by estimates derived from Kolhe et al. (2014). 144 This study used the NHS costing system’s relative value units that capture cost information associated with several cost items including LoS on wards, drugs, physiotherapy, radiology and medical staff costs.
Unit costs were obtained from standard sources and also considered those suggested in the manufacturer submissions. Where necessary, costs were adjusted to 2019–20 prices using standard sources. 146 The daily cost of cUTIs treated on general medical wards was derived from the weighted average cost of non-elective short stay for kidney or UTIs with/without interventions (LA04H–LA04S). The daily cost of HAP/VAP treated on general medical wards was derived from the cost of non-elective short-stay bronchopneumonia with or without interventions (DZ23H–DZ23N). The daily cost of ICU was assumed to be the weighted average cost of non-specific, general adult critical care (CCU01) with zero to six organs supported (XC01Z–XC07Z), assuming that ventilation cost is reflected in the organ support costs. The daily cost of HDU was assumed to be the weighted average cost of medical adult patients in critical care (CCU03) with zero to six organs supported (XC01Z–XC07Z). Weighting was based on the overall volume of each type of organ support reported for the NHS. The daily cost of isolation was derived from Knight et al. (2018),145 and included the cost of gloves, aprons and infectious waste stream. It was assumed that all patients would be subject to isolation measures as they are either highly suspected of having or confirmed to have an MDR infection. One-off costs of stock disposal are not included as these are assumed to apply equally to all patients.
Following discharge, patients’ long-term costs are determined by their health state. Patients alive without a history of AKI, or with recovered renal function, experience no further costs. Patients with irreversible renal failure (i.e. CKD) face a weighted average cost that reflects the CKD-severity distribution in England and requirement for dialysis. Kerr et al. (2012)142 estimated the annual per patient NHS expenditure on CKD direct care, dialysis and transplants. The mean annual cost of direct CKD care per patient not on dialysis (i.e. antihypertensive drugs, primary care tests and consultations, nephrology consultations and cost due to excess incidence of cardiovascular events) was estimated at £278, while the annual cost of CKD-related care for a patient on dialysis was estimated at £31,933. These costs are similar to those proposed by Shionogi who estimate that patients with nephrotoxicity not experiencing dialysis face a cost of £173 in year 1 and £89 from year 2 onwards (based on assumed resource use), and £24,000–29,000 for those receiving dialysis (source unclear). As for quality of life, 2% of the diagnosed CKD population were estimated to be receiving dialysis based on UK data. 142 The clinical advisors to EEPRU for this project indicated that this may be an overestimate, but use of a lower value is unlikely to substantially change the results of the modelling. Our clinical advisors expected that, in the highly comorbid group considered within the HVCSs, transplant would be rare; the costs of transplant were not, therefore, included in the CKD cost estimates. This results in a weighted average cost of CKD of £911 per annum in 2019–20 prices.
We did not include differential rates of discharge to long-term care facilities in the base-case analysis as no evidence was found comparing UK usage of care among those with and without AKI that adjusted for differences between patients with and without AKI. US data suggest that AKI is associated with an elevated risk of discharge to long-term care even with adjustment for other predictive factors. Liangos (2006)147 found that 8.9% of patients without AKI will be discharged to long-term care, and this is elevated to 17.8% in those with an AKI (reflecting an adjusted OR of 2.2, 95% CI 2.1 to 2.2). We combine this with information on the costs of long-term care and model a scenario based on this. We use a weekly cost of £1049 (average of private sector nursing home, and local-authority own-provision residential care for older people146) and apply this for the lifetime of the patient. This is likely to be an overestimate as some patients may be discharged from long-term care and the full cost of this care may not fall on the NHS/Personal Social Services (PSS) budgets.
We did not include the cost of end-of-life or palliative care as this was considered unlikely to substantially influence model outcomes.
Drug acquisition costs were based on the cost of the daily dose derived from published sources,148,149 the daily doses reported by WHO Collaboration Centre for Drug Statistics Methodology150 and the treatment duration derived from published literature. 149,151–153 When more than one formulation or pack size was available, we based costs on the largest pack size and IV formulations. When the treatment duration was provided as a range, we used the longest duration, to reflect the high severity of infections. When more than one AM was available for treatment in a particular setting (e.g. colistin or aminoglycosides for the treatment of HAP/VAP in MDS), the most expensive treatment was chosen to reflect that often combination or higher doses of therapy may be used. In the ES, patients who require a treatment switch following availability of their susceptibility results are assumed to receive 5 days of treatment, whereas those who do not require a treatment switch receive the full course. The unit cost of all comparators is shown in Appendix 7, Drug acquisition costs, Table 51. The drug acquisition costs used in the model are summarised in Table 22.
Colistin/aminoglycoside-based treatment in ES | nca-based ES | Colistin/aminoglycoside-based treatment in MDS (£) | nca-based MDS (£) | Salvage (£) | |
---|---|---|---|---|---|
MBL Enterobacterales | Full course: £163.56 (colistin + fosfomycin) Five days: £90.66 |
N/A for empiric | 232.30 (amikacin) | 298.20 (tigecycline) | 397.78 |
MBL P. aeruginosa | Full course: £163.56 (colistin + fosfomycin)** Five days: £90.66 |
Full course: £9.66 (fosfomycin) Five days: £9.66 |
153.90 (colistin) | 9.66 (fosfomycin) | 397.78 |
Model outputs and uncertainty analysis
Per patient lifetime costs, QALYs and NHEs are presented for each subgroup described in Table 14. For the subgroups of patients eligible for treatment in the MDS, incremental results are presented for the comparison of the overall MDS cohort who receive tailored therapy with the new drug available to the overall cohort who receive tailored therapy under existing treatment options only. For patients eligible for treatment in the ES, incremental results are presented for the pathway including cefiderocol as an empiric treatment, and the pathway including cefiderocol as an MDS treatment, each compared to the treatment pathways including only existing AMs. These estimates represent the INHEs offered by cefiderocol over and above existing therapeutic options.
Calculation of NHEs requires a measure of health opportunity cost in order to convert additional healthcare costs (or savings) to health foregone (or accrued). We present estimates of NHEs using a measure of health opportunity cost of £20,000/QALY as specified in the NICE scope for this evaluation,156 with scenarios presented using £15,000 to reflect empirical estimates of health opportunity cost used by the Department of Health and Social Care (see e.g. Department of Health and Social Care)157 and £30,000/QALY to reflect the upper bound of the approval norm used by NICE in its technology appraisal process. 158
Results are presented using the base-case assumptions and data sources outlined above. In addition, a series of scenarios is generated to address uncertain assumptions and reflect alternative plausible evidence sources. Parameter uncertainty is quantified using PSA, the results of which are presented as distributions of INHEs.
Modelling direct population net health effects in high-value clinical scenario
Two key drivers of estimates of population-level INHEs are the size of the affected population, and the efficacy of AMs in this population. Both drivers are expected to vary over time. Increasing rates of resistance to carbapenems (due to a MBL mechanism) will increase the population that could benefit from treatment with a newer AM. For cefiderocol, and potentially for the comparators, it is anticipated that resistance will change over time, with some of this change driven by changes in rates of AM use. The focus of this section is to describe the methods used to obtain quantitative estimates of changes in the affected population and AM efficacy over time. These estimates are used to generate predictions of the total population-level INHEs for cefiderocol over 20 years. This time horizon was chosen pragmatically to explore the long-term value of cefiderocol while avoiding additional uncertainties associated with very long-term population-level predictions.
There are four main aims of this section:
-
Predict how the number of people in each HVCS will change in the future.
-
Predict how rates of resistance to existing AMs will change within the HVCSs in the future if cefiderocol is not used (‘current practice’ scenario).
-
Predict how resistance will increase over time for cefiderocol within the HVCSs.
-
Predict the impact, if any, on resistance of reducing current levels of AM use due to the introduction of cefiderocol in the HVCSs.
There is a degree of overlap in the above aims. For example, aims 2–4 each involve the prediction of how resistance to an AM will change over time. In addition, for aims 1 and 2, the evidence sources were time-series data for the HVCSs. These time series were made available by PHE, and these were analysed using time-series methods. For aims 3 and 4, a range of potential evidence sources was considered. These sources included the published literature and publicly available surveillance data, and in general were for a population that was more broadly defined than the HVCSs. Evidence for a broader patient population was considered as it included evidence on both AM use and AM resistance, and so allowed for an estimate of how these two factors interact (this evidence was not available for the population of interest). As there are distinct modelling challenges associated with each aim, they are discussed in turn. A brief overview is presented here, with more details provided in Appendix 7, Further details on modelling direct population net health effects in high-value clinical scenario.
Predicting the future sizes of the high-value clinical scenarios
The objective of this analysis was to statistically model changes in the number of patients within the HVCS over time, to inform a quantitative forecast of the number of patients presenting in the HVCS over the next 20 years.
Data on the number of infections over time for the mechanisms of interest were provided as a time series by PHE. Two populations were included:
-
Enterobacterales with a MBL mechanism
-
Pseudomonas aeruginosa with a MBL mechanism.
Data were supplied for invasive infections which are predominantly infections where the specimen sample relates to a BSI or cerebrospinal fluid infection. It was assumed that, for each pathogen mechanism of interest, the trends in population size for invasive infections generalise to the HVCSs. This was considered reasonable by the clinical advisors to the project. The small number of invasive infections made it challenging to reliably identify if there was a trend in the growth of the HVCSs. As such, this analysis is supplemented by a secondary analysis which looks at trends in the number of screening isolates. These isolates are from screening specimen sites. Screening samples were broadly categorised as samples from swabs, wounds and the lower gastrointestinal tract. It includes potential infections as well as isolates from people who do not have infections but may be colonised by a MDR pathogen. These screening isolates were only used to confirm or refute the potential presence of a trend rather than inform the growth estimates as they may be influenced by screening policy changes over time which may not feed through to changes in identified infections. Data on both invasive infections and screening isolates were obtained from the Antimicrobial Resistanceand Healthcare-Associated Infections (AMRHAI) national reference laboratory. These data were provided by PHE as monthly counts and are available from 2004 (P. aeruginosa: from 2003) to April 2021. During 2018, guidance on which samples should be sent to AMRHAI changed, and charges were introduced. This led to a gradual ‘artificial’ decrease in referrals. Further detail on the nature of this data set is provided in Report Supplementary Material 1.
Due to small numbers, data on invasive infections were aggregated to quarterly for analyses and restricted to October 2012 onwards. The last observations used were for March 2018 (inclusive), as after this point the observed numbers decreased. For screening isolates numbers were larger, so monthly data were used. For these, the first observation was set to be the first time point for which there were no future months with zero counts (April 2013 for Enterobacterales and November 2014 for P. aeruginosa).
Time-series (state-space exponential smoothing) models were used to forecast the isolate data. For the invasive isolates, the use of other time-series models was also considered. Further details on the models considered and the justification and implementation of the state-space models is provided in Appendix 7, Further details on modelling direct population net health effects in high-value clinical scenario. Three state-space models were considered. These varied with regard to the assumptions made about any long-term trends in the growth of the HCVS:
-
no growth (no trend)
-
growth in the short term that in the long term changes to no growth (a ‘damped trend’ model; the degree of dampening is estimated from the data and influences how quickly the growth tends to zero)
-
persistent growth (trend that is not damped).
Within-sample goodness-of-fit statistics for the three models and the two data sets are provided in Appendix 7, Goodness of fit of forecast models, Table 52. Estimates of population growth are provided in Figure 13 (estimates are not shown for P. aeruginosa as the best-fitting model was always one without a trend). The two isolate data sets are very different with regard to absolute numbers. Figure 13 shows the change in population size over time for both the data set of invasive infections and the screening isolates.
FIGURE 13.
Change in population size over time (top pane = invasive isolates, bottom = screening isolates). MBL, number of isolates with Enterobacterales metallo-beta-lactamases.

For the invasive isolates, for both the Enterobacterales and P. aeruginosa populations, the best-fitting model (based on within-sample fit) is one without a trend. Of the two models that include a trend, the trend model provided the best fit for the Enterobacterales population, while the damped trend model provided the best fit for the P. aeruginosa populations. Visually, there was no evidence of a trend for the P. aeruginosa, while for the Enterobacterales, there was a potential trend.
For the screening isolates, the best model for the P. aeruginosa was again one without a trend, while for the Enterobacterales, it was a model with a non-damped trend. However, for the screening isolates, it is unclear if long-term increases in Enterobacterales reflect genuine increases or the results of increased testing. It is also unclear if any genuine increases would persist into the future. For Enterobacterales, the largest relative change was for the screening isolates with a non-damped trend and the smallest (non-constant) change was for the invasive isolates with a damped trend model. As long-term estimates were very sensitive to the choice of model, and there were little statistical grounds to choose between the two models, both the damped trend and trend models for invasive isolates were considered within the decision-analytic modelling for MBL Enterobacterales. It was assumed that there was no trend in the future size of P. aeruginosa.
Details on how the estimates of future change in the HVCSs were used in the economic model are provided in Appendix 7, Further details on modelling direct population net health effects in high-value clinical scenario.
Predicting future rates of resistance for current practice
The objective of this analysis was to characterise historical changes in resistance to existing AMs among patients with MBL Enterobacterales and MBL P. aeruginosa to inform a quantitative forecast of how resistance might change in the future.
This analysis used time-series data provided by PHE, obtained from the same evidence sources as described in the previous subsection (i.e. the AMRHAI national reference unit). Analyses were restricted to comparators used in the economic model. Resulting data were available for:
Enterobacterales with MBL:
-
aminoglycosides (gentamicin, amikacin, tobramycin)
-
aztreonam
-
colistin
-
tigecycline
Pseudomonas aeruginosa with MBL
-
colistin.
For AM classes with evidence from multiple AMs, the most resistant result was retained in the supplied data. It is not expected that retaining the least resistant result would have a noticeable impact on estimates of resistance over time. As already described, isolates that were reported as ‘intermediate’ resistant were assumed to represent resistant isolates for the purpose of this analysis. Hence, any tested isolate was either categorised as ‘susceptible’ or ‘resistant’ for this analysis. Combining ‘intermediate’ and ‘resistant’ categories was based on advice from clinical advisors. It is, however, noted that current EUCAST guidance is to combine ‘intermediate’ and ‘susceptible’ when only two categories are used. 159 The methods used to generate forecasts are broadly the same as those considered in the previous section, and are discussed in more detail in Appendix 7, Further details on modelling direct population net health effects in high-value clinical scenario. Due to the sparsity of the available evidence, trends in the resistance (or susceptibility) to comparator AMs were not incorporated into the decision-analytic model (for further details, see Appendix 7, Further details on modelling direct population net health effects in high-value clinical scenario, Table 53).
Predicting future resistance trajectories for cefiderocol
The time-series data on susceptibility provided by PHE (and detailed in the previous subsection) do not include cefiderocol. In the absence of evidence for the drug of interest, trajectories for the development of resistance were identified for other AMs. Due to a lack of evidence, the searching to identify these trajectories were not restricted to the HVCS populations. Evidence linking these resistance trajectories to quantity of AM use was also sought. This was to enable a link with the economic modelling; differences in levels of cefiderocol use (e.g. in response to an increase in the HVCS populations over time or when comparing use in the ES with use in the MDS) are expected to be associated with differences in the rate at which resistance is gained. To emphasise that the evidence used is for a different drug and patient population, it is referred to as ‘external AM use-resistance data’. Including an association between cefiderocol use and the development of resistance means that scenarios with increased levels of cefiderocol use will not automatically result in better long-term outcomes. There is a large body of literature demonstrating that AM use is associated with subsequent resistance. 160–162 As there are no historic data on how resistance develops for cefiderocol (in either the PHE data described in the previous section or identified in the susceptibility searches of Clinical evidence), any resistance trajectories used in the economic modelling will be subject to considerable uncertainty. This is particularly pertinent as the relationship between AM use and resistance has been shown to vary by both type of AM and geographical setting, and in some situations, there is no apparent relationship. 161,163,164 In the company submission for cefiderocol, it was contended that the development of resistance may be lower for cefiderocol than historically observed for other AMs (see section 2.3.5 of the company submission82). Conversely, as an AM with broad coverage, the development of resistance to cefiderocol may be similar to other broad-spectrum AMs (which is typically quicker than the development of resistance for narrow-spectrum AMs). This emphasises the importance of identifying the robustness of estimates of population-level INHE to different potential resistance trajectories.
Two approaches were used to identify external AM use-resistance data that may inform the use-resistance association. First, the entire database of studies that was used during the reviewing process (for both CAZ-AVI and cefiderocol) was searched. Studies were filtered to include those which included ‘use’, ‘usage’, ‘volume’ or ‘consumption’, and these were searched for any relevant evidence. In addition, to identify any English studies (which may use evidence from PHE, or the online portal ‘fingertips’), a Web of Science search was conducted with the terms ‘(AM* OR antibiotic* OR resistan*) AND (fingertip OR “Public health England”)’. These searches were complemented by any studies that were identified via other reviewing activities or already known to the study team. As a result, three studies were identified that, while not using data in the public domain, provided information on a use-resistance relationship. 165–167 Details of these studies are provided in Appendix 7, Further details on modelling direct population net health effects in high-value clinical scenario, Table 54. These existing studies informed the de novo analyses reported here by suggesting that autoregressive integrated moving average (ARIMA) models would be suitable time-series models for capturing use-resistance associations, with a lag of 1 year between use and resistance when using annual data.
In addition, several studies used publicly available surveillance data. 163,168–172 These data were reanalysed for this project to identify potentially useful associations. For this project, there were two types of data of interest:
-
English data on AM use and AM resistance, from the ‘AMR local indicators profile’. 173
-
European data on AM use and AM resistance from the European AM Resistance Surveillance Network (EARS-Net) and European Surveillance of AM Consumption Network (ESAC-Net), respectively. 174,175 These are available as annual data.
Further details on these evidence sources are provided in Report Supplementary Material 3. For cefiderocol, increases in resistance will be from a low starting point. Observed trajectories for external evidence which also showed an increase from a low starting point were only identified for the European data and so this was used in subsequent analyses.
Thirty countries from the European Union contribute data to EARS-Net on AM resistance for up to eight pathogens. 176 These data were further filtered based on the following criteria:
-
Pathogen is included in the HVCSs (E. coli as an Enterobacterales and P. aeruginosa).
-
Data were available for both AM use and AM resistance (cephalosporins of all types, and carbapenems).
-
Countries with at least 5000 isolates were tested, baseline resistance (average over the first 3 years of available data) was < 3% (Enterobacterales) or < 15% (P. aeruginosa), with at least 10 years of observations for carbapenems and 15 years of observations for cephalosporins (these did not have to be consecutive).
This resulted in the following 23 pathogen–drug–country combinations:
-
Pseudomonas aeruginosa, carbapenems: Finland, France, Ireland, Netherlands, Norway, Slovenia, Sweden
-
E. coli, carbapenems: France, Greece, Netherlands, Norway
-
E. coli, cephalosporins: Bulgaria, Croatia, Estonia, Finland, France, Greece, Ireland, Luxembourg, Malta, Norway, Slovenia, Sweden.
For these countries, ARIMA models were used to estimate the impact of increasing AM use (defined daily doses per 1000 inhabitants per day) in a given year on resistance to that AM in the following year. The results of these analyses are shown in Appendix 7, Further details on modelling direct population net health effects in high-value clinical scenario, Table 55. Of the 23 combinations considered:
-
Just under half provided a significant association (12/23; P. aeruginosa = 4/7, E. coli = 2/4 for carbapenems and 6/12 for cephalosporins).
-
Of the 12 significant associations, 7 were positive associations (increasing use led to an increase in resistance), while 5 were negative (decreasing use led to an increase in resistance). Four of the negative associations were for E. coli cephalosporins, the remaining one was for P. aeruginosa.
Hence, this analysis resulted in up to seven significant positive associations that could be used to link increases in AM use to AM resistance in the economic model. Increases in AM use are driven by increases in the eligible population over time.
Projections of expected usage for cefiderocol from Extrapolation from high-value clinical scenario to expected usage were linked to these estimates of the relationship between usage and resistance to predict emergence of resistance to cefiderocol over time. Even under more extreme usage predictions and the strongest associations between usage and resistance emergence, this predicted small absolute increases in resistance up to 0.04% and 0.16% over 20 years for the MBL Enterobacterales and P. aeruginosa populations, respectively (see Report Supplementary Material 3 for more details). This may represent an underestimate of the potential for resistance emergence for two reasons. Firstly, the spread of MDR infections is influenced by international travel and the ‘importation’ of MDR pathogens. Resistance emergence may, therefore, be influenced by cefiderocol usage outside the UK which is not accounted for in these projections.
Secondly, the relationships between usage and resistance characterised in the available data reflect all tested isolates in the community and hospital settings. Resistance emergence may be much higher within the HVCSs where usage will be concentrated. For this reason, EEPRU has conducted a range of scenario analyses to characterise the potential emergence of resistance to cefiderocol. These were informed by considering the absolute increases in resistance for the drug–pathogen combinations and countries discussed above, where there was a statistically significant increase (see Report Supplementary Material 3 for more details). The highest absolute increase in resistance (an annual absolute increase of 1.65%, leading to a projected 20-year increase of 33%) was used to bound these analyses. The second largest increase was 0.95% per year (19% over 20 years). Based on these considerations, EEPRU ran analyses with resistance emergence reaching 1%, 5%, 10% and 30% at 20 years. It is noted that the upper scenario may be very extreme.
Of note, this analysis was focused on data sets which demonstrated an increase in resistance over time. Hence, any significant associations between AM use and decreasing resistance were not explored. As an alternative to an ARIMA model, a dynamic differential equations model was also developed. This was designed to incorporate AM use and resistance, as well as the spontaneous loss or gain of resistance over time as well as the impact of deaths. Details of this model are provided in Report Supplementary Material 3; when evaluated in a simulation study, it was shown to provide biased parameter estimates. This was potentially due to the non-identifiability of the model (due to the number of potential AM drivers considered), so this model was not considered further.
As a face-validity check of the estimates of AM use employed in the model, these were compared to hospital inpatient drug use as reported in the 2019/20 ESPAUR report. 6 This provided an estimate of 2.4 DDD/1000 inhabitants for all AMs used in an inpatient setting. Drug use during the first year of the economic model for both CAZ-AVI and cefiderocol (combining results from both evaluations) for the sites cUTI, IAIs, HAP/VAP and BSIs (all four in Enterobacterales, P. aeruginosa, and Stenotrophomonas) was estimated to be 0.00018 DDD/1000 inhabitants, hence representing 0.01% of all hospital inpatient AM use. This estimate, as an upper bound on the potential use of both cefiderocol and CAZ-AVI, was felt by the modelling team to have face validity.
Predicting the impact of reduced drug use on resistance
Introducing cefiderocol (compared with the situation when it is not available) may lead to a reduced use of comparator AMs. As the economic model includes an association between increased use of cefiderocol and increased resistance (as described in the previous subsection), then intuitively a decrease in AM use would be expected to lead to a decrease in resistance. However, AM use in the population of interest is only one of a multitude of potential drivers for increases in AM resistance. Other potential drivers include the number of invasive procedures, AM use in other countries, environmental factors and AM use in animals. 177,178 The existing evidence on the effect of reduced AM use on AM resistance is mixed,179 with findings including no decrease, a decrease and even an increase in AM resistance. 180–182 Hence, while the introduction of a new AM is expected to lead to an increase in resistance over time, reducing AM use has less predictable effects on resistance. Due to the heterogeneity in the existing literature and the lack of evidence for the population and AMs of interest, it was assumed that reductions in the use of existing AMs did not lead to reductions in resistance over time.
Extrapolation from high-value clinical scenario to expected usage
An important part of understanding the value of cefiderocol is understanding the range of patients in whom it is expected to be used. This is also relevant to understanding how resistance to cefiderocol is likely to emerge over time (as higher usage is likely to contribute to higher resistance). To inform this assessment, we provide a qualitative description of the range of ways (outside of the HVCSs) that cefiderocol is expected to be used. This is informed by discussions with our clinical advisors, the manufacturer submission for cefiderocol, and input by other stakeholders during the NICE process to identify patient groups in whom cefiderocol may offer significant improvements in HRQoL and mortality compared to existing therapies.
Following this, for those areas of usage considered by the clinical advisors and study team to be the most significant in terms of population size and potential impact on INHEs, we have quantified the likely size of the populations who would receive cefiderocol. This is based on data from PHE, where available, and supplemented by data from the literature and expert opinion where necessary. These estimates are also compared to those provided by Shionogi in their manufacturer submission. These estimates are then used to rescale the population-level INHEs from the HVCSs.
Areas of expected usage
Infection sites and patient characteristics
Outside of the HVCSs, the following infection sites were considered to be most important in driving expected usage and gains in NHEs: BSI and IAI. Our clinical advisors emphasised the importance of cefiderocol in treating BSIs. The incremental value of cefiderocol (and AMs in general) in IAI is less clear as the quality of surgical procedures used to manage IAI was considered more important than the choice of AM and identifying MDR infections is more challenging. The clinical advisors also emphasised the importance of cefiderocol in treating patients who are immunocompromised (e.g. haematology, transplant), patients with cystic fibrosis and patients with burn injuries who are predisposed to acquiring resistant infections. In immunocompromised patients, BSIs are of particular concern, while in patients with cystic fibrosis, chronic respiratory infections are of particular concern. Patients with a higher propensity for renal complications and those with renal impairment may receive more significant benefits from cefiderocol, as renal complications may rule out or increase the toxicity of agents that remain effective in treating MDR infections (i.e. colistin, aminoglycosides).
MDR pathogens/mechanisms
Outside of the HVCSs, the following pathogen-mechanism combinations were discussed as relevant areas for usage for cefiderocol:
-
non-MBL P. aeruginosa
-
Stenotrophomonas (which is inherently MBL)
-
OXA-40/24, -51, -58, -143 and MBL Acinetobacter
-
pathogens with serine carbapenemases (e.g. OXA-48, KPC) or non-carbapenemase causes of carbapenem resistance (e.g. porin and efflux pump mechanisms).
Our clinical advisors considered that patients with non-MBL P. aeruginosa had other effective treatment options available and that this was not, therefore, a priority area of usage for cefiderocol, but that Stenotrophomonas and OXA-40/24, -51, -58, -143 and MBL Acinetobacter were potentially important areas of usage, though the latter two groups were small. Use of cefiderocol in patients with other serine carbapenemases (e.g. OXA-48, KPC) or non-carbapenemase causes of carbapenem resistance (porin and efflux pump mechanisms) was not generally considered a priority by our clinical advisors due to the availability of other effective treatment options. The exception to this was infections that were MDR due to multiple types of carbapenem resistance (e.g. serine, porin and efflux pump) in whom cefiderocol may represent an important treatment option.
Empiric usage
During the course of these evaluations, there was substantial debate about the appropriate definition of the ES. Stakeholders were broadly aligned that the risk-based ES should be driven by the severity of the clinical scenario rather than the site of infection alone.
The manufacturer and the clinical advisors to this project presented differing perspectives on how to define a patient as at high risk of carbapenem resistance for the purposes of identifying patients that might appropriately receive risk-based empiric treatment with cefiderocol. As documented throughout this report, the clinical advisors to this project considered that it was appropriate to restrict usage in the ES to patients with a high risk of an infection caused by MBL P. aeruginosa or MBL Enterobacterales where this high risk was based on one of three factors:
-
The patient was previously hospitalised in a healthcare setting with high prevalence of Enterobacterales or P. aeruginosa with MBL.
-
There is an outbreak of infection with Enterobacterales or P. aeruginosa with MBL on a ward where the patient has stayed during their current admission.
-
Previous cultures (taken during the current or previous hospital stays) show that the patient was previously colonised/infected by Enterobacterales or P. aeruginosa with MBL.
This view was based on the desire to restrict usage to those in whom the benefit was most significant, thus controlling the emergence of resistance. The clinical advisors also expressed concerns that a broader definition could lead to stewardship challenges.
The manufacturer considered a broader definition of patients at high risk of a drug-resistant infection. This included patients at risk of resistance due to ‘international travel and immunosuppression’, and patients considered at risk of a range of types of carbapenem resistance ‘including all classes of beta-lactamases (e.g. serine β-lactamase, metallo-β-lactamase), porin channels or efflux pumps related resistance mechanisms, alone or in combination’. The manufacturer noted that, for many patients, the specific type of carbapenem resistance may be unknown and there may just be a general suspicion of carbapenem resistance. The manufacturer emphasised that, for this group, cefiderocol was particularly relevant due to its broad coverage.
The clinical advisors considered that usage under a broader suspicion of resistance should only be considered in exceptional cases. The appropriateness of a wider definition of empiric usage is, in principle, a question that could be addressed empirically, by assessing the health benefits of a more inclusive definition, against the health costs of treating more patients who do not have a resistant infection with cefiderocol and, therefore, contributing to higher levels of long-term resistance. This trade-off was not addressed quantitively by EEPRU or the manufacturer, largely reflecting the difficulties in accurately quantifying the long-term implications of different levels of usage for the emergence of resistance to cefiderocol.
Population size estimates produced by the manufacturer
Shionogi estimates that there are 5000 infections caused by MBL-producing pathogens per year in England. The manufacturer estimates that 750 infections caused by MBL-producing pathogens are critical and could be identified as high risk of resistance. The manufacturer does not present an estimate of the number of infections that would be treated with cefiderocol in the MDS. The company’s estimate of the population size is based on uncertain evidence and a series of assumptions. The estimates are presented in Appendix 7, Manufacturer assessment of population size, Tables 56 and 57, and further discussed in Appendix 7, Manufacturer assessment of population size.
Quantitative extrapolation to expected usage
Current population sizes
The aim was to estimate the number of infections in the HVCSs and other important areas of expected usage. Based on feedback from our clinical advisors, the majority of cefiderocol use in HAP/VAP and BSIs was expected to be in the ES and the majority of cefiderocol use in cUTI and IAI was expected to be in the MDS. Furthermore, cefiderocol was also expected to be used in certain hard-to-treat infections caused by Stenotrophomonas. We therefore set out to estimate the number of patients with the following characteristics:
-
HAP/VAP and BSIs with suspected infection caused by Enterobacterales with MBL or P. aeruginosa with MBL, according to the criteria outlined in Population, intervention, comparison, outcomes, study designs for high-value clinical scenarios
-
cUTIs and IAIs caused by Enterobacterales with MBL or P. aeruginosa with MBL, as confirmed by resistance mechanism testing
-
HAP/VAP, cUTIs, BSIs and IAIs caused by Stenotrophomonas, resistant to other treatment options.
The current population size was derived from SGSS data (AMR module) supplied by PHE. SGSS is a national database of laboratory data provided by approximately 98% of hospital microbiology laboratories in England. 6 It contains resistance mechanism and antibiotic susceptibility testing for all submitted isolates. We analyse data for the period between October 2020 and April 2021 as from October 2020, reporting of acquired carbapenemase-producing GNB by laboratories has became mandatory.
The SGSS data set includes anonymised patient ID, specimen type, species, referral location, laboratory, resistance mechanism tested and mechanism results. The site of infection was not available directly; instead, it was inferred from the specimen types.
Clinical advisors to the project highlighted that there is considerable uncertainty in the categorisation of infection sites according to the specimen type. To reflect this uncertainty, we explored two separate classifications in scenario analyses, shown in Table 23. The classification in Scenario 1 was derived by PHE, based on a set of specimens that map directly to infection sites. The scenario excluded all specimens from female patients in cUTIs except nephrostomy specimens, and all sputum samples. The sputum samples were removed following a clinical review (discussed in more detail in ESPAUR report 20216) because a large number of sputum samples are considered to be contaminants without further evidence of clinical infection. The clinical advisors to EEPRU considered this classification to be conservative and a broader classification in Scenario 2 was derived with guidance from clinical advisors. Scenario 2 included sputum samples, and urine samples from both male and female patients where the medical requestor is ‘acute’ care, as a proxy for hospitalised patients. The scenario is likely to capture other relevant infections excluded from Scenario 1 but may include some specimens that do not relate to the infection types of interest.
HAP/VAP | cUTIs | BSIs | IAIs | |
---|---|---|---|---|
Specimen types in scenario I1 | Lower respiratory tract (bronchial) | Urine/kidney specimens from all male patients, irrespective of setting (urine, CSU, MSU, urinary catheter, suprapubic aspirate, bladder, kidney, urethra, urine/kidney, spa, ureter, urethral swab, EMU, ileal/bladder conduit, perinephric, first void, pus-associated kidney/urinary tract); nephrostomy specimens in male and female patients |
Blood samples (blood, plasma, dried blood spot, haematoma, cord blood, fetal blood) | Wound specimens (surgical and traumatic wounds) |
Additional specimen types in scenario I2 | Lower respiratory tract (alveolar lavage, trachea, BAL, chest, lung, lower respiratory tract, tracheal aspirate), sputum (sputum, endotracheal secretions, endotracheal aspirate, endotracheal tube, induced sputum), swab (lung swab) | Urine/kidney specimens in Scenario 1 in all hospitaliseda patients (both male and female), upper genital tract in male and female hospitaliseda patients | Heart/heart valve (heart, heart valve, mitral valve), intravascular line (TIP-NOS, arterial line/tip, Hickman line, CVP line tip, aortic valve, Venflon, aorta, haemodialysis access, arteriovenous shunt), pacemaker, catheter swab, aortic tissue, heart valve prosthesis (cardiac prosthesis, heart valve prosthesis), vascular graft (vascular graft), liver/bile (bile, gall bladder), hip tissue, hip swab, skin/wound (pressure sore), bone (bone, bone/joint, vertebra), bone marrow, bone pin/plate (prosthesis pin, bone pin/plate, prosthesis plate), joint prosthesis (artificial joint), intervertebral disc (intervertebral disc), IUCD, peritoneum, foreign body, implant NOS, CSF shunt (ventriculoatrial valve), bone biopsy sample | None |
In the data set, repeated entries were only removed if they were directly repeated for the same patient, species, specimen, referral location, laboratory, mechanism and mechanism results. However, it is possible that reported numbers included multiple entries from the same infectious episode if multiple specimen samples were analysed (e.g. on different days). Furthermore, samples were likely to be tested for multiple resistance mechanisms – specimens tested for MBL Enterobacterales included tests for IMP, VIM and NDM, and tests for MBL P. aeruginosa included tests for IMP, VIM, DIM and NDM. When multiple resistance mechanisms were tested, each mechanism was recorded as an individual entry. When deriving the current population size for this report, the number of MBL Enterobacterales and MBL P. aeruginosa samples tested were divided by 3 and 4, respectively, under the assumption that each sample was tested for each resistance mechanism. Finally, on advice from clinical advisors, the number of specimens positive for Stenotrophomonas were multiplied by 0.15 to reflect the fact that the advisors estimated that approximately 15% of infections caused by Stenotrophomonas would be eligible to receive cefiderocol. The majority (85%) of Stenotrophomonas infections would not be eligible for treatment with cefiderocol as they may not require treatment with AMs at all or may be effectively treated with other AMs. Patients with Stenotrophomonas infections receiving cefiderocol were assumed to do so within the MDS.
The number of specimens tested for MBL was used to approximate the population size in ES. This reflects an assumption that all of the mechanism testing conducted was initiated following high suspicion of that resistance mechanism by the treating clinicians for the reasons specified in Population, intervention, comparison, outcomes, study designs for high-value clinical scenarios. The number of isolates confirmed to have the resistance mechanism was used to approximate the population size in the MDS. The derived population sizes are shown in Table 24. The specimen types included did not impact on the number of BSIs and IAIs.
HAP/VAP (tested) | cUTIs (confirmed positive) | BSIs (tested) | IAIs (confirmed positive) | HAP/VAP (confirmed positive) | BSIs (confirmed positive) | ||
---|---|---|---|---|---|---|---|
MBL Enterobacterales | Scenario P1 | 25 | 62 | 155 | 51 | N/A | N/A |
Scenario P2 | 157 | 84 | 155 | 51 | N/A | N/A | |
Pseudomonas MBL | Scenario P1 | 6 | 17 | 13 | 12 | N/A | N/A |
Scenario P2 | 87 | 10 | 13 | 12 | N/A | N/A | |
Stenotrophomonas (MDS) | Scenario P1 | N/A | 64 | N/A | 38 | 100 | 63 |
Scenario P2 | N/A | 63 | N/A | 38 | 547 | 63 |
It should be noted that the sum of population sizes for individual infection sites may overestimate the total population size, if the same infection presents at multiple sites. For example, BSIs are often sequelae of other infections. If a BSI develops from HAP/VAP following unsuccessful treatment with cefiderocol, it would likely be treated with an alternative AM, despite having the resistance mechanism of interest.
The estimates in Table 24 are associated with considerable uncertainty due to uncertainty in the completeness of the SGSS data set (labs may not submit all specimens to SGSS), uncertainty in how accurately specimen types represent the infection sites of interest, uncertainty about whether all tested patients would fall within our defined target population for empiric treatment and the potential double counting of samples from the same infectious episode.
To provide an alternative estimate of the population size, we conducted a survey (previously described in Sources of evidence) about the number of HAP/VAP infections eligible for treatment in the ES. The survey targeted infectious disease specialists and collected information about the participants’ place of work (number of hospital beds, and the number of other infectious disease specialists), and the number of suspected and confirmed HAP/VAP infections caused by MBL Enterobacterales and MBL P. aeruginosa they encountered per annum. The survey was disseminated to infectious disease consultants and microbiologists who were members of the BSAC, to clinical advisors to the project and to experts recommended by the clinical advisors. The infection numbers were scaled to country-level estimates using the number of hospital beds per infectious disease specialist (derived from the survey responses) and the unweighted average number of hospital beds in England for four quarters in 2020–21. 187
In total, 25 participants started the survey, of which 9 provided information required to estimate the total number of patients eligible for treatment in ES in England. The estimates varied considerably between experts (with responses implying 0–33,554 suspected and 0–26,843 confirmed infections in England). The weighted average (9356 suspected and 6669 confirmed infections) was considered to be implausibly high by clinical advisors to the project, possibly because of higher survey take up among experts who are more likely to encounter the infections of interest and these estimates were not, therefore, taken forward to the decision analytic modelling.
The population size over 20 years was derived by applying the year-on-year population growth detailed in Modelling direct population net health effects in high-value clinical scenario to the current annual population size (see Table 24). The population size estimates are used to rescale the estimates of patient-level INHEs and are presented in Appendix 8, Figure 46. Four scenarios are used to model the eligible population over time. Scenario P1G1 is the most conservative, as it uses the conservative baseline number of infections (Scenario P1 in Table 24), and the population growth derived from a time-series model with a damped trend (see Predicting the future sizes of the high-value clinical scenario for details). Scenario P2G2 is the least conservative, as it uses the larger baseline number of infections (Scenario P2 in Table 24), and the population growth derived from a time series without a damped trend (see Predicting the future sizes of the high-value clinical scenario for details). When using the model with a damped trend, the total population size across all sites of infection increased from between 605 and 1280 (P1G1 and P2G1) in year 1 to between 1175 and 2269 in year 20. The model with the non-damped trend increased the total population size substantially, from between 605 and 1280 (P1G2 and P2G2) in year 1 to between 2553 and 4508 in year 20.
In addition, we derive estimates of expected total drug usage for cefiderocol as these influence some of the scenarios relating to resistance emergence (see Predicting future resistance trajectories for cefiderocol). Expected usage of cefiderocol was derived by adjusting the population size for the proportion of patients eligible for treatment with cefiderocol. In the ES, all infections were assumed to be eligible for empiric treatment with cefiderocol. In the MDS, infections confirmed to have the relevant resistance mechanisms in Table 24 were adjusted for the proportion of patients who are not susceptible to non-colistin/aminoglycoside-based treatment but were susceptible to cefiderocol. Susceptibility of infections caused by Enterobacterales and P. aeruginosa with MBL were derived using the evidence described in Clinical parameters – susceptibility evidence. Susceptibility of Stenotrophomonas infections was derived from the average susceptibility of Enterobacterales with MBL and P. aeruginosa with MBL infections, weighted by the proportion of infections caused by each microorganism (in Table 24). When deriving expected usage, susceptibility was assumed to be static over time for simplicity, as susceptibility changes over time were expected to have a small impact on usage.
The total expected usage over 20 years is shown in Table 25.
HAP/VAP (MBL Enterobacterales) | HAP/VAP (Pseudomonas MBL) | HAP/VAP (Stenotrophonomas) | cUTI (MBL Enterobacterales) | cUTI (Pseudomonas MBL) | cUTI (Stenotrophonomas) | BSI (MBL Enterobacterales) | BSI (Pseudomonas MBL) | BSI (Stenotrophonomas) | BSI (MBL Enterobacterales) | IAI (Pseudomonas MBL) | IAI (Stenotrophonomas) | |
---|---|---|---|---|---|---|---|---|---|---|---|---|
Scenario P1G1 | 830 | 111 | 618 | 125 | 339 | 351 | 5110 | 266 | 365 | 104 | 237 | 215 |
Scenario P1G2 | 1362 | 111 | 1014 | 204 | 339 | 514 | 8388 | 266 | 564 | 170 | 237 | 319 |
Scenario P2G1 | 5186 | 1740 | 2743 | 170 | 203 | 352 | 5110 | 266 | 351 | 104 | 237 | 207 |
Scenario P2G2 | 8511 | 1740 | 3781 | 278 | 203 | 546 | 8388 | 266 | 542 | 170 | 237 | 307 |
Extrapolation of INHEs between populations
Population-level INHEs were derived by multiplying patient-level INHEs by the population size. Patient-level INHE, derived from the model described in Modelling direct patient net health effects in high-value clinical scenario, was conditional on the site of infection, the pathogen mechanism and the treatment setting (ES or MDS). Patient-level INHEs for cUTIs in the MDS and for HAP/VAP in the ES were estimated by the model, for all infections caused by Enterobacterales with MBL and P. aeruginosa with MBL. Patient-level INHEs in BSI and IAIs were assumed to be the same as in HAP/VAP and cUTIs, respectively, based on feedback from our clinical advisors. BSI and HAP/VAP are both severe infections where cefiderocol is expected to be used predominantly empirically. Although the consequences of IAI can be more severe than the consequences of cUTI as they are very difficult to treat (requiring a combination of ABs and surgery), on the other hand, the benefits of cefiderocol may be smaller due to the complexity of treating these infections and the lesser role of AMs compared to other treatment modalities in their management. Patient-level INHEs in infections caused by Stenotrophomonas were derived from INHEs in Enterobacterales with MBL and P. aeruginosa with MBL, weighted by the proportion of infections caused by each microorganism (in Table 24), based on feedback from our clinical advisors. All Stenotrophomonas were assumed to be treated in the MDS, again based on feedback from our clinical advisors.
Population-level INHEs in years 1–20 were discounted at an annual rate of 3.5% to account for the delayed start of treatment.
Probabilistic analysis
The parameters included in the probabilistic analysis were chosen pragmatically. The analysis incorporated uncertainty in the patient-level INHE (as described in Modelling direct patient net health effects in high-value clinical scenario) and uncertainty in the population growth. The probabilistic analysis did not reflect uncertainty in the current population size; instead, this was explored in the scenario analyses outlined above. Expected usage and the link between this and resistance was not made probabilistic for simplicity and due to the challenges in characterising with any accuracy the uncertainty around emergence of resistance, again this was explored via scenario analyses outlined in Modelling direct population net health effects in high-value clinical scenario.
Additional elements of value for new antimicrobials
The literature on the economic evaluation of AMs has described the different sources of value associated with these products. 21,122 In EEPRU’s earlier work on evaluation methods,21 the principles by which each of these ‘elements of value’ can be reflected in models focused on estimating the impact of new products on population NHEs were discussed.
In Additional elements of value relevant to antimicrobials, we present a summary of how the different elements of value are conceptualised in the literature, within the manufacturer submission and how they are understood by our clinical advisory group. We summarise the extent to which each element of value is reflected in the quantitative assessments of value for the HVCSs or quantitative evidence presented in the manufacturer submission. For each element of value for which a quantitative assessment was not conducted, we provide a discussion of the extent to which that element of value is likely to be quantitively important in influencing the assessment of population-level INHEs for cefiderocol. This is based on evidence from the literature, evidence presented in the manufacturer submission and the views of our clinical advisors.
Validation
To ensure the appropriateness of the decision problem, scope of the decision model, model structure and evidence used, we consulted extensively: with microbiologists and clinicians involved in treating serious drug-resistant infections and in related research; those with expertise in transmission modelling; and experts in specific types of evidence. Given the complexity of the appraisal and the multiple components of the work this required, approximately 10 separate calls on different aspects of the work.
A technical validation of the data analyses, synthesis and decision-analytic modelling conducted by EEPRU was conducted. This comprised a review of the code by a second reviewer.
Chapter 8 Results of quantification of value
This section reproduces and adapts, with permission, content previously published as part of the study protocol. 1
Direct patient net health effects in high-value clinical scenarios
Metallo-beta-lactamases Enterobacterales, empiric setting, hospital-acquired pneumonia/ventilator-associated pneumonia
The base-case results are shown in Table 26 for patients correctly suspected as having MBL Enterobacterales (herein ‘with MBL Enterobacterales’), those wrongly suspected of having MBL Enterobacterales (herein ‘without MBL Enterobacterales’) and in the average patient suspected to have MBL Enterobacterales in the ES (whose outcomes are a weighted average of those with and without MBL Enterobacterales). There is no non-colistin/aminoglycoside-based treatment combination in the PICOS for this patient population.
E1 | E2ca | E3ca | E1–E2ca | E1–E3ca | |
---|---|---|---|---|---|
Patients with MBL Enterobacterales | |||||
Summary of in-hospital outcomes (proportions) across both lines of treatment available | |||||
Death | 0.399 | 0.403 | 0.403 | −0.004 | −0.003 |
Survival no AKI | 0.473 | 0.428 | 0.428 | 0.046 | 0.045 |
Survival AKI | 0.127 | 0.169 | 0.169 | −0.042 | −0.042 |
Survival CKD | 0.000 | 0.000 | 0.001 | 0 | 0 |
Economic outcomes (all discounted) | |||||
Treatment costs | £101 | £179 | £178 | −£78 | −£77 |
AKI costs hospital | £1954 | £2285 | £2283 | −£331 | −£329 |
Other costs hospital | £21,301 | £16,319 | £16,301 | £4982 | £5000 |
Long-term costs | £566 | £569 | £570 | −£3 | −£4 |
Total costs | £23,922 | £19,352 | £19,331 | £4570 | £4591 |
Life years | 2.58 | 2.52 | 2.52 | 0.06 | 0.06 |
QALYs | 1.81 | 1.77 | 1.77 | 0.04 | 0.04 |
Per person NHE | 0.615 | 0.803 | 0.806 | −0.188 | −0.191 |
Patients without MBL Enterobacterales | |||||
Summary of in-hospital outcomes (proportions) across both lines of treatment available | |||||
Death | 0.351 | 0.403 | 0.403 | −0.052 | −0.052 |
Survival no AKI | 0.524 | 0.428 | 0.428 | 0.096 | 0.096 |
Survival AKI | 0.125 | 0.169 | 0.169 | −0.044 | −0.044 |
Survival CKD | 0.000 | 0.000 | 0.000 | 0 | 0 |
Economic outcomes (all discounted) | |||||
Treatment costs | £30 | £179 | £179 | −£149 | −£149 |
AKI costs hospital | £1672 | £2285 | £2285 | −£612 | −£612 |
Other costs hospital | £16,736 | £16,319 | £16,319 | £417 | £417 |
Long-term costs | £609 | £569 | £569 | £40 | £40 |
Total costs | £19,048 | £19,352 | £19,352 | −£304 | −£304 |
Life years | 2.80 | 2.52 | 2.52 | 0.28 | 0.28 |
QALYs | 1.97 | 1.77 | 1.77 | 0.19 | 0.19 |
Per person NHE | 1.013 | 0.803 | 0.803 | 0.209 | 0.209 |
All patients presenting in the ES | |||||
Total costs | £19,800 | £19,352 | £19,349 | £447 | £451 |
QALYs | 1.94 | 1.77 | 1.77 | 0.17 | 0.17 |
Per person NHE | 0.952 | 0.803 | 0.803 | 0.148 | 0.147 |
Among patients with MBL Enterobacterales, cefiderocol is associated with lower susceptibility (67% for cefiderocol compared to 96% for colistin/aminoglycoside-based therapy) but improved safety. Overall, this results in slightly higher QALYs for cefiderocol (incremental difference 0.04). The safety advantage delivers a substantial QALY gain due to the reduced mortality associated with AKI in the short and long term. The lower susceptibility associated with cefiderocol results in a longer LoS, this overwhelms AKI-related cost savings and drives the £4600 incremental costs associated with cefiderocol. The patient-level INHE is −0.19 QALYs when comparing cefiderocol with colistin/aminoglycoside-based therapy in patients with MBL Enterobacterales.
Among patients without MBL Enterobacterales, cefiderocol and colistin/aminoglycoside-based therapy are assumed to offer similar susceptibility but cefiderocol offers improved safety. This results in a patient-level INHE of 0.21 QALYs for cefiderocol compared to colistin/aminoglycoside-based therapy. This is driven by the QALY gain due to the reduced mortality associated with AKI in the short and long term and a small cost saving associated with reduced risk of AKI.
In the average ES patient suspected of having MBL Enterobacterales, the use of cefiderocol in the ES is associated with a patient-level INHE of 0.15 QALYs compared to colistin/aminoglycoside-based therapy. This reflects that the safety gains associated with avoiding colistin/aminoglycoside-based therapy across patients offset the lower susceptibility associated with cefiderocol in patients with MBL Enterobacterales.
Restricting the use of cefiderocol to patients who fail empiric treatment and require treatment in the MDS results in very similar INHE benefit as existing therapy. This is attributable to a number of factors. Many patients can be treated effectively in the ES with existing treatments or die prior to reaching the MDS (i.e. not all patients progress to the MDS), many patients do not have MBL Enterobacterales and are not, therefore, eligible to receive cefiderocol in the MDS and among those with MBL Enterobacterales, the majority (91%) are susceptible to a non-colistin-based treatment option and, therefore, do not receive cefiderocol in the model.
There is a large degree of parameter uncertainty around the patient-level INHEs of cefiderocol. The distribution of patient-level INHEs is shown in Figure 14. This reflects uncertainty in the probability a patient has MBL Enterobacterales, the relative susceptibility of these treatment options, their safety and the benefits of avoided AKIs.
FIGURE 14.
Distribution of patient-level INHEs of cefiderocol compared to colistin/aminoglycoside-based therapy: MBL Enterobacterales HAP/VAP ES (2000 simulations).
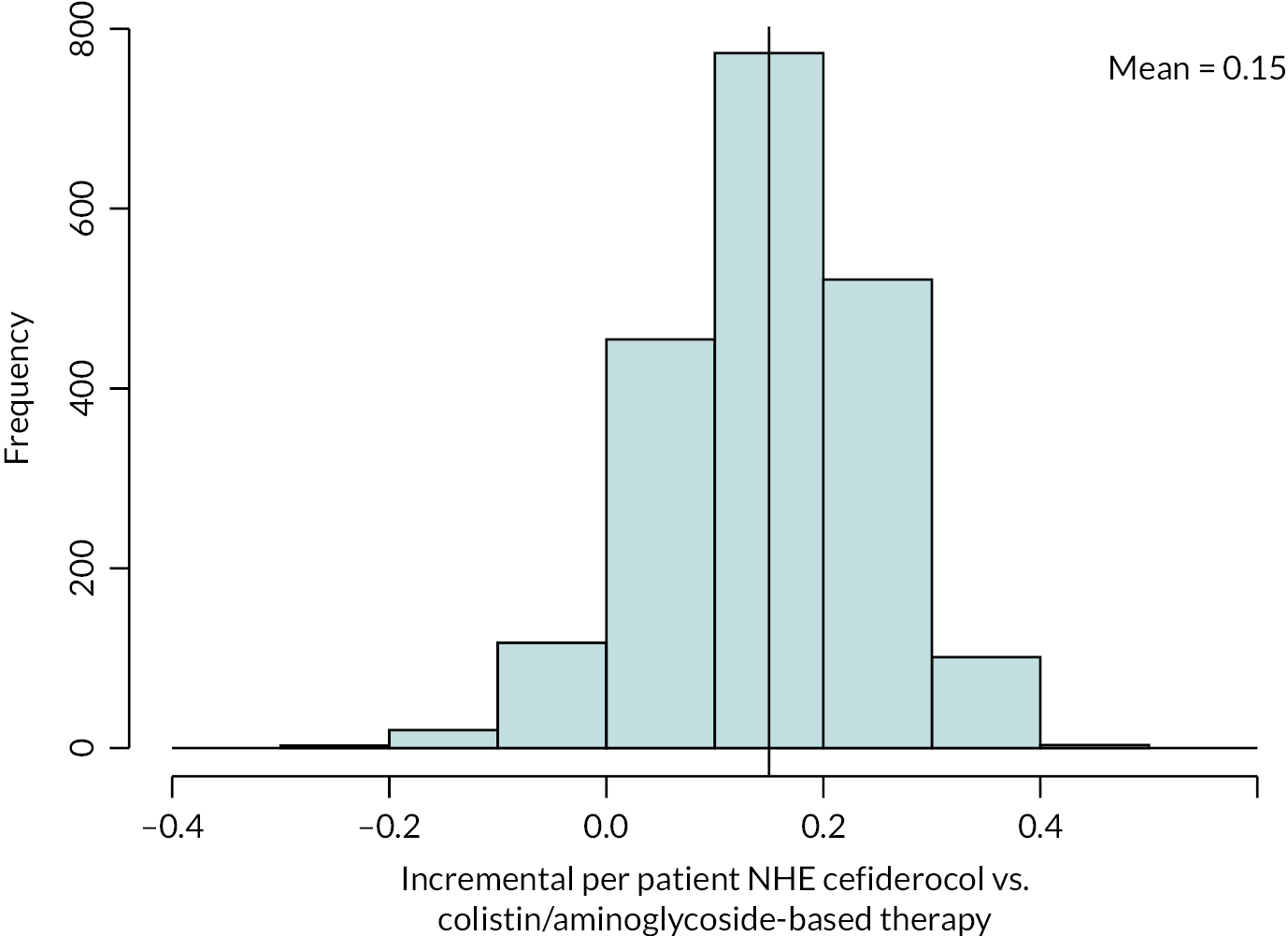
Scenario analyses that modified the base-case INHE by more than 10% (and three scenario analyses requested by NICE marked by a) are shown in Table 27. The main areas of uncertainty relate to the probability that a patient has MBL Enterobacterales, the susceptibility scenarios, the impact of colistin/aminoglycoside-based therapy on AKI risk and its long-term implications and long-term survival following discharge from hospital.
Scenario name | Base-case value/assumption | Scenario value/assumption | Optimal cefiderocol use | Patient-level INHE of cefiderocol |
---|---|---|---|---|
Base case | - | - | Empiric (E1) | 0.168 |
p_bug_survey | Probability patient has MBL Enterobacterales is 0.15 | Probability patient has MBL Enterobacterales is 0.71 based on BSAC survey data | Reserve for use in MDS (E3ca) | 0.002 |
p_bug_0 | Probability patient has MBL Enterobacterales is 0.15 | Probability patient has MBL Enterobacterales is 0.00 | Empiric (E1) | 0.228 |
p_bug_10 | Probability patient has MBL Enterobacterales is 0.15 | Probability patient has MBL Enterobacterales is 0.10 | Empiric (E1) | 0.189 |
p_bug_20 | Probability patient has MBL Enterobacterales is 0.15 | Probability patient has MBL Enterobacterales is 0.20 | Empiric (E1) | 0.150 |
p_bug_30 | Probability patient has MBL Enterobacterales is 0.15 | Probability patient has MBL Enterobacterales is 0.30 | Empiric (E1) | 0.111 |
p_bug_40 | Probability patient has MBL Enterobacterales is 0.15 | Probability patient has MBL Enterobacterales is 0.40 | Empiric (E1) | 0.072 |
p_bug_50 | Probability patient has MBL Enterobacterales is 0.15 | Probability patient has MBL Enterobacterales is 0.50 | Reserve for use in MDS (E3ca) | 0.033 |
p_bug_60 | Probability patient has MBL Enterobacterales is 0.15 | Probability patient has MBL Enterobacterales is 0.60 | Reserve for use in MDS (E3ca) | 0.002 |
p_bug_70 | Probability patient has MBL Enterobacterales is 0.15 | Probability patient has MBL Enterobacterales is 0.70 | Reserve for use in MDS (E3ca) | 0.002 |
p_bug_80 | Probability patient has MBL Enterobacterales is 0.15 | Probability patient has MBL Enterobacterales is 0.80 | Reserve for use in MDS (E3ca) | 0.002 |
p_bug_90 | Probability patient has MBL Enterobacterales is 0.15 | Probability patient has MBL Enterobacterales is 0.90 | Reserve for use in MDS (E3ca) | 0.003 |
p_bug_100 | Probability patient has MBL Enterobacterales is 0.15 | Probability patient has MBL Enterobacterales is 1.00 | Reserve for use in MDS (E3ca) | 0.003 |
S1 | Susceptibility based on NMA of EUCAST studies | Susceptibility based on NMA of CLSI studies | Empiric (E1) | 0.208 |
S3 | Susceptibility based on NMA of EUCAST studies | PHE data, with cefiderocol and fosfomycin data from separate cefiderocol and fosfomycin networks (CLSI studies) | Empiric (E1) | 0.229 |
p_AKI_Chien | Probability of AKI with colistin/aminoglycoside therapy based on Sisay (2021) (0.45) | Probability of AKI with colistin/aminoglycoside therapy based on Chien (2020) (0.32) | Empiric (E1) | 0.128 |
OR_AKI_Wagenlehner | OR comparing AKI for colistin/aminoglycoside-based therapy to non-colistin/aminoglycoside-based therapy from all studies analysis in Chien (2020) (1.81) | OR comparing AKI for colistin/aminoglycoside-based therapy to non-colistin/aminoglycoside-based therapy from all studies analysis in Wagenlehner (2021) (2.23) | Empiric (E1) | 0.234 |
OR_AKI_ChienRIFLE | OR comparing AKI for colistin/aminoglycoside-based therapy to non-colistin/aminoglycoside-based therapy from all studies analysis in Chien (2020) (1.81) | OR comparing AKI for colistin/aminoglycoside-based therapy to non-colistin/aminoglycoside-based therapy from RIFLE criteria studies analysis in Chien (2020) (1.61) | Empiric (E1) | 0.128 |
OR_AKI_death_halved | OR of mortality for AKI compared with no AKI derived from Kerr (2014) (5.11) | OR of mortality for AKI compared with no AKI halved (2.56) | Empiric (E1) | 0.124 |
double.ckd.risk | Risk of CKD as observed in Bucaloiu (2012) | Risk of CKD doubled to reflect potential higher propensity for CKD in HVCS | Empiric (E1) | 0.136 |
loglogistic | Log-normal model fit to CARBAR survival data | Log-logistic model fit to CARBAR survival data | Empiric (E1) | 0.136 |
Weibull | Log-normal model fit to CARBAR survival data | Weibull model fit to CARBAR survival data | Empiric (E1) | 0.095 |
lt.care | No costs of long-term care | Costs of discharge to long-term care | Empiric (E1) | 0.174 |
thresh15a | Cost-effectiveness threshold £20,000 | Cost-effectiveness threshold £15,000 | Empiric (E1) | 0.159 |
thresh30a | Cost-effectiveness threshold £20,000 | Cost-effectiveness threshold £30,000 | Empiric (E1) | 0.176 |
dr1.5a | Discount rate for costs and benefits 3.5% | Discount rate for costs and benefits 1.5% | Empiric (E1) | 0.196 |
The results are very sensitive to the proportion of people with MBL Enterobacterales. When this proportion is 60% or more, the preferred treatment pathway is no longer to use cefiderocol empirically but to use colistin/aminoglycoside-based therapy empirically due to its susceptibility advantage among those with MBL Enterobacterales and reserve cefiderocol for use in the MDS (this is associated with small INHE benefits as few patients both reach the MDS and require cefiderocol treatment).
The INHE associated with cefiderocol use increases to 0.21–0.23 when the CLSI breakpoints are used within the NMA (susceptibility Scenarios S1 and S3), as this resulted in higher susceptibility to cefiderocol of 87% under Scenario 1 and 98% under Scenario 3.
The results were sensitive to assumptions about AKI risk including the use of alternative scenarios for absolute AKI risk and the increase in AKI risk associated with colistin/aminoglycoside-based therapy, as well as the short- and long-term consequences of AKIs. Across these scenarios, the patient-level INHEs varied between 0.12 and 0.23 QALYs. Interestingly, scenarios that increased (reduced) the post-30-day consequences of AKI for mortality reduced (increased) the patient-level INHE. This suggests that survival duration following discharge from hospital is more important than differences in mortality between patients with and without history of AKI. This is further confirmed by the sensitivity of the model results to the parametric survival model used to predict long-term survival post discharge. In particular, use of the Weibull model reduced the patient-level INHE to 0.10.
Changing the cost-effectiveness threshold had a small effect on the model results and using a lower discount rate of 1.5% for costs and health outcomes increases the patient-level INHEs to 0.20.
Metallo-beta-lactamases Enterobacterales, microbiology-directed setting, hospital-acquired pneumonia/ventilator-associated pneumonia and complicated urinary tract infection
The base-case results are shown in Table 28 for patients with MBL Enterobacterales in the MDS who have HAP/VAP or cUTI. The advantages of cefiderocol are smaller in the MDS as, once susceptibility results are known, many patients (91%) can be treated with a non-colistin/aminoglycoside-based option to which they are susceptible and do not receive cefiderocol. The patient-level INHE associated with cefiderocol are driven by avoided safety issues related to use of colistin and aminoglycosides in those susceptible to these agents (7% of the MDS cohort), and gains in efficacy and safety for those who are resistant to all available treatment options (1% of the MDS cohort). Overall, the patient-level INHE associated with using cefiderocol in the MDS are 0.02 QALYs for cUTI and HAP/VAP.
MDS pathway with cefiderocol | MDS pathway without cefiderocol | Incremental values | |
---|---|---|---|
HAP/VAP | |||
Summary of in-hospital outcomes (proportions) across both lines of treatment available | |||
Death | 0.374 | 0.378 | −0.004 |
Survival no AKI | 0.496 | 0.49 | 0.007 |
Survival AKI | 0.129 | 0.132 | −0.002 |
Survival CKD | 0 | 0 | 0 |
Economic outcomes (all discounted) | |||
Treatment costs | £280 | £295 | −£15 |
AKI costs hospital | £1673 | £1712 | −£39 |
Other costs hospital | £34,755 | £34,822 | −£67 |
Long-term costs | £590 | £587 | £3 |
Total costs | £37,297 | £37,415 | −£117 |
Life years | 2.69 | 2.67 | 0.02 |
QALYs | 1.89 | 1.87 | 0.02 |
Per person NHE | 0.024 | 0.003 | 0.021 |
cUTI | |||
Summary of in-hospital outcomes (proportions) across both lines of treatment available | |||
Death | 0.126 | 0.13 | −0.004 |
Survival no AKI | 0.646 | 0.638 | 0.008 |
Survival AKI | 0.228 | 0.232 | −0.004 |
Survival CKD | 0 | 0 | 0 |
Economic outcomes (all discounted) | |||
Treatment costs | £280 | £295 | −£15 |
AKI costs hospital | £1673 | £1712 | −£39 |
Other costs hospital | £17,370 | £17,427 | −£57 |
Long-term costs | £830 | £827 | £3 |
Total costs | £20,152 | £20,261 | −£108 |
Life years | 3.71 | 3.69 | 0.02 |
QALYs | 2.61 | 2.59 | 0.02 |
Per person NHE | 1.599 | 1.579 | 0.021 |
There is a lower degree of uncertainty around the patient-level INHEs of cefiderocol compared to the existing treatment options in the MDS. The distribution of patient-level INHEs is shown in Figure 15.
FIGURE 15.
Distribution of patient-level INHEs of introducing cefiderocol in to the MDS compared to existing therapies: (a) MBL Enterobacterales HAP/VAP; and (b) MBL Enterobacterales cUTI (2000 simulations).

