Notes
Article history
The research reported in this issue of the journal was funded by PGfAR as project number RP-PG-0407-10500. The contractual start date was in July 2008. The final report began editorial review in July 2014 and was accepted for publication in June 2015. As the funder, the PGfAR programme agreed the research questions and study designs in advance with the investigators. The authors have been wholly responsible for all data collection, analysis and interpretation, and for writing up their work. The PGfAR editors and production house have tried to ensure the accuracy of the authors’ report and would like to thank the reviewers for their constructive comments on the final report document. However, they do not accept liability for damages or losses arising from material published in this report.
Declared competing interests of authors
none
Permissions
Copyright statement
© Queen’s Printer and Controller of HMSO 2016. This work was produced by Coid et al. under the terms of a commissioning contract issued by the Secretary of State for Health. This issue may be freely reproduced for the purposes of private research and study and extracts (or indeed, the full report) may be included in professional journals provided that suitable acknowledgement is made and the reproduction is not associated with any form of advertising. Applications for commercial reproduction should be addressed to: NIHR Journals Library, National Institute for Health Research, Evaluation, Trials and Studies Coordinating Centre, Alpha House, University of Southampton Science Park, Southampton SO16 7NS, UK.
Chapter 1 Introduction
Violence-related morbidity is a key public health problem1 resulting in major concern among the public and policy-makers in the UK. Interventions to prevent violence are no longer the sole responsibility of criminal justice agencies2 and mental health services have become increasingly involved in initiatives to reduce violence. However, prevention in the field of mental health is underdeveloped and almost exclusively operates at secondary and tertiary levels. This means that mental health professionals are restricted to secondary preventative measures, such as early detection of violence risk with the aim of prompt intervention to prevent violence occurring among service users, or tertiary prevention, including measures aimed to reduce the impact on the public of future violence from those who have already been identified as a potential risk on the basis of their previous behaviour. At present, tertiary-level strategies focus on reducing the impact of identified risk factors, including psychiatric morbidity, usually after violence has occurred.
Violence prevention is not recognised as a primary function in all mental health services. Furthermore, it tends to be just one of several goals even within secure mental health services. This means that the primary prevention of violence (which consists of actions and measures aimed at inhibiting the emergence of environmental, economic, social and behavioural conditions, cultural patterns of living, etc., known to increase the risk of violence) is not yet perceived as a core focus of mental health services. Public mental health equivalents of primary prevention through personal and communal efforts, such as enhancing nutritional status, immunising against communicable diseases and eliminating environmental risks, have not yet been developed. Risk assessment, which is designed to facilitate risk management in mental health and the criminal justice system, is of increasing importance but represents the equivalent of secondary and tertiary levels of prevention. By implication, risk management by mental health professionals for violence involves targeted, rather than population, interventions with individuals who are recognised as being of high risk. 3
Compton et al. 4 have argued that the population approach to prevention can be applied in mental health. Universal preventative interventions or population interventions target a whole population or the general public. 5 Such interventions benefit everyone in the population, regardless of their risk for violence. These might include public service announcements or media campaigns to prevent substance abuse, legislation to increase the legal drinking age or more serious penalties for violent behaviour and carrying weapons. Selective preventative interventions target individuals or subgroups of the population whose risk of violence is significantly higher than average. A high-risk group may be identified by psychological, biological or social risk factors. An intervention can include lifestyle modification to avoid situations in which individuals encounter or become involved in violence or, as indicated by our programme, an intervention for boys who witness violence in the family home (see Chapter 7, Study 1).
Indicated preventative interventions target particularly high-risk individuals – those with risk factors, or a condition, that identify them as being at high risk of future violence. At the present time, much of the debate around risk assessment in mental health and the criminal justice system has focused on the accuracy of identifying high-risk individuals. This is primarily because of the implications for these individuals if judged to be at high risk. Interventions available for those who are considered to be at exceptionally high risk, for example psychopathy, are often very limited. Interventions can include prolonged detention, more severe sentencing in court and detention and treatment in hospital against an individual’s will.
Development of risk assessment has been led primarily by clinical psychologists over the past 30 years. As the law has turned to behavioural and medical sciences to improve accuracy in the assessment and management of violence, specialist instruments (or ‘tools’) have been developed for the prediction and management of certain kinds of serious violence and criminal offending. 6 It has been estimated that > 120 risk assessment instruments have been developed and promoted for use in mental health services and the criminal justice system, with competing claims of superiority with regard to accuracy. 7 However, our programme has questioned the superiority of the predictive accuracy of any one tool over another. 8 Furthermore, few of these instruments lead easily to the second and essential component of a risk assessment – a plan of risk management. Bridging to risk management is described as the key component. 9 However, few currently available risk assessment tools either link to or incorporate risk management strategies.
Changes to the original aims of the programme
The theoretical basis of the research programme underwent major changes over the 5-year period. Unexpected findings conflicted with established views of how risk should be assessed, as described in the international literature. These had determined the basis of the original application and its aims. By the time the programme began, risk assessment for violence had become divided into two main approaches: actuarial risk assessment (ARA) and structured professional judgement (SPJ). Adherents to these approaches had become increasingly in opposition to each other. Our original application was largely committed to the actuarial approach to develop our new instruments during the programme. ARA instruments provide numerical probabilities of future violence, at different subsequent time intervals from a baseline measurement. This is a predictive method. Adherents to the alternative approach, SPJ, have argued that an actuarial approach is inappropriate for risk assessment because probabilities based on the group average method do not apply to the individual being assessed. 10 Assessors are encouraged instead to rate individual risk items according to their presence or absence and then formulate their risk-level rating according to a global, clinical understanding of the risk posed by the individual, followed by possible scenarios that could result in violence that might occur in the future.
By the time the programme started, the UK criminal justice system and the NHS mental health systems had proceeded in opposite directions. Risk assessment within the criminal justice system is currently based on a series of actuarial measures developed from large data sets of offenders. Ratings typically determine level of security and intensity of supervision and are provided to sentencers in courts to assist with sentencing or to parole boards to help determine parole. These are administered by offender managers (probation and prison staff). This method provides a highly efficient and economic method of assessing risk and can be carried out with minimal training. Probabilities of an individual’s future offending can be determined using computerised data derived from routine ratings by Ministry of Justice staff. However, our programme increasingly began to question the accuracy of these measures, corresponding to doubt expressed by previous investigators,10,11 particularly the high percentage of individuals incorrectly classified as at risk of further offending or not at risk.
Mental health services had meanwhile adopted SPJ, specifically the use of the Historical, Clinical, Risk Management-20 (HCR-20). 12 The authors of this instrument are Canadian. Over the time span of the programme, intensive training for NHS staff as well as staff in other European countries had begun. Local initiatives for risk assessment previously introduced by NHS trusts were replaced by SPJ, which became the risk assessment of choice in mental health services. For some NHS services, completion of the HCR-20 for each patient had become essential to receive funding from commissioners.
To carry out a risk assessment such as the HCR-20, staff require intensive training. Completion of the instrument can take over an hour. Clinical teams in mental health services typically complete the assessment together, with a member of the team filling in the forms. These are then filed as a hard copy in case notes or entered in electronic format. Different services have opted for different time spans over which the SPJ should be repeated, although in most cases this is on a single occasion during hospital admission. There is little indication that SPJ continues routinely after discharge. This is when service users are most likely to encounter new or previously demonstrated risk factors and when intervention is most urgently required if there is an indication of impending violence.
Structured professional judgement also provides no clinical advice on how, when or indeed whether or not to intervene; instead, it considers whether or not the service user is a risk and encourages the rater to imagine what situations might occur to increase risk. The strategy of management is then for the clinician to determine.
The costs of using SPJ methods include fees for training. European trainers now increasingly run courses, with less reliance on employing North American teachers. More recently, however, new versions of SPJ have become increasingly expensive and it is necessary to buy manuals from North America. Trainers in North America and Europe have also developed their own commercial interests. There is therefore a need for the NHS to develop new approaches to risk assessment and develop its own products. This became a key aim of our research by the end of the programme with our development of new Bayesian networks.
The programme of research therefore progressed through a series of phases. It initially became necessary to ‘deconstruct’ earlier approaches to risk assessment that had been applied within the actuarial approach, but also within SPJ. At an early stage we discovered that the statistical model that we had chosen to develop our risk assessment instrument, and which had appeared to show considerable promise before we started, conveyed no benefits whatsoever. Furthermore, it was likely to result in an instrument with poorer accuracy than if we had used conventional statistical methods. This corresponded to the disappointing findings from the US MacArthur risk study,13–15 the largest and most expensive ever conducted, which had used a similar statistical method. This US programme had ultimately failed in its aim to develop a new method of risk assessment with superior accuracy for patients discharged from psychiatric hospitals in the USA. 13–15 We had intended to develop a similar method of risk assessment in our programme and compare it with the McArthur Classification of Violence Risk (COVR) instrument. 13
The reasons for this failure are now entirely clear from our research. As we learned more about the shortcomings of risk assessment, particularly the actuarial approach, it became necessary to substantially revise our approach. Our original aims and objectives for the programme therefore changed. At the same time, we also became increasingly sceptical about the SPJ approach. SPJ had evolved during the early stages of our programme, exemplified in the change from the HCR-20 version 2 (HCR-20v2)16 to the HCR-20 version 3 (HCR-20v3),17 which we had agreed to validate in our programme with the authors of the instrument. Whereas proponents of the SPJ approach were highly critical of the rival actuarial method of adding scores from risk items, this method had previously been widely used in the rating of the HCR-20v2. It was now prohibited for clinical use in the new third version and assessors were instructed to make overall correlations of ‘high’, ‘medium’ or ‘low’ future risk based on their global perception following the assessment. Nevertheless, all empirical research using SPJ continues to rely on producing numerical scores of risk based on individual items. Validation of SPJ instruments therefore continues to depend on actuarial prediction methods, most commonly using the area under the receiver operating characteristic (ROC) curve (AUC) statistic, to determine their accuracy of prediction of future violence. Although we have applied this method in our programme, we have also investigated its considerable limitations and proposed alternatives.
One additional limitation became apparent from our research: the HCR-20 and other SPJ instruments are ‘checklists’. They do not allow for the multidimensional approach that is necessary for a comprehensive risk assessment. They depend on a ‘compartmentalised’ assessment of a predetermined number of areas. These are fixed and not determined by an individual’s previous longitudinal history. The HCR-20v3 has been improved and considerably expanded in an attempt to incorporate more information on risk and encourages assessors to use their own judgement and identify additional risks. However, the included items are not covered in a manner similar to clinical history taking in mental health services. For a clinical assessment, individual components such as criminal history require a greater depth of understanding if the links are to be made between a previous history of antisocial behaviour and associated risk and predictive factors. The HCR-20 does not capture the potential synergistic effects of these different components and ultimately relies on clinical experience and the expertise of the clinician rater. Finally, SPJ does not impel the rater to intervene when a risk factor is clearly present or indicate which factors should be targeted for intervention on the basis of an established causal link between the factor and violent outcome, and these limitations are the motives for moving from a risk assessment to effective risk management. They became our primary aim by the end of our programme.
Finally, and most importantly, there is currently no evidence that SPJ can predict violence more accurately than an ARA instrument. 8 Furthermore, there is currently no evidence that either an ARA instrument or an assessment based on SPJ can prevent violence. The only randomised controlled trial (RCT) of a SPJ instrument, the Short-Term Assessment of Risk and Treatability (START), failed to demonstrate any improvement in clinical outcome or violence reduction compared with management as usual. 18 Although our programme provides a third alternative to ARA instruments and SPJ, a RCT is clearly the next phase of research required; it will be necessary to test our Bayesian models in the future against current clinical practice.
A theoretical model of risk pathway to violence
This report presents a new approach to risk assessment and risk management (Figure 1) that could be incorporated into clinical practice. It is based on a model of assessment that we have empirically tested, but is also derived from clinical experience. It is based on a longitudinal approach that aims to capture the evolution of risk over time when an offender is released from custody or a service user is discharged from hospital. Clinical risk assessment of an individual would proceed through each of the five stages in Figure 1. This would be based on previous behaviour and a full assessment of an individual’s previous history, including current circumstances and reason for the assessment, detailed assessment of previous and more recent violent and criminal behaviour, family history and developmental history from childhood to the present day. Although Figure 1 shows that the first stage of the assessment is to make a diagnosis, clinicians would point out that this is usually completed towards the end of a clinical assessment. It would include assessment of current and previous mental state to attain a formal diagnosis of mental disorder, including personality disorder and history of substance misuse. Figure 1 is therefore the basis of a risk formulation. This would follow a full clinical assessment. It also forms the basis of our programme of research.
FIGURE 1.
Theoretical model of risk pathway to violence.
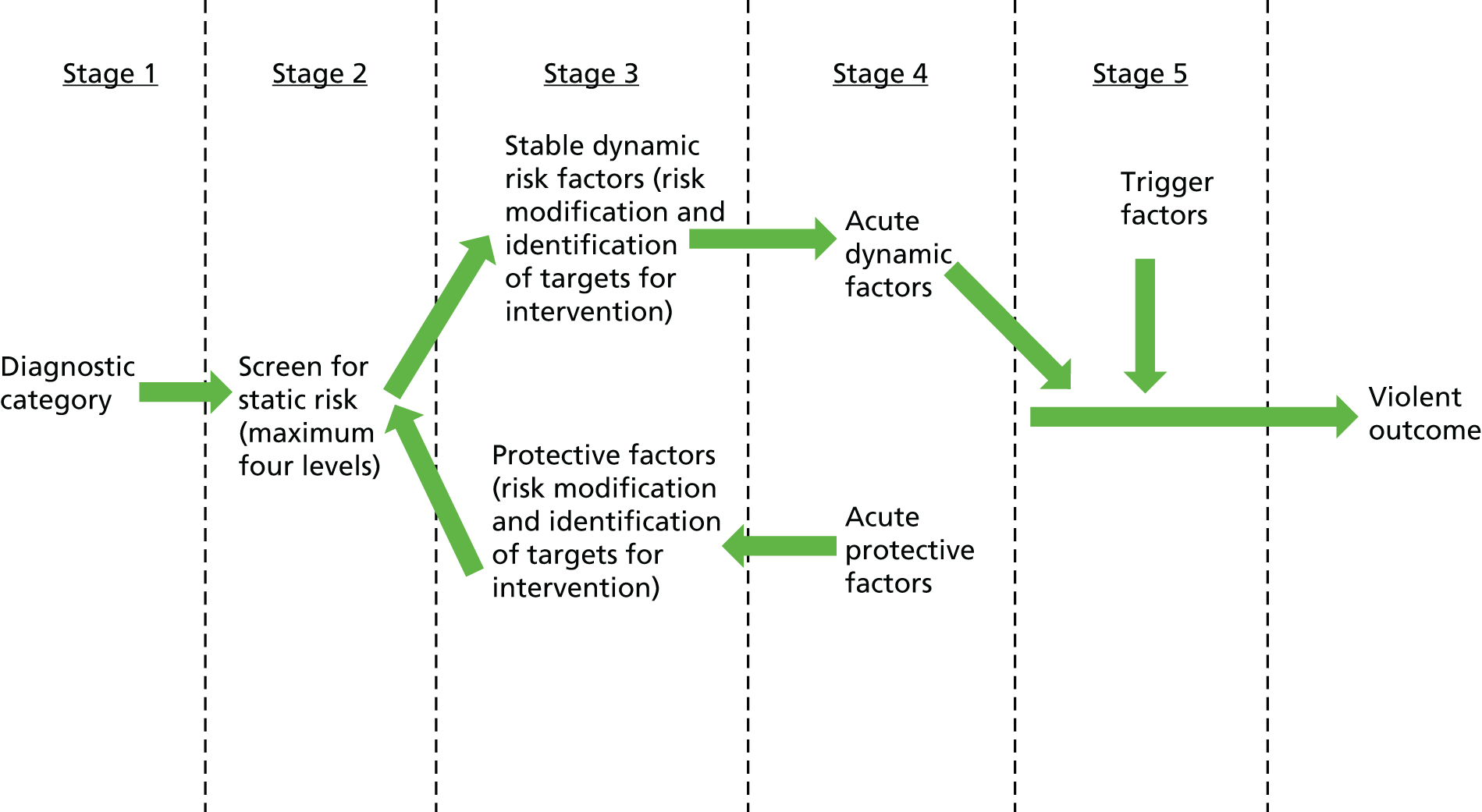
Figure 1 therefore begins with the establishment of a psychiatric diagnosis at the outset together with the level of static risk for future violence, based on actuarial measures. We have already referred to the limitations of the actuarial approach but it is necessary to demonstrate these in this programme report in coming to this conclusion. We believe that an actuarial measure of risk has some value but this is limited. We shall explain the limits and the importance of combining actuarial measures with dynamic risk assessment. A clinician may wish to know the score of risk based on previous offending and this may result in a more in-depth profiling of an individual’s criminal history, but should not be the basis of major decisions such as release from hospital or prison. It is also of some limited value when screening offenders to indicate when an in-depth clinical assessment is required. However, when combined with dynamic factors, a static or actuarial measure of risk can provide a more accurate assessment and indicate dynamic risk factors that will be targeted for intervention.
Stage 3 requires an assessment of ongoing dynamic risk factors, which are changeable and should be identified as targets for future intervention. In our previous research we identified the importance of protective factors and the interaction that can occur between protective factors and risk factors. 19 However, protective factors were not an aim of the programme and are not described in detail in this report.
In stage 4, the individual may encounter acute risk factors that have a direct influence on subsequent behaviour, for example acute intoxication or involvement in a group or gang, which have a more immediate bearing on violent outcome.
At stage 5, certain trigger factors, which have an immediate and causal effect on the violent outcome, may be encountered. Alternatively, the violence may have been planned for some time, although a sudden triggering factor can occur in certain cases when planning has been present.
In developing this model we have shifted considerably from our original goal, which was to develop predictive measures. Our programme of research changed to the identification of causal risk factors because only causal factors should be targets for intervention. A risk factor may be highly predictive of future violence but, if it is not causal, attempts to modify it will not prevent future violence from occurring. In contrast, we have observed that causal risk factors may have no predictive ability in estimating the probability of future violence. This is particularly the case for a dynamic risk factor that shows fluctuations of intensity, such as the presence of anger as a result of delusions. 20,21
Aim
Our overall aim was to carry out a programme of linked research studies aimed at improving the quality of clinical risk management of individuals identified as being at high risk of harm to the public.
Section A of our report examines both static risk factors and psychiatric diagnosis (relating to stages 1 and 2 of our theoretical model in Figure 1). We shall show that risk of violence in terms of cross-sectional associations at the population level differs according to both diagnostic category and demographic factors.
Section B of our report describes the development of a risk assessment tool for patients presenting to general psychiatric services based on a first-episode cohort of patients with psychoses. This section describes a model based on multilevel modelling, a sophisticated statistical approach to multiple measures over a prolonged period. This is a prototype for further development. Section B covers stages 1–4 of the model.
Section C of our report describes the Validation of new Risk Assessment Instruments for Use with Patients Discharged from Medium Secure Services (VoRAMSS) study, a prospective follow-up of patients discharged from medium secure units (MSUs) across England. In this section, which covers stages 1–4 of the model, we test the accuracy of prediction of violent behaviour of standardised risk assessment instruments, ARA instruments and SPJ over the 12 months following discharge. We also validate the Medium Security Recidivism Assessment Guide (MSRAG),22 an actuarial instrument that we developed previously. We shall demonstrate that all ARA instruments and SPJ have shortcomings if validated using predictive methods and that dynamic factors are essential measures because they directly influence the violent behaviour. Nevertheless, we describe a new way of using ARA instruments in conjunction with dynamic measures that can improve accuracy.
Section D describes the development of four new ARA instruments for violent, robbery, drug-related and acquisitive offending. We have developed a computerised version of each as well as a version that can be used by clinicians with pencil and paper. Although ARA instruments clearly have their limitations, we show that combining them with dynamic factors shows a highly promising method that can be adapted and used in clinical practice. We then developed a dynamic risk assessment instrument to combine with these ARA instruments and validated it using a very large data set provided to us by the National Offender Management Service (NOMS). In the criminal justice system, offender managers use ARA instruments because they are economical, are less time-consuming and do not require intensive training. It is not possible to routinely use SPJ because of the number of offenders who must be assessed and the consequent size of caseloads. The instruments that we have developed are therefore of primary usefulness to the work of criminal justice personnel, especially probation officers. Because probation officers routinely complete Offender Assessment System (OASys) ratings on their clients that are computerised, our model can now be adapted for routine use for improved risk assessment, with important implications for risk management.
Section E describes the development of a Bayesian network to assess risk and identify the key dynamic risk factors for preventative interventions to guide risk management. Bayesian networks were chosen for this section of the programme because they are used to identify factors that are causal. They can provide actuarial measures of risk as well, but are better suited to our main aim of establishing causal associations. This final and most important part of our programme has established the basis for further development in the field of risk assessment and management. We have developed models that have operated successfully and have been used in their preliminary prototypic form by clinicians. They are at the stage of development before programming of a computerised application (app) for use by a clinician on a tablet in the field. Following this stage, the models will be ready for comparison with standard SPJ in a RCT to assess their effectiveness for violence prevention.
Table 1 summarises the studies, populations and outcomes presented in this report.
General population | Adult psychiatric population | Adult forensic population | Prisoners | |
---|---|---|---|---|
Study | Psychiatric morbidity among adults living in private households in England, Wales and Scotland (n = 8880) | First-episode psychosis: baseline (n = 409), follow-up (n = 389) | VoRAMSS study: baseline (n = 409), 6-month follow-up (n = 387), 12-month follow-up (n = 344) | PCS: pre release (n = 1717), post release (n = 1004) |
APMS (n = 7403) | ||||
Men’s Modern Lifestyle Survey: main (n = 3247), ethnic minority booster (n = 1540), low social class booster (n = 1002), Hackney, London, booster (n = 883), Glasgow East booster (n = 789) | NOMS (n = 53,800) | |||
Location in report | Section A | Section B | Sections C and E | Section D |
Outcome | Identification of risk factors for violence in the general population | Development of sex-specific static and dynamic instruments for the assessment and management of violence risk | Validation of static and dynamic risk instruments for violence; development of a Bayesian model for the assessment and management of offending behaviour | Development and validation of static and dynamic risk assessment instruments; development of a Bayesian model for the assessment and management of offending behaviour |
Section A Epidemiology of risk factors for violence in Great Britain
Chapter 2 Demography and typology of violence
Background
The public health impact of mental health on violence depends on the base rate of violence in the general population. This may ultimately influence whether targeted ‘high-risk’ or large-scale ‘population’ strategies are chosen for violence prevention. 3 In geographical locations with low violence rates, the proportion of violence attributed to mentally disordered people may appear high and efforts to contain their violence will achieve public health and political prominence. However, in locations with high base rates, more relevant risk factors may include weapon availability, substance misuse and gang violence, and being young, male, single and of low social class are the strongest risk factors for violence, irrespective of psychiatric morbidity. Demographic and social factors are prominent in most risk assessment instruments for violence and criminality. Factors such as younger age and criminological variables are contained in most tools and these, together with childhood factors indicating early onset of violence behaviour and substance misuse, appear to be the most predictive individual items for future violence. For some instruments, these are the only predictive items and clinical factors may have little predictive ability. 23 Nevertheless, there is a consensus that mental disorder is related to violence24–31 and that it increases the risk of violence over the lifespan. 32–36 Questions remain, however, over the size of the contribution from those with mental disorder to the overall level of violence within the general population and also over which disorders make the greatest contribution. The predictive ability of mental health variables to determine future violence will be dealt with in subsequent chapters. This current chapter will outline the association between mental health and violence at the population level in Great Britain, the size of the problem and whether or not the public health approach to violence is appropriate.
The public health problem of violence has generated less interest here than in the USA. 37,38 The UK has a relatively low rate of homicide. However, the high annual medical and social costs of injury from deliberate harm are highlighted by investigations in accident and emergency (A&E) departments in the UK. 39 Violence is a major public health problem that affects millions of people across the UK. The Crime Survey for England and Wales (CSEW)40 estimated that just over 2 million violent incidents were committed against adults in 2011/12. Over the same period, police recorded around 762,500 violence against the person offences in England and Wales. 41 A further 53,665 sexual offences were recorded. Just under half of violent incidents recorded by the police resulted in injury, as did half of those reported by adults to the CSEW. The difference between the number of violent incidents reported through the CSEW and the number of offences recorded by police shows that many incidents of violence are not recorded in the criminal justice system. Nevertheless, violence resulting in injury often requires treatment and there were 34,713 emergency hospital admissions for violence in England and Wales in 2010/11. 42 Rates were highest among young males and among those with increasing levels of socioeconomic deprivation. 43 For every hospital admission for violence it is estimated that a further 10 assault victims require treatment in A&E. A&E assault attendances peak at night over the weekend and are often related to alcohol. There were large increases in the levels of violence across England in the 1990s and early 2000s. However, more recent data suggest that the trend has reversed and that violence is decreasing. 44
Study 1: the demography of violence among adults in Great Britain
Objectives
The objectives of study 1 were to:
-
investigate the demographic characteristics of adults in the UK general population who report violence
-
construct a typology of violence among men and women based on behavioural characteristics, including victims and the location of the violence.
Methods
Data from two national cross-sectional surveys and the commissioned second Men’s Modern Lifestyle Survey (MMLS) were used to identify high-risk behaviours, including violence, and demographic, psychiatric, lifestyle and service use correlates.
National surveys of psychiatric morbidity in the UK
People aged 16–74 years were sampled in the National Household Psychiatric Morbidity Survey (NHPMS) in 2000, details of which have been described previously. 45 This was a two-phase survey. 46 Computer-assisted interviews in person were carried out by Office for National Statistics interviewers. The small users Postcode Address File (www.poweredbypaf.com) was used as the sampling frame and the Kish grid method47 was applied to systematically select one person in each household. A total of 8886 adults completed the first-phase interview reported here, a response rate of 69.5%, and 8397 (94.5%) of these completed all sections of the questionnaire. Among non-respondents, 24% refused and 6.5% were non-contacts in the household. There was no information on psychiatric status of non-respondents to enable analysis of whether or not their omission resulted in biased estimates of the prevalence of violence. However, weighting procedures that were applied throughout the analyses took into account the proportions of non-respondents according to age, sex and region. This was to ensure a sample that was representative of the national population, compensating for sampling design and non-respondents in the standard error (SE) of the prevalence, and to control for the effects of selecting an individual per household.
The Adult Psychiatric Morbidity Survey (APMS) in 200748 sampled adults aged ≥ 16 years living in private households in England. In this survey, data were obtained from English adults only. The survey was commissioned by the NHS Health & Social Care Information Centre and was carried out by the National Centre for Social Research in collaboration with the University of Leicester. Field work was carried out between October 2006 and December 2007. A multistage stratified probability sampling design was adopted based on the small user Postcode Address File. One adult aged ≥ 16 years was selected from each household for interview using the Kish grid method. 47 Phase 1 data were collected by lay interviewers. A total of 7403 adults completed first-phase interviews, representing 57% of those eligible and originally approached. There is no information regarding the mental health status of non-respondents. However, data were again weighted to account for non-response. Sample weights were also assigned to take into account different probabilities in household selection. All models were corrected for area clusters based on postcodes. Of the 7403 participants, 7369 completed the violence self-report questions.
Men’s Modern Lifestyle Survey
Violence and high-risk behaviour at the population level disproportionately involves young men. However, young men are the least likely demographic group to voluntarily access health services, especially when confronted with emotional and social problems. 49,50 To investigate in more detail violence and use of services by young men, together with implications for risk management, we commissioned our own survey for the programme. This was carried out by ICM Research (www.icmunlimited.com).
The second MMLS was carried out in 2011. The MMLS was based on random location methodology, an advanced form of quota sampling shown to reduce the biases introduced when interviewers choose a location to sample from. Individual sampling units (census areas of 150 households each) were randomly selected within British regions, in proportion to their population. The basic survey derived a representative sample of young men (aged 18–34 years) from England, Scotland and Wales (n = 3247). In addition, there were four boost surveys. First, young black and minority ethnic men were selected from output areas with a minimum of 5% black and minority ethnic inhabitants (n = 1540) and young men from lower social grade D or E were selected from output areas with a minimum of 30 men aged 18–64 years in these social grades (n = 1002); The final boost surveys were based on output areas in two locations characterised by high levels of deprivation, the London borough of Hackney (n = 883) and Glasgow East (n = 789). The same sampling principles applied to each survey type.
Topics in the survey not included in the NHPMS or APMS included leisure activities, weight and exercise, use of pornography, enhanced information on antisocial and criminal behaviours and attitudes, including violence, harassment and stalking, gang membership and attitudes to accessing health-care services. Respondents completed the pencil and paper questionnaire in privacy and were paid £5 for participation.
Violence module
Participants in all surveys answered questions about the presence of violent behaviour. An affirmative answer to the question ‘Have you ever been in a physical fight, assaulted or deliberately hit anyone in the past 5 years?’ was followed by questions that qualified the violent events, including frequency, whether there were injuries or whether the act was committed while intoxicated. Additional questions assessed the identity of victims and the locations of the incidents.
Measurement of psychiatric morbidity
The Psychosis Screening Questionnaire (PSQ)51 was used to screen participants for psychosis, with a positive screening being one in which three or more criteria were met. Questions from the Structured Clinical Interview for DSM-IV Axis II Personality Disorders (SCID-II) screening questionnaire52,53 identified antisocial personality disorder (ASPD) and borderline personality disorder (BPD) in all surveys.
In the MMLS, the Hospital Anxiety and Depression Scale (HADS)54 was used to define anxiety and depression, based on a score of ≥ 11 in the past week. In the NHPMS and APMS, the Clinical Interview Schedule Revised Version (CIS-R)55 was used to obtain the prevalence of common mental disorders in the week preceding the interview. These were combined into two categories of anxiety disorder and depressive disorder.
The principal instrument used to assess alcohol misuse was the Alcohol Use Disorders Identification Test (AUDIT),56,57 which defines hazardous drinking (a score of ≥ 8), alcohol misuse (a score of ≥ 16) and alcohol dependence (a score of ≥ 20). A number of questions designed to measure drug use were included in the NHPMS and APMS. Positive responses to any of five questions measuring drug dependence for a series of different substances over the previous year were combined to produce categories of drug dependence according to drug type. 45 Scores of ≥ 6 on the Drug Use Disorders Identification Test (DUDIT)58 were used to identify drug misuse and scores of ≥ 20 were used to identify drug dependence in the MMLS.
Analysis of data
Outcome data, including self-reported violent behaviour towards others and violent and sexual victimisation, including domestic violence, were examined in relation to measures of demography, general health, service use, common mental disorders, personality disorder, psychosis screen, adult attention deficit hyperactivity disorder (ADHD) and substance use, among others. Weighted analyses were used to account for the sampling procedure. Risks were measured by odds ratios (ORs) in all cross-sectional data. Multivariate statistical models were used to handle covariates among multiple outcomes of lifestyle and behaviour. Random-effects models were employed when area variation was thought to have a substantial effect on the outcome of interest.
Results
The weighted prevalence of severity and type, victims and location of violence in the three UK surveys (NHPMS 2000, APMS 2007 and MMLS 2011) is summarised in Table 2.
Violence outcomes | NHPMS 2000, n (%) | APMS 2007, n (%) | MMLS 2011, n (%) |
---|---|---|---|
All participants, Na | 8382 | 7393 | 5240 |
Any violence | 982 (11.7) | 614 (8.3) | 1681 (32.1) |
Violence when intoxicated | 422 (5.0) | 263 (3.6) | 765 (14.9) |
Repeated violence (five or more incidents) | 237 (2.8) | 98 (1.3) | 255 (5.0) |
Victim injured | 333 (4.0) | 172 (2.3) | 720 (13.8) |
Perpetrator injured | 310 (3.7) | 204 (2.8) | 713 (13.7) |
Police involved | 254 (3.0) | 177 (2.4) | 410 (7.9) |
Minor violence | 408 (4.9) | 247 (3.3) | 374 (7.2) |
Gang fights | NA | NA | 266 (5.3) |
Victim of violence | |||
Intimate partner | 137 (1.6) | 115 (1.6) | 201 (3.9) |
Family member | 63 (0.8) | 91 (1.2) | 223 (4.3) |
Friend | 180 (2.1) | 132 (1.8) | 437 (8.4) |
Someone known | 316 (3.8) | 195 (2.6) | 483 (9.3) |
Stranger | 484 (5.8) | 300 (4.1) | 737 (14.1) |
Police | 53 (0.6) | NA | 131 (2.5) |
Location of violent incident | |||
Own home | 168 (2.0) | 123 (1.7) | 235 (4.5) |
Someone else’s home | 76 (0.9) | 61 (0.8) | 281 (5.4) |
Street/outdoors | 555 (6.6) | 354 (4.8) | 855 (16.4) |
Bar/pub | 358 (4.3) | 183 (2.5) | 622 (11.9) |
Workplace | 81 (1.0) | 21 (0.3) | 60 (1.2) |
At sporting event | NA | NA | 360 (7.2) |
The MMLS survey, which was restricted to men aged 18–34 years, reported the highest prevalence of violence in all categories, including violence when intoxicated, victim and perpetrator injured and violence repetition (i.e. five or more incidents). However, Table 2 also shows that, when the NHPMS and APMS are compared, the prevalence of all levels of severity and types of violence, victims and violence in specific locations was lower in the 2007 survey than in the 2000 survey among both men and women (except for violence against intimate partners and family members).
In terms of victims, violence towards strangers was the most prevalent category in the NHPMS, AMPS and MMLS. The rate of intimate partner violence (IPV) was similar in both household surveys and was higher among young men.
In each survey the most common location of violent incidents was in the street or outdoors. This was followed by violence in a pub or bar and violence in the respondent’s own home.
Age
Age was subsequently included as an adjustment in all models of association with violence in our studies. The mean age of violent men was 31.0 [standard deviation (SD) 11.6] years in the NHPMS, 32.0 (SD 12.7) years in the AMPS and 24.6 (SD 5.0) years in the MMLS, compared with 47.4 (SD 14.9) years, 52.7 (SD 17.7) years and 25.7 (SD 5.1) years, respectively, for non-violent men. Violent men were significantly younger than non-violent men in each survey (p < 0.001). The mean age of violent women was 30.1 (SD 9.9) years in the NHPMS and 31.4 (SD 11.8) years in the AMPS whereas the mean age of non-violent women was 46.3 (SD 15.4) years and 52.2 (18.5) years respectively. Violent women were significantly younger than non-violent women in each survey (p < 0.001).
Figure 2 shows the prevalence of self-reported violence in the last 5 years by age group (10-year age bands) for the two household surveys. In both surveys the prevalence of violence decreased with increasing age. This linear decrease was significant for both surveys (p < 0.001).
FIGURE 2.
Prevalence of any violence in the past 5 years in the UK by age group and survey.
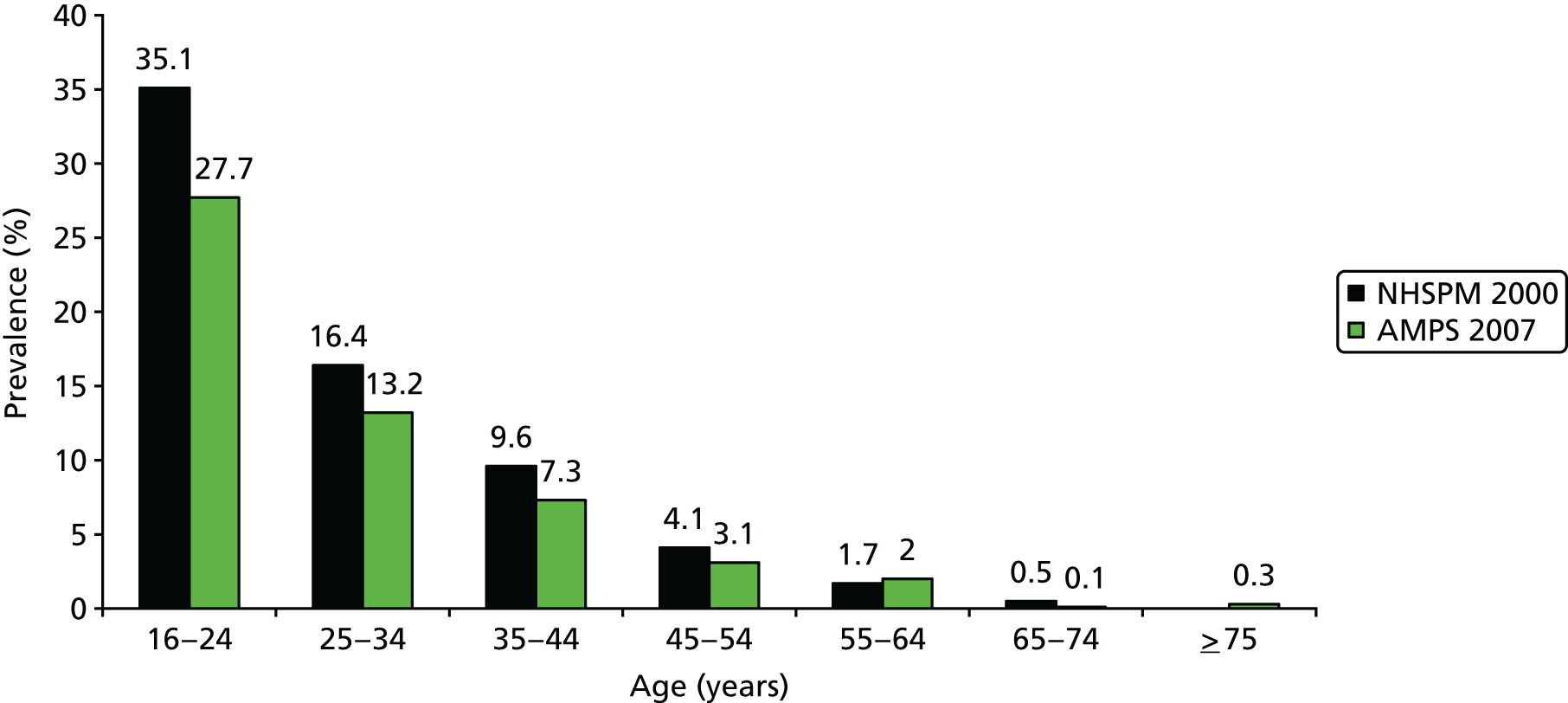
The MMLS has a more restricted age range (18–34 years), which was divided into four age groups. Among those aged 18–20 years the prevalence of violence was 39.4%, among those aged 22–25 years prevalence was 34.8%, among those aged 26–29 years it was 28.5% and among the oldest age group of 30–34 years it was 27.3%. The linear trend for the effect of age on the prevalence of violence was highly significant (p < 0.001). Age was inversely associated with violence in all three surveys. Adjusted associations between age and any violence (Table 3) indicate that, compared with the youngest age group, increasing age exerts a protective effect on violence.
Demographic characteristics | NHPMS 2000 | APMS 2007 | ||
---|---|---|---|---|
OR (95% CI) | AORa (95% CI) | OR (95% CI) | AORa (95% CI) | |
Sex | ||||
Female | Reference | Reference | Reference | Reference |
Male | 3.74 (3.12 to 4.49)*** | 4.23 (3.39 to 5.27)*** | 2.94 (2.36 to 3.66)*** | 2.87 (2.22 to 3.71)*** |
Age group (years) | ||||
16–34 | Reference | Reference | Reference | Reference |
35–54 | 0.24 (0.20 to 0.29)*** | 0.32 (0.26 to 0.40)*** | 0.23 (0.18 to 0.29)*** | 0.29 (0.22 to 0.38)*** |
55–74 | 0.04 (0.03 to 0.06)*** | 0.06 (0.04 to 0.08)*** | 0.05 (0.03 to 0.08)*** | 0.06 (0.03 to 0.09)*** |
≥ 75 | No data | No data | 0.01 (0.00 to 0.05)*** | 0.02 (0.01 to 0.07)*** |
Marital status | ||||
Married/widowed/cohabiting | Reference | Reference | Reference | Reference |
Single | 6.60 (5.46 to 7.97)*** | 2.27 (1.83 to 2.83)*** | 5.34 (4.24 to 6.72)*** | 1.78 (1.35 to 2.35)*** |
Separated/divorced | 2.53 (1.96 to 3.26)*** | 2.66 (2.03 to 3.49)*** | 1.63 (1.16 to 2.28)** | 2.03 (1.38 to 3.00)*** |
Social class | ||||
I | Reference | Reference | Reference | Reference |
II | 1.37 (0.78 to 2.41) | 2.11 (1.15 to 3.87)* | 1.38 (0.57 to 3.32) | 1.73 (0.66 to 4.51) |
IIIM | 2.10 (1.19 to 3.70)* | 3.87 (2.10 to 7.13)*** | 2.26 (0.94 to 5.41) | 2.93 (1.12 to 7.66)* |
IIINM | 3.34 (1.93 to 5.78)*** | 4.22 (2.31 to 7.70)*** | 4.10 (1.75 to 9.63)** | 4.57 (1.81 to 11.54)** |
IV | 3.13 (1.81 to 5.43)*** | 5.05 (2.78 to 9.14)*** | 3.26 (1.36 to 7.84)** | 3.64 (1.39 to 9.52)** |
V | 2.60 (1.37 to 4.94)** | 4.65 (2.35 to 9.21)*** | 4.21 (1.67 to 10.62)** | 6.15 (2.22 to 17.03)*** |
Ethnicity | ||||
White | Reference | Reference | Reference | Reference |
Black | 1.38 (0.85 to 2.26) | 0.82 (0.44 to 1.50) | 1.13 (0.58 to 2.18) | 0.86 (0.43 to 1.69) |
South Asian | 0.59 (0.29 to 1.20) | 0.33 (0.14 to 0.76)** | 0.81 (0.42 to 1.57) | 0.45 (0.21 to 0.97)* |
Other | 1.30 (0.72 to 2.37) | 1.18 (0.61 to 2.30) | 1.05 (0.58 to 2.18) | 0.77 (0.38 to 1.57) |
Employment | ||||
Employed | Reference | Reference | Reference | Reference |
Unemployed | 2.60 (1.84 to 3.66)*** | 1.20 (0.78 to 1.84) | 3.08 (1.90 to 5.00)*** | 0.99 (0.57 to 1.74) |
Sex
Just over half of the participants in the NHPM 2000 and APMS 2007 surveys were women. Table 4 provides the frequencies and proportions of all violent outcomes by sex. Among men, the most prevalent violent outcome in 2000 was violence when intoxicated, towards strangers and taking place in the streets or outdoors and in bars/pubs. The same pattern was observed in 2007, but with a lower prevalence.
Violence outcomes | NHPMS 2000 | APMS 2007 | ||
---|---|---|---|---|
Men | Women | Men | Women | |
Any violence | 749 (18.0) | 233 (5.5) | 441 (12.3) | 173 (4.6) |
Violence when intoxicated | 361 (8.7) | 61 (1.4) | 194 (5.4) | 69 (1.8) |
Repeated violence (five or more incidents) | 193 (4.6) | 44 (1.0) | 82 (2.3) | 16 (0.4) |
Victim injured | 287 (6.9) | 46 (1.1) | 132 (3.7) | 40 (1.0) |
Perpetrator injured | 245 (5.9) | 65 (1.5) | 149 (4.2) | 55 (1.4) |
Police involved | 202 (4.8) | 52 (1.2) | 129 (3.6) | 48 (1.3) |
Minor violence | 282 (6.8) | 126 (3.0) | 174 (4.8) | 73 (1.9) |
Victim of violence | ||||
Intimate partner | 47 (1.1) | 90 (2.1) | 39 (1.1) | 76 (2.0) |
Family member | 31 (0.7) | 32 (0.8) | 56 (1.6) | 35 (0.9) |
Friend | 144 (3.5) | 36 (0.9) | 92 (2.6) | 41 (1.1) |
Someone known | 252 (6.1) | 64 (1.5) | 144 (4.0) | 51 (1.4) |
Stranger | 435 (10.4) | 48 (1.1) | 249 (6.9) | 51 (1.3) |
Police | 47 (1.1) | 6 (0.1) | 26 (0.7) | 9 (0.2) |
Location of violent incident | ||||
Own home | 65 (1.6) | 104 (2.5) | 53 (1.5) | 70 (1.8) |
Someone else’s home | 54 (1.3) | 22 (0.5) | 40 (1.1) | 21 (0.6) |
Street/outdoors | 455 (10.9) | 100 (2.4) | 262 (7.3) | 92 (2.4) |
Bar/pub | 307 (7.4) | 51 (1.2) | 136 (3.8) | 47 (1.2) |
Workplace | 71 (1.7) | 9 (0.2) | 15 (0.4) | 6 (0.2) |
Other | 123 (3.0) | 22 (0.5) | 78 (2.2) | 17 (0.5) |
The highest prevalence among women was for minor violence, IPV and violence taking place in the home. The prevalence of self-reported IPV was higher among women than among men. However, men were overall three times more likely to have engaged in any violence than women in both the NHPMS and the APMS (see Table 4).
Marital status
Marital status was coded according to three combined categories: (1) married, widowed or cohabiting, (2) single and (3) separated or divorced. In all statistical models category (1) was the reference group against which other categories were contrasted to estimate risk for violence. The prevalence of violence in the NHPMS was 4.9% among those who were married/cohabiting/widowed, 25.4% among those who were single and 11.6% among those who were separated or divorced. The prevalence of violence in the AMPS was 4.6% among those who were married/cohabiting/widowed, 20.4% among those who were single and 7.2% among those who were separated or divorced. The prevalence of violence in the MMLS was 26.6% among those who were married/cohabiting/widowed, 35.0% among those who were single and 36.1% among those who were separated or divorced. Tables 2 and 4 show the unadjusted and adjusted findings of the effects of marital status on violence, respectively.
Being single or separated/divorced was associated with a higher prevalence of violence throughout. In the household surveys, the likelihood of violence was approximately twofold among single and separated/divorced respondents. The odds of violence increased by approximately 50% among single and divorced young men in the MMLS.
Ethnicity
Ethnic groups recorded in the surveys were reclassified as white, black (originating from Africa or the West Indies), South Asian and ‘other’. In the NHSPM the prevalence of violence was 11.7% among white participants, 15.5% among black participants, 7.3% among South Asian participants and 14.7% among participants from other ethnic groups. In the AMPS the prevalence of violence was 8.3% among white participants, 9.3% among black participants, 6.9% among South Asian participants and 8.7% among participants from other ethnic groups. In the MMLS the prevalence of violence was 36.9% among white participants, 31.1% among black participants, 19.2% among South Asian participants and 21.1% among participants from other ethnic groups. The adjusted demographic models indicate that the South Asian ethnicity group was protective for any violence compared with white respondents in both household surveys (see Table 3). All black and minority ethnic groups in the MMLS were less likely to report violence than white respondents (Table 5).
Demographic characteristics | MMLS 2011 | |
---|---|---|
OR (95% CI) | AORa (95% CI) | |
Age group (years) | ||
18–24 | Reference | Reference |
25–34 | 0.65 (0.58 to 0.73)*** | 0.71 (0.62 to 0.82)*** |
Marital status | ||
Married/cohabiting | Reference | Reference |
Single | 1.48 (1.30 to 1.69)*** | 1.27 (1.09 to 1.47)** |
Separated/divorced | 1.56 (1.15 to 2.12)** | 1.50 (1.09 to 2.07)* |
Social class | ||
I and II | Reference | Reference |
IIIM/IIINM | 1.06 (0.86 to 1.31) | 0.97 (0.78 to 1.21) |
IV and V | 1.30 (1.05 to 1.61)* | 1.19 (0.95 to 1.48) |
Unemployed | 1.53 (1.25 to 1.87)*** | 1.21 (0.97 to 1.50) |
Ethnicity | ||
White | Reference | Reference |
Black | 0.77 (0.65 to 0.91)** | 0.77 (0.65 to 0.92)** |
South Asian | 0.41 (0.34 to 0.48)** | 0.41 (0.34 to 0.49)*** |
Other | 0.46 (0.27 to 0.78)** | 0.49 (0.28 to 0.86)* |
Immigration
Among the young men in the MMLS, 740 (14.0%) reported being born outside the UK. Of these, 99 (13.4%) reported any violence in the past 5 years; this compared with 1346 (30.6%) of those who were born in the UK. Those who were not born in the UK were significantly less likely to report any violence in a univariate model [OR 0.46, 95% confidence interval (CI) 0.36 to 0.58; p < 0.001] and after adjusting for all other demographic characteristics (OR 0.54, 95% CI 0.42 to 0.69; p < 0.001).
Social class and unemployment
Social class was based on the UK Registrar General’s classification,59 which was chosen because it uses the most recent occupation of head of household: I – professional, II – managerial, IIINM – skilled non-manual, IIIM – skilled manual, IV – partly skilled and V – unskilled. This classification provides an indicator of various domains including income, education and level of responsibility at work. 60,61
Table 6 shows the results for each category of social class (with class I as the reference) by sex. Among men in the NHPMS, all other categories of lower social class increased the risk for violence compared with class I. Participants from social classes IIINM and below were four times as likely to report violence in the past 5 years and social class V was associated with a fivefold increase in violence. However, among women, only social classes IIIM and V were associated with an increase in reported violence.
Social class | n (%) | OR (95% CI) | AORa (95% CI) |
---|---|---|---|
NHPMS 2000 | |||
Men | |||
I | 22 (5.3) | Reference | Reference |
II | 126 (10.2) | 1.71 (1.04 to 2.81)* | 1.94 (1.16 to 3.24)* |
IIINM | 136 (27.5) | 5.71 (3.46 to 9.43)*** | 4.62 (2.74 to 7.79)*** |
IIIM | 214 (18.1) | 3.32 (2.05 to 5.38)*** | 4.02 (2.43 to 6.64)*** |
IV | 127 (22.3) | 4.31 (2.61 to 7.12)*** | 4.40 (2.61 to 7.42)*** |
V | 45 (27.7) | 5.76 (3.24 to 10.23)*** | 5.08 (2.75 to 9.36)*** |
Women | |||
I | 22 (5.3) | Reference | Reference |
II | 40 (3.6) | 1.58 (0.41 to 6.06) | 3.06 (0.68 to 13.74) |
IIINM | 71 (4.8) | 2.14 (0.57 to 8.06) | 3.74 (0.84 to 16.57) |
IIIM | 22 (6.8) | 3.11 (0.79 to 12.27) | 5.79 (1.25 to 26.92)* |
IV | 66 (9.0) | 4.22 (1.12 to 15.93)* | 7.22 (1.62 to 32.14)** |
V | 11 (4.0) | 1.76 (0.42 to 7.37) | 4.65 (0.94 to 22.95) |
All | |||
I | 21 (5.3) | Reference | Reference |
II | 166 (7.1) | 1.37 (0.86 to 2.17) | 2.11 (1.30 to 3.42)** |
IIINM | 207 (10.4) | 2.10 (1.33 to 3.31)** | 3.87 (2.38 to 6.28)*** |
IIIM | 236 (15.6) | 3.34 (2.12 to 5.26)*** | 4.22 (2.62 to 6.81)*** |
IV | 192 (14.8) | 3.13 (1.98 to 4.96)*** | 5.05 (3.10 to 8.21)*** |
V | 56 (12.6) | 2.60 (1.55 to 4.35)*** | 4.65 (2.66 to 8.12)*** |
APMS 2007 | |||
Men | |||
I | 8 (3.2) | Reference | Reference |
II | 66 (6.0) | 1.96 (0.93 to 4.12) | 2.03 (0.92 to 4.50) |
IIINM | 61 (14.8) | 5.34 (2.51 to 11.36)*** | 3.96 (1.77 to 8.86)** |
IIIM | 142 (14.3) | 5.13 (2.48 to 10.60)*** | 6.16 (2.84 to 13.37)*** |
IV | 73 (15.5) | 5.65 (2.68 to 11.92)*** | 4.72 (2.12 to 10.48)*** |
V | 32 (26.2) | 10.92 (4.86 to 24.54)*** | 10.77 (4.45 to 26.07)*** |
Women | |||
I | 4 (3.6) | Reference | Reference |
II | 36 (3.1) | 0.84 (0.29 to 2.43) | 0.99 (0.34 to 2.90) |
IIINM | 47 (4.3) | 1.19 (0.42 to 3.38) | 1.33 (0.46 to 3.87) |
IIIM | 14 (4.9) | 1.39 (0.44 to 4.33) | 1.56 (0.49 to 5.03) |
IV | 38 (5.9) | 1.68 (0.58 to 4.82) | 1.69 (0.57 to 4.97) |
V | 6 (3.3) | 0.91 (0.25 to 3.31) | 1.45 (0.36 to 5.79) |
All | |||
I | 12 (3.3) | Reference | Reference |
II | 102 (4.5) | 1.38 (0.75 to 2.53) | 1.73 (0.91 to 3.29) |
IIINM | 108 (7.1) | 2.26 (1.23 to 4.15)** | 2.93 (1.54 to 5.60)** |
IIIM | 156 (12.2) | 4.10 (2.25 to 7.47)*** | 4.57 (2.42 to 8.63)*** |
IV | 111 (10.0) | 3.26 (1.77 to 5.99)*** | 3.64 (1.91 to 6.95)*** |
V | 38 (12.5) | 4.21 (2.16 to 8.22)*** | 6.15 (2.98 to 12.73)*** |
Certain differences were observed in the APMS. Social class was not significantly associated with violence among women; however, among men in social class V, the risk associated with violence was increased 10-fold.
There was a statistically significant trend in prevalence of violence according to lower social class for men (p < 0.001) and women (p < 0.001). However, in the NHPMS, we observed an unexpected 17.3% increase in the prevalence of violence among men from social class II to social class IIINM. This increase was statistically significant (10.2% vs. 27.5% respectively; p < 0.001). This contrasted with a 1.2% non-significant increase in self-reported violence among women from social class II to social class IIINM (3.6% vs. 4.8% respectively; p = 0.14). Compared with other categories, those men in social classes II and IIINM were younger (82.9% were aged 16–34 years vs. 67.1%; p < 0.008), more were single (81.1% vs. 56.6%; p < 0.001) and more were living with their parents (34.0% vs. 11.8%, p < 0.001). In addition, fewer lived in rented accommodation (25.0% vs. 40.1%, p < 0.01) and as a couple (21.9% vs. 51.7%, p < 0.001). Violent men in social class IIINM were approximately three times more likely to be single and living with their parents than violent men in other social classes.
Discussion
Sex and age differences in violent behaviour identified in our study are in accordance with a previous meta-analysis. 62 Physical aggression is more common among men than women at all ages and this is consistent across cultures, appearing from early childhood onwards and showing a peak between 20 and 30 years of age. However, studies that have measured anger did not show sex differences. Higher levels of indirect aggression among females, such as expressions of anger, are limited to later childhood and adolescence, but tend to vary according to the methods of measurement and were not included in this study.
Sexual selection theory hypothesises that the origin of greater male physical aggression in human evolutionary history is a consequence of unequal parental investment, leading to greater male than female reproductive competition and, therefore, overt aggression. 63 This is thought to be the psychological accompaniment of physical sex differences such as size, strength and longevity. 62 Evolutionary analyses have identified different degrees of risk that an individual is prepared to take during a conflict as a crucial difference between the sexes. Greater variation in male and female reproductive success among mammals leads to more intense male competition. Selection favours high-risk strategies (even when mortality rates are high) if the reward of victory is high and the consequence of losing is little or no chance of reproducing. 64 This theoretical approach suggests that sex differences and physical aggression will be largest when reproductive competition is highest, for example during young adulthood, and can include higher risk and escalated forms of aggression, such as those involving death or severe injury. Our findings are in agreement with this theory in demonstrating that men in early adulthood were more likely to engage in severe violence against strangers and people known to them. Weissfeld65 has argued that boys compete in this way to form dominance orders or hierarchies. This has been compared with the behaviour of other primates and is thought to be important for providing access to resources, including reproductive success in social animals. Hierarchies are based on dominance and the use of aggression, which is stable over time, together with high dominance. This appears to rank with certain other attributes, including personality.
All levels of severity of violence and all victim types were more prevalent among men than women, except violence against intimate partners and family. Women were approximately twice as likely to report an intimate partner as a victim than men. However, national surveys have shown that women who are married or cohabiting are more likely to report fear of bodily injury, actual injuries and the use of medical, mental health and criminal justice system services as a result of IPV than men. 66 Similarly, substantially more women report that they have experienced sexual violence from an intimate partner,67 which was not included in this study. Nevertheless, Dutton and Nicholls68 have argued that the sex disparity in injuries from domestic violence is less than originally portrayed by feminist theory. In a review of studies,69 high levels of unilateral intimate violence by women towards both men and women have been observed. Furthermore, men report their own victimisation less often than women and do not view female violence against them as a crime. As a result, male victimisation by female partners is under-reported in crime victim surveys.
Married men are less likely to commit crimes, including violence. However, there are questions of selection and confounding when studying this relationship. Samson et al. 70 carried out a study of high-risk boys followed up prospectively from adolescence to age 32 years. They found that being married was associated with an average reduction of approximately 35% in the odds of committing a crime compared with not being married. Previous research has also indicated that marriage is a key turning point in desistance from crime. 71 The establishment of a good relationship is thought to facilitate this. Correspondingly, Bersani et al. 72 found that marriage reduced offending, including violent offending, for both men and women in the Netherlands. Laub et al. 73 argue that a close relationship acts protectively on crime and violence by the formation of social bonds and an investment process in the relationship. It is the quality of the marital bond that affects this. However, the influence is gradual and is cumulative over time. Our finding that divorce and separation were strongly associated with violence in this study corresponds to this. However, the processes leading to the breakdown of a relationship are likely to be complex and could reflect an individual’s tendency to violence or even be the result of violence towards the intimate partner.
The association between violent crime and single marital status could be explained by social factors in the lives of young people and was independent of age. The move to social independence among young men is important. Although many young men now remain in the parental home, this was not found to have a protective effect among young men in Great Britain. 74 Furthermore, violence when intoxicated observed among young men (see Chapter 6, Study 2) and fighting with strangers can be construed as one example among a series of hedonistic and negative social behaviours (including hazardous drinking, drug misuse, sexual risk taking and non-violent antisocial behaviour) exhibited by single young men without the responsibilities of providing their own accommodation or supporting dependent children or the ameliorating effects on their behaviour of living with a female partner. It has been questioned whether or not this lifestyle has become more prevalent among some men within the context of increasing prolongation of early adulthood and when it now takes longer to obtain a full-time job that pays sufficiently to support a family. 75 US research has indicated that many young people in their early 20s have not become fully adult according to their own subjective assessment and do not perceive themselves as either ready or able to perform these roles. A comparison of census data in the USA from the years 1960 and 200076 demonstrated that fewer men aged 40 years in 2000 than in 1960 had completed all the major transitions of leaving home, finishing school, becoming financially independent, getting married and having a child. Furthermore, young people remaining at home now receive more substantial financial aid from their parents than previous generations. 75 Not having to provide accommodation or support dependents means a relatively higher disposable income and more leisure time, possibly associated with higher-risk activities, including violence.
We did not find differences in self-reported violence in our surveys between black and minority ethnic subgroups and white study participants. Being of South Asian origin appeared to convey a protective effect. This was before adjusting for other demographic factors, most importantly social class. This finding is in marked contrast to the number of stop and search encounters and convictions for violent crime in black and minority ethnic populations and proportions of people imprisoned from a black and minority ethnic origin in the UK. Similarly, the lack of an association between immigration and violence in this study is consistent with the lack of an association between immigration and crime in the UK. 77 The continuous reduction in the number of overall property crimes in England and Wales since 2002 has occurred in the face of an increasing foreign-born population, but there is no evidence to suggest that rising migration causes a decline in crime rates. The foreign-born proportion of the population is also unrelated to violent crime according to most recent research findings. 78
It has long been established that the strength of the relationship between social class and violence varies significantly, but depends primarily on the measure of social class. 79 Our finding that in 2000 there was a higher than expected prevalence of violence among men from social classes II and IIINM is most likely explained by the characteristics of the men in these two social groups. For example, an unexpectedly large number of men still living in the parental home, not having children or not being involved in a relationship contributed substantially to the association between social class IIINM and violent behaviour.
It is thought that unemployment has a key part to play in violence and that lack of routine activity and the economic effects of being unemployed increase the risk of crime. However, research has indicated that violent crime, as opposed to burglary and theft, is pro-cyclical: higher in good times when unemployment is lower. It has been argued that alcohol consumption, which is higher in good times and more strongly related to violence, is a key determinant. 80 Nevertheless, the association between violence and unemployment is highly complex and other associated factors are highly relevant. Lack of finances is important because labour markets are important sources of status and the focus of struggles over norms of fairness and a validated identity. Unemployment can be relevant to violence when it intersects with collective identities: masculinity, race or ethnicity or religion. When there are no structured institutional mechanisms for unemployed people to express complaints and press for improvement, the chances are increased that there may be one or other type of violence. This may also apply to IPV. At the international level, the contribution of the labour market structure and opportunities and relations to violent conflicts cannot be understood in isolation from the broader structural and policy features of a society. 81
Study 2: a typology of violent persons in the population
Aims and objective
The aims of study 2 were to:
-
Identify groups, or subtypes, of people in the population of Great Britain according to their patterns of self-reported violent behaviour. We included measures of severity and frequency, their victims and the location of their violence to determine subtypes.
-
Validate these subtypes according to their differing demographic characteristics.
The overall objective was to create a typology for investigating the associations with psychiatric morbidity in subsequent studies in this section of the report.
Methods
Participants
We used a combined sample of men and women drawn from the first phase of the NHPMS 2000 and the APMS 2007 (see Study 1). Design and sampling procedures have previously been described. 45,82 As each of the surveys employed the same measures of demography and violence outcomes, we conducted joint analyses of individual-level data. All analyses on violence typologies were carried out separately by sex.
Measures
We used the self-reported measures of violence described in study 1. Social class was based on the UK Registrar General’s classification,59 which uses the most recent occupation of the head of the household. Sociodemographic covariates included age in 20-year bands, marital status, ethnicity and employment.
Latent class analysis
We used latent class analysis (LCA) to explore whether or not individuals could be classified into a set of latent variables based on their endorsement of the violence indicators. Membership of these subgroups, often called latent classes, is defined by the specific set of responses to a series of observed characteristics. This approach allowed us to describe the relationships of the variables as they combined into classes that defined groups of people within a sample or population.
Latent class analysis was used to empirically define participant groups based on their violent behaviours profile and explore the existence of typologies of violence. Decisions regarding the most appropriate model were led by statistical indicators and clinical considerations. The default estimator was the robust maximum likelihood (MLR). However, MLR may lead to the presence of a problem called local maxima. To fully avoid this, all LCA models were estimated with different random starting values: we used 2000 random starts at the initial stage and 200 optimisations at the final stage. Models were inspected to ensure that the log-likelihood value for each model was successfully replicated several times (an indication of low probability of local maxima). We gave priority to this rule in selecting our final latent class model.
After selecting the classes that fitted best by sex, we described them in terms of the aforementioned demographic characteristics.
All analyses were performed using Mplus software (Muthéu and Muthéu, Los Angeles, CA, USA) for Windows OS version 7.11.
Results
Typology of violence among men
To identify the constructs in each class (classification) included in Table 7, we established indicators with probabilities of < 0.29 (low probability), from 0.30 to 0.59 (moderate probability) and > 0.60 (high probability). We classified men into the following classes: class 1 – ‘no violence’; class 2 – ‘minor violence’, characterised by fights with strangers, persons known and friends, in the street or in bars, with few incidents or only one incident and in which no-one is injured; class 3 – ‘violence towards known persons/family’, characterised by more serious violence resulting in injuries to the victim and perpetrator, involving a range of different victims but mainly persons known, friends, family members and intimate partners and in a range of locations, mainly in the street or in bars but also in their own or another’s home; class 4 – ‘fighting with strangers’, characterised by fights almost exclusively with strangers taking place in the street or in bars, often when intoxicated and leading to injury to the victim or perpetrator (one in five men in class 4 had been involved in multiple violent incidents with strangers); and class 5 – ‘serious repetitive violence’, characterised by multiple incidents of violence, usually when intoxicated, resulting in injuries to multiple victims and in multiple locations and including family and intimate partners (see Table 7).
Violence indicators | Class 1 (N = 6583; 84.8%), n (%) | Class 2 (N = 453; 5.8%), n (%) | Class 3 (N = 296; 3.8%), n (%) | Class 4 (N = 308; 4.0%), n (%) | Class 5 (N = 121; 1.6%), n (%) |
---|---|---|---|---|---|
Repeated violence (five or more incidents) | 1 (0.0) | 66 (14.6) | 43 (14.5) | 63 (20.6) | 102 (84.5) |
Violent when intoxicated | 0 (0.0) | 155 (34.2) | 142 (47.8) | 158 (51.3) | 100 (83.5) |
Victim injured | 4 (0.1) | 0 (0.0) | 138 (46.4) | 157 (51.1) | 121 (100.0) |
Perpetrator injured | 0 (0.0) | 0 (0.0) | 172 (58.0) | 137 (44.6) | 85 (70.4) |
Minor violence | 0 (0.0) | 453 (100.0) | 0 (0.0) | 1 (0.3) | 2 (1.7) |
IPV | 0 (0.0) | 25 (5.6) | 37 (12.6) | 0 (0.0) | 23 (19.2) |
Towards a family member | 0 (0.0) | 31 (6.8) | 39 (13.3) | 3 (0.9) | 14 (11.7) |
Towards a friend | 0 (0.0) | 86 (19.0) | 84 (28.5) | 16 (5.3) | 49 (40.9) |
Towards someone known | 0 (0.0) | 134 (29.5) | 177 (59.8) | 2 (0.7) | 83 (68.7) |
Towards a stranger | 0 (0.0) | 237 (52.3) | 31 (10.6) | 308 (100.0) | 108 (89.2) |
In the home | 0 (0.0) | 34 (7.4) | 58 (19.5) | 0 (0.0) | 27 (22.2) |
In someone else’s home | 0 (0.0) | 26 (5.7) | 33 (11.3) | 13 (4.1) | 22 (18.3) |
In the street/outdoors | 5 (0.1) | 206 (45.5) | 182 (61.4) | 212 (68.8) | 111 (92.2) |
In a bar/pub | 0 (0.0) | 144 (31.9) | 76 (25.7) | 112 (36.5) | 110 (91.2) |
In the workplace | 2 (0.0) | 30 (6.7) | 15 (5.0) | 15 (4.8) | 25 (20.7) |
Several models of latent subgroups defined by violence indicators were estimated for men and women. Complex sampling and weights were considered in the development of the latent class models. Model fit and information criteria for LCA model selection are included in Table 8 for men and Table 9 for women. Model fit indices favoured the five-class model in men. A three-class model provided the best fit to the data in women.
Model | Log-likelihood | Replicated log-likelihood | AIC | BIC | aBIC | VLMR-LRT p-value | Entropy |
---|---|---|---|---|---|---|---|
Class 1 | 17590.6 | Yes | 35211.3 | 35313.9 | 35266.3 | NA | NA |
Class 2 | 11163.4 | Yes | 22388.8 | 22601.0 | 22502.5 | < 0.0001 | 0.99 |
Class 3 | 10638.0 | Yes | 21370.1 | 21691.8 | 21542.4 | < 0.0001 | 0.99 |
Class 4 | 10484.1 | Yes | 21094.2 | 21525.4 | 21325.2 | < 0.0001 | 0.99 |
Class 5 | 10392.1 | Yes | 20816.1 | 21356.8 | 21105.8 | 0.0002 | 0.98 |
Model | Log-likelihood | Replicated log-likelihood | AIC | BIC | aBIC | VLMR-LRT p-value | Entropy |
---|---|---|---|---|---|---|---|
Class 1 | 9320.7 | Yes | 18671.3 | 18777.6 | 18729.9 | NA | NA |
Class 2 | 5360.1 | Yes | 10782.2 | 11001.9 | 10903.4 | < 0.0001 | 0.99 |
Class 3 | 5145.5 | Yes | 10385.1 | 10718.1 | 10568.8 | < 0.0001 | 0.99 |
Table 10 shows that members of class 5 (‘serious repetitive violence’) were younger than members of the other classes, with no men in the older age group (55–74 years), no black men and predominantly white and single men. Most were employed in occupations from social classes IIIM and IIINM. Class 4 showed similar demographic characteristics, with significantly fewer Asian men and fewer separated or divorced men. Class 3 had the largest proportion of separated or divorced men and one-quarter were economically inactive. Class 2 showed few differences from class 1 (non-violent men), except that more were younger and single.
Demographic characteristics | Violence typologies (latent classes) | ||||
---|---|---|---|---|---|
Class 1 (%) (reference) | Class 2 (%) | Class 3 (%) | Class 4 (%) | Class 5 (%) | |
Age group (years) | |||||
16–34 (reference) | 27.3 | 75.3 | 70.9 | 69.2 | 82.7 |
35–54 | 40.4 | 21.9a | 24.0a | 26.6a | 17.3a |
55–74 | 32.3 | 2.8a | 5.1a | 4.2a | 0.0a |
Ethnicity | |||||
White (reference) | 90.8 | 88.6 | 93.4 | 95.7 | 96.7 |
Black | 2.7 | 5.1 | 2.5 | 1.6 | 0.0a |
South Asian | 4.0 | 3.1 | 2.6 | 1.0a | 2.3 |
Other | 2.5 | 3.3 | 1.5 | 1.7 | 1.1 |
Social class | |||||
I and II (reference) | 42.8 | 23.4 | 17.9 | 23.6 | 10.6 |
IIIM and IIINM | 40.5 | 49.0b | 54.7b | 48.9b | 70.3b |
IV and V | 16.7 | 27.6b | 27.4b | 27.6b | 19.1b |
Marital status | |||||
Married or cohabiting (reference) | 67.9 | 27.0 | 36.3 | 35.8 | 26.7 |
Single | 24.3 | 66.9b | 54.6 | 57.2b | 67.4b |
Separated/divorced | 7.8 | 6.1b | 9.0b | 7.0b | 6.0 |
Employment | |||||
Employed (reference) | 70.2 | 74.8 | 66.2 | 81.8 | 73.5 |
Unemployed | 3.1 | 8.5 | 8.5 | 6.5 | 4.6 |
Inactive economically | 26.7 | 16.7 | 25.3b | 11.7 | 22.0 |
We carried out a further investigation to see to what extent IPV had determined the classes. We found that only 24 (3.1%) men reported that they were uniquely violent towards their intimate partner, indicating that IPV had little effect in determining the classes among men.
Typology of violence among women
As with men, we established that indicators with a probability < 0.29 as low, from 0.30 to 0.59 as moderate probability and above 0.60 as high probability among women. Table 11 shows that class 1 was characterised by ‘no violence’ and had a prevalence of 94.9%. Class 2 (‘general violence’) was characterised by a range of different victims, mainly persons known and strangers, but also family members, intimate partners and friends, with violence occurring usually in the street or in a bar, but also in the perpetrator’s own or someone else’s home. This class resembled class 3 in men. Class 3 (‘intimate family violence’) was characterised by violence occurring exclusively in the home and involving intimate partners and family members. It usually involved minor violence and if a participant was injured it was usually the female perpetrator. Class 2 included the highest proportion of women of young age (16–34 years) (83%) followed by class 3 (58.3%). Ethnic composition was similar across the classes. Class 2 had significantly higher proportions of women in lower social classes. Women in classes 2 and 3 were mainly single, with more women in class 3 separated or divorced. There was no association with employment status (Table 12).
Violence indicators | Class 1 (N = 7608; 94.9%), n (%) | Class 2 (N = 282; 3.5%), n (%) | Class 3 (N = 124; 1.5%), n (%) |
---|---|---|---|
Repeated violence (five or more incidents) | 0 (0.0) | 35 (12.4) | 25 (20.0) |
Violent when intoxicated | 0 (0.0) | 108 (38.2) | 22 (18.2) |
Victim injured | 0 (0.0) | 76 (27.1) | 9 (7.5) |
Perpetrator injured | 0 (0.0) | 88 (31.2) | 32 (25.7) |
Minor violence | 0 (0.0) | 119 (42.4) | 79 (64.3) |
IPV | 0 (0.0) | 35 (12.6) | 98 (79.3) |
Towards a family member | 0 (0.0) | 68 (24.2) | 31 (25.1) |
Towards a friend | 0 (0.0) | 76 (27.1) | 0 (0.0) |
Towards someone known | 0 (0.0) | 115 (40.7) | 0 (0.0) |
Towards a stranger | 0 (0.0) | 99 (35.2) | 0 (0.0) |
In the home | 0 (0.0) | 50 (17.6) | 124 (100.0) |
In someone else’s home | 0 (0.0) | 36 (12.9) | 7 (5.9) |
In the street/outdoors | 0 (0.0) | 176 (62.4) | 17 (13.6) |
In a bar/pub | 0 (0.0) | 93 (33.1) | 5 (3.9) |
In the workplace | 0 (0.0) | 14 (5.0) | 1 (0.6) |
Demographic characteristics | Violence typologies (latent classes) | ||
---|---|---|---|
Class 1 (%) (reference) | Class 2 (%) | Class 3 (%) | |
Age group (years) | |||
16–34 (reference) | 30.2 | 83.0 | 58.3 |
35–54 | 37.8 | 15.9a | 36.1a |
55–74 | 32.0 | 1.1a | 5.6a |
Ethnicity | |||
White (reference) | 92.8 | 90.4 | 93.1 |
Black | 2.4 | 3.0 | 4.5 |
South Asian | 2.8 | 2.0 | 1.0 |
Other | 2.1 | 4.7 | 1.4 |
Social class | |||
I and II (reference) | 33.6 | 18.9 | 31.5 |
IIIM and IIINM | 42.5 | 44.8b | 39.6 |
IV and V | 23.9 | 36.4b | 29.0 |
Marital status | |||
Married or cohabiting (reference) | 68.4 | 20.3 | 35.7 |
Single | 21.1 | 68.7b | 42.1b |
Separated/divorced | 10.5 | 11.0b | 22.1b |
Employment | |||
Employed (reference) | 57.4 | 62.5 | 70.6 |
Unemployed | 2.1 | 5.2 | 1.5 |
Inactive economically | 40.6 | 32.3 | 27.8 |
Discussion
We identified five classes of violent men, which we further validate against categories of psychiatric morbidity and other psychopathology in Chapters 3–9 of this section of the report. Class 2 included men who had become involved in minor violence, usually on a ‘one-off’ basis. Apart from being young and single (the most common risk factors for becoming involved in violence), there was little to differentiate them from men who were not violent in the population.
Men in class 3 (‘violence towards known persons/family’) engaged in more serious violence than men in class 2: they and their victims became injured. Violence involved victims known to the perpetrators, including friends, family and intimate partners, and occurred in a range of different locations. The higher prevalence of divorced and separated men in this group and the finding that one-quarter were economically inactive suggests that they were poor at sustaining relationships and friendships and that many were dependent on state benefits.
Men in class 4 (‘fighting with strangers’) were involved in more serious violence leading to injuries, almost exclusively with people previously unknown to them, sometimes in multiple violent encounters. The narrower range of characteristics suggests a lifestyle in which potential violent altercations with other young men are likely to occur, such as in bars or encounters in the street, or at sporting activities, and/or that these men actively seek such altercations, finding violence exciting and a personal challenge.
Class 5 was the most distinct subgroup, characterised by serious violence to the widest range of victims. Men in this class had similar altercations with strangers as in class 4, but their violence also extended to their friends, family members and intimate partners, in multiple different locations. Similar observations have previously been made for those in the community with a diagnosis of ASPD. 83 Because the study was cross-sectional, it could not be investigated whether a subgroup will eventually mature or ‘burn out’. This might explain the concentration of class 5 men in the youngest age group. However, although class 5 appeared to be the most serious public health risk to others as a result of violence, and their repetitive violence suggested the probability of greater psychological dysfunction and psychiatric morbidity (which will be investigated in subsequent chapters), other characteristics, such as a concentration of these men in social classes IIINM and IIIM and the absence of black men from this class, were of considerable interest.
Intimate partner violence did not determine a unique group among men as observed with class 3 women. Very few men in the study reported that their violence was uniquely directed against partners. For men, IPV was, therefore, an indication of a general violent disposition, extending to strangers and others outside of the home. For women, the situation was different. A considerably larger proportion reported that their violence was exclusively directed against partners or family members. This violence was largely minor, with no-one being injured. If someone was injured in the violent altercation, it was most likely the woman herself, most probably by her male partner. It was noteworthy that the social class of class 3 women was not significantly different from that of non-violent women.
Class 2 women were younger and of a lower social class and engaged in violence towards a range of different victims, but most commonly persons known to them. There was a similarity to class 3 and to a lesser extent class 5 among men. This group, exhibiting violence with similarities to men’s violence, is therefore of considerable interest. It is hypothesised that, to become violent and antisocial, women must surmount a higher threshold of risk than men and are therefore more severely afflicted (‘threshold/paradox’ hypothesis). 84 The female threshold is presumed to be raised by the sex role socialisation of women against aggression at the level of culture. The risk over this threshold is presumed to come from psychobiological or developmental factors at the level of the individual. If more severe aetiology is found for women, then the inference can be made that a higher threshold for women exists. 85,86
Chapter 3 Psychiatric morbidity and violence
Background
It has long been assumed that there is a link between major mental illness and violence. This belief is prevalent across cultures: community surveys show that the general population often associates mental disorder with violence. More recently, however, a lack of association has been reported in certain studies of mentally disordered offenders, particularly for schizophrenia. 87,88 The same conclusion was reached in a meta-analysis of variables associated with recidivism among mentally disordered offenders,89 which found that the average association between psychosis and violence was small and negative across 11 studies that reported on psychosis and that demographic factors and previous violence were the strongest risk factors for future violence.
The uncertainty extends to other diagnoses, including anxiety and depression. Coid and Ullrich90 found an association between increased levels of violence and anxiety when comorbid with ASPD. However, it remains unclear if anxiety is important as a risk factor at the general population level. Depressive disorder is not thought of as a common risk factor and depression may act as a factor that reduces the risk of violence for some individuals. Douglas et al. 91 have suggested that severe mental disorder might be causally associated with violence. In this case, symptoms of mental disorder might provide a clear motivation for violence or interfere with the ability to manage interpersonal conflict. On the other hand, severe mental disorder might be a consequence of violence. This stress of perpetrating violence could trigger the onset of mental disorder in people who are so predisposed. Finally, severe disorder may be a simple correlate of violence. The association between the two may be statistical rather than causal, the result of links with some third variable such as stressful life events, lack of social support, personality traits, substance use or victimisation. Douglas et al. 91 suggested that, if this explanation was true, it would be expected that there would be no clear time-related or statistical association between severe mental disorder and psychosis, at least after controlling for potential confounding factors.
Study 1: anxiety disorder
Objectives
The objectives of this study were to:
-
Investigate the associations between anxiety disorder and self-reported violence towards others. We investigated associations between five individual categories of anxiety disorder in the International Statistical Classification of Diseases and Related Health Problems, Tenth Edition (ICD-10),92 and a combined category.
-
Investigate the associations between anxiety disorder and the classes of violence identified in the population in Chapter 2 (see Study 2).
Methods
Sample
For the purposes of this analysis we combined two data sets, the APMS 2007 and the NHPMS 2000, to provide a total of 15,734 subjects. 45,49
Definition and assessment
Anxiety disorders in the combined category included generalised anxiety disorder, panic disorder, mixed anxiety disorder and depression, obsessive–compulsive disorder and phobias. These were assessed using the CIS-R55 and were coded according to ICD-10 criteria. The CIS-R assesses 14 neurotic syndromes by asking respondents two screening questions in each of the sections, namely somatic symptoms, fatigue, concentration and forgetfulness, sleep problems, irritability, worry about physical health, depression, depressive ideas, worry, anxiety, phobias, panic, compulsions and obsessions. For positive responses, further questions were asked to ascertain the duration, frequency and severity of the neurotic symptomatology. These were rated from 0 to 4, except for depression, which is rated from 0 to 5. Mixed anxiety and depressive disorder was diagnosed when a threshold score of 12 on the CIS-R was reached without the diagnostic criteria for depression or any anxiety disorder being met.
The violent outcomes module questions have been previously described in Chapter 2 (see Violence module).
Associations between anxiety disorders and any violence in the past 5 years were examined. Risks were measured using ORs. Weighted analyses were used to account for the sampling procedure in the surveys, as described in Chapter 2.
Results
Table 13 shows that 1596 (10.1%) individuals in the combined survey samples reported any violence in the past 5 years. Violence was more common among men than women, among younger adults and among those from lower socioeconomic groups.
Covariate | Any violence in the past 5 years (n = 1596; 10.1%) | Anxiety classification (n = 2437; 15.3%) | ||
---|---|---|---|---|
n (%) reported | AOR (95% CI) | n (%) reported | AOR (95% CI) | |
Sex | ||||
Female | 406 (5.1) | Reference | 1479 (18.3) | Reference |
Male | 1190 (15.4) | 3.16 (2.68 to 3.72)*** | 958 (12.2) | 0.53 (0.47 to 0.59)*** |
Age group (years) | ||||
16–34 | 1175 (22.4) | Reference | 849 (16.0) | Reference |
35–54 | 371 (6.3) | 0.34 (0.28 to 0.40)*** | 1048 (17.6) | 1.21 (1.06 to 1.39)** |
55–74 | 51 (1.1) | 0.07 (0.05 to 0.09)*** | 540 (11.5) | 0.79 (0.68 to 0.92)** |
Marital status | ||||
Married | 480 (4.7) | Reference | 1411 (13.7) | Reference |
Single | 973 (23.4) | 1.74 (1.46 to 2.08)*** | 679 (16.1) | 0.96 (0.83 to 1.11) |
Divorced/separated | 144 (9.9) | 2.09 (1.67 to 2.63)*** | 346 (23.5) | 1.58 (1.39 to 1.81)*** |
Social class | ||||
I and II | 302 (5.6) | Reference | 725 (13.3) | Reference |
IIIM and IIINM | 708 (11.3) | 2.11(1.75 to 2.53)*** | 990 (15.5) | 1.15 (1.03 to 1.29)* |
IV and V | 398 (12.6) | 2.44 (1.97 to 3.04)*** | 578 (18.0) | 1.27 (1.11 to 1.45)** |
Ethnicity | ||||
White | 1463 (10.2) | Reference | 2177 (14.9) | Reference |
Black | 49 (12.1) | 0.95 (0.60 to 1.50) | 73 (17.6) | 1.15 (0.84 to 1.56) |
Indian subcontinent | 36 (7.0) | 0.47 (0.26 to 0.84)* | 85 (16.6) | 1.39 (0.98 to 1.97) |
Other | 40 (11.2) | 0.92 (0.55 to 1.54) | 75 (20.4) | 1.27 (0.89 to 1.81) |
ASPD | 246 (46.2) | 3.09 (2.29 to 4.17)*** | 195 (35.9) | 2.36 (1.83 to 3.05)*** |
Drug dependency | 276 (48.4) | 2.47 (1.86 to 3.29)*** | 193 (33.8) | 2.04 (1.56 to 2.67)*** |
Alcohol dependency | 371 (35.3) | 2.36 (1.89 to 2.95)*** | 313 (29.4) | 2.37 (1.96 to 2.86)*** |
Psychosis | 7 (14.0) | 0.72 (0.20 to 2.63) | 42 (77.3) | 14.59 (6.37 to 33.43)*** |
We found that 2437 (15.3%) individuals met ICD-10 criteria for anxiety disorders. Anxiety disorders were significantly more common among females, those who were aged > 55 years and those who were divorced.
Table 14 shows that, following adjustments for demographics (age, sex, marital status, ethnicity and social class), any violence was significantly associated with anxiety disorder, phobia, obsessional and compulsive disorder, mixed anxiety and depression, but not panic disorder.
Exposure | n (%) violent | AORa (95% CI) | AORb (95% CI) |
---|---|---|---|
Anxiety (any) | 394 (24.7) | 2.44 (2.06 to 2.89)*** | 1.91 (1.59 to 2.29)*** |
Panic disorder | 22 (1.4) | 1.38 (0.80 to 2.38) | 0.99 (0.55 to 1.79) |
Any phobia | 66 (4.1) | 3.03 (2.02 to 4.54)*** | 2.08 (1.32 to 3.27)** |
OCD | 42 (2.6) | 2.98 (1.88 to 4.72)*** | 2.13 (1.29 to 3.54)** |
GAD | 103 (6.5) | 2.18 (1.63 to 2.91)*** | 1.77 (1.29 to 2.42)*** |
MADD | 91 (5.7) | 2.11 (1.51 to 2.94)*** | 1.90 (1.31 to 2.75)** |
Depression | 79 (4.9) | 2.33 (1.67 to 3.24)*** | 1.42 (0.96 to 2.09) |
When further adjusted for alcohol, drug dependency and psychiatric comorbidity (ASPD, psychosis and depression), any violence remained significantly associated with a combined category of any anxiety disorder, phobic disorder, obsessive–compulsive disorder, generalised anxiety disorder and mixed anxiety and depression, but not panic disorder or depression.
Table 15 shows that, when different subtypes of violence were examined, anxiety disorder (combining the previously described five categories of anxiety disorders and excluding depression) was significantly associated with violence while intoxicated, minor violence, repetitive violence and violence that resulted in a victim sustaining injury after adjusting for demographic factors. However, following adjustment for demographic factors, anxiety disorder was not significantly associated with violence in the workplace.
Outcomes | n (%) violent | AORa (95% CI) | AORb (95% CI) |
---|---|---|---|
Any violence | 394 (16.5) | 2.00 (1.73 to 2.32)*** | 1.91 (1.59 to 2.29)*** |
Violence while intoxicated | 192 (8.0) | 2.28 (1.87 to 2.78)*** | 1.76 (1.36 to 2.28)*** |
Severity of violence | |||
Minor violence | 130 (5.4) | 1.40 (1.12 to 1.76)** | 1.57 (1.22 to 2.03)** |
Five or more violent incidents | 95 (3.9) | 2.25 (1.66 to 3.04)*** | 1.97 (1.38 to 2.81)*** |
Victim injured | 139 (5.8) | 2.20 (1.74 to 2.78)*** | 1.77 (1.30 to 2.42)*** |
Perpetrator injured | 149 (6.2) | 2.36 (1.86 to 3.00)*** | 1.65 (1.24 to 2.21)** |
Police involved | 130 (5.4) | 2.50 (1.94 to 3.21)*** | 1.82 (1.35 to 2.44)*** |
Victim of violence | |||
Intimate partner | 122 (5.1) | 5.47 (4.16 to 7.20)*** | 3.55 (2.62 to 4.82)*** |
Family member | 44 (1.8) | 2.27 (1.47 to 3.51)*** | 1.90 (1.15 to 3.16)* |
Friend | 76 (3.2) | 1.82 (1.29 to 2.57)*** | 1.66 (1.10 to 2.51)* |
Known person | 128 (5.4) | 1.92 (1.49 to 2.48)*** | 1.67 (1.23 to 2.28)** |
Stranger | 183 (7.6) | 1.76 (1.42 to 2.18)*** | 1.60 (1.22 to 2.08)** |
Police | 26 (1.1) | 2.29 (1.34 to 3.90)** | 1.23 (0.65 to 2.32) |
Location of violent incident | |||
Own home | 120 (5.0) | 4.05 (3.10 to 5.29)*** | 2.80 (2.07 to 3.79)*** |
Someone else’s home | 51 (2.1) | 3.36 (2.12 to 5.31)*** | 2.58 (1.52 to 4.38)*** |
Bar/pub | 136 (5.7) | 2.31 (1.91 to 2.79)*** | 2.28 (1.81 to 2.88)*** |
Street | 254 (10.6) | 1.93 (1.52 to 2.46)*** | 1.41 (1.05 to 1.90)* |
Workplace | 19 (0.8) | 1.31 (0.73 to 2.37) | 0.98 (0.55 to 1.75) |
Significant associations were also found, following adjustments, with any phobia, obsessive–compulsive disorder, generalised anxiety disorder and mixed anxiety and depressive disorder.
When further adjusting for the same demographic factors together with alcohol and drug dependency and other psychiatric morbidity (ASPD, psychosis and depression), anxiety disorder remained significantly associated with all measures of violence except violence involving police as a victim.
Violence classes and anxiety disorder
Latent class models included five violence classes for men and three violence classes for women, as described in Chapter 2 (see Study 2).
We used multinomial logistic regression models to estimate associations between the latent classes and anxiety, which are shown for men and women in Tables 16 and 17 respectively. These revealed significant associations with anxiety disorder for all classes exhibiting violence compared with the non-violent class for both men and women after adjustments. Serious repetitive violence among men and IPV among women showed the strongest associations with anxiety disorder after adjustments.
Violence typologies | Anxiety, n (%) | RRRa (95% CI) | RRRb (95% CI) |
---|---|---|---|
No violence | 697 | Reference | Reference |
Minor violence | 63 (14.0) | 1.68 (1.18 to 2.39)** | 1.54 (1.07 to 2.23)* |
Violence towards known persons | 71 (23.9) | 2.85 (1.94 to 4.20)*** | 1.98 (1.31 to 2.99)* |
Fighting with strangers | 72 (23.5) | 2.46 (1.67 to 3.62)*** | 1.88 (1.24 to 2.85)** |
Serious repetitive violence | 39 (31.8) | 4.68 (2.72 to 8.04)*** | 2.63 (1.43 to 4.86)** |
Violence typologies | Anxiety, n (%) | RRRa (95% CI) | RRRb (95% CI) |
---|---|---|---|
No violence | 1310 (17.2) | Reference | Reference |
General violence | 94 (33.5) | 2.18 (1.57 to 3.03)*** | 1.74 (1.23 to 2.46)** |
Intimate/family violence | 51 (41.5) | 3.23 (2.15 to 4.84)*** | 2.87 (1.88 to 4.39)*** |
Discussion
We found a strong, independent association between anxiety disorder and violence in the general population of Great Britain. Anxiety disorder would not at first appear to be a likely risk factor for violence. It might be expected that anxious people would avoid situations leading to violence. However, a state of anxiety can also be seen as corresponding to a fight or flight response, with hyperarousal, or an acute stress response as a physiological reaction to a harmful event, attack or threat. 93 This primes the individual for fighting or fleeing. 94 Alternatively, aggression and anxiety can both result from poor emotional regulation and heightened emotional reactivity. 95 However, it is also necessary to differentiate between fear and anxiety. A behavioural inhibition system regulating responsiveness to aversive stimuli and associated with fear contrast and have differing effects on behavioural activating systems, leading to excessive emotionality, including anxiety, and subsequent violence. 96,97 The association we observed with all subcategories of anxiety disorder except for panic disorder therefore indicates the importance of a chronic state of anxiety for violence, rather than sudden extreme anxiety presenting intermittently in the form of panic attacks.
We found independent associations between anxiety disorder and all levels of violence severity. Because these findings were adjusted for other psychiatric morbidity, both the wide range of victims we observed and the levels of seriousness, including multiple incidents, confirmed the importance of a chronic state of anxiety. Anxiety disorder was also associated with each of the classes of violent individuals we identified in Chapter 2 (see Study 2). However, the strongest associations observed at the population level were for IPV, particularly among women in class 3.
These associations between anxiety disorder and IPV at the population level represent a novel finding and require further investigation. However, high levels of anxiety disorder have been observed in samples of people arrested for IPV, both men and women. 98–101 Anxiety disorder in these studies was one among several mental disorders associated with IPV. A specific role of anxiety in IPV has been described through its effects on anxious attachment in adult romantic relationships, with potentially destructive effects on relationships among highly anxious individuals. Anxious attachment is defined as uncertainty regarding the availability of attachment figures and is thought to develop when infants receive a pattern of inconsistent care from their attachment figure, becoming unsure regarding the availability of the caregiver, particularly in times of need. Children with anxious ambivalent attachment exhibit approach-avoidance behaviours towards their caregivers when distressed, mixing needs for comfort and support with emotional arousal and strong expressions of anger. People who are anxiously attached in adulthood to their partner tend to develop sexual behaviours that are less rewarding. When they are distressed, they exaggerate the severity of their adversities, become obsessed with thoughts of being abandoned by their partner and display intense negative emotions. 102,103
Intimate partner violence is thought more likely to occur when there is an inappropriate matching of partners, particularly the ‘mispairing’ of a male partner who is avoidant of making attachments with an anxious female partner, a combination thought to increase the risk of both male and female violence. 104 Avoidant men may respond to the behaviour of anxious women with violence and women then respond with violence as a self-protective behaviour. Alternatively, a woman with high attachment anxiety may view her partner’s violence as an act of rejection and respond to activation of her fears of abandonment with violence towards her partner.
Study 2: depressive disorder
Objective
The objectives of this study were to:
Methods
Sample
For the purposes of this analysis, we combined two data sets, the APMS 2007 and the NHPMS 2000, giving a total of 15,734 subjects.
Definition and assessment
Depressive symptoms were assessed using the CIS-R with depression diagnosed according to ICD-10 criteria, as described in the previous section for anxiety disorders (see Study 1). Depressive symptoms that were ongoing and which had occurred during the preceding month were included in this measure.
Associations between depression and any violence in the past 5 years were examined. Risks were measured using ORs. Weighted analyses were used to account for the sampling procedure in the surveys as described in Chapter 2.
Results
We found 441 (2.3%) people meeting criteria for depressive disorder. Table 18 shows that depression was significantly more common among those who were middle-aged, those who were single or divorced and those from lower socioeconomic groups.
Covariate | Any violence in the past 5 years (n = 1596; 10.1%) | Depression (n = 441; 2.76%) | ||
---|---|---|---|---|
n (%) reported | OR (95% CI) | n (%) reported | OR (95% CI) | |
Sex | ||||
Female | 406 (5.1) | Reference | 253 (3.1) | Reference |
Male | 1190 (15.4) | 3.44 (2.91 to 4.07)*** | 188 (2.4) | 0.83 (0.65 to 1.06) |
Age group (years) | ||||
16–34 | 1175 (22.4) | Reference | 129 (2.4) | Reference |
35–54 | 371 (6.3) | 0.33 (0.28 to 0.39)*** | 217 (3.6) | 1.77 (1.33 to 2.35)** |
55–74 | 51 (1.1) | 0.07 (0.05 to 0.09)*** | 95 (2.0) | 1.29 (0.93 to 1.79) |
Marital status | ||||
Married/cohabiting | 480 (4.7) | Reference | 217 (2.1) | Reference |
Single | 973 (23.4) | 1.76 (1.47 to 2.10)*** | 132 (4.0) | 1.63 (1.24 to 2.14)*** |
Divorced/separated | 144 (9.9) | 2.00 (1.59 to 2.51)*** | 91 (6.2) | 2.21 (1.70 to 2.87)*** |
Social class | ||||
I and II | 302 (5.6) | Reference | 99 (1.8) | Reference |
IIIM and IIINM | 708 (11.3) | 2.10 (1.74 to 2.53)*** | 180 (2.8) | 1.40 (1.08 to 1.81)** |
IV and V | 398 (12.6) | 2.42 (1.95 to 3.01)*** | 133 (4.1) | 1.88 (1.41 to 2.50)*** |
Ethnicity | ||||
White | 1463 (10.2) | Reference | 386 (2.7) | Reference |
Black | 49 (12.1) | 0.94 (0.59 to 1.50) | 18 (4.5) | 1.30 (0.72 to 2.36) |
Indian subcontinent | 36 (7.0) | 0.46 (0.26 to 0.81)** | 15 (2.8) | 1.12 (0.57 to 2.26) |
Other | 40 (11.2) | 0.91 (0.54 to 1.54) | 14 (3.9) | 1.49 (0.82 to 2.70) |
ASPD | 246 (46.2) | 2.81 (2.07 to 3.82)*** | 40 (7.3) | 1.22 (0.77 to 1.93) |
Drug dependency | 276 (48.4) | 2.31 (1.73 to 3.08)*** | 43 (7.4) | 1.53 (0.91 to 2.56) |
Alcohol dependency | 371 (35.3) | 2.19 (1.75 to 2.74)*** | 70 (6.6) | 1.65 (1.16 to 2.35)** |
Psychosis | 7 (14.0) | 0.52 (0.15 to 1.78) | 16 (28.4) | 2.09 (0.91 to 4.83) |
Anxiety | 394 (16.5) | 1.90 (1.59 to 2.29)*** | 266 (10.9) | 7.42 (5.80 to 9.49)*** |
When adjusting for demographics (sex, age, marital status and ethnicity), any violence in the past 5 years remained associated with depression (OR 2.37, 95% CI 1.70 to 3.30; p < 0.001). However, when further adjusting for the same demographic factors, substance misuse and other psychiatric morbidity (anxiety, psychosis, ASPD), depression was no longer significantly associated with violence (OR 1.33, 95% CI 0.89 to 1.97; p = 0.164).
Table 19 shows the associations between depression and seriousness, victims and location of violence. After adjusting for demographics (sex, age, marital status and ethnicity), depression was associated with violence while intoxicated, repetitive violence, violence that resulted in a victim sustaining an injury, violence in which the perpetrator sustained an injury, violence that required police intervention, violence in the perpetrator’s own home or in the street, IPV and violence against a friend, known person and stranger. However, after adjusting for other psychiatric morbidity, depression remained significantly associated only with violence in the perpetrator’s own home.
Outcomes | n (%) violent | AORa (95% CI) | AORb (95% CI) |
---|---|---|---|
Any violence | 79 (18.9) | 2.16 (1.66 to 2.81)*** | 1.42 (0.96 to 2.09) |
Violence while intoxicated | 35 (8.3) | 2.08 (1.42 to 3.05)** | 1.10 (0.63 to 1.93) |
Severity of violence | |||
Minor violence | 25 (5.9) | 1.50 (0.92 to 2.44) | 1.28 (0.74 to 2.20) |
Five or more violent incidents | 20 (4.6) | 2.40 (1.46 to 3.92)** | 1.09 (0.50 to 2.38) |
Victim injured | 26 (6.1) | 2.06 (1.31 to 3.23)** | 1.09 (0.54 to 2.20) |
Perpetrator injured | 30 (7.2) | 2.41 (1.60 to 3.61)** | 1.11 (0.59 to 2.09) |
Police involved | 29 (6.9) | 2.81 (1.87 to 4.21)*** | 1.32 (0.75 to 2.34) |
Victim of violence | |||
Intimate partner | 24 (5.6) | 3.96 (2.60 to 6.02)*** | 1.24 (0.67 to 2.29) |
Family | 7 (1.7) | 1.79 (0.84 to 3.85) | 0.89 (0.37 to 2.13) |
Friend | 17 (4.0) | 2.14 (1.18 to 3.88)* | 1.38 (0.59 to 3.25) |
Known person | 26 (6.2) | 2.07 (1.27 to 3.36)* | 1.07 (0.54 to 2.13) |
Stranger | 33 (7.7) | 1.66 (1.10 to 2.51)* | 1.05 (0.62 to 1.79) |
Police | 5 (1.2) | 2.22 (0.88 to 5.61) | 0.64 (0.22 to 1.85) |
Location of violent incident | |||
Own home | 35 (8.2) | 5.29 (3.60 to 7.77)*** | 2.35 (1.42 to 3.90)** |
Someone else’s home | 5 (1.3) | 1.47 (0.74 to 2.93) | 0.62 (0.27 to 1.41) |
Bar/pub | 24 (5.7) | 1.79 (1.10 to 2.90) | 0.85 (0.42 to 1.73) |
Street | 48 (11.4) | 2.20 (1.56 to 3.10)*** | 1.31 (0.78 to 2.19) |
Workplace | 7 (1.6) | 2.68 (1.08 to 6.65) | 2.20 (0.83 to 5.83) |
Violence classes and depression
Latent class models derived five violence classes for men and three violence classes for women in this joint data set as described in Chapter 2 (see Study 2). Tables 20 and 21 show the prevalence of depression among men and women in the different violence classes respectively. Class 5 in men, the serious repetitive violence class, and class 3 in women, IPV, showed the highest prevalence of depression.
Violence typologies | Depression, n (%) | RRRa (95% CI) | RRRb (95% CI) |
---|---|---|---|
No violence | 137 (2.1) | Reference | Reference |
Minor violence | 10 (2.3) | 1.21 (0.50 to 2.87) | 0.94 (0.40 to 2.23) |
Violence towards known persons | 15 (4.9) | 2.40 (1.20 to 4.78) | 1.21 (0.54 to 2.67) |
Fighting with strangers | 9 (3.0) | 1.51 (0.64 to 3.54) | 0.86 (0.36 to 2.06) |
Serious repetitive violence | 11 (9.4) | 4.20 (1.64 to 10.76)* | 1.63 (0.41 to 6.48) |
Violence typologies | Depression, n (%) | RRRa (95% CI) | RRRb (95% CI) |
---|---|---|---|
No violence | 210 (2.8) | Reference | Reference |
General violence | 20 (7.2) | 2.46 (1.35 to 4.47)** | 1.63 (0.80 to 3.34) |
Intimate/family violence | 13 (10.6) | 3.63 (2.09 to 6.31)*** | 2.15 (1.17 to 3.96)** |
Table 20 shows that, following adjustments for demographics, only class 5 among men (serious repetitive violence) was associated with depression. However, following further adjustments for other psychiatric morbidity, this was no longer significant. Table 21 shows the same analyses for the three violent classes in women. A strong association with depression remained among women who were violent towards intimate partners following adjustments for demographics and other psychiatric morbidity.
Discussion
This study found few independent associations between depression and violence. The findings indicate that the associations initially observed following adjustments for demography were explained by other comorbid psychopathology among depressed people who were violent. However, an independent association was observed between class 3 women who were violent towards their partners and members of their family and this remained significant following adjustments for comorbidity. This finding was supported by the only other independent association that we observed, with violence in the perpetrator’s home, corresponding to the location where violence against these victims was most likely to occur.
An epidemiological study previously found that depression was associated with an increased risk of violent behaviour in the US population. 24 However, this survey did not adjust for comorbid psychopathology. Furthermore, because no independent measure of violence was originally used at the fieldwork stage, criteria items for ASPD were used instead and ASPD could not, therefore, be used as an adjustment. Nevertheless, other epidemiological studies have argued that depression is associated with an increased risk of violent behaviour towards a spouse,105,106 in agreement with our findings. Koh et al. 107 found that patients with depression demonstrated more anger and expressions of anger than patients with anxiety disorders. However, our representative community sample suggested that the association with anxiety disorder was stronger than the association with depressive disorder when the outcome was actual violence.
These findings do not support the psychoanalytical theory that conflicts about anger play a central role in the development of depression. Anger in people with depression is thought to stem from narcissistic vulnerability, a sensitivity to perceived or actual loss or rejection. These angry reactions are then thought to cause intrapsychic conflicts through the onset of guilt and fear that angry feelings will disrupt relationships. These conflicts lead to anger being directed inwards and further lowered self-esteem, creating a vicious cycle. Defence mechanisms that are triggered are ineffective in managing these conflicts and are thought to further prevent the appropriate expression of anger. 108 Certain features of this psychodynamic formulation show some correspondence with our observations for IPV, but for anxiety disorder rather than depression (see Study 1, Discussion). Furthermore, the anger (or proneness to violence) thought to stem from a narcissistic vulnerability in this study could be considered a comorbid form of psychopathology, such as the narcissistic personality components of ASPD, as the key factor leading to violence, and not depression.
Study 3: psychosis
Objectives
The objectives of this study were to investigate:
-
the prevalence of violent behaviour in a large representative sample of the adult population of Great Britain associated with psychosis and symptoms of psychosis
-
the independent associations between psychosis and psychotic symptoms and characteristics of violence, victim types and locations of violence
-
the independent associations between psychosis and symptoms of psychosis and the classes of violence identified in the population.
Methods
Sample
For the purposes of this analysis we combined two data sets, the NHPMS 2000 and the APMS 2007, to provide a total of 15,734 subjects.
The PSQ was used to assess the experience of five common symptoms of psychosis: hypomania, thought interference, paranoid delusions, strange experiences and auditory and visual hallucinations. 51 We used a cut-off point of three or more symptoms using the PSQ to identify a categorical diagnosis of psychosis.
Statistical analyses
Weighted (n) frequencies and proportions were reported on all categorical variables. Group associations between psychosis and violence were established using binary logistic regression with the OR as the measure of magnitude. Multiple categorical predictor covariates were assigned a reference category against which other categories were contrasted.
All statistical analyses were adjusted by including covariates in each model simultaneously. Adjustments were carried out for demographic factors, drug dependency, alcohol dependency, ASPD and anxiety disorders. To adjust for the effects of selecting one individual per household and the under-representation of certain subgroups, and to account for any deviation from selecting a simple random sample, all estimates were weighted. Details of the procedures used in weighting have previously been described. 45 All models employed robust SEs to adjust for clustering of individuals within postcodes. All analyses were performed using Stata version 13 (StataCorp LP, College Station, TX, USA).
Results
Demographic characteristics
Of 15,743 respondents, 1596 (10.1%) reported violence in the past 5 years. Table 22 shows that sociodemographic factors of male sex, marital status other than married and social class lower than I and II were significantly associated with violence, whereas age > 34 years was protective. The weighted count and prevalence of psychosis in this joint sample was 55 (0.34%). Separated marital status and black ethnicity were demographic factors associated with an increased risk of psychosis. Anxiety disorder was significantly associated with psychosis in adjusted models.
Covariate | Any violence in the past 5 years (n = 1596; 10.1%) | Psychosis (n = 55; 0.3%) | ||
---|---|---|---|---|
n (%) | AORa (95% CI) | n (%) | AORa (95% CI) | |
Sex | ||||
Female | 406 (5.1) | Reference | 28 (0.4) | Reference |
Male | 1190 (15.4) | 3.41 (2.89 to 4.04)*** | 27 (0.3) | 0.99 (0.52 to 1.89) |
Age group (years) | ||||
16–34 | 1175 (22.4) | Reference | 20 (0.4) | Reference |
35–54 | 371 (6.3) | 0.33 (0.28 to 0.39)*** | 28 (0.5) | 3.35 (1.02 to 10.98)* |
≥ 55 | 51 (1.1) | 0.06 (0.05 to 0.09)*** | 8 (0.2) | 2.18 (0.51 to 9.43) |
Marital status | ||||
Married/cohabiting | 480 (4.7) | Reference | 19 (0.2) | Reference |
Single | 973 (23.4) | 1.80 (1.51 to 2.14)*** | 22 (0.5) | 2.10 (0.68 to 6.49) |
Separated/divorced | 144 (9.9) | 2.03 (1.62 to 2.56)*** | 14 (1.0) | 3.90 (1.81 to 8.40)*** |
Social class | ||||
I and II | 302 (5.6) | Reference | 12 (0.2) | Reference |
IIIM and IIINM | 708 (11.3) | 2.11 (1.76 to 2.54)*** | 10 (0.2) | 0.72 (0.31 to 1.68) |
IV and V | 398 (12.6) | 2.41 (1.94 to 3.00)*** | 19 (0.6) | 1.76 (0.77 to 4.02) |
Ethnicity | ||||
White | 1463 (10.2) | Reference | 36 (0.3) | Reference |
Black | 49 (12.1) | 0.91 (0.57 to 1.44) | 12 (2.8) | 8.34 (3.29 to 21.13)*** |
Indian subcontinent | 36 (7.0) | 0.46 (0.26 to 0.81)** | 4 (0.8) | 2.14 (0.62 to 7.39) |
Other | 40 (11.2) | 0.91 (0.54 to 1.52) | 0 (0.0) | Collapsed |
Drug dependency | 276 (48.4) | 2.32 (1.75 to 3.09)*** | 8 (1.5) | 1.46 (0.44 to 4.85) |
Alcohol dependency | 371 (35.3) | 2.20 (1.76 to 2.75)*** | 10 (1.0) | 1.75 (0.60 to 5.09) |
Anxiety disorder | 375 (16.2) | 1.86 (1.55 to 2.24)*** | 39 (1.6) | 10.74 (4.89 to 23.57)*** |
ASPD | 246 (46.2) | 2.85 (2.10 to 3.87)*** | 5 (1.0) | 1.25 (0.37 to 4.24) |
Associations of psychosis with violence
Table 23 shows the unadjusted and adjusted associations of psychosis with level of severity of violence, victim types and locations of reported violence. Before adjustment, psychosis as a categorical construct showed associations only with violence towards a friend and in an unspecified location. After adjustments there were no associations between psychosis and violence in the household population.
Outcomes | n (%) violent | OR (CI 95%) | AORa (CI 95%) |
---|---|---|---|
Any violence | 1596 (10.1) | 1.54 (0.61 to 3.86) | 0.54 (0.16 to 1.84) |
Violence while intoxicated | 685 (4.3) | 1.44 (0.46 to 4.51) | 0.56 (0.19 to 1.61) |
Severity of violence | |||
Minor violence | 655 (4.2) | 1.10 (0.21 to 5.67) | 0.21 (0.03 to 1.60) |
Five or more violent incidents | 335 (2.1) | 1.71 (0.53 to 5.54) | 1.49 (0.50 to 4.48) |
Victim injured | 505 (3.2) | 1.70 (0.47 to 6.21) | 0.62 (0.14 to 2.76) |
Perpetrator injured | 514 (3.3) | 1.59 (0.44 to 5.81) | 0.49 (0.13 to 1.90) |
Police involved | 431 (2.7) | 2.12 (0.55 to 8.22) | 1.60 (0.34 to 7.49) |
Victim of violence | |||
Intimate partner | 252 (1.6) | 1.17 (0.28 to 4.92) | 0.46 (0.11 to 1.83) |
Family member | 153 (1.0) | 1.00 (1.00 to 1.00) | 1.00 (1.00 to 1.00) |
Friend | 312 (2.0) | 3.89 (1.07 to 14.20)* | 0.58 (0.10 to 3.37) |
Person known | 511 (3.2) | 1.19 (0.24 to 5.76) | 0.23 (0.04 to 1.31) |
Stranger | 783 (5.0) | 1.55 (0.44 to 5.51) | 1.08 (0.19 to 6.27) |
Police | 88 (0.6) | 2.22 (0.30 to 16.33) | 1.69 (0.18 to 15.64) |
Other | 108 (0.7) | 1.79 (0.24 to 13.16) | 2.54 (0.33 to 19.30) |
Location of violent incident | |||
Own home | 292 (1.9) | 1.23 (0.29 to 5.15) | 0.56 (0.15 to 2.07) |
Someone else’s home | 138 (0.9) | 4.19 (0.84 to 20.98) | 0.55 (0.07 to 4.69) |
Street | 909 (5.8) | 1.51 (0.48 to 4.77) | 0.87 (0.20 to 3.91) |
Bar/pub | 541 (3.4) | 1.91 (0.61 to 6.01) | 0.81 (0.29 to 2.26) |
Workplace | 101 (0.6) | 2.27 (0.31 to 16.70) | 1.59 (0.23 to 11.12) |
Other | 241 (1.5) | 5.37 (1.51 to 19.15)** | 1.35 (0.16 to 11.30) |
Individual psychotic symptoms and characteristics of violence
Analyses of the associations between all violent outcomes and each psychotic symptom in the PSQ were carried out (Table 24). There were no associations between hypomania and violence. Thought insertion significantly increased the risk for any reported violence, violence in which the police were involved and violence towards a stranger. The paranoid delusions item showed the largest number of associations – with any violence, violence when intoxicated, five or more violent incidents, victim injured, violence against a known person, violence occurring in the street and violence occurring in a bar or pub. Strange experiences doubled the risk of IPV. Hallucinations were not associated with any of the violent outcomes.
Outcomes | Hypomania, AORa (95% CI) | Thought insertion, AORa (95% CI) | Paranoid delusions, AORa (95% CI) | Strange experiences, AORa (95% CI) | Hallucinations, AORa (95% CI) |
---|---|---|---|---|---|
Any violence | 0.97 (0.37 to 2.56) | 1.85 (1.05 to 3.26)* | 1.75 (1.03 to 2.96)* | 1.03 (0.68 to 1.56) | 0.92 (0.48 to 1.74) |
Violence while intoxicated | 0.20 (0.02 to 2.23) | 1.50 (0.64 to 3.47) | 1.90 (1.07 to 3.35)* | 1.12 (0.65 to 1.95) | 1.46 (0.63 to 3.34) |
Severity of violence | |||||
Minor violence | 0.76 (0.16 to 3.65) | 1.51 (0.68 to 3.38) | 1.03 (0.50 to 2.12) | 0.69 (0.37 to 1.30) | 0.55 (0.16 to 1.87) |
Five or more violent incidents | 0.58 (0.08 to 4.16) | 1.60 (0.73 to 3.51) | 3.59 (1.83 to 7.05)*** | 0.55 (0.29 to 1.05) | 2.25 (0.89 to 5.72) |
Victim injured | 1.63 (0.45 to 5.81) | 0.69 (0.20 to 2.33) | 2.15 (1.13 to 4.09)* | 0.97 (0.50 to 1.87 | 1.34 (0.51 to 3.47) |
Perpetrator injured | 1.65 (0.57 to 4.77) | 1.29 (0.53 to 3.17) | 1.42 (0.77 to 2.63) | 0.91 (0.51 to 1.62) | 1.83 (0.87 to 3.86) |
Police involved | 1.25 (0.34 to 4.57) | 3.07 (1.44 to 6.56)** | 1.36 (0.72 to 2.57) | 1.43 (0.80 to 2.55) | 1.21 (0.53 to 2.77) |
Victim of violence | |||||
Intimate partner | 1.26 (0.39 to 4.03) | 1.16 (0.51 to 2.64) | 1.44 (0.69 to 2.99) | 2.04 (1.08 to 3.85)* | 0.82 (0.28 to 2.36) |
Family member | 1.57 (0.29 to 8.32) | 2.71 (0.85 to 8.61) | 1.41 (0.55 to 3.63) | 1.16 (0.41 to 3.29) | 2.40 (0.72 to 7.97) |
Friend | 1.41 (0.16 to 12.22) | 1.12 (0.32 to 3.90) | 2.01 (0.83 to 4.87) | 1.17 (0.54 to 2.52) | 0.76 (0.18 to 3.13) |
Person known | 0.93 (0.16 to 5.52) | 0.64 (0.22 to 1.83) | 1.96 (1.05 to 3.65)* | 0.79 (0.40 to 1.54) | 1.10 (0.40 to 2.99) |
Stranger | 0.67 (0.17 to 2.65) | 2.91 (1.31 to 6.45)** | 1.45 (0.79 to 2.68) | 0.82 (0.48 to 1.39) | 1.27 (0.58 to 2.79) |
Police | 2.32 (0.25 to 21.56) | 3.32 (0.71 to 15.49) | 1.50 (0.47 to 4.83) | 1.28 (0.46 to 3.54) | 2.25 (0.66 to 7.73) |
Other | 1.67 (0.19 to 14.25) | 3.17 (0.81 to 12.36) | 2.75 (0.83 to 9.06) | 0.90 (0.28 to 2.92) | 1.05 (0.15 to 7.21) |
Location of violent incident | |||||
Own home | 1.80 (0.61 to 5.34) | 1.35 (0.63 to 2.92) | 0.99 (0.50 to 1.96) | 1.19 (0.65 to 2.18) | 1.82 (0.73 to 4.54) |
Someone else’s home | 0.62 (0.08 to 5.05) | 1.45 (0.34 to 6.23) | 1.68 (0.58 to 4.85) | 0.84 (0.31 to 2.27) | 1.09 (0.24 to 4.94) |
Street | 1.12 (0.33 to 3.74) | 1.03 (0.46 to 2.30) | 1.84 (1.00 to 3.37)* | 0.90 (0.55 to 1.47) | 1.51 (0.72 to 3.13) |
Bar/pub | 0.57 (0.12 to 2.78) | 1.20 (0.44 to 3.28) | 2.22 (1.25 to 3.95)** | 0.86 (0.46 to 1.63) | 0.89 (0.33 to 2.41) |
Workplace | 1.24 (0.15 to 10.54) | 0.75 (0.17 to 3.34) | 1.26 (0.26 to 6.16) | 0.77 (0.26 to 2.30) | 1.57 (0.38 to 6.41) |
Other | 1.00 (1.00 to 1.00) | 3.56 (0.97 to 13.09) | 3.09 (1.21 to 7.86)* | 0.53 (0.21 to 1.37) | 1.73 (0.49 to 6.10) |
Violence classes and psychosis
Latent class models derived five violence classes for men and three violence classes for women (see Chapter 2, Study 2). Tables 25 and 26 show the associations between these classes and psychosis for men and women respectively.
Violence typologies | Psychosis, n (%) | RRRa (95% CI) | RRRb (95% CI) |
---|---|---|---|
No violence | 22 (0.3) | Reference | Reference |
Minor violence | 2 (0.4) | 0 (0 to 0) | 0 (0 to 0) |
Violence towards known persons | 1 (0.2) | 0.70 (0.10 to 5.03) | 0.43 (0.06 to 2.80) |
Fighting with strangers | 2 (0.6) | 3.33 (0.37 to 29.63) | 2.15 (0.25 to 18.49) |
Serious repetitive violence | 1 (0.5) | 3.36 (0.35 to 31.89) | 2.03 (0.17 to 23.92) |
Violence typologies | Psychosis, n (%) | RRRa (95% CI) | RRRb (95% CI) |
---|---|---|---|
No violence | 24 (0.3) | Reference | Reference |
General violence | 2 (0.8) | 1.22 (0.24 to 6.29) | 0.62 (0.13 to 3.01) |
Intimate/family violence | 0 (0.0) | 0 (0 to 0) | 0 (0 to 0) |
The ‘no violence’ class had the lowest prevalence of psychosis (0.3%). Prevalences were < 1% for all classes and only marginally increased above the population base rate (0.3%). No significant associations were found between the latent classes and psychosis.
Violence typologies and individual psychotic symptoms
The frequencies and percentages of individual psychotic symptoms observed for each violence typology class are provided in Table 27 for men and Table 28 for women.
Psychotic symptoms (PSQ)a | Class | ||||
---|---|---|---|---|---|
No violence, n (%) | Minor violence, n (%) | Violence towards known persons, n (%) | Fighting with strangers, n (%) | Serious repetitive violence, n (%) | |
Hypomania, 0.7% | 43 (0.7) | 5 (1.0) | 1 (0.5) | 3 (0.9) | 0 (0.0) |
Thought insertion, 0.9% | 55 (0.8) | 4 (1.0) | 4 (1.2) | 6 (2.1) | 3 (2.4) |
Paranoid delusions, 2.1% | 95 (1.4) | 14 (3.1) | 20 (6.9) | 12 (3.8) | 20 (16.7) |
Strange experiences, 3.0% | 165 (2.5) | 14 (3.2) | 15 (5.0) | 22 (7.0) | 21 (16.9) |
Hallucinations, 0.8% | 42 (0.6) | 4 (0.9) | 2 (0.6) | 7 (2.4) | 5 (4.5) |
Psychotic symptoms (PSQ)a | Class | ||
---|---|---|---|
No violence, n (%) | General violence, n (%) | Intimate/family violence, n (%) | |
Hypomania, 0.6% | 38 (0.5) | 6 (2.2) | 3 (2.3) |
Thought insertion, 1.0% | 66 (0.9) | 11 (3.9) | 4 (3.3) |
Paranoid delusions, 1.4% | 86 (1.1) | 20 (7.0) | 2 (1.6) |
Strange experiences, 3.3% | 216 (2.8) | 27 (9.6) | 13 (10.4) |
Hallucinations, 1.0% | 74 (1.0) | 5 (1.9) | 2 (1.3) |
In men, both ‘paranoid delusions’ and ‘strange experiences’ appear over-represented in the classes ‘violence towards known persons’ and ‘serious repetitive violence’. Association tests after adjusting for the other psychotic symptoms showed that ‘paranoid delusions’ was the only psychotic symptom associated with any violence classes, namely ‘violence towards known persons’ and ‘serious repetitive violence’ (Table 29).
Psychotic symptoms (PSQ) | Class | ||||
---|---|---|---|---|---|
No violence, RRRa (95% CI) | Minor violence, RRRa (95% CI) | Violence towards known persons, RRRa (95% CI) | Fighting with strangers, RRRa (95% CI) | Serious repetitive violence, RRRa (95% CI) | |
Hypomania | Reference | 0.88 (0.12 to 6.32) | 0.73 (0.10 to 5.37) | 1.18 (0.25 to 5.48) | 0 (0 to 0) |
Adjustedb | Reference | 0.76 (0.09 to 6.19) | 0.55 (0.05 to 5.52) | 0.99 (0.19 to 5.03) | 0 (0 to 0) |
Thought insertion | Reference | 1.46 (0.54 to 3.96) | 1.44 (0.47 to 4.43) | 2.67 (0.89 to 8.01) | 5.23 (1.28 to 21.32)* |
Adjustedb | Reference | 1.61 (0.49 to 5.29) | 1.14 (0.35 to 3.74) | 1.35 (0.43 to 4.26) | 2.06 (0.48 to 8.76) |
Paranoid delusions | Reference | 1.77 (0.74 to 4.25) | 4.43 (2.14 to 9.16)*** | 2.57 (1.15 to 5.75)* | 15.22 (6.57 to 35.27)*** |
Adjustedb | Reference | 1.43 (0.53 to 3.88) | 2.69 (1.16 to 6.25)* | 1.06 (0.46 to 2.46) | 4.68 (1.62 to 13.51)** |
Strange experiences | Reference | 0.84 (0.38 to 1.88) | 0.94 (0.43 to 2.03) | 2.67 (1.47 to 4.84)** | 5.26 (2.56 to 10.78)*** |
Adjustedb | Reference | 0.58 (0.24 to 1.42) | 0.39 (0.15 to 1.00) | 1.33 (0.68 to 2.60) | 1.20 (0.45 to 3.21) |
Hallucinations | Reference | 0.46 (0.06 to 3.62) | 0.88 (0.17 to 4.53) | 3.93 (1.46 to 10.53)** | 7.00 (1.50 to 32.64)* |
Adjustedb | Reference | 0.37 (0.04 to 3.18) | 0.54 (0.08 to 3.59) | 2.29 (0.86 to 6.08) | 2.18 (0.43 to 11.12) |
For women, the most salient psychotic symptom observed in the classes ‘general violence’ and ‘intimate/family violence’ was ‘strange experiences’ (see Table 28). However, after adjusting for the other psychotic symptoms, only ‘thought insertion’ significantly increased the likelihood of membership of the class ‘general violence’ (Table 30).
Psychotic symptoms (PSQ) | Class | ||
---|---|---|---|
No violence, RRRa (95% CI) | General violence, RRRa (95% CI) | Intimate/family violence, RRRa (95% CI) | |
Hypomania | Reference | 1.21 (0.23 to 6.47) | 3.43 (1.06 to 11.07)* |
Adjustedb | Reference | 1.20 (0.21 to 6.75) | 3.08 (0.93 to 10.22) |
Thought insertion | Reference | 4.94 (2.33 to 10.47)*** | 3.32 (1.23 to 8.91)* |
Adjustedb | Reference | 3.73 (1.54 to 9.07)** | 2.63 (0.89 to 7.77) |
Paranoid delusions | Reference | 4.95 (2.40 to 10.19)*** | 1.20 (0.44 to 3.30) |
Adjustedb | Reference | 2.31 (0.87 to 6.11) | 0.45 (0.13 to 1.55) |
Strange experiences | Reference | 2.54 (1.43 to 4.53)** | 3.29 (1.59 to 6.81)** |
Adjustedb | Reference | 1.24 (0.56 to 2.77) | 2.13 (0.89 to 5.12) |
Hallucinations | Reference | 1.41 (0.52 to 3.80) | 1.20 (0.27 to 5.38) |
Adjustedb | Reference | 0.43 (0.13 to 1.42) | 0.44 (0.09 to 2.25) |
Discussion
Epidemiological studies have demonstrated that the key risk factors for violence among those with psychosis are the same as those among the general population, after adjustment for comorbid psychopathology. 83,109–112 Considerable emphasis has been placed on the possibility that most of the associations (or indeed the entire association) between psychotic illness and violence are the result of co-occurring substance misuse disorder. 88,110 We did not find a significant association between violence in general in the population and our categorical measure of psychosis after adjustments, including for substance dependence. Prior to adjustments we found an association with violence towards friends and violence in ‘other’ locations, but these associations were no longer present following adjustments. Furthermore, there was considerable change in the odds of association and, although no longer significant, there was a trend for psychosis to have a negative association with several violent outcomes.
Examining the CIs, the power to detect an association in this sample was low. Only 0.3% of the population was classified as psychotic using the PSQ. This is most likely because we chose a high cut-off point of three out of five PSQ items. We have previously investigated the association in the 2000-household sample using a lower cut-off point of two or more items. 83 However, the prevalence of those screening positive was still lower than might be expected at 0.6%. Furthermore, after adjustments in this previous study, we did not find associations with any of the outcomes, including levels of violence severity, victim types or locations of the violence, except for a significant association with five or more violent incidents. 30 Taken together, our current findings would tend to confirm our previous findings that comorbid psychopathology is the explanatory variable in a pathway between a categorical diagnosis of psychotic illness and violence.
More recently, several researchers have begun to reconsider the association between violence and psychosis and the observation that the risk of violence is higher when the symptoms of the psychosis are active. 113–119 However, associations between psychotic symptoms and violence at the population level have not been firmly established. Earlier studies challenged the classical ‘syndrome’ approach and promoted the investigation of single symptoms. 120,121 By investigating individual symptoms, we confirmed a much stronger association with violence, but only for symptoms of thought insertion, strange experiences and paranoid delusions. In general, the strongest odds of association and the largest number of outcomes were observed in the associations between violence and paranoid delusions. Paranoid delusions were associated with violence when intoxicated, repetitive violence (five or more incidents) and injury to a victim. There was a specific association with persons known and these incidents were more likely to occur in the street and in a bar/pub as well as in ‘other’ locations. Paranoid delusions were also independently associated with membership of class 5 for men (‘serious repetitive violence’) and class 3 for women (‘intimate/family violence’).
Our findings therefore correspond to those of previous studies showing that, among a range of psychotic symptoms, persecutory delusions are most strongly associated with violence in community surveys. 111,122–124 However, the findings relating to hallucinations, thought interference and external influences or control were inconsistent in these studies. The considerable methodological heterogeneity of studies, previously observed in meta-analyses of clinical psychosis, is likely to explain this. 91
Among the other symptoms, we found that thought insertion had an independent association with any violence, violent incidents that were serious enough for the police to become involved and violent incidents involving a stranger. There was no association between the violence classes among men and thought insertion. However, this symptom was associated with the general violence class (class 2) among women.
Strange experiences did not show an association with any of the levels of violence severity or locations. This symptom was found to show an association only with IPV. There were also no associations between strange experiences and the classes of violence for men or for women in this sample.
Chapter 4 Personality disorders
Background
Most mental health professionals believe that there is a strong relationship between personality disorders and violence. In the past, this has led to reluctance to accept patients with this diagnosis. 125 Longitudinal studies have provided strong evidence of personality disorders representing a significant risk for future violence. 30,126,127 Personality disorder symptoms were found to be even stronger predictors of violence than overall diagnosis. In particular, items from personality disorders included in cluster A and cluster B disorders corresponded to violence in the community. Overall, paranoid, narcissistic, passive–aggressive personality disorder symptoms correlated significantly with violence. These findings remained significant after controlling for Axis I disorders and demography. However, it was of significant interest that these researchers did not find any associations between violence and BPD.
These findings were generally confirmed in a survey of adults in households in Great Britain, with those with cluster B disorders (including ASPD, BPD and narcissistic personality disorder) being 10 times as likely to be violent as those without. 30 However, it is probable that a single cluster B disorder, ASPD, had primarily accounted for the raised risk. One possibility considered by previous researchers is that clinical and forensic studies that have a high prevalence of BPD are likely to have sampled a more severe form of this condition than is studied in community samples. An elevated risk for violence might be evident only in prisoners and in hospitalised patients with severe conditions. These are typically characterised by poor emotional regulation and impulse control leading to behaviour that in turn results in hospitalisation. 126,127 Nevertheless, Roberts and Coid128 did not find an association between violent offending and BPD in a representative sample of UK prisoners. Conduct disorder and adult antisocial symptoms using continuous scores demonstrated the highest and most frequent associations with criminal behaviour including violence. ASPD was thought to demonstrate extensive overlap with the criminological construct of the career criminal. 129,130 Previous studies of offending behaviour among individuals with ASPD have consistently shown an association with crimes involving financial gain, including burglary and theft, robbery and firearm offences,131 and violent offences. 132–134
It is unsurprising that ASPD is found to be associated with violence because certain criteria for diagnosing ASPD actually include violent behaviour. The essential feature of ASPD is a pervasive pattern of disregard for, and violation of, the rights of others that begins in childhood or early adolescence and continues into adulthood. For the diagnosis to be given, the individual must be at least 18 years of age and have had a history of some symptoms of conduct disorder before the age of 15 years. Conduct disorder involves a repetitive and persistent pattern of behaviour in which the basic rights of others or major age-appropriate societal norms or rules are violated. 135 BPD is defined as a pervasive pattern of instability of interpersonal relationships, self-image and affects and marked impulsivity, beginning by early adulthood and present in a variety of contexts. 135 It might be expected that criteria for BPD, which include unstable and intense interpersonal relationships, affective instability because of a marked reactivity of mood, and inappropriate, intense anger or difficulty controlling anger, might be associated with violent behaviour despite previous studies failing to find this association. In this chapter we report an investigation of the associations of ASPD and BPD with violence in a large representative sample of the population of Great Britain. Although the DSM-IV Axis II Personality Disorders (SCID-II) screening questionnaire includes 10 personality disorder categories, only ASPD and BPD have consistently been included in the National Household Surveys. Furthermore, these two personality disorders are the only personality disorders that are currently within National Institute for Health and Care Excellence (NICE) guidelines for treatment and management. 136,137
Study 1: antisocial personality disorder
Objectives
The objectives of the study were to investigate:
-
the prevalence of self-reported violence associated with ASPD in a large representative sample of the household population of Great Britain
-
the independent associations of ASPD with different characteristics of violence, victim types and locations of violent behaviour
-
the association between ASPD and comorbid psychiatric disorder and violence
-
the association between ASPD and latent classes of violence among men and women.
Methods
Sample
For the purpose of this analysis we combined two data sets, the NHPMS 2000 and the APMS 2007, to provide a total of 15,734 subjects.
Antisocial personality disorder was identified using the SCID-II screen. 53 Participants gave ‘yes’ or ‘no’ responses to questions administered on a laptop computer. The ASPD category of the Diagnostic and Statistical Manual of Mental Disorders, Fourth edition (DSM-IV) Axis II138 was created by manipulating cut-off points to increase levels of agreement, measured by the kappa coefficient, between both individual criteria and clinical diagnoses. 52
Analysis
Weighted (n) frequencies and proportions were reported on all categorical variables. Group associations between ASPD and violence were established using binary logistic regression with the OR as the measure of magnitude. Multiple categorical predictor covariates were assigned a reference category against which other categories were contrasted.
All statistical analyses were adjusted by including covariates in each model simultaneously. Adjustments were carried out for demographic factors, depression, drug dependency, alcohol dependency, screening positive for psychosis (three or more symptoms on the PSQ) and anxiety disorders. To adjust for the effects of selecting one individual per household and under-representation of certain subgroups, and to account for any deviation from selecting a simple random sample, all estimates were weighted. Details of the procedures used in weighting have been described previously. 45,82 All models employed robust SEs to adjust for clustering of individuals within postcodes. All analyses were performed using Stata.
Results
Demographic characteristics
Of the 15,734 respondents, 1596 (10.1%) reported any violence in the past 5 years. Table 31 shows that male sex, marital status other than married and social class lower than I and II were significantly associated with violence, whereas age > 34 years was protective. The overall prevalence of ASPD in the sample was 3.4%. The older age group (≥ 55 years) showed a significantly lower rate of ASPD. Being male, separated or divorced and from social classes IV and V showed significantly increased associations with ASPD. Anxiety disorders and drug and alcohol dependency were also significantly associated with ASPD in adjusted models.
Covariate | Any violence in the past 5 years (n = 1596; 10.1%) | ASPD (n = 542; 3.4%) | ||
---|---|---|---|---|
n (%) | AORa (95% CI) | n (%) | AORa (95% CI) | |
Sex | ||||
Female | 406 (5.1) | Reference | 127 (1.6) | Reference |
Male | 1190 (15.4) | 3.57 (3.02 to 4.21)*** | 414 (5.3) | 3.30 (2.52 to 4.31)*** |
Age group (years) | ||||
16–34 | 1175 (22.4) | Reference | 320 (6.1) | Reference |
35–54 | 371 (6.3) | 0.33 (0.28 to 0.39)*** | 182 (3.1) | 0.67 (0.51 to 0.90)** |
≥ 55 | 51 (1.1) | 0.06 (0.05 to 0.08)*** | 40 (0.9) | 0.25 (0.17 to 0.36)*** |
Marital status | ||||
Married/cohabiting | 480 (4.7) | Reference | 209 (2.1) | Reference |
Single | 973 (23.4) | 1.78 (1.49 to 2.13)*** | 265 (6.3) | 1.26 (0.92 to 1.72) |
Separated/divorced | 144 (9.9) | 2.09 (1.66 to 2.63)*** | 68 (4.7) | 1.77 (1.29 to 2.43)*** |
Social class | ||||
I and II | 302 (5.6) | Reference | 139 (2.6) | Reference |
IIIM and IIINM | 708 (11.3) | 2.11 (1.76 to 2.53)*** | 227 (3.6) | 1.24 (0.95 to 1.61) |
IV and V | 398 (12.6) | 2.47 (1.99 to 3.06)*** | 147 (4.6) | 1.60 (1.18 to 2.17)** |
Ethnicity | ||||
White | 1463 (10.2) | Reference | 496 (3.4) | Reference |
Black | 49 (12.1) | 0.90 (0.57 to 1.44) | 14 (3.5) | 0.90 (0.47 to 1.73) |
Indian subcontinent | 36 (7.0) | 0.44 (0.25 to 0.78)** | 9 (1.8) | 0.47 (0.18 to 1.26) |
Other | 40 (11.2) | 0.90 (0.54 to 1.51) | 17 (4.6) | 1.19 (0.56 to 2.52) |
Drug dependency | 276 (48.4) | 2.93 (2.22 to 3.86)*** | 159 (27.8) | 6.12 (4.32 to 8.67)*** |
Alcohol dependency | 371 (35.3) | 2.38 (1.91 to 2.96)*** | 170 (16.1) | 2.47 (1.80 to 3.39)*** |
Anxiety disorder | 375 (16.2) | 2.00 (1.67 to 2.40)*** | 190 (8.1) | 2.61 (2.00 to 3.40)*** |
Psychosis | 7 (14.0) | 0.52 (0.16 to 1.70) | 5 (10.7) | 1.20 (0.36 to 3.99) |
Main associations of antisocial personality disorder with violence
Table 32 shows unadjusted and adjusted associations of ASPD with violent outcomes, victim types and locations of reported violence. The only violent outcomes that ASPD did not show associations with following adjustments were minor violence, violence towards a friend and violence occurring in someone else’s home.
Outcomes | n (%) | OR (CI 95%) | AORa (CI 95%) |
---|---|---|---|
Any violence | 1596 (10.1) | 8.57 (6.89 to 10.66)*** | 2.81 (2.07 to 3.82)*** |
Violence while intoxicated | 685 (4.3) | 11.40 (8.82 to14.74)*** | 3.14 (2.21 to 4.45)*** |
Severity of violence | |||
Minor violence | 655 (4.2) | 2.52 (1.81 to 3.52)*** | 1.03 (0.67 to 1.58) |
Five or more violent incidents | 335 (2.1) | 10.54 (7.48 to 14.84)*** | 2.77 (1.75 to 4.37)*** |
Victim injured | 505 (3.2) | 12.19 (9.23 to 16.11)*** | 3.98 (2.74 to 5.77)*** |
Perpetrator injured | 514 (3.3) | 9.93 (7.50 to 13.14)*** | 3.04 (2.08 to 4.45)*** |
Police involved | 431 (2.7) | 10.44 (7.72 to 14.11)*** | 3.17 (2.19 to 4.60)*** |
Victim of violence | |||
Intimate partner | 252 (1.6) | 9.99 (7.02 to 14.23)*** | 3.98 (2.40 to 6.60)*** |
Family member | 153 (1.0) | 6.80 (4.00 to 11.56)*** | 3.08 (1.66 to 5.71)*** |
Friend | 312 (2.0) | 5.68 (3.83 to 8.42)*** | 1.40 (0.83 to 2.35) |
Person known | 511 (3.2) | 8.17 (6.01 to 11.10)*** | 2.52 (1.65 to 3.84)*** |
Stranger | 783 (5.0) | 7.36 (5.62 to 9.64)*** | 2.16 (1.54 to 3.04)*** |
Police | 88 (0.6) | 26.07(15.63 to 43.48)*** | 7.69 (4.25 to13.90)*** |
Other | 108 (0.7) | 7.49 (4.23 to 13.26)*** | 3.24 (1.43 to 7.32)** |
Location of violent incident | |||
Own home | 292 (1.9) | 6.85 (4.71 to 9.96)*** | 2.73 (1.63 to 4.55)*** |
Someone else’s home | 138 (0.9) | 7.05 (4.05 to 12.28)*** | 2.15 (1.00 to 4.65) |
Street | 909 (5.8) | 8.64 (6.78 to 11.01)*** | 2.60 (1.87 to 3.62)*** |
Bar/pub | 541 (3.4) | 10.29 (7.75 to 13.67)*** | 2.90 (2.02 to 4.16)*** |
Workplace | 101 (0.6) | 8.58 (4.90 to 15.05*** | 3.47 (1.90 to 6.32)*** |
Other | 241 (1.5) | 6.23 (3.93 to 9.89)*** | 2.03 (1.12 to 3.66)* |
Impact of coexisting disorders on the association of antisocial personality disorder with any violence
We performed logistic regression analyses stratified by comorbid categories of psychiatric morbidity (presence/absence) to examine the impact of specific co-occurring disorders on the association of ASPD with any reported violence. Table 33 shows the results of these adjusted analyses. ASPD was significantly associated with any violence both in the absence and in the presence of comorbid drug dependency, alcohol dependency, psychosis and anxiety disorders. The odds of association for violence and APSD in the presence of each co-occurring disorder were higher than the odds in the absence of the co-occurring disorder. However, these differences were not statistically significant.
Comorbid category | Without comorbidity | With comorbidity |
---|---|---|
Psychosis | 2.81 (2.07 to 3.82)*** | 5.46 (1.62 to 18.35)** |
Drug dependency | 2.66 (1.88 to 3.77)*** | 5.55 (3.24 to 9.52)*** |
Alcohol dependency | 2.79 (1.97 to 3.96)*** | 3.72 (2.24 to 6.18)*** |
Anxiety disorder | 2.85 (1.95 to 4.19)*** | 3.50 (2.27 to 5.42)*** |
Violence classes and antisocial personality disorder
Latent class models derived five violence classes for men and three violence classes for women in this joint data set (see Chapter 2, Study 2). Tables 34 and 35 show the associations between individuals with ASPD and the violence classes for men and for women respectively.
Violence typologies | ASPD, n (%) | RRRa (95% CI) | RRRb (95% CI) |
---|---|---|---|
No violence | 223 (3.4) | Reference | Reference |
Minor violence | 34 (7.6) | 1.76 (1.10 to 2.81)* | 1.35 (0.81 to 2.24) |
Violence towards known persons | 55 (18.6) | 4.26 (2.75 to 6.62)*** | 2.33 (1.37 to 3.98)** |
Fighting with strangers | 55 (18.0) | 4.47 (2.89 to 6.89)*** | 3.00 (1.87 to 4.80)*** |
Serious repetitive violence | 46 (37.7) | 11.59 (6.34 to 21.21)*** | 5.33 (2.77 to 10.27)*** |
Violence typologies | ASPD, n (%) | RRRa (95% CI) | RRRb (95% CI) |
---|---|---|---|
No violence | 74 (1.0) | Reference | Reference |
General violence | 40 (14.3) | 7.18 (4.18 to 12.34)*** | 4.55 (2.54 to 8.14)*** |
Intimate/family violence | 13 (10.5) | 6.51 (2.85 to 14.88)*** | 4.70 (1.78 to12.38)** |
Among men, the ‘no violence’ class had the lowest prevalence of ASPD at 3.4%, followed by ‘minor violence’ at 7.6%. In contrast, the ‘serious repetitive violence’ class had an ASPD prevalence of 37.7%. Using the ‘no violence’ class as a reference and after adjustment for demographics and depression, alcohol dependency, drug dependency, anxiety and psychosis, all other classes of violence were significantly associated with an increased risk for ASPD with the exception of ‘minor violence’ (see Table 34).
Female distribution of ASPD across the classes was 1.0% for the ‘no violence’ class, 14.3% for the ‘general violence’ class and 10.5% for the ‘intimate/family violence’ class. Multinomial logistic regression models to estimate associations between violence latent classes and ASPD showed significant associations, after adjustments, for both violent classes (see Table 35).
Discussion
The demographic characteristics of those in the general population of Great Britain who reported violence in the past 5 years were very similar to the demographic characteristics of those who received a diagnosis of ASPD. In both cases they were more likely to be male, younger, single or separated, of lower social class and with comorbid drug dependency, alcohol dependency and anxiety disorder. Of those with ASPD, 1 in 10 reported that they had been violent in the past 5 years, which corresponds to the somewhat higher prevalence of 14% reported in a meta-analysis by Yu et al. ,139 although this study contained a larger proportion of men and men in clinical and prison settings.
