Notes
Article history
The research reported in this issue of the journal was funded by PGfAR as project number RP-PG-0707-10031. The contractual start date was in January 2009. The final report began editorial review in September 2014 and was accepted for publication in October 2015. As the funder, the PGfAR programme agreed the research questions and study designs in advance with the investigators. The authors have been wholly responsible for all data collection, analysis and interpretation, and for writing up their work. The PGfAR editors and production house have tried to ensure the accuracy of the authors’ report and would like to thank the reviewers for their constructive comments on the final report document. However, they do not accept liability for damages or losses arising from material published in this report.
Declared competing interests of authors
Jack Cuzick reports grants from AstraZeneca for being on the advisory board, and funding of the prevention trial outside the submitted work.
Permissions
Copyright statement
© Queen’s Printer and Controller of HMSO 2016. This work was produced by Evans et al. under the terms of a commissioning contract issued by the Secretary of State for Health. This issue may be freely reproduced for the purposes of private research and study and extracts (or indeed, the full report) may be included in professional journals provided that suitable acknowledgement is made and the reproduction is not associated with any form of advertising. Applications for commercial reproduction should be addressed to: NIHR Journals Library, National Institute for Health Research, Evaluation, Trials and Studies Coordinating Centre, Alpha House, University of Southampton Science Park, Southampton SO16 7NS, UK.
Chapter 1 Introduction and background
Breast cancer causes 11,684 deaths per year in the UK; in the age group 35–50 years, it causes more deaths than any other medical condition,1 and the highest number of quality life-years are lost in this age group. In 2011, there were 50,285 breast cancer diagnoses in the UK. 1 Currently, screening through the NHS Breast Screening Programme (NHSBSP), with 3-yearly mammography, is targeted at women aged 47–73 years. Women aged 40–49 years with a ≥ 3% risk in that decade are also eligible for annual mammography, but currently these women are assessed by family history alone. A smaller group of women who carry mutations in BRCA1 (breast cancer 1 gene)/BRCA2 (breast cancer 2 gene), or who have an 8% risk aged 30–39 years or a 12% risk aged 40–49 years, can be assessed for magnetic resonance imaging (MRI) screening. 2 In the UK, 10–11% of women are projected to develop breast cancer in their lifetime and treatment is becoming increasingly expensive, with newer, complex chemotherapy regimens and drugs such as Herceptin® (Genentech Inc., San Francisco, CA, USA). Although the number of breast cancer deaths has decreased in many Western countries, the incidence of the disease is continuing to rise. In particular, in countries with historically low incidence, breast cancer rates are rising rapidly, making it now the world’s most prevalent cancer. 3 The increase in incidence is almost certainly related to dietary and reproductive patterns associated with Western lifestyles. Indeed, there is evidence from genetic studies in the USA, Iceland and the UK of a threefold increased incidence, in the general population and in those at the highest level of risk with BRCA1/BRCA2 mutations, in the past 80 years. 2,4,5 BRCA1 and BRCA2 are high-penetrance genes mutations, which are carried by around 1 in 400 women in the outbred UK population but by as many as 1 in 40 women of Ashkenazi Jewish origin. 4–6 Women who carry mutations in these genes have a risk of breast cancer to 70 years of up to 85%,4–6 but population-based studies have shown lower risk estimates of 40–50%. 5 Targeted screening and prevention strategies would, potentially, create huge savings to the NHS and increase the quality and length of life for many women. Although preventative measures based on chemotherapy and lifestyle change are possible, these are not feasible on a population basis, in part because of the difficulties of identifying women in the general population who are at increased risk. Tamoxifen and raloxifene are now approved by the National Institute for Health and Care Excellence (NICE) as chemoprevention to be offered to high-risk women (10-year risk of ≥ 8%). 2
Unlike most screening programmes in other countries, which typically use 1- or 2-yearly intervals, the interval between mammograms in the NHSBSP is 3 years; possibly partly as a result of this, 40% of tumours arise in the interval between mammograms. These cancers have a poorer prognosis and reduce the potential effectiveness of the programme. 6,7 Identifying women likely to develop interval cancers and offering them tailored screening and preventative interventions may be a way to reduce the incidence of interval cancers. There is evidence to suggest that women at high risk of breast cancer are more likely to develop interval cancers. The Swedish 2-county study8 showed that women with family history of breast cancer were significantly more likely to develop breast cancer in the interval between 2-yearly screens than equivalent women with no family history. High mammographic breast density (MD) also considerably increases the risk of developing interval breast cancer. 9,10 A screening programme adapted to risk may, therefore, improve the effectiveness and efficiency of the NHSBSP. For women at very low risk of developing breast cancer, the screening interval might be extended, thereby potentially safely reducing the number needing to be screened.
Evans and Howell3 state that there are two main types of risk assessment: the chances of developing breast cancer over a given time span, including the lifetime, and the chances of there being a mutation in a known high-risk gene such as BRCA1 or BRCA2. While some risk assessment models are aimed primarily at solving one of these questions, many also have an output for the other. For example, the Tyrer–Cuzick11 model was developed to assess breast cancer risk over time, but does have a read-out for BRCA1/BRCA2 probability for the individual (text reproduced with permission from Evans and Howell. 3 © BioMed Central 2007). Breast cancer risk algorithms which aim to predict risk over a given time span generally include a combination of known risk factors, such as a family history of the disease and reproductive and hormonal history. Although current models perform well at predicting the overall number of breast cancer cases arising in a particular population, they are poor at identifying specific individuals. 4 To assess breast cancer risks over time as accurately as possible, all known risk factors for breast cancer need to be assessed.
Risk factors
Family history of breast cancer in relatives12
-
Age at onset of breast cancer.
-
Bilateral disease.
-
Degree of relationship to family member (first or greater).
-
Multiple cases in the family (particularly on one side).
-
Other related early-onset tumours (e.g. ovary, sarcoma).
-
Number of unaffected individuals (large families with many unaffected relatives will be less likely to harbour a high-risk gene mutation).
Hormonal and reproductive risk factors
Hormonal and reproductive factors have been recognised for a long time to have an important role in breast cancer development. Prolonged exposure to endogenous oestrogens is an adverse risk factor for breast cancer. Early menarche and late menopause increase breast cancer risk, as they prolong exposure to oestrogen and progesterone. 13–22
Long-term combined hormone replacement therapy (HRT) treatment (> 5 years) after the menopause is associated with a significant increase in risk. However, shorter-term treatments may still be associated with risk to those with a family history of breast cancer. 14 In a large meta-analysis, the risk appeared to increase cumulatively by 1–2% per year but disappear within 5 years of cessation. 15 Oestrogen-only HRT has a risk that appears much lower, and it may be risk neutral. 16–19 A meta-analysis also suggested that both during current use of the combined oral contraceptive and 10 years post use, there may be a 24% increase in risk of breast cancer. 13
A woman’s age at first pregnancy influences the relative risk (RR) of breast cancer, as pregnancy transforms breast parenchymal cells into a more stable state, potentially resulting in less proliferation in the second half of the menstrual cycle. As a result, early first pregnancy offers some protection, while women having their first child over the age of 30 years have double the risk of women delivering their first child under the age of 20 years, and these are likely to be similar in those at highest risk from a BRCA1/BRCA2 mutation. 20,21
Mammographic breast density
It has been shown that increased breast density not only is associated with an elevated risk of breast cancer but is the largest risk factor after age. 23–26 The difference in risk between women with extremely dense, as opposed to predominantly fatty, breasts is approximately four- to sixfold. 26 Assuming that the association between breast density and breast cancer risk is causal, MD is the single assessable risk factor with the largest population attributable risk and may also have a substantial heritable component. 25,26
If MD is to be used to estimate breast cancer risk, it is necessary to identify the optimal method of MD assessment, in terms of both practicality and feasibility of incorporation into routine practice, and accuracy of risk prediction. The assessment of breast density from mammograms has generally been provided by the subjective visual evaluation of an expert. Computer-based methods have also been developed in an attempt to make the assessment of MD more quantitative; however, many of the older computer-based methods, such as Cumulus (Sunnybrook Health Sciences Centre, Toronto, ON, Canada), still rely on some subjectivity. More recently developed computer-based methods have aimed to determine the true volumes of dense and fatty tissue from digital mammograms. As these methods are automated and require no subjective input, they are by far the most practical methods for wider use.
Genetic factors
Mutations in breast cancer genes such as BRCA1 and BRCA2 are too infrequent to affect risk prediction appreciably in the models for the general population. However, recently identified single nucleotide polymorphisms (SNPs) in many genes and outside genes (n = 77),27 which individually confer small changes in risk, may prove useful in predicting larger differences in risk when considered together. Four Genome-Wide Association Studies (GWASs),8–10,28 published before our programme grant, found common genetic variants (SNPs) each carried by 28–44% of the population were associated with a 1.07–1.26 RR of breast cancer. These variants linked to four genes [FGFR2 (fibroblast growth factor receptor 2), TOX3 (TOX high-mobility group box family member 3), MAP3K1 (mitogen-activated protein kinase kinase kinase 1, E3 ubiquitin protein ligase) and LSP1 (lymphocte-specific protein 1)] confer as much as a 1.17–1.64 risk if two copies are carried. When combined in an individual, they give higher than additive risk of breast cancer. 29 Another variant, CASP8 (caspase 8, apoptosis-related cysteine peptidase), is associated with reduced breast cancer risk. 30 There are now 77 genetic variants associated with breast cancer risk, but their application requires further validation and assessment of interactions. Therefore, to improve the accuracy of existing risk prediction models, it is necessary to investigate validated SNPs as they are discovered, and, where possible, incorporate these genetic factors into the best performing risk models.
Other risk factors
A number of other risk factors for breast cancer are being further validated. Obesity, diet and exercise are probably interlinked. 31,32 Other risk factors such as alcohol intake have a fairly small effect, and protective factors such as breastfeeding are also of small effect unless a number of years of total feeding have taken place. None of these factors is currently incorporated into available risk assessment models.
Risk models
In a comparison of the Gail (National Cancer Institute; www.cancer.gov/bcrisktool), Claus (www4.utsouthwestern.edu/breasthealth/cagene/default.asp), Ford (BRCAPRO) (BayesMendel Lab, Harvard University, Boston, MA, USA; http://bcb.dfci.harvard.edu/bayesmendel/index.php) and Tyrer–Cuzick (TC) (version 6; Professor Jack Cuzick, Centre for Cancer Prevention, London, UK) risk prediction models, using observed data from 3170 women with a family history of breast cancer in the UK, we showed that the TC model performed best. 13 We identified a need to reassess these models in larger numbers of women and also to compare the BOADICEA (Breast and Ovarian Analysis of Disease Incidence and Carrier Estimation Algorithm; Cambridge University, http://ccge.medschl.cam.ac.uk/boadicea/) model alongside these models in the family history population. There has not been a large-scale study comparing risk models in the general population, and, therefore, we aimed to assess the TC and Gail models in the general population. The Claus and BRCAPRO models are unsuitable for population prediction, as they are entirely based on family history risk factors.
Improving the risk models
Current risk prediction models are based on combinations of risk factors and have good overall predictive power, but are still weak at predicting which particular women will develop the disease. New risk prediction methods are likely to come from examination of a range of high-risk genes as well as SNPs in several genes associated with lower risks. 8 This was married in a prediction programme with other known risk factors to provide a far more accurate individual prediction.
The incorporation of density into the standard risk prediction models is associated with some improvement in risk prediction,33,34 and three publications suggest that adding breast density improves the Gail risk model. 35–37 Therefore, to improve the accuracy of the best performing risk model in each population of women (family history and general population), it is necessary to incorporate MD. We used a number of different density assessments to determine which adds most to the precision of breast cancer risk estimation.
Economic evidence supporting the incorporation of risk prediction into the NHS Breast Screening Programme
There is a substantial economic evidence base that has been generated to support the introduction of breast screening programmes, in general. To date, however, there is no economic evidence to support that using a risk-based screening programme will be an effective use of limited health-care resources. To understand the potential impact of introducing a risk-based screening programme, preliminary economic analysis was conducted to identify relevant costs and benefits. A decision-analytic model was constructed to represent the options for screening strategies for women at increased risk of breast cancer who have been identified using the best performing risk model. A systematic review summarised existing economic modelling research in this clinical area and used to inform the development of a model structure. 38–41 Earlier work of the programme informed necessary changes to the model structure, clinical pathways, data sources, etc. An expert panel (project leads, geneticists, oncologists, patient representatives) refined the modelling structure. Data from PROCAS (Predicting the Risk of Cancer At Screening) [UK Clinical Research Network identification number (UKCRN-ID) 8080] and systematic reviews and assimilation of published data were used to inform the model inputs.
Overarching research questions and aims
The overall aim of this project was to improve risk prediction and early detection of breast cancer, for women who have a family history of the disease and for those in the NHSBSP. To achieve this, it is necessary to first identify the best performing model in each population and then improve the precision of the best performing model by incorporating MD (assessed using the optimal method) and new genetic modifiers of risk, SNPs (where possible). This will enable better individualised risk prediction and allow women access to appropriate risk-management strategies and screening intervals. We also aimed to conduct a preliminary economic analysis to identify the relevant incremental costs and benefits associated with introducing a risk-based screening programme on a national level. As the report is largely based on two cohort studies, we have used STROBE (Strengthening the Reporting of Observational Studies in Epidemiology) guideline reporting recommendations where possible.
Chapter 2 concentrates on the population with a family history of breast cancer. Our original proposal was to update our earlier study, based on just over 3000 women screened for breast cancer between 1987 and 2002, which had shown that the TC model and a Manual approach were most predictive. As outlined in the application, it has been possible to rerun these models in nearly 10,000 women, with almost an eightfold increased power and with over 400 detected breast cancers. In addition to the Gail, TC and Manual models, we have been able to assess the newer BOADICEA model in a subset of women. In addition, as proposed, MD has been assessed in a case–control study (see Chapter 4). This was able to assess a visual assessment score (VAS) in all patients for whom mammograms were available. In addition, two automatic models were assessable on those who had digital mammograms.
In Chapter 3, we report on a large-scale analysis of SNPs undertaken in a number of populations, as proposed in the application. At the time of application, only eight or nine SNPS were assessable, but in 2010 this rose to 18, and these 18 were used in all the analyses using a PRS. We have assessed the SNP18 in four populations:
-
We proposed to extended our analysis to include all female BRCA1/BRCA2 carriers (n = 850 at that time) and assess the SNP18 for interactions and modifier effects. As above, the proposed research was extended to test 925 proven BRCA1 or BRCA2 female carriers to assess impact on breast cancer risk.
-
The second proposed analysis was to test the SNPs in 1400 high-risk BRCA1-/BRCA2-negative breast cancer cases and compare this with 500 matched control samples. The variants will be weighted for their individual effects in heterozygous and homozygous states and assessed for interactions with other variants. This analysis was deemed unnecessary, as we obtained funding (from the Genesis Breast Cancer Prevention Appeal) for obtaining saliva deoxyribonucleic acid (DNA) in 10,000 women from our population-based PROCAS study. We carried out testing of > 5000 women in PROCAS and have extended the modelling to assess the effects of using 67 SNPs.
-
The testing of DNA from 58 breast cancer phenocopies (women who are negative for the family BRCA1/BRCA2 mutation) was likewise proposed to be assessed for the variants in a weighted analysis to identify whether or not significantly more of the high-risk alleles are carried by those women to account for their increased risk of breast cancer. SNP18 was run on these women.
-
We proposed using data from the first three analyses to develop a weighted score to assess the predictive value of the combined group of validated variants in predicting which women developed breast cancer in our familial screening programme. We proposed using the incident cancers and matching with controls on a 1 : 3 basis randomly selected from our family history clinic (FHC) for the presence or absence of familial BRCA1/BRCA2 mutation plus all other currently used risk factors (menarche, parity, age, family history and breast disease). Women were recruited from our FHCs to provide blood samples for DNA analysis or to give their permission to use stored DNA. The matching of controls was changed (with statistical advice from Professor Cuzick) to matching for just age and type of mammogram.
Chapter 4 concentrates on our large-population PROCAS study. In this study, which was the largest recruiting portfolio study nationally, we recruited > 53,000 women and reached our target of 600 prospective breast cancers. As outlined in the application, we have shown that it was feasible to collect risk information from women attending mammography through the NHSBSP and have already given risk feedback to nearly 800 women at high and low risk. We have shown that, as anticipated, MD adds substantially to the predictive precision of the risk models TC and Gail. We were hopeful that automatic measures of density carried out on digital mammograms would be at least equivalent to the gold-standard Cumulus or VAS, but this was not the case, and more work is therefore required to develop a better digital method. A VAS-adjusted TC model was shown to improve risk prediction and identified 70% of the population at < 3.5% 10-year risk who were at very low risk of a high-stage cancer.
Finally, in Chapter 5, three studies are reported. The first study was a systematic review of published economic evaluations relevant to breast screening and summarised the current economic evidence base. Study 2 explored the potential out-of-pocket expenses incurred by women attending a national breast screening programme. Study 3 structured a decision-analytic model to conduct a preliminary cost-effectiveness analysis to identify the relevant costs and patient benefits if a risk-based breast screening programme was introduced into clinical practice.
Chapter 2 Project 1: improvement of risk prediction algorithms for women at high risk of breast cancer
Introduction
Risk factors for breast cancer
Family history
Family history can be by far the most significant factor in predisposition. Although at extremes of age the RR can be huge, the RR in a 35-year-old woman who carries a BRCA1/BRCA2 mutation is higher than the risk in a 75-year-old in the general population. About 4–5% of breast cancer is thought to be due to the inheritance of a high-risk dominant cancer-predisposing gene. 42,43 Hereditary factors play a part in a proportion of the rest (up to 27% of breast cancer from twin studies44,45), but these factors are harder to pin down. Nonetheless, lower-risk genes are now being identified from association studies. There are no external markers of risk (no phenotype) to help to identify those who carry a faulty gene, except in very rare cases, such as those with Cowden disease. 46 To assess the likelihood of there being a predisposing gene in a family, it is necessary to assess the family tree. Inheritance of a germline mutation or deletion of a predisposing gene causes the disease at a young age and, often, if the individual survives, cancer in the contralateral breast. Some gene mutations may give rise to susceptibility to other cancers, such as ovarian cancer, sarcomas and colon cancer. 47–51 Multiple primary cancers in one individual or related early-onset cancers in a pedigree are, therefore, suggestive of a predisposing gene. To illustrate the importance of age, it is thought that > 25% of breast cancer at < 30 years of age is due to a mutation in a high-risk dominant gene, whereas < 1% of the disease at > 70 years of age is so caused. 43 Therefore, the important features in a family history are:
-
age at onset of breast cancer
-
bilateral disease
-
degree of relationship to family member (first or greater)
-
multiple cases in the family (particularly on one side)
-
other related early-onset tumours
-
number of unaffected individuals (large families are more informative).
There are very few families in whom it is possible to be certain of dominant inheritance; however, where four first-degree relatives have early-onset or bilateral breast cancer, the risk for a sister or daughter of inheriting a susceptibility gene is close to 50%. Epidemiological studies have shown that approximately 80% of mutation carriers develop breast cancer in their lifetime. Therefore, unless there is significant family history on both sides of the family, the maximum risk counselled is 40–45%. Breast cancer genes can be inherited through the father, and a dominant history on the father’s side of the family would give at least a 20–28% lifetime risk to his daughters.
Other risk factors
The main emphasis in the past has been on hormonal and reproductive factors. Essentially, a woman is most protected by never ovulating. Breast cancer is, therefore, very uncommon in those with Turner’s syndrome. The next best protection is proffered by ovulating as few times as possible before a first pregnancy. A late menarche and early first pregnancy is most protective. Pregnancy transforms breast parenchymal cells into a more stable state, where proliferation in the second half of the cycle is less. There is now good evidence that current use of the oral contraceptive, and for 10 years post use, results in around a 24% increase in risk. 13 The oestrogen element of the pill, although suppressing ovulation, will still stimulate the breast cells. With a greater number of women delaying their first pregnancy by using the pill, particularly women in professional classes, who may in any case be more predisposed, breast cancer incidence is continuing to climb. An early menopause is protective, again probably by reducing the exposure of the breast to oestrogen and progesterone. Other factors, such as the number of pregnancies and breastfeeding, may have a small protective effect. Hormone replacement is another area that was previously the subject of intense debate. Long-term treatment (> 10 years) after the menopause is associated with a significant increase in risk. However, shorter treatments may still be associated with risk to those with a family history of breast cancer. 14 In a large meta-analysis,15 the risk appeared to increase cumulatively by 1–2% per year, but disappear within 5 years of cessation. It is becoming clear that the risk from combined oestrogen/progesterone HRT is greater than that from oestrogen only. 16,17,19 Interestingly, the increase in risk is lower in overweight women, but these women are likely to already be at increased risk from endogenous hormone production, with a RR of 2 for women who have gained ≥ 20 kg since the age of 18 years. 31,32
It is important to emphasise that these factors do not have an all-or-nothing effect on the breast, but may alter risks by a factor of 2 at the extremes. 52 Many women who have all of these unfavourable factors will not develop breast cancer, and some, particularly if they have a germline mutation, will develop the disease even if all factors are favourable. Diet may also play a part, with those who have a diet low in animal fats from dairy produce and red meat being marginally less likely to develop the disease. Perhaps the greatest risk is attached to women who, on biopsy, are found to have proliferative disease such as atypical ductal hyperplasia. 53,54
Risk estimation
Where there is no dominant family history, risk estimation is based on large epidemiological studies, which give 1.5- to 3-fold increased risks with family history of a single affected relative. 42,43 Clinicians must be careful to differentiate between lifetime and age-specific risks. Some studies quote ninefold or greater risk associated with bilateral disease in a mother or if the woman herself has proliferative breast disease. However, if these at-risk individuals are followed up for many years, the risk returns towards normal levels. 53 Clearly, if one uses these risks and multiplies them on a lifetime incidence of 1 in 8–12, some women will apparently have a > 100% chance of having the disease. The risks do not multiply and may not even add. Perhaps the best way to assess risk is to take the strongest risk factor, which in our case is nearly always family history. If risk is assessed on this alone, minor adjustments can be made for other factors. It is arguable whether or not these other factors will have a large effect on an 80% penetrant gene other than to speed up or delay the onset of breast cancer. Therefore, we can only really assume an effect on the non-hereditary element of the risk. Although studies do point to an increase in risk in family history cases associated with some factors, these may just represent an earlier expression of the gene. Generally, therefore, we will arrive at risks between 40% and 8–10%, although lower risks are occasionally given. Higher risks are applicable only when a woman at 40% genetic risk is shown to have a germline mutation, to have inherited a high-risk allele or to have proliferative breast disease.
Several methods based on currently known risk factors have been devised to predict risk of breast cancer in the clinic and in the general population. 55 Some depend on family history alone (e.g. the Claus and Ford risk evaluation models) and others depend on hormonal and reproductive factors in addition to family history (e.g. the Gail and TC models). Outside risk assessment clinics, where most women have sufficiently strong family histories to have a probability of harbouring mutations in BRCA1, BRCA2 and TP53 (tumour protein p53) genes, it is probable that models in which as many risk factors as possible are combined may be preferable. After all, only 10% of breast cancer occurs in the context of a first-degree family history of breast cancer. The Gail model accurately predicted the number of cancers in the Nurses’ Health Study,56 but in our FHC, the TC model, which depends on the extent of family history and several endocrine factors, showed a better prediction than those that used fewer risk factors. 57 Our own clinical manual assessment was, nonetheless, as good as TC and significantly better than the other computer-based models. 57 Although these models have reasonably good predictive power for the number of cancer cases likely to be seen in a population, they have low discriminatory accuracy, in that they cannot positively identify which particular woman will develop breast cancer. 55,57 This is not surprising, given that most of the inherited component of at least 27% from twin studies44,45 would not be identified from family history and the risk model cannot predict who has and who has not inherited any genetic factors in a particular family. At present, most of the known non-family history risk factors are not included in risk models. In particular, perhaps the greatest factor apart from age, MD,58 is not yet included. Further studies are in progress to determine whether or not the inclusion of additional factors into existing models, such as MD, weight gain31 and serum steroid hormone measurements,59 will improve prediction. These are not straightforward additions, as there may be significant interactions between risk factors. Although breast density is an independent risk factor for BRCA1 and BRCA2 cancer risk,60 the density itself may be heritable and not increase risk in a similar way to the context of family history of breast cancer alone.
Women who are at increased risk for breast cancer can be identified on the basis of their individual risk factors. However, this approach does not permit the combination of multiple risk factors or the calculation of a woman’s lifetime probability of breast cancer. In breast cancer FHCs in Europe, the Claus tables43 were frequently employed to assess lifetime risk and risk in a given decade (Table 1). However, these did not take into account risk modification from hormonal and reproductive factors or from having many unaffected female relatives.
Age (years) | 20–29 BC in mother or sister | 30–39 | 40–49 | 50–59 | 60–69 | 70–79 |
---|---|---|---|---|---|---|
29 | 0.007 | 0.005 | 0.003 | 0.002 | 0.002 | 0.001 |
39 | 0.025 | 0.017 | 0.012 | 0.008 | 0.006 | 0.005 |
49 | 0.062 | 0.044 | 0.032 | 0.023 | 0.018 | 0.015 |
59 | 0.116 | 0.086 | 0.064 | 0.049 | 0.040 | 0.035 |
69 | 0.171 | 0.130 | 0.101 | 0.082 | 0.070 | 0.062 |
79 | 0.211 | 0.165 | 0.132 | 0.110 | 0.096 | 0.088 |
Lifetime risk | 1 in 5 | 1 in 6 | 1 in 8 | 1 in 9 | 1 in 10 | 1 in 12 |
Therefore, multivariate risk models have been introduced. These models allow the determination of a woman’s composite RR for breast cancer as well as her cumulative lifetime risk adjusted for several risk factors. Such models, therefore, provide an individualised breast cancer risk assessment, which is an essential component of the risk–benefit analysis from which decisions regarding the implementation of frequent surveillance, chemoprevention or risk-reducing surgery can be made.
In 2003, we published a study designed to compare the predictive value of the Gail, Claus, Ford and TC risk assessment models using a cohort of 3151 women attending the Family History Evaluation and Screening Programme. 57 The study used a read-out from the Cyrillic computer program [version 3.0; www.exetersoftware.com/cat/cyrillic/cyrillic.html (accessed 30 March 2004)]. 61 These computerised models were also compared with risk assessment undertaken by clinicians based on Claus tables with adjustment for other risk factors, particularly hormonal factors (the Manual model). This showed that although the TC and Manual models were accurate at predicting breast cancer risk over time, the Claus, Gail and Ford models substantially underestimated risk (Table 2). Our 2003 report57 remains the only large-scale effort to validate risk assessment models in a family history setting. We now have more than fourfold follow-up time and over sixfold the number of prospective cancers to validate risk prediction models. We are also in a position to validate the BOADICEA model for the first time.
RR at extremes | Gail | Claus | BRCAPRO/Ford | TC | BOADICEA | |
---|---|---|---|---|---|---|
Prediction | ||||||
Amir et al.57 validation study ratioa | 0.48 | 0.56 | 0.49 | 0.81 | Not assessed | |
95% CI | 0.37 to 0.64 | 0.43 to 0.75 | 0.37 to 0.65 | 0.62 to 1.08 | Not assessed | |
Personal information | ||||||
Age (20–70) | 30 | Yes | Yes | Yes | Yes | Yes |
BMI | 2 | No | No | No | Yes | No |
Alcohol intake (0–4 units) daily | 1.24 | No | No | No | No | No |
Hormonal/reproductive factors | ||||||
Age at menarche | 2 | Yes | No | No | Yes | No |
Age at first live birth | 3 | Yes | No | No | Yes | No |
Age at menopause | 4 | No | No | No | Yes | No |
HRT use | 2 | No | No | No | Yes | No |
OCP use | 1.24 | No | No | No | No | No |
Breastfeeding | 0.8 | No | No | No | No | No |
Plasma oestrogen | 5 | No | No | No | No | No |
Personal breast disease | ||||||
Breast biopsies | 2 | Yes | No | No | Yes | No |
Atypical ductal hyperplasia | 3 | Yes | No | No | Yes | No |
Lobular carcinoma in situ | 4 | No | No | No | Yes | No |
Breast density | 6 | No | No | No | No | No |
Family history | ||||||
First-degree relatives | 3 | Yes | Yes | Yes | Yes | Yes |
Second-degree relatives | 1.5 | No | Yes | Yes | Yes | Yes |
Third-degree relatives | No | No | No | No | Yes | |
Age of onset of breast cancer | 3 | No | Yes | Yes | Yes | Yes |
Bilateral breast cancer | 3 | No | No | Yes | Yes | Yes |
Ovarian cancer | 1.5 | No | No | Yes | Yes | Yes |
Male breast cancer | 3–5 | No | No | Yes | No | Yes |
We have now expanded our follow-up in the FHC to nearly three times the size and twice the average years. To reassess the Manual model, we have updated the Claus tables to reflect modern breast cancer incidence in the UK. We show that the Manual model is accurate and convenient for use in the busy clinic, but that TC and BOADICEA also appear to predict cancers accurately.
Study 1a: cohort study
Methods
Study population
Since 1987, 10,177 women have been assessed in the FHC at the University Hospital of South Manchester on the basis of their family history of breast and other cancers and in terms of their hormonal and reproductive factors. These women completed a comprehensive breast cancer risk assessment, which was analysed and archived to a database. Breast examination and mammography were also carried out.
Archived information includes demographic details, family pedigree, including any history of cancer (current age or age of death of any relative, type of cancer and age at diagnosis), reproductive history (age at menarche, age at first pregnancy, duration of episodes of lactation and age at menopause if applicable), history of benign breast disease (including number of benign biopsies), artificial oestrogen exposure (duration of oral contraceptive pill usage, hormone replacement or fertility drugs) and morphometric information (height and weight). In addition, the database stores an absolute lifetime risk calculated using the Manual model.
The database also contains information regarding all breast cancer diagnoses in the population until at least August 2011. All women previously assessed in whom vital and tumour status was not available as of August 2011 and whose address showed residency within the North-West regional boundaries were checked for such information on the North West Cancer Intelligence Service (NWCIS). The NWCIS data, in combination with tumour and vital status from the database, were used as the observed numbers of breast cancers for the purpose of comparison. Follow-up was censored if women had left the area at the time of breast cancer diagnosis, at the date of risk-reducing mastectomy or at the date of last mammogram if this was after 1 August 2011.
Study tools
Manual risk calculation (the Manual model) was used to calculate risk in the clinic. The Manual model uses the Claus tables43 and curves52,62,63 to calculate a heterozygote and lifetime risk (which includes a population risk element). Families fulfilling Breast Cancer Linkage Consortium (BCLC) criteria or with a proven BRCA1 or BRCA2 mutation64 were given risks based on the penetrance and 10-year risks from our regional penetrance estimates. 5 All other women were given lifetime risks, with 10-year risks calculated from the equivalent figure given in the Claus tables after a clinician’s modification for hormonal and reproductive factors. Those women with average risk factors, such as menarche aged 12–13 years and first pregnancy aged 23–27 years, had no alteration to the genetic risk-based assessment. However, those with unfavourable factors, such as menarche aged < 12 years and late first pregnancy and nulliparity, would go up a risk category, say from 1 in 4 to 1 in 3 lifetime risk, and those with favourable factors of a late menarche and early first pregnancy would drop to 1 in 5, equivalent to a 20–30% alteration. More significant alterations would occur with a very early menopause which, aged 40 years, could halve the risk. A more detailed review of our Manual model can be found elsewhere. 52,62,63 The output of these lifetime risks was used to calculate the expected numbers of breast cancers.
The original Claus tables were based on invasive breast cancer incidence rates for the 1970s and 1980s in the USA. 43 We therefore updated these to reflect modern incidence rates for the 1990s and 2000s in the UK (Table 3). The increase in breast cancer incidence in recent times was discussed in NICE guidance,2 in which 2001 incidence rates from the Office for National Statistics2 predicted a risk to age 80 years for the average woman as 10.7%, whereas Claus tables predict a lower risk for women with a single first-degree relative affected > 60 years of age (see Table 2). Minor upwards adjustments were made only to values with a risk to age 80 years of < 25% to reflect the increase in risk in the last 20 years (see Table 3). The 3% 10-year risk for women in their forties with a single first-degree relative affected aged 30–39 is equivalent to the version 6 TC read-out for a typical pedigree (Figure 1).
Age (years) | 20–29 | 30–39 | 40–49 | 50–59 | 60–69 | 70–79 |
---|---|---|---|---|---|---|
29 | 0.007 | 0.005 | 0.003 | 0.002 | 0.002 | 0.001 |
39 | 0.028 | 0.024 | 0.018 | 0.012 | 0.010 | 0.008 |
49 | 0.065 | 0.054 | 0.042 | 0.033 | 0.028 | 0.025 |
59 | 0.126 | 0.096 | 0.074 | 0.067 | 0.057 | 0.050 |
69 | 0.181 | 0.144 | 0.116 | 0.102 | 0.090 | 0.082 |
79 | 0.231 | 0.195 | 0.162 | 0.140 | 0.126 | 0.118 |
Lifetime risk | 1 in 4 | 1 in 5 | 1 in 6–7 | 1 in 7 | 1 in 8 | 1 in 9 |
FIGURE 1.
Tyrer–Cuzick read-out giving 10-year risk aged 40 years for a woman with a single first-degree relative with breast cancer.

Inclusion criteria
Only first-ever invasive [International Classification of Diseases, Tenth Edition (ICD-10)65 C50] or ductal carcinoma in situ (DCIS) breast cancer diagnosis, from time of initial assessment to date of last follow-up (usually 1 August 2011), was assessed for the entire cohort; all prevalent cancers were excluded to exclude lead time bias. Mutation carriers and those testing negative for a BRCA1, BRCA2 or TP53 mutation were included as gene carriers only if they were ascertained by the clinic as such. This avoids the bias of those developing breast cancer being more likely to be tested in follow-up. As such, final identified gene carrier status was not considered owing to testing bias, as breast cancers were preferentially tested.
Statistical analysis
The predicted risk during the follow-up period was compared against the observed numbers of breast cancers (database and NWCIS data). To express the predicted risk in terms of the follow-up period, projections of the absolute 10-year risk were obtained from the models. These were then converted, first into an annual risk (division by 10) and then into a follow-up risk by multiplying by the number of years of follow-up. Follow-up was taken as the period of time from initial assessment with clinical breast examination and mammogram to the most recent examination or 1 August 2011, whichever was the later. The expected number (E) of breast cancers in the cohort was calculated as the sum of these predicted risks. The E was then compared with the observed number (O) of women with breast cancer. A Poisson model was used to obtain 95% confidence intervals (CIs) of the ratio of expected versus observed (E : O) numbers. 66
Risk models
Gail model
-
Absolute risks of 1–20 years (including and excluding competing mortality) were calculated using the code available from www.cancer.gov/bcrisktool.
-
Ethnicity was taken to be ‘white’ for all probands, as 98% were of white origin in the Family History Risk Study (FH-Risk).
-
Very little information was available about benign disease, and no information was available on the number of biopsies. In total, 23 of the probands had a previous hyperplasia diagnosis. These were all taken to have one previous biopsy.
-
A summary of the other risk factors (age, number of first-degree relatives, age at menarche and age at first child) is in Table 4.
Percentiles | Age (years) | FDR (n) | Family size (n) | Age at menarche (years) | Age at first birth (years) |
---|---|---|---|---|---|
Other | N/A = 1257 | N/A = 4220, none = 1042 | |||
1% | 20 | 0 | 3 | 9 | 16 |
25% | 33 | 1 | 14 | 12 | 21 |
50% | 39 | 1 | 20 | 13 | 25 |
75% | 45 | 1 | 27 | 14 | 28 |
99% | 63 | 3 | 61 | 17 | 38 |
Tyrer–Cuzick model
-
Absolute risks of 1–20 years (no competing mortality), based on version 6.
-
The family history information used included age, diagnosis and age of breast cancer, bilateral breast cancer and ovarian cancer for the following relatives:
-
mother
-
sisters and half-sisters
-
daughters
-
aunt, maternal and paternal
-
grandmother, maternal and paternal
-
nieces (from brother or sister)
-
cousins (from uncles and aunts).
-
-
Affected father and brother status was also included.
-
No BRCA test results were used, but they will be incorporated later.
-
Similar to the Gail model, there was very little information on benign disease.
-
In addition to the Gail model factors, information on height and weight was used (Table 5).
Percentiles | Height (m) | Weight (kg) | BMI (kg/m2) |
---|---|---|---|
Missing value, n | 2008 | 3349 | 3460 |
1% | 1.49 | 46.3 | 17.9 |
25% | 1.57 | 58.1 | 21.8 |
50% | 1.63 | 64.4 | 24.0 |
75% | 1.68 | 73.0 | 27.3 |
99% | 1.80 | 112.5 | 42.2 |
BOADICEA model
The BOADICEA model used the same family history information as TC; 10- to 20-year risks were generated. Unfortunately, BOADICEA risks could be generated only for individuals from families with only one proband (Table 6). Therefore, output was possible on only 6717 women.
Number of probands | Count | Per cent | Cancer | Cancer per cent |
---|---|---|---|---|
1 | 6717 | 84.0 | 210 | 50.5 |
2 | 895 | 11.2 | 102 | 24.5 |
3 | 226 | 2.8 | 33 | 7.9 |
4 | 84 | 1.1 | 23 | 5.5 |
5 | 35 | 0.4 | 19 | 4.6 |
6 | 15 | 0.2 | 14 | 3.4 |
7 | 7 | 0.1 | 3 | 0.7 |
8 | 4 | 0.1 | 5 | 1.2 |
9 | 2 | 0.0 | 3 | 0.7 |
10 | 2 | 0.0 | 1 | 0.2 |
11 | 2 | 0.0 | 0 | 0.0 |
12 | 2 | 0.0 | 0 | 0.0 |
13 | 1 | 0.0 | 1 | 0.2 |
40 | 1 | 0.0 | 2 | 0.5 |
Results
Manual model
The analysis of the whole study population was carried out on data from 8824 women [age range 14.5–81.3 years; interquartile range (IQR) 33.9–46.0 years; median 39.5 years at entry] (Table 7). Forty prevalent cancers (15 carcinomas in situ) were excluded (rate 40/8824 = 4.5 per 1000). The mean time of follow-up was 9.71 years (range 0.10–26.00 years). During follow-up, 406 first incident breast cancers were diagnosed (372 invasive, 34 carcinomas in situ) in 85,726.9 woman-years, giving an incidence of 4.7 cancers per 1000 women per year (95% CI 4.3 to 5.2) (Table 8). The expected incidence based on 9.71 years of follow-up for a woman aged 39.5 years would be 1.5 per 1000 in the local region based on NWCIS rates.
Risk | Number | Median age at entry (years) | Range of age at entry (years) | Prospective breast cancers | Invasive/ductal | Invasive lobular | Carcinoma in situ |
---|---|---|---|---|---|---|---|
All | 8824 | 39.5 | 16.9–81.3 | 446 | 382 | 15 | 49 |
Very high | 1447 | 38.05 | 16.5–77.4 | 105 | 89 | 1 | 15 |
High | 2659 | 39.0 | 16.9–72.0 | 140 | 115 | 6 | 19 |
Moderate | 3518 | 39.4 | 14.8–81.3 | 149 | 131 | 4 | 14 |
Average | 1200 | 43.6 | 16.9–76.7 | 52 | 47 | 4 | 1 |
Lifetime risk | Number (prevalence only) | Prevalence cancers on initial assessment | Years’ follow-up | Predicted BC | Actual BC | Rate/1000 | O : E (95% CI) |
---|---|---|---|---|---|---|---|
Manual risk prediction: twentiesa | |||||||
Average (8–16%) | 116 | 0/116 | 401.9 | 0.09 | 0 | – | |
Moderate (17–29%) | 436 | 1/436 | 1630 | 1.12 | 0 | – | |
High (30–39%) | 258 | 0/258 | 838 | 1.1 | 1 | 1.2 | 0.91 (0.02 to 5.07) |
Very high (≥ 40%) | 192 | 1/192 | 651 | 1.25 | 2 | 3.1 | 1.60 (0.19 to 5.78) |
Total | 1002 | 2/1002 | 3520.9 | 3.56 | 3 | 0.84 (0.17 to 2.46) | |
Lifetime risk | Number (prevalence only) | Prevalence cancers on initial screen | Years’ follow-up | Predicted BC | Actual BC | Rate/1000 | O : E ratio (95% CI) |
Manual risk prediction: thirtiesb | |||||||
Average (8–16%) | 436 (9) | 0/150 | 2323 | 1.84 | 1 | 0.4 | 0.54 (0.01 to 3.03) |
Moderate (17–29%) | 1636 (55) | 2/1276 | 7986 | 14.7 | 14 | 1.8 | 0.95 (0.52 to 1.60) |
Moderate (20–29%) | 1007 | 4788 | 9.79 | 9 | 1.9 | 0.92 (0.42 to 1.75) | |
High (30–39%) | 1326 (72) | 2/1208 | 5387.7 | 16.2 | 14 | 2.6 | 0.86 (0.47 to 1.45) |
Very high (≥ 40%) | 762 (33) | 6/653 | 3427.7 | 17.5 | 21 | 5.8 | 1.20 (0.74 to 1.83) |
Very high (40–50%) | 681 | 3132 | 13.4 | 18 | 5.7 | 1.34 (0.80 to 2.12) | |
Total | 4160 (169) | 10/3287 | 18,943 | 50.2 | 50 | 1.00 (0.74 to 1.31) | |
Lifetime risk | Number (prevalence only) | Prevalence cancers on initial screen | Years’ follow-up | Predicted BC | Actual BC | Rate/1000 | O : E (95% CI) |
Manual risk prediction: forties | |||||||
Average (8–16%) | 811 (5) | 0/323 | 4591 | 8.3 | 12 | 2.6 | 1.45 (0.75 to 2.53) |
Average (12–16%) | 468 | 2181 | 4.57 | 6 | 2.75 | 1.31 (0.48 to 2.86) | |
Moderate (17–29%) | 2307 (62) | 9/1174 | 13,144.2 | 47.4 | 50 | 3.8 | 1.05 (0.78 to 1.39) |
Moderate (17–24%) | 1609 | 9223.6 | 29.75 | 31 | 3.25 | 1.04 (0.71 to 1.48) | |
Moderate (17–19%) | 941 | 5469.5 | 16.41 | 17 | 3.1 | 1.04 (0.60 to 1.66) | |
Moderate (20–24%) | 669 | 3754.1 | 13.34 | 14 | 3.7 | 1.05 (0.57 to 1.76) | |
Moderate (25–29%) | 698 | 3920.6 | 17.65 | 19 | 4.8 | 1.08 (0.65 to 1.68) | |
High (30–39%) | 1913 (48) | 4/777 | 9845.6 | 57.3 | 51 | 5.2 | 0.89 (0.66 to 11.7) |
Very high (≥ 40%) | 820 (17) | 5/375 | 3933.8 | 34.8 | 33 | 8.1 | 0.95 (0.65 to 1.33) |
Total | 5851 (132) | 18/2649 | 31,514.6 | 147.8 | 146 | 0.99 (0.83 to 1.16) | |
Lifetime risk | Number (prevalence only) | Prevalence cancers on initial screen | Years’ follow-up | Predicted BC | Actual BC | Rate/1000 | O/E (95% CIs) |
Manual risk prediction: fifties | |||||||
Average (8–16%) | 812 (6) | 1/114 | 5390 | 16.8 | 27 | 5.0 | 1.61 (1.06 to 2.34) |
Moderate (17–29%) | 1652 (10) | 3/244 | 9664 | 52.3 | 48 | 5.0 | 0.92 (0.68 to 1.22) |
High (30–39%) | 1011 (21) | 2/238 | 4838.4 | 39.3 | 48 | 9.99 | 1.22 (0.90 to 1.62) |
Very high (≥ 40%) | 458 (11) | 4/141 | 2190.5 | 23.7 | 21 | 9.6 | 0.89 (0.55 to 1.35) |
Total | 3933 | 10/737 | 22,082.9 | 132.1 | 144 | 1.09 (0.92 to 1.28) | |
Lifetime risk | Number (prevalence only) | Prevalence cancers on initial screen | Years’ follow-up | Predicted BC | Actual BC | Rate/1000 | O/E (95% CIs) |
Manual risk prediction: sixties | |||||||
Average (8–16%) | 504 (6) | 1/59 | 2697 | 9.3 | 6 | 2.2 | 0.65 (0.24 to 1.40) |
Moderate (17–29%) | 670 (20) | 0/46 | 3255 | 18.4 | 21 | 6.44 | 1.14 (0.71 to 1.74) |
High (30–39%) | 318(8) | 0/30 | 1360 | 11 | 18 | 13.2 | 1.64 (0.97 to 2.59) |
Very high (≥ 40%) | 177 (5) | 0/34 | 716.5 | 7.0 | 10 | 14 | 1.43 (0.69 to 2.63) |
Total | 1669 | 1/169 | 8028.5 | 45.4 | 55 | 1.21 (0.91 to 1.58) | |
Lifetime risk | Number (prevalence only) | Prevalence cancers on initial screen | Years’ follow-up | Predicted BC | Actual BC | Rate/1000 | O/E (95% CIs) |
Manual risk prediction: seventies and older | |||||||
Average (8–16%) | 139 | 0/6 | 707.9 | 2.8 | 3 | 2.8 | 1.07 (0.22 to 3.13) |
Moderate (17–29%) | 120 | 0/2 | 556.8 | 2.67 | 2 | 3.6 | 0.75 (0.09 to 2.71) |
High (30–39%) | 47 | 0/4 | 149 | 0.82 | 2 | 13.4 | 2.43 (0.30 to 8.81) |
Very high (≥ 40%) | 29 | 1/9 | 80 | 0.57 | 2 | 25 | 3.51 (0.42 to 12.67) |
Total | 334 | 1/21 | 1493.7 | 6.86 | 9 | 1.31 (0.60 to 2.49) | |
Lifetime risk | Number (prevalence only | Prevalence cancers on initial screen | Years’ follow-up | Predicted BC | Actual BC (carcinoma in situ) | Predicted lifetime risk to 80 (70) | O/E (95% CIs) |
Manual risk prediction: all ages | |||||||
Average (8–16%) | 1200 | 3 | 16,068.9 | 39.13 | 49 (1) | 15.1% | 1.25 (0.93 to 1.66) |
Moderate (17–29%) | 3518 | 14 | 36,239.9 | 135.5 | 135 (10) | 20.2% | 1.00 (0.84 to 1.18) |
High (30–39%) | 2659 | 6 | 22,442.1 | 125.6 | 134 (14) | 43.8% (30.4%) | 1.07 (0.89 to 1.26) |
Very high (≥ 40%) | 1447 | 17 | 10,976 | 84.9 | 88 (9) | 63% (38%) | 1.04 (0.83 to 1.28) |
Total | 8824 | 40 | 855,726.9 | 385.1 | 406 | 1.05 (0.95 to 1.16) |
Eighteen female carriers contributed risk in their twenties, 81 contributed risk in their thirties, 37 contributed risk in their forties and 26, 18 and 8, respectively, contributed risk in the subsequent three decades. In total, eight breast cancers occurred, with 8.40 expected [observed vs. expected (O : E) 0.99, 95% CI 0.43 to 1.96] using rates from our regional penetrance estimates. 66 Of the 406 breast cancers that occurred, 203 (50%) had no upwards or downwards variation in their genetic-based risk based on reproductive risk factors by the study clinicians (DGE and AH), while 68 (17%) had a downwards change and 135 (33%) had an upwards change in risk. Of women without breast cancer, 42% had no variation in their genetic-based risk based on reproductive risk factors, while 39% had a downwards change and 19% had an upwards change.
The expected against observed counts for all breast cancers are shown in Table 9 divided into risk categories as defined by NICE guidelines. 2 These O : E ratios are close to 1.0 across all risk categories, especially for women in their forties. A further breakdown of risk for the forties group shows good discrimination around the 3% 10-year threshold for annual mammography set by NICE. The 12–16% lifetime risk category, which predominantly includes a single first-degree relative aged in their forties with breast cancer, has a 10-year risk of 2.75%, below the 3% threshold, whereas the 17–19% category, which predominantly includes women with a single first-degree relative aged in their thirties with breast cancer, has a 10-year risk of 3.1% above the threshold. The only risk and age category that statistically differed from 1.0 was the near population (average) risk group in their fifties, in whom there was a significant underestimate of the breast cancers that occurred (O : E 1.61, 95% CI 1.06 to 2.34).
Model risk 10-year (%) | n | Person-years | Observed | Expected | O : E | 95% CI | p-valuea |
---|---|---|---|---|---|---|---|
Overall | 9527 | 97,958 | 416 | 382.9 | 1.09 | 0.98 to 1.20 | 0.092 |
Overall | 9527 | 97,958 | 416 | 412.5 | 1.01 | 0.91 to 1.11 | 0.863 |
0–1 | 1175 | 12,481 | 21 | 11.0 | 1.92 | 1.19 to 2.93 | 0.006 |
0–1 | 1223 | 12,272 | 20 | 9.9 | 2.02 | 1.23 to 3.12 | 0.004 |
1–2 | 1652 | 17,917 | 39 | 34.9 | 1.12 | 0.80 to 1.53 | 0.446 |
1–2 | 1603 | 16,326 | 48 | 30.8 | 1.56 | 1.15 to 2.07 | 0.004 |
2–3 | 1669 | 17,691 | 53 | 51.3 | 1.03 | 0.77 to 1.35 | 0.780 |
2–3 | 1518 | 15,433 | 59 | 44.0 | 1.34 | 1.02 to 1.73 | 0.029 |
3–4 | 1504 | 15,702 | 69 | 59.3 | 1.16 | 0.91 to 1.47 | 0.217 |
3–4 | 1070 | 10,445 | 49 | 37.9 | 1.29 | 0.96 to 1.71 | 0.074 |
4–5 | 1102 | 11,303 | 64 | 53.4 | 1.20 | 0.92 to 1.53 | 0.150 |
4–5 | 1682 | 18,062 | 79 | 84.7 | 0.93 | 0.74 to 1.16 | 0.587 |
5–8 | 1734 | 16,591 | 106 | 105.4 | 1.01 | 0.82 to 1.22 | 0.922 |
5–8 | 1536 | 16,469 | 94 | 100.5 | 0.94 | 0.76 to 1.14 | 0.550 |
8–12 | 516 | 4556 | 40 | 43.0 | 0.93 | 0.67 to 1.27 | 0.760 |
8–12 | 565 | 5720 | 45 | 58.4 | 0.77 | 0.56 to 1.03 | 0.088 |
12+ | 175 | 1717 | 24 | 24.7 | 0.97 | 0.62 to 1.45 | 1.000 |
12+ | 330 | 3232 | 22 | 46.3 | 0.48 | 0.30 to 0.72 | < 0.001 |
Computer models
Follow-up was for a median of 10.2 years (IQR 5.0–15.5 years; range 0.1–26.0 years). A total of 9527 women had assessable follow-up and 416 breast cancers occurred in 97,958 years of follow-up. 382.9 cancers were expected by O : E ratio 1.09 (95% CI 0.98 to 1.20) with TC and 412.5 were expected by O : E ratio 1.01 (95% CI 0.91 to 1.11) with Gail. However, although Gail accurately predicted the number of cancers, its calibration was substantially worse than TC. The observed and expected risks are tabulated in Table 9, based on 10-year absolute risk groups from TC and Gail. Gail significantly overestimates risk in those with risks in the lowest three categories (0–3% 10-year) risk and significantly overestimates risk in the highest risk category (> 12% 10-year risk). In contrast, TC significantly underestimated risk only in the lowest risk category (0–1% 10-year risk). Figure 2 shows the distribution of 10-year risk at baseline from the Gail model (includes competing risks) and the TC model (does not).
FIGURE 2.
Baseline risks. (a) Histogram of 10-year risk distribution in this cohort at baseline from TC and Gail models; and (b) individual risk prediction comparison (Spearman’s correlation coefficient 0.79).

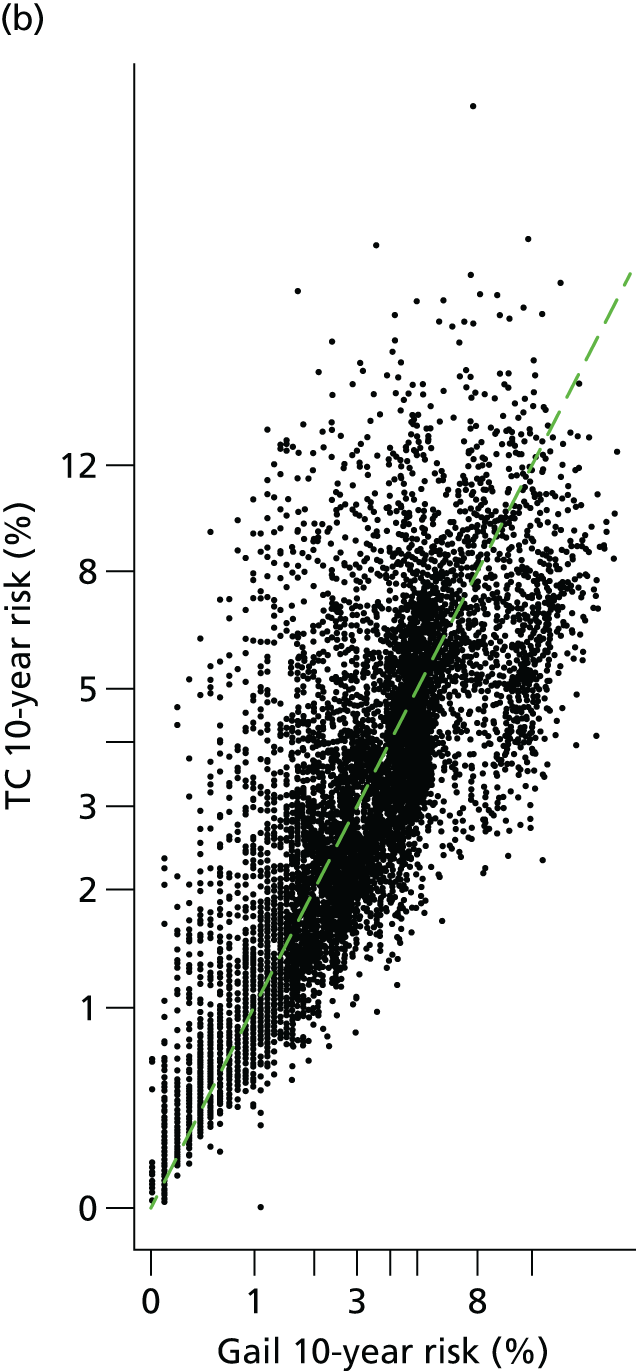
Figure 3 shows observed risk from Kaplan–Meier estimates by 10-year risk quintile. There is overlap of the quintiles with Gail, but good separation with TC.
FIGURE 3.
Observed risk by 10-year risk at baseline quintile (Q1 = bottom quintile, Q5 = top quintile). (a) Gail; and (b) TC.


Figure 4 shows the same, but with emphasis on the spread of risk between the upper and lower quintiles of TC and Gail.
FIGURE 4.
Comparison of the spread of observed risk from the upper and lower quintile groups at baseline. Q, quintile.

Figure 5 again shows the upper and lower quintiles, but adds the expected risk from those groups based on the two models. There is much better overlap in the TC plot than in Gail.
FIGURE 5.
Comparison of observed and expected risk through follow-up time from the upper and lower quintile 10-year risk groups from the (a) TC and (b) Gail risk models.