Scenario analyses that modified the base-case deterministic INHE by more than 10% (and three scenario analyses requested by NICE marked by a) are shown in Table 29. The main areas of uncertainty relate to the susceptibility scenarios and long-term survival following discharge from hospital.
Scenario name | Base-case value/assumption | Scenario value/assumption | Patient-level INHE of cefiderocol: HAP/VAP | Patient-level INHE of cefiderocol: cUTI |
---|---|---|---|---|
Base case | - | - | 0.023 | 0.022 |
S1 | Susceptibility based on NMA of EUCAST studies | Susceptibility based on NMA of CLSI studies | 0.011 | 0.011 |
S3 | Susceptibility based on NMA of EUCAST studies | PHE data, with cefiderocol and fosfomycin data from separate cefiderocol and fosfomycin networks (CLSI studies) | 0.017 | 0.017 |
S4 | Susceptibility based on NMA of EUCAST studies | PHE data (cefiderocol from EUCAST NMA, excludes fosfomycin) | 0.056 | 0.056 |
OR_AKI_Wagenlehner | OR comparing AKI for colistin/aminoglycoside-based therapy to non-colistin/aminoglycoside-based therapy from all studies analysis in Chien (2020) (1.81) | OR comparing AKI for colistin/aminoglycoside-based therapy to non-colistin/aminoglycoside-based therapy from all studies analysis in Wagenlehner (2021) (2.23) | 0.020 | 0.020 |
OR_AKI_ChienRIFLE | OR comparing AKI for colistin/aminoglycoside-based therapy to non-colistin/aminoglycoside-based therapy from all studies analysis in Chien (2020) (1.81) | OR comparing AKI for colistin/aminoglycoside-based therapy to non-colistin/aminoglycoside-based therapy from RIFLE criteria studies analysis in Chien (2020) (1.61) | 0.027 | 0.027 |
OR_AKI_death_halved | OR of mortality for AKI compared to no AKI derived from Kerr (2014) (5.11) | OR of mortality for AKI compared to no AKI halved (2.56) | 0.020 | 0.020 |
double.ckd.risk | Risk of CKD as observed in Bucaloiu (2012) | Risk of CKD doubled to reflect potential higher propensity for CKD in HVCS | 0.019 | 0.019 |
abs.increase | ORs on mortality associated with nephrotoxicity from Bucaloiu (2012) are applied multiplicatively to underlying risk in HVCS | Absolute risk increases in Bucaloiu (2012) are assumed to apply | Change < 10% relative to base case | 0.023 |
all.aki.lt | Base-case assumptions with respect to long-term effects of AKI | Applying a range of alternative assumptions to model the long-term effects of AKI | Change < 10% relative to base case | 0.023 |
loglogistic | Log-normal model fit to CARBAR survival data | Log-logistic model fit to CARBAR survival data | 0.020 | 0.020 |
Weibull | Log-normal model fit to CARBAR survival data | Weibull model fit to CARBAR survival data | 0.016 | 0.016 |
lt.care | No costs of long-term care | Costs of discharge to long-term care | Change < 10% relative to base case | 0.023 |
thresh15a | Cost-effectiveness threshold £20,000 | Cost-effectiveness threshold £15,000 | 0.025 | 0.024 |
thresh30a | Cost-effectiveness threshold £20,000 | Cost-effectiveness threshold £30,000 | 0.020 | 0.021 |
dr1.5a | Discount rate for costs and benefits 3.5% | Discount rate for costs and benefits 1.5% | 0.025 | 0.025 |
The patient-level INHEs were considerably higher when susceptibility was informed using PHE data for comparators (Scenario S4) reaching 0.06 QALYs for both cUTI and HAP/VAP due to the lower proportion of individuals susceptible to a non-aminoglycoside/colistin-based option in this scenario. Interestingly, when the NMA of susceptibility data that included studies using the CLSI breakpoints (Scenario S1), and when the CLSI NMA results for cefiderocol are combined with the PHE data for comparators (Scenario S3), the patient-level INHEs reduce to 0.01 in Scenario S1 and 0.02 in Scenario S3 for both cUTI and HAP/VAP. In these scenarios, although susceptibility to cefiderocol is higher, more individuals are susceptible to a non-aminoglycoside/colistin-based option, thus reducing the proportion of patients who receive cefiderocol in the MDS.
The other scenario which had a large impact on results was the use of the Weibull model to inform long-term mortality which reduced the patient-level INHEs to 0.02 in both HAP/VAP and cUTI.
Metallo-beta-lactamases P. aeruginosa, empiric setting, hospital-acquired pneumonia/ventilator-associated pneumonia
The base-case results are shown in Table 30 for patients with MBL P. aeruginosa, those without MBL P. aeruginosa and in the average patient suspected to have MBL P. aeruginosa in the ES.
E1 | E2nca | E2ca | E3nca | E3ca | E1–E2nca |
E1–E2ca | |
---|---|---|---|---|---|---|---|
Patients with MBL P. aeruginosa | |||||||
Summary of in-hospital outcomes (proportions) across both lines of treatment available | |||||||
Death | 0.352 | 0.496 | 0.401 | 0.459 | 0.397 | −0.143 | −0.048 |
Survival no AKI | 0.522 | 0.362 | 0.43 | 0.404 | 0.432 | 0.161 | 0.092 |
Survival AKI | 0.125 | 0.142 | 0.169 | 0.137 | 0.17 | −0.017 | −0.044 |
Survival CKD | 0.000 | 0.000 | 0.001 | 0.000 | 0.001 | 0.000 | 0.000 |
Economic outcomes (all discounted) | |||||||
Treatment costs | £15 | £131 | £167 | £14 | £156 | −£116 | −£152 |
AKI costs hospital | £1684 | £2607 | £2270 | £2315 | £2255 | −£923 | −£586 |
Other costs hospital | £16,599 | £28,276 | £15,930 | £27,331 | £15,795 | −£11,676 | £669 |
Long-term costs | £608 | £481 | £571 | £514 | £575 | £127 | £37 |
Total costs | £18,906 | £31,495 | £18,938 | £30,175 | £18,781 | −£12,589 | −£32 |
Life years | 2.79 | 2.13 | 2.53 | 2.30 | 2.54 | 0.66 | 0.26 |
QALYs | 1.96 | 1.50 | 1.78 | 1.62 | 1.79 | 0.47 | 0.18 |
Per person NHE | 1.015 | −0.079 | 0.831 | 0.107 | 0.849 | 1.094 | 0.184 |
Patients without MBL P. aeruginosa | |||||||
Summary of in-hospital outcomes (proportions) across both lines of treatment available | |||||||
Death | 0.349 | 0.349 | 0.401 | 0.349 | 0.401 | 0 | −0.052 |
Survival no AKI | 0.526 | 0.526 | 0.43 | 0.526 | 0.43 | 0 | 0.096 |
Survival AKI | 0.125 | 0.125 | 0.169 | 0.125 | 0.169 | 0 | −0.044 |
Survival CKD | 0.000 | 0.000 | 0.001 | 0.000 | 0.001 | 0.000 | 0.000 |
Economic outcomes (all discounted) | |||||||
Treatment costs | £12 | £22 | £167 | £22 | £167 | −£10 | −£155 |
AKI costs hospital | £1663 | £1663 | £2270 | £1663 | £2270 | £0 | −£608 |
Other costs hospital | £16,313 | £16,313 | £15,930 | £16,313 | £15,930 | £0 | £383 |
Long-term costs | £611 | £611 | £571 | £611 | £571 | £0 | £40 |
Total costs | £18,599 | £18,609 | £18,938 | £18,609 | £18,938 | −£10 | −£339 |
Life years | 2.81 | 2.81 | 2.53 | 2.81 | 2.53 | 0.00 | 0.28 |
QALYs | 1.97 | 1.97 | 1.78 | 1.97 | 1.78 | 0.00 | 0.19 |
Per person NHE | 1.042 | 1.041 | 0.831 | 1.041 | 0.831 | 0 | 0.211 |
All patients presenting in the ES | |||||||
Total costs | £18,642 | £20,412 | £18,938 | £20,228 | £18,916 | −£1770 | −£296 |
QALYs | 1.97 | 1.91 | 1.78 | 1.92 | 1.78 | 0.07 | 0.19 |
Per person NHE | 1.038 | 0.884 | 0.831 | 0.910 | 0.834 | 0.153 | 0.207 |
Cefiderocol is associated with similar susceptibility to colistin/aminoglycoside-based therapy but improved safety in both individuals with and without MBL P. aeruginosa. The patient-level INHE gain is, therefore, similar in patients with MBL P. aeruginosa, without MBL P. aeruginosa and the overall ES population at 0.18–0.21 QALYs. The safety advantage delivers a small cost saving as cost savings associated with reduced rates of AKI are offset by longer time spent in hospital for patients receiving cefiderocol as preventing AKIs lowers early in-hospital mortality thus prolonging hospital stay. The safety advantage delivers a substantial QALY gain due to the reduced mortality associated with AKI in the short and long term.
Among patients with MBL P. aeruginosa, cefiderocol is associated with improved susceptibility and comparable safety to non-colistin/aminoglycoside-based therapy. The large difference in susceptibility between these comparators (99% vs. 28%) drives a large gain in patient-level INHE of 1.09 QALYs in this group. This reflects both the substantial QALY gain associated with this improved susceptibility (0.47) and the significant cost saving associated with a reduced LoS (£12,600). In patients without MBL P. aeruginosa, cefiderocol and non-colistin/aminoglycoside-based therapy offer similar efficacy and safety.
In the average ES patient suspected of having MBL Pseudomonas, use of cefiderocol in the ES is associated with a patient-level INHE gain of 0.21 QALYs compared to colistin/aminoglycoside-based therapy and of 0.15 QALYs compared to non-colistin/aminoglycoside-based therapy.
Restricting the use of cefiderocol to patients who fail empiric treatment and require treatment in the MDS is associated with a smaller NHE benefit than the use of cefiderocol in the ES. This reflects that those without MBL P. aeruginosa (86% of the cohort) are not eligible to receive cefiderocol in the MDS.
There is a large degree of parameter uncertainty around the patient-level INHEs of cefiderocol. The distribution of patient-level INHEs is shown in Figure 16. This reflects uncertainty in the probability individuals have MBL Pseudomonas, the relative susceptibility of these treatment options, their safety and the benefits of avoided AKIs. The cluster of values around 0 in the comparison of cefiderocol to non-colistin/aminoglycoside therapy is driven by samples where both susceptibility to non-colistin/aminoglycoside-based therapy is high and the difference in mortality between those treated successfully in the ES and MDS is minimal.
FIGURE 16.
Distribution of patient-level INHEs of cefiderocol in MBL P. aeruginosa HAP/VAP ES compared to (a) non-colistin/aminoglycoside-based therapy and (b) colistin/aminoglycoside-based therapy and (2000 simulations).
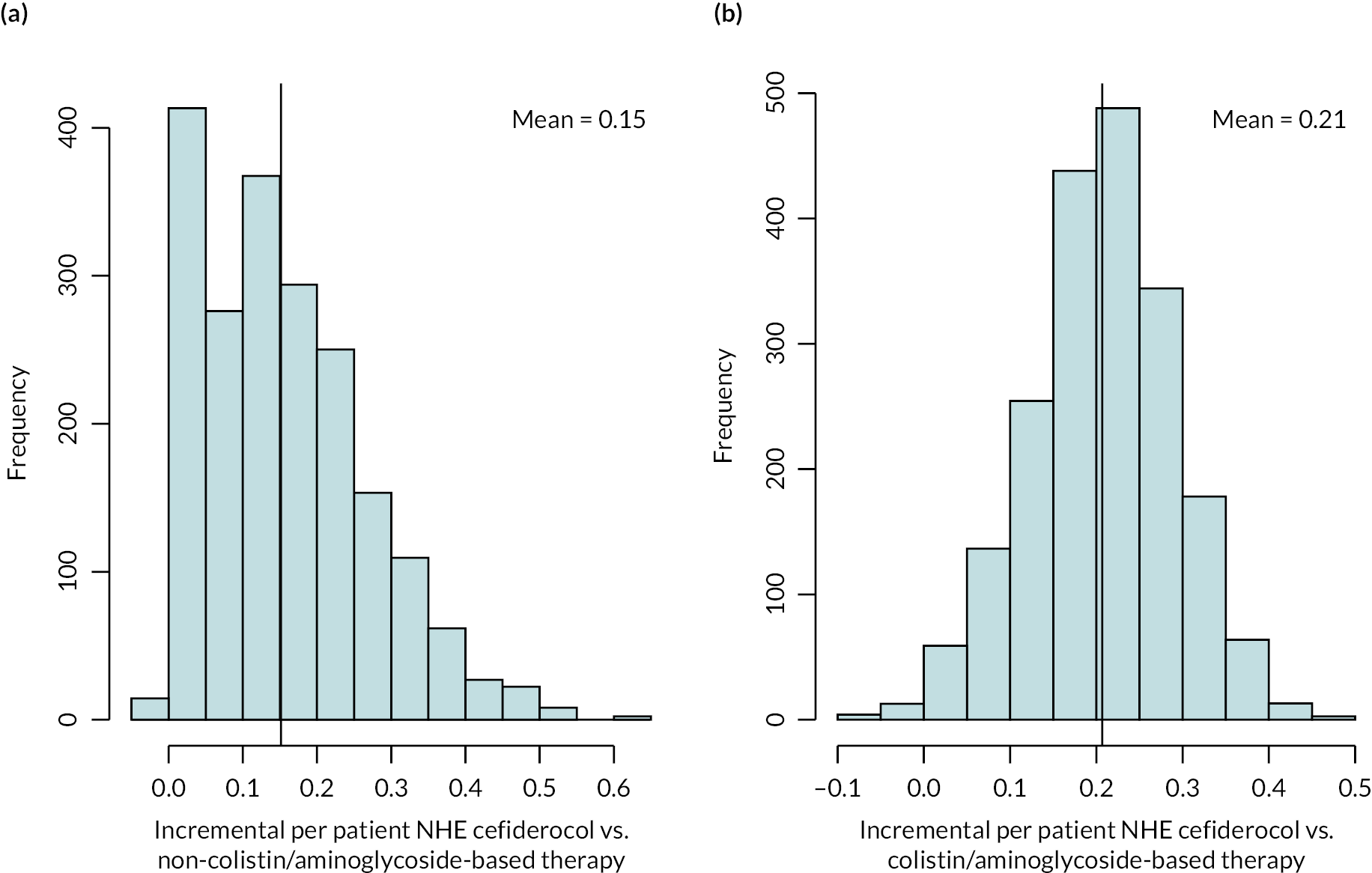
Scenario analyses that modified the deterministic base-case INHE by more than 10% (and three scenario analyses requested by NICE marked by a) are shown in Table 31. The scenarios which had the most marked effect on the study results were the likelihood that an individual has MBL P. aeruginosa and the susceptibility scenarios.
Scenario name | Base-case value/assumption | Scenario value/assumption | Best existing treatment | Patient-level INHE of cefiderocol |
---|---|---|---|---|
Base case | - | - | Non-colistin/amino-based | 0.145 |
p_bug_survey | Probability patient has MBL P. aeruginosa is 0.14 | Probability patient has MBL Enterobacterales is 0.71 based on BSAC survey data | Colistin/amino-based | 0.210 |
p_bug_0 | Probability patient has MBL P. aeruginosa is 0.14 | Probability patient has MBL P. aeruginosa is 0.00 | Non-colistin/amino-based | 0.000 |
p_bug_10 | Probability patient has MBL P. aeruginosa is 0.14 | Probability patient has MBL P. aeruginosa is 0.10 | Non-colistin/amino-based | 0.105 |
p_bug_20 | Probability patient has MBL P. aeruginosa is 0.14 | Probability patient has MBL P. aeruginosa is 0.20 | Colistin/amino-based | 0.211 |
p_bug_30 | Probability patient has MBL P. aeruginosa is 0.14 | Probability patient has MBL P. aeruginosa is 0.30 | Colistin/amino-based | 0.221 |
p_bug_40 | Probability patient has MBL P. aeruginosa is 0.14 | Probability patient has MBL P. aeruginosa is 0.40 | Colistin/amino-based | 0.218 |
p_bug_50 | Probability patient has MBL P. aeruginosa is 0.14 | Probability patient has MBL P. aeruginosa is 0.50 | Colistin/amino-based | 0.216 |
p_bug_60 | Probability patient has MBL P. aeruginosa is 0.14 | Probability patient has MBL P. aeruginosa is 0.60 | Colistin/amino-based | 0.213 |
p_bug_70 | Probability patient has MBL P. aeruginosa is 0.14 | Probability patient has MBL P. aeruginosa is 0.70 | Colistin/amino-based | 0.211 |
p_bug_80 | Probability patient has MBL P. aeruginosa is 0.14 | Probability patient has MBL P. aeruginosa is 0.80 | Colistin/amino-based | 0.208 |
p_bug_90 | Probability patient has MBL P. aeruginosa is 0.14 | Probability patient has MBL P. aeruginosa is 0.90 | Colistin/amino-based | 0.206 |
p_bug_100 | Probability patient has MBL P. aeruginosa is 0.14 | Probability patient has MBL P. aeruginosa is 1.00 | Colistin/amino-based | 0.203 |
S1 | Susceptibility based on NMA of EUCAST studies | Susceptibility based on NMA of CLSI studies | Non-colistin/amino-based | 0.026 |
S3 | Susceptibility based on NMA of EUCAST studies | PHE data, with cefiderocol and fosfomycin data from separate cefiderocol and fosfomycin networks (CLSI studies). | Non-colistin/amino-based | 0.007 |
Weibull | Log-normal model fit to CARBAR survival data | Weibull model fit to CARBAR survival data | Non-colistin/amino-based | 0.123 |
thresh15a | Cost-effectiveness threshold £20,000 | Cost-effectiveness threshold £15,000 | Non-colistin/amino-based | 0.174 |
thresh30a | Cost-effectiveness threshold £20,000 | Cost-effectiveness threshold £30,000 | Non-colistin/amino-based | 0.117 |
dr1.5a | Discount rate for costs and benefits 3.5% | Discount rate for costs and benefits 1.5% | Non-colistin/amino-based | 0.154 |
When the probability the patient has MBL P. aeruginosa was > 0.14 (the base case), the patient-level INHE increases as the preferred comparator switches to colistin/aminoglycosides and the safety advantage of cefiderocol is substantial. Two of the susceptibility scenarios reduced the patient-level INHEs associated with cefiderocol to < 0.03 QALYs. These scenarios (S1, S3) used CLSI breakpoints and found non-colistin/aminoglycoside-based therapy (specifically fosfomycin monotherapy) to offer high levels of susceptibility (86–96%).
Metallo-beta-lactamases P. aeruginosa, microbiology-directed setting, hospital-acquired pneumonia/ventilator-associated pneumonia and complicated urinary tract infection
The base-case results are shown in Table 32 for patients with MBL P. aeruginosa in the MDS who have HAP/VAP or cUTI. The advantages of cefiderocol in the MDS are relatively high in patients with MBL P. aeruginosa as most patients are only susceptible to colistin/aminoglycoside-based treatment. The gains in patient-level NHE associated with cefiderocol are driven by avoided safety issues related to the use of colistin and aminoglycosides in those susceptible to these agents (71% of the MDS cohort). Overall, the patient-level gains in NHE associated with using cefiderocol in the MDS are 0.15 for both HAP/VAP and cUTI.
MDS pathway with cefiderocol | MDS pathway without cefiderocol | Incremental values | |
---|---|---|---|
HAP/VAP | |||
Summary of in-hospital outcomes (proportions) | |||
Death | 0.373 | 0.404 | −0.031 |
Survival no AKI | 0.497 | 0.431 | 0.066 |
Survival AKI | 0.129 | 0.164 | −0.035 |
Survival CKD | 0.000 | 0.000 | 0.000 |
Economic outcomes (all discounted) | |||
Treatment costs | £6 | £114 | −£108 |
AKI costs hospital | £1667 | £2126 | −£459 |
Other costs hospital | £34,724 | £34,755 | −£31 |
Long-term costs | £591 | £568 | £23 |
Total costs | £36,987 | £37,563 | −£576 |
Life years | 2.69 | 2.52 | 0.18 |
QALYs | 1.89 | 1.77 | 0.12 |
Per person NHE | 0.043 | −0.108 | 0.151 |
cUTI | |||
Summary of in-hospital outcomes (proportions) | |||
Death | 0.125 | 0.149 | −0.024 |
Survival no AKI | 0.648 | 0.562 | 0.086 |
Survival AKI | 0.228 | 0.289 | −0.062 |
Survival CKD | 0.000 | 0.000 | 0.000 |
Economic outcomes (all discounted) | |||
Treatment costs | £6 | £114 | −£108 |
AKI costs hospital | £1667 | £2126 | −£459 |
Other costs hospital | £17,345 | £17,375 | −£30 |
Long-term costs | £831 | £819 | £12 |
Total costs | £19,848 | £20,434 | −£586 |
Life years | 3.72 | 3.55 | 0.17 |
QALYs | 2.61 | 2.49 | 0.12 |
Per person NHE | 1.618 | 1.471 | 0.147 |
The uncertainty around the patient-level INHEs of cefiderocol compared to the existing treatment options in the MDS is shown in Figure 17 and is similar to that observed in the ES.
FIGURE 17.
Distribution of INHEs of introducing cefiderocol into the MDS compared to existing therapies: (a) MBL P. aeruginosa HAP/VAP and (b) MBL P. aeruginosa cUTI (2000 simulations).

Scenario analyses that modified the base-case INHE by more than 10% (and three scenario analyses requested by NICE marked by a) are shown in Table 33. The scenarios which had the most marked effect on results both in HAP/VAP and in cUTI were the susceptibility scenarios, scenarios relating to the rate and consequences of AKI, long-term survival following discharge from hospital and the costs of discharge to long-term care.
Scenario name | Base-case value/assumption | Scenario value/assumption | Patient-level INHE of cefiderocol: HAP/VAP | Patient-level INHE of cefiderocol: cUTI |
---|---|---|---|---|
Base case | - | - | 0.174 | 0.170 |
S1 | Susceptibility based on NMA of EUCAST studies | Susceptibility based on NMA of CLSI studies | 0.035 | 0.034 |
S3 | Susceptibility based on NMA of EUCAST studies | PHE data, with cefiderocol and fosfomycin data from separate cefiderocol and fosfomycin networks (CLSI studies). | 0.009 | 0.009 |
S4 | Susceptibility based on NMA of EUCAST studies | NMA of EUCAST studies, absolute colistin susceptibility values from SIDERO WT | 0.253 | 0.254 |
p_AKI_Chien | Probability of AKI with colistin/aminoglycoside therapy based on Sisay (2021) (0.45) | Probability of AKI with colistin/aminoglycoside therapy based on Chien (0.32) | 0.144 | 0.140 |
OR_AKI_Wagenlehner | OR comparing colistin/aminoglycoside-based therapy to non-colistin/aminoglycoside-based therapy from all studies analysis in Chien (2020) (1.81) | OR comparing colistin/aminoglycoside-based therapy to non-colistin/aminoglycoside-based therapy from all studies analysis in Wagenlehner (2021) (2.23) | 0.224 | 0.218 |
OR_AKI_ChienRIFLE | OR comparing colistin/aminoglycoside-based therapy to non-colistin/aminoglycoside based therapy from all studies analysis in Chien (2020) (1.81) | OR comparing colistin/aminoglycoside-based therapy to non-colistin/aminoglycoside based therapy from RIFLE criteria studies analysis in Chien (2020) (1.61) | 0.145 | 0.140 |
OR_AKI_death_halved | OR of mortality for AKI compared to no AKI derived from Kerr (2014) (5.11) | OR of mortality for AKI compared to no AKI halved (2.56) | 0.136 | 0.131 |
double.ckd.risk | Risk of CKD as observed in Bucaloiu (2012) | Risk of CKD doubled to reflect potential higher propensity for CKD in HVCS | 0.151 | 0.148 |
abs.increase | ORs on mortality associated with nephrotoxicity from Bucaloiu (2012) are applied multiplicatively to underlying risk in HVCS | Absolute risk increases in Bucaloiu (2012) are assumed to apply | Change < 10% relative to base case | 0.158 |
all.aki.lt | Base-case assumptions with respect to long-term effects of AKI | Applying a range of alternative assumptions to model the long-term effects of AKI | Change < 10% relative to base case | 0.158 |
loglogistic | Log-normal model fit to CARBAR survival data | Log-logistic model fit to CARBAR survival data | 0.151 | 0.148 |
Weibull | Log-normal model fit to CARBAR survival data | Weibull model fit to CARBAR survival data | 0.120 | 0.116 |
lt.care | No costs of long-term care | Costs of discharge to long-term care included | Change < 10% relative to base case | 0.197 |
thresh15a | Cost-effectiveness threshold £20,000 | Cost-effectiveness threshold £15,000 | 0.185 | 0.180 |
thresh30a | Cost-effectiveness threshold £20,000 | Cost-effectiveness threshold £30,000 | 0.164 | 0.159 |
dr1.5a | Discount rate for costs and benefits 3.5% | Discount rate for costs and benefits 1.5% | 0.197 | 0.193 |
When susceptibility was informed by the CLSI NMA (Scenario S1) and when the CLSI NMA results are combined with the PHE data (Scenario S3), the patient-level INHEs decreased to 0.03 in Scenario S1 and 0.01 in Scenario S3 for both cUTI and HAP/VAP. In these scenarios, although susceptibility to cefiderocol is slightly higher, more individuals are susceptible to a non-aminoglycoside/colistin-based option, thus reducing the proportion of patients who receive cefiderocol in the MDS. The opposite is the case in Scenario S4 where, although susceptibility to cefiderocol is slightly lower than the base case, fewer individuals are susceptible to a non-aminoglycoside/colistin-based option, thus increasing the proportion of patients who receive cefiderocol in the MDS. Therefore, in this scenario, INHE is higher.
The results varied according to the impact of colistin/aminoglycoside-based therapy on AKI risk and the long-term consequences of AKI with these scenarios resulting in patient-level NHE gains of 0.13–0.22 for HAP/VAP and cUTI.
Use of a Weibull model for long-term survival reduced the patient-level INHE to 0.12 in both HAP/VAP and cUTI.
Furthermore, inclusion of the costs of discharge to long-term care increases the INHE in cUTI to 0.20 QALYS.
Direct population net health effects in high-value clinical scenario and broader areas of expected usage
Figure 18 shows the population-level INHE over 20 years derived using alternative assumptions about the population size (based on different categorisation of specimen types), population growth (derived with different models for the population growth predictions) and resistance emergence (reaching 1%, 10% and 30% at 20 years – 5% scenario not shown for parsimony). Population-level INHE declines year on year in scenarios where the discount rate exceeds the rate of population growth in all periods; rises and then declines in scenarios where the population growth rate exceeds the discount rate in earlier periods but then falls below the discount rate in later periods; and rises year on year in scenarios where the rate of population growth exceeds the discount rate. Table 34 shows the total discounted population-level INHE aggregated over the 20-year period.
FIGURE 18.
Population-level INHE (QALYs) over 20 years based on two population size scenarios. P1: baseline population based on PHE categorisation of infection sites; P2: baseline population based on clinical advisors’ categorisation of infection sites; G1: damped growth rate; G2: growth rate not damped; R1: 1% resistance after 20 years; R2: 10% resistance after 20 years; R3: 30% resistance after 20 years. (a) PHE categorisation. (b) Expert-guided categorisation of specimen types.


