Notes
Article history
The research reported in this issue of the journal was funded by PGfAR as project number RP-PG-0407-10314. The contractual start date was in January 2009. The final report began editorial review in March 2015 and was accepted for publication in April 2016. As the funder, the PGfAR programme agreed the research questions and study designs in advance with the investigators. The authors have been wholly responsible for all data collection, analysis and interpretation, and for writing up their work. The PGfAR editors and production house have tried to ensure the accuracy of the authors’ report and would like to thank the reviewers for their constructive comments on the final report document. However, they do not accept liability for damages or losses arising from material published in this report.
Declared competing interests of authors
none
Permissions
Copyright statement
© Queen’s Printer and Controller of HMSO 2017. This work was produced by Hemingway et al. under the terms of a commissioning contract issued by the Secretary of State for Health. This issue may be freely reproduced for the purposes of private research and study and extracts (or indeed, the full report) may be included in professional journals provided that suitable acknowledgement is made and the reproduction is not associated with any form of advertising. Applications for commercial reproduction should be addressed to: NIHR Journals Library, National Institute for Health Research, Evaluation, Trials and Studies Coordinating Centre, Alpha House, University of Southampton Science Park, Southampton SO16 7NS, UK.
Chapter 1 Introduction
Linked electronic health records and learning health systems
The global political importance of data
People increasingly leave digital ‘traces’ relevant to health in multiple different information systems as they interact with health and other services and go about their everyday lives. The potential for such data to inform, and potentially transform, our understanding of health and disease has been recognised by world leaders. For example, George Osborne, the UK Chancellor of the Exchequer from 2010 to 2016, said in a Science Speech to the Royal Society in November 2013 that the UK has ‘some of the world’s best and most complete data-sets in healthcare’ (© Crown copyright; contains public sector information licensed under the Open Government Licence v3.0). 1 Following the 2015 State of the Union address, the White House signalled an initiative that would ‘catalyse a new era of data-based and more precise medical treatment’ (reproduced under a Creative Commons Attribution 3.0 License). 2
Data tapestry
The electronic record relevant to health is diverse. This ‘data tapestry’ of information (Figure 1) spans records in primary care and in hospitals, structured data (codes, values) and unstructured data (text). It includes information on genomics, through to social media, the sensed self and wider social determinants of disease. Deriving information and knowledge from one or more sources of these rapidly developing sources of health-relevant data might be of great benefit to inform improvements in care and outcomes of disease.
FIGURE 1.
The tapestry of potentially high-value information sources that may be linked to an individual for use in health care. CPT, current procedural terminology; ECG, electrocardiogram; EPA, US Environmental Protection Agency; GIS, geographic information system; GPS, global positioning system; ICD-9, International Classification of Diseases, Ninth Edition; LOINC, Logical Observation Identifiers Names and Codes; NDC, National Drug Code; OTC, over the counter; SNP, single nucleotide polymorphism. Reproduced with permission from Weber et al. Finding the missing link for big biomedical data. JAMA 2014;311:2479–80. 3 Copyright © 2014 American Medical Association. All rights reserved.
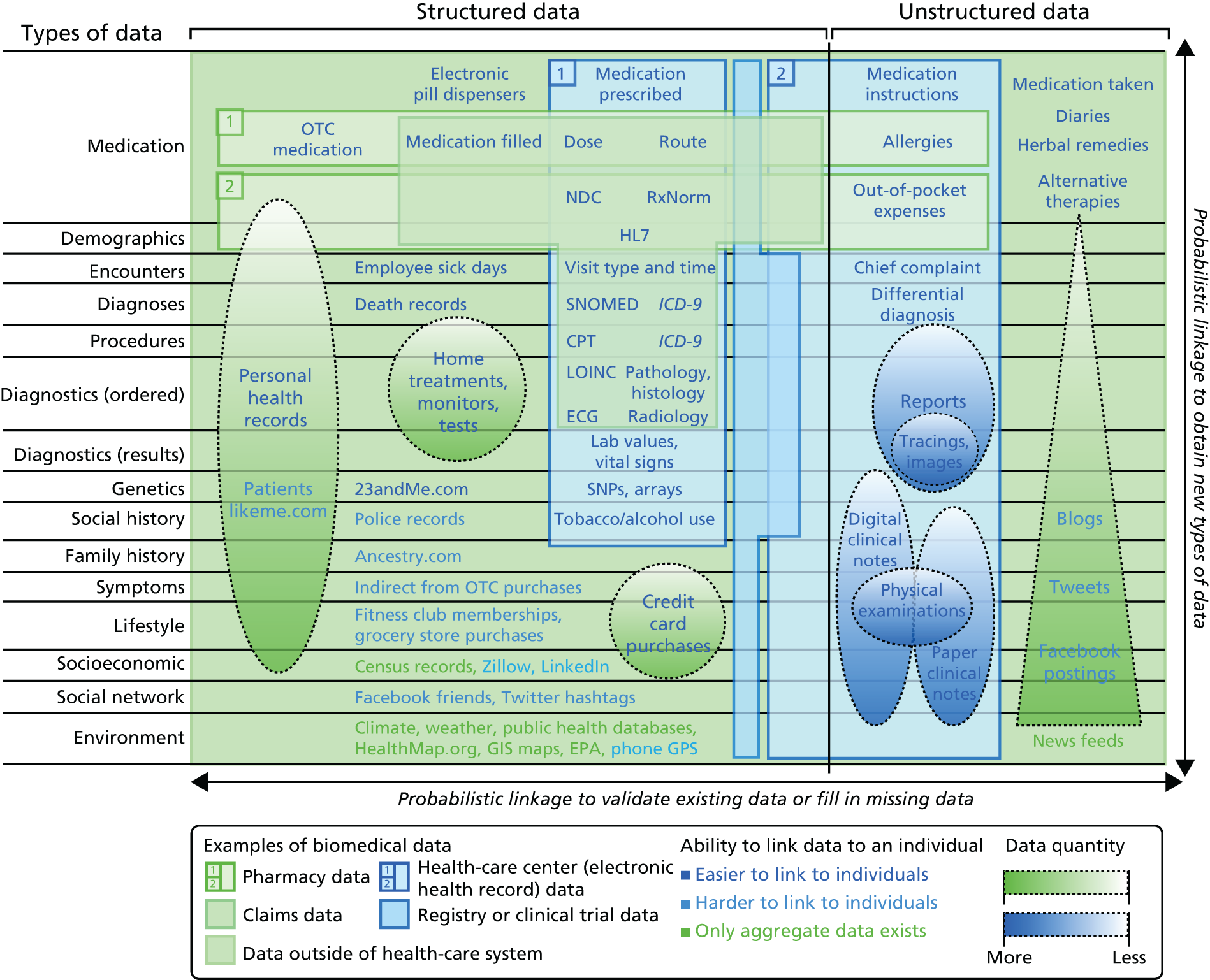
Translational gap
One model that links the process of science and improvements in health care and that has attracted considerable attention in the UK is the translational model, as laid out, for example, in the Cooksey Review. 4 This identified two gaps in the translation of biomedical science to health care, construed as an essentially linear process. The first translational gap is the generation of new therapies to improve health care based on biomedical science. Epidemiological research using electronic health records (EHRs) can help to close the first translational gap by enabling researchers to quantify the need for new therapies, identify risk factors and (with genomic data) suggest potential new drug targets. Randomised clinical trials with follow-up through EHRs can be cheaper and simpler than conventional investigator-led trials and may be particularly helpful for increasing the evidence base for non-drug interventions.
However, it is in the second translational gap, that is, the introduction of new therapies into clinical practice, on which EHR-based research programmes may have the greatest impact.
For example, new prognostic risk scores may help interventions to be targeted most appropriately, decision support tools embedded in clinical systems may help to improve clinical decision-making and health economic analyses can ensure that treatments are managed in a cost-effective way.
Learning health systems
A second, more recent, model linking data, information and knowledge to improvements in health care comes from the concept of a learning health system5 in which science and informatics, involving the real-time access to knowledge and digital capture of the care and outcomes, are a central component.
Electronic health records: what does the UK contribute?
Although there may be a strong scientific and health system case for making much better use of the diversity of the ‘data tapestry’, in reality, existing research efforts have been largely focused on structured data collected as part of an interaction with one or more components of the health system.
The UK is one of the few countries in the world in which a picture of the patient journey can be traced through EHRs spanning primary care, hospitals and, ultimately, death registries. The UK has the potential to address research questions that would currently not be possible in Denmark and Sweden (Table 1), countries that do have outstanding national registries but that have not brought national primary care records to research in the same way as is possible in the UK.
Country | National or regional | Primary and ambulatory care data available for research linkages |
---|---|---|
UK | National | CPRD,6 accessed through Academic Health Sciences Networks |
Sweden | National | Primary care is organised regionally; national initiatives in SwedeHeart7 and the National Registry of Secondary Prevention |
Denmark | National | Register of Medicinal Product Statistics8,9 |
Canada | Regional | Ontario, Institute for Clinical Evaluative Sciences10 |
Regional | Ontario Health Insurance Plan Physician claims database | |
USA | National | Medicare (for people aged ≥ 65 years) (see www.medicare.gov/) |
National | Million Veteran Program (see www.research.va.gov/mvp/veterans.cfm) | |
Regional | Mayo Clinic11 | |
Regional | Rochester Epidemiology Project, Olmsted County (see http://rochesterproject.org/) | |
Regional | Kaiser Permanente California Research Program on Genes, Environment, and Health12 | |
Regional | Intermountain Healthcare13 | |
Republic of Korea | National | National health insurance claims database from the Health Insurance Review & Assessment Service14 |
A combination of features in the UK underpin the potential of EHRs:
Free at point of use
+
unique health identifier
+
≈ every citizen registered with a general practitioner (GP)
+
≈ every general practice uses EHRs
+
wide range of linkage possibilities, including to the national heart attack registry [Myocardial Ischaemia National Audit Project (MINAP)].
Linkage of EHR and registry data, which are commonly held separately across multiple sources, with bespoke phenotypic and genetic information can generate a unique platform to explore why diseases occur and progress, to investigate quality of care and to identify opportunities to improve health outcomes. This new data revolution has attracted substantial investment and promotion with initiatives to improve access to and use of linked EHR data, which will serve to decrease the burden of obtaining data in a research-ready format and encourage research collaboration.
In what senses are linked electronic health records ‘big data’?
One widely used definition of big data concerns the 4 Vs: volume, variety, veracity and velocity. EHR data are potentially ‘big data’ in every sense; not only do they cover a large number of people (entire populations) with a large amount of information [e.g. 2 million patients, 5 billion rows of data in our ClinicAl disease research using LInked Bespoke studies and Electronic health Records (CALIBER) data platform, which forms the foundation of this programme] per patient, but the data are highly varied and complex, with different ways of coding information (e.g. by means of International Classification of Diseases and Read codes). The data validity (‘veracity’) is simultaneously a concern for clinical care and for research. Clearly, having data in real time (‘velocity’) can be crucial for clinical decision support. Harnessing EHR data for research requires a deep understanding of the health-care system from which the data originated, as well as the statistical and computing skills to analyse large data sets.
In 2011 the UK government published a Strategy for UK Life Sciences,16 which placed EHR research as a central part of the strategy to accelerate health research in the UK. The UK is unique in being the only country with national cardiovascular disease (CVD) registries and primary care data (including important information on cardiovascular risk factors and their management in the community) available at a scale for research. The recent report on personalised health care by the National Information Board and Genomics England17 urged the NHS to transform its use of information in order to provide better care as well as to enable better research. This will require a substantial increase in the maturity of EHRs in the UK as well as a framework to make these data available for research.
Current state of electronic health records in UK hospitals relevant to quality and outcomes research
Although UK general practices have, via the Clinical Practice Research Datalink (CPRD)6 and other initiatives, contributed EHR data that have led to > 1000 peer-reviewed publications, the use of data within hospitals beyond the simple Hospital Episode Statistics (HES) returns to generate useful knowledge has led to very few publications. One of the reasons for this is that UK hospitals, like those in Europe, have been at a low level of maturity, according, for example, to the Healthcare Information Management Systems Society classification (Table 2). This is changing rapidly in the UK in light of the emerging strategy from the National Information Board and NHS Transformation programmes such as the Genomics England-led 100,000 Genomes Project. 19
Stage | Cumulative capabilities | Percentage of hospitals |
---|---|---|
Stage 7 | Complete electronic medical record; continuity of care documents to share data; data warehousing feeding outcomes reports, quality assurance and business intelligence; data continuity with emergency department, ambulatory and outpatient | 0.3 |
Stage 6 | Physician documentation interaction with full clinical decision support and closed loop medication administration | 2.5 |
Stage 5 | Full complement of picture archiving and communication systems displaces film-based images | 29.5 |
Stage 4 | Computerised physician order entry in at least one clinical service area or for medication (ePrescribing) | 6.7 |
Stage 3 | Nursing/clinical documentation (flow sheets) | 5.3 |
Stage 2 | Clinical data repository | 34.5 |
Stage 1 | Laboratory, radiology and pharmacy information systems | 7.9 |
Stage 0 | No laboratory, radiology or pharmacy information systems | 13.3 |
However, part of the government’s plan for making health-care information available for research, the care.data programme,20 aroused national anxieties about patient confidentiality and had to be suspended pending further public consultation. This illustrates the sensitivities that must be respected in EHR research. Technical solutions such as data pseudonymisation and secure computer workspaces (‘safe havens’) can enable sensitive patient data to be safely used for research, but it is vital to involve patients and the public in balancing privacy concerns against the benefits of research.
Cardiovascular disease
People at risk of, or with, CVDs increasingly leave digital ‘traces’ relevant to health in multiple different information systems in different parts of the health-care and health systems. The potential for such data to inform, and potentially transform, our understanding of cardiovascular health and disease is the focus of this programme.
Cardiovascular diseases: current public health impact
Cardiovascular diseases remain the leading cause of premature death in the UK and the developed world. 21 Between 1990 and 2010 there were only minor improvements (3% decrease) in years lived with disability from coronary heart disease (CHD),22 and CVD is estimated to cost the NHS about £15.7B a year – 21% of the total NHS budget. 23 Preventing the onset or progression of CVDs remains an important national priority. Interventions can be targeted at different time points along the patient journey: before disease manifests (by risk factor modification), during initial presentation of disease (with prompt and accurate diagnosis, delivery of effective care on referral and admission to hospital) and after discharge from hospital and beyond (optimising secondary prevention). Temporal changes in CVD management have been driven by the availability of new treatment targets [e.g. National Institute for Health and Care Excellence (NICE) guidance and Joint British Societies recommendations on the prevention of CVD guidance] and availability and use of effective treatments for CVDs. 24 Increases in the number of prescriptions of lipid-lowering drugs and number of percutaneous coronary interventions (PCIs) carried out in the UK, together with changes in CVD risk assessment and prevalence, have contributed towards decreases in CVD mortality over the past decade, although the extent to which improved mortality can be attributed to changes in major risk factors, such as smoking, blood pressure (BP) and cholesterol, or specific CVD treatments, remains unclear.
Concerns of a ‘broken pipeline’ in cardiovascular research
There is widespread concern that current models of discovering new interventions, evaluation in trials and implementation in clinical practice take too long (an average of 17 years), are too costly (US$5–11B for each new licensed drug) and are too risky for investors. There is a major need for new research models in general and trial models in particular in cardiovascular research, for several reasons. First, because major unmet needs remain [e.g. acute myocardial infarction (AMI) 1-year mortality is still 20% despite five proven secondary preventative medications]. Second, because late drug failures occurring within Phase III trials (each costing US$50M–100M) are particularly problematic and have been recently seen for drugs that raise high-density lipoprotein (HDL) cholesterol and for heart rate-lowering agents [ivabradine (Procoralan®, Servier, Neuilly-sur-Seine, France)]. Third, non-drug interventions, based on clinical algorithms and decision support, are of growing importance in CVD but are rarely trialled at all. Fourth, growing use of antiplatelet and anticoagulant drugs is associated with major side effects (bleeding is the second most common cause of iatrogenic hospital admission). Fifth, rare CVDs are undertrialled, partly owing to difficulties in recruitment. Finally, the observational evidence that leads to trials often comes from primary prevention settings; however, the populations in which drugs are first trialled are often in secondary preventative and high-risk settings. Planning a trial can be complex because of the lack of suitable populations from which to estimate accurate event rates and select the most appropriate end points. It is too slow and costly to evaluate feasibility, and opportunities are frequently missed to collect valuable data that will adequately inform late-phase trials. It is inefficient to collect clinical data separately for trials and for clinical care, and the process of randomisation and outcome ascertainment is not integrated into the pathway of clinical care. Trial participants are highly selected, which may limit the generalisability of the trial results, and clinical outcomes in clinical care may not be as promising as suggested by the trials.
Electronic health records data opportunities in coronary disease
It is precisely because hospital information systems have historically been unfit for any purpose relating to understanding the quality and outcomes of care that professional societies have organised themselves and established post hoc collections of data that populate disease registries.
There are very few countries (possibly only the UK and Sweden) that have national mandatory ongoing heart attack registries in which every hospital in the country returns data on care and outcome. MINAP is one of the only national registries recording continuous data from every hospital that manages acute coronary syndrome (ACS) patients. The information it provides about clinical phenotypes is unavailable from any other national source and has shown that the reduced rates of heart attack in the early part of this century have been largely confined to ST-segment elevation acute myocardial infarction (STEMI), whereas rates of non-ST-segment elevation acute myocardial infarction (NSTEMI) have remained static. 25 This detailed epidemiology is less easily available for other manifestations of CVD, such as abdominal aortic aneurysm and ischaemic stroke, because disease-specific registries either have not been developed or have become nationwide (all hospitals) only in the past 5 years. 26 This represents an important gap in our knowledge, and it is not clear whether or not the risk factors and changing epidemiology for CHD can be generalised to other manifestations of CVD.
In the UK, rates of hospitalised AMI (heart attack) and coronary death can be extracted from national data sets such as HES and the Office for National Statistics (ONS) death registry. Richer data on CVDs and procedures are available from national cardiovascular registries. The national registry of percutaneous intervention was originally managed by the British Cardiovascular Interventional Society, and the British Cardiovascular Society and the Royal College of Physicians originally managed the MINAP heart attack registry. In 2010, the Healthcare Quality Improvement Partnership handed responsibility for the six cardiovascular registries to the newly established National Institute for Cardiovascular Outcomes Research (NICOR), which now not only has responsibility for the annual audit reports but also makes the registry data available to research groups. 27
The overarching aim of CALIBER is to harness diverse data sources to undertake novel research in CVDs, including opportunities improving care. Our research programme adopted the ‘patient journey’ concept to explore missed opportunities in primary prevention, diagnosis, acute care and secondary prevention (Figure 2). By harnessing the potential of linked EHRs, we seek to provide valuable information to guide clinical decision-making and health policy-making, with the potential to improve quality of care and outcomes for patients.
FIGURE 2.
Overview of the 33 studies in our programme: the patient journey. SCAD, stable coronary artery disease.
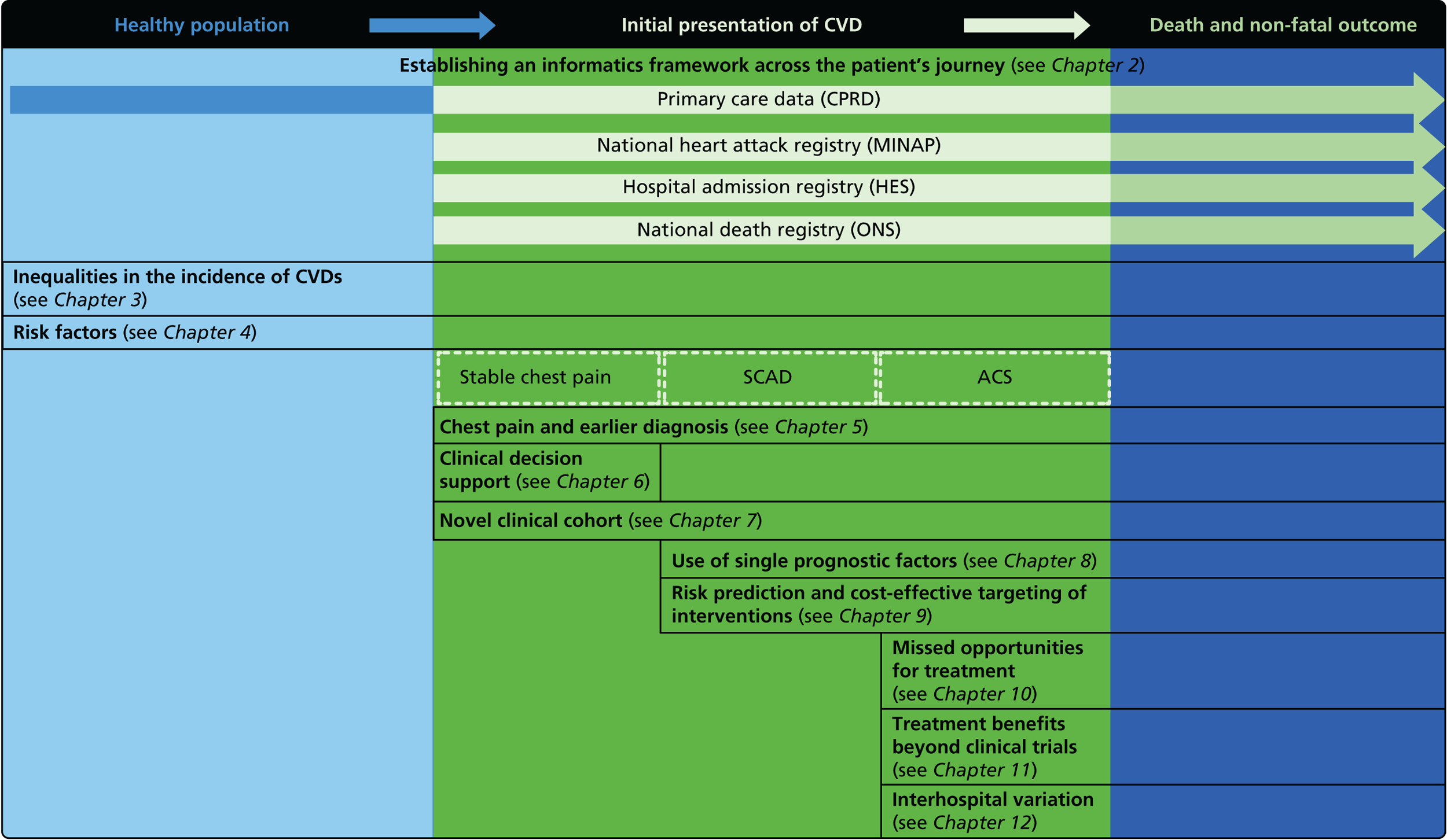
Owing to the evolution of coronary atherosclerosis and symptomatic manifestations (angina, heart attack), which can be viewed as chronic, long-term conditions, our research programme adopted the ‘patient journey’ concept to explore missed opportunities in primary prevention, diagnosis, acute care and secondary prevention. In particular, we have attempted to exploit the potential offered by linked EHRs spanning the patient journey through primary care, the hospital system and ultimately to death. By identifying the nature and scale of such missed opportunities over time, we seek to provide valuable information to guide clinical decision-making and health policy-making, with the potential to improve quality of care and outcomes for patients. A summary of our main findings and research recommendations for 33 studies included in our programme can be found in Table 3.
Main research findings | Research recommendations |
---|---|
Chapter 2 | |
Study 1: we established the CALIBER data resource. We successfully built tools to link coded data on diagnoses, symptoms, drugs and procedures, and clinically recorded biomarkers across primary care, secondary care and disease registries for almost 2 million people and curated ≈ 600 EHR disease and risk factor phenotyping algorithms based on linked EHR data15 |
|
Study 2: we assessed the completeness and diagnostic validity of recording AMI events in primary care, hospital care, disease registry and national mortality records. Across CALIBER’s four linked sources, we found that each source misses a substantial proportion of events; therefore, linking multiple sources is important. AMI recorded in these sources, in terms of risk factor profiles and mortality at 1 year was found to be valid28 | Further research is required to:
|
Study 3: we established the PROGRESS partnership to promote high-quality prognostic research leading to patient benefit. We highlighted opportunities to improve the design, conduct, analysis and reporting of prognosis research in 24 recommendations29–33 | The PROGRESS recommendations include:
|
Chapter 3 | |
Study 4: we investigated a central question in CVD – what is the first CVD to present across different ages, sexes, ethnicities and levels of deprivation? We found important differences that conventional epidemiological approaches based on small consented studies have eluded. These include that heart attack and stroke account for the minority of presentations, that women are less likely to get some CVDs than men (e.g. subarachnoid haemorrhage), the strength and shape of association of age with many CVDs varies substantially, and those of South Asian ethnicity experience substantially increased hazard of stable and unstable coronary presentations compared with white people34,35 | Estimates of national and global burden of CVDs need to take account of the modern epidemic in which heart failure, PAD and other chronic diseases are becoming more common than AMI and stroke. Male sex, older age, South Asian ethnicity and low social deprivation, although associated with many CVDs, are not associated with all, and this heterogeneity has implications for research into risk prediction, aetiology and prognosis |
Study 5: there was a discordance between incidence of SCAD, which was markedly higher among those of South Asian ethnicity than for those of white ethnicity, and prognosis which was significantly better in South Asians36 | Large-scale EHR cohort studies with detailed measures over time of clinical risk, and patient care identified through linkage of primary care, ACS registry, hospitalisation and cause-specific death data are warranted to better understand how South Asian ethnicity influences the onset and progression of coronary and other CVDs. Such studies will allow longitudinal patterns of risk and missed opportunities to lower risk before admission and after discharge to be evaluated |
Chapter 4 | |
Study 6: among 34,198 patients with type 2 diabetes mellitus, we found that type 2 diabetes differs in its associations with 12 CVDs, with its greatest impact on PAD and heart failure, but type 2 diabetes was protective against abdominal aortic aneurysm37 | In clinical trial design, selection of primary end points is crucial and should reflect the mechanism of action of the intervention and, where appropriate, the public health burden of CVDs in the 21st century. This would suggest a growing importance of trials with end points of heart failure and PAD |
Study 7: in this contemporary study of 1.25 million adults, we found that despite the availability of modern preventative medication, the lifetime burden of hypertension on incidence of subsequent CVDs remains considerable. Notably, SBP and DBP were found to differ in their associations with 12 CVDs and at different ages38 | New approaches are required to mitigate the excess CVD risk that remains despite modern BP-lowering therapy combinations. New and existing clinical trials investigating the effectiveness of BP-lowering strategies should include other common initial CVD presentations as the primary outcome |
Study 8: in a cohort of individuals with newly diagnosed hypertension, we identified high levels of undertreatment among hypertensives. Consultation rates were significantly lower among those whose BP was not reduced to target (manuscript in preparation)39 | New approaches are required to close the second translational gap in BP lowering. This may include research on clinical decisions support for multiple drug classes to achieve target BP levels and other interventions, as well as understanding how best to lower absolute cardiovascular risk |
Chapter 5 | |
Study 9: we identified high levels of failure to diagnose angina at first presentation with chest pain, which led to poor prognosis among this group (manuscript in preparation)40 | Contemporary prognostic models should be developed and validated based on the characteristics of patients presenting with unspecified chest pain so those at risk of cardiovascular events can be more accurately identified |
Study 10: we found that first AMI, especially with STEMI, was heralded by previous symptomatic atherosclerotic disease, one or more cardiovascular risk factors, or chest pain41 | Further research is warranted to better characterise the phenotypes, causes and prognosis of unheralded STEMI and NSTEMIs to improve the usability of EHR for research. Analyses comparing AMI patients with a control group without AMI, including a comparison of missed opportunities for care in measuring and controlling elevated risk are warranted |
Study 11: we developed an open source free-text data mining tool (the FMA) for extracting diagnoses and causes of death from unstructured text in EHRs, which showed high precision and recall on a test data set from CPRD, and can be used to extract uncoded diagnosis or symptom information for EHR research. The tool may be useful in aiding early identification of CVD42 | Machine learning and NLP approaches to examining the large corpus of unstructured (free-text) data that exists in primary care and hospital records should be developed in order to identify opportunities for earlier diagnosis, add phenotypic information and safety signals |
Study 12: we developed a data mining tool (the S3CM algorithm) to extract diagnoses and investigation results from unstructured text in EHRs by semisupervised machine learning, which outperformed comparator programs on data-mining tasks43 | Further testing of our semisupervised machine learning tool should be conducted using other EHR data sets, such as discharge letters and electronic hospital notes |
Chapter 6 | |
Study 13: the OMA CDSS was used in 84% of RACPC consultations. OMA was found to have a negligible impact on decision-making in the hospital chest pain clinic, with qualitative data suggesting that clinicians found OMA useful in confirming their decision-making44,45 | Based on our understanding of the ways in which clinicians interacted with a computerised CDSS, there is a need to develop new tools to aid clinical decision-making with a greater likelihood of being adopted in practice |
Chapter 7 | |
Study 14: we established a novel consented clinical cohort of 3345 patients being investigated for new-onset chest pain with linked phenotypic, genetic and biomarker data. 4C is a resource for researchers investigating onset of SCAD and its progression to CVD events (manuscript in preparation)46 | There is a need for embedding genetic information in hospital EHR at scale in order to carry out a wide range of translational research, from discovery efforts, through drug repurposing and embedded pharmacogenetics for patient safety. Collaborative research based on individual patient data should be encouraged to strengthen prognostic model development and external validation of models |
Chapter 8 | |
Study 15: among two study populations (patients with new-onset stable angina and no previous ACS and patients with a first AMI) thresholds in both men and women were identified below which the clinically available biomarker haemoglobin showed a linear inverse relationship with increased mortality47 | New clinical trials are warranted to assess whether or not haemoglobin levels are causal and whether or not clinicians should intervene to increase haemoglobin levels |
Study 16: elevated heart rate was associated with increased risk of heart failure and cardiovascular death and all-cause mortality in patients with SCAD (manuscript in preparation)48 | In designing randomised trials, the target population, the choice of composition of the primary end point and the likely rate at which the end point accrues can all be informed by the examination of linked EHRs |
Study 17: published evidence of associations between levels of the novel circulating marker of inflammation CRP levels and risk of cardiovascular events was of variable quality, and our data suggest that the degree of association may have been overstated49 | New clinical cohorts are needed with published pre-specified statistical analytic protocols and prospective registration of studies, which can be pooled to evaluate the true usefulness of the role of CRP in prognosis in SCAD |
Study 18: our systematic review and meta-analysis from > 190,000 individuals experiencing 20,000 events showed that Ch9p21, a novel genetic factor, was associated with increased risk of first but not subsequent cardiovascular events50 | More work should be carried out to determine the effect of mechanistic differences for the differential genetic effect, to enhance understanding of the underlying reasons. Our findings argue for a consortium of studies set in individuals with established CHD to better understand the genomic susceptibility to subsequent CHD events and also to perform detailed analysis, including assessment for selection biases, which is not possible with literature-based meta-analyses |
Study 19: we found that changes in BP in people with diabetes and SCAD predict risk. SBP in type 2 diabetes patients with stable angina declined over time. Lower values of SBP significantly increased risk of CVD outcomes (manuscript in preparation)51 | Methods for dynamic risk prediction in which the patterns of change of clinically measured biomarkers can be incorporated into clinical decision support models in clinical practice are required |
Chapter 9 | |
Study 20: we present validated prognostic models developed with 5-year risk predictions for estimating risk of all-cause mortality and coronary outcomes based on clinical parameters that are commonly available in all people with SCAD52 | Further external validation in external data sets and recalibration for different populations and time periods is required. Economic evaluation is needed to establish the appropriateness of using the prognostic model in practice |
Study 21: our cost-effectiveness studies using linked EHR data showed high use of primary care and frequent hospitalisations in patients with SCAD, who incurred considerable ongoing costs. Five-year and lifetime costs varied according to CVD risk, which may be predicted from baseline patient-level data. Based on published data53,54 | Extend models such as the model presented here to explicitly capture the trajectory of prognostic risk factors over time, to address broader questions around the cost-effectiveness of interventions that target underlying risk factors |
Chapter 10 | |
Study 22: we compared short-term mortality outcomes among patients with a first AMI admission using nationwide outcome registries in Sweden and the UK. Our findings, which took into account measures of case-mix and in-hospital treatment measures, showed that short-term mortality after AMI was markedly higher in the UK than in Sweden and suggest that > 10,000 early deaths would have been prevented or delayed had UK patients experienced the care of their Swedish counterparts55 | International harmonisation of detailed measures of quality of care in clinical registries, such as pathways of care, is needed to identify and evaluate additional patient-level health-care factors that are not measured here and which might explain differences between countries. Outcomes should be compared with those in other countries, such as France, Hungary, Poland and the USA, through assessment of national registries. Linkage to national EHRs will extend comparisons to include ambulatory care before and after admission for heart attack, non-fatal events and longer-term outcome |
Study 23: we identified that high numbers of patients hospitalised with STEMI who were eligible for nine evidence-based care components did not receive all components, and that these patients went on to experience higher rates of mortality56 | Outcomes research specifically designed to follow care into the primary care setting is warranted to quantify the full implication of CMOCs |
Study 24: we carried out analyses of data from national registries in the UK, Sweden and the USA. International comparisons identified significant differences in use of surgical interventions and use of some secondary prevention medications in the UK57 | Further studies are needed to better explain patterns of clinical practice within and outside the UK, such as rates of coronary angiography and PCI |
Study 25: in a study to determine the extent to which GPs offer recommended smoking cessation advice and pharmacological interventions to smokers after discharge from hospital with ACS, we found that fewer than one-quarter of smokers received smoking cessation interventions in the 3 months following discharge. Those who quit experienced fewer cardiac events than those who continued smoking58 | Future research, both in larger prospective epidemiological studies and in RCTs, of the promotion of general practice smoking cessation interventions, the association between interventions and cessation and the effects on long-term clinical outcomes is warranted |
Chapter 11 | |
Study 26: our study using linked CALIBER data to compare the effectiveness of drugs used for secondary prevention of CVD after AMI showed that use of fewer than five classes of secondary prevention drugs was associated with increased mortality. Omission of beta-blockers or statins from the discharge prescription was associated with a marked increase in the hazard of death in the first 30 days, with only minor reductions in the effect size during the first year and beyond (based on unpublished data) | Combinations of drugs that are recommended lifelong but have not been evaluated in RCTs should be investigated in observational studies for associations with fatal and non-fatal outcomes over the remaining life expectancy |
Study 27: we found that use of a beta-blocker after AMI in adults with COPD was associated with lower subsequent mortality. Improved survival was observed for use of beta-blockers started either at the time of hospital admission for AMI or before an AMI59 | A RCT is justified to examine the effectiveness of beta-blockers after AMI in patients with COPD |
Study 28: we used linked CALIBER data and a self-controlled case series design to measure the association between use of PPIs and a range of harmful outcomes in patients using clopidogrel and aspirin. Our findings suggest that drug interaction between PPIs and clopidogrel does not result in clinical harm60 | Methods are required by which a learning health system can interrogate the safety of drugs and drug–drug interactions in a more timely and more scaled fashion |
Study 29: in this first study to use linked registries to determine persistence of clopidogrel treatment after AMI in primary care, we found that discontinuation of clopidogrel after AMI is common and is associated with adverse outcomes61 | Further research, incorporating methods of causal inference, is required to fully investigate the extent to which the association between clopidogrel discontinuation and adverse events we identified was causal or was confounded by the influence of unrecorded comorbidities or bleeding events |
Chapter 12 | |
Study 30: in this study comparing mortality among hospitals in the UK, we observed that short-term mortality varied substantially between hospitals. Up to four severely underperforming hospitals were identified with high confidence but there was considerable uncertainty in adjusted mortality rates (based on unpublished data) | Further research is required to obtain real-time risk-adjusted, treatment-adjusted estimates of between-hospital variation in mortality and a wider range of non-fatal outcomes |
Study 31: we carried out a preliminary volume–outcome analysis of patients with AMI admitted to all hospitals in England and Wales. We found no clear relationship between the number of patients admitted to individual hospitals or the quality of BP management in primary care and 30-day mortality rates (based on unpublished data) | Further work is necessary to assess the distribution of AMI patients across hospitals and other hospital characteristics that contribute to patient outcomes |
Study 32: international comparisons using AMI disease registry data in Sweden and the UK showed that hospital-level treatment varied between the two countries. Short-term mortality was greater in the UK than in Sweden (9.7% vs. 8.4%, respectively)62 | Opportunities for more ambitious comparisons along the care pathway should be exploited by linkage to national EHRs, that would permit analysis of non-fatal events before and after hospital admission and longer-term outcomes |
Study 33: our qualitative study in 10 hospitals identified five processes that appeared to facilitate good care for NSTEMI patients: (1) early specialist assessment; (2) active patient monitoring and appropriate action; (3) cross-disciplinary communication; (4) co-ordinated discharge and initiation of secondary prevention prior to discharge; and (5) visible cardiology leadership63–65 | Mixed-methods research is required to inform actions to reduce hospital variations in care and outcomes |
Public and user group involvement
Electronic health records are increasingly considered to belong to individual patients. The National Information Board recommended online access to the full primary care record by April 2015. 17 In CALIBER we have had an active public and user involvement group which has had biannual meetings since 2006. Following the INVOLVE guidelines,66 this group represents a high level of user and lay participation in research, which includes contributing to the design and conduct of research. The group includes people relatively new to the ‘patient journey’ and others with many years’ experience of being cared for within the UK NHS. Our programme has benefited greatly from the group’s engagement, which has informed the development of studies from inception through to analysis and interpretation of findings. Central to the vision of the Farr Institute of Health Informatics Research is the need to transform the relationship between the patient, their health record and its uses for clinical care and translational research. The Farr Patient and Public Working Group meets biannually and is actively involved in national health informatics research network projects, research and events which are also open to lay members of the public.
Introduction to chapters
Chapter 2 (studies 1–3): the ClinicAl disease research using LInked Bespoke studies and Electronic health Records platform
We have established a framework for studying CVD across the patient journey, introducing novel approaches and scrutinising underlying methodology.
-
Linked EHRs: to map the patient journey, we exploited the previously untapped resource of the EHR for research purposes and, for the first time, linked data across primary care (CPRD), hospital admission (HES), a disease-specific registry (MINAP) and the national death registry (ONS). The resulting CALIBER data set comprises > 2 million patient records. The governance and analytic challenges were considerable, requiring a series of permissions, a contractual relationship with the Medicines and Healthcare products Regulatory Agency, engagement of a trusted third party to perform the linkage, safe storage of the linked data sets, development of detailed algorithms to define clinical phenotypes of interest and, finally, application of complex analytic strategies to take account of the volume of data, missing data and hidden confounders.
-
Promoting high-quality prognostic research leading to patient benefit: in order to establish a high-quality framework for prognosis research, we created the international PROGnosis RESearch Strategy (PROGRESS) partnership, with representatives from academia and medical journal editors, to evaluate the field of prognosis research and make specific recommendations on improving the quality and impact of prognosis research.
Clinical context of diagnosis, risk prediction and treatment of coronary diseases: synopses of studies
The studies in the chapters that follow are a combination of synopses of previously published (n = 26) and currently unpublished (n = 15) studies and are summarised in Table 3 and Figure 2. We have included more detail for the unpublished studies. A summary of the published papers relating to individual studies is presented in Appendix 1.
Primary prevention of disease
In principle, CHD is largely preventable with behaviour change (including smoking cessation, increasing physical activity and lowering saturated fat intake) and, in some cases, pharmaceutical intervention to lower BP or low-density lipoprotein cholesterol. 67 In the landmark INTERHEART study conducted in 52 countries, just nine potentially modifiable risk factors were found to account for > 90% of the population attributable risk of AMI in all regions of the world. 68
Primary prevention is thus an important public health priority in the UK. National public smoke-free legislation introduced in 2006 saw an almost immediate decline in rates of STEMI,69 and the NHS Health Check Programme introduced 2 years later identifies individuals at risk of disease development for whom preventative strategies are deemed appropriate. 70 The contemporary focus on disease prevention is reflected in the 2014 NICE guideline,71 which has now dramatically widened its recommendations for statin therapy to include all people with a 10-year risk of heart attack or stroke that is ≥ 10%. 71 The Joint British Societies 2014 guideline,24 which also emphasises lifetime risk assessment, further widens access to risk-lowering strategies to many young people at risk of disease in later life. 24 In practice, there are many missed opportunities for primary prevention; this is the focus of studies in Chapters 3 and 4 of this report.
Chapter 3 (studies 4–5): inequalities in health care by demographic determinants of cardiovascular disease
In this chapter we present a novel analysis of inequalities and their associations with age, sex, ethnicity and socioeconomic deprivation across these 12 CVD conditions. We also unravel the complex relationships between incidence and prognosis of CHD among people of South Asian ethnicity living in the UK and analyse factors driving the excess coronary mortality among South Asian groups.
Chapter 4 (studies 6–8): prevention
Reported failures to meet national guideline targets and treatment opportunities to optimise risk reduction among people without established disease represent an important missed opportunity for protecting patients against CVD. We have investigated risk factor modification among healthy populations and people at risk of coronary disease, specifically evaluating if guideline targets for BP reduction are being achieved in clinical practice, as well as opportunities for risk reduction in people with diabetes mellitus. We also investigate the association between two major risk factors (BP and type 2 diabetes) and 12 different presentations of CVD.
Importance of studying first presentation of cardiovascular disease
The Department of Health’s 2013 Cardiovascular Outcomes strategy72 has recommended management of CVD as a single family of diseases. An initial CVD event affecting head (stroke), abdomen (aneurysm) or legs [peripheral arterial disease (PAD)] shows some common risk factors and usually leads to initiation of lifelong secondary prevention medication. The pattern of initial presentation of CVD is changing, with a decrease in relative frequency of AMI and stroke. The study of the initial presentation of other CVDs has been difficult because of the need for large cohorts with detailed clinical follow-up, covering hospital and ambulatory care. However, linked EHR data provide the statistical scale and clinical resolution necessary to carry out this research. 73
Initial presentation with stable chest pain
Securing a firm diagnosis of coronary artery disease (CAD) is important to reduce the number of unnecessary invasive tests on the one hand and inappropriate discharges on the other hand. Early diagnosis of patients is a high priority for policy-makers, as evidenced by the widespread introduction of rapid access chest pain clinics (RACPCs) for patients with suspected coronary disease. A central requirement for early diagnosis is that primary care physicians are able to recognise early symptoms and signs of disease and respond appropriately with prompt referral for specialist assessment. However, there is considerable diagnostic uncertainty surrounding the diagnosis of chest pain and we investigate this in Chapters 5 and 6. RACPCs are effective at identifying patients with angina74 but, in study 9, we found that during follow-up nearly one-third of cardiac events occurred in patients who had not been given a diagnosis of angina. As well as becoming more common, there are substantial uncertainties in how to manage such patients, including how to identify them and stratify them according to risk. Development of new-onset chest pain is a crucial intervention point, and identification of those at risk is a major clinical and research challenge.
Chapter 5 (studies 9–12): diagnosis and first presentation
These studies identify important missed opportunities at the point of initial presentation of disease and opportunities for early diagnosis.
-
Incidence of chest pain and heralding of AMI. We have investigated missed opportunities for diagnosis of chest pain in RACPCs, as well as the extent and nature of STEMI and NSTEMI heralding and its impact on primary and secondary prevention of ACS.
-
Mining free text in clinical notes to improve research on symptoms. Primary care EHRs contain clinician-entered records of patient symptoms as well as formal diagnoses. It is important to study these symptoms in order to be able to improve primary care decision and early diagnosis, but many of the symptoms are entered as free text rather than using medical terminology codes. We have developed tools that may help to extract information from unstructured free text in EHRs.
Chapter 6 (study 13): tools to improve assessment
We conducted a pilot evaluation of a clinical decision support system (CDSS) aimed at optimising use of angiography (and thereby revascularisation), secondary preventative medication, lifestyle interventions and referral to cardiac rehabilitation in RACPCs, to determine whether or not a large trial was justified. The study built on our earlier simulation showing that a tool incorporating contemporary appropriateness criteria could contribute positively to clinical judgement in guiding investigation of patients with suspected angina.
-
Piloting a CDSS in a RACPC. We developed a patient-specific computerised CDSS, piloting its use in four RACPCs, to model the effectiveness of the intervention and determine the need for a definitive trial powered on CVD events.
-
Qualitative ethnographic study. To determine the utility of the tool in day-to-day practice, we conducted an ethnographic study based on interviews with clinicians and observation of clinical practice.
Consented cohorts recruited in clinical settings make important contributions towards addressing translational research questions in prognosis research.
Chapter 7 (study 14): clinical cohort
We have established a new cohort of patients with suspected stable coronary artery disease (SCAD), the Clinical Cohorts in Coronary disease Collaboration (4C), consisting of genetic, biomarker, clinical and phenotypic data, linked to outcome data from national registries. The cohort was established as a resource to encourage collaboration among researchers through sharing of individual patient data, to strengthen prognostic model development and external validation of data.
Stable coronary artery disease
Better diagnosis and improved survival is leading to growing numbers of patients with SCAD, spanning two patient populations: those who have survived an AMI and are in the early very high-risk period, arbitrarily defined as the first 6 months after an AMI event, and patients who have never had an AMI but who have been diagnosed with coronary disease after coronary angiography or documented with myocardial ischaemia; the latter patients usually present initially as having ‘chronic stable angina’. SCAD is of growing importance for several reasons. Investigation of SCAD, independently of AMI, is important because the distinction of CAD phenotypes may yield causal mechanistic insights relevant to public health;75,76 angina pectoris is one of the most common initial presentations of coronary disease, yet it is commonly excluded from such aggregates, and little is known about factors determining disease progression. Furthermore, compared with ACS, there has been much less research on outcomes and quality of care for SCAD.
Among patients with SCAD, RACPCs now provide prompt patient access to specialist consultation and prescription of antiplatelet and statin therapy to stabilise atherothrombotic disease and protect against coronary events. Meanwhile, the options for correcting symptoms have been extended by the recent introduction of novel antianginal drugs such as ivabradine and ranolazine (Ranexa®, Menarini, Florence, Italy) which, together with rapidly changing drug-eluting stent technology, allow many patients with coronary disease to lead an active life free from ischaemic symptoms. 77,78
Chapter 8 (studies 15–19): biomarkers and prognostic indicators
Single biomarkers have traditionally lacked incremental predictive value to be considered for clinical use. We have evaluated the use of biomarkers as prognostic indicators to improve targeting of clinical interventions in SCAD. Using evidence syntheses and analyses of linked CALIBER data, we have examined the strength of the evidence base supporting the use of established and novel prognostic factors in SCAD, and evaluated the general quality of evidence being generated in prognostic factor research.
Chapter 9 (studies 20–21): prognostic and economic modelling
Improved survival after ACS and an ageing population have resulted in a large population of SCAD patients, with high health-care resource use. Validated prognostic tools and information on resource use and costs based on data collected as part of usual clinical care could assist in clinical management of SCAD patients and policy-making. We have developed and validated prognostic models, which we have used to examine health-care resource utilisation and costs, and to evaluate the cost-effectiveness of treatments.
-
Development, validation and impact of a risk prediction model. We have used linked CALIBER data sets to develop a prognostic model for SCAD, and validated the model using data from an independent study.
-
Health-care costs, resource use and decision models using a risk prediction model. We have combined resource use estimates derived from CALIBER data and prognostic models to model the outcomes and lifetime health-care costs of patients with SCAD.
Acute myocardial infarction treatments
The decline in the population burden of CHD has been driven partly by novel drug and device therapies, for which clinical efficacy has been established in randomised trials. 79 Thus, reperfusion strategies in AMI have moved away from thrombolytic therapy towards percutaneous intervention, together with potent dual antiplatelet regimens that now include adenosine diphosphate receptor antagonists such as clopidogrel and ticagrelor (Brilique®, AstraZeneca plc, London, UK). 80 This has seen UK hospital mortality rates for AMI drop to 9%,47 and ongoing antiplatelet therapy, statins and inhibitors of the renin angiotensin system provide protection against recurrent coronary events and left ventricular remodelling. Nevertheless, AMI 1-year mortality is still 20% despite five proven secondary preventative medications (study 26 in this report) and patients remain at high risk of further CVD events and death. In Chapter 10, we examine the extent of missed opportunities for treatment after hospitalisation for AMI, and go on in Chapter 11 to investigate further opportunities to improve outcomes after AMI.
Chapter 10 (studies 22–25): hospital and post-discharge care
A key aim of our research programme was to determine the cumulative impact of missed opportunities for improving patient outcomes after hospitalisation for AMI, across a spectrum of the most common symptomatic cardiovascular presentations. We anticipated the evolving interest in outcomes research and recognised that the management of CVD is an ongoing commitment for clinicians that spans the patient journey from initial presentation through hospitalisations to death.
-
Missed opportunities in hospital. We used linked CALIBER data to evaluate the impact of missed opportunities in hospital for patients with ACS. We also report, for the first time, international treatment and outcome comparisons in collaboration with investigators in the USA and Sweden.
-
Missed opportunities after discharge from hospital. We examined opportunities for smoking cessation, which substantially reduces the risk of recurrent AMI and coronary death, in the community after discharge from hospital, recognising that sustained lifestyle modification and secondary prevention treatment is a key guideline recommendation in patients who have experienced AMI.
Chapter 11 (studies 26–29): ‘real-world’ evidence
We have used EHR data to explore treatment benefits beyond clinical trials, examining the efficacy of specific drug combinations for secondary prevention, the safety and effectiveness of beta-blockers in patients with chronic obstructive pulmonary disease (COPD), and the clinical consequences of clopidogrel’s interaction with proton pump inhibitors (PPIs).
Hospital and clinician performance
The importance of applying evidence-based treatments equitably has been recognised through the development of regularly updated guidelines published in the national society journals of Europe and the USA. In the UK these have been complemented by landmark policy initiatives such as the National Service Framework for Coronary Heart Disease in 2000,81 which focused on disease management in the hospital sector, and the Quality and Outcomes Framework in 2004, which introduced an incentive programme to enhance treatment in primary care. 82
Recognition that a major purpose of treatment is to improve patient outcomes has led policy-makers to shift their attention towards the monitoring of performance not only of hospitals but also of individual clinicians. 72 Methods of evaluating hospital performance have been the subject of debate but the need was brought into sharp focus by the Mid-Staffordshire NHS Foundation Trust scandal in 2009, when it became clear that mortality rates in preceding years had been higher than expected yet no action had been taken by those charged with the supervision and regulation of the hospital. 83 One of the main recommendations of the 2013 Francis Report83 was that the ‘Department of Health should set up a working group to review the use of comparative hospital mortality statistics’ for identifying underperformance (© Crown copyright 2013; contains public sector information licensed under the Open Government Licence v3.0). We await the working group’s report but, meanwhile, individual clinicians are increasingly being held accountable for the outcomes of patients under their care, particularly clinicians engaged in surgical and interventional procedures (e.g. http://scts.org/modules/surgeons/default.aspx and www.bcis.org.uk/).
This focus on performance is central to the UK Government’s 2013 transparency in health-care agenda, which not only includes commitments to publish more clinician-level data but also to link clinical data from primary care to hospital data systems in order ‘to allow new insights into the quality of services and better understanding of the way services interact’. 84 These commitments relate directly to some of the achievements of our research programme.
International comparisons can provide additional insight into performance, and have highlighted poor performance within the UK health system. 22 The extent to which this applies to CVD is not yet clear. Variation in outcomes between hospitals for ACS patients is the subject of our studies in Chapter 12.
Chapter 12 (studies 30–33): hospital variation
Using EHR data and in-depth qualitative studies, we have examined the impact of individual hospitals on the outcomes of AMI patients.
-
Hospital-level variation in outcomes after AMI. For the first time, we have analysed interhospital variation in 30-day mortality adjusted for case-mix and hospital treatment, and examined its potential to identify and rank underperforming hospitals. In collaboration with Swedish investigators, we have compared interhospital mortality variations in the UK and Sweden.
-
Qualitative analysis of hospital processes of care. We carried out qualitative studies examining processes of care potentially associated with favourable outcomes.
Chapter 2 Establishing a framework to study cardiovascular diseases across the patient journey: underlying methodology and approaches
Abstract
Background
Electronic health records are generated at an unprecedented scale during patients’ interactions with the NHS. The UK is unique in being able to trace a complete patient journey through coded EHRs spanning primary care, hospitals, disease registries and, ultimately, death registries. Large-scale linkage of EHRs in CVD has major challenges, and the quality of prognosis research, which provides crucial evidence for translating research findings into clinical practice, is poor.
Objectives
(1) To establish an EHR-based research platform and data resource in CVD (CALIBER) and (2) to develop a framework for exemplary prognosis research.
Methods
(1) We linked coded EHR data from four sources spanning primary care (CPRD), secondary care (HES) and national registry databases (MINAP and the ONS death registry). (2) We established the international PROGRESS partnership.
Results
(1) Through CALIBER, we linked and curated data covering almost 2 million people, and curated ≈ 600 EHR disease and risk factor phenotyping algorithms based on linked EHR data. (2) We made 24 recommendations to address challenges and opportunities across four prognosis research themes.
Conclusions
The CALIBER platform promotes the transparent and scalable use of EHR data, enabling the wealth of information in UK EHRs to be exploited for research purposes. Our CALIBER data resource and recommendations for improving the quality of prognosis research provide a framework for high-quality CVD research.
Development, feasibility and validation of our data linkage platform: ClinicAl disease research using LInked Bespoke studies and Electronic health Records
Patients’ interactions with the health-care system generate unprecedented amounts of structured and unstructured EHR data that are stored in disparate clinical information systems. There, rich national EHR data sources are increasingly being linked for translational research in the USA and Europe with the potential to have a positive impact on medical care and research. In the UK, a unique 10-digit identifier is assigned to each patient on their interaction with the NHS, allowing the complete patient journey to be traced through EHRs spanning primary care, hospitals, disease registries and, ultimately, death registries.
These features have provided a platform for the CALIBER programme, led from the Farr Institute (London, UK, under the directorship of Harry Hemingway), which has linked EHRs from four key sources: the CPRD, HES, MINAP and the ONS death registry. CALIBER is a research platform that aims to meet the need for a structured approach to EHR phenotyping algorithm development for epidemiological research using national linked data, while also providing a central online repository for the storage and distribution of algorithms, documentation (flow charts, free-text description, pseudocode) and metadata. The platform contains EHR data on 2 million people and provides a unique platform for investigating a range of important issues in CVD diagnosis, management and prevention, and health system performance. Our findings have important implications for clinicians and clinical decision-making, for health policy and for shaping future research.
Study 1: establishing the ClinicAl disease research using LInked Bespoke studies and Electronic health Records data resource
This study is based on the peer-reviewed paper by Denaxas et al. 15
Surprisingly little is known about the initial presentation of CVD in general practice and its long-term management through to death. However, the UK is the only country in the world where all the components of this patient journey are recorded electronically at national scale in primary care, hospital and death registries. Moreover, all patients in the UK are uniquely identified by a 10-digit NHS number, providing a reliable and robust identifier for record linkage and data sharing while preserving patient confidentiality. This has provided the opportunity to develop a programme of CVD research using linked bespoke studies and EHRs (CALIBER) led from the Farr Institute. 15 The linkage was carried out in October 2010 by a trusted third party, using a deterministic match between NHS number, date of birth and sex. Overall, 96% of patients with a valid NHS number were successfully matched.
Linked data sources
The four linked data sets that we used are shown in Figure 3, which illustrates the longitudinal, population-based nature of CALIBER data sets. Each source captures a different aspect of the patient journey:
-
CPRD contains longitudinal primary care coded data on symptoms, diagnosis, prescriptions, investigations, referrals and vaccinations. 85 Data are recorded prospectively as part of clinical care and are not subject to recall bias or differential bias related to outcome. Diagnostic data are coded using Read codes, which map to Systematic Nomenclature of Medicine – Clinical Terms. Of the 684 primary care practices in CPRD (13.6 million patients), 226 consented to data linkage across the CALIBER data sources. These practices contained 3.9% of the population of England in 2006.
-
HES is a national data set of all admissions to NHS hospitals in the UK (see www.hscic.gov.uk/hes). Diagnoses are recorded using the International Statistical Classification of Diseases and Health-Related Problems, Tenth Edition (ICD-10) and procedures using the Office of Population Censuses and Surveys’ Classification of Interventions and Procedures version 4.
-
MINAP is a national registry of patients admitted to all acute hospitals in England and Wales with ACS events (n = 230). For each admission, MINAP records patient demographic characteristics, limited medical history, clinical features and investigations, drug treatment (pre, during and post admission) and final diagnosis, including differentiation into STEMI, NSTEMI and unstable angina. Data undergo annual quality assessments. A descriptive review of the MINAP registry has been published. 86
-
The ONS provides data on cause-specific mortality for all residents of the UK. 87 Cause-specific mortality data are extracted from death certificates and recorded using ICD-10. Small-area measures of social deprivation are recorded using the 2007 Index of Multiple Deprivation (IMD)88 and Townsend scores.
FIGURE 3.
Longitudinal nature of four linked primary care and disease registry data sources in the CALIBER data resource. AAA, abdominal aortic aneurysm; ACEI, angiotensin-converting enzyme inhibitor; ECG, electrocardiogram; MI, myocardial infarction. Reproduced from Denaxas et al. 15 Published by Oxford University Press on behalf of the International Epidemiological Association. © The Author 2012. This is an Open Access article distributed under the terms of the Creative Commons Attribution License (http://creativecommons.org/licenses/by-nc/3.0/), which permits non-commercial reuse, distribution, and reproduction in any medium, provided the original work is properly cited.
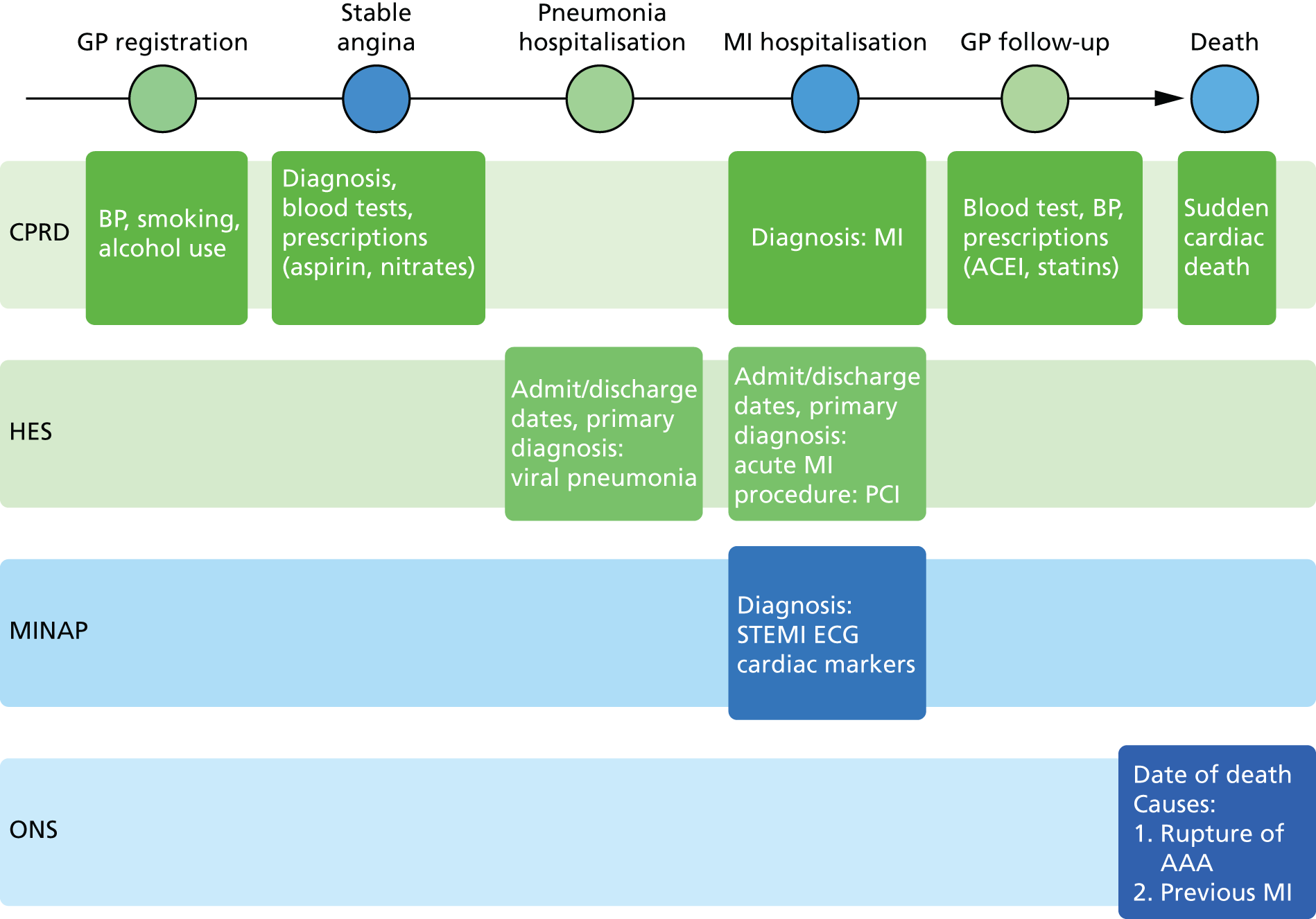
Converting raw data to research-ready data: curated common data model
Important challenges remain in relation to converting raw EHR data into research-ready variables. The manner and reason for the generation, capture and recording of EHR data vary substantially between health-care settings. In addition, different medical classification systems are often used for different data sources and, consequently, clinical information may be recorded in multiple sources but at different levels of clinical detail. Integrating data from these different sources is therefore complex, but doing so is advantageous for research and consequently for clinical purposes. For example, our research illustrated how linkage of EHR data sources substantially improves the identification of AMI cases.
The complexities of EHR data mean that a structured method for combining diverse data sources and creating EHR ‘phenotypes’ is needed, but no internationally recognised framework currently exists. An EHR phenotyping algorithm translates these clinical requirements into queries that leverage multiple linked EHR sources, identify relevant patients and extract disease onset, severity and subphenotype information. Defining phenotyping algorithms for a study (disease end points, disease start points, exclusions, covariates and risk factors) consists of identifying relevant codes for diagnosis (e.g. diagnosis of cancer) or risk factors (e.g. recording of smoking status) and defining how they should be combined. However, these definitions, on which research findings are based, are poorly described in published literature, with the exception of cases in which the curation of phenotyping algorithms occurs upstream by the data provider [e.g. electronic medical records and genomics network (eMERGE)].
We created and evaluated a framework (Figure 4) for transforming raw EHR data into research-ready phenotypes in a collaborative and transparent manner by involving domain experts across disciplines (epidemiology, computer science, public health, health informatics) and using a centralised repository for their distribution and curation. Here, we illustrate the steps involved in creating a phenotype using the example of atrial fibrillation (Figure 5). The framework consists of multiple iterative steps from initial phenotype definition with domain experts to algorithm implementation, validation and distribution to the scientific community. All CALIBER studies are registered in the public domain on Clinicaltrials.gov, and their analytic protocol is made available for download.
FIGURE 4.
Development of a phenotype algorithm using the CALIBER programme. Reproduced from Morley et al. 89 © 2014 Morley et al. This is an open-access article distributed under the terms of the Creative Commons Attribution License (http://creativecommons.org/licenses/by/4.0), which permits unrestricted use, distribution, and reproduction in any medium, provided the original author and source are credited.

FIGURE 5.
Flow diagram illustrating the CALIBER phenotype for atrial fibrillation. AF, atrial fibrillation; DVT, deep-vein thrombosis; PE, pulmonary embolism. Reproduced from Morley et al. 89 © 2014 Morley et al. This is an open-access article distributed under the terms of the Creative Commons Attribution License (http://creativecommons.org/licenses/by/4.0), which permits unrestricted use, distribution, and reproduction in any medium, provided the original author and source are credited.

To aid the process of creating, validating and disseminating EHR phenotyping algorithms for epidemiological research, we created the CALIBER data portal (www.caliberresearch.org/portal), an interactive online repository of phenotyping algorithms. All algorithms are displayed on the portal, where registered external researchers can view and comment on them. A number of metadata fields is recorded for each algorithm and is made available. The data portal contains phenotyping algorithms that leverage multiple EHR data sources in order to define > 90 diseases and related risk factors. The data portal also facilitates the transportability of the developed algorithms on national EHR data from different countries where subtle differences might exist in the manner clinical information is recorded. Algorithms available through the data portal can be utilised for performing international comparisons between health systems (e.g. England and the USA) or act as the base on which phenotyping algorithms reflecting the local health system are created.
The CALIBER portal operates a collaboration-driven data-sharing model and prioritises collaborations based on scientific-added value, capacity development and sustainable funding. Details on CALIBER and how to access CALIBER resources are available at www.ucl.ac.uk/health-informatics/caliber.
Strengths and limitations of the data platform
The CALIBER portal spans ambulatory and hospitalised care in large population samples, permitting a ‘higher resolution’ approach to cardiovascular epidemiology in three respects:
-
resolution of broad CVD aggregates (e.g. CVD, CHD) into distinct disease phenotypes in different vascular beds:
-
cerebral circulation – transient ischaemic attack, ischaemic stroke, subarachnoid haemorrhage, intracerebral haemorrhage
-
peripheral circulation – PAD, abdominal aortic aneurysm
-
coronary circulation – stable angina, unstable angina, AMI (STEMI and NSTEMI), unheralded CHD death, heart failure, cardiac arrest/sudden coronary death
-
-
sufficient temporal resolution to distinguish whether a first event (e.g. heart attack or stroke) was the first manifestation of CVD or if it was preceded by any of a range of non-fatal events
-
sufficient number of events and patients to generate accurate estimates of risk.
The main limitations of any EHR research platform relate to data quality:
-
Missing data. Data completeness is variable [e.g. 82.6% of people have ≥ 1 body mass index (BMI) measurement but only 44.9% have ≥ 1 cholesterol measurement]. Developments in imputation within longitudinal cohorts may offer a partial solution. 90
-
CALIBER makes use of coded data from multiple national EHR sources. A plethora of unstructured data exist in secondary care that are recorded within hospital information systems but remain inaccessible for research. This is mainly due to the lack of a centralised manner in which these data can be extracted, linked and utilised at scale. Significant national initiatives such as the National Institute for Health Research (NIHR) Health Informatics Collaborative (HIC) aim to create informatics infrastructure that will provide means for accessing these phenotypically rich data sources.
-
An important aspect of EHR phenotype development is validation, preferably against a ‘gold standard’ (such as a manual review of case notes). We could not validate the developed phenotypes in this manner as, for the CALIBER programme, the initiation and funding of a separate study is required for recontacting participants or clinicians to confirm diagnoses or review records. Previous studies, however, have shown the high degree of reliability of the data. 91
-
Currently, restrictive confidentiality legislation and information governance constraints in England prevent the use of anonymised clinical text on a large scale. Electronic free text in secondary care does not get captured or extracted in any form by HES and is thus not available for research. In primary care, free text from GPs contributing to CPRD is downloaded and held on government servers at CPRD, and is not readily available at scale to researchers based in universities (researchers can get access to very small corpora of text which has to be manually anonymised, a process that requires substantial resources and does not scale). Consequently, our approach to developing phenotyping algorithms has limited ability to fully utilise data elements extracted from clinical text as others have done.
Study 2: completeness and diagnostic validity of recording acute myocardial infarction events in primary care, hospital care, disease registry and national mortality records
This study is based on the peer-reviewed paper by Herrett et al. 28 Sections of the text have been reproduced/adapted from the original article; Copyright © Herrett et al. 28 2013. This is an Open Access article distributed in accordance with the Creative Commons Attribution Non Commercial (CC BY-NC 3.0: https://creativecommons.org/licenses/by-nc/3.0/) license.
Introduction
We assessed the concordance, completeness and diagnostic validity of AMI recording across CPRD, HES, MINAP and ONS in order to ensure the robustness of the data linkage. Our specific aims in this study were to compare the incidence, recording, agreement of dates and codes, risk factors and all-cause mortality of AMI.
Methods
Study population and follow-up
We identified records of AMI in CPRD, HES and ONS. In MINAP, STEMI and NSTEMI were identified using hospital discharge diagnosis, markers of myocardial necrosis and coded electrocardiogram (ECG) findings, in accordance with the international definition of AMI. 92 The study period was 1 January 2003 to 31 March 2009 (when all record sources were concurrent) and confined to patients who had been registered with their general practice for at least 1 year. We selected the first record of AMI during the patient’s study period as the index event and considered AMI records in the other data sources as representing the same event if they were dated within 30 days of the index event. Follow-up after a record of AMI was for death as recorded in the ONS death registry. We categorised patients as having fatal or non-fatal AMI according to whether or not they died of any cause within 7 days of AMI.
Agreement in recording
If the time difference between the earliest date of AMI in different sources was ≤ 30 days we considered that the records of AMI in the different sources agreed. An AMI recorded > 30 days after the earliest date was considered a new event and was not included, ensuring that each patient appeared only once in the analysis.
Statistical analysis
We estimated population-based incidence rates of fatal and non-fatal AMI using the denominator of all adults in the CALIBER primary care population aged ≥ 18 years (2.2 million people), followed up for a mean 4.1 years between 2003 and 2009. We used each of the data sources separately and together to identify incident AMI. We compared patients with fatal and non-fatal AMI identified in the four data sources for risk factors and coexisting conditions recorded in primary care. We produced cumulative incidence curves for coronary and non-coronary mortality for patients recorded in each data source and compared mortality using a Cox proportional hazards model adjusted for age and sex. We would expect patients who survived 7 days after AMI to be recorded in CPRD, MINAP and HES, and we assessed agreement between these three sources in a Venn diagram. For patients who died within 7 days, we examined the proportion recorded by each source but did not compare agreement across all four sources, as we would not expect the hospital discharge data and disease registry to record patients who died before reaching hospital. In patients who did not have a record of AMI in one or more data sources, we looked for other codes that may have been used to describe the event. In MINAP, we looked for admission diagnoses of any ACS. In CPRD and HES, we sought other ACS, coronary disease, chest pain or other cardiac diagnoses (e.g. atrial fibrillation, heart failure, cardiac arrest). In CPRD we also examined codes indicating contact with secondary care. Where none of these codes was recorded, we tabulated all recorded codes in the 30 days before and after the date of AMI to see if there were any relevant codes that we had overlooked. We performed a logistic regression analysis to establish whether age, sex, deprivation, rate of primary care consultation, year of AMI or mortality at 30 days explained suboptimal recording of AMI in CPRD, HES or MINAP sources. We calculated the positive predictive value (PPV) of CPRD or HES diagnoses of AMI among patients who also had a record in MINAP.
Results
Some 21,482 patients had a record of fatal or non-fatal AMI recorded in any of the four data sources. Single-source crude estimates for incident AMI per 100,000 patient-years are shown in Figure 6. The crude incidence of AMI was 25% lower using only CPRD and 50% lower using only MINAP compared with using all three sources.
FIGURE 6.
Crude incidence of fatal and non-fatal AMI estimated using different combinations of data from primary care (CPRD), hospital admissions (HES), disease registry (MINAP) and death registry (ONS). MI, myocardial infarction. Reproduced from Herrett et al. 28 Copyright © 2013, British Medical Journal Publishing Group. This is an Open Access article distributed under the terms of the Creative Commons Attribution License (http://creativecommons.org/licenses/by-nc/3.0/), which permits non-commercial reuse, distribution, and reproduction in any medium, provided the original work is properly cited.
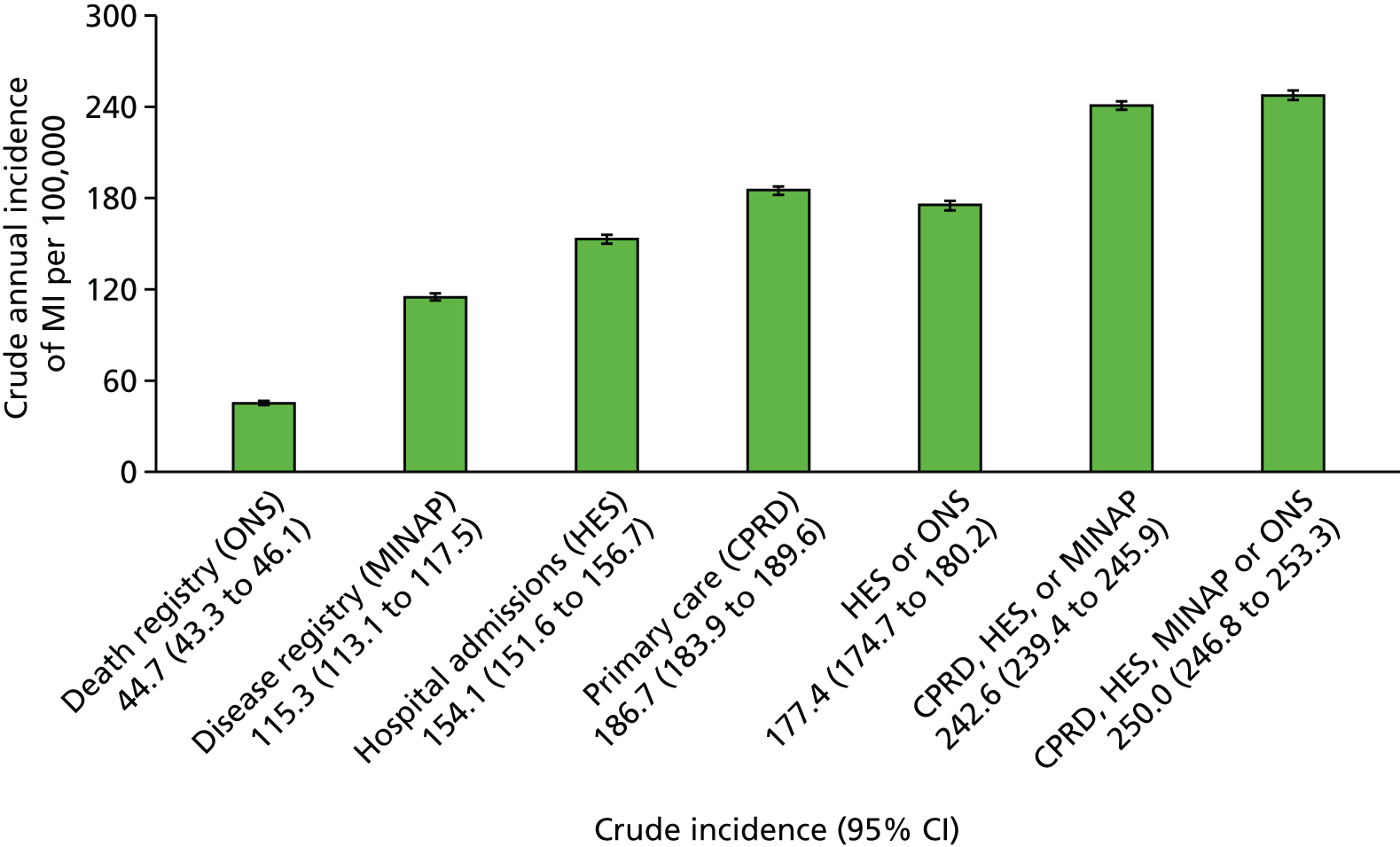
Cardiovascular risk factors and comorbidity
Cohorts identified from CPRD, HES and MINAP had a similar prevalence of cardiovascular risk factors and comorbidities. However, compared with patients recorded only in MINAP or HES, those with AMI recorded only in CPRD were, on average, 2 years younger, more likely to be current smokers and in the most deprived quintile for socioeconomic status (p < 0.001 for these comparisons). Patients recorded in ONS were older than patients in the other sources and had a higher burden of risk factors reflecting their age. However, other demographic characteristics and cardiovascular risk factors were broadly similar across patients recorded in primary care and hospital care sources.
Death after acute myocardial infarction
Crude 30-day mortality was lower in MINAP [hazard ratio (HR) 10.8%, 95% confidence interval (CI) 10.2% to 11.4%] than in HES (HR 13.9%, 95% CI 13.3% to 14.4%) or CPRD (HR 14.9%, 95% CI 14.4% to 15.5%) (Figure 7a). At 1 year, however, mortality was similar in all three groups, at around 20% (Figure 7b).
FIGURE 7.
Kaplan–Meier curves. (a) Kaplan–Meier curves showing 30-day all-cause mortality, stratified by record source (CPRD, HES and MINAP) in 20,819 patients. CPRD, n = 15,819; HES, n = 13,831; and MINAP, n = 10,351. MIs recorded by the ONS are not shown, as they are, by definition, fatal on the date of AMI; and (b) Kaplan–Meier curves showing 12-month all-cause mortality, stratified by record source (CPRD, HES and MINAP) in 20,819 patients. MI, myocardial infarction. Reproduced from Herrett et al. 28 Copyright © 2013, British Medical Journal Publishing Group. This is an Open Access article distributed under the terms of the Creative Commons Attribution License (http://creativecommons.org/licenses/by-nc/3.0/), which permits non-commercial reuse, distribution, and reproduction in any medium, provided the original work is properly cited.
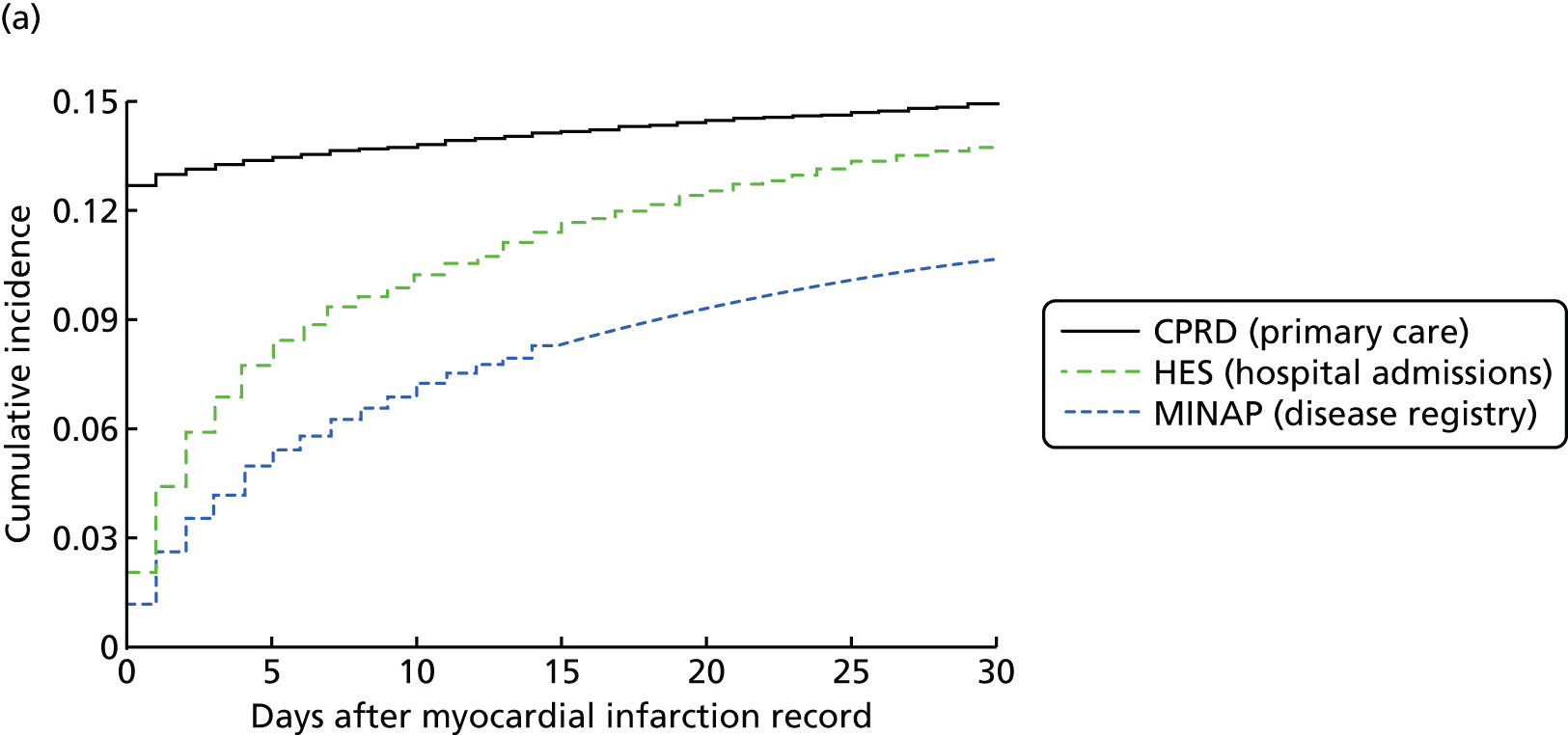

In the linked data, patients with AMI recorded in only one source had higher mortality than those recorded in more than one source (age- and sex-adjusted HR 2.29, 95% CI 2.17 to 2.42; p < 0.001); those recorded only in CPRD had the highest mortality on the first day but the lowest mortality thereafter, compared with HES and MINAP (adjusted HR for coronary mortality 0.49, 95% CI 0.40 to 0.60; p < 0.001; and for other causes 0.57, 95% CI 0.49 to 0.67; p < 0.001). Of the 3518 patients with AMI recorded in any of the four sources who died of any cause within 7 days, 54.4% (n = 1914) had an AMI code recorded in CPRD within 30 days. The underlying cause of death recorded in ONS was AMI in 2924 patients (83.0%). Of fatal AMIs identified by ONS, only 36.7% (n = 1072) were recorded in HES and 17.1% (n = 498) in MINAP within 30 days, but 55.9% (n = 1631) were recorded in CPRD.
Non-fatal acute myocardial infarction
Among the 17,964 patients with at least one record of non-fatal AMI, 13,380 (74.5%) were recorded in CPRD, 12,189 (67.9%) in HES and 9438 (52.5%) in MINAP. Overall, 5561 (31.0%) patients had the event recorded in all three sources and 11,482 (63.9%) patients had it recorded in at least two sources (Figure 8). When we extended the recording window from 30 to 90 days, the proportion recorded in all three sources increased only slightly, to 32.0% (n = 5747). Only 25.1% (3364/13,380) of the non-fatal AMIs recorded in CPRD stated the type (STEMI or NSTEMI), compared with 100% in MINAP.
FIGURE 8.
Number and percentage of records recorded in primary care (CPRD), hospital care (HES) and disease registry (MINAP) for non-fatal AMI across the three sources (n = 17,964 patients). Reproduced from Herrett et al. 28 Copyright © 2013, British Medical Journal Publishing Group. This is an Open Access article distributed under the terms of the Creative Commons Attribution License (http://creativecommons.org/licenses/by-nc/3.0/), which permits non-commercial reuse, distribution, and reproduction in any medium, provided the original work is properly cited.
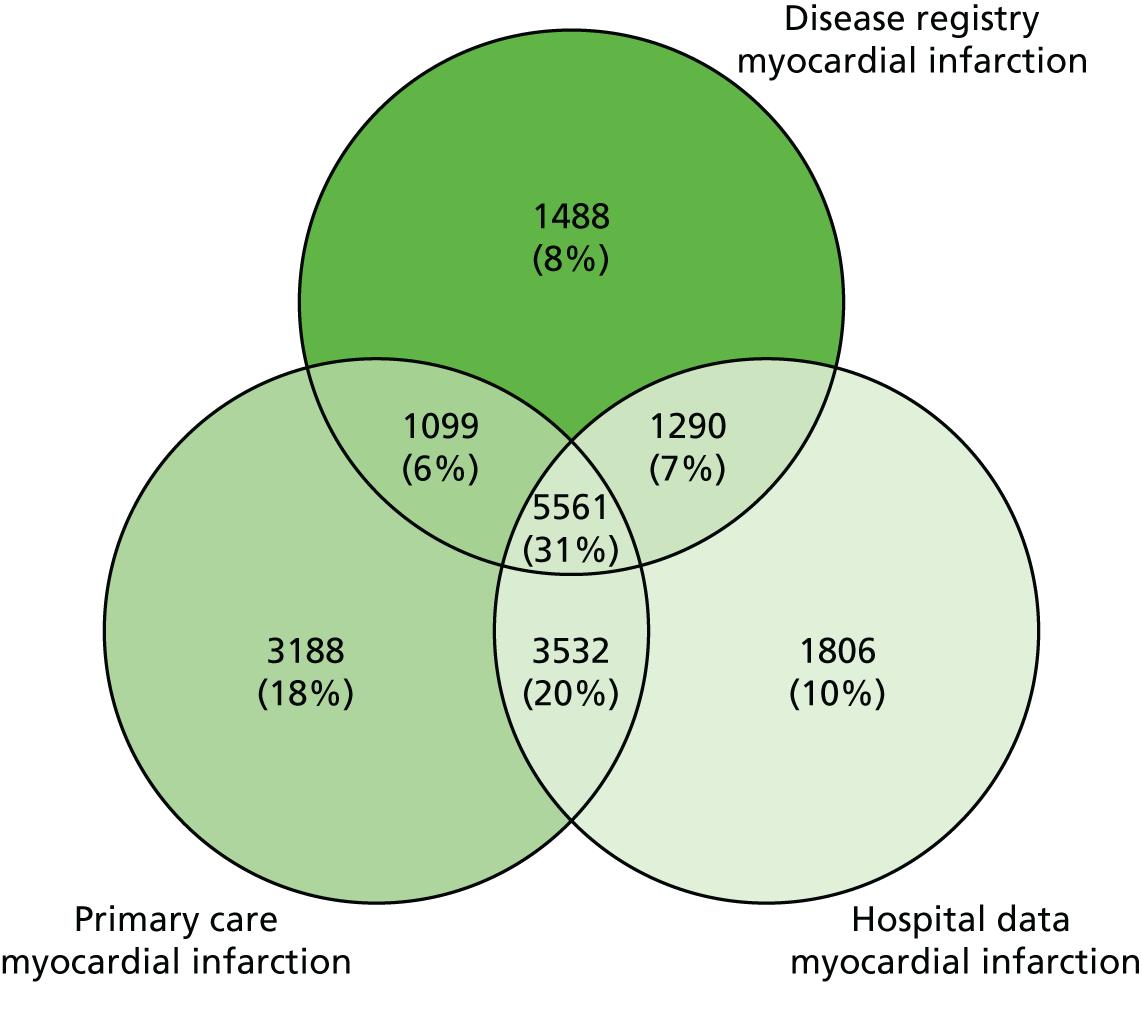
Positive predictive value
For CPRD or HES patients with an associated record in MINAP, the PPV of the AMI diagnosis (the probability that the diagnosis in MINAP was AMI rather than unstable angina or a non-cardiac diagnosis) was 92.2% (95% CI 91.6% to 92.8%; 6660/7224) in CPRD and 91.5% (95% CI 90.8% to 92.1%; 6851/7489) in HES (Table 4). Eighty-five per cent of patients recorded in CPRD and HES (7386/8707) had a record of raised cardiac markers and half (3766/8707) had a record of ST-segment elevation on electrocardiography.
Information in MINAP | Source of AMI record | ||
---|---|---|---|
CPRD | HES | CPRD and HES | |
Number of patients | 7224 | 7489 | 6006 |
Electrocardiographic findings, n (%) | |||
ST-segment elevation | 3373 (46.7) | 3455 (46.1) | 3062 (51.0) |
Other abnormality | 2337 (32.4) | 2485 (33.2) | 1816 (30.2) |
Normal | 389 (5.4) | 429 (5.7) | 306 (5.1) |
Not recorded | 1125 (15.6) | 1120 (15) | 822 (13.7) |
Cardiac markers, n (%) | |||
Raised | 6149 (85.1) | 6358 (84.9) | 5121 (85.3) |
Normal | 368 (5.1) | 411 (5.5) | 299 (5.0) |
Missing | 707 (9.8) | 720 (9.6) | 586 (9.8) |
Peak troponin | |||
Level recorded, n (%) | 6109 (84.6) | 6357 (84.9) | 5029 (83.7) |
Median (IQR), ng/ml | 2.03 (0.47–10.0) | 2.04 (0.45–10.2) | 2.34 (0.53–11.6) |
CALIBER diagnosis | |||
STEMI, n (%) | 3386 (46.9) | 3441 (46.0) | 3064 (51.0) |
NSTEMI, n (%) | 3274 (45.3) | 3410 (45.5) | 2497 (41.6) |
Unstable angina, n (%) | 384 (5.3) | 425 (5.7) | 312 (5.2) |
Other, n (%) | 180 (2.5) | 213 (2.8) | 133 (2.2) |
Positive predictive value (95% CI) for AMI | 92.2 (91.6 to 92.8) | 91.5 (90.8 to 92.1) | 92.6 (91.9 to 93.3) |
Discussion
In comparing records of one major disease event – AMI – across CALIBER’s four linked sources, we found that each source misses a substantial proportion of events. However, our data confirm the validity of AMI recorded in these sources, in terms of risk factor profiles and mortality at 1 year. Taken together, these findings support the wider use of linkage of multiple record sources by clinicians, policy-makers and researchers in order to maximise diagnostic capture.
Fatal acute myocardial infarction
Both CPRD and ONS can be used to capture fatal AMI occurring out of hospital among people without a record in HES or MINAP. ONS is the most useful source for research, identifying AMI as the cause of death in 83.0% of patients who died within 7 days with AMI recorded in any of the data sources. These figures agree with the Oxford Record Linkage Study where, among 5686 patients admitted to hospital with AMI, 85.2% who died within 30 days had AMI recorded as the underlying cause of death. 93
Non-fatal acute myocardial infarction
Only one-third of non-fatal AMIs were recorded in all three data sources (primary care, hospital admissions and disease registry) and two-thirds in at least two sources. CPRD was the single most complete source of non-fatal AMI records, missing one-quarter of recorded cases, with HES missing one-third and MINAP missing nearly half of recorded cases (see Figure 8). This agrees with the results of other two-source comparisons of AMI recording in Scotland, Australia, Denmark and the Netherlands, all of which showed that combining sources provided the highest estimates of incidence. 94–97 Despite the low sensitivity of CALIBER’s individual data sources, the diagnostic validity of AMI records in CPRD and HES was high, as reflected by a PPV of > 90% compared with the MINAP gold standard, based on the international definition of AMI. Our results using cross-referencing of EHRs in 20,000 patients are consistent with previous manual approaches to validation in primary care, which cross-reference a few hundred patients with disease diagnoses recorded using Read codes against anonymised free text, death certificates, paper medical records or hospital discharge summaries, or questionnaires to GPs. 86,98–104 Our much larger sample size, however, allowed us to evaluate individual Read terms that are used to record AMI. This type of validation has not been done previously for AMI and may be relevant to other common conditions that can be recorded using a variety of codes, such as stroke. 105
Hospital admission data
To our knowledge, no studies have examined the PPV of ICD-10-coded AMI diagnosis in hospital admission data (HES) against an ongoing disease registry (MINAP). We found that 62.8% of non-fatal AMIs recorded in CPRD and 72.6% recorded in MINAP were also in the HES record. This is consistent with a single EHR source (HES ICD-10 I21 and I22) capturing 53% of AMIs in an investigator-led cohort with active follow-up. 106
Disease registry and maximising true positives
The strengths of MINAP lie in the fact that its diagnostic records (troponin values, ECG findings, and cardiologist diagnosis of STEMI and NSTEMI), offering validated end points electronically for all hospitals in England and Wales, are not available in other sources. An AMI recorded in MINAP is thus an EHR gold standard and may be important for detecting end points in cohort studies and trials, in which false positives can dilute any observed effect and reduce the power of a study. Furthermore, in such studies it has been shown that avoiding false positives is more important than avoiding false negatives. 107 Validation of AMIs recorded by primary care and hospital admissions against those recorded by the disease registry showed a PPV of > 90%, making them suitable for detecting end points in cohort studies and trials, where poor end-point resolution can dilute any observed effect and reduce the power of a study. The PPV was not 100% because some AMI records in primary care may actually have been related to unstable angina or chest pain of unknown cause.
Limitations
Our data were from a sample of 244 English general practices contributing to CPRD. However, patients in practices that participated in CALIBER are representative of the UK population and also of CPRD as a whole in terms of age, social deprivation, BMI and prescription of key drugs. 108 Hospital admissions with linked primary care data were also representative of all admissions to hospital in England in terms of the distribution of age, sex and diagnostic group. 109 The UK Life Sciences Strategy16 aims to increase the proportion of the UK population available for research through the CPRD. 6
A second limitation concerns the generalisability of our findings for the quality of primary care data. Practices contributing data to CPRD are advised of recording guidelines and their data are accepted only when they meet standards of data completeness, so they are likely to record disease events better than practices that do not contribute. Our estimates of agreement may therefore be higher than for practices that do not contribute to CPRD.
Third, our validation of HES and CPRD AMIs against MINAP was limited to the subset of patients with a MINAP record, and caution must be exercised in extending these conclusions to patients with AMIs without a MINAP record.
Implications for research using ClinicAl disease research using LInked Bespoke studies and Electronic health Records data
Our findings show that by linking EHRs we not only provide a longitudinal component with which to map the CVD journey from first diagnosis to death, but also enrich the data sources and maximise capture of AMI with a high level of diagnostic validity. Assuming this extends to other manifestations of CVD, our findings provide reassurance that the CALIBER programme has the scale and diagnostic validity necessary for national EHR research.
Clinical and policy implication
We propose much wider use of linked record sources in commissioning and in research to estimate disease occurrence and outcome, because of the biases inherent in using only one source of records. Our findings underscore the importance of international initiatives to accelerate availability of linked data in the USA, Europe and elsewhere (see www.research.va.gov/mvp/ and https://healthy.kaiserpermanente.org). 110 Going forward, a national strategy for biomedical informatics is required based on a single record for a single health-care event that is propagated in multiple record systems. Efforts to reform the process of death certification are already under way,111 but this needs to be broadened to include other health records. Primary care records could be improved by recording AMI only for its occurrence rather than repeated entries for consultations related to a history of AMI.
Future research
Several lines of research are warranted by our findings. First, research is required to understand how the coding of EHR data can be improved. Second, more extensive cross-referencing is required against additional sources of information on AMI. These include self-reported AMI (which may be less dependent on specific settings in the health system) and manual review of all the available local case records (paper and electronic). Investigation of uncoded electronic free text in the primary care record will enhance its data yield and is a key part of both our research and that of the UK Biobank cohort (n = 500,000). 107,112 Investigators are beginning to recognise the value of linking bespoke cohorts and trials with the primary care record and also with national disease registries, such as MINAP, for the capture and validation of ischaemic end points. 113
Conclusions
Our findings show that by linking EHRs we not only provide a longitudinal component with which to map the CVD journey from first diagnosis to death but also enrich the data sources and maximise capture of AMI with a high level of diagnostic validity. Assuming this extends to other manifestations of CVD, our findings provide reassurance that the CALIBER programme has the scale and diagnostic validity necessary for national EHR research.
PROGnosis RESearch Strategy
In an era when more people are surviving disease than ever before, researchers are increasingly focused on the study of prognosis, which provides crucial evidence for translating research findings into clinical practice. However, prognosis research studies often fall far short of the high standards required in other fields such as therapeutic trials. Serious flaws in the design, conduct and reporting of prognosis studies need to be addressed, and the clinical value of reliable prognostic evidence needs to be more widely recognised.
Hemingway et al. 114 and von Elm et al. 115 have highlighted that the field of prognosis research, which spans quality of care and outcomes research, prognostic factor research and prognostic models, faces multiple methodological challenges. These include the proliferation of small studies, lack of biomarker replication, lack of systematic evaluations of the quality of research on a single prognostic biomarker and a dearth of studies that provide clinically actionable decision support.
To address this issue, we established the PROGRESS partnership, with representatives from academia and medical journals. Our aim was to create a more coherent and rigorous framework for prognosis research, with specific recommendations on how the field can be developed and broaden its impact. Key principles have been summarised in a series of articles in medical journals and on the PROGRESS website (http://progress-partnership.org/). 29–33
Study 3: the PROGnosis RESearch Strategy partnership
This study is based on peer-reviewed papers by Hemingway et al. ,29 Hingorani et al. ,30 Riley et al. ,31 Steyerberg et al. 32 and Peat et al. 33
In 2012 we established the PROGRESS initiative, which seeks to promote high-quality prognostic research leading to patient benefit. The partnership was set up to analyse research into quality-of-care outcomes, prognostic factors, risk prediction models and predictors of differential treatment response. We outlined a framework for prognosis research, published as a series of British Medical Journal (BMJ) and PLOS Medicine articles with recommendations across five key areas: (1) fundamental prognosis research – the course of health-related conditions in the context of current care; (2) prognostic factor research – studies of specific factors such as biomarkers associated with prognosis; (3) prognostic model research – development, validation and impact of statistical models that predict individual risk of a future outcome; (4) stratified medicine research – use of prognostic information to tailor treatment decisions to an individual or group of individuals with similar characteristics; and (5) transparency of research – the role of reporting, data sharing, study registration and protocols. 29–33 Our recommendations were designed to improve the design, conduct, analysis and reporting of prognosis research, which will be crucial if prognosis research is ultimately to improve patient outcomes. We made 24 recommendations to address challenges and opportunities for prognosis research, including the introduction of new programmes of methodological and empirical prognosis research exploiting EHRs to define, phenotype and follow up people with different health-related conditions (recommendation 3)29 and the establishment of a comprehensive set of clinical cohorts recruiting people with specified health-related condition(s), including diagnosed disease and symptoms as platforms for addressing a wide range of prognosis research questions (recommendation 14). 29
In many diseases, the most intensively studied prognostic factors are biomarkers. There is potential for identifying prognostic factors, from their use within current clinical practice to their implications for randomised trial design and developing new interventions. With a suitable prognostic model, decision-makers can use multiple prognostic factors to improve the targeting of interventions on the basis of patients’ predicted individual risk of subsequent outcomes.
Many prognostic models have been described, yet few are used or implemented in clinical practice. They are often developed from inadequate data, inappropriately analysed and poorly reported, and few models are evaluated for their impact on health outcomes (Figure 9). We outlined several key steps to make prognostic models more useful, including improved development of models, validation, evaluation of impact on clinical practice and outcomes, updating and clinical use. In addition, any prognostic models are used without clear evidence of their impact, whereas other well-developed and validated models are not used. We encourage researchers to support collaborative efforts to share individual patient data, to improve model development and external validation. With biomarker and -omics research generating a wealth of possible new factors to incorporate into models, the extent to which new markers add value to existing models needs to be assessed.
FIGURE 9.
Four reviews cataloguing prognostic model development, validation and impact assessment in published articles. Reproduced from Steyerberg et al. 32 © 2013 Steyerberg et al. 32 This is an open-access article distributed under the terms of the Creative Commons Attribution License (http://creativecommons.org/licenses/4.0), which permits unrestricted use, distribution, and reproduction in any medium, provided the original author and source are credited.
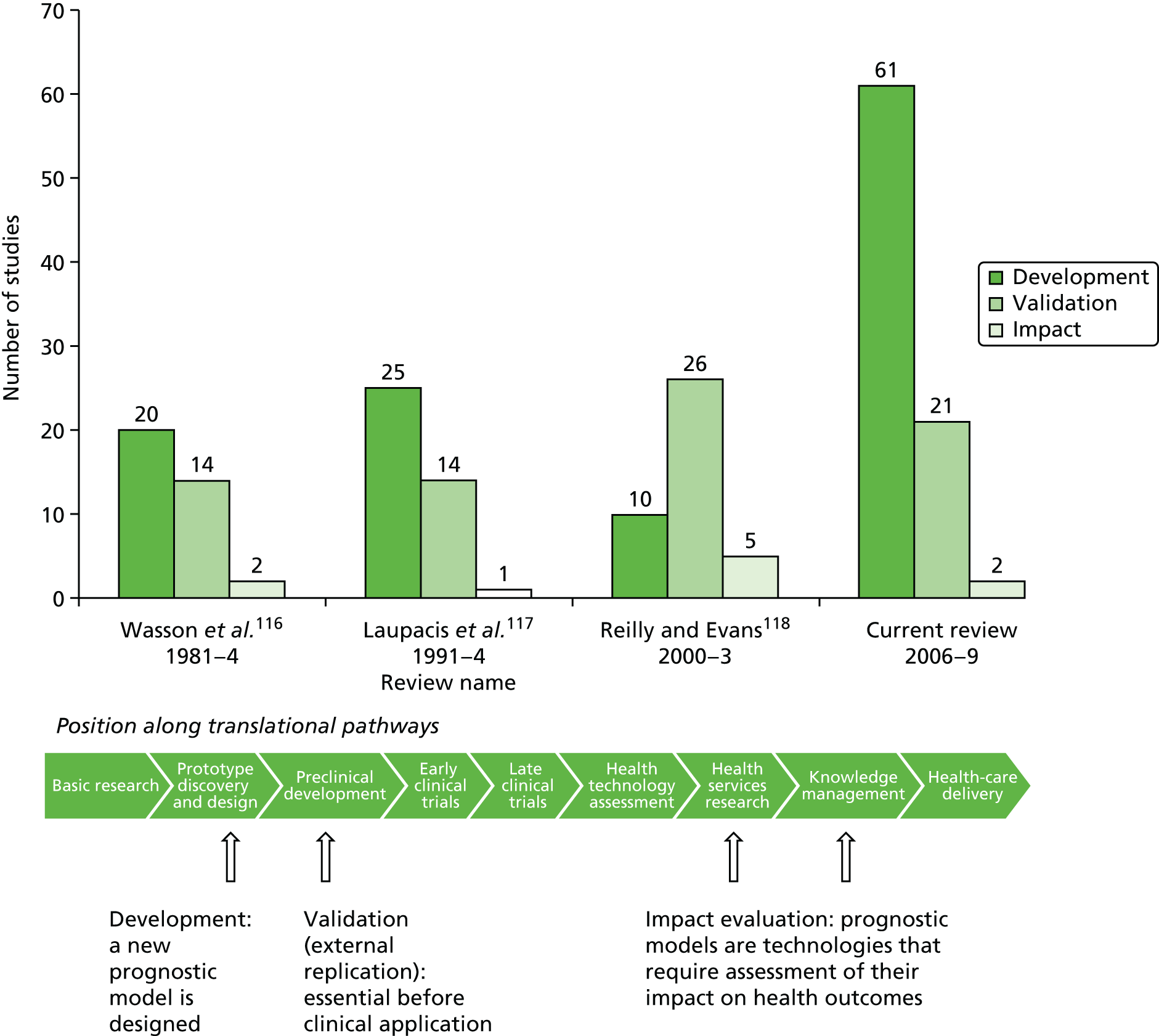
Chapter 3 Inequalities in the incidence of 12 cardiovascular diseases across the patient journey
Abstract
Background
Understanding how CVDs first present is vital to organise primary and secondary prevention efforts, but little is known about CVDs other than AMI, stroke and coronary death. UK South Asian populations are particularly affected by CHD but it is unclear if this reflects a higher incidence of disease, worse prognosis or both.
Objectives
(1) To identify how the development of 12 CVDs is affected by age, sex, ethnicity and socioeconomic deprivation; and (2) to examine the complex relationships between incidence and prognosis of CHD among South Asian populations.
Methods
(1) An analysis of CALIBER linked data; and (2) an analysis of cohort data and a meta-analysis of published data.
Results
(1) Acute myocardial infarction, stroke and coronary death collectively account for just 34% of initial cardiovascular events. The strength and shape of the association of CVDs differed with age, and the risk of different CVDs differed by sex. Initial presentation of CVDs showed considerable heterogeneity with deprivation. Those of South Asian ethnicity were at substantially increased risk of all coronary presentations compared with white patients. (2) CHD incidence among those of South Asian ethnicity was markedly higher than among white patients, but their prognosis was significantly better.
Conclusions
These are the only contemporary, large-scale incidence estimates of a wide range of fatal and non-fatal cerebrovascular, cardiac and peripheral CVDs. Our findings are crucial for understanding the global burden of CVDs. Our findings argue against the use of composite measures of cardiovascular health, which may mask important variability across conditions.
The studies in this chapter relate to people without established disease (healthy population) (Figure 10).
FIGURE 10.
Overview of the 33 studies in our programme: population without established disease.