The overall performance of the models is compared in Table 10. The log-likelihood of the predictions was used as a primary performance measure to compare models. Using this performance measure, TC performed much better than Gail: on a likelihood radio chi-squared scale the difference was 98.3.
Model | IQR | HR | LR-CHI | Ch | Cg | Log-likelihood |
---|---|---|---|---|---|---|
Gail 10-year risk | 1.7–5.0 | 1.64 | 62.29 | 0.62 | 0.61 | –2001.2 |
TC 10-year risk | 1.7–5.0 | 2.13 | 118.01 | 0.66 | 0.65 | –1952.1 |
Assessment of BOADICEA
The BOADICEA model could be assessed only in the families with only one proband. This limited the analysis to 6268 single-proband families. One hundred and ninety-seven breast cancers occurred in 70,293 years of follow-up. BOADICEA predicted 203.59 breast cancers, with good discrimination across the risk groups (Table 11).
10-year risk category (%) | Number | Observed | Expected | E : O | 95% CI |
---|---|---|---|---|---|
< 1 | 972 | 11 | 10.25 | 0.93 | 0.52 to 1.68 |
1–2 | 1391 | 33 | 30.8 | 0.93 | 0.66 to 1.31 |
2–3 | 1553 | 52 | 49.67 | 0.96 | 0.73 to 1.25 |
3–4 | 1103 | 42 | 45.64 | 1.09 | 0.80 to 1.47 |
4–5 | 701 | 33 | 35.33 | 1.07 | 0.76 to 1.51 |
5–8 | 424 | 20 | 23.95 | 1.20 | 0.77 to 1.86 |
> 8 | 124 | 6 | 7.95 | 1.33 | 0.60 to 2.95 |
Total | 6268 | 197 | 203.59 | 1.03 | 0.90 to 1.19 |
Assessment of the Ford model (BRCAPRO)
Assessment of this model was abandoned. It was the worst performing model in our previous analysis and it proved not possible to run the analyses on time owing to difficulties with running the pedigrees through the model.
Discussion
Accurate individualised breast cancer risk assessment is essential for the provision of risk–benefit analysis prior to the initiation of any surveillance or preventative interventions. We have extended our original study57 with a longer follow-up and greater number of incident cancers (sixfold power) to confirm that manual risk assessment and the TC model remain accurate tools for women with a family history of breast cancer. We also provide updated Claus tables for current use in the UK. The expected-to-observed ratios with the Manual model are all very close to 1.0, particularly for women in their forties, for whom discrimination between moderate and near population risk is even more important for risk stratification in order to implement annual mammography. NICE2 has set a 3% 10-year risk aged 40 years as the threshold for annual mammography in England and Wales, and the Manual model accurately predicts this based on the adjusted Claus tables. The TC model (see Figure 1), Claus model (as accessed on version CancerGene667) and BOADICEA68,69 also predict this accurately, with a 10-year risk of 3.1%, 3% and 3%, respectively, compared with the 3.1% observed for women who typically had this type of pedigree. However, when the same pedigree is input to Gail and BRCAPRO (FORD) (as accessed on CancerGene version 6; The University of Texas Southwestern Medical Center at Dallas and the BayesMendel Group, www4.utsouthwestern.edu/breasthealth/cagene/), the 10-year risks were < 3.0%, at 2.7% and 1.7%, respectively. Previously, we showed that the Gail, Claus and Ford models all significantly underestimated risk. 57 In particular, the Gail, Claus and Ford models all underestimated risk in women with a single first-degree relative affected with breast cancer, while the TC and the Manual models were both accurate in this subgroup. The new outputs from the Claus model, in particular, suggest that modifications have been made to this to provide data similar to those in the tables and this might now provide a more accurate assessment. Our modifications to the Claus tables provide more accurate assessment for current breast cancer risks.
When risks in other age groups are assessed, only the very high-risk group exceeds the 10-year 3% risk from age 30 years and the group as a whole falls short of the 8% 10-year risk previously deemed as the threshold for MRI screening in the thirties. 2,70 Indeed, when gene carriers are excluded, the 10-year risk drops to 5.7% in those at very high risk (lifetime 40–50%) of breast cancer. The American College of Surgeons recommends MRI screening in women with a lifetime risk of breast cancer as low as 20–25%. 71 The incidence rates in this risk group are 20–25% of those deemed cost-effective by NICE at 8% 10-year risk in the thirties and 20% 10-year risk in the forties. 2 Thus, American College of Surgeons screening for moderate-risk women based on the cost-effectiveness analysis is likely to cost in excess of US$120,000 per quality-adjusted life-year (QALY). 70 In fact, NICE has now dropped the breast cancer risk threshold in favour of a simple mutation-based eligibility, as work from the MARIBS study showed that those testing negative for BRCA1 and BRCA2 dropped below the breast cancer risk thresholds. 72 This was possible as NICE dropped the genetic testing threshold to a 10% likelihood of identifying a mutation and included unaffected women for the first time in the eligibility. 2
The question of which risk model to use in the family history setting is a problem, as the models often give quite different read-outs. 73,74 To our knowledge there has been only one other assessment of breast cancer risk prediction in the family history setting, this time in North America, which again showed that TC substantially outperformed the Gail model. 75 In this study, the Gail model, as in our original series,57 predicted cancer rates half of those that actually occurred. This is, perhaps, not surprising, as Gail does not include second-degree relatives or the age at breast cancer onset in first-degree relatives. Nonetheless, in the current update Gail predicted the number of breast cancers accurately, but not the proportions in each risk category. Changes have been made to the model since 2003 to increase the rates expected with a family history, but its discrimination compared with TC remains poor. Surprisingly, our interrogation of the Claus model showed that a maternal grandmother with breast cancer was ignored but a maternal aunt was included in assessing risk. This may be because the original Claus tables did not have a read-out for grandmothers. 43 Using the Manual model, we would include a grandmother in the same way that we would include an aunt in the tables. 52,62,63 It is likely, therefore, that the Claus model would predict lower incidence than the Manual model use of tables where there is an affected maternal grandmother. Nearly 1500 of our women had an affected maternal grandmother. As yet, there has been no formal validation of the BOADICEA model for its breast cancer risk output. We did consider using the Claus ‘extended’ model to incorporate ovarian and other cancers, but this would have meant reassessing > 8000 women. 76
Although the core risk assessment in the present study was adjusted Claus tables, there were upwards or downwards alterations owing to non-familial risk factors in > 50% of women. This was usually by only one or less than one order in the odds ratios (ORs) that we usually quote for women. For instance, an upwards variation from 25% or 1 in 4 lifetime risk usually was to either 3 in 10 (30%) or 1 in 3 (33%). It is of note that the predominant variation in those with cancer was an upwards variation, whereas in those without cancer it was downwards. This demonstrates the added value of adding hormonal risk factor information, which is currently not incorporated into the Claus model, BRCAPRO (Ford) or BOADICEA.
There are some potential weaknesses in the present study. Although screening is likely to increase the incidence of breast cancer through early detection, most of this effect will be offset by excluding the prevalent cases. Moreover, the Gail and TC models have been derived from a screened population. The subanalysis carried out on each risk group does reduce power to discriminate between models and, thus, for some estimates CIs are wide. We have not compared the Manual model directly with the computer models in this present study, other than in specific examples. The primary aim was to determine whether or not adjustments to the Claus tables to reflect modern incidence rates of breast cancer provided an accurate risk prediction. The longer mean follow-up data and the large study size also mitigate this potential weakness. We have included both invasive breast cancers and in situ disease. Exclusion of in situ disease would not have substantially affected the results; the E : O ratio would move from 0.95 to 1.03 with the Manual model. It would also have made the TC model more accurate. The other main issue is the alteration in risk in the Manual model from the ‘genetic prediction’ based on hormonal and reproductive factors. This study is, therefore, not a pure output of modified Claus tables and the modification by two experienced clinicians (DGE and AH) may not be entirely reproducible. Given that screening is likely to be recommended in women at moderate and high risk, we believe that our Manual model and the TC models are the most appropriate in the family history setting. The Manual model can be used quickly and gives similar read-out to the TC, which provides the most useful and accurate tool for clinicians involved in breast cancer risk assessment. 57
Conclusions
Manual risk prediction which adds hormonal and reproductive factors remains an accurate approach to breast cancer risk estimation when used in conjunction with adjusted Claus tables provided here. This approach would be reasonable as a back-up to use of validated tools such as TC or as a stand-alone in a busy clinic and where incidence rates of breast cancer are similar, such as North America, northern Europe and Australasia.
Study 1b: expected versus observed ovarian cancers (FH-Risk cohort)
This section has been reproduced from Ovarian cancer among 8005 women from a breast cancer family history clinic: no increased risk of invasive ovarian cancer in families testing negative for BRCA1 and BRCA2. Ingham SL, Warwick J, Buchan I, Sahin S, O’Hara C, Moran A, Howell A, Evans DG. J Med Genet vol. 50, pp. 368-372, 2013,77 with permission from BMJ Publishing Group Ltd.
The Breast Cancer FHC in South Manchester was established in 1987 and includes information (demographic, pedigree, screening and disease symptomatology) on individuals and families with a family history of breast and ovarian cancer. Families in the North-West region of England who have a family history of breast or ovarian cancer are referred to the FHC from their GP surgeries. Women who attend the FHC have a detailed family tree elicited with all first-, second- and, if possible, third-degree relatives. The genetic status of all family members is recorded (BRCA1, BRCA2 and negative results) if testing has occurred. Details of all tested and untested patients and relatives are entered into a FileMaker Pro 7 database (FileMaker Inc., Santa Clara, CA, USA).
This was a retrospective study, and only women in the family history database (FileMaker Pro 7) were included. Their data were verified against medical records, NHS Summary Care Records and the NWCIS. All cases of ovarian cancers were confirmed by means of hospital/pathology records, Regional Cancer Registries (from 1960) or death certification. The date the patient first attended the FHC was considered the ascertainment date. Follow-up was censored on 31 December 2010, date of ovarian cancer, date of oophorectomy or date of death (obtained from death certification either directly or via the NWCIS). Patients were excluded if they had ovarian cancer or oophorectomy prior to being seen in the FHC.
Statistics
Patients were grouped by genetic status and by ovarian cancer type (invasive epithelial or borderline). Person-years at risk analyses were performed to assess expected ovarian cancers in the family history population using data from the NWCIS. The expected numbers of cases for each genetic status (BRCA1, BRCA2, BRCA negative and BRCA untested) were calculated. Observed-to-expected ratios were assessed for statistical significance using the common method from Clayton and Hills based on the Poisson assumption. Confidence limits for RR were based on the Byar’s approximation of the exact Poisson distribution.
Results
We have assessed risk of ovarian cancer in 8005 women from 895 families from time of referral without ovarian cancer to our FHC. A total of 1613 women from breast cancer families that had tested negative for BRCA1/BRCA2 were followed for a total of 17,589 years between 0.04 and 25 years and checked against a cancer registry for ovarian cancer incidence. Data were censored at ovarian cancer diagnosis, oophorectomy or death. During follow-up, only one invasive epithelial ovarian cancer occurred, although two borderline tumours were diagnosed. Expected rates for this cohort from cancer registry data were 2.99 epithelial ovarian tumours, with 2.74 for invasive cancer and 0.25 for borderline tumours. The RR of developing invasive ovarian cancer in this group was 0.37 (95% CI 0.01 to 2.03), compared with the general population. The upper confidence limit for invasive RR was 2.03 and for borderline tumours was 28.90 (Table 12). The 351 women who were from BRCA2 breast cancer families had a total follow-up of 3230.47 years between 0.02 and 23.72 years. These years of follow-up to ovarian cancer, oophorectomy or death showed no borderline tumours, but five invasive epithelial tumours were recorded. Expected rates for women from BRCA2 positive families were 0.319 ovarian tumours, including 0.300 invasive cancers and 0.032 borderline tumours (see Table 12). The RR was 16.67 (95% CI 5.41 to 38.89) (see Table 12) for invasive cancers in BRCA2.
BRCA status | n | Observed cancers | Expected cancers | RR | 95% CI |
---|---|---|---|---|---|
BRCA1 | |||||
Invasive epithelial | 310 | 13 | 0.26 | 50.00 | 26.62 to 85.50 |
Borderline tumour | 0 | 0.03 | 0 | 0 to 122.960 | |
BRCA2 | |||||
Invasive epithelial | 351 | 5 | 0.300 | 16.67 | 5.41 to 38.89 |
Borderline tumour | 0 | 0.032 | 0 | 0 to 122.960 | |
BRCA negative | |||||
Invasive epithelial | 1613 | 1 | 2.74 | 0.37 | 0.01 to 2.03 |
Borderline tumour | 2 | 0.25 | 8.00 | 0.97 to 28.90 | |
BRCA untested | |||||
Invasive epithelial | 5731 | 9 | 9.07 | 0.99 | 0.45 to 1.88 |
Borderline tumour | 3 | 0.84 | 3.57 | 0.74 to 10.44 |
Thirteen cancers were identified in the cohort of 310 women whose families had tested positive for BRCA1 in 1981.60 years of follow-up, of which all 13 were invasive epithelial tumours and none were borderline cancers. The expected rate of invasive tumours was 0.26, which, as expected, was higher than the expected rate of borderline cancers (0.03). The RR for the invasive cancers was 50.00, although with wide CIs (95% CI 26.62 to 85.50) (see Table 12).
From families untested for BRCA1/BRCA2, 5731 women had 58,904 years of follow-up and 14 ovarian tumours were diagnosed. There were nine epithelial ovarian cancers, two malignant germ cell tumours and three borderline tumours. Using the same average invasive ovarian tumour rates of 0.154 per 1000 as in the untested cohort, we would have expected 9.07 invasive ovarian cancers and, using a similar analysis, 0.84 borderline tumours. The RR for invasive cancers was calculated as 0.99 (95% CI 0.45 to 1.88), increasing to RR 3.57 for borderline tumours (95% CI 0.72 to 10.44) (see Table 12).
Discussion
The present study from our FH-Risk cohort has shown that in a large cohort of women from breast cancer families testing negative for BRCA1/BRCA2, there was no increased risk of ovarian cancer. There was a non-significant (95% CI 0.97 to 28.90) increased risk of borderline tumour, and an apparently non-significant reduced risk of invasive epithelial ovarian cancer, with only one cancer occurring when 2.74 were expected. This study provides strong evidence to support the counselling of women whose breast cancer-only family tests negative for BRCA1/BRCA2 that they are not at increased risk of ovarian cancer, although indications are that counselling in ovarian cancer risk does need to continue in women whose families have tested BRCA1/BRCA2 positive. Statistically significant increased risk was seen in both BRCA1 and BRCA2 families: RR 50.0 (95% CI 26.62 to 85.50) and RR 16.67 (95% CI 5.41 to 38.89), respectively.
Our study contained only 167 women who had developed breast cancer and who personally had tested negative for BRCA1/BRCA2, but support that these women may also be reassured comes from a large Swedish study78 that found that their founder mutation, BRCA1 3171 ins5, explains the excess of ovarian cancer after breast cancer in 2600 women in their region. The authors estimated that BRCA1 gene mutations were associated with about 80–85% of the estimated 63 excess cases of ovarian cancer diagnosed after breast cancer.
The only other prospective study mainly in unaffected women showed that, during 2534 woman-years of follow-up, one case of ovarian cancer was diagnosed, when 0.66 was expected (standard incidence ratio = 1.52, 95% CI 0.02 to 8.46). 79 This study used questionnaires to families and did not verify diagnoses against a cancer registry as we have done. Our study also has over five times the follow-up. Nonetheless, both studies show no overall evidence of any increased risk.
Although data from the BCLC estimated that close to 100% of families with two or more ovarian cancers in addition to breast cancer (at least 2 < 60 years) had mutations in BRCA1/BRCA2,64 recent results have shown that three of eight (37.5%) of the mutations in RAD51D (RAD51 paralog D) were in families with two or more ovarian cancers that fulfilled BCLC criteria. 80 However, the frequency of RAD51C (RAD51 paralog C) and RAD51D mutations was only 1.3%81 and 0.9%,80 respectively, in breast ovary kindreds negative for pathogenic mutations in BRCA1 and BRCA2. Furthermore, although the initial study on RAD51C81 suggested that mutations might be high risk for both breast and ovarian cancer, the RAD51D study estimated that the risk was high only for ovarian cancer, with a non-significant increased breast cancer risk of less than twofold (1.37, 95% CI 0.92 to 2.05; p = 0.64)). 80 Neither study found mutations in breast cancer-only kindreds (0/737), supporting the lack of a strong link with breast cancer. 80,81
If BRCA1/BRCA2 mutations account for the great majority of the inherited link between breast and ovarian cancer, the main factor affecting the ability to confidently exclude risk of ovarian cancer in families testing negative is the sensitivity of the testing. Tests on most cancer-predisposing genes are limited, in that they do not screen the intronic areas outside the intron–exon boundaries, and nor do they screen for positional effects of mutations in other genes that can affect genes at a distance, such as EPCAM (epithelial cell adhesion molecule) mutations and MSH2 (MutS homolog 1). 82 There are relatively few papers that adequately assess the sensitivity of BRCA1/BRCA2 mutation testing. Simply using a panel of found mutations and assessing different screening tests does not address the overall sensitivity. 83 The tests can be assessed only against a gold standard, such as gene sequencing, which, in any case, does not screen the introns. It is first necessary to identify families with a very high a priori probability of BRCA1/BRCA2 involvement, such as breast/ovarian families fulfilling BCLC criteria or such families with a Manchester score84 of ≥ 40. We have previously shown that sequencing plus multiplex ligation dependent probe amplification (MLPA) identified mutations in 58 out of 65 (89%) families with both breast and ovarian cancer and a Manchester score of ≥ 40. 85 Breast cancer phenocopies can reduce the sensitivity of tests, as around 6% of tests of breast cancers in families with mutations are mutation negative;86 thus, true sensitivity could be closer to 95%. Even if one takes the lower sensitivity estimate, this would reduce the excess risk of ovarian cancer in a breast cancer-only family by ninefold. The true likelihood of a missed mutation can be estimated from our testing of 2009 breast cancer-only families, of whom only 240 (11.9%: 100 BRCA1; 140 BRCA2) had mutations identified by sequencing plus MLPA. Allowing for a Bayesian calculation, no more than 1.5% of these breast cancer-only families would have had a missed mutation.
There are some potential limitations to the present study. Not all participants remained in active follow-up, and a small proportion may have moved out of the north-west of England and an ovarian cancer could have been missed. Despite the large numbers of women, the confidence limits are still quite wide and include a twofold RR of invasive ovarian cancer. Nevertheless, the data are now strongly supportive of informing women from breast cancer-only families who test negative for BRCA1/BRCA2 that their risks of ovarian cancer are not increased.
In conclusion, this, the largest prospective follow-up of a BRCA-negative cohort, has demonstrated that there is no evidence of an increased risk of invasive ovarian cancer in families who have tested negative for BRCA1/BRCA2.
Study 2: FH-Risk case–control study
Recruitment
A total of 320 cases and 980 controls were recruited between November 2010 and September 2012 to the MD study. A further series of cases and controls were available who had blood DNA but no assessable mammogram for a cancer. All cases have been matched to three controls, with two extra controls recruited. Samples of DNA have been obtained on 426 women affected with breast cancer and 1275 controls. Direct consent for FH-Risk was obtained for 320 cases and 980 controls (Figure 6). A further 38 who had been affected with breast cancer and had subsequently died having previously supplied a blood sample were also included.
FIGURE 6.
Uptake to the FH-Risk study over a 2-year period. (a) Cumulative uptake to FH-Risk – cases; and (b) cumulative uptake to FH-Risk – controls.
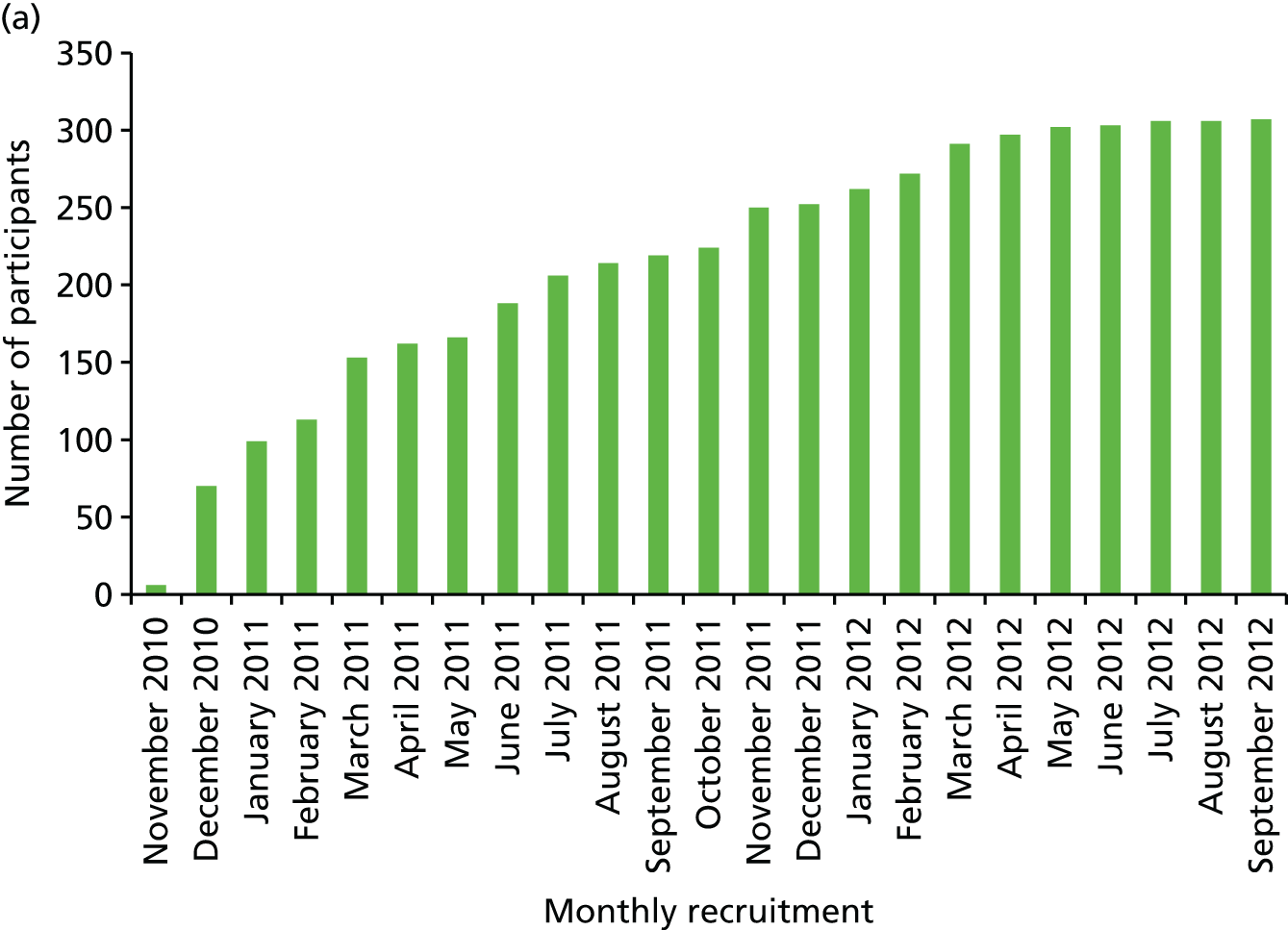
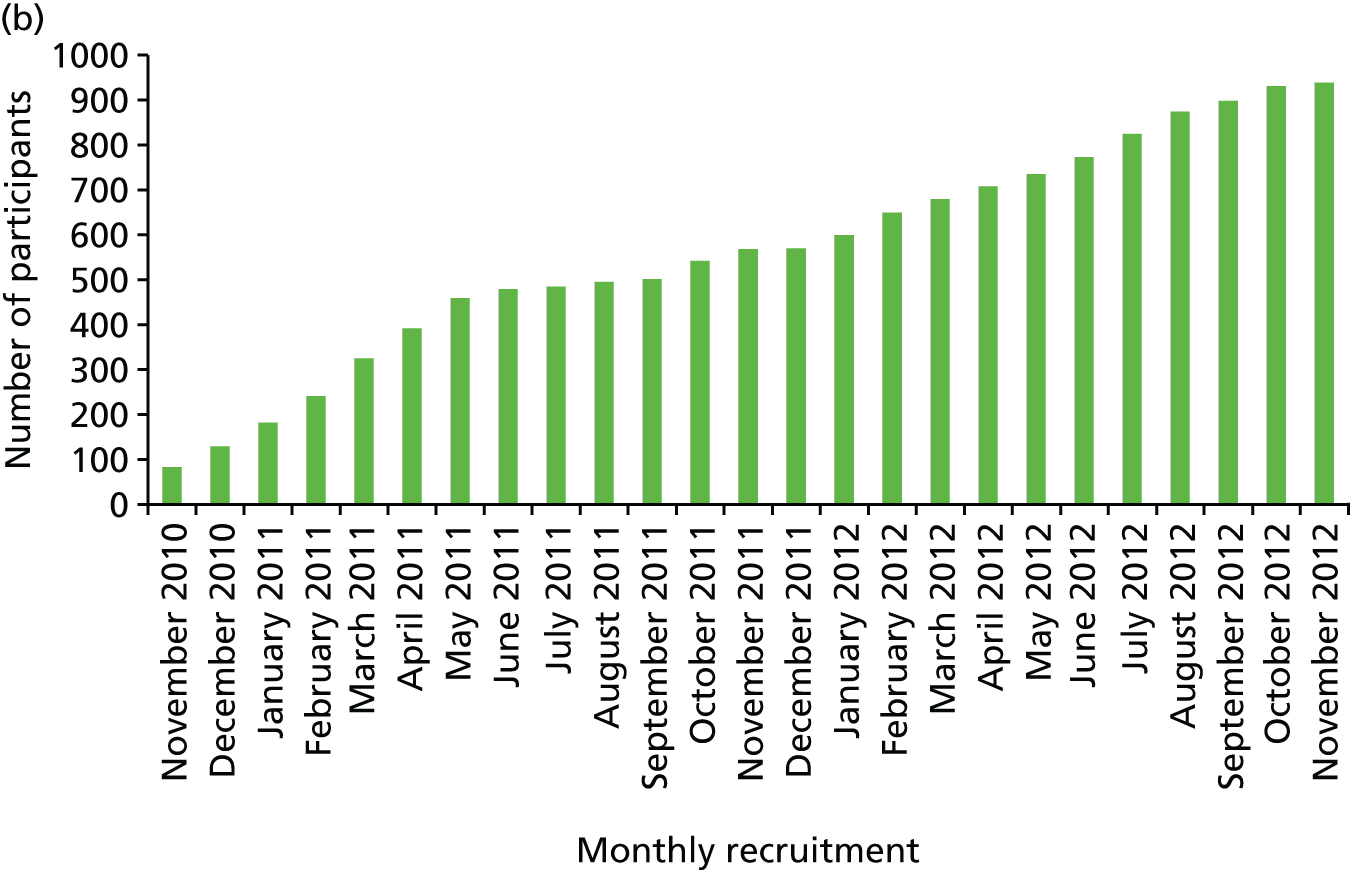
Methods
Cases and controls were matched on a 1 : 3 basis, with age at assessable mammogram being the matching criterion. When sufficient controls were unavailable, matching was carried out on a 1 : 1 basis. Controls did not have cancer at last follow-up and cases had to have been diagnosed after an assessable mammogram or have an assessable mammogram at date of cancer diagnosis and no cancer in the contralateral breast. Controls were also matched for type of mammogram, either digital or analogue. The questionnaire data were updated to include clothes size and alcohol intake as well as ensuring that data were held on age at menarche and age at first full-term pregnancy. The TC model was used to incorporate an adjustment for MD using VASs and two digital methods [Volpara™ (version 1.4.5; Volpara Solutions, Wellington, New Zealand) and Quantra™ (version 2.0; Hologic, Inc., Marlborough, MA, USA)]. For breast cancer cases with no assessable mammogram but in whom blood DNA was available, controls were also recruited for the DNA SNP study in Chapter 3.
Mammographic density analysis
We carried out case–control analyses of data from women who developed breast cancer prior to, or while, attending the FHC, along with matched controls, with the aim of investigating the association of MD with case–control status. It was necessary to group the cases into those in whom the cancer was detected in a film mammogram (either at time of diagnosis or prior to diagnosis) (n = 82) and those in whom the cancer was detected by full-field digital mammography (FFDM) (either at time of diagnosis or prior to diagnosis) (n = 48). The number of cases in this substudy was limited by the availability of Cumulus data. The two groups were analysed separately. Cases were matched to controls who did not develop breast cancer, and were imaged using a similar modality. Controls were identified from the FHC at the time of recruitment. Matching was performed on the basis of age at which the mammogram was taken, with film mammograms matched to a single film control and FFDM mammograms matched to three digital controls. Film mammograms were digitised using a Vidar CAD-PRO® (Vidar Systems Corporation, Hemdon, VA, USA) digitiser to enable Cumulus analysis.
The MD assessments were made in the contralateral breast of cancer cases matched to the same breast in the controls. Although visual assessment using VAS and Cumulus reading were carried out on both digital and analogue mammograms, these were not comparable, as the appearance of images from the two modalities differs. VAS assessments were made by two readers drawn from a pool of 12 on a pragmatic basis, with at least one reader being a consultant radiologist or a breast physician. Scores were averaged across readers and views for the breast of interest. Cumulus was undertaken by a single trained and validated reader (JS) who assessed a single mediolateral oblique (MLO) view, with cases and controls presented in random order. The Cumulus assessor was blinded to case–control status, and undertook assessment of the analogue and digital groups in different sessions.
The demographic characteristics were reported as number and percentage by case–control status. Comparisons of categorical data were made using the chi-squared test. For those variables where the data were ordinal, a chi-squared test for trend was also conducted. Continuous variables were assessed by means of an unpaired sample t-test when the distribution was normally distributed or by the Mann–Whitney U-test when the distribution was not normally distributed. The analysis of the relationship between density methods and case–control status was performed using conditional logistic regression [in Statistical Product and Service Solutions (SPSS) version 20; IBM Corporation, Armonk, NY, USA] owing to the matched nature of the data set. The univariate associations were performed initially, and the multivariate associations were subsequently performed adjusting for breast area. Factors not associated in univariate analysis were not assessed in multivariate analyses.
Results
The SNP results are in Chapter 3. The FH-Risk case–control study was also used to assess incorporation of MD into risk assessment. The study was affected by the policy of destroying analogue mammograms after 3 years and by the fact that no raw data from digital mammograms were saved. Therefore, only 320 cases had an assessable mammogram. The controls were matched for age and type of mammogram but, owing to hospital policy of destroying mammograms, the analogue controls were from a much later era (see Table 13).
Density results: analogue (film) mammograms
Table 13 shows the demographic characteristics of women with film mammograms. The majority were parous and had a body mass index (BMI) in the normal range; mean BMI was approximately 24.5 kg/m2. There were very few current or previous users of HRT (< 10% of cancer cases and < 5% of controls) based on data available at the time of referral; however, the average age was approximately 43 years and the majority of women had not yet been through the menopause at the time of referral. Fifty-six per cent of cancers were in the left breast, and 44% in the right breast. The year of mammogram ranged from 1988 to 2007, with more of the control subjects than the case subjects having recent images. Matching on age alone appeared to be adequate in the analogue film group, as there were no significant differences between cases and controls for other important parameters such as BMI, parity, HRT use and menopausal status.
Characteristics | Case subjects (N = 82), n (%) | Control subjects (N = 82), n (%) | p-value |
---|---|---|---|
Age (years) | |||
< 38 | 24 (29.3) | 23 (28.0) | |
38–42 | 17 (20.7) | 21 (25.6) | |
43–47 | 19 (23.2) | 18 (22.0) | |
≥ 48 | 22 (26.8) | 20 (26.8) | 0.904 |
Mean (SD) | 43.7 (8.1) | 43.3 (7.9) | 0.795 |
Menopausal status | |||
Premenopausal | 57 (69.5) | 67 (81.7) | |
Started | 7 (8.5) | 2 (2.4) | |
Finished | 16 (19.5) | 10 (12.2) | |
Unknown | 2 (2.4) | 3 (3.7) | 0.160 |
BMI (kg/m2) | |||
< 25 | 48 (58.5) | 40 (48.8) | |
25–29 | 24 (29.3) | 25 (30.5) | |
≥ 30 | 5 (6.1) | 9 (11.0) | |
Unknown | 5 (6.1) | 8 (9.8) | 0.461 |
Mean (SD) | 24.3 (3.8) | 24.8 (4.5) | 0.486 |
Parity | |||
Nulliparous | 13 (15.9) | 19 (23.2) | |
Parous | 68 (82.9) | 62 (75.6) | |
Unknown | 1 (1.2) | 1 (1.2) | 0.375 |
HRT use | |||
Never/unknown | 74 (90.2) | 78 (95.1) | |
Previous/current | 8 (9.8) | 4 (4.9) | 0.231 |
Year of mammogram | |||
1988–92 | 11 (13.4) | 1 (1.2) | |
1993–7 | 24 (29.3) | 3 (3.7) | |
1998–2002 | 21 (25.6) | 18 (22.0) | |
2003–7 | 26 (31.7) | 60 (73.2) | 0.000 |
Table 14 shows the analysis of breast density measures made in women with film mammograms. The results show no association between MD, as measured by VAS, and risk of developing breast cancer. The only significant association with risk was in women with Cumulus percentage density of 41–50% with an OR of 4.16 (95% CI 1.32 to 13.06) compared with those in the lowest quartile (< 27% density). Those with percentage density of ≥ 50% also had an increased risk of breast cancer (OR 3.19, 95% CI 0.99 to 10.31), which approached statistical significance. Adjustment for breast area increased the ORs slightly, but there were no changes in statistical significance.
Density assessment | Case subjects (N = 82), n (%) | Control subjects (N = 82), n (%) | OR (95% CI) | OR (95% CI) (adjusting for cumulus breast area) |
---|---|---|---|---|
VAS | ||||
< 30 | 23 (28.0) | 20 (24.4) | 1.00 (referent) | |
30–46 | 16 (19.5) | 24 (29.3) | 0.57 (0.24 to 1.37) | |
47–60 | 21 (25.6) | 21 (25.6) | 0.93 (0.42 to 2.09) | |
≥ 60 | 22 (26.8) | 17 (20.7) | 1.19 (0.49 to 2.87) | |
Mean (SD) | 46.1 (20.8) | 43.6 (20.9) | ||
Cumulus dense area (mm3) | ||||
Mean (SD) | 205,688 (92,817) | 193,095 (90,939) | ||
< 140,000 | 17 (20.7) | 23 (28.0) | 1.00 (referent) | 1.00 (referent) |
140,001–190,000 | 22 (26.8) | 20 (24.4) | 1.59 (0.66 to 3.85) | 1.65 (0.66 to 4.14) |
190,001–250,000 | 21 (25.6) | 18 (22.0) | 1.76 (0.67 to 4.70) | 2.10 (0.69 to 6.36) |
≥ 250,000 | 22 (26.8) | 21 (25.6) | 1.52 (0.60 to 3.88) | 2.03 (0.67 to 6.17) |
Cumulus percentage density | ||||
Mean (SD) | 41.0 (15.3) | 37.0 (17.9) | ||
< 27 | 13 (15.9) | 24 (29.3) | 1.00 (referent) | 1.00 (referent) |
28–40 | 24 (29.3) | 24 (29.3) | 2.48 (0.81 to 7.58) | 2.47 (0.73 to 8.43) |
41–50 | 24 (29.3) | 15 (18.3) | 4.16 (1.32 to 13.06) | 4.41 (1.15 to 16.99) |
≥ 50 | 21 (25.6) | 19 (23.2) | 3.19 (0.99 to 10.31) | 3.43 (0.89 to 13.20) |
Density results: digital mammograms
Table 15 shows the demographic characteristics of women with FFDM mammograms. More case subjects were premenopausal (88% cases; 66% controls); this difference was not statistically significant. There was, however, a significant difference between BMI categories for the cases and controls. The cases had a lower mean BMI (24.2 kg/m2) than the controls (26.5 kg/m2), although it should be noted that BMI was not recorded for 22% of controls and 14% of cases. Fifty-six per cent of the cancers were in the right breast and 44% were in the left breast. There were very few current or previous users of HRT (< 5%) based on data available at the time of referral; however, the average age was approximately 46 years. The year of mammogram ranged from 2006 to 2012, with more of the control subjects than the case subjects having recent images.
Characteristics | Case subjects (N = 48), n (%) | Control subjects (N = 144), n (%) | p-value |
---|---|---|---|
Age (years) | |||
< 39 | 11 (23.4) | 35 (24.5) | |
39–46 | 15 (31.9) | 38 (26.6) | |
47–50 | 10 (21.3) | 32 (22.4) | |
> 50 | 11 (23.4) | 38 (26.6) | 0.911 |
Mean (SD) | 45.7 (8.1) | 45.9 (8.2) | 0.859 |
Menopausal status | |||
Premenopausal | 40 (83.3) | 95 (66.0) | |
Started | 2 (4.2) | 19 (13.2) | |
Finished | 5 (10.4) | 22 (15.3) | |
Unknown | 1 (2.1) | 8 (5.6) | 0.124 |
BMI (kg/m2) | |||
< 25 | 26 (54.2) | 55 (38.2) | |
25–29 | 10 (20.8) | 37 (25.7) | |
≥ 30 | 5 (10.4) | 20 (13.9) | |
Unknown | 7 (14.6) | 32 (22.2) | 0.280 |
Mean (SD) | 24.2 (4.0) | 26.5 (6.5) | 0.036 |
Parity | |||
Nulliparous | 7 (14.6) | 29 (20.1) | |
Parous | 40 (83.3) | 113 (78.5) | |
Unknown | 1 (2.1) | 2 (1.4) | 0.667 |
HRT use | |||
Never/unknown | 45 (93.8) | 139 (96.5) | |
Previous/current | 3 (6.3) | 5 (3.5) | 0.404 |
Year of mammogram | |||
2006 | 5 (10.4) | 0 (0.0) | |
2007 | 10 (20.8) | 3 (2.1) | |
2008 | 12 (25.0) | 6 (4.2) | |
2009 | 13 (27.1) | 9 (6.3) | |
2010 | 6 (12.5) | 41 (28.5) | |
2011 | 1 (2.1) | 67 (46.5) | |
2012 | 1 (2.1) | 18 (12.5) | 0.000 |
Table 16 shows the analysis of breast density measures made in women with digital mammograms. The results show no significant association between MD and risk of developing breast cancer for density measured by Cumulus (both percentage density and dense area), but a significant association for density measured by VAS following adjustment for menopausal status, breast area and BMI. The ORs for Cumulus tended to be higher after adjusting for BMI, menopausal status and breast area; however, none was statistically significant.
Density assessment | Case subjects (N = 48), n (%) | Control subjects (N = 144), n (%) | OR (95% CI) | OR (95% CI) (adjusting for Cumulus breast area, menopausal status and BMI) |
---|---|---|---|---|
VAS | ||||
Mean (SD) | 38.99 (18.1) | 34.37 (19.2) | ||
< 21 | 9 (18.8) | 37 (25.7) | 1.00 (referent) | 1.00 (referent) |
21–35 | 13 (27.1) | 39 (27.1) | 1.38 (0.54 to 3.54) | 15.80 (1.24 to 200.69) |
36–48 | 12 (25.0) | 35 (24.3) | 1.40 (0.50 to 3.90) | 18.44 (1.30 to 261.01) |
≥ 49 | 14 (29.2) | 33 (22.9) | 1.76 (0.67 to 4.66) | 7.81 (0.62 to 98.80) |
Cumulus dense area (mm3) | ||||
Mean (SD) | 75,552 (37,763) | 74,164 (39,933) | ||
< 48,000 | 9 (22.0) | 37 (26.4) | 1.00 (referent) | 1.00 (referent) |
48,001–70,000 | 12 (29.3) | 33 (23.6) | 1.36 (0.52 to 3.54) | 1.99 (0.46 to 8.73) |
70,001–96,000 | 9 (22.0) | 34 (24.3) | 1.22 (0.41 to 3.67) | 2.23 (0.44 to 11.33) |
≥ 96,000 | 11 (26.8) | 36 (25.7) | 1.22 (0.44 to 3.41) | 1.86 (0.35 to 9.93) |
Cumulus percentage density | ||||
Mean (SD) | 36.2 (19.6) | 33.8 (18.3) | ||
< 19 | 11 (26.8) | 33 (23.6) | 1.00 (referent) | 1.00 (referent) |
19–32 | 7 (17.1) | 38 (27.1) | 0.53 (0.17 to 1.67) | 1.15 (0.16 to 8.04) |
33–48 | 11 (26.8) | 35 (25) | 0.99 (0.38 to 2.56) | 3.58 (0.54 to 23.59) |
≥ 49 | 12 (29.3) | 34 (24.3) | 1.19 (0.45 to 3.18) | 3.39 (0.34 to 33.32) |
Discussion
In women with film mammograms, Cumulus was the only method that showed significant association with breast cancer risk. Use of Cumulus for mammograms acquired in this way is well established, and the inclusion of prior mammograms as well as contralateral breast of diagnostic mammograms provides a similar methodology to published validation of Cumulus for breast cancer risk. 26,87,88 In a study in which mammograms (contralateral breast for cancers, and matched controls) were assigned to density classes, the RR for women in the highest density group associated with radiologist allocation was higher than computer-based allocation using interactive thresholding. 26 However, in this Family History analogue mammography substudy, VAS assessments were not related to cancer risk; this may be due, in part, to interobserver variability dominating any genuine effect in a small evaluation. The failure to match on date of mammogram is also an important shortcoming in this study. It is of interest that in the density analysis of the larger PROCAS screening study (see Chapter 4), and in the subgroup of family history cases with digital screening mammograms which were matched with controls on a 1 : 3 basis, VAS provided the most predictive MD method. Visual assessment recorded on VAS, following adjustment for BMI, menopausal status and Cumulus breast area, was the only method significantly associated with an increased risk of breast cancer in the digital group. For the other methods, the ORs tended to be higher after adjusting for menopausal status and breast area, but did not show statistical significance. For the VAS measures, the CIs for the OR were very wide, reflecting the limited number of cases. Significance was achieved only following the inclusion of breast area as measured by Cumulus in the model.
These results are consistent with previously published work showing that the association between breast cancer and MD is higher in an asymptomatic population. 33 In this substudy we were unable to assess volumetric breast density; in analogue mammograms, the Manchester Stepwedge method can be applied prospectively only, and in the digital group only seven cases/controls were amenable to processing by Quantra and Volpara (with GE Healthcare images and raw data).
The small number of cases for which both Cumulus and VAS were available is a limitation of this substudy. Furthermore, Cumulus was performed on a single mammographic image (MLO), whereas VAS assessment used the mean across both views [MLO and craniocaudal (CC)]; ideally, we would have liked to have had Cumulus for both mammographic views for the contralateral breast. A further limitation is that the covariate information was collected at the time of referral, which was very often not the same time as the mammogram was acquired. Of all FH-Risk mammograms (both digital and film), approximately 25% were taken at the same time as referral to the clinic, 5% were taken within 1 year of referral and the remaining 70% were taken over 1 year after referral. For those with a larger interval, BMI, HRT use and menopausal status may be inaccurate.
Publications resulting from work in Chapter 2
Ingham SL, Warwick J, Buchan I, Sahin S, O’Hara C, Moran A, et al. Ovarian cancer among 8005 women from a breast cancer family history clinic: no increased risk of invasive ovarian cancer in families testing negative for BRCA1 and BRCA2. J Med Genet 2013;50:368–72. 77
Evans DG, Ingham S, Dawe S, Roberts L, Lalloo F, Brentnall AR, et al. Breast cancer risk assessment in 8824 women attending a family history evaluation and screening programme. Fam Cancer 2014;13:189–96. 89
Chapter 3 Project 2: assessment of predictive value of new genetic variants
Introduction
Deoxyribonucleic acid testing
Over the years there has been growing epidemiological evidence that women with a family history of breast cancer are at an increased risk of developing the disease. One of the early large studies investigating familial breast cancer risk (the Cancer and Steroid Hormone case–control study) showed that the RR of breast cancer was 2.1 in females with a first-degree relative affected by breast cancer. 90 In addition, the study predicted that the underlying genetic susceptibility was due to one or more, rare, highly penetrant autosomal dominant genes. The Cancer and Steroid Hormone study provided epidemiological evidence that familial breast cancers develop at a relatively younger age, in comparison with non-familial breast cancers, there are more cases of bilateral breast cancer in familial clusters, and, in familial clusters, cases of ovarian cancer are often seen in relatives of women with breast cancer. 90 In addition, there was evidence that there was an association between male breast cancer and familial risk of breast cancer, with female relatives of affected males at a two- to threefold increased risk compared with the general population risk. 91 The increased risk of breast cancer in relatives of probands affected by breast cancer potentially could be attributed to shared environmental and/or genetic factors. However, evidence from twin studies indicates that the majority of the excess familial risk is due to an inherited predisposition. One study estimated that individuals who have a monozygotic twin who has had a diagnosis of breast cancer have a lifetime breast cancer risk of 33%. Another twin study showed that 27% of breast cancer was attributable to inherited factors,44 demonstrating that single high-risk dominant genes which were estimated to cause around 4–5% of breast cancer could account for only a fraction of the familial component.
Genes increasing breast cancer susceptibility
The epidemiological evidence for familial predisposition to breast cancer led to extensive searches for genes which underlie this susceptibility. Three classes of breast cancer susceptibility genes have been identified so far from these studies: high-, moderate- and low-penetrance genes, according to the level of breast cancer risk they confer and the prevalence of disease-causing variants in the population. The level of penetrance describes the likelihood that an individual who carries a mutation in a gene or a particular genetic variant will develop breast cancer as a result of the presence of that mutation/variant. Mutations in high-penetrance genes such as BRCA1 and BRCA2 confer around a 10- to 20-fold increased risk of breast cancer over that of non-mutation carriers (approximating to a 40–80% lifetime risk). The group of genes described as moderate penetrance confer an approximately two- to three fold increased risk (equating to a 20–36% lifetime risk). The loci described here of low penetrance confer a RR of less than 1.3-fold. It is possible that other breast cancer susceptibility genes exist which may confer RR of breast cancer which do not comply with these descriptions. The proportion of familial component of breast cancer identified in 2008, when we applied for funding for the National Institute for Health Research (NIHR) programme grant, and that currently identified (2014) are shown in Figures 7 and 8. 92 BRCA1 and BRCA2 account for a large proportion of high-risk predisposition.
FIGURE 7.
Proportion of the familial excess risk accounted for by known genes or variants in 2008. ATM, ATM serine/threonine kinase; BRIP1, BRCA1-interacting protein C-terminal helicase 1; CDH1, cadherin 1; CHEK2, checkpoint kinase 2; PALB2, partner and localizer of BRCA2; PTEN, phosphatase and tensin homolog; STK11, serine/threonine kinase 11.
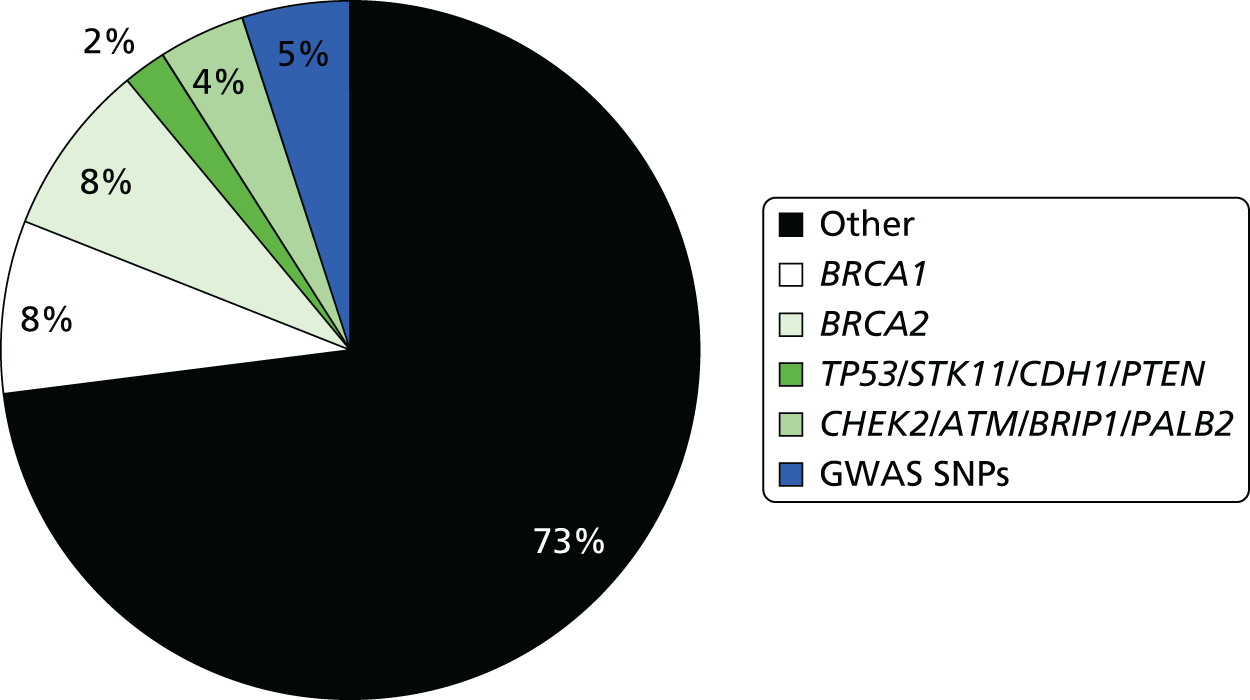
FIGURE 8.
Proportion of the familial excess risk accounted for by known genes or variants in 2014. ATM, ATM serine/threonine kinase; BRIP1, BRCA1-interacting protein C-terminal helicase 1; CDH1, cadherin 1; CHEK2, checkpoint kinase 2; PALB2, partner and localizer of BRCA2; PTEN, phosphatase and tensin homolog; STK11, serine/threonine kinase 11; ICOG, International Collaborative Oncological Gene-environment Study.
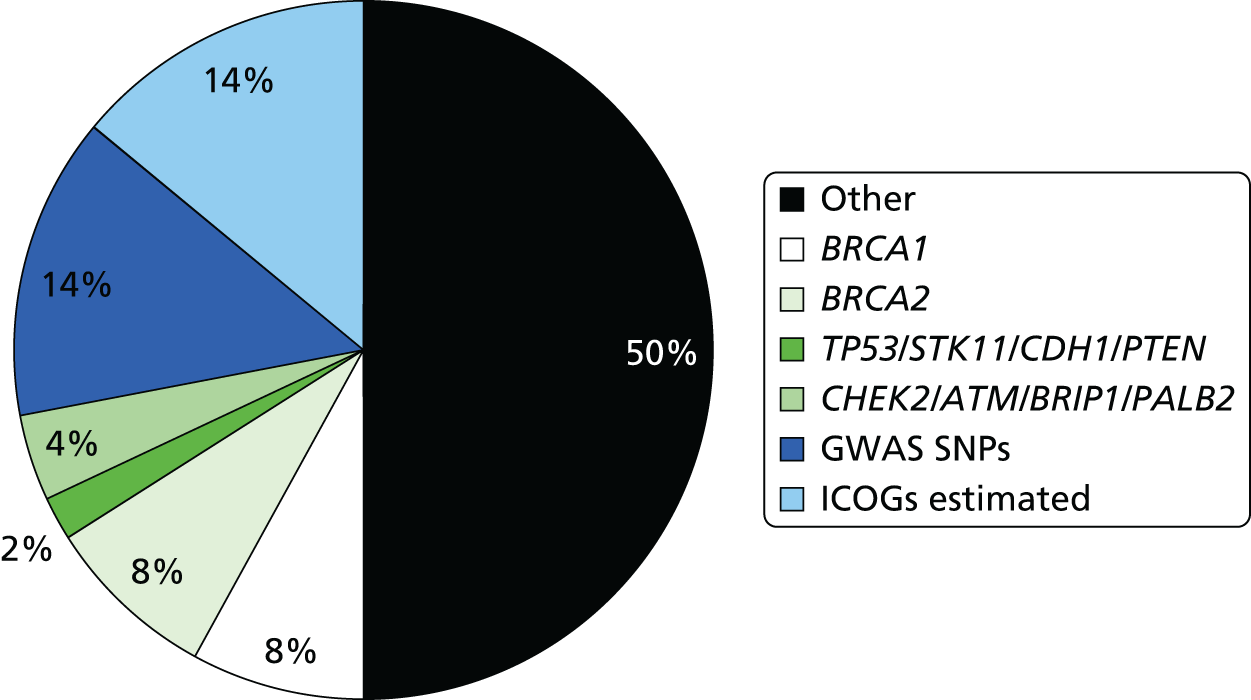
High-penetrance breast cancer susceptibility genes
The identification of pathogenic mutations in BRCA1 and BRCA2 in the last decade of the twentieth century was a major advance in the understanding of breast cancer susceptibility. Mutations in BRCA1 and BRCA2 are relatively rare, with a combined frequency of around 0.2% in outbred populations. 92 The associated phenotype is inherited in an autosomal dominant manner. At the cellular level, however, they act as recessive genes. Breast and ovarian cancer cells are homozygous for mutations in these genes owing to somatic loss of the wild-type allele. 93,94 Mutation carriers have a 10- to 20-fold increased risk of breast cancer and are at an increased risk of ovarian and other cancers. 95,96 Pathogenic mutations in BRCA1 and BRCA2 account for approximately 16% of familial breast cancer. 27,92–95
BRCA1
In 1990, genetic linkage analysis of families with multiple cases of early-onset breast cancer led to the localisation of this, the first breast cancer susceptibility gene, to chromosome 17q12–q21. 97 Following this, an international collaborative study coordinated by the BCLC confirmed linkage to chromosome 17q, and BRCA1 was cloned in 1994. 47
BRCA2
Following evidence from a study which showed that only 45% of families with multiple cases of breast cancer were linked to the BRCA1 locus, it became clear that BRCA1 was not the only breast cancer susceptibility gene. 98 As a result of this, the presence of at least one further breast cancer susceptibility gene was confirmed by the absence of linkage to BRCA1 of families with early-onset female breast cancers and at least one case of male breast cancer. 21 A genome-wide linkage search was performed using families which included male breast cancer cases and multiple-case families which were not linked to BRCA1. This led to the localisation of BRCA2 to chromosome 13q12–q13 in 199499 and the cloning of the BRCA2 gene the following year. 48,100
TP53 and other high-penetrance genes
TP53 is a high-penetrance gene, mutations in which cause Li–Fraumeni syndrome. 49,101 Patients with germline mutations in TP53 may have a family history of a number of cancer types including leukaemia, soft tissue sarcomas, brain tumours and osteosarcomas. Female TP53 mutation carriers have an 18-fold RR of being diagnosed with breast cancer before the age of 45 years, compared with the general population, and > 50% of TP53 mutation carriers are diagnosed with cancer before the age of 30 years. 102 TP53 germline mutations are, however, considerably rarer than BRCA1 and BRCA2103 mutations.
There are three other genes also thought to confer high risks of breast cancer: mutations in PTEN (phosphatase and tensin homolog) cause Cowden disease,46 mutations in LKB1/STK11 (serine/threonine kinase 11) cause Peutz–Jeghers syndrome104 and mutations in CDH1 (cadherin 1, type 1) cause hereditary diffuse gastric cancer. 105 The precise risks associated with these gene mutations are not known.
The hunt for other high-penetrance breast cancer genes
It is likely that all of these high-penetrance genes together account for no more than about 20% of the familial risk of breast cancer (see Figure 7); therefore, genome-wide linkage analyses have been performed on large numbers of families affected by breast cancer without a BRCA1 or BRCA2 mutation, with the aim of identifying further high-penetrance breast cancer susceptibility genes. Although no such genes have yet been identified through these studies, their existence cannot be excluded. 106 If other high-penetrance genes do exist, only a small number of breast cancer families are likely to be due to mutations in these genes. In 2001, Antoniou et al. 107 performed a large population-based study which suggested that a number of common, moderate- to low-penetrance genes with additive effects could account for familial cases not caused by BRCA1 or BRCA2. Subsequently, rare moderate penetrance and common low-penetrance breast cancer susceptibility alleles were identified.
Breast cancer susceptibility genes with moderate penetrance
Text in this section has been reused with permission from Evans DG. Genetic Predisposition and Breast Screening. In Benson JR, Gui G, Tuttle TM, editors. Early BreastCancer: From Screening to Multidisciplinary Management. 3rd edn. CRC Press; 2013. pp. 17–27. 108
In order to explain the 80% of familial breast cancer risk which is not attributable to the high-risk breast cancer genes discussed previously, candidate genes have been investigated through large case–control studies. These genes were selected for mutational analysis because the proteins they encode have been shown to be involved in the same biological pathways as BRCA1 and BRCA2. Such studies have yielded at least four genes which each confer an approximately twofold risk of breast cancer in mutation carriers.
CHEK2
The first rare moderate-penetrance breast cancer susceptibility gene to be identified was CHEK2 (checkpoint kinase 2). This gene encodes a cell cycle checkpoint protein kinase that phosphorylates p53 and BRCA1 and is involved in DNA repair. 109,110 It was demonstrated that CHEK2*1100delC, a frameshift mutation leading to truncated protein which affects its kinase activity, was present in 1.1% (18/1620) of healthy individuals but was present in 5.5% (55/1071) of familial breast cancer cases (p = 0.00000003). The RR of breast cancer in carriers of the CHEK2*1100delC allele was estimated to be 2.2-fold. 110
ATM
This was the second moderate-penetrance breast cancer gene for which there was strong molecular evidence. The possibility that ATM (ATM serine/threonine kinase) could be a breast cancer susceptibility gene was first proposed over 20 years ago when epidemiologists suggested that relatives of patients with an autosomal recessive condition called ataxia-telangiectasia which predisposes to cancer in childhood, particularly lymphoid cancers, had an increased risk of breast cancer. 111 The ATM gene was then mapped and identified by positional cloning. 112,113 ATM is a protein kinase with multiple complex functions, including a central role in DNA double-strand break repair in concert with BRCA1/BRCA2. The ATM protein has been demonstrated to phosphorylate the BRCA1, p53 and CHEK2 proteins. 114
The role of ATM in breast cancer susceptibility has been investigated in multiple studies. The largest of these, which identified 12 mutations in 443 familial breast cancer cases and two in healthy controls (p = 0.0047), has shown that the RR of breast cancer in monoallelic ATM mutation carriers is 2.37;115 however, certain mutations may cause high-risk susceptibility.
BRIP1
In 2006, BRIP1 (BRCA1-interacting protien C-terminal helicase 1), a BRCA1-interacting helicase (also known as BACH1), was also shown to be a rare moderate-penetrance breast cancer susceptibility allele. This gene was investigated as a candidate gene because it is a binding partner of BRCA1 and, thus, is implicated in some of the activities of BRCA1 in DNA repair. 116,117 Truncating mutations in this gene are rarer than in CHEK2 or ATM. In a case–control study, nine mutations were identified in 1212 familial breast cancer cases, compared with two in 2081 healthy controls (p = 0.003); the RR of breast cancer in monoallelic carriers of BRIP1 mutations is 2.0. 117 Interestingly, almost simultaneously, BRIP1 was identified as the gene responsible for Fanconi anaemia (complementation group J) in biallelic mutation carriers of the gene. 117 BRIP1 was the second gene found to predispose to breast cancer in monoallelic mutation carriers and to cause Fanconi anaemia in biallelic mutation carriers; BRCA2 was the first such breast cancer susceptibility gene. 118
PALB2
The fourth rare moderate-penetrance breast cancer susceptibility gene to be identified, PALB2 (partner and localizer of BRCA2), has also been demonstrated to predispose to breast cancer in monoallelic mutation carriers and to cause Fanconi anaemia (complementation group N) in biallelic mutation carriers. 119,120 PALB2 encodes a protein which interacts with BRCA2. In a familial breast cancer case–control study, 10 truncating mutations were identified in 923 individuals with breast cancer, but no mutations were identified in 1084 healthy controls (p = 0.0004). The RR of breast cancer associated with a mutation in PALB2 was estimated at 2.3-fold. 121
Each of these moderate-penetrance genes makes up a relatively small proportion of the overall familial risk of breast cancer (see Figure 7). Compared with the 16–20% of familial risk accounted for by mutations in BRCA1 and BRCA2, in 2008, it was estimated that the currently identified moderate-penetrance breast cancer susceptibility genes accounted for only 2.3% of the familial component of risk. 122 In keeping with findings in BRCA1 and BRCA2, most of the pathogenic mutations in these genes lead to premature protein truncation through nonsense codons or translational frameshifts. A very small number are possibly due to missense sequence variants. The moderate-penetrance breast cancer susceptibility genes each harbour multiple different rare pathogenic mutations.
The features of moderate-penetrance breast cancer susceptibility genes differ from those of high-penetrance genes in several ways. There are differences in the extent of segregation of disease-causing mutations with breast cancer. In breast cancer families which are due to mutations in BRCA1 or BRCA2, the mutation and disease status usually occur together in individuals, that is, family members who carry the mutation will usually be the individuals who are affected by breast and/or ovarian cancer. However, it has been suggested that phenocopies (women with breast cancer but without the familial mutation) can constitute around 24% of breast cancer cases in a family; this is based on data from families known to carry a BRCA1 or BRCA2 mutation. 86 In breast cancer families due to moderate-penetrance breast cancer genes where the risks are two- to threefold, disease-causing mutations often do not segregate with the disease. This is due to the lower penetrance of the mutations in these genes, which means that most mutation carriers do not actually develop breast cancer. Other relevant factors are the high rate of sporadic breast cancer, and the likelihood that familial breast cancer clusters without mutations in BRCA1 or BRCA2 are probably due to a random aggregation of susceptibility alleles in many different genes.
In contrast to BRCA1 and BRCA2, there is no strong evidence for the occurrence of other cancers, or an early age at onset of breast cancer, in heterozygous carriers of mutations in CHEK2, ATM, BRIP1 and PALB2. A possible reason for this could be the lack of sufficient identified cases to provide evidence of these features.
Neurofibromatosis type 1
Women with the inherited tumour-prone condition neurofibromatosis type 1 are now thought to be at moderately increased risk of developing breast cancer. 123
The currently identified high- and moderate-risk genes are shown in Table 17.
Disease gene | Location | Tumours | Tumour age | Lifetime risk (%) | Birth incidence of mutations | Life expectancy |
---|---|---|---|---|---|---|
CHEK2 | 22q | Breast cancer | > 25 years | 20 | 1 in 200 | ?normal |
ATM | 11q | Breast cancer | > 25 years | 20 | 1 in 200 | ?normal |
BRIP | Breast cancer | > 25 years | 20 | 1 in 1000 | ?normal | |
PALB2 | Breast cancer | > 25 years | 20–40 | < 1 in 1000 | ?normal | |
NF1 | 17q | Neurofibroma | First year | 100 | 1 in 2600 | 54–72 years |
Glioma | First year | 12 | ||||
Breast cancer | > 25 years | 17 | ||||
PTENCowden | 10q | Breast cancer | > 25 years | 60 | 1 in 200,000–250,000 | Reduced in women |
Thyroid | 30 years | 10 | ||||
PJS | 19p | GI malignancy | 20 years | 60 | 1 in 25,000 | 58 years |
Breast | > 25 years | 40 | ||||
LFS/TP53 | 17p | Sarcoma | First year | 80 | 1 in 30,000 | Severely reduced |
Breast cancer (women) | > 16 years | 95 | ||||
Gliomas | First year | 20 | ||||
HDGC/CDH1 | 16q | Gastric | > 16 years | 70–80 | Very rare | Reduced |
Breast (women) | > 35 years | 20–40 | ||||
BRCA2 | 13q | Breast/ovary (women) | > 18 years | 40–90 | 1 in 800 | 68 years |
Prostate (men) | > 30 years | 20 | ||||
Pancreas | > 30 years | 5 | ||||
BRCA1 | 17q | Breast (women) | > 18 years | 60–90 | 1 in 1000 | 62 years |
Ovary | > 20 years | 40–60 |
Breast cancer susceptibility genes and loci with low penetrance
The identification of genes and loci leading to low-penetrance breast cancer susceptibility required an alternative strategy from the linkage studies employed in the discovery of genes like BRCA1 and BRCA2 because, by their nature, they do not give rise to the large multiple-case families required for this. Therefore, other methodologies have been utilised in the discovery of these loci. In the past, this has usually been through association studies which have investigated candidate genes related to the biology of normal breast tissue or breast cancer. More recently, the availability of high-resolution linkage disequilibrium maps and comprehensive sets of tagging SNPs that cover most common sequence variants has led to the successful completion of multiple GWASs. The benefits of this study design are that the approach is not biased and does not rely on knowledge of prior function. It also minimises the likelihood of failing to identify important variants in genes which have not previously been studied. Although it has been speculated for some time that a proportion of familial breast cancer risk is due to common low-penetrance loci, these have only recently been identified. In the past, only small numbers of cases and controls were utilised in targeted association studies, but, when these studies were expanded, preliminary associations were usually not confirmed. The recent results in this field are due to large-scale, multinational collaborations which have given rise to thousands of cases and controls, resulting in the statistical power to detect small effects, thereby overcoming the limitations of earlier small studies. 124
By 2008, when we applied for funding, 10 susceptibility loci had been reported for which there was statistically robust evidence. 8–10,28,30,125–129 Five of these loci were in regions that include known protein-encoding genes. The genes are CASP8,30 FGFR2,8,9 TNRC9 (trinucleotide repeat-containing 9),8,10 MAP3K1 and LSP1. 8 Some of these loci also include other protein-encoding genes, and it is possible that the association with breast cancer could be due to these, or even to regions which currently are not known to include biologically relevant sequences.
The SNP at 6q22 was identified through cases and controls from the relatively genetically isolated Ashkenazi Jewish population;125 the RR of breast cancer conferred by this SNP in other populations may differ. The group that has recently identified the breast cancer susceptibility locus at 6q25 has provided evidence that common loci can confer varying risks of breast cancer dependent on the population studied. The group found that the RR of breast cancer in the population of Chinese women studied was 1.36 or 1.59, respectively, for the G>A heterozygous and homozygous variants at this locus. However, in the European population studied the association was weaker and the RRs conferred were 1.11 and 1.35, respectively. 28
Investigations studying the subtypes of breast tumour associated with these susceptibility loci have revealed that certain loci, particularly in FGFR2, TNRC9, 8q24, 2q35 and 5p12, are more commonly associated with oestrogen receptor-positive disease than oestrogen receptor-negative disease. 128 These findings suggest that breast tumours of these two subtypes might have differing aetiologies relating to genetic background, as has already been indicated by findings in breast tumours of BRCA1 and BRCA2 mutation carriers. In 2009, two further susceptibility loci were identified. 130
In 2010, a larger GWAS was published of 582,886 SNPs in 3659 breast cancer cases with a family history of breast cancer and 4897 controls. 131 This validated the existing SNPs and identified five further SNPs, bringing the total to 18 validated SNPs. Turnbull published new ORs and allele frequencies allowing application of these SNPs in clinical practice. 131 The SNPs and the allele frequencies are shown in Table 18.
SNP | Gene/locus | Published risk alleles, RAFs and per-allele ORs for developing breast cancer | Genotype frequencies among the 478 who provided DNA samples | ||||
---|---|---|---|---|---|---|---|
Risk allele | RAF131 | OR131 | No risk alleles (%) | One risk allele (%) | Two risk alleles (%) | ||
rs2981579 | FGFR2 | T | 0.42 | 1.43 | 35 | 50 | 15 |
rs10931936 | CASP8 | C | 0.74 | 0.88 | 8 | 37 | 55 |
rs3803662 | TOX3 | T | 0.26 | 1.3 | 55 | 38 | 7 |
rs889312 | MAP3K | C | 0.28 | 1.22 | 51 | 39 | 10 |
rs13387042 | 2q | A | 0.49 | 1.21 | 25 | 52 | 23 |
rs1011970 | CDKN2A | T | 0.17 | 1.09 | 70 | 28 | 2 |
rs704010 | 10q22 | A | 0.39 | 1.07 | 38 | 48 | 14 |
rs1156287 | COX11 | A | 0.71 | 1.1 | 8 | 36 | 56 |
rs11249433 | NOTCH | C | 0.42 | 1.08 | 35 | 45 | 20 |
rs614367 | 11q13 | T | 0.15 | 1.15 | 72 | 25 | 3 |
rs10995190 | 10q21 | G | 0.85 | 1.16 | 2 | 23 | 75 |
rs4973768 | 3p24 | T | 0.47 | 1.16 | 32 | 48 | 20 |
rs3757318 | ESR1 | A | 0.07 | 1.3 | 89 | 11 | 0 |
rs1562430 | 8q24 | G | 0.42 | 0.85 | 41 | 43 | 16 |
rs8009944 | RAD51L1 | A | 0.75 | 0.88 | 7 | 41 | 52 |
rs909116 | LSP1 | T | 0.53 | 1.17 | 21 | 51 | 28 |
rs9790879 | 5p12 | C | 0.40 | 1.1 | 34 | 51 | 15 |
rs713588 | 10q | A | 0.60 | 0.86 | 16 | 48 | 36 |
Since the Turnbull report,131 a major international initiative, the International Collaborative Oncological Gene-Environment Study (ICOGS),132 was initiated, culminating in multiple reports in Nature Genetics in March 2013. These brought the number of identified validated SNPs to only 77132–134 but boosted the ‘known’ familial component of breast cancer attributed to SNPs to only 14% (see Figure 7). 27 Evidence from the GWAS data nonetheless suggests that other, rarer SNPs may contribute as much as 41% of heritability, leaving only 24% as ‘missing heritability’. 27
The RR of breast cancer conferred by the common low-penetrance loci ranges from 1.04 to 1.43 in European populations (some SNPs are protective, giving ORs of 0.85–0.88, equivalent to an increase in risk for the common allele of 1.13–1.18; see Table 18). The risks are small, but, as the population prevalence of each allele is high, their contribution to the familial risk of breast cancer, in European populations, is relatively large. It has been estimated that if the attributable risks of the loci characterised by Turnbull et al. , and the breast cancer susceptibility variant in CASP8, were combined, they would account for 10% of the familial risk of breast cancer in European populations. It is postulated that there may be up to hundreds of further common low-penetrance breast cancer susceptibility alleles yet to be identified which confer small risks of breast cancer, which are most likely less than those conferred by the variants so far identified. An important and interesting area of ongoing research is in identifying the role of the loci described above in the development of breast cancer. Some work has already been performed in this field to investigate possible pathways by which SNPs in FGFR2 could be causative for breast cancer, but much remains to be learnt regarding the mechanism by which common low-penetrance SNPs lead to cancer formation.
Methods
In our application to NIHR in 2008, we were aware of 10 SNPs that could be assessed. Our plan at that time was to:
-
assess BARD1 (Cys557Ser) variant
-
assess common ATM variants
-
assess five genes from the GWAS
-
assess variant in the type I TGF-ss receptor, TGFBR1*6 A, which may account for ≈5% of all breast cancer
-
add other validated SNPs as they are found.
Frequencies of the variants will be compared with a regional control sample set of 500 women matched for ethnicity and age.
The necessity of identifying a control population was negated by the publication of allele frequencies in the Turnbull data,131 many of which derived from Manchester. Pragmatically, the research plan changed in 2010 to include all of the SNPs identified in the Turnbull et al. study.
We initially proposed analyses in four studies assessing impact of SNPs in different situations, the fourth assessing the predictive value from the other three.
-
We had already validated seven gene variants previously associated with increased breast cancer risk (BARD1, TOX3, FGFR2, MAP3K1, LSP1, CASP8 and 8q) in ≈200 female BRCA1 and ≈200 BRCA2 carriers, confirming increased risk of breast cancer conferred by variants in FGFR2 and TOX3 and the protective effect of the minority allele in CASP8 in BRCA1/BRCA2-positive individuals. 135 We proposed to extend our analysis to include all female carriers (n = 850 at that time) and assess the variants for interactions and modifier effects. New variants were proposed to be added as they were identified. As above, the proposed research was extended to test 925 proven BRCA1 or BRCA2 female carriers for the 18 SNPs identified by Turnbull et al. 131
-
The second proposed analysis was to test the SNPs in 1400 high-risk BRCA1/BRCA2-negative breast cancer cases and compare this with 500 matched control samples. The variants were to be weighted for their individual effects in heterozygous and homozygous states and assessed for interactions with other variants. This analysis was deemed unnecessary as we obtained funding (from the Genesis breast cancer prevention appeal) for obtaining saliva DNA in 10,000 women from our population-based PROCAS study. We were, thus, able to assess the predictive value of SNPs for non-BRCA-related breast cancer.
-
Testing of DNA from 58 breast cancer phenocopies (women who are negative for the family BRCA1/BRCA2 mutation) was, likewise, proposed to be assessed for the variants in a weighted analysis to identify whether or not significantly more of the high-risk alleles are carried by those women to account for their increased risk of breast cancer. DNA from phenocopies was tested for the 18 SNPs identified by Turnbull et al. 131
-
We proposed using data from the first three analyses to develop a weighted score to assess the predictive value of the combined group of validated variants in predicting which women developed breast cancer in our familial screening programme. We proposed using the incident cancers and matching with controls on a 1 : 3 basis randomly selected from our FHC for presence or absence of familial BRCA1/BRCA2 mutation, plus all other currently used risk factors (menarche, parity, age, family history and breast disease). Women were recruited from our FHCs to provide blood samples for DNA analysis or to give permission to use stored DNA. Matching of controls was changed (with statistical advice) to matching for just age and type of mammogram. It was felt that overmatching would not allow for the examination of differences in the other risk factors being assessed. Matching was still kept at a 1 : 3 cases-to-controls basis and tested for the 18 SNPs identified by Turnbull et al. 131
Deoxyribonucleic acid testing
The DNA was extracted from blood or saliva samples provided by women attending the genetic clinics at St Mary’s Hospital or the FHC at Wythenshawe hospital.
BRCA1/BRCA2 testing
Using DNA Sanger sequencing and MLPA analysis, BRCA1 and BRCA2 mutations were identified in women referred with breast or ovarian cancer; relatives of these identified BRCA1/BRCA2 mutation carriers were then also offered targeted screening for the family-specific genetic mutation.
Single nucleotide polymorphism testing
Women were typed for 18 SNPs that have been shown to be associated with breast cancer risk in the general population [FGFR2, CASP8, TOX3, MAP3K, 2q, CDKN2A (cyclin-dependent kinase inhibitor 2A), 10q22, COX11 (COX11 cytochrome c oxidase copper chaperone), NOTCH, 11q13, 10q21, SLC4A7 (solute carrier family 4, sodium bicarbonate cotransporter, member 7), 6q25.1, 8q24, RAD51L1, LSP1, 5p12 and 10q], as risks were identical for two SNPs at the same locus; the nineteenth SNP was dropped. 131 Using the published per SNP ORs and risk allele frequencies (RAFs) from Turnbull et al. 131 (e.g. FGFR2 per-allele OR is 1.43 with RAF of 0.42; see Table 18), we calculated the OR for each of the three SNP genotypes (no risk alleles, one risk allele and two risk alleles), assuming independence. To obtain an overall breast cancer risk score for each woman, we multiplied the ORs for each of her 18 genotypes together. As a subset of three SNPs (TOX3, 2q and 6q25.1) has now been validated to be associated with increased risk of breast cancer in BRCA1 mutation carriers,136 and another subset of nine SNPs (FGFR2, TOX3, MAP3K, 2q, 1p11.2, SLC4A7, 6q25.1, LSP1 and 5p12) in BRCA2 mutation carriers,136,137 we repeated the analysis using only these SNPs. Finally, we calculated an alternative overall breast cancer risk score based on a combination of SNPs validated to increase risk in BRCA1/BRCA2 mutation carriers and SNPs not yet validated in BRCA1/BRCA2 mutation carriers, that is, all of the original 18 breast cancer susceptibility SNPs, except for those that have been shown to have no association with breast cancer risk in BRCA1/BRCA2 mutation carriers.
Statistical analysis
Age at the development of breast cancer (time from date of birth to the date of diagnosis in cases) or from date of birth to the date of last follow-up (if not a case) was analysed using survival analysis with the censor variable set at 1 for breast cancer cases and 0 otherwise. Cumulative hazard curves were calculated using the Nelson–Aalen estimator136 and the Cox proportional hazards137 model was used to assess the relationship between breast cancer risk and overall breast cancer risk score (which was split into quintiles and entered into the model as an ordinal categorical variable) and calculate the HRs. The proportional hazards assumption was assessed graphically by plotting –ln(–ln(survival)) versus ln(time) for each of the five risk groups and checking to see that the curves are parallel.
Methods: study 1 – BRCA1/BRCA2
Families with individuals with breast and/or ovarian cancer have been screened for mutations in BRCA1/BRCA2 since 1996 in the Manchester region in north-west England, encompassing ≈5 million people. Women with a family history of breast/ovarian cancer who attend specialist genetic clinics in these two regions have a detailed three-generation family tree constructed. The date the first family member was referred to the genetic service was considered as the family ascertainment date. If a BRCA1/BRCA2 mutation is identified, further attempts are made to ensure that all individuals relevant to discussions on risk are represented on the family tree. All cases of breast/abdominal cancers are confirmed by means of hospital/pathology records, regional Cancer Registries (from 1960) or death certification. Once a family-specific pathogenic BRCA1/BRCA2 mutation is identified, predictive testing is offered to all blood relatives.
The details of all tested relatives and first-degree untested female relatives were entered onto a FileMaker Pro 7 database. The initial individual in whom a mutation was identified was designated the ‘index’ case, with all other individuals being classified according to their position in the pedigree compared with a proven mutation carrier. All women reaching 20 years were entered on the database even if they were untested for a mutation. The exception was for mothers of a mutation carrier when it was clear that the mutation was paternally inherited (i.e. there was no maternal family history but a very convincing paternal history of breast/ovarian cancer). A total of 807 index cases were studied. Date of birth and date of last follow-up, breast cancer status, ovarian cancer status, dates of diagnoses and date of death (if applicable), gene mutation identified in the family, the individuals relationship to a known mutation carrier and their mutation status were entered.
All identified mutation carriers from whom DNA was available were assessed for breast cancer incidence. Women were censored at risk-reducing mastectomy last follow-up/death or at the event of breast cancer, whichever was the soonest. The predictive ability of the Cox models was assessed using Harrell’s C concordance statistic. 138,139 The type I error (α) was set at 5%. Thus, this analysis assessed the impact of the multiplicative SNP genotypes on penetrance of breast cancer. We estimate that about half of our patient samples have been used in analysis of SNPs in CIMBA (Consortium of Investigators of Modifiers of BRCA1/BRCA2). Ethics approval for the study was through the North Manchester Research Ethics Committee (08/H1006/77) and the University of Manchester Ethics Committee (08229).
Methods: study 2 – PROCAS (UKCRN-ID 8080)
A subset of the PROCAS population was invited to attend drop-in days and provide a saliva sample for DNA extraction. Women giving their consent were provided with an Oragene kit (DNA Genotek Inc., Ottawa, ON, Canada) to collect a saliva sample. DNA was extracted in accordance with the manufacturer’s protocols and 18 known validated breast cancer SNPs (only one for each genetic locus) associated with breast cancer were typed (see Table 18). Genotyping was performed as multiplexed assays using the Sequenom® MassARRAY™ iPLEX™ Gold system (Agena Bioscience GmbH, Hamburg, Germany), thereby reducing the costs and time associated with the genetic analysis to sample sets of 384 being analysed and scored in < 5 days. Chips were run on a MALDI-TOF mass spectrometer (Wickham Laboratories Ltd, Gosport, UK) and the mass automatically converted to the allele call. One of the SNPs (rs10931936) was genotyped using a TaqMan® (ThermoFisher Scientific, Waltham, MA, USA) SNP genotyping allelic discrimination assay (C___2960444_10). Reactions were performed at standard conditions and analysed using SDS software (The WERCS, Latham, NY, USA). Quality checks including duplicate samples, water and positive controls were undertaken. Using the published estimates of per-allele ORs for breast cancer from the most recent GWAS131 for the majority of the SNPs (all except rs713588), we calculated the RR of developing breast cancer for each genotype. For example, for FGFR2, the published RAF of the risk allele T is 0.42 and the per-allele OR is 1.43. The frequency of genotypes TT, TC and CC is, therefore, 0.17 (0.422), 0.49 (2 × 0.42 × 0.58) and 0.34 (0.582), respectively, the average population risk relative to genotype CC is 1.39 (0.17 × 1.432 + 0.49 × 1.43 + 0.34 × 1.00) and the risk relative to the general population for each of the three genotypes is 1.47 (1.432/1.39), 1.03 (1.43/1.39) and 0.72 (1/1.39), respectively. We then calculated a combined risk score for each woman, based on her SNPs, by multiplying her individual risk ratios together.
Methods: study 3 – phenocopies in BRCA1/BRCA2
Families including individuals with breast and/or ovarian cancer have been screened for mutations in BRCA1/BRCA2 since 1996 in the overlapping regions of Manchester and Birmingham in mid/north-west England, encompassing ≈10 million people. The methods used were as in study 1 but only women with breast cancer who tested negative for the family mutation were the main point of the analysis. The resultant combined series is referred to as the M6-ICE (Inherited Cancer in England) study.
Women with breast or ovarian cancer who tested negative for the family mutation were defined as phenocopies. In 90% of cases, at least two independent blood draws from every phenocopy have been genotyped to firmly establish negative mutation status. Only first-degree relatives of proven pathogenic mutation carriers were included in the study.
An analysis was undertaken assessing prospective breast cancer risk in individuals testing negative for the family mutation using date of ascertainment of the family by the genetic service as the start date. Standard incidence ratios were derived using age- and year-specific data from the population-based NWCIS, as previously described. 44 Follow-up was censored at 1 July 2011 or date of breast cancer, date of death or date of bilateral risk reducing breast surgery, whichever was the earliest. Person-years at risk analyses were performed to assess expected cancers in the general female population using data from the NWCIS. O : E ratios were assessed for statistical significance using the common method from Clayton and Hills based on the Poisson assumption. 100 A subset of women testing negative for the family mutation was part of an assessment programme, FH-Risk, for which we had ethics approval to check details against the NWCIS for cancer incidence. This was carried out in September 2011. A final analysis was carried out using date of testing of unaffected first-degree relatives as the start date.
An assessment of the strength of family history of breast cancer was also included by summating the BRCA2 element of the Manchester scoring system for each affected family member. 49 This system scores breast cancers in the direct lineage based on age at diagnosis, giving higher scores for earlier age at diagnosis. An assessment was also made of close breast cancer family history (first-degree relative and second-degree relative) using diagnosis < 40 years in a first-degree relative, < 50 years in at least two relatives (including a first-degree relative) or at least three relatives (including a first-degree relative) diagnosed at < 60 years as a surrogate for increased ‘breast cancerness’.
Deoxyribonucleic acid testing for single nucleotide polymorphisms
Methods were as per Methods: study 2 – PROCAS (UKCRN-ID 8080) using the SNP18 score.
Methods: study 4 – FH-Risk case–control
The cases and controls were matched on a 1 : 3 basis with age at assessable mammogram being the matching criterion. The controls did not have cancer at last follow-up and cases had to have been diagnosed after an assessable mammogram or have an assessable mammogram at date of cancer diagnosis and no cancer in the contralateral breast. The controls were also matched for type of mammogram, either digital or analogue. The questionnaire data were updated to include clothes size and alcohol intake as well as ensuring that data were held on age at menarche and age at first full-term pregnancy. The TC model was used to incorporate an adjustment for MD using the VAS and two digital methods (Volpara and Quantra). For breast cancer cases with no assessable mammogram but in whom blood DNA was available, controls were also recruited for the DNA SNP study.
Recruitment was between 1987 and 2012. Follow-up was until 2013, median 8.4 years in the present study. The primary end point was diagnosis of invasive breast cancer or DCIS (ICD-1065 codes C-50 and D-05.1). The cases that accrued were identified by BRCA1-negative controls who attended the clinic but were not diagnosed with breast cancer to last follow-up and were matched approximately 3 : 1 to the non-BRCA1 cases on age at breast cancer diagnosis or last mammogram prior to diagnosis (cases), with age at last follow-up or mammogram (controls). As many BRCA1-confirmed participants were genotyped as was possible. A 18 SNP polygenic score was assessed for predictive performance. This was calculated relative to the population, based on the RAFs in Table 18, so that the mean risk for each SNP in the population was 1.0. The OR estimates were taken from Turnbull et al. 131 when not available. The TC breast cancer risk model was used to calculate expected risk from breast cancer on the basis of family histories of the disease and other phenotypic factors, as described in the analysis of the complete family history cohort. 57
Analysis was carried out separately for BRCA1 and non-BRCA1 carriers, and when combined. Hardy–Weinberg equilibrium for each SNP was tested by assessing the observed number of homozygotes against the expected number using a binomial distribution. Age and SNP score distributions were summarised by percentiles. The numbers of failed assays were reported by SNP, as well as the distribution for individual samples. Assay failures were ignored in the SNP score by imputing a population RR of 1.0 when they occurred. Analysis of baseline risk factors (age) and time to diagnosis was undertaken by assuming that the controls were representative of the complete cohort controls. A plot of observed versus expected RR from the SNP score was obtained using a binary regression (normal) kernel smoother, with bandwidth set by hand. Unconditional logistic regression models were used to assess the performance of the SNP score by fitting a model with a single covariate for the log-RR, 10-year absolute risk from age, and 10-year absolute risk from age and the SNP score. The main measure of prediction power was the likelihood-ratio χ2 (degrees of freedom = 1) statistic from the model. The area under the receiver operating characteristic curve was used as a secondary measure of discrimination. Kaplan–Meier survival estimates of risk groups were obtained, weighting by the sampling fraction of controls in the cohort. The SNP18 and TC RRs were compared using a scatterplot.
Results
Study 1: BRCA1/BRCA2
Text in this section has been reused with permission from Ingham SL, Warwick J, Byers H, Lalloo F, Newman WG, Evans DGR. Is multiple SNP testing in BRCA2 and BRCA1 female carriers ready for use in clinical practice? Results from a large Genetic Centre in the UK. Clinical Genetics 2013, 84:1, 37–42, © 2012 John Wiley & Sons A/S. Published by John Wiley & Sons Ltd. 140
A total of 480 and 445 women with a confirmed pathogenic mutation in BRCA1 and BRCA2, respectively, were identified from the records of the Department of Genetic Medicine (Table 19). Of the 480 BRCA1 mutation carriers, 462 were included in the analyses (18 failed DNA samples); 58% (268/462) to date have developed breast cancer. Estimates of the HRs (each quintile relative to the highest risk group, quintile 1) and the corresponding 95% CIs are also shown (Table 20). There was no difference in age at the development of breast cancer between the risk groups (overall breast cancer risk score split into quintiles) based on the 18 SNPs (p = 0.25; see Table 20). There was little separation between the cumulative hazard curves (Figure 9). Basing our risk score on only the three SNPs that have been validated in BRCA1 carriers, or the three validated SNPs, plus six that have not yet been validated in BRCA1 mutation carriers, did not lead to better discrimination; there was no difference in age at the development of breast cancer between the risk groups (p = 0.12 and p = 0.92, respectively; see Table 20) and there was no distinct separation between the corresponding cumulative hazard curves (Figure 10). The Harrell’s C-statistic (the probability that when one of two subjects is observed to develop breast cancer before other one that develops breast cancer second has the lower of the predicted HRs) for the 18, three and nine SNPs based overall breast cancer risk scores was 0.54, 0.51 and 0.48, respectively (i.e. the models have poor predictive ability).
Gene | Carrier | Breast cancer | Number of carriers |
---|---|---|---|
BRCA1 | Index | Yes | 211 |
No | 44 | ||
First-degree relative | Yes | 52 | |
No | 125 | ||
Second-degree relative | Yes | 7 | |
No | 11 | ||
Third-degree relative | Yes | 0 | |
No | 7 | ||
Fourth-degree relative | Yes | 0 | |
No | 1 | ||
Fifth-degree relative | Yes | 1 | |
No | 1 | ||
BRCA2 | Index | Yes | 200 |
No | 33 | ||
First-degree relative | Yes | 69 | |
No | 118 | ||
Second-degree relative | Yes | 5 | |
No | 10 | ||
Third-degree relative | Yes | 8 | |
No | 2 | ||
Fourth-degree relative | Yes | 0 | |
No | 0 | ||
Fifth-degree relative | Yes | 0 | |
No | 0 |
Overall breast cancer risk score | Category | Median age (years) at the development of breast cancer (95% CI) | HR (95% CI) | p-value | Harrell’s C |
---|---|---|---|---|---|
18 SNPsa | Quintile 1 (highest risk) | 47.01 (44.4 to 49.98) | 1.00 (1.00) | 0.25 | 0.54 |
Quintile 2 | 46.35 (43.21 to 49.50) | 1.09 (0.75 to 1.59) | |||
Quintile 3 | 51.34 (43.89 to 63.94) | 0.85 (0.56 to 1.27) | |||
Quintile 4 | 44.33 (40.89 to 50.01) | 1.24 (0.85 to 1.80) | |||
Quintile 5 (lowest risk) | 45.46 (43.06 to 47.59) | 1.20 (0.82 to 1.75) | |||
3 SNPsb | Quintile 1 (highest risk) | 44.64 (42.35 to 47.90) | 1.00 (1.00) | 0.12 | 0.51 |
Quintile 2 | 44.50 (43.04 to 48.62) | 0.97 (0.69 to 1.36) | |||
Quintile 3 | 51.10 (41.67 to 62.50) | 0.70 (0.41 to 1.21) | |||
Quintile 4 | 48.42 (45.46 to 52.06) | 0.74 (0.54 to 1.03) | |||
Quintile 5 | 45.91 (39.38 to 54.47) | 0.94 (0.60 to 1.47) | |||
9 SNPsc | Quintile 1(highest risk) | 46.60 (44.28 to 48.62) | 1.00 (1.00) | 0.92 | 0.48 |
Quintile 2 | 45.51 (43.06 to 50.18) | 1.12 (0.77 to 1.61) | |||
Quintile 3 | 45.85 (44.05 to 54.24) | 0.83 (0.56 to 1.23) | |||
Quintile 4 | 44.31 (41.08 to 49.50) | 1.11 (0.76 to 1.62) | |||
Quintile 5 | 48.42 (43.23 to 51.90) | 0.99 (0.68 to 1.45) |
FIGURE 9.
Cumulative hazard of developing breast cancer in 480 BRCA1 mutation carriers, by risk group (overall breast cancer risk score split into quintiles), based on the 18 SNPs from Turnbull et al. 131 Source: reproduced with permission from Ingham SL, Warwick J, Byers H, Lalloo F, Newman WG, Evans DGR. Is multiple SNP testing in BRCA2 and BRCA1 female carriers ready for use in clinical practice? Results from a large Genetic Centre in the UK. Clinical Genetics 2013, 84:1, 37–42, © 2012 John Wiley & Sons A/S. Published by John Wiley & Sons Ltd. 140
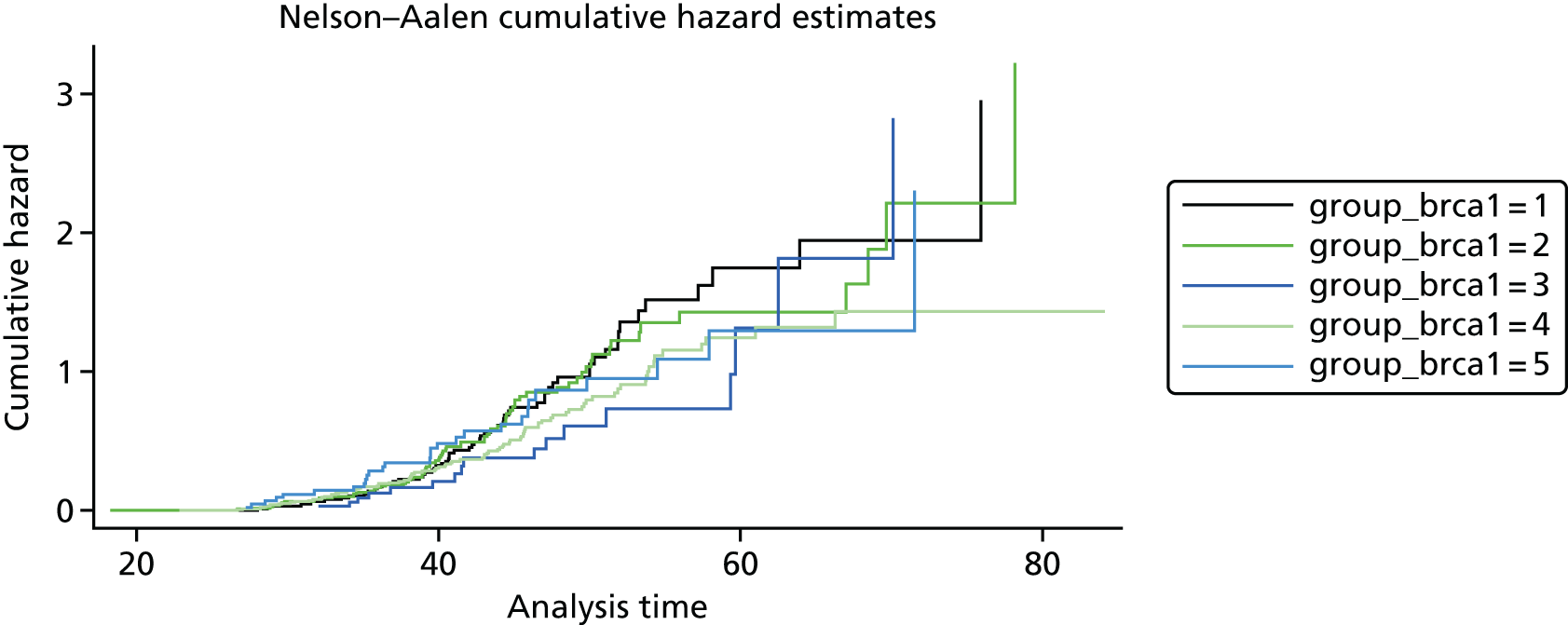
FIGURE 10.
Cumulative hazard of developing breast cancer in 480 BRCA1 mutation carriers, by risk group (overall breast cancer risk score split into quintiles), based on the three validated SNPs from Antoniou et al. 136 and the six SNPs from Turnbull et al. 131 that have not yet been validated in BRCA1 mutation carriers. Source: reproduced with permission from Ingham SL, Warwick J, Byers H, Lalloo F, Newman WG, Evans DGR. Is multiple SNP testing in BRCA2 and BRCA1 female carriers ready for use in clinical practice? Results from a large Genetic Centre in the UK. Clinical Genetics 2013, 84:1, 37–42, © 2012 John Wiley & Sons A/S. Published by John Wiley & Sons Ltd. 140

Of the 445 BRCA2 carriers, 280 had developed breast cancer by the cut-off date of 1 December 2010 for follow-up and data extraction. The HRs (each quintile relative to quintile 1, the highest-risk group) and corresponding 95% CIs are given in Table 21. There was a significant difference in age at the development of breast cancer between the risk groups (overall breast cancer risk score split into quintiles) based on the 18 SNPs (p < 0.001; see Table 21) and clear trend for reducing hazard with reducing overall breast cancer risk score (increasing quintile). The cumulative hazard curves (Figure 11) illustrate that there was clear differentiation between the risk groups. It should be noted, however, that breast cancer cases arise across a wide range of ages in each quintile (as indicated by the position of the steps in the cumulative hazard curves) and that the predictive ability of the model was, therefore, only modest (Harrell’s C = 0.59). The alternative breast cancer risk scores (based on 9, 5 and 15 SNPs, respectively) also lead to risk groups with significant differences in age at the development of breast cancer (p < 0.001 for all). The cumulative hazard curves (Figure 12) suggest that the overall breast cancer risk scores based on 15 SNPs might be slightly better than those based on the original 18 SNPs as a measure of breast cancer risk in BRCA2 mutation carriers, but Harrell’s C-statistic for this model was also 0.59, suggesting that in terms of predictive ability it offers no improvement.
Overall breast cancer risk score | Category | Median age (years) at the development of breast cancer (95% CI) | HR | 95% CI | p-value | Harrell’s C |
---|---|---|---|---|---|---|
18 SNPsa | Quintile 1 (highest risk) | 43.31 (39.67 to 49.64) | 1.00 | 1.00 | p < 0.001 | 0.59 |
Quintile 2 | 46.78 (43.65 to 48.11) | 0.97 | 0.68 to 1.38 | |||
Quintile 3 | 49.66 (45.26 to 53.79) | 0.66 | 0.46 to 0.95 | |||
Quintile 4 | 49.37 (46.22 to 57.08) | 0.63 | 0.44 to 0.91 | |||
Quintile 5 (lowest risk) | 52.46 (49.1 to 58.60) | 0.47 | 0.33 to 0.69 | |||
9 SNPsb | Quintile 1 (highest risk) | 45.16 (42.23 to 49.26) | 1.00 | 1.00 | p < 0.001 | 0.58 |
Quintile 2 | 46.55 (42.53 to 48.93) | 1.01 | 0.71 to 1.45 | |||
Quintile 3 | 46.24 (44.16 to 48.92) | 0.94 | 0.67 to 1.33 | |||
Quintile 4 | 53.87 (48.11 to 60.90) | 0.51 | 0.35 to 0.75 | |||
Quintile 5 (lowest risk) | 53.79 (50.11 to 58.6) | 0.49 | 0.33 to 0.71 | |||
5 SNPsc | Quintile 1 (highest risk) | 46.27 (42.78 to 49.65) | 1.00 | 1.00 | p < 0.001 | 0.57 |
Quintile 2 | 46.67 (41.28 to 49.64) | 1.00 | 0.70 to 1.42 | |||
Quintile 3 | 46.78 (42.88 to 48.93) | 1.01 | 0.71 to 1.43 | |||
Quintile 4 | 52.36 (49.11 to 58.51) | 0.56 | 0.38 to 0.81 | |||
Quintile 5 (lowest risk) | 52.08 (46.28 to 59.06) | 0.57 | 0.38 to 0.84 | |||
15 SNPsd | Quintile 1 (highest risk) | 45.16 (41.28 to 49.26) | 1.00 | 1.00 | p < 0.001 | 0.59 |
Quintile 2 | 45.26 (42.78 to 47.16) | 1.05 | 0.74 to 1.49 | |||
Quintile 3 | 48.19 (44.3 to 51.99) | 0.75 | 0.52 to 1.07 | |||
Quintile 4 | 53.79 (48.78 to 59.06) | 0.52 | 0.36 to 0.75 | |||
Quintile 5 (lowest risk) | 54.53 (49.11 to 58.51) | 0.48 | 0.33 to 0.70 |
FIGURE 11.
Cumulative hazard of developing breast cancer in 445 BRCA2 mutation carriers by risk group (overall breast cancer risk score split into quintiles) based on the 18 SNPs from Turnbull et al. 131 Source: reproduced with permission from Ingham SL, Warwick J, Byers H, Lalloo F, Newman WG, Evans DGR. Is multiple SNP testing in BRCA2 and BRCA1 female carriers ready for use in clinical practice? Results from a large Genetic Centre in the UK. Clinical Genetics 2013, 84:1, 37–42, © 2012 John Wiley & Sons A/S. Published by John Wiley & Sons Ltd. 140

FIGURE 12.
Cumulative hazard of developing breast cancer in 445 BRCA2 mutation carriers, by risk group (overall breast cancer risk score split into quintiles), based on the nine validated SNPs from Antoniou et al. 136 and the six SNPs from Turnbull et al. 131 that have not yet been validated in BRCA2 mutation carriers. Source: reproduced with permission from Ingham SL, Warwick J, Byers H, Lalloo F, Newman WG, Evans DGR. Is multiple SNP testing in BRCA2 and BRCA1 female carriers ready for use in clinical practice? Results from a large Genetic Centre in the UK. Clinical Genetics 2013, 84:1, 37–42, © 2012 John Wiley & Sons A/S. Published by John Wiley & Sons Ltd. 140

Study 2: PROCAS population testing
As part of the PROCAS study, women were invited to provide a saliva DNA sample for testing of the 18 SNPs. A total of 9958 saliva samples have now been obtained, including samples from 398 women with breast cancer after recruitment and 454 with prevalent breast cancers. Genotyping has been carried out on 6954 women. Initial analysis was performed on the first 993 cases to assess interaction between SNP score and TC risk. The overall risk score for each woman (her estimated RR of developing breast cancer compared with that of the general population) was obtained by multiplying her individual per-allele RRs together. The interquartile range (IQR) for this SNP-based estimate of breast cancer RR was 0.68–1.29, whereas for the same 993 women the TC estimate of breast cancer risk, relative to the population average of 2.7%, was 0.84–1.45. The IQR of the corresponding TC estimates of absolute breast cancer risk was 2.28–3.93%. If it was assumed that every woman has a 10-year absolute risk of developing breast cancer of 2.7% (i.e. roughly the population average), adding the SNPs information gave modified individual risk estimates with an IQR of 1.78–3.48%. Using the individual risk estimates from the TC model instead of the population mean to represent the underlying risk led to modified individual risk estimates with an IQR of 1.83–4.41. The distribution of these SNP-based risk estimates together with those obtained from the TC model are shown in Figure 13. The addition of SNPs to the TC model broadened the distribution of risk estimates so that fewer individuals are in the average-risk category (Figure 14). Furthermore, greater numbers were assigned to both the highest- and lowest-risk categories, suggesting that the incorporation of SNPs information into the TC model may lead to better discrimination. Overall, in the 993 who provided a DNA sample, there was no correlation between the SNP-based risk estimates and those from the TC model (ρ = 0.02), between the SNP-based risk estimates and MD (ρ = –0.09), or between the risk estimates from the TC model and MD (ρ = 0.07). A scatterplot showing lack of correlation is shown in Figure 15. Overlap of highest 10% risk on 18 SNP, VAS and TC is shown in Figure 16. This shows only a random association, with only 1 out of 993 being high risk on all three, as would be expected by chance.
FIGURE 13.
Predicted breast cancer risk from the TC model (based on classic breast cancer risk factors), and predicted breast cancer risk based on the SNPs alone (assuming that each woman has average breast cancer risk) for the 993 women who provided DNA samples.