Baseline population | Pop. growth rate | Change in resistance | HAP/VAP (MBL CPE) | HAP/VAP (P. aeruginosa MBL) | HAP/VAP (Sten.) | cUTI (MBL CPE) | cUTI (P. aeruginosa MBL) | cUTI (Sten.) | BSI (MBL CPE) | BSI (P. aeruginosa MBL) | BSI (Sten.) | IAI (MBL CPE) | IAI (P. aeruginosa MBL) | IAI (Sten.) | Total |
---|---|---|---|---|---|---|---|---|---|---|---|---|---|---|---|
PHE categories of specimen types (Scenario P1) | Model with damped effect (G1) | 1% (R1) | 98 | 12 | 52 | 32 | 43 | 72 | 604 | 28 | 54 | 27 | 30 | 41 | 1093 |
5% (R2) | 96 | 12 | 51 | 31 | 42 | 71 | 589 | 27 | 53 | 26 | 29 | 40 | 1067 | ||
10% (R3) | 93 | 11 | 49 | 30 | 41 | 69 | 570 | 27 | 51 | 25 | 29 | 39 | 1034 | ||
30% (R4) | 80 | 10 | 41 | 25 | 37 | 60 | 495 | 23 | 44 | 21 | 26 | 34 | 896 | ||
Model without damped effect (G2) | 1% (R1) | 153 | 12 | 82 | 50 | 43 | 102 | 945 | 28 | 80 | 42 | 30 | 58 | 1625 | |
5% (R2) | 149 | 12 | 79 | 49 | 42 | 99 | 918 | 27 | 78 | 40 | 29 | 57 | 1579 | ||
10% (R3) | 144 | 11 | 75 | 46 | 41 | 96 | 884 | 27 | 75 | 39 | 29 | 55 | 1522 | ||
30% (R4) | 122 | 10 | 61 | 38 | 37 | 83 | 749 | 23 | 64 | 31 | 26 | 47 | 1291 | ||
Clinical advisors’ categories of specimen types (Scenario P2) | Model with damped effect (G1) | 1% (R1) | 613 | 185 | 794 | 44 | 26 | 53 | 604 | 28 | 54 | 27 | 30 | 41 | 2499 |
5% (R2) | 598 | 180 | 777 | 42 | 25 | 52 | 589 | 27 | 53 | 26 | 29 | 40 | 2438 | ||
10% (R3) | 579 | 174 | 755 | 41 | 25 | 50 | 570 | 27 | 51 | 25 | 29 | 39 | 2365 | ||
30% (R4) | 502 | 151 | 669 | 34 | 22 | 43 | 495 | 23 | 44 | 21 | 26 | 34 | 2064 | ||
Model without damped effect (G2) | 1% (R1) | 959 | 185 | 1059 | 68 | 26 | 79 | 945 | 28 | 80 | 42 | 30 | 58 | 3559 | |
5% (R2) | 932 | 180 | 1033 | 66 | 25 | 77 | 918 | 27 | 78 | 40 | 29 | 57 | 3462 | ||
10% (R3) | 897 | 174 | 1001 | 63 | 25 | 74 | 884 | 27 | 75 | 39 | 29 | 55 | 3343 | ||
30% (R4) | 760 | 151 | 874 | 51 | 22 | 63 | 749 | 23 | 64 | 31 | 26 | 47 | 2861 |
Hospital-acquired pneumonia/ventilator-associated pneumonia and BSIs, and MBL Enterobacterales infections are the key drivers of population-level benefit consistently across all scenarios due to the population size. The impact of the population size is evident when comparing results across different scenarios, where different categorisations of specimen types (which determine the baseline number of infections) have the greatest impact, changing the total 20-year population-level INHE from between 896 and 1625 to between 2064 and 3559 QALYs. Population growth scenarios and the resistance emergence scenario where 30% of the population is resistant after 20 years have similar impacts on population-level INHE. Resistance between 1% and 10% results in similar total INHE. The Stenotrophomonas populations account for 20–38% of the total population-level INHE depending on the scenario, due to their large population size. Of note, when the EEPRU clinical advisors’ categorisation of infection sites is used, the current number of HAP/VAP Stenotrophomonas infections in which cefiderocol would be relevant increases to 547 (compared to 100 under the PHE classification). This introduces an additional element of uncertainty as no clinical evidence reviews or economic modelling were conducted for Stenotrophomonas.
We also estimated how much of the value of cefiderocol accrues to patients initiating treatment in the first 10 years of use, as this is the period of the contract for the delinked payment. Full results are presented in Appendix 8, Table 58. Across scenarios relating to baseline population, population growth and resistance emergence, the proportion of value that accrues in the first 10 years of use is 41–57%. The proportion of value accruing in the first 10 years is less than might be expected for other pharmaceuticals. For a pharmaceutical where population size is expected to be stable over time, we would expect 59% of the value to accrue in the first 10 years.
Following a request from NICE, we also assessed the impact on the population-level results of using a 1.5% discount rate. These results reflect an assumption of zero emergence of resistance to cefiderocol and are intended to give an indication of the broad effect of a lower discount rate. Across the scenarios relating to population size and population growth, the 20-year population-level INHE ranged from 1527 to 5057 when using a 1.5% discount rate. This indicates a substantive increase compared to the results observed using a 3.5% discount rate.
There is a large degree of uncertainty around the population-level INHEs of cefiderocol. The distribution of population-level INHEs for two population size scenarios (P1G1, P2G2) under a scenario of no resistance emergence to cefiderocol is shown in Figure 19. The distribution of population-level INHE reflects the patient-level INHE parameter uncertainty discussed in Metallo-beta-lactamasesEnterobacterales,empiric setting, hospital-acquired pneumonia/ventilator-associated pneumonia, Metallo-beta-lactamasesEnterobacterales,microbiology-directed setting, hospital-acquired pneumonia/ventilator-associated pneumonia and complicated urinary tract infection, Metallo-beta-lactamasesP. aeruginosa,empiric setting, hospital-acquired pneumonia/ventilator-associated pneumonia and Metallo-beta-lactamasesP. aeruginosa,microbiology-directed setting, hospital-acquired pneumonia/ventilator-associated pneumonia and complicated urinary tract infection as well as uncertainty in the rate of population growth over time. The difference in dispersion (range) between two histograms indicates the uncertainty in population growth between the two scenarios.
FIGURE 19.
Distribution of total population-level INHEs of cefiderocol (2000 simulations). P1G1: baseline population (point estimate) based on PHE categorisation of infection sites, growth rate damped (uncertain); P2G2: baseline population (point estimate) based on clinical advisors’ categorisation of infection sites, growth rate not damped (uncertain).