Study 4: inequalities in the presentation of cardiovascular diseases, by age, sex, ethnicity and deprivation
This study is based on the peer-reviewed papers by Pujades-Rodriguez et al. 34 and George et al. 35
Introduction
An important principle in CVD management is that the first lifetime diagnosis indicates the failure of primary prevention and the need for measures to prevent subsequent events. The decades-long emphasis given to the prevention of AMI and stroke is reflected in the significant declines in their incidence in developed countries. 119 Incidence rates for angina, heart failure and other chronic CVD presentations do not appear to have similarly declined. 119–121 Consequently, the spectrum of initial lifetime presentations of CVD in contemporary practice is likely to have changed, compared with the end of the last century. Cohort studies that report only fatal end points (‘final presentations’)122 are less informative for primary preventative efforts than those that investigate initial lifetime presentations, and studies that include non-fatal events have generally focused on AMI and stroke while ignoring other CVDs. 123–126
Large-scale studies that evaluate the first lifetime diagnosis in different ages, sexes, socioeconomic groups and ethnicities across a wide range of acute and chronic CVDs including both fatal and non-fatal presentations are overdue.
Fundamental unanswered questions about initial lifetime CVD presentation arise. First, what is the relative frequency of different CVDs as they affect different ages, sexes, ethnicities and socioeconomic groups in contemporary practice? Second, what is the lifetime risk of the most common CVDs? Lifetime risks provide an intuitive measure of the burden of each disease in the population, relevant to public health policy and risk communication. Lifetime risks have recently been reported for CHD and CVD aggregates127–129 and heart failure,130 but rarely for a wide range of initial presentations of CVDs. 38 Third, is male sex an equally strong risk factor common to all CVDs, or does the association differ across a range of diseases?
The lack of large, contemporary, population-based cohorts with detailed clinical follow-up spanning hospital and ambulatory care has hindered the study of the initial presentation of a wide range of acute and chronic CVDs. It has been suggested that EHR data might be ‘meaningfully reused’131 to create ‘mega cohorts’ for such research. 132 We studied a contemporary, population-based cohort based on linked EHRs across primary, secondary, disease registry and death records to address these questions.
Methods
The CALIBER study population was 1,937,360 patients for age, sex and deprivation analyses, and 1,068,318 for ethnicity analyses. For ethnicity, patients were categorised as white, black, South Asian or other/mixed. Deprivation was measured by the IMD (2007 revision),88 derived from postcode of residence. Patients were categorised into quintiles, where the first quintile indicated the least deprived and the fifth quintile indicated the most deprived group. Primary end points for the analysis were the initial presentation of fatal and non-fatal CVDs across the CALIBER data sources. CVD presentations studied were stable angina, unstable angina, AMI, heart failure, a composite of ventricular arrhythmia, cardioversion, cardiac arrest or sudden cardiac death (SCD), transient ischaemic attack, subarachnoid haemorrhage, intracerebral haemorrhage, ischaemic stroke, abdominal aortic aneurysm and PAD. Data analysis was based on Cox proportional hazards models.
Results
Follow-up was for a median of 5.5 years, during which 114,859 subjects experienced one of the 12 CVDs (52.3% in men). AMI, stroke and coronary death accounted for less than one-third of the total CVD presentations recorded in CALIBER.
Age
The strength and shape of the association of CVDs with age (Figure 11) varied from predominantly linear (in angina and non-fatal AMI) to strongly quadratic (unheralded coronary death, stroke, abdominal aortic aneurysm), and from weak (subarachnoid haemorrhage, unstable angina and cardiac arrest-SCD) to very strong (heart failure and abdominal aortic aneurysm). 35
FIGURE 11.
Age-adjusted HRs of men vs. women for initial presentation of 12 different CVDs among a population of 1.93 million adults. HRs are derived from Cox proportional hazards models stratified by general practice and adjusted for baseline age (linear and quadratic terms) and interaction between sex and baseline age. MI, myocardial infarction. Reproduced from George et al. 35 Copyright © 2015, The Authors. Circulation is published on behalf of the American Heart Association, Inc., by Wolters Kluwer. This is an open access article under the terms of the Creative Commons Attribution License (http://creativecommons.org/licenses/4.0), which permits use, distribution, and reproduction in any medium, provided that the original work is properly cited.

Sex
The most frequent initial CVD presentation was non-fatal AMI for men (17% of total CVD) and stable angina for women (12% of total CVD) (Figure 12). The cumulative lifetime incidence of CVD was 51.3% for men and 41.2% for women. Men were more likely than women to experience non-fatal AMI (7.1% vs. 3.9%) and abdominal aortic aneurysm (2.4% vs. 0.9%). Men experienced conditions affecting the myocardium, PAD and abdominal aortic aneurysm around 10 years earlier than women. However, the lifetime incidence of heart failure (8%), transient ischaemic attack (5%), ischaemic stroke (3%) and intracerebral haemorrhage (1%) was similar in men and women.
FIGURE 12.
Proportion of total initial lifetime presentations of CVDs by disease type in men and women. Columns in descending order by proportion of events in men aged 30–39 years. Number of events displayed at base of column. MI, myocardial infarction; NOS, not otherwise specified. Reproduced from George et al. 35 Copyright © 2015 The Authors. Circulation is published on behalf of the American Heart Association, Inc., by Wolters Kluwer. This is an open access article under the terms of the Creative Commons Attribution License (http://creativecommons.org/licenses/4.0), which permits use, distribution, and reproduction in any medium, provided that the original work is properly cited.
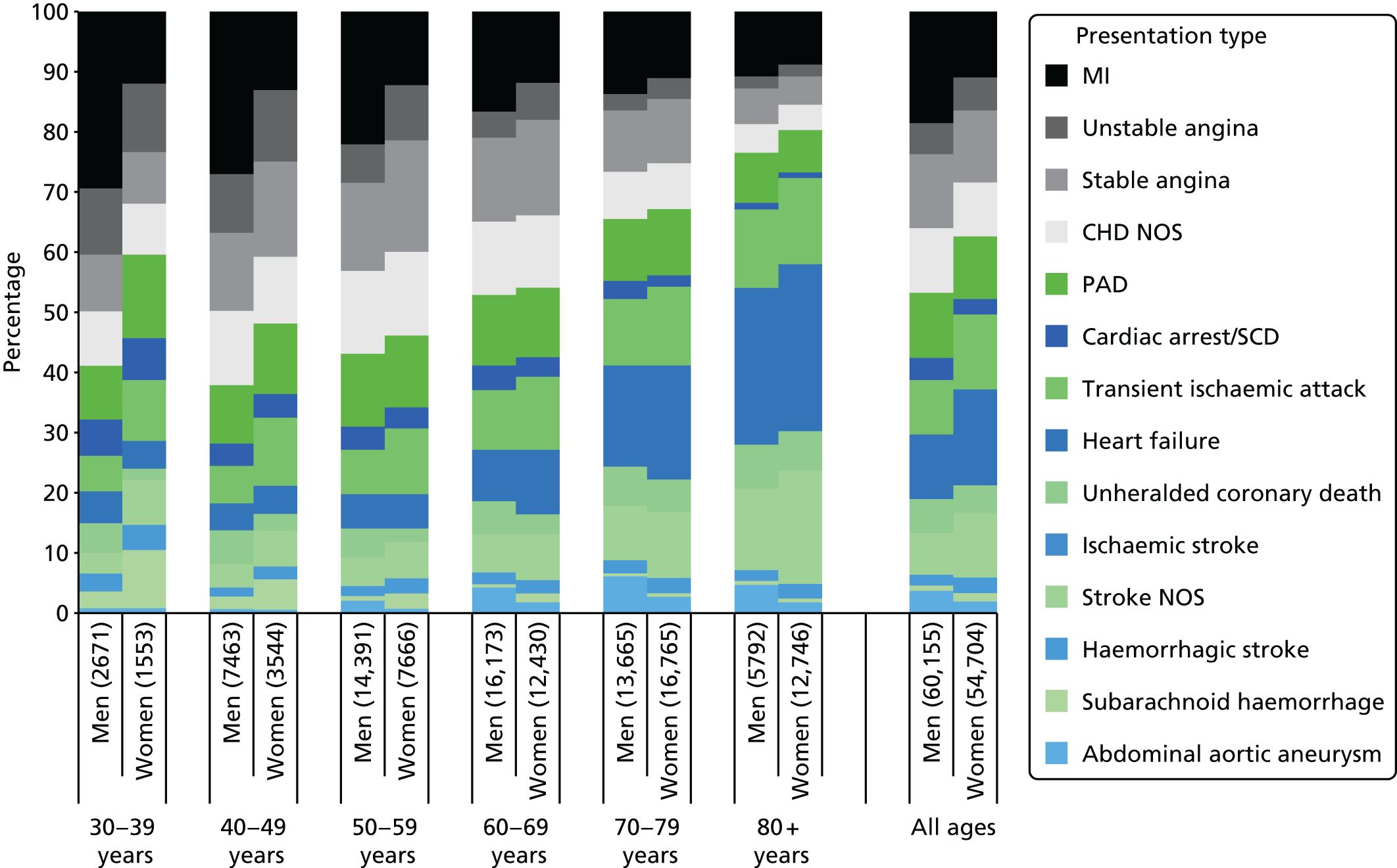
Apart from subarachnoid haemorrhage (HR men vs. women 0.69; 95% CI 0.59 to 0.79), all presentations showed a positive association with male sex (Figure 13). However, the strength of this association varied markedly: for transient ischaemic attack, intracerebral haemorrhage and unstable angina, age-adjusted HRs for male sex were < 1.5; for stable angina, ischaemic stroke, PAD, heart failure and cardiac arrest/SCD they were between 1.5 and 2.0; and for abdominal aortic aneurysm, AMI and unheralded coronary death they were between 3.6 and 5.0.
FIGURE 13.
Hazard ratios of men vs. women for initial lifetime presentation of 12 different CVDs among a population of 1.93 million adults. The vertical grey dotted line corresponds to the HR of the composite CVD end point. Reproduced from George et al. 35 Copyright © 2015 The Authors. Circulation is published on behalf of the American Heart Association, Inc., by Wolters Kluwer. This is an open access article under the terms of the Creative Commons Attribution License (http://creativecommons.org/licenses/4.0), which permits use, distribution, and reproduction in any medium, provided that the original work is properly cited.

Ethnicity
South Asian ethnicity was associated with a substantially increased hazard of initial presentation with coronary presentations compared with white ethnicity (stable angina: HR 1.67, 95% CI 1.52 to 1.84; unstable angina: HR 1.82, 95% CI 1.56 to 2.13; and AMI: HR 1.67, 95% CI 1.49 to 1.87). Conversely, black ethnicity appeared to be protective, with HRs for initial presentation with coronary presentations consistently < 1.0. There was a tendency towards a greater hazard of initial presentation with ischaemic stroke and intracerebral haemorrhage in people of black ethnicity, and in both black and South Asian populations hazards of subarachnoid haemorrhage and PAD were lower than in white populations.
Deprivation
Associations of deprivation with initial presentations of CVDs showed considerable heterogeneity in both women and men. In women (Figure 14), socioeconomic deprivation showed no association with abdominal aortic aneurysm. In men too there was no association with abdominal aortic aneurysm and no association with stable angina, cardiac arrest-SCD, subarachnoid haemorrhage or transient ischaemic attack. For other CVDs, hazard increased linearly with deprivation quintile, the increase being comparable between the sexes for unstable angina, AMI and heart failure, but steeper in men for PAD, ischaemic stroke and intracerebral haemorrhage.
FIGURE 14.
Hazard ratios for the association between the initial presentation of 12 CVDs and socioeconomic deprivation adjusted for cardiovascular risk factors measured at baseline in women. Reference: least deprived quintile. MI, myocardial infarction; Q, quintile. Reproduced from Pujades-Rodriguez et al. 34 © 2014 Pujades-Rodriguez et al. 34 This is an open-access article distributed under the terms of the Creative Commons Attribution License (http://creativecommons.org/licenses/4.0), which permits unrestricted use, distribution, and reproduction in any medium, provided the original author and source are credited.
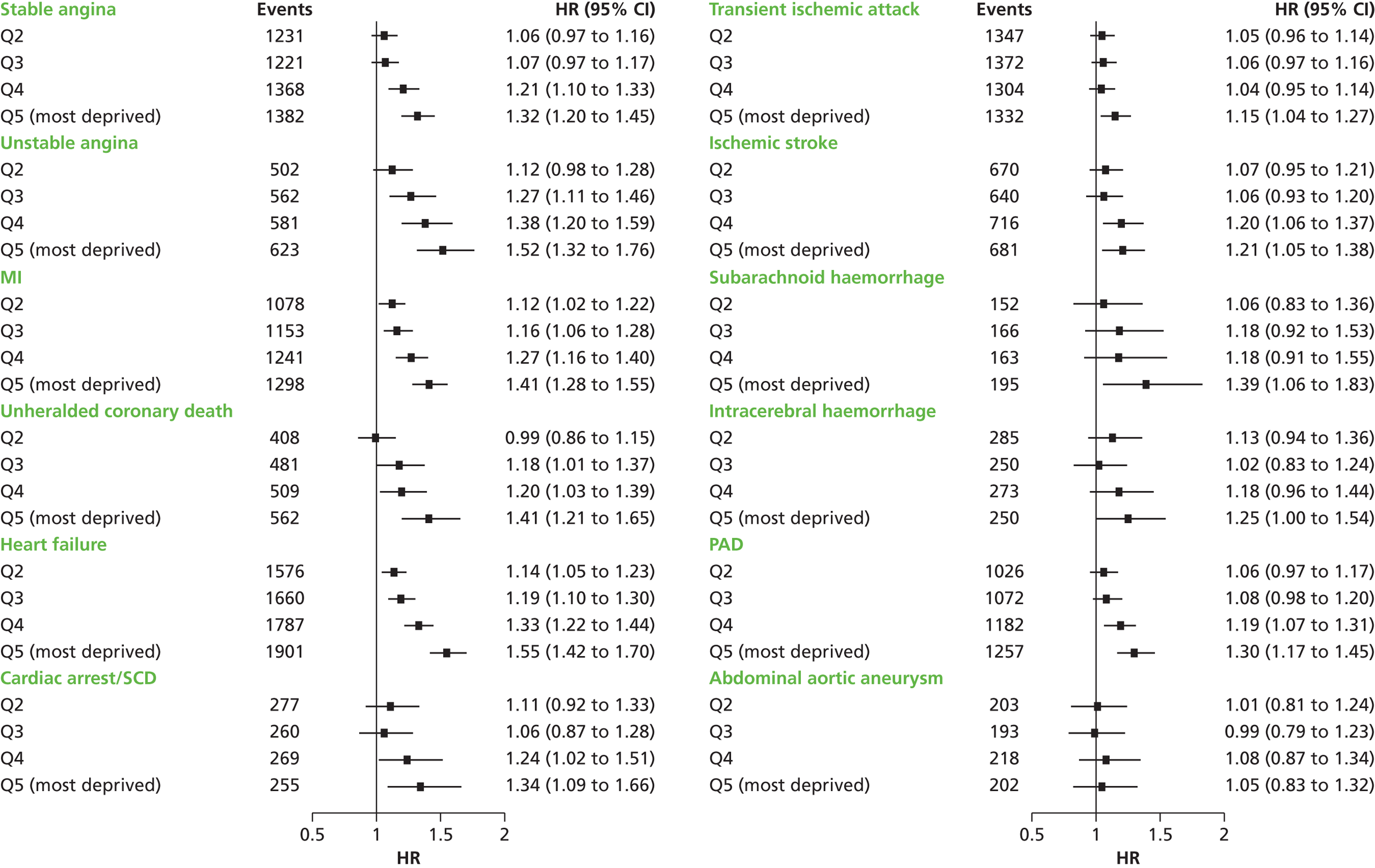
Differences in lifetime risk between the least and most deprived quintiles were greatest for PAD in both women (4.3% vs. 5.8%) and men (4.6% vs. 7.8%). However, in both sexes, differences were small or non-existent for cardiac arrest-SCD, transient ischaemic attack, abdominal aortic aneurysm, and ischaemic and intracerebral haemorrhage.
Discussion
By linking EHRs, this study has analysed > 100,000 initial CVD presentations arising in a cohort of nearly 2 million people, providing sufficient scale to distinguish multiple types of CVD and assess inequalities in the risk of initial presentation according to age, sex, ethnicity and levels of deprivation. Through the use of linked EHRs, we were able to capture diseases first presenting in primary care and were not confined to hospitalised cases. Our cohort is population based, with > 99% of the English population estimated to be registered with a family physician,133 unlike other recent large recent cohort studies, such as the UK Biobank,107 which had response rates of < 10%. 134 The size of cohort possible with this EHR platform – nearly 2 million people – allows us to include serious but less common events such as abdominal aortic aneurysm and subarachnoid haemorrhage, as well as to have sufficient events in women to study sex differences reliably. We were able to follow up actual events in a clinically meaningful 5-year time frame, similar to the time horizon of randomised controlled trials (RCTs). More broadly, we demonstrate the potential of linked EHR cohorts to complement bespoke, investigator-led cohorts. 73 The UK Biobank,107,112 the Research Program for Genes Environment and Health in Kaiser12 and the ‘precision’ medicine initiatives135 all place a major emphasis on specific disease types and follow-up through health records. Furthermore, such large EHR cohorts hold out the promise of a lower cost to research funders for data collection, the intrinsic clinical relevance of ‘real-world’ data, the opportunities to study diseases with higher specificity given the cohort sizes possible, and the prospect for researchers and clinicians to work across the boundaries that currently impede the translation of new discoveries into public health benefit. 73
Although AMI and ischaemic stroke are the most commonly used end points in trials, they collectively account for just 34% of initial cardiovascular events. The majority of cardiovascular presentations are heart failure, angina, transient ischaemic attack and PAD, which are rarely evaluated individually in primary prevention studies.
Despite recent progress in prevention, there is still a 45% lifetime risk of any CVD between the ages of 30 and 95 years, with men at greater risk than women (51.3% vs. 41.2%). However, the effect of male sex varies significantly between the 12 different CVD presentations. Men are actually less likely to experience subarachnoid haemorrhage and show similar incidence levels to women for several conditions. Male sex showed a moderate association with five presentations and a strong association with abdominal aortic aneurysm, AMI and unheralded coronary death. However, because men typically experience some CVDs at a younger age, the lifetime incidence of several presentations (heart failure, transient ischaemic attack, ischaemic stroke and intracerebral haemorrhage) was similar in men and women.
Differences in the risk of CVDs associated with South Asian and black ethnicity were striking but often affected different vascular beds. Thus, the heightened risk of presenting with coronary disease among South Asian than white populations contrasted with the reduced risk for people of black ethnicity who were more subject to haemorrhagic stroke. However, those of both South Asian and black ethnicity had a reduced risk of presenting with PAD than people of white ethnicity, which was consistent with the findings of a recent meta-analysis. 136
Similarly, we found that simple associations between local socioeconomic deprivation and broad categories of CVD do not hold true for more granular CVD classifications, particularly in men. Age was an important influence, with associations between socioeconomic deprivation and CVDs falling with increasing age. Hence, the cardiovascular health risk linked to local socioeconomic deprivation falls most heavily on younger people, and on young women in particular.
Our findings suggest that future research on the primary prevention of CVDs should take account of current patterns of disease presentation and redress the imbalance of previous literature, which has focused overwhelmingly on heart attack and stroke. Estimates of national and global burden of CVDs need to take account of the modern epidemic in which heart failure, PAD and other chronic diseases are becoming more common than AMI and stroke. Male sex, older age, South Asian ethnicity and low levels of social deprivation, although associated with some CVDs, are not associated with all, and this heterogeneity has implications for research into risk prediction, aetiology and prognosis.
Our findings have implications for the design and interpretation of observational studies, randomised trials and meta-analyses investigating the primary prevention of CVDs. Because the fundamental risk factors of age and sex have such heterogeneous associations with different CVDs, and most studies are sufficiently powered to examine only CVD aggregates, it is important to account for the relative proportion of each disease in the composite end point in meta-analysis. These proportions have been shown to differ substantially between studies. 137 Despite an extensive literature on the underlying biological and behavioural pathways by which sex may influence aggregates of CVD and CHD, there is a lack of mechanism studies that investigate why sex has such heterogeneous associations with different CVDs.
Conclusions
These inequalities in CVD development support the use of separate risk models for women and men and for different ethnic groups. They argue against the use of composite measures of cardiovascular health, which may mask important variability across conditions. The findings suggest that policies and interventions targeting local socioeconomic deprivation are likely to have the greatest impact on specific forms of CVD, such as heart failure and PAD, and on women. Whether or not the complex inequalities in CVD development are associated with inequalities in CVD outcomes is an important question that we begin to address in the following study, which focuses on ethnicity and its association with incidence and prognosis of CHD.
Study 5: acute coronary disease – incidence and prognosis of acute coronary syndromes in South Asian and white populations
This study is based on a peer-reviewed paper by Zaman et al. 36
Introduction
Data presented in study 4 point strongly to greater disease incidence as a key contributor to the higher coronary death rate in South Asian people than in white people living in the UK. Whether or not a worse prognosis among people with established coronary disease also contributes to this higher death rate remains unresolved. Understanding the relative importance of incidence and prognosis to health outcomes would provide important information to guide the most appropriate public health interventions. To generate a clearer picture, this study adopted a two-pronged strategy. A systematic review and meta-analysis was carried out to synthesise the evidence on coronary disease incidence and prognosis in South Asian populations. In addition, MINAP data were used to generate a contemporary cohort of South Asian patients from England and Wales, to examine possible factors affecting prognosis in this group.
Methods
The systematic review was based on an expert search strategy of the published literature in MEDLINE (PubMed) from inception until January 2012 to identify papers on incidence or prognosis in people of South Asian ancestry specifically related to coronary disease. Three reviewers independently extracted information according to a predetermined protocol. The new ACS cohort was based on consecutively collected patients from 230 hospitals in England and Wales recorded in MINAP between 2004 and 2008. The primary outcome measures were age-adjusted mortality at 1 year and at 30 days.
Results
Incidence
The meta-analysis was based on data for 111,555 people of South Asian ethnicity and 4,197,923 white people included in 11 eligible studies. It showed that South Asian participants had a higher incidence of coronary disease than white people (HR 1.35, 95% CI 1.30 to 1.40). This was consistent for the two cohorts examining incidence of fatal coronary disease and the studies examining incidence of non-fatal (alone or with fatal) coronary disease (Figure 15).
FIGURE 15.
Incidence of coronary disease in South Asian compared with white individuals: meta-analysis of 11 incidence populations including 111,555 South Asians. Estimates are HRs unless otherwise indicated. A, age; D, diabetes; F, female; F/NF MI, fatal/non-fatal myocardial infarction; G, sex; H, hypertension; L, dyslipidaemia; M, male; S, smoking; UKPDS, UK Prospective Diabetes Study. a, Odds ratio; b, incidence ratio. Reproduced from Zaman et al. 36 Copyright © 2013 BMJ Publishing Group Ltd and the British Cardiovascular Society. This is an Open Access article distributed under the terms of the Creative Commons Attribution License (http://creativecommons.org/licenses/by-nc/3.0/) which permits non-commercial reuse, distribution, and reproduction in any medium, provided the original work is properly cited.

Prognosis
By contrast, an analysis of 12 prognosis populations including 14,531 South Asian and 274,977 white patients showed that South Asian individuals had a better prognosis than white people (HR 0.78, 95% CI 0.74 to 0.82). This more favourable prognosis was particularly apparent in bigger, more recent studies on acute clinical presentations (Figure 16). In the ACS cohort, diabetes was markedly more common in South Asian than in white populations (42.4% vs. 16.9%) and had greater impact on mortality. Even so, prognosis was superior for South Asian people overall and in most subgroups analysed, including those with diabetes (HR 0.78, 95% CI 0.71 to 0.86), those of older age (≥ 80 years of age; HR 0.72, 95% CI 0.63 to 0.83) and those living in areas of highest social deprivation (HR 0.73, 95% CI 0.65 to 0.82). This superior prognosis was seen for patients of Indian, Pakistani and Bangladeshi origin. It was also seen over the 30-day time frame (HR 0.85, 95% CI 0.77 to 0.94, after adjustments). An analysis of the MINAP cohort showed that South Asian patients with ACS were more likely than white patients to receive revascularisation and to be discharged on four secondary prevention medications.
FIGURE 16.
Prognosis of coronary disease in South Asian individuals compared with white people: meta-analysis of 12 prognosis populations including 14,531 people of South Asian ethnicity. Estimates are HRs unless otherwise indicated. A, age; ACRE, Appropriateness of Coronary REvascularisation; D, diabetes; G, sex; H, hypertension; I, IMD; L, dyslipidaemia; P, phenotype of ACS; S, smoking. a, Odds ratio. Reproduced from Zaman et al. 36 Copyright © 2013 BMJ Publishing Group Ltd and the British Cardiovascular Society. This is an Open Access article distributed under the terms of the Creative Commons Attribution License (http://creativecommons.org/licenses/by-nc/3.0/) which permits non-commercial reuse, distribution, and reproduction in any medium, provided the original work is properly cited.
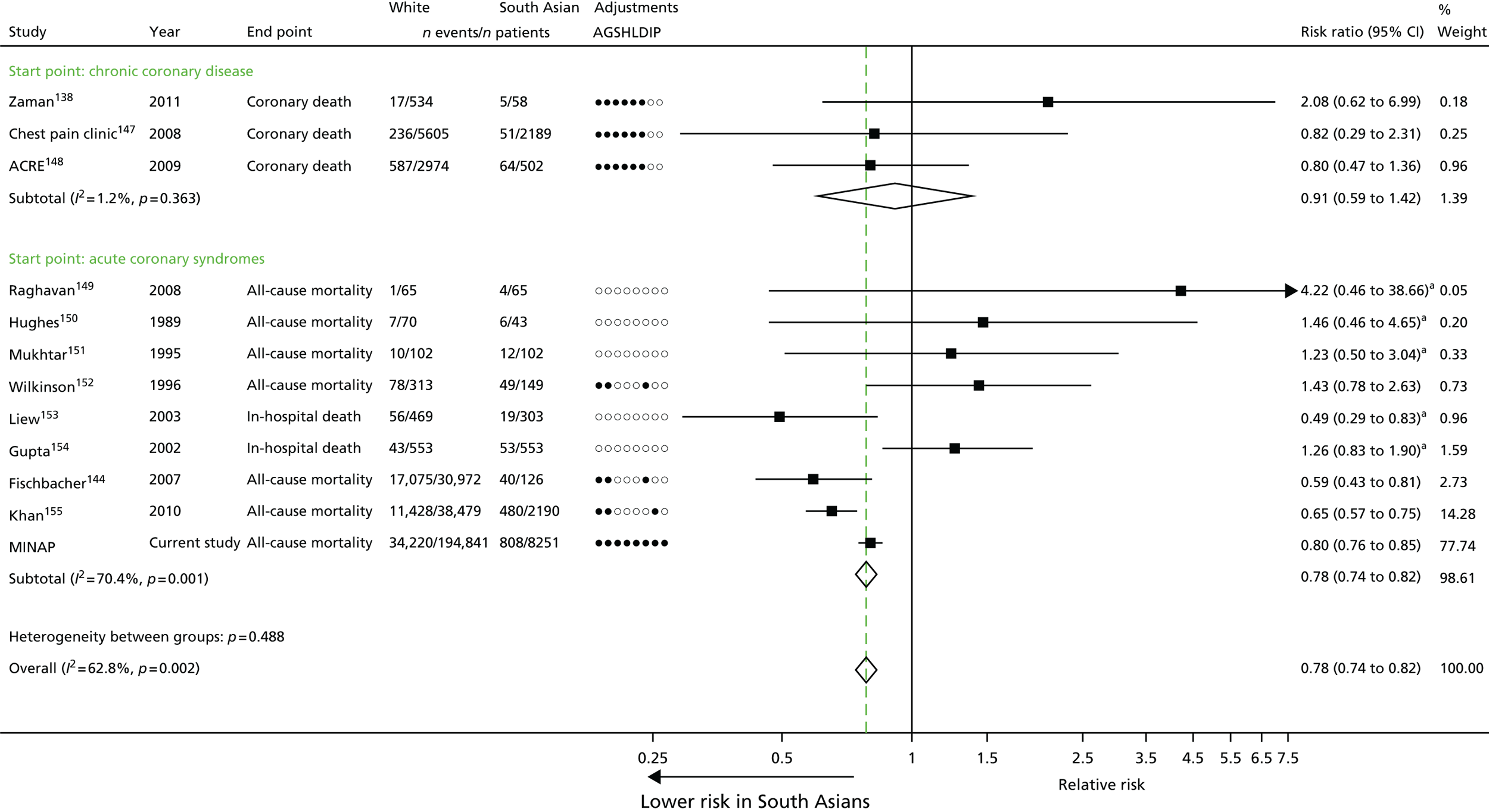
Discussion
The evidence synthesis suggests that South Asian ethnicity has different effects on the incidence and prognosis of coronary disease. Although South Asian patients show a markedly higher incidence than white patients, after adjustment for age, risk factors and disease severity, their prognosis is significantly better.
This study confirms ethnic inequality in the risk of developing coronary disease, consistent with our CALIBER analyses in study 4. The present meta-analysis confirmed that South Asian patients with SCAD were at no prognostic disadvantage. Indeed, among South Asian patients with ACS, prognosis was significantly better than for white patients. There was no evidence of undertreatment, with South Asian patients being more likely to be prescribed secondary prevention medications at discharge and more likely to have undergone coronary angiography. This may reflect awareness on the part of physicians of the great coronary disease risk in South Asian groups, or a more general awareness in the health system of factors relevant to the health of South Asian individuals.
Large cohort studies identified through linkage of primary care, ACS registry, hospitalisation and cause-specific death data are warranted to better understand how South Asian ethnicity influences the onset and progression of coronary disease and other CVDs. Such studies will allow longitudinal patterns of risk, and missed opportunities to lower risk before admission and after discharge, to be evaluated.
Conclusions
Although South Asian populations experience health inequalities in coronary disease, this study suggests that this reflects a higher incidence of disease rather than deficiencies in service provision affecting prognosis. The findings therefore suggest that public health measures to tackle ethnic inequalities in coronary mortality should focus on primary prevention and reducing the anomalous incidence of disease in South Asian populations.
Chapter 4 Risk factor modification among people without established cardiovascular disease
Abstract
Background
Reported failures to meet national guideline targets and treatment opportunities to optimise risk reduction among people without established disease represent an important missed opportunity for protecting patients against CVD.
Objectives
(1) To investigate and compare associations between type 2 diabetes and the risk of 12 initial cardiovascular presentations in men and women; (2) to investigate the association between BP and 12 presentations of CVDs; and (3) to identify the proportion of hypertensive patients attaining BP control according to national targets and factors associated with attainment of targets.
Methods
An analysis of CALIBER linked data.
Results
(1) Type 2 diabetes had the greatest impact on PAD and heart failure but was protective against abdominal aortic aneurysm. (2) There was substantial heterogeneity in associations with BP across different CVDs and ages, and the lifetime burden of hypertension was considerable. (3) Twenty per cent of newly diagnosed hypertensive patients did not achieve guideline-recommended reductions in BP, partly reflecting underuse of antihypertensive drugs and longer time periods between diagnosis and initiation of treatment. Consultation rates were significantly lower among those whose BP was not reduced to target (6.9 vs. 34 consultations per year).
Conclusions
This study confirms that there are high levels of undertreatment among hypertensive patients. The lifetime burden of hypertension remains substantial, despite modern therapy. More vigorous clinical management could increase the numbers achieving BP targets. In patients with type 2 diabetes, more rigorous glycaemic control could have benefits for cardiovascular health.
The studies in this chapter relate to people without established disease (healthy population) (see Figure 10)
Introduction
The CALIBER platform has enabled us to examine the use of risk-reduction strategies in primary care that are likely to impact on later cardiovascular outcomes. The benefits of multiple risk-reduction measures have been unequivocally demonstrated, informing the development of national and international clinical guidelines. Failure to meet national guideline targets, or to optimise treatments in individuals in advance of disease, may represent important missed opportunities to protect patients against CVD.
To illustrate the role of CALIBER, we examined whether or not guideline targets for BP reduction were being achieved in clinical practice and identified factors associated with ineffective BP control. Hypertension is a well-established and common CVD risk factor and is straightforward to identify and treat. Failure to achieve guideline BP targets would therefore be an important missed opportunity to reduce the impact of CVD.
We also focused on one important patient group, namely individuals with type 2 diabetes, to assess whether or not effective glycaemic control was associated with improved cardiovascular outcomes. We took advantage of the scale of the CALIBER data set to explore associations between type 2 diabetes and 12 distinct subtypes of CVD, to provide a more nuanced view of the impact of this common disease on different CVD presentations.
Study 6: diabetes, quality of care and opportunities for risk reduction
This study is based on the peer-reviewed paper by Shah et al. 37
Introduction
In the UK and globally, there is an epidemic of type 2 diabetes and growing concern over the contemporary impact of type 2 diabetes on a wide range of macrovascular consequences. The association of type 2 diabetes with CHD and stroke is well established156 but its wider associations across the range of CVDs affecting the myocardium and cerebral, abdominal and peripheral vasculature are less well documented. No previous studies have been sufficiently large to compare these associations and to estimate less common outcomes reliably. It is not clear, therefore, to what extent patients with diabetes are at risk of different CVDs, or whether or not risks are affected by glycaemic control as reflected by glycated haemoglobin (HbA1c) levels. The possibility that these treatment opportunities are being missed prompted this cohort study, which used the CALIBER database of linked EHRs to investigate and compare associations between type 2 diabetes and future risk of 12 of the most common initial cardiovascular presentations in men and women.
Methods
The study period was January 1997 to March 2010, and patients were eligible for inclusion if they were at least 30 years of age. We excluded patients with a prior history of CVD, and patients with type 1 diabetes or diabetes of uncertain type. We defined patients as having type 2 diabetes based on coded diagnoses recorded in CPRD or HES on or before study entry. We assessed glycaemic control by the mean HbA1c from 3 years before to 3 years after study entry. The primary end point was the first record of one of the following 12 cardiovascular presentations in any of the data sources: stable angina, unstable angina, AMI, unheralded coronary death, heart failure, transient ischaemic attack, ischaemic stroke, subarachnoid haemorrhage, intracerebral haemorrhage, PAD, abdominal aortic aneurysm and a composite ‘arrhythmia or sudden cardiac death’ consisting of cardioversion, ventricular arrhythmia, implantable cardioverter defibrillator, cardiac arrest or SCD.
Results
The study cohort included 1,887,062 patients without diabetes and 34,198 patient with type 2 diabetes. The use of statins and antihypertensive medication was higher in patients with type 2 diabetes and increased over time. Patients with type 2 diabetes were approximately twice as likely to have black or South Asian ethnicity as those without diabetes (Table 5).
Characteristics at baseline | Women | Men | ||
---|---|---|---|---|
No diabetes | Type 2 diabetes | No diabetes | Type 2 diabetes | |
Number of patients | 955,655 | 15,805 | 931,407 | 18,393 |
Age (years), mean (SD) | 48.2 (16.2) | 64.7 (14.4) | 45.4 (14) | 60.8 (13) |
Social deprivation (% in most deprived quintile) | 19.4 | 27.3 | 20.3 | 23.3 |
Ethnicity (%) | ||||
White | 90.8 | 81.8 | 90.5 | 82.4 |
South Asian | 2.6 | 7.7 | 3.0 | 8.0 |
Black | 3.0 | 6.3 | 3.1 | 5.2 |
Other | 3.5 | 4.2 | 3.4 | 4.5 |
Smoking (%) | ||||
Current | 17.6 | 14.4 | 23.7 | 20.6 |
Ex | 11.4 | 18.5 | 11.8 | 29.9 |
Never | 67.9 | 64.9 | 58.7 | 45.3 |
SBP (mmHg), mean (SD)a | 127 (19.6) | 141 (19.7) | 133 (17.2) | 139 (17.6) |
DBP (mmHg), mean (SD)a | 77.1 (10.3) | 79.5 (10.1) | 80.3 (10.0) | 80.5 (10.0) |
Total cholesterol (mmol/l), mean (SD)a | 5.54 (1.14) | 5.08 (1.22) | 5.39 (1.11) | 4.77 (1.16) |
HDL cholesterol (mmol/l), mean (SD)a | 1.57 (0.45) | 1.34 (0.39) | 1.28 (0.37) | 1.14 (0.33) |
BMI (kg/m2), mean (SD)a | 26.0 (5.59) | 30.5 (6.76) | 26.5 (4.43) | 29.3 (5.43) |
Random glucose (mmol/l), mean (SD)a | 5.35 (1.16) | 10.4 (4.93) | 5.56 (1.29) | 10.7 (4.78) |
Fasting glucose (mmol/l), mean (SD)a | 5.14 (0.75) | 8.72 (3.53) | 5.31 (0.79) | 9.1 (3.51) |
Glycaemic controlb | ||||
HbA1c recorded (%) | 1.3 | 77.1 | 1.3 | 78.5 |
HbA1c (mmol/l), mean (SD) | 44.3 (14.7) | 59.3 (18) | 46.9 (16.3) | 60.5 (18.1) |
Diabetes treatment in year before study entry (%) | ||||
Diabetes treated using diet alone | 29.0 | 29.7 | ||
Metformin | 0.1 | 48.8 | 0 | 47.6 |
Sulphonylurea | 0 | 39.5 | 0 | 40.9 |
Insulin | 0 | 12.3 | 0 | 10.2 |
Thiazolidinedione | 0 | 6.1 | 0 | 6.7 |
DPP4 inhibitor, meglitinide derivative or GLP-1 agonist | 0 | 1.0 | 0 | 1.0 |
Cardiovascular preventative treatment in year prior to study entry (%) | ||||
Statin | 1.9 | 35.7 | 2.1 | 36.3 |
Any antihypertensive medication | 16.3 | 59.5 | 10.6 | 53.8 |
ACEI | 6.2 | 33.4 | 4.7 | 34.4 |
ARB | 0.8 | 8.6 | 0.6 | 6.9 |
Beta-blocker | 5.7 | 17.4 | 3.7 | 14.8 |
We analysed 113,638 non-fatal and fatal cardiovascular events over 11.6 million patient-years of follow-up (median follow-up 5.5 years). PAD and heart failure were the most common end points among patients with type 2 diabetes (16.2% and 14.1%, respectively) (Figure 17).
FIGURE 17.
Distribution of initial presentations of 12 CVDs in people with and without type 2 diabetes and no history of CVD. MI, myocardial infarction. Reproduced from Shah et al. 37 Copyright © 2015 Shah et al. This is an open-access article distributed under the terms of the Creative Commons Attribution License (http://creativecommons.org/licenses/by/4.0/), which permits unrestricted use, distribution, and reproduction in any medium, provided the original author and source are credited. Published by Elsevier Ltd.

In multiply adjusted Cox models (Figure 18), there were strong positive associations between type 2 diabetes and PAD (HR 2.98, 95% CI 2.76 to 3.22), ischaemic stroke (HR 1.72, 95% CI 1.52 to 1.95), stable angina (HR 1.62, 95% CI 1.49 to 1.77), heart failure (HR 1.56, 95% CI 1.45 to 1.69) and non-fatal AMI (HR 1.54, 95% CI 1.42 to 1.67). In contrast, type 2 diabetes was inversely associated with abdominal aortic aneurysm (HR 0.46, 95% CI 0.35 to 0.59) and subarachnoid haemorrhage (HR 0.48, 95% CI 0.26 to 0.89). There was no statistically significant association with arrhythmia/SCD (HR 0.95, 95% CI 0.76 to 1.19).
FIGURE 18.
Association of type 2 diabetes with 12 CVDs in patients aged ≥ 30 years. MI, myocardial infarction. Reproduced from Shah et al. 37 Copyright © 2015 Shah et al. This is an open-access article distributed under the terms of the Creative Commons Attribution License (http://creativecommons.org/licenses/by/4.0/), which permits unrestricted use, distribution, and reproduction in any medium, provided the original author and source are credited. Published by Elsevier Ltd.

The risk of CVDs associated with the presence of type 2 diabetes was highest for patients with a HbA1c of > 58 mmol/mol or those in whom the HbA1c had not been recorded (Figure 19). Patients with type 2 diabetes and a HbA1c of < 48 mmol/mol had an increased risk of PAD (HR 1.82, 95% CI 1.52 to 2.18) and ischaemic stroke (HR 1.53, 95% CI 1.18 to 1.98), but no greater risk of any of the other CVDs.
FIGURE 19.
Association of type 2 diabetes with initial presentation of 12 CVDs by level of glycaemic control. MI, myocardial infarction. Reproduced from Shah et al. 37 Copyright © 2015 Shah et al. This is an open-access article distributed under the terms of the Creative Commons Attribution License (http://creativecommons.org/licenses/by/4.0/), which permits unrestricted use, distribution, and reproduction in any medium, provided the original author and source are credited. Published by Elsevier Ltd.
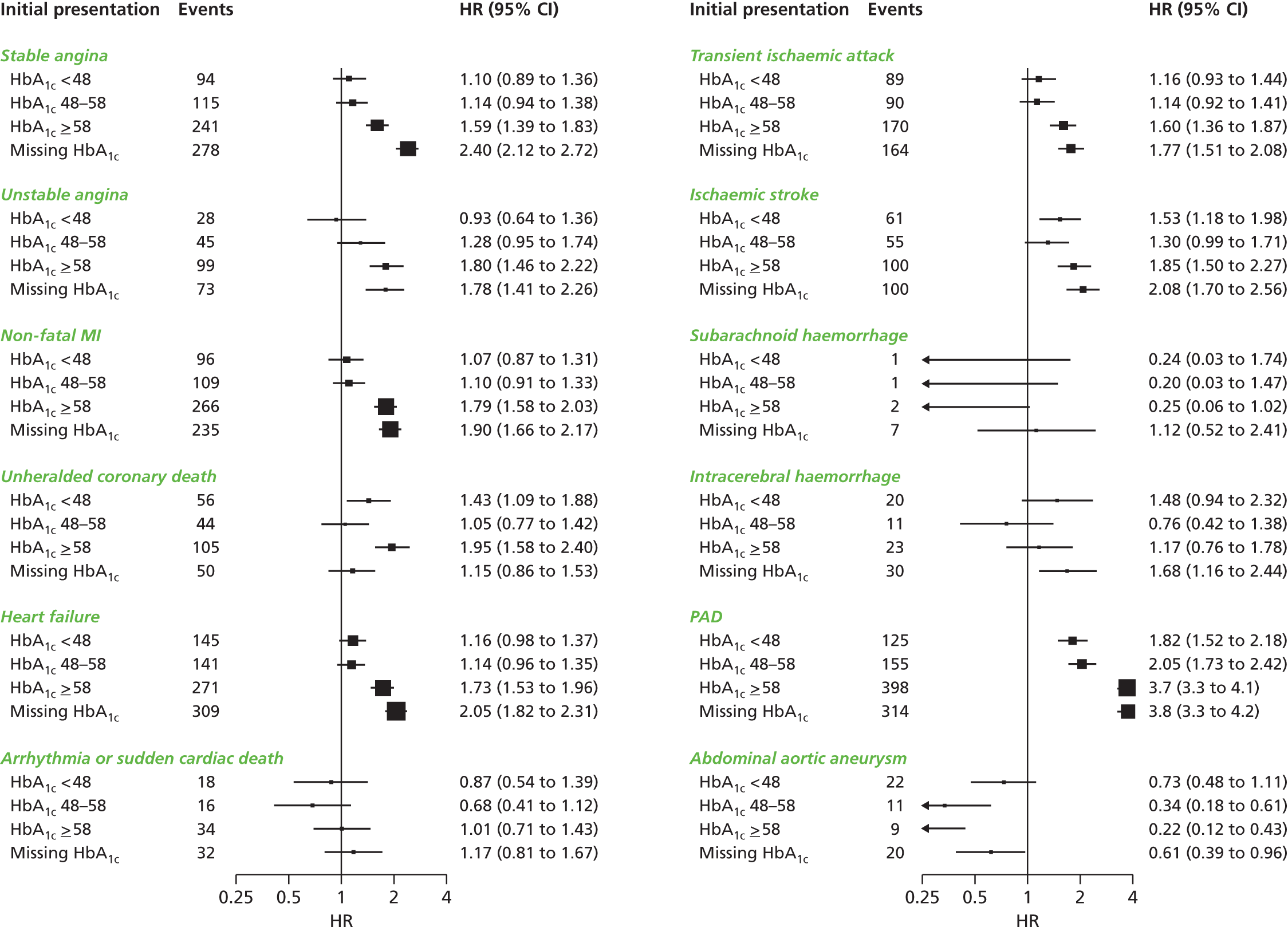
Adjusted HRs for different initial presentations of CVDs associated with type 2 diabetes, adjusted for age, sex, BMI, level of deprivation, HDL cholesterol, total cholesterol, systolic blood pressure (SBP), smoking status and statin and antihypertensive drug prescriptions. Two non-specific components of the primary end point are not shown in the figure for simplicity (they are imprecisely recorded versions of one of the 12 initial presentations): coronary disease not further specified (HR 1.58, 95% CI 1.45 to 1.73; p < 0.0001); stroke not further specified (HR 1.64, 95% CI 1.48 to 1.81; p < 0.0001).
Discussion
This population-based, contemporary study of nearly 2 million individuals with a median follow-up of 5.5 years provides strong evidence that associations between type 2 diabetes and 12 specific CVD outcomes differ in terms of direction and magnitude of effects. The most frequent cardiovascular presentations were PAD and heart failure. For those CVD presentations associated with type 2 diabetes, the risk is greatest when glycaemic control is poor, as reflected by a HbA1c of > 58 mmol/mol. Our findings support the use of clinically collected real-world data from EHRs in studying risk of different CVDs among people with type 2 diabetes, and to complement findings from smaller, less contemporary, investigator-led cohort studies, and suggest that intensifying glycaemic control in type 2 diabetes provides an important opportunity for reducing the risk of major cardiovascular complications.
A key finding of our study was that PAD was among the most common first presentations of CVD, which is consistent with the increase in prevalence between 2000 and 2010 found in a recent systematic review. 157 Furthermore, of all the 12 diseases studied, PAD showed the strongest association with type 2 diabetes, with an adjusted HR of 3, which is consistent with previous studies. 157 Heart failure was also among the most common first presentations, with possible mechanisms including prolonged hypertension, chronic hyperglycaemia, microvascular disease, glycosylation of myocardial proteins, diabetic nephropathy and autonomic neuropathy. 156
Conversely, type 2 diabetes appeared protective against abdominal aortic aneurysm and subarachnoid haemorrhage, consistent with previous reports from cross-sectional screening studies158–160 and case–control studies. 161,162 Potential mechanisms for diabetic protection against aneurysmal disease, which might have therapeutic implications, include diabetes-mediated changes in the vascular extracellular matrix, such as glycation of the extracellular matrix and covalent cross-linking between elastin and collagen in the aortic wall. 163
Our finding that levels of HbA1c were associated with the risk of many CVDs strengthens the case for the causal relevance of blood glucose. It also provides support for management strategies directed at intensifying glycaemic control in type 2 diabetes, although targets should probably fall short of ‘normalising’ HbA1c levels in light of the negative findings with respect to cardiovascular death and non-fatal stroke and AMI in the Action to Control Cardiovascular Risk in Diabetes (ACCORD) trial. 164
The differences between relative risks of different CVDs in patients with type 2 diabetes have implications for clinical risk assessment and trial design. Our findings point to the importance of considering chronic disease end points such as heart failure, PAD and stable angina in the primary end point because they are common, have a high morbidity burden and might have different treatment effects from AMI or stroke.
Conclusions
This study has shown heterogeneous associations between type 2 diabetes and 12 different CVDs, the most frequent presentations with PAD, heart failure and angina, reinforcing guideline recommendations for treating type 2 diabetes patients with statins. Guidelines also recommend angiotensin-converting enzyme inhibitors (ACEIs) to protect against microvascular complications but our data suggest that these drugs may also have a role in protecting against left ventricular remodelling and progressive heart failure. Our finding that the risk of many CVDs increases substantially with increasing HbA1c concentrations identifies enhanced glycaemic control as a potentially important opportunity for improving outcomes in these patients.
Study 7: blood pressure and incidence of 12 cardiovascular diseases
This study is based on the peer-reviewed paper by Rapsomaniki et al. 38 Sections of the text have been reproduced/adapted from the original article. Copyright © 2014 Rapsomaniki et al. 38 This is an open Access article distributed under the terms of the Creative Commons Attribution License (http://creativecommons.org/licenses/by/4.0/), which permits unrestricted use, distribution, and reproduction in any medium, provided the original author and source are credited.
Introduction
Hypertension is a major risk factor for all forms of major CVD events and the leading risk factor for overall recent global burden of disease. 165 A meta-analysis of 61 cohorts containing individual data for 1 million adults aged between 40 and 89 years recruited between 1950 and 1990 reported log-linear associations between SBP and diastolic blood pressure (DBP) and ischaemic heart disease and stroke mortality. 122 The study found no apparent threshold below which any further reduction in risk is observed, down to a BP of 115/75 mmHg. However, no previous study has compared the associations of BP across a wide range of incident CVDs, across a broad age range (among people aged < 40 years of age) and a wider range of BP values (including < 115/75 mmHg). In this novel contemporary study using the CALIBER linked data set, we set out to investigate the association between BP and 12 different presentations of CVD. Study findings could help to refine strategies for primary prevention and inform the design of future clinical trials.
Methods
Using CALIBER linked EHR data, we selected 1.25 million patients from 225 general practices in England registered between January 1997 and March 2010. Patients were eligible for inclusion if they were aged ≥ 30 years at study entry, had been registered with their practice for at least 1 year before the index date, had not been diagnosed with CVD and had at least one baseline BP measurement. Baseline BP, recorded during consultations in primary care, was based on mean SBP or DBP readings taken within 2 years of the index date. Where multiple BP measurements were available, an average reading was calculated. In sensitivity analyses, we used the BP measurement recorded closest to the index date. Hypertension was defined as baseline BP of ≥ 140/90 mmHg, a recorded diagnosis of hypertension, or evidence of repeat prescriptions (at least two monthly packs) for BP-lowering drugs, including thiazide diuretics, beta-blockers, ACEIs, angiotensin receptor blockers (ARBs) or calcium channel blockers. The most recent BP measurement recorded in the year before the index date was used for covariate adjustments. Missing covariate values were imputed using multiple imputation using the ‘mice’ algorithm in the statistical package R version 15.2 (The R Foundation for Statistical Computing, Vienna, Austria). Patients were censored on the date of first CVD presentation, death from other causes, deregistration from the practice or final practice data collection, whichever occurred first. Initial presentation of CVD was defined as 12 CVDs diagnosed in primary or secondary care, or death, and total CVD (all 12 CVDs combined). HRs were based on disease-specific Cox models with time since study entry as the timescale, adjusted for baseline age (linear and quadratic term) and stratified by sex and primary care practice.
Results
Among 1,258,006 patients included in the cohort, a total of 83,098 initial CVD presentations were recorded over a median of 5.2 years’ follow-up. SBP and DBP showed a range of associations with different CVD outcomes (Figure 20). A 20-mmHg rise in SBP showed marked associations with stable angina (HR 1.41, 95% CI 1.36 to 1.46), subarachnoid haemorrhage (HR 1.43, 95% CI 1.25 to 1.63) and intracerebral haemorrhage (HR 1.44, 95% CI 1.32 to 1.58). DBP showed substantially weaker associations with stable angina, PAD, AMI and with total CVD. The strongest associations of all CVDs were observed for abdominal aortic aneurysm: with DBP (HR 1.45, 95% CI 1.34 to 1.56) and mean arterial pressure (HR per 10 mmHg 1.61, 95% CI 1.48 to 1.75). It also showed the weakest association with SBP (HR 1.08, 95% CI 1.00 to 1.17) per 20 mmHg, and was the only CVD presentation where the association with higher pulse pressure was reversed (HR per 10 mmHg 0.91, 95% CI 0.86 to 0.98). AMI had a stronger association with SBP in women than in men (p < 0.0001), but associations with other CVD presentations were similar in both sexes.
FIGURE 20.
Forest plot of HRs and 95% CIs for 12 CVDs per 20/10-mmHg increase in SBP or DBP, adjusted for age and sex. SBP is represented by a black box, DBP is represented by a green box. Vertical dashed lines correspond to the associations of SBP (black) or DBP (green) with total CVD. Adjustments include age, quadratic age and stratification by sex and primary care practice. CIs are Bonferroni corrected (13 end points × 2 variables = 26 tests). MI, myocardial infarction. Reproduced from Rapsomaniki et al. 38 Copyright © 2014 Rapsomaniki et al. This is an open-access article distributed under the terms of the Creative Commons Attribution License (http://creativecommons.org/licenses/by/4.0/), which permits unrestricted use, distribution, and reproduction in any medium, provided the original author and source are credited.

Associations with both SBP and DBP decreased with age for all outcomes at varying rates for different CVD presentations (Figure 21).
FIGURE 21.
Forest plots of HRs and 95% CIs for 12 CVDs for 20/10-mmHg changes in BP in different age groups, adjusted for age and sex. Models included continuous age, age group, interaction between BP and age group (which is the association reported in the forest plot), and stratification by sex and primary care practice. CIs are Bonferroni corrected (3 age groups × 12 end points = 36 tests). MI, myocardial infarction. Reproduced from Rapsomaniki et al. 38 Copyright © 2014 Rapsomaniki et al. This is an open-access article distributed under the terms of the Creative Commons Attribution License (http://creativecommons.org/licenses/by/4.0/), which permits unrestricted use, distribution, and reproduction in any medium, provided the original author and source are credited.

The shape of associations for SBP and DBP showed substantial variation across different CVDs and ages. Between the ages of 30 and 79 years, we observed linear associations between SBP and DBP with stable angina, AMI, unstable angina, unheralded coronary death and subarachnoid haemorrhage. Risk was lowest among those with a SBP of 90–114 mmHg and was significantly lower than among those with a higher SBP of 115–129 mmHg. Associations with other CVDs were mostly log-linear, with little or no reduction in risk for BP of < 130/85 mmHg. However, modest increases in either SBP or DBP of > 130/85 mmHg were associated with large increases in risk, especially for unheralded coronary death, heart failure, ischaemic stroke, intracerebral haemorrhage and PAD.
The observed association between SBP and subarachnoid haemorrhage or abdominal aortic aneurysm disappeared in people aged ≥ 60 years of age. Among people aged ≥ 80 years of age (men and women), SBP was strongly associated with stable angina, AMI, intracerebral haemorrhage and PAD.
Receipt of BP-lowering medication at baseline did not decrease the associations with SBP and DBP. Hypertensive patients (diagnosed as having a BP of ≥ 140/90 mmHg or being in receipt of BP-lowering drugs) developed CVD 5 years earlier than patients without hypertension (95% CI 4.8 to 5.2) and had a lifetime risk of overall CVD at 30 years of age of 63.3% (95% CI 62.9% to 63.8%) compared with non-hypertensive people (HR 46.1%, 95% CI 45.5% to 46.8%).
The mean number of CVD-free life-years lost associated with hypertension was 5.0 years (95% CI 4.8 to 5.4 years) from 30 years of age, 3.4 years (95% 3.3 to 3.6 years) from 60 years and 1.6 years (95% CI 1.5 to 1.7 years) from 80 years (Figure 22). The largest proportions of CVD-free years of life lost associated with hypertension at index 30 years of age were attributable to stable angina (22%), unstable angina (21%) and AMI (15%). However, from an index age of 80 years, stable angina and heart failure accounted for most (19% each) of all CVD-free years of life lost, followed by unstable angina (15%), AMI (12%) and ischaemic stroke (10%).
FIGURE 22.
Years of life lost to CVD associated with hypertension at index ages of 30, 60, and 80 years, adjusted for sex, smoking, diabetes, and total and HDL cholesterol. A total of 83,098 CVD events occurred. MI, myocardial infarction. Reproduced from Rapsomaniki et al. 38 Copyright © 2014 Rapsomaniki et al. This is an open-access article distributed under the terms of the Creative Commons Attribution License (http://creativecommons.org/licenses/by/4.0/), which permits unrestricted use, distribution, and reproduction in any medium, provided the original author and source are credited.
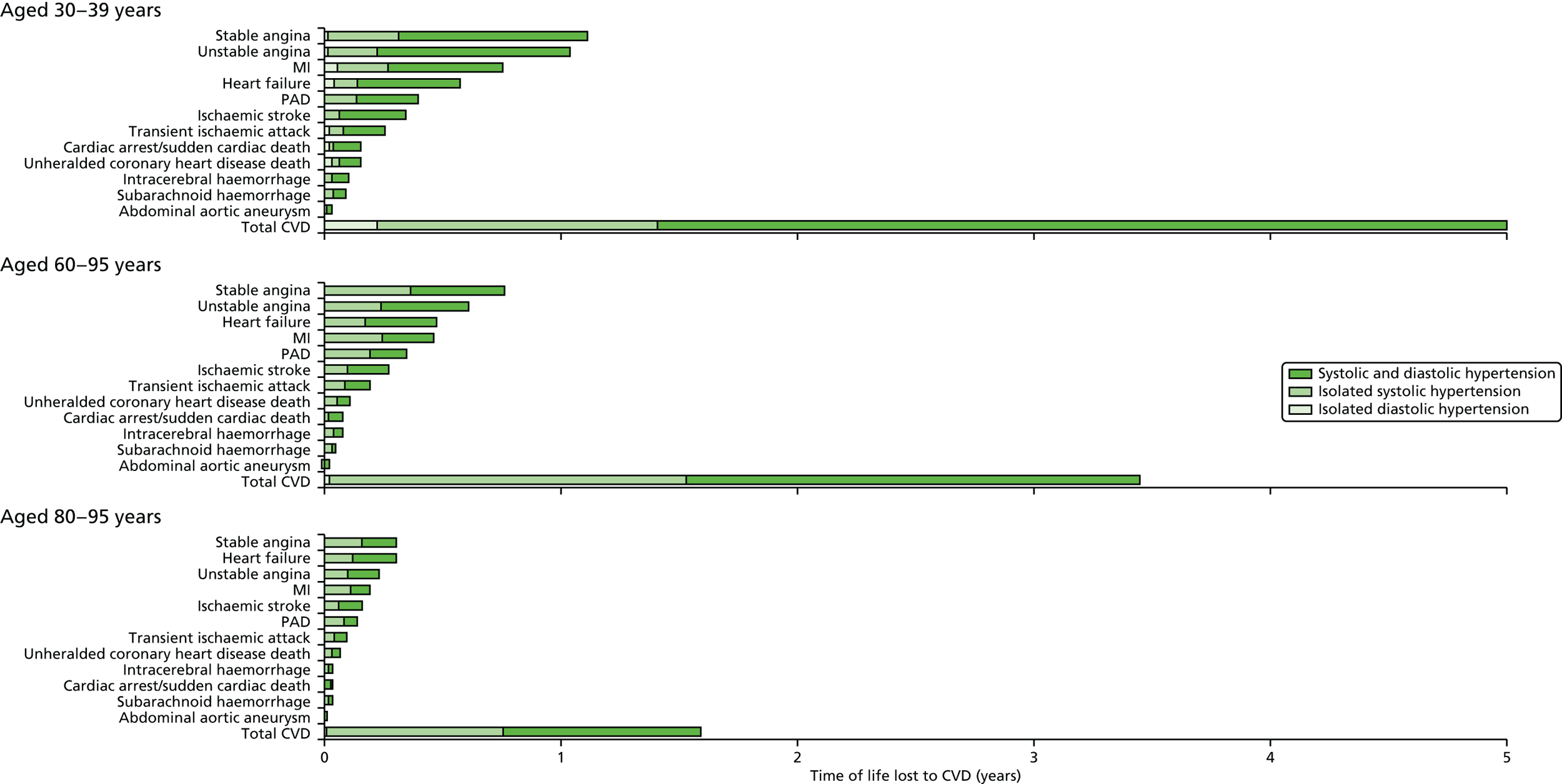
Discussion
In this contemporary study of 1.25 million adults, we show that, despite availability of modern preventative medication, the lifetime burden of hypertension remains considerable. Our findings challenge previous assumptions that BP has strong associations with incidence of all CVDs and across a wide age range, and that diastolic and systolic associations are concordant.
Our study has important advantages over previous research. First, we report associations of BP across 12 CVDs in a validated EHR linkage setting, including younger adults and a lower range of BP values than in other studies. Second, our findings are generalisable to the general population (99% of the UK population are registered with a primary care practice) and focus on measurements obtained as part of clinical practice, and, as such, are clinically relevant.
Our findings have important clinical implications. In this study, we show the high burden of CVDs such as stable and unstable angina, and heart failure in older age (≥ 80 years). The lifetime burden of BP in relation to different CVDs that we observed in this study can be used to inform decision-making and extend counselling treatments of patients, as emphasised in recent published national and international guidance. 24,166 Our findings also emphasise an unmet need, in the form of improved BP management and better management of other CVD risk factors as part of global risk estimation, to reduce risks of CVD.
Our findings can be used to help inform the design of new clinical trials and to aid interpretation of existing trials to assess new BP-lowering strategies. Although heart failure and PAD are observed to be common initial presentations of CVD, they are included less frequently in the primary outcome of BP-lowering trials. 167
Conclusions
Our novel, contemporary, high-resolution study showed that SBP and DBP show heterogeneous associations across a wide range of CVDs and at different ages. Our findings have implications for the design of new trials and preventative strategies to address the substantial contemporary unmitigated lifetime burden of hypertension.
Study 8: control of blood pressure
This manuscript by Pujades-Rodriguez et al. is in preparation. 39
Introduction
The value of risk factor modification for protecting against CVD has long been recognised and is written into all international guidelines. In the landmark INTERHEART study, just nine potentially modifiable risk factors were found to account for > 90% of the population-attributable risk of AMI in all regions of the world. 68 Among these risk factors, hypertension is particularly important because it is common, easily diagnosed and readily treatable.
In patients with diagnosed hypertension, guidelines set treatment targets to optimise risk reduction, with the recent report from the Eighth Joint National Committee (JNC 8) recommending < 140 mmHg for SBP (< 150 mmHg for patients aged ≥ 60 years without diabetes and chronic kidney disease) and < 90 mmHg for DBP. 168 Historically, however, there is evidence that guideline targets for BP reduction are not consistently achieved in clinical practice,169–171 although contemporary data for primary care populations are unavailable. A continuing failure to meet guideline targets would represent an important missed opportunity for protecting patients against CVD. We have used the CALIBER data set to analyse the scale of this missed opportunity in contemporary UK practice, with the primary objectives of describing (1) the proportion of hypertensive patients attaining BP control according to JNC 8 targets168 and (2) factors associated with attainment of BP targets.
Methods
We analysed a cohort of all individuals prospectively and newly identified with high BP among individuals aged ≥ 18 years, registered in the general practices contributing data to the CALIBER data set between January 1997 and March 2010 (the administrative censoring date of the CPRD database). Individuals had a minimum of 1 year of follow-up after practice registration, no prior history of CVD and a minimum of 6 months of follow-up since their high BP diagnosis. Individuals with drug-induced hypertension and those with pregnancy-induced hypertension were excluded (Figure 23).
FIGURE 23.
CALIBER patient inclusion flow diagram.

High BP was defined as the record of three or more systolic or diastolic high BP readings within a 1-year period, or two or more systolic or diastolic high BP readings within a 6-month period in CPRD. High BP measurements were defined as in the 2014 JNC 8 guidelines168 for patient management, that is, ≥ 140/90 mmHg for individuals aged ≤ 60 years and ≥ 150/90 mmHg for individuals aged ≥ 60 years. The date of diagnosis of high BP was the earliest date of the last BP reading that identified the individual as having high BP (e.g. the date of the third reading when defined as three or more systolic or diastolic high BP readings within 1 year). In sensitivity analyses, high BP was defined as the record of two or more systolic or diastolic high BP readings within a 1-year period.
Results
Of the 196,212 patients with newly diagnosed high BP, 156,587 (79.8%) attained BP control during follow-up (Table 6). The median yearly consultation rate was higher in patients who reached BP control than in those who did not (6.9 vs. 34 per year). BP-lowering medication was prescribed to 95,972 (48.9%) individuals during a median of 8.0 months after diagnosis, with the proportion receiving treatment being higher in those who did achieve BP control than in those who did not (56.3% vs. 19.9%). Lifestyle interventions were more frequently recorded for patients who achieved BP control.
Characteristics at baseline | BP control attained (N = 156,587) | BP control not attained (N = 39,625) |
---|---|---|
Age (years), median (IQR) | 55 (44–65) | 49 (41–57) |
Women, n (%) | 90,342 (57.7) | 16,883 (42.6) |
IMD in quintiles, n (%) | ||
1 (least deprived) | 33,531 (21.5) | 8527 (21.6) |
5 (most deprived) | 25,944 (16.6) | 6941 (17.6) |
Ethnicity, n (%) | ||
White | 81,171 (93.7) | 15,737 (92.6) |
Asian | 2444 (2.8) | 434 (2.5) |
Afro-Caribbean | 18,513 (2.1) | 536 (3.2) |
General practice registration | ||
Duration of registration (years), median (IQR) | 13.1 (6.4–22.5) | 12.3 (5.5–20.7) |
Any GP consultation rate in previous year, median (IQR) | 5 (3–9) | 4 (3–7) |
Hypertension diagnosis | ||
1998–2003 | 81,603 (52.1) | 12,268 (31.0) |
2004–6 | 47,590 (30.4) | 11,739 (29.6) |
2007–9 | 27,394 (17.5) | 15,618 (39.4) |
SBP (mmHg), mean (SD) | 154 (17.0) | 152 (15.1) |
DBP (mmHg), mean (SD) | 91 (9.8) | 91 (9.4) |
Hypertension grade | ||
Grade 1 (≥ 140/90 mmHg) | 89,453 (57.1) | 25,741 (65.0) |
Grade 2 (≥ 160/100 mmHg) | 48,909 (31.2) | 10,603 (26.8) |
Grade 3 (≥ 180/110 mmHg) | 18,225 (11.6) | 3281 (8.3) |
Cardiovascular risk factors | ||
Smoking, n (%) | ||
Current | 19,499 (15.1) | 7122 (21.0) |
Former | 31,614 (24.5) | 7992 (23.5) |
Never | 77,876 (60.4) | 18,877 (55.5) |
BMI (kg/m2), mean (SD) | 28.4 (5.6) | 29.3 (6.0) |
Underweight (< 18.5) | 597 (0.8) | 154 (0.9) |
Normal weight (18.5–24.9) | 16,657 (23.5) | 3352 (19.0) |
Overweight (25.0–29.9) | 27,386 (38.6) | 6416 (36.3) |
Obese I (30.0–34.9) | 16,315 (23.0) | 4556 (25.8) |
Obese II (35.0–39.9) | 6602 (9.3) | 1976 (11.2) |
Obese III (≥ 40) | 3438 (4.8) | 1211 (6.9) |
Total cholesterol (mmol/l), mean (SD) | 5.7 (1.1) | 5.7 (1.1) |
Serum creatinine (mg/l), mean (SD) | 85.7 (17.8) | 85.9 (17.1) |
Medication prescribed, n (%) | ||
Statins | 5560 (3.6) | 764 (1.9) |
Antiplatelet drugs | 3454 (2.2) | 335 (0.9) |
Comorbidities, n (%) | ||
Diabetes | 9772 (6.2) | 827 (2.1) |
PAD | 1381 (0.9) | 204 (0.5) |
COPD | 39,908 (25.5) | 9161 (23.1) |
Renal disease | 199 (0.1) | 78 (0.2) |
Factors significantly associated with BP control (Table 7) in the fully adjusted model included female sex [odds ratio (OR) 2.34, 95% CI 2.25 to 2.43], BP-lowering treatment (OR 4.90, 95% CI 4.48 to 5.35), statin medication (OR 1.78, 95% CI 1.58 to 2.003) and diabetes (OR 2.53, 95% CI 2.32 to 2.77). Patients diagnosed earlier during the study period were also more likely to achieve BP control than those diagnosed later. BP control was less likely to be achieved in patients with more severe grades of hypertension (grade 2: OR 0.79, 95% CI 0.75 to 0.82; grade 3: OR 0.59, 95% CI 0.54 to 0.63, compared with grade 1), those with abnormal weight (lower or higher than normal) and those who did not receive thiazides as BP-lowering medication.
Characteristics at baseline | OR adjusted for age and sex (95% CI) | OR fully adjusted (95% CI) |
---|---|---|
Age (years)* | 1.02 (1.02 to 1.03) | 1.01 (1.01 to 1.01) |
Women vs. men* | 1.93 (1.89 to 1.98) | 2.34 (2.25 to 2.43) |
Most vs. least deprived quintile (IMD) | 0.94 (0.89 to 0.98) | 0.97 (0.90 to 1.04) |
Year of diagnosis | ||
1998–2003 | 3.67 (3.57 to 3.78) | 2.89 (2.76 to 3.03) |
2004–6 | 2.32 (2.25 to 2.39) | 2.16 (2.06 to 2.26) |
Hypertension grade (vs. grade 1) | ||
Grade 2* | 1.21 (1.17 to 1.24) | 0.79 (0.75 to 0.82) |
Grade 3 | 1.35 (1.30 to 1.41) | 0.59 (0.54 to 0.63) |
Smoking (vs. never)* | ||
Ex-smoker | 0.99 (0.96 to 1.03) | 1.14 (1.09 to 1.19) |
Current smoker | 0.76 (0.74 to 0.79) | 0.86 (0.81 to 0.90) |
BMI (kg/m2) (vs. 18.5–24.9)* | ||
Underweight (< 18.5) | 0.60 (0.50 to 0.73) | 0.70 (0.57 to 0.85) |
Obese III (≥ 40.0) | 0.69 (0.63 to 0.74) | 0.54 (0.49 to 0.59) |
BP-lowering medication prescribed* | 5.25 (5.11 to 5.40) | 4.90 (4.48 to 5.35) |
First drug (vs. thiazides)* | ||
ACEIs | 0.74 (0.70 to 0.79) | 0.87 (0.78 to 0.96) |
Calcium blockers | 0.70 (0.65 to 0.76) | 0.83 (0.74 to 0.94) |
Beta-blockers | 0.73 (0.67 to 0.80) | 0.78 (0.67 to 0.91) |
ARBs | 0.95 (0.81 to 1.11) | 0.99 (0.78 to 1.27) |
Centrally acting | 0.77 (0.63 to 0.93) | 0.94 (0.63 to 1.42) |
Alpha-blockers | 0.90 (0.71 to 1.15) | 0.62 (0.45 to 0.84) |
Time between diagnosis and first prescription (vs. no medication)* | ||
Time to first drug prescription* | ||
≤ 1 month | 4.11 (3.93 to 4.29) | 5.17 (4.80 to 5.57) |
1–3 months | 5.48 (5.11 to 5.87) | 6.08 (5.44 to 6.80) |
3–12 months | 4.85 (4.57 to 5.14) | 4.52 (4.13 to 4.95) |
13–36 months | 5.84 (5.53 to 6.17) | 4.71 (4.34 to 5.11) |
3–13 years | 7.00 (6.61 to 7.41) | 4.90 (4.48 to 5.35) |
Statin medication* | 1.79 (1.66 to 1.93) | 1.78 (1.58 to 2.00) |
Comorbidities vs. none | ||
Diabetes* | 3.43 (3.19 to 3.69) | 2.53 (2.32 to 2.77) |
PAD* | 1.41 (1.22 to 1.64) | 1.14 (0.88 to 1.47) |
COPD* | 1.05 (1.02 to 1.08) | 1.15 (1.10 to 1.20) |
Discussion
In this population-based CALIBER cohort of patients presenting with newly diagnosed hypertension, BP control according to JNC 8168 targets was achieved in about 20% of patients. Patients in whom BP control was achieved were more likely to have grade 2 and 3 than grade 1 hypertension, to visit their doctor more often and to receive BP-lowering treatment and statins. The longer the delay between diagnosis of hypertension and the prescription of treatment, the less likely patients were to achieve control.
Importantly, despite the availability of a range of effective antihypertensive drug classes, the BP targets set by the JNC 8168 were not achieved in one in five hypertensive patients. The failure to optimally reduce cardiovascular risk in such a large proportion of patients must contribute significantly to the CVD burden, given its direct association with BP across a wide spectrum of measurements. 122,172 The relative underuse of antihypertensive drugs and delays between diagnosis and initiation of treatment are making a major contribution to this missed opportunity to reduce cardiovascular risk. Nevertheless, our data show that other factors, beyond utilisation of antihypertensive drugs, are also important, including higher rates of obesity and smoking, and lower rates of prescription of secondary prevention drugs, particularly statins, among patients who did not achieve BP control.
Conclusions
This study confirms high levels of undertreatment among hypertensive patients and shows that the missed opportunity to reduce cardiovascular risk includes a range of lifestyle factors that are all potentially amenable to modification. However, successful treatment of hypertension requires vigilant clinical supervision, and it was notable that consultation rates were significantly lower among patients whose BP was not treated to target (although we cannot be sure that frequent consultation to monitor BP and other cardiovascular risk factors would lead to more effective risk reduction). If the 20% of hypertensive patients who fail to achieve JNC 8168 targets were treated more vigorously in terms of BP reduction and lifestyle modification, the dividend for cardiovascular morbidity and mortality would be substantial.
Chapter 5 Initial presentation with stable chest pain: opportunities for earlier diagnosis
Abstract
Background
Delayed diagnosis in patients with symptoms suggestive of ischaemic heart disease is a common missed opportunity for treatment.
Objectives
(1) To determine the incidence of chest pain according to diagnostic type and the extent and nature of AMI heralding; and (2) to develop automated methods for identifying relevant texts and extracting coded information from unstructured free text in EHRs.
Methods
An analysis of CALIBER linked data. In a subset of CALIBER patients we developed and tested two free-text data-mining tools: the freetext matching algorithm (FMA) and a novel machine-learning algorithm [the ‘Semi-supervised Set Covering Machine’ (S3CM)].
Results
(1) Among 172,181 patients with a first recorded label of chest pain, we identified high levels (72%) of failure to attribute a cause for chest pain at first presentation, and many members of this group went on to experience cardiovascular events. (2) Among patients experiencing a first AMI, only 14% of STEMI events and 9% of NSTEMI events were unheralded. (3) FMA achieved high precision and recall. (4) The S3CM algorithm outperformed comparator programs on data-mining tasks.
Conclusions
(1) Failure to attribute a cause to chest pain is leading to missed opportunities for primary or secondary prevention of disease. Most AMIs are heralded by prior atherosclerotic disease or cardiovascular risk factors. (2) Further development of natural language processing algorithms such as FMA and S3CM may improve the usability of EHR for research on CVDs and symptoms.
The CALIBER programme provides a unique opportunity to explore the events leading up to an AMI, as it contains data from primary care (risk factors and consultations prior to the event) and secondary care (hospital admission and disease registry data). The primary care data in CALIBER includes access to limited samples of unstructured free text entered by GPs, and we have developed tools to make the free text a useful data source for research. However, detailed information about patients’ hospital admissions are not currently collected at scale; future work on hospital informatics should aim to address this.
The studies in this chapter relate to patients presenting for the first time with stable chest pain (Figure 24).
FIGURE 24.
Overview of the 33 studies in our programme: initial presentation with stable chest pain.
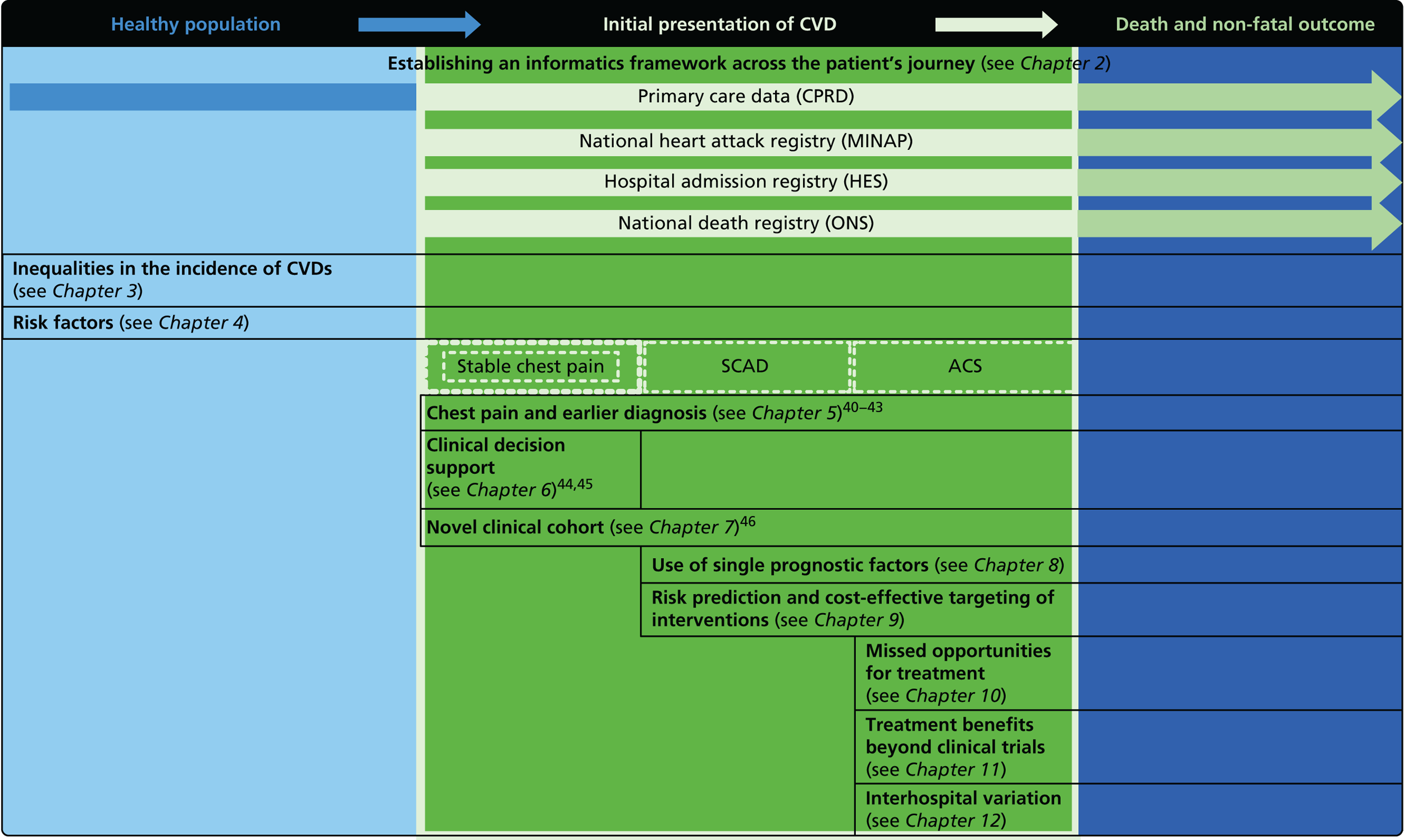
Missed opportunities in primary care
Early diagnosis, particularly in patients with life-threatening disorders, is now a high priority for policy-makers, as evidenced by the 2-week referral standard for patients with suspected cancer and the widespread introduction of RACPCs for patients with suspected coronary disease. 173,174 A central requirement for early diagnosis is that primary care physicians, who are usually the first port of call, are able to recognise early symptoms and signs of disease and respond appropriately with prompt referral for specialist assessment. This requirement is not, however, always met, with patients often receiving benign reassurance at first presentation, only to find later that their symptoms were portentous of something more serious. 74
To explore this issue, we used the CALIBER platform to explore the assessment of chest pain in RACPCs. We also examined the later incidence of cardiovascular events in patients given a diagnosis of angina or unattributed chest pain.
‘Early warning signs’ of impending AMI would provide opportunities for intervention with more intensive preventative strategies. The degree to which STEMI and NSTEMI are heralded by atherosclerotic disease or prodromal symptoms, or cardiovascular risk factors, is unclear, although it has been suggested that STEMI in particular often occurs ‘out of the blue’. The CALIBER platform has enabled us to examine this issue by evaluating prospectively collected information on the onset of atherosclerotic disease (in coronary, cerebral and peripheral circulations), cardiovascular risk factors and prodromal chest pain in advance of AMI.
Study 9: trajectories of care determined by the categorisation of chest pain at first presentation
A paper by Jordan et al. is in preparation. 40
Introduction
Cardiac diagnosis that is delayed after first symptomatic presentation or not made until the advent of a major complication represents a clear missed opportunity for treatment and occurs not uncommonly in the patient presenting with cardiac chest pain. Thus, in a cohort analysis of patients attending a RACPC we have previously reported that about one-third of major events (non-fatal AMI or coronary death) during follow-up occurred in patients reassured with a non-cardiac diagnosis. 74 We have now undertaken a more detailed analysis using the CALIBER data set, with the aim of determining:
-
the annual distribution of chest pain presentations in primary and secondary care according to diagnostic type (unattributed to a cause, attributed to non-coronary cause, recorded as angina)
-
the common short-term (6-month) trajectories of presented chest pain in primary and secondary care
-
the annual incidence of cardiovascular events in patients with a first chest pain presentation.
Methods
We included only patients with a first recorded label of chest pain (cause unattributed or attributed to non-coronary cause) or angina code or at least two anti-anginal drugs in primary or secondary care between 2002 and 2009. This first recorded label was defined as the index presentation. No patient who had a prior record of chest pain or of any prior cardiovascular event was included. Follow-up was for a median of 3.3 years [interquartile range (IQR) 1.6–5.5 years].
Results
Clinical characteristics of the 172,181 patients meeting inclusion criteria are shown in Table 8. There was a preponderance of women, who constituted 54% of the group, with no significant change across index years. Only 5% of the group received a diagnosis of angina, with the majority of chest pain presentations being ‘unattributed’. There was a significant difference in the distribution of chest pain categories across index years, rates of unattributed and non-coronary chest pain increasing from 2002 to 2009, while rates of diagnosed angina diminished (p < 0.001) (Figure 25). Deprivation quintile had no apparent effect on index chest pain presentations in contrast to age, the proportion of unattributed and non-coronary presentations tending to diminish as angina presentations increased with advancing age.
Characteristic | Chest pain unattributed, n (%) | Chest pain non-coronary, n (%) | Angina, n (%) |
---|---|---|---|
Male | 59,036 (74) | 16,607 (21) | 4104 (5) |
Female | 65,652 (71) | 22,625 (24) | 4156 (4) |
Age (years) | |||
18–44 | 53,900 (72) | 20,159 (27) | 563 (< 1) |
45–64 | 46,498 (75) | 12,694 (20) | 2869 (5) |
65–74 | 14,032 (71) | 3588 (18) | 2158 (11) |
≥ 75 | 10,258 (65) | 2791 (18) | 2670 (17) |
Deprivation quintile | |||
1: least deprived | 25,698 (72) | 8328 (23) | 1568 (4) |
2 | 25,331 (72) | 7995 (23) | 1643 (5) |
3 | 24,825 (72) | 7664 (22) | 1765 (5) |
4 | 24,660 (73) | 7460 (22) | 1714 (5) |
5: most deprived | 23,738 (72) | 7632 (23) | 1545 (5) |
Year of index date | |||
2002 | 14,384 (70) | 4500 (22) | 1594 (8) |
2003 | 15,370 (72) | 4723 (22) | 1327 (6) |
2004 | 16,493 (73) | 5062 (22) | 1139 (5) |
2005 | 16,228 (73) | 5007 (23) | 989 (4) |
2006 | 15,826 (73) | 4862 (23) | 874 (4) |
2007 | 15,753 (73) | 4889 (23) | 847 (4) |
2008 | 15,477 (73) | 5064 (24) | 723 (3) |
2009 | 15,157 (72) | 5125 (24) | 767 (4) |
FIGURE 25.
Type of chest pain diagnosis by year among CALIBER patients with a first recorded label of chest pain.
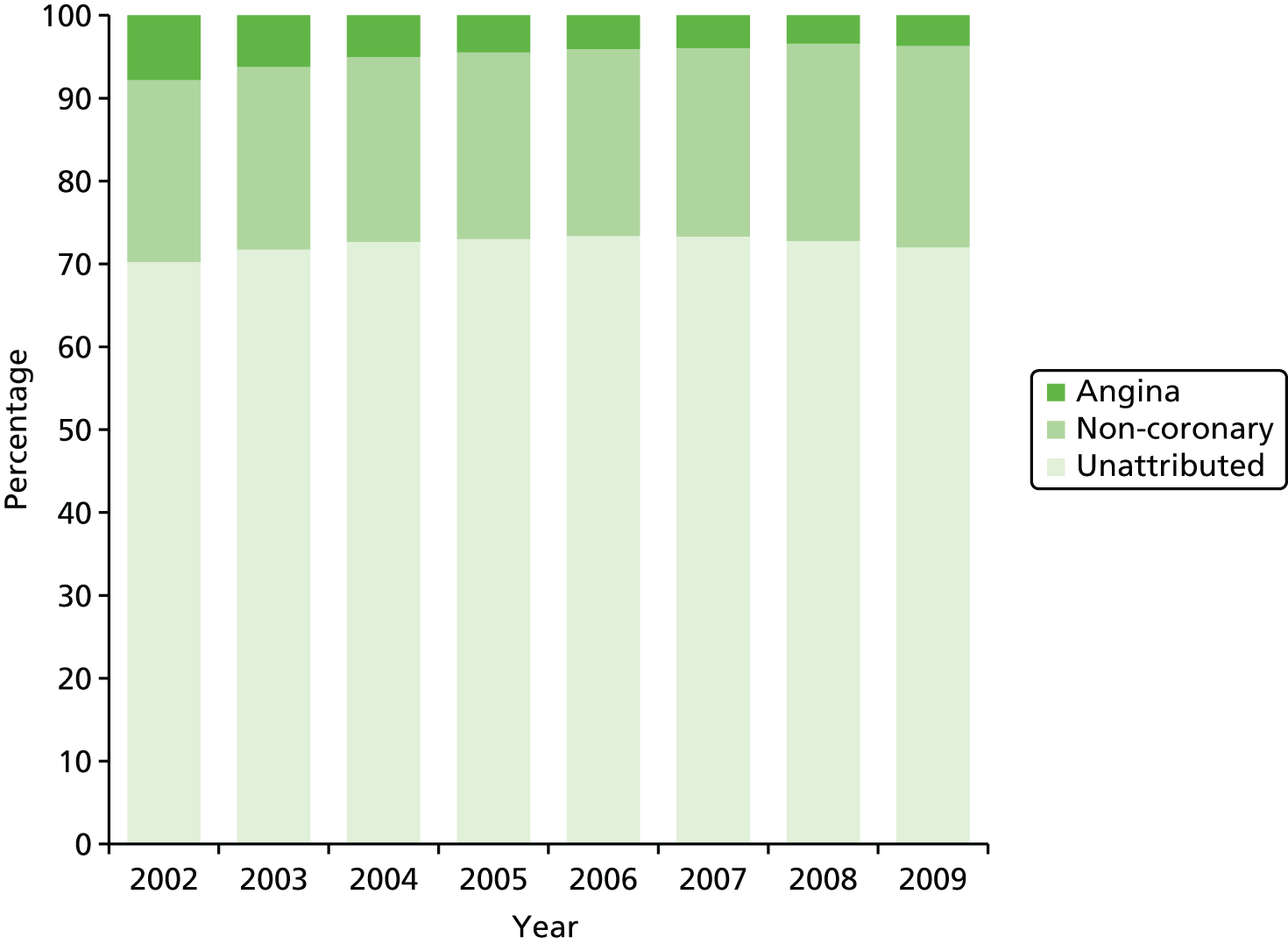
Figure 26a and b shows follow-up consultations for chest pain by month after index diagnoses of unattributed and non-cardiac chest pain, respectively. Less than 25% of patients in either diagnostic group attended for follow-up consultations during the first 6 months, most commonly in the first month. For the majority of these patients, the consultation resulted in no modification of the index diagnosis, with < 1% per month of all patients in either diagnostic group converting to a diagnosis of angina.
FIGURE 26.
Number of follow-up consultations for chest pain by diagnosis and by month after index diagnosis of (a) unattributed chest pain and (b) non-coronary chest pain. Data are proportions (%) of patients attending for consultation stratified by diagnosis.
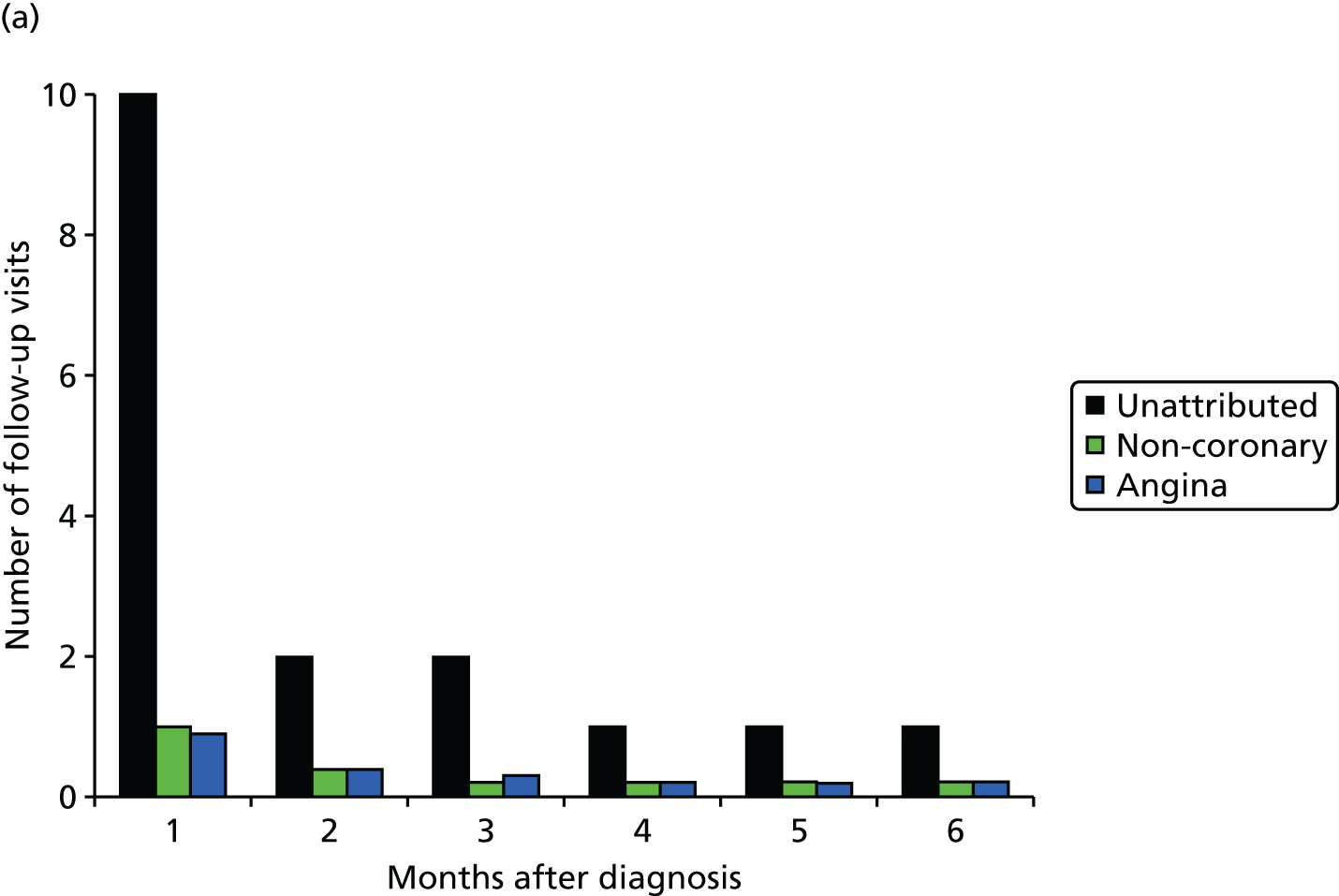

The incidence of cardiovascular events during follow-up for patients with index diagnoses of non-coronary chest pain and unattributed chest pain was < 10% and < 20%, respectively. Patients with an index diagnosis of angina were considerably older and 62% experienced cardiovascular events during follow-up (Table 9).
Patient characteristics | Chest pain unattributed (N = 124,689) | Chest pain non-coronary (N = 39,232) | Angina (N = 8260) |
---|---|---|---|
Age (years), mean (SD) | 49 (17) | 46 (17) | 67 (14) |
Angina, n (%) | 8167 (7) | 681 (2) | |
AMI, n (%) | 2148 (2) | 277 (< 1) | 745 (9) |
Coronary heart disease not otherwise specified, n (%) | 5893 (5) | 539 (1) | 3059 (37) |
Other cardiovascular, n (%)a | 4931 (4) | 1129 (3) | 1292 (16) |
Discussion
The annual incidence of recorded chest pain from 2002 to 2009 has shown progressive reductions in rates of diagnosed angina as rates of unattributed and non-coronary chest pain have increased. Our data emphasise how trajectories of care and cardiovascular outcomes are largely predetermined by the initial diagnostic categorisation of chest pain. This initial categorisation was generally stable and in only a very small proportion of patients was it modified during follow-up consultations in the first 6 months. Although the data were generally reassuring with regard to the prognostic validity of the first recorded chest pain category, substantial numbers of patients with unattributed chest pain went on to experience cardiovascular events, including AMI and coronary death. In a significant proportion of these cases it must be assumed that failure to diagnose angina at first presentation resulted in an inappropriate trajectory of care, representing an important missed opportunity to provide treatment and lifestyle advice to protect against the progression of CVD.
Failure to attribute a cause for chest pain at first presentation occurred in > 70% of cases and it was in this group that prognostic consequences were particularly telling, with an 8% incidence of cardiovascular events during follow-up, accounting for more than four times the absolute number of events compared with the angina group. This has important clinical implications, indicating a need for contemporary prognostic models based on the characteristics of patients presenting with unspecified chest pain so that those at risk of cardiovascular events can be more accurately identified.
Conclusions
Only 5% of patients assessed for chest pain receive a diagnosis of angina and this proportion has been falling over the past decade. One in 12 of the > 70% of patients diagnosed with unattributed chest pain went to experience a cardiovascular event; indeed, more events occurred in this group than in the group diagnosed with angina. A failure to identify patients with chest pain of cardiac origin at first presentation is an important missed opportunity for initiating preventative treatment and reducing the incidence of CVD.
Study 10: heralding of acute myocardial infarction – opportunities for primary and secondary prevention
This study is based on the peer-reviewed paper by Herrett et al. 41 Sections of the text have been adapted from the original article; copyright © 2013, SAGE Publications. This open access article is distributed under the terms of the Creative Commons Attribution License (http://creativecommons.org/licenses/by/4.0/), which permits unrestricted use, distribution, and reproduction in any medium, provided the original author and source are credited.
Introduction
In the weeks or months prior to AMI, heralding by major risk factors, established atherosclerotic disease or prodromal symptoms provide an important opportunity to intervene with preventative strategies. Studies that have retrospectively evaluated medical history suggest that risk factors and prior atherosclerotic disease are common in people experiencing AMI, particularly NSTEMI, but they may underestimate the burden of disease and may poorly reflect the timing of prodromal symptoms. 175–179 CALIBER’s linked registries permit, for the first time, an evaluation of the extent and nature of STEMI and NSTEMI heralding using prospectively collected information on the onset of atherosclerotic disease (in coronary, cerebral and peripheral circulations), cardiovascular risk factors and prodromal chest pain.
Methods
We included patients aged ≥ 18 years experiencing a first AMI between 1 January 2003 and 31 December 2008, with at least 1 year of prior observation in CPRD and at least one consultation during that period. Atherosclerotic disease, risk factors or chest pain recorded in MINAP or CPRD at any time prior to AMI were defined as ‘heralding’ the AMI. The earliest record of atherosclerotic disease in CPRD was taken as the date of onset (dates were not recorded in MINAP). Risk factors investigated were smoking, hypertension, dyslipidaemia and diabetes (diagnosed diabetes or insulin prescription) and were defined by codes in CPRD or MINAP. We also determined whether or not patients had been prescribed BP-lowering, lipid-lowering or antiplatelet medications in the 6 months before AMI. The frequency of primary care consultations for chest pain was assessed in patients without diagnosed atherosclerotic disease.
Results
There were 8174 eligible patients with a first AMI. Figure 27 shows that only 14% (95% CI 13% to 16%) of STEMIs and 9% (95% CI 9% to 10%) of NSTEMIs were unheralded by prior atherosclerotic disease, one or more cardiovascular risk factors or chest pain, leaving 14% (95% CI 13% to 16%) unheralded by these factors.
FIGURE 27.
Percentage of patients with previous atherosclerotic disease and risk factors in patients experiencing first STEMI (n = 3780) and NSTEMI (n = 4394). Reproduced from Herrett et al. 41 Copyright © The European Society of Cardiology 2013. Published by SAGE. This article is distributed under the terms of the Creative Commons Attribution License (www.creativecommons.org/licenses/by-nc/3.0/), which permits non-commercial reuse, distribution, and reproduction in any medium, provided the original work is attributed as specified on the SAGE and Open Access page (www.uk.sagepub.com/aboutus/openaccess.htm).
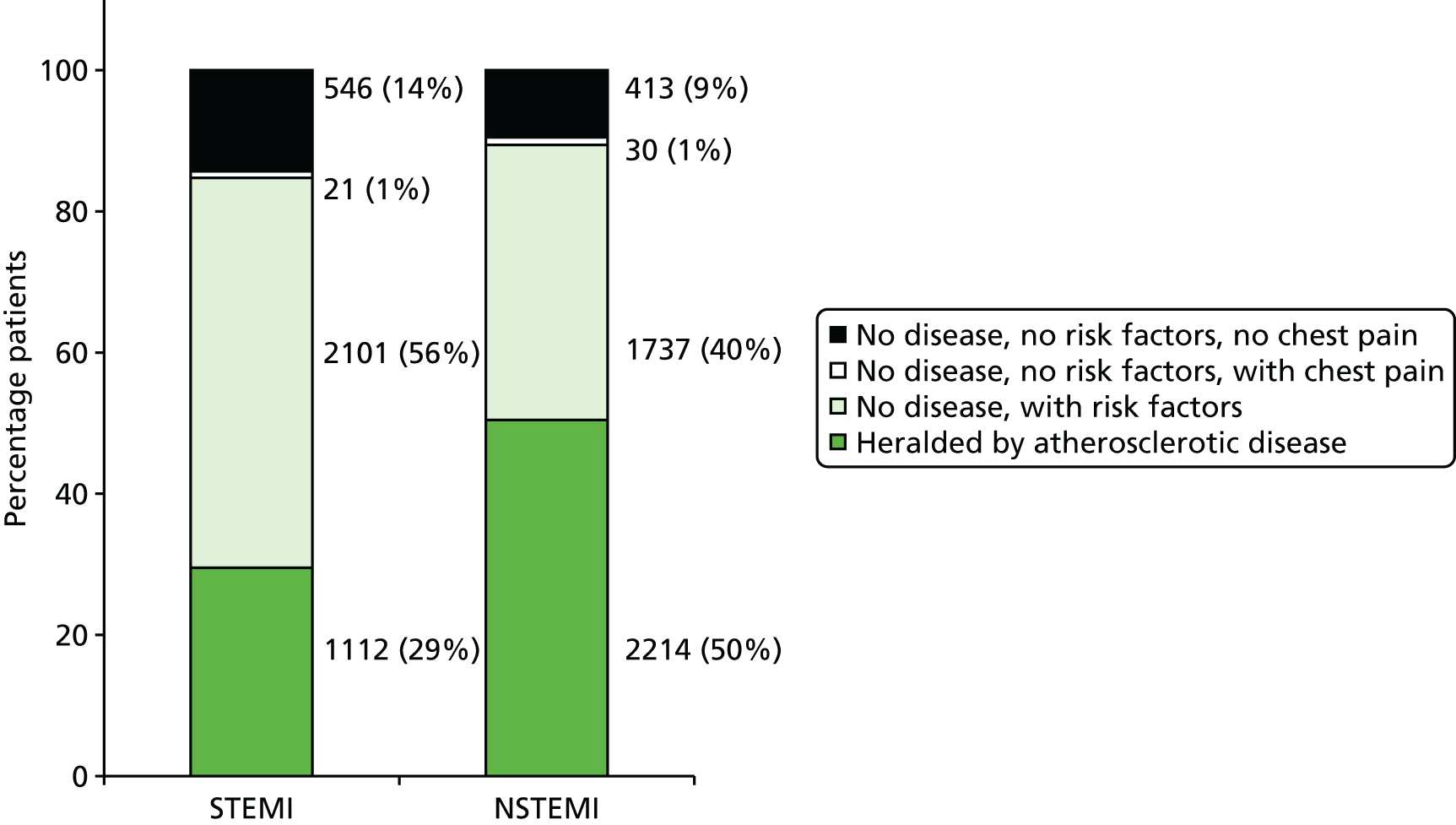
Some 41% of patients had previously diagnosed atherosclerotic disease (Table 10), more of whom had NSTEMI than STEMI (50% vs. 29%). Coronary disease was the most common presentation in the year before AMI, recorded in 21% of STEMI and 41% of NSTEMI patients, with rates rising rapidly in the 3 months before AMI (Figure 28a). A similar pattern was observed for chest pain consultations in patients without diagnosed atherosclerotic disease (Figure 28b). Among patients with previously diagnosed atherosclerotic disease, 87% were being prescribed one or more of aspirin, statins and BP-lowering treatment in the 6 months before AMI, but only 34% were receiving all three forms of medication. Some 59% of AMIs were unheralded by previously diagnosed atherosclerotic disease; 79% of these patients had one or more risk factors (a record of diabetes, hypertension, dyslipidaemia, current smoking, or a prescription for statins, BP-lowering medication or antiplatelet medication in the 6 months before AMI). This was the same in STEMI (79%) and NSTEMI (80%) patients. AMI was unheralded by all the factors discussed in 546 (14%) STEMI patients and 413 (9%) NSTEMI patients. These patients were more likely to be younger [median age 67 years (IQR 58–77 years ) vs. 71 years (IQR 60–80 years)], male (72% vs. 63%) and to have a lower rate of primary care consultation prior to AMI (median four vs. seven consultations per year) than those with heralded infarction.
Presentation | STEMI (n = 3780) | NSTEMI (n = 4394) | p-valuea | ||||
---|---|---|---|---|---|---|---|
n (%) | Standardised prevalence (95% CI) | Median disease duration (IQR) | n (%) | Standardised prevalence (95% CI) | Median disease duration (IQR) | ||
Any atherosclerotic disease | 1112 (29.4) | 32.0 (30.5 to 33.5) | 6.2 (2.2–11.7) | 2214 (50.4) | 47.2 (45.8 to 48.5) | 7.6 (3.2–13.4) | < 0.001 |
Coronary disease | 788 (20.8) | 22.7 (21.3 to 24) | 4.5 (1–8.9) | 1795 (40.9) | 38.2 (36.8 to 39.5) | 4.2 (1.1–9.3) | < 0.001 |
Stable angina | 587 (15.5) | 16.9 (15.7 to 18.2) | 6.3 (1.4–11.4) | 1442 (32.8) | 30.8 (29.5 to 32.1) | 7.2 (2.5–13.2) | < 0.001 |
Unstable angina | 46 (1.2) | 1.4 (1.0 to 1.9) | 4.6 (1.8–7.9) | 172 (3.9) | 3.8 (3.2 to 4.3) | 2.7 (0.3–6.9) | < 0.001 |
PCI or CABG | 99 (2.6) | 2.6 (2.1 to 3.1) | 6.5 (1.5–10.7) | 281 (6.4) | 6.4 (5.7 to 7.2) | 7.4 (2.0–13.1) | < 0.001 |
Coronary heart disease not otherwise specified | 404 (10.7) | 11.7 (10.6 to 12.7) | 7.3 (2.8–12.2) | 969 (22.1) | 20.5 (19.4 to 21.7) | 8.1 (3.5–13.7) | < 0.001 |
Heart failure | 142 (3.8) | 4.6 (3.9 to 5.4) | 4.5 (1.5–9.5) | 498 (11.3) | 9.9 (9.1 to 10.7) | 4.1 (1.2–7.9) | < 0.001 |
Cardiac arrest | 3 (0.1) | 0.1 (0 to 0.1) | 0.1 (0–8.3) | 7 (0.2) | 0.2 (0.0 to 0.3) | 2.3 (0.4–18.8) | 0.277 |
Other atherosclerotic disease | 537 (13.9) | 15.6 (14.4 to 16.8) | 4.8 (1.8–9.3) | 1036 (23.6) | 21.7 (20.5 to 22.8) | 5.6 (2.6–9.7) | < 0.001 |
Cerebrovascular disease | 276 (6.9) | 9.5 (8.5 to 10.5) | 5.3 (2.2–11.3) | 554 (12.6) | 12.6 (11.7 to 13.5) | 6.1 (2.8–10.9) | < 0.001 |
PAD | 261 (6.9) | 7.7 (6.8 to 8.6) | 4.4 (1.7–8.4) | 565 (12.9) | 12.0 (11.1 to 13.0) | 6.1 (2.9–10.5) | < 0.001 |
Unknown initial presentationb | 6 (0.2) | 0.2 (0.0 to 0.3) | 15.8 (12.7–17.6) | 23 (0.5) | 0.5 (0.3 to 0.8) | 4.4 (2.1–8.1) | 0.009 |
FIGURE 28.
Rates of (a) coronary diagnosis and (b) chest pain consultations in the months leading to first STEMI and NSTEMI, with 95% CIs among CALIBER patients. Each time point covers a 1-month time band (0–1 months, 1–2 months, etc.). Part (b) represents chest pain consultations just in patients without previously diagnosed atherosclerotic disease; although these patients have not received a coronary disease diagnosis, they may well be heralded by possible coronary symptoms. Reproduced from Herrett et al. 41 Copyright © The European Society of Cardiology 2013. Published by SAGE. This article is distributed under the terms of the Creative Commons Attribution License (www.creativecommons.org/licenses/by-nc/3.0/), which permits non-commercial reuse, distribution, and reproduction in any medium, provided the original work is attributed as specified on the SAGE and Open Access page (www.uk.sagepub.com/aboutus/openaccess.htm).