FIGURE 14.
Predicted breast cancer risk from the TC model (based on classic breast cancer risk factors), and predicted breast cancer risk from the TC model (crudely adjusted to take account of individual SNPs) for the 993 women who provided DNA samples.
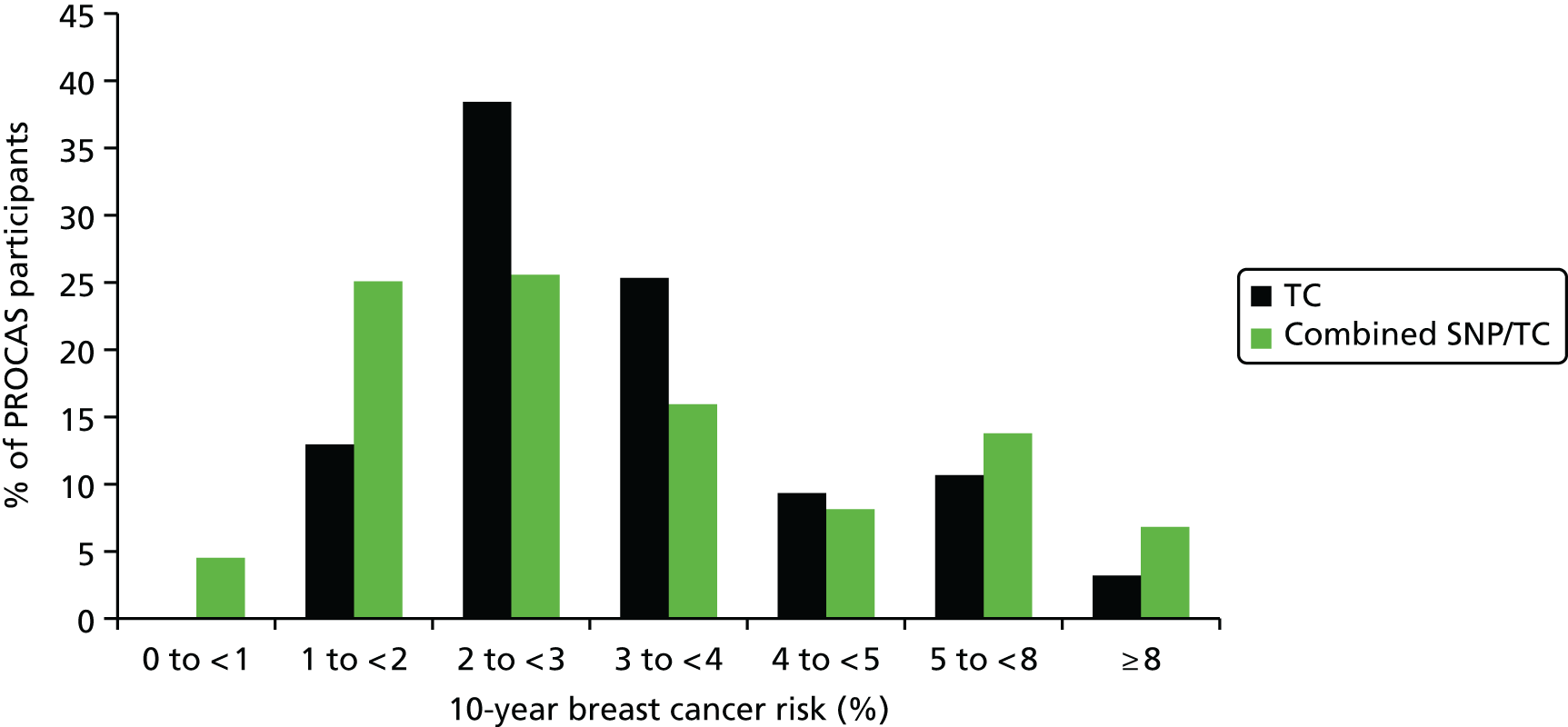
FIGURE 15.
Scatterplot showing correlation between TC and SNP RR.
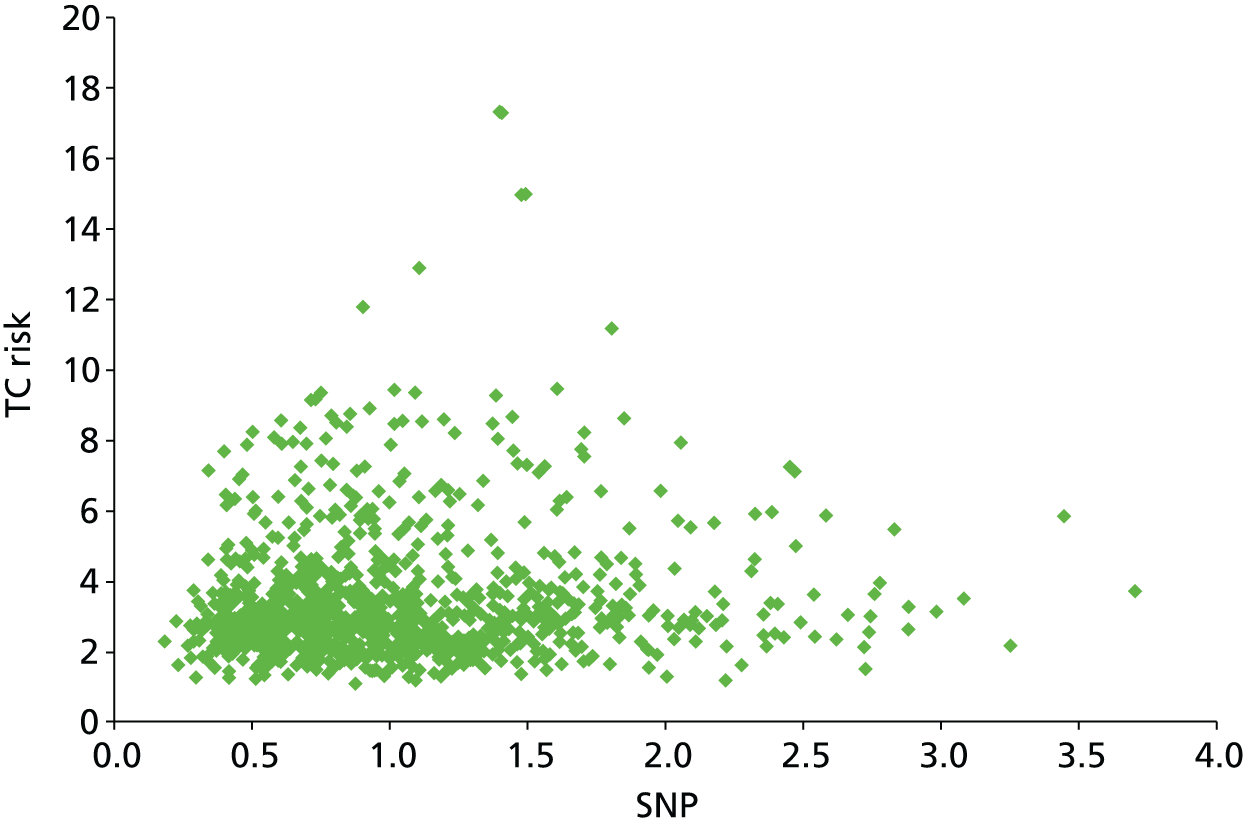
FIGURE 16.
Venn diagram of overlap of highest 10% risk from 993 women with SNP, TC score and VAS density.
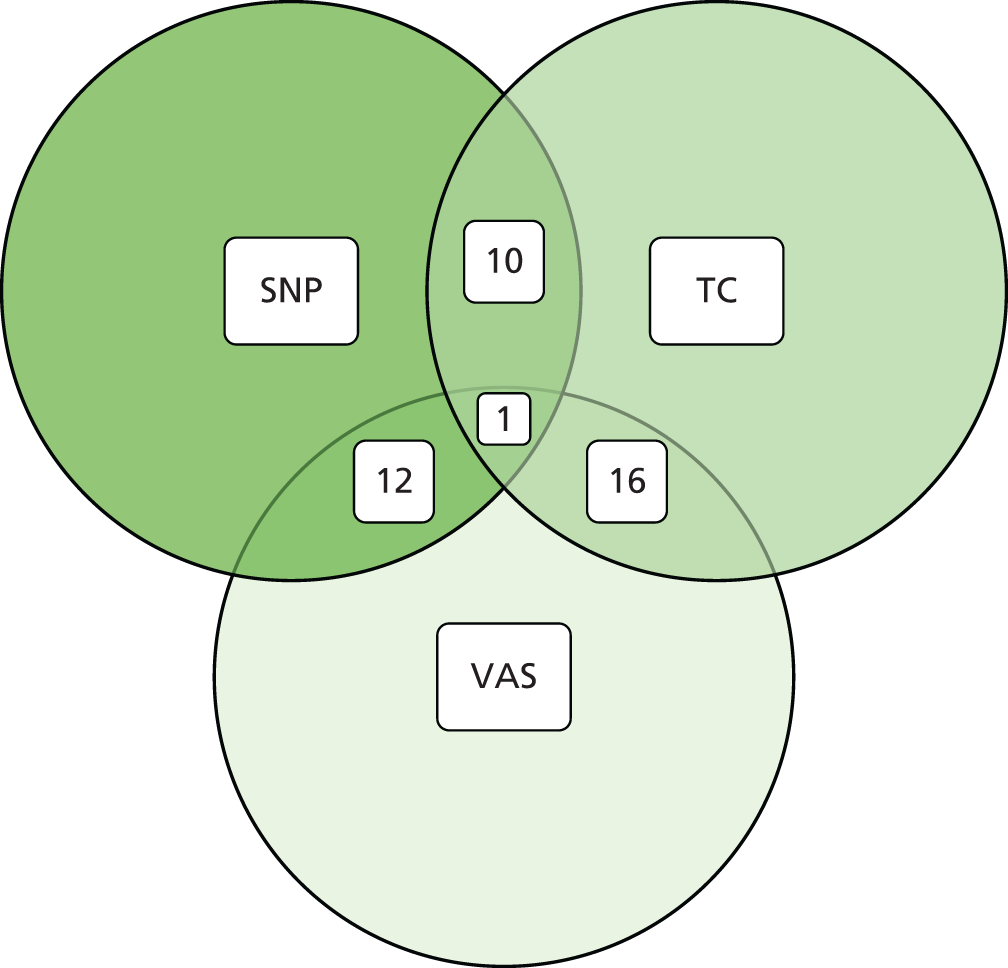
We have genotyped 6954 women from PROCAS, including 673 with breast cancer. There was a clear increase in overall predicted RR from the PRS in those with breast cancer. Median (mean) RR was 1.00 (1.15), compared with 0.90 (1.02) for those without. The distribution was also shifted to the right in all categories (Figure 17).
FIGURE 17.
Ten-year risk in those with breast cancer compared with those without based on PRS from SNP18. BC, breast cancer.
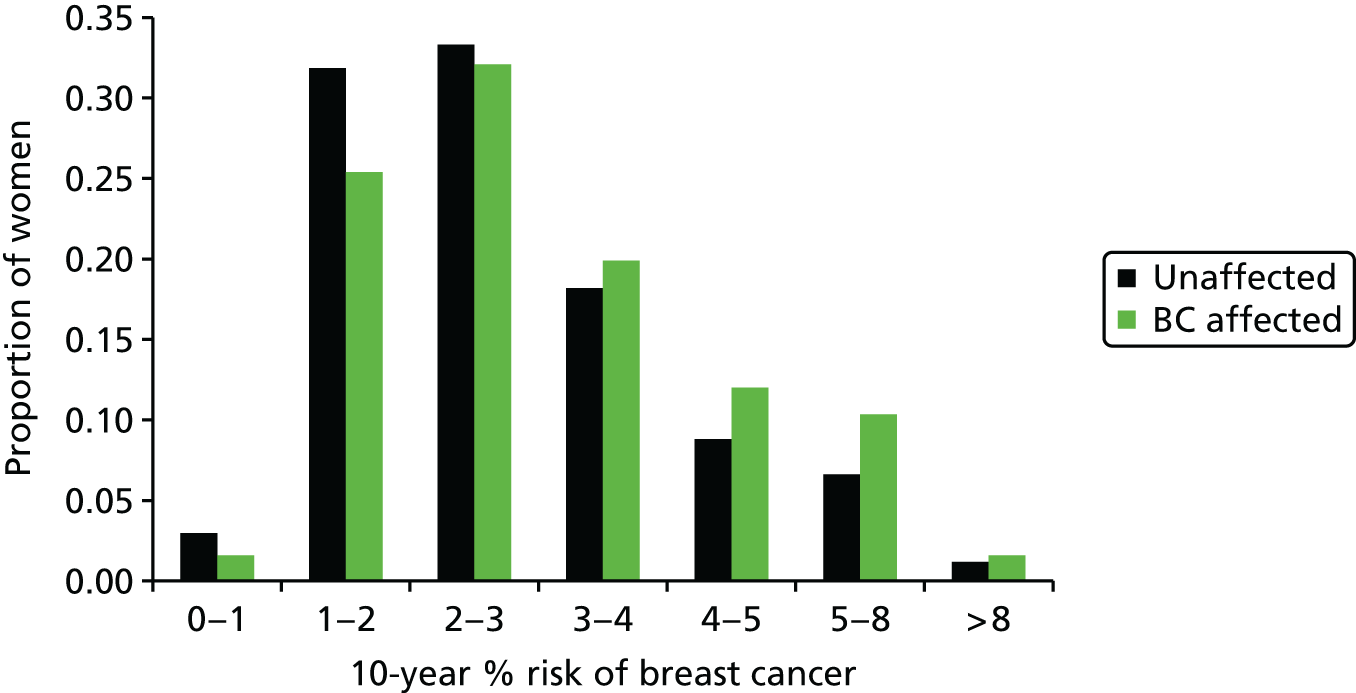
We next investigated how a polygenic SNP score based on these SNPs would compare with classical risk factors, and how much information it might add to risk assessment.
Our analysis was based on simulated SNP scores from 100,000 women with population allele frequencies for the 67 SNPs identified,27 and treating them as independent so that a combined risk score can be obtained by multiplying their RRs; and the TC risk model risk predictions from the first 10,000 women enrolled to the PROCAS study (predicting risk of breast cancer at screening) in Manchester, UK. 141 The outcome measure was the 10-year RR of developing breast cancer.
Histograms are shown in Figure 18 for the TC model, the SNP score using 18 genes previously published141 but with risks updated from Latif et al. ,135 the full set of 67 SNPs, and a combined TC + SNP67 distribution assuming independence. Initial evaluations have shown that the TC model and SNP18 scores appear to be independent. 141
FIGURE 18.
Predicted 10-year RR and corresponding absolute 10-year risk for a woman aged 50 years using classical factors (TC), the 18 SNPs in Turnbull et al. ,131 the 67 ICOGS SNPs (SNP67), and both combined (TC + SNP67). The phenotypic markers are from 10,000 women of routine-screening age (46–70 years) in the UK.

Of particular interest is the > 8% 10-year risk group, for whom NICE guidelines in the UK advise offering the preventative use of tamoxifen. 142 The SNP score was less able than the TC model to identify women at high risk (0.02% for SNP18, 0.37% for SNP67 and 0.77% for the TC model). However, adding SNP67 to TC gave a substantial increment to 2.85%, and similar effects were seen in the 5–8% 10-year risk group (1.72%, 4.28%, 6.64% and 8.17%, respectively), in which the number of women identified is much greater. It is also noticeable that the SNPs identified more low-risk women than the TC model, which mainly uses uncommon high-risk phenotypes.
Study 3: phenocopies
We undertook an analysis first to assess the potential increased risk in those testing negative for BRCA1 or BRCA2. This analysis was not part of the original data analysis plan, but fed into the SNP analysis that was funded. Among 809 families with a proven pathogenic mutation (428 BRCA1 or 381 BRCA2), 290 first-degree female relatives with breast cancer have undergone genetic testing following the identification of the family mutation. Forty-nine (17%) first-degree relatives with breast cancer tested negative for the family mutation. Ninety-five breast cancers occurred in first-degree relatives after the family ascertainment date and 21 (22%) of these tested negative. Of those who underwent predictive testing for the family mutation as unaffected individuals but who have subsequently developed breast cancer, 8 out of 42 (19%) have tested negative.
Prospective analysis
In total 279 female first-degree relatives tested negative for the family BRCA1 mutation and 250 tested negative for BRCA2. Two women (BRCA1) who had undergone bilateral risk-reducing breast surgery prior to ascertainment in the genetics service were excluded from the analysis, as were 27 women who had developed breast cancer prior to family ascertainment and one woman who died prior to family ascertainment (BRCA2). Thus, 17 breast cancers from BRCA1 families and 13 breast cancers from BRCA2 families were excluded from the prospective analysis. Following family ascertainment, seven (2.5%) cases of breast cancer occurred in the remaining 262 women testing negative for the familial BRCA1 mutation and 14 (5.9%) occurred in 237 women testing negative for their familial BRCA2 mutation.
Using a pragmatic recent date of follow-up (30 June 2011), assuming notification of breast cancers, the rates were 2.17 per 1000 (in 3217 years) in BRCA1 non-carriers, with a median age at ascertainment of 36.4 years, and 5.3 per 1000 (in 2634 years) in BRCA2 non-carriers, with a median age at ascertainment of 35.9 years (see Table 2). Using a person-years at risk analysis, 3.95 cancers would have been expected in the cohort of BRCA1 women and 3.06 in BRCA2. The O : E ratio was, therefore, 1.77 (95% CI 0.71 to 3.65) for the BRCA1 group and 4.57 (95% CI 2.50 to 7.67) for the BRCA2 group. The difference between the observed and expected values for BRCA2 was statistically significant: p < 0.0001. This analysis does not allow for any testing bias of those developing breast cancer. We are aware of 15 breast cancers in untested first-degree relatives, of which two or three (using the phenocopy rate of 17%) would be expected to have tested negative. However, we cannot be sure that we were informed of breast cancers in relatives who were not known to the service, perhaps because they were estranged or were living abroad.
Sufficient DNA was available to test 36 first-degree relative phenocopies for the 18 validated SNPs. Testing was also carried out on 445 BRCA2 mutation carriers (280 affected and 165 unaffected with breast cancer) and 462 BRCA1 carriers, 185 family history breast cancers testing negative for BRCA1/BRCA2 from full mutation testing and 421 population female controls from the NHSBSP in the PROCAS trial, as described above. The mean RR for the 18 SNPs was 1.27 for 22 first-degree relative BRCA2 phenocopies (range 0.82–3.17; median 1.18) and 1.31 (range 0.82–3.17) for the 18 first-degree relatives diagnosed aged < 60 years. The BRCA1 phenocopy RR was 1.24 (range 0.41–2.63) for 14 first-degree relatives and 1.13 for 12 aged < 60 years at diagnosis. In the 280 affected BRCA2 mutation carriers, the mean RR was 1.165 (range 0.29–5.09; median 1.01), with a RR of 0.993 (0.33–3.36; median 0.86) for the 165 unaffected carriers. In the 268 affected BRCA1 mutation carriers, the mean RR was 1.07 (range 0.24–4.35; median 0.93), with a RR of 1.11 (0.265–3.79; median 0.956) for the 194 unaffected individuals. Among 185 family history-positive breast cancers without BRCA1/BRCA2 mutations, the RR was 1.24 (range 0.37–4.62; median 1.10). The mean score in a series of 421 control samples from the general female population was 1.04 (range 0.24–4.3; median 0.93).
Study 4: FH-Risk
Samples of DNA have been obtained from 426 women affected with breast cancer and 1275 controls. Direct consent for FH-Risk was obtained for 320 cases and 980 controls (Figure 19). A further 38 who had been affected with breast cancer and had subsequently died having previously supplied a blood sample were also included. In addition, a further 68 BRCA1/BRCA2 mutation carriers and 295 who had tested negative for a family mutation as part of study 1 were included, as they were part of the FHC. DNA was tested in 1701 individuals and 18SNP RR scores were generated as previously described. In view of the results from study 1, 67 BRCA1 mutation breast cancer-affected carriers and 189 unaffected BRCA1 carriers were excluded from further evaluation. The distribution of RRs for unaffected and affected controls is shown in Figure 20. Although overall distribution of RR was very similar, a significantly higher proportion of cases, 33 out of 359 (9.2%), had RRs > 2, compared with controls: 56 out of 1079 (5.2%) (p = 0.01).
FIGURE 19.
Uptake to the FH-Risk study over a 2-year period. (a) Cumulative uptake to FH-Risk – cases; and (b) cumulative uptake to FH-Risk – controls.


FIGURE 20.
Distribution of RRs from 18SNP scores in FH-Risk cases and controls. BC, breast cancer.

Prospective analysis
A second analysis was carried out only using prospective breast cancers and excluding cancers detected on first screen (prevalent).
In this analysis, of 1415 women genotyped who attended the FHC and were breast cancer free at baseline, 199 were BRCA1 carriers. There were 306 cases, including 45 BRCA1 carriers.
The median year of enrolment for cases was 1999 (IQR 1995–2004); it was 2002 (IQR 1996–2007) for controls. The median age at matching was 50 years in cases and controls.
Single nucleotide polymorphism 18 score
-
Table 22 shows the SNPs assayed and their failure rates. A total of 1148 (94.4%) of the non-BRCA1 samples were successful for all 18 SNPS; the numbers with 1, 2, 3, 4 and 5+ failures were, respectively, 41 (3.4%), 17 (1.4%), 5 (0.4%), 3 (0.2%) and 2 (0.2%), with a maximum of 6/18. The tests for Hardy–Weinberg equilibrium for each SNP are in Table 22.
-
Tables 23 and 24 show quantiles of the SNP score and age at baseline in cases and controls by BRCA1 test result.
-
Table 25 shows the results from logistic regression fits of the log SNP score, log 10-year absolute risk from age at recruitment, and log 10-year absolute risk from age at recruitment and the SNP score. It shows that adding the SNP score to age rates was useful for non-BRCA1 participants, but that neither age at baseline nor the SNP score was predictive for BRCA1 carriers.
-
Graphs are used to show the performance of the SNP score and age at recruitment in Figure 21.
-
Excluding BRCA1 cases and controls, the predicted risk from the SNP score was 82% (95% CI 45% to 121%) of expected. See also the histogram in Figure 21a and the binary regression plot in Figure 22.
SNP | Locus | Allele | RAF | RR | Fail | Ph control | Ph case | O/E control | O/E case |
---|---|---|---|---|---|---|---|---|---|
rs614367 | 11q13 | T | 15 | 1.21 | 1 (< 1%) | 0.589 | 0.953 | 0.99 | 1.00 |
rs704010 | 10q22 | A | 38 | 1.08 | 0 (0%) | 0.736 | 0.231 | 0.99 | 1.07 |
rs713588 | 10q | A | 44 | 0.98 | 3 (< 1%) | 0.448 | 0.772 | 1.02 | 1.01 |
rs889312 | MAP3K | C | 28 | 1.12 | 5 (< 1%) | 0.659 | 0.621 | 1.01 | 0.97 |
rs909116 | LSP1 | T | 53 | 1.17 | 2 (< 1%) | 0.149 | 0.854 | 1.05 | 1.01 |
rs1011970 | CDKN2A | T | 17 | 1.06 | 1 (< 1%) | 0.354 | 0.442 | 1.02 | 0.97 |
rs1156287 | COX11 | G | 29 | 0.91 | 26 (2%) | 0.366 | 0.481 | 0.97 | 1.03 |
rs1562430 | 8q24 | A | 58 | 1.17 | 5 (< 1%) | 0.428 | 0.568 | 1.02 | 1.03 |
rs2981579 | FGFR2 | T | 40 | 1.27 | 1 (< 1%) | 0.718 | 0.119 | 0.99 | 0.90 |
rs3757318 | ESR1 | A | 7 | 1.16 | 2 (< 1%) | 0.706 | 0.957 | 1.00 | 1.00 |
rs3803662 | TOX3 | T | 26 | 1.24 | 6 (< 1%) | 0.425 | 0.142 | 0.98 | 0.92 |
rs4973768 | 3p24 SLC | T | 47 | 1.10 | 4 (< 1%) | 0.751 | 0.104 | 1.01 | 0.90 |
rs8009944 | RAD51L1 | A | 75 | 0.88 | 3 (< 1%) | 0.391 | 0.945 | 1.02 | 0.99 |
rs9790879 | 5p12 | C | 40 | 1.10 | 0 (0%) | 0.793 | 0.051 | 0.99 | 0.88 |
rs10995190 | 10q21 | A | 16 | 0.86 | 0 (0%) | 0.536 | 0.964 | 0.99 | 1.00 |
rs11249433 | 1p11.2 | C | 40 | 1.09 | 20 (2%) | 0.700 | 0.355 | 1.01 | 1.05 |
rs13387042 | 2q35 | A | 49 | 1.14 | 32 (3%) | 0.669 | 0.243 | 1.01 | 1.07 |
rs10931936 | CASP8 | C | 74 | 0.88 | 2 (< 1%) | 0.096 | 0.857 | 0.96 | 1.01 |
Quantiles | |||||
---|---|---|---|---|---|
5% | 25% | 50% | 75% | 95% | |
Control | 0.56 | 0.76 | 0.99 | 1.28 | 1.84 |
Case | 0.59 | 0.82 | 1.07 | 1.39 | 2.08 |
BRCA1 | 0.53 | 0.76 | 0.95 | 1.22 | 2.06 |
BRCA1 – control | 0.51 | 0.74 | 0.96 | 1.25 | 2.08 |
BRCA1 – case | 0.57 | 0.78 | 0.92 | 1.13 | 1.59 |
Not BRCA | 0.57 | 0.78 | 1.02 | 1.33 | 1.90 |
Not BRCA – control | 0.56 | 0.77 | 1.00 | 1.29 | 1.79 |
Not BRCA – case | 0.59 | 0.84 | 1.10 | 1.42 | 2.10 |
Quantiles | |||||
---|---|---|---|---|---|
5% | 25% | 50% | 75% | 95% | |
Control | 28 | 34 | 39 | 44 | 54 |
Case | 29 | 35 | 42 | 48 | 56 |
BRCA1 | 25 | 31 | 36 | 42 | 53 |
BRCA1 – control | 25 | 31 | 37 | 42 | 52 |
BRCA1 – case | 27 | 32 | 35 | 42 | 53 |
Not BRCA | 28 | 35 | 40 | 45 | 55 |
Not BRCA – control | 28 | 35 | 39 | 44 | 54 |
Not BRCA – case | 29 | 36 | 43 | 49 | 56 |
OR | OR 95% CI | LR-CHI | AUC | AUC 95% CI | |
---|---|---|---|---|---|
SNP | 1.39 | 1.16 to 1.66 | 13.4 | 0.56 | 0.52 to 0.60 |
Age | 1.39 | 1.19 to 1.62 | 18.0 | 0.58 | 0.54 to 0.62 |
Age + SNP | 1.97 | 1.51 to 2.59 | 25.6 | 0.59 | 0.56 to 0.63 |
SNP (BRCA1) | 0.90 | 0.58 to 1.38 | 0.2 | 0.53 | 0.44 to 0.61 |
Age (BRCA1) | 1.01 | 0.65 to 1.56 | 0.0 | 0.50 | 0.41 to 0.59 |
SNP + age (BRCA1) | 0.91 | 0.53 to 1.54 | 0.1 | 0.49 | 0.39 to 0.58 |
FIGURE 21.
The SNP18 results for BRCA1-negative cases and controls. (a) Histograms of the SNP score RR distribution in cases and controls; and (b) histograms of absolute 10-year risk based on age at recruitment and the SNP RR. Plot (c) shows the ROCs; and (d) is the estimated cumulative risk (weighted by sampling fraction) by 10-year absolute risk quantile based on age and the SNP score. AUC, area under the curve.




FIGURE 22.
Observed vs. expected RR from SNP18 in BRCA1-negative samples.

Tyrer–Cuzick model and single nucleotide polymorphism 18 score
-
Figure 23 shows that there was very little correlation between the SNP18 score and projected 10-year RR from the TC model. SNP18 had a median RR of 1.00 in controls; it was 2.47 for TC.
-
Figure 24 shows the results of combining the TC model and SNP18.
-
Table 26 provides a tabular summary of combining the SNP18 score with TC in this cohort. There is a substantial improvement in the LR-c2 seen when adding SNPs to TC by treating them as independent.
FIGURE 23.
Tyrer–Cuzick vs. SNP18, BRCA1-negative cases and controls.

FIGURE 24.
Tyrer–Cuzick × SNP18 results, BRCA1-negative cases and controls. (a) Histograms of the TC 10-year risk in cases and control; and (b) histograms of TC × SNP18 10-year risk. Plot (c) shows receiver operating characteristic curve; and (d) is the estimated cumulative risk (weighted by sampling fraction) by 10-year absolute risk quantile. AUC, area under the curve.


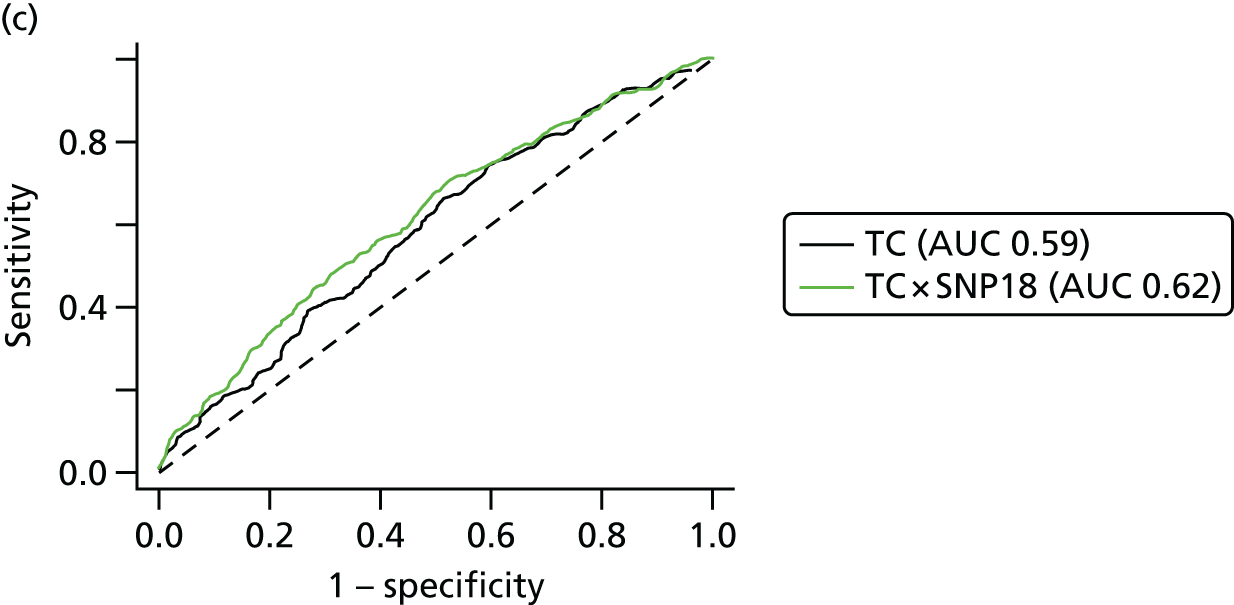
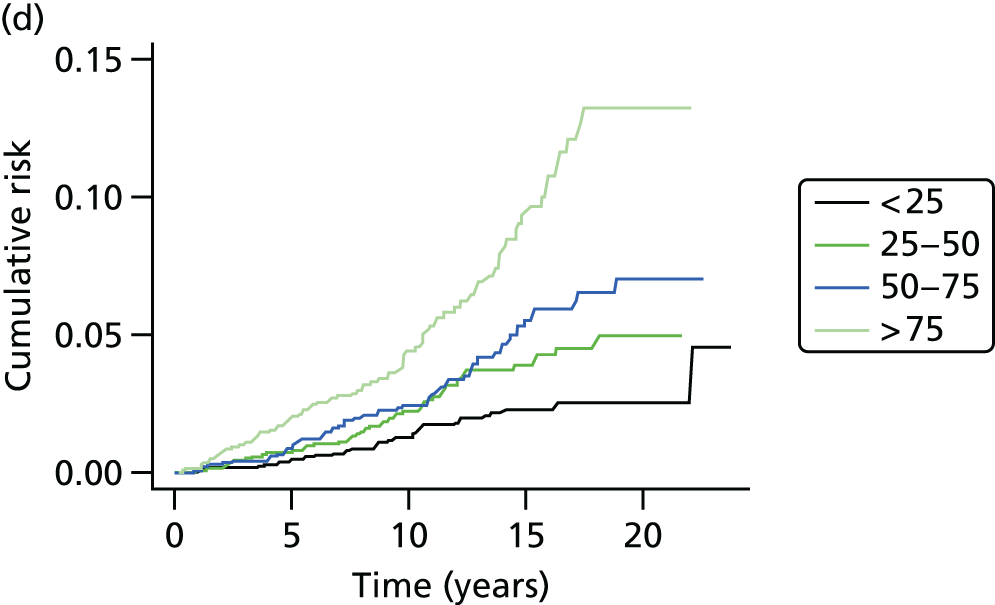
IQR (controls) | OR | 95% CI | AUC | 95% CI | LR-CHI2 | |
---|---|---|---|---|---|---|
SNP RR | 0.77–1.29 | 1.53 | 1.26 to 1.87 | 0.58 | 0.54 to 0.62 | 18.4 |
TC 10-year | 2.29–5.68 | 1.57 | 1.30 to 1.91 | 0.59 | 0.55 to 0.63 | 22.8 |
TC + SNP 10-year | 2.17–5.82 | 1.74 | 1.45 to 2.09 | 0.62 | 0.58 to 0.66 | 37.7 |
Discussion
Overall, it has been possible to show some utility in using SNPs to predict breast cancer risk. This is not only for women in the general population, but also for those with a family history and also, in particular, for BRCA2 mutation carriers.
Study 1: BRCA1/BRCA2 carriers
The recent publications from CIMBA have suggested that breast cancer susceptibility SNPs could be used to provide BRCA2, and possibly BRCA1, mutation carriers with more detailed information as to their breast cancer risk. 27,132 The present study has confirmed that in BRCA2 mutation carriers there is a strong relationship between a woman’s SNPs profile and the age at which she develops breast cancer, but suggests that in BRCA1 mutation carriers the SNPs do not add further discriminatory information. Furthermore, it seems that in BRCA2 mutation carriers the overall breast cancer risk scores derived from the CIMBA-validated SNPs only with the adjusted weightings for BRCA2136 are no better at predicting risk than the weightings from all 18 SNPs from the general population of breast cancer in Turnbull et al. ,131 including the nine SNPs validated in the CIMBA study and three SNPs that have not been shown to increase risk in BRCA2 mutation carriers.
In BRCA1 mutation carriers, the median age at the development of breast cancer varies with risk group, but there was no trend for later age at diagnosis with lower risk score (see Table 19). Furthermore, there was considerable overlap between the 95% CIs for the respective risk groups. There are a number of possible explanations for this. First, it may be that the RAFs used are inappropriate as they relate to the general population rather than to BRCA1 carriers. Second, as we saw no improvement in the predictive ability of our risk model when only BRCA1-validated SNPs (TOX3, 2q, 6q25.1) were included, it may be that too small a proportion of the variation in time to the development of breast cancer in this population is explained by the SNPs that have been validated in BRCA1 carriers so far (i.e. we need to find more BRCA1-validated SNPs or increase the study size considerably). Third, it may be that in BRCA1 carriers the modifying effect of the SNPs (individually or jointly) on age at the development of breast cancer is very much smaller than in BRCA2 carriers or the general population so that, again, our study lacks sufficient power.
In BRCA2 mutation carriers, however, for the overall breast cancer risk scores based on either 18 or 15 SNPs, there was a clear trend for an increased age at diagnosis with decreasing risk scores (see Table 2). For example, in the highest-risk group, 7% of women (based on 18 SNPs) had developed breast cancer by the age of 30 years and 92% had developed breast cancer by the age of 70 years, whereas in the lowest-risk group the corresponding figures were 2% and 77%, respectively. Thus, there is clearly information in the overall breast cancer risk score that might be useful to both clinicians and women with BRCA2 mutations. Our results on cumulative incidence must be interpreted with caution, as there is substantial testing bias towards young women affected with breast cancer. Unfortunately, we do not have access to samples on a large proportion of unaffected close relatives and can test only those who have come forward for clinical predictive testing. As a result, the cumulative risks to 70 years would be predicted to be substantially lower in an unaffected woman tested aged 20 years. In reality, the predicted risks can be used from the range provided by Antoniou et al. ,136,137 and even this may widen as more validated SNPs are added. The performance of the Cox model to predict the order in which a pair of women would develop breast cancer was disappointing but not surprising, given the overlap between the risk groups as regards age at diagnosis.
Estimates of breast cancer risk can vary considerably between family-based studies5,64 and population-based studies,143–145 especially for BRCA2. This difference in risk could be partly explained by low-risk genetic variants, such as the SNPs identified in GWAS. The current analysis validates the work of CIMBA and suggests that use of SNPs with the BRCA2-related weightings137 or the Turnbull weightings131 can be used to advise women within a range of breast cancer lifetime risks of between 42% and 96%. Clinicians could use the SNP multiplicative risk alongside family history of breast cancer and other risk factors to give a better indication of where their risk is likely to lie in this range. We have previously shown that decisions regarding risk-reducing surgery are driven by counselled risk. 146 Although some of the present sample set was used to derive the initial large-scale study of validation in BRCA2,137 we are not aware of any genetics centres using SNPs. Validation in a smaller overlapping set does show that the risk differences are meaningful even between the bottom and top 20% of samples. Therefore, we believe the time is right to consider the use of SNP-adjusted penetrance estimates and that this is likely to affect decision-making in women with BRCA2 mutations.
Conclusions
The 18 validated breast cancer susceptibility SNPs identified through GWAS96 differentiate the risks of breast cancer between women with BRCA2 mutations, but not between those with BRCA1 mutations. There is little difference in the altered risks when validated susceptibility SNPs or a mixture of validated and unvalidated SNPs are factored in. Although further studies would be useful in confirming and refining the risk effects of the SNPs, it may now be appropriate to use these SNPs to help women with BRCA2 mutations make maximally informed decisions about their management options.
Study 2: PROCAS (population modelling)
In order to assess whether or not there are substantial interactions between existing risk factors including family history and MD and the 18SNP gene test, it was necessary to model this using population data. This is particularly important, as family history clearly has a strong genetic component, and MD also is thought to have a 60% heritable component. We carried this out on nearly 1000 samples (n = 993) and showed that the 18SNP test was effectively independent of existing risk factors in TC and MD as measured by a VAS.
The range of 10-year risks identified by the TC programme in the first 10,000 women in PROCAS is quite narrow, with 43.2% of all women having a 10-year risk of between 2% and 3%. 141 These risks were calculated from family history information as well as from standard reproductive risk factors, but when risk information from SNPs was added to that from the TC model, a wider spread was generated, suggesting that adding SNPs to the TC model might lead to better discrimination. Two other studies, using seven147 and 15 susceptibility SNPs,148 also found no association between being assessed as high risk using one of the established breast cancer risk prediction models, such as the Gail149,150 and TC models,11 and being assessed as high risk based on SNPs. The addition of SNP information to the Gail model led to better discrimination,147,151 although the magnitude of the improvement was small; for one study,147 the area under the curve (AUC) increased from 0.557 to 0.594 with the addition of SNPs to the classic Gail risk model (p < 0.001) and, for the other, adding seven SNPs to the National Cancer Institute’s Breast Cancer Risk Assessment Tool increased the AUC from 0.607 to 0.632. 151 Although significant, these effects are relatively modest and still result in AUCs which are not of a high value. The fact that there is little association between the three methods of assessing breast cancer risk (percentage dense area, TC model and SNPs) is promising, as it suggests that the addition of MD and SNPs to the TC model may lead to improved overall performance (by adding new, independent, information). The issue of how information on MD and SNPs might be used to further develop the TC model will be explored in detail, and the predictive ability of the expanded model assessed, when the data from this study are suitably mature and a sufficient number of breast cancers have occurred.
We have also shown that the assessment of MD can be incorporated into routine screening practice. It is likely that addition of MD to standard risk factors and DNA testing will further improve the precision of risk assessment, although, ideally, this will involve an automated measure of MD that can be made on digital mammograms that are now carried out in many areas of the NHSBSP. Ultimately, the incorporation of risk SNP results and MD into current risk prediction models will require further ongoing research and the maturation of prospective data from this and other cohorts. The performance of this, and existing risk prediction models, is currently being assessed, as the PROCAS study now has sufficient follow-up data. However, we are hoping to boost the number of prospective breast cancers with DNA above the current 398, and some cases are yet to be genotyped.
We also carried out modelling work to assess the potential impact of moving from the 18SNP test to a 67SNP test. Our data suggest that, although the spread in towards high risk currently achieved by SNP67 is not as large as that obtained from classical phenotypic markers, SNPs may add substantially to classic factors when used together.
Study 3: phenocopies
In a prospective analysis of the excess risk of breast cancer in women testing negative for a family BRCA1/BRCA2 mutation, we found excess risk to be confined to BRCA2 non-carriers with an observed to expected ratio of 4.57-fold (95% CI 2.50 to 7.67; p < 0.0001) (O : E in BRCA1 non-carriers 1.77). 152 Increased risk was seen especially in BRCA2 families with high incidence of breast cancer (Manchester BRCA2 score of > 10), potentially implicating unlinked genetic modifiers causing this excess. Genotyping of 18 breast cancer susceptibility SNPs defined a RR of 1.31 for BRCA2 breast cancer phenocopies with a breast cancer diagnosis aged < 60 years. In this case, unaffected women in BRCA2 mutation-positive families might be expected to have a protective profile with a SNP RR < 1.0. However, we did not find this, suggesting that there is a bias towards higher allele frequencies of risk SNPs in the BRCA2 mutation-positive families. This then infers that selection of families for mutation screening also selects for higher SNP scores, irrespective of the subsequent BRCA2 mutation status. For BRCA1, there was little effect of the SNPs, with affected carriers having a lower RR than unaffected individuals. The 18 SNPs appear to contribute to the higher rate of breast cancer for those testing negative in BRCA2 families, although there must be other factors involved. At present, the 18 SNPs are considered to account for no more than 10% of the familial component of breast cancer. 27
FH-Risk single nucleotide polymorphisms
This analysis has suggested that a SNP18 score may be added to the TC model by treating them as independent, for individuals who have not tested positive for BRCA1 mutations. Some points for discussion include the following. The study design, matching by age at diagnosis or last mammogram prior to diagnosis, differs from the International Breast Cancer Intervention Study I (IBIS-I) case–control study for SNPs, in which controls were matched by age at baseline and length of follow-up. An advantage of the present case–control design is that age at baseline is not matched out, so one may estimate the performance of risk models that include age as a factor.
Overall SNP18 improves the AUC with TC from 0.59 to 0.62 and, therefore, in non-BRCA1-related risk appears to add useful discrimination.
Conclusions
Our study in four different populations has shown that addition of the SNP18 improves the discrimination of breast cancer risk. This appears particularly effective in non-BRCA family history-positive cases (FH-Risk) and in the general population (PROCAS). However, our assessment has shown that this is not yet useful in BRCA1 carriers. This may be because SNP18 includes only a small number of SNPs that are associated with oestrogen receptor-negative breast cancer. The overall effects of adding SNPs to risk models are still relatively modest, with AUC scores which are still relatively low. Modelling as part of our study has shown that further benefit may be achieved by adding the additional SNPs with lower RRs identified in 2013 as part of the ICOGS initiative.
Publications resulting from work in Chapter 3
Evans DG, Ingham SL, Buchan I, Woodward ER, Byers H, Howell A, et al. Increased rate of phenocopies in all age groups in BRCA1/BRCA2 mutation kindred, but increased prospective breast cancer risk is confined to BRCA2 mutation carriers. Cancer Epidemiol Biomarkers Prev 2013;22:2269–76. 152
Howell A, Astley S, Warwick J, Stavrinos P, Sahin S, Ingham S, et al. Prevention of breast cancer in the context of a national breast screening programme. J Intern Med 2012;271:321–30. 153
Evans DG, Warwick J, Astley SM, Stavrinos P, Sahin S, Ingham SL, et al. Assessing individual breast cancer risk within the UK National Health Service Breast Screening Programme: a new paradigm for cancer prevention. Cancer Prev Res (Phila) 2012;5:943–51. 141
Ingham SL, Warwick J, Byers H, Lalloo F, Newman WG, Evans DG. Is multiple SNP testing in BRCA2 and BRCA1 female carriers ready for use in clinical practice? Results from a large Genetic Centre in the UK. Clin Genet 2013;84:37–42. 140
Brentnall AR, Evans DG, Cuzick J. Distribution of breast cancer risk from SNPs and classical risk factors in women of routine screening age in the UK. Br J Cancer 2014;110:827–8. 154
Brentnall AR, Evans DG, Cuzick J. Value of phenotypic and single-nucleotide polymorphism panel markers in predicting the risk of breast cancer. J Genet Syndr Gene Ther 2013;4. 155
Chapter 4 PROCAS: Predicting Risk of Breast Cancer at Screening
Introduction
Individual breast cancer risk is highly variable, and yet the interval for mammography in the NHSBSP is the same (3-yearly) for all women regardless of risk. The only exception is women who identify themselves through cancer genetic services and are assessed as high risk according to the 2013 updated NICE guidance. These women are now entitled to annual screening between the ages of 50 and 59 years, although this is still not fully embedded in the NHSBSP. During the 2011–12 screening round, overall NHSBSP coverage was 77.0%. 156 Uptake of routine invitations for women aged 50–70 years was 73.1%,156 with comparatively lower uptake (67.7%) in the 71–74 years age group, including the programme’s extension to women aged 71–73 years. 156 A total of 15,749 women aged ≥ 45 years had cancers detected by the screening programme in 2011–12, a rate of 8.1 cases per 1000 women screened, with the cancer detection rate being highest among women aged > 70 years (13.9 per 1000 women screened). 156 Uptake to breast screening in Greater Manchester is typically slightly lower than the national average, with 69.5% of eligible women screened during the 2011–12 screening round.
The Greater Manchester NHSBSP covers five main areas of Greater Manchester: Tameside, Oldham, Salford, Manchester and Trafford. In each of these areas there are several local screening sites. Attendance at screening varies broadly, both across each main area and by local screening site. Table 27 shows the overall uptake in each screening area, along with figures for the sites with the lowest and highest uptake in each area.
Area | Average uptake across all sites (%) | Site with lowest uptake (%) | Site with highest uptake (%) |
---|---|---|---|
Manchester | 58 | 52 | 65 |
Oldham | 66 | 28 | 75 |
Tameside | 72 | 50 | 87 |
Trafford | 71 | 49 | 78 |
Salford | 66 | 44 | 90 |
Evidence suggests that approximately 10% of women of screening age are likely to be at moderate or high risk of breast cancer. Although those with a significant family history of breast cancer may be aware that they are at increased risk, a large number of women without significant family history may be at increased risk because of lifestyle factors and be unaware of this risk. Furthermore, with the exception of those who request referral to a family history or genetics clinic, there is currently no way for women to receive risk assessment and access appropriate risk-based surveillance and preventative interventions.
Risk factors
There are a number of breast cancer risk factors, which fall broadly into the following categories: family history, hormonal and reproductive risk factors, genetic factors, lifestyle risk factors and MD.
Family history of breast cancer in relatives12
When assessing risk based on family history of breast cancer, the following factors must be taken into consideration: degree of relationship (first degree or greater); whether the cancer is unilateral or bilateral; age at onset of breast cancer; number of cases in the family and whether or not they occur on the same side; other related early-onset tumours (e.g. ovarian); and number of unaffected relatives (large families with many unaffected relatives will be less likely to harbour a high-risk gene mutation).
Hormonal and reproductive risk factors
Exposure to endogenous oestrogens is an important risk factor for breast cancer. Early age at menarche (aged < 12 years) and late menopause (aged > 53 years) increase breast cancer risk by increasing years of oestrogen and progesterone exposure. 13–21
Long-term combined HRT use after the menopause (> 5 years) is associated with a significant increase in breast cancer risk, although the risk from oestrogen-only HRT appears much lower and may be risk neutral. 16–19 The results of a meta-analysis suggest that HRT appears to increase risk cumulatively by 1–2% per year, although the risk disappears within 5 years of cessation. 15 A meta-analysis suggested that both during current use of the combined oral contraceptive and 10 years post use, there may be a 24% increase in risk of breast cancer. 13
The age at first full-term pregnancy influences the RR of breast cancer, with early first full-term pregnancy offering some protection by transforming breast parenchymal cells into a more stable state, potentially resulting in less proliferation in the second half of the menstrual cycle. Women having their first full-term pregnancy over the age of 30 years have double the risk of women who had their first full-term pregnancy under the age of 20 years, and this is the case for both women in the general population and those at highest risk from a BRCA1/BRCA2 mutation. 20,21
Genetic factors
Mutations in breast cancer genes such as BRCA1 and BRCA2 are too infrequent to affect risk prediction appreciably in the models for the general population. However, recently identified SNPs in many genes and outside genes (n = 77),27 which individually confer small changes in risk, may prove useful in predicting larger differences in risk when considered together. Four GWASs,8–10,27 published before our programme grant, found common genetic variants (SNPs) each carried by 28–44% of the population associated with a 1.07–1.26 RR of breast cancer. These variants, linked to four genes (FGFR2, TOX3, MAP3K1 and LSP1), confer as much as a 1.17–1.64 risk if two copies are carried. When combined in an individual, they give higher than additive risk of breast cancer. 29 Another variant, CASP8, is associated with reduced breast cancer risk. 30 There are now 77 genetic variants associated with breast cancer risk, but their application requires further validation and assessment of interactions. Therefore, to improve the accuracy of existing risk prediction models, it is necessary to investigate validated SNPs as they are discovered, and, where possible, incorporate these genetic factors into the best-performing risk models.
Lifestyle risk factors
Expert reports estimate that 15–40% of breast cancer may be preventable by lifestyle change: weight control, exercise and reduced alcohol intake. 157,158 These estimations are consistent with recent cohort studies which report 25–30% less breast cancer among women who adhere to cancer prevention recommendations, that is, those who are a healthy weight (BMI of 18.5–25 kg/m2), limit alcohol (< 1–2 units per day) and engage in regular moderate or vigorous physical activity (100–200 minutes per week), than among women who do not follow these recommendations. 159–161 We and others have demonstrated that losing and maintaining a weight loss of ≥ 5%, either before or after the menopause, reduces post-menopausal breast cancer risk by 25–40% in the general population. 162–164 Weight control also appears to be important for reducing the risk of post-menopausal breast cancer among women at moderate risk with a family history with low-penetrance genetic variants (15–30% lifetime risk),159,160,165 and among high-risk BRCA1/BRCA2 carriers (up to 60–80% lifetime risk). BMI is the only lifestyle factor that is currently incorporated into any risk model.
Mammographic density
The relationship between MD and breast cancer risk was first established using subjective categorical classifications, based on descriptors or on the percentage of the breast area occupied by radio-opaque (dense) connective and epithelial tissue, as opposed to the radiolucent fatty component of the breast. 23,26 Women with high density were found to have a risk 4–6 times higher than that of those with predominantly fatty breasts. 33 Subsequently, semiautomated methods were developed to quantify more accurately the percentage area of dense tissue. In particular, Cumulus software34 became the accepted gold standard for quantification of density for research purposes. Cumulus is an interactive program in which an operator selects a threshold to separate dense from fatty regions. It has never been widely adopted in routine clinical practice as it requires a skilled operator and is time-consuming, but Cumulus measurements have been shown to relate strongly to breast cancer risk. 26 In the USA, the most widely used categorisation of density is the American College of Radiology’s BI-RADS (Breast Imaging Reporting and Data System), a subjective visual assessment into four classes which aims to identify mammograms in which the sensitivity of mammography is reduced because of MD. 166 In the UK, the use of BI-RADS is not common, and other techniques such as estimation of percentage density using a VAS have been successfully related to risk of cancer. 167
Subjective visual estimates of MD and thresholding methods suffer from the limitation that the assessment is in two dimensions, as the mammogram is a projection image of the three-dimensional structure of the breast. The same volume of dense tissue could, therefore, give rise to different density estimates, depending on compression and imaging. To overcome this and reduce dependence on imaging parameters, researchers developed methods to calculate the three-dimensional volumes of fatty and dense fibroglandular tissue in digitised film mammograms, either by a process of calibration involving the acquisition of images of, for example, a calibrated stepwedge168,169 or by modelling the physics of the imaging process. 170 With the advent of FFDM, it has become possible to routinely quantify the volumes of dense fibroglandular and fatty tissue in the breast, using models of the imaging process and information from the Digital Imaging and Communications in Medicine (DICOM) header associated with the images. The first two commercial products to achieve this were Quantra, from Hologic, and Volpara, from Volpara Solutions. Although the body of research associating such methods to risk is not as extensive as that for visual and semiautomated methods because of the limited longitudinal FFDM data available, these methods have been shown to correlate with BI-RADS and Cumulus, and agree with MR-based assessments of volumes of fat and gland. 171–175
Risk estimation models
Breast cancer risk is generally assessed using models that include a combination of the previously stated risk factors. 11,22,149,150 Breast cancer risk models perform well at predicting the overall number of breast cancer cases arising in a particular population, but are poor at identifying the specific individuals. 22
In the USA, the Gail model is widely used. 149,150 Until recently, the two most frequently used models were the Gail model and the Claus model. More recently, in the UK, the TC and BOADICEA models have been used.
Gail model
The Gail model was originally designed to determine eligibility for the Breast Cancer Prevention Trial, and has since been modified (in part to adjust for race)11,22 and made available on the National Cancer Institute website (http://bcra.nci.nih.gov/brc/q1.htm). The model has been validated in a number of settings and probably works best in general assessment clinics,176 where family history is not the main reason for referral. The Gail model is based on age, first-degree family history, the number of surgical biopsies of the breast and reproductive factors such as age at menarche, age at first pregnancy and age at menopause. The major limitation of the Gail model is the inclusion of only first-degree relatives, which results in underestimating risk in the 50% of families with cancer in the paternal lineage, and also takes no account of age at onset of breast cancer. 57,176 As a result, it performed less well in our own validation set from a FHC (Table 28), substantially underestimating risk overall and in most subgroups groups assessed.
RR at extremes | Gail | Claus | BRCAPRO/ Ford | TC | BOADICEA | |
---|---|---|---|---|---|---|
Prediction | ||||||
Amir et al.57 validation study ratioa | 0.48 | 0.56 | 0.49 | 0.81 | Not assessed | |
95% CI | 0.37 to 0.64 | 0.43 to 0.75 | 0.37 to 0.65 | 0.62 to 1.08 | Not assessed | |
Personal information | ||||||
Age (20–70) | 30 | Yes | Yes | Yes | Yes | Yes |
BMI | 2 | No | No | No | Yes | No |
Alcohol intake (0–4 units) daily | 1.24 | No | No | No | No | No |
Hormonal/reproductive factors | ||||||
Age at menarche | 2 | Yes | No | No | Yes | No |
Age at first live birth | 3 | Yes | No | No | Yes | No |
Age at menopause | 4 | No | No | No | Yes | No |
HRT use | 2 | No | No | No | Yes | No |
OCP use | 1.24 | No | No | No | No | No |
Breastfeeding | 0.8 | No | No | No | No | No |
Plasma oestrogen | 5 | No | No | No | No | No |
Personal breast disease | ||||||
Breast biopsies | 2 | Yes | No | No | Yes | No |
Atypical ductal hyperplasia | 3 | Yes | No | No | Yes | No |
Lobular carcinoma in situ | 4 | No | No | No | Yes | No |
Breast density | 6 | No | No | No | No | No |
Family history | ||||||
First-degree relatives | 3 | Yes | Yes | Yes | Yes | Yes |
Second-degree relatives | 1.5 | No | Yes | Yes | Yes | Yes |
Third-degree relatives | No | No | No | No | Yes | |
Age of onset of breast cancer | 3 | No | Yes | Yes | Yes | Yes |
Bilateral breast cancer | 3 | No | No | Yes | Yes | Yes |
Ovarian cancer | 1.5 | No | No | Yes | Yes | Yes |
Male breast cancer | 3–5 | No | No | Yes | No | Yes |
Claus model
Three years after the publication of the Claus model, lifetime risk tables for most combinations of affected first- and second-degree relatives were published. 43 Although these do not give figures for some combinations of relatives, such as for mother and maternal grandmother, an estimation of this risk can be garnered by using the mother–maternal aunt combination. An expansion of the original Claus model estimates breast cancer risk in women with a family history of ovarian cancer. 177 The major drawback of the Claus model is that it does not include any of the non-hereditary risk factors, and agreement between the Gail and Claus models has been shown to be relatively poor. 178–180 Although the tables make no adjustments for unaffected relatives, the computerised version is able to reduce the likelihood of the ‘dominant gene’ with increasing number of affected women. However, the tables give consistently higher risk figures than the computer model, suggesting either that a population risk element is not added back into the calculation or that the adjustment for unaffected relatives is made from the original averaged figure rather than assuming that each family will have already had an ‘average’ number of unaffected relatives. 57 The latter appears to be the likely explanation, as inputting families with zero unaffected female relatives gives risk figures close to the Claus table figure. Another potential drawback of the Claus tables is that these reflect risks for women in the 1980s in the USA. These are lower than the current incidence in both North America and most of Europe. As such, an upwards adjustment of 3–4% for lifetime risk is necessary for lifetime risks < 20%. Our own validation of the Claus computer model showed that it substantially underestimated risks in the FHC. However, manual use of the Claus tables provided accurate risk estimation (see Table 28). A modified version of the Claus model has now been validated as ‘Claus extended’, by adding risk for bilateral disease, ovarian cancer and three or more affected relatives. 76
BRCAPRO
Parmigiani et al. 181 developed a Bayesian model that incorporated published BRCA1 and BRCA2 mutation frequencies, cancer penetrance in mutation carriers, cancer status (affected, unaffected or unknown) and age of the consultee’s first- and second-degree relatives. An advantage of this model is that it includes information on both affected and unaffected relatives. In addition, it provides estimates for the likelihood of finding either a BRCA1 or a BRCA2 mutation in a family. An output that calculates breast cancer risk using the likelihood of BRCA1/BRCA2 can be utilised. None of the non-hereditary risk factors can yet be incorporated into the model (see Table 28). The major drawback from the breast cancer risk assessment aspect is that no other ‘genetic’ element is allowed for. 15 Therefore, in breast cancer-only families it will underestimate risk. As a result, BRCAPRO produced the least accurate breast cancer risk estimation from our FHC validation. It predicted only 49% of the breast cancers that actually occurred in the screened group of 1900 women. 57
Tyrer–Cuzick model
Until recently, no single model integrated family history, surrogate measures of endogenous oestrogen exposure and benign breast disease in a comprehensive fashion. The TC model, based partly on a data set acquired from IBIS-I and other epidemiological data, has now done this. 11 The major advantage over the Claus model and BRCAPRO is that the model allows for the presence of multiple genes of differing penetrance. It does give a read-out of BRCA1/BRCA2, but also allows for a lower-penetrance BRCAX. As can be seen in Table 28, the TC model addresses many of the pitfalls of the previous models: significantly, the combination of extensive family history, endogenous oestrogen exposure and benign breast disease (atypical hyperplasia). In our original validation, the TC model performed by far the best at breast cancer risk estimation. 57
Model validation
In a previous study, the goodness of fit and discriminatory accuracy of the above four models was assessed using data from 1317 women. The main analysis was on data from 1933 women attending our Family History Evaluation and Screening Programme in Manchester, UK, who underwent ongoing screening, of whom 52 developed cancer. 57 All models were applied to these women over a mean follow-up of 5.27 years to estimate risk of breast cancer. The ratios of expected to observed numbers of breast cancers (95% CI) were 0.48 (0.37 to 0.64) for Gail, 0.56 (0.43 to 0.75) for Claus, 0.49 (0.37 to 0.65) for Ford and 0.81 (0.62–1.08) for TC (see Table 28). The accuracy of the models for individual cases was evaluated using receiver operating characteristic curves. These showed that the AUC was 0.735 for Gail, 0.716 for Claus, 0.737 for Ford and 0.762 for TC. The TC model was the most consistently accurate model for prediction of breast cancer. Gail, Claus and Ford all significantly underestimated risk, although with a manual approach the accuracy of Claus tables may be improved by making adjustments for other risk factors (‘Manual method’) by subtracting from the lifetime risk for a positive endocrine risk factor (e.g. a lifetime risk may change from 1 in 5 to 1 in 4 with late age of first pregnancy). The Gail, Claus and BRCAPRO models all underestimated risk, particularly in women with a single first-degree relative affected with breast cancer. TC and the Manual model were both accurate in this subgroup. Conversely, all of the models accurately predicted risk in women with multiple relatives affected with breast cancer (i.e. two first-degree relatives and one first-degree plus two other relatives). This implies that the effect of a single affected first-degree relative is higher than may have been previously thought. The Gail model is likely to have underestimated in this group, as it does not take into account age of breast cancer, and most women in our single first-degree relative category had a relative diagnosed at < 40 years of age. The Ford, TC and Manual models were the only models to accurately predict risk in women with a family history of ovarian cancer. As these were the only models to take account of ovarian cancer in their risk assessment algorithm, this confirmed that ovarian cancer has a significant effect on breast cancer risk.
The Gail, Claus and BRCAPRO models all significantly underestimated risk in women who were nulliparous or whose first live birth occurred after the age of 30 years. Moreover, the Gail model appeared to increase risk with pregnancy under the age of 30 years in the familial setting. It is not clear why such a modification to the effects of age at first birth should be made, unless it is as a result of modifications to the model made after early results suggested an increase with BRCA1/BRCA2 mutation carriers. However, the Gail model has determined an apparent increase in risk with early first pregnancy and it would appear to be misplaced from our results, and from subsequent studies published on BRCA1/BRCA2. Furthermore, the Gail, Claus and BRCAPRO models also underestimated risk in women whose menarche occurred after the age of 12 years. The TC and Manual models accurately predicted risk in these subgroups. These results suggest that age at first live birth also has an important effect on breast cancer risk, while age at menarche perhaps has a lesser effect. The effect of pregnancy under the age of 30 years appeared to reduce risk by 40–50%, compared with an older first pregnancy or late age/nulliparity, whereas at the extremes of menarche there was only a 12–14% effect. Our study remains the only one to validate risk models prospectively and, clearly, further such studies are necessary to gauge the accuracy of these and newer models. Indeed, the tendency to modify models to adapt for new risk factors without prospective validation in an independent data set is a problem, and can lead to erroneous risk prediction.
BOADICEA
Using segregation analysis, a group in Cambridge, UK, has derived a susceptibility model (BOADICEA) in which susceptibility is explained by mutations in BRCA1 and BRCA2 together with a polygenic component reflecting the joint multiplicative effect of multiple genes of small effect on breast cancer risk. 182 The group has shown that the overall familial risks of breast cancer predicted by the model are close to those observed in epidemiological studies. The predicted prevalences of BRCA1 and BRCA2 mutations among unselected cases of breast and ovarian cancer were also consistent with observations from population-based studies. The group also showed that its predictions were closer to the observed values than those obtained using the Claus model and BRCAPRO. The predicted mutation probabilities and cancer risks in individuals with a family history can now be derived from this model. Early validation studies have been carried out on mutation probability but not yet on cancer risk prediction. 183
Model selection in NHS Breast Screening Programme
The Claus, BRCAPRO and BOADICEA models are unsuitable for population prediction, as they are entirely based on family history risk factors. Therefore, the models being assessed as part of this project are Gail and TC.
National Institute for Health and Care Excellence guidelines for moderate- and high-risk women
Women are considered to be at moderate risk of breast cancer if they have a 10-year risk of between 5% and 8%, as measured by TC, and at high risk if they have a 10-year risk of ≥ 8%. Current NICE guidelines for management of women at risk of familial breast cancer recommend that women between the ages of 50 and 59 years who are at increased risk but do not have a BRCA1/BRCA2 mutation be offered annual mammography and advice about menstrual, reproductive, hormonal and lifestyle risk factors. 142 Chemoprevention with tamoxifen or raloxifene is also recommended for those at increased familial risk of breast cancer. 142
Methods
Collection of risk information
Risk information was collected by running a large-scale, regional study entitled PROCAS within the Greater Manchester NHSBSP. Recruitment was carried out in two phases: for the first 3 years of recruitment, all women invited for breast screening were sent an invitation to participate in the PROCAS study; the second phase of recruitment involved inviting only those who had not previously attended screening in the area. As screening is triennial, this meant that all women attending screening during the recruitment period were invited once during this time.
A two-page questionnaire (see Appendix 1) was devised to collect the risk information required to calculate individual breast cancer risk. Family history information, including number and ages of sisters, current age or age at death of mother and details of any relatives affected by breast or ovarian cancer, was collected. It was not possible to collect information on unaffected second-degree relatives, as this would have required a much longer questionnaire, which we believe would have deterred women from participating. Hormonal risk factors, namely age at menarche, menopausal status, HRT use and parity, were collected. The following lifestyle information was also collected: current BMI, BMI aged 20 years, clothes size, alcohol consumption and exercise habits.
Women were mailed the questionnaire and a consent form in the interval between receiving the call for screening and attendance, in five screening areas of Greater Manchester. Women were consented to the study when they attended their screening appointment. The vast majority of participants were consented by a radiographer, rather than by a dedicated member of the study team. Completed consent forms and questionnaires were sent to the study team, based at the University Hospital of South Manchester, where the questionnaire data were entered onto a study database and a 10-year TC risk score for each individual was automatically produced. Participants were also asked to indicate whether or not they wished to be informed of their individual risk of breast cancer.
Uptake to screening in the Greater Manchester NHSBSP across all screening sites during the first phase of recruitment was 68%, and overall uptake to PROCAS across all sites during this time was 37%. There was wide variation in uptake to screening and to PROCAS in the various screening sites, as demonstrated in Table 29.
Site | Main area | % uptake to screening | % uptake PROCAS |
---|---|---|---|
Longsight | Manchester | 53.93 | 23.76 |
Lower Broughton | Salford | 61.24 | 27.49 |
Kath Lock | Manchester | 64.08 | 28.93 |
Little Hulton Health Centre | Salford | 63.39 | 29.04 |
North Manchester General Hospital | Manchester | 55.42 | 29.10 |
Clayton | Manchester | 63.59 | 29.71 |
Eccles | Salford | 67.37 | 30.91 |
Harpurhey | Manchester | 69.48 | 31.78 |
Pendleton Gateway | Salford | 62.75 | 33.51 |
Partington | Trafford | 63.47 | 33.94 |
Gorton | Manchester | 63.88 | 34.23 |
Withington Community Hospital | Manchester | 34.36 | 34.36 |
Tameside | Tameside | 73.75 | 34.59 |
Crickett’s Lane | Tameside | 73.53 | 34.80 |
Disabled clinics | Manchester | 81.80 | 35.57 |
Seymour Grove | Trafford | 64.03 | 36.35 |
Hyde | Tameside | 74.03 | 36.66 |
Royton | Oldham | 81.58 | 37.30 |
Irlam | Salford | 75.01 | 38.14 |
Trafford General Hospital | Trafford | 79.48 | 38.32 |
Pendlebury | Salford | 73.41 | 38.34 |
Wythenshawe | Manchester | 63.90 | 38.40 |
Ann Street | Manchester | 74.95 | 41.42 |
Walkden | Salford | 76.66 | 44.14 |
Stalybridge | Tameside | 77.44 | 45.26 |
Uppermill | Oldham | 80.97 | 45.58 |
Glossop | Tameside | 79.25 | 47.61 |
Bodmin Road | Manchester | 78.25 | 52.34 |
Oldham Integrated Care Centre | Oldham | 75.48 | 56.19 |
In the second phase of recruitment, uptake to screening across all sites was 58% and uptake to PROCAS was 47%. As recruitment phase 2 involved recruiting only those attending screening for the first time, it is not possible to report uptake by site, as these data are gathered collectively for all attendees and so cannot be obtained for specific groups of women. It also means that there will be a significantly higher number of younger participants than in recruitment phase 1.
Demographics of PROCAS participants
Tables 30 and 31 show the demographics of the PROCAS participants recruited in each recruitment phase. During recruitment to phase 1, the proportion of women in age groups 50–54, 55–59 and 60–64 years was 20–25%; fewer women were in the 65–69 age group (17%) and in the under-50 (7%) and over-70 age groups (6%). The majority were white (91%) and almost 4% did not report their ethnicity. Initial TC scores were low (< 2), average (2–4), moderate (5–7) and high (≥ 8) for 19.6%, 70.6%, 8.6% and 1.2%, respectively. As expected in phase 2, the proportion of women recruited were younger, with 43% aged < 50 years and 49% aged 50–54 years, and the majority were white (89%). Equally high proportions in both phases stated a preference to be informed of their risk.
Demographic | Number | Percentage | Percentage invited |
---|---|---|---|
Age (years) | |||
< 50 | 3448 | 7.01 | 6.41 |
50–54 | 12,533 | 25.47 | 26.32 |
55–59 | 10,721 | 21.79 | 21.36 |
60–64 | 11,093 | 22.54 | 20.90 |
65–69 | 8488 | 17.25 | 17.39 |
≥ 70 | 2923 | 5.94 | 7.62 |
Ethnicity | |||
White | 44,836 | 91.12 | 82.8 GMRa |
Black or black British | 506 | 1.03 | 2.76 GMRa |
Asian or Asian British | 647 | 1.31 | 10.15 GMRa |
Mixed | 238 | 0.48 | 2.26 GMRa |
Jewish | 451 | 0.92 | 1.2 GMRa |
Other | 710 | 1.44 | 1 GMRa |
Data not known | 1818 | 3.69 | |
10-year TC risk | |||
0–1 | 87 | 0.18 | |
> 1–2 | 9572 | 19.45 | |
> 2–3 | 21,298 | 43.28 | |
> 3–4 | 9625 | 19.56 | |
> 4–5 | 3780 | 7.68 | |
> 5–8 | 4242 | 8.62 | |
> 8 | 602 | 1.22 | |
Preference to be informed of risk | |||
Yes | 46,767 | 95.04 | |
No | 2439 | 4.96 |
Demographic | Number | Percentage | Percentage invited for first screen |
---|---|---|---|
Age (years) | |||
< 50 | 1868 | 42.55 | 27.14 |
50–54 | 2144 | 48.84 | 43.14 |
55–59 | 212 | 4.83 | 13.26 |
60–64 | 103 | 2.35 | 8.49 |
65–69 | 43 | 0.98 | 5.99 |
≥ 70 | 20 | 0.46 | 1.98 |
Ethnicity | |||
White | 3909 | 89.04 | 82.8 GMRa |
Black or black British | 97 | 2.21 | 2.76 GMRa |
Asian or Asian British | 144 | 3.28 | 10.15 GMRa |
Mixed | 31 | 0.71 | 2.26 GMRa |
Jewish | 22 | 0.50 | 1.2 GMRa |
Other | 110 | 2.51 | 1 GMRa |
Data not known | 77 | 1.75 | |
10-year TC risk | |||
0–1 | 7 | 0.16 | |
> 1–2 | 820 | 18.68 | |
> 2–3 | 1945 | 44.31 | |
> 3–4 | 892 | 20.32 | |
> 4–5 | 303 | 6.90 | |
> 5–8 | 378 | 8.61 | |
> 8 | 45 | 1.03 | |
Preference to be informed of risk | |||
Yes | 4244 | 96.67 | |
No | 146 | 3.33 |
The higher percentage of Asian participants in phase 2 may simply reflect the ethnicity spread to younger ages in those of Asian origin in the UK and Greater Manchester. The NHSBSP does not have ethnicity data to determine which ethnicities have been invited for screening.
Table 32 shows further characteristics of the whole PROCAS population. Approximately one-third of the PROCAS population were in the normal BMI category range; this was a greater proportion than expected from the general population (p < 0.0001). However, the PROCAS population, although containing fewer overweight women (p < 0.0001), also contained a larger proportion of obese women (p < 0.0001) (Table 33).
Characteristic | Number (%) of participants |
---|---|
BMI (kg/m2) | |
Underweight (< 18.5) | 389 (0.73) |
Normal weight (18.5–24.9) | 18,536 (34.58) |
Overweight (25.0–29.9) | 17,760 (33.14) |
Obese (≥ 30) | 13,273 (24.76) |
Unknown or not stated | 3638 (6.79) |
Menopausal status | |
Premenopausal | 5338 (9.96) |
Perimenopausal | 9486 (17.70) |
Postmenopausal | 36,143 (67.44) |
Unknown or not stated | 2629 (4.90) |
Parity | |
Nulliparous | 6719 (12.53) |
Parous | 46,675 (87.09) |
Unknown or not stated | 202 (0.38) |
HRT use | |
Current user/within last 5 years | 10,228 (19.08) |
Never taken/over 5 years ago | 42,784 (79.83) |
Unknown or not stated | 584 (1.09) |
Alcohol intake | |
0 units per week | 14,678 (27.39) |
1–2 units per week | 7725 (14.41) |
3–6 units per week | 10,462 (19.52) |
7–14 units per week | 12,454 (23.24) |
15–27 units per week | 4846 (9.04) |
≥ 28 units per week | 1017 (1.90) |
Does drink alcohol but amount not stated | 1545 (2.88) |
Unknown or not stated | 869 (1.62) |
Exercisea | |
Inactive (≤ 659 minutes/week) | 43,412 (81.00) |
Moderately inactive (660–779 minutes/week) | 1089 (2.03) |
Moderately active (780–1679 minutes/week) | 4414 (8.24) |
Active (≥ 1680 minutes/week) | 2204 (4.11) |
Unknown or not stated | 2477 (4.62) |
Number of affected FDRs | |
0 | 46,742 (87.21) |
1 | 6369 (11.88) |
2 | 450 (0.84) |
3 | 31 (0.06) |
≥ 4 | 4 (0.01) |
IMD score | |
Mean | 24.53 |
BMI (kg/m2) | PROCAS population (of those who provided BMI data) | General population of Greater Manchester |
---|---|---|
Underweight (< 18.5) | 0.8% | 1.5% |
Normal weight (18.5–24.9) | 37.1% | 33.8% |
Overweight (25.0–29.9) | 35.5% | 40.7% |
Obese (≥ 30) | 26.6% | 24.0% |
Approximately two-thirds of women in the PROCAS study were postmenopausal, with a further 18% being perimenopausal. Nineteen per cent reported being current or previous users (within the last 5 years) of HRT. The majority were parous (87%) and did not have an affected first-degree relative (87%).
The national UK average alcohol consumption for women aged ≥ 45 years in 2009 was 8.5 units per week. 185 Assuming that the alcohol intake was in the middle of the range and that those drinking over 28 units averaged 35 units, the average intake of PROCAS women was 6.3 units daily, a little lower than the national average (see Table 32). The majority of women were inactive (81%) as defined by the EPIC study. 22
The mean Index of Multiple Deprivation score for all women in the PROCAS population was 24.53. 186 This ranged from 13.09 in Trafford to 38.44 in the Manchester district, where a higher Index of Multiple Deprivation score indicates a higher level of deprivation.
Risk feedback
All participants recruited to the study were asked to specify whether or not they wished to be informed of their personal 10-year TC breast cancer risk. Participants were advised that the majority of women would receive their risk via letter at the end of the study, but that all women who were found to be at high risk of breast cancer (≥ 8% 10-year TC risk) and a small number of those at low and moderate risk would receive an invitation for a risk feedback appointment with a study clinician experienced in risk communication. All participants had at least two opportunities to opt out of receiving personal breast cancer risk information: first at the time of initial consent (by not ticking a box labelled ‘I wish to know my risk’) and later by contacting the study co-ordinator. Those who received an invitation for a risk appointment were also given a further opportunity to opt out of receiving their risk by declining an appointment.
The majority of women recruited to PROCAS have received their risk feedback via letter in 2014 and 2015. This has been done as part of an externally funded study, which has allowed us to work directly with participants to co-design a letter that effectively communicates individual breast cancer risk while minimising negative psychological impact; to explore the acceptability of receiving this information; and to explore intentions to change behaviour. However, all women found to be at high risk of breast cancer (TC 10-year risk of ≥ 8%) and a proportion of those at moderate (TC 10-year risk of 5–7.99%) and low risk (TC 10-year risk of ≤ 1.5%) have received their risk feedback either in person or via telephone.
In total, to June 2014, 984 participants across the low-, moderate- and high-risk categories were invited for a risk feedback appointment and 687 (69.82%) participants attended their appointment. Uptake of risk feedback varied across the different risk categories, with highest uptake among those at highest risk. Pairwise comparisons showed that there were significant differences between attendance in those in the high- and moderate-risk categories versus those in the low-risk category (p < 0.001, p < 0.005, respectively) (Table 34).
Original risk category | Number who were invited | Number (%) who attended | Proportion receiving telephone counselling (%) | Number (%) who declined appointment | Number (%) with no response to invitation | Number (%) who did not attend a scheduled appointment |
---|---|---|---|---|---|---|
Low risk (≤ 1.5% 10-year TC risk) | 192 | 105 (54.69) | 67 | 25 (13.02) | 56 (29.17) | 6 (3.13) |
Moderate risk (5–7.99% 10-year TC risk) | 95 | 69 (72.63) | 60 | 7 (7.37) | 17 (17.89) | 2 (2.11) |
High risk (≥ 8% 10-year TC risk) | 689 | 513 (74.46) | 55 | 53 (7.69) | 115 (16.69) | 8 (1.16) |
It became apparent during the risk feedback process that information provided by participants on their PROCAS questionnaire was not always accurate. As a result, participants’ risk often changed following a risk consultation, and in some cases participants’ risk changed to such an extent that they no longer remained in the same risk category. Table 35 shows how the numbers of participants in each risk category change following risk counselling. It is apparent that there were fewer changes in risk categorisation in those who were originally assessed as being low risk. This is to be expected because the majority of errors are with recording of relatives’ cancer diagnoses and the low-risk participants are very unlikely to have had any affected relatives. The greatest proportion of changes in risk occurred in those originally assessed as having a 10-year TC risk of ≥ 8%. This is largely because the PROCAS questionnaire, owing to space restrictions, did not collect information on unaffected female relatives, which is an important factor in risk prediction.
Original 10-year TC risk category | Number (%) of participants in each 10-year TC risk category following risk feedback | |||
---|---|---|---|---|
≤ 1.5% | 1.5–4.99% | 5–8% | > 8% | |
≤ 1.5% (n = 105) | 89 (84.76) | 16 (15.24) | 0 | 0 |
5–7.99% (n = 69) | 0 | 9 (13.04) | 54 (78.26) | 6 (8.70) |
≥ 8% (n = 513) | 0 | 19 (3.70) | 166 (32.36) | 328 (63.94) |
The process of risk feedback, although part of the planned PROCAS study, was not part of the originally funded programme grant. Therefore, full analyses for all participants recruited to PROCAS are still ongoing. However, based on the first 40,000 participants, we are able to report that referral for 18-monthly screening was offered to 330 women, of whom two declined, and referrals from primary care providers have been received for 260 women, with 23 already receiving screening through the FHC. Thus, 283 out of 330 women (86%) have commenced additional breast screening. Three breast cancers have now been detected on the interval 18-monthly breast screen: (1) a multifocal DCIS with an 8 mm and 4 mm focus of invasive grade 2 node-negative invasive ductal carcinoma in a 57-year-old; (2) a 51-year-old with a 15 mm grade 2 node-negative invasive ductal carcinoma; and (3) a 54-year-old with a 7 mm grade 1 node-negative invasive ductal carcinoma. Of the first 40,000 participants, 10 of the 575 with ≥ 8% 10-year risk were identified with breast cancer on the first mammogram; three of the five detected subsequently were picked up on the interval mammogram; one was an interval cancer before risk counseling and the other was picked up on the 3-year mammogram. Therefore, 15 (2.6%) cancers have occurred in those with a TC ≥ 8% 10-year risk. Six cancers (2.6%) have occurred in the 232 women with a 5–8% 10-year risk with ≥ 60% density: four at prevalent screen and two on the 3-year mammogram. Only nine breast cancers (0.64%) have occurred in the 1395 women with < 1.5% 10-year risks. After confirmation of the high-risk category, there have been 15 out of 441 (3.4%) who developed breast cancer.
Reattendance at screening
Of the first 40,000 participants, for high-risk women attending their risk feedback appointment the reattendance rate at the next 3-year screening was 93% (200/215). However, this rose to 99% (200/202) for those actually invited (six were aged over 70 years and therefore were not invited, one died and six had moved area). For low-risk women the reattendance rate was 81% (43/53). In addition, we were able to assess reattendance in those women who did not have risk feedback. Of those due to have a further mammogram, 112 out of 143 (78%) had reattended. Overall reattendance at the next 3-yearly screen was 411 out of 454 (90.5%) for those risk counselled, with high-risk reattendance significantly higher (p = 0.0006; p < 0.0001 for those invited) than for usual reattendance rates but reattendance rates for low-risk and those not counselled were not significantly lower (p = 0.65 and 0.065). Figures from the 2012–13 Greater Manchester NHSBSP showed that among women who attended their previous mammogram and whose last screen was within the last 5 years, 39,058 were invited and 32,925 (84.3%) attended. Overall reattendance at the next 3-yearly screen for women who attended their risk appointments over all three risk categories (high, moderate and low) was 90.5%. Reattendance was significantly higher for high-risk women invited for feedback (p = 0.015) than usual reattendance rates in Greater Manchester, but was not significantly lower for low-risk women and non-counselled women.
Risk perception
Of the first 40,000 participants, 253 out of 459 (55%) high-risk women and 56 out of 100 (56%) low-risk women filled in the risk perception questionnaire. Thirty-one high-risk women had previously attended the FHC for risk assessment between 1991 and 2011 (median 1997), some 1–20 years prior to their risk assessment in PROCAS. There was a clear trend for high-risk women to ascribe higher levels of risk to themselves than did low-risk women, and previously counselled women had more accurate risk perceptions for themselves and the general population than low-risk women. Women expressed risk in both descriptive categories and ORs as being higher in the high-risk group, although their estimates of the population risk were similar. Only a minority (37% high, 29% low) gave the ‘correct’ current lifetime risk range for the general population of 1 in 8–10, apart from those seen previously in the FHC (57%). 185
There have been no untoward adverse events in women at high risk that we are aware of, and women were content to receive their risk information and keen to take action despite 86% learning their risk for the first time. Women at low risk were pleased to be informed of their risk, but only one (1%) expressed a desire to cease screening.
The present study has shown that it is possible to collect and feedback risk information to women at both high and low risk of breast cancer from a large population-based mammography screening programme. We believe that this is the first study to both assess and feed back breast cancer risk information on a population basis on this scale. Women at high risk were more likely than those at low risk to perceive that they were high risk before risk counselling. This is most likely due to the almost certain presence of a family history of breast cancer in those at high risk and the absence of this in those at low risk. Accordingly, both attendance at risk counselling (69% vs. 52%) and reattendance at the subsequent mammography screen were significantly higher in women counselled about their high risk than in those told about their low risk (p < 0.0001). Indeed, high-risk women were more likely than the entire screening population to reattend. Low-risk women, the majority of whom had received prior screening, were reluctant to discontinue screening. It is reassuring to screening programmes, which are judged by reattendance rate, that there was not a significant drop-off in attendance at the subsequent screen when such a programme as PROCAS is introduced.
Women at high lifetime risk of breast cancer are now recommended in the UK to be offered annual mammography screening between 40 and 60 years of age. 142 There was a high take-up of the offer of additional screening in high-risk women in PROCAS; for the 14% not referred for extra screening by their GP, we are aware that this decision not to refer might have been the GP’s rather than the woman’s. It is reassuring that not only does the TC programme reliably identify women at high risk (the 2.6% detection rate can be considered to represent a 3-year period, including lead time: thus, 0.9% annually), but > 1% have been detected with extremely good prognosis stage 1 cancers at the interval screen. Although these numbers are small for the interval screens, they represent an extremely high rate. As all three of the cancers occurred in women in their fifties, even the grade 1 cancer would probably have presented during the woman’s lifetime and might not be considered an ‘overdiagnosis’.
This study has also assessed risk perception. Unlike many previous studies such as our own that were based mainly on women coming forward concerned about their breast cancer risks owing to family history,187–189 the present study addresses risk perception in women at either end of the risk spectrum from the general population, the great majority of whom had not been assessed previously. Risk perception was, as reported previously, not overly accurate;187,189 however, high-risk women were significantly more likely than low-risk women to assess their risks as above average in both a verbal and an OR format. Perception of population risk was not statistically significantly different between the two previously uncounselled groups. However, those seen previously in the FHC had better overall risk perceptions, as we have reported before. 188
There are some limitations to the present study. Although the study represents sampling of the whole screening population, only 43% of those screened joined the study. This could have biased the population to women with higher risks. A survey alongside our FHC did not suggest that this was the case, with the proportion identified as moderate risk from family history alone not being higher than those already identified in the 40–49 years age group in our region. 190 We have not conducted formal assessment of the impact of risk information on anxiety and intention to change behaviour, although funding has been sought for this and this is planned in a new prospective arm. There were some inaccuracies in women’s filling-in of the questionnaires, particularly in relation to bilateral disease and the timing of menopause. In future, using an online version with prompts and pop-up questions to confirm these areas is likely to improve accuracy. Certainly, if only a paper questionnaire is used, confirming details in those whose management will change is important, as this may change risk category substantially. This means that, for those identified at high risk, further assessment is necessary. This is likely in practice, as women identified at high risk from questionnaire data would usually be offered referral.
Measurement of mammographic density in PROCAS
In the initial proposal, we planned to undertake visual assessment of mammograms using three breast density measures: VASs, Cumulus thresholding and the Manchester Stepwedge (for mammograms recorded on film).
Assessment using VASs had previously been employed by mammographic film readers in Manchester in CADET (Computer-Aided Detection Evaluation Trial),191 and a significant association with cancer risk was found. 167 Cumulus thresholding has been widely used in research studies and the relationship with risk of developing cancer is well established. 26,87 However, the research that underpins these relationships was based on analysis of film mammograms, and the NHSBSP was in the process of a transition to FFDM at the time the PROCAS study commenced. Risk relationships for digital mammography are at an early stage because of a lack of longitudinal data. The appearance of digital mammograms differs significantly from that of FFDM images, despite the application of post-processing. VAS and Cumulus are both area-based methods; they are assessments of a two-dimensional image of a three-dimensional structure. Consequently, they provide estimates of the proportion of the breast area occupied by dense fibroglandular tissue, and the estimates can vary depending on the way in which the breast is positioned. The third method we proposed to use was one we developed ourselves and which was evaluated in a pilot trial. 168 This involved the analysis of digitised film mammograms by imaging a calibrated stepwedge alongside the breast. Preliminary evaluation of our own method and a similar one developed by colleagues in California192 suggests that calibration techniques provide risk information. Such methods were particularly attractive in the context of the NHSBSP, as an automatic read-out could be obtained from digitised mammograms, which could be integrated with risk information by appropriate computer programs.
As PROCAS progressed, two other breast density methods became available to us. These were both designed for use with FFDM images, provided that the raw (‘for processing’) data were available. The first of these is Quantra, from Hologic, which we obtained in July 2010. We started to run Volpara from Volpara Solutions in September 2010. Both of these methods were based on the work of Highnam and Brady,170 originally for film mammograms, which models the physics of the imaging process and enables the computation of volumetric breast density.
Visual estimation of percentage density using visual assessment scores
Screening mammograms are routinely reviewed by pairs of readers, with arbitration by a further pair, if required. Reader pairs generally comprise a consultant radiologist or a breast physician working with an advanced practitioner radiographer, but pairings are pragmatic, with the proviso of a maximum of one advanced practitioner radiographer in a pair. In analogue (film) mammography, the mammograms are displayed on an illuminated viewer, and for digital mammography, the images are presented on high-resolution monitors. In PROCAS, readers assess MD at the time of reading the screening films, recording estimates for all four views on a single paper form containing four 10-cm horizontal VASs, labelled 0% and 100% at the left and right ends, respectively. The two readers complete VAS forms independently. The forms are digitised and processed by custom software which reads the patient identification number, finds the positions of the scales and marks, converts them to percentage densities and outputs the results in a spreadsheet. As visual assessment is subjective, it suffers from intra- and interobserver variability. To improve consistency between readers, we developed a method for correcting values adjusting for each reader. 193
Cumulus thresholding
Cumulus thresholding is applicable to both digitised film mammograms and FFDM images; for film mammograms we used a Vidar CAD-PRO digitiser. Cumulus software was obtained for use in this project, and training was undertaken in January 2010 by one of us (RW) (see Contributions of authors) who had been trained by the Toronto team that developed the software. Although several readers were trained, assessment using Cumulus was undertaken by a single reader (JS), whose performance was validated on test sets of data developed for this purpose by RW and the Toronto team. It takes approximately 1 day to analyse 200 images, so a single MLO view (the contralateral breast for cancer cases) was analysed for each woman in the FH-Risk cohort and a case–control set in the PROCAS-screening women. Analysis involves delineating the pectoral muscle and adjusting thresholds to identify the breast area and glandular component. The method produces measures of breast area, dense tissue area and hence fat area and area-based percentage density. As Cumulus is based on an operator’s assessment, it is subject to intra- and interobserver variability.
Manchester Stepwedge
The Manchester Stepwedge method is applicable only to digitised mammograms on which the calibrated aluminium stepwedge and thickness markers (on the breast compression plate) have been imaged. Calibration data were obtained for each analogue mammography unit used in PROCAS. To analyse the images, digitisation was undertaken using a Vidar CAD-PRO digitiser. An operator then ran custom software which locates the stepwedge and the compression markers in each image, providing an opportunity to review the automated detections and correct them as necessary. Analysis of the distance between pairs of markers in the mammogram enables accurate measurement of compressed breast thickness, taking into account tilt of the compression plate. The brightness of each pixel in the mammogram can be matched to the stepwedge image and, with the compressed thickness and calibration data, this enables computation of the thickness of fibroglandular tissue. The method accounts for differences in compression, plate tilt, imaging parameter changes and the drop-off in breast thickness where the breast loses contact with the compression plate. It outputs a measure of the volume of fat and gland in the breast and hence percentage density by volume.
Quantra
Quantra, from Hologic, is applicable to FFDM images obtained on Hologic 2D Systems (Hologic, Inc., Marlborough, MA, USA), GE 2D Systems (GE Healthcare Life Sciences, Buckinghamshire, UK) or Siemens Mammomat Novation Systems (Siemens Medical Solutions USA, Inc., Malvern, PA, USA) for which the raw (‘for processing’) data are available. It is a fully automated method that uses a model of the physics of the imaging process along with data from the DICOM image header to calculate the thickness of fibroglandular tissue at each pixel position in the image. It provides values for each screening view, each breast and per patient, giving the volume of the breast in cubic centimetres, volume of fibroglandular tissue (in cubic centimetres), percentage density by volume, a BI-RADS-like score and the area of dense tissue as a percentage of breast area. During the course of PROCAS, the version of software changed, and we are now using version 2.0.
Volpara
Volpara, from Volpara Solutions, is able to process images from a range of manufacturers (Hologic, GE, Siemens and Fuji). It is a fully automated method in which knowledge of tissue attenuation coefficients, the physics of the imaging process and information in the DICOM header are used to compute glandular thickness at each pixel position. Volpara uses a relative physics model which reduces the need for accurate imaging physics data, but depends on locating a suitable fatty reference area within the image. 172,194 Volpara outputs the fibroglandular tissue volume, total breast tissue volume, percentage of density by volume, and a Volpara Density Grade correlated with BI-RADS. The software developed during the course of the PROCAS study, with the most recent version used being 1.4.5.
Mammogram data in PROCAS
The mammogram data used in the PROCAS study have been obtained using analogue mammography and two different types of FFDM system [Fischer Senoscan (Carestream Health Inc., Rochester, NY, USA) and GE Essential (GE Medical Systems Ltd, Chalfont St Giles, UK)]. At the outset of the study, the raw digital data were not collected. Table 36 gives a summary of the data available for analysis and the MD methods available for each data source.
System | Number of cases | VAS | Quantra | Volpara | Stepwedge | Cumulus |
---|---|---|---|---|---|---|
Analogue | 6787 | ✓ | ✓ | ✓ | ||
Fischer | 1724 | ✓ | ||||
GE (no raw data) | 4200 | ✓ | ||||
GE (raw data) | 38,861 | ✓ | ✓ | ✓ | ✓ |
Figure 25 shows the mammographic percentage density distributions for VAS, Quantra and Volpara for all women recruited to the PROCAS study. In total, 50,831 women had VAS MD assessment with a mean percentage density of 27.4, compared with 38,706 women who had Volpara measurement and mean density of 7.05 and 36,014 women who had Quantra measurement and a mean density of 12.01.
FIGURE 25.
Measurement of density assessment for VAS, Volpara and Quantra. (a) Mean = 27.396, SD = 17.079, n = 50,831; (b) mean = 7.05, SD = 4.037, n = 38,706; and (c) mean = 12.01, SD = 7.113, n = 36,014. SD, standard deviation.