An additional scenario (presented in Appendix 8, Additional scenario: contraindications to colistin and aminoglycosides) was provided to reflect the population-level benefit when a proportion of patients (20–40%) who, despite being susceptible to colistin/aminoglycosides, would instead be given salvage therapy due to the risk of colistin/aminoglycoside toxicity, in the absence of cefiderocol. The results of this scenario are presented in detail in Appendix 8, Additional scenario: contraindications to colistin and aminoglycosides with patient-level INHE shown in Appendix 8, section Additional scenario: contraindications to colistin and aminoglycosides, Table 59. Population-level INHE (see Appendix 8, Additional scenario: contraindications to colistin and aminoglycosides, Table 61 and Figure 47) increased from a base-case range of 856–2995 to 1616–5566 QALYs if 20% of patients who are susceptible to colistin/aminoglycosides do not receive these treatments due to toxicity concerns. This increases to 2383–8138 if the proportion of patients increases to 40%.
These expected population-level INHEs may overestimate the total INHE for several reasons. Firstly, the 20–40% proportion of patients who would not be given colistin/aminoglycosides because of toxicity fears in the absence of cefiderocol is high compared to the assessment of the clinical advisors consulted by EEPRU. Secondly, the scenario assumes that outcomes in patients who can and cannot take colistin/aminoglycosides are comparable, when, in practice, patients who cannot take colistin may have poorer prognoses than patients who can. Thirdly, the scenario assumes patients would be contraindicated to colistin and aminoglycosides, but clinical advisors to EEPRU (and consultation comments from the British Infection Association) suggested that most of the concern is about colistin.
Finally, the scenario results represent the benefit in HVCS when, in the ES, all patients with suspected infection are treated with colistin/aminoglycosides. In the EEPRU base case, the empiric treatment of MBL P. aeruginosa infections with non-colistin/aminoglycoside therapy had a higher patient-level net benefit (and lower incremental benefit of cefiderocol) than treatment with colistin/aminoglycosides, suggesting that, without cefiderocol, non-aminoglycosides are the preferred empiric treatment.
Results showing the combined effect of uncertainty at the patient and population level are presented in Appendix 8, Combined effect of uncertainties at the patient and population level, Table 62.
Additional elements of value relevant to antimicrobials
Conceptualisation of additional elements of value
The conceptualisation of each additional element of value, and how these additional elements of value may influence the INHEs associated with cefiderocol are presented in Table 35. These reflect the different viewpoints on the additional elements of value found in the literature, presented by the manufacturer and discussed by the clinical advisors and other stakeholders of this project.
Element of value | What this represents | Specific pathways to INHEsa |
---|---|---|
Enablement value | Impact on population health from additional medical procedures being possible as a result of cefiderocol being available to manage otherwise resistant infections with few alternative treatment options |
|
Diversity value | Impact on population health over time as a result of cefiderocol being available and adding to the range of treatments currently available. This can result in a reduction in selection pressure on and resistance to other available treatments, hence retaining their effectiveness for longer |
|
Insurance value | Insurance value is presented in the literature in different ways.21 One relates to the impact on population health over time as a result of cefiderocol being ‘held back’ in reserve until resistance to existing treatments effectively eliminates the latter as options. Resistance to cefiderocol would be limited due to being used less A second meaning is that cefiderocol would ameliorate a potentially catastrophic situation where multidrug resistance becomes so widespread that cefiderocol is the only option across a large number of clinical scenarios. This is a low-probability but high-consequence outcome |
|
Transmission value | The impact on population health over time as a result of cefiderocol reducing the rate of transmission of a given pathogen from patients treated with that product to other individuals, potentially reducing the rate of resistant infections |
|
Spectrum value | Benefits of cefiderocol replacing broad-spectrum AMs and the problems associated with their overuse: potential collateral damage to the human microbiome resulting in a greater chance of developing resistance to AMs used in the future |
|
Importance and quantification of additional elements of value
Enablement value
Improved treatment of pre- and postoperative MDR infections is included within our HVCSs and expected usage projections. There is some uncertainty as to whether the full benefits of treatment of preoperative infections are reflected within our analysis. We assume that all patients who are alive at 30 days experience the same survival. If, however, the speed of resolution of an infection influences whether a procedure or treatment can go ahead, then it is possible that 30-day survival is longer for patients whose infection resolves more quickly as they may be more likely to receive procedures. The magnitude of this effect is uncertain due to uncertainties about the number of patients who experience infections preoperatively, the impact of infection duration on the likelihood that operations will go ahead, and the implications of operations not going ahead (which will depend on the type of procedure and whether the procedure is not conducted at all or delayed).
Enablement value may be also realised if the risk of MDR infection and clinicians’ ability to treat an MDR infection influences a decision about whether to bring a patient in for a procedure. An example of this scenario was provided by our clinical advisory group, whereby if an MDR infection is known to be circulating in a haematology unit, certain patients may not receive planned procedures or treatments. This is particularly likely to apply for patients in whom existing antibiotics for MDR infections are not an option. Here, the specific example of myeloma patients was highlighted as such patients are predisposed to renal impairment which rules out key effective treatments for MDR infections such as colistin. There is uncertainty with respect to the number of patients who would be affected as this would depend on both the number of patients whose treatment would be impacted by an outbreak and the frequency of outbreaks in key units such as haematology. There is also uncertainty about the consequences for patients not receiving planned therapy, as this will depend on the nature of the procedure/treatment and whether therapy is not received at all or delayed. These effects are not captured within the EEPRU modelling or any quantitative assessments submitted by the manufacturer.
A related way in which enablement value may be realised is if the availability of effective treatments for MDR infections allows wards to be kept open in the face of outbreaks. EEPRU considers it unlikely that cefiderocol would have this effect as most patients with drug-resistant infections do have alternative (albeit more toxic) treatment options – namely colistin. These effects are not captured within the EEPRU modelling or any quantitative assessments submitted by the manufacturer.
A final way in which enablement value may be realised is by use of cefiderocol freeing up healthcare resources. For example, use of cefiderocol may reduce time in hospital (alleviating pressure on beds), including time in the ICU/HDU. This may be particularly important where patients with MDR infections consume additional resources and staff time due to the need for additional infection control procedures including isolation measures. Any freed-up resources can then be repurposed to provide care for other patients within the hospital. To the extent possible, the impact of cefiderocol on resource use has been captured in the EEPRU modelling. When calculating the INHEs of cefiderocol, we have translated cost savings to health benefits using standard measures of health opportunity cost (which allow monetary savings in healthcare resources to be translated to health gains across the NHS).
Diversity value
Our clinical advisors indicated that, within the HVCSs, diverse prescribing strategies (e.g. randomly allocating patients with similar clinical indications to different treatments) were unlikely to be appropriate given the lack of safe and effective alternative treatments. They were not supportive of the use of cefiderocol in broader populations as part of a diverse prescribing strategy due to the desire to reduce emergence of resistance to cefiderocol and concerns that the evidence for diverse prescribing was uncertain. This is in contrast to the views of the manufacturer who emphasised the potential role of cefiderocol in OXA and KPC populations where, due to the availability of other effective treatment options, it could be used as part of a diverse prescribing strategy. Diverse prescribing strategies were not, therefore, included in our quantitative assessments of population-level INHEs.
There is uncertainty about how reduced use of existing agents (e.g. colistin) due to availability of the cefiderocol will contribute to the emergence of resistance to these drugs. Due to these uncertainties, this was not reflected in the EEPRU modelling. If the reduced use of existing agents reduces resistance to existing drugs within areas of expected usage for cefiderocol, this will reduce the INHEs associated with cefiderocol. However, if resistance to existing agents reduces outside areas of expected usage for cefiderocol, this will increase the INHEs associated with cefiderocol. Given the potential for these countervailing effects, and the wide range of factors driving resistance to existing drugs, this is not expected to have a large impact on INHEs.
Insurance value
The scenarios modelled can be considered to reflect this form of insurance value as they involve heavily restricting usage to preserve long-term effectiveness. It is generally agreed that the value of cefiderocol will depend on the trajectory of emergence of MDR infections over time. Within the HVCSs, we have used statistical forecasting methods and explored uncertainty around these to understand the possibility that cefiderocol results in the avoidance of significant/catastrophic health losses. This is presented as distributions of population-level INHEs to inform the committee’s deliberations about whether avoidance of these extreme events should be considered differentially to other forms of health losses.
There is uncertainty around whether these distributions adequately reflect the uncertainty around high-consequence/low-probability outcomes. There are several reasons why this may not fully quantify longer-term benefits:
-
We may see higher levels of resistance to existing drugs within the areas of expected usage over time.
-
We may see MDR pathogens, against which the new drugs are effective, emerge that are currently rare or even unknown.
-
We may see pathogens that are currently treatable with existing therapies (and are not, therefore, included in the areas of expected usage) become resistant.
If one or more of these factors emerge, they would be expected to occur in the long term and quite possibly beyond 20 years.
Conducting quantitative modelling of these effects is unavoidably highly speculative. However, on request from the NICE committee, EEPRU developed an additional scenario to explore the magnitude of these effects. The scenario aimed to assess the effect on INHEs of cefiderocol in case of emergence of multiresistant pathogens against which cefiderocol is the only effective treatment and, in that product’s absence, clinicians would be forced to use multidrug salvage therapy. A simple Excel tool was developed to explore the potential magnitude of these effects informed by parameters obtained from the NICE Committee. This showed an additional benefit of 0.8–58.4 QALYs, which is low relative to other expected benefits of cefiderocol, as estimated by the main analysis (896–3559 QALYs). This reflects the low likelihood of the emergence of a highly resistant strain and the low number of individuals affected by the strain in the medium term. Further details of the methods and results of this additional analysis are presented in Appendix 8, Additional analysis to reflect insurance value. The patient-level INHE used within this analysis and other relevant parameters are shown in Appendix 8, Additional analysis to reflect insurance value, Tables 63 and 64, and the population-level results are shown in Appendix 8, Additional analysis to reflect insurance value, Table 65.
Transmission value
Our clinical advisors indicated that the direction of effect of introduction of cefiderocol on transmission was uncertain, but that, overall, the magnitude of effect was expected to be small. This reflects the fact that introducing a new effective drug for the treatment of MDR infections has a number of countervailing effects. If the drugs reduce time in hospital, this is expected to reduce transmission. However, among MDR patients with poor prognosis, more effective treatments may, feasibly, increase the time spent in hospital by reducing mortality. In addition, where the use of the new drugs reduces mortality, this will increase the number of people returning colonised to the community, as cefiderocol was considered unlikely to eradicate colonisation by the clinical advisors to this project. This may contribute to increased transmission in the community or via further hospitalisations in this highly comorbid population.
The key drivers of transmission of MBL Enterobacterales and MBL P. aeruginosa are broad and driven by transmission in populations beyond the HVCSs (e.g. colonised individuals in the community and in the hospital, and importation of drug resistance from abroad), making this a challenging area to model. Given the views of our clinical advisors that this would not be a key driver of population-level INHE and these modelling challenges, we did not attempt to quantify transmission value using transmission modelling.
To support the committee in its decision-making we do, however, provide a summary of the impact of each drug on time in hospital and time alive post discharge. Briefly, cefiderocol led to a short reduction in the hospital LoS (< 1 day on average), and increased the length of life by 7–84 days (0.02–0.23 years) depending on the subgroup. We note that time post discharge is likely to include further periods spent in hospital given the patient population though we did not quantify these.
A number of advisors discussed the substantial impact of outbreaks of MDR infections in terms of disrupting healthcare provision and incurring large costs due to the need for more extensive infection control measures. However, no evidence was provided that cefiderocol would substantially impact on the likelihood of an outbreak or its spread. The possibility of outbreaks leading to large numbers of cases and the additional potential value of cefiderocol as a treatment in this scenario is discussed under insurance value.
Spectrum value
Our clinical advisors and other stakeholders did not consider spectrum value to be a significant consideration for cefiderocol which has a broad spectrum of activity. Therefore, this was not considered in our quantitative assessments of population-level INHE. The manufacturer, however, notes that:
cefiderocol is also expected to have low impact on the gut microbiota and cause minimal collateral damage to patients due to its narrow aerobic Gram-negative spectrum and predominantly renal clearance with negligible excretion into faeces. Therefore, it would likely not contribute to further disruption of the protective gut microflora and would not add to selective pressure for persistence of MDR and carbapenem-resistant pathogens in the GI tract or contribute to CDI. 81
Summary of additional elements of value
Table 36 summarises where EEPRU has been able to quantify the additional elements of value and, for those elements where this has not been feasible, provides an indication of their likely importance. Overall, EEPRU considers that the main areas of uncertainty are enablement value, transmission value and insurance value. EEPRU considers it unlikely that transmission value is a significant driver of population-level INHE, though this remains an area of uncertainty. EEPRU considers that it is possible that, by treating preoperative infections and offering the possibility of an effective low-toxicity option for treating MDR infections, cefiderocol will facilitate additional or at least more prompt receipt of required treatments/procedures for certain groups. EEPRU considers that the magnitude of these population-level INHE remain highly uncertain.
Element of value | Specific pathways to INHEs | Quantified in HVCS? EEPRU assessment of importance if not quantified |
---|---|---|
Enablement value |
|
Quantified in HVCSs and extrapolation to expected usage |
|
Partially quantified in HVCSs and extrapolation to expected usage (area of uncertainty) | |
|
Potential significant driver of population INHEs (area of uncertainty) | |
|
Unlikely to be significant driver of population INHEs | |
|
Quantified in HVCS | |
Diversity value |
|
Diverse prescribing not considered appropriate for cefiderocol |
|
Unlikely to be significant driver of population INHEs | |
Insurance value |
|
Quantified in HVCS |
|
Quantified in HVCS (though no differential valuation applied), and in an additional scenario | |
Transmission value |
|
Unlikely to be significant driver of population INHEs (area of uncertainty) |
Spectrum value |
|
Unlikely to be significant driver of population INHEs |
Chapter 9 Discussion of quantitative assessment of value
Table 37 summarises the patient-level INHEs for cefiderocol in the HVCSs. Across subgroups, there is a high degree of uncertainty surrounding the benefits of cefiderocol.
ES HAP/VAP | MDS HAP/VAP | MDS cUTI | |
---|---|---|---|
MBL Enterobacterales | 0.15 (0.00–0.23) | 0.02 (0.01–0.06) | 0.02 (0.01–0.06) |
MBL P. aeruginosa | 0.15 (0.00–0.22) | 0.15 (0.01–0.25) | 0.15 (0.01–0.25) |
For HAP/VAP patients treated empirically with cefiderocol due to suspected MBL Enterobacterales, cefiderocol is associated with lower susceptibility but improved safety compared to colistin/aminoglycoside-based treatment in those who are correctly suspected of having MBL Enterobacterales (patient-level INHE −0.19), and the same susceptibility but improved safety in those who have infections caused by other pathogens/mechanisms (patient-level INHE 0.21). As the proportion of patients who have MBL Enterobacterales increases, the patient-level INHEs, therefore, reduce dramatically. Conversely, if the CLSI breakpoints are used to determine susceptibility, the patient-level INHEs increase to 0.21 QALYs reflecting the higher susceptibility to cefiderocol in this scenario. Scenarios examining a larger effect of colistin/aminoglycoside-based treatment on AKI risk, which reduce the implications of AKI for short-term mortality and shorten long-term survival for this patient group, also markedly affect the results (patient-level INHE 0.12–0.23).
For HAP/VAP patients treated empirically with cefiderocol due to suspected MBL P. aeruginosa, cefiderocol is associated with comparable susceptibility and improved safety compared to colistin/aminoglycoside-based therapy and improved susceptibility compared to non-colistin/aminoglycoside-based therapy. The most significant source of uncertainty in this population is the effectiveness of non-colistin/aminoglycoside-based treatment. The synthesis of evidence using CLSI breakpoints indicated susceptibility to fosfomycin is similar to cefiderocol and, therefore, there is limited advantage of using cefiderocol, though we note that CLSI breakpoints are less relevant to the UK and that fosfomycin does not have established breakpoints for P. aeruginosa making links with clinical outcomes more tenuous.
For patients treated in the MDS, the advantage of cefiderocol is much higher for MBL P. aeruginosa than MBL Enterobacterales as the latter patient group has a higher probability of being susceptible to a non-colistin/aminoglycoside-based treatment, and hence not requiring treatment with cefiderocol. There is a large degree of uncertainty in the patient-level INHE in the MBL P. aeruginosa population. This reflects the differences across scenarios in the susceptibility to non-colistin/aminoglycoside-based treatments. This is much higher when the CLSI breakpoint studies are synthesised, and much lower when the baseline colistin susceptibility is set at the value from SIDERO WT.
In addition to these uncertainties, the modelling approach makes a number of important assumptions which were not amenable to sensitivity analysis or scenario testing within the scope of this project: (1) patients with intermediate resistance are assumed to respond as per those with resistant infections; (2) patients receiving multi-AM regimens perform as well if they are susceptible to one component, two components or more components of the regimen; (3) the use of meropenem in MBL Enterobacterales and MBL P. aeruginosa confers no clinical benefit; (4) patients’ AM susceptibility remains stable between the empiric and MDSs; and (5) patients who are suspected to have MBL Enterobacterales and MBL P. aeruginosa who have another pathogen mechanism are broadly susceptible and experience the same outcomes regardless of the choice of empiric treatment. We also did not account for uncertainties relating to the availability or usage of other agents in the future – for example, since the current project was completed, evidence has emerged that supports a role for CAZ-AVI combined with aztreonam in MBL Enterobacterales. 188,189
Due to the scope of work required to produce the population-level estimates of INHE, comprehensive reviews were not possible for all parameters, and it is possible that additional evidence was missed. In particular, we were reliant on existing systematic reviews and meta-analyses to quantify the safety implications of alternative treatments. A preferred approach would have been to conduct a de novo systematic review and synthesis tailored to the current decision problem; however, this was not feasible. There were limitations to the evidence underpinning the model and, in particular, the surrogacy relationships between susceptibility and mortality/hospitalisation were informed by a combination of evidence of associations at the individual patient level, and structured expert elicitation.
We were unable to select a base case for the population-level results. Population-level results are, therefore, presented for two different approaches to estimating current infection numbers (based on different methods to classify infections from clinical specimen sites), two alternative approaches to forecasting increases in infections over time (based on whether observed trends are assumed to persist indefinitely or not) and three different trajectories with respect to resistance emergence (1%, 5% and 10% at 20 years).
These results are summarised in Table 38. These indicate that assumptions about baseline population size and growth are strong drivers of population-level INHEs, which vary from 896 to 3559 QALYs depending on the scenario. The results are particularly sensitive to the assumption about which clinical specimen sites are indicative of HAP/VAP, with the more conservative definition provided by PHE indicating 131 HAP/VAP-suspected MBL Enterobacterales or MBL P. aeruginosa, or confirmed Stenotrophomonas would be eligible to receive cefiderocol per annum; and the broader definition provided by our clinical advisors indicating that 791 patients with HAP/VAP infections would be eligible. Of note, a substantial part of the value of cefiderocol (20–38% depending on scenario) is generated among patients with Stenotrophomonas who were outside of the HVCSs considered by EEPRU. Departures from the base-case assumptions in the patient-level model also had substantial effects on population-level INHEs, as did the additional scenario where, without cefiderocol, a proportion of patients cannot take colistin and other aminoglycoside treatments and, therefore, without the new drug, would receive multidrug salvage therapy.
Baseline population | Population growth rate | Predicted patients initiating cefiderocol over 20 years | Range of population-level INHEs across resistance scenarios 1%, 5% and 10% at 20 years (base-case assumptions used for patient-level model) |
---|---|---|---|
PHE categorisation of infection sites | Model with damped trends | 8671 | 896–1093 |
Model with persistent trends | 13,488 | 1291–1625 | |
Clinical advisors’ categorisation of infection sites | Model with damped trends | 16,669 | 2064–2499 |
Model with persistent trends | 24,969 | 2861–3559 |
The population size estimates used to generate the estimates of population-level INHEs are subject to considerable uncertainties relating to the completeness of the national data, how accurately specimen types represent the infection sites of interest, whether all tested patients would fall within the HVCS populations for empiric treatment, the potential double counting of samples from the same infectious episode and the inherent uncertainties in forecasting population size over time.
In addition, estimates of population-level INHEs were generated using a number of strong assumptions about how evidence can be generalised between settings. Namely, that patient-level INHE of cefiderocol in patients with BSIs can be approximated based on outcomes in HAP/VAP patients, and that the patient-level INHE of cefiderocol in patients with IAIs can be proxied by that in patients with cUTIs. These assumptions were based on discussions with clinical experts.
Table 39 summarises where EEPRU has been able to quantify the additional elements of value and, for those elements where this has not been feasible, provides an indication of their likely importance. Overall, EEPRU considers that the main areas of uncertainty are enablement value and transmission value. EEPRU considers it unlikely that transmission value is a significant driver of population-level INHE, though this remains an area of uncertainty. EEPRU considers that it is possible that, by treating preoperative infections and offering the possibility of an effective low-toxicity option for treating MDR infections, cefiderocol will facilitate additional or at least more prompt receipt of required treatments/procedures for certain groups. EEPRU considers that the magnitude of these population-level INHE remains highly uncertain.
Element of value | Summary of importance in modifying quantitative estimates of population INHEs,a indicates areas of high uncertainty |
---|---|
Enablement value | Benefits of improved treatment of postoperative infections quantified Benefits of improved treatment of preoperative infections partially quantifieda Benefits of increasing number of procedures that can go ahead not quantifieda Benefits of keeping wards open during MDR infection outbreaks unlikely to be a significant driver of population INHEs Benefits of reduced use of hospital resources quantified |
Diversity value | Unlikely to be a significant driver of population INHEs |
Insurance value | Benefit in HVCSs quantified. Benefit in novel, hypothetical outbreaks highly uncertain. |
Transmission value | Unlikely to be a significant driver of population INHEsa |
Spectrum value | Unlikely to be a significant driver of population INHEs |
Equality, diversity and inclusion: we sought to identify data sources that were representative of the patients within the HVCSs and used nationally representative data wherever possible. This research emphasised the high level of comorbidities among people with drug-resistant infections; further work should ensure that research in these populations reflects these comorbidities.
Patient and public involvement: there was no patient or public involvement for this research.
Conclusion
The quantitative assessment of value in this report indicates that cefiderocol is associated with a base-case population-level INHE across its areas of expected usage of 896–3559 QALYs over 20 years. These quantitative assessments of value were informed by a series of interlinked decision-analytic models informed by evidence collated via systematic reviews of the literature and evidence synthesis, additional national data provided by PHE, structured expert elicitation and, where necessary, assumptions informed by clinical opinion.
This work has provided quantitative estimates of the value of cefiderocol within its areas of expected usage within the NHS. The results indicate that the maximum amount the NHS could pay for cefiderocol was £18–71 million if the health lost as a result of making these payments rather than funding other NHS services is not to exceed the health benefits of using this AM. The high level of uncertainty could be addressed via further research (see Recommendations for future research). An important question is whether this and other post-approval evidence should inform adjustments to payments. Although this would better align payments with value delivered to the NHS, it would add additional uncertainty to payments to manufacturers in a context where investment conditions are already considered unfavourable. Further research is required to assess the potential costs and benefits of conditional reimbursement in this context. A broader and important question is ‘what would represent the “optimal” scope of usage for cefiderocol?’ Further methodological and quantitative work is required to address this question.
Chapter 10 Recommendations for future research
Our recommendations for further research (in priority order) are:
-
Data linkage studies linking patient-level clinical, prescribing and laboratory data
Evaluations of new AMs, and indeed of many anti-AMR interventions, would benefit from linked national-level clinical, prescribing and laboratory (microbiology) data. This would facilitate improved estimates of the relationship between in vitro susceptibility to an AM treatment and relevant outcomes in people with MDR infections. It would also provide improved estimates of the patterns of resistance across the UK to identify likely areas of expected usage, the number of patients with specific types of MDR infections and emerging threats.
-
Post-approval routine randomisation to assess clinical effectiveness
-
The reliance on in vitro susceptibility evidence (linked to other data sources) to infer clinical effectiveness and safety is a major source of uncertainty in the current evaluation. The lack of relevant clinical data for cefiderocol in MDR infections is problematic not only within the current evaluation which was designed to inform payment but also for clinicians who need to trade-off the risks and benefits of alternative therapeutic choices. The use of post-approval routine randomisation (e.g. using novel trial designs190) should be supported to improve the evidence base informing AM choice for MDR infections.
-
Improved methods for the conduct, review and synthesis of susceptibility studies
Susceptibility study conduct, reporting and appraisal could be improved by the development of reporting guidelines (similar to those provided by PRISMA and CONSORT) and quality assessment tools. To inform appropriate choices when synthesising susceptibility evidence, further research is warranted to assess the comparability of different laboratory methods and clinical breakpoints, and the appropriateness of applying newer breakpoints to historical data.
-
Methods to quantify the enablement benefits of new AMs
An important potential benefit of new antibiotics is in ensuring that patients’ infections are managed so that planned medical procedures and treatments unrelated to these infections can go ahead. Data collection [e.g. data from the UK Antimicrobial Registry (UKAR) on occurrence of procedures following cefiderocol] and decision models should be developed to assess the magnitude of these benefits.
-
Methods for quantifying the benefits and costs associated with different usage scenarios
An important question is: how extensively should new AMs be used? More extensive use may deliver benefits to a wider set of patients in the short term but will be associated with higher levels of resistance emergence over time. Further work is required to quantify the nature of these trade-offs; this requires decision modelling and further clinical and epidemiological research to quantify the relationship between AM usage and resistance emergence.
-
Methods for quantifying the benefits and costs of conditional reimbursement
Post-approval evidence is likely to improve estimates of the value of new AMs. In principle, this could inform revisions to payment levels over time. Further research is required to assess the appropriate design of conditional reimbursement approaches for AMs, and whether the benefits of aligning payment with value exceed the disbenefits in terms of exposing manufacturers to additional revenue risk and potentially disincentivising investment in AM development.
Additional information
Contributions of authors
Beth Woods (https://orcid.org/0000-0002-7669-9415) (Senior Research Fellow) was jointly responsible for the day-to-day management of the project and development of the work elements. She led the design of the economic model, and conducted modelling for the patient-level and extrapolation components of the model and was responsible for drafting these sections of the report, as well as the discussion.
Laetitia Schmitt (https://orcid.org/0000-0003-1052-488X) (Research Fellow) designed and conducted the economic modelling for patient-level outcomes.
Dina Jankovic (https://orcid.org/0000-0002-9311-1409) (Research Fellow) conducted the expert elicitation, the literature searches that informed the structure of the patient-level economic model with Laetitia Schmitt and Claire Rothery, conducted modelling of population-level outcomes, drafted the relevant sections of the report and performed model validation.
Benjamin Kearns (https://orcid.org/0000-0001-7730-668X) (Research Fellow) designed and conducted the economic modelling for the population-level component of the model and was responsible for drafting these sections of the report.
Alison Scope (https://orcid.org/0000-0003-1604-1758) (Research Fellow) contributed to the design and conduct of the systematic review of clinical efficacy, drafted some of the clinical review sections of the report and reviewed other sections of the clinical review.
Shijie Ren (https://orcid.org/0000-0003-3568-7124) (Senior Research Fellow) designed and conducted the network meta-analysis and was responsible for drafting those sections of the report.
Tushar Srivastava (https://orcid.org/0000-0002-5961-9348) (Research Associate) contributed to literature searches for the economic modelling and the analyses for the population-level component.
Chu Chang Ku (https://orcid.org/0000-0002-4719-117X) (Research Associate) contributed to modelling for both the patient-level and population-level components.
Jean Hamilton (https://orcid.org/0000-0003-3326-9842) (Research Fellow) designed and conducted the network meta-analysis and was responsible for drafting those sections of the report.
Claire Rothery (https://orcid.org/0000-0002-7759-4084) (Senior Research Fellow) designed the literature searches that informed the model structure and provided support with scoping of the project and drafting of the report.
Laura Bojke (https://orcid.org/0000-0001-7921-9109) (Professor) led the design and conduct of the expert elicitation and was responsible for drafting those sections of the report.
Mark Sculpher (https://orcid.org/0000-0003-3746-9913) (Professor) was jointly responsible for the day-to-day management of the project and development of the work elements. He was responsible for drafting specific report sections.
Sue Harnan (https://orcid.org/0000-0002-9318-9206) (Senior Research Fellow) was responsible for the day-to-day management of the systematic review of clinical efficacy data, designed and conducted the systematic review of clinical efficacy data and was responsible for drafting the clinical efficacy review sections of the report, including the discussion.
Acknowledgements
We are grateful for the support of our clinical advisors: Professors David Jenkins, William Hope, Philip Howard, Colm Leonard, Mark Wilcox; and Drs Alan Ashwoth and Andrew Bentley. Thanks for modelling advice to Professor Mark Jit and Dr Gwen Knight; and for comments on drafts, we thank Professor Allan Wailoo, Dr James Fotheringham, Professor Simon Dixon, Rita Neves De Faria and Professor Stephen Palmer. We are also grateful for support from Sarah Gerver and Rebecca Guy at UK Health Security Agency (formerly Public Health England). Finally, we thank Marrissa Martyn-St-James, Kath Devlin, Kay Fountain, Tim Glover, Donna Davis, Ruth Helstrip, Liz Mclintock, Steph Richards and Alex Rollinger for their support in developing this report. Any errors are the responsibility of the authors.
Data-sharing statement
The only primary data collection for this study related to the expert elicitation; ethics approval for this element of the study does not permit data sharing. All queries should be sent to the corresponding author.
Ethics statement
Ethics approval for the expert elicitation exercise was granted from the University of York Department of Health Sciences Research Governance Committee (reference number: HSRGC/2021/448/G; date of approval: 14 May 2021). No other activities required primary data collection, so no further ethics approval was required.
Information governance statement
The University of York is committed to handling all personal information in line with the UK Data Protection Act (2018) and the General Data Protection Regulation (EU GDPR) 2016/679. Under the Data Protection legislation, the University of York is the Data Controller, and you can find out more about how we handle personal data, including how to exercise your individual rights and the contact details for our Data Protection Officer here (www.york.ac.uk/records-management/dp/).
Disclosure of interests
Full disclosure of interests: Completed ICMJE forms for all authors, including all related interests, are available in the toolkit on the NIHR Journals Library report publication page at https://doi.org/10.3310/YGWR4511.
Primary conflicts of interest: All authors declare funding for the current work from the National Institute for Health and Care Research (NIHR) Policy Research Programme, conducted through the Policy Research Unit in Economic Methods of Evaluation in Health and Social Care Interventions, PR-PRU-1217-20401. Beth Woods sits on the Board of the York Health Economics Consortium, a private sector company (role not remunerated) and the HTA Clinical Evaluation and Trials Committee 2020–4. Claire Rothery sits on the HTA Commissioning Committee 2021–25. Laura Bojke sat on the NICE Technology Appraisals committee B 2019–22, she is also a member of HS&DR Committee, NIHR 2019 to present. Mark Sculpher has been a paid consultant to various life sciences companies, none relating to antimicrobials.
References
- Harnan SE, Woods B, Schmitt L, Kearns B, Srivastava T, Scope A, et al. Protocol for the technology evaluation of cefiderocol for treating severe aerobic Gram-negative bacterial infections. Health Technol Assess 2021. www.nice.org.uk/guidance/hte2/history (accessed 21 February 2024).
- Hawkey PM, Warren RE, Livermore DM, McNulty CA, Enoch DA, Otter JA, et al. Treatment of infections caused by multidrug-resistant gram-negative bacteria: report of the British Society for Antimicrobial Chemotherapy/Healthcare Infection Society/British Infection Association Joint Working Party. J Antimicrob Chemother 2018;73:iii2-78.
- Munita JM, Arias CA. Mechanisms of antibiotic resistance. Microbiol Spectr 2016;4:1-24.
- Hawkey PM. Multidrug-resistant Gram-negative bacteria: a product of globalization. J Hosp Infect 2015;89:241-7.
- McNulty CAM, Boyle P, Nichols T, Clappison P, Davey P. Don’t wear me out – the public’s knowledge of and attitudes to antibiotic use. J Antimicrob Chemother 2007;59:727-38.
- English Surveillance Programme for Antimicrobial Utilisation and Resistance (ESPAUR) Report 2019 to 2020. London: UK Health Security Agency; 2020.
- Hughes S, Gilchrist M, Heard K, Hamilton R, Sneddon J. Treating infections caused by carbapenemase-producing Enterobacterales (CPE): a pragmatic approach to antimicrobial stewardship on behalf of the UKCPA Pharmacy Infection Network (PIN). JAC-Antimicrob Resist 2020;2.
- World Health Organization . WHO Publishes List of Bacteria for Which New Antibiotics Are Urgently Needed 2017.
- World Health Organization . Global Priority List of Antibiotic-Resistant Bacteria to Guide Research, Discovery, and Development of New Antibiotics 2017.
- Tan X, Kim HS, Baugh K, Huang Y, Kadiyala N, Wences M, et al. Therapeutic options for metallo-β-lactamase-producing Enterobacterales. Infect Drug Resist 2021;14:125-42.
- Ito A, Kohira N, Bouchillon SK, West J, Rittenhouse S, Sader HS, et al. In vitro antimicrobial activity of S-649266, a catechol-substituted siderophore cephalosporin, when tested against non-fermenting Gram-negative bacteria. J Antimicrob Chemother 2016;71:670-7.
- Ito A, Nishikawa T, Matsumoto S, Yoshizawa H, Sato T, Nakamura R, et al. Siderophore cephalosporin cefiderocol utilizes ferric iron transporter systems for antibacterial activity against Pseudomonas aeruginosa. Antimicrob Agents Chemother 2016;60:7396-401.
- Portsmouth S, van Veenhuyzen D, Echols R, Machida M, Ferreira JCA, Ariyasu M, et al. Cefiderocol versus imipenem-cilastatin for the treatment of complicated urinary tract infections caused by Gram-negative uropathogens: a phase 2, randomised, double-blind, non-inferiority trial. Lancet Infect Dis 2018;18:1319-28.
- Wunderink RG, Matsunaga Y, Ariyasu M, Clevenbergh P, Echols R, Kaye KS, et al. Cefiderocol versus high-dose, extended-infusion meropenem for the treatment of Gram-negative nosocomial pneumonia (APEKS-NP): a randomised, double-blind, phase 3, non-inferiority trial. Lancet Infect Dis 2021;21:213-25.
- Bassetti M, Echols R, Matsunaga Y, Ariyasu M, Doi Y, Ferrer R, et al. Efficacy and safety of cefiderocol or best available therapy for the treatment of serious infections caused by carbapenem-resistant Gram-negative bacteria (CREDIBLE-CR): a randomised, open-label, multicentre, pathogen-focused, descriptive, phase 3 trial. Lancet Infect Dis 2021;21:226-40.
- Tsuji M, Hackel M, Echols R, Yamano Y, Sahm DF. In Vitro Activity of Cefiderocol Against Globally Collected Carbapenem-Resistant Gram-Negative Bacteria Isolated from Urinary Tract Source: SIDERO-CR-2014/2016. Poster presented at: IDWeek, Arlington, VA; 2017.
- Nguyen S. In Vitro Antibacterial Activity of Cefiderocol against a Multi-National Collection of Carbapenem Non-Susceptible Gram-Negative Bacteria Isolated from Respiratory, Blood, Skin/Soft Tissue and Urinary Sources of Infection: SIDERO-WT-2014-2017. New Orleans, LA: ASM; 2017.
- Zhanel GG, Golden AR, Zelenitsky S, Wiebe K, Lawrence CK, Adam HJ, et al. Cefiderocol: a siderophore cephalosporin with activity against carbapenem-resistant and multidrug-resistant Gram-negative bacilli. Drugs 2019;79:271-89.
- Shionogi . European Medicines Agency. Annex I: Summary of Product Characteristics Fetcroja 1g n.d. https://ema.europa.eu/en/documents/product-information/fetcroja-epar-product-information_en.pdf (accessed 16 May 2024).
- National Institute for Health and Care Excellence . Cefiderocol for Treating Severe Drug-Resistant Gram-Negative Bacterial Infections n.d. www.nice.org.uk/about/what-we-do/life-sciences/scientific-advice/models-for-the-evaluation-and-purchase-of-antimicrobials/cefiderocol (accessed 16 May 2024).
- Rothery C, Woods B, Schmitt L, Claxton K, Palmer S, Sculpher M. Framework for Value Assessment of New Pharmaceuticals: Implications of Alternative Funding Arrangements for NICE Appraisal. EEPRU; 2018.
- Public Health England . Framework of Actions to Contain Carbapenemase-Producing Enterobacterales 2020.
- Public Health England . UK Standards for Microbiology Investigations: Detection of Bacteria With Carbapenem-Hydrolysing β-Lactamases (Carbapenemases) 2020.
- European Committee on Antimicrobial Susceptibility Testing . Standard Operating Procedure: Setting Breakpoints for New Antimicrobial Agents 2019. www.eucast.org/fileadmin/src/media/PDFs/EUCAST_files/EUCAST_SOPs/EUCAST_SOP_1.3_Setting_breakpoints_new_agents_20191023.pdf (accessed 16 May 2024).
- Harnan SE, Cooper K, Jones SL, Jones E. Pruning and prioritising: a case study of a pragmatic method for managing a rapid systematic review with limited resources. Evid Policy J Res Debate Pract 2015;11:589-601.
- Uttley L, Whiteman BL, Woods HB, Harnan S, Philips ST, Cree IA. Early Cancer Detection Consortium . Building the evidence base of blood-based biomarkers for early detection of cancer: a rapid systematic mapping review. EBioMedicine 2016;10:164-73.
- Kaltenthaler E, Tappenden P, Paisley S, Squires H. NICE DSU Technical Support Document 13: Identifying and Reviewing Evidence to Inform the Conceptualisation and Population of Cost-Effectiveness Models. Sheffield: Decision Support Unit, ScHARR, University of Sheffield; 2011.
- Hoy D, Brooks P, Woolf A, Blyth F, March L, Bain C, et al. Assessing risk of bias in prevalence studies: modification of an existing tool and evidence of interrater agreement. J Clin Epidemiol 2012;65:934-39.
- Munn Z, Moola S, Lisy K, Riitano D, Tufanaru C. JBI Manual for Evidence Synthesis. JBI; 2024.
- Hinneburg I. ROBINS-1: a tool for assessing risk of bias in non-randomised studies of interventions. Med Monatsschr Pharm 2017;40:175-7.
- Sterne JA, Savović J, Page MJ, Elbers RG, Blencowe NS, Boutron I, et al. RoB 2: a revised tool for assessing risk of bias in randomised trials. BMJ 2019;366.
- Peterson J, Welch V, Losos M, Tugwell P. The Newcastle-Ottawa Scale (NOS) for Assessing the Quality of Nonrandomised Studies in Meta-analyses. Ottawa: Ottawa Hospital Research Institute; 2011.
- Shionogi . Candor Simulation – Retrospective Analysis of Cefiderocol and Comparators by Population PK PD Simulation 2021.
- Shionogi . Response to EEPRU’s Data Request Relating to SIDERO-WT 2021. www.nice.org.uk/guidance/hte2/documents/agenda-4 (accessed 28 February 2024).
- Shionogi . Response to EEPRU’s Data Request Relating to SIDERO-CR 2021. www.nice.org.uk/guidance/hte2/documents/agenda-4 (accessed 28 February 2024).
- Albano M, Karau MJ, Schuetz AN, Patel R. Comparison of agar dilution to broth microdilution for testing in vitro activity of cefiderocol against Gram-negative Bacilli. J Clin Microbiol 2020;59.
- Biagi M, Vialichka A, Jurkovic M, Wu T, Shajee A, Lee M, et al. Activity of cefiderocol alone and in combination with levofloxacin, minocycline, polymyxin B, or trimethoprim-sulfamethoxazole against multidrug-resistant Stenotrophomonas maltophilia. Antimicrob Agents Chemother 2020;64.
- Delgado-Valverde M, Del Carmen Conejo M, Serrano L, Fernandez-Cuenca F, Pascual A. Activity of cefiderocol against high-risk clones of multidrug-resistant Enterobacterales, Acinetobacter baumannii, Pseudomonas aeruginosa and Stenotrophomonas maltophilia. J Antimicrob Chemother 2020;75:1840-9.
- Ghazi IM, Monogue ML, Tsuji M, Nicolau DP. Humanized exposures of cefiderocol, a siderophore cephalosporin, display sustained in vivo activity against siderophore-resistant Pseudomonas aeruginosa. Pharmacology 2018;101:278-84.
- Ghazi IM, Monogue ML, Tsuji M, Nicolau DP. Pharmacodynamics of cefiderocol, a novel siderophore cephalosporin, in a Pseudomonas aeruginosa neutropenic murine thigh model. Int J Antimicrob Agents 2018;51:206-12.
- Golden AR, Adam HJ, Baxter M, Walkty A, Lagace-Wiens P, Karlowsky JA, et al. In vitro activity of cefiderocol, a novel siderophore cephalosporin, against Gram-negative bacilli isolated from patients in Canadian Intensive Care Units. Diagn Microbiol Infect Dis 2020;97.
- Hackel MA, Tsuji M, Yamano Y, Echols R, Karlowsky JA, Sahm DF. In vitro activity of the siderophore cephalosporin, cefiderocol, against carbapenem-nonsusceptible and multidrug-resistant isolates of Gram-negative bacilli collected worldwide in 2014 to 2016. Antimicrob Agents Chemother 2018;62:e01968-17.
- Hackel MA, Tsuji M, Yamano Y, Echols R, Karlowsky JA, Sahm DF. Reproducibility of broth microdilution MICs for the novel siderophore cephalosporin, cefiderocol, determined using iron-depleted cation-adjusted Mueller-Hinton broth. Diagn Microbiol Infect Dis 2019;94:321-5.
- Hackel MA, Tsuji M, Yamano Y, Echols R, Karlowsky JA, Sahma DF. In vitro activity of the siderophore cephalosporin, cefiderocol, against a recent collection of clinically relevant gram-negative Bacilli from North America and Europe, including carbapenem-nonsusceptible isolates (SIDERO-WT-2014 study). Antimicrob Agents Chemother 2017;61.
- Hsueh SC, Lee YJ, Huang YT, Liao CH, Tsuji M, Hsueh PR. In vitro activities of cefiderocol, ceftolozane/tazobactam, ceftazidime/avibactam and other comparative drugs against imipenem-resistant Pseudomonas aeruginosa and Acinetobacter baumannii, and Stenotrophomonas maltophilia, all associated with bloodstream infections in Taiwan. J Antimicrob Chemother 2019;74:380-6.
- Huband MD, Ito A, Tsuji M, Sader HS, Fedler KA, Flamm RK. Cefiderocol MIC quality control ranges in iron-depleted cation-adjusted Mueller-Hinton broth using a CLSI M23-A4 multi-laboratory study design. Diagn Microbiol Infect Dis 2017;88:198-200.
- Iregui A, Khan Z, Landman D, Quale J. Activity of cefiderocol against Enterobacterales, Pseudomonas aeruginosa, and Acinetobacter baumannii endemic to medical centers in New York City. Microb Drug Resist 2020;26:722-6.
- Ito A, Nishikawa T, Ota M, Ito-Horiyama T, Ishibashi N, Sato T, et al. Stability and low induction propensity of cefiderocol against chromosomal AmpC b-lactamases of Pseudomonas aeruginosa and Enterobacter cloacae. J Antimicrob Chemother 2018;73:3049-52.
- Johnston BD, Thuras P, Porter SB, Clabots C, Johnsona JR. Activity of cefiderocol, ceftazidime-avibactam, and eravacycline against extended-spectrum cephalosporin-resistant Escherichia coli clinical isolates (2012-20017) in relation to phylogenetic background, sequence type 131 subclones, blaCTX-M genotype, and coresistance. Diagn Microbiol Infect Dis 2021;100.
- Karlowsky JA, Hackel MA, Tsuji M, Yamano Y, Echols R, Sahm DF. In vitro activity of cefiderocol, a siderophore cephalosporin, against Gram-negative Bacilli isolated by clinical laboratories in North America and Europe in 2015–2016: SIDERO-WT-2015. Int J Antimicrob Agents 2019;53:456-66.
- Katsube T, Echols R, Arjona Ferreira JC, Krenz HK, Berg JK, Galloway C. Cefiderocol, a siderophore cephalosporin for Gram-negative bacterial infections: pharmacokinetics and safety in subjects with renal impairment. J Clin Pharmacol 2017;57:584-91.
- Katsube T, Echols R, Wajima T. Pharmacokinetic and pharmacodynamic profiles of cefiderocol, a novel siderophore cephalosporin. Clin Infect Dis 2019;69:S552-8.
- Katsube T, Saisho Y, Shimada J, Furuie H. Intrapulmonary pharmacokinetics of cefiderocol, a novel siderophore cephalosporin, in healthy adult subjects. J Antimicrob Chemother 2019;74:1971-4.
- Kawaguchi N, Katsube T, Echols R, Wajima T. Population pharmacokinetic analysis of cefiderocol, a parenteral siderophore cephalosporin, in healthy subjects, subjects with various degrees of renal function, and patients with complicated urinary tract infection or acute uncomplicated pyelonephritis. Antimicrob Agents Chemother 2018;62.
- Kawaguchi N, Katsube T, Echols R, Wajima T. Population pharmacokinetic and pharmacokinetic/pharmacodynamic analyses of cefiderocol, a parenteral siderophore cephalosporin, in patients with pneumonia, bloodstream infection/sepsis, or complicated urinary tract infection. Antimicrob Agents Chemother 2021;65.
- Kawai A, McElheny CL, Iovleva A, Kline EG, Sluis-Cremer N, Shields RK, et al. Structural basis of reduced susceptibility to ceftazidime-avibactam and cefiderocol in Enterobacter cloacae due to AmpC R2 loop deletion. Antimicrob Agents Chemother 2020;64.
- Matsumoto S, Singley CM, Hoover J, Nakamura R, Echols R, Rittenhouse S, et al. Efficacy of cefiderocol against carbapenem-resistant Gram-negative bacilli in immunocompetent-rat respiratory tract infection models recreating human plasma pharmacokinetics. Antimicrob Agents Chemother 2017;61.
- Nath S, Moussavi F, Abraham D, Landman D, Quale J. In vitro and in vivo activity of single and dual antimicrobial agents against KPC-producing Klebsiella pneumoniae. J Antimicrob Chemother 2018;73:431-6.
- Paul Morris C, Bergman Y, Tekle T, Fissel JA, Tamma PD, Simner PJ. Cefiderocol antimicrobial susceptibility testing against multidrug-resistant Gram-negative bacilli: a comparison of disk diffusion to broth microdilution. J Clin Microbiol 2021;59.
- Pybus CA, Felder-Scott C, Obuekwe V, Greenberg DE. Cefiderocol retains antibiofilm activity in multidrug-resistant Gram-negative pathogens. Antimicrob Agents Chemother 2021;65.
- Pybus CA, Greenberg DE. Cefiderocol retains anti-biofilm activity in MDR Gram-negative pathogens. Open Forum Infect 2019;6:S323-4.
- Rolston KVI, Gerges B, Shelburne S, Aitken SL, Raad I, Prince RA. Activity of cefiderocol and comparators against isolates from cancer patients. Antimicrob Agents Chemother 2020;64.
- Sanabria C, Migoya E, Mason JW, Stanworth SH, Katsube T, Machida M, et al. Effect of cefiderocol, a siderophore cephalosporin, on QT/QTc interval in healthy adult subjects. Clin Ther 2019;41:1724-36.e4.
- Sato T, Ito A, Ishioka Y, Matsumoto S, Rokushima M, Kazmierczak KM, et al. Escherichia coli strains possessing a four amino acid YRIN insertion in PBP3 identified as part of the SIDERO-WT-2014 surveillance study. JAC-Antimicrob Resist 2020;2.
- Talan DA, Takhar SS, Krishnadasan A, Mower WR, Pallin DJ, Garg M, et al. Emergence of extended-spectrum beta-lactamase urinary tract infections among hospitalized emergency department patients in the United States. Ann Emerg Med 2021;77:32-43.
- Tsiplakou S, Papaioannou V, Trikka-Graphakos E, Charalampakis N, Sereti C, Lebessi E, et al. Activity of cefiderocol (S-649266) against carbapenem-resistant Gram-negative bacteria collected from inpatients in Greek hospitals. J Antimicrob Chemother 2017;72:1704-8.
- Dobias J, Denervaud-Tendon V, Poirel L, Nordmann P. Activity of the novel siderophore cephalosporin cefiderocol against multidrug-resistant Gram-negative pathogens. Eur J Clin Microbiol Infect Dis 2017;36:2319-27.
- Jacobs MR, Abdelhamed AM, Good CE, Rhoads DD, Hujer KM, Hujer AM, et al. ARGONAUT-I: Activity of cefiderocol (s-649266), a siderophore cephalosporin, against Gram-negative bacteria, including carbapenem-resistant nonfermenters and Enterobacteriaceae with defined extended-spectrum beta-lactamases and carbapenemases. Antimicrob Agents Chemother 2019;63.
- Mushtaq S, Sadouki Z, Vickers A, Livermore DM, Woodford N. In vitro activity of cefiderocol, a siderophore cephalosporin, against multidrug-resistant Gram-negative bacteria. Antimicrob Agents Chemother 2020;64.
- Ito A, Sato T, Ota M, Takemura M, Nishikawa T, Toba S, et al. In vitro antibacterial properties of cefiderocol, a novel siderophore cephalosporin, against Gram-negative bacteria. Antimicrob Agents Chemother 2018;62.
- Kresken M, Korte-Berwanger M, Gatermann SG, Pfeifer Y, Pfennigwerth N, Seifert H, et al. In vitro activity of cefiderocol against aerobic Gram-negative bacterial pathogens from Germany. Int J Antimicrob Agents 2020;56.
- Bleibtreu A, Dortet L, Bonnin RA, Wyplosz B, Sacleux SC, Mihaila L, et al. Susceptibility testing is key for the success of cefiderocol treatment: a retrospective Cohort study. Microorganisms 2021;9.
- Falcone M, Tiseo G, Nicastro M, Leonildi A, Vecchione A, Casella C, et al. Cefiderocol as rescue therapy for Acinetobacter baumannii and other carbapenem-resistant Gram-negative infections in ICU patients. Clin Infect Dis 2020;17:2021-4.
- Haller S, Kramer R, Becker K, Bohnert JA, Eckmanns T, Hans JB, et al. Extensively drug-resistant Klebsiella pneumoniae ST307 outbreak, north-eastern Germany, June to October 2019. Euro Surveill 2019;24.
- Oliva A, Ceccarelli G, De Angelis M, Sacco F, Miele MC, Mastroianni CM, et al. Cefiderocol for compassionate use in the treatment of complicated infections caused by extensively and pan-resistant Acinetobacter baumannii. J Glob Antimicrob Resist 2020;23:292-6.
- Shields RK, Iovleva A, Kline EG, Kawai A, McElheny CL, Doi Y. Clinical evolution of AmpC-mediated ceftazidime-avibactam and cefiderocol resistance in Enterobacter cloacae complex following exposure to cefepime. Clin Infect Dis 2020;71:2713-6.
- Zingg S, Nicoletti GJ, Kuster S, Junker M, Widmer A, Egli A, et al. Cefiderocol for extensively drug-resistant Gram-negative bacterial infections: real-world experience from a case series and review of the literature. Open Forum Infect 2020;7.
- Johnston BD, Thuras P, Porter SB, Anacker M, VonBank B, Vagnone PS, et al. Activity of cefiderocol, ceftazidime-avibactam, and eravacycline against carbapenem-resistant Escherichia coli isolates from the United States and international sites in relation to clonal background, resistance genes, coresistance, and region. Antimicrob Agents Chemother 2020;64.
- Kazmierczak KM, Tsuji M, Wise MG, Hackel M, Yamano Y, Echols R, et al. In vitro activity of cefiderocol, a siderophore cephalosporin, against a recent collection of clinically relevant carbapenem-non-susceptible Gram-negative bacilli, including serine carbapenemase- and metallo-beta-lactamase-producing isolates (SIDERO-WT-2014 Study). Int J Antimicrob Agents 2019;53:177-84.
- Kohira N, West J, Ito A, Ito-Horiyama T, Nakamura R, Sato T, et al. In vitro antimicrobial activity of a siderophore cephalosporin, S-649266, against Enterobacteriaceae clinical isolates, including carbapenem-resistant strains. Antimicrob Agents Chemother 2016;60:729-34.
- Longshaw C, Manissero D, Tsuji M, Echols R, Yamano Y. In vitro activity of the siderophore cephalosporin, cefiderocol, against molecularly characterized, carbapenem-non-susceptible Gram-negative bacteria from Europe. JAC Antimicrob Resist 2020;2.
- Shionogi . National Institute of Health and Care Excellence, Antimicrobial Health Technology Evaluation: Cefiderocol for Treating Severe Aerobic Gram-Negative Bacterial Infections n.d. www.nice.org.uk/guidance/hte2/history (accessed 21 February 2024).
- Chakraborty T, Sadek M, Yao Y, Imirzalioglu C, Stephan R, Poirel L, et al. Cross-border emergence of Escherichia coli producing the carbapenemase NDM-5 in Switzerland and Germany. J Clin Microbiol 2021;59.
- Kaase M, Pfennigwerth N, Lange F, Anders A, Gatermann SG. Molecular epidemiology of VIM-1 producing Escherichia coli from Germany referred to the National Reference Laboratory. Int J Med Microbiol 2015;305:784-9.
- Livermore DM, Warner M, Mushtaq S, Doumith M, Zhang J, Woodford N. What remains against carbapenem-resistant Enterobacteriaceae? Evaluation of chloramphenicol, ciprofloxacin, colistin, fosfomycin, minocycline, nitrofurantoin, temocillin and tigecycline. Int J Antimicrob Agents 2011;37:415-9.
- Ojdana D, Gutowska A, Sacha P, Majewski P, Wieczorek P, Tryniszewska E. Activity of ceftazidime-avibactam alone and in combination with ertapenem, fosfomycin, and tigecycline against carbapenemase-producing Klebsiella pneumoniae. Microb Drug Resist 2019;25:1357-64.
- Perry JD, Naqvi SH, Mirza IA, Alizai SA, Hussain A, Ghirardi S, et al. Prevalence of faecal carriage of Enterobacteriaceae with NDM-1 carbapenemase at military hospitals in Pakistan, and evaluation of two chromogenic media. J Antimicrob Chemother 2011;66:2288-94.
- Cuba GT, Rocha-Santos G, Cayô R, Streling AP, Nodari CS, Gales AC, et al. In vitro synergy of ceftolozane/tazobactam in combination with fosfomycin or aztreonam against MDR Pseudomonas aeruginosa. J Antimicrob Chemother 2020;75:1874-8.
- Aires CA, Pereira PS, de Araujo CF, Chagas TP, Oliveira JC, Buonora SN, et al. Multiclonal expansion of Klebsiella pneumoniae isolates producing NDM-1 in Rio de Janeiro, Brazil. Antimicrob Agents Chemother 2017;61.
- Sonnevend A, Ghazawi A, Darwish D, Barathan G, Hashmey R, Ashraf T, et al. In vitro efficacy of ceftazidime-avibactam, aztreonam-avibactam and other rescue antibiotics against carbapenem-resistant Enterobacterales from the Arabian Peninsula. Int J Infect Dis 2020;99:253-9.
- Vasoo S, Cunningham SA, Cole NC, Kohner PC, Menon SR, Krause KM, et al. In vitro activities of ceftazidime-avibactam, aztreonam-avibactam, and a panel of older and contemporary antimicrobial agents against carbapenemase-producing Gram-negative Bacilli. Antimicrob Agents Chemother 2015;59:7842-6.
- Jahan S, Davis H, Ashcraft DS, Pankey GA. Evaluation of the in vitro interaction of fosfomycin and meropenem against metallo-β-lactamase-producing Pseudomonas aeruginosa using Etest and time-kill assay. J Investig Med 2021;69:371-6.
- Public Health England . English Surveillance Programme for Antimicrobial Utilisation and Resistance (ESPAUR) Report 2018 to 2019 2020.
- Kohira N, Hackel MA, Ishioka Y, Kuroiwa M, Sahm DF, Sato T, et al. Reduced susceptibility mechanism to cefiderocol, a siderophore cephalosporin, among clinical isolates from a global surveillance programme (SIDERO-WT-2014). J Glob Antimicrob Resist 2020;22:738-41.
- European Committee on Antimicrobial Susceptibility Testing . Breakpoint Tables for Interpretation of MICs and Zone Diameters 2021. www.eucast.org/fileadmin/src/media/PDFs/EUCAST_files/Breakpoint_tables/v_11.0_Breakpoint_Tables.pdf (accessed 22 October 2021).
- Brown DFJ, Wootton M, Howe RA. Antimicrobial susceptibility testing breakpoints and methods from BSAC to EUCAST. J Antimicrob Chemother 2015;71:3-5.
- Dias S, Welton NJ, Sutton AJ, Ades A. NICE DSU Technical Support Document 2: A Generalised Linear Modelling Framework for Pairwise and Network Meta-analysis of Randomised Controlled Trials. London: National Institute for Health and Care Excellence (NICE); 2014.
- Dias S, Welton NJ, Sutton AJ, Caldwell DM, Lu G, Ades AE. NICE DSU Technical Support Document 4: Inconsistency in Networks of Evidence Based on Randomised Controlled Trials. Sheffield, UK: NICE Decision Support Unit; 2011.
- Daly C, Downing BC, Welton NJ. A Practical Guide to Inconsistency Checks in Bayesian Network Meta-analysis. Bristol, UK; n.d.
- EUnetHTA. Authoring Team . Cefiderocol (Fetcroja®) for the Treatment of Infections Due to Aerobic Gram-Negative Organisms in Adults With Limited Treatment Options 2020. www.eunethta.eu (accessed 16 May 2024).
- Bassetti M, Echols R, Matsunaga Y, Portsmouth S, Ariyasu M, Toyoizumi K, et al. Efficacy and safety of cefiderocol and best available therapy in patients with serious infections caused by carbapenem-resistant Gram-negative infections: results of the pathogen-focused phase 3 CREDIBLE-CR study. Open Forum Infect 2020;7:S652-3.
- Bassetti M, Ariyasu M, Binkowitz B, Nagata TD, Echols RM, Matsunaga Y, et al. Designing a pathogen-focused study to address the high unmet medical need represented by carbapenem-resistant Gram-negative pathogens – the international, multicenter, randomized, open-label, phase 3 CREDIBLE-CR study. Infect Drug Resist 2019;12:3607-23.
- Mushtaq S, Sadouki Z, Vickers A, Livermore D, Woodford N. In-vitro activity of cefiderocol against multidrug-resistant Enterobacterales, Pseudomonas aeruginosa and Acinetobacter baumannii isolates from the UK. Access Microbiology 2020;2.
- Mushtaq S, Sadouki Z, Vickers A, Livermore DM, Woodford N. In Vitro Activity of Cefiderocol against Extensively Drug-resistant Pseudomonas aeruginosa and Acinetobacter baumannii from the UK 2019.
- Bojke L, Soares M, Claxton K, Colson A, Fox A, Jackson C, et al. Developing a reference protocol for structured expert elicitation in health-care decision-making: a mixed-methods study. Health Technol Assess (Winchester, England) 2021;25:1-124.
- Chang W, Cheng J, Allaire J, Xie Y, McPherson J. Package ‘Shiny’ 2015. https://cran.r-project.org/web/packages/shiny/index.htm (accessed 16 May 2024).
- O’Hagan A, Buck CE, Daneshkhah A, Eiser JR, Garthwaite PH, Jenkinson DJ, et al. Uncertain Judgements: Eliciting Experts’ Probabilities. Chichester, UK: John Wiley; 2006.
- Lopes S, Franceschini M, Han Y, Green W, Dymond A, Gill A. Economic Evaluation of Cefiderocol for the Treatment of Carbapenem Resistant Infections in the United States 2020.
- Tichy E, Torres A, Bassetti M, Kongnakorn T, Di Virgilio R, Irani P, et al. Cost-effectiveness comparison of ceftazidime/avibactam versus meropenem in the empirical treatment of hospital-acquired pneumonia, including ventilator-associated pneumonia, in Italy. Clin Ther 2020;42:802-17.
- Kongnakorn T, Wagenlehner F, Falcone M, Tichy E, Di Virgilio R, Baillon-Plot N, et al. Cost-effectiveness analysis of ceftazidime/avibactam compared to imipenem as empirical treatment for complicated urinary tract infections. Int J Antimicrob Agents 2019;54:633-41.
- Nguyen CP, Dan Do TN, Bruggemann R, Ten Oever J, Kolwijck E, Adang EMM, et al. Clinical cure rate and cost-effectiveness of carbapenem-sparing beta-lactams vs. meropenem for Gram-negative infections: a systematic review, meta-analysis, and cost-effectiveness analysis. Int J Antimicrob Agents 2019;54:790-7.
- Simon MS, Sfeir MM, Calfee DP, Satlin MJ. Cost-effectiveness of ceftazidime-avibactam for treatment of carbapenem-resistant Enterobacteriaceae bacteremia and pneumonia. Antimicrob Agents Chemother 2019;63.
- Chen GJ, Pan SC, Foo J, Morel C, Chen WT, Wang JT. Comparing ceftolozane/tazobactam versus piperacillin/tazobactam as empiric therapy for complicated urinary tract infection in Taiwan: a cost-utility model focusing on Gram-negative bacteria. J Microbiol Immunol Infect 2019;52:807-15.
- Mewes JC, Pulia MS, Mansour MK, Broyles MR, Nguyen HB, Steuten LM. The cost impact of PCT-guided antibiotic stewardship versus usual care for hospitalised patients with suspected sepsis or lower respiratory tract infections in the US: a health economic model analysis. PLOS ONE 2019;14.
- Nelson RE, Ray W, Rubin MA, Schweizer M. Evaluating the Cost-effectiveness of Decolonization for Prevention of MRSA Infections using a Dynamic Transmission Model. Antimicrobial Resistance and Infection Control Conference: 5th International Conference on Prevention and Infection Control, ICPIC 2019;8.
- Gordon J, Darlington O, McEwan P, Lumley M, Taie A, Hicks M, et al. Estimating the value of new antimicrobials in the context of antimicrobial resistance: development and application of a dynamic disease transmission model. PharmacoEcon 2020;38:857-69.
- Wagner AP, Enne VI, Livermore DM, Craig JV, Turner DA. INHALE Study Group . Review of health economic models exploring and evaluating treatment and management of hospital-acquired pneumonia and ventilator-associated pneumonia. J Hosp Infect 2020;106:745-56.
- Edwards SJ, Wordsworth S, Clarke MJ. Treating pneumonia in critical care in the United Kingdom following failure of initial antibiotic: a cost-utility analysis comparing meropenem with piperacillin/tazobactam. Eur J Health Econ 2012;13:181-92.
- Grau S, Alvarez-lerma F, del Castillo A, Neipp R, Rubio-terrés C. Cost-effectiveness analysis of the treatment of ventilator-associated pneumonia with linezolid or vancomycin in Spain. J Chemother 2005;17:203-11.
- Kongnakorn T, Mwamburi M, Merchant S, Akhras K, Caro JJ, Nathwani D. Economic evaluation of doripenem for the treatment of nosocomial pneumonia in the US: discrete event simulation. Curr Med Res Opin 2010;26:17-24.
- Kauf TL, Prabhu VS, Medic G, Borse RH, Miller B, Gaultney J, et al. Cost-effectiveness of ceftolozane/tazobactam compared with piperacillin/tazobactam as empiric therapy based on the in-vitro surveillance of bacterial isolates in the United States for the treatment of complicated urinary tract infections. BMC Infect Dis 2017;17.
- Schaffer SK, West P, Towse A, Henshall C, Mestre-Ferrandiz J, Masterton R, et al. Assessing the Value of New Antibiotics: Additional Elements of Value for Health Technology Assessment Decisions. London: Office of Health Economics; 2017.
- Medicines and Healthcare products Regulatory Agency . Aminoglycosides (Gentamicin, Amikacin, Tobramycin, and Neomycin): Increased Risk of Deafness in Patients With Mitochondrial Mutations 2021. www.gov.uk/drug-safety-update/aminoglycosides-gentamicin-amikacin-tobramycin-and-neomycin-increased-risk-of-deafness-in-patients-with-mitochondrial-mutations (accessed 16 May 2024).
- Pfizer . National Institute of Health and Care Excellence, Antimicrobial Health Technology Evaluation: Ceftazidime With Avibactam for Treating Severe Aerobic Gram-Negative Bacterial Infections n.d. www.nice.org.uk/guidance/hte1/history (accessed 21 February 2024).
- Tumbarello M, De Pascale G, Trecarichi EM, Spanu T, Antonicelli F, Maviglia R, et al. Clinical outcomes of Pseudomonas aeruginosa pneumonia in intensive care unit patients. Intensive Care Med 2013;39:682-92.
- Mushtaq S, Garello P, Vickers A, Woodford N, Livermore DM. Activity of cefepime/zidebactam (WCK 5222) against ‘problem’ antibiotic-resistant Gram-negative bacteria sent to a national reference laboratory. J Antimicrob Chemother 2021;76:1511-22.
- Livermore DM, Nicolau DP, Hopkins KL, Meunier D. Carbapenem-resistant Enterobacterales, carbapenem resistant organisms, carbapenemase-producing Enterobacterales, and carbapenemase-producing organisms: terminology past its ‘sell-by date’ in an era of new antibiotics and regional carbapenemase epidemiology. Clin Infect Dis 2020;71:1776-82.
- Sisay M, Hagos B, Edessa D, Tadiwos Y, Mekuria AN. Polymyxin-induced nephrotoxicity and its predictors: a systematic review and meta-analysis of studies conducted using RIFLE criteria of acute kidney injury. Pharmacol Res 2021;163.
- Chien H-T, Lin Y-C, Sheu C-C, Hsieh K-P, Chang J-S. Is colistin-associated acute kidney injury clinically important in adults? A systematic review and meta-analysis. Int J Antimicrob Agents 2020;55.
- Kerr M, Bedford M, Matthews B, O’Donoghue D. The economic impact of acute kidney injury in England. Nephrol Dial Transplant 2014;29:1362-8.
- Wagenlehner F, Lucenteforte E, Pea F, Soriano A, Tavoschi L, Steele VR, et al. Systematic review on estimated rates of nephrotoxicity and neurotoxicity in patients treated with polymyxins. Clin Microbiol Infect 2021;27:671-86.
- Oliota AF, Penteado ST, Tonin FS, Fernandez-Llimos F, Sanches AC. Nephrotoxicity prevalence in patients treated with polymyxins: a systematic review with meta-analysis of observational studies. Diagn Microbiol Infect Dis 2019;94:41-9.
- Palacios-Baena ZR, Giannella M, Manissero D, Rodríguez-Baño J, Viale P, Lopes S, et al. Risk factors for carbapenem-resistant Gram-negative bacterial infections: a systematic review. Clin Microbiol Infect 2020;27:228-35.
- Open VIE Ltd. UK Draft Study Report. CARBAR . A retrospective study to evaluate the epidemiology, standard of care, outcomes and resource use associated with patients who have or are at risk of, infection with carbapenem non-susceptible Gram-negative organisms of interest (Enterobacterales, Pseudomonas spp., Stenotrophomonas spp. and Acinetobacter spp 2020.
- Merrick B, Tan MKI, Bisnauthsing K, Goldenberg SD. Healthcare resource use in hospitalized patients with carbapenem-resistant Gram-negative infections. J Hosp Infect 2021;110:7-14.
- Guyot P, Ades A, Ouwens MJ, Welton NJ. Enhanced secondary analysis of survival data: reconstructing the data from published Kaplan-Meier survival curves. BMC Med Res Methodol 2012;12:1-13.
- Latimer N. NICE DSU Technical Support Document 14: Survival Analysis For Economic Evaluations Alongside Clinical Trials – Extrapolation With Patient-Level Data NICE Decision Support Unit. 2011.
- Bucaloiu ID, Kirchner HL, Norfolk ER, Hartle JE, II, Perkins RM. Increased risk of death and de novo chronic kidney disease following reversible acute kidney injury. Kidney Int 2012;81:477-85.
- Ara R, Brazier JE. Populating an economic model with health state utility values: moving toward better practice. Value Health 2010;13:509-18.
- Hadjiat Y, Serrie A, Treves R, Chomier B, Geranton L, Billon S. Pain associated with health and economic burden in France: results from recent National Health and Wellness Survey data. ClinicoEcon 2018;10:53-65.
- Wyld M, Morton RL, Hayen A, Howard K, Webster AC. A systematic review and meta-analysis of utility-based quality of life in chronic kidney disease treatments. PLOS Med 2012;9.
- Kerr M, Bray B, Medcalf J, O’Donoghue DJ, Matthews B. Estimating the financial cost of chronic kidney disease to the NHS in England. Nephrol Dial Transplant 2012;27:iii73-80.
- Muscedere JG, Shorr AF, Jiang X, Day A, Heyland DK. The adequacy of timely empiric antibiotic therapy for ventilator-associated pneumonia: an important determinant of outcome. J Crit Care 2012;27:322.e7-322.e14.
- Kolhe NV, Eldehni MT, Selby NM, McIntyre CW. The reimbursement and cost of acute kidney injury: a UK hospital perspective. Nephron Clin Pract 2014;126:51-6.
- Knight GM, Costelloe C, Deeny SR, Moore LSP, Hopkins S, Johnson AP, et al. Quantifying where human acquisition of antibiotic resistance occurs: a mathematical modelling study. BMC Med 2018;16.
- Curtis L, Burns A. Unit Costs of Health & Social Care. Canterbury: PSSRU, University of Kent; 2020.
- Liangos O, Wald R, O’Bell JW, Price L, Pereira BJ, Jaber BL. Epidemiology and outcomes of acute renal failure in hospitalized patients: a national survey. Clin J Am Soc Nephrol 2006;1:43-51.
- Department of Health and Social Care . Drugs and Pharmaceutical Electronic Market Information Tool (eMIT) 2021. www.gov.uk/government/publications/drugs-and-pharmaceutical-electronic-market-information-emit (accessed 16 May 2024).
- National Institute for Health and Care Excellence (NICE) . British National Formulary 2021. https://bnf.nice.org.uk/ (accessed 16 May 2024).
- World Health Organisation . WHO Collaboration Centre for Drug Statistics Methodology 2021. https://apps.who.int/whocc/Detail.aspx?Y4LL80197gk3R/zebVRpCw== (accessed 8 February 2024).
- Kallel H, Hergafi L, Bahloul M, Hakim A, Dammak H, Chelly H, et al. Safety and efficacy of colistin compared with imipenem in the treatment of ventilator-associated pneumonia: a matched case–control study. Intensive Care Med 2007;33:1162-7.
- Hawkey PM, Warren RE, Livermore DM, McNulty CAM, Enoch DA, Otter JA, et al. Treatment of infections caused by multidrug-resistant Gram-negative bacteria: report of the British Society for Antimicrobial Chemotherapy/Healthcare Infection Society/British Infection Association Joint Working Party. J Antimicrob Chemother 2018;73:iii2-78.
- Renascience Pharma Ltd . Renapime 1g Powder for Solution for Injection Infusion: SmPC n.d. www.medicines.org.uk/emc/product/9671/smpc (accessed 8 February 2024).
- National Institute for Health and Care Excellence (NICE) . BNF: British National Formulary – NICE 2021 2021. https://bnf.nice.org.uk/ (accessed 16 May 2024).
- Drugs and Pharmaceutical Electronic Market Information Tool (eMIT). 2021.
- National Institute for Health and Care Excellence . Antimicrobial Health Technology Evaluation: Ceftazidime With Avibactam for Treating Severe Aerobic Gram-Negative Bacterial Infections: Final Scope London (National Institute for Health and Care Excellence) 2021. www.nice.org.uk/about/what-we-do/life-sciences/scientific-advice/models-for-the-evaluation-and-purchase-of-antimicrobials/ceftazidime-with-avibactam (accessed 17 October 2021).
- Department of Health and Social Care . Impact Assessment 9553: 2018 Statutory Scheme – Branded Medicines Pricing 2018.
- National Institute for Health and Care Excellence . Guide to the Methods of Technology Appraisal 2013 2013.
- EUCAST . New S, I and R Definitions 2021. www.eucast.org/newsiandr/ (accessed 16 May 2024).
- Costelloe C, Metcalfe C, Lovering A, Mant D, Hay AD. Effect of antibiotic prescribing in primary care on antimicrobial resistance in individual patients: systematic review and meta-analysis. BMJ 2010;340.
- Bell BG, Schellevis F, Stobberingh E, Goossens H, Pringle M. A systematic review and meta-analysis of the effects of antibiotic consumption on antibiotic resistance. BMC Infect Dis 2014;14:1-25.
- Bakhit M, Hoffmann T, Scott AM, Beller E, Rathbone J, Del Mar C. Resistance decay in individuals after antibiotic exposure in primary care: a systematic review and meta-analysis. BMC Med 2018;16:1-19.
- Jeffrey B, Aanensen DM, Croucher NJ, Bhatt S. Predicting the future distribution of antibiotic resistance using time series forecasting and geospatial modelling. Wellcome Open Res 2020;5.
- Organisation for Economic Co-operation and Development . Stemming the Superbug Tide: Just a Few Dollars More n.d. https://oecd.org/health/stemming-the-superbug-tide-9789264307599-en.htm (accessed 8 February 2024).
- Ortiz-Brizuela E, Caro-Vega Y, Bobadilla-del-Valle M, Leal-Vega F, Criollo-Mora E, Luis BL, et al. The influence of hospital antimicrobial use on carbapenem-non-susceptible Enterobacterales incidence rates according to their mechanism of resistance: a time-series analysis. J Hosp Infect 2020;105:757-65.
- Gharbi M, Moore LS, Gilchrist M, Thomas CP, Bamford K, Brannigan ET, et al. Forecasting carbapenem resistance from antimicrobial consumption surveillance: lessons learnt from an OXA-48-producing Klebsiella pneumoniae outbreak in a West London renal unit. Int J Antimicrob Agents 2015;46:150-6.
- Berger P, Pascal L, Sartor C, Delorme J, Monge P, Ragon CP, et al. Generalized additive model demonstrates fluoroquinolone use/resistance relationships for Staphylococcus aureus. Eur J Epidemiol 2004;19:453-60.
- McDonnell L, Armstrong D, Ashworth M, Dregan A, Malik U, White P. National disparities in the relationship between antimicrobial resistance and antimicrobial consumption in Europe: an observational study in 29 countries. J Antimicrob Chemother 2017;72:3199-204.
- Pouwels KB, Freeman R, Muller-Pebody B, Rooney G, Henderson KL, Robotham JV, et al. Association between use of different antibiotics and trimethoprim resistance: going beyond the obvious crude association. J Antimicrob Chemother 2018;73:1700-7.
- Colson AR, Megiddo I, Alvarez-Uria G, Gandra S, Bedford T, Morton A, et al. Quantifying uncertainty about future antimicrobial resistance: comparing structured expert judgment and statistical forecasting methods. PLOS ONE 2019;14.
- Durham L, Ge M, Cuccia A, Quinn J. Modeling antibiotic resistance to project future rates: quinolone resistance in Escherichia coli. Eur J Clin Microbiol Infect Dis 2010;29:353-6.
- Goossens H, Ferech M, Vander Stichele R, Elseviers M, Group EP. Outpatient antibiotic use in Europe and association with resistance: a cross-national database study. Lancet 2005;365:579-87.
- Johnson AP, Muller-Pebody B, Budd E, Ashiru-Oredope D, Ladenheim D, Hain D, et al. Improving feedback of surveillance data on antimicrobial consumption, resistance and stewardship in England: putting the data at your Fingertips. J Antimicrob Chemother 2017;72:953-6.
- European Centre for Disease Prevention and Control . European Surveillance of Antimicrobial Consumption Network (ESAC-Net) 2021. www.ecdc.europa.eu/en/about-us/partnerships-and-networks/disease-and-laboratory-networks/esac-net (accessed 16 May 2024).
- European Centre for Disease Prevention and Control . European Antimicrobial Resistance Surveillance Network (EARS-Net) 2021. https://ecdc.europa.eu/en/about-us/networks/disease-networks-and-laboratory-networks/ears-net-data (accessed 16 May 2024).
- European Centre for Disease Prevention and Control . Antimicrobial Resistance in the EU EEA (EARS-Net) – Annual Epidemiological Report for 2019 2020. www.ecdc.europa.eu/en/publications-data/surveillance-antimicrobial-resistance-europe-2019 (accessed 16 May 2024).
- Morel CM, Alm RA, Årdal C, Bandera A, Bruno GM, Carrara E, et al. A one health framework to estimate the cost of antimicrobial resistance. Antimicrob Resist Infect Control 2020;9:1-14.
- Chatterjee A, Modarai M, Naylor NR, Boyd SE, Atun R, Barlow J, et al. Quantifying drivers of antibiotic resistance in humans: a systematic review. Lancet Infect Dis 2018;18:e368-78.
- The effect of antibiotic restriction programs on prevalence of antimicrobial resistance: a systematic review and meta-analysis. Open Forum Infect 2021;8.
- Holmes AH, Moore LS, Sundsfjord A, Steinbakk M, Regmi S, Karkey A, et al. Understanding the mechanisms and drivers of antimicrobial resistance. Lancet 2016;387:176-87.
- Enne VI, Livermore DM, Stephens P, Hall LM. Persistence of sulphonamide resistance in Escherichia coli in the UK despite national prescribing restriction. Lancet 2001;357:1325-8.
- Nasrin D, Collignon PJ, Roberts L, Wilson EJ, Pilotto LS, Douglas RM. Effect of β lactam antibiotic use in children on pneumococcal resistance to penicillin: prospective cohort study. BMJ 2002;324:28-30.
- Point Prevalence Survey of Healthcare-Associated Infections and Antimicrobial Use in European Acute Care Hospitals. Stockholm: ECDC; 2013.
- National Institute for Health and Care Excellence . Pneumonia in Adults: Diagnosis and Management (CG.191) 2014. https://nice.org.uk/guidance/cg191 (accessed 8 February 2024).
- Specialist Pharmacy Service (SPS) . Prescribing Outlook 2020.
- Castanheira M, Deshpande LM, Costello A, Davies TA, Jones RN. Epidemiology and carbapenem resistance mechanisms of carbapenem-non-susceptible Pseudomonas aeruginosa collected during 2009–11 in 14 European and Mediterranean countries. J Antimicrob Chemother 2014;69:1804-14.
- Public Health England . AMR Local Indicators 2021. https://fingertips.phe.org.uk/profile/amr-local-indicators (accessed 16 May 2024).
- Paul M, Carrara E, Retamar P, Tängdén T, Bitterman R, Bonomo RA, et al. European Society of Clinical Microbiology and Infectious Diseases (ESCMID) guidelines for the treatment of infections caused by multidrug-resistant Gram-negative bacilli (endorsed by European Society of Intensive Care Medicine). Clin Microbiol Infect 2022;28:521-47.
- Tamma P, Aitken S, Bonomo R, Mathers A, van Duin D, Clancy C. Infectious Diseases Society of America Antimicrobial-Resistant Treatment Guidance: Gram-Negative Bacterial Infections Infectious Diseases Society of America 2022. www.idsociety.org/practice-guideline/amr-guidance/#null (accessed 16 May 2024).
- Walker AS, White IR, Turner RM, Hsu LY, Yeo TW, White NJ, et al. Personalised randomised controlled trial designs – a new paradigm to define optimal treatments for carbapenem-resistant infections. Lancet Infect Dis 2021;21:e175-81.
- Harnan SE, Tappenden P, Essat M, Gomersall T, Minton J, Wong R, et al. Measurement of exhaled nitric oxide concentration in asthma: a systematic review and economic evaluation of NIOX MINO, NIOX VERO and NObreath. Health Technol Assess 2015;19:1-330.
- Montori VM, Wilczynski NL, Morgan D, Haynes RB, Hedges T. Optimal search strategies for retrieving systematic reviews from Medline: analytical survey. BMJ 2005;330.
- National Institute of Health and Care Excellence . Diabetic Foot Infection: Antimicrobial Prescribing. NICE Evidence Reviews Collection 2019.
- Li L, Smith HE, Atun R, Tudor Car L. Search strategies to identify observational studies in MEDLINE and Embase. Cochrane Database Syst Rev 2019;3.
- Ayiku L, Levay P, Hudson T, Craven J, Barrett E, Finnegan A, et al. The medline UK filter: development and validation of a geographic search filter to retrieve research about the UK from OVID medline. Health Info Libr J 2017;34:200-16.
- Ayiku L, Levay P, Hudson T, Craven J, Finnegan A, Adams R, et al. The Embase UK filter: validation of a geographic search filter to retrieve research about the UK from OVID Embase. Health Info Libr J 2019;36:121-33.
- Bassetti M, Rello J, Blasi F, Goossens H, Sotgiu G, Tavoschi L, et al. A systematic review on the impact of appropriate versus inappropriate initial antibiotic therapy on the outcomes of patients with severe bacterial infections. Int J Antimicrob Agents 2020;56.
- Arber M, Garcia S, Veale T, Edwards M, Shaw A, Glanville JM. Performance of Ovid Medline search filters to identify health state utility studies. Int J Technol Assess Health Care 2017;33:472-80.
- Katsube T, Wajima T, Ishibashi T, Arjona Ferreira JC, Echols R. Pharmacokinetic/pharmacodynamic modeling and simulation of cefiderocol, a parenteral siderophore cephalosporin, for dose adjustment based on renal function. Antimicrob Agents Chemother 2017;61:e01381-16.
- Zasowski EJ, Bassetti M, Blasi F, Goossens H, Rello J, Sotgiu G, et al. A systematic review of the effect of delayed appropriate antibiotic treatment on the outcomes of patients with severe bacterial infections. Chest 2020;158:929-38.
- Herkel T, Uvizl R, Doubravska L, Adamus M, Gabrhelik T, Htoutou Sedlakova M, et al. Epidemiology of Hospital-Acquired Pneumonia: Results of a Central European Multicenter, Prospective, Observational Study Compared With Data from the European Region 2016;160:448-55.
- Pena C, Gómez-Zorrilla S, Oriol I, Tubau F, Dominguez M, Pujol M, et al. Impact of multidrug resistance on Pseudomonas aeruginosa ventilator-associated pneumonia outcome: predictors of early and crude mortality. Eur J Clin Microbiol Infect Dis 2013;32:413-20.
- Amaral AC, Holder MW. Timing of antimicrobial therapy after identification of ventilator-associated condition is not associated with mortality in patients with ventilator-associated pneumonia: a cohort study. PLOS ONE 2014;9.
- Hyndman RJ, Athanasopoulos G. Forecasting: Principles and Practice: OTexts. Melbourne, Australia: Otexts; 2018.
- Liboschik T, Fokianos K, Fried R. tscount: an R package for analysis of count time series following generalized linear models. J Stat Softw 2017;82:1-51.
- Aliabadi S, Anyanwu P, Beech E, Jauneikaite E, Wilson P, Hope R, et al. Effect of antibiotic stewardship interventions in primary care on antimicrobial resistance of Escherichia coli bacteraemia in England (2013–18): a quasi-experimental, ecological, data linkage study. Lancet Infect Dis 2021;21:1689-700.
- Kearns B, Stevenson MD, Triantafyllopoulos K, Manca A. Generalized linear models for flexible parametric modeling of the hazard function. Med Decis Making 2019;39:867-78.
- Escherichia coli (E. coli): Guidance, Data and Analysis. 2017.
- Sharland M, Pulcini C, Harbarth S, Zeng M, Gandra S, Mathur S, et al. 21st WHO Expert Committee on Selection and Use of Essential Medicines . Classifying antibiotics in the WHO Essential Medicines List for optimal use – be AWaRe. Lancet Infect Dis 2018;18:18-20.
- Bennett Institute for Applied Data Science . OpenPrescribing.Net 2021. https://openprescribing.net/ (accessed 16 May 2024).
- Davey P, Brown E, Charani E, Fenelon L, Gould IM, Holmes A, et al. Interventions to improve antibiotic prescribing practices for hospital inpatients. Cochrane Database Syst Rev 2013.
- Bhattacharya A, Hopkins S, Sallis A, Budd EL, Ashiru-Oredope D. A process evaluation of the UK-wide Antibiotic Guardian campaign: developing engagement on antimicrobial resistance. J Public Health 2017;39:e40-7.
- McKenzie E, Gardner Jr ES. Damped trend exponential smoothing: a modelling viewpoint. Int J Forecast 2010;26:661-5.
- Spicknall IH, Foxman B, Marrs CF, Eisenberg JN. A modeling framework for the evolution and spread of antibiotic resistance: literature review and model categorization. Am J Epidemiol 2013;178:508-20.
Appendix 1 Search strategies
Clinical searches
Number of records retrieved
Cefiderocol clinical searches
Term group(s): Cefiderocol AND filter
Filters: Exclusions filter (MEDLINE, EMBASE)
Limits: None
Ovid MEDLINE(R) and Epub Ahead of Print, In-Process, In-Data-Review and Other Non-Indexed Citations, Daily and Versions(R) 1946 to March 05, 2021 (searched via the Ovid SP platform)
8 March 2021
# | Searches | Results |
---|---|---|
1 | cefiderocol.mp. | 160 |
2 | fetroja.mp. | 4 |
3 | fetcroja.mp. | 0 |
4 | rsc-649266.mp. | 0 |
5 | or/1-4 | 160 |
6 | Case report.tw. | 328,791 |
7 | Letter/ | 1,125,503 |
8 | Historical article/ | 362,469 |
9 | 6 or 7 or 8 | 1,800,153 |
10 | exp Animals/ | 23,873,090 |
11 | Humans/ | 19,076,531 |
12 | 10 not (10 and 11) | 4,796,559 |
13 | 9 or 12 | 6,547,157 |
14 | 5 not 13 | 143 |
Embase 1974–5 March 2021 (searched via the Ovid SP platform)
8 March 2021
# | Searches | Results |
---|---|---|
1 | cefiderocol.mp. | 281 |
2 | fetroja.mp. | 9 |
3 | fetcroja.mp. | 1 |
4 | rsc-649266.mp. | 0 |
5 | or/1-4 | 281 |
6 | Case study/ | 76,989 |
7 | Case report.tw. | 442,512 |
8 | Abstract report/ or letter/ | 1,190,878 |
9 | editorial.pt. | 686,858 |
10 | (case$ and series).tw. | 282,645 |
11 | animal/ | 1,510,117 |
12 | human/ | 21,955,778 |
13 | 11 not (11 and 12) | 1,106,000 |
14 | or/6-10,13 | 3,696,820 |
15 | 5 not 14 | 257 |
CRD database (searched via the University of York CRD platform)
8 March 2021
# | Searches | Results |
---|---|---|
1 | (cefiderocol) | 0 |
2 | (fetroja) | 0 |
3 | (fetcroja) | 0 |
4 | (rsc-649266) | 0 |
Fosfomycin search strategy
Searched using PubMed on 26 August 2021, from database inception.
Search number | Search term used in PubMed | Terms PubMed searched | Hits |
---|---|---|---|
1 | Fosfomycin | ‘fosfomycin’[MeSH Terms] OR ‘fosfomycin’[All Fields] OR ‘fosfomycine’[All Fields] | 3802 |
2 | (metallo beta lactamase) OR (MBL) | (‘metallo’[All Fields] AND (‘beta lactamases’[MeSH Terms] OR ‘beta lactamases’[All Fields] OR (‘beta’[All Fields] AND ‘lactamase’[All Fields]) OR ‘beta lactamase’[All Fields])) OR (‘mol biol los angel’[Journal] OR ‘mbl’[All Fields]) | 8805 |
3 | ((susceptibility) OR (resistance)) OR (antibiogram) OR ((susceptib*) OR (resistan*)) OR (AM susceptibility[MeSH Terms]) | ‘susceptib*’[All Fields] OR ‘resistan*’[All Fields] OR ((‘anti infective agents’[Pharmacological Action] OR ‘anti infective agents’[MeSH Terms] OR (‘anti infective’[All Fields] AND ‘agents’[All Fields]) OR ‘anti infective agents’[All Fields] OR ‘AM’[All Fields] OR ‘AMs’[All Fields] OR ‘AMly’[All Fields]) AND ‘disease susceptibility’[MeSH Terms]) OR (‘disease susceptibility’[MeSH Terms] OR (‘disease’[All Fields] AND ‘susceptibility’[All Fields]) OR ‘disease susceptibility’[All Fields] OR ‘susceptibilities’[All Fields] OR ‘susceptibility’[All Fields] OR ‘susceptible’[All Fields] OR ‘susceptibles’[All Fields] OR ‘susceptive’[All Fields] OR ‘susceptivity’[All Fields] OR (‘resist’[All Fields] OR ‘resistance’[All Fields] OR ‘resistances’[All Fields] OR ‘resistant’[All Fields] OR ‘resistants’[All Fields] OR ‘resisted’[All Fields] OR ‘resistence’[All Fields] OR ‘resistences’[All Fields] OR ‘resistent’[All Fields] OR ‘resistibility’[All Fields] OR ‘resisting’[All Fields] OR ‘resistive’[All Fields] OR ‘resistively’[All Fields] OR ‘resistivities’[All Fields] OR ‘resistivity’[All Fields] OR ‘resists’[All Fields]) OR (‘microbial sensitivity tests’[MeSH Terms] OR (‘microbial’[All Fields] AND ‘sensitivity’[All Fields] AND ‘tests’[All Fields]) OR ‘microbial sensitivity tests’[All Fields] OR ‘antibiogram’[All Fields] OR ‘antibiograms’[All Fields])) | 1,733,217 |
4 | #1 and #2 and #3 | 84 | |
5 | (review, systematic[MeSH Terms]) OR (systematic review) | ((‘review’[Publication Type] OR ‘review literature as topic’[MeSH Terms] OR ‘review’[All Fields]) AND ‘classification’[MeSH Terms]) OR (‘systematic review’[Publication Type] OR ‘systematic reviews as topic’[MeSH Terms] OR ‘systematic review’[All Fields]) | 241,211 |
6 | #1 and #3 and #5 | 30 | |
7 | #4 and #5 | 113 |
Cefiderocol cost-effectiveness analysis models
Term group(s): Cefiderocol AND filter
Filters: Economic (MEDLINE, EMBASE), exclusion filter (EMBASE)
Limits: None
Ovid MEDLINE(R) and Epub Ahead of Print, In-Process, In-Data-Review and Other Non-Indexed Citations, Daily and Versions(R) 1946–26 February 2021 (searched via the Ovid SP platform)
1 March 2021
# | Searches | Results |
---|---|---|
1 | cefiderocol.mp. | 160 |
2 | fetroja.mp. | 4 |
3 | fetcroja.mp. | 0 |
4 | rsc-649266.mp. | 0 |
5 | or/1-4 | 160 |
6 | exp ‘Costs and Cost Analysis’/ | 242,835 |
7 | Economics/ | 27,294 |
8 | exp Economics, Hospital/ | 24,969 |
9 | exp Economics, Medical/ | 14,242 |
10 | Economics, Nursing/ | 4002 |
11 | exp models, economic/ | 15,443 |
12 | Economics, Pharmaceutical/ | 2971 |
13 | exp ‘Fees and Charges’/ | 30,592 |
14 | exp Budgets/ | 13,800 |
15 | budget*.tw. | 30,546 |
16 | ec.fs. | 431,631 |
17 | cost*.ti. | 125579 |
18 | (cost* adj2 (effective* or utilit* or benefit* or minimi*)).ab. | 157179 |
19 | (economic* or pharmacoeconomic* or pharmaco-economic*).ti. | 50,939 |
20 | (price* or pricing*).tw. | 42,703 |
21 | (financial or finance or finances or financed).tw. | 97,358 |
22 | (fee or fees).tw. | 18,704 |
23 | (value adj2 (money or monetary)).tw. | 2515 |
24 | quality-adjusted life years/ | 12,949 |
25 | (qaly or qalys).af. | 11,325 |
26 | (quality adjusted life year or quality adjusted life years).af. | 19,387 |
27 | or/6-26 | 801,858 |
28 | 5 and 27 | 0 |
MEDLINE costs filter from Harnan et al191 (see appendix 13, available from: www.ncbi.nlm.nih.gov/books/NBK321808/). Copyright © Queen’s Printer and Controller of HMSO (2015).
Embase 1974–26 February 2021 (searched via the Ovid SP platform)
1 March 2021
# | Searches | Results |
---|---|---|
1 | cefiderocol.mp. | 278 |
2 | fetroja.mp. | 9 |
3 | fetcroja.mp. | 1 |
4 | rsc-649266.mp. | 0 |
5 | or/1-4 | 278 |
6 | ‘cost benefit analysis’/ | 87,111 |
7 | ‘cost effectiveness analysis’/ | 158,540 |
8 | economics/ | 241,957 |
9 | health economics/ | 33,700 |
10 | pharmacoeconomics/ | 7505 |
11 | fee/ | 14,329 |
12 | budget/ | 30,564 |
13 | budget$.tw. | 40,639 |
14 | cost$.ti. | 168,111 |
15 | (cost$ adj2 (effective$ or utilit$ or benefit$ or minimi$)).ab. | 218,259 |
16 | (economic$ or pharmacoeconomic$ or pharmaco-economic$).ti. | 64,563 |
17 | (price$ or pricing$).tw. | 60,859 |
18 | (financial or finance or finances or financed).tw. | 135,326 |
19 | (fee or fees).tw. | 25,728 |
20 | (value adj2 (money or monetary)).tw. | 3455 |
21 | health care quality/ | 247,699 |
22 | quality adjusted life year/ | 28,517 |
23 | (qaly or qalys).tw. | 21,188 |
24 | (quality adjusted life year or quality adjusted life years).tw. | 20,472 |
25 | or/6-24 | 1,102,354 |
26 | letter.pt. | 1,185,036 |
27 | editorial.pt. | 691,062 |
28 | historical article.pt. | 0 |
29 | or/26-28 | 1,876,098 |
30 | 25 not 29 | 1,021,484 |
31 | animals/ | 1,253,461 |
32 | humans/ | 13,458,185 |
33 | 31 not (31 and 32) | 965,742 |
34 | 30 not 33 | 1,010,813 |
35 | 5 and 34 | 3 |
EMBASE costs filter: Adapted from Harnan et al. 191 (see appendix 13, available from: www.ncbi.nlm.nih.gov/books/NBK321808/). Copyright © Queen’s Printer and Controller of HMSO (2015).
CRD database (searched via the University of York CRD platform)
1 March 2021
# | Searches | Results |
---|---|---|
1 | (cefiderocol) | 0 |
2 | (fetroja) | 0 |
3 | (fetcroja) | 0 |
4 | (rsc-649266) | 0 |
Web of Science – Conference proceedings index (searched via the Clarivate Analytics platform)
1 March 2021
# | Searches | Results |
---|---|---|
# 1 | TOPIC: (cefiderocol) | 8 |
# 2 | TOPIC: (fetroja) | 0 |
# 3 | TOPIC: (fetcroja) | 0 |
# 4 | TOPIC: (rsc-649266) | 0 |
# 5 | #4 OR #3 OR #2 OR #1 | 8 |
Non-clinical evidence
Systematic searches were conducted from March until July 2021 to identify non-clinical evidence for relating to the evaluation.
The following electronic databases were searched from database inception:
-
MEDLINE and Epub Ahead of Print, In-Process, In-Data-Review and Other Non-Indexed Citations, Daily and Versions: Ovid, 1946 to Present
-
EMBASE: Ovid, 1980 to present
-
The University of York CRD platform:
-
DARE: CRD, 1994–2015
-
HTA Database: CRD, 1989–2018
-
NHS EED: CRD, 1972–2015.
-
Number of records retrieved
Focused antimicrobial resistance models search
Term group(s): Focused AM resistance AND modelling AND filter
Filters: Pragmatic and unvalidated economic filter (MEDLINE, EMBASE)
Limits: 2011–present, English language
Ovid MEDLINE(R) and Epub Ahead of Print, In-Process, In-Data-Review and Other Non-Indexed Citations, Daily and Versions(R) 1946–31 March 2021 (searched via the Ovid SP platform)
1 April 2021
# | Searches | Results |
---|---|---|
1 | ((AM or antibiotic or antibacterial) and resistan*).mp. | 148,175 |
2 | (model* or ‘population dynamic*’ or simulat*).ti. | 718,508 |
3 | 1 and 2 | 2671 |
4 | limit 3 to yr=‘2011 -Current’ | 1901 |
5 | limit 4 to english language | 1884 |
6 | Cost-benefit analysis/ | 83,842 |
7 | Economic value of life/ | 5741 |
8 | Quality-adjusted life years/ | 13,042 |
9 | exp models, economic/ | 15,508 |
10 | cost utilit$.tw. | 4939 |
11 | cost benefit$.tw. | 11,329 |
12 | cost minim$.tw. | 1563 |
13 | cost effect$.tw. | 143,618 |
14 | economic evaluation$.tw. | 12,455 |
15 | or/6-14 | 213,673 |
16 | 5 and 15 | 26 |
EMBASE 1974–31 March 2021 (searched via the Ovid SP platform)
1 April 2021
# | Searches | Results |
---|---|---|
1 | ((AM or antibiotic or antibacterial) and resistan*).mp. | 298,764 |
2 | (model* or ‘population dynamic*’ or simulat*).ti. | 863,662 |
3 | 1 and 2 | 4531 |
4 | limit 3 to yr=‘2011 -Current’ | 3042 |
5 | ‘cost benefit analysis’/ | 86,983 |
6 | Economic value of life/ | 145,299 |
7 | quality adjusted life year/ | 28,664 |
8 | exp economic model/ | 2513 |
9 | cost utilit$.tw. | 7843 |
10 | cost benefit$.tw. | 15,750 |
11 | cost minim$.tw. | 2664 |
12 | cost effect$.tw. | 198,907 |
13 | economic evaluation$.tw. | 17,713 |
14 | (‘quality adjusted life year*’ or qaly or qalys).tw. | 26,170 |
15 | or/5-14 | 433,603 |
16 | 4 and 15 | 67 |
CRD database (searched via the University of York CRD platform)
1 April 2021
# | Searches | Results |
---|---|---|
1 | (((AM or antibiotic or antibacterial) and resistan*)) | 459 |
2 | ((model* or ‘population dynamic*’ or simulat*)):TI | 1554 |
3 | #1 AND #2 | 8 |
5 | (#3) FROM 2011 TO 2021 | 2 |
Broad OXA-48 Metallo-beta-lactamases search for database dredging
Term group(s): Mechanisms (OXA-48, NDM, VIM, IMP) AND Germ (enterobacteria, E. coli, K. pneumonia, P. aeruginosa) AND filters.
Filters: Reviews, RCTs, observational studies filter (MEDLINE, EMBASE).
Limits: None.
Ovid MEDLINE(R) and Epub Ahead of Print, In-Process, In-Data-Review and Other Non-Indexed Citations, Daily and Versions(R) 1946–29 March 2021 (searched via the Ovid SP platform)
7 April 2021
# | Searches | Results |
---|---|---|
1 | (oxa-48 or ‘oxa 48’ or oxacillinase-48 or ‘oxacillinase 48’).tw. | 1202 |
2 | ((‘new delhi’ or ndm or ‘verona integrated-encoded’ or vim or imipenemase or imp) and (mbl or ‘metallo-beta-lactamase’ or ‘metallo beta lactamase’)).tw. | 1867 |
3 | 1 or 2 | 2969 |
4 | Enterobacteriaceae/ | 19,296 |
5 | Escherichia coli/ | 271,295 |
6 | Klebsiella pneumoniae/ | 14,859 |
7 | Pseudomonas aeruginosa/ | 43,940 |
8 | (enterobact* or enterobacteriaceae or ‘escherichia coli’ or ‘e. coli’ or ‘klebsiella pneumoniae’ or ‘k. pneumoniae’ or ‘Pseudomonas aeruginosa’ or ‘Pseudomonas aeruginosa’).tw. | 399,190 |
9 | or/4-8 | 495,144 |
10 | 3 and 9 | 2507 |
11 | (MEDLINE or systematic review).tw. or meta analysis.pt. | 312,794 |
12 | Randomized Controlled Trial.pt. | 526,445 |
13 | Controlled Clinical Trial.pt. | 94,120 |
14 | Clinical Trial.pt. | 528,138 |
15 | exp Clinical Trials as Topic/ | 354,862 |
16 | Placebos/ | 35,413 |
17 | Random Allocation/ | 105,006 |
18 | Double-Blind Method/ | 163,341 |
19 | Single-Blind Method/ | 29,950 |
20 | Cross-Over Studies/ | 49,836 |
21 | ((random$ or control$ or clinical$) adj3 (trial$ or stud$)).tw. | 1,322,185 |
22 | (random$ adj3 allocat$).tw. | 38,452 |
23 | placebo$.tw. | 223,839 |
24 | ((singl$ or doubl$ or trebl$ or tripl$) adj (blind$ or mask$)).tw. | 179,179 |
25 | (crossover$ or (cross adj over$)).tw. | 90,152 |
26 | (‘phase 3’ or ‘phase three’).tw. | 16,453 |
27 | or/12-26 | 2,134,299 |
28 | animals/ not humans/ | 4,776,462 |
29 | 27 not 28 | 2,002,988 |
30 | Observational Studies as Topic/ | 6077 |
31 | Observational Study/ | 95,871 |
32 | Epidemiologic Studies/ | 8608 |
33 | exp Case-Control Studies/ | 1,155,597 |
34 | exp Cohort Studies/ | 2,110,104 |
35 | Cross-Sectional Studies/ | 359,015 |
36 | Controlled Before-After Studies/ | 605 |
37 | Historically Controlled Study/ | 196 |
38 | Interrupted Time Series Analysis/ | 1184 |
39 | Comparative Study.pt. | 1,886,769 |
40 | case control$.tw. | 136,201 |
41 | case series.tw. | 81,917 |
42 | (cohort adj (study or studies)).tw. | 231,371 |
43 | cohort analy$.tw. | 8925 |
44 | (follow up adj (study or studies)).tw. | 50,873 |
45 | (observational adj (study or studies)).tw. | 119,734 |
46 | longitudinal.tw. | 263,046 |
47 | prospective.tw. | 604,957 |
48 | retrospective.tw. | 582,233 |
49 | or/30-48 | 4,760,829 |
50 | 10 and 11 | 11 |
51 | 10 and 29 | 80 |
52 | 10 and 49 | 311 |
Embase 1974–6 April 2021 (searched via the Ovid SP platform)
7 April 2021
# | Searches | Results |
---|---|---|
1 | (oxa-48 or ‘oxa 48’ or oxacillinase-48 or ‘oxacillinase 48’).tw. | 1483 |
2 | ((‘new delhi’ or ndm or ‘verona integrated-encoded’ or vim or imipenemase or imp) and (mbl or ‘metallo-beta-lactamase’ or ‘metallo beta lactamase’)).tw. | 2156 |
3 | 1 or 2 | 3502 |
4 | Enterobacteriaceae/ | 24,817 |
5 | Escherichia coli/ | 355,829 |
6 | Klebsiella pneumoniae/ | 44,139 |
7 | Pseudomonas aeruginosa/ | 102,141 |
8 | (enterobact* or enterobacteriaceae or ‘escherichia coli’ or ‘e. coli’ or ‘klebsiella pneumoniae’ or ‘k. pneumoniae’ or ‘Pseudomonas aeruginosa’ or ‘Pseudomonas aeruginosa’).tw. | 446,239 |
9 | or/4-8 | 573,320 |
10 | 3 and 9 | 3045 |
11 | (meta-analysis or systematic review).tw. | 352,331 |
12 | Randomization/ | 90,999 |
13 | Placebo/ | 367,151 |
14 | Double Blind Procedure/ | 183,893 |
15 | Single Blind Procedure/ | 42,628 |
16 | Crossover Procedure/ | 66,858 |
17 | ((random$ or control$ or clinical$) adj3 (trial$ or stud$)).tw. | 1,846,260 |
18 | (random$ adj3 allocat$).tw. | 48,159 |
19 | placebo$.tw. | 325,978 |
20 | ((singl$ or doubl$ or trebl$ or tripl$) adj (blind$ or mask$)).tw. | 251,245 |
21 | (crossover$ or (cross adj over$)).tw. | 112,515 |
22 | or/12-21 | 2,272,133 |
23 | nonhuman/ not human/ | 4,810,057 |
24 | 22 not 23 | 2,173,105 |
25 | Clinical study/ | 157,356 |
26 | Case control study/ | 171,323 |
27 | Family study/ | 26,257 |
28 | Longitudinal study/ | 153,994 |
29 | Retrospective study/ | 1,061,177 |
30 | comparative study/ | 895,931 |
31 | Prospective study/ | 678,405 |
32 | Randomized controlled trials/ | 201,238 |
33 | 31 not 32 | 670,835 |
34 | Cohort analysis/ | 693,427 |
35 | cohort analy$.tw. | 14,434 |
36 | (Cohort adj (study or studies)).tw. | 338,607 |
37 | (Case control$ adj (study or studies)).tw. | 146,583 |
38 | (follow up adj (study or studies)).tw. | 66,194 |
39 | (observational adj (study or studies)).tw. | 188,213 |
40 | (epidemiologic$ adj (study or studies)).tw. | 111,182 |
41 | (cross sectional adj (study or studies)).tw. | 248,198 |
42 | case series.tw. | 114,881 |
43 | prospective.tw. | 921,226 |
44 | retrospective.tw. | 972,633 |
45 | or/25-30,33-44 | 4,373,011 |
46 | 10 and 11 | 13 |
47 | 10 and 24 | 80 |
48 | 10 and 45 | 382 |
CRD database (searched via the University of York CRD platform)
30 March 2021
# | Searches | Results |
---|---|---|
1 | ((oxa-48 or ‘oxa 48’ or oxacillinase-48 or ‘oxacillinase 48’)) | 0 |
2 | (((‘new delhi’ or ndm or ‘verona integrated-encoded’ or vim or imipenemase or imp) and (mbl or ‘metallo-beta-lactamase’ or ‘metallo beta lactamase’))) | 0 |
Focused long-term outcomes search
Term group(s): (carbapenem resistance OR mechanisms) AND [sites (UTI/HAPVAP)] AND filters.
Filters: UK (MEDLINE, EMBASE), Europe (unvalidated).
Limits: 2010-present, English language.
Ovid MEDLINE(R) and Epub Ahead of Print, In-Process, In-Data-Review and Other Non-Indexed Citations, Daily and Versions(R) 1946–10 June 2021 (searched via the Ovid SP platform)
11 June 2021
# | Searches | Results |
---|---|---|
1 | (carbapenem-resistan* or ‘carbapenem resistan*’ or carbapenemase).tw. | 10,189 |
2 | (carbapenem* and (non-susceptib* or ‘non susceptib*’ or nonsusceptib*)).tw. | 674 |
3 | (oxa-48* or ‘oxa 48*’ or oxacillinase-48* or ‘oxacillinase 48*’ or blaoxa-48* or ‘blaoxa 48*’).tw. | 1595 |
4 | ((‘new delhi’ or ndm or ‘verona integrated-encoded’ or vim or imipenemase or imp) and (mbl or ‘metallo-beta-lactamase’ or ‘metallo beta lactamase’)).tw. | 1900 |
5 | or/1-4 | 11,737 |
6 | (cohort* or longitudinal or prospective or retrospective or follow-up or ‘follow up’ or long-term or ‘long term’ or year).tw. | 4,211,288 |
7 | (mortality or death* or survival).tw. | 2,271,430 |
8 | Urinary Tract Infections/ | 39,976 |
9 | urinary tract infection*.tw. | 42,419 |
10 | (uti or utis or cuti or cutis).tw. | 17,460 |
11 | exp Pneumonia/ | 178,125 |
12 | pneumon*.tw. | 202,270 |
13 | exp Intensive Care Units/ | 91,189 |
14 | ((hospital* or ventilator* or icu or intensive care) adj3 (acquired or associat*)).tw. | 49,009 |
15 | Pneumonia, Ventilator-Associated/ | 3704 |
16 | (hap or vap).tw. | 10,159 |
17 | (11 or 12) and (13 or 14) | 17,397 |
18 | 8 or 9 or 10 or 15 or 16 or 17 | 91,038 |
19 | 5 and 6 and 7 and 18 | 160 |
20 | limit 19 to english language | 154 |
21 | limit 20 to yr=‘2010 -Current’ | 146 |
22 | exp Great Britain/ | 374,892 |
23 | (national health service* or nhs*).ti,ab,in. | 220,908 |
24 | (english not ((published or publication* or translat* or written or language* or speak* or literature or citation*) adj5 english)).ti,ab. | 40,760 |
25 | (gb or ‘g.b’. or britain* or (british* not ‘british columbia’) or uk or ‘u.k’. or united kingdom* or (england* not ‘new england’) or northern ireland* or northern irish* or scotland* or scottish* or ((wales or ‘south wales’) not ‘new south wales’) or welsh*).ti,ab,jw,in. | 2,187,630 |
26 | (bath or ‘bath’s’ or ((birmingham not alabama*) or (‘birmingham’s’ not alabama*) or bradford or ‘bradford’s’ or brighton or ‘brighton’s’ or bristol or ‘bristol’s’ or carlisle* or ‘carlisle’s’ or (cambridge not (massachusetts* or boston* or harvard*)) or (‘cambridge’s’ not (massachusetts* or boston* or harvard*)) or (canterbury not zealand*) or (‘canterbury’s’ not zealand*) or chelmsford or ‘chelmsford’s’ or chester or ‘chester’s’ or chichester or ‘chichester’s’ or coventry or ‘coventry’s’ or derby or ‘derby’s’ or (durham not (carolina* or nc)) or (‘durham’s’ not (carolina* or nc)) or ely or ‘ely’s’ or exeter or ‘exeter’s’ or gloucester or ‘gloucester’s’ or hereford or ‘hereford’s’ or hull or ‘hull’s’ or lancaster or ‘lancaster’s’ or leeds* or leicester or ‘leicester’s’ or (lincoln not nebraska*) or (‘lincoln’s’ not nebraska*) or (liverpool not (new south wales* or nsw)) or (‘liverpool’s’ not (new south wales* or nsw)) or ((london not (ontario* or ont or toronto*)) or (‘london’s’ not (ontario* or ont or toronto*)) or manchester or ‘manchester’s’ or (newcastle not (new south wales* or nsw)) or (‘newcastle’s’ not (new south wales* or nsw)) or norwich or ‘norwich’s’ or nottingham or ‘nottingham’s’ or oxford or ‘oxford’s’ or peterborough or ‘peterborough’s’ or plymouth or ‘plymouth’s’ or portsmouth or ‘portsmouth’s’ or preston or ‘preston’s’ or ripon or ‘ripon’s’ or salford or ‘salford’s’ or salisbury or ‘salisbury’s’ or sheffield or ‘sheffield’s’ or southampton or ‘southampton’s’ or st albans or stoke or ‘stoke’s’ or sunderland or ‘sunderland’s’ or truro or ‘truro’s’ or wakefield or ‘wakefield’s’ or wells or westminster or ‘westminster’s’ or winchester or ‘winchester’s’ or wolverhampton or ‘wolverhampton’s’ or (worcester not (massachusetts* or boston* or harvard*)) or (‘worcester’s’ not (massachusetts* or boston* or harvard*)) or (york not (‘new york*’ or ny or ontario* or ont or toronto*)) or (‘york’s’ not (‘new york*’ or ny or ontario* or ont or toronto*))))).ti,ab,in. | 1,514,463 |
27 | (bangor or ‘bangor’s’ or cardiff or ‘cardiff’s’ or newport or ‘newport’s’ or st asaph or ‘st asaph’s’ or st davids or swansea or ‘swansea’s’).ti,ab,in. | 60165 |
28 | (aberdeen or ‘aberdeen’s’ or dundee or ‘dundee’s’ or edinburgh or ‘edinburgh’s’ or glasgow or ‘glasgow’s’ or inverness or (perth not australia*) or (‘perth’s’ not australia*) or stirling or ‘stirling’s’).ti,ab,in. | 223,983 |
29 | (armagh or ‘armagh’s’ or belfast or ‘belfast’s’ or lisburn or ‘lisburn’s’ or londonderry or ‘londonderry’s’ or derry or ‘derry’s’ or newry or ‘newry’s’).ti,ab,in. | 28,507 |
30 | or/22-29 | 2,749,551 |
31 | (exp africa/ or exp americas/ or exp antarctic regions/ or exp arctic regions/ or exp asia/ or exp oceania/) not (exp great britain/ or europe/) | 3,021,384 |
32 | 30 not 31 | 2,615,096 |
33 | 21 and 32 | 10 |
34 | (europe* or austria* or belgium* or ‘czech republic*’ or france* or paris* or germany* or berlin* or ireland* or greece* or athens* or hungary* or italy* or rome* or netherlands* or luxembourg* or poland* or portugal* or scandinav* or denmark* or estonia* or finland* or iceland* or norway* or sweden* or ‘slovak republic*’ or slovenia* or spain* or switzerland* or turkey* or israel*).ti,ab,tw. | 905,468 |
35 | 21 and 34 | 17 |
36 | 33 or 35 | 23 |
MEDLINE UK search filter: Ayiku et al. 195 Copyright © Health Libraries Group (2017).
EMBASE 1974–10 June 2021 (searched via the Ovid SP platform)
11 June 2021
# | Searches | Results |
---|---|---|
1 | (carbapenem-resistan* or ‘carbapenem resistan*’ or carbapenemase).tw. | 13,503 |
2 | (carbapenem* and (non-susceptib* or ‘non susceptib*’ or nonsusceptib*)).tw. | 1006 |
3 | (oxa-48* or ‘oxa 48*’ or oxacillinase-48* or ‘oxacillinase 48*’ or blaoxa-48* or ‘blaoxa 48*’).tw. | 2084 |
4 | ((‘new delhi’ or ndm or ‘verona integrated-encoded’ or vim or imipenemase or imp) and (mbl or ‘metallo-beta-lactamase’ or ‘metallo beta lactamase’)).tw. | 2210 |
5 | or/1-4 | 15,369 |
6 | (cohort* or longitudinal or prospective or retrospective or follow-up or ‘follow up’ or long-term or ‘long term’ or year).tw. | 6,159,657 |
7 | (mortality or death* or survival).tw. | 3,257,266 |
8 | urinary tract infection/ | 108,436 |
9 | urinary tract infection*.tw. | 63,504 |
10 | (uti or utis or cuti or cutis).tw. | 29,713 |
11 | exp pneumonia/ | 330,487 |
12 | pneumon*.tw. | 280,722 |
13 | exp intensive care unit/ | 217,620 |
14 | ((hospital* or ventilator* or icu or intensive care) adj3 (acquired or associat*)).tw. | 75,142 |
15 | ventilator associated pneumonia/ | 11,398 |
16 | (hap or vap).tw. | 14,412 |
17 | (11 or 12) and (13 or 14) | 37,422 |
18 | 8 or 9 or 10 or 15 or 16 or 17 | 175,174 |
19 | 5 and 6 and 7 and 18 | 413 |
20 | limit 19 to english language | 400 |
21 | limit 20 to yr=‘2010 -Current’ | 386 |
22 | United Kingdom/ | 391,825 |
23 | (english not ((published or publication* or translat* or written or language* or speak* or literature or citation*) adj5 english)).ti,ab. | 48,212 |
24 | (gb or ‘g.b’. or britain* or (british* not ‘british columbia’) or uk or ‘u.k’. or united kingdom* or (england* not ‘new england’) or northern ireland* or northern irish* or scotland* or scottish* or ((wales or ‘south wales’) not ‘new south wales’) or welsh*).ti,ab,jx,in,ad. | 3,336,942 |
25 | (bath or ‘bath’s’ or ((birmingham not alabama*) or (‘birmingham’s’ not alabama*) or bradford or ‘bradford’s’ or brighton or ‘brighton’s’ or bristol or ‘bristol’s’ or carlisle* or ‘carlisle’s’ or (cambridge not (massachusetts* or boston* or harvard*)) or (‘cambridge’s’ not (massachusetts* or boston* or harvard*)) or (canterbury not zealand*) or (‘canterbury’s’ not zealand*) or chelmsford or ‘chelmsford’s’ or chester or ‘chester’s’ or chichester or ‘chichester’s’ or coventry or ‘coventry’s’ or derby or ‘derby’s’ or (durham not (carolina* or nc)) or (‘durham’s’ not (carolina* or nc)) or ely or ‘ely’s’ or exeter or ‘exeter’s’ or gloucester or ‘gloucester’s’ or hereford or ‘hereford’s’ or hull or ‘hull’s’ or lancaster or ‘lancaster’s’ or leeds* or leicester or ‘leicester’s’ or (lincoln not nebraska*) or (‘lincoln’s’ not nebraska*) or (liverpool not (new south wales* or nsw)) or (‘liverpool’s’ not (new south wales* or nsw)) or ((london not (ontario* or ont or toronto*)) or (‘london’s’ not (ontario* or ont or toronto*)) or manchester or ‘manchester’s’ or (newcastle not (new south wales* or nsw)) or (‘newcastle’s’ not (new south wales* or nsw)) or norwich or ‘norwich’s’ or nottingham or ‘nottingham’s’ or oxford or ‘oxford’s’ or peterborough or ‘peterborough’s’ or plymouth or ‘plymouth’s’ or portsmouth or ‘portsmouth’s’ or preston or ‘preston’s’ or ripon or ‘ripon’s’ or salford or ‘salford’s’ or salisbury or ‘salisbury’s’ or sheffield or ‘sheffield’s’ or southampton or ‘southampton’s’ or st albans or stoke or ‘stoke’s’ or sunderland or ‘sunderland’s’ or truro or ‘truro’s’ or wakefield or ‘wakefield’s’ or wells or westminster or ‘westminster’s’ or winchester or ‘winchester’s’ or wolverhampton or ‘wolverhampton’s’ or (worcester not (massachusetts* or boston* or harvard*)) or (‘worcester’s’ not (massachusetts* or boston* or harvard*)) or (york not (‘new york*’ or ny or ontario* or ont or toronto*)) or (‘york’s’ not (‘new york*’ or ny or ontario* or ont or toronto*))))).ti,ab,in,ad. | 2,582,812 |
26 | (bangor or ‘bangor’s’ or cardiff or ‘cardiff’s’ or newport or ‘newport’s’ or st asaph or ‘st asaph’s’ or st davids or swansea or ‘swansea’s’).ti,ab,in,ad. | 105817 |
27 | (aberdeen or ‘aberdeen’s’ or dundee or ‘dundee’s’ or edinburgh or ‘edinburgh’s’ or glasgow or ‘glasgow’s’ or inverness or (perth not australia*) or (‘perth’s’ not australia*) or stirling or ‘stirling’s’).ti,ab,in,ad. | 355745 |
28 | (armagh or ‘armagh’s’ or belfast or ‘belfast’s’ or lisburn or ‘lisburn’s’ or londonderry or ‘londonderry’s’ or derry or ‘derry’s’ or newry or ‘newry’s’).ti,ab,in,ad. | 48,430 |
29 | or/22-28 | 4,048,950 |
30 | (exp ‘arctic and antarctic’/ or exp oceanic regions/ or exp western hemisphere/ or exp africa/ or exp asia/) not (united kingdom/ or europe/) | 3,102,680 |
31 | 29 not 30 | 3,833,270 |
32 | 21 and 31 | 25 |
33 | (europe* or austria* or belgium* or ‘czech republic*’ or france* or paris* or germany* or berlin* or ireland* or greece* or athens* or hungary* or italy* or rome* or netherlands* or luxembourg* or poland* or portugal* or scandinav* or denmark* or estonia* or finland* or iceland* or norway* or sweden* or ‘slovak republic*’ or slovenia* or spain* or switzerland* or turkey* or israel*).ti,ab,tw. | 1,633,082 |
34 | 21 and 33 | 52 |
35 | 32 or 34 | 72 |
EMBASE UK search filter: Ayiku et al. 196 Copyright © Health Libraries Group (2019).
CRD database (searched via the University of York CRD platform)
11 June 2021
# | Searches | Results |
---|---|---|
1 | ((carbapenem-resistan* or ‘carbapenem resistan*’ or carbapenemase)) | 5 |
2 | ((carbapenem* and (non-susceptib* or ‘non susceptib*’ or nonsusceptib*))) | 0 |
3 | ((oxa-48* or ‘oxa 48*’ or oxacillinase-48* or ‘oxacillinase 48*’ or blaoxa-48* or ‘blaoxa 48*’)) | 0 |
4 | (((‘new delhi’ or ndm or ‘verona integrated-encoded’ or vim or imipenemase or imp) and (mbl or ‘metallo-beta-lactamase’ or ‘metallo beta lactamase’))) | 0 |
5 | ((cohort* or longitudinal or prospective or retrospective or follow-up or ‘follow up’ or long-term or ‘long term’ or year)) | 29,687 |
6 | ((mortality or death* or survival)) | 16,968 |
7 | #1 AND #5 AND #6 | 0 |
Focused clinical outcomes search
Search terms adapted from Bassetti et al. (2021): Sites (UTI/HAPVAP) AND (inappropriate OR appropriate antibiotics)/susceptibility AND hospitalisation AND filter.
Filters: UK.
Limits: MEDLINE only, 2007–present.
Ovid MEDLINE(R) and Epub Ahead of Print, In-Process, In-Data-Review and Other Non-Indexed Citations, Daily and Versions(R) 1946–30 June 2021 (searched via the Ovid SP platform)
1 July 2021
# | Searches | Results |
---|---|---|
1 | urinary tract infection/ | 40,171 |
2 | urinary tract infection*.tw. | 42,550 |
3 | (uti or utis or cuti or cutis).tw. | 17,530 |
4 | exp pneumonia/ | 182,723 |
5 | pneumon*.tw. | 202,985 |
6 | exp intensive care unit/ | 91,779 |
7 | ((hospital* or ventilator* or icu or intensive care) adj3 (acquired or associat*)).tw. | 49,262 |
8 | ventilator associated pneumonia/ | 3730 |
9 | (hap or vap).tw. | 10,187 |
10 | (4 or 5) and (6 or 7) | 17,538 |
11 | 1 or 2 or 3 or 8 or 9 or 10 | 91,372 |
12 | ((inappropriat$ or inadequat$ or ineffectiv$ or discordan$ or incorrect$ or appropriat$ or adequate$ or concordan$) and (antibiotic$ or anti-biotic$ or AM$ or anti-microbial$ or antibacterial$ or anti-bacterial$ or bacteriocid$ or antimycobacterial$ or anti-mycobacterial$)).ti. | 1302 |
13 | ((inappropriat$ or inadequat$ or ineffectiv$ or discordan$ or incorrect$ or appropriat$ or adequate$ or concordan$) adj3 (antibiotic$ or anti-biotic$ or AM$ or anti-microbial$ or antibacterial$ or anti-bacterial$ or bacteriocid$ or antimycobacterial$ or anti-mycobacterial$)).ab,kf. | 16,750 |
14 | 12 or 13 | 17,382 |
15 | exp Hospitalization/ | 259,764 |
16 | exp Hospitals/ or exp Hospital Units/ | 395,569 |
17 | (hospital$ or inhospital$).ti,ab,kf,hw. | 1,709,507 |
18 | secondary care/ or tertiary healthcare/ or ((secondary or tertiary) adj (care or healthcare or health care)).ti,ab,kf. | 61,580 |
19 | (ward or wards or infirmary or infirmaries).ti,ab,kf. | 67,375 |
20 | (inpatient$ or in-patient).ti,ab,kf. | 184,282 |
21 | (ER or ERs or emergency room$1 or emergency department$1 or ED or EDs or casualty department$1 or ‘accident and emergency’ or ‘A&E’ or ‘A & E’ or triage).ti,ab,kf. | 316,488 |
22 | (admission$1 or admitted$1 or readmission$1 or readmitted$1).ti,ab,kf. | 424,729 |
23 | (nosocomial or healthcare associated or health care associated or ventilator associated).ti,ab,kf. | 45,058 |
24 | exp Critical Care/ | 61,100 |
25 | exp Intensive Care Units/ | 91,779 |
26 | (acute care or critical care or critically ill or critical illness$).ti,ab,kf. | 106,880 |
27 | (high dependency adj2 (care or unit$1)).ti,ab,kf. | 955 |
28 | intensive care.ti,ab,kf. | 161,143 |
29 | intensive therapy unit$1.ti,ab,kf. | 646 |
30 | recovery room$.ti,ab,kf. | 3442 |
31 | (ITU or ICU or CCU or CICU or CITU or HDU or ITUs or ICUs or CCUs or CICUs or CITUs or HDUs).ti,ab,kf. | 71,336 |
32 | (level 2 care or level 3 care or level two care or level three care).ti,ab,kf. | 41 |
33 | or/15-32 | 2,397,151 |
34 | 11 and 14 and 33 | 1226 |
35 | limit 34 to yr=‘2007 -Current’ | 889 |
36 | exp Great Britain/ | 375,996 |
37 | (national health service* or nhs*).ti,ab,in. | 222,142 |
38 | (english not ((published or publication* or translat* or written or language* or speak* or literature or citation*) adj5 english)).ti,ab. | 40,948 |
39 | (gb or ‘g.b’. or britain* or (british* not ‘british columbia’) or uk or ‘u.k’. or united kingdom* or (england* not ‘new england’) or northern ireland* or northern irish* or scotland* or scottish* or ((wales or ‘south wales’) not ‘new south wales’) or welsh*).ti,ab,jw,in. | 2,194,256 |
40 | (bath or ‘bath’s’ or ((birmingham not alabama*) or (‘birmingham’s’ not alabama*) or bradford or ‘bradford’s’ or brighton or ‘brighton’s’ or bristol or ‘bristol’s’ or carlisle* or ‘carlisle’s’ or (cambridge not (massachusetts* or boston* or harvard*)) or (‘cambridge’s’ not (massachusetts* or boston* or harvard*)) or (canterbury not zealand*) or (‘canterbury’s’ not zealand*) or chelmsford or ‘chelmsford’s’ or chester or ‘chester’s’ or chichester or ‘chichester’s’ or coventry or ‘coventry’s’ or derby or ‘derby’s’ or (durham not (carolina* or nc)) or (‘durham’s’ not (carolina* or nc)) or ely or ‘ely’s’ or exeter or ‘exeter’s’ or gloucester or ‘gloucester’s’ or hereford or ‘hereford’s’ or hull or ‘hull’s’ or lancaster or ‘lancaster’s’ or leeds* or leicester or ‘leicester’s’ or (lincoln not nebraska*) or (‘lincoln’s’ not nebraska*) or (liverpool not (new south wales* or nsw)) or (‘liverpool’s’ not (new south wales* or nsw)) or ((london not (ontario* or ont or toronto*)) or (‘london’s’ not (ontario* or ont or toronto*)) or manchester or ‘manchester’s’ or (newcastle not (new south wales* or nsw)) or (‘newcastle’s’ not (new south wales* or nsw)) or norwich or ‘norwich’s’ or nottingham or ‘nottingham’s’ or oxford or ‘oxford’s’ or peterborough or ‘peterborough’s’ or plymouth or ‘plymouth’s’ or portsmouth or ‘portsmouth’s’ or preston or ‘preston’s’ or ripon or ‘ripon’s’ or salford or ‘salford’s’ or salisbury or ‘salisbury’s’ or sheffield or ‘sheffield’s’ or southampton or ‘southampton’s’ or st albans or stoke or ‘stoke’s’ or sunderland or ‘sunderland’s’ or truro or ‘truro’s’ or wakefield or ‘wakefield’s’ or wells or westminster or ‘westminster’s’ or winchester or ‘winchester’s’ or wolverhampton or ‘wolverhampton’s’ or (worcester not (massachusetts* or boston* or harvard*)) or (‘worcester’s’ not (massachusetts* or boston* or harvard*)) or (york not (‘new york*’ or ny or ontario* or ont or toronto*)) or (‘york’s’ not (‘new york*’ or ny or ontario* or ont or toronto*))))).ti,ab,in. | 1,520,233 |
41 | (bangor or ‘bangor’s’ or cardiff or ‘cardiff’s’ or newport or ‘newport’s’ or st asaph or ‘st asaph’s’ or st davids or swansea or ‘swansea’s’).ti,ab,in. | 60,441 |
42 | (aberdeen or ‘aberdeen’s’ or dundee or ‘dundee’s’ or edinburgh or ‘edinburgh’s’ or glasgow or ‘glasgow’s’ or inverness or (perth not australia*) or (‘perth’s’ not australia*) or stirling or ‘stirling’s’).ti,ab,in. | 224,761 |
43 | (armagh or ‘armagh’s’ or belfast or ‘belfast’s’ or lisburn or ‘lisburn’s’ or londonderry or ‘londonderry’s’ or derry or ‘derry’s’ or newry or ‘newry’s’).ti,ab,in. | 28,660 |
44 | or/36-43 | 2,757,556 |
45 | (exp africa/ or exp americas/ or exp antarctic regions/ or exp arctic regions/ or exp asia/ or exp oceania/) not (exp great britain/ or europe/) | 3,038,160 |
46 | 45 not 44 | 2,902,099 |
47 | 35 and 46 | 172 |
48 | (susceptib$ and (antibiotic$ or anti-biotic$ or AM$ or anti-microbial$ or antibacterial$ or anti-bacterial$ or bacteriocid$ or antimycobacterial$ or anti-mycobacterial$)).ti. | 10,075 |
49 | (susceptib$ adj3 (antibiotic$ or anti-biotic$ or AM$ or anti-microbial$ or antibacterial$ or anti-bacterial$ or bacteriocid$ or antimycobacterial$ or anti-mycobacterial$)).ab,kf. | 27,690 |
50 | 48 or 49 | 32,247 |
51 | 11 and 33 and 50 | 1563 |
52 | 46 and 51 | 520 |
53 | limit 52 to yr=‘2007 -Current’ | 425 |
Utilities search: Charlson Comorbidity Index
Search terms: CCI and utility filter.
Filters: Health State Utility Value filter by Arber et al. (2017).
Limits: MEDLINE, English language.
Ovid MEDLINE(R) and Epub Ahead of Print, In-Process, In-Data-Review and Other Non-Indexed Citations, Daily and Versions(R) 1946–12 July 2021
13 July 2021
# | Searches | Results |
---|---|---|
1 | Quality-Adjusted Life Years/ | 13,500 |
2 | Value of Life/ | 5752 |
3 | (qaly* or qald* or qale* or qtime*).ti,ab,kf. | 12,063 |
4 | (quality adjusted or adjusted life year*).ti,ab,kf. | 18,964 |
5 | disability adjusted life.ti,ab,kf. | 3946 |
6 | daly*1.ti,ab,kf. | 3468 |
7 | ((index adj3 wellbeing) or (quality adj3 wellbeing) or qwb).ti,ab,kf. | 868 |
8 | (multiattribute* or multi attribute*).ti,ab,kf. | 1013 |
9 | (utility adj3 (score*1 or scoring or valu* or measur* or evaluat* or scale*1 or instrument*1 or weight or weights or weighting or information or data or unit or units or health* or life or estimat* or elicit* or disease* or mean or cost* or expenditure*1 or gain or gains or loss or losses or lost or analysis or index* or indices or overall or reported or calculat* or range* or increment* or state or states or status)).ti,ab,kf. | 37,081 |
10 | utility.ab./freq = 2 | 19,465 |
11 | utilities.ti,ab,kf. | 7876 |
12 | disutili*.ti,ab,kf. | 515 |
13 | (HSUV or HSUVs).ti,ab,kf. | 84 |
14 | health*1 year*1 equivalent*1.ti,ab,kf. | 40 |
15 | (hye or hyes).ti,ab,kf. | 75 |
16 | (hui or hui1 or hui2 or hui3).ti,ab,kf. | 1679 |
17 | (illness state*1 or health state*1).ti,ab,kf. | 7144 |
18 | (euro qual or euro qual5d or euro qol5d or eq-5d or eq5-d or eq5d or euroqual or euroqol or euroqual5d or euroqol5d).ti,ab,kf. | 12,834 |
19 | (eq-sdq or eqsdq).ti,ab,kf. | 1 |
20 | (short form* or shortform*).ti,ab,kf. | 37,135 |
21 | (sf36* or sf 36* or sf thirtysix or sf thirty six).ti,ab,kf. | 23,718 |
22 | (sf6 or sf 6 or sf6d or sf 6d or sf six or sfsix or sf8 or sf 8 or sf eight or sfeight).ti,ab,kf. | 3519 |
23 | (sf12 or sf 12 or sf twelve or sftwelve).ti,ab,kf. | 5294 |
24 | (sf16 or sf 16 or sf sixteen or sfsixteen).ti,ab,kf. | 30 |
25 | (sf20 or sf 20 or sf twenty or sftwenty).ti,ab,kf. | 344 |
26 | (15D or 15-D or 15 dimension).ti,ab,kf. | 5601 |
27 | (standard gamble* or sg).ti,ab,kf. | 11,912 |
28 | (time trade off*1 or time tradeoff*1 or tto or timetradeoff*1).ti,ab,kf. | 2046 |
29 | or/1-28 | 160,013 |
30 | (‘charlson comorbidity index’ or ‘charlson index’ or (cci and (comorbid* or ‘co morbid*’ or multimorbid* or ‘multi morbid*’))).mp. | 8444 |
31 | 29 and 30 | 387 |
32 | limit 31 to english language | 368 |
Appendix 2 Risk of bias assessment tool
Table 40 shows the bespoke risk of bias assessment tool developed as part of this project to assess the in vitro susceptibility studies.
Questions | Score Low risk Unclear risk High risk |
---|---|
1. Target population | |
Is the target population of the study broadly appropriate to the HVCS? Consider:
|
|
Were isolates selected based on resistance to comparators?
|
|
Was there appropriate inclusion or exclusion of isolates with co-carriage of other significant mechanisms, as per HVCS?
|
|
Were all isolates tested for the pathogen mechanism of interest in a standard way, and does this match the HVCS?
|
|
Was the beta-lactamase test appropriate?
|
|
Were data collected over an appropriate time period? Consider
|
|
Target population overall judgement
|
|
2. Sampling strategy | |
Were isolates sampled from the target population in an appropriate way?
|
|
NB
|
|
Sampling strategy overall judgement If any item scores high risk or unclear risk, the overall judgement should be high or unclear risk, respectively If all items score low risk, the overall judgement should be low risk |
|
3. Outcome measurement | |
Was susceptibility measured in an appropriate, standard way? Consider:
|
|
|
|
|
|
Does the study demonstrate selective analysis reporting, with respect to S, I and R? Susceptibility testing reports either S, I and R, or where no I category is defined by the guideline group, just S and R. Selective analysis reporting may occur where I is reported as S or R inappropriately for all treatments. Inappropriate would depend on the review question, in our context this would be to report I and S as one category. |
|
Were S, I and R reported consistently for all treatments?
|
|
Outcome measurement overall judgement
|
|
4. Missing data | |
Is there a risk of bias from missing data? Were all isolates tested for all treatments? Where this isn’t the case, is it likely that missingness was associated with treatment outcome? Where some isolates were not tested for some treatments, and reasons were not provided, score unclear risk of bias. Where some isolates were not tested for some treatments, and the reasons for this were due to expected susceptibility, score high risk of bias. |
|
Missing data overall judgement
|
Appendix 3 Reviews 1 and 2
Review 1: randomised controlled trials
Based on RCT evidence, what is the comparative effectiveness of the intervention and comparators in patients with cUTI or HAP/VAP caused by Enterobacterales or P. aeruginosa MBL infections?
Of the 5313–15,33–81,199 studies included in the key characteristics mapping, three were RCTs (APEKS cUTI,13 APEKS NP,14 CREDIBLE CR15). Details of these three RCTs were examined against the inclusion and exclusion criteria presented in the PICOS (see Table 2 of the main report). All three RCTs recruited patients with infections caused by GNO. In APEKs-cUTI13 these were described as patients at risk of MDR Enterobacterales and P. aeruginosa, in APEKs-NP14 infections caused by any pathogen were eligible, while in CREDIBLE-CR15 patients with known carbapenem-resistant infections were included. The comparator in the three trials varied, including imipenem-cilastatin (APEKs-cUTI13), meropenem (APEKs-NP14) and BST [standard of care with either a polymyxin-based or non-polymyxin-based regimen as determined by the investigator and consisting of 1–3 marketed antibacterial agent(s) (CREDIBLE-CR15)]. APEKS cUTI13 and APEKS NP14 aimed to recruit patients who were expected to be responsive to the study treatments, based on the treating physician’s judgement or known susceptibility, and since both included carbapenems within the comparator arm, patients with known CR infections were excluded from these two trials. Outcome data were not reported for MBLs separately (APEKs-cUTI,13 APEKs-NP14), meaning these trials have low relevance to the HVCSs.
The third trial (CREDIBLE-CR15) had an objective to provide evidence potentially relating to the target population (MBLs), since it recruited those with a CR infection. Although clinical outcomes for the sites of interest were presented separately, the sample size was small (n = 59 NP and n = 22 cUTI) and provided only data relevant to the microbiology setting in that all pathogens were susceptible to cefiderocol. In their company submission (see section 2.3.4.1 of the company submission),80 Shionogi presented numerical data on all-cause mortality for MBL patients in CREDBLE-CR. 15 However, these data were based on a small number of patients (16 in the cefiderocol arm, 7 in the BST arm) and were therefore not used in the evaluation due to the chance of baseline imbalances introducing bias. Consequently, across the trials the populations were largely carbapenem-susceptible infections, or were based on very small numbers in non-stratified subgroups, and therefore had low relevance or low quality with respect to the HVCSs.
Although the RCTs have low relevance to the HVCSs due to the low numbers of CR infections, it is important to establish that cefiderocol is an effective treatment in the sites of interest (HAP/VAP and cUTI). The three trials (APEKS NP,14 APEKS cUTI,13 CREDIBLE CR15) at these sites reported similar or non-inferior efficacy (see Table 41) between cefiderocol and comparator arms, as determined by the primary outcome measure [composite of clinical and microbiological response (CREDIBLE-CR15)]; 14-day all-cause mortality (APEKS NP14) or clinical cure (APEKS cUTI13). The safety of cefiderocol is addressed by Review 6 (see Clinical evidence).
Author (date) acronym | Country | Total N | Intervention (n), comparator (n) | Site of infection | Pathogen(s) | MBL patients | Limitations in terms of HVCS | Data for Q3b?a | Primary outcome |
---|---|---|---|---|---|---|---|---|---|
Portsmouth et al. (2018) APEKs-cUTI13 | Multicentre; multinational; not UK | 452 | Cefiderocol (n = 303) imipenem/cilastatin (n = 149) | cUTI | MDR GNO; EC; KP; P. aeruginosa; Proteus mirabilis; Enterobacter clocae complex; others | N/R | CR patients were excluded. | N/R | Composite of clinical and microbiological outcomes at ToC Cefiderocol: 183/252 (73%) Imipenem-cilastatin: 65/119 (55%) Adjusted treatment difference 18.58% (95% CI 8.23 to 28.92; p = 0.0004) |
Wunderink et al. (2021) APEKs-NP14 | Multicentre; multinational; | 300 | Cefiderocol (n = 148), meropenem (n = 152) | HAP/VAP/HCAP, cUTI or BSI/sepsis | GNO; Any eligible: A. baumannii; K. pneumoniae; P. aeruginosa; S. maltophilia; Acinetobacter nosocomialis; Enterobacter cloacae; E. coli | N/R | CR patients were excluded. | Meropenem non-susceptible data. | 14-day all-cause mortality Cefiderocol: 18/145 (12.4%) Meropenem: 17/146 (11.6%) Adjusted treatment difference 0.8%, 95% CI –6.6 to 8.2; p = 0.002 |
Bassetti et al. (2019, 2020) CREDIBLE-CR15 | Multicentre; multinational; including Europe | 152 | Cefiderocol (n = 101), BAT (n = 51)b | HAP/VAP/HCAP, cUTI or BSI/sepsis | GNO; Any eligible: A. baumannii; K. pneumoniae; P. aeruginosa; S. maltophilia; Acinetobacter nosocomialis; Enterobacter cloacae; E. coli | Cefiderocol (n = 16); BAT (n = 7) Includes IMP cefiderocol (n = 2); BAT (n = 3) NDM cefiderocol (n = 10); BAT (n = 5) VIM cefiderocol (n = 4); BAT (n = 0). | Only relevant to the MD setting – all pathogens susceptible to cefiderocol. Small samples in sites of interest (n = 59 in NP and n = 22 in cUTI) | N/R | CC at ToC (HAP/VAP/HCAP) Cefiderocol: 20/40 (50%; 33.8–66.2) BAT: 10/19 (53%; 28.9–75.6) CC at ToC (BSI/sepsis) Cefiderocol: 10/23 (43%; 23.2–65.5) BAT: 6/14 (43%; 17.7–71.1) MC at ToC (cUTI) Cefiderocol: 9/17 (53%; 27.8–77.0) BAT: 1/5 (20%; 0.5–71.6) |
Review 2: Observational studies
Based on observational studies, what is the comparative effectiveness of the intervention and comparators in patients with cUTI or HAP/VAP caused by Enterobacterales or P. aeruginosa MBL infections?
Since the RCTs13–15 did not recruit or report outcomes for subgroups of patients with Enterobacterales or P. aeruginosa MBL infections, and were largely in patients susceptible to carbapenems, Approach 2 was considered. Of the 5313–15,33–81,199 studies included in the key characteristics mapping, six were observational studies72–77 reporting treatment with cefiderocol. Details of these six studies72–77 were examined against the inclusion and exclusion criteria presented in the PICOS (see Table 2 of the main report). All six observational studies were excluded.
Table 42 presents the reasons for exclusion. Of the six72–77 observational studies, only three73,74,77 reported outcomes for patients with MBL infections. However, all reported infections across a range of sites, and it was not possible to separate out patients with cUTI or HAP/VAP. In addition, none of the studies reported data for a comparator, and as such it would have been necessary to obtain patient-level data for at least one study in order to perform any (adjusted) form of synthesis. Given the timescales of the project this could not be achieved. All studies were of a small sample size (range from n = 2–17 patients, with the majority including 10 or fewer patients) and were highly heterogeneous in terms of key characteristics that are prognostic and expected to modify treatment response (e.g. site, pathogen/bug, treatment line), limiting the conclusions that could be drawn from them and increasing the likely uncertainty associated with any synthesis performed.
Author (date) | Country | Site of infection | Intervention | Comparator | Pathogen(s) | Mechanism | Sample size | Reasons for exclusion |
---|---|---|---|---|---|---|---|---|
Falcone et al. (2020)73 | Italy | Bacteremia or VAP | Cefiderocol | No comparator | CR KP, AB, S. maltophilia |
AB + NDM-producing KP (n = 1) NDM-producing KP (n = 1) NDM-producing KP + S. malthophilia (n = 1) |
10 | Small case series |
Oliva et al. (2020)75 | Italy | VAP; BSI; spinal implant infection and lung empyema caused by MRSA | Cefiderocol | No comparator | XDR/PDR AB |
N/R | 3 | Small case series |
Shields et al. (2020)76 | USA | VAP; cholangitis | Cefiderocol | No comparator | CR PA |
N/R | 2 | Small case series |
Zingg et al. (2020)77 | Switzerland | Acute osteomyelitis; postoperative implant-associated surgical site infection; pleural empyema | Cefiderocol | No comparator | XDR GNO | Case 1: AB (OXA-23); E. cloacae (KPC); PA (VIM) Case 2: AB (OXA-40, NDM) Case 3: AB (OXA-23, OXA-58) |
3 | Small case series |
Bleibtreu et al. (2021)72 | France | Respiratory tract infections (RTI, n = 10); intra-abdominal (n = 2); osteo-articular (n = 2), skin-and-skin structure (n = 1), and urinary tract (n = 1) | Cefiderocol | No comparator | MDR GNO PA | Carbapenemase-producing PA (n = 9), AB (n = 2), KP (n = 1), and Enterobacter hormaechei (n = 1). | 12 | Case series; only 1 patient in site of interest |
Haller et al. (2019)74 | Germany | Six cases presented clinical symptoms (sepsis, pneumonia, UTI), 11 colonised | Cefiderocol | No comparator | KP (ST307) | OXA-48 (and NDM-1) | 17 | Case series; site N/R; 11 cases were colonised; most had severe underlying diseases |
Appendix 4 Susceptibility synthesis methods and sensitivity analysis results
Statistical model for the network meta-analysis
The data are presented as the total number susceptible rik out of the total number of isolates, nik, for patients arm k of study i. The data generation process is assumed to follow a Binomial likelihood such that,
where pikrepresents the probability of an event in arm k of trial i. The probabilities are modelled on the logit scale as
where the μi are trial-specific baselines, representing the log-odds of response in the baseline treatment. The trial-specific treatment effects, δi,1k are log-ORs of response for the treatment in arm k, relative to the baseline treatment.
For the REs model, the trial-specific treatment effects, δi,1k, are assumed to arise from a common REs distribution:
where dti1tik represents the mean effect of the treatment in arm k of study i,tik, compared to the treatment in arm 1 of study i,tik, and τ2 represents the between-study variance in treatment effects (heterogeneity) which is assumed to be the same for all treatments.
The model was completed by specifying prior distributions for the parameters. Wherle-wrape there were sufficient sample data, conventional non-informative prior distributions were used:
-
trial-specific baseline, μi~N(0,1002)
-
treatment effects relative to reference treatment, d1k~N(0,1002)
-
between-study SD of treatment effects, τ~U(0,3)
Summary of network meta-analyses
Table 43 summarises the measures of model fit for the NMAs.
Model description | Number of studies | Absolute model fit | Model comparison | Heterogeneity | |
---|---|---|---|---|---|
DP | TRD | DIC | SD (95 % CrI) | ||
SIDERO, fosfomycin and PHE studies | |||||
EUCAST breakpoint MBL Enterobacterales (base-case model) | 8 | 35 | 33.50 | 188.54 | 1.45 (0.93 to 2.35) |
EUCAST breakpoint MBL Enterobacterales (UME model) | 8 | 35 | 33.14 | 187.76 | 1.08 (0.67 to 1.82) |
EUCAST breakpoint P. aeruginosa MBL (base-case model) |
3 | 11 | 9.3 | 40.00 | 0.87 (0.04 to 2.76) |
Sensitivity analysis network meta-analysis results
European Committee on Antimicrobial Susceptibility Testing breakpoint, only studies that report cefiderocol data (SIDERO)
Metallo-beta-lactamases Enterobacterales using European Committee on Antimicrobial Susceptibility Testing breakpoints and only studies that report cefiderocol data (SIDERO)
Two studies34,35 contributed to the NMA of MBL Enterobacterales infections with EUCAST breakpoint for SIDERO studies only, considering a total of two comparators, and the full network diagram is shown in Figure 20.
FIGURE 20.
Network diagram of all studies contributing to the NMA (MBL Enterobacterales with EUCAST breakpoint for SIDERO studies only).
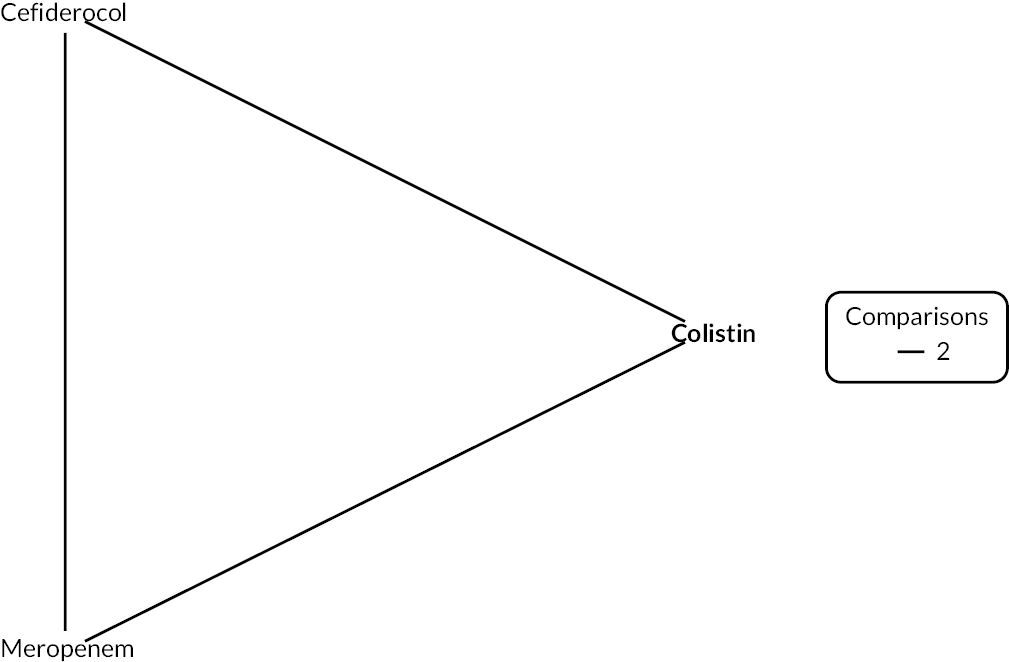
FIGURE 21.
Forest plot of OR vs. colistin for MBL Enterobacterales with EUCAST breakpoint (SIDERO studies only). PB, probability being the best treatment.