Discussion
This study shows how important opportunities for the primary or secondary prevention of arterial disease are either being missed or failing to protect against AMI. In patients with previously diagnosed atherosclerotic disease or risk factors, AMI represents the unmet potential of secondary or primary prevention, respectively. In the majority of cases, prior atherosclerotic disease was diagnosed long in advance of both STEMI and NSTEMI, providing an extended period during which secondary prevention could be implemented. Our data describing the use of secondary prevention measures in the 6 months before AMI showed that most patients with diagnosed atherosclerotic disease were receiving one of either statins, aspirin or BP-lowering drugs, but only one-third were receiving all three, indicating that there are likely to be missed opportunities for secondary prevention in this group.
Coronary disease was the most common pre-AMI presentation, but 10% of both STEMIs and NSTEMIs were heralded by PAD and/or cerebrovascular disease alone. This emphasises the importance of further efforts to improve secondary prevention following diagnoses in the cerebral and peripheral arteries in order to prevent an important proportion of AMI. The high prevalence of risk factors in both STEMI and NSTEMI highlights the importance of tackling the widely reported missed opportunities for the implementation of existing interventions that are known to be effective.
In addition, the categorisation of continuous measures in this analysis may have been an oversimplification of cardiovascular risk. Although a binary indicator is simple to interpret in studies and a useful basis on which to prescribe treatment, it does not reflect the continuum of risk over the full range of measurements. A more detailed investigation of these risk factors might reveal borderline raised risk in many patients, and lowering BP and lipids in those not diagnosed as hypertensive or dyslipidaemic may also prevent AMI. However, the implications of our analysis are limited by a lack of comparison to AMI-free controls. Such a comparison may allow further conclusions to be drawn from these data.
Clinical experience and retrospective studies have long suggested that AMI might be preceded by premonitory symptoms of chest pain presenting to a GP or ambulatory care. 180,181 Our study extends knowledge in several respects. First, we confirmed this association with prospective data. Second, we found that there were increases in coronary disease diagnoses and chest pain consultations in both STEMI and NSTEMI. This is in contrast to the widely held view that STEMI is usually of sudden onset. Third, we showed that the increases were specific to coronary diagnoses and chest pain, rather than to disease in cerebral or peripheral circulations, suggesting a local rather than systemic pro-thrombotic state.
Further research is warranted to better characterise the phenotypes, causes and prognoses of unheralded STEMI and NSTEMIs to improve the usability of EHRs for research. Analyses comparing AMI patients with a control group without AMI, including a comparison of missed opportunities for care in measuring and controlling elevated risk, are needed.
Conclusions
New-onset chest pain, even in the absence of major risk factors or prior atherosclerotic disease, may identify patients at risk of imminent AMI. Interestingly, a further CALIBER analysis has shown that chest pain and other ischaemic presentations in the 7 days preceding AMI reduces the risk of death in the first week by more than one-third, perhaps owing to a natural preconditioning effect. 182 Nevertheless, the opportunity for careful triage of these patients, in accordance with the recommendations of recent guidelines,183 should not be missed to ensure that high-risk individuals can be identified and treated to prevent progression to AMI.
Missed opportunities for earlier diagnosis: a role for free-text data mining?
It is important to determine if opportunities for early diagnosis are being missed, and the unstructured free-text notes of EHRs provide a good starting point from which to answer this question. A large volume of clinical information in primary care is not coded within the EHR but stored as free text, isolating it from researchers. It is often in uncoded free text that the apparently ‘trivial’ presenting symptoms, signs and test results of life-threatening disease are recorded before a coded diagnosis is arrived at. 43,184–186
This uncoded free text is, therefore, a potentially rich source of diagnostic information, and there is considerable interest in methods of accessing it for research purposes. We have developed algorithms to extract useful information from free-text fields of EHRs as a step towards identifying early symptoms of CVD before a formal diagnosis is made.
Study 11: a freetext matching algorithm for extracting diagnoses and causes of death from unstructured text in electronic health records
This study is based on the peer-reviewed paper by Shah et al. 42
Introduction
Electronic health records research to date has predominantly used coded data, which are readily available for analysis, but free-text fields may contain important additional information. 43,184–186 Manual review of free-text records is time-consuming, so there has been interest in developing software algorithms to extract diagnoses and other clinical information from free text. Development of such software algorithms is challenging, however, as clinical text contains complex language structures and specialist terminology, as well as context-specific abbreviations. Some algorithms have been developed to extract information about specific subjects, such as smoking status, heart failure or family history. 187–191 Other programs have wider functionality but have tended not to be widely used outside the laboratories that developed them. 190 The linked CPRD record in CALIBER includes coded information but also free-text fields containing information recorded by GPs or derived from hospital letters. One potentially valuable use of free-text information is in investigations of cause of death, which are often recorded in free-text fields. This study describes the development of a software tool for extracting information about cause of death, as well as a selection of other clinical information, from free-text fields in CPRD.
Methods
The FMA was developed to extract diagnoses, dates, durations, laboratory results and heart rate and blood measures from free-text fields. Using lookup tables and synonyms, the program aims to match samples of free text to the standard Read codes used by GPs (Figure 29) (www.connectingforhealth.nhs.uk/systemsandservices/data/uktc/readcodes). The EHRs of a random sample of 3310 patients who died in 2001 were manually analysed to identify causes of death. The initial version of the program was developed to extract causes of death from these data, and iterations of the program were tested on random samples of several hundred free-text entries. The prototype program was developed in Visual Basic with a Microsoft Access® 2000 (Microsoft Corporation, Redmond, WA, USA) user interface to facilitate reviewing. Following this development phase, FMA was tested on two samples of free text, from the CPRD (1000 texts associated with death in 2001) and 1000 general texts from a CAD study. Results were compared with those generated by the US Library of Medicine’s MetaMap program and manual review (the gold standard). 192
FIGURE 29.
Flow chart showing the stages of matching samples of text used by GPs in primary care using the FMA program and analysis of text. OXMIS, Oxford Medical Information System. Reproduced from Shah et al. 42 © 2012 Shah et al. ; licensee BioMed Central Ltd. This article is published under license to BioMed Central Ltd. This is an Open Access article distributed under the terms of the Creative Commons Attribution License (http://creativecommons.org/licenses/by/2.0), which permits unrestricted use, distribution, and reproduction in any medium, provided the original work is properly cited.

Results
When tested on the 1000 texts associated with death, FMA successfully matched 683 out of 735 positive diagnoses, achieving a precision (PPV) of 98.4% (95% CI 97.2% to 99.2%) and a recall (sensitivity) of 92.9% (95% CI 90.8% to 94.7%). On the general sample, FMA successfully identified 346 of the 447 positive diagnoses, achieving a precision of 91.5% (95% CI 88.3% to 94.1%) and a recall of 77.4% (95% CI 73.2% to 81.2%). Its performance was similar to that of MetaMap (Table 11).
Performance criterion | Algorithm | |||
---|---|---|---|---|
FMA | MetaMap | |||
Deatha (Read/OXMISb) | Generala (Read/OXMISb) | Generala (Read/OXMISb) | Generala (full readb) | |
Number of text | 1000 | 1000 | 1000 | 1000 |
Number of words | 7534 | 25,981 | 25,981 | 25,981 |
Positive diagnoses detected in free text | ||||
True positives | 683 | 346 | 286 | 273 |
False positives | 11 | 32 | 126 | 18 |
False negatives | 52 | 101 | 161 | 174 |
Precision, % (95% CI) | 98.4 (97.2 to 99.2) | 91.5 (88.3 to 94.1) | 69.4 (64.7 to 73.8) | 93.8 (90.4 to 96.3) |
Recall, % (95% CI) | 92.9 (90.8 to 94.7) | 77.4 (73.2 to 81.2) | 64.0 (59.3 to 68.4) | 61.1 (56.4 to 65.6) |
F-score | 0.96 | 0.84 | 0.67 | 0.74 |
Strictly defined precision for 625 positive diagnoses (best term and correct attribute) | ||||
Number strictly correct | 625 | 315 | 260 | 247 |
Precision, % (95% CI) | 90.1 (87.6 to 92.2) | 83.3 (79.2 to 86.9) | 63.1 (58.2 to 67.8) | 84.9 (80.2 to 88.8) |
Precision of non-diagnosis positive concepts | ||||
True positives | 84 | 304 | 295 | 453 |
False positives | 2 | 22 | 55 | 41 |
Precision, % (95% CI) | 97.7 (91.9 to 99.7) | 93.3 (90.0 to 95.7) | 84.3 (80.0 to 87.9) | 91.7 (88.9 to 94.0) |
Overall precision of positive concepts detected (diagnostic and non-diagnostic) | ||||
True positives | 767 | 650 | 581 | 726 |
False positives | 13 | 54 | 181 | 59 |
Precision, % (95% CI) | 98.3 (97.2 to 99.1) | 92.3 (90.1 to 94.2) | 76.2 (73.1 to 79.2) | 92.5 (90.4 to 94.2) |
Discussion
Through the analysis of free text in CPRD, FMA was able to correctly assign Read terms of death in 93% of cases, achieving a precision of > 90% on various types of free text. FMA also proved capable of extracting information about test results such as pulse rate. FMA may provide a valuable tool for extracting information from EHRs for research, reducing the need for manual review and overcoming confidentiality concerns over access to free text for researchers. This could potentially generate important additional information; in the 2001 CPRD data, for example, almost 20% of causes of death are recorded in free-text rather than coded form. FMA can also extract useful supporting information such as timing and suddenness of death.
The code of FMA is available in an open-access repository, under the GNU General Public Licence (see https://github.com/anoopshah/freetext-matching-algorithm) to enable use and modification of the algorithm by other researchers and to facilitate further collaborative development.
Machine learning and natural language processing approaches to examining the large corpus of unstructured (free-text) data that exist in primary care and hospital records should be developed in order to identify opportunities for earlier diagnosis and to add phenotypic information and safety signals.
Finally, as well as research on EHRs, real-time natural language processing systems could support enhanced coding in clinical practice. Such systems are recommended in the Connecting for Health NHS Common User Interface guidance,193 to enable clinicians to enter information in a natural form that is automatically converted into the appropriate codes.
Conclusions
The FMA is an open source, free-text data-mining tool, which showed high precision and recall on a test data set from the CPRD and can be used to extract uncoded diagnosis or symptom information for EHR research.
Study 12: extracting diagnoses and investigation results from unstructured text in electronic health records by semisupervised machine learning
This study is based on the peer-reviewed paper by Wang et al. 43
Introduction
In developing our FMA we were able to match samples of free text to the standard Read codes used by GPs, thereby coding the samples to make them available for research. The analysis of clinical text is challenging, however, because of its specialist terminology, potentially complex language structures and use of context-specific abbreviations. In this study we adopted a different approach termed ‘machine learning’, in which we do encode detailed language rules by hand, but allow a learning computer algorithm to derive its own rules based on some training data. 187,194,195 In some studies, tools that incorporate machine learning components have been shown to perform better than purely rule-based or pattern-matching approaches. 196–198
Here, we describe the development of a machine learning algorithm (S3CM) related to previous models developed by Rosales et al. 199 to determine whether or not clinical free text contains specific information of interest. Its ability to detect coronary angiogram test results and a diagnosis of ovarian cancer was compared with a fully supervised predecessor system,200 the transductive support vector machine (TSVM),201 and FMA. 42 A potential advantage of S3CM was that it did not require a perfectly labelled training set, which means that the existing Read codes associated with each free text could be used as the label, rather than requiring time-consuming manual labelling of the training data set.
Methods
We tested S3CM on free-text records in CPRD that might contain coronary angiogram results. Just 4.2% of CPRD records have angiogram results coded, but a much larger number have codes indicating that an angiogram was performed. The results of these ‘missing’ angiograms may be available in free-text fields. A second task was to detect a suspected or definite diagnosis of ovarian cancer. This cancer is difficult to diagnose, and clinicians may note down suspicions before a formal diagnosis is recorded. An analysis of these earlier thoughts would provide useful insight into clinical decision-making processes.
The algorithms were trained using texts with Read codes relevant to the two tasks. The performance of S3CM and the other tools was compared with the gold standard of manual review by a specialist clinician.
Results
In the training set for the coronary angiogram task, only 60% of records with an angiogram result Read code actually had angiogram results in the free-text field. Nevertheless, presented with unlabelled texts, S3CM achieved 87% recall with 64% precision. It outperformed both the fully supervised SCM (60% precision, 78% recall) and the TSVM (3% precision, 2% recall). Similarly, in the ovarian cancer diagnosis task, S3CM outperformed both SCM and TSVM, detecting 303 out of 353 diagnoses (86% recall, 74% precision). FMA achieved greater precision (85%) but lower recall (62%). In a test set, S3CM identified 99% of patients with ovarian cancer, even though only 82% had a formally coded diagnosis.
Discussion
The S3CM algorithm was developed as a semisupervised learning algorithm for the classification of clinical test records. It has shown promising performance in two unrelated pilot tasks, even though the training data set for the first of these tests was far from perfect. One advantage of S3CM is that training examples can be obtained simply through the use of diagnostic codes rather than by manual review. Moreover, because it does not depend on a subject-specific knowledge base or set of linguistic rules, it is, in theory, applicable to other subject areas (and languages). Its main disadvantage is that it will not detect particularly complex or rare forms of language. Misspellings and abbreviations may also present issues.
Further testing of our semisupervised machine-learning tool should be conducted using other EHR data sets, such as discharge letters and electronic hospital notes.
Conclusions
The tool is likely to be of most value in applications in which diagnostic or other information is typically recorded in free-text form. It is semi-automatic and easy to run, and may have most use as a way of winnowing large data sets for more detailed review. It would also be suitable for use on other sources of data, such as electronic hospital notes, and is sufficiently flexible to be used across multiple disease areas, requiring only a small number of labelled data for training.
Chapter 6 Initial presentation with stable chest pain: opportunities using a clinical decision support system – the Optimising the Management of Angina programme
Abstract
Background
Evidence from EHR- and investigator-led studies suggests that angina is not being adequately diagnosed in RACPCs and potential cardiac-related chest pain may be being inappropriately investigated. CDSSs provide an approach for guiding appropriate investigation and initial treatment decisions, but their integration into hospital information systems and usual practice remains challenging.
Objectives
To assess the feasibility, use and outcomes of a web-based CDSS [Optimising the Management of Angina (OMA)] in RACPCs.
Methods
Patients from three UK NHS RACPCs were recruited before and after the introduction of OMA. We carried out an ethnographic study of OMA use.
Results
Integration of the CDSS with hospital information systems and the clinical workflow was hindered by multiple technical and procedural problems. OMA was used in 84% of consultations. There were no differences in medication prescribing or investigation within 6 months between groups using OMA and delivering usual care. Qualitative data suggested that clinicians found OMA useful in confirming rather than guiding their decision-making, but it had no impact on the assessment of the most difficult-to-interpret cases. Difficulties implementing the CDSS and a strong belief in the primacy of clinicians’ judgements were associated with OMA’s lack of influence.
Conclusions
The OMA programme had a negligible effect on the decision-making behaviour of clinicians in RACPCs, and a trial powered on cardiac events was deemed unwarranted. Insight into the ways in which clinicians interacted with OMA could inform development of new tools to aid clinical decision-making with a greater likelihood of being adopted in practice.
The studies in this chapter relate to patients presenting for the first time with stable chest pain (see Figure 24).
Introduction
The reliable diagnosis of angina is essential for ensuring that patients receive the most appropriate treatments for symptoms and the prevention of cardiovascular events. There is growing evidence, including from our CALIBER-based study reported above (study 6), that angina is currently being underdiagnosed, even in specialist centres such as RACPCs. Assessment of chest pain is difficult, and the diagnosis of angina could potentially be enhanced by a suitable CDSS. Drawing on recommendations in clinical guidelines and a behaviour-change framework, we have developed a CDSS to support chest pain assessment and angina diagnosis, with associated training and support, namely the OMA programme. After encountering technical difficulties integrating with the hospital information system in each hospital, we designed the CDSS as a web-based tool that could integrate with the clinical care workflow with minimal data duplication and additional resource requirements.
To assess its impact on clinical decision-making behaviour and its acceptability to clinicians, we carried out a pilot study of OMA in RACPCs. We adopted a mixed-methods approach, combining a quantitative study of OMA use and its impact on clinical decision-making, alongside a qualitative study exploring clinicians’ attitudes to and interactions with the tool. The study was planned as a pilot to inform the feasibility and design of a RCT of the OMA CDSS, powered to detect differences in cardiovascular event.
Study 13: Optimising the Management of Angina pilot study
This study is based on the peer-reviewed papers by Cramer et al. 44 and Johnson et al. 45
Introduction
Rapid access chest pain clinics may incorrectly diagnose some patients, and not all of those patients diagnosed with angina receive appropriate secondary prevention measures. For example, in a cohort of 8762 patients followed for 3 years after attending a chest pain clinic, 33.1% of all cardiovascular events occurred in patients with a diagnosis of non-cardiac chest pain. 74 This study also reported underprescription of evidence-based treatments for angina: of those patients receiving a diagnosis of angina, only 28% were taking a statin. The need for better decision-making for patients presenting with new-onset stable chest pain was highlighted in the 2010 NICE chest pain guidelines,202 which recommended investigation decisions based on the pre-test probability of coronary disease.
The OMA CDSS is a web-based computerised version of the NICE Clinical Guideline (CG)95,202 with additional guidance provided on investigation and prescribing decisions derived from a RAND (RAND Corporation, Santa Monica, CA, USA) Delphi panel of 11 experts in the fields of general cardiology, imaging, radiology, general practice, chest pain clinics, clinical pharmacology and biomarkers/inflammation. The panel was convened in December 2009, and panel members were asked to rate the appropriateness of investigation and risk-lowering medication in patients with suspected stable angina pectoris attending RACPCs. Members rated the appropriateness (inappropriate, uncertain or appropriate) of 2400 hypothetical patient-specific indications for exercise ECG, coronary computerised tomography (CT), functional imaging and invasive coronary angiography and initiation of the risk-lowering medications aspirin, beta-blocker, ACEI and statin. This involves eliciting decision thresholds using pre-test probability (diagnostic) and 10-year risk (prognostic) scores and categorical clinical information.
Optimising the Management of Angina clinicians entered the following data into the CDSS: age, sex, typicality of chest pain, resting ECG result and cardiovascular risk factors (smoking status, diabetes and hyperlipidaemia). These data are used to calculate the individual’s pre-test probability for coronary heart disease and to give investigation recommendations in line with the NICE guideline.
The OMA programme (Figure 30) is a complex intervention delivered at the clinic level. The program has three stages: preparation, training and clinic tools. The aim of the preparation and training stages is to facilitate the use of the clinic tools and, in particular, the CDSS. The components of the intervention were developed with attention to the behavioural change domains identified by Michie et al. 203
FIGURE 30.
Summary of the OMA intervention. Source: reproduced from Johnson et al. 45 © Johnson et al. 2015. This is an open-access article distributed under the terms of the Creative Commons Attribution License (http://creativecommons.org/licenses/by/4.0/), which permits unrestricted use, distribution, and reproduction in any medium, provided the original author and source are credited.
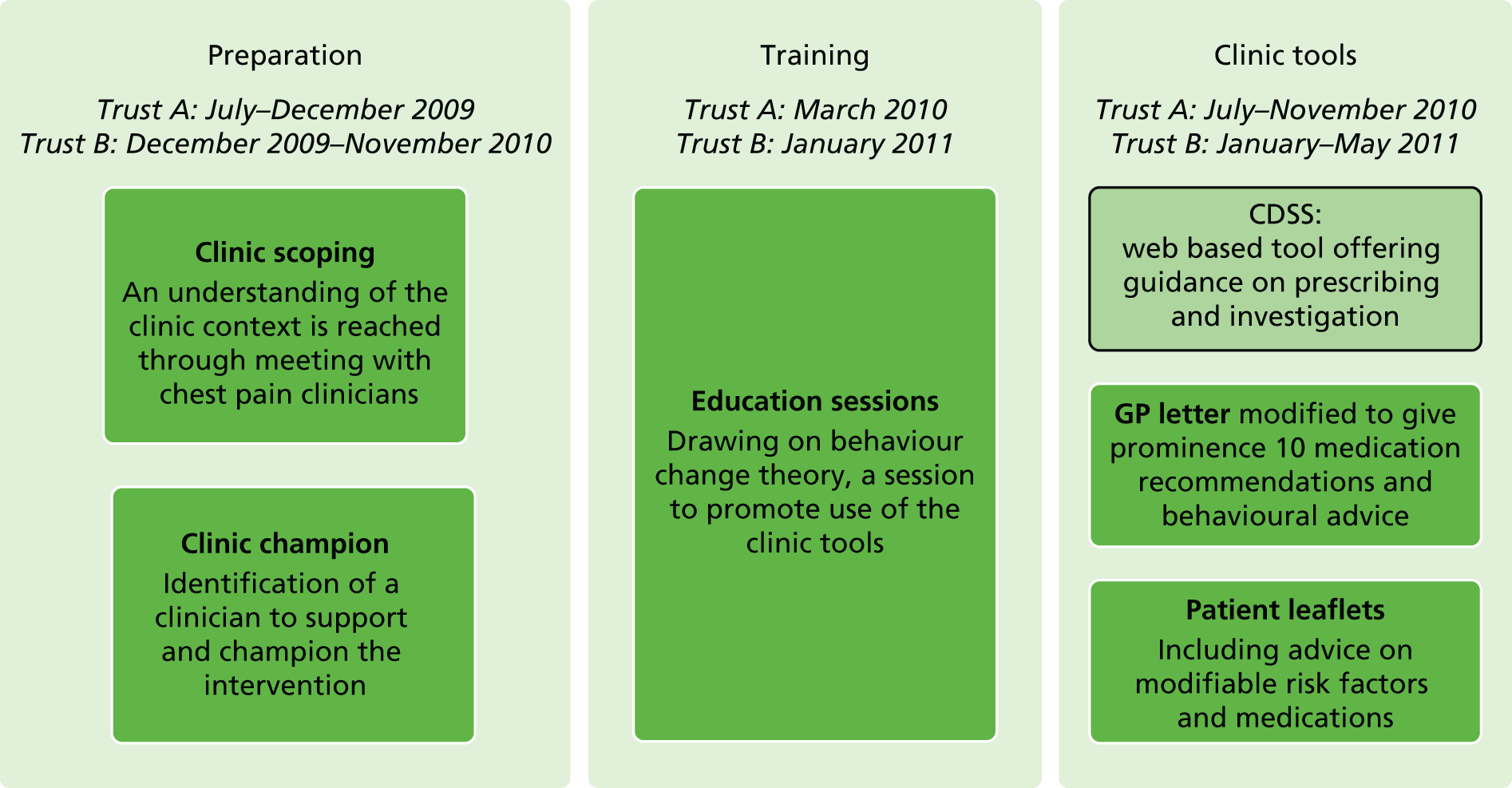
Clinical decision support systems can be effective at modifying clinician behaviour,204 with four factors predicting improved clinical practice. We planned the design of OMA to incorporate all of these components, that is, provision of decision support at the time and place of decision-making, provision of recommendations rather than just assessments, computer-based decision support and real-time provision of decision support to clinicians during the consultation. However, less is known about the effect of CDSSs on patient outcomes, and what evidence does exist is conflicting. A systematic review205 of studies evaluating the effectiveness of computer-based guideline implementation systems providing real-time interaction during consultations in ambulatory care settings identified a lack of evidence of an effect of these systems on patient outcomes and conflicting evidence of effect on process outcomes.
A meta-regression of 162 randomised trials of CDSSs206 identified several factors that could explain why some systems succeed in terms of clinician behaviour change and others fail. Systems were more likely to be successful if they required practitioners to provide reasons when over-riding advice and if they provided advice concurrently to patients and practitioners. A linked editorial207 argued for an approach including qualitative contextual evaluation and observation to further our understanding of the factors that make CDSSs successful.
Implementation of CDSSs is a complex intervention. The Medical Research Council (MRC) framework208 for the development of complex interventions promotes a focus on feasibility and pilot work, using a mixture of qualitative and quantitative methods where appropriate, so that full evaluations, preferably in RCTs, are conducted only on interventions that are feasible and acceptable, and that have a likelihood of improving outcomes.
Methods
In this study we used a mixed-methods approach to answer the following research questions:
-
Is delivery and use of the OMA CDSS feasible in chest pain clinics?
-
In what proportion of consultations is the OMA CDSS used?
-
Are the OMA CDSS recommendations for investigation and medication followed?
-
Does the investigation and medication treatment of participants differ before and after delivery of the OMA programme, and is this likely to impact on outcomes?
Quantitative methods were used to answer questions 2–4. Qualitative ethnographic methods were used to answer question 1, and to help explain the quantitative results.
Setting and study population
We recruited patients from one chest pain clinic in a provincial city centre (A) in two phases: (1) before and (2) after the delivery of the OMA programme. A second chest pain clinic in a suburban hospital (B) was chosen to provide a comparison site. We were unable to deliver the OMA programme at hospital B as clinics did not have web access, which was required to use the CDSS. Therefore, the intervention was delivered at a third chest pain clinic in a suburban hospital (C). Hence, clinic A recruited patients before and after delivery of the OMA programme, clinic B recruited participants before the delivery of the OMA programme and clinic C recruited patients after delivery of the OMA programme only. Where the OMA programme was not delivered, patients received usual care.
Patients were eligible for the study if they had been referred to the chest pain clinic by their GP. We excluded patients who had a previous history of CVD and patients who did not speak English. Participants were invited to provide consent for the study team to access their hospital and primary care records through their GP.
Quantitative data
Baseline patient characteristics
Age, sex, prescribed medications [antiplatelet (aspirin or clopidogrel), statins and beta-blockers] and cardiovascular risk factors (smoking status, hyperlipidaemia and diabetes) were determined for each participant at baseline. Data on age and sex were taken from consent forms. Medication prescriptions current on the day of chest pain clinic were manually extracted from the primary care EHR by two researchers. Cardiovascular risk factor data were taken from the CDSS database where used, and from the chest pain clinic record, GP referral letter, or primary care electronic record for all other participants.
The CDSS database stored data on chest pain that were not routinely collected as part of standard clinical care. The CDSS stored the classification of the chest pain according to the Diamond and Forrester criteria209 (typical, atypical or non-anginal), ECG findings and cardiovascular risk factors.
For participants for whom the CDSS was not used during a consultation at the clinic, CDSS rules were applied retrospectively on patient data during the analysis stage. Resting ECGs were reported as normal or abnormal independently by two clinical researchers and disagreements were resolved by a consultant cardiologist. Typicality of chest pain was determined primarily from the clinic description of the participant’s chest pain, with the addition of general practice referral information where the clinic description was insufficient. Clinic descriptions of chest pain were considered by two clinical cardiologists and discussed by the research team where they were unable to reach a decision. If agreement could not be reached, patients were excluded from analyses requiring typicality of chest pain. Data from the CDSS and the clinical record were managed in a Microsoft Access database.
Process outcomes at 6 months
Participants were followed up for at least 6 months. Prescriptions of antiplatelet medication (aspirin or clopidogrel), beta-blockers and statins at 6 months were extracted from the electronic primary care record. Cardiac investigations (CT calcium scoring, CT coronary angiography, stress echocardiography, myocardial perfusion scanning, angiography/angioplasty, cardiac magnetic resonance imaging) performed in the 6 months after consultation were manually extracted from the hospital EHR by trained researchers.
Qualitative data
To examine how the CDSS was used in practice and how it might impact on clinicians’ diagnostic decision-making and patient management, we carried out an ethnographic study in clinic A 2 months before and after the introduction of the CDSS (Table 12). This included observations of chest pain consultations and testing, interviews with clinicians and patients, and a focus group with clinicians at the end of the pilot.
Data type | Data type | Number | Sites |
---|---|---|---|
Observational | Post-intervention consultations | 40 | Clinic A |
Self-reported | Field interviews immediately following observation of CDSS | 25 | Clinic A |
Clinician interviews | 5 | Clinic A (4) and clinic C (1)a | |
Clinician focus group | 1 | Clinic A |
Quantitative analysis
We compared the management of patients seen before (clinic A, phase 1, and clinic B) and after (clinic A, phase 2, and clinic C) receipt of the OMA programme. For the period after the receipt of the programme, we compared patients managed with and without the use of the CDSS, giving three groups for analysis: (1) before OMA; (2) after OMA, CDSS used; and (3) after OMA, CDSS not used. We determined that the CDSS had been used when data had been entered into the CDSS database. At baseline (date of consultation), we compared age, sex, prescribed medications (antiplatelet medication, statins and beta-blockers) and risk factors (smoking status, diabetes and hyperlipidaemia).
The proportions of patients for whom there was concordance of investigation choice and medication prescribed with CDSS guidance at 6 months were reported, using the CDSS recommendations for patients for whom the CDSS was used, and what the CDSS would have recommended for patients for whom the CDSS was not used.
For each study group, we compared the prescription of statins, beta-blockers and antiplatelet medication at 6 months after consultation. We calculated the mean number of medication classes prescribed for each CDSS recommendation (no medications, statin and beta-blocker, and aspirin, statin and beta-blocker). Concordance of investigation choice with the guidance of the CDSS was assessed by determining the pattern of first investigations within 6 months for each study group.
National Institute for Health and Care Excellence CG95202 does not give investigation recommendations for individuals with a pre-test probability of coronary disease of > 90%. A consensus panel of 12 experts in cardiovascular care recommended angiography for patients with a pre-test probability of > 90%. The expert panel also provided medication recommendations for the CDSS, based on each patient’s pre-test probability.
Qualitative analysis
Interview and consultation transcripts and observational notes were imported into qualitative data analysis software [ATLAS.ti version 5.0 (Scientific Software Development, Berlin, Germany) and NVivo (QSR International, Warrington, UK)], coded and analysed thematically using the constant comparative method. 210 Analyses of the interview and focus group data focused on identifying advantages and limitations of using the CDSS in practice from clinicians’ points of view. Coding categories were iteratively developed into themes that were further interpreted in the context of the literature on the role of CDSSs in clinical decision-making and on professional behaviour change in clinical practice.
The analysis of the consultation data proceeded in two stages. First, transcripts and field notes (FNs) for the observed post-intervention consultations were linked to clinical and diagnostic information from patients’ medical records and to the recommendations for drug treatment and investigative procedures generated by the CDSS. Cases were indexed with the help of NVivo qualitative software to record clinicians’ classification of patients’ chest pain on the CDSS (typical, atypical or non-anginal), whether or not their decision matched the CDSS recommendation and, in cases of mismatch, whether or not clinicians had opted for different investigations from those recommended by the CDSS.
In a second step, the resulting typology of decision scenarios was used to guide a more detailed qualitative examination of the consultation processes and contextual factors that had contributed to clinical decision-making in each case based on the available ethnographic material. This included an analysis of patients’ provision of medical history, patients’ treadmill performance, clinicians’ explanations to patients, clinicians’ ‘think alouds’ delivered to researchers after consultations and researchers’ observations of clinicians’ interaction with the CDSS. Insights gained from the case-by-case analysis of clinicians’ use and non-use of the CDSS in practice were then synthesised with the themes that emerged from the analysis of clinicians’ views based on the interview and focus group data.
Technical implementation
Originally, we had planned to deploy the OMA decision support tool by embedding it in the EHR systems of each participating chest pain clinic. This would enable the seamless interaction with clinicians during the routine clinical workflow and the extraction of data items directly from EHRs with minimal intervention.
We performed an initial scoping exercise that discovered and documented the different EHR systems (and their respective components) used in the three chest pain clinics. We discovered significant variation within and across clinics on the manner in which data were recorded and the EHR systems used to record information. In the vast majority of cases, the EHR systems did not provide a software layer enabling programmatic access by external software modules, thus rendering the ability to embed a decision support tool directly in the system unfeasible. The option of contacting the system vendors and adding this was not considered, as it was outside the scope of this project and would require significant resources. As a result, the decision support tool was developed and deployed as an online application accessible through web browser software.
The decision support tool was hosted on a secure university server behind a firewall and access was protected using dedicated firewall rules and a Secure Sockets Layer certificate. Each user had two authentication credentials (password and personal identification number) and the internet protocol addresses of their host institutions had to be verified and added to the firewall prior to account activation. The DST was developed on a Linux (The Linux Foundation, San Francisco, CA, USA) platform using Perl 5.8 (The Perl Foundation, Walnut, CA, USA), Apache (Apache Software Foundation, Los Angeles, CA, USA), MySQL (Cupertino, CA, USA), Yahoo User Interface (Verizon, Basking Ridge, NJ, USA) and CGI (CGI Group, Montreal, QC, Canada). Despite deploying the tool via the web, significant challenges were still encountered. There was significant variation in the browsers and browser versions both within and across hospital trusts, making the development of a uniform tool challenging. In addition, constant liaison with NHS Information Technology was required to whitelist access to the tool despite the fact that no patient-sensitive identifiers were ever stored or transmitted when using it.
Results
Quantitative study
We recruited 294 patients to the quantitative study between November 2009 and May 2011 (Figure 31), 291 of whom were followed up for 6 months. The web-based CDSS was used in the chest pain clinic in two of the pilot sites, clinics A and C.
FIGURE 31.
Flow diagram showing recruitment of patients attending RACPCs to the OMA programme. a, Trust 1, Clinic A Before OMA and Trust 2, Clinic B; b, Trust 1, Clinic A After OMA and Trust 2, Clinic C. Reproduced from Johnson et al. 45 © Johnson et al. 2015. This is an open-access article distributed under the terms of the Creative Commons Attribution License (http://creativecommons.org/licenses/by/4.0/), which permits unrestricted use, distribution, and reproduction in any medium, provided the original author and source are credited.

Baseline characteristics and clinical decision support system use
The baseline characteristics of recruited patients are shown in Table 13. Of the 183 consultations in a clinic after delivery of the OMA programme, the CDSS was used in 154 (84%).
Characteristics at baseline | Before OMA (n = 106) (seen in clinic which had not received OMA programme)a | After OMA (n = 179) (seen in clinic which had received OMA programme)b |
---|---|---|
Age (years), mean (SD) | 59.4 (11.0) | 59.0 (11.6) |
Sex, n (%) | ||
Male | 52 (49) | 97 (54) |
Female | 54 (51) | 82 (45) |
Risk factors, n/N (%)c | ||
Smoking | 43/106 (41) | 59/179 (33) |
Diabetes | 9/106 (8) | 20/179 (11) |
Hyperlipidaemia | 34/106 (32) | 62/179 (35) |
Medications current at chest pain clinic, n/N (%) | ||
Antiplateletd | 23/106 (22) | 34/179 (19) |
Beta-blocker | 5/106 (5) | 17/179 (9) |
Statin | 26/106 (25) | 37/179 (21) |
Typicality of chest pain, n/N (%) | ||
Typical | 20/106 (19) | 46/179 (26) |
Atypical | 17/106 (16) | 28/179 (16) |
Non-cardiac | 69/106 (65) | 105/179 (59) |
There were no clear differences in either medications at 6 months or first investigation within 6 months between the before and after OMA groups, suggesting that the OMA CDSS did not change prescribing practice or patterns of investigation. Furthermore, clinicians’ decisions were often in conflict with OMA recommendations. Among patients for whom OMA recommended no investigation, 86% of patients did not receive an investigation in clinics before the OMA intervention, and the same percentage did not receive an investigation in clinics trained in the use of OMA. However, among patients for whom the OMA CDSS would recommend an investigation, 50% of patients underwent any investigation before the OMA programme and just 59% underwent any investigation after OMA training.
Qualitative findings
In this section, we describe the range of perceptual and contextual factors that contributed to clinicians using the CDSS in a manner different from that which was originally intended. We begin by outlining obvious structural and practical barriers that limited clinicians’ ability to use the CDSS or to implement its recommendations. We then consider the extent and direction of clinicians’ disagreements with CDSS recommendation based on the full sample of observed post-intervention consultations in clinic A. Finally, we move on to a more detailed examination of the meanings that clinicians attached to different stages of their interaction with the CDSS in terms of its required patient-specific input, its underlying algorithms and the interpretation and contextualisation of its outputs. Our aim here is to provide an explanatory account of clinicians’ engagement with the CDSS that probes beyond the appearance of intervention non-compliance to generate a better understanding of the extent to which clinicians’ decision-making processes within the chest pain clinic are amenable to being supported by CDSS technology in principle. Verbatim participant quotations on the role of computerised decision support in clinical decision-making and clinicians’ perceptions about possible uses of the OMA CDSS in clinical practice are presented in Appendices 2 and 3, respectively.
We present our findings from the analysis of clinicians’ self-reported and observational data in an integrated manner.
Structural and practical barriers to use of the clinical decision support system as intended
Several structural barriers prevented the implementation of the CDSS recommendations, including clinicians’ lack of authority to prescribe medication and the non-availability of specific investigative procedures at the hospital site. Two members of staff at the observed site were not able to prescribe medications. Chest pain clinicians who were authorised prescribers rarely made use of the facility for the direct issuing of medication from the hospital pharmacy instituted to facilitate prescribing. Reasons cited for this were lack of habit, GPs having a better grasp of how medication fitted with patients’ existing drug regimen and additional cost to the hospital trust. At the time of the pilot, calcium scoring was not available, and CT angiogram became available only half-way through the pilot.
Practical barriers to the integration of the CDSS into the workflow of the clinic included computer glitches, such as the program suddenly freezing up and the loss of data already entered. Although rare, these occurrences caused frustration among clinicians. Clinicians also had to complete separate electronic records with similar information to that requested by the CDSS, which may have felt like an avoidable duplication of effort.
The extent and direction of clinicians’ disagreement with clinical decision support system recommendations
Observational data on clinicians’ decision-making and use of the CDSS were available for 37 patients (Table 14). Clinicians disagreed with CDSS recommendations about investigative procedures in almost half of all observed cases. Clinician disagreement encompassed both more and fewer investigations than recommended by the CDSS. Researcher observations confirmed that in all these cases, clinicians stuck to their original decision instead of considering changes in light of the CDSS output. Indeed, there was a broad consensus among clinicians, articulated in interviews and the focus group, that clinical decision-making should not be driven by technology and that clinicians should remain the final arbiters of any decisions to be implemented.
CDSS recommendation | Investigation done | ||
---|---|---|---|
n | Done | Percentage done (95% CI) | |
Before OMA CDSS recommendation (investigation or no investigation) followed in 78 out of 106 cases (74%, 95% CI 65% to 82%) | |||
Investigation | 36 | 18 | 50 (34 to 66) |
No investigation | 70 | 10 | 14 (6 to 22) |
After OMA CDSS recommendation (investigation or no investigation) followed in 134 out of 179 cases (75%, 95 CI 69% to 81%) | |||
Investigation | 74 | 44 | 59 (48 to 70) |
No investigation | 105 | 15 | 14 (7 to 21) |
Clinicians described the possible benefits to themselves of using the OMA CDSS mainly in terms of providing reassurance and supporting a systematic approach to conducting consultations. This orientation towards the CDSS as ‘decision feedback’ rather than ‘decision support’ was also reflected in the timing of clinicians’ engagement with the CDSS. In two-thirds of the observed cases, clinicians used the CDSS after they had communicated an independently made decision to the patient and the patient had left the room.
Looking at individual cases of clinicians’ agreement and disagreement with the CDSS recommendations, clinicians were most likely to agree with the CDSS recommendation of ‘no further investigation’ when pain was categorised as ‘non-anginal’ (15/19 cases). For typical angina symptoms, clinicians also expressed agreement with CDSS recommendations in theory, although, in practice, they occasionally ‘downgraded’ the recommended methods of investigation when they felt that a more aggressive approach was not in the patient’s best interest or was clinically unwarranted.
Clinicians were most likely to disagree with the CDSS when pain had been categorised as ‘atypical’ (12/18 cases). When the CDSS was being developed, patients presenting with atypical pain had been seen as the category for which computerised decision support had most to offer in terms of supplementing clinician expertise. It is therefore of particular interest that, in practice, clinicians regarded CDSS recommendations as most reliable and trustworthy – but probably also least needed – when case histories were typical for angina or clearly non-anginal.
Problems with the entry of patient information into the clinical decision support system
Clinicians felt that there were a number of problems relating to how patient information had to be entered into the CDSS. These included (1) classifying patient-reported symptoms according to Diamond and Forrester criteria,209 (2) an inability to update previously entered information and (3) concern over entering information about risk information in a binary format as ‘present’ or ‘absent’ rather than in a more graded manner.
Concerns over available clinical information not used by the clinical decision support system
The results of patients’ exercise treadmill test (ETT) performance were not taken into account in the CDSS. However, from observations and interviews there was evidence of the continued impact of the ETT on clinicians’ decision-making in terms of upgrading or downgrading the investigative procedures indicated by NICE guidelines on the basis of patient history (pain classification and risk factors). Clinicians freely admitted their unwillingness to do away with the additional information that they felt that they could gain from ETT results; one clinician explained that she would use the CDSS only after she had interpreted the ETT results so as not to ‘cloud her judgement’. At the same time, clinicians acknowledged the limitations of the ETT’s diagnostic value. We have explored the use of the ETT in the chest pain clinic in a previously published paper. 44
Discussion
Clinical decision support systems are often considered one of the most important rationales for developing flexible and extendable hospital EHR systems. The long-term viability and sustainability of the CDSS requires modular access to the underlying EHR system and ongoing, bidirectional data flows between research and care components. In this study, we attempted to develop, implement and evaluate a CDSS in a clinical setting lacking a modular EHR system that can foster CDSS integration – a situation that is still widespread within both primary care and secondary physician information systems in the NHS. Our initial technical scoping exercise revealed significant variation both within and across hospitals, which rendered the option of creating an integrated CDSS unfeasible. As a result, we built and deployed a system that was accessible through the internet and required minimal interaction with the underlying hospital information systems.
Through our mixed-methods study, we have developed an in-depth understanding of the feasibility and plausibility of the proposed OMA CDSS, and compared process outcomes in patients managed in clinics before and after delivery of the OMA programme. The OMA CDSS, delivered within the OMA programme, has been shown not to be feasible in its present form and, in line with MRC guidance, these findings do not support a trial of the OMA programme.
Although the CDSS was used in 84% of consultations for which it was available, it had little impact on medication prescribing at 6 months and first investigation within 6 months, and we have, therefore, not carried out an expected value of information analysis. Of those patients recommended an investigation by the CDSS, 50% of patients before OMA and 59% of patients after OMA did not receive any investigation, and it is possible that this potential underinvestigation could lead to incorrect diagnoses of non-cardiac chest pain. The range of investigations requested by clinicians was more limited than recommended by the CDSS, with invasive angiography being the most commonly undertaken investigation.
The qualitative analysis describes how the CDSS was used in practice, highlighting structural and practical difficulties, issues with data input and how the data are processed by the CDSS problems with matching of all clinically available information to the CDSS algorithm, and difficulties with implementation of the CDSS recommendations.
The strengths of this study lie in its mixed-methods approach. The quantitative study used both hospital and primary care records to allow follow-up of patients at 6 months, and high rates of follow-up were achieved (99% at 6 months). The qualitative study was extensive and was conducted by experienced ethnographers who were familiar with the chest pain clinic setting. Although the quantitative data suggested that the CDSS was used, the qualitative data were essential in developing a more complete understanding of how it was used and provided multiple examples of difficulties in practice. Linking qualitative data to clinical and diagnostic information helped to make sense of the ways in which the CDSS was used and recommendations followed. The ethnographic method uses several sources of data (interviews, observations, focus groups), allowing comparisons to be made between what clinicians say they do and what they actually do.
The qualitative data collection was limited by the inclusion of observations after the delivery of the OMA programme in only one site, although we were able to interview one clinician from the second site at which the OMA programme was delivered. Each of the researchers involved in collection of qualitative data was involved in the development of the OMA programme.
For patients for whom the CDSS was not available or, when available, was not used during the clinical consultation, the assessment of cardiovascular risk factors was retrospectively made using routinely collected data for which there was more than one source (GP referral and hospital record). Data were collected from the GP referral only when it was not available in the hospital clinic record. We found disagreement between the sources for the presence of hyperlipidaemia and for smoking status, which may affect the comparison of the groups at baseline.
For the purposes of populating the CDSS for this group of patients, the clinic data were used to determine the typicality of chest pain. The complexity of categorising chest pain based on a patient’s description has been described before. 211 We found labelling chest pain typicality to be difficult, which resonated with the difficulties the chest pain clinicians described. Given that typicality is central to the pre-test probability of coronary disease and, hence, to CDSS recommendations, the difficulties experienced by both the research team and chest pain clinicians are important.
The OMA CDSS is a component of the OMA programme, which includes preparation and education stages. This study focuses on the CDSS, but clearly the other components of the OMA programme may have an important impact on the way in which the CDSS is received and used.
We have measured medication prescription in general practice 6 months after consultation, as a proxy for the use of secondary prevention medication. Clearly, there are many factors affecting whether or not medication is continued at 6 months. For example, medications may be prescribed for new indications or may be stopped following investigations that rule out coronary disease.
Tools to aid decision-making are introduced into the clinical workplace, a dynamic and complex system in which technologies, people and organisational routines dynamically interact. The OMA CDSS was introduced at a time of transition, NICE CG95202 having been published 4 months earlier. At this time, the ETT had a central role in chest pain clinic routines, and our main qualitative study site did not routinely use CT or CT coronary angiography for the diagnosis of angina. Although our original plan had been to develop a CDSS with both medication and investigation guidance taken from our expert panel, and allowing the incorporation of exercise test findings, given the primacy of NICE as a source of national guidance, it seemed prudent to incorporate NICE guidance. However, the absence of a test that was an entrenched part of chest pain clinic practice added to the difficulties of the clinicians in using the CDSS. Chest pain clinics are unlikely to be able to incorporate guidance immediately, and it is possible that the CDSS would have had more impact on clinic processes when clinics were more able to follow guidance.
Although largely based on national guidance, the CDSS did not ‘fit’ with established clinical practice in several ways. Gaining entry to the guideline requires clear categorisation of a patient, in this case through entering clinical data, which results in an estimate of a patient’s pre-test probability of significant CAD. This categorisation is dependent on multiple inputs, including typicality of chest pain and risk factors. The CDSS requires data entry in a dichotomised way, in contrast to the contextualised and nuanced information available to the clinician. Use of the CDSS could be viewed as a series of interactions during which the clinician first decides how to fit clinical inputs to the CDSS and subsequently considers how to act on the CDSS outputs, given the clinical context and structural factors, such as the availability of particular investigations. Each of these interactions requires the clinician to balance the requirements of the tool and the breadth of information available within the clinical context. Actions contrary to CDSS recommendations could be seen not as ‘non-compliance’ but as rational decision-making under conditions of uncertainty.
The privileging of clinical expertise in decision-making
Superficially, our findings suggest a failure to integrate the CDSS into the chest pain clinic workflow and reluctance among clinicians to engage with computerised decision support. Indeed, resistance to professional behaviour change and distrust of new technologies are previously cited factors for past failures in CDSS implementation. 212 The finding that the CDSS did not alter clinicians’ decision-making about investigative and prescription medication means that it is unlikely that the OMA intervention would result in more appropriate treatment and improved patient outcomes.
Rather than read clinicians’ behaviour as intervention non-compliance, however, we should aim to understand it as reasoned action within complex real-life settings. Clinical decision-making does not occur in a vacuum of probabilistic reasoning, but is steered to at least some extent by concerns over professional accountability, the threat of litigation if mistakes are made, concerns over scarce resources and so on. Our qualitative analysis of rich ethnographic data collected in one of the intervention pilot sites has enabled a more in-depth understanding of how clinicians engaged with the CDSS in specific decision scenarios, why they felt confident to over-rule its recommendations, and importantly, what might need to change to make the CDSS relevant and helpful to their decision-making process. These investigations have highlighted important factors such as clinicians’ fears about missing things and erring on the side of caution when deciding on further investigative procedures, and entrenched ways of practising, with a reluctance to abandon established diagnostic approaches.
Conclusions
Our pilot study suggests that the OMA intervention has no significant impact on the decision-making behaviour of clinicians in chest pain clinics, and significant challenges were encountered when attempting to integrate it with standard clinical workflows and hospital EHR systems. It is not feasible in its current form and should not be evaluated further. The mixed-methods approach that we adopted, including qualitative ethnographic methods, has provided an in-depth understanding of the ways in which clinicians interacted with a CDSS. This understanding could support the development of new tools to aid clinical decision-making with a greater likelihood of being adopted in practice and with higher integration with hospital EHR systems.
Chapter 7 Stable chest pain and stable coronary artery disease: a novel cohort – the Clinical Cohorts in Coronary disease Collaboration
Abstract
Background
Cohorts recruited in clinical settings make important contributions to addressing translational research questions in prognosis research. Few large, well-characterised cohorts are available to support research into CVD.
Objectives
To establish a contemporary, prospective clinical cohort of patients with linked phenotypic, genetic and biomarker data as a resource.
Methods
Consecutive patients undergoing investigation for new-onset, non-acute chest pain were recruited between 2009 and 2014 from four UK NHS hospitals. Participants provided blood samples for biomarker and genetic analysis, and completed a health questionnaire at baseline. Detailed clinical information was manually extracted from hospital electronic databases. Participants are being followed up for CVD events, and health-related quality of life was assessed using a postal questionnaire (mean follow-up of 469 days).
Results
We enrolled 3345 consecutive patients {63.1% men; mean age 58.0 years [standard deviation (SD) ± 12.5 years]}. Blood samples were collected for 84% of patients, baseline questionnaire data for 89% of patients and clinical data for 99% of patients.
Conclusions
The Clinical Cohorts in Coronary disease Collaboration is a contemporary consented genetic bioresource linked to hospital EHRs, questionnaire data and health outcomes. Significant challenges remain for automatically extracting the entire patient EHR from health information systems and, as a result, a wealth of data remain inaccessible for research. The design, development and provision of common infrastructure, data standards and services that will allow researchers to access and exploit such data are crucial for expanding 4C in the future. Related initiatives such as the NIHR HIC will provide best practices and guidelines for doing so.
The studies in this chapter relate to patients presenting for the first time with stable chest pain (see Figure 24).
Introduction
Despite their value in translational research, few large, prospective clinical cohorts have been established in SCAD. To provide a new resource for prognosis research, we have established a novel, contemporary clinical cohort, the 4C, which includes a genetic and biomarker resource linked to hospital EHRs, questionnaire data and health outcomes. Using a combination of -omic, clinical and phenotypic data, 4C will support research on the causes of SCAD and its main complication, ACS, and the complex interactions between genetic and environmental determinants of post-AMI outcomes. 4C forms part of GENIUS-CHD,213 an international consortium of 63 clinical cohorts containing CHD patients and deoxyribonucleic acid (DNA) to associate genetic variants with subsequent events. The consortium was established in 2014 and contains data from cohorts from 17 countries, including > 270,000 patients – the largest effort of its kind studying determinants of risk for subsequent CHD events. This study describes the rationale and design of the 4C cohort, and baseline characteristics of patients.
Study 14: establishment of a novel consented clinical cohort and blood resource (the Clinical Cohorts in Coronary disease Collaboration) – study design and baseline characteristics
This manuscript by Fitzpatrick et al. 46 is in preparation.
Introduction
Consented cohorts recruited in clinical settings make important contributions to addressing translational research questions in prognosis research. 29–32 However, despite their potential advantages, large prospective clinical cohorts are lacking across all disease areas, and only a handful exist for CAD. 214–217
There is a stark contrast between the significant scientific interest in and the lack of clinically useful novel biomarkers. New technologies such as mass spectrometry and nuclear magnetic resonance spectroscopy for measuring metabolites (metabolomics) or proteins (proteomics) have the potential to provide early insight into the aetiology of CAD and, thus, better predictive performance. However, the value of existing circulating biomarkers, or panels of biomarkers, for predicting or mediating disease prognosis has yet to be proven.
Although in its early stages, the adoption of information technology in health care and biosciences – ‘clinical informatics’ – is gaining rapid momentum and is predicted to revolutionise the way -omics data linked to phenotypes recorded within EHRs will drive translation of scientific discovery into clinical practice and patient benefit. In the USA, consented biorepositories linked to EHRs are increasingly successfully being used for translational research at scale. 218 For example, BioVU (Vanderbilt University Medical Centre, Nashville, TN, USA)219 is a biorepository containing over 200,000 DNA samples extracted from discarded blood linked to the full hospital EHR of consented patients attending the Vanderbilt University Medical Centre.
To advance research through collaboration, 4C was established in the UK in 2009 as a resource in which DNA and biomarker samples were obtained at the time of presentation with chest pain and linked to detailed phenotypic data obtained from EHRs and participant self-completed questionnaires. We sought to explore and assess the current potential of setting up a comparable consented research platform by collecting DNA samples and to quantify the extent to which diverse NHS hospital information systems are accessible for extracting secondary care data (structured and unstructured) for research purposes at scale.
Methods
Design and setting
Prospective, observational cohort study in which participants were recruited from RACPCs, cardiac catheter laboratories and coronary angiogram pre-assessment clinics in four ethnically diverse NHS hospitals: the Heart Hospital, University College London Hospitals NHS Foundation Trust; the London Chest Hospital and Newham University Hospital, Barts Health NHS Trust; and the Bristol Heart Institute, University Hospitals Bristol NHS Foundation Trust.
Study cohort
Eligibility
Consecutive patients undergoing evaluation for chest pain at RACPCs or elective invasive coronary angiography at participating hospitals were approached for inclusion (Figure 32). Patients were not eligible for inclusion if they were diagnosed with structural heart disease, cardiomyopathy or arrhythmia, or were referred for ablation or placement of devices (e.g. pacemakers). No patients were excluded on the basis of age or coexisting conditions. Trained researchers identified eligible patients by checking clinic appointment databases and angiogram procedure lists on a daily basis.
FIGURE 32.
Clinical Cohorts in Coronary disease Collaboration study flow diagram.
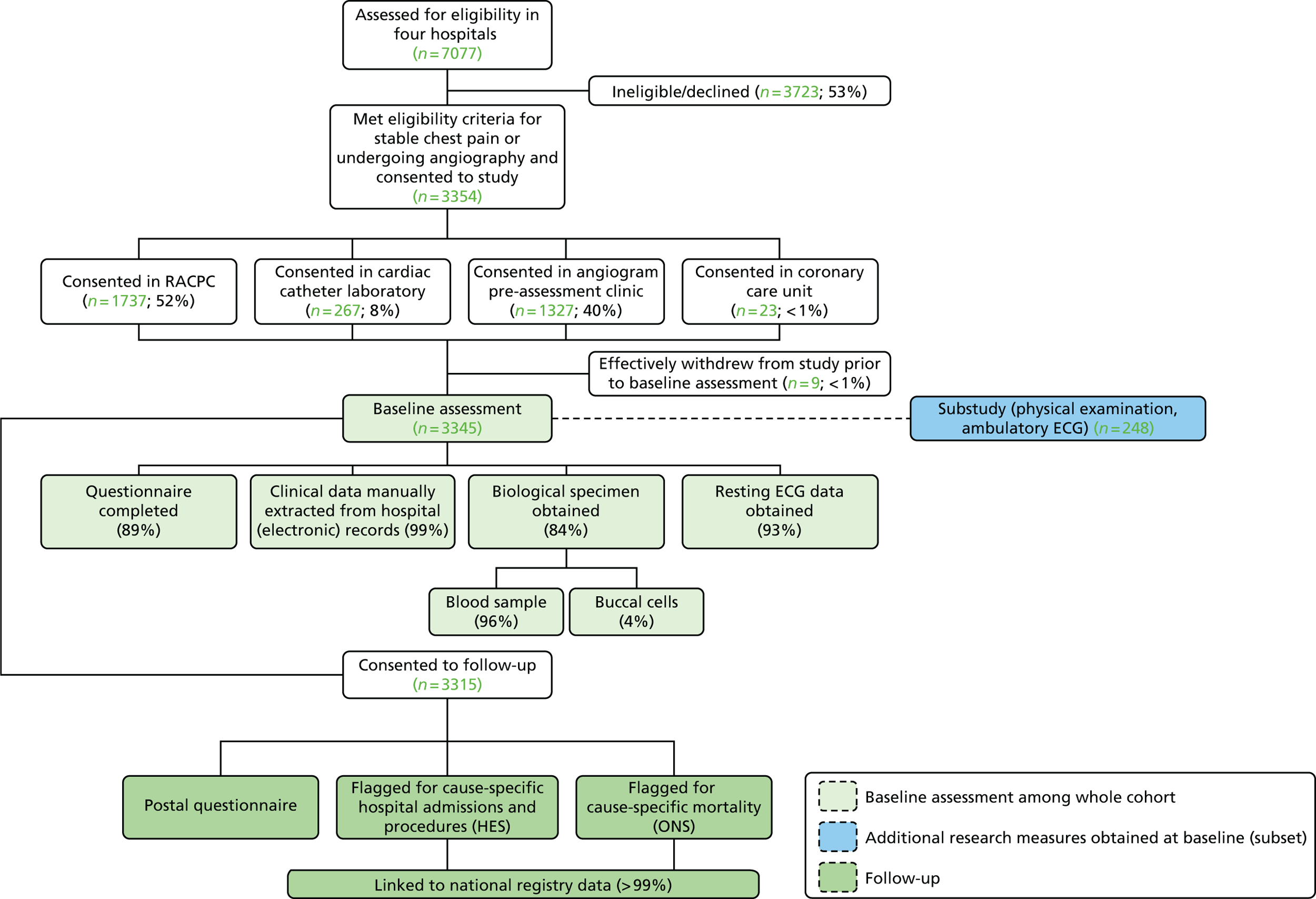
Participant consent
Patients were approached directly by a researcher at the hospital at the time of their clinic appointment. Informed consent was sought for (1) completion of a baseline health questionnaire; (2) provision of a blood sample for long-term storage and analysis of DNA and biomarkers; (3) extraction of patient hospital data; and (4) linkage of patient data with national EHR data sources (e.g. coded hospitalisations and procedures, emergency admissions and cause-specific mortality) (see Appendix 4).
Baseline data collection and measures
Measures were piloted and refined prior to commencement of the study. Three unique sources of data contributed to the participant baseline assessment, which took place during patients’ scheduled hospital appointments.
Collection of research measures
A non-fasting blood sample was obtained from consenting participants, including those in whom a diagnosis of angina was subsequently ruled out, by staff trained in venepuncture. A total of 20.0 ml of blood was drawn to enable a wide range of research measures to be obtained. Samples were processed in accordance with protocol and aliquoted into 0.5-ml 2-D barcoded cryovial tubes suitable for long-term cryopreservation. Each cryovial was individually logged in two unlinked databases using a single-tube quick response code reader, placed in a 96-position rack and stored locally at –20 °C. PAXgene [PAXgene Blood RNA System (PreAnalytiX, Switzerland)] ribonucleic acid tubes were stored as whole blood at –20 °C. DNA was collected using a buccal swab from consenting participants who did not provide a venous blood sample. All samples were transported in temperature-controlled shipping boxes for long-term storage at a biobank at –80 °C (see Appendix 5).
DNA was extracted from 2556 individual participant samples, and genotyping of 51 CHD-specific single-nucleotide polymorphisms identified in Coronary ARtery DIsease Genome wide Replication and Meta-analysis (CARDIoGRAM PlusC4D)220 was carried out at a company with specialised services for extraction of DNA and genotyping, in accordance with specification.
Hydrogen-1 nuclear magnetic resonance spectroscopy was used to quantify 216 metabolites in serum from 2323 participants.
Patients completed a health questionnaire (average completion time of 5–10 minutes), which included questions on education, ethnicity, general health functioning [EuroQol-5 Dimensions (EQ-5D)],221 functional chest pain (Rose angina questionnaire),222 angina severity [Canadian Cardiovascular Society (CCS) classification],223 and symptoms of anxiety [Generalized Anxiety Disorder 7-item scale (GAD-7)]224 and depression [Patient Health Questionnaire-9 (PHQ-9)]225 at baseline (see Appendix 6).
Additional measures obtained from a subset of patients for research use included a 60-minute physical examination [anthropometric measures, lung function, aortic BP, pulse wave (augmentation) index, pulse wave velocity and ankle brachial index] and 31-hour ambulatory ECG, and tri-axial accelerometry and sleep measures. Substudy participants also completed an extended health questionnaire which included questions on diet and sleep.
Collection of information collected as part of standard clinical care
Trained extractors reviewed hospital databases and recorded detailed clinical information on standardised case report forms (see Appendix 7). Depending on the clinic or hospital, different clinical systems were searched to manually extract data that could not be downloaded or exported as a data file (Table 15). Information included reason(s) for referral, history of chest pain, physician-recorded risk factors for CAD, previously diagnosed medical history and test results. The resting ECG and ETT had to be removed from the notes or ordered from medical records and photocopied. The manual extraction of clinical biochemistry results from the hospital systems proved burdensome and was abandoned.
Measure | Hospital 1a | Hospital 2a | Hospital 3a | Hospital 4a | ||||
---|---|---|---|---|---|---|---|---|
Data available for electronic download | Method of collection | Data available for electronic download | Method of collection | Data available for electronic download | Method of collection | Data available for electronic download | Method of collection | |
Clinical biochemistry results | No | Manual extraction of results from clinical system | No | Not done | No | Not done | Yes | Not done |
Resting ECG | No | Manual photocopying of paper ECG from notes on the ward or off-site from medical records store, or manual extraction of data from clinical database | No | Manual photocopying of paper ECG from notes on the ward or off-site from medical records store, or manual extraction of data from clinical database | No | Manual printing of paper ECG from ECG machine in clinic or manual extraction of data from clinical database | No | Manual photocopying of paper ECG from notes or manual extraction of data from clinical database |
ETT | No | Manual printing of report and paper trace | No | Not done | No | Manual extraction of information from database | No | Not done |
Clinical risk factors | No | Manual extraction of information from the GP referral letter | No | Manual extraction of information from the GP referral letter | No | Manual extraction of information from the GP referral letter and database | No | Manual extraction of information from the GP referral letter |
Medical history | No | Manual extraction of information from the GP referral letter | No | Manual extraction of information from the GP referral letter | No | Manual extraction of information from the GP referral letter and database | No | Manual extraction of information from the GP referral letter |
Symptoms and clinical examination | No | Manual extraction of information from clerking sheet | No | Manual extraction of information from GP referral letter, angiogram report and discharge letter | No | Manual extraction of information from discharge letter and database | No | Manual extraction of information from discharge letter and database |
Diagnosis | No | Manual extraction of information from clerking sheet discharge summary | No | Manual extraction of information from discharge letter | No | Manual extraction of information from discharge letter, angiogram report and database | No | Manual extraction of information from discharge letter and database |
Coronary angiogram results | No | Manual extraction of information from clinical system | No | Manual extraction of information from clinical system | No | Manual extraction of information from clinical system | No | Manual extraction of information from clinical system |
Cardiac investigations/imaging | No | Manual extraction from clinical systems and imaging reports | No | Manual extraction from clinical systems and imaging reports | No | Manual extraction from clinical systems and imaging reports | No | Manual extraction from clinical systems and imaging reports |
Medical management | No | Manual extraction of information from discharge summary | No | Manual extraction from discharge summary | No | Manual extraction from angiogram report and discharge summary | No | Manual extraction from discharge summary |
Data were then manually entered onto a research database at the study co-ordinating centre, cleaned and coded ready for analysis.
Electronic health records linkages for participant follow-up
We linked consented individuals with secondary care data from HES, a national data warehouse of administrative data containing ICD-10-coded hospital diagnoses and OPCS4-coded procedures. We additionally linked participants with mortality data from the ONS, which provided ICD-10-coded cause-specific mortality data. The linkages were conducted via the NHS Health and Social Care Information Centre (formerly known as the NHS Information Centre) and patients were identified using their NHS number (a unique 10-digit numeric identifier for the health-care system), sex, date of birth and postcode.
Postal questionnaire participant follow-up
A postal follow-up questionnaire was sent to study participants (mean follow-up of 469 days) after recruitment to assess general health status (EQ-5D), functional status (Rose Angina Questionnaire,222 Seattle Angina Questionnaire),226 symptoms of depression (Patient Health Questionnaire-2) and anxiety (GAD-7),224 and use of nitrate medication.
Clinical outcomes
The primary end point is a pre-specified composite of cardiovascular mortality, non-fatal AMI, non-fatal stroke and PAD. The secondary outcomes are angina status (CCS classification, Seattle Angina Questionnaire, nitrate use) and general health functioning (EQ-5D) from the follow-up questionnaire.
Study approvals
The study protocol was approved by the London City Road and Hampstead National Research Ethics Committee (reference 11/LO/1621) and local NHS Research and Development committees.
Results
Challenges obtaining data from hospital databases
We identified many challenges to the collection of data from hospital electronic databases in a research-ready format. We accessed multiple clinical systems containing different sources of information but we were unable to download or export data as electronic files at any of the hospitals.
Baseline data
Between 9 July 2009 and 9 January 2014, 3345 participants [63.1% men; mean age (± SD) 58.8 years (± 12.5 years)] were enrolled in the study. Detailed clinical information was manually extracted from hospital EHRs 6 months after the baseline assessment, to allow time for procedure and test results to be uploaded. Approximately half (52%) of the participants were recruited from RACPCs and 48% were recruited from angiography pre-assessment clinics and cardiac catheter laboratories. Ethnicity was available for 98.5% of participants, with 54.8% describing themselves as white, 23.1% describing themselves as South Asian (Indian, Pakistani and Bangladeshi) and 11% describing themselves as black (Table 16).
Baseline characteristics | Alla (N = 3315) | Men (n = 2089) | Women (n = 1226) | p < 0.05b |
---|---|---|---|---|
Symptoms on presentation (%) | ||||
Chest pain | 95.5 | 95.5 | 95.5 | NS |
Shortness of breath | 33.4 | 31.2 | 37.2 | 0.001 |
Characterisation of chest pain (%) | < 0.001 | |||
Typical | 34.8 | 38.8 | 38.6 | |
Atypical | 45.5 | 42.4 | 50.4 | |
Non-cardiac | 19.7 | 18.8 | 21.2 | |
Cardiac history (%) | ||||
Ischaemic/coronary heart disease | 29.0 | 34.0 | 20.6 | < 0.001 |
AMI | 11.3 | 14.1 | 6.4 | < 0.001 |
Unstable angina | 0.9 | 1.1 | 0.5 | NS |
Heart failure | 2.4 | 2.7 | 1.8 | NS |
Risk factors for CAD | ||||
Smoking status (current/ex/non) (%) | 19.1/26.0/28.9 | 21.8/30.6/23.8 | 14.6/18.3/37.4 | < 0.001 |
Family history of coronary disease (%) | 31.3 | 30.0 | 33.5 | NS |
Hypercholesterolaemia (%) | 52.5 | 53.2 | 51.7 | NS |
Hypertension (%) | 66.2 | 66.5 | 69.2 | NS |
Type 1 diabetes (%) | 0.7 | 0.7 | 0.7 | NS |
Type 2 diabetes (%) | 22.4 | 24.3 | 19.3 | 0.001 |
BMI (kg/m2), mean (SD) | 29.5 (± 5.5) | 29.1 (± 4.9) | 30.5 (± 6.5) | < 0.001 |
Pre-test probability of CAD reported for stable angina patient (%) | NS | |||
Low | 3.4 | 3.4 | 3.5 | |
Medium | 18.5 | 16.6 | 22.4 | |
High | 78.1 | 80.0 | 74.1 | |
Investigations performed (index procedure) (%) | ||||
Invasive coronary angiogram | 54.9 | 60.0 | 47.1 | < 0.001 |
Coronary CT angiogram | 6.3 | 5.5 | 7.7 | 0.013 |
Angiogram assessment (%) | < 0.001 | |||
Normal angiogram | 28.0 | 11.0 | 15.2 | |
Non-obstructive CAD | 12.3 | 22.0 | 40.4 | |
One diseased vessel | 21.2 | 22.0 | 20.3 | |
Two diseased vessels | 23.5 | 26.9 | 16.4 | |
Three diseased vessels | 14.9 | 18.5 | 7.8 | |
Medication on referral (%) | ||||
Aspirin/antiplatelet medication | 19.8 | 21.5 | 16.9 | 0.001 |
Beta-blocker | 14.5 | 16.4 | 11.3 | < 0.001 |
ACEI | 12.6 | 13.9 | 10.4 | 0.004 |
Statin | 23.2 | 25.1 | 20.1 | 0.001 |
Glyceryl trinitrate | 8.1 | 7.4 | 9.3 | 0.049 |
Long-acting nitrate | 6.2 | 6.9 | 4.9 | 0.018 |
Calcium channel blocker | 10.2 | 9.7 | 11.1 | NS |
ARB | 4.3 | 4.3 | 4.5 | NS |
Outcome (%) | 0.229 | |||
No treatment | 54.4 | 52.6 | 57.5 | |
Medical management | 19.6 | 20.2 | 18.5 | |
PCI | 19.2 | 20.2 | 17.5 | |
Coronary artery bypass surgery | 3.9 | 3.9 | 3.9 | |
Further investigation | 1.4 | 3.1 | 1.6 |
Of the 3345 participants recruited into the study, 3315 (99.1%) granted full access to their medical records. Chest pain was the presenting symptom in 94.5% of the participants, of whom 33.4% complained of associated shortness of breath (31.2% for men and 37.2% for women; p = 0.001).
Risk factors
Smoking status was recorded for 74% of participants, of whom 19.1% were current smokers. Men were more likely than women to smoke or be ex-smokers (21.8% and 30.6%, respectively, vs. 14.6% and 18.3%, respectively). Participants were defined as being hypertensive if they fulfilled any of the following criteria: (1) previous diagnosis of hypertension was recorded on referral or during appointment at the time of recruitment; (2) another diagnosis from which hypertension can be inferred was recorded (e.g. chronic kidney disease due to hypertension); (3) SBP measurement recorded up to 3 months prior to recruitment was ≥ 140 mmHg and/or DBP was ≥ 0 mmHg; or (4) participants were taking BP-lowering medication on referral. Following these criteria, 68.1% of the cohort was defined as hypertensive at baseline. Using criteria 1, 2 and 4, 27.6% of the cohort was classified as being diabetic, with a higher percentage of men diagnosed with diabetes than women (29.2% vs. 25.0%; p = 0.008). Of these, 22.4% were recorded as having type 2 diabetes, whereas 0.7% of the cohort presented with type 1 diabetes. A previous diagnosis of hypercholesterolaemia was recorded for 52.5% of the cohort, with no significant difference between men and women (53.2% vs. 51.7%, respectively). A BMI of ≥ 30 kg/m2 was recorded for only 37.4% of the cohort, with a significantly higher percentage of women than men recorded as being obese (43.5% vs. 34.1%; p < 0.001). A positive family history of coronary disease was present in 31.3% of participants, with women presenting a higher percentage of first-degree relatives with history of CAD than men (33.5% vs. 30.0%, p = 0.035).
Cardiac history
A positive history of ischaemic heart disease (IHD) was recorded for 29% of patients, with a significantly higher percentage of men than women (34.0% vs. 20.6%; p < 0.001) with a previous diagnosis; unsurprisingly, more men had previous coronary angiograms than women (21.0% vs. 13.2%; p < 0.001). Similarly, history of previous AMI was higher among men than women (14.1% vs. 6.4%; p < 0.001), with 17.5% of men having had a previous PCI (vs. 7.9% of women; p < 0.001) and 7.4% having had previous coronary artery bypass surgery (vs. 3.0% of women; p < 0.001).
Physical measurements
Baseline heart rate was obtained from resting ECGs performed on the day of the participants’ appointment and is available for 67.5% of the participants. Women had a significantly higher heart than men [74.5 ± 13.6 beats per minute (b.p.m.) vs. 71.6 ± 14.6 b.p.m.; p < 0.001]. SBP and DBP were obtained from referral letters and GP correspondence up to 3 months before recruitment or discharge letters from RACPC on the date of recruitment. Both SBP and DBP were significantly lower in men than in women (130.0 ± 19.8 mmHg and 77.9 ± 11.7 mmHg, respectively for men vs. 133.8 ± 21.0 mmHg and 79.0 ± 11.6 mmHg, respectively for women; p < 0.001).
Investigation
The initial diagnosis provided by the clinician or nurse assigned to the patient was stable angina in 51.5% of the cohort for whom this information was recorded. Surprisingly, 41.4% of the participants were initially diagnosed as having non-CAD chest pain, of whom a higher percentage were women (51.7% vs. 36.0% for men). However, only 56.1% of our cohort had information available on initial diagnosis.
The overall assessment of diseased vessels, obtained for 61.1% of the cohort, was based on the angiogram assessment of severity or percentage of luminal diameter stenosis, with number of diseased vessels classified according to vascular territory of the three major coronaries. The following criteria were then applied: (1) no evidence of disease in any of the territories was classified as normal; (2) non-obstructive disease (e.g. mild disease or irregularities, ‘no significant disease’, ‘no flow limiting disease’) classified as less than moderate (obstruction ≤ 50%) in severity, was considered non-obstructive CAD; (3) evidence of moderate (50–69%) or worse (severe: ≥ 70%) disease in any one of the three territories was classified as one diseased vessels; (4) evidence of moderate or worse disease in any two territories or the left main stem was classified as two diseased vessels; and (5) evidence of moderate or worse in all three territories was classified as three diseased vessels. Applying these criteria, 28.0% of our cohort was classified as having a normal angiogram, 12.3% as having non-obstructive CAD, 21.2% as having one diseased vessel, 23.5% as having two diseased vessels and 14.9% as having three diseased vessels. Women had a higher percentage of normal coronaries than men (40.4% vs. 22.0%; p < 0.05), whereas men had more coronary angiograms classified as three diseased vessels than women (18.4% vs. 7.7%; p < 0.05). These findings are consistent with the higher percentage of men presenting risk factors and a previous history of CAD (IHD, AMI).
Management
Medical management was the opted treatment for 16.4% of participants, whereas a subsequent PCI was required for 19.2%. A smaller percentage of cases were recommended for coronary artery bypass graft (CABG) (4.6%).
Sex differences
In our cohort, men were more likely than women to smoke, be hypertensive and diabetic, have previous diagnoses of IHD and/or AMI, and have had a previous interventional procedure (PCI or CABG surgery). The index investigation also showed that men were more likely to have a higher number of vessels obstructed and were more likely to require CAD-specific treatment than women.
Baseline health questionnaire
A total of 2981 participants (89%) completed a baseline health questionnaire (Table 17). Three-quarters (76.4%) of participants reported pain/discomfort problems (73.6% of men vs. 81.3% of women; p < 0.001), and nearly half (45.6%) reported problems with anxiety/depression (42.3% of men vs. 51.6% of women; p < 0.001). However, only 14.4% of participants reported problems with self-care (14.5% of men vs. 16% of women; p < 0.001). More than three-quarters of participants (78.4%) were classified as experiencing exertional chest pain based on the Rose Angina Questionnaire, with no significant difference between men and women (77.2% vs. 80.4%, respectively). Many participants (60.7%) reported moderate limitations in daily living activities or an inability to perform any activity without angina or angina at rest (CCS classification III or IV). Women were more likely than men to report depression and anxiety based on the PHQ-9 and GAD-7 assessment questionnaires.
Baseline health questionnaire | Alla (n = 2981) (%) | Men (n = 1897) (%) | Women (n = 1084) (%) | p < 0.05b |
---|---|---|---|---|
Functional health status (EQ-5D)221 | ||||
Mobility | < 0.001 | |||
Respondents reporting no problems | 60.4 | 64.3 | 53.6 | |
Respondents reporting any problem | 39.6 | 35.7 | 46.4 | |
Self-care | NS | |||
Respondents reporting no problems | 85.6 | 86.5 | 84.0 | |
Respondents reporting any problem | 14.4 | 14.5 | 16.0 | |
Usual activities | < 0.001 | |||
Respondents reporting no problems | 64.2 | 68.2 | 57.1 | |
Respondents reporting any problem | 35.8 | 31.8 | 42.9 | |
Pain/discomfort | < 0.001 | |||
Respondents reporting no problems | 23.6 | 26.4 | 18.7 | |
Respondents reporting any problem | 76.4 | 73.6 | 81.3 | |
Anxiety/depression | < 0.001 | |||
Respondents reporting no problems | 54.4 | 57.7 | 48.4 | |
Respondents reporting any problem | 45.6 | 42.3 | 51.6 | |
Rose Angina Questionnaire222 | NS | |||
Exertional chest pain | 78.4 | 77.2 | 80.4 | |
Non-cardiac chest pain | 21.6 | 22.8 | 19.6 | |
CCS classification223 | < 0.001 | |||
I | 16.8 | 19.1 | 12.7 | |
II | 22.6 | 21.5 | 24.6 | |
III | 10.5 | 11.2 | 9.2 | |
IV | 50.2 | 48.3 | 53.6 | |
Mental health | ||||
Depression (PHQ-9)225 | < 0.001 | |||
None | 46.7 | 49.9 | 40.9 | |
Mild | 28.2 | 26.6 | 31.2 | |
Moderate | 14.9 | 14.0 | 16.6 | |
Moderate/severe | 6.2 | 5.5 | 7.3 | |
Severe | 4.0 | 4.0 | 4.0 | |
Anxiety (GAD-7)224 | 0.002 | |||
None | 53.6 | 51.0 | 46.1 | |
Mild | 23.2 | 24.0 | 25.5 | |
Moderate | 15.1 | 16.7 | 19.6 | |
Severe | 8.1 | 8.3 | 8.7 |
Single-nucleotide polymorphism genotyping
Minor allele frequencies were replicated from published data (Figure 33).
FIGURE 33.
Genotyping of single-nucleotide polymorphisms in the 4C cohort and replication of allele frequencies from published data (CARDIoGRAM PlusC4D). A total of 51 single-nucleotide polymorphisms were successfully genotyped. Minor allele frequencies as expected from published/reported data.

Metabolic profiling
1H-NMR spectroscopy was used to resource 225 cardiovascular-related metabolites in human serum. These included (1) lipoprotein lipids consisting of lipids, serum albumin and albumin-bound fatty acid resonances; (2) low-molecular-weight molecules, pyruvic acid, amino acids, ketone bodies and creatinine; and (3) serum constituents with detailed molecular information on serum lipid extracts including free and esterified cholesterol, sphingomyelin and omega-3 fatty acids. A total of 2323 samples were successfully analysed, and 2025 patients underwent angiography (1816 invasive coronary angiograms and 209 coronary CT angiograms).
Interim follow-up data for hospital admissions and procedures and mortality
A high proportion of consented participants were successfully linked to hospital admission and procedure data in HES (96.7% of patients sent for matching to date). In addition, 99% of participants were linked successfully with the ONS national death registry and 84 incident deaths were recorded [with 25 recorded as CHD-related deaths (ICD-10 codes I20–25) as the underlying cause of death] (Table 18).
Follow-up | ONS (mortality) | HES (non-fatal diagnoses and procedures)a |
---|---|---|
Patients who consented to follow-up (of 3345 recruited to 4C), N (%) | 3315 (99.1) | 3315 (99.1) |
Patients sent for matching, n (%) | 3315 (100) | 867 (26.2)a |
Patients matched to record, n (%) | 3311 (99.9) | 839 (96.7)a |
Follow-up (months), median (IQR) | 20 (14–26) | 326 (155–563) |
Observed participants with end point from ONS, n (%) | ||
All-cause deaths | 84 (2.5) | – |
Cause of death registered | 54 (64.3) | – |
CHD-related deaths (ICD-10 codes I20–25)b | 25 (46.3) | – |
Non-CHD CVD-related deaths (ICD-10 codes: I010–I99, excluding I200–I259)c | 9 (16.7) | – |
Non-CVD deaths | 20 (37.3) | – |
Awaiting cause of death registration | 30 (35.7) | – |
Observed participants with end point from HES, n (%) | ||
Non-fatal AMI + non-fatal stroke + PAD | – | 27 (3.2) |
Approximate annual risk (%) | 1.3 | 3.6 |
Discussion
Although researchers carrying out large-scale studies were previously limited to utilising EHR data from single sources, the potential to access data spanning the entire translational cycle now exists, with new opportunities to link clinical health-care data with other sources of electronic information and bespoke data on a large scale. To exploit these new opportunities, we established the 4C cohort, a contemporary, prospective cohort of 3345 patients undergoing investigation for SCAD, with blood and questionnaire data linked to the hospital EHRs, who are being followed up for a range of disease end points recorded in national registry databases. The cohort was established as a resource to encourage collaborative research based on individual patient data, in order to strengthen prognostic model development and external validation.
We faced two significant challenges during the conduct of our study. First, despite attempts to co-ordinate permissions from NHS organisations to undertake clinical research with the introduction of the NIHR Co-ordinated System for Permissions in 2008, we experienced significant delays in obtaining the required approvals [mean NHS Trust site-specific approval time was 85 days (range 50–115 days) across four sites], delaying recruitment of sites and influencing participant accrual targets. Health Research Authority plans to unify the approvals process for research in the UK came into effect at the end of 2015 (see www.hra.nhs.uk/), and it remains to be seen whether or not efforts to reduce the time it takes to approve research are successful, in the same way that regulatory approval of clinical trials was expedited following a highly critical Academy of Medical Sciences report on the effect of delays on approvals on scientific discovery. 227 Contractual negotiations, human resources checks and governance relating to cross-site working within NHS hospital trusts further impacted on recruitment and must also be reviewed. 228
A second challenge was an inability to download data electronically from the hospital EHR, resulting in time-consuming manual collection and recording of clinical data. The lack of efficient means to access or transfer EHR data, including discharge summaries and biochemistry results stored on separate clinical systems, greatly increased the time and resulting financial cost required to collect complete data.
The design, development and provision of common infrastructure, data standards and services are required to allow researchers to seamlessly interact and extract clinical data for research purposes across hospitals. An example of such an initiative is the NIHR-funded HIC health informatics programme. Across five participating Biomedical Research Centres (University College London Hospitals, Imperial College Healthcare, Guy’s and St Thomas’ NHS Foundation Trust, Oxford University Hospitals and Cambridge University Hospitals), the HIC aims to enable the collaborative sharing of NHS data collected and captured as part of usual clinical care to facilitate more effective clinical research. HIC investigators have developed a common data model that is used to extract clinical data (retrospectively and prospectively) from hospital information systems across five scientific themes (viral hepatology, ACS, ovarian cancer, renal transplantation and clinical care). The extracted data are catalogued through a common metadata catalogue and shared via the use of secure safe havens that are accredited to international information security standards such as International Organization for Standardization (ISO) 27001:2013. The HIC approach has demonstrated how robust e-infrastructure and health information modelling approaches are required in order to enable the extraction of clinical data for research from hospitals and to tackle the challenges associated with diverse vendors and information systems being used within and across trusts. A streamlined technical approach also enables researchers to effectively address generic challenges related to data sharing, information governance and patient confidentiality more effectively through the use of standards.
In his State of the Union address in 2015, US President Barack Obama emphasised the power of big data in his personalised medicine initiative. 2 The new era of ‘big data’ brings unprecedented opportunities for prognosis research. Proposals to link clinical data systems locally and embed patient consent for research into clinical care would mitigate the complexities of obtaining complete data and recruiting patients in busy clinical settings, and thereby increase the utility of such cohorts. High-throughput, high-quality research will also be expedited by ambitious discovery projects that are linking EHR data to genomic data, such as the 100,000 Genomes Project,19 and large international EHR research platforms such as the eMERGE network,229 the Danish National Biobank Registry230 and the UK Biobank. 107 However, complex issues, such as how to repurpose EHR data for research (acquisition of ‘research-ready’ data) and extract accurate phenotypes from EHRs, will require careful consideration.
Strengths and limitations
The 4C study adopted rigorous standards for data collection. A wealth of bespoke phenotypic information, including self-reported health functioning, and quality-of-life and clinical data from the EHR, has been collected and is linked to biomarker and genetic data. More than 99% of participants have been linked to comprehensive, nationally collected data on hospitalisations, procedures and mortality.
This study has limitations. Half of the patients approached for inclusion in the study were either ineligible or declined to participate, and low response rates were more marked in hospitals serving an ethnically diverse population, so selection bias must be considered. Sex differences should take into account the fact that a higher percentage of men were recruited into the study, raising questions about whether or not there are sex differences in health-seeking behaviour that could partially account for more severe obstructive CAD cases among men or if there are, in fact, underlying differences in the aetiology, diagnosis, management and treatment of CAD between men and women that are not yet fully understood. EHR data quality varied significantly between hospital information systems and data were often missing or incomplete, which required manual adjudication and validation by trained staff. In particular, we found a high proportion of missing data in the EHR, so under-reporting of medical history, risk factors for coronary disease and clinical symptoms is likely. Finally, in the UK, there is currently no centralised conduit, with national coverage, for linking and extracting primary care data at scale for research. We attempted to link consented participants with primary care resources but that involved going to individual GP practices and performing manual queries and data extraction operations – a route that is both unscalable and cost inefficient. As a result, we were unable to obtain the primary care EHR for our participants but it is foreseen that substantial investment in this area (e.g. CPRD) will make this process feasible in the future.
Conclusions
The 4C cohort is a novel contemporary DNA and plasma resource linked to detailed information collected from the hospital EHR and questionnaire data and health outcomes. Significant challenges remain for automatically extracting the entire patient EHR from health information systems and, as a result, a wealth of data remains inaccessible for research. The design, development and provision of common infrastructure, data standards and services that will allow researchers to access and exploit such data are crucial for expanding 4C in the future, driven by best-practice initiatives such as the NIHR HIC.
Chapter 8 Stable coronary artery disease: use of single prognostic factors
Abstract
Background
The scope and impact of prognosis research and EHR research (in primary and secondary care, and in disease and procedure registries) are intimately related. Guidelines recommend measuring a wide range of clinical factors, but there are uncertainties in the reliability of individual biomarkers and their clinical usefulness in predicting clinical outcomes.
Objectives
To examine evidence supporting the use of five established and novel prognostic factors for predicting the progression of disease and outcomes among SCAD patients.
Methods
An analysis of CALIBER data and literature-based systematic reviews and meta-analyses.
Results
(1) Haemoglobin below 13.5 g/dl for men and 12.8 g/dl for women was associated with increased mortality. (2) Heart rate of > 87 b.p.m. was associated with increased hazard of heart failure and mortality. (3) Published evidence of associations between C-reactive protein (CRP) levels and risk of cardiovascular events was of variable quality, and the degree of association may have been overstated. (4) Pooled data showed that Ch9p21 was convincingly associated with increased risk of first but not subsequent cardiovascular events. (5) SBP in type 2 diabetes patients diagnosed with stable angina declined over time. Lower values of SBP significantly increased the risk of all-cause mortality, non-fatal AMI or non-fatal stroke, as did a greater decline in the rate of change of SBP.
Conclusions
Some biomarkers appear to be useful prognostic factors in SCAD, although poor methodology impairs the interpretation of many study findings.
The studies in this chapter relate to patients presenting for the first time with SCAD (Figure 34).
FIGURE 34.
Overview of the 33 studies in our programme: initial presentation with SCAD.

Introduction
The era of ‘big data’ heralds opportunities to conduct large-scale studies in prognosis research arising from linking EHRs. Prognostic factors for SCAD are measurements associated with the risk of subsequent clinical outcomes, such as (further) heart attack or other CVD, or death. As such, prognostic factors are a specific subset of biomarkers that are related over time to such end points. Although the term ‘risk factors’ is commonly used in the setting of disease onset, prognostic factors are related to disease progression. There are several reasons why the reliable identification of a prognostic factor may be important:
-
It may improve the accuracy of prognostic models, enabling clinicians to assess patient risk more effectively and providing policy-makers with more effective planning tools.
-
It may provide insight into causal processes or mechanisms in disease progression, including some that may suggest new interventions (or new uses for existing interventions).
-
Even if not causally implicated, a prognostic factor may be useful in multivariate risk prediction models, if it is associated with outcomes independently of other prognostic factors.
-
It might be useful on its own as a way to monitor disease progression. For this use, prognostic factors need to be assessed at multiple time points.
The risk factors for the incidence of stable angina and AMI have not been previously compared in large-scale studies. We have demonstrated that associations between the initial presentation of 12 different CVDs and BP,231 smoking,232 diabetes,37 resting heart rate and BMI vary in terms of presence, strength and shape of association. Our findings signify that these are not the same diseases and challenge the widely held assumptions that risk factors have strong associations with the occurrence of all CVDs.
In the studies that follow, we have evaluated a range of potential prognostic factors. These included measurements made in clinical practice that are not currently used prognostically, namely a molecular measure (the blood test haemoglobin) and a physiological measure (heart rate), two of the most widely performed and cheapest clinical measures available. We also evaluated two ‘novel’ markers that are under evaluation and not used in normal clinical practice (the genetic marker Ch9p21) or that are used to a varying degree in clinical practice (CRP). The European Society of Cardiology (ESC) recommends measuring a wide range of clinical factors, including haemoglobin and heart rate, both of which have been incorporated in prognostic models. 233 However, current models are not in routine clinical use, and additional informative biomarkers would be valuable.
Study 15: clinically available biomarkers – threshold haemoglobin levels and prognosis of stable coronary artery disease
This study is based on the peer-reviewed paper by Shah et al. 47 Sections of the text have been reproduced/adapted from the original article; © 2011 Shah et al. 47 This is an open-access article distributed under the terms of the Creative Commons Attribution License (https://creativecommons.org/licenses/by/4.0/).
Introduction
Given the known links between myocardial hypoxia and later acute coronary events, low haemoglobin levels may be a useful prognostic biomarker in SCAD. Furthermore, haemoglobin levels are routinely monitored, and well-established interventions exist to boost haemoglobin levels.
Previous studies have identified an association between low haemoglobin levels and mortality in patients with CAD. 234,235 However, these studies have not been able to characterise the shape of the relationship between haemoglobin levels and mortality, or to determine whether or not threshold levels exist. In addition, they did not examine sex differences and did not distinguish patients with a history of either angina or AMI.
Methods
A retrospective cohort study using CPRD data was carried out. Among CPRD patients aged 35–90 years, we defined two study populations on the basis of coded diagnoses between 2001 and 2006: first 20,131 patients with new-onset stable angina and no prior ACS and second, 14,171 patients with an AMI with survival beyond 7 days. The date of the earliest stable angina or AMI diagnosis for each patient was designated the ‘index date’. For the stable angina population, we included patients with a code for angioplasty or coronary artery bypass but not angina only if they had evidence of previous angina symptoms (e.g. a code for chest pain or a nitrate prescription; the date of the earliest such entry was taken as the index date). We excluded patients with ACS within 7 days of the index date because their initial presentation may have been unstable rather than stable angina. From both populations we excluded patients who died within 7 days of the index date, patients with other cardiac conditions prior to the index date (myocarditis, endocarditis, pericarditis, valve disease or congenital cardiac abnormalities) and patients who were pregnant less than 3 months before the index date. We estimated the glomerular filtration rate from serum creatinine using the four-variable Modification of Diet in Renal Disease formula. Follow-up data were available for a mean of 3.2 years. We analysed data using semiparametric Cox regression with multiple adjustment.
We carried out a systematic review, which involved searching MEDLINE and EMBASE databases between 1966 and November 2008 for prospective studies in which blood biomarkers were measured in patients with stable angina or at least 2 weeks after ACS, and a relative risk for mortality or coronary events by haemoglobin concentration was reported with a p-value or CI. Two reviewers reviewed article titles and abstracts for eligibility and independently abstracted data from eligible articles using a pre-defined coding protocol. Studies with less than 1-year follow-up were excluded. Two reviewers independently abstracted data from eligible articles, with disagreements resolved by consensus, or adjudication by a third reviewer if required.
Results
Patients with lower haemoglobin tended to be older and were more likely to have diabetes, renal impairment, cancer, lower mean corpuscular volume and to be taking iron supplements and nitrates (p < 0.001 for all) (Figures 35 and 36).
FIGURE 35.
Adjusted HRs for death for patients with stable angina or AMI by haemoglobin concentration. (a) Schematic diagram of haemoglobin threshold model for adjusted HRs for mortality; (b) women with stable angina; (c) men with stable angina; (d) women with AMI; and (e) men with AMI. Reproduced from Shah et al. 47 © 2011 Shah et al. 47 This is an open-access article distributed under the terms of the Creative Commons Attribution License (http://creativecommons.org/licenses/by/4.0/), which permits unrestricted use, distribution, and reproduction in any medium, provided the original author and source are credited.

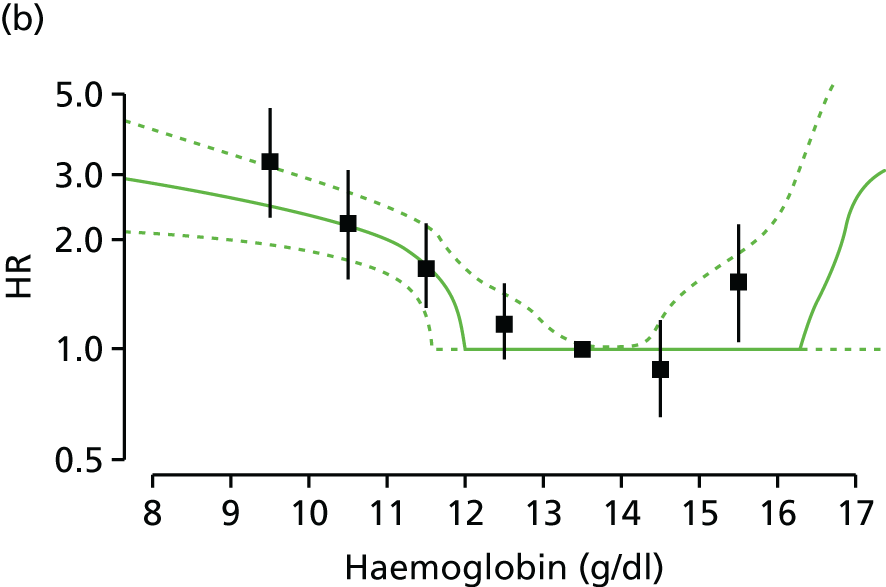

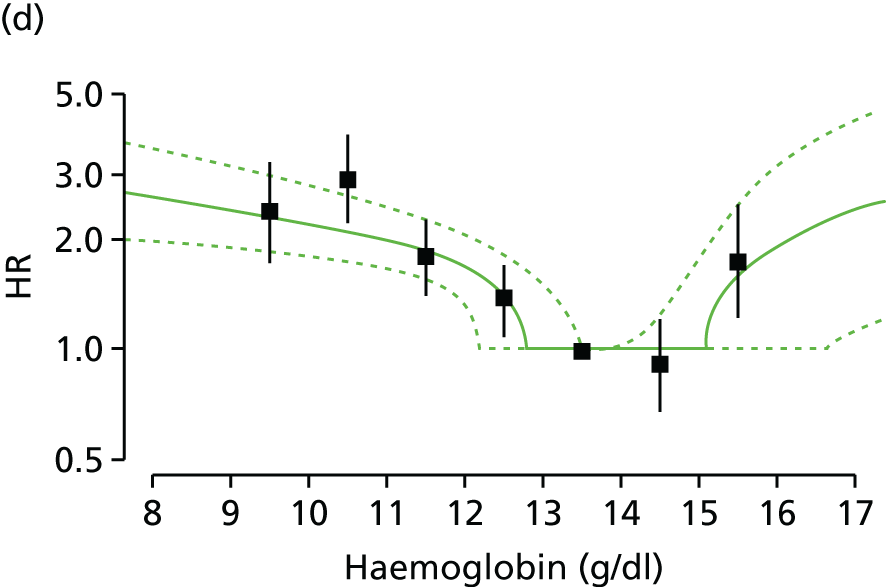
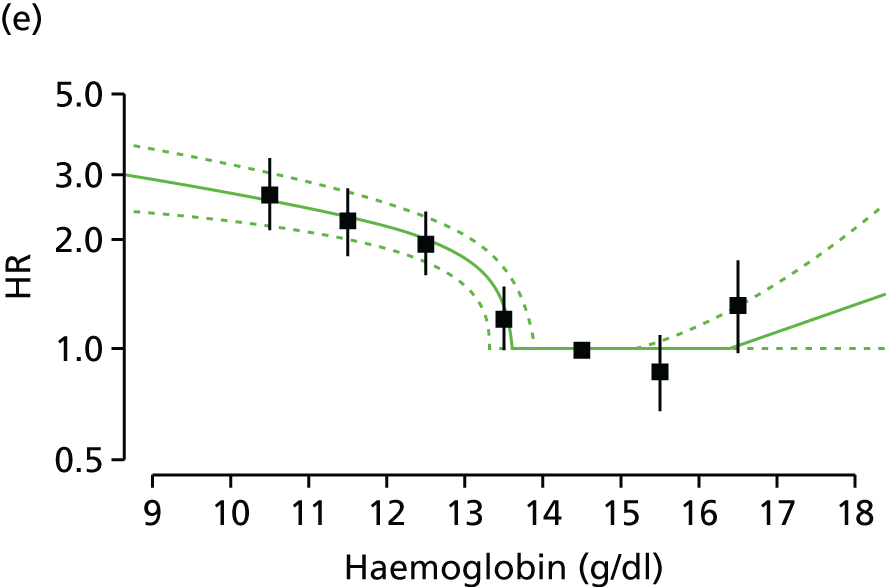
In both men and women, thresholds were identified below which mortality showed a linear inverse relationship with haemoglobin levels (Figures 36 and 37).
FIGURE 36.
Multiply adjusted Kaplan–Meier curves showing the probability in (a) women and (b) men with new-onset stable angina according to haemoglobin level. Adjustment was done by stratification on linear predictors from a Cox model, which included age, eGFR, SBP, total cholesterol, family history of diabetes, stroke and comorbidity (Charlson Index), but not sex or haemoglobin. Reproduced from Shah et al. 47 © 2011 Shah et al. 47 This is an open-access article distributed under the terms of the Creative Commons Attribution License (http://creativecommons.org/licenses/by/4.0/), which permits unrestricted use, distribution, and reproduction in any medium, provided the original author and source are credited.