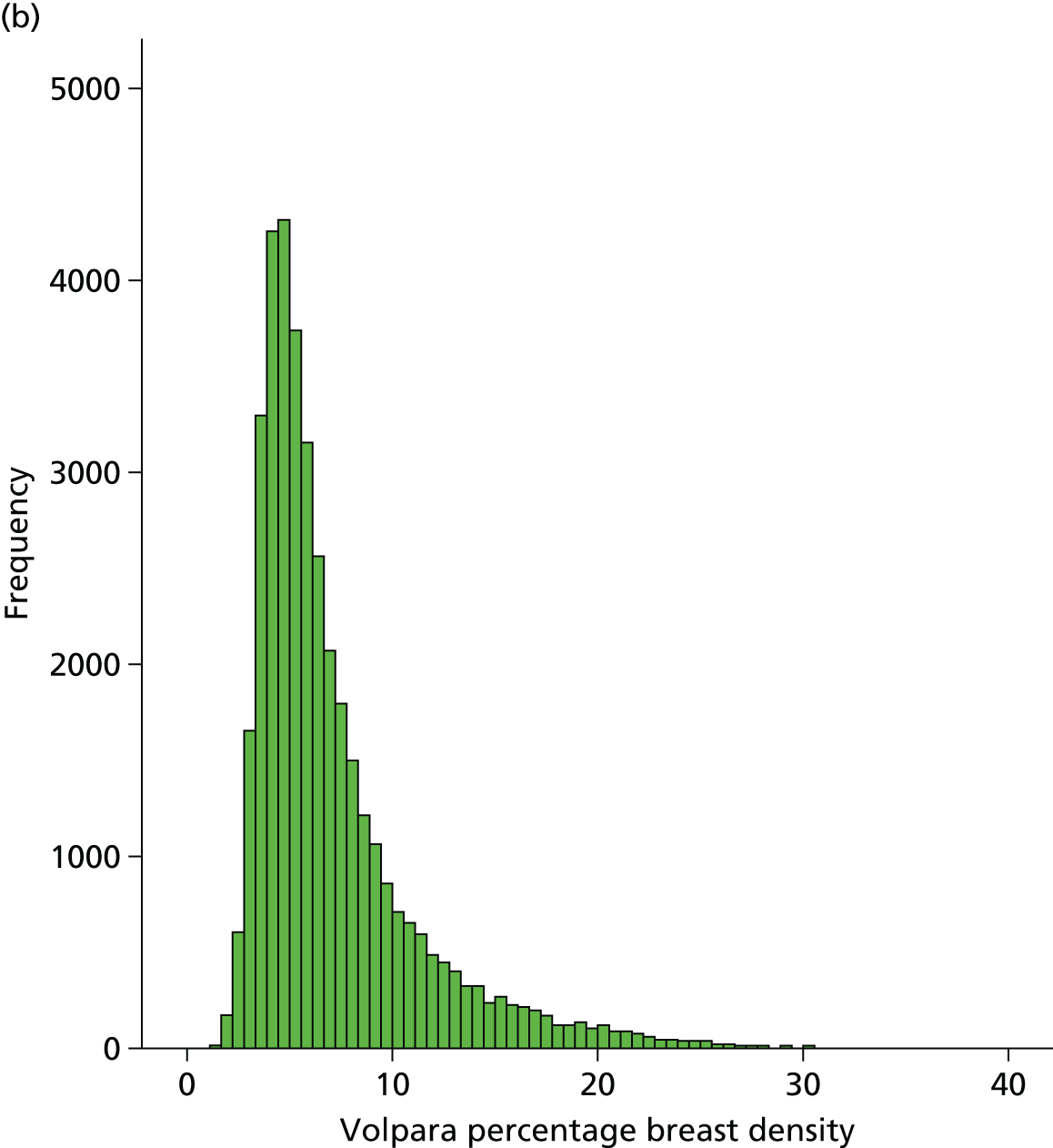
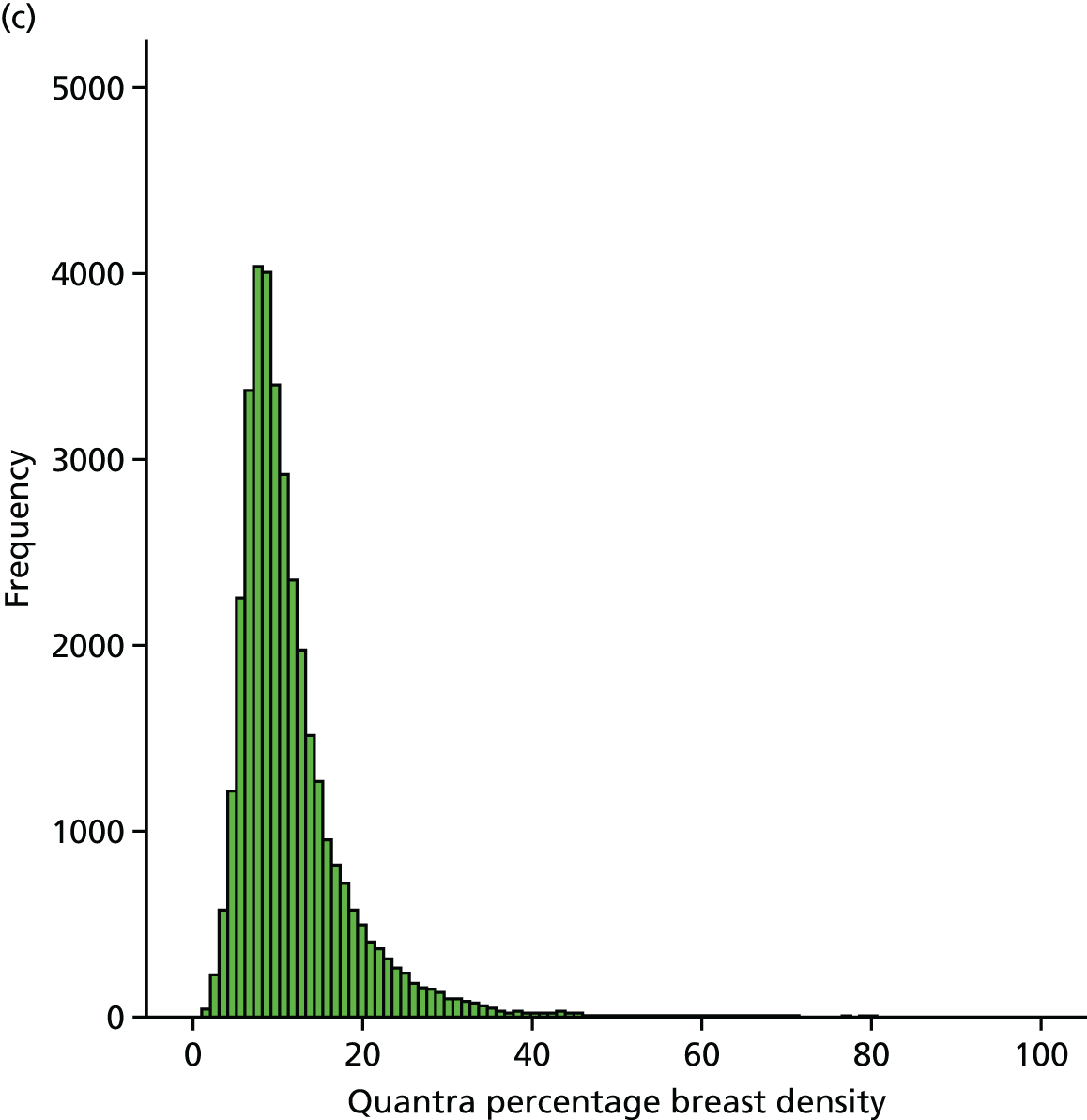
Analysis of density data
We have undertaken a number of different analyses of MD data in PROCAS. The most comprehensive is a cohort analysis comparing the density of cancer cases with that of non-cancer cases. We have also undertaken a more rigorous case–control study which compares the density of the contralateral breast of women with unilateral screen-detected cancer in FFDM images with the density of the same breast in matched controls. Ideally, we would have evaluated density in the prior mammograms (the screening mammograms prior to cancer detection) as this would have provided a genuine assessment of the ability of MD to predict cancer. Within PROCAS this is not currently possible for digital data, as insufficient women have had a cancer at their second screen, or in the interval following the first screen; however, as the data become available we will undertake this analysis. The contralateral breasts of women with unilateral cancers are used as a surrogate for the priors, and MD is assessed by all available methods. Priors are when a previous mammogram is available before the mammogram which detected the cancer. We present a small case–control analysis of the film priors of cancer cases compared with matched controls.
We have also undertaken analyses of the impact of HRT, parity and menopausal status on volumetric MD; of the inter- and intraobserver variability in visual assessment of MD;195 of the relationship of MD to ethnicity;196 and of factors affecting density assessment. 197 These analyses are described below, along with a method that we have developed which enables the adjustment of visual assessments to compensate for differences in practice between observers. 193 Finally, we have evaluated the potential of using automated measures of breast volume to predict self-reported weight and BMI;198 the acquisition of weight data is problematic, and an automated, objective approach would be helpful.
Cohort study
Aim
The aim of this study was to compare the MD of women who developed breast cancer with that of those who did not, and hence to evaluate the performance of the different density assessment methods (VAS, Volpara and Quantra) employed in the PROCAS study.
Methods
Design
The study design was a large cohort study of women from the Greater Manchester area who were invited for breast cancer screening from October 2009 to June 2014. Cases were those who developed breast cancer while taking part in the PROCAS study, while controls were those who did not develop breast cancer while taking part in the PROCAS study. Density was assessed using all mammographic views. Mammograms were mainly analogue in the initial 12-month period (October 2009–September 2010), switching to completely digital thereafter (October 2010–June 2014). The date of entry to the study was the date of mammogram at study entry. Although the study was notified of breast cancers by the three methods below, a determined ‘last date of follow-up’ was not possible, as the study could not be certain that a participant was not affected by breast cancer on a certain date using the case ascertainment method.
Identification of cases
Cancer cases were identified by one of three methods:
-
Matching the PROCAS data set to the Somerset Cancer Registry. The Somerset Cancer Registry is a ‘real-time’ database that collects information about the patient journey. 199
-
Matching with the NWCIS database, a histological database of breast cancer diagnoses, for cancers diagnosed in the north-west. 200
-
Notifications from participants that they had been diagnosed with breast cancer.
The three sources of information were cross-checked and all cancer diagnoses were validated.
Inclusion criteria
To enable processing with Volpara and Quantra, all women in this study had to have GE digital screening mammograms with raw (‘for processing’) image data as well as MD assessment on VAS. Mammograms were mainly analogue in the initial 12-month period (October 2009–September 2010), switching to completely digital thereafter (October 2010–June 2014).
Exclusion criteria
All women with a previous diagnosis of cancer were excluded from this study, as were those who did not have MD assessment by all three methods. These were the only exclusion criteria.
Outcome
The outcome for this study was the development of breast cancer by June 2014.
Density assessment
Mammographic density for the first mammogram while taking part in the PROCAS study was assessed by an area-based method (VAS) and volumetric methods (Volpara and Quantra). The VAS measures were made by two independent readers per case, drawn from a pool of 17 readers, and averaged. Version 1.4.5 of Volpara and version 2.0 of Quantra were used to obtain volumetric density data. For Volpara, the mean density across the four views was used, while for Quantra the maximum was used, in accordance with recommended practice.
Statistical analysis
In order to examine the relationship between density methods and case–control status, analysis was performed using logistic regression (in SPSS). Univariate associations were performed using quartiles for each density measure, with the lowest quartile as the referent category. Further multivariate associations were performed adjusting for age, menopausal status and BMI.
Results
In total, 33,543 women had MD assessment by all three density measures, of whom 401 had a previous diagnosis of cancer and were, therefore, excluded from this particular study. This left 33,142 women, of whom 437 developed breast cancer (1.32%).
Table 37 shows the number and percentage of cases and controls in each quartile for each density measure, as well as the univariate and multivariate associations. In the univariate analysis, all density measures, with the exception of Volpara percentage density, were associated with an increased risk of developing breast cancer. The strongest association was for VAS, with those in the highest quartile having twice the odds of developing breast cancer of those in the lowest quartile. Corresponding odds for Volpara dense volume, Quantra dense volume and Quantra percentage density were in the region of 1.5–1.7. Further adjustment for age, menopausal status and BMI made the associations with the third quartile of Volpara of dense volume and second quartile of Quantra percentage density non-significant, but the highest quartile of Volpara became statistically significant (OR 1.60, 95% CI 1.15 to 2.23). The other associations with Volpara and Quantra were of similar magnitude to those in the univariate analysis. On the other hand, the ORs for VAS increased further after adjustment for age, menopausal group and BMI, with those in the highest quartile having an OR of developing cancer of 2.75 compared with those in the lowest quartile.
Mammography density assessment | Controls, n (%) | Cases, n (%) | Univariate, OR (95% CI) | Multivariate (adjusted for age, BMI and menopausal status), OR (95% CI) |
---|---|---|---|---|
VAS | ||||
< 14 | 8373 (25.6) | 68 (15.6) | 1.00 (referent) | 1.00 (referent) |
14–23 | 7758 (13.7) | 107 (24.5) | 1.70 (1.25 to 2.31) | 2.04 (1.48 to 2.81) |
24–36 | 8166 (25.0) | 117 (26.8) | 1.76 (1.31 to 2.38) | 2.05 (1.48 to 2.84) |
> 36 | 8408 (25.7) | 145 (33.2) | 2.12 (1.59 to 2.84) | 2.75 (1.99 to 3.81) |
Volpara dense volume (cc) | ||||
< 35 | 7863 (24.0) | 82 (18.8) | 1.00 (referent) | 1.00 (referent) |
36–46 | 8673 (26.5) | 112 (25.6) | 1.24 (0.93 to 1.65) | 1.19 (0.89 to 1.61) |
46–61 | 8264 (25.3) | 115 (26.3) | 1.33 (1.00 to 1.77) | 1.31 (0.97 to 1.78) |
> 61 | 7905 (24.2) | 128 (29.3) | 1.55 (1.18 to 2.05) | 1.57 (1.15 to 2.14) |
Volpara percentage density | ||||
< 4.4 | 8073 (24.7) | 96 (22.0) | 1.00 (referent) | 1.00 (referent) |
4.4–5.7 | 8260 (25.3) | 112 (25.6) | 1.14 (0.87 to 1.50) | 1.19 (0.89 to 1.60) |
5.8–8.2 | 8167 (25.0) | 112 (25.6) | 1.15 (0.88 to 1.52) | 1.33 (0.98 to 1.81) |
> 8.2 | 8199 (25.1) | 117 (26.8) | 1.20 (0.92 to 1.58) | 1.60 (1.15 to 2.23) |
Quantra dense volume (cc) | ||||
< 60 | 8123 (24.8) | 81 (18.5) | 1.00 (referent) | 1.00 (referent) |
60–87 | 8046 (24.6) | 105 (24.0) | 1.31 (0.98 to 1.75) | 1.33 (0.98 to 1.80) |
88–129 | 8477 (25.9) | 118 (30.4) | 1.40 (1.05 to 1.86) | 1.47 (1.08 to 1.99) |
> 129 | 8059 (24.6) | 133 (30.4) | 1.66 (1.25 to 2.19) | 1.72 (1.24 to 2.38) |
Quantra percentage density | ||||
< 8 | 6924 (21.2) | 69 (15.8) | 1.00 (referent) | 1.00 (referent) |
8–10 | 10,507 (32.1) | 139 (31.8) | 1.33 (0.99 to 1.78) | 1.24 (0.92 to 1.68) |
11–13 | 6620 (20.2) | 99 (22.7) | 1.50 (1.10 to 2.04) | 1.50 (1.09 to 2.06) |
> 13 | 8654 (26.5) | 130 (29.7) | 1.51 (1.12 to 2.02) | 1.67 (1.22 to 2.27) |
Discussion
In the PROCAS cohort, for whom VAS assessment and the two volumetric methods were used, VAS showed the strongest associations with the development of breast cancer, but all methods showed some associations. For the cancer cases, the image including the cancer was included in the analysis. We have established that in the majority of cases the difference in volumetric density between mammographic images with cancer and the opposite (cancer-free) breast is small,197 but the inclusion of diagnostic images showing cancer might have slightly increased the average density of the cases.
One limitation of this analysis is that it includes a mix of prior and diagnostic mammograms; however, owing to the transition of mammography from film screen to digital during the course of the PROCAS project and the necessity of using FFDM images for this study, most of the cancers are diagnostic mammograms. Until we have a larger temporal data set, we are unable to comment on the way in which density changes prior to and at the time of diagnosis, but this will remain a longer-term aim. As the questionnaire was administered at the time of initial mammography in PROCAS, the covariate information may be less accurate for those women with cancers detected as interval cancers or at a subsequent screen.
In this analysis we carried out adjustment for a limited number of factors (age, BMI and menopausal status); however, further adjustment for other factors such as HRT and parity will be an important next step. Another issue with these data is that for Volpara the mean density assessed across the four views was used, while for Quantra the maximum was used. It would be interesting to evaluate both of these strategies on both volumetric methods to establish which produces the most predictive estimates of density.
Case–control study
Aim
The aim of this study was to compare MD in the contralateral breast of screen-detected cancers at the time of diagnosis with that of matched controls and hence to evaluate the performance of different density assessment methods employed in the PROCAS study.
Methods
Design
The study design was a case–control study, in which cases were those who developed unilateral breast cancer during their initial screening round while taking part in the PROCAS study. Cases were matched to controls whose mammograms were deemed cancer free at both the initial and the subsequent screening rounds. For controls, the mammograms from the initial screening round were analysed.
Inclusion criteria
To enable processing with Volpara and Quantra all women had GE digital screening mammograms with raw (‘for processing’) image data. For analysis with Cumulus, processed (‘for presentation’) images were required. For inclusion as a cancer case, breast cancer was identified at the first screen following recruitment to the PROCAS study. For inclusion as a control, a cancer-free screening mammogram subsequent to the initial screen in the PROCAS study was required. These criteria ensured that that risk information was current for the mammograms analysed, and that the control mammograms were unlikely to show early signs of cancer.
Exclusion criteria
All women with a previous diagnosis of cancer were excluded from the study. Cancer cases were excluded if they had bilateral breast cancer or unknown laterality. Women with breast implants and those with unacceptable values for BMI calculated from self-reported height and weight (< 10 kg/m2 or > 60 kg/m2) were also excluded from analysis.
Questionnaire data
PROCAS questionnaire data were used to obtain age, menopausal status and self-reported height and weight.
Identification of cases
Cancer cases were identified by one of three methods:
-
Matching the PROCAS data set to the Somerset Cancer Registry. The Somerset Cancer Registry is a ‘real-time’ database that collects information about the patient journey. 199
-
Matching with the NWCIS database, a histological database of breast cancer diagnoses, for cancers diagnosed in the north-west. 200
-
Notifications from participants that they had been diagnosed with breast cancer.
The three sources of information were cross-checked and all cancer diagnoses were validated.
Cancers were categorised into those detected at the initial screen in PROCAS and those detected at a subsequent screen, and then those which were obtained on a GE system and had raw data were identified. A total of 324 cancer cases were identified who matched the inclusion and exclusion criteria.
Matching
Controls were identified from the existing PROCAS study database. As they were required to have two screens in the PROCAS study, women with two screening appointments more than 180 days apart were identified. These women were then matched to the PROCAS data set on NHS number and date of the initial mammogram after recruitment to PROCAS, and the exclusion criteria listed above were applied.
Cancer cases were matched to three controls on the basis of age (within 6 months), menopausal status (premenopausal, perimenopausal, postmenopausal or unknown), HRT use (current, never or previous) and BMI categories (underweight < 18.5 kg/m2, normal weight 18.5–24 kg/m2, overweight 25–29 kg/m2 and obese > 30 kg/m2). When an exact match was not possible, the matching criteria were relaxed, for example age matched within 1 year or BMI matched to the next category.
Density assessment
Mammographic density was assessed by area-based methods (VAS and Cumulus) and volumetric methods (Volpara and Quantra). The VAS measures were made by two independent readers per case, drawn from a pool of 17 readers. Cumulus was undertaken by a single trained and validated reader (JS) who assessed a single MLO view of 180 cancers and 540 controls, presented in random order in four batches, each containing approximately 50 cancers and 150 matched controls. The assessor was blinded to case–control status. Version 1.4.5 of Volpara and version 2.0 of Quantra were used to obtain volumetric density data.
Statistical analysis
The data were merged into a single database for statistical analysis. The demographic characteristics were reported as number and percentage by case–control status. Comparisons of categorical data were made using the chi-squared test. For those variables for which the data were ordinal, a chi-squared test for trend was also conducted. Continuous variables were assessed by means of an unpaired sample t-test when the distribution was normally distributed or by the Mann–Whitney U-test when the distribution was not normally distributed.
To examine the relationship between density methods and case–control status, analysis was performed using conditional logistic regression (in SPSS) owing to the matched nature of the data set. Univariate associations were performed initially, and multivariate associations were performed adjusting for breast area (for Cumulus) and for breast volume (for Volpara and Quantra).
Results
Table 38 shows the composition of the case–control data set. There was no significant difference between cases and controls in any of the descriptors listed, apart from the TC risk score computed at entry to the PROCAS study, which was significantly higher for cases than for controls (p < 0.05).
Parameter assessment | Control subjects (N = 972), n (%) | Case subjects (N = 324), n (%) |
---|---|---|
Age (years) | ||
< 50 | 53 (5.5) | 19 (5.9) |
50–54 | 252 (25.9) | 82 (25.3) |
55–59 | 154 (15.8) | 52 (16.0) |
60–64 | 234 (24.1) | 78 (24.1) |
65–69 | 199 (20.5) | 67 (20.7) |
≥ 70 | 80 (8.2) | 26 (8.0) |
Mean (SD) | 59.99 (7.05) | 60.02 (7.17) |
Menopausal status | ||
Perimenopausal | 116 (11.9) | 38 (11.7) |
Postmenopausal | 717 (73.8) | 241 (74.4) |
Premenopausal | 96 (9.9) | 33 (10.2) |
Unknown | 43 (4.4) | 12 (3.7) |
HRT | ||
Never | 583 (60.0) | 212 (65.4) |
Previous | 335 (34.5) | 92 (28.4) |
Current | 54 (5.6) | 18 (5.6) |
BMI (kg/m2) | ||
< 25 | 281 (28.9) | 92 (28.4) |
25–29 | 342 (35.2) | 112 (34.6) |
≥ 30 | 287 (29.5) | 95 (29.3) |
Unknown | 62 (6.4) | 25 (7.7) |
Mean (SD) | 28.41 (5.71) | 28.08 (5.19) |
Ethnic origin | ||
Asian/Asian British | 6 (0.6) | 3 (0.9) |
Black/black British | 11 (1.1) | 0 (0) |
Jewish | 10 (1) | 1 (0.3) |
Mixed | 6 (0.6) | 3 (0.9) |
Other | 15 (1.5) | 9 (2.8) |
White | 917 (94.3) | 300 (92.6) |
Initial TC | ||
Mean (SD) | 3.13 (1.42) | 3.53 (2.29) |
Alcohol | ||
No | 254 (26.1) | 76 (23.5) |
Yes | 702 (72.2) | 240 (74.1) |
Unknown | 16 (1.6) | 8 (2.5) |
Any children | ||
No | 112 (11.5) | 40 (12.3) |
Yes | 859 (88.4) | 284 (87.7) |
Biopsy of breast | ||
No | 794 (81.7) | 254 (78.4) |
Yes | 151 (15.5) | 57 (17.6) |
Not known | 27 (2.8) | 13 (4.0) |
The mean age of the women was 60 years. Seventy-four per cent were postmenopausal, and 94% indicated that they were not current users of HRT. The mean BMI was approximately 28 kg/m2 (overweight), with about one-third of women in each of the BMI categories. The majority of women declared their ethnicity as white. In the cancer group, and hence in the controls, there was an equal split with regard to the laterality of the cancer.
Table 39 shows univariate analysis of MD measured by the area-based methods.
Parameter assessment | Control subjects (N = 972), n (%) | Case subjects (N = 324), n (%) | Univariate analysis, OR (95% CI) |
---|---|---|---|
VAS | |||
< 12.5 | 263 (27.7) | 45 (14.1) | 1.00 (referent) |
12.5–22 | 261 (27.4) | 85 (26.6) | 1.98 (1.32 to 2.98) |
23–35 | 215 (22.6) | 75 (23.4) | 2.23 (1.45 to 3.43) |
≥ 36 | 212 (22.3) | 115 (35.9) | 3.59 (2.37 to 5.43) |
Mean (SD) | 23.69 (15.1) | 29.89 (16.65) | |
Cumulus percentage density | |||
Mean (SD) | 21.2 (12.81) | 24.06 (13.46) | |
< 12 | 148 (27.4) | 40 (22.2) | 1.00 (referent) |
12–19 | 129 (23.9) | 39 (21.7) | 1.18 (0.71 to 1.95) |
20–29 | 140 (25.9) | 47 (26.1) | 1.42 (0.84 to 2.39) |
≥ 30 | 123 (22.8) | 54 (30.0) | 1.93 (1.12 to 3.34) |
Cumulus dense area (mm3) | |||
Mean (SD) | 54,907 (33,440) | 60,832 (32,439) | |
< 32,000 | 144 (26.7) | 35 (19.4) | 1.00 (referent) |
32,000–50,799 | 136 (25.2) | 44 (24.4) | 1.37 (0.82 to 2.29) |
50,800–72,999 | 133 (24.6) | 50 (27.8) | 1.60 (0.97 to 2.64) |
≥ 72,300 | 127 (23.5) | 51 (28.3) | 1.76 (1.04 to 2.95) |
Density measured using VAS was significantly associated with cancer status, and showed a dose–response relationship with increasing density (χ2 trend 33.3; p = 0.000). Those in the highest quartile of dense area and percentage density for Cumulus had an increased likelihood of cancer (OR 1.76 and 1.93, respectively), compared with those in the lowest quartile. Adjustment for breast area made little difference to the ORs for Cumulus dense area and Cumulus percentage density. For Cumulus dense area, the OR for the highest category became 1.87, with 95% CI 1.10 to 3.19. For Cumulus percentage density, the OR for the highest density group, following adjustment for breast area, was 1.80 with 95% CI 1.03 to 3.15. Table 40 shows univariate analysis of the volumetric MD measures.
Parameter assessed | Control subjects (N = 972), n (%) | Case subjects (N = 324), n (%) | Univariate analysis, OR (95% CI) |
---|---|---|---|
Volpara gland volume (cm3) | |||
< 35 | 249 (25.8) | 63 (19.7) | 1.00 (referent) |
35–46 | 254 (26.3) | 93 (29.2) | 1.46 (1.02 to 2.10) |
47–60 | 229 (23.7) | 74 (23.2) | 1.33 (0.90 to 1.96) |
≥ 60 | 233 (24.1) | 89 (27.9) | 1.56 (1.05 to 2.3) |
Mean (SD) | 50.6 (23.51) | 54.97 (28.67) | |
Volpara breast density (%) | |||
< 4 | 211 (21.9) | 42 (13.2) | 1.00 (referent) |
4–5.49 | 280 (29.0) | 95 (29.9) | 1.88 (1.23 to 2.86) |
5.5–7 | 253 (26.2) | 95 (29.9) | 2.15 (1.39 to 3.32) |
≥ 7 | 220 (22.8) | 86 (27) | 2.33 (1.46 to 3.72) |
Mean (SD) | 6.52 (3.58) | 7.25 (4.1) | |
Quantra gland volume (cm3) | |||
Mean (SD) | 112.3 (74.3) | 111.9 (76.3) | |
< 70 | 264 (27.3) | 98 (30.6) | 1.00 (referent) |
70–94 | 204 (21.1) | 73 (22.8) | 0.95 (0.66 to 1.37) |
95–134 | 251 (26.0) | 66 (20.6) | 0.71 (0.49 to 1.02) |
≥ 135 | 248 (25.6) | 83 (25.9) | 0.86 (0.59 to 1.27) |
Quantra breast density (%) | |||
Mean (SD) | 12.2 (6.73) | 12.3 (6.67) | |
< 9 | 270 (27.9) | 95 (29.7) | 1.00 (referent) |
9–10 | 205 (21.2) | 59 (18.4) | 0.84 (0.57 to 1.22) |
11–14 | 268 (27.7) | 94 (29.4) | 0.99 (0.70 to 1.40) |
≥ 15 | 224 (23.2) | 72 (22.5) | 0.91 (0.62 to 1.33) |
Volpara percentage density showed an association with cancer status and a dose–response relationship with increasing density (χ2 trend 9.2; p = 0.002). The relationship with Volpara gland volume was less clear.
Adjustment for breast volume for Volpara increased the OR of the highest percentage density group to 2.61 with 95% CI 1.55 to 4.39, and for Volpara gland volume the OR of the highest group was increased to 1.72 with 95% CI 1.12 to 2.64. There was no association between MD measured by Quantra and cancer status, even after adjustment for breast volume.
Discussion of mammographic density measures
We performed a matched case–control analysis using the contralateral breast images of women with unilateral breast cancer to determine which MD method showed the strongest association with the presence of cancer. Much of the literature on MD and risk is based on relative, area-based measures applied to film mammograms, but in the PROCAS study the vast majority of mammograms are FFDM images and hence are amenable to processing by automated volumetric density software. This had the potential additional benefits of allowing absolute rather than relative density measures, which should be less susceptible to change in weight, which has previously been associated with a change in the fatty content of the breast. 201 Such methods enable objective measurement that is independent of observer bias and imaging parameters, and is reproducible and feasible on a large scale. However, in this analysis, subjective assessment by mammographic readers demonstrated the strongest relationship with cancer, despite known interobserver variability. 202
Volumetric measures fared less well, with the exception of percentage density measured using Volpara. Commercial volumetric measures were developed to fulfil a need for density assessment in the USA, where readers in many states are obliged by law to inform women of their MD; most readers currently use the subjective BI-RADS categorisation, but FDA-approved volumetric methods are an attractive alternative in a litigious environment. Volumetric measures are thus used most often not to identify risk of developing cancer, but to identify women for whom mammography is less effective. However, the volumetric software from both manufacturers is evolving to quantify density more accurately in response to the drive for personalised screening. It is possible that the relationship of dense tissue to fat in area-based measures is more strongly related to cancer risk than that in volumetric measures, and that using volumes of fat and gland independently and in different proportions may provide improved risk prediction. Furthermore, manufacturers of volumetric density software have recently begun to output area-based measures of density. Our results also indicate that correcting MD measures for breast volume may help in strengthening the association with cancer.
Visual assessments made by the readers in the PROCAS study are subject to inter- and intraobserver variability. 202 The VAS density readings were undertaken in a pragmatic fashion at the time of radiological assessment of the images rather than in a carefully managed, artificial environment. Despite this, the average VAS reading from the pair of readers was found to be associated with cancer, with the OR increasing for higher density estimates. VAS reading is relatively time-consuming and required subsequent automated analysis of the VAS forms to convert the markers into percentages. It would, however, be straightforward to computerise the process, with readers sliding a cursor to indicate percentage. As such, VAS was chosen for incorporation into the best-performing prediction model for the purposes of this report. VAS was also available on all subjects.
The semiautomated thresholding approach showed some relationship with cancer, but was not as effective as either VAS reading or Volpara percentage density. All of the Cumulus assessments took place in a limited time period by a single observer blinded to case–control status, but this was a considerable time after reader training and validation, and the images were acquired using FFDM, unlike those used for validation. Cumulus is impractical for large-scale use, as it is labour intensive and requires a skilled operator.
Density case–control study of film priors using the Manchester Stepwedge and visual assessment score
Aim
The aim of this case–control study was to compare MD in the screening round prior to detection of breast cancer using a case–control methodology. Differences were compared using an area-based (VAS) and a volumetric-based (Manchester Stepwedge) measure.
Methods
The study design was case–control, in which cases were those who developed unilateral breast cancer after their first screening round while taking part in the PROCAS study. Cases, therefore, had to have a ‘normal’ screen prior to developing breast cancer during their second screen or between screens. Cases were matched to controls who were deemed to be cancer free at both the initial and the subsequent screening rounds. For cases and controls, the mammograms from the initial screening round were analysed.
To enable processing with the Manchester Stepwedge, only data from women who were imaged using analogue mammography with the stepwedge calibration object in position at the first screen following recruitment to the PROCAS study were included. All women with a previous diagnosis of cancer were excluded from the study. Cancer cases were excluded if they had bilateral breast cancer or unknown laterality. Controls were also identified from the PROCAS study database as women with two screening appointments more than 180 days apart, and an initial PROCAS film mammogram showing a stepwedge imaged alongside the breast. For controls, the subsequent screening mammogram was read as cancer free. Women with breast implants and those with infeasible values for BMI calculated from self-reported height and weight (< 10 kg/m2 or > 60 kg/m2) were also excluded from analysis. Questionnaire data were used to obtain age, menopausal status and self-reported height and weight.
Cancer cases were matched to one control on the basis of age (within 1 year), menopausal status (premenopausal, perimenopausal, postmenopausal or unknown), HRT use (current, never or previous) and BMI categories (underweight < 18.5 kg/m2, normal weight 18.5–24 kg/m2, overweight 25–29 kg/m2 and obese > 30 kg/m2). When an exact match was not possible, the matching criteria were relaxed, for example age matched within 18 months or BMI matched to the next category.
Density assessment
Mammographic density of cases was assessed in the prior mammogram of the breast that developed breast cancer; for controls, density of the same breast as that of their matched case was used. The VAS measures were made by two independent readers per case, drawn from a pool of 17 readers. The Manchester Stepwedge software was used to produce results of volumetric breast densities for both groups of patients. The programme enabled the operator to identify the stepwedge and the positions of radio-opaque markers along the edges of the mammogram. These data were used along with calibration data to estimate the thickness of dense tissue at all points in the compressed breast image. The software outputs breast volume, dense volume and percentage of dense tissue (dense volume as a proportion of breast volume)
Statistical analysis
Demographic characteristics were reported as number and percentage by case–control status. Comparisons of categorical data were made using the chi-squared test. For those variables where the data was ordinal, a chi-squared test for trend was also conducted. Continuous variables were assessed by means of an unpaired sample t-test when the distribution was normally distributed or by the Mann–Whitney U-test when the distribution was not normally distributed.
To examine the relationship between density methods and case–control status, analysis was performed using conditional logistic regression (in SPSS) owing to the matched nature of the data set.
Results
In total, 104 women with analogue mammograms developed breast cancer during the course of the study. Forty-four of these were diagnosed at their first screen while taking part in the PROCAS study and were therefore not eligible for this particular case–control study. The remaining 60 women were eligible for inclusion; however, following exclusion of those for whom there was no calibrated stepwedge on the mammograms and those with missing analogue mammograms, the available sample was 49 women. For one further subject, the software failed, and this subject was subsequently excluded from the study.
Women were matched to one control, and Table 41 shows the demographic characteristics for cases and controls. The matching criteria were adequate, with women in the case and control groups being of similar age (mean approximately 59 years) and BMI (mean approximately 27 kg/m2), and with similar proportions of women who were postmenopausal (65% both groups) and current users of HRT (23% of cases and 25% of controls). Study participants were also similar with regard to other characteristics, including parity (approximately 85% in each group were parous), initial TC score (mean: cases 3.02, controls 2.84; p = 0.46), ethnicity, previous breast biopsies and year of mammogram.
Characteristic | Case subjects (N = 49), n (%) | Control subjects (N = 49), n (%) | p-value |
---|---|---|---|
Age (years) | |||
< 55 | 14 (29.2) | 13 (27.1) | |
55–59 | 8 (16.7) | 12 (25.0) | |
60–64 | 14 (29.2) | 11 (22.9) | |
≥ 65 | 12 (25.0) | 12 (25.0) | 0.754 |
Unknown | |||
Mean (SD) | 59.3 (6.80) | 58.9 (6.71) | 0.775 |
Menopausal status | |||
Premenopausal | 3 (6.3) | 4 (8.3) | |
Perimenopausal | 8 (16.7) | 8 (16.7) | |
Postmenopausal | 31 (64.6) | 31 (64.6) | |
Unknown | 6 (12.5) | 5 (10.4) | 0.972 |
BMI (kg/m2) | |||
< 25 | 19 (39.6) | 20 (41.7) | |
25–29 | 18 (37.5) | 17 (35.4) | |
≥ 30 | 8 (16.7) | 9 (18.8) | |
Unknown | 3 (6.3) | 2 (4.2) | 0.958 |
Mean (SD) | 26.9 (5.04) | 26.7 (4.95) | 0.920 |
Parity | |||
Nulliparous | 6 (12.5) | 6 (12.5) | |
Parous | 41 (85.4) | 42 (87.5) | |
Unknown | 1 (2.1) | 0 (0.0) | 0.603 |
HRT use | |||
Never/unknown | 19 (39.6) | 19 (39.6) | |
Previous | 18 (37.5) | 17 (35.4) | |
Current | 11 (22.9) | 12 (25.0) | 0.965 |
Year of mammogram | |||
2009 | 9 (18.80) | 14 (29.20) | |
2010 | 39 (81.20) | 34 (70.80) | 0.232 |
Initial TC | |||
Mean (SD) | 3.02 (1.22) | 2.84 (1.27) | 0.464 |
Ethnic origin | |||
White | 43 (89.6) | 46 (95.8) | |
Other/unknown | 5 (10.4) | 2 (4.2) | 0.453 |
Biopsy of breast | |||
Yes | 11 (22.92) | 6 (12.5) | |
No/unknown | 37 (77.08) | 42 (87.5) | 0.182 |
Table 42 shows the number and percentages of cancer and control subjects for each density method. Density methods were split into quartiles, with the lowest quartile used as the referent group. There were no statistically significant associations with any density method; however, the VAS for the CC view did approach statistical significance for the second quartile (OR 3.24, 95% CI 0.99 to 10.54). We did not adjust for any other factors.
Mammographic density measure | Case subjects (N = 49), n (%) | Control subjects (N = 49), n (%) | Univariate, OR (95% CI) | p-value |
---|---|---|---|---|
VAS | ||||
< 16 | 11 (22.9) | 14 (29.2) | 1.00 (referent) | |
16–28 | 11 (22.9) | 9 (18.8) | 1.54 (0.49 to 4.82) | |
28–44 | 16 (33.3) | 12 (25.0) | 1.65 (0.57 to 4.81) | |
≥ 45 | 10 (20.8) | 13 (27.1) | 0.91 (0.27 to 3.06) | 0.677 |
Mean (SD) | 33.4 (21.5) | 30.8 (19.8) | 0.515 | |
Median | 29.3 | 29.0 | 0.587 | |
VAS (CC view) | ||||
< 15 | 8 (16.7) | 15 (31.3) | 1.00 (referent) | |
15–29 | 17 (35.4) | 9 (18.8) | 3.24 (0.99 to 10.54) | |
30–43 | 11 (22.9) | 12 (25.0) | 1.61 (0.51 to 5.05) | |
≥ 44 | 12 (25.0) | 12 (25.0) | 1.80 (0.49 to 6.62) | 0.201 |
Mean (SD) | 33.6 (21.5) | 30.2 (19.7) | 0.424 | |
Median | 28.8 | 27.8 | 0.538 | |
Stepwedge breast volume (cm3) | ||||
< 550 | 12 (25.0) | 12 (25.0) | 1.00 (referent) | |
550–749 | 17 (35.4) | 8 (16.7) | 1.88 (0.45 to 7.87) | |
750–1049 | 11 (22.9) | 14 (29.2) | 0.47 (0.09 to 2.47) | |
≥ 1050 | 8 (16.7) | 14 (29.2) | 0.29 (0.05 to 1.70) | 0.155 |
Mean (SD) | 784.63 (360.5) | 933.25 (553.25) | 0.122 | |
Median | 668.4 | 868.6 | 0.215 | |
Stepwedge gland volume (cm3) | ||||
< 20 | 11 (22.9) | 10 (20.8) | 1.00 (referent) | |
20–34 | 12 (25.0) | 15 (31.3) | 0.72 (0.23 to 2.19) | |
35–54 | 12 (25.0) | 11 (22.9) | 1.03 (0.32 to 3.3) | |
≥ 55 | 13 (27.1) | 11 (22.9) | 1.03 (0.34 to 3.08) | 0.901 |
Mean (SD) | 50.22 (50.53) | 44.45 (39.47) | 0.535 | |
Median | 37.0 | 32.1 | 0.667 | |
Stepwedge breast density (%) | ||||
< 2 | 13 (27.08) | 11 (22.9) | 1.00 (referent) | |
2–4.49 | 11 (22.92) | 12 (25.0) | 0.81 (0.25 to 2.63) | |
4.5–8 | 12 (25.00) | 11 (22.9) | 0.94 (0.32 to 2.78) | |
≥ 9 | 12 (25.00) | 14 (29.2) | 0.71 (0.22 to 2.29) | 0.939 |
Mean (SD) | 8.07 (10.95) | 6.35 (5.74) | 0.336 | |
Median | 4.74 | 4.77 | 0.436 |
Discussion
These data are interesting because by using the prior mammograms of cancer rather than the contralateral mammogram at time of diagnosis the density data genuinely assess risk of developing cancer. However, the numbers are very small and no density measure achieved statistical significance.
There were limitations of the method that affected the viability of some of the results. With regard to the markers which are used to measure compressed breast thickness, ideally two pairs should be located to enable the measurement of paddle tilt. In most cases the software identified at least two pairs of markers, but in some instances incomplete or poorly located pairs were identified (e.g. when a marker coincided with a patient identification label). This produces inaccurate thickness estimates and hence errors in density assessment.
Ideally, we would have liked to have analysed MLO views as well as CC views with the stepwedge method, but the process of digitisation and analysis is time-consuming, and we decided to perform an initial investigation of a single view in the first place. We did, however, analyse VAS results for both mammographic views and for the CC view alone to enable comparison with the stepwedge method. VAS for a single view approached statistical significance for those in the second quartile. We would also ideally match with more controls to increase the power to detect a significant effect. These analyses do not correct for other factors such as BMI. 203 Although we did not correct for BMI explicitly, data were matched on BMI category.
The data set contained both interval (n = 11) and screen-detected cancers (n = 38). Had any signs of abnormality been missed when the prior mammogram was first read, this might have had an impact on the density at the initial screen.
From these data we are unable to predict the presence of cancer from MD, with either area-based or volumetric density methods. However, the evaluation of a larger set of images is required; previous research has demonstrated the ability of visual and computer-assisted density assessment to predict later cancers in more extensive data sets. 167,204 Longitudinal assessment (such as that employed by Kerlikowske et al. ,205 but using continuous objective density assessment) may also be important.
The stepwedge method has previously been evaluated in a screening population,206 and was found to be a feasible but time-consuming method of obtaining volumetric estimates from film mammograms. The main drawback of the technique is that the stepwedge and markers have to be imaged at the time of mammography, and images without these objects cannot be analysed. The numbers of cancers evaluable in the study were too small to make any meaningful evaluation. As it is now possible to calibrate digital mammograms without using a stepwedge, this method is unlikely to be used in the future.
The relationship between volumetric and area-based mammographic density to age and hormonal factors
Introduction and aims
Percentage breast density estimated visually or assessed by computer-assisted area-based measures declines with age, menopausal status and parity and increases with current HRT use. 58,207–211 Automated volumetric density measurement methods, including Quantra and Volpara, remove subjectivity; it is important to determine how these methods relate to age and endocrine changes, and here we describe these associations. For comparison we also present VAS measurements.
Methods
Women undergoing routine screening in the NHSBSP who agreed to enter the PROCAS study completed questionnaires concerning personal information, including weight, height, parity, menopausal status and HRT use.
There were originally 50,929 subjects, of whom 731 were excluded owing to a previous diagnosis of cancer, as were a further 506 with a current diagnosis of cancer. At the time of analysis, volumetric density measures were available for Quantra (23,253) and Volpara (11,947) subjects. From these cohorts, a further 1692 (7.3%) and 826 (6.9%) were excluded from the Quantra and Volpara groups, respectively, because they had a BMI outside the range 17.5–60 kg/m2. A further 188 and 95 subjects were excluded because their average breast density distribution had not returned a reading. Finally, a further three in each group were excluded owing to discrepancies in the reporting of ‘ever used HRT’ and ‘still on HRT’. Thus, the final numbers of subjects in this substudy were 21,370 in the Quantra data set and 11,023 in the Volpara 1.4.0 data set.
Results
Descriptive statistics for the subjects for each of the two density methods are shown in Table 43. Density significantly declined with age (p < 0.001) by both methods. Figure 26 shows plots of gland volume and percentage density for Quantra and Volpara. Both methods show a decline in volumetric density until the 56–60 years age group for percentage density, and a further decline in gland volume until the 61–65 years age group is seen only with Volpara.
Characteristic | Quantra (N = 21,370), n (%) | Volpara (N = 11,023), n (%) |
---|---|---|
Age (years) | ||
< 50 | 2187 (10.2) | 1540 (14.0) |
51–55 | 5401 (25.3) | 2373 (21.5) |
56–60 | 4814 (22.5) | 2377 (21.6) |
61–65 | 4736 (22.2) | 2565 (23.3) |
66–70 | 3273 (15.3) | 1799 (16.3) |
> 70 | 959 (4.5) | 369 (3.3) |
Mean (SD) | 59.0 (6.6) | 58.8 (6.8) |
BMI (kg/m2) | ||
Mean (SD) | 27.6 (5.5) | 27.9 (5.5) |
Menopausal status | ||
Premenopausal | 1474 (6.9) | 996 (9.0) |
Perimenopausal | 2624 (12.3) | 1341 (12.2) |
Postmenopausal | 15,222 (71.2) | 7550 (68.5) |
Missing | 2050 (9.6) | 1136 (10.3) |
Current HRT use | ||
No | 19,735 (92.3) | 10,296 (93.4) |
Yes | 1635 (7.7) | 727 (6.6) |
FIGURE 26.
Plots of gland volume and percentage MD by volume by age for Quantra and Volpara. (a) Gland volume vs. age (Quantra); (b) gland volume vs. age (Volpara); (c) percentage density vs. age (Quantra); and (d) percentage density vs. age (Volpara).
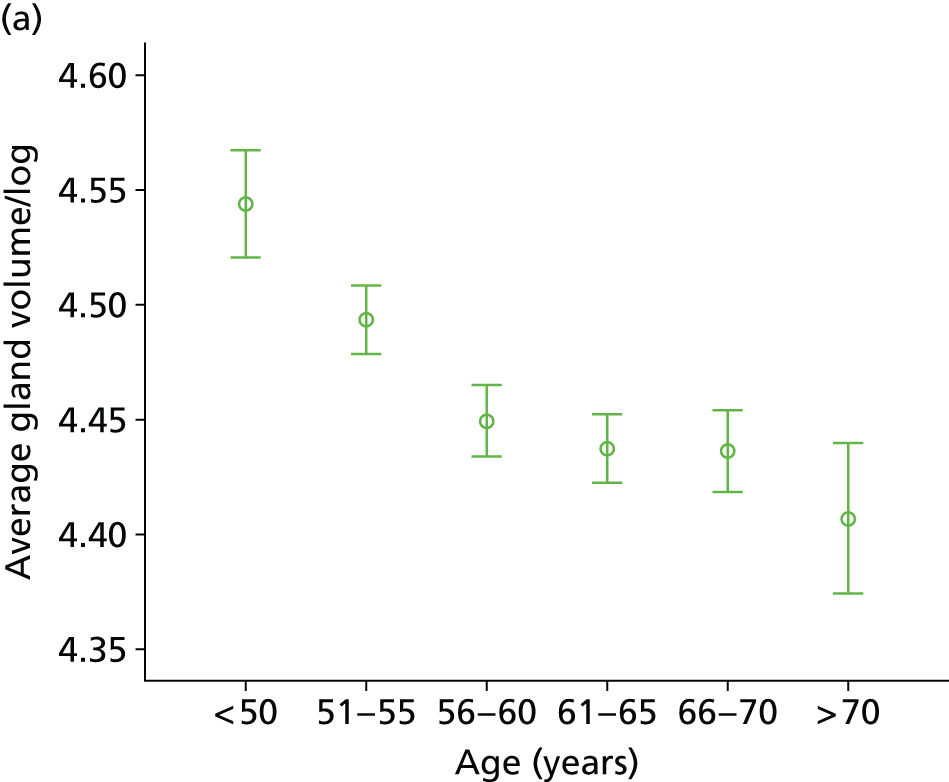


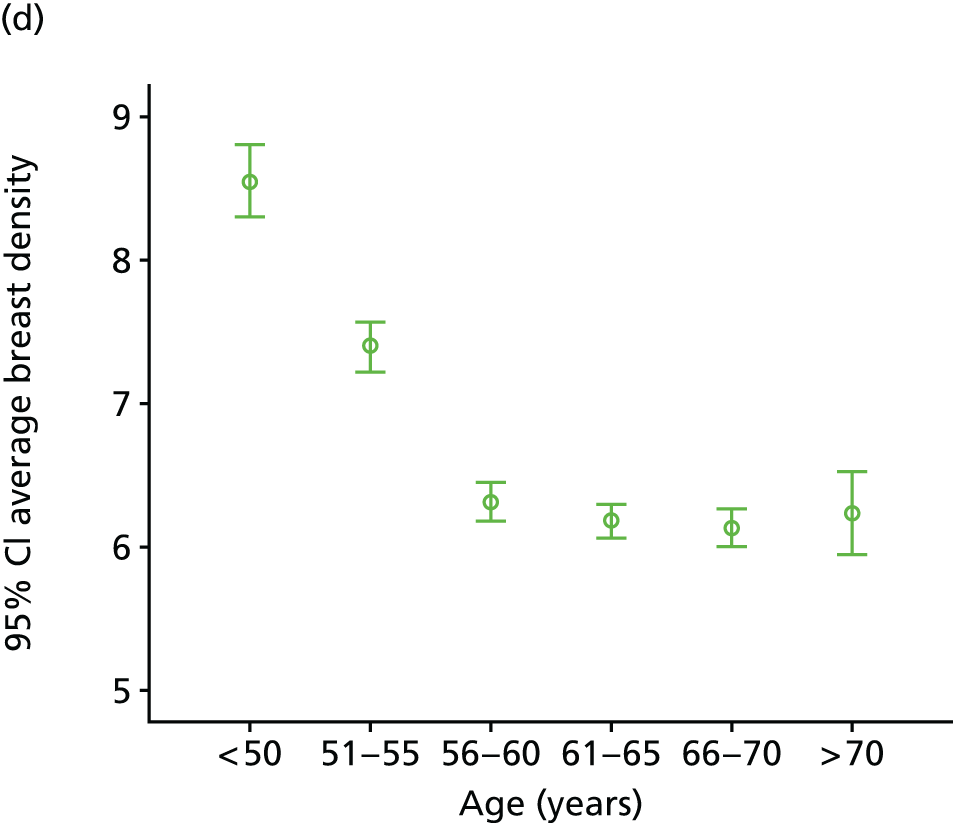
The effect of hormonal factors on glandular volume is illustrated in Figure 27. In women aged < 50 years the mean percentage density by Quantra was 19.23 for premenopausal women and 16.29 for postmenopausal women (p < 0.05), and in women aged 51–55 years these figures were 18.06 and 15.72, respectively (p < 0.05). For density measured by Volpara, the corresponding figures are 7.74 and 6.64 (p < 0.05) for women aged < 50 years, and 7.54 and 6.00 (p < 0.05) for women aged 51–55 years.
FIGURE 27.
Plots of gland volume and percentage MD by age and menopausal status, HRT use and parity for Quantra and Volpara. Gland volume vs. menopausal status by age group by (a) Quantra and (b) Volpara (black, premenopausal; blue, perimenopausal; green, postmenopausal); gland volume vs. HRT use by age group by (c) Quantra and (d) Volpara (green, never/ever used; black, current use); and gland volume vs. parity by age group by (e) Quantra and (f) Volpara (green, no children; black, children).
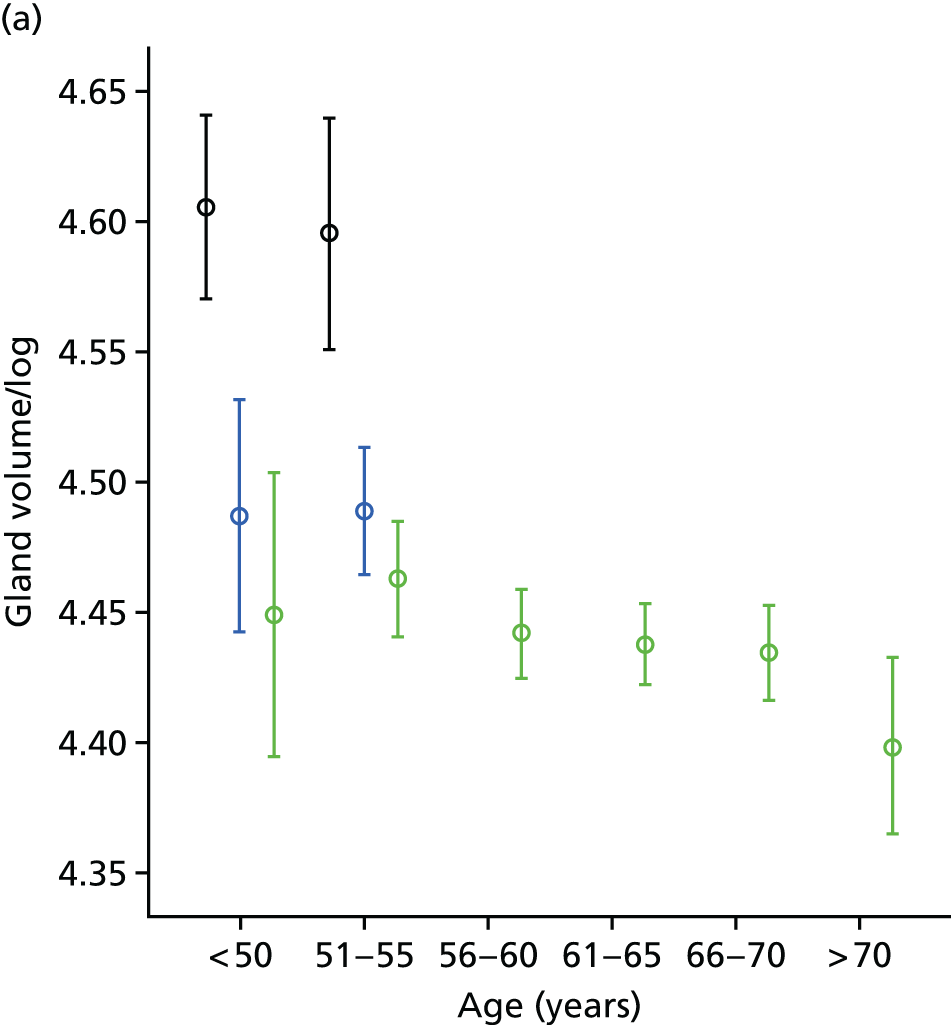