The relative susceptibility for each comparator relative to colistin is shown in Figure 21. The model fitted the data well, with a total residual deviance of 5.17, which was close to the number of data points included in the analysis of 6. The between-study SD was 0.55 (95% CrI 0.03 to 2.59), which indicates high heterogeneity. Cefiderocol was associated with a lower susceptibility relative to colistin (OR 0.33, 95% CrI 0.06 to 1.65), but the result was not statistically significant. Cefiderocol also had a 6% probability of being the most effective treatment; median rank 2. Meropenem was associated with lower susceptibility than colistin, and the result was not statistically significant. For all comparators the high between-study SD results in wide 95% PrI.
The sensitivity analysis restricting to comparators specific to the pathogen produced a very similar OR for cefiderocol (0.33, 95% CrI 0.039 to 2.916). A plot could not be generated for this analysis as, after removal of meropenem, only cefiderocol and colistin remained in the network.
Metallo-beta-lactamases P. aeruginosa using European Committee on Antimicrobial Susceptibility Testing breakpoints and only studies that report cefiderocol data (SIDERO)
Two studies contributed to the NMA of P. aeruginosa MBL infections with EUCAST breakpoint for SIDERO studies only,34,35 considering a total of 2 comparators, and the full network diagram is shown in Figure 22. One study (SIDERO WT data request) contained zero susceptibility counts and therefore had a continuity correction applied prior to synthesis.
FIGURE 22.
Network diagram of all studies contributing to the NMA (P. aeruginosa MBL with EUCAST breakpoint for SIDERO studies only).