The only associations with violence outcomes, including measures of severity, victim types and locations of the violence, that were not significant were those with minor violence and violence towards friends, occurring in someone else’s home. This corresponds to the highly versatile quality of criminal offending among prisoners with ASPD and the extensive overlap with the criminological construct of the career criminal. 128,130 Previous studies of offending behaviour have shown associations with crimes involving financial gain, including burglary and theft, robbery and firearm offences, together with violent offences and drug offences. Criminal versatility is a component of psychopathy. 140 It is possible that individuals in the community with ASPD come into contact with multiple potential victims through their varied criminal activity. On the other hand, it could be argued that personality traits of deceitfulness, impulsivity, irritability and aggressiveness, reckless disregard for safety of self and others, consistent irresponsibility and lack of remorse mean that they are prone to violently victimise many individuals whom they come into contact with, in multiple locations, irrespective of whether or not they are engaged in criminal activities.
We found that co-occurring drug dependence, psychosis, alcohol dependence and anxiety all increased the odds of association with violence among those with ASPD. However, the difference between those with and those without this comorbid diagnosis was not statistically significant. This would suggest that co-occurring disorders can increase risk but that the risk of violence as a result of the personality disorder itself is already high, so that most of the violent behaviour associated with the diagnosis is explained by ASPD.
Antisocial personality disorder did not discriminate men involved in minor violence (class 2) from those who were not involved in violence. However, classes 3–5 were significantly associated with a diagnosis of ASPD, particularly class 5 (‘serious repetitive violence’), in which more than one-third of men received an ASPD diagnosis. This provides further support that class 5 contains individuals who exhibit early-onset persistent antisocial behaviour, corresponding to Moffitt’s141 classification.
Although it might be expected that class 2 among women (‘general violence’) would have a stronger association with ASPD, it was of some interest that 1 in 10 women involved in IPV as an exclusive category of violent behaviour received a diagnosis of ASPD. However, whether this represents a subgroup of women who have a violent disposition resulting in them becoming particularly aggressive towards their partners and members of their family or whether these women form partnerships with aggressive and antisocial men with whom they come into frequent conflict with cannot be determined by this study.
Study 2: borderline personality disorder
Objectives
The aims of the study were to investigate:
-
the prevalence of self-reported violence associated with BPD in a combined data set representative of the household population of Great Britain
-
the association of BPD with different characteristics of violence, victim types and locations after adjusting for sociodemographic factors and comorbid psychiatric morbidity
-
the impact of comorbid psychiatric disorder on the association of BPD with violence
-
whether or not potential associations between BPD and violence in the household population are sex dependent.
Methods
Sample
For the purpose of this analysis, we combined two data sets, the NHPMS 2000 and the APMS 2007, to provide a total of 15,734 subjects.
Borderline personality disorder was identified using the SCID-II screen. 53 Participants gave ‘yes’ or ‘no’ responses to specific questions derived from the diagnostic criteria administered on a laptop computer. The BPD category of DSM-IV Axis II138 was created by manipulating cut-off points to increase levels of agreement, measured by the kappa coefficient, between both individual criteria and clinical diagnoses.
Statistical analyses
Weighted (n) frequencies and proportions were reported on all categorical variables. Group associations between BPD and violence were established using binary logistic regression with the OR as the measure of magnitude. Multiple categorical predictor covariates were assigned a reference category against which other categories were contrasted.
We quantified the associations between positive BPD classification and the violence typologies described in Chapter 2 (see Study 2).
All statistical analyses were adjusted by including covariates in each model simultaneously. Adjustments were made for demographic factors, drug dependence, alcohol dependence, positive psychosis screening, ASPD and anxiety disorders. To adjust for the effects of selecting one individual per household and under-representation of certain subgroups, and to account for any deviation from selecting a simple random sample, all variance estimates were weighted. Details of the procedures used in weighting have been described previously. 45,82 All models employed robust SEs to adjust for clustering of individuals within postcodes. All analyses were performed using Stata.
Results
Demographic characteristics
Of 15,734 respondents, 1596 (10.1%) reported any violence in the past 5 years. The overall prevalence of BPD in the sample was 1.4%. Table 36 shows that the older age groups (35–54 years and ≥ 55 years) had significantly lower rates of BPD. Sex was not associated with BPD-positive screening. However, in adjusted models, being separated or divorced and being in the lowest social classes were all significantly associated with BPD. Anxiety disorders, drug and alcohol dependence, psychosis and ASPD were also all significantly associated with BPD in adjusted models.
Covariate | Any violence in the past 5 years (n = 1596; 10.1%) | BPD (n = 220; 1.5%) | ||
---|---|---|---|---|
n (%) | AORa (95% CI) | n (%) | AORa (95% CI) | |
Sex | ||||
Female | 406 (5.1) | Reference | 124 (1.6) | Reference |
Male | 1190 (15.4) | 3.41 (2.89 to 4.04)*** | 96 (1.3) | 0.66 (0.46 to 1.02) |
Age groups (years) | ||||
16–34 | 1175 (22.4) | Reference | 138 (2.8) | Reference |
35–54 | 371 (6.3) | 0.33 (0.28 to 0.39)*** | 70 (1.3) | 0.56 (0.38 to 0.83)** |
≥ 55 | 51 (1.1) | 0.06 (0.05 to 0.09)*** | 12 (0.3) | 0.21 (0.11 to 0.38)*** |
Marital status | ||||
Married/cohabiting | 480 (4.7) | Reference | 73 (0.8) | Reference |
Single | 973 (23.4) | 1.79 (1.50 to 2.14)*** | 111 (2.8) | 1.27 (0.81 to 2.00) |
Separated/divorced | 144 (9.9) | 2.05 (1.63 to 2.58)*** | 35 (2.5) | 1.71 (1.08 to 2.70)* |
Social class | ||||
I and II | 302 (5.6) | Reference | 48 (0.9) | Reference |
IIIM and IIINM | 708 (11.3) | 2.11 (1.75 to 2.54)*** | 87 (1.5) | 1.35 (0.90 to 2.02) |
IV and V | 398 (12.6) | 2.42 (1.95 to 3.01)*** | 63 (2.1) | 1.71 (1.10 to 2.66)* |
Ethnicity | ||||
White | 1463 (10.2) | Reference | 201 (1.5) | Reference |
Black | 49 (12.1) | 0.92 (0.58 to 1.46) | 7 (2.0) | 1.21 (0.47 to 3.10) |
Indian subcontinent | 36 (7.0) | 0.46 (0.26 to 0.81)** | 7 (1.5) | 1.16 (0.27 to 4.90) |
Other | 40 (11.2) | 0.90 (0.53 to 1.51) | 4 (1.4) | 0.32 (0.08 to 1.38) |
Drug dependency | 276 (48.4) | 2.31 (1.74 to 3.08)*** | 53 (9.8) | 2.74 (1.59 to 4.70)*** |
Alcohol dependency | 371 (35.3) | 2.21 (1.77 to 2.77)*** | 73 (7.2) | 2.84 (1.81 to 4.44)*** |
Anxiety disorder | 375 (16.2) | 1.87 (1.55 to 2.24)*** | 158 (7.3) | 10.76 (7.31 to 15.83)*** |
Psychosis | 7 (14.0) | 0.53 (0.16 to 1.79) | 12 (28.7) | 7.20 (2.80 to 18.49)*** |
ASPD | 246 (46.2) | 2.84 (2.09 to 3.86)*** | 57 (11.3) | 2.92 (1.73 to 4.91)*** |
Main associations of borderline personality disorder with violence
Table 37 shows unadjusted and adjusted associations of BPD with all violent outcomes, victim types and locations of reported violence. Following adjustments, BPD was significantly associated with only IPV and violence in the respondent’s own home. Furthermore, logistic regression analyses stratified by sex revealed no associations between BPD and violence for women or men when carried out separately (p > 0.05).
Outcomes | Violent total, n (%) | Violent within BPD group, n (%) | BPD, OR (95% CI) | BPD, AORa (95% CI) |
---|---|---|---|---|
Any violence | 1596 (10.1) | 80 (36.5) | 5.39 (3.87 to 7.49)*** | 1.50 (0.94 to 2.41) |
Violence while intoxicated | 685 (4.3) | 52 (23.9) | 7.38 (5.01 to10.88)*** | 1.62 (0.90 to 2.91) |
Severity of violence | ||||
Minor violence | 655 (4.2) | 17 (7.6) | 1.96 (1.07 to 3.59)* | 0.92 (0.46 to 1.84) |
Five or more violent incidents | 335 (2.1) | 25 (11.3) | 6.48 (3.92 to10.70)*** | 1.65 (0.83 to 3.29) |
Victim injured | 505 (3.2) | 26 (11.7) | 4.13 (2.59 to 6.60)*** | 0.72 (0.38 to 1.37) |
Perpetrator injured | 514 (3.3) | 37 (17.0) | 6.34 (4.10 to 9.80)*** | 1.25 (0.66 to 2.38) |
Police involved | 431 (2.7) | 32 (14.5) | 6.60 (4.21 to 10.36)*** | 1.44 (0.76 to 2.73) |
Victim of violence | ||||
Intimate partner | 252 (1.6) | 32 (14.7) | 11.79 (7.53 to 18.46)*** | 1.92 (1.04 to 3.54)* |
Family member | 153 (1.0) | 8 (3.7) | 3.87 (1.84 to 8.17)*** | 1.34 (0.52 to 3.43) |
Friend | 312 (2.0) | 22 (11.1) | 5.96 (3.45 to10.30)*** | 1.46 (0.64 to 3.32) |
Person known | 511 (3.2) | 27 (12.3) | 4.39 (2.68 to 7.20)*** | 1.00 (0.51 to 1.97) |
Stranger | 783 (5.0) | 34 (15.7) | 3.76 (2.38 to 5.92)*** | 0.99 (0.53 to 1.83) |
Police | 88 (0.6) | 7 (3.0) | 6.12 (2.45 to15.31)*** | 0.97 (0.22 to 4.22) |
Other | 108 (0.7) | 5 (2.2) | 3.49 (1.05 to11.59)* | 0.46 (0.09 to 2.36) |
Location of violent incident | ||||
Own home | 292 (1.9) | 32 (14.7) | 9.98 (6.35 to15.67)*** | 2.17 (1.19 to 3.95)* |
Someone else’s home | 138 (0.9) | 9 (4.2) | 5.58 (2.53 to12.32)*** | 0.95 (0.31 to 2.89) |
Street | 909 (5.8) | 53 (24.0) | 5.52 (3.84 to 7.93)*** | 1.34 (0.77 to 2.33) |
Bar/pub | 541 (3.4) | 37 (16.8) | 6.13 (3.94 to 9.52)*** | 1.51 (0.80 to 2.85) |
Workplace | 101 (0.6) | 4 (1.8) | 3.01 (1.00 to 9.06)* | 1.22 (0.37 to 4.01) |
Other | 241 (1.5) | 11 (5.0) | 3.54 (1.60 to 7.81)** | 0.95 (0.33 to 2.77) |
Impact of coexisting disorders on the association of borderline personality disorder with any violence
We performed logistic regression analyses stratified by comorbid psychiatric morbidity (presence/absence) to examine the impact of specific co-occurring disorders on the association of BPD with any reported violence. Table 38 shows the results of these adjusted analyses. BPD was not significantly associated with reports of any violence in the absence of any comorbid disorders, except for ASPD. Associations with violence were present when BPD was specifically comorbid with drug dependency, alcohol dependency and anxiety disorders (see Table 38). Despite significant associations in the presence of these comorbid disorders, the increased levels that we observed were not statistically significant.
Comorbid category | Without comorbidity, AORa (95% CI) | With comorbidity |
---|---|---|
Drug dependence | 1.39 (0.80 to 2.42) | 2.58 (1.17 to 5.70)* |
Psychosis | 1.49 (0.92 to 2.40) | 0.77 (0.27 to 2.22) |
Alcohol | 1.19 (0.67 to 2.12) | 2.93 (1.33 to 6.46)** |
Anxiety | 1.26 (0.54 to 2.94) | 2.42 (1.38 to 4.24)** |
ASPD | 1.84 (1.13 to 3.01)* | 1.41 (0.67 to 2.98) |
Violence classes and borderline personality disorder
Latent class models derived five violence classes for men and three violence classes for women in this joint data set. (see Chapter 2, Study 2.) Tables 39 and 40 report associations between individuals with BPD and the violence classes for men and women respectively.
Violence typologies | BPD, n (%) | RRRa (95% CI) | RRRb (95% CI) |
---|---|---|---|
No violence | 45 (0.7) | Reference | Reference |
Minor violence | 9 (2.0) | 2.06 (0.76 to 5.55) | 1.31 (0.46 to 3.72) |
Violence towards known persons | 22 (7.7) | 6.22 (2.72 to 14.23)*** | 2.32 (0.92 to 5.86) |
Fighting with strangers | 9 (3.1) | 3.32 (1.18 to 9.32) | 1.22 (0.41 to 3.69) |
Serious repetitive violence | 11 (9.9) | 8.81 (3.40 to 22.80)*** | 2.02 (0.67 to 6.10) |
Violence typologies | BPD, n (%) | RRRa (95% CI) | RRRb (95% CI) |
---|---|---|---|
No violence | 94 (1.3) | Reference | Reference |
General violence | 22 (8.2) | 3.30 (1.80 to 6.03)*** | 1.40 (0.66 to 2.97) |
Intimate/family violence | 7 (5.8) | 3.09 (1.42 to 6.72)** | 1.17 (0.44 to 3.11) |
For men, the ‘no violence’ class 1 had the lowest prevalence of BPD at 0.7%, followed by ‘minor violence’ at 2.0%. The ‘serious repetitive violence’ class had a BPD prevalence of 9.9%. Using the ‘no violence’ class as a reference, none of the remaining classes was significantly associated with BPD in the fully adjusted model (see Table 39).
Female distribution of BPD across the classes was 1.3% for the ‘no violence’ class, 8.2% for the ‘mild/moderate violence’ class and 5.8% for the ‘intimate/family violence’ class. Multinomial logistic regression models to estimate associations between violence latent classes and BPD showed no significant associations after full adjustment (see Table 39).
Discussion
Borderline personality disorder is the category of personality disorder that is most commonly found among patients in mental health services. There has been more research carried out into BPD, particularly into its treatment, than into any other Axis II disorder. In the past, the condition was considered to be associated with difficulties in management, particularly on an inpatient basis, and in this chapter we have described how personality disorders, including BPD, were excluded from many services. There is no information to indicate whether improvements in treatment have resulted in mental health professionals becoming more comfortable with the treatment of patients with BPD. However, more are now treated in the community with psychological therapies than in the past and avoiding hospitalisation is considered an important goal. Although there are several features of BPD that might be expected to result in violence, and thereby cause difficulties in treatment and management, our study did not show an increased risk of violence among members of the general household population with a diagnosis of BPD.
We found in this large representative sample that there was no difference in the prevalence of BPD between men and women. However, as with violence in the population, the diagnosis was uncommon among older people. It was more common among those who were separated and those of lower social classes. BPD was highly comorbid with other conditions, including psychosis and anxiety disorder, as well as with substance dependence and ASPD. Our study revealed that, when those with BPD do behave violently, it is most likely to be driven by comorbid conditions, including drug and alcohol dependency and anxiety disorder, rather than by the personality disorder. Because BPD was not a factor in determining the general level of violence in the household population, it did not discriminate between the classes of violence for either men or women. The only association that we found between violence and BPD was for IPV and violence occurring in the person’s own home. This corresponds to the demographic finding that this condition was more common among those who were separated and to criterion 2 of the DSM-IV BPD diagnosis: ‘a pattern of unstable and intense interpersonal relationships characterised by alternating between extremes of idealisation and devaluation’. 138 It may also correspond to criterion 1, ‘frantic efforts to avoid real or imagined abandonment’, resulting in altercations with partners who threaten to leave.
Chapter 5 Neurodevelopmental disorder and violence
Part of this chapter was first published in González R, Kallis C, Coid JW. Attention deficit hyperactivity disorder and violence in the population of England: does comorbidity matter? PLOS ONE 2013;8:e75575. It is reused here under the terms of the Creative Commons Attribution (CC BY) licence.
Background
Reports describing high rates of neuropsychiatric abnormalities among death row inmates, forensic psychiatric inpatients and others with a history of violence have led in the past to assertions that evidence of brain-behavioural impairment may mitigate or excuse criminal behaviour. 142–145 It has been argued that there is an episodic dyscontrol syndrome related to minimal brain dysfunction and complex partial seizures146 and that violently recidivistic criminals are men who are likely to have abnormal brain biology. 147 Neuroimaging studies suggest that the brain areas associated with violent and impulsive acts are located in the prefrontal cortex and medial temporal regions. 148 However, although a higher level of brain abnormalities tends to be found in individuals who are violent, particularly those who are repetitively violent, there is no established cause of association between brain pathology and violent behaviour.
An alternative approach is to consider violence as one form of antisocial behaviour that may be understood as a disorder having neurodevelopmental origins that, alongside autism, hyperactivity and dyslexia, shows strong male preponderance, early childhood onset, subsequent persistence and low prevalence in the population. These features were described by Moffitt142 in early-onset, persistent antisocial behaviour. Moffitt85 later argued that one form of antisocial behaviour, the early-onset persistent type, is a neurodevelopmental disorder. The other form of antisocial behaviour, afflicting females as well as males, is common and emerges in the context of social relationships.
Chapter 4 examined the strong association between violence and individuals with ASPD, who fulfil many of the criteria of Moffitt’s141 early-onset persistent subtype (see Study 1). In this chapter we examine associations between violence and childhood neurodevelopmental disorders and proxy measures of neurodevelopmental disorders at the population level among adults. When investigating intelligence, it could be rightly argued that this is not an accurate measure of neuropsychological development as much as educational and social functioning and at a highly complex level. However, taking a simplistic view, a high score for intelligence can be considered to reflect a high level of functioning and might therefore be presumed to have a negative association with neuropsychological deficits that might lead to violent behaviour at the population level.
Childhood neurodevelopmental disorders have been studied in relation to violent criminality using population-based registers of child and adolescent mental health services in Stockholm, Sweden. 149 No association with violent crime could be observed for autistic spectrum disorders (ASDs), although ADHD showed an elevated risk of committing a violent crime.
Study 1: intelligence and violence
Objectives
The objectives of this study were to investigate:
-
the association between total intelligence quotient (IQ) score and violent behaviour in the general population to identify the risk or protective effects of a verbal measure of IQ
-
potential direct associations between total IQ score and violent behaviour in the general population by adjusting for demographic factors that are linked to both IQ and violence perpetration
-
whether or not there are mean differences in IQ score according to a violence typology for men and women.
Methods
Sample
For the purpose of this study we combined two data sets, the NHPMS 2000 and the APMS 2007, to provide a sample of 15,734 men and women.
Intellectual functioning
Intellectual functioning was estimated using the National Adult Reading Test (NART),150 a proxy measure of pre-morbid IQ that includes 50 words printed in order of increasing difficulty. Originally developed to predict Wechsler Adult Intelligence Scale (WAIS) IQ scores,151 the NART was subsequently restandardised to predict WAIS-Revised IQ scores. Acceptable construct validity152 and high correlations with measures of IQ have been consistently reported for the NART. 153,154
Statistical analyses
As each of the surveys employed the same measures of IQ, demography and violence outcomes, we conducted joint analyses of individual-level data.
Weighted frequencies and proportions were reported for categorical predictors and outcomes. We estimated their impact on violence using logistic regression models, with ORs as indicators of the magnitude of associations.
All models are presented (1) unadjusted and (2) adjusted for social class, sex, age, marital status and ethnicity. Demographic variables significantly associated with either exposure (IQ) or outcome (violence) were included as confounders in multivariable models.
The IQ total mean score differences between the latent violence classes were estimated through analysis of variance (ANOVA) with Bonferroni post hoc tests.
To adjust for the effects of selecting one individual per household and under-representation of certain subgroups, and to account for any deviation from selecting a simple random sample, probability weights were used. All models employed robust SEs to adjust for clustering of individuals within postcodes. To control for differences between the two sources of data, survey was included as a fixed factor on model estimates.
Results
Demographic characteristics
All age categories above 16–34 years were protective for ‘any violence’. Participants from the Indian subcontinent were also less likely to report perpetration of violence. Being male, single or separated or divorced and from social classes lower than I and II singificantly increased the likelihood of violence (Table 41).
Covariate | Any violence in the past 5 years (n = 1596; 10.1%) | IQ total score | ||
---|---|---|---|---|
n (%) reported | AORa (95% CI) | Mean (SD) | βb | |
Sex | ||||
Male | 1190 (15.4) | 3.78 (3.23 to 4.43)*** | 103.0 (16.6) | –0.45 |
Female | 406 (5.1) | Reference | 103.1 (15.4) | Reference |
Age group (years) | ||||
16–34 | 1175 (22.4) | Reference | 98.0 (15.0) | Reference |
35–54 | 371 (6.3) | 0.31 (0.26 to 0.37)*** | 105.0 (15.3) | 7.36*** |
55–74 | 49 (1.2) | 0.06 (0.04 to 0.08)*** | 104.6 (16.3) | 7.08*** |
≥ 75 | 2 (0.3) | 0.02 (0.01 to 0.07)*** | 103.5 (17.1) | 5.99*** |
Marital status | ||||
Married | 480 (4.7) | Reference | 104.4 (15.6) | Reference |
Single | 973 (23.4) | 2.05 (1.73 to 2.43)*** | 99.7 (16.3) | –6.13*** |
Separated/divorced | 144 (9.9) | 2.47 (1.99 to 3.08)*** | 102.5 (15.8) | –2.11*** |
Social class | ||||
I and II | 302 (5.6) | Reference | 110.7 (13.5) | Reference |
IIIM and IIINM | 708 (11.3) | 2.20 (1.84 to 2.62)*** | 101.3 (15.1) | –9.86*** |
IV and V | 398 (12.6) | 2.58 (2.09 to 3.18)*** | 95.0 (15.3) | –15.69*** |
Ethnicity | ||||
White | 1463 (10.2) | Reference | 103.3 (15.8) | Reference |
Black | 49 (12.1) | 0.83 (0.53 to 1.31) | 92.9 (16.9) | –8.68*** |
Indian subcontinent | 36 (7.0) | 0.38 (0.21 to 0.66)** | 96.0 (16.1) | –6.48*** |
Other | 40 (11.2) | 0.94 (0.57 to 1.54) | 100.1 (15.8) | –3.49** |
Table 41 also shows differences in IQ score according to each sociodemographic variable. There were no differences in IQ by sex. Age was positively associated with IQ score. Being single or separated/divorced, from social classes lower than I and II and from any ethnic group other than white were all associated with a lower IQ score.
Main associations of intellectual functioning with violence outcomes
Total IQ score showed significant protective associations with all violent outcomes. The higher the IQ score, the less likely the individual was to report violence (Table 42). Most of these findings remained significant after adjusting for all sociodemographic characteristics, with certain exceptions: associations with minor violence, violence towards family members or strangers and violence in the home or the workplace were no longer significantly associated with IQ score after adjustment.
Outcomes | n (%) violent | OR (95% CI) | AORa (95% CI) |
---|---|---|---|
Any violence | 1596 (10.1) | 0.97 (0.97 to 0.98)*** | 0.99 (0.98 to 0.99)*** |
Violence while intoxicated | 685 (4.3) | 0.97 (0.97 to 0.98)*** | 0.99 (0.98 to 1.00)** |
Severity of violence | |||
Minor violence | 655 (4.2) | 0.98 (0.97 to 0.98)*** | 0.99 (0.99 to 1.00) |
Five or more violent incidents | 335 (2.1) | 0.97 (0.96 to 0.97)*** | 0.99 (0.98 to 1.00)* |
Victim injured | 505 (3.2) | 0.97 (0.96 to 0.98)*** | 0.99 (0.98 to 1.00)* |
Perpetrator injured | 514 (3.3) | 0.97 (0.96 to 0.98)*** | 0.99 (0.98 to 1.00)* |
Police involved | 431 (2.7) | 0.97 (0.96 to 0.98)*** | 0.98 (0.98 to 0.99)*** |
Victim of violence | |||
Intimate partner | 252 (1.6) | 0.97 (0.96 to 0.98)*** | 0.98 (0.97 to 0.99)*** |
Family member | 153 (1.0) | 0.97 (0.96 to 0.99)*** | 0.99 (0.98 to 1.01) |
Friend | 312 (2.0) | 0.96 (0.95 to 0.97)*** | 0.98 (0.97 to 0.99)** |
Known person | 511 (3.2) | 0.96 (0.95 to 0.97)*** | 0.98 (0.97 to 0.99)*** |
Stranger | 783 (5.0) | 0.98 (0.97 to 0.98)*** | 1.00 (0.99 to 1.00) |
Police | 88 (1.0) | 0.95 (0.94 to 0.97)*** | 0.96 (0.95 to 0.98)*** |
Location of violent incident | |||
Own home | 292 (1.9) | 0.98 (0.97 to 0.99)*** | 0.99 (0.99 to 1.00) |
Someone else’s home | 138 (0.9) | 0.97 (0.96 to 0.98)*** | 0.99 (0.98 to 1.01) |
Bar/pub | 541 (3.4) | 0.97 (0.96 to 0.97)*** | 0.98 (0.97 to 0.99)*** |
Street/outdoors | 909 (5.8) | 0.97 (0.97 to 0.98)*** | 0.99 (0.98 to 1.00)** |
Workplace | 101 (0.6) | 0.99 (0.97 to 1.00)* | 1.00 (0.99 to 1.02) |
Violence classes and intelligence quotient
There was variation in the mean IQ score according to the violence typologies for men and women. In men, the mean IQ score was 99.9 (SD 25.9) for those in the ‘no violence’ class, 95.7 (SD 20.1) for those in the ‘minor violence’ class, 93.0 (SD 18.7) for those in the ‘violence towards known persons’ class, 97.5 (SD 20.9) for those in the ‘fighting with strangers’ class and 93.7 (SD 14.6) for those in the ‘serious repetitive violence’ class. For women, the mean IQ score was 100.0 (SD 24.0) for those in the ‘no violence’ class, 92.3 (SD 15.9) for those in the ‘general violence’ class and 100.2 (SD 18.7) for those in the ‘intimate/family violence class’. There were significant differences in mean IQ score between the classes for both men (F = 7.2, p < 0.001) and women (F = 13.1, p < 0.001). Post hoc tests revealed that men in the ‘violence towards known persons’ class had a significantly lower mean IQ score than men in the ‘no violence’ class (p < 0.01). Women in the ‘general violence’ class had a significantly lower mean IQ score than those in the ‘no violence’ and ‘intimate/family violence’ classes (p < 0.01).
Discussion
An extensive body of research has previously linked low IQ score and intellectual disability to delinquency, serious crime and interpersonal violence. 32,155–157 Our findings therefore correspond in showing a negative association with all violent outcomes except minor violence, violence towards family members and strangers and violence in the home or in the workplace. This would imply that a higher IQ score conveys a protective effect against violence, independent of demography. This corresponds to evidence on the protective effects of above-average intelligence and intact cognition on both general health158 and antisocial behaviours. 159
Only two classes of the violence typology were significantly associated with lower IQ score than in the general population. Nevertheless, these are of considerable interest in the context of our findings reported in other chapters. Class 4 in men (‘violence towards known persons’) was associated with impaired social functioning in that these individuals were unemployed and economically inactive. A proportion were also dependent on drugs and alcohol and more had shown difficulty in maintaining close relationships.
Class 2 (‘general violence’) in women showed the lowest mean IQ score of any subgroup, with the mean score also being lower than those of the male classes. This group showed some similarities to classes 4 and 5 (‘serious repetitive violence’) among men in that women appeared to be socially dysfunctional. Intellectual functioning was hence identified as an important distinguishing characteristic of this subgroup.
Study 2: attention deficit hyperactivity disorder and violence
Objectives
The objectives of this study were to:
-
estimate the prevalence of self-reported violence associated with ADHD and its severity and victims and the location of violent incidents
-
investigate the independent associations between the symptom dimensions of ADHD: inattention and hyperactivity/impulsivity
-
investigate the associations of ADHD with a typology of violence in the general population.
Methods
Sample
For the purpose of this analysis we used a single data set, the APMS 2007, which recruited adults aged ≥ 16 years living in private households in England.
Measures
The survey included the six-item version of the Adult Self-Report Scale (ASRS),160 based on DSM-IV diagnostic criteria as a measure of ADHD. The ASRS has strong concordance with clinical diagnoses, with a reported AUC of 0.90. Four items are related to inattention and two to hyperactivity. Items are based on a 5-point Likert scale. We used the ASRS-6 scoring and classification methods, with a binary cut-off point of ≥ 13. 161 To explore the relative contributions of ADHD symptoms, the sum of the response scores was calculated for the inattention items (items 1–4) and the hyperactivity items (items 5 and 6).
Statistical analyses
Weighted frequencies and proportions were reported for all categorical variables. Group associations were established using binary logistic regression with the OR as the measure of magnitude. Multiple categorical predictor covariates were assigned a reference category against which other categories were contrasted.
Two approaches examined the associations of ADHD with dependent binary measures of violence. In the first approach the exposure was a binary measure of ADHD, based on a cut-off point of ≥ 13. 160 In the second approach we estimated hyperactivity and inattention scores as an alternative to the ADHD categorical classifications using symptom dimension scores.
Additionally, we quantified the associations between ADHD classification and the violence typologies described in Chapter 2 (see Study 2).
All statistical analyses were adjusted by including covariates in each model simultaneously. Adjustments were made for demographic factors, ASPD, drug dependence, alcohol dependence and anxiety disorders. To adjust for the effects of selecting one individual per household and under-representation of certain subgroups, and to account for any deviation from selecting a simple random sample, all variance estimates were weighted. Details of the procedures used in weighting have been described previously. 45,82 All models employed robust SEs to adjust for clustering of individuals within postcodes. All analyses were performed using Stata.
Results
The overall prevalence of ADHD in the sample was 5.7%. The prevalence of any violence in the past 5 years was 8.4%. The older age group (≥ 55 years) had a significantly lower rate of ADHD. Being male increased the association with ADHD. Anxiety disorders, ASPD, psychotic symptoms and alcohol dependence were significantly associated with ADHD (Table 43).
Covariate | Any violence in the past 5 years (n = 614; 8.4%) | ADHD (n = 424; 5.7%) | ||
---|---|---|---|---|
n (%) reported | AORa (95% CI) | n (%) reported | AORa (95% CI) | |
Sex | ||||
Female | 173 (4.6) | Reference | 305 (5.4) | Reference |
Male | 441 (12.3) | 3.14 (2.42 to 4.07)*** | 219 (6.1) | 1.41 (1.09 to 1.81)** |
Age group (years) | ||||
16–34 | 448 (19.9) | Reference | 189 (8.3) | Reference |
35–54 | 141 (5.4) | 0.32 (0.24 to 0.42)*** | 171 (6.5) | 1.01 (0.74 to 1.38) |
≥ 55 | 25 (1.0) | 0.06 (0.04 to 0.10)*** | 64 (2.6) | 0.47 (0.32 to 0.68)*** |
Marital status | ||||
Married/cohabiting | 235 (4.6) | Reference | 225 (4.4) | Reference |
Single | 340 (20.4) | 1.61 (1.20 to 2.16)** | 157 (9.4) | 1.10 (0.79 to 1.53) |
Divorced/separated | 39 (7.2) | 1.70 (1.14 to 2.54)** | 42 (7.6) | 1.16 (0.82 to 1.64) |
Social class | ||||
I and II | 114 (4.3) | Reference | 110 (4.2) | Reference |
IIIM and IIINM | 264 (9.5) | 2.10 (1.55 to 2.84)*** | 158 (5.6) | 1.27 (0.93 to 1.73) |
IV and V | 149 (10.5) | 2.15 (1.51 to 3.08)*** | 101 (7.1) | 1.40 (0.99 to 1.98) |
Ethnicity | ||||
White | 550 (8.3) | Reference | 366 (5.5) | Reference |
Black | 21 (9.3) | 0.87 (0.43 to 1.76) | 22 (9.9) | 1.67 (0.89 to 3.14) |
Indian subcontinent | 19 (6.9) | 0.50 (0.23 to 1.08) | 10 (3.7) | 0.66 (0.29 to 1.50) |
Other | 19 (8.7) | 0.78 (0.37 to 1.63) | 20 (9.4) | 1.35 (0.66 to 2.76) |
ASPD | 80 (41.1) | 2.26 (1.40 to 3.64)** | 45 (22.6) | 1.72 (1.02 to 2.91)* |
Drug dependency | 111 (44.2) | 3.36 (2.18 to 5.16)*** | 46 (18.1) | 1.64 (0.93 to 2.91) |
Alcohol dependency | 106 (24.5) | 1.38 (0.95 to 2.00) | 74 (17.0) | 1.70 (1.09 to 2.65)* |
Anxiety disorder | 165 (15.2) | 2.07 (1.54 to 2.80)*** | 228 (20.8) | 6.89 (5.37 to 8.85)*** |
Psychosis | 23 (24.5) | 1.77 (0.83 to 3.81) | 33 (33.6) | 3.13 (1.78 to 5.53)*** |
Main associations of attention deficit hyperactivity disorder with violence
Table 44 shows the numbers of respondents who reported the different violence outcomes. Following adjustment, an association with ADHD was observed only for having been involved in any violence in the past 5 years and in violent events considered minor.
Outcomes | n (%) violent | ADHD, AORa (95% CI) | Hyperactivity, ORb (95% CI) | Inattention, ORb (95% CI) |
---|---|---|---|---|
Any violence | 614 (8.4) | 1.75 (1.14 to 2.68)* | 1.15 (1.08 to 1.23)*** | 1.06 (1.02 to 1.11)** |
Violence while intoxicated | 263 (3.6) | 1.34 (0.72 to 2.48) | 1.15 (1.04 to 1.26)** | 1.03 (0.97 to 1.10) |
Severity of violence | ||||
Minor violence | 247 (3.3) | 2.54 (1.48 to 4.34)** | 1.18 (1.07 to 1.30)** | 1.11 (1.05 to 1.18)** |
Five or more violent incidents | 98 (1.3) | 1.42 (0.49 to 4.15) | 1.16 (0.96 to 1.40) | 1.01 (0.92 to 1.11) |
Victim injured | 172 (2.3) | 0.88 (0.37 to 2.14) | 1.10 (0.98 to 1.24) | 0.99 (0.92 to 1.06) |
Perpetrator injured | 204 (2.8) | 1.10 (0.55 to 2.20) | 1.06 (0.95 to 1.18) | 1.00 (0.94 to 1.07) |
Police involved | 177 (2.4) | 1.19 (0.56 to 2.55) | 1.15 (1.02 to 1.28)* | 0.97 (0.90 to 1.04) |
Victim of violence | ||||
Intimate partner | 115 (1.6) | 1.52 (0.70 to 3.28) | 1.16 (1.01 to 1.32)* | 1.05 (0.97 to 1.14) |
Family member | 91 (1.2) | 1.77 (0.70 to 4.44) | 1.26 (1.08 to 1.46)** | 1.04 (0.94 to 1.16) |
Friend | 132 (1.8) | 1.53 (0.60 to 3.89) | 1.18 (1.02 to 1.37)* | 1.06 (0.97 to 1.16) |
Person known | 195 (2.6) | 1.16 (0.57 to 2.36) | 1.10 (0.98 to 1.23) | 1.03 (0.95 to 1.11) |
Stranger | 300 (4.1) | 1.15 (0.64 to 2.08) | 1.07 (0.97 to 1.18) | 1.04 (0.99 to 1.10) |
Location of violent incident | ||||
Own home | 123 (1.7) | 1.75 (0.87 to 3.52) | 1.21 (1.06 to 1.39)** | 1.02 (0.94 to 1.11) |
Someone else’s home | 61 (0.8) | 1.25 (0.49 to 3.17) | 1.21 (1.05 to 1.40)* | 0.93 (0.83 to 1.03) |
Bar/pub | 183 (2.5) | 1.50 (0.74 to 3.05) | 1.17 (1.05 to 1.31)** | 1.01 (0.93 to 1.10) |
Workplace | 21 (0.3) | 2.79 (0.83 to 9.39) | 1.25 (1.01 to 1.54)* | 1.14 (0.98 to 1.32) |
Attention deficit hyperactivity disorder symptom dimensions and violence: hyperactivity and inattention
To estimate the independent contributions of hyperactivity and inattention, we developed adjusted models replacing the ADHD binary variable with continuous scores of hyperactivity and inattention. The inattention and hyperactivity scores were entered in models simultaneously (to adjust for each other). Table 44 shows that inattention may be associated with any violence, but specifically only with minor violence. However, there were additional independent associations with hyperactivity, including any violence, violence while intoxicated and police involvement. Direct associations with hyperactivity were also observed for specific victim types (intimate partner, family members and friends) and locations of violence (in the participant’s or another person’s home, in a bar/pub or in the workplace). However, hyperactivity was not associated with more severe violence leading to injury to victim or perpetrator and was associated with minor violence.
Violence classes and attention deficit hyperactivity disorder
Latent class models derived five violence classes for men and three violence classes for women in the 2007 APMS. Development of these LCAs is described in detail in Chapter 2. Tables 45 and 46 include the associations between individuals with ADHD and the violence classes for men and women respectively.
Violence typologies | ADHD, n (%) | RRRa (95% CI) | RRRb (95% CI) |
---|---|---|---|
No violence | 150 (4.8) | Reference | Reference |
Minor violence | 28 (16.1) | 3.51 (1.88 to 6.56)*** | 1.29 (0.46 to 3.63) |
Violence towards known persons | 18 (16.3) | 2.10 (0.93 to 4.76) | 2.23 (0.88 to 5.62) |
Fighting with strangers | 17 (14.2) | 2.96 (1.40 to 6.25)** | 1.23 (0.41 to 3.70) |
Serious repetitive violence | 6 (19.1) | 2.74 (0.84 to 9.01) | 1.87 (0.62 to 5.67) |
Violence typologies | ADHD, n (%) | RRRa (95% CI) | RRRb (95% CI) |
---|---|---|---|
No violence | 181 (5.0) | Reference | Reference |
General violence | 16 (12.6) | 1.67 (0.76 to 3.68) | 1.42 (0.68 to 2.99) |
Intimate/family violence | 7 (17.0) | 3.50 (1.62 to 7.52)** | 1.14 (0.42 to 3.04) |
For men, the ‘no violence’ class had the lowest prevalence of ADHD at 4.8%; this was followed by ‘fighting with strangers’ at 14.2%, ‘minor violence’ at 16.1%, ‘violence towards known persons’ at 16.3% and ‘serious repetitive violence’ at 19.1%. With the ‘no violence’ class as a reference, none of the remaining classes was significantly associated with ADHD after adjustment for psychopathology (see Table 45).
The female distribution of ADHD across the classes was 5.0% for the ‘no violence’ class, 12.6% for the ‘general violence’ class and 17.0% for the ‘intimate/family violence’ class. Multinomial logistic regression models implemented to estimate associations between violence latent classes and ADHD revealed no significant associations after adjustment for psychopathology (see Table 46).
Discussion
In this study we aimed to examine the main effects of ADHD among individuals involved in violent incidents, as well as its impact on the severity, frequency, targets and locations of violence. After adjustments, ADHD appeared to be only moderately associated with violence, with the significant association observed being between ADHD and violent incidents classified as minor.
After examining two continuous measures using symptom dimensions, inattention demonstrated little or no impact on violence in the population and involved only minor incidents. On the other hand, hyperactivity was directly associated with violent incidents that resulted in police involvement, with reports of victims in close relationships and with violence taking place in the perpetrator’s or someone else’s home as well as in pubs and bars.
This finding is consistent with two other epidemiological studies that have reported significant associations between retrospective measures of ADHD and violent outcomes. 162,163 Our findings also provide validation of what has been evidenced by small study samples in forensic settings, which indicate an association between ADHD symptoms and violent breaches of discipline and offending. 164,165 However, in the general population, our findings suggest that direct associations of ADHD are restricted to violence towards those in intimate relationships and relatives. ADHD did not discriminate between the violence classes that we identified in Chapter 2 (see Study 2).
This study confirmed the importance of the association of hyperactivity with behavioural disturbance and impulsivity. Hyperactivity has been linked to an increased risk of aggression among adults. 166 This corresponds to the view that aggression and violence stem from deficient self-regulatory processes, including response disinhibition, failure to delay gratification, and emotional reactivity and dysregulation. 167–169
Study 3: autism and violence
Objective
The objective of this study was to investigate the prevalence of self-reported violence associated with autism and associated spectrum disorders in the household population.
Methods
Sample
The sample was drawn from the APMS 2007, a national survey of psychiatric morbidity among adults aged ≥ 16 years living in households in England in 2007. A total of 7403 participants completed the survey (response rate 57.0%). The design and sampling procedures have previously been described. 45,82
Autism was diagnosed in this sample using the Autism Diagnostic Observation Schedule module-4 (ADOS-4), which provides a face-to-face assessment of current respondent behaviours for an autistic disorder. 170 Non-specific pervasive developmental disorder (PDD) and autism classifications can be derived from ADOS-4 algorithm scores of ≥ 7 and ≥ 10 respectively. 171
Statistical analyses
Weighted frequencies and proportions were reported for all categorical variables. Group associations between autism and violence were established using binary logistic regression with the OR as the measure of magnitude. Multiple categorical predictor covariates were assigned a reference category against which other categories were contrasted.
All statistical analyses were adjusted by including covariates in each model simultaneously. Adjustments were made for demographic factors, alcohol dependence, psychosis, drug dependence, ASPD, depression and anxiety disorders. To adjust for the effects of selecting one individual per household and under-representation of certain subgroups, and to account for any deviation from selecting a simple random sample, all estimates were weighted. Details of the procedures used in weighting have been described previously. 82 All models employed robust SEs to adjust for clustering of individuals within postcodes. All analyses were performed using Stata.
Results
Demographic characteristics
Of 7361 respondents (weighted), 614 (8.4%) reported any violence in the past 5 years. Table 47 shows that the sociodemographic factors of male sex, marital status other than married and social classes lower than I and II were significantly associated with violence risk. Any age category above 34 years was protective.
Covariate | Any violence in the past 5 years (n = 614; 8.4%) | Autism (n = 21; 0.3%) | ||
---|---|---|---|---|
n (%) reported | AORa (95% CI) | n (%) reported | AORa (95% CI) | |
Sex | ||||
Female | 173 (4.6) | Reference | 4 (0.1) | Reference |
Male | 441 (12.3) | 3.17 (2.45 to 4.11)*** | 17 (0.5) | 4.12 (1.14 to 14.85)* |
Age groups (years) | ||||
16–34 | 448 (19.9) | Reference | 11 (0.5) | Reference |
35–54 | 141 (5.4) | 0.32 (0.24 to 0.42)*** | 2 (0.1) | 0.22 (0.04 to 1.20) |
≥ 55 | 25 (1.0) | 0.06 (0.04 to 0.10)*** | 7 (0.3) | 0.80 (0.16 to 4.03) |
Marital status | ||||
Married/cohabiting | 235 (4.6) | Reference | 10 (0.2) | Reference |
Single | 340 (20.4) | 1.60 (1.19 to 2.15)** | 8 (0.5) | 0.71 (0.11 to 4.52) |
Divorced/separated | 40 (7.2) | 1.73 (1.16 to 2.58)** | 2 (0.4) | 2.11 (0.70 to 6.40) |
Social class | ||||
I and II | 114 (4.3) | Reference | 3 (0.1) | Reference |
IIIM and IIINM | 264 (9.5) | 2.08 (1.54 to 2.82)*** | 8 (0.3) | 2.24 (0.61 to 8.27) |
IV and V | 149 (10.5) | 2.15 (1.51 to 3.07)*** | 5 (0.4) | 3.34 (0.75 to 14.81) |
Ethnicity | ||||
White | 550 (8.3) | Reference | 21 (0.3) | Reference |
Black | 21 (9.3) | 0.89 (0.44 to 1.82) | 0 (0.0) | No observations |
Indian subcontinent | 19 (6.9) | 0.50 (0.23 to 1.07) | 0 (0.0) | No observations |
Other | 19 (8.7) | 0.76 (0.36 to 1.61) | 0 (0.0) | No observations |
Alcohol dependence | 106 (24.5) | 1.38 (0.94 to 2.01) | 1 (0.3) | 0.66 (0.08 to 5.16) |
Psychosis | 3 (10.0) | 0.73 (0.10 to 5.35) | 0 (0.0) | No observations |
Drug dependence | 111 (44.2) | 3.30 (2.14 to 5.10)*** | 0 (0.0) | No observations |
Anxiety disorder | 165 (15.2) | 2.07 (1.53 to 2.80)*** | 4 (0.4) | 1.52 (0.55 to 4.23) |
ASPD | 80 (41.1) | 2.30 (1.42 to 3.72)** | 4 (2.2) | 2.85 (0.39 to 20.68) |
Depressive episode | 35 (16.3) | 1.23 (0.74 to 2.06) | 2 (1.0) | 3.95 (0.70 to 22.14) |
The overall weighted prevalence of autism in the sample in phase 1 of the two-phase survey was 0.3%. Being male was the only sociodemographic factor associated with autism.
Main associations of drug dependence with violence
There were no observations with categorically defined diagnosis of autism that reported any violence in the past 5 years associated with drug dependence. Using the less strict cut-off score for PDD of ≥ 7 revealed only two participants who were violent respondents.
Discussion
The prevalence of autism in the sample was very low, resulting in a problem of statistical power when searching for associations with violence. However, because there have been a series of media stories suggesting that there might be a link between violence and individuals with ASD (particularly Asperger’s syndrome) in cases of high-school shootings in the USA, it was important to observe that no cases of ASD were found. Only two cases were found in the population sample when the cut-off score was lowered to allow detection of more cases. This would suggest that there is little evidence for an association between ASD at the population level and violence. This is consistent with previous findings. 149
It has been observed that as the prevalence of autism spectrum disorders has increased, attention has shifted towards consideration of these disorders in adolescence and adulthood, as well as the public health repercussions for this population. The social and emotional deficits within these disorders could be relevant to certain cognitive features of criminal and violent behaviour that is not intended by the violent individual, for example impulsive behaviour triggered by stressful environments. Because of media attention over ASD and serious criminal acts, not always with clear evidence that the perpetrator actually has ASD, judicial and legislative state systems in the USA have begun to develop policies that lack an evidence base. 172 This has fortunately not occurred in the UK. Overall, there are thought to be three deficits characteristic of individuals with ASDs that might have a bearing on violent and criminal behaviour. These include theory of mind, emotional regulation and moral reasoning. However, there is no clear evidence base for these at present. More importantly, a key component of ASD is poor social interaction and social withdrawal. Because violence can be considered a highly ‘social’ activity, or at least it occurs most commonly in social settings, it should not be surprising that our study has failed to demonstrate an association between autism and violence.
Chapter 6 Substance dependence and violence
Background
Substance use is among the most consistently reported risk factor for violent behaviour. 173,174 Substance misuse is prevalent both among those in the general population reporting violence175 and among offenders convicted for violence. 176,177 In the NHPMS 2000, the highest percentage of violent incidents and the highest population-attributable risk were explained by individuals engaging in hazardous drinking followed by drug misuse. 83 Clinical studies of alcohol use have confirmed strong, if complex, associations with violence. 178 Furthermore, the high annual medical and social costs of injury from deliberate harm are highlighted by measures taken in A&E departments in the UK. These correlate with unemployment, poverty and, in particular, expenditure on alcohol. 179,180
Associations with substance misuse are highly complex. For example, Arseneault et al. 181 have argued that, because of involvement in the illegal economy of drugs markets, young people who are dependent on drugs must rely on violence to solve problematic transactions with dealers and others involved in drug-related social interactions. However, the high level of violence reported by drug-misusing individuals suggests that additional mechanisms must be operating in this association. 83 Furthermore, many drug misusers are also alcohol misusers and individuals with a propensity to violence may be inclined to abuse alcohol and drugs. The chemical effects of drugs and alcohol, including disinhibition, are thought by many to explain the association with violence, and many violent people attempt to explain their violence in terms of these chemical effects.
Because the associations are complex, we restricted our two studies of substance misuse reported in this chapter to the most severe level of dependence on drugs and alcohol.
Study 1: drug dependence
Objectives
The objectives of this study were to investigate:
-
the prevalence of self-reported violence associated with drug dependence in the household population of Great Britain
-
the associations between the characteristics of violence, victim types and locations of violence, after adjusting for sociodemographic factors, and drug dependence
-
the distribution of drug dependence and associations with a typology of violence in the general population.
Methods
Sample
For the purposes of this analysis we combined two data sets, the APMS 2007 and the NHPMS 2000, to provide a sample of 15,734 participants.
Definitions and assessment
Questions about drug use were asked in a computer-assisted self-completion interview. This included lifetime experience of 13 different types of illicit drugs together with patterns of use over the last year. Five questions derived from the Diagnostic Interview Schedule (DIS)182 were used to measure substance dependence.
Statistical analyses
Weighted frequencies and proportions were reported for all categorical variables. Group associations between drug dependence and violence were established using binary logistic regression with the OR as the measure of magnitude. Multiple categorical predictor covariates were assigned a reference category against which other categories were contrasted. The violence typologies for men and women described in Chapter 2 were regressed onto drug dependence using multinomial logistic regression.
All statistical analyses were adjusted by including covariates in each model simultaneously. Adjustments were made for demographic factors, psychosis, alcohol dependence, ASPD, depression and anxiety disorders. To adjust for the effects of selecting one individual per household and under-representation of certain subgroups, and to account for any deviation from selecting a simple random sample, all estimates were weighted. Details of the procedures used in weighting have been described previously. 45,82 All models employed robust SEs to adjust for clustering of individuals within postcodes. All analyses were performed using Stata.
Results
Demographic characteristics
Of the 15,734 respondents, 1596 (10.1%) reported any violence in the past 5 years. Table 48 shows that male sex, marital status other than married and social class lower than I and II were significantly associated with violence risk, whereas any age category > 34 years and being of Indian/Asian ethnicity were protective. The overall weighted prevalence of drug dependence in the sample was 3.6%. Male sex, marital status other than married and social class lower than I and II increased risk associations with drug dependence. Having an anxiety disorder or ASPD or alcohol dependence were directly associated with drug dependence in adjusted models.
Covariate | Any violence in the past 5 years (n = 1596; 10.1%) | Drug dependence (n = 573; 3.6%) | ||
---|---|---|---|---|
n (%) | AORa (95 CI) | n (%) | AORa (95 CI) | |
Sex | ||||
Female | 406 (5.1) | Reference | 181 (2.3) | Reference |
Male | 1190 (15.4) | 3.50 (2.95 to 4.14)*** | 392 (5.0) | 1.75 (1.35 to 2.27)*** |
Age groups (years) | ||||
16–34 | 1175 (22.4) | Reference | 446 (8.5) | Reference |
35–54 | 371 (6.3) | 0.31 (0.26 to 0.37)*** | 98 (1.6) | 0.27 (0.19 to 0.36)*** |
≥ 55 | 51 (1.1) | 0.06 (0.04 to 0.08)*** | 29 (0.6) | 0.17 (0.11 to 0.26)*** |
Marital status | ||||
Married/cohabiting | 480 (4.7) | Reference | 132 (1.3) | Reference |
Single | 973 (23.4) | 1.85 (1.55 to 2.20)*** | 389 (9.3) | 2.38 (1.78 to 3.18)*** |
Divorced/separated | 144 (9.9) | 2.05 (1.63 to 2.58)*** | 52 (3.6) | 2.01 (1.38 to 2.93)*** |
Social class | ||||
I and II | 302 (5.6) | Reference | 118 (2.2) | Reference |
IIIM and IIINM | 708 (11.3) | 2.14 (1.78 to 2.57)*** | 251 (3.9) | 1.60 (1.20 to 2.15)** |
IV and V | 398 (12.6) | 2.46 (1.98 to 3.05)*** | 151 (4.7) | 1.76 (1.27 to 2.43)** |
Ethnicity | ||||
White | 1463 (10.2) | Reference | 517 (3.6) | Reference |
Black | 49 (12.1) | 0.91 (0.57 to 1.45) | 23 (5.5) | 1.07 (0.57 to 1.99) |
Indian subcontinent | 36 (7.0) | 0.45 (0.26 to 0.79)** | 8 (1.6) | 0.53 (0.18 to 1.53) |
Other | 40 (11.2) | 0.91 (0.55 to 1.52) | 23 (6.3) | 1.67 (0.90 to 3.13) |
Psychosis | 7 (14.0) | 0.51 (0.17 to 1.55) | 8 (15.8) | 1.49 (0.46 to 4.77) |
Alcohol dependence | 371 (35.3) | 2.39 (1.92 to 2.96)*** | 186 (17.5) | 2.98 (2.21 to 4.03)*** |
Anxiety disorder | 375 (16.2) | 1.87 (1.54 to 2.26)*** | 182 (7.7) | 2.03 (1.52 to 2.72)*** |
ASPD | 246 (46.2) | 3.43 (2.57 to 4.60)*** | 159 (29.4) | 6.45 (4.59 to 9.06)*** |
Depressive episode | 79 (18.9) | 1.51 (1.02 to 2.22)* | 43 (9.8) | 1.41 (0.77 to 2.56) |
Main associations of drug dependence with violence
Table 49 shows unadjusted and adjusted associations of drug dependence with all key measures of violence. Drug dependence was significantly associated with all violent outcomes in univariate models (all p < 0.001). After adjustments, drug dependence was still an important source of risk for most outcomes of violence, including incidents of violence while intoxicated, repeated violence, violence leading to injuries and violence in which the police were involved.
Outcomes | n (%) violent | OR (CI 95%) | AORa (CI 95%) |
---|---|---|---|
Any violence | 1596 (10.1) | 9.87 (7.88 to 12.35)*** | 2.32 (1.74 to 3.10)*** |
Violence while intoxicated | 685 (4.4) | 14.63 (11.40 to 18.78)*** | 2.61 (1.89 to 3.62)*** |
Severity of violence | |||
Minor violence | 655 (4.2) | 3.17 (2.23 to 4.50)*** | 1.03 (0.67 to 1.56) |
Five or more violent incidents | 335 (2.1) | 11.49 (8.14 to 16.22)*** | 2.36 (1.53 to 3.62)*** |
Victim injured | 505 (3.2) | 11.40 (8.61 to 15.10)*** | 2.17 (1.49 to 3.17)*** |
Perpetrator injured | 514 (3.3) | 10.61 (7.94 to 14.19)*** | 1.99 (1.33 to 2.97)*** |
Police involved | 431 (2.7) | 11.42 (8.58 to 15.19)*** | 2.65 (1.85 to 3.80)*** |
Victim of violence | |||
Iintimate partner | 252 (1.6) | 7.05 (4.88 to 10.18)*** | 1.77 (1.07 to 2.92)* |
Family member | 153 (1.0) | 4.10 (2.22 to 7.58)*** | 1.04 (0.50 to 2.14) |
Friend | 312 (2.0) | 11.46 (7.86 to 16.70)*** | 3.18 (2.02 to 5.01)*** |
Person known | 511 (3.2) | 9.91 (7.26 to 13.52)*** | 2.65 (1.77 to 3.95)*** |
Stranger | 783 (5.0) | 7.95 (6.20 to 10.21)*** | 1.64 (1.19 to 2.25)** |
Police | 88 (0.6) | 14.80 (8.56 to 25.59)*** | 2.35 (1.13 to 4.87)* |
Other | 108 (0.7) | 5.97 (3.25 to 10.98)*** | 1.52 (0.60 to 3.82) |
Location of violent incident | |||
Own home | 292 (1.9) | 7.18 (5.06 to 10.19)*** | 2.33 (1.44 to 3.77)*** |
Someone else’s home | 138 (0.9) | 9.84 (5.91 to 16.37)*** | 2.52 (1.33 to 4.79)** |
Street | 909 (5.8) | 9.92 (7.77 to 12.67)*** | 2.18 (1.59 to 3.00)*** |
Bar/pub | 541 (3.4) | 9.52 (7.19 to 12.61)*** | 1.72 (1.20 to 2.48)** |
Workplace | 101 (0.6) | 5.64 (2.94 to 10.84)*** | 1.60 (0.76 to 3.37) |
Other | 241 (1.5) | 7.40 (4.80 to 11.43)*** | 2.41 (1.35 to 4.29)** |
Drug dependence also significantly increased the likelihood of violence towards intimate partners, friends, persons known, strangers and the police and the likelihood of violence taking place in all locations, with the exception of the workplace.
Violence classes and drug dependence
Latent class models derived five violence classes for men and three violence classes for women in this joint data set. The development of these LCAs is described in detail in Chapter 2. Tables 50 and 51 show the associations between individuals with drug dependence and the violence classes for men and women respectively.
Violence typologies | Drug dependence, n (%) | RRRa (95% CI) | RRRb (95% CI) |
---|---|---|---|
No violence | 169 (2.6) | Reference | Reference |
Minor violence | 48 (10.6) | 1.95 (1.22 to 3.13)** | 1.57 (0.96 to 2.58) |
Violence towards known persons | 72 (24.4) | 6.38 (4.09 to 9.94)*** | 3.63 (2.21 to 5.94)*** |
Fighting with strangers | 54 (17.4) | 2.98 (1.83 to 4.86)*** | 1.67 (0.98 to 2.83) |
Serious repetitive violence | 48 (39.8) | 11.16 (6.31 to 19.72)*** | 4.73 (2.61 to 8.56)*** |
Violence typologies | Drug dependence, n (%) | RRRa (95% CI) | RRRb (95% CI) |
---|---|---|---|
No violence | 127 (1.7) | Reference | Reference |
General violence | 43 (15.4) | 4.94 (3.02 to 8.07)*** | 3.16 (1.84 to 5.43)*** |
Intimate/family violence | 9 (7.0) | 2.01 (0.87 to 4.65) | 1.06 (0.43 to 2.60) |
In men, the ‘no violence’ class had the lowest prevalence of drug dependence at 2.6%. The highest prevalence of drug dependence was observed for class 5 (‘serious repetitive violence’) at 39.8%. Following adjustments, drug dependence was associated with more than a threefold increase in the odds of violence towards known persons and almost a fivefold increase of belonging in the odds of serious repetitive violence (see Table 50).
The female distribution of drug dependence across the classes was 1.7% for the ‘no violence’ class, 15.4% for the ‘general violence’ class and 7.0% for the ‘intimate/family violence’ class. Multinomial logistic regression models to estimate associations between violence latent classes and drug dependence showed a threefold increase in the odds of general violence compared with no violence (see Table 51).
Discussion
We found that the demographic characteristics of drug-dependent individuals in these household surveys were very similar to the demographic characteristics of those who were violent. As with individuals who report violence, drug-dependent individuals tend to be younger men who are single or separated from their partner, from lower social classes and with comorbid alcohol dependence and ASPD. The odds of reporting any violence in the past 5 years were more than doubled with drug dependence and it was unsurprising, in view of their dependence on drugs, that individuals were more likely to report violence when intoxicated. All levels of severity of violence were increased, including multiple incidents, incidents in which a victim was injured and incidents in which the perpetrator was injured. More importantly, individuals with drug dependence were more likely to report violence in which the police became involved. All victim types were reported except for members of the family. Friends and persons known showed the highest odds of association together with the police.
Because we do not have details of individual incidents, it cannot be confirmed whether these violent incidents corresponded to intoxication with drugs, situations in which drugs were bought and sold or situations in which altercations with drug dealers or customers had occurred or whether certain individuals had a pre-existing violent propensity that predisposed them both to violence and to abusing drugs to the extent that they became dependent on them. It is probable that all of these possibilities, in different combinations, had an effect on the associations with drug dependence. The level of police involvement with drug-dependent individuals in the study would suggest that they had regularly come into conflict with others and that the police had become involved. Alternatively, the police had become involved because of their drug-taking or other criminal activities such as theft and burglary, to obtain money for drugs. The strong association with friends and persons known to these individuals suggested that violence had occurred in a social milieu with other drug-abusing individuals, outside of the home. Nevertheless, risks of violence towards an intimate partner were also increased among this subgroup. On the other hand, the lack of association with other family members might suggest that drug misuse had caused these individuals to be estranged from close family members or that they were unable to support or maintain a family themselves.
Following adjustments, drug dependence was not found to be associated with classes 2 (‘minor violence’) and 4 (‘fighting with strangers’) among men. The strong association with serious repetitive violence among men (class 5) corresponded to the findings in Chapter 2 (see Study 2). Drug dependence may partly explain the wide range of potential victims of men in this class, with the risks increased for friends and persons known in the context of buying, selling and misusing drugs. The association with class 3 (‘violence towards known persons’) is also of considerable interest. Nearly one-quarter of these individuals were dependent on drugs. The higher level of divorced and separated men in this group and the finding that one-quarter were economically inactive, leading them to be poor at sustaining relationships and friendships, with many dependent on state benefits, may be partly explained by their drug dependence.
Similarities between women from class 2 and men from classes 3 and 5 were previously observed in Chapter 2 (see Study 2). More than one in six of this class of women were dependent on drugs.
There are four basic explanatory models for the relationship between drug use and violence: (1) substance use causes violence; (2) violence leads to substance use; (3) the relationship is reciprocal; and (4) the relationship is spurious, that is, it is coincidental or explained by a set of common causes. 183 Each model may be applicable to different subgroups of the population or to different incidents of drug-related violence. Unfortunately, a cross-sectional method does not allow exploration of these possibilities in any depth in this chapter. Nevertheless, these explanations are likely to have contributed to the findings.
Study 2: alcohol misuse
Objectives
The objectives this study were to:
-
investigate the prevalence of self-reported violence associated with alcohol dependence in a combined data set representative of the household population of Great Britain
-
investigate the independent associations of alcohol dependence with characteristics of violence, victim types and locations of violence
-
investigate the associations of alcohol dependence with a typology of violence in the general population.
Methods
Sample
The combined sample was drawn from the first phase of the NHPMS 2000 and the APMS 2007. The total sample included 15,734 men and women. Alcohol dependence was identified by a score of ≥ 20 on the AUDIT. 184
Statistical analyses
Weighted frequencies and proportions were reported for all categorical variables. Group associations between alcohol dependence and violence were established using binary logistic regression with the OR as the measure of magnitude. Multiple categorical predictor covariates were assigned a reference category against which other categories were contrasted. The violence typology for men and women described in Chapter 2 (see Study 1) were regressed on alcohol dependence using multinomial logistic regression.
All statistical analyses were adjusted by including covariates in each model simultaneously. Adjustments were made for demographic factors, psychosis, drug dependence, ASPD, depression and anxiety disorders. To adjust for the effects of selecting one individual per household and under-representation of certain subgroups, and to account for any deviation from selecting a simple random sample, all estimates were weighted. Details of the procedures used in weighting have been described previously. 45,82 All models employed robust SEs to adjust for clustering of individuals within postcodes. All analyses were performed using Stata.
Results
Demographic characteristics
Of the 15,734 respondents, 1596 (10.1%) reported any violence in the past 5 years. Table 52 shows that male sex, marital status other than married and social class lower than I and II were significantly associated with violence risk. Any age category > 34 years and being of Asian/Indian ethnicity were protective.
Covariate | Any violence in the past 5 years (n = 1596; 10.1%) | Alcohol dependence (n = 1065; 6.7%) | ||
---|---|---|---|---|
n (%) reported | AORa (95% CI) | n (%) reported | AORa (95% CI) | |
Sex | ||||
Female | 406 (5.1) | Reference | 248 (3.1) | Reference |
Male | 1190 (15.4) | 3.70 (3.14 to 4.36)*** | 817 (10.4) | 3.73 (3.13 to 4.45)*** |
Age group (years) | ||||
16–34 | 1175 (22.4) | Reference | 602 (11.4) | Reference |
35–54 | 371 (6.3) | 0.32 (0.27 to 0.38)*** | 368 (6.2) | 0.73 (0.60 to 0.89)** |
≥ 55 | 51 (1.1) | 0.06 (0.04 to 0.08)*** | 95 (2.0) | 0.29 (0.22 to 0.38)*** |
Marital status | ||||
Married/cohabiting | 480 (4.7) | Reference | 376 (3.7) | Reference |
Single | 973 (23.4) | 1.90 (1.59 to 2.26)*** | 558 (13.3) | 2.31 (1.91 to 2.79)*** |
Divorced/separated | 144 (9.9) | 2.12 (1.69 to 2.66)*** | 131 (8.9) | 2.17 (1.74 to 2.72)*** |
Social class | ||||
I and II | 302 (5.6) | Reference | 314 (5.8) | Reference |
IIIM and IIINM | 708 (11.3) | 2.10 (1.75 to 2.53)*** | 464 (7.3) | 1.18 (0.97 to 1.45) |
IV and V | 398 (12.6) | 2.38 (1.92 to 2.95)*** | 227 (7.1) | 1.05 (0.84 to 1.31) |
Ethnicity | ||||
White | 1463 (10.2) | Reference | 1015 (7.0) | Reference |
Black | 49 (12.1) | 0.85 (0.53 to 1.36) | 15 (3.6) | 0.38 (0.20 to 0.70)** |
Indian subcontinent | 36 (7.0) | 0.43 (0.25 to 0.75)** | 8 (1.6) | 0.22 (0.09 to 0.52)** |
Other | 40 (11.2) | 0.86 (0.51 to 1.46) | 20 (5.6) | 0.63 (0.34 to 1.16) |
Psychosis | 7 (14.0) | 0.50 (0.15 to 1.69) | 10 (19.1) | 1.66 (0.63 to 4.37) |
Drug dependence | 371 (35.3) | 2.63 (1.99 to 3.47)*** | 186 (32.5) | 2.83 (2.08 to 3.83)*** |
Anxiety disorder | 276 (48.4) | 1.92 (1.60 to 2.32)*** | 294 (12.5) | 2.23 (1.82 to 2.72)*** |
ASPD | 246 (46.2) | 3.14 (2.33 to 4.22)*** | 170 (31.4) | 2.48 (1.81 to 3.39)*** |
Depression | 79 (18.9) | 1.53 (1.05 to 2.24)* | 70 (16.0) | 1.80 (1.24 to 2.61)** |
The overall weighted prevalence of alcohol dependence in the sample was 6.7%. Being male and of single or divorced/separated marital status increased the risk associations with alcohol dependence. Any age category > 34 years and being of Asian/Indian or black ethnicity had protective associations with alcohol dependence. Having an anxiety disorder, depression or ASPD or being dependent on drugs was associated with alcohol dependence in adjusted models and therefore highly comorbid in the study population.
Main associations of alcohol dependence with violence
Table 53 shows the unadjusted and adjusted associations of alcohol dependence with all key violent outcomes, victim types and locations of reported violence. Alcohol dependence was significantly associated with all violent outcomes in univariate models (all p < 0.001). After adjustments, alcohol dependence was still an important risk correlate for most descriptors of violence, including violence while intoxicated, repeated violence, violence leading to injuries and violence in which the police were involved.
Outcomes | n (%) violent | OR (CI 95%) | AORa (CI 95%) |
---|---|---|---|
Any violence | 1596 (10.1) | 5.90 (4.97 to 7.00)*** | 2.19 (1.75 to 2.74)*** |
Violence while intoxicated | 685 (4.3) | 11.09 (9.02 to 13.63)*** | 3.86 (2.98 to 5.01)*** |
Severity of violence | |||
Minor violence | 655 (4.2) | 2.91 (2.22 to 3.82)*** | 1.27 (0.92 to 1.75) |
Five or more violent incidents | 335 (2.1) | 6.95 (5.05 to 9.56)*** | 1.88 (1.27 to 2.78)** |
Victim injured | 505 (3.2) | 6.32 (4.91 to 8.13)*** | 2.00 (1.44 to 2.78)*** |
Perpetrator injured | 514 (3.3) | 7.25 (5.75 to 9.14)*** | 2.68 (2.00 to 3.59)*** |
Police involved | 431 (2.7) | 5.64 (4.29 to 7.41)*** | 1.81 (1.30 to 2.53)*** |
Victim of violence | |||
Intimate partner | 252 (1.6) | 4.96 (3.61 to 6.82)*** | 2.29 (1.49 to 3.52)*** |
Family member | 153 (1.0) | 3.05 (1.82 to 5.11)*** | 1.48 (0.77 to 2.84) |
Friend | 312 (2.0) | 5.65 (4.04 to 7.90)*** | 1.68 (1.11 to 2.53)* |
Person known | 511 (3.2) | 4.38 (3.34 to 5.73)*** | 1.47 (1.05 to 2.06)* |
Stranger | 783 (5.0) | 6.72 (5.38 to 8.40)*** | 2.23 (1.68 to 2.95)*** |
Police | 88 (0.6) | 9.00 (5.42 to 14.95)*** | 2.37 (1.30 to 4.32)** |
Other | 108 (0.7) | 2.92 (1.62 to 5.28)*** | 0.98 (0.46 to 2.08) |
Location of violent incident | |||
Own home | 292 (1.9) | 3.86 (2.83 to 5.27)*** | 1.68 (1.11 to 2.53)* |
Someone else’s home | 138 (0.9) | 4.51 (2.66 to 7.66)*** | 1.22 (0.67 to 2.23) |
Street/outdoors | 909 (5.8) | 5.68 (4.59 to 7.02)*** | 1.88 (1.43 to 2.46)*** |
Bar/pub | 541 (3.4) | 9.73 (7.73 to 12.26)*** | 3.41 (2.54 to 4.59)*** |
Workplace | 101 (0.6) | 3.93 (2.20 to 7.00)*** | 1.28 (0.71 to 2.32) |
Other | 241 (1.5) | 2.96 (1.87 to 4.67)*** | 0.85 (0.48 to 1.50) |
Alcohol dependence also significantly increased the likelihood of violence towards intimate partners, friends, persons known, strangers and the police and the likelihood of violence taking place in all locations with the exception of someone else’s home and in the workplace.
Violence classes and alcohol dependence
Latent class models derived five violence classes for men and three violence classes for women in this joint data set. Development of these LCAs is described in detail in Chapter 2. Tables 54 and 55 include the associations between individuals with alcohol dependence and the violence classes for men and women respectively.
Violence typologies | Alcohol n (%) | RRRa (95% CI) | RRRb (95% CI) |
---|---|---|---|
No violence | 494 (7.5) | Reference | Reference |
Minor violence | 82 (18.2) | 1.77 (1.24 to 2.54)** | 1.57 (1.08 to 2.28)* |
Violence towards known persons | 91 (30.8) | 4.17 (2.89 to 6.01)*** | 3.10 (2.13 to 4.52)*** |
Fighting with strangers | 86 (28.0) | 3.13 (2.15 to 4.55)*** | 2.45 (1.65 to 3.63)*** |
Serious repetitive violence | 55 (45.5) | 5.90 (3.59 to 9.69)*** | 3.59 (2.07 to 6.21)*** |
Violence typologies | Alcohol, n (%) | RRRa (95% CI) | RRRb (95% CI) |
---|---|---|---|
No violence | 193 (2.5) | Reference | Reference |
General violence | 38 (13.4) | 2.68 (1.63 to 4.41)*** | 1.57 (0.87 to 2.84) |
Intimate/family violence | 15 (12.1) | 3.14 (1.71 to 5.77)*** | 1.99 (0.99 to 3.98) |
In men, the ‘no violence’ class had the lowest prevalence of alcohol dependence at 7.5%. The highest prevalence of alcohol dependence was observed for class 5 (‘serious repetitive violence’) at 45.5%. After adjustments, alcohol dependence was associated with more than a threefold increase in the odds of violence towards known persons and serious repetitive violence. Alcohol dependence was also associated with minor violence and fighting with strangers (see Table 54).
The female distribution of alcohol dependence across the classes was 2.5% for the ‘no violence’ class, 13.4% for the ‘general violence’ class and 12.1% for the ‘intimate/family violence’ class. Adjusted multinomial logistic regression models to estimate associations between violence latent classes and alcohol dependence revealed no significant associations for the violence classes compared with the ‘no violence’ class (see Table 55).
Discussion
The demographic associations with both violence and alcohol dependence in the general population were similar and corresponded in part to those observed for drug dependence. Violent and alcohol-dependent individuals tended to be male, younger, single or separated and with comorbid drug dependence, anxiety disorder and ASPD. However, the association that we observed for drug dependence with lower social class was not observed for alcohol dependence. Furthermore, being of black and Indian subcontinent ethnic origin appeared to be protective for alcohol dependence and also for violence among individuals originating from the Indian subcontinent. As with drug dependence, it was an unsurprising finding that alcohol dependence was associated with violence when intoxicated. However, the odds of association were higher for dependence on alcohol than for dependence on drugs. Alcohol dependence was not associated with minor violent incidents and was strongly associated with more severe altercations with others, violence involving injuries and police involvement. The somewhat stronger association with the perpetrator being injured than with the victim being injured may correspond to previous observations in cases of homicide that, when a fight has occurred in which one of the participants is killed (typically an altercation between strangers when intoxicated in or outside a bar), the deceased is subsequently found to have consumed a higher level of alcohol than the person charged with the murder. 185 In general, studies of victims of violence in Denmark186 and the Netherlands187 have shown that between 40% and 50% of victims had been drinking, although Shepherd et al. 188 found that 74% of male victims in a British study had been drinking.
Victims of those with alcohol dependence were similar to victims of those with drug dependence, but with a stronger level of association for IPV. There was no association with violence towards family members and the level of violence towards friends and persons known showed a weaker association than for drug dependence, but with a strong association for violence towards strangers and police involvement. The locations of violence were fewer than for drug dependence, with violence occurring in the home of perpetrators, which would correspond to IPV, but also in the street/outdoors or in a bar/pub.
Alcohol misuse and its relationship to violence, particularly binge drinking, have generated increasing public and political concern in the UK following a marked increase in the number of licensed premises selling alcohol over the past 25 years189 and legislative changes relaxing the laws relating to the sale of alcohol. Research into alcohol-related disorder highlights the concentration of violent and public order offences in urban areas with high densities of licensed premises, which peaks at weekends. 44 This has emerged within the planned regeneration of certain inner-urban areas in the UK but where there is competition among licensed premises designed to accommodate large numbers of drinkers, resulting in cheaper alcohol. The financial resources available to the alcohol industry in the UK (facilitating more effective litigation to overcome objections of residents and regulations of local authorities), coupled with an inherent culture of binge drinking, have compounded these problems. 190
In a previous study83 of violence and psychiatric morbidity in the household population of Great Britain, the highest percentage of incidents and the high population-attributable risk were explained by individuals who engaged in hazardous drinking (27%). The prevalence of alcohol dependence in this previous study (involving one of the two data sets included in the present study) was a similar 7% of the population. However, many more of the population engage in hazardous drinking and therefore hazardous drinkers accounted for 56% of violent incidents over the study period. Nevertheless, the relatively small percentage of alcohol-dependent individuals was associated with a substantial 29% of all violent events. This would indicate that a targeted approach to alcohol-dependent individuals who are prone to become involved in violence might have a major impact on the overall level of violence in the population. 74
Alcohol dependence was associated with all classes of violence among men, but the associations with alcohol dependence were no longer significant following adjustments among women. These were surprising findings and did not correspond to the observation in study 1 that women involved in general violence (class 2) showed a significant association with drug dependence. Two factors may partly explain these differences. First, the relative proportions of men and women who are drug and alcohol dependent differs, with a larger proportion of men dependent on alcohol relative to women compared with those dependent on drugs. Second, we have previously referred to the ‘threshold’ hypothesis and the notion that women who develop antisocial behaviour surmount a higher threshold of risk than men and are therefore more severely afflicted. 86 We earlier showed that women were found to have raised thresholds of risk from affective and anxiety disorders and personality disorder. However, men in the general population of Great Britain were found to surmount a lower threshold of risk specifically from hazardous drinking and alcohol dependence than violent women. It was thought that the explanation for these raised thresholds of risk from heavy drinking could be explained primarily by the drinking culture of men in Great Britain. Many more men than women drank heavily. In this context it is probable that a large number of additional factors associated with heavy alcohol misuse, but not investigated in this study, characterised men but not women to account for these sex differences. However, these were not associated with drug dependence, with both men and women appearing to show relatively similar associations with violence.
Chapter 7 Childhood maltreatment and adult victimisation
Background
There is a strong overlap between violence and victimisation. Perpetrators and victims share significant characteristics and behaviours and are often the same people. 191–198 Posick199 has pointed out that, traditionally, research on violence has considered two separate groups of individuals: one group that harms others (offenders or perpetrators of violence) and one group that is harmed by others (victims). However, their common characteristics suggest that it is difficult to understand either violence or violent victimisation without understanding both.
Although many violent incidents occur with little or no contribution on the part of the victim, social interactions between the participants during violent incidents often suggest that there are many similarities and that victims can contribute to their own injury through their behaviour. Furthermore, individuals who are violent towards others also have a tendency to put themselves at greater risk.
Hinderlang193 has described how early systems of law and politics can provide a backdrop for a violent cycle of attack and retaliation. In some cultures it is considered necessary that victims seek revenge for wrongs committed against them or their family. In these social settings, a person may be the victim of an attack one day and the perpetrator the next. Ethnographic research on violence and disadvantaged neighbourhoods reveals that some individuals are enmeshed in the violent culture of offending and victimisation through retaliation. 152,200,201 This is particularly the case among gangs in such neighbourhoods. 202 Posick199 has described victims and offenders as similar groups of individuals in such contexts, who are involved in a ‘cycle of violence’. The social setting may be the link between offending and victimisation. Who becomes a victim and who becomes an offender within a particular violent event may be based on the immediate social interaction. The initial offender may become the ultimate victim and vice versa. The difference between victims and offenders in such interactions may be obscure and both parties may escalate the violent situation by trying to save face through acting aggressively. 203 Some individuals may act tough and resort to violence if disrespected. However, because there are many individuals in certain locations, there are likely to be some ‘winners’ (who will be seen as perpetrators) and some ‘losers’ (who become victims). The next time similar individuals meet, roles may be reversed. 204
A further body of research that has proceeded independently but which shows close parallels with the above studies of adult perpetrator–victim interactions is the child to adult ‘cycle of violence’ hypothesis. This posits that being abused or neglected as a child increases the risk for delinquency, adult criminal behaviour and violent criminal behaviour. 205 Although abused and neglected children are generally at ‘high risk’ for social problems, not all succumb to these and protective factors can intervene in a child’s development, leading some to be ‘resilient’. These include dispositional attributes, environmental conditions, biological predispositions and positive events that mitigate against early negative experiences. 206 However, recent research was unable to confirm the cycle of violence hypothesis207 and a literature review has also highlighted methodological inconsistencies across studies. 205
In this chapter we first investigate a wider range of childhood exposures and psychopathology in adulthood than in previous studies to test the cycle of violence hypothesis. In addition to childhood experiences of physical abuse, sexual abuse and neglect, we also include witnessing domestic violence and being the victim of bullying. In the second study of victimisation and violence perpetration in adulthood, the cross-sectional methods used ultimately prevented us from examining the relationship between victims and perpetrators. Our investigation is, therefore, limited to both of these experiences as reported by the same individuals, with the intention of measuring their association at the population level. Study 2 therefore provides a basis for future epidemiological investigation.
Study 1: childhood maltreatment and perpetration of violence in adulthood
Objectives
The objectives of the study were to investigate:
-
whether or not direct associations exist between different types of early maltreatment and violence among young adult men in a representative national sample of young adult men
-
whether or not there is a linear increase in the proportion of violence associated with multiple forms of maltreatment
-
the associations between child maltreatment and a typology of violence in the general population.
Methods
Data collection
The second MMLS was a cross-sectional survey of young adult men aged 18–34 years (n = 5400) carried out in 2011 in Great Britain. The sampling methods and components, and measures of the survey, are described in Chapter 2 (see Study 1).
Measures
Early maltreatment was defined by affirmative responses to self-reported experiences before 16 years of age, including sexual abuse/assault, physical abuse, neglect, having been bullied, witnessing violence in the home and witnessing parents or carers fighting.
Statistical analyses
For descriptive purposes, absolute (n) and relative (%) frequencies were reported for all dichotomous/polytomous categorical variables.
We initially investigated associations between early maltreatment and violence in the past 5 years and estimated their independent effects by including all forms of maltreatment simultaneously. To estimate the impact of exposure to multiple adverse childhood events, we combined the binary maltreatment variables and plotted their frequencies against any violence in the past 5 years and explored whether or not there was a significant linear trend.
To control for differences between samples, survey type was included as a covariate in all analyses. We also used robust SEs to account for correlations within survey areas because of clustering within postcodes. An α level of 0.05 was adopted throughout. All analyses were performed in Stata.
Results
Demography and sampling
The weighted final sample included 5400 men aged 18–34 years, of whom 1360 (25.2%) reported being bullied, 585 (10.8%) reported witnessing violence at home, 571 (10.6%) reported witnessing parents/carers fighting, 135 (2.5%) reported experiencing sexual abuse, 337 (6.2%) reported experiencing physical abuse and 272 (5.0%) reported having being neglected before 16 years of age (Table 56).
Covariate | Bullied, n (%) | Witnessed violence at home, n (%) | Witnessed parents/carers fighting, n (%) | Sexual abuse, n (%) | Physical abuse, n (%) | Neglect, n (%) |
---|---|---|---|---|---|---|
All participants | 1360 (25.2) | 585 (10.8) | 571 (10.6) | 135 (2.5) | 337 (6.2) | 272 (5.0) |
Age group (years) | ||||||
18–24 (reference) | 513 (37.7) | 194 (33.1) | 215 (37.7) | 42 (31.3) | 114 (33.8) | 103 (37.7) |
25–34 | 847 (62.3) | 391 (66.9)** | 356 (62.3) | 93 (68.7) | 223 (66.2) | 169 (62.3) |
Marital status | ||||||
Married/cohabiting (reference) | 450 (33.2) | 190 (32.8) | 191 (33.8) | 36 (27.4) | 120 (35.9) | 89 (33.2) |
Single | 850 (62.7) | 358 (61.9) | 345 (61.1) | 89 (67.4) | 188 (56.4) | 160 (59.4) |
Divorced/separated | 55 (4.1) | 31 (5.3) | 29 (5.1) | 7 (5.2) | 26 (7.7)* | 20 (7.4)* |
Social class | ||||||
I and II (reference) | 147 (11.2) | 51 (9.1) | 48 (8.7) | 20 (15.4) | 34 (10.6) | 32 (11.9) |
IIIM and IIINM | 362 (27.7) | 119 (21.1) | 119 (21.4) | 37 (29.0) | 70 (21.5) | 56 (21.0) |
IV and V | 302 (23.1) | 137 (24.1) | 139 (25.0) | 19 (14.6)*a | 75 (23.0) | 62 (23.5) |
Unemployed/NC | 496 (37.9) | 259 (45.8)* | 250 (45.0)* | 53 (41.0) | 147 (44.9) | 116 (43.6) |
Ethnicity | ||||||
White (reference) | 988 (72.7) | 443 (75.8) | 445 (77.9) | 72 (53.4) | 238 (70.6) | 156 (57.3) |
Black | 184 (13.5) | 87 (14.9) | 74 (13.0) | 31 (22.7) | 58 (17.4) | 61 (22.4)* |
Asian and other | 187 (13.8)*a | 54 (9.3)**a | 52 (9.1)***a | 32 (23.9) | 40 (12.0)*a | 55 (20.3) |
Non-UK born | 114 (8.6)**a | 53 (9.3) | 57 (10.3) | 32 (24.4) | 38 (11.6) | 38 (14.5) |
Survey type | ||||||
Main (reference) | 603 (44.4) | 218 (37.3) | 229 (40.2) | 48 (35.8) | 125 (37.1) | 78 (28.7) |
BME | 182 (13.4)**a | 66 (11.3) | 68 (11.9) | 18 (13.2)*a | 35 (10.3) | 39 (14.3) |
DE | 197 (14.5) | 82 (14.1) | 97 (17.0) | 15 (10.8) | 55 (16.4) | 45 (16.4)* |
Hackney | 161 (11.8)**a | 66 (11.2) | 47 (8.3)*a | 42 (31.0) | 66 (19.5) | 72 (26.4)** |
Glasgow East | 216 (15.9) | 153 (26.2)*** | 129 (22.6) | 12 (9.1) | 56 (16.7) | 39 (14.2) |
Table 57 shows that, after adjusting for all other maltreatment types, those of Asian and ‘other’ ethnicity were less likely to report witnessing their parents fighting but more likely to report neglect. Men who were not born in the UK were less likely to report having been bullied. Compared with those in higher social classes (I and II), men from social classes IV and V were less likely to report sexual abuse. Older age was associated with reports of witnessing violence in the home.
Covariate | Being bullied, AOR (95% CI) | Witness violence at home, AOR (95% CI) | Witness parents/carers fighting, AOR (95% CI) | Sexual abuse, AOR (95% CI) | Physical abuse, AOR (95% CI) | Neglect, AOR (95% CI) |
---|---|---|---|---|---|---|
Age group (years) | ||||||
18–24 (reference) | – | – | – | – | – | – |
25–34 | 1.04 (0.88 to 1.24) | 1.56 (1.16 to 2.08)** | 0.83 (0.64 to 1.07) | 1.32 (0.79 to 2.19) | 0.91 (0.65 to 1.27) | 0.76 (0.53 to 1.09) |
Marital status | ||||||
Married/cohabiting (reference) | – | – | – | – | – | – |
Single | 1.06 (0.89 to 1.28) | 1.12 (0.83 to 1.53) | 0.82 (0.62 to 1.07) | 1.63 (0.98 to 2.70) | 0.69 (0.47 to 1.00) | 0.82 (0.55 to 1.24) |
Divorced/separated | 0.96 (0.64 to 1.44) | 0.99 (0.49 to 2.02) | 0.84 (0.40 to 1.75) | 0.93 (0.37 to 2.31) | 1.73 (0.92 to 3.27) | 1.48 (0.72 to 3.05) |
Social class | ||||||
I and II (reference) | – | – | – | – | – | – |
IIIM and IIINM | 1.14 (0.85 to 1.53) | 1.05 (0.65 to 1.71) | 0.94 (0.62 to 1.44) | 1.01 (0.50 to 2.06) | 0.86 (0.48 to 1.54) | 0.83 (0.48 to 1.43) |
IV and V | 0.90 (0.66 to 1.22) | 1.29 (0.77 to 2.18) | 1.19 (0.78 to 1.80) | 0.41 (0.19 to 0.88)* | 0.92 (0.53 to 1.61) | 0.97 (0.55 to 1.71) |
Unemployed/NC | 1.02 (0.77 to 1.37) | 1.42 (0.86 to 2.35) | 1.26 (0.84 to 1.89) | 0.89 (0.45 to 1.75) | 1.43 (0.80 to 2.55) | 1.27 (0.74 to 2.19) |
Ethnicity | ||||||
White (reference) | – | – | – | – | – | – |
Black | 1.01 (0.75 to 1.37) | 1.32 (0.77 to 2.29) | 0.62 (0.38 to 1.02) | 1.77 (0.90 to 3.47) | 0.75 (0.42 to 1.34) | 1.84 (0.99 to 3.41) |
Asian and other | 0.82 (0.59 to 1.13) | 0.65 (0.37 to 1.14) | 0.39 (0.22 to 0.68)** | 1.74 (0.90 to 3.34) | 0.70 (0.37 to 1.34) | 2.04 (1.21 to 3.46)** |
Non-UK born | 0.62 (0.47 to 0.80)*** | 0.85 (0.52 to 1.38) | 1.39 (0.90 to 2.15) | 1.74 (0.89 to 3.37) | 1.06 (0.61 to 1.87) | 0.99 (0.61 to 1.60) |
Survey type | ||||||
Main (reference) | – | – | – | – | – | – |
BME | 0.63 (0.44 to 0.90)* | 0.88 (0.46 to 1.69) | 1.24 (0.68 to 2.25) | 0.37 (0.15 to 0.91)* | 0.89 (0.39 to 2.00) | 0.93 (0.46 to 1.89) |
DE | 0.95 (0.74 to 1.21) | 0.83 (0.57 to 1.21) | 1.31 (0.93 to 1.85) | 0.84 (0.39 to 1.81) | 1.10 (0.70 to 1.75) | 1.68 (1.05 to 2.70)* |
Hackney | 0.60 (0.45 to 0.80)*** | 0.82 (0.50 to 1.35) | 0.62 (0.38 to 1.03) | 1.89 (1.11 to 3.21)* | 2.02 (1.13 to 3.61)* | 2.74 (1.55 to 4.85)** |
Glasgow East | 0.76 (0.60 to 0.97)* | 2.07 (1.53 to 2.81)*** | 1.13 (0.79 to 1.62) | 0.47 (0.21 to 1.04) | 0.88 (0.55 to 1.39) | 1.11 (0.68 to 1.80) |
Early maltreatment and violence
Table 58 shows the frequencies of violent outcomes and associations between early maltreatment and the violent outcomes before adjusting for the other types of maltreatment but after adjustments for demography. Having been a victim of bullying and physical abuse and having witnessed violence in the home showed positive associations with all outcomes except minor violence. Witnessing parents/carers fighting was associated with all outcomes except violence towards an unspecified victim type (‘other’). Sexual abuse was associated with all outcomes except minor violence and violence towards a person known and an unspecified victim type (‘other’). Experiencing physical abuse was associated with all outcomes except minor violence. Experiencing neglect was associated with all outcomes except minor violence and violence towards an unspecified victim type (‘other’).
Outcomes | Being bullied, n (%) | Witnessed violence at home, n (%) | Witnessed parents/carers fighting, n (%) | Sexual abuse, n (%) | Physical abuse, n (%) | Neglect, n (%) |
---|---|---|---|---|---|---|
Any violence | 607 (45.0)*** | 388 (66.4)*** | 346 (60.8)*** | 75 (55.8)*** | 214 (63.6)*** | 167 (61.9)*** |
Violence while intoxicated | 322 (24.3)*** | 234 (41.7)*** | 208 (38.0)*** | 37 (29.9)*** | 134 (42.0)*** | 94 (36.4)*** |
Severity | ||||||
Minor violence | 117 (8.7) | 45 (7.8) | 61 (10.8)* | 8 (6.1) | 24 (7.0) | 18 (6.6) |
Repeated violence | 111 (8.4)*** | 89 (16.2)*** | 72 (13.4)*** | 23 (17.5)*** | 61 (19.0)*** | 43 (16.9)*** |
Victim injured | 270 (20.1)*** | 217 (37.2)*** | 188 (33.3)*** | 31 (23.1)*** | 120 (35.8)*** | 91 (33.7)*** |
Perpetrator injured | 331 (24.6)*** | 219 (37.6)*** | 178 (31.4)*** | 42 (31.5)*** | 132 (39.5)*** | 89 (33.3)*** |
Police involved | 180 (13.4)*** | 126 (21.6)*** | 111 (19.6)*** | 33 (25.0)*** | 91 (27.2)*** | 62 (23.2)*** |
Victim of violence | ||||||
Intimate partner | 104 (7.7)*** | 85 (14.6)*** | 70 (12.4)*** | 27 (20.2)*** | 54 (16.2)*** | 55 (20.7)*** |
Family member | 98 (7.3)*** | 100 (17.2)*** | 89 (15.7)*** | 16 (12.2)*** | 51 (15.2)*** | 37 (13.8)*** |
Friend | 205 (15.2)*** | 122 (20.9)*** | 127 (22.4)*** | 27 (20.2)*** | 85 (25.4)*** | 65 (24.1)*** |
Person known | 210 (15.6)*** | 142 (24.3)*** | 138 (24.4)*** | 16 (11.7) | 71 (21.3)*** | 57 (21.4)*** |
Stranger | 287 (21.3)*** | 184 (31.5)*** | 185 (32.7)*** | 32 (23.9)*** | 114 (34.1)*** | 85 (31.5)*** |
Police | 59 (4.4)*** | 51 (8.8)*** | 40 (7.1)*** | 9 (6.6)*** | 34 (10.1)*** | 22 (8.1)*** |
Other | 37 (2.7)* | 21 (3.7)* | 14 (2.5) | 5 (3.7) | 15 (4.6)*** | 7 (2.6) |
Location of violent incident | ||||||
Own home | 114 (8.5)*** | 106 (18.1)*** | 84 (14.8)*** | 15 (11.4)** | 59 (17.5)*** | 41 (15.3)*** |
Someone else’s home | 113 (8.4)*** | 110 (18.9)*** | 96 (17.0)*** | 23 (17.3)*** | 60 (18.0)*** | 47 (17.7)*** |
Street/outdoors | 353 (26.3)*** | 228 (39.0)*** | 214 (37.8)*** | 38 (28.6)*** | 128 (38.1)*** | 100 (37.3)*** |
Bar/pub | 255 (19.0)*** | 174 (29.8)*** | 168 (29.6)*** | 34 (25.7)*** | 99 (29.4)*** | 80 (29.8)*** |
Workplace | 30 (2.2)*** | 16 (2.7)** | 16 (2.8)*** | 8 (5.8)*** | 15 (4.5)*** | 11 (4.0)** |
Table 59 shows the independent associations between self-reported maltreatment before 16 years of age and all violence outcomes following adjustment for other forms of maltreatment. Sexual abuse was not associated with any of the violent outcomes. Having been bullied was associated with violence while intoxicated, incidents in which the perpetrator was injured and incidents in which the police were involved. Being bullied was also related to violence towards intimate partners, friends and known persons and violence in the home, in the street, in bars/pubs and in the workplace.
Outcomes | Being bullied, AOR (95% CI) | Witnessed violence at home, AOR (95% CI) | Witnessed parents/carers fighting, AOR (95% CI) | Sexual abuse, AOR (95% CI) | Physical abuse, AOR (95% CI) | Neglect, AOR (95% CI) |
---|---|---|---|---|---|---|
Any violence | 1.43 (1.20 to 1.70)*** | 2.93 (2.21 to 3.89)*** | 1.67 (1.28 to 2.17)*** | 0.94 (0.52 to 1.70) | 1.55 (1.12 to 2.13)** | 1.75 (1.22 to 2.52)** |
Violence while intoxicated | 1.51 (1.23 to 1.85)*** | 2.52 (1.85 to 3.41)*** | 1.78 (1.32 to 2.38)*** | 0.82 (0.44 to 1.54) | 1.96 (1.37 to 2.80)*** | 1.45 (1.00 to 2.11)* |
Severity | ||||||
Repeated violence | 1.30 (0.93 to 1.81) | 2.42 (1.54 to 3.80)*** | 1.38 (0.90 to 2.10) | 1.27 (0.69 to 2.36) | 2.25 (1.44 to 3.50)*** | 1.40 (0.87 to 2.24) |
Victim injured | 1.10 (0.88 to 1.38) | 2.59 (1.94 to 3.46)*** | 1.57 (1.17 to 2.11)** | 0.76 (0.40 to 1.45) | 1.82 (1.27 to 2.61)** | 1.62 (1.12 to 2.34)* |
Perpetrator injured | 2.00 (1.60 to 2.50)*** | 2.60 (1.90 to 3.56)*** | 1.32 (0.97 to 1.79) | 1.00 (0.57 to 1.74) | 1.79 (1.26 to 2.53)** | 1.15 (0.77 to 1.71) |
Police involved | 1.40 (1.07 to 1.83)* | 1.83 (1.24 to 2.68)** | 1.28 (0.88 to 1.87) | 1.71 (0.94 to 3.11) | 2.30 (1.52 to 3.48)*** | 1.55 (0.98 to 2.46) |
Minor violence | 1.26 (0.96 to 1.65) | 0.82 (0.52 to 1.30) | 1.61 (1.07 to 2.43)* | 0.90 (0.41 to 1.98) | 0.75 (0.43 to 1.31) | 0.96 (0.55 to 1.69) |
Victim of violence | ||||||
Intimate partner | 1.67 (1.18 to 2.36)** | 3.08 (1.84 to 5.14)*** | 1.69 (1.04 to 2.76)* | 1.89 (0.93 to 3.85) | 1.29 (0.77 to 2.16) | 2.23 (1.40 to 3.55)*** |
Family member | 1.30 (0.88 to 1.92) | 3.66 (2.32 to 5.78)*** | 2.24 (1.44 to 3.47)*** | 0.85 (0.40 to 1.81) | 1.38 (0.80 to 2.36) | 1.24 (0.72 to 2.13) |
Friend | 1.85 (1.43 to 2.38)*** | 1.32 (0.92 to 1.90) | 2.17 (1.57 to 3.01)*** | 1.09 (0.61 to 1.96) | 1.56 (1.06 to 2.28)* | 1.62 (1.07 to 2.47)* |
Person known | 1.57 (1.23 to 2.00)*** | 1.89 (1.32 to 2.71)*** | 1.90 (1.34 to 2.69)*** | 0.62 (0.32 to 1.23) | 1.14 (0.77 to 1.69) | 1.60 (1.04 to 2.45)* |
Stranger | 1.25 (1.00 to 1.57) | 1.60 (1.18 to 2.17)** | 1.96 (1.46 to 2.63)*** | 0.82 (0.46 to 1.47) | 1.65 (1.14 to 2.40)** | 1.53 (1.05 to 2.24)* |
Police | 1.30 (0.87 to 1.94) | 2.95 (1.68 to 5.19)*** | 1.01 (0.56 to 1.82) | 1.00 (0.41 to 2.42) | 2.07 (1.20 to 3.59)** | 1.17 (0.62 to 2.21) |
Other | 1.50 (0.88 to 2.55) | 1.63 (0.86 to 3.09) | 0.64 (0.33 to 1.21) | 1.39 (0.52 to 3.67) | 2.51 (1.32 to 4.78)** | 0.76 (0.34 to 1.72) |
Location of violent incident | ||||||
Own home | 1.50 (1.07 to 2.09)* | 4.08 (2.59 to 6.43)*** | 1.59 (1.04 to 2.45)* | 0.74 (0.36 to 1.49) | 1.70 (1.04 to 2.77)* | 1.06 (0.63 to 1.81) |
Someone else’s home | 1.12 (0.81 to 1.54) | 2.69 (1.84 to 3.94)*** | 1.67 (1.11 to 2.50)* | 1.79 (0.80 to 4.01) | 1.45 (0.92 to 2.30) | 1.46 (0.90 to 2.38) |
Street/outdoors | 1.56 (1.28 to 1.90)*** | 2.10 (1.55 to 2.85)*** | 1.77 (1.34 to 2.32)*** | 0.79 (0.45 to 1.37) | 1.40 (0.99 to 1.98) | 1.61 (1.13 to 2.31)** |
Bar/pub | 1.36 (1.08 to 1.72)** | 1.83 (1.30 to 2.58)*** | 1.91 (1.40 to 2.59)*** | 1.22 (0.68 to 2.22) | 1.21 (0.84 to 1.74) | 1.79 (1.25 to 2.57)** |
Workplace | 1.94 (1.05 to 3.58)* | 1.09 (0.38 to 3.09) | 1.52 (0.58 to 4.01) | 1.90 (0.67 to 5.40) | 2.00 (0.74 to 5.44) | 1.43 (0.58 to 3.50) |
Having witnessed violence in the home was independently associated with all violent outcomes except minor violence, all victim types except friends and all locations except the workplace. Having witnessed parents/carers fighting was independently associated with violence while intoxicated, victim injury and minor violence, all victim types except the police and all locations except the workplace. Physical abuse was independently associated with all violent outcomes except minor violence, violence towards a friend, a stranger and the police and violence in the home. Neglect was independently associated with violence while intoxicated, victim injury, IPV, violence to friends, persons known and strangers and violence in the street/outdoors and in bars or pubs.
Multiple forms of maltreatment and violence
Figure 3 shows the change in the proportion of violence responders by number of early maltreatment types. Multiple forms of maltreatment were endorsed by young men in the sample. There was evidence of a linear trend in these weighted proportions (F = 71.2; p < 0.001).
FIGURE 3.
Impact of multiple maltreatment types on any violence in the last 5 years among young men.
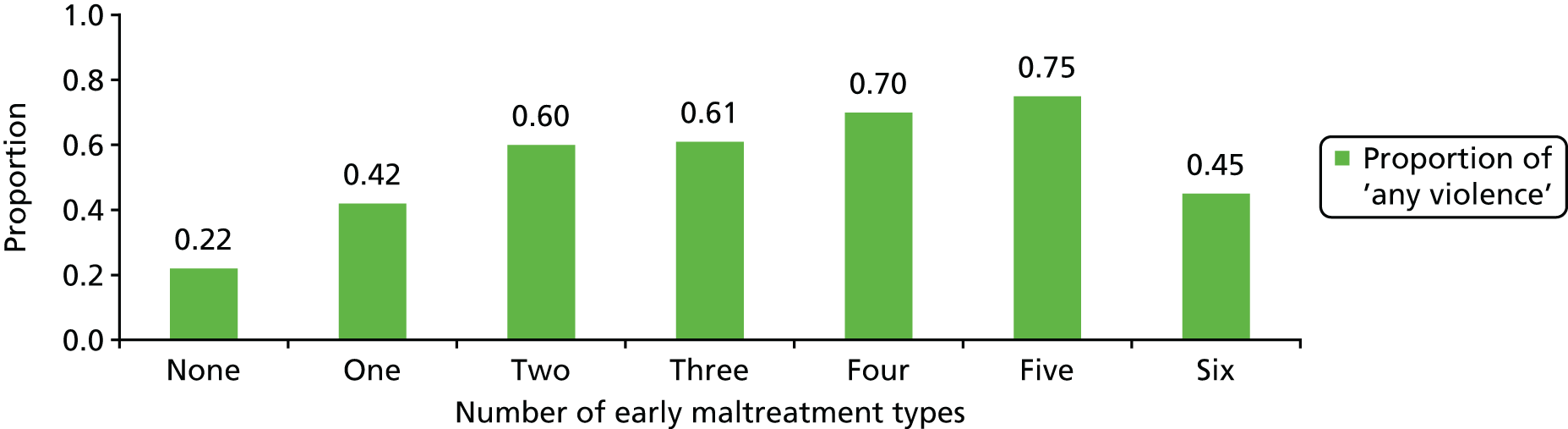
Discussion
We confirmed that child maltreatment is a strong predictor of violent behaviour in adulthood among young adult men in the UK population. Our survey also revealed some important epidemiographic trends that require investigation. First, there were important demographic differences among those who reported early maltreatment. We examined typical demographic factors in the survey. However, we included four boost surveys: black and minority ethnic men, men of lower social class who were unemployed, the London borough of Hackney and Glasgow East. Hackney and Glasgow East contained very unusual populations with concentrations of severe socioeconomic deprivation. Hackney has one of the largest black and minority ethnic populations in the UK. Our findings therefore suggest the possibility that demographic and cultural influences, together with a concentration of social problems that affect children, are found together and interact in certain geographical areas.
The findings do not explain the mechanism but it would appear that men of South Asian and other black and minority ethnic origin, together with those who were not born in the UK, were less likely to report bullying in childhood. Similarly, significantly fewer men from the black and minority ethnic and Hackney boost surveys reported bullying before the age of 15 years. It is of interest that black and minority ethnic men reported less perpetration of violence towards others and perpetration/victimisation in adulthood (see Study 2).
Examining each subcategory of early maltreatment, men in the older age range (25–34 years) reported more childhood maltreatment than younger men (18–24 years). Whether there has been a change in the level of violence over time within families in the UK or whether this finding represents recall bias cannot be evaluated from this cross-sectional survey, but this is an important question for future research. However, the finding that more unemployed men had witnessed violence in the home and their parents/carers fighting suggested that these childhood experiences or associated disadvantage had a detrimental effect on later life success. It was of some interest that significantly more men from Glasgow East, the most socioeconomically deprived area of the UK, and with an almost entirely white population, reported the highest levels of violence in the home during childhood, suggesting the importance of area-level effects.
Difficulties in sustaining relationships in adulthood may be linked to physical abuse and neglect from parents/carers during childhood. Neglect was reported significantly more often by men of black ethnicity, men of lower social class, the unemployed and men from Hackney. Asian and other black and minority ethnic men appeared significantly less likely to report physical abuse, suggesting different parenting practices among different ethnic subgroups.
Examining adult outcomes of violence, lack of an association between childhood sexual abuse and violence in adulthood was the most striking observation. Sexual abuse has often been combined with other forms of maltreatment in previous studies, which prevents conclusions being drawn on the independent effects on Iater violence. However, childhood sexual abuse has been found to increase the risk of adult psychopathology208,209 and is associated with self-harm210,211 and with an increased risk of repeated sexual and other forms of victimisation (rather than perpetration) in adulthood, in samples that have included women. 212–214
Witnessing violence at home showed the strongest odds of association with adult outcomes of violence and a range of victims of violence. The range of different victims would explain the corresponding range of different locations of violence. However, childhood physical abuse was also independently associated with a range of different victims and more serious forms of violence towards others. A literature review has concluded that early physical abuse is the most consistent predictor of youth violence, particularly when compounded by additional forms of maltreatment. 215
Finally, we observed that the greater the number of early types of maltreatment experienced, the greater the proportion of men reporting violence towards others in adulthood. When all six forms of maltreatment were reported the above trend ceased to exist. This may be because of the small numbers of men in this subgroup and lack of power. Nevertheless, the linear trend observed suggests a dose–response relationship between childhood maltreatment and violence in adulthood, suggesting a causal relationship that requires confirmation in longitudinal studies.
Study 2: childhood maltreatment, victimisation and violence perpetration in adulthood
Objectives
The objectives of this study were to:
-
investigate the prevalence of victims of violence, violent perpetrators and victims/perpetrators in a representative sample of young men aged 18–34 years
-
compare victims and perpetrators with young men who either had never been a victim of violence or had acted violently in the past 5 years
-
identify characteristics of victims and perpetrators of violence.
Methods
Data collection
The second MMLS was a cross-sectional survey of young adult men aged 18–34 years (n = 5400) carried out in 2011 in Great Britain. This survey is described in Chapter 2 (see Study 1).
Measures
Early maltreatment and adversity was defined by affirmative responses to self-reported experiences before the age of 16 years, including having been taken to local authority care, sexual abuse/assault, physical abuse, neglect, having been bullied, witnessing violence in the home and witnessing parents or carers fighting.
Participants were classified based on their self-report of ever acting violently towards another person or ever having been a victim of violence. They were then divided into four groups: no violence, victim of violence, perpetrator of violence and victims/perpetrators.
Statistical analyses
For descriptive purposes, absolute (n) and relative (%) frequencies were reported for all dichotomous/polytomous categorical variables.
We investigated associations between demographic characteristics, mental health problems and a series of childhood adversities and mutually exclusive categories of victims of violence, perpetrators of violence and victims/perpetrators, with no violence as the reference. We fitted multinomial logistic regression models with categories of victimisation and perpetration regressed on all demographic correlates, mental health problems and childhood adversity.
To control for differences between samples, survey type was included as a covariate in all analyses. We also used robust SEs to account for correlations within survey areas because of clustering within postcodes. An α level of 0.05 was adopted throughout. All analyses were performed in Stata.
Results
Demography and sampling
The weighted final sample included 5400 men aged 18–34 years, of whom 2751 (54.1%) had not been violent in the past 5 years and had not been a victim of violence, 161 (3.2%) reported that they had been a victim but not a perpetrator of violence, 1491 (29.3%) reported perpetration of violence but not victimisation and 680 (13.4%) reported both victimisation and perpetration of violence in adulthood (Table 60).
Survey type | No violence, n (%) | Victim, n (%) | Perpetrator, n (%) | Victim/perpetrator, n (%) |
---|---|---|---|---|
Main | 950 (48.7) | 59 (3.0) | 669 (34.3) | 272 (14.0) |
BME | 709 (69.3) | 25 (2.5) | 241 (23.6) | 48 (4.7) |
DE | 277 (44.8) | 16 (2.5) | 216 (34.8) | 111 (17.8) |
Hackney | 464 (64.7) | 21 (2.9) | 174 (24.2) | 59 (8.2) |
Glasgow East | 350 (45.3) | 41 (5.3) | 191 (24.7) | 191 (24.7) |
Total sample | 2751 (54.1) | 161 (3.2) | 1491 (29.3) | 680 (13.4) |
Victims of violence were less likely to be single and were over-represented in the Glasgow East boost survey (Tables 61 and 62). Perpetrators were less likely to be from social classes IV and V and more were born in the UK. In contrast, South Asian and other ethnic groups and men from Hackney and Glasgow East were less likely to be perpetrators. Victims/perpetrators were significantly older, divorced or separated, from lower social classes and unemployed and more likely to be born in the UK. Victims/perpetrators were under-represented in Hackney but were over-represented in Glasgow East.
Demographic characteristics | No violence, n (%) | Victim, n (%) | Perpetrator, n (%) | Victim/perpetrator, n (%) |
---|---|---|---|---|
All participants | 2751 (54.1) | 161 (3.2) | 1491 (29.3) | 680 (13.4) |
Age group (years) | ||||
18–24 | 1076 (39.1) | 65 (40.1) | 572 (38.4) | 218 (32.1) |
25–34 | 1675 (60.9) | 97 (59.9) | 919 (61.7) | 462 (67.9) |
Marital status | ||||
Married/cohabiting/widowed | 902 (33.3) | 62 (38.8) | 504 (34.0) | 218 (32.1) |
Single | 1727 (63.7) | 90 (56.5) | 915 (61.7) | 422 (62.2) |
Divorced/separated | 82 (3.0) | 8 (4.7) | 64 (4.3) | 39 (5.7) |
Social class | ||||
I and II | 338 (12.9) | 17 (10.4) | 192 (13.7) | 49 (7.6) |
IIIM and IIINM | 717 (27.4) | 50 (31.3) | 365 (26.0) | 167 (25.6) |
IV and V | 709 (27.1) | 33 (20.7) | 344 (24.5) | 174 (26.6) |
Unemployed | 852 (32.6) | 60 (37.6) | 502 (35.8) | 263 (40.2) |
Non-UK born | 467 (17.3) | 16 (9.9) | 151 (10.4) | 43 (6.5) |
Ethnicity | ||||
White | 1526 (55.6) | 114 (70.5) | 1035 (69.5) | 573 (84.3) |
Black | 414 (15.1) | 21 (13.0) | 221 (14.9) | 66 (9.7) |
South Asian and other | 805 (29.3) | 27 (16.6) | 234 (15.7) | 41 (6.0) |
Covariates | No violence (reference) | Victim, RRRa (95% CI) | Perpetrator, RRRa (95% CI) | Victim/perpetrator, RRRa (95% CI) |
---|---|---|---|---|
Age group (years) | ||||
18–24 | Reference | – | – | – |
25–34 | – | 0.82 (0.55 to 1.22) | 1.05 (0.89 to 1.25) | 1.44 (1.16 to 1.78)** |
Marital status | ||||
Married/cohabiting/widowed | Reference | – | – | – |
Single | – | 0.62 (0.39 to 0.97)* | 0.95 (0.79 to 1.14) | 1.06 (0.84 to 1.33) |
Divorced/separated | – | 1.23 (0.52 to 2.93) | 1.30 (0.86 to 1.98) | 1.71 (1.05 to 2.78)* |
Social class | ||||
I and II | Reference | – | – | – |
IIIM and IIINM | – | 1.24 (0.65 to 2.33) | 0.79 (0.61 to 1.04) | 1.44 (0.97 to 2.15) |
IV and V | – | 0.81 (0.43 to 1.54) | 0.78 (0.61 to 1.00)* | 1.53 (1.02 to 2.30)* |
Unemployed | – | 1.27 (0.67 to 2.42) | 0.96 (0.74 to 1.25) | 1.86 (1.25 to 2.77)** |
Non-UK born | – | 0.80 (0.35 to 1.81) | 0.74 (0.57 to 0.97)* | 0.67 (0.46 to 0.98)* |
Ethnicity | ||||
White | Reference | – | – | – |
Black | – | 1.01 (0.44 to 2.32) | 1.05 (0.77 to 1.41) | 0.86 (0.55 to 1.34) |
South Asian + other | – | 0.69 (0.27 to 1.78) | 0.64 (0.48 to 0.85)** | 0.30 (0.18 to 0.50)*** |
Survey type | ||||
Main | Reference | – | – | – |
BME | – | 0.82 (0.30 to 2.22) | 0.68 (0.48 to 0.96)* | 0.51 (0.30 to 0.87)* |
DE | – | 0.93 (0.50 to 1.73) | 1.15 (0.91 to 1.47) | 1.36 (0.99 to 1.88) |
Hackney | – | 0.88 (0.40 to 1.92) | 0.66 (0.48 to 0.89)** | 0.59 (0.35 to 0.98)* |
Glasgow East | – | 1.90 (1.18 to 3.07)** | 0.77 (0.60 to 0.99)* | 1.64 (1.23 to 2.19)** |
Categories of victimisation and mental health problems
After adjusting for demographics and other psychopathology, victims of violence reported experiencing long-standing mental health problems and admission to inpatient services and suffered from anxiety and psychotic symptoms. Drug dependence, alcohol dependence and depression were not significantly endorsed by victims of violence (Table 63).
Exposures | No violence (reference) | Victim, RRRa (95% CI) | Perpetrator, RRRa (95% CI) | Victim/perpetrator, RRRa (95% CI) |
---|---|---|---|---|
Long-standing problems | – | 4.26 (2.38 to 7.62)*** | 2.50 (1.71 to 3.66)*** | 5.67 (3.82 to 8.41)*** |
Adjustedb | – | 3.50 (1.65 to 7.42)** | 1.65 (1.07 to 2.52)* | 2.86 (1.76 to 4.65)*** |
Admission to psychiatric hospital | – | 4.06 (1.65 to 9.97)** | 2.01 (1.27 to 3.18)** | 4.63 (2.95 to 7.28)*** |
Adjustedb | – | 3.42 (1.07 to 10.95)* | 1.40 (0.86 to 2.31) | 2.65 (1.50 to 4.67)** |
Anxiety disorder | – | 2.01 (1.22 to 3.32)** | 1.91 (1.48 to 2.46)*** | 3.57 (2.73 to 4.68)*** |
Adjustedb | – | 2.05 (1.16 to 3.65)* | 1.59 (1.22 to 2.07)** | 2.32 (1.69 to 3.19)*** |
Depression | – | 0.96 (0.57 to 1.63) | 0.77 (0.59 to 0.99)* | 0.88 (0.61 to 1.25) |
Adjustedb | – | 0.74 (0.43 to 1.29) | 0.60 (0.45 to 0.81)** | 0.49 (0.32 to 0.73)*** |
Psychosis | – | 6.03 (2.44 to 14.89)*** | 7.91 (4.32 to 14.50)*** | 14.09 (7.12 to 27.91)*** |
Adjustedb | – | 3.72 (1.32 to 10.52)* | 3.89 (2.09 to 7.26)*** | 5.37 (2.57 to 11.24)*** |
Alcohol dependence | – | 1.80 (1.01 to 3.22)* | 2.50 (1.82 to 3.44)*** | 3.83 (2.79 to 5.25)*** |
Adjustedb | – | 1.17 (0.59 to 2.29) | 1.67 (1.23 to 2.25)** | 2.03 (1.41 to 2.92)*** |
Drug dependence | – | 3.07 (0.60 to 15.84) | 16.91 (7.37 to 38.80)*** | 38.57 (16.30 to 91.26)*** |
Adjustedb | – | 2.21 (0.40 to 12.23) | 10.88 (4.52 to 26.22)*** | 21.60 (8.61 to 54.17)*** |
Depression was under-represented among perpetrators of violence. All other mental health indicators were significantly increased among this group, with the exception of admission to inpatient services.
All mental health and psychopathology indicators were significantly more prevalent in the victim/perpetrator group. These men had the strongest odds of association with anxiety, psychosis and alcohol and drug dependence (see Table 63). Depression was less prevalent in this group.
Categories of victimisation and childhood adversities
After adjusting for demographics and other psychopathology, victims of violence were more likely to report having been bullied, witnessing violence in the home and having experienced physical abuse and neglect before the age of 16 years (Table 64). These men showed the strongest associations with having been bullied and experiencing physical abuse and neglect among all comparison groups.
Exposures | No violence (reference) | Victim, RRRa (95% CI) | Perpetrator, RRRa (95% CI) | Victim/perpetrator, RRRa (95% CI) |
---|---|---|---|---|
Taken to local authority | – | 1.92 (0.64 to 5.73) | 3.76 (2.41 to 5.87)*** | 7.41 (4.70 to 11.70)*** |
Adjustedb | – | 1.20 (0.38 to 3.76) | 2.98 (1.82 to 4.88)*** | 3.87 (2.22 to 6.77)*** |
Been bullied | – | 7.21 (4.85 to 10.73)*** | 1.89 (1.56 to 2.28)*** | 5.92 (4.79 to 7.32)*** |
Adjustedb | – | 5.25 (3.60 to 7.66)*** | 1.50 (1.23 to 1.83)*** | 3.69 (2.91 to 4.67)*** |
Witnessed violence in the home | – | 6.60 (3.94 to 11.06)*** | 3.75 (2.77 to 5.08)*** | 13.32 (9.99 to 17.77)*** |
Adjustedb | – | 2.35 (1.31 to 4.22)** | 1.79 (1.28 to 2.51)** | 4.32 (2.99 to 6.24)*** |
Witnessed parents/carers fighting | – | 4.82 (2.74 to 8.47)*** | 4.16 (3.15 to 5.48)*** | 9.12 (6.88 to 12.09)*** |
Adjustedb | – | 1.39 (0.76 to 2.54) | 2.51 (1.85 to 3.40)*** | 2.20 (1.51 to 3.21)*** |
Sexual abuse | – | 7.32 (3.24 to 16.53)*** | 2.52 (1.36 to 4.68)** | 10.51 (5.90 to 18.72)*** |
Adjustedb | – | 1.23 (0.40 to 3.77) | 0.93 (0.41 to 2.12) | 1.59 (0.64 to 3.95) |
Physical abuse | – | 15.46 (8.01 to 29.84)*** | 3.68 (2.41 to 5.64)*** | 17.97 (11.78 to 27.40)*** |
Adjustedb | – | 4.87 (2.53 to 9.38)*** | 1.71 (1.05 to 2.78)* | 3.93 (2.35 to 6.58)** |
Neglect | – | 9.54 (4.69, 19.41)*** | 4.47 (2.90, 6.90)*** | 10.15 (6.56, 15.69)*** |
Adjustedb | – | 2.77 (1.17 to 6.55)* | 2.18 (1.35 to 3.53)** | 1.79 (1.01 to 3.16)* |
All childhood adversity indicators were significantly increased among perpetrators of violence except for experiencing sexual abuse before the age of 16 years.
All childhood adversity indicators were significantly increased in the victim/perpetrator group except for experiencing sexual abuse before the age of 16 years. Among the four groups, victims/perpetrators showed the strongest associations with having been taken to local authority care and witnessing violence in the home (see Table 64).
Discussion
We found that few young adult men fitted the stereotype of an ‘innocent victim’ of violence, reporting victimisation but never having perpetrated violence towards others. However, most men had neither perpetrated violence nor been the victim of violence.
The distribution of men in our four categories across the components of the survey, particularly the additional boost surveys, is of some interest. For example, although men in the London borough of Hackney were found to have high levels of multiple problems, there was a high prevalence of those who reported no involvement in violence, which is of some interest. This is most likely a reflection of the unusual nature of the white men in the borough, many of whom were employed and in skilled occupations and of a higher social class, together with the relatively high proportion of men of Asian origin. This would suggest that a relatively small proportion of men in the borough are involved in violence as well as in repetitive and severe violence. In contrast, fewer men from the Glasgow East sample reported that they had been neither a victim nor a perpetrator of violence. We found that victimisation was highest in this subsample. In contrast, our representative UK sample of black and minority ethnic men was consistently less likely to report victimisation or perpetration. These trends were also confirmed following adjustments for demographic characteristics. Our findings suggest that men who were both victims and perpetrators tended to be older, divorced or separated, unemployed or of low social class and born in the UK and were unlikely to be of South Asian or other ethnic origin. Furthermore, victims/perpetrators were more likely to report long-standing mental health problems, admission to psychiatric hospital, anxiety disorder, symptoms of psychosis, alcohol dependence and drug dependence and were less likely to report depression. When examining their experiences of childhood maltreatment and poor care, victims/perpetrators showed the strongest association with being taken into local authority care and with witnessing violence in the family home. They were also more likely to report being bullied, witnessing parents/carers fighting, physical abuse and neglect. These findings suggest a constellation of maltreatment in childhood preceding violence and violent victimisation in adulthood, associated with poor physical and mental health in adulthood. Our findings suggest that this pattern or life course trajectory may be most prevalent in areas characterised by concentrated socioeconomic deprivation.
It was of some interest that men who reported being perpetrators of violence but not having experienced violent victimisation themselves made up a relatively large proportion of the overall sample. Furthermore, they were less likely to be participants from the boost surveys of the highly deprived inner-city areas of Hackney and Glasgow East. They were also less likely to be of black and minority ethnic origin, particularly South Asian and other, and were likely to be non-UK born and from social classes IV and V. They were more likely to report long-standing mental health problems than men who had not been involved in violence and report higher levels of anxiety disorder, psychotic symptoms, alcohol dependence and drug dependence. However, this group’s mental health problems did not result in them being admitted to a psychiatric hospital and they were less likely to be depressed. It is probable that individuals in the victim/perpetrator group were more reckless and impulsive than the perpetrator-only subgroup and thereby prone to become victims when they engaged in violent altercations with others. It is also possible that they carried out more violent assaults leading to a greater statistical chance that they would ultimately become a victim. Alternatively, their higher level of psychopathology, particularly substance dependence, may have rendered them more vulnerable during violent altercations to becoming a victim of violence. Ultimately, however, the cross-sectional method used in this study does not allow us to determine these possibilities.
In the case of the smallest group of individuals, victims and not perpetrators of violence, certain stereotyped notions of victimised men may well have applied. Victims were less likely to be single men. The finding that they were more likely to be found in the boost sample from Glasgow East could reflect a generally increased level of risk for violent victimisation when living in that geographical location. However, the strength of the odds of association between certain childhood factors suggested that there was a strong continuity in the risk of repeated victimisation from these childhood experiences to victimisation in adulthood. Men who were victims but not perpetrators showed stronger associations with being bullied in childhood, physical abuse and neglect than either perpetrators or victims/perpetrators. They were also more likely to report violence in the home during childhood than men who were not involved in violence. In adulthood, they showed the strongest associations with long-standing mental health problems, admission to a psychiatric hospital and psychotic symptoms and anxiety disorder, suggesting either vulnerability to adult victimisation as a result of their psychopathology or psychopathology as a result of their victimisation. However, being a victim was not specifically associated with alcohol dependence or drug dependence.
Finally, our study has confirmed that one of the best predictors of future victimisation is past victimisation. 216 Studies of sexual abuse among women show that those who have been abused as a child are two to three times more likely to be sexually assaulted later in life. 217 Our findings confirmed that men who have been physically abused and neglected in childhood are more likely to be violent towards others in adulthood but also to become victims themselves. However, we did not find an association between sexual abuse in childhood and adult victimisation. Finkelhor et al. 218 has argued that the re-victimisation and repeated victimisation literature has been limited because there is a tendency to consider victimisation in somewhat narrow terms, typically because studies have examined the recurrence of only one or a few kinds of victimisation, for example sexual abuse or violent crime. However, victimisation of one type, such as physical maltreatment, may create vulnerability for other kinds of victimisation such as bullying by peers or sexual victimisation. This would suggest that childhood maltreatment may act as a vulnerability for more than one type of victimisation in adulthood.
Another limitation to the re-victimisation literature is that it has tended to view victimisation as an event rather than a condition. Finkelhor et al. 218 argue that victimisation has often been treated as an unusual, individual event of a particularly traumatic nature; however, many victimisations are ongoing, as studies on bullying, child abuse and IPV make clear. Furthermore, studies of peer relationships among young children have increasingly suggested that some children become entrapped in the victimisation condition in which they are subjected to repeated attacks of different types from different children. 219,220 This type of victimisation proneness continues for years. Our findings suggest that this may certainly be the case for men who were victims/perpetrators but also for those who were victims. This suggests that an important focus of further investigation should be on the persistence of victimisation as a condition rather than simply the recurrence of certain kinds of victimisation events. Our findings also indicate that the ‘condition’ of revictimisation described by Finkelhor et al. 218 shows a strong association with psychiatric morbidity.
Chapter 8 Social deprivation and violence
Background
Almost two decades have passed since the World Health Assembly declared violence to be a major public health concern. 1 Numerous studies on the causes and consequences of violence have been carried out. One mechanism suggested as being responsible for violent behaviour is the influence of an individual’s neighbourhood of residence. Empirical research has consistently shown that intentional injuries are more prevalent among young people, in particular men, and people from adverse socioeconomic backgrounds. 221–223 There is also growing concern that people living in disadvantaged neighbourhoods experience a heightened risk of exposure to violence. 224,225
A study carried out in Scotland demonstrated that an increase in mortality as a result of assault was most pronounced in men living in the most deprived quintile of areas. 226 A Welsh study on youth violence showed that injury increased with increasing deprivation in cities and their feeder towns. 227 A retrospective review of NHS emergency department computer records demonstrated a very strong relationship between material deprivation and the risk of assault. 224 Finally, the ratio of the median rate of intentional to unintentional injuries increased steeply with economic deprivation in a study carried out in the USA. 228
Objectives
The objectives of this study were therefore to investigate whether or not:
-
socioeconomic deprivation in a representative sample of young men in Great Britain was associated with violent behaviour and type of violence
-
socioeconomic deprivation in a representative sample of young men in Great Britain demonstrated a relationship with specific individuals as victims of violence
-
specific locations where violent incidents occurred differed depending on level of socioeconomic deprivation.
Methods
The sample under study was the second MMLS, described in Chapter 2. However, to investigate the effects of socioeconomic deprivation on violent outcome it was not possible to include the booster samples, as we wanted to include a representative sample of the underlying population. For subsequent analyses only the representative main survey with 2046 study participants was utilised.
Measures
The outcome measures of violence used in this study are described in Chapter 2.
Acorn (http://acorn.caci.co.uk/downloads/Acorn-user-guide.pdf) is a segmentation tool that categorises the UK’s population into demographic types and provides information about the level of socioeconomic deprivation by analysing significant social factors and population behaviour. Categories of Acorn were coded as ordinal variables (from 1 to 5, indicating an increase in socioeconomic deprivation) and were:
-
Affluent achievers These are some of the most financially successful people in the UK. They live in wealthy, high-status rural, semirural and suburban areas of the country. Middle-aged or older people predominate as many have grown-up children no longer living at home and are wealthy retired. Some neighbourhoods contain large numbers of well-off families with school-aged children, particularly the more suburban locations.
-
Rising prosperity These are generally younger, well-educated and mostly prosperous people living in major towns and cities. Most are single or couples, with some yet to start a family and others having younger children. Often these are highly educated younger professionals moving up the career ladder.
-
Comfortable communities This category contains much of the average Great Britain, whether in the suburbs, smaller towns or the countryside. All life stages are represented in this category. Many areas have mostly stable families and families with grown-up children no longer living at home, especially in suburban or semi-rural locations. There are also comfortably off pensioners living in retirement areas around the coast or in the countryside and sometimes younger couples just starting out in their lives together.
-
Financially stretched This category contains a mix of traditional areas of Great Britain. Housing is often terraced or semi-detached, with a mix of lower-value owner-occupied housing and homes rented from the council or housing associations, including social housing developments specifically for the elderly. This category also includes student term-time areas. There tends to be fewer traditional married couples than usual and more single parents and single, separated and divorced people than the national average. Incomes tend to be well below the national average. Although some in this category have reasonably well-paid jobs, more people are in lower-paid administrative, clerical, semiskilled and manual jobs. Apprenticeships and O levels are the most common educational qualifications in this category. The levels of unemployment are above the national average as are the proportions of people claiming other benefits.
-
Urban adversity This category contains the most deprived areas of large and small towns and cities across the UK. Household incomes are low and nearly always below the national average. The number of people having difficulties with debt or having been refused credit is double the national average. The number claiming Jobseeker’s Allowance and other benefits is also well above the national average. Qualification levels are low and those in work are likely to be employed in semiskilled or unskilled occupations. The housing is a mix of low-rise estates, with terraced and semidetached houses, and purpose-built flats, including high-rise blocks. Properties tend to be small and there may be overcrowding. Over half of the housing is rented from the local council or a housing association. There is some private renting.
Statistical analyses
For descriptive purposes, absolute (n) and relative (%) frequencies were reported for dichotomous/polytomous categorical variables, means and SDs for variables on interval/ratio level.
Binary logistic regression was performed to examine the effects of socioeconomic deprivation (ordinal measure) on violent outcome (dichotomous). Appropriate weights were included in all analyses and analyses were adjusted for clustering within postcodes. No demographic variables were adjusted for in these analyses as the measure of socioeconomic deprivation was derived from factors including age, ethnicity, social class, education and employment status.
Results
The weighted mean age of the sample was 26.1 years. The majority of the sample was white (n = 1796, 88%) and nearly half of the sample was single (n = 819, 40.3%). In total, 11% (n = 229) had not achieved any educational qualifications. The distribution across social classes was as follows: 283 (13.8%) high, 627 (30.6%) medium and 449 (21.9%) low. Approximately one-third of the sample was not classifiable because of unemployment (310, 15.2%) or for other reasons (n = 378, 18.5%). The distribution of study participants across the different Acorn categories was as follows: (1) affluent achievers 14.8% (n = 302), (2) rising prosperity 15.1% (n = 308), (3) comfortable communities 28.1% (n = 574), (4) financially stretched 19.9% (n = 408) and (5) urban adversity 22.2% (n = 455). The highest prevalence was in the middle category.
Approximately one-third of the sample (n = 622, 31.7%) reported any violent behaviour in the past 5 years.
The weighted absolute and relative frequencies of types and victims of violence and location of violent incidents in each Acorn category of socioeconomic deprivation are provided in Table 65.
Outcomes | Acorn category 1,a n (%) | Acorn category 2,a n (%) | Acorn category 3,a n (%) | Acorn category 4,a n (%) | Acorn category 5,a n (%) |
---|---|---|---|---|---|
Any violence | 70 (24.0) | 75 (24.9) | 185 (33.4) | 139 (36.2) | 152 (35.4) |
Violence while intoxicated | 36 (12.4) | 36 (12.1) | 99 (17.9) | 82 (21.7) | 83 (19.8) |
Severity of violence | |||||
Repeated violence (five or more times) | 10 (3.5) | 8 (2.7) | 26 (4.7) | 22 (5.9) | 30 (7.1) |
Victim injured | 29 (9.9) | 38 (12.5) | 95 (17.1) | 67 (17.6) | 82 (19.0) |
Perpetrator injured | 26 (8.9) | 40 (13.4) | 84 (15.2) | 59 (15.4) | 73 (16.9) |
Police involved | 18 (6.1) | 25 (8.4) | 53 (9.6) | 42 (10.8) | 52 (12.1) |
Minor violence | 23 (8.0) | 19 (6.3) | 45 (8.1) | 42 (10.9) | 32 (7.5) |
Victim of violence | |||||
Intimate partner | 8 (2.6) | 7 (2.4) | 17 (3.0) | 14 (3.6) | 21 (4.8) |
Family member | 3 (0.9) | 6 (2.1) | 29 (5.3) | 18 (4.7) | 22 (5.1) |
Friend | 13 (4.6) | 22 (7.4) | 51 (9.2) | 30 (7.9) | 44 (10.1) |
Someone known | 24 (8.0) | 27 (8.9) | 61 (10.9) | 52 (13.5) | 42 (9.8) |
Stranger | 42 (14.2) | 45 (14.9) | 107 (19.3) | 75 (19.4) | 88 (20.3) |
Police | 7 (2.2) | 7 (2.3) | 13 (2.4) | 18 (4.7) | 10 (2.3) |
Location of violent incident | |||||
Own home | 3 (1.0) | 6 (2.0) | 27 (4.8) | 18 (4.7) | 28 (6.5) |
Someone else’s home | 6 (2.1) | 8 (2.8) | 25 (4.5) | 17 (4.5) | 23 (5.4) |
Street/outdoors | 41 (13.9) | 45 (14.8) | 124 (22.4) | 92 (23.8) | 84 (19.4) |
Bar/pub | 33 (11.3) | 38 (12.6) | 84 (15.1) | 65 (17.0) | 66 (15.3) |
Workplace | 3 (0.9) | 1 (0.4) | 11 (1.9) | 4 (0.9) | 10 (2.1) |
Other | 15 (5.2) | 15 (4.8) | 24 (4.3) | 16 (4.3) | 21 (4.9) |
To assure that associations of deprivation with violence were not accounted for by psychopathology, we tested whether or not there was an increase in the prevalence of mental illness with increasing deprivation. Of all mental disorders included (psychosis, anxiety, depression, alcohol abuse and dependence, drug abuse and dependence and ASPD), none was significantly associated with level of socioeconomic deprivation (p ≥ 0.05).
Type of violence
Apart from minor violence, all other types of violence were significantly associated with increasing level of socioeconomic deprivation as follows: any violence – adjusted OR (AOR) 1.16 (95% CI 1.07 to 1.26; p < 0.001), violence while intoxicated – AOR 1.18 (95% CI 1.07 to 1.30; p < 0.001), repeated violence (five or more times) – AOR 1.26 (95% CI 1.07 to 1.50; p = 0.006), victim injured – AOR 1.19 (95% CI 1.07 to 1.32; p = 0.001), perpetrator injured – AOR 1.16 (95% CI 1.04 to 1.28; p = 0.005) and police involved – AOR 1.18 (95% CI 1.04 to 1.34; p = 0.009).
Victims of violence
Level of socioeconomic deprivation predicted violence against a family member (AOR 1.33, 95% CI 1.13 to 1.57; p = 0.001), friends (AOR 1.16, 95% CI 1.03 to 1.31; p = 0.017) and strangers (AOR 1.12, 95% CI 1.02 to 1.23; p = 0.022). IPV (AOR 1.20, 95% CI 0.97 to 1.48; p = 0.093) and violence against someone known (AOR 1.08, 95% CI 0.96 to 1.21; p = 0.206) and the police (AOR 1.09, 95% CI 0.88 to 1.34; p = 0.426) were not associated with socioeconomic deprivation.
Location of violent incidents
Locations of violence significantly predicted by socioeconomic deprivation were the perpetrator’s home (AOR 1.44, 95% CI 1.21 to 1.71; p < 0.001), someone else’s home (AOR 1.24, 95% CI 1.05 to 1.47; p = 0.014) and streets/locations outdoors (AOR 1.12, 95% CI 1.03 to 1.22; p = 0.010). No association was found with bars/pubs (AOR 1.10, 95% CI 0.99 to 1.22; p = 0.072), the workplace (AOR 1.26, 95% CI 0.93 to 1.70; p = 0.135) and other locations (AOR 1.09, 95% CI 0.88 to 1.34; p = 0.426).
Discussion
Our findings confirm that level of socioeconomic deprivation is associated with risk of violence towards others in a representative sample of young British men. However, this association was found only with more serious forms of violence indicated by repetition, injury of perpetrator and/or victim and police involvement. In total, 8% of young men living in the most affluent parts of the country reported committing minor violent acts in the past 5 years, a similar prevalence to that among those living in the most deprived areas. This may correspond to the lack of association with IPV, indicating that violence towards spouses is independent of socioeconomic status. These findings contradict previous research in which socioeconomic deprivation on the national229 and international230 level was associated with IPV. However, those samples included a substantial number of older participants aged > 34 years whereas the age range of participants in the current study was restricted. Minor violence and IPV may be more common among younger men irrespective of level of deprivation.
Violence while intoxicated was more likely to occur in areas with increased deprivation. However, there was no association between substance abuse/dependence and level of deprivation that could explain the higher prevalence. Furthermore, violence occurring in pubs or bars did not demonstrate a significant association with deprivation level. There is substantial evidence that binge drinking is a strong and consistent risk factor for violence. 231 One explanation could be that binge drinking occurs more often in those exposed to higher socioeconomic deprivation, increasing the likelihood of violent incidents. Further research is necessary to investigate this hypothesis.
With increasing levels of socioeconomic deprivation victims of violence were more likely to be family members, friends and strangers, corresponding to the locations where violence occurred (own home, someone else’s home and on the streets/outdoors). The findings of a recent study suggest that there are spatiotemporal patterns of injury related to violence depending on the time of the day. 232 During the day, the locations where incidents happened and the residence of the victims were similar. During the night, however, there was a shift in the locations of violent incidents towards certain areas whereas the residence of the victims remained unchanged.
Our findings suggest that exposure to socioeconomic deprivation is associated with serious violent behaviour and that violent victimisation is not restricted to those known by the perpetrators. Although it cannot be concluded on the basis of these data that socioeconomic deprivation causes serious violence in those exposed, intervention strategies should consider contextual factors in their aim to reduce violence on the population level.
Chapter 9 Risk taking and violence
Background
The largest contributors to morbidity and mortality in adolescence are not disease and illness but behaviours such as unsafe driving, experimentation with alcohol, tobacco and illicit drugs, involvement in crime and unsafe sex. 233 Early adolescence (typically 10–14 years of age) is a critical developmental period when risk taking typically emerges. 234 However, by early adulthood many of these behaviours have receded, associated with the establishment of close emotional bonds in relationships and successful entry into the labour market. 235 However, a subgroup persist in risk-taking behaviour and this is associated with poorer physical and mental health. 236,237 Although there have been studies in developed countries of women found to be taking risks,238 substantially more men than women engage in risk taking. Among the risk-taking behaviours, violence, particularly violence involving weapons, conveys the greatest chances of infliction of serious harm. However, risk taking is also thought to involve appetitive processes in which positive rewards from the behaviour are pursued and enhanced by the risk-taking individual. 239–241 Models of risk taking therefore include the notion that people are differentially prone to take risks because of a stable, underlying difference in their risk-taking propensity. 96,234,242–245
Objectives
The objectives of this study were to:
-
investigate whether or not subgroups exist and identify a typology of risk-taking behaviour among young adult men using self-reported behaviours from the domains of substance misuse, deliberate self-harm, reckless driving, high-risk sexual behaviour and risks to long-term physical health
-
investigate the associations between subtypes of risk taking in adulthood and self-reported violence
-
investigate the associations between subtypes of risk taking in adulthood and psychiatric morbidity.
Methods
Latent class analysis can identify groups or classes in the population according to their endorsement of observed characteristics. It follows the assumption of an underlying unobserved categorical variable that separates a population into subgroups. The classes are multidimensional, as they are defined by various indicators. This approach allows description of the relationships of variables, as they combine into classes that define groups of people within a sample or population.
High-risk behaviours for violent outcomes were selected from a range of previously investigated variables from the domains of alcohol misuse, drug misuse, self-harm, reckless driving, HIV infection risk, lack of exercise and heavy drinking. 233 LCA was used to empirically define participant groups based on these risk behaviours and explore the associations of the classification scheme with violence outcomes. Decisions regarding the most appropriate model are led by statistical indicators and by conceptual considerations. We used the default estimator, which is a MLR. However, maximum likelihood may lead to the presence of a problem called local maxima. To avoid this, all LCA models were estimated with different random starting values: we used 1000 random starts at the initial stage and 100 optimisations at the final stage. Models were inspected to ensure that the log-likelihood value for each model was successfully replicated several times (an indication of low probability of local maxima).
The LCA models were then evaluated using several model fit indicators: log-likelihood, Akaike information criterion (AIC), Bayesian information criterion (BIC) and sample size adjusted BIC (aBIC). The Vuong–Lo–Mendell–Rubin likelihood ratio test (VLMR-LRT) and the entropy measure, additional important indicators of model fit, were also considered in our analysis. Higher entropy indicates overall better classification of participants into their classes.
Associations between the derived classes of multiple high risks and sociodemographic factors were estimated by fitting multinomial logistic regression models with all demographic variables entered simultaneously. Associations between the derived classes of multiple high risks and outcomes were assessed using logistic regression models, with the highest prevalence class as the reference group.
This analysis was performed using Mplus software for Windows OS version 7.11.
Results
Table 66 shows the prevalence and frequency of high-risk behaviours among young men in the population (n = 5400). The majority of men do not take health risks. The most prevalent risks included lack of exercise and substance misuse. Less than 5% of men took high risks for sexually transmitted infections (STIs).
High-risk behaviours | No, n (%) | Yes, n (%) |
---|---|---|
Alcohol misuse | 4223 (83.2) | 853 (16.8) |
Drug misuse | 4122 (82.9) | 849 (17.1) |
Self-harm | 4752 (93.3) | 342 (6.7) |
Suicide attempt | 4810 (93.6) | 329 (6.4) |
Reckless driving | 4434 (88.1) | 598 (11.9) |
STI risk | 4680 (96.8) | 156 (3.2) |
Lack of exercise | 4260 (81.7) | 955 (18.3) |
Heavy smoker | 4496 (85.9) | 737 (14.1) |
None | 2122 (45.0) | – |
Table 67 shows the associations between each high-risk behaviour and any reported violence in the past 5 years. Following adjustments, all high-risk behaviours were significantly associated with violence except for lack of exercise, which decreased the risk of violence, and STI risk, which was no longer significant. Table 67 shows that there was a threefold increase in risk of violence associated with drug misuse.
High-risk behaviours | Any violence in the last 5 years | ||
---|---|---|---|
n (%) | OR (95% CI) | AORa (95% CI) | |
Alcohol misuse | 476 (29.9) | 3.34 (2.71 to 4.12)*** | 1.76 (1.38 to 2.23)*** |
Drug misuse | 570 (36.2) | 5.94 (4.84 to 7.30)*** | 3.19 (2.49 to 4.07)*** |
Self-harm | 210 (12.9) | 3.77 (2.84 to 4.99)*** | 1.56 (1.08 to 2.26)* |
Suicide attempt | 209 (12.9) | 4.32 (3.20 to 5.85)*** | 1.98 (1.30 to 3.01)** |
Reckless driving | 397 (25.2) | 5.52 (4.47 to 6.83)*** | 2.63 (2.03 to 3.42)*** |
STI risk | 82 (5.2) | 2.24 (1.44 to 3.48)*** | 0.75 (0.48 to 1.15) |
Lack of exercise | 282 (17.2) | 0.83 (0.69 to 0.99)* | 0.57 (0.45 to 0.71)*** |
Heavy smoker | 346 (21.0) | 2.11 (1.76 to 2.54)*** | 1.49 (1.16 to 1.90)** |
Latent classes
We tested five models of identified subgroups defined by risk factors in the population. Model fit and information criteria for LCA model selection are provided in Table 68. All indicators of parsimony favour the models with four and five classes, with relatively similar entropy indices. Models also replicated the log-likelihood, providing evidence of the non-existence of random classes. We chose the four-class model because of its parsimony and fit indices; the five-class model was not chosen because of its lack of theoretical interpretation.
Model | Log-likelihood | Replicated log-likelihood | AIC | BIC | aBIC | VLMR-LRT p-value | Entropy |
---|---|---|---|---|---|---|---|
1C | 14178.6 | Yes | 28373.1 | 28425.8 | 28400.4 | NA | NA |
2C | 13118.5 | Yes | 26270.9 | 26382.9 | 26328.9 | < 0.001 | 0.742 |
3C | 12940.7 | Yes | 25933.3 | 26104.6 | 26022.0 | < 0.001 | 0.792 |
4C | 12890.3 | Yes | 25850.5 | 26081.1 | 25969.9 | 0.008 | 0.800 |
5C | 12841.9 | Yes | 25771.9 | 26061.8 | 25921.9 | < 0.001 | 0.786 |
Results from the LCA of the eight high-risk behaviours are shown in Table 68 and Figure 4. The classes were distributed as follows: overall low risk (class 1) 78.4%, high substance misuse (class 2) 13.6%, high self-harm (class 3) 5.6% and multiple high risks (class 4) 2.4%.
FIGURE 4.
Latent classes of multiple high-risk behaviours and substance use disorders.