For stable angina patients, the lower threshold was 11.9 g/dl (95% CI 11.5 to 13.5 g/dl) for women and 13.1 g/dl (95% CI 13.0 to 13.4 g/dl) for men. For men with AMI, this threshold was 13.5 g/dl (95% CI 13.2 to 13.9 g/dl). For the AMI population, the threshold for women with AMI was slightly lower, at 12.8 g/dl (95% CI 12.1 to 13.5 g/dl) compared with 13.5 g/dl (95% CI 13.2 to 13.9 g/dl) for men. The shape and strength of the association was similar in both sexes, and in both AMI and angina patients.
A total of 29.5% of male AMI patients with subthreshold haemoglobin levels were at statistically significantly greater risk of death (HR 2.00, 95% CI 1.76 to 2.29).
The systematic review and meta-analysis identified 10 published studies reporting just 1127 end points in total, not one of which examined risk thresholds.
Discussion
This study has reported more data than all previous studies combined (3174 deaths vs. 1127 events), providing the first estimates of haemoglobin level thresholds associated with a markedly enhanced risk of death. As well as identifying risk thresholds, the study indicates that, contrary to previous assumptions, the relationship between risk and haemoglobin levels is not linear.
Thresholds are higher than might have been anticipated, placing large numbers of patients (15–30%) in a high-risk category. Elevated risk associated with subthreshold haemoglobin levels was seen in both women and men, and in both AMI and angina patients, with risk being more pronounced in AMI patients. Attributable risk calculations suggest that the link between haemoglobin levels and mortality could be responsible for up to one-quarter of deaths after AMI. This level of risk is similar to that identified for LDL cholesterol and fibrinogen. There was some evidence of an association between high haemoglobin levels and poor outcomes, but the number of patients affected was small.
New clinical trials to assess whether or not haemoglobin levels are causal and whether or not clinicians should intervene to increase haemoglobin levels are warranted.
Conclusions
Our findings provide support for clinical trials to evaluate strategies to increase haemoglobin levels in patients with SCAD, through either iron supplementation or more aggressive treatments, such as intravenous iron infusions or erythropoiesis-stimulating agents. Further studies are needed to assess the potential prognostic value of haemoglobin in stratifying SCAD patients.
Study 16: clinically available biomarkers – heart rate as a physiological prognostic biomarker for stable coronary artery disease
This manuscript by Archangelidi et al. 48 is in preparation.
Introduction
The relationship between resting heart rate and CVD and mortality has been extensively investigated and acknowledged in patients with SCAD236 and in the general population. However, findings remain controversial. In 2008, two large trials of ivabradine, a pure heart rate-lowering agent without other known cardiovascular effects, showed controversial effects related to risk reduction for both stable and unstable coronary disease in patients with CAD and chronic heart failure,237 and in patients without reduced left ventricular function with SCAD;237 these results indicated conflicting scenarios for the mechanisms through which an increased heart rate could be involved in an increased risk of CVD. 238
Contemporary data regarding elevated heart rate and prognosis of CAD patients are derived from clinical trials or angiographic registries that are collected solely for research purposes and are confined to high-risk patients238–240 or those admitted for ACS,240,241 and not among the general population. 236,238 These data are biased by the selective nature of the populations studied and might compromise external validity. 233 In addition, much of the evidence draws on the effect of heart rate-lowering medication and not the actual heart rate marker on cardiovascular end points. 237,242,243 The majority of studies have also failed to examine potential differences in heart rate effects on cardiovascular events between men and women, probably owing to limited sample sizes and subsequent restricted statistical power. 240,244–246 The preponderance of composite cardiovascular end points in the published literature indicates the need for a study that will investigate the association of heart rate and specific cardiovascular events in a SCAD population.
Using linked EHRs we investigated the prognosis of SCAD in patients with low or elevated heart rate, using real-world data in a general population. The aim of this study was to examine the relationship between resting heart rate and a range of previously unexplored future cardiovascular phenotypes and non-cardiac complications, as well as cerebrovascular events, in a large population of patients with suspected or proven SCAD. We also aimed to determine the shape of any associations and to explore potential differences in the associations between men and women.
Methods
Data sources
Linked CALIBER data. CPRD data were used to obtain heart rate measurements, demographic variables and other risk factors.
Study design
Observational prospective cohort study.
Study population
A cohort of 2.23 million people covering approximately 5% of the UK population was drawn from patients registered with their general practice between January 1997 and March 2010. Participants were eligible for inclusion if they were aged ≥ 30 years at study entry (‘index date’), had been registered with the practice ≥ 1 year prior to the index date, had at least one prior diagnosis of CVD (any time prior to the index date, or in case of its absence, any time after the index date) and had at least one recorded heart rate measurement after the index date. Where patients had a non-fatal or fatal CVD event prior to and after the index date, we used the date of the event after the index date. Participants were censored on the date of leaving the practice, last submission of data, death or end-point occurrence.
Heart rate
Heart rate (in b.p.m.) was prospectively collected by general practice staff. The method of heart rate measurement was not recorded in the database, although values are likely to have been obtained through palpation at the wrist, auscultation of the heart and readings from an automated BP device or ECG.
Cardiovascular risk factors
Information on CVD risk factors was obtained from CPRD, as recorded during consultations in primary care. The most recent measurement (or prescription) recorded in CPRD up to 1 year before or on the date of study entry (first heart rate measurement after the index date) was used to define baseline covariates. Socioeconomic status was derived from the ONS using the IMD,88 which was divided into five categories (quintiles), whereby the first quintile corresponds to the least deprived and the fifth quintile to the most deprived groups. The most recent smoking record before the index date was used to classify participants as never, ex or current smokers.
End points
The primary end points were the first presentation of non-fatal or fatal CVDs after the heart rate measurement date across the four data sources. CVD outcomes included stable angina, AMI, heart failure, a composite of cardiac arrest plus ventricular fibrillation plus SCD, a composite of cerebrovascular diseases including ischaemic stroke plus subarachnoid haemorrhage plus intracerebral haemorrhage, PAD and CVD mortality and all-cause mortality. Diagnoses were identified using ICD-10 codes for hospital data (HES) and mortality data (ONS), from Read Codes for primary care data (CPRD) and bespoke variables in the ACS registry (MINAP).
Statistical analysis
Heart rate was divided into sex-specific quintiles (with quintile 1 as the reference). In sensitivity analyses we used heart rate as a continuous variable of a 10-b.p.m. increase. Differences in risk factors according to heart rate quintiles were tested using the F-statistic from analysis of variance or its non-parametric version (Kruskal–Wallis test) and the chi-squared statistic, as appropriate. The association between heart rate and each end point was assessed using Cox proportional hazard models with time since study entry as the timescale, adjusting for baseline age and sex and stratified by primary care practice. Covariates were added in the models in two steps (sex- and age-adjusted) and were adjusted for common CVD factors (SBP, smoking, BMI, diabetes, HDLs, total serum cholesterol and IMD classification).
Change in heart rate was classified as either downwards (a fall of one, two or three quintiles), upwards (one, two or three quintiles higher) or stable. This risk represented the hazard of experiencing a cardiovascular event when the proportion of quintile changes increased (i.e. whether or not downwards or upwards jumps in heart rate quintiles affect risk). Statistical analyses were performed using Stata® version 13 (StataCorp LP, College Station, TX, USA) and R version 3.0.3.
Results
Baseline characteristics
The cohort consisted of 51,703 patients (44% women) with heart rate measurements available at baseline (Table 19). Participants experienced 21,918 cardiovascular events (including 9960 atrial fibrillation and 4216 bleeding events) during 132,359.7 person-years of follow-up (mean follow-up of 2.56 years per patient). Women were less likely than men to have experienced an AMI event prior to their inclusion in the study (p < 0.05). Compared with quintile group 1 (heart rate < 65 b.p.m.), patients in quintile group 5 (heart rate > 83 b.p.m.) were more commonly female, current smokers, hypertensive and diabetic, and had larger proportions of people in the top deprivation quintile (p < 0.05). Fewer patients in quintile group 5 were receiving cardiovascular medications, including beta-blockers, than in quintile 1. Participants who had a past unstable angina episode and were stabilised were more likely to be white, hypertensive and diabetic than participants with past stable angina or AMI, who received more beta-blocker medication and had higher BP or antiarrhythmic medication (p < 0.05).
Baseline characteristics | Q1 (20–60 b.p.m.) | Q2 (61–68 b.p.m.) | Q3 (68.5–75 b.p.m.) | Q4 (75.5–82 b.p.m.) | Q5 (82.5–240 b.p.m.) | Total, n (%) | |||||
---|---|---|---|---|---|---|---|---|---|---|---|
Mean | SD | Mean | SD | Mean | SD | Mean | SD | Mean | SD | ||
Demographic factors | |||||||||||
Age at entry (years) | 71.5 | 10.6 | 71.6 | 11.2 | 72.6 | 11.5 | 73.1 | 11.7 | 72.5 | 11.8 | 51,703 (100) |
Female (%) | 33.46 | 40.2 | 46.3 | 50.4 | 52.7 | 22,809 (44.1) | |||||
White ethnicity (%) | 79.7 | 79.7 | 79.9 | 80.4 | 80.9 | 37,980 (80.1) | |||||
Deprivation (most deprived quintile) (%) | 16.5 | 18.7 | 19.9 | 21.1 | 24.3 | 10,300 (19.98) | |||||
Clinical factors | |||||||||||
Heart rate (continuous) (b.p.m.) | 55.4 | 5.3 | 65.2 | 2.2 | 71.6 | 1.6 | 78.8 | 1.8 | 94.0 | 12.0 | 51,703 (100) |
SBP (mmHg) | 138.3 | 20.0 | 137.4 | 19.6 | 138.2 | 19.8 | 138.5 | 19.6 | 138.9 | 20.7 | 44,297 (85.6) |
DBP (mmHg) | 75.5 | 10.7 | 76.3 | 10.5 | 76.9 | 10.6 | 77.4 | 10.5 | 78.6 | 11.4 | 44,297 (85.6) |
HDL (mmol/l) | 1.3 | 0.4 | 1.3 | 0.4 | 1.3 | 0.4 | 1.4 | 0.4 | 1.4 | 0.5 | 26,461 (51.2) |
LDL (mmol/l) | 2.4 | 0.8 | 2.4 | 0.8 | 2.5 | 0.9 | 2.5 | 0.9 | 2.6 | 0.9 | 21,381 (41.0) |
Total cholesterol (mmol/l) | 4.4 | 1.0 | 4.5 | 1.1 | 4.6 | 1.1 | 4.6 | 1.1 | 4.7 | 1.1 | 35,078 (67.8) |
Triglycerides (mmol/l) | 1.54 | 0.87 | 1.58 | 1.08 | 1.60 | 1.09 | 1.61 | 0.97 | 1.66 | 1.23 | 26,382 (51.0) |
Creatinine (µmol/l) | 103.3 | 35.6 | 101.9 | 42.3 | 102.2 | 41.8 | 103.1 | 121.7 | 101.6 | 42.1 | 37,083 (71.7) |
Haemoglobin (g/dl) | 13.6 | 1.5 | 13.5 | 1.6 | 13.4 | 1.6 | 13.4 | 1.6 | 13.3 | 1.7 | 28,096 (54.3) |
Lymphocytes (109/l) | 2.00 | 0.89 | 2.05 | 1.49 | 2.04 | 1.20 | 2.05 | 1.44 | 2.05 | 1.24 | 25,181 (48.7) |
Neutrophils (109/l) | 4.35 | 1.66 | 4.43 | 1.71 | 4.60 | 1.96 | 4.69 | 1.92 | 5.01 | 2.18 | 25,213 (48.7) |
Behavioural/environmental factors | |||||||||||
Non-smokers (%) | 38.4 | 39.4 | 41.5 | 41.7 | 39.5 | 19,713 (95.2) | |||||
Ex-smoker (%) | 50.32 | 47.6 | 44.3 | 43.5 | 43.3 | 22,670 (95.2) | |||||
Current smoker (%) | 10.32 | 11.9 | 12.7 | 13.1 | 15.3 | 6177 (95.2) | |||||
BMI (kg/m2) | 27.9 | 4.8 | 28.3 | 5.3 | 28.1 | 5.4 | 28.3 | 5.7 | 28.6 | 6.2 | 23,928 (46.2) |
Family history of CHD (%) | 2.45 | 2.14 | 1.96 | 1.70 | 1.44 | 1013 (1.96) | |||||
Type 2 diabetes (%) | 10.1 | 11.2 | 11.8 | 11.8 | 14.3 | 51,703 (100) | |||||
Current alcohol drinker (%) | 60.3 | 57.3 | 55.1 | 54.6 | 52.4 | 9367 (18) | |||||
Hypertensive (%) | 5.3 | 5.1 | 6.2 | 6.4 | 8.8 | 2791 (6.3) | |||||
Medication (%) | |||||||||||
Beta-blockers | 76.5 | 60.1 | 45.4 | 36.5 | 28.8 | 26,212 (50.7) | |||||
Anti-anginal medicationa | 68.0 | 65.2 | 65.3 | 64.2 | 66.0 | 34,040 (65.8) | |||||
BP medication | 78.5 | 76.1 | 74.6 | 72.2 | 73.8 | 38,871 (75.2) |
Heart rate and presentation of cardiovascular diseases
Age- and sex-adjusted analyses showed a significantly increased hazard of heart failure, cardiovascular and all-cause death and atrial fibrillation in quintile 5 compared with quintile 1 (Figure 37). An increased risk of stroke and bleeding events was also identified. These associations remained after adjusting for sex, age, smoking, SBP, diabetes, cholesterol, HDL, BMI and IMD. Strong associations were found between high heart rate and hazard of heart failure independently of the type of previous coronary event (for overall coronary event types HR 2.42, 95% CI 2.19 to 2.68). Association with the risk of stroke was also found for participants in quintile 5 (HR 1.25, 95% CI 1.10 to 1.43) and for people who experienced a previous stable angina event (HR 1.28, 95% CI 1.11 to 1.48), but not in people who experienced an AMI or unstable angina, probably owing to the smaller number of cases and the subsequent loss of statistical power. Similarly, bleeding and atrial fibrillation events were higher in people in quintile 5 (HR 1.21, 95% CI 1.10 to 1.33) than in people in quintile 1 (HR 1.58, 95% CI 1.49 to 1.68). The association with atrial fibrillation was present when taking into account the different subtypes of prior stable coronary events. No association was found between heart rate and AMI, PAD, stable angina or SCD, the last of which perhaps as a result of the limited number of events.
FIGURE 37.
Multivariable-adjusted HRs for the association between heart rate (top vs. bottom quintile) and the risk of eight CVDs, atrial fibrillation and bleeding events in CALIBER patients. MI, myocardial infarction.

Increased risk of heart failure, AMI and stable angina were found among participants with increased heart rate quintile changes, irrespective of their direction (upwards or downwards). However, the small sample size resulted in low power and such effects were significant only at the 10% level (results not shown).
Effects of age and sex
We found a slightly higher increase in the risk of stroke among women (HR 1.36, 95% CI 1.13 to 1.64) as opposed to among men (HR 1.12, 95% CI 0.92 to 1.37) in quintile 5. However, at lower heart rate values these associations were reversed, with men in quintile 4 having a greater risk of stroke (HR 1.35, 95% CI 1.13 to 1.62); for women this association was not statistically significant. A higher risk of atrial fibrillation was found in men in quintiles 4 and 5 (HR 1.28, 95% CI 1.18 to 1.39 and HR 1.73, 95% CI 1.59 to 1.88, respectively) than in women, with risk restricted to heart rate values > 87 b.p.m. (HR 1.39, 95% CI 1.27 to 1.53). Higher risk of heart failure was similar between men and women in quintiles 4 and 5, as was the risk for a 10-b.p.m. increase in heart rate. For every 10-b.p.m. heart rate increase, the risk for CVD and all-cause mortality was attenuated in people aged > 80 years. A mild but consistent decrease of heart failure, atrial fibrillation and bleeding risk was also found in people aged > 80 years, with the risk of heart failure falling for those aged > 60 years.
Discussion
In this prospective study, we report an association between elevated heart rate and risk of cardiovascular end points in patients with SCAD. Our study confirms previous reports that elevated heart rate is a predictor of mortality and heart failure in patients with SCAD. 236,238,239
However, our results also extend these observations to cerebrovascular events, atrial fibrillation and complications such as bleeding events. We found no association between increased heart rate and AMI, stable angina, SCD or PAD, whereas a strong association was identified with heart failure, cardiovascular death and all-cause mortality. An increased risk of stroke, atrial fibrillation and bleeding events was also found in both univariate and multivariate analyses in people with heart rates of > 87 b.p.m. in quintile 5 compared with people in quintile 1 (< 65 b.p.m.).
The effects of heart rate-lowering medication on mortality in patients with CAD have been extensively demonstrated. 247–249 However, the association between resting heart rate values (without the mediation of heart rate medication in analyses) and specific cardiovascular end points and atrial fibrillation has been underinvestigated. The lack of association between heart rate and CHD found in this study contrasts with results from previous post hoc analyses of trials with heart rate-lowering agents (e.g. ivabradine), suggesting that it would improve outcomes in patients with SCAD. 250
In patients with heart failure, the reduction in heart rate with ivabradine has been shown to improve clinical outcomes, beyond the improvements observed with beta-blockers. 251 The benefits of lowering heart rate in patients with heart failure but not in those with SCAD may reflect the fact that an elevated heart rate is attributable to different pathophysiological mechanisms in these two conditions,252 such as neurohormonal activation in patients with heart failure and further ventricular remodelling. Sympathetic activation and progressive disruption of autonomic balance may also be important in the pathogenesis of atrial fibrillation after coronary artery bypass grafting. 253,254 White et al. 255 found a significant increase in the incidence of atrial fibrillation among patients in whom the administration of beta-adrenoceptor antagonists was ceased compared with those whose drug treatment was continued after surgery. Further evidence of a mechanistic link of heart rate with atrial fibrillation comes from genome-wide association studies that have identified heart rate-increasing alleles at five loci associated with both increased and decreased risk of atrial fibrillation. 256
Published studies have suggested a threshold effect of heart rate on mortality outcomes, above which risk increases steeply. Data from the Coronary Artery Surgery Study (CASS) registry suggested an increased risk of all-cause mortality for a heart rate of > 77 b.p.m. and of CVD death at a heart rate of > 83 b.p.m.,236 whereas other studies have supported a slightly lower threshold of > 70 b.p.m. 246 Data from the ivabradine for patients with stable coronary artery disease and left-ventricular systolic dysfunction (BEAUTIFUL) study have suggested that the increase in death and heart failure outcomes rose continuously at ≥ 70 b.p.m., whereas the relationship was less pronounced for AMI and revascularisation. 238 Our study provides evidence for a similar shape in risk as the BEAUTIFUL study, with a steep increase in risk for heart failure and cardiovascular mortality at > 70 b.p.m., a threshold significantly lower than the majority of the available studies. A similar steep increase was also found for atrial fibrillation events above a heart rate of 70 b.p.m., whereas the risk of SCD shows an increase at higher heart rate values (> 90 b.p.m.).
Heart rate is one of the most easily accessible biomarkers; however, rates of adequate heart rate control appear to be low in current clinical practice, potentially representing a missed therapeutic opportunity. In designing randomised trials, the target population, the choice of composition of the primary end point and the likely rate at which the end point accrues can all be informed by the examination of linked EHRs. Future clinical trials on heart rate-specific medications, such as ivabradine, should refine our understanding of the potential role for specific heart rate lowering in the treatment and prevention of people at early stages of heart failure and resting tachycardia.
Conclusions
Resting heart rate is an easily accessible biomarker in primary and secondary care patients with heterogeneous associations with CVDs. Higher resting heart rates increase the hazard of heart failure events, stroke in women, atrial fibrillation and bleeding events, without substantially affecting the hazard of vascular events in the coronary arterial beds. Our findings show how disaggregation of CVD into specific phenotypes can help to disentangle the underlying disease mechanisms, with implications for clinical practice and the design and interpretation of clinical trials.
Study 17: novel biomarkers – circulating marker of inflammation (evaluation of the quality of research using C-reactive protein in stable coronary artery disease)
This study is based on the peer-reviewed paper by Hemingway et al. 49
Introduction
Prognostic biomarkers have great potential in translational medicine research and could support clinical decision-making. However, based mainly on experience in cancer, concerns have been raised about the quality of prognostic biomarker research. 31 In particular, evidence syntheses have highlighted issues with publication bias and the selective reporting of results. 257,258 In addition, few evaluations have assessed the quality of research evidence related to individual biomarkers.
It is unclear whether or not similar biases affect the evidence base for biomarkers in CVD. To address this question, we systematically analysed the evidence related to use of CRP as a prognostic biomarker in SCAD. CRP has been widely studied in this context, and its measurement is recommended in US259 and European260 clinical guidelines (although actual clinical practice varies widely).
We undertook a systematic review, meta-analysis and metaregression of published evidence on CRP. Our key aims were to assess the quality of published evidence, the extent to which reported associations are independent of other prognostic factors, the scale of any bias attributable to small study size, any possible sources of heterogeneity and whether or not papers report clinically useful figures on the ability of CRP measurements to distinguish patients who experience a coronary event from those who do not.
Methods
As no agreed criteria exist for assessing reporting quality, we developed a 17-item list based on previous work in CVD and cancer (including the REMARK reporting guidelines). Notably, this list also included whether or not studies reported a reference to a study protocol or statistical protocol specified in advance of data collection.
MEDLINE (1966–2009) and EMBASE (1980–2009) were searched and selected prospective studies of patients with stable coronary disease, reporting a relative risk for the association of CRP with death and non-fatal cardiovascular events. We identified relevant papers and assessed individual papers according to the 17-item checklist. Two reviewers independently abstracted data from eligible articles (n = 116) using a predefined coding protocol. Individual item disagreement between the two reviewers was resolved by consensus or, rarely, adjudication by a third reviewer. Reported relative risk, ORs or HRs were extracted from each eligible paper.
Statistical analysis
Relative risk estimates were converted to a standard scale of effect, in which the highest third in the CRP distribution was compared with the lowest third. The I2-statistic was used to assess statistical heterogeneity among studies. Funnel plots and quantitative methods (including Egger’s test) were used to assess small-study publication bias.
Results
Of 1566 articles, 83 studies met inclusion criteria, covering 61,684 patients and 6485 events.
The median number of study-quality items reported was seven out of a possible 17, with no increase between 1997 and 2009. Just two studies reported a study protocol; none reported a statistical analysis protocol (Figure 38). The meta-analysis generated a relative risk for the top versus bottom third of the CRP distribution of 1.97 (95% CI 1.78 to 2.17), although the size of effect varied widely (I2 = 79.5) (Figure 39). Just 13 studies adjusted for standard risk factors; adjustment lowered relative risk to 1.65 (95% CI 1.39 to 1.96). Ten different methods were used to compare CRP levels, with use of continuous measures giving lower relative risks.
FIGURE 38.
Quality of individual reports (n = 17 items, n = 83 studies) included in our systematic review of CRP as a prognostic biomarker in SCAD based on REMARK guidelines. Reproduced from Hemingway et al. 49 © 2010 Hemingway et al. This is an open-access article distributed under the terms of the Creative Commons Attribution License (http://creativecommons.org/licenses/by/4.0/), which permits unrestricted use, distribution, and reproduction in any medium, provided the original author and source are credited.
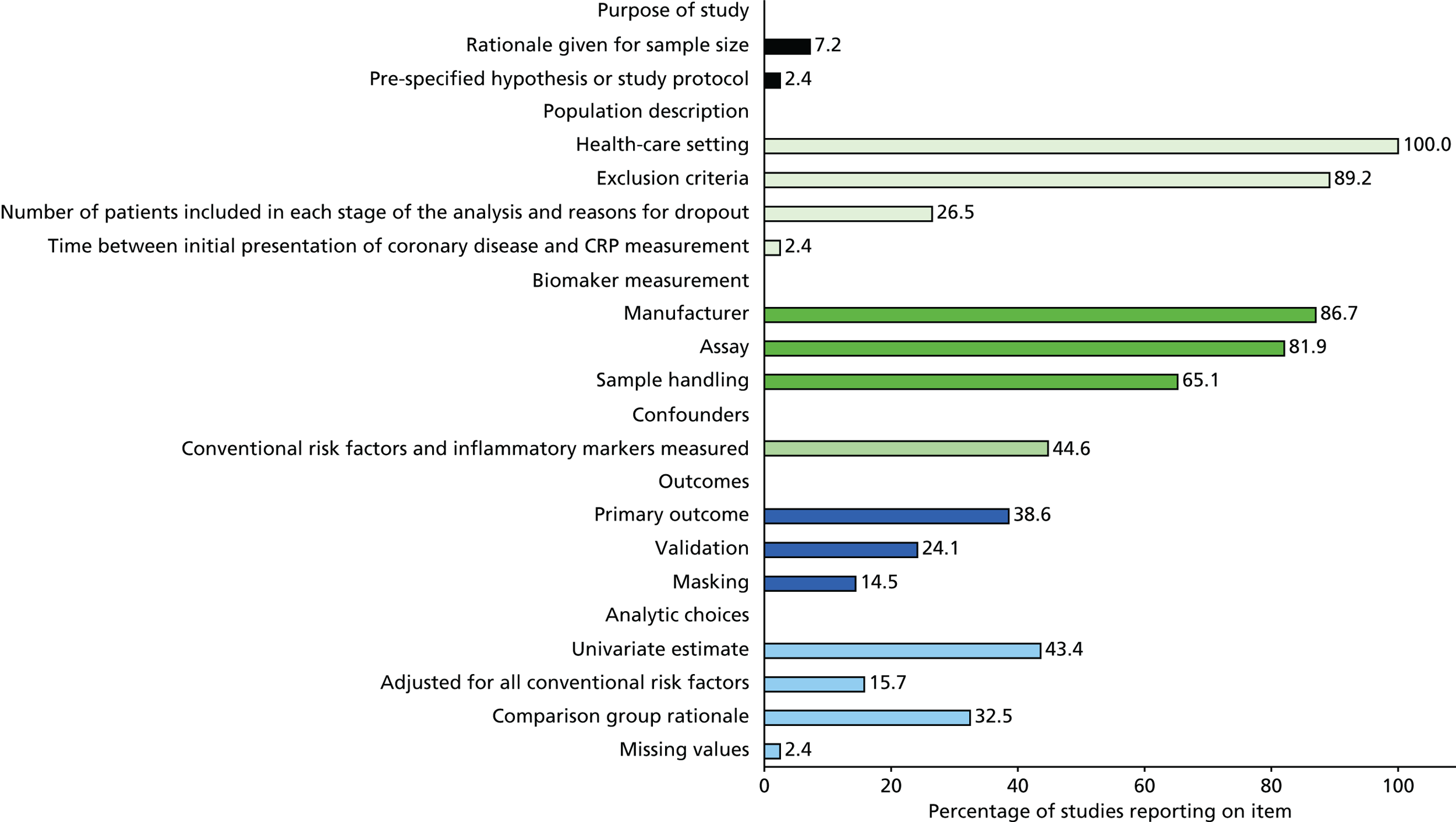
FIGURE 39.
Forest plot of the effect of CRP on prognosis among patients with SCAD based on 83 studies included in our systematic review and meta-analysis. For example, Jánoskuti et al. 264 adjusted for age, sex, smoking and obesity and three other non-conventional risk factors, but not for diabetes. a, Four studies did not report the number of events; b, five studies made no adjustments at all; one (Minoretti 2006313) adjusted for age and sex. A, age; D, diabetes; G, sex; L, lipids; O, obesity; S, smoking; Wt, study weight percentage from random-effects meta-analysis. Reproduced from Hemingway et al. 49 © 2010 Hemingway et al. 49 This is an open-access article distributed under the terms of the Creative Commons Attribution License (http://creativecommons.org/licenses/by/4.0/), which permits unrestricted use, distribution, and reproduction in any medium, provided the original author and source are credited.
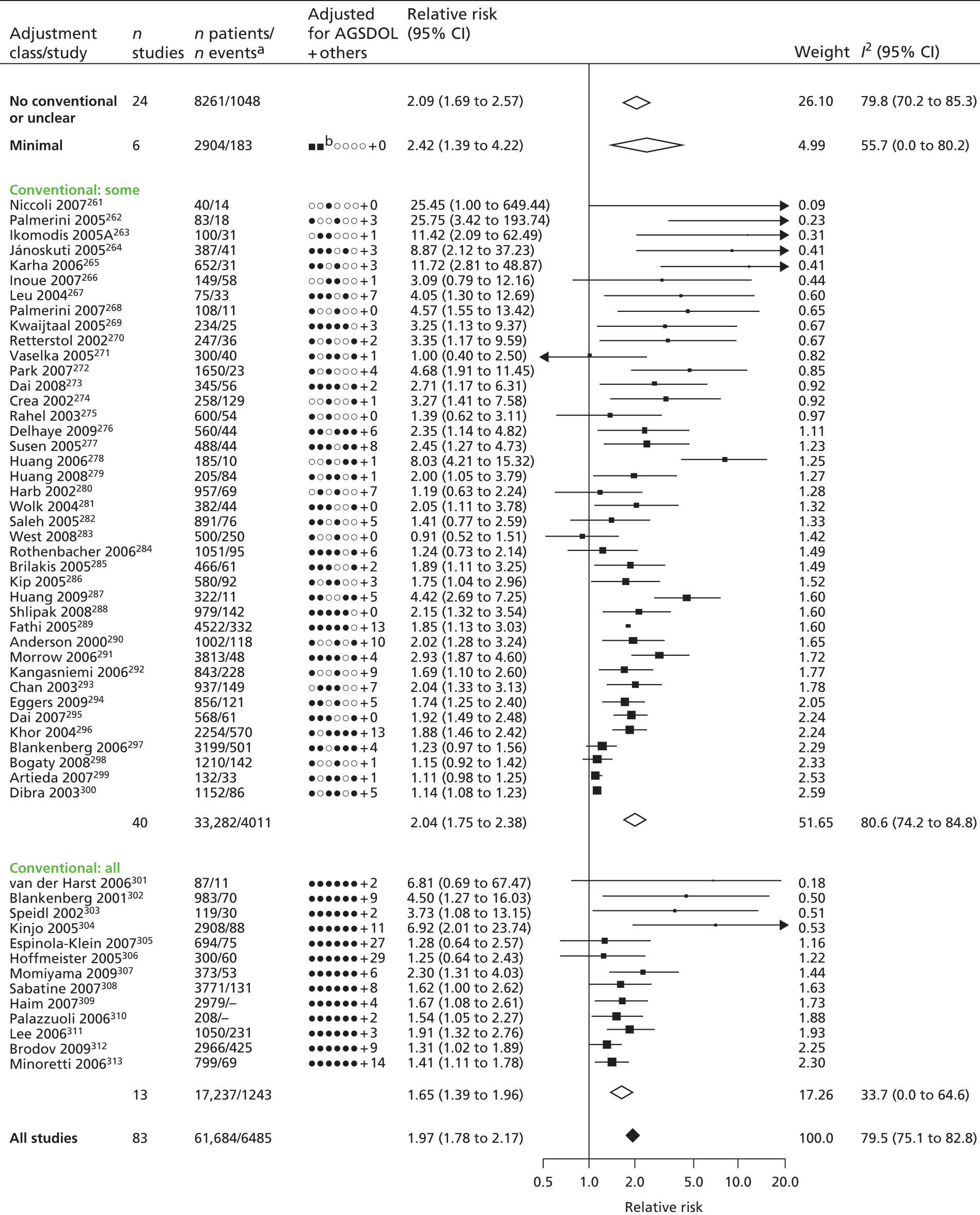
Funnel plots and quantitative analyses provided strong evidence for publication bias (Figure 40). Further adjustment for publication bias reduced the relative risk to 1.19 (95% CI 1.13 to 1.25). Just two studies reported a measure of discrimination (c-statistic). When data from 20 suitable studies were pooled, the detection rate was calculated as 31% for a 10% false-positive rate, giving a c-statistic value of 0.61 (95% CI 0.57 to 0.66).
FIGURE 40.
Funnel plot showing extent of publication bias for studies included in our systematic review and meta-analysis of CRP as a prognostic biomarker in SCAD, with contours showing different levels of study significance. MA, meta-analysis. Reproduced from Hemingway et al. 49 © 2010 Hemingway et al. 49 This is an open-access article distributed under the terms of the Creative Commons Attribution License (http://creativecommons.org/licenses/by/4.0/), which permits unrestricted use, distribution, and reproduction in any medium, provided the original author and source are credited.
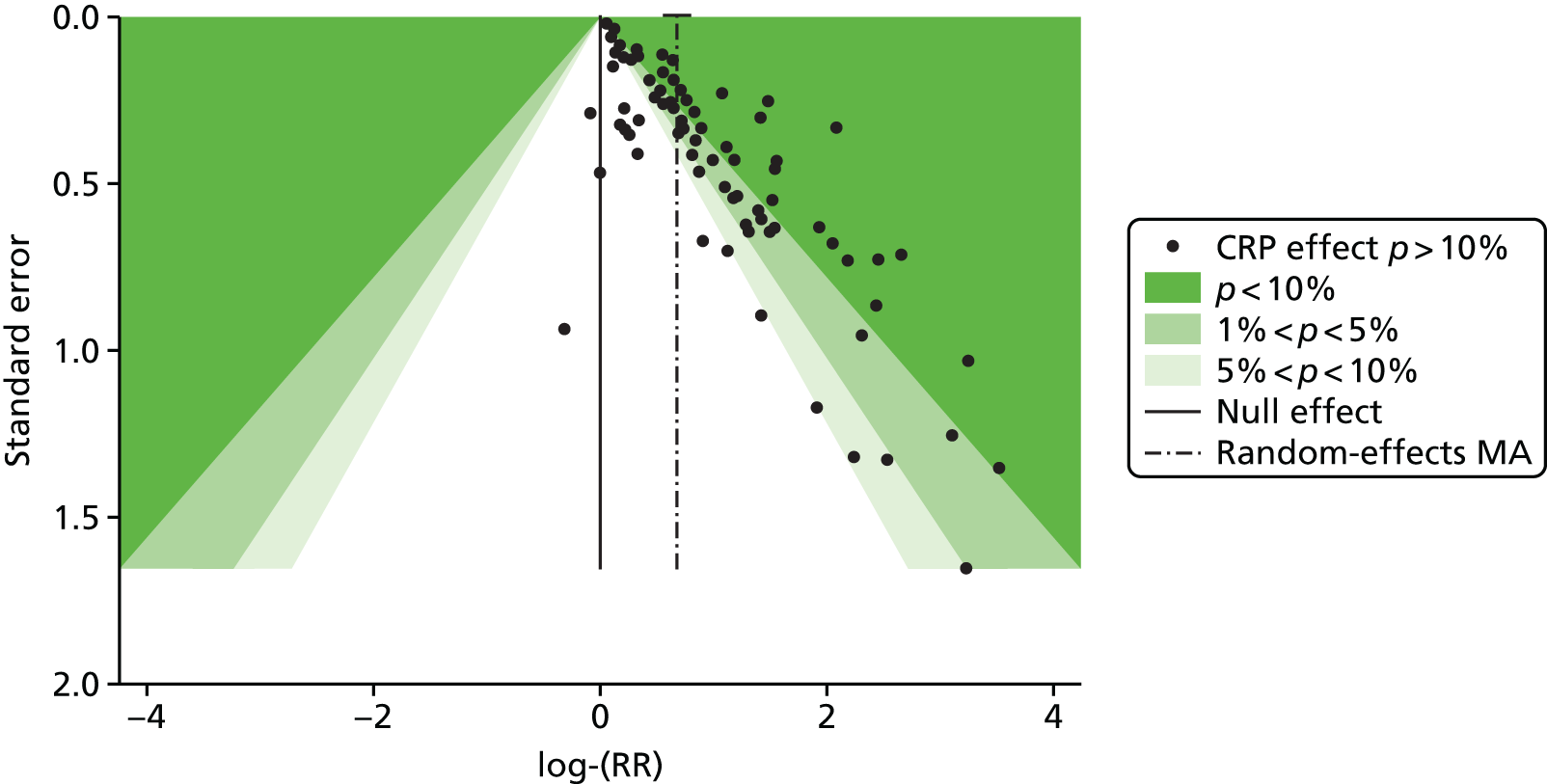
Discussion
This large-scale systematic review and meta-analysis identified significant shortcomings in the evidence base on CRP as a prognostic biomarker, particularly the absence of a pre-specified statistical analysis protocol and strong evidence of publication bias having a sizeable impact on association.
There are no agreed comprehensive criteria for measuring the quality of study reports. The REMARK reporting guidelines for tumour prognostic markers provide a useful start, but are not currently in a form that lend themselves to measurement and omit reference to statistical analytic protocols.
Our review suggests that there is a need for new clinical cohorts with published pre-specified statistical analytic protocols and prospective registration of studies, which can be pooled to evaluate the true usefulness of the role of CRP in prognosis in SCAD. Better reporting is required and existing guidelines require development across disease areas and formalisation into data extraction tools.
Along with a widespread failure to adjust for potential confounders, the findings call into question the reported association between CRP and prognosis of patents with SCAD. Furthermore, the discriminatory potential of CRP was so low as to offer little clinically useful information.
Conclusions
The findings of this evaluation suggest that current CG recommendations on the measurement of CRP need to be revised. More generally, they highlight major flaws in the design of studies on prognostic biomarkers. There is a need for enhanced reporting and, in particular, for the registration of studies and statistical analysis protocols.
Study 18: novel genetic factors – the 9p21 chromosomal locus and risk of coronary disease events
This study is based on the peer-reviewed paper by Patel et al. 50
Introduction
Chromosomal locus 9p21 (Ch9p21) is a well-established genetic risk factor for CHD. Several lines of evidence have implicated Ch9p21 in risk of first coronary events,314 but it is less clear whether or not it also influences susceptibility to further coronary events, with conflicting results from mostly small-scale studies.
The distinction is important, as the predictive value of genetic variants at Ch9p21 may differ for first and subsequent coronary events. In addition, differences would also imply that the mechanisms of disease may not be the same in first and subsequent coronary events, which would have important implications for secondary prevention and drug development.
To address this question, we carried out a systematic review and meta-analysis to synthesise the evidence relating to Ch9p21 and the risk of first and subsequent coronary events, and to identify any sources of bias that could influence associations.
Methods
We searched PubMed and EMBASE from inception to 30 June 2013 for studies reporting associations between genetic variants at Ch9p21 and CHD up to 30 June 2013. Two reviewers extracted information, and discrepancies were resolved by consensus. We also searched studies reporting associations of genetic risk scores with CHD, as they may have incorporated data on the Ch9p21 locus [thus, our search strategy encompassed terms capturing the genetic locus (9p21 or 9p21.3), genetic risk score and CHD].
Prospective cohorts and nested case–control or cohort studies were included in the review. We excluded case–control or cross-sectional studies of Ch9p21 and CHD, editorials and reviews.
Two authors independently extracted data and any discrepancies were resolved by consensus.
Statistical analysis
The size of effect was quantified as HRs per Ch9p21 risk allele. Funnel plots and quantitative analyses (Egger’s test) were used to examine small-study bias. The possibility of survival bias was examined by comparison of risk allele frequencies in CHD-free population cohorts and in clinical cohorts.
Results
Of 327 papers identified, 25 satisfied inclusion criteria. As well as these data, from 33 cohorts, we added data from two unpublished studies. After the elimination of data from four cohorts reporting genetic results in a recessive format, we analysed 31 cohorts in total, covering 193,372 individuals and 20,100 coronary events.
In the 16 cohorts reporting first events, 15,664 events occurred among a population of 168,209. The pooled HR for Ch9p21 was 1.19 (95% CI 1.17 to 1.22) per risk allele. In cohorts of individuals who had already experienced CHD, 4436 events occurred among a population of 25,163. The pooled HR for Ch9p21 for subsequent events was 1.01 (95% CI 0.97 to 1.06) per risk allele. Funnel plots revealed no evidence of small-study bias (Figure 41). Risk allele frequencies were similar in population and clinical cohorts, arguing against any survival bias.
FIGURE 41.
Small-study bias illustrated by funnel plots of the association of Ch9p21 (per risk allele) with (a) first and (b) subsequent coronary heart disease events in 31 studies included in our systematic review and meta-analysis. Reproduced from Patel et al. 50 Copyright © 2014 American College of Cardiology Foundation. Published by Elsevier Inc. This article is available under the terms of the Creative Commons Attribution License (CC BY). You may copy and distribute the article, create extracts, abstracts and new works from the article, alter and revise the article, text or data mine the article and otherwise reuse the article commercially (including reuse and/or resale of the article) without permission from Elsevier.

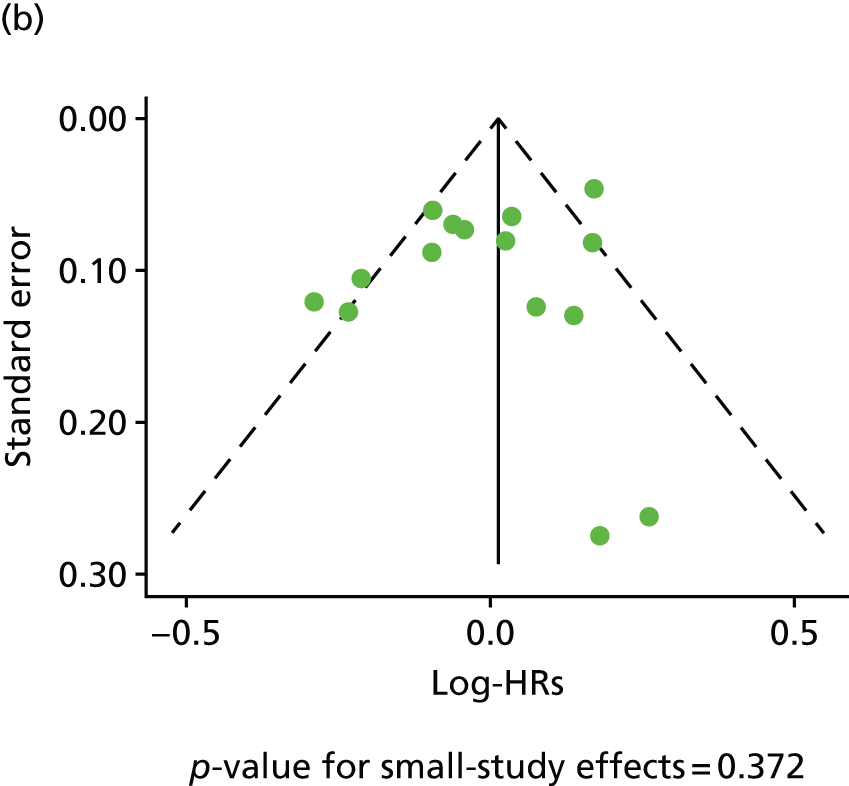
Discussion
This systematic review and meta-analysis of data from more than 190,000 individuals, experiencing 20,000 events, has confirmed that genetic variants at Ch9p21 are associated with initial CHD events. However, it found no evidence to suggest that Ch9p21 contributes to the risk of further events in patients with established CHD. There was no evidence to suggest that this differential association reflected a lack of power or publication or survival bias.
Our study has important research and clinical implications. First, our findings suggest that future genetic association studies should be cautious in assuming that genetic variants have similar effects for both first and subsequent CHD events. Second, further research into mechanistic differences that may account for the differential genetic effect is warranted to enhance understanding of the underlying reasons for this. Our findings point to the need for a consortium of studies among individuals with established CHD to better understand the genomic susceptibility for subsequent CHD events and to carry out analysis, including assessment for selection biases, which is not possible with literature-based meta-analyses. More research is warranted to determine the effect of mechanistic differences for the differential genetic effect, to enhance understanding of the underlying reasons.
Conclusions
Our findings suggest that disease processes may differ between first and subsequent coronary events, with Ch9p21 playing a part only in the former. The precise role of Ch9p21 in disease is difficult to assess, as the molecular mechanisms affected by Ch9p21 genetic variants are unclear. A better understanding of Ch9p2’s role could help to clarify how pathogenic processes differ between first and subsequent experiences of CHD.
The findings call for caution in extrapolating the results of genetic association studies from first presentation of CHD to subsequent events. In addition, they raise questions about the use of genetic testing to assess risk – direct-to-consumer and physician-ordered testing options exist, but are unlikely to provide useful information in patients with existing disease.
Study 19: patient journey approaches to prognostic factors – clinically available biomarkers (changes in blood pressure in people with diabetes and stable coronary artery disease and dynamic risk prediction)
This manuscript by Crowther et al. is in preparation. 51
Introduction
Biomarker levels, such as BP, are recorded in clinical practice at more than one time point, often as a monitoring tool to indicate patient status, as well as clinical event data such as time to AMI, stroke or death. Extensive studies in CVD have been conducted to assess the role of one or more biomarkers measured at a single time point in predicting clinical events, such as death from cardiovascular causes. 49,315–317 Repeated measurements have only recently received attention, demonstrating that variation in BP during follow-up is independently (of baseline BP) associated with risk of adverse events, such as stroke and AMI. 318 Many biomarkers, such as SBP and DBP, are now collected as part of usual care over time, and this vast resource of information, existing in registry sources such as CPRD, is yet to be fully explored. 319 Furthermore, as data linkage becomes more common,320 there are great opportunities for the development of real-time dynamic risk prediction models. 321
Through a joint modelling approach, we can both directly model a biomarker profile over time, accounting for measurement error and capturing between-patient variability, and directly link aspects of the biomarker profile to the time to event. This methodological approach is now well established, minimising bias and improving efficiency in estimates of association between longitudinal and clinical event data. 322–324 Alternatively, a joint model can be thought of modelling a biomarker profile over time, adjusting for informative drop-out, often owing to death.
In this study, our aim was to determine the extent to which current values of BP, trend over time or within-person variability adds information beyond a single baseline value in the prediction of death among people with stable angina and type 2 diabetes, AMI and stroke. We studied people with angina and type 2 diabetes as a high-risk population in whom clinical guidelines recommend close BP monitoring. Despite this, little is known about patterns of BP change (e.g. whether or not it increases with age as in the general population).
Methods
Patient population
The study cohort was derived from the CALIBER linked data set. 15 The study period was January 1998 to March 2010, and individuals were eligible if they had a pre-existing diagnosis of type 2 diabetes and subsequently entered the study cohort at diagnosis of stable angina. Study participants also had a BP recording in the year prior to diagnosis of stable angina (baseline measure of BP).
Covariates
Covariates available for analysis include age at baseline (in years) and sex. In all analyses, age was centred at 68 years.
End points
The combined primary end points were SBP, measured repeatedly, and the time to composite end point of all-cause mortality, non-fatal AMI or non-fatal stroke. Baseline SBP was defined as the measurement at, or within 1 year prior to, a diagnosis of stable angina. All subsequent SBP measurements were included prior to an event or censoring; however, for any patients with > 20 SBP measures, we sampled 20 observations at random.
Statistical analysis
Exploratory plots were used to summarise the trend in SBP over time, using a non-parametric smoother. Joint longitudinal survival plots were also used to investigate differences in the average SBP trend prior to being censored or experiencing the event, reversing the timescale by taking away each patient’s censoring or event time. 325
To inform the joint model, we fitted linear mixed-effects models to the repeated measures of SBP, investigating the trajectory using polynomials of time. We adjusted for age and sex at baseline, and investigated any interactions between age, sex and time. We also fitted Weibull survival models for the time to composite end point, including the observed repeated SBP measures as a time-varying covariate. We adjusted for age and sex at baseline and any interactions. Non-linear associations in continuous covariates (SBP and age) were investigated using polynomials. We used predicted plots of the estimated HR to summarise the association between SBP and the risk of death, AMI or stroke.
We then fitted a full joint model, combining a linear mixed-effects model and a Weibull time-to-event model, investigating the association between the time to death, AMI or stroke, and the current value and rate of change in SBP. We plotted conditional survival predictions to indicate how a patient-specific SBP profile can be used to predict survival probabilities, based on the fitted joint model. 326
In all analyses, the timescale is derived by taking away each patient’s date of study entry from the date of SBP measurement.
All analyses were conducted using Stata version 13.1.
Results
Baseline characteristics of study participants
The study cohort included 5246 patients with type 2 diabetes diagnosed with stable angina at study entry, and consisted of 2920 men and 2326 women. The mean age at baseline was 69.8 years (SD 11.4 years) in women and 67.6 years (SD 10.5 years) in men. Mean SBP at baseline was 143.8 mmHg (SD 21.0 mmHg) in women and 141.3 mmHg (SD 18.9 mmHg) in men. A total of 85,428 SBP measurements were available for analysis, with a median number of SBP measures of 12 (range 1–336) in women and 11 (range 1–131) in men; however, for any patients with > 20 measures, we sampled 20 at random from those available. This led to a total of 61,507 repeated SBP measurements being available for analysis.
Exploratory analysis
Figure 42 shows the observed SBP values for a patient with 336 repeated measures, illustrating the trajectory of SBP over time. Figure 43 shows the overall trend in observed SBP profiles for all patients.
FIGURE 42.
Observed SBP for a CALIBER patient with type 2 diabetes and stable angina with 336 repeated measurements of BP.

FIGURE 43.
Non-parametric smoothed estimates of observed SBP among CALIBER patients with type 2 diabetes and stable angina. (a) Time from diagnosis as the timescale; and (b) adjusted timescale, with each patient’s censoring/event time taken away from the measurement times.


Figure 43a shows a non-parametric smoother of SBP from time since diagnosis of stable angina, separately for patients who were censored at the end of follow-up, and those who experienced the composite end point. In Figure 43b we use the same non-parametric smoother of SBP, but adjust the timescale by taking away each patient’s observed censoring or event time. This gives an indication of any differences in the trend of SBP over time in the period leading up to an event, compared with patients who are censored. Figure 43b indicates that patients who experience the composite end point have a pronounced decline in SBP in the 2–3 years prior to an event, which could imply that the rate of change of SBP over time could play an important part in the risk of composite end point.
Profile of systolic blood pressure over time
We observed an estimated population-level mean SBP at baseline of 141.089 mmHg for a male aged 68 years. There is an estimated average decrease in SBP of –0.831 mmHg per year, again for a male aged 68 years. For patients aged 68 years, a female has an estimated 2.664 mmHg higher SBP than a male at baseline (Table 20).
Covariate | Estimate | p-value | 95% CI |
---|---|---|---|
Time | –0.0831 | < 0.001 | –0.0930 to –0.0732 |
Age (centred) | 0.0051 | 0.036 | 0.0003 to 0.0103 |
Female | 0.2664 | < 0.001 | 0.1882 to 0.3446 |
Age female | 0.0145 | < 0.001 | 0.0073 to 0.0217 |
Intercept | 14.1089 | < 0.001 | 14.0518 to 14.1659 |
Random effects | |||
sd(time) | 0.2022 | 0.1916 to 0.2133 | |
sd(Intercept) | 1.4728 | 1.4372 to 1.5092 | |
corr(time,intercept) | –0.5659 | –0.6021 to –0.5273 | |
sd(Residual) | 1.5428 | 1.5336 to 1.5521 |
Modelling the time to death, acute myocardial infarction or stroke
For a female as opposed to a male of the same age at baseline and at the same value of SBP, the risk of an event is reduced by 17.2% (95% CI 8.9% to 24.8%) (Table 21).
Parameter | Log-HR | p-value | 95% CI |
---|---|---|---|
SBP | –0.0704 | < 0.001 | –0.1020 to –0.0389 |
SBP2 | 0.0345 | < 0.001 | 0.0287 to 0.0403 |
SBP3 | –0.0021 | 0.001 | –0.0034 to –0.0009 |
Age | 0.0554 | < 0.001 | 0.0503 to 0.0605 |
Age2 | 0.0004 | 0.032 | 0.0000 to 0.0605 |
Female | –0.1888 | < 0.001 | –0.2848 to –0.0928 |
Intercept | –2.5811 | < 0.001 | –2.6798 to –2.4824 |
log-(gamma) | –0.0780 | < 0.001 | –0.1179 to –0.0380 |
Interpretation of covariate effects is complicated with the introduction of polynomial terms of SBP and age. Figure 44 shows the non-linear relationship between SBP and the risk of composite end point.
FIGURE 44.
Estimated HR for the effect of SBP on the risk of composite end point, relative to a reference value of SBP of 140 mmHg in CALIBER patients with type 2 diabetes and stable angina.

Joint modelling of the profile of systolic blood pressure and time to death, acute myocardial infarction or stroke
The results from the full joint model, linking repeatedly measured SBP and the time to composite end point are presented in Table 22.
Parameter | Coefficient | p-value | 95% CI |
---|---|---|---|
Longitudinal | |||
Time | –0.0951 | < 0.001 | –0.1061 to –0.0842 |
Age | 0.0046 | 0.070 | –0.0004 to 0.0097 |
Female | 0.2710 | < 0.001 | 0.1927 to 0.3494 |
Age × female | 0.0138 | < 0.001 | 0.0066 to 0.0211 |
Intercept | 14.1143 | < 0.001 | 14.0570 to 14.1717 |
Survival | |||
Association (current value) | –0.1382 | < 0.001 | –0.1869 to –0.0894 |
Association (slope) | –1.0552 | < 0.001 | –1.4820 to –0.6285 |
Age | 0.0560 | < 0.001 | 0.0509 to 0.0612 |
Age2 | 0.0005 | 0.005 | 0.0001 to 0.0008 |
Female | –0.1574 | 0.002 | –0.2549 to –0.0600 |
Intercept | –0.6142 | 0.070 | –1.2786 to 0.0501 |
log-(gamma) | –0.0971 | < 0.001 | –0.1386 to –0.0556 |
Random effects | |||
sd(time) | 0.2048 | 0.1942 to 0.2161 | |
sd(intercept) | 1.4805 | 1.4448 to 1.5173 | |
corr(time,intercept) | –0.5717 | –0.6075 to –0.5336 | |
sd(residual) | 1.5423 | 1.5331 to 1.5516 |
We found highly statistically significant associations (p < 0.001) between the risk of composite end point and both the current value of SBP and the rate of change. Both association coefficients are negative, indicating that lower values of SBP increase the risk of an event, and a greater decline in the rate of change of SBP increases a patient’s risk.
This is illustrated in Figure 45, which shows predicted event probabilities for two patients of the same sex and similar age at baseline, conditional on patient-specific SBP profiles. Patient A shows a slightly increasing trend in SBP over time compared with patient B, who has a decreasing trend in SBP. Subsequently, patient A has an improved event probability prediction compared with patient B.
FIGURE 45.
Predicted event probabilities conditional on patient-specific SBP profiles among CALIBER patients with type 2 diabetes and stable angina for (a) patient A and (b) patient B.


Discussion
This linked EHR-based study of type 2 diabetes patients diagnosed with stable angina provides strong evidence of a declining trend in SBP following diagnosis. This is in contrast to a generally increasing trend among a healthy population. The current value and rate of change of SBP over time are strongly associated with the risk of death, AMI or stroke, indicating that lower values of SBP and a declining trend in SBP may have an important role in identifying patients at increased risk.
This is the first study to use repeated measures of SBP, both to model its trend over time adjusting for dropout and to investigate how time-dependent aspects such as the current value and rate of change are associated with risk of death, AMI or stroke. The joint model results provide a platform for dynamic risk prediction, whereby individual patients’ risk profiles can be updated when new measurements become available. To date, all current prediction models for cardiovascular risk use single baseline measurements for all modelled covariates (i.e. measurements taken at the start of follow-up). Methods for dynamic risk prediction in which the patterns of change of clinically measured biomarkers can be incorporated into clinical decision support models in clinical practice are required.
Conclusions
Patients with type 2 diabetes and diagnoses of stable angina have an overall decreasing trend in SBP over time. The current value and rate of change of SBP over time are both strongly associated with the risk of death, AMI or stroke, indicating that lower values of SBP and a declining trend in SBP may have an important role in identifying patients at increased risk.
Joint modelling provides the ability to investigate trends in a biomarker over time, accounting for measurement error and dropout (often as a result of death) or, alternatively, to assess the association between a biomarker profile (or aspects of the trajectory such as rate of change) and the time to event of interest.
Chapter 9 Stable coronary artery disease: risk prediction and cost-effective targeting of interventions
Abstract
Background
Real-world data based on linked EHRs can contribute important prognostic information in unselected patients. Improved survival after ACS and in an ageing population have resulted in a large population of patients with SCAD who require high health-care resource use. Validated prognostic models could assist in clinical management of SCAD patients and, in combination with information on resource use and costs, provide valuable tools for policy-makers.
Objectives
To develop prognostic models, examine health-care use and costs, and to provide a framework to evaluate the cost-effectiveness of treatments in SCAD patients.
Methods
Prognostic models and 5-year risk predictions were developed, and lifetime health-care costs were calculated using CALIBER data.
Results
Prognostic models achieved a C-index of 0.811 for all-cause mortality and 0.778 for non-fatal AMI or coronary death. The strongest predictors were initial diagnosis, CVD comorbidities and biomarker levels. Five-year and lifetime costs were £9181 (57% CVD related) and £107,052 (60% CVD related), respectively, for lowest-risk patients, versus £21,872 (72% CVD related) and £38,606 (72% CVD related) for highest-risk patients. A new treatment with 20% hazard reduction for AMI, ischaemic stroke or CVD death would be cost-effective if priced at < £61 per year for the lowest-risk group and < £657 per year for the highest-risk group.
Conclusions
Validated prognostic models could be used to support the clinical management of SCAD patients. Cost modelling can inform resource allocation decisions and be used to assess the cost-effectiveness of new treatments.
The studies in this chapter relate to patients presenting for the first time with SCAD (see Figure 34).
Introduction
Electronic health records research provides new opportunities to use clinically recorded information to identify candidate prognostic factors, which can then be incorporated into multivariate prognostic models. Our PROGRESS initiative recommended new programmes of methodological and empirical prognosis research exploiting EHRs to define, phenotype and follow up people with different health-related conditions. 29 We have developed prognostic models using a large contemporary and representative population of UK patients with SCAD within the linked CALIBER data resource, validated the models using data from an independent study, and assessed their clinical usefulness in terms of life-years saved had they been implemented.
An important use of validated prognostic factors is the development of models that predict the risk of future coronary or other health-related events. The prognosis for SCAD patients is topical, with new treatments327 and new applications of existing treatments328 currently undergoing Phase III trials in this patient population. With policy-makers and the clinical community233,329–331 increasingly turning their attention to SCAD, risk equations have recently been published for this population. 52 These emerging prognostic models are of potential value in clinical practice, helping to stratify patients and guide choice of treatment, and more widely in public health, providing insight into the probable future burdens of disease.
As the number of people with SCAD continues to increase, it is important that policy-makers are able to estimate the probable future health-care research usage of this group of patients. We have used our prognostic model to address the limitations of previous attempts to model costs, providing estimates of medium-term and lifetime health-care costs and health outcomes of patients with SCAD, stratified according to their initial levels of risk. This modelling also provides a valuable tool for assessing the cost-effectiveness of new interventions.
Study 20: risk prediction – development and validation of a prognostic model
This study is based on the peer-reviewed paper by Rapsomaniki et al. 52
Introduction
Stable coronary artery disease is becoming increasingly common, as a result of an ageing population and enhanced survival after ACS. In the USA alone, it affects approximately 7% of the population. 332 Guidelines produced by the American College of Cardiology Foundation (ACCF)329 and American Heart Association (AHA)329 recommend that patients should be stratified into high-, intermediate- and low-risk groups on the basis of their annual mortality risk, a classification that should then guide further investigation and choice of treatment. However, prognostic tools developed to date have typically been based on data collected in clinical trials rather than ‘real-world’ data gathered as part of clinical practice. 260,329,333 Furthermore, despite recommendations in guidelines, models have not assessed both all-cause mortality and coronary events, and no model has been validated using an independent data set. None has yet been recommended for clinical use.
To address these gaps, we used the CALIBER platform to develop prognostic models consistent with the recommendations made in clinical guidelines. Using CALIBER linked data, we sought to identify associations between outcomes and a wide range of clinical and other measures. Prognostically useful factors were incorporated into prognostic models for all-cause mortality and non-fatal AMI or coronary death, and models were assessed to quantify the accuracy of their predictions and their clinical usefulness – in terms of life-years saved had they been implemented. The performance of prognostic models was validated using an independent data set.
Methods
Candidate prognostic factors were identified from recent guidelines on the management of SCAD and included demographic factors, disease subtype, nitrate use, previous experience of heart surgery, history of other conditions, various physiological measures, psychosocial factors and standard clinically assessed biomarkers.
Prognostic models were developed according to the checklist in the Transparent Reporting of a Model for Individual Prognosis or Diagnosis (TRIPOD) guidelines. Five-year risk predictions were developed, extending annual mortality risks to < 5% (low), 5–14% (intermediate) and > 15% (high), and a net reclassification improvement was calculated for each factor added to the model. The potential clinical impact of each model was estimated by calculating the number of additional years of life saved had the model been implemented. Models were validated using data from 4020 patients in the Appropriateness of Coronary REvascularisation (ACRE) study, which followed patients from 1996/97 to 2004. 334
Results
Of 102,023 patients identified, 44.7% had stable angina, 21.5% had another CHD, 13.1% had unstable angina, 4.7% had STEMI, 6.7% had NSTEMI and 9.4% had unclassified AMI. The 5-year mortality for the group as a whole was 20.6% (95% CI 20.3% to 20.9%) but varied markedly among disease subtypes. The 5-year risk for non-fatal AMI or coronary death was 9.7% (95% CI 9.4% to 9.9%). Factors with prognostic value included age, sex, diagnosis, socioeconomic deprivation, smoking, hypertension, diabetes, lipid levels, heart failure, PAD, atrial fibrillation, stroke, chronic kidney disease, chronic pulmonary disease, liver disease, cancer, depression, anxiety, heart rate, creatinine levels, white cell count and haemoglobin levels (Table 23).
Prognostic factors | All-cause mortality, HRa (95% CI) | Non-fatal AMI or coronary death, HRb (95% CI) |
---|---|---|
Sociodemographic characteristics | ||
Age in men, per year | 1.065 (1.063 to 1.067) | 1.046 (1.043 to 1.048) |
Age in women, per year | 1.081 (1.078 to 1.083) | 1.065 (1.061 to 1.069) |
Women vs. men | 0.204 (0.162 to 0.257) | 0.158 (0.112 to 0.221) |
Most deprived quintile, yes vs. no | 1.151 (1.111 to 1.192) | 1.117 (1.060 to 1.178) |
SCAD diagnosis and severity | ||
Other CHD vs. stable angina | 1.024 (0.982 to 1.067) | 1.180 (1.107 to 1.257) |
Unstable angina vs. stable angina | 1.021 (0.970 to 1.075) | 1.323 (1.232 to 1.421) |
NSTEMI vs. stable angina | 1.298 (1.238 to 1.360) | 2.373 (2.217 to 2.539) |
STEMI vs. stable angina | 1.083 (1.006 to 1.166) | 1.940 (1.750 to 2.149) |
PCI in last 6 months, yes vs. no | 0.651 (0.605 to 0.699) | 0.702 (0.642 to 0.768) |
CABG in last 6 months, yes vs. no | 0.516 (0.469 to 0.566) | 0.424 (0.370 to 0.486) |
Previous/current AMI, yes vs. no | 1.136 (1.095 to 1.179) | 1.399 (1.330 to 1.472) |
Use of nitrates, yes vs. no | 1.152 (1.118 to 1.188) | 1.405 (1.342 to 1.470) |
CVD risk factors | ||
Ex-smoker vs. never | 1.110 (1.065 to 1.157) | 1.094 (1.008 to 1.188) |
Current smoker vs. never | 1.315 (1.245 to 1.389) | 1.215 (1.126 to 1.311) |
Hypertension, present vs. absent | 0.965 (0.929 to 1.001) | 1.064 (1.000 to 1.131) |
Diabetes, present vs. absent | 1.203 (1.160 to 1.248) | 1.387 (1.315 to 1.463) |
Total cholesterol per 1-mmol/l increase | 1.012 (0.983 to 1.042) | 1.061 (1.029 to 1.094) |
HDL per 0.5-mmol/l increase | 1.006 (0.987 to 1.025) | 0.910 (0.879 to 0.942) |
CVD comorbidities | ||
Heart failure, present vs. absent | 1.543 (1.495 to 1.593) | 1.181 (1.089 to 1.281) |
PAD, present vs. absent | 1.286 (1.234 to 1.340) | 1.085 (1.031 to 1.142) |
Atrial fibrillation, present vs. absent | 1.280 (1.236 to 1.326) | 0.952 (0.887 to 1.021) |
Stroke, present vs. absent | 1.329 (1.277 to 1.382) | 1.138 (0.925 to 1.401) |
Non-CVD comorbidities | ||
Chronic kidney disease, present vs. absent | 1.116 (1.058 to 1.178) | 1.085 (1.031 to 1.142) |
COPD, present vs. absent | 1.150 (1.114 to 1.187) | 1.081 (1.089 to 1.281) |
Cancer, present vs. absent | 1.377 (1.324 to 1.432) | 0.952 (0.887 to 1.021) |
Chronic liver disease, present vs. absent | 1.631 (1.443 to 1.842) | 1.138 (0.925 to 1.401) |
Psychosocial characteristics | ||
Depression at diagnosis, present vs. absent | 1.179 (1.135 to 1.225) | 1.059 (0.998 to 1.124) |
Anxiety at diagnosis, present vs. absent | 1.172 (1.116 to 2.231) | 1.015 (0.937 to 1.100) |
Biomarkers | ||
Heart rate per 10-b.p.m. increase | 1.098 (1.04 to 1.112) | 1.069 (1.030 to 1.110) |
Creatinine per 30-µmol/l increase | 1.065 (1.051 to 1.080) | 1.087 (1.064 to 1.110) |
White cell count per 15 × 109/l increase | 1.120 (1.106 to 1.135) | 1.111 (1.088 to 1.135) |
Haemoglobin per 1.5-g/dl increase | 0.758 (0.724 to 0.794) | 0.822 (0.800 to 0.845) |
C-index | ||
Internal cross-validation | 0.811 (0.806 to 0.816) | 0.778 (0.770 to 0.785) |
External validation (ACRE study) | 0.735 (0.715 to 0.755) | 0.718 (0.700 to 0.736) |
Models incorporating these factors achieved a C-index of 0.811 for all-cause mortality (95% CI 0.806 to 0.816) and 0.778 for non-fatal AMI or coronary death (95% CI 0.770 to 0.785) (i.e. when comparing two patients, the model correctly predicted who would have an earlier event 81.1% of the time). The strongest predictors were initial diagnosis, CVD comorbidities and biomarker levels (Figure 46).
FIGURE 46.
Potential clinical impact of using CALIBER risk models for clinical evaluation of patients with SCAD: evaluation of prediction performance on incremental addition of different sets of predictors, using sociodemographic characteristics as the reference model. (a) All-cause mortality model; (b) non-fatal MI or coronary death model. a, Based on guideline definition of low (< 1%), intermediate (1–3%) and high (> 3%) annual risk; b, assuming that management is associated with HR 0.8 and is cost-effective at high risk. MI, myocardial infarction; NRI, net reclassification index. Reproduced from Rapsomaniki et al. 52 Copyright © 2014, © The author 2013. Published by Oxford University Press on behalf of the European Society of Cardiology. This is an open-access article distributed under the terms of the Creative Commons Attribution License (http://creativecommons.org/licenses/by-nc/3.0/), which permits non-commercial re-use, distribution, and reproduction in any medium, provided the original work is properly cited.

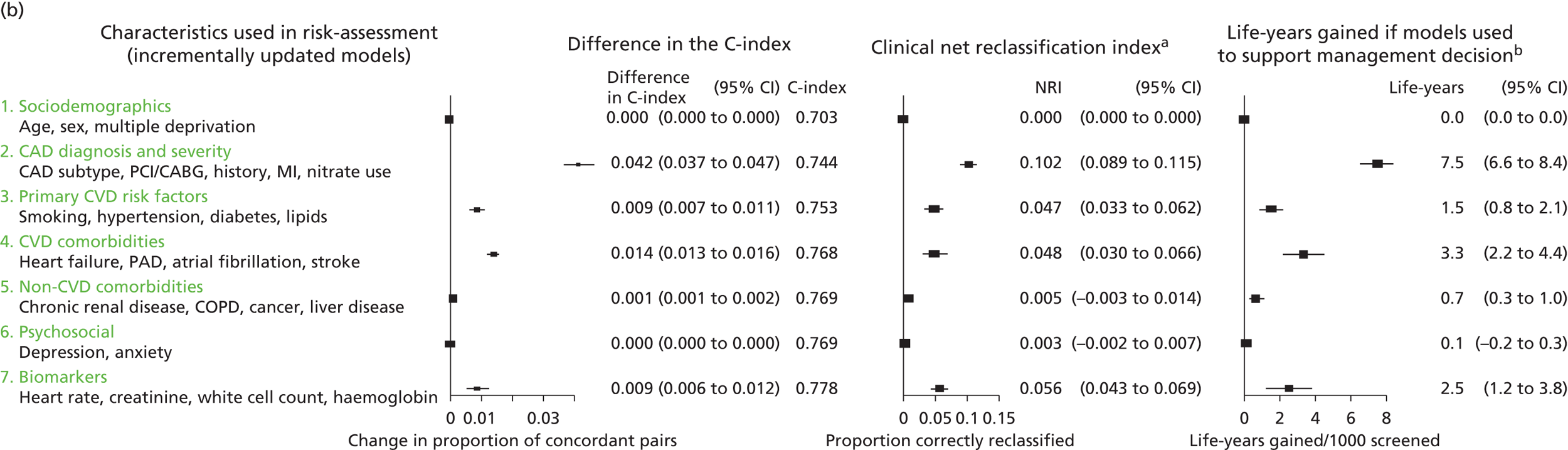
If the models had been used to identify patients at high risk (3% annual mortality), and a management programme with a HR of 0.8 had been implemented, an additional 13–16 life-years or 15–18 coronary-free years could have been achieved per 1000 patients screened, in comparison with models based only on sex, age and deprivation (Figure 47).
FIGURE 47.
Contribution of each variable included in the CALIBER model for predicting all-cause mortality in SCAD, assessed by the decrease in the C-index on its removal from the complete model. MI, myocardial infarction; TCHOL, total cholesterol. Reproduced from Rapsomaniki et al. 52 Copyright © 2014, © The author 2013. Published by Oxford University Press on behalf of the European Society of Cardiology. This is an open-access article distributed under the terms of the Creative Commons Attribution License (http://creativecommons.org/licenses/by-nc/3.0/), which permits non-commercial reuse, distribution, and reproduction in any medium, provided the original work is properly cited.
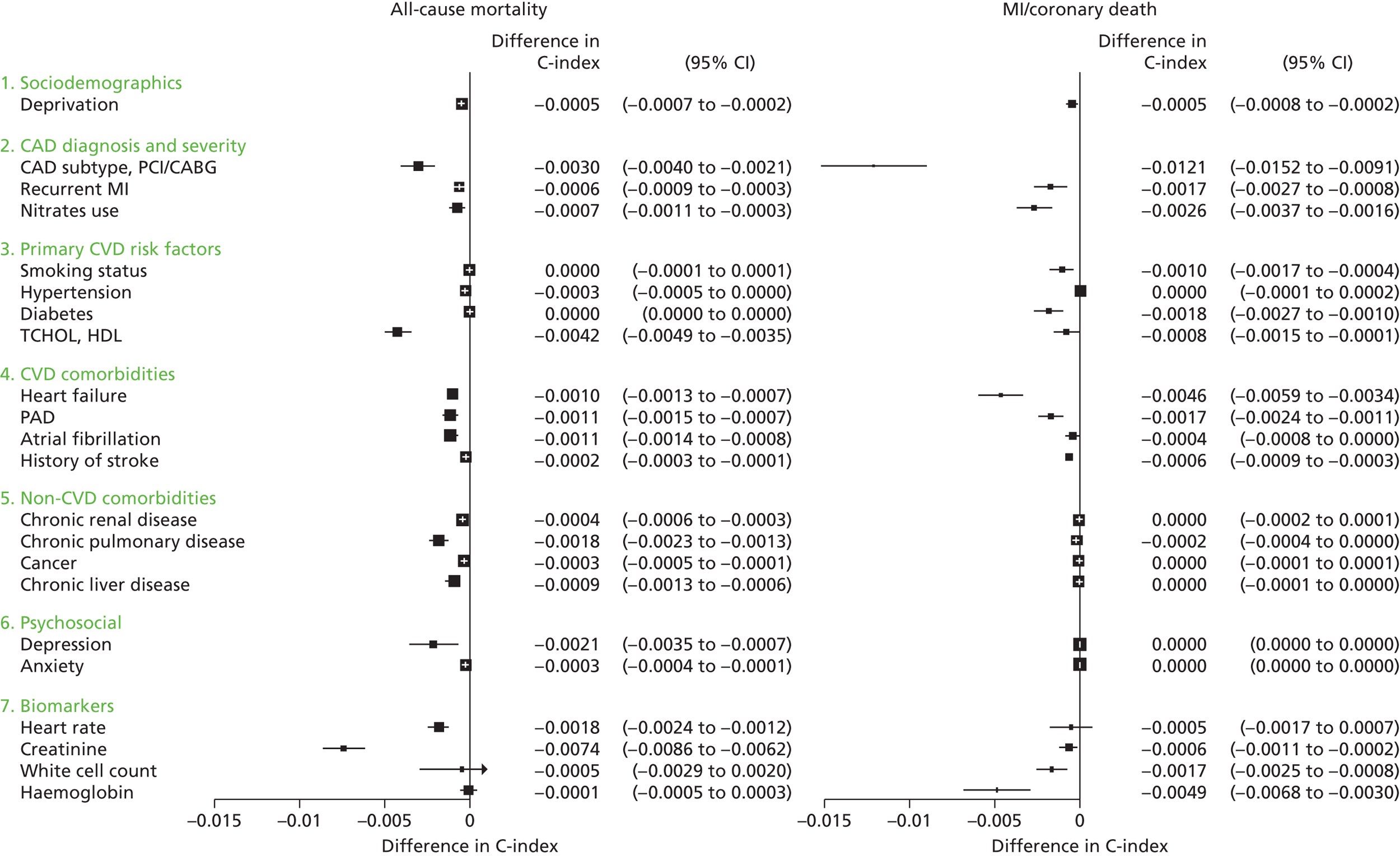
In the ACRE validation, 872 deaths and 1238 non-fatal AMI or coronary deaths were seen during 7.5 years of follow-up. In this data set, the model’s C-index for discrimination was 0.735 for all-cause mortality (95% CI 0.715 to 0.755) and 0.718 for non-fatal AMI or coronary death (95% CI 0.700 to 0.736).
Discussion
In this population-based study of > 100,000 SCAD patients, prognostic models based on widely available clinical data could identify patients at high risk of long-term mortality and coronary events with a high degree of accuracy.
The models showed good calibration and discrimination, when applied to both original source data and an independent data set. Their relevance to clinical decision-making was confirmed through calculations demonstrating significant benefits had they been applied in practice to stratify patients. As there is no cost involved in acquiring new data, use of the models is likely to be highly cost-effective.
The models have been developed to be consistent with ACCF/AHA definitions and guidelines. 329 The findings support use of the models in early stages of stepwise risk assessment, to identify patients for intensive management or further investigation. Further external validation in external data sets and recalibration for different populations and time periods is required. An economic evaluation is needed to establish the appropriateness of using the prognostic model in practice.
Conclusions
We present validated prognostic models for estimating the risk of all-cause mortality and coronary outcomes based on clinical parameters that are commonly available in all people with SCAD. These models can be implemented alongside further medical investigations to support medical decision-making. Further independent evaluation is required in different settings, including different EHR systems, health-care organisations and geographic locations to guide use in clinical practice. A risk calculator is available online (www.caliberresearch.org/model).
Study 21: resource use, costs and health outcomes in patients with stable coronary artery disease using linked electronic health record data
This study is based on the peer-reviewed papers by Asaria et al. ,53 and Walker et al. 54
Introduction
Stable coronary arterial disease patients vary widely in their risk of subsequent AMI or coronary death (about 10-fold, between top and bottom deciles of risk). 52 Understanding how drug use, procedures, primary care consultations and hospital admissions vary according to baseline risk may contribute to clinical decision-making for SCAD patients and policy-making.
However, existing studies on resource use and costs in SCAD have important limitations. Studies have been based on populations with disease and do not reflect contemporary and standard clinical practice; for example, they use selected small samples from trials undergoing non-standard care. 335 Despite the need to understand the long-term course of SCAD, previous studies have taken AMI as a starting point336 and use overly simplistic models. We are not aware of any published study using data collected as part of usual care that has evaluated resource utilisation and costs according to baseline cardiovascular risk, and longer-term and lifetime implications of SCAD have not been reported accurately elsewhere.
The majority of models estimating the costs and health effects of CVD have focused on primary prevention,337,338 and have made predictions over relatively short time horizons. 339 Hence, they are unable to capture lifetime costs and health effects, are based on selected samples (potentially biasing baseline risk and cost estimates, and limiting their generalisability) and fail to model all relevant end points and their interdependence. 335
Population-based contemporary linked EHRs could be used to provide reliable estimates of the lifetime cost and quality-adjusted life expectancy of patients with this important chronic condition. Using CALIBER linked data, we set out to (1) determine health-care utilisation and the associated costs in the first year with SCAD and in the year following a first non-fatal event (AMI, ischaemic or haemorrhagic stroke), (2) identify predictors of costs in the first year of SCAD and (3) model patients with SCAD, estimating their baseline risk of experiencing further CVD events and then predicting both costs and key health outcomes over the lifetime of these patients stratified by their baseline CVD risk. This model will allow us both to understand the cost implications of managing this growing population under current standards of care and to evaluate the cost-effectiveness of new treatment strategies, potentially differentiated by risk group.
Methods
Study population
The analysis was based on 94,966 SCAD patients in CALIBER identified between January 2001 and March 2010. Eligible patients were those with a diagnosis of stable angina, history of AMI, CABG or PCI prior to the start of the study period, or a diagnosis of ACS within the study period (unstable angina or AMI). Patients with prior ACS were defined as stable if they had survived > 6 months following the acute event.
Health-care utilisation
Health-care use extracted from the data set included primary care consultations, pharmaceutical prescriptions, inpatient stays (which included procedure costs) and diagnostic tests. Primary care consultations included all contact between the patient and health-care professionals captured in the CPRD. Prescription data were available from the CPRD. Inpatient stays extracted from HES were based on Healthcare Resource Group codes and defined as CVD or non-CVD related. Diagnostic tests, but not outpatient consultations, were also extracted from HES; the latter were not extracted owing to the absence of outpatient HES data linkage.
Resource use
Costs were calculated from the perspective of the UK NHS in UK pounds sterling based on 2011/12 prices. Costs were calculated by combining health-care use with associated unit costs that were taken from published UK sources. 340–342 For hospitalisations, costs were calculated based on finished consultant episodes in HES.
Health-care resource use was estimated from the CALIBER data set. A panel was constructed using a 90-day cycle length for SCAD patients capturing resource use in terms of hospital episodes, use of drugs, diagnostic tests and primary care costs. Costs were attached to this resource use using the NHS Reference Costs,341 NHS Prescription Cost Analysis342 and Personal Social Services Research Unit costs for primary care340 data sets.
Model
A state transition model was developed to capture the natural history of SCAD patients based on previous models in CVD335 and clinical advice. The model (Figure 48) focuses on predicting the first non-fatal CVD event that SCAD patients are likely to experience as well as CVD and non-CVD mortality. The model has a lifetime time horizon and uses a 90-day cycle length to capture the time-varying and age-dependent nature of risks, costs and health-related quality of life. Costs and health-related quality of life were attached to model states and were adjusted for patient covariates at baseline as well as age and time post event in the model.
FIGURE 48.
State transition model capturing the natural history of SCAD in CALIBER patients. MI, myocardial infarction. Reproduced from Asaria et al. 53 Copyright © 2016, BMJ Publishing Group Ltd and the British Cardiovascular Society. This is an open-access article distributed under the terms of the Creative Commons Attribution License (http://creativecommons.org/licenses/by/4.0/), which permits unrestricted use, distribution, and reproduction in any medium, provided the original author and source are credited.

End points
The median follow-up of SCAD patients in CALIBER was 4.2 years (IQR 1.9–6.9 years), during which 16,783 deaths and 8203 coronary outcomes were observed. The primary clinical end points in the model were first occurrences of non-fatal AMI, ischaemic stroke and haemorrhagic stroke, as well as CVD and non-CVD mortality. Other clinical end points were CVD and non-CVD mortality following a non-fatal event. These were combined to produce the primary economic outputs from the model, which were expected survival duration, quality-adjusted life-years (QALYs) total and CVD-specific costs, each predicted over the remaining lifetime of the patient. The model was also used to produce estimates of event rates and disease progression over time stratified by baseline CVD risk.
Statistical modelling of risk equations
A range of parametric survival models (generalised gamma, log-normal, Weibull, exponential) was estimated for each of the end points, and the best fitting parametric model for each end point was selected. Key prognostic factors included in the models were based on those used by Rapsomaniki et al. 52 and included demographic measures (age, sex, ethnicity, social deprivation), SCAD subtype (stable angina, other CHD, unstable angina, AMI, STEMI, NSTEMI), use of long-acting nitrates, whether CABG or PCI was performed in the 6 months following CAD diagnosis, previous AMI, smoking, BP, diagnosis of hypertension, diabetes, lipids, CVD comorbidities (heart failure, PAD, atrial fibrillation, stroke), non-CVD comorbidities [chronic renal disease, COPD, cancer, chronic liver disease, psychosocial factors (depression, anxiety)] and clinically assessed biomarkers (heart rate, white cell count, haemoglobin, creatinine).
Risk equations for the six subsequent events (CVD and non-CVD mortality following non-fatal AMI, ischaemic stroke and haemorrhagic stroke) were estimated in a similar way. Owing to the greatly reduced numbers of events observed, these were based only on sex and age at the time of the non-fatal event.
Non-CVD mortality beyond the maximum follow-up in the CALIBER data set (10 years) was based on age- and sex-specific non-CVD mortality from national life tables.
Risk equations were combined using a competing risks framework to account for the interdependence of the risks. 343 Models were estimated using R (version 3.1.0) and the R package flexsurv (version 0.3).
Health-related quality of life
EuroQol-5 Dimensions scores for the UK344 were used to calculate age-, condition- and event-specific health-related quality of life and included in the model to calculate QALYs.
Statistical analysis
Estimates of health-care utilisation and costs are presented for the first year with SCAD and the first year following a non-fatal event (either AMI, ischaemic or haemorrhagic stroke), with results reported in terms of mean (SD) or median (IQR). Patients who were censored (i.e. those for whom the data are incomplete for the year but who did not die) were excluded from the analysis.
We used ordinary least squares regression analysis to estimate the impact of baseline covariates on costs in the first year. Covariates were based on those used in the prognostic model for SCAD patients described above, on the assumption that predictors of costs were likely linked to prognostic indicators. Models were fitted on five multiply imputed data sets and estimates combined using Rubin’s rules. 345
Panel data methods with time-invariant covariates were used to estimate patient costs over each 90-day period, as well as the impact of events (non-fatal AMI, ischaemic and haemorrhagic stroke, CVD- and non-CVD-related death) on the costs in the period of the event and subsequent periods if the event was non-fatal. These costs were then combined with the state transition Markov model to estimate costs over a longer period.
Given that the model was designed to be used with a heterogeneous population, results were produced by risk groups. The 5-year baseline CVD risk for each SCAD patient was calculated based on the estimated risk equations, and patients were ranked and grouped into 10 equally sized risk groups based on this calculation. Model results were calculated at the mean covariate values across patients within each risk group.
The model was used to calculate life expectancy, QALYs, 5-year and lifetime total health-care costs, and CVD-specific health-care costs for standard care, as well as for indicative new treatments that are assumed to reduce CVD hazards by 10%, 20%, 30% and 40% (including hazards of AMI, ischaemic stroke or CVD death). The results were used to estimate the maximum price that could be charged for the new treatments in each of the risk groups assuming a cost-effectiveness threshold of £20,000 per QALY as used by NICE. 346
The model was evaluated probabilistically by means of a Monte Carlo simulation run for 1000 iterations in order to incorporate and characterise the uncertainty in the model inputs. The model was run for each of the 10 profiles evaluated against each of the five treatment scenarios using R (version 3.1.0) on the N8 HPC supercomputer [N8 HPC Centre of Excellence, funded by the N8 consortium and the Engineering and Physical Sciences Research Council (EPSRC) (Grant No. EP/K000225/1), Universities of Leeds and Manchester].
Results
Health-care utilisation
Table 24 reports health-care utilisation in the first year with SCAD and in the first year following a non-fatal event (AMI, ischaemic or haemorrhagic stroke). In the first year with SCAD, 26.9% of patients (n = 23,801) were hospitalised for CVD, and those who were hospitalised had an average 1.9 stays (spells) in hospital. In the year following a non-fatal event, 76% of patients (n = 5613) were hospitalised for CVD and spent an average of 2.4 stays in hospital. In the first year with SCAD, patients had an average of 10.8 primary care appointments, which increased to 13.6 in the first year following a non-fatal event. In the first year with SCAD, 88% of patients were taking cardiovascular medication, which increased to 90% in the year following a non-fatal event.
Health-care utilisation | Mean (SD) | Median (IQR) |
---|---|---|
Health-care utilisation in first year (n = 85,605) | ||
Hospitalisations | ||
Percentage hospitalised | 0.38 (0.48) | 0 (0–1) |
Percentage hospitalised for CVD | 0.27 (0.44) | 0 (0–1) |
Inpatient stays | 0.87 (4.42) | 0 (0–1) |
Inpatient stays for CVD | 0.5 (2.55) | 0 (0–1) |
With any hospitalisation (n = 32,160) | ||
Inpatient stays | 2.33 (6.98) | 1 (1–2) |
With any hospitalisation for CVD (n = 23,081) | ||
Inpatient stays for CVD | 1.86 (4.64) | 1 (1–2) |
Primary care consultations | 10.77 (10.86) | 8 (0–30) |
Number of diagnostic tests | 31.25 (38.73) | 22 (2–44) |
Drugs | ||
Percentage of patients on any drugs | 0.91 (0.28) | 1 (1–1) |
Percentage of patients on CVD drugs | 0.88 (0.32) | 1 (1–1) |
Health-care utilisation in year following non-fatal event (n = 7762) | ||
Hospitalisations | ||
Percentage hospitalised | 0.76 (0.42) | 1 (1–1) |
Percentage hospitalised for CVD | 0.72 (0.45) | 1 (0–1) |
Inpatient stays | 2.16 (5.97) | 1 (1–3) |
Inpatient stays for CVD | 1.71 (4.72) | 1 (0–2) |
With any hospitalisation (n = 5936) | ||
Inpatient stays | 2.83 (6.69) | 2 (1–3) |
With any hospitalisation for CVD (n = 5613) | ||
Inpatient stays for CVD | 2.37 (5.41) | 2 (1–3) |
Primary care consultations | 13.57 (14.84) | 10 (0–38) |
Number of diagnostic tests | 40.42 (45.87) | 31 (4–58) |
Drugs | ||
Percentage of patients on any drugs | 0.91 (0.28) | 1 (1–1) |
Percentage of patients on CVD drugs | 0.9 (0.3) | 1 (1–1) |
Costs
Table 25 reports costs for hospitalisations, primary care consultations, diagnostic tests and drugs in patients in the first year with SCAD and in the first year following a non-fatal event (AMI, ischaemic or haemorrhagic stroke). Average total health-care costs in the first year with SCAD were £3127 per patient, of which 70.2% (£2194) was related to CVD, increasing to £9239 per patient in the year following a non-fatal event, of which 84.5% (£7809) was CVD related. The majority of health-care costs were driven by hospitalisations (64% in first year with SCAD and 83% in the year following a non-fatal event). Costs in all categories were highly skewed, with mean costs considerably higher than the median.
Cost | Mean (SD) (£) | Median (IQR) (£) |
---|---|---|
Costs in first year (n = 85,605) | ||
Total costs | ||
Total cost | 3127 (6096.61) | 1147 (506–3145) |
Total CVD cost | 2194 (4628.17) | 734 (367–1818) |
Hospitalisations | ||
Inpatient costs | 2012 (5629.07) | 0 (0–1606) |
Inpatient CVD costs | 1481 (4488.98) | 0 (0–695) |
Primary care costs | 466 (463.27) | 361 (0–1291) |
Diagnostic test costs | 141 (231.49) | 70 (0–537) |
Drugs | ||
All drug costs | 508 (1546.28) | 209 (0–1698) |
CVD drug costs | 105 (113.48) | 84 (0–272) |
Costs in first year following an event (n = 7762) | ||
Total costs | ||
Total cost | 9239 (11,684.65) | 5942 (2119–12,229) |
Total CVD cost | 7809 (10,367.94) | 4817 (1367–10,372) |
Hospitalisations | ||
Inpatient costs | 7643 (11,029.41) | 4393 (561–10,261) |
Inpatient CVD costs | 6863 (10,232.13) | 3926 (0–9333) |
Primary care costs | 585 (603.095) | 447 (192–794) |
Diagnostic test costs | 222 (305.015) | 124 (27–287) |
Drugs | ||
All drug costs | 789 (2932.95) | 287 (124–671) |
CVD drug costs | 139 (161.26) | 108 (46–182) |
Cost predictors in the first year with stable coronary artery disease
Non-CVD-related comorbidities had the largest impact on costs, with a history of renal disease having the largest increment of £2432 per patient. Of possible SCAD diagnoses, NSTEMI had the largest impact on cost, with an increment of £742 per patient compared with stable angina. Women incurred significantly lower costs than men, with a decrement of £652 per patient.
Estimated 90-day period and event costs
Panel data methods were used to calculate 90-day period costs and impacts of events on costs. For example, the background total health-care costs for a male, aged 69 years, with no comorbidities, would be £349 in the first 90 days, increasing by £9 for each subsequent 90-day period. If the patient has a non-fatal AMI she or he would incur an incremental cost (on top of the normal period cost of £372) of £4817 in the 90 days following the AMI, with the incremental costs decreasing in subsequent 90-day periods until 360 days, after which there is an incremental cost of £426 per 90 days, suggesting significant ongoing lifetime costs of events. Also of note, the costs of death from CVD- and non-CVD-related causes are £1402 and £1583, respectively.
Lifetime costs and outcomes
There was large variation in CVD risk between the lowest- and highest-risk groups, with an absolute difference in risk of 40% over 5 years. The risk of clinical events was positively correlated with age and higher levels of CVD risk factors such as hypertension and diabetes, as well as with higher prevalence of CVD comorbidities. There were no obvious trends in the key modifiable CVD risk factors such as cholesterol, HDL and heart rate in this population of clinically managed patients.
The modelled progression of CVD over time by risk group is shown in Figure 49. Higher-risk groups were predicted to have much higher levels of CVD mortality than lower-risk groups, who were predicted to remain event free for a much longer period.
FIGURE 49.
Progression of disease over time by baseline CVD risk group among CALIBER patients with SCAD. (a) Lowest risk; (b) decile 2; (c) decile 3; (d) decile 4; (e) decile 5; (f) decile 6; (g) decile 7; (h) decile 8; (i) decile 9; and (j) highest risk. MI, myocardial infarction. Reproduced from Asaria et al. 53 Copyright © 2016, BMJ Publishing Group Ltd and the British Cardiovascular Society. This is an open-access article distributed under the terms of the Creative Commons Attribution License (http://creativecommons.org/licenses/by/4.0/), which permits unrestricted reuse, distribution, and reproduction in any medium, provided the original work is properly cited.
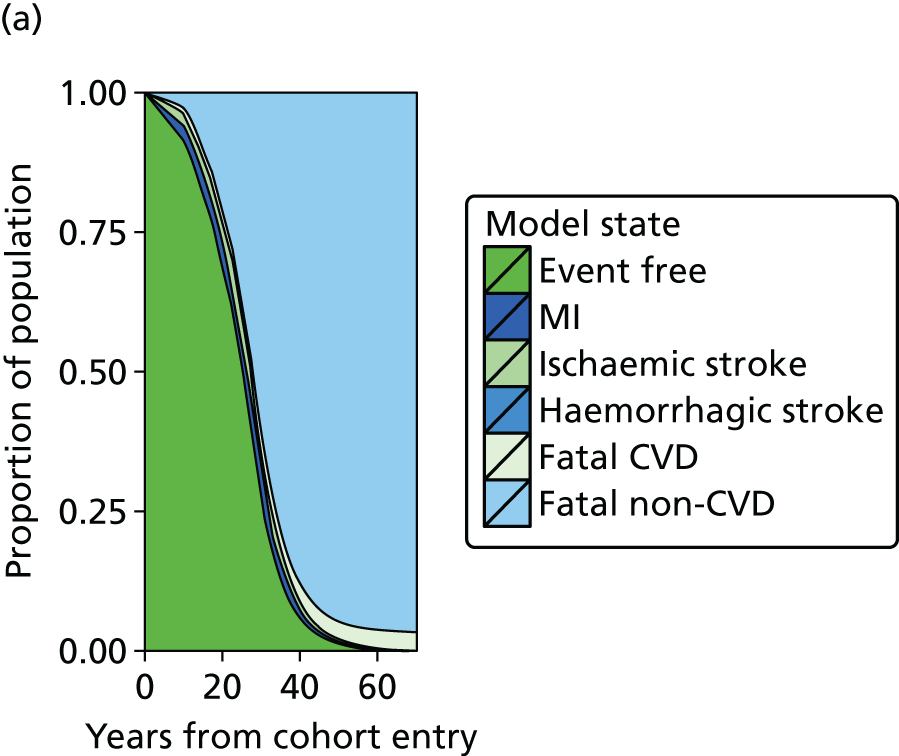

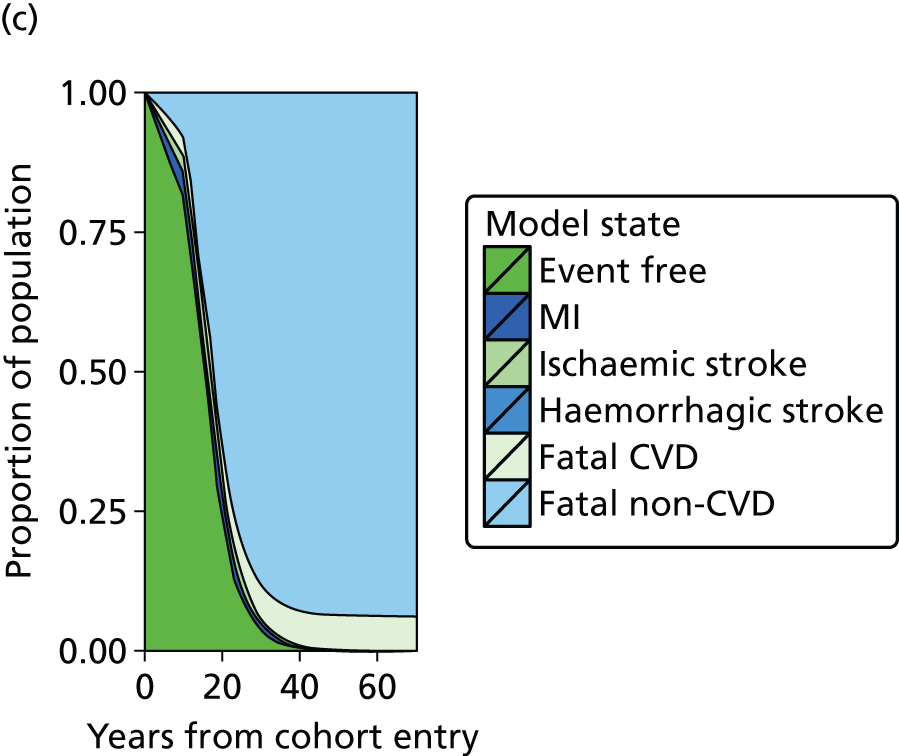
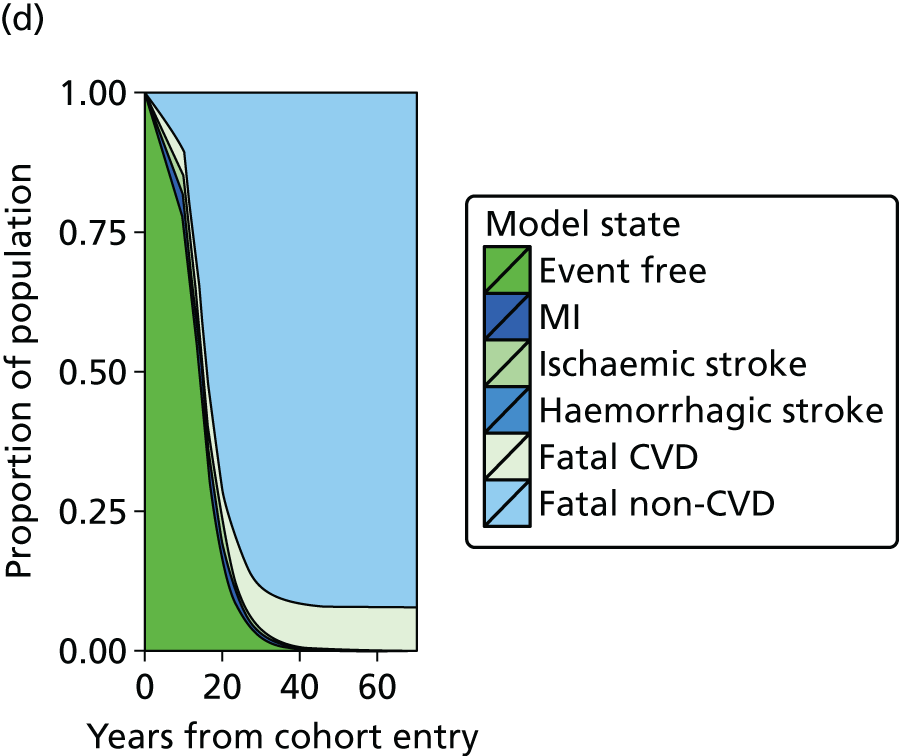

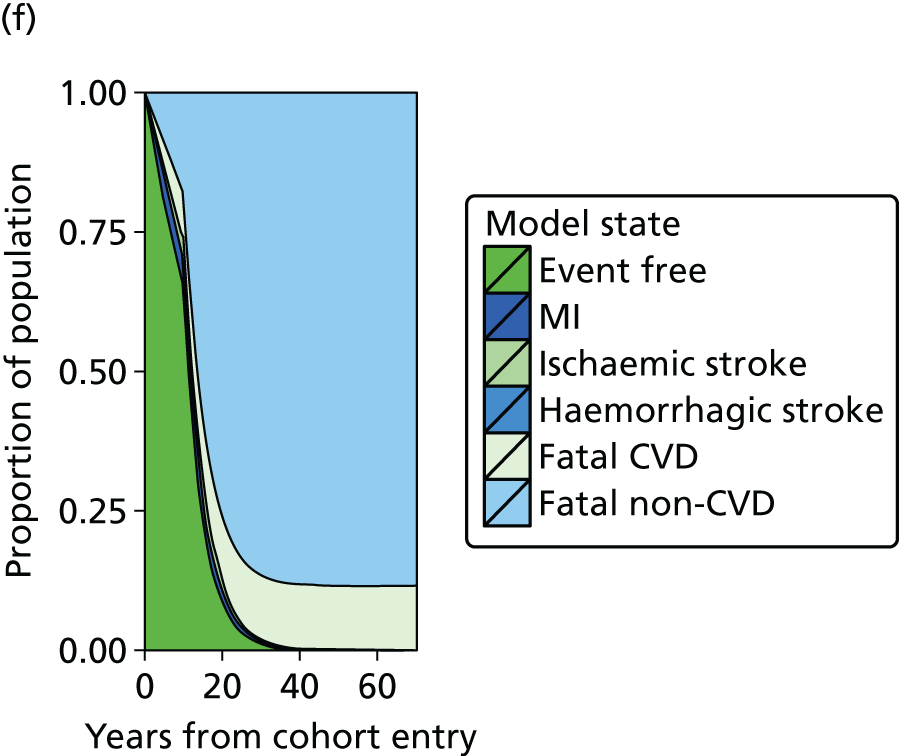



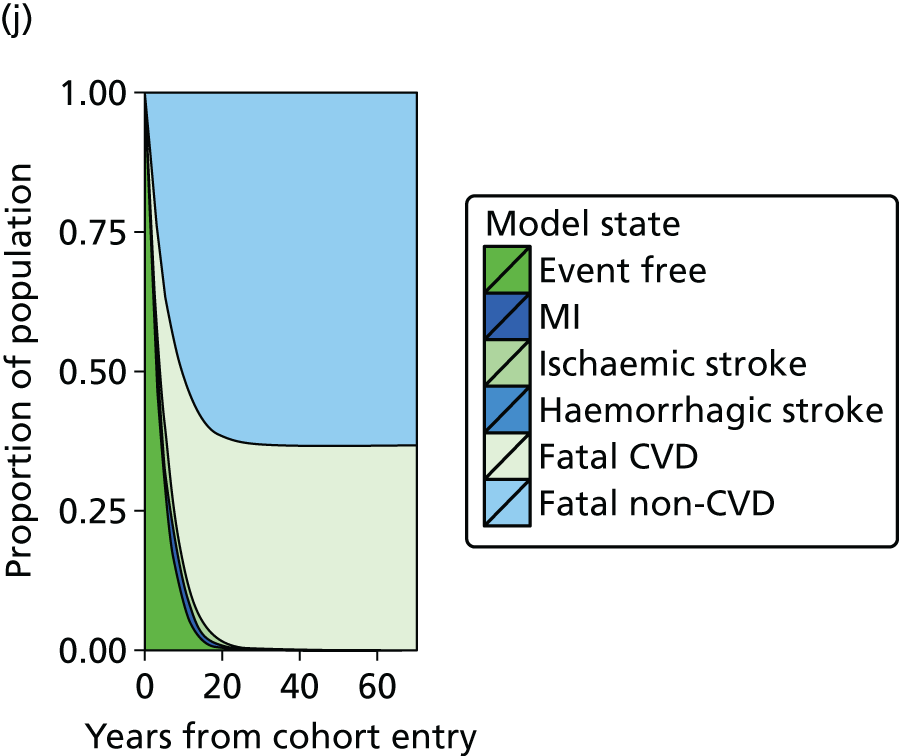
Estimated costs over time for stable coronary artery disease patients
A patient with SCAD representative of the lowest-risk group (5-year cardiovascular risk of 3.43% and a life expectancy of 26.9 years) would have expected undiscounted costs of £9181 over 5 years, of which 57% would be CVD related, and undiscounted lifetime costs of £107,052, of which 60% would be CVD related. A representative patient of the highest-risk group (5-year cardiovascular risk of 43.7% and a life expectancy of 5.3 years) would have expected undiscounted costs of £21,872 over 5 years, of which 72% would be CVD related, and lifetime undiscounted costs of £38,606, of which 72% would be CVD related.
Figure 50 shows the predicted total and CVD-related costs over a 25-year period for average characteristics of risk groups 1 (lowest risk), 4, 7 and 10 (highest risk). Higher-risk patients with SCAD have higher initial costs, which are overtaken by the lower-risk patients as a result of greater mortality in the higher-risk groups, resulting in less time to accrue costs.
FIGURE 50.
(a) Expected total health-care costs and (b) CVD-related health-care costs over time for patients in cardiovascular risk groups 1 (lowest-risk group), 4, 7 and 10 (highest-risk group) in CALIBER patients with SCAD. Reproduced from Walker et al. 54 © 2016 Walker et al. 54 Published by Oxford University Press on behalf of the European Society of Cardiology. This is an open-access article distributed under the terms of the Creative Commons Attribution License (http://creativecommons.org/licenses/by/4.0/), which permits unrestricted reuse, distribution, and reproduction in any medium, provided the original work is properly cited.
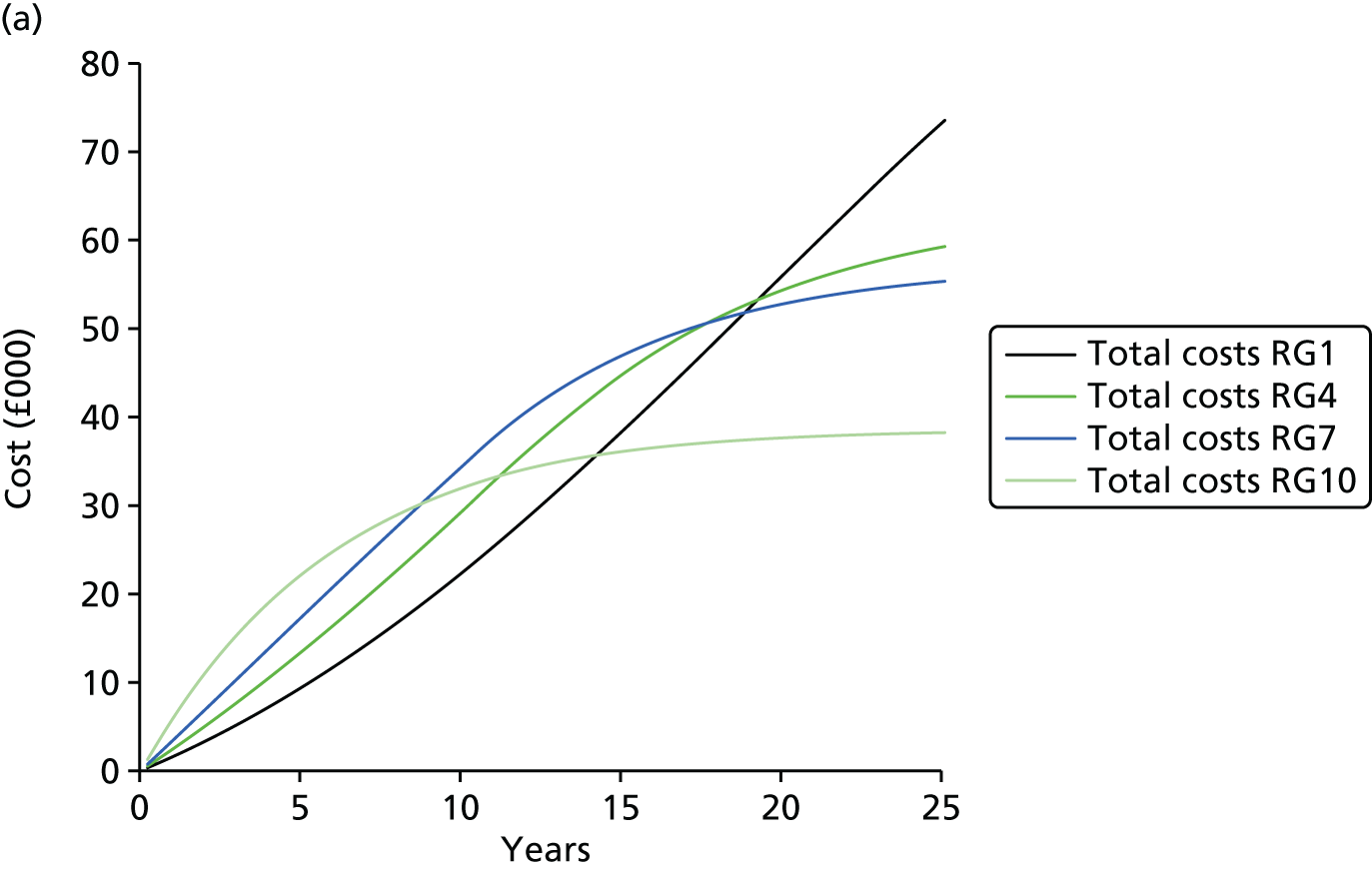

Estimated costs and outcomes over time for stable coronary artery disease patients
Summary model results by risk group are shown in Table 26. Lower-risk patients were estimated to have both greater remaining QALYs and greater health-care costs. Combining these factors, we observed that, under standard care, mean total costs per QALY over the remaining lifetime in the lowest-risk group are projected to be £5581, compared with £11,806 in the highest-risk group.
Model results split by 5-year risk of composite CVD event | ||||||||||
---|---|---|---|---|---|---|---|---|---|---|
Estimated costs and outcomes | Risk group (95% CI) | |||||||||
Lowest risk | 2 | 3 | 4 | 5 | 6 | 7 | 8 | 9 | Highest risk | |
Life-years | 26.81 (26.63 to 26.98) | 19.62 (19.48 to 19.80) | 17.34 (17.18 to 17.53) | 15.63 (15.47 to 15.84) | 14.26 (14.08 to 14.49) | 13.03 (12.83 to 13.28) | 11.92 (11.69 to 12.21) | 10.48 (10.21 to 10.84) | 8.52 (8.19 to 8.94) | 5.51 (5.09 to 6.02) |
QALYs | 19.11 (18.06 to 19.93) | 13.97 (13.26 to 14.54) | 12.29 (11.66 to 12.80) | 11.01 (10.45 to 11.48) | 9.97 (9.44 to 10.41) | 9.03 (8.53 to 9.45) | 8.13 (7.65 to 8.53) | 6.99 (6.54 to 7.40) | 5.50 (5.09 to 5.89) | 3.34 (3.01 to 3.72) |
Total costs (£1000s) | 117 (65 to 168) | 81 (55 to 108) | 73 (54 to 92) | 68 (54 to 83) | 65 (53 to 76) | 62 (54 to 71) | 61 (55 to 69) | 59 (54 to 65) | 54 (49 to 60) | 43 (38 to 49) |
CVD costs (£1000s) | 72 (29 to 114) | 52 (30 to 74) | 48 (31 to 64) | 45 (33 to 58) | 43 (34 to 53) | 42 (35 to 50) | 42 (36 to 48) | 41 (37 to 46) | 38 (34 to 43) | 31 (27 to 35) |
Time to first event (years) | 24.55 (24.31 to 24.76) | 17.80 (17.64 to 17.95) | 15.62 (15.47 to 15.75) | 13.98 (13.85 to 14.11) | 12.67 (12.54 to 12.8) | 11.49 (11.36 to 11.62) | 10.43 (10.29 to 10.57) | 9.00 (8.85 to 9.15) | 7.06 (6.91 to 7.22) | 4.07 (3.90 to 4.23) |
AMI infarction as primary end point (%) | 6.00 (5.55 to 6.49) | 7.11 (6.73 to 7.49) | 8.06 (7.72 to 8.43) | 8.94 (8.61 to 9.29) | 9.84 (9.50 to 10.15) | 10.70 (10.39 to 11.01) | 11.59 (11.28 to 11.90) | 12.33 (12.01 to 12.64) | 12.89 (12.57 to 13.22) | 14.3 (13.87 to 14.73) |
Ischaemic stroke as primary end point (%) | 5.51 (5.01 to 6.06) | 5.70 (5.34 to 6.11) | 6.06 (5.73 to 6.43) | 6.39 (6.07 to 6.74) | 6.80 (6.48 to 7.11) | 7.37 (7.05 to 7.68) | 8.29 (7.95 to 8.63) | 9.31 (8.96 to 9.68) | 10.07 (9.72 to 10.43) | 9.97 (9.58 to 10.38) |
Haemorrhagic stroke as primary end point (%) | 0.67 (0.48 to 0.89) | 0.67 (0.54 to 0.81) | 0.71 (0.59 to 0.82) | 0.72 (0.62 to 0.84) | 0.74 (0.65 to 0.84) | 0.76 (0.67 to 0.86) | 0.79 (0.70 to 0.89) | 0.78 (0.69 to 0.88) | 0.7 (0.61 to 0.81) | 0.48 (0.40 to 0.57) |
CVD mortality (%) | 4.48 (3.45 to 5.55) | 6.60 (5.45 to 7.51) | 8.52 (7.22 to 9.47) | 10.39 (8.97 to 11.44) | 12.63 (11.07 to 13.85) | 15.48 (13.78 to 17.07) | 20.17 (18.17 to 22.63) | 26.29 (23.61 to 30.18) | 34.46 (30.65 to 39.32) | 45.95 (41.34 to 50.07) |
Non-CVD mortality (%) | 95.46 (94.40 to 96.49) | 93.40 (92.49 to 94.55) | 91.48 (90.53 to 92.78) | 89.60 (88.56 to 91.03) | 87.37 (86.15 to 88.93) | 84.52 (82.93 to 86.22) | 79.83 (77.37 to 81.83) | 73.71 (69.82 to 76.39) | 65.54 (60.68 to 69.35) | 54.05 (49.93 to 58.66) |
Policy implications
Using these results, we calculated the maximum price that the NHS should be willing to pay for new treatments targeted at each risk group that reduce CVD hazards by between 10% and 40% (Figure 51). This maximum price increases with both increasing baseline risk and with the higher relative risk-reduction impact of treatments.
FIGURE 51.
Theoretical maximum price of new treatments by risk for cardiovascular events among CALIBER patients with SCAD. MI, myocardial infarction. Reproduced from Asaria et al. 53 Copyright © 2016, BMJ Publishing Group Ltd and the British Cardiovascular Society. This is an open-access article distributed under the terms of the Creative Commons Attribution License (http://creativecommons.org/licenses/by/4.0/), which permits unrestricted reuse, distribution, and reproduction in any medium, provided the original work is properly cited.