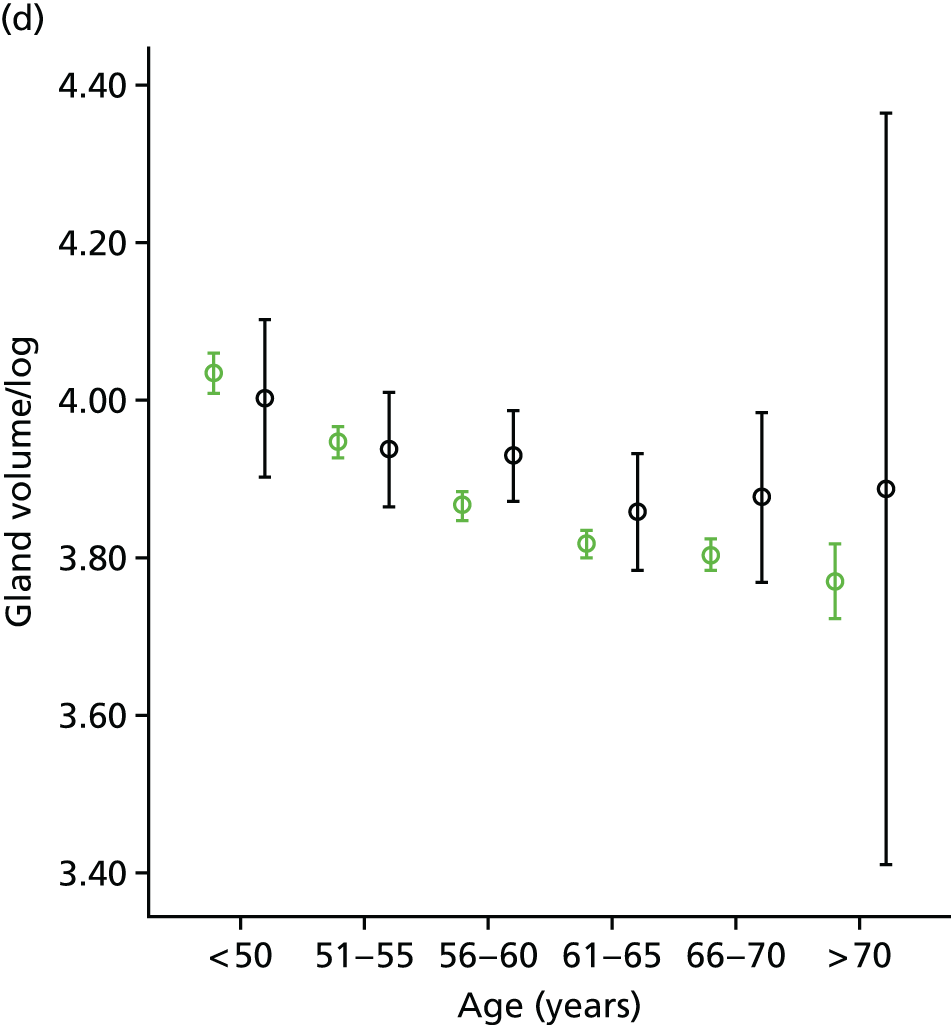


Current HRT use was associated with significantly higher percentage density (p < 0.05) for those over 50 years (Volpara) and from 51 to 70 years (Quantra). Nulliparity was associated with higher density at all ages (p < 0.05 for Quantra above the age of 50 years and for Volpara for age 51–65 years).
Conclusion
These data indicate that volumetric percentage density by both methods is related to age and endocrine factors in the same directions as area-based methods. In women under the age of 55 years, density was significantly higher for premenopausal than postmenopausal women. For those aged over 50 years, current use of HRT also showed an increase in density, as did nulliparity.
Ethnicity and mammographic density
Introduction and aims
Increased mobility of the world population has resulted in many countries having a diverse ethnic mix, now apparent in the breast screening age group in Greater Manchester. 212 Ethnicity is related to risk of breast cancer, with women of white ethnicity being more likely than women in other racial groups to develop breast cancer. 213 In one study, approximately 141 per 100,000 women of white ethnic origin were found to have developed the disease, compared with 119 per 100,000 for African American women, 96 per 100,000 for Asian American women, 90 per 100,000 for Hispanic/Latina women and 50 per 100,000 for Native American/Alaskan native women. 214
Published data relating MD to ethnicity have yielded mixed results. A UK study of 428 symptomatic patients using Quantra showed significant differences between white, Asian and black women, but did not control for any confounding factors such as age or HRT use. 215 White, Hispanic, Asian, Native American and black women participated in a study of 28,501 women in a breast-screening programme in Washington, USA. 216 After adjusting for age, differences in MD were found between Native American and white women, and between white and Asian women. However, when BMI, HRT use, menopausal status and parity were taken into account, the difference between Native American and white women was no longer significant. More recent research in similar ethnic groups evaluated the breast density of 442 women. 217 African American women were found to have higher density than Asian American women after adjusting for BMI, family history, menstrual and reproductive factors. In this work, Asian American and white women were found to have similar MDs. In contrast with this, a British study found that Asian women had significantly lower breast density, assessed using Wolfe grades, than Caucasian participants. 218 However, in a study of 15,292 women of Asian, white, African American and other (Native American and Caribbean) racial backgrounds, no significant differences were found when confounding factors, including bra size, were taken into account. 219 The picture is, thus, unclear; previous studies have evaluated different populations using a variety of methodologies, including subjective assessment of density.
Use of automated digital volumetric measurement of breast density may offer advantages over visual and semiautomated methods, including objectivity, reproducibility, suitability for population-based studies, resolution and the ability to assess absolute, rather than relative, breast density. 220 Regardless of the degree of association with risk, the identification of women with high MD is important because the detection of cancers using conventional mammography is more difficult in this case,221 and it may be appropriate to use alternative screening methodologies.
The aims of this substudy are to determine whether or not there is an association between MD and ethnicity in the PROCAS cohort.
Method
Data were used in this substudy from women for whom GE FFDM images with raw (‘for processing’) image data and questionnaire data on ethnicity, date of birth, HRT use, weight and height were available. Records for all non-white British or Irish participants recruited to PROCAS before 15 June 2011 were examined, and the first 1038 white British or Irish PROCAS participants with suitable data were also included. Women diagnosed with cancer at the time of screening and women with previous breast cancers were excluded.
Mammograms were analysed using Hologic’s Quantra software, which provided measures of breast volume, glandular volume and percentage density by volume for the left and right breasts. These were averaged to provide a single measure of each type per woman.
Questionnaire data were extracted from the PROCAS study database. BMI was calculated from self-reported height and weight. One-way analysis of variance was used to determine whether or not a relationship existed between the breast density measures and ethnicity. Pairwise comparisons were carried out on each ethnic group versus white British/Irish (the largest group which was used as a reference) using Scheffé’s test. A general linear model was used to further investigate the link between average breast density and ethnicity while adjusting for HRT use, BMI and age. Univariate analysis of the variables was performed and pairwise comparisons were done using Bonferroni’s test.
Ethnicities
The ethnic categories available for participants to select on the questionnaire were Asian or Asian British – Bangladeshi, Indian, Pakistani, Chinese; black or black British – African or Caribbean; Jewish origin; Jewish Ashkenazi; mixed – white and black African/Asian/black Caribbean; white – British or Irish; and other – please specify. Women were instructed to ‘please tick all that apply’ on the questionnaire. In subsequent analysis, the Jewish Ashkenazi women were included in the ‘Jewish origin’ category.
Results
The age of participants ranged from 46 to 74 years. The mean BMI for all the ethnic groups in the study was > 25 kg/m2, in the overweight range. Mean ages and BMIs for each group are shown in Table 44.
Ethnicity | Number analysed | Mean BMI (kg/m2) (SD) | Mean age (years) (SD) |
---|---|---|---|
White British or Irish | 1038 | 27.36 (5.49) | 58.8 (6.99) |
Black or black British | 71 | 29.49 (4.60) | 57.9 (7.36) |
Asian or Asian British | 76 | 26.24 (4.64) | 57.5 (6.75) |
Jewish origin | 89 | 25.52 (4.22) | 60.1 (6.87) |
Mixed | 30 | 29.75 (5.83) | 56.7 (6.29) |
Others | 48 | 25.50 (4.66) | 58.8 (6.37) |
All | 1352 | 27.45 (5.37) | 58.7 (6.96) |
Just over one-third of the women in the study had ever used HRT. Usage was highest in the Jewish group and lowest in women of black origin and those of Asian or mixed race (Table 45). The mean age of women who reported ever using HRT (61.41 years) was significantly higher than that of women who had never used it (57.19 years) (p < 0.01). This is likely to relate to the menopausal status of the individuals.
Ethnicity | Ever used HRT (%) |
---|---|
White British or Irish | 37.5 |
Black or black British | 23.5 |
Asian or Asian British | 26.7 |
Jewish | 42.9 |
Mixed | 26.7 |
Others | 35.4 |
All ethnicities | 36.2 |
The volumetric MDs of women in the different ethnic groups are presented in Table 46, along with fat and gland volumes.
Ethnicity | Gland volume (cm3) | Breast volume (cm3) | Volumetric density (%) | |||
---|---|---|---|---|---|---|
Mean | SD | Mean | SD | Mean | SD | |
White | 101.9 | 58.9 | 642.4 | 363.2 | 16.9 | 6.5 |
Black or black British | 126.4 | 72.2 | 777.8 | 442.5 | 17.1 | 5.9 |
Asian or Asian British | 78.9 | 47.9 | 454.8 | 259.2 | 18.3 | 6.0 |
Jewish origin | 100.7 | 50.2 | 544.5 | 260.9 | 19.6 | 7.5 |
Mixed | 117.0 | 45.5 | 694.6 | 293.5 | 17.3 | 3.9 |
Others | 97.5 | 63.3 | 596.3 | 450.1 | 18.1 | 6.4 |
Using Scheffé’s test, slight differences were observed in the average volumetric density in all ethnic groups. However, only women of Jewish ethnic origin had significantly higher breast density than the white British or Irish population (p = 0.012).
Once adjusted for age, BMI and HRT use, the results showed that the difference between average breast density of the Jewish participants and that of the white British or Irish women was of only borderline significance (p = 0.053).
Discussion
Investigation of the relationship between breast density and ethnicity, although facilitated by the availability of automated methods of measuring density, remains difficult because of the many confounding factors such as the possible impact of a change in lifestyle on second-generation immigrants, and wide variations between definitions of ethnic groups. This substudy is the first that has specifically compared the volumetric breast density of Jewish women with that of white British or Irish women; this comparison is particularly interesting because of the known difference in genetic susceptibility to breast cancer of Ashkenazi Jewish women. 222 The high rate of HRT use found in this group is of interest.
The population studied is unlikely to be representative of women of screening age in Greater Manchester, as attendance at screening is not uniform across all ethnic groups, with women of non-white origin less likely to present for screening. 223 Further, the sample was selected from the PROCAS study on a pragmatic basis aimed at maximising the proportion of non-white British participants. The mobile units used for screening relocate to facilitate access, and the uptake of screening and the proportion of women consenting to take part in PROCAS vary according to location, with lower rates in less affluent areas of the city.
The work reported here uses Quantra for MD assessment. This holds several potential advantages over visual and semiautomated methods including objectivity, reproducibility, suitability for population-based studies, resolution and the ability to assess absolute, rather than relative, breast density. 220 Regardless of the degree of association with risk, the identification of subgroups of women with high MD is important because the detection of cancers using conventional mammography is more difficult in this case,221 and it may be appropriate to use alternative screening methodologies.
In this sample we found that the only ethnicities for which, after adjusting for potential confounding factors, there was some limited evidence of a difference in breast density were white British or Irish and Jewish women. This is in contrast to recently reported data from the UK, which found that Asian women had lower breast density as measured by Quantra; however, that research was carried out in a symptomatic population rather than a screening population, and did not adjust for confounding factors such as age and BMI. 215 Quantra also provides data on volume of glandular tissue in the breast. This may be more reliable than percentage density, as it is affected less by the weight of the women at the time of imaging. 201
Using breast volume as a surrogate for weight/body mass index
Introduction
Mammographic density is one of the strongest modifiable risk factors for breast cancer and is usually reported as a relative measure, describing the proportion of the breast area or volume occupied by radio-dense tissue. However, there is evidence that when women gain weight, they gain breast fat and hence gain breast volume. 201 This results in a decrease in percentage breast density and hence a decrease in the apparent risk of developing breast cancer, despite the gain in weight conferring an actual increase in risk for postmenopausal women. Similarly, loss of weight (which is beneficial in terms of breast cancer risk) leads to an increase in percentage density and apparent risk. For this reason, breast density measurements made for the purpose of assessing cancer risk are often corrected for BMI or weight as well as for age. 211,224
As weight is not routinely recorded at mammographic screening, and the weight of many women changes between screens, it would be advantageous to find a surrogate measure that could be used to correct relative density measures. It has been proposed that the breast volume or fat volume computed by commercial breast density software could be used in this way. 225 The aim of this evaluation198 is to determine whether or not this would be appropriate.
Method
We used PROCAS questionnaire data, including height and weight at the time of screening, and the corresponding digital screening mammograms were analysed with Quantra (version 1.3) and Volpara (version 1.3.1). Quantra outputs total breast volume and dense tissue volume for both breasts, combining data from the CC and MLO views. A single average total breast volume was calculated for each case. The fat volume was obtained by subtracting the dense tissue volume from the total breast volume. Volpara gave measures of average breast volume and average fat volume from both the left and the right breasts. It also provided average total breast volume and average fat volume for each case.
Women were excluded if they had had a previous breast cancer or tissue biopsy, if essential data were missing or invalid or if their recorded clothes size was unrealistic for their calculated BMI. We thus analysed data from 7398 women, out which 500 data sets were set aside for evaluation, leaving a sample of 6898 data sets. Weight ranged from 36 kg to 172 kg and BMI ranged from 15.17 kg/m2 to 62.38 kg/m2, with 95.7% of women declaring themselves to be white British or Irish.
To test the significance of a possible association between weight or BMI and the volumetric breast measures, Pearson’s correlation was used. This was run as a two-tailed test, and a correlation coefficient (r) > 0.4 was taken as a positive correlation between two variables. Owing to the large population size, an additional criterion of r > 0.7 was used to indicate a significant association, in this instance the correlation squared (r2) is 0.49, which would indicate that almost half of the variation in ‘true’ BMI (or weight) between women could be explained by the prediction model. Linear regression was used to produce predictive models for weight and BMI from the sample population; these were applied to the test set data in order to predict weight and BMI for these 500 women. Predicted values were compared with self-reported weight and BMI values and analysed by calculating intraclass correlation (ICC).
Finally, to increase the data available for testing, the predictive models were applied to the entire data set available and histograms were plotted to show the differences between self-reported and calculated weights and BMIs.
Results
All volumetric breast measurements showed a positive correlation with either weight or BMI (Table 47). Figure 28 shows an example plot for weight versus Volpara breast volume. 198 In general the points on the graphs become more scattered with increasing volumetric measurements, suggesting that predictive models may perform better for women of lower weight/BMI.
Breast density measure | Weight or BMI | Coefficient value | p-value |
---|---|---|---|
Quantra breast volume | Weight | 0.688 | < 0.001 |
Quantra fat volume | Weight | 0.695 | < 0.001 |
Volpara breast volume | Weight | 0.709 | < 0.001 |
Volpara fat volume | Weight | 0.712 | < 0.001 |
Quantra breast volume | BMI | 0.701 | < 0.001 |
Quantra fat volume | BMI | 0.710 | < 0.001 |
Volpara breast volume | BMI | 0.724 | < 0.001 |
Volpara fat volume | BMI | 0.728 | < 0.001 |
FIGURE 28.
Self-reported weight plotted against breast volume measured by Volpara.

For illustration, self-reported and predicted values for the minimum, maximum and mean self-reported weights and BMIs in a separate group of women were obtained and are presented for Quantra in Table 48 and for Volpara in Table 49.
Variable | Minimum | Maximum | Mean | SD |
---|---|---|---|---|
Self-reported weight (kg) | 48 | 121 | 72.95 | 14.88 |
Weight (kg) predicted from breast volume | 55 (14.58) | 114 (5.78) | 72.62 (0.45) | 10.31 |
Weight (kg) predicted from fat volume | 55 (14.58) | 111 (8.26) | 72.83 (0.16) | 10.51 |
Calculated BMI (kg/m2) | 19.79 | 47.71 | 27.97 | 5.37 |
BMI (kg/m2) predicted from breast volume | 21.13 (6.77) | 44.57 (6.58) | 28.17 (0.72) | 4.05 |
BMI (kg/m2) predicted from fat volume | 21.16 (6.92) | 41.44 (13.14) | 27.68 (1.04) | 3.82 |
Variable | Minimum | Maximum | Mean | SD |
---|---|---|---|---|
Self-reported weight (kg) | 49 | 132 | 72.90 | 13.85 |
Weight (kg) predicted from breast volume | 56 (14.29) | 125 (5.30) | 73.32 (0.58) | 11.47 |
Weight (kg) predicted from fat volume | 56 (14.29) | 123 (6.82) | 72.94 (0.05) | 11.31 |
Calculated BMI (kg/m2) | 19.49 | 53.15 | 27.96 | 5.45 |
BMI (kg/m2) predicted from breast volume | 21.37 (9.64) | 46.58 (12.36) | 27.80 (0.57) | 4.17 |
BMI (kg/m2) predicted from fat volume | 21.35 (9.54) | 45.98 (13.49) | 27.66 (1.07) | 4.11 |
Intraclass correlation was used to assess agreement between predicted and self-reported values. For all predicted values, the ICCs indicated moderate agreement. For weight, the ICC ranged between 0.609 and 0.634. The lowest ICC agreement was obtained when using the values predicted using Volpara breast volume data, for which the ICC = 0.609 (95% CI 0.522 to 0.683; p < 0.001). The highest ICC agreement was obtained when using the values predicted using Quantra fat volume data, for which the ICC = 0.634 (95% CI 0.573 to 0.689; p < 0.001).
For BMI, the ICC ranged between 0.594 and 0.629. This indicates a slightly weaker agreement between these predicted values and self-reported values than for weight. The lowest ICC agreement between self-reported and predicted values was obtained with values predicted using Volpara breast volume data, for which the ICC = 0.594 (95% CI 0.505 to 0.671; p < 0.001). The highest ICC was the same for two of the sets of predicted values analysed against self-reported values, the values predicted using Quantra breast volume data and those predicted using Quantra fat volume data, for which the ICC = 0.629 (95% CI 0.567 to 0.685; p < 0.001).
Frequency histograms showing the difference between actual (self-reported) and predicted weight and BMI values and those calculated by applying the predictive models to the entire data set are shown in Figure 29.
FIGURE 29.
Frequency histograms (a) showing the difference between actual (self-reported) and predicted weight for Volpara; (b) showing the difference between actual (self-reported) and predicted weight for Quantra; (c) showing the difference between actual (self-reported) and predicted BMI for Volpara; and (d) showing the difference between actual (self-reported) and predicted BMI for Quantra.
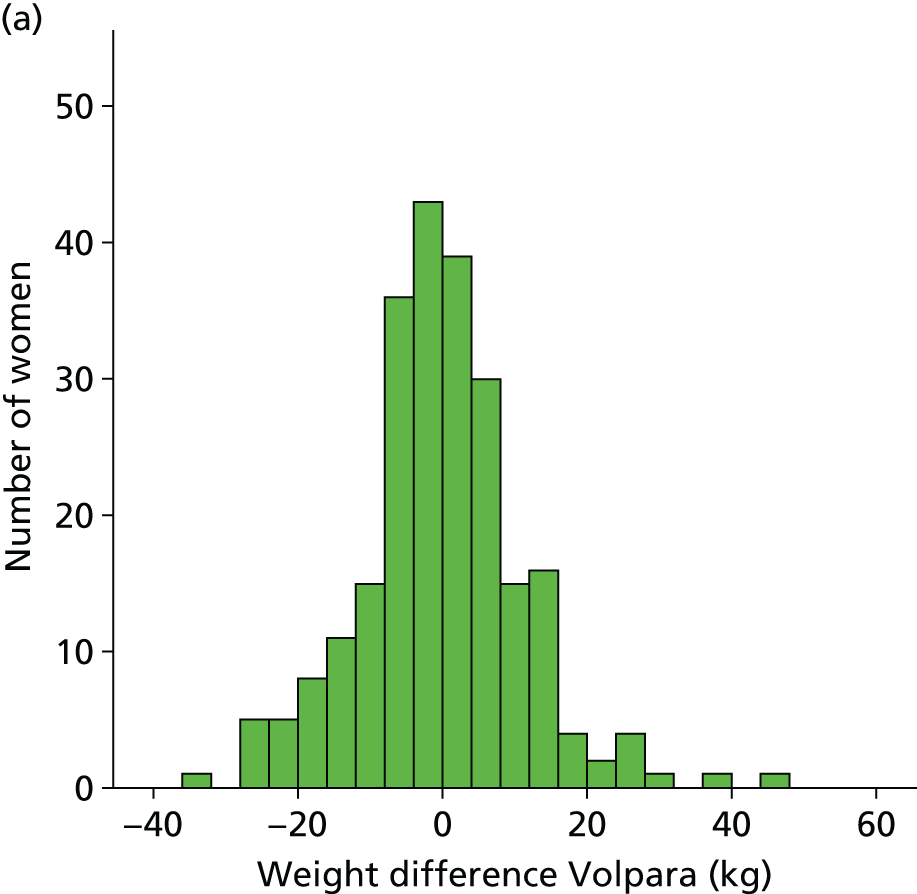
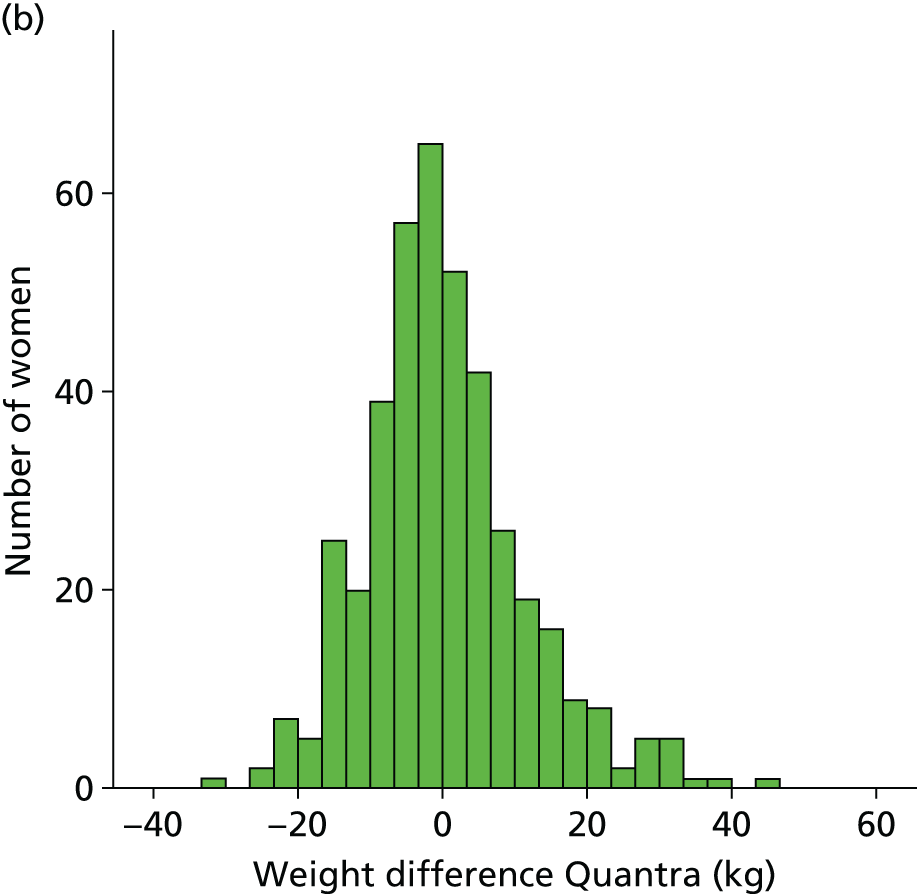

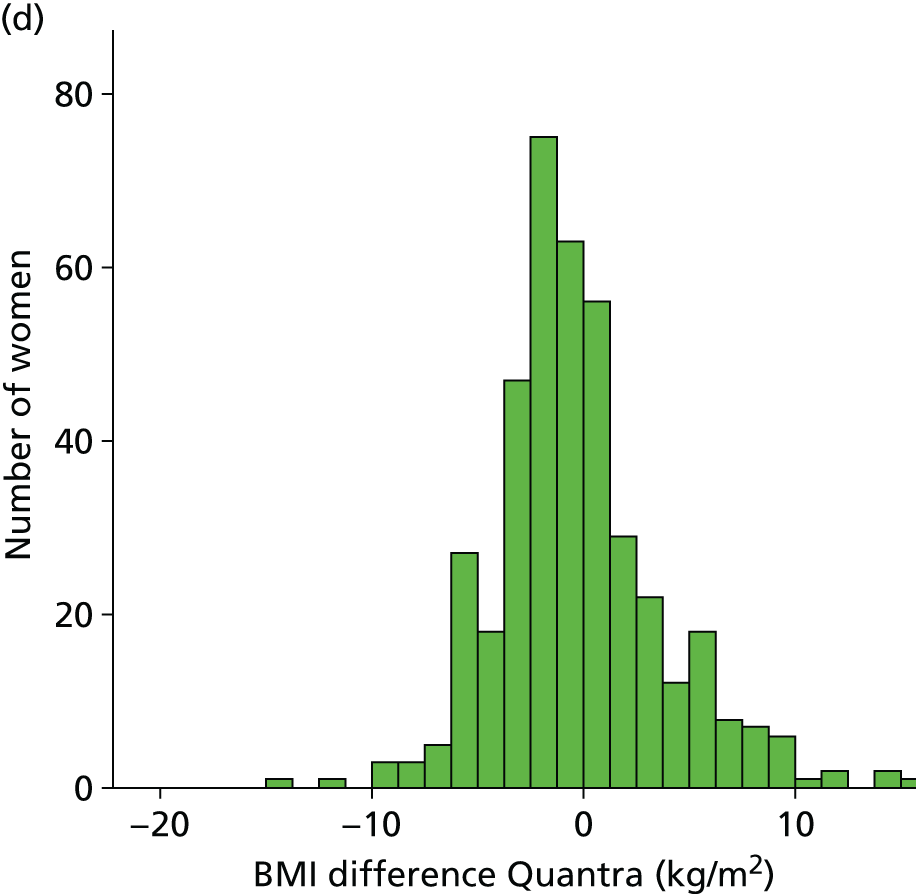
Discussion
One of the limitations of this work is that we have assessed the volumetric measures against self-reported, rather than measured, values. It is known that self-reported data are subject to errors, particularly in people who are overweight,226 and this could potentially have contributed to the greater spread of data seen with increasing weight. For similar reasons, it is unsurprising that prediction of BMI was less successful than prediction of weight as the calculation of BMI involved the use of two self-reported data items, weight and height. It is, however, difficult to obtain measured values for weight and height in a screening setting, in which appointments are short and space and privacy on mobile units are limited, and so the use of self-reported data is a pragmatic solution. Should risk-adapted screening be introduced, it is likely that many of the data collected will be self-reported, and verification will only be implemented should women fall into high-risk groups, or on the borderline between average and high risk.
Our results indicate that volumetric breast measurements made from mammograms are not an adequate surrogate for self-reported weight and BMI in models of individual risk. A correlation of 0.634 does not provide evidence of accurate prediction (the correlation squared is only 0.40, which can be interpreted as the proportion of variation in ‘true’ BMI between women explained by the prediction model – this is not a large proportion). However, volumetric measurements could be used as a sanity check on self-reported data, rather than asking about clothes size, for example, which is itself error-prone because of variations in size between clothing manufacturers. They could also be used in cases where women fail to provide self-reported data.
Repeatability of visual assessment score assessment of mammographic density
Visual assessment of MD is the only method that was applicable to all mammograms in PROCAS. This is a subjective, relative, area-based method, and in PROCAS it was implemented as a visual estimate of percentage density by an expert observer recorded on a VAS. This form of MD estimation has been shown to have a strong association with risk of breast cancer when both the MLO and CC views of the breast are used,167 and there is evidence that measurements of the relative area of dense tissue are more predictive of breast cancer than categorical measurements of MD. 33 However, the inherent subjectivity of visual assessment is of concern when it is applied for risk stratification and the reliability of identifying individuals at increased or reduced risk of breast cancer is important. Although there is a literature on the variability of categorical assessment of MD by observers,205,227 or using computer-assisted thresholding methods such as Cumulus,204 there was a lack of data on variability of visual assessment recorded on a continuous scale until we undertook this analysis for the PROCAS study. 202
Aim
The aim of this substudy was to examine the repeatability of breast density assessment using VASs.
Method
Seven of the PROCAS VAS density assessors (five consultant radiologists and two breast physicians) each repeated the assessment of breast density for 100 sets of mammograms that they had previously assessed for MD during the PROCAS study. The level of agreement between the original and repeat assessments was investigated.
The readers had between 2 and > 10 years’ experience of MD assessment using VAS and had all completed between 3000 and 7604 density assessments in 2011, with the exception of the reader with > 10 years’ experience, who had undertaken 661 VAS assessments that year.
A set of 100 cases was selected for each reader by randomly sampling 10 sets of mammograms from each decile of the distribution of VAS density as assessed in the PROCAS study by that reader during the period May 2010–May 2011. Selection in this manner ensured the inclusion of cases across a range of densities, and an interval of at least 12 months between the initial assessment of the images and the repeat assessment undertaken for this substudy in May and June 2012. The mammograms were cases that had been read as normal, and were from women without a previous history of breast surgery of any kind, or previous breast biopsy. All of the mammogram images were produced by GE Senographe Essential FFDM systems (GE Healthcare Ltd, Chalfont St Giles, UK); in PROCAS we have observed differences in mean visually assessed density between different types of mammograms (GE Digital, Fischer Digital and analogue), so for consistency we used images from a single platform.
Both the initial MD estimation undertaken for the PROCAS study and the repeat assessment for this substudy took place under similar conditions. Readers viewed the MLO and CC images of both breasts and marked their density estimates for each view on a single paper form with a set of four 10-cm VASs, labelled 0% and 100% at the ends. The forms were scanned and density estimates were converted into numeric values using custom software. All assessment took place in the same clinical reporting room, with images displayed on Planar Dome E5 5MP self-calibrating monitors (Ampronix Imaging Technology, Irvine, CA, USA). Readers were blinded to the assessment of other readers and to their own previous assessments.
Agreement between the initial and repeat sets of density results for each reader was examined using the Bland–Altman limits of agreement framework. 228,229 Differences between paired readings were plotted against their mean, with horizontal lines indicating the mean difference and 1.96 standard deviations (SDs) above and below the mean difference. These lines represent the limits of agreement, between which 95% of differences are expected to lie. 229 This approach is often used when the level of agreement between two distinct methods of measurement is evaluated. In this substudy, we treated pairs of density assessments as replicates by the same method, that is, repeat observations at different times. We can thus also examine a reader’s coefficient of repeatability, 2.77 sw, where sw is the within-subject SD, estimated by the square root of the residual mean square in a one-way analysis of variance. 229 Replicates by the same method are expected to be within one coefficient of repeatability of each other for 95% of subjects.
Results
In Table 50 we present a summary of the differences between the initial (‘old’) density assessments of each reader and those (‘new’) assessments produced for this repeatability substudy. Results are shown by mammographic view for each of the seven readers, along with estimates of the 95% limits of agreement, within-subject SD and coefficient of repeatability. Six of the seven readers have positive mean differences for all views. This indicates, on average, an increase in density estimates between the initial assessment and repeat assessment. For the seventh reader, on average, a decrease in density estimates was observed.
Reader | View | Difference: new – old | Limits of agreement | Within-subject SD | Coeffient of repeatability | |||
---|---|---|---|---|---|---|---|---|
Mean | SD | Max abs | Lower | Upper | ||||
1 | RCC | 2.28 | 7.37 | 30 | –12.16 | 16.72 | 5.43 | 15.05 |
RMLO | 0.38 | 7.43 | 34 | –14.18 | 14.94 | 5.23 | 14.50 | |
LCC | 3.10 | 7.27 | 28 | –11.15 | 17.35 | 5.57 | 15.43 | |
LMLO | 0.34 | 7.38 | 32 | –14.12 | 14.80 | 5.20 | 14.40 | |
2 | RCC | 5.78 | 8.99 | 28 | –11.84 | 23.40 | 7.53 | 20.88 |
RMLO | 4.97 | 8.55 | 27 | –11.78 | 21.72 | 6.96 | 19.30 | |
LCC | 5.55 | 9.41 | 29 | –12.89 | 23.99 | 7.70 | 21.33 | |
LMLO | 4.97 | 9.09 | 26 | –12.85 | 22.79 | 7.30 | 20.23 | |
3 | RCC | 1.81 | 11.76 | 65 | –21.24 | 24.86 | 8.37 | 23.21 |
RMLO | 2.75 | 12.14 | 52 | –21.05 | 26.55 | 8.76 | 24.28 | |
LCC | 1.92 | 11.75 | 64 | –21.10 | 24.94 | 8.37 | 23.21 | |
LMLO | 2.83 | 12.18 | 64 | –21.04 | 26.70 | 8.80 | 24.39 | |
4 | RCC | 14.66 | 13.21 | 62 | –11.24 | 40.56 | 13.92 | 38.60 |
RMLO | 10.19 | 12.84 | 56 | –14.98 | 35.36 | 11.56 | 32.03 | |
LCC | 14.11 | 13.43 | 60 | –12.22 | 40.44 | 13.74 | 38.09 | |
LMLO | 13.24 | 13.87 | 61 | –13.95 | 40.43 | 13.52 | 37.49 | |
5 | RCC | 3.54 | 10.37 | 43 | –16.79 | 23.87 | 7.72 | 21.39 |
RMLO | 2.47 | 12.79 | 47 | –22.60 | 27.54 | 9.17 | 25.41 | |
LCC | 2.85 | 9.88 | 42 | –16.51 | 22.21 | 7.24 | 20.06 | |
LMLO | 2.12 | 12.08 | 49 | –21.56 | 25.80 | 8.63 | 23.93 | |
6 | RCC | –1.29 | 10.55 | 33 | –21.96 | 19.38 | 7.48 | 20.72 |
RMLO | –2.18 | 10.01 | 30 | –21.80 | 17.44 | 7.21 | 19.98 | |
LCC | –0.77 | 10.55 | 31 | –21.44 | 19.90 | 7.44 | 20.62 | |
LMLO | –1.58 | 10.17 | 29 | –21.52 | 18.36 | 7.24 | 20.08 | |
7 | RCC | 2.14 | 12.99 | 42 | –23.33 | 27.61 | 9.27 | 25.69 |
RMLO | 1.83 | 13.70 | 47 | –25.03 | 28.69 | 9.73 | 26.97 | |
LCC | 2.26 | 12.70 | 42 | –22.63 | 27.15 | 9.08 | 25.16 | |
LMLO | 1.45 | 13.30 | 44 | –24.62 | 27.52 | 9.41 | 26.10 |
Reader 4, who had only 2 years’ experience reading using VAS, showed the widest limits of agreement (–13.95 to 40.43 for the left mammogram lateral oblique view) and largest coefficient of repeatability (38.60 for the right CC view). The sizes of the mean differences for reader 4 (10.19 to 14.66 percentage points) are considerably larger than those for the other six readers (0.34 to 5.78 percentage points). Reader 1 (the most experienced VAS reader with the lowest annual VAS workload) showed the narrowest limits of agreement (–11.15 to 17.35, left CC view) and smallest coefficient of repeatability (14.40, left mammogram lateral oblique view).
Scatterplots of the new and old estimates, and Bland–Altman plots of the differences between them, are presented for all readers in Figures 30 and 31. There is little variation between the different mammographic views within readers. The high proportion of points above the lines of perfect concordance in Figure 30 shows the increase from the initial to repeat assessments by most readers, while Figure 31 illustrates the variation around the mean difference between the estimates for each reader.
FIGURE 30.
Scatterplots of new (y-axes) and old (x-axes) density estimates by reader and view. All axes range from 0% to 100%. Solid diagonal lines are the lines of perfect concordance. 202 LCC, left CC; LMLO, left mammogram lateral oblique; RCC, right CC; RMLO, right mammogram lateral oblique.

FIGURE 31.
Bland–Altman plots of the difference (new – old) against the mean of new and old readings. Solid horizontal lines are the mean differences and dashed horizontal lines are the 95% limits of agreement. 202 LCC, left CC; LMLO, left mammogram lateral oblique; RCC, right CC; RMLO, right mammogram lateral oblique.

Discussion
We have investigated the repeatability of MD assessment by visual assessment recorded on VASs. Six of the seven readers’ density estimates were higher at the repeat assessment; this may at least partly be explained by the effect of the gradual transition from screen film mammography to digital mammography during the period of investigation. Screen film mammography was still being used during the initial PROCAS assessments of density, so at that time readers were assessing images from both modalities. We have observed that visual assessments of film mammograms tend to be higher, on average, than those of digital mammograms. It is possible that once the film comparator was removed (as was the case by the time of the repeat assessments) the readers may have realigned their baseline for digital mammography and hence increased their estimates.
Variation around the mean difference between current and previous readings was considerable, with 95% of differences expected to fall, at best, within 14.25 percentage points of the mean. Such a high degree of variability is problematic where MD is used to identify individuals at increased risk of breast cancer or where visual assessment of breast density is used to predict response to a preventative intervention. For example, a 12- to 18- month reduction in visual breast density of at least 10 percentage points was found to be a predictor of a reduction in risk of breast cancer in a study of tamoxifen as a preventative treatment in high-risk women. 230 This result was obtained using a single, highly experienced, observer and the level of intraobserver variability found in our pragmatic study would not be clinically acceptable in this context. However, it is worth noting that a previous study using synthetic mammograms found that reader accuracy in assessing change in VAS breast density could be improved by viewing the current images and priors simultaneously. 231
Interobserver variability of visual assessment score assessment and correction method
Introduction
Interobserver variability is inherent in the visual assessment of breast density, and was investigated in PROCAS using a common set of images assessed by 12 readers. 195 To account for the differences, we developed a method for adjusting readers’ estimates. 193
Methods
To assess interobserver variability, the MD of 120 screening cases with GE Senographe Essential FFDMs was independently assessed by 12 experienced mammographic readers (consultant radiologists, breast physicians and advanced practitioner radiographers). Assessment was performed using a separate VAS for each projection (CC and MLO) of each breast. These were scanned and converted to percentages, and then averaged. A Cumulus density result was also produced for each image by a trained and validated user. The level of agreement between readers was assessed using Bland–Altman limits of agreement228 and the concordance correlation coefficient (CCC). The VAS percentage densities were also converted to BI-RADS breast composition categories [(1) < 25% glandular; (2) 25–50% glandular; (3) 51–75% glandular; and (4) > 75% glandular] and agreement on this ordinal scale was measured with Cohen’s weighted kappa.
We developed a two-stage method to adjust different observers’ estimates of breast density in order to make them comparable. 193 First, results from all observers are transformed onto the same distribution. Individual readers produce VAS density results on their own distribution; we compute the empirical cumulative distribution function (ECDF) separately for each view by each reader, and then construct the overall ECDF by averaging the individual ECDFs, weighting each reader equally. We transform an original ‘raw’ VAS by a reader to its position in the ECDF of the reader, and then transform that position in the overall ECDF back to the 0–100% density scale.
Different readers perform assessment on different sets of cases, and each case is assessed by two readers. The second stage of the process is to account for any differences in case mix seen by different observers, exploiting differences in pairwise assessment after the first stage of the process. We can then estimate a correction factor for each reader to deal with differences in case mix.
We applied this two-stage approach to 12 experienced mammographic readers assessing a total of 13,694 screening cases from the PROCAS study. We then investigated the effect of using the adjustment method on risk stratification by examining the numbers of women who would be reclassified into a different risk group after adjustment.
Results
This section contains text reproduced with permission from Sergeant JC, Walshaw L, Wilson M, Seed S, Barr N, Beetles U, et al. ‘Same task, same observers, different values: the problem with visual assessment of breast density’, Proc SPIE 8673, Medical Imaging 2013: Image Perception, Observer Performance, and Technology Assessment, 86730T, March 28 2013. 202
The greatest difference between any two readers’ estimates for the same case was 67.75 percentage points, while the mean difference between two readers ranged from 0.76 to 28.58 percentage points. The 95% limits of agreement between pairs of readers were –6.96 to 18.62 at their narrowest and –59.13 to 1.97 at their widest. Pairwise CCC values ranged from 0.44 to 0.92, while the overall CCC across the 12 readers was 0.70. Pairwise kappa values for the BI-RADS classification ranged from 0.37 to 0.84, with a mean of 0.65.
An illustration of the variability of reader estimations of density is shown in Figure 32. Here, the readings of each of the 12 readers are plotted against each other, and against the Cumulus estimates of density. Figure 33 shows Bland–Altman plots illustrating pairings with the poorest and best agreement. For Cumulus, the widest 95% limits of agreement were for observer 9 (–3.94 to 49.71) and the narrowest were for observer 3 (3.29 to 32.55); these are shown in Figure 34. CCC values ranged from 0.48 to 0.87 and kappa values ranged from 0.40 to 0.80.
FIGURE 32.
Scatterplot matrix of density results by observers (labelled 1–12) and Cumulus, with all axes scaled 0–100% and lines of perfect concordance shown.

FIGURE 33.
Example Bland–Altman plots for (a) the pair of readers with the widest 95% limits of agreement (observers 1 and 8); and (b) the pair with the narrowest 95% limits of agreement (observers 2 and 7).
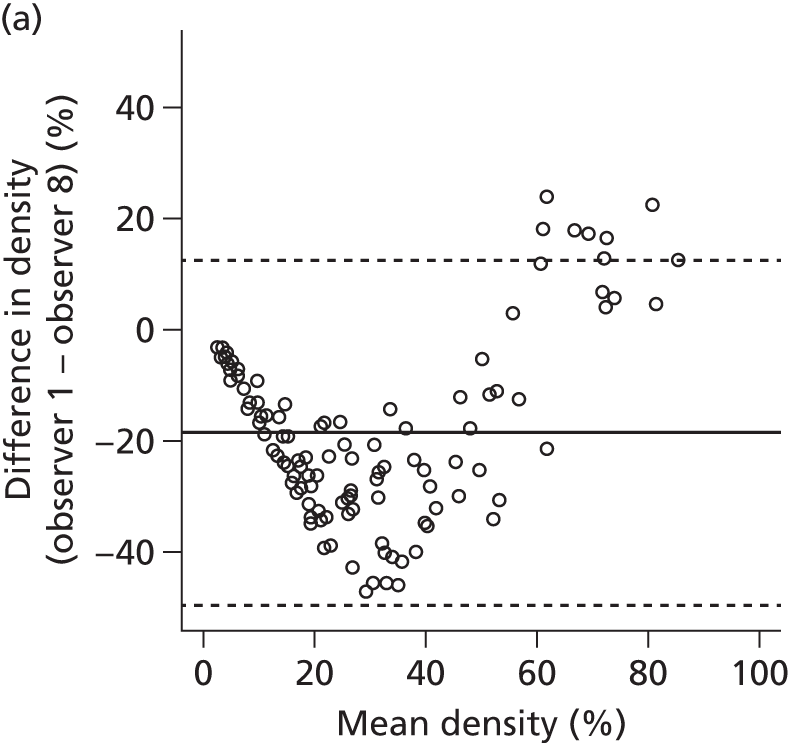
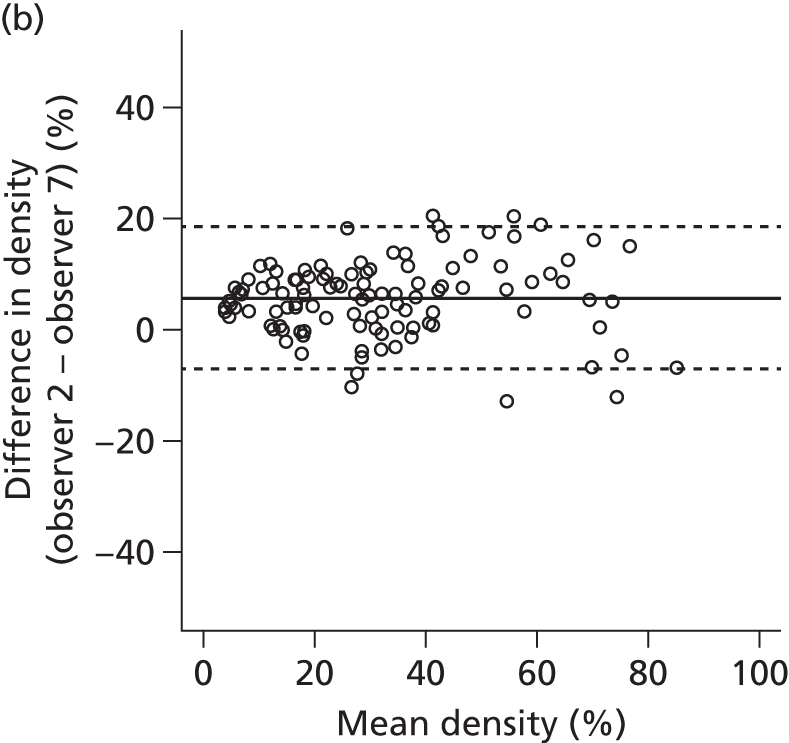
FIGURE 34.
Example Bland–Altman plots for (a) the widest 95% limits of agreement with Cumulus (observer 9); and (b) the narrowest 95% limits of agreement with Cumulus (observer 3).
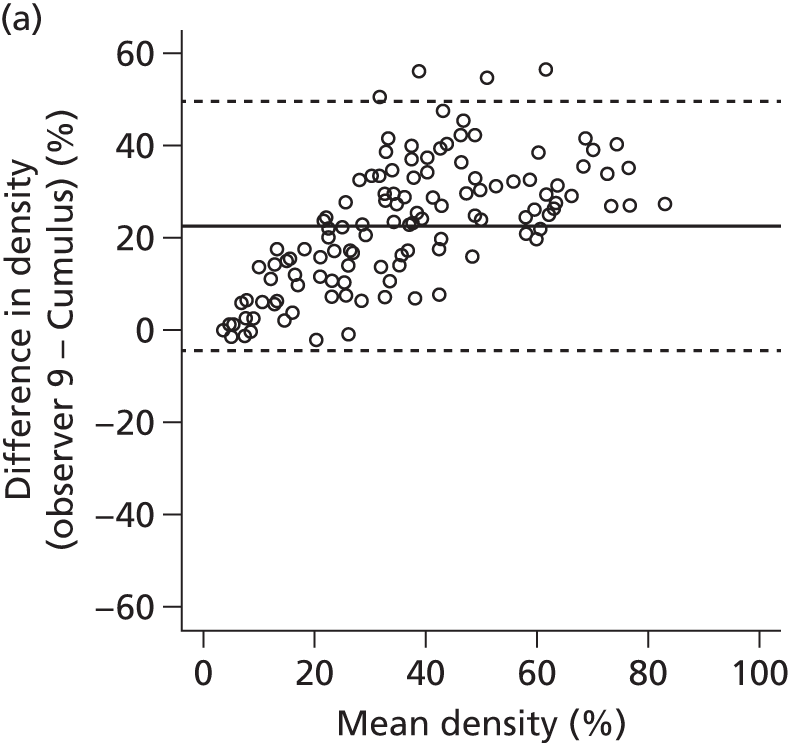

We applied the two-stage density adjustment approach to 13 experienced mammographic readers assessing a total of 13,694 screening cases. Figure 35 shows box plots of the readers’ estimates of MD for the cases they read. A scatterplot matrix of pairwise density assessments corresponding to these is shown in Figure 36.
FIGURE 35.
Box plots showing reader VASs for 13 readers, each reading a subset of 13,694 screening cases.
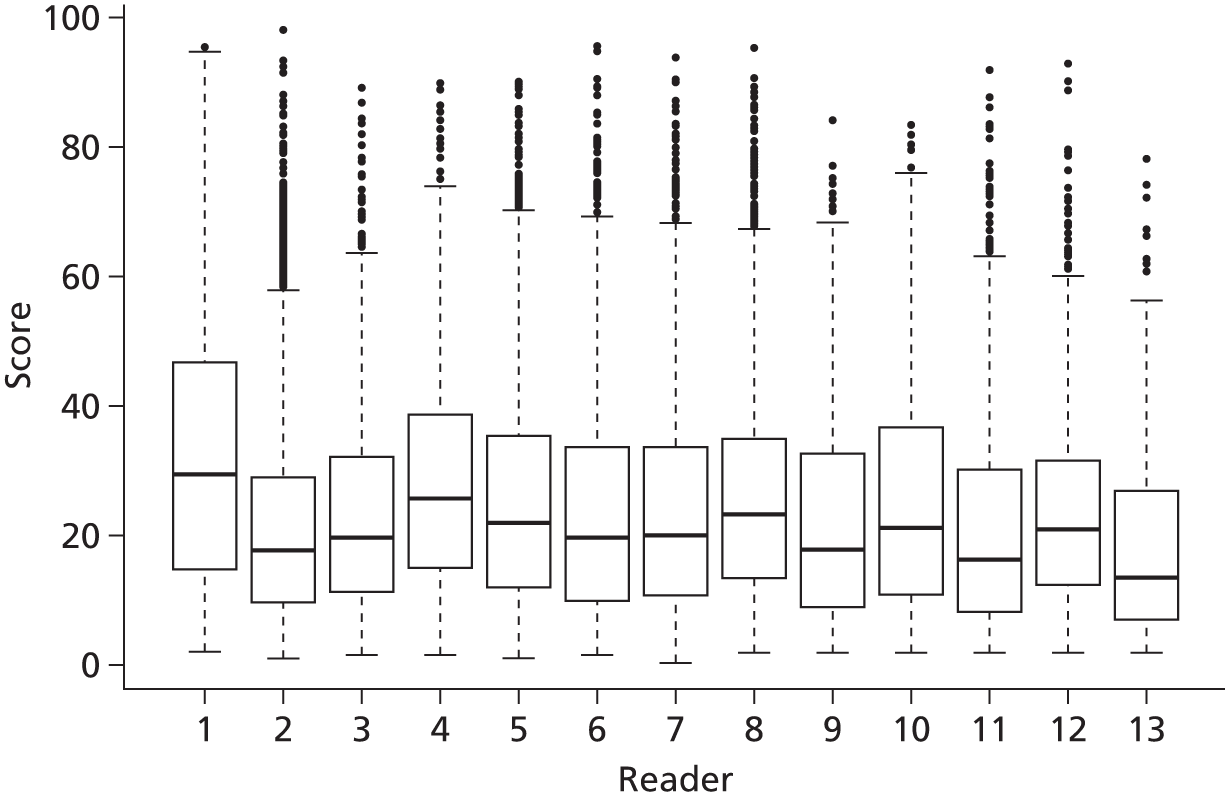
FIGURE 36.
A scatterplot matrix of pairwise density assessments corresponding to the assessments in Figure 35. Blank cells in the scatterplot matrix indicates readers who never assessed the same mammograms. All axes are scaled 0–100% and lines of perfect concordance are shown.

In PROCAS, women are categorised as having a high risk of developing breast cancer if their 10-year risk as computed by a validated family history-based risk model is between 5% and 8% and their breast density is at least 46%, the 90th percentile observed in the study population. In this substudy, 1125 of the 13,694 women had a 10-year breast cancer risk of at least 5% but < 8%. Of these, 126 had initial breast density estimates of at least 46%, and were therefore classified as high risk. Following VAS density adjustment, 147 women were classified as high risk. Of these, 35 women were reclassified (3.5% of those initially classified as non-high risk). Fourteen women who were initially classified as high risk had their risk category reduced after adjustment (11.1% of those initially classified as high risk). This is illustrated in the reclassification table (Table 51).
Before adjustment | After adjustment | ||
---|---|---|---|
High risk | Non-high risk | Total | |
High risk | 112 | 14 | 126 |
Non-high risk | 35 | 964 | 999 |
Total | 147 | 978 | 1125 |
Discussion
Substantial lack of agreement was found between readers visually assessing percentage breast density, and between the readers and Cumulus assessments. This study demonstrates the need for reader harmonisation, either by standardised training before reading commences or by adjustment of results after reading has taken place, should VAS density assessment be used for risk stratification.
Adjustment of VAS estimates of percentage breast density to take account of interobserver variation thus had a substantial effect on which women were classified as being at high risk of developing breast cancer owing to the combination of their 10-year risk estimate and breast density. If VAS assessment of density is to be used to help assess cancer risk in order to inform screening strategies and preventative interventions, adjustment must be considered.
Selection of best performing risk prediction model in PROCAS and incorporation of density
Aim
To assess, using incident and prevalent breast cancers identified on the first mammography screen as part of PROCAS, the predictive performance and characteristics of risk stratification categories (1) from the TC and Gail risk models; (2) from MD; and (3) when combined. The aim is to assess their rank-ordering performance at baseline.
Methods
Data
Sample selection
As of 3 March 2014 there were 53,184 women and 632 confirmed breast cancers (DCIS and invasive) that occurred after enrolment. The following exclusions were made for this report:
-
769 who had a previous diagnosis of breast cancer (22 cancers in PROCAS)
-
nine who had a bilateral breast cancer diagnosis, because the breast density measurement would be affected
-
45 who had missing data on the side of breast cancer diagnosis
-
2400 who had no visual assessment of breast density available (eight cancers)
-
14 who were older than 73 years at enrolment (no cancers)
-
nine with BMI of > 80 kg/m2 and 22 with BMI of < 10 kg/m2 (one cancer).
This left 49,916 women who were breast cancer free at baseline, of whom 547 were diagnosed with breast cancer after enrolment into PROCAS and had a valid breast density measurement.
Tyrer–Cuzick risk model
The 10-year absolute risk from version 6.0 of the algorithm was applied to data as provided on the questionnaires before quality control was complete.
Gail risk model
The 10-year absolute risk (which includes competing mortality) was obtained using the code from the NRI website in April 2014. 232 It was applied to data as provided on the questionnaires. Number of first-degree relatives is based on only mothers and sisters. Ethnicity was taken to be white unless reported as black, when that was used. The number of previous biopsies was not recorded in the questionnaire; it was taken to be 1 if the woman had reported a previous biopsy of her breast.
Breast density
Mean percentage density was obtained from two readers and eight views. For cancers this was obtained from the mean of the scores on the contralateral breast.
Statistical methods
Age, BMI, TC and Gail 10-year risk, and breast density were tabulated by percentiles. The relationship between density and age and BMI was assessed. To combine breast density with the TC and Gail absolute risks, a residual was obtained by fitting a linear regression of density against age and BMI, for those with BMI information. The model also included a term for type of mammogram (digital or film), because visual density measurements from digital images are known to be systematically less than those from film. The difference between that expected given age and BMI and that observed was used as a measure of breast density that is by definition independent of age and BMI and zero when the women has mean density for her BMI (if known) and age. Logistic regression was used to assess the significance of TC and the density residual (DR), and to calibrate the RR from the density measure. The calibrated RR from VAS was combined with the TC score to produce TC + density absolute 10-year risks. Tables showing the breakdown of cancers in 1–2%, 2–3%, 3–5%, 5–8% and ≥ 8% 10-year risk groups showed the stage, grade and lymph node positivity data by risk group for both TC and TC + density predictions. To compare against the TC groups, a table with the same number of women in each group was produced.
Results
Table 52 summarises the distribution of age, BMI, the TC 10-year risk and VAS density by case status. Breast cancer cases had marginally higher BMI but were well matched on age. Breast cancer cases also had higher overall 10-year risk based on the TC and Gail models.
Factor | Breast cancer | n | Quantiles | ||||
---|---|---|---|---|---|---|---|
5% | 25% | 50% | 75% | 95% | |||
Age | No | 49,369 | 49.0 | 52.0 | 58.0 | 64.0 | 70.0 |
Yes | 547 | 49.0 | 53.0 | 60.0 | 65.0 | 70.0 | |
BMI | No | 45,640 | 20.5 | 23.6 | 26.5 | 30.4 | 38.2 |
Yes | 503 | 21.0 | 24.1 | 26.9 | 30.5 | 38.8 | |
Gail 10-year | No | 49,369 | 2.2 | 2.9 | 3.5 | 4.3 | 7.0 |
Yes | 547 | 2.4 | 3.0 | 3.7 | 4.5 | 7.5 | |
TC 10-year | No | 49,369 | 1.6 | 2.1 | 2.7 | 3.5 | 6.1 |
Yes | 547 | 1.7 | 2.3 | 2.9 | 3.9 | 6.8 | |
Density | No | 49,369 | 5.8 | 13.6 | 24.4 | 37.8 | 60.5 |
Yes | 547 | 8.2 | 18.2 | 28.0 | 40.8 | 66.9 |
Figure 37 shows that MD was strongly influenced by both age and BMI.
FIGURE 37.
Box plots of visually assessed MD against age (years) for (a) breast cancers diagnosed; and (b) the complete cohort; and box plots of visually assessed MD against BMI (kg/m2) for (c) breast cancers diagnosed; and (d) the complete cohort.




Table 53 also shows that density was affected by the type of mammogram. Old analogue film mammograms were associated with higher VASs than digital mammograms.
Factor | Group | n | Quantiles | ||||
---|---|---|---|---|---|---|---|
5% | 25% | 50% | 75% | 95% | |||
Age | < 50 | 4306 | 8.5 | 20.9 | 34.0 | 47.7 | 70.1 |
50–59 | 24,038 | 6.0 | 14.5 | 25.8 | 39.2 | 62.5 | |
≥ 60 | 21,572 | 5.4 | 12.1 | 21.4 | 33.6 | 54.4 | |
BMI | < 20 | 1573 | 12.8 | 26.4 | 39.8 | 56.9 | 80.1 |
20–25 | 15,817 | 8.6 | 19.9 | 31.4 | 44.9 | 67.2 | |
25–30 | 16,421 | 6.1 | 13.9 | 23.6 | 35.9 | 55.1 | |
≥ 30 | 12,332 | 4.5 | 9.0 | 15.8 | 26.8 | 45.9 | |
Unknown | 3773 | 5.6 | 13.2 | 23.6 | 37.0 | 59.5 | |
Mammogram type | Film | 6723 | 6.0 | 15.0 | 27.2 | 43.1 | 71.0 |
Fisher | 1665 | 6.2 | 16.0 | 27.9 | 44.5 | 66.8 | |
Digital | 40,826 | 5.8 | 13.4 | 23.8 | 36.8 | 57.8 | |
Unknown | 702 | 6.8 | 13.1 | 25.9 | 43.2 | 65.0 |
A linear model was fitted by regressing density on age, BMI and type of mammogram (digital or not). The DR was the difference between the expected value of density from this model and that observed. In other words, the DR is interpreted as the difference between a woman’s density and the average density for women of the same age and BMI. For example, if DR = +10, then the woman has density of 10 percentage points more than would be expected based on her age and BMI.
Table 54 shows summary results of using TC and Gail with the DR to predict case status in the cohort. The Gail model only achieved an AUC c-statistic of 0.54, while TC was better, at 0.57. Adding DR to the models significantly improved the discrimination to a c-statistic of 0.58 for Gail and 0.60 for TC. Furthermore, adding DR to each model increased the ORs between upper and lower quantiles in both models.
Risk prediction algorithm | OR (95% CI) | Lower 25th | Upper 75th | AUC | ΔLR-C2 | p-value |
---|---|---|---|---|---|---|
Gail 10-year | 1.12 (1.05 to 1.19) | 2.90 | 4.30 | 0.54 | 12.3 | 4.47e-04 |
DR (with Gail) | 1.42 (1.28 to 1.58) | –11.81 | 9.43 | 0.58 | 39.7 | < 0.0001 |
TC 10-year | 1.18 (1.11 to 1.25) | 2.12 | 3.48 | 0.57 | 30.7 | < 0.0001 |
DR (with TC) | 1.41 (1.26 to 1.56) | –11.81 | 9.43 | 0.60 | 37.9 | < 0.0001 |
The multivariate ORs are given for the difference between the upper 75th and lower 25th quantiles. The p-value is from the stepwise likelihood-ratio test for each predictor (TC or Gail first, then density); similarly, the AUCs are when TC or Gail are used alone, and then when the DR is added.
Figure 38 compares the receiver operating characteristic curves showing the improved performances with density added as DR.
FIGURE 38.
Receiver operator characteristic curves showing performance of Gail and TC models with and without DR.
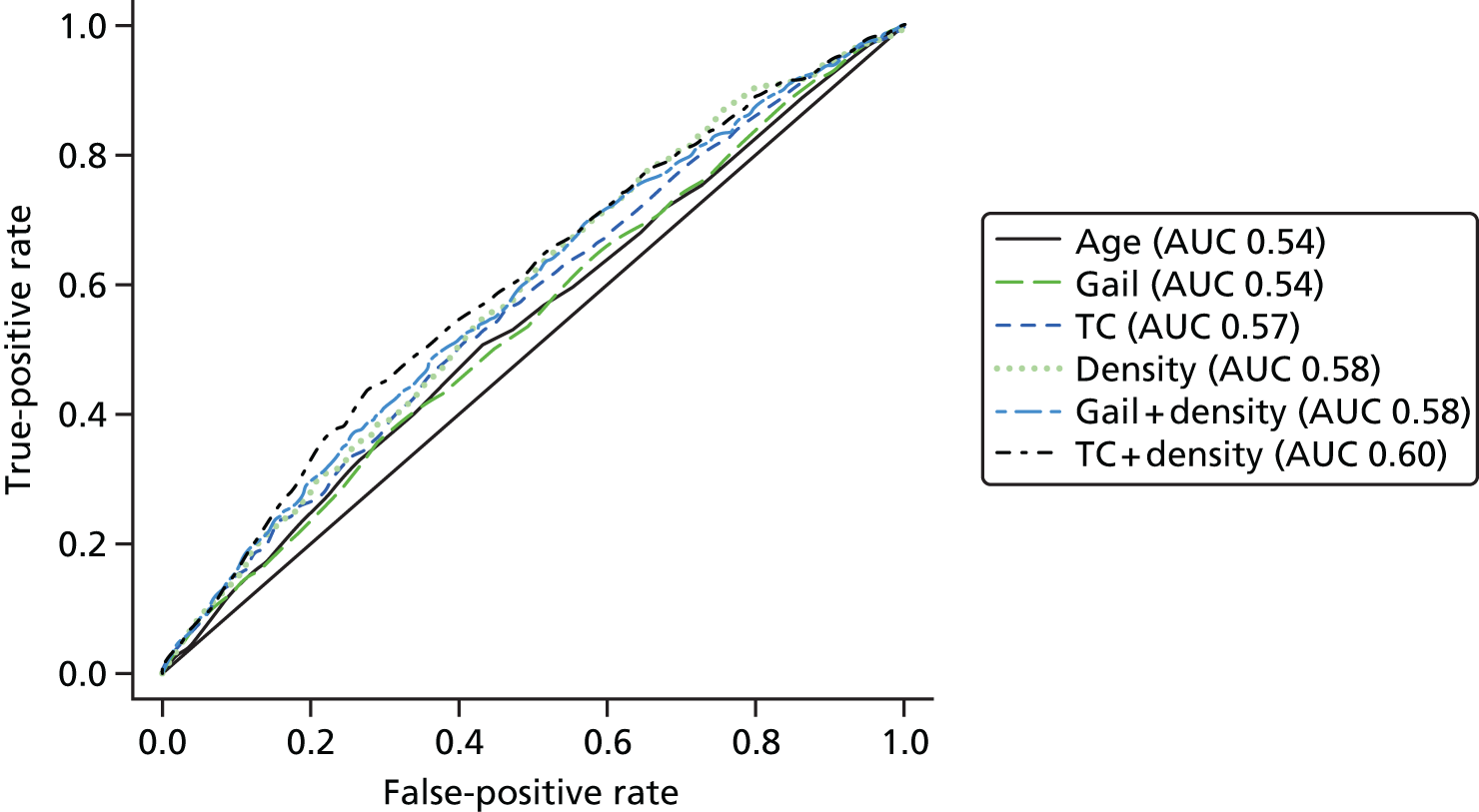
Figure 39 shows the histogram of ORs from fitted logistic regression models with (1) TC alone, (2) DR alone, and (3) TC and the DR combined.
FIGURE 39.
Cancers and risk scores for Gail and TC.
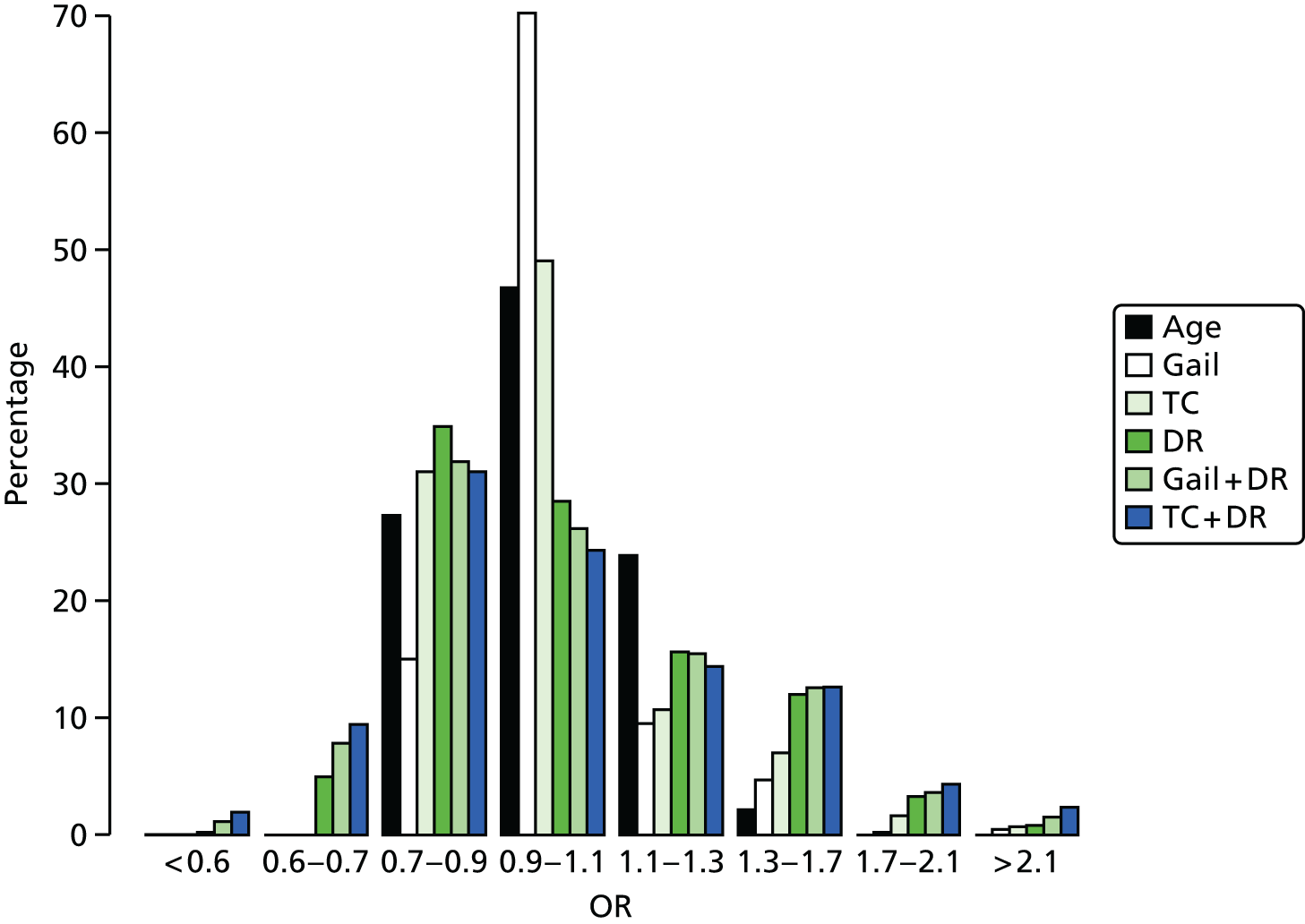
Figure 40 shows a scatterplot with cancers marked in red against the 10-year risk score from Gail and TC.
FIGURE 40.
Gail and TC predictions (green X = breast cancer diagnosed).