The average classification probabilities for the four latent classes were 0.805 for class 1, 0.763 for class 2, 0.926 for class 3 and 0.827 for class 4.
Figure 4 shows the four latent classes identified in the population of young men aged 18–34 years. Class 1 includes more than three-quarters of the men and this group has a low prevalence of all risks except risks for future health (lack of exercise and heavy smoking), although the latter were at a much lower prevalence than in classes 2–4.
Class 2 was characterised primarily by alcohol and drug misuse and heavy smoking. In addition, men in this class took less exercise and reported a higher prevalence of reckless driving. Fewer were of South Asian or ‘other’ ethnic origin and non-UK born and more were single and unemployed than in class 1 (Table 69).
Demographic characteristics | Violence typologies (latent classes) | |||
---|---|---|---|---|
Class 1,a n (%) (reference) | Class 2,a n (%) | Class 3,a n (%) | Class 4,a n (%) | |
Age group (years) | ||||
18–24 (reference) | 1619 (38.5) | 285 (38.9) | 113 (37.3) | 42 (33.3) |
25–34 | 2587 (61.5) | 446 (61.1) | 190 (62.7) | 85 (66.7)b |
Ethnicity | ||||
White (reference) | 2502 (59.6) | 560 (76.6) | 239 (79.2) | 62 (92.8) |
Black | 630 (15.0) | 97 (13.3) | 24 (8.1)c | 47 (37.0)b |
South Asian and other | 1064 (25.4) | 74 (10.1)c | 38 (12.7)c | 18 (14.2) |
Non-UK born | 654 (15.9) | 36 (5.0)c | 39 (12.8) | 7 (5.9)c |
Single | 2585 (62.1) | 484 (67.2)b | 204 (67.6) | 75 (59.0) |
Unemployed | 1522 (37.0) | 324 (46.3)b | 150 (50.7)b | 68 (53.9)b |
Class 3 was characterised primarily by high level of self-harm. In addition, men in this class were less likely to be of black and minority ethnic origin and more likely to be unemployed than men in class 1 (see Table 69).
Class 4 was characterised by multiple high risks at a higher prevalence than in the other classes. In addition, men in this class were significantly older, more likely to be black, less likely to be non-UK born and more likely to be unemployed than men in class 1 (see Table 69).
Associations between classes of risk takers and violent outcomes
Subsequent analyses of violent outcomes and class membership (see Figure 4) used the low-risk group (class 1) as a reference. The results for the main violent outcomes are shown in Tables 70 and 71. Each of the three classes of risk-taking men was significantly associated with each of the violent outcomes except for minor violence in the case of classes 3 and 4.
Outcomes | Class membership (weighted percentages) | |||
---|---|---|---|---|
Class 1,a n (%) | Class 2,a n (%) | Class 3,a n (%) | Class 4,a n (%) | |
Any violence | 951 (23.2) | 468 (64.4) | 151 (51.7) | 109 (86.6) |
Violence while intoxicated | 322 (7.9) | 292 (42.2) | 73 (25.8) | 78 (67.1) |
Severity of violence | ||||
Repetitive violence | 86 (2.1) | 97 (14.3) | 29 (9.9) | 43 (36.9) |
Victim injured | 379 (9.3) | 231 (32.0) | 66 (22.6) | 45 (36.8) |
Perpetrator injured | 315 (7.7) | 245 (34.0) | 82 (28.3) | 70 (57.9) |
Police involved | 178 (4.4) | 132 (18.2) | 58 (19.8) | 43 (35.0) |
Minor violence | 267 (6.5) | 74 (10.2) | 24 (8.4) | 9 (7.2) |
Gang fights | 78 (2.0) | 113 (16.3) | 19 (6.7) | 55 (47.1) |
Victim of violence | ||||
Intimate partner | 43 (1.0) | 82 (11.4) | 26 (9.0) | 50 (41.1) |
Family member | 77 (1.9) | 93 (12.8) | 27 (9.2) | 27 (21.9) |
Friend | 195 (4.8) | 156 (21.6) | 42 (14.3) | 44 (36.5) |
Someone known | 259 (6.3) | 149 (20.6) | 52 (18.0) | 23 (19.2) |
Stranger | 405 (9.9) | 217 (30.0) | 70 (24.0) | 45 (37.0) |
Police | 47 (1.2) | 55 (7.7) | 15 (5.2) | 14 (11.3) |
Other | 54 (1.3) | 15 (2.0) | 14 (4.9) | 9 (7.1) |
Location of violent incident | ||||
Own home | 76 (1.9) | 98 (13.5) | 32 (10.9) | 30 (24.3) |
Someone else’s home | 100 (2.5) | 117 (16.2) | 30 (10.4) | 34 (27.7) |
Outdoors/in the street | 460 (11.3) | 256 (35.5) | 88 (30.2) | 51 (42.4) |
Bar/pub | 302 (7.4) | 222 (30.7) | 49 (16.9) | 49 (40.4) |
Workplace | 28 (0.7) | 14 (2.0) | 9 (3.0) | 9 (7.1) |
Sporting event | 150 (3.8) | 132 (19.0) | 23 (8.0) | 54 (46.9) |
Outcomes | Class membership | Intraclass contrasts | |||||
---|---|---|---|---|---|---|---|
Class 1a (reference) | Class 2a OR (95% CI) | Class 3a OR (95% CI) | Class 4a OR (95% CI) | Class 4 – class 3 OR (95% CI) | Class 4 – class 2 OR (95% CI) | Class 3 – class 2 OR (95% CI) | |
Any violence | Reference | 6.26 (5.06 to 7.74)*** | 3.11 (2.33 to 4.14)*** | 19.70 (11.28 to 34.39)*** | 6.34 (3.47 to 11.60)*** | 3.15 (1.78 to 5.56)*** | 0.50 (0.36 to 0.69)*** |
Violence while intoxicated | Reference | 8.07 (6.52 to 10.00)*** | 3.09 (2.20 to 4.36)*** | 22.93 (14.57 to 36.08)*** | 7.41 (4.35 to 12.64)*** | 2.84 (1.78 to 4.52)*** | 0.38 (0.27 to 0.54)*** |
Severity of violence | |||||||
Repetitive violence | Reference | 7.71 (5.56 to 10.68)*** | 4.52 (2.74 to 7.46)*** | 18.82 (12.63 to 28.04)*** | 4.16 (2.43 to 7.12)*** | 2.44 (1.61 to 3.69)*** | 0.59 (0.35 to 0.98)* |
Victim injured | Reference | 4.56 (3.68 to 5.63)*** | 2.46 (1.75 to 3.47)*** | 7.28 (4.98 to 10.63)*** | 2.96 (1.81 to 4.82)*** | 1.60 (1.07 to 2.38)* | 0.54 (0.37 to 0.78)*** |
Perpetrator injured | Reference | 5.76 (4.50 to 7.37)*** | 4.21 (3.04 to 5.82)*** | 13.30 (9.09 to 19.47)*** | 3.16 (2.00 to 4.98)*** | 2.31 (1.54 to 3.46)*** | 0.73 (0.51 to 1.04) |
Police involved | Reference | 4.62 (3.58 to 5.97)*** | 3.67 (2.49 to 5.41)*** | 12.86 (8.42 to 19.65)*** | 3.51 (2.07 to 5.93)*** | 2.78 (1.77 to 4.38)*** | 0.79 (0.52 to 1.20) |
Minor violence | Reference | 1.49 (1.11 to 2.00)** | 1.26 (0.79 to 2.03) | 0.99 (0.51 to 1.90) | 0.78 (0.35 to 1.74) | 0.66 (0.33 to 1.31) | 0.85 (0.49 to 1.46) |
Gang fights | Reference | 9.16 (6.48 to 12.96)*** | 2.91 (1.56 to 5.44)*** | 24.65 (15.97 to 38.06)*** | 8.47 (4.33 to 16.58)*** | 2.69 (1.75 to 4.13)*** | 0.32 (0.17 to 0.59)*** |
The three classes differed significantly in terms of their degree of association with violence. For any violence in the last 5 years, the multiple high-risk group (class 4) showed a significantly higher level of association than the substance misuse group (class 2). However, the substance misuse group was more likely to report violence than the self-harm group (class 3). The same differential patterns between the classes were observed for the following outcomes: violent acts when intoxicated, violence repetition, victim injured and involvement in gang fights (see Table 71).
Table 72 shows that the substance misuse (class 2) and self-harm (class 3) groups did not differ from each other in terms of the prevalence of IPV, violence towards a family member or violence towards the police. The multiple high-risk group (class 4) showed a stronger association with all victims of violence than the substance misuse group, except for someone known. The latter group had stronger associations than the self-harm group with both violence towards a friend and violence towards a stranger.
Violence targets | Class membership | Intraclass contrasts | |||||
---|---|---|---|---|---|---|---|
Class 1a (reference) | Class 2a OR (95% CI) | Class 3a OR (95% CI) | Class 4a OR (95% CI) | Class 4 – class 3 OR (95% CI) | Class 4 – class 2 OR (95% CI) | Class 3 – class 2 OR (95% CI) | |
Intimate partner | Reference | 12.32 (7.80 to 19.45)*** | 7.97 (4.29 to 14.79)*** | 37.50 (22.31 to 63.04)*** | 4.71 (2.53 to 8.75)*** | 3.04 (1.98 to 4.68)*** | 0.65 (0.37 to 1.14) |
Family member | Reference | 7.17 (5.03 to 10.23)*** | 4.23 (2.43 to 7.37)*** | 12.73 (7.56 to 21.46)*** | 3.01 (1.56 to 5.81)** | 1.78 (1.06 to 2.97)* | 0.59 (0.34 to 1.02) |
Friend | Reference | 4.98 (3.88 to 6.41)*** | 2.98 (2.00 to 4.43)*** | 9.98 (6.72 to 14.82)*** | 3.35 (2.03 to 5.54)*** | 2.00 (1.33 to 3.02)*** | 0.60 (0.40 to 0.90)* |
Someone known | Reference | 3.51 (2.77 to 4.44)*** | 2.37 (1.62 to 3.47)*** | 4.39 (2.82 to 6.84)*** | 1.85 (1.07 to 3.21)* | 1.25 (0.79 to 1.98) | 0.67 (0.45 to 1.01) |
Stranger | Reference | 3.84 (3.09 to 4.77)*** | 2.25 (1.59 to 3.17)*** | 7.74 (5.07 to 11.80)*** | 3.44 (2.10 to 5.63)*** | 2.01 (1.30 to 3.13)** | 0.59 (0.40 to 0.85)** |
Police | Reference | 5.56 (3.68 to 8.40)*** | 2.97 (1.53 to 5.78)** | 12.57 (6.78 to 23.30)*** | 4.23 (1.91 to 9.35)*** | 2.26 (1.25 to 4.09)** | 0.53 (0.28 to 1.03) |
Other | Reference | 1.25 (0.65 to 2.39) | 2.56 (1.23 to 5.34)* | 4.65 (2.11 to 10.23)*** | 1.81 (0.69 to 4.75) | 3.73 (1.47 to 9.49)** | 2.06 (0.86 to 4.90) |
Table 73 shows that, in most locations, violence was more prominent among classes 2 (substance misuse), 3 (self-harm) and 4 (multiple risks). All locations for violence were more prevalent among class 4 than among classes 2 and 3 (self-harm). Violence in own home, in someone else’s home, outdoors/in the street, in a bar/pub and at sporting events was more commonly reported by class 2 than by class 3.
Location of violent incident | Class membership | Intraclass contrasts | |||||
---|---|---|---|---|---|---|---|
Class 1a (reference) | Class 2a OR (95% CI) | Class 3a OR (95% CI) | Class 4a OR (95% CI) | Class 4 – class 3 OR (95% CI) | Class 4 – class 2 OR (95% CI) | Class 3 – class 2 OR (95% CI) | |
Own home | Reference | 6.50 (4.64 to 9.12)*** | 4.17 (2.47 to 7.04)*** | 13.15 (8.03 to 21.53)*** | 3.15 (1.67 to 5.98)*** | 2.02 (1.24 to 3.29)** | 0.64 (0.38 to 1.08) |
Someone else’s home | Reference | 7.12 (5.28 to 9.61)*** | 4.20 (2.56 to 6.87)*** | 13.22 (8.12 to 21.53)*** | 3.15 (1.69 to 5.87)*** | 1.86 (1.15 to 3.00)* | 0.59 (0.36 to 0.96)* |
Outdoors/in the street | Reference | 4.44 (3.61 to 5.47)*** | 2.79 (2.06 to 3.79)*** | 6.91 (4.74 to 10.08)*** | 2.47 (1.57 to 3.89)*** | 1.56 (1.04 to 2.32)* | 0.63 (0.45 to 0.88)** |
Bar/pub | Reference | 5.46 (4.27 to 6.99)*** | 2.11 (1.43 to 3.10)*** | 8.19 (5.43 to 12.35)*** | 3.89 (2.31 to 6.55)*** | 1.50 (0.97 to 2.31) | 0.39 (0.26 to 0.58)*** |
Workplace | Reference | 2.53 (1.16 to 5.52)* | 2.90 (1.04 to 8.06)* | 14.26 (6.29 to 32.30)*** | 4.92 (1.49 to 16.21)** | 5.63 (2.19 to 14.44)*** | 1.14 (0.36 to 3.63) |
Sporting event | Reference | 6.28 (4.69 to 8.39)*** | 2.39 (1.48 to 3.87)*** | 13.74 (9.22 to 20.48)*** | 5.75 (3.30 to 10.02)*** | 2.19 (1.46 to 3.28)*** | 0.38 (0.24 to 0.62)*** |
Early adversity and childhood maltreatment
Tables 74 and 75 show the associations between class membership (see Figure 4) and reported experiences of early adversity and maltreatment before the age of 16 years. Classes 2–4 (substance misuse, self-harm and multiple risks, respectively) were each significantly more likely to report these factors than class 1 (low risk). The odds of association were higher for class 4, with significant differences from classes 2 and 3 for all childhood factors except for sexual abuse and being in local authority care compared with class 3. There were no significant differences between class 3 and class 2 except for being bullied in childhood, which was significantly higher in class 3.
Early adversity, childhood maltreatment, mental health and criminality | Class membership (weighted percentages) | |||
---|---|---|---|---|
Class 1,a n (%) | Class 2,a n (%) | Class 3,a n (%) | Class 4,a n (%) | |
Early adversity and childhood maltreatment | ||||
Being bullied | 892 (21.2) | 252 (34.5) | 150 (49.4) | 65 (51.0) |
Witnessed violence in the home | 267 (6.4) | 187 (25.5) | 79 (26.1) | 52 (40.8) |
Witnessed parents fighting | 297 (7.1) | 163 (22.3) | 77 (25.5) | 34 (26.7) |
Sexual abuse | 56 (1.3) | 33 (4.5) | 27 (8.9) | 20 (15.9) |
Physical abuse | 139 (3.3) | 111 (15.2) | 48 (15.8) | 40 (31.3) |
Neglect | 117 (2.8) | 77 (10.5) | 40 (13.2) | 38 (30.1) |
Serious life-threatening injury | 59 (1.4) | 36 (4.9) | 21 (7.0) | 23 (17.8) |
Local authority care | 81 (2.0) | 68 (9.7) | 36 (12.4) | 26 (23.5) |
Mental health | ||||
Anxiety | 404 (9.9) | 178 (24.7) | 132 (44.5) | 92 (73.8) |
Depression | 385 (9.5) | 115 (15.9) | 59 (19.8) | 33 (27.5) |
Psychosis | 46 (1.1) | 28 (3.9) | 39 (13.4) | 37 (30.1) |
ASPD | 178 (4.5) | 331 (48.6) | 75 (27.5) | 94 (87.9) |
Pathological gambling | 65 (1.8) | 77 (11.4) | 21 (7.5) | 68 (57.2) |
Problem use of pornography | 22 (0.6) | 40 (5.7) | 11 (3.9) | 32 (26.4) |
Stalking | 59 (1.4) | 44 (6.1) | 12 (3.9) | 41 (34.5) |
Criminal history | ||||
Conviction ever | 254 (6.4) | 257 (37.3) | 78 (27.7) | 51 (44.5) |
Ever in prison | 74 (1.8) | 104 (14.3) | 30 (9.8) | 32 (25.4) |
Gang membership | 9 (0.3) | 52 (8.7) | 7 (2.8) | 39 (45.3) |
Friends encouraged crime | 186 (4.7) | 219 (32.2) | 42 (15.7) | 76 (65.3) |
Friends encouraged drug use | 464 (11.8) | 426 (61.8) | 96 (34.6) | 92 (75.6) |
Early adversity and child maltreatment | Class membership | Intraclass contrasts | |||||
---|---|---|---|---|---|---|---|
Class 1a (reference) | Class 2a OR (95% CI) | Class 3a OR (95% CI) | Class 4a OR (95% CI) | Class 4 – class 3 OR (95% CI) | Class 4 – class 2 OR (95% CI) | Class 3 – class 2 OR (95% CI) | |
Being bullied | Reference | 1.75 (1.44 to 2.14)*** | 2.95 (2.21 to 3.94)*** | 4.77 (3.46 to 6.58)*** | 1.62 (1.08 to 2.41)* | 2.72 (1.89 to 3.91)*** | 1.68 (1.22 to 2.33)** |
Witnessed violence in the home | Reference | 4.47 (3.54 to 5.65)*** | 3.71 (2.65 to 5.19)*** | 10.77 (7.16 to 16.19)*** | 2.90 (1.77 to 4.77)*** | 2.41 (1.57 to 3.69)*** | 0.83 (0.58 to 1.19) |
Witnessed parents fighting | Reference | 3.45 (2.70 to 4.40)*** | 2.97 (2.12 to 4.16)*** | 6.84 (4.50 to 10.41)*** | 2.31 (1.41 to 3.78)*** | 1.98 (1.27 to 3.10)** | 0.86 (0.59 to 1.25) |
Sexual abuse | Reference | 3.94 (2.34 to 6.64)*** | 6.85 (3.92 to 11.98)*** | 13.27 (7.44 to 23.66)*** | 1.94 (0.95 to 3.95) | 3.37 (1.76 to 6.43)*** | 1.74 (0.93 to 3.23) |
Physical abuse | Reference | 4.71 (3.43 to 6.45)*** | 4.35 (2.91 to 6.50)*** | 11.19 (7.09 to 17.68)*** | 2.57 (1.54 to 4.31)*** | 2.38 (1.52 to 3.71)*** | 0.92 (0.62 to 1.38) |
Neglect | Reference | 5.18 (3.69 to 7.28)*** | 4.90 (3.15 to 7.63)*** | 11.11 (6.95 to 17.76)*** | 2.27 (1.29 to 3.99)** | 2.14 (1.35 to 3.40)** | 0.95 (0.60 to 1.50) |
Serious life-threating injury | Reference | 4.19 (2.66 to 6.60)*** | 4.43 (2.41 to 8.16)*** | 12.97 (7.55 to 22.28)*** | 2.92 (1.44 to 5.96)** | 3.10 (1.70 to 5.66)*** | 1.06 (0.58 to 1.94) |
Local authority care | Reference | 4.67 (3.21 to 6.82)*** | 5.24 (3.09 to 8.88)*** | 9.46 (5.40 to 16.57)*** | 1.81 (0.94 to 3.46) | 2.02 (1.18 to 3.47)* | 1.12 (0.67 to 1.87) |
Psychiatric morbidity
Table 74 shows the associations between class membership (see Figure 4) and measures of psychiatric morbidity. Classes 2–4 (substance misuse, self-harm and multiple risks respectively) were all associated with a significantly higher prevalence of anxiety disorder, depression, psychosis, ASPD, pathological gambling, problem use of pornography and stalking than the low-risk group (class 1). Class 4 showed a significantly stronger association with diagnoses of anxiety disorder, ASPD, pathological gambling and stalking than both class 2 and class 3 and a significantly stronger association with psychosis than class 2. When comparing classes 3 and 2, class 3 had significantly stronger associations with anxiety disorder, depression and psychosis, whereas class 2 had a significantly stronger association with ASPD.
Criminal history
Table 74 shows the associations between class membership (see Figure 4) and measures of criminal history. All classes were significantly associated with ever having received a criminal conviction, ever having been in prison, having friends who encouraged drug use, having friends who encouraged crime and self-reported gang membership compared with class 1 (low risk).
Class 4 (multiple risks) was significantly more likely to report previous convictions, imprisonment, friends encouraging drug use and crime and gang membership than either class 3 (self-harm) or class 2 (substance abuse).
Table 74 also shows that class 2 (substance abuse) were significantly more likely to report all criminal history outcomes than class 3 (self-harm).
Adverse health and service use
Tables 76 and 77 show the associations between class membership and measures of self-reported physical and mental health problems and health service use. Compared with the low-risk group (class 1), classes 2 (substance abuse) and 4 (multiple risks) showed significant associations with all variables except for obesity.
Health and health service use | Class membership (weighted percentages) | |||
---|---|---|---|---|
Class 1,a n (%) | Class 2,a n (%) | Class 3,a n (%) | Class 4,a n (%) | |
Fair/poor health | 395 (9.5) | 139 (19.2) | 75 (25.3) | 32 (25.5) |
Accident leading to injury | 724 (17.7) | 196 (27.8) | 111 (38.1) | 63 (51.3) |
Accident leading to another being injured | 215 (5.3) | 70 (10.0) | 25 (8.5) | 51 (41.4) |
Currently taking medication (physical health problem) | 229 (5.6) | 78 (11.0) | 62 (21.2) | 42 (33.4) |
Attended A&E | 469 (12.0) | 129 (18.6) | 87 (31.7) | 58 (47.5) |
Obesity | 420 (12.4) | 78 (13.5) | 31 (12.2) | 9 (9.5) |
STI | 212 (5.8) | 168 (25.1) | 44 (15.6) | 69 (57.4) |
Seen mental health professional (past 12 months) | 255 (6.2) | 87 (12.2) | 103 (35.3) | 52 (42.6) |
Currently taking medication (mental health problem) | 93 (2.3) | 55 (7.9) | 68 (23.1) | 34 (28.0) |
Hospitalised for psychiatric care | 89 (2.2) | 33 (4.7) | 55 (19.2) | 39 (31.7) |
Health outcomes | Class membership | Intraclass contrasts | |||||
---|---|---|---|---|---|---|---|
Class 1a (reference) | Class 2a OR (95% CI) | Class 3a OR (95% CI) | Class 4a OR (95% CI) | Class 4 – class 3 OR (95% CI) | Class 4 – class 2 OR (95% CI) | Class 3 – class 2 OR (95% CI) | |
Fair/poor health | Reference | 1.87 (1.45 to 2.40)*** | 3.16 (2.31 to 4.33)*** | 2.97 (1.93 to 4.58)*** | 0.94 (0.57 to 1.56) | 1.59 (0.99 to 2.54) | 1.69 (1.18 to 2.42)** |
Accident leading to injury | Reference | 1.90 (1.52 to 2.39)*** | 2.69 (1.97 to 3.67)*** | 5.96 (4.11 to 8.64)*** | 2.22 (1.39 to 3.52)*** | 3.13 (2.10 to 4.67)*** | 1.41 (0.99 to 2.02) |
Accident leading to another being injured | Reference | 2.21 (1.59 to 3.08)*** | 1.56 (0.95 to 2.54) | 9.01 (6.20 to 13.08)*** | 5.79 (3.34 to 10.03)*** | 4.07 (2.69 to 6.16)*** | 0.70 (0.41 to 1.20) |
Current taking medication (physical health problem) | Reference | 1.68 (1.22 to 2.33)** | 4.11 (2.75 to 6.15)*** | 6.90 (4.37 to 10.90)*** | 1.68 (0.95 to 2.97) | 4.10 (2.41 to 6.97)*** | 2.44 (1.53 to 3.89)*** |
Attended A&E | Reference | 1.46 (1.13 to 1.87)** | 3.39 (2.45 to 4.70)*** | 4.88 (3.37 to 7.05)*** | 1.44 (0.92 to 2.25) | 3.35 (2.23 to 5.03)*** | 2.33 (1.61 to 3.38)*** |
Obesity | Reference | 1.11 (0.81 to 1.53) | 1.18 (0.76 to 1.82) | 0.55 (0.26 to 1.15) | 0.46 (0.20 to 1.06) | 0.49 (0.22 to 1.08) | 1.06 (0.64 to 1.75) |
STI | Reference | 4.86 (3.67 to 6.43)*** | 2.83 (1.84 to 4.35)*** | 14.74 (9.76 to 22.26)*** | 5.21 (2.98 to 9.09)*** | 3.03 (1.95 to 4.71)*** | 0.58 (0.38 to 0.90)* |
Mental health professional (12 m) | Reference | 1.74 (1.27 to 2.38)*** | 7.68 (5.53 to 10.67)*** | 9.27 (5.99 to 14.34)*** | 1.21 (0.74 to 1.97) | 5.33 (3.29 to 8.64)*** | 4.42 (2.94 to 6.62)*** |
Current taking medication (mental health problem) | Reference | 2.75 (1.84 to 4.12)*** | 13.55 (8.98 to 20.45)*** | 11.91 (6.87 to 20.67)*** | 0.88 (0.48 to 1.60) | 4.33 (2.35 to 7.98)*** | 4.93 (3.10 to 7.83)*** |
Hospitalised for psychiatric care | Reference | 1.88 (1.13 to 3.11)* | 10.92 (6.87 to 17.35)*** | 17.77 (10.62 to 29.75)*** | 1.63 (0.93 to 2.84) | 9.48 (5.02 to 17.90)*** | 5.82 (3.34 to 10.16)*** |
Members of class 4 were more likely to report having been involved in an accident leading to themselves and another person being injured and to have had a STI, but the other variables did not discriminate between classes 4 and 3. However, compared with class 2, members of class 4 were significantly more likely to report accidents leading to themselves and others being injured, that they were currently taking medication for both physical and mental health problems, that they had attended an A&E department, that they had had a STI and that they had consulted a professional for mental health problems in the past year or had been in a psychiatric hospital. When comparing classes 3 and 2, members of class 2 were more likely to report fair/poor physical health, that they were currently taking medication for physical health problems, that they had attended an A&E department, that they were taking medication for mental health problems, that they had consulted a health-care professional for mental health problems in the past year and that they had been in a psychiatric hospital. However, they were less likely to report that they had had a STI.
Discussion
We found that, by early adulthood, most young men are not actively engaged in taking risks with their future health or carrying out activities that result in a risk of harm to others. However, approximately one-quarter of young men in the population studied do take these risks and the risk factors are generally intercorrelated across the population. We created a typology based on three classes of risk factors. We found that class 2 was characterised primarily by substance misuse, class 3 by self-harm and class 4 by multiple high risks from all domains. Class 4 showed a higher prevalence of both substance misuse and self-harm behaviours than either class 2 or class 3. Class 4 was the smallest, but exceptionally high-risk, subgroup.
Class 4, multiple risk takers, are of particular interest and, of all subgroups of young men, place the highest burden of care on the health services. Men in class 4 were more likely to be injured in accidents, injure others, receive treatment in an A&E department, take medication for their physical health and report their health as fair/poor. They were also considerably more likely to acquire a STI and to consult a mental health professional, take psychotropic medication and report hospitalisation for psychiatric treatment. This subgroup had experienced multiple forms of childhood maltreatment and adversity and the majority has ASPD, indicating early onset of antisocial behaviour persisting into adulthood and corresponding to these experiences. The finding that members of class 4 were significantly older was unexpected. This would suggest that persistence of risk-taking behaviour had continued together with a series of criminal and violent behaviours and was associated with high levels of substance abuse.
Moffitt141 proposed a theoretical framework that makes specific predictions about risk and protective factors related to early-onset conduct disorder. Class 4 would appear to correspond to the proposed life course-persistent (or early-onset) group. This has its origins in both neurological deficits and exposure to environmental risk, such as poor parenting and parental antisocial behaviour. Neurological deficits are thought to lead to the child becoming vulnerable to poor parenting from caretakers. These early risk factors start the child on a trajectory of increasing acts and behaviours that escalate through adolescence and persist into adulthood. Our finding that persistent risk-taking is closely associated with persistent criminal and violent behaviour into the late 20s and early 30s suggests that this is a particularly severe subgroup in whom persistence of ASPD is associated with an underlying risk-taking propensity.
Previous research emphasises the importance of protective factors over the life course and the importance of accumulated adverse events over time. 246 Our study did not include protective factors but demonstrated an accumulation of early adverse events during childhood and mental health problems in adulthood that are likely to be closely inter-related and result in persistence, including anxiety and psychotic symptoms as well as substance misuse.
It has been suggested that young people who take risks are less amenable to positive protection effects as they get older if they continue to take risks. 248 For example, individuals who are still involved in violence at age 21 years are the most entrenched and committed offenders and are less amenable to change. Life-course studies of offending and associated risk taking suggest that hazard rates of these behaviours are not dynamic. 247 By sorting individuals into categories of behaviour over time, there is little evidence that the occurrence of protective factors at later time points corresponds to positive changes in behaviour for entrenched individuals. Nevertheless, employment and partner satisfaction continue to be recommended as targets for intervention when violence continues among older offenders, with confirmed effectiveness. 248
The persistence of risk taking therefore questions the purpose of these behaviours for the individual if it results in multiple adverse outcomes in adulthood, including injury, illnesses and hospitalisation. Our findings suggest an appetitive, or at least purposeful, component. This could be the result of early trauma and possible neurological deficits in these individuals. The reduced association with depression following adjustments is therefore interesting in this context. Risk taking can be construed as a displacement activity and mechanism for enhancing self-esteem in childhood, together with a means of coping with depression in adulthood to reduce the deleterious effects of a negative environment at both stages of life, including childhood maltreatment, educational failure and later unemployment and lack of a supportive emotional relationship. However, in this cross-sectional study we could not ultimately determine the direction of the association.
A longitudinal study of self-harming behaviour among adolescents shows that it resolves spontaneously in the majority of people. 249 This would indicate that class 3 represents a poor prognostic group, with self-harm persisting into adulthood. Persistence into adulthood was associated with symptoms of anxiety and depression, antisocial behaviour, high-risk alcohol use, cannabis use and cigarette smoking,249 as observed for class 3, but, in an extreme form, with multiple, other high-risk behaviours,250 as observed in class 4. In this current study, non-suicidal self-injury and suicidal behaviour were closely associated, corresponding to other studies in the community and in prisons,250–252 with additional associations observed with heavy drinking and sexual behaviours. 253 A large-scale community survey of adolescents found that those who reported non-suicidal self-injury were more depressed and hopeless, had experienced childhood physical abuse, had less parental connectedness and had run away from home. 254 Suicidal ideation and self-harm behaviour are also found to be associated with high levels of impulsivity and risk taking,255 together with high-risk use of the internet, which is closely related to symptoms of depression in studies of adolescents. 256
The distinction between classes 3 and 4 was shown in a study of self-harm among young offenders. 257 Self-harm was found to be strongly associated with a wide range of risk-taking behaviours and was considered to be a distinct epidemiological profile from that of the general population. 257 Class 4 would correspond more to this profile because it is associated with a wider range of risk-taking behaviours, higher rates of psychiatric morbidity, substance misuse and social risk factors.
The differentiation between class 2 and class 4 appears to be dependent on the level of substance misuse in the sample and its association with a wide range of other high-risk behaviours. Class 4 would appear to include men with the highest levels of substance misuse and the widest range of other behaviours, whereas class 2 appears to be restricted primarily to those with a high-level use of substances, including tobacco. A large longitudinal study of UK children followed up until 15 years of age found that smoking, alcohol use and antisocial behaviour were associated with an increased risk of morbidity and mortality. 258 At 15 years old the most prevalent behaviours were physical inactivity (74%), antisocial and criminal behaviour (42%) and hazardous drinking (34%). 258 This previous study found that boys and girls engaged in a similar number of behaviours, but that antisocial and criminal behaviours, cannabis use and vehicle-related risk behaviours (the latter observed in association with class 2 in the present study) were more prevalent among boys. Tobacco smoking, self-harm and physical inactivity were more prevalent among girls.
Finally, persistence into adulthood of substance misuse and associated risk taking may be determined by the differential development of neural circuitry and its association with both impulsive and compulsive behaviour. 259,260 This requires further investigation in the classes that we have identified (see Figure 4). The underlying biological mechanisms may underlie compulsive, impulsive and addictive behaviours and therefore may be related to the ‘appetitive’ aspects of risk-taking behaviour. 261,262
Chapter 10 Health service use and violence
Background
The importance of treatment, and access to treatment, for those with mental disorders who are violent has become increasingly recognised in recent years. This has significant implications for the successful management of violence, particularly in at-risk populations. 263,264 However, delivery of successful treatment for mental disorders is not straightforward; often those showing the highest need for treatment are also those least likely to have access to, or seek contact with, services offering such treatment. 265,266 In this chapter we consider the relationship between mental disorders, use of treatment services and violence within the UK population.
Objectives
The objectives of this chapter were to:
-
describe patterns of mental health and physical health service use in the UK household population and examine the associations of service use with violence
-
investigate whether or not mental health service use is associated with a differential risk of violence for those with a mental disorder.
Methods
Participants
For this study we analysed data on the use of services for both mental and physical health problems. We used data from the NHPMS 2000 and APMS 2007. As each of the surveys employed the same measures of demography, psychiatric morbidity, service use and violence outcomes, we conducted joint analyses of individual-level data. The total sample for the study included 15,973 men and women.
In both surveys, participants were asked a series of questions about their use of services for mental and physical health problems over the previous 2 weeks [contact with a general practitioner (GP)], quarter (inpatient or outpatient service use, use of day services), year (contact with a GP, use of medication) and lifetime (being a psychiatric inpatient).
Statistical analysis
Weighting was used to control for the under-representation of various demographic groupings in the surveys (young men, ethnic minorities, lower social classes). 45,82 The prevalence of key variables (service use and violence) was analysed in both surveys and the total sample and then the associations between, first, psychiatric morbidity and service use and, second, service use and violence were analysed using logistic regression with ORs and 95% CIs. Robust SE estimates were used to account for correlations with survey areas that may have resulted from postcode randomisation.
Throughout the analysis, adjustments were made for demographic factors and, when associations with psychiatric morbidity were explored, comorbidity. For example, analysis of psychotic illness would be adjusted for anxiety, depression, alcohol and drug misuse and personality disorder.
All analyses were performed using Stata version 13.1.
Results
Prevalence of service use
The reported use of health services for physical and mental health problems is presented in Tables 78 and 79. Table 78 shows the prevalence of different types of service use in the preceding quarter, whereas Table 79 shows the prevalence of different types of service use in the preceding 12 months. In both tables, differences between the surveys based on a chi-square test are noted after the combined prevalences; differences between men and women are noted after the individual survey findings. Significantly more matching variables between surveys were available for the last quarter outcomes. These variables were therefore used for the analysis of associations with violence; the exception was variables relating to seeing a GP, which were available for only the preceding 2 weeks. These variables relating to seeing a GP, together with the reporting of other service use in the previous quarter (see Table 78), were combined to give estimates of service use in the preceding quarter.
Service use, last quarter | Combined sample (n = 15,983) | NHPMS 2000 | APMS 2007 | ||||||||||||||
---|---|---|---|---|---|---|---|---|---|---|---|---|---|---|---|---|---|
Total (n = 7393) | Men (n = 3592) | Women (n = 3801) | Total (n = 8580) | Men (n = 4285) | Women (n = 4295) | ||||||||||||
n | % | p-value | n | % | n | % | n | % | p-value | n | % | n | % | n | % | p-value | |
Physical health care | |||||||||||||||||
Seen GPa | 1959 | 12.3 | ** | 988 | 11.5 | 397 | 9.3 | 591 | 13.8 | ** | 971 | 13.1 | 406 | 11.3 | 565 | 14.9 | ** |
Inpatient stay | 444 | 2.8 | 221 | 2.6 | 106 | 2.6 | 115 | 2.7 | 223 | 3.0 | 105 | 2.9 | 118 | 3.1 | |||
Outpatient stay | 2955 | 18.5 | 1561 | 18.2 | 725 | 16.9 | 836 | 19.5 | ** | 1394 | 18.9 | 610 | 17.0 | 784 | 20.6 | ** | |
Day activity | 39 | 0.5 | 18 | 0.4 | 21 | 0.5 | No data | ||||||||||
Community care | 240 | 3.0 | 115 | 2.7 | 125 | 2.9 | No data | ||||||||||
Medication (non-psychotropic)b | 6163 | 38.6 | 3644 | 42.5 | 1253 | 29.2 | 1793 | 41.8 | ** | 3365 | 39.2 | 1357 | 31.7 | 2008 | 46.8 | ** | |
Mental health care | |||||||||||||||||
Seen GPa | 304 | 1.9 | ** | 136 | 1.6 | 49 | 1.0 | 86 | 2.0 | ** | 168 | 2.3 | 65 | 1.8 | 103 | 2.7 | * |
Inpatient stay | 14 | 0.1 | 9 | 0.1 | 5 | 0.1 | 3 | 0.1 | 6 | 0.1 | 5 | 0.1 | 1 | 0.1 | |||
Outpatient stay | 124 | 0.8 | 59 | 0.7 | 27 | 0.6 | 32 | 0.7 | 65 | 0.9 | 37 | 1.0 | 29 | 0.8 | |||
Day activity | 6 | 0.1 | 2 | 0.1 | 4 | 0.1 | No data | ||||||||||
Community care | 39 | 0.5 | 20 | 0.5 | 19 | 0.5 | No data | ||||||||||
Psychotropic medicationb | 968 | 6.1 | 509 | 5.9 | 169 | 3.9 | 340 | 7.9 | ** | 459 | 6.2 | 148 | 4.1 | 311 | 8.2 | ** |
Service use, last 12 months | Combined sample (n = 15,983) | NHPMS 2000 | APMS 2007 | ||||||||||||||
---|---|---|---|---|---|---|---|---|---|---|---|---|---|---|---|---|---|
Total (n = 7393) | Men (n = 3592) | Women (n = 3801) | Total (n = 8580) | Men (n = 4285) | Women (n = 4295) | ||||||||||||
n | % | p-value | n | % | n | % | n | % | p-value | n | % | n | % | n | % | p-value | |
Physical health care | |||||||||||||||||
Seen GP | 9761 | 61.1 | 5204 | 60.7 | 2445 | 57.1 | 2758 | 64.2 | ** | 4557 | 61.6 | 2068 | 57.6 | 2489 | 65.5 | ** | |
Inpatient stay | No data | 767 | 10.4 | 328 | 9.1 | 439 | 11.6 | ** | |||||||||
Community/outpatient | No data | No data | |||||||||||||||
Mental health care | |||||||||||||||||
Seen GP | 1823 | 11.4 | 1005 | 11.7 | 341 | 8.0 | 665 | 15.5 | ** | 817 | 11.1 | 255 | 7.1 | 562 | 14.8 | ** | |
Inpatient staya | 373 | 2.3 | 210 | 2.5 | 96 | 2.2 | 114 | 2.7 | 163 | 2.2 | 69 | 1.9 | 94 | 2.5 | |||
Community/outpatient | No data | 490 | 6.6 | 210 | 5.9 | 280 | 7.4 | * |
A significantly greater proportion of individuals reported going to see their GP for physical health issues in the preceding 2 weeks in the 2007 sample than in the 2000 sample (OR 1.16, 95% CI 1.04 to 1.29; p = 0.004). The proportion of individuals seeing their GP for mental or emotional difficulties in the preceding 2 weeks was also greater in the 2007 sample (OR 1.45, 95% CI 1.15 to 1.83; p = 0.002). However, this finding was not replicated when participants were asked about health service use in the previous year as a whole. All other service use variables showed a comparable prevalence between the surveys, when matching data were available.
Table 80 shows the associations between clinical and demographic factors and service use in the preceding quarter. In a fully adjusted regression model, only age, sex, anxiety disorder and depression were significantly linked with physical service use. Being female (AOR 1.71, 95% CI 1.58 to 1.85; p < 0.001) and older were associated with increased service use after adjustment, as were anxiety (AOR 1.78, 95% CI 1.59 to 2.00; p < 0.001) and depression (AOR 1.45, 95% CI 1.05 to 1.99; p = 0.023). ASPD also showed a trend towards significance at the α = 0.05 level.
Covariate | Physical health service use (last quarter) | Mental health service use (last quarter) | ||||||
---|---|---|---|---|---|---|---|---|
n (%) | AORa | 95% CI | p-value | n (%) | AORa | 95% CI | p-value | |
Sex | ||||||||
Female | 4419 (54.6) | Reference | 772 (9.5) | Reference | ||||
Male | 3250 (41.3) | 0.60 | 0.55 to 0.64 | < 0.001 | 394 (5.0) | 0.51 | 0.43 to 0.59 | < 0.001 |
Age group (years) | ||||||||
16–34 | 1881 (35.5) | Reference | 256 (4.8) | Reference | ||||
35–54 | 2618 (43.8) | 1.37 | 1.24 to 1.42 | < 0.001 | 516 (8.6) | 1.78 | 1.39 to 2.28 | < 0.001 |
≥ 55 | 3432 (73.0) | 5.13 | 4.59 to 5.74 | < 0.001 | 393 (8.4) | 2.18 | 1.21 to 1.77 | < 0.001 |
Marital status | ||||||||
Married/cohabiting | 5517 (53.6) | Reference | 734 (7.1) | Reference | ||||
Single | 1607 (38.2) | 1.02 | 0.92 to 1.14 | 0.701 | 226 (5.4) | 0.85 | 0.67 to 1.09 | 0.205 |
Divorced/separated | 807 (54.7) | 1.04 | 0.92 to 1.16 | 0.554 | 206 (14.0) | 1.50 | 1.25 to 1.80 | < 0.001 |
Social class | ||||||||
I and II | 2596 (47.7) | Reference | 304 (5.6) | Reference | ||||
IIIM and IIINM | 3247 (50.9) | 1.07 | 0.98 to 1.17 | 0.131 | 482 (7.6) | 1.29 | 1.09 to 1.52 | 0.004 |
IV, V and VI | 1708 (52.4) | 1.08 | 0.97 to 1.20 | 0.171 | 305 (9.4) | 1.46 | 1.21 to 1.77 | < 0.001 |
Ethnicity | ||||||||
White | 7356 (50.5) | Reference | 1088 (7.5) | Reference | ||||
Black | 173 (41.8) | 0.88 | 0.68 to 1.14 | 0.344 | 27 (6.5) | 0.67 | 0.40 to 1.11 | 0.117 |
South Asian | 178 (34.7) | 0.78 | 0.59 to 1.03 | 0.076 | 18 (3.6) | 0.30 | 0.12 to 0.75 | 0.010 |
Other | 167 (45.5) | 1.23 | 0.92 to 1.66 | 0.166 | 15 (4.0) | 0.53 | 0.27 to 1.05 | 0.068 |
Drug dependency | 206 (35.9) | 0.85 | 0.66 to 1.10 | 0.224 | 81 (14.1) | 2.34 | 1.57 to 3.50 | < 0.001 |
Alcohol dependency | 302 (40.6) | 0.95 | 0.77 to 1.18 | 0.654 | 84 (11.2) | 1.53 | 1.07 to 2.19 | 0.02 |
Anxiety disorder | 1397 (59.9) | 1.78 | 1.59 to 2.00 | < 0.001 | 443 (23.7) | 4.75 | 4.07 to 5.55 | < 0.001 |
Depression | 168 (59.6) | 1.45 | 1.05 to 1.99 | 0.023 | 151 (53.7) | 8.09 | 5.62 to 11.62 | < 0.001 |
Psychosis | 23 (70.0) | 1.62 | 0.70 to 3.76 | 0.262 | 28 (82.9) | 51.2 | 15.3 to 171.8 | < 0.001 |
ASPD | 164 (46.7) | 1.32 | 0.99 to 1.75 | 0.056 | 39 (11.3) | 0.98 | 0.62 to 1.56 | 0.94 |
Patterns of association with mental health service use appeared more multifactorial than for physical service use. Female sex and older age were still significant predictors of mental health service use, but so too was being divorced or separated and being in social classes III or IV–VI. Additionally, being of South Asian ethnicity was negatively associated with use of mental health services after adjustment for other demographic factors and the presence of mental disorder. As expected, the presence of all mental disorders was positively associated with the use of mental health services, except for ASPD, which showed no association after adjustment.
Associations with violence
Associations with violent behaviour of those using health services in the previous quarter are presented in Table 81. The associations are presented first unadjusted and then adjusted for possible confounding variables based on the analysis above.
Outcomes | Physical health service use | Mental health service use | ||||
---|---|---|---|---|---|---|
n (%) | OR (95% CI) | AORa (95% CI) | n (%) | OR (95% CI) | AORb (95% CI) | |
Any violence | 591 (7.6) | 0.56 (0.50 to 0.64)*** | 1.08 (0.93 to 1.25) | 148 (13.0) | 1.36 (1.11 to 1.66)** | 1.56 (1.18 to 2.05)** |
Violence while intoxicated | 214 (2.7) | 0.45 (0.37 to 0.55)*** | 0.88 (0.71 to 1.10) | 59 (5.2) | 1.22 (0.90 to 1.65) | 1.27 (0.80 to 2.02) |
Severity of violence | ||||||
Minor violence | 229 (2.9) | 0.53 (0.43 to 0.66)*** | 0.97 (0.77 to 1.21) | 58 (5.1) | 1.27 (0.2 to 1.75) | 1.88 (1.27 to 2.79)** |
Five or more incidents | 131 (1.7) | 0.65 (0.49 to 0.86)** | 1.34 (0.99 to 1.81) | 32 (2.8) | 1.38 (0.92 to 2.09) | 1.36 (0.81 to 2.29) |
Victim injured | 191 (2.5) | 0.61 (0.49 to 0.76)*** | 1.23 (0.96 to 1.57) | 44 (3.9) | 1.23 (0.86 to 1.77) | 1.06 (0.65 to 1.72) |
Perpetrator injured | 211 (2.7) | 0.80 (0.56 to 0.87)** | 1.29 (1.02 to 1.64)* | 52 (4.5) | 1.46 (1.07 to 1.99)* | 1.20 (0.79 to 1.83) |
Police involved | 172 (2.2) | 0.67 (0.53 to 0.84)** | 1.18 (0.92 to 1.52) | 50 (4.4) | 1.73 (1.25 to 2.40)** | 1.55 (1.02 to 2.34)* |
Victim of violence | ||||||
Intimate partner | 142 (1.8) | 1.32 (0.99 to 1.74) | 1.60 (1.19 to 2.14)** | 56 (4.9) | 3.76 (2.74 to 5.16)*** | 2.13 (1.46 to 3.11)*** |
Family | 71 (0.9) | 0.87 (0.58 to 1.30) | 1.41 (0.93 to 2.14) | 13 (1.2) | 1.23 (0.67 to 2.23) | 0.63 (0.30 to 1.33) |
Friend | 88 (1.2) | 0.40 (0.29 to 0.55)*** | 0.82 (0.59 to 1.12) | 26 (2.2) | 1.15 (0.72 to 1.83) | 1.38 (0.70 to 2.73) |
Person known | 190 (2.4) | 0.59 (0.47 to 0.75)*** | 1.16 (0.89 to 1.50) | 47 (4.1) | 1.30 (0.90 to 1.87) | 1.25 (0.77 to 2.03) |
Stranger | 270 (3.5) | 0.62 (0.43 to 0.62)*** | 1.09 (0.88 to 1.34) | 56 (4.9) | 0.99 (0.72 to 1.36) | 1.09 (0.71 to 1.68) |
Police | 35 (0.5) | 0.68 (0.41 to 1.13) | 1.34 (0.78 to 2.34) | 14 (1.2) | 2.44 (1.29 to 4.64)** | 1.75 (0.77 to 3.99) |
Other | 36 (0.5) | 0.50 (0.31 to 0.82)** | 0.96 (0.57 to 1.62) | 6 (0.6) | 0.81 (0.37 to 1.78) | 0.84 (0.31 to 2.29) |
Location of violent incident | ||||||
Own home | 161 (2.1) | 1.27 (0.97 to 1.65) | 1.60 (1.21 to 2.12)** | 62 (5.5) | 3.63 (2.69 to 4.90)*** | 2.04 (1.42 to 2.95)*** |
Someone else’s home | 53 (0.7) | 0.63 (0.40 to 0.97)* | 1.05 (0.68 to 1.63) | 19 (1.6) | 2.02 (1.19 to 3.42)** | 1.78 (0.96 to 3.30) |
Street | 317 (4.1) | 0.52 (0.44 to 0.62)*** | 1.02 (0.84 to 1.24) | 87 (7.6) | 1.38 (1.06 to 1.79)* | 1.48 (1.03 to 2.13)* |
Bar/pub | 191 (2.5) | 0.55 (0.44 to 0.68)*** | 1.09 (0.86 to 1.37) | 47 (4.1) | 1.21 (0.85 to 1.73) | 1.19 (0.73 to 1.94) |
Workplace | 39 (0.5) | 0.63 (0.39 to 1.03) | 1.13 (0.68 to 1.89) | 10 (0.9) | 1.37 (0.57 to 3.33) | 1.50 (0.46 to 4.89) |
Other | 88 (1.3) | 0.58 (0.40 to 0.84)** | 1.26 (0.85 to 1.87) | 15 (1.3) | 0.83 (0.46 to 1.50) | 1.03 (0.50 to 2.15) |
Service use for physical health problems showed many significant associations with violence. However, following adjustment, significant associations were found for violence involving injury to the perpetrator, IPV and violence in the perpetrator’s home.
Use of services for mental or emotional health was positively associated with violence in this analysis following adjustment. It was not linked to violence when intoxicated nor to any form of severe violence (i.e. repeated violence or violence resulting in injury). However, it was associated with minor violence that did not lead to injury to any party, although the police were more likely to be involved. Mental health service users were also more than twice as likely as those not using services to be involved in IPV and violence in the home and were also more likely to be involved in street violence.
Service use compared with non-service use
A final analysis was conducted to differentiate patterns of violence between those individuals with mental disorder who made use of services and those who screened positive for one or more mental disorder (anxiety disorder, alcohol or drug dependence, psychosis or ASPD) but who did not use services for mental or emotional problems.
A total of 3325 individuals (20.8% of the total sample) screened positive for one or more mental disorders. Of these, only 631 (19.0%) reported any service use within the last quarter, meaning that 2695 individuals (16.9% of the total sample) met caseness criteria for a mental disorder but had not recently accessed services. This group was defined as having an ‘unmet need’ for service use. The two groups (service use and unmet need) were compared on demography, prevalence of mental disorder and violence. Those in the unmet need group were more likely to be male (AOR 3.51, 95% CI 2.58 to 4.79; p < 0.001) and younger in age and less likely to be divorced or separated (AOR 0.67, 95% CI 0.56 to 0.83; p < 0.001); they were considerably more likely to be of South Asian origin (AOR 6.51, 95% CI 1.40 to 30.4; p = 0.017) and less likely to be of social classes III (AOR 0.75, 95% CI 0.58 to 0.97; p = 0.029) or IV–VI (AOR 0.62, 95% CI 0.46 to 0.82; p = 0.001); and they were also more likely to suffer from a higher number of mental disorders (Wilcoxon z = 2.17, p = 0.030) and to have been involved in violence in the past 5 years (AOR 1.39, 95% CI 1.09 to 1.77; p = 0.009).
The ORs of associations with violence for those who used services and those who did not are presented in Table 82. The analysis was adjusted for demography and all other mental disorders. Within this sample, no individual who screened positive for the presence of a psychotic disorder had not sought treatment within the last quarter and so the odds of violence in this group could not be calculated.
Mental disorder | Used services, AORa (95% CI) | No service use, AORa (95% CI) |
---|---|---|
Drug dependence | 2.89 (1.37 to 6.14)** | 1.56 (1.09 to 2.24)* |
Psychosis | 0.58 (1.62 to 2.11) | – |
Alcohol dependence | 2.57 (1.31 to 5.04)** | 1.79 (1.27 to 2.52)** |
Anxiety | 0.73 (0.36 to 1.45) | 1.03 (0.73 to 1.45) |
ASPD | 3.60 (1.37 to 9.42)** | 2.31 (1.57 to 3.40)*** |
Anxiety disorder showed no independent association with violence for either group, but for all other mental disorders the odds for involvement with violence were lower in the group who did not use services.
Discussion
This chapter considers associations between making use of health services and involvement in violence. A distinction was made between use of services for physical health problems and use of services for mental health problems and a further analysis was conducted to explore the effect of receiving treatment compared with not receiving treatment for those with a mental disorder.
Service use for physical health problems was not associated with violence generally and was found to be protective of most forms of severe violence before adjustment. Violence requires significant physical resources and those seeking treatment for physical problems may find themselves limited in opportunities or physical ability to be violent. Indeed, the only form of serious violence directly associated with use of physical health services after adjustment was violence resulting in injury to the perpetrator (i.e. the respondent). Logically, those who are injured as a result of violence – regardless of who initiated the violence – will make use of physical health services; no such finding was observed for mental health service use following injury.
Although physical service use was not associated with violence, of those who were violent, those receiving physical services were more likely than those not using services to have been involved in violence against a partner and to be violent in the home. These two findings are likely to be related, given that IPV most commonly takes place at home and is itself one of the most common forms of violence in the UK, accounting for between 16% and 25% of all violent crime. 267 The association with physical health service use may be spurious or it may be related to a link between physical ill health and confinement to the home setting, increasing domestic disharmony. However, it should be understood in the context of there being no overall association between service use for physical health and violence.
Use of health services for mental and emotional difficulties was positively associated with involvement in violence in the preceding 5 years. This finding, although interesting and consistent with the results of previous research,264,265 is harder to interpret given the differing reporting periods of the outcome variables, with service use measured over the previous quarter and violence over the previous 5 years. It is not possible to say whether the violence was committed as a result of mental disorder or vice versa. It is possible that an incident of violence led an individual to seek treatment for a mental disorder when previously he or she may not have done so.
Finally, although not using mental health services was associated with higher odds of violence than using mental health services, associations between mental disorder and violence were stronger in the group who used services than in the group who did not. This suggests that risk factors other than mental disorder are the major drivers of violence in those who do not use mental health services. This is perhaps to be expected from the demographic composition of the group within this sample, which tended to consist of younger men who would naturally be at a higher risk of violence on the evidence of most previous studies of criminality and violence. Alternatively, there may be a role for comorbidity within the propensity for individuals to use services and/or commit violence.
Chapter 11 Impact of violence on health-care costs
Background
It is likely that violence will result in high costs in relation to the victim. However, it is unclear whether or not violent behaviour in the general population places a heavy burden on health and social services in relation to the perpetrator.
Objective
The objective of this study was to measure and compare the service use and costs for three specific groups: (1) a representative sample of the population, (2) those who have committed acts of violence and (3) those who have not committed acts of violence.
Methods
Analyses were conducted to assess the impact of violent behaviour on resource use. The perspective was that of the health-care system, with the addition of some social care services. The analyses proceeded in two stages. First, an estimate was made of the overall service use and costs over a retrospective period for members of the survey. Second, we examined the impact of violence on service costs. This was performed using univariate and multivariate analyses.
Resource use
Resource use data were based on information collected as part of the NHPMS 2000. This yielded a total sample of 8580 participants. Details have been reported previously. 45 In addition to demographic and clinical data, the survey collected information on a range of health-care services that participants used over 1 year and 3 months preceding the date of the survey. The service use information collected included the numbers of contacts with GPs, days spent in hospital, outpatient visits, day care attendances and contacts with community-based professionals such as community psychologists, community psychiatrists, community psychiatric nurses and social workers.
Data were collected for different time periods but for these analyses and for the purposes of comparability resource use data recorded for the 3 months preceding the survey date were used. When a service had been used but the quantity or the contact duration was missing, the median values from those who provided this information were used. In addition, when no information was collected (as for the number of outpatient visits for general health), an external source was used to estimate the number of contacts.
Unit costs
All unit costs, in UK pounds sterling, were estimated at 2012–13 prices and collected from sources in the public domain. The unit costs are summarised in Table 83. Costs per unit of measurement for each type of service (such as GP visits, inpatient days, outpatient attendances) were taken from Curtis. 268 The NHS Schedule of Reference Costs was used to estimate the costs of psychiatric inpatient days and outpatient attendances (in addition to Curtis268). Unit costs for orthopaedic physicians and osteopaths were taken from the Bupa website [www.bupa.co.uk (accessed 16 February 2016)] and the General Osteopathic Council website [www.osteopathy.org.uk (accessed 16 February 2016)] respectively. Unit costs for some services (such as self-help/support groups) were not identified and other services were used as proxies for these (see Table 83).
Service | Unit cost (£) | Source | Comments |
---|---|---|---|
GP: psychiatric and non-psychiatric | |||
GP (per surgery consultation) | 34 | Curtis268 | |
Inpatient: non-psychiatric | |||
Inpatient (bed-days) | 598 | Curtis268 | |
Inpatient: psychiatric | |||
Acute psychiatric ward (bed-days) | 430 | Curtis268 | |
A&E (care contact) | 204 | NHS reference costs 2012–13269 | |
Rehabilitation ward (bed-days) | 595 | NHS reference costs 2012–13269 | |
General medical ward (bed-days) | 430 | Curtis268 | |
Outpatient: non-psychiatric | |||
Outpatient (appointment) | 135 | Curtis268 | |
Outpatient: psychiatric | |||
A&E (care contact) | 204 | NHS reference costs 2012–13269 | |
Psychiatric outpatient department (attendance) | 100 | Curtis268 | |
Hospital outpatient department (attendance) | 100 | Curtis268 | |
Alcohol clinic (attendance) | 104 | NHS reference costs 2012–13269 | |
Bupa outpatient (attendance) | 100 | Curtis268 | Unit cost not found. PSSRU 2013 unit cost for the weighted average of all adult outpatient attendances for mental health services was used as a proxy |
Psychotherapist (attendance) | 100 | Curtis268 | Unit cost not found. PSSRU 2013 unit cost for the weighted average of all adult outpatient attendances for mental health services was used as a proxy |
Alternative therapy centre (attendance) | 100 | Curtis268 | Unit cost not found. PSSRU 2013 unit cost for the weighted average of all adult outpatient attendances for mental health services was used as a proxy |
Physiotherapist (attendance) | 50 | NHS reference costs 2012–13 | |
Day services: psychiatric | |||
Community mental health centre (hour) | 36 | Curtis268 | |
Day activity centre (session) | 38 | Curtis268 | |
Sheltered workshop (hour) | 11 | Curtis268 | Taken from PSSRU 2009/10 (£9.80).270 Cost inflated to 2013 unit cost (£11) |
Cardiac rehabilitation (appointment) | 265 | NHS reference costs 2012–13269 | |
Community care: psychiatric | |||
Psychiatrist (contact) | 145 | NHS reference costs 2012–13269 | Unit cost not found. NHS reference costs 2012–13269 unit cost for other specialist mental health services was used as a proxy |
Psychologist (contact) | 134 | Curtis268 | |
Community psychiatric nurse (contact) | 65 | Curtis268 | |
Social worker (contact) | 159 | Curtis268 | |
Self-help/support group (session) | 30 | Curtis268 | Unit cost not found. PSSRU 2013 unit cost for private sector day care for people with mental health problems was used as proxy |
Home help/home-care worker (contact) | 24 | Curtis268 | |
Outreach worker/family support (hour) | 49 | Curtis268 | |
Community chiropodist (hour) | 30 | Curtis268 | |
Community midwife (contact) | 68 | NHS reference costs 2012–13269 | |
Community physiotherapist (hour) | 30 | Curtis268 | |
Community speech and language therapist (hour) | 30 | Curtis268 | |
District nurse (hour) | 60 | Curtis268 | |
Health visitor (hour) | 61 | Curtis268 | |
Community occupational therapist (hour) | 30 | Curtis268 | |
General practice nurse | 44 | Curtis268 | |
Hospital psychiatric nurse (hour) | 84 | Curtis268 | |
Orthopaedic physician (session) | 75 | www.bupa.co.uk | |
Osteopath (session) | 50 | www.osteopathy.org.uk | |
Macmillan nurse (contact) | 60 | NHS reference costs 2012–13269 | Unit cost not found. NHS reference costs 2012–13269 unit cost for other specialist nursing was used as a proxy |
Parentcraft (session) | 83 | NHS reference costs 2012–13269 | |
Support group (session) | 30 | Curtis268 | |
School-based nurse (contact) | 46 | NHS reference costs 2012–13269 | |
Rheumatology nurse (contact) | 45 | NHS reference costs 2012–13269 | Unit cost not found. NHS reference costs 2012–13269 unit cost for arthritis nursing was used as a proxy |
Asthma and respiratory nurse (contact) | 75 | NHS reference costs 2012–13269 | |
Continence nurse (contact) | 85 | NHS reference costs 2012–13269 | |
Diabetic nurse (contact) | 70 | NHS reference costs 2012–13269 | |
Stoma nurse (contact) | 43 | NHS reference costs 2012–13269 |
Cost calculations
Costs were categorised into five groups: GP costs, inpatient costs, outpatient costs, day services costs and community care costs. Each category was further grouped into psychiatric and non-psychiatric costs. The definition of ‘psychiatric’, taken from the survey, was any service use related to a mental health problem as well as a combination of a mental health and a physical health complaint. ‘Non-psychiatric’ was defined as any service use related to a physical complaint only. The reason for this grouping of service costs was to see whether or not violence was more likely to affect psychiatric care costs than non-psychiatric care costs.
Service costs were derived by combining service use with the appropriate national unit costs. Costs were calculated for the period of 3 months preceding the survey date for all services except for GP care for which the cost was extrapolated from 2 weeks preceding the survey date to 3 months preceding the survey date.
Analyses
Service use over the 3-month period was described by the numbers of participants with and without contact with each service sector, the mean numbers of contacts for those who used these services and the mean cost for each participant. These data were presented for those with self-reported violence and those with no self-reported violence.
The impact of violence on service costs was estimated using ordinary least squares regression. Separate models were run for each service category and each measure of violence adjusting for age, sex, social class, marital status and employment. We also separately report models adjusting for psychiatric comorbidity. Given the non-normality of the cost distribution, the CIs around the regression coefficients were generated using non-parametric bootstrap methods. This involved random sampling with replacement 1000 times from the original data set and generating percentile CIs from these 1000 samples. 271 A significance level of 5% was used and statistical analyses were performed using Stata version 12.
Results
Service use
The numbers of participants using services at least once over the 3 months prior to the survey date are provided in Table 84. Of the overall sample, 18.9% were in contact with non-psychiatric outpatient services. A similar contact rate was observed for the ‘any violence’ and ‘no violence’ samples. Those reporting violence were more likely to have had a psychiatric inpatient admission, although this was still rare. Most of the other differences between the groups were small.
Service sector | n (%) with contact | n (%) no contact | |
---|---|---|---|
Psychiatric | Non-psychiatric | ||
GPa | |||
Total sample | 156 (1.8) | 979 (11.4) | 7445 (86.8) |
Any violence | 25 (3.1) | 72 (9.0) | 707 (87.9) |
No violence | 127 (1.7) | 870 (11.5) | 6566 (86.8) |
Inpatient | |||
Total sample | 11 (0.1) | 248 (2.9) | 8231 (95.9) |
Any violence | 4 (0.5) | 25 (3.1) | 775 (96.4) |
No violence | 6 (0.08) | 213 (2.8) | 7344 (97.1) |
Outpatient | |||
Total sample | 71 (0.8) | 1624 (18.9) | 6885 (80.2) |
Any violence | 13 (1.6) | 145 (18.0) | 646 (80.4) |
No violence | 50 (0.66) | 1431 (18.9) | 6082 (80.4) |
Day activity | |||
Total sample | 52 (0.6) | 0 | 8528 (99.3) |
Any violence | 7 (0.9) | 0 | 797 (99.1) |
No violence | 37 (0.5) | 0 | 7526 (99.5) |
Community care | |||
Total sample | 332 (3.9) | 0 | 8248 (96.1) |
Any violence | 45 (5.6) | 0 | 759 (94.4) |
No violence | 270 (3.6) | 0 | 7293 (96.4) |
Table 85 details the intensity of service use by participants. The data in the table relate only to those who actually use the service, that is, zero values are omitted. Psychiatric services were used more often than non-psychiatric services. The mean numbers of contacts with GPs and days in hospital for psychiatric reasons were higher for participants who reported violent behaviour than for those reporting no violence. In addition, participants who reported violent behaviour had fewer contacts with outpatient, day activity and community care services for psychiatric reasons (2.6, 3.9 and 6.2 respectively) than the overall sample (3.4, 15.4 and 12.9 respectively) and those reporting no violence (3.8, 17.2 and 12.5 respectively).
Service sector | Mean (SD) number of contacts | |
---|---|---|
Psychiatric | Non-psychiatric | |
GPa | ||
Total sample | 8.5 (4.6) | 7.8 (3.9) |
Any violence | 9.8 (7.2) | 9.1 (5.2) |
No violence | 8.5 (4.6) | 7.8 (3.9) |
Inpatient | ||
Total sample | 13.8 (26.7) | 6.5 (11.3) |
Any violence | 18.8 (39.8) | 3.9 (5.9) |
No violence | 11.7 (15.5) | 6.6 (11.8) |
Outpatient | ||
Total sample | 3.4 (4.0) | 1.28 (1.59)b |
Any violence | 2.6 (2.9) | 1.28 (1.59)b |
No violence | 3.8 (4.5) | 1.28 (1.59)b |
Day activity | ||
Total sample | 15.4 (15.8) | – |
Any violence | 3.9 (1.9) | – |
No violence | 17.2 (15.8) | – |
Community care | ||
Total sample | 12.9 (24.6) | – |
Any violence | 6.2 (5.8) | – |
No violence | 12.5 (24.8) | – |
Total costs
Table 86 provides details of the costs by service group. The mean costs in the table relate to the whole sample, that is, with zero values included. The mean cost for GP contacts was highest if the contacts were for non-psychiatric reasons. A similar result was also observed for inpatient and outpatient services.
Service sector | Mean (SD) cost (£) of service | |
---|---|---|
Psychiatric | Non-psychiatric | |
GPa | ||
Total sample | 4.6 (39.1) | 31.2 (96.9) |
Any violence | 7.7 (51.3) | 28.4 (104.3) |
No violence | 4.4 (37.5) | 31.1 (96.1) |
Inpatient | ||
Total sample | 10.6 (642.2) | 112.1 (1321.7) |
Any violence | 68.4 (1888.7) | 72.1 (732.0) |
No violence | 4.7 (297.7) | 111.4 (1354.9) |
Outpatient | ||
Total sample | 3.1 (49.0) | 40.2 (92.2) |
Any violence | 4.9 (51.1) | 38.5 (90.7) |
No violence | 2.8 (49.0) | 40.3 (92.5) |
Day activity | ||
Total sample | 13.7 (294.1) | 0 |
Any violence | 2.4 (37.2) | 0 |
No violence | 11.7 (255.3) | 0 |
Community care | ||
Total sample | 32.4 (582.0) | 0 |
Any violence | 38.1 (388.1) | 0 |
No violence | 26.3 (509.2) | 0 |
For all service groups, when the contact was for psychiatric reasons, with the exception of day activities, the mean cost of care was higher for those reporting violent behaviour than for those who did not report violent behaviour. The opposite was the case for services use for non-psychiatric reasons.
The total cost (i.e. the summation of all individual service costs) was not separated into psychiatric compared with non-psychiatric services (as some are explicitly psychiatric). The mean cost of all services was £217.65 (SD £1677.21) for the total sample, £229.88 (SD £2072.29) for those reporting any violence and £202.70 (SD £1529.63) for those not reporting any violence.
Regression analysis
The results of the regression analysis are reported in Table 87 (adjusted for sex, age, social class, marital status, employment status and psychiatric comorbidity) and Table 88 (adjusted for sex, age, social class, marital status and employment status only).
Covariate | Observed (95% CI); SE (£) | ||||||||
---|---|---|---|---|---|---|---|---|---|
Total costs | GP non-psychiatric cost | GP psychiatric cost | Inpatient non-psychiatric cost | Inpatient psychiatric cost | Outpatient non-psychiatric cost | Outpatient psychiatric cost | Day care services cost | Community care cost | |
Any violence | 48.63 (–86.37 to 232.01); 85.65 | 0.36 (–0.95 to 1.80); 0.71 | 0.04 (–0.48 to 0.64); 0.29 | 5.39 (–52.85 to 79.37); 33.69 | 72.15 (–14.21 to 249.87); 81.16 | 6.23 (–1.08 to 13.81); 3.85 | 0.25 (–4.05 to 4.77); 2.23 | –21.88 (–39.07 to –8.21); 7.81 | 13.91 (–56.79 to 25.53); 21.17 |
Violent while intoxicated | 141.15 (–130.33 to 583.44); 202.38 | 0.51 (–1.40 to 3.20); 1.11 | 0.41 (–0.54 to 1.42); 0.52 | 7.53 (–53.96 to 91.16); 38.06 | 187.00 (–13.82 to 637.27); 199.12 | 3.35 (–7.55 to 15.42); 5.9 | –0.16 (–5.22 to 5.02); 2.6 | –23.02 (–49.36 to 2.44); 12.29 | –34.47 (–96.82 to 18.80); 29.83 |
Five or more violent incidents | 311.31 (–93.61 to 1010.07); 305.61 | 2.66 (–0.58 to 6.26); 1.80 | 0.15 (–1.03 to 1.69); 0.68 | 17.15 (–42.87 to 101.94); 38.40 | 305.54 (–18.01 to 1073.59); 319.68 | 12.95 (–1.50 to 28.38); 7.6 | –4.30 (–11.37 to 2.06); 3.45 | –28.36 (–49.96 to –10.81); 10.35 | 5.52 (–73.80 to 119.45); 49.63 |
Minor violence | –27.27 (–130.40 to 120.31); 63.97 | –0.61 (–1.90 to 0.86); 0.72 | 0.13 (–0.59 to 0.96); 0.40 | 40.20 (–53.92 to 166.99); 58.87 | –16.09 (–42.69 to –1.46); 11.24 | –6.62 (–14.57 to 0.94); 3.88 | –1.81 (–5.19 to 1.27); 1.63 | –15.24 (–27.87 to 5.64); 5.73 | –27.22 (–26.36 to –3.89); 13.15 |
Victim injured | 191.73 (–104.99 to 723.32); 230.06 | 0.96 (–1.24 to 3.68); 1.24 | 0.16 (–0.66 to 1.25); 0.51 | 16.81 (–58.23 to 115.72); 45.02 | 217.61 (–13.46 to 739.37); 232.84 | 12.42 (0.30 to 26.43); 6.67 | 4.64 (–3.41 to 15.72); 4.98 | –19.82 (–36.98 to –5.74); 7.9 | –41.04 (–76.31 to 11.49); 16.5 |
Respondent injured | 216.95 (–68.87 to 728.75); 219.60 | 1.24 (–1.16 to 3.92); 1.34 | 0.44 (–0.58 to 1.67); 0.58 | 2.64 (–58.69 to 93.26); 40.82 | 221.31 (–8.47 to 758.45); 230.41 | 19.95 (7.68 to 32.36); 6.64 | –0.31 (–5.55 to 5.48); 2.77 | –24.83 (–44.32 to –8.63); 9.33 | –3.49 (–62.20 to 63.78); 32.4 |
Police involved in the incident | 288.02 (–54.47 to 876.67); 257.43 | 2.03 (–0.71 to 5.18); 1.49 | 0.12 (–0.84 to 1.40); 0.57 | 41.47 (–39.09 to 162.56); 50.85 | 242.23 (–10.79 to 761.04); 237.81 | 12.82 (–0.60 to 28.26); 7.25 | 1.50 (–4.41 to 7.76); 3.03 | –16.57 (–34.55 to –1.54); 8.56 | 4.42 (–55.66 to 94.21); 39.55 |
Covariate | Observed (95% CI); SE (£) | ||||||||
---|---|---|---|---|---|---|---|---|---|
Total costs | GP non-psychiatric cost | GP psychiatric cost | Inpatient non-psychiatric cost | Inpatient psychiatric cost | Outpatient non-psychiatric cost | Outpatient psychiatric cost | Day care services cost | Community care cost | |
Any violence | 86.12 (–41.83 to 247.93); 76.10 | 1.04 (–0.14 to 2.34); 0.64 | 0.75 (0.24 to 1.40); 0.29 | –0.68 (–58.78 to 72.47); 34.71 | 64.63 (–9.08 to 219.60); 69.87 | 10.78 (3.81 to 17.95); 3.65 | 3.44 (0.04 to 8.28); 2.08 | –10.76 (–20.81 to –2.81); 4.64 | 16.92 (–31.50, to 51.95) 16.46 |
Violent while intoxicated | 163.69 (–56.96 to 520.92); 158.56 | 1.30 (–0.43 to 3.33); 0.97 | 1.35 (0.43 to 2.46); 0.52 | –16.64 (–82.75 to 57.86); 36.59 | 156.87 (–8.59 to 508.99); 163.57 | 8.36 (–1.50 to 19.75); 5.47 | 3.61 (0.06 to 8.03); 2.05 | –8.00 (–19.53 to 3.09); 5.64 | 16.85 (–12.51 to 59.37); 18.40 |
Five or more violent incidents | 372.12 (–19.45 to 1033.20); 292.19 | 3.57 (0.37 to 7.11); 1.77 | 1.18 (–0.08 to 2.80); 0.72 | 10.38 (–59.89 to 96.06); 39.80 | 296.45 (–7.43 to 951.00); 298.01 | 19.18 (4.78 to 35.68); 7.89 | 1.94 (–1.36 to 6.40); 2.00 | –9.30 (–18.56 to –1.23); 4.26 | 48.73 (–14.45 to 163.79); 48.67 |
Minor violence | –4.57 (–108.01 to 145.41); 65.88 | –0.31 (–1.74 to 0.99); 0.71 | 0.45 (–0.23 to 1.36); 0.40 | 37.77 (–60.60 to 182.08); 63.96 | –15.89 (–42.46 to –0.83); 12.30 | –4.20 (–11.67 to 3.00); 3.77 | –0.20 (–2.37 to 2.87); 1.34 | –10.20 (–16.58 to –4.53); 3.16 | –11.99 (–27.84 to 4.31); 8.16 |
Victim injured | 219.59 (–46.40 to 674.43); 200.89 | 1.74 (–0.49 to 4.33); 1.21 | 0.99 (0.09 to 2.24); 0.55 | 5.76 (–73.58 to 100.60); 43.28 | 209.30 (–9.44 to 646.00); 196.99 | 17.27 (5.84 to 30.13); 6.44 | 8.21 (0.63 to 20.49); 5.25 | –9.20 (–19.32 to –0.43); 4.70 | –6.47 (–23.75 to 11.97); 8.93 |
Respondent injured | 258.79 (–7.07 to 716.74); 201.71 | 2.13 (–0.14 to 4.63); 1.25 | 1.34 (0.31 to 2.64); 0.62 | –6.67 (–73.87 to 78.30); 38.60 | 205.44 (–4.02 to 661.61); 206.58 | 23.49 (12.42 to 38.97); 6.93 | 3.61 (–0.65 to 8.83); 2.51 | –11.15 (–19.66 to –3.93); 4.11 | 38.59 (–9.48 to 105.61); 29.16 |
Police involved in the incident | 312.23 (–5.25 to 820.93); 226.66 | 2.74 (0.18 to 6.15); 1.47 | 0.92 (–0.09 to 2.26); 0.59 | 32.96 (–51.90 to 136.86); 49.97 | 229.18 (–6.26 to 744.82); 232.45 | 17.37 (4.06 to 31.08); 7.06 | 5.05 (–0.19 to 11.50); 3.04 | –6.80 (–17.56 to 5.22); 5.77 | 30.82 (–20.44 to 117.83); 37.88 |
Individuals who reported injuries to the victim or to themselves had outpatient non-psychiatric costs that were on average £12.42 and £19.95 higher, respectively, than for those with no such violence reported (see Table 87). Any violence was related to day care activity costs that were on average £21.88 lower than for those reporting no violence. Similar findings were observed for five or more violent incidents, the respondent being injured, the victim being injured or police involvement. Minor violence and the victim being injured were significantly associated with lower community service costs. No significant violence predictors were observed for total costs, GP psychiatric and non-psychiatric costs, inpatient non-psychiatric costs and outpatient psychiatric costs.
With psychiatric morbidity not controlled for, any violence was significantly associated with higher GP psychiatric and outpatient psychiatric and non-psychiatric costs and lower day care activity costs (see Table 88). Violence while intoxicated was associated with increased GP and outpatient psychiatric costs. Reporting more than five violent acts was significantly associated with higher GP and outpatient non-psychiatric costs. Minor violence was associated with lower psychiatric inpatient and day care costs. Violence in which the victim was injured was associated with increased GP psychiatric and outpatient psychiatric and non-psychiatric costs. Day care activity and community service costs were reduced for this group. If the respondent was injured then GP psychiatric and outpatient non-psychiatric costs were significantly increased whereas day care activity costs were reduced. Finally, if police involvement was reported then GP and outpatient non-psychiatric costs were increased.
Conclusions
The key strengths of these analyses were that service use and costs were derived from a nationally representative sample and disaggregated into subsets of costs (psychiatric and non-psychiatric) and the impact of violence variables on service costs were then examined in these subsets of costs. The analyses found that participants who reported violent behaviour used more GP and inpatient services for psychiatric reasons but had less contact with outpatient, day care and community care services.
From the multivariate analyses, no violence variable was found to be a statistically significant predictor of total costs despite it appearing to have a strong impact when univariate analyses were conducted. The likely explanation for this is that, at the aggregated level, the direct impact of violence cannot be distinguished from the impact of other demographic and clinical characteristics. Violence predicted lower costs for day care services. This is consistent with the result showing that individuals reporting violence were less likely to have day care contacts than those not reporting violence. Use of community services also appeared to be adversely affected by violence. This suggests that, although violence does not seem to affect access to health care, it does seem to reduce access to more ‘social’ services such as day care and community services. The health-care consequences of such reduced access should be studied.
The study found violence to be correlated with psychiatric comorbidity. In the first set of regression analyses, which controlled for psychiatric comorbidity (see Table 87), only a few violence variables were found to be statistically significant predictors of costs. However, in the second regression analysis in which psychiatric comorbidity was not controlled for (see Table 88), the number of violence variables that were statistically significant predictors of costs doubled. There is likely to be a complex relationship between violence and psychiatric morbidity and further work on the additional link with costs should also be a research priority.
There are a number of limitations of these analyses. First, the survey did not collect extensive information on GP contacts (focusing on the previous 2 weeks may be inadequate) and no information was available on the number of non-psychiatric outpatient visits. Extrapolating GP contacts to 3 months is likely to have resulted in an underestimate for some participants and an overestimate for others. For outpatient contacts, an external source was used as a proxy for this information and the same figure was applied across the whole sample. Second, although the sample was large the number of participants in the inpatient psychiatric group was relatively small and this may have restricted the analytical power of the analysis conducted. Third, the cost of medication was not considered in the analysis although individuals with a psychiatric condition are likely to be on some form of medication. If these costs were to be included the service costs would have been higher than the costs estimated, but it is unclear what any association with violence would be. Fourth, the analysis was based on self-report data of service use and this may have been subject to some inaccuracies. Likewise, self-reported violence may not always have been accurate.
In conclusion, this component of the study has shown that there is not a clear-cut impact of violence on health-care costs. Some service costs do seem to be increased, whereas access to other services seems to be reduced if violence occurs and this results in cost reductions.
Section B Severe mental illness and risk of violence
Chapter 12 Incidence cases of psychosis
Background
Prediction, prevention and punishment of violence frequently dominate public discussion and require significant public resources. Rates of violent crime rise and fall and are related to numerous factors. However, there remains a particular fear of violence perpetrated by those with mental illness, especially those diagnosed with schizophrenia, major depression or bipolar disorder. Studies show that up to 75% of the public believe that people with a mental illness are dangerous. 273,274 Media coverage of mental illness most often focuses on violence and crime,275–277 therefore encouraging fear of the mentally ill within our communities. 278,279 People with mental illness are among the most stigmatised groups in society280,281 and may internalise such stigma, resulting in reduced self-esteem and self-efficacy. 282 A perception that people with mental illness are inherently violent undoubtedly contributes to this well-documented stigma. 283
However, violence to others is a leading public health concern. To the extent that mental illness raises the risk for violence, those with mental illness in the community will be victimised. Often, when mentally ill individuals are violent, the victims of their violence are family members, who therefore may bear a disproportionate risk of victimisation and personal suffering. 284
It is widely expected by policy-makers and the public that assessment of violence risk in patients with mental illness should be a core skill and responsibility of mental health professionals. Violence risk assessment plays an important role in mental health law worldwide and ‘dangerousness to others’ is a key criterion for civil and forensic commitment in most jurisdictions. Imposition of tort liability on mental health professionals who negligently fail to predict, manage and prevent a patient’s violence towards others has become common.
The correct identification of those at risk for future violence is, therefore, of utmost importance to:
-
protect the public and
-
minimise additional stigmatisation among those with mental illness.
However, despite major developments and improvements in the assessment of risk for future violence, currently available risk assessment instruments still suffer from many shortcomings.
Accuracy of the prediction of future violence
State-of-the-art risk assessment instruments can be divided into two groups:
-
actuarial instruments
-
SPJ.
Actuarial instruments such as the Violence Risk Appraisal Guide (VRAG)285 or the Static-99286 have been developed on the basis of risk factors that are empirically related to violent behaviour. Risk factors included in these instruments are predominantly static but relatively simple to code. The codings of the items relating to risk factors are added up according to a fixed algorithm and conclusions with regard to the level of risk are based on the total score.
Structured professional judgement instruments such as the HCR-20287 are administered by experienced mental health professionals utilising a standardised checklist that contains empirically derived historical and dynamic risk factors for violence. The final risk judgement, however, is not based on a fixed algorithm but on expert decision-making. Risk factors are critically examined, combined and integrated to reach a conclusion. However, to validate these SPJ instruments, scores have to be assigned to allow a classification of level of risk that then can be tested using appropriate methods.
It is accepted that structured risk assessment instruments (ARA instruments and SPJ) outperform clinical judgement in the accuracy of the prediction of violent behaviour. 288,289 However, it has been previously demonstrated that most of these instruments achieve only a fair level of predictive accuracy (AUC values of 0.7) in comparison studies between more than one instrument. 8 Furthermore, most items in three instruments in one study [Psychopathy Checklist – Revised (PCL-R), VRAG, HCR-20] were not independently predictive of future violence and their predictive power was based on only a small number of their items. 23 Clinicians should be aware of these limitations and be critical when using either an actuarial or a SPJ instrument if the intention is to carry out a comprehensive assessment of risk on which to base subsequent risk management or treatment interventions. Moreover, the percentage correctly classified (PCC), which reflects the percentage of cases correctly classified (true positive and true negative) in the prediction of violence using either actuarial or SPJ instruments, is usually around 60%. 290 This implies that if a clinician relies on classification of risk based on these instruments, in approximately 40% of cases this classification will be wrong.
Causal compared with predictive models of risk for future violence
Most research carried out in the field of violence risk assessment utilises a predictive approach to either identify risk factors for violence or assess the predictive accuracy of instruments. Prediction requires temporal ordering of exposure (risk factors) and outcome (violence). Risk factors measured at some time point are investigated to see whether or not they are associated with violence occurring in a subsequent time window. This time window can cover a few weeks, months or several years. With regard to static, unchangeable risk factors, the choice of time frame following assessment should not matter. Static risk factors should equally predict violence occurring within the subsequent month and violence occurring within the subsequent year. However, when choosing the subsequent time frame for violent outcome after assessment of dynamic risk it is rarely taken into consideration that dynamic risk factors vary over time. Symptoms of mental illness are dynamic in nature and fluctuate. When investigating the association between mental illness and violent behaviour it is therefore essential to establish that a person was symptomatic when the violent incident occurred. Predictors derived from studies measuring symptoms or diagnoses at various points over the lifetime and comparing them with self-report or criminal records over extended periods cannot establish valid associations. Furthermore, because acute psychotic symptoms may present for relatively short periods, predictors that are identified over the lifespan may not be specific for psychosis and may apply equally to incidents of violence among the general (non-psychotic) population.
In the large National Epidemiologic Survey on Alcohol and Related Conditions (NESARC), it was demonstrated that the incidence of violence was higher for people with severe mental illness, but only significantly for those with co-occurring substance abuse and/or dependence. 112 Multivariate analyses revealed that severe mental illness alone did not predict future violence; it was associated instead with historical, clinical, dispositional and contextual factors. However, most of these factors were more prevalent in people with severe mental illness.
However, reanalysis of NESARC data produced completely different results and revealed a positive (moderate) association between major mental illness and violence, emphasising that temporal closeness between dependent (violence) and independent (symptoms of mental illness) variables is key when investigating these relationships. 117
The MacArthur Violence Risk Assessment Study (MVRAS) has been one of the most influential studies on the association between mental illness and violence. A key finding of this study was that delusions do not predict violence among recently discharged psychiatric patients and this conclusion had a profound negative impact on research in this field. 20 Reanalysis confirmed that delusions (present in the past 10 weeks) are not predictors of subsequent violent behaviour (in the following 10 weeks). Redefinition of the time frame of occurrence considering temporal proximity, however, revealed strong associations between violence and delusional beliefs implying threat to the individual. 21 These results supported the findings of a previous study in which delusions of conspiracy and being spied on and persecutory delusions (implying threat to the symptomatic individual) were associated with serious violent behaviour in patients presenting with a first episode of psychotic illness. 291 However, in both studies the pathway from delusional beliefs towards violence was not direct; the key explanatory variable was anger as a result of delusions. These findings suggest that, when investigating the association between symptoms of mental illness and psychosis, different and complex pathways have to be taken into consideration.
Rationale for constructing a new instrument
Based on the shortcomings of currently available risk assessment instruments we considered it crucial to develop a new instrument addressing the problems and issues identified in recent research in the field of violence risk. Our main focus was identification of symptoms of mental illness that are causal, dynamic risk factors for violence in those with affective and non-affective psychotic illness and that are amenable to treatment in adult forensic and general psychiatric settings. We also aimed to create a static risk instrument for those with psychosis to inform clinicians about their patients’ propensity towards future violence. These static, historical factors may be unchangeable but can guide clinicians in their decision-making process.
Previous research has shown that risk factors differ substantially in men and women. It appears that clinical risk factors predict violence in women with sufficient accuracy, whereas criminogenic and criminal history variables are better at identifying men at risk for violence. 292 We therefore considered it essential to develop two modules to address sex differences in risk for violent behaviour.
Most importantly, we aimed not only to sensitise clinicians to symptom constellations in their patients that increase the risk of violence but also to advise action if a patient presents with these symptoms. Currently available ARA instruments classify patients only with regard to the level of risk for violence and SPJ instruments aim to help clinicians to understand the risk for violence in their patients. However, administration of risk assessment instruments (considering that these assessments are sometimes very time-consuming and require extensive training of those who administer them) should lead to appropriate management and, as a consequence, to a reduction in violent behaviour. The only study, however, that investigated whether or not administration of a SPJ instrument led to actual prevention of criminal and violent recidivism came to the conclusion ‘that the primary goal of preventing recidivism was not reached through risk assessment embedded in shared decision-making’ (p. 365). 18
Objectives
Our objectives were to:
-
identify symptoms of mental illness that are causal risk factors in those patients with psychosis and amenable to treatment in forensic and general psychiatric settings
-
develop a static risk instrument for future violence to inform about patients’ propensities for future violence.
Method
Study design and sample
Baseline study
The East London First Episode Psychosis Study (ELFEPS)291 was carried out between December 1996 and December 2000 in the London boroughs of City and Hackney, Tower Hamlets and Newham. All those aged 16–84 years living in the study area who made contact with mental health services (including adult community health teams, inpatient units, forensic services, learning disability services, adolescent mental health services and drug and alcohol units) because of a first episode of any probable psychotic disorder were identified and screened. Initial inclusion criteria were based on a World Health Organization (WHO) study293 and the Ætiology and Ethnicity in Schizophrenia and Other Psychoses (AESOP) study. 294 Methods used by Cooper et al. 295 were used to minimise leakage and identify patients missed by screening. Patients who passed the screen underwent a battery of assessments. The Schedules for Clinical Assessment in Neuropsychiatry (SCAN)296 make up a set of instruments used to assess adult major psychiatric disorders. Three clinical research fellows carried out the data collection and were trained in the SCAN interview by taking a course approved by the WHO. Prestudy reliability was established using independent ratings of videotaped interviews. ICD-10 and DSM-IV diagnoses were allocated by consensus agreement between the principal investigator (JWC) and the research team.
Overall, 490 individuals were recruited [City and Hackney, n = 167 (34.1%); Tower Hamlets, n = 166 (33.9%); Newham, n = 157 (32.0%)]. More than half of those recruited were men [n = 302 (61.6%)] and the sample was ethnically diverse [white, n = 179 (36.5%); black, n = 165 (33.7%); Asian, n = 117 (23.9%); other, n = 29 (5.9%)]. Approximately half of the study participants were not born in the UK [n = 243 (49.6%)]. The mean age of the sample at baseline was 30.5 years (SD 10.1 years).
The most prevalent consensus diagnosis at baseline was schizophrenia (34.3%). Schizotypal personality disorder was diagnosed in 0.4% of the sample, delusional disorder in 5.9%, acute/transient psychosis in 9.6%, schizoaffective disorder in 18.6% and other non-affective psychosis in 6%. Approximately 24% of the study participants presented with affective psychosis including unipolar (14%), bipolar (10.4%) and other (0.8%) affective psychoses.
Follow-up study
Data collection commenced in January 2010 and was finished on 30 June 2013. The study was granted Section 251 (NHS Act 2006297) approval from the National Information Governance Board (NIGB) to gather data without the consent of the baseline study participants. The design was a retrospective case note study that aimed to cover 10 years after the initial assessment at baseline.
We utilised a multitude of resources including medical records in 31 primary and 20 secondary care trusts across England, the NHS databases SPINE and RIO to identify if and when participants exited the NHS and the death register to identify the proportion of participants who died during follow-up and their cause of death. The Police National Computer (PNC), an operational police database containing criminal histories of all offenders in England, Wales and Scotland, was searched in January 2012 by the Ministry of Justice to gather information on criminal convictions and cautions of the sample.
Data were collected by research assistants and clinical studies officers from the Primary Care Research Network and the Mental Health Research Network.
We obtained complete 10-year follow-up data for 74% of the sample and complete 5-year follow-up data for 81% of the sample. At least 1 year of follow-up data were available for 95.1% of the sample. Thirty-four participants (6.9%) died at some point during the follow-up period. Causes of death included eight suicides and in three cases the cause of death was unascertainable. The majority of the deceased died of natural causes. Loss to follow-up mostly occurred because baseline participants had exited the NHS (mostly to return to their country of origin). In a few cases the GP surgery where a patient was registered refused access to his or her medical records.
Measures
Static risk factors
Static risk factors were assessed at baseline using a standardised interview schedule with operational definitions for all ratings.
Symptoms of mental illness: dynamic risk factors
The OPerational CRITeria checklist (for psychotic and affective symptoms) (OPCRIT) system298 was administered to collect information on a broad range of symptoms of mental illness. The checklist was specifically designed for the needs of empirical research and demonstrated good reliability and validity. 299 Episodes were dated and differentiated by a 2-month symptom-free interval for affective symptoms and a 6-month symptom-free interval for psychotic symptoms. Symptom domains relevant for the study and coded were appearance and behaviour (e.g. bizarre behaviour, catatonia), speech and form of thought (formal thought disorder), affect and associated features, abnormal beliefs and ideas (delusions) and abnormal perceptions (hallucinations).
Outcome
The MacArthur Community Violence Instrument (MCVI)300 was rated based on case notes in primary and secondary care. Actions were considered to constitute serious violence if they were:
-
batteries that resulted in physical injury or involved the use of a weapon
-
sexual assaults
-
threats made with a weapon in hand.
Batteries not resulting in injury of the victim were considered as minor violence.
Further outcome data were derived from convictions and cautions recorded in the PNC, an operational police database containing criminal histories of all offenders in England, Wales and Scotland. For categorisation of violent offences, we used offences in the Home Office’s Standard List301 for definition of violence (committed) plus threats to commit such an offence for England, Wales and Scotland.
To ensure sufficient statistical power, violent incidents derived from medical records and PNC data were combined into one outcome measure: ‘violent behaviour’. Because of small numbers, it was not possible to differentiate minor and serious violence.
Statistical analyses
For descriptive purposes, absolute (n) and relative (%) frequencies were reported for dichotomous/polytomous categorical variables and means and SDs were reported for variables at the interval/ratio level.
To ensure sufficient statistical power it was decided to divide the 10-year follow-up into 6-month windows. This resulted in up to 20 repeated measurements per study participant. All analyses were stratified to account for sex differences.
Static risk instrument
All static risk factors were binary and their predictive accuracy was assessed using the ROC using the ‘somersd’ command and ‘lincom’ for statistical significance in Stata. ROC plots display the areas of pairs of ‘sensitivity’ and ‘1 – specificity’ for each score. Predictive accuracy is quantified in a value known as the AUC. The AUC is equal to the probability that a randomly chosen violent person will score higher on the measure in question than a randomly chosen non-violent person. AUC values can range from 0.50 (no discrimination) to 1.00 (perfect discrimination); values exceeding 0.70 are considered large, with acceptable AUCs indicating greater predictive accuracy.
In a first step AUC values were computed for each of the 20 time windows to investigate their stability over time. The static risk model was then developed utilising the first 6-month time window after the baseline assessment. To avoid shrinkage when applying the instrument to a different, external sample we decided to keep the model as simple as possible. Based on a forward selection process the highest AUC values were identified and subsequently added up. The magnitude of the AUC value, sensitivity, specificity and PCC were utilised to identify the optimal cut-off point. The stability of the predictive accuracy over time was then tested by adding 6-month time windows cumulatively.
Dynamic risk factors
To take advantage of the longitudinal study design, multilevel modelling was applied. These models account for dependence of data collected longitudinally by modelling the relatedness of repeated measurements within the same individual as random effects. Unlike other approaches, mixed-effect (multilevel) models do not require that data are complete for individuals at each time point or require imputation of data, which may result in bias. 302 By making use of all available data, multilevel models are therefore particularly powerful in longitudinal studies in which the data are often incomplete.
Logistic mixed-effect models (‘melogit’ in Stata) were applied to investigate associations between symptoms of mental illness and violent outcome. Data from all 20 study periods were included in the analyses. To estimate the effects of exposure on outcome over the entire study period regardless of time point, we included time as a covariate. The logistic mixed-effect models therefore provided a single estimate (OR), 95% CIs and a significance value of the relationship between symptoms and violence over the entire course of the study.
To ensure temporal proximity between dependent and independent variables, we investigated the associations between exposure and outcome occurring in the same time period.
As the mixed-model approach resulted in a substantial increase in statistical power we decided to adopt a conservative approach in the selection of confounding variables. All static risk factors were entered separately and tested in the total, male and female samples (Table 89). As subsequent moderation analyses required the inclusion of the total sample, variables significantly associated with violence in the total sample were adjusted for throughout, including age < 35 years, black ethnic origin, no educational qualifications, poverty and parental discord before the age of 15 years, history of violent behaviour leading to contact with services, threatening or annoying behaviour leading to contact with services, violent and non-violent offending, family history of criminal behaviour, a diagnosis of schizophrenia at first presentation to services, high level of trait anger, long duration of untreated psychosis, conduct disorder before the age of 15 years and drug use in the past year.
Static risk factors | Total | Men | Women | ||||||
---|---|---|---|---|---|---|---|---|---|
AORa | 95% CI | p-value | AORa | 95% CI | p-value | AORa | 95% CI | p-value | |
Demography | |||||||||
Male sex | 2.56 | 1.61 to 4.07 | < 0.001 | ||||||
Age < 35 years | 2.58 | 1.53 to 4.35 | < 0.001 | 2.74 | 1.47 to 5.12 | 0.002 | 1.86 | 0.76 to 4.57 | 0.177 |
Black ethnicity | 1.33 | 0.86 to 2.06 | 0.206 | 1.48 | 0.90 to 2.44 | 0.126 | 0.73 | 0.31 to 1.75 | 0.480 |
Single marital status | 1.54 | 1.00 to 2.37 | 0.050 | 1.27 | 0.75 to 2.15 | 0.384 | 1.24 | 0.57 to 2.69 | 0.586 |
UK born | 1.45 | 0.95 to 2.22 | 0.083 | 1.24 | 0.76 to 2.03 | 0.388 | 1.65 | 0.77 to 3.57 | 0.200 |
Asylum seeker/refugee | 0.91 | 0.48 to 1.72 | 0.772 | 0.81 | 0.41 to 1.61 | 0.546 | 0.75 | 0.17 to 3.37 | 0.705 |
No educational qualifications | 1.77 | 1.15 to 2.72 | 0.010 | 1.66 | 1.01 to 2.73 | 0.045 | 1.73 | 0.77 to 3.84 | 0.182 |
Three or more moves of residence | 1.33 | 0.85 to 2.08 | 0.207 | 1.52 | 0.92 to 2.51 | 0.104 | 0.79 | 0.33 to 1.89 | 0.591 |
Not worked in the past year | 1.01 | 0.65 to 1.55 | 0.976 | 1.20 | 0.73 to 1.97 | 0.463 | 0.77 | 0.34 to 1.74 | 0.526 |
Childhood adversities before 15 years of age | |||||||||
In care/adopted/fostered | 0.45 | 0.19 to 1.05 | 0.065 | 0.63 | 0.24 to 1.68 | 0.360 | 0.25 | 0.04 to 1.45 | 0.123 |
Poverty | 1.74 | 1.06 to 2.87 | 0.030 | 1.69 | 0.96 to 2.96 | 0.068 | 1.56 | 0.58 to 4.18 | 0.373 |
Parental discord | 2.40 | 1.51 to 3.80 | < 0.001 | 2.58 | 1.53 to 4.35 | < 0.001 | 1.65 | 0.67 to 4.08 | 0.278 |
Cruelty/physical abuse | 1.38 | 0.69 to 2.72 | 0.361 | 1.84 | 0.86 to 3.94 | 0.119 | 0.51 | 0.10 to 2.54 | 0.415 |
Sexual abuse | 0.58 | 0.21 to 1.62 | 0.298 | 1.67 | 0.52 to 5.43 | 0.392 | – | ||
Criminogenic factors | |||||||||
History of violent behaviour leading to contact with services | 2.48 | 1.62 to 3.79 | < 0.001 | 2.22 | 1.37 to 3.60 | 0.001 | 2.02 | 0.87 to 4.72 | 0.101 |
History of threatening/annoying behaviour leading to contact with services | 2.70 | 1.70 to 4.28 | < 0.001 | 2.49 | 1.43 to 4.34 | 0.001 | 2.16 | 0.98 to 4.77 | 0.057 |
History of violent offending | 3.74 | 2.14 to 6.53 | < 0.001 | 2.65 | 1.46 to 4.81 | 0.001 | 8.18 | 1.98 to 33.82 | 0.004 |
History of non-violent offending | 2.73 | 1.76 to 4.24 | < 0.001 | 2.23 | 1.38 to 3.59 | 0.001 | 1.80 | 0.44 to 7.41 | 0.417 |
Family history of criminal behaviour | 1.96 | 1.01 to 3.81 | 0.048 | 2.17 | 1.04 to 4.54 | 0.040 | 1.15 | 0.28 to 4.70 | 0.845 |
Clinical factors | |||||||||
Schizophrenia at first presentation | 1.69 | 1.10 to 2.60 | 0.016 | 1.31 | 0.80 to 2.16 | 0.283 | 2.63 | 1.23 to 5.64 | 0.013 |
High trait impulsiveness | 1.76 | 0.89 to 3.48 | 0.104 | 1.89 | 0.93 to 3.83 | 0.077 | – | – | – |
High trait anger | 2.81 | 1.51 to 5.21 | 0.001 | 3.09 | 1.62 to 5.91 | 0.001 | 0.35 | 0.03 to 3.60 | 0.373 |
Long duration of untreated psychosis | 0.21 | 0.05 to 0.86 | 0.030 | 0.27 | 0.06 to 1.14 | 0.075 | – | – | – |
Conduct disorder | 1.98 | 1.18 to 3.33 | 0.010 | 1.78 | 1.03 to 3.07 | 0.038 | 0.53 | 0.08 to 3.45 | 0.509 |
Alcohol abuse past year | 0.97 | 0.48 to 1.92 | 0.921 | 0.60 | 0.29 to 1.26 | 0.181 | 4.05 | 0.74 to 22.10 | 0.106 |
Drug use past year | 1.79 | 1.18 to 2.70 | 0.006 | 1.40 | 0.86 to 2.28 | 0.175 | 1.69 | 0.75 to 3.76 | 0.203 |
Family history of severe mental illness | 1.43 | 0.91 to 2.24 | 0.123 | 1.25 | 0.75 to 2.09 | 0.393 | 1.52 | 0.65 to 3.57 | 0.333 |
Family history of substance abuse | 1.26 | 0.63 to 2.48 | 0.514 | 1.24 | 0.61 to 2.54 | 0.556 | 0.32 | 0.03 to 3.27 | 0.337 |
To account for co-occurrence, dynamic risk factors from the same symptom domain were adjusted for each other.
Mediation analyses to identify indirect pathways were carried out by testing the required triangle associations: statistically significant relationship between (1) independent (dynamic risk factor: symptom of mental illness) and dependent (violence) variables; (2) independent and hypothesised mediator (affect) variables; and (3) mediator and dependent variables. By comparing standardised regression coefficients from models with and without mediator as a covariate,303 we estimated the proportion of direct effects that were mediated and tested their significance using bootstrapped SEs and CIs (using 1000 repetitions). This method is preferred over other tests for significant indirect effects, such as the Sobel test, because it is less conservative and does not require normality assumptions to be met. 304
Moderation analyses were performed to investigate effect modification. This was applied when testing sex differences in the association between dynamic risk factors and violence and the effects of static risk level. A multiplicative term was included in the statistical models.
All statistical analyses were conducted in Stata SE.
An alpha level of p < 0.05 was adopted throughout.
Results
Violent outcome
Violent behaviour demonstrated great fluctuation over the 10-year follow-up (Figure 5). Unsurprisingly, the prevalence of violent behaviour was consistently higher among men. For both sexes prevalence was highest in the first 6 months following inclusion in the study.
FIGURE 5.
Violent behaviour across 10 years of follow-up.
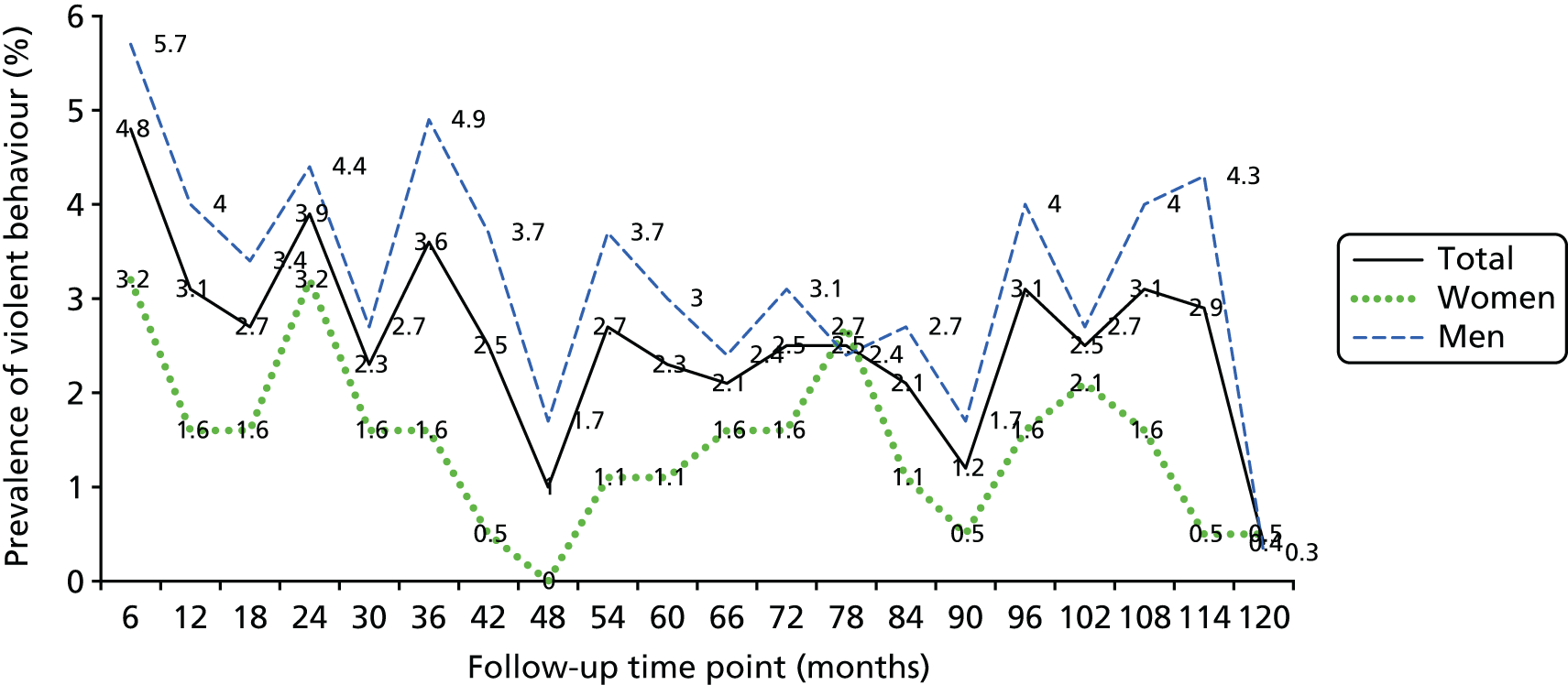
Static risk instrument
Overall, 27 static factors from four domains were included. The demography domain covered age < 35 years, black ethnicity, single marital status, UK born, asylum seeker, no educational qualifications, three or more moves of residence in the past year and not worked in past year. Childhood adversities covered care/adopted/fostered, poverty, parental discord, cruelty/physical abuse and sexual abuse. Criminogenic variables were a history of violent offending leading to contact, a history of threatening/annoying behaviour leading to contact, a history of violent offending, a history of non-violent offending and a history of family criminal behaviour. In the clinical domain, the variables covered were schizophrenia at first presentation, high trait impulsiveness, high trait anger, long duration of untreated psychosis, conduct disorder, alcohol abuse in the past year, drug use in the past year, a family history of mental illness and a family history of substance abuse.
In a first step we calculated AUC values for each 6-month window for each of the static factors. As can be seen in Tables 90 and 91, there was great variation across time and between sexes.
Static risk factors | Months | |||||||||||||||||||
---|---|---|---|---|---|---|---|---|---|---|---|---|---|---|---|---|---|---|---|---|
6 | 12 | 18 | 24 | 30 | 36 | 42 | 48 | 54 | 60 | 66 | 72 | 78 | 84 | 90 | 96 | 102 | 108 | 114 | 120 | |
Demography | ||||||||||||||||||||
Age < 35 years | 0.48 | 0.58 | 0.63 | 0.47 | 0.63 | 0.63 | 0.58 | 0.62 | 0.63 | 0.63 | 0.63 | 0.48 | 0.63 | 0.63 | 0.62 | 0.57 | 0.56 | 0.58 | 0.57 | 0.62 |
Black ethnicity | 0.55 | 0.49 | 0.58 | 0.52 | 0.54 | 0.61 | 0.52 | 0.49 | 0.47 | 0.61 | 0.66 | 0.54 | 0.54 | 0.51 | 0.42 | 0.66 | 0.38 | 0.65 | 0.47 | 0.32 |
Single marital status | 0.48 | 0.57 | 0.47 | 0.56 | 0.51 | 0.47 | 0.50 | 0.49 | 0.51 | 0.58 | 0.57 | 0.44 | 0.59 | 0.47 | 0.56 | 0.43 | 0.52 | 0.47 | 0.55 | 0.14 |
UK born | 0.50 | 0.55 | 0.41 | 0.47 | 0.65 | 0.54 | 0.57 | 0.38 | 0.62 | 0.72 | 0.55 | 0.35 | 0.57 | 0.46 | 0.61 | 0.54 | 0.47 | 0.63 | 0.46 | 0.21 |
Asylum seeker | 0.53 | 0.50 | 0.61 | 0.41 | 0.49 | 0.47 | 0.48 | 0.60 | 0.48 | 0.43 | 0.51 | 0.50 | 0.43 | 0.56 | 0.53 | 0.54 | 0.49 | 0.47 | 0.48 | 0.43 |
No educational qualifications | 0.42 | 0.59 | 0.59 | 0.51 | 0.64 | 0.54 | 0.56 | 0.38 | 0.62 | 0.42 | 0.63 | 0.42 | 0.64 | 0.59 | 0.61 | 0.49 | 0.46 | 0.58 | 0.56 | 0.71 |
Three or more moves of residence in the past year | 0.58 | 0.58 | 0.58 | 0.42 | 0.55 | 0.62 | 0.49 | 0.67 | 0.64 | 0.77 | 0.33 | 0.40 | 0.55 | 0.58 | 0.52 | 0.49 | 0.45 | 0.55 | 0.53 | 0.32 |
Not worked in the past year | 0.54 | 0.51 | 0.39 | 0.67 | 0.50 | 0.40 | 0.57 | 0.55 | 0.47 | 0.58 | 0.39 | 0.51 | 0.37 | 0.60 | 0.52 | 0.50 | 0.48 | 0.40 | 0.64 | 0.73 |
Childhood adversities before 15 years of age | ||||||||||||||||||||
In care/adopted/fostered | 0.45 | 0.54 | 0.45 | 0.50 | 0.53 | 0.60 | 0.46 | 0.46 | 0.46 | 0.53 | 0.45 | 0.45 | 0.46 | 0.52 | 0.45 | 0.45 | 0.52 | 0.50 | 0.45 | 0.45 |
Poverty | 0.54 | 0.57 | 0.46 | 0.65 | 0.54 | 0.53 | 0.44 | 0.56 | 0.54 | 0.61 | 0.56 | 0.54 | 0.54 | 0.65 | 0.59 | 0.56 | 0.51 | 0.42 | 0.43 | 0.38 |
Parental discord | 0.61 | 0.60 | 0.46 | 0.70 | 0.61 | 0.68 | 0.49 | 0.89 | 0.64 | 0.75 | 0.55 | 0.38 | 0.53 | 0.64 | 0.48 | 0.55 | 0.57 | 0.56 | 0.48 | 0.38 |
Cruelty/physical abuse | 0.49 | 0.50 | 0.45 | 0.50 | 0.45 | 0.45 | 0.45 | 0.45 | 0.50 | 0.45 | 0.45 | 0.53 | 0.53 | 0.65 | 0.66 | 0.51 | 0.59 | 0.60 | 0.56 | 0.46 |
Sexual abuse | 0.48 | 0.52 | 0.48 | 0.48 | 0.48 | 0.48 | 0.48 | 0.48 | 0.53 | 0.48 | 0.48 | 0.56 | 0.63 | 0.48 | 0.59 | 0.48 | 0.49 | 0.53 | 0.54 | 0.49 |
Criminogenic factors | ||||||||||||||||||||
History of violence leading to contact | 0.61 | 0.48 | 0.63 | 0.57 | 0.53 | 0.69 | 0.57 | 0.64 | 0.62 | 0.45 | 0.74 | 0.52 | 0.60 | 0.76 | 0.41 | 0.59 | 0.50 | 0.63 | 0.52 | 0.31 |
History of threatening/annoying behaviour leading to contact | 0.61 | 0.55 | 0.54 | 0.52 | 0.60 | 0.63 | 0.57 | 0.49 | 0.62 | 0.45 | 0.59 | 0.68 | 0.67 | 0.60 | 0.57 | 0.61 | 0.41 | 0.62 | 0.51 | 0.66 |
History of violent offending | 0.55 | 0.48 | 0.63 | 0.49 | 0.58 | 0.58 | 0.64 | 0.60 | 0.58 | 0.58 | 0.61 | 0.50 | 0.51 | 0.49 | 0.43 | 0.43 | 0.43 | 0.57 | 0.64 | 0.43 |
History of non-violent offending | 0.49 | 0.51 | 0.53 | 0.49 | 0.70 | 0.62 | 0.59 | 0.67 | 0.74 | 0.55 | 0.68 | 0.55 | 0.62 | 0.58 | 0.74 | 0.55 | 0.59 | 0.61 | 0.47 | 0.32 |
Family history of criminal behaviour | 0.53 | 0.54 | 0.46 | 0.61 | 0.60 | 0.51 | 0.51 | 0.46 | 0.51 | 0.60 | 0.46 | 0.60 | 0.53 | 0.52 | 0.46 | 0.45 | 0.58 | 0.55 | 0.56 | 0.45 |
Clinical factors | ||||||||||||||||||||
Schizophrenia at first presentation | 0.44 | 0.47 | 0.61 | 0.55 | 0.58 | 0.53 | 0.66 | 0.80 | 0.50 | 0.52 | 0.64 | 0.29 | 0.60 | 0.76 | 0.40 | 0.41 | 0.43 | 0.53 | 0.45 | 0.80 |
High trait impulsiveness | 0.52 | 0.53 | 0.51 | 0.55 | 0.52 | 0.54 | 0.54 | 0.44 | 0.49 | 0.44 | 0.61 | 0.66 | 0.59 | 0.57 | 0.44 | 0.44 | 0.70 | 0.49 | 0.44 | 0.44 |
High trait anger | 0.62 | 0.48 | 0.44 | 0.54 | 0.51 | 0.54 | 0.60 | 0.61 | 0.59 | 0.58 | 0.53 | 0.44 | 0.66 | 0.70 | 0.64 | 0.61 | 0.57 | 0.58 | 0.54 | 0.44 |
Long duration of untreated psychosis | 0.47 | 0.47 | 0.47 | 0.47 | 0.47 | 0.47 | 0.52 | 0.47 | 0.47 | 0.54 | 0.47 | 0.47 | 0.54 | 0.47 | 0.47 | 0.47 | 0.47 | 0.47 | 0.47 | 0.47 |
Conduct disorder | 0.53 | 0.56 | 0.39 | 0.44 | 0.54 | 0.58 | 0.54 | 0.39 | 0.65 | 0.38 | 0.48 | 0.46 | 0.75 | 0.64 | 0.69 | 0.56 | 0.58 | 0.62 | 0.59 | 0.39 |
Alcohol abuse in the past year | 0.42 | 0.47 | 0.43 | 0.48 | 0.43 | 0.48 | 0.53 | 0.43 | 0.53 | 0.43 | 0.43 | 0.58 | 0.58 | 0.43 | 0.63 | 0.48 | 0.56 | 0.47 | 0.48 | 0.43 |
Drug use in the past year | 0.47 | 0.47 | 0.54 | 0.48 | 0.59 | 0.70 | 0.52 | 0.56 | 0.63 | 0.58 | 0.56 | 0.29 | 0.65 | 0.60 | 0.52 | 0.44 | 0.47 | 0.69 | 0.57 | 0.72 |
Family history of mental illness | 0.44 | 0.55 | 0.40 | 0.54 | 0.69 | 0.66 | 0.58 | 0.83 | 0.63 | 0.61 | 0.64 | 0.46 | 0.61 | 0.51 | 0.41 | 0.36 | 0.57 | 0.40 | 0.56 | 0.31 |
Family history of substance abuse | 0.44 | 0.66 | 0.44 | 0.49 | 0.65 | 0.57 | 0.59 | 0.43 | 0.48 | 0.43 | 0.43 | 0.50 | 0.43 | 0.56 | 0.53 | 0.43 | 0.56 | 0.52 | 0.53 | 0.42 |
Static risk factors | Months | |||||||||||||||||||
---|---|---|---|---|---|---|---|---|---|---|---|---|---|---|---|---|---|---|---|---|
6 | 12 | 18 | 24 | 30 | 36 | 42 | 48 | 54 | 60 | 66 | 72 | 78 | 84 | 90 | 96 | 102 | 108 | 114 | 120 | |
Demography | ||||||||||||||||||||
Age < 35 years | 0.55 | 0.49 | 0.49 | 0.66 | 0.66 | 0.41 | 0.16 | – | 0.66 | 0.16 | 0.66 | 0.66 | 0.56 | 0.66 | 0.66 | 0.49 | 0.49 | 0.66 | 0.15 | 0.65 |
Black ethnicity | 0.65 | 0.34 | 0.34 | 0.42 | 0.34 | 0.35 | 0.85 | – | 0.35 | 0.85 | 0.51 | 0.34 | 0.45 | 0.35 | 0.35 | 0.52 | 0.35 | 0.52 | 0.86 | 0.35 |
Single marital status | 0.58 | 0.44 | 0.63 | 0.62 | 0.80 | 0.29 | 0.28 | – | 0.54 | 0.29 | 0.46 | 0.63 | 0.48 | 0.28 | 0.28 | 0.62 | 0.62 | 0.62 | 0.28 | 0.29 |
UK born | 0.66 | 0.42 | 0.42 | 0.33 | 0.59 | 0.50 | 0.25 | – | 0.50 | 0.75 | 0.75 | 0.75 | 0.44 | 0.24 | 0.75 | 0.58 | 0.58 | 0.58 | 0.75 | 0.75 |
Asylum seeker | 0.56 | 0.46 | 0.63 | 0.46 | 0.46 | 0.46 | 0.47 | – | 0.47 | 0.47 | 0.46 | 0.46 | 0.46 | 0.46 | 0.46 | 0.63 | 0.46 | 0.46 | 0.47 | 0.46 |
No educational qualifications | 0.75 | 0.58 | 0.41 | 0.32 | 0.40 | 0.49 | 0.73 | – | 0.48 | 0.73 | 0.57 | 0.74 | 0.54 | 0.74 | 0.74 | 0.57 | 0.40 | 0.57 | 0.75 | 0.74 |
Three or more moves of residence in the past year | 0.44 | 0.51 | 0.70 | 0.43 | 0.53 | 0.36 | 0.86 | – | 0.36 | 0.36 | 0.53 | 0.53 | 0.46 | 0.36 | 0.36 | 0.36 | 0.53 | 0.53 | 0.36 | 0.36 |
Not worked in the past year | 0.68 | 0.51 | 0.33 | 0.41 | 0.15 | 0.66 | 0.15 | – | 0.40 | 0.65 | 0.49 | 0.49 | 0.35 | 0.65 | 0.65 | 0.31 | 0.31 | 0.48 | 0.65 | 0.65 |
Childhood adversities before age 15 years | ||||||||||||||||||||
In care/adopted/fostered | 0.43 | 0.43 | 0.44 | 0.43 | 0.43 | 0.43 | 0.43 | – | 0.43 | 0.43 | 0.60 | 0.43 | 0.43 | 0.43 | 0.43 | 0.43 | 0.43 | 0.43 | 0.43 | 0.93 |
Poverty | 0.62 | 0.42 | 0.59 | 0.42 | 0.42 | 0.42 | 0.92 | – | 0.42 | 0.42 | 0.76 | 0.58 | 0.41 | 0.92 | 0.42 | 0.58 | 0.42 | 0.58 | 0.42 | 0.92 |
Parental discord | 0.60 | 0.40 | 0.57 | 0.57 | 0.91 | 0.40 | 0.40 | – | 0.65 | 0.40 | 0.57 | 0.40 | 0.60 | 0.40 | 0.40 | 0.56 | 0.56 | 0.57 | 0.40 | 0.90 |
Cruelty/physical abuse | 0.45 | 0.45 | 0.63 | 0.54 | 0.63 | 0.45 | 0.45 | – | 0.45 | 0.45 | 0.45 | 0.45 | 0.45 | 0.45 | 0.45 | 0.45 | 0.62 | 0.45 | 0.45 | 0.45 |
Sexual abuse | 0.45 | 0.45 | 0.45 | 0.45 | 0.45 | 0.45 | 0.45 | – | 0.45 | 0.45 | 0.45 | 0.45 | 0.45 | 0.45 | 0.45 | 0.45 | 0.45 | 0.45 | 0.45 | 0.45 |
Criminogenic factors | ||||||||||||||||||||
History of violence leading to contact | 0.58 | 0.38 | 0.71 | 0.63 | 0.54 | 0.88 | 0.38 | – | 0.88 | 0.88 | 0.38 | 0.38 | 0.48 | 0.88 | 0.38 | 0.54 | 0.37 | 0.54 | 0.88 | 0.38 |
History of threatening/annoying behaviour leading to contact | 0.55 | 0.24 | 0.75 | 0.67 | 0.75 | 0.75 | 0.75 | – | 0.75 | 0.75 | 0.24 | 0.41 | 0.65 | 0.75 | 0.24 | 0.75 | 0.58 | 0.41 | 0.75 | 0.25 |
History of violent offending | 0.49 | 0.49 | 0.66 | 0.58 | 0.83 | 0.49 | 0.49 | – | 0.49 | 0.49 | 0.49 | 0.49 | 0.59 | 0.49 | 0.49 | 0.66 | 0.66 | 0.49 | 0.49 | 0.49 |
History of non-violent offending | 0.47 | 0.47 | 0.47 | 0.47 | 0.64 | 1.47 | 0.47 | – | 0.47 | 0.47 | 0.64 | 0.46 | 0.57 | 0.46 | 0.46 | 0.63 | 0.46 | 0.46 | 0.47 | 0.46 |
Family history of criminal behaviour | 0.56 | 0.46 | 0.46 | 0.46 | 0.63 | 0.46 | 0.46 | – | 0.46 | 0.46 | 0.46 | 0.46 | 0.56 | 0.46 | 0.46 | 0.63 | 0.45 | 0.63 | 0.46 | 0.46 |
Clinical factors | ||||||||||||||||||||
Schizophrenia at first presentation | 0.56 | 0.53 | 0.52 | 0.69 | 0.69 | 0.61 | 0.35 | – | 0.60 | 0.35 | 0.86 | 0.69 | 0.65 | 0.35 | 0.34 | 0.34 | 0.85 | 0.86 | 0.34 | 0.85 |
High trait impulsiveness | 0.47 | 0.47 | 0.48 | 0.48 | 0.48 | 0.48 | 0.48 | – | 0.48 | 0.48 | 0.48 | 0.48 | 0.48 | 0.48 | 0.48 | 0.48 | 0.48 | 0.48 | 0.48 | 0.48 |
High trait anger | 0.47 | 0.47 | 0.47 | 0.47 | 0.47 | 0.47 | 0.47 | – | 0.47 | 0.47 | 0.47 | 0.64 | 0.47 | 0.47 | 0.48 | 0.48 | 0.48 | 0.48 | 0.47 | 0.48 |
Long duration of untreated psychosis | 0.48 | 0.48 | 0.47 | 0.47 | 0.47 | 0.47 | 0.47 | – | 0.47 | 0.47 | 0.47 | 0.47 | 0.48 | 0.48 | 0.48 | 0.48 | 0.48 | 0.48 | 0.47 | 0.48 |
Conduct disorder | 0.57 | 0.47 | 0.47 | 0.46 | 0.46 | 0.46 | 0.46 | – | 0.46 | 0.46 | 0.46 | 0.46 | 0.46 | 0.46 | 0.46 | 0.46 | 0.46 | 0.63 | 0.46 | 0.46 |
Alcohol abuse in the past year | 0.59 | 0.49 | 0.49 | 0.49 | 0.49 | 0.49 | 0.49 | – | 0.49 | 0.49 | 0.66 | 0.49 | 0.49 | 0.49 | 0.49 | 0.49 | 0.49 | 0.49 | 0.49 | 0.99 |
Drug use in the past year | 0.46 | 0.53 | 0.52 | 0.44 | 0.69 | 0.35 | 0.35 | – | 0.60 | 0.85 | 0.69 | 0.69 | 0.45 | 0.35 | 0.85 | 0.51 | 0.68 | 0.51 | 0.85 | 0.85 |
Family history of mental illness | 0.64 | 0.47 | 0.30 | 0.47 | 0.29 | 0.38 | 0.12 | – | 0.37 | 0.62 | 0.46 | 0.46 | 0.53 | 0.63 | 0.63 | 0.29 | 0.46 | 0.63 | 0.63 | 0.13 |
Family history of substance abuse | 0.53 | 0.53 | 0.53 | 0.53 | 0.53 | 0.53 | 0.53 | – | 0.53 | 0.53 | 0.54 | 0.36 | 0.54 | 0.54 | 0.54 | 0.54 | 0.54 | 0.54 | 0.54 | 0.54 |
Scrutinising the AUC values derived for the first 6-month window resulted in the selection of five static risk factors for men: high trait anger, history of threatening/annoying behaviour leading to contact with services, parental discord before 15 years of age, history of violence leading to contact with services and three or more moves of residence in the past year. Inclusion of the last variable resulted in a decrease in the AUC value and it was therefore removed from the algorithm.
As can be seen in Table 92, the highest accuracy was achieved when including four static risk factors and utilising a cut-off point of ≥ 2, resulting in acceptable sensitivity and specificity of the static instrument. The achieved AUC value remained relatively stable in cumulative analyses (0.63–0.70) and remained significant throughout.
Static risk factors included | AUC (95% CI) | Cut-off point | Sensitivity (%) | Specificity (%) | Correctly classified (%) |
---|---|---|---|---|---|
High level of trait anger | 0.61 (0.49 to 0.74) | ≥ 0 | 100.0 | 0.0 | 6.1 |
≥ 1 | 33.3 | 89.2 | 85.8 | ||
High level of trait anger, history of threatening behaviour leading to contact | 0.67 (0.54 to 0.81) | ≥ 0 | 100.0 | 0.0 | 6.1 |
≥ 1 | 86.7 | 34.2 | 37.4 | ||
≥ 2 | 33.3 | 90.5 | 87.0 | ||
High level of trait anger, history of threatening behaviour leading to contact, history of violent behaviour leading to contact | 0.66 (0.51 to 0.81) | ≥ 0 | 100.0 | 0.0 | 6.1 |
≥ 1 | 86.7 | 29.1 | 32.7 | ||
≥ 2 | 40.0 | 78.7 | 76.3 | ||
≥ 3 | 33.3 | 95.7 | 91.8 | ||
High level of trait anger, history of threatening behaviour leading to contact, history of violent behaviour leading to contact, parental discord before 15 years of age | 0.68 (0.54 to 0.83) | ≥ 0 | 100.0 | 0.0 | 6.1 |
≥ 1 | 86.7 | 27.0 | 30.6 | ||
≥ 2 | 73.3 | 60.0 | 60.8 | ||
≥ 3 | 33.3 | 84.8 | 81.6 | ||
≥ 4 | 20.0 | 97.0 | 92.2 |
Application of this algorithm resulted in 111 men (42.5%) being classified as having a high risk for violence in the first 6 months after assessment.
In the female sample, initially seven static risk factors were considered: no educational qualifications, not having worked in the past year, UK born, no family history of mental disorder (the variable ‘family history of mental disorder’ demonstrated an inverse association and was therefore recoded), poverty before 15 years of age, black ethnicity and parental discord before 15 years of age. The final two variables did not increase the predictive accuracy of the instrument and were therefore excluded.
As can be seen in Table 93, the highest accuracy was achieved when including five variables. A cut-off point of ≥ 4 appeared to be the best choice to achieve sufficient accuracy.
Static risk factors included | AUC (95% CI) | Cut-off point | Sensitivity (%) | Specificity (%) | Correctly classified (%) |
---|---|---|---|---|---|
No educational qualifications | 0.76 (0.72 to 0.80) | ≥ 0 | 100.0 | 0.0 | 3.5 |
≥ 1 | 100.0 | 51.8 | 53.5 | ||
No educational qualifications, not worked in past year | 0.81 (0.78 to 0.85) | ≥ 0 | 100.0 | 0.0 | 2.9 |
≥ 1 | 100.0 | 25.9 | 28.1 | ||
≥ 2 | 100.0 | 62.7 | 63.7 | ||
No educational qualifications, not worked in past year, UK born | 0.87 (0.77 to 0.98) | ≥ 0 | 100.0 | 0.0 | 2.9 |
≥ 1 | 100.0 | 13.9 | 16.4 | ||
≥ 2 | 100.0 | 46.4 | 48.0 | ||
≥ 3 | 80.0 | 85.5 | 85.4 | ||
No educational qualifications, not worked in past year, UK born, no family history of mental disorder | 0.91 (0.82 to 1.00) | ≥ 0 | 100.0 | 0.0 | 2.9 |
≥ 1 | 100.0 | 4.22 | 7.0 | ||
≥ 2 | 100.0 | 21.1 | 23.4 | ||
≥ 3 | 100.0 | 54.2 | 55.6 | ||
≥ 4 | 80.0 | 91.6 | 91.2 | ||
No educational qualifications, not worked in past year, UK born, no family history of mental disorder, poverty before 15 years of age | 0.94 (0.90 to 0.98) | ≥ 0 | 100.0 | 0.0 | 2.9 |
≥ 1 | 100.0 | 3.0 | 5.9 | ||
≥ 2 | 100.0 | 18.8 | 21.2 | ||
≥ 3 | 100.0 | 47.9 | 49.4 | ||
≥ 4 | 100.0 | 86.1 | 86.5 | ||
≥ 5 | 20.0 | 98.8 | 96.5 |
Over the 10-year follow-up period there was substantial variation in the predictive accuracy of the static instrument for women (0.65–0.94). There was a significant drop in accuracy after inclusion of a further 6 months and when predicting violence over an 18-month time frame the lower CI of the AUC value dropped below 0.5 and was no longer significant. Stabilisation occurred from 24 months onwards with moderate AUC values, which were significant throughout the follow-up period.
Application of the algorithm for static risk resulted in 30 women (16.6%) being classified as having a high risk for violence within the first 6 months after assessment.
Both algorithms constituted the static part of the risk assessment instrument (see Appendix 1).
Dynamic risk factors
Overall, 29 dynamic factors were considered as dynamic risk factors for violent outcome: appearance and behaviour (disorganised/abnormal behaviour, recklessness, reduced need for sleep, increased sociability, agitation, retardation/loss of energy); speech and form of thought (disorganised thinking: speech, disorganised thinking: formal thought disorder, pressured speech/thoughts racing); delusions (persecutory delusions, grandiose delusions, delusions of reference, delusions of external control, delusions of guilt); hallucinations (third-person hallucinations, commentary voices, abusive/persecutory voices, other auditory hallucinations); affect (restricted/blunted affect, inappropriate affect, elation, irritability, depression, anhedonia, excessive guilt, suicidal ideation); and unspecific symptoms (sleeping problems, changes in appetite, poor concentration).
Following adjustments, six dynamic factors demonstrated a significant association with violence in men. These were agitation, pressured speech/thoughts racing, persecutory delusions, abusive/persecutory voices, irritability and changes in appetite (Table 94).
Symptoms of mental illness | Total | Men | Women | ||||||
---|---|---|---|---|---|---|---|---|---|
AORa | 95% CI | p-value | AORa | 95% CI | p-value | AORa | 95% CI | p-value | |
Appearance and behaviour | |||||||||
Disorganised/abnormal behaviourb | 1.27 | 0.79 to 2.05 | 0.323 | 0.97 | 0.55 to 1.70 | 0.913 | 2.65 | 0.93 to 7.58 | 0.070 |
Recklessness | 1.26 | 0.65 to 2.42 | 0.490 | 1.29 | 0.59 to 2.78 | 0.525 | 1.52 | 0.44 to 5.33 | 0.511 |
Reduced need for sleep | 1.51 | 0.71 to 3.22 | 0.287 | 1.48 | 0.55 to 3.97 | 0.440 | 1.40 | 0.40 to 4.85 | 0.596 |
Increased sociability | 2.21 | 0.83 to 5.86 | 0.113 | 2.97 | 0.97 to 9.05 | 0.056 | 0.46 | 0.04 to 4.80 | 0.513 |
Agitation | 4.71 | 3.07 to 7.25 | < 0.001 | 4.74 | 2.92 to 7.69 | < 0.001 | 2.78 | 0.98 to 7.84 | 0.054 |
Retardation/loss of energy | 0.90 | 0.54 to 1.50 | 0.681 | 0.66 | 0.33 to 1.29 | 0.222 | 1.54 | 0.64 to 3.71 | 0.335 |
Speech and form of thought | |||||||||
Disorganised thinking: speechb | 1.89 | 1.18 to 3.04 | 0.009 | 0.96 | 0.53 to 1.74 | 0.898 | 8.52 | 3.66 to 19.82 | < 0.001 |
Disorganised thinking: formal thought disorder | 1.40 | 0.78–2.54 | 0.261 | 1.39 | 0.68–2.84 | 0.364 | 1.41 | 0.45 to 4.41 | 0.560 |
Pressured speech/thoughts racing | 3.25 | 1.99 to 5.32 | < 0.001 | 3.73 | 2.08 to 6.69 | < 0.001 | 2.10 | 0.86–5.07 | 0.100 |
Delusions | |||||||||
Persecutory delusionsb | 3.33 | 2.31 to 4.81 | < 0.001 | 2.64 | 1.71 to 4.09 | < 0.001 | 6.86 | 3.48 to 13.51 | < 0.001 |
Grandiose delusionsb | 1.65 | 0.96 to 2.81 | 0.068 | 1.34 | 0.71 to 2.53 | 0.369 | 2.62 | 1.04 to 6.55 | 0.040 |
Delusions of referenceb | 1.32 | 0.71 to 2.44 | 0.375 | 1.02 | 0. 45 to 2.31 | 0.972 | 1.15 | 0.38 to 3.52 | 0.805 |
Delusions of external control | 1.28 | 0.62 to 2.61 | 0.507 | 1.23 | 0.53 to 2.85 | 0.638 | 1.43 | 0.35 to 5.85 | 0.621 |
Delusions of guilt | 1.06 | 0.16 to 7.02 | 0.955 | 0.90 | 0.06 to 13.06 | 0.940 | 0.70 | 0.04 to 11.83 | 0.805 |
Hallucinations | |||||||||
Third-person hallucinationsb | 1.07 | 0.55 to 2.07 | 0.840 | 0.80 | 0.35 to 1.85 | 0.606 | 1.96 | 0.65 to 5.92 | 0.235 |
Commentary voices | 3.59 | 1.27 to 10.14 | 0.016 | 1.34 | 0.26 to 6.84 | 0.729 | 4.92 | 1.21 to 20.05 | 0.026 |
Abusive/persecutory voices | 2.66 | 1.63 to 4.34 | < 0.001 | 3.00 | 1.71 to 5.26 | < 0.001 | 1.38 | 0.49 to 3.87 | 0.546 |
Other auditory hallucinationsb | 1.99 | 1.26 to 3.16 | 0.003 | 1.46 | 0.84 to 2.54 | 0.179 | 3.83 | 1.68 to 8.72 | 0.001 |
Affect | |||||||||
Restricted/blunted affect | 1.05 | 0.64 to 1.72 | 0.861 | 1.16 | 0.64 to 2.08 | 0.631 | 0.80 | 0.31 to 2.09 | 0.650 |
Inappropriate affectb | 1.50 | 0.90 to 2.51 | 0.118 | 1.04 | 0.55 to 1.99 | 0.898 | 3.19 | 1.28 to 7.95 | 0.013 |
Elation | 1.47 | 0.86 to 2.51 | 0.155 | 1.44 | 0.75 to 2.77 | 0.276 | 1.08 | 0.41 to 2.85 | 0.871 |
Irritability | 4.45 | 2.93 to 6.75 | < 0.001 | 4.24 | 2.60 to 6.93 | < 0.001 | 5.39 | 2.09 to 13.93 | < 0.001 |
Depression | 1.17 | 0.71 to 1.92 | 0.547 | 1.00 | 0.55 to 1.84 | 0.987 | 1.13 | 0.41 to 3.15 | 0.809 |
Anhedonia | 0.66 | 0.30 to 1.44 | 0.292 | 0.88 | 0.34 to 2.27 | 0.792 | 0.71 | 0.17 to 2.98 | 0.641 |
Excessive guilt | 0.56 | 0.06 to 5.27 | 0.608 | – | – | – | 2.65 | 0.25 to 27.89 | 0.416 |
Suicidal ideation | 2.14 | 1.18 to 3.87 | 0.012 | 1.72 | 0.81 to 3.68 | 0.159 | 2.11 | 0.75 to 5.96 | 0.157 |
Unspecific symptoms | |||||||||
Sleeping problems | 1.48 | 0.91 to 2.41 | 0.116 | 1.46 | 0.83 to 2.56 | 0.190 | 1.52 | 0.56 to 4.12 | 0.415 |
Changes in appetite | 2.31 | 1.38 to 3.87 | 0.001 | 2.35 | 1.28 to 4.32 | 0.006 | 1.71 | 0.64 to 4.55 | 0.282 |
Poor concentrationb | 2.04 | 1.28 to 3.26 | 0.003 | 1.57 | 0.89 to 2.74 | 0.117 | 3.35 | 1.29 to 8.68 | 0.013 |
In the female sample, eight dynamic factors were significant risk factors for violent behaviour: disorganised thinking (speech), persecutory delusion, grandiose delusions, commentary voices, other auditory hallucinations, inappropriate affect, irritability and poor concentration (see Table 94).
Moderation analyses: sex × dynamic risk factor interactions
We observed significant sex interactions for disorganised/abnormal behaviour, disorganised thinking (speech), persecutory delusions, grandiose delusions and delusions of reference, third-person hallucinations and other auditory hallucinations, inappropriate affect and poor concentration (see Table 94). These significant interaction terms indicated that the effects of the dynamic risk factors on violent outcome were stronger in women than in men.
Mediation analyses: affect
Of the eight affective states under study, only irritability was significantly associated with violent outcome in men (see Table 94) and qualified as a potential mediator. In a first step we investigated whether or not irritability was associated with other non-affective symptoms identified as positively related to violent outcome. As can be seen in Table 95, irritability was significantly associated with all symptoms. The strongest effect was observed with agitation.
Symptoms of mental illness | OR | 95% CI | p-value |
---|---|---|---|
Agitation | 44.59 | 25.45 to 78.12 | < 0.001 |
Pressure of speech/thoughts racing | 17.34 | 8.97 to 33.49 | < 0.001 |
Persecutory delusions | 23.70 | 14.27 to 39.35 | < 0.001 |
Abusive/persecutory voices | 11.20 | 5.79 to 21.69 | < 0.001 |
Changes in appetite | 4.27 | 2.37 to 7.67 | < 0.001 |
Subsequent inclusion of the mediator in the statistical model (Figure 6) resulted in a non-significant pathway for pressure of speech (percentage of total effect mediated: 23.2%), persecutory delusions (percentage of total effect mediated: 60.8%), abusive voices (percentage of total effect mediated: 34.3%) and changes in appetite (percentage of total effect mediated: 34.3%). The only remaining direct pathway was from agitation to violence (percentage of total effect mediated: 26.2%).
FIGURE 6.
Mediation analyses: irritability – men. Figures represent AORs. Figures in parentheses are ORs after the inclusion of the mediator variable in the statistical model. **p < 0.01; ***p < 0.001.
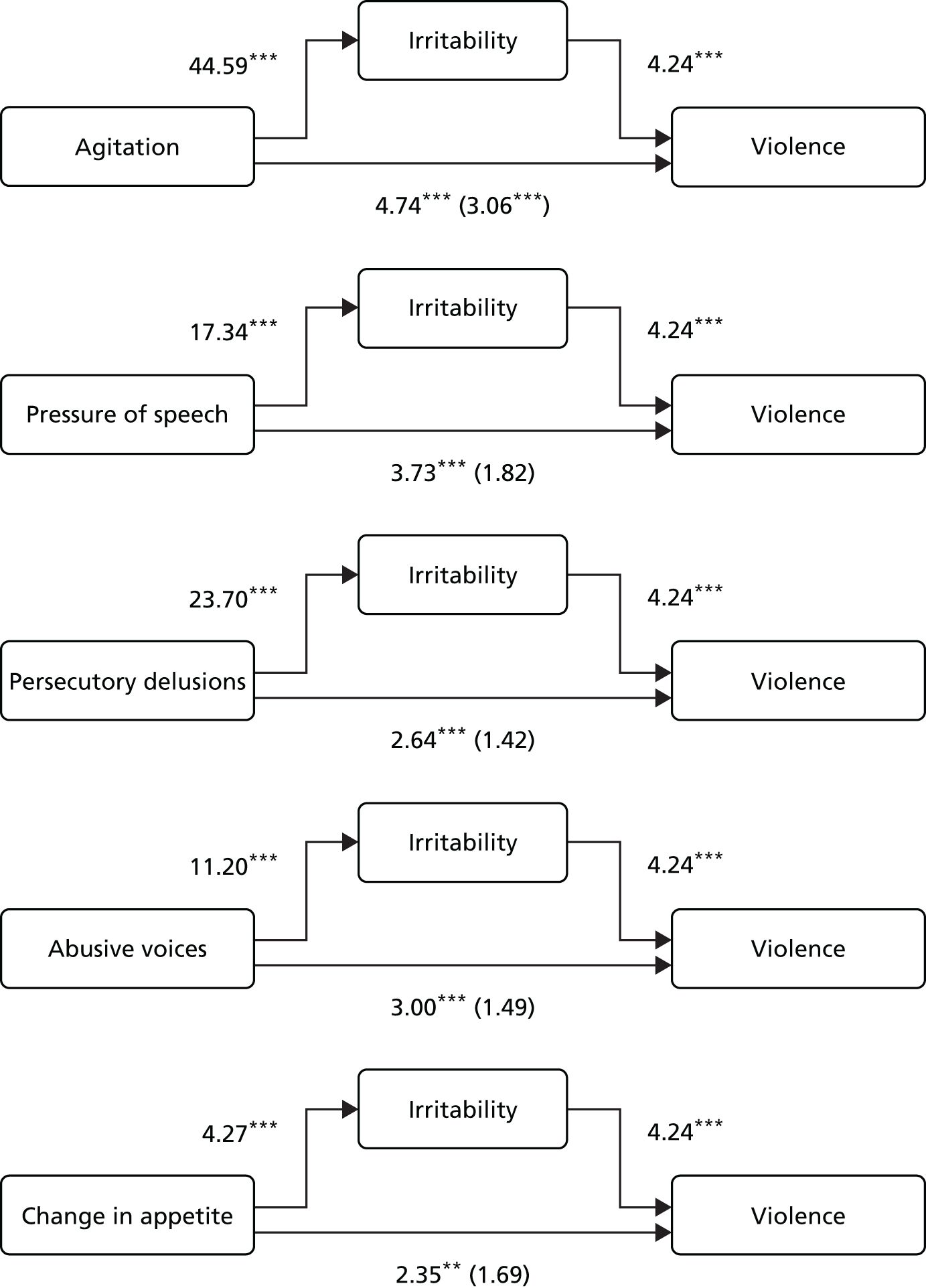
In women, both inappropriate affect and irritability were associated with violence and therefore qualified as potential mediator variables. Inappropriate affect was significantly associated with disorganised thinking (speech), persecutory and grandiose delusions and other auditory hallucinations (Table 96). Irritability demonstrated a significant relationship with disorganised thinking (speech), persecutory delusions, other auditory hallucinations and poor concentration (see Table 96).
Symptoms of mental illness | OR | 95% CI | p-value |
---|---|---|---|
Inappropriate affect | |||
Disorganised thinking: speech | 15.24 | 5.02 to 46.24 | < 0.001 |
Persecutory delusions | 8.09 | 3.43 to 19.07 | < 0.001 |
Grandiose delusions | 15.06 | 3.78 to 59.99 | < 0.001 |
Commentary voices | – | – | – |
Other auditory hallucinations | 14.28 | 2.97 to 68.60 | 0.001 |
Poor concentration | 1.44 | 0.52 to 4.04 | 0.483 |
Irritability | |||
Disorganised thinking: speech | 6.32 | 2.53 to 15.77 | < 0.001 |
Persecutory delusions | 64.08 | 24.95 to 164.60 | < 0.001 |
Grandiose delusions | 1.42 | 0.38 to 5.31 | 0.603 |
Commentary voices | 1.99 | 0.12 to 31.65 | 0.627 |
Other auditory hallucinations | 3.02 | 1.11 to 8.24 | 0.031 |
Poor concentration | 11.26 | 5.03 to 25.19 | < 0.001 |
Subsequent mediation analyses revealed a direct pathway from disorganised thinking (speech), persecutory delusions and other auditory hallucinations to violent behaviour. There was some attenuation in the ORs (percentage of the total effect mediated: 24.5%, 41.3% and 8.0%, respectively). The association, however, was still significant after inclusion of the two mediators (Figure 7).
FIGURE 7.
Mediation analyses: inappropriate affect and irritability – women. Figures represent AORs. Figures in parentheses are ORs after the inclusion of the mediator variable in the statistical model. *p < 0.05; **p < 0.01; ***p < 0.001.