The relative susceptibility for each comparator relative to colistin is shown in Figure 23. The model fitted the data well, with a total residual deviance of 4.38, which was close to the number of data points included in the analysis of 6. The between-study SD was 0.96 (95% CrI 0.04 to 2.82), which indicates high heterogeneity. Cefiderocol was associated with a lower susceptibility relative to colistin (OR 0.49, 95% CrI 0.03 to 5.29), but the result was not statistically significant. Cefiderocol also had a 24% probability of being the most effective treatment; median rank 2. Meropenem was associated with no susceptibility. For all comparators, the high between-study SD results in wide 95% PrI.
FIGURE 23.
Forest plot of OR vs. colistin for P. aeruginosa MBL with EUCAST breakpoint (SIDERO studies only). PB, probability being the best treatment.

The network for the sensitivity analysis restricting to comparators specific to the pathogen was identical to the original network, since there were no data for comparators not in-scope for the pathogen.
European Committee on Antimicrobial Susceptibility Testing breakpoint with fosfomycin studies only
Five studies80–82,83–89,92–94 contributed to the NMA of MBL Enterobacterales infections with EUCAST breakpoint for fosfomycin studies only, considering a total of seven comparators, and the full network diagram is shown in Figure 24. Two studies84,86 contained 100% susceptibility counts for one or more of the included comparators and therefore had a continuity correction applied prior to synthesis.
FIGURE 24.
Network diagram of all studies contributing to the NMA (MBL Enterobacterales with EUCAST breakpoint for fosfomycin studies only).

The relative susceptibility for each comparator relative to colistin is shown in Figure 25a. The model fitted the data well, with a total residual deviance of 21.37, which was close to the number of data points included in the analysis of 23. The between-study SD was 2.04 (95% CrI 1.20 to 2.91), which indicates extremely high heterogeneity. Fosfomycin was associated with a lower susceptibility relative to colistin (OR 0.24, 95% CrI 0.02 to 3.09), but the result was not statistically significant. Fosfomycin also had a 10% probability of being the most effective treatment; median rank 2. The remainder of the treatments were associated with lower susceptibility than colistin, and the results were not statistically significant. For all comparators, the high between-study SD results in wide 95% PrI.
FIGURE 25.
Forest plot of OR vs. colistin for MBL Enterobacterales with EUCAST breakpoint (fosfomycin studies only). (a) All comparators; and (b) only comparators specific to the pathogen. PB, probability being the best treatment.

The sensitivity analysis restricting to comparators specific to the pathogen produced a very similar OR for fosfomycin (0.23, 95% CrI 0.02 to 2.36). The plot is displayed in Figure 25b.
There was only one study88 included in the NMA of P. aeruginosa MBL infections with EUCAST breakpoint for fosfomycin studies only. No synthesis was performed.
Clinical Laboratory Standards Institute breakpoints sensitivity analysis
Appendix 4, Metallo-beta-lactamasesEnterobacteralesnetwork including all studies using Clinical Laboratory Standards Institute breakpoints details the Enterobacterales network, while Appendix 4, Metallo-beta-lactamasesP. aeruginosanetwork including all studies using Clinical Laboratory Standards Institute breakpoints details the P. aeruginosa network.
Metallo-beta-lactamases Enterobacterales network including all studies using Clinical Laboratory Standards Institute breakpoints
Six studies34,35,78,89,90,91 contributed to the NMA of MBL Enterobacterales infections with CLSI breakpoint for SIDERO and fosfomycin studies, considering a total of eight comparators, and the full network diagram is shown in Figure 26. Four studies (SIDERO CR data request, Johnston 2020,78 Aires 201789 and Sonnevend 202090) contained either zero susceptibility counts for one or more of the included comparators and therefore had a continuity correction applied prior to synthesis.
FIGURE 26.
Network diagram of all studies contributing to the NMA (MBL Enterobacterales with CLSI breakpoint for SIDERO and fosfomycin studies).

The relative susceptibility for each comparator relative to colistin is shown in Figure 27. The model fitted the data well, with a total residual deviance of 32.81, which was close to the number of data points included in the analysis of 33. The between-study SD was 2.38 (95% CrI 1.70 to 2.96), which indicates extremely high heterogeneity. Cefiderocol was associated with a higher susceptibility relative to colistin (OR 5.11, 95% CrI 0.38 to 71.34), but the result was not statistically significant. Cefiderocol was also associated with a 58% probability of being the most effective treatment; median rank 1. The remainder of the treatments, expect for fosfomycin and tigecycline, were associated with a lower susceptibility. But none of the results were statistically significant. For all comparators, the high between-study SD results in wide 95% PrI.
FIGURE 27.
Forest plot of OR vs. colistin for MBL Enterobacterales with CLSI breakpoint (SIDERO and fosfomycin studies). PB, probability being the best treatment.

Inconsistency checking was performed using the UME model. The model fits the data well and the DIC was similar to the base-case NMA model (33 data points vs. 33.04 total residual deviance). The estimated between-study SD is slightly smaller from the UME model compared to the base-case NMA model, but it still indicates extremely high heterogeneity (SD: 1.93 with 95% CrI 1.14 to 2.89). The deviance plot (see Appendix 4, Summary of network meta-analyses) indicates that the colistin arm from Johnston (2020) has an improvement in the fit when using the UME model. Additional NMA was conducted excluding the colistin arm from Johnston (2020).
Six studies contributed to the NMA of MBL Enterobacterales infections with CLSI breakpoint for SIDERO and fosfomycin studies without Johnston (2020) colistin arm, considering a total of eight comparators, and the full network diagram is shown in Figure 28. Three studies (SIDERO CR data request, Aires 201789 and Sonnevend 202090) contained either zero susceptibility counts for one or more of the included comparators and therefore had a continuity correction applied prior to synthesis.
FIGURE 28.
Network diagram of all studies contributing to the NMA (MBL Enterobacterales with CLSI breakpoint for SIDERO and fosfomycin studies without Johnston 2020 colistin arm).
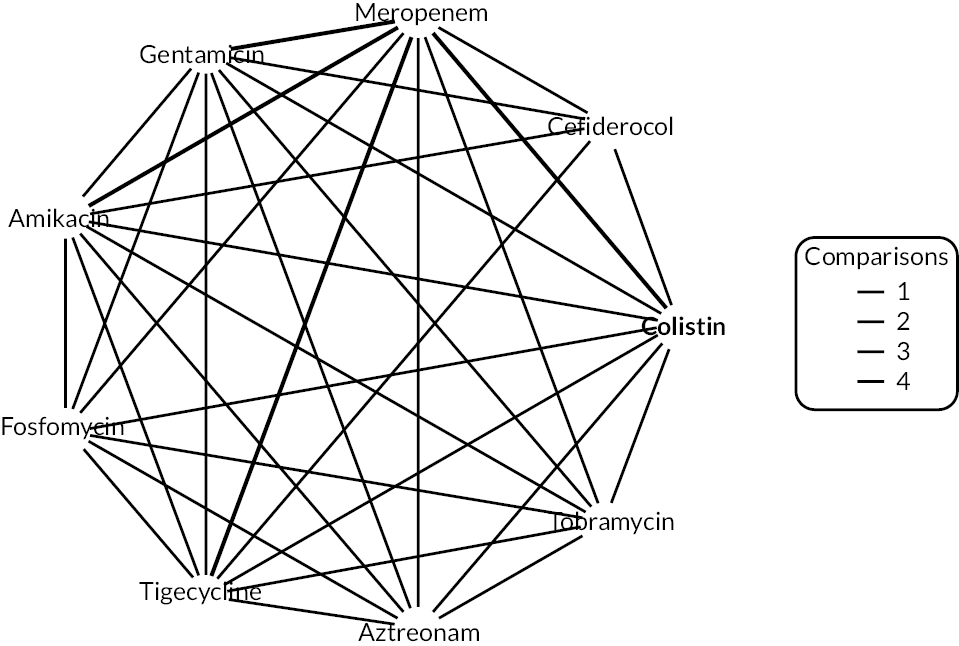
The relative susceptibility for each comparator relative to colistin is shown in Figure 29. The model fitted the data well, with a total residual deviance of 31.46 being close to the number of data points included in the analysis, which was 32. The between-study SD was 1.75 (95% CrI 1.14 to 2.67), which indicates extremely high heterogeneity. Cefiderocol was associated with a higher susceptibility relative to colistin (OR 1.38, 95% CrI 0.16 to 12.05), but the result was not statistically significant. Cefiderocol was also associated with a 50% probability of being the most effective treatment; median rank 1. The remainder of the treatments were associated with a lower susceptibility. But none of the results were statistically significant. For all comparators, the high between-study SD results in wide 95% PrI.
FIGURE 29.
Forest plot of OR vs. colistin for MBL Enterobacterales with CLSI breakpoint (SIDERO and fosfomycin studies without Johnston 2020 colistin arm). (a) All comparators, without Kohira (2016)80; (b) all comparators with Kohira (2016)80 included; and (c) only comparators specific to the pathogen. PB, probability being the best treatment.

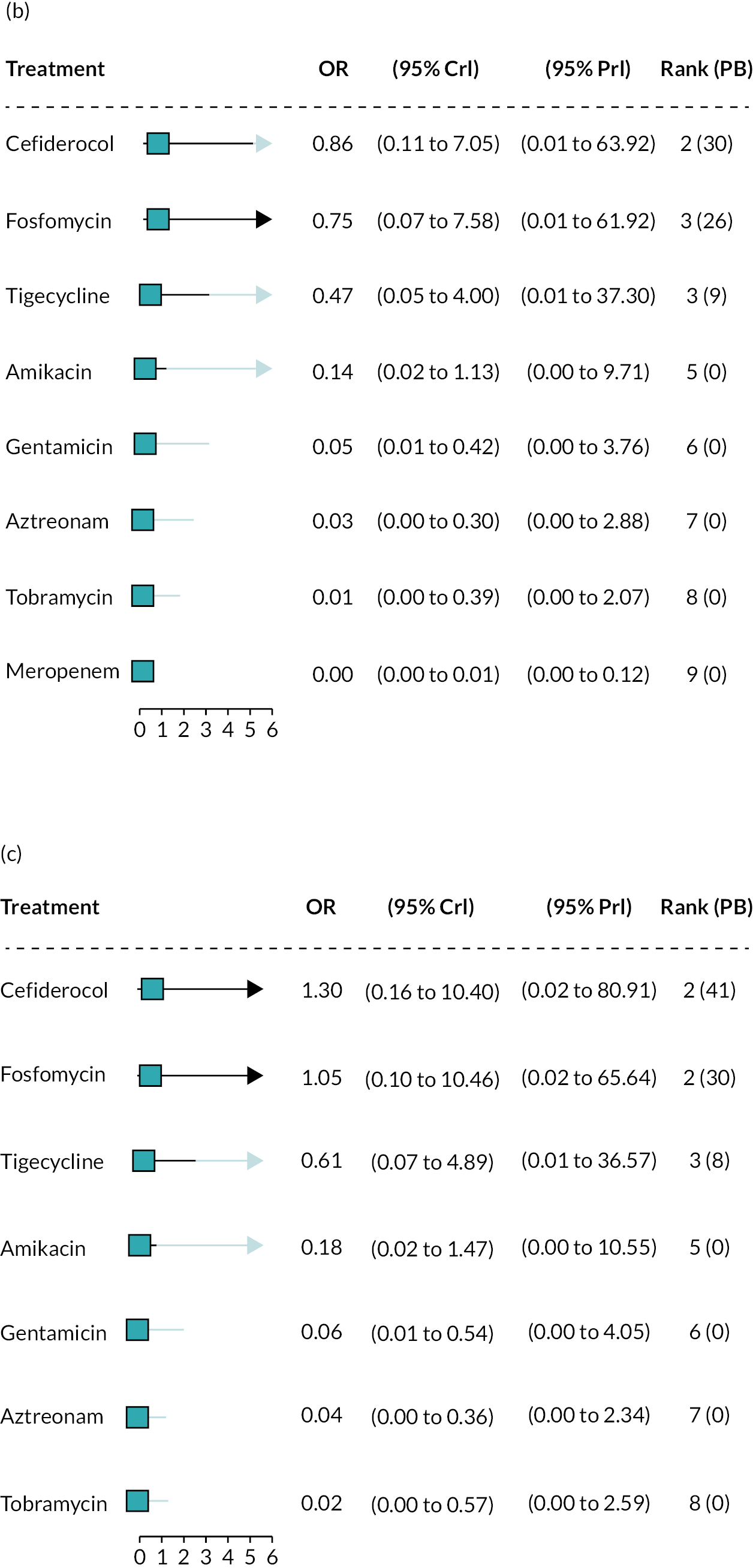
When the missing study Kohira (2016)94 was included in the analysis, the OR for cefiderocol was 0.86 (0.11 to 7.05) (see Figure 29b).
When the network was restricted to comparators specific to the pathogen, the OR for cefiderocol was very similar to the original analysis (OR 1.30, CrI 0.16 to 10.40), but fosfomycin’s OR indicated susceptibility higher relative to colistin, rather than lower (see Figure 29c).
Metallo-beta-lactamases P. aeruginosa network including all studies using Clinical Laboratory Standards Institute breakpoints (no data for PHE)
Three studies34,35,92 contributed to the NMA of P. aeruginosa MBL infections with CLSI breakpoint for SIDERO and fosfomycin studies, considering a total of five comparators, and the full network diagram is shown in Figure 30. All three studies contained either 0% or 100% susceptibility counts for one or more of the included comparators and therefore had a continuity correction applied prior to synthesis.
FIGURE 30.
Network diagram of all studies contributing to the NMA (P. aeruginosa MBL with CLSI breakpoint for SIDERO and fosfomycin studies).

The relative susceptibility for each comparator relative to colistin is shown in Figure 31. The model fitted the data well, with a total residual deviance of 9.23, which was close to the number of data points included in the analysis of 11. The between-study SD was 0.98 (95% CrI 0.04 to 2.82) which indicates high heterogeneity. Cefiderocol was associated with a statistically significant higher susceptibility relative to colistin (OR 71.34, 95% CrI 4.33 to 5934.35). Cefiderocol was also associated with a 99% probability of being the most effective treatment; median rank 1. The remainder of the treatments were associated with a lower susceptibility. But none of the results were statistically significant. For all treatments, the high between-study SD results in wide 95% PrI. The result for cefiderocol was still statistically significant using PrI.
FIGURE 31.
Forest plot of OR vs. colistin for P. aeruginosa MBL with CLSI breakpoint (SIDERO) and fosfomycin studies (CLSI data not reported for PHE). (a) All comparators; and (b) only comparators specific to the pathogen. PB, probability being the best treatment.

When the network was restricted to comparators specific to the pathogen, the OR for cefiderocol was similar to the original analysis (OR 64.19, CrI 4.28 to 3047.07), as were the ORs for the comparators.
Clinical Laboratory Standards Institute breakpoint including only studies reporting cefiderocol data
Metallo-beta-lactamases Enterobacterales, Clinical Laboratory Standards Institute breakpoints only studies that report cefiderocol data
Three studies34,35,78 contributed to the NMA of MBL Enterobacterales infections with CLSI breakpoint using only studies that report cefiderocol data, considering a total of five comparators, and the full network diagram is shown in Figure 32. Two studies (SDIERO CR data request, Johnston 2020)78 contained zero susceptibility counts for one or more of the included comparators and therefore had a continuity correction applied prior to synthesis.
FIGURE 32.
Network diagram of all studies contributing to the NMA (MBL Enterobacterales with CLSI breakpoint for SIDERO studies only).
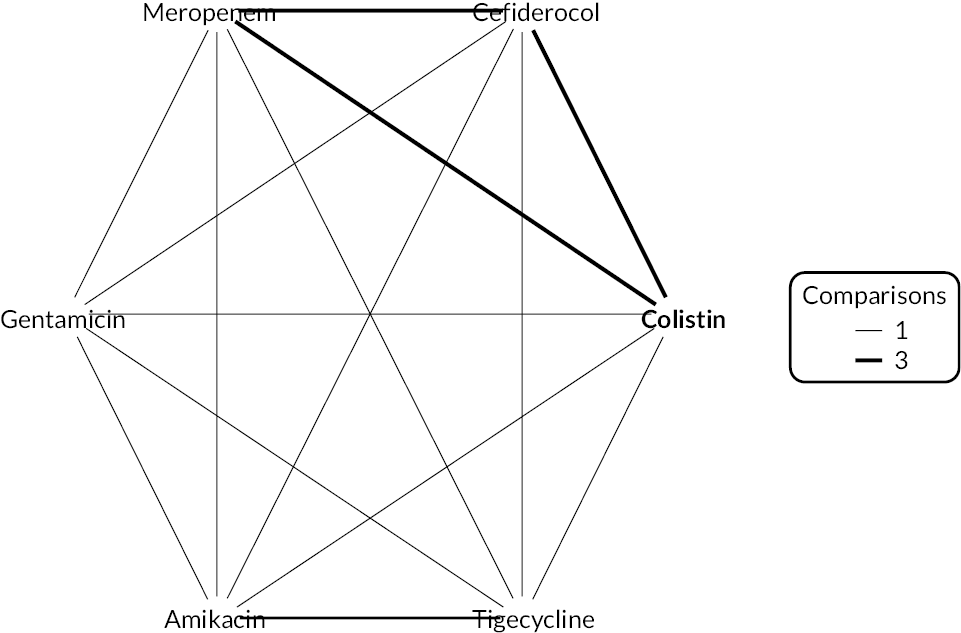
The relative susceptibility for each comparator relative to colistin is shown in Figure 33. The model fitted the data well, with a total residual deviance of 11.92, which was close to the number of data points included in the analysis of 12. The between-study SD was 2.56 (95% CrI 1.59 to 2.98), which indicates extremely high heterogeneity. Cefiderocol was associated with a higher susceptibility relative to colistin (OR 15.70, 95% CrI 0.83 to 320.72), but the result was not statistically significant. Cefiderocol also had an 8% probability of being the most effective treatment; median rank 2. The remainder of the treatments, except for meropenem, were also associated with a higher susceptibility. Only the result for tigecycline was statistically significant. For all comparators, the high between-study SD results in wide 95% PrI. The plot is provided in Figure 33a.
FIGURE 33.
Forest plot of OR vs. colistin for MBL Enterobacterales with CLSI breakpoint (SIDERO studies only). (a) All comparators included in the network; and (b) only comparators specific to the pathogen included in the network. PB, probability being the best treatment.

The sensitivity analysis restricting to comparators specific to the pathogen produced a very similar OR for cefiderocol (16.76, 95% CrI 1.19 to 285.30). The plot is provided in Figure 33b.
Metallo-beta-lactamases P. aeruginosa, Clinical Laboratory Standards Institute breakpoints (SIDERO studies only)
Two studies34,35 contributed to the NMA of P. aeruginosa MBL infections with CLSI breakpoint for SIDERO studies only, considering a total of two comparators, and the full network diagram is shown in Figure 34. All two studies (SIDERO WT data request and SIDERO CR data request) contained zero susceptibility counts for one or more of the included comparators and therefore had a continuity correction applied prior to synthesis.
FIGURE 34.
Network diagram of all studies contributing to the NMA (P. aeruginosa MBL with CLSI breakpoint for SIDERO studies only).

The relative susceptibility for each comparator relative to colistin is shown in Figure 35. The model fitted the data well, with a total residual deviance of 4.75, which was close to the number of data points included in the analysis of six. The between-study SD was 1.15 (95% CrI 0.06 to 2.87), which indicates extremely high heterogeneity. Cefiderocol was associated with a statistically significant higher susceptibility relative to colistin (OR 66.73, 95% CrI 3.61 to 3284.37). Cefiderocol also had a 100% probability of being the most effective treatment; median rank 1. Meropenem was associated with no susceptibility. For all comparators, the high between-study SD results in wide 95% PrI.
FIGURE 35.
Forest plot of OR vs. colistin for P. aeruginosa MBL with CLSI breakpoint (SIDERO studies only). PB, probability being the best treatment.

The network for the sensitivity analysis restricting to comparators specific to the pathogen was identical to the original network, since there were no data for comparators not in scope for the pathogen.
Clinical Laboratory Standards Institute breakpoint with fosfomycin studies only
Three studies89,90,91 contributed to the NMA of Enterobacterales MBL infections with CLSI breakpoint for fosfomycin studies only, considering a total of seven comparators, and the full network diagram is shown in Figure 36. Two studies89,90 contained zero susceptibility counts for one or more of the included comparators and therefore had a numerical adjustment applied prior to synthesis.
FIGURE 36.
Network diagram of all studies contributing to the NMA (MBL Enterobacterales with CLSI breakpoint for fosfo studies only).

The relative susceptibility for each comparator relative to colistin is shown in Figure 37a. The model fitted the data well, with a total residual deviance of 21.72 being close to the number of data points included in the analysis, which was 23. The between-study SD was 1.34 (95% CrI 0.67 to 2.50), which indicates extremely high heterogeneity. Fosfomycin was associated with a lower susceptibility relative to colistin (OR 0.52, 95% CrI 0.06 to 4.01), but the result was not statistically significant. Fosfomycin was also associated with a 23% probability of being the most effective treatment; median rank 2. The remainder of the treatments were also associated with lower susceptibility than colistin, and the results were not statistically significant. For all comparators, the high between-study SD results in wide 95% PrI.
FIGURE 37.
Forest plot of OR vs. colistin for MBL Enterobacterales with CLSI breakpoint (fosfomycin studies only). (a) All comparators; and (b) only comparators specific to the pathogen.

The sensitivity analysis restricting to comparators specific to the pathogen produced a very similar OR for fosfomycin (0.59, 95% CrI 0.12 to 2.64). The plot is displayed in Figure 37b.
There was only one study92 included in the NMA of P. aeruginosa MBL infections with CLSI breakpoint for fosfomycin studies only. No synthesis was performed.
Inconsistency checks
Deviance plot for accessing inconsistency
Figures 38 and 39 show the deviance plots used to assess inconsistency.
FIGURE 38.
Deviance plot for the NMA with MBL Enterobacterales (EUCAST breakpoint for SIDERO and fosfomycin and PHE studies).