Tables 55 and 56 show TC and Gail predictions broken down by cancer detection. Neither TC nor Gail was predictive of a higher proportion of higher stage, grade or lymph node involvement.
10-year BC risk | N (%) | BC, n (%) | Stage, n | High, n (%) | Grade, n | High, n (%) | LN, n | Yes, n (%) |
---|---|---|---|---|---|---|---|---|
< 1% | 98 (0.2) | 0 (0.0) | 0 | 0 (0.0) | 0 | 0 (0) | 0 | 0 (0) |
1–2% | 9415 (19.1) | 71 (0.8) | 64 | 7 (10.9) | 65 | 15 (23) | 64 | 12 (19) |
2–3.5% | 27712 (56.1) | 298 (1.1) | 273 | 20 (7.3) | 267 | 52 (19) | 266 | 39 (15) |
3.5–5% | 7322 (14.8) | 98 (1.3) | 81 | 9 (11.1) | 85 | 20 (24) | 81 | 16 (20) |
5–8% | 4225 (8.6) | 63 (1.5) | 59 | 9 (15.3) | 59 | 14 (24) | 55 | 11 (20) |
≥ 8% | 597 (1.2) | 17 (2.8) | 17 | 1 (5.9) | 16 | 2 (12) | 16 | 3 (19) |
Total | 49369 (100.0) | 547 (1.1) | 494 | 46 (9.3) | 492 | 103 (21) | 482 | 81 (17) |
10-year BC risk | N (%) | BC, n (%) | Stage, n | High, n (%) | Grade, n | High, n (%) | LN, n | Yes, n (%) |
---|---|---|---|---|---|---|---|---|
< 1% | 0 (0.0) | 0 (0.0) | 0 | 0 (0.0) | 0 | 0 (0) | 0 | 0 (0) |
1–2% | 877 (1.8) | 6 (0.7) | 5 | 0 (0.0) | 5 | 3 (60) | 5 | 0 (0) |
2–3.5% | 22,823 (46.2) | 228 (1.0) | 205 | 19 (9.3) | 204 | 46 (23) | 203 | 33 (16) |
3.5–5% | 18,385 (37.2) | 215 (1.2) | 195 | 18 (9.2) | 194 | 36 (19) | 189 | 31 (16) |
5–8% | 6375 (12.9) | 79 (1.2) | 70 | 4 (5.7) | 71 | 12 (17) | 67 | 9 (13) |
≥ 8% | 909 (1.8) | 19 (2.1) | 19 | 5 (26.3) | 18 | 6 (33) | 18 | 8 (44) |
Total | 49,369 (100.0) | 547 (1.1) | 494 | 46 (9.3) | 492 | 103 (21) | 482 | 81 (17) |
Table 57 does the same analysis for the DR, by restricting the number of women in each group to be the same as the absolute risk groups from Tables 53 and 54. Density does appear predictive of increased proportions of higher-stage cancers.
10-year BC risk | N (%) | BC, n (%) | Stage, n | High, n (%) | Grade, n | High, n (%) | LN, n | Yes, n (%) |
---|---|---|---|---|---|---|---|---|
1 (low) | 97 (0.2) | 1 (1.0) | 1 | 0 (0.0) | 1 | 0 (0) | 1 | 0 (0) |
2 | 9436 (19.1) | 50 (0.5) | 43 | 1 (2.3) | 41 | 8 (20) | 41 | 2 (5) |
3 | 27,692 (56.1) | 318 (1.1) | 286 | 25 (8.7) | 287 | 61 (21) | 280 | 46 (16) |
4 | 7319 (14.8) | 101 (1.4) | 94 | 12 (12.8) | 92 | 19 (21) | 92 | 17 (18) |
5 | 4222 (8.6) | 66 (1.6) | 59 | 8 (13.6) | 60 | 14 (23) | 57 | 16 (28) |
6 (high) | 603 (1.2) | 11 (1.8) | 11 | 0 (0.0) | 11 | 1 (9) | 11 | 0 (0) |
Total | 49,369 (100.0) | 547 (1.1) | 494 | 46 (9.3) | 492 | 103 (21) | 482 | 81 (17) |
Tables 58 and 59 show how the prediction from Tables 53 and 54 change when density is combined with TC and Gail. Combining Gail and TC means that the proportion of low-risk cancers that have high stage (stages 2b and 3) is substantially lower. Only 18 out of 272 (6.6%) breast cancers occurring in women with a MD-adjusted TC score of < 3.5% 10-year risk had high stage at diagnosis, whereas 28 out of 222 (12.6%) of those with TC risks of > 3.5% in 10 years had high-stage cancers (p = 0.029). If we assume that the inclusion of prevalent cases adds a soujourn time of 2.5 years and that overall follow-up is, on average, 4 years, then in women with average or below-average risks (< 3.5% 10-year risk) the chances of getting a high-stage cancer with 3-yearly NHSBSP screening is 18 in 4 × 34,670 = 1.3 per 10,000 per year, compared with 28 in 4 × 14,699 = 4.76 per 10,000 annually (p < 0.001). TC picked out only 29.8% of the population as having a 10-year risk of > 3.5%. While adjusting the Gail score with density also predicted higher-stage cancers, 49.8% of the population was deemed to have a 10-year risk of > 3.5%. Thirty-five high-stage cancers occurred in 333 (10.5%) women at < 3.5% 10-year risk, compared with only 11 in 191 (5.8%) (p = 0.077).
10-year BC risk | N (%) | BC, n (%) | Stage, n | High, n (%) | Grade, n | High, n (%) | LN, n | Yes, n (%) |
---|---|---|---|---|---|---|---|---|
< 1% | 414 (0.8) | 2 (0.5) | 2 | 0 (0.0) | 2 | 0 (0) | 2 | 1 (50) |
1–2% | 12,642 (25.6) | 89 (0.7) | 78 | 3 (3.8) | 72 | 12 (17) | 75 | 7 (9) |
2–3.5% | 21,614 (43.8) | 208 (1.0) | 192 | 15 (7.8) | 194 | 42 (22) | 189 | 29 (15) |
3.5–5% | 8434 (17.1) | 134 (1.6) | 116 | 15 (12.9) | 120 | 26 (22) | 117 | 26 (22) |
5–8% | 4863 (9.9) | 84 (1.7) | 77 | 10 (13.0) | 77 | 16 (21) | 73 | 13 (18) |
8%+ | 1402 (2.8) | 30 (2.1) | 29 | 3 (10.3) | 27 | 7 (26) | 26 | 5 (19) |
Total | 49,369 (100.0) | 547 (1.1) | 494 | 46 (9.3) | 492 | 103 (21) | 482 | 81 (17) |
10-year BC risk | N (%) | BC, n (%) | Stage, n | High, n (%) | Grade, n | High, n (%) | LN, n | Yes, n (%) |
---|---|---|---|---|---|---|---|---|
< 1% | 1 (0.0) | 0 (0.0) | 0 | 0 (0.0) | 0 | 0 (0) | 0 | 0 (0) |
1–2% | 3429 (6.9) | 20 (0.6) | 17 | 0 (0.0) | 18 | 6 (33) | 17 | 1 (6) |
2–3.5% | 21,381 (43.3) | 194 (0.9) | 174 | 11 (6.3) | 171 | 43 (25) | 170 | 21 (12) |
3.5–5% | 14,077 (28.5) | 165 (1.2) | 147 | 17 (11.6) | 150 | 25 (17) | 144 | 30 (21) |
5–8% | 8350 (16.9) | 130 (1.6) | 119 | 14 (11.8) | 118 | 22 (19) | 117 | 22 (19) |
≥ 8% | 2131 (4.3) | 38 (1.8) | 37 | 4 (10.8) | 35 | 7 (20) | 34 | 7 (21) |
Total | 49,369 (100.0) | 547 (1.1) | 494 | 46 (9.3) | 492 | 103 (21) | 482 | 81 (17) |
Density was associated with stage grade and nodal status, which drives the pattern seen in Tables 60 and 61. Table 60 shows a clear rising proportion of cancers with increasing DR. Further data on the cancers are given in Tables 61–65.
DR | N (%) | High stage, n (%) |
---|---|---|
< –20 | 19 (4) | 0 (0) |
–20 to –10 | 74 (15) | 4 (5) |
–10 to 0 | 129 (26) | 10 (8) |
0 to 10 | 112 (23) | 12 (11) |
10 to 20 | 71 (14) | 12 (17) |
≥ 20 | 89 (18) | 8 (9) |
Age (years) | N (%) | High stage, n (%) |
---|---|---|
< 50 | 34 (7) | 3 (9) |
50–55 | 120 (24) | 14 (12) |
55–60 | 81 (16) | 7 (9) |
60–65 | 121 (24) | 17 (14) |
65–70 | 104 (21) | 4 (4) |
≥ 70 | 34 (7) | 1 (3) |
Test | OR (95% CI) | p-value |
---|---|---|
Grade | ||
DR | 1.01 (0.76 to 1.33) | 0.961 |
Age (10-year) | 0.66 (0.48 to 0.91) | 0.012 |
Stage | ||
DR | 1.53 (1.04 to 2.24) | 0.030 |
Age ≥ 65 years | 0.28 (0.09 to 0.66) | 0.009 |
LN+ | ||
DR | 1.44 (1.06 to 1.94) | 0.019 |
Age ≥ 65 years | 0.47 (0.09 to 0.66) | 0.017 |
DR | N (%) | LN+, n (%) |
---|---|---|
< –20 | 19 (4) | 1 (5) |
–20 to –10 | 73 (15) | 8 (11) |
–10 to 0 | 123 (26) | 17 (14) |
0 to 10 | 111 (23) | 22 (20) |
10 to 20 | 70 (15) | 15 (21) |
≥ 20 | 86 (18) | 18 (21) |
Age (years) | N (%) | LN+, n (%) |
---|---|---|
< 50 | 34 (7) | 4 (12) |
50–55 | 115 (24) | 28 (24) |
55–60 | 81 (17) | 11 (14) |
60–65 | 117 (24) | 24 (21) |
65–70 | 104 (22) | 11 (11) |
≥ 70 | 31 (6) | 3 (10) |
Age (years) | N (%) | High grade, n (%) |
---|---|---|
< 50 | 34 (7) | 9 (26) |
50–55 | 116 (24) | 32 (28) |
55–60 | 85 (17) | 19 (22) |
60–65 | 121 (25) | 21 (17) |
65–70 | 103 (21) | 18 (17) |
≥ 70 | 33 (7) | 3 (9) |
These tables also show that breast cancers diagnosed after the age of 65 years were less likely to be high stage with lymph node involvement, and were of lower grade.
Discussion
The results from our analyses of prospective breast cancers in PROCAS indicate that TC, Gail and density are predictive for a screening population, and that density may be combined with a prediction model. TC appeared to perform better than Gail and, in particular, has good discriminatory value for overall prediction and high-stage prediction when combined with density. More cancers occurred with Gail and TC in higher-risk groups, but little difference by risk group was identified in predicting the probability of high stage, grade or lymph node status given a cancer diagnosis based on Gail or TC. High density was linked to higher stage and lymph node involvement. Although the addition of density significantly improved the AUCs for both Gail and TC, the overall AUC scores were still modest.
We have previously shown that TC is accurate in risk assessment57 in the FHC setting and the present study has shown that it predicts accurately in the general population. A number of breast cancer risk models have been developed in the past 25 years. 22 These incorporate known genetic, reproductive and other risk factors to a greater or lesser extent (see Table 28). Gail et al. 149,150 described a risk assessment model, which focuses primarily on non-genetic risk factors with limited information on family history. A model of RRs for various combinations of the utilised risk factors (see Table 28) was developed from case–control data from the Breast Cancer Detection Demonstration Project. Individualised breast cancer probabilities from information on RRs and the baseline hazard rate are generated. These calculations take into account competing risks and the interval of risk. The data depend on having periodic breast surveillance. The Gail model was originally designed to determine eligibility for the Breast Cancer Prevention Trial, and has since been modified (in part to adjust for race) and made available on the National Cancer Institute website. 232 The model has been validated in a number of settings and probably works best in general assessment clinics, in which family history is not the main reason for referral,149,150,176 although it should also be useful in general population screening programmes. The major limitation of the Gail model is the inclusion of only first-degree relatives, which results in underestimating risk in the 50% of familial risk with cancer in the paternal lineage and also takes no account of age at onset of breast cancer.
The Claus model177 and BRCAPRO181 are primarily genetic models calculating a likelihood of either a putative high-risk dominant gene177 or BRCA1/BRCA2. 181 Breast cancer risks are imputed from this calculation. As such, given the rarity of BRCA1/BRCA2 or the putative dominant gene in the Claus model, these models are useful only in the familial setting and are not relevant to the current study. BOADICEA69 is another model primarily developed to assess genetic risk, but has been validated in a population-based series of breast cancers. Although the inclusion of non-genetic risks is anticipated, these are not yet available in the online model.
The TC model,11 based partly on a data set acquired from IBIS-I and other epidemiological data, incorporates both familial and non-genetic risk factors in a comprehensive way. 11 The major advantage over the Claus model and BRCAPRO is that the model allows for the presence of multiple genes of differing penetrance. It does give a read-out of BRCA1/BRCA2, but also allows for a lower-penetrance BRCAX. As such, the TC model addresses many of the pitfalls of the previous models: significantly, the combination of extensive family history, endogenous oestrogen exposure and benign breast disease (atypical hyperplasia). It is unsurprising, therefore, that the model performs better than the simpler Gail model, this being particularly so in the familial setting57 and now, as we have shown, in the population setting.
Mammographic density is the single assessable risk factor with the largest population attributable risk and also has a substantial heritable component. 233,234 The difference in risk between women with extremely dense, as opposed to predominantly fatty, breasts is approximately four- to sixfold. 235 The incorporation of MD into standard risk prediction models has been associated with some improvement in precision of risk prediction. 34,35 Here we have shown that adding an adjusted MD score to Gail and TC not only improves the discrimination significantly, but also predicts higher-stage cancers. It is likely that our results also indicate that TC should replace Gail in North America, as a study from Canada has shown that TC substantially outperformed the Gail model. 75 The authors applied 10-year absolute risk of breast cancer, using prospective data from 1857 women, over a mean follow-up length of 8.1 years, of whom 83 developed cancer. The 10-year risks assigned by Gail and TC differed, with ranges at the extremes of 0.001% and 79.5%. The mean Gail- and TC-assigned risks of 3.2% and 5.5%, respectively, were lower than the cohort’s 10-year cumulative probability of developing breast cancer of 6.25%. Agreement between assigned and observed risks was better for TC [HL X4(2) = 7.2, p-value 0.13] than for Gail, with Gail significantly underpredicting cancers (p < 0.001). The TC model also showed better discrimination (AUC = 69.5%, 95% CI 63.8% to 75.2%) than the Gail model (AUC = 63.2%, 95% CI 57.6% to 68.9%). In almost all covariate-specific subgroups, Gail mean risks were significantly lower than the observed risks, while IBIS-I risks showed generally good agreement with observed risks, even in the subgroups of women considered at average risk (e.g. no family history of breast cancer, BRCA1/BRCA2 mutation negative).
A further study from Marin County using data from 12,843 participants, of whom 203 had developed breast cancer during a 5-year period, showed that TC achieved an AUC of 0.65 (95% CI 0.61 to 0.68), compared with 0.62 (95% CI 0.59 to 0.66) for Gail and 0.60 (95% CI 0.56 to 0.63) for BRCAPRO. The corresponding estimated expected versus observed ratios for the models were 1.08 (95% CI 0.95 to 1.25), 0.81 (95% CI 0.71 to 0.93) and 0.59 (95% CI 0.52 to 0.68). In women with an age at first birth of > 30 years, the AUC for the TC, Gail and BRCAPRO models was 0.69 (95% CI 0.62 to 0.75), 0.63 (95% CI 0.56 to 0.70) and 0.62 (95% CI 0.56 to 0.68), and the E : O ratio was 1.15 (95% CI 0.89 to 1.47), 0.81 (95% CI 0.63 to 1.05) and 0.53 (95% CI 0.41 to 0.68), respectively.
The current study has, therefore, shown that TC is a reliable model for risk prediction in a UK general population screening programme. The discriminatory value is significantly improved by incorporating an adjustment for age and BMI-adjusted MD. These results have implications for national breast screening programmes as it appears effective to offer 3-yearly mammography screening in around 70% of the female population aged 47–73 years of age and the higher rates of high-stage cancers in women with above-average risk would justify an 18-monthly interval screen, which may downstage such cancers.
Publications arising from work in Chapter 4
Sergeant JC, Wilson M, Barr N, Beetles U, Boggis C, Bundred S, et al. Inter-observer agreement in visual analogue scale assessment of percentage breast density. Breast Cancer Res 2013;15(Suppl. 1):17. 195
Sergeant JC, Walshaw L, Wilson M, Seed S, Barr N, Beetles U, et al. ‘Same task, same observers, different values: the problem with visual assessment of breast density’, Proc SPIE 8673, Medical Imaging 2013: Image Perception, Observer Performance, and Technology Assessment, 86730T, March 28 2013. 202
Sperrin M, Bardwell L, Sergeant JC, Astley S, Buchan I. Correcting for rater bias in scores on a continuous scale, with application to breast density. Stat Med 2013;32:4666–78. 193
Hashmi S, Sergeant JC, Morris J, Whiteside S, Stavrinos P, Evans DG, et al. Ethnic variation in volumetric breast density. In Maidment ADA, Bakic PR, Gavenonis, S, editors. Breast Imaging 11th International Workshop. Berlin Heidelberg: Springer-Verlag; 2012. pp. 127–33. 196
O’Donovan E, Sergeant J, Harkness E, Morris J, Wilson M, Lim Y, et al. Use of Volumetric Breast Density Measures for the Prediction of Weight and Body Mass Index. In Fujita H, Hara T, Muramatsu C, editors. Breast Imaging 12th International Workshop. Berlin: Springer-Verlag; 2014. pp. 282–9. 198
Evans DG, Brentnall AR, Harvie M, Dawe S, Sergeant J, Stavrinos P, et al. Breast cancer risk in young women in the national breast screening programme: implications for applying NICE guidelines for additional screening and chemoprevention. Cancer Prev Res (Phila) 2014;7:993–1001. 190
Chapter 5 Cost-effectiveness
This section reports the aims, methods and results of three studies used to address the overarching research question outlined in the original proposal to understand the relative cost-effectiveness of using risk-based algorithms to inform the appropriate screening intervals in the NHSBSP. The early work in the programme was used to provide a mechanism (risk-based algorithm) for dividing a population of women into distinct categories that predicted their risk of breast cancer, which was then used to infer prespecified screening intervals to use as part of a risk-based national breast screening programme. The risk prediction studies, PROCAS and the study designed to assess the predictive value of new genetic variants (reported in Chapters 2, 3 and 4, respectively) informed the development of a risk-based algorithm which is used to select the appropriate screening interval for women participating in a national breast screening programme.
Aim
The focus of the cost-effectiveness work was to identify the relevant costs and patient benefits affected by using risk-based algorithms to inform the appropriate length of screening interval compared with the current NHSBSP.
Objectives
There were two initial objectives:
-
to identify and critically appraise the existing economic evidence supporting the use of a national breast screening programme and individual screening modalities in the UK
-
to identify the potential incremental costs and patient benefits associated with a risk-based screening programme as part of the NHSBSP and identify the expected cost-effectiveness.
At the request of external reviewers, a third objective was added:
-
to quantify the impact of women attending the UK breast screening programme on their out-of-pocket expenses.
These three objectives were addressed using three distinct studies:
-
systematic review of economic evaluations of breast screening programmes and screening modalities
-
quantifying the impact of a national breast screening programme on women’s out-of-pocket expenses
-
preliminary model-based cost-effectiveness analysis of a risk-based screening strategy for breast cancer as part of the NHSBSP.
The aim, methods and results from these three studies are now described.
Study 1: systematic review of economic evaluations of breast screening programmes and screening modalities
Background
A key stage in structuring an economic model, suitable to inform resource allocation decisions, is to identify and critically appraise existing published economic evaluations related to the intervention (breast screening programmes) of interest.
Aim
The primary aim is to identify published articles which have conducted an economic evaluation of breast cancer screening programmes and to summarise and critically appraise these studies.
Objectives of review
-
Identify the number of published economic evaluations of breast cancer screening programmes.
-
Identify the comparators, specifically if an option of no screening was evaluated and, if not, what screening intervention comparator was used.
-
Identify the interventions evaluated and classify according to screening modality, age range and screening interval and studies which have examined risk-based screening interventions.
-
Classify the evaluations as either trial or model based.
Methods
A systematic review was conducted of economic evaluations relevant to breast screening in a general population of women and published in peer-reviewed journals.
Literature search
The electronic databases Ovid MEDLINE, Ovid EMBASE and NHS Economic Evaluations Database (accessed via the Centre for Reviews and Dissemination databases) were systematically searched to identify economic evaluations published in peer-reviewed journals. Electronic search strategies were designed for each respective database and combined index and free-text terms for breast cancer screening and associated screening modalities (e.g. mammography) with the Centre for Reviews and Dissemination economics filter (see Appendices 2–4). In addition, the reference lists of relevant studies were hand-searched to identify further studies meeting the inclusion criteria. All searches were performed on 30 January 2014.
Inclusion criteria and study selection for critical review
Two reviewers (IJ and EG) independently screened all retrieved titles and abstracts to identify the studies eligible for inclusion in the critical review. Studies were included if they met the inclusion criteria summarised in Table 66.
Aspect of study | Inclusion criteria |
---|---|
Study design | Full economic evaluationa |
Population | Adult women in the general population |
Intervention and comparator | Breast screening programme, change to a breast screening programme or screening modality included as either intervention or comparator |
Type | Cost per unit of effectiveness; cost per QALY; cost–benefit analysis |
Outcomes | Cost and direct impact on effectiveness (cases detected; cancer deaths prevented) and/or patient benefits (life-years gained, QALYs) |
Availability | English; full text |
Data collection and extraction
Data were extracted from the identified studies by two reviewers (Sean Gavan and KP) using a structured data collection form. The de novo data extraction form was developed based on the CHEERS (Consolidated Health Economic Evaluation Reporting Standards)237 checklist and supplemented by criteria suggested by Karnon et al. 238
The data extracted encompassed:
-
viewpoint – societal/health system/health service/payer
-
type of alternative (technology/screening programme/screening interval)
-
comparator (current screening programme or no screening)
-
study population (eligible age)
-
type of evaluation (model or trial based)
-
model type: decision tree/Markov model
-
whether or not calibration was performed
-
whether or not model validation was performed
-
uncertainty analysis included (one way and/or probabilistic)
-
method used to estimate effectiveness and data source (meta-analysis/RCT/cohort study/published studies)
-
whether or not screening was linked to treatment
-
primary measure of benefit (clinical effectiveness/life-years/QALYs)
-
resources included
-
price year (currency)
-
time horizon (discount rate)
-
incremental cost-effectiveness ratio results
-
key parameters driving cost-effectiveness.
Results were tabulated and then summarised in a narrative synthesis.
Results
This section reports the main findings from the systematic review of published economic evaluations of breast screening programmes.
Study identification
Figure 41 summarises the study identification and inclusion process. The search of electronic databases identified 2959 published studies, which were screened for eligibility. A total of 71 studies were identified and included in the review. A further 15 studies may be added at a later date if the hard copies of the manuscripts can be sourced.
FIGURE 41.
Study identification and inclusion. BSP, breast screening programme.
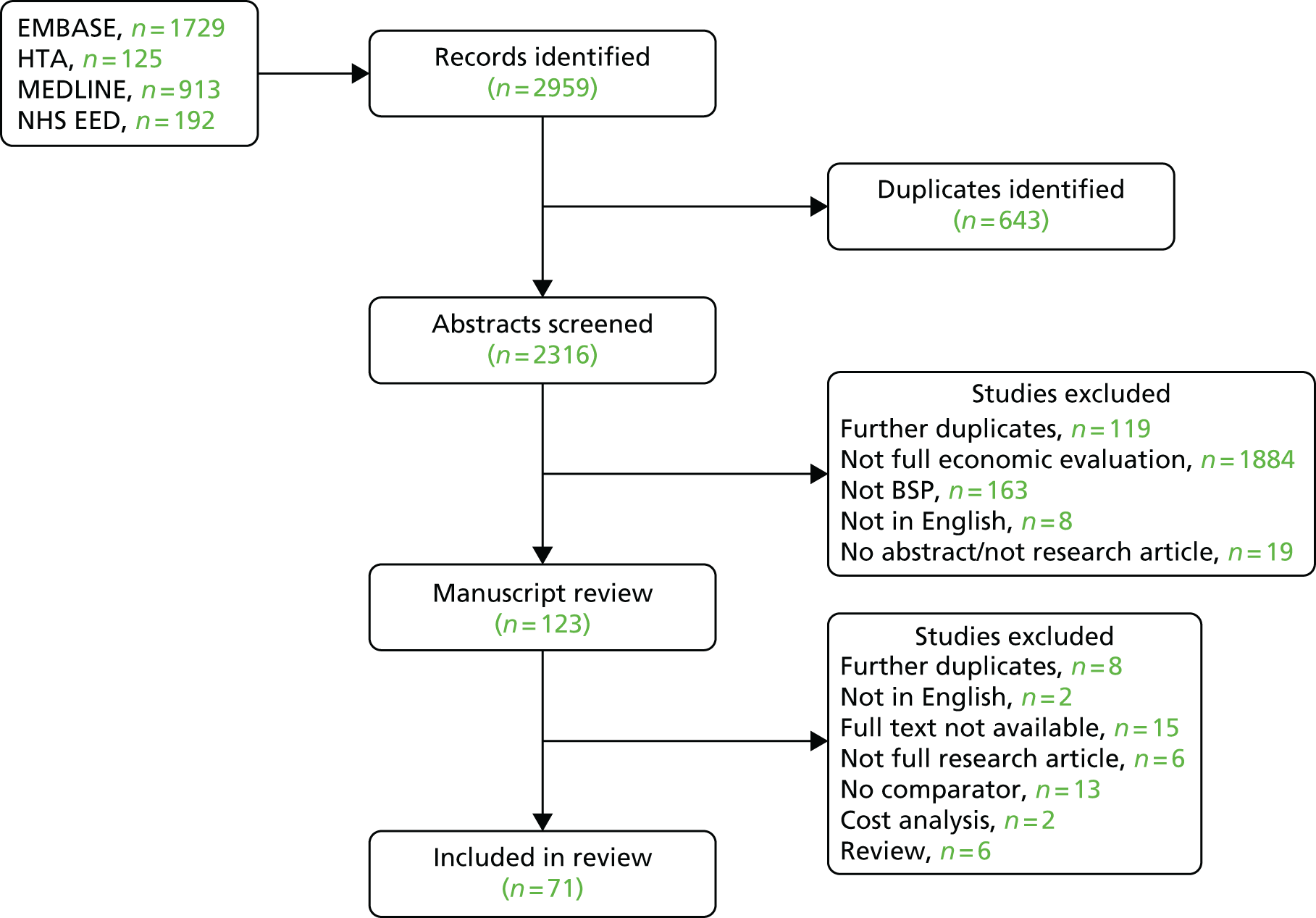
Table 67 provides an overview of these studies. The full data extraction table is available from the authors on request. Three other key modelling studies were identified: Tan et al. ,310 the Wisconsin simulation model311 and Breastscreen Australia Evaluation (2009). 312 Tan et al. 310 developed a Markov model to characterise breast cancer progression but did not attach cost data, and this study is not, therefore, an economic evaluation. The Wisconsin model311 was developed as part of the National Cancer Institute Cancer Intervention and Surveillance Modelling Network. It is a patient-level simulation model, continually updated, that uses data from Wisconsin State, USA. The model simulates breast cancer in a population over time, generating cancer registry-like data sets. The model uses parametric input assumptions about natural history, screening and treatment, and can be used to evaluate the relative cost-effectiveness of different breast screening-related policies. The Australian cost-effectiveness analysis312 compared the current BreastScreen Australia programme and various alternative screening scenarios with a hypothetical no-screening scenario using a Markov model. BreastScreen Australia actively targets all women aged 50–69 years; however, women aged 40–49 years and > 70 years are also eligible to attend. The model consists of two separate components: the natural history of disease component and the BreastScreen Australia screening pathway. These models were not included in the review as they were not published in a peer-reviewed journal, but they are mentioned here as they were subsequently used to inform the final model structure.
Author (year), country | Relevant alternatives | Type of evaluation |
---|---|---|
Ahern (2009),239 USA | Viewpoint: not reported Type of alternative: screening intervals (10 strategies) Comparator: no screening Study population (eligible age): women in USA (40–79 years) |
Model Cost–utility analysis |
Arveux (2003),240 France | Viewpoint: not reported Type of alternative: screening programme Comparator: no screening Study population (eligible age): women in France (50–65 years) |
Model based Cost-effectiveness analysis |
Baker (1998),241 UK | Viewpoint: societal/health system/health service/payer Type of alternative: screening programme (seven policies) Comparator: no screening Study population (eligible age): women (50–65 years) |
Model Cost-effectiveness analysis |
Boer (1995),242 the Netherlands | Viewpoint: not reported Type of alternative: screening interval Comparator: current 2-year interval screening Study population (eligible age): women in the Netherlands (aged 51–75 years) |
Model based Cost–utility analysis |
Boer (1998),243 UK | Viewpoint: not reported Type of alternative: screening interval (two strategies) Comparator: 3-year interval screening for women aged 50–64 years Study population (eligible age): women in the UK (50–69 years) |
Model based Cost-effectiveness analysis |
Boer (1999),244 the Netherlands | Viewpoint: health system Type of alternative: screening interval (three strategies) Comparator: 2-year interval screening for women aged 50–64 years Study population (eligible age): women in the Netherlands (50–96 years) |
Model based Cost-effectiveness analysis |
Brancato (2007),245 Italy | Viewpoint: not reported Type of alternative: technology Comparator: no ultrasonography Study population (eligible age): women who have self-referred to receive an ultrasonography, within 1 month of receiving a negative mammography (29–85 years) |
Retrospective cohort based Cost-effectiveness analysis |
Brown (1996),246 UK | Viewpoint: health service and patients Type of alternative: technology (consensus or non-consensus double mammography reading) Comparator: single reading of mammography Study population (eligible age): women in the UK (50–64 years) |
Prospective cohort based Cost-effectiveness analysis |
Bryan (1995),247 UK | Viewpoint: health service and patients Type of alternative: technology (two-view) screening Comparator: one-view screening Study population (eligible age): women in the UK (50–64 years) |
Prospective cohort based Cost-effectiveness analysis |
Burnside (2001),248 USA/Sweden | Viewpoint: not reported Type of alternative: screening programme (two countries): USA Comparator: screening programme in Sweden Study population (eligible age): women in the USA (40–79 years) |
Model based Cost-effectiveness analysis |
Cairns (1998),249 UK | Viewpoint: not reported Type of alternative: technology (double reading) Comparator: current practice – single reading of X-rays Study population (eligible age): women in Scotland (age unreported) |
Retrospective cohort based Cost-effectiveness analysis |
Carles (2011),250 Spain (Catalonia) | Viewpoint: national health system Type of alternative: screening programme (20 strategies) Comparator: not reported explicitly Study population (eligible age): women in Spain (40–79 years) |
Model based Cost-effectiveness analysis |
Caumo (2011),251 Italy | Viewpoint: health service Type of alternative: technology (arbitration of double reading) Comparator: current practice in Florence in 2005 Study population (eligible age): women (not reported) |
Prospective cohort based Cost-effectiveness analysis |
Ciatto (1995),252 Italy | Viewpoint: health service Type of alternative: technology (double reading) Comparator: single reading Study population (eligible age): women (50–70 years) |
Prospective cohort based Cost-effectiveness analysis |
Clarke (1991),253 UK | Viewpoint: health service Type of alternative: screening programme Comparator: no screening Study population (eligible age): women (not reported explicitly) |
Trial based Cost-effectiveness analysis |
Clarke (1998),254 Australia | Viewpoint: societal Type of alternative: screening programme (mobile van) Comparator: fixed screening unit Study population (eligible age): women (50–69 years) |
Model based Cost–benefit analysis |
de Gelder (2009),255 Switzerland | Viewpoint: not reported explicitly Type of alternative: screening (mammography and % of opportunistic: five strategies) Comparator: no screening Study population (eligible age): women (50–69 years) |
Model based Cost-effectiveness analysis |
de Koning (1991),256 the Netherlands | Viewpoint: not reported Type of alternative: screening interval and age groups (five strategies) Comparator: no screening Study population (eligible age): women (varied: 50–70 years for comparator) |
Model based Cost-effectiveness analysis |
Feig (1995),257 USA | Viewpoint: not reported Type of alternative: screening programme Comparator: not reported explicitly Study population (eligible age): women (40–49 years) |
Prospective-cohort based Cost-effectiveness analysis |
Garuz (1997),258 Spain | Viewpoint: not reported Type of alternative: screening programme Comparator: do nothing Study population (eligible age): women (50–65 years) |
Model based Cost-effectiveness analysis |
Groenewoud (2007),259 the Netherlands | Viewpoint: not reported Type of alternative: technology (double reading/referral strategies) Comparator: current referral strategy Study population (eligible age): women (50–74 years) |
Model based Cost-effectiveness analysis |
Gryd-Hansen (2000),260 Denmark | Viewpoint: societal Type of alternative: screening programme Comparator: current practice Study population (eligible age): women (varied) |
Model based Cost–benefit analysis |
Guerriero (2011),261 UK | Viewpoint: health service Type of alternative: technology (computer aided detection – CAD) Comparator: current (double reading) practice Study population (eligible age): women (50–70 years) |
Trial-based Cost-effectiveness analysis |
Hall (1992),262 Australia | Viewpoint: not reported explicitly Type of alternative: screening programme Comparator: no screening Study population (eligible age): women (45–69 years) |
Model based Cost-effectiveness analysis |
Henderson (2012),263 USA | Viewpoint: payer Type of alternative: technology (digital mammography) Comparator: single film mammography Study population (eligible age): women (≥ 65 years) |
Retrospective cohort based Cost-effectiveness analysis |
Hunter (2004),264 Canada | Viewpoint: health service Type of alternative: screening programme from age 40 Comparator: current screening (50–69 years) Study population (eligible age): women (40–69 years) |
Model based Cost-effectiveness analysis |
Johnston (1999),265 UK | Viewpoint: health service Type of alternative: technology (two-view mammography) Comparator: current practice (one view) Study population (eligible age): women (50–64 years) |
Prospective cohort based Cost-effectiveness analysis |
Kang (2013),266 Korea | Viewpoint: government payer Type of alternative: screening programme Comparator: no screening (non-attenders) Study population (eligible age): women (≥ 40 years) |
Retrospective cohort based Cost-effectiveness analysis |
Kerlikowske (1999),267 USA | Viewpoint: not reported Type of alternative: screening age Comparator: screening between 50 and 69 years Study population (eligible age): women (≥ 65 years) |
Model based Cost-effectiveness analysis |
Lee (2009),268 Korea | Viewpoint: health system Type of alternative: screening interval and age range Comparator: current screening Study population (eligible age): women (35–75 years) |
Model based Cost-effectiveness analysis |
Leivo (1999),269 Finland | Viewpoint: societal Type of alternative: screening programme Comparator: no screening Study population (eligible age): women (50–59 years) |
Retrospective cohort based Cost-effectiveness analysis |
Leivo (1999),270 Finland | Viewpoint: societal Type of alternative: technology (double reading) Comparator: single reading Study population (eligible age): women (50–59 years) |
Retrospective cohort based Cost-effectiveness analysis |
Lindfors (1995),271 USA | Viewpoint: payer Type of alternative: screening interval and age bands Comparator: no screening Study population (eligible age): women (varied age bands between 40 and 79 years) |
Model based Cost-effectiveness analysis |
Lindfors (2006),272 USA | Viewpoint: societal Type of alternative: technology (with and without computer aided detection) Comparator: no screening Study population (eligible age): women (40–79 years) |
Model based Cost-effectiveness analysis |
Madan (2010),273 UK | Viewpoint: health service Type of alternative: screening age Comparator: no screening Study population (eligible age): women (47–49 years) |
Model based Cost-effectiveness analysis |
Mandelblatt (2005),274 USA | Viewpoint: societal Type of alternative: screening age Comparator: screening age 50 to 70 years Study population (eligible age): women (50–70 years, 79 years or lifetime) |
Model based Cost-effectiveness analysis |
Melnikow (2013),275 USA | Viewpoint: health system Type of alternative: technology (digital or film mammography)/screening interval(annual or every 2 years)/screening age (start 40 or 50 years) Comparator: no screening Study population (eligible age): women (40–64 years) |
Model based Cost-effectiveness analysis |
Mooney (1982),276 UK | Viewpoint: societal Type of alternative: screening programme (6 strategies) Comparator: not reported explicitly Study population (eligible age): women (40–59 years) |
Retrospective cohort based Cost-effectiveness analysis |
Moskowitz (1979),277 USA | Viewpoint: not reported Type of alternative: screening programme Comparator: no screening Study population (eligible age): women (not reported) |
Retrospective cohort based Cost-effectiveness analysis |
Moskowitz (1987),278 USA | Viewpoint: societal Type of alternative: screening programme Comparator: no screening Study population (eligible age): women (not reported) |
Retrospective cohort based Cost-effectiveness analysis |
Moss (2001),279 UK | Viewpoint: health service Type of alternative: screening age Comparator: current screening (50–64 years) Study population (eligible age): women (50–69 years) |
Retrospective cohort based Cost-effectiveness analysis |
Neeser (2007),280 Switzerland | Viewpoint: health system insurance Type of alternative: screening programme Comparator: opportunistic screening Study population (eligible age): women (baseline age 40–70 years) |
Model based Cost-effectiveness analysis |
Nguyen (2013),281 Vietnam | Viewpoint: payer Type of alternative: screening programme Comparator: no screening Study population (eligible age): women (40 years) |
Model based Cost-effectiveness analysis |
Norum (1999),282 Norway | Viewpoint: societal Type of alternative: screening programme Comparator: no screening Study population (eligible age): women (50–69 years) |
Model based Cost-effectiveness analysis |
Nutting (1994),283 USA | Viewpoint: payer Type of alternative: technology (mammography) Comparator: current practice of clinical breast examination Study population (eligible age): Native American women (≥ 50 years) |
Model based Cost-effectiveness analysis |
Ohnuki (2006),284 Japan | Viewpoint: health-care payer Type of alternative: technology and screening age Comparator: no screening (clinical breast examination) Study population (eligible age): women (30–79 years) |
Model based Cost-effectiveness analysis |
Okonkwo (2008),285 India | Viewpoint: not reported Type of alternative: technology, screening interval and screening age Comparator: no screening (clinical breast examination) Study population (eligible age): women (40–70 years; varied) |
Model based Cost-effectiveness analysis |
Okubo (1991),286 Japan | Viewpoint: payer Type of alternative: technology (mammography ± physical examination) Comparator: no screening Study population (eligible age): women (30–80 years) |
Model based Cost-effectiveness analysis |
Pharoah (2013),287 UK | Viewpoint: health service Type of alternative: screening programme Comparator: no screening Study population (eligible age): women (50–85 years) |
Model based Cost-effectiveness analysis |
Rojnik (2008),288 Slovenia | Viewpoint: health system Type of alternative: screening programme (36 strategies varied by interval and start age) Comparator: no screening Study population (eligible age): women (≥ 40 years) |
Model based Cost-effectiveness analysis |
Rosenquist (1994),289 USA | Viewpoint: payer Type of alternative: technology (mammography) Comparator: no screening (clinical observation) Study population (eligible age): women (40–49 years) |
Model based Cost-effectiveness analysis |
Rosenquist (1998),290 USA | Viewpoint: societal Type of alternative: screening interval and start age Study population (eligible age): women (40–79 years; varied) |
Model based Cost-effectiveness analysis |
Salzmann (1997),291 USA | Viewpoint: payer Type of alternative: screening start age Comparator: no screening Study population (eligible age): women (40–69 years) |
Model based Cost-effectiveness analysis |
Schousboe (2011),292 USA | Viewpoint: payer Type of alternative:a screening programme, screening interval, screening age Comparator: no screening Study population (eligible age): women (40–79 years; varied) |
Model based Cost-effectiveness analysis |
Shen (2005),293 USA | Viewpoint: health system Type of alternative: technology (mammography), screening interval, screening aged (48 strategies) Comparator: no screening Study population (eligible age): women (40–79 years) |
Model based Cost-effectiveness analysis |
Souza (2013),294 Brazil | Viewpoint: health system Type of alternative: screening technology (film or digital mammography), screening interval, screening age (seven strategies) Comparator: no screening (usual care) Study population (eligible age): women (40–69 years; varied) |
Model based Cost-effectiveness analysis |
Stout (2006),295 USA | Viewpoint: societal Type of alternative: screening interval, screening age (11 strategies) Comparator: no screening Study population (eligible age): women (40–80 years; varied) |
Model based Cost-effectiveness analysis |
Szeto (1996),296 New Zealand | Viewpoint: health system Type of alternative: technology (mammography), screening interval, screening age (four strategies) Comparator: no screening Study population (eligible age): women (45–69 years; varied) |
Model based Cost-effectiveness analysis |
Taylor (2005),297 UK | Viewpoint: health service Type of alternative: technology (computer aided detection) Comparator: no computer aided detection Study population (eligible age): women (50 years) |
Model based Cost-effectiveness analysis |
Tosteson (2008),298 USA | Viewpoint: societal and payer Type of alternative:a technology (digital mammography), age-targeted screening, breast density-targeted screening Comparator: film mammography Study population (eligible age): women (≥ 40 years; varied) |
Model based Cost-effectiveness analysis |
Van Dyck (2012),299 UK | Viewpoint: not reported explicitly Type of alternative:a screening programme (stratification by genetic test and risk calculator) Comparator: current screening programme Study population (eligible age): women (50–69 years) |
Model based Cost-effectiveness analysis |
van Ineveld (1993),300 Spain/France/UK/the Netherlands | Viewpoint: health service Type of alternative: screening programme (different countries) Comparator: not reported explicitly Study population (eligible age): women (50–70 years) |
Model based Cost-effectiveness analysis |
Wald (1995),301 UK | Viewpoint: societal Type of alternative: technology (two view mammography; two strategies) Comparator: current programme (one view mammography) Study population (eligible age): women (50– 64 years) |
Trial based Cost-effectiveness analysis |
Wang (2009),302 Australia | Viewpoint: societal Type of alternative: technology (digital mammography) Comparator: film mammography Study population (eligible age): pre- or perimenopausal women with dense breasts (< 50 years) |
Model based Cost-effectiveness analysis |
Warmerdam (1997),303 Germany | Viewpoint: health system Type of alternative: screening programme (two ‘quality’ strategies) Comparator: baseline current screening programme Study population (eligible age): women (50– 69 years) |
Model based Cost-effectiveness analysis |
Wong (2007),304 Hong Kong | Viewpoint: societal Type of alternative: screening programme, screening age Comparator: no screening Study population (eligible age): women (40–79 years; varied) |
Model based Cost-effectiveness analysis |
Wong (2010),305 Hong Kong | Viewpoint: societal Type of alternative: screening programme, screening age Comparator: no screening Study population (eligible age): women (40–79 years; varied) |
Model based Cost-effectiveness analysis |
Wong (2012),306 Hong Kong | Viewpoint: societal Type of alternative: screening programme, treatment strategies (five strategies) Comparator: current screening programme Study population (eligible age): women (40–79 years; varied) |
Model based Cost-effectiveness analysis |
Woo (2007),307 Hong Kong | Viewpoint: societal Type of alternative: screening programme, screening interval, screening age (18 strategies) Comparator: no screening Study population (eligible age): women (40–74 years) |
Model based Cost-effectiveness analysis |
Zelle (2012),308 Ghana | Viewpoint: health system Type of alternative: screening programme, technology (16 strategies) Comparator: current practice (10% coverage) Study population (eligible age): women (40–69 years; varied) |
Model based Cost-effectiveness analysis |
Zelle (2013),309 Peru | Viewpoint: health system Type of alternative: screening programme, technology (94 strategies) Comparator: current practice Study population (eligible age): women (40–69 years; varied) |
Model based Cost-effectiveness analysis |
Study overview
There were a total of 71 economic evaluations included in the review. Of these, 52 were model-based evaluations, 10 were retrospective cohort-based evaluations, six were prospective cohort-evaluations and three were trial-based evaluations. The majority (n = 69) conducted cost-effectiveness analysis and there were two cost–benefit analyses.
The review covered studies up to the end of December 2013 and the first study277 was published in 1979. The analyses were based in a number of countries including the USA (n = 18239,257,263,267,271,272,274,275,277,278,283,289–293,295,298), the UK (n = 15241,243,246,247,249,253,261,265,273,276,279,287,297,299,303), the Netherlands (n = 4242,244,256,259), Hong Kong (n = 4304–307), Australia (n = 3254,262,302), Italy (n = 3245,251,252), Japan (n = 2284,286), Korea (n = 2266,268), Finland (n = 2269,270), Spain (n = 2250,258) and Switzerland (n = 2255,280); and there was one study in each of France,240 Brazil,294 Ghana,308 Germany,303 New Zealand,296 Vietnam,281 Peru,309 Norway,282 Slovenia,288 Canada,264 India285 and Denmark. 260 One study300 compared programmes across four countries (the UK, the Netherlands, Spain and France) and one study248 compared programmes across two countries (the USA and Sweden).
The number of different interventions and varied jurisdictions for the analyses limits the comparability between the programmes and ability to provide an overall statement of relative cost-effectiveness owing to issues with generalisability. 313 Van Ineveld et al. 300 explicitly concluded, in their comparison of breast screening programmes for four European countries (the UK, France, Spain and the Netherlands), that ‘no uniform policy recommendations for breast cancer screening can be made for all countries in the European Community’. In terms of the remaining 15 analyses that focused on the UK, eight studies concluded that the programme or technology was potentially cost-effective but that more robust evidence was needed before a definitive conclusion could be reached,243,249,254,287,299 five studies suggested an optimal programme based on relative cost-effectiveness242,246,247,279,301 and two studies, both evaluating computer-aided detection, concluded that the technology was not likely to be a cost-effective use of resources but that more evidence was needed to support this conclusion. 292,298
Interventions and comparators
A number of interventions were evaluated in the identified studies: introducing a screening programme using mammography; using a mobile screening unit; different screening programmes that may vary by screening modality, screening interval or screening age; screening interval; screening age; and new technologies (two-view reading, double reading, computer-aided detection, digital mammography, ultrasonography). Some studies included multiple interventions for evaluation and hence reported a range of strategies. One study309 reported that 94 strategies were evaluated. Two studies248,300 compared screening programmes across countries. Three studies explicitly covered some form of stratified screening strategy (risk and/or density based). 292,298,299
A study had to state that a comparator was included in the analysis to be defined as an economic evaluation and hence to be eligible for the review. However, on some occasions, a comparator, although alluded to, was not reported explicitly. On the occasions when a comparator was reported explicitly, those used were no screening, opportunistic screening and the existing screening programme or technology (in terms of modality, screening interval and screening age).
Study population
The age range of the women included in the eligible study population depended on the country base for the analysis and the screening programme under evaluation. A number of studies specifically evaluated the choice of age range for the screening programme in terms of the impact on the relative costs and outcomes. Not every study reported the eligible age range for the screening programme. The earliest reported starting age for a screening programme that was evaluated was 30 years (Japan) and the oldest included age was 85 years (UK), with one study evaluating lifetime screening (USA).
The majority of the modelling studies described pre-clinical disease incidence and disease progression rates, which were sourced from longitudinal observational databases. For some studies, historical incidence data for breast cancer were incorporated into the model because either opportunistic screening or a population-based screening programme was already available in the study setting.
Validation and calibration
Validation and calibration of decision-analytic and mathematical models of screening interventions are important aspects to include in the design of a robust model-based economic analysis. Validation has always been recognised to be a key component of developing a model structure which accurately reflects the stated decision problem. 314 In contrast, calibration emerged into recognition as a key component around 2010 onwards. 315 However, owing to word constraints set by journal article lengths, the process of model validation or calibration is not generally reported in detail.
Three studies described a method of model validation. de Koning et al. 256 compare the predicted results with the actual results from the first 7500 women screened in the Netherlands in 1989. The study presents six model outputs: attendance rate, positive screens, biopsy, breast cancer detection rate, positive predictive value, and malignant diagnosis with biopsy and lymph node metastases. The predicted estimates from the model match very closely those observed. However, it is worth noting that the outputs chosen for validation represent a short time period. The performance of the model, in terms of long-term predictions, cannot be ascertained. Both Schousboe et al. 292 and the Australian Department of Health Review (not included in the review as not in a peer-reviewed journal) compare the predicted age-adjusted breast cancer incidence and mortality data for their respective countries.
Stout et al. 295 was the only identified study that explicitly described a process of model calibration for unobservable input parameters. The study utilised breast cancer incidence data from the USA to calibrate a lag time parameter representing the period between the start of biological growth and the detection of a tumour. In addition, the model parameter determining the growth rate of a tumour is also calibrated. In furthering the robustness of their model, once calibrated the model outputs were validated using cancer registry data. This study remains the only robust description of modelling calibration and validation for any of the studies identified.
Overview of key model-based evaluations
There were 52 model-based evaluations. The most commonly reproduced model structure was the Microsimulation Screening Analysis (MISCAN) model, which was used in nine studies. The MISCAN model was applied in a range of country settings outside the Netherlands, for which it was originally designed, but in all cases these models still relied on Dutch data to populate key aspects of the model structure. The MISCAN model is not publicly available. Therefore, a range of model types including decision trees, Markov state transition models, individual patient-level simulation models and mathematical models were used in the studies identified. In general, each individual study developed a de novo model relevant to a specific research question.
Overview of evaluations of stratified screening strategies
Three studies explicitly stated that they conducted a model-based analysis of a stratified screening strategy. 292,298,299 Two of these studies were based in the USA292,298 and one was UK based. 299 These studies are now described with their key findings and relevance to the UK setting.
Tosteson298 used a discrete event simulation (DES) model with a lifetime horizon, taking a societal and Medicare perspective relevant to the US setting. The model was the Wisconsin breast cancer epidemiology simulation model. 311 All-digital mammography was compared with targeted mammography interventions. Mammography screening was targeted using different strategies: age (women < 50 years); and age and density targeted (women < 50 years or women ≥ 50 years with dense breasts). The model structure and data sources were relevant to US women, which limits the relevance of the findings of this study to the UK setting. The authors concluded that all-digital was not a cost-effective option when compared with film mammography. Age-targeted mammography was identified to be a relatively cost-effective use of resources but density-targeted mammography was of uncertain added value, especially in older women (those aged ≥ 65 years). The findings were sensitive to a number of assumptions but, most importantly, the accuracy of the screening modality in older women with non-dense breasts.
Schousboe et al. 292 used a five-state Markov model with a lifetime horizon, taking the US payers’ perspective and using data from a US population of women. The study perspective and source of data limit the relevance of the findings from this study to the UK setting. The Markov model was also overly simplistic in terms of capturing the potentially relevant health states and pathways of care likely to be influenced by a stratified screening programme, and was not viewed as a potentially useful starting point for the model-based analysis proposed as part of this programme. This study did not define a single stratified screening intervention per se but rather used observational data to identify what factors influenced relative cost-effectiveness of a breast screening programme. The authors concluded that mammography screening (offered every 3–4 years, biennially or annually) should be stratified based on key criteria: woman’s age; breast density (defined using BI-RADS categories 1–4); history of breast biopsy; family history of breast cancer; and beliefs about the potential benefits and harms of screening. The findings were sensitive to the cost of digital mammography and the assumed prevalence of dense breasts in a population.
Van Dyck et al. 299 used four ‘what-if’ scenarios structured around pathways depicted by decision trees and populated with data from the UK population. The study perspective, time horizon, nature and source of model inputs or method of analysis were not reported in the main body of the text. Supplementary materials were provided but did not give additional information, except that reference was made to using a Markov model alongside the decision tree reported in the main paper. The interventions proposed involved stratifying women aged ≥ 50 years by family history and genetic testing using electronic health records, a SNP-based test [BRCA1, BRCA2, TP53, FGFR2, TNRCP, MAP3K1, LSP1, CHECK2, gene(ATM), BRIP1, PALB2] and a breast cancer risk calculator (it was not stated explicitly which one was used). These interventions were combined and used pre screen to create two risk profiles (high and low, but risk levels were not reported). The estimated incremental costs and benefits were not reported and the key drivers of relative cost-effectiveness were not stated. The data inputs and method of analysis were not reported sufficiently for this study to provide a useful starting point for any subsequent analysis.
Discussion
The main aim of an economic evaluation is to examine the available evidence on the costs and effectiveness of health technologies in order to provide decision-makers with the relevant information to assess the technology’s ability to provide value for money to the payer. However, this may require information to be gathered from outside the traditional evidence base of a randomised controlled trial (RCT). 316 For example, trial evidence may not examine all the relevant comparators or it may have a short study period which does not examine long-term costs or effects. Models have been exploited in economic evaluation as a means to synthesise the relevant sources of information that are available, extrapolate results into the future and provide estimates of costs and effects in the absence of direct observation.
Broadly, four classifications of modelling approaches are described in the breast screening literature: decision trees, state-transition, mathematical and DES. The MISCAN model was developed by van Oortmarssen et al. 317 and is widely reported in the literature. The model is a specifically developed computer simulation package to, first, assess the clinical impact of different screening policies. The model has also been applied to prostate and colorectal cancer screening programmes.
The MISCAN model structure was used as an individual patient sampling model in nine of the identified studies. The natural history of this model is defined in terms of the size of the tumour, where distinction is made between pre-clinical, clinical and screen-detected cancers. This follows closely the general modelling approach for screening programmes presented by Karnon et al. 238 The advantage of this modelling approach is that it allows a patient pathway to vary between those who attend screening and those who do not and, where appropriate, account for differences in the patient characteristics, such as disease progression and incidence. Movement from one phase to another is also determined by the treatment an individual is assumed to receive based on the characteristics of the tumour in terms of size.
In contrast, some models, such as the ones used by Carles et al. 250 and Salzmann et al. ,291 present comparatively simple four-state model structures. Both of the studies fail to explicitly describe and justify the model structures presented. It is questionable if these structures capture the underlying essentials of the breast disease process and screening interventions. Madan et al. 273 provided a unique approach in their model of the addition of an extra screening round to the English NHSBSP. Although the authors do not explicitly state the model type, it can be inferred from the model structure that a decision-tree-type model is used, representing the only identified study to take such an approach. The premise of their study is to provide a ‘rapid-response’ economic analysis and argue that decision-makers may be willing to trade-off precision of results estimates for a faster turnaround time with producing and analysing a model.
None of the identified studies described the process of defining the model structure. The process for defining a model structure as described by Karnon et al. 238 may be considered as a second-order issue when presenting higher-level methods and results. If this is the case, and combined with limited amount of space in which studies are reported in academic journals, it is perhaps understandable that this feature is not included in the identified studies. This may also be because some of the models reported were ‘borrowed’ from previous studies and directly applied to the current research question. Therefore, the authors were not involved in the construction phase of the model and hence were not able to define the process of defining the model structure. All of the studies fail to report a justification for the choice of modelling technique employed. Again, this may be a result of the limited space available and the relative importance authors place on including this justification, compared with presenting the detail of the results from a model.
Concluding remarks
This review has identified a substantial number of published economic evaluations relevant to breast screening programmes, the technologies used and modifications to the programme in terms of screening interval and age groups appropriate for screening. None of the identified 52 models provides an ‘off-the-shelf’ model that is fit for the purpose of answering the research question posed by PROCAS. Therefore, it will be necessary to construct a de novo model. The process suggested by Karnon et al. 238 will prove useful to inform the general concepts of how to model in the context of breast screening. The MISCAN model cannot be used directly as it is not publicly available, but will inform the approach taken to including cumulative incidence and unobserved parameters. This approach will be supplemented by the modelling proposed by Tan et al. ,220 a study which, although not an economic evaluation, provides some useful information.
Study 2: quantifying the impact of a national breast screening programme on women’s out-of-pocket expenses
Background
In the UK, guidance published by NICE41 recommends using the NHS and social services perspective in economic evaluations. The NHS perspective was, therefore, suggested as the relevant viewpoint for the proposed economic evaluation of the risk-stratified breast screening programme. Some published economic evaluations of mammography-based breast screening programmes, such as the one by Brown et al. ,246 have used a broader perspective to include the costs incurred by women attending the screening in the UK. The study by Brown et al. used a survey-based approach to identify and quantify the travel and time costs for the women to attend the screening van. The study identified costs for a sample of 132 women who attended screening in 1992 and found that the mean private cost of assessment was £43.53 (SD £30.29), which included a mean travel cost of £10.74 and mean time cost of £32.75. Knowledge of this study might have prompted reviewers of the original PROCAS proposal to suggest that the study design also include an evaluation of the private assessment costs incurred by the women.
Aim
To identify the private assessment cost incurred by women when attending a breast screening programme.
Study objectives
This study has two objectives:
-
to estimate the distance travelled by women attending the NHSBSP van
-
to identify the self-reported distance travelled and time taken to attend the NHSBSP van.
Method
This study used two approaches to estimate and identify distance travelled and time to attend the screen: (1) survey-based self-report and (2) geographical distance calculation. The methods for these two approaches are now described.
Survey of PROCAS participants
An online survey (available from Ian Jacob on request) was used to identify PROCAS participants’ self-reported travel and time costs by focusing on four key aspects related to attending the breast screening appointment: the journey to the breast screening centre; the time at the breast screening centre; whether or not they had any companions for their visit; and whether or not they were responsible for caring for children or older people at home. The survey was piloted in a sample of women to check the wording of the questions. The final survey was administered using the QUALTRICS online survey tool (www.qualtrics.com).
Sampling frame
This aspect of the study used a stratified purposive sampling approach. At the time of invitation (February 2014), the PROCAS study sample was 53,605. From this eligible sample, women who had attended their screening mammogram within the previous 6 months were identified as potentially eligible survey respondents. Respondents who had died or received a diagnosis of breast cancer were excluded from the eligible sample, which gave a potential sample size of 51,540, of whom 2350 had attended for screening in the last 6 months. The aim was to target a representative sample from each of the 14 active screening sites, and the final target sample size was 600 owing to financial constraints. Not all screening locations are active in the Greater Manchester area at the same time, and 14 screening sites were active at the time of the survey. A weighted sample by number of participants in each of these sites was asked to complete the survey (n = 594). An invitation to participate in the online survey was posted to each of these identified participants. No reminder was sent.
Table 68 shows the total number of women who were eligible for screening, the number recruited to the PROCAS study and the number invited to participate in the survey by screening van location.
Screening location | Total | PROCAS participants | Invited to complete survey |
---|---|---|---|
Total | 53,605 | 2350 | 594 |
Ann Street, Manchester | 1747 | 0 | 0 |
Ashton Primary Care Centre, Tameside | 2521 | 0 | 0 |
Bodmin Road, Manchester | 7884 | 0 | 0 |
Clayton/Newton Heath, Manchester | 1634 | 131 | 39 |
Cricketts Lane, Tameside | 466 | 0 | 0 |
Eccles, Salford | 1294 | 0 | 0 |
Elizabeth Hall | 4385 | 0 | 0 |
Failsworth, Manchester | 1 | 0 | 0 |
Glossop, Tameside | 2463 | 635 | 160 |
Gorton, Manchester | 1005 | 0 | 0 |
Harpurhey, Manchester | 1333 | 0 | 0 |
Hyde, Tameside | 2053 | 90 | 24 |
Irlam, Salford | 751 | 0 | 0 |
Kath Lock, Manchester | 221 | 0 | 0 |
Little Hulton, Salford | 343 | 0 | 0 |
Longsight, Manchester | 1072 | 68 | 23 |
Lower Broughton, Salford | 588 | 52 | 10 |
Nightingale Centre, Manchester | 330 | 2 | 1 |
Nightingale Car Park, Manchester | 569 | 544 | 124 |
North Manchester, Manchester | 593 | 0 | 0 |
Northenden, Manchester | 606 | 0 | 0 |
Oldham | 12 | 0 | 0 |
Oldham Integrated Care Centre, Oldham | 2882 | 412 | 119 |
Other | 14 | 0 | 0 |
Partington, Trafford | 263 | 0 | 0 |
Pendlebury, Salford | 1396 | 128 | 29 |
Pendleton Gateway, Salford | 1691 | 83 | 20 |
Royal Oldham, Oldham | 2 | 0 | 0 |
Royton, Oldham | 185 | 0 | 0 |
Seymour Grove, Trafford | 1246 | 1 | 1 |
Stalybridge, Tameside | 2656 | 21 | 6 |
Trafford General, Trafford | 2618 | 29 | 5 |
Uppermill, Oldham | 1509 | 0 | 0 |
Walkden, Salford | 2220 | 0 | 0 |
Withington Community Hospital, Manchester | 3615 | 154 | 33 |
Wythenshawe, Manchester | 1436 | 0 | 0 |
Geographical distance calculation
This method uses data collected from PROCAS study participants’ stated home addresses with postcodes and the known postcode location of the relevant NHSBSP van. Figure 42 shows the location of the screening van sites in Manchester.
FIGURE 42.
Screening van sites in Manchester.
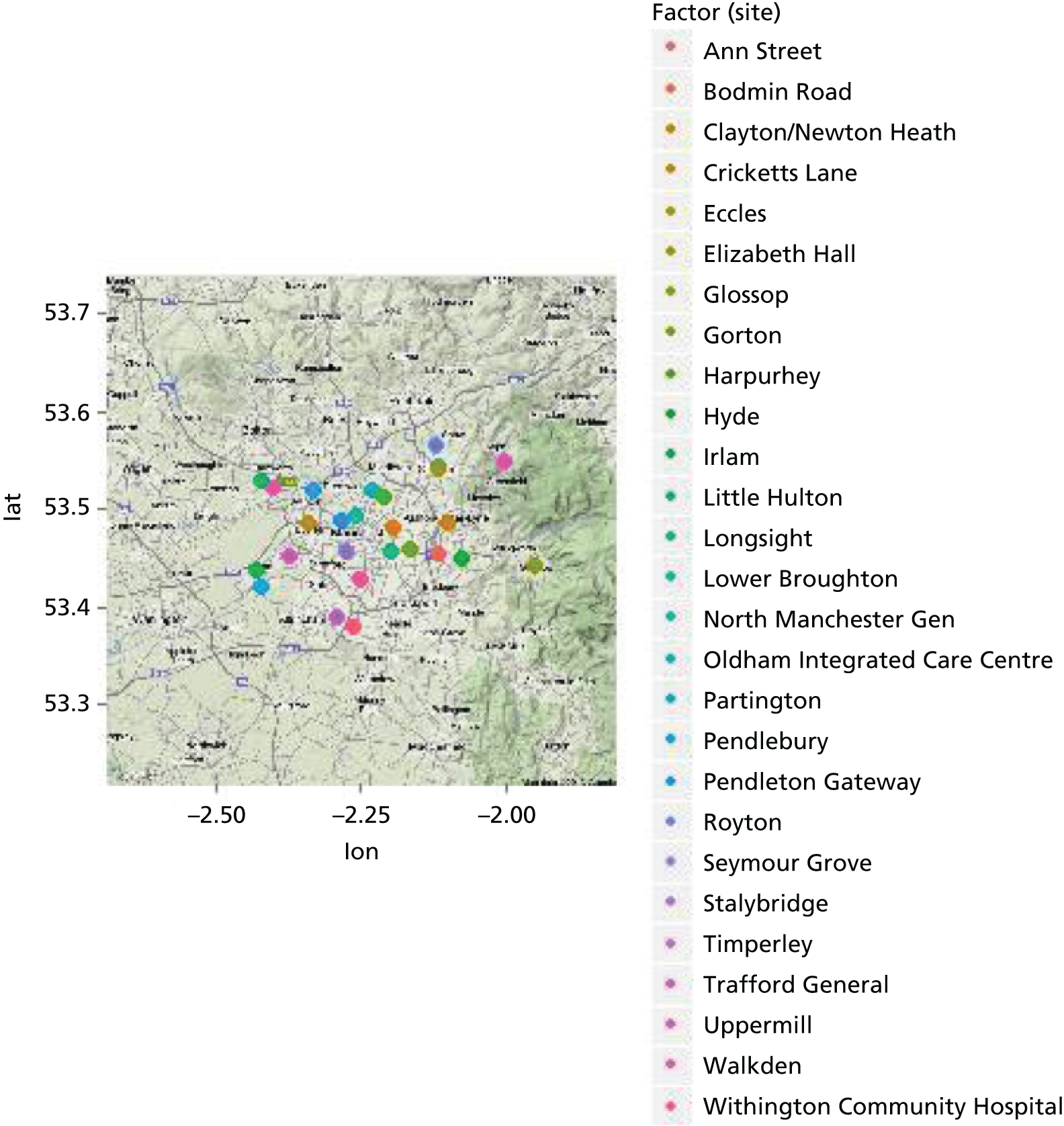
The distance between these two postcode-location points was then calculated using Google Maps (Google Inc., Mountain View, CA, USA) and the code ‘ggmap’ in the statistical software package R (The R Foundation for Statistical Computing, Vienna, Austria). The distance calculation involved estimating the distance from the home postcode location to the screening site postcode.
Sampling frame
This aspect of the study used the addresses of all PROCAS participants who attended the van for a screening visit recruited up to March 2013. This equated to a sample size of 50,016 women.
Results
This section reports the results from the two approaches used to generate estimates of travel distance for the study participants to the screening van and the time taken to attend screening.
Survey of PROCAS participants
A total of 175 respondents started to complete the survey and are included in the analysis, which represents a response rate of 29% (175/594). Two of these women stated that they were eligible for free public transport.
Table 69 summarises the responses to the questions included in the survey to identify out-of-pocket expenses.
Question | Percentage (n) of respondents (N = 175) |
---|---|
Where did you travel from? | |
From home | 75% (131) |
From work | 25% (43) |
Other: from the gym | 0.5% (1) |
How did you travel? | |
Car | 81% (141) |
Walked | 11% (19) |
Bus | 6% (10) |
Taxi | 2% (3) |
Other: unreported | 0.5% (1) |
Where would you have been if you were not on the screening van? | |
At work | 61% (106) |
Looking for work | 0.5% (1) |
Leisure activities | 5% (9) |
Doing housework | 24% (42) |
Sick leave | 1% (2) |
Childcare, caring for relative/friend or voluntary work | 2% (3) |
Some ‘other’ activity | 6% (10) |
Did not answer | 1% (2) |
The majority (75%, n = 131) of women travelled to the screening van from home and the most common mode of travel was car (81%, n = 141). Twenty-nine per cent (n = 51) did not return to their original starting location after their visit to the screening van but travelled on to a different location, for example they returned to work or went shopping.
Eleven (6%) women reported paying a fare to travel to the screening van, which was a mean of £4.00 (range £2.10 to £8.00).
The mean time taken to travel to the screening van was 18 minutes (range 1–90 minutes). One woman did not answer this question.
The mean distance travelled to the screening van was 4.08 miles (range 0–17 miles). Seven of the women reported a travel distance of zero miles.
The mean time that women reported spending at the screening van, including waiting and screening time, was 36 minutes (range 10–240 minutes). Two women did not answer this question.
The majority of women (61%, n = 106) reported that they would have been at work had they not been at the screening van. For the women who reported taking time off work, the mean reported time away from work was 2 hours and 23 minutes (range 3 minutes to 8 hours and 30 minutes). Four women did not answer this question. Seven per cent of women (n = 12) reported that they had lost earnings as a result of being away from work.
Some women (17%, n = 29) reported they had a companion with them when they visited the screening van. Just one woman reported that this person accompanied them because of their poor health. A very small percentage of women (2%, n = 4) needed to get someone to look after children/dependants while they were at the screening visit, which took a mean of 1 hour and 55 minutes (range 40 minutes to 4 hours). No one had to pay for this care.
A small percentage of women, 13% (n = 22), reported that they needed to pay other costs while attending the screening visit. Two women did not answer this question. The reported additional expense was parking, with the exception of one woman who reported paying for petrol. The mean reported additional cost was £2.83 (range £1.20 to £5.00).
Attaching unit costs
The cost of women’s time was estimated using the median gross weekly earnings for women (including full-time and part-time workers) for 2013, which is £327.5318 for an assumed 37-hour week. This equated to a cost of £2.66 (range 15 pence to £13.28) for travel time and £5.31 (range £1.48 to £35.41) for screening time.
Using the government-advised mileage rate for a 2000cc petrol car, which is 24 pence per mile,319 the distance travelled equates to 98 pence (range £0 to £4.08).
Geographical distance calculation
This study used data for 50,016 women recruited to PROCAS. The mean estimated distance travelled was 1.48 miles, which would take a mean of 5.57 minutes to travel.
Discussion
This study has identified relatively low travel costs and travel time for women to attend the breast screening programme, which is not surprising given that the service is available in screening vans close to women’s homes. There was a large variation in the reported time it took for women to be screened, including the waiting time.
There is no agreed method for estimating patients’ assessment costs for attending a screening programme. The two approaches used here are not likely to produce the same estimates of travel distance because the self-report survey asked women to use the actual starting location on the day they attended the screening van. However, the geographical distance travelled method assumed that the women travelled from their home to the screening van.
Limitations
This study has a number of limitations. The self-report survey was limited by the relatively low response rate and subsequent small sample size. However, this sample size was still larger than the sample used in the study previously reported by Brown et al. 246 The survey could also have been biased as a result of the use of self-reporting and relying on reliable recall of the actual day of visiting the screening van. We tried to minimise the impact of poor recall by asking women to complete the survey within 6 months of their screening visit.
The screening van locations rotate around the Greater Manchester area, and hence it was not possible to sample from every location in the area. We also had to limit the sampling frame to exclude women without a subsequent diagnosis of cancer. The potential bias from limiting the sampling frame in these two ways is not known.
Concluding remarks
This study has shown that it was valid to exclude patient assessment costs from the eocnomic evaluation and use the NHS perspective for identifying and quantifying resource use and costs.
Study 3: preliminary model-based cost-effectiveness analysis of a risk-based screening strategy for breast cancer as part of the NHS Breast Screening Programme
Background
There is still considerable uncertainty about who should receive mammographic screening, at what age and at what interval. When it was first introduced, the existing NHSBSP was informed by economic evidence. 320 In theory, any changes to existing programmes should also be informed by economic evidence, as modifications are likely to alter the balance of relative costs and patient benefits. The risk-based screening programme developed as part of PROCAS will ideally save money by concentrating resources where they are needed most while also avoiding unnecessary screening in women at low risk of developing cancer. It is, however, necessary to identify the incremental costs and benefits associated with the proposed risk-based screening programme. Generating this evidence base will help decision-makers to understand what factors will help to facilitate an effective use of the finite health-care budget allocated to the provision of a national screening programme.
Owing to the timing of the work in the programme grant, the proposed risk-based screening intervention developed as part of PROCAS was still in its initial stages when the work on the economic modelling was started. It was decided to develop a preliminary economic model, rather than produce a definitive model, to identify the potentially relevant costs and patient benefits for a risk-based strategy compared with the existing screening programme. The draft model would be structured in such a way to allow it to be repopulated as and when data from the PROCAS cohort become available over time.
Aim
This study aimed to identify the incremental costs and patient benefits associated with using risk-based screening strategies for breast cancer.
Method
A preliminary model-based cost-effectiveness analysis was conducted. A systematic review did not identify any relevant model-based evaluations. Therefore, a de novo decision-analytic model, taking the NHS perspective over a lifetime horizon, was structured to compare risk-based breast screening with the current screening programme. The risk stratification was assumed to be provided using a risk prediction algorithm (developed during projects 1 and 2).
Model structure
A range of mathematical and simulation modelling techniques has been used to evaluate breast cancer screening policies. These models include simple decision trees, cohort Markov models and more complex modelling approaches that aim to overcome the Markovian assumption of exponential transition rates between health states. These decision-analytic models provide a structure for ordering and synthesising information from a wide range of sources, which may include primary and secondary data analysis alongside less robust evidence such as expert opinion. The ultimate aim of which is to explore the impacts of screening policies in terms of effectiveness (incidence and mortality) and costs over the lifetime of a screening cohort.
Before constructing a model, it is important to be clear about the nature of the problem and define the objectives, scope and context of the study. Table 70 describes the objectives and scope of this preliminary model-based evaluation.
Element | Statement |
---|---|
Decision problem/decision objective | To evaluate a risk-based screening strategy to inform an interval policy, as proposed by the PROCAS study in the context of the NHSBSP |
Research and policy context | The preliminary analysis will be used to inform the data needed to produce a model-based cost per unit of effectiveness. The preliminary analysis may also help to inform future developments of NHSBSP screening policies |
Perspective | Health care/NHS |
Target population | A cohort of English women aged 50 years for whom a 10-year or lifetime risk of developing breast cancer will determine their risk category and screening interval |
Health outcomes | Breast cancer incidence Breast cancer mortality Life-years gained QALYs |
Strategies/comparators | Risk-based screening interval (as determined by PROCAS developed risk-based algorithm) Current NHSBSP screening policy (universal 3-year interval) |
Time horizon | Lifetime |
Model approach
The selected modelling approach used in this preliminary analysis was informed by a review of existing model-based evaluations of breast screening programmes and also current methods and approaches informed by the more general literature on how to conduct economic analyses of cancer screening programmes. A general cancer screening model structure, as presented by Karnon et al. ,238 formed the framework for identifying the relevant modelling structure and approach (Figure 43). In this description there is a distinction made between the model structure and the modelling approaches (or techniques) used to inform the final structure described in the following section. The framework proposed by Karnon et al. defined two broad cancer states, non-invasive and invasive, which interact with screening. Moving from this general framework to a breast cancer-specific model requires representation of disease progression. Karnon et al. also suggested natural history of disease modelling as the preferred analytic approach, where the disease is described from the point of progression, at which it becomes detectable, and subsequent death. It was argued that this approach permits the evaluation of screening options that have not been observed in primary research where only estimates of test sensitivity and specificity are required to assess these options. The systematic review (see study 1) identified two broad approaches when representing the natural history of breast cancer: (1) tumour size and (2) tumour stage.
Several modelling approaches (or techniques) are available to evaluate breast cancer screening. Broadly, three classifications of models are described in the general modelling literature including decision trees, state-transition models (Markov) and individual sampling models (DES). In reviewing modelling studies of screening programmes, Karnon et al. 238 also distinguished an additional classification of ‘complex mathematical models’. Decision tree models are not useful as a single approach in this context, as they are overly simplistic and restrictive when evaluating screening programmes, but they may be combined with other structures to represent the complexity of the process being modelled.
The cohort Markov model is the most commonly employed technique when modelling the economic impact of health-care interventions over time. This also appears to be the most common approach taken when examining screening programmes. This is a state-transition approach that requires the definition and representation of disease and screening programme in terms of mutually exclusive (health) states. The time horizon of the model is split into cycles of equal length, at the end of which a cohort of individuals may move to another health state and remain in the same state. This process continues until the entire cohort reaches an absorbing state (e.g. death). Movement between these states is determined by transition probabilities, which are conditional on the current health state but may also vary according to the overall time in the model (Figure 44).
FIGURE 44.
An example of a state-transition model.

Individual event-based models provide an alternative and perhaps more flexible modelling technique, an example of which is DES. This type of model asks what and when is the next event for the participant at the point in which they experience their current event. This approach is distinguished from a state-transition model in which this question is asked at regularly occurring intervals. The participants in a DES model structure can retain a memory of previous events and individual characteristics which then can influence their pathway through the model. Barton et al. 292 catalogue four different DES modelling techniques which can be used to determine which event occurs and the timing of the events and discuss the advantages and disadvantages of (1) the time-slice approach; (2) determining first event and then time; (3) determining first time and then event; and (4) sampling times for each possible event and taking the minimum.
State-transition models require transition probabilities between each of the allowed subsequent states. For example, the rate of transition between DCIS to clinically invasive breast cancer per cycle length or the transition probability of ‘other cause mortality’ (from any health state), which may be age dependent. This is distinguished from time-to-event parameters in an event-based model, which are typically derived from fitting parametric survival curves to observed data (e.g. Kaplan–Meier curves).
The natural history of breast cancer can be described in more complex mathematical models, such as those presented by Baker241 and updates to the family of MISCAN models. 317 Using this method, the authors describe input parameters as continuous variables that change over time, the parameters of which are specified in mathematical functions which may be solved either analytically or numerically (by simulation). The type of data required by these more complex models are similar to those of event-based models, but are generally used in order to better describe the pre-clinical phases of the disease.
Evaluating an individual risk-based screening policy means that there is a need to incorporate an element of within-cohort heterogeneity in terms of breast cancer risk and also to understand how to set the defined screening intervals based on estimated risk. Increased risk for subgroups of the cohort would, in turn, mean that each defined risk category faces differing breast cancer rates. Previously published models assumed that all women face the same risk of developing disease; incorporating heterogenity based on risk in other model structures would result in cumbersome structures. The MISCAN model may have proved a useful starting point for this preliminary economic analysis of a risk stratified screening programme. However, three factors meant that using the MISCAN model was not practical or feasible. The MISCAN model is not publicy available and would have required collaboration with the model developers. This was explored but did not prove to be a practical solution. The MISCAN model is populated from US data (the Surveilance, Epidemiology and End Results program), which makes it of limited direct relevance to the UK population without further intepretation of the relevance of these data. In addition, the MISCAN model would have still required extensive modification to make it relevant to evaluate a risk-based screening strategy for the UK context.
A de novo model was, therefore, developed [hereafter termed the Manchester Breast Screening Model (MBSM)]. The MBSM developed for this takes into account the advantages and disadvantages of each published model type (see the systematic review in study 1) and the policy question under evaluation. The MSBM needed to be able to incorporate four components:
-
a decision-tree based structure to represent the choice between a risk-based screening programme and current practice (Figure 45)
-
a DES to represent the care pathway in the screening programmes (Figure 46; box C)
-
a state-transition structure to represent development of a tumour (see Figure 46; boxes A and B)
-
a survival analysis approach to represent subsequent progression to death.
Table 71 summarises the key events represented in the model.
FIGURE 45.
The intervention and comparator pathways included in the MBSM.

FIGURE 46.
The MBSM.