Discussion
In this study we address a fundamental gap in knowledge: what is the clinical, health service and economic burden associated with SCAD? Using data from a large, contemporary and representative population of UK patients with SCAD, we have shown that substantial health-care costs are likely to be incurred as a result of improved ACS survivorship and the ageing population. Moreover, 5-year and lifetime costs vary according to CVD risk, which may be predicted from baseline patient-level data.
Stable coronary artery disease patients might be considered to have ‘fallen off the radar’ of clinical interest: they are no longer in cardiac rehabilitation (mainly offered to those immediately after AMI or CABG), no longer on dual antiplatelet therapy (maximum current recommendation for therapy is 12 months) and, in most cases, discharged from specialist care, with prescribing adherence to secondary preventative medication waning. SCAD guidelines give little guidance about how such patients should be followed up in health care or how they should be appropriately risk stratified. 233,329 Our results clearly highlight the unmet need and the shortfalls of current approaches: they experience high use of primary care and frequent hospitalisations, incurring considerable ongoing costs. The number of primary care consultations, a mean of 10.76 per patient in the first year with SCAD, is higher than previous estimates for the average population (5.5 per year). 347 In the first year with SCAD, 37% of patients were hospitalised (26.9% for a CVD). This is markedly higher than recent estimates for the general population in one area of the UK (14.9%)348 and higher than that in the general population for similar age groups (23.4% and 21.2% of 60- to 74-year-old males and females, respectively, based on HES data).
Health-care utilisation is insufficient as a metric of the impact on the health-care system. It is also important to consider the costs associated with those resources to the NHS. Average costs of £3127 in the first year with SCAD are markedly higher than those in patients with no chronic conditions (£293) but comparable with other chronic conditions (e.g. diabetes £3036). 348 High costs in the first year following a non-fatal event (£9239) reflect the health-care resources required to treat that event.
We provide here, for the first time, estimates of lifetime health-care costs. In low-risk patients (5-year CVD risk of 3%), remaining lifetime health-care costs were £117,000 and remaining lifetime QALYs were 19.11 years; in the highest-risk group (5-year CVD risk of 44%), remaining lifetime health-care costs were £43,000 and remaining lifetime QALYs were 3.34 years. This is important because it illustrates and quantifies the general phenomenon that the highest costs per patient occur in the highest-risk patients and are concentrated towards the end of life.
Importantly, we provide new information on the price that the NHS should be willing to pay for new treatments to reduce CVD risk in SCAD patients. A new treatment giving a 20% hazard reduction in cardiovascular events would be cost-effective if priced < £61 per year in the lowest-risk group and £657 per year in the highest-risk group. The relatively high cost and modest efficacy of the newer treatments currently completing Phase III trials suggests that they should be targeted at the highest-risk patient groups in order to be cost-effective.
Our model is the first comprehensive lifetime model based on long-term EHR data modelling the full range of CVD end points and accounting for the interdependence of CVD risks in the SCAD population. We have demonstrated that such data can be used to model the long-term costs and health effects of chronic conditions. Furthermore, the size of such data sets (number of patients and the number of years of follow-up) and their scope (number of end points and risk factors captured) enable increasingly biologically plausible models of such conditions to be built.
The model allows policy-makers to quantify and understand both the health and the cost burden of SCAD, and serves as a basis for evaluating the cost-effectiveness of new treatments aiming to reduce CVD risk in this population. Our results suggest that, for the vast majority of SCAD patients, it is likely that low-cost interventions to improve adherence to existing secondary prevention drugs should be prioritised over high-cost new treatments. It is also notable from our results that, even among the groups with the highest CVD risk, more patients are predicted to die of non-CVD-related than CVD-related causes, highlighting the vital role of primary care in the holistic management of both cardiovascular and non-cardiovascular risk for these patients.
As the data sets underpinning this analysis are further enriched in terms of both length of follow-up and breadth of linked data sets, it will become feasible for models such as ours to be extended to capture the trajectory of prognostic risk factors over time. This would allow us to go beyond a focus solely on treatments that directly impact on CVD risk to address broader questions around the cost-effectiveness of interventions that target these underlying risk factors.
This study has limitations. First, outpatient appointment costs cannot currently be linked from HES, so we were unable to include them. As a result, all estimates are underestimates of the total health-care costs of this population. Second, the estimation of costs and consequences involved extrapolation over longer time periods than is currently available. This extrapolation is reflected in the uncertainty around the model predictions. Third, health-related quality-of-life data were not recorded in CALIBER and were drawn from external studies. Finally, changes in prognostic risk factors over time were not explicitly modelled; instead, the equations underpinning our model are informed by the baseline values of these risk factors.
Conclusions
Patients with SCAD account for significant use of health-care resources and incur substantial health-care costs, which can be predicted by individual 5-year CVD risk profiles. We provide a model for estimating the lifetime health-care costs and health outcomes of patients with SCAD. This model lends itself to informing decisions on commissioning, regulatory approval and the ongoing evaluation of new interventions. It also demonstrates the value of utilising linked EHRs for economic evaluation purposes. Improved ACS survivorship and an ageing UK population will require targeted resource allocation in anticipation of burgeoning demand.
Chapter 10 Acute myocardial infarction: missed opportunities for treatment during and after hospitalisation
Abstract
Background
Disease registries have been invaluable in collecting information about a specific disease and its treatment in order to drive audit and research, thus improving the quality of care. AMI is exceptional among diseases in being one of the most ‘registered’, and in being a disease for which health-care interventions have significantly improved prognosis over the years.
Objectives
To evaluate missed opportunities for care in hospital and after discharge for AMI using CVD registries in the UK and other countries.
Methods
Analyses of data from national AMI registries in the UK, Sweden and the USA.
Results
(1) International comparisons identified significant differences in use of surgical interventions and use of some secondary prevention medications in the UK. Short-term mortality after AMI was markedly higher in the UK than in Sweden. (2) More than half of hospitalised STEMI patients eligible for nine evidence-based care components did not receive all components. Greater numbers of missed opportunities for care were associated with higher mortality. (3) Only 23% of smokers received smoking cessation interventions in the 3 months following discharge. Those who ceased smoking experienced fewer cardiac events than those who continued smoking.
Conclusions
Despite guideline recommendations, major opportunities to improve cardiovascular outcomes for ACS patients are being missed, during hospital stays and after discharge. International comparisons may identify key areas that could be targeted to improve outcomes in the UK.
The studies in this chapter relate to patients presenting for the first time with ACS (Figure 52).
FIGURE 52.
Overview of the 33 studies in our programme: presentation with ACS.
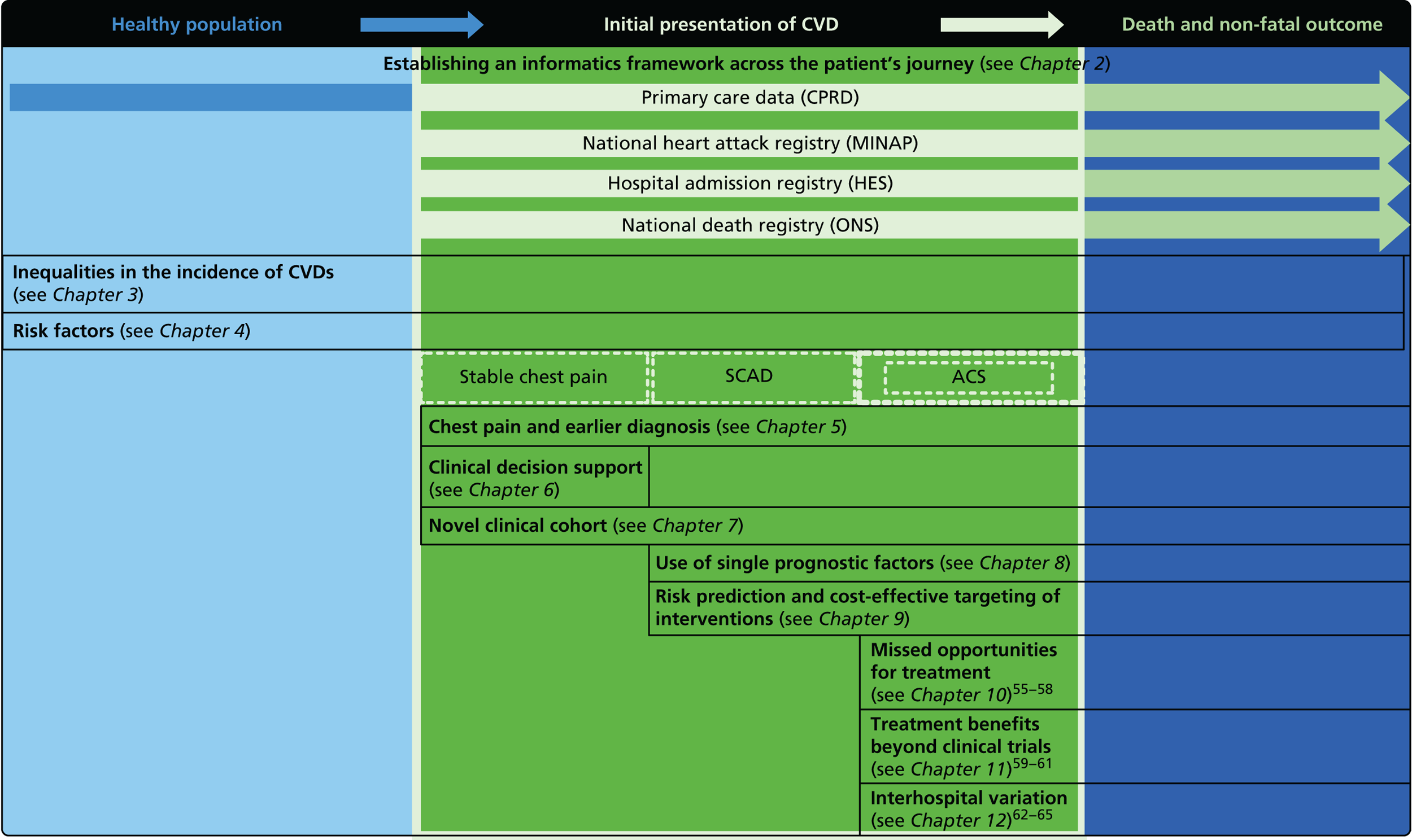
Introduction
Abundant evidence has been gathered from RCTs on the most appropriate management of patients after AMI. Recommended practice is incorporated into national and international guidelines, but implementation of these guidelines is frequently imperfect; even without the introduction of new treatments, optimisation of existing treatments could significantly reduce coronary mortality. 349
We have used the UK’s national ACS registry, MINAP, to investigate the extent to which guidelines are being followed in practice, and what impact non-adherence has on survival. We have also examined one specific recommendation after discharge, for smokers experiencing an AMI to be offered smoking-cessation advice and support.
We have further taken advantage of comprehensive national disease registries in the UK and Sweden, and a selective but large US registry, to compare practices in the three countries. For Sweden and the UK, we were also able to analyse how these differences translated into difference in outcome after AMI.
Study 22: comparative effectiveness of systems of care for acute myocardial infarction – a comparison of short-term survival using nationwide outcome registries in Sweden and the UK
This study is based on the peer-reviewed paper by Chung et al. 55
Introduction
Our comparative analysis of NSTEMI management in the UK, Sweden and the USA highlighted apparent underuse of invasive procedures and beta-blockers in the UK. How these potential missed opportunities for care influenced prognosis, however, could not be determined owing to the absence of studies that simultaneously examine care and outcomes. Additional uncertainty was introduced by differences in the hospitals contributing data to the national registries, with those in the USA being a self-selected subset of hospitals contributing on a voluntary basis, in contrast to the UK and Sweden where data contribution is mandated across all hospitals. Indeed, the UK and Sweden are the only countries in the world with continuous national ACS registries. These are linked to mortality records and therefore offer a unique opportunity for comparative effectiveness research between whole health systems, incorporating both treatment and outcome data. Until now, studies have not standardised the mortality of patients in one country by the case mix in another, resulting in international comparison studies between health systems.
Our objectives in the present study were (1) to evaluate the validity of comparing data from these two nationwide clinical registries; (2) to compare changes over time in the proportions of patients receiving effective interventions during hospitalisation and at discharge between 2004 and 2010; (3) to compare crude and case-mix-standardised 30-day mortality between the two countries, and in clinically important subgroups; (4) to estimate and compare time trends in case-mix-standardised mortality in Sweden and the UK; and (5) to explore the contribution of clinical care to any mortality difference.
Methods
The study population was drawn from all hospitals that provide AMI care in Sweden (n = 86) and the UK (n = 242), and contribute data on consecutive patients to Swedish Web-system for Enhancement and Development of Evidence-based care in Heart disease Evaluated According to Recommended Therapies/Register of Information and Knowledge about Swedish Heart Intensive care Admissions (SWEDEHEART/RIKS-HIA) and MINAP, respectively. Patients with a first AMI admission between 1 January 2004 and 31 December 2010 were included. Fields in SWEDEHEART/RIKS-HIA and MINAP were compared, leading to selection of 17 case-mix measures and 14 in-hospital treatment measures (Table 27). The primary clinical outcome was all-cause mortality within 30 days after hospital admission, as recorded in Swedish and UK national death registries (data available for 99.3% and 98.7% of patients, respectively).
Case mix and treatment | Sweden | UK | ||
---|---|---|---|---|
n | % (95% CI) | n | % (95% CI) | |
STEMI | 38,432 | 32.1 (31.8 to 32.3) | 157,418 | 40.3 (40.1 to 40.4) |
Age (years), mean (SD) | 71.2 (12.3) | 69.5 (13.6) | ||
Female | 43,512 | 36.3 (36.1 to 36.6) | 135,664 | 34.8 (34.7 to 34.9) |
AMI severity, median (Q1, Q2) | ||||
SBP (mmHg) | 145 (125, 165) | 139 (120, 158) | ||
Heart rate (b.p.m.) | 78 (65, 93) | 79 (66, 94) | ||
Troponin I (µg/l) | 4.2 (0.8, 18.0) | 4.4 (0.8, 21.7) | ||
Troponin T (µg/l) | 0.7 (0.2, 2.3) | 0.65 (0.2, 2.3) | ||
Risk factor | ||||
Current smoking | 25,085 | 23.3 (23.0 to 23.5) | 104,522 | 29.5 (29.3 to 29.6) |
History of diabetes | 26,992 | 22.7 (22.4 to 22.9) | 65,458 | 17.6 (17.4 to 17.7) |
History of hypertension | 53,155 | 45.2 (44.9 to 45.5) | 173,342 | 47.3 (47.2 to 47.5) |
CVD history | ||||
Heart failure | 10,859 | 9.7 (9.5 to 9.8) | 18,944 | 5.3 (5.2 to 5.4) |
Cardiac arrest prior to admission | 1578 | 1.3 (1.3 to 1.4) | 8478 | 2.3 (2.2 to 2.3) |
Cerebrovascular disease | 9816 | 10.1 (9.9 to 10.3) | 30,091 | 8.5 (8.4 to 8.5) |
Myocardial infarction | 26,526 | 22.4 (22.1 to 22.6) | 67,346 | 18.3 (18.1 to 18.4) |
Pre-hospital treatment | ||||
Antiplatelet monotherapy | 43,485 | 36.6 (36.3 to 36.6) | 98,247 | 26.4 (26.3 to 26.6) |
Antiplatelet dual therapy | 4788 | 4.0 (3.9 to 4.1) | 10,931 | 2.9 (2.9 to 3) |
PCI | 9475 | 8.0 (7.8 to 8.2) | 19,473 | 5.4 (5.4 to 5.5) |
CABG | 9192 | 7.7 (7.6 to 7.9) | 17,383 | 4.8 (4.8 to 4.9) |
Hospital treatment | ||||
STEMI patients | ||||
Total reperfusion treatment | 27,354 | 71.2 (70.7 to 71.6) | 118,880 | 76.6 (76.4 to 76.8) |
Pre-hospital fibrinolysis | 1533 | 4.1 (3.9 to 4.4) | 13,903 | 9.3 (9.2 to 9.5) |
In-hospital fibrinolysisa | 4539 | 11.8 (11.5 to 12.1) | 84,112 | 54.2 (54.0 to 54.5) |
Primary PCI | 22,773 | 59.3 (58.8 to 59.8) | 34,695 | 22.4 (22.2 to 22.6) |
Delay: symptom to fibrinolysis (minutes), median (Q1, Q3) | 177 (108, 322) | 150 (94, 285) | ||
Delay: symptom to primary PCI (minutes), median (Q1, Q3) | 198 (129, 365) | 199 (140, 328) | ||
NSTEMI and STEMI patients | ||||
Coronary intervention other than primary PCI | 34,288 | 28.6 (28.4 to 28.9) | 58,492 | 17.3 (17.2 to 17.5) |
IV glycoprotein IIb/IIIa receptor inhibitors | 24,993 | 21.0 (20.8 to 21.2) | 28,389 | 8.6 (8.5 to 8.7) |
Anticoagulants | 87,271 | 73.2 (73.0 to 73.5) | 283,344 | 83.0 (82.9 to 83.1) |
Hospitalisation (days), medium (Q1, Q3) | 5 (3, 7) | 6 (3, 10) | ||
Discharge medication | ||||
Antiplatelet monotherapy | 30,409 | 27 (26.8 to 27.3) | 103,218 | 34.2 (34.1 to 34.4) |
Antiplatelet dual therapy | 76,099 | 67.6 (67.3 to 67.9) | 183,753 | 60.9 (60.8 to 61.1) |
Beta-blocker | 99,779 | 88.7 (88.5 to 88.9) | 231,505 | 78.2 (78 to 78.3) |
ACEI or ARB | 63,102 | 56.2 (55.9 to 56.5) | 242,300 | 82.3 (82.2 to 82.5) |
Statin | 89,767 | 79.7 (79.5 to 79.9) | 276,335 | 92.8 (92.7 to 92.9) |
Statistical analysis
We compared mortality outcomes using Kaplan–Meier survival curves. We modelled 30-day mortality for both countries using the 17 case-mix variables, and then applied the Sweden model to the UK AMI population to estimate the case-mix-standardised relative risk of observed versus expected UK 30-day mortality, for each study year and all years. In propensity matching, we estimated the propensity of being a STEMI patient and the propensity of being a NSTEMI patient in Sweden and the UK based on logistic regression models of the 17 case-mix variables, and then matched patients based on the propensity score. Case-mix models incorporated a random effect for each participant hospital. The extent of missing values was examined and then managed by multiple imputation. We explored the extent to which mortality differences between countries might be explained by differences in medical care by standardisation with case-mix and in-hospital treatment and also by estimating the ‘what if’ scenario of UK having had the same use of primary PCI and beta-blocker medication as Sweden from year 2004 onwards, assuming treatment benefits reported in clinical trials.
Results
Eligible patients with AMI included 119,786 from Sweden and 391,077 from the UK (see Table 27). There was a lower proportion of STEMI in Sweden (32% vs. 40%) but indices of infarct severity were similar in Sweden and the UK. In patients with STEMI, primary PCI was more common in Sweden (59% vs. 22%) compared with the UK, where fibrinolysis was used more frequently. In both countries, use of primary PCI increased with time, whereas use of fibrinolysis decreased (Figure 53).
FIGURE 53.
Use of reperfusion. (a) Use of primary PCI; (b) use of fibrinolysis to treat STEMI in Sweden and the UK, by year. Reproduced from Chung et al. 55 Copyright © 2014 Chung et al. This is an open-access article distributed under the terms of CC BY. Published by Elsevier Ltd. This document may be redistributed and reused, subject to certain conditions.
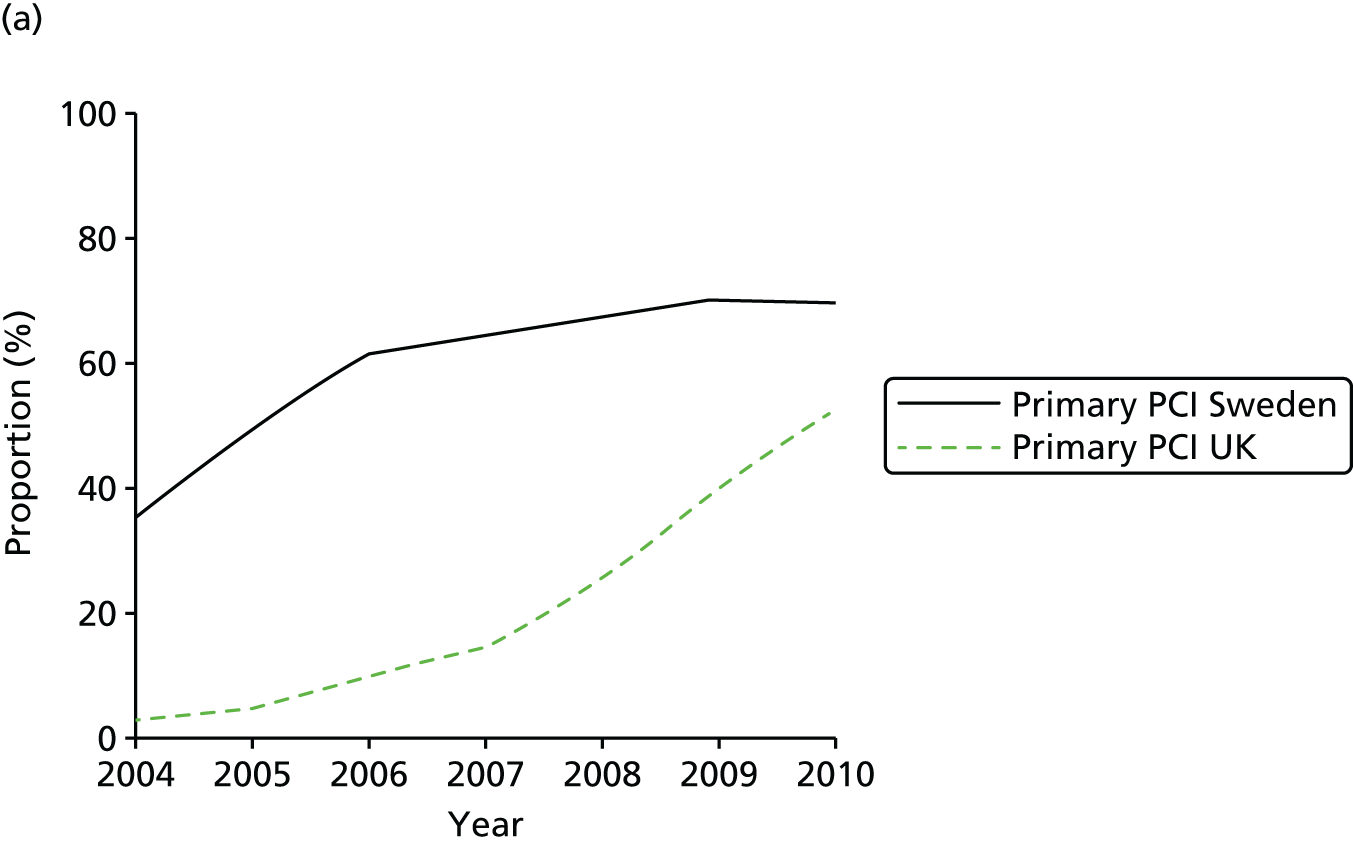

For patients surviving to hospital discharge, Swedish patients were more likely than UK patients to be prescribed dual antiplatelet therapy (68% vs. 61%) and beta-blockers (89% vs. 78%), but less likely to be prescribed ACEIs or ARBs (56% vs. 82%) and statins (80% vs. 93%). The cumulative 30-day mortality was 7.6% (95% CI 7.4% to 7.7%) in Sweden and 10.5% (95% CI 10.4% to 10.6%) in the UK (Figure 54).
FIGURE 54.
Kaplan–Meier curves for cumulative mortality at 30 days after admission with AMI in Sweden and the UK. Reproduced from Chung et al. 55 Copyright © 2014 Chung et al. This is an open-access article distributed under the terms of CC BY. Published by Elsevier Ltd. This document may be redistributed and reused, subject to certain conditions.

In the UK, 30-day mortality was consistently higher in strata defined by measures of infarct severity (troponins, heart rate and BP), age, sex, calendar year, smoking and diabetes status. Standardising the 30-day mortality of UK patients using the Swedish case mix reduced forecast mortality to 7.7% (95% CI 7.3% to 8.2%) with a standardised mortality ratio of 1.37 (95% CI 1.30 to 1.45), corresponding to an estimated 11,263 (95% CI 9620 to 12,827) more deaths in the UK between 2004 and 2010. However, standardised mortality ratios decreased progressively from 1.47 (95% CI 1.38 to 1.58) in 2004 to 1.20 (95% CI 1.12 to 1.29), p-value for trend 0.01 in 2010 (Figure 55). Propensity-matched analyses gave similar findings.
FIGURE 55.
Thirty-day mortality of UK patients admitted in each study year, standardised according to the Swedish case-mix model. p = 0.01 for linear trend across years for the relative risks. Reproduced from Chung et al. 55 Copyright © 2014 Chung et al. This is an open-access article distributed under the terms of CC BY. Published by Elsevier Ltd. This document may be redistributed and reused, subject to certain conditions.
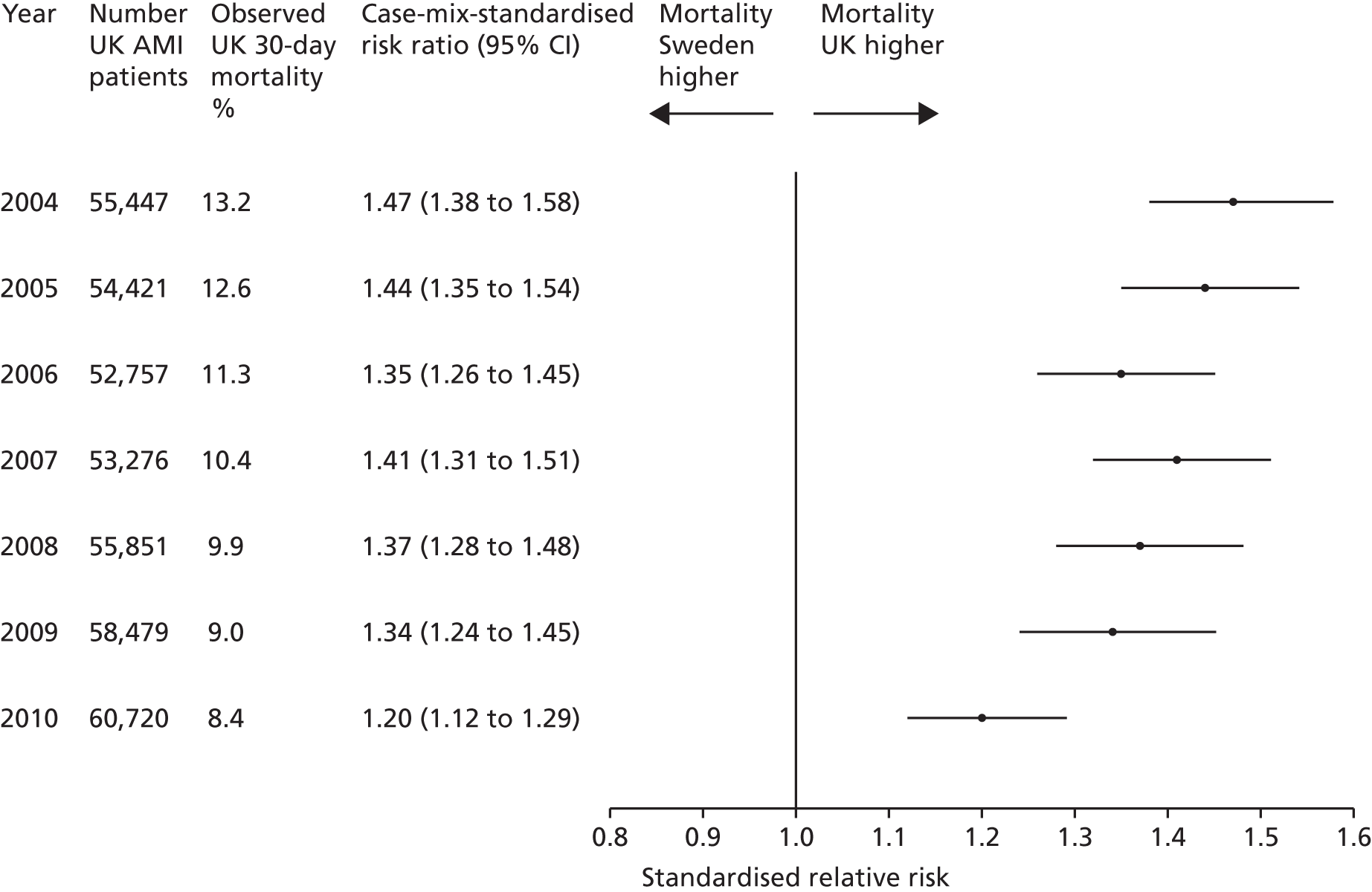
Discussion
We found greater cumulative 30-day mortality after AMI in the UK (10.5%) than in Sweden (7.6%). Case-mix standardisation attenuated the mortality difference. The findings suggest that > 10,000 early deaths would have been prevented or delayed had UK patients experienced the care of their Swedish counterparts. We believe that the comparison between Sweden and the UK was valid because, for both countries, we captured consecutive AMI admissions across all hospitals, using common data definitions, and we showed that the diagnosis of AMI was comparable, with similar troponin values and similar propensity to make a diagnosis.
Variation in the way patients were managed in the UK and in Sweden may have contributed to the 30-day mortality differences. Use of primary PCI for STEMI patients in the UK lagged behind Sweden; the rate in 2010 in the UK (53%) was similar to that of Sweden in 2005. Primary PCI is more effective than fibrinolysis and was incorporated into US and European guidelines in 2004 and 2005, respectively; however, the UK did not have a national policy for primary PCI until 2008. This probably explains the rapid increase in primary PCI at that time, although only in 2011–12 did rates exceed 90%. 350 The use of evidence-based secondary prevention medication showed a mixed pattern, with statins and ACEIs/ARBs being more commonly prescribed in the UK, whereas beta-blocker use on discharge was more common in Sweden. The mortality gap between Sweden and the UK decreased over time, from a standardised mortality ratio of 1.47 in 2004 to 1.20 in 2010, consistent with a narrowing of gaps in treatment differences. Other unmeasured aspects of health care, which probably contributed to the mortality gap, include the dose, timing and adherence to drugs, differences in operator experience, shared and specialty care pathways, use of decision support tools and organisational culture. 351
This study comparing the delivery of health care in different health-care systems has demonstrated differences in short-term outcomes for AMI patients; however, many patients remain at increased risk beyond this period. We analysed linked EHR and disease registry data in four countries {England (CALIBER), Sweden [Health Outcomes, Life Expectancy, Resource Use and Costs in Patients With a History of Myocardial Infarction and Additional Risk Factors for Atherothrombosis (HELICON)], the US (Medicare) and France [Echantillon Généraliste des Bénéficiaires (EGB)]} to investigate longer-term CVD risk among 140,880 patients surviving to the first anniversary of their heart attack. Our study found that cumulative risk of AMI, stroke, CVD death, all-cause mortality and hospitalised bleeding remained high for 3 years across all four countries, and predictors of risk had similar strong positive associations with the outcomes in each country. This work further highlights the power and generalisability of prognosis research that utilises the EHRs across different health-care systems. 352 Linkage to national EHRs will extend comparisons to include ambulatory care before and after admission for heart attack, non-fatal events and longer-term outcome.
Policy initiatives are required to identify, understand and reduce gaps in treatments and outcomes between health systems. We suggest that progress towards this goal is achievable, as mortality differences are narrowing and are, therefore, reversible. The study suggests a novel approach of between-country comparison; to date, quality outcome initiatives have emphasised within-country, within-system metrics. For Sweden, these results provide further evidence of the value of sustained, system-wide quality improvement initiatives, including public reporting of mortality outcomes at hospital level. For the UK, these results suggest the value of learning from systems that appear to be better performing.
International harmonisation of detailed measures of quality of care in clinical registries, such as pathways of care, is needed to identify and evaluate additional patient-level health-care factors that are not measured here that might explain differences between countries. Outcomes should be compared with those in other countries, such as France, Hungary, Poland and the USA, through assessment of national registries.
Limitations include the possibility of unmeasured confounders and the potential for patients not captured in national registries – who are at higher risk – to distort the findings. However, such missed cases may be less common in Sweden, where nationwide registries have a more established history than in the UK, suggesting the mortality difference may actually be wider.
Conclusions
In conclusion, clinically important differences in the care and outcomes of AMI are observed between Sweden and the UK. International comparisons of care and outcome registries may inform new research and policy initiatives to improve the quality of health systems.
Study 23: mortality and missed opportunities along the pathway of care for ST-segment elevation acute myocardial infarction – a national cohort study
This study is based on the peer-reviewed paper by Simms et al. 56 Sections of the text have been adapted from the original article; Copyright © 2015, © SAGE Publications.
Introduction
There are many guideline-recommended acute interventions for patients with ACSs. Monitoring adherence to guidelines is important in order to continually improve the quality of clinical care, and the MINAP registry is invaluable in this respect. In this study we used MINAP to evaluate the association between cumulative missed opportunities for care (CMOCs) and mortality in patients hospitalised with STEMI.
Methods
The MINAP study population was drawn from 121,567 consecutive patients aged ≥ 18 years presenting for the first time with STEMI to hospitals in England and Wales from 1 January 2007 to 31 December 2010 and surviving to be discharged. Mortality data (30-day and 1-year all-cause) were obtained from the ONS. We identified nine guideline-recommended care opportunities recorded in MINAP along the pathway of STEMI care: (1) the recording of a pre-hospital ECG; (2) use of acute aspirin; (3) timely coronary reperfusion (within 120 minutes or thrombolysis within 60 minutes of the call for help); (4) the individual prescriptions of aspirin; (5) the individual prescription of thienopyridine inhibitor; (6) the individual prescription of beta-blockers; (7) the individual prescription of an ACEI or ARB; (8) the individual prescription of statins; and (9) enrolment into a cardiac rehabilitation programme at the time of discharge from hospital. 353–355 A CMOC score was calculated for each patient, based on the number of these nine components that were not provided. To overcome instability due to small numbers, patients were categorised into CMOC groups of 0, 1, 2, 3 and ≥ 4 missed care opportunities.
Statistical analysis
Fixed-effects univariable logistic regression models were built to identify possible factors associated with ineligibility for care and model estimates expressed as ORs with 95% CIs. Similar models were fitted to quantify the relative risk of missing ‘upstream’ interventions (pre-hospital ECG and timely reperfusion) on the number of missed ‘downstream’ (hospital and cardiac rehabilitation) interventions. The association between CMOC and mortality was quantified using mixed-effects models. We adjusted for case mix using variables from the Global Registry of Acute Coronary Event (GRACE) risk score356,357 and patient characteristics. These models were then used to estimate risk-adjusted mortality rates.
Results
A total of 112,286 patients were discharged alive from hospital following STEMI, of whom 62,267 were eligible for all nine care interventions. Of the 31,187 (50.1%) eligible for all nine care interventions who had complete data, 49.4%, 36.4%, 11.5%, 1.9% and 0.8% had CMOC scores of 0, 1, 2, 3 and ≥ 4, respectively (Table 28). No patient eligible for all care opportunities missed more than eight components. Patients with higher CMOC scores more frequently were older and female. Patients also had a greater burden of comorbidity, higher GRACE risk scores and less frequently received coronary angiography.
Patient characteristics | CMOC group (for patients eligible for all nine care components) | Unadjusted relative risk of CMOC, IRR (95% CI)a | ||||
---|---|---|---|---|---|---|
0, n = 15,416 (49.4%) | 1, n = 11,356 (36.4%) | 2, n = 3,571 (11.5%) | 3, n = 606 (1.9%) | ≥ 4, n = 238 (0.8%) | ||
Age (years), mean (SD), per 10 years | 61.7 (± 11.9) | 63.5 (± 13.0) | 64.4 (± 13.5) | 67.9 (± 14.2) | 71.5 (± 15.7) | 1.11 (1.10 to 1.12) |
Female (%) | 21.3 | 24.8 | 29.0 | 34.3 | 40.1 | 1.23 (1.19 to 1.27) |
SBP (mmHg) mean (± SD) | 133.7 (± 26.0) | 135.8 (± 27.0) | 137.3 (± 27.3) | 135.4 (± 27.7) | 132.7 (± 29.7) | 1.02 (1.01 to 1.02) |
Heart rate (b.p.m.), mean (± SD) | 74.6 (± 17.8) | 75.9 (± 18.6) | 77.6 (± 19.3) | 77.1 (± 20.5) | 78.7 (± 21.0) | 1.03 (1.03 to 1.04) |
Previous AMI (%) | 10.3 | 13.2 | 15.8 | 20.0 | 24.6 | 1.28 (1.23 to 1.32) |
Previous angina (%) | 11.5 | 13.7 | 15.8 | 22.6 | 27.3 | 1.24 (1.20 to 1.29) |
Previous revascularisation (%) | 0.7 | 1.0 | 1.6 | 2.1 | 2.7 | 1.45 (1.29 to 1.63) |
Diabetes (%) | 9.4 | 11.9 | 16.3 | 15.0 | 15.3 | 1.26 (1.21 to 1.31) |
Hypertension (%) | 36.8 | 42.0 | 46.6 | 50.6 | 43.7 | 1.19 (1.15 to 1.22) |
Peripheral vascular disease (%) | 2.0 | 2.5 | 2.5 | 3.1 | 7.3 | 1.22 (1.12 to 1.33) |
Stroke (%) | 2.9 | 4.1 | 4.5 | 8.6 | 10.7 | 1.35 (1.26 to 1.44) |
COPD (%) | 6.9 | 7.6 | 8.3 | 11.6 | 10.6 | 1.12 (1.07 to 1.18) |
Chronic renal failure (%) | 0.9 | 1.6 | 2.2 | 2.7 | 5.1 | 1.50 (1.37 to 1.66) |
Chronic heart failure (%) | 0.7 | 1.0 | 1.4 | 3.4 | 3.7 | 1.56 (1.39 to 1.75) |
Mini-GRACE risk score, mean (SD), per 1 unit | 105.1 (± 24.4) | 107.5 (± 26.2) | 108.6 (± 27.0) | 115.8 (± 27.9) | 120.0 (± 32.0) | 1.04 (1.03 to 1.04) |
Hospital survival of cardiac arrest (%) | 7.0 | 5.8 | 5.0 | 5.7 | 6.9 | 0.89 (0.84 to 0.94) |
Referred for coronary catheterisation or PPCI (%) | 89.8 | 87.1 | 83.6 | 75.6 | 60.6 | 0.72 (0.70 to 0.75) |
Crude 30-day mortality (%) | 0.53 | 0.97 | 0.94 | 2.07 | 5.41 | 1.56 (1.38 to 1.77) |
Crude 1-year mortality (%) | 3.21 | 5.41 | 5.68 | 10.57 | 22.75 | 1.51 (1.43 to 1.61) |
Figure 56 shows that acute aspirin therapy was the least frequent contributor to CMOC, whereas pre-hospital ECG and timely reperfusion were commonly included in CMOC. Both of these upstream interventions independently predicted further downstream missed opportunities [pre-hospital ECG: incident rate ratio (IRR) 1.64, 95% CI 1.58 to 1.70; and timely reperfusion: IRR 9.94, 95% CI 9.51 to 10.40].
FIGURE 56.
Profile of each missed opportunity along the pathway of STEMI care, by CMOC group for patients eligible for all nine care opportunities in MINAP. Reproduced with permission from Simms et al. 56 © 2015, © SAGE Publications.
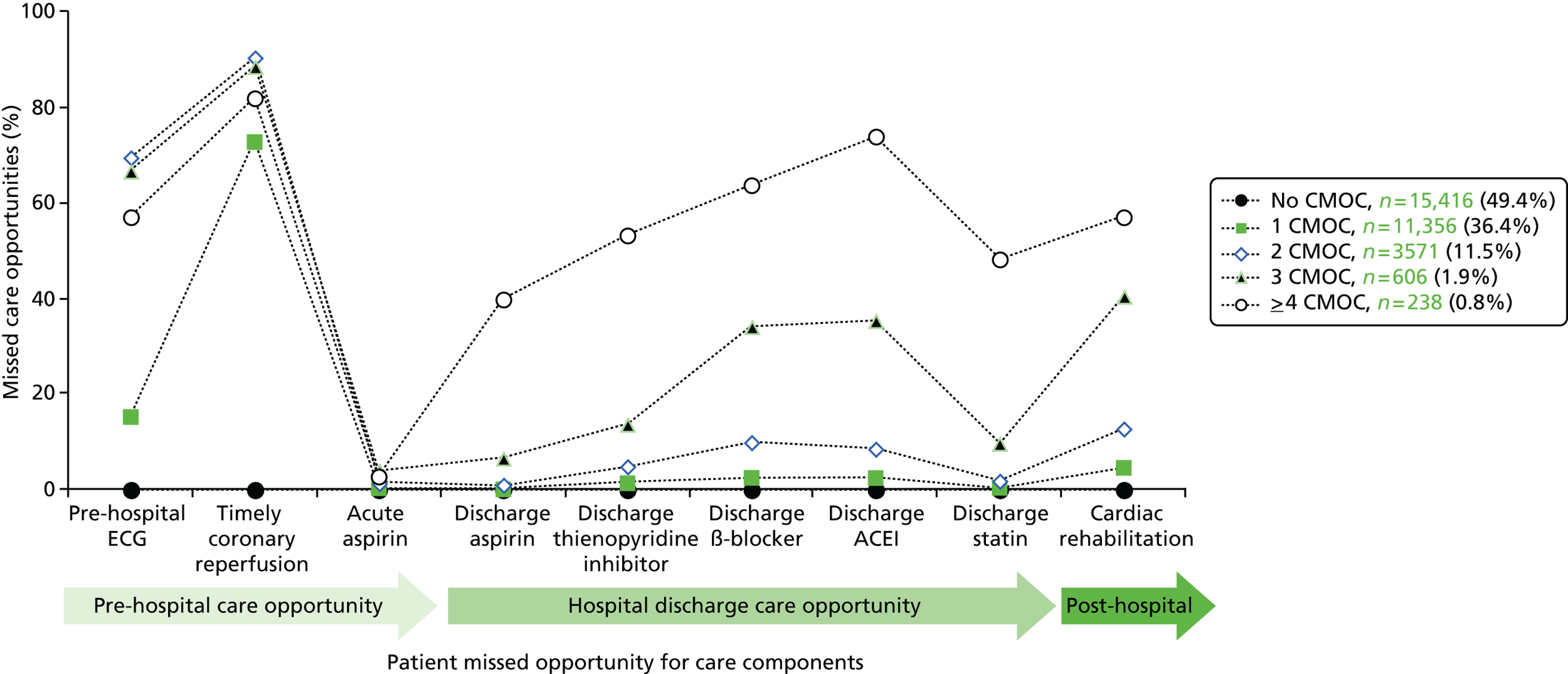
Hospitals with ≤ 30 cardiology beds had significantly higher CMOC scores than those with > 50 beds. Hospital volume of STEMI was negatively associated with CMOC score but there was no significant association between CMOC score and whether or not hospitals had on-site coronary angiography/PCI facilities.
Risk-adjusted mortality rates rose with increasing CMOC (Figure 57). Kaplan–Meier survival estimates stratified by CMOC also showed a positive association between CMOC and mortality (Figure 58), and this was confirmed by the risk-adjusted Cox analysis (Figure 59).
FIGURE 57.
Crude 30-day and 1-year mortality rates and 95% CIs by number of ineligible care for patients eligible for nine evidence-based care components in MINAP.
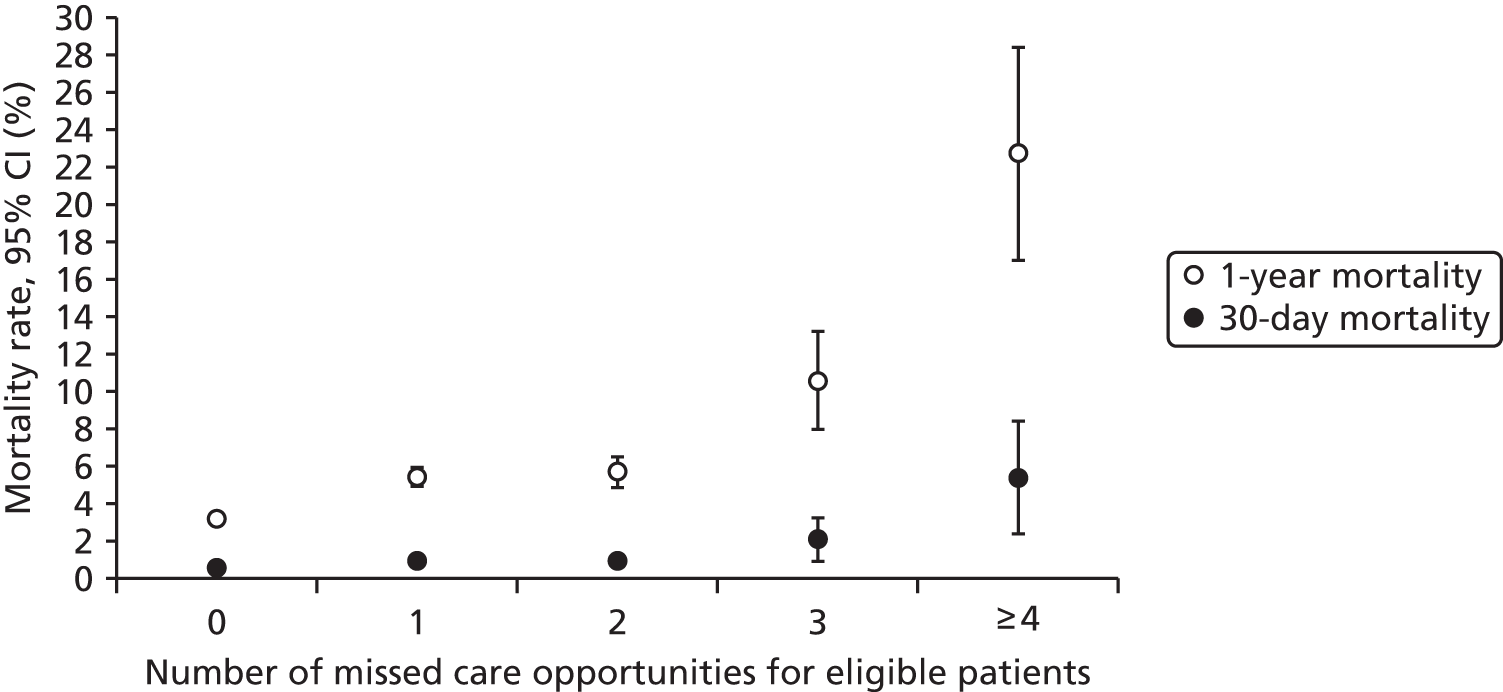
FIGURE 58.
Kaplan–Meier unadjusted survival estimates for patients with STEMI, stratified by CMOC group, for patients eligible for all nine evidence-based care components in MINAP. Reproduced with permission from Simms et al. 56 © 2015, © SAGE Publications.
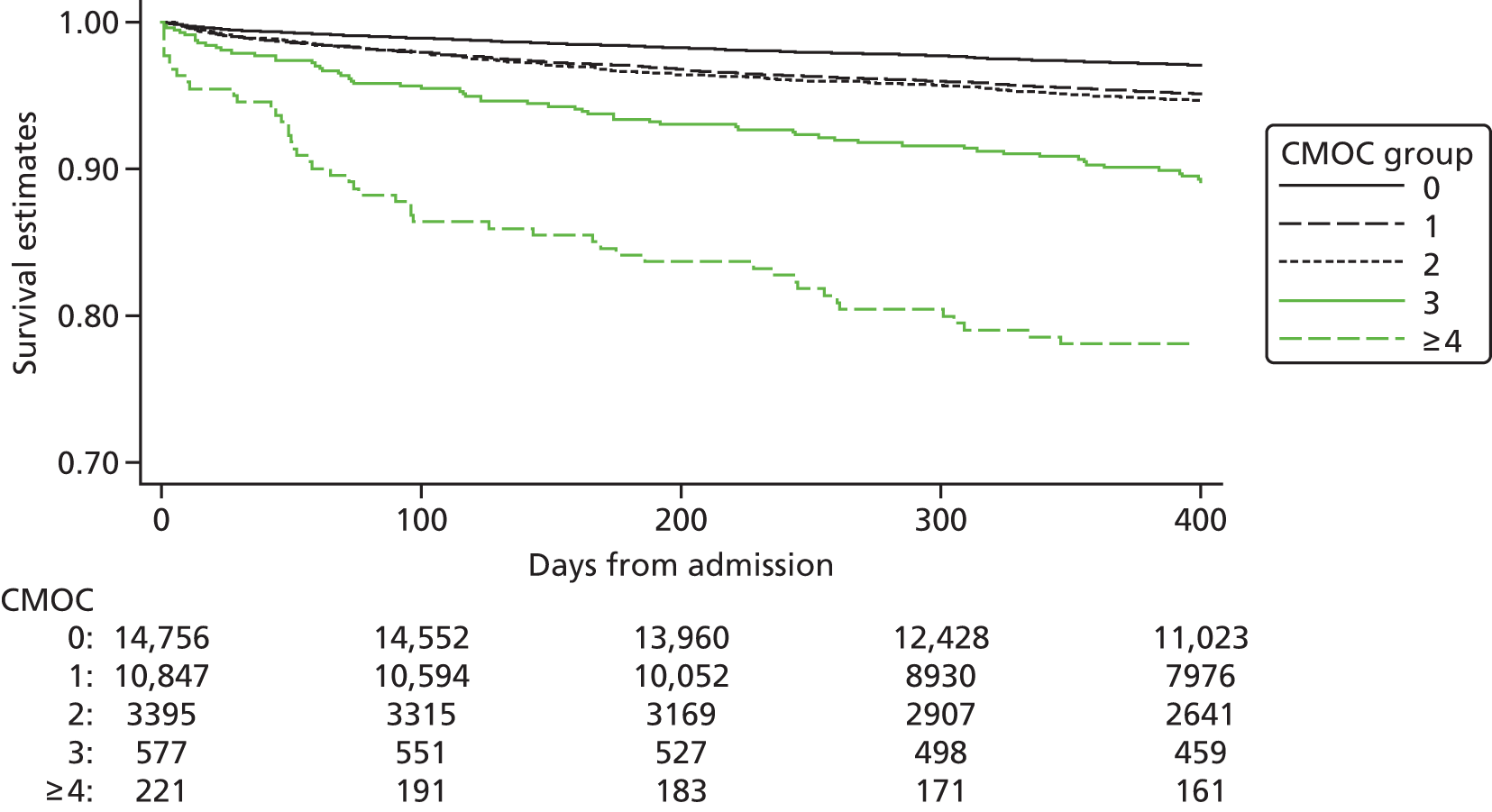
FIGURE 59.
Forest plot of adjusted risk of 30-day and 1-year mortality, by CMOC group, for patients eligible for all nine evidence-based care components in MINAP. Reproduced with permission from Simms et al. 56 © 2015, © SAGE Publications.

Discussion
This study shows that missed opportunities during the patient journey extend beyond primary care and are common following hospital admission in patients with STEMI. More than half of this high-risk group failed to receive at least one of nine components of care recommended in national guidelines. We found a significant relationship between CMOC score and early mortality, which remained significant after 1 year. The data suggest that CMOC represents an important target for improving quality of care and outcomes.
Patients with higher CMOC scores were at greater baseline risk and had more comorbid conditions. Failure to provide upstream interventions – pre-hospital ECG and timely coronary reperfusion – significantly predicted downstream missed opportunities. The association between CMOC score and mortality remained strong, despite adjustment for case mix and invasive treatments, suggesting that it was driven more by hospital factors than the risk characteristics of the patients. Thus, our data identified underprovision of designated cardiology beds and larger catchment populations as hospital factors significantly associated with CMOC. However, hospital associations with CMOC were complex, with low-volume centres reporting more missed components of the care pathway. Overall, our findings suggest that adequately resourced, high-throughput, high-volume hospitals (heart attack centres) with appropriate facilities may offer superior care and may, therefore, deliver better outcomes. Outcomes research specifically designed to follow care into the primary care setting is warranted to quantify the full implication of CMOCs.
Conclusions
Of patients with STEMI eligible for nine evidence-based care components, over half did not receive all components. Missed opportunities for care were significantly associated with early- and longer-term mortality. Outcomes for STEMI would likely improve if CMOC was reduced and physicians provided all components of the pathway of care to their patients.
Study 24: international comparisons of the management of patients with non-ST-segment elevation acute myocardial infarction
This study is based on the peer-reviewed paper by McNamara et al. 57 Sections of the text have been reproduced/adapted from the original article. Copyright © 2014 The Authors. Published by Elsevier Inc. This is an open-access article distributed under the terms of the Creative Commons Attribution License (http://creativecommons.org/licenses/by/3.0/).
Introduction
Our analysis of missed opportunities for care along CALIBER’s patient journey has focused largely on deviations from guideline recommendations in the management of patients with CVD, both in primary care and hospital settings. However, registry-based research offers an alternative means of evaluating care through the comparison of management strategies in different countries. To date, there have been few international comparisons of care for patients with NSTEMI, and none that reflects the recent changes in clinical management recommended in international guidelines.
We have established a collaboration with investigators in Sweden and the USA in order to compare patterns of in-hospital treatment and use of interventional diagnostic and therapeutic procedures among patients admitted with NSTEMI across three national clinical registries: MINAP in the UK, SWEDEHEART/RIKS-HIA in Sweden and Acute Coronary Treatment and Intervention Outcomes Network Registry (ACTION) in the USA. 7,358 SWEDEHEART/RIKS-HIA and MINAP cover all hospitals providing care for ACS in Sweden and the UK, respectively; ACTION is a voluntary registry that includes patients in a large but self-selected group of hospitals in the USA. Data validity checks in SWEDEHEART/RIKS-HIA, MINAP and ACTION have demonstrated agreement rates of 96.1%, 89.5% and 89.7%, respectively. 7,86,359
Methods
The study population was drawn from all hospitals providing AMI care in England and Wales (236 hospitals, 137,009 patients), Sweden (74 hospitals, 45,069 patients) and a voluntary subset of hospitals, most with the capability to perform PCI, in the USA (500 hospitals, 147,438 patients). We included patients admitted between 1 January 2007 and 31 December 2010, and aged ≤ 30 years with first recorded NSTEMI. The distribution of case-mix (demographics, past history and presentation characteristics) and treatment variables was compared in the UK, Sweden and the USA. Recognising the difference between nationwide and voluntary registries, we conducted a sensitivity analysis, comparing results of NSTEMI patients attending PCI hospitals only. We defined PCI hospitals as those performing at least 24 procedures in a calendar year.
Results
Patients were youngest in the USA, where rates of smoking, diabetes, hypertension, prior heart failure and prior AMI were higher than in Sweden or the UK (Table 29). Prior use of medications, PCI and CABG was also higher in the USA. Angiography and PCI were performed more often in the USA and Sweden than in the UK, but the rate of increase in these procedures from 2007 to 2010 was greatest in the UK (Table 30). Similarly, PCI was performed more in the USA. Prescription of dual antiplatelet therapy was similar across the countries. UK physicians prescribed beta-blockers at discharge least often but statins and ACEIs/ARBs most often.
Characteristics at baseline | Total NSTEMI | ||
---|---|---|---|
UK | Sweden | USA | |
Number of patients | 137,009 | 45,069 | 147,438 |
Number of participating hospitals | 236 | 74 | 500 |
Demographics | |||
Age (years), median (IQR) | 73 (62–82) | 73 (64–82) | 67 (56–78) |
Female sex (%) | 37.5 | 37.6 | 38.7 |
Risk factors (%) | |||
Current smoker | 23 | 19.6 | 29.6 |
Diabetes | 21.7 | 25.0 | 35.4 |
Hypertension | 53.1 | 50.6 | 76.3 |
History of CVD (%) | |||
Heart failure | 7.0 | 11.5 | 16.9 |
AMI | 22.8 | 27.8 | 28.8 |
Treatment prior to hospital admission | |||
Single antiplatelet (%) | 30.4 | 42.2 | 39.4 |
Dual antiplatelet (%) | 4.3 | 4.4 | 13.4 |
Beta-blocker (%) | 28.8 | 44.4 | 44.2 |
ACEI or ARB (%) | 38.3 | 37.3 | 42.5 |
Statin (%) | 44.8 | 32.3 | 43.5 |
PCI (%) | 7.5 | 12.3 | 25.0 |
CABG (%) | 7.2 | 10.4 | 19.0 |
SBP (mmHg), median (IQR) | 140 (121–160) | 149 (130–168) | 145 (125–166) |
Heart rate (b.p.m.), median (IQR) | 80 (68–96) | 79 (66–94) | 83 (70–99) |
Haemoglobin (g/dl), median (IQR) | 13.5 (12.0–14.8) | 13.7 (12.5–14.8) | 13.7 (12.2–14.9) |
Creatinine (mmol/l), median (IQR) | 95 (79–119) | 86 (72–107) | 97.2 (79.6–123.8) |
Troponin, median (IQR)a | |||
Troponin T (ng/ml) | 0.33 (0.13–0.91) | 0.39 (0.14–1.09) | 0.1 (0.03–0.4) |
Troponin I (ng/ml) | 1.9 (0.39–7.59) | 2.0 (0.48–7.2) | 0.4 (0.1–1.8) |
Initial ECG or ECG determining treatment (%) | |||
Transient ST-segment elevation | 2.7 | 4.0 | 2.7 |
ST-segment depression | 30.3 | 34.3 | 23.8 |
Other T-wave abnormalitiesb | 29.8 | 14.9 | 14.1 |
No ST-segment abnormalitiesc | 37.2 | 46.8 | 59.4 |
Treatment | Data set (country) | Year of hospital admission (%) | ||||
---|---|---|---|---|---|---|
2007 | 2008 | 2009 | 2010 | 2007–10 | ||
In-hospital angiography | MINAP (UK) | 22.9 | 27.9 | 35.5 | 38.8 | 31.8 |
RIKS-HIA (Sweden) | 60.4 | 62.7 | 66.8 | 69.8 | 64.8 | |
ACTION (USA) | 72.4 | 75.2 | 76.6 | 78.3 | 76.0 | |
In-hospital PCI | MINAP (UK) | 16.5 | 20.0 | 23.4 | 26.1 | 21.8 |
RIKS-HIA (Sweden) | 39.0 | 40.2 | 42.7 | 45.9 | 41.9 | |
ACTION (USA) | 41.8 | 43.3 | 44.2 | 45.5 | 43.9 | |
Beta-blocker at discharge | MINAP (UK) | 73.1 | 74.9 | 76.5 | 78.7 | 76.0 |
RIKS-HIA (Sweden) | 88.8 | 88.6 | 88.2 | 89.0 | 88.6 | |
ACTION (USA) | 90.1 | 89.4 | 89.1 | 89.1 | 89.4 | |
Antiplatelet at discharge (Any) | MINAP (UK) | 94.2 | 94.0 | 94.7 | 95.4 | 94.6 |
RIKS-HIA (Sweden) | 93.6 | 94.4 | 95.0 | 94.9 | 94.5 | |
ACTION (USA) | 95.0 | 95.1 | 95.6 | 95.7 | 95.4 | |
Antiplatelet at discharge (Dual) | MINAP (UK) | 74.9 | 74.6 | 76.4 | 78.6 | 76.2 |
RIKS-HIA (Sweden) | 63.2 | 66.9 | 70.6 | 72.4 | 68.1 | |
ACTION (USA) | 64.7 | 65.1 | 66.9 | 68.0 | 66.4 | |
Statin at discharge | MINAP (UK) | 91.2 | 91.0 | 91.5 | 92.1 | 91.5 |
RIKS-HIA (Sweden) | 79.7 | 81.4 | 82.7 | 83.6 | 81.1 | |
ACTION (USA) | 82.0 | 83.7 | 85.4 | 85.9 | 84.5 | |
ACEI or ARB at discharge | MINAP (UK) | 77.1 | 77.5 | 79 | 80.6 | 78.7 |
RIKS-HIA (Sweden) | 65.5 | 68.3 | 69.9 | 72.1 | 68.8 | |
ACTION (USA) | 65.9 | 64.5 | 64.3 | 63.9 | 64.5 |
Discussion
This comparison of ongoing national registries showed important differences in the application of guideline-recommended treatments in the UK compared with in Sweden and the USA, despite close similarities in case mix. The data suggest missed opportunities for care on a national scale reflected in the UK’s less aggressive use of interventional procedures for NSTEMI, although the gap with Sweden and the USA narrowed over time. In contrast, secondary prevention medications (except beta-blockers) were more commonly prescribed in the UK than in Sweden or the USA.
Clinical trials have previously shown wide variation in practice among countries or groups of countries in the management of AMI patients. 360–362 However, management patterns within a clinical trial do not necessarily reflect management in usual clinical practice. 363 Clinical registries address some of the selection bias and are valuable resources for comparison and for assessing trends in treatment. 363–367 The finding of similarly high PCI rates in an unselected (SWEDEHEART/RIKS-HIA) and a selected (ACTION) registry makes the differences seen in other aspects of NSTEMI care more informative. It suggests that US procedure rates are not being driven by fee for service and that Sweden’s increased emphasis on system-wide quality improvement is bearing fruit. 368,369 The lower rates of interventional management in the UK provide further evidence of slow implementation of emerging technologies, although the steeper increase in in-hospital procedure rates between 2007 and 2010 suggests that concerted efforts to increase PCI capability have been effective. 370
The lower use of beta-blockers in the UK than in the USA and Sweden was accompanied by higher use of statins and cannot, therefore, be driven by economic factors or by delayed implementation (the evidence for beta-blockers in AMI pre-dates that for statins). One possibility is that UK patients had more perceived contraindications, such as COPD, although our CALIBER study (see study 27 below) has shown that beta-blockers are beneficial and safe in patients with COPD who present with AMI. 59
Further studies are warranted to better explain patterns of clinical practice within and outside the UK, such as rates of coronary angiography and PCI.
Conclusions
Differences exist in the acute management of NSTEMI among patients in national registries from the UK, Sweden and the USA. Specifically, the use of invasive procedures such as angiography and PCI and of beta-blockers on discharge was higher in the USA and Sweden than in the UK. Differences are becoming smaller but need to be understood if areas for improvement are to be identified.
Study 25: smoking cessation interventions following acute coronary syndrome – a missed opportunity?
This study is based on the peer-reviewed paper by Boggon et al. 58 Sections of the text have been adapted from the original article; © The European Society of Cardiology.
Introduction
In mapping the CVD journey in CALIBER, we had the unique opportunity to examine patient care in the community after discharge from hospital, recognising that sustained lifestyle modification and secondary prevention treatment is a key guideline recommendation in patients who have experienced AMI. Perhaps the most important lifestyle modification is smoking cessation, which substantially reduces the risk of recurrent AMI and coronary death. 371 Recommendations are for GPs to offer smoking cessation advice and specific treatment to all smokers after ACS,330 but the extent to which this opportunity to reduce mortality is missed has not been clarified. To answer this question, we used linked CALIBER records to analyse the extent of smoking cessation advice and pharmaceutical interventions for smoking cessation provided by GPs after AMI and to describe patient outcomes stratified by smoking status.
Methods
We studied 4834 patients aged ≥ 30 years discharged alive from hospital after AMI between 1 January 2002 and 30 September 2009. Patients were followed to death, transfer out of the general practice or the last data collection date. Smoking interventions recorded in CPRD were classified as follows: (1) prescribed pharmacological interventions for smoking cessation; (2) GP smoking cessation advice or referral to stop-smoking clinic (without pharmaceutical interventions); and (3) no GP intervention.
Major clinical outcomes were explored in patients who smoked prior to their ACS, stratified by quitting status. Outcomes included death, recurrent AMI, stroke, heart failure and major adverse cardiac events, defined as death, recurrent ACS, CABG or target lesion revascularisation.
Results
Characteristics of patients are shown in Table 31. Of the 4834 patients, 33% were non-smokers, 44% were ex-smokers and 20% were smokers.
Characteristics | Non-smokers (N = 1611) | Ex-smokers (N = 2122) | Smokers (N = 965) | Unknown (N = 136) | Total (N = 4834) |
---|---|---|---|---|---|
Men, n (%) | 743 (46.1) | 1437(67.7) | 690 (71.5) | 81 (59.6) | 2951(61.0) |
Age (years) at ACS hospitalisation, n (%) | |||||
30–39 | 6 (0.4) | 6 (0.3) | 23 (2.4) | 4 (2.9) | 39 (0.8) |
40–49 | 42 (2.6) | 41 (1.9) | 139 (14.4) | 9 (6.6) | 231 (4.8) |
50–59 | 149 (9.2) | 145 (6.8) | 248 (25.7) | 19 (14.0) | 561 (11.6) |
60–69 | 214 (13.3) | 395 (18.6) | 233 (24.1) | 20 (14.7) | 862 (17.8) |
70–79 | 468 (29.1) | 700 (33.0) | 204 (21.1) | 24 (17.6) | 1396 (28.9) |
≥ 80 | 732 (45.4) | 835 (39.3) | 118 (12.2) | 60 (44.1) | 1745 (36.1) |
Alcohol consumption, n (%) | |||||
Non-drinker | 310 (19.2) | 214 (10.1) | 109 (11.3) | 1 (0.7) | 634 (13.1) |
Ex-drinker | 164 (10.2) | 244 (11.5) | 96 (9.9) | 1 (0.7) | 505 (10.4) |
Drinker | 955 (59.3) | 1527 (72.0) | 657 (68.1) | 6 (4.4) | 3145 (65.1) |
Unknown | 182 (11.3) | 137 (6.5) | 103 (10.7) | 128 (94.1) | 550 (11.4) |
SBP (mmHg) mean (median) | 139.1 (140.0) | 138.3 (139.0) | 137.8 (138.0) | 143.1 (140.0) | 138.6 (139.0) |
Total/HDL cholesterol, n (%) | |||||
≤ 7 | 971 (60.3) | 1530(72.1) | 498 (51.6) | 19 (14.0) | 3018 (62.4) |
≥ 7 | 29 (1.8) | 25 (1.2) | 39 (4.0) | 0 (0.0) | 93 (1.9) |
Unknown | 611 (37.9) | 567 (26.7) | 428 (44.4) | 117 (86.0) | 1723 (35.6) |
Deprivation (IMD) quintile, n (%) | |||||
0 (least deprived) | 287 (17.8) | 328 (15.5) | 84 (8.7) | 17 (12.5) | 716 (14.8) |
1 | 378 (23.5) | 465 (21.9) | 158 (16.4) | 32 (23.5) | 1033 (21.4) |
2 | 351 (21.8) | 444 (20.9) | 203 (21.0) | 31 (22.8) | 1029 (21.3) |
3 | 317 (19.7) | 441 (20.8) | 237 (24.6) | 30 (22.1) | 1025 (21.3) |
4 (most deprived) | 278 (17.3) | 444 (20.9) | 283 (29.3) | 26 (19.1) | 1031 (21.3) |
Peak troponin (ng/ml), mean (median) | 5.3 (0.8) | 6.8 (0.8) | 6.6 (0.8) | 5.0 (0.9) | 6.2 (0.8) |
In the 3 months following discharge, over three-quarters of smokers received no smoking intervention, despite 99% of patients having had some contact with their GP (Table 32). The 320 (33%) smokers who quit in the first 3 months after ACS experienced significantly lower rates of death and major adverse cardiac events than non-quitters (Figure 60).
Intervention | Coded data, n (%) | Free text, n (%) | Combined, n (%) |
---|---|---|---|
Pharmacological | 141 (14.6) | 42 (4.4) | 143 (14.8) |
Advice/referral | 4 (0.4) | 107 (11.1) | 82 (8.5) |
None | 820 (85.0) | 816 (84.6) | 740 (76.7) |
FIGURE 60.
Relative rate of major clinical outcomes comparing smokers who quit in the first 3 months after ACS compared with people who continued to smoke in CALIBER. MACE, major acute coronary events. Reproduced from Boggon et al. 58 © 2014 SAGE publications. This article is distributed under the terms of the Creative Commons Attribution License (www.creativecommons.org/licenses/by-nc/3.0/), which permits non-commercial use, reproduction and distribution of the work without further permission provided the original work is attributed as specified on the SAGE and Open Access page (www.uk.sagepub.com/aboutus/openaccess.htm).
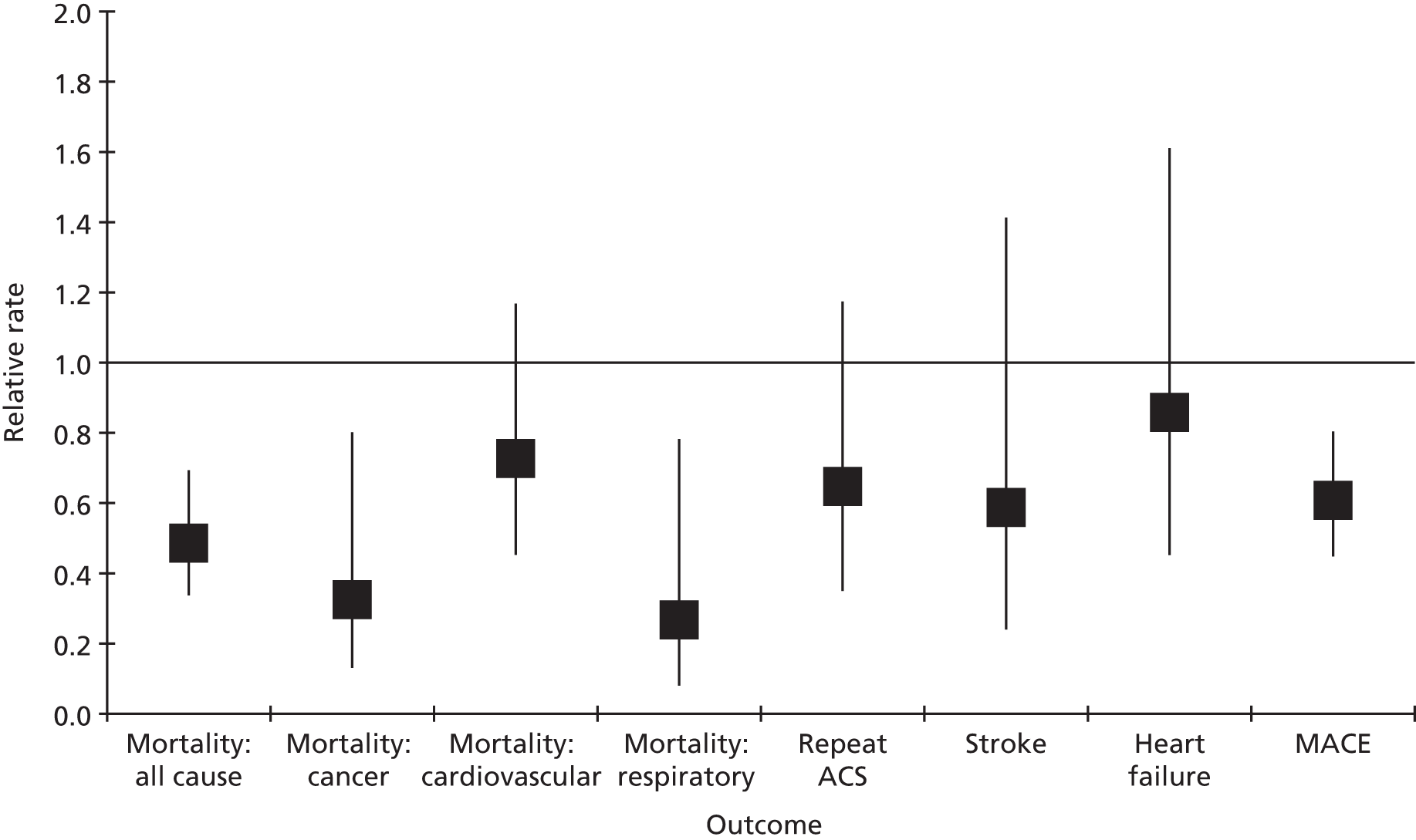
Discussion
This CALIBER study provides a unique longitudinal perspective on smoking cessation interventions after hospitalisation for AMI. The results show that only a minority of patients received interventions for smoking cessation and a large number continued to smoke. This missed opportunity for care is important because patients who continued to smoke had a significantly worse prognosis than those who quit.
In the first 3 months after ACS, when motivation to stop smoking was likely to be high, only 23% of smokers received smoking cessation interventions. The benefits of smoking cessation interventions are well established372–375 and the demonstrated failure of adherence to guideline recommendations for smoking cessation interventions no doubt contributed to the high proportion of smokers who failed to quit after ACS and who were thereby exposed to an increased risk of adverse outcomes.
To further understand the association between interventions and cessation and the effects on long-term clinical outcomes, future research into the promotion of GP smoking cessation interventions in both larger prospective epidemiological studies and RCTs is warranted.
This study has limitations. Smoking cessation advice may have been provided without being recorded in the patient record. 376 GP recording of smoking status and of advice against smoking may have changed following the introduction in 2004 of the Quality and Outcomes Framework contract, which offers financial incentives for the recording of smoking status and advice. 377,378
Conclusions
This CALIBER study provides evidence that smoking is common among those patients hospitalised for ACS. However, despite NICE guideline recommendations, few GPs offer smoking cessation advice, referral to smoking clinics, or pharmacological intervention in the 3 months following ACS discharge. Given the lower rates of major clinical outcomes, including mortality and major adverse cardiac events, among those who quit smoking following ACS, there remains a significant opportunity for GPs to actively engage smokers in cessation interventions.
Chapter 11 Acute myocardial infarction: evidence of treatment benefits beyond those provided by clinical trials – further opportunities to improve outcomes
Abstract
Background
Guidelines recommending use of secondary prevention drugs after AMI are based on results from clinical trials. However, trials do not provide evidence of long-term effectiveness of treatments or impact of interactions between them. Analysis of EHRs may help address these questions.
Objectives
To examine the use, timing, potential drug interactions and long-term effectiveness of secondary prevention medications after AMI.
Methods
An analysis of CALIBER linked data.
Results
(1) Among patients who had experienced an AMI, prognosis was most favourable in patients given a discharge prescription including all five classes of secondary prevention drugs. Use of fewer drugs was associated with increased mortality, particularly within the first 30 days and where beta-blockers, ACEIs and statins were omitted. (2) Use of a beta-blocker during and before AMI admission in patients with COPD was associated with reduced mortality. (3) Among AMI patients receiving clopidogrel plus aspirin, use of a PPI was associated with increased risk of AMI and death, as was use of drugs not predicted to interact with clopidogrel. A case series found no evidence that PPIs increased risk of death. (4) Discontinuation of clopidogrel after AMI is common and is associated with adverse outcomes.
Conclusions
‘Real-world’ data can provide important information beyond that generated by clinical trials to guide prescribing decisions.
The studies in this chapter relate to patients presenting for the first time with ACS (see Figure 52).
Introduction
Electronic health record observational data are a key resource for evaluating benefits and harms. The characteristics and richness of CPRD data (the world’s largest computerised database of anonymised longitudinal clinical records from primary care) have been significant in informing regulatory pharmacovigilance decision-making. Novel statistical methods such as self-controlled case series, where cases act as their own controls and, thus, where the effect of fixed confounders is eliminated, may be useful in active surveillance of drug safety.
Randomised clinical trials have led to recommendations for a range of secondary prevention drugs in AMI patients. However, the relative effectiveness of these drugs is unknown and the need for extended treatment beyond 2–3 years has never been tested. Treatment recommendations, therefore, are to some extent pragmatic and have been made in an attempt to ensure that no patient is denied the opportunity of benefiting from these drugs. Pragmatism is also required in dealing with recruitment biases and explicit exclusion criteria of clinical trials, which lead to important differences in the characteristics of trial participants compared with real-world populations. 379,380 Guideline groups will often generalise trial results to include a broader swathe of patients, but the potential exists for important missed treatment opportunities if specific patient groups that might otherwise have benefited are excluded from treatment. Thus, beta-blockers are recommended for secondary prevention after AMI, but there are concerns that they might exacerbate reversible airways obstruction, leading to treatment being withheld in many of these patients. This might represent a missed treatment opportunity adversely affecting prognosis. 381
The use of surrogate outcomes in drug studies can cause further difficulty when it comes to clinical decision-making, an important recent example being reports of an interaction of PPIs with clopidogrel, reducing its antiplatelet activity. 382–393 This has led to suggestions that PPIs should not be used after AMI, even though these drugs are of proven value for gastric protection. 394
We have taken advantage of the MINAP data set to examine the relative effectiveness of beta-blockers and other drugs for secondary prevention after AMI. We have also re-examined the use of beta-blockers for COPD patients, using the CALIBER data set to examine the effects of these drugs on mortality after AMI. We then used CALIBER to determine whether or not use of PPIs alongside clopidogrel affects prognosis after AMI.
Study 26: comparative effectiveness of drugs used for secondary prevention of cardiovascular disease after acute myocardial infarction
This study is based on previously unpublished data by A Timmis (Farr Institute of Health Informatics Research and Barts Health NHS Trust), O Nicholas (Department of Epidemiology and Public Health, University College London), S-C Chung (Farr Institute of Health Informatics Research) and H Hemingway (Farr Institute of Health Informatics Research) 2015.
Introduction
Guideline recommendations for secondary prevention drugs after AMI include aspirin, clopidogrel, beta-blockers, ACEIs and statins. 353,395,396 For all but clopidogrel, the recommendation is for life-long treatment, on the assumption that benefits are additive and efficacy remains undiminished in the longer term. However, none of the trials was designed to measure the additive effects of treatment or the relative efficacy of the various drugs. Moreover, follow-up in these trials was rarely longer than 3 years and often shorter. It is open to question, therefore, whether indefinite treatment is necessary or whether some of the recommended drugs might be discontinued, with potential benefits for patient safety and health-care costs.
A number of observational studies have suggested that the benefits of secondary prevention drugs after AMI are additive, mortality declining with the number prescribed. However, these studies are likely to be confounded by healthy user bias and are too small to answer the question of comparative effectiveness of specific drug combinations, with most enrolling fewer than 6000 patients. 397–401 The MINAP registry is substantially larger and has provided, for the first time, the opportunity to evaluate the comparative effectiveness of aspirin, clopidogrel, beta-blockers, ACEIs and statins individually and in all combinations.
Methods
We included patients aged ≥ 30, with a first STEMI or NSTEMI in the period 1 January 2004 to 6 August 2009 (n = 316,648). MINAP records if patients were discharged on aspirin, clopidogrel, an ACEI or ARB (conflated as ACEI in this study), a beta-blocker and a statin. Primary outcomes were survival from discharge to 30 days; survival from 30 days after discharge to 1 year; and survival beyond 1 year.
Statistical analysis
We analysed the effects on mortality of five classes of secondary prevention drugs prescribed individually and in all possible combinations using survival analysis to allow patients lost to follow-up to contribute information. For analysis, we imputed missing covariate data (comprising 21% of the overall amount of covariate data missing or not) independently for STEMI and NSTEMI, using Gibbs sampling to generate a 600 long chain from which we selected 10 hypothetical data sets from the last 300. 402 We used model covariates for imputation with the addition of angiography. Models were fitted to each data set using maximum likelihood.
Results
The study group comprised 287,615 patients discharged from hospital with diagnoses of STEMI (39%) or NSTEMI (61%). Rates of omission of secondary prevention drugs were 10% and 11% for aspirin and statins, increasing to 21% and 26% for ACEIs and beta-blockers, respectively, and to 45% for clopidogrel (Table 33). Patients in whom any of the secondary prevention drugs were omitted were older than the mean age of the group, more frequently female and more likely to have a discharge diagnosis of NSTEMI.
Characteristics | All (n = 287,615, mean (% recorded) | Patients (%) with discharge medication omitted: observed (imputed) | ||||
---|---|---|---|---|---|---|
Beta-blocker | ACEI | Statin | Aspirin | Clopidogrel | ||
Missing data for discharge drugs | 0.11 | 0.11 | 0.10 | 0.10 | 0.44 | |
Proportion | 0.26 (0.26) | 0.21 (0.21) | 0.11 (0.11) | 0.10 (0.10) | 0.25 (0.45) | |
Age (years) | 68.9 (100) | 73.1 (72.7) | 72.4 (72.0) | 74.1 (73.8) | 72.2 (71.9) | 72.2 (70.0) |
Female | 0.34 (100) | 0.41 (0.40) | 0.40 (0.40) | 0.43 (0.42) | 0.39 (0.39) | 0.38 (0.36) |
Ethnicity non-white | 0.05 (86) | 0.04 (0.03) | 0.04 (0.04) | 0.03 (0.03) | 0.04 (0.03) | 0.04 (0.03) |
IMD | 23 (90) | 23 (23) | 23 (22) | 22 (22) | 23 (23) | 22 (23) |
NSTEMI | 0.61 (100) | 0.71 (0.72) | 0.76 (0.76) | 0.77 (0.78) | 0.74 (0.75) | 0.61 (0.61) |
STEMI | 0.39 (100) | 0.29 (0.28) | 0.24 (0.24) | 0.23 (0.22) | 0.26 (0.25) | 0.39 (0.39) |
ECG abnormal | 0.92 (92) | 0.92 (0.92) | 0.90 (0.90) | 0.92 (0.91) | 0.92 (0.92) | 0.94 (0.93) |
Current smoker | 0.31 (92) | 0.27 (0.26) | 0.25 (0.24) | 0.22 (0.22) | 0.23 (0.23) | 0.26 (0.29) |
Diabetes | 0.18 (94) | 0.21 (0.20) | 0.18 (0.18) | 0.18 (0.18) | 0.21 (0.21) | 0.19 (0.18) |
Hypertension | 0.47 (94) | 0.47 (0.47) | 0.46 (0.46) | 0.47 (0.47) | 0.50 (0.49) | 0.48 (0.46) |
SBP (mmHg) | 142 (85) | 140 (140) | 139 (139) | 140 (140) | 140 (140) | 140 (141) |
Heart rate (b.p.m.) | 82 (86) | 85 (85) | 84 (84) | 86 (86) | 86 (86) | 85 (83) |
Left ventricular function < 50% | 0.19 (18) | 0.20 (0.44) | 0.19 (0.40) | 0.21 (0.45) | 0.21 (0.45) | 0.22 (0.43) |
Troponin (top quintile) | 0.13 (44) | 0.10 (0.12) | 0.08 (0.10) | 0.08 (0.10) | 0.09 (0.11) | 0.11 (0.16) |
Glucose (mmol/l) | 8.2 (59) | 8.4 (8.3) | 8.1 (8.0) | 8.2 (8.2) | 8.3 (8.3) | 8.3 (8.3) |
Haemoglobin (mmol/l) | 13.6 (32) | 13.3 (13.3) | 13.2 (13.2) | 13.4 (13.2) | 13.3 (13.2) | 13.2 (13.6) |
Creatinine (mmol/l) | 104.7 (34) | 109.7 (110.1) | 116.1 (117.9) | 109.2(111.1) | 109.7(109.5) | 112.3(106.8) |
Cholesterol (mmol/l) | 5.2 (72) | 4.9 (4.9) | 5.0 (4.9) | 4.9 (4.9) | 4.9 (4.8) | 5.0 (5.1) |
AMI | 0.19 (95) | 0.22 (0.22) | 0.21 (0.21) | 0.21 (0.21) | 0.23 (0.23) | 0.19 (0.20) |
Other | 0.34 (85) | 0.42 (0.42) | 0.40 (0.41) | 0.41 (0.42) | 0.43 (0.44) | 0.38 (0.37) |
Aspirin | 0.60 (93) | 0.56 (0.56) | 0.56 (0.56) | 0.53 (0.53) | 0.43 (0.44) | 0.58 (0.59) |
Beta-blocker | 0.28 (60) | 0.13 (0.14) | 0.28 (0.29) | 0.26 (0.27) | 0.28 (0.29) | 0.28 (0.29) |
ACEI or ARB | 0.33 (60) | 0.36 (0.36) | 0.21 (0.21) | 0.34 (0.35) | 0.37 (0.37) | 0.35 (0.34) |
Statin | 0.40 (61) | 0.41 (0.41) | 0.40 (0.40) | 0.25 (0.25) | 0.43 (0.43) | 0.39 (0.39) |
Previous PCI | 0.05 (91) | 0.05 (0.05) | 0.05 (0.05) | 0.04 (0.05) | 0.05 (0.05) | 0.04 (0.04) |
Previous CABG | 0.05 (92) | 0.05 (0.05) | 0.05 (0.05) | 0.05 (0.05) | 0.06 (0.06) | 0.05 (0.05) |
Arrival time (hours) | 2.8 (82) | 2.8 (2.8) | 2.8 (2.9) | 2.8 (2.8) | 2.8 (2.8) | 2.7 (2.8) |
CCU | 0.56 (99) | 0.47 (0.47) | 0.44 (0.45) | 0.42 (0.42) | 0.47 (0.47) | 0.54 (0.56) |
Reperfusion therapy | 0.84 (62) | 0.84 (0.58) | 0.79 (0.61) | 0.82 (0.65) | 0.85 (0.62) | 0.81 (0.51) |
IIb/IIIa inhibition | 0.08 (82) | 0.05 (0.06) | 0.05 (0.06) | 0.05 (0.06) | 0.05 (0.05) | 0.03 (0.06) |
In-hospital CABG | 0.02 (85) | 0.02 (0.02) | 0.03 (0.03) | 0.03 (0.03) | 0.03 (0.04) | 0.04 (0.03) |
Heparin | 0.95 (75) | 0.94 (0.94) | 0.94 (0.94) | 0.92 (0.93) | 0.89 (0.90) | 0.91 (0.96) |
Bleeding | 0.02 (89) | 0.02 (0.02) | 0.02 (0.02) | 0.02 (0.02) | 0.04 (0.04) | 0.03 (0.02) |
Admitted under cardiologist | 0.55 (98) | 0.62 (0.59) | 0.61 (0.58) | 0.64 (0.60) | 0.61 (0.60) | 0.59 (0.60) |
The Kaplan–Meier landmark analysis showed that in each of the three time periods (first 30 days, 31 days to 1 year, beyond 1 year) there was progressive worsening of crude and adjusted survival as the number of omitted secondary prevention drugs increased (Figure 61). Only the group with all five secondary prevention drugs omitted deviated from the dose–response relationship, exhibiting a survival trajectory intermediate between two and three omitted drugs.
FIGURE 61.
Kaplan–Meier mortality curves stratified by number of omitted secondary prevention drugs prescribed at discharge at 30 days and 1 year, and prevalence of omission compared with no omitted drugs among patients with a first STEMI or NSTEMI in MINAP.

Adjusted HRs for mortality varied according to which drug was omitted from the discharge prescription, tending to be higher for omission of either a beta-blocker, an ACEI or a statin compared with omission of aspirin or clopidogrel (Table 34).
Omitted drug | 0–30 days, HR (99% CI) | 30 days to 1 year, HR (99% CI) | ≥ 1 year, HR (99% CI) | p-value |
---|---|---|---|---|
Beta-blocker | 1.43 (1.33 to 1.54) | 1.32 (1.26 to 1.38) | 1.36 (1.30 to 1.45) | 0.849 |
ACEI | 1.49 (1.37 to 1.62) | 1.22 (1.17 to 1.28) | 1.09 (1.05 to 1.15) | < 0.001 |
Statin | 1.49 (1.35 to 1.64) | 1.20 (1.13 to 1.28) | 1.27 (1.19 to 1.38) | 0.940 |
Aspirin | 1.18 (1.07 to 1.30) | 1.07 (1.02 to 1.13) | 1.05 (1.00 to 1.12) | 0.121 |
Clopidogrel | 1.00 (0.92 to 1.07) | 1.01 (0.97 to 1.05) | 1.05 (1.00 to 1.09) | 0.988 |
Hazard ratios for drug omissions diminished sharply beyond the first 30 days, although this was less marked for beta-blockers or statins. When patients were grouped by the number of omitted drugs, Cox analysis showed a dose–response relationship of increasing hazard for 30-day mortality but, within each group, HRs varied according to which drugs were omitted (Figure 62a). Compared to patients discharged on all five secondary prevention drugs, those with single omission of clopidogrel showed a similar mortality hazard (HR 1.00, 95% CI 0.92 to 1.07), whereas those with single omission of aspirin showed a small excess hazard (HR 1.18, 95% CI 1.07 to 1.30). Single omissions of beta-blockers, ACEIs or statins were associated with a greater hazard, all with point estimates > 1.33. This pattern repeated itself as the number of omitted secondary prevention drugs increased, with combinations of omission that included aspirin and clopidogrel tending to be associated with a lower hazard of 30-day mortality than combinations that included beta-blockers, ACEIs or statins. The pattern was similar for mortality between 30 days and 1 year but, beyond 1 year, associations between specific combinations of discharge drug omissions and hazard of death were less clear (Figure 62b).
FIGURE 62.
Adjusted HRs and 99% CIs for death at 30 days and beyond 1 year associated with omission of combinations of secondary prevention medications among patients with a first STEMI or NSTEMI in MINAP. (a) Death at 30 days; and (b) death beyond 1 year.


Discussion
The relationship between the number of secondary prevention drugs omitted from the discharge prescription of patients with AMI and subsequent mortality depends on which drugs are omitted. Effect sizes were greatest in the first 30 days and were most evident for omission of beta-blockers, ACEIs or statins, which increased the hazard of death by > 40%. HRs tended to decrease in the longer term, particularly for omission of ACEIs, but for omission of beta-blockers and statins the hazard of death remained > 27% after 1 year of follow-up. Omission of aspirin from the discharge prescription caused only a small increase in the hazard of death, the point estimate dropping below 10% after the first 30 days, whereas omission of clopidogrel had little discernible effect on mortality.
The present study confirmed an association between the number of prescribed secondary prevention drugs and mortality,397–401 which persisted after full adjustment for all covariates. However, the size of this national cohort allowed us to analyse for the first time the effects of all possible combinations of drug omissions on mortality. The data showed important class effects, omissions of antiplatelet agents having less effect on the dose–response relationship than other secondary prevention drugs, particularly beta-blockers and statins. This was most evident for mortality in the first 30 days, when treatment effects were least likely to be distorted by non-adherence and the influence of new comorbidities, but the pattern remained perceptible at 1 year and beyond.
We found that single omission of aspirin from the discharge prescription of patients who received all other secondary prevention drugs was associated with a 30-day mortality increase of about 13%, falling to < 5% beyond the first year. Moreover, combinations of omitted drugs that included aspirin tended to increase the hazard of death less than combinations including beta-blockers and statins. The data reflect aspirin’s short-term protective effect, demonstrated in the Second International Study of Infarct Survival (ISIS-2) trial,403 but also highlight the caution that others have counselled404 in generalising randomised trial data beyond parameters of patient selection and treatment duration. Thus, patients randomised to aspirin in ISIS-2 received just 6 weeks of treatment and, although sustained benefits for this group have been reported,405 our own landmark analysis shows significant diminution of benefit in the longer term.
Unlike aspirin, omission of beta-blockers or statins from the discharge prescription was associated with a marked increase in the hazard of death in the first 30 days, with only minor reductions in the effect size during the first year and beyond. Our findings provide a strong case for long-term treatment with these drugs after AMI. Omission of ACEIs also increased the early hazard of death but, unlike beta-blockers and statins, the effect size diminished sharply beyond 1 year, when combinations of discharge prescriptions that omitted ACEIs were associated with a < 10% increase in the hazard of death. Our study provides no mechanistic insights, but it is possible to speculate that the pattern of diminishing hazard we found with omission of ACEIs from the discharge prescription reflects the protection they provide against left ventricular remodelling, which has its main impact during the first few months after AMI. 406
We were unable to demonstrate any association of clopidogrel omission with increased mortality and, like aspirin, combinations of omitted drugs that included clopidogrel tended to increase the hazard of death less than combinations including beta-blockers and statins. This is consistent with clinical trials that have shown reductions in composite end points for patients randomised to clopidogrel without clear effects on death from cardiovascular or non-cardiovascular causes. 407 However, in the current era of primary PCI, there is good evidence that clopidogrel can protect against stent thrombosis in the first year after AMI, although guideline recommendations to discontinue treatment beyond the first year appear borne out by our findings.
Combinations of drugs that are recommended lifelong but have not been evaluated in RCTs should be investigated in observational studies for associations with fatal and non-fatal outcomes over the remaining life expectancy.
Conclusions
Our findings remind clinicians and guideline groups that, although randomised trials are the most robust means of evaluating the efficacy of specific treatments, information about comparative effectiveness is available only from trials that randomise patients to two or more different agents. Examples include ISIS-2, which showed comparable effectiveness for streptokinase and aspirin,403 and Gruppo Italiano per lo Studio della Sopravvivenza nell’Infarto Miocardico (GISSI)-3, which showed effectiveness limited to lisinopril, not transdermal nitrate. 408
Our study highlights the variable consequences of specific drug omissions and reminds clinicians of the need to set thresholds for omitting beta-blockers or statins from the discharge prescription at a high level in order to avoid substantial long-term increases in the hazard of death. Our study also shows that the diminution of effectiveness apparent for all secondary prevention drugs in the longer term appears more marked for aspirin and ACEIs. Nevertheless, small reductions in the hazard of death beyond 1 year persist for both these drug classes, and our data do not provide a basis for recommending their discontinuation.
This study confirms that in patients with AMI, a discharge prescription that includes all five classes of secondary prevention drugs is associated with the most favourable prognosis, but suggests that beta-blockers and statins contribute the greatest protection against death, particularly in the longer term. The effectiveness of all drug classes tends to diminish with time but this is particularly marked for aspirin and ACEIs.
Study 27: do beta-blockers reduce mortality after acute myocardial infarction in adults with chronic obstructive pulmonary disease?
This study is based on the peer-reviewed paper by Quint et al. 59 Sections of the text have been reproduced/adapted from the original article. Copyright © 2013, British Medical Journal Publishing Group. This is an open-access article distributed under the terms of Creative Commons Attribution License (https://creativecommons.org/licenses/by-nc/3.0/). This document may be redistributed and reused, subject to certain conditions.
Introduction
Patients with COPD have lower survival after AMI than patients without COPD. 406,409–412 Historical concerns that beta-blockers might be harmful in COPD probably contribute to their underuse in AMI, even though these concerns have been challenged by recent evidence. 413 Hence, the underuse of beta-blockers in COPD patients with AMI may be an important missed opportunity to improve cardiovascular outcomes. We used the CALIBER linked data sets to quantify the association between COPD and mortality after AMI to investigate whether or not the use and timing of prescription of beta-blockers in patients with COPD after a first AMI was associated with improved survival, and to identify factors related to the use of beta-blockers in patients with COPD.
Methods
We included patients with COPD experiencing their first AMI from 1 January 2003 to 31 December 2008 as recorded in MINAP. Patients were defined as having COPD if the Read-coded diagnosis was recorded in CPRD before or after AMI. Two or more exacerbations per year were defined as frequent exacerbations. 414 Information on COPD and drug prescriptions was obtained from CPRD. The primary outcome was all-cause mortality after AMI in patients with COPD stratified by beta-blocker prescription during hospital admission. We used Cox proportional hazards models to produce adjusted HRs to determine whether or not mortality differed by prescription of a beta-blocker.
Results
We included 1063 COPD patients with a MINAP record of AMI during the study period. Over half (55%) were never prescribed a beta-blocker and 23% were on a beta-blocker before AMI. The remainder (22%), who were prescribed a beta-blocker at the time of AMI, were younger and less likely to have a history of hypertension, cerebrovascular disease, PAD, heart failure, dyslipidaemia or angina before their AMI. They were also more likely to have infrequent exacerbations and were less likely to have been prescribed diuretics before their AMI. Of the beta-blockers prescribed during hospital admission, 93.8% were cardioselective.
After adjustment for history of smoking and sex, and stratification by age, patients with COPD prescribed a beta-blocker during hospital admission for AMI and those prescribed a beta-blocker before an AMI had better survival than those never prescribed a beta-blocker (HR 0.45, 95% CI 0.34 to 0.60; p < 0.001; and 0.72, 95% CI 0.57 to 0.90; p = 0.004, respectively) (Figure 63).
FIGURE 63.
Kaplan–Meier estimated survival and 95% CIs after AMI in patients with COPD prescribed a beta-blocker before MI, during hospital admission for myocardial infarction or never, excluding deaths on day of diagnosis, in MINAP. MI, myocardial infarction. Reproduced from Quint et al. 59 Copyright © 2013, British Medical Journal Publishing Group. This is an open-access article distributed under the terms of the Creative Commons Attribution License (http://creativecommons.org/licenses/by-nc/3.0/), which permits others to distribute, remix, adapt, build upon this work non-commercially, and license their derivative works on different terms, provided the original work is properly cited.