FIGURE 39.
Deviance plot for the NMA with MBL Enterobacterales (CLSI breakpoint for SIDERO and fosfomycin studies only).
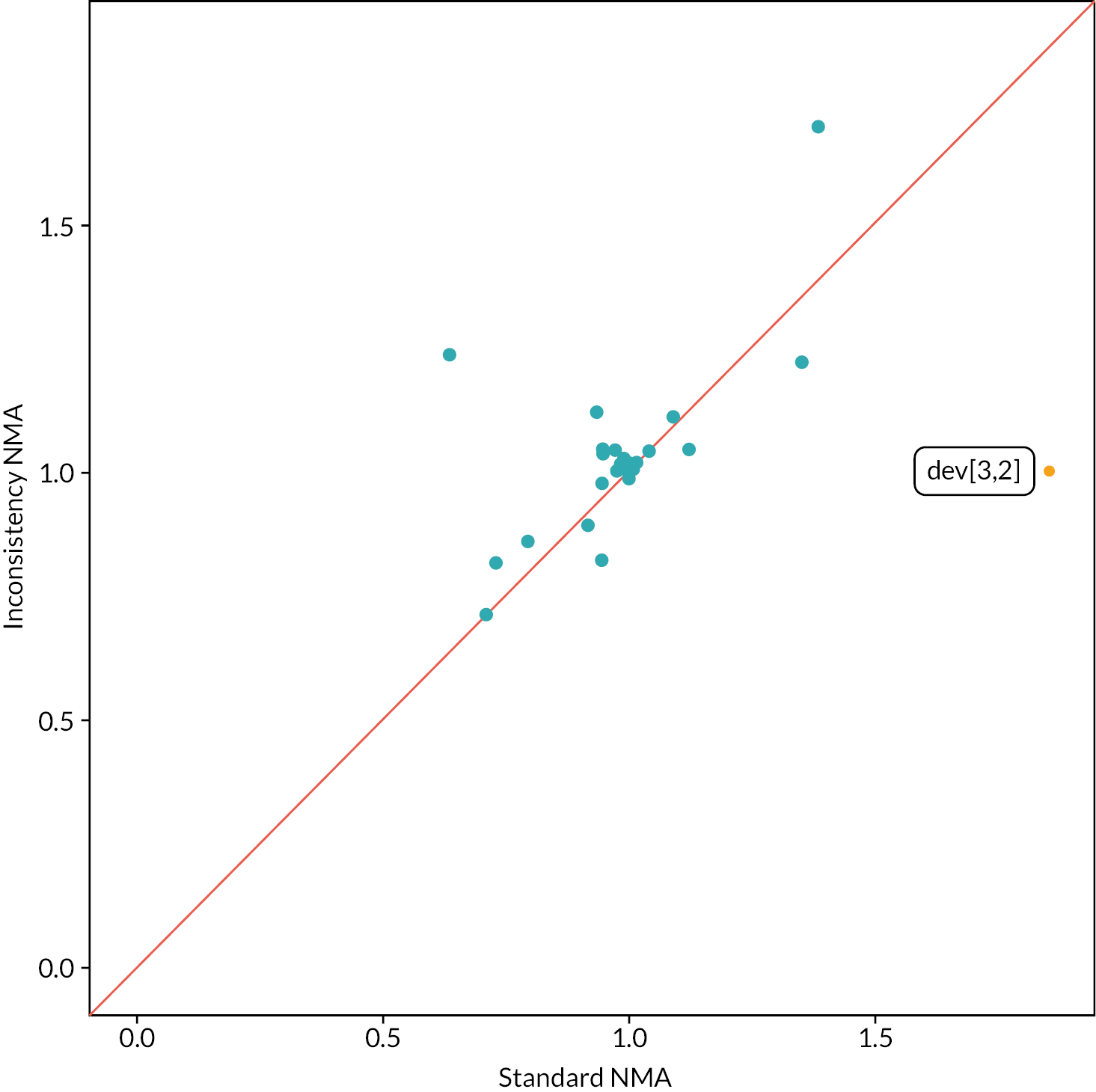
Appendix 5 Methods and results for reviews 4 and 5
Review question 4
Methods
Of the two proposed approaches, only approach A was taken forward. In this approach, it is assumed that clinical outcomes would be similar regardless of the treatment received, conditional upon susceptibility. This review included studies of any design linking susceptibility (to any antibiotic) to clinical outcomes in cUTI or HAP/VAP caused by any pathogen mechanism. Three approaches were used to identify evidence relating to this question.
-
A systematic review update of Bassetti et al. (2020). 197
-
Searching and screening of additional databases and review of studies included within Bassetti et al. (2020). 197
-
Review of the RCTs identified in Review 1 for any subgroup data.
The three approaches are described here in more detail:
-
Bassetti et al. (2020)197 systematically reviewed the impact of appropriate and inappropriate antibacterial therapy on clinical outcomes of patients with severe bacterial infections, where appropriate therapy was defined as treatment with an antibiotic the isolate was susceptible to. The review was assessed for quality and relevance (see Report Supplementary Material 1) and was judged to be of good quality and suitable for updating. The original review covered the period from 2007 (to ensure clinical practices were contemporary) and the searches were performed in 2018. For the update, given resource and time constraints the search strategy was restricted to terms relating to the UK (since clinical practice may differ in other countries), the sites of interest (cUTI, HAP/VAP) and to remove terms relating to treatment delay, which were included in Bassetti et al. (2020)197 to address a separate review question (the effect of delayed appropriate antibiotic therapy) addressed in Zasowski et al. (2020). 200 The adapted search strategy was run from 2007 to June 2021, to capture any new studies, as well as any studies the adapted strategy identified that were missed by the previous review (between 2007 and 2018). It was further noted that the original search strategy did not include search terms relating to susceptibility, and therefore an additional search, using this search term, was conducted to capture any additional studies from 2007 onwards. The search strategies are presented in Appendix 1, section Focused clinical outcomes search and were run in Ovid MEDLINE and Epub Ahead of Print, In-Process, In-Data-Review and Other Non-Indexed Citations, Daily and Versions: Ovid, 1946–Present.
-
In addition to the update of Bassetti et al. (2020),197 a number of other approaches were taken to identify relevant studies (more detail is provided in Report Supplementary Material 1):
-
A large database (3172 references) was created, based on search terms for the mechanisms of resistance relevant to the two concurrent EEPRU evaluations relating to cefiderocol and CAZ-AVI (namely MLB, NDM, VIM and IMP). This database was then searched using a series of keywords and phrases to identify relevant studies. The search strategy is presented in Appendix 1, Broad OXA-48 Metallo-beta-lactamases search for database dredging.
-
Screening, citation searching and reference checking of studies retrieved by a search for cost-effectiveness models (66 references) (see Appendix 1, Focused antimicrobial resistance models search).
-
Keyword search of the Endnote library provided by Shionogi as part of the EEPRU evaluation of cefiderocol (1261 references).
-
Screening the list of key references provided by Shionogi as part of the EEPRU evaluation of cefiderocol (45 references).
-
Keyword search of references provided by Pfizer as part of the EEPRU evaluation of CAZ-AVI (299 references).
-
Screening the studies included in two systematic review articles provided by Shionogi as part of the EEPRU evaluation of cefiderocol (Zasowski et al. 2020;200 Bassetti et al. 2020). 197
-
-
In addition to the two previous approaches, the RCTs identified for the intervention were examined for any additional data relating to this question (see Appendix 3, Review 1: randomised controlled trials).
Identified studies were assessed for relevance against pre-specified inclusion criteria listed in Table 44.
Results
-
Systematic review update of Bassetti et al. (2020).
The searches for the systematic review update yielded 172 citations; the screening process did not result in any studies that met the inclusion criteria.
-
Searching and screening additional databases.
Eight studies were extracted in total, of which four and five studies reported outcomes in patients with cUTIs and HAP/VAP, respectively. None of the studies were conducted in the UK. None of the studies on patients with cUTIs included patients who received microbiology-directed treatment. In studies on patients with HAP/VAP, three studies only included patients receiving empiric treatment,125,143,201 one study202 included patients both on microbiology-directed and empiric treatment (it did not report outcomes conditional on the setting) and one study203 did not report whether treatment was microbiology-directed of empiric. Of the three studies conducted solely in the ES, one reported ICU mortality, hospital mortality, mechanical ventilation, LoS and ICU LoS,143 one study reported 30-day mortality only201 and one reported Kaplan–Meier curves for 30-day mortality. 125
-
Review of the RCTs identified in Review 1 for any subgroup data.
The RCTs were also examined for data on clinical outcomes contingent on susceptibility to treatment. No analyses were identified in these RCTs.
Item | Inclusion criteria | Exclusion criteria |
---|---|---|
Population | cUTI or HAP/VAP Any infective pathogen |
Other sites |
Exposure | Treatment with any antibiotic that the isolate is susceptible to | Treatment with an antibiotic that the isolate has intermediate susceptibility to |
Comparison | Treatment with any antibiotic that the isolate is not susceptible to (resistant or intermediate/increased exposure) | No comparison provided |
Outcomes | Mortality, hospitalisation, LoS, BSI or other subsequent infections, HRQoL | Short-term outcomes such as clinical cure |
Setting | MDS or ES UK studies (only applied to search update) |
Not UK (only applied to search update) |
Study design | Experimental or observational studies that assessed susceptibility to treatment prospectively or retrospectively | Published prior to 2010 |
Review question 5
What is the long-term risk of mortality (and other outcomes) for patients with carbapenem-resistant cUTI or HAP/VAP?
Methods
The previous reviews did not identify any long-term mortality data. Given the paucity of data in this area, the scope of this review question was widened to include any carbapenem-resistant infections treated with any treatment, under the assumption these data could be generalised to MBL Enterobacterales and P. aeruginosa infections. A focused search was conducted to identify UK studies reporting long-term (> 3 months) mortality and other outcomes such as hospitalisation, subsequent infection, costs and adverse events for patients with carbapenem-resistant [including MDR and extensively drug-resistant (XDR)] cUTI or HAP/VAP. The search strategy comprised terms for (Carbapenem Resistance OR mechanisms) AND [sites (UTI/HAPVAP)] AND filters. The search scope was limited using terms for the UK, and the search was run from 2010 to ensure clinical practices were contemporary. Since no UK studies were identified, the search was expanded to include studies from Europe. The search strategy is presented in Appendix 1, Focused long-term outcomes search. Studies were assessed for eligibility against the inclusion criteria in Table 45 by one reviewer.
Item | Inclusion criteria | Exclusion criteria |
---|---|---|
Population | CR, XDR or MDR cUTI or HAP/VAP infections | Infections at sites other than cUTI or HAP/VAP |
Exposure | Any treatment or no treatment | |
Outcomes | Mortality measured more than 3 months after treatment Other long-term outcomes such as hospitalisations, subsequent infections, costs, adverse events |
Outcomes measured at or before 3 months after treatment |
Setting | UK, expand to Europe if no UK studies | |
Study design | Experimental or observational studies or data sets | Studies published prior to 2010 |
Results
The electronic database searches, following the removal of duplicates, identified 76 records relating to long-term outcomes for patients with carbapenem-resistant cUTI or HAP/VAP. After examination of the title and abstracts, 76 records were excluded because they did not meet the inclusion criteria.
Appendix 6 Structured expert elicitation
Description of elicited parameters
We required outcomes for patients with HAP, VAP and cUTIs caused by carbapenem-resistant Gram-negative bacteria. We were only interested in outcomes following microbiology-directed treatment for patients with an infection caused by Enterobacterales with an OXA-48 or MBL resistance mechanism, or Pseudomonas with a MBL resistance mechanism.
Outcomes were elicited depending on whether the infectious pathogen is susceptible to treatment. Therefore, outcomes only depend on whether a patient is susceptible to treatment or not, and not to the specific treatment given. The outcomes we were interested in were 30-day mortality, LoS in hospital and the type of ward these patients would stay on in hospital.
As background information we provided experts with several related studies (see Report Supplementary Material 2). In these studies, infecting pathogens were not confirmed to be susceptible to the antibiotics administered (cefiderocol or CAZ-AVI); however, in our assessment, they are likely to have been susceptible.
For HAP, VAP and cUTI, both for susceptible and not-susceptible patients, the following questions were asked of experts:
-
Question 1. In this patient population, what proportion of patients will still be alive 30 days after starting microbiology-directed treatment?
-
Question 2. In the patient population described at the top of the page, what will be the average LoS?
-
Question 3. In the patient population described at the top of the page, what proportion of hospital stay would be spent on each of the following wards? This number should represent the average for all such patients, regardless of their outcome.
Protocol for elicitation
The following sections describe the details of the elicitation exercise, according to the elements as described in the MRC elicitation guidance.
Selecting the quantities (preparation and design stage)
The choice of quantity considered the following three objectives:50 fitness for purpose, directly observable and homogeneity in the quantities elicited. Eliciting the same summaries throughout will reduce the burden of training. 203
For question 1 the quantities elicited relate to the proportion of patients with an event at a certain time. Question 2 relates to a continuous outcome, LoS, which, in principle, can take values up to ∞. Question 3 relates to the proportionate split of LoS between the three types of wards – general ward, HDU and ICU. As the total proportion must sum to 100, these quantities were not elicited with uncertainty, and instead a mean proportion elicited.
Methods to encode judgements (preparation and design stage)
Either the Chips and Bins method or a Bisection method have been shown to work equally well in healthcare elicitation. The Chips and Bins approach, however, is viewed as less complex and easier to complete by healthcare professionals, and so this method is used here.
Experts were first asked to express the range for their beliefs, the minimum, which is the value such that the experts believe that there is a 1% probability that the proportion is less than that value, and the maximum, a value, such that the experts believe that there is a 1% probability that the proportion is more than that value. Grids were then generated based on this range and experts were asked to place ‘chips’ on this grid to represent their beliefs.
Validation (preparation and design stage)
At the end of each task, experts were given a qualitative summary of their responses. If experts felt that these did not represent their views, they were encouraged to revise their responses. Experts also had an opportunity to revise their responses following the feedback round (see below).
Selecting experts (preparation and design stage)
The models developed for this project span across HAP, VAP and cUTI and also relate to likely outcomes depending on susceptibility to treatment. Therefore, there are multiple types of experts relevant for this task. Here we have included hospital consultants, microbiologists and pharmacists as experts. As part of the task, experts were asked to identify which of these disciplines they worked in. Experts were not expected to have any normative skills. Experts were recruited using recommendation from peers.
Pilot exercise (preparation and design stage)
The wording of the questions was piloted for clarity and adequacy. The draft exercise was sent to a lead clinician and feedback sought. Following feedback the questions were modified, specifically the wording of the questions.
Training and preparation for experts (preparation and design stage)
A narrated PowerPoint training session was delivered to experts prior to the task. The training session described the objectives of the elicitation exercise, clarified concepts such as uncertainty, familiarised the experts with the quantities elicited, described and explained the impact of bias and heuristics, and trained experts on the methods of elicitation used. A recorded version of the training slides was also sent to the experts following the session and also key details from this repeated in the task itself.
Experts were also reminded throughout the SEE that they were to elicit uncertainty on their estimate rather than thinking about variability across this heterogeneous group of patients.
Level of elicitation (elicitation stage)
Each expert elicited their judgements individually without interaction with other experts. Eliciting judgements individually reduced the risk of estimates being biased by a subset of experts. In the SEE elicitation literature, there are concerns that experts may not feel confident in eliciting judgements individually; however, the experts in this SEE process elicited their beliefs on a condition that they encounter regularly in general practice. Concerns regarding individual-level elicitation and lower confidence among experts generally arise when dealing with problems/technologies or conditions that are new or unknown to the experts.
Mode of administration (elicitation stage)
The elicitation exercise was administered via an application in SHINY. The task was delivered remotely, due to current restrictions on face-to-face meetings. Experts were offered the opportunity to complete the exercise remotely alongside one of the team. E-mail contacts were given to provide any support needed.
Feedback to experts and revision (elicitation stage)
Once experts expressed their beliefs and completed each question, they were presented with graphical feedback of what their estimates looked like. Experts were able to see how the grid looked once they had placed all of their chips on it. In addition, once experts had completed the grid, a summary of their answers was relayed to them. This provided the following information:
Your answers imply that (example quantities given):
-
There is a 17% probability that the proportion of patients is between 19% and 20%.
-
There is a 50% probability that the proportion of patients is between 20% and 21%.
-
There is a 33% probability that the proportion of patients is between 21% and 22%.
Following the individual elicitation, beliefs were then aggregated using linear opinion pooling. This overall distribution was then relayed back to experts, and they were given the opportunity to revise their own beliefs on the histograms they previously completed. This approach has been shown to generate less biased parameters when the quantities elicited are unknown to the experts. Following this revision, expert’s beliefs were aggregated using the same approach, linear opinion pooling, and the final parameter values determined.
Opportunity for interaction (elicitation stage)
Given the individual level of elicitation that was chosen, there was no opportunity for interaction between the experts. The revision stage was done remotely so experts did not interact with each other.
Feedback from experts on process (elicitation stage)
Qualitative feedback on the elicitation process was collected from the experts, including rationales for their responses. This was collected during the task using free text boxes. This form of validation helps to highlight if experts understood the task and responded as best they could.
If/how to aggregate (aggregation, analysis and post elicitation)
As an individual level of elicitation was chosen, mathematical aggregation was applied to generate the distributions, specifically linear opinion pooling using equal weighting of experts. First a probability distribution was fitted to each expert’s beliefs from the histogram and then these were pooled, assuming that each expert contributed equally to the group overall distribution.
This overall distribution was then relayed back to experts, and they were given the opportunity to revise their own beliefs. Following this revision, expert’s beliefs were aggregated using the same approach, linear opinion pooling, and the final parameter values determined.
Fit to distribution (aggregation, analysis and post elicitation)
A Beta distribution was fitted to expert’s distributions for question 1 as these relate to proportions. For question 2 a log-normal distribution was fitted. Question 3 only asked for point estimates so not fitting was required.
Data protection and anonymity (aggregation, analysis and post elicitation)
Experts were asked to give their opinions individually (not in groups). The information provided, including personal details, is kept anonymous and confidential, stored securely and only accessed by those carrying out the study.
Results
Eleven experts agreed to take part in the elicitation task and took part in the training. Of these 11, 9 experts attempted the task. The experts included medical consultants (n = 2), microbiologists (n = 5), ICU consultants (n = 1) and pulmonary consultants (n = 1). Seven experts completed the task, while two terminated it before answering all questions. Responses from the two experts who terminated the task before answering all questions were included in the analysis for all outcomes where they provided an estimate for both susceptible and not susceptible populations. Following the elicitation task, experts were sent group summaries and asked if they would like to revise their responses. Only two experts stated that they reviewed the group summaries, and one adjusted their initial responses in light of group summaries.
Two experts indicated that the probability of survival was lower in patients who were susceptible to treatment than those who were not susceptible, for two sites of infection. This was judged to be implausible, and so the two experts were removed from the sample in the base case. Ultimately, five experts’ judgements were therefore included within the analysis.
Group summaries – base case
The group summaries on 30-day mortality (see Figure 40) indicate that survival is the lowest for VAP patients and highest for cUTI patients, and that susceptibility to treatment increases the probability of survival, for all three sites of infection. The group summaries on LoS (see Figure 41) indicate that the LoS is the shortest in patients with cUTIs and the longest for patients with VAP. For all three sites of infection, susceptibility to treatment decreased the LoS.
FIGURE 40.
Thirty-day survival. P, proportion.

FIGURE 41.
Expected LoS.
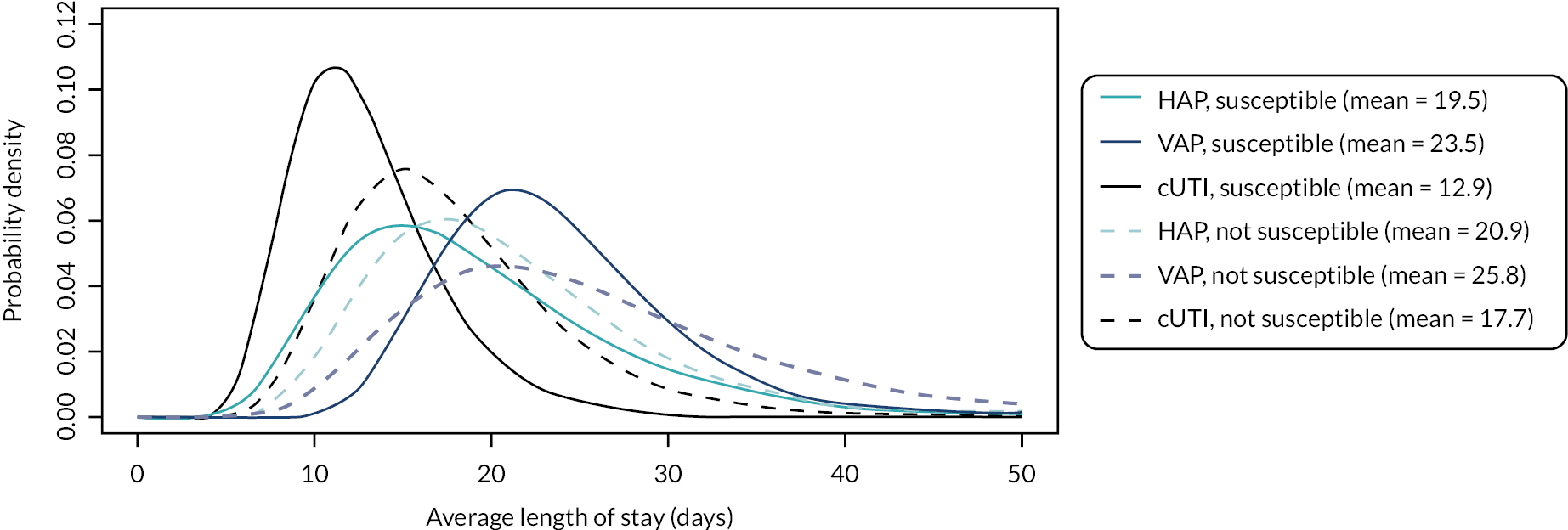
The group summaries about the proportion of time spent on different types of wards are shown in Table 46. The summaries indicate that patients with VAP spend the most time in ICU and the least time on general medical wards, followed by HAP, then cUTIs. Furthermore, patients who are susceptible to treatment are expected to spend more time on the general medical ward and less on ICU and HDU, for all three sites of infection.
ICU | HDU | General medical ward | |
---|---|---|---|
HAP, susceptible | 24.3 | 19.0 | 56.7 |
VAP, susceptible | 60.0 | 13.3 | 26.7 |
cUTI, susceptible | 15.0 | 17.0 | 68.0 |
HAP, not susceptible | 39.3 | 20.7 | 40.0 |
VAP, not susceptible | 66.7 | 15.8 | 17.5 |
cUTI, not susceptible | 23.3 | 18.3 | 58.3 |
In the model, outcomes of HAP and VAP were modelled together, and so experts’ priors on outcomes were pooled. When pooling the priors, outcomes for HAP and VAP were weighted by their relative occurrence in Tumbarello et al. (2013) – 0.283 (28/99) for HAP and 0.717 (71/99) for VAP. Tumbarello was chosen as the study where participants were the most representative of patients in our HVCS, that reported the proportion of patients with HAP that was ventilator-associated.
The pooled priors are shown in Figures 42 and 43 and Table 47.
FIGURE 42.
Thirty-day survival with HAP/VAP combined. P, proportion.

FIGURE 43.
Expected LoS with HAP/VAP combined.
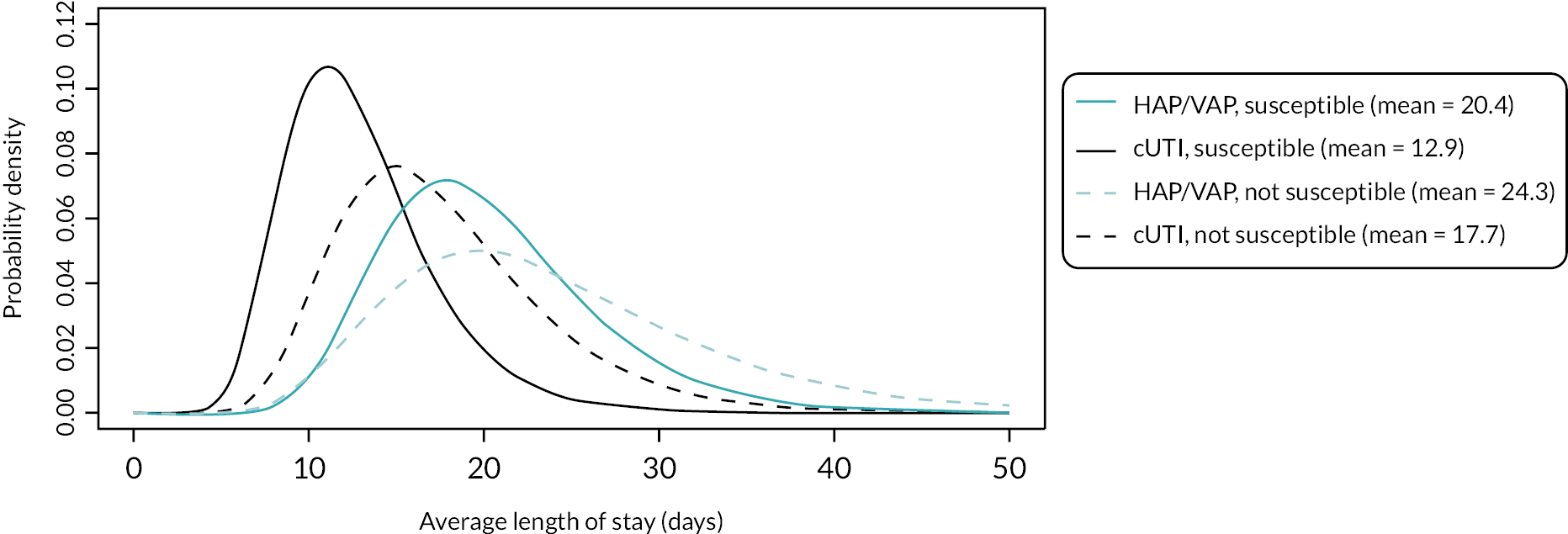
ICU | HDU | General medical ward | |
---|---|---|---|
HAP/VAP, susceptible | 49.90 | 14.94 | 35.16 |
cUTI, susceptible | 15.00 | 17.00 | 68.00 |
HAP/VAP, not susceptible | 58.92 | 17.21 | 23.86 |
cUTI, not susceptible | 23.33 | 18.33 | 58.33 |
Group summaries – all experts included
Results with all priors, including those that indicated that survival would be lower in susceptible patients, are shown in Figures 44 and 45, and Table 48. Overall, the priors indicate the same relative differences between outcomes and sites of infection.
FIGURE 44.
Thirty-day mortality – all experts. P, proportion.

FIGURE 45.
Expected LoS – all experts.

ICU | HDU | General medical ward | |
---|---|---|---|
HAP, susceptible | 23.56 | 21.22 | 55.22 |
VAP, susceptible | 62.86 | 14.29 | 22.86 |
cUTI, susceptible | 13.57 | 16.00 | 70.43 |
HAP, not susceptible | 36.00 | 22.00 | 42.00 |
VAP, not susceptible | 68.57 | 16.43 | 15.00 |
cUTI, not susceptible | 21.43 | 18.57 | 60.00 |
Validation of experts’ estimates
We explored alternative sources of evidence to inform LoS in the model, in order to validate the elicitation results. In particular, we considered two UK-based studies that reported LoS in patients with carbapenem-resistant organisms, CARBAR134 and Merrick,135 and the study by Muscedere143 that was used to derive the relative reduction in the LoS associated with appropriate empiric therapy in the ES (see Resource use and costs for details).
The mean LoS in CARBAR134 was 47.2 days. The median LoS in Muscedere143 in patients who received appropriate and inappropriate empiric treatment was 27.9 and 42.2 days, respectively. This was estimated to equate to the mean LoS of 43.1 days and 85.7 days, respectively (see Resource use and costs for details). The LoS in both studies was considerably longer than experts’ estimates (~20 and ~24 days from the start of microbiology-directed treatment in susceptible and resistant patients, respectively). However, the LoS in both studies was measured from hospital admission, rather than from the start of microbiology-directed treatment following infection onset.
CARBAR reported that the average time between hospitalisation and infection was 8 days (median) for all patients, 16.8 days (mean) time for infections diagnosed from sputum samples and 13.9 days for UTI-related samples. In addition, the median time between infection onset and microbiology-directed treatment in CARBAR was 5 days. Assuming that 13 (8 + 5) to 21.8 (16.8 + 5) days passed between admission and administration of microbiology-directed treatment, the LoS from the start of microbiology-directed treatment in CARBAR (25.4–34.2 days) was comparable to experts’ estimates. Muscedere did not report the time between admission and infection onset, and so could not be directly compared to experts’ estimates. In Merrick,135 the median LoS after infections caused by carbapenem-resistant organisms was 24 days, comparable to the mean estimates from experts. The authors did not report the mean.
Appendix 7 Further detail on methods for economic evaluation
Incorporating susceptibility evidence into the economic model
Evidence on conditional susceptibilities
In general, the review of susceptibility studies described in Clinical evidence (and subsequent NMA) provided evidence on absolute susceptibility to a given AM (or in statistical language, the marginal susceptibility). To use evidence on susceptibility in the economic modelling, information on conditional susceptibility is required. This required evidence takes two different forms depending on the treatment setting. In the ES many treatments are combinations of two AMs. For this, evidence is required on the susceptibility to one AM in the combination treatment, conditional on being resistant to the other AM in the combination (so collectively this evidence allows for a derivation of overall susceptibility to the combination treatment). In the MDS interest lies in the proportion of patients who are susceptible to at least one AM in a given group (where the groupings are one of ‘colistin or an aminoglycoside’, ‘a different AM’ or ‘no AMs’). Here the required evidence is again for susceptibility to an AM given resistance to other AMs, but now this resistance could be to multiple AMs. These two settings are discussed in turn, followed by a discussion of issues specific to cefiderocol.
The evidence used to inform estimates and assumptions about conditional susceptibilities was obtained from two primary sources. The first was the review of susceptibility studies described in Clinical evidence (Approach 3). The second was de novo data requests, as described in Report Supplementary Material 1.
Empiric setting
Two options were considered:
-
Assume independence of absolute susceptibilities when determining overall susceptibility to combination treatments. Under this assumption, the susceptibility of a given isolate to a given AM is the same irrespective of what other AMs the isolate is susceptible to. With this assumption, obtain overall susceptibility to two AMs, the following equation is used:
-
Overall susceptibility = susceptibility to AM1 + (1 – susceptibility to AM1) * susceptibility to AM2.
-
In other words, it is assumed that those not susceptible to AM1 have the same susceptibility to AM2 as the whole sample.
-
-
Use observed evidence on overall susceptibility. This includes evidence on conditional susceptibility (susceptibility to an AM given resistance to another AM). Isolate-level data were available from one source: a de novo data request from PHE. Under this second approach ‘susceptibility to AM2’ becomes ‘susceptibility to AM2 given resistance to AM1’.
The second approach will provide more nuanced estimates of overall susceptibility to combination treatments by accounting for cross-resistance. However, it is restricted to AM combinations for which there is evidence and is reliant on smaller samples of susceptibility data. In particular, the NMA of susceptibility evidence does not provide any evidence on overall or conditional susceptibility. In contrast, the first approach may be used with the NMA results and any other studies. The key assumption of the first approach is that of independence of absolute susceptibility. To assess the credibility of this assumption, analyses of the isolate-level data were performed.
Among the CPE population, evidence from PHE includes two of the three combination treatments listed in the PICOS. These were colistin with tigecycline and colistin with aztreonam; there were no data for colistin with fosfomycin. There were decreases in susceptibility when assessing conditional values for all the drugs. For example, the absolute susceptibility for tigecycline was 70%, while conditional on being resistant to colistin it was 60%. However, numbers were generally small, and none of the decreases were statistically significant when a two-sided z-test for a difference in proportions was performed. For the Pseudomonas population, there was no evidence for colistin with fosfomycin.
Microbiology-directed setting
In the MDS (for which it is assumed that individuals will receive any AM to which they are susceptible), one approach would be to also assume independence of susceptibilities when deriving susceptibility groups (susceptible to a non-colistin/aminoglycoside AM, susceptible to only colistin or an aminoglycoside and not susceptible to any AM). The appropriateness of this assumption for the first group was checked using data from PHE (which includes all the comparators apart from fosfomycin. Assuming independence results in 77% of patients being in the non-colistin/aminoglycoside group, compared with the true value of 75%. While these numbers are very similar, they are only for two AMs (due to a lack of evidence), and it is unclear if the assumption of independence will hold for additional AMs. Hence, the assumption of independence was not employed when deriving susceptibility for the groups. Instead, the PHE data were used to calculate the likely overestimate when assuming independence. Hence, given the above numbers, the true value is likely to be 97% (75/77) of the value obtained when assuming independence. As the NMA evidence does not capture dependencies among AMs, these estimates were first combined to obtain susceptibility groups assuming independence. The scaling factor from the PHE data was then applied to adjust for the likely over-estimate due to assuming independence. The same method was used to derive adjusted values for the second susceptibility group (with the third susceptibility group obtained by noting that the sum across the three groups had to sum to 100%).
Cefiderocol
There is limited evaluation in the literature of the susceptibility to cefiderocol of isolates that were resistant to other treatments. In a study by Johnson et al. overall susceptibility to cefiderocol was 92%, with decreased susceptibility among isolates that were resistant to an aminoglycoside (88% and 81% for these resistant to gentamicin and amikacin, respectively). However, the Johnson et al. study was of multiple resistance mechanisms, not just MBLs; for both cefiderocol and aminoglycosides susceptibility was statistically significantly reduced among isolates with a MBL mechanism (cefiderocol from 92% to 70%, gentamicin from 55% to 19% and amikacin from 78% to 48%) compared to susceptibility among all isolates. Hence, in this study, resistance to an aminoglycoside may be confounded by an increased prevalence of MBLs. In the Kazmierczak et al. study, the MIC 90 for cefiderocol was 4 μg/ml in the overall population and 2 μg/ml among colistin-resistant isolates, suggesting little impact of colistin resistance on cefiderocol resistance. In response to a data request, Shinogi provided evidence on susceptibility to cefiderocol conditional on resistance to other AMs. Susceptibility to cefiderocol was broadly unaffected by resistance to non-toxic AMs (those in the PICOS, excluding colistin or aminoglycosides). There was some evidence of a reduced susceptibility to cefiderocol among isolates resistant to all other AMs, but this was based on small numbers. Due to the uncertainty and lack of evidence to inform the effect of resistance on cefiderocol susceptibility, it was decided to assume that susceptibility to cefiderocol is independent of resistance to other AMs.
Scenario analyses for susceptibility evidence
For the base-case analysis it was assumed that conditional susceptibilities were the same as absolute susceptibilities. This assumption was relaxed in the following scenario analyses:
-
Scaling conditional susceptibility: with this scaling factor informed by PHE data, where available. For example, if in the PHE data, the conditional susceptibility to tigecycline among isolates that were resistant to colistin was 10% lower than the absolute susceptibility to tigecycline, then the absolute susceptibility to tigecycline obtained from the NMA was reduced by 10% to obtain the conditional susceptibility.
-
For the CPE-MBL population, use of only PHE data. As there is no PHE evidence for fosfomycin, this scenario assumed that fosfomycin was not used.
As susceptibility to colistin was almost 100% in the base case for the Pseudomonas population, an additional scenario was explored which used the SIDERO-WT study for colistin susceptibility. This study was chosen as it reported the lowest colistin susceptibility of the EUCAST studies identified (80.9%).
In a further scenario analysis, evidence from just CLSI studies was used. For both the base-case analysis of EUCAST studies and the scenario of CLSI studies, additional scenarios were explored. For these additional scenarios, PHE data were used for all AMs apart from cefiderocol and fosfomycin. Evidence for these two AMs was obtained from their own network (keeping the cefiderocol and fosfomycin networks separate). These scenarios were motivated by noting that literature searches had only been conducted for cefiderocol and fosfomycin, so it may not be reasonable to obtain estimates for the other AMs from the NMA.
Parametric survival modelling of CARBAR
Table 49 summarises the assessment of internal and external validity of the parametric survival analysis of all-cause mortality from CARBAR.
Distribution | AIC | Visual assessment of fit | Comparison with external data and assessment of face validity |
---|---|---|---|
Exponential | 953 | Poor | No convergence with general population mortality |
Weibull | 935 | Moderate | Converges towards general population mortality but annual probability of death always greater |
Gompertz | 952 | Poor | Converges with general population mortality at 9 years |
Log-logistic | 938 | Moderate | Converges with general population mortality at 15 years |
Log-normal | 953 | Moderate | Converges with general population mortality at 13 years |
Generalised gamma | 933 | Poor | Rapidly accelerating mortality and divergence with general population mortality |
Health-related quality-of-life weights by comorbidity level
Table 50 shows SF-6D utilities by CCI, and the distribution of people within each CCI score within the CARBAR study.
CCI-score | SF-6D score140 | Proportion of people within each CCI score (CARBAR) (%) |
---|---|---|
CCI 0 | 0.729 | 20 |
CCI 1–2 | 0.667 | 31 |
CCI 3–4 | 0.621 | 21 |
CCI 5+ | 0.615 | 28 |
Drug acquisition costs
Table 51 shows the drug costs used in the model.
AM | Price | Daily dose | Cost per day | Cost per course of treatment (treatment duration in days) | Cost per 5 days of treatment |
---|---|---|---|---|---|
Colistimethate sodium | £18.00 (10 × 1MU vial)154 | 9MU | £16.20 | £153.9 (9.5 days151) | £81.00 |
Aminoglycosides (gentamicin) | £10.97 (20 × 360 mg/120 ml solution for infusion bags)155 | 0.24 g | £10.97 | £76.79 (maximum IV treatment 7 days154) | £54.85 |
Aminoglycosides (amikacin) | £38.72 (5 × 500 mg/2 ml vials)155 | Maximum dose 1.5 g | £23.23 | £232.30 (10 days154) |
£116.15 |
Aminoglycosides (tobramycin) | £10.69 (1 × 240 mg/6 ml solution for injection vials)155 | 0.24 g | £10.69 | £74.83 (maximum IV treatment 7 days154) | £53.45 |
Tigecycline | £106.52 (10 × 50 mg vials)155 | 0.1 g | £21.30 | £298.20 (14 days154) | £106.5 |
Fosfomycin | £4.86 (1 × 3 g sachet)154 | 3 g (1 sachet)154 | £4.86 | £9.66 (2 doses2) | £9.66 |
Fluoroquinolones (ciprofloxacin) | £5.02 (10 × 400 mg/200 ml infusion)155 | 1.2 g | £1.51 | £10.57 (7 days154) | £7.55 |
Fluoroquinolones (levofloxacin) | £20.95 (10 × 500 mg/100 ml infusion bags)155 | 0.5 g | £2.10 | £29.40 (14 days154) | £10.5 |
Cephalosporins (cefepime) | £70.00 (10 × 1 g vial)154 | 4 g | £28.00 | £280.00 (10 days) | £140.00 |
Cephalosporins (ceftriaxone) | £5.25 (10 × 1 g vial)155 | 4 g | £2.10 | £29.40 (14 days154 | £10.5 |
Aztreonam | £18.82 (2 g powder for solution for injection) 154 | 4 g | £37.64 | £263.48 (7 days, assumed) | £188.2 |
Goodness of fit of forecast models
Table 52 shows the goodness-of-fit statistics (AIC, for which lower values indicate better fit) for the alternative forecasting models.
Model: Enterobacterales-MBL | Invasive isolates | Screening isolates |
---|---|---|
No trend | 93.49 | 192.95 |
Damped trend | 97.71 | 193.81 |
Trend | 95.08 | 185.76 |
Model: P. aeruginosa | Invasive isolates | Screening isolates |
No trend | 443.55 | 134.49 |
Damped trend | 444.75 | 136.87 |
Trend | 447.93 | 141.23 |
Further details on modelling direct population net health effects in high-value clinical scenario
Predicting the future sizes of the high-value clinical scenario
Time-series data were provided by PHE. This included evidence on changes over time in both invasive infection isolates and screening isolates. Neither isolate type (invasive infections and screening) are the same as the isolate type included in the HVCS (all infections). Of the two types available, the invasive infections were the most similar to all infections, so were the primary focus of analyses. Screening isolates were considered in secondary analyses. Data were supplied from the Reference Laboratory provided by the AMRHAI national reference unit, with data available until April 2021.
Further details on the analyses of invasive infections and screening isolates are provided in the subsequent subsections.
Time-series models
Time-series methods were used to generate future predictions of the population size. Three classes of model were considered:
-
Exponential smoothing (state-space) models. 204 This models variation in the data via variation in latent (unobserved) states representing a level (average) and trend. For extrapolations, predictions of these states are informed by all the available data, with more weight given to more recent observations and less weight given to older observations. The weight given to older observations decreases based on an exponential function, with the amount of decay estimated from the data. Use of this model assumes that extrapolations of (the logarithm of) the population follow a linear model. An alternative assumption is that the trend in the linear model is successively ‘damped’ over time so that eventually it becomes zero, and extrapolations become constant. This dampening can help to avoid forecasts becoming too large. Hence, three exponential smoothing models were considered; a trend model, a damped-trend model and a model with no trend.
-
ARIMA models. 204 These model the autocorrelations in the data. Unlike exponential smoothing models, ARIMA models do not incorporate a trend. Instead, they assume that after differencing the data (calculating the differences between observations; this is potentially repeated multiple times) there is no trend.
-
Generalised linear models for count time series data. 205 Poisson and negative binomial models were considered, with a logarithmic link for both. Hence, for both models, it is assumed that the logarithm of the counts follows a linear model. These models may be viewed as extending standard regression models to account for correlations among observations.
All models were fitted in R version 4.0.2, using the ‘forecast’ package for both exponential smoothing and ARIMA models, and the ‘tscount’ package for the generalised linear models. 204,205 The exponential smoothing and ARIMA models are for Gaussian (normally distributed) outcomes. Count data are not normally distributed, and due to the small numbers involved in the analysis the normal distribution would not be a good approximation. Instead, the logarithm of the data was taken prior to fitting the exponential smoothing and ARIMA models.
Point estimates from the three model types were generally very similar, as were model diagnostics (which included visual goodness of fit, statistical significance of the autocorrelation function, the distribution of residuals and the Ljung–Box test). Initially none of the models identified a trend in the time series, with forecasts being set to either the last observed value or an average of the observed data. As such, subsequent analyses focused on exponential smoothing models, for the following reasons:
-
The ability to specify models that include a trend (in contrast to ARIMA models which do not have an explicit trend parameter).
-
Having analytical formulae to express uncertainty in forecasts (which was not available for the generalised linear models).
Exponential smoothing models with both damped and undamped additive trends were considered. The error type (additive or multiplicative) was chosen by the fitting software (based on model goodness of fit), as was a Box–Cox transformation.
Incorporating forecasts in the economic model
To incorporate the extrapolations within the economic model, these were converted into year-on-year relative changes; that is, the relative change in year ‘t’ was calculated as the forecast in year ‘t + 1’ divided by the forecast in year ‘t’. For PSA, forecasts were obtained using the following process:
-
Obtain the mean and SD, both on the log-scale, at each time point. For example, to obtain forecasts for 20 years, 20 pairs of mean and SD are obtained.
-
Use these values to sample a value from a log-normal distribution. Hence, for a 20-year forecast, for a single iteration of the PSA, 20 samples are obtained; one for each year where each year has its own unique mean and SD.
Within a single iteration of the PSA the same random number was used for sampling. Different random numbers were used across PSA iterations. This ensured that trends in forecast were retained in the PSA.
Predicting future rates of resistance for current practice
Two options were considered for which data to use:
-
Forecast counts of both ‘susceptible’ (or ‘resistant’) as well as the denominator (susceptible plus resistant) and use the outputs from these forecasts to estimate future percentages of susceptibility or resistance. To reduce the noise in the data, forecasts would focus on the numerator for which there is the highest counts (e.g. for drugs to which isolates are mainly susceptible, the forecast would be counts of susceptible isolates).
-
Forecast the percentage susceptible (or resistant) directly.
An advantage of the first approach is that the data to be forecast (counts) are of the same type as the data forecast in the previous section, so the models of that section can also be considered. The main disadvantage of the first approach is that it ignores any correlations among the numerator and denominator, whereas by definition these are correlated. The second approach removes the need to consider correlations but has the main limitation it ignores evidence on the denominator (number of tests), which varies over time. As such, the second approach will give equal weight to each time point, even if some are based on a larger number of tests.
Prior to generating forecasts, exploratory modelling of the susceptibility data was undertaken to visually assess if there was likely to be a trend in the available data. Due to the typically small numbers and high variation observed in the susceptibility data, a visual approach to identifying a trend was taken in preference to significance testing. A Poisson generalised additive model was used, with the number of susceptible tests as the outcome and the number of tests as the offset (so allowing for a derivation of the susceptibility rate). This statistical approach is consistent with a recent publication of susceptibility data, with a further improvement to make the statistical model more flexible and so less prone to model misspecification (by using a generalised additive model instead of a generalised linear model). 206,207
Graphs for each AM are provided in Report Supplementary Material 3. Table 53 provides an overview of any trends in susceptibility using data from PHE. To add additional context, information on any trends in AM prescribing in secondary care in the time period 2015–19 (obtained from the ESPAUR report) is also included.
AM | Trends in susceptibility (PHE data) | Trends in prescribing (ESPAUR report) |
---|---|---|
CPE-MBL population | ||
Aminoglycosides | Potential increasing susceptibility, but due to uncertainty data are also consistent with no trend | Increase of 10.7% and 22.3% in inpatient and outpatient wards, respectively (2015–9, statistical significance not stated) |
Aztreonam | Potential increasing susceptibility, but due to uncertainty data are also consistent with no trend | No evidence provided |
Colistin | No trend | Increase from 15.8 to 25.2 defined daily doses per 1000 admissions (2015–9, statistical significance not stated) |
Tigecycline | Potential increasing susceptibility, but due to uncertainty data are also consistent with no trend | Significant increase in tetracyclines |
Pseudomonas population | ||
Colistin | No trend | Increase from 15.8 to 25.2 defined daily doses per 1000 admissions (2015–9, statistical significance not stated) |
In summary, there was no trend for colistin susceptibility for either population. For the other three AMs in the CPE-MBL population, it was unclear if susceptibility was increasing over time or not. Due to the large uncertainty in the susceptibility data (due to both small numbers and being restricted to invasive infections), it was decided that for the base-case analysis no trend would be used.
Predicting future resistance trajectories for cefiderocol
Supporting evidence
An overview of the studies identified via literature searches is provided in Table 54.
Study | Design | Population | AMs | Association |
---|---|---|---|---|
Ortiz-Brizuela (2020)165 | ARIMA models with lags between 1 and 12 months | Carbapenem-non-susceptible Enterobacterales treated in a hospital setting in Mexico City between July 2013 and December 2018. N = 451 | Resistance for three populations: carbapenem-non-susceptible Enterobacterales, CPE, and OXA-232 CPE. Evaluated for 17 AMs (DDD per 100 hospital patient-days) | For each population, a positive association was only found for piperacillin-tazobactam at a 6-month lag |
Gharbi (2015)166 | ARIMA models. Considered multiple yearly lags (not stated) | An outbreak of K. pneumoniae with OXA-48 in a London renal unit, January 2008–April 2010, N = 13 | Meropenem consumption (DDD per 100 occupied bed-days) | One-year lag had the largest correlation, with a coefficient from the ARIMA model of 1.07 (95% CI 0.10 to 2.05) |
Berger (2004)167 | Generalised additive model. Tested monthly lags | Staphylococcus aureus treated in hospitals in France, July 1997–June 2000. N = 1116 | Fluoroquinolone (DDD per 1000 days of hospitalisation) | The best fit was with a 4-month lag. Increasing use from the 25th–75th percentile had a relative risk of 1.27 (95% CI 1.13 to 1.42) |
While these studies were not used to estimate the link between AM use and AM resistance, they informed the approach to subsequent analysis. Two model types were used to assess the relationship between use and resistance: ARIMA models and generalised additive models. Of these, only the former are time-series models in the sense that they can capture autocorrelations within the data. Hence, this model type was retained for the de novo analyses reported here. With regard to the time lag to use, findings from the studies suggest that for monthly data a lag of 4–6 months would be appropriate, while for annual data a 1-year lag should be used.
When performing a de novo analysis, two types of publicly available evidence were available:
-
English data on AM use and AM resistance, from the ‘AMR local indicators profile’173
-
European data on AM use and AM resistance from the EARS-Net and ESAC-Net, respectively. 174,175
The England-specific data are made publicly available by PHE via the Fingertips database. 187 Data on resistance are available for E. coli bacteraemia for four AMs: gentamicin, ciprofloxacin, piperacillin/tazobactam and cephalosporins. Reporting of E. coli has been mandatory for NHS acute trusts since June 2011, and Fingertips provides quarterly data since the last quarter of 2015. 208 Data on AM use cover both primary and secondary care. For primary care, data are available for both the total number of AM prescriptions and the total number of prescriptions of broad-spectrum AMs, defined as cephalosporins, fluoroquinolones and co-amoxiclav. Secondary care AM use is available for the total number of AM prescriptions, the number of carbapenems prescriptions and the number of prescriptions for each of the World Health Organization’s access, watch, reserve categories. 209 An alternative data source for AM prescriptions is OpenPrescribing.net. 210 This provides information on primary care prescriptions for the last 5 years in England. This source does not include secondary care prescriptions but does include some of the drugs that are included in the Fingertips resistance data (gentamicin, ciprofloxacin and piperacillin/tazobactam).
Thirty countries from the European Union contribute data to EARS-Net on AM resistance for up to eight pathogens. 176 The analyses reported here focused on three pathogens that overlapped with those in the HVCS: E. coli, K. pneumoniae (as Enterobacterales) and P. aeruginosa. There was initially no restriction on the time periods, countries or AMs considered. The AMs for which resistance data are available are E. coli (aminoglycosides, aminopenicillins, carbapenems, fluoroquinolones and cephalosporins), K. pneumoniae (aminoglycosides, carbapenems, fluoroquinolones and cephalosporins) and P. aeruginosa (aminoglycosides, carbapenems, ceftazidime, fluoroquinolones and piperacillin-tazobactam).
Data on AM consumption (defined daily doses per 1000 inhabitants per day) were obtained from ESAC-Net, which provides use in both the community and hospitals. 174 Data are drawn from a variety of sources; for example, AM use in acute hospitals is based on a point-prevalence survey, while both sales and reimbursement data could contribute to overall estimates of use. Defined daily doses were developed by the World Health Organization Collaborating Centre for Drug Statistics Methodology and are the average maintenance dose per day for a drug when used in its main adult indication. There were two AMs for which surveillance data on both consumption and resistance were available: cephalosporins and carbapenems, hence analyses were restricted to these. Data for cephalosporins included first-, second-, third- and fourth-generation cephalosporins, as well as ‘other cephalosporins and penems’.
The general aim was to identify trajectories of resistance to existing AMs, and for to assess the association with AM use. This would then provide a set of potential use-resistance trajectories which could then be applied to cefiderocol, for which levels of use would be estimated from the economic model. A two-stage approach was employed. In the first stage, resistance trajectories were visualised to identify any trajectories for which resistance started at a low level. Trajectories were retained even if there was no apparent trend in resistance over time. This was because existing evidence suggested that for some AMs there may be no association between use and resistance. 163 Within the England-specific data, there were no clear examples of when resistance increased from a low baseline. Hence, subsequent analyses were restricted to the European surveillance data.
A visual inspection of the two Enterobacterales pathogens showed that low initial levels of resistance were more common for E. coli than K. pneumoniae, hence only the former was retained. For E. coli, an initial filter was applied to only retain countries for which at least 5000 isolates were tested, and baseline resistance (average over the first 3 years of available data) was < 3%. For P. aeruginosa countries were retained if at least 5000 isolates were tested, and baseline resistance was < 15%. As a result, 37 countries were retained (27 for E. coli and 10 for P. aeruginosa). After visually examining plots of AM use and AM resistance for these countries, it was decided to further filter the list of countries by restricting the evidence for carbapenems to countries with at least 10 non-zero observations for both AM use and AM resistance. For cephalosporins at least 15 non-zero observations were required, due to the large list of retained countries. This resulted in the following 23 pathogen–drug–country combinations:
-
Pseudomonas aeruginosa, carbapenems: Finland, France, Ireland, Netherlands, Norway, Slovenia, Sweden
-
Escherichia coli, carbapenems: France, Greece, Netherlands, Norway
-
Escherichia coli, cephalosporins: Bulgaria, Croatia, Estonia, Finland, France, Greece, Ireland, Luxembourg, Malta, Norway, Slovenia, Sweden.
For these countries, time-series models were used to assess the association between drug use in 1 year and resistance in the following year. This was achieved by fitting ARIMA models for which resistance over time was the outcome, and the lagged time series of drug use was the predictor. The regression coefficient for this predictor provides inferences: if it is significantly different to zero, this suggests that there is an association between AM use and resistance, with positive coefficients indicating that an increase (decrease) in use will lead to an increase (decrease) in resistance in the following year. Conversely, a negative coefficient indicates that an increase (decrease) in use will lead to a decrease (increase) in resistance in the following year. An overview of the coefficients for each retained country is provided in Table 55. Corresponding graphs are provided in Report Supplementary Material 3.
Country | Coefficient (standard error) |
Interpretation |
---|---|---|
P. aeruginosa, carbapenems | ||
Finland | −71.92 (63.7) |
Not significant |
France | 100.4 (108.79) |
Not significant |
Ireland | −0.67 (22.62) |
Not significant |
Netherlands | 295.97 (13.89) |
Significant: increase in use → increase in resistance |
Norway | −337.17 (123.8) |
Significant negative association: both an increase in use (→ a decrease in resistance) and a decrease in use (→ an increase in resistance) were observed (the option of having separate coefficients for these two negative associations was not explored) |
Slovenia | 358.2 (26.32) |
Significant: increase in use → increase in resistance |
Sweden | 180.51 (14.06) |
Significant: increase in use → increase in resistance |
E. coli, carbapenems | ||
France | 1.07 (0.32) |
Significant: increase in use → increase in resistance |
Greece | 7.06 (0.71) |
Significant: increase in use → increase in resistance |
Netherlands | −5.5 (3.25) |
Not significant |
Norway | −1.21 (0.91) |
Not significant |
E. coli, cephalosporins | ||
Bulgaria | 5.78 (1.16) |
Significant increase in use → increase in resistance |
Croatia | 0.69 (0.76) |
Not significant |
Estonia | 10.11 (1.59) |
Significant increase in use → increase in resistance |
Finland | −0.88 (1.62) |
Not significant |
France | −1.11 (0.64) |
Not significant |
Greece | 0.18 (0.67) |
Not significant |
Ireland | −2.03 (1.59) |
Not significant |
Luxembourg | −2.08 (0.93) |
Significant: decrease in use → increase in resistance |
Malta | 1.31 (0.77) |
Not significant |
Norway | −27.69 (2.27) |
Significant: decrease in use → increase in resistance |
Slovenia | −11.29 (3.71) |
Significant: decrease in use → increase in resistance |
Sweden | −12.63 (2.01) |
Significant: decrease in use → increase in resistance |
In summary, of the 23 combinations considered:
-
Just under half provided a significant association (12/23; P. aeruginosa = 4/7, E. coli = 2/4 for carbapenems and 6/12 for cephalosporins).
-
Of the 12 significant associations, 7 were positive associations (increasing use led to an increase in resistance), while 5 were negative (decreasing use led to an increase in resistance). Four of the negative associations were for E. coli-cephalosporins, the remaining one was for P. aeruginosa.
Of note, this analysis was focused on data sets which demonstrated an increase in resistance over time. Hence, any significant associations between AM use and decreasing resistance were not explored.
Based on this, we decided to explore three associations between increasing AM use and resistance:
-
no association
-
a weak positive association
-
a strong positive association.
There were four significant positive associations from the E. coli analyses, ranging from 1.07 (France, carbapenems) to 10.11 (Estonia, cephalosporins). Hence, these values were used to represent weak and strong associations for the CPE population, respectively. For the P. aeruginosa population, values of 180.51 (Sweden) and 358.2 (Slovenia) were used, respectively.
Use-resistance association: statistical models considered
Time-series model
An ARIMA time-series model was used because, in contrast to exponential smoothing models, software exists to fit models that include covariate effects. This provides the time-series version of a linear regression for which the outcome is the rate of resistance, and the dependent variable is AM use over time. 204
An advantage of using time-series methods (in preference to regression models) is that they capture autocorrelations among the data; that is, observations closer together in time are likely to be more similar than observations further apart in time. Incorporating this temporal structure is of particular importance when producing estimates of future values (extrapolations). In general, the further into the future predictions are required, the more uncertain they will be. This extrapolation uncertainty is accommodated by time-series models, but not standard regression models.
A key property of time-series methods is that predictions of the future are based on the assumption that trends observed in the historical data will continue into the future. External factors may alter these trends and hence lead to inaccurate forecasts. For example, an increased use or effectiveness of AM stewardship strategies/campaigns may lead to a reduced rate of resistance gain. 211 This may apply to both the AMs evaluated here and existing AMs such as carbapenems. UK examples of stewardship campaigns include the ‘Antibiotic Guardians’ and the Quality Premium. 206,212 Use of a damped-trend model can partly mitigate against this, as it successively reduces the extrapolated trend as the extrapolated time horizon increases. There is also empirical evidence from the literature that long-term forecasts from a time-series model with a damped trend will generally outperform similar models without a damped trend. 213
Differential equations model
A de novo model was developed to link the rate of change in AM resistance to AM use and other factors: natural mutations leading to resistance, loss of resistance (reflecting ‘fitness’ cost) and deaths among people with a resistant infection. This model was developed to provide a more comprehensive quantification of the differing potential drivers of AM resistance. Model conceptualisation was informed by both an existing review-based modelling framework214 and a new literature search. Report Supplementary Material 3 provides details on both the model specification and the supporting literature search.
Due to the relatively large number of parameters in the model, there was a danger that some of the parameters may lack identifiability (cannot be estimated from the available data). To explore this possibility, a simulation study was conducted. This study (reported in the Report Supplementary Material 3) had two objectives: first to identify the sample size required and second to quantify any bias in parameter estimates. This suggested that approximately 15 observations were required, and that while estimates of rates of natural resistance gain and loss were unbiased, there was a persistent under-estimation of the effect of AM use on AM resistance. Due to this bias, the differential equations model was not pursued further.
Model of no association
The sensitivity analysis exploring no relationship between AM use and resistance was motivated by existing literature demonstrating no, or very weak, association in certain settings. 163,164 This is likely to be because there are many drivers of resistance beyond AM use. This includes use in other populations (including other countries) as well as natural mutations. Hence, it may be that relative to these other drivers, use in the populations of interest plays a minimal role, so does not need to be explicitly modelled.
Manufacturer assessment of population size
The manufacturer’s calculation of population size is presented in Tables 56 and 57.
Parameter | Value | Source |
---|---|---|
Infections caused by Gram-negative pathogens in England | 200,000 | Estimate based on the annual rate of HAIs in England (243,746183 and 300,000184) and the assumption that the majority of HAIs are caused by Gram-negative bacteria |
Proportion of Gram-negative infections that are carbapenem resistant | 8% | Specialist Pharmacy Service185 |
Proportion of carbapenem-resistant infections that produce MBL | 33% | ESPAUR report6 data on MBL rates in Enterobacterales; Castanheira et al.186 data on MBL rates among carbapenem-producing P. aeruginosa |
Total number of infections caused by MBL-producing pathogens | 5000 | Calculation |
Parameter | Value | Source |
---|---|---|
Total number of infections caused by MBL-producing pathogens | 5000 | See. Tables 56 |
Proportion of infections considered critical | 30% | Proportion of infections that are respiratory infections or BSIs among all healthcare infections183 |
Proportion of infections that would be considered at high suspicion of MBL production in the ES | 50% | Expert opinion |
Total number of infections caused by MBL-producing pathogens that would be eligible for treatment in the ES | 750 | Calculation |
In addition, the manufacturer provides a range of examples of increasing infection rates and rates of resistance which they assess as indicative of an annual growth rate in the number of patients eligible for cefiderocol of 5% per annum.
The company’s estimate of the population size is based on uncertain evidence and a series of assumptions outlined in Tables 56 and 57. The resulting estimate is uncertain, reflecting the sparsity of evidence about the prevalence of infections caused by pathogens with MBL. Similarly, the company’s estimate of the annual growth rate is a rough estimate, higher than the growth rate in BSIs in general, and higher than the rate of increase in resistance in P. aeruginosa or Enterobacterales infections which the company cites. The mark-up is based on qualitative arguments and is therefore considered to be highly uncertain.
The company’s estimate represents the total number of MBL infections. In clinical practice, it is likely that not all MBL infections would be identified by clinicians as high risk of being MBL or confirmed as MBL. As result, 750 infections are likely to be an overestimate of the number of infections that would be treated with cefiderocol in clinical practice in the ES.
Appendix 8 Additional results from economic evaluation
Predicted population size over time
Figure 46 shows the base-case scenarios for population size over time by pathogen and infection site.
FIGURE 46.
Population size over time. P1G1: baseline population based on PHE categorisation of infection sites, damped growth rate; P1G2: baseline population based on PHE categorisation of infection sites, growth rate not damped; P2G1: baseline population based on clinical advisors’ categorisation of infection sites, damped growth rate; P2G2: baseline population based on clinical advisors’ categorisation of infection sites, growth rate not damped.