The pathway from grandiose delusions and poor concentration to violent behaviour was no longer significant after inclusion of the mediator variable. The percentage of the total effect mediated was 29.5% and 64.1%, respectively (Figure 8).
FIGURE 8.
Mediation analyses: inappropriate affect and irritability – women. Figures represent AORs. Figures in parentheses are ORs after the inclusion of the mediator variable in the statistical model. *p < 0.05; ***p < 0.001.
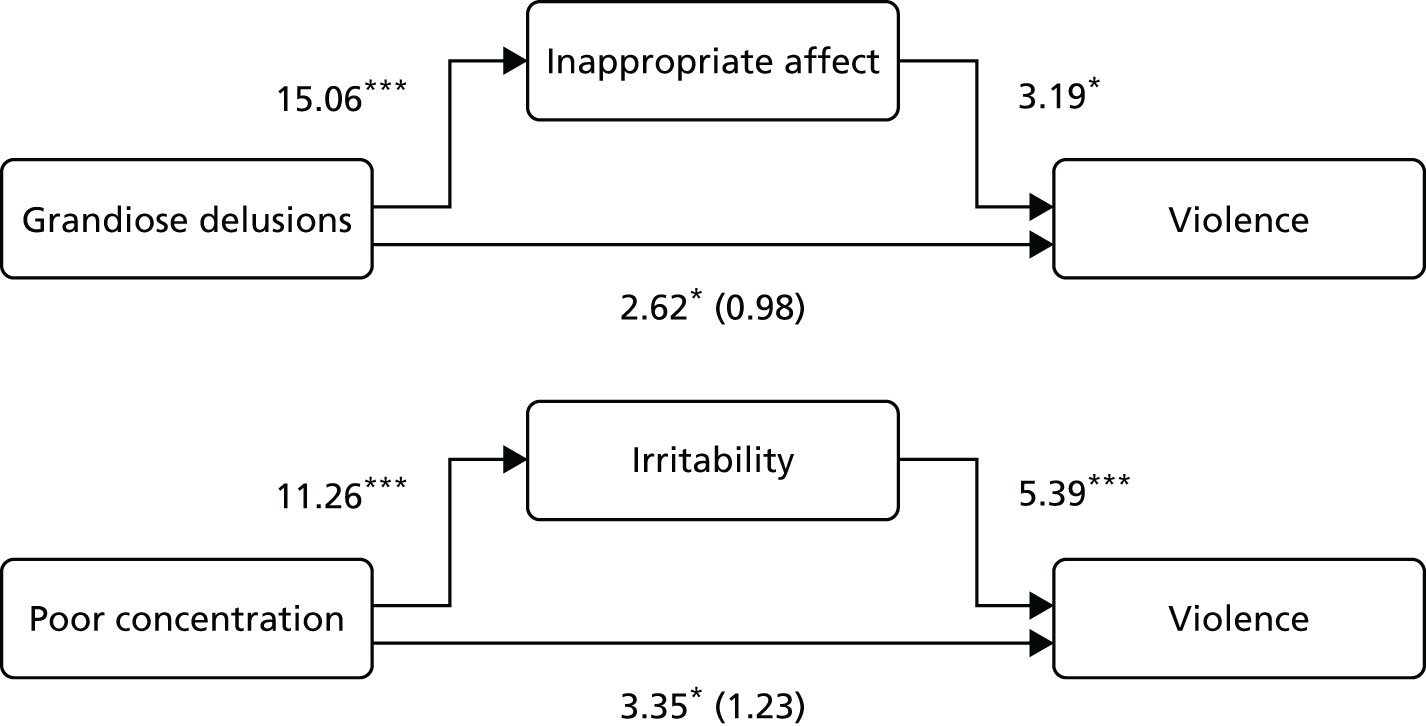
Moderation analyses: static × dynamic risk factor interactions
Interaction terms of high level of static risk × dynamic risk factors were modelled to identify whether the effects of dynamic factors on violent outcome differed significantly depending on level of static risk derived from the static risk assessment instrument described earlier. As in previous analyses, these models were run separately in men and women.
In men, retardation and loss of energy had a different effect on violent outcome depending on the level of static risk (AOR 0.18, 95% CI 0.04 to 0.75; p = 0.018). If retardation and loss of energy were present, there was no increase in the likelihood of violence irrespective of static risk. Static risk in the absence of this dynamic factor showed a positive association with violent outcome. Abusive, persecutory voices were associated with violence only when the patient was classified as being at high risk for violence (AOR 3.58, 95% CI 1.21 to 10.56; p = 0.021). At a low level of risk, abusive and persecutory voices were not related to violent outcome.
In women, the only significant interaction term was found with agitation (AOR 0.22, 95% CI 0.05 to 0.98; p = 0.047). Agitation was associated with violence irrespective of high or low static risk. The association of high static risk and violence, however, was significantly lower in the absence of agitation.
Discussion
Our findings confirm those of previous studies that violence in those with psychosis is higher at first presentation than following treatment. 116,305 They also confirm that there is a higher prevalence of violent behaviour in men than in women throughout the whole follow-up period.
Men and women with psychotic illness, however, do not differ only with regard to the frequency of their engagement in violent behaviours. As previously demonstrated, risk factors for violence differed markedly between men and women and the pathways towards violence were distinct as well. 292 Risk factors identified in this population without consideration of sex differences may therefore lead to incorrect conclusions.
Static risk
The static risk factors identified as relevant for future violence did not show any overlap between men and women.
Of the 27 static risk factors under study, only four were relevant risk factors for violence in men in the 6 months following assessment. These included a high level of trait anger, a history of violent and threatening/annoying behaviours leading to contact with services and parental discord before the age of 15 years. Apart from trait anger, none of the clinical history variables, such as diagnosis at first presentation or alcohol/drug abuse, predicted violence with sufficient accuracy in the 6 months following assessment. Furthermore, the four static risk factors were predictive only at a moderate level of accuracy and the PCC demonstrated that in about 40% of cases these men were misclassified. However, this is unsurprising and reflects the inaccuracy inherent in a multitude of actuarial and (even) SPJ instruments. 21,23,24 Almost 43% of men were classified as being at high risk for violence within the following 6 months with a prevalence of violence of 6%. Over time these static risk factors remained remarkably stable. Throughout the 10-year follow-up period they were significant predictors and the magnitude of the AUC values did not fall below 0.60.
In women, 5 out of 27 static risk factors predicted violence in the subsequent 6 months. In total, 17% of the women were classified as being at high risk for violence within the following 6 months, with a base rate of violence of approximately 3%. The AUC value was excellent and only 24% of the women were misclassified, with a rate of correct identification of high-risk women of 100%. However, none of the risk factors was related to clinical history variables but instead included no educational qualifications, not having worked in the past year, UK born, no family history of mental disorder and poverty before the age of 15 years. After this excellent prediction of short-term violence, the AUC values dropped significantly after adding a further 6 months to the prediction period and dropped further when predicting violence 18 months after assessment (being no longer significant). From 2 years onwards the AUC values stabilised and demonstrated similar predictive accuracy to those for men.
It appears that in both men and women static risk factors tend to identify those with a propensity towards violence irrespective of their history of illness or other past clinical characteristics. However, the higher precision of correct identification of women in the short term suggests that they form a more homogeneous group with regard to their predisposition to engage in violent behaviours.
Dynamic risk
The findings of this study emphasise that investigation of dynamic risk for violent behaviour requires a thorough consideration of pathways towards violence and the application of appropriate statistical methods. This approach may not result in findings on the (questionable) accuracy of prediction (as in the static risk approach) but informs clinicians about causal relationships of specific symptom constellations as long as they are present. Moreover, dynamic risk factors are changeable and therefore amenable to intervention. Appropriate management of these should therefore result in fewer violent incidents.
As in the static risk module, our analyses revealed significant differences between men and women. At first glance there appeared to be some overlap of dynamic risk factors in both sexes. Significant dynamic causal risk factors for men in the first step of analyses were agitation, pressure of speech/thoughts racing, persecutory delusions, abusive/persecutory voices, irritability and changes in appetite. In women, dynamic causal risk factors included disorganised thinking (speech), persecutory delusions, grandiose delusions, commentary voices, other auditory hallucinations, inappropriate affect, irritability and poor concentration.
In previous studies using clinical samples we have demonstrated that there is a strong association between persecutory delusions, or delusions implying threat to the individual, and violent behaviour. 21,291 However, this pathway was not direct but was explained by angry affect as a result of the delusional beliefs. In both men and women, persecutory delusions were a risk factor for violent behaviour, with the effect being significantly stronger for women. We did not have a measure of anger in this study but irritability shares common features with angry affect and served as a proxy measure. Irritability was related to both persecutory delusions and violence in men and women. However, mediation analyses revealed that the pathways were different dependent on sex. The association between persecutory delusions and violence was no longer significant and substantially explained by irritability in men. In women there was some attenuation in the magnitude of the effect, but persecutory delusions still demonstrated a direct association with violence after inclusion of the mediator (irritability). This has important treatment implications. To prevent violence in psychotic men, treatment of irritability or anger is of utmost importance. In women, however, treatment of irritability will reduce the risk but only to some degree. Their persecutory delusions need attention and, if unresponsive to treatment or non-compliant, women will be at higher risk for violence even if their irritability has abated.
Irritability was the only affective state in men associated with violent behaviour. Furthermore, it was a significant mediator and explained not only the association with persecutory delusions but also the association with pressure of speech/thoughts racing and abusive/persecutory voices. Agitation was the only symptom that demonstrated a direct pathway towards violent outcome. Irritability and agitation in men with psychotic illness are therefore the most important symptoms that should be treated to reduce the risk of violence.
In women, inappropriate affect was another causal risk factor for violence and a further important mediator. However, the associations with disorganised thinking (speech) and other auditory hallucinations were still significant (although attenuated) after inclusion of both affective states. The association of grandiose delusions with violence was explained by inappropriate affect and the relationship of poor concentration with violence was explained by irritability. In women, risk factors for violence were irritability and inappropriate affect but attention should also be paid to persecutory delusions, disorganised thinking (speech) and auditory hallucinations such as third-person hallucinations, command hallucinations, neutral voices and non-verbal auditory hallucinations.
Interactions of static and dynamic risk
We identified few symptom constellations in which the association between dynamic risk factors and violent outcome was dependent on the level of static risk assessed with our static risk algorithms. The pattern was again different in men and women.
In men, abusive and persecutory voices were risk factors for violence only when the patient was classified as being at high static risk. In women, agitation demonstrated a significant interaction with static risk and should be considered relevant irrespective of high or low static risk.
Conclusions
There are remarkable differences in the risk for violence between men and women with psychotic illness. Symptoms of psychosis are more strongly associated with violent behaviour in women whereas affective states play a more important role in men. Inherent propensity towards violence as measured by static risk has little influence on the association of clinically relevant dynamic risk factors and engagement in violence. Sex differences in risk for violence require further investigation and should be considered in management and treatment to reduce violent incidents.
Construction/audit of the instrument
The findings of this study were presented at two consultant meetings in adult forensic and general psychiatry (Centre for Mental Health in Newham and John Howard Centre in Hackney, London). The consultants were invited to comment on the theoretical and analytical approach of the instrument. Based on their comments a first version of the risk assessment instrument was constructed including two modules, one for men and one for women, that incorporated a static and a dynamic risk assessment. The instrument was then sent out for audit and further comments about its usefulness and practicability.
Overall, the feedback was very positive. The majority of consultants considered the instrument to be very useful. According to the consultants, there was variation with regard to the time it would take to gather the information, which ranged from 5 to 30 minutes. However, considering that administration and rating of the HCR-20287 can take hours we believe that the time to code the instrument can be fitted into clinical practice.
There were requests regarding a more precise definition of the time frame in which risk is elevated and how often the instrument should be administered. These comments were taken into consideration and the instrument was amended accordingly (see Appendix 1).
Mental illness and violence: service users’ perspectives
Katherine
My name is Katherine Barrett and I have been a mental health service user for 23 years. During this time, I have been in hospitals and crisis houses. In 2000, I became very unwell after a year of psychotherapy at the Tavistock. I met with a crisis team regularly between 1999 and 2007 when I was a revolving door patient. My recovery process took time and involved taking medication, returning to work, stopping taking my medication, becoming unwell again, taking months off work, seeing the crisis team, taking the medication again and – slowly – getting back to better health and maintaining it.
I am a qualified teacher and experienced a lot of stigma in my past teaching posts because of my mental illness. Since 2008, I have been taking medication every day, so my quality of life has improved. However, I experience side effects of the medication that are sometimes difficult to cope with but I know that it is necessary to maintain my health.
Through service user involvement, I now do some work that involves my past experience of mental illness and I have been involved in various research projects.
Violence committed by a mentally ill person is a very sensitive subject because of the stigma surrounding it. The media coverage does not help. The media often portray mental illness horrifically. This adds to the stigma already associated with mental illness and people who read the articles can become fearful and biased towards people with mental health problems.
But violence does happen in those with mental illness. I have been confronted with this issue when I was participating in the approved mental health professionals (AMHPs) re-warranting panels. The AMHPs often go to someone’s house with the police if the person has a history of violence. Depending on how they behave and how the team assesses the risk of aggressive and violent behaviour, they can end up being sectioned and taken away from their home to hospital in a police van. This can destroy community relationships for the service user and can be very traumatic for them. However, I have also witnessed violence on acute wards. Acute inpatient wards are difficult places to be. Once I observed a women shouting and pulling over a bookcase. On another occasion a women was punching a police officer as he carried her to her room. I saw a drunken man who became sick in a corridor. He was wandering around without his top and was shouting. Acute wards are distressing enough because you are sick. Having to face up to violent behaviour increases the distress. It is such a difficult situation to find yourself in and makes you feel frightened, worried, anxious and sad. None of the team on the ward talked to me about these incidents. I think a mental health practitioner should be involved if you witness violence on an acute ward. It is very difficult to handle the situation on your own.
So why do mentally unwell people become violent? I believe some are violent irrespective of their mental illness. It may be because of their personality. Others may be violent because they are frustrated or it may be the way that they have dealt with things in the past and it has worked. Others, however, are violent because they feel that they are persecuted or that there are voices telling them to do so. For these people it is very difficult to accept their own violent behaviour when the voices, the feelings of being persecuted, have gone away. Depending on how serious their violent behaviour was, this can be a very distressing experience and extremely difficult to cope with, knowing that they have hurt someone else badly.
David
My name is David Hindle. It is now 12 years since I lost my full-time job at the age of 44 because of being unable to continue because of mental ill health. It was totally devastating, but looking back it was the right thing for me. It enabled me to start a journey of recovery without a key pressure in my life. Although I was originally diagnosed as suffering from depression, this was later changed to bipolar affective disorder. Although for me the lows prevailed, there have also been times of agitation, anger and sometimes elation.
For me having something to do is important, but I have to do it at my own pace and try to avoid stress. Through various activities I started to rebuild my shattered confidence and self-esteem. I became involved in various service user activities, seeking to improve local services. Eventually I pulled back on some of those activities and started to work with the Mental Health Research Network. That led me around 5 years ago to start to be actively involved in mental health research. Through that I have gained various opportunities to carry out research.
I find instinctively the issue of thinking about violence and mental health together as tricky. One reason for this is that such an association does in my mind feed into a stigmatising stereotyping of those who do have mental health issues, particularly psychosis. It can also feel like focusing on the issue of safety/risk rather than looking at the whole person. It reminds me of the negative headlines sometimes seen in the media. I have, however, been around in the mental health system long enough to appreciate that at times mentally ill people, if not appropriately treated, can behave violently.
In one of my current roles as an Associate Hospital Manager I sit on Managers’ Panels. It often strikes me how difficult it is to judge the issue of the likelihood of future violence, despite that issue sometimes being a key part on which a clinician is making a recommendation for continued detention or a community treatment order. Sometimes, these decisions can appear arbitrary and not grounded in a realistic assessment of risk.
We both have been involved in the First Episode Psychosis Follow-Up study and sat on the steering group panel of the VoRAMSS study (see Section C). We believe that the research output from this programme is very important to tackle the extremely sensitive issue of violence in those with mental illness. Considering both sides we believe that it is important for clinicians to have good accurate tools to help them make good clinical decisions about the likelihood of violence. It can be a means whereby some patients who are unnecessarily kept in inpatient wards, because of unfounded concerns about their risk of violence, could instead be discharged. On the other hand, it can be used as a way of ensuring better support and treatment for those who may be at risk of violence, thereby helping to aid recovery. We appreciate the aim to identify risk factors that are changeable. Focusing on factors that cannot be changed will not help the patients concerned. But treating symptoms that are causally related to violent behaviour will help to prevent such incidents, therefore helping the service user as well.
Section C The validation of new risk assessment instruments for use with patients discharged from medium secure services
Chapter 13 A follow-up of patients discharged from medium secure services in England and Wales
Background
Accuracy of violence risk assessment and good risk management are key objectives for both public protection and quality of care among patients discharged from medium secure units. Methods of risk assessment have developed significantly over the past two decades. 306 Currently, the preferred method in UK mental health services is SPJ, which combines the use of empirically validated instruments or guidelines with professional knowledge and discretion. 307 This allows for risk assessment to be a dynamic process and ensures that important personal/individual factors are not overlooked. It also means that research has an important role to play in externally validating risk assessment measures with different samples. 308
Until recently, the focus of risk assessment has been mainly on factors that may increase the likelihood of future violence. Risk management strategies have concentrated on reducing the impact of these problem areas. Recently, assessment of protective factors has been shown to be a valuable addition to the risk management process. 309
Risk assessment and management are vital components of discharge planning, transfer between different levels of security and care reviews. 307 Key transition points, such as discharge from inpatient settings, are considered to be times of elevated risk and vulnerability and present challenges in terms of continuity of care and risk management, because care is often passed from one service to another. 310 In forensic mental health services, clinical decisions on risk are made at all stages of the service user’s pathway through services.
Over the last two decades, the concept of violence risk assessment in mentally disordered service users has gained considerable public, political and clinical attention. A relatively small yet significant number of incidents involving people with mental illness have received considerable media attention. 311–313 Recommendations from inquiries following such events have also been widely publicised, highlighting procedural failings or missed opportunities for prevention. 314 This has left a strong impression of the potential dangerousness to the public from individuals who have various forms of mental disorder and has engendered a lack of public confidence in the ability of mental health services to manage risk adequately. 315
Evidence suggests that the percentage of the public who believe that people with a mental illness are prone to violence is increasing. 316 However, research has demonstrated that, over an 11-year period, approximately 9% of homicides in England and Wales were found to have been committed by individuals who had an abnormal mental state310 and that the proportion of people with a mental illness committing criminal homicide has been decreasing. 317 Nonetheless, given the grave consequences of some isolated incidents and the immeasurable impact on victims’ families and communities, it is perhaps not surprising that risk assessment and management have become a central focus for mental health policy and practice.
The Criminal Justice Act (CJA) 2003318 introduced legislation requiring multiagency risk assessment of all offenders who pose a serious risk of harm to the public. Multiagency public protection arrangements obligate local criminal justice agencies to work in partnership to assess and manage the risk posed by violent offenders in their area. The introduction of extended sentences for certain violent or sexual offences (Legal Aid, Sentencing and Punishment of Offenders Act 2012319) and other legislation reliant on an assessment of ‘dangerousness’ (CJA 2003318) has also elevated the role of risk assessment and management in forensic settings for public protection purposes. Risk assessments may be used in court or at hearings and may influence legal decisions on sentencing, further detention or release, whereas risk management is a vital aspect of sentence planning. 320
In contemporary forensic mental health services, risk assessments are key to risk management formulation, planning and decision-making, which can ultimately prevent violent behaviour and reduce victimisation. 307 Assessing risk of violence is especially important when planning the discharge of mentally disordered offenders from secure psychiatric services so that suitable placements and multiagency support packages can be put in place. 321 Clinically, there is a difficult balance to be achieved in risk assessment and care planning between promoting recovery for the service user and protecting others from harm. Overly restrictive measures could limit opportunities for rehabilitation and recovery as well as infringe on an individual’s freedom. 322 Failure to manage risk effectively, on the other hand, could result in serious harm. 311
To provide continuity of care between inpatient and community services, the Care Programme Approach (CPA) was introduced in 1991 as a framework for the co-ordination of effective mental health care for people with complex needs in contact with secondary mental health services in the UK. 323 The CPA outlines basic requirements that should be in place for all service users. These include the formulation of a care plan, an appointed worker who co-ordinates the implementation of the care plan and regular reviews. 323 Risk management is a core element of the CPA. A best practice guide for risk management324 in mental health services and a series of tools to inform local policies were disseminated as part of the government’s National Mental Health Risk Management Programme. 324 The value of a structured, evidence-based and consistent yet individualised approach was emphasised. Interdisciplinary and interagency communications were highlighted as essential and a collaborative positive approach with a focus on recovery was promoted. 307
Methods of risk assessment
Despite government advice clearly encouraging multiagency collaboration, communication and consistency across services,307 there are still vast disparities in approaches to risk assessment between agencies and disciplines, which may serve to make this task more difficult. 325–327
There has also been substantial debate and controversy about the ability of mental health professionals to predict violence, with one review concluding that clinicians’ predictions about future violence were accurate on only around one in three occasions. 328 One of the reasons suggested for this is the low base rate of violence. It is difficult to predict violent behaviour with any degree of certainty, especially if the violence is serious and therefore rare. The most common inaccuracy is a false-positive outcome, in which violence is predicted but does not occur. 329,330 Further, prediction formulae are unable to control for fluctuations in mood, mental state or behaviour. 331,332
In a sample of 959 service users discharged from UK medium secure services (MSSs) over a 12-month period, Maden et al. 333 observed a violent reconviction rate of only 6% over a 24-month follow-up period. Their recommendations included less focus on violence prediction and more on screening for violence to identify those at highest risk to prioritise risk management interventions for this group. The variety of risk assessment methods and tools used, coupled with a lack of consistency in training and quality assurance within and between services, complicates the task of successful multiagency risk management yet further. 334,335 Methods of assessment have developed significantly over the past two decades and different approaches including clinical opinion, SPJ and statistical or actuarial techniques have been the subject of much debate and research.
Clinical opinion or unstructured professional judgement
The ‘first-generation’ approach to violence risk assessment was that of clinical opinion. This involves clinicians evaluating risk based on their own professional experience and allows them complete freedom to apply their discretion and personal intuition to each case. There is generally no structured guidance on which information should be considered as part of the assessment and this has therefore also been described as unstructured professional judgement. 289 It has been criticised on several grounds, including poor violence prediction accuracy and low inter-rater reliability,336–338 but others have highlighted the advantage of being able to consider factors that may be specific to individuals. 339 In addition, in contrast to Monahan’s328 findings, later research suggests that the ability of clinicians to predict violence is significantly better than chance. 340,341
Actuarial methods
Actuarial risk assessment is, in contrast, a structured method that involves a calculation of the level of risk by coding or weighting each case according to a set of predetermined factors or rules. The factors are often static, such as demographics or past convictions, and are chosen as they are known or thought to be correlated with violence risk. Actuarial methods appear to offer increased consistency in risk assessment289,342 but critics have identified that crucial case-specific dynamic factors (e.g. threats of violence, deteriorating mental state) cannot be usefully considered in actuarial assessments, leading to potentially important indicators of violent behaviour being overlooked. 331,343
Structured professional judgement
Both clinical and actuarial approaches have further advantages and disadvantages and the debate as to which approach is most relevant to clinical practice is complex. However, the current consensus appears to be that a combination of clinical and actuarial approaches is warranted, whereby the clinical task of assessing and managing violence risk is validated by the SPJ approach. 307,344 In the SPJ approach, the emphasis is on developing evidence-based guidelines or frameworks that promote systematisation and consistency yet are flexible enough to account for case-specific influences and the context in which assessments are conducted. SPJ methods have generally been adopted more readily in clinical practice than actuarial measures alone as these methods can promote transparency and accountability yet encourage the use of professional discretion and the basis of the guidelines is rooted in scientific knowledge evaluation of a model of violence. 331 In addition, this approach moves away from the research priority of violence prediction and supports instead the clinical reality of building a comprehensive plan for violence risk management and violence prevention. 345,346
Protective factors
Whereas extensive research has attempted to identify the factors most associated with an increase in violence risk in various populations and situations, limited attention has been paid to those factors that may reduce it. De Vogel et al. 347 sought to right this balance and complement existing SPJ risk assessment measures with the development of a measure that assesses various factors thought to reduce or mitigate violence risk. Their tool, the Structured Assessment of Protective Factors for Violence Risk (SAPROF), includes positive and predominantly dynamic factors, therefore supporting one of the best practice principles of violence risk management, that it ‘must be built on a recognition of the service user’s strengths and should emphasise recovery’. 307 The SAPROF has recently been validated in a sample of Dutch forensic psychiatric service users. 348 It showed good inter-rater reliability and both the total SAPROF score and final risk judgement predicted non-recidivism. When combined with the HCR-20,287 it increased the predictive validity of violent recidivism in comparison with the HCR-20 used alone.
Rationale for the current study
Borum349 identified the strengths of the SPJ approach but called for the development of new assessment tools, clinical guidelines for their application and training to move clinical risk assessment practice forward.
There are many SPJ measures available and more are being developed, but it is vital that their use is validated through research before their application to new samples and clinical practice. 308
The most frequently used SPJ guideline for violence risk assessment is the HCR-20. 287 The HCR-20 has been widely tested in forensic services in the UK and there is promising evidence to support its use. 321,350 However, the HCR-20 has recently been further developed into version 3 (HCR-20v3), which requires further validation in UK samples.
Previous research has demonstrated that the majority of offenders with mental illness in medium secure hospitals were admitted under the Mental Health Act 1983351 legal classification of mental illness. 352–354 Most actuarial measures and SPJ guidelines have been developed among samples of prisoners and service users without consideration of mental disorders or among samples with a variety of mental disorders including personality disorders. Therefore, it is likely that existing SPJs may not be suitable for the majority of mentally disordered offenders detained in UK MSSs. A recent study by Hickey et al. 22 attempted to address this dilemma by developing the MSRAG as an actuarial risk prediction instrument based on a sample of mentally disordered offenders with a major psychotic disorder discharged from MSSs. If the MSRAG is to have any practical clinical utility, it is essential to externally validate this instrument to compare it with existing instruments using new samples. This study, therefore, investigated the predictive validity of the HCR-20v3 and the MSRAG with a sample of discharged offenders from NHS medium secure pathways in England and Wales.
It has also been noted in previous studies that certain factors mitigate against the likelihood of future violence and it has been hypothesised that those discharged from MSSs are less likely to be violent if additional community support and supervision is provided. 22,321 Although the knowledge of putative violence risk factors and the development of violence risk assessments has grown enormously, hardly any attention has been paid thus far to aspects that may compensate for or mitigate against risk. This study examined how levels of support post discharge impact on violent outcomes. The SAPROF was also evaluated in this sample to enable further analysis of the role of protective factors. 347
Previous research studies attempting to validate risk factors and risk assessment instruments have been beset by methodological problems, which have threatened the validity and reliability of the findings. A number of crucial, methodological problems have been noted in this area. 355 First, predictor variables in violence risk assessment research tend to be very narrow and inconsistent. Second, the criterion variables tend to be weak in that the reliance in many studies is on official records that tend to overlook violence that occurred which did not precipitate intervention by a government agency. 356 Finally, another crucial weakness of previous research is the problem of selection bias.
In prospective validation studies that address the methodological problems highlighted above, the generalisability of the findings remains limited by the fact that in all studies there is a significant minority of at least 30% of potential participants who refuse to participate. 300,321 It is also likely that those service users who do not consent are the group who are most at risk of being non-compliant and antisocial and who are, therefore, more likely to be violent post discharge. As a result, the data that new instruments are based on are imperfect. This could seriously impact on the validity of any instrument derived from research and, therefore, the full benefits of the research in developing evidence-based approaches to reduce the risk of community violence may be lost.
This study adopted a confidential inquiry approach to overcome some of the methodological problems experienced in previous research while continuing to protect the legal rights and anonymity of the participants. All service users discharged within the study period were included subject to Section 251 of the NHS Act 2006. 297 This maximised the validity and reliability of the findings. Violent outcome was measured using three sources. PNC records were used to detect cautions or convictions for violent offences during follow-up. The MCVI300 was also used during interview with a collateral informant (a professional who had worked closely with the service user during the follow-up period, the care co-ordinator or a social supervisor when possible). This ensured that any violent incidents that may have occurred but which did not result in contact with the criminal justice system were also captured. The interview was also supplemented by a review of clinical records.
There is also little information on what risk management systems exist in health-care services for service users and offenders with mental disorders in the UK. There is little hard evidence to show the way that risk assessment and management might be effectively implemented in practice. Implications for clinical practice were therefore considered throughout the course of this body of work and results will be disseminated to key stakeholders. In summary, public protection from violent, sexual and other high-risk behaviours is increasingly expected from health-care professionals and services that they work for. These problems place a considerable expectation on health-care services and some specialist mental health services are specifically designed to reduce risk. Risk assessment and risk management are key methods used by professionals. Risk is highest in community settings where monitoring and supervision are limited and therefore risk is more difficult to manage. New risk management interventions are required with a community focus. Research methods and statistical procedures have been developed to provide clinical tools to help health-care professionals in risk management. Our research using a confidential inquiry approach aimed to build on these developments. As far as we are aware, these methods have not been previously applied to UK or international populations.
The purpose of the current research was to build on previous research38 validating new ARA instruments in MSS samples;306,307 validating new SPJ guidelines in medium secure forensic samples; and identifying and validating measures of protective factors for violence. 308
Objectives
The objectives of the study were to:
-
describe the characteristics of patients discharged from MSSs
-
identify their location following discharge at 6 and 12 months
-
compare patients who were violent following discharge with those who were not violent.
Methods
Sample
The study team was notified of all discharges from a medium secure NHS pathway in England and Wales during a 12-month period (1 September 2010 to 31 August 2011). All service users discharged during this time from participating MSUs and low secure units (LSUs) were eligible for inclusion in the study, except for those aged < 18 years or > 65 years. Independent sector hospitals were excluded, as were any units that specialised in learning disabilities. In total, 32 MSUs and 21 LSUs directly linked to the medium secure discharge pathway participated. In this study, NHS units were selected as they are subject to similar governance and regulations and this would enhance consistency across sites.
Ethics
The National Research Ethics Service – North West – Preston Committee (09/H1016/126) and the University of Manchester Ethics Committee granted ethical approval. Site-specific approvals were also obtained from the relevant NHS trusts responsible for each MSU or LSU. All researchers had NHS research passports and letters of access.
All participating staff were provided with a staff information sheet. During the initial telephone contact, researchers gave a full explanation of the study rationale, relevant approvals and the nature of staff involvement. Written consent from staff was obtained by researchers in each case, prior to participation. Approval from the NIGB under Section 251 of the NHS Act 2006297 to access information about NHS service users without their consent was sought and granted.
A trial of consent was undertaken at two sampled MSUs (Edenfield, Manchester, and Guild Lodge, Preston). Details of the study and expectations of participants were explained to service users approaching discharge (on pre-discharge wards). The study was described as one that might be carried out in the future and it was suggested to service users that they were being asked if they would consider taking part if it were to go ahead, rather than actually committing to participation in an active study. They were then given the opportunity to think about whether or not they would participate and asked to indicate this on a consent form, which was posted back to the research team once completed. The response rate to the trial was rather low. Of 24 pre-discharge service users identified at the Edenfield, five replied (four gave consent and one refused). Of 70 potential participants at Guild Lodge, eight replied (two gave consent and six refused). The research team felt that the trial confirmed the need for Section 251 approval to achieve a full and meaningful sample.
Pathways
While engaging with all of the NHS MSSs throughout England and Wales for the purposes of this research, it became apparent that the structure of these services is very complex. For example, many MSUs have the equivalent of low secure care and even open units integrated into their MSS pathway. At these units, service users therefore pass through low secure wards or areas as part of their care pathway to the community. These service users, on discharge to the community, were recruited to the study as MSU discharges, even though they had left the equivalent of low secure and/or open unit care. In contrast, other MSUs have only medium secure provisions on site. They then have LSUs on separate sites to which they regularly discharge service users as part of their care pathway, in preparation for moving on to the community. Therefore, to be consistent in recruitment and standardise the sample, it became clear that those who were discharged from a MSS pathway, rather than just from a MSU, needed to be included. In some cases, this meant recruiting at the point of LSU discharge to the community, where service users had transferred from medium secure services. Only then could all service users be captured at a similar point along their pathway from medium secure care.
Individual service care pathways
Pathways were identified using a questionnaire that was sent out to link people at all participating MSUs. This enabled mapping of the regular care pathways of each service and specifically identified LSUs that formed part of those care pathways. Results from all sites were collated and half of these low secure sites were selected for inclusion, using a stratified sampling technique. The LSUs were stratified according to the nature of their relationship to the MSU: whether they were one of many LSUs that a MSU regularly discharged to or a single LSU to which most MSU service users were discharged as part of the main care pathway. Within these groups, LSUs were further stratified by their size according to the number of beds at both their unit and the MSU that discharged to them. Within these groups, 50% (n = 21) were then randomly sampled for inclusion in the study. Only 50% were included for practical reasons relating to resources and logistics. For those units selected, the relevant site approvals were sought.
Participants were deemed eligible for inclusion if they had been discharged from one of the sampled pathway LSUs to the community during the recruitment phase and had been in conditions of medium security immediately before being in the LSU, without interruption. Therefore, LSU service users had to be on a clear care pathway directly from medium secure services and be discharged to the community to be eligible.
Participants were discharged to one of four types of location: high secure unit (HSU), LSU, prison [Her Majesty’s Prison (HMP)] or the community and/or step-down open units. Therefore, all discharge locations other than a HSU, LSU or HMP were the community and/or step-down open units. As such, the definition of ‘community’ encompassed various types of accommodation, including independent tenancies, supported accommodation, hostels, open rehabilitation wards and open psychiatric units.
Procedure
The prospective cohort follow-up design was modelled on a similar study conducted by Doyle and Dolan321 although all discharges were included in the study regardless of consent.
A link person was identified at each of the participating sites. These link people acted as single points of contact throughout the course of the study and worked with the research team to develop a discharge notification system. In the majority of cases, this involved an e-mail from the link person to the study team with the date and destination of discharge, followed by a telephone call to supply identifiable details. At the end of the recruitment phase, participating units were asked to supply a list of all service users discharged during the 12-month period. Researchers then used this information to check that no one eligible for inclusion in the study had been missed and that no service users with multiple discharges had been duplicated in the sample. Research staff received training in the application of the relevant risk assessment tools from qualified trainers. Inter-rater reliability checks were conducted throughout the data collection period to ensure the correct application of the measures, accuracy of ratings and consistency between the ratings of researchers.
Part 1: baseline
Time of discharge acted as baseline. There were 788 discharges in the 12-month baseline period. All service users discharged into the community were assessed at baseline (n = 409). Baseline assessments consisted of:
-
an interview with a member of staff who knew the service user well in the 6 months prior to discharge
-
a review of each individual’s full historical clinical record.
Interviews were organised and conducted over the telephone whereas records were reviewed at the relevant unit in person by research staff. The link people helped to facilitate this process.
Measures
Demographic and personal information was collected for each individual in relation to sex, age, ethnicity, index offence, primary mental health diagnosis, length of stay as an inpatient and medication.
Information required to rate the following measures was also gleaned from a combination of the interview and a review of records.
Medium Secure Recidivism Assessment Guide
The MSRAG is a 12-item actuarial violence risk assessment tool designed in two parts to predict acquisitive recidivism and serious offending recidivism by service users discharged into the community for up to 2 years post discharge. 22 Each of the items has a simple 3-point rating (–1, 0, +1) or binary rating (–1, +1).
Historical, Clinical, Risk Management-20 items
The HCR-20v2 is a broadband violence risk assessment instrument with potential applicability to a variety of settings. 287 The conceptual scheme of the HCR-20 aligns risk markers into past, present and future. Ten historical factors relate to the past, five clinical items reflect current dynamic correlates of violence and five risk management items focus on situational post-assessment factors that may increase or mitigate risk. The items are scored as 0, 1 or 2. The HCR-20 is sensitive to change as the C (clinical) and R (risk) items are dependent on current functioning and context and act as a risk barometer. It has proved reliable in numerous research studies.
The HCR-20v3 is in the latter stages of validation and this study is expected to contribute to the evidence base for the instrument. Version 3 retains many of the core features of version 2 but changes have been made to the structure to reflect updates in professional and empirical literature and to improve the application of the instrument in clinical practice. Version 3 includes some new items of which several have been subdivided into multiple parts. This means the rater needs to consider 41 items in total. Item indicators are also provided to improve the reliability of the ratings and a relevance rating is now provided to allow the rater to code whether the risk factor is causally relevant to the risk of violence. The completion of a psychopathy checklist is no longer required for the HCR-20v3.
The Structured Assessments of Protective Factors for Violence Risk
The SAPROF is a guideline developed to measure protective factors for violence risk. 347 There are 17 items covering internal, motivational and external factors that have been found to protect against violence. Each item is rated on a 3-point scale (0, 1 or 2) and scores range between 0 and 34.
Positive and Negative Syndrome Scale
The Positive and Negative Syndrome Scale (PANSS) is a 30-item rating instrument that evaluates the presence/absence and severity of positive, negative and general psychopathology of schizophrenia. 357 All 30 items are rated on a 7-point scale (1 = absence, 7 = extreme).
The PANSS was completed using information gathered from the staff interview and records review about the behaviour of the service users 1 month prior to discharge.
An interview schedule was devised that included each item of the SAPROF, HCR-20v3 and PANSS. Researchers explained each tool, item and the range of response options to participating staff, who then provided a rating on each item or subitem based on their knowledge of the service user in the 6 months prior to discharge (or 1 month for the PANSS). This method was based on previous research357 in which measures such as the Brief Psychiatric Rating Scale (BPRS) have been reliably rated using only information from collateral informants, their observations of service users and information noted in case records. This was, therefore, considered acceptable for research purposes. 300,358
Data collection sheets similar to the interview schedule (but with the addition of the MSRAG and historical items of the HCR-20v3) were used to gather information and record ratings during the review of clinical records and the PNC by researchers.
Two scores per service user were generated, from the record review and the interview with the collateral informant. A composite score for each subitem and item was created in each case, based on the presence or absence from one or both information sources.
The Hare Psychopathy Checklist: Screening Version
The Hare Psychopathy Checklist: Screening Version (PCL:SV) has 12 items divided into two subsections: part 1 – interpersonal and affective; and part 2 – social deviance. 359 Each item is scored on a 3-point scale (0 = no/item does not apply, 1 = maybe applies and 2 = yes, definitely applies). Total scores can range from 0 to 24.
The PCL:SV was rated once for all cases at 12 months’ follow-up as recommended in a previous study of this type,360 using information from both the interview and the records review. At interview, questions usually asked of the service user under assessment were asked of the collateral informant in relation to the service user instead.
Part 2: follow-up
All participants who were discharged to the community at baseline (n = 409) were eligible to be followed up at 6 and 12 months post discharge. The follow-up assessments consisted of a review of clinical records and an interview with the community social supervisor or care co-ordinator involved in each individual’s care during the relevant 6-month period.
Community follow-up measures
Violence
Violence was defined by combining the definitions of ‘violence’ and ‘other aggressive acts’ from the MCVI. Therefore, the definition of violence in this study included ‘sexual assaults; assaultive acts that involved the use of a weapon; or threats made with a weapon in hand’ (pp. 154–5),300 as well as all acts of battery, regardless of whether or not they resulted in injury. Verbal threats alone were not included.
MacArthur Community Violence Instrument
The MCVI consists of 18 questions focusing on violent incidents. 300 Information gathered includes acts committed by the individual in question and acts committed by others in which he or she was the aggrieved or injured party.
Mental health staff completed the MCVI within the follow-up interview and this was used to detect any violent incidents in which service users were involved as either victims or perpetrators. If a violent incident had taken place, further details were gathered in relation to the following: when it happened in relation to discharge; the type of location; the relationship between those involved; and whether or not anyone involved was known to have been using substances or to have been symptomatic at the time of the incident.
Details of any violent incidents found during the review of clinical records were also noted and cross-matched with information obtained at interview.
Police National Computer
Details of criminal convictions in the 12 months post discharge were gathered using data from the PNC. Data provided included offence dates and a brief description of what had actually happened to constitute the offence. This enabled researchers to detect violent convictions or cautions relating only to offences actually committed in the relevant follow-up period that fit the definition of violence. Information from all sources (interview, notes and PNC) was combined to ascertain whether or not participants had been involved in any violent incidents during follow-up.
Community support
Detailed information about the frequency and type of informal and professional support available to each individual was gathered at interview and during the clinical records review. A formula developed in a previous study332 was then used to quantify levels of community support available in each case. Staff from mental health and social services who were in direct contact with the service users in the community were assigned a value depending on their role and responsibilities, with the highest value being assigned to the most highly qualified member of staff and vice versa (i.e. responsible medical officers = 3; other mental health professionals, e.g. community psychiatric nurses and social workers = 2; and unqualified support staff = 1). The frequency of contact was also assigned a value, with higher frequencies being given higher scores (e.g. less than monthly = 1, daily = 7). An overall score for community support for each service user was then calculated by multiplying the frequency value by the value assigned to each person and totalling for all staff in contact with each service user.
Information on current medication, treatment in the community and current living situation was ascertained at 6 and 12 months post discharge for each participant, alongside details of employment, structured activities, substance use, contact with criminal justice agencies and any periods of rehospitalisation. The same information was sought from both sources.
Analysis
Data were analysed using IBM SPSS for Windows version 20 (IBM Corporation, Armonk, NY, USA) and Stata.
Descriptive statistics of age, sex, ethnic origin, diagnoses, source of referral and legal status are reported. T-tests were used to investigate the difference in mean scores on the various scales between violent and non-violent groups. Chi-squared analyses were used to establish differences in prevalence of violence post discharge based on sex, legal status, ethnic origin, history of substance use, diagnosis of major mental illness or diagnosis of personality disorder. ROC analyses were used to examine the predictive validity of instruments. Pearson’s r correlation coefficient was used to specify the correlation between ratings on measures and the frequency of violence. Logistic regression analysis was used to examine the effect of sex and age on the association of the measures with violence.
Inter-rater reliability
The inter-rater reliability on all scales was calculated between four raters on 20 cases. Each of the raters was trained and supervised in the use of all scales. The intraclass correlations are provided in Table 97 and demonstrate a high level of agreement on all scales and subscales.
Scale | Intraclass correlation average measuresa | Intraclass correlation single measuresa |
---|---|---|
HCR-20v3 | ||
Total | 0.916 | 0.732 |
Historical total | 0.910 | 0.716 |
Clinical total | 0.900 | 0.692 |
Risk management total | 0.926 | 0.757 |
SAPROF | ||
Total | 0.980 | 0.925 |
Internal | 0.957 | 0.848 |
Motivational | 0.977 | 0.913 |
External | 0.904 | 0.702 |
MSRAG | ||
Total | 0.959 | 0.855 |
Acquisitive | 0.971 | 0.893 |
Serious | 0.932 | 0.775 |
PANSS total | 0.932 | 0.775 |
Results
There were 788 eligible service users discharged during the study period, of whom 409 (52%) were discharged to the community, 197 (25%) were discharged to low security, 159 (20%) were discharged to prison and 23 (3%) were discharged to high security.
Sample
The sample for this study included all those discharged to community settings. The frequency of discharge by originating MSU is shown in Table 98.
Unit name | n (%) |
---|---|
North London Forensic Service | 28 (6.8) |
Bracton Centre | 32 (7.8) |
River House | 26 (6.4) |
Bridge House | 6 (1.5) |
Three Bridges | 17 (4.2) |
Orchard | 5 (1.2) |
John Howard Centre | 21 (5.1) |
Shaftesbury Clinic | 7 (1.7) |
Ashen Hill | 12 (2.9) |
Brockfield House | 5 (1.2) |
Trevor Gibbens Unit | 9 (2.2) |
Norvic Clinic | 14 (3.4) |
Ravenswood | 21 (5.1) |
Marlborough House | 7 (1.7) |
Oxford Clinic | 3 (0.7) |
Butler Clinic | 6 (1.5) |
Fromeside | 14 (3.4) |
Caswell Clinic | 9 (2.2) |
Hatherton Centre | 12 (2.9) |
Reaside | 47 (11.5) |
Ardenleigh | 3 (0.7) |
Arnold Lodge | 9 (2.2) |
Bamburgh | 9 (2.2) |
Roseberry Park/Hutton Centre | 7 (1.7) |
Humber Centre | 2 (0.5) |
Green Trees | 2 (0.5) |
Newton Lodge | 7 (1.7) |
Wathwood | 3 (0.7) |
Edenfield Centre | 22 (5.4) |
Guild Lodge | 24 (5.9) |
Ty Llwellyn | 5 (1.2) |
Scott Clinic | 15 (3.7) |
Numbers of discharges varied from unit to unit. The greatest number of discharges was from Reaside (Birmingham and Solihull NHS Foundation Trust, n = 47), with the fewest from Green Trees and the Humber Centre (Humber Mental Health Teaching NHS Trust, n = 2). Units located in the vicinity of large cities had the greatest discharge numbers, with four London-based units discharging 20+ service users within the study period (North London Forensic Service, Bracton Centre, River House and John Howard Centre) alongside Ravenswood (Portsmouth), Guild Lodge (Preston) and the Edenfield Centre (Manchester).
Sample characteristics
General demographic information was gathered for the 409 service users who were discharged to community settings. The characteristics of the sample are provided in Table 99.
Variable | Variable type | n (%) |
---|---|---|
Age (years) | Mean (SD), median | 37.63 (9.74), 38 |
Male median | 38 | |
Female median | 39 | |
Length of stay (days) | Mean (SD), median, min.–max. | 879.11 (974.45), 391, 7–7299 |
Sex | Male | 365 (89.2) |
Female | 44 (10.8) | |
Ethnic origin | White | 244 (59.7) |
Asian | 24 (5.9) | |
Mixed heritage | 27 (6.6) | |
Black | 104 (25.4) | |
Chinese or other | 1 (0.2) | |
Unknown | 9 (2.2) | |
Primary mental health diagnosisa | Schizophrenia | 267 (65.3) |
Schizoaffective disorder | 52 (12.7) | |
Mania/bipolar | 29 (7.1) | |
Anxiety disorder | 3 (0.7) | |
Personality disorder | 7 (1.7) | |
Substance use | 1 (0.2) | |
Depression | 5 (1.2) | |
No diagnosis | 19 (4.6) | |
Other diagnoses | 26 (6.4) | |
Legal status | Restricted (37/41) | 178 (43.5) |
Community treatment order | 118 (28.9) | |
Section 3 | 6 (1.5) | |
Absolute discharge | 2 (0.5) | |
Informal | 24 (5.9) | |
Other | 9 (2.2) | |
Unknown | 72 (17.6) | |
Source of referral | Community | 83 (20.3) |
Prison | 161 (39.4) | |
Non-forensic | 36 (8.8) | |
HSU | 29 (7.1) | |
MSU | 56 (13.7) | |
LSU | 10 (2.4) | |
A&E | 3 (0.7) | |
Other | 28 (6.8) | |
Unknown | 3 (0.7) |
Community settings
Those discharged to the community went to a range of settings with varied levels of professional support available. Of these, 123 (30.1%) were discharged to independent addresses, 240 (58.7%) to staffed supported accommodation and 46 (11.2%) to other open units.
Follow-up
At 6 months post discharge, the follow-up collateral interview and notes review were completed for 387 (95%) of 409 discharges, with 22 participants lost at this stage. At 12 months post discharge, 344 (89%) of the remaining 387 participants were successfully followed up, with a further 43 lost.
Prevalence of community violence over 6 months post discharge
Prevalence of community violence was obtained from three sources: the PNC, the MCVI interview with the collateral informant and the review of clinical records. PNC data were requested for all of the community sample and 369 records (90.2%) were successfully reviewed, with the remainder unable to be traced using the details available.
Information from all sources was combined to detect violent incidents that met the study operational definition of violence. At 6 months post discharge, 54 of 387 participants (14.0%) successfully followed up had committed at least one violent incident. According to the PNC alone, only six (1.6%) were violent; in all cases in which violence was detected by the PNC it was also detected by either interview with the collateral informant or case record review.
Non-violent individuals tended to be older (mean 38.42 years, SD 9.73 years) than violent individuals (mean years 34.28, SD 8.69 years) and this difference was significant (p = 0.003). There was also a significant difference in prevalence of violence based on length of stay (p = 0.018). Those who were not violent during follow-up had been resident in medium secure care for longer before discharge (mean 957.59 days, SD 1018.74 days) than those who were violent (mean 615.44 days, SD 701.63 days).
As shown in Table 100, a greater proportion of men than women were violent but this result was not significant. There was, however, a significant difference in prevalence of violence based on legal status. A smaller proportion of those conditionally discharged under Section 37/41 (Mental Health Act 1983361) were violent than those discharged under other sections.
Variable | Violent (n = 54), n (%) | Non-violent (n = 333), n (%) | χ2 | df | p-value |
---|---|---|---|---|---|
Sex | |||||
Male | 51 (14.8) | 293 (85.2) | 1.96 | 1 | 0.161 |
Female | 3 (7.0) | 40 (93.0) | |||
Diagnosis | |||||
Major mental illness | 49 (13.7) | 309 (86.3) | 0.28 | 1 | 0.595 |
No major mental illness | 5 (17.2) | 24 (82.8) | |||
Ethnicity | |||||
White British/Irish/any other white background | 28 (12.0) | 205 (88.0) | 1.83 | 1 | 0.176 |
Other ethnic groups | 26 (16.9) | 128 (83.1) | |||
Personality disorder | |||||
History of personality disorder | 12 (15.4) | 66 (84.6) | 0.167 | 1 | 0.683 |
No history of personality disorder | 42 (13.6) | 267 (86.4) | |||
Legal status at discharge | |||||
Section 37/41 | 17 (9.7) | 159 (90.3) | 4.958 | 1 | 0.026 |
Other | 37 (17.5) | 174 (82.5) | |||
Substance use | |||||
History of serious substance use | 38 (14.8) | 219 (85.2) | 0.442 | 1 | 0.506 |
No history of serious substance use | 16 (12.3) | 114 (87.7) |
Of 54 individuals who were violent by 6 months post discharge, information from a collateral informant was available in 43 cases. Just over 42% of these 43 cases were described as symptomatic at the time of the incident, with 58% not experiencing symptoms of mental illness at the time, according to the interviewee.
Prevalence of community violence over 12 months post discharge
The same procedure was applied to detect the prevalence of violence by 12 months post discharge. By this stage, 87 (22.5%) participants had been violent according to information gathered from interview and file review (Table 101). Again, the PNC level of detection was lower, with only 11 (2.8%) cases having recorded violent convictions or cautions. However, in three cases, the violent incidents on the PNC had not been detected by interview or case record review. In addition, interestingly, the PNC detected violence committed by one individual who was not able to be followed up by the study team as they had disengaged with services.
Variable | Violent (n = 54), n (%) | Non-violent (n = 333), n (%) | χ2 | df | p-value |
---|---|---|---|---|---|
Sex | |||||
Male | 81 (23.5) | 263 (76.5) | 2.02 | 1 | 0.155 |
Female | 6 (14.0) | 37 (86.0) | |||
Diagnosis | |||||
Major mental illness | 81 (22.6) | 277 (77.4) | 0.06 | 1 | 0.810 |
No major mental illness | 6 (20.7) | 23 (79.3) | |||
Ethnicity | |||||
White British/Irish/any other white background | 47 (20.2) | 186 (79.8) | 1.79 | 1 | 0.181 |
Other ethnic groups | 40 (26.0) | 114 (74.0) | |||
Personality disorder | |||||
History of personality disorder | 17 (21.8) | 61 (78.2) | 0.03 | 1 | 0.871 |
No history of personality disorder | 70 (22.7) | 239 (77.3) | |||
Legal status at discharge | |||||
Section 37/41 | 27 (15.3) | 149 (84.7) | 9.44 | 1 | 0.002 |
Other | 60 (28.4) | 151 (71.6) | |||
Substance use | |||||
History of serious substance use | 62 (24.1) | 195 (75.9) | 1.19 | 1 | 0.276 |
No history of serious substance use | 25 (19.2) | 105 (80.8) |
Differences between the violent and non-violent groups based on age and length of stay remained significant at 12 months post discharge. The non-violent group was again older on average (mean 39.08, SD 9.50 years) than the violent group (mean 33.56 years, SD 9.13 years) (p < 0.001). Similarly, those who were not violent had remained as an inpatient for longer before discharge (mean 1003.99 days, SD 1051.10 days) than those who were violent (mean 585.25 days, SD 628.13 days) (p < 0.001).
Table 101 shows that a significant difference in the prevalence of violence based on legal status remained at 12 months post discharge. Again, a smaller proportion of those conditionally discharged under Section 37/41 (Mental Health Act 1983361) were violent than those discharged under other sections. No significant differences in violent outcome were identified in relation to sex, ethnicity, history of serious substance use, diagnosis of major mental illness or presence of personality disorder.
By 12 months post discharge, information from a collateral informant was available for 64 of the 87 violent individuals. At this stage, 47% were described as symptomatic at the time of the incident.
Frequency of violence during the first 6 months post discharge
The 54 people who were violent in the first 6 months following discharge committed a total of 99 violent acts. Thirty-three people had committed a single act of violence and the highest number of violent acts committed by a single person was eight.
Frequency of violence by 12 months post discharge
By 12 months’ follow-up, the 87 people who had been violent had committed a total of 176 violent acts. Fifty-one people had committed a single act of violence, with the highest number of acts committed by a single person rising to 12.
The association between frequency of post-discharge violence at 6 months and 12 months and scores on all scales and subscales was investigated. There was a significant correlation between all of the scales and post-discharge violence at 6 months. The SAPROF total score had the strongest correlation at 6 months. At 12 months all scales were significantly correlated with post-discharge violence except for the PANSS negative subscale. The SAPROF total score was again the strongest correlate with violence compared with other scales (Table 102).
Scale | Violence at 6 months post discharge | Violence at 12 months post discharge |
---|---|---|
HCR-20v3 | ||
Total | 0.231*** | 0.243*** |
Historical | 0.144** | 0.139** |
Clinical | 0.218*** | 0.243*** |
Risk management | 0.181*** | 0.194*** |
SAPROF | ||
Total | –0.318*** | –0.311*** |
Internal | –0.262*** | –0.270*** |
Motivational | –0.278*** | –0.271*** |
External | –0.204*** | –0.187*** |
MSRAG | ||
Acquisitive offending | 0.123* | 0.140** |
Serious offending | 0.105* | 0.116* |
PANSS | ||
Total | 0.199*** | 0.177*** |
Positive | 0.162*** | 0.152** |
Negative | 0.115** | 0.088 |
General | 0.176** | 0.153** |
Aggression | 0.296*** | 0.288*** |
Severity of violence
All violent incidents recorded complied with our operational definition of violence. The majority of incidents detected using the MCVI during interview with a collateral informant were of a less serious nature. Of 112 incidents detected at 12 months’ follow-up using this method, only 16 were categorised as hitting someone with a fist or beating them up and eight related to the use of knives, guns or lethal weapons. The remainder (78.6%) therefore fell into categories covering slaps, kicks and bites. No incidents of homicide or acts of a violent sexual nature were detected by interview.
The 11 people who committed violent offences during follow-up according to the PNC were convicted of a total of 28 such offences. The most severe was an individual convicted of nine violent offences, which included five counts of rape and one of grievous bodily harm (GBH) with intent. All nine offences related to the same episode of violence. Five of the 11 committed common assault or battery. Other offences included were incidents of kidnap, false imprisonment, robbery and affray and violent disorder, in which threats were made with a weapon in hand during the commission of the offence.
Risk assessment measures and violence at 6 months post discharge
Those in the violent group scored significantly higher than those in the non-violent group across all of the subscales and on the overall total score for the HCR-20v3, MSRAG and PANSS (Table 103). Likewise, those in the violent group scored significantly lower than those in the non-violent group on all of the subscales and the overall total score of the SAPROF.
Scale | Violent, mean (SD) | Non-violent, mean (SD) | t | df | p-value | Mean difference | 95% CI |
---|---|---|---|---|---|---|---|
HCR-20v3 | |||||||
Total | 26.56 (5.10) | 21.75 (6.39) | –5.25 | 385 | < 0.001 | –4.80 | –6.59 to –3.01 |
Historical | 14.39 (3.22) | 12.91 (3.70) | –2.77 | 385 | 0.006 | –1.48 | –2.52 to –0.43 |
Clinical | 6.70 (2.27) | 4.48 (2.63) | –5.87 | 385 | < 0.001 | –2.22 | –2.97 to –1.48 |
Risk management | 5.46 (1.99) | 4.36 (2.04) | –3.71 | 385 | < 0.001 | –1.10 | –1.69 to –0.52 |
SAPROF | |||||||
Total | 20.94 (4.44) | 25.22 (4.69) | 6.25 | 385 | < 0.001 | 4.27 | 2.93 to 5.62 |
Internal | 5.87 (1.96) | 7.17 (1.77) | 4.93 | 385 | < 0.001 | 1.30 | 0.78 to 1.82 |
Motivational | 8.57 (2.74) | 10.85 (2.67) | 5.78 | 385 | < 0.001 | 2.27 | 1.50 to 3.05 |
External | 6.50 (1.19) | 7.20 (1.44) | 3.39 | 385 | 0.001 | 0.70 | 0.29 to 1.10 |
MSRAG | |||||||
Acquisitive offending | 6.93 (0.28) | 6.10 (0.11) | –2.71 | 384 | 0.007 | –0.83 | –1.43 to –0.23 |
Serious offending | 6.19 (0.28) | 5.42 (0.11) | –2.66 | 384 | 0.008 | –0.76 | –1.33 to –0.20 |
PANSS | |||||||
Total | 69.15 (28.77) | 54.04 (18.90) | –5.01 | 385 | < 0.001 | –15.11 | –21.03 to –9.18 |
Positive | 14.89 (7.46) | 11.18 (5.22) | –4.53 | 385 | < 0.001 | –3.71 | –5.32 to –2.10 |
Negative | 14.24 (7.54) | 12.30 (5.97) | –2.13 | 385 | 0.034 | –1.94 | –3.73 to 0.15 |
General | 31.98 (12.39) | 25.62 (8.52) | –4.74 | 385 | < 0.001 | –6.36 | –9.00 to –3.72 |
Aggression | 8.04 (4.40) | 4.94 (2.58) | –7.30 | 385 | < 0.001 | –3.10 | –3.94 to –2.27 |
Risk assessment measures and violence at 12 months post discharge
Table 104 shows that, in line with the findings at 6 months post discharge, there were significant differences in scores on all of the subscales and the total scores for the HCR-20v3, SAPROF and MSRAG between violent and non-violent groups. Those who were violent scored significantly higher for risk factors and significantly lower for protective factors. Those who were violent scored higher on mental state symptoms across all subscales of the PANSS with the exception of the negative symptoms subscale.
Scale | Violent, mean (SD) | Non-violent, mean (SD) | t | df | p-value | Mean difference | 95% CI |
---|---|---|---|---|---|---|---|
HCR-20v3 | |||||||
Total | 25.75 (5.72) | 21.46 (6.32) | –5.68 | 385 | < 0.001 | –4.29 | –5.77 to –2.80 |
Historical | 14.31 (3.23) | 12.77 (3.72) | –3.49 | 385 | 0.001 | –1.54 | –2.40 to –0.67 |
Clinical | 6.24 (2.48) | 4.37 (2.60) | –5.96 | 385 | < 0.001 | –1.87 | –2.49 to –1.25 |
Risk management | 5.20 (2.07) | 4.32 (2.02) | –3.55 | 385 | < 0.001 | –0.88 | –1.37 to –0.39 |
SAPROF | |||||||
Total | 22.08 (5.18) | 25.36 (4.54) | 5.74 | 385 | < 0.001 | 3.28 | 2.15 to 4.40 |
Internal | 6.23 (1.93) | 7.21 (1.78) | 4.45 | 385 | < 0.001 | 0.98 | 0.55 to 1.41 |
Motivational | 9.23 (2.97) | 10.91 (2.62) | 5.09 | 385 | < 0.001 | 1.68 | 1.03 to 2.32 |
External | 6.62 (1.45) | 7.24 (1.39) | 3.63 | 385 | < 0.001 | 0.62 | 0.28 to 0.96 |
MSRAG | |||||||
Acquisitive offending | 6.98 (0.22) | 5.99 (0.12) | –3.93 | 384 | < 0.001 | –0.98 | –1.48 to –0.49 |
Serious offending | 6.22 (0.20) | 5.33 (0.11) | –3.77 | 384 | < 0.001 | –0.89 | –1.33 to –0.20 |
PANSS | |||||||
Total | 64.55 (26.23) | 53.71 (18.83) | –4.30 | 385 | < 0.001 | –10.84 | –15.80 to –5.88 |
Positive | 13.89 (6.77) | 11.06 (5.22) | –4.14 | 385 | < 0.001 | –2.83 | –4.17 to –1.48 |
Negative | 13.61 (7.08) | 12.27 (5.96) | –1.77 | 385 | 0.078 | –1.34 | –2.83 to 0.15 |
General | 29.91 (11.39) | 25.53 (8.51) | –3.90 | 385 | < 0.001 | –4.38 | –6.59 to –2.17 |
Aggression | 7.15 (3.95) | 4.85 (2.57) | –6.42 | 385 | < 0.001 | –2.30 | –3.00 to –1.59 |
Predictive validity of measures
Table 105 shows that all of the risk subscales and total scores significantly predicted 6-month post-discharge violence, apart from the PANSS negative symptoms subscale. The SAPROF total scale and motivational subscale, the HCR-20v3 total scale and clinical subscale and PANSS aggression subscale had the highest AUC scores.
Scale | AUC | SE | p-value | 95% CI |
---|---|---|---|---|
HCR-20V3 | ||||
Total | 0.729 | 0.035 | < 0.001 | 0.660 to 0.799 |
Historical | 0.627 | 0.037 | 0.003 | 0.553 to 0.700 |
Clinical | 0.741 | 0.034 | < 0.001 | 0.674 to 0.809 |
Risk management | 0.666 | 0.038 | < 0.001 | 0.592 to 0.740 |
SAPROF | ||||
Total | 0.763 | 0.030 | < 0.001 | 0.705 to 0.822 |
Internal | 0.691 | 0.039 | < 0.001 | 0.616 to 0.767 |
Motivational | 0.740 | 0.032 | < 0.001 | 0.678 to 0.802 |
External | 0.661 | 0.037 | < 0.001 | 0.588 to 0.733 |
MSRAG | ||||
Acquisitive offending | 0.611 | 0.042 | 0.008 | 0.528 to 0.693 |
Serious offending | 0.605 | 0.044 | 0.015 | 0.520 to 0.691 |
PANSS | ||||
Total | 0.678 | 0.042 | < 0.001 | 0.597 to 0.760 |
Positive | 0.676 | 0.040 | < 0.001 | 0.599 to 0.754 |
Negative | 0.570 | 0.046 | 0.099 | 0.480 to 0.660 |
General | 0.675 | 0.039 | < 0.001 | 0.598 to 0.753 |
Aggression | 0.723 | 0.042 | < 0.001 | 0.641 to 0.806 |
At 12 months post discharge, the PANSS negative symptoms subscale remained the only measure that did not significantly predict violence. All of the other subscales showed AUC values of > 0.6 and were significant (Table 106).
Scale | AUC | SE | p-value | 95% CI |
---|---|---|---|---|
HCR-20v3 | ||||
Total | 0.703 | 0.032 | < 0.001 | 0.640 to 0.766 |
Historical | 0.626 | 0.032 | < 0.001 | 0.563 to 0.690 |
Clinical | 0.703 | 0.031 | < 0.001 | 0.641 to 0.764 |
Risk management | 0.628 | 0.033 | < 0.001 | 0.563 to 0.693 |
SAPROF | ||||
Total | 0.693 | 0.031 | < 0.001 | 0.632 to 0.754 |
Internal | 0.648 | 0.031 | < 0.001 | 0.583 to 0.713 |
Motivational | 0.673 | 0.034 | < 0.001 | 0.613 to 0.733 |
External | 0.623 | 0.031 | < 0.001 | 0.556 to 0.690 |
MSRAG | ||||
Acquisitive offending | 0.633 | 0.034 | < 0.001 | 0.566 to 0.699 |
Serious offending | 0.628 | 0.034 | < 0.001 | 0.562 to 0.694 |
PANSS | ||||
Total | 0.640 | 0.035 | < 0.001 | 0.571 to 0.709 |
Positive | 0.649 | 0.033 | < 0.001 | 0.583 to 0.714 |
Negative | 0.554 | 0.037 | 0.132 | 0.481 to 0.626 |
General | 0.630 | 0.035 | < 0.001 | 0.561 to 0.698 |
Aggression | 0.685 | 0.033 | < 0.001 | 0.616 to 0.755 |
Controlling for age and sex
The scales were further analysed using logistic regression to investigate the univariate association of each of them for post-discharge violence at 12 months. The procedure was repeated with age and sex added as covariates to see if the scales remained associated with violence. Table 107 shows the ORs for the measures before and after age and sex were added. All of the scales remained associated with violence when age and sex were controlled for.
Scale | Step 1: univariate analysis | Step 2: covariates age and sex added | ||||
---|---|---|---|---|---|---|
OR | 95% CI | p-value | AOR | 95% CI | p-value | |
HCR-20v3 | 1.126 | 1.017 to 1.176 | < 0.001 | 1.122 | 1.012 to 1.174 | < 0.001 |
MSRAG acquisitive offending | 1.270 | 1.122 to 1.438 | < 0.001 | 1.173 | 1.028 to 1.338 | 0.017 |
MSRAG serious offending | 1.267 | 1.115 to 1.438 | < 0.001 | 1.154 | 1.006 to 1.323 | 0.040 |
SAPROF | 0.875 | 0.832 to 0.920 | < 0.001 | 0.864 | 0.818 to 0.913 | < 0.001 |
PANSS total | 1.022 | 1.011 to 1.033 | < 0.001 | 1.026 | 1.014 to 1.037 | < 0.001 |
Professional support
A calculation was used in this study to record the level of professional support received by discharged service users in the community. A higher score for professional contact indicated a greater frequency of contact with mental health professionals or contact with more senior staff or a combination of the two.
Mean scores for professional support were not significantly different between the violent (mean 16.45, SD 6.82) and non-violent (mean 15.47, SD 6.11) groups at 6 months post discharge [t (377) = –1.08; p = 0.281].
Similarly, mean scores for professional support were not significantly different between the violent (mean 13.78, SD 6.53) and non-violent (mean 13.90, SD 6.18) groups at 12 months post discharge [t (335) = –0.135, p = 0.892]. However, the mean score had decreased at 12 months for both the violent group and the non-violent group, demonstrating that level and frequency of contact with professionals had reduced between the two time points. The violent group had a higher average score than the non-violent group at 6 months’ follow-up. By 12 months post discharge, the average scores were very similar but the non-violent group scored higher, although the difference was not significant.
Social support
One of the protective factors in the SAPROF relates to the presence of prosocial support for the service user. Of 409 service users discharged to the community, 229 (56%) had prosocial support that was deemed to be ‘clearly present’. The remaining 180 (44%) did not have support or had support that was only partially or somewhat present. Chi-square analysis showed that a significantly greater proportion of those without tangible prosocial support than those with prosocial support were violent in the 6 months following discharge (n = 31, 18.6% vs. n = 23, 10.5%; p = 0.023). By 12 months there was no significant difference in prevalence of violence based on the presence of social support (n = 123, 74.3% vs. n = 176, 80.0%; p = 0.180).
Discussion
This is the first prospective study based on a whole cohort of discharged service users from NHS MSSs in England and Wales. Comparisons over time can be drawn using the most recent similar study of all discharges from MSUs from 1 April 1997 to 31 March 1998. 333
The sample size in this study was smaller, with fewer people discharged during the 12-month recruitment period: 788 compared with 959. 333 This could be because slightly fewer units participated in this study (as independent units were excluded), but it is also of note that length of stay prior to discharge was considerably longer in this sample (median 391 days vs. 206 and 259 days for men and women respectively362). Service users were also older at discharge overall in this study. Bed numbers in MSUs have increased significantly since 1998 and these findings therefore indicate that MSUs are detaining service users for longer. 363
Of particular interest are the differences in the pathways out of medium secure services. In previous studies, approximately 10% of all MSU discharges went to prison,362,364 whereas in this study the figure was 20%. This may reflect developments in the provision of mental health care within prison environments and therefore greater opportunities for individuals with mental illness to receive treatment in these settings. 365 Future research should seek to investigate this pathway further, including implications for standards of care, costs and outcomes on release from prison.
During the recruitment phase it also became apparent that the structure of the national medium secure estate is very complex, with some ‘medium secure’ services incorporating wards, units or even houses in their grounds, with conditions reflecting those of low secure or open units. When present, these areas appear to serve a rehabilitative purpose, preparing service users for discharge directly to community locations without the need for a ‘step-down’ facility. The development of such facilities could provide a further explanation for the increase in average length of stay, as service users remain classified as being within medium secure care throughout their admission, even if they spend the latter period of their stay in low secure or open conditions.
The care pathways described by MSUs without these provisions usually involved discharge to one or several LSUs, with service users no longer being MSS users on discharge. Definitions are therefore somewhat blurred as to what constitutes a MSU or a LSU and at what point a service user ceases to become a MSS user. The presence and utilisation of ‘open’ rehabilitation units, and a host of other terms such as ‘ward in the community’ and ‘supported accommodation’, serve to potentially confuse matters further. A review of secure services is therefore recommended, with the aim of clarifying, defining and perhaps standardising the various pathways and terminology employed. Service users, their families, researchers and mental health-care providers could all benefit from a clearer understanding of the service pathway and specific milestones. 366
Prevalence of violence
The prevalence of violence in this study was considerably higher than that found in studies that relied on official records only. 333,367 PNC records detected only a 2.8% base rate of violence and there were only three violent incidents that were detected by the PNC but which were not reported either by the collateral informant during interview or in the case records. It would appear that most violent incidents committed during follow-up did not lead to conviction. The present study therefore adds further weight to the suggestion that rates of violence may be significantly underestimated if official records alone are relied on. Information should be sought from multiple sources where possible. 321,356 However, the prevalence of violence at 6 months was slightly higher than that reported for the forensic sample in the study by Doyle and Dolan321 (12%), despite their use of three sources, including self-report.
The low rate of violent reconvictions may be a result of the type of violence committed, with violence being mostly low level such as slapping, shoving and punching. However, it may also reflect the location of incidents or the relationship between the victim and the perpetrator. Others have shown that when violent incidents occur between service users or when staff or family members are victims, incidents are under-reported and rarely result in conviction. 368–370 This is also thought to be the case for incidents occurring within specialist units or hostel-type accommodation, where low-level incidents may be dealt with ‘in-house’ and are often not formally recorded. 371 Nevertheless, there was evidence of violent behaviour of a serious nature that did not result in conviction. Further information about the nature of violent incidents, the mechanisms used to deal with them in various settings and how these factors relate to outcomes (including the frequency of further incidents) could be used to inform best practice in this aspect of risk management.
The highest number of violent incidents committed by one individual during follow-up was 12. The vast majority of service users were violent on only one or two occasions. This is in line with previous findings, including those of Monahan et al. 300 and Doyle and Logan. 346
Severity of violence
Most violent incidents detected were of a relatively minor nature and did not result in criminal convictions. These findings are consistent with those of previous research. 300,346 Of those convicted, only one individual was found to have committed serious sexual violence and a further four committed assaults of a severity that led to a conviction of either GBH or robbery. This equates to a rate of conviction for very serious offences of 1 in 77, which is considerably lower than that in the study by Coid et al. ,367 who reported that one in eight men was reconvicted of a grave offence during follow-up, although the follow-up period averaged 6 years. Follow-up of the sample over an extended period of time is recommended using the method detailed in this study. This would provide information regarding risk and protective factors relevant to more long-term outcomes following discharge and perhaps relating to more serious violence, if more of the most severe incidents were detected.
Sex differences
A greater proportion of men than women were violent, which contradicts previous findings that there is little difference between sexes or that women are slightly more likely to be violent in secure hospital settings353 or to reoffend. 338,353,372 However, this result was not significant and caution should be applied when interpreting these findings, as only a small number of women were recruited to the study. Female sex was not found to be a confounding factor for the predictive validity of the scales.
Levels of support
No significant differences were found between the violent group and the non-violent group in relation to frequency and level of professional contact received during follow-up. By 12 months post discharge, levels of professional contact had reduced and were very similar for both groups. However, at 6 months post discharge, the violent group had a higher mean score for professional contact, suggesting that this group had more frequent contact with qualified staff on average than the non-violent group. These findings mirror those of Doyle et al. ,332 who devised the method employed for calculating professional support in this study. They appear to contradict the hypothesis that increased professional support would act as a protective factor against violent outcome.
However, as suggested by Doyle et al. ,332 increased support for the violent group could paradoxically be attributed to a response from services to a crisis period or a perceived increase in risk indicators that preceded the violent behaviour. This result could therefore be interpreted as support services correctly identifying a time of increased risk, even if their input was not enough to ultimately prevent a violent incident from occurring. The lack of available information on the perceived value of professional support according to the service users themselves is a potential limitation of the present study. Without input from service users, the perceived quality of the support, which may be of more importance than the quantity, could not be evaluated in the context of violent outcomes, as was the case in previous research. 332 More research needs to be carried out to develop valid and reliable measures of professional and social support in the community.
The legal status of service users was found to have a significant relationship with violent outcome at both stages of follow-up. Those subject to restrictions under Section 37/41 of the Mental Health Act 1983361 were significantly less likely to be violent. Others have reported similar findings, which suggests that the nature of this section (the implementation of specific conditions on discharge and the power to recall if they are breached) may act as a protective factor in itself. 321,367 When considered alongside findings in relation to professional support, this paints a slightly confused and inconclusive picture and further investigation into the impact of these factors is warranted.
Informal or social support could not be reliably scored in the same way as professional support without the involvement of service users themselves. It is unlikely that collateral informants could or would have been able to provide sufficiently accurate information about the frequency and nature of any support that service users received from family, friends and peers. The only measure of social support used was item 13 of the SAPROF (pro-social and supportive network), which stipulates that it relates to family and friends rather than professional support or intimate relationships. A significantly greater proportion of those for whom a pro-social support network was deemed to be not present, or only partially so, were violent in the first 6 months post discharge.
By 12 months’ follow-up there was no significant difference in levels of social support between violent and non-violent groups. These findings indicate that social support networks could play an important role in protecting against violence but that the precise mechanism and active ingredients of that support remain unclear. Future research should aim to include reliable information from service users or their families to build a more detailed picture of which specific elements of social support are most protective, similar to previous studies of psychosocial factors that influence violence. 373
Additional factors
Age was found to be related to violent outcome at both 6 and 12 months post discharge. Older service users were significantly less likely to be violent than their younger counterparts. This reflects the findings of other studies300,346,367,374 and lends support to the theory that violent offending reduces with increasing age. 89,375–377
As reported previously, those who had spent longer in a MSS prior to discharge were also significantly less likely to be violent during follow-up. 333,367 This could be interpreted as the positive result of having had enough time to settle in to the environment, engage in or complete various therapeutic interventions and make adequate preparations for discharge. This result could also have been influenced by the relationship between age and violence, as those who have stayed for long periods in secure care are likely to be older on discharge than those who have stayed for shorter periods. Further, increased length of stay has been found to be associated with detention under Section 37/41 (Mental Health Act 1983361) and therefore a greater likelihood of discharge to a supported environment,378 both factors that may in themselves be protective. A more detailed investigation of all factors involved is required before causal relationships can be identified.
No significant differences were found between the violent group and the non-violent group in relation to psychiatric diagnosis. This is consistent with findings from Monahan et al. 300 and Doyle and Dolan. 321 Specifically, no significant relationship was found between a personality disorder diagnosis and violence, despite others having reported personality disorder as a robust violence risk factor. 379–381
No significant differences were found between the violent group and the non-violent group based on history of serious substance misuse, despite others having suggested previously that substance use is a major risk factor for violence in the mentally ill. 36,382–384 However, this could be explained by the low rate of violence in the sample, coupled with the very high prevalence of substance use across the violent and non-violent groups. A greater proportion of those with a history of serious substance use than those without had been violent by both 6 and 12 months post discharge, but three-quarters of this group were not found to be violent at all within the follow-up periods. A more detailed analysis of the relationship between these factors is necessary, along with exploration of other potentially confounding factors that may have an influence. Substance use during follow-up could also be investigated further to assess its impact as a dynamic variable.
Limitations of the study
Some of the strengths and limitations of the study have already been mentioned earlier in the discussion but the remainder will now be addressed. The use of Section 251 of the NHS Act 2006 allowed the use of a whole and therefore representative sample, thus avoiding the limitations experienced by others who have previously sought consent from service users. 355 However, this also made it harder to control for possible confounding factors, including treatment offered, engagement and compliance. 321
Lack of service user consent also meant that those involved could not be consulted or interviewed. Information relating to key factors such as symptoms and experiences of support was reliant on knowledge of third parties such as primary nurses, care co-ordinators and responsible clinicians. Although staff involved were recruited only if they had worked closely with service users at the relevant time point and were confident that they knew the cases well, the perspective of the service users themselves could have added value. Doyle and Dolan321 identified that self-report, especially in relation to impulsiveness and anger, may have clinical utility in identifying those at subsequent risk of violence. Nevertheless, on balance, the findings using the method employed in this study are likely to be more accurate, given the expected rate of non-consent demonstrated in the trial of consent.
In addition, at each stage of follow-up, the sample was reduced in size as cases could not be followed up for various reasons. Although 95% and 89% were followed up at 6 and 12 months, respectively, it could be argued that, in terms of violent outcome, some of the most interesting cases, for example those who disengaged with services or who had no further input from mental health services, were not included in the study. PNC data identified only one such individual who had been convicted of a violence offence during follow-up, but there could have been individuals who were violent at a subconviction level. PNC data were potentially limited by the time restrictions within the study protocol. They were considered 6 months after the final 12-month follow-up date to allow the longest possible time for any incidents during follow-up to have reached conviction. However, given the complexities of some investigations or delays in reporting offences, it could be the case that some incidents had not yet reached an outcome. Repeated analysis of PNC data at a later date for this sample could resolve this issue.
A further limitation was the way in which measures were rated and coded. For example, the PANSS is designed for use as an interview, with the service user being rated rather than information being gathered from third-party sources. Previous research has validated the latter method in relation to similar measures, including the BPRS. 300,358 However, the authors are not aware of the PANSS having been used like this previously. In addition, the SAPROF and HCR-20v3 were scored in an actuarial way, with scores totalled for each subscale and overall. Although the methods employed here have been accepted for research purposes, they do not reflect the usual clinical application of the measures.
Similarly, the rating of measures by trained researchers rather than by qualified clinicians could be seen as a limitation of the findings. However, all research staff received training from qualified trainers and, in the case of the HCR-20v3, from the authors themselves. In addition, De Vogel and De Ruiter385 identified good inter-rater reliability for the HCR-20v3 between clinicians and researchers; it was in the interpretation of scores that they differed. Therefore, given the excellent inter-rater reliability achieved and the similarity of the findings to those of previous studies, the methods used are considered to have been suitable for the purposes of the research.
Violent outcome was defined in binary terms (yes or no) for the majority of the analyses, regardless of severity. It is potentially limiting to assess violent incidents in this way as those who have committed multiple and/or severe acts of violence are classified with those who commit only one minor act. Although the ability of the measures to predict the frequency of violence was examined, the severity of violence was not accounted for. Results were therefore based only on the ability of measures to detect the presence, absence and frequency of violent behaviour during follow-up, rather than on the identification of those at risk of the most severe or serious violence. Nonetheless, all violence reported in this study involved a physical assault and/or involved a threat with a weapon in hand.
Chapter 14 Validation of the predictive ability of risk assessment instruments for patients discharged from medium secure services
Background
A substantial proportion of patients in MSSs suffer from major psychotic disorders. The MSRAG was constructed to measure the risk of acquisitive and serious reoffending in patients with schizophrenia and delusional disorder. 22 It is used to assess static predictor variables and was designed for use by clinicians during the stage of rehabilitation before discharge into the community. The instrument was created in a subsample of 935 patients with schizophrenia and other non-affective psychoses. Data were collected retrospectively and sources included medium secure medical records, central records from the Home Office Mental Health Unit and medical records from high secure and some general adult psychiatric hospitals. The outcomes to be predicted were criminal offending. Two categories were examined: acquisitive recidivism (theft, stealing, burglary or fraud post discharge) and serious offending recidivism (actual or threatened violence against the person, sexual offences, aggravated burglary, abduction, arson or weapons offences post discharge). The instrument was constructed and validated in a sample of 1344 patients who were admitted to secure services from 7 of 14 regional health authorities in England between 1989 and 1993 and discharged before the end of 1998.
The predictive efficacy of the acquisitive recidivism scale was 0.89 in the development sample and 0.88 in the validation subsample. The serious offence scale showed a predicted efficacy of 0.79 in the development sample and 0.76 in the validation subsample. The validation figures were comparable with those achieved with the development subsample, suggesting that the MSRAG was valid. 22
Part of the original aim of this programme was to test the validity of a new MSRAG using a tree model. However, previous studies that have compared logistic regression, classification and regression tree, and neural network models according to their predictive validity have shown inconsistent results when attempting to demonstrate the superiority of any one model. 386 These three models were tested in a prospective sample of UK male prisoners386 who were followed up for a mean of 3.3 years after release. Using the HCR-20 items as predictors and using violent reconvictions as outcome, a multivalidation procedure was used to reduce the sampling error and report predictive accuracy. The overall accuracy of the three models varied between 0.59 and 0.67, with an overall AUC range of 0.65–0.72. Although the performance of neural networks was slightly better than that of the logistic regression and classification and regression tree models, it did not demonstrate a statistically significant improvement. It was thought that this experiment had failed to demonstrate the superiority of a tree model over logistic regression as was originally intended in the programme. The research team therefore decided to dispense with the proposed study. There were additional concerns that tree modelling would result in overfitting and a less accurate predictive instrument than could be achieved using conventional methods. We determined that logistic regression should be the method of choice for further investigation. 387
In this chapter, we validate the MSRAG in a new sample of medium secure patients discharged into the community. We also compare the predictive accuracy of the MSRAG with that of other instruments.
Objectives
Following the description of violent and non-violent service users in Chapter 13, the objectives of this study were to:
-
validate the predictive accuracy of the MSRAG and compare this accuracy with that of other instruments for predicting violent behaviour
-
compare the predictive accuracy of the MSRAG with that of other instruments for criminal convictions for acquisitive and serious offending
-
investigate the item-specific predictive accuracy for violence for all instruments included in the study.
Methods
A detailed description of the sample used is provided in Chapter 13.
The primary risk assessment instrument of interest in this study was the MSRAG. 22 Each of the two parts of the instrument (acquisitive and serious offending recidivism) consists of two separate six-item scales. The items in each scale are scored using either a 3-point rating (–1, 0, +1) or a 2-point rating (–1, +1).
Additional instruments included in this study and which were compared with the MSRAG are based on SPJ checklist scores. Information was collected as described in Chapter 13. These instruments included the HCR-20v3, SAPROF (scales were reversed to measure risk for comparative analysis) and PANSS. In our analysis we included the total score and the score for each subscale for each instrument.
We included additional actuarial instruments that were scored using information from medical records and the PNC. These included the Risk Matrix 2000 for Violence (RM2000V),388 the Offender Group Reconviction Scale, version 2 (OGRS2)389 and the Pencil and Paper Instrument for Violence (PIV). Finally, we included the PCL:SV,359 which has been used extensively as a risk assessment tool for violence in previous publications, although it was originally developed as a screen for the diagnosis of psychopathy.
The main outcomes of interest were binary outcomes for any violence within 6 months (54 patients, 14.0%) and 12 months (87 patients, 22.5%) following discharge.
Additional outcomes based on PNC criminal records were included to validate the predictive accuracy of the main instrument of interest (MSRAG) according to the outcomes it had been originally constructed for: acquisitive offending convictions and serious offending convictions. In total, 12 former inpatients (3.2%) were convicted of acquisitive offending within 6 months of discharge and 15 patients (4.0%) were convicted for the same type of offence within 12 months of discharge. Furthermore, nine patients (2.4%) were convicted of serious offending within 6 months of discharge and 19 patients (5.1%) were convicted for the same offence within 12 months of discharge.
Finally, we included convictions for any offence to obtain an estimate of predictive accuracy for overall offending behaviour. In total, 29 individuals (7.8%) were convicted of an offence (any) within 6 months of discharge and 48 individuals (12.9%) were convicted of an offence (any) within 12 months of discharge.
To estimate the predictive ability of the risk assessment instruments, AUC values were used. This provides an overall measure of discrimination between violent and non-violent patients. Among instruments used to identify violent cases, an instrument with a higher AUC is likely to have a better screening performance than an instrument with a lower AUC. The instrument with the higher AUC will have a higher specificity (percentage of correctly identified non-violent cases) for a given sensitivity (percentage of correctly identified violent cases).
We initially estimated AUCs for the total scale and subscale of each instrument and tested whether each AUC was significantly different from 0.5 (no discrimination). We subsequently compared each MSRAG scale AUC (acquisitive recidivism, serious offending recidivism) separately, comparing them with other scales and subscales.
In addition to AUC estimates, we used the conventional median split for each of the scales and subscales to obtain several statistical parameters we used to further assess their predictive accuracy. These included sensitivity, specificity, positive predictive value (PPV), negative predictive value (NPV) and PCC.
Finally, we investigated item-specific predictive validity for certain instruments. We identified items that were predictive of the outcome of interest by testing whether or not the item-specific AUC was significantly different from 0.5 (no discrimination).
Results
Any violence within 6 and 12 months
For any violence within 6 months following discharge, AUC estimates with corresponding 95% CIs and p-values for the significance test that AUC = 0.5 test against 0.5, as well as additional statistical parameters, are shown in Table 108. The highest AUC value was found for SAPROF (reverse) total scale. The total HCR-20v3 scale, clinical HCR-20v3 scale and SAPROF motivational (reverse) scale showed similar levels of accuracy. The only other scale with an AUC value of ≥ 0.7 (good predictive accuracy) was the PANSS aggression scale.
Measure | AUC | 95% CI | p-value | Sensitivity (%) | Specificity (%) | PPV (%) | NPV (%) | PCC |
---|---|---|---|---|---|---|---|---|
MSRAG | ||||||||
Acquisitive recidivism | 0.63 | 0.55 to 0.72 | 0.002 | 59.3 | 53.3 | 17.1 | 88.9 | 54.1 |
Serious offending recidivism | 0.63 | 0.54 to 0.72 | 0.003 | 48.2 | 71.1 | 21.3 | 89.4 | 67.9 |
HCR-20v3 | ||||||||
Historical | 0.64 | 0.56 to 0.71 | < 0.001 | 70.4 | 53.2 | 19.6 | 91.7 | 55.6 |
Clinical | 0.75 | 0.68 to 0.82 | < 0.001 | 77.8 | 66.7 | 27.5 | 94.9 | 68.2 |
Risk management | 0.67 | 0.60 to 0.75 | < 0.001 | 74.1 | 55.3 | 21.2 | 92.9 | 57.9 |
Total | 0.75 | 0.68 to 0.82 | < 0.001 | 81.5 | 60.7 | 25.1 | 95.3 | 63.6 |
SAPROF | ||||||||
Total (reverse) | 0.76 | 0.70 to 0.82 | < 0.001 | 75.9 | 63.1 | 25.0 | 94.2 | 64.9 |
Internal (reverse) | 0.68 | 0.60 to 0.77 | < 0.001 | 63.0 | 67.6 | 23.9 | 91.8 | 66.9 |
Motivational (reverse) | 0.74 | 0.68 to 0.81 | < 0.001 | 79.6 | 63.4 | 26.1 | 95.1 | 65.6 |
External (reverse) | 0.64 | 0.57 to 0.72 | < 0.001 | 48.2 | 74.5 | 23.4 | 89.9 | 70.8 |
PANSS | ||||||||
Positive | 0.69 | 0.60 to 0.77 | < 0.001 | 70.4 | 61.0 | 22.6 | 92.7 | 62.3 |
Negative | 0.57 | 0.48 to 0.67 | 0.125 | 55.6 | 58.6 | 17.9 | 89.0 | 58.1 |
General | 0.68 | 0.60 to 0.76 | < 0.001 | 63.0 | 58.0 | 19.5 | 90.6 | 58.7 |
Aggression | 0.72 | 0.63 to 0.80 | < 0.001 | 74.1 | 56.5 | 21.6 | 93.1 | 58.9 |
Total | 0.68 | 0.59 to 0.77 | < 0.001 | 72.2 | 55.0 | 20.6 | 92.4 | 57.4 |
RM2000V | 0.59 | 0.52 to 0.67 | 0.020 | 65.3 | 51.9 | 18.4 | 90.0 | 53.8 |
OGRS2 | 0.60 | 0.52 to 0.68 | 0.017 | 57.1 | 51.2 | 16.7 | 87.5 | 52.1 |
PIV | 0.59 | 0.51 to 0.68 | 0.031 | 47.1 | 68.3 | 20.0 | 88.5 | 65.3 |
PCL:SV | 0.59 | 0.50 to 0.68 | 0.046 | 63.0 | 55.0 | 18.5 | 90.2 | 56.1 |
For the scales of primary interest (MSRAG acquisitive and serious reoffending) the AUCs showed a moderate level of accuracy. However, compared with the AUC for the MSRAG acquisitive recidivism scale, the AUC for the SAPROF (reverse) total scale was significantly higher (p = 0.010). The same conclusion can be reached when comparing the MSRAG acquisitive recidivism scale with the total HCR-20v3 scale (p = 0.007), the clinical HCR-20v3 scale (p = 0.013) and the SAPROF (reverse) motivational scale (p = 0.043). However, the MSRAG acquisitive recidivism scale was not significantly different from the PANSS aggression scale (p = 0.169). Similarly, the MSRAG serious offending scale AUC was significantly lower than the SAPROF (reverse) total scale (p = 0.008), total HCR-20v3 scale (p = 0.006), clinical HCR-20v3 scale (p = 0.015) and SAPROF (reverse) motivational scale (p = 0.039) AUCs. The PANSS aggression scale AUC was not significantly different from the MSRAG serious offending scale AUC (p = 0.172).
Table 108 shows that the HCR-20v3 total scale had the highest sensitivity using median splits. On the other hand, the SAPROF (reverse) external scale had the highest specificity. The highest PPV was obtained by median split of the HCR-20v3 clinical subscale and the highest NPV was obtained by median split of the HCR-20v3 total scale. Finally, the HCR-20v3 clinical subscale showed the highest PCC for the combined outcome of violence (clinically reported and from the PNC).
For any violence within 12 months of discharge, AUC estimates and 95% CIs and p-values for the test against 0.5 are shown in Table 109. There were only three instruments with an AUC ≥ 0.7: the SAPROF (reverse) total scale, the total HCR-20v3 scale and the clinical HCR-20v3 scale. All other instruments failed to reach a level of good predictive accuracy for the same outcome.
Measure | AUC | 95% CI | p-value | Sensitivity (%) | Specificity (%) | PPV (%) | NPV (%) | PCC |
---|---|---|---|---|---|---|---|---|
MSRAG | ||||||||
Acquisitive recidivism | 0.63 | 0.56 to 0.70 | < 0.001 | 63.2 | 55.9 | 29.4 | 83.9 | 57.5 |
Serious offending recidivism | 0.63 | 0.56 to 0.70 | < 0.001 | 44.8 | 72.2 | 32.0 | 81.8 | 66.1 |
HCR-20v3 | ||||||||
Historical | 0.63 | 0.56 to 0.69 | < 0.001 | 66.7 | 54.7 | 29.9 | 85.0 | 57.4 |
Clinical | 0.70 | 0.63 to 0.77 | < 0.001 | 65.5 | 68.0 | 37.3 | 87.2 | 67.4 |
Risk management | 0.62 | 0.55 to 0.69 | 0.001 | 66.7 | 56.3 | 30.7 | 85.4 | 58.7 |
Total | 0.70 | 0.64 to 0.77 | < 0.001 | 73.6 | 63.0 | 36.6 | 89.2 | 65.4 |
SAPROF | ||||||||
Total (reverse) | 0.70 | 0.63 to 0.76 | < 0.001 | 64.4 | 64.0 | 34.2 | 86.1 | 64.1 |
Internal (reverse) | 0.65 | 0.58 to 0.72 | < 0.001 | 52.9 | 68.0 | 32.4 | 83.3 | 64.6 |
Motivational (reverse) | 0.68 | 0.62 to 0.75 | < 0.001 | 62.1 | 63.0 | 32.7 | 85.1 | 62.8 |
External (reverse) | 0.62 | 0.55 to 0.68 | 0.001 | 44.8 | 76.0 | 35.1 | 82.6 | 69.0 |
PANSS | ||||||||
Positive | 0.66 | 0.59 to 0.73 | < 0.001 | 64.4 | 62.7 | 33.3 | 85.8 | 63.0 |
Negative | 0.55 | 0.47 to 0.62 | 0.221 | 52.9 | 59.3 | 27.4 | 81.3 | 57.9 |
General | 0.63 | 0.55 to 0.70 | 0.001 | 57.5 | 58.7 | 28.7 | 82.6 | 58.4 |
Aggression | 0.69 | 0.62 to 0.76 | < 0.001 | 67.8 | 58.0 | 31.9 | 86.1 | 60.2 |
Total | 0.64 | 0.57 to 0.71 | < 0.001 | 65.5 | 56.0 | 30.2 | 84.9 | 58.1 |
RM2000V | 0.64 | 0.57 to 0.70 | < 0.001 | 71.1 | 55.2 | 31.0 | 87.1 | 58.7 |
OGRS2 | 0.65 | 0.58 to 0.71 | < 0.001 | 65.8 | 54.6 | 29.8 | 84.5 | 57.1 |
PIV | 0.66 | 0.59 to 0.73 | < 0.001 | 56.8 | 72.9 | 38.3 | 85.0 | 69.2 |
PCL:SV | 0.62 | 0.55 to 0.69 | 0.001 | 64.4 | 57.3 | 30.4 | 84.7 | 58.9 |
The AUCs for the MSRAG scales were again of a moderate level, using the median split for the acquisitive recidivism scale and the serious offending recidivism scale.
Compared with the AUC for the MSRAG acquisitive recidivism scale, only the total HCR-20v3 scale AUC was significantly higher (p = 0.045). The SAPROF (reverse) total scale and the clinical HCR-20v3 scale AUCs were not significantly different from the MSRAG acquisitive recidivism scale AUC (p-values 0.114 and 0.088 respectively). The MSRAG serious offending recidivism scale AUC was significantly lower (p = 0.049) than the total HCR-20v3 scale AUC, but not significantly different from the SAPROF (reverse) total scale (p = 0.106) and the clinical HCR-20v3 scale (p = 0.091) AUCs.
Table 109 shows that the HCR-20v3 total scale had the highest sensitivity and the SAPROF (reverse) external scale had the highest specificity. In addition, the PIV had the highest PPV and the HCR-20v3 total scale had the highest NPV. Finally, the highest PCC was obtained using the PIV.
Acquisitive offending
Table 110 shows the estimates of predictive accuracy for convictions for acquisitive offending within 6 months of discharge. The highest AUCs were for the MSRAG serious offending recidivism and acquisitive recidivism scales.
Measure | AUC | 95% CI | p-value | Sensitivity (%) | Specificity (%) | PPV (%) | NPV (%) | PCC (%) |
---|---|---|---|---|---|---|---|---|
MSRAG | ||||||||
Acquisitive recidivism | 0.89 | 0.79 to 0.98 | < 0.001 | 91.7 | 52.0 | 6.0 | 99.5 | 53.3 |
Serious offending recidivism | 0.90 | 0.80 to 0.99 | < 0.001 | 91.7 | 69.8 | 9.2 | 99.6 | 70.5 |
HCR-20v3 | ||||||||
Historical | 0.67 | 0.49 to 0.85 | 0.064 | 75.0 | 50.6 | 4.8 | 98.4 | 51.3 |
Clinical | 0.65 | 0.50 to 0.81 | 0.053 | 66.7 | 60.3 | 5.3 | 98.2 | 60.5 |
Risk management | 0.71 | 0.52 to 0.91 | 0.032 | 75.0 | 50.0 | 4.8 | 98.4 | 50.9 |
Total | 0.72 | 0.53 to 0.92 | 0.023 | 75.0 | 55.0 | 5.3 | 98.5 | 55.6 |
SAPROF | ||||||||
Total (reverse) | 0.71 | 0.54 to 0.89 | 0.018 | 75.0 | 56.9 | 5.5 | 98.6 | 57.5 |
Internal (reverse) | 0.68 | 0.51 to 0.86 | 0.043 | 66.7 | 62.8 | 5.6 | 98.3 | 62.9 |
Motivational (reverse) | 0.70 | 0.53 to 0.86 | 0.020 | 75.0 | 56.7 | 5.5 | 99.0 | 57.3 |
External (reverse) | 0.71 | 0.59 to 0.83 | 0.001 | 50.0 | 70.8 | 5.4 | 97.7 | 70.2 |
PANSS | ||||||||
Positive | 0.57 | 0.41 to 0.74 | 0.382 | 50.0 | 55.3 | 3.6 | 97.1 | 55.1 |
Negative | 0.58 | 0.45 to 0.71 | 0.224 | 50.0 | 55.8 | 3.6 | 97.1 | 55.6 |
General | 0.63 | 0.48 to 0.79 | 0.091 | 66.7 | 55.3 | 4.7 | 98.0 | 55.6 |
Aggression | 0.67 | 0.49 to 0.84 | 0.066 | 66.7 | 53.1 | 4.5 | 98.1 | 53.5 |
Total | 0.63 | 0.46 to 0.80 | 0.135 | 66.7 | 51.1 | 4.3 | 98.1 | 51.1 |
RM2000V | 0.74 | 0.62 to 0.86 | < 0.001 | 83.3 | 51.3 | 5.6 | 99.0 | 52.4 |
OGRS2 | 0.86 | 0.77 to 0.94 | < 0.001 | 100.0 | 52.2 | 7.0 | 100.0 | 54.0 |
PIV | 0.81 | 0.72 to 0.90 | < 0.001 | 75.0 | 67.2 | 7.1 | 98.8 | 67.5 |
PCL:SV | 0.45 | 0.26 to 0.64 | 0.622 | 50.0 | 52.5 | 3.4 | 97.0 | 52.4 |
Using the median split, the MSRAG acquisitive recidivism and MSRAG serious offending recidivism scales showed the highest sensitivity. However, the highest specificity was obtained using the SAPROF (reverse) external scale. The MSRAG serious offending recidivism scale had the highest PPV and the OGRS2 had the highest NPV. Finally, the MSRAG serious offending recidivism scale showed the highest PCC.
Estimates of predictive accuracy for acquisitive offending convictions within 12 months of discharge are shown in Table 111. As with the estimates for acquisitive offending within 6 months, the MSRAG acquisitive recidivism and MSRAG serious offending recidivism scales showed the highest AUC values.
Measure | AUC | 95% CI | p-value | Sensitivity (%) | Specificity (%) | PPV (%) | NPV (%) | PCC |
---|---|---|---|---|---|---|---|---|
MSRAG | ||||||||
Acquisitive recidivism | 0.87 | 0.79 to 0.96 | < 0.001 | 93.3 | 52.4 | 7.7 | 99.5 | 54.1 |
Serious offending recidivism | 0.87 | 0.79 to 0.96 | < 0.001 | 86.7 | 70.1 | 11.0 | 99.2 | 71.0 |
HCR-20v3 | ||||||||
Historical | 0.68 | 0.53 to 0.83 | 0.019 | 73.3 | 50.7 | 6.1 | 97.8 | 52.0 |
Clinical | 0.67 | 0.53 to 0.81 | 0.017 | 66.7 | 61.0 | 6.6 | 98.0 | 60.8 |
Risk management | 0.68 | 0.50 to 0.85 | 0.049 | 66.7 | 50.1 | 5.3 | 97.3 | 51.0 |
Total | 0.73 | 0.56 to 0.90 | 0.007 | 73.3 | 55.2 | 6.4 | 98.0 | 56.1 |
SAPROF | ||||||||
Total (reverse) | 0.73 | 0.58 to 0.87 | 0.003 | 80.0 | 57.4 | 7.3 | 99.1 | 58.3 |
Internal (reverse) | 0.68 | 0.53 to 0.83 | 0.018 | 60.0 | 62.8 | 6.3 | 97.4 | 62.6 |
Motivational (reverse) | 0.71 | 0.58 to 0.85 | 0.002 | 80.0 | 57.1 | 7.3 | 99.5 | 58.1 |
External (reverse) | 0.70 | 0.58 to 0.82 | 0.001 | 53.3 | 71.2 | 7.2 | 97.3 | 70.4 |
PANSS | ||||||||
Positive | 0.56 | 0.41 to 0.72 | 0.419 | 46.7 | 55.2 | 4.2 | 96.1 | 55.0 |
Negative | 0.55 | 0.42 to 0.67 | 0.483 | 46.7 | 55.7 | 4.2 | 96.1 | 55.4 |
General | 0.62 | 0.47 to 0.77 | 0.115 | 66.7 | 55.5 | 6.0 | 98.0 | 56.0 |
Aggression | 0.66 | 0.50 to 0.82 | 0.046 | 66.7 | 53.2 | 6.1 | 97.4 | 54.1 |
Total | 0.61 | 0.45 to 0.77 | 0.175 | 66.7 | 51.0 | 5.4 | 97.3 | 51.3 |
RM2000V | 0.74 | 0.64 to 0.85 | < 0.001 | 86.7 | 52.0 | 7.2 | 99.0 | 53.2 |
OGRS2 | 0.86 | 0.79 to 0.93 | < 0.001 | 100.0 | 52.7 | 9.0 | 100.0 | 55.0 |
PIV | 0.83 | 0.75 to 0.90 | < 0.001 | 80.0 | 68.1 | 9.5 | 99.1 | 68.3 |
PCL:SV | 0.49 | 0.33 to 0.65 | 0.866 | 53.3 | 53.1 | 4.5 | 96.4 | 53.1 |
The OGRS2 scale had the highest sensitivity and the SAPROF (reverse) external scale had the highest specificity. The MSRAG serious offending recidivism scale showed the highest PPV and the OGRS2 scale had the highest NPV. Finally, the MSRAG serious offending recidivism scale showed the highest PCC.
Serious offending
Table 112 shows the estimates of predictive accuracy for serious offending convictions within 6 months of discharge. The SAPROF (reverse) external scale had the highest AUC, which was significantly higher than the MSRAG acquisitive recidivism scale AUC and the MSRAG serious offending recidivism scale AUC.
Measure | AUC | 95% CI | p-value | Sensitivity (%) | Specificity (%) | PPV (%) | NPV (%) | PCC |
---|---|---|---|---|---|---|---|---|
MSRAG | ||||||||
Acquisitive recidivism | 0.67 | 0.52 to 0.82 | 0.031 | 67.0 | 51.7 | 3.3 | 98.4 | 51.4 |
Serious offending recidivism | 0.63 | 0.47 to 0.78 | 0.105 | 56.0 | 68.3 | 4.2 | 98.4 | 68.0 |
HCR-20v3 | ||||||||
Historical | 0.68 | 0.57 to 0.79 | 0.002 | 89.1 | 51.1 | 4.3 | 99.5 | 52.1 |
Clinical | 0.74 | 0.63 to 0.84 | < 0.001 | 89.1 | 61.0 | 5.3 | 99.5 | 61.3 |
Risk management | 0.77 | 0.64 to 0.90 | < 0.001 | 89.0 | 50.1 | 4.2 | 99.5 | 51.1 |
Total | 0.81 | 0.71 to 0.91 | < 0.001 | 89.1 | 55.1 | 5.1 | 100.0 | 56.1 |
SAPROF | ||||||||
Total (reverse) | 0.83 | 0.73 to 0.93 | < 0.001 | 88.1 | 57.0 | 5.1 | 100.0 | 73.3 |
Internal (reverse) | 0.70 | 0.54 to 0.86 | 0.012 | 78.0 | 63.1 | 5.3 | 99.1 | 63.2 |
Motivational (reverse) | 0.75 | 0.61 to 0.88 | 0.001 | 78.0 | 57.0 | 4.2 | 99.0 | 57.9 |
External (reverse) | 0.87 | 0.79 to 0.96 | < 0.001 | 89.0 | 72.3 | 7.2 | 100.0 | 72.0 |
PANSS | ||||||||
Positive | 0.54 | 0.39 to 0.69 | 0.574 | 56.0 | 55.4 | 3.0 | 98.1 | 55.4 |
Negative | 0.39 | 0.18 to 0.59 | 0.269 | 33.3 | 55.4 | 2.0 | 97.1 | 55.3 |
General | 0.51 | 0.34 to 0.68 | 0.931 | 44.4 | 55.0 | 2.4 | 98.4 | 54.3 |
Aggression | 0.57 | 0.39 to 0.76 | 0.425 | 67.0 | 53.0 | 3.4 | 99.0 | 53.2 |
Total | 0.50 | 0.33 to 0.67 | 0.985 | 67.0 | 50.4 | 3.2 | 98.4 | 51.1 |
RM2000V | 0.73 | 0.57 to 0.89 | 0.004 | 78.0 | 51.0 | 4.0 | 99.0 | 52.2 |
OGRS2 | 0.75 | 0.61 to 0.88 | < 0.001 | 78.0 | 51.2 | 4.0 | 99.0 | 52.1 |
PIV | 0.75 | 0.63 to 0.87 | < 0.001 | 78.0 | 67.4 | 6.1 | 99.2 | 67.2 |
PCL:SV | 0.62 | 0.45 to 0.79 | 0.156 | 67.0 | 53.0 | 3.4 | 99.0 | 53.2 |
Table 112 also shows that the highest sensitivity was shown by three scales: HCR-20v3 historical, HCR-20v3 clinical, and HCR-20v3 total. The highest specificity (72.3%) was shown by the SAPROF (reverse) external scale. The same scale also showed the highest PPV. The HCR-20v3, SAPROF (reverse) total and SAPROF (reverse) external scales showed the maximum possible value for NPV. The highest PCC was obtained by median split of the SAPROF (reverse) total scale.
The analysis for predictive accuracy of serious offending convictions within 12 months of discharge is shown in Table 113. The SAPROF (reverse) total scale showed the highest AUC, which was significantly higher than the MSRAG acquisitive recidivism scale AUC (p = 0.009) and the MSRAG serious offending recidivism scale AUC (p = 0.001).
Measure | AUC | 95% CI | p-value | Sensitivity (%) | Specificity (%) | PPV (%) | NPV (%) | PCC |
---|---|---|---|---|---|---|---|---|
MSRAG | ||||||||
Acquisitive recidivism | 0.65 | 0.54 to 0.76 | 0.006 | 68.4 | 51.6 | 7.1 | 97.0 | 52.4 |
Serious offending recidivism | 0.60 | 0.48 to 0.71 | 0.109 | 47.4 | 68.6 | 7.6 | 96.0 | 67.5 |
HCR-20v3 | ||||||||
Historical | 0.65 | 0.56 to 0.74 | 0.001 | 74.0 | 51.0 | 8.0 | 97.3 | 52.2 |
Clinical | 0.70 | 0.60 to 0.79 | < 0.001 | 74.0 | 61.2 | 9.3 | 98.0 | 62.2 |
Risk management | 0.73 | 0.62 to 0.84 | < 0.001 | 74.0 | 50.4 | 7.4 | 97.3 | 52.1 |
Total | 0.76 | 0.67 to 0.85 | < 0.001 | 80.0 | 56.1 | 9.0 | 98.0 | 57.8 |
SAPROF | ||||||||
Total (reverse) | 0.82 | 0.75 to 0.90 | < 0.001 | 84.2 | 58.1 | 10.0 | 99.0 | 59.4 |
Internal (reverse) | 0.75 | 0.65 to 0.86 | < 0.001 | 68.4 | 64.0 | 9.2 | 97.4 | 64.1 |
Motivational (reverse) | 0.75 | 0.64 to 0.85 | < 0.001 | 68.4 | 57.0 | 7.9 | 97.1 | 57.5 |
External (reverse) | 0.79 | 0.68 to 0.90 | < 0.001 | 74.0 | 73.2 | 13.1 | 98.1 | 72.6 |
PANSS | ||||||||
Positive | 0.60 | 0.47 to 0.72 | 0.122 | 58.0 | 56.0 | 6.6 | 96.1 | 56.1 |
Negative | 0.46 | 0.30 to 0.62 | 0.609 | 36.8 | 55.2 | 4.2 | 94.2 | 54.3 |
General | 0.59 | 0.46 to 0.72 | 0.194 | 52.6 | 55.0 | 6.0 | 96.0 | 55.3 |
Aggression | 0.60 | 0.45 to 0.75 | 0.190 | 58.0 | 42.1 | 6.2 | 96.0 | 53.2 |
Total | 0.57 | 0.43 to 0.71 | 0.342 | 58.0 | 50.4 | 6.0 | 96.0 | 51.0 |
RM2000V | 0.72 | 0.61 to 0.82 | < 0.001 | 82.4 | 51.0 | 7.8 | 98.3 | 53.2 |
OGRS2 | 0.70 | 0.59 to 0.80 | < 0.001 | 82.4 | 52.1 | 8.1 | 98.3 | 54.0 |
PIV | 0.74 | 0.65 to 0.84 | < 0.001 | 74.0 | 68.0 | 11.0 | 98.0 | 68.3 |
PCL:SV | 0.65 | 0.52 to 0.77 | 0.022 | 74.0 | 54.0 | 8.1 | 97.4 | 55.0 |
The highest sensitivity in this analysis was shown by median split of the SAPROF (reverse) total scale and the highest specificity, as well as the highest PPV, was shown by the SAPROF (reverse) external scale. The highest NPV was achieved by the SAPROF (reverse) total scale and the highest PCC by the SAPROF (reverse) external scale.
Any criminal convictions at follow-up
Table 114 shows the results of the analysis of predictive accuracy for any convictions in the first 6 months since discharge. The SAPROF (reverse) total scale showed the highest AUC but this was not significantly different from either the MSRAG acquisitive recidivism scale AUC (p = 0.445) or the MSRAG serious offending recidivism scale AUC (p = 0.417).
Measure | AUC | 95% CI | p-value | Sensitivity (%) | Specificity (%) | PPV (%) | NPV (%) | PCC |
---|---|---|---|---|---|---|---|---|
MSRAG | ||||||||
Acquisitive recidivism | 0.73 | 0.64 to 0.83 | < 0.001 | 72.4 | 52.5 | 11.5 | 95.7 | 54.1 |
Serious offending recidivism | 0.73 | 0.63 to 0.83 | < 0.001 | 65.5 | 70.6 | 16.0 | 96.0 | 70.2 |
HCR-20v3 | ||||||||
Historical | 0.68 | 0.58 to 0.77 | < 0.001 | 83.0 | 52.5 | 12.8 | 97.3 | 55.3 |
Clinical | 0.69 | 0.60 to 0.78 | < 0.001 | 72.4 | 62.1 | 14.1 | 96.4 | 63.0 |
Risk management | 0.72 | 0.62 to 0.83 | < 0.001 | 83.0 | 52.0 | 13.0 | 97.3 | 54.3 |
Total | 0.75 | 0.65 to 0.85 | < 0.001 | 76.0 | 57.0 | 13.0 | 97.2 | 58.1 |
SAPROF | ||||||||
Total (reverse) | 0.79 | 0.70 to 0.88 | < 0.001 | 83.0 | 59.2 | 14.6 | 97.6 | 61.0 |
Internal (reverse) | 0.71 | 0.61 to 0.81 | < 0.001 | 72.4 | 65.0 | 14.8 | 96.5 | 65.3 |
Motivational (reverse) | 0.74 | 0.64 to 0.83 | < 0.001 | 79.3 | 58.6 | 13.9 | 97.1 | 60.2 |
External (reverse) | 0.78 | 0.70 to 0.86 | < 0.001 | 65.5 | 73.2 | 17.1 | 96.2 | 72.6 |
PANSS | ||||||||
Positive | 0.58 | 0.48 to 0.69 | 0.118 | 55.2 | 56.0 | 9.6 | 93.7 | 56.0 |
Negative | 0.49 | 0.39 to 0.59 | 0.877 | 41.4 | 55.4 | 7.3 | 91.8 | 54.3 |
General | 0.59 | 0.48 to 0.69 | 0.097 | 58.6 | 55.7 | 10.1 | 94.1 | 56.1 |
Aggression | 0.65 | 0.55 to 0.76 | 0.004 | 72.4 | 54.5 | 11.9 | 96.0 | 56.0 |
Total | 0.59 | 0.48 to 0.69 | 0.111 | 65.5 | 51.3 | 10.2 | 94.6 | 52.4 |
RM2000V | 0.75 | 0.66 to 0.83 | < 0.001 | 82.8 | 53.0 | 13.3 | 97.2 | 55.4 |
OGRS2 | 0.80 | 0.73 to 0.86 | < 0.001 | 93.1 | 54.4 | 15.5 | 98.9 | 57.5 |
PIV | 0.79 | 0.73 to 0.86 | < 0.001 | 75.9 | 69.4 | 17.3 | 97.1 | 69.9 |
PCL:SV | 0.60 | 0.49 to 0.72 | 0.079 | 65.6 | 53.9 | 10.7 | 94.9 | 54.8 |
Table 114 also shows that OGRS2 scale had the highest sensitivity and the SAPROF (reverse) external scale the highest specificity. The highest PPV was obtained by a median split of the PIV scale and the highest NPV by the OGRS2 scale. Finally, the highest PCC was achieved by the SAPROF (reverse) external scale.
The results for any convictions within 12 months of discharge are shown in Table 115. Similar to the results in Table 114, the SAPROF (reverse) total scale had the highest AUC, but this was not significantly better at predicting the outcome of interest than the MSRAG acquisitive recidivism scale (p = 0.180) or the MSRAG serious offending recidivism scale (p = 0.197).
Measure | AUC | 95% CI | p-value | Sensitivity (%) | Specificity (%) | PPV (%) | NPV (%) | PCC |
---|---|---|---|---|---|---|---|---|
MSRAG | ||||||||
Acquisitive recidivism | 0.71 | 0.63 to 0.78 | < 0.001 | 72.9 | 54.1 | 19.2 | 93.0 | 56.5 |
Serious offending recidivism | 0.71 | 0.63 to 0.78 | < 0.001 | 60.4 | 72.0 | 24.4 | 92.4 | 70.5 |
HCR-20v3 | ||||||||
Historical | 0.63 | 0.55 to 0.72 | 0.002 | 70.8 | 52.8 | 18.2 | 92.4 | 55.1 |
Clinical | 0.65 | 0.57 to 0.73 | < 0.001 | 64.6 | 63.0 | 20.5 | 92.3 | 63.2 |
Risk management | 0.67 | 0.58 to 0.76 | < 0.001 | 68.8 | 51.9 | 17.5 | 91.8 | 54.0 |
Total | 0.69 | 0.61 to 0.78 | < 0.001 | 70.8 | 57.7 | 19.8 | 93.0 | 59.4 |
SAPROF | ||||||||
Total (reverse) | 0.78 | 0.71 to 0.85 | < 0.001 | 79.6 | 61.1 | 23.2 | 95.2 | 63.4 |
Internal (reverse) | 0.71 | 0.64 to 0.79 | < 0.001 | 64.6 | 65.7 | 21.8 | 92.6 | 65.6 |
Motivational (reverse) | 0.72 | 0.65 to 0.80 | < 0.001 | 72.9 | 59.9 | 21.2 | 93.7 | 61.6 |
External (reverse) | 0.73 | 0.65 to 0.80 | < 0.001 | 60.4 | 74.7 | 26.1 | 92.7 | 72.8 |
PANSS | ||||||||
Positive | 0.60 | 0.52 to 0.68 | 0.020 | 56.3 | 56.8 | 16.2 | 89.8 | 56.8 |
Negative | 0.47 | 0.39 to 0.56 | 0.557 | 37.5 | 54.6 | 10.9 | 85.5 | 52.4 |
General | 0.59 | 0.51 to 0.68 | 0.031 | 58.3 | 56.5 | 16.6 | 90.2 | 56.7 |
Aggression | 0.63 | 0.54 to 0.72 | 0.004 | 64.6 | 54.9 | 17.5 | 91.3 | 56.2 |
Total | 0.58 | 0.49 to 0.67 | 0.066 | 60.4 | 51.5 | 15.6 | 89.8 | 52.7 |
RM2000V | 0.75 | 0.68 to 0.81 | < 0.001 | 84.8 | 55.2 | 21.7 | 96.1 | 59.0 |
OGRS2 | 0.76 | 0.69 to 0.83 | < 0.001 | 89.1 | 56.4 | 23.6 | 97.2 | 60.7 |
PIV | 0.77 | 0.70 to 0.84 | < 0.001 | 72.9 | 71.6 | 27.6 | 94.7 | 71.8 |
PCL:SV | 0.62 | 0.52 to 0.71 | 0.015 | 66.7 | 55.3 | 18.1 | 91.8 | 56.7 |
From Table 115 we can see that the scale with highest sensitivity was the OGRS2 scale and the scale with the highest specificity was the SAPROF (reverse) external scale. Furthermore, the SAPROF (reverse) external scale showed the highest PPV and the OGRS2 scale the highest NPV. Finally, the highest PCC was obtained using the SAPROF (reverse) external scale.
Item-specific prediction of any violence
The next step in our analysis was to investigate item-specific predictive accuracy for any violence within 6 months and 12 months following discharge. Table 116 shows that, for MSRAG items in the acquisitive recidivism scale, only two items – number of prior offences and subject to a restricted hospital order – performed significantly better than chance for predicting any violence within 6 months since discharge. Similarly, using the scale for serious offending recidivism, number of prior serious offences and subject to a hospital restriction order performed significantly better than chance for the same outcome. The results for any violence within 12 months since discharge (see Table 116) were similar to those described for 6 months. Using the acquisitive recidivism scale, three items – number of prior offences and being subject to a restriction order but also age at index offence – were significant predictors of any violence. Similarly, using the serious offending recidivism scale, number of prior serious offences, subject to a restriction order and age at index offence had AUC values that were significantly higher than 0.5.
MSRAG items | Within 6 months | Within 12 months | ||||
---|---|---|---|---|---|---|
AUC | 95% CI | p-value | AUC | 95% CI | p-value | |
Acquisitive recidivism | ||||||
Number of prior offences | 0.61 | 0.54 to 0.68 | 0.002 | 0.61 | 0.55 to 0.67 | < 0.001 |
Any prior custodial sentences | 0.52 | 0.45 to 0.58 | 0.635 | 0.54 | 0.48 to 0.59 | 0.162 |
Acquisitive index offence | 0.51 | 0.46 to 0.56 | 0.720 | 0.49 | 0.45 to 0.53 | 0.576 |
Age at index offence | 0.55 | 0.47 to 0.62 | 0.228 | 0.58 | 0.51 to 0.64 | 0.021 |
Subject to a restricted hospital order | 0.57 | 0.50 to 0.65 | 0.040 | 0.58 | 0.52 to 0.64 | 0.012 |
Admitted to MSU from a high secure hospital | 0.50 | 0.47 to 0.53 | 0.961 | 0.51 | 0.48 to 0.53 | 0.591 |
Serious offending recidivism | ||||||
Number of prior custody sentences | 0.54 | 0.45 to 0.62 | 0.376 | 0.55 | 0.48 to 0.61 | 0.169 |
Number of prior serious offences | 0.58 | 0.52 to 0.64 | 0.012 | 0.60 | 0.55 to 0.65 | < 0.001 |
Acquisitive index offence | 0.51 | 0.46 to 0.56 | 0.720 | 0.49 | 0.45 to 0.53 | 0.576 |
Age at index offence | 0.54 | 0.47 to 0.62 | 0.240 | 0.57 | 0.51 to 0.64 | 0.023 |
Subject to a restricted hospital order | 0.58 | 0.51 to 0.65 | 0.030 | 0.58 | 0.52 to 0.64 | 0.007 |
Admitted to MSU from a high secure hospital | 0.50 | 0.47 to 0.54 | 0.890 | 0.51 | 0.48 to 0.53 | 0.509 |
We then investigated the predictive accuracy of HCR-20v3 items for any violence within 6 and 12 months. Table 117 shows that, using the historical scale, only 4 out of 10 items (other antisocial behaviour, traumatic experiences, violent attitudes and treatment or supervision response) were significant predictors of violence within 6 months. Using the clinical scale, apart from symptoms of major mental disorder, all items were significantly predictive of violence within 6 months. The only risk management scale item that was predictive of any violence within 6 months of discharge was treatment or supervision response. Table 117 shows that there were four historical items that were predictive of any violence within 12 months following discharge: other antisocial behaviour, traumatic experiences, violent attitudes and treatment or supervision response. Similar to the findings for violence within 6 months, all clinical items apart from symptoms of major mental disorder were significant predictors at 12 months. Similarly, in the risk management scale, treatment or supervision response but also stress or coping were significantly predictive of any violence at 12 months.
HCR-20v3 items | Within 6 months | Within 12 months | ||||
---|---|---|---|---|---|---|
AUC | 95% CI | p-value | AUC | 95% CI | p-value | |
Historical scale | ||||||
H1: violence | 0.51 | 0.45 to 0.57 | 0.842 | 0.51 | 0.46 to 0.56 | 0.644 |
H2: other antisocial behaviour | 0.59 | 0.51 to 0.67 | 0.021 | 0.59 | 0.53 to 0.66 | 0.004 |
H3: relationships | 0.50 | 0.43 to 0.58 | 0.925 | 0.49 | 0.42 to 0.55 | 0.654 |
H4: employment | 0.53 | 0.45 to 0.61 | 0.444 | 0.52 | 0.46 to 0.59 | 0.466 |
H5: substance use | 0.54 | 0.47 to 0.61 | 0.303 | 0.54 | 0.49 to 0.60 | 0.134 |
H6: major mental disorder | 0.48 | 0.43 to 0.52 | 0.317 | 0.49 | 0.46 to 0.52 | 0.666 |
H7: personality disorder | 0.56 | 0.48 to 0.64 | 0.130 | 0.54 | 0.48 to 0.61 | 0.210 |
H8: traumatic experiences | 0.60 | 0.52 to 0.68 | 0.010 | 0.63 | 0.57 to 0.70 | < 0.001 |
H9: violent attitudes | 0.60 | 0.52 to 0.68 | 0.013 | 0.58 | 0.52 to 0.65 | 0.009 |
H10: treatment or supervision response | 0.60 | 0.54 to 0.67 | 0.002 | 0.58 | 0.52 to 0.64 | 0.006 |
Clinical scale | ||||||
C1: insight | 0.64 | 0.57 to 0.71 | < 0.001 | 0.62 | 0.55 to 0.68 | < 0.001 |
C2: violent ideation or intent | 0.62 | 0.55 to 0.70 | 0.001 | 0.64 | 0.58 to 0.70 | < 0.001 |
C3: symptoms of major mental disorder | 0.51 | 0.43 to 0.59 | 0.795 | 0.50 | 0.44 to 0.57 | 0.915 |
C4: instability | 0.66 | 0.58 to 0.74 | < 0.001 | 0.64 | 0.58 to 0.70 | < 0.001 |
C5: treatment or supervision response | 0.63 | 0.55 to 0.71 | 0.002 | 0.60 | 0.53 to 0.66 | 0.005 |
Risk management scale | ||||||
R1: professional services and plans | 0.50 | 0.45 to 0.55 | 0.861 | 0.53 | 0.48 to 0.57 | 0.214 |
R2: living situation | 0.55 | 0.48 to 0.63 | 0.176 | 0.54 | 0.48 to 0.61 | 0.188 |
R3: personal support | 0.55 | 0.46 to 0.63 | 0.269 | 0.54 | 0.47 to 0.61 | 0.262 |
R4: treatment or supervision response | 0.66 | 0.59 to 0.74 | < 0.001 | 0.61 | 0.55 to 0.68 | < 0.001 |
R5: stress or coping | 0.57 | 0.49 to 0.64 | 0.086 | 0.57 | 0.51 to 0.63 | 0.022 |
In addition, we investigated the predictive accuracy of individual SAPROF (reversed) items for any violence within 6 and 12 months of discharge. Table 118 shows that, for any violence within 6 months since discharge, three of the internal factors (empathy, coping and self-control) were significant predictors. With the exception of life goals, all other motivational factors were predictive of 6-month violence. Among the external factors, three items (social network, professional care and external control) were predictive of violence within 6 months.
SAPROF (reverse) items | Within 6 months | Within 12 months | ||||
---|---|---|---|---|---|---|
AUC | 95% CI | p-value | AUC | 95% CI | p-value | |
Internal factors | ||||||
Intelligence | 0.55 | 0.48 to 0.63 | 0.176 | 0.54 | 0.48 to 0.60 | 0.182 |
Secure attachment in childhood | 0.56 | 0.49 to 0.64 | 0.101 | 0.55 | 0.49 to 0.62 | 0.088 |
Empathy | 0.62 | 0.54 to 0.69 | 0.003 | 0.59 | 0.53 to 0.66 | 0.005 |
Coping | 0.62 | 0.55 to 0.70 | 0.001 | 0.60 | 0.54 to 0.66 | 0.002 |
Self-control | 0.66 | 0.59 to 0.73 | < 0.001 | 0.62 | 0.56 to 0.68 | < 0.001 |
Motivational factors | ||||||
Work | 0.65 | 0.60 to 0.71 | < 0.001 | 0.62 | 0.57 to 0.67 | < 0.001 |
Leisure activities | 0.62 | 0.55 to 0.69 | 0.002 | 0.57 | 0.51 to 0.63 | 0.021 |
Financial management | 0.62 | 0.55 to 0.69 | 0.001 | 0.59 | 0.53 to 0.65 | 0.002 |
Motivation for treatment | 0.62 | 0.55 to 0.70 | 0.001 | 0.58 | 0.52 to 0.64 | 0.007 |
Attitudes towards authority | 0.67 | 0.60 to 0.74 | < 0.001 | 0.64 | 0.58 to 0.70 | < 0.001 |
Life goals | 0.57 | 0.50 to 0.65 | 0.058 | 0.55 | 0.49 to 0.61 | 0.114 |
Medication | 0.58 | 0.51 to 0.64 | 0.019 | 0.55 | 0.50 to 0.61 | 0.035 |
External factors | ||||||
Social network | 0.60 | 0.52 to 0.67 | 0.014 | 0.56 | 0.49 to 0.62 | 0.074 |
Intimate relationship | 0.52 | 0.47 to 0.57 | 0.431 | 0.51 | 0.46 to 0.55 | 0.779 |
Professional care | 0.49 | 0.48 to 0.50 | 0.014 | 0.50 | 0.48 to 0.51 | 0.705 |
Living circumstances | 0.56 | 0.49 to 0.62 | 0.112 | 0.57 | 0.52 to 0.63 | 0.010 |
External control | 0.59 | 0.52 to 0.66 | 0.009 | 0.59 | 0.54 to 0.65 | 0.001 |
For any violence within 12 months of discharge (see Table 118), the same internal factors predictive for 6-month violence were again predictive: empathy, coping and self-control. Similarly, all motivational factors except life goals were significant predictors of any violence within 12 months of discharge. Finally, among the external factors, only living circumstances and external control were predictive of violence at 12 months.
We finally tested the predictive accuracy of PANSS items for any violence within 6 and 12 months (Table 119). For any violence within 6 months, the following positive items were predictive: conceptual disorganisation, excitement, grandiosity, suspiciousness/persecution and hostility. None of the negative items was predictive. Among the general items, anxiety, depression, unco-operativeness, unusual thought content, poor attention, lack of judgement and insight, poor impulse control and preoccupation were significant predictors. In addition, all aggression items were predictive for any violence within 6 months of discharge. For violence at 12 months the positive items excitement, grandiosity, suspiciousness/persecution and hostility were predictive. Among negative items, the only predictive item was poor rapport. The general items unco-operativeness, unusual thought content, poor attention, lack of judgement and insight and poor impulse were predictive of any violence within 12 months. Finally, as with any violence within 6 months, all aggression items were predictive of 12-month violence.
PANSS items | Within 6 months | Within 12 months | ||||
---|---|---|---|---|---|---|
AUC | 95% CI | p-value | AUC | 95% CI | p-value | |
Positive | ||||||
Delusions | 0.56 | 0.49 to 0.63 | 0.109 | 0.56 | 0.50 to 0.62 | 0.061 |
Conceptual disorganisation | 0.59 | 0.51 to 0.66 | 0.019 | 0.55 | 0.50 to 0.61 | 0.073 |
Hallucinatory behaviour | 0.50 | 0.44 to 0.57 | 0.875 | 0.50 | 0.45 to 0.55 | 0.895 |
Excitement | 0.62 | 0.55 to 0.70 | 0.001 | 0.60 | 0.54 to 0.65 | 0.001 |
Grandiosity | 0.58 | 0.50 to 0.65 | 0.040 | 0.58 | 0.52 to 0.64 | 0.010 |
Suspiciousness/persecution | 0.60 | 0.52 to 0.68 | 0.015 | 0.57 | 0.51 to 0.63 | 0.027 |
Hostility | 0.68 | 0.60 to 0.76 | < 0.001 | 0.66 | 0.60 to 0.72 | < 0.001 |
Negative | ||||||
Blunted affect | 0.50 | 0.43 to 0.58 | 0.970 | 0.52 | 0.46 to 0.58 | 0.587 |
Emotional withdrawal | 0.47 | 0.40 to 0.54 | 0.452 | 0.46 | 0.41 to 0.52 | 0.200 |
Poor rapport | 0.58 | 0.50 to 0.66 | 0.053 | 0.58 | 0.51 to 0.64 | 0.018 |
Passive/apathetic social withdrawal | 0.54 | 0.46 to 0.62 | 0.354 | 0.52 | 0.45 to 0.58 | 0.625 |
Difficulty in abstract thinking | 0.58 | 0.50 to 0.66 | 0.052 | 0.57 | 0.50 to 0.63 | 0.052 |
Lack of spontaneity and flow | 0.54 | 0.47 to 0.61 | 0.298 | 0.52 | 0.46 to 0.58 | 0.463 |
Stereotyped thinking | 0.55 | 0.48 to 0.63 | 0.172 | 0.54 | 0.48 to 0.60 | 0.184 |
General | ||||||
Somatic concern | 0.52 | 0.45 to 0.59 | 0.596 | 0.51 | 0.45 to 0.56 | 0.748 |
Anxiety | 0.59 | 0.51 to 0.67 | 0.035 | 0.56 | 0.49 to 0.63 | 0.072 |
Guilt feelings | 0.48 | 0.42 to 0.55 | 0.628 | 0.50 | 0.44 to 0.55 | 0.917 |
Tension | 0.57 | 0.49 to 0.65 | 0.082 | 0.52 | 0.46 to 0.58 | 0.492 |
Mannerisms and posturing | 0.55 | 0.49 to 0.61 | 0.079 | 0.54 | 0.49 to 0.58 | 0.106 |
Depression | 0.60 | 0.52 to 0.67 | 0.014 | 0.56 | 0.50 to 0.62 | 0.066 |
Motor retardation | 0.46 | 0.42 to 0.50 | 0.052 | 0.47 | 0.43 to 0.51 | 0.107 |
Unco-operativeness | 0.68 | 0.60 to 0.76 | < 0.001 | 0.65 | 0.58 to 0.71 | < 0.001 |
Unusual thought content | 0.60 | 0.52 to 0.67 | 0.009 | 0.58 | 0.52 to 0.64 | 0.006 |
Disorientation | 0.52 | 0.48 to 0.56 | 0.376 | 0.52 | 0.49 to 0.55 | 0.228 |
Poor attention | 0.58 | 0.50 to 0.66 | 0.048 | 0.57 | 0.50 to 0.63 | 0.039 |
Lack of judgement and insight | 0.67 | 0.60 to 0.75 | < 0.001 | 0.61 | 0.54 to 0.68 | 0.002 |
Disturbance of volition | 0.57 | 0.50 to 0.63 | 0.046 | 0.55 | 0.50 to 0.61 | 0.052 |
Poor impulse control | 0.71 | 0.63 to 0.78 | < 0.001 | 0.68 | 0.62 to 0.74 | < 0.001 |
Preoccupation | 0.58 | 0.50 to 0.65 | 0.037 | 0.55 | 0.49 to 0.61 | 0.122 |
Active social avoidance | 0.53 | 0.46 to 0.61 | 0.394 | 0.48 | 0.43 to 0.54 | 0.608 |
Aggression | ||||||
Anger | 0.67 | 0.59 to 0.75 | < 0.001 | 0.66 | 0.59 to 0.72 | < 0.001 |
Difficulty in delaying gratification | 0.69 | 0.62 to 0.77 | < 0.001 | 0.66 | 0.60 to 0.73 | < 0.001 |
Affective liability | 0.69 | 0.61 to 0.77 | < 0.001 | 0.63 | 0.57 to 0.69 | < 0.001 |
Discussion
The MSRAG showed considerable shrinkage in its predictive accuracy for violent behaviour at 6 months and 12 months following discharge in this validation sample. AUC values were now poor at predicting the violent outcomes using the serious offending recidivism and acquisitive recidivism scales. However, because the MSRAG was originally standardised on serious offending behaviour measured using criminal convictions and over a considerably longer period of, on average, 6 years, it may have been unrealistic to expect the instrument to perform well on a different outcome from the one it was originally designed to measure. Nevertheless, the shrinkage could simply be the result of the instrument performing poorly on a new validation sample. This has previously been observed with other instruments, such as the COVR, which was marketed as a compact disc read-only memory (CD-ROM) to be used by clinicians to assess risk in patients discharged from psychiatric facilities. 13
We had originally intended to purchase the COVR and compare it with the MSRAG and other instruments. However, by the time our programme had started, it had become clear that the COVR performed particularly poorly. We subsequently examined the method used to develop the COVR and determined that the classification tree model used did not adequately explain certain items that might not appear in new samples or patient populations. These may have been unique to the original sample and therefore unlikely to have good predictive power in a new sample. Subsequent validation showed considerable shrinkage in the predictive power of the COVR. 13,14 McCusker15 has argued that the clinical use of the instrument was questionable, the construction sample had fitted the data too specifically (a problem we believed had been exacerbated by use of tree modelling) and environmental influences would really determine future accuracy.
Our findings would therefore question whether or not we had encountered similar problems shown in the development of the COVR in the USA. The analysis of individual items suggests that part of the problem may have been explained by the fact that not all items were predictive of the outcomes under study. This was a problem shown with items in the HCR-20v3 scales, particularly the historical scale. However, when compared with other actuarial instruments, including the RM2000V, OGRS2, PIV and PCL:SV, the MSRAG performed better, with a higher AUC value at 6 months. At 12 months after discharge, the performance of all static instruments was approximately the same.
When comparing the MSRAG with the HCR-20v3, it is appropriate to compare it with the historical scale, which consists of static items (the clinical and risk scales are used to measure dynamic items). Our findings showed that the predictive accuracy of the HCR-20v3 historical scale and the MSRAG were similar, at both 6 months and 12 months. However, AUC values for the clinical scale were considerably better. This was an unexpected finding. Previous studies examining the predictive ability of the clinical scale using previous versions of the HCR-20 have tended to find that both the clinical and the risk scales have poorer predictive ability than the historical scale. 8 Nevertheless, most of these studies used an outcome of violent behaviour measured over a longer period than 6 or 12 months, as in this study. These findings would suggest that clinical items, and to a lesser extent management items, were more relevant measures of risk in the short term, certainly over a period of 6 months. Management items were less accurate by 12 months. Considering the implications of these findings, it could be argued that it is inappropriate to use clinical and management items as a predictive scale for future behaviour. Instead of this approach, individual items should be identified as risk factors that should be modified to prevent subsequent violence by the clinicians responsible for the patients care, but not used to ‘predict’ future violence.
Items in these two subscales are ‘dynamic’ in the sense that the behaviour that was measured before release is likely to have continued and therefore is likely to have been predictive using the statistical method of the study. However, at a later date, some of these items might well change and, possibly, recede. This would explain why other studies, over longer periods, have found that clinical and risk management scales do not have predictive ability or have poor ability in the longer term. If this were the case in our sample, it would, in turn, question whether or not it is appropriate to validate dynamic measures such as the clinical and management scales of the HCR-20v3 using the predictive method that we employed in this study. Nevertheless, all other researchers in the field, including those who have developed the HCR-20 through its previous versions, have used this same method for validation. 390
When considering the SAPROF, the instrument demonstrated a small decline in predictive ability from 6 months to 12 months. Nevertheless, the total score showed that it had superior predictive ability to the MSRAG. On the other hand, the scoring of the SAPROF was not carried out according to its original design. The instrument is intended to advise clinicians of strengths in a patient’s clinical history and items that are protective for future violent behaviour. The scoring in this study is therefore artificial in reversing the scores so that patients with fewer protective factors score higher, thereby indicating that they are at greater risk.
Previous studies suggest that symptoms of severe mental illness are not particularly good predictors of future violent behaviour, especially over the longer term. 20 In the short term, aggressive behaviour and thinking performed moderately well as predictors. However, symptoms of severe mental illness are also dynamic. A score on different PANSS scales, including positive, negative and general symptoms, may or may not correspond to violent behaviour over the longer term. For example, it has been observed that delusions are not predictive of future violence among patients discharged from US psychiatric hospitals. 21 However, although not predictive, delusions appear to cause violence when the violence is measured during the same time period as the delusions. 21,291
Screening
So far, we have compared the predictive accuracy of the MSRAG with that of other instruments using AUC values. We found that it performed similarly to the RM2000V, OGRS2, PIV and PCL:SV, at a low level of predictive accuracy. When compared with the historical scale of the HCR-20v3, the scale that contains static or historical items similar to those of actuarial instruments, its performance was the same. The MSRAG was exceeded only by the clinical scale of the HCR-20v3, the total scale of the HCR-20,v3 which included the clinical scale items, and the SAPROF motivational scale and total scale, the latter including the motivational scale items. However, when we applied statistics used more commonly in epidemiological studies of screening instruments that are used to measure the accuracy of current classifications, different patterns emerged. Differences between PPV and NPV were less apparent and all total and subscales of the instruments performed similarly. The MSRAG (serious offending) appeared to be relatively less specific than most instruments for any violent behaviour at 6 months and 12 months, but had higher sensitivity. When the percentage of all subjects correctly classified was considered (i.e. those predicted to be violent who were violent and those predicted not to be violent who were not violent), the MSRAG performed as well as any screen in the study.
It can be argued that it is inappropriate to attempt to predict violent behaviour using the acquisitive recidivism scale of the MSRAG. The serious offending recidivism scale would be considered closer in terms of intended outcome. It is therefore reassuring that the serious offending recidivism scale performed better than the acquisitive recidivism scale. Examining the PCC, the MSRAG serious offending recidivism scale performed as accurately as the HCR-20v3 clinical scale and nearly as accurately as the SAPROF external scale.
Finally, when comparing all instruments, the PPV was low. These instruments do not perform well in terms of accurately classifying those who actually behave violently at 6 months and 12 months. There was some small improvement at 12 months. However, when considering the PCC, our findings suggest that approximately one in three cases will be incorrectly classified as violent or not violent by these instruments. This has important implications because it indicates that the use of such instruments to make major decisions, including discharge and extended sentencing, would not be justified solely on the basis of the ratings of any instrument included in the study.
Acquisitive offending
If the aim of the study was to predict future acquisitive offending then the MSRAG performed better than any instrument or subscale included in the study. AUC values were superior to those of all other instruments, although they were only slightly higher than those for the OGRS2 (the OGRS2 was specifically designed to measure risk of general offending and was originally standardised on a large sample of prisoners). However, when examining the conventional measures of accuracy, OGRS2 showed 100% sensitivity and MSRAG showed 91.7% sensitivity. All instruments performed well for NPV but the PPV was low for all instruments. Relying on the PCC as the final arbiter of whether an instrument should be used to classify a patient, the SAPROF (reverse) external scale and the MSRAG serious reoffending scale classified the largest number of patients accurately for future acquisitive convictions. However, in both cases approximately 30% of patients would have been misclassified, similar to our findings for violence.
These findings, although initially discouraging with regard to the use of instruments to classify patients, are nevertheless of considerable importance. The MSRAG serious offending scale classifies acquisitive offending more accurately than the acquisitive recidivism scale. It is worth noting that the HCR-20v3 historical scale is specifically designed to assess risk for future violence. However, the AUC value for the historical scale was slightly higher for predicting acquisitive offending than for predicting violence at 12 months, with the PCC similar in each case. When considering these findings, it has to be questioned why instruments that are specifically designed to predict violent behaviour should perform better, or as well, for predicting future acquisitive offending.
Previous research has demonstrated that the PCL-R predicts both violence and general recidivism. 391–393 Furthermore, the VRAG, despite being developed to predict violence, also predicts general recidivism. 394 Both instruments are highly correlated, with some items in common. 395
Criminological research has demonstrated that frequent, violent offenders are indistinguishable from frequent, non-violent offenders and that involvement in violence is primarily a function of increasing offence frequency, that is, there is no tendency to specialise in violence. Farrington et al. 396 examined a large sample of offenders and found a small but significant degree of specialising in offending. However, this was superimposed on a considerable amount of versatility of offending behaviour. In this US sample, offending careers showed that the most specialised offences were not violence, but running away from home, burglary, motor vehicle theft, liquor violations, incorrigibility, curfew, truancy and drugs. Reviewing the Cambridge Study in Delinquent Development, Farrington397 concluded that there is significant continuity in aggression and violence from childhood to adulthood, spanning a time period of nearly a quarter of a century in the sample studied. It was clear that boys who had been aggressive in childhood or adolescence tended to be more deviant in adulthood. They were found later to be living in worse home circumstances, more likely to be in conflict with, and violent towards, their wife or cohabitee, more likely to be unemployed, more likely to be a heavier smoker and drinker, more likely to be a drunk driver and drug taker and to have committed more offences (including violence). Farrington believed that this continuity was probably not specific to aggression and violence but a part of a general continuity of antisocial and deviant behaviour from childhood to adulthood. These findings question whether or not it is possible to develop a risk assessment instrument that specifically and exclusively measures risk for future violence, especially if based on samples of offenders.
It must be questioned why the predictive method of validation using AUC values is applied to both SPJ and ARA instruments. This method is arguably more suitable for ARA instruments because the purpose of these is limited to classifying individuals more simply into levels of risk. SPJ instruments are intended to guide clinical decision-making and clinicians are instructed not to sum the total scores of the items in each scale (historical, clinical and risk management). However, in this study we did this in contravention of the recommendation. On the other hand, the developers of the HCR-20v3 and other SPJ instruments have used the same method of validation and continue to do so. The difficulty in interpreting our findings would suggest that to continue to do so is methodologically unsound. For a risk factor to be successfully modified to prevent risk it must be causally related to violence, the outcome of interest. The validation method that we and other researchers have employed does not identify causal relationships between either items or scores on instruments and violent outcome. An item may be predictive but that does not mean that it is causal. Paradoxically, the item may be poorly predictive of future violence and yet it may have a strong causal relationship if occurring in close temporal proximity to the violence. If SPJ instruments are to be validated in the future, then new methods must be developed for this purpose. Ultimately, the test of whether the time-consuming and expensive process of completing a SPJ assessment can prevent a single act of violence must be determined by a RCT. There is currently no evidence that completing an ARA instrument or a SPJ instrument can achieve this.
Serious offending (convictions)
The fact that the MSRAG was standardised on official criminal records may explain why it performs relatively well compared with other instruments when using conventional statistics for classification. However, AUC values would suggest that it performs poorly and only the SAPROF and HCR-20v3 total scale scores achieved good predictive accuracy at 6 months, with a decline in accuracy for the HCR-20v3 total score scale at 12 months. However, our findings revealed a problem when evaluating accuracy. Whereas the MSRAG performed poorly using AUC values, by 12 months the serious offending scale outperformed all other instruments except for the PIV scale and the SAPROF external scale on the basis of the PCC.
Any offending
The paradoxical findings observed for serious offending can also be observed when all convictions are combined. Whereas AUC values compare for the MSRAG serious offending scale and SAPROF and HCR-20v3, the PCC using MSRAG exceeded the two SPJ scales. For any offending, other actuarial instruments show improved AUC values over the MSRAG. However, the serious offending scale outperformed these instruments on the basis of PCC.
Predictability of individual items of the Medium Security Recidivism Assessment Guide
We finally investigated the predictive ability of the MSRAG in terms of individual items included in certain other instruments in the study (HCR-20v3, SAPROF and PANSS). Our aim was to examine which individual items predicted any violence at 6 months and 12 months after discharge. We found that only two items were predictive in the MSRAG acquisitive recidivism scale and that these were poor predictors: number of prior criminal offences and being subject to a hospital restriction order. These same items were also predictive for the MSRAG serious offending scale at 6 months. By 12 months there had been some improvement in their predictive accuracy and age at index offence leading to hospitalisation had also become significant. Nevertheless, the AUC values still indicated a poor predictive ability of these individual items.
When examining the predictive efficacy of the HCR-20v3 historical scale, only four items showed predictive ability and this was at a low level (AUC values all < 0.60). There was no improvement over 12 months in contrast to the MSRAG. The HCR-20v3 clinical scale included four items that were predictive, but none of these achieved a ‘moderate’ AUC value of 0.7. Treatment or supervision response on the risk management scale was significant at both time points and by 12 months stress or coping became significant. However, both predictors would still be considered ‘poor’ in terms of an AUC value.
When considering the predictive ability of the SAPROF scale, considerably more items achieved statistical significance than for the other instruments. There was no improvement by 12 months and, as with the other instruments, none of the items achieved a measure of predictive efficacy that could be considered ‘moderate’ based on AUC values.
If an AUC value of ≥ 0.7 is considered moderate predictive ability and a value of ≥ 0.8 is considered good predictive ability, then only poor impulse control at 6 months used in the PANSS achieved a moderate level of prediction. By 12 months, excitement, grandiosity, suspiciousness/persecution, hostility, poor rapport, unco-operativeness, unusual thought content, poor attention, lack of judgement and insight, poor impulse control, anger, difficulty in delaying gratification, and affective liability were all predictive. However, none of these items achieved an AUC value of > 0.7.
Chapter 15 Dynamic effects of risk assessment instruments for patients discharged from medium secure services
Background
We have previously demonstrated that the predictive ability of most risk assessment instruments is explained by a limited number of their items. 23 This means that, if the intention is to measure predictive accuracy, the number of items can be reduced while still maintaining accuracy in prediction. However, such an exercise serves little purpose in the preliminary stages of developing dynamic risk assessment instruments. It is essential to test whether or not dynamic items have a causal relationship with violence and are factors that should be targeted for intervention. In this chapter we carry out a preliminary investigation of individual dynamic risk and protective factors from the HCR-20v3 and SAPROF and their associations with violence. The long-term objective is to identify candidate causal risk factors. These should undergo further investigation using methods that establish causal associations.
More recently, our research has shown that the predictive accuracy of different risk assessment instruments differs considerably according to diagnosis. 398 We found that, for patients with severe personality disorder (particularly psychopathy), risk assessment instruments performed poorly. For psychopathy their accuracy was no better than tossing a coin. It therefore follows that the dynamic risk and protective factors that are of relevance to violent outcome may also differ according to diagnosis. This would correspond to clinical observations that the risk factors for a patient with severe personality disorder may be very different from those for a patient with schizophrenia.
Objectives
The objectives of this study were to:
-
investigate the association between dynamic items and concurrent violence to identify items with candidate causal effects that might be helpful as offender management targets
-
examine these associations according to the presence or absence of major mental illness and the presence or absence of personality disorder.
Methods
The sample under study as well as exposure and outcome measures have been described in detail in Chapters 13 and 14.
For the statistical analysis we used multilevel logistic regression models to estimate simultaneously for both time periods (from baseline to 6 months and from 6 months to 12 months) the effect of each dynamic item (quantified by OR) on concurrent violence. We adjusted our analysis only for time period to obtain the univariate overall effect of each item on violence over the same period. By using multilevel models we increased the power of detecting an item effect on violence and at the same time adjusted for patient-specific unobserved heterogeneity (random effect).
In addition to the analysis for the overall sample, we carried out subgroup analysis based on presence/absence of major mental illness. The definition of major mental illness was based on a primary diagnosis for one of the following: schizophrenia, schizoaffective disorder, mania/bipolar disorder, delusional disorder, severe depressive episode with psychotic symptoms, first-episode psychosis and affective psychosis. Of 387 patients, 349 (90.2%) had major mental illness and 38 (9.8%) were without major mental illness.
We also performed additional subgroup analysis based on presence/absence of personality disorder. In total, 76 patients (19.6%) had personality disorder as a primary or co-occurring condition and 311 (80.4%) had no personality disorder.
Results
The results for the analysis of HCR-20v3 dynamic items (clinical and risk management scales) for the total sample are shown in Table 120. Apart from the item R1 (professional services and plans), all items were significantly associated with concurrent violence. The largest OR corresponded to item R5 (stress or coping), followed by the OR for item C2 (violent ideation or intent).
HCR-20v3 items | AOR | 95% CI | p-value |
---|---|---|---|
Clinical scale | |||
C1: insight | 4.37 | 2.54 to 7.54 | < 0.001 |
C2: violent ideation or intent | 7.48 | 4.11 to 13.62 | < 0.001 |
C3: symptoms of major mental disorder | 3.18 | 2.07 to 4.88 | < 0.001 |
C4: instability | 5.95 | 3.76 to 9.42 | < 0.001 |
C5: treatment or supervision response | 3.26 | 2.25 to 4.72 | < 0.001 |
Risk management scale | |||
R1: professional services and plans | 1.46 | 0.78 to 2.73 | 0.235 |
R2: living situation | 2.14 | 1.36 to 3.36 | 0.001 |
R3: personal support | 1.89 | 1.24 to 2.88 | 0.003 |
R4: treatment or supervision response | 3.53 | 2.25 to 5.53 | < 0.001 |
R5: stress or coping | 9.35 | 4.63 to 18.92 | < 0.001 |
The association between SAPROF items (reverse direction) and violence over the same time period are reported In Table 121. Among internal factors, we found that lack of empathy, lack of coping and lack of self-control were associated with violence, whereas low intelligence level and lack of secure attachment in childhood were not. All motivational factors were associated with violence. However, among external factors, only lack of social network was significantly associated with concurrent violence.
SAPROF (reverse) items | AOR | 95% CI | p-value |
---|---|---|---|
Internal factors | |||
Intelligence | 1.54 | 0.85 to 2.78 | 0.153 |
Secure attachment in childhood | 1.46 | 0.99 to 2.15 | 0.054 |
Empathy | 3.45 | 2.28 to 5.22 | < 0.001 |
Coping | 5.56 | 3.25 to 9.49 | < 0.001 |
Self-control | 8.28 | 4.65 to 14.73 | < 0.001 |
Motivational factors | |||
Work | 1.74 | 1.15 to 2.64 | 0.009 |
Leisure activities | 2.97 | 2.00 to 4.41 | < 0.001 |
Financial management | 2.26 | 1.47 to 3.46 | < 0.001 |
Motivation for treatment | 3.45 | 2.19 to 5.46 | < 0.001 |
Attitudes towards authority | 4.15 | 2.73 to 6.32 | < 0.001 |
Life goals | 2.64 | 1.69 to 4.10 | < 0.001 |
Medication | 2.76 | 1.77 to 4.30 | < 0.001 |
External factors | |||
Social network | 2.29 | 1.48 to 3.54 | < 0.001 |
Intimate relationship | 0.96 | 0.60 to 1.54 | 0.878 |
Professional care | 1.39 | 0.43 to 4.52 | 0.581 |
Living circumstances | 0.91 | 0.58 to 1.42 | 0.664 |
External control | 0.89 | 0.52 to 1.54 | 0.687 |
The PANNS item effects on concurrent violence for the overall sample are presented in Table 122. All positive items were significantly associated with violence. Among the negative items, apart from blunted affect all other items were significantly associated with violence. Among general items, those not associated with violence included somatic concern, guilt feelings, depression, motor retardation and disorientation. Finally, all aggression items were significantly associated with violence.
PANSS items | AOR | 95% CI | p-value |
---|---|---|---|
Positive | |||
Delusions | 1.67 | 1.30 to 2.16 | < 0.001 |
Conceptual disorganisation | 1.82 | 1.40 to 2.37 | < 0.001 |
Hallucinatory behaviour | 1.37 | 1.07 to 1.76 | 0.012 |
Excitement | 2.02 | 1.51 to 2.71 | < 0.001 |
Grandiosity | 1.64 | 1.33 to 2.03 | < 0.001 |
Suspiciousness/persecution | 1.97 | 1.56 to 2.49 | < 0.001 |
Hostility | 2.35 | 1.89 to 2.94 | < 0.001 |
Negative | |||
Blunted affect | 1.25 | 0.97 to 1.62 | 0.091 |
Emotional withdrawal | 1.40 | 1.10 to 1.79 | 0.007 |
Poor rapport | 1.58 | 1.29 to 1.93 | < 0.001 |
Passive/apathetic social withdrawal | 1.43 | 1.16 to 1.75 | 0.001 |
Difficulty in abstract thinking | 1.28 | 1.06 to 1.54 | 0.010 |
Lack of spontaneity and flow | 1.25 | 1.02 to 1.54 | 0.031 |
Stereotyped thinking | 1.27 | 1.03 to 1.56 | 0.024 |
General | |||
Somatic concern | 1.29 | 0.99 to 1.67 | 0.055 |
Anxiety | 1.49 | 1.20 to 1.85 | < 0.001 |
Guilt feelings | 1.24 | 0.94 to 1.62 | 0.123 |
Tension | 1.79 | 1.38 to 2.32 | < 0.001 |
Mannerisms and posturing | 2.12 | 1.38 to 3.26 | 0.001 |
Depression | 1.17 | 0.90 to 1.51 | 0.238 |
Motor retardation | 0.54 | 0.23 to 1.27 | 0.159 |
Unco-operativeness | 2.22 | 1.77 to 2.79 | < 0.001 |
Unusual thought content | 1.87 | 1.45 to 2.41 | < 0.001 |
Disorientation | 1.53 | 0.93 to 2.54 | 0.096 |
Poor attention | 1.47 | 1.17 to 1.86 | 0.001 |
Lack of judgement and insight | 2.00 | 1.56 to 2.55 | < 0.001 |
Disturbance of volition | 1.80 | 1.39 to 2.33 | < 0.001 |
Poor impulse control | 2.78 | 2.06 to 3.75 | < 0.001 |
Preoccupation | 1.69 | 1.37 to 2.09 | < 0.001 |
Active social avoidance | 1.46 | 1.18 to 1.81 | < 0.001 |
Aggression | |||
Anger | 2.62 | 2.03 to 3.37 | < 0.001 |
Difficulty in delaying gratification | 2.10 | 1.66 to 2.66 | < 0.001 |
Affective liability | 2.21 | 1.72 to 2.84 | < 0.001 |
Table 123 shows the association between HCR-20v3 items and violence for the subgroups with and without major mental illness. In the subgroup without major mental illness, fewer clinical scale items were significantly associated with violence [C1 (insight), C2 (violent ideation or intent) and C5 (treatment or supervision response)]. Among risk management scale items, only item R4 (treatment or supervision response) was associated with the outcome of interest. In contrast, in the subgroup with major mental illness, apart from item R1 (professional services and plans), all items were associated with violence.
HCR-20v3 items | Without major mental illness (n = 38) | With major mental illness (n = 349) | ||||
---|---|---|---|---|---|---|
AOR | 95% CI | p-value | AOR | 95% CI | p-value | |
Clinical scale | ||||||
C1: insight | 6.79 | 1.51 to 30.51 | 0.012 | 4.16 | 2.31 to 7.49 | < 0.001 |
C2: violent ideation or intent | 12.91 | 1.14 to 146.05 | 0.039 | 6.94 | 3.80 to 12.67 | < 0.001 |
C3: symptoms of major mental disorder | 1.85 | 0.60 to 5.76 | 0.285 | 3.37 | 2.12 to 5.37 | < 0.001 |
C4: instability | 28.45 | 0.82 to 986.45 | 0.064 | 5.45 | 3.45 to 8.61 | < 0.001 |
C5: treatment or supervision response | 4.99 | 1.64 to 15.19 | 0.005 | 3.02 | 2.03 to 4.50 | < 0.001 |
Risk management scale | ||||||
R1: professional services and plans | 3.09 | 0.85 to 11.29 | 0.088 | 1.15 | 0.55 to 2.42 | 0.715 |
R2: living situation | 4.31 | 0.95 to 19.51 | 0.058 | 1.91 | 1.17 to 3.13 | 0.010 |
R3: personal support | 2.12 | 0.53 to 8.40 | 0.286 | 1.86 | 1.19 to 2.91 | 0.007 |
R4: treatment or supervision response | 9.23 | 1.10 to 77.63 | 0.041 | 3.18 | 1.99 to 5.08 | < 0.001 |
R5: stress or coping | 12.45 | 0.99 to 156.59 | 0.051 | 8.98 | 4.35 to 18.56 | < 0.001 |
The results from the analysis of the effects of SAPROF items (reverse) on concurrent violence in the subgroups with and without major mental illness are shown in Table 124. For patients without major mental illness, lack of empathy, lack of coping and lack of self-control were internal factors associated with violence. Among motivational factors, lack of leisure activities, lack of motivation for treatment and lack of life goals were associated with the outcome of interest. Finally, none of the external factors was associated with violence. For patients with major mental illness, among the internal factors, lack of empathy, lack of coping and lack of self-control were associated with violence. Furthermore, all motivational factors were associated with violence whereas the only external factor associated with violence was lack of social network.
SAPROF (reverse) items | Without major mental illness (n = 38) | With major mental illness (n = 349) | ||||
---|---|---|---|---|---|---|
AOR | 95% CI | p-value | AOR | 95% CI | p-value | |
Internal factors | ||||||
Intelligence | 3.61 | 0.68 to 19.26 | 0.133 | 1.39 | 0.74 to 2.63 | 0.304 |
Secure attachment in childhood | 2.98 | 0.65 to 13.74 | 0.161 | 1.33 | 0.88 to 2.00 | 0.173 |
Empathy | 3.95 | 1.19 to 13.07 | 0.024 | 3.42 | 2.18 to 5.37 | < 0.001 |
Coping | 6.04 | 1.57 to 23.20 | 0.009 | 5.57 | 3.08 to 10.09 | < 0.001 |
Self-control | 15.54 | 2.13 to 113.32 | 0.007 | 7.95 | 4.29 to 14.76 | < 0.001 |
Motivational factors | ||||||
Work | 6.02 | 0.71 to 50.88 | 0.099 | 1.58 | 1.03 to 2.42 | 0.037 |
Leisure activities | 6.16 | 1.13 to 33.65 | 0.036 | 2.76 | 1.83 to 4.15 | < 0.001 |
Financial management | 2.74 | 0.89 to 8.40 | 0.078 | 2.14 | 1.34 to 3.42 | 0.001 |
Motivation for treatment | 4.98 | 1.52 to 16.30 | 0.008 | 3.29 | 1.98 to 5.46 | < 0.001 |
Attitudes towards authority | 3.33 | 0.86 to 12.86 | 0.081 | 4.28 | 2.73 to 6.69 | < 0.001 |
Life goals | 3.24 | 1.17 to 9.00 | 0.024 | 2.61 | 1.59 to 4.28 | < 0.001 |
Medication | 1.49 | 0.61 to 3.63 | 0.377 | 3.25 | 1.88 to 5.60 | < 0.001 |
External factors | ||||||
Social network | 0.89 | 0.22 to 3.61 | 0.874 | 2.57 | 1.62 to 4.06 | < 0.001 |
Intimate relationship | 0.40 | 0.09 to 1.91 | 0.253 | 1.14 | 0.68 to 1.93 | 0.614 |
Professional care | 4.01 | 0.08 to 210.98 | 0.492 | 1.27 | 0.36 to 4.49 | 0.715 |
Living circumstances | 1.52 | 0.47 to 4.95 | 0.487 | 0.83 | 0.51 to 1.36 | 0.468 |
External control | 0.73 | 0.14 to 3.77 | 0.710 | 0.86 | 0.47 to 1.57 | 0.627 |
The associations between PANSS items and violence over the same time period for patients with and without major mental illness are reported in Table 125. For patients without major mental illness, among the positive items, delusions and excitement were associated with violence. None of the negative items was associated with violence. Among the general items, unco-operativeness and poor impulse control were associated with violence and, among the aggression items, all items were associated with violence. For patients with major mental illness, all positive and negative items were associated with concurrent violence. Among general items, those not significantly associated with the outcome of interest were somatic concern, guilt feelings, depression and motor retardation. Finally, all aggression items were significantly associated with violence.
PANSS items | Without major mental illness (n = 38) | With major mental illness (n = 349) | ||||
---|---|---|---|---|---|---|
AOR | 95% CI | p-value | AOR | 95% CI | p-value | |
Positive | ||||||
Delusions | 3.09 | 1.06 to 8.97 | 0.038 | 1.67 | 1.27 to 2.19 | < 0.001 |
Conceptual disorganisation | 1.20 | 0.56 to 2.56 | 0.631 | 1.95 | 1.45 to 2.61 | < 0.001 |
Hallucinatory behaviour | 2.08 | 0.95 to 4.55 | 0.067 | 1.38 | 1.05 to 1.79 | 0.019 |
Excitement | 2.74 | 1.36 to 5.53 | 0.005 | 2.00 | 1.45 to 2.74 | < 0.001 |
Grandiosity | 1.24 | 0.61 to 2.52 | 0.561 | 1.70 | 1.36 to 2.14 | < 0.001 |
Suspiciousness/persecution | 1.73 | 0.90 to 3.34 | 0.103 | 2.06 | 1.59 to 2.66 | < 0.001 |
Hostility | 3.97 | 0.70 to 22.56 | 0.120 | 2.32 | 1.85 to 2.92 | < 0.001 |
Negative | ||||||
Blunted affect | 0.82 | 0.28 to 2.40 | 0.717 | 1.32 | 1.00 to 1.74 | 0.048 |
Emotional withdrawal | 1.52 | 0.63 to 3.70 | 0.355 | 1.42 | 1.10 to 1.84 | 0.007 |
Poor rapport | 1.81 | 0.90 to 3.67 | 0.098 | 1.60 | 1.29 to 1.99 | < 0.001 |
Passive/apathetic social withdrawal | 1.12 | 0.63 to 1.99 | 0.711 | 1.48 | 1.18 to 1.84 | 0.001 |
Difficulty in abstract thinking | 0.85 | 0.39 to 1.85 | 0.686 | 1.34 | 1.10 to 1.63 | 0.004 |
Lack of spontaneity and flow | 0.95 | 0.47 to 1.93 | 0.893 | 1.31 | 1.05 to 1.63 | 0.018 |
Stereotyped thinking | 0.89 | 0.40 to 1.97 | 0.773 | 1.31 | 1.06 to 1.64 | 0.014 |
General | ||||||
Somatic concern | 2.46 | 0.78 to 7.79 | 0.125 | 1.26 | 0.96 to 1.65 | 0.097 |
Anxiety | 2.03 | 0.90 to 4.57 | 0.086 | 1.44 | 1.15 to 1.81 | 0.001 |
Guilt feelings | 1.70 | 0.54 to 5.29 | 0.362 | 1.19 | 0.89 to 1.58 | 0.237 |
Tension | 0.76 | 0.20 to 2.93 | 0.693 | 1.93 | 1.45 to 2.58 | < 0.001 |
Mannerisms and posturing | – | – | – | 2.28 | 1.42 to 3.65 | 0.001 |
Depression | 1.27 | 0.66 to 2.43 | 0.476 | 1.14 | 0.86 to 1.52 | 0.353 |
Motor retardation | – | – | – | 0.64 | 0.27 to 1.51 | 0.305 |
Unco-operativeness | 2.37 | 1.28 to 4.41 | 0.006 | 2.23 | 1.74 to 2.86 | < 0.001 |
Unusual thought content | 1.18 | 0.49 to 2.85 | 0.708 | 2.00 | 1.50 to 2.66 | < 0.001 |
Disorientation | 0.40 | 0.04 to 4.48 | 0.459 | 1.74 | 1.02 to 2.97 | 0.043 |
Poor attention | 0.95 | 0.42 to 2.13 | 0.901 | 1.55 | 1.20 to 2.00 | 0.001 |
Lack of judgement and insight | 1.68 | 0.91 to 3.10 | 0.095 | 2.06 | 1.57 to 2.71 | < 0.001 |
Disturbance of volition | 0.64 | 0.10 to 4.23 | 0.640 | 1.92 | 1.45 to 2.53 | < 0.001 |
Poor impulse control | 2.38 | 1.28 to 4.45 | 0.006 | 2.92 | 2.05 to 4.16 | < 0.001 |
Preoccupation | 0.84 | 0.30 to 2.40 | 0.746 | 1.79 | 1.42 to 2.26 | < 0.001 |
Active social avoidance | 1.06 | 0.54 to 2.09 | 0.866 | 1.52 | 1.20 to 1.92 | < 0.001 |
Aggression | ||||||
Anger | 3.73 | 1.80 to 7.71 | < 0.001 | 2.59 | 1.96 to 3.42 | < 0.001 |
Difficulty in delaying gratification | 2.79 | 1.62 to 4.80 | < 0.001 | 2.03 | 1.58 to 2.61 | < 0.001 |
Affective liability | 2.67 | 1.26 to 5.67 | 0.011 | 2.21 | 1.69 to 2.90 | < 0.001 |
The estimates for HCR-20v3 items for patients with and without personality disorder are reported in Table 126. With the exception of item R1 (professional services and plans), all items were significantly associated with the outcome of interest for patients without personality disorder. Among patients with personality disorder, for the clinical scale items, with the exception of item C3 (symptoms of major mental disorder), all items were associated with violence. The risk management items significantly associated with violence were R4 (treatment or supervision response) and R5 (stress or coping).
HCR-20v3 items | Without personality disorder (n = 311) | With personality disorder (n = 76) | ||||
---|---|---|---|---|---|---|
AOR | 95% CI | p-value | AOR | 95% CI | p-value | |
Clinical scale | ||||||
C1: insight | 3.93 | 2.23 to 6.92 | < 0.001 | 8.99 | 1.27 to 63.55 | 0.028 |
C2: violent ideation or intent | 7.25 | 3.78 to 13.88 | < 0.001 | 8.10 | 2.04 to 32.14 | 0.003 |
C3: symptoms of major mental disorder | 3.48 | 2.15 to 5.63 | < 0.001 | 1.89 | 0.66 to 5.37 | 0.234 |
C4: instability | 6.28 | 3.79 to 10.39 | < 0.001 | 4.74 | 1.64 to 13.71 | 0.004 |
C5: treatment or supervision response | 3.33 | 2.18 to 5.09 | < 0.001 | 3.08 | 1.39 to 6.80 | 0.006 |
Risk management scale | ||||||
R1: professional services and plans | 1.54 | 0.76 to 3.08 | 0.228 | – | – | – |
R2: living situation | 2.34 | 1.43 to 3.82 | 0.001 | 1.55 | 0.44 to 5.44 | 0.493 |
R3: personal support | 1.68 | 1.05 to 2.68 | 0.031 | 4.57 | 0.84 to 24.75 | 0.078 |
R4: treatment or supervision response | 3.31 | 2.02 to 5.42 | < 0.001 | 4.67 | 1.55 to 14.09 | 0.006 |
R5: stress or coping | 9.53 | 4.40 to 20.64 | < 0.001 | 8.67 | 1.56 to 48.12 | 0.014 |
The effects of the SAPROF (reverse) items are shown in Table 127. Without personality disorder, the internal factors lack of empathy, lack of coping and lack of self-control were all associated with violence. With the exception of lack of work, all other motivational factors were associated with violence whereas, among the external factors, only lack of social network was associated with violence. similarly, for those with personality disorder, the internal factors lack of empathy, lack of coping and lack of self-control were all associated with violence. Among the motivational factors, all were associated with violence apart from lack of work and lack of leisure activities. None of the external factors was associated with violence.
SAPROF (reverse) items | Without personality disorder (n = 311) | With personality disorder (n = 76) | ||||
---|---|---|---|---|---|---|
AOR | 95% CI | p-value | AOR | 95% CI | p-value | |
Internal factors | ||||||
Intelligence | 1.34 | 0.72 to 2.51 | 0.353 | 3.17 | 0.56 to 18.08 | 0.194 |
Secure attachment in childhood | 1.37 | 0.90 to 2.09 | 0.142 | 2.34 | 0.63 to 8.76 | 0.205 |
Empathy | 3.11 | 2.03 to 4.76 | < 0.001 | 8.58 | 1.67 to 44.02 | 0.010 |
Coping | 5.14 | 2.85 to 9.27 | < 0.001 | 8.37 | 2.18 to 32.09 | 0.002 |
Self-control | 7.33 | 3.96 to 13.56 | < 0.001 | 16.96 | 3.28 to 87.69 | 0.001 |
Motivational factors | ||||||
Work | 1.42 | 0.92 to 2.21 | 0.115 | 7.79 | 0.75 to 80.57 | 0.085 |
Leisure activities | 2.89 | 1.92 to 4.35 | < 0.001 | 5.09 | 0.83 to 31.32 | 0.079 |
Financial management | 2.07 | 1.28 to 3.36 | 0.003 | 2.98 | 1.19 to 7.49 | 0.020 |
Motivation for treatment | 3.27 | 1.96 to 5.45 | < 0.001 | 4.35 | 1.49 to 12.68 | 0.007 |
Attitudes towards authority | 3.85 | 2.49 to 5.95 | < 0.001 | 7.41 | 1.83 to 29.98 | 0.005 |
Life goals | 2.42 | 1.49 to 3.91 | < 0.001 | 3.74 | 1.27 to 11.02 | 0.017 |
Medication | 2.66 | 1.60 to 4.42 | < 0.001 | 3.18 | 1.26 to 8.04 | 0.014 |
External factors | ||||||
Social network | 2.35 | 1.47 to 3.75 | < 0.001 | 2.28 | 0.71 to 7.40 | 0.168 |
Intimate relationship | 0.91 | 0.55 to 1.50 | 0.703 | 1.25 | 0.34 to 4.57 | 0.738 |
Professional care | 1.30 | 0.38 to 4.42 | 0.678 | – | – | – |
Living circumstances | 0.98 | 0.62 to 1.55 | 0.928 | – | – | – |
External control | 0.82 | 0.45 to 1.49 | 0.520 | – | – | – |
Table 128 shows the estimates for PANSS items in subgroups with and without personality disorder. For those without personality disorder, all positive items were associated with violence. With the exception of blunted affect, all other negative items were associated with violence. Among the general items, the only items not significantly associated with violence were guilt feelings, depression and motor retardation. All aggression outcomes were associated with violence over the same time period. For those with personality disorder, the only positive items associated with violence were suspiciousness/persecution and hostility. None of the negative items was associated with violence and the only general items associated with violence were unco-operativeness, lack of judgement and insight and poor impulse control. All aggression items were associated with violence.
PANSS items | Without personality disorder (n = 311) | With personality disorder (n = 76) | ||||
---|---|---|---|---|---|---|
AOR | 95% CI | p-value | AOR | 95% CI | p-value | |
Positive | ||||||
Delusions | 1.79 | 1.33 to 2.40 | < 0.001 | 1.29 | 0.76 to 2.17 | 0.349 |
Conceptual disorganisation | 1.91 | 1.43 to 2.54 | < 0.001 | – | – | – |
Hallucinatory behaviour | 1.42 | 1.06 to 1.91 | 0.020 | 1.23 | 0.72 to 2.11 | 0.448 |
Excitement | 1.92 | 1.43 to 2.60 | < 0.001 | – | – | – |
Grandiosity | 1.77 | 1.40 to 2.23 | < 0.001 | 1.30 | 0.72 to 2.32 | 0.384 |
Suspiciousness/persecution | 2.00 | 1.55 to 2.58 | < 0.001 | 1.96 | 1.08 to 3.56 | 0.027 |
Hostility | 2.27 | 1.81 to 2.85 | < 0.001 | 3.58 | 1.17 to 10.93 | 0.025 |
Negative | ||||||
Blunted affect | 1.20 | 0.91 to 1.58 | 0.189 | 1.61 | 0.70 to 3.71 | 0.263 |
Emotional withdrawal | 1.47 | 1.12 to 1.91 | 0.005 | 1.10 | 0.56 to 2.14 | 0.785 |
Poor rapport | 1.57 | 1.25 to 1.97 | < 0.001 | 1.78 | 0.97 to 3.28 | 0.064 |
Passive/apathetic social withdrawal | 1.48 | 1.18 to 1.85 | 0.001 | 1.31 | 0.74 to 2.32 | 0.361 |
Difficulty in abstract thinking | 1.31 | 1.07 to 1.60 | 0.008 | 1.13 | 0.67 to 1.88 | 0.650 |
Lack of spontaneity and flow | 1.31 | 1.05 to 1.63 | 0.018 | 1.01 | 0.53 to 1.91 | 0.977 |
Stereotyped thinking | 1.32 | 1.06 to 1.64 | 0.015 | 1.21 | 0.58 to 2.55 | 0.608 |
General | ||||||
Somatic concern | 1.49 | 1.10 to 2.02 | 0.010 | 0.71 | 0.31 to 1.62 | 0.416 |
Anxiety | 1.50 | 1.19 to 1.89 | 0.001 | – | – | – |
Guilt feelings | 1.26 | 0.94 to 1.69 | 0.121 | – | – | – |
Tension | 1.86 | 1.41 to 2.47 | < 0.001 | 1.57 | 0.75 to 3.31 | 0.233 |
Mannerisms and posturing | 2.18 | 1.40 to 3.40 | 0.001 | – | – | – |
Depression | 1.09 | 0.81 to 1.47 | 0.569 | 1.57 | 0.69 to 3.58 | 0.287 |
Motor retardation | 0.71 | 0.31 to 1.63 | 0.416 | – | – | – |
Unco-operativeness | 2.19 | 1.71 to 2.80 | < 0.001 | 2.48 | 1.36 to 4.54 | 0.003 |
Unusual thought content | 1.86 | 1.43 to 2.43 | < 0.001 | – | – | – |
Disorientation | 1.98 | 1.04 to 3.77 | 0.039 | 1.03 | 0.36 to 2.92 | 0.963 |
Poor attention | 1.47 | 1.14 to 1.89 | 0.003 | – | – | – |
Lack of judgement and insight | 1.93 | 1.49 to 2.48 | < 0.001 | 2.63 | 1.10 to 6.29 | 0.029 |
Disturbance of volition | 1.79 | 1.37 to 2.34 | < 0.001 | – | – | – |
Poor impulse control | 2.77 | 1.97 to 3.90 | < 0.001 | 3.10 | 1.59 to 6.03 | 0.001 |
Preoccupation | 1.71 | 1.37 to 2.13 | < 0.001 | – | – | – |
Active social avoidance | 1.57 | 1.24 to 2.00 | < 0.001 | 1.11 | 0.64 to 1.91 | 0.715 |
Aggression | ||||||
Anger | 2.52 | 1.91 to 3.33 | < 0.001 | 3.36 | 1.72 to 6.55 | < 0.001 |
Difficulty in delaying gratification | 2.05 | 1.60 to 2.62 | < 0.001 | 2.86 | 1.18 to 6.96 | 0.020 |
Affective liability | 2.16 | 1.66 to 2.80 | < 0.001 | 2.95 | 1.27 to 6.86 | 0.012 |
Discussion
We included items from the clinical and risk management scales of the HCR-20v3 in these analyses because they may have a causal relationship with the outcome of any violence over the 12 months following discharge from medium secure services. Although we cannot establish causality on the basis of the analysis in this chapter, our method provides a means of identifying candidate items for future investigation of causality. Our findings contrast with those in Chapter 14 where it was observed that symptoms of mental disorder (C3) were not predictive in the clinical scale and where professional services and plans, living situation, personal support and stress or coping were not predictive in the risk management scale. All items in the clinical scale were measured in close temporal proximity to the violent outcome and showed high levels of association when estimated using AORs. Similarly, all risk items except professional services and plans now showed strong associations. It was of considerable interest that stress or coping (R5) showed the highest odds of association. These findings suggest that a patient who is stressed following discharge, is unable to cope, shows clinical signs of instability, is known to have violent thoughts or intentions, has a poor treatment or supervision response and is displaying active symptoms of major mental disorder is at high risk of violent behaviour. However, this occurs only at a time when these risk factors are in operation. Preventing violence could be achieved by targeting these factors following discharge if it can subsequently be established that they are causal risk factors for violence. For example, it might be necessary to transfer a patient to a more suitable living situation if his or her current circumstances result in increased stress and an inability to cope. It would be necessary to provide more support, ensure that the patient is receiving treatment, particularly treatment to prevent active symptoms of major mental disorder, and institute a treatment intervention designed to reduce violent ruminations or thoughts of harming others. It might be necessary to return the patient to a more secure environment if there is sufficient evidence of violent intent and a violent assault is thought probable.
Motivation for treatment, attitude towards authority and poor empathy appeared to be factors that were strongly associated with violent behaviour, as measured by the reversed SAPROF items. However, for many of these factors, the AORs were relatively lower than those observed for the HCR-20v3. Overall, our findings suggest that there is little to be gained by the process of reversing SAPROF scores that cannot be measured by the use of the HCR-20v3. We would not recommend further use of this method because the SAPROF is not intended to measure risk. Future investigations should concentrate instead on the reduction in violence and its association with protective factors measured using the SAPROF, together with the interaction between scores on the SAPROF for protection with scores of risk measured using other instruments.
The findings in Chapter 14 that delusions had no predictive ability for future violence as measured with the PANSS but that they were significantly associated with violence if they occur in close temporal proximity corresponds to recent research with other samples. 21,291 However, the strength of the associations were low in this sample. We previously found that the association between delusions and violence was mediated by anger related to the delusional beliefs. All positive symptoms were found to have some degree of association with violence, together with certain negative symptoms in this study. However, few of the associations observed using the PANSS had an OR > 2.0 following adjustments (hostility, excitement, mannerisms and posture, co-operativeness, poor impulse control, anger, difficulty in delaying gratification and affective liability). Not all of these symptoms are necessarily caused by severe mental illness, even though they were measured using the PANSS. Several could be secondary to personality disorder, which was present in a subgroup of the sample. Finally, a limitation of the method is that we did not adjust the risk factors, protective factors and symptoms for each other.
Are dynamic risk factors specifically associated with violence according to diagnosis?
Diagnosis was found to exert an important effect on whether or not the risk factors we studied were associated with violent outcome. In patients with a primary diagnosis of major mental illness, analysis of the HCR-20v3 showed strong associations with all items in the clinical scale and strong associations with treatment or supervision response and stress or coping in the risk management scale. There were weaker associations with living situation and personal support and no significant association with professional services and plans. Among the subgroup of patients who did not have a primary diagnosis of major mental illness, symptoms of major mental disorder and instability in the clinical scale were no longer significant. Insight, violent ideation or intent and treatment or supervision response showed stronger odds of association, although the CIs were wide. Treatment or supervision response showed a strong association in the risk management scale, but all other items were now no longer significant.
A somewhat similar pattern emerged when the sample was stratified to include only patients with a primary or secondary diagnosis of personality disorder. Once again, of the items in the clinical scale, symptoms of major mental disorder were no longer a dynamic risk factor. Insight and violent ideation or intent were again the strongest risk factors associated with violence for this subgroup, although the CIs were wide.
With regard to the risk management scale, there were insufficient cases to investigate professional services and plans, but stress or coping difficulties, treatment or supervision response and personal support all showed strong associations, although the CIs were wide. Living situation was no longer a significant risk factor for patients with personality disorder.
For the SAPROF, for those with a diagnosis of personality disorder, the major associations (but with wide CIs) were with poor empathy, poor coping and most importantly poor self-control, antiauthority attitudes and poor motivation. Taken together, these findings suggest that the dynamic factors associated with violence among patients with personality disorder show some overlap with but are inherently different from those for major mental illness.
Because the PANSS measures symptoms of major mental illness, it is unsurprising that, when using this instrument, some of the most dramatic contrasts can be observed between patients with major mental illness, those with no major mental illness and those with a diagnosis of personality disorder. For those with no diagnosis of major mental illness, only delusions and excitement among the positive symptoms were associated with violence. There were no negative symptoms associated with violence. Among the general symptoms, there were strong associations with anger, difficulty in delaying gratification and affective liability. These corresponded to factors associated with violence among the subgroup with a primary diagnosis of major mental illness. The latter findings also corresponded closely to findings using the aggression subscale of the PANSS among patients with a diagnosis of personality disorder. However, there were few positive symptoms associated with violence, except grandiosity and suspiciousness/persecution, and there were no negative symptoms associated with violence in patients with personality disorder. Of the general symptoms, unco-operativeness, lack of judgement and insight and poor impulse control (which might be considered features of the personality disorder rather than symptoms) were associated with violence.
These findings confirm that very different dynamic factors may have a causal relationship with violence among patients with personality disorder and those with major mental illness. This may apply whether or not the personality disorder is comorbid with the major mental illness. However, findings also confirm the importance of paying closer attention in clinical assessments to whether positive symptoms of psychosis are active following discharge among those with severe mental illness. The process of carrying out a risk assessment on patients with and without severe mental illness in similar samples, and with and without personality disorder, is likely to require different measures to ensure accuracy because the key risk factors are likely to differ. 398,399 These findings partly explain why instruments that are developed for patients with mental illness do not perform as accurately in individuals with personality disorder.
Chapter 16 Moderating the effects of post-discharge dynamic factors on levels of static risk
Background
It has become increasingly clear that the process of validating risk assessment instruments using a predictive method and comparing their accuracy conveys little benefit for clinical practice. The use of AUC values leads to claims of accuracy that are not fulfilled when conventional statistics for screening are applied. If clinicians use these instruments to make important decisions, then their decisions are likely to be wrong in approximately one-third of cases. It is argued that no instrument should be used as the sole basis for making such important decisions. SPJ should be used to structure such decision-making. However, it then must be questioned why the predictive method continues to be used to validate SPJ instruments, especially if their ability to outperform ARA instruments is questionable. One alternative is to investigate whether or not it is possible to classify patients more accurately using a different method. This should not involve a two-phase process of screening because this is unlikely to bring improvements in predictive accuracy. On the other hand, we demonstrated in Chapter 15 that a key component of SPJ instruments, and their advantage over actuarial instruments, is that they contain dynamic factors. If these are used in a method in which they truly act in a dynamic manner, it may be possible to identify targets for intervention because they have causal associations. This still leaves the unanswered question over whether or not actuarial assessments of risk, including the MSRAG and HCR-20v3 historical scales, still retain value in a risk assessment.
One possibility is that dynamic factors that occur post discharge have a moderating effect on the risk of future violence as estimated using an actuarial instrument. If the estimated level of static risk is substantially changed in the presence of a dynamic factor, then this may provide very useful information for a clinician when carrying out risk management subsequently. If the level of static risk measured using the percentage of patients correctly classified changes on the basis of a dynamic factor being present, then a clinician may need to discriminate in the first stage of assessment between levels of static risk, measured with an ARA instrument, and then modify the clinical decision accordingly.
When the MSRAG was developed we investigated whether or not post-discharge dynamic factors demonstrated interactive effects. 22 We calculated the recidivism rates for individuals in each risk band defined by the MSRAG and took into consideration additional dynamic risk factors. We found that, in relation to the static measure of acquisitive recidivism, the rates changed substantially when there was poor adherence to either supervision during the initial period following discharge or a medication regime. Patients were initially classified into ‘low’, ‘medium’, ‘high’ or ‘very high’ risk according to the MSRAG. The effects of these dynamic factors differed between these four levels of static risk. It was further demonstrated that good adherence to either the supervision or the medication regime did not appear to interact with the effects of the static risk to reduce the risk of recidivism. However, discharge to a general psychiatric hospital, thereby increasing the time spent as an inpatient prior to discharge into the community, did appear to moderate the effects of static risk for those at high risk, reducing their acquisitive recidivism rate from 49% to 33%.
On examining the serious offending recidivism scale, poor adherence to the early period of supervision or an initial medication regime appeared to moderate the effects of static risk for those at high and very high risk, with their risk of recidivism being substantially increased. Those among the low- and medium-risk categories who co-operated with supervision showed little difference from those in the same subgroups who were poor at adhering to the supervision regime. However, among the high-risk group, 38% of patients reoffended violently among those who adhered to supervision, whereas 64% reoffended violently among those whose supervision co-operation was poor. Among the very high-risk group, half of patients who co-operated with supervision nevertheless reoffended violently; however, among those in this group whose adherence to supervision was poor, this risk of conviction increased to 100% within 6 years. Similar observations were made for adherence to medication prescribed during the initial months following discharge. 22
These findings give some indication of how an actuarial score of risk might be usefully applied in clinical practice. The measure would appear to have little benefit with regard to a clinician’s decision-making unless used in conjunction with a dynamic factor that is known to change the level of risk. The same would apply for protective factors if it could be estimated to what extent the presence or absence of the protective factors reduces the risk of future violence.
In this preliminary study we attempted to replicate the findings of Hickey et al. 22 We used a simple method of demonstrating change in risk by producing a descriptive ‘look-up’ table that might be used by clinicians to estimate the risk of violence in their patients over a 12-month period after discharge from a MSU. We have not provided a more detailed and deeper level of statistical analysis at this stage and these findings should be considered preliminary. Nevertheless, we propose that this method should be further refined and developed in future investigations to incorporate both static and dynamic measures of risk into clinical decision-making.
Objectives
The objectives of this study were to:
-
investigate the moderating effects of dynamic risk items from the HCR-20v3 on levels of static risk using the MSRAG
-
investigate the moderating effects of dynamic risk items from the HCR-20v3 on levels of static risk using the historical scale of the HCR-20v3
-
investigate the moderating effects of protective items from the SAPROF on levels of static risk using the MSRAG
-
investigate the moderating effects of protective items from the SAPROF on levels of static risk using the historical scale of HCR-20v3.
Methods
To investigate the moderating effects of dynamic factors measured at baseline on violence rates, based on static risk levels, we included dynamic items derived from the HCR-20v3 (clinical and risk management scales) and protective factors from the SAPROF. Each dynamic item from the HCR-20v3 and protective factor from the SAPROF had three possible values: not present, partially present and present. The outcome of interest was a binary indicator for any violence within 12 months following discharge.
Static risk levels were based on the MSRAG serious offending scale and the HCR-20v3 historical scale. The MSRAG serious offending scale was categorised according to Hickey et al. 22 but with a combined high-risk and very high-risk category: cases with a score of 0–4 were classified as ‘low’ risk, those with a score of 5–7 were classified as ‘medium’ risk and those with a score of 8–11 were classified as ‘high’ risk. For the HCR-20v3 historical scale, we created three risk levels using sample-based tertiles: cases with a score of 0–12 were classified as ‘low’ risk, those with a score of 13–15 were classified as ‘medium’ risk and those with a score of 16–20 were classified as ‘high’ risk.
To identify the moderating effects of dynamic factors, in a similar way to Hickey et al. ,22 we compared the prevalence of any violence within 12 months following discharge for each combination of static risk level and dynamic item value.
Results
The findings of this study are presented in Tables 129–136. These tables include the numbers of patients in the different categories to determine the prevalence for each category. For the purposes of describing the moderating effects, it is preferable to use Tables 133–136, which show only the percentages, and these will be discussed below.
HCR-20v3 dynamic items | Violence, n (%) | ||
---|---|---|---|
MSRAG serious offending scale (N = 386) | |||
Low risk (n = 122; 31.6%) | Medium risk (n = 201; 52.1%) | High risk (n = 63; 16.3%) | |
Any violence within 12 months since discharge (n = 87; 22.5%) | 18 (14.8) | 44 (21.9) | 25 (39.7) |
C1: insight | |||
Not present | 1 (5.9) | 3 (13.6) | 1 (14.3) |
Partially present | 7 (13.0) | 17 (19.8) | 5 (22.7) |
Present | 10 (19.6) | 24 (25.8) | 19 (55.9) |
C2: violent ideation or intent | |||
Not present | 4 (5.8) | 13 (15.7) | 2 (15.4) |
Partially present | 10 (27.8) | 2 (17.0) | 10 (45.5) |
Present | 4 (23.5) | 19 (40.4) | 13 (46.4) |
C3: symptoms of major mental disorder | |||
Not present | 6 (14.6) | 13 (19.4) | 8 (47.1) |
Partially present | 7 (16.3) | 7 (12.3) | 5 (22.7) |
Present | 5 (13.2) | 24 (31.2) | 12 (50.0) |
C4: instability | |||
Not present | 3 (4.6) | 7 (8.3) | 3 (16.7) |
Partially present | 8 (25.0) | 16 (25.8) | 12 (48.0) |
Present | 7 (28.0) | 21 (38.2) | 10 (50.0) |
C5: treatment or supervision response | |||
Not present | 9 (14.5) | 8 (11.9) | 6 (28.6) |
Partially present | 7 (14.3) | 20 (21.3) | 11 (40.7) |
Present | 2 (18.2) | 16 (40.0) | 8 (53.3) |
R1: professional services and plans | |||
Not present | 17 (16.8) | 33 (20.5) | 16 (34.0) |
Partially present | 1 (7.2) | 7 (25.9) | 8 (53.3) |
Present | 0 (0.0) | 4 (30.8) | 1 (100.0) |
R2: living situation | |||
Not present | 11 (20.0) | 15 (17.2) | 7 (26.9) |
Partially present | 7 (12.1) | 24 (26.1) | 16 (51.6) |
Present | 0 (0.0) | 5 (22.7) | 2 (33.3) |
R3: personal support | |||
Not present | 7 (17.0) | 12 (20.3) | 5 (27.8) |
Partially present | 8 (13.6) | 13 (15.9) | 13 (46.4) |
Present | 3 (13.6) | 19 (31.7) | 7 (41.2) |
R4: treatment or supervision response | |||
Not present | 5 (15.6) | 4 (12.9) | 2 (22.2) |
Partially present | 4 (6.6) | 19 (19.4) | 5 (25.0) |
Present | 9 (31.0) | 21 (29.2) | 18 (53.0) |
R5: stress or coping | |||
Not present | 1 (20.0) | 0 (0.0) | 0 (0.0) |
Partially present | 8 (11.4) | 9 (11.3) | 5 (26.3) |
Present | 9 (19.2) | 35 (30.2) | 20 (47.6) |
HCR-20v3 dynamic items | Violence n (%) | ||
---|---|---|---|
HCR-20v3 historical scale (N = 387) | |||
Low risk (n = 152; 39.3%) | Medium risk (n = 128; 33.1%) | High risk (n = 107; 27.6%) | |
Any violence within 12 months since discharge (n = 87; 22.5%) | 22 (14.5) | 33 (25.8) | 32 (29.9) |
C1: insight | |||
Not present | 3 (12.0) | 1 (9.1) | 1 (10.0) |
Partially present | 6 (9.5) | 10 (19.2) | 13 (27.1) |
Present | 13 (20.3) | 22 (33.9) | 18 (36.7) |
C2: violent ideation or intent | |||
Not present | 7 (8.0) | 6 (12.8) | 6 (19.4) |
Partially present | 10 (23.3) | 13 (25.5) | 9 (25.7) |
Present | 5 (23.8) | 14 (46.7) | 17 (41.5) |
C3: symptoms of major mental disorder | |||
Not present | 8 (13.8) | 9 (23.1) | 10 (34.5) |
Partially present | 6 (11.3) | 8 (23.5) | 5 (14.3) |
Present | 8 (19.5) | 16 (29.1) | 17 (39.5) |
C4: instability | |||
Not present | 4 (4.6) | 6 (11.8) | 3 (10.3) |
Partially present | 8 (21.1) | 17 (40.5) | 11 (28.2) |
Present | 10 (38.5) | 10 (28.6) | 18 (46.2) |
C5: treatment or supervision response | |||
Not present | 9 (11.8) | 7 (17.1) | 7 (20.6) |
Partially present | 16 (10.2) | 18 (29.0) | 14 (28.6) |
Present | 7 (41.2) | 8 (32.0) | 11 (45.8) |
R1: professional services and plans | |||
Not present | 19 (15.0) | 27 (25.5) | 20 (26.0) |
Partially present | 2 (12.5) | 4 (28.6) | 10 (38.5) |
Present | 1 (11.1) | 2 (25.0) | 2 (50.0) |
R2: living situation | |||
Not present | 13 (16.7) | 7 15.9) | 13 (27.7) |
Partially present | 8 (12.7) | 22 (32.8) | 17 (33.3) |
Present | 1 (9.1) | 4 (23.5) | 2 (22.2) |
R3: personal support | |||
Not present | 7 (10.9) | 8 (26.7) | 9 (36.0) |
Partially present | 7 (11.5) | 14 (23.7) | 13 (26.5) |
Present | 8 (29.6) | 11 (28.2) | 10 (30.3) |
R4: treatment or supervision response | |||
Not present | 8 (16.3) | 2 (14.3) | 1 (11.1) |
Partially present | 4 (6.1) | 16 (23.9) | 8 (17.0) |
Present | 10 (27.0) | 15 (31.9) | 23 (45.1) |
R5: stress or coping | |||
Not present | 1 (11.1) | 0 (0.0) | 0 (0.0) |
Partially present | 9 (10.5) | 9 (18.0) | 4 (11.8) |
Present | 12 (21.1) | 24 (31.6) | 28 (38.9) |
SAPROF protective factors | Violence, n (%) | ||
---|---|---|---|
MSRAG serious offending scale (N = 386) | |||
Low risk (n = 122; 31.6%) | Medium risk (n = 201; 52.1%) | High risk (n = 63; 16.3%) | |
Any violence within 12 months since discharge (n = 87; 22.5%) | 18 (14.8) | 44 (21.9) | 25 (39.7) |
1. Intelligence | |||
Not present | 5 (27.8) | 6 (26.1) | 6 (75.0) |
Partially present | 8 (12.3) | 28 (19.3) | 17 (36.2) |
Present | 5 (12.8) | 10 (30.3) | 2 (25.0) |
2. Secure attachment in childhood | |||
Not present | 4 (21.1) | 9 (25.7) | 3 (27.3) |
Partially present | 4 (11.8) | 18 (27.3) | 12 (54.6) |
Present | 10 (14.5) | 17 (17.0) | 10 (33.3) |
3. Empathy | |||
Not present | 3 (50.0) | 5 (38.5) | 6 (60.0) |
Partially present | 8 (17.4) | 23 (25.3) | 7 (31.8) |
Present | 7 (10.0) | 16 (16.5) | 12 (38.7) |
4. Coping | |||
Not present | 2 (40.0) | 2 (22.2) | 2 (40.0) |
Partially present | 9 (20.5) | 25 (27.8) | 14 (45.2) |
Present | 7 (9.6) | 17 (16.7) | 9 (33.3) |
5. Self-control | |||
Not present | 0 (0.0) | 4 (57.1) | 1 (50.0) |
Partially present | 7 (25.9) | 23 (37.1) | 8 (38.1) |
Present | 11 (11.8) | 17 (12.9) | 16 (40.0) |
6. Work | |||
Not present | 12 (17.4) | 34 (30.6) | 17 (44.7) |
Partially present | 3 (14.3) | 7 (21.2) | 5 (31.3) |
Present | 3 (9.4) | 3 (5.3) | 3 (33.3) |
7. Leisure activities | |||
Not present | 4 (57.1) | 6 (37.5) | 2 (33.3) |
Partially present | 5 (17.9) | 14 (26.4) | 6 (35.3) |
Present | 9 (10.3) | 24 (18.2) | 17 (42.5) |
8. Financial management | |||
Not present | 3 (60.0) | 2 (25.0) | 4 (80.0) |
Partially present | 5 (20.8) | 14 (32.6) | 8 (36.4) |
Present | 10 (10.8) | 28 (18.7) | 13 (36.1) |
9. Motivation for treatment | |||
Not present | 2 (50.0) | 1 (16.7) | 4 (66.7) |
Partially present | 7 (26.9) | 10 (21.7) | 10 (55.6) |
Present | 9 (9.8) | 33 (22.2) | 11 (28.2) |
10. Attitudes towards authority | |||
Not present | 4 (100.0) | 6 (40.0) | 1 (20.0) |
Partially present | 8 (30.8) | 15 (28.3) | 13 (52.0) |
Present | 6 (6.5) | 23 (17.3) | 11 (33.3) |
11. Life goals | |||
Not present | 3 (27.3) | 5 (18.5) | 5 (71.4) |
Partially present | 4 (14.3) | 15 (26.8) | 8 (38.1) |
Present | 11 (13.3) | 24 (20.3) | 12 (34.3) |
12. Medication | |||
Not present | 0 (0.0) | 2 (25.0) | 1 (50.0) |
Partially present | 5 (33.3) | 8 (28.6) | 7 (46.7) |
Present | 13 (12.3) | 34 (20.6) | 17 (37.0) |
13. Social network | |||
Not present | 2 (25.0) | 8 (28.6) | 7 (87.5) |
Partially present | 2 (5.6) | 15 (25.4) | 9 (32.1) |
Present | 14 (18.0) | 21 (18.4) | 9 (33.3) |
14. Intimate relationship | |||
Not present | 15 (17.2) | 36(21.1) | 20 (37.0) |
Partially present | 3 (15.0) | 4 (25.0) | 3 (75.0) |
Present | 0 (0.0) | 4 (28.6) | 2 (40.0) |
15. Professional care | |||
Not present | – | – | – |
Partially present | 0 (0.0) | 0 (0.0) | 1 (50.0) |
Present | 18 (14.9) | 44 (22.2) | 24 (39.3) |
16. Living circumstances | |||
Not present | 3 (27.3) | 4 (25.0) | 5 (62.5) |
Partially present | 2 (13.3) | 12 (35.3) | 6 (42.9) |
Present | 13 (13.5) | 28 (18.5) | 14 (34.2) |
17. External control | |||
Not present | 1 (50.0) | 1 (33.3) | 1 (50.0) |
Partially present | 5 (20.0) | 15 (33.3) | 11 (57.9) |
Present | 12 (12.6) | 28 (18.3) | 13 (31.0) |
SAPROF protective factors | Violence, n (%) | ||