After adjustment for age, sex, history of smoking, and frequency of exacerbations, survival analysis with cardiac and non-cardiac death as the outcome showed an improved survival in those prescribed a beta-blocker than those not prescribed a beta-blocker during the hospital admission for AMI (HR for cardiac deaths 0.57, 95% CI 0.38 to 0.86; p = 0.03; and non-cardiac deaths 0.49, 95% CI 0.32 to 0.75; p = 0.01). Among patients prescribed a beta-blocker during hospital admission, 203 (87.1%) were still taking it at 6 months, 185 (79.4%) at 1 year and 142 (60.9%) at 2 years.
Discussion
This is the largest study of the effect of beta-blockers on survival after AMI in patients with COPD. 414–416 Use of a beta-blocker after a first AMI was associated with lower subsequent mortality, a benefit that persisted after adjustment for age and cardiovascular comorbidities. Only 38.6% of people with COPD received a beta-blocker during the hospital admission for AMI, however, indicating an important missed opportunity for improving care. Despite earlier studies reporting improved survival after AMI in COPD patients prescribed beta-blockers,415 the reluctance to prescribe remains a worldwide phenomenon that cannot be attributed to problems with tolerability, our own data showing that nearly 80% of patients prescribed a beta-blocker were still taking it at 1 year.
The lower mortality in COPD patients prescribed a beta-blocker was due to a reduction in both cardiac and non-cardiac deaths, consistent with previous suggestions that their benefits extend beyond cardiac effects. 416,417 There is evidence that beta-blockers do not worsen lung function in patients with COPD,416,417 so severity of disease or lack of spirometry should not deter their prescription in AMI, particularly use of cardioselective agents. A RCT is justified to examine the effectiveness of beta-blockers after AMI in patients with COPD.
The main limitation of our study is that residual confounding, including confounding by contraindications, cannot be excluded, and there is also the risk of healthy user bias.
Conclusions
Our data suggest that beta-blockers should be used more widely in patients with COPD who have had an AMI. Low rates of use represent an important missed opportunity for treatment. Safety to date is good in these patients, but further evaluation of the safety of beta-blockers in this high-risk group might be required to change current prescribing practice.
Study 28: clopidogrel and its interaction with proton pump inhibitors
This study is based on the peer-reviewed paper by Douglas et al. 60 Sections of the text have been reproduced/adapted from the original article. Copyright © 2012, British Medical Journal Publishing Group. Copyright © 2012, British Medical Journal Publishing Group. This is an open-access article distributed under the terms of the Creative Commons Attribution License (http://creativecommons.org/licenses/by-nc/2.0/), which permits use, distribution, and reproduction in any medium, provided the original work is properly cited, the use is non commercial and is otherwise in compliance with the license.
Introduction
In patients with AMI receiving dual antiplatelet therapy with aspirin and clopidogrel, the risk of gastric bleeding can be reduced by coprescription of a PPI. However, PPIs inhibit cytochrome P450 2C19, the enzyme that converts clopidogrel to its active form. This interaction reduces clopidogrel activity as measured by platelet activation studies, but whether or not this has important clinical effects has been hard to determine, with conflicting evidence from outcome studies. 382–393 We used CALIBER linked data sets to conduct two observational studies to investigate this possible drug interaction, the first using a traditional cohort design, and the second using a self-controlled case series design to eliminate the fixed confounding between people that can affect case–control or cohort designs. 418
Methods
Patients were selected if they were recorded in the CPRD from 1 January 2003 onwards and had at least 12 months between first registration and first recorded prescription for clopidogrel. Patients also had to be concurrently prescribed aspirin as recorded in the database. Follow-up was censored at the earliest of stopping treatment with clopidogrel, stopping treatment with aspirin, occurrence of an outcome of interest, death, transfer out of the practice, last data collection date for the practice or 31 July 2009. Follow-up time was then classified according to whether or not the patient was receiving a PPI as recorded in the database. Treatment with aspirin was assumed to be continuous but for clopidogrel and PPI exposure, where possible, we calculated the anticipated length of each prescription based on pack size and dosing instructions. We undertook an observational cohort study and a self-controlled case series. The primary outcome was a composite end point of all-cause mortality or incident AMI.
Results
Observational cohort study
We identified 24,471 patients receiving clopidogrel in combination with aspirin, of whom 9111 (37%) also received a PPI from the date of first clopidogrel prescription. The crude HR for the primary outcome was 1.41 (95% CI 1.31 to 1.52) during PPI exposure relative to non-exposure, reducing to 1.37 (95% CI 1.27 to 1.48) after adjustment for age, sex, BMI, smoking, alcohol, diabetes, PAD, CHD, ischaemic stroke and cancer. Exposure to ranitidine, a non-PPI antacid, produced similar adjusted hazards for the primary outcome of 1.20 (95% CI 0.99 to 1.46), as did exposure to strong (paroxetine or fluoxetine) and weak (citalopram) 2C19-inhibiting antidepressants (1.42, 95% CI 1.17 to 1.72 and 1.52, 95% CI 1.32 to 1.76, respectively) (Figure 64).
FIGURE 64.
Association between PPIs, ranitidine, paroxetine/fluoxetine, or citalopram and the composite end point of all-cause mortality or incident AMI among patients receiving dual antiplatelet therapy with aspirin and clopidogrel: a cohort analysis and self-controlled case series analysis. Relative risk expressed as HR for cohort analysis and IRR for self-controlled case series. MI, myocardial infarction. Reproduced from Douglas et al. 60 Copyright © 2012, British Medical Journal Publishing Group. This is an open-access article distributed under the terms of the Creative Commons Attribution License (http://creativecommons.org/licenses/by-nc/2.0/), which permits use, distribution, and reproduction in any medium, provided the original work is properly cited, the use is non commercial and is otherwise in compliance with the license.

Self-controlled case series
Exposure to a PPI concurrently with clopidogrel and aspirin gave an IRR of 0.75 (95% CI 0.55 to 1.01), adjusted for age. For the secondary exposures of interest, the IRR for AMI was 0.57 (95% CI 0.31 to 1.06) for ranitidine, 1.65 (95% CI 0.87 to 3.15) for paroxetine/fluoxetine and 0.84 (95% CI 0.49 to 1.45) for citalopram.
Discussion
The large sample size available within the CALIBER data set has allowed us to resolve the conflicting results of smaller studies that have investigated the clinical consequences of interactions between clopidogrel and PPIs. The cohort analysis of > 24,000 patients receiving clopidogrel and aspirin showed that exposure to PPIs was associated with an increased risk of all outcomes including AMI and all-cause mortality. However, comparable results were seen with other drug exposures, including drugs that would not be expected to interact with clopidogrel (such as ranitidine and citalopram), suggesting that the association is unlikely to be causal but driven by differences between PPI users and non-users. Consistent with this were the results of the within-person study design, which removed the influence of differences between people and showed no association between PPI exposure and AMI. Thus, a plausible explanation for these results is that the increased risk of both vascular and non-vascular harmful outcomes seen in patients receiving PPIs and other long-term drugs could be caused by confounding between people.
Our overall findings are consistent with several studies that failed to find an association between PPIs and less favourable outcomes in clopidogrel users. 383,387–393 Of note, the case–control study by Valkhoff et al. 393 did find a harmful association but when comparisons were restricted to former or current users of PPIs, the association disappeared, again suggesting a biased result driven by confounding between people. The only randomised trial designed to specifically answer this question was stopped early and so is underpowered and unable to rule out a clinically relevant effect. 392 The available results, however, did not point towards any harmful association.
Our findings point to the need for new methods by which a learning health system can interrogate the safety of drugs and drug–drug interactions in a more timely and more scaled fashion.
Conclusions
The drug interaction between PPIs and clopidogrel does not result in clinical harm. PPIs should continue to be seen as important prophylactic drugs in patients on dual antiplatelet therapy with aspirin and clopidogrel who are at high risk of gastrointestinal bleeding.
Study 29: discontinuation of clopidogrel after acute coronary syndrome – frequency, predictors and clinical consequences
This study is based on the peer-reviewed paper by Boggon et al. 61 Sections of the text have been reproduced/adapted from the original article; published on behalf of the European Society of Cardiology. All rights reserved. © The Author 2011. The online version of this article has been published under an open-access model; the Journal, Learned Society and Oxford University Press are attributed as the original place of publication.
Introduction
Guideline recommendations are for dual antiplatelet therapy with aspirin and clopidogrel to continue for 12 months after AMI (NSTEMI and STEMI) with aspirin monotherapy thereafter. In patients who do not fill their prescriptions for clopidogrel early after hospital discharge, the risk of recurrent thrombotic events is significantly increased. 419 This takes no account of the potential risk associated with discontinuation of treatment later after discharge, although there is little information available about patient adherence to clopidogrel therapy after AMI. The extent to which patients are non-adherent to dual antiplatelet therapy in the 12 months following AMI represents an important missed opportunity to optimise care and reduce recurrent events. In the present study we have used CALIBER data to document relationships between clopidogrel discontinuation and coronary events in the 12 months after NSTEMI and STEMI. Our specific objectives were (1) to define the extent to which clopidogrel treatment (initiated in hospital) is continued in primary care, (2) to identify factors predictive of clopidogrel discontinuation and (3) to analyse the hazard of death or recurrent AMI associated with clopidogrel discontinuation.
Methods
We studied 7543 patients aged ≥ 40 years, with a MINAP-recorded diagnosis of NSTEMI or STEMI (ACS) who were discharged from hospital to home from 2003 to 2009. Logistic regression was used to determine the characteristics associated with GP prescribing of clopidogrel within 3 months of hospital discharge. Characteristics included the in-hospital presentation (BP and heart rate), treatment strategy (reperfusion strategy and coronary intervention), diagnosis recorded in MINAP, demographics (age, sex, socioeconomic status, calendar year and geographical region), medical history (diabetes and angina/AMI), prior GP prescribing (clopidogrel, aspirin and warfarin), and lifestyle behaviours (alcohol consumption, smoking status and BMI) recorded in the General Practice Research Database (GPRD), and prior hospitalisations (all-cause and cardiovascular related) recorded in HES. If there were no records of BMI, smoking status or alcohol consumption, indicators of missingness were used. ORs and 95% CIs were calculated. As statins are routinely prescribed following ACS, information on statin discontinuation was used to compare clopidogrel discontinuation with another evidence-based treatment. Discontinuation of clopidogrel or statin was defined as no prescriptions for the medication within a period defined as the estimated duration of the prescription plus a grace period of 91 days. Cox regression was used to assess the same set of characteristics for association with clopidogrel discontinuation within the first year of discharge, with the additional time-dependent characteristic of any bleeding within the treatment period. Clopidogrel prescription on hospital discharge was included only in the MINAP data set after 2005 and had no duration information associated with it, so this parameter was not analysed in this study. Bleeding was defined as any bleeding event (major or minor) in the patient’s GPRD or HES record. HRs and 95% CIs were calculated.
Patients were followed for up to 1 year for incidences of subsequent AMI (as recorded in MINAP, GPRD or HES) or death (as recorded in ONS). Descriptive Cox regression was used to identify the characteristics associated with these outcomes with clopidogrel treatment following discharge entered into the model as a time-dependent covariate, categorised as follows: no treatment, 0–3 months’ use, 3–11 months’ use, 11+ months’ use and discontinued use. This allowed a patient to move from one categorisation of clopidogrel exposure to another over time.
Results
Only 68.6% of the 4108 patients with NSTEMI and 62.8% of the 2915 patients with STEMI received a primary care clopidogrel prescription in the first 3 months. Clopidogrel discontinuation after the first prescription was substantially more frequent compared with statins, both for NSTEMI and for STEMI (Figure 65).
FIGURE 65.
Discontinuation of clopidogrel and statins in primary care after NSTEMI or STEMI in MINAP. Reproduced from Boggon et al. 61 Published on behalf of the European Society of Cardiology. All rights reserved. © The Author 2011. The online version of this article has been published under an open-access model. Users are entitled to use, reproduce, disseminate, or display the open-access version of this article for non-commercial purposes provided that the original authorship is properly and fully attributed; the Journal, Learned Society and Oxford University Press are attributed as the original place of publication with correct citation details given.
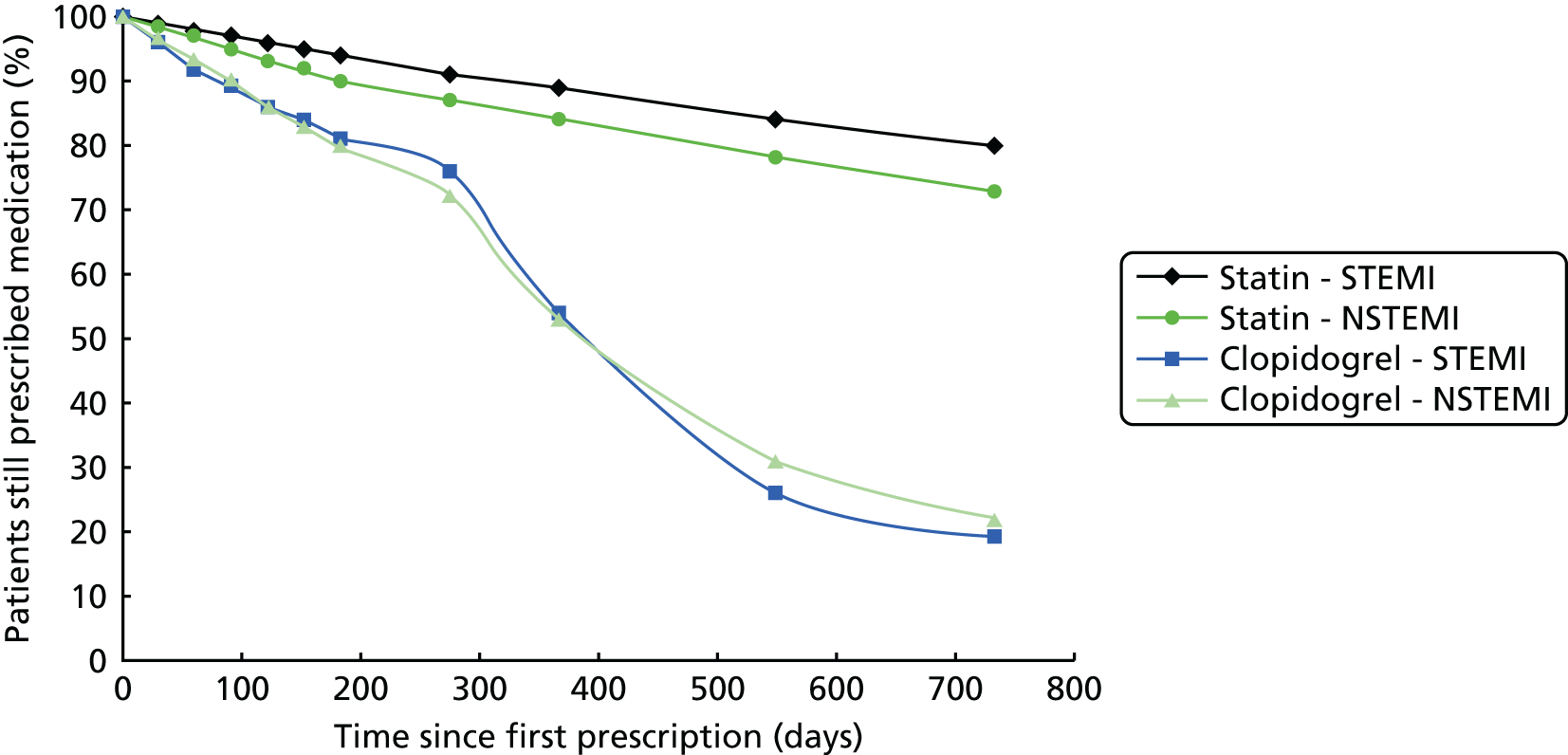
Clopidogrel prescribing
The adjusted odds of receiving a primary care clopidogrel prescription (see Figure 65) in the first 3 months increased from 1.45 (95% CI 1.11 to 1.89) in 2004 to 5.78 (95% CI 4.20 to 7.96) in 2009. The odds were higher in younger age groups and decreased progressively with advancing age (for age 70–79 years, 0.64, 95% CI 0.47 to 0.85; for age ≥ 80 years, 0.52, 95% CI 0.39 to 0.71) compared with age 40–49 years. In patients managed with percutaneous intervention during the hospital admission, the odds of receiving a clopidogrel prescription were substantially higher (6.19, 95% CI 4.80 to 7.99) than for patients in whom no coronary intervention was performed. Clopidogrel prescribing increased over time from 35% in 2003 to 79% in 2009.
Clopidogrel discontinuation during first year
The adjusted HR for clopidogrel discontinuation was higher in patients aged ≥ 80 years (HR 1.50, 95% CI 1.16 to 1.94), and those who had a bleeding event after leaving hospital (HR 1.34, 95% CI 1.03 to 1.73). Comparing patients who did and did not undergo in-hospital revascularisation, the adjusted hazard for clopidogrel discontinuation was higher following CABG (HR 1.82, 95% CI 0.99 to 3.35) than PCI (HR 0.72, 95% CI 0.63 to 0.83).
Bleeding complications
In patients prescribed clopidogrel in primary care within 3 months of discharge, the rates of bleeding per 100 patient-years were similar following NSTEMI (12.03, 95% CI 10.62 to 13.64) and STEMI (10.55, 95% CI 8.96 to 12.42).
Death and non-fatal acute myocardial infarction
In patients prescribed clopidogrel in primary care, the rate of death and non-fatal AMI in the first year post discharge (per 100 person-years) was reduced by > 50% in NSTEMI (from HR 48.4, 95% CI 44.4 to 52.8, to HR 22.4, 95% CI 20.5 to 24.5) and STEMI (from HR 23.6, 95% CI 20.7 to 26.8, to HR 11.7, 95% CI 10.1 to 13.6). The Cox analysis (Figure 66) confirmed that clopidogrel prescribing during the first year following discharge was associated with a lower hazard of death or non-fatal AMI than for patients who received no prescriptions. Clopidogrel discontinuation at any time within 12 months was independently associated with an increased risk of death or AMI both compared with untreated patients (HR 1.45, 95% CI 1.22 to 1.73) and with those patients persisting with clopidogrel treatment (HR 2.62, 95% CI 2.17 to 3.17).
FIGURE 66.
Characteristics associated with death or AMI in the first year after discharge among patients with a first-recorded diagnosis of STEMI or NSTEMI in MINAP. Data are HRs (95% CIs) adjusted for age, sex, discharge diagnosis, socioeconomic status, medical history of angina/AMI, calendar year and geographical region. a, These analyses are restricted to those prescribed clopidogrel. Modified from Boggon et al. 61 Published on behalf of the European Society of Cardiology. All rights reserved. © The Author 2011. The online version of this article has been published under an open-access model. Users are entitled to use, reproduce, disseminate, or display the open-access version of this article for non-commercial purposes provided that the original authorship is properly and fully attributed; the Journal, Learned Society and Oxford University Press are attributed as the original place of publication with correct citation details given.
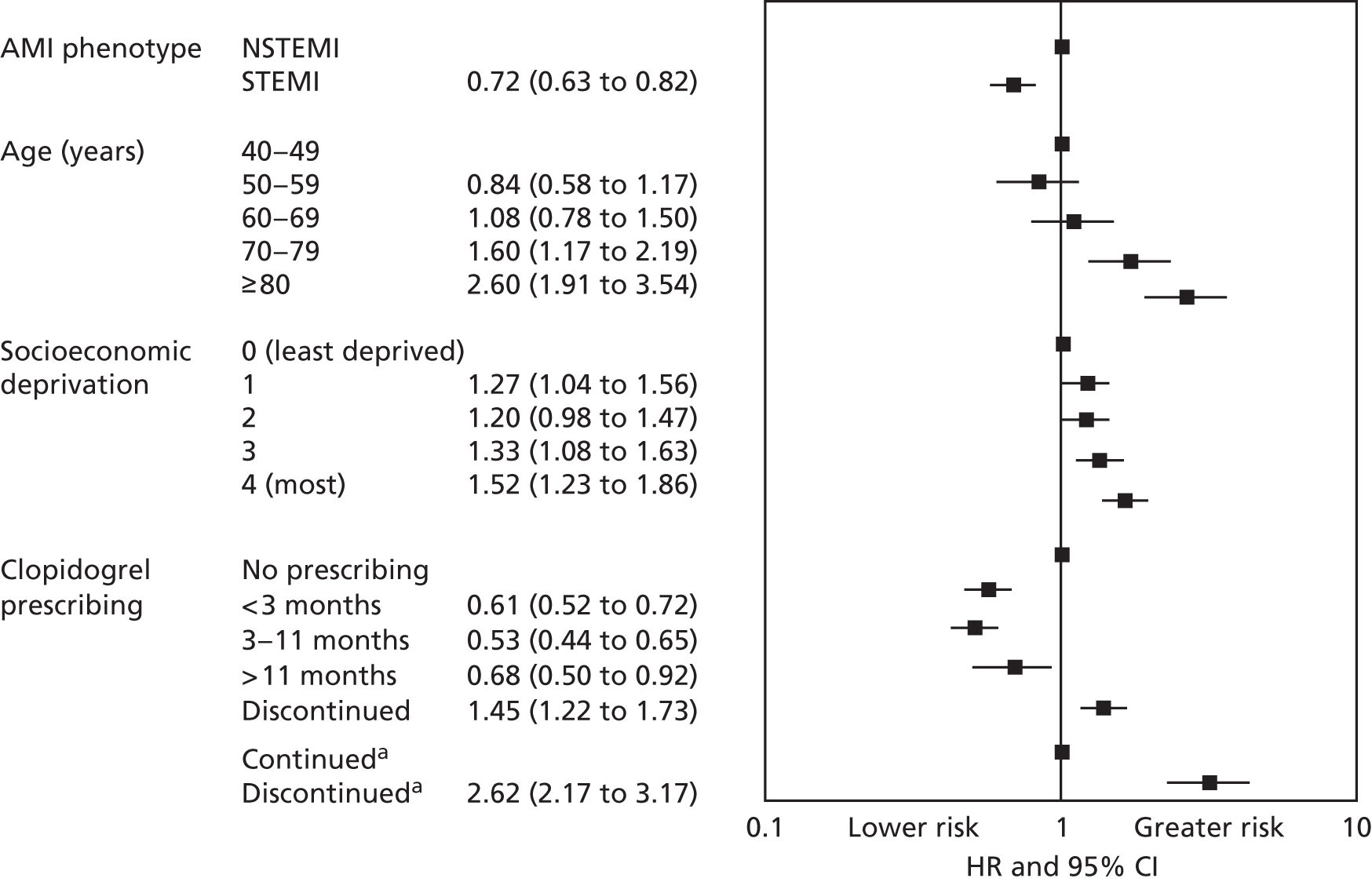
Discussion
This study shows that failure to complete a 12-month course of clopidogrel after AMI is common and represents an important missed opportunity to reduce mortality and recurrent thrombotic events after AMI. Clopidogrel adherence, as reflected by prescription rates, declines more steeply compared with statins in the first 12 months and is followed by an even steeper decline thereafter (see Figure 66), consistent with guideline recommendations. 330,420
Guideline recommendations are for clopidogrel to continue for 12 months in patients with NSTEMI and STEMI treated with drug-eluting stents. 330,420
However, we found that in around half of all patients with NSTEMI, prescription of clopidogrel was discontinued within the first 12 months after discharge from hospital. The trajectory of decline for clopidogrel prescription was steeper than for statins, suggesting that it was driven by factors additional to general compliance issues, including underprescription in older age groups and bleeding complications, which were more frequent than observed in the original randomised trial. 407
The main finding in the present study was the association between clopidogrel discontinuation in the first 12 months and the outcome following either NSTEMI or STEMI. Clopidogrel prescription during this period was associated with a reduction in the hazard of death or non-fatal AMI compared with patients who received no prescription, consistent with trial evidence of clopidogrel’s prognostic benefit in patients with ACS. 407 Importantly, among those patients prescribed clopidogrel who then had it discontinued, the hazard of death or non-fatal AMI was significantly greater than for patients who continued to receive a clopidogrel prescription.
Our findings are consistent with those of Ho et al. ,419 who reported that any delay in filling clopidogrel prescriptions after hospital discharge in patients treated with drug-eluting stents was associated with nearly twice the rate of coronary events during follow-up for 22 months. Our findings are also consistent with other observational studies in US and Australian populations where discontinuation of clopidogrel therapy within 1 year of stenting was associated with worse outcomes. 421–423 Further research, incorporating methods of causal inference, is required to fully investigate the extent to which the association between clopidogrel discontinuation and adverse events we identified was causal or was confounded by the influence of unrecorded comorbidities or bleeding events.
Our study has important limitations. Data on hospital clopidogrel prescribing, both during the admission and on discharge, were not comprehensively recorded and was not included in the study. Although GP prescription information was robust, we do not know if clopidogrel was collected or taken by individual patients or was prescribed by a third party. This limited the focus of the study to clopidogrel prescriptions within primary care. Also, we cannot determine whether or not the association between clopidogrel discontinuation and adverse events was causal or was confounded by the influence of unrecorded comorbidities or bleeding events. 407,422–424 Healthy user bias is difficult to avoid in studies of this type; however, similar healthy user bias will apply to prescribing of statins, which showed a slower decline in prescribing than clopidogrel. 424 Although our outcome analysis was adjusted for baseline factors that included prior coronary events and hospitalisations (all-cause and cardiovascular), we do not know if the patients who discontinued clopidogrel were predisposed to adverse outcomes through other mechanisms.
Conclusions
This is the first study to use linked registries to determine the prognostic associations of clopidogrel discontinuation in patients discharged from hospital after NSTEMI and STEMI. Our data show that discontinuation of clopidogrel during the first 12 months is common, particularly in older patients, and is associated with an increased risk of death or recurrent AMI. If the association between clopidogrel discontinuation and death or AMI is causal, ensuring that adherence to treatment is maintained in all patients for a full 12 months after discharge from hospital represents an important opportunity to improve cardiovascular outcomes in this high-risk group.
Chapter 12 Acute myocardial infarction: variation between hospitals in short-term mortality outcomes
Abstract
Background
Variation in patient outcomes between hospitals is ubiquitous across a range of diseases. No studies have compared outcomes after AMI across hospitals adjusted for case mix.
Objectives
(1) To assess interhospital variation in mortality following AMI, adjusted for case mix, and whether or not variation can help to identify and rank underperforming hospitals. (2) To examine the impact of hospital characteristics and quality of primary care on mortality after AMI. (3) To compare interhospital variation across different health-care systems. (4) To identify processes of care that may contribute to variation in outcomes between hospitals.
Methods
Analysis of UK and Swedish AMI disease registry data, and an ethnographic study of AMI care in the UK.
Results
(1) Thirty-day mortality varied substantially between UK hospitals. Up to four severely underperforming hospitals could be identified with high confidence, although there was considerable uncertainty in adjusted mortality rates. (2) We found no clear relationship between the number of patients admitted to individual hospitals or the quality of BP management in primary care and 30-day mortality rates for AMI patients. (3) Interhospital variation in 30-day mortality was greater in the UK than in Sweden, with the lowest mortality in hospitals adhering to guideline-recommended procedures and treatments. (4) We identified five processes that appeared to facilitate good care.
Conclusions
Variation in hospital performance was only partly explained by differences in case mix and use of guideline-recommended treatments. A systematic quality improvement programme, akin to that implemented in Sweden, may reduce variation in practice and patient outcomes across UK hospitals.
The studies in this chapter relate to patients presenting for the first time with ACS (see Figure 52).
Introduction
Whereas the previous chapters in this report have focused on individual patients, in this chapter we investigate clustering at the level of the hospital and investigate opportunities for intervention at a different locus. National quality improvement registries are an important component of EHRs. Variation in patient outcomes between hospitals has been a consistent finding across a broad range of common diseases425,426 and has attracted interest from policy-makers because of the insight it provides into overall system performance. 81 Understanding hospital variation in mortality for AMI is particularly important because effective drug and procedural interventions are available to improve the quality of care. Policy-makers might choose to deliver these interventions at a national level to reduce variation across the hospital service or at hospital level by applying management strategies used in top-performing hospitals to those with less favourable outcomes.
Measuring hospital performance is a central component of the UK Government’s transparency agenda, which increasingly calls for process measures of performance to be complemented by hard outcome indicators. 84 Indeed, Dr Foster has been publishing mortality data using the Hospital Standardised Mortality Ratio (HSMR) for every UK hospital since 2001. 427 This has revealed widespread variation in death rates between English hospitals but is based on HES and is limited by lack of information on case-mix factors and emergency treatment, making performance comparisons between hospitals difficult to interpret.
Compared with HES, MINAP provides a much richer source of data for patients admitted to UK hospitals with AMI,86 enabling us to provide a more nuanced view of interhospital variation in care. We carried out two novel studies using MINAP data to analyse variation in outcomes between hospitals and to provide insight into the performance of the UK’s NHS. The first study provides a detailed view of variation in short-term mortality between hospitals in England and Wales, adjusted for case mix, and has important implications for efforts to identify underperformance and rank hospital performance. The second compares variation across hospitals in England and Wales with that seen in Sweden, to provide an international benchmark and to explore the role of interhospital variation in national differences in short-term mortality.
To investigate the extent to which differences between hospitals are explained by factors open to change in NHS, we carried out a preliminary volume–outcome analysis of patients with AMI admitted to all hospitals in England and Wales to investigate the impact of hospital characteristics and quality of primary care on 30-day mortality after AMI.
As we found that interhospital variation in mortality is not wholly explained by difference in case mix or use of guideline-recommended treatments, we have also sought to identify factors affecting the quality of care delivery that might influence outcomes. We adopted an ethnographic approach, examining NSTEMI care in 10 hospitals with contrasting levels of 30-day mortality. Through interviews with health-care workers and patients, and observation of daily practice, we sought to identify processes of care that might influence adherence to guidelines and contribute to interhospital variation in mortality.
Study 30: interhospital variation in mortality – UK hospitals
This study is based on previously unpublished data by O Nicholas (Department of Epidemiology and Public Health, University College London) and A Timmis (Farr Institute of Health Informatics Research and Barts Health NHS Trust), 2015.
Introduction
Existing research into interhospital variation in patient outcomes after AMI has major limitations. We found no previous studies of interhospital variation in 30-day mortality that included detailed case-mix and treatment data from all hospitals in one country. Investigation has often been restricted to subsets of hospitals contributing data to voluntary registries, which may not be representative of other hospitals within the same health-care system. 428–432 In other studies, attention focused on hospitals grouped by academic status, geographical region or receipt of achievement awards, not on individual hospitals within these groupings. 431,433,434 Selection at patient level often means that whole segments of the population are excluded, with many studies confining their analyses to patients aged ≥ 65 years428,430,432 or to STEMI patients. 429,431,433 Across all studies, patient-level data are sparse, with some studies providing information about demographic factors only435 and most providing little information about infarct severity. 429–433,435–438 Information about in-hospital treatments is nearly always absent428,430,432,434,435,437 or, when available, has not been used to inform individual hospital performance. 429,431,433
We have sought to address these limitations using data from the UK, one of the few countries in which all hospitals participate in a national registry of patients admitted with AMI (MINAP), which records data on case mix, treatment and mortality. 86 We have chosen 30-day mortality for patients treated within individual hospitals as the outcome of interest because it is sufficiently early after the index AMI to capture the influence that hospital effects might have on outcomes without confounding by later factors such as non-concordance with treatment and new comorbidities. We aimed to determine (1) the extent of clinically important interhospital variation in 30-day mortality after admission for AMI; (2) the extent to which the variation is explained by case mix, treatments received in hospital and other hospital-level factors; (3) whether or not underperforming hospitals can be reliably identified; and (4) whether or not hospitals can be reliably ranked by 30-day mortality.
Methods
Patient population
Using the MINAP registry, we selected patients aged ≥ 30 years, with a first STEMI or NSTEMI in the period 1 January 2004 to 6 August 2009 (n = 316,648). AMI was defined by the discharge diagnosis recorded in MINAP, based on the consensus definitions of the Joint ESC/American College of Cardiology for AMI subtypes,439 raised cardiac biomarkers (creatine kinase or troponin) with or without ST-segment elevation on the resting ECG. All case-mix, treatment and hospital-level factors listed in Table 35 were obtained from the MINAP registry.
Case-mix, treatment and hospital factors | Patients (N = 316,648) | Hospitals (N = 236) | |
---|---|---|---|
Observed, means or proportions (% recorded) | Observed, median (first, third quartile) | Imputed, median (first, third quartile) | |
Case mix | |||
Demographics | |||
Age (years) | 69.9 (100) | 69.7 (67.8, 71.6) | 69.7 (67.8, 71.5) |
Female | 0.35 (100) | 0.35 (0.31, 0.38) | 0.35 (0.31, 0.38) |
Ethnicity non-white | 0.05 (85) | 0.02 (0.01, 0.07) | 0.02 (0.01, 0.06) |
IMD | 22.7 (90) | 20.0 (16.0, 28.0) | 21.1 (16.6, 27.4) |
AMI phenotype and ECG | |||
NSTEMI | 0.61 (100) | 0.63 (0.51, 0.71) | 0.63 (0.51, 0.71) |
STEMI | 0.39 (100) | 0.37 (0.29, 0.49) | 0.37 (0.29, 0.49) |
ECG abnormal | 0.93 (92) | 0.94 (0.91, 0.97) | 0.93 (0.91, 0.96) |
Risk factors | |||
Current smoker | 0.30 (91) | 0.30 (0.26, 0.34) | 0.29 (0.25, 0.34) |
Diabetes | 0.18 (93) | 0.18 (0.16, 0.20) | 0.18 (0.16, 0.20) |
Hypertension | 0.47 (93) | 0.47 (0.43, 0.51) | 0.47 (0.43, 0.51) |
Haemodynamics | |||
SBP (mmHg) | 141 (85) | 141 (138, 143) | 140 (139, 143) |
Heart rate (b.p.m.) | 8.3 (85) | 82 (80, 84) | 82 (80, 84) |
Left ventricular function < 50% | 0.40 (17) | 0.42 (0.33, 0.54) | 0.42 (0.40, 0.44) |
Blood markers | |||
Pooled troponin I or T upper quintile | 0.13 (43) | 0.07 (0.01, 0.22) | 0.14 (0.10, 0.19) |
Glucose (mmol/l) | 8.3 (58) | 8.4 (8.1, 8.6) | 8.3 (8.1, 8.4) |
Haemoglobin (g/dl) | 13.5 (31) | 13.6 (13.3, 13.9) | 13.6 (13.5, 13.8) |
Creatinine (mmol/l) | 107.7 (35) | 105.9 (101.4, 112.2) | 105.3 (102.0, 108.7) |
Cholesterol (mmol/l) | 5.1 (70) | 5.1 (5.0, 5.3) | 5.1 (4.9, 5.1) |
History of CVD | |||
AMI | 0.19 (94) | 0.18 (0.16, 0.21) | 0.19 (0.16, 0.21) |
Other | 0.35 (84) | 0.35 (0.30, 0.40) | 0.36 (0.31, 0.40) |
Prior treatment | |||
Aspirin | 0.59 (53) | 0.59 (0.51, 0.65) | 0.59 (0.51, 0.65) |
Beta-blocker | 0.27 (59) | 0.24 (0.21, 0.28) | 0.25 (0.23, 0.28) |
ACEI or ARB | 0.34 (59) | 0.31 (0.27, 0.36) | 0.31 (0.28, 0.35) |
Statin | 0.39 (60) | 0.36 (0.32, 0.42) | 0.37 (0.34, 0.41) |
Previous revascularisation | |||
PCI | 0.05 (90) | 0.05 (0.03, 0.07) | 0.05 (0.04, 0.07) |
CABG | 0.05 (91) | 0.05 (0.04, 0.06) | 0.05 (0.04, 0.06) |
Delay | |||
Time from symptom onset to arrival (hours) | 2.7 (81) | 2.7 (2.3, 3.1) | 2.7 (2.5, 3.1) |
Treatment and complications | |||
Acute management | |||
Reperfusion therapy | 0.80 (44) | 0.79 (0.68, 0.88) | 0.76 (0.70, 0.82) |
IIb/IIIa inhibition | 0.07 (82) | 0.04 (0.01, 0.10) | 0.04 (0.02, 0.09) |
CABG | 0.02 (86) | 0.01 (0.00, 0.02) | 0.01 (0.01, 0.02) |
Heparin | 0.95 (74) | 0.97 (0.94, 0.98) | 0.96 (0.94, 0.98) |
Discharge medication | |||
Aspirin | 0.90 (90) | 0.93 (0.88, 0.95) | 0.92 (0.88, 0.96) |
Clopidogrel | 0.75 (56) | 0.78 (0.65, 0.86) | 0.76 (0.69, 0.82) |
Beta-blocker | 0.74 (89) | 0.75 (0.69, 0.81) | 0.75 (0.70, 0.86) |
ACEI or ARB | 0.79 (89) | 0.81 (0.75, 0.87) | 0.81 (0.75, 0.86) |
Statin | 0.89 (90) | 0.92 (0.87, 0.95) | 0.92 (0.88, 0.96) |
Complications | |||
Bleeding | 0.02 (88) | 0.01 (0.01, 0.03) | 0.01 (0.01, 0.03) |
Hospital-level factors | |||
Admitted to CCU | 0.55 (99) | 0.57 (0.46, 0.74) | 0.57 (0.45, 0.74) |
Admitted under cardiologist | 0.56 (98) | 0.66 (0.33, 0.81) | 0.65 (0.35, 0.81) |
Cases per year | 192 | 171 (90, 255) | a |
Case mix
A simple summary of case mix comprised age, admission heart rate and admission SBP, as previously validated in MINAP and other studies. 440,441 A more comprehensive measure of case mix included demographic factors [age, sex, self-reported ethnic group and the IMD at lower-layer super output area,88 AMI phenotype (STEMI, NSTEMI)], risk factors (smoking status, diabetes, hypertension), cardiac history (previous angina, heart failure, ACS, cerebrovascular disease), previous PCI, previous CABG, prior cardioprotective drugs (aspirin, beta-blocker, ACEI, statin) and admission concentrations of haemoglobin, creatinine, glucose and cholesterol.
In-hospital treatment
We included data on acute medications common to STEMI and NSTEMI (aspirin before or at the time of admission, thienopyridine, heparin), reperfusion therapy (for STEMI only, thrombolysis or primary PCI), time from pain onset to reperfusion therapy, angiography during admission (for NSTEMI only) and discharge medications (aspirin, thienopyridine, beta-blocker, ACEI, statin).
Hospital-level factors
We included data on the number of prior ACSs, the proportion of patients admitted under a cardiologist and the availability of an on-site catheter laboratory. All hospital-level factors were calculated per hospital per year, and each patient record was amended to include the relevant statistic for the year of admission.
Primary outcome
The primary outcome was all-cause mortality at 30 days after hospital admission.
Statistical analysis
We compared 30-day mortality for individual hospitals with mean 30-day mortality across the study population. We chose a mortality ≥ 40% above average as reflecting clinically important hospital underperformance, being equivalent in terms of risk to adding ≥ 5 years to the age of every patient according to our fully adjusted model. We modelled interhospital variation in 30-day mortality taking account of observed case-mix, treatment and hospital-level factors listed in Table 35. We treated observed factors as fixed effects (whether at the hospital or patient level) and allowed for random effects at the hospital level. We included all hospitals, no matter how small the number of patients, as our random-effects modelling using empirical Bayes ensures shrinkage to the mean of outlying but uncertain estimates. We successively introduced case-mix and treatment variables into the model to assess their contribution to apparent mortality variation between hospitals. We compared the likelihood of our posterior estimates of random effects under the assumption of little variance (SD ≤ 0.07, equivalent under our fully adjusted model to 1 year of age) with the assumption of greater variance (SD > 0.07) to evaluate the likelihood of true variation. Our modelling used parametric survival, allowing for different survival trajectories for STEMI and NSTEMI, with calendar-year-specific hazards, proportional hazards for the effects of covariates, and hospital-level random effects split into a constant component and a 30-day component in order to adjust for unmodelled factors that might otherwise bias 30-day assessment. We assumed secondary prevention drugs to take effect from discharge and not before. For adjusted funnel plots, we plotted estimated hazard against the precision of the estimate, where the precision was taken to be 1.96 over the 95% interval size all squared. 442 For year-by-year distributions of estimated hospital hazards, we combined calendar-year-specific hazards with estimated hospital hazards. For analysis we imputed missing covariate data (comprising 23% of all covariate data) independently for STEMI and NSTEMI, making the assumption that covariates appearing in the fully adjusted model together with the logarithm of time to death or censoring443 had a multivariate normal distribution444 with a linear trend with respect to calendar time in mean. To this end we employed Gibbs sampling to generate a 600-long chain from which we selected 10 hypothetical data sets from the last 300. 402 We used model covariates for imputation with the addition of angiography. Models were fitted to each data set using maximum likelihood. All analyses were carried out in Matlab version 7.14 (Mathworks, Natick, MA, USA) and software written in C++ version 11 (Intel, Santa Clara, CA, USA).
Results
Study population
We identified 316,648 patients experiencing a first STEMI or NSTEMI between 1 January 2004 and 6 August 2009. During that period, we estimated a 47% (95% CI 39% to 55%) improvement in 30-day mortality, representing an average annual improvement of 6.7% (95% CI 5.7% to 7.6%).
Case-mix, treatment and hospital-level factors
Interhospital variation in factors used for modelling the primary outcome is shown in Table 35. Differences between average and interhospital variability of factors based on non-missing observations and observations that included imputed data were small, and we used the imputed data to maximise patient inclusion in the modelling process. Interhospital variations in case-mix, treatment and hospital-level factors were often large, as reflected in the IQRs across all hospitals, NSTEMI for example accounting for ≤ 51% and ≥ 71% of cases in lower- and upper-quartile hospitals. Rates of reperfusion therapy also varied, ranging from ≤ 68% to ≥ 88% in lower- and upper-quartile hospitals. Similar variation was seen in hospital-level factors, with the proportion of patients receiving cardiac care unit treatment ranging from ≤ 46% to ≥ 74% in lower- and upper-quartile hospitals. Imputation of data preserved interhospital variation in covariates.
Variation in 30-day mortality
Crude 30-day mortality for the whole study population was 9.4% (IQR 6.5–11.2%) but among the 3760 patients with complete data for all 39 case-mix variables used for modelling it was 0.9% (IQR 0.0–7.7%), reflecting the 23% missing covariate data. This necessitated the use of imputed data sets to generate adjusted models of hospital variation. The SD of log-hazard across all hospitals was 0.33 (95% CI 0.31 to 0.35), hospitals with a 30-day mortality > 1 SD either side of the average adding or subtracting at least 28% [1-exp (–0.33)] to the average risk, depending on whether performance was bad or good. In 34 hospitals (14.4%, 95% CI 11.9% to 17.4%) mortality was ‘excessive’, as defined by a mortality of ≥ 40% above average. Adjustment for case mix, whether ‘simple’ (age, heart rate and SBP) or ‘full’, and then for treatment- and hospital-level factors, reduced hospital variation in 30-day mortality. Thus, the SD of log-hazard across all hospitals was 0.24 (95% CI 0.23 to 0.25) in the fully adjusted model, significantly exceeding our assumption of no variation (p < 0.001) – hospitals with a 30-day mortality > 1 SD either side of the average adding or subtracting at least 21% [1-exp (–0.24)] to the average risk. In the fully adjusted model, 15 hospitals (6.4%, 95% CI 4.7% to 8.5%) met the ≥ 40% criterion for excessive 30-day mortality which, given an estimated HR of 1.07 for each year of age, was equivalent to adding at least 5 years of age to each patient treated in underperforming hospitals (Figure 67).
FIGURE 67.
Hospital variation in 30-day mortality after AMI in models adjusting for case-mix, treatment and hospital-level factors from MINAP. Data are for all 236 hospitals in England and Wales, 413,642 patients.

Hospital ranking
Hospitals were ranked by the fully adjusted point estimates of their 30-day mortality hazards (Figure 68). The 95% CIs around these estimates were wide, and across most of the hospitals in England and Wales there was considerable uncertainty about the rankings, making it difficult to draw robust inferences about relative performance. This is further illustrated in Figure 68b, which shows the probability of any particular hospital being among the 15 worst-performing hospitals with an excess 30-day mortality of ≥ 40%. For the 11 lowest-ranked hospitals, the chances were greater than evens of any of them being among the 15 worst performers. Greater certainty about severe underperformance was possible for two hospitals, in which the probability of being ranked among the worst performers exceeded 90%. The funnel plot (Figure 69) indicated 12 hospitals lying above the line of ≥ 40% excess risk, with four outside the 99% confidence limit for underperformance.
FIGURE 68.
Hospitals ranked by (a) performance and (b) probability of being in the worst-performing 6% (15 out of 236) of hospitals in MINAP. Data are median hospital log-hazard for 30-day mortality with 95% (lines) and 99% (dots) fully adjusted CIs.


FIGURE 69.
Funnel plot of estimated hospital performance for AMI 30-day mortality in MINAP. Each circle represents one hospital with 95% (grey lines) and 99% (black lines) control limits. Hospitals above the horizontal line have > 1.4 times worse mortality than the average across all hospitals, with a probability ranging from 0.54 to 1.

Discussion
Our study is nationally comprehensive in terms of hospitals included (100%), and in terms of recording of acute and discharge treatments, we showed that the 30-day mortality rate for over 300,000 patients with AMI varied substantially between hospitals. Unlike previous studies, we used a metric comprehensible to patients and policy-makers, and showed that about one in 20 hospitals had an excess mortality equivalent to increasing the age of patients they cared for by at least 5 years. Up to two severely underperforming hospitals could be identified with high levels of confidence, but for most hospitals ranking estimates based on adjusted 30-day mortality were imprecise and not a reliable indication of performance.
The rich patient-level data available within MINAP that were used to model the primary outcome showed considerable variation between hospitals in terms of case mix but also of emergency management. The importance of case-mix adjustment for understanding hospital variation has been repeatedly emphasised,81,436,445,446 the data confirming substantial interhospital differences in major determinants of outcome such as age, rates of diabetes and proportions of current smokers. Unlike many previous studies of hospital variation, we were also able to examine emergency treatment delivered in hospital and secondary prevention drugs prescribed at discharge. Again, substantial differences between hospitals were found, particularly in rates of reperfusion therapy, but also in the key secondary prevention drugs recommended in international guidelines. Some have argued that process measures such as these provide advantages for evaluating hospital performance – being a direct measure of the standard of care and less likely to generate institutional stigma than mortality estimates. 447
Despite wide differences between hospitals in case mix and emergency care, patient-level treatment and hospital-level factors explained only a proportion of the absolute variation in mortality, reducing by 50% the estimated number of underperforming hospitals. We found that adjusting for treatment appeared to explain some variation when the case-mix adjustment was simple (based on a small number of factors) and explained more when case-mix adjustment was full. When we used all the available data to account for case mix, further adjustment for treatment had no impact on variation in 30-day mortality, highlighting the limitations of studies of interhospital variation that have taken only partial account of case-mix factors. Much of the interhospital variation we identified remains unexplained and must be attributed to unmeasured or poorly measured factors. Unmeasured patient-level aspects of care include dose, timing and compliance with prescribed medication, behavioural interventions (e.g. smoking cessation) and genetic differences. Unmeasured hospital-level factors include process measures and hospital characteristics that other investigators have associated with interhospital variability but were unavailable for modelling in the present study. 351
Notably, although we identified serious interhospital variation in 30-day mortality, we could confidently identify only up to four hospitals that were underperforming according to our criteria. For all other hospitals, the uncertainty around individual hospital mortality estimates, as reflected by wide overlapping CIs, made specific hospital rankings imprecise as a measure of performance, confirming the limitations of hospital league tables. 448 The failure of treatment factors to explain more than a small part of the variability in hospital 30-day mortality suggests that policy-makers may need to look at organisational and other hospital-level factors to identify the main determinants of underperformance.
The variation in 30-day mortality between hospitals in England and Wales cannot be ignored and requires strategies to restore prognostic equity. Our results suggest that a strategy confined to targeting up to four underperforming hospitals that can be identified with confidence would have a very limited impact on AMI mortality at a population level, and should be combined with a strategy of system-wide quality improvement across all hospitals, analogous to the Rose population-wide, as opposed to a risk-based, prevention strategy. 449 We found little evidence that differences in case-mix or emergency management between hospitals made substantial contribution to differences in performance, and identified a need for qualitative research to examine organisational culture within hospitals, there being evidence that a culture supportive of efforts to improve AMI care is associated with better performance within selected US hospitals. 351
An important question, not resolved by our study, is whether or not the interhospital variation in mortality within England and Wales is generalisable to other settings or is a function of the health-care system itself, driven by postcode lottery or other organisational factors. The finding of similar variation in outcomes from studies in the USA does not resolve the question because studies included only selected hospitals and not the entire hospital base. Only Sweden has a unified health-care system in which, as in England and Wales, all hospitals participate in a national registry of patients admitted with AMI (SWEDEHEART) recording data on case mix, treatment and mortality. 7
We have taken up this important opportunity for an international comparison and report our findings for study 32.
Our study has important limitations that are inherent in any large registry analysis. In modelling the primary outcome, we were restricted to data recorded in the MINAP registry. Although this includes a broad range of demographic, case-mix and treatment factors, we were limited to those factors conventionally recorded as part of quality of care. These factors only partially explained the interhospital outcome differences we observed, suggesting that the data recorded in MINAP and other registries needs to be expanded to capture more relevant quality measures, including a broader range of hospital-level factors. We were also limited by missing data in 23% of data entries. Absence of data can itself influence mortality estimates,450 as reflected in the reduced crude mortality rate we observed in the subset of patients with complete case-mix data used for survival modelling. This emphasises the importance of the imputation process we adopted in the present study, which maximised patient inclusion in the modelling process while producing only modest changes in average values for case-mix and treatment factors.
Further research is required to obtain real-time risk-adjusted, treatment-adjusted estimates of between-hospital variation in mortality and a wider range of non-fatal outcomes.
Conclusions
In using national registry data to assess hospital-level mortality for patients with AMI in England and Wales, we have shown for the first time serious variation between hospitals, about one in 20 of which has an excess mortality of ≥ 40%. Underperformance was not explained by differences in case-mix or treatment factors, and qualitative work is now needed to determine the extent to which the organisational culture within hospitals, or other patient-level factors, might have contributed. Promotion of prognostic equity across all hospitals represents an important opportunity to reduce coronary mortality substantially in England and Wales.
Study 31: extent to which differences between hospitals are explained by factors open to change in the NHS – impact of hospital characteristics and quality of primary care on 30-day mortality after acute myocardial infarction
This study is based on previously unpublished data by S-C Chung (Farr Institute of Health Informatics Research) and A Timmis (Farr Institute of Health Informatics Research and Barts Health NHS Trust), 2015.
Introduction
Case-mix and treatment factors influencing prognosis early after AMI are well recognised55 but there is less information about the way in which hospital characteristics might be influential. There is a suggestion that outcomes might be better in large-volume primary PCI units but, as NICE commented in the 2013 myocardial infarction guideline,451 the quality of data is poor and still leaves the question open. Indeed, in that guideline, NICE identified volume–outcome relationships as one of its main research recommendations.
Similarly, few data are available on how the quality of primary care influences early hospital outcomes after AMI. In study 10 we have already reported a sharp increase in primary care consultations in the weeks preceding hospital admission with ACS and how this provides a potential opportunity to implement treatment to protect against progression to coronary occlusion. However, we have no information available about those patients in whom AMI is prevented or the treatment they received.
Hypertension is a well-established risk factor for CVD and is the most prevalent treatable cause of vascular events. 452 The management and control of high BP is therefore one of the most important components of primary and secondary strategies for prevention of cardiovascular mortality and morbidity at patient level. 453 However, uncertainty remains in the association between hospital AMI mortality and individual BP control.
The target measurement for BP control recommended in JNC 8 guidelines for the management of high BP in adults is < 150/90 mmHg for patients aged ≥ 60 years and < 140/90 mmHg for those aged ≤ 60 years, and for patients diagnosed with diabetes or with chronic kidney disease (regardless of age). 168 The development of JNC 8 was based on results from clinical trials. In actual clinical practise, it is unclear whether or not hospital outcome of AMI survival is associated with BP control target defined by JNC 8.
In the absence of hospital characteristics and primary prevention on 30-day mortality after AMI, our objectives in this study were, first, to investigate the hospital volume–outcome association in AMI care and second, to investigate the association between hospital AMI outcome and BP control in primary care. For each patient, BP control was indicated by the mean of all the BP measurements during the study period, and mean deviation of these measures from the control target defined by JNC 8.
Methods
National registry for hospital care for acute coronary syndrome
To investigate the hospital volume–outcome association in AMI care, we used MINAP registry data. MINAP included information on case-mix, treatment and in-hospital outcome of patients admitted to all acute hospital units providing AMI care in England and Wales (n = 242). Eligible patients were identified by age at admission of at least 30 years and admitted to AMI hospital between 1 January 2004 and 31 December 2010. The diagnosis of AMI was based on guidelines from the ESC, American College of Cardiology and American Heart Association. 454 For multiple admissions of the same patient, we used the earliest record. We identified 17 case-mix measures in MINAP, which included demographic factors (age, sex, year of hospitalisation), risk factors (smoking, history of diabetes and hypertension), AMI severity (troponin I or T concentration, SBP and heart rate at admission to hospital and cardiac arrest), history of prior heart failure, cerebrovascular disease and AMI, procedure and medication use prior to hospitalisation (antiplatelet treatment with aspirin, clopidogrel or both, previous PCI and CABG).
Linked primary care, secondary care and patient outcome data
To investigate the association between primary care and hospital AMI outcome, we used CALIBER data and administrative health data from primary care, secondary care and disease registries. We used linked CALIBER data to investigate whether or not BP control may be associated with hospital AMI outcome. Inclusion criteria were incident hypertensive patients defined according to the JNC 8 guidelines168 who developed an AMI during study follow-up, patients aged ≥ 18 years registered with CPRD practices consenting to data linkage with at least 1 year of ‘up to standard’ general practice follow-up, and followed on or after 1 January 1997 with at least 1 year of study follow-up between study inclusion and 25 March 2010. AMI diagnoses were identified in CPRD, HES, ONS and MINAP records. Patients were excluded if they had prior history of atherosclerotic disease, or history of hypertension before study inclusion or if there was < 6 months since diagnosis with hypertension. Patients with pregnancy-induced hypertension were eligible for inclusion 9 months after the date of diagnosis. Patients with drug-induced hypertension were eligible for inclusion 6 months after the date of diagnosis.
Follow-up
The study period was between 1 January 1997 and 25 March 2010, which was the administrative censoring date of the database. Patient follow-up commenced on the date when the patient first met all the inclusion criteria and ended on the exit date from the cohort (last practice visit, deregistration, death).
Blood pressure measurement and study outcome
Baseline BP was defined as BP at study entry. For each eligible patient, we calculated mean BP over repeated measures the patient acquired during study follow-up. We calculated deviation of each follow-up BP measurement in relation to BP control targets recommended in the JNC 8 guidelines168 (< 140/90 mmHg or < 150/90 for patients aged ≥ 60 years without diabetes and chronic kidney disease).
Target BP control was defined in relation to BP values recommended in the JNC 8 guidelines168 for management of adults with high BP (< 140/90 mmHg or < 150/90 for patients aged ≥ 60 years without diabetes and chronic kidney disease). For each eligible patient, we calculated the deviation of each BP measurement in relation to recommended values, and mean BP value over repeated measures.
Mortality outcomes
The primary clinical outcome was all-cause mortality within 30 days of hospitalisation.
Statistical analysis
For hospital volume–outcome association in AMI care, we compared the crude hospital proportions of case mix, treatments and 30-day mortality by year of hospitalisation. Because the hospital proportions were not normally distributed, the IQR was used to describe the observed variation.
The association between unadjusted hospital 30-day mortality and hospital admission volume was initially investigated graphically, then by correlation analysis. To account for patient case mix, we constructed generalised mixed models for each of the guideline-recommended treatment and 30-day mortality using the 17 variables described above. Using these models, the predicted probability of receiving guideline-recommended treatments and death at 30 days after hospital admission was estimated for each patient and summarised at the hospital level to obtain the expected hospital proportions. For each hospital, the observed mortality was divided by expected hospital 30-day mortality to obtain the HSMR. Hospital case-mix-standardised 30-day mortality rates were determined by multiplying each HSMR by the crude AMI 30-day mortality rate of the study population. The association between case-mix-standardised hospital treatment proportions and standardised hospital mortality was estimated by correlation analysis. The analysis was stratified by year of hospitalisation.
For the preliminary analysis to investigate the association between patient BP control and hospital AMI outcome, we categorised study hospitals according to AMI hospital mortality quartiles. The distribution of patient baseline BP, mean BP over repeated measures during study period, mean difference from BP control target over repeated measures taken during study period were summarised according to quartiles of hospitals.
Analyses were performed using SAS version 9.0 (SAS Institute, Cary, NC, USA).
Results
Hospital volume–outcome association in acute myocardial infarction care
Hospital-level case mix
A lower median proportion of STEMI patients were observed in 2010 (27.5%) than in 2007 (40.2%) and 2004 (38%). Compared with 2004, in 2010 there was a higher hospital proportion of patients with a history of diabetes, but a lower hospital proportion of smokers. In 2010, there was a greater hospital proportion of patients admitted with prior treatment of PCI or CABG (Table 36).
Case-mix variables | Year | ||
---|---|---|---|
2004 | 2007 | 2010 | |
Hospital number | 233 | 228 | 225 |
% STEMI, median (IQR) | 38 (23.8) | 40.2 (25.9) | 27.5 (30.5) |
Age (years), mean (SD) | 69.4 (3.7) | 69.2 (4.4) | 69.9 (4.4) |
% Female (IQR) | 34.3 (7.9) | 33.7 (7.3) | 34.7 (8.6) |
MI severity, median (IQR) | |||
SBP (mmHg) | 140 (7.5) | 139 (7) | 139 (7) |
Heart rate (b.p.m.) | 78.3 (4) | 79.5 (3.5) | 79 (4) |
Troponin I (ng/ml) | 7 (16) | 4.3 (7.8) | 2.3 (3.4) |
Troponin T (ng/ml) | 0.9 (2) | 0.7 (1.4) | 0.8 (3.5) |
Risk factor, median (IQR) | |||
% Current smoking | 30.8 (9) | 29.5 (10.9) | 26.6 (10.4) |
% History of diabetes | 16.1 (5.9) | 16.7 (6.2) | 19.4 (5.8) |
% History of hypertension | 43.5 (9.4) | 47.8 (11.8) | 49.7 (10.7) |
History of CVD, median (IQR) | |||
% Heart failure | 5.2 (5.1) | 4 (4.6) | 4.7 (4.8) |
% Previous AMI | 20 (6.5) | 17.1 (6.3) | 17.3 (6.7) |
% Cardiac arrest | 1.8 (2.1) | 1.8 (1.7) | 1.9 (2.6) |
% Stroke | 7.6 (5.4) | 7.5 (5.1) | 8.9 (5.1) |
Treatment prior to admission, median (IQR) | |||
% Single antiplatelet | 20.1 (19.6) | 26.9 (15) | 22.1 (19.5) |
% Combined antiplatelet | 0 (0) | 2.7 (3.8) | 2.1 (3.4) |
% PCI | 3.4 (4.4) | 5.6 (4.7) | 6.1 (4.7) |
% CABG | 3.9 (2.5) | 4.4 (3.1) | 5.4 (3.1) |
We found a weak positive correlation between hospital volume and unadjusted 30-day mortality (Figure 70), hospitals with higher volumes tending to have a higher mortality (Pearson correlation coefficient = 0.14, p = 0.03). The association attenuated after standardised for case-mix factors. However, the annualised analysis indicated that the positive association was largely confined to the early study period before 2008 (2006 and 2007) and disappeared thereafter, such that no association between hospital volume and outcome was apparent in more recent years (Table 37).
FIGURE 70.
Relationship between hospital volume (number of patients admitted with AMI between 2004 and 2010) and hospital 30-day mortality in MINAP.
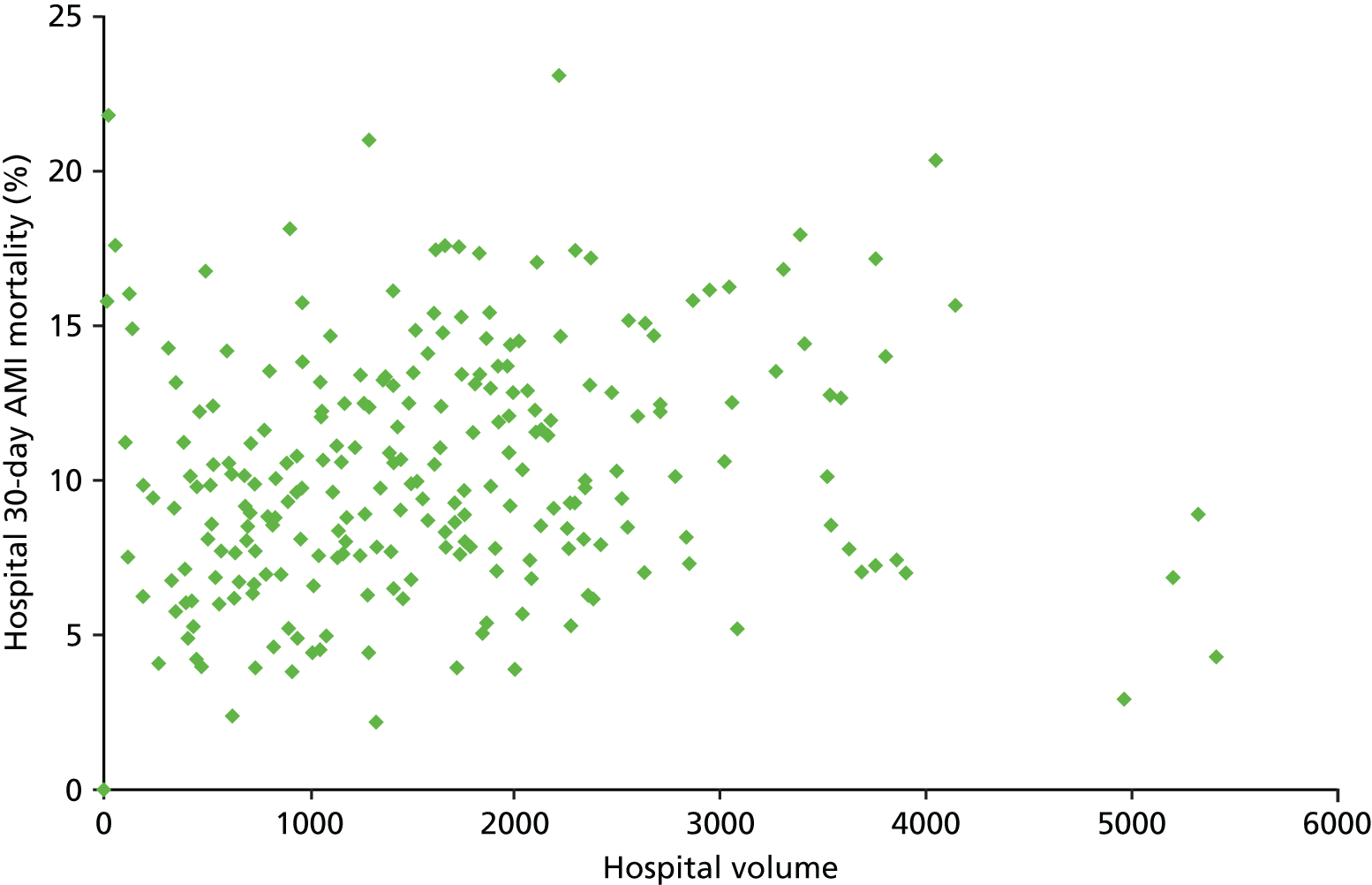
Year | Hospital volume and unadjusted hospital 30-day mortality | Hospital volume and standardised hospital 30-day mortality | ||
---|---|---|---|---|
Correlation coefficient | p-value | Correlation coefficient | p-value | |
2004 | 0.28455 | < 0.0001 | –0.03348 | 0.6111 |
2005 | 0.01813 | 0.7841 | –0.10995 | 0.0955 |
2006 | 0.3206 | < 0.0001 | 0.15108 | 0.0211 |
2007 | 0.2468 | 0.0002 | 0.16118 | 0.0148 |
2008 | 0.04499 | 0.4963 | 0.05311 | 0.4218 |
2009 | 0.06114 | 0.356 | 0.12801 | 0.0525 |
2010 | –0.03241 | 0.6287 | 0.08630 | 0.1972 |
2004–10 | 0.13996 | 0.0295 | 0.09013 | 0.1622 |
Primary care and hospital acute myocardial infarction outcome
A total of 196,212 incident hypertensive patients were identified. The average follow-up time was 5 years and 3 months, and 2825 hypertensive patients (1.4%) who developed AMI during follow-up (mean follow-up 5.83 years) were included in the analysis. We did not identify any differences in baseline BP at diagnosis, mean BP or variation from target BP by hospital AMI mortality quartile during the study period (Table 38).
Hospital grouped by volume-weighted 30-day AMI mortality (n) | Patients (N) | BP at study entry (mmHg), mean (SD) | BP during study period (mmHg), mean (SD) | Difference of BP measure and control target (mmHg), mean (SD) | |||
---|---|---|---|---|---|---|---|
SBP | DBP | SBP | DBP | SBP | DBP | ||
< 17.4% (67) | 704 | 160.6 (18.5) | 90.2 (10.8) | 144.4 (13.6) | 81.4 (7.6) | –1.4 (13.3) | –8.6 (7.6) |
≥ 17.4% to < 23.9% (36) | 676 | 161.2 (17.8) | 90.4 (10.6) | 144.7 (14.2) | 81.3 (7.7) | –1.5 (13.9) | –8.7 (7.7) |
≥ 23.9% to < 33.3% (46) | 702 | 162.4 (19.0) | 89.9 (10.8) | 147.1 (13.7) | 81.9 (7.5) | 0.6 (13.7) | –8.1 (7.5) |
≥ 33.3% (66) | 743 | 162.2 (18.7) | 90.2 (11.0) | 147.6 (14.2) | 82.4 (7.7) | 1.3 (14.1) | –7.6 (7.7) |
Overall (215) | 2825 | 161.6 (18.5) | 90.2 (10.8) | 146 (14) | 81.8 (7.6) | –0.2 (13.8) | –8.2 (7.6) |
Discussion
In this preliminary volume–outcome analysis of patients with AMI admitted to all hospitals in England and Wales, we found no clear relationship between the number of patients admitted to individual hospitals and 30-day mortality rates. Indeed, the tendency was for outcomes to be somewhat worse in high-volume centres. The positive association between hospital volume and case-mix-adjusted 30-day mortality, however, was weak and largely confined to the early study period before 2008 when rates of primary PCI were relatively low. Later in the study period, huge changes in management strategies for AMI were established in the UK, with increasing rates of primary PCI for patients with STEMI who were largely managed in heart attack centres, and a slower trend towards more aggressive interventional management of patients with NSTEMI. In this environment, a large proportion of patients with ACS are either admitted directly or transferred to heart attack centres.
In this preliminary analysis, we found no relationship between the quality of BP management in primary care and 30-day mortality for patients with AMI. Research on hospital AMI care has frequently been blinded to primary care for AMI and truncated at hospital discharge, owing to the unavailability of primary health-care data. As AMI is a chronic long-term condition, initiating from atherosclerosis during the teenage years and continuing to long-term rehabilitation after hospitalisation, by enabling the availability of data, information and knowledge of patient care, the study shows the capacity for using linked EHRs for an integrated understanding of AMI care.
Next, steps should be to describe the time in BP control as defined in JNC 8 guidelines,168 assess the association between yearly time in BP control and the onset of other fatal and non-fatal CVD outcomes, assess the association between deviation of SBP and DBP in relation to recommended target values and the onset of CVD outcomes. Factors associated with yearly duration of time in therapeutic range are to be identified and adjusted in the observed association.
Conclusions
Our finding of a neutral volume–outcome relationship after 2008 is unsurprising if it reflects an even spread of expertise across those centres. Further work is necessary to assess the distribution of AMI patients across hospitals and other hospital characteristics that contribute to patient outcomes.
Study 32: interhospital variation in mortality – international comparisons
This study is based on the peer-reviewed paper by Chung et al. 62
Introduction
To date, interhospital variation in the treatment and outcomes of AMI have been analysed only within individual countries. Although this has provided information about national scope for health system improvement, it has been uninformative about system performance relative to other countries. This represents an important missed opportunity for learning from other health-care systems and for international benchmarking of performance. In this study we compare for the first time interhospital variation in treatment and outcomes of patients with AMI in Sweden and the UK, the only two countries in the world with continuous national AMI registries in which participation is mandated in all hospitals. 7,86 The comparison was facilitated and made more relevant by the similarity of the health systems in the two countries, both of which provide universal care that is free at the point of contact. Our earlier study identified a significant difference in 30-day mortality after AMI between Sweden and the UK,55 and we now seek to determine if this is reflected in differences in interhospital variation in AMI care and outcomes between the two countries. Our specific objectives were to compare Sweden and the UK regarding interhospital variation in (1) the use of AMI guideline-recommended treatment and (2) crude and case-mix-adjusted 30-day mortality.
Methods
Eligible hospitals and patients
All hospital units providing AMI care in Sweden (n = 87) and in England and Wales (n = 242) were included in the study. Eligible patients were aged ≥ 30 years and admitted between 1 January 2004 and 31 December 2010. AMI diagnosis was based on guidelines from the ESC/American College of Cardiology/American Heart Association. 454 For multiple admissions of the same patient, we used the earliest record. The data validation7,86 quality and comparability of variable definitions regarding AMI diagnosis, patient case mix, evidence-based hospital treatment strategies and discharge medication variables in SWEDEHEART/RIKS-HIA and MINAP are described in our earlier study (study 16). 55
Case-mix variables
The 17 case-mix measures included demographic factors (age, sex, admission year), risk factors (smoking, history of diabetes and hypertension), AMI severity (troponin, admission SBP, admission heart rate), history of prior heart failure (cardiac arrest admission, cerebrovascular disease, or AMI), procedure and medication use prior to hospital admission (antiplatelet treatment with aspirin, clopidogrel or both, PCI, CABG).
Guideline-recommended treatment
Guideline-recommended treatment for STEMI patients358,455–458 included reperfusion therapy (primary PCI, any fibrinolysis therapy) and medication for AMI patients surviving at hospital discharge, including the use of antiplatelet therapy (any or combined antiplatelet medication), beta-blockers, ACEIs or ARBs, and statins.
Mortality outcomes
The primary clinical outcome was all-cause mortality within 30 days after hospital admission. Each country has a national unique identifier, which was used to link patients with the National Population Registry (in Sweden) and the ONS death registry (in the UK) to ascertain vital status and date of death (available for 99.3% and 98.7% of patients, respectively).
Statistical analysis
We compared the crude hospital proportions of case mix, treatment and 30-day mortality during the period 2004–10 and by admission year in Sweden and the UK. Hospital rates for treatment and 30-day mortality were derived from patient data and weighted by hospital volume. Given that hospital rates were not normally distributed, IQR was used for describing the observed variation. To standardise patient case mix, we constructed a generalised mixed model with the 17 case-mix variables described above. The model incorporated hospital random effect to account for clustering of patient data at hospital level. Predicted mortality of each patient was summarised by admitted hospitals to obtain the estimated hospital 30-day mortality based on case-mix model. The estimated mortality was divided by the observed hospital 30-day mortality to obtain the standardised mortality ratio of each hospital, and hospital case-mix-standardised mortality was determined by multiplying the standardised mortality ratio by the crude AMI 30-day mortality of the country. To assess the association of hospital treatment variation with AMI 30-day mortality risk, we summarised volume-weighted hospital 30-day mortality by hospital guideline-recommended treatment use quartiles, where hospitals were classified by the percentage of treatment use in admitted patients into the lowest (first quartile), medium–low (second quartile), medium–high (third quartile) and highest (fourth quartile).
We developed a generalised mixed model, adding the variables of hospital treatment quartiles to the 17 case-mix variables, to compare the case-mix-adjusted risk ratios between hospital treatment use quartiles and mortality risk in Sweden and the UK. The model incorporated hospital random effect to control for clustering of patient data at the hospital level. The numbers of patients with missing data were evaluated and managed by multiple imputation. Analyses were performed by SAS version 9.0 in London using secure remote access with a common protocol.
Results
Hospital-level case mix
Hospital-level measures of infarct severity, including heart rate, SBP and troponin were comparable for Sweden and the UK (Table 39). A higher proportion of Swedish patients were female, with a history of diabetes or heart disease. Although the proportion of patients with STEMI at hospital level was greater in the UK than in Sweden during 2004–7, it was more comparable in 2010 (31.7% ± 14.6% vs. 33.3% ± 24.4%, respectively).
Variable | Sweden | UK | ||||
---|---|---|---|---|---|---|
2004 | 2007 | 2010 | 2004 | 2007 | 2010 | |
Hospital number | 75 | 76 | 81 | 233 | 228 | 225 |
% STEMI, median (IQR) | 31.2 (8) | 27.8 (12.3) | 31.3 (11.2) | 38 (23.8) | 40.2 (25.9) | 27.5 (30.5) |
Age (years), mean (SD) | 72.2 (3.1) | 71.2 (3.2) | 70.7 (2.2) | 69.4 (3.7) | 69.2 (4.4) | 69.9 (4.4) |
% Female (IQR) | 37.6 (6.7) | 36.9 (6) | 35.1 (7.1) | 34.3 (7.9) | 33.7 (7.3) | 34.7 (8.6) |
AMI severity, median (IQR) | ||||||
% SBP (mmHg) | 144.3 (10) | 145 (10) | 145 (8.5) | 140 (7.5) | 139 (7) | 139 (7) |
% Heart rate (b.p.m.) | 77 (5) | 77 (5) | 78 (4) | 78.3 (4) | 79.5 (3.5) | 79 (4) |
% Troponin I (ng/ml) | 4.4 (6.4) | 3.7 (5) | 3.1 (3.4) | 7 (16) | 4.3 (7.8) | 2.3 (3.4) |
% Troponin T (ng/ml) | 1 (2.7) | 0.7 (0.6) | 0.8 (1.6) | 0.9 (2) | 0.7 (1.4) | 0.8 (3.5) |
Risk factor, median (IQR) | ||||||
% Current smoking | 22.2 (6.3) | 22.4 (6.1) | 23.1 (6.6) | 30.8 (9) | 29.5 (10.9) | 26.6 (10.4) |
% History of diabetes | 23.7 (4.4) | 22.2 (5.9) | 22.3 (5.7) | 16.1 (5.9) | 16.7 (6.2) | 19.4 (5.8) |
% History of hypertension | 42.4 (8.9) | 46.5 (9.1) | 49.1 (7.6) | 43.5 (9.4) | 47.8 (11.8) | 49.7 (10.7) |
History of CVD, median (IQR) | ||||||
% Heart failure | 12.5 (6.2) | 9.3 (6.7) | 6.7 (4.1) | 5.2 (5.1) | 4 (4.6) | 4.7 (4.8) |
% Previous AMI | 29.4 (6) | 21.5 (5.1) | 18.7 (4.1) | 20 (6.5) | 17.1 (6.3) | 17.3 (6.7) |
% Cardiac arrest | 0.9 (1.6) | 1.2 (1.2) | 1.4 (1.9) | 1.8 (2.1) | 1.8 (1.7) | 1.9 (2.6) |
% Stroke | 7 (14.3) | 10 (4.3) | 8.6 (3.7) | 7.6 (5.4) | 7.5 (5.1) | 8.9 (5.1) |
Treatment prior to admission, median (IQR) | ||||||
% Single antiplatelet | 41.1 (7.4) | 37 (6.8) | 33.5 (7.1) | 20.1 (19.6) | 26.9 (15) | 22.1 (19.5) |
% Combined antiplatelet | 3 (3) | 3.8 (2.5) | 3.3 (2.7) | 0 (0) | 2.7 (3.8) | 2.1 (3.4) |
% PCI | 6.8 (3) | 7.6 (4.4) | 8.4 (3.6) | 3.4 (4.4) | 5.6 (4.7) | 6.1 (4.7) |
% CABG | 7.2 (3.3) | 7.8 (3.3) | 7.4 (3.2) | 3.9 (2.5) | 4.4 (3.1) | 5.4 (3.1) |
Hospital-level treatment
Hospital use of primary PCI was higher (61.9% vs. 34.9%) and variation was lower (IQR 16.1% vs. 50.7%) in Sweden than in the UK. In both countries, the increasing use of primary PCI reduced interhospital variation between 2004 and 2010 (Figure 71), as reflected in reductions of IQRs from 41.6% to 12.8% in Sweden and from 29.2% to 17.3% in the UK. The use of any reperfusion therapy for STEMI patients was higher in UK hospitals owing to greater use of thrombolytic therapy and, although interhospital variation in the use of reperfusion therapy decreased in Sweden between 2004 and 2010, it increased in the UK.
FIGURE 71.
Hospital variation in implementation (per cent, median and IQR) of treatment for AMI by year in (a) Sweden (SWEDEHEART/RIKS-HIA registry) and (b) the UK (MINAP). Reproduced from Chung et al. 62 © 2015 British Medical Journal Publishing Group. This is an open-access article distributed under the terms of the Creative Commons Attribution License (http://creativecommons.org/licenses/by/4.0/), which permits others to distribute, remix, adapt and build upon this work, provided the original work is properly cited.


Hospital variation in the pre-discharge prescription of secondary prevention drugs was lowest for antiplatelet drugs in both Sweden (IQR 4.7% in 2004 and 2.9% in 2010) and the UK (IQR 4.8% in 2004 and 4.2% in 2010). Beta-blocker prescription was higher in Sweden than in the UK, with less variation in Sweden (IQR 4.9%) than in the UK (IQR 10.1%). Conversely, use of ACEIs/ARBs and statins was greater in UK than in Swedish hospitals with somewhat greater variation in Sweden (11.6% and 11.9%, respectively) than in the UK (10.1% and 4.8%, respectively).
Interhospital variation in 30-day mortality
During the study period, crude hospital 30-day mortality in Sweden (7%) was lower than in the UK (10.1%) and showed less variation (IQR 2.1–5.7%). Both mortality and interhospital variation fell between 2004 and 2010, from 9.3% (IQR 3%) to 6.5% (IQR 2.8%) in Sweden and from 12.8% to 7.6% (IQR 5.5–7.5%) in the UK (Figure 72). In Sweden, hospital variation in 30-day mortality was greater for STEMI (IQR 2.5%) than for NSTEMI (IQR 1.8%), in contrast to the UK, where interhospital variation was greater for NSTEMI (IQR 5.4–7.1%). However, hospital case-mix proportions showed that patients with NSTEMI were older, more commonly female and had a greater prevalence of chronic disease. After adjustment, the hospital distribution of standardised 30-day mortality in Sweden (8.4%) was lower than in the UK (9.7%) and showed less variation (IQR 2.6–3.5%) (Figure 73).
FIGURE 72.
Variation in hospital AMI 30-day mortality (per cent, median and first and third quartiles) by year in (a) Sweden (SWEDEHEART/RIKS-HIA registry) and (b) the UK (MINAP). Reproduced from Chung et al. 62 © 2015 British Medical Journal Publishing Group. This is an open-access article distributed under the terms of the Creative Commons Attribution License (http://creativecommons.org/licenses/by/4.0/), which permits others to distribute, remix, adapt and build upon this work, provided the original work is properly cited.