Total population incremental net health effect across the first 10 years of usage
Table 58 shows the INHE associated with initiating cefiderocol within the first 10 years of the population-level model.
Baseline population | Pop. growth rate | Change in resistance (%) | HAP/VAP (CPE MBL) | HAP/VAP (Pseud. MBL) | HAP/VAP (Sten.) | cUTI (CPE MBL) | cUTI (Pseud. MBL) | cUTI (Sten.) | BSI (CPE MBL) | BSI (Pseud. MBL) | BSI (Sten.) | IAI (CPE MBL) | IAI (Pseud. MBL) | IAI (Sten.) | Total | Proportion of 20-year INHE (%) |
---|---|---|---|---|---|---|---|---|---|---|---|---|---|---|---|---|
PHE categories of specimen types (Scenario P1) | Model with damped effect G1) | 1 (R1) | 49 | 7 | 26 | 16 | 25 | 37 | 301 | 17 | 28 | 13 | 17 | 21 | 557 | 51 |
5 (R2) | 48 | 7 | 26 | 16 | 25 | 37 | 298 | 16 | 27 | 13 | 17 | 21 | 551 | 52 | ||
10 (R3) | 48 | 7 | 25 | 15 | 24 | 36 | 293 | 16 | 27 | 13 | 17 | 20 | 541 | 52 | ||
30 (R4) | 45 | 6 | 23 | 14 | 23 | 34 | 275 | 15 | 25 | 12 | 16 | 19 | 507 | 57 | ||
Model without damped effect (G2) | 1 (R1) | 60 | 7 | 32 | 20 | 25 | 44 | 370 | 17 | 33 | 16 | 17 | 25 | 666 | 41 | |
5 (R2) | 59 | 7 | 31 | 19 | 25 | 43 | 366 | 16 | 33 | 16 | 17 | 25 | 657 | 42 | ||
10 (R3) | 58 | 7 | 31 | 19 | 24 | 43 | 359 | 16 | 32 | 16 | 17 | 24 | 646 | 42 | ||
30 (R4) | 54 | 6 | 28 | 17 | 23 | 40 | 335 | 15 | 30 | 15 | 16 | 23 | 602 | 47 | ||
Clinical advisors’ categories of specimen types (Scenario P2) | Model with damped effect G1) | 1 (R1) | 306 | 108 | 419 | 22 | 15 | 27 | 301 | 17 | 28 | 13 | 17 | 21 | 1294 | 52 |
5 (R2) | 302 | 107 | 414 | 21 | 15 | 27 | 298 | 16 | 27 | 13 | 17 | 21 | 1278 | 52 | ||
10 (R3) | 297 | 105 | 408 | 21 | 15 | 26 | 293 | 16 | 27 | 13 | 17 | 20 | 1258 | 53 | ||
30 (R4) | 279 | 98 | 386 | 19 | 14 | 25 | 275 | 15 | 25 | 12 | 16 | 19 | 1183 | 57 | ||
Model without damped effect (G2) | 1 (R1) | 376 | 108 | 479 | 27 | 15 | 32 | 370 | 17 | 33 | 16 | 17 | 25 | 1515 | 43 | |
5 (R2) | 371 | 107 | 474 | 26 | 15 | 32 | 366 | 16 | 33 | 16 | 17 | 25 | 1498 | 43 | ||
10 (R3) | 365 | 105 | 467 | 26 | 15 | 32 | 359 | 16 | 32 | 16 | 17 | 24 | 1474 | 44 | ||
30 (R4) | 340 | 98 | 440 | 24 | 14 | 29 | 335 | 15 | 30 | 15 | 16 | 23 | 1379 | 48 |
Additional scenario: contraindications to colistin and aminoglycosides
Background
The analysis in the EEPRU base case was based on a proportion of patients being resistant to existing therapies other than colistin/aminoglycosides. In which case it was assumed that, in the absence of cefiderocol, colistin/aminoglycosides would be administered to patients. The negative health effects and additional costs of renal toxicity associated with these products were explicitly modelled in assessing the patient-level INHEs of cefiderocol compared with existing therapies. Based on the results of the NMA of EUCAST studies, 82% of patients shown to have MBL P. aeruginosa in the ES were resistant to existing therapies other than colistin/aminoglycosides. For patients with suspected MBL Enterobacterales, all patients were assumed to receive colistin/aminoglycosides empirically. In the MDS, 9% of patients with MBL Enterobacterales, and 72% of patients with MBL P. aeruginosa were resistant to existing therapies other than colistin/aminoglycosides.
Consultees have indicated that, in terms of existing therapies (i.e. in a world without cefiderocol), there is a proportion of patients who would not receive colistin/aminoglycosides, even if no other effective therapy was available. This would be due to a patient’s high clinical risk of renal toxicity. For such patients, it can be assumed that they would only receive salvage therapy. The size of this subgroup of patients with an absolute contraindication to colistin/aminoglycosides was considered small by EEPRU’s clinical advisors. The Committee has requested a scenario which considers the magnitude of population-level INHEs for this subgroup using the Committee’s assumptions about the size of the cohort as a proportion of those estimated for the HVCSs in the report.
Methods
The scenario aimed to reflect the benefit of cefiderocol in patients who cannot take colistin and other aminoglycoside treatments and, therefore, without the new drug, would receive multidrug salvage therapy.
Patient-level benefit
For this scenario, the patient-level INHEs in those who can take colistin (the EEPRU base case) and those who cannot are shown in Table 59. In the ES, the incremental patient-level benefit of cefiderocol in HAP/VAP was derived by combining the EEPRU base-case and the insurance value scenario in Appendix 8, Combined effect of uncertainties at the patient and population level. In patients who were treated empirically and who were later confirmed to have an infection caused by the pathogens of interest (15% of patients with suspected MBL Enterobacterales and 14% of patients with suspected MBL P. aeruginosa, as per Table 19 of the report), outcomes were derived from the insurance value scenario in Appendix 8, Combined effect of uncertainties at the patient and population level, assuming that, without cefiderocol, all patients received ineffective empiric treatment. The incremental benefit of cefiderocol in this subgroup was 1.90 and 2.30 QALYs per person for MBL Enterobacterales and MBL P. aeruginosa, respectively. In patients who were treated empirically and who were later confirmed not to have an infection caused by the pathogens of interest (85% of patients with suspected MBL Enterobacterales and 86% of patients with suspected MBL P. aeruginosa), outcomes with colistin and with salvage therapy were assumed to be the same (0.21 QALYs for both pathogens, as per Tables 26 and 30 of the report).
In the MDS, without cefiderocol, patients who cannot take colistin/aminoglycosides were assumed to receive multidrug salvage therapy. The incremental benefit of cefiderocol was derived in the insurance value scenario (1.031 for HAP/VAP and 1.032 for cUTIs in Table 59). The net benefit was the same for both pathogens, as their susceptibility was assumed to be the same (90%).
HAP/VAP ESa | HAP/VAP MDSb | cUTI, MDSc | |
---|---|---|---|
Base case | CPE: 0.147 PsA: 0.207 |
CPE: 0.021 PsA: 0.151 |
CPE: 0.021 PsA: 0.147 |
New scenario | CPE: 0.462 PsA: 0.503 |
1.031 | 1.032 |
Population-level benefit
The population-level benefit was derived from the updated patient-level INHE, and the extrapolation parameters are shown in Table 60. In the ES, the patient-level benefit of cefiderocol in HAP/VAP was extrapolated to the population with HAP/VAP and BSI caused by MBL Enterobacterales and MBL P. aeruginosa, as in the base-case population-level model in main report. In the MDS, the patient-level benefit of cefiderocol in HAP/VAP was extrapolated to HAP/VAP and BSI caused by Stenotrophomonas, while the patient-level benefit in cUTI was extrapolated to all cUTI and IAI, as in the base-case population-level model in main report.
User-defined parameter | Base case (range) |
---|---|
Probability of event (emergence of highly resistant strains) | 100% |
Time of event (from now) | 0 years |
The number of patients affected in the first year | See Table 24 of the report |
The annual growth in the number of infections (from baseline) | CPE: 5.5% or 19.1% PsA: 0% Stenotrophomonas: 2.7% or 9.5% |
Analysis time horizon (years) | 20 |
Population annual discount rate | 3.5% |
The initial population size was site, setting and pathogen specific, derived as described base-case analysis in the main report. Two different scenarios for the initial population size were explored derived from different classifications of specimen samples in SGSS data set (Scenarios P1 and P2 in Table 24 of the main report). The population growth rate was assumed to be the same across all sites of infection and settings, but pathogen specific. It was approximated using the population size in year 1 and year 20 (shown in Figure 46), assuming a constant rate of increase between those two time points. Two scenarios for the population growth rate were explored derived assuming damped and non-damped population growth trends (Scenarios G1 and G2 in Figure 46) – these correspond to 5.5% and 19.1% annual increase on baseline in the Excel tool for MBL Enterobacterales infections. No growth was assumed for MBL P. aeruginosa infections, as per base case in the main report. For Stenotrophomonas infections, the growth rate was the unweighted average of Enterobacterales and P. aeruginosa infections (2.7% and 9.5% in scenarios G1 and G2, respectively).
The overall benefit was derived by averaging the total INHE from the base case and the new scenario, weighted by the proportion of the total treated population who are susceptible to colistin/aminoglycosides but would be given salvage therapy due to colistin/aminoglycoside toxicity.
Considering the lack of empirical evidence, the NICE Committee suggested a plausible range (20–40%) for the proportion of patients who, despite being susceptible to colistin/aminoglycosides, would instead be given salvage therapy due to colistin/aminoglycoside toxicity, in the absence of cefiderocol.
In the ES (HAP/VAP and BSIs caused by MBL Enterobacterales and MBL P. aeruginosa), this represents 20–40% of the total treated population when colistin/aminoglycosides are used empirically.
In the MDS (HAP/VAP and BSIs caused by Stenotrophomonas and all cUTI and IAI), the scenario is assumed to be applicable to 20–40% of the patients who were not susceptible to non-colistin/aminoglycoside therapy assuming that all such patients would be considered for colistin/aminoglycoside therapy. The susceptible proportions were 9% (100–91%) of Enterobacterales and 72% (100–28%) of P. aeruginosa, as per Table 16 of the report, and 40.5% of Stenotrophomonas assuming the susceptibility was the weighted average of the former two pathogens.
Therefore, the proportion of the total sample in the MDS who would be in this subgroup was between 1.8% (= 0.2*9%) and 3.6% (= 0.4*9%) for Enterobacterales, between 14.4% (= 0.2*72%) and 28.8% (= 0.4*72%) for PsA and between 8.1% (= 0.2*40.5%) and 16.2% (0.4*40.5%) for MDS Stenotrophomonas.
Results
Figure 47 shows how the total expected INHE changes with the proportion of patients who cannot take colistin, compared to the EEPRU base case. In summary, reflecting the outcomes of patients who cannot take colistin/aminoglycosides increases the benefit of cefiderocol, and the benefit increases with the proportion of such patients. The absolute increase in INHE in this scenario increases with the population size, as shown by the orange solid and dashed lines (representing a scenario with a higher patient population) diverging more than the blue solid and dashed lines.
FIGURE 47.
Change in total population-level INHE with varying proportion of patients who cannot take colistin, derived from different assumptions about the population size. P1G1: baseline population (point estimate) based on PHE categorisation of infection sites, growth rate damped; P2G2: baseline population (point estimate) based on clinical advisors’ categorisation of infection sites, growth rate not damped.

Table 61 shows the breakdown of the population-level INHE for each pathogen and site of infection for a range of proportions of patients who cannot take colistin/aminoglycosides, compared to the EEPRU base case. The change in INHE compared to the base case is higher in the MDS (all cUTI and IAI, and HAP/VAP and BSI with Stenotrophomonas) than the ES (HAP/VAP and BSI with MBL Enterobacterales and MBL P. aeruginosa) because the patient-level benefit of cefiderocol in patients who cannot take colistin is higher in the MDS than the ES (shown in Table 61).
Pathogen | Site of infection | Proportion of susceptible patients who cannot take colistin/aminoglycosides | ||
---|---|---|---|---|
0% (base case)b | 20% | 40% | ||
MBL Enterobacterales | HAP/VAP | 79–775 | 115–1207 | 150–1639 |
cUTI | 28–59 | 50–143 | 73–226 | |
BSI | 448–764 | 677–1198 | 906–1632 | |
IAI | 23–36 | 46–78 | 68–119 | |
MBL P. aeruginosa | HAP/VAP | 12–185 | 17–274 | 22–363 |
cUTI | 31–19 | 57–31 | 83–44 | |
BSI | 28–28 | 45–45 | 61–61 | |
IAI | 22–22 | 49–49 | 76–76 | |
Stenotrophomonas | HAP/VAP | 48–925 | 191–2003 | 334–3081 |
cUTI | 57–64 | 148–207 | 246–349 | |
BSI | 48–72 | 134–201 | 219–330 | |
IAI | 32–46 | 89–131 | 145–217 | |
Total | 856–2995 | 1616–5566 | 2383–8138 |
These expected population-level INHE may overestimate the total INHE for several reasons. Firstly, the 20–40% proportion of patients who would not be given colistin/aminoglycosides because of toxicity fears in the absence of cefiderocol is high compared to the assessment of the clinical advisors consulted by EEPRU. Secondly, the scenario assumes that outcomes in patients who can and cannot take colistin/aminoglycosides are comparable, when, in practice, patients who cannot take colistin may have poorer prognoses than patients who can. Thirdly, the scenario assumes patients would be contraindicated to colistin and aminoglycosides, but clinical advisors to EEPRU (and consultation comments from the British Infection Association) suggested that most of the concern is about colistin.
Finally, the scenario results represent the benefit in HVCS when, in the ES, all patients with suspected infection are treated with colistin/aminoglycosides. In the EEPRU base case, the empiric treatment of MBL P. aeruginosa infections with non-colistin/glycoside therapy had a higher patient-level net benefit (and lower incremental benefit of cefiderocol) than treatment with colistin/aminoglycosides, suggesting that, without cefiderocol, non-aminoglycosides are the preferred empiric treatment. The base-case results reflect this lower incremental benefit of cefiderocol achieved when only non-colistin/aminoglycosides are used are first-line empiric treatment.
The benefit of cefiderocol generated by patients who are susceptible to, but who cannot take, colistin/aminoglycosides in the ES is likely to be lower than the estimates if empiric treatment does not include colistin/aminoglycosides. This is because it would only apply to < 1% of the total sample of patients in this setting [20–40% of the 1.8% (0.2 × 9%) who have the infection but are not susceptible to the empiric treatment with non-aminoglycosides]. When MBL P. aeruginosa infections are treated with non-colistin/aminoglycosides in the ES, the total net benefit of cefiderocol (derived using values in column 1 for rows 5 and 7, and columns 2 and 4 for all other rows in) was 1594–5461 QALYs if 20% of susceptible patients cannot take colistin/aminoglycosides, and 2339–7927 QALYs if that proportion is 40%.
Combined effect of uncertainties at the patient and population level
Patient-level scenario analyses that modified the total base-case population-level INHE by more than 10% are shown in Table 62. The results are presented as the range based on most and least conservative assumptions about the population size (Scenarios P1G1 and P2G2) and assuming zero resistance emergence. The scenarios assume that, where applicable, the same assumptions apply across populations, for example if a certain assumption is considered more appropriate for HAP/VAP ES patients, it is also considered more appropriate for BSI ES patients.
Base-case value/assumption | Scenario value/assumption | HAP/VAP Enterobacterales (ES) | HAP/VAP P. aeruginosa (ES) | HAP/VAP Sten. (MDS) | cUTI Enterobacterales (MDS) | cUTI P. aeruginosa (MDS) | cUTI Sten. (MDS) | BSI Enterobacterales (ES) | BSI P. aeruginosa (ES) | BSI Sten. (MDS) | IAI Enterobacterales (MDS) | IAI P. aeruginosa (MDS) | IAI Sten. (MDS) | Total, k l |
---|---|---|---|---|---|---|---|---|---|---|---|---|---|---|
- | - | 99–966 | 12–186 | 53–1065 | 32–69 | 26–43 | 73–79 | 608–952 | 28–28 | 54–81 | 27–42 | 30–30 | 41–59 | 1100–3583 |
Probability patient has MBL Enterobacterales is 0.15, MBL Pseudo. is 0.14 | Probability patient has MBL is 0.71 based on BSAC survey data | 01–12 | 17–269 | 53–1065 | 32–69 | 26–43 | 73–79 | 8–12 | 41–41 | 54–81 | 27–42 | 30–30 | 41–59 | 420–1785 |
Probability patient has MBL Enterobacterales is 0.15, MBL Pseudo. is 0.14 | Probability patient has MBL is 0.30 | 65–637 | 18–282 | 53–1065 | 32–69 | 26–43 | 73–79 | 401–628 | 43–43 | 54–81 | 27–42 | 30–30 | 41–59 | 880–3041 |
Probability patient has MBL Enterobacterales is 0.15, MBL Pseudo. is 0.14 | Probability patient has MBL is 0.40 | 42–413 | 18–279 | 53–1065 | 32–69 | 26–43 | 73–79 | 260–407 | 43–43 | 54–81 | 27–42 | 30–30 | 41–59 | 716–2593 |
Probability patient has MBL Enterobacterales is 0.15, MBL Pseudo. is 0.14 | Probability patient has MBL is 0.50 | 19–189 | 18–276 | 53–1065 | 32–69 | 26–43 | 73–79 | 119–187 | 42–42 | 54–81 | 27–42 | 30–30 | 41–59 | 551–2145 |
Probability patient has MBL Enterobacterales is 0.15, MBL Pseudo. is 0.14 | Probability patient has MBL is 0.60 | 01–10 | 17–273 | 53–1065 | 32–69 | 26–43 | 73–79 | 06–10 | 42–42 | 54–81 | 27–42 | 30–30 | 41–59 | 419–1786 |
Probability patient has MBL Enterobacterales is 0.15, MBL Pseudo. is 0.14 | Probability patient has MBL is 0.70 | 01–12 | 17–269 | 53–1065 | 32–69 | 26–43 | 73–79 | 08–12 | 41–41 | 54–81 | 27–42 | 30–30 | 41–59 | 420–1785 |
Probability patient has MBL Enterobacterales is 0.15, MBL Pseudo. is 0.14 | Probability patient has MBL is 0.80 | 1–14 | 17–266 | 53–1065 | 32–69 | 26–43 | 73–79 | 9–14 | 41–41 | 54–81 | 27–42 | 30–30 | 41–59 | 421–1786 |
Probability patient has MBL Enterobacterales is 0.15, MBL Pseudo. is 0.14 | Probability patient has MBL is 0.90 | 2–15 | 17–263 | 53–1065 | 32–69 | 26–43 | 73–79 | 10–15 | 40–40 | 54–81 | 27–42 | 30–30 | 41–59 | 422–1784 |
Susceptibility (Enterobacterales and PsA) based on NMA of EUCAST studies | Susceptibility based on NMA of CLSI studies | 122–1194 | 02–34 | 25–275 | 16–33 | 5–9 | 21–28 | 751–1177 | 5–5 | 19–28 | 13–20 | 6–6 | 12–18 | 1001–2823 |
Susceptibility (Enterobacterales and PsA) based on NMA of EUCAST studies | Susceptibility based on PHE data, with cefiderocol and fosfomycin data from separate cefiderocol and fosfomycin networks (CLSI studies) | 134–1316 | 1–9 | 40–213 | 25–53 | 1–2 | 21–34 | 828–1297 | 1–1 | 23–34 | 21–32 | 2–2 | 13–18 | 1111–3010 |
Susceptibility (Enterobacterales and PsA) based on NMA of EUCAST studies | MBL Enterobacterales susceptibility based on PHE data (cefiderocol from NMA) | 95–934 | 12–186 | 130–1389 | 80–171 | 26–43 | 108–141 | 588–921 | 28–28 | 96–142 | 67–105 | 30–30 | 63–90 | 1340–4163 |
Susceptibility (Enterobacterales and PsA) based on NMA of EUCAST studies | PsA MBL susceptibility based on NMA of EUCAST studies, absolute colistin susceptibility values from SIDERO WT | 99–966 | 12–180 | 53–1448 | 32–69 | 38–64 | 97–99 | 608–952 | 27–27 | 67–99 | 27–42 | 45–45 | 54–77 | 1185–4042 |
Probability of AKI with colistin/aminoglycoside therapy based on Sisay (2021) (0.45) | Probability of AKI with colistin/aminoglycoside therapy based on Chien (0.32) | 75–732 | 11–171 | 47–891 | 29–62 | 76–46 | 105–109 | 460–721 | 26–26 | 47–69 | 24–38 | 54–54 | 60–86 | 1018–3001 |
OR comparing AKI for colistin/aminoglycoside-based therapy to non-colistin/aminoglycoside-based therapy from all studies analysis in Chien (2020) (1.81) | OR from all studies analysis in Wagenlehner (2021) (2.23) | 137–1341 | 12–185 | 62–1344 | 38–82 | 66–110 | 147–155 | 844–1322 | 28–28 | 67–100 | 32–50 | 77–77 | 85–122 | 1647–4864 |
OR comparing AKI for colistin/aminoglycoside-based therapy to non-colistin/aminoglycoside-based therapy from all studies analysis in Chien (2020) (1.81) | OR from RIFLE criteria studies analysis in Chien (2020) (1.61) | 75–732 | 12–188 | 47–896 | 29–61 | 26–43 | 70–75 | 460–721 | 29–29 | 47–69 | 24–38 | 30–30 | 39–56 | 905–2921 |
Risk of CKD as observed in Bucaloiu (2012) | Risk of CKD doubled to reflect potential higher propensity for CKD in HVCS | 80–782 | 11–174 | 46–925 | 28–61 | 37–22 | 64–70 | 492–770 | 27–27 | 48–70 | 24–37 | 26–26 | 36–51 | 919–3015 |
Log-normal model fit to CARBAR survival data | Log-logistic model fit to CARBAR survival data | 80–783 | 11–174 | 46–925 | 28–61 | 21–35 | 61–68 | 492–771 | 27–27 | 48–70 | 24–37 | 25–25 | 35–50 | 912–3012 |
Log-normal model fit to CARBAR survival data | Weibull model fit to CARBAR survival data | 56–545 | 10–158 | 38–739 | 23–49 | 55–33 | 79–80 | 343–537 | 24–24 | 38–57 | 19–30 | 38–38 | 44–63 | 768–2352 |
OR of mortality for AKI compared to no AKI derived from Kerr (2014) (5.11) | OR of mortality for AKI compared to no AKI halved (2.56) | 73–710 | 11–179 | 45–850 | 28–59 | 45–27 | 72–76 | 447–699 | 27–27 | 45–66 | 23–36 | 32–32 | 41–58 | 889–2819 |
Population growth impacts population-level INHE to a greater extent than scenarios in the patient-level model, as the variation in the total INHE across different population size scenarios of 896–3559 QALYs (the base-case range in Table 34) is more substantial than the variation across different rows in the table (e.g. 419–1647 QALYs in the more conservative scenario about the population size).
The main areas of uncertainty relate to the probability that a patient has MBL, the susceptibility scenarios, the impact of colistin/aminoglycoside-based therapy on AKI risk and long-term mortality post-hospital discharge. These were the most impactful scenarios in patient-level results for MBL Enterobacterales in ES (see Metallo-beta-lactamasesEnterobacterales,empiric setting, hospital-acquired pneumonia/ventilator-associated pneumonia), the setting with the greatest population size.
Additional analysis to reflect insurance value
The patient-level benefit was derived by adapting the model. Specifically, we assumed that, in patients with these new highly resistant infections, existing therapies are no longer effective. In this model, this was achieved by setting the susceptibility for all comparators to zero. Under this illustrative scenario, no safety differences are assumed as it is expected that, if treatments become completely ineffective, no treatment or only safe AMs will be used. Furthermore, the susceptibility for cefiderocol is set to 90% (an estimate broadly reflecting the susceptibility across different scenarios in the report), and maintained at this level over the long term, although we note that this is likely to overestimate INHEs as susceptibility to cefiderocol may be expected to wane over time.
In the ES, we assume everyone gets cefiderocol or non-colistin/aminoglycosides (comparator), then 5 days later they move into the MDS, and switch to the treatment they are susceptible to. For both cefiderocol and the comparator, 85% of the patients are assumed not to have the target multidrug-resistant infection (as in the base-case modelling for MBL Enterobacterales) and these patients receive something else. When cefiderocol is available, the 15% who do have that target infection receive either cefiderocol (for 90% who are susceptible) or salvage therapy (for the 10% not susceptible to cefiderocol). When cefiderocol is not available, 100% of patients receive salvage therapy (the comparator). In MDS we assume 90% cefiderocol/10% salvage therapy (when cefiderocol is available) or 100% salvage therapy (comparator). The results represent the lifetime patient-level INHE of cefiderocol relative to multidrug salvage therapy expressed in QALYs.
To derive population-level benefits, patient-level INHE is multiplied by the expected population size over the relevant time horizon and the probability of this scenario occurring. The patient-level INHE is assumed to remain constant over time. The population size is increased over time at a constant rate relative to baseline. Population benefits over time are discounted to reflect the delay in benefits received. Note that the relevant population here is not the same as the expected population in the EEPRU base case as these assumed to be entirely different pathogens.
The hypothetical nature of the scenario means that there is no formal evidence to inform the extrapolation parameters and, as result, the parameter ranges were provided by the NICE Committee. However, given the highly speculative nature of the analysis, EEPRU provided a flexible Excel-based tool, with user-defined parameters, to support Committee deliberations in assessing the potential additional long-term health effects that may result from cefiderocol usage.
The modifiable parameters in the Excel tool included the six extrapolation parameters: the probability of emergence of the highly resistant strains; the time of the first event; the number of patients affected in the first year; the annual growth rate in the number of infections (constant, relative to baseline); the analysis time horizon; and the discount rate.
In addition, the user could specify the site of infection and treatment setting reflected in the results (HAP/VAP ES, HAP/VAP MDS and cUTI microbiology-directed setting), or an alternative patient-level INHE reflecting the impact of these highly resistant infections in an alternative population.
The parameter estimates to use in the model were sought from the Committee.
The patient-level INHEs in the base case and as used in this additional scenario, expressed in QALYs per patient, are shown in Table 64. In summary, assuming all specific existing treatment options have zero effectiveness increases the patient-level INHE for all sites and settings. The increase is greatest in the MDS, as all patients benefit from treatment with cefiderocol, compared to the ES where only a proportion of people have the suspected pathogen mechanism.
User-defined parameter | Base case (range) |
---|---|
Probability of event (emergence of highly resistant strains) | 1% (0.5–5%) |
Time of first event (from now) | 10 years (5–15 years) |
The number of patients affected in the first year | 25 individuals (25–100) |
The annual growth in the number of infections (from baseline) | 20% (3–30%) |
Analysis time horizon (years) | 50 (20–50) |
Population discount rate | 3.5% |
Base case | New scenario | |
---|---|---|
HAP/VAP, ES | CPE: 0.147 (ca) PsA: 0.153 (nca); 0.207 (ca) |
0.280 |
HAP/VAP, MDS | CPE: 0.021 PsA: 0.151 |
1.031 |
cUTI, MDS | CPE: 0.021 PsA: 0.147 |
1.032 |
Value/assumption in base case | Value/assumption in sensitivity analyses | Total number of patients | Total INHE (QALYs) conditional on event occurring | Expected INHE (QALYs) |
---|---|---|---|---|
Base case, parameter values shown in | 4720 | 1460 | 14.6 | |
Probability of event = 1% | Probability of event = 0.5% | 4720 | 1460 | 7.3 |
Probability of event = 1% | Probability of event = 5% | 4720 | 1460 | 73.0 |
Event occurs in 10 years | Event occurs in 5 years | 6195 | 2180 | 21.8 |
Event occurs in 10 years | Event occurs in 15 years | 3245 | 870 | 8.7 |
Number of patients in year 1 = 25 | Number of patients in year 1 = 100 | 18,880 | 5839 | 58.4 |
Population growth = 20% | Population growth = 3% | 1388 | 452 | 4.5 |
Population growth = 20% | Population growth = 30% | 6680 | 2053 | 20.5 |
Analysis time horizon = 50 years | Analysis time horizon = 20 years | 145 | 79 | 0.8 |
The population-level INHE (assuming patient-level benefit in cUTIs – the site with the highest patient-level INHE) is shown in for a range of population-related scenarios. Overall, the benefit is relatively low (between 0.8 and 58.4 QALYs) compared to the expected benefit estimated for the main analysis (between 896 and 3559 QALYs).
List of abbreviations
- AKI
- acute kidney injury
- AM
- antimicrobial
- AmpC
- ampicillinase C
- AMR
- antimicrobial resistance
- AMRHAI
- antimicrobial resistance and healthcare-associated infections
- AST
- antimicrobial susceptibility testing
- ATLAS
- antimicrobial testing leadership and surveillance
- BAT
- best available therapy
- BSAC
- British Society for Antimicrobial Chemotherapy
- BSIs
- bloodstream infections
- CAZ-AVI
- ceftazidime-avibactam
- CCI
- Charlson Comorbidity Index
- CAZ-AVI
- ceftazidime–avibactam
- CKD
- chronic kidney disease
- CLSI
- Clinical Laboratory Standards Institute
- CPE
- carbapenemases-producing Enterobacterales
- CRD
- Centre for Reviews and Dissemination
- CRE
- carbapenem-resistant Enterobacterales
- CrI
- credible intervals
- cUTI
- complicated urinary tract infection
- DIC
- deviance information criterion
- DIM
- Dutch imipenemase
- EEPRU
- Policy Research Unit in Economic Methods of Evaluation in Health and Social Care Interventions
- EKHUFT
- East Kent Hospitals University NHS Foundation Trust
- ES
- empiric setting
- ESBL
- extended-spectrum β-lactamase
- ESPAUR
- English Surveillance Programme for Antimicrobial Utilisation and Resistance
- EUCAST
- European Committee on Antimicrobial Susceptibility Testing
- GES
- Guiana extended-spectrum β-lactamase
- GNB
- Gram-negative bacteria
- GNO
- Gram-negative organisms
- HAP
- hospital-acquired pneumonia
- HDU
- high-dependency unit
- HES
- Hospital Episode Statistics
- HRQoL
- health-related quality of life
- HTA
- Health Technology Assessment
- HVCS
- high-value clinical scenario
- IAIs
- intra-abdominal infections
- ICU
- intensive care unit
- IMP
- imipenemase
- INHE
- incremental net health effect
- IPD
- individual patient data
- KPC
- Klebsiella pneumoniae carbapenemase
- LoS
- length of stay
- MBL
- metallo-beta-lactamases
- MDR
- multidrug resistant
- MDS
- microbiology-directed setting
- MIC
- minimum inhibitory concentration
- NDM
- New Delhi metallo-beta-lactamases
- NHE
- net health effects
- NHS EED
- NHS Economic Evaluation Database
- NICE
- National Institute for Health and Care Excellence
- NMA
- network meta-analysis
- OR
- odds ratios
- OXA
- oxacillinase
- PB
- probability of being the best treatment
- PICOS
- population, intervention, comparison, outcomes, study designs
- PCR
- polymerase chain reaction
- PD
- pharmacodynamic
- PHE
- Public Health England
- PK
- pharmacokinetic
- PrI
- prediction intervals
- PRISMA
- Preferred Reporting Items for Systematic Reviews and Meta-Analyses
- PSA
- probabilistic sensitivity analysis
- QALY
- quality-adjusted life-year
- RCT
- randomised controlled trial
- RE
- random effect
- RIFLE
- risk, injury, failure, loss and end-stage renal disease
- RR
- relative risk
- SD
- standard deviation
- SGSS
- second-generation surveillance system
- SPM
- Sao Paulo metallo-beta-lactamases
- TSD
- technical support document
- UME
- unrelated mean effects
- UTI
- urinary tract infections
- VAP
- ventilator-associated pneumonia
- VIM
- verona integrated-encoded metallo-beta-lactamases
- WHO
- World Health Organization
- XDR
- extensively drug resistant
Notes
Supplementary material can be found on the NIHR Journals Library report page (https://doi.org/10.3310/YGWR4511).
Supplementary material has been provided by the authors to support the report and any files provided at submission will have been seen by peer reviewers, but not extensively reviewed. Any supplementary material provided at a later stage in the process may not have been peer reviewed.