Model component | Associated events |
Natural history | Onset indolent DCIS |
Onset aggressive DCIS | |
Tumour growth from 1 mm | |
Tumour growth from 11 mm | |
Tumour growth from 21 mm | |
Tumour growth from 51 mm | |
Screening | Screening mammogram |
Do not attend screening mammogram | |
Further assessment from positive screening result | |
Symptomatic presentation to GP | |
Treatment | Diagnosis of breast cancer |
Treatment for breast cancer | |
Mortality | Death from other causes |
Death from breast cancer |
Figure 45 also illustrates how the risk categories informed by the PROCAS-developed risk-based algorithm were linked with the relevant defined screening interval. There were no published data on the relevant risk categories or associated screening interval. Therefore, expert judgement was used to define the relevant risk categories identified in PROCAS and the suggested relevant screening interval. Two clinicians (a geneticist and a breast oncologist) and an imaging scientist provided their expert opinion collectively to produce the six risk categories and screening intervals.
The face validity of the MBSM was confirmed using input from all members of the programme grant research team and also supplemented with expert opion elicited during a separate 1-day (December 2013) workshop (funded by Genesis). The expert workshop was attended by 12 experts representing academics, clinicians and imaging scientists with an interest in breast screening programmes. This included individuals from the national screening committees and academics with previous experience of evaluating screening programmes.
Informing model input parameters
The approaches to informing the model input parameters are now described.
Ten-year and lifetime risk of invasive breast cancer
Incorporating risk of being diagnosed with breast cancer is a key parameter input. There were no published data reporting the proportion of women falling into each risk category (10-year or lifetime) following screening. The estimated 10-year risk was taken from the application of the IBIS-I breast cancer risk evaluation tool11 for the PROCAS study population who were aged 50 years at the time of enrolment (Figure 47). The x-axis on Figure 47 shows the estimated probability associated with each risk category. This was the assumed age at which their risk of breast cancer was estimated. The average 10-year risk is lower for this sample age when compared with the entire PROCAS study population which comprised a range of ages (Table 72).
FIGURE 47.
Empirical distribution of estimated 10-year breast cancer risk.
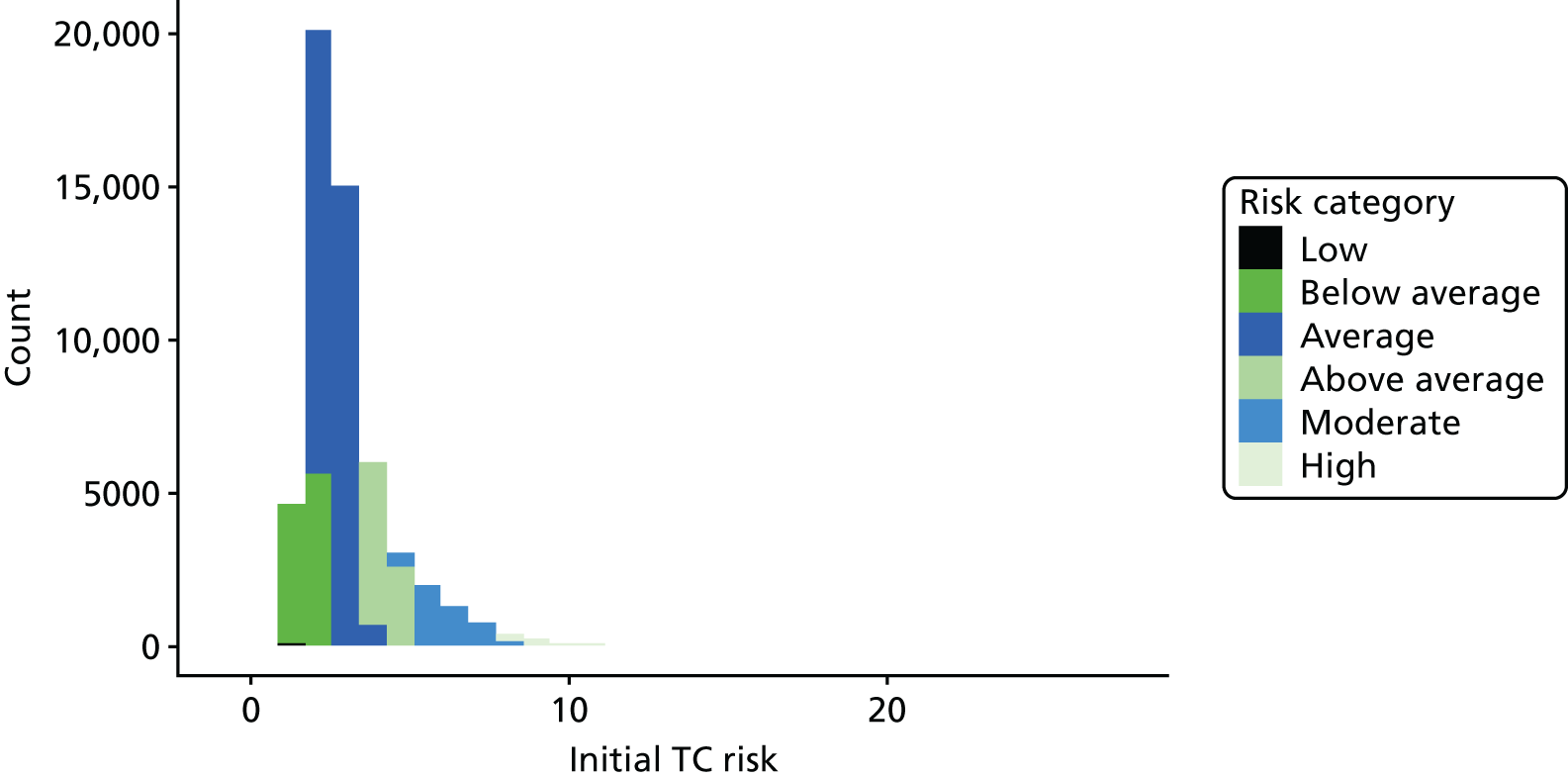
n | Minimum | First quantile | Median | Mean | Third quantile | Maximum | |
---|---|---|---|---|---|---|---|
Entire sample | 53,590 | 0.590 | 2.120 | 2.670 | 3.062 | 3.480 | 26.320 |
Sample aged 50 years | 3254 | 0.880 | 2.120 | 1.650 | 2.951 | 3.300 | 17.310 |
To incorporate individual risk into the MBSM, the estimated 10-year risk for each individual was adjusted to reflect lifetime risk. Owing to lack of data, it was necessary to assume that the RR at 10 years for each individual in comparison with the average population risk as estimated by the IBIS-I tool remained constant throughout the lifetime of an individual.
A range of parametric distributions were fitted to the adjusted lifetime risk of the PROCAS study population, the aim of which was to identify the most appropriate distribution, and its associated parameters, which we could use as a direct input parameter.
Two goodness-of-fit statistics were used to select the most appropriate distribution. The Kolmogorov–Smirnov test measures the fitted cumulative distribution to the input data and is calculated as the largest absolute difference between the cumulative distributions for the input data and fitted distribution. A small p-value indicated that the sample is highly unlikely, and, therefore, the fit should be rejected. Conversely, a high p-value indicated the sample is very likely and would be repeated and, therefore, the fit should not be rejected. The Anderson–Darling statistics can be interpreted in the same way. The best-fitting distribution is selected based on the highest estimated value. For the observed data, the Pearson type V distribution was identified as having the highest p-value and, therefore, this distribution was selected for the model (Table 73).
Distribution | Kolmogorov–Smirnov | p-value | Anderson–Darling | p-value |
---|---|---|---|---|
Beta | 0.117 | 0 | 73.6 | 0 |
Erlang | 0.101 | 0 | 53.3 | 0 |
Exponential | 0.376 | 0 | 620 | 0 |
Gamma | 0.101 | 0 | 53.2 | 0 |
Log-normal | 0.0709 | 1.10 × 10–14 | 23.6 | 0 |
Normal | 0.156 | 0 | 147 | 0 |
Pearson type V | 0.0477 | 7.05 × 10–7 | 8.12 | 0 |
Pearson type VI | 0.0676 | 2.23 × 10–13 | 21.3 | 0 |
Weibull | 0.131 | 0.00 | 127 | 0 |
For comparative purposes, the worst (Weibull) and best (Pearson type V) distributions for the probability density function, cumulative density function, quantile–quantile plot, probability–probability plot and box plots are shown in Appendix 5.
As part of the goodness-of-fit procedure, the estimated parameters for the Pearson type V distribution were estimated, which were then used in the model.
Incidence of invasive and in situ breast cancer
The incidences of invasive (ICD-1065 C50) and in situ (ICD-1065 D05) breast cancer were obtained from the Office for National Statistics for the years 2009–11. 321 These rates represent observed breast cancer dignosis incorporating the effectiveness of the current univeral screening programme (3-year interval, age range 47–73 years). An empirical distribution was formed (Figure 48), representing the age at which a diagnosis is likely to occur. The combined incidence rates for both invasive and in situ carcinoma were used to provide a single age at which clinical diagnosis will occur. This allows specification of the type of DCIS (indolent or aggressive), taking account of the assumption in the model structure that all breast cancer tumours onset in a DCIS phase.
FIGURE 48.
Incidence of invasive and in situ breast cancer UK.