FIGURE 73.
Hospital variation in case-mix-standardised 30-day mortality (%) in the UK (MINAP) and Sweden (SWEDEHEART/RIKS-HIA registry) between 2004 and 2010. Reproduced from Chung et al. 62 © 2015 British Medical Journal Publishing Group. This is an open-access article distributed under the terms of the Creative Commons Attribution License (http://creativecommons.org/licenses/by/4.0/), which permits others to distribute, remix, adapt and build upon this work, provided the original work is properly cited.
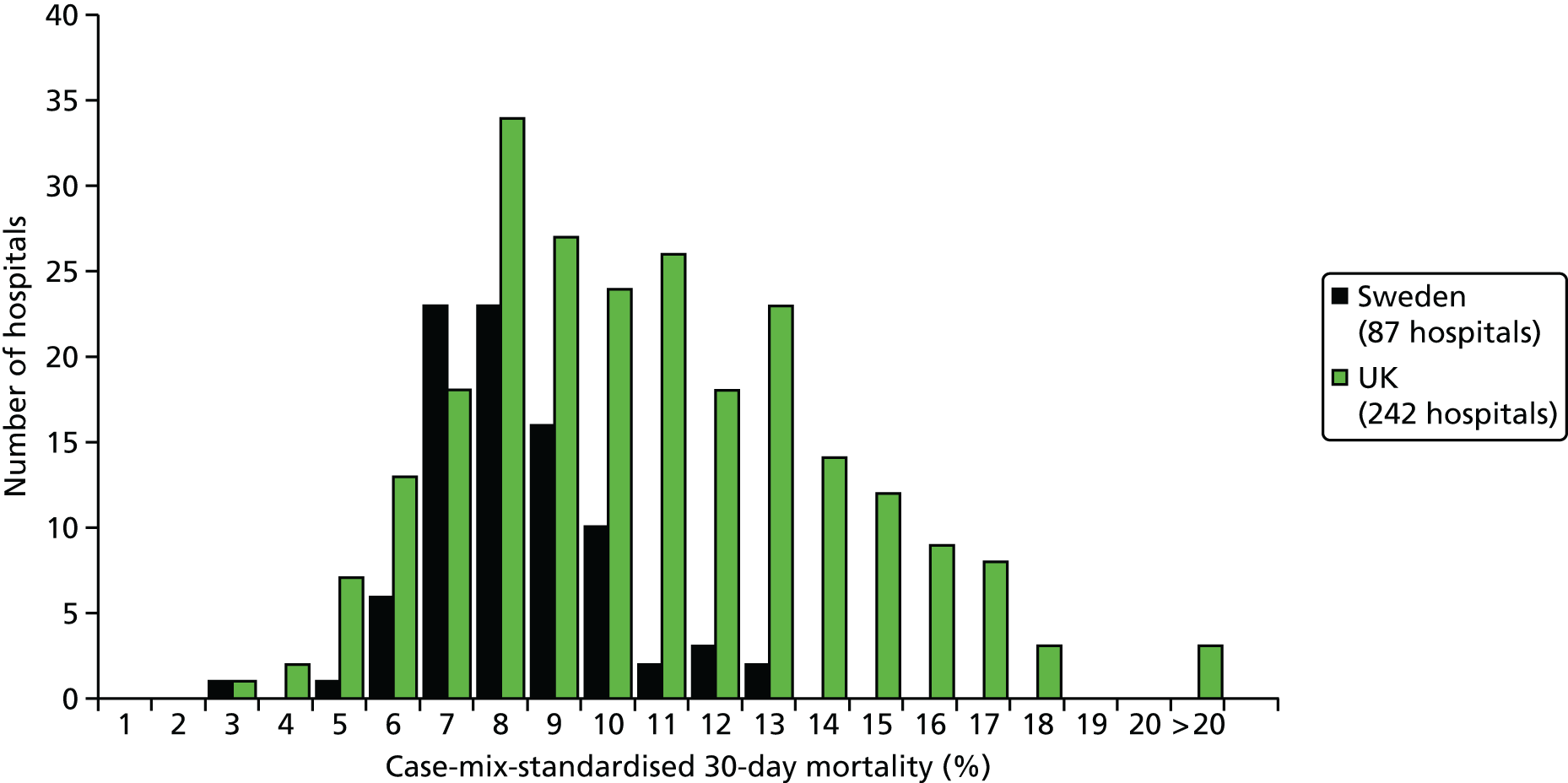
Thirty-day mortality by hospital treatment quartiles
In both Sweden and the UK, lower volume-weighted 30-day mortality was reported in hospitals using guideline-recommended treatments more often. The difference was most notable for treatment of STEMI with primary PCI, where comparison of lowest- (Q1) and highest-quartile (Q4) hospitals showed volume-weighted 30-day mortality rates of 10.7% (95% CI 10.1% to 11.3%) versus 6.6% (95% CI 6% to 7.1%) in Sweden and 12.7% (95% CI 12.4% to 13%) versus 5.8% (95% CI 5.1% to 6.4%) in the UK. In both Sweden and the UK, there was a similar trend for diminishing odds of 30-day mortality for patients with STEMI as hospital primary PCI use increased, with a comparison of Q4 and Q1 hospitals showing an odds of 0.69 (95% CI 0.6 to 0.78) in Sweden and 0.63 (95% CI 0.56 to 0.7) in the UK. Absolute risk differences between Q1 and Q4 hospitals for any reperfusion and discharge medication were lower for Sweden than the UK. For example, differences in volume-weighted STEMI mortality between Q1 and Q4 hospitals for any reperfusion use were 3.4% in Sweden and 6.4% in the UK. For discharge medications, the Q1–4 differences for Sweden and the UK were, respectively, 3.3% and 5.3% for any antiplatelet drug, 3.7% and 5.2% for dual antiplatelet therapy, 0.7% and 5.1% for beta-blockers, 2.6% and 3.2% for ACEIs/ARBs, and 3.7% and 5.3% for statins. If Q1 hospitals had the same volume-weighted mortality rate as Q4 hospitals, more than 2000 lives in Sweden and 11,000 lives in the UK would have be saved or prolonged. After standardisation by patient case mix, differences in excess deaths between treatment use quartiles were smaller, but similar trends remained (Figure 74).
FIGURE 74.
Estimated case-mix-adjusted AMI 30-day mortality and OR by hospital treatment quartiles, in the UK (MINAP) and Sweden (SWEDEHEART/RIKS-HIA registry). Reproduced from Chung et al. 62 © 2015 British Medical Journal Publishing Group. This is an open-access article distributed under the terms of the Creative Commons Attribution License (http://creativecommons.org/licenses/by/4.0/), which permits others to distribute, remix, adapt and build upon this work, provided the original work is properly cited.
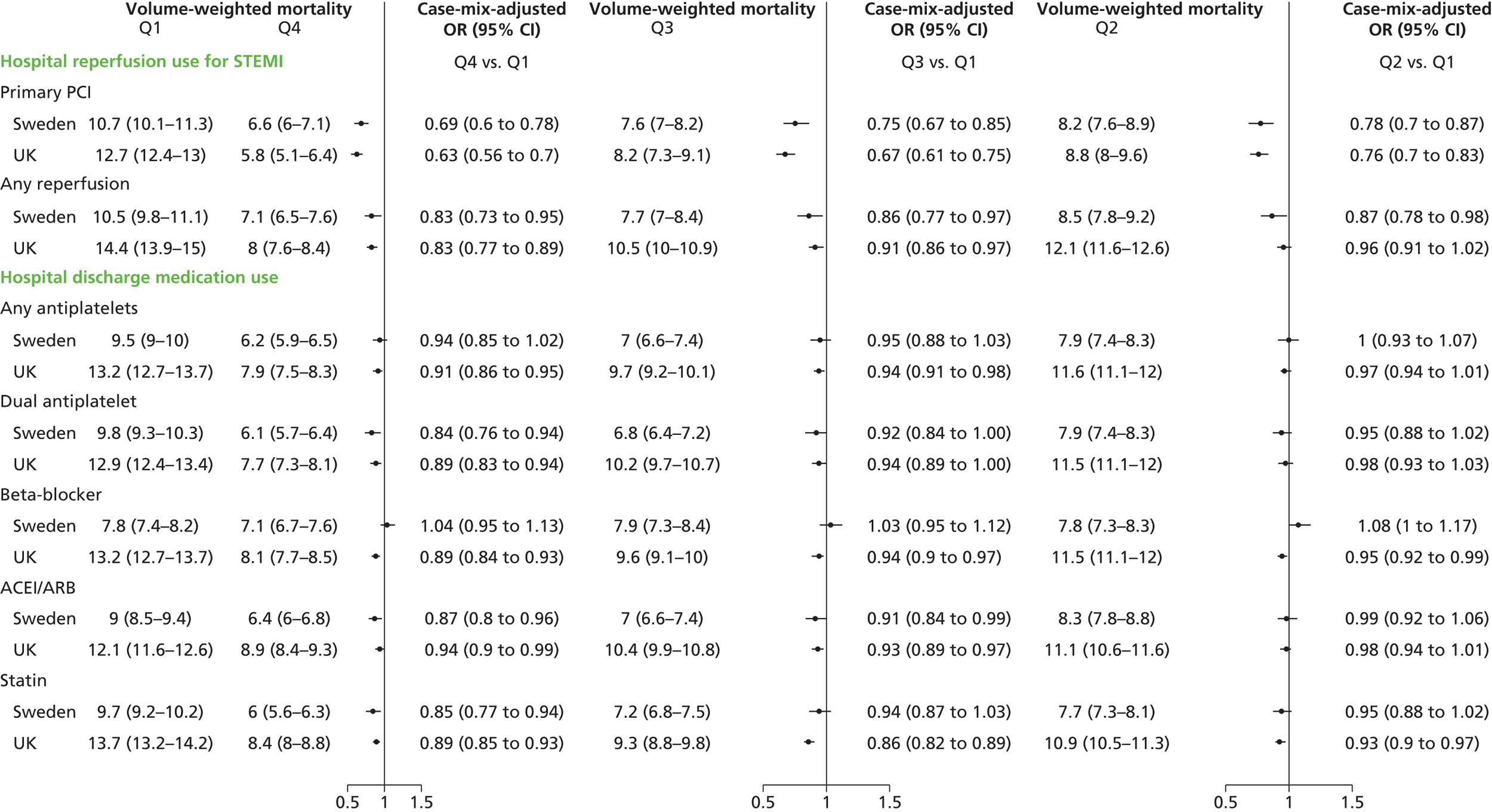
Discussion
This is the first hospital-level international comparison of the characteristics, treatment and outcomes of patients with AMI admitted to all hospitals across Sweden and the UK. In both countries, hospital practice variation and hospital 30-day mortality were inversely related to the use of guideline-recommended treatments, with hospitals in the upper use quartiles showing less practice variation and lower 30-day mortality. A comparative analysis showed that interhospital variation in the use of primary PCI was greater in the UK than in Sweden and was associated with greater variation in 30-day mortality. The data suggest that more consistent health care across all hospitals not only would reduce practice variation but also would deliver improved outcomes.
Treatment variation
UK hospitals showed a distinct temporal trend in the use of reperfusion therapy, with the clinical consensus appearing to favour thrombolytic therapy from 2004 to 2007, with an increasing use of primary PCI thereafter, but not matching Sweden’s use until 2010. The switch towards increasing UK hospital use of primary PCI in 2008 was associated with a significant reduction in interhospital practice variation and corresponded with the launch of a national policy initiative the same year favouring primary PCI. In Sweden, reductions in hospital variation in the use of primary PCI diminished in 2006, reflecting a national consensus that had moved away from thrombolytic therapy at least 2 years earlier than in the UK. This 2-year delay in the implementation of primary PCI in the UK appears to have had salutary consequences, judging by the favourable 30-day mortality odds for STEMI patients receiving treatment in high- versus low-use hospitals. Thus, our data suggest that reducing hospital variation in primary PCI use has the potential to lower STEMI mortality in the UK and Sweden by 37% and 31%, respectively.
Interhospital variation in the use of guideline-recommended secondary prevention therapy was less marked than variation in reperfusion therapy in both Sweden and the UK. It was particularly low for any antiplatelet drugs, with interhospital variation in both countries of < 4% associated with the smallest mortality variation between the lowest- (Q1) and highest-quartile (Q4) hospitals for use of these drugs. The association of low levels of interhospital variation in any antiplatelet drug treatment with low mortality variation between hospitals may provide a useful benchmark for other secondary prevention drugs. If variations between Swedish and UK hospitals in the prescription of all secondary prevention drugs were reduced to the levels recorded for any platelet drugs, important reductions in mortality variation could be achieved.
Mortality variation
It was a major finding of this study that hospital-level 30-day mortality for patients with AMI was not only higher in the UK but also showed greater variation than in Sweden. Thus, the distribution of case-mix-standardised 30-day mortality for UK hospitals was broader based and shifted to the right compared with Swedish hospitals. Our data suggest that variations in hospital treatment explained about half of the variation in hospital mortality in Sweden, where antiplatelet drugs and statins probably had an important role, and 27% of the variation in hospital mortality in the UK, where primary PCI use, dual antiplatelet therapy and beta-blockers appeared more important. Although risk differences between hospital quartiles for secondary prevention drugs were smaller than for reperfusion therapy, reducing variation in the use of secondary prevention drugs has greater potential to reduce mortality, as they benefit all patients with AMI, whereas reducing variation in reperfusion therapy would benefit only STEMI patients.
Policy implications
Our study provides no information about what, beyond variations in hospital treatment, might drive the residual mortality variation between hospitals. Other investigators have reported that multiple factors might be involved, including hospital structure (staff expertise, hospital volume, resources),438,459–463 processes of care (treatment protocol, problem-solving)351,459,461,463 and organisational culture. 351,438,463 We found that more patients in both Sweden and the UK were admitted to hospitals in the lowest treatment use quartiles but, although volume-weighted 30-day mortality was higher in these hospitals, differences in the case-mix-adjusted odds of 30-day mortality between quartiles was less striking, suggesting that factors other than underuse of guideline-recommended treatments are contributing to the mortality excess in low-quartile hospitals. Indeed, it is possible that the low use of guideline-recommended treatment in such hospitals is ‘the canary in the coal mine’, which signals difficulties in implementing high-quality care. These implementation difficulties might include patient vulnerability and other hospital-level and regional factors, emphasising the importance of looking beyond the prescription of guideline-recommended drugs in developing quality improvement programmes. In this scenario, quality improvement might best be achieved not only by targeting underperforming hospitals, but also by the development of system-wide initiatives that aim to deliver equitable management across all national hospitals from time of admission through to discharge and beyond. This no doubt accounted for the success of the UK’s National Service Framework for Coronary Heart Disease in 2000,81 but Sweden has a more comprehensive nationwide programme of quality improvement that includes public reporting of hospital mortality, rapid diffusion of new technologies,464 more complete use of evidence-based practice,465 and a more established system for evaluating and reporting the quality and outcomes of care. 466 This system-wide approach is likely to account, at least in part, for the reduced variation in hospital care of patients with AMI in Sweden. Our results suggest that a national UK strategy aimed at increasing use of guideline-recommended treatments in Q1 hospitals, combined with system-wide quality improvement, could reduce hospital variation in care to Swedish levels, with a leftward shift of the distribution of case-mix-standardised 30-day hospital mortality.
Our study has limitations. First, the contribution that unmeasured case-mix factors make to variability in hospital outcomes cannot be determined. However, it is unlikely to be large because case-mix standardisation gave comparable results to crude hospital proportions. Second, we could not evaluate international differences in management AMI care prior to hospital admission, which may potentially influence in-hospital treatment and outcomes. However, this is unlikely to explain higher variation in the UK, because we found that time from symptom onset to admission, an indicator of pre-hospital management, was similar to that in Sweden. 55 Third, national registries do not capture all patients admitted with AMI, and this may be more common in the UK than in Sweden. Patients who are not captured tend to be older and not admitted under the care of a cardiologist, and this could lead to an underestimation of the true hospital care and outcome differences. Finally, missing values may introduce bias in our case-mix model. However, we have previously reported that estimates for the association between case-mix variables and 30-day mortality, based on multiple imputed data, were verified by estimates based on complete-case analysis. 55
International comparisons are an integral component of contemporary prognosis research. 29 They are informative about the relative performance of health-care systems and further comparisons with other national registries are now needed, including those from the USA,358 China467 and New Zealand. 468 In these countries, participation in registries is often voluntary, and research will be required to understand the impact of hospital selection. Opportunities for more ambitious comparisons along the care pathway are provided by linkage to national EHRs, permitting the analysis of non-fatal events before and after hospital admission and longer-term outcomes. Our findings also have policy implications for reducing interhospital variability in health care, with further work necessary to determine the competing benefits of targeting underperforming hospitals or developing a national programme of quality improvement for implementation across all hospitals.
Conclusions
This study has shown that interhospital variation in guideline-recommended treatment is greater in the UK than in Sweden. This is associated with, and may partly account for, the higher hospital 30-day mortality in the UK. A system-wide national programme modelled on Sweden’s quality improvement programme has the potential to reduce practice variation if implemented across all UK hospitals, with reductions in hospital 30-day mortality towards Swedish levels.
Study 33: variation in coronary care study – a qualitative study on hospital processes and patient experience
Three manuscripts are in preparation. 63–65
Introduction
Investigations of hospitals with poor outcomes, exemplified by the Francis Report,83 have sparked growing concern about the quality of nursing and medical care in the NHS. Furthermore, our investigations indicate that interhospital variation in mortality is only partly explained by patient characteristics and differences in treatment or other hospital-level factors, suggesting that other aspects of care are having a significant impact on patient outcomes.
A better understanding of the processes of cardiac care associated with improved patient outcomes could provide important information to guide hospital and commissioning policy. One of the few studies examining care processes for patients with AMIs351 identified a range of characteristics that distinguished high- from low-performing hospitals in the USA, including the presence of clinical champions, empowered nursing staff and engaged pharmacists, strong communication and co-ordination across disciplines and departments, and greater involvement of senior managers. This study, however, was based on interview data without observations of care and excluded hospitals that did not carry out primary PCI.
Although NICE420 recommends coronary angiography within 96 hours depending on the GRACE risk assessment,469,470 previous studies have shown that delivery on these recommendations is inconsistent between hospitals. In part, this may reflect the need for complex collaboration between emergency staff and follow-on cardiac services and co-ordinated nursing support. 471,472 Using an ethnographic approach, we have examined the management of NSTEMI in 10 hospitals with contrasting levels of 30-day mortality, to identify processes of care that might influence guideline adherence and contribute to variation in outcomes between hospitals. We focused on NSTEMI care because this condition is more common and has a worse prognosis than STEMI, with more initial diagnostic uncertainty and a less well-defined management pathway. Moreover, STEMI patients have benefited from national initiatives in the UK to reduce the time to primary PCI.
Methods
Study design
We undertook an ethnographic study, comprising observations of NSTEMI care, interviews with clinical staff and patients and scrutiny of medical records.
Hospital sample
We used MINAP to identify a sample of 30 hospitals in the top or bottom third for 30-day case-mix-adjusted mortality. We selected a purposive sample of eight hospitals, four from the top tertile and four from the bottom, and with variation in coronary interventional facilities, teaching status, volume of cardiac admissions and geography. Two pilot hospitals from the middle tertile were added to the sample to refine the fieldwork. The research team was blinded to hospital performance status until after the analysis of ethnographic data. Only one of the hospitals that were approached declined to take part and another was chosen to replace it. We obtained initial permission from the clinical lead for cardiology in each hospital and then sought verbal assent followed by written consent before observations and interviews of staff and patients. Study procedures were approved by local Research Ethics Committees (reference 10/H0107/75).
Data collection
At each hospital, we collected data from (1) observation of the care of a sample of NSTEMI patients from admission through to discharge; (2) interviews with clinicians and managers at each site; (3) interviews with patients and family members, and telephone interviews 30 days after discharge; and (4) patients’ medical records. Observations and interviews were carried out during a 2-week period of intensive fieldwork at each hospital (June 2011 to August 2012), in relevant emergency departments, medical assessment units, coronary care units, catheter laboratories and cardiac and general medical wards, focusing on the processes of admission, diagnosis, treatment and discharge. Patient participants were purposefully selected to represent a diversity of age, sex and clinical situation. Staff participants were selected to represent maximum variation of roles but similar types of staff were involved across all sites. Interviews were audio-recorded where permission was given and observational data were collected in detailed paper FNs.
Data analysis
In our analysis we aimed to articulate care processes – from initial hospital presentation of patients with a possible NSTEMI through to their discharge – that appeared to facilitate good-quality care, as represented in NICE guidelines and the perceptions of clinicians and patients who we interviewed. Interview topic guides and observations were informed by experiences at the two pilot sites. Analysis using constant comparison,210 looking for similarities and differences in the data, was used to identify and explore aspects of hospital organisation and processes. Strong themes emerged in core areas. Discussion of themes with the wider team brought multiple disciplinary perspectives to the analysis. Once the core processes had been agreed, the researcher collecting the primary data ranked each hospital with regard to each process. These rankings were based on perceived adherence to NICE guidance in the care of individual patients, with cross-checking between interview and observational data. The rankings for each process were based on data sets for the hospitals taken as a whole; we used all the data sources and incorporated factors such as overall team working and formal organisational mechanisms on, for example, how actively NSTEMI patients were searched for, as well as the contributions of exceptional individuals. We were not able to adequately represent different care for unusual groups of patients in the rankings but have referred to this in the main text. Using a similar method, a second researcher independently ranked each hospital as a whole (i.e. not broken down by care process) and there was broad agreement in overall rankings. Patients and staff participants are represented by codes P1, P2, etc. and S1, S2, etc., respectively. The different sources of data are designated interview (INT), observation (OB), audio-recording (AR) and FNs.
Results
Study hospitals and sample characteristics
The 10 participating hospitals varied in their organisation of care for NSTEMI patients (Table 40). Table 41 describes the study recruitment and type of data collected from 199 hospital staff, 68 patients and 732 hours of observation.
Characteristic | Hospital | |||||||||
---|---|---|---|---|---|---|---|---|---|---|
1 (pilot) | 2 (pilot) | 3 | 4 | 5 | 6 | 7 | 8 | 9 | 10 | |
Teaching status | Teaching (tertiary) | Non-medium teaching | Teaching (tertiary) | Non-medium teaching | Teaching (tertiary) | Non-teaching | Teaching (tertiary) | Non-teaching | Teaching (tertiary) | Non-teaching |
Volume of cardiac admissions 2008 (low/medium/high)a | Medium | Medium | High | Low | High | Low | Low | High | High | Low |
Region | West | West | South | North | East | North | West | North | North | South |
Type of AMI patients | STEMI and NSTEMI | NSTEMI only | STEMI and NSTEMI | NSTEMI only | STEMI and NSTEMI | NSTEMI only | STEMI and NSTEMI | NSTEMI only | STEMI and NSTEMI | NSTEMI only |
Primary PCI? | Yes | No | Yes | No | Yes | No | Yes | No | Yes | No |
Type of intervention available | Angiogram, angioplasty, CABG | Angiogram, angioplasty, CABG | Angiogram, angioplasty | Angiogram, angioplasty | Angiogram, angioplasty | Angiogram, angioplasty | Angiogram, angioplasty, CABG | Angiogram, angioplasty, CABG | Angiogram, angioplasty, CABG | |
Catheter laboratories on site (n) | 4 | 0 | 2 | 2 | 3 | 1 | 3 | 1 | 6 | 2 |
Type of cardiology link person (total n) | ACS nurse (1) | ACS nurse (1) | ACS nurse (1) + cardiologist of the week (1) | Thrombolysis nurses (2) | Cardiac matron (1) + senior sisters (2) + junior sisters (4) + on-call senior house officer (1) | Chest pain nurses (5) | ACS nurses (4) (not as key A&E link but for interhospital transfers) | ACS nurses (5) | Unclear/no ACS nurses (only used registrars and senior house officers) | Unclear/no ACS nurses |
Number of link people on duty at any one time | 1 | 1 | 2 | 2 | 4 | 2 | 2 | 2 | 0 | 0 |
Risk score used by emergency department | TIMI/GRACE | TIMI | None | None | GRACE | No data | TIMI | Own design | TIMI | None |
Type of data collection | Hospitals | Total (N) | |||||||||
---|---|---|---|---|---|---|---|---|---|---|---|
1 | 2 | 3 | 4 | 5 | 6 | 7 | 8 | 9 | 10 | ||
Patients recruited | 13 | 1 | 8 | 7 | 8 | 7 | 6 | 6 | 7 | 5 | 68 |
Interviews with patients | 7 | 1 | 6 | 7 | 8 | 7 | 5 | 4 | 4 | 4 | 53 |
Staff recruited | 36 | 14 | 15 | 14 | 21 | 20 | 26 | 13 | 20 | 20 | 199 |
Staff interviews | 13 | 5 | 12 | 13 | 17 | 12 | 12 | 16 | 21 | 21 | 142 |
Total hours on site | 75 | 23 | 81 | 84 | 88 | 80 | 88 | 80 | 73 | 60 | 732 |
Key non-ST-segment elevation myocardial infarction care processes
We identified five processes that appeared to facilitate good care. These were (1) early specialist assessment; (2) active patient monitoring and appropriate action; (3) cross-disciplinary communication; (4) co-ordinated discharge and initiation of secondary prevention prior to discharge; and (5) visible cardiology leadership. Underpinning these five processes was a perception by clinicians of responsibility for and ‘ownership’ of the process of care for NSTEMI patients. We have ordered the five processes by their temporal trajectory in NSTEMI care from initial hospital entry through to discharge. None of the hospitals in the sample had all the care processes in place for all patients, although one hospital stood out as having most of them (hospital 5).
Early specialist assessment
Although NICE guidelines for NSTEMI patients begin at diagnosis, the diagnostic process is a crucial stage, which directs care and determines further treatment. Only a minority of hospitals in our sample had a system of early specialist filtering by the cardiology team. For example, in hospital 3, staff talked about the lack of early cardiology involvement in triaging possible NSTEMI patients:
We are developing a number of different strategies which allow us to . . . improve the cardiology interface with general medicine . . . Not all of it is as actively managed as we’d like it to be . . . most of the ACS cases go to the general medical wards and then we have to extract them.
S57, director of cardiology, hospital 3, INT: AR
Most, but not all, hospitals used lists of troponin measurements as a method of identifying patients with a NSTEMI that had been missed or that was not diagnosable on admission. According to the ACS nurses in hospital 8, although a laborious task, checking troponin lists was an essential failsafe.
It is frustrating I can visit 10 different wards in a day and none of them turn out for me . . . but I may find that one of those patients has come in with a cardiac presentation or had a cardiac event whilst they’ve been in and I may find that nobody else has noticed that they had a raised troponin and ECG changes . . . but there’s only one way to do it.
S147, cardiac nurse, hospital 8, INT: AR
Active patient monitoring and appropriate action
The status or stability of NSTEMI patients can quickly change, so it is vital that they are monitored closely, with investigation and treatment changed when appropriate. Active patient monitoring and response could be achieved in various ways. For example, specialist cardiac nurses help to support a patient’s care not only by identifying possible NSTEMI patients but also by prescribing, chasing up test results, acting as a liaison or advocate and by nursing on specialist cardiac wards. One example of an integrated cardiac nurse role was found in hospital 5, which had twice-daily consultant-led ward rounds accompanied by a registrar and an experienced cardiac nurse acting as a ‘progress chaser’ (19 January 2012, OB: FN). Hospital 5 also had overnight specialist registrar cover.
At night the cardiac patients are seen, reviewed and medications appropriate started and plans made on the night shift so that group of patients aren’t waiting until 8 o’clock for a consultant to see them before a plan starts . . . and the night registrar does the [8 a.m.] triage round because he’s the one that knows the patients, and if they don’t know the patients there’s trouble . . . also if we had any patients on [cardiac ward] that then become unwell you’ve got a cardiac registrar that’s reviewing them, you haven’t [got] a junior doctor who’s not sure.
S97, cardiac matron, hospital 5, INT: AR
Monitoring in other hospitals was sometimes less effective. Hospital 4 had remote telemetry, that is, cardiac monitoring of patients on diverse wards with data screens at a central nursing station. The monitoring task was allotted to a small proportion of skilled and experienced nurses in the coronary care unit, whose direct care responsibility for patients on the unit sometimes limited their capacity to act on remote telemetry data.
If you cannot spare anybody to go running off without endangering the unit, then you have to keep going through the channels of phone calls . . . and looking at a monitor of a patient in real time that you can see is getting progressively worse, in front of your eyes . . . you know there’s nothing you can do . . . That patient would be dying . . . you start phoning the wards. If you can get through straight away, brilliant. But there have been a couple of occasions where you are phoning against an engaged signal on all the numbers you know.
S73, senior staff nurse, coronary care unit, hospital 4, INT: AR
In some hospitals patient monitoring and appropriate action was restricted to a subset of patients. For example, in hospital 5 there was a formal policy that patients aged > 85 years should go to medicine of the elderly wards and not be under the care of cardiologists unless they were particularly ‘bright’ (25 January 2012, OB: FN) or already known to the cardiology service. One 87-year-old patient was admitted with chest pain and shortness of breath. She was given a diagnosis of NSTEMI and cared for on a medicine for the elderly ward. She continued to have chest pain but, to her frustration and the frustration of nurses involved in her care, she was not reviewed by a cardiologist nor did she have the investigations specified in her management plan. After waiting 7 days, she asked to be discharged and died at home 5 days later (P34, hospital 5, OB: FN and patient notes).
Cross-disciplinary communication
Non-ST-segment elevation myocardial infarction care involves multiple disciplines, including catheter and pathology laboratory services, radiographers, specialist nursing, pharmacy and other clinical specialities for co-occurring conditions. Co-ordination of cross-disciplinary care is essential to facilitate diagnosis, risk assessment and further investigations. Good cross-disciplinary communication was evident in hospital 5.
What used to happen was we’d find that the junior doctors would forget to put patients on the list [for coronary angiography] which is obviously dangerous. Because if they’re not on the list we don’t know about them. And also what happened was the admissions unit would send a cardiology patient to where the bed was, so it might not be in cardiology it might be at the other end of the hospital . . . and we put nurses in charge . . . we put in these nurses, speciality nurses with cardiology experience . . . nurse prescribers, and they then take responsibility for the flow of the patients, so we decide where the patients go not medical staff, so we have ownership, so we deal with who comes in the front door.
S97, cardiac matron, hospital 5, INT: AR
In hospital 5 there were also examples of these skilled cardiac nurses, who were able to challenge doctors’ decisions.
Nurse calls for a registrar from cardiac ward. She queries a drug for a patient. Daltoparin prescribed, but, the patient is anaemic. The registrar says this is a really good call by the nurse, she is completely right. He makes a note to ask the consultant who wrote it up, and tells nurse not to give it yet. Another drug written up, potassium, but patient’s level is raised so nurse didn’t give it. Another good call says the doctor.
30 January 2012, hospital 5, OB: FN
An example of poor cross-disciplinary communication comes from hospital 6, where the cardiology staff had limited control over and poor access to the catheter laboratory, which they perceived as inhibiting appropriate angiography and angioplasty.
Although the cardiac lab[oratory] has got cardiology tech[nician]s and cardiology consultants, it has radiology nurses and radiology radiographers and they’re situated in the radiology department. So radiology gets to call the shots on what’s happening on the ground, which is potentially difficult for cardiology because there are many processes down there that could be better . . .
S120, consultant cardiologist, hospital 6, INT: AR
Co-ordinated discharge and secondary prevention
Secondary prevention medication and cardiac rehabilitation are the most effective means of reducing future cardiac events and reducing post-AMI morbidity and mortality in NSTEMI patients. 349 In our study, only a minority of hospitals began discharge planning and secondary prevention early in a patient’s stay, despite the priority given to this in NICE guidance. For example, in hospital 9, staff talked frankly about NSTEMI patients not being prioritised for rehabilitation services.
This position in rehab[ilitation] is absolutely appalling . . . they will build up a rehab team and then for whatever reason go through cost-cutting exercise and then the rehab team is cut to virtually nothing and the losers on that, they are not the STEMIs, they are the NSTEMIs, every single time.
S161, staff nurse, hospital 9, INT: AR
Most hospitals experienced delays with discharge paperwork and discharge medication. For example, one patient in hospital 4, aged 82 years (P27), was discharged with two medications missing and without her planned angiogram. Only hospital 5 had developed a comprehensive discharge system, enabled by nurse prescribers, but it excluded patients who were not under the care of cardiologists, including most patients aged > 85 years. In hospital 5 a dedicated cardiac pharmacist and technician worked with clinicians checking medications every day in anticipation of discharge. Each patient had a 30-day supply of discharge drugs in a locked bedside box to minimise delay. Discharged patients left the cardiac ward with a date 1 week later for multidisciplinary cardiac rehabilitation, which included a cardiac pharmacist.
In many hospitals without a comprehensive discharge system, the relatively brief in-patient episode (5–6 days on average) for NSTEMI patients,25 as well as pressures of staffing, resources and prioritising of STEMI patients, meant that follow-up largely became the responsibility of general practice. For example, in hospital 6, although there was a cardiac pharmacist, she was not fully integrated into a cardiac team or linked to the rehabilitation services:
In a lot of cases, it may be more prudent to say, ‘GP to review in a week’s time’ and amend a dose. We’ve done that on a few occasions where, we’ve needed a bed . . . an important part of medicines is the discharge counselling of patients, because we get a lot of rebound patients that come in, and you go through a drug history with them . . . the supplies we’ve supplied them with have run out, they’ve reverted back to what they had at home, which has been a wrong combination of medicines or the wrong dose . . . And making sure they have a good understanding of what’s changed . . . it’s something that’s not readily passed over to patients . . . you see more than 50 patients a day. I can’t get round and counsel them all.
S109, cardiac pharmacist, hospital 6, INT: AR
Visible cardiology leadership
Generally, those hospitals that appeared to provide good NSTEMI care had visible cardiology leadership that drove the management of cardiac patients and championed cardiology within the whole hospital. This leadership responded to problems and difficulties as they arose, as well as engaged with changes in technology, interventions and new evidence about effective care. In one hospital, the leadership was a recognisable set of individuals. Staff could approach these individuals with concerns or ideas, and the leadership team had sufficient resources and authority to act. For example, hospital 5 had a leadership team of three people who had led the cardiac department through big changes.
Cardiac matron explains that when the current nurse training was first introduced it was all wrong and they had to start again slowly from scratch with the staff more on board. Taken 12 years to get to this level of flexibility and been very hard in the last 24–18 months, but now working very well.
31 January 2012, hospital 5, OB: FN
Hospitals that did not have a visible cardiology leadership struggled with threats of catheter laboratory closure (hospital 4), catheter laboratory access restrictions (hospitals 2, 4 and 6), restrictions on expansion (hospitals 6, 8 and 10) or poor co-ordination of cardiac care with other key departments, such as accident and emergency (hospitals 3, 9 and 10). Staff in one cardiac department were particularly frustrated about their current role in cardiac care and lack of consultation.
[Ambulance] crews are confused about where to take people and at what time of day. Hardly any ACS patients in CCU [coronary care unit] now. There are more chronic conditions and a lot of care of the elderly, which they say is time-consuming and deskilling. Staff describe ward as dumping ground. Staff feel sad and demoralised. Staff nurse says no one has informed the staff officially that this is what is happening. There has been no meeting, no official letters, no word at all.
11 August 2011, hospital 2, OB: FN
Table 42 summarises our ranking of the hospitals for each of the care processes. Although some hospitals had consistently good or poor processes, most demonstrated a mixed pattern.
Characteristic | Hospital | |||||||||
---|---|---|---|---|---|---|---|---|---|---|
1 (pilot) | 2 (pilot) | 3 | 4 | 5 | 6 | 7 | 8 | 9 | 10 | |
Early specialist assessment | 10a | 5 | 9 | 1 | 8 | 4 | 2 | 7 | 6 | 3 |
Active patient monitoring and appropriate action | 10 | 7 | 8 | 1 | 9 | 4 | 5 | 6 | 2 | 3 |
Cross-disciplinary collaborative communication | 9 | 5 | 6 | 2 | 10 | 1 | 7 | 8 | 3 | 4 |
Co-ordinated discharge and secondary prevention | 7 | 2 | 6 | 1 | 10 | 5 | 3 | 8 | 9 | 4 |
Visible cardiology leadership | 9 | 5 | 8 | 3 | 10 | 4 | 6 | 7 | 2 | 1 |
Totals | 45 | 24 | 37 | 8 | 47 | 18 | 23 | 36 | 22 | 15 |
30-day mortalityb | Middle tertile | Middle tertile | Lowest tertile | Lowest tertile | Top tertile | Top tertile | Lowest tertile | Lowest tertile | Top tertile | Top tertile |
Discussion
We have identified five major care processes that appeared to facilitate good NSTEMI care that was consistent with NICE guidance420 (Table 43), as well as the perceptions of clinicians and patients, summarising evidence for each of the key processes and the related NICE guidance.
Key process | Examples from interviews and observational data illustrating how key processes facilitated or inhibited good-quality care | Link to good-quality care as specified in NICE CG94420 |
---|---|---|
1. Early specialist assessment | Cardiology are in and out of A&E all the time, they lead A&E on policy and clinical work. Low risk patients all have 1 hour & 8 hour Troponin T tests. They may go to the A&E observation unit to wait for the results, or they may be sent into MAU [medical assessment unit] if the A&E team think they should see a cardiologist sooner rather than later14 September 2011, hospital 1 OB: FNNSTEMI patients may not be identified in several different ways without specialist early intervention:Interviewer:When does cardiology get involved?Respondent:It depends . . . It’s just a bit hit and miss, if the patient’s there and the consultant’s on the floor . . . If it’s a not particularly straightforward they can ask one of the, between 9 to 5, one of the cardiology team to pop down and see the patient just to get a bit more information on it. It depends which teams, it might be the gastroenterologist team that day or the cardiology team, or the rheumatologist team, it dependsInterviewer:When does the cardiologist get called in if someone is thought to be ACS . . . where do they start to come into the pathway?Respondent:. . . it may be the A&E department, the majority of time it may be when they’ve gone through either to [secondary assessment units] to await the second Trop[onin] T . . . If it’s a definitely NSTEMI they will be referred to the cardiology, who will pick them up for further investigations such as angiographyS77, hospital 4, INT: AR | Focus is on early identification of patients with NSTEMI and assessment of risk |
2. Active patient monitoring and appropriate action | Only five hospitals out of the 10 used GRACE or TIMI risk scoring to inform management of NSTEMI patients (see Table 40)I try and catch people down in A&E and EAU [emergency assessment unit] before they go to the wards . . . I go through the diagnosis and look to see if there’s anybody with chest pain . . . [A&E staff] they’ll catch me and say . . . I’ve got this patient on 5b I’d like you to have a look for me . . . mopping up any loose ends as well because often they’re seen by junior doctors and then seen by more senior doctors . . . you’ll come in and take the patient if you feel that there’s a need. So you’re not just, the patients aren’t just relying on being recognised by the medical doctor . . . And if tests are needed then I will expedite those tests . . . It’s all about being proactive . . . I can get things movingS11, cardiac specialist nurse, Hospital 3, INT: ARNo link system of prioritising multimorbid patients into cardiology beds, and cardiac patients may not see a cardiologist for several days due to the infrequency of ward rounds. This happened to two patients in the study who went home without their promised angiograms after being kept waiting on non-specialist wards. No evidence of any scoring being used30 November 2012, hospital 4, OB: FN | Use risk assessment to guide clinical management and balance the benefit of a treatment against any risk of related adverse events in the light of this assessment Perform angiography as soon as possible for patients who are clinically unstable or at high ischaemic risk |
3. Cross-disciplinary collaborative communication | Timely response to requests from nursing staff to assess NSTEMI patients and make decisions about angiography was challenging in some hospitals with a high turnover of junior doctors:Interviewed senior cardiologist. He says junior doctors are made aware when they come into cardiology that the nurses are highly skilled and know the patients better than they do, if they call it’s because it’s needed. Usually a flurry of emails when the new juniors come in, & sometimes they have to be taken aside and advised this is how the department works best25 January 2012, hospital 5, OB: FN. . . the doctors change every few months so sometimes you feel you go back to square one with them again when you have to, you phone and say ‘oh I’m really worried about this patient, their blood pressure’s this, their ECG is this, can you come and see them?’. And they’ll fire another load of questions back at you, but once they know the team up here and they know our skills and knowledge, then they respond so it’s just every time you get a new tranche of doctors you have to sort of go through that, just for the first few daysS26, nurse, hospital 2, INT: AR | Offer coronary angiography (with follow-on PCI if indicated) within 96 hours of first admission to hospital to patients who have an intermediate or higher risk of adverse cardiovascular events if they have no contraindications to angiography (such as active bleeding or comorbidity) |
4. Co-ordinated discharge and secondary prevention | Respondent A:Here we’re part of their team so when they’re deciding who they’re discharging they tell me or they tell [name]. So . . .Respondent B:So instead of them getting it all ready and think ‘oh god we need to tell pharmacy’. . .Respondent A:They’re telling us when they’re deciding which ones are going home so they’re highlighting it on the board so that we can see straight away . . . it’s finding out who the co-ordinator is . . . then I will visit their lockers, and make they’re got everything, make sure that everything that’s written on the drug chart is right before they even start the [to take out medications]S81 and S82, cardiology team, hospital 5, INT: ARRespondent:I think an important part of medicines is the discharge counselling of patients, because we get a lot of rebound patients that come in, um, and you go through a drug history with them, and you find that, because the supplies we’ve supplied them with have run out, they’ve reverted back to what they had at home, which has been a wrong combination of medicines or the wrong dose and there’s overlapping . . . making sure [patients] have a good understanding of what’s changed while they’re in hospital, because they lose all control of their tablets and doses while they’re in hospital – somebody else does it – so it’s that education [that’s needed] that’s not readily passed over to patients . . . I will try and counsel as many patients as I can on, on what medicines they’re on, and what changes we’ve made . . . [But] you see more than 50 patients a day. Um, so I can’t get round and counsel them all . . .Interviewer:So, so where does rehab care come into that? Do you, do you link up with rehab care, at all?Respondent:I haven’t had the opportunityS109, cardiology team, hospital 6, INT: AR | Before discharge offer patients advice and information about:
|
5. Visible cardiology leadership | [A senior nurse] has always been at the forefront of development, she always put cardiology forward for anything she’s very motivating and she’s an absolutely brilliant leader . . . we did [a] pilot on this ward and we rolled it out across the ward, and the data from that proved that it was going to be a cost-saving exercise . . . and that system proved beyond all doubt that it is a very much faster system to discharge patients . . . you’re part of the team anyway and the leadership here makes it go out of the way to make sure you feel part of the team . . . and just not one person . . . Dr [name] everybody . . . I think we are quite an exception we always were, whatever important people are visiting the trust, they are brought to cardiologyS81 and S82, cardiology team, hospital 5, INT: AR. . . we do feel collectively as a cardiology department that we are being restrained . . . we feel that we’re being held back not only with our elective numbers but with our treat and return for whatever reason, be it political whatever I don’t know . . . They’d rather have work done at the tertiary centres . . . for PCIs it’s [number] so that’s our planned amount, for diagnostic angiograms its [number] . . . we could do more . . . [But] say we go over the magic [number] then will we be penalised because we’ve used X number of stents at £600, £700, £400 a stent and all the consumables that go with that . . . So it’s kind of juggling it around and making sure that we number one get that target and do it in a safe manner for the patients . . . why are they kind of insinuating that we’re overachieving and that we’re doing more work that we should be doing . . . we as an organisation seem unsure what they want from usS79, senior nurse, hospital 4, INT: AR | When the role of revascularisation or the revascularisation strategy is unclear, resolve this by discussion involving an interventional cardiologist, cardiac surgeon and other health-care professionals relevant to the needs of the patient |
Early specialist assessment and appropriate action
Early specialist assessment was essential for achieving an early diagnosis, not missing NSTEMI patients, directing care and determining further treatment. It was especially important for patients with multiple morbidities or unusual presentations. Early specialist cardiological assessment ensures that patients at medium or highest risk are monitored more closely,473 placed in a cardiac ward and prioritised for timely intervention, such as PCI within 24–96 hours, consistent with NICE guidelines.
Active patient monitoring
There were good examples of cardiac nurses with broad roles, twice-daily specialist ward rounds and specialist night cover. NICE guidelines emphasise that treatments should be introduced at the first sign of instability and interventions brought forward when unstable symptoms do not respond to treatment. Active monitoring is required to identify the patients at highest risk. 474,475
Cross-disciplinary communication
Early intervention in NSTEMI patients saves lives,476,477 requiring timely cardiac catheterisation, as recommended in NICE354 and other478 guidelines. Catheter laboratories often have to fit NSTEMI patients around the needs of elective stable chest pain diagnostic procedures, which requires effective cross-disciplinary communication.
Co-ordinated discharge and secondary prevention
Patients with NSTEMI can usually be discharged 24 hours after coronary intervention (3–4 days after admission),479 which leaves little time for organising guideline-recommended interventions, such as lifestyle advice and enrolment in cardiac rehabilitation. Early planning is, therefore, crucial but is often not enacted.
Visible cardiology leadership
Patients who are cared for in specialist cardiology wards or under cardiological supervision typically have better outcomes. 480 In order to achieve this level of supervision, there must be visible and accessible cardiology leadership.
Organisational culture
Each of these five dimensions may be influenced by the organisational culture at the NHS hospital trust level, at the clinical directorate level or at the ward level. We acknowledge that our approach did not explicitly focus on these contextual influences, partly because these have been more commonly studied elsewhere.
Strengths and limitations
A major strength of this study was our ability to observe, as well as to interview, staff and patients about the delivery of care. 481,482 Another strength was that we were blinded to the estimates of 30-day post-admission mortality for AMI patients. A major limitation was that we were unable to link our findings with variation in the fully adjusted 30-day mortality outcomes for our hospital sample because the parallel statistical analysis resulted in a model with such wide CIs around mortality estimates for most hospitals that we could not draw robust inferences about individual hospital performance. Another limitation was that the ranking of hospitals by implementation of the care processes was conducted in detail only by one fieldworker, although the overall ranking of hospitals was consistent with a second researcher’s assessment.
Relationship to other studies
Our findings are consistent with, and extend other research on, NSTEMI care. For example, Curry et al. 351 identified ‘empowered nurses’ as a key characteristic of top-performing hospitals, a finding confirmed by other studies. 472,483,484 In our study, specialist cardiac nurses featured in all of the five key processes we identified as underpinning high-quality NSTEMI care. Curry et al. 351 did not highlight early initial assessment, but the importance we ascribe to this process is consistent with studies by investigators in Canada485 and England. 486,487 Better information about AMI for patients is a key finding of several studies483,488–490 and a core feature of good-quality discharge. The importance of strong interdisciplinary communication and co-ordination has also been emphasised. 491,492
Implications for health service practice
Our findings reinforce key messages of the independent inquiry into care at the Mid-Staffordshire NHS Foundation Trust hospitals83 with regard to misdiagnosis, in particular, failure to follow up investigations, poor discharge management and lack of communication between staff about what needs to be done. Ethnography is the appropriate method for studying hospital culture, a major concern of the Francis Report83 and of the Keogh Review of hospital mortality,493 which highlighted poor-quality of care in 14 UK hospitals. Findings from the application of ethnographic methods can inform quality improvement, with the caveat that the depth and detail of our data were based on contracts of confidentiality and the trust of participating staff, which would be more difficult to achieve in the context of external quality monitoring. One use of qualitative data from ethnographic evaluations could be quantitative categorisation and ranking (see Table 1) that would complement measures of hospital outcomes such as mortality or readmission.
Public and user involvement in the design and conduct of the study
This study retained the expertise of two public and user group representatives (DT and JW) throughout, who attended and contributed to all the steering meetings. Their input remained useful and grounded in patient concerns. They helped to keep the focus on identifying actionable hospital-level factors rather than on factors that could not be changed, thereby increasing the possible relevance of the study for patient benefit. The representatives also helped to remind us of the importance of discharge and cardiac rehabilitation, and this has led to us considering future research topics on cardiac rehabilitation. To this end, our public and user group representatives have also reviewed some early thoughts and plans for future plans and projects. With more expertise in statistical methods, DT and JW were both helpful in pointing out where our qualitative methodology was not being explained clearly or where we were not justifying our approach sufficiently.
Conclusions
Our ethnographic method applied across hospital sites allowed us to characterise features of hospital culture that supported or undermined the quality of care for NSTEMI patients. We identified five processes that underpin good-quality hospital care for patients with NSTEMI. Better management of these processes is likely to improve the quality of care and outcomes for patients with NSTEMI.
Chapter 13 Conclusions and implications for clinical practice and further research
This NIHR programme illustrates the current potential of emerging ‘big data’ arising from linking EHRs at different stages of a patient’s journey (see Figure 75) to offer important, actionable insights into the diagnosis, risk stratification and cost-effective treatment of people at risk of, or with, angina, SCAD or AMI.
Our research programme set out to address new opportunities for improving quality of care and outcomes for patients with SCAD and ACS, by assembling and exploiting anonymised data resources integrated across the patient journey, and at different levels of care spanning primary and secondary acute and ambulatory care. We provide exemplars of an approach that has potential application across different disease areas, including those with the largest health-care burden (e.g. cancer, dementia, respiratory conditions, musculoskeletal conditions and mental health) as well as rare diseases. EHRs are, in many respects, agnostic to disease or clinical specialty and these features can be further exploited. Our findings should be interpreted within the context of temporal changes in the risk and management of CVDs over the past decade; for example, the availability of new medications, and different treatment targets and competing risks of CVD mortality and events among different populations. Our research findings (summarised in Table 3) and our approach using linked EHRs have informed national and international public health policy (Table 44), clinical guidelines and the development of underpinning methods, which have subsequently been implemented, and which have had an important impact on health care and our capacity to carry out research for patient benefit. We have published 25 papers (see Table 3) in high-impact journals based on analyses from our CALIBER data platform and we have used qualitative methods, systematic reviews and data from our novel, bespoke cohort to address our aims. We leveraged £39M from 10 funders, including NIHR, for EHR research and the establishment of the Farr Institute of Health Informatics Research in 2013 comprising four dedicated centres across the UK, including Farr London (directed by Harry Hemingway).
Policy report | Report contents/recommendation(s) | Farr Institute of Health Informatics Research action/response |
---|---|---|
Bloor C, Sriskandarajah R, Croxford V, White H. Strategy for UK Life Sciences. London: Department for Business, Innovation and Skills; 201216 | Launch of cross-funder call for centres in e-health | Investigators on this programme were successful in the competition and established the Farr Institute London |
Department for Business, Innovation & Skills. Strategy for UK Life Sciences One Year On. London: Department for Business, Innovation & Skills; 2012494 and Strengthening the UK’s Capability in Health Informatics Research Conference Report; 2013495 | Funding of £19M from a consortium of 10 UK government and charity research funders led by the MRC was awarded to establish four e-health research centres based in London, Manchester, Dundee and Swansea (Farr Institute of Health Informatics Research) | |
Speech by the Chancellor of the Exchequer to the Royal Society1 leading to MRC capital award via the Department for Business, Innovation & Skills | The speech made several references to ‘big data’. In his speech, George Osborne said that the UK has ‘some of the world’s best and most complete data-sets in healthcare’, and ‘the UK is well placed for the big data revolution’ (© Crown copyright; contains public sector information licensed under the Open Government Licence v3.0).1 Big health data was one of the eight great technologies quoted in the speech | Established the physical headquarters of Farr London and e-infrastructure including ISO27001-accredited data safe haven for handling patient-identifiable data |
Academy of Medical Sciences. Realising the Potential of Stratified Medicine. London: Academy of Medical Sciences; 2013496 | The Farr Institute was named in Recommendation 3 of the report for consistent policy on governance for all research safe havens that support data sharing and harmonisation across biomedical and health informatics centres | N/A |
Academy of Medical Sciences. Data in Safe Havens. London: Academy of Medical Sciences; 2014497 | The report named the Farr Institute, highlighting cross-centre collaboration underpinned by consistent governance, with independent review and audit, across sites | We participated in the workshop convened by the Academy in partnership with the MRC and the Wellcome Trust, on 24 March 2014 |
Association of the British Pharmaceutical Industry. Big Data Road Map. London: Association of the British Pharmaceutical Industry; 2013498 | The Farr Institute is named in multiple places in the report and made recommendations to the Farr in research around the life cycle of a drug, and training and capacity development | We held the Farr ABPI forum in December 2014 with a constant memorandum of understanding for future collaboration Farr addresses the gap in well-trained data scientists |
HSCIC. Information and Technology for Better Care 2015–20 Draft Report for Consultation. Leeds: HSCIC; 2015499 | One of the five major objectives outlined in the draft report is to ‘Make better use of health and care information’ by maximising the value and benefits that data can provide, and minimising the administrative burden of data collection on care providers with the overall vision for the UK to ‘be seen as one of the best places globally to conduct research and the collection and use of the nation’s care data will have made a substantial contribution to the development of health and social care services’ (Copyright © 2016, Re-used with the permission of the Health and Social Care Information Centre, also known as NHS Digital. All rights reserved) | We are currently co-ordinating a Farr response to the HSCIC strategy in the light of the Partridge review500 and response to care data |
CPRD collaboration with the Farr Institute | N/A | CPRD, along with the Farr Institute, are to develop a memorandum of understanding between Farr and CPRD for research for patient benefit |
Caulfield M, Davies DK, Fowler T, Mahon-Pearson J, Turnbull C, Woods K. Genomics England Clinical Interpretation Partnership (GeCIP): Guidance for Expressions of Interest to Form Domains Within GeCIP. London: Genomics England; 2014501 | GeCIP supports the 100,000 Genomes project,19 a NHS transformation programme to sequence 100,000 genomes from patients with rare inherited disorders, cancer and infectious diseasesIt is anticipated that the scientific activities undertaken within GeCIP will include . . . capitalising upon electronic health records research infrastructure, such as, the Farr Institute . . . to build upon and add value to the clinical, laboratory, and health records data. The evaluation of whole genome sequence data in the context of rich and extended phenotypes and exposures derived from electronic health records. To do this it is expected that GeCIP members will . . . engage with existing infrastructure, such as . . . the Farr InstituteReproduced with permission501 | Farr London (directed by Harry Hemingway) has led a national effort to harness the interpretation of the EHRs. This includes work on people with rare cardiac diseases such as inherited cardiomyopathy |
National Information Board. Personalised Health and Care 2020. Using Data and Technology to Transform Outcomes for Patients and Citizens. A Framework for Action. National Information Board; 201417 | Our objective is to establish England as one of the world’s leading centres for innovation in digital health and care services© Crown copyright; contains public sector information licensed under the Open Government Licence v3.0 | The Farr Institute’s efforts are central to the delivery of Genomics England and personalised care medicine |
Department of Health. Cardiovascular Disease Outcomes Strategy: Improving Outcomes for People with or at Risk of Cardiovascular Disease. London: Department of Health; 201372 | The NHS Commissioning Board and Public Health England will look to establish a cardiovascular intelligence network (CVIN) bringing together epidemiologists, analysts, clinicians and patient representatives. The CVIN, working with the HSCIC, will bring together existing CVD data and identify how to use it best© Crown copyright 2013; contains public sector information licensed under the Open Government Licence v3.0 | We are working with the National Clinical Director for Heart Disease for NHS England and the Chairperson of the NCVIN, Professor Huon Grey, and the Director for Knowledge & Intelligence (Northern and Yorkshire) at Public Health England, Professor Brian Ferguson, to contribute insights from the CALIBER programme into the NCVIN |
In this chapter, we summarise the impact and implications of our NIHR-funded research in the following areas:
-
the capacity to use EHRs to understand and improve quality and outcomes of care
-
the evidence from EHRs used in clinical practice guidelines from NICE and professional societies
-
research governance, methods and training
-
media, journals and national recognition
-
recommendations for future EHR research.
Capacity to use electronic health records to understand and improve quality and outcomes of care
The huge translational potential of the UK’s EHRs for enhancing our understanding and management of CVDs, developing risk models and personalised treatment strategies, and running low-cost, high-volume clinical trials has been recognised by central government in its policy announcements and funding commitments. 72 However, prior to our NIHR research programme (awarded in 2008), the culture was very different, with concerns voiced by practising clinicians and researchers that the data quality of NHS record sources did not make them fit for research purposes. What research existed was mainly confined to single disease or procedure registries, or to HES data. There had been no research linking several sources of data in order to paint a detailed picture of the patient journey.
Our CALIBER research programme has contributed, along with other initiatives, to realising this potential. There has been a marked change in research culture, whereby interrogation of EHRs is now seen as integral to our understanding of the quality and outcomes of care of CVD, and for identifying and quantifying the importance of potential missed opportunities of care.
Evidence of our contribution to this ‘new culture’ comes from a variety of sources, detailed in the following section.
Our cognate set of research findings
Our detailed results are summarised in Table 3.
Fitness for purpose: data quality in the Electronic Health Record
An overarching finding across studies in this programme is that the data have sufficient clinical validity to provide a useful signal in clinical epidemiological research. This is an essential pre-requisite if these data are to form a ‘scaffold’ on which to build further research programmes. One illustration of this is the relationship between SBP and the risk of heart attack (Figure 75). The linear relationships that have been shown by combining multiple individually small, high-cost consented cohorts, is replicated in a single larger cohort, where recruitment, BP measurement and follow-up of (a wide range of) outcomes is zero cost for the research funder. At the same time, efforts by the Royal College of Physicians, among other institutions, to improve the quality of discharge summaries, for example, are important. The linear increase in risk is seen across a broad range of BP measurements.
FIGURE 75.
Systolic blood pressure recorded in primary care and hazard of a first AMI among a cohort of 1.25 million people in CALIBER initially free from CVD. (a) Age 30–59 years; and (b) age 60–79 years. Reproduced in part from Rapsomaniki et al. 38 Copyright © 2014 Rapsomaniki et al. This is an open-access article distributed under the terms of the Creative Commons Attribution License, which permits unrestricted use, distribution, and reproduction in any medium, provided the original author and source are credited.
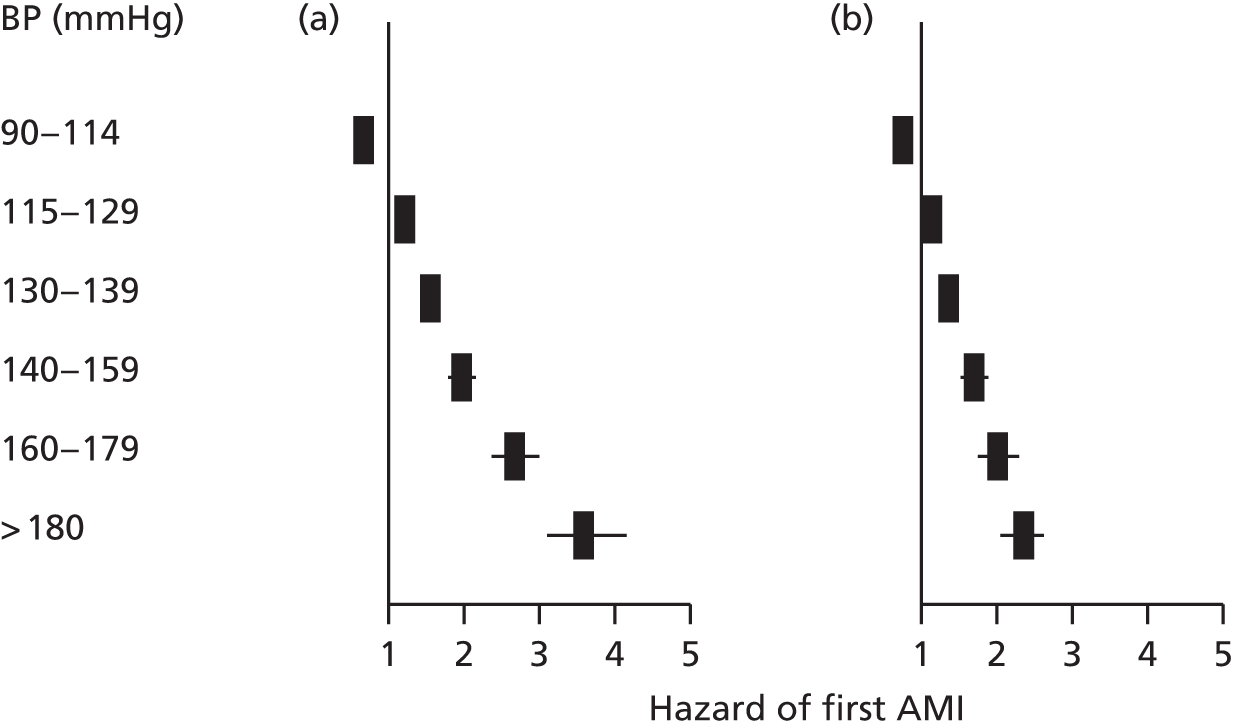
Situating our findings in a translational framework
Our health systems need to innovate new interventions, to evaluate them in clinical trials and to ensure that the implementation of effective treatments in clinical practice is rapid and complete. Our exemplars illustrate key stages along this pathway in the ‘prequel’ to trials, trial conduct and the subsequent implementation of findings (‘sequel’) (Figure 76). From the perspective of the pharmaceutical industry, the Association of the British Pharmaceutical Industry (ABPI) Big Data Road Map498 highlighted the importance of EHRs in the life cycle of a drug. Thus, we present findings on the unmet need, namely clinical areas in which new drugs or new applications of existing drugs can be targeted. The use of CALIBER phenotyping approaches is informing approaches in discovery efforts such as the UK Biobank. 107 We illustrate how the EHRs can examine associations across a wide range of disease end points and have the statistical scale and clinical resolution to identify those end points that are likely to be the most important for inclusion in the primary end points of trials. We provide evidence on the validity of drug targets, for example heart rate-lowering drugs. We demonstrate how risk prediction models may be developed and validated, and used to stratify patient selection and inform funders about real-world cost-effectiveness at different levels of treatment benefit. Thus, at early and late phases of translation EHRs may provide opportunities to address the first and second translational blocks.
FIGURE 76.
Exploiting diverse EHRs for clinical research exemplars driving design, conduct and implementation of clinical trials. HCM, hypertrophic cardiomyopathy; HDL-C, high-density lipoprotein cholesterol; HR, heart rate; SUSAR, suspected unexpected serious adverse reaction.

Situating our findings in the context of a learning health-care system502
In the 2015 State of the Union address, Barack Obama announced a new personalised medicine initiative:
Tonight I launch a New Precision Medicine Initiative . . . to give all of us access to the personalized information we need to keep ourselves and our families healthier. [The Initiative will] catalyze a new era of data-based and more precise medical treatment.
White House Fact Sheet2
The situation of the health-care system today is that data are largely inaccessible, which results in missed opportunities and waste (Figure 77).
FIGURE 77.
Missed opportunities and waste in the current health-care system. Figure reprinted, with permission, from Best Care at Lower Cost: The Path to Continuously Learning Health Care in America, 2013 by the National Academy of Sciences, Courtesy of The National Academies Press, Washington, DC. 502