---|---|---|---|
HCR-20v3 historical scale (N = 387) | |||
Low risk (n = 152; 39.3%) | Medium risk (n = 128; 33.1%) | High risk (n = 107; 27.6%) | |
Any violence within 12 months since discharge (n = 87; 22.5%) | 22 (14.5) | 33 (25.8) | 32 (29.9) |
1. Intelligence | |||
Not present | 4 (25.0) | 7 (38.9) | 6 (40.0) |
Partially present | 11 (12.2) | 20 (22.2) | 22 (28.2) |
Present | 7 (15.2) | 6 (30.0) | 4 (28.6) |
2. Secure attachment in childhood | |||
Not present | 3 (21.4) | 2 (9.5) | 11 (36.7) |
Partially present | 8 (20.5) | 15 (37.5) | 11 (25.6) |
Present | 11 (11.1) | 16 (23.9) | 10 (29.4) |
3. Empathy | |||
Not present | 2 (28.6) | 6 (66.7) | 6 (46.2) |
Partially present | 9 (18.0) | 13 (22.0) | 16 (31.4) |
Present | 11 (11.6) | 14 (23.3) | 10 (23.3) |
4. Coping | |||
Not present | 1 (20.0) | 1 (16.7) | 4 (50.0) |
Partially present | 11 (22.9) | 21 (32.8) | 16 (30.2) |
Present | 10 (10.1) | 11 (19.0) | 12 (26.1) |
5. Self-control | |||
Not present | – | 1 (20.0) | 4 (66.7) |
Partially present | 9 (32.1) | 16 (38.1) | 13 (31.7) |
Present | 13 (10.5) | 16 (19.8) | 15 (25.0) |
6. Work | |||
Not present | 17 (19.8) | 21 (30.4) | 25 (39.7) |
Partially present | 2 (8.0) | 8 (34.8) | 5 (21.7) |
Present | 3 (7.3) | 4 (11.1) | 2 (9.5) |
7. Leisure activities | |||
Not present | 3 (30.0) | 1 (14.3) | 8 (66.7) |
Partially present | 4 (12.9) | 14 (35.9) | 7 (25.0) |
Present | 15 (13.5) | 18 (22.0) | 17 (25.4) |
8. Financial management | |||
Not present | 2 (50.0) | 2 (33.3) | 5 (55.6) |
Partially present | 8 (24.2) | 9 (27.3) | 10 (43.5) |
Present | 12 (10.4) | 22 (24.7) | 17 (22.7) |
9. Motivation for treatment | |||
Not present | 1 (20.0) | 3 (42.9) | 3 (75.0) |
Partially present | 6 (23.1) | 11 (32.4) | 10 (33.3) |
Present | 15 (12.4) | 19 (21.8) | 19 (26.0) |
10. Attitudes towards authority | |||
Not present | 3 (60.0) | 4 (40.0) | 4 (44.4) |
Partially present | 10 (34.5) | 15 (39.5) | 11 (29.7) |
Present | 9 (7.6) | 14 (17.5) | 17 (27.9) |
11. Life goals | |||
Not present | 3 (21.4) | 2 (15.4) | 8 (44.4) |
Partially present | 6 (20.7) | 11 (25.6) | 10 (30.3) |
Present | 13 (11.9) | 20 (27.8) | 14 (25.0) |
12. Medication | |||
Not present | 0 (0.0) | 0 (0.0) | 1 (100.0) |
Partially present | 6 (37.5) | 9 (40.9) | 5 (25.0) |
Present | 16 (12.1) | 24 (23.5) | 24 (28.6) |
13. Social network | |||
Not present | 4 (36.4) | 5 (26.3) | 8 (57.1) |
Partially present | 5 (12.8) | 11 (26.8) | 10 (23.3) |
Present | 13 (12.8) | 17 (25.0) | 14 (28.0) |
14. Intimate relationship | |||
Not present | 17 (14.5) | 28 (26.7) | 26 (28.6) |
Partially present | 3 (16.7) | 3 (20.0) | 4 (57.1) |
Present | 2 (11.8) | 2 (25.0) | 2 (22.2) |
15. Professional care | |||
Not present | – | – | – |
Partially present | 1 (33.3) | 0 (0.0) | 0 (0.0) |
Present | 21 (14.1) | 33 (26.2) | 32 (30.2) |
16. Living circumstances | |||
Not present | 1 (9.1) | 6 (40.0) | 5 (55.6) |
Partially present | 4 (18.2) | 10 (41.7) | 6 (35.3) |
Present | 17 (14.3) | 17 (19.1) | 21 (25.9) |
17. External control | |||
Not present | 1 (33.3) | 1 (50.0) | 1 (50.0) |
Partially present | 6 (18.8) | 14 (45.2) | 11 (42.3) |
Present | 15 (12.8) | 18 (19.0) | 20 (25.3) |
HCR-20v3 dynamic items | Violence, n (%) or % | ||
---|---|---|---|
MSRAG serious offending scale (N = 386) | |||
Low risk (n = 122; 31.6%) | Medium risk (n = 201; 52.1%) | High risk (n = 63; 16.3%) | |
Any violence within 12 months since discharge (n = 87; 22.5%) | 18 (14.8) | 44 (21.9) | 25 (39.7) |
C1: insight | |||
Not present | 5.9 | 13.6 | 14.3 |
Partially present | 13.0 | 19.8 | 22.7 |
Present | 19.6 | 25.8 | 55.9 |
C2: violent ideation or intent | |||
Not present | 5.8 | 15.7 | 15.4 |
Partially present | 27.8 | 17.0 | 45.5 |
Present | 23.5 | 40.4 | 46.4 |
C3: symptoms of major mental disorder | |||
Not present | 14.6 | 19.4 | 47.1 |
Partially present | 16.3 | 12.3 | 22.7 |
Present | 13.2 | 31.2 | 50.0 |
C4: instability | |||
Not present | 4.6 | 8.3 | 16.7 |
Partially present | 25.0 | 25.8 | 48.0 |
Present | 28.0 | 38.2 | 50.0 |
C5: treatment or supervision response | |||
Not present | 14.5 | 11.9 | 28.6 |
Partially present | 14.3 | 21.3 | 40.7 |
Present | 18.2 | 40.0 | 53.3 |
R1: professional services and plans | |||
Not present | 16.8 | 20.5 | 34.0 |
Partially present | 7.2 | 25.9 | 53.3 |
Present | 0.0 | 30.8 | 100.0 |
R2: living situation | |||
Not present | 20.0 | 17.2 | 26.9 |
Partially present | 12.1 | 26.1 | 51.6 |
Present | 0.0 | 22.7 | 33.3 |
R3: personal support | |||
Not present | 17.0 | 20.3 | 27.8 |
Partially present | 13.6 | 15.9 | 46.4 |
Present | 13.6 | 31.7 | 41.2 |
R4: treatment or supervision response | |||
Not present | 15.6 | 12.9 | 22.2 |
Partially present | 6.6 | 19.4 | 25.0 |
Present | 31.0 | 29.2 | 53.0 |
R5: stress or coping | |||
Not present | 20.0 | 0.0 | 0.0 |
Partially present | 11.4 | 11.3 | 26.3 |
Present | 19.2 | 30.2 | 47.6 |
HCR-20v3 dynamic items | Violence, n (%) or % | ||
---|---|---|---|
HCR-20v3 historical scale (N = 387) | |||
Low risk (n = 152; 39.3%) | Medium risk (n = 128; 33.1%) | High risk (n = 107; 27.6%) | |
Any violence within 12 months since discharge (n = 87; 22.5%) | 22 (14.5) | 33 (25.8) | 107 (27.6) |
C1: insight | |||
Not present | 12.0 | 9.1 | 10.0 |
Partially present | 9.5 | 19.2 | 27.1 |
Present | 20.3 | 33.9 | 36.7 |
C2: violent ideation or intent | |||
Not present | 8.0 | 12.8 | 19.4 |
Partially present | 23.3 | 25.5 | 25.7 |
Present | 23.8 | 46.7 | 41.5 |
C3: symptoms of major mental disorder | |||
Not present | 13.8 | 23.1 | 34.5 |
Partially present | 11.3 | 23.5 | 14.3 |
Present | 19.5 | 29.1 | 39.5 |
C4: instability | |||
Not present | 4.6 | 11.8 | 10.3 |
Partially present | 21.1 | 40.5 | 28.2 |
Present | 38.5 | 28.6 | 46.2 |
C5: treatment or supervision response | |||
Not present | 11.8 | 17.1 | 20.6 |
Partially present | 10.2 | 29.0 | 28.6 |
Present | 41.2 | 32.0 | 45.8 |
R1: professional services and plans | |||
Not present | 15.0 | 25.5 | 26.0 |
Partially present | 12.5 | 28.6 | 38.5 |
Present | 11.1 | 25.0 | 50.0 |
R2: living situation | |||
Not present | 16.7 | 15.9 | 27.7 |
Partially present | 12.7 | 32.8 | 33.3 |
Present | 9.1 | 23.5 | 22.2 |
R3: personal support | |||
Not present | 10.9 | 26.7 | 36.0 |
Partially present | 11.5 | 23.7 | 26.5 |
Present | 29.6 | 28.2 | 30.3 |
R4: treatment or supervision response | |||
Not present | 16.3 | 14.3 | 11.1 |
Partially present | 6.1 | 23.9 | 17.0 |
Present | 27.0 | 31.9 | 45.1 |
R5: stress or coping | |||
Not present | 11.1 | 0.0 | 0.0 |
Partially present | 10.5 | 18.0 | 11.8 |
Present | 21.1 | 31.6 | 38.9 |
SAPROF protective factors | Violence, n (%) | ||
---|---|---|---|
MSRAF serious offending scale (N = 386) | |||
Low risk (n = 122; 31.6%) | Medium risk (n = 201; 52.1%) | High risk (n = 63; 16.3%) | |
Any violence within 12 months since discharge (n = 87; 22.5%) | 18 (14.8) | 44 (21.9) | 25 (39.7) |
1. Intelligence | |||
Not present | 27.8 | 26.1 | 75.0 |
Partially present | 12.3 | 19.3 | 36.2 |
Present | 12.8 | 30.3 | 25.0 |
2. Secure attachment in childhood | |||
Not present | 21.1 | 25.7 | 27.3 |
Partially present | 11.8 | 27.3 | 54.6 |
Present | 14.5 | 17.0 | 33.3 |
3. Empathy | |||
Not present | 50.0 | 38.5 | 60.0 |
Partially present | 17.4 | 25.3 | 31.8 |
Present | 10.0 | 16.5 | 38.7 |
4. Coping | |||
Not present | 40.0 | 22.2 | 40.0 |
Partially present | 20.5 | 27.8 | 45.2 |
Present | 9.6 | 16.7 | 33.3 |
5. Self-control | |||
Not present | 0.0 | 57.1 | 50.0 |
Partially present | 25.9 | 37.1 | 38.1 |
Present | 11.8 | 12.9 | 40.0 |
6. Work | |||
Not present | 17.4 | 30.6 | 44.7 |
Partially present | 14.3 | 21.2 | 31.3 |
Present | 9.4 | 5.3 | 33.3 |
7. Leisure activities | |||
Not present | 57.1 | 37.5 | 33.3 |
Partially present | 17.9 | 26.4 | 35.3 |
Present | 10.3 | 18.2 | 42.5 |
8. Financial management | |||
Not present | 60.0 | 25.0 | 80.0 |
Partially present | 20.8 | 32.6 | 36.4 |
Present | 10.8 | 18.7 | 36.1 |
9. Motivation for treatment | |||
Not present | 50.0 | 16.7 | 66.7 |
Partially present | 26.9 | 21.7 | 55.6 |
Present | 9.8 | 22.2 | 28.2 |
10. Attitudes towards authority | |||
Not present | 100.0 | 40.0 | 20.0 |
Partially present | 30.8 | 28.3 | 52.0 |
Present | 6.5 | 17.3 | 33.3 |
11. Life goals | |||
Not present | 27.3 | 18.5 | 71.4 |
Partially present | 14.3 | 26.8 | 38.1 |
Present | 13.3 | 20.3 | 34.3 |
12. Medication | |||
Not present | 0.0 | 25.0 | 50.0 |
Partially present | 33.3 | 28.6 | 46.7 |
Present | 12.3 | 20.6 | 37.0 |
13. Social network | |||
Not present | 25.0 | 28.6 | 87.5 |
Partially present | 5.6 | 25.4 | 32.1 |
Present | 18.0 | 18.4 | 33.3 |
14. Intimate relationship | |||
Not present | 17.2 | 21.1 | 37.0 |
Partially present | 15.0 | 25.0 | 75.0 |
Present | 0.0 | 28.6 | 40.0 |
15. Professional care | |||
Not present | – | – | – |
Partially present | 0.0 | 0.0 | 50.0 |
Present | 14.9 | 22.2 | 39.3 |
16. Living circumstances | |||
Not present | 27.3 | 25.0 | 62.5 |
Partially present | 13.3 | 35.3 | 42.9 |
Present | 13.5 | 18.5 | 34.2 |
17. External control | |||
Not present | 50.0 | 33.3 | 50.0 |
Partially present | 20.0 | 33.3 | 57.9 |
Present | 12.6 | 18.3 | 31.0 |
SAPROF protective factors | Violence, n (%) | ||
---|---|---|---|
HCR-20v3 historical scale (N = 387) | |||
Low risk (n = 152; 39.3%) | Medium risk (n = 128; 33.1%) | High risk (n = 107; 27.6%) | |
Any violence within 12 months since discharge (n = 87; 22.5%) | 22 (14.5) | 33 (25.8) | 32 (29.9) |
1. Intelligence | |||
Not present | 25.0 | 38.9 | 40.0 |
Partially present | 12.2 | 22.2 | 28.2 |
Present | 15.2 | 30.0 | 28.6 |
2. Secure attachment in childhood | |||
Not present | 21.4 | 9.5 | 36.7 |
Partially present | 20.5 | 37.5 | 25.6 |
Present | 11.1 | 23.9 | 29.4 |
3. Empathy | |||
Not present | 28.6 | 66.7 | 46.2 |
Partially present | 18.0 | 22.0 | 31.4 |
Present | 11.6 | 23.3 | 23.3 |
4. Coping | |||
Not present | 20.0 | 16.7 | 50.0 |
Partially present | 22.9 | 32.8 | 30.2 |
Present | 10.1 | 19.0 | 26.1 |
5. Self-control | |||
Not present | – | 20.0 | 66.7 |
Partially present | 32.1 | 38.1 | 31.7 |
Present | 10.5 | 19.8 | 25.0 |
6. Work | |||
Not present | 19.8 | 30.4 | 39.7 |
Partially present | 8.0 | 34.8 | 21.7 |
Present | 7.3 | 11.1 | 9.5 |
7. Leisure activities | |||
Not present | 30.0 | 14.3 | 66.7 |
Partially present | 12.9 | 35.9 | 25.0 |
Present | 13.5 | 22.0 | 25.4 |
8. Financial management | |||
Not present | 50.0 | 33.3 | 55.6 |
Partially present | 24.2 | 27.3 | 43.5 |
Present | 10.4 | 24.7 | 22.7 |
9. Motivation for treatment | |||
Not present | 20.0 | 42.9 | 75.0 |
Partially present | 23.1 | 32.4 | 33.3 |
Present | 12.4 | 21.8 | 26.0 |
10. Attitudes towards authority | |||
Not present | 60.0 | 40.0 | 44.4 |
Partially present | 34.5 | 39.5 | 29.7 |
Present | 7.6 | 17.5 | 27.9 |
11. Life goals | |||
Not present | 21.4 | 15.4 | 44.4 |
Partially present | 20.7 | 25.6 | 30.3 |
Present | 11.9 | 27.8 | 25.0 |
12. Medication | |||
Not present | 0.0 | 0.0 | 100.0 |
Partially present | 37.5 | 40.9 | 25.0 |
Present | 12.1 | 23.5 | 28.6 |
13. Social network | |||
Not present | 36.4 | 26.3 | 57.1 |
Partially present | 12.8 | 26.8 | 23.3 |
Present | 12.8 | 25.0 | 28.0 |
14. Intimate relationship | |||
Not present | 14.5 | 26.7 | 28.6 |
Partially present | 16.7 | 20.0 | 57.1 |
Present | 11.8 | 25.0 | 22.2 |
15. Professional care | |||
Not present | – | – | – |
Partially present | 33.3 | 0.0 | 0.0 |
Present | 14.1 | 26.2 | 30.2 |
16. Living circumstances | |||
Not present | 9.1 | 40.0 | 55.6 |
Partially present | 18.2 | 41.7 | 35.3 |
Present | 14.3 | 19.1 | 25.9 |
17. External control | |||
Not present | 33.3 | 50.0 | 50.0 |
Partially present | 18.8 | 45.2 | 42.3 |
Present | 12.8 | 19.0 | 25.3 |
Moderating effects of dynamic risk factors on static risk
Table 133 shows the moderating effects of five clinical and five dynamic items from the HCR-20v3 on the percentage of patients who behave violently over a 12-month period, stratified by categories of low, medium and high risk measured using the MSRAG serious offending scale. For most dynamic items, their presence increased the risk of violence for each of the three categories. Exceptions to this trend included symptoms of major mental disorder, professional services and plans, personal support and stress and coping for the low-risk category and living situation for all three categories. It appeared that the trend for increasing risk according to the absence, partial presence or presence of certain dynamic factors was less marked for patients in the low-risk category than for those in the other categories.
In most cases, when the dynamic factor was not present, there was a gradient of risk of violence from low through medium to high risk, consistent with the static measures. However, this gradient was not observed for living situation or for stress or coping. Instead, a general trend was observed such that, when the dynamic item was present, compared with when partially or not present, there was an increase in the level of risk.
For certain dynamic factors, the increase in risk when the dynamic factor was present was substantially greater in the high-risk category than in the low-risk and medium-risk categories. This included insight, instability, professional services and plans, treatment or supervision response and stress or coping.
Table 134 shows the same trends for most items, but the table also shows that, when using the HCR-20v3 historical scale to classify patients into low, medium and high risk, the moderating effects of the dynamic factors were not as marked for certain dynamic items as when using the MSRAG serious offending scale to classify patients into low, medium and high risk, although for other dynamic factors the moderating effects appeared to be higher.
Moderating effects of protective factors on static risk
Table 135 shows the moderating effects of the SAPROF protective factors on violent outcome among patients classified according to the MSRAG serious offending scale. In general, the presence of a protective item resulted in a decline in the risk of violence, measured by the percentage who were subsequently found to be violent, and this trend can be observed from not present through to partially present and present for most of the dynamic items. However, for a number of these protective factors (secure attachment in childhood, empathy, coping, leisure activities, financial management, motivation for treatment, attitude towards authority, intimate relationships and external control), the fall in the percentage who were violent was greater in the low-risk subgroup than in other subcategories. For a smaller number of protective factors (intelligence, self-control, life goals, medication, social network and living circumstances), the fall in risk was greater for the high-risk subgroup.
These same trends were observed in Table 136, which shows the moderating effects of the SAPROF protective factors on violent outcome among patients classified according to the HCR-20v3 historical scale. As with risk factors, classification using the MSRAG appeared to be more discriminating than classification using the HCR-20v3 historical scale in terms of showing the moderating effects of the SAPROF protective factors. The trends were generally similar, with no major differences observed, but in certain cases the moderating effects appeared to be greater using the MSRAG classification.
Discussion
The findings of this chapter are preliminary and descriptive. We are not aware of any previous studies that have demonstrated the moderating effect of dynamic items on static measures other than that by Hickey et al. 22 The findings in this study would appear to replicate those of the previous study and a considerably wider range of dynamic factors have been investigated than were included previously.
The general trends observed were that, if certain dynamic risk factors occur, then the moderating effect is greater on patients who are classified as high risk than among those classified as low or medium risk. Conversely, the moderating effects of protective factors appear to be greater among those classified as low risk, although certain protective factors appear to be more important for high-risk patients, the most notable being compliance with prescribed medication, supportive social network and living circumstances.
There are severe limitations in making decisions based on a crude categorisation into low, medium and high risk. However, we believe that clinicians who must make decisions about whether or not to discharge a patient from hospital who is classified as high risk on the MSRAG should be interested to know that such a patient will have a low probability of approximately 11% of becoming violent if insight is good. However, this probability would rise to > 50% if insight is poor. Similarly, > 25% of those in the high-risk category are likely to be violent in the absence of the risk factor, good treatment or supervision response, which rises to > 50% if they have a poor treatment or supervision response. For the risk factor professional services and plans, high-risk patients show an increase from a worrying one-third behaving violently when this risk factor is absent to 100% behaving violently when this risk factor is present.
Examining protective factors, some of the internal measures are problematic with regard to effect modification as these appear to be static measures. For example, intelligence cannot be improved and neither can secure attachment in childhood. However, our findings would suggest that risk of violence becomes considerably greater among patients with lower intelligence in the high-risk subgroup. In terms of changeable dynamic protective factors, improved financial management appears to have a major impact among both low- and high-risk patients. Improving life goals, compliance with medication, attempting to provide a more supportive, non-criminal social network, improving living circumstances and better external control all reduce risk and this effect is maximised in the subgroup categorised as being at high risk using static measures.
Section D Development and validation of new instruments for static and dynamic risk assessment
Chapter 17 Construction and validation of new static risk assessment instruments
Background
Static risk factors are factors that do not change or which change in only one direction. 400 Examples of these risk factors include age, which increases over time, and past criminal offences, which are fixed. Therefore, clinical interventions over time are unable to reduce future criminal offending if they are restricted to targeting a static risk factor. 401 One reason that ARA instruments have been frequently developed is that they consist primarily of risk factors that are easier to measure than dynamic risk factors (see Chapter 2). 402
Actuarial risk assessment instruments estimate the likelihood of reoffending by allocating numerical values to risk factors that are linked to offending. These values are subsequently combined using a statistical algorithm to produce a total score. 7 The primary advantage when using actuarial instruments is the systematic approach that ensures reliability and enables individual scores to be directly compared with each other.
The overall validation results, however, have gradually shown that all validated actuarial instruments perform similarly to each other, with a ceiling effect of approximately 0.70, a value derived from the commonly applied AUC. 8,306 These findings mean that choosing the best tool to guide practice is difficult.
The reason why similar performance is observed among many of these actuarial instruments is that they may be measuring a general underlying construct of criminality. Kroner et al. 403 demonstrated this by placing the items from four frequently used instruments [the PCL-R,404 Level of Service Inventory, Revised (LSI-R),405,406 VRAG407 and General Statistical Information on Recidivism (GSIR)408] into a coffee can and shaking them out to generate four ‘instruments’ consisting of random items; these were then compared with the original instruments. They concluded that the original instruments were no better at predicting reoffending than the new, randomly chosen and assembled coffee can measures.
Because actuarial instruments are generalisable to other outcomes and have a similar prediction performance, Coid et al. 23 proposed that many items found in three risk assessment instruments were redundant and that only a few items contributed to the overall predictive power of the instruments. If only a few items are able to predict future offending among these instruments, particularly in different populations, it would be more effective to focus on a few salient static risk factors to predict offending. Because of these findings, we have developed new static ARA instruments. In this chapter, we describe the development of eight static risk instruments created to predict the following criminal outcomes: drug, acquisitive, robbery and violence convictions. Assessing risk according to specific offences has been advocated by Duwe409 because certain offences such as violence are more costly to society than other offences. This suggestion was previously made by Singh et al. 7 In this chapter the construction of these instruments is first addressed. An external validation is then used to assess the predictive validity of the new instruments.
Study 1: development of a static instrument to predict violence
Objective
The objective was to create a new accurate ARA instrument using static variables to assess the risk of violent convictions among released prisoners.
Methods
The outcome of interest was a binary indicator that is equal to 1 for cases with at least one conviction for an offence related to violence following release from prison and equal to 0 otherwise. Violent offences in this study included homicide (murder, manslaughter and infanticide), major violence (attempted murder, GBH and wounding), minor violence (assault, affray, use of an offensive weapon and threats) and weapon possession.
Based on previous research, the static predictors considered for the static instrument included age and previous convictions for violence, robbery, acquisitive crime and sexual offences. The predictors were included in the model sequentially, starting with the static risk predictor with the highest AUC. To avoid including redundant predictors that would result in poor predictive ability, we combined predictors in a logistic regression model to improve the AUC.
Howard and Dixon410 observed that ‘a point or two on the standard AUC measure translate to substantive improvements in the management and treatment of thousands of offenders every year’ (p.143). Based on this study we used an increase in AUC of 0.01 as the criterion for including an additional predictor in the static instrument.
As the AUC estimate is likely to be optimistic, we also report the leave-one-out cross-validation AUC (LOOCV AUC). Bautista et al. 411 mention that the LOOCV method ‘can obtain a high benefit from small samples in order to validate prediction rules’ (p. 237). This type of internal validation AUC estimate relies on obtaining the predicted probability of the outcome using regression coefficients (weights) from a logistic model constructed on all other cases in the analysis sample. We used the LOOCV AUC estimates for comparisons between our instruments and other well-established instruments such as the OGRS2, VRAG, RM2000V and HCR-20 total score.
In addition to predictive accuracy, we also included the Hosmer–Lemeshow (H–L) chi-square statistic to measure goodness of fit for logistic regression models. This statistic is also used as a measure of calibration of the predicted probabilities. The H–L statistic is based on a comparison between predicted and actual outcome rates for grouped data. We implemented a procedure that created the maximum number of groups with an expected count of at least five in each group to ensure validity of the corresponding test.
Using the conventional median split approach, we estimated the classification accuracy of our instruments. Specifically, under the assumption that all cases will have a score above the median, we estimated the PPV and NPV. In addition, we estimated the PCC, which is the key parameter of interest for classification accuracy.
Results
A total of 1380 men from the Prisoner Cohort Study (PCS)412,413 were interviewed in phase 1 and had available PNC criminal records. These cases were used to create static risk instruments for future violence.
A total of 802 PCS men who were interviewed in phase 2 and who had available PNC criminal records were included in the analysis for creating a dynamic risk model to investigate the relationship between static instruments, dynamic factors and future violence after release from prison.
For each of the factors included, the differences in prevalence between the total sample, the phase 1 analysis sample and the phase 2 analysis sample were relatively small. For example, mean age was similar between the three groups (overall 30.82 years, phase 1 30.74 years and phase 2 31.42 years). Therefore, it is reasonable to expect that the predictive accuracy of a static risk instrument will be similar for each group.
Of the 1380 cases included in the phase 1 analysis sample, 434 (31.4%) were convicted of an offence related to violence following release from prison. In the phase 2 analysis sample, there were 240 (29.9%) among 802 individuals with the same type of conviction.
The predictor for violence with the largest univariate AUC was the number of previous convictions (denoted by prcvio) for violence, with an AUC of 0.68. The next predictor included was age. By adding the number of previous convictions for violence and age in a logistic model, the AUC from the estimated probabilities increased to 0.76.
Including the number of previous convictions for acquisitive crimes (predictor with the third-largest univariate AUC and denoted by prcbtf) increased the multivariate AUC to 0.77. Previous convictions for robbery and previous convictions for sexual offences did not improve the predictive accuracy of the static instrument and therefore were not included in the final version of this instrument.
Based on the results described, we obtained the Computerised Instrument for Violence (CIV), which contains three predictors: previous convictions for violence, age and previous convictions for acquisitive crime. To obtain the probability of having at least one conviction for violence, we calculated the linear score (denoted by S) using the constant of the model and the predictor values, which are weighted by the corresponding regression coefficients. In this case, the equation was as follows:
The corresponding probability (denoted by Pr) for this outcome was obtained using the equation:
The AUC for this instrument was 0.77 (95% CI 0.74 to 0.80; p < 0.001), which indicates good predictive accuracy (AUC > 0.7). The H–L statistic for the same model was 96.03 [84 degrees of freedom (df); p = 0.174], which means that the model fits the data adequately. The corresponding LOOCV AUC was 0.76 (95% CI 0.74 to 0.79; p < 0.001), which suggests that the predictive accuracy of the model would be expected to be good for other similar samples.
Finally, we compared the LOOCV AUC estimate for the CIV with the AUCs for other well-established instruments currently used for risk assessment. The CIV LOOCV was significantly better than the OGRS2 (AUC 0.73; p = 0.002), VRAG (AUC 0.71; p < 0.001), RM2000V (AUC 0.69; p < 0.001) and HCR-20 total score (AUC 0.65; p < 0.001).
Using the median split for CIV cross-validated probabilities, the classification accuracy estimates were 47.7% for the PPV and 84.8% for the NPV. The main parameter of classification accuracy (PCC) was 66.2%, which is similar to the corresponding PCCs for other instruments (61.3% for the HCR-20 total, 66.7% for the RM2000V, 64.1% for the VRAG and 65.3% for the OGRS2).
Risk levels for violence based on the Computerised Instrument for Violence
To simplify the interpretation of the CIV output for future use in clinical practice, we created indicative bands for the predicted probabilities that correspond to actual levels of risk for future violence. We rescaled the LOOCV probabilities using their minimum and maximum values to obtain risk measures with a range from 0 to 100. Subsequently, we divided the rescaled range into four bands so that the actual reconviction rates represent low, moderate, high and very high levels of risk.
The results for the suggested bands are shown in the Table 137. Even though the rate for a moderate level of risk appears too small, it is nevertheless three times higher than the corresponding rate for a low level of risk.
Risk level | Range | n | Prevalence (%) | Reconviction rate (%) |
---|---|---|---|---|
Low | 0–10 | 242 | 17.5 | 6.2 |
Moderate | 11–30 | 415 | 30.1 | 18.6 |
High | 31–60 | 615 | 44.6 | 43.1 |
Very high | 61–100 | 108 | 7.8 | 71.3 |
Individuals in the high-risk level have a 43.1% reconviction rate, which is almost seven times as high as the corresponding rate for those with a low level of risk. Finally, for the very high level of risk, the reconviction rate was 71.3%. This was more than 10 times higher than the low-level rate.
As the banded risk level for violence was to be included in the dynamic model (using the phase 2 sample), it was important to investigate whether or not the bands based on the phase 1 sample could be used in the phase 2 sample.
The findings show that the increases in reconviction rate between levels in the phase 2 sample were similar to those in the phase 1 sample. We therefore concluded that the banded risk levels for violence could be incorporated in the dynamic model for violence.
Even though banding of rescaled probabilities is based on subjective cut-off points, the comparisons between levels of risk for violence indicated that the risk differences between levels are substantial and can provide a simple indicator of risk for violence in a clinical setting.
The final coding sheet for the CIV can be found in Appendix 2.
Pencil and Paper Instrument for Violence
An alternative method for assessing the risk of violence in a clinical setting when there is no access to a computer would be to use a paper version of the CIV. We created categorical versions of the predictors included in the CIV based on the method of Gagliardi et al. 414
This method relies on assigning a score for each level of a categorical predictor according to the relationship between the estimate reconviction rate at each level and the 99% CI for baseline rate for violence baseline rate. The baseline rate in the phase 1 sample was 31.5% and the 99% CI was 28.3 to 34.8.
A score of > 1 is assigned if the violence rate at a predictor level is greater than the 99% CI, a score of 0 is assigned if the violence rate is within this interval and a score of –1 is assigned if the violence rate is below the 99% CI. In addition to the exploratory analysis required for continuous predictors with a large number of values, it is also possible to expand the score to allow for large differences in reconviction rates between predictor levels.
The Pencil and Paper Instrument for Violence (PIV) and corresponding statistical properties are shown in Table 138.
Variables | Recidivism (%) | Score |
---|---|---|
Number of previous convictions for violence (prcvioc) | ||
0 | 18.2 | –1 |
1 | 30.4 | 0 |
2–5 | 42.4 | 1 |
≥ 6 | 65.9 | 2 |
Age (years) (agec) | ||
≤ 21 | 47.3 | 2 |
22–25 | 37.1 | 1 |
26–39 | 29.8 | 0 |
40–45 | 17.2 | –1 |
≥ 46 | 4.0 | –2 |
Number of previous convictions for acquisitive crime (prcbtfc) | ||
0–2 | 19.2 | –1 |
3–12 | 34.6 | 0 |
≥ 13 | 42.6 | 1 |
Our comparison of AUC values for the PIV and CIV indicated that their accuracy levels were not statistically different (p = 0.242). When we compared other established actuarial instruments for violence, the AUC for the PIV was significantly higher than that for the OGRS2 (AUC 0.73; p = 0.014), VRAG (AUC 0.71; p = 0.003), RM2000V (AUC 0.69; p < 0.001) and HCR-20 total score (AUC 0.65; p < 0.001).
The classification accuracy estimates for the PIV scores were 48.5% for the PPV and 84.8% for the NPV. The PCC was 67.1%, almost equal to that for the CIV.
The final coding sheet for the PIV can be found in Appendix 3.
Discussion
The objective of this study was to create a simple static instrument for predicting violent convictions for prisoners who are close to their discharge date from prison. Based on our results, we have shown that it is possible to create parsimonious instruments that have significantly better discrimination ability (based on the AUC statistic) than other well-established instruments. In addition, the computer-based model has an adequate fit and the corresponding cross-validated probabilities are well calibrated. In addition, the classification accuracy results (mainly the PCC) indicate that both the CIV and the PIV are comparable with other instruments in our study.
The internal validation results for classification accuracy suggest that, even though discrimination accuracy is sufficiently high (AUC > 0.70), prediction accuracy is moderate for both the CIV and the PIV and there are therefore limitations when using these instruments in clinical practice. For those cases with scores above the median, further information would be required before identifying them as definitive high-risk individuals with subsequent requirements for offender management interventions to reduce the future risk of violence.
Our proposed instruments might therefore be used as a simple first step in a long and complex process to reduce violent reoffending for those individuals who are released from prison. Further research would be necessary to estimate the potential benefits of identifying high-risk individuals for offender management systems and the individuals who are screened.
Study 2: development of a static instrument to predict robbery
Objective
The objective was to create a new, accurate ARA instrument using static variables to assess the risk of robbery convictions among released prisoners.
Methods
Using a similar method to the static model for any violence described in the previous section, we constructed the corresponding static instrument for any robbery. The outcome of interest was a binary indicator that is equal to 1 for cases with at least one conviction for an offence related to robbery following release from prison and equal to 0 otherwise. Offences related to any robbery included aggravated burglary in addition to robbery.
As with the model for violence, the static predictors that we considered for the static instrument for robbery included age and previous convictions for violence, robbery, acquisitive crime and sexual offences.
Results
A total of 1380 cases were included in the phase 1 analysis sample, of whom 133 (9.6%) were convicted of an offence of robbery or an offence related to robbery after release from prison. In the phase 2 analysis sample, 77 (9.6%) of 802 individuals had the same type of conviction.
The predictor with the largest univariate AUC from the predictors considered for robbery was the log-transformed number of previous convictions + 1 (so that the resulting log-transformed predictor was equal to zero for no previous convictions) for robbery and aggravated burglary (denoted by lprcrobb). The AUC for this predictor was 0.65. By adding the predictor mentioned above and age (second predictor in terms of univariate AUC) in a logistic regression model the AUC increased to 0.70. Finally, by including the log-transformed number of previous convictions for acquisitive crime + 1 (denoted by lprcbtf), the AUC increased further to 0.72. Previous convictions for violence and previous convictions for sexual offences did not improve the predictive accuracy of the static instrument and were therefore not included.
The Computerised Instrument for Robbery (CIR) contains the three predictors described above. To obtain the probability of having at least one conviction for robbery, we calculated the linear score (denoted by S) using the constant of the model and the predictor values, which are weighted by the corresponding regression coefficients. In this case, the equation was as follows:
The corresponding probability (denoted by Pr) for this outcome was obtained using the following equation:
The apparent AUC for the CIR was 0.72 (95% CI 0.68 to 0.76), which indicates good predictive accuracy (AUC > 0.7). The H–L statistic for this model was 26.03 (26 df; p = 0 351) and this suggested that the model fits the data adequately. The LOOCV AUC for the CIR was 0.71 (95% CI 0.68 to 0.77; p < 0.001); this result suggested that the predictive accuracy of the CIR was anticipated to be good for similar samples.
The LOOCV AUC for the CIR was subsequently compared with the AUCs for other well-established instruments designed mainly for predicting general offending (OGRS2) and violence (VRAG, RM2000V and HCR-20 total score). The reason why we compared the CIR with instruments designed to assess violence was that robbery can be seen as a combination of violent and acquisitive crimes. It is, therefore, possible that instruments for assessing violence might be able to predict reconvictions that are partially related to violence (i.e. robbery).
Our results show that the CIR was significantly better than the OGRS2 (AUC 0.67; p = 0.024). It was also better than the VRAG (AUC 0.64; p = 0.003), RM2000V (AUC 0.63; p < 0.001) and HCR-20 total score (AUC 0.60; p < 0.001).
The classification accuracy estimates for CIR cross-validated probabilities were 13.5% for the PPV and 94.1% for the NPV. The main estimate of interest, the PCC, was 53.7%, which is similar to the corresponding estimates for other instruments in this study (57.1% for the HCR-20 total score, 60.8% for the RM2000V, 55.4% for the VRAG and 53.7% for the OGRS2).
Risk levels for robbery based on the Computerised Instrument for Robbery
To simplify the interpretation of the CIR output in clinical practice, we used the method described in study 1 to create risk bands for the CIR. To be specific, we divided the rescaled range for the LOOCV predicted probabilities (range 0–100) into four bands such that the actual reconviction rates would represent low, moderate, high and very high levels of risk for robbery.
The results for the suggested bands are shown in Table 139.
Level | Range | n | Prevalence (%) | Reconviction rate (%) |
---|---|---|---|---|
Low | 0–10 | 480 | 34.8 | 3.5 |
Moderate | 11–20 | 533 | 28.6 | 9.2 |
High | 21–30 | 217 | 15.7 | 14.3 |
Very high | 31–100 | 150 | 10.9 | 24.0 |
Similar comparisons with low level of risk should be considered for the interpretation of reconviction rates for high and very high levels of risk. Individuals in the high-risk level had a 14.3% reconviction rate, which is more than four times as high as the rate for the low-level group. For the very high level, the reconviction rate was 24.0%, which was more than six times higher than the low-level rate.
As the banded risk level for robbery was to be included in the dynamic model (using the phase 2 sample), we investigated whether or not the bands based on the phase 1 sample could be used in the phase 2 sample as expected from the descriptive analysis presented earlier.
The findings show that the increases in reconviction rate between levels in the phase 2 sample were similar to those in the phase 1 sample. This means that the banded risk levels for robbery can be incorporated in the dynamic model for robbery.
The final coding sheet for the CIR is provided in Appendix 4.
Paper Instrument for Robbery
Another method for assessing risk of robbery when access to a computer is not possible would be to use a paper version of the CIR. We created categorical versions of the predictors as for the PIV.
The Paper Instrument for Robbery (PIR) and its corresponding statistical properties are shown in Table 140.
Variables | Recidivism (%) | Score |
---|---|---|
Number of previous convictions for robbery (prcrobbc) | ||
0 | 6.2 | –1 |
1 | 14.9 | 0 |
2+ | 21.3 | 1 |
Age (years) (agerbc) | ||
≤ 21 | 14.8 | 2 |
22–29 | 11.2 | 1 |
30–39 | 9.2 | 0 |
40–45 | 3.5 | –1 |
≥ 46 | 0.0 | –2 |
Number of previous convictions for acquisitive crime (prcbtfcb) | ||
0–2 | 5.4 | –1 |
3–12 | 9.4 | 0 |
≥ 13 | 15.0 | 1 |
The comparison of accuracy between the PIR and the CIR using AUC values indicated that they were not statistically different (p = 0.232). On the other hand, the AUC for the PIR was significantly higher than the corresponding CIR LOOCV AUC (p = 0.013).
This difference might be explained by the fact that the PIR was not cross-validated (as this task would require the extremely time-consuming construction of 1380 versions of the PIR) and the PIR might be slightly optimistic. The CIR AUC was not statistically different from the PIR AUC indicating that the PIR AUC is unlikely to be overoptimistic. The PIR should, therefore, be expected to work well in other similar samples.
Comparing the PIR with other established instruments for both general offending and violence, the AUC for the PIR was significantly higher than those for the OGRS2 (AUC 0.67; p = 0.002), VRAG (AUC 0.64; p < 0.001), RM2000V (AUC 0.63; p < 0.001) and HCR-20 total score (AUC 0.60; p < 0.001).
The classification accuracy estimates for the PIR scores were 17.9% for the PPV and 95.1% for the NPV. The PCC was 67.0%, which is higher than the corresponding estimate for CIR cross-validated probabilities (55.3%).
The final coding sheet for the PIR is provided in Appendix 5.
Discussion
The main aim of this study was to create computer-based and paper static instruments for predicting robbery convictions after release from prison. We are not aware of any previous instruments that have been specifically developed to assess the risk of robbery. We believe that it will be of considerable interest to criminal justice professionals. Using the AUC, we have shown that the discrimination accuracy of both the CIR and the PIR is sufficiently high (AUC > 0.70) and that these instruments are significantly better than other comparable instruments.
Classification accuracy based on the median split indicated that the CIR PCC is similar to that of other instruments and that PIR accuracy is much higher than that of the other instruments in this study. Possible explanations for PIR classification superiority might be slight overfitting of the data or the fact that the median split might be a more suitable cut-off point for the PIR than the CIR and the other instruments included. External validation might help to elucidate this issue.
As with the use of risk instruments for violence, risk instruments for robbery might be helpful in identifying high-risk individuals for further investigation to prioritise cases for offender management interventions. As with other screening measures with low to moderate accuracy, it is unlikely that high-risk identification could lead to measures such as prolonged incarceration and failure to secure parole. Our measures should be regarded as the first step in a complex process of finding the optimal pathway for dealing with high-risk individuals to maximise benefit for both offender management systems and the screened individuals.
Study 3: development of a static instrument to predict drug-related offences
Objective
The objective was to create a new, accurate ARA instrument using static variables to assess the risk of drug-related convictions among released prisoners.
Methods
Using the methodological approaches utilised previously to create the static instruments for violence and robbery, we constructed the corresponding static instrument for drugs. The outcome of interest was a binary indicator that is equal to 1 for cases with at least one conviction for an offence related to drugs following release from prison and equal to 0 otherwise.
As with the models for violence and robbery, the static predictors that we considered for the static instrument for drugs included age and previous convictions for violence, robbery, acquisitive crime and sexual offences.
Results
A total of 1380 cases were included in the phase 1 analysis sample, of whom 289 (20.9%) were convicted of an offence related to drugs after release from prison. In the phase 2 analysis sample, 155 (19.3%) of 802 individuals had the same type of conviction.
The predictor with the largest univariate AUC from the predictors considered was the log-transformed number of previous convictions + 1 (so that the resulting log-transformed predictor is equal to zero for no previous convictions) for drugs (denoted by lprcdrug). The AUC for this predictor was 0.64. By including age (second predictor in terms of univariate AUC) and the predictor mentioned above in a logistic regression model, the AUC increased to 0.72. Finally, by including the log-transformed number of previous convictions for acquisitive crime + 1 (denoted by lprcbtf), the AUC increased further to 0.73. Previous convictions for violence, robbery and sexual offences were not included as they did not increase the predictive accuracy of the static instrument.
The Computerised Instrument for Drugs (CID) contains the three predictors mentioned above. To obtain the probability of having at least one conviction for drugs, we calculated the linear score (denoted by S) using the constant of the model and the predictor values, which are weighted by the corresponding regression coefficients. In this case, the equation was as follows:
The corresponding probability (denoted by Pr) for this outcome was obtained using the following equation:
The apparent AUC for the CID was 0.73 (95% CI 0.71 to 0.76; p < 0.001), which indicates good predictive accuracy (AUC > 0.7). The H–L statistic for this model was 52.98 (55 df; p = 0.552), which means that the model fit was adequate. The LOOCV AUC for the CID was 0.73 (95% CI 0.70 to 0.76; p < 0.001). This result indicated that the predictive accuracy of the CID can be expected to be good for similar samples.
The LOOCV AUC for the CID was compared with the AUCs for other well-established instruments designed for predicting general offending (OGRS2) and violence (VRAG, RM2000V and HCR-20 total score).
Our results show that the CID was significantly better than the OGRS2 (AUC = 0.68; p = 0.001). It was also better than the VRAG (AUC 0.64; p < 0.001), RM2000V (AUC 0.62; p < 0.001) and HCR-20 total score (AUC 0.61; p < 0.001).
The classification accuracy parameters estimates for CID cross-validated probabilities were 32.5% for the PPV and 90.5% for the NPV. For the PCC the estimate was 61.6%, which is comparable to the corresponding parameter estimates for the other instruments considered (58.1% for the HCR-20 total score, 61.1% for the RM2000V, 58.4% for the VRAG and 58.9% for the OGRS2).
Risk levels for drugs based on the Computerised Instrument for Drugs
To simplify the interpretation of the CID output in clinical practice, we used the method described earlier to create risk bands for the CIR. We divided the rescaled range for the LOOCV predicted probabilities into four bands such that the actual reconviction rates represent low, moderate, high and very high levels of risk for drug-related offences.
The results for the suggested bands are shown in Table 141.
Level | Range | n | Prevalence (%) | Reconviction rate (%) |
---|---|---|---|---|
Low | 0–20 | 498 | 36.1 | 6.0 |
Moderate | 21–40 | 550 | 39.8 | 24.6 |
High | 41–70 | 277 | 20.1 | 36.1 |
Very High | 71–100 | 55 | 4.0 | 43.6 |
Individuals in the high-risk level had a 36.1% reconviction rate, which was six times as high as the rate for the low-level group. For the very high level, the reconviction rate was 43.6%, more than seven times higher than the low-level rate.
As the banded risk level for drugs was to be included in the dynamic model (using the phase 2 sample), we investigated whether or not the bands based on the phase 1 sample could be used in the phase 2 sample as expected from the descriptive analysis presented earlier for study 1 and study 2.
The findings show that the increases in reconviction rate between levels in the phase 2 sample were similar to those in the phase 1 sample. This means that the banded risk levels for drugs can be incorporated in the dynamic model for drugs.
The final coding sheet for the CID is provided in Appendix 6.
Paper Instrument for Drugs
Another method for assessing risk of crime related to drug offences when access to a computer is not possible would be to use a paper version of the CID. We created categorical versions of the predictors as for the PIV and the PIR.
The Paper Instrument for Drugs (PID) and its corresponding statistical properties are shown in Table 142.
Variables | Recidivism (%) | Score |
---|---|---|
Number of previous convictions for drugs (prcdrugc) | ||
0 | 14.6 | –1 |
1 | 31.4 | 0 |
≥ 2 | 40.7 | 1 |
Age (years) (agerdc) | ||
≤ 19 | 34.5 | 2 |
20–30 | 25.9 | 1 |
31–37 | 18.6 | 0 |
38–45 | 12.1 | –1 |
≥ 46 | 0.7 | –2 |
Number of previous convictions for acquisitive crime (prcbtfcd) | ||
0–2 | 14.3 | –1 |
3–12 | 22.3 | 0 |
≥ 13 | 27.4 | 1 |
A comparison between PID and CID apparent probabilities using AUC values indicated that they were not statistically different (p = 0.169). Similarly, the AUC for the PID was not significantly different from the corresponding CID LOOCV AUC (p = 0.460).
Comparing the PID with other established instruments for general offending and violence, the AUC for the PID was significantly higher than that for the OGRS2 (AUC 0.68; p = 0.007), VRAG (AUC 0.64; p < 0.001), RM2000V (AUC 0.62; p < 0.001) and HCR-20 total score (AUC 0.61; p < 0.001).
The classification accuracy estimates for the PID scores were 35.7% for the PPV and 86.6% for the NPV. For the PCC, the estimate was 69.4%, which is slightly higher than the corresponding estimate for CID cross-validated probabilities (61.6%).
The final coding sheet for the PID is provided in Appendix 7.
Discussion
We have shown that it is feasible to create parsimonious instruments for predicting drug-related convictions following release from prison based on information easily obtainable from criminal records. Discrimination accuracy for both the CID and the PID was sufficiently high (AUC > 0.70) and significantly higher than that for the other instruments considered in this study.
Classification accuracy was low (61.6%) for the CID and moderate (69.4%) for the PID. As in the previous study on robbery convictions (see Study 2), it is unclear why the PID was more accurate than the CID. As the AUCs for both instruments are very similar, it is possible that the median split is more suitable for the PID than the CID or that there might be slight overfitting of the data for the PID. External validation might help to confirm whether or not the PID is more accurate in another sample.
Screening instruments for drug-related convictions could help in identifying potential high-risk individuals who could benefit from offender management interventions to reduce their future risk of drug reconvictions. Before applying any corrective measures based on screening results, it would be necessary to conduct further investigations to avoid mislabelling an individual as high risk with all the possible consequences of doing unnecessary harm to this individual and wasting valuable resources in counterproductive offender management interventions.
Study 4: development of a static instrument to predict acquisitive offences
Objective
The objective was to create a new, accurate ARA instrument using static variables to assess the risk of acquisitive convictions among released prisoners.
Method
In addition to the static instruments described earlier in this chapter, we constructed the corresponding static instrument for acquisitive crime. The outcome of interest was a binary indicator that was equal to 1 for cases with at least one conviction for an offence related to acquisitive offending after release from prison and equal to 0 otherwise. The static predictors considered for the static instrument for acquisitive crime included age and previous convictions for violence, robbery, acquisitive crime and sexual offences.
Results
Of the 1380 cases included in the phase 1 analysis sample, 524 (38.0%) were convicted for an offence related to acquisitive offending after release from prison. In the phase 2 analysis sample, 303 (37.8%) of 802 individuals had the same type of conviction.
The predictor with the largest univariate AUC of the predictors considered for acquisitive crime was the log-transformed number of previous convictions + 1 for acquisitive crime (denoted by lprcbtf). The AUC for this predictor was 0.72. By including age (second predictor in terms of univariate AUC) and the predictor mentioned above in a logistic regression model, the AUC increased to 0.79. Other predictors related to previous convictions for other types of offences were not included as they did not increase the predictive accuracy of the static instrument.
To establish whether or not the combination of predictors mentioned above gave well-calibrated predicted probabilities, we estimated the H–L statistic for apparent (complete sample) and LOOCV probabilities. For the apparent probabilities, the H–L statistic was 108.98 (97 df; p = 0.191), which indicated that these probabilities and the corresponding logistic model used to estimate them fitted the data adequately.
On the other hand, the H–L statistic for the LOOCV probabilities was 150.69 (97 df; p < 0.001), which suggested that the model will not fit the data adequately for similar samples. Therefore, it was necessary to add further predictors into the model that would give well-calibrated probabilities (both apparent and cross-validated) even if the improvement in the AUC was < 0.01.
Adding the number of previous convictions for drugs (denoted by prcdrug) gave an AUC of 0.79. The H–L statistic for the apparent probabilities was 109.53 (97 df; p = 0.181) and the corresponding statistic for the cross-validated probabilities was 118.75 (97 df; p = 0.066). These results showed that the additional predictor (prcdrug) helped to create an instrument with good predictive accuracy and well-calibrated probabilities for the analysis sample and for other similar samples.
The Computerised Instrument for Acquisitive Crime (CIA) contained three predictors mentioned above. To obtain the probability of having at least one conviction for acquisitive offences, we calculated the linear score (denoted by S) using the constant of the model and the predictor values, which were weighted by the corresponding regression coefficients. In this case, the equation was as follows:
The corresponding probability (denoted by Pr) for this outcome was obtained by the following equation:
The apparent AUC for the CIA was 0.79 (95% CI 0.77 to 0.82; p < 0.001) and the predictive accuracy was good as the AUC was > 0.7. The H–L statistic for this model was 109.53 (97 df; p = 0.181), which meant that the model fit was adequate. The LOOCV AUC for the CIA was 0.79 (95% CI 0.76 to 0.81; p < 0.001). This result indicated that the predictive accuracy of the CIA should be expected to be good for similar samples.
The LOOCV AUC for the CIA was compared with the AUCs for other well-established instruments designed mainly for predicting general offending (OGRS2) and violence (VRAG, RM2000V and HCR-20 total score).
Our results show that the CIA was significantly better than the OGRS2 (AUC 0.76; p = 0.014). It was also better than the VRAG (AUC 0.71; p < 0.001), RM2000V (AUC 0.70; p < 0.001) and HCR-20 total score (AUC 0.67; p < 0.001).
The classification accuracy estimates for the CIA cross-validated probabilities were 57.8% for PPV and 81.9% for NPV. For the PCC the estimate was 69.9%, which is similar to the corresponding parameter estimates for the other instruments considered (62.2% for the HCR-20 total score, 66.1% for the RM2000V, 64.6% for the VRAG and 68.7% for the OGRS2).
Risk levels for acquisitive offending based on the Computerised Instrument for Acquisitive Crime
To simplify the interpretation of the CIA output in clinical practice, we used the method described in study 1 to create risk bands. We divided the rescaled range for the LOOCV predicted probabilities into four bands such that the reconviction rates represent low, moderate, high and very high levels of risk for acquisitive crime.
The results for the suggested bands are shown in Table 143.
Level | Rangea | n | Prevalence (%) | Reconviction rate (%) |
---|---|---|---|---|
Low | 0–30 | 532 | 38.6 | 13.9 |
Moderate | 31–50 | 298 | 21.6 | 34.9 |
High | 51–70 | 286 | 20.7 | 55.2 |
Very high | 71–100 | 264 | 19.1 | 71.2 |
There were 286 individuals in the high-risk level (20.7% of the phase 1 sample) with a reconviction rate of 55.2%, which was almost four times as high as the rate for the low-level group. For the very high level, the reconviction rate was 71.2%, which was more than five times higher than the low-level rate.
As the banded risk level for acquisitive crime was to be included in the dynamic model (using the phase 2 sample), we investigated whether or not the bands based on the phase 1 sample could be used in the phase 2 sample as expected from the descriptive analysis presented earlier in previous studies in this chapter.
The findings show that the increases in reconviction rate between levels in the phase 2 sample were similar to the those in the phase 1 sample. This means that the banded risk levels for acquisitive crime can be incorporated in the corresponding dynamic model.
The final coding sheet for the CIA is provided in Appendix 8.
Paper Instrument for Acquisitive Crime
Another method for assessing risk of acquisitive crime when access to a computer is not possible would be to use a paper version of the risk instrument. We created categorical versions of the predictors as for the PIV and the PIR.
The Paper Instrument for Acquisitive Crime (PIA) and the corresponding statistical properties are shown in Table 144.
Variables | Recidivism (%) | Score |
---|---|---|
Number of previous convictions for acquisitive crime (prcbtfca) | ||
0 | 12.0 | –2 |
1 | 22.8 | –1 |
2–7 | 35.7 | 0 |
8–16 | 50.4 | 1 |
≥ 17 | 60.4 | 2 |
Age (years) (agerac) | ||
≤ 21 | 50.2 | 2 |
22–30 | 45.9 | 1 |
31–37 | 35.6 | 0 |
38–42 | 26.4 | –1 |
≥ 43 | 7.3 | –2 |
Number of previous convictions for drugs (prcdrugcad) | ||
0 | 31.2 | 0 |
≥ 1 | 54.5 | 1 |
Comparison in terms of AUC values between the PIA and the CIA probabilities indicated that they were not statistically different (p = 0.056). Similarly, the AUC for the PIA was not significantly different from the corresponding CIA LOOCV AUC (p = 0.191).
When we compared the PIA with other established instruments for general offending and violence, the AUC for the PIA was not significantly higher than that for the OGRS2 (AUC 0.76; p = 0.104). On the other hand, PIA AUC was significantly higher than the corresponding AUC for VRAG (AUC 0.71; p < 0.001), RM2000V (AUC 0.70; p < 0.001) and HCR-20 total score (AUC 0.67; p < 0.001).
The classification accuracy estimates for the PIA scores were 60.7% for the PPV and 80.9% for the NPV. For the PCC, the estimate was 71.7%, which is similar to the estimate for CIA cross-validated probabilities (69.9%).
The final coding sheet for the PIA is found in Appendix 9.
Discussion
Both the CIA and the PIA risk instruments were created to predict convictions for acquisitive offending after being discharged from prison. Assessment of discrimination accuracy indicated that both the CIA and the PIA were significantly better than most other instruments in this study and that their accuracy was sufficiently high (AUC > 0.70).
Classification accuracy was moderate to high for both instruments (close to 70%). This means that 30% of the cases are misclassified and that further information is required to verify that screen-positive cases (with scores above the median) are actually high-risk cases that require offender management interventions.
We need to emphasise that, despite the limitations of the static instruments (mainly because of misclassification rates of 30%), they still have the potential to help experts in offender management in a first step, which is to prioritise their efforts and resources on released offenders with a high propensity for future reconvictions. Further research is required to estimate the potential benefit of using risk instruments in the offender management system as a whole and for each individual assessed.
Study 5: validation of eight new static instruments to predict reoffending
Objectives
The objectives of this study were to:
-
examine the extent of predictive accuracy of the eight new static risk assessment instruments in a new sample of released prisoners
-
compare their predictive ability with that of other ARA instruments used in the criminal justice system and in clinical practice.
Methods
The external validation sample was provided by NOMS. The original anonymised data set included 53,800 cases. As there was enough power, listwise deletion was used, resulting in 45,577 (86.6%) men and 7066 (13.4%) women, a total of 52,643 individuals. These individuals were randomly selected prisoners who were released from prison for the first time between the years 2005 and 2007. Information regarding the sample was obtained from records held in the OASys and the PNC until 31 December 2012. Because of multiple OASys assessments, only the first assessment was used to create the study variables.
The objectives of this study were to compare the predictive accuracies of the eight static instruments and currently available risk assessment instruments used in practice in another sample. To measure the extent of predictive accuracy, we investigated discrimination and calibration. Discrimination measures the overall performance of an instrument – how well the instrument can discriminate between those who reoffended and those who did not reoffend. The most common performance indicator used to assess discrimination is the AUC. The second aspect of predictive validity measured was calibration. Calibration measures how well an instrument’s prediction of risk is in agreement with the actual observed risk. 415 The PPV and NPV are a form of calibration. They assess how well that instrument is able to separate those who were rated as high risk and went on to reoffend (PPV) from those who were rated as low risk and did not go on to reoffend (NPV). 415
Although the predictive values and PCC (see Study 1) are a form of calibration, H–L goodness-of-fit tests were also conducted to further investigate the extent to which these new instruments were globally calibrated. If the chi-squared test is significant, this means that the instrument is not well calibrated.
Measures
Outcome
The outcome measures were obtained from convictions recorded in the PNC. These convictions were for violence, drug, robbery and acquisitive offences committed after the respondents’ release and until 31 December 2012. The average time of risk for the sample was 6.52 years (SD 0.88 years, range 5.01–9.62 years).
Risk instruments
Four risk assessment instruments that are currently used were compared with the eight new static instruments that we created in Studies 1–4. The instruments used for comparison were the Offender Assessment System General reoffending Predictor (OGP),416 the Offender Assessment System Violent reoffending Predictor (OVP),417 the Offender Group Reconviction Scale, version 3 (OGRS3),418 and the RM2000V. 419
The OGP focuses on non-violent general reoffending with the exception of sexual offending. 418 The OGP combines seven static and dynamic risk factors to create a maximum weighted score of 100. Inclusive in the OGP is the OGRS3, which is the only static risk measure.
The OVP predicts homicide/assault, threats/harassment, weapon possession, robbery and aggravated burglary, criminal damage and public order reoffending. Similar to the OGP, the OVP consists of static and dynamic factors that are weighted to produce a possible maximum score of 100. However, unlike the OGP, the OVP includes 12 items, five of which are static risk factors such as age and the number of sanctions for violent-type offences.
The RM2000V includes three items to predict violent reoffending: age when the offender is released (age at commencement of risk), court appearances for violence that led to conviction and any burglaries. The score range for the RM2000V is 0–9.
The OGRS3 predicts reoffending solely on the basis of three static risks: age, sex and criminal history. These static risks are separated into six variables and together they estimate the likelihood that offenders will be reconvicted within 2 years of release.
Results
Total sample
Table 145 shows the results of the external validation for the total sample. In the total NOMS sample (n = 52,643), the constructed static instruments correctly classified the respondents within a range of 64–75%. According to the AUC values, the CIV and PIV were significantly different from the other instruments, with differences ranging from 0.02 to 0.06 (all p < 0.001). With the exception of OVP (PPV 69.26), the PPVs of the CIV and PIV were higher than those of the other instruments (CIV PPV 60.58; PIV PPV 64.87).
Instruments | AUC | 95% CI | PPV (%) | NPV (%) | PCC |
---|---|---|---|---|---|
Violence | |||||
CIV | 0.69 | 0.69 to 0.70 | 60.58 | 67.64 | 65.9 |
PIV | 0.68 | 0.68 to 0.69 | 64.87 | 63.97 | 64.1 |
OGRS3 | 0.64 | 0.64 to 0.64 | 52.94 | 66.27 | 62.3 |
OVP | 0.67 | 0.67 to 0.68 | 69.26 | 63.53 | 64.1 |
OGP | 0.63 | 0.63 to 0.64 | 50.91 | 66.34 | 61.2 |
RM2000V | 0.65 | 0.64 to 0.65 | 48.84 | 76.02 | 59.2 |
Robbery | |||||
CIR | 0.73 | 0.72 to 0.74 | 11.17 | 96.54 | 75.2 |
PIR | 0.71 | 0.70 to 0.72 | 10.75 | 96.70 | 72.6 |
OGRS3 | 0.65 | 0.64 to 0.66 | 8.91 | 96.10 | 70.3 |
OVP | 0.64 | 0.63 to 0.65 | 12.05 | 95.27 | 87.8 |
OGP | 0.66 | 0.65 to 0.67 | 8.85 | 96.35 | 67.2 |
RM2000V | 0.63 | 0.62 to 0.64 | 7.17 | 97.53 | 41.5 |
Drug-related offences | |||||
CID | 0.71 | 0.71 to 0.72 | 51.08 | 76.75 | 70.3 |
PID | 0.70 | 0.70 to 0.71 | 51.36 | 74.77 | 70.3 |
OGRS3 | 0.65 | 0.64 to 0.65 | 43.61 | 75.42 | 66.0 |
OVP | 0.55 | 0.54 to 0.55 | 32.68 | 70.04 | 66.7 |
OGP | 0.67 | 0.66 to 0.67 | 44.64 | 77.01 | 66.2 |
RM2000V | 0.59 | 0.59 to 0.60 | 35.04 | 77.69 | 51.2 |
Acquisitive offences | |||||
CIA | 0.80 | 0.80 to 0.80 | 82.00 | 60.92 | 66.2 |
PIA | 0.78 | 0.78 to 0.79 | 79.60 | 60.48 | 65.9 |
OGRS3 | 0.74 | 0.74 to 0.75 | 77.47 | 61.82 | 66.5 |
OVP | 0.59 | 0.59 to 0.60 | 63.04 | 51.50 | 52.5 |
OGP | 0.76 | 0.76 to 0.77 | 77.92 | 64.25 | 68.8 |
RM2000V | 0.66 | 0.65 to 0.66 | 59.43 | 65.93 | 61.9 |
All offences | |||||
CIV | 0.67 | 0.66 to 0.67 | 63.93 | 60.49 | 61.4 |
PIV | 0.68 | 0.68 to 0.68 | 68.70 | 57.45 | 58.8 |
CID | 0.66 | 0.66 to 0.67 | 61.01 | 59.52 | 59.9 |
PID | 0.67 | 0.66 to 0.67 | 64.69 | 58.87 | 60.0 |
CIR | 0.69 | 0.69 to 0.69 | 65.55 | 61.03 | 62.2 |
PIR | 0.67 | 0.66 to 0.67 | 63.42 | 61.32 | 61.9 |
CIA | 0.71 | 0.71 to 0.71 | 67.86 | 61.80 | 63.3 |
PIA | 0.70 | 0.69 to 0.70 | 65.94 | 61.40 | 62.6 |
OGRS3 | 0.70 | 0.70 to 0.71 | 68.10 | 63.83 | 65.1 |
OVP | 0.63 | 0.63 to 0.64 | 69.34 | 56.73 | 57.9 |
OGP | 0.71 | 0.71 to 0.72 | 67.42 | 65.29 | 66.0 |
RM2000V | 0.63 | 0.62 to 0.63 | 54.07 | 68.22 | 59.4 |
For future robbery convictions, the predictive validity of the CIR and PIR achieved an AUC of 0.73 and 0.71, respectively, with the CIR correctly classifying more individuals into their respective risk groups than the PIR (75% vs. 73%). Although the OVP correctly classified about 88% of individuals, it did not have a high discrimination accuracy (AUC 0.64). The differences between the existing instruments and each of the constructed instruments were significant at p < 0.001.
For predicting drug offences, the CID and PID were similar in predictive accuracy and in PCC (AUC ≈0.70 and PCC ≈70%). Compared with the four available risk instruments, the CID and PID had significantly higher predictive accuracy. Although the CID and PID had the highest PPVs (CID 51.08%; PID 51.36%), they did not have the highest NPVs (CID 76.75%; PID 74.77%).
For acquisitive offences, the CIA and PIA achieved AUCs of 0.80 and 0.78 respectively. Compared with the available risk assessment instruments for predicting future acquisitive offences, the CIA and PIA showed significantly different discrimination accuracy.
For all offences, the CIA and OGP showed the highest AUCs (0.71), with the OGP correctly classifying 66.0% and the CIA correctly classifying 63.3%. With the exception of the OGP, the AUC of the CIA was significantly different from AUC of the OGRS3, and OVP.
Male released prisoners
There was a similar pattern of findings for the male sample (n = 45,577). For violence, the CIV correctly classified 65.07% of the male sample and achieved an AUC of 0.69. Compared with the AUCs of all other instruments used in practice, the AUC of the CIV was significantly different (p < 0.001). The PIV correctly classified 63.03% of the male sample, with a similar PPV and NPV (65.32% and 62.72%). The AUC of the PIV was also significantly different from the AUCs of the other instruments used in current practice.
The CID and PID had the highest PCCs compared with the other instruments used in current practice (70.17% and 70.08% respectively). They also achieved the highest AUCs compared with other instruments (CID AUC 0.71, PID AUC 0.70; each comparison p < 0.001).
The CIR and PIR obtained the highest AUCs compared with the other risk assessment instruments used in current practice (CIR AUC 0.73, PIR AUC 0.71) and were significantly different from the other instruments. For example, compared with the RM2000V, the AUC of the CIR was 0.11 higher and the AUC of the PIR was 0.09 higher (both p < 0.001). Although these instruments had a high PCC, they had a low PPV (11.62% and 11.09% respectively).
The CIA and PIA both obtained the highest AUCs (0.80 and 0.78 respectively) and these were significantly different from those of the other instruments at the p < 0.001 level. Both constructed instruments also had the highest PPVs (81.16% and 78.59% respectively) but had lower PCCs than the OGP (69.08%) and the OGRS3 (67.59%).
Similar to the overall sample, the OGP and the CIA had the highest AUCs (0.71) when predicting any future offence. Compared with the AUC of the CIA, the AUCs of the OGRS3 (p = 0.02), OVP and RM2000V (p < 0.001) were significantly lower.
Female released prisoners
For the female sample, the CIV and PIV obtained AUCs of 0.70 for violence and had higher NPVs than PPVs; they also correctly classified more women than the other instruments. For example, the PIV had a NPV of 71.87%, a PPV of 61.43% and a PCC of 70.83%, whereas the OVP had a NPV of 69.91%, a PPV of 64.16% and a PCC of 69.69%.
With the exception of the OGP (p = 0.152), the CID and PID had the highest AUCs (0.70) of all of the instruments (p < 0.001); both instruments also had a low PPV (about 46%) and a moderate NPV (about 77%) and correctly classified about 71% of individuals.
For robbery, the CIR had an AUC of 0.72 and this value was significantly higher than that for all instruments used in practice. For the PIR, its AUC (0.71) was significantly higher than that for all other instruments used in practice except for the RM2000V (p = 0.144).
The AUCs for the CIA and PIA were the highest of all of the instruments. The AUC of the CIA (0.82) was significantly higher than the AUCs of the other instruments used in practice (all p < 0.001). In addition, compared with the AUC of the PIA (0.81), with the exception of CIA, the other instruments all had significantly lower AUCs (all p < 0.001). The PPV for both the CIA and the PIA was high (86.88% and 85.88% respectively) and the CIA and PIA correctly classified 63.27% and 62.20% of individuals respectively.
Examining any future offence, the OGP performed the best with the highest AUC (0.74) and the highest PCC (67.10%). Among all of the newly constructed instruments studied, the CIA had the highest AUC (0.73) and highest percentage classified (63.94%). The AUC of the CIA was significantly higher than that of the OVP and the RM2000V (both p < 0.001).
The results of the H–L global calibration tests show that none of these instruments was well calibrated in the external validation sample. The test for each instrument had a very high chi-square value and a fairly wide discrepancy between the overall percentages observed and the expected outcome rates. For example, between the OGP percentage observed and the OGP percentage expected in the total sample there was a 31.7% difference (observed 39.4%, expected 51.9%; χ2 = 7988.07; p < 0.001). In the female sample, however, the CIV achieved the same percentage observed and percentage expected (31.4%) and could be considered to be well calibrated. However, although the overall percentages appear to be equal, the individual risk groups reveal large discrepancies between the percentages for observed and expected. Therefore, the CIV for women was also not well calibrated (χ2 = 74.77; p < 0.001).
Discussion
The new instruments that were created in studies 1–4 of this chapter were externally validated in the present study. The purpose of externally validating these new static instruments was to assess the extent of their applicability in a different population. The results show that the new risk assessment instruments perform just as well, if not better, than the existing risk assessment instruments. With regard to the AUCs, the new instruments tended to obtain higher values, regardless of sex, for discrimination accuracy. The second aspect of predictive accuracy measured was calibration. 415 The results obtained point to poor calibration for not only the new instruments but also the existing instruments studied. The new instruments generally performed as well as their available counterparts according to the predictive values (PPVs and NPVs). However, these values, as well as the PCC, revealed low accuracy. The H–L tests indicate low-level global calibration when externally validated. What this means is that these instruments are not very accurate in detecting the actual observed risk. From the findings, it seems that this is an issue for all of the instruments studied. Nevertheless, the strength of these new instruments is that discrimination accuracy was improved.
The validation of the new instruments addresses several issues with the use of actuarial static risk instruments in practice: parsimony, predictive accuracy and general use. The use of a few items, as observed in each of the new instruments, shows equivalent, if not better, discrimination performance in predicting each specific offence outcome. When separating the analyses by sex, the new instruments also demonstrated similar predictive ability, although the new instruments had slightly higher predictive ability in the female sample. The new instruments demonstrated that only a small number of items are needed in an actuarial instrument and that they can be used in the clinical setting to quickly identify high-risk individuals.
Kallis et al. 420 used a similar approach in developing similar static screening tools. They proposed the use of screens for predicting future violence, created from just two static risk factors: age and previous violent convictions. The purpose of these screens would be to initially identify those who may be at high risk and refer them for further assessment. The benefits of using these items were that they were easily obtainable from the PNC and they addressed the issues of length and specific outcomes in the use of risk assessment.
In practice, because speed and reliability are considered valuable in a static actuarial instrument, more complex methods would need justification. 421 The present study, therefore, recognises the reality of clinical and criminal justice environments and has validated new instruments that take into account the needs of these environments. These new instruments advocate accessibility, parsimony and efficiency, characteristics that complement the needs of clinical practice.
Chapter 18 Development of a dynamic risk assessment for violence
Background
In Chapter 17 we described the development of risk assessment instruments composed of static risk factors. However, static risk instruments are only moderately accurate in the prediction of future violence. Unlike static risk factors, dynamic risk factors are defined by their ability to change throughout the life course. Examples of these factors include unemployment and peer group influences. 422 The pace of change for these risk factors may also vary and they have therefore been further divided into two groups:423 stable dynamic risk factors, which only gradually change with time (e.g. personality traits), and any acute dynamic risk factors (e.g. drug use), which may change on a day-to-day basis. 115
In practice, understanding change in dynamic risk factors is important for assessing the effectiveness of intervention programmes and pinpointing specific individual causal mechanisms. 424 From a methodological standpoint, however, dynamic risk factors are difficult to measure because of their changeability. 402 It is thought that static risk factors may be helpful for longer-term predictions, whereas dynamic risk factors may be helpful for short-term predictions. 401 Dynamic factors also show promise in modifying and managing risk and therefore prospectively measuring dynamic risk factors during a short time period is preferable.
It is ultimately most important to identify dynamic risk factors that have causal rather than predictive associations. Static risk instruments may be useful for grouping individuals by risk level and this will give clinicians some idea of the required intensity of the intervention necessary to prevent future offending. 425 Skeem and Mulvey426 defined this classification as ‘risk status’. However, it is of limited clinical usefulness for treating and monitoring an individual, other than for the identification of level of risk. At the same time, risk status can change if dynamic risk factors change. Further investigation is therefore needed into the relationship between static and dynamic risk for future intervention, pinpointing criminogenic needs, and to identify causal dynamic risk factors. 421
Study 1: a comparison of the effects of dynamic factors on four offending outcomes (violence, robbery, drugs and acquisitive crime)
Objectives
The objectives of this study were to:
-
investigate the associations between dynamic factors following release from prison and offending behaviour (violence, robbery, drugs and acquisitive crime) over 12 months following release
-
guide probation officers and other clinicians in their management of offenders after release by identifying suitable dynamic factors to target that will reduce the risk of offending behaviour.
Methods
For each key offending behaviour (violence, robbery, drugs and acquisitive crime), the outcome variable was a binary indicator for having at least one conviction and/or self-reported criminal behaviour over 12 months following release. For the purpose of study 1, we included self-reported criminal behaviour entered by the subject into a laptop computer, together with information on reoffending over the same time period from PNC records. For this study, the PCS analysis sample was analysed with 754 men and women because they had all necessary data for this analysis. In total, 151 cases had at least one violent conviction and/or self-reported violence (20.0%). There were 14 individuals (1.9%) with at least one robbery offence, 77 (10.2%) with drugs offences and 100 (13.3%) with acquisitive crime offences.
We used logistic regression separately for each of the outcomes described above to estimate the AOR for the association between the dynamic factor and the outcome of interest. The OR was adjusted for length of time from release to phase 2 interview, length of time since being released from prison to end of follow-up period, the location of the interview (prison or community) and sex. To ensure that the association between the dynamic factor and the outcome of interest was not the result of an association with one of the other three outcomes, we included each of the other three outcome indicators separately as adjusting variables.
The dynamic factors were divided into domains based on content. These domains included accommodation, social environment, coping/daily living, leisure time, employment/education, depression/self-harm/anxiety, psychosis, alcohol use, drug use, life events, treatment, compliance with supervision, victimisation, thoughts of violence, thoughts of previous offending and attitudes to crime (Table 146). A total of 125 dynamic factors were examined in 16 domains.
Dynamic factors | n |
---|---|
Accommodation | |
Homelessness | 80 |
Hostel | 143 |
No address | 75 |
Own accommodation | 398 |
Frequent address change | 46 |
Dissatisfied with accommodation | 168 |
Area unsafe | 131 |
Local problems | 576 |
Evicted | 61 |
Social environment | |
Living alone | 173 |
Living with parents | 200 |
Living with partner | 159 |
Socially isolated | 12 |
Living with friends | 25 |
Criminal network | 544 |
Family/friends unsupportive | 183 |
Coping/daily living | |
Difficulties with daily living | 227 |
Behind paying bills | 112 |
Services cut off | 28 |
Borrowing money from others | 357 |
Threats to person/family because of debt | 17 |
Borrowing money from friends | 57 |
No work/no benefits | 62 |
Low income | 174 |
Illegal earnings | 21 |
General financial difficulties | 385 |
Financial difficulties with managing household | 123 |
Coping difficulties scale score (last quartile) | 200 |
High stress score (last quartile) | 170 |
Leisure time | |
Unstructured leisure time | 234 |
Hanging around | 231 |
Frequent visits to bars/pubs | 115 |
Frequent betting | 119 |
Employment/education | |
No employment/education | 357 |
Unemployed – looking for work | 279 |
Unemployed – not looking for work | 78 |
Living off crime | 5 |
Sacked from jobs | 21 |
Disagreements at work | 61 |
Depression/self-harm/anxiety | |
Depressed (HADS) | 61 |
Tedium vitae | 179 |
Anxiety (HADS) | 163 |
Considered suicide | 129 |
Suicide attempt | 29 |
Other self-harm | 29 |
Service use (mental health) | 207 |
Non-compliance to therapeutic interventions | 125 |
Anger (STAXI) last quartile | 170 |
Treatment | |
Not attended treatment mental disorder | 72 |
Not attended treatment substance misuse | 84 |
Not attended therapies | 71 |
Compliance with supervision | |
Missed appointments with probation officer | 207 |
Warning letter from probation officer | 162 |
Victimisation | |
Victim of theft/burglary | 45 |
Victim of violence/threats | 123 |
Victimisation threats | 70 |
Victim other | 70 |
Thoughts of violence | |
Thoughts of violence | 111 |
Frequency of thoughts (at least twice a month) | 101 |
Thoughts of harming others more than once a week | 62 |
Thinking of the same ways of hurting others | 27 |
Thinking of different ways of hurting others | 68 |
Violent thoughts about the same victims | 41 |
Violent thoughts about different victims | 57 |
Thoughts becoming serious | 24 |
Likely to meet victim | 38 |
Psychosis | |
Psychosis (two or more symptoms on PSQ) | 55 |
Psychosis (three or more symptoms on PSQ) | 15 |
Hypomania | 494 |
Thought insertion | 164 |
Paranoid delusions | 295 |
Strange experiences | 190 |
Hallucinations | 66 |
PSQ ≥ 1 + STAXI score of ≥ 5 | 48 |
PSQ ≥ 1 + non-compliance with therapeutic interventions | 39 |
Major psychosis + non-compliance | 29 |
Alcohol use | |
Hazardous drinking (score of ≥ 8 on AUDIT) | 280 |
Alcohol use disorder (score of ≥ 16 on AUDIT) | 93 |
Alcohol dependence (score of ≥ 20 on AUDIT) | 62 |
Drug use | |
Drug use (any) | 408 |
Cannabis use | 362 |
Amphetamine use | 67 |
Sedative use | 66 |
Cocaine powder use | 143 |
Crack cocaine use | 98 |
Heroin use | 94 |
Methadone use | 37 |
Any opiates use | 99 |
Hallucinogens use | 13 |
Ecstasy use | 104 |
Solvents use | 9 |
Any drug dependence | 184 |
Cannabis dependence | 90 |
Stimulants dependence | 13 |
Cocaine dependence | 39 |
Crack cocaine dependence | 43 |
Heroin dependence | 72 |
Opiate dependence | 76 |
Volatile substance dependence | 3 |
Tranquilliser dependence | 14 |
Hallucinogen dependence | 1 |
Ecstasy dependence | 22 |
Methadone dependence | 19 |
Injected drugs | 47 |
Life events | |
Serious illness | 56 |
Serious injury | 33 |
Assaulted | 61 |
Death of someone elsea | 17 |
Other deathb | 89 |
Separation/divorce | 41 |
Problem friend/family/neighbour | 63 |
Redundant/sacked | 43 |
Looked for work unsuccessfully | 281 |
Financial crisis | 75 |
Items stolen | 73 |
At least one life event | 289 |
Thoughts of previous offending | |
Thoughts of offences similar to the index offence | 43 |
Frequency of thoughts (at least once a day) | 8 |
People remind of offencec | 14 |
Thoughts of contacting a previous victim | 52 |
Attitudes to crime | |
OK to steal if very poor | 256 |
OK to steal from the rich | 218 |
OK to steal from shops that make lots of money | 214 |
Sometimes OK to break the law | 285 |
Attitudes towards crime total score (last quartile) | 193 |
Results
Among the accommodation domain factors, homelessness was significantly associated with offences related to violence (AOR 1.87, 95% CI 1.02 to 3.43; p = 0.045) and drugs (AOR 2.37, 95% CI 1.09 to 5.12; p = 0.029), having no address was related only to drug offences (AOR 4.62, 95% CI 2.24 to 9.54; p < 0.001), frequent address change was related to violence (AOR 2.45, 95% CI 1.15 to 5.22; p = 0.020), robbery (AOR 6.42, 95% CI 1.45 to 28.37; p = 0.014) and acquisitive offences (AOR 2.49, 95% CI 1.08 to 5.73; p = 0.032) and being dissatisfied with accommodation was related to acquisitive crimes (AOR 2.11, 95% CI 1.23 to 3.59; p = 0.006). Furthermore, local problems were related to violence only (AOR 1.72, 95% CI 1.01 to 2.93; p = 0.046) but eviction was related to both violence (AOR 2.71, 95% CI 1.45 to 5.12; p = 0.002) and drug offences (AOR 2.55, 95% CI 1.14 to 5.72; p = 0.023).
From the social environment domain, living with a partner was associated with violence (AOR 1.77, 95% CI 1.12 to 2.82; p = 0.015), living with friends was associated with acquisitive crime (AOR 3.62, 95% CI 1.24 to 10.55; p = 0.019), criminal network was associated with violence (AOR 2.66, 95% CI 1.48 to 4.80; p = 0.001) and acquisitive crime (AOR 3.48, 95% CI 1.59 to 7.63; p = 0.002) and unsupportive family/friends was associated with violence (AOR 1.67, 95% CI 1.05 to 2.66; p = 0.029) and robbery (AOR 8.52, 95% CI 1.94 to 37.43; p = 0.005).
A number of coping/daily living domain factors were also related to criminal behaviour after release from prison. Difficulties with daily living were associated with robbery (AOR 4.05, 95% CI 1.03 to 15.87; p = 0.045), services cut off was related to violence (AOR 3.22, 95% CI 1.32 to 7.84; p = 0.010), borrowing money was related to violence (AOR 2.22, 95% CI 1.44 to 3.43; p < 0.001), drug offences (AOR 2.79, 95% CI 1.46 to 5.33; p = 0.002) and acquisitive crime (AOR 2.92, 95% CI 1.69 to 5.06; p < 0.001), threats to person/family because of debt was related to drug offences (AOR 6.06, 95% CI 1.64 to 22.42; p = 0.007) and money from friends was related to robbery (AOR 9.04, 95% CI 2.17 to 37.64; p = 0.002). Low income was associated with acquisitive crime (AOR 1.92, 95% CI 1.10 to 3.34; p = 0.022), illegal earnings were related to robbery (AOR 9.02, 95% CI 1.90 to 42.89; p = 0.006) and acquisitive crime (AOR 12.23, 95% CI 2.96 to 50.53; p = 0.001), financial difficulties were related to violence (AOR 2.13, 95% CI 1.36 to 3.34; p = 0.001), drug offences (AOR 3.55, 95% CI 1.72 to 7.31; p = 0.001) and acquisitive crime (AOR 3.42, 95% CI 1.89 to 6.20; p < 0.001), financial difficulties with managing the household were related to violence (AOR 1.68, 95% CI 1.01 to 2.80; p = 0.046) and acquisitive crime (AOR 1.95, 95% CI 1.10 to 3.46; p = 0.022), general coping difficulties were related to violence (AOR 2.60, 95% CI 1.63 to 4.14; p < 0.001) and acquisitive crime (AOR 1.89, 95% CI 1.06 to 3.38; p = 0.031) and a high stress score was related to violence (AOR 2.44, 95% CI 1.57 to 3.81; p < 0.001).
Of the leisure time dynamic factors, hanging around was related to drug offences (AOR 1.79, 95% CI 1.01 to 3.16; p = 0.046) and frequent visits to bar/pubs (AOR 2.20, 95% CI 1.30 to 3.72; p = 0.003) and frequent betting (AOR 1.71, 95% CI 1.02 to 2.88; p = 0.044) were related to violence only. From the ‘employment/education’ domain, getting sacked was related to drug offences (AOR 3.28, 95% CI 0.05 to 10.27; p = 0.041) and disagreements at work were related to violence only (AOR 2.95, 95% CI 1.48 to 5.89; p = 0.002).
Of the depression, self-harm and anxiety factors, increased anxiety (measured by the HADS) was related to violence (AOR 1.96, 95% CI 1.24 to 3.10; p = 0.004) and drug offences (AOR 1.86, 95% CI 1.02 to 3.42; p = 0.044), considering suicide was related to violence (AOR 2.00, 95% CI 1.23 to 3.24; p = 0.005) and acquisitive crime (AOR 1.83, 95% CI 1.04 to 3.24; p = 0.037), non-compliance to therapeutic interventions was related to drug offences (AOR 2.50, 95% CI 1.21 to 5.16; p = 0.013), anger [measured by the State–Trait Anger Expression Inventory (STAXI)427] was associated with violence (AOR 2.14, 95% CI 1.35 to 3.39; p = 0.001) and drug offences (AOR 2.01, 95% CI 1.08 to 3.74; p = 0.027) and experiencing tedium vitae was related to violence (AOR 1.69, 95% CI 1.0 to 2.64; p = 0.022).
From the psychosis domain, having at least two symptoms on the PSQ was related to violence (AOR 3.18, 95% CI 1.60 to 6.33; p = 0.001) and acquisitive crime (AOR 2.22, 95% CI 1.01 to 4.86; p = 0.047), paranoid delusions were related to violence (AOR 3.67, 95% CI 2.38 to 5.66; p < 0.001) and drug offences (AOR 1.92, 95% CI 1.05 to 3.49; p = 0.034) and having strange experiences were related to violence (AOR 2.60, 95% CI 1.68 to 4.02; p < 0.001). Having a combination of at least one PSQ symptom and a score of at least 5 on the STAXI was associated with violence (AOR 5.49, 95% CI 2.67 to 11.29; p < 0.001) whereas having a combination of at least one PSQ symptom and non-compliance with therapeutic interventions was related to both violence (AOR 2.66, 95% CI 1.18 to 6.01; p = 0.019) and drug offences (AOR 3.03, 95% CI 1.13 to 8.14; p = 0.027).
In the alcohol use domain, those individuals with either hazardous drinking (AOR 3.53, 95% CI 2.31 to 5.40; p < 0.001) or alcohol dependence (AOR 2.65, 95% CI 1.53 to 4.59; p = 0.001) were more likely to commit crimes related to violence. When we compared alcohol-dependent prisoners (AUDIT score of ≥ 20) with all other prisoners (AUDIT score of < 20), we found that alcohol-dependent prisoners were more likely to commit acquisitive crimes (AOR 2.50, 95% CI 1.22 to 5.12; p = 0.012); hazardous drinking was also related to acquisitive crimes (AOR 1.99, 95% CI 1.20 to 3.29; p = 0.008).
In the drug dependence domain, violence, drug offences and acquisitive crimes were significant outcomes of any drug use (AOR 4.89, 95% CI 2.85 to 8.40; p < 0.001; AOR 21.49, 95% CI 5.09 to 90.78; p < 0.001; and AOR 2.53, 95% CI 1.36 to 4.71; p = 0.004 respectively), cannabis use (AOR 2.61, 95% CI 1.66 to 4.08; p < 0.001; AOR 9.71, 95% CI 4.02 to 23.48; p < 0.001; and AOR 1.78, 1.03 to 3.07; p = 0.038 respectively), cocaine powder use (AOR 3.82, 95% CI 2.36 to 6.19; p < 0.001; AOR 3.13, 95% CI 1.66 to 5.92; p < 0.001; and AOR 3.83, 95% CI 2.22 to 6.63; p < 0.001 respectively), crack cocaine use (AOR 2.01, 95% CI 1.14 to 3.54; p = 0.016; AOR 2.77, 95% CI 1.38 to 5.57; p = 0.004; and AOR 4.66, 95% CI 2.52 to 8.63; p < 0.001 respectively) and ecstasy use (AOR 4.97, 95% CI 2.97 to 8.33; p < 0.001; AOR 2.51, 95% CI 1.30 to 4.84; p = 0.006; and AOR 2.00, 95% CI 1.09 to 3.67; p = 0.026 respectively). Amphetamine use was related to violence (AOR 2.25, 95% CI 1.20 to 4.22; p = 0.011) and acquisitive crimes (AOR 3.27, 95% CI 1.65 to 6.47; p = 0.001) and sedative use, heroin use and any opiate use were related to drug offences (AOR 3.43, 95% CI 1.66 to 7.08; p = 0.001; AOR 3.57, 95% CI 1.85 to 6.89, p < 0.001; and AOR 3.53, 95% CI 1.83 to 6.84; p < 0.001 respectively) and acquisitive crime (AOR 5.26, 95% CI 2.71 to 10.19; p < 0.001; AOR 3.01, 95% CI 1.66 to 5.47; p < 0.001; and AOR 2.87, 95% CI 1.58 to 5.21, p = 0.001 respectively). Methadone use was related to drug-related crimes only (AOR 2.96, 95% CI 1.11 to 7.92; p = 0.031). Any drug dependence was related to all four types of offences (violence AOR 2.70, 95% CI 1.69 to 4.32, p < 0.001; robbery AOR 11.77, 95% CI 1.85 to 74.98; p = 0.009; drug offences AOR 5.57, 95% CI 3.01 to 10.31; p < 0.001; and acquisitive crime AOR 3.51, 95% CI 2.05 to 6.01; p < 0.001). Cannabis dependence was related to violence (AOR 2.55, 95% CI 1.45 to 4.48; p = 0.001), robbery (AOR 7.48, 95% CI 1.86 to 30.07; p = 0.005) and drug offences (AOR 3.09, 95% CI 1.60 to 5.98; p = 0.001) and dependence on stimulants was related to robbery (AOR 18.15, 95% CI 2.03 to 162.14; p = 0.009). Dependence on each of the following substances was related to both drugs and acquisitive offences: powder cocaine (AOR 4.98, 95% CI 1.98 to 12.55; p = 0.001 and AOR 2.45, 95% CI 1.02 to 5.87; p = 0.045 respectively), crack cocaine (AOR 3.46, 95% CI 1.33 to 8.99; p = 0.011 and AOR 5.99, 95% CI 2.40 to 14.93; p < 0.001 respectively), heroin (AOR 3.27, 95% CI 1.60 to 6.68; p = 0.001 and AOR 3.36, 95% CI 1.77 to 6.40; p < 0.001 respectively), opiates (AOR 3.15, 95% CI 1.55 to 6.42; p = 0.002 and AOR 3.18, 95% CI 1.68 to 6.03; p < 0.001 respectively) and tranquillisers (AOR 5.82, 95% CI 1.63 to 20.78; p = 0.007; of and AOR 7.71, 95% CI 2.01 to 29.67; p = 0.003 respectively). Ecstasy dependence was associated with violence (AOR 11.90, 95% CI 3.08 to 45.92; p < 0.001) and drug-related offences (AOR 5.55, 95% CI 1.75 to 17.65; p = 0.004) and injecting drugs was related to violence (AOR 2.12, 95% CI 1.04 to 4.32; p = 0.038) and acquisitive offences (AOR 6.01, 95% CI 2.89 to 12.49; p < 0.001).
From the domain of life events, being assaulted was related to violence (AOR 10.30, 95% CI 5.39 to 19.67; p < 0.001) as well as being made redundant or sacked (AOR 3.76, 95% CI 1.83 to 7.71; p < 0.001). Financial crisis was more likely to be related to drug offences (AOR 2.34, 95% CI 1.09 to 5.01; p = 0.028), and, finally, having at least one life event was related significantly with violent offences (AOR 2.38, 95% CI 1.57 to 3.60; p < 0.001).
In the treatment domain, not attending treatment for a mental disorder was related to violence (AOR 2.01, 95% CI 1.09 to 3.72; p = 0.025) and not attending treatment for substance misuse was related to robbery (AOR 5.33, 95% CI 1.08 to 26.34; p = 0.040).
In the compliance with supervision domain, missing appointments with a probation officer was related to violence (AOR 2.27, 95% CI 1.46 to 3.52; p < 0.001), whereas receiving a warning letter from a probation officer was related to violence (AOR 1.90, 95% CI 1.18 to 3.05; p = 0.008), robbery (AOR 12.93, 95% CI 2.70 to 61.92; p = 0.001) and acquisitive crimes (AOR 2.37, 95% CI 1.38 to 4.05; p = 0.002).
In the victimisation domain, being the victim of theft/burglary was related to violence (AOR 2.72, 95% CI 1.30 to 5.70; p = 0.008) and becoming a victim of violence/threats was associated with violence (AOR 16.85, 95% CI 10.03 to 28.31; p < 0.001) and acquisitive offences (AOR 1.92, 95% CI 1.02 to 3.61; p = 0.044). Being a victim of threats and other types of victimisation were both related to violence (AOR 4.19, 95% CI 2.30 to 7.62, p < 0.001 and AOR 3.09, 95% CI 1.70 to 5.63, p < 0.001 respectively).
In the thoughts of violence domain, thinking of violence was associated with violent (AOR 3.84, 95% CI 2.32 to 6.38; p < 0.001), drug-related (AOR 2.42, 95% CI 1.24 to 4.72; p = 0.009) and acquisitive (AOR 1.87, 95% CI 1.03 to 3.41; p = 0.041) offences; having violent thoughts at least twice per month was related to violence (AOR 3.64, 95% CI 2.15 to 6.16; p < 0.001) and drug offences (AOR 2.78, 95% CI 1.41 to 5.49; p = 0.003); and having thoughts of harming others more than once per week was related to violence (AOR 3.23, 95% CI 1.67 to 6.26; p < 0.001), robbery (AOR 5.34, 95% CI 1.19 to 23.90; p = 0.029) and drug offences (AOR 3.51, 95% CI 1.60 to 7.67; p = 0.002). Thinking of different ways of hurting others was related to violence (AOR 3.99, 95% CI 2.16 to 7.39; p < 0.001), robbery (AOR 6.34, 95% CI 1.30 to 30.85; p = 0.022) and drug offences (AOR 4.55, 95% CI 2.15 to 9.63; p < 0.001) and having violent thoughts about different victims was related to violence (AOR 6.45, 95% CI 3.29 to 12.64; p < 0.001) and drug offences (AOR 4.41, 95% CI 1.94 to 10.02; p < 0.001). Being likely to meet a previous victim was related to violence (AOR 5.21, 95% CI 2.37 to 11.47; p < 0.001) and drug offences (AOR 3.72, 95% CI 1.41 to 9.80; p = 0.008).
In the thoughts of previous offending domain, thoughts of offences similar to the index offence were related to robbery (AOR 4.84, 95% CI 1.07 to 21.88; p = 0.040) and acquisitive crimes (AOR 2.79, 95% CI 1.20 to 6.46; p = 0.017) and thoughts of contacting a previous victim were related to violence (AOR 4.68, 95% CI 2.36 to 9.30; p < 0.001) and robbery (AOR 8.74, 95% CI 1.79 to 42.70; p = 0.007).
Finally, in the attitudes to crime domain, reporting that it is OK to steal if very poor was associated with violent (AOR 2.89, 95% CI 1.88 to 4.45; p < 0.001), drug (AOR 1.99, 95% CI 1.10 to 3.60; p = 0.022) and acquisitive (AOR 2.58, 95% CI 1.56 to 4.29; p < 0.001) offences. All other pro-criminal attitudes, as well as a high score on the criminal attitudes scale, were related to only violent and acquisitive offences.
Table 147 summarises the number of significant dynamic factors in each domain for each outcome.
Domain | Violence | Robbery | Drug offences | Acquisitive crimes |
---|---|---|---|---|
Accommodation | 4 | 1 | 2 | 2 |
Social environment | 3 | 1 | 0 | 2 |
Coping/daily living | 6 | 3 | 3 | 6 |
Leisure time | 2 | 0 | 1 | 0 |
Employment/education | 1 | 0 | 1 | 0 |
Depression/self-harm/anxiety | 4 | 0 | 3 | 1 |
Psychosis | 4 | 0 | 3 | 2 |
Alcohol use | 2 | 0 | 0 | 2 |
Drug use | 10 | 14 | 17 | 16 |
Life events | 3 | 0 | 0 | 0 |
Treatment | 1 | 1 | 0 | 0 |
Compliance with supervision | 2 | 0 | 0 | 1 |
Victimisation | 4 | 0 | 0 | 1 |
Thoughts of violence | 6 | 2 | 7 | 1 |
Thoughts of previous offending | 1 | 2 | 0 | 1 |
Attitudes to crime | 5 | 0 | 1 | 5 |
Total | 58 | 24 | 38 | 40 |
Discussion
We found that multiple dynamic factors from all domains showed associations with violent behaviour over the 12 months following release from prison. This contrasted with the results for robbery and, to a lesser extent, drug and acquisitive reconvictions. These findings are important because they question whether or not our main outcome under study, violence, should be considered a simple, unitary construct. It is probable that many studies in the field of behavioural sciences are limited by the highly heterogeneous nature of violence.
Violence
We found that violent behaviour in the 12 months following release was associated with dynamic risk factors in the area of accommodation, including homelessness, frequent address change, the prisoner reporting problems in the local area and eviction. These factors are likely to have strong associations with, and correspond to factors in, the coping/daily living domain, including services being cut off, borrowing money from others, financial difficulties, financial difficulties with managing the household, a high score on the coping difficulties scale and a high stress score. They also corresponded to prisoners reporting that their family and friends were unsupportive.
The strong association between violence and becoming involved in a criminal network (which was strongly associated with pro-criminal attitudes) suggested that some of the violent incidents may have occurred during the commission of other crimes or in the context of a criminal, social milieu in which violence was common. It was surprising that frequent betting did not feature among the dynamic variables for the other crime categories. If betting had led to debt it might be expected that an offender would report to acquisitive crime. However, this could reflect a criminal milieu. Alternatively, violent altercations could have resulted from gambling.
Frequenting bars and pubs corresponded with alcohol use and the severity of alcohol misuse was associated with violence. We have discussed the associations between alcohol misuse and violence in greater detail in Section A.
We also demonstrated that anxiety disorder is associated with violence in the general population of the UK in Section A. Because clinical depression was not associated with violence in this study or in the general population, reporting suicidal thoughts could correspond to impulsivity in these offenders and difficulty with coping with stress, rather than being a component of clinical depression. A high level of anger corresponds to a propensity towards violence as a feature of personality disorder but was also associated with psychotic symptoms in this sample. We also found that paranoid delusions, strange experiences and non-compliance with treatment were all associated with violence over the 12 months. We have previously shown that delusions are causally associated with violence in other samples and that this is mediated through anger resulting from the delusions. 21. 291
Our findings also correspond to those in Section A showing that drug misuse is associated with violence. However, in this study, cannabis use appeared to be among the drugs most strongly associated. This finding questions if cannabis is a drug of choice for individuals with violent tendencies and whether or not using cannabis helps contain and control violent urges. On the other hand, heavy cannabis use may be common in social settings where criminal activities are also common. It was therefore of some interest that a wider range of drugs was found to be associated at the level of misuse rather than at a more severe level of dependence.
Being assaulted would correspond to an individual subsequently considering and then actively taking revenge on the person who assaulted them. Being made redundant or sacked would correspond to poor work performance, which could be explained by underlying instability and impulsiveness in these individuals.
Poor compliance with treatment and supervision may also correspond to impulsiveness and antiauthoritarian attitudes, together with an associated propensity to violent behaviour when under stress. It was also of considerable importance that violent individuals in the sample had experienced multiple forms of victimisation, which may have resulted in violent retaliation.
Strong associations of violence with ruminative thoughts of violence and considering different ways of hurting others and hurting different victims were unsurprising. Furthermore, the importance of considering the likelihood of meeting a previous victim was highlighted by our findings. In some cases, this involved former intimate partners.
Robbery
A considerably narrower range of dynamic factors was associated with robbery during the 12 months following release. Prisoners later convicted of robbery appeared to have returned to a criminal milieu in which they were receiving money from friends, which they would probably be required to pay back through criminal activities. They had frequent changes of address and perceived their family and friends as unsupportive. They also appeared to have become dependent on drugs after leaving prison, particularly cannabis and stimulants. It is probable that robberies reflected their need to pay for drugs, particularly stimulants. However, stimulant use might also reflect stimulus-seeking qualities of these individuals in terms of behaviour and personality.
There are few psychiatric or psychological studies of individuals who commit robbery. However, the criminological literature suggests that, among prisoners, robbers tend to be of the highest status and are highly volatile. 428 We have observed that those convicted of robbery have higher PCL-R scores, indicating higher levels of psychopathic personality traits. This probably explained their poor compliance with probation supervision, including missing appointments with their probation officers and reporting that they had received warning letters. It might also explain their failure to attend treatment for substance misuse.
Drug misuse
It was unsurprising that the strongest associations that we found for drug offences were for drug misuse and dependence with a range of different drugs in the 12 months following release. These offenders had unstable accommodation, their leisure time was spent ‘hanging around’ (possibly selling or obtaining drugs) and they were unable to work consistently. Borrowing money to buy drugs had probably resulted in threats towards them and their family as a result of debts, corresponding to their reported financial difficulties. Unfortunately, they were not compliant with offers of therapeutic intervention.
It was of interest that a number of drug offenders had experienced paranoid delusions and high levels of anger. This may have been a pharmacological effect of their drug misuse and could have explained ruminations of violence towards others. On the other hand, violence towards others was more likely explained by the context of selling drugs to others. Some of these former prisoners may have been considering violent retaliation towards those who had failed to pay them for drugs.
Acquisitive offending
Acquisitive offending was also strongly associated with drug misuse and dependence. This was independent of the other three categories of offending and indicates the need for many who abuse drugs to obtain money to pay for them through crimes such as burglary and theft.
Acquisitive offenders had frequent changes of address and were dissatisfied with their current accommodation. Because many were living with friends, it is reasonable to assume that their friends were part of a criminal network from whom they borrowed money, as a result of which they were living off illegal earnings. They had a low income, had financial difficulties and were poor at coping. Their impulsiveness was suggested by the fact they had considered suicide (while not having a depressive illness). Many were drinking heavily, sometimes to the level of alcohol dependence. They also abused a range of drugs and some were dependent on drugs, particularly opiates. It is probable that, in many cases, their acquisitive offending was to pay for drugs. Some appeared to be injecting drugs. It is unsurprising that they had pro-criminal attitudes towards theft and law-breaking.
Study 2: development of a Dynamic Risk Instrument for Violence
Objective
The objective of this study was to construct the Dynamic Risk Instrument for Violence (DRIV) to aid probation officers and other clinicians in risk management.
Methods
The construction approach for this tool was based on the principle that, at the first stage of offender management, each domain should be investigated separately to reduce the complexity of management interventions across multiple domains. To keep the instrument as simple to use as possible, we decided to prioritise the strongest dynamic factor for violence in each domain (in terms of the AOR magnitude) because these are more likely to lead to a larger reduction in future violence.
Results
For the accommodation domain, clinical management intended to lead to a reduction in violence would begin by addressing the effects on the released prisoner of evictions (AOR 2.71, 95% CI 1.43 to 5.12; p = 0.002) followed by frequent address change (AOR 2.45, 95% CI 1.15 to 5.22; p = 0.020), homelessness (AOR 1.87, 95% CI 1.02 to 3.43; p = 0.045) and finally problems in the local area (AOR 1.72, 95% CI 1.01 to 2.93; p = 0.046). Social environment risks were criminal networks (AOR 2.66, 95% CI 1.48 to 4.80; p = 0.001), living with a partner (AOR 1.77, 95% CI 1.12 to 2.82; p = 0.015) and unsupportive family and friends (AOR 1.67, 95% CI 1.05 to 2.66; p = 0.029).
Within the coping/daily living domain, services having been cut off was the strongest dynamic factor (AOR 3.22, 95% CI 1.32 to 7.84; p = 0.010). Following that, coping difficulties (AOR 2.60, 95% CI 1.63 to 4.14; p < 0.001), high levels of stress (AOR 2.44, 95% CI 1.57 to 3.81; p < 0.001), borrowing money (AOR 2.22, 95% CI 1.44 to 3.43; p < 0.001), general financial difficulties (AOR 2.13, 95% CI 1.36 to 3.34; p = 0.001) and financial difficulties managing the household (AOR 1.68, 95% CI 1.01 to 2.80; p = 0.046) were also significant. The effects of these factors and the links between them and the risk of criminal behaviour should be investigated. Within the leisure time domain, frequent visits to bars/pubs (AOR 2.20, 95% CI 1.30 to 3.72; p = 0.003) followed by frequent betting (AOR 1.71, 95% CI 1.02 to 2.88; p = 0.044) indicated factors that could be targeted for successful management of violent offending. For employment/education, only disagreements at work (AOR 2.95, 95% CI 1.48 to 5.89; p = 0.002) would need to be considered for violence management.
Within the depression/self-harm/anxiety domain, reported anger (AOR 2.14, 95% CI 1.35 to 3.39; p = 0.001), thoughts of suicide (AOR 2.00, 95% CI 1.23 to 3.24; p = 0.005), anxiety (AOR 1.96, 95% CI 1.24 to 3.10; p = 0.004) and experiencing tedium vitae (AOR 1.69, 95% CI 1.08 to 2.64; p = 0.022) would merit further investigation. Within the psychosis domain, the combination of at least one PSQ symptom and a score of at least 5 on the STAXI showed the strongest association with violence (AOR 5.49, 95% CI 2.67 to 11.29; p < 0.001). This was followed by paranoid delusions (AOR 3.67, 95% CI 2.38 to 5.66; p < 0.001), at least two PSQ symptoms (AOR 3.18, 95% CI 1.60 to 6.33; p = 0.001), the combination of at least one PSQ symptom and non-compliance with therapeutic interventions (AOR 2.66, 95% CI 1.18 to 6.01; p = 0.019) and finally having strange experiences (AOR 2.60, 95% CI 1.68 to 4.02; p < 0.001).
Within the alcohol use domain, identifying cases with an AUDIT score of ≥ 8 (AOR 3.53, 95% CI 2.31 to 5.40; p < 0.001) and an AUDIT score of ≥ 16 (AOR 2.65, 95% CI 1.53 to 4.59; p = 0.001) confirmed the association between alcohol use problems and violence.
Within the drug use domain, ecstasy dependence would be the first priority for investigation for managing future violent behaviour (AOR 11.90, 95% CI 3.08 to 45.92; p < 0.001). Other drug-related behaviours meriting further investigation were ecstasy use (AOR 4.97, 95% CI 2.97 to 8.33; p < 0.001), any drug use (AOR 4.89, 95% CI 2.85 to 8.40; p < 0.001), cocaine powder use (AOR 3.82, 95% CI 2.36 to 6.19; p < 0.001), any drug dependence (AOR 2.70, 95% CI 1.69 to 4.32; p < 0.001), cannabis use (AOR 2.61, 95% CI 1.66 to 4.08; p < 0.001), cannabis dependence (AOR 2.55, 95% CI 1.45 to 4.48; p = 0.001), amphetamine use (AOR 2.25, 95% CI 1.20 to 4.22; p = 0.011), injecting drugs (AOR 2.12, 95% CI 1.04 to 4.32; p = 0.038) and crack cocaine use (AOR 2.01, 95% CI 1.14 to 3.54; p = 0.016).
Important factors from the life events domain that were likely to influence violent offending were being assaulted (AOR 10.30, 95% CI 5.39 to 19.67; p < 0.001) followed by being made redundant/sacked (AOR 3.76, 95% CI 1.83 to 7.71; p < 0.001) and having at least one life event (AOR 2.38, 95% CI 1.57 to 3.60; p < 0.001).
Failing to attend treatment for a mental disorder (AOR 2.01, 95% CI 1.09 to 3.72; p = 0.025), missed appointments with probation officers (AOR 2.27, 95% CI 1.46 to 3.52; p < 0.001) and warning letters from probation officers (AOR 1.90, 95% CI 1.18 to 3.05; p = 0.008) were all dynamic factors associated with violence. Victimisation events related to violence (AOR 16.85, 95% CI 10.03 to 28.31; p < 0.001), threats (AOR 4.19, 95% CI 2.30 to 7.62; p < 0.001), theft/burglary (AOR 2.72, 95% CI 1.30 to 5.70; p = 0.008) and other types of victimisation (AOR 3.09, 95% CI 1.70 to 5.63; p < 0.001) were all important to further violent offending.
Several factors within the thoughts of violence domain were identified: thoughts related to different victims (AOR 6.45, 95% CI 3.29 to 12.64; p < 0.001) followed by the likelihood of meeting a previous victim (AOR 5.21, 95% CI 2.37 to 11.47; p < 0.001), thinking about different ways of hurting others (AOR 3.99, 95% CI 2.16 to 7.39; p < 0.001), general thoughts of violence (AOR 3.84, 95% CI 2.32 to 6.38; p < 0.001), thinking of violence at least twice a month (AOR 3.64, 95% CI 2.15 to 6.16; p < 0.001), thoughts of harming others more than once a week (AOR 3.23, 95% CI 1.67 to 6.26; p < 0.001) and thoughts of contacting a previous victim (AOR 4.68, 95% CI 2.36 to 9.30; p < 0.001) were all associated with violent offending.
Finally, within the pro-criminal attitudes domain, believing that it is OK to steal if very poor (AOR 2.89, 95% CI 1.88 to 4.45; p < 0.001) followed by believing that it is OK to steal from the rich (AOR 3.86, 95% CI 2.50 to 5.97; p < 0.001), that it is OK to steal from shops that make lots of money (AOR 3.37, 95% CI 2.17 to 5.23; p < 0.001) and that it is sometimes OK to break the law (AOR 2.86, 95% CI 1.86 to 4.40; p < 0.001) and finally an overall high score on the criminal attitudes scale (AOR 4.26, 95% CI 2.73 to 6.66; p < 0.001) were associated with violent offending.
Table 148 summarises the dynamic factors for violence in the DRIV.
Domain | Factor |
---|---|
Accommodation | Evicteda |
Frequent address change | |
Homelessness | |
Local problems | |
Social environment | Criminal networka |
Living with partner | |
Family/friends unsupportive (first quartile) | |
Coping/daily living | Services cut offa |
Coping difficulties scale score (last quartile) | |
High stress score (last quartile) | |
Borrowing money | |
General financial difficulties | |
Financial difficulties with managing household | |
Leisure time | Frequent visits to bars/pubsa |
Frequent betting | |
Employment/education | Disagreements at worka |
Depression/self-harm/anxiety | Anger (STAXI) last quartilea |
Considered suicide | |
Anxiety (HADS) | |
Tedium vitae | |
Psychosis | PSQ ≥ 1 + STAXI score of ≥ 5a |
Paranoid delusions | |
Psychosis (PSQ2+) | |
PSQ ≥ 1 + non-compliance with therapeutic interventions | |
Strange experiences | |
Alcohol use | Hazardous drinking (AUDIT score of ≥ 8)a |
Alcohol use disorder (AUDIT score of ≥ 16) | |
Drug use | Ecstasy dependencea |
Ecstasy use | |
Drug use (any) | |
Cocaine powder use | |
Any drug dependence | |
Cannabis use | |
Cannabis dependence | |
Amphetamine use | |
Injected drugs | |
Crack cocaine use | |
Life events | Assaulteda |
Redundant/sacked | |
No life events | |
Treatment | Not attended treatment for a mental disordera |
Compliance with supervision | Missed appointments with probation officera |
Warning letter from probation officer | |
Victimisation | Victim of violence/threatsa |
Victimisation threats | |
Victim other | |
Victim of theft/burglary | |
Thoughts of violence | Different victimsa |
Likely to meet victim | |
Different ways of hurting others | |
Thoughts of violence | |
Frequency of thoughts (at least two times a month) | |
Thoughts of harming others more than once a week | |
Thoughts of previous offending | Thoughts of contacting a previous victima |
Attitudes towards crime | OK to steal if very poora |
OK to steal from the rich | |
OK to steal from shops that make lots of money | |
Sometimes OK to break the law | |
Attitudes towards crime total score (last quartile) |
The final coding sheet for the DRIV is found in Appendix 10.
Discussion
The findings of this study are at a preliminary stage with regard to the construction of the DRIV. Certain items are likely to have shown overlap, for example being evicted and frequent address change, and it is probable that the overall number of items can and should be reduced. Furthermore, certain items at first appear not to be consistent with being a risk factor. For example, living with a partner might appear to be an unlikely risk factor in the context of what should be a supportive social environment. However, we have previously observed the heterogeneity of outcomes in study 1. Returning to a social environment where a former prisoner had lived with a partner to whom he had previously been violent would be considered a very different type of risk factor than is initially implied by living with partner. Similarly, in Section A we observed that violence towards partners, particularly among men, tends to be a feature of a generalised tendency to violence and these men have a range of different potential victims. In this sample of prisoners, many of whom may have ASPD, a partner is one among several potential victims.
The perception that family and friends are unsupportive might be better placed among the coping and daily living factors. Among these factors, financial difficulties probably overlaps with and supersedes financial difficulties with managing a household.
Further refinement is also required for the psychosis domain in view of previous findings21,291 that the association between anger and paranoid delusions is a key area of risk. Further analysis may also prove that certain risk factors in this domain ultimately prove redundant.
Despite these limitations, and the over-inclusiveness of our preliminary version of the DRIV, many of these items could be easily measured by clinicians involved in the supervision of offenders following their release into the community. However, there are certain problems for probation officers in that they do not routinely take certain measurements or are not trained to take them, for example for psychosis, anxiety disorder and depression. Diagnosis is considered the professional responsibility of mental health professionals. On the other hand, the measures in this study were taken by employees of a surveying company who were also not trained clinicians. Screens were successfully administered as self-report instruments on a laptop computer. The equivalent instrument administered by probation staff is the OASys. However, this does not include validated mental health measures.
An additional limitation at this preliminary stage is that, although identifying candidate variables with a high likelihood of association with violence, the instrument does not tell the probation officer or clinician how to intervene in the sense of specifying what intervention to make. Certain dynamic risk factors require active, ongoing treatment interventions. Others may not be willingly reported by the prisoner following release and may not be observed. Others, however, are risk factors that might easily be anticipated, such as housing difficulties. However, the clinician or probation officer may not have the resources to successfully intervene. Nevertheless, this preliminary version of the DRIV provides an important first step and is the basis for our subsequent analyses.
Study 3: testing the associations between dynamic risk factors and violence according to level of static risk
Objective
The objective of this study was to investigate the associations between the dynamic factors described in study 1 and violence after release from prison (within a year after release from prison) for three levels of static risk (low, medium and high).
Methods
The first step in the analysis was to identify suitable static risk levels using the PIV described earlier (see Chapter 17, Study 1). Using the Gagliardi et al. 414 method for identifying suitable cut-off points for PIV score, we identified three subgroups: low, medium and high risk.
For each static risk subgroup, we used logistic regression to estimate the association between each dynamic factor (for a list of these factors see Table 149) and violent offending. The OR for each dynamic factor was adjusted for length of time from release to phase 2 interview, length of time out of prison following release from prison, location of interview (prison or community), sex and robbery, drugs and acquisitive offences. The dynamic factors were divided into domains as described in detail in study 1 of this chapter.
Results
Based on the Gagliardi et al. 414 method, the overall sample prevalence of violence was 20.5% and the corresponding 99% CI for this estimate was 16.8% to 24.6%. Using the estimate prevalence for each PIV value, we identified three static risk levels: low risk (PIV 0–3), medium risk (PIV 4–5) and high risk (PIV 6–9). The corresponding sample size and violence prevalence for each static risk subgroup were as follows: low risk, 290 cases (9.4%); medium risk, 284 cases (23.7%); and high risk, 180 cases (33.5%).
Low-risk group
We found that a total of 32 factors were significantly related to violence. Specifically, we found that all of the items in the attitudes to crime domain were significantly related to violence. In addition, the following items were also significantly related to violence: being evicted (AOR 5.61, 95% CI 1.21 to 26.03; p = 0.028), having family and friends who were perceived as unsupportive (AOR 3.61, 95% CI 1.29 to 10.07; p = 0.014), borrowing money (AOR 3.77, 95% CI 1.39 to 10.25; p = 0.009), experiencing general financial difficulties (AOR 2.80, 95% CI 1.03 to 7.59; p = 0.043), experiencing financial difficulties with managing the household (AOR 4.32, 95% CI 1.29 to 14.41; p = 0.017), having a high level of stress (AOR 3.49, 95% CI 1.32 to 9.25; p = 0.012), being unemployed and looking for work (AOR 3.15, 95% CI 1.19 to 8.37; p = 0.021), experiencing tedium vitae (AOR 4.06, 95% CI 1.57 to 10.53; p = 0.004), experiencing paranoid delusions (AOR 4.19, 95% CI 1.53 to 11.44; p = 0.005), having strange experiences (AOR 5.15, 95% CI 1.91 to 13.92; p = 0.001), experiencing a psychotic symptom (PSQ) and having a high STAXIS score (AOR 8.67, 95% CI 1.80 to 41.89; p = 0.007), engaging in hazardous drinking (AOR 3.11, 95% CI 1.17 to 8.27; p = 0.023), any drug use (AOR 2.82, 95% CI 1.03 to 7.68; p = 0.043), use of sedatives (AOR 6.28, 95% CI 1.06 to 37.34; p = 0.043), use of cocaine powder (AOR 4.38, 95% CI 1.36 to 14.14; p = 0.013), use of crack cocaine (AOR 6.30, 95% CI 1.67 to 23.70; p = 0.006), any drug dependence (AOR 6.04, 95% CI 1.84 to 19.89; p = 0.003), heroin dependence (AOR 5.51, 95% CI 1.43 to 21.16), opiate dependence (AOR 5.13, 95% CI 1.37 to 19.20; p = 0.015), having injected drugs (AOR 9.48, 95% CI 1.85 to 48.56; p = 0.007), being assaulted (AOR 19.63, 95% CI 5.62 to 68.53; p < 0.001), being made redundant/sacked (AOR 9.23, 95% CI 2.24 to 38.09; p = 0.002), not attending therapies (AOR 12.42, 95% CI 1.98 to 78.06; p = 0.007), being a victim of violence/threats (AOR 47.78, 95% CI 12.14 to 188.14; p < 0.001), having violent thoughts at least twice a month (AOR 3.68, 95% CI 1.12 to 12.05; p = 0.031), thinking of different victims (AOR 8.16, 95% CI 1.73 to 38.36; p = 0.008) and contacting the previous victim (AOR 9.02, 95% CI 2.31 to 35.21; p = 0.002).
Medium-risk group
A total of 34 factors were related to violence in the medium-risk group: becoming homeless (AOR 3.71, 95% CI 1.48 to 9.30; p = 0.005), having a frequent address change (AOR 3.40, 95% CI 1.06 to 10.94; p = 0.040), living in an unsafe area (AOR 3.36, 95% CI 1.57 to 7.16; p = 0.002), having a high level of coping difficulties (AOR 2.55, 95% CI 1.25 to 5.20; p = 0.010), having a high stress level (AOR 2.03, 95% CI 1.03 to 3.99; p = 0.041), having disagreements at work (AOR 3.13, 95% CI 1.14 to 8.65; p = 0.027), suffering from anxiety (AOR 2.20, 95% CI 1.12 to 4.32; p = 0.023), engaging in other types of self-harm (AOR 4.93, 95% CI 1.10 to 22.16; p = 0.037), having a high level of anger (AOR 2.18, 95% CI 1.07 to 4.43; p = 0.032), scoring ≥ 2 for psychosis (AOR 7.76, 95% CI 2.71 to 22.25; p < 0.001), experiencing paranoid delusions (AOR 4.30, 95% CI 2.14 to 8.63; p < 0.001), having strange experiences (AOR 2.38, 95% CI 1.22 to 4.66; p = 0.011), hallucinations (AOR 2.67, 95% CI 1.11 to 6.44; p = 0.029), engaging in hazardous drinking (AOR 2.33, 95% CI 1.23 to 4.41; p = 0.010), any drug use (AOR 5.28, 95% CI 2.08 to 13.41; p < 0.001), cannabis use (AOR 2.69, 95% CI 1.30 to 5.54; p = 0.008), cocaine powder use (AOR 3.94, 95% CI 1.91 to 8.17; p < 0.001), ecstasy use (AOR 3.44, 95% CI 1.62 to 7.34; p = 0.001), cannabis dependence (AOR 3.49, 95% CI 1.51 to 8.07; p = 0.003) and being assaulted (AOR 6.12, 95% CI 2.32 to 16,17; p < 0.001). Other risk factors related to violence included having a psychotic symptom and high STAXI score (AOR 5.42, 95% CI 1.81 to 16.23; p = 0.003), missed appointments with probation officer (AOR 1.99, 95% CI 1.03 to 3.84; p = 0.041), warning letter from probation officer (AOR 2.28, 95% CI 1.14 to 4.57; p = 0.020), being victim of violence or threats (AOR 9.07, 95% CI 4.34 to 18.96; p < 0.001), threats of victimisation (AOR 3.36, 95% CI 1.32 to 8.57; p = 0.011), other types of victimisation (AOR 5.86, 95% CI 2.18 to 15.79; p < 0.001), violent thoughts (AOR 4.08, 95% CI 1.86 to 8.95; p < 0.001), frequent violent thoughts (AOR 3.42, 95% CI 1.52 to 7.68; p = 0.003), thinking with different ways of hurting others (AOR 4.00, 95% CI 1.50 to 10.62; p = 0.005), thinking of hurting different victims (AOR 7.25, 95% CI 2.39 to 21.96; p < 0.001), likely to meet victim (AOR 4.66, 95% CI 1.44 to 15.01; p = 0.010), sometimes OK to break the law (AOR 2.53, 95% CI 1.26 to 5.06; p = 0.009) and attitudes toward crime total score (last quartile) (AOR 3.23, 95% CI 1.58 to 6.62; p = 0.001). Living with parents has a negative association with violence (AOR 0.47, 95% CI 0.23 to 0.98; p = 0.045).
High-risk group
A total of 34 risk factors were significantly related to violence within the high-risk group: getting behind with paying bills (AOR 3.95, 95% CI 1.37 to 11.40; p = 0.011), having a high level of coping difficulties (AOR 4.26, 95% CI 1.69 to 10.69), having a high stress score (AOR 3.74, 95% CI 1.46 to 9.59; p = 0.006), frequenting bars/pubs (AOR 2.51, 95% CI 1.01 to 6.26; p = 0.048), frequent betting (AOR 2.67, 95% CI 1.11 to 6.45; p = 0.029), disagreements at work (AOR 5.52, 95% CI 1.12 to 27.21; p = 0.036), suffering from anxiety disorder (AOR 3.19, 95% CI 1.22 to 8.30; p = 0.018), considering suicide (AOR 6.15, 95% CI 1.82 to 20.71; p = 0.003), suffering from paranoid delusions (AOR 3.75, 95% CI 1.66 to 8.51; p = 0.002), having strange experiences (AOR 3.17, 95% CI 1.34 to 7.52; p = 0.009), scoring highly on the PSQ and STAXI (AOR 5.81, 95% CI 1.36 to 24.84; p = 0.018), engaging in hazardous drinking (AOR 3.91, 95% CI 1.72 to 8.92; p = 0.001), having alcohol use disorder (AUDIT score of ≥ 16) (AOR 3.41, 95% CI 1.33 to 8.76; p = 0.011), any drug use (AOR 3.17, 95% CI 1.08 to 9.27; p = 0.035), use of cocaine powder (AOR 4.21, 95% CI 1.69 to 10.50; p = 0.002), use of crack cocaine (AOR 4.95, 95% CI 1.67 to 14.67; p = 0.004), ecstasy use (AOR 5.06, 95% CI 2.11 to 12.09; p < 0.001), any drug dependence (AOR 2.35, 95% CI 1.05 to 5.25; p = 0.037), being assaulted (AOR 18.38, 95% CI 3.72 to 90.93; p < 0.001), having a problem with a friend/family/neighbour (AOR 6.40, 95% CI 1.66 to 24.66; p = 0.007), having at least one life event (AOR 3.74, 95% CI 1.72 to 8.16; p = 0.001), becoming a victim of violence/threats (AOR 19.24, 95% CI 6.86 to 53.95; p < 0.001), being victimised with threats of violence (AOR 11.98, 95% CI 2.98 to 48.20; p < 0.001), having violent thoughts (AOR 4.57, 95% CI 1.75 to 11.92; p = 0.002), having violent thoughts at least twice a month (AOR 4.40, 95% CI 1.64 to 11.83; p = 0.003), having thoughts of harming others at least once a week (AOR 8.16, 95% CI 2.22 to 29.99; p = 0.002), thinking of different ways of hurting others (AOR 5.85, 95% CI 1.86 to 18.42; p = 0.003), thinking of different victims (AOR 5.57, 95% CI 1.78 to 17.41; p = 0.003), having a high likelihood of meeting a previous victim (AOR 6.32, 95% CI 1.20 to 33.26; p = 0.029), contacting the previous victim (AOR 4.73, 95% CI 1.49 to 14.97; p = 0.008), believing that it is OK to steal if very poor (AOR 2.64, 95% CI 1.18 to 5.87; p = 0.018) or from the rich (AOR 2.80, 95% CI 1.29 to 6.08; p = 0.009) and a high score on the criminal attitudes scale (AOR 2.32, 95% CI 1.06 to 5.10; p = 0.036). Suffering from hypomania (AOR 0.38, 95% CI 0.15 to 0.99; p = 0.047) was the only factor that showed a negative association with violence.
Table 149 summarises the number of important dynamic factors within each domain for each risk level.
Domain | Low risk | Medium risk | High risk |
---|---|---|---|
Accommodation | 1 | 3 | 0 |
Social environment | 1 | 1 | 0 |
Coping/daily living | 4 | 2 | 3 |
Leisure time | 0 | 0 | 2 |
Employment/education | 1 | 1 | 1 |
Depression/self-harm/anxiety | 1 | 3 | 2 |
Psychosis | 3 | 5 | 4 |
Alcohol use | 1 | 1 | 2 |
Drug use | 8 | 5 | 5 |
Life events | 2 | 1 | 3 |
Treatment | 1 | 0 | 0 |
Compliance with supervision | 0 | 2 | 0 |
Victimisation | 1 | 3 | 2 |
Thoughts of violence | 2 | 5 | 6 |
Thoughts of previous offending | 1 | 0 | 1 |
Attitudes to crime | 5 | 2 | 3 |
Discussion
In Section C we studied the effects of three static levels of risk (low, medium and high) on dynamic risk factors in a sample of patients discharged from medium secure hospital facilities. We found important risk modification effects. Dynamic factors showed different patterns of association according to different levels of static risk. Similar findings emerged from this study. However, the differentiation between levels of static risk was not as strong in this present study of released prisoners as in the study of discharged psychiatric patients.
The effects of several dynamic risk factors on associated violent behaviour was similar for each level of static risk, most notably psychotic symptoms including paranoid delusions, strange experiences and the presence of a psychotic symptom at the same time as a high level of anger measured using the STAXI. Similarly, hazardous drinking appeared to exert similar effects at each static level of risk, together with drug misuse, including any misuse of drugs, and specifically using cocaine. Being physically assaulted also appeared to be an important dynamic risk factor associated with violence for all three levels of static risk, together with thoughts of violence twice a month or more and thinking of harming different victims.
The dynamic variables in this study differed from those in Section C where we used structured assessments and where some items were composite measures. It is also possible that sample differences explained the differing levels of impact. However, in Section C we used a descriptive approach to demonstrate the effects of static risk and our presentation of these data in the present study is not directly comparable. Nevertheless, our findings in the present study suggest that certain risk factors among released prisoners have powerful effects on subsequent violence that are not modified by static risk factors.
Certain other dynamic factors appeared to operate differently and according to the level of static risk. For example, problems in the domains of accommodation and social environment did not appear to have an effect on prisoners with a high level of static risk. Prisoners rated as low static risk showed stronger associations with violence if they reported that their family and friends were unsupportive and if they had difficulties with coping and daily living, including borrowing money, general financial difficulties and difficulties with managing household finances. Problems with unemployment appeared to be associated with violence only in the low-risk group. Similarly, heroin and other opiate dependence appeared to be a dynamic risk factor among the low-risk group but not among the medium-risk or high-risk group and corresponded to injecting drugs in this group. These represent important findings that require further investigation because it would at first seem more probable that these risk factors would have a greater impact on released prisoners in the high-risk group, measured using static factors. This questions whether or not ‘threshold’ effects are possible, a situation in which dynamic variables are not to increase risk beyond a level, already explained by a high level of static risk.
Study 4: identification of differential associations between dynamic factors and violence according to psychiatric diagnosis
Objective
The objective of this study was to investigate the associations between dynamic factors and violent offending described in study 1 for subgroups defined by psychiatric morbidity and personality disorder.
Methods
Psychiatric diagnoses considered in this study included schizophrenia and delusional disorder, depressive disorder, drug dependence and alcohol dependence. Personality disorders included were ASPD, BPD and psychopathy (PCL-R score of ≥ 25).
As with studies 1 and 3 in this chapter, we used logistic regression models separately for each dynamic factor in each subgroup. The ORs were adjusted (AORs) for the same confounding variables described in studies 1 and 3 and the psychiatric diagnoses and personality disorders included in this study. The only exception was the psychopathy subgroup. Because it was not included in models for other psychiatric diagnoses, for the psychopathy subgroup analysis, ASPD and BPD were not included as confounding variables.
Results
Table 150 provides a summary of the numbers of significant dynamic factors overall and within each domain for each psychiatric diagnosis and personality disorder.
Domain | Schizophrenia (n = 95) | Depressive disorder (n = 289) | Drug dependence (n = 291) | Alcohol dependence (n = 166) | ASPD (n = 433) | BPD (n = 154) | Psychopathy (n = 120) |
---|---|---|---|---|---|---|---|
Accommodation | 1 | 0 | 1 | 1 | 0 | 1 | 2 |
Social environment | 1 | 1 | 2 | 0 | 0 | 2 | 0 |
Coping/daily living | 1 | 1 | 4 | 1 | 4 | 1 | 1 |
Leisure time | 0 | 1 | 0 | 1 | 1 | 0 | 0 |
Employment/education | 0 | 0 | 0 | 0 | 1 | 0 | 0 |
Depression/self-harm/anxiety | 3 | 0 | 1 | 0 | 2 | 1 | 1 |
Psychosis | 2 | 4 | 3 | 4 | 4 | 3 | 3 |
Alcohol use | 0 | 1 | 1 | 2 | 1 | 2 | 0 |
Drug use | 7 | 3 | 6 | 2 | 6 | 2 | 3 |
Life events | 0 | 2 | 3 | 3 | 2 | 1 | 1 |
Treatment | 0 | 0 | 1 | 0 | 0 | 0 | 0 |
Compliance with supervision | 0 | 0 | 0 | 0 | 0 | 0 | 1 |
Victimisation | 2 | 3 | 3 | 2 | 3 | 2 | 3 |
Thoughts of violence | 3 | 4 | 6 | 5 | 6 | 6 | 4 |
Thoughts of previous offending | 0 | 1 | 1 | 0 | 1 | 0 | 0 |
Attitudes to crime | 0 | 5 | 4 | 0 | 5 | 5 | 2 |
Total | 20 | 26 | 36 | 21 | 36 | 26 | 21 |
Schizophrenia
There were 95 cases in this subgroup. Out of these 95 cases, 92 had information on violent outcome. Thirty out of these 95 cases had either convictions for or self-reported violence within 1 year of their release from prison. The corresponding prevalence of violence was 32.6%.
A total of 20 factors (19 risk factors and one protective factor) were significantly related to violence among those experiencing schizophrenia. The risk factors were living in an unsafe area (AOR 102.85, 95% CI 3.20 to 3305.03; p = 0.009), having unsupportive family/friends (AOR 5.36, 95% CI 1.03 to 27.82; p = 0.046), having a high stress score (AOR 7.29, 95% CI 1.51 to 35.25; p = 0.013), experiencing tedium vitae (AOR 6.89, 95% CI 1.30 to 36.63; p = 0.023), considering suicide (AOR 6.49, 95% CI 1.11 to 38.09; p = 0.038), having a high level of anger (AOR 6.31, 95% CI 1.01 to 39.29; p = 0.048), experiencing paranoid delusions (AOR 40.49, 95% CI 2.84 to 577.69; p = 0.006) and strange experiences (AOR 7.30, 95% CI 1.29 to 41.45; p = 0.025), use of cocaine powder (AOR 14.74, 95% CI 2.20 to 98.82; p = 0.006), heroin use (AOR 9.82, 95% CI 1.15 to 83.91; p = 0.037), use of any opiates (AOR 10.64, 95% CI 1.35 to 83.61; p = 0.025), use of ecstasy (AOR 11.33, 95% CI 1.62 to 79.43; p = 0.015), dependence on heroin (AOR 10.88, 95% CI 1.22 to 97.07; p = 0.033) or opiates (AOR 11.70, 95% CI 1.43 to 95.67; p = 0.022), being a victim of theft/burglary (AOR 46.22, 95% CI 2.23 to 957.43; p = 0.013), being a victim of violence/threats (AOR 8.73, 95% CI 1.56 to 48.67; p = 0.013), having violent thoughts (AOR 9.15, 95% CI 1.32 to 63.61; p = 0.025), having violent thoughts at least twice a month (AOR 13.92, 95% CI 1.82 to 106.20; p = 0.011) and thinking of different ways of hurting others (AOR 30.09, 95% CI 2.63 to 343.88; p = 0.006). The use of sedatives (AOR 0.02, 95% CI 0.00 to 0.46; p = 0.015) was the only protective factor against violence for those experiencing major psychosis.
Depressive disorder
There were 289 individuals within the depressive disorder subgroup. Out of these 289 individuals, 283 had information on violent outcome. The prevalence of violence was 22.6% (64 out of 283 cases).
Twenty-seven risk factors for violence were identified for those suffering from depressive disorder: living with a partner (AOR 2.36, 95% CI 1.09 to 5.13; p = 0.029), having services cut off (AOR 3.90, 95% CI 1.09 to 13.98; p = 0.037), having coping difficulties (AOR 2.28, 95% CI 1.03 to 5.06; p = 0.043), frequenting bars/pubs (AOR 3.12, 95% CI 1.22 to 7.99; p = 0.018), scoring high for psychosis on the PSQ (AOR 3.93, 95% CI 1.19 to 13.03; p = 0.025), experiencing paranoid delusions (AOR 4.70, 95% CI 2.10 to 10.53; p < 0.001), having strange experiences (AOR 3.26, 95% CI 1.47 to 7.23; p = 0.004), experiencing a psychotic symptom (PSQ) and having a high STAXIS score (AOR 4.77, 95% CI 1.36 to 16.71; p = 0.015), hazardous drinking (AOR 4.09, 95% CI 1.93 to 8.69; p < 0.001), using any drugs (AOR 3.66, 95% CI 1.47 to 9.12; p = 0.005), cocaine powder use (AOR 3.61, 1.55 to 8.41; p = 0.003), ecstasy use (AOR 4.29, 95% CI 1.73 to 10.67; p = 0.002), being assaulted (AOR 5.70, 95% CI 2.08 to 15.57; p = 0.001), having at least one life event (AOR 2.72, 95% CI 1.32 to 5.62; p = 0.007), being a victim of violence/threats (AOR 16.13, 95% CI 6.56 to 39.62; p < 0.001), experiencing victimisation through threats (AOR 3.73, 95% CI 1.52 to 9.16; p = 0.004), being a victim of some other crime (AOR 3.60, 95% CI 1.38 to 9.39; p = 0.009), having thoughts of violence (AOR 2.74, 95% CI 1.13 to 6.63; p = 0.025), thinking of different ways to hurt others (AOR 3.22, 95% CI 1.10 to 9.42; p = 0.033), thinking of different victims (AOR 7.08, 95% CI 2.21 to 22.69; p = 0.001), having a high likelihood of meeting a former victim (AOR 9.46, 95% CI 2.12 to 42.17; p = 0.003), contacting the previous victim (AOR 4.53, 95% CI 1.32 to 15.53; p = 0.016) and all items in the attitudes to crime domain.
Drug dependence
Drug dependence was identified in 291 cases. Out of these 291 cases, 285 had information on violent outcome. In this subgroup of 285 cases, 82 cases (28.8%) were violent within a year of release from prison.
Thirty-five risk factors and one protective factor were significantly related to violence among the drug-dependent group. The risk factors were being evicted (AOR 2.76, 95% CI 1.17 to 6.55; p = 0.021), living with a partner (AOR 2.14, 95% CI 1.13 to 4.07; p = 0.020), difficulties with daily living (AOR 2.09, 95% CI 1.08 to 4.06; p = 0.030), borrowing money (AOR 2.03, 95% CI 1.04 to 3.94; p = 0.037), a high level of coping difficulties (AOR 2.08, 95% CI 1.02 to 4.27; p = 0.045) and stress (AOR 2.25, 95% CI 1.20 to 4.23; p = 0.012), considering suicide (AOR 2.15, 95% CI 1.03 to 4.50; p = 0.042), experiencing paranoid delusions (AOR 3.74, 95% CI 1.93 to 7.23; p < 0.001), scoring highly on the PSQ plus STAXI (AOR 6.76, 95% CI 2.08 to 21.99; p = 0.002), scoring highly on the PSQ plus non-compliance with therapy (AOR 3.59, 95% CI 1.06 to 12.14; p = 0.040), hazardous drinking (AOR 2.26, 95% CI 1.20 to 4.24; p = 0.011), the use of any drugs (AOR 7.43, 95% CI 2.11 to 26.09; p = 0.002), cocaine powder use (AOR 2.65, 95% CI 1.37 to 5.13; p = 0.004), ecstasy use (AOR 3.46, 95% CI 1.73 to 6.92; p < 0.001), dependence on any drugs (AOR 2.76, 95% CI 1.43 to 5.35; p = 0.003), cannabis dependence (AOR 2.63, 95% CI 1.24 to 5.59; p = 0.012), ecstasy dependence (AOR 8.70, 95% CI 2.09 to 36.13; p = 0.003), being assaulted (AOR 14.74, 95% CI 5.15 to 42.22; p < 0.001), being made redundant/sacked (AOR 3.41, 95% CI 1.31 to 8.91; p = 0.012), having at least one life event (AOR 2.27, 95% CI 1.23 to 4.19; p = 0.009), not attending treatment for a mental disorder (AOR 2.59, 95% CI 1.08 to 6.23; p = 0.033), being a victim of violence/threats (AOR 18.21, 95% CI 7.97 to 41.61; p < 0.001), experiencing victimisation from threats (AOR 5.36, 95% CI 2.17 to 13.23; p < 0.001) and being a victim of some other crime (AOR 4.12, 95% CI 1.58 to 10.74; p = 0.004), having violent thoughts (AOR 3.37, 95% CI 1.63 to 6.96; p = 0.001), having violent thoughts at least twice a month (AOR 3.27, 95% CI 1.54 to 6.96; p = 0.002), having thoughts of harming others more than once a week (AOR 4.27, 95% CI 1.59 to 11.43; p = 0.004), thinking of different ways of hurting others (AOR 3.71, 95% CI 1.52 to 9.06; p = 0.004), thinking of different victims (AOR 5.11, 95% CI 1.87 to 13.93; p = 0.001), having a high likelihood of meeting the victim (AOR 4.16, 95% CI 1.27 to 13.64; p = 0.019), contacting the previous victim (AOR 3.90, 95% CI 1.40 to 10.88; p = 0.009), believing that it is OK to steal from the rich (AOR 3.05, 95% CI 1.59 to 5.86; p = 0.001) and from shops that make a lot of money (AOR 3.02, 95% CI 1.56 to 5.87; p = 0.001), believing that it is sometimes OK to break the law (AOR 1.92, 95% CI 1.03 to 3.59; p = 0.041) and having a high score on the criminal attitudes scale (AOR 2.84, 95% CI 1.48 to 5.45; p = 0.002). Living with one’s parents (AOR 0.45, 95% CI 0.22 to 0.91; p = 0.026) was the only protective factor against violence within this subgroup.
Alcohol dependence
The subgroup of alcohol-dependent released prisoners included 166 individuals. One hundred and sixty-one of these had information on violent outcome. Out of these 161 cases, 40 (24.8%) were violent within a year of release from prison.
For the alcohol-dependent group, 20 risk factors and one protective factor were significantly related to future violence. The risk factors were frequent address changes (AOR 6.98, 95% CI 1.59 to 30.62; p = 0.010), a high level of coping difficulties (AOR 3.38, 95% CI 1.02 to 11.18; p = 0.046), frequenting bars/pubs (AOR 4.30, 95% CI 1.26 to 14.75; p = 0.020), experiencing paranoid delusions (AOR 5.09, 95% CI 1.78 to 14.57; p = 0.002), having strange experiences (AOR 3.70, 95% CI 1.24 to 11.05; p = 0.019), scoring highly on the PSQ plus STAXI (AOR 4.63, 95% CI 1.01 to 21.23; p = 0.049), hazardous drinking (AOR 5.21, 95% CI 1.64 to 16.57; p = 0.005) and alcohol use disorder (AOR 2.75, 95% CI 1.01 to 7.50; p = 0.049), use of cocaine powder (AOR 4.07, 95% CI 1.28 to 12.89; p = 0.017) and ecstasy (AOR 4.13, 95% CI 1.40 to 12.14; p = 0.010), being assaulted (AOR 8.67, 95% CI 1.88 to 39.98; p = 0.006), being made redundant/sacked (AOR 41.16, 95% CI 2.45 to 692.01; p = 0.010) and having at least one life event (AOR 2.63, 95% CI 1.02 to 6.78; p = 0.046), being a victim of violence/threats (AOR 7.38, 95% CI 2.54 to 21.42; p < 0.001) and being a victim of some other crime (AOR 5.60, 95% CI 1.55 to 20.24; p = 0.009), having violent thoughts (AOR 3.66, 95% CI 1.29 to 10.39; p = 0.015), having violent thoughts at least twice a month (AOR 3.08, 95% CI 1.07 to 8.86; p = 0.037), thinking of different ways to hurt others (AOR 5.02, 95% CI 1.33 to 18.94; p = 0.017), thinking of different victims (AOR 9.54, 95% CI 2.26 to 40.22; p = 0.002) and having a high likelihood of meeting a victim (AOR 9.22, 95% CI 2.01 to 42.34; p = 0.004). Scoring ≥ 3 on the PSQ (AOR 0.01, 95% CI 0.00 to 0.31; p = 0.010) was the only protective factor against violence for this subgroup.
Antisocial personality disorder
There were 433 released prisoners with ASPD. Out of these 433 cases, 422 had information on violent outcome. From these 422, 122 (28.9%) were violent within 1 year of release from prison.
A total of 36 risk factors were relevant to the prediction of future violence for those classified as having ASPD: borrowing money (AOR 2.24, 95% CI 1.30 to 3.84; p = 0.004), having financial difficulties (AOR 2.10, 95% CI 1.19 to 3.71; p = 0.011), having a high level of coping difficulties (AOR 2.73, 95% CI 1.49 to 4.99; p = 0.001) and stress (AOR 1.84, 95% CI 1.08 to 3.13; p = 0.026), frequenting bars/pubs (AOR 2.61, 95% CI 1.40 to 4.85; p = 0.002), having disagreements at work (AOR 3.49, 95% CI 1.50 to 8.12; p = 0.004), suffering from anxiety disorder (AOR 1.95, 95% CI 1.13 to 3.37; p = 0.017), having a high level of anger (AOR 1.73, 95% CI 1.01 to 2.98; p = 0.046), scoring ≥ 2 for psychosis (AOR 2.33, 95% CI 1.03 to 5.26; p = 0.042), experiencing paranoid delusions (AOR 3.63, 95% CI 2.13 to 6.19; p < 0.001), having strange experiences (AOR 2.75, 95% CI 1.61 to 4.69; p < 0.001), scoring high on the PSQ plus STAXI (AOR 4.91, 95% CI 1.97 to 12.22; p = 0.001), hazardous drinking (AOR 3.23, 95% CI 1.90 to 5.47; p < 0.001), using any drugs (AOR 4.65, 95% CI 2.20 to 9.84; p < 0.001), cannabis use (AOR 2.14, 95% CI 1.22 to 3.76; p = 0.008), cocaine powder use (AOR 3.25, 95% CI 1.81 to 5.82; p < 0.001), ecstasy use (AOR 4.81, 95% CI 2.62 to 8.84; p < 0.001), dependence on any drugs (AOR 1.85, 95% CI 1.05 to 3.25; p = 0.033) and ecstasy (AOR 13.89, 95% CI 2.80 to 68.97; p = 0.001), being assaulted (AOR 8.63, 95% CI 3.85 to 19.38; p < 0.001) and having at least one life event (AOR 1.94, 95% CI 1.18 to 3.20; p = 0.009), being a victim of violence/threats (AOR 11.52, 95% CI 6.23 to 21.32; p < 0.001), experiencing victimisation from threats (AOR 3.45, 95% CI 1.64 to 7.28; p = 0.001), being a victim of some other crime (AOR 3.71, 95% CI 1.74 to 7.88; p = 0.001), having violent thoughts (AOR 3.37, 95% CI 1.87 to 6.06; p < 0.001), having violent thoughts at least twice a month (AOR 3.23, 95% CI 1.76 to 5.93; p < 0.001), having thoughts of harming others at least once a week (AOR 3.34, 95% CI 1.57 to 7.12; p = 0.002), thinking of different ways of hurting others (AOR 3.19, 95% CI 1.58 to 6.46; p = 0.001), having different victims (AOR 4.60, 95% CI 2.15 to 9.82; p < 0.001), having a high likelihood of meeting a previous victim (AOR 4.79, 95% CI 1.93 to 11.84; p = 0.001), contacting the previous victim (AOR 3.80, 95% CI 1.72 to 8.39; p = 0.001), believing that it is OK to steal if very poor (AOR 2.09, 95% CI 1.24 to 3.52; p = 0.006), from the rich (AOR 2.90, 95% CI 1.73 to 4.87; p < 0.001) and from shops that make lots of money (AOR 3.03, 95% CI 1.78 to 5.15; p < 0.001), believing that it is sometimes OK to break the law (AOR 2.58, 95% CI 1.51 to 4.39; p < 0.001) and having a high score on the criminal attitudes scale (AOR 3.54, 95% CI 2.07 to 6.06; p < 0.001).
Borderline personality disorder
Among 154 patients with BPD, 148 had information on violent outcome. From these 148 cases, 49 (33.1%) were violent within 1 year of release from prison.
Twenty-five risk factors and one protective factor were associated with violence in the BPD subgroup. The 20 risk factors were frequent address changes (AOR 8.52, 95% CI 1.82 to 39.96; p = 0.007), living with a partner (AOR 3.34, 95% CI 1.18 to 9.44; p = 0.023) and having unsupportive family and friends (AOR 3.34, 95% CI 1.23 to 9.08; p = 0.018), having a high level of coping difficulties (AOR 3.66, 95% CI 1.23 to 10.88; p = 0.020), anxiety disorder (AOR 2.72, 95% CI 1.10 to 6.71; p = 0.030), experiencing paranoid delusions (AOR 4.85, 95% CI 1.72 to 13.67; p = 0.003), having strange experiences (AOR 5.17, 95% CI 1.95 to 13.72; p = 0.001), scoring highly on the PSQ plus STAXI (AOR 8.18, 95% CI 2.15 to 31.06; p = 0.002), having a high level of hazardous drinking (AOR 4.94, 95% CI 1.84 to 13.25; p = 0.001) and alcohol use disorder (AOR 4.25, 95% CI 1.37 to 13.25; p = 0.013), use of ecstasy (AOR 7.38, 95% CI 2.02 to 26.98; p = 0.003), having problems with a friend/family/neighbour (AOR 5.23, 95% CI 1.26 to 21.67; p = 0.022), being a victim of violence/threats (AOR 11.76, 95% CI 3.70 to 37.36; p < 0.001) and a victim of some other crime (AOR 18.51, 95% CI 3.49 to 98.23; p = 0.001), having violent thoughts (AOR 6.03, 95% CI 2.21 to 16.48; p < 0.001), having these thoughts at least twice a month (AOR 4.96, 95% CI 1.81 to 13.58; p = 0.002), having thoughts of harm for more than a week (AOR 3.86, 95% CI 1.19 to 12.49; p = 0.024), thinking of different ways of hurting others (AOR 5.55, 95% CI 1.75 to 17.61; p = 0.004), thinking of different victims (AOR 8.13, 95% CI 2.45 to 27.05; p = 0.001), having a high likelihood of meeting a previous victim (AOR 12.79, 95% CI 2.44 to 67.13; p = 0.003) and all items in the attitudes to crime domain. Tranquilliser dependence (AOR 0.03, 95% CI 0.00 to 0.71; p = 0.029) was the only protective factor against violence for those diagnosed with BPD.
Psychopathy
A total of 120 individuals in our sample had a PLC-R score of at least 25 and were identified as psychopaths. One hundred and sixteen had information on violent outcome. Of these 116 cases, 35 (30.2%) were violent within 1 year of release from prison.
For men and women who scored ≥ 25 on the PCL-R, 19 risk factors and two protective factors were predictive of violence. The risk factors were frequent address changes (AOR 10.88, 95% CI 1.04 to 114.28; p = 0.047), having a high stress score (AOR 5.90, 95% CI 1.79 to 19.50; p = 0.004), anxiety disorder (AOR 4.38, 95% CI 1.31 to 14.73; p = 0.017), experiencing paranoid delusions (AOR 3.76, 95% CI 1.12 to 12.64; p = 0.032), scoring highly on the PSQ plus STAXI (AOR 7.29, 95% CI 1.18 to 45.09; p = 0.033), having major psychosis and non-compliance with therapeutic treatment (AOR 44.42, 95% CI 1.54 to 1283.07; p = 0.027), using any drugs (AOR 5.99, 95% CI 1.23 to 29.11; p = 0.027) and ecstasy (AOR 4.26, 95% CI 1.15 to 15.77; p = 0.030), being assaulted (AOR 7.33, 95% CI 1.71 to 31.49; p = 0.007), receiving a warning letter from the probation officer (AOR 4.36, 95% CI 1.24 to 15.37; p = 0.022), being a victim of theft/burglary (AOR 48.18, 95% CI 3.67 to 633.01; p = 0.003), being a victim of violence/threats (AOR 11.42, 95% CI 2.93 to 44.48; p < 0.001), being a victim of some other crime (AOR 12.31, 95% CI 2.30 to 65.94; p = 0.003), having violent thoughts (AOR 7.00, 95% CI 2.08 to 23.56; p = 0.002) and having them at least twice a month (AOR 4.57, 95% CI 1.37 to 15.19; p = 0.013), thinking of different ways of hurting others (AOR 5.17, 95% CI 1.21 to 22.06; p = 0.027) and having a high likelihood of meeting a previous victim (AOR 25.86, 95% CI 1.87 to 357.66; p = 0.015), believing that it is OK to steal from shops that make lots of money (AOR 4.38, 95% CI 1.29 to 14.80; p = 0.018) and having a high score on the criminal attitudes scale (AOR 3.99, 95% CI 1.19 to 13.33; p = 0.025). Owning their own accommodation (AOR 0.11, 95% CI 0.03 to 0.45; p = 0.002) and methadone use (AOR 0.06, 95% CI 0.00 to 0.94; p = 0.045) were protective against violence among psychopaths.
Discussion
Categorisation into diagnostic groupings proved less discriminating in terms of effect modification of violence than had been expected. We controlled for each diagnostic category in our analyses so that the outcomes were independent of each other. However, there appeared to be considerable similarity in the risk factors across each diagnostic group. Psychotic symptoms, such as paranoid delusions, and associations with anger were seen in several diagnostic groups and were not restricted to those with schizophrenia. This corresponds to our findings in Section A that paranoid delusions are common in the general population and are associated with violence. Nevertheless, paranoid delusions among those with schizophrenia showed the highest odds of association. We have previously found that prisoners with a diagnosis of schizophrenia in the PCS who do not receive treatment either in prison or following release are three times as likely to be violent after release. Furthermore, their violence is associated with the re-emergence of paranoid delusions after leaving prison. 263 This is likely to account for the substantial odds of association that we found between paranoid delusions and violence in the subsample with schizophrenia. However, in other categories, this corresponded to the importance of paranoid delusions/delusional beliefs occurring independently. We found paranoid delusions in association with conditions such as depressive disorder, drug and alcohol dependence, ASPD, BPD and psychopathy.
An association with all diagnostic groups of thoughts of and ruminations about violence, particularly when occurring frequently and when thinking of different ways of harming others, was unsurprising. One problem with these measures is that it is unclear whether these thoughts had been shaped by actual experiences following release into the community, for example being assaulted or threatened with assault, and as a result the offender was considering violent retaliation, or whether they were part of a desire for retaliation because of delusional beliefs of persecution. Actual violent victimisation, however, appeared to be a common risk factor across all diagnoses.
With regard to drug misuse and dependence, prisoners with schizophrenia appeared to have a stronger association with dependence on opiates than might have been expected. However, a high prevalence of drug and alcohol dependence has previously been observed in a representative sample of the prison population. Prisoners with schizophrenia also have higher levels of psychopathy and substance misuse than other prisoners and appear to be a highly unstable group with a high risk of reoffending (including violence) on release. 429 Our findings therefore suggest that a constellation of risk factors, including substance dependence, are important in increasing the risk of violence among released prisoners with schizophrenia. Among this group, difficulties from high levels of stress (which may relate to the re-emergence of symptoms), the perception that they live in an unsafe area, with a lack of support from family and friends, high levels of anger and thoughts of harming themselves are important risk factors for violence.
The pattern of risk factors for the depressive disorder group appeared generally to be similar to the pattern for other diagnostic categories. However, there was some suggestion that individual victim characteristics, possibly relating to violence towards partners, had influenced some of these findings. It would be necessary to investigate in greater depth the victims of this subgroup and specifically whether or not IPV was related to depressive disorder, as suggested in Section A.
A criminal attitude and resumption of drug misuse, particularly cocaine and ecstasy use, and becoming dependent on drugs again following release were important among those who had been diagnosed as drug dependent prior to imprisonment. Similarly, it was unsurprising that resumption of heavy drinking was a key risk factor among those who had previously been diagnosed with alcohol dependence before release.
Among those with ASPD, there appeared to be a greater number of risk factors than for other diagnostic groups. These included both similar factors to those associated with other diagnostic groups and particular problems with anxiety and anger, as well as having a criminal attitude. The importance of comorbid anxiety in ASPD has been demonstrated in a community sample. 90
Borderline personality disorder was associated with violence in the context of frequent changes of address, having a partner but perceiving lack of support from family and friends, having a high level of coping difficulties and having anxiety disorder. Violence among these individuals also appeared to be influenced by psychotic symptoms, heavy drinking and threats or experiences of victimisation.
The risk factors that we found were associated with psychopathy did not discriminate this group from other diagnostic categories. It is possible that the PCL-R cut-off point of 25 was too low. This had been used to ensure adequate statistical power in investigating the properties of the preliminary version of the DRIV. We previously observed that there were fewer dynamic risk factors associated with psychopathy at a higher cut-off point. It will be important to reanalyse the data for psychopathy at a higher cut-off point in view of the poor performance of static instruments in association with psychopathy measured using a PCL-R level of ≥ 30 that we have previously observed and which is currently unexplained. 398
Chapter 19 External validation of a dynamic risk assessment instrument for violence
Background
In clinical practice, understanding change in dynamic risk factors is important for the assessment of intervention programme effectiveness and for identifying specific individual causal mechanisms. 424 In the previous chapter we introduced the DRIV, a new dynamic risk assessment instrument. The development of the DRIV was based on the need to improve risk management rather than improve risk prediction. Dynamic risk instruments are essential in risk assessment because dynamic risk factors are changeable and may be amenable to clinical intervention. Because it would require a new study of clinical interviews in a large number of offenders, we carried out an external validation using measures in a large data set comparing OASys measures that are routinely collected by probation officers. We established that our dynamic measures in the PCS represented many of the same or similar items in the OASys. We then carried out an external validation using OASys items as proxy measures for our dynamic items in the PCS.
Study 1: validation of the main effects of dynamic risk factors for violence (National Offender Management Service data set)
Objective
The objective of this study was to investigate whether dynamic risk factors identified from the PCS correspond to dynamic risk factors identified in a large data set (NOMS) of released prisoners with regard to their association with violence.
Methods
Two data sets were used in this study: the PCS and a sample obtained from NOMS. The PCS sample consists of 1717 offenders who were required to meet the following criteria: (1) serving a prison sentence of ≥ 2 years for a violent or sexual principal offence; (2) at least 18 years old; and (3) had 1 year left of their sentence to serve. The PCS sample was interviewed in two phases: the first-phase interview was 6–12 months before their release and the second-phase interview occurred about an average of 22.9 months after their release.
The NOMS sample consisted of 53,800 prisoners. These individuals were randomly selected general prisoners who were released from prison for the first time between 2005 and 2007. Information regarding the sample was obtained from records held in the OASys until 31 December 2012. Because of multiple OASys assessments, only the first assessment was used to create the study’s variables.
Preliminary investigation had previously identified that 42 items in the NOMS data set corresponded to those items contained in the PCS. Table 151 shows the 42 identified proxy and PCS items. These 42 items consisted of 32 measures in six domains. It should be noted that only 32 of the risk factors were truly ‘dynamic’; the remaining 10 risk factors either (1) had an item that was identified in phase 1 of the PCS or (2) had a NOMS item that specified an event prior to being taken into custody. Because these items referred to events prior to the assessments, it was decided that they should be excluded as they did not correspond conceptually to their item counterparts. Furthermore, some of these risk factors were represented by more than one item, for example accommodation status had four individual items representing it (permanent independent housing, bail/probation hostel, supported housing and no fixed abode). When risk factors were represented by more than one item, these items were analysed separately. Forty-three corresponding items (for 32 dynamic measures mentioned earlier) were finally considered dynamic (after excluding the non-dynamic items).
Comparable items | Definition of variable | |
---|---|---|
NOMS | PCS | |
Accommodation | ||
1. Accommodation status | Permanent independent housing | Own house or flat/a house rented from the council/a house or flat rented privately |
Bail/probation hostel | A shelter or hostel | |
Supported housing | A room or bedsit | |
No fixed abode | No fixed address/sleeping rough | |
2. Cohabitation | Parent | Living with my parents |
Relative | Living with other members of my family | |
Friend | Living with friends | |
Partner | Living with my husband/wife or partner | |
Alone | Living alone | |
3. Transient accommodation | Currently of no fixed abode or in transient accommodation | And looking at this list what best describes the type of place you are living in at the moment? (1) A hospital, (2) no fixed address, (3) sleeping rough/on the streets |
4. Unsuitability of accommodation | Quality of residence, appropriateness of living arrangements, overcrowding, relationships with rest of household | Thinking about where you are living at the moment, how satisfied are you with it? (very satisfied to dissatisfied) |
5. Unsuitability of location of accommodation | Local level of criminal activity, access to criminal contacts | Area has strangers moving in and out/causing trouble |
Employment and finances | ||
6. Employment status | Unemployed | Unemployed and looking/not looking for work |
Full-time employed or self-employed/part-time employed or self-employed/temporary or casual work | Working full-time or part-time/self-employed | |
7. Main source of income | Wages (self/partner/other) | Earnings or wages from job (full-/part-time) |
State benefits | Unemployment benefit or income support | |
No income source | No earnings from job/no disability allowance/no unemployment benefit or income support | |
8. Bad financial situation | Lack of financial stability, no regular source of income | Services cut off, borrowing money |
9. Poor financial management | Budgeting skills, provision to meet bills/essentials, savings, loans | Difficulty managing money |
10. Illegal earnings | Illegal earnings are a source of income | Earnings from illegal activities |
11. Severe impediment as a result of budgeting | Impossible demands or strained financial situation as a result of external factors | Difficulty with budgeting and paying bills |
Relationships | ||
12. Current poor relationship with family | Relationships with parents, siblings, grandparents and any other family members/step-family members with whom he or she has regular contact | Perceives friends/family as unsupportive/uncaring |
13. Current poor relationship with partner | Level of support, mutual respect/affection, strength of relationship . . . | Separation and divorce |
14. Partner has criminal record | Current partner has criminal record | Some family have criminal records |
15. Evidence of domestic violence | Includes threats and psychological abuse | In a relationship and threatened violence |
16. Poor community integration | Attachments to inviduals or community groups. Organised activities not linked to offending | And have you belonged to any of the groups, clubs or organisations on this list since you left prison? |
17. Easily influenced by criminal associates | Are most offences committed with others? Spend large amount of time with other offenders | Read some statements about your family and friends. This time I would like you to tell me whether you agree or disagree with each statement: (1) some of my family or friends have criminal convictions, (2) offered me drugs since release, (3) asked me to get money or goods through crime since release, (4) suggested we commit crime together since release, (5) got me into a fight with other people since release |
Drugs and alcohol | ||
18. Drug use | Drugs ever misused (in custody or community) | Whether or not taken since release: (1) cannabis, (2) amphetamines, (3) cocaine/coke, (4) crack, (5) ecstasy, (6) heroin, (7) LSD, (8) methadone/physeptone, (9) tranquillisers, (10) glues/solvents/gas/aerosols |
19. Main drug used in last 6 months | Cannabis | Cannabis |
Heroin | Heroin | |
20. Current drug use | Current drug noted (class A drug) | Current drug use (class A) |
21. Level of main drug use | Level of use of main drug | (1) How many times have you used (specify drug) since leaving prison? (2) Since leaving prison, have you used every day for 2 weeks or more? |
22. No motivation to tackle drug misuse | Evidence of problems recognised; recognises or is motivated to reduce drug dependency | Whether tried to cut down on (1) cannabis, (2) amphetamines, (3) cocaine/coke, (4) crack, (5) ecstasy, (6) heroin, (7) LSD (lysergic acid diethylamide), (8) methadone/physeptone, (9) tranquillisers, (10) glues/solvents/gas/aerosols |
23. Drug use and obtaining drugs a major activity | Drug use and obtaining drugs a major activity | Dependency on (1) cannabis, (2) stimulants, (3) cocaine, (4) crack, (5) heroin, (6) volatile substances, (7) tranquillisers, (8) hallucinogens, (9) ecstasy, (10) methadone, (11) opiates |
24. No motivation to tackle alcohol misuse | Recognises or is motivated to reduce alcohol consumption and whether capable of change | Needed a drink first thing in the morning in last 3 months/drinking led to memory loss in last 3 months |
Mental well-being | ||
25. Difficulties coping | Evidence of emotional instability or emotional stress; whether becomes easily upset, feels low or anxious | Stress in past month: (1) upset because of something that happened unexpectedly, (2) felt unable to control important things in life, (3) felt nervous or stressed, successfully dealt with irritating hassles of everyday life, (4) effectively coping with important changes occurring in your life, (5) confident in ability to handle personal problems, (6) felt things were going your way, (7) could not cope with all the things that you had to do, (8) could control irritations in your life, (9) felt on top of things, (10) made angry by things that had no control over, (11) thinking about the things that you have to get done, (12) been able to control the way you spend your time, (13) had so many difficulties that you would not be able to overcome them |
26. Current psychological problems | Psychological dysfunction or symptoms diagnosed by GP | Whether seen as an outpatient/day patient for mental health problems |
27. Social isolation | Have social networks outside of family and friends that they interact with on a daily basis | Not counting people you live with, how often have you done any of the following things since release? (1) Seen friends, (2) spoken to neighbours, (3) spoken to friends on the phone |
28. Recurrent suicidal or self-mutilating behaviour | Self-harm, attempted suicide, suicidal thoughts or feelings | (1) Deliberately harm self, (2) whether thought about taking own life since release, (3) whether made an attempt to take own life since release |
29. Current psychiatric problems | Psychiatric illness or symptoms diagnosed by GP | (1) Prescribed medication for mental health problems since release, (2) prescribed injections for mental health problems since release |
Personality and attitudes | ||
30. Poor problem-solving skills | Have insight into areas of life that are problematic | (1) Whether appropriate for male to hit partner, (2) whether appropriate for female to hit partner |
31. Pro-criminal attitudes | Express attitudes supportive of criminal behaviour in general? | (1) Whether OK to steal if very poor, (2) whether OK to steal from the rich, (3) whether OK to steal from shops that make lots of money, (4) whether OK to break the law |
32. Negative attitude towards supervision | Past experience of supervision; view supervision favourably? | Probation officer understanding |
Items that merit further investigation | ||
33. Negative attitude to education and training | Attitude towards education, feelings about schools, motivation to attend education courses | Did you get any educational qualifications? |
34. Main drug used before custody | Heroin | Heroin |
Cannabis | Cannabis | |
Crack | Crack | |
35. Ever injected drugs | Ever injected drugs | Whether ever injected drugs since release |
36. Violent behaviour related to drugs | Any official record or self-report of violent behaviour when under the influence of drugs | Pattern of offences related to periods of drug abuse/dependence |
37. Violent behaviour related to alcohol misuse | Official record or self-report of violent behaviour when drinking | Pattern of offences related to periods of alcohol abuse/dependence |
38. Poor ability to recognise problems | Insight into areas in life that are problematic | Lacks insight |
39. Problems in achieving goals | Illogical? Employs inappropriate strategies; fails to set goals in all areas of life | Lacks realistic goals |
40. Does not understand other people’s views | Unable to interpret social situations or form acceptable relationships with authority | Callous; lacks remorse; parasitic lifestyle |
41. Poor concrete/abstract thinking | Rigid dogmatic views or has difficulty in thinking in general terms | Plans lack feasibility |
42. Does not understand motivation | How well does offender recognise which of their attitudes, beliefs, emotions . . .? | Lacks insight |
The methods and strategy of analysis for this study were as follows. First, a subsample of the PCS (n = 574), who also had assessments from the OASys, was used to examine how well the PCS dynamic items corresponded with the OASys items. Many of these items, however, were not inherently dichotomous. Because the outcome measure was binary and a measure of effect size (which corresponded to the presence or absence of an item) was prioritised, all explanatory items were dichotomised to obtain an OR. For the OASys items belonging to the NOMS data set, most of the items were coded on a scale of 0–2 (‘not a problem’, ‘some problems’ and ‘significant problems’, respectively, with an item). The binary version recoded these items as 0, ‘no problems’ (initially coded as 0), and 1, ‘some problems’ to ‘significant problems’ (originally coded as 1 or 2). For the proxy items from the PCS, variables that were measured on a scale were dichotomised into the top 25% (problematic; coded as 1) and the remainder (coded as 0).
Next, the NOMS data set (n = 53,800) was combined with the PCS sample (n = 1004 from phase 2) according to their corresponding dynamic risk factors. The combined sample totalled 54,804 individuals. The purpose of this combined data set was to (1) examine the strength of the relationship between each item and violence and (2) identify whether or not the proxy items were similar to the PCS items in their association with violence. With this data set, the main effects between the dynamic risk factor and violence (committed within 1 year) were examined. The outcome of violence in this study was any violent criminal conviction within 12 months of release.
Results
Table 152 shows the level of similarity between the corresponding PCS and NOMS items as measured using kappa coefficients and ORs. The first column of results displays the kappa coefficient, a statistical test of reliability. 430 The purpose of this analysis was to establish the inter-rater agreement between the items. The primary disadvantage of using the kappa coefficient in 2 × 2 tables, however, is that it is misleading; its maximum possible value may be < 1 if the row and column totals are different. This is a common problem because it is difficult to predict the base rate and match the selection ratio to it. 431 Therefore, in column 3, using the relative improvement over chance (RIOC; according to Farrington and Loeber,432 the RIOC equation is as follows: corrected κ = κ/maximum κ). 431 According to Landis and Koch,433 a kappa effect size of 0.40–0.59 is considered moderate; 0.60–0.79 is considered substantial; and ≥ 0.80 is considered outstanding. With the corrected kappas, 18 of the 43 dynamic risk factors433 had at least moderate effect size. Out of these 18 factors, three had substantial effect size [numbers 2 (parent), 34 (heroin) and 35].
PCS and NOMS items | κ | Max. κ | Corrected κ | Effect size | OR | 95% CI |
---|---|---|---|---|---|---|
Accommodation | ||||||
1. Accommodation status | ||||||
Independent housing | 0.385 | 0.936 | 0.41 | * | 5.16*** | 3.30 to 8.07 |
Hostel | 0.514 | 0.896 | 0.57 | * | 16.29*** | 8.97 to 29.55 |
Supported housing | –0.032 | 0.811 | –0.04 | 0.53 | 0.07 to 4.08 | |
No fixed abode | 0.238 | 0.752 | 0.32 | 13.03*** | 3.44 to 49.40 | |
2. Cohabitation | ||||||
Parent | 0.568 | 0.865 | 0.66 | ** | 18.17*** | 10.70 to 30.85 |
Relative | 0.366 | 0.637 | 0.57 | * | 17.33*** | 7.44 to 40.38 |
Friend | 0.086 | 0.661 | 0.13 | 2.96 | 0.93 to 9.49 | |
Partner | 0.43 | 0.933 | 0.46 | * | 7.43*** | 4.71 to 11.73 |
Alone | 0.285 | 0.868 | 0.33 | 4.00*** | 2.51 to 6.37 | |
3. Transient accommodation | 0.119 | 0.379 | 0.31 | 4.74*** | 1.91 to 11.78 | |
4. Unsuitability of accommodation | 0.136 | 0.763 | 0.18 | 1.95** | 1.29 to 2.93 | |
5. Unsuitability of location of accommodation | 0.128 | 0.759 | 0.17 | 1.85** | 1.18 to 2.88 | |
Employment and finances | ||||||
6. Employment status | ||||||
Unemployed | 0.218 | 0.763 | 0.29 | 2.60*** | 1.73 to 3.91 | |
Employed | 0.393 | 0.815 | 0.48 | * | 7.61*** | 4.52 to 12.82 |
7. Main source of income | ||||||
Wage | 0.334 | 0.741 | 0.45 | * | 5.14*** | 3.23 to 8.18 |
Benefits | 0.319 | 0.808 | 0.39 | 4.33*** | 2.79 to 6.72 | |
No income | 0.046 | 0.644 | 0.07 | 1.96 | 0.54 to 7.07 | |
8. Bad financial situation | 0.102 | 0.491 | 0.21 | 1.97** | 1.25 to 3.11 | |
9. Poor financial management | 0.106 | 0.839 | 0.13 | 1.55* | 1.09 to 2.22 | |
10. Illegal earnings | 0.264 | 0.572 | 0.46 | * | 7.45*** | 3.95 to 14.05 |
11. Severe impediment to budgeting | 0.068 | 0.97 | 0.07 | 1.37 | 0.90 to 2.08 | |
Relationships | ||||||
12. Current poor relationship with family | 0.17 | 0.829 | 0.21 | 2.38*** | 1.52 to 3.71 | |
13. Current poor relationship with partner | 0.054 | 0.569 | 0.09 | 1.82 | 0.79 to 4.22 | |
14. Partner has criminal record | 0.005 | 0.185 | 0.03 | 1.13 | 0.54 to 2.38 | |
15. Evidence of domestic violence | 0.113 | 0.455 | 0.25 | 3.22* | 1.29 to 8.08 | |
16. Poor community integration | 0.035 | 0.601 | 0.06 | 1.61* | 1.10 to 2.35 | |
17. Easily influenced by criminal associates | 0.051 | 0.247 | 0.21 | 2.28* | 1.17 to 4.45 | |
Drugs and alcohol | ||||||
18. Drug use | 0.439 | 0.767 | 0.57 | * | 9.14*** | 5.84 to 14.32 |
19. Main drug used in last 6 months | ||||||
Cannabis | 0.33 | 0.797 | 0.41 | * | 4.27** | 1.43 to 12.76 |
Heroin | 0.355 | 0.762 | 0.47 | * | 5.83** | 1.66 to 20.52 |
20. Current drug use | 0.176 | 0.441 | 0.40 | * | 5.95*** | 2.98 to 11.86 |
21. Level of main drug use | 0.112 | 0.496 | 0.23 | 2.27** | 1.35 to 3.80 | |
22. No motivation to tackle drug misuse | 0.179 | 0.902 | 0.20 | 2.39*** | 1.57 to 3.64 | |
23. Drug use and obtaining drugs a major activity | 0.29 | 0.907 | 0.32 | 3.74*** | 2.51 to 5.56 | |
24. No motivation to tackle alcohol misuse | 0.1 | 0.286 | 0.35 | 9.03** | 2.40 to 33.99 | |
Mental well-being | ||||||
25. Difficulties coping | 0.061 | 0.35 | 0.17 | 1.76* | 1.07 to 2.87 | |
26. Current psychological problems | 0.171 | 0.393 | 0.44 | * | 8.25*** | 3.60 to 18.89 |
27. Social isolation | 0.117 | 0.89 | 0.13 | 1.67** | 1.15 to 2.42 | |
28. Recurrent suicidal or self-mutilating behaviour | 0.351 | 0.686 | 0.51 | * | 7.16*** | 3.11 to 16.50 |
29. Current psychiatric problems | 0.246 | 0.737 | 0.33 | 3.82*** | 2.41 to 6.06 | |
Personality and attitudes | ||||||
30. Poor problem-solving skills | 0.12 | 0.77 | 0.16 | 1.90** | 1.21 to 2.97 | |
31. Pro-criminal attitudes | 0.07 | 0.488 | 0.14 | 1.67* | 1.00 to 2.78 | |
32. Negative attitude towards supervision | 0.196 | 0.61 | 0.32 | 3.46*** | 2.09 to 5.71 | |
Items that merit further investigation | ||||||
33. Negative attitude to education and training | 0.098 | 0.7 | 0.14 | 1.98* | 1.10 to 3.56 | |
34. Main drug used before custody | ||||||
Heroin | 0.365 | 0.605 | 0.60 | ** | 11.36*** | 3.91 to 33.05 |
Cannabis | 0.002 | 0.346 | 0.01 | 1.03 | 0.19 to 5.51 | |
Crack | 0.002 | 0.708 | 0.00 | 1.02 | 0.12 to 8.72 | |
35. Ever injected drugs | 0.365 | 0.613 | 0.60 | ** | 14.88*** | 7.35 to 30.10 |
36. Violent behaviour related to drugs | 0.418 | 0.726 | 0.58 | * | 7.96*** | 5.10 to 12.44 |
37. Violent behaviour related to alcohol misuse | 0.523 | 0.975 | 0.54 | * | 10.68*** | 7.00 to 16.29 |
38. Poor ability to recognise problems | 0.083 | 0.85 | 0.10 | 1.55 | 1.00 to 2.41 | |
39. Problems in achieving goals | 0.226 | 0.897 | 0.25 | 3.49*** | 2.1 to 5.66 | |
40. Does not understand other people’s views | 0.163 | 0.793 | 0.21 | 2.36*** | 1.51 to 3.67 | |
41. Poor concrete/abstract thinking | 0.121 | 0.957 | 0.13 | 2.05** | 1.23 to 3.43 | |
42. Does not understand motivation | 0.017 | 0.744 | 0.02 | 1.11 | 0.67 to 1.83 |
In addition, ORs and their CIs are provided in the final two columns of Table 152. ORs give an indication of the strength of the relationship between the corresponding PCS and NOMS items. In contrast to the kappa coefficients, all corresponding items were highly related to each other, with the exception of supportive home environment, living with friends, having no income, severe problem with budgeting, current relationship with partner and partner having a criminal record.
Table 153 summarises number of the significant main effects of items for the PCS and NOMS. The relationship between each corresponding item and violent convictions within 1 year was examined separately by sample. Compared with the NOMS sample, the PCS had fewer significant corresponding items associated with violent convictions. The difference in number of significant items between the two data sets might be partly explained by the very large sample size for the NOMS. Seven items that were significant for both the PCS and the NOMS were no income (PCS OR 2.52, 95% CI 1.31 to 4.86, p = 0.006; NOMS OR 1.21, 95% CI 1.08 to 1.35, p = 0.001), poor financial management (PCS OR 2.01, 95% CI 1.16 to 3.49, p = 0.013; NOMS OR 1.32, 95% CI 1.25 to 1.40, p < 0.001), easily influenced by criminal associates (PCS OR 2.39, 95% CI 1.35 to 4.21, p = 0.003; NOMS OR 1.17, 95% CI 1.09 to 1.25, p < 0.001), main drug used (PCS OR 3.97, 95% CI 1.85 to 8.52, p < 0.001; NOMS OR 1.43, 95% CI 1.34 to 1.54, p < 0.001), drug use and obtaining drugs a major activity (PCS OR 2.18, 95% CI 1.38 to 3.44, p = 0.001; NOMS OR 0.94, 95% CI 0.88 to 0.99, p = 0.024), poor problem-solving skills (PCS OR 3.35, 95% CI 1.49 to 7.51, p = 0.003; NOMS OR 1.45, 95% CI 1.37 to 1.54, p < 0.001) and pro-criminal attitudes (PCS OR 3.23, 95% CI 1.82 to 5.75, p < 0.001; NOMS OR 1.36, 95% CI 1.25 to 1.48, p < 0.001).
Domain | Total number of items | PCS (n = 1717) | NOMS (n = 53,800) |
---|---|---|---|
Accommodation | 12 | 0 | 10 |
Employment and finances | 9 | 2 | 9 |
Relationships | 6 | 2 | 7 |
Drugs and alcohol | 8 | 0 | 5 |
Mental well-being | 5 | 0 | 5 |
Personality and attitudes | 3 | 3 | 4 |
Total | 43 | 7 | 40 |
Several factors were found to be protective for the NOMS sample in terms of having negative associations with violent reoffending: presently living at home (OR 0.83, 95% CI 0.78 to 0.88; p < 0.001) or in a hostel (OR 0.55, 95% CI 0.45 to 0.67; p < 0.001), living with either a parent (OR 0.89, 95% CI 0.84 to 0.95; p < 0.001), a relative (OR 0.88, 95% CI 0.81 to 0.95; p = 0.001), a partner (OR 0.64, 95% CI 0.60 to 0.69; p < 0.001) or alone (OR 0.91, 95% CI 0.85 to 0.98, p = 0.015), being employed (OR 0.78, 95% CI 0.72 to 0.84; p < 0.001), having a wage (OR 0.92, 95% CI 0.86 to 0.99; p = 0.022), living off illegal earnings (OR 0.75, 95% CI 0.70. to 0.80; p < 0.001), using heroin in the past 6 months (OR 0.85, 95% CI 0.79 to 0.92; p < 0.001) and obtaining drugs a major activity (OR 0.94, 95% CI 0.88 to 0.99; p = 0.024). Unlike the NOMS sample, no significant protective factors were identified in the PCS sample.
After examining the main effects, interactions were finally included in a model that included both corresponding dynamic items from both data sets. For example, the PCS item and NOMS item for independent housing and an interaction term [independent housing × sample type (e.g. PCS or NOMS)] was inserted into a model. The aim of the interaction term was to determine whether or not a specific dynamic risk factor was significantly different in one sample from the other. If the interaction term was non-significant, this meant that the individual items that represent the dynamic risk factor were similar across the two samples. The analysis revealed that 33 out of the 43 interaction effects were non-significant, meaning that the individual items were similar between the two groups. Factors that were different from each other and which therefore had a significant interaction term were cohabiting with a relative (p = 0.036) or partner (p = 0.042), main source of income benefits (p = 0.002), no income (p = 0.029), illegal earnings (p = 0.040), drug use (p = 0.009), drug use and obtaining drugs a major activity (p < 0.001), social isolation (p = 0.022) and having a pro-criminal attitude (p = 0.004)
Discussion
We validated the dynamic risk factors that we had previously identified in the PCS data set by using proxy dynamic factors from the OASys in the NOMS data set. Initially, 32 items were selected as probable corresponding dynamic risk factors. These were selected because they showed a moderate level of similarity between items and across samples. The similarity between items was demonstrated by the kappa coefficients and the ORs, whereas the similarity across samples was supported by the interaction terms. Although the proxy items identified in the external validation sample (NOMS) were similar to the items in the internal validation sample (PCS; according to the ORs), not all of the relationships between 1-year violence and the dynamic risk factors were similar across the samples. However, most translated across the samples.
Protective factors were also identified; however, some of these factors, although they were protective against 1-year violence, were not conducive to pro-social skills in an offender and well-being. For example, it would be inappropriate to encourage released prisoners to obtain illegal earnings and use heroin for the sake of decreasing their risk for violence. These findings probably indicated that living off illegal earnings and heroin use were risk factors for other types of offending behaviour and that released prisoners who engaged in these particular criminal activities during the follow-up period were less likely to be violent. However, these possibilities cannot be confirmed from the analysis carried out in this study.
The inconsistency between the two samples in terms of significant dynamic risk factors may be attributable to the characteristics of the internal validation sample. Compared with the external validation sample, the internal validation sample had low power. This may explain why the majority of the associations found between the dynamic risk factors and 1-year violence were significant in the external sample. Because the PCS also contained items found in the OASys, it was thought that the NOMS data set was the best available sample to validate on. Informed by the interaction terms, these dynamic risk factors seem promising and the next study will conduct further external validation.
Study 2: second external validation of interactions between static and dynamic risk factors for violence
Objectives
The primary aim of this study was to externally validate the 32 dynamic risk factors using static risk levels. The specific objectives were to:
-
investigate the level of agreement between the PCS and the NOMS dynamic risk items
-
investigate the strength of the relationship between each dynamic risk factor and violence
-
investigate whether or not the NOMS items were similar to the corresponding PCS items in their associations with violence.
Methods
The present study used similar methods to those in the previous study. The data sets included phase 2 of the PCS (n = 991) (only 991 of 1004 respondents in the PCS phase 2 were included in this particular study because they had complete information for the PIV measure) and the NOMS (n = 52,808 because of listwise deletion). The outcome measure for this study was violent convictions within 1 year obtained from PNC. The samples, which were combined to form one data set, were then stratified according to risk level. The total sample included 53,799 individuals.
To determine risk level, the PIV (see Chapter 17, Study 1) was used to separate the combined sample at three cut-off points: 0 = low risk (PCS n = 547; NOMS n = 25,007; total n = 25,554), 1 = medium risk (PCS n = 166; NOMS n = 11,466; total n = 11,632) and 2 = high risk (PCS n = 278; NOMS n = 16,335; total n = 16,613).
The main effects for each sample (PCS or NOMS) were examined within each risk level. Subsequently, an interaction term was included into a model with both the main effects (dynamic risk factor for PCS and NOMS) to determine whether or not the relationship between the risk factor and violence was significantly different between the samples.
Results
Table 154 summarises the numbers of significant main effects and non-significant interaction effects by domain and risk level.
Domain | Total number of items | Low risk | Medium risk | High risk | ||||||
---|---|---|---|---|---|---|---|---|---|---|
PCS | NOMS | Non-significant interactions | PCS | NOMS | Non-significant interactions | PCS | NOMS | Non-significant interactions | ||
Accommodation | 12 | 1 | 9 | 6 | 1 | 4 | 9 | 0 | 5 | 11 |
Employment and finances | 9 | 2 | 8 | 7 | 0 | 3 | 9 | 1 | 3 | 7 |
Relationships | 6 | 1 | 4 | 5 | 0 | 4 | 6 | 0 | 4 | 6 |
Drugs and alcohol | 7 | 3 | 4 | 2 | 0 | 6 | 5 | 0 | 6 | 4 |
Mental well-being | 5 | 0 | 5 | 5 | 0 | 5 | 4 | 0 | 5 | 5 |
Personality and attitudes | 3 | 0 | 2 | 3 | 0 | 1 | 3 | 1 | 2 | 2 |
Total | 42 | 7 | 32 | 28 | 1 | 23 | 36 | 2 | 25 | 35 |
Low-risk group
Because of stratification, there was less power in the PCS and some main effects were not estimated. For example, within cohabitation, the main effects for living with a relative and living alone were absent. Within the PCS, a total of seven factors only were significantly related to violence: current drug use (OR 13.76, 95% CI 1.76 to 101.36; p < 0.001) followed by evidence of domestic violence (OR 13.16, 95% CI 2.24 to 77.15; p < 0.01), illegal earnings (OR 10.81, 95% CI 2.67 to 43.77; p < 0.001), poor financial management (OR 10.00, 95% CI 2.17 to 46.13; p < 0.01), drugs a major activity (OR 6.48, 95% CI 2.45 to 17.16; p < 0.001), unsuitability of accommodation (OR 3.85, 95% CI 1.22 to 12.18; p < 0.01) and lack of motivation to tackle drug misuse (OR 3.28, 95% CI 1.04 to 10.30; p < 0.05). Drugs as a major activity was a significant risk factor for the PCS sample but not for the NOMS sample. Illegal earnings was a risk factor for violence in the PCS sample but it was a significant protective factor in the NOMS sample (PCS OR 10.81 vs. NOMS OR 0.63; p < 0.001).
Many of the dynamic risk factors were significantly associated with violence within the NOMS sample. Thirty-two of the 43 dynamic items were salient. Within the accommodation domain, 9 out of 12 items were significant and four of these were protective factors. Within this domain the strongest risk factor was the unsuitability of accommodation (OR 1.52, 95% CI 1.36 to 1.70; p < 0.001) and the strongest protective factor was living with a relative (OR 0.81, 95% CI 0.69 to 0.95; p = 0.001). Eight of the nine dynamic factors within the employment and finances domain were significantly related to violence. This was in contrast to the PCS sample in which only two of the nine dynamic factors were significantly related to violence. Two of these dynamic factors were protective: being employed (OR 0.81, 95% CI 0.70 to 0.94; p = 0.004) and having illegal earnings (OR 0.63, 95% CI 0.54 to 0.74; p < 0.001). The two strongest risk factors within the employment and finances domain were being on benefits (OR 2.04, 95% CI 1.83 to 2.28; p < 0.001) and having no income (OR 1.46, 95% CI 1.19 to 1.80; p < 0.001).
Within the relationships domain, four of the six dynamic factors were significant and they were all risk factors: evidence of domestic violence (OR 2.10, 95% CI 1.88 to 2.36; p < 0.001), poor current relationship with partner (OR 2.07, 95% CI 1.82 to 2.35; p < 0.001), poor current relationship with family (OR 1.78, 95% CI 1.57 to 2.02; p < 0.001) and poor community integration (OR 1.45, 95% CI 1.29 to 1.64; p < 0.001). Four out of eight items were significant in the drugs and alcohol domain and served as risk factors. The strongest risk factor was using cannabis in the last 6 months (OR 2.01, 95% CI 1.71 to 2.36; p < 0.001). For mental well-being, all dynamic factors were significant, with having difficulties with coping being the strongest risk factor (OR 2.08, 95% CI 1.84 to 2.35; p < 0.001). Finally, two of the three factors within the personality and attitudes domain were salient, with negative attitudes towards supervision having the strongest association (OR 1.59, 95% CI 1.37 to 1.85; p < 0.001).
After including the interaction terms, 28 of the 43 factors were similar across the samples. Specifically, six of the factors were similar within the accommodation domain, seven were similar within the employment and finances domain, five were similar within the relationships domain, two were similar within the drugs and alcohol domain, five were similar within the mental well-being domain and three were similar within the personality and attitudes domain.
Medium-risk group
For the medium-risk group, the PCS sample had only one risk factor: living with a relative (OR 4.29, 95% CI 1.02 to 18.12; p = 0.048). In contrast, living with a relative served as protective factor in the NOMS sample (OR 0.74, 95% CI 0.62 to 0.87; p < 0.01). The interaction term for this model was significant, meaning that the relationship between living with a relative and violence was significantly different between the samples.
For the NOMS sample, 23 items were significantly related to violence, of which nine were protective and 14 were risk factors. Within the accommodation domain, unsuitability of accommodation was a risk factor (OR 1.19, 95% CI 1.05 to 1.34; p = 0.005), whereas the strongest protective factor was living with a parent (OR 0.81, 95% CI 0.72 to 0.92; p = 0.001). Nine of these factors were similar across samples and, because of low power in the PCS, two interaction terms were unavailable.
Two risk factors and one protective factor were identified within the employment and finances domain. Being unemployed (OR 1.17, 95% CI 1.03 to 1.34; p = 0.018) and on benefits (OR 1.43, 95% CI 1.27 to 1.60; p < 0.001) predicted violence within 1 year, whereas living on illegal earnings was protective against violence (OR 0.60, 95% CI 0.51 to 0.69; p < 0.001). Although there were significant relationships for NOMS, all of the interactions were non-significant, meaning that these items did not vary across samples.
For relationships, the strongest risk factor for the medium-risk group was a poor current relationship with a partner (OR 1.56, 95% CI 1.35 to 1.80; p < 0.001). Being easily influenced by criminal associates, however, was protective for violent convictions (OR 0.84, 95% CI 0.73 to 0.97; p = 0.015). The interactions for each of these factors across samples were non-significant.
In the drugs and alcohol domain, four protective factors were significantly related to violence. The strongest association was with current drug use (OR 0.84, 95% CI 0.73 to 0.97; p = 0.017). Two risk factors within this domain significantly predicted violence: cannabis use within the last 6 months (OR 1.56, 95% CI 1.34 to 1.81; p < 0.001) and poor motivation to tackle alcohol misuse (OR 1.99, 95% CI 1.77 to 2.23; p < 0.001). Five of the eight factors were similar across samples, as indicated by their non-significant p-values.
All five dynamic factors within mental well-being were significant and were risk factors. The strongest risk factor was having difficulties with coping (OR 1.55, 95% CI 1.35 to 1.78; p < 0.001). The interaction terms for four of these factors were non-significant. The fifth factor estimate was not obtained because of low power in the PCS. For the personality and attitudes domain, problem-solving was the only salient risk factor (OR 1.19, 95% CI 1.06 to 1.34; p = 0.003). However, all three factors were similar across the samples.
High-risk group
Two risk factors were the only salient factors found for the high-risk PCS sample. Having no income (OR 2.65, 95% CI 1.13 to 6.26; p = 0.026) and pro-criminal attitudes (OR 4.38, 95% CI 1.81 to 10.62; p = 0.001) predicted violence within 1 year.
The high-risk group in the NOMS sample were at risk for violence if they had transient accommodation (OR 1.10, 95% CI 1.01 to 1.19; p = 0.035), unsuitable accommodation (OR 1.15, 95% CI 1.06 to 1.25; p = 0.001) or an unsuitable location of accommodation (OR 1.12, 95% CI 1.03 to 1.22; p = 0.005), whereas they were protected from violence if they were living with their partner (OR 0.68, 95% CI 0.62 to 0.75; p < 0.001) or were living in a hostel (OR 0.53, 95% CI 0.39 to 0.73; p < 0.001). However, all interaction terms in the accommodation domain were non-significant with the exception of unsuitability of location of accommodation.
In the NOMS sample, if a high-risk released prisoner was unemployed (OR 1.13, 95% CI 1.03 to 1.25; p = 0.014) or on benefits (OR 1.35, 95% CI 1.25 to 1.47; p < 0.001), this increased his/her risk for violence. Illegal earnings, however, was a protective factor (OR 0.64, 95% CI 0.59 to 0.71; p < 0.001). Seven of the nine factors were similar across samples and this was exclusive of being on benefits.
In the relationships domain, a current poor relationship with family (OR 1.35, 95% CI 1.23 to 1.49; p < 0.001) or with a partner (OR 1.27, 95% CI 1.14 to 1.41; p < 0.001), evidence of domestic abuse (OR 1.29, 95% CI 1.18 to 1.40; p < 0.001) and poor community integration (OR 1.17, 95% CI 1.07 to 1.26; p < 0.001) also increased the risk for violence within this group. For the drugs and alcohol domain, a total of two risk factors and four protective factors were significant in this group. The strongest risk factor was lack of motivation to tackle alcohol abuse (OR 2.03, 95% CI 1.87 to 2.20; p < 0.001) and the strongest protective factor was a high level of main drug use (OR 0.85, 95% CI 0.79 to 0.92; p < 0.001).
All factors were significant within the mental well-being domain in the NOMS high-risk sample. Each of these factors served as a risk factor, with difficulties with coping being the strongest risk factor (OR 1.40, 95% CI 1.27 to 1.54; p < 0.001). For the personality and attitudes domain, risk factors in the NOMS high-risk group included poor problem-solving skills (OR 1.17, 95% CI 1.08 to 126; p < 0.001) and a negative attitude towards supervision (OR 1.10, 95% CI 1.00 to 1.20; p = 0.039). The remainder of the interaction terms were non-significant, with the exception of pro-criminal attitudes.
Discussion
Within each risk group classified according to low, medium or high static risk, the external validation showed that the majority of the NOMS items did not differ from the PCS items across the groups. However, for some items it was not possible to determine similarity across samples because the internal sample had low power following stratification into risk groups. In addition, few significant risk and protective factors were identified in the internal sample compared with the external sample. The primary limitation of this study was, therefore, the relatively small internal validation sample size.
Nevertheless, despite the small sample size for internal validation, both NOMS and PCS items performed similarly across the groups. Our study provides supporting evidence that items from the PCS are applicable for use in a different sample and for different risk groups.
Factors relevant to violence in the short term (convictions in the 12 months following release) varied between the groups, particularly within the external validation sample. The direction of these factors, however, remained the same in each risk group. For example, being on benefits was a risk factor for violence in each risk group. Although the factors varied in their level of association between groups, similar factors were identified. For all three risk groups, living with a partner and living on illegal earnings were protective of violence. Fourteen similar risk factors were identified across the risk groups: having unsuitable accommodation, being unemployed, being on benefits, having a current poor relationship with family, having a current poor relationship with a partner, evidence of domestic abuse, using cannabis in the last 6 months, having no motivation to tackle alcohol misuse, having difficulties with coping, having current psychological problems, having current psychiatric problems, social isolation, having recurrent suicidal thoughts and having poor problem-solving skills. A strong risk factor common to all groups was problems with mental well-being. Within this domain, all items were significantly predictive of short-term violence. We have previously demonstrated the importance of symptoms of psychosis and anxiety disorder in Section A, Chapter 3, Study 1.
For the medium- and high-risk groups, use of drugs was protective of violence. This highlights the fact that, despite the highly unfavourable nature of certain risk factors and their association with other types of offending behaviour, they may be protective for violence. However, it is important to put these factors into context. Preoccupation with drugs might be protective of violence because the individual is less likely to become violent when using drugs, for example when heavily intoxicated with opiates on a regular basis. On the other hand, some offenders may become involved in violent altercations in drug deals.
These findings indicate the importance of identifying dynamic risk factors that are associated with different offending outcomes, for example acquisitive crimes, drug offences, robbery and those offences specific to violence. However, the next stage of investigation following the confirmation that these risk factors can be used in different samples is to identify which factors are causal. Douglas and Skeem115 have suggested that interpersonal relationships, impulsivity, negative affect, particularly anger, antisocial attitudes, psychosis, treatment alliance and adherence and substance use problems are highly important to future investigation of causality, corresponding to our findings. However, we have additionally identified the importance of poor mental health as a key component for further investigation.
Section E Development of a multistage, multimodel system for risk assessment and management of offending behaviour using Bayesian networks
Chapter 20 Development of a Bayesian network for the risk management of violent prisoners
Background
Context
Contemporary violence risk assessment tools such as the HCR-209 or Violence Risk Scale (Wong S, Gordon A. The Violence Risk Scale. Saskatoon, SK: University of Saskatchewan; 1999–2003: unpublished manuscript) offer a structured alternative to a purely clinical assessment of an offender’s or a forensic mental patient’s level of risk of future violence. Functioning as they do on predictive efficacy (i.e. the power of the tool to predict future violent outcome based on an empirically derived base of risk-related items) they suffer from the dual restrictions of both not attending to causal pathways to violence434 and an inherent limitation or ‘ceiling’ in their predictive power with samples other than that used for the initial creation/validation. 8
Further, and more importantly in a climate where clinical time and expertise are at a premium to services, instruments intended for the assessment of violence risk do not necessarily contribute directly to the management of the risk they assess. They may do so in a broad sense, that is, indicating that some interventions may be more beneficial in reducing future violence for those in a high-risk group of offenders than those in a low-risk group (and vice versa). However, specific recommendations about management and – perhaps more importantly – a causal explanation for these recommendations cannot be inferred from an instrument designed primarily to guide risk assessment.
In this chapter we describe the underpinning theory, construction and initial validation of a prototype causally based decision support tool for the management of violence risk among released prisoners using Bayesian networks. 435 Chapter 21 applies a similar concept and methodology to the creation of a risk management tool for discharged forensic prisoners, who are likely to be at risk of violence but who have potentially different causal drivers for their violence from prisoners and hence require different management strategies. Chapter 22 then describes a clinical utility pilot performed with clinicians from different disciplines to gather initial opinions about the potential utility and applications of the approach adopted here in the management of offenders and forensic patients.
Bayesian networks
Bayesian networks, sometimes also called belief networks or causal probabilistic networks, are graphical models that encode the probabilistic relationships among variables of interest. The term ‘Bayesian’ comes from the reliance on Bayes’ theorem for calculating probabilities. The Bayes’ rule is nothing more than a simple equation that specifies how to calculate conditional probabilities. 436 They are also called networks because of the directed graph that each Bayesian network represents, with nodes representing variables (which can rely on data and/or expert judgements) and arcs representing causal/influential relationships between variables. Bayesian probability provides a way for rational real-world reasoning. It reflects knowledge, which can be personal or based on hard evidence, and any belief about uncertainty is assumed to be provisional on experience or data gained to date; this is what we call prior probability. Prior probability is then updated by new experience or data to provide a new disposition about the uncertainty; this is known as posterior probability.
However, probability is not only about numbers, but also about causal reasoning. 437 This is where networks become useful. One of the main reasons why Bayesian probability has gained in popularity is because Bayes’ theorem supports reasoning about causal propositions. 435
Bayesian networks may be used to identify relationships in the data. However, in this study, causal relationships between variables of interest (i.e. the causal network structure) are solely determined by clinical judgement. The Bayesian network is given this predetermined structure as an input for causal analysis.
Some important advantages of Bayesian networks over the standard statistical regression techniques used within this area of research are:
-
In a Bayesian network, inference propagates through a (causally) structured variable network (Figures 9 and 10), as opposed to typical linear regression techniques (Figure 11).
-
Inference can be performed from cause to effect, as in standard predictive models (e.g. inferring from association between a disease and a test result), but unlike other approaches inference can also be performed from effect to cause (e.g. measuring the risk of actually having the disease after having observed a positive test result).
-
This unique capability (i.e. inferring from effect to cause) provides radically improved decision support as it enables extensive ‘what if’ analysis. Figures 9 and 10 demonstrate how prior probabilities are updated to posterior probabilities after having observed evidence for three of the network variables, whereas Figure 12 demonstrates how each unobserved variable is ranked, in terms of sensitivity (or impact), to the specified target variable (in this case violence).
FIGURE 9.
A Bayesian network model with prior probabilities.