In situ carcinoma and invasive carcinoma
To reflect that a certain number of in situ carcinomas (DCIS) will never progress to invasive disease, two types of DCIS, indolent and aggressive, were defined. It was assumed that indolent DCIS will never progress but aggressive DCIS will progress through a defined natural history model. The probability of aggressive DCIS was informed by Tan et al. 310
Breast cancer stage
Breast cancer stage was based on the tumour node metastasis (TNM) staging system and matched the tumour size at diagnosis to stage.
Onset lag period
Current breast cancer incidence represents the age at which diagnosis occurs. However, it is not possible to directly observe the age at which the tumour is initiated in an individual. In the model, a tumour onset lag parameter was defined to adjust observed breast cancer incidence to reflect the onset of a tumour. This parameter was informed from a published breast cancer natural history model. 322 To reflect the uncertainty with this variable, a uniform distribution was assumed based on the reported range for the estimated lag period.
Tumour growth
The rates of growth of preclinical cancers is also an unobservable parameter. The approach presented in Tan et al. 310 was taken to estimate this parameter in which tumour growth is defined as a series of events classified by the size in millimeters. Each of the sojourn times was taken from Tan et al. 310 and used to estimate individual growth rates for each participant. Each sojourn time was assumed to follow an exponential distribution.
Mortality
The model incorporates two events for mortality: death due to other causes and death due to breast cancer. The probability of dying from other causes was modelled as an age-dependent probability distribution which was informed by Office for National Statistics death registrations for England and Wales by single year of age for females. 323 To reflect more recent mortality rates, we use the period 2003–12. These data were used to construct an empirical distribution which allows the sampling of age-conditional rates. Figure 49 shows the comparison between all-cause mortality for the entire period (1963–2012; see black line) and the period used to inform our model (green line). Figure 50 shows the same comparison of data plotted as a cumulative probability mortality distribution.
FIGURE 49.
Smoothed empirical probability mortality distribution.
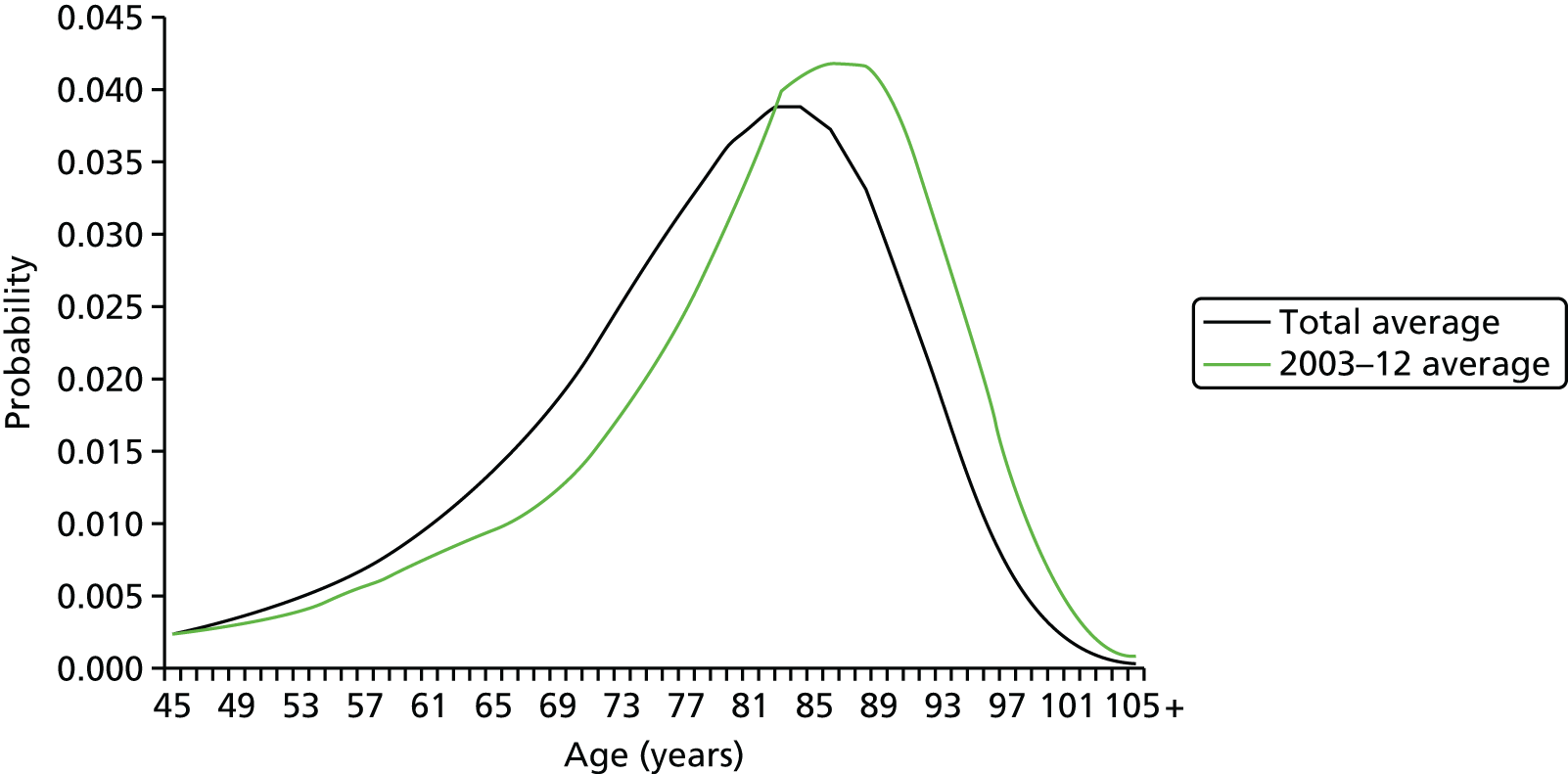
FIGURE 50.
Smoothed empirical cumulative probability mortality distribution.
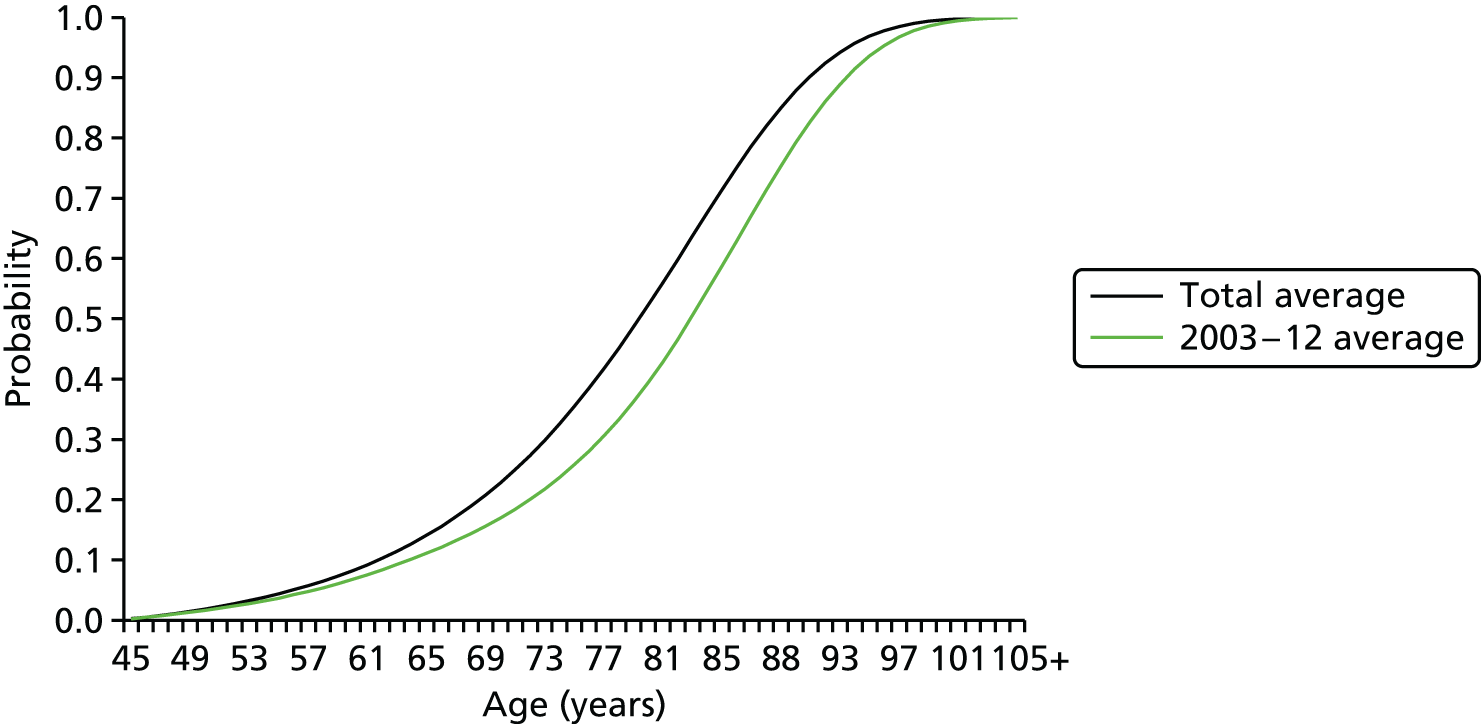
The relative survival of screening participants who are subsequently diagnosed with breast cancer based on tumour diameter was modelled, using data reported by the National Cancer Intelligence Network;324 these data are matched to the tumour size at diagnosis within the model (Table 74).
Tumour diameter (mm) | Relative survival |
---|---|
0–10 | 0.91 |
11–20 | 0.818 |
12–50 | 0.6165 |
≥ 51 | 0.48 |
Mammography screening test performance
The performance of mammography screening is defined in terms of sensitivity and specificity. The sensitivity of mammography screening depends on the size of the tumour (Table 75). This parameter was informed by the study by Tan et al. 310
Sensitivity | DCIS (mm) | 0.88 |
---|---|---|
≤ 10 | 0.90 | |
11–20 | 0.91 | |
21–50 | 0.92 | |
≥ 51 | 0.93 | |
Specificity | 0.95 |
Costs associated with the screening, diagnosis and treatment of breast cancer in the UK
The associated costs for screening were estimated from the reported programme cost from the NHSBSP. The programme screened 1.94 million women aged ≥ 45 years, which equated to approximately £49 per woman screened. However, the year for these cost data was not reported in the original data source. Once a diagnosis of breast cancer has occurred, it is classified according to TNM stage. The UK relevant costs by stage were taken from Dolan et al. 325 and inflated to current prices. These were the only available data and, ideally, more recent treatment costs would make the estimates more up to date.
Health-related quality of life
To simplify the calculation of QALYs between events in the model, age-dependent utility values were not incorporated. Instead, an average breast cancer free utility value between the ages of 45 and 100 years was defined as 0.79. This was informed by age-specific UK general population utility values, which came from the EQ-5D. 326
Utility values associated with breast cancer were informed by a published literature review and synthesised meta-analysis. 327 The review defined utilities in terms of early breast cancer and metastatic breast cancer. These were then matched based on the stage at which the breast cancer is diagnosed (Table 76). Peasgood et al. 327 identified a large number of utility values. A total of 13 databases were searched, and 49 papers providing 476 unique utility values were identified. A variety of methods were used to elicit the utility values, and these were found to vary significantly between valuation methods and depending on who conducted the valuation. The identified utility values were grouped into six categories: screening-related states; preventative states; adverse events in breast cancer and its treatment; non-specific breast cancer; metastatic breast cancer states; and early breast cancer states. The large number of values identified for metastatic breast cancer and early breast cancer states enabled data to be synthesised using metaregression. This method used utility as the dependent variables with study characteristics as independent variables allowing the estimation of pooled utility values. The authors used two separate models for early and metastatic breast cancer as these health states differ considerably, which requires different control variables to be used in the metaregression. Table 77 shows three models together with the overall mean estimated utility value. Model 1 was weighted by the inverse of the SD, model 2 was weighted by sample size using all available utility values and model 3 was weighted by sample size but drops any values that may not be recognised as utility scores.
Model parameter | Value |
---|---|
Utility cancer free | 0.85 |
Utility early breast cancer | 0.679 |
Utility metastatic breast cancer | 0.638 |
Lifetime risk of breast cancer | Pearson V |
α = 7.83851 | |
β = 0.64014 | |
Sojourn time aggressive DCIS | Exponential |
β = 0.01 | |
Sojourn time ≤ 10 mm tumour | Exponential |
β = 0.80 | |
Sojourn time 11–20 mm | Exponential |
β = 2.40 | |
Sojourn time 21–50 mm | Exponential |
β = 6.40 | |
Sojourn time ≥ 51 mm | Exponential |
β = 0.40 | |
Onset lag period | Uniform |
a = 1.5 | |
b = 4 | |
All-cause mortality | Empirical distribution |
Breast cancer relative survival | 0–10 mm = 0.91 |
11–20 mm = 0.818 | |
12–50 mm = 0.615 | |
51 mm = 0.48 | |
Sensitivity | |
DCIS | 0.88 |
≤ 10 mm | 0.90 |
11–20 mm | 0.91 |
21–50 mm | 0.92 |
≥ 51mm | 0.93 |
Specificity | 0.95 |
Cost screening mammography | £49 |
Cost recall assessment | £320 |
Cost symptomatic presentation | £486 |
Cost breast cancer treatment | |
Stage 1 | £12,571 |
Stage 2 | £10,694 |
Stage 3 | £9293 |
Stage 4 | £15,546 |
Model 1 | Model 2 | Model 3 | Mean | |
---|---|---|---|---|
Early breast cancer states | 0.725 | 0.663 | 0.648 | 0.679 |
Metastatic breast cancer states | 0.641 | 0.64 | 0.632 | 0.638 |
Discounting
To present costs and benefits in present values, it is necessary to apply a discount rate. In line with current recommendations published by NICE, all costs and benefits were discounted at 3.5% per year. 328
Two approaches were taken to calculate discounted costs and discounted QALYs because the analysis aims to identify the incremental costs and benefits of risk-based screening intervals over the lifetime of a population of women. Approach 1 takes account of the instantaneous events in the screening programme and approach 2 takes account of discounting between these events.
The first approach deals with costs or QALYs accrued at a single time point, such as the cost of attending subsequent screening mammograms. The standard discounting formula is applied (see Equation 1):
To calculate the discounted costs or QALYs which were accrued between two events, for example the QALYs between two screening mammograms, an alternative discounting formula was applied (see Equation 2):
where Vab is the value of costs or QALYs accrued at a constant rate between the times of events a and b. The instantaneous discount rate (IDR) is equivalent to the discount rate (DR) from Equation 1, given by Equation 3:
Summary of model input parameters
Table 76 summarises the model input parameters.
Modelling assumptions
The key assumptions taken in our model are:
-
Death attributable to breast cancer occurs only following the initiation of breast cancer onset in the model.
-
Following a test positive mammogram, screen recall assessment happens immediately.
-
Following a confirmed diagnosis of breast cancer, treatment follows immediately.
-
Only women who have a progressing preclinical tumour will present symptomatically [i.e. women without a breast tumour will not present symptomatically outside a screening programme (e.g. ‘worried well’ women)].
-
Once women reach the maximum age range for the screening programme, no further screening mammograms take place (i.e. once women reach the maximum screening age they will not request a screening appointment)
-
Women with indolent DCIS will not progress on to subsequent breast cancer natural history states.
-
Only one diagnosis can occur in the lifetime of a woman.
-
Following the initial screening appointment where screening interval based on risk is determined, no further adjustments are made to this risk-based interval.
-
The TC estimated risk is a perfect measure of risk in our population.
-
The risk of being diagnosed with breast cancer does not change over time [e.g. when women change lifestyle factors that may effect this risk (i.e. smoking cessation)].
-
All women enter the model at the age of 45 years rather than 50 years. This was necessary to allow time for the tumour to progress to a detectable size at the age of 50 years, which would mirror current evidence. In addition, to account for prevalence of breast cancer at the initial screening appointment; tumour onset may have occurred subsequent to 45 years of age.
-
There is a lag period, taken from the literature, which shifts the observed incidence of breast cancer under the current screening policy to younger ages. The age distribution of onset remains the same.
-
There is independence between the risk of developing breast cancer and the rate at which breast cancer progresses.
Results
Results from the preliminary economic model are now presented. These results are indicative only and represent an initial analysis of the data.
Costs
The estimated total costs and discounted cost per screening participant are shown in Table 78.
Total costs | Difference | Total discounted costs | Difference | |
---|---|---|---|---|
Current | £463.89 | £297.16 | ||
Risk based | £735.78 | £271.88 | £463.75 | £166.53 |
Life-years
The estimated total life-years per screening participant are shown in Table 79.
Life-years | Difference | |
---|---|---|
Current | 34.804 | |
Risk based | 34.858 | 0.054 |
Quality-adjusted life-years
The estimated total QALYs per screening participant are shown in Table 80.
Discounted QALYS | Difference | |
---|---|---|
Current | 0.808 | |
Risk based | 1.023 | 0.215 |
Incremental quality-adjusted life-year
The estimated total incremental cost-effectiveness ratio for the proposed risk-based screening policy compared with the current universal screening policy in shown in Table 81.
Cost (£) | QALYs | |
---|---|---|
Current | 297.16 | 0.808 |
Risk based | 463.75 | 1.023 |
Difference | 166.58 | 0.215 |
ICER | £773.63 per QALY |
Discussion
This study conducted a preliminary economic analysis model to represent a risk-based breast screening strategy compared with the current NHSBSP. The model was populated to show some indicative results from introducing a risk-based screeing programme with subsequent targeted screening intervals. The model-based evaluation was, as anticipated, limited by the availability of key data inputs. Key data are needed before a sufficiently robust base case analysis can be conducted.
In the absence of these data, it was not logical to perform sensitivity analysis on a base case that was not sufficiently advanced. Conducting a sensitivity analysis at this stage of the model development would only indicate what we already know, which is that there are key parameter inputs that were not sufficiently informed. Ideally, we would want to use a combination of probabilistic and one-way sensitivity analyses to understand joint uncertainty in the model parameters together with key structural assumptions that are driving relative cost-effectiveness. Three remaining analyses are in progress: model calibration to confirm whether or not the estimated unobservable natural history parameters accurately reflect observed incidence of in situ and invasive breast cancer; (2) probabilistic sensitivity analysis to identify the joint parameter uncertainty in the model; and (3) expected value of perfect information will be calculated to quantify the value of future research.
Concluding remarks
This preliminary model-based cost-effectiveness analysis study has identified the relevant costs and patient benefits associated with introducing a risk-based stratified screening programme. Using the available data, the risk-based screening programme appears to be a potentially cost-effective option, but this result is uncertain because of the limitations in the available data. The current level of uncertainty would suggest that it is not rational to recommend the introduction of a risk-based breast screening programme at a national level.
General discussion
A substantial body of economic evidence has been developed to inform the inception and modification of breast screening programmes in the UK and globally. This study conducted a systematic review, which confirmed this extensive evidence base but failed to identify definitive evidence to support the cost-effectiveness of existing screening programmes. Subsequent modifications to a breast screening programme should also be informed by economic evidence. This study started to look at the implications of introducing a risk-based stratified screening programme by structuring a preliminary model-based cost-effectiveness analysis to identify the incremental costs and benefits compared with the current national breast screening programme.
The model-based analysis assumed the NHS perspective. A survey to understand the patient assessment costs of attending a screening programme was conducted, and confirmed that the patient costs incurred were relatively low. The results from this survey were consistent with those reported by Brown et al. 246 This finding provided reassurance that taking the NHS perspective for the economic analysis was reasonable. The NHS viewpoint is also suggested to be most appropriate given the lack of agreement on the methods to incorporate a broader perspective, and current national guidelines for economic analysis suggest that the NHS perspective is appropriate. 328
The economic analysis suggested that if the assumptions and model inputs used in the preliminary analysis are correct then risk-based stratified screening could be a cost-effective use of health-care resources. The estimated cost per QALY was below the nationally agreed threshold range of £20,000 per QALY used by NICE to guide whether or not interventions are likely to be cost-effective. However, this finding should be interpreted with extreme caution. The availability of data necessary to understand the impact of introducing a risk-based screening programme was low in terms of relevance to the research question. In particular, the identified key data requirements included information on the predictive value of the risk-based algorithm; empirical data to support the identified relevant risk categories and associated screening interval; estimated lifetime risk for each individual is needed to replace the current extrapolation of 10-year risk data to incorporate individual risk into the MBSM; whether or not the duration of the preclinical screen-detectable period (sojourn time) is linked with the algorithm predicted risk category; and the cost of treatment linked with identified risk category.
A number of key assumptions were made to generate an estimate of cost-effectiveness, which are likely to drive the observed incremental costs and benefits of the intervention compared with current practice. One key assumption that needs further evaluation using a prospective follow-up study is that the method used to create the risk stratifications, the TC risk algorithm, generates a perfectly sensitive and specific measure of risk in the population of women. There were no data to support or refute this assumption available for this economic analysis but expert opinion suggested that this was a valid approach to take. Generating robust evidence to identify the predictive value of risk-scoring algorithms will be a common challenge as health-care systems embrace the move towards personalised medicine. 329
A further key assumption was the assumed independence between the estimated risk of developing breast cancer and subsequent rate at which breast cancer progresses. This has implications for the treatment strategies used by clinicians and the overall survival of women. There are an increasing number of personalised medicines in the area of breast cancer emerging into practice. However, these currently focus on the genetic variation or the presence of specific biomarkers in the breast tumour. 330 It is likely that, as risk-scoring algorithms become used in practice, clinicians will evolve their prescribing behaviour to personalise the treatment regimens and more aggressively treat women at a high risk of breast cancer. This is an area for future research.
Overdiagnosis is a key topic for continued debate when evaluating the impact of national breast screening programmes. Overdiagnosis describes cancers picked up and treated as a result of screening which would not have been diagnosed in a woman’s lifetime had screening not taken place. Duffy et al. 331 estimated the absolute benefits of a national breast screening programme to be 8.8 and 5.7 breast cancer deaths prevented per 1000 women screened for 20 years starting at age 50 years, from the Swedish Two-County Trial and screening programme in England, respectively. The corresponding estimated numbers of cases overdiagnosed per 1000 women screened for 20 years were, respectively, 4.3 and 2.3 per 1000. The issue of overdiagnosis is an important caveat for any screening programme and it is not clear whether using a risk-based strategy will inflate or decrease the number of overdiagnosed cases of breast cancer or the associated impact on relative cost-effectiveness.
There are a number of limitations with the assumed cost of the screening programme. The component costs of the assumed £96M cost per year is not known. It is likely that the number of women screened is increasing over time, which would mean that £96M per year will be an underestimate of the current costs. Furthermore, this figure does not include non-attenders, which may affect the total cost. Overall, the published figure is likely to be an underestimate of the total NHSBSP cost, but there are no other data sources to verify this figure. This is an area for future research.
Implications for policy
This study has developed a preliminary economic model, which is indicative of the relative cost-effectiveness of the risk-based stratified screening programme compared with the current UK screening programme. The model has indicated some key parameters and assumptions that drive the observed estimate of cost-effectiveness. Based on the current uncertainties in the evidence base, it is necessary for further work to be carried out. This study was designed from a UK perspective and it is outside its scope to try and draw comparison across other EU countries. The model provides a conceptual basis for identifying the relative costs and benefits of a risk-based strategy, but further data, and subsequent sensitivity analysis, are required before definitive conclusions can be made.
Future research
The model-based analysis has identified some key uncertainties in the evidence base needed to generate robust estimates of the relative cost-effectiveness of a risk-based breast screening programme.
-
The accuracy and predictive value of the algorithm (TC) used to generate the risk subgroups in the population of women.
-
Information on the link between the estimated risk of developing breast cancer and the rate at which breast cancer progresses.
-
The observed uptake rate of a risk-based screening programme together with an understanding of the factors driving the observed uptake rate and relationship with women’s perception of their perceived risk of developing breast cancer.
-
The actual cost of the current national breast screening programme.
-
The cost of treatment for breast cancer, taking into account current treatment modalities, and the potential link between risk category and selection of treatment.
-
The resources and costs used, for a national programme, to inform women of their individual risk of developing cancer and the mechanism needed to target them towards the appropriate screening interval. Subsequent modifications to the risk-based algorithm and associated screening interval could be evaluated using the proposed MBSM.
Conclusion
This preliminary model-based cost-effectiveness analysis has shown that using a risk-based stratified breast screening programme may potentially be a cost-effective use of resources. This result should be interpreted with extreme caution, as there were limitations in the data available to populate the model inputs and the estimated relative cost-effectiveness is associated with extensive uncertainty. Additional research is needed to reduce the current uncertainty in the evidence base to produce a reliable base-case analysis, and then subsequent sensitivity analyses are needed to identify the key drivers of the relative cost-effectiveness of a risk-based breast screening programme.
Chapter 6 Patient and public involvement
Patient and public involvement contribution to study design and study management
The involvement of patients and the public has been vital in the design, set-up and management of our programme grant. When devising our initial research plan, the opinions of patients in our FHC were used to inform the initial study design. We found that 98% of women seen in our FHC wanted to know their precise breast cancer risk. As the overall purpose of this programme grant is to improve personalised breast cancer risk prediction, we needed to ensure that women would opt to receive this information.
In 2008, prior to the start of the grant, a public forum was set up, during which our research plans were discussed with women from the local area, not necessarily those women with a family history of breast cancer. Feedback from this session supported the views expressed by our FHC patients, so it was clear that women from both the screening population and the FHC population would welcome this research, as it would give them the opportunity to be informed of their risks and access information about how to manage their risk.
Wendy Watson, the director of the Hereditary Breast Cancer Helpline, has acted as patient representative throughout this programme grant. In 2011, Fiona Harrison, a previous FHC patient who participated in the IBIS-I breast cancer prevention study, became involved as a patient representative on a related Research for Patient Benefit grant being run by our team. As the two grants were related, Fiona also became involved in this programme grant and, along with Wendy, has offered advice on study design, participant literature, feedback of risk information and dissemination of study results. In addition, both Fiona and Wendy have attended the regular 2-monthly programme management meetings, which last, on average, 3 hours.
As part of International Women’s Day in March 2013, we held an evening event for women about breast cancer prediction and prevention. Thirty-one women invited (out of 100) from our FHC and PROCAS study attended a series of three presentations about current research into breast cancer risk prediction and how women can reduce their risk of breast cancer and other diseases by following a healthy diet and lifestyle, and in some instances through drug prevention. After the talks, we invited women to form small groups and initiated discussion of issues surrounding lifestyle change, and how women could be encouraged and supported to make positive changes to their current diet and exercise behaviours to minimise their risk of breast cancer and other diseases. The conclusions from each group were presented by the facilitators at the end. Women in general gave strong support to the concept of introducing preventative measures based on risk but were more sceptical about reducing screening in women found to be at low risk. The International Women’s Day event has helped to shape our plans for future research projects.
Impact of patient and public involvement
Although it was not funded in this programme grant, as part of PROCAS we offered all participants the opportunity to receive their personalised risk information.
A working group of researchers and patient representatives was set up to develop the risk feedback letter. Through the working group, we developed a short information leaflet for the FH-Risk study, which reminded women about the aims of the study and how SNPs contribute to breast cancer risk. Once approved by the ethics board, this information leaflet was sent out, along with a letter that informed women of whether or not their breast cancer risk had changed since their original risk assessment at the FHC. We found that the newly developed information leaflet reduced the number of queries the research team received from women on the study.
Although we have given risk feedback to all women at high risk on the PROCAS study and a proportion of the women at low risk in person, we are yet to send out risk feedback to the majority of women who will be receiving this information by letter. Following extensive discussions with our patient representatives and the working group, it was decided that it was necessary to conduct a full study to explore, in depth, how best to communicate risk while minimising the potentially negative psychological impact of receiving risk information by letter.
Challenges of patient and public involvement and lessons learnt
Both of our patient representatives are involved in our other ongoing research projects as well as our proposals for future research. Their input has been invaluable; however, we have highlighted some ways we could improve our patient and public involvement. First, as both our patient representatives have been heavily involved in many of our research projects they have become expert representatives and we felt that it was important to have some input from lay people. In addition, both of our patient representatives have a family history of breast cancer, and although part of this programme grant is focused on this population of women, the PROCAS study is being run in the general screening population. We decided that it would be beneficial to have input from public representatives who do not have as much knowledge of familial breast cancer risk. We also felt that it was important to have input from a greater number of patient and public representatives, from more diverse backgrounds. Finally, we were aware that the way in which we engage with our patient representatives does not utilise their skills in the best way possible, because this occurs mainly in the context of formal investigator meetings.
From our International Women’s Day forum we realise that it may be difficult to ‘sell’ a reduction or removal of mammography screening to women identified at low risk as part of a risk stratified screening approach. However, women attending were likely to be strong ‘believers’ in the benefits rather than disbenefits of mammography screening.
In 2014 we sought funding to set up a formal patient and public panel, involving women from diverse ethnic and social backgrounds. The patient and public panels include women from both the FHC and the general population (NHSBSP). The panel is led by Fiona Harrison and one of the research team (Louise Donnelly) and meets separately from the bimonthly investigators’ meetings, allowing a more informal forum for discussion. The function of the panel is to review new project ideas, funding applications and patient information, and to assist the research team in developing research through a patient lens. The panel will meet up to four times per year, but have also reviewed intended studies via e-mail. After a panel meeting, Fiona Harrison feeds back the outcome of the panel at the next investigators’ meeting. We have found this to be a more effective utilisation of our patient representative’s skills, knowledge and input. We have secured external funding for our patient and public panel from Genesis Breast Cancer Prevention Appeal. This allows us to hold regular sessions, involve more members, as appropriate, and develop training for the panel members.
Chapter 7 Conclusions
This report covers a 5-year programme of work linking risk prediction, screening and prevention in the familial and population settings. The vast majority of the goals set out in the original grant application from 2008 have been fulfilled, with many transcended. The three large bodies of work in Chapters 2–4 are enhanced by the preliminary cost-effectiveness analysis presented in Chapter 5. The project outlined in Chapter 2 has shown that risk prediction is accurate using a number of models in the FHC setting. In a study of nearly 10,000 women, there was broad accuracy in terms of the number of predicted cancers in total, as well as over various risk groupings. Although a Manual model incorporating adapted Claus tables was accurate,318 this involved a clinician’s adjustment for non-familial risk factors and may not be transposable to all routine practice. TC was clearly superior to the Gail model, but direct comparisons with the BOADICEA model were not possible across the whole population. On the basis of this research, the use of either TC or BOADICEA appears appropriate in the FHC setting, as recommended already by the recent NICE guidelines for familial breast cancer. Work in the FH-Risk cohort also showed that the increased risk of ovarian cancer was confined to families with BRCA1 and BRCA2 mutations and that women with a breast cancer-only family history testing negative for BRCA1/BRCA2 can be reassured that they are not at increased risk of ovarian cancer. 319 The addition of SNP testing using a PRS improved the accuracy of TC in the familial setting, as shown in Chapter 3, with an AUC c-statistic of 0.6. Further work in Chapter 3 showed that using a SNP18 PRS was not accurate for BRCA1 families but had good predictive value for BRCA2 and non-BRCA2 familial breast cancer. 320 We also showed that SNPs may partly explain the additional risk of breast cancer in those testing negative for a known family BRCA2 mutation. 332 We showed that, using a TC score adjusted for PRS, the breast cancer risks were much more stretched out over a larger range, with many more identified at lower levels of risk. 321,333 The PRS also significantly improved breast cancer risk prediction by TC in the general screening population aged 46–73 years of age. Although further research is required to validate more recent SNPs, it appears that use of a PRS-adjusted TC has good utility in both the general population and in familial clinics and that using further recently identified SNPs will improve risk precision. 322,323
In Chapter 4 we assessed all of the various measures of MD. A VAS on a linear scale by radiologists was shown to have good predictive value in determining breast cancer risk and was shown to be superior to Cumulus and automatic measures in 324 breast cancers and 972 controls. Although VAS was the best performing measure, we have noted significant inter- and intraobserver variations, although these can be corrected. 324–326 Addition of MD from VAS in the form of a DR adjusted for age, menopausal status and BMI also significantly improved TC in the PROCAS population of > 50,000 women. Use of a DR adjustment to the best performing risk prediction model, TC, substantially improved risk prediction in the general population for high-stage breast cancer. The 30% of the population with above average risk (> 3.5% 10-year risk) had a rate of high-stage cancers 3.5-fold that of those at average and below average risk (4.7 per 10,000 vs. 1.3 per 10,000). This allows better risk stratification such that women at high and moderate risk will potentially gain better access to NICE-approved additional surveillance and preventative strategies such as chemoprevention with tamoxifen or raloxifene. 329 The research also indicates that 3-yearly screening is sufficient for ≈70% of the population with average/below-average risks, but that more frequent screening may be justified in those with a MD-adjusted 10-year risk of > 3.5%.
The research has also shown that it is feasible to collect breast cancer risk information321,333 from women attending breast screening through the NHSBSP and that most women wish to know their risk. Ninety-five per cent of women indicated that they wanted risk information and 75% of high-risk women invited for a telephone or clinic appointment received their risks. Importantly, 99% of those invited for their next mammogram reattended.
Chapter 5 reported the preliminary model-based cost-effectiveness analysis that identified the relevant incremental costs and benefits of introducing a risk-based screening strategy with associated screening intervals. Using existing available data, the economic analysis suggested that a risk-based stratified breast screening programme may potentially be a cost-effective use of resources, but this result should be interpreted with extreme caution. There were several limitations in the data available to populate the model inputs and the estimated relative cost-effectiveness is associated with extensive uncertainty. Additional research is needed to reduce the current uncertainty in the evidence base to produce a reliable base-case analysis and then subsequent sensitivity analyses are needed to identify the key drivers of the relative cost-effectiveness of a risk-based breast screening programme.
The body of research from this programme grant over a 5-year period has already resulted in 11 peer-reviewed publications318–329,332,333 and we have a further eight already in preparation. This research will inform NICE guidelines on familial breast cancer, particularly in terms of the use of risk prediction models, and will inform clinical practice over use of SNPs in the familial and general population settings. We have shown that it is feasible to undertake risk provision in the setting of the NHSBSP and that it is possible to deliver reasonably accurate risks to women that can inform future risk stratification to enhance personalised medicine including extra screening and NICE-approved chemoprevention. Nonetheless, further work is necessary to improve risk precision, particularly in low-risk groups.
Implications for practice
A number of conclusions can be considered for potential inclusion in current practice:
-
For more accurate breast cancer risk assessment, the inclusion of data from multiple SNP testing and MD could be considered in conjunction with a validated risk assessment model such as BOADICEA or TC, based on work in both FH-Risk and PROCAS.
-
Risk-stratified screening could be considered in the general population, even if this identifies just those at sufficient risk for NICE-approved enhanced screening. Further studies are needed to confirm if the higher stage identified in the above-average risk group is validated.
-
Population assessment of breast cancer risk could be considered for eligibility for NICE-approved chemoprevention.
Research recommendations (in priority order)
Research recommendations require two separate but linked work streams: (a) improved risk prediction (research recommendations 2, 3 and 5) and (b) better integration of risk prediction into services and patient experience (research recommendations 1, 4 and 6).
-
A pilot study of breast risk provision in real time in the NHSBSP using an online system as preference and receipt of results in 2 weeks. A randomised study may be required to assess whether simple letters to women explaining their risks and, if indicated, access to further services through their GP may require an additional use of personnel to provide risk information to those at high and possibly moderate risk.
-
Development of a better risk prediction automatic MD model. This is required as the best performing measure was VAS and for full automation better performance of an automatic measure is required.
-
Validation studies of SNP67 in the screening population and FHCs using prospective cohorts.
-
Population of a model-based cost-effectiveness analysis using model inputs from data relevant to a risk-based stratified population, such as the predictive value of the risk-based algorithm; the selected relevant risk categories and associated screening interval; estimated lifetime risk for each identified risk category; duration of the preclinical screen-detectable period (sojourn time); the cost of the screen including providing risk-information and subsequent mammography; and the cost of treating identified breast cancers.
-
Randomised controlled studies to show whether increased screening frequency will downstage breast cancers in women predicted by MD and TC to have a risk of > 3.5% is effective or whether further strategies such as tomosynthesis or MRI may be required.
-
Impact of risk assessment on women in population screening programmes.
Acknowledgements
Contributions of authors
D Gareth Evans designed the study, undertook the analysis of SNPs and the assessment of risk models, and contributed to data collection and analysis and the writing of the manuscript.
Susan Astley designed the study, conducted the analysis of MD, and contributed to data analysis and the writing of the manuscript.
Paula Stavrinos contributed to data collection and the writing of the manuscript.
Elaine Harkness contributed to data analysis and the writing of the manuscript.
Louise S Donnelly contributed to the writing of the manuscript.
Sarah Dawe contributed to data collection.
Ian Jacob undertook the cost-effectiveness analysis and data analysis.
Michelle Harvie took part in manuscript writing and editing.
Jack Cuzick designed the study and contributed to data analysis.
Adam Brentnall undertook the analysis of SNPs and the assessment of risk models, conducted the analysis of MD and contributed to data analysis.
Mary Wilson took part in manuscript writing and editing.
Fiona Harrison took part in manuscript writing and editing.
Katherine Payne undertook the cost-effectiveness analysis and contributed to data analysis and the writing of the manuscript.
Anthony Howell designed the study.
All authors edited and approved the manuscript.
All people who contributed to the work in the report
University Hospital of South Manchester NHS Foundation Trust
Breast radiology and radiography staff at the Nightingale University Hospital of South Manchester NHS Foundation Trust between October 2009 and April 2014, including:
Dr Ursula Beetles
Dr Megan Bydder
Professor Anil Jain
Dr Anthony Maxwell
Dr Yit Yoong Lim
Dr Sara Bundred
Dr Nicky Barr
Professor Caroline Boggis
Dr Soujanya Gadde
Valerie Reece
Elizabeth Lord
Claire Mercer
Alix Hartley.
PROCAS and FH-Risk research administrative team
Lynne Fox.
Jake Southworth.
Jill Fox.
Helen Ruane.
Sarah Grannell.
Ellie Tinning.
Faiza Idries.
Rosemary Greenhalgh.
Julia Wiseman.
University of Manchester
Dr Sarah Ingham.
Helen Byers.
Dr William Newman.
Dr Jamie Sergeant.
Professor Iain Buchan.
Dr Ewan Gray.
Imperial College London
Dr Jane Warwick.
University of Cambridge
Dr Ruth Warren.
We would also like to acknowledge all of the women who have participated in these studies.
Publications
Evans DG, Warwick J, Astley SM, Stavrinos P, Sahin S, Ingham SL, et al. Assessing individual breast cancer risk within the UK National Health Service Breast Screening Programme: a new paradigm for cancer prevention. Cancer Prev Res (Phila) 2012;5:943–51.
Hashmi S, Sergeant JC, Morris J, Whiteside S, Stavrinos P, Evans DG, et al. Ethnic variation in volumetric breast density. In Maidment ADA, Bakic PR, Gavenonis S, editors. Breast Imaging 11th International Workshop. Berlin Heidelberg: Springer-Verlag; 2012. pp. 127–33.
Howell A, Astley S, Warwick J, Stavrinos P, Sahin S, Ingham S, et al. Prevention of breast cancer in the context of a national breast screening programme. J Intern Med 2012;271:321–30.
Brentnall AR, Evans DG, Cuzick J. Value of phenotypic and single-nucleotide polymorphism panel markers in predicting the risk of breast cancer. J Genet Syndr Gene Ther 2013;4:202.
Evans DG, Ingham SL, Buchan I, Woodward ER, Byers H, Howell A, et al. Increased rate of phenocopies in all age groups in BRCA1/BRCA2 mutation kindred, but increased prospective breast cancer risk is confined to BRCA2 mutation carriers. Cancer Epidemiol Biomarkers Prev 2013;22:2269–76.
Ingham SL, Warwick J, Buchan I, Sahin S, O’Hara C, Moran A, et al. Ovarian cancer among 8005 women from a breast cancer family history clinic: no increased risk of invasive ovarian cancer in families testing negative for BRCA1 and BRCA2. J Med Genet 2013;50:368–72.
Ingham SL, Warwick J, Byers H, Lalloo F, Newman WG, Evans DG. Is multiple SNP testing in BRCA2 and BRCA1 female carriers ready for use in clinical practice? Results from a large genetic centre in the UK. Clin Genet 2013;84:37–42.
Sergeant JC, Walshaw L, Wilson M, Seed S, Barr N, Beetles U, et al. ‘Same task, same observers, different values: the problem with visual assessment of breast density’, Proc SPIE 8673, Medical Imaging 2013: Image Perception, Observer Performance, and Technology Assessment, 86730T, March 28 2013.
Sergeant JC, Wilson M, Barr N, Beetles U, Boggis C, Bundred S, et al. Inter-observer agreement in visual analogue scale assessment of percentage breast density. Breast Cancer Res 2013;15(Suppl. 1):17.
Sperrin M, Bardwell L, Sergeant JC, Astley S, Buchan I. Correcting for rater bias in scores on a continuous scale, with application to breast density. Stat Med 2013;32:4666–78.
Brentnall AR, Evans DG, Cuzick J. Distribution of breast cancer risk from SNPs and classical risk factors in women of routine screening age in the UK. Br J Cancer 2014;110:827–8.
Evans DG, Brentnall AR, Harvie M, Dawe S, Sergeant J, Stavrinos P, et al. Breast cancer risk in young women in the national breast screening programme: implications for applying NICE guidelines for additional screening and chemoprevention. Cancer Prev Res (Phila) 2014;7:993–1001.
Evans DG, Ingham S, Dawe S, Roberts L, Lalloo F, Brentnall AR, et al. Breast cancer risk assessment in 8,824 women attending a family history evaluation and screening programme. Fam Cancer 2014;13:189–96.
O’Donovan E, Sergeant J, Harkness E, Morris J, Wilson M, Lim Y, et al. Use of Volumetric Breast Density Measures for the Prediction of Weight and Body Mass Index. In Fujita H, Hara T, Muramatsu C, editors. Breast Imaging 12th International Workshop. Berlin Heidelberg: Springer-Verlag; 2014. pp. 282–9.
Data sharing statement
Data can be obtained from the corresponding author on request.
Disclaimers
This report presents independent research funded by the National Institute for Health Research (NIHR). The views and opinions expressed by authors in this publication are those of the authors and do not necessarily reflect those of the NHS, the NIHR, CCF, NETSCC, PGfAR or the Department of Health. If there are verbatim quotations included in this publication the views and opinions expressed by the interviewees are those of the interviewees and do not necessarily reflect those of the authors, those of the NHS, the NIHR, NETSCC, the PGfAR programme or the Department of Health.
References
- Cancer Research UK Statistics For Breast Cancer . Cancer Incidence for Common Cancers n.d. www.cancerresearchuk.org/cancer info/cancerstats/incidence/commoncancers/2011 (accessed 17 June 2014).
- McIntosh A, Shaw C, Evans G, Turnbull N, Bahar N, Barclay M, et al. Clinical Guidelines and Evidence Review for The Classification and Care of Women at Risk of Familial Breast Cancer. London: National Collaborating Centre for Primary Care/University of Sheffield; 2004.
- Evans DG, Howell A. Breast cancer risk-assessment models. Breast Cancer Res 2007;9. http://dx.doi.org/10.1186/bcr1750.
- King MC, Marks JH, Mandell JB. New York Breast Cancer Study Group . Breast and ovarian cancer risks due to inherited mutations in BRCA1 and BRCA2. Science 2003;302:643-6. http://dx.doi.org/10.1126/science.1088759.
- Evans DG, Shenton A, Woodward E, Lalloo F, Howell A, Maher ER. Penetrance estimates for BRCA1 and BRCA2 based on genetic testing in a Clinical Cancer Genetics service setting: risks of breast/ovarian cancer quoted should reflect the cancer burden in the family. BMC Cancer 2008;8. http://dx.doi.org/10.1186/1471-2407-8-155.
- Evans DG. The new genetics: prediction and prevention of breast cancer. Adv Breast Cancer 2006;3:71-5.
- Howell A, Sims AH, Ong KR, Harvie MN, Evans DG, Clarke RB. Mechanisms of disease: prediction and prevention of breast cancer-cellular and molecular interactions. Nat Clin Pract Oncol 2005;2:635-46. http://dx.doi.org/10.1038/ncponc0361.
- Easton DF, Pooley KA, Dunning AM, Pharoah PD, Thompson D, Ballinger DG, et al. Genome-wide association study identifies novel breast cancer susceptibility loci. Nature 2007;447:1087-93. http://dx.doi.org/10.1038/nature05887.
- Hunter DJ, Kraft P, Jacobs KB, Cox DG, Yeager M, Hankinson SE, et al. A genome-wide association study identifies alleles in FGFR2 associated with risk of sporadic postmenopausal breast cancer. Nat Genet 2007;39:870-4. http://dx.doi.org/10.1038/ng2075.
- Stacey SN, Manolescu A, Sulem P, Rafnar T, Gudmundsson J, Gudjonsson SA, et al. Common variants on chromosomes 2q35 and 16q12 confer susceptibility to estrogen receptor-positive breast cancer. Nat Genet 2007;39:865-9. http://dx.doi.org/10.1038/ng2064.
- Tyrer J, Duffy SW, Cuzick J. A breast cancer prediction model incorporating familial and personal risk factors. Stat Med 2004;23:1111-30. http://dx.doi.org/10.1002/sim.1668.
- Lalloo F, Evans DG. Familial breast cancer. Clin Genet 2012;82:105-14. http://dx.doi.org/10.1111/j.1399-0004.2012.01859.x.
- Collaborative Group on Hormonal Factors in Breast Cancer . Breast cancer and hormonal contraceptives: collaborative reanalysis of individual data on 53,297 women with breast cancer and 100,239 women without breast cancer from 54 epidemiological studies. Lancet 1996;347:1713-27. http://dx.doi.org/10.1016/S0140-6736(96)90806-5.
- Steinberg KK, Thacker SB, Smith J, Stroup DF, Zack MM, Flanders WD, et al. A meta-analysis of the effect of estrogen replacement therapy on the risk of breast cancer. JAMA 1991;265:1985-90. http://dx.doi.org/10.1001/jama.1991.03460150089030.
- Collaborative Group on Hormonal Factors in Breast Cancer . Breast cancer and hormone replacement therapy: collaborative reanalysis of data from 51 epidemiological studies of 52,705 women with breast cancer and 108,411 women without breast cancer. Lancet 1997;350:1047-59. http://dx.doi.org/10.1016/S0140-6736(97)08233-0.
- Ross RK, Paganini-Hill A, Wan PC, Pike MC. Effect of hormone replacement therapy on breast cancer risk: estrogen versus estrogen plus progestin. J Natl Cancer Inst 2000;92:328-32. http://dx.doi.org/10.1093/jnci/92.4.328.
- Schairer C, Lubin J, Troisi R, Sturgeon S, Brinton L, Hoover R. Menopausal estrogen and estrogen-progestin replacement therapy and breast cancer risk. JAMA 2000;283:485-91. http://dx.doi.org/10.1001/jama.283.4.485.
- Writing Group for the Women’s Health Initiative Investigators . Risks and benefits of estrogen plus progestin in healthy postmenopausal women. JAMA 2002;288:321-33. http://dx.doi.org/10.1001/jama.288.3.321.
- Beral V. Million Women Study Collaborators . Breast cancer and hormone-replacement therapy in the Million Women Study. Lancet 2003;362:419-27. http://dx.doi.org/10.1016/S0140-6736(03)14596-5.
- Antoniou AC, Shenton A, Maher ER, Watson E, Woodward E, Lalloo F, et al. Parity and breast cancer risk among BRCA1 and BRCA2 mutation carriers. Breast Cancer Res 2006;8. http://dx.doi.org/10.1186/bcr1630.
- Andrieu N, Goldgar DE, Easton DF, Rookus M, Brohet R, Antoniou AC, et al. Pregnancies, breast-feeding, and breast cancer risk in the International BRCA12 Carrier Cohort Study (IBCCS). J Natl Cancer Inst 2006;98:535-44. http://dx.doi.org/10.1093/jnci/djj132.
- Amir E, Freedman OC, Seruga B, Evans DG. Assessing women at high risk of breast cancer: a review of risk assessment models. J Natl Cancer Inst 2010;102:680-91. http://dx.doi.org/10.1093/jnci/djq088.
- Wolfe JN. Breast patterns as an index of risk for developing breast cancer. AJR AM J Roentgenology 1976;126:1130-7. http://dx.doi.org/10.2214/ajr.126.6.1130.
- Wolfe JN. Risk for breast cancer development determined by mammographic parenchymal pattern. Cancer 1976;35:2486-92. http://dx.doi.org/10.1002/1097-0142(197605)37:5<2486::AID-CNCR2820370542>3.0.CO;2-8.
- Boyd NF, O’Sullivan B, Fishell E, Simor I, Cooke G. Mammographic signs as risk factors for breast cancer. Br J Cancer 1982;45:185-93. http://dx.doi.org/10.1038/bjc.1982.32.
- Boyd NF, Byng JW, Jong RA, Fishell EK, Little LE, Miller AB, et al. Quantitative classification of mammographic densities and breast cancer risk: results from the Canadian National Breast Screening Study. J Natl Cancer Inst 1995;87:670-5. http://dx.doi.org/10.1093/jnci/87.9.670.
- Eccles SA, Aboagye EO, Ali S, Anderson AS, Armes J, Berditchevski F, et al. Critical research gaps and translational priorities for the successful prevention and treatment of breast cancer. Breast Cancer Res 2013;15. http://dx.doi.org/10.1186/bcr3493.
- Zheng W, Long J, Gao YT, Li C, Zheng Y, Xiang YB, et al. Genome-wide association study identifies a new breast cancer susceptibility locus at 6q25.1. Nat Genet 2009;41:324-8. http://dx.doi.org/10.1038/ng.318.
- Pharoah PD, Antoniou AC, Easton DF, Ponder BA. Polygenes, risk prediction, and targeted prevention of breast cancer. N Engl J Med 2008;358:2796-803. http://dx.doi.org/10.1056/NEJMsa0708739.
- Cox A, Dunning AM, Garcia-Closas M, Balasubramanian S, Reed MW, Pooley KA, et al. A common coding variant in CASP8 is associated with breast cancer risk. Nat Genet 2007;39:352-8. http://dx.doi.org/10.1038/ng1981.
- Huang Z, Hankinson SE, Colditz GA, Stampfer MJ, Hunter DJ, Manson JE, et al. Dual effects of weight gain on breast cancer risk. JAMA 2000;278:1407-11. http://dx.doi.org/10.1001/jama.1997.03550170037029.
- Harvie M, Hooper, Howell A. Central obesity and breast cancer risk: a systematic review. Obes Rev 2003;4:157-73. http://dx.doi.org/10.1046/j.1467-789X.2003.00108.x.
- McCormack VA, dos Santos Silva I. Breast density and parenchymal patterns as markers of breast cancer risk: a meta-analysis. Cancer Epidemiol Biomarkers Prev 2007;15:1159-69. http://dx.doi.org/10.1158/1055-9965.EPI-06-0034.
- Chen J, Pee D, Ayyagari R, Graubard B, Schairer C, Byrne C, et al. Projecting absolute invasive breast cancer risk in white women with a model that includes mammographic density. J Natl Cancer Inst 2006;98:1215-26. http://dx.doi.org/10.1093/jnci/djj332.
- Barlow WE, White E, Ballard-Barbash R, Vacek PM, Titus-Ernstoff L, Carney PA, et al. Prospective breast cancer risk prediction model for women undergoing screening mammography. J Natl Cancer Inst 2006;98:1204-14. http://dx.doi.org/10.1093/jnci/djj331.
- Tice JA, Cummings SR, Smith-Bindman R, Ichikawa L, Barlow WE, Kerlilowske K. Using clinical factors and mammographic breast density to estimate breast cancer risk: development and validation of a new predictive model. Ann Intern Med 2008;148:337-47. http://dx.doi.org/10.7326/0003-4819-148-5-200803040-00004.
- Kaufhold J, Thomas JA, Eberhard JW, Galbo CE, Gonzalez Trotter DE. A calibration approach to glandular tissue composition estimation in digital mammography. Med Phys 2002;29:1867-80. http://dx.doi.org/10.1118/1.1493215.
- Rosman DS, Kaklamani V, Pasche B. New insights into breast cancer genetics and impact on patient management. Curr Treat Options Oncol 2007;8:61-73. http://dx.doi.org/10.1007/s11864-007-0021-5.
- NHS Economic Evaluation Database Handbook (NHS EED). York: University of York; 2007.
- Philips Z, Bojke L, Sculpher M, Claxton K, Golder S. Good practice guidelines for decision-analytic modelling in health technology assessment: a review and consolidation of quality assessment. Pharmacoeconomics 2006;24:355-71. http://dx.doi.org/10.2165/00019053-200624040-00006.
- Guide to the Methods of Technology Appraisal (Reference N0515). London: NICE; 2004.
- Newman B, Austin MA, Lee M, King M. Inheritance of human breast cancer: evidence for autosomal dominant transmission in high-risk families. Proc Natl Acad Sci USA 1988;85:3044-8. http://dx.doi.org/10.1073/pnas.85.9.3044.
- Claus EB, Risch N, Thompson WD. Autosomal dominant inheritance of early onset breast cancer. Cancer 1994;73:643-51. http://dx.doi.org/10.1002/1097-0142(19940201)73:3<643::AID-CNCR2820730323>3.0.CO;2-5.
- Lichtenstein P, Holm NV, Verkasalo PK, Lliadou A, Kaprio J, Koskenvuo M, et al. Environmental and heritable factors in the causation of cancer analyses of cohorts of twins from Sweden, Denmark, and Finland. N Engl J Med 2000;343:78-85. http://dx.doi.org/10.1056/NEJM200007133430201.
- Peto J, Mack TM. High constant incidence in twins and other relatives of women with breast cancer. Nat Genet 2000;26:411-14. http://dx.doi.org/10.1038/82533.
- Liaw D, Marsh DJ, Li J, Dahia PL, Wang SI, Zheng Z, et al. Germline mutations of the PTEN gene in Cowden disease, an inherited breast and thyroid cancer syndrome. Nat Genet 1997;16:64-7. http://dx.doi.org/10.1038/ng0597-64.
- Miki Y, Swensen J, Shattuck-Eidens D, Futreal PA, Harshman K, Tavtigian S, et al. A strong candidate for the breast and ovarian cancer gene BRCA1. Science 1994;266:66-71. http://dx.doi.org/10.1126/science.7545954.
- Wooster R, Bignell G, Lancaster J, Swift S, Seal S, Mangion J, et al. Identification of the breast cancer susceptibility gene BRCA2. Nature 1995;378:789-92. http://dx.doi.org/10.1038/378789a0.
- Malkin D, Li FP, Strong LC, Fraumeni JF, Nelson CE, Kim DH, et al. Germ line p53 mutations in a familial syndrome of breast cancer, sarcomas, and other neoplasms. Science 1990;250:1233-8. http://dx.doi.org/10.1126/science.1978757.
- Scott RJ, McPhillips M, Meldrum CJ, Fitzgerald PE, Adams K, Spigelman AD, et al. Hereditary nonpolyposis colorectal cancer in 95 families: differences and similarities between mutation-positive and mutation-negative kindreds. Am J Hum Genet 2001;68:118-27. http://dx.doi.org/10.1086/316942.
- Meijers-Heijboer H, Wijnen J, Vasen H, Wasielewski M, Wagner A, Hollestelle A, et al. The CHEK2 1100delC mutation identifies families with a hereditary breast and colorectal cancer phenotype. Am J Hum Genet 2003;72:1308-14. http://dx.doi.org/10.1086/375121.
- Evans DGR, Fentiman IS, McPherson K, Asbury D, Ponder BAJ, Howell A. Familial breast cancer. Brit Med J 1994;308:183-7. http://dx.doi.org/10.1136/bmj.308.6922.183.
- Dupont WD, Page DL. Relative risk of breast cancer varies with time since diagnosis of atypical hyperplasia. Hum Pathol 1989;20:723-5. http://dx.doi.org/10.1016/0046-8177(89)90063-4.
- Skolnick MH, Cannon-Albright LA, Goldgar DE, Ward JH, Marshall CJ, Schumann GB, et al. Inheritance of proliferative breast disease in breast cancer kindreds. Science 1990;250:1715-21. http://dx.doi.org/10.1126/science.2270486.
- Freedman AN, Seminara D, Gail MH, Hartge P, Colditz GA, Ballard-Barbash R, et al. Cancer risk prediction models: a workshop on development, evaluation, and application. J Natl Cancer Inst 2005;97:715-23. http://dx.doi.org/10.1093/jnci/dji128.
- Rockhill B, Spiegelman D, Byrne C, Hunter DJ, Colditz GA, . Validation of the Gail et al. model of breast cancer risk prediction and implications for chemoprevention. J Natl Cancer Inst 2001;93:358-66. http://dx.doi.org/10.1093/jnci/93.5.358.
- Amir E, Evans DG, Shenton A, Lalloo F, Moran A, Boggis C, et al. Evaluation of breast cancer risk assessment packages in the family history evaluation and screening programme. J Med Genet 2003;40:807-14. http://dx.doi.org/10.1136/jmg.40.11.807.
- Boyd NF, Lockwood GA, Martin LJ, Knight JA, Byng JW, Yaffe MJ, et al. Mammographic densities and breast cancer risk. Cancer Epidemiol Biomarkers Prev 1998;7:1133-44. http://dx.doi.org/10.3233/bd-1998-103-412.
- Key TJ, Appleby PN, Reeves GK, Roddam A, Dorgan JF, Longcope C, et al. Body mass index, serum sex hormones, and breast cancer risk in postmenopausal women. J Natl Cancer Inst 2003;95:1218-26. http://dx.doi.org/10.1093/jnci/djg022.
- Mitchell G, Antoniou AC, Warren R, Peock S, Brown J, Davies R, et al. Mammographic density and breast cancer risk in BRCA1 and BRCA2 mutation carriers. Cancer Res 2006;66:1866-72. http://dx.doi.org/10.1158/0008-5472.CAN-05-3368.
- Cyrillic 3.0 Pedigree Software n.d. www.exetersoftware.com/cat/cyrillic/cyrillic.html (accessed 30 March 2004).
- Evans DGR, Lalloo F. Risk assessment and management of high risk familial breast cancer. J Med Genet 2002;39:865-71. http://dx.doi.org/10.1136/jmg.39.12.865.
- Evans DGR, Kerr B, Lalloo F, Evans DGR, Kerr B, Lalloo F, et al. Risk Assessment and Management In Cancer Genetics. Oxford: Oxford University Press; 2005.
- Ford D, Easton DF, Stratton M, Narod S, Goldgar D, Devilee P, et al. genetic heterogeneity and penetrance analysis of the BRCA1 and BRCA2 genes in breast cancer families. Am J Hum Genet 1998;62:676-89. http://dx.doi.org/10.1086/301749.
- International Classification of Diseases. Geneva: WHO; 2010.
- Breslow NE, Day NE. Statistical Methods in Cancer Research Vol II. The Design and Analysis of Cohort Studies (IARC) Scientific Publication No 82. Oxford: Oxford University Press; 1987.
- Collaborative Group on Hormonal Factors in Breast Cancer . Familial breast cancer: collaborative reanalysis of individual data from 52 epidemiological studies including 58 209 women with breast cancer and 101 986 women without the disease. Lancet 2001;358:1389-99. http://dx.doi.org/10.1016/S0140-6736(01)06524-2.
- Centre for Cancer Genetic Epidemiology, Department of Public Health and Primary Care, University of Cambridge . BOADICEA n.d. https://pluto.srl.cam.ac.uk/cgi-bin/bd2/v2/bd.cgi 2010 (accessed 9 August 2013).
- Antoniou AC, Cunningham AP, Peto J, Evans DG, Lalloo F, Narod SA. The BOADICEA model of genetic susceptibility to breast and ovarian cancers: updates and extensions. Br J Cancer 2008;98. http://dx.doi.org/10.1038/sj.bjc.6604411.
- Norman RP, Evans DG, Easton DF, Young KC. The cost-utility of magnetic resonance imaging for breast cancer in BRCA1 mutation carriers aged 30–49. Eur J Health Econ 2007;8:137-44. http://dx.doi.org/10.1007/s10198-007-0042-9.
- Saslow D, Boetes C, Burke W, Harms S, Leach MO, Lehman CD, et al. American Cancer Society guidelines for breast screening with MRI as an adjunct to mammography. CA Cancer J Clin 2007;57:75-89. http://dx.doi.org/10.3322/canjclin.57.2.75.
- Evans DGR, Lennard F, Pointon LJ, Ramus SJ, Gayther SA, Sodha N, et al. On behalf of The UK study of MRI screening for breast cancer in women at high risk (MARIBS). Eligibility for MRI screening in the UK: effect of strict selection criteria and anonymous DNA testing on breast cancer incidence in the MARIBS study. Cancer Epidemiol Biomarkers Prev 2009;18:2123-31. http://dx.doi.org/10.1158/1055-9965.EPI-09-0138.
- Ozanne EM, Drohan B, Bosinoff P, Semine A, Jellinek M, Cronin C, et al. Which risk model to use? Clinical implications of the ACSMRI screening guidelines. Cancer Epidemiol Biomarkers Prev 2013;22:146-9. http://dx.doi.org/10.1158/1055-9965.EPI-12-0570.
- Jacobi CE, de Bock GH, Siegerink B, van Asperen CJ. Differences and similarities in breast cancer risk assessment models in clinical practice: which model to choose?. Breast Cancer Res Treat 2009;115:381-90. http://dx.doi.org/10.1007/s10549-008-0070-x.
- Quante AS, Whittemore AS, Shriver T, Strauch K, Terry MB. Breast cancer risk assessment across the risk continuum: genetic and nongenetic risk factors contributing to differential model performance. Breast Cancer Res 2012;14. http://dx.doi.org/10.1186/bcr3352.
- van Asperen CJ, Jonker MA, Jacobi CE, van Diemen-Homan JE, Bakker E, Breuning MH, et al. Risk estimation for healthy women from breast cancer families: new insights and new strategies. Cancer Epidemiol Biomarkers Prev 2004;13:87-93. http://dx.doi.org/10.1158/1055-9965.EPI-03-0090.
- Ingham SL, Warwick J, Buchan I, Sahin S, O’Hara C, Moran A, et al. Ovarian cancer among 8005 women from a breast cancer family history clinic: no increased risk of invasive ovarian cancer in families testing negative for BRCA1 and BRCA2. J Med Genet 2013;50:368-72. http://dx.doi.org/10.1136/jmedgenet-2013-101607.
- Einbeigi Z, Enerbäck C, Wallgren A, Nordling M, Karlsson P. BRCA1 gene mutations may explain more than 80% of excess number of ovarian cancer cases after breast cancer – a population based study from the Western Sweden Health Care region. Acta Oncol 2010;49:361-7. http://dx.doi.org/10.3109/02841860903521095.
- Kauff ND, Mitra N, Robson ME, Hurley KE, Chuai S, Goldfrank D, et al. Risk of ovarian cancer in BRCA1 and BRCA2 mutation-negative hereditary breast cancer families. J Natl Cancer Inst 2005;97:1382-4. http://dx.doi.org/10.1093/jnci/dji281.
- Meindl A, Hellebrand H, Wiek C, Erven V, Wappenschmidt B, Niederacher D, et al. Germline mutations in breast and ovarian cancer pedigrees establish RAD51C as a human cancer susceptibility gene. Nat Genet 2010;42:410-14. http://dx.doi.org/10.1038/ng.569.
- Loveday C, Turnbull C, Ramsay E, Hughes D, Ruark E, Frankum JR, et al. Germline mutations in RAD51D confer susceptibility to ovarian cancer. Nat Genet 2011;43:879-82. http://dx.doi.org/10.1038/ng.893.
- Ligtenberg MJ, Kuiper RP, Chan TL, Goossens M, Hebeda KM, Voorendt M, et al. Heritable somatic methylation and inactivation of MSH2 in families with Lynch syndrome due to deletion of the 3′ exons of TACSTD1. Nat Genet 2009;41:112-17. http://dx.doi.org/10.1038/ng.283.
- Gerhardus A, Schleberger H, Schlegelberger B, Gadzicki D. Diagnostic accuracy of methods for the detection of BRCA1 and BRCA2 mutations: a systematic review. Eur J Hum Genet 2007;15:619-27. http://dx.doi.org/10.1038/sj.ejhg.5201806.
- Evans DGR, Eccles DM, Rahman N, Young K, Bulman M, Amir E, et al. A new scoring system for the chances of identifying a BRCA1/2 mutation outperforms existing models including BRCAPRO. J Med Genet 2004;41:474-80. http://dx.doi.org/10.1136/jmg.2003.017996.
- Evans GR, Lalloo F. Development of a scoring system to screen for BRCA1/2 mutations. Methods Mol Biol 2010;653:237-47. http://dx.doi.org/10.1007/978-1-60761-759-4_14.
- Smith A, Moran A, Boyd MC, Bulman M, Shenton A, Smith L, et al. Phenocopies in BRCA1 and BRCA2 families: evidence for modifier genes and implications for screening. J Med Genet 2007;44:10-5. http://dx.doi.org/10.1136/jmg.2006.043091.
- Byng JW, Boyd NF, Fishell E, Jong RA, Yaffe MJ. The quantitative analysis of mammographic densities. Phys Med Biol 1994;39:1629-38. http://dx.doi.org/10.1088/0031-9155/39/10/008.
- Vachon CM, Brandt KR, Ghosh K, Scott CG, Maloney SD, Carston MJ, et al. Mammographic breast density as a general marker of breast cancer risk. Cancer Epidemiol Biomarkers Prev 2007;16:43-9. http://dx.doi.org/10.1158/1055-9965.EPI-06-0738.
- Evans DG, Ingham S, Dawe S, Roberts L, Lalloo F, Brentnall AR, et al. Breast cancer risk assessment in 8,824 women attending a family history evaluation and screening programme. Fam Cancer 2014;13:189-96. http://dx.doi.org/10.1007/s10689-013-9694-z.
- Claus EB, Risch N, Thompson WD. Genetic analysis of breast cancer in the cancer and steroid hormone study. Am J Hum Genet 1991;48:232-42.
- Anderson DE, Badzioch MD. Familial breast cancer risks. Effects of prostate and other cancers. Cancer 1993;72:114-19. http://dx.doi.org/10.1002/1097-0142(19930701)72:1<114::AID-CNCR2820720122>3.0.CO;2-0.
- Peto J, Collins N, Barfoot R, Seal S, Warren W, Rahman N, et al. Prevalence of BRCA1 and BRCA2 gene mutations in patients with early-onset breast cancer. J Natl Cancer Inst 1999;91:943-9. http://dx.doi.org/10.1093/jnci/91.11.943.
- Neuhausen SL, Marshall CJ. Loss of heterozygosity in familial tumors from three BRCA1-linked kindreds. Cancer Res 1994;54:6069-72.
- Collins N, McManus R, Wooster R, Mangion J, Seal S, Lakhani SR, et al. Consistent loss of the wild type allele in breast cancers from a family linked to the BRCA2 gene on chromosome 13q12–13. Oncogene 1995;10:1673-5.
- Anglian Breast Cancer Study Group . Prevalence and penetrance of BRCA1 and BRCA2 mutations in a population-based series of breast cancer cases. Br J Cancer 2000;83:1301-8. http://dx.doi.org/10.1054/bjoc.2000.1407.
- Thompson D, Easton DF. Cancer incidence in BRCA1 mutation carriers. J Natl Cancer Inst 2002;94:1358-65. http://dx.doi.org/10.1093/jnci/94.18.1358.
- Hall JM, Lee MK, Newman B, Morrow JE, Anderson LA, Huey B, et al. Linkage of early-onset familial breast cancer to chromosome 17q21. Science 1990;250:1684-9. http://dx.doi.org/10.1126/science.2270482.
- Easton DF, Bishop DT, Ford D, Crockford GP. Genetic linkage analysis in familial breast and ovarian cancer: results from 214 families. The Breast Cancer Linkage Consortium. Am J Hum Genet 1993;52:678-701.
- Wooster R, Neuhausen SL, Mangion J, Quirk Y, Ford D, Collins N, et al. Localization of a breast cancer susceptibility gene, BRCA2, to chromosome 13q12–13. Science 1994;265:2088-90. http://dx.doi.org/10.1126/science.8091231.
- Tavtigian SV, Simard J, Rommens J, Couch F, Shattuck-Eidens D, Neuhausen S, et al. The complete BRCA2 gene and mutations in chromosome 13q-linked kindreds. Nat Genet 1996;12:333-7. http://dx.doi.org/10.1038/ng0396-333.
- Srivastava S, Zou ZQ, Pirollo K, Blattner W, Chang EH. Germ-line transmission of a mutated p53 gene in a cancer-prone family with Li-Fraumeni syndrome. Nature 1990;348:747-9. http://dx.doi.org/10.1038/348747a0.
- Garber JE, Goldstein AM, Kantor AF, Dreyfus MG, Fraumeni JF, Li FP. Follow-up study of twenty-four families with Li-Fraumeni syndrome. Cancer Res 1991;51:6094-7.
- Lalloo F, Varley J, Ellis D, O’Dair L, Pharoah P, Evans DGR, et al. Family history is predictive of pathogenic mutations in BRCA1, BRCA2 and TP53 with high penetrance in a population based study of very early onset breast cancer. Lancet 2003;361:1101-2. http://dx.doi.org/10.1016/S0140-6736(03)12856-5.
- Hearle N, Schumacher V, Menko FH, Olschwang S, Boardman LA, Gille JJ, et al. Frequency and spectrum of cancers in the Peutz-Jeghers syndrome. Clin Cancer Res 2006;12:3209-15. http://dx.doi.org/10.1158/1078-0432.CCR-06-0083.
- Pharoah PD, Guilford P, Caldas C. Incidence of gastric cancer and breast cancer in CDH1 (E-cadherin) mutation carriers from hereditary diffuse gastric cancer families. Gastroenterology 2001;121:1348-53. http://dx.doi.org/10.1053/gast.2001.29611.
- Smith P, McGuffog L, Easton DF, Mann GJ, Pupo GM, Newman B, et al. A genome wide linkage search for breast cancer susceptibility genes. Genes Chromosomes Cancer 2006;45:646-55. http://dx.doi.org/10.1002/gcc.20330.
- Antoniou AC, Pharoah PD, McMullan G, Day NE, Ponder BA, Easton D. Evidence for further breast cancer susceptibility genes in addition to BRCA1 and BRCA2 in a population-based study. Genet Epidemiol 2001;21:1-18. http://dx.doi.org/10.1002/gepi.1014.
- Evans DG, Benson JR, Gui G, Tuttle TM. Early Breast Cancer: From Screening to Multidisciplinary Management. Boca Raton, FL: CRC Press; 2013.
- Meijers-Heijboer H, van den Ouweland A, Klijn J, Wasielewski M, de Snoo A, Oldenburg R, et al. Low-penetrance susceptibility to breast cancer due to CHEK2(*)1100delC in noncarriers of BRCA1 or BRCA2 mutations. Nat Genet 2002;31:55-9. http://dx.doi.org/10.1038/ng879.
- Ahn J, Urist M, Prives C. The Chk2 protein kinase. DNA Repair (Amst) 2004;3:1039-47. http://dx.doi.org/10.1016/j.dnarep.2004.03.033.
- Gatti RA, Berkel I, Boder E, Braedt G, Charmley P, Concannon P, et al. Localization of an ataxia-telangiectasia gene to chromosome 11q22–23. Nature 1988;336:577-80. http://dx.doi.org/10.1038/336577a0.
- Savitsky K, Bar-Shira A, Gilad S, Rotman G, Ziv Y, Vanagaite L, et al. A single ataxia telangiectasia gene with a product similar to PI-3 kinase. Science 1995;268:1749-53. http://dx.doi.org/10.1126/science.7792600.
- Shiloh Y. The ATM-mediated DNA-damage response: taking shape. Trends Biochem Sci 2006;31:402-10. http://dx.doi.org/10.1016/j.tibs.2006.05.004.
- Renwick A, Thompson D, Seal S, Kelly P, Chagtai T, Ahmed M, et al. ATM mutations that cause ataxia-telangiectasia are breast cancer susceptibility alleles. Nat Genet 2006;38:873-5. http://dx.doi.org/10.1038/ng1837.
- Cantor SB, Bell DW, Ganesan S, Kass EM, Drapkin R, Grossman S, et al. BACH1, a novel helicase-like protein, interacts directly with BRCA1 and contributes to its DNA repair function. Cell 2001;105:149-60. http://dx.doi.org/10.1016/S0092-8674(01)00304-X.
- Peng M, Litman R, Jin Z, Fong G, Cantor SB. BACH1 is a DNA repair protein supporting BRCA1 damage response. Oncogene 2006;25:2245-53. http://dx.doi.org/10.1038/sj.onc.1209257.
- Seal S, Thompson D, Renwick A, Elliott A, Kelly P, Barfoot R, et al. Truncating mutations in the Fanconi anemia J gene BRIP1 are low-penetrance breast cancer susceptibility alleles. Nat Genet 2006;38:1239-41. http://dx.doi.org/10.1038/ng1902.
- Howlett NG, Taniguchi T, Olson S, Cox B, Waisfisz Q, De Die-Smulders C, et al. Biallelic inactivation of BRCA2 in Fanconi anemia. Science 2002;297:606-9. http://dx.doi.org/10.1126/science.1073834.
- Levitus M, Waisfisz Q, Godthelp BC, de Vries Y, Hussain S, Wiegant WW, et al. The DNA helicase BRIP1 is defective in Fanconi anemia complementation group. J Nat Genet 2005;37:934-5. http://dx.doi.org/10.1038/ng1625.
- Reid S, Schindler D, Hanenberg H, Barker K, Hanks S, Kalb R, et al. Biallelic mutations in PALB2 cause Fanconi anemia subtype FA-N and predispose to childhood cancer. Nat Genet 2007;39:162-4. http://dx.doi.org/10.1038/ng1947.
- Rahman N, Seal S, Thompson D, Kelly P, Renwick A, Elliott A, et al. PALB2, which encodes a BRCA2-interacting protein, is a breast cancer susceptibility gene. Nat Genet 2007;39:165-7. http://dx.doi.org/10.1038/ng1959.
- Stratton MR, Rahman N. The emerging landscape of breast cancer susceptibility. Nat Genet 2008;40:17-22. http://dx.doi.org/10.1038/ng.2007.53.
- Sharif S, Moran A, Huson SM, Iddenden R, Shenton A, Howard E, et al. Women with Neurofibromatosis 1 (NF1) are at a moderately increased risk of developing breast cancer and should be considered for early screening. J Med Genet 2007;44:481-4. http://dx.doi.org/10.1136/jmg.2007.049346.
- Breast Cancer Association Consortium . Commonly studied single-nucleotide polymorphisms and breast cancer: results from the Breast Cancer Association Consortium. J Natl Cancer Inst 2006;98:1382-96. http://dx.doi.org/10.1093/jnci/djj374.
- Gold B, Kirchhoff T, Stefanov S, Lautenberger J, Viale A, Garber J, et al. Genome-wide association study provides evidence for a breast cancer risk locus at 6q22.33. Proc Natl Acad Sci U S A 2008;105:4340-5. http://dx.doi.org/10.1073/pnas.0800441105.
- Stacey SN, Manolescu A, Sulem P, Thorlacius S, Gudjonsson SA, Jonsson GF, et al. Common variants on chromosome 5p12 confer susceptibility to estrogen receptor-positive breast cancer. Nat Genet 2008;40:703-6. http://dx.doi.org/10.1038/ng.131.
- Fletcher O, Johnson N, Gibson L, Coupland B, Fraser A, Leonard A, et al. Association of genetic variants at 8q24 with breast cancer risk. Cancer Epidemiol Biomarkers Prev 2008;17:702-5. http://dx.doi.org/10.1158/1055-9965.EPI-07-2564.
- Garcia-Closas M, Chanock S. Genetic susceptibility loci for breast cancer by estrogen receptor status. Clin Cancer Res 2008;14:8000-9. http://dx.doi.org/10.1158/1078-0432.CCR-08-0975.
- Meyer KB, Maia AT, O’Reilly M, Teschendorff AE, Chin SF, Caldas C, et al. Allele-specific up-regulation of FGFR2 increases susceptibility to breast cancer. PLOS Biol 2008;6. http://dx.doi.org/10.1371/journal.pbio.0060108.
- Ahmed S, Thomas G, Ghoussaini M, Healey CS, Humphreys MK, Platte R, et al. Newly discovered breast cancer susceptibility loci on 3p24 and 17q23.2. Nat Genet 2009;41:585-90. http://dx.doi.org/10.1038/ng.354.
- Turnbull C, Ahmed S, Morrison J, Pernet D, Renwick A, Maranian M, et al. Genome-wide association study identifies five new breast cancer susceptibility loci. Nat Genet 2010;42:504-7. http://dx.doi.org/10.1038/ng.586.
- Melchor L, Benitez J. The complex genetic landscape of familial breast cancer. Hum Genet 2013;132:845-63. http://dx.doi.org/10.1007/s00439-013-1299-y.
- Michailidou K, Hall P, Gonzalez-Neira A, Ghoussaini M, Dennis J, Milne RL, et al. Large-scale genotyping identifies 41 new loci associated with breast cancer risk. Nat Genet 2013;45:353-61. http://dx.doi.org/10.1038/ng.2563.
- Sakoda LC, Jorgenson E, Witte JS. Turning of COGS moves forward findings for hormonally mediated cancers. Nat Genet 2013;45:345-8. http://dx.doi.org/10.1038/ng.2587.
- Latif A, Hadfield KD, Roberts SA, Shenton A, Lalloo F, Black GC, et al. Breast cancer susceptibility variants alter risks in familial disease. J Med Genet 2010;47:126-31. http://dx.doi.org/10.1136/jmg.2009.067256.
- Antoniou AC, Beesley J, McGuffog L, Sinilnikova OM, Healey S, Neuhausen SL, et al. Common breast cancer susceptibility alleles and the risk of breast cancer for BRCA1 and BRCA2 mutation carriers: implications for risk prediction. Cancer Res 2010;70:9742-54. http://dx.doi.org/10.1158/0008-5472.CAN-10-1907.
- Antoniou AC, Kartsonaki C, Sinilnikova OM, Soucy P, McGuffog L, Healey S, et al. Common alleles at 6q25.1 and 1p11.2 are associated with breast cancer risk for BRCA1 and BRCA2 mutation carriers. Hum Mol Genet 2011;20:3304-21. http://dx.doi.org/10.1093/hmg/ddr226.
- Nelson W. Hazard plotting for incomplete failure data. J Qual Technol 1969;1:27-52.
- Cox DR. Regression models and life-tables. J Roy Stat Soc B Met 1972;34:187-220.
- Ingham SL, Warwick J, Byers H, Lalloo F, Newman WG, Evans DG. Is multiple SNP testing in BRCA2 and BRCA1 female carriers ready for use in clinical practice? Results from a large genetic centre in the UK. Clin Genet 2013;84:37-42. http://dx.doi.org/10.1111/cge.12035.
- Evans DGR, Warwick J, Astley SM, Stavrinos P, Sahin S, Ingham S, et al. Assessing individual breast cancer risk within the UK National Health Service Breast Screening Program: a new paradigm for cancer prevention. Cancer Prev Res 2012;5:943-51. http://dx.doi.org/10.1158/1940-6207.CAPR-11-0458.
- Familial Breast Cancer: Classification and Care of People at Risk of Familial Breast Cancer and Management of Breast Cancer and Related Risks in People with a Family History of Breast Cancer. London: National Collaborating Centre for Cancer; 2013.
- Chen S, Iversen ES, Friebel T, Finkelstein D, Weber BL, Eisen A, et al. Characterization of BRCA1 and BRCA2 mutations in a large United States sample. J Clin Oncol 2006;24:863-71. http://dx.doi.org/10.1200/JCO.2005.03.6772.
- Warner E, Foulkes W, Goodwin P, Meschino W, Blondal J, Paterson C, et al. Prevalence and penetrance of BRCA1 and BRCA2 gene mutations in unselected Ashkenazi Jewish women with breast cancer. J Natl Cancer Inst 1999;91:1241-7. http://dx.doi.org/10.1093/jnci/91.14.1241.
- Hopper JL, Southey MC, Dite GS, Jolley DJ, Giles GG, McCredie MR, et al. Population-based estimate of the average age-specific cumulative risk of breast cancer for a defined set of protein-truncating mutations in BRCA1 and BRCA2. Australian Breast Cancer Family Study. Cancer Epidemiol Biomarkers Prev 1999;8:741-7.
- Evans DG, Lalloo F, Ashcroft L, Shenton A, Clancy T, Baildam AD, et al. Uptake of risk reducing surgery in unaffected women at high risk of breast and ovarian cancer is risk, age and time dependent. Cancer Epid Biomarkers Prev 2009;18:2318-24. http://dx.doi.org/10.1158/1055-9965.EPI-09-0171.
- Mealiffe ME, Stokowski RP, Rhees BK, Prentice RL, Pettinger M, Hinds DA. Assessment of clinical validity of a breast cancer risk model combining genetic and clinical information. J Natl Cancer Inst 2010;102:1618-27. http://dx.doi.org/10.1093/jnci/djq388.
- Comen E, Balistreri L, Gönen M, Dutra-Clarke A, Fazio M, Vijai J, et al. Discriminatory accuracy and potential clinical utility of genomic profiling for breast cancer risk in BRCA-negative women. Breast Cancer Res Treat 2011;127:479-87. http://dx.doi.org/10.1007/s10549-010-1215-2.
- Gail MH, Brinton LA, Byar DP, Corle DK, Green SB, Schairer C, et al. Projecting individualized probabilities of developing breast cancer for white females who are being examined annually. J Natl Cancer Inst 1989;81:1879-86. http://dx.doi.org/10.1093/jnci/81.24.1879.
- Costantino JP, Gail MH, Pee D, Anderson S, Redmond CK, Benichou J, et al. Validation studies for models projecting the risk of invasive and total breast cancer incidence. J Natl Cancer Inst 1999;91:1541-8. http://dx.doi.org/10.1093/jnci/91.18.1541.
- Gail MH. Value of adding single-nucleotide polymorphism genotypes to a breast cancer risk model. J Natl Cancer Inst 2009;101:959-63. http://dx.doi.org/10.1093/jnci/djp130.
- Evans DG, Ingham SL, Buchan I, Woodward ER, Byers H, Howell A, et al. Increased rate of phenocopies in all age groups in BRCA1/BRCA2 mutation kindred, but increased prospective breast cancer risk is confined to BRCA2 mutation carriers. Cancer Epidemiol Biomarkers Prev 2013;22:2269-76. http://dx.doi.org/10.1158/1055-9965.EPI-13-0316-T.
- Howell A, Astley S, Warwick J, Stavrinos P, Sahin S, Ingham S, et al. Prevention of breast cancer in the context of a national breast screening programme. J Intern Med 2012;271:321-30. http://dx.doi.org/10.1111/j.1365-2796.2012.02525.x.
- Brentnall AR, Evans DG, Cuzick J. Distribution of breast cancer risk from SNPs and classical risk factors in women of routine screening age in the UK. Br J Cancer 2014;110:827-8. http://dx.doi.org/10.1038/bjc.2013.747.
- Brentnall AR, Evans DG, Cuzick J. Value of phenotypic and single-nucleotide polymorphism panel markers in predicting the risk of breast cancer. J Genet Synd Gene Ther 2013;4.
- Health and Social Care Information Centre (HSCIC) . Breast Screening Programme England 2011–2012 [NS] n.d. www.hscic.gov.uk/catalogue/PUB10339 (accessed May 2014).
- Parkin DM, Boyd L, Walker LC. 16. The fraction of cancer attributable to lifestyle and environmental factors in the UK in 2010. Br J Cancer 2011;105. http://dx.doi.org/10.1038/bjc.2011.489.
- World Cancer Research Fund/American Institute for Cancer Research . Continuous Update Project Report. Food, Nutrition, Physical Activity, and the Prevention of Breast Cancer 2010. www.dietandcancerreport.org/cancer_resource_center/downloads/cu/Breast-Cancer-2010-Report.pdf (accessed May 2014).
- Gramling R, Lash TL, Rothman KJ, Cabral HJ, Silliman R, Roberts M, et al. Family history of later-onset breast cancer, breast healthy behavior and invasive breast cancer among postmenopausal women: a cohort study. Breast Cancer Res 2010;12. http://dx.doi.org/10.1186/bcr2727.
- Petracci E, Decarli A, Schairer C, Pfeiffer RM, Pee D, Masala G, et al. Risk factor modification and projections of absolute breast cancer risk. J Natl Cancer Inst 2011;103:1037-48. http://dx.doi.org/10.1093/jnci/djr172.
- Catsburg C, Miller AB, Rohan TE. Adherence to cancer prevention guidelines and risk of breast cancer. Int J Cancer 2014;135:2444-52. http://dx.doi.org/10.1002/ijc.28887.
- Harvie M, Howell A, Vierkant RA, Kumar N, Cerhan JR, Kelemen LE, et al. Association of gain and loss of weight before and after menopause with risk of postmenopausal breast cancer in the Iowa women’s health study. Cancer Epidemiol Biomarkers Prev 2005;14:656-61. http://dx.doi.org/10.1158/1055-9965.EPI-04-0001.
- Eliassen AH, Colditz GA, Rosner B, Willett WC, Hankinson SE. Adult weight change and risk of postmenopausal breast cancer. JAMA 2006;296:193-201. http://dx.doi.org/10.1001/jama.296.2.193.
- Teras LR, Goodman M, Patel AV, Diver WR, Flanders WD, Feigelson HS. Weight loss and postmenopausal breast cancer in a prospective cohort of overweight and obese US women. Cancer Causes Control 2011;22:573-9. http://dx.doi.org/10.1007/s10552-011-9730-y.
- Cecchini RS, Costantino JP, Cauley JA, Cronin WM, Wickerham DL, Land SR, et al. Body mass index and the risk for developing invasive breast cancer among high-risk women in NSABP P-1 and STAR breast cancer prevention trials. Cancer Prev Res (Phila) 2012;5:583-92. http://dx.doi.org/10.1158/1940-6207.CAPR-11-0482.
- Sickles EA, D’Orsi CJ, Bassett LW, Appleton CM, Berg WA, Burnside ES. ACR BI-RADS® Atlas, Breast Imaging Reporting and Data System. Reston, VA: American College of Radiology; 2013.
- Duffy SW, Nagtegaal ID, Astley SM, Gillan MGC, McGee MA, Boggis CRM, et al. Visually assessed breast density, breast cancer risk and the importance of the craniocaudal view. Breast Cancer Res 2008;10. http://dx.doi.org/10.1186/bcr2123.
- Diffey J, Hufton A, Astley S, Astley S, Brady M, Rose C, et al. Digital Mammography 8th International Workshop. Berlin: Springer-Verlag; 2006.
- Pawluczyk O, Augustine BJ, Yaffe MJ, Rico D, Yang J, Mawdsley GE. A volumetric method for estimation of breast density in digitised screen-film mammograms. Med Phys 2003;30:352-64. http://dx.doi.org/10.1118/1.1539038.
- Highnam RP, Brady M. Mammographic Image Analysis (Computational Imaging and Vision). Dordrecht: Kluwer Academic Publishers; 1999.
- Jeffreys M, Harvey J, Highnam R, Martí J, Oliver A, Freixenet J, et al. Digital Mammography 10th International Workshop. Berlin: Springer-Verlag; 2010.
- Highnam R, Brady M, Yaffe MJ, Karssemeijer N, Harvey J, Martí J, et al. Digital Mammography 10th International Workshop. Berlin: Springer-Verlag; 2010.
- Ciatto S, Bernadi D, Calabrese M, Durando M, Gentilini MA, Mariscotti G, et al. A first evaluation of breast radiological density assessment by QUANTRA software as compared to visual classification. Breast 2012;21:503-6. http://dx.doi.org/10.1016/j.breast.2012.01.005.
- Wang J, Azziz A, Fan B, Malkov S, Klifa C, Newitt D, et al. Agreement of mammographic measures of volumetric breast density to MRI. PLOS ONE 2013;8. http://dx.doi.org/10.1371/journal.pone.0081653.
- Kontos D, Bakic PR, Acciavatti RJ, Conant EF, Maidment ADA, Martí J, et al. Digital Mammography 10th International Workshop. Berlin: Springer-Verlag; 2010.
- Euhus DM, Leitch AM, Huth JF, Peters GN. Limitations of the Gail model in the specialized breast cancer risk assessment clinic. Breast 2002;8:23-7. http://dx.doi.org/10.1046/j.1524-4741.2002.08005.x.
- Claus EB, Risch N, Thompson WD. The calculation of breast cancer risk for women with a first degree family history of ovarian cancer. Breast Cancer Res Treat 1993;28:115-20. http://dx.doi.org/10.1007/BF00666424.
- McTiernan A, Kuniyuki A, Yasui Y, Bowen D, Burke W, Culver JB, et al. Comparisons of two breast cancer risk estimates in women with a family history of breast cancer. Cancer Epidemiol Biomarkers Prev 2001;10:333-8.
- McGuigan KA, Ganz PA, Breant C. Agreement between breast cancer risk estimation methods. J Natl Cancer Inst 1996;88:1315-17. http://dx.doi.org/10.1093/jnci/88.18.1315.
- Tischkowitz M, Wheeler D, France E, Chapman C, Lucassen A, Sampson J, et al. A comparison of methods currently used in clinical practice to estimate familial breast cancer risks. Ann Oncol 2000;11:451-4. http://dx.doi.org/10.1023/A:1008396129543.
- Parmigiani G, Berry DA, Aquilar O. Determining carrier probabilities for breast cancer susceptibility genes BRCA1 and BRCA2. Am J Hum Genet 1998;62:145-8. http://dx.doi.org/10.1086/301670.
- Antoniou AC, Pharoah PP, Smith P, Easton DF. The BOADICEA model of genetic susceptibility to breast and ovarian cancer. Br J Cancer 2004;91:1580-90. http://dx.doi.org/10.1038/sj.bjc.6602175.
- Simard J, Dumont M, Moisan AM, Gaborieau V, Malouin H, Durocher F, et al. Evaluation of BRCA1 and BRCA2 mutation prevalence, risk prediction models and a multistep testing approach in French-Canadian families with high risk of breast and ovarian cancer. J Med Genet 2007;44:107-21. http://dx.doi.org/10.1136/jmg.2006.044388.
- Public Health England . Local Obesity Prevalence n.d. www.noo.org.uk/LA/obesity_prev (accessed May 2014).
- Cancer Research UK . Breast Cancer Statistics n.d. www.cancerresearchuk.org/cancer-info/cancerstats/types/breast/?script=true (accessed May 2014).
- Department for Communities and Local Government . English Indices of Deprivation 2010 n.d. www.gov.uk/government/uploads/system/uploads/attachment_data/file/6871/1871208.pdf.
- Evans DGR, Burnell LD, Hopwood P, Howell A. Perception of risk in women with a family history of breast cancer. Br J Cancer 1993;67:612-14. http://dx.doi.org/10.1038/bjc.1993.112.
- Evans DGR, Blair V, Greenhalgh R, Hopwood P, Howell A. The impact of genetic counselling on risk perception in women with a family history of breast cancer. Br J Cancer 1994;70:934-8. http://dx.doi.org/10.1038/bjc.1994.423.
- Hopwood P, Shenton A, Lalloo F, Evans DGR, Howell A. Risk perception and cancer worry: an exploratory study of the impact of genetic risk counselling in women with a family history of breast cancer. J Med Genet 2001;38. http://dx.doi.org/10.1136/jmg.38.2.139.
- Evans DGR, Brentnall AR, Harvie M, Dawe S, Sergeant JC, Stavrinos P, et al. Breast cancer risk in young women in the National Breast Screening Programme: implications for applying NICE guidelines for additional screening and chemoprevention. Cancer Prev Res (Phila) 2014;7:993-1001. http://dx.doi.org/10.1158/1940-6207.CAPR-14-0037.
- Gilbert FJ, Astley SM, Gillan MG, Agbaje OF, Wallis MG, James J, et al. Single reading with computer-aided detection for screening mammography. N Engl J Med 2008;359:1675-84. http://dx.doi.org/10.1056/NEJMoa0803545.
- Malkov S, Wang J, Kerlikowske K, Cummings SR, Shepherd JA. Single X-ray absorptiometry method for the quantitative mammographic measure of fibroglandular tissue volume. Med Phys 2009;36:5525-36. http://dx.doi.org/10.1118/1.3253972.
- Sperrin M, Bardwell L, Sergeant JC, Astley S, Buchan I. Correcting for rater bias in scores on a continuous scale, with application to breast density. Stat Med 2013;32:4666-78. http://dx.doi.org/10.1002/sim.5848.
- Van Engeland S, Snoeren PR, Huisman H, Boetes C, Karssemeijer N. Volumetric breast density estimation from full field digital mammograms. IEEE Trans Med Imaging 2006;25:273-82. http://dx.doi.org/10.1109/TMI.2005.862741.
- Sergeant JC, Wilson M, Barr N, Beetles U, Boggis C, Bundred S, et al. Inter-observer agreement in visual analogue scale assessment of percentage breast density. Breast Cancer Res 2013;15. http://dx.doi.org/10.1186/bcr3517.
- Hashmi S, Sergeant JC, Morris J, Whiteside S, Stavrinos P, Evans DG, et al. Breast Imaging 11th International Workshop. Berlin: Springer-Verlag; 2012.
- Beattie L, Harkness E, Bydder M, Sergeant J, Maxwell A, Barr N, et al. Breast Imaging 12th International Workshop. Berlin: Springer-Verlag; 2014.
- O’Donovan E, Sergeant J, Harkness E, Morris J, Wilson M, Lim Y, et al. Breast Imaging 12th International Workshop. Berlin: Springer-Verlag; 2014.
- NHS Musgrove Park Hospital . Somerset Cancer Registry n.d. www.musgroveparkhospital.nhs.uk/wards-and-departments/departments-services/somerset-cancer-register/ (accessed May 2014).
- Public Health England . North West Cancer Teams, National Cancer Registration Service and Cancer Knowledge and Intelligence n.d. www.nwcis.nhs.uk/ (accessed May 2014).
- Patel HG, Astley SM, Hufton AP, Harvie M, Hagan K, Marchant TE, et al. Digital Mammography 8th International Workshop. Berlin Heidelberg: Springer-Verlag; 2006.
- Sergeant JC, Walshaw L, Wilson M, Seed S, Barr N, Beetles U, et al. Same task, same observers, different values: the problem with visual assessment of breast density. Proc. SPIE 8673, Medical Imaging 2013: Image Perception, Observer Performance, and Technology Assessment, 86730 T n.d. http://proceedings.spiedigitallibrary.org/proceeding.aspx?articleid7673826 (accessed 28 March 2013).
- Boyd NF, Martin LJ, Sun L, Guo H, Chiarelli A, Hislop G, et al. Body size, mammographic density, and breast cancer risk. Cancer Epidemiol Biomarkers Prev 2006;15:2086-92. http://dx.doi.org/10.1158/1055-9965.EPI-06-0345.
- Boyd NF, Guo H, Martin LJ, Sun L, Stone J, Fishell E, et al. Mammographic density and the risk and detection of breast cancer. N Engl J Med 2007;356:227-36. http://dx.doi.org/10.1056/NEJMoa062790.
- Kerlikowske K, Ichikawa L, Miglioretti DL, Buist DSM, Vacek PM, Smith-Bindman R, et al. Longitudinal measurement of clinical mammographic breast density to improve estimation of breast cancer risk. J Natl Cancer Inst 2007;99:386-95. http://dx.doi.org/10.1093/jnci/djk066.
- Diffey J, Hufton A, Astley S, Mercer C, Maxwell A, Krupinski E. 9th International Workshop. Berlin: Springer-Verlag; 2008.
- Byrne C, Schairer C, Wolfe J, Parekh N, Salane M, Brinton LA, et al. Mammographic features and breast cancer risk: effects with time, age, and menopause status. J Natl Cancer Inst 1995;87:1622-9. http://dx.doi.org/10.1093/jnci/87.21.1622.
- Wolfe JN. Breast parenchymal patterns and their changes with age. Radiology 1976;121:545-52. http://dx.doi.org/10.1148/121.3.545.
- Boyd N, Martin L, Stone J, Little L, Minkin S, Yaffe M. A longitudinal study of the effects of menopause on mammographic features. Cancer Epidemiol Biomarkers Prev 2002;11:1048-53.
- Greendale GA, Reboussin BA, Slone S, Wasilauskas C, Pike MC, Ursin G. Postmenopausal hormone therapy and change in mammographic density. J Natl Cancer Inst 2003;95:30-7. http://dx.doi.org/10.1093/jnci/95.1.30.
- Rutter CM, Mandelson MT, Laya MB, Taplin S. Changes in breast density associated with initiation, discontinuation, and continuing use of hormone replacement therapy. JAMA 2001;285:171-6. http://dx.doi.org/10.1001/jama.285.2.171.
- Manchester City Council . Indices of Multiple Deprivation 2010: Analysis for Manchester n.d. www.manchester.gov.uk/downloads/download/414/research_and_intelligence_population_publications_deprivation (accessed 11 July 2013).
- Chlebowski RT, Chen Z, Anderson GL, Rohan T, Aragaki A, Lane D, et al. Ethnicity and breast cancer: factors influencing differences in incidence and outcome. J Natl Cancer Inst 2005;97:439-48. http://dx.doi.org/10.1093/jnci/dji064.
- Smigal C, Jemal A, Ward E, Cokkinides V, Smith R, Howe HL, et al. Trends in breast cancer by race and ethnicity: update 2006. CA Cancer J Clin 2006;56:169-87. http://dx.doi.org/10.3322/canjclin.56.3.168.
- Tzias D, George S, Wilkinson L, Mehta R, Lobo C, Hainsworth A, et al. Correlation of ethnicity with breast density as assessed by Quantra™. Breast Cancer Res 2011;13. http://dx.doi.org/10.1186/bcr2951.
- El-Bastawissi AY, White E, Mandelson MT, Taplin S. Variation in mammographic breast density by race. Ann Epidemiol 2001;11:257-63. http://dx.doi.org/10.1016/S1047-2797(00)00225-8.
- Chen Z, Wu AH, Gauderman WJ, Bernstein L, Ma H, Pike MC, et al. Does mammographic density reflect ethnic differences in breast cancer incidence rates?. Am J Epidemiol 2004;159:140-7. http://dx.doi.org/10.1093/aje/kwh028.
- Turnbull AE, Kapera L, Cohen MEL. Mammographic parenchymal pattern in Asian and Caucasian women attending for screening. Clin Radiol 1993;48:38-40. http://dx.doi.org/10.1016/S0009-9260(05)80105-9.
- del Carmen MG, Hughes KS, Halpern E, Rafferty E, Kopans D, Parisky YR, et al. Racial differences in mammographic breast density. Cancer 2003;98:590-6. http://dx.doi.org/10.1002/cncr.11517.
- Hartman K, Highnam R, Warren R, Jackson V, Krupinski E. 9th International Workshop. Berlin: Springer-Verlag; 2008.
- Rosenberg RD, Hunt WC, Williamson MR, Gilliland FD, Wiest PW, Kelsey CA, et al. Effects of age, breast density, ethnicity, and estrogen replacement therapy on screening mammographic sensitivity and cancer stage at diagnosis: review of 183,134 screening mammograms in Albuquerque, New Mexico. Radiology 1998;209:511-18. http://dx.doi.org/10.1148/radiology.209.2.9807581.
- Struewing JP, Hartge P, Wacholder S, Baker SM, Berlin M, McAdams M, et al. The risk of cancer associated with specific mutations of BRCA1 and BRCA2 among Ashkenazi Jews. N Engl J Med 1997;336:1401-8. http://dx.doi.org/10.1056/NEJM199705153362001.
- Goel MS, Wee CC, McCarthy EP, Davis RB, Ngo-Metzger Q, Phillips RS. Racial and ethnic disparities in cancer screening: the importance of foreign birth as a barrier to care. J Gen Intern Med 2003;18:1028-35. http://dx.doi.org/10.1111/j.1525-1497.2003.20807.x.
- Nutine L, Sergeant JC, Morris J, Stavrinos P, Evans DG, Howell T, et al. Breast Imaging 11th International Workshop. Berlin: Springer-Verlag; 2012.
- Ellison-Loschmann L, McKenzie F, Highnam R, Cave A, Walker J, Jeffreys M. Age and ethnic differences in volumetric breast density in New Zealand women: a cross-sectional study. PLOS ONE 2013;8. http://dx.doi.org/10.1371/journal.pone.0070217.
- Rowland ML. Self-reported weight and height. Am J Clin Nutr 1990;52:1125-33.
- Jinnan G, Warren R, Warren-Forward H, Forbes JF. Reproducibility of visual assessment on mammographic density. Breast Cancer Res Treat 2008;108:121-7. http://dx.doi.org/10.1007/s10549-007-9581-0.
- Bland JM, Altman DG. Statistical methods for assessing agreement between two methods of clinical measurement. Lancet 1986;327:307-10. http://dx.doi.org/10.1016/S0140-6736(86)90837-8.
- Bland JM, Altman DG. Measuring agreement in method comparison studies. Stat Methods Med Res 1999;8:135-60. http://dx.doi.org/10.1191/096228099673819272.
- Cuzick J, Warwick J, Pinney E, Duffy SW, Cawthorn S, Howell A, et al. Tamoxifen-induced reduction in mammographic density and breast cancer risk reduction: a nested case–control study. J Natl Cancer Inst 2011;103:744-52. http://dx.doi.org/10.1093/jnci/djr079.
- Astley S, Swayamprakasam C, Berks M, Sergeant J, Morris J, Wilson M, et al. Assessment of change in breast density: reader performance using synthetic mammographic images. Proc. SPIE 8318, Medical Imaging 2012: Image Perception, Observer Performance, and Technology Assessment, 831810 2012:810-31.
- National Cancer Institute . Breast Cancer Risk Assessment Tool n.d. www.cancer.gov/bcrisktool/ (accessed May 2014).
- Boyd NF, Dite GS, Stone J, Gunasekara A, English DR, McCredie MR, et al. Heritability of mammographic density, a risk factor for breast cancer. N Engl J Med 2002;347:886-94. http://dx.doi.org/10.1056/NEJMoa013390.
- Pankow JS, Vachon CM, Kuni CC, King RA, Arnett DK, Grabrick DM, et al. Genetic analysis of mammographic breast density in adult women: evidence of a gene effect. J Natl Cancer Inst 1997;89:549-56. http://dx.doi.org/10.1093/jnci/89.8.549.
- Santen R, Boyd N, Chlebowski RT, Cummings S, Cuzick J, Dowsett M, et al. Critical assessment of new risk factors for breast cancer: considerations for development of an improved risk prediction model. Endocr Relat Cancer 2007;14:169-87. http://dx.doi.org/10.1677/ERC-06-0045.
- Drummond MF, Sculpher MJ, Torrance GW, O’Brien BJ, Stoddart GL. Methods for the Economic Evaluation of Health Care Programmes. Oxford: Oxford University Press; 2005.
- Husereau D, Drummond M, Petrou S, Carswell C, Moher D, Greenberg D, et al. Consolidated health economic evaluation reporting standards (CHEERS) statement. BMC Med 2013;11. http://dx.doi.org/10.1186/1741-7015-11-80.
- Karnon J, Goyder E, Tappenden P, McPhie S, Towers I, Brazier J, et al. A review and critique of modelling in prioritising and designing screening programmes. Health Technol Assess 2007;11. http://dx.doi.org/10.3310/hta11520.
- Ahern CH, Shen Y. Cost-effectiveness analysis of mammography and clinical breast examination strategies: a comparison with current guidelines. Cancer Epidemiol Biomarkers Prev 2009;18:718-25. http://dx.doi.org/10.1158/1055-9965.EPI-08-0918.
- Arveux P, Wait S, Schaffer P. Building a model to determine the cost-effectiveness of breast cancer screening in France. Eur J Cancer Care 2003;12:143-53. http://dx.doi.org/10.1046/j.1365-2354.2003.00373.x.
- Baker RD. Use of a mathematical model to evaluate breast cancer screening policy. Health Care Manage Sci 1998;1:103-13. http://dx.doi.org/10.1023/A:1019046619402.
- Boer R, de Koning HJ, van Oortmarssen GJ, van der Maas PJ. In search of the best upper age limit for breast cancer screening. Eur J Cancer 1995;31:2040-43. http://dx.doi.org/10.1016/0959-8049(95)00457-2.
- Boer R, de Koning H, Threlfall A, Warmerdam P, Street A, Friedman E, et al. Cost effectiveness of shortening screening interval or extending age range of NHS breast screening programme: computer simulation study. BMJ 1998;317:376-9. http://dx.doi.org/10.1136/bmj.317.7155.376.
- Boer R, de Koning HJ, van der Maas PJ. A longer breast carcinoma screening interval for women age older than 65 years?. Cancer 1999;86:1506-10. http://dx.doi.org/10.1002/(SICI)1097-0142(19991015)86:8<1506::AID-CNCR17>3.0.CO;2-2.
- Brancato B, Bonardi R, Catarzi S, Iacconi C, Risso G, Taschini R, et al. Negligible advantages and excess costs of routine addition of breast ultrasonography to mammography in dense breasts. Tumori 2007;93:562-6.
- Brown J, Bryan S, Warren R. Mammography screening: an incremental cost effectiveness analysis of double versus single reading of mammograms. BMJ 1996;312:809-12. http://dx.doi.org/10.1136/bmj.312.7034.809.
- Bryan S, Brown J, Warren R. Mammography screening: an incremental cost effectiveness analysis of two view versus one view procedures in London. J Epidemiol Community Health 1995;49:70-8. http://dx.doi.org/10.1136/jech.49.1.70.
- Burnside E, Belkora J, Esserman L. The impact of alternative practices on the cost and quality of mammographic screening in the United States. Clinical Breast Cancer 2001;2:145-52. http://dx.doi.org/10.3816/CBC.2001.n.019.
- Cairns J, van der Pol M. Cost-effectiveness of non-consensus double reading. Breast 1998;7:243-6. http://dx.doi.org/10.1016/S0960-9776(98)90088-1.
- Carles M, Vilaprinyo E, Cots F, Gregori A, Pla R, Román R, et al. Cost-effectiveness of early detection of breast cancer in Catalonia (Spain). BMC Cancer 2011;11. http://dx.doi.org/10.1186/1471-2407-11-192.
- Caumo F, Brunelli S, Tosi E, Teggi S, Bovo C, Bonavina G, et al. On the role of arbitration of discordant double readings of screening mammography: experience from two Italian programmes. Radiol Med 2011;116:84-91. http://dx.doi.org/10.1007/s11547-010-0606-0.
- Ciatto S, Del Turco MR, Morrone D, Catarzi S, Ambrogetti D, Cariddi A, et al. Independent double reading of screening mammograms. J Med Screen 1995;2:99-101.
- Clarke PR, Fraser NM. Economic Analysis of Screening for Breast Cancer: Report for Scottish Home and Health Department. Edinburgh: Scottish office; 1991.
- Clarke PM. Cost–benefit analysis and mammographic screening: a travel cost approach. J Health Econ 1998;17:767-87. http://dx.doi.org/10.1016/S0167-6296(98)00031-9.
- De Gelder R, Bulliard J, de Wolf C, Fracheboud J, Draisma G, Schopper D, et al. Cost-effectiveness of opportunistic versus organised mammography screening in Switzerland. Eur J Cancer 2009;45:127-38. http://dx.doi.org/10.1016/j.ejca.2008.09.015.
- De Koning HJ, van Ineveld BM, van Oortmarssen GJ, de Haes JC, Collette HJ, Hendriks JH, et al. Breast cancer screening and cost-effectiveness; policy alternatives, quality of life considerations and the possible impact of uncertain factors. Int J Cancer 1991;49:531-7. http://dx.doi.org/10.1002/ijc.2910490410.
- Feig SA. Mammographic screening of women aged 40–49 years. Benefit, risk, and cost considerations. Cancer 1995;76:2097-106. http://dx.doi.org/10.1002/1097-0142(19951115)76:10+<2097::AID-CNCR2820761332>3.0.CO;2-B.
- Garuz R, Forcen T, Cabases J, Antonanzas F, Trinxet C, Rovira J, et al. Economic evaluation of a mammography-based breast cancer screening programme in Spain. Eur J Public Health 1997;7:68-76. http://dx.doi.org/10.1093/eurpub/7.1.68.
- Groenewoud JH, Otten JDM, Fracheboud J, Draisma G, van Ineveld BM, Holland R, et al. Cost-effectiveness of different reading and referral strategies in mammography screening in the Netherlands. Breast Cancer Res Treat 2007;102:211-18. http://dx.doi.org/10.1007/s10549-006-9319-4.
- Gyrd-hansen D. Cost–benefit analysis of mammography screening in Denmark based on discrete ranking data. Int J Technol Assess Healthcare 2000;3:811-21. http://dx.doi.org/10.1017/S0266462300102089.
- Guerriero C, Gillan MGC, Cairns J, Wallis MG, Gilbert FJ. Is computer aided detection (CAD) cost effective in screening mammography? A model based on the CADET II study. BMC Health Serv Res 2011;11. http://dx.doi.org/10.1186/1472-6963-11-11.
- Hall J, Gerard K, Salkeld G, Richardson J. A cost utility analysis of mammography screening in Australia. Soc Sci Med 1992;34:993-1004. http://dx.doi.org/10.1016/0277-9536(92)90130-I.
- Henderson LM, Hubbard RA, Onega TL, Zhu W, Buist DSM, Fishman P, et al. Assessing health care use and cost consequences of a new screening modality: the case of digital mammography. Med Care 2012;50:1045-52. http://dx.doi.org/10.1097/MLR.0b013e318269e0d1.
- Hunter DJW, Drake SM, Shortt SED, Dorland JL, Tran N. Simulation modeling of change to breast cancer detection age eligibility recommendations in Ontario, 2002–2021. Cancer Detect Prev 2004;28:453-60. http://dx.doi.org/10.1016/j.cdp.2004.08.003.
- Johnston K, Brown J. Two view mammography at incident screens: cost effectiveness analysis of policy options. BMJ 1999;319:1097-102. http://dx.doi.org/10.1136/bmj.319.7217.1097.
- Kang MH, Park E-C, Choi KS, Suh M, Jun JK, Cho E. The National Cancer Screening Program for breast cancer in the Republic of Korea: is it cost-effective?. Asian Pac J Cancer Prev 2013;14:2059-65. http://dx.doi.org/10.7314/APJCP.2013.14.3.2059.
- Kerlikowske K. Continuing screening mammography in women aged 70 to 79 years: impact on life expectancy and cost-effectiveness. JAMA 1999;282:2156-63. http://dx.doi.org/10.1001/jama.282.22.2156.
- Lee SY, Jeong SH, Kim YN, Kim J, Kang DR, Kim H-C, et al. Cost-effective mammography screening in Korea: high incidence of breast cancer in young women. Cancer Sci 2009;100:1105-11. http://dx.doi.org/10.1111/j.1349-7006.2009.01147.x.
- Leivo T, Salminen T, Sintonen H, Tuominen R, Auermo K, Partanen K, et al. Incremental cost-effectiveness of double-reading mammograms. Br Cancer Res Treat 1999;54:261-7. http://dx.doi.org/10.1023/A:1006136107092.
- Leivo T, Sintonen H, Tuominen R, Hakama M, Pukkala E, Heinonen OP. The cost-effectiveness of nationwide breast carcinoma screening in Finland, 1987–1992. Cancer 1999;86:638-46. http://dx.doi.org/10.1002/(SICI)1097-0142(19990815)86:4<638::AID-CNCR12>3.0.CO;2-H.
- Lindfors KK, Rosenquist CJ. The cost-effectiveness of mammographic screening strategies. JAMA 1995;274:881-4. http://dx.doi.org/10.1001/jama.1995.03530110043033.
- Lindfors KK, McGahan MC, Rosenquist CJ, Hurlock GS. Computer-aided detection of breast cancer: a cost-effectiveness study. Radiology 2006;239:710-17. http://dx.doi.org/10.1148/radiol.2392050670.
- Madan J, Rawdin A, Stevenson M, Tappenden P. A rapid-response economic evaluation of the UK NHS Cancer Reform Strategy breast cancer screening program extension via a plausible bounds approach. Value Health 2010;13:215-21. http://dx.doi.org/10.1111/j.1524-4733.2009.00667.x.
- Mandelblatt JS, Schechter CB, Yabroff KR, Lawrence W, Dignam J, Extermann M, et al. Toward optimal screening strategies for older women. Costs, benefits, and harms of breast cancer screening by age, biology, and health status. J Gen Inter Med 2005;20:487-96. http://dx.doi.org/10.1111/j.1525-1497.2005.0116.x.
- Melnikow J, Tancredi DJ, Yang Z, Ritley D, Jiang Y, Slee C, et al. Program-specific cost-effectiveness analysis: breast cancer screening policies for a safety-net program. Value Health 2013;16:932-41. http://dx.doi.org/10.1016/j.jval.2013.06.013.
- Mooney G. Breast cancer screening. A study in cost-effectiveness analysis. Soc Sci Med 1982;16:1277-83. http://dx.doi.org/10.1016/0277-9536(82)90071-5.
- Moskowitz M, Fox SH. Cost analysis of aggressive breast cancer screening. Radiology 1979;130:253-6. http://dx.doi.org/10.1148/130.1.253.
- Moskowitz M. Cost–benefit determinations in screening mammography. Cancer 1987;60:1680-3. http://dx.doi.org/10.1002/1097-0142(19871001)60:1+<1680::AID-CNCR2820601206>3.0.CO;2-W.
- Moss SM, Brown J, Garvican L, Coleman DA, Johns LE, Blanks RG, et al. Routine breast screening for women aged 65–69: results from evaluation of the demonstration sites. Br J Cancer 2001;85:1289-94. http://dx.doi.org/10.1054/bjoc.2001.2047.
- Neeser K, Szucs T, Bulliard J-L, Bachmann G, Schramm W. Cost-effectiveness analysis of a quality-controlled mammography screening program from the Swiss statutory health-care perspective: quantitative assessment of the most influential factors. Value Health 2007;10:42-53. http://dx.doi.org/10.1111/j.1524-4733.2006.00143.x.
- Nguyen LH, Laohasiriwong W, Stewart JF, Wright P, Nguyen YTB, Coyte PC. Cost-effectiveness analysis of a screening program for breast cancer in Vietnam. Value Health Regional Issues 2013;2:21-8. http://dx.doi.org/10.1016/j.vhri.2013.02.004.
- Norum J. Breast cancer screening by mammography in Norway. Is it cost-effective?. Ann Oncol 1999;10:197-203. http://dx.doi.org/10.1023/A:1008376608270.
- Nutting PA, Calonge BN, Iverson DC, Green LA. The danger of applying uniform clinical policies across populations: the case of breast cancer in American Indians. Am J Public Health 1994;84:1631-6. http://dx.doi.org/10.2105/AJPH.84.10.1631.
- Ohnuki K, Kuriyama S, Shoji N, Nishino Y, Tsuji I, Ohuchi N. Cost-effectiveness analysis of screening modalities for breast cancer in Japan with special reference to women aged 40–49 years. Cancer Sci 2006;97:1242-7. http://dx.doi.org/10.1111/j.1349-7006.2006.00296.x.
- Okonkwo QL, Draisma G, der Kinderen A, Brown ML, de Koning HJ. Breast cancer screening policies in developing countries: a cost-effectiveness analysis for India. J Natl Cancer Inst 2008;100:1290-300. http://dx.doi.org/10.1093/jnci/djn292.
- Okubo I, Glick H, Frumkin H, Eisenberg JM. Cost-effectiveness analysis of mass screening for breast cancer in Japan. Cancer 1991;67:2021-9. http://dx.doi.org/10.1002/1097-0142(19910415)67:8<2021::AID-CNCR2820670802>3.0.CO;2-L.
- Pharoah PDP, Sewell B, Fitzsimmons D, Bennett HS, Pashayan N. Cost effectiveness of the NHS breast screening programme: life table model. BMJ 2013;346. http://dx.doi.org/10.1136/bmj.f2618.
- Rojnik K, Naveršnik K, Mateovic T. Probabilistic Cost-effectiveness modeling of different breast cancer screening policies in Slovenia. Value Health 2008;11:139-48. http://dx.doi.org/10.1111/j.1524-4733.2007.00223.x.
- Rosenquist CJ, Lindfors KK. Screening mammography in women aged 40–49 years: analysis of cost-effectiveness. Radiology 1994;191:647-50. http://dx.doi.org/10.1148/radiology.191.3.8184041.
- Rosenquist CJ, Lindfors KK. Screening mammography beginning at age 40 years: a reappraisal of cost-effectiveness. Cancer 1998;82:2235-40. http://dx.doi.org/10.1002/(SICI)1097-0142(19980601)82:11<2235::AID-CNCR19>3.0.CO;2-V.
- Salzmann P, Kerlikowske K, Phillips K. Cost-effectiveness of extending screening mammography guidelines to include women 40 to 49 years of age. Ann Intern Med 1997;127:1013-22. http://dx.doi.org/10.7326/0003-4819-127-11-199712010-00001.
- Schousboe JT, Kerlikowske K, Loh A, Cummings SR. personalizing mammography by breast density and other risk factors for breast cancer: analysis of health benefits and cost-effectiveness. Ann Intern Med 2011;155:10-2. http://dx.doi.org/10.7326/0003-4819-155-1-201107050-00003.
- Shen Y, Parmigiani G. A model-based comparison of breast cancer screening strategies: mammograms and clinical breast examinations. Cancer Epidemiol Biomarker Prev 2005;14:529-32. http://dx.doi.org/10.1158/1055-9965.EPI-04-0499.
- Souza FH, Polanczyk CA. Is age-targeted full-field digital mammography screening cost-effective in emerging countries? A micro simulation model. Springerplus 2013;2. http://dx.doi.org/10.1186/2193-1801-2-366.
- Stout NK, Rosenberg MA, Trentham-Dietz A, Smith MA, Robinson SM, Fryback DG. Retrospective cost-effectiveness analysis of screening mammography. J Natl Cancer Inst 2006;98:774-82. http://dx.doi.org/10.1093/jnci/djj210.
- Szeto KL, Devlin NJ. The cost-effectiveness of mammography screening: evidence from a microsimulation model for New Zeland. Health Policy 1996;38:101-15. http://dx.doi.org/10.1016/0168-8510(96)00843-3.
- Taylor P, Champness J, Johnston K, Potts H. Impact of computer-aided detection prompts on screening mammography. Health Technol Assess 2005;9. http://dx.doi.org/10.3310/hta9060.
- Tosteson ANA, Stout NK, Fryback DG, Acharyya S, Herman BA, Hannah LG, et al. Cost-effectiveness of digital mammography breast cancer screening. Ann Intern Med 2008;148:1-10. http://dx.doi.org/10.7326/0003-4819-148-1-200801010-00002.
- Van Dyck W, Gassull D, Vértes G, Jain P, Palaniappan M, Schulthess D, et al. Unlocking the value of personalised healthcare in Europe – breast cancer stratification. Health Policy Technol 2012;1:63-8. http://dx.doi.org/10.1016/j.hlpt.2012.04.006.
- Van Ineveld BM, van Oortmarssen GJ, de Koning HJ, Boer R, van der Maas PJ. How cost-effective is breast cancer screening in different EC countries?. Eur J Cancer 1993;29A:1663-8. http://dx.doi.org/10.1016/0959-8049(93)90100-T.
- Wald NJ, Murphy P, Major P, Parkes C, Townsend J, Frost C. UKCCCR multicentre randomised controlled trial of one and two view mammography in breast cancer screening. BMJ 1995;311:1189-93. http://dx.doi.org/10.1136/bmj.311.7014.1189.
- Wang S, Merlin T, Kreisz F, Craft P, Hiller JE. Cost and cost-effectiveness of digital mammography compared with film-screen mammography in Australia. Aust N Z J Public Health 2009;33:430-6. http://dx.doi.org/10.1111/j.1753-6405.2009.00424.x.
- Warmerdam PG, de Koning HJ, Boer R, Beemsterboer PM, Dierks ML, Swart E, et al. Quantitative estimates of the impact of sensitivity and specificity in mammographic screening in Germany. J Epidemiol Community Health 1997;51:180-6. http://dx.doi.org/10.1136/jech.51.2.180.
- Wong IOL, Kuntz KM, Cowling BJ, Lam CLK, Leung GM. Cost effectiveness of mammography screening for Chinese women. Cancer 2007;110:885-95. http://dx.doi.org/10.1002/cncr.22848.
- Wong IOL, Kuntz, Cowling BJ, Lam CL, Leung GM. Cost-effectiveness analysis of mammography screening in Hong Kong Chinese using state-transition Markov modelling. Hong Kong Med J 2010;16:38-41.
- Wong IOL, Tsang JWH, Cowling BJ, Leung GM. Optimizing resource allocation for breast cancer prevention and care among Hong Kong Chinese women. Cancer 2012;118:4394-403. http://dx.doi.org/10.1002/cncr.27448.
- Woo PPS, Kim JJ, Leung GM. What is the most cost-effective population-based cancer screening program for Chinese women?. J Clin Oncol 2007;25:617-24. http://dx.doi.org/10.1200/JCO.2006.06.0210.
- Zelle SG, Nyarko KM, Bosu WK, Aikins M, Niëns LM, Lauer JA, et al. Costs, effects and cost-effectiveness of breast cancer control in Ghana. Trop Med Int Health 2012;17:1031-43. http://dx.doi.org/10.1111/j.1365-3156.2012.03021.x.
- Zelle SG, Vidaurre T, Abugattas JE, Manrique JE, Sarria G, Jeronimo J, et al. Cost-effectiveness analysis of breast cancer control interventions in Peru. PLOS ONE 2013;8. http://dx.doi.org/10.1371/journal.pone.0082575.
- Tan KHX, Simonella L, Wee HL, Roellin A, Lim Y-W, Lim W-Y, et al. Quantifying the natural history of breast cancer. Br J Cancer 2013;109:2035-43. http://dx.doi.org/10.1038/bjc.2013.471.
- National Cancer Institute . Cancer Intervention and Surveillance Modeling Network (CISNET) n.d. http://cisnet.cancer.gov/profiles (accessed 27 June 2014).
- Breastscreen Australia Evaluation: Economic Evaluation and Modelling Study. Canberra, ACT; 2009.
- Drummond M, Barbieri M, Cook J, Glick HA, Lis J, Malik F, et al. Transferability of economic evaluations across jurisdictions: ISPOR Good Research Practices Task Force Report. Value Health 2009;12:409-18. http://dx.doi.org/10.1111/j.1524-4733.2008.00489.x.
- Eddy DM, . Model transparency and validation: a report of the ISPOR-SMDM modeling good research practices Task Force-7. Value Health 2012;15:843-50. http://dx.doi.org/10.1016/j.jval.2012.04.012.
- Vanni T, Karnon J, Madan J, White RG, Edmunds WJ, Foss AM, et al. Calibrating models in economic evaluation: a seven-step approach. PharmacoEconomics 2011;29:35-49. http://dx.doi.org/10.2165/11584600-000000000-00000.
- Buxton MJ, Drummond MF, Van Hout BA, Prince RL, Sheldon TA, Szucs T, et al. Modelling in ecomomic evaluation: an unavoidable fact of life. Health Econ 1997;6:217-27. http://dx.doi.org/10.1002/(SICI)1099-1050(199705)6:3<217::AID-HEC267>3.0.CO;2-W.
- Van Oortmarssen GJ, Habbema JD, Lubbe JT, van der Maas PJ. A model-based analysis of the HIP project for breast cancer screening. Int J Cancer 1990;46:207-13. http://dx.doi.org/10.1002/ijc.2910460211.
- Annual Survey of Hours and Earnings, 2013 Provisional Results. ONS; 2013.
- HM Revenue & Customs . Company Cars – Advisory Fuel Rates from 1 June 2014 n.d. www.hmrc.gov.uk/cars/advisory_fuel_current.htm (accessed May 2014).
- Forrest P. Breast Cancer Screening: Report to the Health Ministers of England, Wales, Scotland &Amp; Northern Ireland 1986. www.cancerscreening.nhs.uk/breastscreen/publications/forrest-report.pdf (accessed May 2014).
- Cancer Statistics Registrations, England (Series MB1), No. 42. ONS; 2011.
- Fryback DG, Stout NK, Rosenberg MA, Trentham-Dietz A, Kuruchittham V, Remington PL. The Wisconsin Breast Cancer Epidemiology Simulation Model. J Natl Cancer Inst Monogr 2006;36:37-4. http://dx.doi.org/10.1093/jncimonographs/lgj007.
- Death Registrations Summary Tables, England and Wales. ONS; 2012.
- National Cancer Intelligence Network . Improved Survival for Screen-Detected Breast Cancer n.d. www.ncin.org.uk/publications/data_briefings/improved_survival_for_screen_detected_breast_cancer (accessed May 2014).
- Dolan P, Torgerson DJ, Wolstenholme J. Costs of breast cancer treatment in the United Kingdom. Breast 1999;8:205-7. http://dx.doi.org/10.1054/brst.1999.0035.
- Kind P, Hardman G, Macran S. UK Population Norms for EQ-5D (No. 172). York: Centre for Health Economics; 1999.
- Peasgood T, Ward SE, Brazier J. Health-state utility values in breast cancer. Expert Rev Pharmacoecon Outcomes Res 2010;10:553-66. http://dx.doi.org/10.1586/erp.10.65.
- Methods Guide for Technology Appraisals. London: NICE; 2013.
- Annemans L, Redekop K, Payne K. Current methodological issues in the economic assessment of personalized medicine. Value Health 2013;16:s20-6. http://dx.doi.org/10.1016/j.jval.2013.06.008.
- Cho SH, Jeon J, Kim SI. Personalized medicine in breast cancer: a systematic review. J Breast Cancer 2012;15:265-72. http://dx.doi.org/10.4048/jbc.2012.15.3.265.
- Duffy S, Tabar L, Olsen AH, Vitak B, Allgood PC, Chen TH, et al. Absolute numbers of lives saved and overdiagnosis in breast cancer screening, from a randomized trial and from the Breast Screening Programme in England. J Med Screen 2010;17:25-30. http://dx.doi.org/10.1258/jms.2009.009094.
- Barton P, Bryan S, Robinson S. Modelling in the economic evaluation of health care: selecting the appropriate approach. J Health Serv Res Policy 2004;9:110-18. http://dx.doi.org/10.1258/135581904322987535.
- Tan SYGL, van Oortmarssen GJ, de Koning HJ, Boer R, Habbema JDF. The MISCAN-Fadia continuous tumor growth model for breast cancer. J Natl Cancer Inst Monogr 2006;36:56-65. http://dx.doi.org/10.1093/jncimonographs/lgj009.
Appendix 1 PROCAS two-page questionnaire
Appendix 2 MEDLINE search filter
-
Economics/
-
exp “Costs and Cost Analysis”/
-
“Value of Life”/
-
Economics, Dental/
-
exp Economics, Hospital/
-
Economics, Medical/
-
Economics, Nursing/
-
Economics, Pharmaceutical/
-
1 or 2 or 3 or 4 or 5 or 6 or 7 or 8
-
(econom$ or cost or costs or costly or costing or price or prices or pricing or pharmacoeconomic$).ti,ab.
-
(expenditure$ not energy).ti,ab.
-
(value adj1 money).ti,ab.
-
budget$.ti,ab.
-
10 or 11 or 12 or 13
-
9 or 14
-
letter.pt.
-
editorial.pt.
-
historical article.pt.
-
16 or 17 or 18
-
15 not 19
-
Animals/
-
Humans/
-
21 not (21 and 22)
-
20 not 23
-
(metabolic adj cost).ti,ab.
-
((energy or oxygen) adj cost).ti,ab.
-
24 not (25 or 26)
-
Breast/ or Breast Neoplasms/ or Breast Self-Examination/ or Carcinoma, Ductal, Breast/ or Breast Diseases/
-
screening.mp. or Mass Screening/
-
28 and 29
-
27 and 30
-
limit 31 to english language
Appendix 3 EMBASE search filter
-
Health Economics/
-
exp Economic Evaluation/
-
exp Health Care Cost/
-
exp Pharmacoeconomics/
-
1 or 2 or 3 or 4
-
(econom$ or cost or costs or costly or costing or price or prices or pricing or pharmacoeconomic$).ti,ab.
-
(expenditure$ not energy).ti,ab.
-
(value adj2 money).ti,ab.
-
budget$.ti,ab.
-
6 or 7 or 8 or 9
-
5 or 10
-
letter.pt.
-
editorial.pt.
-
note.pt.
-
12 or 13 or 14
-
11 not 15
-
(metabolic adj cost).ti,ab.
-
((energy or oxygen) adj cost).ti,ab.
-
((energy or oxygen) adj expenditure).ti,ab.
-
17 or 18 or 19
-
16 not 20
-
exp Animal/
-
exp Animal Experiment/
-
Nonhuman/
-
(rat or rats or mouse or mice or hamster or hamsters or animal or animals or dog or dogs or cat or cats or bovine or sheep).ti,ab.
-
22 or 23 or 24 or 25
-
exp Human/
-
exp Human Experiment/
-
27 or 28
-
26 not (26 and 29)
-
21 not 30
-
exp breast cancer/ or exp breast tumor/ or exp breast/ or exp breast carcinoma/ or exp breast metastasis/ or exp breast self examination/
-
mass screening/ or screening/ or cancer screening/ or screening test/
-
32 and 33
-
31 and 34
-
limit 35 to english language
Appendix 4 NHS Economic Evaluation Database and Health Technology Assessment search filter
1 MeSH DESCRIPTOR Breast EXPLODE ALL TREES
2 MeSH DESCRIPTOR Breast Diseases EXPLODE ALL TREES
3 MeSH DESCRIPTOR Breast Neoplasms EXPLODE ALL TREES
4 MeSH DESCRIPTOR Breast Self-Examination EXPLODE ALL TREES
5 MeSH DESCRIPTOR Carcinoma, Ductal, Breast EXPLODE ALL TREES
6 #1 OR #2 OR #3 OR #4 OR #5
7 MeSH DESCRIPTOR Early Detection of Cancer EXPLODE ALL TREES
8 MeSH DESCRIPTOR Mass Screening EXPLODE ALL TREES
9 #7 OR #8
10 #6 AND #9
11 * IN NHSEED
12 * IN HTA
13 #10 AND #11
14 #10 AND #12search filters
Appendix 5 Examples of parametric distributions potentially fitted to the adjusted lifetime risk of the PROCAS study population
To display the goodness of fit of the parameteric distribution chosen to represent the lifetime risk of developing breast cancer, we display plots comparing the worst (Weibull) and best (Pearson type V) distributions. Each of these plots is used to assess how closely the input data for risk and parametric distributions agree. Fitted density displays the empirical distribution of the input data in comparison with the parametric distributions. The comparatively larger peak for the Pearson type V clearly indicates an improved fit. Fitted distribution displays the cumulative probability distribution. The quantile–quantile (Q–Q) plots the quantiles and the probability–probability (P–P) plots the cumulative distribution; the goodness of fit for both of these plots is represented by deviations from the 45-degree line. Finally, we display the box plots for the quartiles for the best and worst distribution and all fitted distributions.
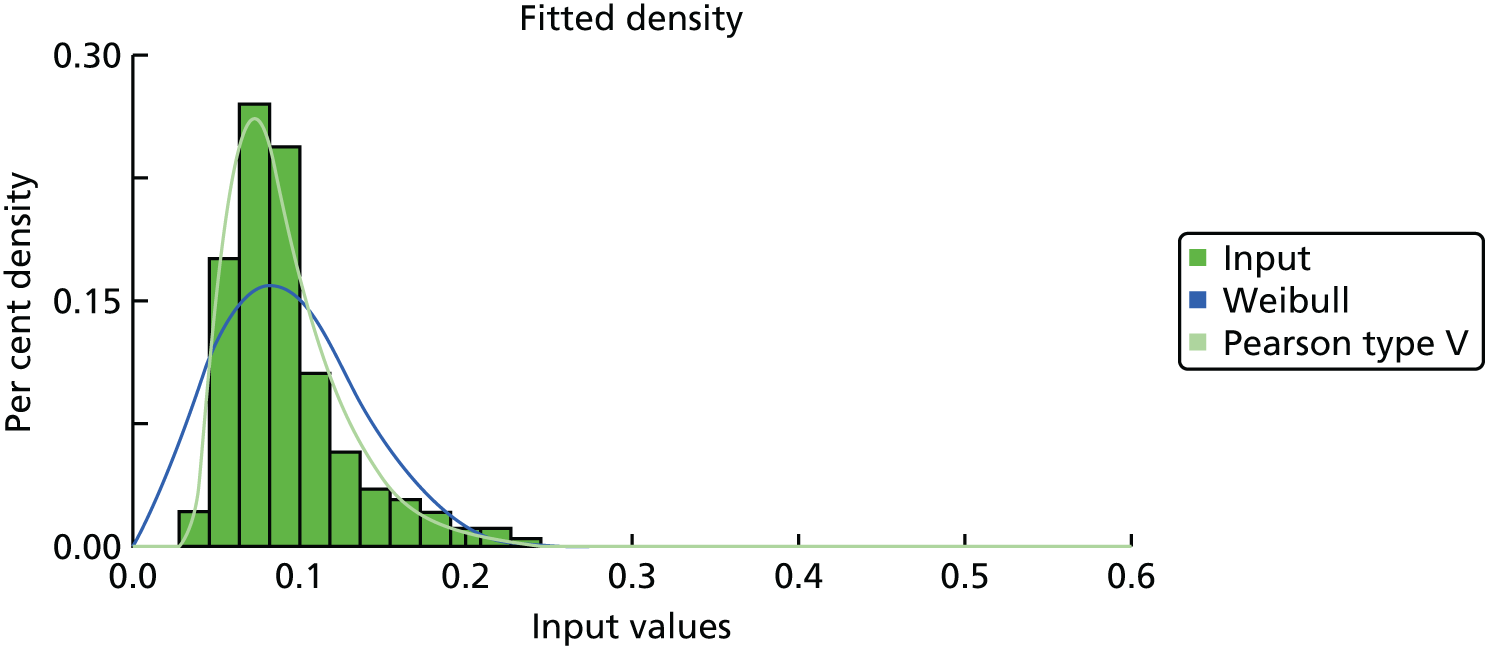
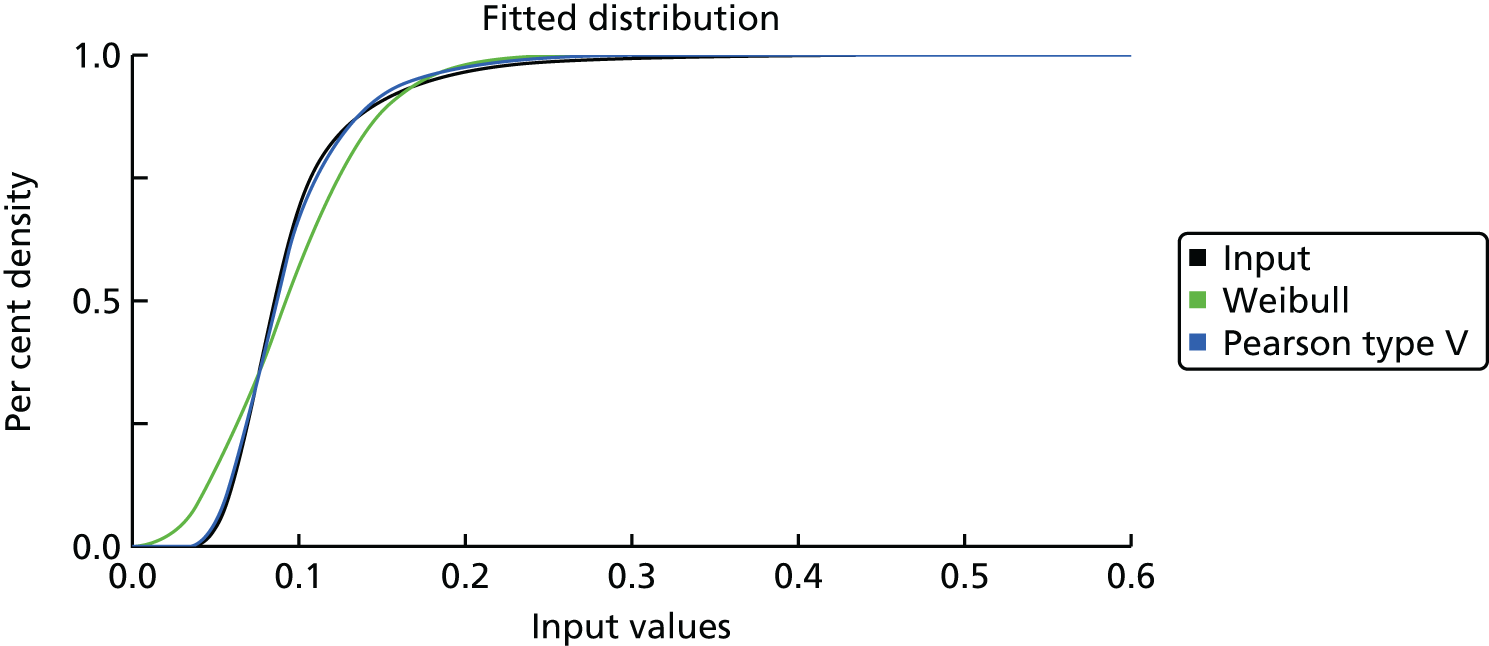
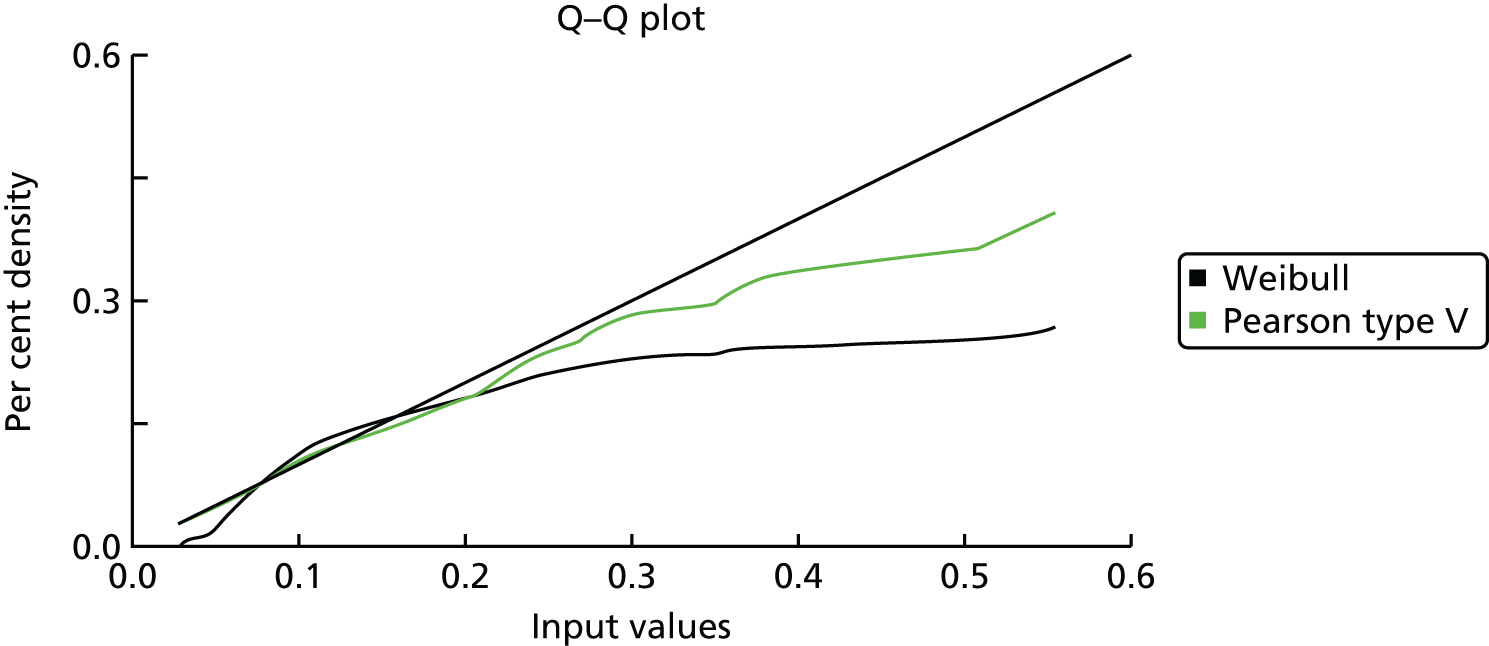
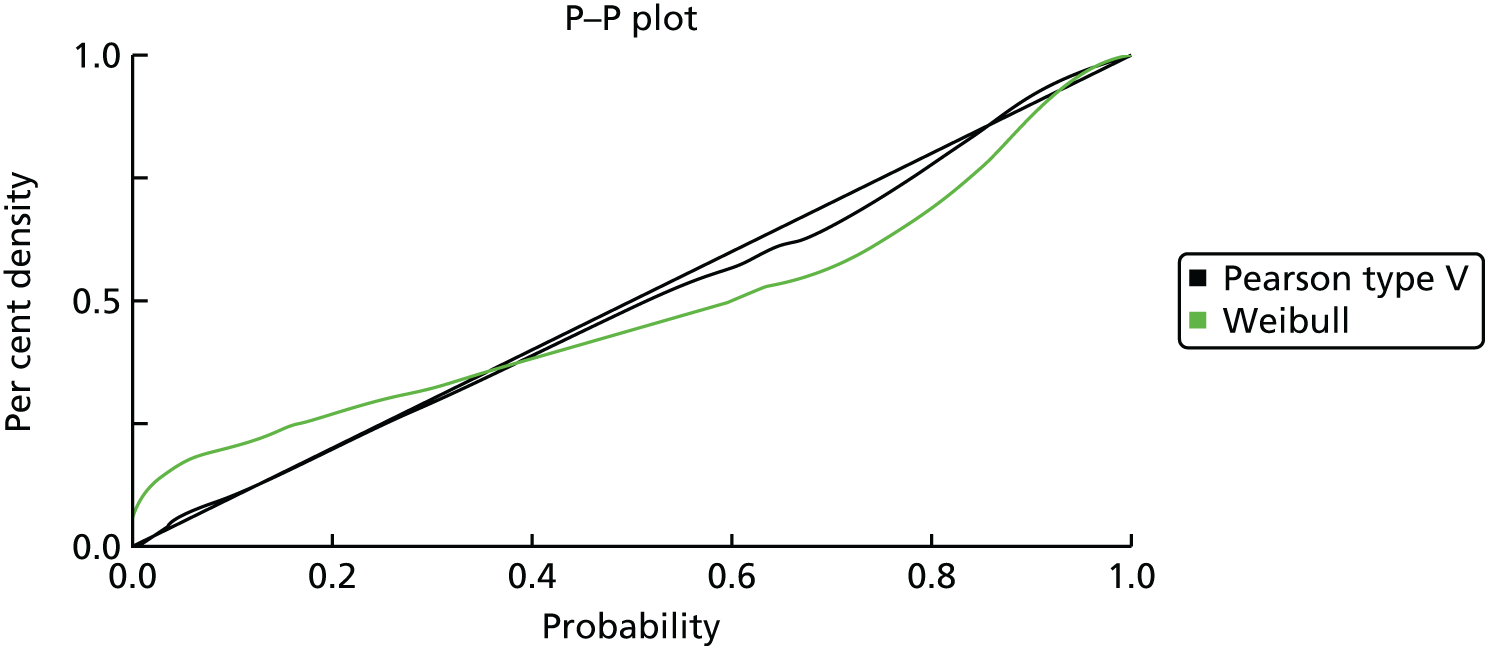

List of abbreviations
- ATM
- ATM serine/threonine kinase
- AUC
- area under the curve
- BCLC
- Breast Cancer Linkage Consortium
- BI-RADS
- Breast Imaging Reporting and Data System
- BMI
- body mass index
- BOADICEA
- Breast and Ovarian Analysis of Disease Incidence and Carrier Estimation Algorithm
- BRCA1
- breast cancer 1 gene
- BRCA2
- breast cancer 2 gene
- BRIP1
- BRCA1-interacting protein C-terminal helicase 1
- CASP8
- caspase 8, apoptosis-related cysteine peptidase
- CC
- craniocaudal
- CCC
- concordance correlation coefficient
- CDH1
- cadherin 1
- CHEK2
- checkpoint kinase 2
- CI
- confidence interval
- CIMBA
- Consortium of Investigators of Modifiers of BRCA1/BRCA2
- DCIS
- ductal carcinoma in situ
- DES
- discrete event simulation
- DICOM
- Digital Imaging and Communications in Medicine
- DNA
- deoxyribonucleic acid
- DR
- density residual
- ECDF
- empirical cumulative distribution function
- E : O
- expected vs. observed
- FFDM
- full-field digital mammography
- FGFR2
- fibroblast growth factor receptor 2
- FHC
- family history clinic
- FH-Risk
- Family History Risk Study
- GWAS
- Genome-Wide Association Study
- HRT
- hormone replacement therapy
- IBIS-I
- International Breast Intervention Study I
- ICC
- intraclass correlation
- ICD-10
- International Classification of Diseases, Tenth Edition
- ICOG
- International Collaborative Oncological Gene-Environment Study
- IQR
- interquartile range
- LSP1
- lymphocte-specific protein 1
- MAP3K1
- mitogen-activated protein kinase kinase kinase 1, E3 ubiquitin protein ligase
- MBSM
- Manchester Breast Screening Model
- MD
- mammographic breast density
- MISCAN
- Microsimulation Screening Analysis
- MLO
- mediolateral oblique
- MLPA
- multiplex ligation dependent probe amplification
- MRI
- magnetic resonance imaging
- NHSBSP
- NHS Breast Screening Programme
- NICE
- National Institute for Health and Care Excellence
- NIHR
- National Institute for Health Research
- NWCIS
- North West Cancer Intelligence Service
- O : E
- observed vs. expected
- OR
- odds ratio
- PALB2
- partner and localizer of BRCA2
- PROCAS
- Predicting the Risk of Cancer At Screening
- PRS
- polygenic risk score
- PTEN
- phosphatase and tensin homolog
- QALY
- quality-adjusted life-year
- RAF
- risk allele frequency
- RR
- relative risk
- SD
- standard deviation
- SNP
- single nucleotide polymorphism
- STK11
- serine/threonine kinase 11
- TC
- Tyrer–Cuzick
- TNM
- tumour node metastasis
- TOX3
- TOX high-mobility group box family member 3
- TP53
- tumour protein p53
- UKCRN-ID
- UK Clinical Research Network identification number
- VAS
- visual assessment score