FIGURE 10.
A Bayesian network model with posterior probabilities after having observed three variables.

FIGURE 11.
A standard statistical regression model.

Causal inference allows for specific risk factors to be targeted for causal intervention for risk management of future reoffending and this makes the model useful in terms of answering complex clinical questions that are based on unobserved evidence. We assume that the interventions causally manipulate (rather than correlate with) specific risk factors. This is the standard assumption for interventions within a Bayesian network framework. Targeted risk factors for intervention have been identified on the basis of clinical judgement. As a result, when indicating an intervention in the Bayesian network, the model takes into consideration the current risk profile of an individual and simulates how the specified intervention is expected to affect the targeted risk factor. Furthermore, it accounts for how the targeted risk factor will further affect other variables within the network and which may be directly or indirectly linked to (and therefore influence) the risk of violence. Figure 12 demonstrates how violence risk management is performed by measuring the impact of different interventions for an individual case.
FIGURE 12.
Violence risk management with interventions. Relevant characteristics of the individual include anxiety, hallucinations, paranoid delusions, cannabis use and dependence, hazardous drinking and alcohol dependence.
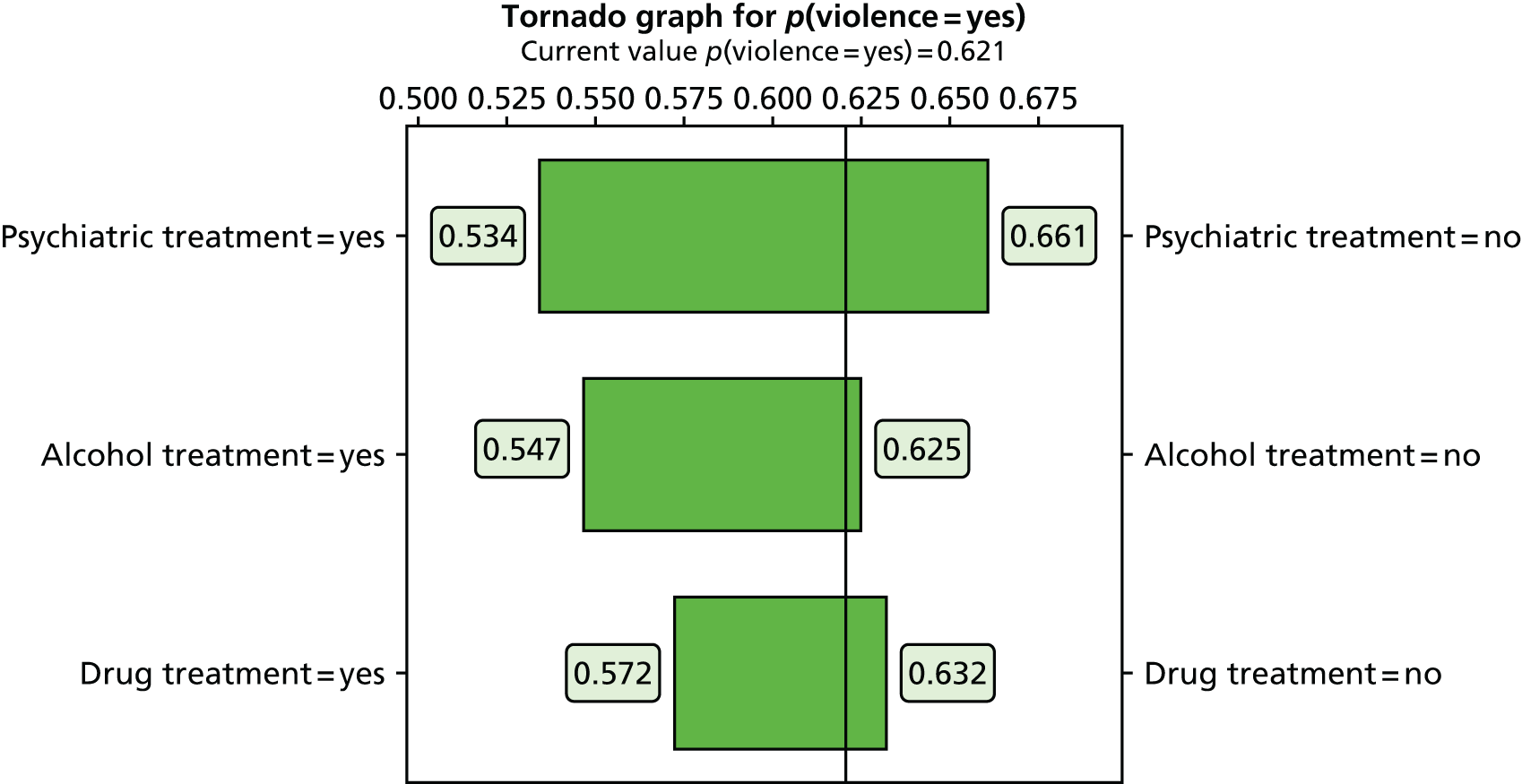
Although Bayesian probability has been around for a long time, it is only in the last decade or so that efficient algorithms and tools that use them have been developed to enable propagation of inference in Bayesian networks. 435 As a result, there has been a recent explosion of interest in Bayesian networks, which have now become powerful tools for decision support systems. Bayesian networks are increasingly recognised as a potentially powerful solution to complex risk assessment problems438,439 and have already been employed to model knowledge with success in many different fields such as computational biology and bioinformatics,440,441 engineering,442 computer science,443 artificial intelligence and machine learning,444,445 law,446 gaming, sports sciences and gambling,447,448 natural sciences,442 medicine449,450 and image processing. 451
Objectives
The objectives of this study were to:
-
outline a methodology for the development of a Bayesian network for the management of violence risk in released offenders
-
utilise this method to model violence risk for prisoners in a Bayesian network
-
further develop the network to incorporate interventions and outcomes
-
examine preliminary evidence for the predictive efficacy of the Bayesian network as a risk assessment measure
-
model potential risk management interventions, such as treatment programmes, within the Bayesian network and explore the evidence for their performance in risk reduction.
Methods
Sample
The sample used for the creation and learning of the Bayesian network was the PCS, described in detail in Section D. 292 The sample included 953 prisoners (778 men and 175 women) assessed after release into the community.
Network structure and specification
The method used for the creation of the Bayesian network for the management of violence risk was expert knowledge elicitation. 452 This method has additional advantages over, for example, data-based learning alone as it allows for greater efficiency of design and parameterisation and greater parsimony of model design.
To apply this method, initially, a review of the literature on risk factors for violence was conducted. A ‘domain expert’ in the field (MF) was paired with a decision scientist (AC) and the decision scientist elicited knowledge from the expert that would enable the construction of the Bayesian network using AgenaRisk software (AgenaRisk Professional version 6.1, Agena, Cambridge, UK). A second clinical expert (JC) was closely involved in the process and provided additional input into the structure of the network, reviewing it at key points in the development. Although it is possible for individual research papers within the literature to demonstrate conflicting evidence, the domain experts considered this information as a whole and attempted to reach a consensus regarding the structure of the network.
To ensure that the experts agreed on the structure of the model, as well as the variables considered for inference, elicitation of expert knowledge was performed over an extensive iterative process between the clinical experts and the decision scientist, whereby the structure of the network was subjectively validated by examining how observations of one node were influencing inferences of another node. The network was built in seven separate domains, each corresponding to an area that could have a potential plausible link to future violence in released prisoners. Each component was then realised individually in terms of nodal structures (subnetworks), which were then connected via causal relationships that might exist between networks (e.g. substance misuse influencing mental illness).
The seven components for which subnetworks were developed are listed below.
-
Criminal attitude This domain encompassed attitudes, dispositions and behaviours that may have positively influenced an individual’s involvement in general crime, which has been linked by a plausible causal process to a greater likelihood of a violent lifestyle because of the necessity of operating outside the scope of the law. 183,453
-
Personality disorder and psychopathy Several personality disorders, including ASPD and BPD, have been linked to violent behaviour and include traits that could be causal drivers of violence, such as impulsivity. 454
-
Socioeconomic and rehabilitation factors Low or unstable socioeconomic status has been associated with violent crime, but causally only in the case of acute stress (e.g. the stress of being made homeless) or in the context of a general ‘strain theory’, by which violence can be explained as the product of multiple overlapping stressors on an individual. From a preventative perspective, evidence shows that protective factors such as stable intimate relationships and appropriate supervised living circumstances for prisoners and patients nearing discharge are important factors in preventing violence. 19,348
-
Mental illness Other studies within this project have considered the ‘intricate’ link between mental illness and violence,112 but also the possibility of causal relationships between specific symptoms and short-term violent outcome (see Section B). 263,291 Therefore, our approach here was to model mental illness in terms of symptoms rather than diagnostic categories.
-
Substance misuse Substance misuse has been found to increase the risk of violence by up to four times in most populations, particularly in individuals suffering from existing mental illness. 383 The relationship between substance abuse and violence may be causative in the sense that some stimulants directly increase aggressive or violent behaviour through their psychopharmacological action;455 substance abuse or dependency stimulates acquisitive violence to fund addictive habits; or use of illegal substances implies involvement in social systems where violence is more likely. 456
-
Treatment response Poor adherence or response to treatment in individuals with severe mental illness is known as a risk factor for violence. 457 The treatment response component is represented by two factors: (1) the responsiveness to any given treatment and (2) the risk of refusing or failing to attend any given therapy.
-
Violence and static risk This component contained established static risk predictors featured in other models. They were not intended to be causal structures (although it is possible that age and sex have some complex causal connection to particular crimes), but rather to provide improved accuracy in situations in which limited information about the preceding six components was available to the clinician, that is, the network would always offer a risk assessment ‘as good as any other measure’.
This was an iterative process and so after an initial, a priori model specification, the network was revised and simplified to reflect causal associations between variables and with violence. Once a final specification had been established, the model was parameterised (i.e. theoretical variables in the causal structure were matched to existing variables within the learning data set) to pair models in the network with those in the PCS sample. After the initial model specification, a simplification process was applied to remove redundant nodes and clarify the structure. Similarly, the parameterisation process may have meant that some theoretical nodes could not be parameterised (e.g. aggression) and therefore served as definitional/synthetic or latent variables inferred from other nodes that could be parameterised to variables within the data set, which would require a further iteration of the model. This process is outlined in Figure 13.
FIGURE 13.
Iterative model design process for the Decision Support for Violence Management in Prisoners Bayesian network.

The full parameterisation of the model based on PCS variables is reproduced in Appendix 11.
Example of network specification and parameterisation
As an example of the network specification (design) and parameterisation process, we used the example of personality disorder. As described in Chapter 4, personality disorders show a complex link with violence, dependent on the nature of the disorder458 and comorbidity. 459 At its most simplistic, however, the relationship can be modelled as simple prediction, as shown in Figure 14, whereby one large latent variable (personality disorder) is related to violence in an unspecified fashion. This is the first step in the construction of the component and tells us little about causal structure or inference.
FIGURE 14.
Step 1: basic predictive link between personality disorder and violence.
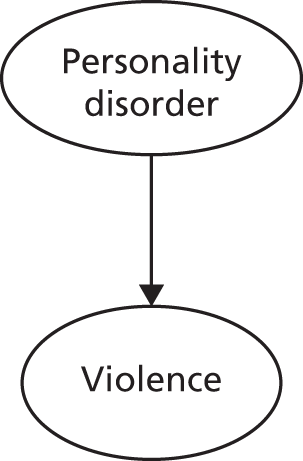
In the second iterative step (Figure 15), the component was iterated such that particular personality disorders with specific traits shown to have a link to violence (criminality, anger, impulsivity, aggressiveness), such as ASPD and BPD, are present in the model to elaborate the specific nature of the relationship between personality disorder and violence.
FIGURE 15.
Step 2: elaborated predictive link between personality disorder and violence including a trait-based structure.

In the third iteration (Figure 16), the model is further elaborated to include hypothesised causal drivers for the variables themselves, including childhoood antecedents of personality disorder and intersections with variables from other domains. Some variables were further differentiated at this point to increase the heuristic potential for the network, for example psychopathy was differentiated into ‘factor 1’ (callous interpersonal style and shallow affect) psychopathy, which has been causally related to poor treatment outcome460 because of deficient emotional learning abilities,454 and ‘factor 2’ (impulsivity and antisocial lifestyle) psychopathy, which is associated with ASPD. 461
FIGURE 16.
Step 3: causal structure for links between personality disorder and other network components, pre-parameterisation.
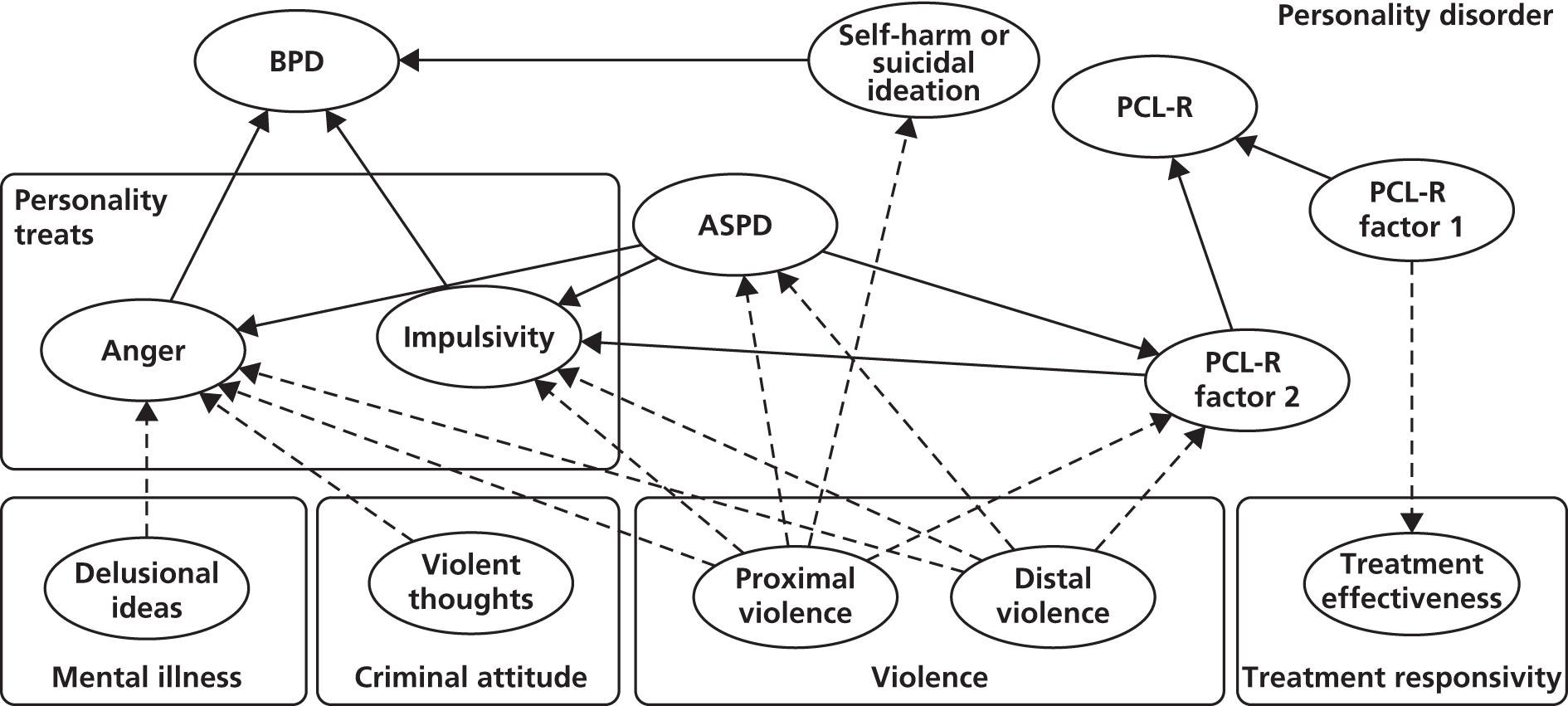
Finally, in a fourth step (Figure 17) the model was parameterised based on available data. When data were not available for a particular node (e.g. aggression, for which no direct measurement existed in the learning data set), it was replaced with a definitional/synthetic or latent concept indicated as a ‘danger level’. In the case of aggression, the aggression ‘danger level’ took information from both the personality disorder and the criminal attitude components and was measured based on the variables impulsivity (with parent nodes ASPD and BPD) and violent thoughts (with parent nodes anger post treatment and victimisation). Violent thoughts in this model were measured as present or absent whereas impulsivity was measured on an ordinal scale of 0–2 depending on severity. The model considers maximum risk to be when violent thoughts are present in conjunction with an impulsivity score of 2 and minimum risk to be when violent thoughts are absent in conjunction with an impulsivity score of 0.
FIGURE 17.
Step 4: simplified overall topography of the Decision Support for Violence Management in Prisoners Bayesian network.

Assessment of predictive validity for violence
Once the network had been constructed, parameterised and ‘learned’ with the PCS data set, its ability to accurately predict violence in the released prisoner data set was assessed using the AUC statistic of a ROC curve.
The performance of the Decision Support for Violence Management in Prisoners (DSVM-P) was then assessed relative to that of other commonly used risk assessments rated in the study sample, such as the HCR-20,287 VRAG407 and PCL-R140. Somer’s d statistic was used to calculate significant differences between AUC values.
Although a naturalistic study of the risk management (intervention efficacy) aspect of the DSVM-P was not within the remit of this project, we were able to perform a sensitivity analysis of the available data on the effects of interventions performed in prison on future commission of violence. These were presented as reductions in the percentage chance of reoffending following intervention, assuming varying degrees of compliance on the behalf of the offender.
Analysis was conducted using AgenaRisk 6.1, R version 3 for Windows (The R Foundation for Statistical Computing, Vienna, Austria) and Stata SE for Windows version 13.1.
Results
Network layout
A simplified topography of the overall design of the completed Bayesian network for violence, the DSVM-P, is given in Figure 17; the full version of this model is available in Constaninou et al. 462 The layout shows the interconnecting nodes for the causal/predictive model but excludes the detail of the modelling of treatment efficacy.
Efficacy as a violence risk assessment tool
Table 155 shows the performance of the DSVM-P in comparison to the performance of other risk assessment instruments when calculated using the PCS sample. The models in this table are based on a ’10-fold’ model of cross-validation whereby predictive accuracy for 10% of the cases in the sample is repeated for the other nine blocks of 10% of cases and then mean AUC values are compared.
Risk assessment instrument | AUC | 95% CI | p-value |
---|---|---|---|
DSVM-P | 0.790 | 0.755 to 0.822 | Reference |
VRAG | 0.717 | 0.682 to 0.753 | 0.004 |
PCL-R | 0.665 | 0.627 to 0.703 | < 0.001 |
HCR-20 | 0.665 | 0.626 to 0.704 | < 0.001 |
The results show that the DSVM-P outperformed the three alternative risk assessment tools within the PCS sample with a high degree of significance.
Evidence for the efficacy of interventions
We subsequently used the DSVM-P to model the expected reduction in the risk of violence for each intervention introduced: psychiatric treatment, anger management, drug misuse treatment and alcohol misuse treatment. This was carried out using sensitivity or ‘what if’ analysis modelling: the probability of violence occurring; the probability of violence occurring during the following 5 years (i.e. time at risk = 1825 days); observable active symptoms for the intervention under analysis; and observable inactive symptoms for the remaining three interventions (with all of the other model factors unknown).
Assuming no intervention (i.e. no treatment/therapy), the results show that psychotic symptoms generate a considerable higher risk for violence (i.e. 42.85%) then hazardous drinking, drug use and anger. When the offender undertakes an intervention (e.g. psychotropic medication), the results suggest little difference between partial responsiveness and full responsiveness to treatment over all four interventions and show that psychiatric treatment can be very effective, with a 42.88% relative reduction in the risk of violent reoffending, followed by alcohol treatment, with a relative risk reduction of 24.43%. However, drug treatment and anger management were less effective (when assuming observable active symptoms only for the intervention under analysis). However, results relating to anger management should be interpreted with caution because of the temporal unreliability of the ‘state’ model used to measure anger levels in sample participants (i.e. the STAXI – state scale427).
Conclusion
In this chapter we have presented the methodology for and preliminary validation of a prototype decision support measure for forensic clinical and criminal justice decision-makers using a Bayesian network. The network included seven components, each relating to a different domain of potential causes for violence, and was learned with an existing data set based on 953 released prisoners. The model showed good predictive efficacy of future violence, outperforming actuarial and SPJ risk assessment tools by 8–9%, which is suggestive of a conceptually valid network. Additionally, it was able to successfully model the impact of interventions conducted within the prisoner sample and vary these dependent on individual prisoners’ engagement and responsivity.
The key potential benefits of the DSVM-P Bayesian network are:
-
It allows for specific risk factors to be targeted for causal intervention for risk management of future reoffending. This makes the model useful in terms of answering complex clinical questions that are based on unobserved evidence.
-
It generates both binary (i.e. yes/no) and multinomial (i.e. expected number of violent convictions) predictive distributions for future violence. Thus, it is able to identify not only those at high risk of violence in the short term but also those at risk of prolific violence in the longer term.
-
It allows flexibility with model inputs because of the Bayesian network framework; it does not require complete information to make a useful prediction.
-
Inference within a Bayesian network propagates through a structured variable network so that inference can be performed from cause to effect as in standard predictive models but, unlike other approaches, can also be performed from effect to cause. This unique capability provides radically improved decision support as it enables the prediction of variables other than violence as the ‘outcome’ of the modelling process.
-
If required (i.e. in future studies or when the DSVM-P is learnt with a different data set), expert knowledge can be easily incorporated for factors that are important for prediction but which are not represented in a validation or ‘learning’ data set.
Limitations
This study had some limitations. Primarily, the DSVM-P was intended to be a decision support tool for professionals working with offenders, but its efficacy cannot be evaluated fully without, first, further development so that the software is usable outside of the AgenaRisk environment and, second, a subsequent trial with ‘live’ cases using a prospective study design, probably against a comparable risk assessment measure. Further suggestions in this regard are detailed in Chapter 23.
The validation study presented above also suffers from two significant restrictions. First, AUCs reported on the whole development sample are likely to be optimistic, especially as the model was optimised for the sample on which it was developed, in which case running the danger of overfitting the model. However, when performing a 10-fold cross-validation the AUC score decreased only slightly, which is not suggestive of model overfitting and suggests that the predictive accuracy of the DSVM-P is expected to be very good for other similar data samples.
Second, other ‘traditional’ risk assessment tools based on predictive models have shown promising validity with their initial validation sample only to find that their predictive efficacy drops when applied to other populations or even different samples from a similar population. 8 The predictive validity of the DSVM-P should therefore be tested with different samples and populations, and possibly iterated further, before it is deployed in ‘live’ settings.
Chapter 21 Development of a Bayesian network for risk management of patients discharged from forensic mental health services
Background
Having completed and successfully validated a Bayesian network for the management of violence risk among released prisoners, as detailed in Chapter 20, we replicated the process of network creation and specification to see whether the process could be duplicated for patients discharged from MSSs in the UK.
Objectives
The objectives of this component of the study were as follows:
-
to utilise the methodology specified in Chapter 20 to develop a Bayesian network suitable for the assessment and management of violence risk among patients discharged from forensic MSSs
-
to provide a preliminary validation of predictive efficacy of this Bayesian network as a risk assessment measure within a sample of discharged MSSs patients
-
to examine the most important drivers of violence risk in the sample.
Methods
Sample
The sample used for this study was the VoRAMSS sample described in greater detail in Section C. The sample used for parameterisation and learning of the network included 386 patients discharged from MSSs in the UK (343 men, 43 women) over a 12-month period. They were assessed at baseline using a battery of risk assessments including the HCR-20,287 SAPROF348 and PANSS357 and were then reassessed at 6 and 12 months following discharge.
Creation and specification of the network
The method used for the creation and specification of the network was procedurally identical to that adopted for the DSVM-P tool described in Chapter 20. There were four exceptions to this.
-
No specific intervention outcome data were available. It was therefore not possible to provide risk management decision support. The only significant intervention variable to be modelled was time in treatment, in this case time spent inside the secure service prior to discharge, observed as a potential protective factor in a meta-analysis by Coid et al. 367
-
The data set used to parameterise and learn the network was the VoRAMSS study sample (see Section C) in place of the PCS. This necessitated the removal of some nodes present in the DSVM-P and the inclusion of other new nodes, many of which were drawn from the risk assessments.
-
To account for the nature of the population, additional modelling work was carried out on symptoms of mental illness and emotion, specifically in terms of symptoms of psychosis derived from the PANSS.
-
As the study used for learning used a three-wave design, the learning and validation of the network proceeded in a two-stage process: first, the baseline risk data were used to learn the network and predict violence at 6 months post discharge and, second, data gathered at 6 months were used to predict violent outcome at 12 months.
As with the DSVM-P network, some nodes were definitional/synthetic or ‘latent’ rather than data based. This was carried out in particular because of the number of variables in the data set that appeared to link directly to violence. Figure 18 shows an example of this process in which there are potentially eight variables with causal risk factors for violence: drug usage (cannabis, cocaine, stimulants and hazardous alcohol use), aggressive attitudes (anger, hostility and lack of self-control) and violent ideation. Modelling these directly to violence would have resulted in (32 × 27) = 1152 possible combinations; this would have been problematic given the number of cases from which the data were to be learned (n = 386). Using three synthetic nodes (aggression, uncontrolled aggression and disinhibition) reduced this complexity to 24 combinations while retaining a plausible causal structure.
FIGURE 18.
Synthetic nodes (dashed circles) introduced to reduce dimensionality and improve definitional relationships.

Analysis of predictive efficacy
As in Chapter 20, ROC analysis was used to compare the predictive efficacy of the MSSs Bayesian network against the risk assessment measures included in the data set, previously published in Doyle et al. ,366 specifically the HCR-20,287 the PANSS357 and the SAPROF. 348 AUCs for the Bayesian network were calculated using LOOCV as in the DSVM-P and predictive accuracy was assessed both for (1) clinician-detected violence – incidents of violence recorded by the researcher from either file notes or clinician interview – and (2) violent convictions post discharge.
For comparative analysis with the SAPROF, which rates items that are protective of violence (negatively associated) rather than those that are predictive of violence, AUCs were calculated for the inverted total score, that is, adjusted score = 34 – total score.
Sensitivity analysis
Once the MSSs Bayesian network had been parameterised and learned using the VoRAMSS data, it was possible to conduct a sensitivity analysis of the network, involving a measurement of the uncertainty within a system or model attributable to particular inputs. 463 In this case, the analysis focused on which nodes proved the most sensitive in determining the key outcome, that is, violence after discharge in patients discharged from MSSs.
This analysis was particularly important in the context of a lack of intervention data as it meant that the network was able to identify major drivers of violence, if not make recommendations about the efficacy of potential treatments or other efforts to mitigate risk. A skilled clinician would still be able to infer what the major risk drivers were for an individual patient and, if they were amenable to change (i.e. dynamic rather than static), take steps to address them.
Results
Network layout
A simplified Bayesian network layout following parameterisation is depicted in Figure 19.
FIGURE 19.
Simplified topography of the Bayesian network for violence risk in discharged forensic mental health patients.
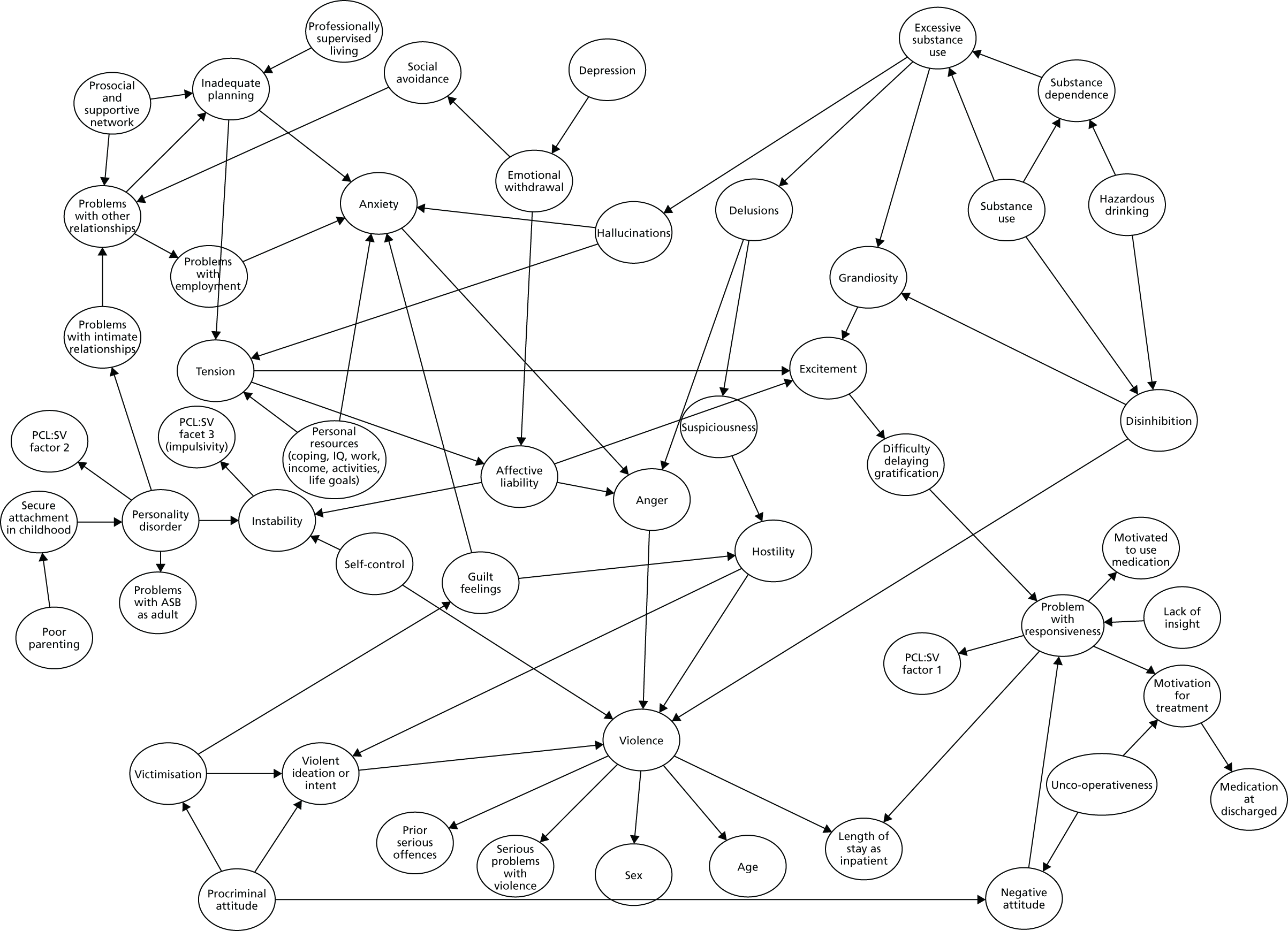
Predictive accuracy as a risk assessment tool
The predictive efficacy of the Bayesian network calculated using AUC values is shown in Tables 156 and 157. Table 156 shows the accuracy of the network at predicting violence as assessed by clinician interview and Table 157 shows the accuracy of the network at predicting reconviction.
Instrument | AUC (0–6 months) | 95% CI | p-value | AUC (0–12 months) | 95% CI | p-value |
---|---|---|---|---|---|---|
HCR-20v3 | 0.728 | 0.658 to 0.797 | 0.667 | 0.701 | 0.638 to 0.765 | 0.863 |
SAPROF | 0.764 | 0.705 to 0.823 | 0.169 | 0.692 | 0.631 to 0.753 | 0.700 |
PANSS total | 0.675 | 0.592 to 0.757 | 0.500 | 0.640 | 0.571 to 0.709 | 0.105 |
PANNS positive | 0.678 | 0.600 to 0.756 | 0.530 | 0.653 | 0.589 to 0.718 | 0.193 |
PANSS negative | 0.562 | 0.472 to 0.653 | 0.006 | 0.549 | 0.478 to 0.620 | 0.000 |
PANSS general | 0.676 | 0.598 to 0.754 | 0.500 | 0.628 | 0.560 to 0.695 | 0.065 |
PANSS aggression | 0.716 | 0.634 to 0.798 | 0.877 | 0.680 | 0.613 to 0.747 | 0.514 |
Bayesian network model | 0.691 | 0.619 to 0.764 | Reference | 0.708 | 0.656 to 0.761 | Reference |
Instrument | AUC (0–6 months) | 95% CI | p-value | AUC (0–12 months) | 95% CI | p-value |
---|---|---|---|---|---|---|
HCR-20v3 | 0.878 | 0.817 to 0.939 | 0.401 | 0.717 | 0.551 to 0.886 | 0.417 |
SAPROF | 0.814 | 0.704 to 0.923 | 0.548 | 0.830 | 0.693 to 0.968 | 0.682 |
PANSS total | 0.625 | 0.417 to 0.833 | 0.019 | 0.605 | 0.446 to 0.764 | 0.038 |
PANNS positive | 0.623 | 0.445 to 0.800 | 0.007 | 0.628 | 0.469 to 0.787 | 0.068 |
PANSS negative | 0.517 | 0.298 to 0.737 | 0.001 | 0.485 | 0.395 to 0.666 | 0.002 |
PANSS general | 0.613 | 0.426 to 0.801 | 0.007 | 0.615 | 0.458 to 0.772 | 0.048 |
PANSS aggression | 0.716 | 0.518 to 0.915 | 0.139 | 0.573 | 0.405 to 0.742 | 0.021 |
Bayesian network model | 0.845 | 0.784 to 0.907 | Reference | 0.797 | 0.710 to 0.884 | Reference |
When considering clinician-reported violence, the Bayesian network showed significantly improved predictive accuracy over the PANSS negative symptom scale at both 6 and 12 months but did not differ significantly from any other risk assessment outcome. However, for violent reconviction, the Bayesian network showed significantly better performance than all PANSS scales except aggression at 6 months and all PANSS scales except positive symptoms at 12 months. AUCs were higher for the Bayesian network than for the HCR-20 for both outcomes at 12 months but were lower at 6 months.
Overall, the Bayesian network model appeared to demonstrate moderate improvements in violence risk assessment compared with the established risk assessment models reported here when employed with the same data set.
Sensitivity analysis
Although an analysis of intervention effectiveness was not possible because of a lack of available modelling data, it was possible to perform a sensitivity analysis to identify which variables were most sensitive for the outcome (violent convictions). The results are presented in Figures 20 and 21, which display tornado plots representing the relative influences of nodes on the outcome.
FIGURE 20.
Sensitivity analysis for violent convictions between 0 and 6 months after release on the basis of nine specified sensitivity nodes. A, age; ANG, anger; G, gender; HST, hostility; LSI, length of stay as inpatient; PSO, previous serious offences; SC, self-control; SPV, serious problems with violence; VII, violent ideation or intent.
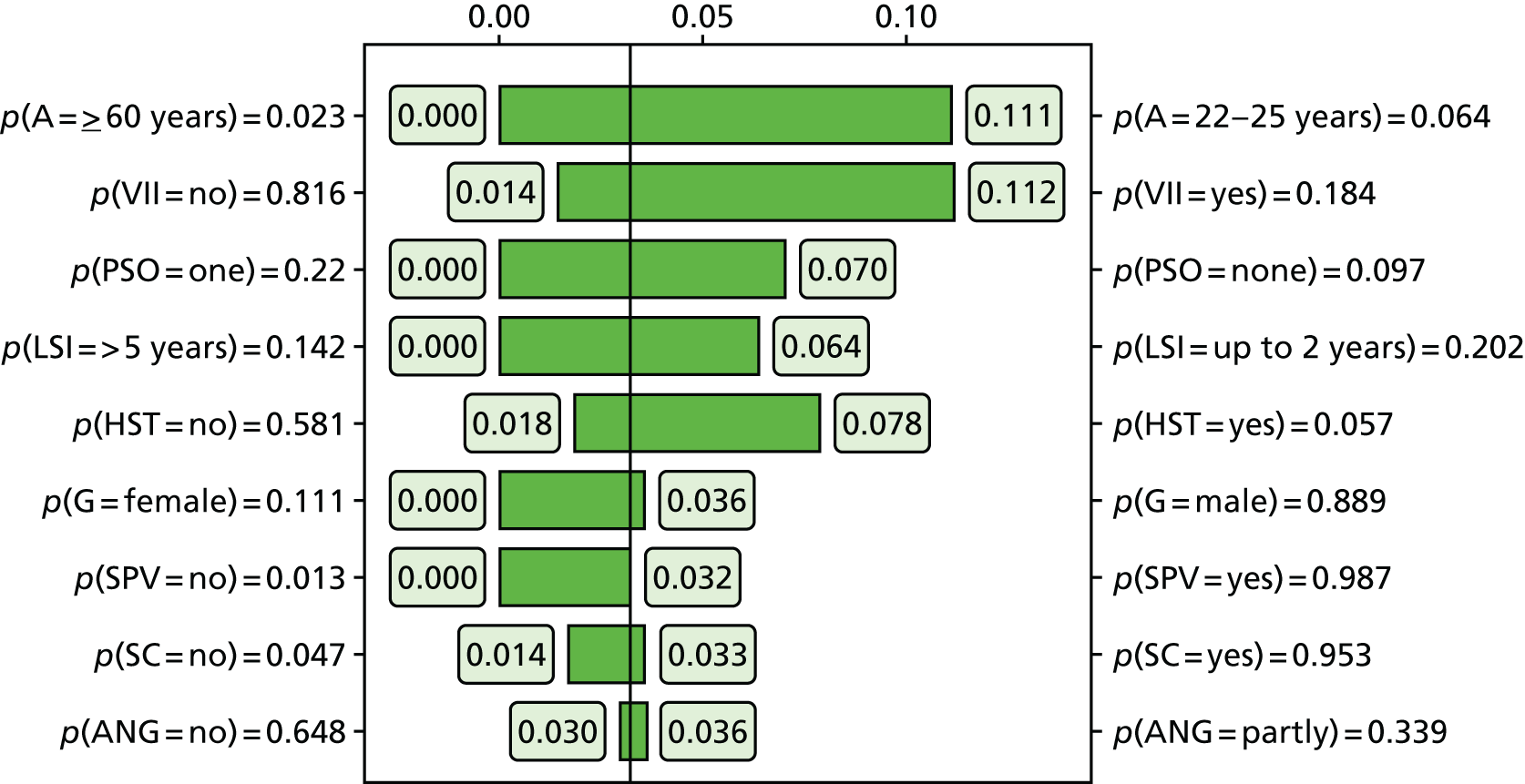
FIGURE 21.
Sensitivity analysis for violent convictions between 6 and 12 months after release on the basis of nine specified sensitivity nodes. A, age; ANG, anger; DIS, disinhibition; G, gender; HST, hostility; LSI, length of stay as inpatient; PSO, previous serious offences; SC, self-control; SPV, serious problems with violence; VII, violent ideation or intent.
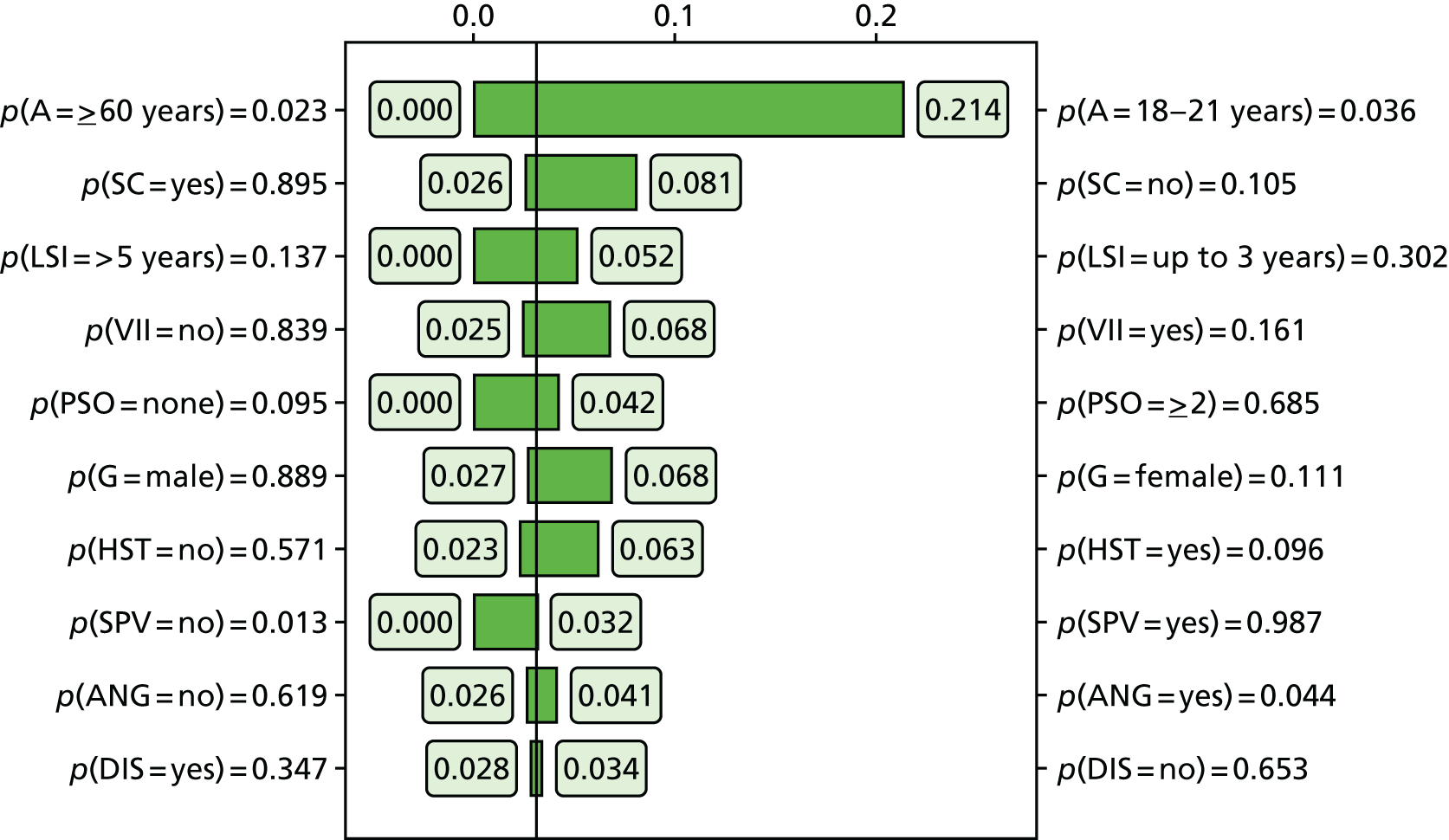
For violent convictions at 0–6 months post release, the most important drivers were age, violent ideation or intent (as a symptom of mental illness), previous serious offences, length of stay within the MSS of ≤ 2 years; and hostility (symptom).
For the period 6–12 months following release, the major violence drivers were significantly different. Age remained the single most sensitive factor but absence of self-control (a protective factor derived from the SAPROF) at 6 months following discharge became far more relevant to violent conviction over this time period; other risk factors such as violent ideation or intent, shorter length of stay in the MSS and previous serious offending remained significant. One counterintuitive finding was that, over this period, female patients appeared to be at higher risk of reconviction than male patients (although this did not have a great influence on the model, accounting for only 3–5% of the uncertainty).
Discussion
The development of a second Bayesian network for the risk assessment and management of violence risk in discharged forensic mental patients generated promising results in a preliminary validation and provided interesting material in terms of the relative influence of dynamic items in violent offending among discharged patients. It showed good to high predictive accuracy on a par with, or exceeding, that of contemporary SPJ risk assessment instruments.
It is of interest that the Bayesian network was more effective at predicting violence at the 12-month stage than at the 6-month stage, particularly when the reverse was true for all other risk assessment models. It is possible that existing risk assessments for violence assume that the dynamic component of their models has a ‘shelf life’ of approximately 6 months, that is, that the dynamic variables are prone to change after this time period causing predictive accuracy to decline over any longer term,115 but this does not explain why the Bayesian network, which also relies heavily on dynamic items, seems to improve in predictive accuracy at the 12-month stage and decline less severely when predicting violent convictions. The answer to this may lie in the sensitivity analysis also produced, which shows the differential influence of risk factors in the periods from discharge to 6 months and from 6 months to 12 months post discharge. Although factors relating to mental illness (violent intent or ideation, hostility, anger) showed considerable influence on the model in the initial 6-month period, during 6–12 months personal resources such as ‘self-control’ have more influence and much of the model sensitivity is explained by static factors such as age and previous offending.
Limitations
This study suffered from a similar limitation as in the DSVM-P study in that a single study sample was used for model parameterisation and cross-validation. Performing cross-validation on a single data set constitutes internal cross-validation. We have not tested how well the model performs for different populations (i.e. external cross-validation). However, this concern is not relevant to comparative assessments of model accuracy as predictive comparisons are made against other models that also suffer from this limitation (i.e. trained and cross-validated with the same data set). Further validation studies with different samples are necessary to establish the generalisable predictive efficacy of the model.
Furthermore, it was not possible to model the impact of specific, targeted interventions beyond generic ‘length of stay’ in MSSs using this data set, for example medication, treatment programmes or simple risk management procedures such as discharge area restrictions, restriction orders or use of supported housing. Additional studies incorporating this level of detail about specific interventions would be required to allow for modelling.
Chapter 22 Clinical utility evaluation of a Bayesian network in forensic settings
Background
Previous chapters have outlined the creation and validation of Bayesian networks as the basis for the development of decision support tools. However, when assessing the utility of such a tool, focus should be on the needs of the professionals who will be applying it within their practice. As such, it would be valuable to obtain feedback from professionals who have experienced use of such a tool to collect evidence pertinent to future development, both in terms of the accuracy and usefulness of the information generated and with the intention of developing a user interface that would make the tool of practical value in day-to-day practice. A clinical utility study was therefore conducted with a sample of professionals working in forensic, clinical or criminal justice roles to explore responses to a prototype version of the DSVM-P.
Objectives
The objectives of this project were to:
-
demonstrate the DSVM-P model to professionals with gatekeeping responsibilities for forensic patients or offenders
-
obtain feedback from professionals about the use value of the DSVM-P and the need for further development to enable the model to have practical utility, specifically:
-
whether or not the software was easy to use and, if not, how it could be improved
-
whether or not clinicians believed that the questions asked by the software were the most important ones for the future management of violence
-
whether or not the software made a decision about violence risk that corresponded to that made by professionals and, if so, to what degree
-
whether or not the recommendations in terms of intervention targets made by the software corresponded to those that the professionals would make given similar information and, if not, whether they were helpful or unhelpful recommendations
-
what the most useful aspects of the software were, including:
-
– whether or not it saves time in making decisions about future management
-
– whether or not it provides clinicians with additional information that is useful in making those decisions
-
– whether it is more or less useful in guiding practice than SPJ models such as the HCR-20. 287
-
-
Methods
Design
This was an evaluation study intended to capture and collate feedback from professionals about a prototype of the DSVM-P violence risk decision support tool. It used both quantitative and qualitative approaches to collect structured feedback on what were deemed to be the most pertinent features of the measure, but also less structured feedback on what areas needed development.
Sample and recruitment
The sample for this study consisted of 20 professional staff – either clinicians or criminal justice staff – with gatekeeping roles (forensic psychiatrists, forensic psychologists or probation staff) for patients or offenders. Participants were recruited purposively at two conferences for medical or psychological professionals with a criminal justice focus. The participants attended a stall where the DSVM-P decision support tool was demonstrated and the purpose and remit of the study were explained to them. They were then offered the opportunity to apply the tool to a clinical case (either one of their own or one of a sample of three artificial cases) and asked to provide feedback about the software’s assessment of risk and recommended interventions, together with feedback about a sensitivity analysis of the likely impact of these interventions (see Chapter 21), and complete a questionnaire about their experience. The total interview typically lasted about 20–30 minutes.
All data were collected anonymously; however, participants were asked to specify their disciplinary affiliation and were invited to leave their e-mail address with the researchers if they were interested in receiving updates on the progress of the development of the measure.
Materials
The materials used in this study were:
-
The DSVM-P decision support tool, deployed onto a laptop computer running the AgenaRisk development software and operated by a researcher (AC or MF). The software was set up in a ‘risk table’ setting showing the risk factors relevant to the individual used to calculate the risk of reoffending, the number of reconvictions and a sensitivity analysis of appropriate interventions, with respondents able to select values for risk variables from drop-down menus. A screenshot of the network is shown in Figure 22.
-
A semistructured questionnaire designed specifically for the study to collect structured feedback on the most useful aspects of the tool in line with the study objectives; identify any shortcomings or lacking features; and elicit feedback on how the tool might be developed further in the future to meet the needs of professionals (see Appendix 12). Structured questionnaire items were coded on a Likert scale from 1 ‘not at all’ to 5 ‘a great deal’.
-
Three sample cases from which professionals could choose to assess the performance of the DSVM-P against their own judgement of risk.
FIGURE 22.
Screen shot of the AgenaRisk software configured to demonstrate the DSVM-P network. AgenaRisk software © 2013 Agena Corp.
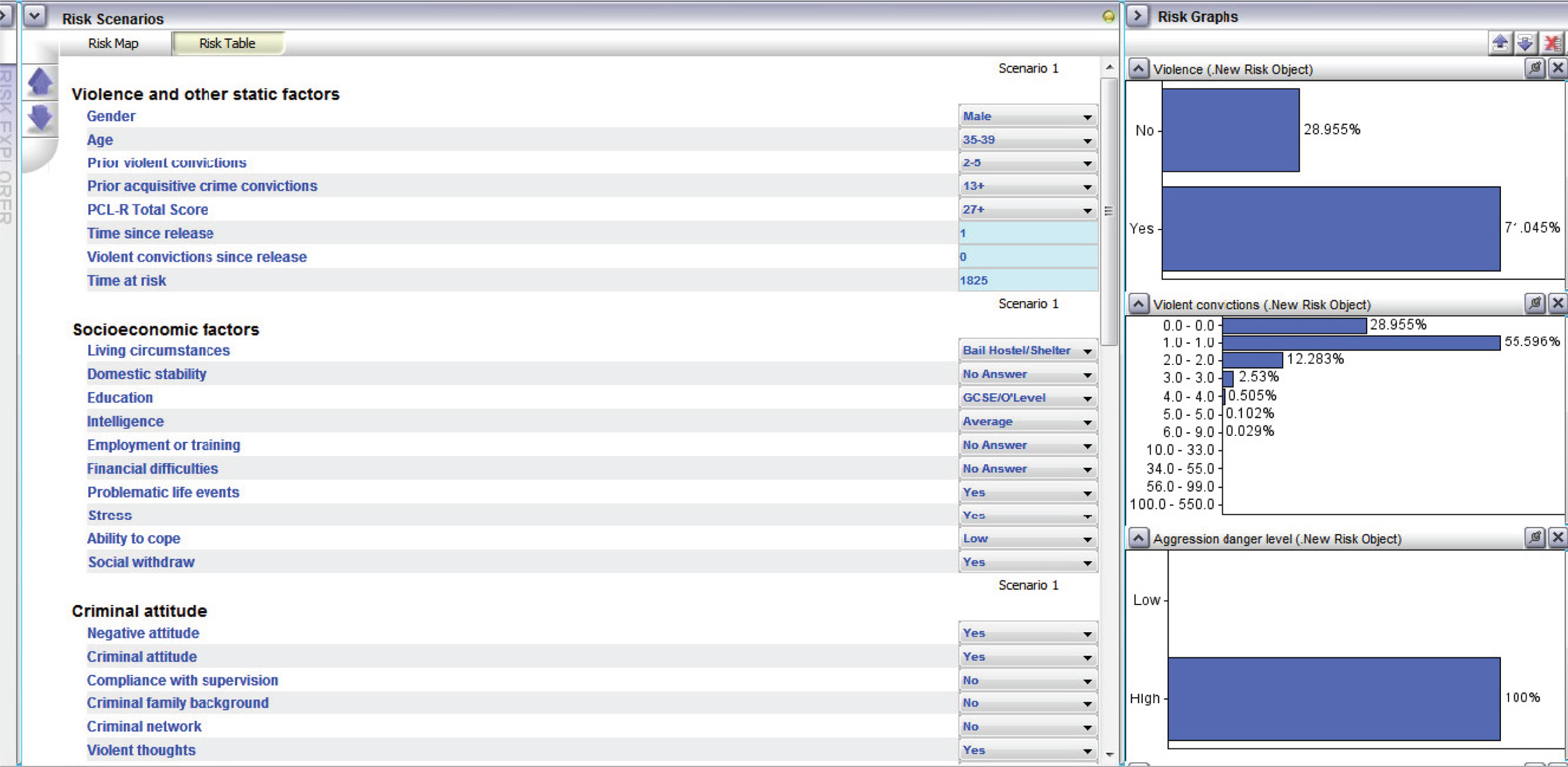
Data analysis
As this was a simple evaluation design to gather feedback on an initial prototype, data analysis was limited to descriptive statistics summarising responses to questionnaire items and a simple thematic synthesis of unstructured feedback items.
Given the small sample size, and the freedom given to respondents to rate their own cases instead of the sample cases, we elected not to use inferential statistics to compare clinician ratings of risk with those generated by the network for the same cases.
Results
Nature of respondents
In total, 15 of 20 individuals (75%) who took part in the demonstration of the software agreed to complete the questionnaire; a further two provided qualitative comments only. The main reason for declining to complete the questionnaire was lack of time (four respondents); one respondent said that they preferred not to complete the questionnaire but would give unstructured verbal feedback, which was recorded on a blank questionnaire form.
Of the respondents, 10 (66%) reported being a psychiatrist, five of whom (33%) were specialised forensic psychiatrists. Five other respondents (33%) identified themselves as psychologists, of whom three were specialised forensic psychologists and the remaining two were clinical psychologists. Of the remaining two respondents, one was as an occupational therapist and one was a probation officer.
Quantitative responses
Responses to the quantitative aspects of the questionnaire were in general positive, with all items being rated on average ≥ 3; these responses are summarised in Figure 23.
FIGURE 23.
Mean responses to DSVM-P questionnaire items with SDs as error bars.
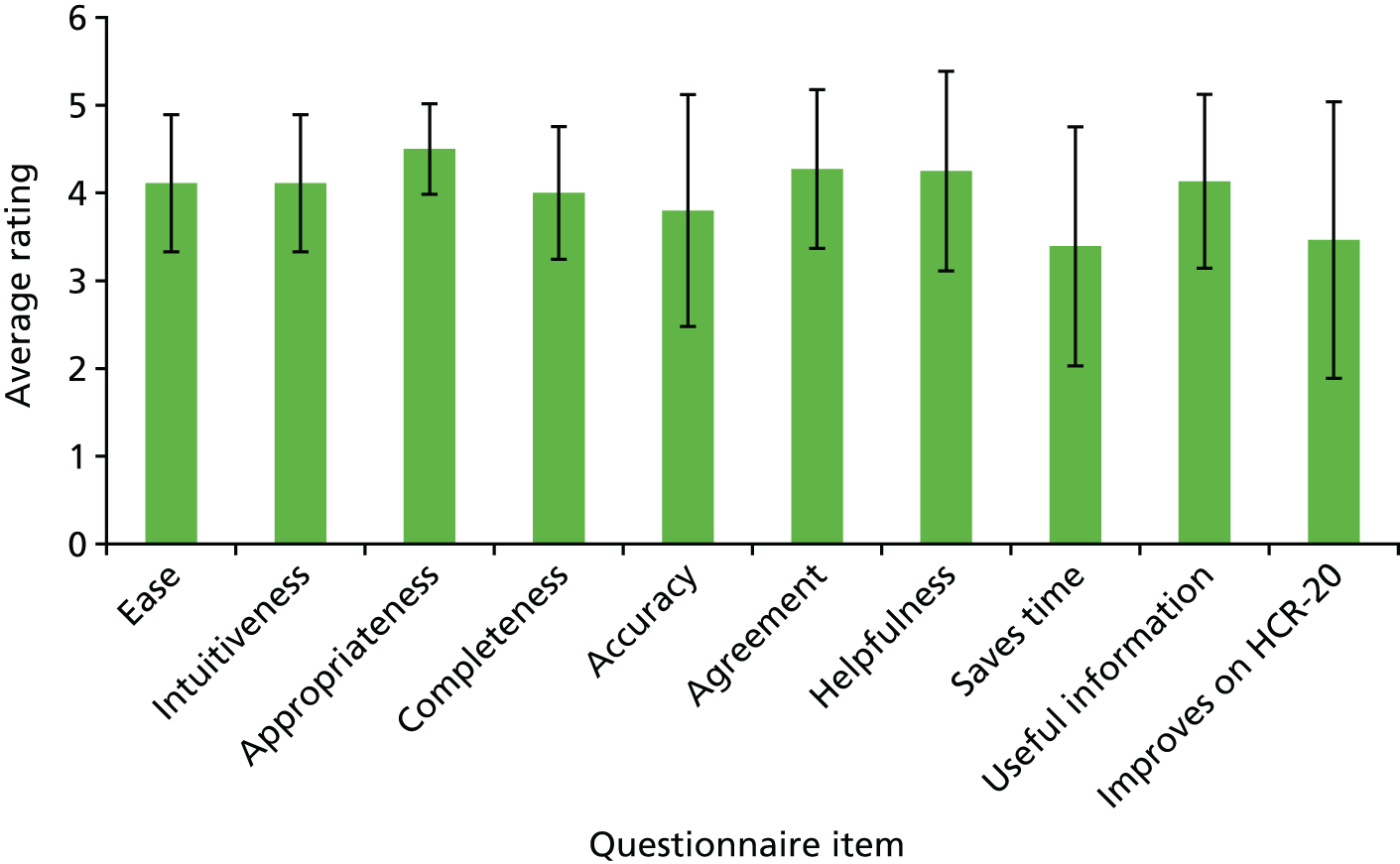
The most highly rated items were item 3 (‘I thought the questions asked by the software were relevant to risk management’; mean 4.50, SD 0.52), item 6 (‘The software recommended similar targets for management to those that I would have’; mean 4.27, SD 0.90) and item 7 (‘The intervention targets identified were helpful’; mean 4.25, SD 1.14). The lowest rated items were item 8 (‘I thought that using the software might save me time in my professional practice’; mean 3.39, SD 1.36) and item 10 (‘I thought that the software was more useful than SPJ tools like the HCR-20’; mean 3.46, SD 1.57). There seemed to be a higher degree of uncertainty about the less positively rated items, as evidenced by higher SDs for item responses.
All respondents endorsed item 3 (relevance of items) and item 4 (‘I thought the software asked all the right questions to enable violence risk management’) with a score of ≥ 3 (‘a fair amount’) and all except one respondent (93% of the sample) rated item 9 (useful information provided) as ≥ 3. In total, 80% of respondents rated the accuracy of the judgement about the risk of the case entered as representing their own judgement ‘a fair amount’ or better.
Qualitative responses
The responses to the unstructured questions provided substantial information about professional perceptions of the potential merits of and problems with the network.
Positive comments included:
Far more useful than existing risk assessment tools.
An easy and simple method to get likelihood of reconviction.
Minimises the need for lengthy assessments of personality (PCL-R etc.).
[It is] fairly quick to answer the risk questions.
[It is helpful] being able to see reconviction change over different time periods.
The ability to make predictions post intervention; we cannot normally estimate drop in risk after individuals complete interventions normally.
Positive comments seemed broadly to endorse the time taken to complete the DSVM-P measure and the high level of information and accuracy it provided. Some of the comments were not entirely accurate, for example the DSVM-P does specifically require a PCL-R and clinical assessments of personality to be completed and relies on these for improved accuracy.
Feedback which suggested that further work might be required was:
What about measurement of institutional violence?
Consider risk scenarios; should consider a sexual offence version.
Can you get the interface right?
Numbers confuse me; explanation of the risk % figure would be helpful.
[What about] circumstances of crime, or trigger?
A limited number of interventions modelled.
I was sceptical about the reliability of the model to make specific predictions of violence for an individual, e.g. 67% likelihood of reconviction is higher than most other predictive tools.
Lack of evidence for medical treatment; no medication.
One individual had also written the following in the margin of the questionnaire next to item 10 asking whether or not the software was more useful than SPJ tools like the HCR-20: ‘Only if completed instead of, not in addition to’.
Some of these comments reflected the developers’ own concerns, specifically with respect to the unfriendliness of the interface and the possible need for additional interpretation of raw probabilities of reoffending (e.g. categorical ratings such as high, medium or low risk). The suggestion that the number of interventions modelled should be expanded, possibly to include medication, was mostly accurate, although the modelling of ‘psychiatric treatment’ (described in Chapter 20) does relate primarily to psychopharmacological treatment rather than psychological interventions.
Other comments seemed to relate to other areas of need within forensic settings that were intentionally not addressed by the DSVM-P, for example suggestions of alternative networks covering institutional violence and/or sexual reoffending. However they do appear to illustrate an enthusiasm for the approach adopted. One comment relating to the inclusion of specific violence triggers and contexts was well taken, but modelling work on this has not yet proved possible with existing data. 464
Discussion
This project obtained feedback from a small sample of ‘likely users’ of a Bayesian network for the risk management of violent offenders, specifically a computer-based prototype version of such a tool. Responses to structured questions eliciting feedback about the prototype were in the majority very positive, with respondents endorsing higher than the median scale value for all items on the questionnaire. However, some respondents expressed reservations about whether or not the software would in fact save them time in their practice, specifically – as illustrated by the responses to unstructured questions – if they were asked to complete it in addition to completing existing risk assessment measures rather than as an alternative. This perhaps reflects a more generalised concern in forensic services over the array of available risk assessment instruments for different populations and outcomes (violence, general offending, sexual offending, institutional violence, etc.) in a climate of increasing pressure on time and resources of skilled and appropriately trained clinicians. In particular, participants did not overwhelmingly endorse the prototype as a replacement for existing standard risk assessment instruments such as the HCR-20.
However, respondents did identify specific strengths and limitations of the DSVM-P prototype. The strengths included the speed of completion; the high accuracy of risk probability (if only prima facie); the detailed recommendations about the efficacy of interventions; and the ability to model risk over different specific time periods. The limitations of the prototype identified included the limited user interface; results that are hard to interpret (e.g. risk probabilities); and the limited modelling of a large potential range of interventions. Some of these restrictions are the result of data limitations (e.g. only four interventions are accurately captured in the PCS data set used to develop the DSVM-P) and others could be modelled more clearly with more development of the network.
Future revisions of the prototype are likely to take the majority of this feedback on board, specifically with respect to the generation of a user interface; wider modelling of potential interventions based on ‘risk targets’, that is, identified drivers of violence risk that should be addressed irrespective of the availability of data relating to suitable intervention(s); and more clinically interpretable feedback from the software, such as categorical risk judgements.
Limitations
This was a simple evaluation study designed to gather feedback and reflections on the possible utility of the DSVM-P from a sample of potential users of the decision support tool. It cannot take the place of a full, naturalistic evaluation of the tool or even an evaluation of inter-rater reliability, exploring the accuracy of the ratings generated by the software relative to those of clinician ‘experts’. However, it has provided important end-user input that will make the future conduct of such studies more productive.
More specifically, the pilot study did not include representatives from the discipline of nursing. Community practice nurses would be the most likely health workers supporting patients on discharge from mental health services and would therefore be among the key potential users of any Bayesian network used with this population.
As stated, this study was an initial evaluation of clinicians’ views on the acceptability of the prototype tool. Further investigative work is needed to ensure that the decision-making processes modelled by the Bayesian network are complementary to those of clinicians and professionals in practical settings, possibly involving further iteration of the model.
Chapter 23 Conclusions and future directions for risk management tools using Bayesian networks
Objectives
In this chapter we summarise the findings of this section, as well as providing some additional validation information for the DSVM-P prototype decision support tool, and consider the future direction of the work performed in this section for the development of Bayesian networks to assist with violence risk management. Our objectives were to:
-
present external validation data for the DSVM-P, the more advanced of the two networks developed because of its ability to provide ‘real-world’ modelling of interventions
-
summarise briefly the work performed on the development of decision support tools for use in violence risk management
-
suggest future directions for research that will build on the existing prototypes and support their deployment and full evaluation of their risk management potential.
Further external validation of the Decision Support for Violence Management in Prisoners measure
A concern that we addressed previously in this section was the danger of reporting inflated predictive ability for the Bayesian networks when cross-validating with the development samples, a concern that applies to all risk assessment measures. Although risk assessment is only a part of the purpose of these decision support tools, we made use of an existing data set from the UK NOMS of 52,643 prisoners, described in more detail in Chapter 19, to enable further validation of the DSVM-P measures with a second sample, as we believed that this would ascertain the predictive validity of the tool outside of the development sample.
Method
To parameterise the DSVM-P to accommodate the significantly smaller number of variables available from the NOMS data set, we excluded a number of nodes from the network and recalculated other nodes as ‘indirectly observed’ or synthetic variables. The results of this reparameterisation are presented in Figure 24.
FIGURE 24.
Simplified topography of the DSVM–P network reparameterised from the NOMS data. Green circles: unobserved variables (no relevant data in the NOMS data set); light green circles: indirectly observable variables (relevant data in the NOMS data set that did not match the exact same information represented by the specific node); white circles: observable variables (NOMS data perfectly matched the variables and states). Solid white circles were those where available data states (e.g. ‘present/absent’) mapped directly into the existing data structure; dotted white circles represent variables that were present but required some amendment of state (e.g. ‘0, 1 or 2’ conflated to ‘0 or 1’).

Following this reparameterisation, we followed an iterative procedure to establish the validity of the DSVM-P with the NOMS sample:
-
The DSVM-P network was ‘trained’ with the PCS data only and was then used to predict violent reconvictions in the NOMS sample.
-
As previous work has shown that risk assessment tools perform differently with men and women in the NOMS sample (see Chapter 17, Study 5), this analysis was then repeated separately with men and women.
-
The DSVM-P was then trained with both the NOMS and the PCS data and used to predict violent reconvictions in the PCS sample.
-
The network trained on both samples was then used to predict violent outcomes in the NOMS sample and in both male and female samples separately.
Results
The results of this analysis are presented in Table 158. We report the AUC and also the PPV, NPV and PCC for each stage of the validation. The results of the initial validation study (see Chapter 20) are included as test 1 for ease of comparison.
Test | Training set | Test set | AUC (95% CI) | PPV | NPV | PCC |
---|---|---|---|---|---|---|
1 | PCS | PCS (cross-validation) | 0.780 (0.745 to 0.815) | 0.469 | 0.857 | 0.727 |
2 | PCS | NOMS | 0.696 (0.691 to 0.700) | 0.528 | 0.736 | 0.670 |
3 | PCS | NOMS (male) | 0.690 (0.685 to 0.695) | 0.528 | 0.729 | 0.662 |
4 | PCS | NOMS (female) | 0.722 (0.709 to 0.735) | 0.529 | 0.772 | 0.724 |
5 | PCS + NOMS | PCS | 0.776 (0.742 to 0.810) | 0.585 | 0.830 | 0.782 |
6 | PCS + NOMS | NOMS | 0.700 (0.696 to 0.705) | 0.605 | 0.699 | 0.685 |
7 | PCS + NOMS | NOMS (male) | 0.696 (0.691 to 0.701) | 0.603 | 0.692 | 0.677 |
8 | PCS + NOMS | NOMS (female) | 0.719 (0.706 to 0.732) | 0.643 | 0.740 | 0.733 |
The first stage of the validation process, training with PCS data only, showed that, although the AUCs were acceptable and better than those of other assessment tools (if only marginally), there was a drop in the predictive accuracy of the DSVM-P with the NOMS sample; The percentage of offenders correctly classified as violently reoffending also fell by 6%. Introducing the NOMS sample itself into the training data set, so allowing the network to learn associations between nodes from the information included in the data set, improved the AUC fractionally and the PCC by 1.5%. The dual learning appeared to cause a large increase in PPV and a small drop in NPV. As found in Section C, risk assessment tools seem to predict violence more accurately with female offenders within the NOMS sample and this trend was also present in the findings for the DSVM-P.
When repeating the original validation study on the PCS data using a network learned from the combined samples, predictive accuracy as measured by the AUC dropped fractionally and non-significantly, although there was a large increase in the PCC, from 73% to 78%.
Discussion
Although the performance of the DSVM-P declined when applied to an external sample, it still demonstrated good accuracy in predicting violence following release and superior predictive accuracy to that of any other risk assessment measure validated with the same sample. The reduction in predictive ability may have been attributable to (1) the heterogeneity of the sample, which contained a far lower proportion of violent or serious offenders than the original PCS development sample, and (2) the necessity of simplifying the network underlying the DSVM-P for use with a more restricted number of variables relating primarily to criminal justice management rather than clinical information.
Conclusions
Summary of the study findings
This section has presented the results of three studies that set out to use a form of causal modelling, specifically Bayesian networks, to develop a new generation of tools for the risk management of offenders. This modelling moved beyond simple prediction of violence and into explanatory modelling of violent outcomes based on interlinked networks of causal factors that can be analysed for sensitivity at the individual level, rather than simply as group averages. The tools focused on the management of violence risk in offenders discharged or released into the community following time in prison or treatment in forensic MSSs. There were a number of key findings of this development and validation process.
-
The two networks developed showed good to excellent predictive accuracy for future violence in their development samples, with AUCs ranging from 0.69 to 0.85.
-
Further external validation showed similar predictive accuracy for the DSVM-P when used with a further, very large, non-development sample. Evidence suggests that ‘training’ of further data into the model tended to improve performance, although this was only in men and subject to diminishing returns.
-
Because of their ability to perform reverse inference (e.g. from effect to cause as well as from cause to effect), Bayesian networks do not require complete information to make inferences, which would be useful in situations in which professionals do not have access to a large amount of information about an individual.
-
The DSVM-P incorporated data on the effectiveness of available interventions (including treatments) and can model this as output to support recommendations about future risk management.
-
The networks are able to identify not only those at high risk of future violence but also violence over multiple time periods (e.g. 6 months, 5 years) and also the frequency of violence over these time periods. This may be useful in distinguishing ‘persistent and prolific’ violent offenders from those for whom there is a shorter-term and more specific risk.
-
The response to the DSVM-P from professionals working in risk management was positive, especially with regard to the level of information returned by the Bayesian network, although they expressed reservations about the existing user interface and how time-consuming it may be to complete.
Future directions for research
Although the findings relating to the performance and clinical utility of the Bayesian network decision support tools have been promising, we accept that it is too early to consider the prototype networks as usable clinical tools. Not only is further, naturalistic validation work required to assess their performance with ‘real’ clinical data, as opposed to their retrospective application to research data sets, but also, for the networks to be of practical utility in supporting professional decision-making, more development work is required to move the decision support algorithms out of the AgenaRisk development environment and make them deployable within applied settings. To this end, we therefore propose three future studies that would establish the viability of the DSVM-P and/or other networks for the decision support of management of risk in forensic and criminal justice settings.
Study 1: feasibility and pilot study of the use of a Bayesian network risk management tool in secure services
This study would apply the DSVM-P or a hybrid network to ‘real’ clinical data, which should be retrospective because of the exploratory nature of the research and the uncertain value of the tool for decision support. It would involve the completion of the DSVM-P prototype at two time points with multiple (40 or ≈10 per modelled intervention) cases taken from a sample of forensic patients discharged within the previous 60 months, by a researcher blinded to their eventual outcome. The first time point would be at admission to services, with data based on psychiatric assessment, and the second time point would be at discharge, based on medical notes and discharge summaries. The sample would be chosen on a random basis but would be stratified by the patients’ involvement in specific interventions (e.g. for drugs, alcohol, psychiatric treatment and anger) during their stay in secure services, so that these could be modelled accurately. The cases would then be followed up using PNC records and violent outcomes would be recorded.
The feasibility of the use of the DSVM-P could then be assessed based on whether or not (1) the tool, completed at admission, would have recommended the same interventions that were undertaken by patients during the course of their stay in services and (2) the tool was able to correctly predict violent reconviction on the basis of clinical data available at discharge.
Study 2: external validation of a Bayesian network for risk assessment of violent offenders
Although the present study has found promising evidence for the superior accuracy of Bayesian networks over actuarial or SPJ tools for the prediction of violence across different samples and populations, the existing validations still suffer from the weaknesses identified by Singh et al. ,465 who showed that the involvement of the original authors in validation studies for risk assessment tools can inflate predictive performance by up to twice that reported by independent investigators. We would therefore support fully an independent study conducted with a large sample of violent offenders to ascertain the performance of the DSVM-P as a risk assessment measure.
Study 3: multistage clinical trial of the Decision Support for Violence Management in Prisoners compared with management as usual for discharged forensic patients
Previous clinical trials of risk assessment tools for the prevention of violence in clinical settings have failed to find a significant effect. 18 However, if a feasibility study (study 1) was able to show promising results for risk management modelling as well as predictive accuracy, there would be an evidence base sufficient to conduct a full, randomised trial of a clinically usable software package based on the DSVM-P (or a similar network adapted to a forensic clinical population, such as the VoRAMSS Bayesian network). The software suite would be developed from the prototype into a form usable in clinical settings (e.g. iPad/Android app) and would be completed pre discharge by clinicians to provide evidence-based support on managing risk in the community. Clinicians would complete the DSVM-P together with a mandated risk assessment measure such as the HCR-20 and would then record their responses to the management recommendations indicated by the DSVM-P – whether or not they agreed with the recommendation (e.g. to move the patient into supported housing) and whether or not it was in fact followed.
The trial would be cluster randomised by service to simplify its deployment and ensure efficient use of resources and patients would be followed up for 24 months post discharge to assess for any violence based on PNC records or clinician report.
Forensic secure settings would be a preferred choice to criminal justice settings because of the increased resources available to complete routine risk assessments [e.g. the HCR-20 or HoNOS-S (Health of the Nation Outcome Scales – Secure version),466 which are mandatory for most forensic services] that could be used to model management as usual. However, with sufficient resources it would be possible to conduct a similar trial with offenders released to probation services.
Chapter 24 Summary and conclusions
Section A
-
Young, single or separated adult men of lower social class are responsible for the majority of violence in the general population. Male-on-male violence is the largest public health problem.
-
Married people, immigrants and people of South Asian origin are less likely to be violent.
-
There are strong independent associations between anxiety disorder, ASPD, alcohol dependence, drug dependence and violence.
-
No associations were observed between depressive disorder, BPD, autism and ASD or psychosis as a categorical diagnosis and violence.
-
Higher IQ conveys a protective effect against violence.
-
Childhood maltreatment is strongly associated with later violence and victimisation in adulthood. There is a dose–response relationship with violence: the greater the number of different types of early maltreatment the more extensive the violence in adulthood.
-
Self-reported violence is associated with level of socioeconomic deprivation measured at small-area level where the violent perpetrator resides. However, this association holds only for more serious violence.
-
Violence is associated with other risk-taking behaviours including substance misuse, deliberate self-harm, reckless driving, high-risk sexual behaviour and taking risks associated with poor long-term physical health.
-
Use of mental health services is associated with violence. However, use of services for physical health problems is not associated with violence. The association with mental health services holds only for minor violence and not serious violence.
-
There is no clear-cut impact of violence on health-care costs at the population level. Although some service costs increase, access to other services is lower among those who are violent and this results in cost reductions.
Section B
-
Five static risk factors of having no educational qualifications, not having worked in the past year, being UK born, having no family history of mental disorder and poverty before the age of 15 years predicted violence with excellent accuracy among women with a first episode of psychosis.
-
Four static risk factors of a high level of trait anger, a history of violent and threatening/annoying behaviours leading to contact with services and parental discord before the age of 15 years predicted violence over a 10-year period with a moderate level of accuracy among men with a first episode of psychosis.
-
Risk factors differ between men and women with psychosis and clinicians should be aware of differing pathways to violence between the sexes when considering preventative interventions.
Section C
-
Static risk assessment instruments for violence perform at a ‘poor’ level of accuracy among patients discharged from MSSs.
-
The clinical and risk management scales of the HCR-20v3 and SAPROF show significantly better predictive ability than the MSRAG and other actuarial scales.
-
The percentage correctly classified as violent or non-violent indicates that all risk assessment instruments misclassify approximately one-third of patients. This indicates that they should not be used as the sole basis for making clinical decisions.
-
Dynamic factors in the HCR-20v3 and SAPROF show stronger associations with future violence in patients with psychosis. This suggests that different instruments are required to assess risk according to diagnosis.
-
A preliminary analysis of moderating effects suggests that a two-stage process, in which patients are initially rated with static measures and subsequently with dynamic risk and protective factors, may improve accuracy in identifying targets for intervention.
Section D
-
Actuarial instruments can be constructed using very few variables but can still accurately measure risk for violence, robbery, drug offences and acquisitive offending. These instruments show a high level of discrimination, measured using the AUC statistic. However, the instruments show poor calibration using the H–L goodness-of-fit statistic. In future studies, new instruments should be tested according to their calibration as well as their discrimination.
-
Dynamic factors show different patterns of association with offending when measured according to different levels of static risk. However, these patterns of association differed between a sample of released prisoners and patients discharged from MSSs. The effects of several dynamic factors were more apparent among released prisoners who had low levels of static risk.
Section E
-
A violence risk management decision support tool for clinicians and criminal justice professionals showed superior predictive accuracy to other risk assessment tools using a Bayesian network. The network outperformed SPJ.
-
Bayesian networks can identify those at risk of violent behaviour over multiple time periods together with those at risk of repeated episodes of violence.
-
A pilot study demonstrated the feasibility of the Bayesian network risk management tool for use by clinicians in secure services. However, clinicians require an improved interface and a reduction in the time taken to complete the ratings. A further developmental phase is needed to improve this new instrument.
Acknowledgements
We want to thank Professor Peter Donnelly and Dr Damien Williams for their invaluable input throughout this programme; NOMS for supplying OASys Data Evaluation and Analysis Team (O-DEAT) data for Section D; Greater Manchester Police for supplying PNC data for Section C; the Ministry of Justice for supplying PNC data for Sections B and D; and ICM Research (Martin Boon) for undertaking the surveying for the MMLS in Section A.
Contributions of authors
Professor Jeremy W Coid is Professor of Forensic Psychiatry and Consultant Psychiatrist. He was the chief investigator for this programme and was responsible for the design, overview and process of the whole programme and for the writing of this report.
Dr Simone Ullrich is Senior Lecturer in Forensic Mental Health. She was principal investigator for Section B and was also involved in the design of the rest of the programme and contributed greatly to the statistical output of other sections. She wrote substantial sections of this report.
Dr Constantinos Kallis is Senior Lecturer in Medical Statistics. He was principal investigator for Section D and was also involved in supervising and advising on statistical methodology for the whole programme. He wrote substantial sections of this report.
Dr Mark Freestone was Principal Investigator for Section E. He also advised on methodology for Section A and wrote substantial sections of this report.
Dr Rafael Gonzalez is a Postdoctoral Research Fellow and psychologist. He performed most of the analysis for Section A and was involved in planning of the methodology for Section D. He also contributed to the drafting of Section A.
Dr Laura Bui is a Postdoctoral Research Fellow. She was involved in the analysis of data for Section D and contributed to the drafting of Section D.
Dr Artemis Igoumenou is NIHR Clinical Lecturer in Forensic Psychiatry. She was involved in analysis of Section A and drafted part of that section.
Dr Anthony Constantinou is a Postdoctoral Research Fellow. He was responsible for the design and implementation of the Bayesian networks under the supervision of Dr William Marsh and Professor Norman Fenton. He also wrote substantial parts of Section E.
Professor Norman Fenton is Professor of Risk Information Management. He was responsible for the supervision of Dr Anthony Constantinou and also contributed to the drafting of Section E.
Dr William Marsh is Lecturer in Computer Science. He contributed to the design of Section E, supervised Anthony Constantinou and contributed to the drafting of Section E.
Professor Min Yang is Professor of Medical Statistics. She contributed to the original design of this programme and also acted as a statistical consultant for the whole programme.
Professor Bianca DeStavola is Professor of Biostatistics. She acted as consultant statistician for Section B and was responsible for advising on the statistical design and drafting of this section. She also gave statistical advice on other sections of this study.
Professor Junmei Hu is Professor of Forensic Psychiatry. She contributed to the design of Section A.
Professor Jenny Shaw was Principal Investigator for Section C. She supervised the execution of this section and contributed substantially to the writing of this section.
Dr Mike Doyle is Honorary Senior Lecturer and Nurse Consultant. He was involved in the supervision of the execution of Section C and in the drafting of that section.
Ms Laura Archer-Power is a Research Assistant. She was involved in the statistical analysis for Section C and in the drafting of that section.
Dr Mary Davoren is an Academic Clinical Fellow. She was involved in the analysis for Section A and drafted parts of that section.
Dr Beatrice Osumili is a Research Associate in Health Economics. She undertook the analysis for and wrote substantial parts of Chapter 11 in Section A.
Professor Paul McCrone is Professor of Health Economics. He was involved in the methodology design and preparation of Chapter 11 in Section A.
Ms Katherine Barrett is a Lay Advisor. She contributed to Section B and C of this report.
Mr David Hindle is a Lay Advisor. He contributed to Section B and C of this report.
Professor Paul Bebbington is Emeritus Professor of Social and Community Psychiatry. He contributed to the design of this programme and advised on the analysis methods for all sections of the programme.
Publications
Journal articles
Coid J, Ullrich S. Antisocial personality disorder and anxiety disorder – a diagnostic variant. J Anxiety Disord 2010;24:452–60.
Coid J, Ullrich S. Antisocial personality disorder is on a continuum with psychopathy. Compr Psychiat 2010;51:426–33.
Coid J, Yang M. Violence and delayed social independence among young adult men. Soc Psychiatry Psychiatr Epidemiol 2010;45:301–8.
Kirkbride J, Coid JW, Morgan C, Fearon P, Dazzan P, Yang M, et al. Translating the epidemiology of psychosis into public mental health: evidence, challenges, and future prospects. J Public Ment Health 2010;9:4–14.
Roberts A, Coid J. Personality disorder and offending behaviour: finding from the national survey of male prisoners in England and Wales. J Forensic Psychiatry Psychol 2010;21:221–37.
Skogen JC, Mykletun A, Ferri CP, Bebbington P, Brugha T, Coid J, et al. Mental and personality disorders and abstinence from alcohol: results from a national household survey. Psychol Med 2010;41:809–18.
Ullrich S, Coid JW. Antisocial personality disorder: stable and unstable subtypes. J Pers Disord 2010;24:171–87.
Ullrich S, Yang M, Coid J. Dangerous and severe personality disorder: an investigation of the construct. Int J Law Psychiatry 2010;33:84–8.
Yang M, Coid J, Tyrer P. Personality pathology recorded by severity: national survey. Br J Psychiatry 2010;197:193–9.
Yang M, Liu Y, Coid J. Applying neural networks and other statistical models to the classification of serious offenders and the prediction of recidivism. Ministry of Justice Research Series 2010;6(10). URL: www.justice.gov.uk/publications/docs/neural-networks-research.pdf (accessed 15 April 2016).
Yang M, Wong SC, Coid JW. The efficacy of violence prediction: a meta-analytic comparison of nine risk assessment tools. Psychol Bull 2010;136:740–67.
Coid JW, Ullrich S. Prisoners with psychosis in England and Wales: diversion to psychiatric inpatient services? Int J Law Psychiatry 2011;34:99–108.
Coid J, Yang M. The impact of psychopathy on violence among the household population of Great Britain. Soc Psychiatry Psychiatr Epidemiol 2011;46:473–80.
Coid JW, Yang M, Ullrich S, Zhang T, Sizmur S, Farrington D, Rodgers R. Most items in structured risk assessment instruments do not predict violence. J Forensic Psychiatr Psychol 2011;22:3–21.
Hu J, Yang M, Huang X, Coid JW. Forensic psychiatry in China. Int J Law Psychiatry 2011;34:7–12.
Liu Y, Yang M, Ramsay M, Li X, Coid JW. A comparison of logistic regression, classification and regression tree, and neural networks models in predicting violent re-offending. J Quant Criminol 2011;27:547–53.
Shah A, Waldron G, Boast N, Coid JW, Ullrich S. Factors associated with length of admission at a medium secure forensic psychiatric unit. J Forensic Psychiatr Psychol 2011;22:496–512.
Ullrich S, Coid J. Protective factors for violence among released prisoners – effects over time and interactions with static risk. J Consult Clin Psychol 2011;79:381–90.
Coid JW, Freestone M, Ullrich S. Subtypes of psychopathy in the British household population: findings from the National Household Survey of Great Britain. Soc Psychiatry Psychiatr Epidemiol 2012;47:879–91.
Coid JW, Ullrich S, Kallis C. Predicting future violence among individuals with psychopathy. Br J Psychiatry 2013;203:387–8.
Coid JW, Ullrich S, Kallis C, Keers R, Barker D, Cowden F, Stamps R. The relationship between delusions and violence: findings from the East London first episode psychosis study. JAMA Psychiatry 2013;70:465–71.
Coid JW, Ullrich S, Keers R, Bebbington P, Destavola BL, Kallis C, et al. Gang membership, violence, and psychiatric morbidity. Am J Psychiatry 2013;170:985–93.
González RA, Kallis C, Coid JW. Adult attention deficit hyperactivity disorder and violence in the population of England: does comorbidity matter? PLOS ONE 2013;8:e75575.
Kirkbride JB, Jackson D, Perez J, Fowler D, Winton F, Coid JW, et al. A population-level prediction tool for the incidence of first-episode psychosis: translational epidemiology based on cross-sectional data. BMJ Open 2013;3:e001998.
Minoudis P, Craissati J, Shaw J, McMurran M, Freestone M, Chaun S, et al. An evaluation of case formulation training and consultation with probation officers. Crim Behav Mental Health 2013;23:252–62.
Yang M, Wong SCP, Coid JW. Violence, mental health and violence risk factors among women in the general population: an epidemiology study based on two national household surveys in the UK. BMC Public Health 2013;13:1020.
Coid JW, Ullrich S, Kallis C. Authors’ reply. Br J Psychiatry 2014;204:240–1.
Doyle M, Coid J, Archer-Power L, Dewa L, Hunter-Didirchsen A, Stevenson R, et al. Discharges to prison from medium secure psychiatric units in England and Wales. Br J Psychiatry 2014;205:177–82.
González RA, Kallis C, Ullrich S, Zhang T, Coid JW. The protective role of higher intellectual functioning on violence in the household population of Great Britain. Pers Individ Dif 2014;61–62:80–5.
Kallis C, Bui L, Yang M, Coid JW. Static screening instruments for risk of minor and major violence: findings from the Prisoner Cohort Study. J Forensic Psychiatry Psychol 2014;25:397–410.
Keers R, Ullrich S, DeStavola BL, Coid JW. Association of violence with emergence of persecutory delusions in untreated schizophrenia. Am J Psychiatry 2014;171:332–9.
Kirkbride JB, Jones PB, Ullrich S, Coid JW. Social deprivation, inequality, and the neighbourhood-level incidence of psychotic syndromes in East London. Schizophr Bull 2014;40:169–80.
Ullrich S, Keers R, Coid JW. Delusions, anger, and serious violence: new findings from the MacArthur Violence Risk Assessment Study. Schizophr Bull 2014;40:1174–81.
Coid J, Yang M, Ullrich S, Hickey N, Kahtan N, Freestone M. Psychiatric diagnosis and differential risks of offending following discharge. Int J Law Psychiatry 2015;38:68–74.
Coid JW, Yang M, Ullrich S, Zhang T, Sizmur S, Farrington D, et al. Improving accuracy of risk prediction for violence: does changing the outcome matter? Int J Forensic Ment Health 2015;14:23–32.
Conference presentations
Coid JW. Comorbidity and Violence in Free and Captive Populations. Annual Meeting of the Royal College of Psychiatrists, Liverpool, UK, 2 June 2009.
Coid J. Deconstructing Static Risk Assessment for Violence. International Association of Forensic Mental Health Services Conference, Edinburgh, UK, 26 June 2009.
Coid J. From Risk Assessment to Risk Management. International Association of Forensic Mental Health Services Conference, Edinburgh, UK, 26 June 2009.
Coid J. Personality Disorder and Risk – What is the Size of the Problem? St. Andrew’s Healthcare Personality Disorder Conference, Basildon, UK, 14 May 2009.
Coid J. Symptoms of Affective and Anxiety Disorder in Borderline Personality Disorder. First International Congress on Borderline Personality Disorder, Berlin, Germany, 3 July 2010.
Coid J. Predicting the Risk of Reconviction among MDOs: The Development of the ‘Medium Security Risk Assessment Guide’ (MSRAG). Division of Forensic Psychology Annual Faculty Conference Royal College of Psychiatrists, Liverpool, 2010.
Coid J. Violence is Fun: Subcultural Risk Factors Explain an Excess of Minor Violence among UK Working Class Men. Congress of the International Federation of Psychiatric Epidemiology, Kaohsiung, Taiwan, 30 March–2 April 2010.
Coid J. Structured Risk Assessment Instruments do not Predict Future Violence among High Risk Individuals. Congress of the International Academy of Law and Mental Health, Berlin, Germany, 17–23 July 2011.
Coid J. Part I: Deconstructing Risk Assessment: Why is the Prediction Accuracy of our Instruments so Poor? and Part II: Reconstructing Risk Assessment. International Association of Forensic Mental Health Services Conference, Barcelona, Spain, 29 June–1 July 2011.
Coid J. The Relationship between Delusions and Violence is Explained by Anger: Findings from the East London First Episode Psychosis Study (ELFEPS). European Congress on Violence in Clinical Psychiatry, Prague, Czech Republic, 19–22 October 2011.
Coid J et al. Dynamic Risk Factors in a New Model of Risk Assessment. International Association of Forensic Mental Health Services Conference, Miami, FL, USA, 22–24 April 2011.
Coid J et al. The Epidemiology of Violence and Risk-taking Among Young Adult Men. International Association of Forensic Mental Health Services Conference, Miami, FL, USA, 22–24 April 2011.
Coid JW. The Epidemiology of Psychopathy. Diagnosis and Treatment of Psychopathy Conference, London, UK, 3 May 2012.
Coid JW. Gang Membership, Violence and Psychiatric Morbidity and Sex and Gang Members. Friday morning teaching session, Institute of Psychiatry, King’s College London, London, UK, 3 May 2013.
Coid JW. Predicting Future Violence Among Individuals with Psychopathy. Advances in Structured Professional Judgement, Institute of Psychiatry, London, UK, 17–18 October 2013.
Coid JW, Keers R, Kallis C, Ullrich S. Anger due to Delusions causes Violence. Royal College of Psychiatry’s Forensic Faculty Annual Residential Conference, Copenhagen, Denmark, 6–8 February 2013.
Coid JW, Keers R, Kallis C, Ullrich S. Antisocial Personality and Anxiety Disorder: Mega-analytic Study of an Antisocial Sub-syndrome. International Congress on the Disorders of Personality, Copenhagen, Denmark, 16–19 September 2013.
Coid JW, Ullrich S, Kallis C, Keers R. Symposium of Four Presentations entitled ‘Delusions and Violence post-MacArthur Violence Risk Assessment Study’. American Psychiatry Association Annual Meeting, San Francisco, CA, USA, 18–22 May 2013.
Kallis C. A Novel Approach for Improving Predictive Accuracy of Prognostic Models for Violent Reoffending. American Statistical Association Conference on Statistical Practice, New Orleans, LA, USA, 21–23 February 2013.
Kallis C. Computer Instrument for Violence (CIV): A New Prognostic Model for Violent Reoffending for UK Serious Offenders. Classification Society 2012 Annual Meeting, Pittsburgh, PA, USA, 14–16 June 2013.
Coid JW, Constantinou A, Freestone M, Kallis C, Bui L. Causal Models for Violence Risk Assessment and Management: A New Paradigm. International Association of Forensic Mental Health Services Conference, Toronto, ON, Canada, 19–22 June 2014.
Coid JW, Ullrich S, Gonzalez R. Gangs, Violence, and Psychiatric Morbidity. International Association of Forensic Mental Health Services Conference, Toronto, ON, Canada, 19–22 June 2014.
Coid JW, Ullrich S, Gonzalez R. Gangs, Violence, and Psychiatric Morbidity. International Congress of the Royal College of Psychiatrists, London, 24–27 June 2014.
Ullrich S, Freestone M, Coid JW. Delusions as Causal Risk Factors for Violence? International Association of Forensic Mental Health Services Conference, Toronto, ON, Canada, 19–22 June 2014.
Data sharing statement
Requests for access to data should be addressed to the corresponding author.
Disclaimers
This report presents independent research funded by the National Institute for Health Research (NIHR). The views and opinions expressed by authors in this publication are those of the authors and do not necessarily reflect those of the NHS, the NIHR, CCF, NETSCC, PGfAR or the Department of Health. If there are verbatim quotations included in this publication the views and opinions expressed by the interviewees are those of the interviewees and do not necessarily reflect those of the authors, those of the NHS, the NIHR, NETSCC, the PGfAR programme or the Department of Health.
References
- Krug EG, Mercy JA, Dahlberg LL, Zwi AB. The world report on violence and health. Lancet 2002;360:1083-8. http://dx.doi.org/10.1016/S0140-6736(02)11133-0.
- Guidance on the Discharge of Mentally Disordered People and their Continuing Care in the Community. London: Department of Health; 1994.
- Rose G. The Strategy of Preventive Medicine. Oxford: Oxford University Press; 1992.
- Compton MT, Koplan C, Oleskey C, Powers RA, Pruitt D, Wissou L, et al. Clinical Manual of Prevention in Mental Health. Washington, DC: American Psychiatric Publishing; 2010.
- Reducing Risk for Mental Disorders: Frontiers for Preventive Intervention Research. Washington, DC: Institute of Medicine; 1994.
- Heilbrun K, Yasuhara K, Shah S, Offo RK, Douglas KS. Handbook of Violence Risk Assessment International Perspective on Forensic Mental Health. New York, NY: Routledge; 2010.
- Singh JP, Grann M, Fazel S. A comparative study of violence risk assessment tools: a systematic review and metaregression analysis of 68 studies involving 25,980 participants. Clin Psychol Rev 2011;31:499-513. http://dx.doi.org/10.1016/j.cpr.2010.11.009.
- Yang M, Wong SC, Coid J. The efficacy of violence prediction: a meta-analytic comparison of nine risk assessment tools. Psychol Bull 2010;136:740-67. http://dx.doi.org/10.1037/a0020473.
- Douglas KS, Blanchard A, Hendry M, Logan C, Johnston L. Risk Assessment and Management: Clinical Guidelines for Effective Practice. New York, NY: Routledge; 2013.
- Hart SD, Michie C, Cooke DJ. Precision of actuarial risk assessment instruments: evaluating the ‘margins of error’ of group v. individual predictions of violence. Br J Psychiatry 2007;198:S60-5. http://dx.doi.org/10.1192/bjp.190.5.s60.
- Cooke DJ, Michie C. Limitations of diagnostic precision and predictive utility in the individual case: a challenge for forensic practice. Law Hum Behav 2010;34:259-74. http://dx.doi.org/10.1007/s10979-009-9176-x.
- Douglas KS, Hart SD, Webber CD, Bulfrage H, Eaves D. HCR-20: Assessing Risk for Violence, Version 3. Burnaby, BC: Mental Health Law and Policy Institute, Simon Fraser University; 2008.
- Monahan J, Streadman H, Appelbaum P, Grisso T, Mulvey E, Roth L, et al. The Classification of Violence Risk. Lutz, FL: Psychological Assessment Resources; 2005.
- Monahan J, Steadman HJ, Appelbaum PS, Grisso T, Mulvey EP, Roth LH, et al. The classification of violence risk. Behav Sci Law 2006;24:721-30. http://dx.doi.org/10.1002/bsl.725.
- McCusker PJ. Issues regarding the clinical use of the Classification of Violence Risk (COVR) assessment instrument. Int J Offender Ther Comp Criminol 2007;51:676-85. http://dx.doi.org/10.1177/0306624X07299227.
- Webster CD, Douglas KS, Eaves D, Hart SD. HCR-20: Assessing Risk for Violence (Version 2). Burnaby, BC: Mental Health, Law and Policy Institute, Simon Fraser University; 1997.
- Douglas KS, Hart SD, Webster CD, Bulfrage H. HCR-20v3: Assessing Risk of Violence – User Guide. Burnaby, BC: Mental Health Law and Policy Institute, Simon Fraser University; 2013.
- Troquete NA, van den Brink RH, Beintema H, Mulder T, van Os TW, Schoevers RA, et al. Risk assessment and shared care planning in out-patient forensic psychiatry: cluster randomised controlled trial. Br J Psychiatry 2013;202:365-71. http://dx.doi.org/10.1192/bjp.bp.112.113043.
- Ullrich S, Coid J. Protective factors for violence among released prisoners – effects over time and interactions with static risk. J Consult Clin Psychol 2011;79:381-90. http://dx.doi.org/10.1037/a0023613.
- Appelbaum PS, Robbins PC, Monahan J. Violence and delusions: data from the MacArthur Violence Risk Assessment Study. Am J Psychiatry 2000;157:566-72. http://dx.doi.org/10.1176/appi.ajp.157.4.566.
- Ullrich S, Keers R, Coid JW. Delusions, anger, and serious violence: new findings from the MacArthur Violence Risk Assessment Study. Schizophr Bull 2014;40:1174-81. http://dx.doi.org/10.1093/schbul/sbt126.
- Hickey N, Yang M, Coid J. The development of the Medium Security Recidivism Assessment Guide (MSRAG): an actuarial risk prediction instrument. Journal of Forensic Psychiatry &Amp; Psychology 2009;20:202-24. http://dx.doi.org/10.1080/14789940802207683.
- Coid JW, Yang M, Ullrich S, Zhang TQ, Sizmur S, Farrington D, et al. Most items in structured risk assessment instruments do not predict violence. J Forensic Psychiatry Psychol 2011;22:3-21. http://dx.doi.org/10.1080/14789949.2010.495990.
- Swanson JW, Holzer CE, Ganju VK, Jono RT. Violence and psychiatric disorder in the community – evidence from the Epidemiologic Catchment Area surveys. Hosp Community Psych 1990;41:761-70. http://dx.doi.org/10.1176/ps.41.7.761.
- Link BG, Andrews H, Cullen FT. The violent and illegal behavior of mental patients reconsidered. Am Sociol Rev 1992;57:275-92. http://dx.doi.org/10.2307/2096235.
- Stueve A, Link BG. Violence and psychiatric disorders: results from an epidemiological study of young adults in Israel. Psychiatr Q 1997;68:327-42. http://dx.doi.org/10.1023/A:1025443014158.
- Wallace C, Mullen P, Burgess P, Palmer S, Ruschena D, Browne C. Serious criminal offending and mental disorder – case linkage study. Br J Psychiatry 1998;172:477-84. http://dx.doi.org/10.1192/bjp.172.6.477.
- Mullen PE, Burgess P, Wallace C, Palmer S, Ruschena D. Community care and criminal offending in schizophrenia. Lancet 2000;355:614-17. http://dx.doi.org/10.1016/S0140-6736(99)05082-5.
- Corrigan PW, Watson AC. Findings from the National Comorbidity Survey on the frequency of violent behavior in individuals with psychiatric disorders. Psychiatry Res 2005;136:153-62. http://dx.doi.org/10.1016/j.psychres.2005.06.005.
- Coid J, Yang M, Roberts A, Ullrich S, Moran P, Bebbington P, et al. Violence and psychiatric morbidity in a national household population – a report from the British Household Survey. Am J Epidemiol 2006;164:1199-208. http://dx.doi.org/10.1093/aje/kwj339.
- Coid J, Yang M, Tyrer P, Roberts A, Ullrich S. Prevalence and correlates of personality disorder in Great Britain. Br J Psychiatry 2006;188:423-31. http://dx.doi.org/10.1192/bjp.188.5.423.
- Hodgins S. Mental disorder, intellectual deficiency, and crime. Evidence from a birth cohort. Arch Gen Psychiatry 1992;49:476-83. http://dx.doi.org/10.1001/archpsyc.1992.01820060056009.
- Hodgins S, Janson CG. Criminality and Violence among the Mentally Disordered. Cambridge: Cambridge University Press; 2002.
- Tiihonen J, Isohanni M, Rasanen P, Koiranen M, Moring J. Specific major mental disorders and criminality: a 26-year prospective study of the 1966 northern Finland birth cohort. Am J Psychiatry 1997;154:840-5. http://dx.doi.org/10.1176/ajp.154.6.840.
- Brennan PA, Mednick SA, Hodgins S. Major mental disorders and criminal violence in a Danish birth cohort. Arch Gen Psychiatry 2000;57:494-500. http://dx.doi.org/10.1001/archpsyc.57.5.494.
- Arseneault L, Moffitt TE, Caspi A, Taylor PJ, Silva PA. Mental disorders and violence in a total birth cohort: results from the Dunedin study. Arch Gen Psychiatry 2000;57:979-86. http://dx.doi.org/10.1001/archpsyc.57.10.979.
- Winett LB. Constructing violence as a public health problem. Public Health Rep 1998;113:498-507.
- Stanistreet D. Violence: developing a policy agenda. J Epidemiol Community Health 1999;53:2-3. http://dx.doi.org/10.1136/jech.53.1.2.
- Bellis MA, Hughes K, Anderson Z, Tocque K, Hughes S. Contribution of violence to health inequalities in England: demographics and trends in emergency hospital admissions for assault. J Epidemiol Community Health 2008;62:1064-71. http://dx.doi.org/10.1136/jech.2007.071589.
- Office for National Statistics . Crime in England and Wales, Year Ending June 2012 n.d. www.ons.gov.uk.
- Taylor P, Bond S. Crimes Detected in England and Wales 2011/12. London: Home Office; 2012.
- Bellis MA, Hughes K, Wood S, Wykes S, Perkins C. National five-year examination of inequalities and trends in emergency hospital admission for violence across England. Inj Prevent 2011;17:319-25. http://dx.doi.org/10.1136/ip.2010.030486.
- Bellis MA, Lowey H, Hughes K, Deacon L, Stansfield J, Perkins C. Variations in risk and protective factors for life satisfaction and mental wellbeing with deprivation: a cross-sectional study. BMC Public Health 2012;12. http://dx.doi.org/10.1186/1471-2458-12-492.
- Bellis MA, Hughes K, Perkins C, Benneth A. Protecting People Promoting Health: A Public Health Approach to Violence Prevention for England. Liverpool: Department of Health; 2012.
- Singleton N, Bumpstead R, O’Brien M. Psychiatric Morbidity Among Adults Living in Private Households, 2000. London: The Stationery Office; 2001.
- Shrout PE, Newman SC. Design of two-phase prevalence surveys of rare disorders. Biometrics 1989;45:549-55. http://dx.doi.org/10.2307/2531496.
- Kish L. Survey Sampling. London: Wiley; 1965.
- McManus S, Meltzer H, Brugha TS, Bebbington PE, Jerkin R. Adult Psychiatric Morbidity in England, 2007: Results of a Household Survey. Leeds: National Centre for Social Research/NHS Information Centre; 2009.
- Biddle L, Gunnell D, Sharp D, Donovan JL. Factors influencing help seeking in mentally distressed young adults: a cross-sectional survey. Br J Gen Pract 2004;54:248-53.
- Oliver MI, Pearson N, Coe N, Gunnell D. Help-seeking behaviour in men and women with common mental health problems: cross-sectional study. Br J Psychiatry 2005;186:297-301. http://dx.doi.org/10.1192/bjp.186.4.297.
- Bebbington P, Nayani T. The psychosis screening questionnaire. Int J Methods Psychiatr Res 1995;5:11-20.
- Ullrich S, Deasy D, Smith J, Johnson B, Clarke M, Broughton N, et al. Detecting personality disorders in the prison population of England and Wales: comparing case identification using the SCID-II screen and the SCID-II clinical interview. J Forensic Psychiatry Psychol 2008;19:301-22. http://dx.doi.org/10.1080/14789940802045182.
- First MB, Gibbon M, Spritzer RL, William JBW, Benjamin LS. Structured Clinical Interview for DSM-IV Axis II Personality Disorders. Washington, DC: American Psychiatric Press; 1997.
- Zigmond AS, Snaith RP. The Hospital Anxiety and Depression Scale. Acta Psychiatr Scand 1983;67:361-70. http://dx.doi.org/10.1111/j.1600-0447.1983.tb09716.x.
- Lewis G, Pelosi AJ. Manual of the Revised Clinical Interview Schedule (CIS-R). London: Institute of Psychiatry; 1990.
- Babor TF, Higgings-Briddle JC, Saunders JB, Monteiro MG. AUDIT: the Alcohol Use Disorders Identification Test: Guidelines for Use in Primary Care. Geneva: WHO; 1992.
- Bohn MJ, Babor TF, Kranzler HR. The Alcohol Use Disorders Identification Test (AUDIT): validation of a screening instrument for use in medical settings. J Stud Alcohol 1995;56:423-32. http://dx.doi.org/10.15288/jsa.1995.56.423.
- Berman AH, Bergman H, Palmstierna T, Schlyter F. Evaluation of the Drug Use Disorders Identification Test (DUDIT) in criminal justice and detoxification settings and in a Swedish population sample. Eur Addict Res 2005;11:22-31. http://dx.doi.org/10.1159/000081413.
- Standard Occupational Classification Volume 3. London: Her Majesty’s Stationery Office; 1991.
- Elovainio M, Ferrie JE, Singh-Manoux A, Shipley M, Batty GD, Head J, et al. Socioeconomic differences in cardiometabolic factors: social causation or health-related selection? Evidence from the Whitehall II Cohort Study, 1991–2004. Am J Epidemiol 2011;174:779-89. http://dx.doi.org/10.1093/aje/kwr149.
- Stringhini S, Berkman L, Dugravot A, Ferrie JE, Marmot M, Kivimaki M, et al. Socioeconomic status, structural and functional measures of social support, and mortality: the British Whitehall II Cohort Study, 1985–2009. Am J Epidemiol 2012;175:1275-83. http://dx.doi.org/10.1093/aje/kwr461.
- Archer J. Sex differences in aggression in real-world settings: a meta-analytic review. Rev Gen Psychol 2004;8:291-322. http://dx.doi.org/10.1037/1089-2680.8.4.291.
- Trivers R, Campbell BB. Sexual Selection and the Descent of Man. Chicago, IL: Aldine; 1972.
- Daly M, Wilson M. Killing the competition: female/female and male/male homicide. Hum Nat 1990;1:81-107. http://dx.doi.org/10.1007/BF02692147.
- Weisfeld G, Archer J. Male Violence. London: Routledge; 1994.
- Tjaden P, Thoennes N. Prevalence and consequences of male to female and female to male intimate partner violence as measured by the National Violence Against Women survey. Violence Against Wom 2000;6:142-61. http://dx.doi.org/10.1177/10778010022181769.
- Black MC, Basile KC, Breiding NJ, Smith SG, Walters ML, Merrick MT, et al. The National Intimate Partner and Sexual Violence Survey (NISVS): 2010 Summary Report. Atlanta, GA: National Center for Injury Prevention and Control, Centers for Disease Control and Prevention; 2011.
- Dutton DG, Nicholls TL. The gender paradigm in domestic violence research and theory: part 1 – the conflict of theory and data. Aggress Violent Behav 2005;10:680-714. http://dx.doi.org/10.1016/j.avb.2005.02.001.
- Kantor GK, Jasinski JL, Jasinski JL, William LM. A Comprehensive Review of 20 Years of Research. Sage; 1998.
- Sampson RJ, Laub JH, Wimer C. Does marriage reduce crime? A counterfactual approach to within-individual causal effects. Criminology 2006;44:465-508. http://dx.doi.org/10.1111/j.1745-9125.2006.00055.x.
- Laub JH, Sampson RJ. Shared Beginnings, Divergent Lives: Delinquent Boys to Age 70. Cambridge, MA: Harvard University Press; 2003.
- Bersani BE, Laub JH, Nieuwbeerta P. Marriage and desistance from crime in the Netherlands: do gender and socio-historical context matter?. J Quant Criminol 2009;25:3-24. http://dx.doi.org/10.1007/s10940-008-9056-4.
- Laub JH, Nagin DS, Sampson RJ. Trajectories of change in criminal offending: good marriages and the desistance process. Am Sociol Rev 1998;63:225-38. http://dx.doi.org/10.2307/2657324.
- Coid J, Yang M. Violence and delayed social independence among young adult British men. Soc Psychiatry Psychiatr Epidemiol 2010;45:301-8. http://dx.doi.org/10.1007/s00127-009-0077-6.
- Settersten RA, Furstenber FF, Rumbaut RG. On the Frontier of Adulthood: Theory, Research and Public Policy. Chicago, IL: University of Chicago Press; 2005.
- Settersten RA, Furstenberg FF, Rumbaut RG. On the Frontier of Adulthood: Theory, Research, and Public Policy. Chicago, IL: University of Chicago Press; 2008.
- Bell B, Fasani F, Machin S. Crime and immigration: evidence from large immigrant waves. Rev Econ Stat 2013;21:1278-90. http://dx.doi.org/10.1162/REST_a_00337.
- Bell B, Machin S. Briefing: Immigration and Crime: Evidence for the UK and other Countries. Oxford: Migration Observatory; 2013.
- Brownfield D. Social class and violent behavior. Criminology 1986;24:421-38. http://dx.doi.org/10.1111/j.1745-9125.1986.tb00384.x.
- Raphael S, Winter-Ebmer R. Identifying the effect of unemployment on crime. J Law Econ 2001;44:259-83. http://dx.doi.org/10.1086/320275.
- Cramer C. Unemployment and Participation in Violence. London: School of Oriental and African Studies; 2010.
- McManus S, Multzer H, Brugha T, Bebbington P, Jenkins R. Adult Psychiatric Morbidity in England, 2007: Results of a Household Survey. Leeds: National Centre for Social Research/NHS Information Centre; 2009.
- Coid J, Yang M, Roberts A, Ullrich S, Moran P, Bebbington P, et al. Violence and psychiatric morbidity in the national household population of Britain: public health implications. Br J Psychiatry 2006;189:12-9. http://dx.doi.org/10.1192/bjp.189.1.12.
- Moffitt TE. Sex Differences in Antisocial Behaviour: Conduct Disorder, Delinquency, and Violence in the Dunedin Longitudinal Study. Cambridge University Press; 2001.
- Moffitt TE. Sex Differences in Antisocial Behaviour: Conduct Disorder, Delinquency, and Violence in the Dunedin Longitudinal Study Series. Cambridge: Cambridge University Press; 2001.
- Yang M, Coid J. Gender differences in psychiatric morbidity and violent behaviour among a household population in Great Britain. Soc Psychiatry Psychiatr Epidemiol 2007;42:599-605. http://dx.doi.org/10.1007/s00127-007-0226-8.
- Monahan J. Mental disorder and violent behavior: perceptions and evidence. Am Psychol 1992;47. http://dx.doi.org/10.1037/0003-066X.47.4.511.
- Fazel S, Gulati G, Linsell L, Geddes JR, Grann M. Schizophrenia and violence: systematic review and meta-analysis. PLOS Med 2009;6. http://dx.doi.org/10.1371/journal.pmed.1000120.
- Bonta J, Law M, Hanson K. The prediction of criminal and violent recidivism among mentally disordered offenders: a meta-analysis. Psychol Bull 1998;123:123-42. http://dx.doi.org/10.1037/0033-2909.123.2.123.
- Coid J, Ullrich S. Antisocial personality disorder and anxiety disorder: a diagnostic variant?. J Anxiety Disord 2010;24:452-60. http://dx.doi.org/10.1016/j.janxdis.2010.03.001.
- Douglas KS, Guy LS, Hart SD. Psychosis as a risk factor for violence to others: a meta-analysis. Psychol Bull 2009;135:679-706. http://dx.doi.org/10.1037/a0016311.
- International Statistical Classification of Diseases and Related Health Problems. Geneva: WHO; n.d.
- Cannon W. Wisdom of the Body. New York, NY: WW Norton; 1932.
- Jansen AS, Nguyen XV, Karpitskiy V, Mettenleiter TC, Loewy AD. Central command neurons of the sympathetic nervous system: basis of the fight-or-flight response. Science 1995;270:644-6. http://dx.doi.org/10.1126/science.270.5236.644.
- Kunimatsu MM, Marsee MA. Examining the presence of anxiety in aggressive individuals: the illuminating role of flight-or-fight mechanisms. Child Youth Care Forum 2012;41:247-58. http://dx.doi.org/10.1007/s10566-012-9178-6.
- Fowles DC, Missel K, Fowles D, Sutkers P. Progress in Experimental Personality and Psychopathology Research 1994: Special Focus on Psychopathology and Antisocial Behaviour – a Developmental Perspective. New York, NY: Springer; 1994.
- Gray JA. The Psychology of Fear and Stress. Cambridge: Cambridge University Press; 1989.
- Shorey RC, Emlmquist J, Nihneman A, Brasfield H, Febres J, Rothman EF, et al. The association between intimate partner violence perpetration, victimisation, and mental health among women arrested for domestic violence. Partner Abuse 2012;3:3-21. http://dx.doi.org/10.1891/1946-6560.3.1.3.
- Shorey RC, Febres J, Brasfield H, Stuart GL. The prevalence of mental health problems in men arrested for domestic violence. J Fam Violence 2012;27:741-8. http://dx.doi.org/10.1007/s10896-012-9463-z.
- Stuart GL, Moore TM, Gordon KC, Ramsey SE, Kahler CW. Psychopathology in women arrested for domestic violence. J Interpers Violence 2006;21:376-89. http://dx.doi.org/10.1177/0886260505282888.
- Mauricio AM, Lopez FG. A latent classification of male batterers. Violence Vict 2009;24:419-38. http://dx.doi.org/10.1891/0886-6708.24.4.419.
- Campbell L, Marshall T. Anxious attachment and relationship processes: an interactionist perspective. J Pers 2011;79:917-47. http://dx.doi.org/10.1111/j.1467-6494.2011.00723.x.
- Campbell L, Simpson JA, Boldry J, Kashy DA. Perceptions of conflict and support in romantic relationships: the role of attachment anxiety. J Pers Soc Psychol 2005;88:510-31. http://dx.doi.org/10.1037/0022-3514.88.3.510.
- Doumas DM, Pearson CL, Elgin JE, McKinley CC. Adult attachment as a risk factor for intimate partner violence: the ‘mispairing’ of partner’s attachment styles. J Interpers Violence 2008;23:616-34. http://dx.doi.org/10.1177/0886260507313526.
- Pan HS, Neidig PH, Oleary KD. Predicting mild and severe husband-to-wife physical aggression. J Consult Clin Psychol 1994;62:975-81. http://dx.doi.org/10.1037/0022-006X.62.5.975.
- Feldbau-Kohn S, Heyman RE, O’Leary K. Major depressive disorder and depressive symptomatology as predictors of husband to wife physical aggression. Violence Vict 1998;13:347-60.
- Koh KB, Kim CH, Park JK. Predominance of anger in depressive disorders compared with anxiety disorders and somatoform disorders. J Clin Psychiatry 2002;63:486-92. http://dx.doi.org/10.4088/JCP.v63n0604.
- Busch FN. Anger and depression. Adv Psychiatr Treat 2009;15:271-8. http://dx.doi.org/10.1192/apt.bp.107.004937.
- Fazel S, Langstrom N, Hjern A, Grann M, Lichtenstein P. Schizophrenia, substance abuse, and violent crime. JAMA 2009;301:2016-23. http://dx.doi.org/10.1001/jama.2009.675.
- Fazel S, Lichtenstein P, Grann M, Goodwin GM, Langstrom N. Bipolar disorder and violent crime: new evidence from population-based longitudinal studies and systematic review. Arch Gen Psychiatry 2010;67:931-8. http://dx.doi.org/10.1001/archgenpsychiatry.2010.97.
- Mojtabai R. Psychotic-like experiences and interpersonal violence in the general population. Soc Psychiatry Psychiatr Epidemiol 2006;41:183-90. http://dx.doi.org/10.1007/s00127-005-0020-4.
- Elbogen EB, Johnson SC. The intricate link between violence and mental disorder: results from the National Epidemiologic Survey on Alcohol and Related Conditions. Arch Gen Psychiatry 2009;66:152-61. http://dx.doi.org/10.1001/archgenpsychiatry.2008.537.
- Junginger J, McGuire L. Psychotic motivation and the paradox of current research on serious mental illness and rates of violence. Schizophr Bull 2004;30:21-30. http://dx.doi.org/10.1093/oxfordjournals.schbul.a007064.
- Skeem JL, Mulvey EP. Psychopathy and community violence among civil psychiatric patients: results from the MacArthur Violence Risk Assessment Study. J Consult Clin Psychol 2001;69:358-74. http://dx.doi.org/10.1037/0022-006X.69.3.358.
- Douglas KS, Skeem JL. Violence risk assessment: getting specific about being dynamic. Psychol Public Policy Law 2005;11:347-83. http://dx.doi.org/10.1037/1076-8971.11.3.347.
- Nielssen O, Large M. Rates of homicide during the first episode of psychosis and after treatment: a systematic review and meta-analysis. Schizophr Bull 2010;36:702-12. http://dx.doi.org/10.1093/schbul/sbn144.
- Van Dorn R, Volavka J, Johnson N. Mental disorder and violence: is there a relationship beyond substance use?. Soc Psychiatry Psychiatr Epidemiol 2012;47:487-503. http://dx.doi.org/10.1007/s00127-011-0356-x.
- Swanson JW, Swartz MS, Van Dorn RA, Elbogen EB, Wagner HR, Rosenheck RA, et al. A national study of violent behavior in persons with schizophrenia. Arch Gen Psychiatry 2006;63:490-9. http://dx.doi.org/10.1001/archpsyc.63.5.490.
- Krakowski MI, Czobor P, Citrome L, Bark N, Cooper TB. Atypical antipsychotic agents in the treatment of violent patients with schizophrenia and schizoaffective disorder. Arch Gen Psychiatry 2006;63:622-9. http://dx.doi.org/10.1001/archpsyc.63.6.622.
- Bentall RP. The illusion of reality: a review and integration of psychological research on hallucinations. Psychol Bull 1990;107:82-95. http://dx.doi.org/10.1037/0033-2909.107.1.82.
- Persons JB. The advantages of studying psychological phenomena rather than psychiatric diagnoses. Am Psychol 1986;41:1252-60. http://dx.doi.org/10.1037/0003-066X.41.11.1252.
- Link BG, Stueve A, Phelan J. Psychotic symptoms and violent behaviors: probing the components of ‘threat/control-override’ symptoms. Soc Psychiatry Psychiatr Epidemiol 1998;33:S55-60. http://dx.doi.org/10.1007/s001270050210.
- Swanson JW, Borum R, Swartz MS, Monahan J. Psychotic symptoms and disorders and the risk of violent behaviour in the community. Crim Behav Ment Health 1996;6:309-29. http://dx.doi.org/10.1002/cbm.118.
- Kinoshita Y, Shimodera S, Nishida A, Kinoshita K, Watanabe N, Oshima N, et al. Psychotic-like experiences are associated with violent behavior in adolescents. Schizophr Res 2011;126:245-51. http://dx.doi.org/10.1016/j.schres.2010.08.028.
- Personality Disorder, No Longer a Diagnosis of Exclusion. London: Department of Health; 2003.
- Johnson JG, Cohen P, Smailes E, Kasen S, Oldham JM, Skodol AE, et al. Adolescent personality disorders associated with violence and criminal behavior during adolescence and early adulthood. Am J Psychiatry 2000;157:1406-12. http://dx.doi.org/10.1176/appi.ajp.157.9.1406.
- Berman ME, Fallon AE, Coccaro EF. The relationship between personality psychopathology and aggressive behavior in research volunteers. J Abnorm Psychol 1998;107:651-8. http://dx.doi.org/10.1037/0021-843X.107.4.651.
- Roberts A, Coid J. Personality disorder and offending behaviour: finding from the national survey of male prisoners in England and Wales. J Forensic Psychiatry Psychol 2010;21:221-37. http://dx.doi.org/10.1080/14789940903303811.
- Coid JW, Tyrer P, Stein G. Personality Disorder Reviewed. London: Royal College of Psychiatrists; 1993.
- Farrington DP. The development of offending and antisocial behaviour from childhood: key findings from the Cambridge Study in delinquent development. J Child Psychol Psychiatry 1995;36:926-64.
- Coid JW, Skodal AE. Psychopathology and Violent Crime. Washington, DC: American Psychiatric Press; 1998.
- Hodgins S. Epidemiologic investigations of the associations between major mental disorder and crime. Methodological limitations and validity of the conclusions. Soc Psychiatry Psychiatr Epidemiol 1998;33:S29-37. http://dx.doi.org/10.1007/s001270050207.
- Hodgins S, Mednick SA, Brennan PA, Schulsinger F, Engberg M. Mental disorder and crime. Evidence from a Danish birth cohort. Arch Gen Psychiatry 1996;53:489-96. http://dx.doi.org/10.1001/archpsyc.1996.01830060031004.
- Rasmussen K, Levander S. Crime and violence among psychiatric patients in a maximum security psychiatric hospital. Crim Justice Behav 1996;23:455-71. http://dx.doi.org/10.1177/0093854896023003003.
- Diagnostic and Statistical Manual of Mental Disorders. Washington, DC: American Psychiatric Association; 2013.
- Borderline Personality Disorder: Treatment and Management. London: NICE; 2009.
- Antisocial Personality Disorder: The NICE Guidelines on Treatment, Management and Prevention. London: British Psychological Society and Royal College of Psychiatrists; 2010.
- Diagnostic and Statistical Manual of Mental Disorders. Washington, DC: American Psychiatric Association; 2000.
- Yu RQ, Geddes JR, Fazel S. Personality disorders, violence, and antisocial behavior: a systematic review and meta-regression analysis. J Pers Disord 2012;26:775-92. http://dx.doi.org/10.1521/pedi.2012.26.5.775.
- Hare RD. Manual of the Psychopathy Checklist – Revised. Toronto, ON: Multi-Health Systems; 2003.
- Moffitt TE. Adolescence-limited and life-course-persistent antisocial-behavior: a developmental taxonomy. Psychol Rev 1993;100:674-701. http://dx.doi.org/10.1037/0033-295X.100.4.674.
- Lewis DO, Pincus JH, Bard B, Richardson E, Prichep LS, Feldman M, et al. Neuropsychiatric, psychoeducational, and family characteristics of 14 juveniles condemned to death in the United States. Am J Psychiatry 1988;145:584-9. http://dx.doi.org/10.1176/ajp.145.5.584.
- Lewis DO, Pincus JH, Feldman M, Jackson L, Bard B. Psychiatric, neurological, and psychoeducational characteristics of 15 death-row inmates in the United States. Am J Psychiatry 1986;143:838-45. http://dx.doi.org/10.1176/ajp.143.7.838.
- Martell DA. Estimating the prevalence of organic brain dysfunction in maximum-security forensic psychiatric patients. J Forensic Sci 1992;37:878-93. http://dx.doi.org/10.1520/JFS12002J.
- Filley CM, Price BH, Nell V, Antionette T, Morgan AS, Bresnahan JF, et al. Toward an understanding of violence: neurobehavioural aspects of unwarranted physical aggression: Aspen Neurobehavioural Conference Consensus Statement. Neuropsychiatry Neuropsychol Behav Neurol 2001;14:1-14.
- Elliott FA. The episodic dyscontrol syndrome and aggression. Neurol Clin 1984;2:113-25.
- Raine A. The Anatomy of Violence: The Biologival Roots of Crime. New York, NY: Random House; 2013.
- Bufkin JL, Luttrell VR. Neuroimaging studies of aggressive and violent behavior: current findings and implications for criminology and criminal justice. Trauma Violence Abuse 2005;6:176-91. http://dx.doi.org/10.1177/1524838005275089.
- Lundström S, Forsman M, Larsson H, Kerekes N, Serlachius E, Langstrom N, et al. Childhood neurodevelopmental disorders and violent criminality: a sibling control study. J Autism Dev Disord 2014;44:2707-16. http://dx.doi.org/10.1007/s10803-013-1873-0.
- Nelson HE, Willison J. National Adult Reading Test (NART): Test Manual (2nd Edition). Windsor: NFER Nelson; 1991.
- Kaufman AS, Lichtenberger EO. Assessing Adolescent and Adult Intelligence. John Wiley & Sons; 2005.
- Singer SI. Homogenous victim offender populations: a review and some research implications. J Crim Law Criminol 1981;72:779-88. http://dx.doi.org/10.2307/1143015.
- Crawford JR, Allan KM, Cochrane RH, Parker DM. Assessing the validity of NART-estimated premorbid IQs in the individual case. Br J Clin Psychol 1990;29:435-6. http://dx.doi.org/10.1111/j.2044-8260.1990.tb00908.x.
- Crawford JR, Deary IJ, Starr J, Whalley LJ. The NART as an index of prior intellectual functioning: a retrospective validity study covering a 66-year interval. Psychol Med 2001;31:451-8. http://dx.doi.org/10.1017/S0033291701003634.
- Frisell T, Pawitan Y, Langstrom N. Is the association between general cognitive ability and violent crime caused by family-level confounders?. PLOS ONE 2012;7. http://dx.doi.org/10.1371/journal.pone.0041783.
- Barker ED, Seguin JR, White HR, Bates ME, Lacourse E, Carbonneau R, et al. Developmental trajectories of male physical violence and theft: relations to neurocognitive performance. Arch Gen Psychiatry 2007;64:592-9. http://dx.doi.org/10.1001/archpsyc.64.5.592.
- Theobald D, Farrington DP. Child and adolescent predictors of male intimate partner violence. J Child Psychol Psychiatry 2012;53:1242-9. http://dx.doi.org/10.1111/j.1469-7610.2012.02577.x.
- Martin LT, Kubzansky LD. Childhood cognitive performance and risk of mortality: a prospective cohort study of gifted individuals. Am J Epidemiol 2005;162:887-90. http://dx.doi.org/10.1093/aje/kwi300.
- Kandel E, Mednick SA, Kirkegaard-Sorensen L, Hutchings B, Knop J, Rosenberg R, et al. IQ as a protective factor for subjects at high risk for antisocial behavior. J Consult Clin Psychol 1988;56:224-6. http://dx.doi.org/10.1037/0022-006X.56.2.224.
- Kessler RC, Adler L, Ames M, Demler O, Faraone S, Hiripi E, et al. The World Health Organization Adult ADHD Self-Report Scale (ASRS): a short screening scale for use in the general population. Psychol Med 2005;35:245-56. http://dx.doi.org/10.1017/S0033291704002892.
- Kessler RC, Adler LA, Gruber MJ, Sarawate CA, Spencer T, Van Brunt DL. Validity of the World Health Organization Adult ADHD Self-Report Scale (ASRS) Screener in a representative sample of health plan members. Int J Methods Psychiatr Res 2007;16:52-65. http://dx.doi.org/10.1002/mpr.208.
- Bernat DH, Oakes JM, Pettingell SL, Resnick M. Risk and direct protective factors for youth violence: results from the National Longitudinal Study of Adolescent Health. Am J Prev Med 2012;43:S57-66. http://dx.doi.org/10.1016/j.amepre.2012.04.023.
- Loeber R, Pardini D, Homish DL, Wei EH, Crawford AM, Farrington DP, et al. The prediction of violence and homicide in young men. J Consult Clin Psychol 2005;73:1074-88. http://dx.doi.org/10.1037/0022-006X.73.6.1074.
- Chang Z, Lichtenstein P, Larsson H. The effects of childhood ADHD symptoms on early-onset substance use: a Swedish twin study. J Abnorm Child Psychol 2012;40:425-35. http://dx.doi.org/10.1007/s10802-011-9575-6.
- Lee SS, Hinshaw SP. Predictors of adolescent functioning in girls with attention deficit hyperactivity disorder (ADHD): the role of childhood ADHD, conduct problems, and peer status. J Clin Child Adolesc Psychol 2006;35:356-68. http://dx.doi.org/10.1207/s15374424jccp3503_2.
- Hofvander B, Stahlberg O, Nyden A, Wentz E, degl’Innocenti A, Billstedt E, et al. Life History of Aggression scores are predicted by childhood hyperactivity, conduct disorder, adult substance abuse, and low cooperativeness in adult psychiatric patients. Psychiatry Res 2011;185:280-5. http://dx.doi.org/10.1016/j.psychres.2010.05.008.
- Barkley RA. The executive functions and self-regulation: an evolutionary neuropsychological perspective. Neuropsychol Rev 2001;11:1-29. http://dx.doi.org/10.1023/A:1009085417776.
- Barkley RA, Fischer M. The unique contribution of emotional impulsiveness to impairment in major life activities in hyperactive children as adults. J Am Acad Child Adolesc Psychiatry 2010;49:503-13.
- Barkley RA. Differential diagnosis of adults with ADHD: the role of executive function and self-regulation. J Clin Psychiatry 2010;71. http://dx.doi.org/10.4088/JCP.9066tx1c.
- Lord C, Volkmar F. Genetics of childhood disorders: XLII. Autism, part 1: dagnosis and assessment in autistic spectrum disorders. J Am Acad Child Adolesc Psychiatry 2002;41:1134-6. http://dx.doi.org/10.1097/00004583-200209000-00015.
- Brugha TS, McManus S, Smith J, Scott FJ, Meltzer H, Purdon S, et al. Validating two survey methods for identifying cases of autism spectrum disorder among adults in the community. Psychol Med 2012;42:647-56. http://dx.doi.org/10.1017/S0033291711001292.
- Lerner MD, Haque OS, Northrup EC, Lawer L, Bursztajn HJ. Emerging perspectives on adolescents and young adults with high-functioning autism spectrum disorders, violence, and criminal law. J Am Acad Psychiatry Law 2012;40:177-90.
- Hoaken PN, Stewart SH. Drugs of abuse and the elicitation of human aggressive behavior. Addict Behav 2003;28:1533-54. http://dx.doi.org/10.1016/j.addbeh.2003.08.033.
- Eronen M, Angermeyer MC, Schulze B. The psychiatric epidemiology of violent behaviour. Soc Psychiatry Psychiatr Epidemiol 1998;33:S13-23. http://dx.doi.org/10.1007/s001270050205.
- Grann M, Fazel S. Substance misuse and violent crime: Swedish population study. BMJ 2004;328:1233-4. http://dx.doi.org/10.1136/bmj.328.7450.1233.
- Shaw J, Hunt IM, Flynn S, Amos T, Meehan J, Robinson J, et al. The role of alcohol and drugs in homicides in England and Wales. Addiction 2006;101:1117-24. http://dx.doi.org/10.1111/j.1360-0443.2006.01483.x.
- Haggard-Grann U, Hallqvist J, Langstrom N, Moller J. The role of alcohol and drugs in triggering criminal violence: a case-crossover study. Addiction 2006;101:100-8. http://dx.doi.org/10.1111/j.1360-0443.2005.01293.x.
- Graham K, Leonard KE, Room R, Wild TC, Pihl RO, Bois C, et al. Current directions in research on understanding and preventing intoxicated aggression. Addiction 1998;93:659-76. http://dx.doi.org/10.1046/j.1360-0443.1998.9356593.x.
- Sivarajasingam V, Shepherd J, Matthews K, Jones S. Trends in violence in England and Wales 1995–2000: an accident and emergency perspective. J Public Health Med 2002;24:219-26. http://dx.doi.org/10.1093/pubmed/24.3.219.
- Hutchinson H, Mageunis P, Shepherd JP, Brown AE. The BAOMS United Kingdom survey of facial injuries, part 1: aetiology and the association with alcohol consumption. Br J Oral Maxillofac Surg 1998;36:3-13. http://dx.doi.org/10.1016/S0266-4356(98)90739-2.
- Arseneault L, Moffit TE, Caspi A, Taylor A. The targets of violence committed by young offenders with alcohol dependence, marijuana dependence and schizophrenia-spectrum disorders: findings from a birth cohort. Crim Behav Ment Health 2002;12:155-68. http://dx.doi.org/10.1002/cbm.493.
- Malgady RG, Rogler LH, Tryon WW. Issues of validity in the Diagnostic Interview Schedule. J Psychiatr Res 1992;26:59-67. http://dx.doi.org/10.1016/0022-3956(92)90016-H.
- White HR, Stoff DM, Brejling J, Maser JD. Handbook of Antisocial Behaviour. New York, NY: John Wiley; 1997.
- Babor TF, Higgings-Briddle JC, Saunders JB, Monteiro M. The Alcohol Use Disorders Identification Test. Geneva: WHO; 2001.
- Strohm RB, Wolfgang ME. The relationship between alcohol and criminal homicide. Q J Stud Alcohol 1956;17:411-25.
- Moller-Madsen B, Dalgaard JB, Charles AV, Grymer F, Hedeboe J, Jensen SE, et al. Alcohol involvement in violence. A study from a Danish community. Z Rechtsmed 1986;97:141-6. http://dx.doi.org/10.1007/BF00204447.
- Kingma J. Alcohol consumption in victims of violence: a trend study for the period 1970–1998. Psychol Rep 2000;87:803-11. http://dx.doi.org/10.2466/PR0.87.7.803-811.
- Shepherd J, Irish M, Scully C, Leslie I. Alcohol consumption among victims of violence and among comparable UK populations. Br J Addict 1989;84:1045-51. http://dx.doi.org/10.1111/j.1360-0443.1989.tb00787.x.
- Liquor Licensing, England and Wales July 2000–June 2001. London: Home Office; 2002.
- Hobbs D, Hadfield P, Lister S. Violence and Governance in the Night-Time Economy. Oxford: Oxford University Press; 2005.
- Broidy LM, Daday JK, Crandall CS, Slklar DP, Jost PF. Exploring demographic structural and behavioural overlap among homicide offenders and victims. Homicide Stud 2006;10:155-80. http://dx.doi.org/10.1177/1088767906288577.
- Gottfredson MR. On the etiology of criminal victimisation. J Crim Law Criminol 1981;74:714-26. http://dx.doi.org/10.2307/1143011.
- Hinderlang MJ. Criminal Victimisation in Eight American Cities: a Descriptive Analysis of Common Theft and Assault. Cambridge, MA: Ballinger; 1976.
- Lauritsen JL, Laub JH, Hough M, Maxfield M. Crime Prevention Studies: Vol. 22. Surveying Crime in the 21st Century. Monsey, NY: Criminal Justice Press; 2007.
- Lauritsen JL, Sampson RJ, Laub JH. The link between offending and victimisation among adolescents. Criminology 1991;29:265-92. http://dx.doi.org/10.1111/j.1745-9125.1991.tb01067.x.
- Schrek CJ, Wright RA, Miller JM. A study of individual and situational antecedents of violent victimisation. Justice Q 2002;19:159-80. http://dx.doi.org/10.1080/07418820200095201.
- Mustaine EE, Tewksbury R. Comparing the lifestyles of victims, offenders, and victim-offenders: a routine activity theory assessment of similarities and differences for criminal incident participants. Sociol Focus 2000;33:339-62. http://dx.doi.org/10.1080/00380237.2000.10571174.
- Wolfgang ME. Patterns in Criminal Homicide. New York, NY: Wiley; 1958.
- Posick C. The overlap between offending and victimisation among adolescents. J Contemp Crim Justice 2013;29:106-24. http://dx.doi.org/10.1177/1043986212471250.
- Anderson SW, Bechara A, Damasio H, Tranel D, Damasio AR. Impairment of social and moral behavior related to early damage in human prefrontal cortex. Nat Neurosci 1999;2:1032-7. http://dx.doi.org/10.1038/12194.
- Harding D. Living the Drama: Community, Conflict, and Culture among Inner City Boys. Chicago, IL: University of Chicago Press; 2010.
- Coid JW, Ullrich S, Keers R, Bebbington P, Destavola BL, Kallis C, et al. Gang membership, violence, and psychiatric morbidity. Am J Psychiatry 2013;170:985-93. http://dx.doi.org/10.1176/appi.ajp.2013.12091188.
- Luckenbill DF. Criminal homicide as a situated transaction. Soc Probl 1977;25:176-86. http://dx.doi.org/10.2307/800293.
- Katz J. Seductions of Crime: Moral and Sensual Attractions in Doing Evil. New York, NY: Basic Books; 1988.
- Widom CS. The cycle of violence. Science 1989;244:160-6. http://dx.doi.org/10.1126/science.2704995.
- Garmezy N, Rabin AI, Aronoff J, Barclay AM, Zucker RA. Further Explorations in Personality. New York, NY: Wiley; 1981.
- Forsman M, Langstrom N. Child maltreatment and adult violent offending: population-based twin study addressing the ‘cycle of violence’ hypothesis. Psychol Med 2012;42:1977-83. http://dx.doi.org/10.1017/S0033291711003060.
- Nelson BS, Wampler KS. Systemic effects of trauma in clinic couples: an exploratory study of secondary trauma resulting from childhood abuse. J Marital Fam Ther 2000;26:171-84. http://dx.doi.org/10.1111/j.1752-0606.2000.tb00287.x.
- Dinwiddie S, Heath AC, Dunne MP, Bucholz KK, Madden PA, Slutske WS, et al. Early sexual abuse and lifetime psychopathology: a co-twin-control study. Psychol Med 2000;30:41-52. http://dx.doi.org/10.1017/S0033291799001373.
- Asgeirsdottir BB, Sigfusdottir ID, Gudjonsson GH, Sigurdsson JF. Associations between sexual abuse and family conflict/violence, self-injurious behavior, and substance use: the mediating role of depressed mood and anger. Child Abuse Negl 2011;35:210-19. http://dx.doi.org/10.1016/j.chiabu.2010.12.003.
- Noll JG, Horowitz LA, Bonanno GA, Trickett PK, Putnam FW. Revictimization and self-harm in females who experienced childhood sexual abuse: results from a prospective study. J Interpers Violence 2003;18:1452-71. http://dx.doi.org/10.1177/0886260503258035.
- Follette VM, Polusny MA, Bechtle AE, Naugle AE. Cumulative trauma: the impact of child sexual abuse, adult sexual assault, and spouse abuse. J Trauma Stress 1996;9:25-3. http://dx.doi.org/10.1002/jts.2490090104.
- Coid J, Petruckevitch A, Feder G, Chung W, Richardson J, Moorey S. Relation between childhood sexual and physical abuse and risk of revictimisation in women: a cross-sectional survey. Lancet 2001;358:450-4. http://dx.doi.org/10.1016/S0140-6736(01)05622-7.
- Messman-Moore TL, Long PJ, Siegfried NJ. The revictimization of child sexual abuse survivors: an examination of the adjustment of college women with child sexual abuse, adult sexual assault, and adult physical abuse. Child Maltreat 2000;5:18-27. http://dx.doi.org/10.1177/1077559500005001003.
- Maas C, Herrenkohl TI, Sousa C. Review of research on child maltreatment and violence in youth. Trauma Violence Abuse 2008;9:56-67. http://dx.doi.org/10.1177/1524838007311105.
- Kilpatrick DG, Acierno R. Mental health needs of crime victims: epidemiology and outcomes. J Trauma Stress 2003;16:119-32. http://dx.doi.org/10.1023/A:1022891005388.
- Arata CM. Child sexual abuse and sexual revictimisation. Clin Psychol Sci Pract 2009;9:135-64. http://dx.doi.org/10.1093/clipsy.9.2.135.
- Finkelhor D, Ormrod RK, Turner HA. Revictimisation patterns in a national longitudinal sample of children and youth. Child Abuse Neglect 2007;31:479-502. http://dx.doi.org/10.1016/j.chiabu.2006.03.012.
- Kochenderfer-Ladd B. Identification of aggressive and asocial victims and the stability of their peer victimisation. Merrill-Palmer Q 2003;49:401-25. http://dx.doi.org/10.1353/mpq.2003.0022.
- Kochenderfer-Ladd B, Ladd GW, Juvonen J, Graham S. Peer Harassment in School: the Plight of the Vulnerable and Victimised. New York, NY: Guilford Press; 2001.
- Singh GK, Yu SM. Trends and differentials in adolescent and young adult mortality in the United States, 1950 through 1993. Am J Public Health 1996;86:560-4. http://dx.doi.org/10.2105/AJPH.86.4.560.
- Cubbin C, LeClere FB, Smith GS. Socioeconomic status and injury mortality: individual and neighbourhood determinants. J Epidemiol Community Health 2000;54:517-24. http://dx.doi.org/10.1136/jech.54.7.517.
- Cubbin C, Pickle LW, Fingerhut L. Social context and geographic patterns of homicide among US black and white males. Am J Public Health 2000;90:579-87. http://dx.doi.org/10.2105/AJPH.90.4.579.
- Howe A, Crilly M. Deprivation and violence in the community: a perspective from a UK Accident and Emergency department. Injury 2001;32:349-51. http://dx.doi.org/10.1016/S0020-1383(01)00015-8.
- Mair JS, Mair M. Violence prevention and control through environmental modifications. Annu Rev Public Health 2003;24:209-25. http://dx.doi.org/10.1146/annurev.publhealth.24.100901.140826.
- Leyland AH, Dundas R. The social patterning of deaths due to assault in Scotland, 1980–2005: population-based study. J Epidemiol Community Health 2010;64:432-9. http://dx.doi.org/10.1136/jech.2009.095018.
- Jones SJ, Sivarajasingam V, Shepherd J. The impact of deprivation on youth violence: a comparison of cities and their feeder towns. Emerg Med J 2011;28:496-9. http://dx.doi.org/10.1136/emj.2009.090282.
- Krieger N, Waterman PD, Chen JT, Soobader MJ, Subramanian SV. Monitoring socioeconomic inequalities in sexually transmitted infections, tuberculosis, and violence: geocoding and choice of area-based socioeconomic measures – the public health disparities geocoding project (US). Public Health Rep 2003;118:240-60.
- Khalifeh H, Hargreaves J, Howard LM, Birdthistle I. Intimate partner violence and socioeconomic deprivation in England: findings from a national cross-sectional survey. Am J Public Health 2013;103:462-72. http://dx.doi.org/10.2105/AJPH.2012.300723.
- Sabina C. Individual and national level associations between economic deprivation and partner violence among college students in 31 national settings. Aggress Behav 2013;39:247-56. http://dx.doi.org/10.1002/ab.21479.
- Fagan J, Tonry M, Norris N. Crime and Justice: A Review of Research. Chicago, IL: University of Chicago Press; 1990.
- Cusimano M, Marshall S, Rinner C, Jiang D, Chipman M. Patterns of urban violent injury: a spatio-temporal analysis. PLOS ONE 2010;5. http://dx.doi.org/10.1371/journal.pone.0008669.
- The Science of Adolescent Risk-Taking: Workshop Report. Washington, DC: Institute of Medicine and National Research Council Committee on the Science of Adolescence; 2011.
- MacPherson L, Reynolds EK, Daughters SB, Wang F, Cassidy J, Mayes LC, et al. Positive and negative reinforcement underlying risk behavior in early adolescents. Prev Sci 2010;11:331-42. http://dx.doi.org/10.1007/s11121-010-0172-7.
- Birkland MS, Leversen I, Torsheim T, Wold B. Development and aging: pathways to adulthood and their precursors and outcomes. Scand J Psychol 2014;55:26-32. http://dx.doi.org/10.1111/sjop.12087.
- Colman I, Wadsworth ME, Croudace TJ, Jones PB. Forty-year psychiatric outcomes following assessment for internalizing disorder in adolescence. Am J Psychiatry 2007;164:126-33. http://dx.doi.org/10.1176/ajp.2007.164.1.126.
- Sourander A, Jensen P, Davies M, Niemela S, Elonheimo H, Ristkari T, et al. Who is at greatest risk of adverse long-term outcomes? The Finnish From a Boy to a Man study. J Am Acad Child Adolesc Psychiatry 2007;46:1148-61. http://dx.doi.org/10.1097/chi.0b013e31809861e9.
- Mahalik JR, Levine Coley R, McPherran Lombardi C, Doyle Lynch A, Markowitz AJ, Jaffee SR. Changes in health risk behaviors for males and females from early adolescence through early adulthood. Health Psychol 2013;32:685-94. http://dx.doi.org/10.1037/a0031658.
- Byrnes JP, Miller DC, Schafer WD. Gender differences in risk taking. Psychol Bull 1999;125:367-83. http://dx.doi.org/10.1037/0033-2909.125.3.367.
- Leigh BC. Peril, chance, adventure: concepts of risk, alcohol use and risky behavior in young adults. Addiction 1999;94:371-83. http://dx.doi.org/10.1046/j.1360-0443.1999.9433717.x.
- Cooper ML, Agocha VB, Sheldon MS. A motivational perspective on risky behaviors: the role of personality and affect regulatory processes. J Pers 2000;68:1059-88. http://dx.doi.org/10.1111/1467-6494.00126.
- Lejuez CW, Read JP, Kahler CW, Richards JB, Ramsey SE, Stuart GL, et al. Evaluation of a behavioral measure of risk taking: the Balloon Analogue Risk Task (BART). J Exp Psychol Appl 2002;8:75-84. http://dx.doi.org/10.1037/1076-898X.8.2.75.
- Lejuez CW, Aklin WM, Jones HA, Richards JB, Strong DR, Kahler CW, et al. The Balloon Analogue Risk Task (BART) differentiates smokers and nonsmokers. Exp Clin Psychopharmacol 2003;11:26-33. http://dx.doi.org/10.1037/1064-1297.11.1.26.
- Zuckerman M. Sensation seeking and sports. Pers Individ Dif 1983;4:285-92. http://dx.doi.org/10.1016/0191-8869(83)90150-2.
- Steinberg L. A social neuroscience perspective on adolescent risk-taking. Dev Rev 2008;28:78-106. http://dx.doi.org/10.1016/j.dr.2007.08.002.
- Hawkins JD, Catalano RF, Miller JY. Risk and protective factors for alcohol and other drug problems in adolescence and early adulthood: implications for substance abuse prevention. Psychol Bull 1992;112:64-105. http://dx.doi.org/10.1037/0033-2909.112.1.64.
- Moffitt TE, Flannery DJ, Vazsonyi AT, Waldman ID. The Cambridge Handbook of Violent Behaviour and Aggression. Cambridge: Cambridge University Press; 2007.
- Bushway SD, Krohn MD, Lizotte AJ, Phillips MD, Schmidt NM. Are risky youth less protectable as they age? The dynamics of protection during adolescence and young adulthood. Justice Q 2013;30:84-116. http://dx.doi.org/10.1080/07418825.2011.592507.
- Moran P, Coffey C, Romaniuk H, Olsson C, Borschmann R, Carlin JB, et al. The natural history of self-harm from adolescence to young adulthood: a population-based cohort study. Lancet 2012;379:236-43. http://dx.doi.org/10.1016/S0140-6736(11)61141-0.
- Tung KY, Chen ML, Wang HJ, Chen GS, Peck M, Yang J, et al. A seven-year epidemiology study of 12,381 admitted burn patients in Taiwan – using the Internet registration system of the Childhood Burn Foundation. Burns 2005;31:S12-17. http://dx.doi.org/10.1016/j.burns.2004.10.006.
- Toprak S, Cetin I, Guven T, Can G, Demircan C. Self-harm, suicidal ideation and suicide attempts among college students. Psychiatry Res 2011;187:140-4. http://dx.doi.org/10.1016/j.psychres.2010.09.009.
- Hawton K, Linsell L, Adeniji T, Sariaslan A, Fazel S. Self-harm in prisons in England and Wales: an epidemiological study of prevalence, risk factors, clustering, and subsequent suicide. Lancet 2014;383:1147-54. http://dx.doi.org/10.1016/S0140-6736(13)62118-2.
- Cheung YT, Wong PW, Lee AM, Lam TH, Fan YS, Yip PS. Non-suicidal self-injury and suicidal behavior: prevalence, co-occurrence, and correlates of suicide among adolescents in Hong Kong. Soc Psychiatry Psychiatr Epidemiol 2013;48:1133-44. http://dx.doi.org/10.1007/s00127-012-0640-4.
- Taliaferro LA, Muehlenkamp JJ, Borowsky IW, McMorris BJ, Kugler KC. Factors distinguishing youth who report self-injurious behavior: a population-based sample. Acad Pediatr 2012;12:205-13. http://dx.doi.org/10.1016/j.acap.2012.01.008.
- Giannetta MM, Betancourt LM, Brodsky NL, Wintersteen MB, Romer D, Giannetta JM, et al. Suicidal ideation and self-harm behavior in a community sample of preadolescent youth: a case–control study. J Adolesc Health 2012;50:524-6. http://dx.doi.org/10.1016/j.jadohealth.2011.09.013.
- Fischer G, Brunner R, Parzer P, Klug K, Durkee T, Carli V, et al. Depression, deliberate self-harm and suicidal behaviour in adolescents engaging in risky and pathological internet use. Prax Kinderpsychol Kinderpsychiatr 2012;61:16-31. http://dx.doi.org/10.13109/prkk.2012.61.1.16.
- Borschmann R, Coffey C, Moran P, Hearps S, Degenhardt L, Kinner SA, et al. Self-harm in young offenders. Suicide Life Threat Behav 2014;44:641-52. http://dx.doi.org/10.1111/sltb.12096.
- MacArthur GJ, Smith MC, Melotti R, Heron J, Macleod J, Hickman M, et al. Patterns of alcohol use and multiple risk behaviour by gender during early and late adolescence: the ALSPAC cohort. J Public Health 2012;34:S20-30. http://dx.doi.org/10.1093/pubmed/fds006.
- Fineberg NA, Potenza MN, Chamberlain SR, Berlin HA, Menzies L, Bechara A, et al. Probing compulsive and impulsive behaviors, from animal models to endophenotypes: a narrative review. Neuropsychopharmacology 2010;35:591-604. http://dx.doi.org/10.1038/npp.2009.185.
- Hollander E. Compulsivity and impulsivity-personal reflections: why now and why here?. CNS Spectr 2014;19:6-7. http://dx.doi.org/10.1017/S1092852913000886.
- Fontenelle LF, Oostermeijer S, Harrison BJ, Pantelis C, Yucel M. Obsessive–compulsive disorder, impulse control disorders and drug addiction: common features and potential treatments. Drugs 2011;71:827-40. http://dx.doi.org/10.2165/11591790-000000000-00000.
- Leeman RF, Potenza MN. Similarities and differences between pathological gambling and substance use disorders: a focus on impulsivity and compulsivity. Psychopharmacology 2012;219:469-90. http://dx.doi.org/10.1007/s00213-011-2550-7.
- Keers R, Ullrich S, Destavola BL, Coid JW. Association of violence with emergence of persecutory delusions in untreated schizophrenia. Am J Psychiatry 2014;171:332-9. http://dx.doi.org/10.1176/appi.ajp.2013.13010134.
- Swanson J, Estroff S, Swartz M, Borum R, Lachicotte W, Zimmer C, et al. Violence and severe mental disorder in clinical and community populations: the effects of psychotic symptoms, comorbidity, and lack of treatment. Psychiatry 1997;60:1-22.
- McNiel DE, Binder RL. Psychiatric emergency service use and homelessness, mental disorder, and violence. Psychiatr Serv 2005;56:699-704. http://dx.doi.org/10.1176/appi.ps.56.6.699.
- Tyrer P, Mitchard S, Methuen C, Ranger M. Treatment rejecting and treatment seeking personality disorders: type R and type S. J Personal Disord 2003;17:263-8. http://dx.doi.org/10.1521/pedi.17.3.263.22152.
- Dodd T, Nicholas S, Povey D, Walker A. Crime in England and Wales, 2003/2004. London: Home Office; 2004.
- Curtis L. Unit Costs of Health and Social Care 2013. Canterbury: Personal Social Services Research Unit, University of Kent; 2013.
- Department of Health . NHS Reference Costs 2012–13 n.d. www.gov.uk/government/publications/nhs-reference-costs-2012-to-2013.
- Curtis L. Unit Costs of Health and Social Care 2010. Canterbury: University of Kent; 2010.
- McCrone P, Menezes PR, Johnson S, Scott H, Thornicroft G, Marshall J, et al. Service use and costs of people with dual diagnosis in South London. Acta Psychiatr Scand 2000;101:464-72. http://dx.doi.org/10.1034/j.1600-0447.2000.101006464.x.
- Wallace P, Haines A, Harrison R, Barber J, Thompson S, Roberts J, et al. Putting telemedicine to the test: design and performance of a multi-centre randomised controlled trial and economic evaluation of joint tele-consultations. BMC Fam Pract 2002;3.
- Pescosolido BA, Monahan J, Link BG, Stueve A, Kikuzawa S. The public’s view of the competence, dangerousness, and need for legal coercion of persons with mental health problems. Am J Public Health 1999;89:1339-45. http://dx.doi.org/10.2105/AJPH.89.9.1339.
- Link BG, Phelan J, Bresnahan M, Sueve A, Pescosolido B. Public conceptions of mental illness: labels, causes, dangerousness, and social distance. Am J Public Health 1999;89:1328-33. http://dx.doi.org/10.2105/AJPH.89.9.1328.
- Nawková L, Nawka A, Adamkova T, Rukavina TV, Holcnerova P, Kuzman MR, et al. The picture of mental health/illness in the printed media in three Central European countries. J Health Commun 2012;17:22-40. http://dx.doi.org/10.1080/10810730.2011.571341.
- Coverdale J, Nairn R, Claasen D. Depictions of mental illness in print media: a prospective national sample. Aust N Z J Psychiatry 2002;36:697-700. http://dx.doi.org/10.1046/j.1440-1614.2002.00998.x.
- Robillard JM, Illes J. A link in the ink: mental illness and criminal responsibility in the press. J Ethics Mental Health 2011;6:S1-7.
- Corrigan PW, Watson AC, Gracia G, Slopen N, Rasinski K, Hall LL. Newspaper stories as measures of structural stigma. Psychiatr Serv 2005;56:551-6. http://dx.doi.org/10.1176/appi.ps.56.5.551.
- Angermeyer MC, Matschinger H. The effect of violent attacks by schizophrenic persons on the attitude of the public towards the mentally ill. Soc Sci Med 1996;43:1721-8. http://dx.doi.org/10.1016/S0277-9536(96)00065-2.
- Corrigan PW, O’Shaughnessy JR. Changing mental illness stigma as it exists in the real world. Aust Psychol 2007;42:90-7. http://dx.doi.org/10.1080/00050060701280573.
- Corrigan PW, Penn DL. Lessons from social psychology on discrediting psychiatric stigma. Am Psychol 1999;54:765-76. http://dx.doi.org/10.1037/0003-066X.54.9.765.
- Watson AC, Corrigan P, Larson JE, Sells M. Self-stigma in people with mental illness. Schizophr Bull 2007;33:1312-18. http://dx.doi.org/10.1093/schbul/sbl076.
- Phelan J, Link B. The growing belief that people with mental illness are violent: the role of the dangerousness criterion for civil commitment. Soc Psychiatry Psychiatr Epidemiol 1998;33:7-12. http://dx.doi.org/10.1007/s001270050204.
- Estroff SE, Swanson JW, Lachicotte WS, Swartz M, Bolduc M. Risk reconsidered: targets of violence in the social networks of people with serious psychiatric disorders. Soc Psychiatry Psychiatr Epidemiol 1998;33:S95-101. http://dx.doi.org/10.1007/s001270050216.
- Rice ME, Harris GT. Violent recidivism: assessing predictive validity. J Consult Clin Psychol 1995;63:737-48. http://dx.doi.org/10.1037/0022-006X.63.5.737.
- Hanson RK, Thornton D. Static-99: Improving Actuarial Risk Assessments for Sex Offenders. Ottawa, ON: Department of the Solicitor General of Canada; 1999.
- Webster C, Douglas K, Eaves D, Hart S. HCR-20: Assessing Risk for Violence, Version 2. Vancouver, BC: Simon Fraser University; 1997.
- Dawes RM, Faust D, Meehl PE. Clinical versus actuarial judgment. Science 1989;243:1668-74. http://dx.doi.org/10.1126/science.2648573.
- Grove WM, Meehl PE. Comparative efficiency of informal (subjective, impressionistic) and formal (mechanical, algorithmic) prediction procedures: the clinical–statistical controversy. Psychol Public Policy Law 1996;2:293-32. http://dx.doi.org/10.1037/1076-8971.2.2.293.
- Troquete NA, van den Brink RH, Beintema H, Mulder T, van Os TW, Schoevers RA, et al. Predictive validity of the Short-Term Assessment of Risk and Treatability for violent behavior in outpatient forensic psychiatric patients. Psychol Assess 2015;27:377-91. http://dx.doi.org/10.1037/a0038270.
- Coid JW, Ullrich S, Kallis C, Keers R, Barker D, Cowden F, et al. The relationship between delusions and violence: findings from the East London first episode psychosis study. JAMA Psychiatry 2013;70:465-71. http://dx.doi.org/10.1001/jamapsychiatry.2013.12.
- Coid J, Yang M, Ullrich S, Zhang T, Sizmur S, Roberts C, et al. Gender differences in structured risk assessment: comparing the accuracy of five instruments. J Consult Clin Psychol 2009;77:337-48. http://dx.doi.org/10.1037/a0015155.
- Jablensky A, Sartorius N, Ernberg G, Anker M, Korten A, Cooper JE, et al. Schizophrenia: manifestations, incidence and course in different cultures. A World Health Organization ten-country study. Psychol Med Monogr Suppl 1992;20:1-97. http://dx.doi.org/10.1017/S0264180100000904.
- Kirkbride JB, Fearon P, Morgan C, Dazzan P, Morgan K, Tarrant J, et al. Heterogeneity in incidence rates of schizophrenia and other psychotic syndromes: findings from the 3-centre AeSOP study. Arch Gen Psychiatry 2006;63:250-8. http://dx.doi.org/10.1001/archpsyc.63.3.250.
- Cooper JE, Goodhead D, Craig T, Harris M, Howat J, Korer J. The incidence of schizophrenia in Nottingham. Br J Psychiatry 1987;151:619-26. http://dx.doi.org/10.1192/bjp.151.5.619.
- Schedules for Clinical Assessment in Neuropsychiatry. Geneva: WHO; 1992.
- NHS Act 2006. London: The Stationery Office; 2006.
- McGuffin P, Farmer A, Harvey I. A polydiagnostic application of operational criteria in studies of psychotic illness. Development and reliability of the OPCRIT system. Arch Gen Psychiatry 1991;48:764-70. http://dx.doi.org/10.1001/archpsyc.1991.01810320088015.
- Brittain PJ, Stahl D, Rucker J, Kawadler J, Schumann G. A review of the reliability and validity of OPCRIT in relation to its use for the routine clinical assessment of mental health patients. Int J Methods Psychiatr Res 2013;22:110-37. http://dx.doi.org/10.1002/mpr.1382.
- Monahan J, Steadman HJ, Silver E, Appelbaum PS, Clark Robbins P, Mulvey EP, et al. Rethinking Risk Assessment. The MacArthur Study of Mental Disorder and Violence. New York, NY: Oxford University Press; 2001.
- Home Office . Standard List n.d. www.gov.uk/government/uploads/system/uploads/attachment_data/file/217713/offence-classifications.xls (accessed 31 July 2014).
- Gueorguieva R, Krystal JH. Move over ANOVA: progress in analyzing repeated-measures data and its reflection in papers published in the Archives of General Psychiatry. Arch Gen Psychiatry 2004;61:310-17. http://dx.doi.org/10.1001/archpsyc.61.3.310.
- MacKinnon DP, Dwyer JH. Estimating mediated effects in prevention studies. Eval Rev 1993;17:144-58. http://dx.doi.org/10.1177/0193841X9301700202.
- Preacher KJ, Hayes AF. Asymptotic and resampling strategies for assessing and comparing indirect effects in multiple mediator models. Behav Res Methods 2008;40:879-91. http://dx.doi.org/10.3758/BRM.40.3.879.
- Large MM, Nielssen O. Violence in first-episode psychosis: a systematic review and meta-analysis. Schizophr Res 2011;125:209-20. http://dx.doi.org/10.1016/j.schres.2010.11.026.
- Skeem JL, Monaham J. Current directions in violence risk assessment. Curr Dir Psychol Sci 2011;20:38-42. http://dx.doi.org/10.1177/0963721410397271.
- Best Practice in Managing Risk. London: Department of Health; 2007.
- Douglas KS, Cox DN. Violence risk assessment: science and practice. Legal Criminol Psych 1999;4:149-84. http://dx.doi.org/10.1348/135532599167824.
- De Vogel V, De Vries Robbe M, De Ruiter C, Bouman YH. Assessing protective factors in forensic psychiatric practice: introducing the SAPROF. Int J Forensic Ment Health 2011;10:171-7. http://dx.doi.org/10.1080/14999013.2011.600230.
- Appleby L, Shaw J, Amos T, McDonnell R, Harris C, McCann K, et al. Suicide within 12 months of contact with mental health services: national clinical survey. BMJ 1999;318:1235-9. http://dx.doi.org/10.1136/bmj.318.7193.1235.
- Ritchie J, Dick D, Lingham R. The Report of the Enquiry into the Care and Treatment of Christopher Clunis. London: HMSO; 1994.
- The Report of the Enquiry into the Care and Treatment of Christopher Clunis. London: NHS London; 2006.
- Walker P. Police ‘missed chance to carry out checks on woman who went on to kill’. The Guardian 2013. www.theguardian.com/uk/2013/mar/04/police-woman-stabbing-nicola-edgington (accessed 8 March 2013).
- Brown T, Fraser K, Morley A. A Report of the Inquiry into the Care and Treatment of Jonathon Crisp. Nunthorpe: Tees Health Authority; 1999.
- Reed J. Risk assessment and clinical risk management: the lessons from recent inquiries. Br J Psychiatry 1997;170:S4-7.
- Prior G. Attitudes to Mental Illness. London: TNS; 2010.
- Taylor PJ, Gunn J. Homicides by people with mental illness: myth and reality. Br J Psychiatry 1999;174:9-14. http://dx.doi.org/10.1192/bjp.174.1.9.
- Criminal Justice Act 2003. London: The Stationery Office; 2003.
- Legal Aid, Sentencing and Punishment of Offenders Act 2012. London: The Stationery Office; 2012.
- White R, Graham H. Working with Offenders: A Guide to Concepts and Practices. Abingdon: Willan; 2012.
- Doyle M, Dolan M. Predicting community violence from patients discharged from mental health services. Br J Psychiatry 2006;189:520-6. http://dx.doi.org/10.1192/bjp.bp.105.021204.
- Steadman HJ, Cocozza JJ. Careers of the Criminally Insane: Excessive Social Control of Deviance. Lexington, MA: Lexington Books; 1974.
- Care Programme Approach London. London: Department of Health; 1990.
- Department of Health, National Risk Management Programme . Best Practice in Managing Risk 2007. www.gov.uk/government/uploads/system/uploads/attachment_data/file/478595/best-practice-managing-risk-cover-webtagged.pdf.
- McIvor G, Kemshall H. Serious Violent and Sexual Offenders: The Use of Risk Assessment Tools in Scotland. Edinburgh: Scottish Executive; 2002.
- Khiroya R, Weaver T, Maden T. Use and perceived utility of structured violence risk assessments in English medium secure forensic units. Psychiatr Bull 2009;33:129-32. http://dx.doi.org/10.1192/pb.bp.108.019810.
- Woods P. Risk assessment and management approaches on mental health units. J Psychiatr Ment Health Nurs 2012;20:1-7. http://dx.doi.org/10.1111/jpm.12022.
- Monahan J. Predicting Violent Behavior – An Assessment of Clinical Techniques. Washington, DC: Sage; 1981.
- Large MM, Ryan CJ, Singh SP, Paton MB, Nielssen OB. The predictive value of risk categorization in schizophrenia. Harv Rev Psychiatry 2011;19:25-33. http://dx.doi.org/10.3109/10673229.2011.549770.
- Fazel S, Singh JP, Doll H, Grann M. Use of risk assessment instruments to predict violence and antisocial behaviour in 73 samples involving 24 827 people: systematic review and meta-analysis. BMJ 2012;345. http://dx.doi.org/10.1136/bmj.e4692.
- Hart SD. The role of psychopathy in assessing risk for violence: conceptual and methodological issues. Legal Criminol Psychol 1998;3:121-37. http://dx.doi.org/10.1111/j.2044-8333.1998.tb00354.x.
- Doyle M, Dolan M, Shaw J, Carter S. The Development of a Practical Risk Assessment Framework for Multidisciplinary Use in Mental Health Services: Final Report. Manchester: University of Manchester; 2008.
- Maden A, Scott F, Burnett R, Lewis GH, Skapinakis P. Offending in psychiatric patients after discharge from medium secure units: prospective national cohort study. BMJ 2004;328. http://dx.doi.org/10.1136/bmj.38075.467569.EE.
- Green B, Carroll A, Brett A. Structured risk assessment in community forensic mental health practice. Australas Psychiatry 2010;18:538-41. http://dx.doi.org/10.3109/10398562.2010.498513.
- Young A, Woodcock R. Forensic risk assessment in mental health practice: part 2. Br J Healthcare Assist 2011;5:89-92. http://dx.doi.org/10.12968/bjha.2011.5.2.89.
- Monahan J, Steadman HJ. Violence and Mental Disorder: Developments in Risk Assessment. Chicago, IL: University of Chicago Press; 1994.
- Meehl PE. Clinical Versus Statistical Prediction. Minneapolis, MN: University of Minnesota; 1954.
- Lidz CW, Mulvey EP, Gardner W. The accuracy of predictions of violence to others. JAMA 1993;269:1007-11. http://dx.doi.org/10.1001/jama.1993.03500080055032.
- Snowden P. Practical aspects of clinical risk assessment and management. Br J Psychiatry 1997;170:S32-4.
- Otto R. Prediction of dangerous behaviour: a review and analysis of ‘second-generation’ research. Forensic Rep 1992;5:103-33.
- Mossman D. Assessing predictions of violence: being accurate about accuracy. J Consult Clin Psychol 1994;62:783-92. http://dx.doi.org/10.1037/0022-006X.62.4.783.
- Monahan J, Steadman HJ, Appelbaum PS, Robbins PC, Mulvey EP, Silver E, et al. Developing a clinically useful actuarial tool for assessing violence risk. Br J Psychiatry 2000;176:312-19. http://dx.doi.org/10.1192/bjp.176.4.312.
- Craig L, Browne K, Stringer I, Beech A. Limitations in actuarial risk assessment of sexual offenders: a methodological note. Br J Forensic Pract 2004;6:16-32. http://dx.doi.org/10.1108/14636646200400003.
- Douglas KS, Ogloff JRP, Hart SD. Evaluation of a model of violence risk assessment among forensic psychiatric patients. Psych Serv 2003;54:1372-9. http://dx.doi.org/10.1176/appi.ps.54.10.1372.
- Hanson RK. Twenty years of progress in violence risk assessment. J Interpers Violence 2005;20:212-17. http://dx.doi.org/10.1177/0886260504267740.
- Doyle M, Logan C. Operationalizing the assessment and management of violence risk in the short-term. Behav Sci Law 2012;30:406-19. http://dx.doi.org/10.1002/bsl.2017.
- De Vogel V, De Ruiter C, Bouman Y, De Vries Robbe M. Structured Assessment of Protective Factors for Violence Risk. Utrecht: Forum Educatief; 2009.
- De Vries Robbe M, De Vogel V, De Spa E. Protective factors for violence risk in forensic psychiatric patients: a retrospective validation study of the SAPROF. Int J Forensic Ment Health 2011;10:178-86. http://dx.doi.org/10.1080/14999013.2011.600232.
- Borum R. Improving the clinical practice of violence risk assessment. Technology, guidelines, and training. Am Psychol 1996;51:945-56. http://dx.doi.org/10.1037/0003-066X.51.9.945.
- Gray NS, Taylor J, Snowden RJ. Predicting violent reconvictions using the HCR-20. Br J Psychiatry 2008;192:384-7. http://dx.doi.org/10.1192/bjp.bp.107.044065.
- Mental Health Act 1984. London: The Stationery Office; 1984.
- Baxter R, Rabe-Hesketh S, Parrott J. Characteristics, needs and reoffending in a group of patients with schizophrenia formerly treated in medium security. J Forens Psychiatry Psychol 1999;10:69-83. http://dx.doi.org/10.1080/09585189908402140.
- Coid J, Kahtan N, Gault S, Jarman B. Women admitted to secure forensic psychiatry services: I. comparison of women and men. J Forens Psychiatry Psychol 2000;11:275-95. http://dx.doi.org/10.1080/09585180050142525.
- Davies S, Clarke M, Hollin C, Duggan C. Long-term outcomes after discharge from medium secure care: a cause for concern. Br J Psychiatry 2007;191:70-4. http://dx.doi.org/10.1192/bjp.bp.106.029215.
- Monahan J. Risk assessment of violence among the mentally disordered: generating useful knowledge. Int J Law Psychiatry 1988;11:249-57. http://dx.doi.org/10.1016/0160-2527(88)90012-X.
- Mulvey EP, Shaw E, Lidz C. Why use multiple sources in research on patient violence in the community?. Crim Behav Ment Health 1994;4:253-8.
- Kay SR, Fiszbein A, Opler LA. The positive and negative syndrome scale (PANSS) for schizophrenia. Schizophr Bull 1987;13:261-76. http://dx.doi.org/10.1093/schbul/13.2.261.
- Shaw J, Senior J, Hayes AF, Roberts A, Evans G, Rennie C. An Evaluation of the Department of Health’s Procedure for the Transfer of Prisoners to and from Hospital under Sections 47 and 48 of the Mental Health Act (1983) Initiative. Manchester: NICE; 2008.
- Hart SD, Cox DN, Hare RD. Hare Psychopathy Checklist: Screening Version (PCL:SV). Toronto, ON: Multi-Health Systems; 1995.
- Skeem JL, Miller JD, Mulvey E, Tiemann J, Monahan J. Using a five-factor lens to explore the relation between personality traits and violence in psychiatric patients. J Consult Clin Psychol 2005;73:454-65. http://dx.doi.org/10.1037/0022-006X.73.3.454.
- Mental Health Act 1983. London: The Stationery Office; 1983.
- Maden A, Skapinakis P, Lewis G, Scott F, Jamieson E. Gender differences in reoffending after discharge from medium-secure units. National cohort study in England and Wales. Br J Psychiatry 2006;189:168-72. http://dx.doi.org/10.1192/bjp.bp.105.014613.
- Rutherford MSD. Facts and Figures on Current Provision. London: Sainsbury’s Centre for Mental Health, Forensic Mental Health Services; 2007.
- Maden A, Rutter S, McClintock T, Friendship C, Gunn J. Outcome of admission to a medium secure psychiatric unit. I. Short- and long-term outcome. Br J Psychiatry 1999;175:313-16. http://dx.doi.org/10.1192/bjp.175.4.313.
- Changing the Outlook: A Strategy for Developing and Modernising Mental Health Services in Prisons. London: Department of Health; 2001.
- Doyle M, Logan C, Ludlow A, Holloway J. Milestones to recovery: preliminary validation of a framework to promote recovery and map progress through the medium secure inpatient pathway. Crim Behav Ment Health 2012;22:53-64. http://dx.doi.org/10.1002/cbm.818.
- Coid J, Hickey N, Kahtan N, Zhang T, Yang M. Patients discharged from medium secure forensic psychiatry services: reconvictions and risk factors. Br J Psychiatry 2007;190:223-9. http://dx.doi.org/10.1192/bjp.bp.105.018788.
- Hodgins S, Alderton J, Cree A, Aboud A, Mak T. Aggressive behaviour, victimization and crime among severely mentally ill patients requiring hospitalisation. Br J Psychiatry 2007;191:343-50. http://dx.doi.org/10.1192/bjp.bp.106.06.029587.
- Solomon PL, Cavanaugh MM, Gelles RJ. Family violence among adults with severe mental illness: a neglected area of research. Trauma Violence Abuse 2005;6:40-54. http://dx.doi.org/10.1177/1524838004272464.
- Anderson A, West SG. Violence against mental health professionals: when the treater becomes the victim. Innov Clin Neurosci 2011;8:34-9.
- Owen C, Tarantello C, Jones M, Tennant C. Violence and aggression in psychiatric units. Psychiatr Serv 1998;49:1452-7. http://dx.doi.org/10.1176/ps.49.11.1452.
- Steadman HJ, Monahan J, Appelbaum PS, Grisso T, Mulvey EP, Roth LH, et al. Violence and Mental Disorder: Developments in Risk Assessment. Chicago, IL: University of Chicago Press; 1994.
- Estroff SE, Zimmer C, Lachicotte WS, Benoit J. The influence of social networks and social support on violence by persons with serious mental illness. Hosp Community Psychiatry 1994;45:669-79. http://dx.doi.org/10.1176/ps.45.7.669.
- Hoptman MJ, Yates KF, Patalinjug MB, Wack RC, Convit A. Clinical prediction of assaultive behavior among male psychiatric patients at a maximum-security forensic facility. Psychiatr Serv 1999;50:1461-6. http://dx.doi.org/10.1176/ps.50.11.1461.
- Gendreau P, Little T, Goggin C. Criminology 1996;34:575-608. http://dx.doi.org/10.1111/j.1745-9125.1996.tb01220.x.
- Hanson RK, Bussiere MT. Predicting relapse: a meta-analysis of sexual offender recidivism studies. J Consult Clin Psychol 1998;66:348-62. http://dx.doi.org/10.1037/0022-006X.66.2.348.
- Hirschi T, Gottfredson M. Age and the explanation of crime. Am J Sociol 1983;89:552-84. http://dx.doi.org/10.1086/227905.
- Brown DW, Anda RF, Tiemeier H, Felitti VJ, Edwards VJ, Croft JB, et al. Adverse childhood experiences and the risk of premature mortality. Am J Prev Med 2009;37:389-96. http://dx.doi.org/10.1016/j.amepre.2009.06.021.
- Widiger TA, Trull TJ, Monahan J, Steadman HJ. Violence and Mental Disorder: Developments in Risk Assessment. Chicago, IL: University of Chicago Press; 1994.
- Nestor PG. Mental disorder and violence: personality dimensions and clinical features. Am J Psychiatry 2002;159:1973-8. http://dx.doi.org/10.1176/appi.ajp.159.12.1973.
- McMurran M. Expert Paper: Personality Disorder. Liverpool: NHS National Programme on Forensic Mental Health Research and Development; 2002.
- Swanson JW, Monahan J, Steadman HJ. Violence and Mental Disorder: Developments in Risk Assessment. Chicago, IL: University of Chicago Press; 1994.
- Steadman HJ, Mulvey EP, Monahan J, Robbins PC, Appelbaum PS, Grisso T, et al. Violence by people discharged from acute psychiatric inpatient facilities and by others in the same neighborhoods. Arch Gen Psychiatry 1998;55:393-401. http://dx.doi.org/10.1001/archpsyc.55.5.393.
- Swartz MS, Swanson JW, Hiday VA, Borum R, Wagner HR, Burns BJ. Violence and severe mental illness: the effects of substance abuse and nonadherence to medication. Am J Psychiatry 1998;155:226-31.
- De Vogel V, De Ruiter C. Differences between clinicians and researchers in assessing risk of violence in forensic psychiatric patients. J Forens Psychiatry Psychol 2004;15:145-64. http://dx.doi.org/10.1080/14788940410001655916.
- Liu Y, Yang M, Ramsey M, Li XS, Coid JW. A comparison of logistic regression, classification and regression tree and neural network models in predicting violent offending. J Quantitative Criminol 2011;27:547-73. http://dx.doi.org/10.1007/s10940-011-9137-7.
- Liu P, Erez A, Nagamani SC, Bi W, Carvalho CM, Simmons AD, et al. Copy number gain at Xp22.31 includes complex duplication rearrangements and recurrent triplications. Hum Mol Genet 2011;20:1975-88. http://dx.doi.org/10.1093/hmg/ddr078.
- Thornton D. Scoring for Risk Matrix 2000.9 SVC 2007. www.birmingham.ac.uk/Documents/college-les/psych/RM2000scoringinstructions.pdf (accessed 31 July 2014).
- Taylor R. Predicting Reconviction for Sexual and Violent Offences Using the Revised Offender Group Reconviction Scale (No. 104) 1999.
- Douglas KS, Reeves KA, Otto RK, Douglas KS. International Perspectives on Forensic Mental Health. New York, NY: Routledge; 2010.
- Hemphill JF, Hare RD, Wong S. Psychopathy and recidivism: a review. Legal Criminol Psych 1998;3:139-70. http://dx.doi.org/10.1111/j.2044-8333.1998.tb00355.x.
- Salekin RT, Rogers R, Sewell KW. A review and meta-analysis of the Psychopathy Checklist and the Psychopathy Checklist-Revised: predictive validity of dangerousness. Clin Psychol Sci Pract 1996;3:203-15. http://dx.doi.org/10.1111/j.1468-2850.1996.tb00071.x.
- Serin RC. Violent recidivism in criminal psychopaths. Law Hum Behav 1996;20:207-16. http://dx.doi.org/10.1007/BF01499355.
- Glover AJJ, Nicholson DE, Hemmati T, Bernfeld GA, Quinsey VL. A comparison of predictors of general and violent recidivism among high-risk federal offenders. Crim Justice Behav 2002;29:235-49. http://dx.doi.org/10.1177/0093854802029003001.
- Belfrage H, Douglas KS. Treatment effects on forensic psychiatric patients measured with the HCR-20 violence risk assessment scheme. Int J Forensic Ment Health 2002;1:25-36. http://dx.doi.org/10.1080/14999013.2002.10471158.
- Farrington DP, Snyder HN, Finnegan TA. Specialization in juvenile-court careers. Criminology 1988;26:461-87. http://dx.doi.org/10.1111/j.1745-9125.1988.tb00851.x.
- Farrington DP, Pepler DJ, Rubin KH. The Development and Treatment of Childhood Aggression. Hillsdale, NJ: Lawrence Erlbaum; 1991.
- Coid JW, Ullrich S, Kallis C. Predicting future violence among individuals with psychopathy. Br J Psychiatry 2013;203:387-8. http://dx.doi.org/10.1192/bjp.bp.112.118471.
- Gray NS, Taylor J, Snowden RJ. Predicting violence using structured professional judgment in patients with different mental and behavioral disorders. Psychiatry Res 2011;187:248-53. http://dx.doi.org/10.1016/j.psychres.2010.10.011.
- Bonta J. Offender risk assessment – guidelines for selection and use. Crim Justice Behav 2002;29:355-79. http://dx.doi.org/10.1177/0093854802029004002.
- Chu CM, Thomas SDM, Ogloff JRP, Daffern M. The short- to medium-term predictive accuracy of static and dynamic risk assessment measures in a secure forensic hospital. Assessment 2013;20:230-41. http://dx.doi.org/10.1177/1073191111418298.
- Gottfredson SD, Moriarty LJ. Statistical risk assessment: old problems and new applications. Crime Delinq 2006;52:178-200. http://dx.doi.org/10.1177/0011128705281748.
- Kroner DG, Mills JF, Reddon JR. A coffee can, factor analysis, and prediction of antisocial behavior: the structure of criminal risk. Int J Law Psychiatry 2005;28:360-74. http://dx.doi.org/10.1016/j.ijlp.2004.01.011.
- Hare RD. The Hare Psychopathy Checklist-Revised. Toronto, ON: Multi-Health System; 1991.
- Gordon V, Williams DJ, Donnelly PD. Exploring the relationship between ADHD symptoms and prison breaches of discipline amongst youths in four Scottish prisons. Public Health 2012;126:343-8. http://dx.doi.org/10.1016/j.puhe.2012.01.004.
- Andrews DA, Guzzo L, Raynor P, Rowe RC, Rettinger LJ, Brews A, et al. Are the major risk/need factors predictive of both female and male reoffending?: a test with the eight domains of the Level of Service/Case Management Inventory. Int J Offender Ther Comp Criminol 2012;56:113-33. http://dx.doi.org/10.1177/0306624X10395716.
- Harris GT, Rice ME, Quinsey VL. Violent recidivism of mentally disordered offenders – the development of a statistical prediction instrument. Crim Justice Behav 1993;20:315-35. http://dx.doi.org/10.1177/0093854893020004001.
- Nufield J. Parole Decision-Making in Canada: Research towards Decision Guidelines. Ottawa, ON: Ministry of Supply and Sercices Canada; 1982.
- Duwe G. The development, validity, and reliability of the Minnesota Screening Tool Assessing Recidivism Risk (MnSTARR). Crim Justice Policy Rev 2014;25:579-613. http://dx.doi.org/10.1177/0887403413478821.
- Howard P, Dixon L. Developing an empirical classification of violent offences for use in the prediction of recidivism in England and Wales. J Aggress Confl Peace Res 2011;3:141-54. http://dx.doi.org/10.1108/17596591111154176.
- Bautista D, Estanislao A, Marti-Bonmati L, Paredes R. Validation of logistic regression models in small samples: application to calvarial lesion diagnosis. J Clin Epidemiol 1999;52:237-41. http://dx.doi.org/10.1016/S0895-4356(98)00165-6.
- Coid J, Yang M, Ullrich S, Zhang T, Roberts R, Roberts C, et al. Predicting and Understanding Risk of Reoffending: The Prisoner Cohort Study 2007. www.crim.cam.ac.uk/people/academic_research/david_farrington/priscomoj.pdf (accessed 31 July 2014).
- Coid J, Ullrich S, Yang M, Zhang T, Roberts A. Measuring Dangerousness and Severity of Personality Disorder: The Prisoner Cohort Study. London: Home Office; 2005.
- Gagliardi GJ, Lovell D, Peterson PD, Jemelka R. Forecasting recidivism in mentally ill offenders released from prison. Law Hum Behav 2004;28:133-55. http://dx.doi.org/10.1023/B:LAHU.0000022319.03637.45.
- Singh JP. Predictive validity performance indicators in violence risk assessment: a methodological primer. Behav Sci Law 2013;31:8-22. http://dx.doi.org/10.1002/bsl.2052.
- Howard P. Improving the Prediction of Reoffending Using the Offender Assessment System 2009. http://webarchive.nationalarchives.gov.uk/20110201125714/http://www.justice.gov.uk/publications/docs/oasys-research-summary-02-09.pdf (accessed 31 July 2014).
- Howard PD, Dixon L. The construction and validation of the OASys Violence Predictor: advancing violence risk assessment in the English and Welsh correctional services. Crim Justice Behav 2012;39:287-30. http://dx.doi.org/10.1177/0093854811431239.
- Howard P, Francis B, Soothill K, Humphreys L. OGRS 3: The Revised Offender Group Reconviction Score. London: Ministry of Justice; 2009.
- Thornton D, Mann R, Webster S, Blud L, Travers R, Friendship C, et al. Distinguishing and combining risks for sexual and violent recidivism. Ann NY Acad Sci 2003;989:225-35. http://dx.doi.org/10.1111/j.1749-6632.2003.tb07308.x.
- Kallis C, Bui L, Yang M, Coid JW. Static screening instruments for risk of minor and major violence. J Forens Psychiatry Psychol 2014;25:397-410. http://dx.doi.org/10.1080/14789949.2014.925136.
- Howard PD, Dixon L. Identifying change in the likelihood of violent recidivism: causal dynamic risk factors in the OASys violence predictor. Law Hum Behav 2013;37:163-74. http://dx.doi.org/10.1037/lhb0000012.
- MacLeod JF, Grove PG, Farrington DP. Explaining Criminal Careers: Implications for Justice Policy. Oxford: Oxford University Press; 2012.
- Hanson RK, Harris AJR. Where should we intervene? Dynamic predictors of sexual offense recidivism. Crim Justice Behav 2000;27:6-35. http://dx.doi.org/10.1177/0093854800027001002.
- Otto RK, Douglas KS, Otto RK, Douglas KS. International Perspectives on Forensic Mental Health. New York, NY: Routledge; 2010.
- Dvoskin JA, Heilbrun K. Risk assessment and release decision-making: toward resolving the great debate. J Am Acad Psychiatry 2001;29:6-10.
- Skeem J, Mulvey E, Buchanan C. Care of the Mentally Disordered Offender in the Community. New York, NY: Oxford University Press; 2002.
- Spielberger CD. Manual for the State–Trait Anger Expression Inventory. Odessa, FL: Psychological Assessment Resources; 1988.
- Bennett T, Brookman F, Brookman F, Maguire M, Pierpoint H, Bennett T. Handbook on Crime. Uffculme: Willan Publishing; 2010.
- Coid J, Ullrich S. Prisoners with psychosis in England and Wales: diversion to psychiatric inpatient services?. Int J Law Psychiatry 2011;34:99-108. http://dx.doi.org/10.1016/j.ijlp.2011.02.003.
- Cohen J. A coefficient of agreement for nominal scales. Educ Psychol Meas 1960;20:37-46. http://dx.doi.org/10.1177/001316446002000104.
- Farrington DP. Early predictors of adolescent aggression and adult violence. Violence Vict 1989;4:79-100.
- Farrington DP, Loeber R. Relative improvement over chance (RIOC) and phi as measures of predictive efficiency and strength of association in 2×2 tables. J Quan Criminol 1989;5:201-13. http://dx.doi.org/10.1007/BF01062737.
- Landis JR, Koch GG. Measurement of observer agreement for categorical data. Biometrics 1977;33:159-74. http://dx.doi.org/10.2307/2529310.
- Duggan C, Howard R, McMurran M, Howard R. Personality, Personality Disorder and Violence. London: Wiley-Blackwell; 2009.
- Fenton NE, Neil M. Risk Assessment and Decision Analysis with Bayesian Networks. Boca Raton, FL: CRC Press; 2012.
- Bayes T. An essay toward solving a problem in the doctrine of chances. Phil Trans 1763;53:370-418. http://dx.doi.org/10.1098/rstl.1763.0053.
- Pearl J. Probabilistic Reasoning in Intelligent Systems: Networks of Plausible Inference. San Mateo, CA: Morgan Kaufmann Publishers; 1988.
- Heckerman D, Mamdani A, Wellman MP. Real-world applications of Bayesian networks – introduction. Commun ACM 1995;38:24-6. http://dx.doi.org/10.1145/203330.203334.
- Jensen FV. An Introduction to Bayesian Networks. New York, NY: Springer; 1996.
- Friedman N, Linial M, Nachman I, Pe’er D. Using Bayesian networks to analyze expression data. J Comput Biol 2000;7:601-20. http://dx.doi.org/10.1089/106652700750050961.
- Jiang X, Neapolitan RE, Barmada MM, Visweswaran S. Learning genetic epistasis using Bayesian network scoring criteria. BMC Bioinformatics 2011;12. http://dx.doi.org/10.1186/1471-2105-12-89.
- Pourret O, Naim P, Marcot B. Bayesian Networks: A Practical Guide to Applications. Chichester: Wiley; 2008.
- Fenton NE, Neil M. Combining evidence in risk analysis using Bayesian networks. Saf Crit Syst Newsl 2004;14:8-13.
- de Campos LM, Fernandez-Luna JM, Huete JF. Bayesian networks and information retrieval: an introduction to the special issue. Inform Process Manag 2004;40:727-33. http://dx.doi.org/10.1016/j.ipm.2004.03.001.
- Koumenides C, Shadbolt N. Combining Link and Content-Based Information in a Bayesian Inference Model for Entity Search n.d. http://dx.doi.org/10.1145/2379307.2379310.
- Kadane JB, Schum DA. A Probabilistic Analysis of the Sacco and Vanzetti Evidence. New York, NY: John Wiley; 1996.
- Constantinou AC, Fenton NE, Neil M. pi-football: a Bayesian network model for forecasting Association Football match outcomes. Knowl-Based Syst 2012;36:322-39. http://dx.doi.org/10.1016/j.knosys.2012.07.008.
- Constantinou AC, Fenton NE, Neil M. Profiting from an inefficient Association Football gambling market: prediction, risk and uncertainty using Bayesian networks. Knowl-Based Syst 2013;50:60-86. http://dx.doi.org/10.1016/j.knosys.2013.05.008.
- Uebersax JS. Breast Cancer Risk Modeling: An Application of Bayes Networks. Spain: RavenPack International SL; 2004.
- Jiang X, Barmada MM, Visweswaran S. Identifying genetic interactions in genome-wide data using Bayesian networks. Genet Epidemiol 2010;34:575-81. http://dx.doi.org/10.1002/gepi.20514.
- Diez FJ, Mira J, Iturralde E, Zubillaga S. DIAVAL, a Bayesian expert system for echocardiography. Artif Intell Med 1997;10:59-73. http://dx.doi.org/10.1016/S0933-3657(97)00384-9.
- Hu X-X, Wang H, Wang S. Using Expert’s Knowledge to Build Bayesian Networks n.d.
- Andrews DA, Bonta J. The Psychology of Criminal Conduct. Cincinatti, OH: Anderson Publishing; 1994.
- Blair RJ. Psychopathy: cognitive and neural dysfunction. Dialogues Clin Neurosci 2013;15:181-90.
- Davis WM. Psychopharmacologic violence associated with cocaine abuse: kindling of a limbic dyscontrol syndrome?. Prog Neuropsychopharmacol Biol Psychiatry 1996;20:1273-300. http://dx.doi.org/10.1016/S0278-5846(96)00126-1.
- Boles SM, Miotto K. Substance abuse and violence – a review of the literature. Aggress Violent Behav 2003;8:155-74. http://dx.doi.org/10.1016/S1359-1789(01)00057-X.
- Witt K, van Dorn R, Fazel S. Risk factors for violence in psychosis: systematic review and meta-regression analysis of 110 studies. PLOS ONE 2013;8. http://dx.doi.org/10.1371/journal.pone.0055942.
- Farrell M, Boys A, Singleton N, Meltzer H, Brugha T, Bebbington P, et al. Predictors of mental health service utilization in the 12 months before imprisonment: analysis of results from a national prisons survey. Aust N Z J Psychiatry 2006;40:548-53. http://dx.doi.org/10.1111/j.1440-1614.2006.01836.x.
- Freestone M, Howard R, Coid JW, Ullrich S. Adult antisocial syndrome co-morbid with borderline personality disorder is associated with severe conduct disorder, substance dependence and violent antisociality. Personal Ment Health 2013;7:11-2. http://dx.doi.org/10.1002/pmh.1203.
- Olver ME, Lewis K, Wong SCP. Risk reduction treatment of high-risk psychopathic offenders: the relationship of psychopathy and treatment change to violent recidivism. Personal Disord 2013;4:160-7. http://dx.doi.org/10.1037/a0029769.
- Coid J, Ullrich S. Antisocial personality disorder is on a continuum with psychopathy. Compr Psychiatry 2010;51:426-33. http://dx.doi.org/10.1016/j.comppsych.2009.09.006.
- Constantinou AC, Freestone M, Marsh W, Fenton NE, Coid J. Risk assessment and risk management of violent reoffending among prisoners. Expert Syst Appl 2015;42:7511-29. http://dx.doi.org/10.1016/j.eswa.2015.05.025.
- Saltelli A, Chan K, Scott M. Sensitivity Analysis. New York, NY: John Wiley; 2000.
- Freestone M, Ullrich S, Coid J. Acute Risk Factors and Triggers for Violence n.d.
- Singh JP, Grann M, Fazel S. Authorship bias in violence risk assessment? A systematic review and meta-analysis. PLOS ONE 2013;8. http://dx.doi.org/10.1371/journal.pone.0072484.
- Sugarman P, Walker L. HoNOS-SECURE Version 2b. London: Royal College of Psychiatrists College Research and Teaching Unit; 2007.
- Coid JW, Ullrich S, Kallis C, Gonzalez R, Bui L, Freestone M, et al. Improving Risk Management in Mental Health Services 2014.
- Office for National Statistics . FOI Request: List of Notifiable Offences 2012–2013 2013. http://webarchive.nationalarchives.gov.uk/20160105160709/http://www.ons.gov.uk/ons/about-ons/business-transparency/freedom-of-information/previous-foi-requests/people--population-and-community/list-of-notifiable-offences-2012---2013/index.html (accessed 31 July 2014).
Appendix 1 Violence in Psychotic Persons instrument version 2

Simone Ullrich,1 Constantinos Kallis,1 Bianca L DeStavola2 and Jeremy W Coid1
1Violence Prevention Research Unit, Queen Mary University of London, London, UK
2Department of Medical Statistics, London School of Hygiene & Tropical Medicine


Introduction
Prediction, prevention and punishment of violence frequently dominate public discussion and require significant public resources. Rates of violent crime rise and fall and are related to numerous factors. However, there remains a particular fear of violence perpetrated by those with mental illness, especially those diagnosed with schizophrenia, major depression or bipolar disorder. Studies show that up to 75% of the public believe that people with mental illness are dangerous. 273,274 Media coverage of mental illness most often focuses on violence and crime,275–277 therefore encouraging fear of the mentally ill within our communities. 278,279 Those with mental illness are among the most stigmatised groups in society280,281 and may internalise such stigma, resulting in reduced self-esteem and self-efficacy. 282 A perception that people with mental illness are violent undoubtedly contributes to this well-documented stigma. 283
However, violence to others is a leading public health concern. To the extent that mental illness raises the risk for violence, persons in the community will be victimised. Often, when mentally ill individuals are violent, the victims of their violence are family members,284 who therefore may bear a disproportionate risk of victimisation and personal suffering.
It is widely expected by policy-makers and the public that assessment of violence risk in patients with mental illness should be a core skill and responsibility of mental health professionals. Violence risk assessment plays an important role in mental health law worldwide and ‘dangerousness to others’ is a key criterion for civil and forensic commitment in most jurisdictions. Imposition of tort liability on mental health professionals who negligently fail to predict, manage and prevent a patient’s violence towards others has become common.
The correct identification of those at risk for future violence is therefore of utmost importance to (1) protect the public and (2) minimise additional stigmatisation among those with mental illness. However, despite major developments and improvements in the assessment of risk for future violence, currently available risk assessment instruments still suffer from many shortcomings.
Accuracy of the prediction of future violence
State of the art risk assessment instruments can be divided into two groups: (1) actuarial instruments and (2) SPJ. Actuarial instruments such as the VRAG285 or the Static-99286 are developed on the basis of risk factors that are empirically related to violent behaviour. Risk factors included in these instruments are predominantly static and unchangeable but relatively simple to code. The codings for the risk factors are added up according to a fixed algorithm and conclusions of level of risk are based on the total score.
Structured professional judgement instruments such as the HCR-20287 are administered by experienced forensic mental health professionals utilising a standardised checklist containing empirically derived historical and dynamic risk factors for violence. The final risk judgement, however, is not based on a fixed algorithm but on expert decision-making. Risk factors are critically examined, combined and integrated to reach a conclusion. However, to validate these SPJ instruments scores have to be assigned to allow a classification of level of risk.
It is accepted that structured risk assessment instruments (actuarial and SPJ) outperform clinical judgement in the accuracy of prediction of violent behaviour. 288,289 However, it has been previously demonstrated that most of these instruments achieve a predictive accuracy around only a moderate area under the ROC curve (AUC) value of 0.7 in comparison studies between more than one instrument. 8 Furthermore, in a study investigating three instruments (PCL-R, VRAG, HCR-20) most items were not independently predictive of future violence and their predictive power was based on only a small number of their items. 23 Clinicians should be aware of these limitations and be critical when using either an actuarial or a SPJ instrument if the intention is to carry out a comprehensive assessment of risk on which to base subsequent risk management or treatment interventions. Moreover, the PCC, which reflects the ratio of true positives/true negatives and false positives/false negatives in the prediction of violence, is usually around 60% using either actuarial or SPJ instruments. 467 This implies that, if a clinician relies on classification of risk based on these instruments, in approximately 40% of cases this classification will be wrong.
Causal compared with predictive models of risk for future violence
Most research carried out in the field of violence risk assessment utilises a predictive approach to either identify risk factors for violence or assess the predictive accuracy of instruments. Prediction requires temporal ordering of exposure (risk factors) and outcome (violence). Risk factors measured at some time point are investigated to see whether they are associated with violence occurring in a subsequent time window. This time window can cover a few weeks, months or several years. With regard to static, unchangeable risk factors, choice of time frame should not matter. Static risk factors should equally predict violence occurring within the subsequent month as violence occurring within the subsequent year. However, when choosing the subsequent time frame for violent outcome after assessment of dynamic risk it is rarely taken into consideration that dynamic risk factors oscillate over time. Symptoms of mental illness are dynamic in nature and fluctuate. Therefore, when investigating the association between mental illness and violent behaviour it is essential to establish that a person was symptomatic when the violent incident occurred. Predictors derived from studies measuring symptoms or diagnoses at various points over the lifetime and comparing them with self-report or criminal records over extended periods cannot establish valid associations. Furthermore, because acute psychotic symptoms may present for relatively short periods, predictors that are identified over the lifespan may not be specific for psychosis and may apply equally to incidents of violence among the general (non-psychotic) population.
In a large epidemiological study on alcohol and related conditions (NESARC)112 it was demonstrated that the incidence of violence was higher for people with severe mental illness, but only significantly for those with co-occurring substance abuse and/or dependence. Multivariable analyses revealed that severe mental illness alone did not predict future violence; it was associated instead with historical, clinical, dispositional and contextual factors. Most of these factors, though, were more prevalent in people with severe mental illness.
However, reanalysis of NESARC data came to completely different conclusions and revealed a positive association between major mental illness and violence, demonstrating that temporal closeness between dependent (violence) and independent (symptoms of mental illness) variables is key when investigating these relationships. 117
The MacArthur Violence Risk Assessment Study (MVRAS) is one of the most influential studies on the association between mental illness and violence. A key finding was that delusions do not predict violence among recently discharged psychiatric patients20 and this conclusion had a profound impact on research in this field. Reanalysis confirmed that delusions (present in the past 10 weeks) are not predictors of subsequent violent behaviour (in the following 10 weeks). Redefinition of the time frame of occurrence considering temporal proximity, however, revealed strong associations between violence and delusional beliefs implying threat to the individual. 21 These results supported the findings of a previous study in which delusions of conspiracy and being spied on and persecutory delusions (implying threat to an individual) were associated with serious violent behaviour in patients presenting with a first episode of psychotic illness. 291 However, in both studies the pathway from delusional beliefs towards violence was not direct; the key explanatory variable was delusional anger. These findings suggest that, when investigating the association between symptoms of mental illness and psychosis, different and complex pathways have to be taken into consideration.
The Violence in Psychotic Persons intrument
Rationale
Based on the shortcomings of currently available risk assessment instruments we considered it crucial to develop a new instrument addressing the problems and issues identified in recent research in the field of violence risk. Our main focus was the identification of symptoms of mental illness that are causal, dynamic risk factors for violence in those with affective and non-affective psychotic illness and which are amenable by treatment in adult forensic and general psychiatric settings. Dynamic risk factors were investigated in a sample of psychotic patients to ensure identification of diagnosis-specific risk factors.
Previous research has shown that risk factors differ substantially in female and male samples. It appears that clinical risk factors predict violence in women with sufficient accuracy whereas criminogenic and criminal history variables are better at identifying men at risk for violence. 292 We therefore developed two modules to address sex differences in risk for violent behaviour.
Most importantly, we aimed not only to sensitise clinicians to symptom constellations in their patients that increase the risk of violence but also to advise action if a patient presents with these symptoms. Currently available risk assessment instruments classify patients only with regard to the level of risk for violence and SPJ instruments aim to help clinicians to understand the risk for violence in their patients. However, administration of risk assessment instruments (considering that these assessments are sometimes very time-consuming) should lead to appropriate management and to a reduction in violent behaviour. The only study, however, that investigated whether or not administration of a SPJ instrument led to actual prevention of criminal and violent recidivism came to the conclusion ‘that the primary goal of preventing recidivism was not reached through risk assessment embedded in shared decision-making’ (p. 365). 18
Despite the problems outlined above we considered it necessary to provide a static instrument for men and women with psychotic illness to identify their propensity to violent behaviour. However, these risk factors are not amenable to intervention and can serve only for informative purposes.
Construction of the instrument
Baseline study
The ELFEPS was carried out between December 1996 and December 2000 in the London boroughs of City and Hackney, Tower Hamlets and Newham. All those aged 16–84 years living in the study area who made contact with mental health services (including adult community health teams, inpatient units, forensic services, learning disability services, adolescent mental health services and drug and alcohol units) because of a first episode of any probable psychotic disorder were identified and screened. Initial inclusion criteria were based on the WHO293 and the AESOP study. 294 Methods in the study by Cooper et al. 295 were used to minimise leakage and identify patients missed by screening. Patients who passed the screen underwent a battery of assessments. The SCAN296 make up a set of instruments used to assess adult major psychiatric disorders. Three clinical research fellows carried out the data collection and were trained in the SCAN interview by taking a course approved by the WHO. Prestudy reliability was established using independent ratings of videotaped interviews. ICD-10 and DSM-IV diagnoses were allocated by consensus agreement between the principal investigator (JWC) and the research team.
Sample description
Overall, 490 individuals were recruited [City and Hackney: n = 167 (34.1%); Tower Hamlets: n = 166 (33.9%); Newham: n = 157 (32.0%)]. More than half were men [n = 302 (61.6%)] and the sample was ethnically diverse [white: n = 179 (36.5%); black: n = 165 (33.7%); Asian: n = 117 (23.9%); other: n = 29 (5.9%)]. Approximately half of the study participants were not born in the UK [n = 243 (49.6%)]. The mean age of the sample at baseline was 30.5 years (SD 10.1 years).
The most prevalent consensus diagnosis at baseline was schizophrenia (Figure 25). Approximately 24% of the study participants presented with affective psychosis including unipolar, bipolar and other affective psychoses.
FIGURE 25.
Distribution of diagnoses at baseline.

Follow-up study
Data collection commenced in January 2010 and was finished on 30 June 2013. The study was granted Section 251 approval from the National Governance Information Board to gather data without consent of the baseline study participants. The design was a retrospective case note study that aimed to cover 10 years after the initial assessment at baseline.
We utilised a multitude of resources including medical records in primary and secondary care across England (Boxes 1 and 2), the databases Spine and RiO to identify if and when participants exited the NHS and the death register to identify the proportion of participants who died during the follow-up and their cause of death. The PNC, an operational police database containing criminal histories of all offenders in England, Wales and Scotland, was searched in January 2012 by the Ministry of Justice to gather information on criminal convictions and cautions of the sample.
Barking and Dagenham PCT.
Bexley PCT.
Blackpool PCT.
Brent and Harrow PCT.
Camden PCT.
City and Hackney Teaching PCT.
Coventry Teaching PCT.
Croydon PCT.
Cumbria Teaching PCT.
Derby City PCT.
Ealing PCT.
East and North Hertfordshire PCT.
Eastern and Coastal Kent PCT.
Gloucestershire PCT.
Haringey Teaching PCT.
Kensington and Chelsea PCT.
Kirklees PCT.
Lewisham PCT.
Medway PCT.
Newham PCT.
NHS North West London PCT.
North East Essex PCT.
North Yorkshire and York PCT.
Peterborough PCT.
Plymouth Teaching PCT.
Redbridge PCT.
South East Essex PCT.
Surrey PCT.
Sutton and Merton PCT.
Tower Hamlets PCT.
Waltham Forest PCT.
West Kent PCT.
Westminster PCT.
PCT, primary care trust.
Barnet, Enfield and Haringey Mental Health NHS Trust.
Barts Health NHS Trust.
Central and North West London NHS Foundation Trust.
Cheshire and Wirrall Partnership NHS Foundation Trust.
Cumbria Partnership NHS Foundation Trust.
East London NHS Foundation Trust.
Isle of Wight NHS Trust.
Kent and Medway NHS Foundation Trust.
Norfolk NHS Foundation Trust.
North East London NHS Foundation Trust.
North Essex NHS Foundation Trust.
Nottinghamshire Healthcare NHS Trust.
Oxleas NHS Foundation Trust.
South Essex Partnership University NHS Foundation Trust.
South London and Maudsley NHS Foundation Trust.
South West London and St George’s Trust.
South West Yorkshire Partnership NHS Foundation Trust.
Suffolk NHS Foundation Trust.
Tees, Esk and Wear Valleys NHS Foundation Trust.
West London Mental Health NHS Trust.
We obtained complete 10-year follow-up data for 74% of the sample and complete 5-year follow-up data for 81% of the sample (Figure 26). Overall, 34 study participants (6.9%) died at some point during the follow-up period. Causes of death included eight suicides and in three cases the cause of death was unascertainable. The majority of the deceased died of natural causes. Loss to follow-up mostly occurred because baseline participants had exited the NHS (mostly to return to their country of origin). In a few cases the GP surgery where a patient was registered refused access to his or her medical records.
FIGURE 26.
Achieved follow-up in years.
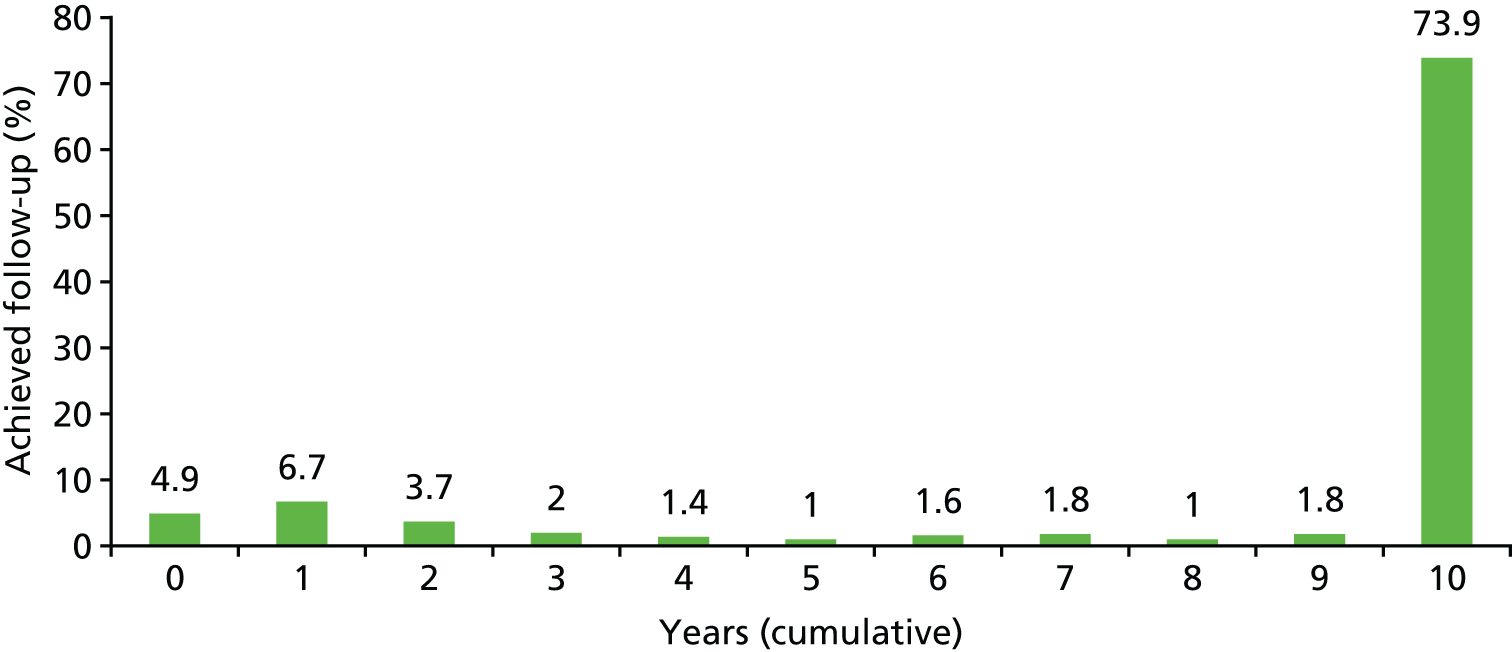
Symptoms of mental illness
The OPCRIT298 was administered to collect information on symptoms of mental illness. The checklist was specifically designed for the needs of empirical research. Episodes were dated and differentiated by a 2-month symptom-free interval for affective symptoms and a 6-month symptom-free interval for psychotic symptoms. Symptom domains relevant for the study and coded were appearance and behaviour (e.g. bizarre behaviour, catatonia), speech and form of thought (formal thought disorder), affect and associated features, abnormal beliefs and ideas (delusions) and abnormal perceptions (hallucinations).
Outcome
The MacArthur Community Violence Interview (MCVI)300 was rated based on case notes in primary and secondary care. Actions were considered to constitute serious violence if they were (1) batteries that resulted in physical injury or involved the use of a weapon; (2) sexual assaults; or (3) threats made with a weapon in hand. Batteries not resulting in injury of the victim were coded as minor violence.
Further outcome data were derived from convictions and cautions recorded in the PNC. For categorisation of violent offences, we used offences in the Home Office’s Standard List468 for definition of violence (committed) plus threats to commit such an offence for England, Wales and Scotland.
To ensure sufficient statistical power, violent incidents derived from medical records and PNC data were combined into one outcome measure: ‘violent behaviour’. Unfortunately, because of small numbers it was not possible to differentiate minor and serious violence.
As can be seen in Figure 27, the prevalence of violence throughout the follow-up was higher in men than in women and violence was highest within the first 6 months after initial assessment.
FIGURE 27.
Violent behaviour across 10 years of follow-up.
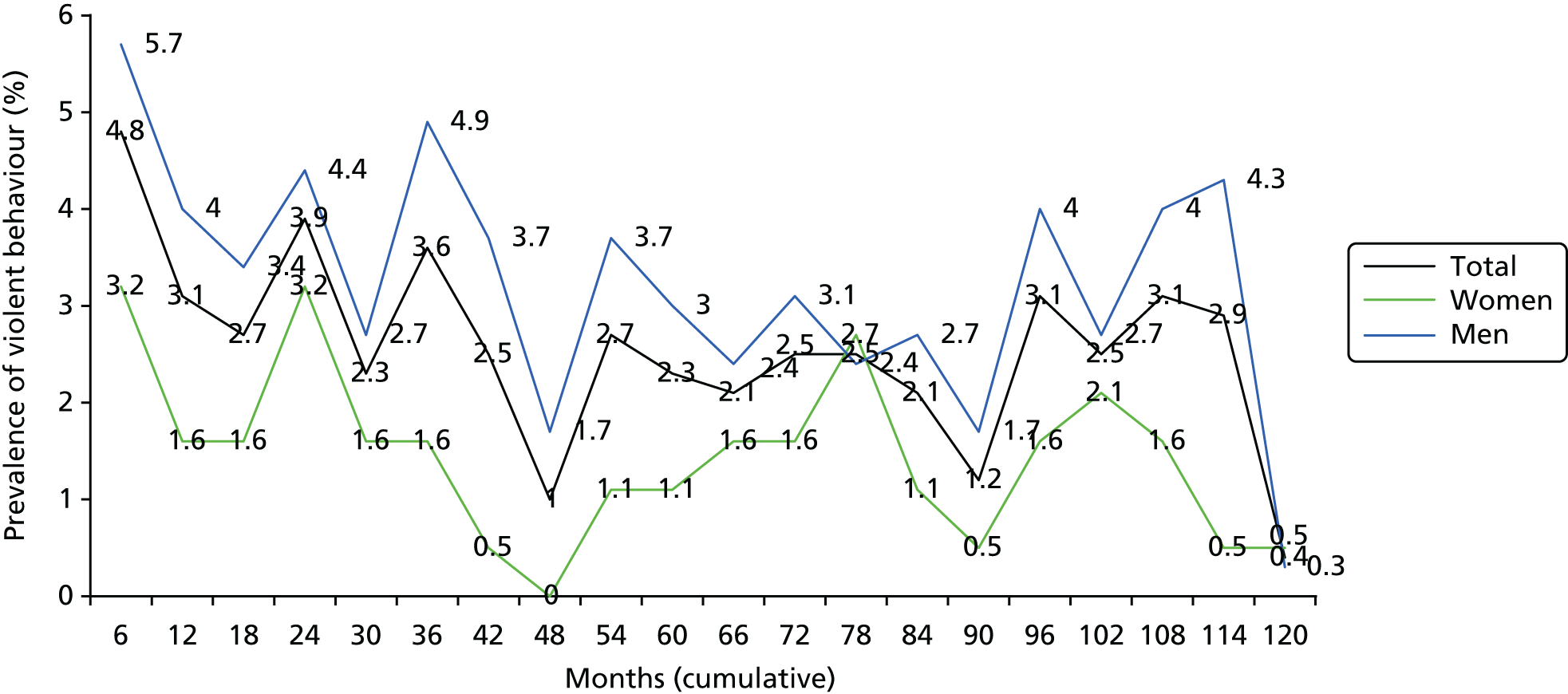
Selection of risk factors: methods and statistics
For the static part of the instrument, variables were selected on the basis of their AUC values. The following variables were included in analyses:
-
demography:
-
male sex
-
age < 35 years
-
black ethnicity
-
single marital status
-
UK born
-
asylum seeker/refugee
-
no educational qualifications
-
three or more moves of residence
-
has not worked in the past year
-
-
childhood adversities before age 15 years:
-
in care/adopted/fostered
-
poverty
-
parental discord
-
cruelty/physical abuse
-
sexual abuse
-
-
criminogenic:
-
history of violent behaviour leading to contact with services
-
history of threatening/annoying behaviour leading to contact with services
-
history of violent offending
-
history of non-violent offending
-
family history of criminal behaviour
-
-
clinical:
-
schizophrenia at first presentation
-
high trait impulsiveness
-
high trait anger
-
long duration of untreated psychosis
-
conduct disorder
-
alcohol abuse past year
-
drug use past year
-
family history of severe mental illness
-
family history of substance abuse.
-
We divided the 10-year follow-up period into 6-months window and observed the AUC values over time. As there was much variation over time we decided to predict violence within 6 months after assessment. To minimise shrinkage when using the instrument in a different sample we opted for a simple model and added the variables identified using a forward selection process. We utilised AUC values and sensitivity (those correctly identified as violent), specificity (those correctly classified and not violent) and PCC to identify the optimal cut-off point. Using a cumulative approach we then tested how stable predictive accuracy proved to be over the complete 10-year follow-up.
As can be seen in Figure 28, the AUC value for women was excellent for the first 6 months after assessment. There was a significant drop after inclusion of a further 6 months and the AUC was no longer significant when violence within the following 18 months was predicted. From 2 years onwards the AUC values stabilised and were significant but were of only moderate magnitude.
FIGURE 28.
Static risk: AUC values over time: women.

For men, the initial AUC value was moderate for the first 6 months after assessment. However, AUCs were more stable across time than in the female sample (Figure 29).
FIGURE 29.
Static risk: AUC values over time: men.

For the dynamic part of the instrument, the following variables were included:
-
appearance and behaviour:
-
disorganised/abnormal behaviour
-
recklessness
-
reduced need for sleep
-
increased sociability
-
agitation
-
retardation, loss of energy
-
-
speech and form of thought:
-
disorganised thinking: speech
-
disorganised thinking: formal thought disorder
-
pressured speech, thoughts racing
-
-
delusions:
-
persecutory delusions
-
grandiose delusions
-
delusions of reference
-
delusions of external control
-
delusions of guilt
-
-
hallucinations:
-
third-person hallucinations
-
commentary voices
-
abusive, persecutory voices
-
other auditory hallucinations
-
-
affect:
-
restricted, blunted affect
-
inappropriate affect
-
elation
-
irritability
-
depression
-
anhedonia
-
excessive guilt
-
suicidal ideation
-
-
unspecified symptoms:
-
sleeping problems
-
changes in appetite
-
poor concentration.
-
Variables were identified as risk factors when they demonstrated a significant association with violent behaviour throughout the follow-up period. Mixed-effects models for repeated measures were performed, adjusting for time and relatedness of the measures. As this method significantly increases statistical power, we opted for a conservative approach and identified potential confounders (static risk factors) using the total sample. Adjustments were made for age, educational qualifications, poverty, parental discord, history of violent behaviour leading to contact with services, history of threatening/annoying behaviour leading to contact with services, history of violent offending, history of non-violent offending, family history of criminal behaviour, schizophrenia at first presentation, high trait anger, long duration of untreated psychosis, conduct disorder and drug use in the past year. The results are shown in Table 159.
Total | Men | Women | |||||||
---|---|---|---|---|---|---|---|---|---|
ORa | 95% CI | p-value | ORa | 95% CI | p-value | ORa | 95% CI | p-value | |
Appearance and behaviour | |||||||||
Disorganised/abnormal behaviourb | 1.27 | 0.79 to 2.05 | 0.323 | 0.97 | 0.55 to 1.70 | 0.913 | 2.65 | 0.93 to 7.58 | 0.070 |
Recklessness | 1.26 | 0.65 to 2.42 | 0.490 | 1.29 | 0.59 to 2.78 | 0.525 | 1.52 | 0.44 to 5.33 | 0.511 |
Reduced need for sleep | 1.51 | 0.71 to 3.22 | 0.287 | 1.48 | 0.55 to 3.97 | 0.440 | 1.40 | 0.40 to 4.85 | 0.596 |
Increased sociability | 2.21 | 0.83 to 5.86 | 0.113 | 2.97 | 0.97 to 9.05 | 0.056 | 0.46 | 0.04 to 4.80 | 0.513 |
Agitation | 4.71 | 3.07 to 7.25 | < 0.001 | 4.74 | 2.92 to 7.69 | < 0.001 | 2.78 | 0.98 to 7.84 | 0.054 |
Retardation, loss of energy | 0.90 | 0.54 to 1.50 | 0.681 | 0.66 | 0.33 to 1.29 | 0.222 | 1.54 | 0.64 to 3.71 | 0.335 |
Speech and form of thought | |||||||||
Disorganised thinking: speechb | 1.89 | 1.18 to 3.04 | 0.009 | 0.96 | 0.53 to 1.74 | 0.898 | 8.52 | 3.66 to 19.82 | < 0.001 |
Disorganised thinking: formal thought disorder | 1.40 | 0.78 to 2.54 | 0.261 | 1.39 | 0.68 to 2.84 | 0.364 | 1.41 | 0.45 to 4.41 | 0.560 |
Pressured speech, thoughts racing | 3.25 | 1.99 to 5.32 | < 0.001 | 3.73 | 2.08 to 6.69 | < 0.001 | 2.10 | 0.86 to 5.07 | 0.100 |
Delusions | |||||||||
Persecutory delusionsb | 3.33 | 2.31 to 4.81 | < 0.001 | 2.64 | 1.71 to 4.09 | < 0.001 | 6.86 | 3.48 to 13.51 | < 0.001 |
Grandiose delusionsb | 1.65 | 0.96 to 2.81 | 0.068 | 1.34 | 0.71 to 2.53 | 0.369 | 2.62 | 1.04 to 6.55 | 0.040 |
Delusions of referenceb | 1.32 | 0.71 to 2.44 | 0.375 | 1.02 | 0. 45 to 2.31 | 0.972 | 1.15 | 0.38 to 3.52 | 0.805 |
Delusions of external control | 1.28 | 0.62 to 2.61 | 0.507 | 1.23 | 0.53 to 2.85 | 0.638 | 1.43 | 0.35 to 5.85 | 0.621 |
Delusions of guilt | 1.06 | 0.16 to 7.02 | 0.955 | 0.90 | 0.06 to 13.06 | 0.940 | 0.70 | 0.04 to 11.83 | 0.805 |
Hallucinations | |||||||||
Third-person hallucinationsb | 1.07 | 0.55 to 2.07 | 0.840 | 0.80 | 0.35 to 1.85 | 0.606 | 1.96 | 0.65 to 5.92 | 0.235 |
Commentary voices | 3.59 | 1.27 to 10.14 | 0.016 | 1.34 | 0.26 to 6.84 | 0.729 | 4.92 | 1.21 to 20.05 | 0.026 |
Abusive, persecutory voices | 2.66 | 1.63 to 4.34 | < 0.001 | 3.00 | 1.71 to 5.26 | < 0.001 | 1.38 | 0.49 to 3.87 | 0.546 |
Other auditory hallucinationsb | 1.99 | 1.26 to 3.16 | 0.003 | 1.46 | 0.84 to 2.54 | 0.179 | 3.83 | 1.68 to 8.72 | 0.001 |
Affect | |||||||||
Restricted, blunted affect | 1.05 | 0.64 to 1.72 | 0.861 | 1.16 | 0.64 to 2.08 | 0.631 | 0.80 | 0.31 to 2.09 | 0.650 |
Inappropriate affectb | 1.50 | 0.90 to 2.51 | 0.118 | 1.04 | 0.55 to 1.99 | 0.898 | 3.19 | 1.28 to 7.95 | 0.013 |
Elation | 1.47 | 0.86 to 2.51 | 0.155 | 1.44 | 0.75 to 2.77 | 0.276 | 1.08 | 0.41 to 2.85 | 0.871 |
Irritability | 4.45 | 2.93 to 6.75 | < 0.001 | 4.24 | 2.60 to 6.93 | < 0.001 | 5.39 | 2.09 to 13.93 | < 0.001 |
Depression | 1.17 | 0.71 to 1.92 | 0.547 | 1.00 | 0.55 to 1.84 | 0.987 | 1.13 | 0.41 to 3.15 | 0.809 |
Anhedonia | 0.66 | 0.30 to 1.44 | 0.292 | 0.88 | 0.34 to 2.27 | 0.792 | 0.71 | 0.17 to 2.98 | 0.641 |
Excessive guilt | 0.56 | 0.06 to 5.27 | 0.608 | – | – | – | 2.65 | 0.25 to 27.89 | 0.416 |
Suicidal ideation | 2.14 | 1.18 to 3.87 | 0.012 | 1.72 | 0.81 to 3.68 | 0.159 | 2.11 | 0.75 to 5.96 | 0.157 |
Unspecific symptoms | |||||||||
Sleeping problems | 1.48 | 0.91 to 2.41 | 0.116 | 1.46 | 0.83 to 2.56 | 0.190 | 1.52 | 0.56 to 4.12 | 0.415 |
Changes in appetite | 2.31 | 1.38 to 3.87 | 0.001 | 2.35 | 1.28 to 4.32 | 0.006 | 1.71 | 0.64 to 4.55 | 0.282 |
Poor concentrationb | 2.04 | 1.28 to 3.26 | 0.003 | 1.57 | 0.89 to 2.74 | 0.117 | 3.35 | 1.29–8.68 | 0.013 |
To test whether or not there were significant sex differences in the association of the dynamic risk factors with violent outcome we performed statistical analyses to test for effect modification by modelling two-way interaction terms (sex × dynamic risk factor). A significant result indicated that observed differences in associations were statistically relevant when comparing men and women.
Interaction terms were also modelled to test whether or not level of risk identified with the static part of the instrument influenced associations of dynamic risk factors with violent outcome.
Based on previous research identifying negative affect as a key variable in the pathway towards violence,21,291 we performed mediation analyses. Affect was considered as a potential mediator when it demonstrated a significant relationship with both (1) violence and (2) symptom. By comparing standardised regression coefficients from models with and without affect as covariate,303 we estimated the proportion of direct effects that were mediated by affect and tested their significance using bootstrapped standard errors and CIs (using 1000 repetitions). This method is preferred over other tests for significant indirect effects such as the Sobel test because it is less conservative and does not require normality assumptions to be met. 304
Coding procedure
The coding of the risk factors needs to be performed after a thorough and systematic examination of all available information including medical records and criminal history and interviews with the patient and/or significant others. However, the information needed to code the static and dynamic part of the Violence in Psychotic Persons (VIPP) instrument is usually obtained in routine clinical assessments and completing the coding sheets should not take longer than 15 minutes.
The static part of the VIPP instrument has to be repeated every 6 months; reliance on previous ratings after a longer time period will result in inaccuracy of the prediction.
Risk factors for the dynamic part of the VIPP instrument should be checked routinely as changes in these symptoms and symptom constellations can result in changes in level of risk for violence.
Both static and dynamic risk factors are coded as ‘0’ if they are absent and ‘1’ if they are present. If information is not available/accessible, no code is given. However, risk assessment is less accurate when information is missing.
In the static part of the VIPP instrument the codings are added up. A total score of ≥ 4 in women and ≥ 2 in men is indicative that an individual’s risk of violence within 6 months after the assessment is increased.
For dynamic factors it is not necessary to add up the codings as the presence of only one risk factor is sufficient to indicate an elevated risk for violence. This is not restricted to a specified time frame, with risk elevated as long as the symptom is present. In this case we would advise that the risk for violence in these patients be reconsidered and appropriate measures be taken to reduce the risk.
Coding sheet: module for women
Name:
Age:
Date of assessment:
Assessed by:
Static risk
Dynamic risk
Coding sheet: module for men
Name:
Age:
Date of assessment:
Assessed by:
Static risk
Dynamic risk
Appendix 2 Coding sheet for the Computerised Instrument for Violence
Name:
Date of assessment:
Assessed by:
Enter predictor values | Enter number |
---|---|
Number of previous convictions for violence (PreVio) | |
Number of previous convictions for acquisitive offence (PreAcq) | |
Age at time of interview in years (Age) | |
Compute score (S) | Enter score |
S = 0.24 × PreVio – 0.09 × Age + 0.02 × PreAcq + 1.11 | |
Compute probability (P) | Enter probability |
P = exp(S)/(1 + exp(S)) | |
Define risk level (RL) based on sample median (0.316) | Enter risk level |
If P > 0.316, risk level = high; if P < = 0.316, risk level = low |
Appendix 3 Coding sheet for the Pencil and Paper Instrument for Violence
Name:
Date of assessment:
Assessed by:
Enter predictor values | Enter number |
---|---|
Number of previous convictions for violence (PreVio) | |
Number of previous convictions for acquisitive offence (PreAcq) | |
Age at time of interview in years (Age) | |
Number of previous convictions for violence levels: PreVioc | Coding score |
0 | –1 |
1 | 0 |
2–5 | 1 |
6+ | 2 |
Enter coding score based on actual PreVioc level | Enter coding score |
PreVioc = | |
Number of previous convictions for acquisitive crime levels: PreAcqc | Coding score |
0–2 | –1 |
3–12 | 0 |
13+ | 1 |
Enter coding score based on actual PreAcqc level | Enter coding score |
PreAcqc = | |
Age at time of interview in years levels: Agec | Coding score |
≤ 21 | 2 |
22–25 | 1 |
26–39 | 0 |
40–45 | –1 |
46+ | –2 |
Enter coding score based on actual Agec level | Enter coding score |
Agec = | |
Compute score (S) | Enter score |
S = PreVioc + Agec + PreAcqc + 4 | |
Define risk level (RL) based on sample median (4) | Enter risk level |
If S > 4, Risk level = high; if S ≤ 4, risk level = low |
Appendix 4 Coding sheet for the Computerised Instrument for Robbery
Name:
Date of assessment:
Assessed by:
Enter predictor values | Enter number |
---|---|
Number of previous convictions for robbery (PreRobb) | |
Number of previous convictions for acquisitive offence (PreAcq) | |
Age at time of interview in years (Age) | |
Compute score (S) | Enter score |
S = 0.65 × log(PreRobb + 1) – 0.06 × Age + 0.32 × log(PreAcq + 1) – 1.39 | |
Compute probability (P) | Enter probability |
P = exp(S)/(1 + exp(S)) | |
Define risk level (RL) based on sample median (0.082) | Enter risk level |
If P > 0.082, risk level = high; if P ≤ 0.082, risk level = low |
Appendix 5 Coding sheet for the Paper Instrument for Robbery
Name:
Date of assessment:
Assessed by:
Enter predictor values | Enter number |
---|---|
Number of previous convictions for robbery (PreRobb) | |
Number of previous convictions for acquisitive offence (PreAcq) | |
Age at time of interview in years (Age) | |
Number of previous convictions for robbery levels: PreRobbc | Coding score |
0 | –1 |
1 | 0 |
2+ | 1 |
Enter coding score based on actual PreRobbc level | Enter coding score |
PreRobbc = | |
Number of previous convictions for acquisitive crime levels: PreAcqc | Coding score |
0–2 | –1 |
3–12 | 0 |
13+ | 1 |
Enter coding score based on actual PreAcqc level | Enter coding score |
PreAcqc = | |
Age at time of interview in years levels: Agec | Coding score |
≤ 21 | 2 |
22–29 | 1 |
30–39 | 0 |
40–45 | –1 |
46+ | –2 |
Enter coding score based on actual Agec level | Enter coding score |
Agec = | |
Compute score (S) | Enter score |
S = PreRobbc + Agec + PreAcqc + 4 | |
Define risk level (RL) based on sample median (4) | Enter risk level |
If S > 4, risk level = high; if S ≤ 4, risk level = low |
Appendix 6 Coding sheet for the Computerised Instrument for Drugs
Name:
Date of assessment:
Assessed by:
Enter predictor values | Enter number |
---|---|
Number of previous convictions for drugs (PreDrugs) | |
Number of previous convictions for acquisitive offence (PreAcq) | |
Age at time of interview in years (Age) | |
Compute score (S) | Enter score |
S = 0.87 × log(PreDrugs + 1) – 0.08 × Age + 0.26 × log(PreAcq + 1) + 0.002 | |
Compute probability (P) | Enter probability |
P = exp(S)/(1 + exp(S)) | |
Define risk level (RL) based on sample median (0.187) | Enter risk level |
If P > 0.187, risk level = high; if P ≤ 0.187, risk level = low |
Appendix 7 Coding sheet for the Paper Instrument for Drugs
Name:
Date of assessment:
Assessed by:
Enter predictor values | Enter number |
---|---|
Number of previous convictions for drugs (PreDrugs) | |
Number of previous convictions for acquisitive offence (PreAcq) | |
Age at time of interview in years (Age) | |
Number of previous convictions for drugs levels: PreDrugsc | Coding score |
0 | –1 |
1 | 0 |
2+ | 1 |
Enter coding score based on actual PreDrugsc level | Enter coding score |
PreDrugsc = | |
Number of previous convictions for acquisitive crime levels: PreAcqc | Coding score |
0–2 | –1 |
3–12 | 0 |
13+ | 1 |
Enter coding score based on actual PreAcqc level | Enter coding score |
PreAcqc = | |
Age at time of interview in years levels: Agec | Coding score |
≤ 19 | 2 |
20–30 | 1 |
31–37 | 0 |
38–45 | –1 |
46+ | –2 |
Enter coding score based on actual Agec level | Enter coding score |
Agec = | |
Compute score (S) | Enter score |
S = PreDrugsc + Agec + PreAcqc + 4 | |
Define risk level (RL) based on sample median (4) | Enter risk level |
If S > 4, risk level = high, if S ≤ 4, risk level = low |
Appendix 8 Coding sheet for the Computer Instrument for Acquisitive Crime
Name:
Date of assessment:
Assessed by:
Enter predictor values | Enter number |
---|---|
Number of previous convictions for drugs (PreDrugs) | |
Number of previous convictions for acquisitive offence (PreAcq) | |
Age at time of interview in years (Age) | |
Compute score (S) | Enter score |
S = 0.08 × PreDrugs – 0.10 × Age + 0.81 × log(PreAcq + 1) + 0.663 | |
Compute probability (P) | Enter probability |
P = exp(S)/(1 + exp(S)) | |
Define risk level (RL) based on sample median (0.369) | Enter risk level |
If P > 0.369, risk level = high; if P ≤ 0.369, risk level = low |
Appendix 9 Coding sheet for the Paper Instrument for Acquisitive Crime
Name:
Date of assessment:
Assessed by:
Enter predictor values | Enter number |
---|---|
Number of previous convictions for drugs (PreDrugs) | |
Number of previous convictions for acquisitive offence (PreAcq) | |
Age at time of interview in years (Age) | |
Number of previous convictions for drugs levels: PreDrugsc | Coding score |
0 | 0 |
1+ | 1 |
Enter coding score based on actual PreDrugsc level | Enter coding score |
PreDrugsc = | |
Number of previous convictions for acquisitive crime levels: PreAcqc | Coding score |
0 | –2 |
1 | –1 |
2–7 | 0 |
8–16 | 1 |
17+ | 2 |
Enter coding score based on actual PreAcqc level | Enter coding score |
PreAcqc = | |
Age at time of interview in years levels: Agec | Coding score |
≤ 21 | 2 |
22–30 | 1 |
31–37 | 0 |
38–42 | –1 |
43+ | –2 |
Enter coding score based on actual Agec level | Enter coding score |
Agec = | |
Compute score (S) | Enter score |
S = PreDrugsc + Agec + PreAcqc + 4 | |
Define risk level (RL) based on sample median (5) | Enter risk level |
If S > 5, risk level = high; if S ≤ 5, risk level = low |
Appendix 10 Coding sheet for the Dynamic Risk Instrument for Violence
For each domain, first enter in the ‘Present (Y/N)’ column Y if risk factor is present and N if risk factor is not present. For risk factors that are present, enter a value in the ‘Priority number’ column according to the number in front of the risk factors that are present (i.e. starting from the risk factor that is present with the smallest number and continuing with the risk factor that is present with the second smallest number). For example, in domain 1 (accommodation), if the factors ‘evicted’ and ‘homelessness’ are absent and the factors ‘frequent address change’ and ‘local problems’ are present, the priority number for ‘frequent address change’ is 1 (first priority) and for ‘local problems’ is 2 (second priority).
Risk factor | AOR | 95% CI | p-value | Present (Y/N) | Priority number |
---|---|---|---|---|---|
Domain 1: accommodation | |||||
1. Evicted | 2.71 | 1.43 to 5.12 | 0.002 | ||
2. Frequent address change | 2.45 | 1.15 to 5.22 | 0.020 | ||
3.Homelessness | 1.87 | 1.02 to 3.43 | 0.045 | ||
4. Local problems | 1.72 | 1.01 to 2.93 | 0.046 | ||
Domain 2: social environment | |||||
1. Criminal network | 2.66 | 1.48 to 4.80 | 0.001 | ||
2. Living with partner | 1.77 | 1.12 to 2.82 | 0.015 | ||
3. Family/friends unsupportive (first quartile) | 1.67 | 1.05 to 2.66 | 0.029 | ||
Domain 3: coping/daily living | |||||
1. Services cut off | 3.22 | 1.32 to 7.84 | 0.010 | ||
2. Coping difficulties score (last quartile) | 2.60 | 1.63 to 4.14 | < 0.001 | ||
3. High stress score (last quartile) | 2.44 | 1.57 to 3.81 | < 0.001 | ||
4. Borrowing money | 2.22 | 1.44 to 3.43 | < 0.001 | ||
5. Financial difficulties | 2.13 | 1.36 to 3.34 | 0.001 | ||
6. Financial difficulties managing household | 1.68 | 1.01 to 2.80 | 0.046 | ||
Domain 4: leisure time | |||||
1. Frequent visits to bars/pubs | 2.20 | 1.30 to 3.72 | 0.003 | ||
2. Frequent betting | 1.71 | 1.02 to 2.88 | 0.044 | ||
Domain 5: employment/education | |||||
1. Disagreements at work | 2.95 | 1.48 to 5.89 | 0.002 | ||
Domain 6: depression/self-harm/anxiety | |||||
1. Anger (STAXI) last quartile | 2.14 | 1.35 to 3.39 | 0.001 | ||
2. Considered suicide | 2.00 | 1.23 to 3.24 | 0.005 | ||
3. Anxiety (HADS) | 1.96 | 1.24 to 3.10 | 0.004 | ||
4. Tedium vitae | 1.69 | 1.08 to 2.64 | 0.022 | ||
Domain 7: psychosis | |||||
1. Paranoid delusions | 5.49 | 2.67 to 11.29 | < 0.001 | ||
2. Psychosis (PSQ 2+) | 3.67 | 2.38 to 5.66 | < 0.001 | ||
3. PSQ = 1 + non-compliance to therapeutic intervention | 3.18 | 1.60 to 6.33 | 0.001 | ||
4. Strange experiences | 2.66 | 1.18 to 6.01 | 0.019 | ||
Domain 8: alcohol use | |||||
1. Hazardous drinking (AUDIT score of ≥ 8) | 3.53 | 2.31 to 5.40 | < 0.001 | ||
2. Alcohol use disorder (AUDIT score of ≥ 16) | 2.65 | 1.53 to 4.59 | 0.001 | ||
Domain 9: drug use | |||||
1. Ecstasy dependence | 11.90 | 3.08 to 45.92 | < 0.001 | ||
2. Ecstasy use | 4.97 | 2.97 to 8.33 | < 0.001 | ||
3. Drug use (any) | 4.89 | 2.85 to 8.40 | < 0.001 | ||
4. Cocaine powder use | 3.82 | 2.36 to 6.19 | < 0.001 | ||
5. Any drug dependence | 2.70 | 1.69 to 4.32 | < 0.001 | ||
6. Cannabis use | 2.61 | 1.66 to 4.08 | < 0.001 | ||
7. Cannabis dependence | 2.55 | 1.45 to 4.48 | 0.001 | ||
8. Amphetamine use | 2.25 | 1.20 to 4.22 | 0.011 | ||
9. Injected drugs | 2.12 | 1.04 to 4.32 | 0.038 | ||
10. Crack cocaine use | 2.01 | 1.14 to 3.54 | 0.016 | ||
Domain 10: life events | |||||
1. Assaulted | 10.30 | 5.39 to 19.67 | < 0.001 | ||
2. Redundant/sacked | 3.76 | 1.83 to 7.71 | < 0.001 | ||
3. At least one life event | 2.38 | 1.57 to 3.60 | < 0.001 | ||
Domain 11: treatment | |||||
1. Not attended treatment for mental disorder | 2.01 | 1.09 to 3.72 | 0.025 | ||
Domain 12: compliance with supervision | |||||
1. Missed appointments with probation officer | 2.27 | 1.46 to 3.52 | < 0.001 | ||
2. Warning letter from probation officer | 1.90 | 1.18 to 3.05 | 0.008 | ||
Domain 13: victimisation | |||||
1. Victim violence/threats | 16.85 | 10.03 to 28.31 | < 0.001 | ||
2. Victimisation threats | 4.19 | 2.30 to 7.62 | < 0.001 | ||
3. Victim other | 3.09 | 1.70 to 5.63 | < 0.001 | ||
4. Victim theft/burglary | 2.72 | 1.30 to 5.70 | 0.008 | ||
Domain 14: thoughts of violence | |||||
1. Different victims | 6.45 | 3.29 to 12.64 | < 0.001 | ||
2. Likely to meet victim | 5.21 | 2.37 to 11.47 | < 0.001 | ||
3. Different ways of hurting others | 3.99 | 2.16 to 7.39 | < 0.001 | ||
4. Thoughts of violence | 3.84 | 2.32 to 6.38 | < 0.001 | ||
5. Frequency of thoughts (at least two times a month) | 3.64 | 2.15 to 6.16 | < 0.001 | ||
6. Thoughts of harm more than once a week | 3.23 | 1.67 to 6.26 | < 0.001 | ||
Domain 15: thoughts of previous offending | |||||
1. Contact previous victim | 4.68 | 2.36 to 9.30 | < 0.001 | ||
Domain 16: attitudes to crime | |||||
1. OK to steal if very poor | 2.89 | 1.88 to 4.45 | < 0.001 | ||
2. OK to steal from the rich | 3.86 | 2.50 to 5.97 | < 0.001 | ||
3. OK to steal from shops that make lots of money | 3.37 | 2.17 to 5.23 | < 0.001 | ||
4. Sometimes OK to break the law | 2.86 | 1.86 to 4.40 | < 0.001 | ||
5. Attitudes towards crime total score (last quartile) | 4.26 | 2.73 to 6.66 | < 0.001 |
Appendix 11 Description of model variables in the Decision Support for Violence Management in Prisoners network
Variable number | Node name | Model component | Node type | Node category | Node states |
---|---|---|---|---|---|
1 | Victimisation | Criminal attitude | Labelled | Observable | No/yes |
2 | Gang member | Labelled | Observable | No/yes | |
3 | Criminal network | Labelled | Observable | No/yes | |
4 | Criminal family background | Labelled | Observable | No/yes | |
5 | Criminal attitude | Labelled | Observable | No/yes | |
6 | Violent thoughts | Labelled | Observable | No/yes | |
7 | Compliance with supervision | Labelled | Observable | No/partial/yes | |
8 | Negative attitude | Labelled | Observable | No/partial/yes | |
9 | Attitude danger level | Labelled | Latent | Low/high | |
10 | Aggression danger level | Criminal attitude/personality disorder | Labelled | Latent | Low/high |
11 | ASPD | Personality disorder | Labelled | Observable | No/yes |
12 | BPD | Labelled | Observable | No/yes | |
13 | Abuse or neglect as a child | Labelled | Observable | No/yes | |
14 | Anger | Labelled | Observable | No/yes | |
15 | Impulsivity | Labelled | Observable | No/partial/yes | |
16 | PCLR factor 1 | ∼TNormal(µ,σ2,0,16) | Observable | 0–16 | |
17 | PCLR factor 2 | ∼TNormal(µ,σ2,0,18) | Observable | 0–18 | |
18 | PCLR facet 3 | ∼TNormal(µ,σ2,0,10) | Observable | 0–10 | |
19 | Anger management | Labelled | Observable intervention | No/yes | |
20 | Anger management given failure | Labelled | Latent | No/yes | |
21 | Anger management post treatment | Labelled | Latent | No/yes | |
22 | Intelligence | Socioeconomic factors | Labelled | Observable | Extremely low/borderline/low average/average/high average/superior |
23 | Living circumstances | Labelled | Observable | Homeless/bail hostel or shelter/living alone/living with partner/living with family or friends/other | |
24 | Education | Labelled | Observable | No/GCSE or O level/A level+/other | |
25 | Stress | Labelled | Observable | No/yes | |
26 | Financial difficulties | Labelled | Observable | No/yes | |
27 | Employment or training | Labelled | Observable | No/yes | |
28 | Problematic life events | Labelled | Observable | No/yes | |
29 | Socially withdraw | Labelled | Observable | No/yes | |
30 | Ability to cope | Labelled | Observable | Low/high | |
31 | Domestic stability | Labelled | Observable | Low/high | |
32 | Social protective level | Labelled | Latent | Low/high | |
33 | Symptoms of mental illness | Mental illness | Labelled | Observable | No/partial/yes |
34 | Depressive symptoms | Labelled | Observable | No/yes | |
35 | Anxiety | Labelled | Observable | No/yes | |
36 | Thought insertion | Labelled | Observable | No/yes | |
37 | Hallucinations | Labelled | Observable | No/yes | |
38 | Strange experiences | Labelled | Observable | No/yes | |
39 | Paranoid delusions | Labelled | Observable | No/yes | |
40 | Psychiatric treatment | Labelled | Observable intervention | No/yes | |
41 | Depressive symptoms post treatment | Labelled | Latent | No/yes | |
42 | Anxiety post treatment | Labelled | Latent | No/yes | |
43 | Thought insertion post treatment | Labelled | Latent | No/yes | |
44 | Hallucinations post treatment | Labelled | Latent | No/yes | |
45 | Strange experiences post treatment | Labelled | Latent | No/yes | |
46 | Paranoid delusions post treatment | Labelled | Latent | No/yes | |
47 | Psychiatric treatment failure | Labelled | Latent | No/yes | |
48 | Mental illness danger level | Labelled | Latent | Low/high | |
49 | Cocaine use before prison sentence | Substance misuse | Labelled | Observable | No/yes |
50 | Cannabis use before prison sentence | Labelled | Observable | No/yes | |
51 | Ecstasy use before prison sentence | Labelled | Observable | No/yes | |
52 | Cocaine use during prison sentence | Labelled | Observable | No/yes | |
53 | Cannabis use during prison sentence | Labelled | Observable | No/yes | |
54 | Ecstasy use during prison sentence | Labelled | Observable | No/yes | |
55 | Cocaine use after release | Labelled | Observable | No/yes | |
56 | Cannabis use after release | Labelled | Observable | No/yes | |
57 | Ecstasy use after release | Labelled | Observable | No/yes | |
58 | Hazardous drinking after release | Labelled | Observable | No/yes | |
59 | Cocaine dependence | Labelled | Observable | No/yes | |
60 | Cannabis dependence | Labelled | Observable | No/yes | |
61 | Ecstasy dependence | Labelled | Observable | No/yes | |
62 | Alcohol dependence | Labelled | Observable | No/yes | |
63 | Drug treatment | Labelled | Observable | No/yes | |
64 | Alcohol treatment | Labelled | Observable intervention | No/yes | |
65 | Cocaine use post treatment | Labelled | Latent | No/yes | |
66 | Cannabis use post treatment | Labelled | Latent | No/yes | |
67 | Ecstasy use post treatment | Labelled | Latent | No/yes | |
68 | Hazardous drinking post treatment | Labelled | Latent | No/yes | |
69 | Drug treatment given failure | Labelled | Latent | No/yes | |
70 | Alcohol treatment given failure | Labelled | Latent | No/yes | |
71 | Any drug dependence | Labelled | Definitional | No/yes | |
72 | Response given drug dependence | Labelled | Latent | No/yes | |
73 | Response given alcohol dependence | Labelled | Latent | No/yes | |
74 | Substance misuse danger level | Labelled | Latent | Low/high | |
75 | Responsiveness to treatment | Treatment responsivity | Labelled | Observable | No/yes |
76 | Refusal or failure to attend therapy | Labelled | Latent | No/yes | |
77 | Previous acquisitive crime convictions | Violence and other static risk factors | Labelled | Observable | 0–2/3–12/13+ |
78 | Previous violent convictions | Labelled | Observable | 0/1/2–5/6+ | |
79 | PCL-R total score | Labelled | Observable | 0–9/10–16/17–26/27+ | |
80 | Age | Labelled | Observable | 18–19/20–21/22–25/26–29/30–34/35–39/40–49/50–59/60+ years | |
81 | Gender | Labelled | Observable | Female/male | |
82 | Time at risk (days) | ∼Uniform(a,b) | Observable | 0–5000 | |
83 | Violent reconvictions rate (step 1) | ∼Beta(a,b) | Latent | 0–1 | |
84 | Violent reconvictions rate (step 2) | Revised ∼Beta | Latent | 0–1 | |
85 | Violent reconvictions rate (step 3) | Revised ∼Beta | Latent | 0–1 | |
86 | Time since initial release (days) | ∼Uniform(a,b) | Observable | 0–5000 | |
87 | Violent convictions since initial release | ∼Binomial(n,p) | Observable | 0-infinity | |
88 | Violent convictions | ∼Binomial(n,p) | Latent | 0-infinity | |
89 | Violence | Labelled | Latent | No/yes |
Appendix 12 Clinical utility questionnaire
Offender risk management decision-support software usability questionnaire
List of abbreviations
- A&E
- accident and emergency
- aBIC
- adjusted Bayesian information criterion
- ADHD
- attention deficit hyperactivity disorder
- ADOS-4
- Autism Diagnostic Observation Schedule module-4
- AESOP
- Ætiology and Ethnicity in Schizophrenia and Other Psychoses
- AIC
- Akaike information criterion
- AMHP
- approved mental health professional
- AOR
- adjusted odds ratio
- APMS
- Adult Psychiatric Morbidity Survey
- app
- application
- ARA
- actuarial risk assessment
- ASD
- autistic spectrum disorder
- ASPD
- antisocial personality disorder
- ASRS
- Adult Self-Report Scale
- AUC
- area under the receiver operating characteristic curve
- AUDIT
- Alcohol Use Disorders Identification Test
- BIC
- Bayesian information criterion
- BPD
- borderline personality disorder
- BPRS
- Brief Psychiatric Rating Scale
- CI
- confidence interval
- CIA
- Computerised Instrument for Acquisitive Crime
- CID
- Computerised Instrument for Drugs
- CIR
- Computerised Instrument for Robbery
- CIS-R
- Clinical Interview Schedule Revised Version
- CIV
- Computerised Instrument for Violence
- CJA
- Criminal Justice Act
- COVR
- Classification of Violence Risk
- CPA
- Care Programme Approach
- CSEW
- Crime Survey for England and Wales
- df
- degree of freedom
- DRIV
- Dynamic Risk Instrument for Violence
- DSM-IV
- Diagnostic and Statistical Manual of Mental Disorders-Fourth Edition
- DSVM-P
- Decision Support for Violence Management in Prisoners
- ELFEPS
- East London First Episode Psychosis Study
- GBH
- grievous bodily harm
- GP
- general practitioner
- HADS
- Hospital Anxiety and Depression Scale
- HCR-20
- Historical, Clinical, Risk Management-20
- HCR-20v2
- Historical, Clinical, Risk Management-20, version 2
- HCR-20v3
- Historical, Clinical, Risk Management-20, version 3
- H–L
- Hosmer–Lemeshow
- HMP
- Her Majesty’s Prison
- HSU
- high secure unit
- ICD-10
- International Statistical Classification of Diseases and Related Health Problems, Tenth Edition
- IPV
- intimate partner violence
- IQ
- intelligence quotient
- LCA
- latent class analysis
- LOOCV AUC
- leave-one-out cross-validation area under the curve
- LSU
- low secure unit
- MCVI
- MacArthur Community Violence Instrument
- MLR
- robust maximum likelihood
- MMLS
- Men’s Modern Lifestyle Survey
- MSRAG
- Medium Security Recidivism Assessment Guide
- MSS
- medium secure service
- MSU
- medium secure unit
- NART
- National Adult Reading Test
- NESARC
- National Epidemiologic Survey on Alcohol and Related Conditions
- NHPMS
- National Household Psychiatric Morbidity Survey
- NIGB
- National Information Governance Board
- NICE
- National Institute for Health and Care Excellence
- NOMS
- National Offender Management Service
- NPV
- negative predictive value
- OASys
- Offender Assessment System
- OGP
- Offender Assessment System General reoffending Predictor
- OGRS2
- Offender Group Reconviction Scale, version 2
- OGRS3
- Offender Group Reconviction Scale, version 3
- OPCRIT
- OPerational CRITeria checklist (for psychotic and affective symptoms)
- OR
- odds ratio
- OVP
- Offender Assessment System Violent reoffending Predictor
- PANSS
- Positive and Negative Syndrome Scale
- PCC
- percentage correctly classified
- PCL-R
- Psychopathy Checklist – Revised
- PCL:SV
- Psychopathy Checklist: Screening Version
- PCS
- Prisoner Cohort Study
- PDD
- pervasive developmental disorder
- PIA
- Paper Instrument for Acquisitive Crime
- PID
- Paper Instrument for Drugs
- PIR
- Paper Instrument for Robbery
- PIV
- Pencil and Paper Instrument for Violence
- PNC
- Police National Computer
- PPV
- positive predictive value
- PSQ
- Psychosis Screening Questionnaire
- RCT
- randomised controlled trial
- RIOC
- relative improvement over chance
- RM2000V
- Risk Matrix 2000 for Violence
- ROC
- receiver operating characteristic
- RRR
- relative risk ratio
- SAPROF
- Structured Assessment of Protective Factors for Violence Risk
- SCAN
- Schedules for Clinical Assessment in Neuropsychiatry
- SCID-II
- Structured Clinical Interview for DSM-IV Axis II Disorders
- SD
- standard deviation
- SE
- standard error
- SPJ
- structured professional judgement
- STAXI
- State–Trait Anger Expression Inventory
- STI
- sexually transmitted infection
- VMR-LRT
- Vuong–Lo–Mendell–Rubin likelihood ratio test
- VoRAMSS
- Validation of New Risk Assessment Instruments for Use with Patients Discharged from Medium Secure Services
- VRAG
- Violence Risk Appraisal Guide
- WAIS
- Wechsler Adult Intelligence Scale
- WHO
- World Health Organization