The vision (Figure 78) is the development of a continuously learning science health-care system that joins together science, evidence and care to provide seamless care that is ‘efficient, delivers increased value, and is continuously innovating and improving in its ability to deliver high value to patients’. 502
FIGURE 78.
Vision for providing best care at lower cost through a continuously learning science health-care system. Figure reprinted, with permission, from Best Care at Lower Cost: The Path to Continuously Learning Health Care in America, 2013 by the National Academy of Sciences, Courtesy of The National Academies Press, Washington, DC. 502
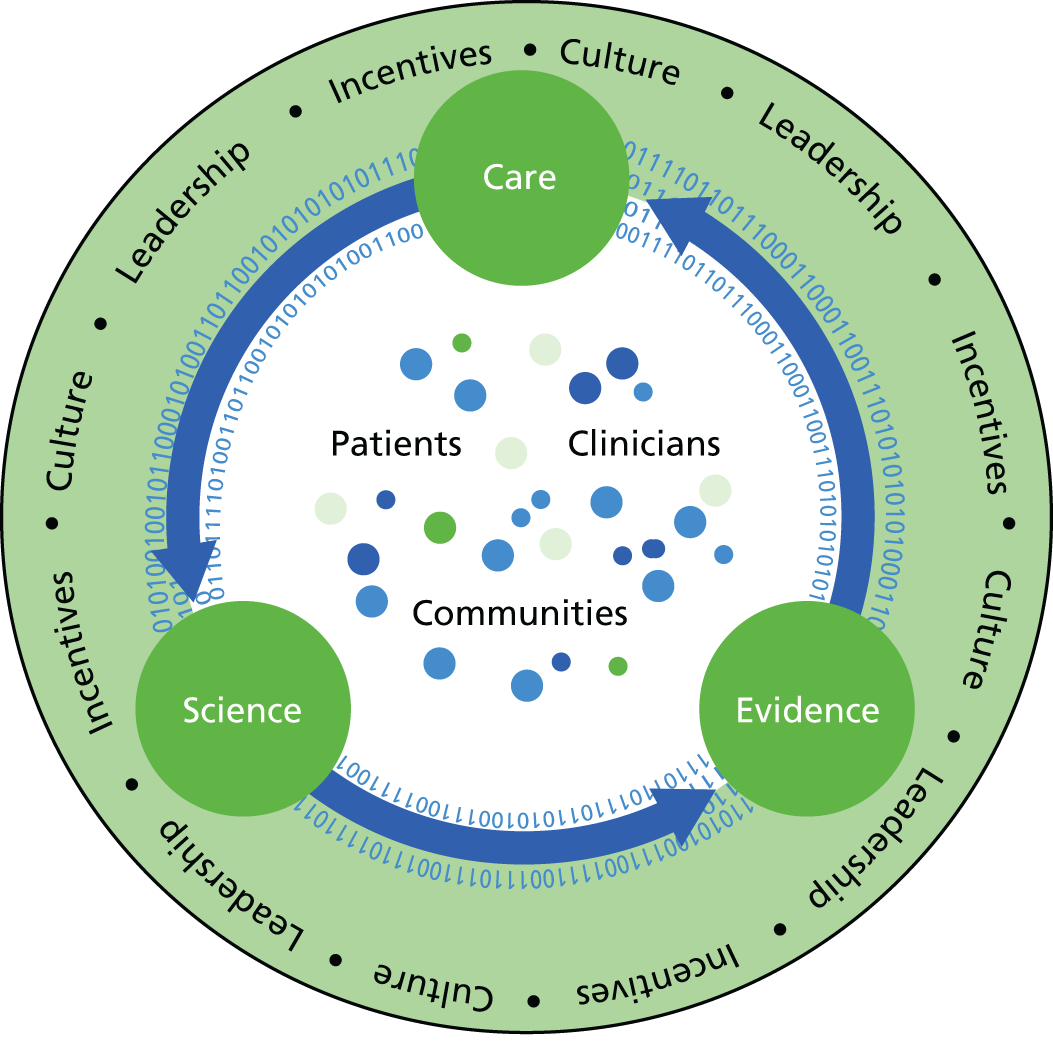
The Farr Institute–Association of British Pharmaceutical Industries forum
In order to enhance the scale and impact of these, and other, exemplars we launched the Farr–ABPI forum with 25 companies and 14 universities represented among the 120 delegates at the first meeting in December 2014. Addressed by the new Life Sciences Minister, George Freeman MP, we announced at this meeting the signing of a memorandum of understanding between ABPI and the Farr Institute to accelerate collaboration both at the pre-competitive and competitive phase.
ClinicAl disease research using LInked Bespoke studies and Electronic health Records
Raw EHR data require significant amounts of pre-processing in order to be transformed into research-ready variables for statistical analysis. We have established a methodological framework and novel approaches using CALIBER data (Figure 79) to address the challenges associated with EHR data. By means of an iterative and collaborative cross-disciplinary framework we created, validated and curated EHR disease and risk factor phenotypes. Farr London is working to achieve data linkages, tools for visualisation and interrogation, and standard statistical approaches, whereby the interrogation of clinical outcomes in relation to drug safety, quality of care and pathways of care over the patient journey becomes an established, high-quality component of clinical research. We have developed and evaluated e-health data based around multiple imputation methodology, which addresses issues in record linkage, missing data and measurement error, as well as methods for causal inference. Research into the design of solid information systems that will harness clinical knowledge and improve the delivery of health services requires close engagement with everyday health care to develop systems that are to be used and are useful. We anticipate that the showcasing of this report will result in the use of the CALIBER platform expanding substantially.
FIGURE 79.
Screenshot of the CALIBER homepage.
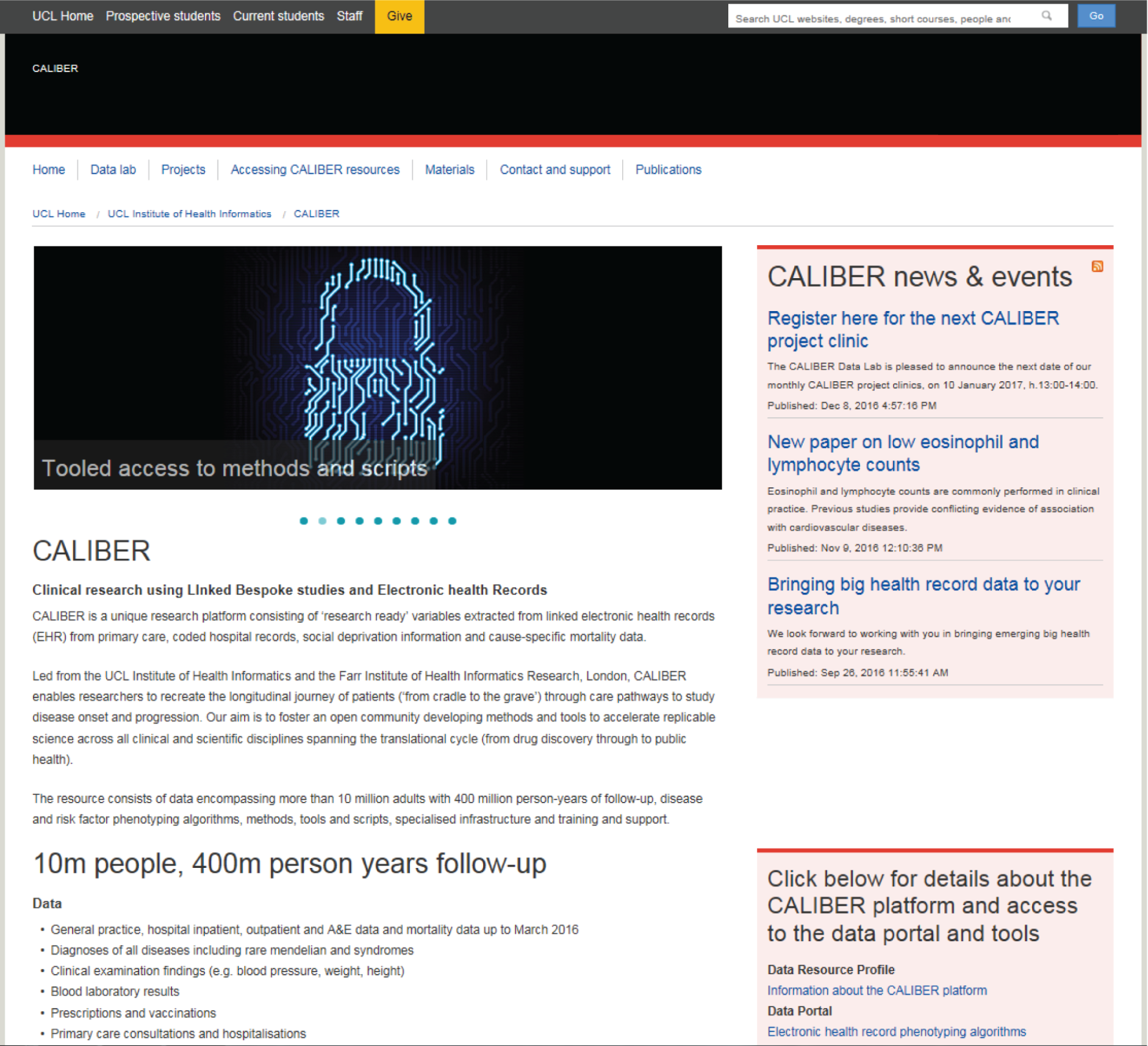
The Myocardial Ischaemia National Audit Project and the National Institute for Cardiovascular Outcomes Research: from audit to research platform
A further indication of the new research culture to which our NIHR programme has contributed is the evolution of the national cardiovascular registries from stand-alone audit tools to linked engines of research. The first step in this evolution was the establishment in 2005 of the MINAP Academic Group (chaired by Adam D Timmis, and including Harry Hemingway and Spiros Denaxas as members), which was charged with developing the research potential of the MINAP heart attack registry by means of the safe distribution of data sets to research groups across the UK. The MINAP Academic Group’s success is reflected in > 60 MINAP papers that have been published in the last 9 years, some of which have been presented in this report. Our group contributed significantly to this large research output and, significantly, established international collaborations with investigators across multiple centres in analysis of pre-hospital thrombolysis503 and with the ACTION (USA)57 and SWEDEHEART (Sweden)55 investigators in ongoing comparative analyses. The outputs from these analyses have provided the first whole-country international comparisons of the management and outcomes of AMI, providing a new benchmark against which to assess service delivery in the UK.
Meanwhile, the new research culture has extended its footprint at NICOR which, following a successful Healthcare Quality Improvement Partnership bid from a group that included Harry Hemingway and Adam D Timmis, now houses all the major national cardiovascular registries [National Adult Cardiac Surgery Audit (NACSA), National Audit of Percutaneous Coronary Intervention, National Audit of Cardiac Rhythm Management (CRM), National Audit of Congenital Heart Disease (CHD), National Heart Failure Audit and MINAP], and is making data available to research groups across the cardiovascular spectrum. These developments contributed to establishment of the Farr Institute of Health Informatics Research.
The Farr Institute of Health Informatics Research
The translational potential of the UK’s EHRs for enhancing our understanding and management of CVDs and other diseases, for developing risk models and personalised treatment strategies, and for running low-cost, high-volume clinical trials has been recognised by central government72 and considerable funds have been made available for EHR research. In 2012, a consortium of 10 UK funders, including NIHR and led by the MRC, awarded £19M for the establishment of four research centres in London, Manchester, Scotland and Wales (the Farr Institute of Health Informatics Research), which were charged with harnessing the research potential of EHRs for research to improve health and health care. In 2013, a further £20M of MRC capital was awarded after the speech by the Chancellor of the Exchequer, George Osborne, to the Royal Society1 to establish physical institutes and e-infrastructure, including ISO27001 data safe havens. 504
The Farr Institute London (under the directorship of Harry Hemingway), in addition to its work in CVDs, has rapidly developing research programmes across diabetes, infection and child and maternal health, spanning the translational cycle and exploiting our nationally leading informatics strengths in areas of major clinical and public health importance to develop more effective treatments, improve drug safety, identify risks to public health and gain insight into the cause and development of diseases by linking anonymised patient records and health research data. The Farr Institute is developing innovative methodological approaches to the health informatics data cycle, which is central to high-quality patient care and translational research, particularly where we can scale innovation across the UK and drive capacity development through the strategic development across partner organisations of training and career opportunities for clinicians, those in technical and enabling roles, and the wide range of academic disciplines relevant to health informatics research, and where we can promote a new culture of engagement between the public, patients, clinicians and researchers based on the joint development of consent models and EHR research to improve patient care and public health.
UK Life Sciences Strategy
A major focus of our NIHR programme and the UK Life Sciences Strategy16 is based on record linkage of national electronic registries recording primary care (CPRD), heart attack (MINAP), hospital admission (HES) and cause-specific mortality (ONS) data. Our record linkage platform was a precursor to two significant developments in the field of linked EHRs. First, the establishment of an informatics platform for research in the form of the CPRD was recommended in the Strategy for UK Life Sciences report. 16 CPRD, a £60M investment by the NIHR and MHRA, was launched in March 2012 and provides access to data that support clinical trials and population observational studies on an unprecedented scale. 494
National Institute for Health Research and the Health Informatics Collaborative
Our programme of research, and the work of the Farr Institute more generally, has directly catalysed other NIHR investments. These include the HIC, an initiative to identify and share data across the five large biomedical research centres in England.
Department of Health Cardiovascular Disease Outcomes Strategy
As recommended in the Department of Health Cardiovascular Disease Outcomes Strategy,72 CVD represents a single family of diseases and conditions linked by common risk factors and the direct effect they have on CVD mortality and morbidity, and they should be managed as such. We used data to deconstruct CVD into 12 different phenotypes and demonstrated heterogeneous associations with BP and other major coronary risk factors. 37,38,232
In recognition of the growing importance of ‘real-world evidence’ from EHR studies, NICE has established a new committee on observational data, of which Harry Hemingway is a member.
Clinical practice guidelines from the National Institute for Health and Care Excellence and professional societies
Research based on EHR has begun to inform clinical guidelines. The research that underpinned our NIHR programme application has informed national guidance in the last 5 years (Table 45). Co-investigator Adam D Timmis chaired – and Chief Investigator Harry Hemingway was a member of – the National Guideline Development Groups on the investigation of chest pain (NICE CG95). 202 Adam D Timmis also chaired the group that produced the management of stable angina guideline (NICE CG126),508 which was published 1 year later, and coauthored the ESC guideline on the management of SCAD. 233
Clinical area | Policy document | Influence on policy | Guideline recommendation | Impact |
---|---|---|---|---|
Investigation of chest pain | NICE CG95202 | AD Timmis chaired and H Hemingway was a member of guideline development group. Citations to our research: Sekhri et al.;505 Zaman et al.147 | Do not use exercise ECG to diagnose or exclude stable angina for people without known CAD1.3.6.5For people with confirmed CAD (for example, previous MI, revascularisation, previous angiography), offer non-invasive functional testing when there is uncertainty about whether chest pain is caused by myocardial ischaemia. . . . An exercise ECG may be used instead of functional imaging1.3.4.8Do not define typical and atypical features of anginal chest pain and nonanginal chest pain differently in men and women1.3.3.2Do not define typical and atypical features of anginal chest pain and non-anginal chest pain differently in ethnic groups1.3.3.3 | ‘The proportion of the study population before and after the guidelines undergoing exercise testing was 50.1% vs 0.0%; for calcium score/CT coronary angiography 0.0% vs 14.7%; for functional imaging 25.6% vs 13.4%; and for invasive coronary angiography 15.3% vs 25.8%’.506 The proportion of patients who did not require further testing was 30.0% before compared with 31.0% after guidelines, a figure that remained unchanged In 2013, it was reported that ‘implementation of the 2010 NICE guidance. . .resulted in a greater proportion of initial discharge to primary care with less frequent outpatient review and similar rates of referral for coronary angiography’507 These two recommendations (arising from Zaman et al.147) for the first time make an unequivocal statement to clinicians that typical anginal pain does not differ by sex and ethnicity. This is important because it has previously been used as a post hoc justification of why rates of procedure use might differ between these groups |
Management of stable angina | NICE CG126508 | Citations to our research: Griffin et al.509 | When either procedure would be appropriate, explain to the person the risks and benefits of PCI and CABG for people with anatomically less complex disease whose symptoms are not satisfactorily controlled with optimal medical treatment. If the person does not express a preference, take account of the evidence that suggests that PCI may be the more cost-effective procedure in selecting the course of treatment1.5.5When either procedure would be appropriate, take into account the potential survival advantage of CABG over PCI for people with multivessel disease whose symptoms are not satisfactorily controlled with optimal medical treatment and who: have diabetes or are over 65 years or have anatomically complex three-vessel disease, with or without involvement of the left main stem1.5.6Discuss the following with people whose symptoms are satisfactorily controlled with optimal medical treatment: their prognosis without further investigation (noting that existing prognostic risk scores have not been validated)1.5.11Offer people optimal drug treatment for the initial management of stable angina. Optimal drug treatment consists of one or two anti-anginal drugs as necessary plus drugs for secondary prevention of cardiovascular disease1.4.1 | Our studies on appropriateness (Griffin et al.509) have been part of a growing evidence base which has led to a slowing in the rate of increase of PCI for SCAD Previous prognostic models have not been validated. We have addressed this gap using an external data set to validate our prognostic models, and demonstrate how real-world clinical data (as distinct from research data) contribute important prognostic information in unselected patients (Rapsomaniki et al.52). Evidence from our study suggests implementation of the CALIBER prognostic models in EHRs in clinical practice would support risk stratification, as recommended in clinical guidelines Our work on secondary prevention showed that omission of secondary prevention drugs from the discharge prescription of patients with AMI has a variable effect depending on the specific drug omissions. The data suggest that beta-blockers and statins are associated with the greatest protection against death that is sustained in the longer term. However, the benefits of continuing treatment with antiplatelet drugs and ACEIs appear to decline beyond the first 12 months |
NICE. Management of Stable Angina. Evidence Update 23510 | Citations to our research: Sutaria et al.511 | Prognostic value of natriuretic peptides: The evidence found by the review comprised early-phase studies of limited quality. There is therefore currently a lack of robust, clinically meaningful evidence for the use of natriuretic peptides in prognosis of stable coronary disease. This is consistent with the absence of recommendations from NICE CG126 for the use of biomarkers in prognosis of stable angina1.3During the development of the Evidence Update, the following evidence uncertainties were identified for the NHS Evidence UK Database of Uncertainties about the Effects of Treatments (UK DUETs)General principles for treating people with stable anginaBiomarkers in SCAD for prognosisWhat is needed?Extending existing systematic reviews | Based on our published findings (Sutaria et al.511), the evidence update recommended extending existing systematic reviews | |
Management of SCAD | Montalescot et al.233 | Coinvestigator Adam D Timmis was a member of the guideline development group and coauthored the guideline Citations to our research: Hemingway et al.49 Sutaria et al.511 |
Biochemical tests: Although there may be some additional prognostic value in other biomarkers, there is insufficient evidence to recommend the routine use of natriuretic peptides, haemostasis markers or genetic testing in the management of patients with SCAD2336.2.1.1 | One important area that we highlight is the need to identify clear clinical terms that can help in decision-making. For example, the term SCAD is used to cover people with a history of myocardial infarction and those without. Furthermore, there is no current consensus on the definition of the time after an AMI at which patients become stable nor what risk should be defined as ‘high’ |
Management of ACS | NICE technology appraisal guidance on ticagrelor (TA236)512 | Making recommendations about the prolonged use of dual antiplatelet therapy after AMI513 | Ticagrelor combined with low-dose aspirin is recommended for up to 1 year as a possible treatment for some people with ACS | Evidence to the committee included unmet need in four countries (USA, UK, France and Sweden), and the extent to which participants selected into the Pegasus trial had different rates of the primary benefit end point (atherothrombolic events) and the primary safety end point (major bleeding)513,514 |
Over the past 50 years, the exercise ECG had been the most widely used non-invasive test for investigating suspected angina in patients. However, our multicentre study of UK chest pain clinics, showing that the exercise ECG added little to risk assessment,505 led to a paradigm change in diagnostic testing, with NICE recommending that the exercise ECG should no longer be used in the investigation of patients with suspected angina. 202 This recommendation has since gone on to change clinical practice. Thus, in 2010, it was reported that:
The proportion of the study population before and after the guidelines undergoing exercise testing was 50.1% vs 0.0%; for calcium score/CT coronary angiography 0.0% vs 14.7%; for functional imaging 25.6% vs 13.4%; and for invasive coronary angiography 15.3% vs 25.8%.
Haq et al. 506
The proportion of patients who did not require further testing was 30.0% before compared with 31.0% after guidelines, a figure that remained unchanged. In 2013, it was reported that ‘implementation of the 2010 NICE guidance . . . resulted in a greater proportion of initial discharge to primary care with less frequent outpatient review and similar rates of referral for coronary angiography’. 507
This shift in clinical practice has led to an increase in the use of CT coronary angiography, as its high diagnostic sensitivity makes it more useful than the exercise ECG as a rule-out test in patients with a low probability of disease. 515 Its use in patients with an intermediate pre-test probability of disease is also increasing based on evidence of superior prognostic performance compared with the exercise ECG. 516
Our research further influenced these NICE guidelines by showing that relationships between typicality of symptoms and prognosis in patients with suspected angina are unaffected by sex and ethnicity. Based on these findings, NICE CG95202 stated: ‘Do not define typical and atypical features of anginal and non-anginal chest pain differently in men and women (or) in ethnic groups.’ Indeed, our research, which was cited in the 2013 ESC guideline on the management of SCAD, has shown that angina is almost as common in women as in men with similar prognostic associations. 517
All the contemporary guidelines for patients with SCAD emphasise the importance of prognosis in guiding management decisions, starting with an evaluation based on simple clinical parameters. However, existing prognostic models have been based on data collected for research purposes rather than the information that clinicians record in real-world practice, and none has been recommended for clinical use. An important potential impact of our work, therefore, has been the presentation of a clinically applicable prognostic model for patients with SCAD that incorporates the broad range of clinical characteristics highlighted by these guidelines, many of which (atrial fibrillation, cancer, liver disease, depression, anxiety and haemoglobin) have not previously been incorporated in prognostic models for SCAD.
Research governance, methods and training
Impact of methodological contributions
Prognosis research findings should be integral to clinical decision-making, health-care policy and discovering and evaluating new approaches to patient management. We have discussed a worrying gap between the potential and actual impact of prognosis research on health, with studies often falling a long way short of the high standards required in other fields, such as therapeutic trials and genetic epidemiology. Our PROGRESS initiative published a series of 24 recommendations as a framework for researching clinical outcomes, to promote high-quality prognosis research leading to patient benefit, which centred on how the field can be developed and its impact broadened,29–32 and we have promoted transparency of research, emphasising measures such as registration of studies, sharing of data and protocols. 33
Following our and others’115 recommendations, the open-access journal PLOS Medicine took the significant step of publishing new guidelines for observational studies to facilitate assessment of the reliability of published observational research. 518 These included: (1) submission of Strengthening the Reporting of OBservational studies in Epidemiology (STROBE; www.strobe-statement.org/index.php?id=available-checklists) or Standards for Reporting Diagnostic Accuracy (STARD; www.equator-network.org/reporting-guidelines/stard/) checklists at the time of submission of manuscripts to the journal; (2) incorporation of data-sharing requirements in PLOS data policy; (3) clear specification of hypotheses, what was done and any deviation from the protocol by researchers; and (4) submission of analytic protocols on submission of the manuscript to the journal, whereby the ‘. . . goal (is) not only to establish these practices for PLOS Medicine but to advocate for such transparency as a standard within the research community’. 518
Training and capacity development
It is widely acknowledged that there is a substantial international shortfall in people trained to make sense of, and analyse, EHR data. The Farr Institute of Health Informatics Research is addressing this concern and will train a new cadre of health informaticians and related methodologists, providing the academic and professional underpinning of world-leading e-health innovation from the UK. We are also committed to offering opportunities in the form of a doctoral training programme, a series of taught courses and career development and leadership development.
Our NIHR programme has supported a number of postgraduate degrees and career development positions with a total of 13 Master of Science dissertations and 12 doctoral awards arising from the programme. Individual awards are listed in Appendix 8.
We have presented our findings at key conferences (see Appendix 9). We held three prognosis research workshop meetings in London in 2011, which were attended by opinion leaders in the field and journal editors and which led to the development of five thematic papers published in the BMJ and PLOS Medicine. The themes were as follows: (1) What is the prognosis of this disease?; (2) Is this a causal factor for disease?; (3) What impact does this risk prediction model have?; (4) Is there treatment-factor interaction?; and (5) What is the case for study registration and analytic protocol publication?
We held short courses on the concepts, methods and clinical application of prognosis research in 2013 and 2014 at our annual international PROGRESS summer school at Keele University. We have also been involved in running courses at the Netherlands Institute for Health Sciences Erasmus Short course programme and, in March 2015, Farr London ran a unique, intensive programme of 13 inter-related short courses spanning a wide range of basic and applied health research applications in the field of EHRs. This training heralded a unique opportunity for high-quality training harnessing EHRs for research for people from a wide range of backgrounds, including health care, epidemiology, biostatistics, health informatics, NHS information technology, bioinformatics, genomics and computer science, and at different career stages.
Media, journals and national awards
Media
Our research has generated media interest across national radio, television and clinical journals. Our work on 1-year survivors of heart attack was featured on ITV’s ‘This Morning’ (September 2014) and in a series of radio broadcasts over 1 day (BBC Newcastle, September 2014).
New scientific journals
Circulation: Quality and Outcomes was the first cardiac journal devoted to the new prognosis research culture. It was launched in 2008, with Harry Hemingway an Editorial Board member, and has played an important part in defining the scope of EHR research and disseminating its outputs. Soon to be launched is the European Heart Journal: Quality of Care and Outcomes, the second journal in this field with Adam D Timmis appointed the founding editor. The involvement of our group in the editorial boards of these new journals is based largely on international recognition of the quality of our EHR research programme.
National awards
Adam D Timmis’ work in utilising electronic registry data for evaluating chest pain clinics resulted in his work being shortlisted for the BMJ Group Award for Outstanding Achievement in Evidence Based Healthcare. Quint et al. 59 were voted runners up in the BMJ UK research paper of the year award (2014) for their work on the effect of beta-blockers on mortality after AMI in adults with COPD (see Chapter 11). The award recognises original UK research that has the potential to contribute significantly to improving health and health care.
Recommendations for future research
We propose three priority directions for further research. First, there is an urgent need to ‘unlock’ more detailed data within hospitals, to expand the coverage of the UK’s 65 million population with primary care data available for research, and to expand and make sustainable the range of record linkages (e.g. to a wider range of disease registries). Second, there should be major expansion in the underlying methods and applications of scaled approaches to using EHR to design and carry out trials and interpret the implementation of trial results. This is important for delivering learning health systems and patient benefit. Third, large-scale, disease agnostic DNA and biological collections linked to such EHRs are required in order to deliver precision medicine and innovate discovery of new drug targets or repurpose existing drugs.
Our work has highlighted a number of priority research questions with the potential to have an impact on patient benefit in two areas:
-
Next steps in realising the potential of EHR research, which is agnostic to disease, relevant across different stages of translation and applicable to both prevention and treatment.
-
CVD-specific clinical questions for patients at risk of, and with, CVD. A summary of these clinically specific recommendations is presented in Table 3.
Research approaches with electronic health records
The CALIBER resource consists of a combination of highly trained staff, data resources and tools, specialised infrastructure, and training and support. CALIBER operates a collaboration-driven data-sharing model and promotes the transparent and scalable use of EHR data for research by utilising a structured approach for developing and validating phenotyping algorithms and providing a central, online repository for the storage and distribution of algorithms, documentation (flow charts, free-text description, pseudocode) and metadata. To date, the resource has supported 51 individual projects with more than 50 published manuscripts and more than 1000 citations across a range of diseases including, but not limited to, multimorbidity, dementia, lower back pain, cancer, type 2 diabetes, infection and epilepsy. Details on CALIBER are available at www.caliberresearch.org/ and information on how to access the CALIBER resource is available at www.ucl.ac.uk/health-informatics.
More research is needed that combines EHRs with other forms of research and data to unlock better health care, both for individuals and the wider population. The development of new programmes of prognosis research exploiting EHRs to define, phenotype and follow up patients, and a comprehensive set of cohorts with established disease should be a made a priority, as the capability to link and analyse ‘big data’ burgeons. Key areas for investment are outlined below.
Hospital informatics
Most data in the NHS reside in hospitals, where they have been inaccessible for research. A wealth of phenotypic data exist locked within individual hospital electronic systems and, as such, remain largely untapped for research use. The quality of blood, imaging and other diagnostic data are all improving, and developments are already under way to enable collaborative sharing of NHS data to facilitate clinical research (e.g. the NIHR HIC). The Genomics England 100,000 Genomes Project – an ambitious discovery project involving the sequencing of 100,000 genomes and linkage of genomic information to EHR data) – makes this a priority area. 19
Structured interoperable electronic health records
Electronic health records system design has been predominantly vendor-led, leading to differences in the way clinical information is structured and difficulties in integrating care records from multiple health-care sites. Although this is primarily a task for the NHS rather than researchers, demonstrations of interoperable EHR will be a major lever for future health research. 519 The openEHR archetypes (openEHR Release 1.0.2 Architecture overview, 2008; see www.openehr.org/releases/1.0.2/architecture/overview.pdf) are a set of clinician-led clinical information models, with an international governance and review framework. Vendors of EHR systems can adopt openEHR archetypes to ensure that patients’ medical records are accessible to their caregivers and are organised in a way that facilitates audit and research.
Wider coverage of greater parts of the population
The majority of the UK’s 65 million population is registered with a GP, and records are nearly all computerised. Efforts are required to ensure that coverage of the population is accessible to researchers up from the current level (e.g. < 10% in CPRD).
Strategic approach to record linkages
Many national sources of health record data remain in silos, unlinked and of limited value for research for patient benefit. Efforts to date for record linkage have been largely ad hoc. This impedes international competitiveness by comparison with, for example, Sweden or Denmark. Specifically in CVD, CPRD, NICOR and HES would be an early priority, with an extension to social care and laboratory databases.
Efforts to understand and improve the quality of electronic health record data
This will involve policy action as well as research cross-referencing multiple structured and unstructured sources of data. Investigation of electronic free text recorded by GPs, and further research to understand how EHR data are coded and how coding may be improved, is required.
Use of electronic health record data in clinical trials to further enhance the translational potential of electronic health record data
There is a major need for new trial models in clinical research. There is widespread concern that existing models of the clinical trial pipeline are ‘broken’ because they are too costly and slow, and because important clinical areas remain undertrialled. EHRs, because of their scale and phenotypic depth, may contribute to solving some of these problems, and may provide wholly new opportunities to understand and improve cardiovascular health. There is a need for new studies to develop and evaluate novel tools for rapidly and efficiently addressing issues related to the design and conduct of trials, including EHR phenotyping (using clinical, imaging, genomic and sensor data), and increase discoverability and accessibility of these data for research purposes (‘prequel to sequel’ approaches to the trials pipeline).
Our research has highlighted the usefulness of EHR data to understanding outcomes in CVD. Use of ‘big data’ should now be extended to investigate how EHRs can understand and improve quality and outcomes of care in other devastating disease areas, such as cancer and dementia.
Cardiovascular disease-specific research recommendations
Our findings also point to a number of specific recommendations for further research, across the patient journey and for different CVD phenotypes.
How does cardiovascular disease first present?
Research efforts should take account of the way in which CVD first presents in the 21st century, with the importance of PAD and heart failure and other chronic conditions (and the decline of AMI and acute stroke).
Incidence of coronary heart disease and influence of health inequalities on outcomes (see Chapter 3)
-
Estimates of national and global burden of CVDs need to take account of the modern epidemic in which heart failure, PAD and other chronic diseases are becoming more common than AMI and stroke. Male sex, older age, South Asian ethnicity and low social deprivation, despite being associated with many CVDs, are not associated with all, and this heterogeneity has implications for research into risk prediction, aetiology and prognosis.
-
Large cohort studies identified through the linkage of primary care, ACS registry, hospitalisation and cause-specific death data are warranted to better understand how South Asian ethnicity influences the onset and progression of coronary disease and other CVDs. Such studies will allow longitudinal patterns of risk, and missed opportunities to lower risk before admission and after discharge, to be evaluated.
Understanding the impact of risk factor modification in cardiovascular diseases deconstructed into 12 different phenotypes (see Chapter 4)
-
Our novel, contemporary, high-resolution studies showed heterogeneous associations for BP across a wide range of CVDs. New and existing clinical trials investigating the effectiveness of BP-lowering strategies should include other common initial CVD presentations as the primary outcome.
-
New approaches are required to close the second translational gap in BP lowering. This may include research on clinical decision support for multiple drug classes to achieve target BP levels and other interventions, as well as understanding how best to lower absolute cardiovascular risk.
Initial presentation of coronary disease and opportunities for earlier diagnosis (see Chapter 5)
-
Contemporary prognostic models should be developed based on the characteristics of patients presenting with unspecified chest pain so that those at risk of cardiovascular events can be more accurately identified.
-
Further research is warranted to better characterise the phenotypes, causes and prognosis of unheralded STEMI and NSTEMIs to improve the usability of EHRs for research. Analyses comparing AMI patients with a control group of patients without AMI, including a comparison of missed opportunities for care in measuring and controlling elevated risk, is warranted.
-
Further development of natural language processing algorithms such as FMA and S3CM may improve the usability of EHR for research on CVDs and symptoms.
Further evaluation of the potential for use of clinical decision support in assessment of angina in chest pain clinics (see Chapter 6)
-
The assessment of chest pain is difficult, and, although the diagnosis of angina may be enhanced by a suitable CDSS, our study showed that incorporating such potentially useful tools into clinical practice is challenging. More research is needed to explore the potential for developing new tools to aid clinical decision-making with a greater likelihood of being adopted in practice.
Intensifying collaborative efforts in prognosis research (see Chapter 7)
-
Collaborative research based on individual patient data should be encouraged, in order to strengthen prognostic model development and external validation. This is timely, with new standards to improve the quality of prognosis research29,518 and new opportunities to link clinical health-care data with other sources of electronic information and bespoke data at scale.
-
Proposals to link clinical data systems locally and embed patient consent for research into clinical care would mitigate the complexities of obtaining complete data and recruiting patients in busy clinical settings, and thereby increase the utility of such cohorts.
Further evaluation of prognostic risk factors (see Chapter 8)
-
With biomarker and -omics research generating a wealth of possible new factors to incorporate into models, the extent to which new markers add value to existing models needs to be evaluated. Improved methodology and further research, including the design of new clinical trials and clinical cohorts, is warranted to assess the incremental prognostic value of biomarkers in risk stratification of patients.
-
We recommend new clinical trials to assess whether or not haemoglobin levels are causal and whether or not clinicians should intervene to increase haemoglobin levels.
-
In designing randomised trials, the target population, the choice of composition of the primary end point and the likely rate at which the end point accrues can all be informed by the examination of linked EHRs.
-
New clinical cohorts are needed with published pre-specified statistical analytic protocols and prospective registration of studies, which can be pooled to evaluate the true usefulness of the role of CRP in prognosis in SCAD.
-
More work should be carried out to determine the effect of mechanistic differences for the differential genetic effect, to enhance understanding of the underlying reasons. Our findings argue for a consortium of studies set in individuals with established CHD to better understand the genomic susceptibility to subsequent CHD events and also to perform detailed analysis, including assessment for selection biases, which is not possible with literature-based meta-analyses.
-
Methods for dynamic risk prediction in which the patterns of change of clinically measured biomarkers can be incorporated into clinical decision support models in clinical practice are required.
Evaluation of prognostic risk models and cost-effective targeting of interventions (see Chapter 9)
-
Further independent evaluation of prognostic models is required in different settings, including different EHR systems, health-care organisations and geographic locations to guide their use in clinical practice.
-
Economic evaluation is needed to establish the appropriateness of using our and other prognostic models in practice. Models should be extended to explicitly capture the trajectory of prognostic risk factors over time, to address broader questions around the cost-effectiveness of interventions that target underlying risk factors.
Opportunities to improve cardiovascular outcomes for acute coronary syndrome patients during and after hospitalisation (see Chapter 10)
-
International harmonisation of detailed measures of quality of care in clinical registries, such as pathways of care, is needed to identify and evaluate additional patient-level health-care factors that are not measured here, which might explain differences between countries.
-
Outcomes should be compared with those in other countries, such as France, Hungary, Poland and the USA, through the assessment of national registries.
-
Linkage to national EHRs will extend comparisons to include ambulatory care before and after admission for heart attack, non-fatal events and longer-term outcomes.
-
Outcomes research specifically designed to follow care into the primary care setting is warranted to quantify the full implication of CMOCs.
-
Further studies are needed to better explain patterns of clinical practice within and outside the UK, such as rates of coronary angiography and PCI.
-
Future research, both in larger prospective epidemiological studies and RCTs, of the promotion of GP smoking cessation interventions, the association between interventions and cessation, and the effects on long-term clinical outcomes is warranted.
Improving outcomes for acute coronary syndrome patients beyond clinical trials (see Chapter 11)
-
We have shown that ‘real-world’ data can provide important information beyond that generated by clinical trials to guide prescribing decisions.
-
Combinations of drugs that are recommended to be taken for the rest of a patient’s life but that have not been evaluated in RCTs should be investigated in observational studies for associations with fatal and non-fatal outcomes over the remaining life expectancy.
-
A RCT is justified to examine the effectiveness of beta-blockers after AMI in patients with COPD.
-
Methods are required by which a learning health system can interrogate the safety of drugs and drug–drug interactions in a timelier and more scaled fashion.
-
Further research, incorporating methods of causal inference, is required to fully investigate the extent to which the association between clopidogrel discontinuation and adverse events we identified was causal or was confounded by the influence of unrecorded comorbidities or bleeding events.
International comparisons (see Chapter 12)
International comparisons of care and outcome registries are needed to identify key areas that could be targeted to improve outcomes and inform new research and policy initiatives to improve the quality of health systems. International comparisons are an integral component of contemporary prognosis research and further comparisons with other national registries, for example in the USA, China and New Zealand, are needed to inform the relative performance of health-care systems. Further work is required to determine the competing benefits of targeting underperforming hospitals or developing a national programme of quality improvement for implementation across all hospitals. In particular:
-
further research is required to obtain real-time, risk-adjusted, treatment-adjusted estimates of between-hospital variation in mortality and a wider range of non-fatal outcomes
-
further work is necessary to assess the distribution of AMI patients across hospitals and other hospital characteristics that contribute to patient outcomes
-
opportunities for more ambitious comparisons along the care pathway should be exploited by linkage to national EHRs, which would permit an analysis of non-fatal events before and after hospital admission and longer-term outcomes
-
mixed-methods research is required to inform actions to reduce hospital variations in care and outcomes.
Acknowledgements
We are extremely grateful to all patients who generously gave their time to participate in the OMA pilot trial and ‘My Healthy Heart’ (4C) study, and to staff in the hospital chest pain clinics and cardiac catheter laboratories who accommodated and assisted our research team during the patient recruitment phase of 4C.
Special thanks to members of our Public and User Involvement Group: Mr David Thomson, Mr John Walsh, Mr Baldeth Maheraj (posthumously), Mrs Joan Maheraj, Mrs Ellie Sanchez and Miss Jean Turner for their guidance, advice and commitment.
Thank you to our NIHR co-investigators for their involvement across the programme: Professor Mark Sculpher, Professor Stephen Palmer and Professor Keith Abrams (lead on the health economics and statistics for study 21); Professor Aroon Hingorani (who provided expert advice on genetic epidemiology and pharmacogenetics for studies 1, 3, 7, 14, 15, 17, 18, 20, 21 and 28); Professor Sandra Eldridge (who provided expert advice on statistical modelling for study 13); Professor Liam Smeeth (who provided expertise in clinical epidemiology and primary care for studies 1, 2, 4, 6, 7, 10, 20, 27 and 28); and Professor Roger Boyle (MINAP Academic Group member: study 1).
We would also like to thank the following people for their invaluable help and guidance:
Mr Innocent Bvekerwa, Ms Katie Ruffles, Ms Eve Harris, Dr Bruna Rubbo, Ms Min Zhao, Dr Olia Archangelidi, Dr James Moss and Dr Andrew Thompson for their commitment and hard work recruiting patients and collecting data for 4C, and Dr Pascal Meier for his support and advice.
Qualitative researchers Dr Rachel Johnson, Dr Helen Cramer, Dr Maggie Evans, Dr Jackie Hughes, Professor Christi Deaton and Ms Katie Juttner, and the Variation in Coronary Care steering committee: Ms Sunita Berry, Ms Ailsa Cameron, Professor Sandra Eldridge, Dr Julie George, Dr Julian Strange, Dr Jenny Tagney, Mr David Thompson and Mr John Walsh.
Dr Abbie Masding, Dr Dionisio Izquierdo, Dr Phil Xiu, Dr Shahed Islam, Ms Emma Threadgold, Ms Rissa Besana and Ms Abby Gill for contributing to the 4C case report form data extraction and coding.
Ms Carla Logon, Ms Arpi Dedegian, Ms Iris Kounenis and Mr Alexios Dallas who assisted with 4C data entry and provided administrative support.
Mr Ian Jones for his help in preparing this report.
Funding
The NIHR Programme Grants for Applied Research programme, with additional funding from the NIHR Research for Patient Benefit (study 33), NIHR Research Methods Fellowship funding (study 19) and Servier (study 16).
Anoop Shah’s Wellcome Fellowship is supported by a Wellcome Trust clinical research training fellowship (0938/30/Z/10/Z).
Contributions of authors
Professor Harry Hemingway (Professor of Clinical Epidemiology, Centre Director, The Farr Institute of Health Informatics Research, London), Professor Gene S Feder (Professor of Primary Health Care, Centre for Academic Primary Care, University of Bristol) and Professor Adam D Timmis (Professor of Cardiology, Barts NHS Trust, London, and The Farr Institute of Health Informatics Research, London) led on conceptualising the programme of research, oversaw the design, methodology, data collection and analysis for all workstreams and contributed to the writing and approval of the final report, based on published papers by the Programme team (see Publications).
Natalie K Fitzpatrick (Research Programme Manager, The Farr Institute of Health Informatics Research, London) co-ordinated the programme, contributed to the design, methodology, data collection and analysis for 4C, and contributed to the writing and approval of the final report.
Dr Spiros Denaxas (Research Fellow, The Farr Institute of Health Informatics Research, London) led on the development of the CALIBER data platform and contributed to the design and methodology of several studies across the programme, and contributed to the writing and approval of the final report.
Dr Anoop D Shah (Clinical Research Fellow, The Farr Institute of Health Informatics Research, London) contributed to the design, methodology and analysis of several studies across the programme, and contributed to the writing and approval of the final report.
Publications
We would like to acknowledge the contribution of the following authors cited in the journal outputs arising from the programme as follows:
Study 1: Denaxas SC, George J, Herrett E, Shah AD, Kalra D, Hingorani AD, et al. Data resource profile: cardiovascular disease research using linked bespoke studies and electronic health records (CALIBER). Int J Epidemiol 2012;41:1625–38.
Study 2: Herrett E,* Shah AD,* Boggon R, Denaxas S, Smeeth L, van Staa T, et al. Completeness and diagnostic validity of recording acute myocardial infarction events in primary care, hospital care, disease registry, and national mortality records: cohort study. BMJ 2013;346:f2350.
Study 3: Hemingway H, Croft P, Perel P, Hayden JA, Abrams K, Timmis A, et al. Prognosis Research Strategy (PROGRESS) 1: a framework for researching clinical outcomes. BMJ 2013;346:e5595.
Study 3: Riley RD, Hayden JA, Steyerberg EW, Moons KG, Abrams K, Kyzas PA, et al. Prognosis Research Strategy (PROGRESS) 2: prognostic factor research. PLOS Med 2013;10:e1001380.
Study 3: Steyerberg EW, Moons KG, van der Windt DA, Hayden JA, Perel P, Schroter S, et al. Prognosis Research Strategy (PROGRESS) 3: prognostic model research. PLOS Med 2013;10:e1001381.
Study 3: Hingorani AD, Windt DA, Riley RD, Abrams K, Moons KG, Steyerberg EW, et al. Prognosis Research Strategy (PROGRESS) 4: stratified medicine research. BMJ 2013;346:e5793.
Study 3: Peat G, Riley RD, Croft P, Morley KI, Kyzas PA, Moons KG, et al. Improving the transparency of prognosis research: the role of reporting, data sharing, registration, and protocols. PLOS Med 2014;11:e1001671.
Study 4: Pujades-Rodriguez M, Timmis A, Stogiannis D, Rapsomaniki E, Denaxas S, Shah A, et al. Socioeconomic deprivation and the incidence of 12 cardiovascular diseases in 1.9 million women and men: implications for risk prediction and prevention. PLOS ONE 2014;9:e104671.
Study 4: George J,* Rapsomaniki E,* Pujades-Rodriguez M, Shah AD, Denaxas S, Herrett E, et al. How does cardiovascular disease first present in women and men? Incidence of 12 cardiovascular diseases in a contemporary cohort of 1,937,360 people. Circulation 2015;132:1320–8.
Study 5: Zaman MJ, Philipson P, Chen R, Farag A, Shipley M, Marmot MG, et al. South Asians and coronary disease: is there discordance between effects on incidence and prognosis? Heart 2013;99:729–36.
Study 6: Shah AD, Langenberg C, Rapsomaniki E, Denaxas S, Pujades-Rodriguez M, Gale CP, et al. Type 2 diabetes and incidence of cardiovascular diseases: a cohort study in 1.9 million people. Lancet Diabetes Endocrinol 2015;3:105–13.
Study 7: Rapsomaniki E, Timmis A, George J, Pujades-Rodriguez M, Shah AD, Denaxas S, et al. Blood pressure and incidence of twelve cardiovascular diseases: lifetime risks, healthy life-years lost, and age-specific associations in 1.25 million people. Lancet 2014;383:1899–911.
Study 10: Herrett E, George J, Denaxas S, Bhaskaran K, Timmis A, Hemingway H, Smeeth L. Type and timing of heralding in ST-elevation and non-ST-elevation myocardial infarction: an analysis of prospectively collected electronic healthcare records linked to the national registry of acute coronary syndromes. Eur Heart J Acute Cardiovasc Care 2013;2:235–45.
Study 11: Shah AD, Martinez C, Hemingway H. The freetext matching algorithm: a computer program to extract diagnoses and causes of death from unstructured text in electronic health records. BMC Med Inform Decis Mak 2012;12:88.
Study 12: Wang Z, Shah AD, Tate AR, Denaxas S, Shawe-Taylor J, Hemingway H. Extracting diagnoses and investigation results from unstructured text in electronic health records by semi-supervised machine learning. PLOS ONE 2012;7:e30412.
Study 13: Cramer H, Evans M, Featherstone K, Johnson R, Zaman MJ, Timmis AD, et al. Treading carefully: a qualitative ethnographic study of the clinical, social and educational uses of exercise ECG in evaluating stable chest pain. BMJ Open 2012;2:e000508.
Study 13: Johnson R, Evans M, Cramer H, Bennert K, Morris R, Eldridge S, et al. Feasibility and impact of a computerised clinical decision support system on investigation and initial management of new onset chest pain: a mixed methods study. BMC Med Inform Decis Mak 2015;15:71.
Study 15: Shah AD, Nicholas O, Timmis AD, Feder G, Abrams KR, Chen R, et al. Threshold haemoglobin levels and the prognosis of stable coronary disease: two new cohorts and a systematic review and meta-analysis. PLOS Med 2011;8:e1000439.
Study 17: Hemingway H, Philipson P, Chen R, Fitzpatrick NK, Damant J, Shipley M, et al. Evaluating the quality of research into a single prognostic biomarker: a systematic review and meta-analysis of 83 studies of C-reactive protein in stable coronary artery disease. PLOS Med 2010;7:e1000286.
Study 18: Patel RS, Asselbergs FW, Quyyumi AA, Palmer TM, Finan CI, Tragante V, et al. Genetic variants at chromosome 9p21 and risk of first versus subsequent coronary heart disease events: a systematic review and meta-analysis. J Am Coll Cardiol 2014;63:2234–45.
Study 20: Rapsomaniki E, Shah A, Perel P, Denaxas S, George J, Nicholas O, et al. Prognostic models for stable coronary artery disease based on electronic health record cohort of 102 023 patients. Eur Heart J 2014;35:844–52.
Study 21: Asaria M, Walker S, Palmer S, Gale CP, Shah AD, Abrams KR, et al. Using electronic health records to predict costs and outcomes in stable coronary artery disease. Heart 2016;102:755–62.
Study 21: Walker S, Sculpher M, Asaria M, Palmer S, Manca A, Timmis A, Hemingway H. Long-term healthcare use and costs in patients with stable coronary artery disease: a population based cohort using linked electronic health records (CALIBER). Eur Heart J Qual Care Clin Outcomes 2016;2:125–40.
Study 22: Chung SC, Gedeborg R, Nicholas O, James S, Jeppsson A, Wolfe C, et al. Acute myocardial infarction: a comparison of short-term survival in national outcome registries in Sweden and the UK. Lancet 2014;383:1305–12.
Study 23: Simms AD, Weston CF, West RM, Hall AS, Batin PD, Timmis A, et al. Mortality and missed opportunities along the pathway of care for ST-elevation myocardial infarction: a national cohort study. Eur Heart J Acute Cardiovasc Care 2015;4:241–53.
Study 24: McNamara RL, Chung SC, Jernberg T, Holmes D, Roe M, Timmis A, et al. International comparisons of the management of patients with non-ST segment elevation acute myocardial infarction in the United Kingdom, Sweden, and the United States: The MINAP/NICOR, SWEDEHEART/RIKS-HIA, and ACTION Registry-GWTG/NCDR registries. Int J Cardiol 2014;175:240–7.
Study 25: Boggon R, Timmis A, Hemingway H, Raju S, Malvestiti FM, Van Staa TP. Smoking cessation interventions following acute coronary syndrome: a missed opportunity? Eur J Prev Cardiol 2014;21:767–73.
Study 27: Quint JK, Herrett E, Bhaskaran K, Timmis A, Hemingway H, Wedzicha JA, Smeeth L. Effect of β blockers on mortality after myocardial infarction in adults with COPD: population based cohort study of UK electronic healthcare records. BMJ 2013;347:f6650.
Study 28: Douglas IJ, Evans SJ, Hingorani AD, Grosso AM, Timmis A, Hemingway H, Smeeth L. Clopidogrel and interaction with proton pump inhibitors: comparison between cohort and within person study designs. BMJ 2012;345:e4388.
Study 29: Boggon R, van Staa TP, Timmis A, Hemingway H, Ray KK, Begg A, et al. Clopidogrel discontinuation after acute coronary syndromes: frequency, predictors and associations with death and myocardial infarction – a hospital registry-primary care linked cohort (MINAP-GPRD). Eur Heart J 2011;32:2376–86.
Study 32: Chung SC, Sundström J, Gale CP, James S, Deanfield J, Wallentin L, et al. Comparison of hospital variation in acute myocardial infarction care and outcome between Sweden and United Kingdom: population based cohort study using nationwide clinical registries. BMJ 2015;351:h3913.
Pasea L, Chung S-C, Pujades-Rodriguez M, Moayyeri A, Denaxas S, Fox KAA, et al. Personalising the decision for prolonged dual antiplatelet therapy: development, validation and potential impact of prognostic models for cardiovascular events and bleeding in myocardial infarction survivors. Eur Heart J 2017; in press.
*Joint first authors.
Manuscripts under review or in preparation contributing to our programme
Study 8: Pujades-Rodriguez M, Chung S-C, Shah AD, Gale CP, Timmis A, Hemingway H. Who achieves blood pressure targets in newly diagnosed hypertensives and what are the associations with cardiovascular diseases in a population-based longitudinal study?
Study 9: Jordan K, Timmis A, Croft P, van der Windt D, Denaxas S, Hayward R, Perel P, Hemingway H. Chest pain symptoms in primary care and their association with long term cardiovascular disease: a prospective electronic health record cohort study.
Study 14: Fitzpatrick NK, Timmis AD, Feder GS, Patel R, Hingorani AD, Moss J, Rubbo B, Zhao M, Bvekerwa I, Archangelidi O, Thompson A, Hemingway H. Establishment of a novel consented clinical cohort and blood resource – the Clinical Cohorts in Coronary disease Collaboration (4C): study design and baseline characteristics.
Study 16: Archangelidi O, Pujades-Rodrigues M, Denaxas S, Timmis A, Hemingway H. Heart rate and the onset and prognosis of atrial fibrillation in an initially healthy general population: a CALIBER study.
Study 19: Crowther M, Shah AD, Abrams K, Lazarides E, Lambert P, Hemingway H. Modelling repeatedly measured systolic blood pressure to predict second cardiovascular event in a cohort of 5,246 type 2 diabetic patients diagnosed with stable angina using linked electronic health records.
Study 33: Deaton C, Johnson R, Hughes J, Evans M, Timmis A, Zaman MJS, et al. Aligning the planets: the role of nurses in the care of patients with non-ST elevation myocardial infarction. Nurs Open 2017;4:49–56.
Study 33: Cramer H, Hughes J, Evans M, Johnson R, Featherstone K, Timmis A, Deaton C, Hemingway H, Zaman MJS, Feder GS. Active monitoring and timely response in acute myocardial infarction: an ethnographic study of NSTEMI care.
Study 33: Cramer H, Featherstone K, Hughes J, Johnson R, Evans M, Deaton C, Timmis AD, Hemingway H, Feder GS. Who does this patient belong to? Working across and against boundaries in (NSTEMI) heart attack care.
Data sharing statement
All available data can be obtained from the corresponding author.
Disclaimers
This report presents independent research funded by the National Institute for Health Research (NIHR). The views and opinions expressed by authors in this publication are those of the authors and do not necessarily reflect those of the NHS, the NIHR, CCF, NETSCC, PGfAR or the Department of Health. If there are verbatim quotations included in this publication the views and opinions expressed by the interviewees are those of the interviewees and do not necessarily reflect those of the authors, those of the NHS, the NIHR, NETSCC, the PGfAR programme or the Department of Health.
References
- HM Treasury and the Rt Hon George Osborne MP . Speech by the Chancellor of the Exchequer, Rt Hon George Osborne MP, to the Royal Society n.d. www.gov.uk/government/speeches/speech-by-the-chancellor-of-the-exchequer-rt-hon-george-osborne-mp-to-the-royal-society (accessed 4 December 2016).
- The White House, Office of the Press Secretary . FACT SHEET: President Obama’s Precision Medicine Initiative 2015. www.whitehouse.gov/the-press-office/2015/01/30/fact-sheet-president-obama-s-precision-medicine-initiative (accessed 4 December 2016).
- Weber GM, Mandl KD, Kohane IS. Finding the missing link for big biomedical data. JAMA 2014;311:2479-80. https://doi.org/10.1001/jama.2014.4228.
- Cooksey DS. A Review of UK Health Research Funding. London: Her Majesty’s Treasury; 2006.
- Krumholz HM. Big data and new knowledge in medicine: the thinking, training, and tools needed for a learning health system. Health Aff 2014;33:1163-70. https://doi.org/10.1377/hlthaff.2014.0053.
- Medicines and Healthcare products Regulatory Agency . Clinical Practice Research Datalink n.d. www.cprd.com/intro.asp (accessed 4 December 2016).
- Jernberg T, Attebring MF, Hambraeus K, Ivert T, James S, Jeppsson A, et al. The Swedish web-system for enhancement and development of evidence-based care in heart disease evaluated according to recommended therapies (SWEDEHEART). Heart 2010;96:1617-21. https://doi.org/10.1136/hrt.2010.198804.
- Lindhardsen J, Ahlehoff O, Gislason GH, Madsen OR, Olesen JB, Svendsen JH, et al. Risk of atrial fibrillation and stroke in rheumatoid arthritis: Danish nationwide cohort study. BMJ 2012;344. https://doi.org/10.1136/bmj.e1257.
- Sørensen R, Hansen ML, Abildstrom SZ, Hvelplund A, Andersson C, Jørgensen C, et al. Risk of bleeding in patients with acute myocardial infarction treated with different combinations of aspirin, clopidogrel, and vitamin K antagonists in Denmark: a retrospective analysis of nationwide registry data. Lancet 2009;374:1967-74. https://doi.org/10.1016/S0140-6736(09)61751-7.
- Gershon AS, Warner L, Cascagnette P, Victor JC, To T. Lifetime risk of developing chronic obstructive pulmonary disease: a longitudinal population study. Lancet 2011;378:991-6. https://doi.org/10.1016/S0140-6736(11)60990-2.
- St Sauver JL, Jacobson DJ, McGree ME, Girman CJ, Klee GG, Lieber MM, et al. Associations between longitudinal changes in serum estrogen, testosterone, and bioavailable testosterone and changes in benign urologic outcomes. Am J Epidemiol 2011;173:787-96. https://doi.org/10.1093/aje/kwq438.
- Risch N, Schaefer C. Kaiser Permanente Research Program on Genes, Environment and Health. Oakland, CA: Kaiser Permanente Division of Research; 2011.
- Intermountain Healthcare . Intermountain Healthcare Cardiovascular Research 2015. http://intermountainhealthcare.org/services/heart/research/pages/home.aspx (accessed 7 December 2016).
- Jang MJ, Bang SM, Oh D. Incidence of pregnancy-associated venous thromboembolism in Korea: from the Health Insurance Review and Assessment Service database. J Thromb Haemost 2011;9:2519-21. https://doi.org/10.1111/j.1538-7836.2011.04518.x.
- Denaxas SC, George J, Herrett E, Shah AD, Kalra D, Hingorani AD, et al. Data resource profile: cardiovascular disease research using linked bespoke studies and electronic health records (CALIBER). Int J Epidemiol 2012;41:1625-38. https://doi.org/10.1093/ije/dys188.
- Bloor C, Sriskandarajah R, Croxford V, White H. Strategy for UK Life Sciences. London: Department for Business, Innovation and Skills; 2012.
- Personalised Health and Care 2020. Using Data and Technology to Transform Outcomes for Patients and Citizens. A Framework for Action. Leeds: National Information Board; 2014.
- Healthcare Information Management Systems Society Europe . Electronic Medical Records Adoption Model (EMRAM) Score Distribution – European Countries n.d. http://himss.eu/sites/himsseu/files/analytics/HE%20EMRAM%20Score%20Distribution%20Q3_2016.pdf (accessed 7 December 2016).
- Genomics England . The 100,000 Genomes Project n.d. www.genomicsengland.co.uk/the-100000-genomes-project/ (accessed 4 December 2016).
- NHS England . The care.Data Programme n.d. www.england.nhs.uk/ourwork/tsd/care-data/ (accessed 27 February 2016).
- Global Burden of Diseases Database. Seattle, WA: Institute for Health Metrics and Evaluation, University of Washington; 2014.
- Murray CJ, Richards MA, Newton JN, Fenton KA, Anderson HR, Atkinson C, et al. UK health performance: findings of the Global Burden of Disease Study 2010. Lancet 2013;381:997-1020. https://doi.org/10.1016/S0140-6736(13)60355-4.
- Luengo-Fernández R, Leal J, Gray A, Petersen S, Rayner M. Cost of cardiovascular diseases in the United Kingdom. Heart 2006;92:1384-9. https://doi.org/10.1136/hrt.2005.072173.
- Joint British Societies recommendations on the prevention of Cardiovascular Disease Board . Joint British Societies’ consensus recommendations for the prevention of cardiovascular disease (JBS3). Heart 2014;100:1-67. https://doi.org/10.1136/heartjnl-2014-305693.
- How the NHS Cares for Patients with Heart Attack. MINAP Annual Public Report April 2012 – March 2013. London: National Institute for Cardiovascular Outcomes Research, University College London; 2013.
- Royal College of Physicians . Sentinel Stroke National Audit Programme n.d. www.strokeaudit.org/ (accessed 4 December 2016).
- Gale CP, Weston C, Denaxas S, Cunningham D, de Belder MA, Gray HH, et al. Engaging with the clinical data transparency initiative: a view from the National Institute for Cardiovascular Outcomes Research (NICOR). Heart 2012;98:1040-3. https://doi.org/10.1136/heartjnl-2012-302469.
- Herrett E, Shah AD, Boggon R, Denaxas S, Smeeth L, van Staa T, et al. Completeness and diagnostic validity of recording acute myocardial infarction events in primary care, hospital care, disease registry, and national mortality records: cohort study. BMJ 2013;346. https://doi.org/10.1136/bmj.f2350.
- Hemingway H, Croft P, Perel P, Hayden JA, Abrams K, Timmis A, et al. Prognosis Research Strategy (PROGRESS) 1: a framework for researching clinical outcomes. BMJ 2013;346. https://doi.org/10.1136/bmj.e5595.
- Hingorani AD, Windt DA, Riley RD, Abrams K, Moons KG, Steyerberg EW, et al. Prognosis Research Strategy (PROGRESS) 4: stratified medicine research. BMJ 2013;346. https://doi.org/10.1136/bmj.e5793.
- Riley RD, Hayden JA, Steyerberg EW, Moons KG, Abrams K, Kyzas PA, et al. Prognosis Research Strategy (PROGRESS) 2: prognostic factor research. PLOS Med 2013;10. https://doi.org/10.1371/journal.pmed.1001380.
- Steyerberg EW, Moons KG, van der Windt DA, Hayden JA, Perel P, Schroter S, et al. Prognosis Research Strategy (PROGRESS) 3: prognostic model research. PLOS Med 2013;10. https://doi.org/10.1371/journal.pmed.1001381.
- Peat G, Riley RD, Croft P, Morley KI, Kyzas PA, Moons KG, et al. Improving the transparency of prognosis research: the role of reporting, data sharing, registration, and protocols. PLOS Med 2014;11. https://doi.org/10.1371/journal.pmed.1001671.
- Pujades-Rodriguez M, Timmis A, Stogiannis D, Rapsomaniki E, Denaxas S, Shah A, et al. Socioeconomic deprivation and the incidence of 12 cardiovascular diseases in 1.9 million women and men: implications for risk prediction and prevention. PLOS ONE 2014;9. https://doi.org/10.1371/journal.pone.0104671.
- George J, Rapsomaniki E, Pujades-Rodriguez M, Shah AD, Denaxas S, Herrett E, et al. How does cardiovascular disease first present in women and men? Incidence of 12 cardiovascular diseases in a contemporary cohort of 1,937,360 people. Circulation 2015;132:1320-8. https://doi.org/10.1161/CIRCULATIONAHA.114.013797.
- Zaman MJ, Philipson P, Chen R, Farag A, Shipley M, Marmot MG, et al. South Asians and coronary disease: is there discordance between effects on incidence and prognosis?. Heart 2013;99:729-36. https://doi.org/10.1136/heartjnl-2012-302925.
- Shah AD, Langenberg C, Rapsomaniki E, Denaxas S, Pujades-Rodriguez M, Gale CP, et al. Type 2 diabetes and incidence of cardiovascular diseases: a cohort study in 1.9 million people. Lancet Diabetes Endocrinol 2015;3:105-13. https://doi.org/10.1016/S2213-8587(14)70219-0.
- Rapsomaniki E, Timmis A, George J, Pujades-Rodriguez M, Shah AD, Denaxas S, et al. Blood pressure and incidence of twelve cardiovascular diseases: lifetime risks, healthy life-years lost, and age-specific associations in 1.25 million people. Lancet 2014;383:1899-911. https://doi.org/10.1016/S0140-6736(14)60685-1.
- Pujades-Rodriguez M, Chung S-C, Shah AD, Gale CP, Timmis A, Hemingway H. Who Achieves Blood Pressure Targets in Newly Diagnosed Hypertensives and What Are the Associations With Cardiovascular Diseases in a Population-Based Longitudinal Study? 2016.
- Jordan K, Timmis A, Croft P, van der Windt D, Denaxas S, Hayward R, et al. Chest Pain Symptoms in Primary Care and Their Association With Long Term Cardiovascular Diseases: A Prospective Electronic Health Record Cohort Study 2016.
- Herrett E, George J, Denaxas S, Bhaskaran K, Timmis A, Hemingway H, et al. Type and timing of heralding in ST-elevation and non-ST-elevation myocardial infarction: an analysis of prospectively collected electronic healthcare records linked to the national registry of acute coronary syndromes. Eur Heart J Acute Cardiovasc Care 2013;2:235-45. https://doi.org/10.1177/2048872613487495.
- Shah AD, Martinez C, Hemingway H. The freetext matching algorithm: a computer program to extract diagnoses and causes of death from unstructured text in electronic health records. BMC Med Inform Decis Mak 2012;12. https://doi.org/10.1186/1472-6947-12-88.
- Wang Z, Shah AD, Tate AR, Denaxas S, Shawe-Taylor J, Hemingway H. Extracting diagnoses and investigation results from unstructured text in electronic health records by semi-supervised machine learning. PLOS ONE 2012;7. https://doi.org/10.1371/journal.pone.0030412.
- Cramer H, Evans M, Featherstone K, Johnson R, Zaman MJ, Timmis AD, et al. Treading carefully: a qualitative ethnographic study of the clinical, social and educational uses of exercise ECG in evaluating stable chest pain. BMJ Open 2012;2. https://doi.org/10.1136/bmjopen-2011-000508.
- Johnson R, Evans M, Cramer H, Bennert K, Morris R, Eldridge S, et al. Feasibility and impact of a computerised clinical decision support system on investigation and initial management of new onset chest pain: a mixed methods study. BMC Med Inform Decis Mak 2015;15. https://doi.org/10.1186/s12911-015-0189-8.
- Fitzpatrick NK, Timmis A, Feder GS, Patel R, Hingorani A, Moss J, et al. Establishment of a Novel Consented Clinical Cohort and Blood Resource – The Clinical Cohorts in Coronary Disease Collaboration (4C): Study Design and Baseline Characteristics 2016.
- Shah AD, Nicholas O, Timmis AD, Feder G, Abrams KR, Chen R, et al. Threshold haemoglobin levels and the prognosis of stable coronary disease: two new cohorts and a systematic review and meta-analysis. PLOS Med 2011;8. https://doi.org/10.1371/journal.pmed.1000439.
- Archangelidi O, Pujades-Rodriguez M, Denaxas S, Timmis A, Hemingway H. Heart Rate and the Onset and Prognosis of Atrial Fibrillation in an Initially Healthy General Population: A CALIBER Study 2016.
- Hemingway H, Philipson P, Chen R, Fitzpatrick NK, Damant J, Shipley M, et al. Evaluating the quality of research into a single prognostic biomarker: a systematic review and meta-analysis of 83 studies of C-reactive protein in stable coronary artery disease. PLOS Med 2010;7. https://doi.org/10.1371/journal.pmed.1000286.
- Patel RS, Asselbergs FW, Quyyumi AA, Palmer TM, Finan CI, Tragante V, et al. Genetic variants at chromosome 9p21 and risk of first versus subsequent coronary heart disease events: a systematic review and meta-analysis. J Am Coll Cardiol 2014;63:2234-45. https://doi.org/10.1016/j.jacc.2014.01.065.
- Crowther M, Shah AD, Abrams K, Lazarides E, Lambert P, Hemingway H. Modelling Repeatedly Measured Systolic Blood Pressure to Predict Second Cardiovascular Event in a Cohort of 5,246 Type 2 Diabetic Patients Diagnosed With Stable Angina Using Linked Electronic Health Records 2016.
- Rapsomaniki E, Shah A, Perel P, Denaxas S, George J, Nicholas O, et al. Prognostic models for stable coronary artery disease based on electronic health record cohort of 102 023 patients. Eur Heart J 2014;35:844-52. https://doi.org/10.1093/eurheartj/eht533.
- Asaria M, Walker S, Palmer S, Gale CP, Shah AD, Abrams KR, et al. Using electronic health records to predict costs and outcomes in stable coronary artery disease. Heart 2016;102:755-62. https://doi.org/10.1136/heartjnl-2015-308850.
- Walker S, Asaria M, Manca A, Palmer S, Gale CP, Shah AD, et al. Long-term healthcare use and costs in patients with stable coronary artery disease: a population-based cohort using linked health records (CALIBER). Eur Heart J Qual Care Clin Outcomes 2016;2:125-40. https://doi.org/10.1093/ehjqcco/qcw003.
- Chung SC, Gedeborg R, Nicholas O, James S, Jeppsson A, Wolfe C, et al. Acute myocardial infarction: a comparison of short-term survival in national outcome registries in Sweden and the UK. Lancet 2014;383:1305-12. https://doi.org/10.1016/S0140-6736(13)62070-X.
- Simms AD, Weston CF, West RM, Hall AS, Batin PD, Timmis A, et al. Mortality and missed opportunities along the pathway of care for ST-elevation myocardial infarction: a national cohort study. Eur Heart J Acute Cardiovasc Care 2015;4:241-53. https://doi.org/10.1177/2048872614548602.
- McNamara RL, Chung SC, Jernberg T, Holmes D, Roe M, Timmis A, et al. International comparisons of the management of patients with non-ST segment elevation acute myocardial infarction in the United Kingdom, Sweden, and the United States: The MINAP/NICOR, SWEDEHEART/RIKS-HIA, and ACTION Registry-GWTG/NCDR registries. Int J Cardiol 2014;175:240-7. https://doi.org/10.1016/j.ijcard.2014.04.270.
- Boggon R, Timmis A, Hemingway H, Raju S, Malvestiti FM, Van Staa TP. Smoking cessation interventions following acute coronary syndrome: a missed opportunity?. Eur J Prev Cardiol 2014;21:767-73. https://doi.org/10.1177/2047487312460517.
- Quint JK, Herrett E, Bhaskaran K, Timmis A, Hemingway H, Wedzicha JA, et al. Effect of β blockers on mortality after myocardial infarction in adults with COPD: population based cohort study of UK electronic healthcare records. BMJ 2013;347. https://doi.org/10.1136/bmj.f6650.
- Douglas IJ, Evans SJ, Hingorani AD, Grosso AM, Timmis A, Hemingway H, et al. Clopidogrel and interaction with proton pump inhibitors: comparison between cohort and within person study designs. BMJ 2012;345. https://doi.org/10.1136/bmj.e4388.
- Boggon R, van Staa TP, Timmis A, Hemingway H, Ray KK, Begg A, et al. Clopidogrel discontinuation after acute coronary syndromes: frequency, predictors and associations with death and myocardial infarction – a hospital registry-primary care linked cohort (MINAP-GPRD). Eur Heart J 2011;32:2376-86. https://doi.org/10.1093/eurheartj/ehr340.
- Chung SC, Sundström J, Gale CP, James S, Deanfield J, Wallentin L, et al. Comparison of hospital variation in acute myocardial infarction care and outcome between Sweden and United Kingdom: population based cohort study using nationwide clinical registries. BMJ 2015;351. https://doi.org/10.1136/bmj.h3913.
- Deaton C, Johnson R, Hughes J, Evans M, Timmis A, Zaman MJS, et al. Aligning the planets: the role of nurses in the care of patients with non-ST elevation myocardial infarction. Nurs Open 2017;4:49-56. https://doi.org/10.1002/nop2.69.
- Cramer H, Hughes J, Evans M, Johnson R, Featherstone K, Timmis A, et al. Active Monitoring and Timely Response in Acute Myocardial Infarction: An Ethnographic Study of NSTEMI Care 2016.
- Cramer H, Featherstone K, Hughes J, Johnson R, Evans M, Deaton C, et al. Who does this patient belong to? Working across and against boundaries in (NSTEMI) heart attack care. Sociol Health Illn n.d.
- Briefing Notes for Researchers: Public Involvement in NHS, Public Health and Social Care Research. London: National Institute for Health Research; 2012.
- Smolina K, Wright FL, Rayner M, Goldacre MJ. Determinants of the decline in mortality from acute myocardial infarction in England between 2002 and 2010: linked national database study. BMJ 2012;344. https://doi.org/10.1136/bmj.d8059.
- Yusuf S, Hawken S, Ounpuu S, Dans T, Avezum A, Lanas F, et al. Effect of potentially modifiable risk factors associated with myocardial infarction in 52 countries (the INTERHEART study): case-control study. Lancet 2011;364:937-52. https://doi.org/10.1016/S0140-6736(04)17018-9.
- Lu L, Mackay DF, Pell JP. Meta-analysis of the association between cigarette smoking and peripheral arterial disease. Heart 2014;100:414-23. https://doi.org/10.1136/heartjnl-2013-304082.
- Putting Prevention First – Vascular Checks: Risk Assessment and Management. London: Department of Health; 2008.
- Statins for the Prevention of Cardiovascular Events. NICE Technology Appraisals TA94. NICE; 2006.
- Cardiovascular Disease Outcomes Strategy: Improving Outcomes for People with or at risk of Cardiovascular Disease. London: Department of Health; 2013.
- Lauer MS. Time for a creative transformation of epidemiology in the United States. JAMA 2012;308:1804-5. https://doi.org/10.1001/jama.2012.14838.
- Sekhri N, Feder GS, Junghans C, Hemingway H, Timmis AD. How effective are rapid access chest pain clinics? Prognosis of incident angina and non-cardiac chest pain in 8762 consecutive patients. Heart 2007;93:458-63. https://doi.org/10.1136/hrt.2006.090894.
- Ritchie MD, Denny JC, Zuvich RL, Crawford DC, Schildcrout JS, Bastarache L, et al. Genome- and phenome-wide analyses of cardiac conduction identifies markers of arrhythmia risk. Circulation 2013;127:1377-85. https://doi.org/10.1161/CIRCULATIONAHA.112.000604.
- Denny JC, Ritchie MD, Basford MA, Pulley JM, Bastarache L, Brown-Gentry K, et al. PheWAS: demonstrating the feasibility of a phenome-wide scan to discover gene-disease associations. Bioinformatics 2010;26:1205-10. https://doi.org/10.1093/bioinformatics/btq126.
- Jones DA, Timmis A, Wragg A. Novel drugs for treating angina. BMJ 2013;347. https://doi.org/10.1136/bmj.f4726.
- Palmerini T, Biondi-Zoccai G, Della Riva D, Stettler C, Sangiorgi D, D’Ascenzo F, et al. Stent thrombosis with drug-eluting and bare-metal stents: evidence from a comprehensive network meta-analysis. Lancet 2012;379:1393-402. https://doi.org/10.1016/S0140-6736(12)60324-9.
- Nabel EG, Braunwald E. A tale of coronary artery disease and myocardial infarction. N Engl J Med 2012;366:54-63. https://doi.org/10.1056/NEJMra1112570.
- Eshaghian S, Shah PK, Kaul S. Advances in antiplatelet treatment for acute coronary syndromes. Heart 2010;96:656-61. https://doi.org/10.1136/hrt.2009.185983.
- National Service Framework for Coronary Heart Disease: Modern Standards and Service Models. London: Department of Health; 2000.
- Quality and Outcomes Framework. Leeds, UK: Health and Social Care Information Centre; 2014.
- Report of the Mid Staffordshire NHS Foundation Trust Public Inquiry. London: The Stationery Office; 2013.
- NHS England . NHS England Announces New Commitments for Increased Openness and Transparency 2013. www.england.nhs.uk/2013/10/31/op-trans/ (accessed 4 December 2016).
- Walley T, Mantgani A. The UK General Practice Research Database. Lancet 1997;350:1097-9. https://doi.org/10.1016/S0140-6736(97)04248-7.
- Herrett E, Smeeth L, Walker L, Weston C. behalf of the MINAP Academic Group . The Myocardial Ischaemia National Audit Project (MINAP). Heart 2010;96:1264-7. https://doi.org/10.1136/hrt.2009.192328.
- NHS Digital . Mortality Data from the Office for National Statistics n.d. http://content.digital.nhs.uk/onsmortality (accessed 27 February 2016).
- ONS . Index of Multiple Deprivation 2007 n.d. https://data.gov.uk/dataset/index_of_multiple_deprivation_imd_2007 (accessed 4 December 2016).
- Morley KI, Wallace J, Denaxas SC, Hunter RJ, Patel RS, Perel P, et al. Defining disease phenotypes using national linked electronic health records: a case study of atrial fibrillation. PLOS ONE 2014;9. https://dx.doi.org/10.1371/journal.pone.0110900.
- Marston L, Carpenter JR, Walters KR, Morris RW, Nazareth I, Petersen I. Issues in multiple imputation of missing data for large general practice clinical databases. Pharmacoepidemiol Drug Saf 2010;19:618-26. https://doi.org/10.1002/pds.1934.
- Herrett E, Thomas SL, Schoonen WM, Smeeth L, Hall AJ. Validation and validity of diagnoses in the General Practice Research Database: a systematic review. Br J Clin Pharmacol 2010;69:4-14. https://doi.org/10.1111/j.1365-2125.2009.03537.x.
- Thygesen K, Alpert JS, Jaffe AS, Simoons ML, Chaitman BR, White HD, et al. Third universal definition of myocardial infarction. Eur Heart J 2012;33:2551-67. https://doi.org/10.1093/eurheartj/ehs184.
- Goldacre MJ, Roberts SE, Griffith M. Place, time and certified cause of death in people who die after hospital admission for myocardial infarction or stroke. Eur J Public Health 2004;14:338-42. https://doi.org/10.1093/eurpub/14.4.338.
- Payne RA, Abel GA, Simpson CR. A retrospective cohort study assessing patient characteristics and the incidence of cardiovascular disease using linked routine primary and secondary care data. BMJ Open 2012;2. https://doi.org/10.1136/bmjopen-2011-000723.
- Boyle CA, Dobson AJ. The accuracy of hospital records and death certificates for acute myocardial infarction. Aust N Z J Med 1995;25:316-23. https://doi.org/10.1111/j.1445-5994.1995.tb01896.x.
- Madsen M, Davidsen M, Rasmussen S, Abildstrom SZ, Osler M. The validity of the diagnosis of acute myocardial infarction in routine statistics: a comparison of mortality and hospital discharge data with the Danish MONICA registry. J Clin Epidemiol 2003;56:124-30. https://doi.org/10.1016/S0895-4356(02)00591-7.
- Merry AH, Boer JM, Schouten LJ, Feskens EJ, Verschuren WM, Gorgels AP, et al. Validity of coronary heart diseases and heart failure based on hospital discharge and mortality data in the Netherlands using the cardiovascular registry Maastricht cohort study. Eur J Epidemiol 2009;24:237-47. https://doi.org/10.1007/s10654-009-9335-x.
- García Rodríguez LA, Varas-Lorenzo C, Maguire A, González-Pérez A. Nonsteroidal antiinflammatory drugs and the risk of myocardial infarction in the general population. Circulation 2004;109:3000-6. https://doi.org/10.1161/01.CIR.0000132491.96623.04.
- van Staa TP, Abenhaim L. The quality of information recorded on a UK database of primary care records: a study of hospitalizations due to hypoglycemia and other conditions. Pharmacoepidemiol Drug Safe 1994;3:15-21. https://doi.org/10.1002/pds.2630030106.
- Meier CR, Jick SS, Derby LE, Vasilakis C, Jick H. Acute respiratory-tract infections and risk of first-time acute myocardial infarction. Lancet 1998;351:1467-71. https://doi.org/10.1016/S0140-6736(97)11084-4.
- Jick H, Jick S, Myers MW, Vasilakis C. Risk of acute myocardial infarction and low-dose combined oral contraceptives. Lancet 1996;347:627-8. https://doi.org/10.1016/S0140-6736(96)91334-3.
- Hammad TA, McAdams MA, Feight A, Iyasu S, Dal Pan GJ. Determining the predictive value of Read/OXMIS codes to identify incident acute myocardial infarction in the General Practice Research Database. Pharmacoepidemiol Drug Saf 2008;17:1197-201. https://doi.org/10.1002/pds.1672.
- Hall GC, Brown MM, Mo J, MacRae KD. Triptans in migraine: the risks of stroke, cardiovascular disease, and death in practice. Neurology 2004;62:563-8. https://doi.org/10.1212/01.WNL.0000110312.36809.7F.
- Johansson S, Wallander MA, Ruigómez A, García Rodríguez LA. Is there any association between myocardial infarction, gastro-oesophageal reflux disease and acid-suppressing drugs?. Aliment Pharmacol Ther 2003;18:973-8. https://doi.org/10.1046/j.1365-2036.2003.01798.x.
- Gulliford MC, Charlton J, Ashworth M, Rudd AG, Toschke AM. the eCRT Research Team . Selection of medical diagnostic codes for analysis of electronic patient records. Application to stroke in a primary care database. PLOS ONE 2009;4. https://doi.org/10.1371/journal.pone.0007168.
- Silver LE, Heneghan C, Mehta Z, Banning AP, Rothwell PM. Substantial underestimation of incidence of acute myocardial infarction by hospital discharge diagnostic coding data: a prospective population-based study. Heart 2009;95.
- UK Biobank: Protocol for a Large-Scale Prospective Epidemiological Resource. Stockport: UK Biobank Coordinating Centre; n.d.
- Boggon R, van Staa TP, Chapman M, Gallagher AM, Hammad TA, Richards MA. Cancer recording and mortality in the General Practice Research Database and linked cancer registries. Pharmacoepidemiol Drug Saf 2013;22:168-75. https://doi.org/10.1002/pds.3374.
- Herrett E. Myocardial Infarction As the First Manifestation of Atherosclerotic Disease 2013.
- Johannesdottir SA, Horváth-Puhó E, Ehrenstein V, Schmidt M, Pedersen L, Sørensen HT. Existing data sources for clinical epidemiology: The Danish National Database of Reimbursed Prescriptions. Clin Epidemiol 2012;4:303-13. https://doi.org/10.2147/CLEP.S37587.
- Death Certification Reforms in England and Wales: Update for Coroners. London: Department of Health; 2013.
- Collins R. What makes UK Biobank special?. Lancet 2012;379:1173-4. https://doi.org/10.1016/S0140-6736(12)60404-8.
- van Staa TP, Goldacre B, Gulliford M, Cassell J, Pirmohamed M, Taweel A, et al. Randomised Evaluations of Accepted Choices in Treatment (REACT) trials: large-scale pragmatic trials within databases of routinely collected electronic healthcare records. Trials 2011;12. https://doi.org/10.1186/1745-6215-12-S1-A104.
- Hemingway H, Riley RD, Altman DG. Ten steps towards improving prognosis research. BMJ 2009;339. https://doi.org/10.1136/bmj.b4184.
- von Elm E, Altman DG, Egger M, Pocock SJ, Gotzsche PC, Vandenbroucke JP. The Strengthening the Reporting of Observational Studies in Epidemiology (STROBE) statement: guidelines for reporting observational studies. PLOS Med 2007;4. https://doi.org/10.1371/journal.pmed.0040296.
- Wasson JH, Sox HC, Neff RK, Goldman L. Clinical prediction rules. Applications and methodological standards. N Engl J Med 1985;313:793-9. https://doi.org/10.1056/NEJM198509263131306.
- Laupacis A, Sekar N, Stiell IG. Clinical prediction rules. A review and suggested modifications of methodological standards. JAMA 1997;277:488-94. https://doi.org/10.1001/jama.1997.03540300056034.
- Reilly BM, Evans AT. Translating clinical research into clinical practice: impact of using prediction rules to make decisions. Ann Intern Med 2006;144:201-9. https://doi.org/10.7326/0003-4819-144-3-200602070-00009.
- Go AS, Mozaffarian D, Roger VL, Benjamin EJ, Berry JD, Borden WB, et al. Heart disease and stroke statistics – 2013 update: a report from the American Heart Association. Circulation 2013;127:e6-e245. https://doi.org/10.1161/CIR.0b013e31828124ad.
- Murphy NF, MacIntyre K, Capewell S, Stewart S, Pell J, Chalmers J, et al. Hospital discharge rates for suspected acute coronary syndromes between 1990 and 2000: population based analysis. BMJ 2004;328:1413-14. https://doi.org/10.1136/bmj.38111.650741.F7.
- Beaglehole R, Ebrahim S, Reddy S, Voûte J, Leeder S. Chronic Disease Action Group . Prevention of chronic diseases: a call to action. Lancet 2007;370:2152-7. https://doi.org/10.1016/S0140-6736(07)61700-0.
- Lewington S, Clarke R, Qizilbash N, Peto R, Collins R. Prospective Studies Collaboration . Age-specific relevance of usual blood pressure to vascular mortality: a meta-analysis of individual data for one million adults in 61 prospective studies. Lancet 2002;360:1903-13. https://doi.org/10.1016/S0140-6736(02)11911-8.
- Anand SS, Islam S, Rosengren A, Franzosi MG, Steyn K, Yusufali AH, et al. Risk factors for myocardial infarction in women and men: insights from the INTERHEART study. Eur Heart J 2008;29:932-40. https://doi.org/10.1093/eurheartj/ehn018.
- D’Agostino RB, Vasan RS, Pencina MJ, Wolf PA, Cobain M, Massaro JM, et al. General cardiovascular risk profile for use in primary care: the Framingham Heart Study. Circulation 2008;117:743-53. https://doi.org/10.1161/CIRCULATIONAHA.107.699579.
- Hyvärinen M, Qiao Q, Tuomilehto J, Söderberg S, Eliasson M, Stehouwer CD. The difference between acute coronary heart disease and ischaemic stroke risk with regard to gender and age in Finnish and Swedish populations. Int J Stroke 2010;5:152-6. https://doi.org/10.1111/j.1747-4949.2010.00423.x.
- Kappert K, Böhm M, Schmieder R, Schumacher H, Teo K, Yusuf S, et al. Impact of sex on cardiovascular outcome in patients at high cardiovascular risk: analysis of the Telmisartan Randomized Assessment Study in ACE-Intolerant Subjects With Cardiovascular Disease (TRANSCEND) and the Ongoing Telmisartan Alone and in Combination With Ramipril Global End Point Trial (ONTARGET). Circulation 2012;126:934-41. https://doi.org/10.1161/CIRCULATIONAHA.111.086660.
- Berry JD, Dyer A, Cai X, Garside DB, Ning H, Thomas A, et al. Lifetime risks of cardiovascular disease. N Engl J Med 2012;366:321-9. https://doi.org/10.1056/NEJMoa1012848.
- Hippisley-Cox J, Coupland C, Robson J, Brindle P. Derivation, validation, and evaluation of a new QRISK model to estimate lifetime risk of cardiovascular disease: cohort study using QResearch database. BMJ 2010;341. https://doi.org/10.1136/bmj.c6624.
- Wilkins JT, Ning H, Berry J, Zhao L, Dyer AR, Lloyd-Jones DM. Lifetime risk and years lived free of total cardiovascular disease. JAMA 2012;308:1795-801. https://doi.org/10.1001/jama.2012.14312.
- Bui AL, Horwich TB, Fonarow GC. Epidemiology and risk profile of heart failure. Nat Rev Cardiol 2011;8:30-41. https://doi.org/10.1038/nrcardio.2010.165.
- Blumenthal D, Tavenner M. The ‘meaningful use’ regulation for electronic health records. N Engl J Med 2010;363:501-4. https://doi.org/10.1056/NEJMp1006114.
- Gaziano JM. The evolution of population science: advent of the mega cohort. JAMA 2010;304:2288-9. https://doi.org/10.1001/jama.2010.1691.
- Improving the Way We Meet the Primary Health Care Needs of the Socially Excluded. London: Social Exclusion Task Force; 2010.
- Manolio TA, Weis BK, Cowie CC, Hoover RN, Hudson K, Kramer BS, et al. New models for large prospective studies: is there a better way?. Am J Epidemiol 2012;175:859-66. https://doi.org/10.1093/aje/kwr453.
- Toward Precision Medicine: Building a Knowledge Network for Biomedical Research and a New Taxonomy of Disease. Washington, DC: National Academy Press; 2011.
- Sebastianski M, Makowsky MJ, Dorgan M, Tsuyuki RT. Paradoxically lower prevalence of peripheral arterial disease in South Asians: a systematic review and meta-analysis. Heart 2014;100:100-5. https://doi.org/10.1136/heartjnl-2013-303605.
- Holmes MV, Perel P, Shah T, Hingorani AD, Casas JP. CYP2C19 genotype, clopidogrel metabolism, platelet function, and cardiovascular events: a systematic review and meta-analysis. JAMA 2011;306:2704-14. https://doi.org/10.1001/jama.2011.1880.
- Zaman MJ, Shipley MJ, Stafford M, Brunner EJ, Timmis AD, Marmot MG, et al. Incidence and prognosis of angina pectoris in South Asians and Whites: 18 years of follow-up over seven phases in the Whitehall-II prospective cohort study. J Public Health 2011;33:430-8. https://doi.org/10.1093/pubmed/fdq093.
- Forouhi NG, Sattar N, Tillin T, McKeigue PM, Chaturvedi N. Do known risk factors explain the higher coronary heart disease mortality in South Asian compared with European men? Prospective follow-up of the Southall and Brent studies, UK. Diabetologia 2006;49:2580-8. https://doi.org/10.1007/s00125-006-0393-2.
- Patel JV, Lim HS, Gunarathne A, Tracey I, Durrington PN, Hughes EA, et al. Ethnic differences in myocardial infarction in patients with hypertension: effects of diabetes mellitus. QJM 2008;101:231-6. https://doi.org/10.1093/qjmed/hcm151.
- Mather HM, Chaturvedi N, Fuller JH. Mortality and morbidity from diabetes in South Asians and Europeans: 11-year follow-up of the Southall Diabetes Survey, London, UK. Diabet Med 1998;15:53-9. https://doi.org/10.1002/(SICI)1096-9136(199801)15:1<53::AID-DIA521>3.0.CO;2-V.
- Khattar RS, Swales JD, Senior R, Lahiri A. Racial variation in cardiovascular morbidity and mortality in essential hypertension. Heart 2000;83:267-71. https://doi.org/10.1136/heart.83.3.267.
- Bellary S, O’Hare JP, Raymond NT, Mughal S, Hanif WM, Jones A, et al. Premature cardiovascular events and mortality in south Asians with type 2 diabetes in the United Kingdom Asian Diabetes Study – effect of ethnicity on risk. Curr Med Res Opin 2010;26:1873-9. https://doi.org/10.1185/03007995.2010.490468.
- Fischbacher CM, Bhopal R, Povey C, Steiner M, Chalmers J, Mueller G, et al. Record linked retrospective cohort study of 4.6 million people exploring ethnic variations in disease: myocardial infarction in South Asians. BMC Public Health 2007;7. https://doi.org/10.1186/1471-2458-7-142.
- U.K. Prospective Diabetes Study Group . Ethnicity and cardiovascular disease: the incidence of myocardial infarction in white, South Asian, and Afro-Caribbean patients with type 2 diabetes (U.K. Prospective Diabetes Study 32). Diabetes Care 1998;21:1271-7. https://doi.org/10.2337/diacare.21.8.1271.
- Nijjar AP, Wang H, Quan H, Khan NA. Ethnic and sex differences in the incidence of hospitalized acute myocardial infarction: British Columbia, Canada 1995–2002. BMC Cardiovasc Disord 2010;10. https://doi.org/10.1186/1471-2261-10-38.
- Zaman MJ, Junghans C, Sekhri N, Chen R, Feder GS, Timmis AD, et al. Presentation of stable angina pectoris among women and South Asian people. CMAJ 2008;179:659-67. https://doi.org/10.1503/cmaj.071763.
- Zaman MJ, Crook AM, Junghans C, Fitzpatrick NK, Feder G, Timmis AD, et al. Ethnic differences in long-term improvement of angina following revascularization or medical management: a comparison between south Asians and white Europeans. J Public Health 2009;31:168-74. https://doi.org/10.1093/pubmed/fdn099.
- Raghavan R, Rahme E, Nedjar H, Huynh T. Long-term prognosis of south Asians following acute coronary syndromes. Can J Cardiol 2008;24:585-7. https://doi.org/10.1016/S0828-282X(08)70640-8.
- Hughes LO, Raval U, Raftery EB. First myocardial infarctions in Asian and white men. BMJ 1989;298:1345-50. https://doi.org/10.1136/bmj.298.6684.1345.
- Mukhtar HT, Littler WA. Survival after acute myocardial infarction in Asian and white patients in Birmingham. Br Heart J 1995;73:122-4. https://doi.org/10.1136/hrt.73.2.122.
- Wilkinson P, Sayer J, Laji K, Grundy C, Marchant B, Kopelman P, et al. Comparison of case fatality in south Asian and white patients after acute myocardial infarction: observational study. BMJ 1996;312:1330-3. https://doi.org/10.1136/bmj.312.7042.1330.
- Liew R, Sulfi S, Ranjadayalan K, Cooper J, Timmis AD. Declining case fatality rates for acute myocardial infarction in South Asian and white patients in the past 15 years. Heart 2006;92:1030-4. https://doi.org/10.1136/hrt.2005.078634.
- Gupta M, Doobay AV, Singh N, Anand SS, Raja F, Mawji F, et al. Risk factors, hospital management and outcomes after acute myocardial infarction in South Asian Canadians and matched control subjects. CMAJ 2002;166:717-22.
- Khan NA, Grubisic M, Hemmelgarn B, Humphries K, King KM, Quan H. Outcomes after acute myocardial infarction in South Asian, Chinese, and white patients. Circulation 2010;122:1570-7. https://doi.org/10.1161/CIRCULATIONAHA.109.850297.
- Ryden L, Grant PJ, Anker SD, Berne C, Cosentino F, Danchin N, et al. ESC Guidelines on diabetes, pre-diabetes, and cardiovascular diseases developed in collaboration with the EASD: the Task Force on diabetes, pre-diabetes, and cardiovascular diseases of the European Society of Cardiology (ESC) and developed in collaboration with the European Association for the Study of Diabetes (EASD). Eur Heart J 2013;34:3035-87. https://doi.org/10.1093/eurheartj/eht108.
- Fowkes FG, Rudan D, Rudan I, Aboyans V, Denenberg JO, McDermott MM, et al. Comparison of global estimates of prevalence and risk factors for peripheral artery disease in 2000 and 2010: a systematic review and analysis. Lancet 2013;382:1329-40. https://doi.org/10.1016/S0140-6736(13)61249-0.
- Nordon IM, Hinchliffe RJ, Loftus IM, Thompson MM. Pathophysiology and epidemiology of abdominal aortic aneurysms. Nat Rev Cardiol 2011;8:92-102. https://doi.org/10.1038/nrcardio.2010.180.
- Kent KC, Zwolak RM, Egorova NN, Riles TS, Manganaro A, Moskowitz AJ, et al. Analysis of risk factors for abdominal aortic aneurysm in a cohort of more than 3 million individuals. J Vasc Surg 2010;52:539-48. https://doi.org/10.1016/j.jvs.2010.05.090.
- Kuivaniemi H, Ryer EJ, Elmore JR, Hinterseher I, Smelser DT, Tromp G. Update on abdominal aortic aneurysm research: from clinical to genetic studies. Scientifica 2014;2014. https://doi.org/10.1155/2014/564734.
- Theivacumar NS, Stephenson MA, Mistry H, Valenti D. Diabetes mellitus and aortic aneurysm rupture: a favorable association?. Vasc Endovascular Surg 2014;48:45-50. https://doi.org/10.1177/1538574413505921.
- Feigin VL, Rinkel GJ, Lawes CM, Algra A, Bennett DA, van Gijn J, et al. Risk factors for subarachnoid hemorrhage: an updated systematic review of epidemiological studies. Stroke 2005;36:2773-80. https://doi.org/10.1161/01.STR.0000190838.02954.e8.
- Shantikumar S, Ajjan R, Porter KE, Scott DJ. Diabetes and the abdominal aortic aneurysm. Eur J Vasc Endovasc Surg 2010;39:200-7. https://doi.org/10.1016/j.ejvs.2009.10.014.
- The Action to Control Cardiovascular Risk in Diabetes Study Group . Effects of intensive glucose lowering in type 2 diabetes. N Engl J Med 2008;358:2545-59. https://doi.org/10.1056/NEJMoa0802743.
- Lim SS, Vos T, Flaxman AD, Danaei G, Shibuya K, Adair-Rohani H, et al. A comparative risk assessment of burden of disease and injury attributable to 67 risk factors and risk factor clusters in 21 regions, 1990–2010: a systematic analysis for the Global Burden of Disease Study 2010. Lancet 2012;380:2224-60. https://doi.org/10.1016/S0140-6736(12)61766-8.
- Goff DC, Lloyd-Jones DM, Bennett G, Coady S, D’Agostino RB, Gibbons R, et al. 2013 ACC/AHA guideline on the assessment of cardiovascular risk: a report of the American College of Cardiology/American Heart Association Task Force on Practice Guidelines. J Am Coll Cardiol 2014;63:2935-59. https://doi.org/10.1016/j.jacc.2013.11.005.
- Turnbull F. Blood Pressure Lowering Treatment Trialists’ Collaboration . Effects of different blood-pressure-lowering regimens on major cardiovascular events: results of prospectively-designed overviews of randomised trials. Lancet 2003;362:1527-35. https://doi.org/10.1016/S0140-6736(03)14739-3.
- James PA, Oparil S, Carter BL, Cushman WC, Dennison-Himmelfarb C, Handler J, et al. 2014 evidence-based guideline for the management of high blood pressure in adults: report from the panel members appointed to the Eighth Joint National Committee (JNC 8). JAMA 2014;311:507-20. https://doi.org/10.1001/jama.2013.284427.
- Berlowitz DR, Ash AS, Hickey EC, Friedman RH, Glickman M, Kader B, et al. Inadequate management of blood pressure in a hypertensive population. N Engl J Med 1998;339:1957-63. https://doi.org/10.1056/NEJM199812313392701.
- Berlowitz DR, Ash AS, Hickey EC, Glickman M, Friedman R, Kader B. Hypertension management in patients with diabetes: the need for more aggressive therapy. Diabetes Care 2003;26:355-9. https://doi.org/10.2337/diacare.26.2.355.
- Borzecki AM, Wong AT, Hickey EC, Ash AS, Berlowitz DR. Hypertension control: how well are we doing?. Arch Intern Med 2003;163:2705-11. https://doi.org/10.1001/archinte.163.22.2705.
- Vasan RS, Larson MG, Leip EP, Evans JC, O’Donnell CJ, Kannel WB, et al. Impact of high-normal blood pressure on the risk of cardiovascular disease. New Engl J Med 2001;345:1291-7. https://doi.org/10.1056/NEJMoa003417.
- National Service Framework for Coronary Heart Disease. London: Department of Health; 2000.
- NICE . Suspected Cancer: Recognition and Referral n.d. www.nice.org.uk/guidance/ng12 (accessed 4 December 2016).
- Yawn BP, Wollan PC, Jacobsen SJ, Fryer GE, Roger VL. Identification of women’s coronary heart disease and risk factors prior to first myocardial infarction. J Womens Health 2004;13:1087-100. https://doi.org/10.1089/jwh.2004.13.1087.
- Pierard LA, Dubois C, Smeets JP, Boland J, Carlier J, Kulbertus HE. Prognostic significance of angina pectoris before first acute myocardial infarction. Am J Cardiol 1988;61:984-7. https://doi.org/10.1016/0002-9149(88)90111-7.
- Cupples LA, Gagnon DR, Wong ND, Ostfeld AM, Kannel WB. Preexisting cardiovascular conditions and long-term prognosis after initial myocardial infarction: the Framingham Study. Am Heart J 1993;125:863-72. https://doi.org/10.1016/0002-8703(93)90182-9.
- Kobayashi Y, Miyazaki S, Itoh A, Daikoku S, Morii I, Matsumoto T, et al. Previous angina reduces in-hospital death in patients with acute myocardial infarction. Am J Cardiol 1998;81:117-22. https://doi.org/10.1016/S0002-9149(97)00884-9.
- Bhatt DL, Peterson ED, Harrington RA, Ou FS, Cannon CP, Gibson CM, et al. Prior polyvascular disease: risk factor for adverse ischaemic outcomes in acute coronary syndromes. Eur Heart J 2009;30:1195-202. https://doi.org/10.1093/eurheartj/ehp099.
- Harper RW, Kennedy G, DeSanctis RW, Hutter AM. The incidence and pattern of angina prior to acute myocardial infarction: a study of 577 cases. Am Heart J 1979;97:178-83. https://doi.org/10.1016/0002-8703(79)90353-3.
- Stowers M, Short D. Warning symptoms before major myocardial infarction. Br Heart J 1970;32:833-8. https://doi.org/10.1136/hrt.32.6.833.
- Herrett E, Bhaskaran K, Timmis A, Denaxas S, Hemingway H, Smeeth L. Association between clinical presentations before myocardial infarction and coronary mortality: a prospective population-based study using linked electronic records. Eur Heart J 2014;35:2363-71. https://doi.org/10.1093/eurheartj/ehu286.
- Skinner JS, Smeeth L, Kendall JM, Adams PC, Timmis A. Chest Pain Guideline Development Group . NICE guidance. Chest pain of recent onset: assessment and diagnosis of recent onset chest pain or discomfort of suspected cardiac origin. Heart 2010;96:974-8. https://doi.org/10.1136/hrt.2009.190066.
- Tate AR, Martin AGR, Ali A, Cassell JA. Using free text information to explore how and when GPs code a diagnosis of ovarian cancer: an observational study using primary care records of patients with ovarian cancer. BMJ Open 2011;1. https://doi.org/10.1136/bmjopen-2010-000025.
- Pakhomov S, Hemingway H, Weston S, Jacobsen S, Rodeheffer R, Roger V. Epidemiology of angina pectoris: role of natural language processing of the medical record. Am Heart J 2007;153:666-73. https://doi.org/10.1016/j.ahj.2006.12.022.
- Pakhomov SV, Buntrock J, Chute CG. Prospective recruitment of patients with congestive heart failure using an ad-hoc binary classifier. J Biomed Inform 2005;38:145-53. https://doi.org/10.1016/j.jbi.2004.11.016.
- Savova GK, Ogren PV, Duffy PH, Buntrock JD, Chute CG. Mayo clinic NLP system for patient smoking status identification. J Am Med Inform Assoc 2008;15:25-8. https://doi.org/10.1197/jamia.M2437.
- Uzuner O, Goldstein I, Luo Y, Kohane I. Identifying patient smoking status from medical discharge records. J Am Med Inform Assoc 2008;15:14-2. https://doi.org/10.1197/jamia.M2408.
- Clark C, Good K, Jezierny L, Macpherson M, Wilson B, Chajewska U. Identifying smokers with a medical extraction system. J Am Med Inform Assoc 2008;15:36-9. https://doi.org/10.1197/jamia.M2442.
- Pakhomov S, Weston SA, Jacobsen SJ, Chute CG, Meverden R, Roger VL. Electronic medical records for clinical research: application to the identification of heart failure. Am J Manag Care 2007;13:281-8.
- Melton GB, Raman N, Chen ES, Sarkar IN, Pakhomov S, Madoff RD. Evaluation of family history information within clinical documents and adequacy of HL7 clinical statement and clinical genomics family history models for its representation: a case report. J Am Med Inform Assoc 2010;17:337-40. https://doi.org/10.1136/jamia.2009.002238.
- Aronson A. MetaMap 2011. https://metamap.nlm.nih.gov/ (accessed 15 June 2011).
- Microsoft and NHS England . NHS Common User Interface Design Guidance – Terminology Matching. Microsoft and NHS England 2007. www.mscui.net/DesignGuide/Pdfs/Design%20Guidance%20--%20Terminology%20--%20Matching.pdf (accessed 4 December 2016).
- Pakhomov S, Shah N, Hanson P, Balasubramaniam S, Smith SA, Smith SA. Automatic quality of life prediction using electronic medical records. AMIA Annu Symp Proc 2008;2008:545-9.
- Li Y, Gorman SL, Elhadad N. Section Classification In Clinical Notes Using Supervised Hidden Markov Model. New York, NY: Association for Computing Machinery; 2010.
- Aronson AR, Bodenreider O, Demner-Fushman D, Fung KW, Lee VK, Mork JG, et al. From Indexing the Biomedical Literature to Coding Clinical Text: Experience with MTI and Machine Learning Approaches. Stroudsburg, PA: Association for Computational Linguistics; 2007.
- Crammer K, Dredze M, Ganchev K, Talukdar PP, Carroll S. Automatic Code Assignment to Medical Text. Stroudsburg, PA: Association for Computational Linguistics; 2007.
- Suominen H, Ginter F, Pyysalo S, Airola A, Pahikkala T, Salanterä S, et al. Machine Learning to Automate the Assignment of Diagnosis Codes to Free-Text Radiology Reports: A Method Description n.d.
- Rosales R, Krishnamurthy P, Rao RB. Semi-Supervised Active Learning for Modeling Medical Concepts from Free Text n.d. https://doi.org/10.1109/icmla.2007.103.
- Marchand M, Taylor JS. The set covering machine. J Mach Learn Res 2003;3:723-46.
- Sindhwani V, Keerthi SS. Large Scale Semi-supervised Linear SVMs. New York, NY: Association for Computing Machinery; 2006.
- Chest Pain of Recent Onset: Assessment and Diagnosis of Recent Onset Chest Pain or Discomfort of Suspected Cardiac Origin. London: NICE; 2010.
- Michie S, Johnston M, Abraham C, Lawton R, Parker D, Walker A. ‘Psychological Theory’ Group . Making psychological theory useful for implementing evidence based practice: a consensus approach. Qual Saf Health Care 2005;14:26-33. https://doi.org/10.1136/qshc.2004.011155.
- Kawamoto K, Houlihan CA, Balas EA, Lobach DF. Improving clinical practice using clinical decision support systems: a systematic review of trials to identify features critical to success. BMJ 2005;330. https://doi.org/10.1136/bmj.38398.500764.8F.
- Heselmans A, Van de Velde S, Donceel P, Aertgeerts B, Ramaekers D. Effectiveness of electronic guideline-based implementation systems in ambulatory care settings – a systematic review. Implement Sci 2009;4. https://doi.org/10.1186/1748-5908-4-82.
- Roshanov PS, Fernandes N, Wilczynski JM, Hemens BJ, You JJ, Handler SM, et al. Features of effective computerised clinical decision support systems: meta-regression of 162 randomised trials. BMJ 2013;346. https://doi.org/10.1136/bmj.f657.
- Lobach DF. The road to effective clinical decision support: are we there yet?. BMJ 2013;346.
- Craig P, Dieppe P, Macintyre S, Michie S, Nazareth I, Petticrew M. Medical Research Council Guidance . Developing and evaluating complex interventions: the new Medical Research Council guidance. BMJ 2008;337.
- Diamond GA, Forrester JS. Analysis of probability as an aid in the clinical diagnosis of coronary-artery disease. N Engl J Med 1979;300:1350-8. https://doi.org/10.1056/NEJM197906143002402.
- Strauss AL, Corbin J. Basics Of Qualitative Research: Grounded Theory Procedures And Techniques. London: Sage Publications; 1998.
- Somerville C, Featherstone K, Hemingway H, Timmis A, Feder GS. Performing stable angina pectoris: an ethnographic study. Soc Sci Med 2008;66:1497-508. https://doi.org/10.1016/j.socscimed.2007.12.010.
- Elwyn G, Rix A, Holt T, Jones D. Why do clinicians not refer patients to online decision support tools? Interviews with front line clinics in the NHS. BMJ Open 2012;2. https://doi.org/10.1136/bmjopen-2012-001530.
- GENIUS-CHD . The Genetics of Subsequent Coronary Heart Disease n.d. www.genius-chd.com (accessed 4 December 2016).
- Oldroyd JC, Cyril S, Wijayatilaka BS, O’Neil A, McKenzie DP, Zavarsek S, et al. Evaluating the impact of depression, anxiety & autonomic function on health related quality of life, vocational functioning and health care utilisation in acute coronary syndrome patients: the ADVENT study protocol. BMC Cardiovasc Disord 2013;13. https://doi.org/10.1186/1471-2261-13-103.
- Vaara S, Nieminen MS, Lokki ML, Perola M, Pussinen PJ, Allonen J, et al. Cohort profile: the Corogene study. Int J Epidemiol 2012;41:1265-71. https://doi.org/10.1093/ije/dyr090.
- Arnold SV, Chan PS, Jones PG, Decker C, Buchanan DM, Krumholz HM, et al. Translational Research Investigating Underlying Disparities in Acute Myocardial Infarction Patients’ Health Status (TRIUMPH): design and rationale of a prospective multicenter registry. Circ Cardiovasc Qual Outcomes 2011;4:467-76. https://doi.org/10.1161/CIRCOUTCOMES.110.960468.
- Rezende PC, Garcia RM, Uchida AH, Costa LM, Scudeler TL, Melo RM, et al. Hypotheses, rationale, design, and methods for evaluation of ischemic preconditioning assessed by sequential exercise tests in diabetic and non-diabetic patients with stable coronary artery disease – a prospective study. BMC Cardiovasc Disord 2013;13. https://doi.org/10.1186/1471-2261-13-117.
- Gottesman O, Kuivaniemi H, Tromp G, Faucett WA, Li R, Manolio TA, et al. The Electronic Medical Records and Genomics (eMERGE) Network: past, present, and future. Genet Med 2013;15:761-71. https://doi.org/10.1038/gim.2013.72.
- Roden DM, Pulley JM, Basford MA, Bernard GR, Clayton EW, Balser JR, et al. Development of a large-scale de-identified DNA biobank to enable personalized medicine. Clin Pharmacol Ther 2008;84:362-9. https://doi.org/10.1038/clpt.2008.89.
- Deloukas P, Kanoni S, Willenborg C, Farrall M, Assimes TL, Thompson JR, et al. Large-scale association analysis identifies new risk loci for coronary artery disease. Nat Genet 2013;45:25-33. https://doi.org/10.1038/ng.2480.
- EuroQol Group . EuroQol – a new facility for the measurement of health-related quality of life. Health Policy 1990;16:199-208. https://doi.org/10.1016/0168-8510(90)90421-9.
- Rose GA. The diagnosis of ischaemic heart pain and intermittent claudication in field surveys. Bull World Health Organ 1962;27:645-58.
- Campeau L. Letter: grading of angina pectoris. Circulation 1976;54:522-3.
- Spitzer RL, Kroenke K, Williams JB, Löwe B. A brief measure for assessing generalized anxiety disorder: the GAD-7. Arch Intern Med 2006;166:1092-7. https://doi.org/10.1001/archinte.166.10.1092.
- Kroenke K, Spitzer RL, Williams JB. The PHQ-9: validity of a brief depression severity measure. J Gen Intern Med 2001;16:606-13. https://doi.org/10.1046/j.1525-1497.2001.016009606.x.
- Spertus JA, Winder JA, Dewhurst TA, Deyo RA, Prodzinski J, McDonell M, et al. Development and evaluation of the Seattle Angina Questionnaire: a new functional status measure for coronary artery disease. J Am Coll Cardiol 1995;25:333-41. https://doi.org/10.1016/0735-1097(94)00397-9.
- A New Pathway for the Regulation and Governance of Health Research. London: Academy of Medical Sciences; 2011.
- Sandy J, Kilpatrick N, Persson M, Bessel A, Waylen A, Ness A, et al. Why are multi-centre clinical observational studies still so difficult to run?. Br Dent J 2011;211:59-61. https://doi.org/10.1038/sj.bdj.2011.570.
- National Human Genome Research Institute . Electronic Medical Records and Genomics (eMERGE) Network n.d. www.genome.gov/27540473/ (accessed 4 December 2016).
- Danish National Biobank n.d. www.biobankdenmark.dk/ (accessed 4 December 2016).
- Rapsomaniki E, Stogiannis D, Emmas C, Chung S, Pasea L, Denaxas S, et al. Health Outcomes in Patients With Stable Coronary Artery Disease Following Myocardial Infarction; Construction of a PEGASUS-TIMI-54 Like Population in UK Linked Electronic Health Records n.d.
- Pujades-Rodriguez M, Rapsomaniki E, George J, Shah AD, Denaxas S, West R, et al. Heterogeneous associations between smoking and a wide range of initial presentations of cardiovascular disease in 1,937,360 people: lifetime risks and implications for risk prediction. Int J Epidemiol 2015;44:129-41. https://doi.org/10.1093/ije/dyu218.
- Montalescot G, Sechtem U, Achenbach S, Andreotti F, Arden C, Budaj A, et al. 2013 ESC guidelines on the management of stable coronary artery disease: the Task Force on the management of stable coronary artery disease of the European Society of Cardiology. Eur Heart J 2013;34:2949-3003. https://doi.org/10.1093/eurheartj/eht296.
- Sabatine MS, Morrow DA, Giugliano RP, Burton PB, Murphy SA, McCabe CH, et al. Association of hemoglobin levels with clinical outcomes in acute coronary syndromes. Circulation 2005;111:2042-9. https://doi.org/10.1161/01.CIR.0000162477.70955.5F.
- Arant CB, Wessel TR, Olson MB, Bairey Merz CN, Sopko G, Rogers WJ, et al. Hemoglobin level is an independent predictor for adverse cardiovascular outcomes in women undergoing evaluation for chest pain: results from the National Heart, Lung, and Blood Institute Women’s Ischemia Syndrome Evaluation Study. J Am Coll Cardiol 2004;43:2009-14. https://doi.org/10.1016/j.jacc.2004.01.038.
- Diaz A, Bourassa MG, Guertin MC, Tardif JC. Long-term prognostic value of resting heart rate in patients with suspected or proven coronary artery disease. Eur Heart J 2005;26:967-74. https://doi.org/10.1093/eurheartj/ehi190.
- Fox K, Ford I, Steg PG, Tendera M, Ferrari R. BEAUTIFUL Investigators . Ivabradine for patients with stable coronary artery disease and left-ventricular systolic dysfunction (BEAUTIFUL): a randomised, double-blind, placebo-controlled trial. Lancet 2008;372:807-16. https://doi.org/10.1016/S0140-6736(08)61170-8.
- Fox K, Ford I, Steg PG, Tendera M, Robertson M, Ferrari R. BEAUTIFUL investigators . Heart rate as a prognostic risk factor in patients with coronary artery disease and left-ventricular systolic dysfunction (BEAUTIFUL): a subgroup analysis of a randomised controlled trial. Lancet 2008;372:817-21. https://doi.org/10.1016/S0140-6736(08)61171-X.
- Kolloch R, Legler UF, Champion A, Cooper-Dehoff RM, Handberg E, Zhou Q, et al. Impact of resting heart rate on outcomes in hypertensive patients with coronary artery disease: findings from the INternational VErapamil-SR/trandolapril STudy (INVEST). Eur Heart J 2008;29:1327-34. https://doi.org/10.1093/eurheartj/ehn123.
- Jensen MT, Kaiser C, Sandsten KE, Alber H, Wanitschek M, Iversen A, et al. Heart rate at discharge and long-term prognosis following percutaneous coronary intervention in stable and acute coronary syndromes – results from the BASKET PROVE trial. Int J Cardiol 2013;168:3802-6. https://doi.org/10.1016/j.ijcard.2013.06.034.
- Parodi G, Bellandi B, Valenti R, Memisha G, Giuliani G, Velluzzi S, et al. Heart rate as an independent prognostic risk factor in patients with acute myocardial infarction undergoing primary percutaneous coronary intervention. Atherosclerosis 2010;211:255-9. https://doi.org/10.1016/j.atherosclerosis.2010.02.017.
- Borer JS, Fox K, Jaillon P, Lerebours G. Ivabradine Investigators Group . Antianginal and antiischemic effects of ivabradine, an I(f) inhibitor, in stable angina: a randomized, double-blind, multicentered, placebo-controlled trial. Circulation 2003;107:817-23. https://doi.org/10.1161/01.CIR.0000048143.25023.87.
- Bangalore S, Steg G, Deedwania P, Crowley K, Eagle KA, Goto S, et al. β-Blocker use and clinical outcomes in stable outpatients with and without coronary artery disease. JAMA 2012;308:1340-9. https://doi.org/10.1001/jama.2012.12559.
- Anselmino M, Ohrvik J, Rydén L. Euro Heart Survey Investigators . Resting heart rate in patients with stable coronary artery disease and diabetes: a report from the euro heart survey on diabetes and the heart. Eur Heart J 2010;31:3040-5. https://doi.org/10.1093/eurheartj/ehq368.
- Ho JE, Larson MG, Ghorbani A, Cheng S, Coglianese EE, Vasan RS, et al. Long-term cardiovascular risks associated with an elevated heart rate: the Framingham Heart Study. J Am Heart Assoc 2014;3. https://doi.org/10.1161/JAHA.113.000668.
- Hartaigh B, Bosch JA, Pilz S, Loerbroks A, Kleber ME, Grammer TB, et al. Influence of resting heart rate on mortality in patients undergoing coronary angiography (from the Ludwigshafen Risk and Cardiovascular Health [LURIC] study). Am J Cardiol 2012;110:515-20. https://doi.org/10.1016/j.amjcard.2012.03.050.
- Pepine CJ, Cohn PF, Deedwania PC, Gibson RS, Handberg E, Hill JA, et al. Effects of treatment on outcome in mildly symptomatic patients with ischemia during daily life. The Atenolol Silent Ischemia Study (ASIST). Circulation 1994;90:762-8. https://doi.org/10.1161/01.CIR.90.2.762.
- Jonas M, Reicher-Reiss H, Boyko V, Shotan A, Mandelzweig L, Goldbourt U, et al. Usefulness of beta-blocker therapy in patients with non-insulin-dependent diabetes mellitus and coronary artery disease. Bezafibrate Infarction Prevention (BIP) Study Group. Am J Cardiol 1996;77:1273-7. https://doi.org/10.1016/S0002-9149(96)00191-9.
- Frank M, Aboyans V, Le Guyader A, Orsel I, Lacroix P, Cornu E, et al. Usefulness of postoperative heart rate as an independent predictor of mortality after coronary bypass grafting. Am J Cardiol 2010;106:958-62. https://doi.org/10.1016/j.amjcard.2010.05.026.
- Fox K, Ford I, Steg PG, Tardif JC, Tendera M, Ferrari R. SIGNIFY Investigators . Ivabradine in stable coronary artery disease without clinical heart failure. N Engl J Med 2014;371:1091-9. https://doi.org/10.1056/NEJMoa1406430.
- Swedberg K, Komajda M, Böhm M, Borer JS, Ford I, Dubost-Brama A, et al. Ivabradine and outcomes in chronic heart failure (SHIFT): a randomised placebo-controlled study. Lancet 2010;376:875-85. https://doi.org/10.1016/S0140-6736(10)61198-1.
- Fox K, Borer JS, Camm AJ, Danchin N, Ferrari R, Lopez Sendon JL, et al. Resting heart rate in cardiovascular disease. J Am Coll Cardiol 2007;50:823-30. https://doi.org/10.1016/j.jacc.2007.04.079.
- Kalman JM, Munawar M, Howes LG, Louis WJ, Buxton BF, Gutteridge G, et al. Atrial fibrillation after coronary artery bypass grafting is associated with sympathetic activation. Ann Thorac Surg 1995;60:1709-15. https://doi.org/10.1016/0003-4975(95)00718-0.
- Leitch JW, Thomson D, Baird DK, Harris PJ. The importance of age as a predictor of atrial fibrillation and flutter after coronary artery bypass grafting. J Thorac Cardiovasc Surg 1990;100:338-42.
- White HD, Antman EM, Glynn MA, Collins JJ, Cohn LH, Shemin RJ, et al. Efficacy and safety of timolol for prevention of supraventricular tachyarrhythmias after coronary artery bypass surgery. Circulation 1984;70:479-84. https://doi.org/10.1161/01.CIR.70.3.479.
- den Hoed M, Eijgelsheim M, Esko T, Brundel BJ, Peal DS, Evans DM, et al. Identification of heart rate-associated loci and their effects on cardiac conduction and rhythm disorders. Nat Genet 2013;45:621-31. https://doi.org/10.1038/ng.2610.
- Kyzas PA, Loizou KT, Ioannidis JP. Selective reporting biases in cancer prognostic factor studies. J Natl Cancer Inst 2005;97:1043-55. https://doi.org/10.1093/jnci/dji184.
- Riley RD, Abrams KR, Sutton AJ, Lambert PC, Jones DR, Heney D, et al. Reporting of prognostic markers: current problems and development of guidelines for evidence-based practice in the future. Br J Cancer 2003;88:1191-8. https://doi.org/10.1038/sj.bjc.6600886.
- Pearson TA, Mensah GA, Alexander RW, Anderson JL, Cannon RO, Criqui M, et al. Markers of inflammation and cardiovascular disease: application to clinical and public health practice: a statement for healthcare professionals from the Centers for Disease Control and Prevention and the American Heart Association. Circulation 2003;107:499-511. https://doi.org/10.1161/01.CIR.0000052939.59093.45.
- Fox K, Garcia MA, Ardissino D, Buszman P, Camici PG, Crea F, et al. Guidelines on the management of stable angina pectoris: executive summary: The Task Force on the Management of Stable Angina Pectoris of the European Society of Cardiology. Eur Heart J 2006;27:1341-81. https://doi.org/10.1093/eurheartj/ehl001.
- Niccoli G, Ferrante G, Mongiardo R, Perfetti M, Belloni F, Burzotta F, et al. Predictive value of preintervention C-reactive protein on clinical outcome after directional coronary atherectomy followed by stent implantation. Cardiovasc Revasc Med 2007;8:156-60. https://doi.org/10.1016/j.carrev.2007.02.002.
- Palmerini T, Marzocchi A, Marrozzini C, Ortolani P, Saia F, Bacchi-Reggiani L, et al. Preprocedural levels of C-reactive protein and leukocyte counts predict 9-month mortality after coronary angioplasty for the treatment of unprotected left main coronary artery stenosis. Circulation 2005;112:2332-8. https://doi.org/10.1161/CIRCULATIONAHA.105.551648.
- Ikonomidis I, Lekakis J, Revela I, Andreotti F, Nihoyannopoulos P. Increased circulating C-reactive protein and macrophage-colony stimulating factor are complementary predictors of long-term outcome in patients with chronic coronary artery disease. Eur Heart J 2005;26:1618-24. https://doi.org/10.1093/eurheartj/ehi192.
- Jánoskuti L, Förhécz Z, Hosszúfalusi N, Kleiber M, Walentin S, Bálint O, et al. High levels of C-reactive protein with low total cholesterol concentrations additively predict all-cause mortality in patients with coronary artery disease. Eur J Clin Invest 2005;35:104-11. https://doi.org/10.1111/j.1365-2362.2005.01465.x.
- Karha J, Bavry AA, Rajagopal V, Henderson MR, Ellis SG, Brener SJ. Relation of C-reactive protein level and long-term risk of death or myocardial infarction following percutaneous coronary intervention with a sirolimus-eluting stent. Am J Cardiol 2006;98:616-18. https://doi.org/10.1016/j.amjcard.2006.03.039.
- Inoue T, Kotooka N, Morooka T, Komoda H, Uchida T, Aso Y, et al. High molecular weight adiponectin as a predictor of long-term clinical outcome in patients with coronary artery disease. Am J Cardiol 2007;100:569-74. https://doi.org/10.1016/j.amjcard.2007.03.062.
- Leu HB, Lin CP, Lin WT, Wu TC, Chen JW. Risk stratification and prognostic implication of plasma biomarkers in nondiabetic patients with stable coronary artery disease: the role of high-sensitivity C-reactive protein. Chest 2004;126:1032-9. https://doi.org/10.1378/chest.126.4.1032.
- Palmerini T, Marzocchi A, Marrozzini C, Reggiani LB, Savini C, Marinelli G, et al. Preoperative C-reactive protein levels predict 9-month mortality after coronary artery bypass grafting surgery for the treatment of left main coronary artery stenosis. Eur J Cardiothorac Surg 2007;31:685-90. https://doi.org/10.1016/j.ejcts.2006.12.029.
- Kwaijtaal M, van Diest R, Bär FW, van der Ven AJ, Bruggeman CA, de Baets MH, et al. Inflammatory markers predict late cardiac events in patients who are exhausted after percutaneous coronary intervention. Atherosclerosis 2005;182:341-8. https://doi.org/10.1016/j.atherosclerosis.2005.02.022.
- Retterstol L, Eikvar L, Bohn M, Bakken A, Erikssen J, Berg K. C-reactive protein predicts death in patients with previous premature myocardial infarction – a 10 year follow-up study. Atherosclerosis 2002;160:433-40. https://doi.org/10.1016/S0021-9150(01)00595-0.
- Veselka J, Procházková S, Duchonová R, Homolová I, Tesar D. Relationship of C-reactive protein to adverse cardiovascular events in patients treated by percutaneous coronary intervention for stable angina pectoris. Int Heart J 2005;46:195-204. https://doi.org/10.1536/ihj.46.195.
- Park DW, Lee CW, Yun SC, Kim YH, Hong MK, Kim JJ, et al. Prognostic impact of preprocedural C reactive protein levels on 6-month angiographic and 1-year clinical outcomes after drug-eluting stent implantation. Heart 2007;93:1087-92. https://doi.org/10.1136/hrt.2006.099762.
- Dai DF, Hwang JJ, Lin JL, Lin JW, Hsu CN, Lin CM, et al. Joint effects of N-terminal pro-B-type-natriuretic peptide and C-reactive protein vs angiographic severity in predicting major adverse cardiovascular events and clinical restenosis after coronary angioplasty in patients with stable coronary artery disease. Circ J 2008;72:1316-23. https://doi.org/10.1253/circj.72.1316.
- Crea F, Monaco C, Lanza GA, Maggi E, Ginnetti F, Cianflone D, et al. Inflammatory predictors of mortality in the Scandinavian Simvastatin Survival Study. Clin Cardiol 2002;25:461-6. https://doi.org/10.1002/clc.4960251005.
- Rahel BM, Visseren FL, Suttorp MJ, Plokker TH, Kelder JC, de Jongh BM, et al. Preprocedural serum levels of acute-phase reactants and prognosis after percutaneous coronary intervention. Cardiovasc Res 2003;60:136-40. https://doi.org/10.1016/S0008-6363(03)00355-9.
- Delhaye C, Sudre A, Lemesle G, Maréchaux S, Broucqsault D, Hennache B, et al. Preprocedural high-sensitivity C-reactive protein predicts death or myocardial infarction but not target vessel revascularization or stent thrombosis after percutaneous coronary intervention. Cardiovasc Revasc Med 2009;10:144-50. https://doi.org/10.1016/j.carrev.2009.01.005.
- Susen S, Sautière K, Mouquet F, Cuilleret F, Chmaït A, McFadden EP, et al. Serum hepatocyte growth factor levels predict long-term clinical outcome after percutaneous coronary revascularization. Eur Heart J 2005;26:2387-95. https://doi.org/10.1093/eurheartj/ehi436.
- Huang W, Chen QW, Lei H, Deng W, Ke DZ. Predictive value of fibrinogen and high-sensitivity C-reaction protein for cardiovascular events in patients with stable coronary artery disease. Zhonghua Xin Xue Guan Bing Za Zhi 2006;34:718-21.
- Huang PH, Lu TM, Wu TC, Lin FY, Chen YH, Chen JW, et al. Usefulness of combined high-sensitive C-reactive protein and N-terminal-probrain natriuretic peptide for predicting cardiovascular events in patients with suspected coronary artery disease. Coron Artery Dis 2008;19:187-93. https://doi.org/10.1097/MCA.0b013e3282f399e2.
- Harb TS, Zareba W, Moss AJ, Ridker PM, Marder VJ, Rifai N, et al. Association of C-reactive protein and serum amyloid A with recurrent coronary events in stable patients after healing of acute myocardial infarction. Am J Cardiol 2002;89:216-21. https://doi.org/10.1016/S0002-9149(01)02204-4.
- Wolk R, Berger P, Lennon RJ, Brilakis ES, Johnson BD, Somers VK. Plasma leptin and prognosis in patients with established coronary atherosclerosis. J Am Coll Cardiol 2004;44:1819-24. https://doi.org/10.1016/j.jacc.2004.07.050.
- Saleh N, Svane B, Hansson LO, Jensen J, Nilsson T, Danielsson O, et al. Response of serum C-reactive protein to percutaneous coronary intervention has prognostic value. Clin Chem 2005;51:2124-30. https://doi.org/10.1373/clinchem.2005.048082.
- West MJ, Nestel PJ, Kirby AC, Schnabel R, Sullivan D, Simes RJ, et al. The value of N-terminal fragment of brain natriuretic peptide and tissue inhibitor of metalloproteinase-1 levels as predictors of cardiovascular outcome in the LIPID study. Eur Heart J 2008;29:923-31. https://doi.org/10.1093/eurheartj/ehn007.
- Rothenbacher D, Koenig W, Brenner H. Comparison of N-terminal pro-B-natriuretic peptide, C-reactive protein, and creatinine clearance for prognosis in patients with known coronary heart disease. Arch Intern Med 2006;166:2455-60. https://doi.org/10.1001/archinte.166.22.2455.
- Brilakis ES, McConnell JP, Lennon RJ, Elesber AA, Meyer JG, Berger PB. Association of lipoprotein-associated phospholipase A2 levels with coronary artery disease risk factors, angiographic coronary artery disease, and major adverse events at follow-up. Eur Heart J 2005;26:137-44. https://doi.org/10.1093/eurheartj/ehi010.
- Kip KE, Marroquin OC, Shaw LJ, Arant CB, Wessel TR, Olson MB, et al. Global inflammation predicts cardiovascular risk in women: a report from the Women’s Ischemia Syndrome Evaluation (WISE) study. Am Heart J 2005;150:900-6. https://doi.org/10.1016/j.ahj.2005.02.002.
- Huang W, Lei H, Liu Q, Ma KH, Qin S, Chang J, et al. Combination of C-reactive protein and cardiac troponin I for predicting adverse cardiac events after sirolimus-eluting stent implantation. Coron Artery Dis 2009;20:245-50. https://doi.org/10.1097/MCA.0b013e32832a1950.
- Shlipak MG, Ix JH, Bibbins-Domingo K, Lin F, Whooley MA. Biomarkers to predict recurrent cardiovascular disease: the Heart and Soul Study. Am J Med 2008;121:50-7. https://doi.org/10.1016/j.amjmed.2007.06.030.
- Fathi RB, Gurm HS, Chew DP, Gupta R, Bhatt DL, Ellis SG. The interaction of vascular inflammation and chronic kidney disease for the prediction of long-term death after percutaneous coronary intervention. Am Heart J 2005;150:1190-7. https://doi.org/10.1016/j.ahj.2005.01.031.
- Anderson JL, Muhlestein JB, Horne BD, Carlquist JF, Bair TL, Madsen TE, et al. Plasma homocysteine predicts mortality independently of traditional risk factors and C-reactive protein in patients with angiographically defined coronary artery disease. Circulation 2000;102:1227-32. https://doi.org/10.1161/01.CIR.102.11.1227.
- Morrow DA, de Lemos JA, Sabatine MS, Wiviott SD, Blazing MA, Shui A, et al. Clinical relevance of C-reactive protein during follow-up of patients with acute coronary syndromes in the Aggrastat-to-Zocor Trial. Circulation 2006;114:281-8. https://doi.org/10.1161/CIRCULATIONAHA.106.628909.
- Kangasniemi OP, Biancari F, Luukkonen J, Vuorisalo S, Satta J, Pokela R, et al. Preoperative C-reactive protein is predictive of long-term outcome after coronary artery bypass surgery. Eur J Cardiothorac Surg 2006;29:983-5. https://doi.org/10.1016/j.ejcts.2006.02.022.
- Chan AW, Bhatt DL, Chew DP, Reginelli J, Schneider JP, Topol EJ, et al. Relation of inflammation and benefit of statins after percutaneous coronary interventions. Circulation 2003;107:1750-6. https://doi.org/10.1161/01.CIR.0000060541.18923.E9.
- Eggers KM, Lagerqvist B, Venge P, Wallentin L, Lindahl B. Prognostic value of biomarkers during and after non-ST-segment elevation acute coronary syndrome. J Am Coll Cardiol 2009;54:357-64. https://doi.org/10.1016/j.jacc.2009.03.056.
- Dai DF, Lin JW, Kao JH, Hsu CN, Chiang FT, Lin JL, et al. The effects of metabolic syndrome versus infectious burden on inflammation, severity of coronary atherosclerosis, and major adverse cardiovascular events. J Clin Endocrinol Metab 2007;92:2532-7. https://doi.org/10.1210/jc.2006-2428.
- Khor LL, Muhlestein JB, Carlquist JF, Horne BD, Bair TL, Maycock CA, et al. Intermountain Heart Collaborative Study Group . Sex- and age-related differences in the prognostic value of C-reactive protein in patients with angiographic coronary artery disease. Am J Med 2004;117:657-64. https://doi.org/10.1016/j.amjmed.2004.06.021.
- Blankenberg S, McQueen MJ, Smieja M, Pogue J, Balion C, Lonn E, et al. Comparative impact of multiple biomarkers and N-Terminal pro-brain natriuretic peptide in the context of conventional risk factors for the prediction of recurrent cardiovascular events in the Heart Outcomes Prevention Evaluation (HOPE) Study. Circulation 2006;114:201-8. https://doi.org/10.1161/CIRCULATIONAHA.105.590927.
- Bogaty P, Boyer L, Simard S, Dauwe F, Dupuis R, Verret B, et al. Clinical utility of C-reactive protein measured at admission, hospital discharge, and 1 month later to predict outcome in patients with acute coronary disease. The RISCA (recurrence and inflammation in the acute coronary syndromes) study. J Am Coll Cardiol 2008;51:2339-46. https://doi.org/10.1016/j.jacc.2008.03.019.
- Artieda M, Cenarro A, Gañán A, Lukic A, Moreno E, Puzo J, et al. Serum chitotriosidase activity, a marker of activated macrophages, predicts new cardiovascular events independently of C-reactive protein. Cardiology 2007;108:297-306. https://doi.org/10.1159/000099099.
- Dibra A, Mehilli J, Braun S, Hadamitzky M, Baum H, Dirschinger J, et al. Association between C-reactive protein levels and subsequent cardiac events among patients with stable angina treated with coronary artery stenting. Am J Med 2003;114:715-22. https://doi.org/10.1016/S0002-9343(03)00183-9.
- van der Harst P, Voors AA, Volbeda M, Buikema H, van Veldhuisen DJ, van Gilst WH. Usefulness of preoperative C-reactive protein and soluble intercellular adhesion molecule-1 level for predicting future cardiovascular events after coronary artery bypass grafting. Am J Cardiol 2006;97:1697-701. https://doi.org/10.1016/j.amjcard.2006.01.030.
- Blankenberg S, Rupprecht HJ, Bickel C, Peetz D, Hafner G, Tiret L, et al. Circulating cell adhesion molecules and death in patients with coronary artery disease. Circulation 2001;104:1336-42. https://doi.org/10.1161/hc3701.095949.
- Speidl WS, Graf S, Hornykewycz S, Nikfardjam M, Niessner A, Zorn G, et al. High-sensitivity C-reactive protein in the prediction of coronary events in patients with premature coronary artery disease. Am Heart J 2002;144:449-55. https://doi.org/10.1067/mhj.2002.124353.
- Kinjo K, Sato H, Sakata Y, Nakatani D, Mizuno H, Shimizu M, et al. Relation of C-reactive protein and one-year survival after acute myocardial infarction with versus without statin therapy. Am J Cardiol 2005;96:617-21. https://doi.org/10.1016/j.amjcard.2005.04.030.
- Espinola-Klein C, Rupprecht HJ, Bickel C, Lackner K, Schnabel R, Munzel T, et al. AtheroGene Investigators . Inflammation, atherosclerotic burden and cardiovascular prognosis. Atherosclerosis 2007;195:e126-34. https://doi.org/10.1016/j.atherosclerosis.2007.01.021.
- Hoffmeister A, Rothenbacher D, Kunze M, Brenner H, Koenig W. Prognostic value of inflammatory markers alone and in combination with blood lipids in patients with stable coronary artery disease. Eur J Intern Med 2005;16:47-52. https://doi.org/10.1016/j.ejim.2004.09.008.
- Momiyama Y, Kawaguchi A, Kajiwara I, Ohmori R, Okada K, Saito I, et al. Prognostic value of plasma high-sensitivity C-reactive protein levels in Japanese patients with stable coronary artery disease: the Japan NCVC-Collaborative Inflammation Cohort (JNIC) Study. Atherosclerosis 2009;207:272-6. https://doi.org/10.1016/j.atherosclerosis.2009.04.015.
- Sabatine MS, Morrow DA, Jablonski KA, Rice MM, Warnica JW, Domanski MJ, et al. Prognostic significance of the Centers for Disease Control/American Heart Association high-sensitivity C-reactive protein cut points for cardiovascular and other outcomes in patients with stable coronary artery disease. Circulation 2007;115:1528-36. https://doi.org/10.1161/CIRCULATIONAHA.106.649939.
- Haim M, Benderly M, Tanne D, Matas Z, Boyko V, Fisman EZ, et al. C-reactive protein, bezafibrate, and recurrent coronary events in patients with chronic coronary heart disease. Am Heart J 2007;154:1095-101. https://doi.org/10.1016/j.ahj.2007.07.026.
- Palazzuoli A, Deckers J, Calabrò A, Campagna MS, Nuti R, Pastorelli M, et al. Brain natriuretic peptide and other risk markers for outcome assessment in patients with non-ST-elevation coronary syndromes and preserved systolic function. Am J Cardiol 2006;98:1322-8. https://doi.org/10.1016/j.amjcard.2006.06.023.
- Lee KW, Hill JS, Walley KR, Frohlich JJ. Relative value of multiple plasma biomarkers as risk factors for coronary artery disease and death in an angiography cohort. CMAJ 2006;174:461-6. https://doi.org/10.1503/cmaj.050880.
- Brodov Y, Behar S, Goldenberg I, Boyko V, Chouraqui P. Usefulness of combining serum uric acid and C-reactive protein for risk stratification of patients with coronary artery disease (Bezafibrate Infarction Prevention [BIP] study). Am J Cardiol 2009;104:194-8. https://doi.org/10.1016/j.amjcard.2009.03.017.
- Minoretti P, Falcone C, Calcagnino M, Emanuele E, Buzzi MP, Coen E, et al. Prognostic significance of plasma osteopontin levels in patients with chronic stable angina. Eur Heart J 2006;27:802-7. https://doi.org/10.1093/eurheartj/ehi730.
- McPherson R, Pertsemlidis A, Kavaslar N, Stewart A, Roberts R, Cox DR, et al. A common allele on chromosome 9 associated with coronary heart disease. Science 2007;316:1488-91. https://doi.org/10.1126/science.1142447.
- Kannel WB, Dawber TR, Kagan A, Revotskie N, Stokes J. Factors of risk in the development of coronary heart disease – six year follow-up experience. The Framingham Study. Ann Intern Med 1961;55:33-50. https://doi.org/10.7326/0003-4819-55-1-33.
- Conroy RM, Pyörälä K, Fitzgerald AP, Sans S, Menotti A, De Backer G, et al. Estimation of ten-year risk of fatal cardiovascular disease in Europe: the SCORE project. Eur Heart J 2003;24:987-1003. https://doi.org/10.1016/S0195-668X(03)00114-3.
- Hippisley-Cox J, Coupland C, Vinogradova Y, Robson J, May M, Brindle P. Derivation and validation of QRISK, a new cardiovascular disease risk score for the United Kingdom: prospective open cohort study. BMJ 2007;335. https://doi.org/10.1136/bmj.39261.471806.55.
- Rothwell PM, Howard SC, Dolan E, O’Brien E, Dobson JE, Dahlöf B, et al. Prognostic significance of visit-to-visit variability, maximum systolic blood pressure, and episodic hypertension. Lancet 2010;375:895-90. https://doi.org/10.1016/S0140-6736(10)60308-X.
- Crowther MJ, Lambert PC, Abrams KR. Adjusting for measurement error in baseline prognostic biomarkers included in a time-to-event analysis: a joint modelling approach. BMC Med Res Methodol 2013;13. https://doi.org/10.1186/1471-2288-13-146.
- Jutte DP, Roos LL, Brownell MD. Administrative record linkage as a tool for public health research. Annu Rev Public Health 2011;32:91-108. https://doi.org/10.1146/annurev-publhealth-031210-100700.
- Taylor JM, Park YF, Ankerst DP, Proust-Lima C, Williams S, Kestin LF, et al. Real-time individual predictions of prostate cancer recurrence using joint models. Biometrics 2013;69:206-13. https://doi.org/10.1111/j.1541-0420.2012.01823.x.
- Wulfsohn MS, Tsiatis AA. A joint model for survival and longitudinal data measured with error. Biometrics 1997;53:330-9. https://doi.org/10.2307/2533118.
- Lawrence Gould A, Boye ME, Crowther MJ, Ibrahim JG, Quartey G, Micallef S, et al. Joint modeling of survival and longitudinal non-survival data: current methods and issues. Report of the DIA Bayesian joint modeling working group. Stat Med 2015;34:2181-95. https://doi.org/10.1002/sim.6141.
- Henderson R, Diggle P, Dobson A. Joint modelling of longitudinal measurements and event time data. Biostatistics 2000;1:465-80. https://doi.org/10.1093/biostatistics/1.4.465.
- Crowther MJ, Abrams KR, Lambert PC. Joint modeling of longitudinal and survival data. Stata J 2013;13:165-84.
- Rizopoulos D. Dynamic predictions and prospective accuracy in joint models for longitudinal and time-to-event data. Biometrics 2011;67:819-29. https://doi.org/10.1111/j.1541-0420.2010.01546.x.
- Wierzbicki AS, Hardman TC, Viljoen A. New lipid-lowering drugs: an update. Int J Clin Pract 2012;66:270-80. https://doi.org/10.1111/j.1742-1241.2011.02867.x.
- Bonaca MP, Bhatt DL, Braunwald E, Cohen M, Steg PG, Storey RF, et al. Design and rationale for the Prevention of Cardiovascular Events in Patients With Prior Heart Attack Using Ticagrelor Compared to Placebo on a Background of Aspirin-Thrombolysis in Myocardial Infarction 54 (PEGASUS-TIMI 54) trial. Am Heart J 2014;167:437-44.e5. https://doi.org/10.1016/j.ahj.2013.12.020.
- Fihn SD, Gardin JM, Abrams J, Berra K, Blankenship JC, Dallas AP, et al. 2012 ACCF/AHA/ACP/AATS/PCNA/SCAI/STS Guideline for the diagnosis and management of patients with stable ischemic heart disease: a report of the American College of Cardiology Foundation/American Heart Association Task Force on Practice Guidelines, and the American College of Physicians, American Association for Thoracic Surgery, Preventive Cardiovascular Nurses Association, Society for Cardiovascular Angiography and Interventions, and Society of Thoracic Surgeons. J Am Coll Cardiol 2012;60:e44-e164. https://doi.org/10.1016/j.jacc.2012.07.013.
- Myocardial Infarction: Secondary Prevention. London: NICE; 2011.
- Myocardial Infarction: Cardiac Rehabilitation and Prevention of Further Cardiovascular Disease. London: NICE; 2013.
- Roger VL, Go AS, Lloyd-Jones DM, Benjamin EJ, Berry JD, Borden WB, et al. Executive summary: heart disease and stroke statistics – 2012 update: a report from the American Heart Association. Circulation 2012;125:188-97. https://doi.org/10.1161/CIR.0b013e3182456d46.
- Henderson RA, O’Flynn N. Guideline Development Group . Management of stable angina: summary of NICE guidance. Heart 2012;98:500-7. https://doi.org/10.1136/heartjnl-2011-301436.
- Hemingway H, Crook AM, Feder G, Banerjee S, Dawson JR, Magee P, et al. Underuse of coronary revascularization procedures in patients considered appropriate candidates for revascularization. N Engl J Med 2001;344:645-54. https://doi.org/10.1056/NEJM200103013440906.
- Briggs A, Mihaylova B, Sculpher M, Hall A, Wolstenholme J, Simoons M, et al. Cost effectiveness of perindopril in reducing cardiovascular events in patients with stable coronary artery disease using data from the EUROPA study. Heart 2007;93:1081-6. https://doi.org/10.1136/hrt.2005.086728.
- Reed SD, Radeva JI, Weinfurt KP, McMurray JJ, Pfeffer MA, Velazquez EJ, et al. Resource use, costs, and quality of life among patients in the multinational Valsartan in Acute Myocardial Infarction Trial (VALIANT). Am Heart J 2005;150:323-9. https://doi.org/10.1016/j.ahj.2004.08.037.
- Lewsey JD, Lawson KD, Ford I, Fox KA, Ritchie LD, Tunstall-Pedoe H, et al. A cardiovascular disease policy model that predicts life expectancy taking into account socioeconomic deprivation. Heart 2015;101:201-8. https://doi.org/10.1136/heartjnl-2014-305637.
- Unal B, Capewell S, Critchley JA. Coronary heart disease policy models: a systematic review. BMC Public Health 2006;6. https://doi.org/10.1186/1471-2458-6-213.
- Barton P, Andronis L, Briggs A, McPherson K, Capewell S. Effectiveness and cost effectiveness of cardiovascular disease prevention in whole populations: modelling study. BMJ 2011;343. https://doi.org/10.1136/bmj.d4044.
- Curtis L. Unit Costs of Health and Social Care 2012. Canterbury: Personal Social Services Research Unit, University of Kent; 2012.
- NHS Reference Costs: Financial Year 2011 to 2012. London: Department of Health; 2012.
- Prescription Cost Analysis – England, 2012. Leeds: Health and Social Care Information Centre; 2012.
- Putter H, Fiocco M, Geskus RB. Tutorial in biostatistics: competing risks and multi-state models. Stat Med 2007;26:2389-430. https://doi.org/10.1002/sim.2712.
- Sullivan PW, Slejko JF, Sculpher MJ, Ghushchyan V. Catalogue of EQ-5D scores for the United Kingdom. Med Decis Making 2011;31:800-4. https://doi.org/10.1177/0272989X11401031.
- White IR, Royston P, Wood AM. Multiple imputation using chained equations: issues and guidance for practice. Stat Med 2011;30:377-99. https://doi.org/10.1002/sim.4067.
- Guide to the Methods of Technology Appraisal 2013. London: NICE; 2013.
- Hippisley-Cox J, Vinogradova Y. Trends in Consultation Rates in General Practice 1995 to 2008: Analysis of the QRESEARCH Database. Leeds: Health and Social Care Information Centre; 2009.
- Kasteridis P, Street A, Dolman M, Gallier L, Hudson K, Martin J, et al. The Importance of Multimorbidity in Explaining Utilisation and Costs across Health and Social Care Settings: Evidence from South Somersets Symphony Project. York: Centre for Health Economics, University of York; 2014.
- Chew DP, Huynh LT, Liew D, Astley C, Soman A, Brieger D. Potential survival gains in the treatment of myocardial infarction. Heart 2009;95:1844-50. https://doi.org/10.1136/hrt.2009.174276.
- Growth of Primary PCI for the Treatment of Heart Attack Patients in England 2008–11. The Role of NHS Improvement and the Cardiac Networks. Leicester: NHS Improvement; 2012.
- Curry LA, Spatz E, Cherlin E, Thompson JW, Berg D, Ting HH, et al. What distinguishes top-performing hospitals in acute myocardial infarction mortality rates? A qualitative study. Ann Intern Med 2011;154:384-90. https://doi.org/10.7326/0003-4819-154-6-201103150-00003.
- Rapsomaniki E, Janzon M, Cohen DJ, Jernberg T, Moore N, Thuresson M, et al. International Comparison of Outcomes Among 140,880 Patients Stable After Acute MI; Real World Evidence from Electronic Health and Administrative Records n.d.
- O’Gara PT, Kushner FG, Ascheim DD, Casey DE, Chung MK, de Lemos JA, et al. 2013 ACCF/AHA guideline for the management of ST-elevation myocardial infarction: a report of the American College of Cardiology Foundation/American Heart Association Task Force on Practice Guidelines. Circulation 2013;127:e362-e425. https://doi.org/10.1161/CIR.0b013e3182742c84.
- Steg PG, James SK, Atar D, Badano LP, Lundqvist CB, Borger MA, et al. ESC Guidelines for the management of acute myocardial infarction in patients presenting with ST-segment elevation. Eur Heart J 2012;33:2569-619. https://doi.org/10.1093/eurheartj/ehs215.
- Spertus JA, Eagle KA, Krumholz HM, Mitchell KR, Normand SL. American College of Cardiology and the American Heart Association Task Force on Performance Measures . American College of Cardiology and American Heart Association methodology for the selection and creation of performance measures for quantifying the quality of cardiovascular care. Circulation 2005;111:1703-12. https://doi.org/10.1161/01.CIR.0000157096.95223.D7.
- Fox KA, Eagle KA, Gore JM, Steg PG, Anderson FA. GRACE and GRACE2 Investigators . The Global Registry of Acute Coronary Events, 1999 to 2009 – GRACE. Heart 2010;96:1095-101. https://doi.org/10.1136/hrt.2009.190827.
- Simms AD, Reynolds S, Pieper K, Baxter PD, Cattle BA, Batin PD, et al. Evaluation of the NICE mini-GRACE risk scores for acute myocardial infarction using the Myocardial Ischaemia National Audit Project (MINAP) 2003–9: National Institute for Cardiovascular Outcomes Research (NICOR). Heart 2013;99:35-40. https://doi.org/10.1136/heartjnl-2012-302632.
- Peterson ED, Roe MT, Rumsfeld JS, Shaw RE, Brindis RG, Fonarow GC, et al. A call to ACTION (Acute Coronary Treatment and Intervention Outcomes Network): a national effort to promote timely clinical feedback and support continuous quality improvement for acute myocardial infarction. Circ Cardiovasc Qual Outcomes 2009;2:491-9. https://doi.org/10.1161/CIRCOUTCOMES.108.847145.
- Messenger JC, Ho KK, Young CH, Slattery LE, Draoui JC, Curtis JP, et al. The National Cardiovascular Data Registry (NCDR) Data Quality Brief: the NCDR Data Quality Program in 2012. J Am Coll Cardiol 2012;60:1484-8. https://doi.org/10.1016/j.jacc.2012.07.020.
- Pfeffer MA, Braunwald E, Moya LA, Basta L, Brown EJ, Cuddy TE, et al. Effect of Captopril on mortality and morbidity in patients with left ventricular dysfunction after myocardial infarction. New Engl J Med 1992;327:669-77. https://doi.org/10.1056/NEJM199209033271001.
- The Global Use of Strategies to Open Occluded Coronary Arteries (GUSTO) IIb investigators . A comparison of recombinant hirudin with heparin for the treatment of acute coronary syndromes. N Engl J Med 1996;335:775-82. https://doi.org/10.1056/NEJM199609123351103.
- Dabbous OH, Anderson FA, Gore JM, Eagle KA, Fox KA, Mehta RH, et al. Outcomes with the use of glycoprotein IIb/IIIa inhibitors in non-ST-segment elevation acute coronary syndromes. Heart 2008;94:159-65. https://doi.org/10.1136/hrt.2006.105783.
- Yusuf S, Flather M, Pogue J, Hunt D, Varigos J, Piegas L, et al. Variations between countries in invasive cardiac procedures and outcomes in patients with suspected unstable angina or myocardial infarction without initial ST elevation. OASIS (Organisation to Assess Strategies for Ischaemic Syndromes) Registry Investigators. Lancet 1998;352:507-14. https://doi.org/10.1016/S0140-6736(97)11162-X.
- Yan AT, Jong P, Yan RT, Tan M, Fitchett D, Chow CM, et al. Clinical trial derived risk model may not generalize to real-world patients with acute coronary syndrome. Am Heart J 2004;148:1020-7. https://doi.org/10.1016/j.ahj.2004.02.014.
- Alpert JS. Are data from clinical registries of any value?. Eur Heart J 2000;21:1399-401. https://doi.org/10.1053/euhj.2000.2265.
- Gitt AK, Bueno H, Danchin N, Fox K, Hochadel M, Kearney P, et al. The role of cardiac registries in evidence-based medicine. Eur Heart J 2010;31:525-9. https://doi.org/10.1093/eurheartj/ehp596.
- McNamara RL. Cardiovascular registry research comes of age. Heart 2010;96:908-10. https://doi.org/10.1136/hrt.2010.198069.
- Carlhed R, Bojestig M, Peterson A, Aberg C, Garmo H, Lindahl B. Quality Improvement in Coronary Care Study Group . Improved clinical outcome after acute myocardial infarction in hospitals participating in a Swedish quality improvement initiative. Circ Cardiovasc Qual Outcomes 2009;2:458-64. https://doi.org/10.1161/CIRCOUTCOMES.108.842146.
- Larsson S, Lawyer P, Garellick G, Lindahl B, Lundström M. Use of 13 disease registries in 5 countries demonstrates the potential to use outcome data to improve health care’s value. Health Aff 2012;31:220-7. https://doi.org/10.1377/hlthaff.2011.0762.
- NHS Improvement . National Roll-Out of Primary PCI for Patients With ST Segment Elevation Myocardial Infarction: An Interim Report 2010.
- Critchley JA, Capewell S. Mortality risk reduction associated with smoking cessation in patients with coronary heart disease: a systematic review. JAMA 2003;290:86-97. https://doi.org/10.1001/jama.290.1.86.
- Ashenden R, Silagy C, Weller D. A systematic review of the effectiveness of promoting lifestyle change in general practice. Fam Pract 1997;14:160-76. https://doi.org/10.1093/fampra/14.2.160.
- Silagy C, Lancaster T, Stead L, Mant D, Fowler G. Nicotine replacement therapy for smoking cessation. Cochrane Database Syst Rev 2004;3. https://doi.org/10.1002/14651858.cd000146.pub2.
- Hughes JR, Stead LF, Hartmann-Boyce J, Cahill K, Lancaster T. Antidepressants for smoking cessation. Cochrane Database Syst Rev 2014;1. https://doi.org/10.1002/14651858.cd000031.pub4.
- Munafò M, Rigotti N, Lancaster T, Stead L, Murphy M. Interventions for smoking cessation in hospitalised patients: a systematic review. Thorax 2001;56:656-63. https://doi.org/10.1136/thorax.56.8.656.
- Ludt S, Petek D, Laux G, van Lieshout J, Campbell SM, Künzi B, et al. Recording of risk-factors and lifestyle counselling in patients at high risk for cardiovascular diseases in European primary care. Eur J Prev Cardiol 2012;19:258-66. https://doi.org/10.1177/1741826711400510.
- Investing in General Practice: The New General Medical Services Contract. London: Department of Health; 2004.
- Coleman T, Lewis S, Hubbard R, Smith C. Impact of contractual financial incentives on the ascertainment and management of smoking in primary care. Addiction 2007;102:803-8. https://doi.org/10.1111/j.1360-0443.2007.01766.x.
- Nallamothu BK, Hayward RA, Bates ER. Beyond the randomized clinical trial: the role of effectiveness studies in evaluating cardiovascular therapies. Circulation 2008;118:1294-303. https://doi.org/10.1161/CIRCULATIONAHA.107.703579.
- Rothwell PM. External validity of randomised controlled trials: ‘to whom do the results of this trial apply?’. Lancet 2005;365:82-93. https://doi.org/10.1016/S0140-6736(04)17670-8.
- Stefan MS, Bannuru RR, Lessard D, Gore JM, Lindenauer PK, Goldberg RJ. The impact of COPD on management and outcomes of patients hospitalized with acute myocardial infarction: a 10-year retrospective observational study. Chest 2012;141:1441-8. https://doi.org/10.1378/chest.11-2032.
- Juurlink DN, Gomes T, Ko DT, Szmitko PE, Austin PC, Tu JV, et al. A population-based study of the drug interaction between proton pump inhibitors and clopidogrel. CMAJ 2009;180:713-18. https://doi.org/10.1503/cmaj.082001.
- Simon T, Verstuyft C, Mary-Krause M, Quteineh L, Drouet E, Méneveau N, et al. Genetic determinants of response to clopidogrel and cardiovascular events. N Engl J Med 2009;360:363-75. https://doi.org/10.1056/NEJMoa0808227.
- Ho PM, Maddox TM, Wang L, Fihn SD, Jesse RL, Peterson ED, et al. Risk of adverse outcomes associated with concomitant use of clopidogrel and proton pump inhibitors following acute coronary syndrome. JAMA 2009;301:937-44. https://doi.org/10.1001/jama.2009.261.
- Aubert RE, Epstein RS, Teagarden JR, Xia F, Yao J, Desta Z, et al. Proton pump inhibitors effect on clopidogrel effectiveness: the Clopidogrel Medco Outcomes Study. Circulation 2008;118.
- Pezalla E, Day D, Pulliadath I. Initial assessment of clinical impact of a drug interaction between clopidogrel and proton pump inhibitors. J Am Coll Cardiol 2008;52:1038-9. https://doi.org/10.1016/j.jacc.2008.05.053.
- Rassen JA, Choudhry NK, Avorn J, Schneeweiss S. Cardiovascular outcomes and mortality in patients using clopidogrel with proton pump inhibitors after percutaneous coronary intervention or acute coronary syndrome. Circulation 2009;120:2322-9. https://doi.org/10.1161/CIRCULATIONAHA.109.873497.
- Dunn SP, Macauley TE, Brennan DM, Campbell CL, Charnigo RJ, Smyth SS, et al. Baseline proton pump inhibitor use is associated with increased cardiovascular events with and without the use of clopidogrel in the CREDO trial. Circulation 2008;118.
- O’Donoghue ML, Braunwald E, Antman EM, Murphy SA, Bates ER, Rozenman Y, et al. Pharmacodynamic effect and clinical efficacy of clopidogrel and prasugrel with or without a proton-pump inhibitor: an analysis of two randomised trials. Lancet 2009;374:989-97. https://doi.org/10.1016/S0140-6736(09)61525-7.
- Zairis MN, Tsiaousis GZ, Patsourakos NG, Georgilas AT, Kontos CF, Adamopoulou EN, et al. The impact of treatment with omeprazole on the effectiveness of clopidogrel drug therapy during the first year after successful coronary stenting. Can J Cardiol 2010;26:e54-7. https://doi.org/10.1016/S0828-282X(10)70008-8.
- Ray WA, Murray KT, Griffin MR, Chung CP, Smalley WE, Hall K, et al. Outcomes with concurrent use of clopidogrel and proton-pump inhibitors: a cohort study. Ann Intern Med 2010;152:337-45. https://doi.org/10.7326/0003-4819-152-6-201003160-00003.
- Bhatt DL, Cryer BL, Contant CF, Cohen M, Lanas A, Schnitzer TJ, et al. Clopidogrel with or without omeprazole in coronary artery disease. N Engl J Med 2010;363:1909-17. https://doi.org/10.1056/NEJMoa1007964.
- Valkhoff VE, ‘t Jong GW, Van Soest EM, Kuipers EJ, Sturkenboom MC. Risk of recurrent myocardial infarction with the concomitant use of clopidogrel and proton pump inhibitors. Aliment Pharmacol Ther 2011;33:77-88. https://doi.org/10.1111/j.1365-2036.2010.04485.x.
- Scheiman JM, Devereaux PJ, Herlitz J, Katelaris PH, Lanas A, Veldhuyzen van Zanten S, et al. Prevention of peptic ulcers with esomeprazole in patients at risk of ulcer development treated with low-dose acetylsalicylic acid: a randomised, controlled trial (OBERON). Heart 2011;97:797-802. https://doi.org/10.1136/hrt.2010.217547.
- Cooper A, Skinner J, Nherera L, Feder G, Ritchie G, Kathoria M, et al. Clinical Guidelines and Evidence Review for Post Myocardial Infarction: Secondary Prevention in Primary and Secondary Care for Patients Following a Myocardial Infarction. London: National Collaborating Centre for Primary Care and Royal College of General Practitioners; 2007.
- Antman EM, Anbe DT, Armstrong PW, Bates ER, Green LA, Hand M, et al. ACC/AHA Guidelines for the management of patients with ST-elevation myocardial infarction – executive summary: a report of the American College of Cardiology/American Heart Association Task Force on Practice Guidelines (Writing Committee to Revise the 1999 Guidelines for the Management of Patients With Acute Myocardial Infarction). J Am Coll Cardiol 2004;44:671-719. https://doi.org/10.1016/j.jacc.2004.07.002.
- Mukherjee D, Fang J, Chetcuti S, Moscucci M, Kline-Rogers E, Eagle KA. Impact of combination evidence-based medical therapy on mortality in patients with acute coronary syndromes. Circulation 2004;109:745-9. https://doi.org/10.1161/01.CIR.0000112577.69066.CB.
- Bauer T, Gitt AK, Jünger C, Zahn R, Koeth O, Towae F, et al. Guideline-recommended secondary prevention drug therapy after acute myocardial infarction: predictors and outcomes of nonadherence. Eur J Cardiovasc Prev Rehabil 2010;17:576-81. https://doi.org/10.1097/HJR.0b013e328338e5da.
- Bramlage P, Messer C, Bitterlich N, Pohlmann C, Cuneo A, Stammwitz E, et al. The effect of optimal medical therapy on 1-year mortality after acute myocardial infarction. Heart 2010;96:604-9. https://doi.org/10.1136/hrt.2009.188607.
- Lahoud R, Howe M, Krishnan SM, Zacharias S, Jackson EA. Effect of use of combination evidence-based medical therapy after acute coronary syndromes on long-term outcomes. Am J Cardiol 2012;109:159-64. https://doi.org/10.1016/j.amjcard.2011.08.024.
- Tay EL, Chan M, Tan V, Sim LL, Tan HC, Cheng YT. Impact of combination evidence-based medical therapy on mortality following myocardial infarction in elderly patients. Am J Geriatr Cardiol 2008;17:21-6. https://doi.org/10.1111/j.1076-7460.2007.07242.x.
- Gelfand AE. Gibbs sampling. J Am Stat Assoc 2000;95:1300-4. https://doi.org/10.1080/01621459.2000.10474335.
- Anon . Randomised trial of intravenous streptokinase, oral aspirin, both, or neither among 17,187 cases of suspected acute myocardial infarction: ISIS-2. ISIS-2 (Second International Study of Infarct Survival) Collaborative Group. Lancet 1988;2:349-60.
- Cleland JG. POPADAD trial. Time for a proper study of aspirin after a vascular event?. BMJ 2008;337. https://doi.org/10.1136/bmj.a2583.
- Baigent C, Collins R, Appleby P, Parish S, Sleight P, Peto R. ISIS-2: 10 year survival among patients with suspected acute myocardial infarction in randomised comparison of intravenous streptokinase, oral aspirin, both, or neither. The ISIS-2 (Second International Study of Infarct Survival) Collaborative Group. BMJ 1998;316:1337-43. https://doi.org/10.1136/bmj.316.7141.1337.
- Abdulla J, Barlera S, Latini R, Kjoller-Hansen L, Sogaard P, Christensen E, et al. A systematic review: effect of angiotensin converting enzyme inhibition on left ventricular volumes and ejection fraction in patients with a myocardial infarction and in patients with left ventricular dysfunction. Eur J Heart Fail 2007;9:129-35. https://doi.org/10.1016/j.ejheart.2006.05.002.
- Yusuf S, Zhao F, Mehta SR, Chrolavicius S, Tognoni G, Fox KK. Clopidogrel in Unstable Angina to Prevent Recurrent Events Trial Investigators . Effects of clopidogrel in addition to aspirin in patients with acute coronary syndromes without ST-segment elevation. N Engl J Med 2001;345:494-502. https://doi.org/10.1056/NEJMoa010746.
- Gruppo Italiano per lo Studio della Sopravvivenza nell’infarto Miocardico . GISSI-3: effects of lisinopril and transdermal glyceryl trinitrate singly and together on 6-week mortality and ventricular function after acute myocardial infarction. Gruppo Italiano per lo Studio della Sopravvivenza nell’infarto Miocardico. Lancet 1994;343:1115-22.
- Wakabayashi K, Gonzalez MA, Delhaye C, Ben-Dor I, Maluenda G, Collins SD, et al. Impact of chronic obstructive pulmonary disease on acute-phase outcome of myocardial infarction. Am J Cardiol 2010;106:305-9. https://doi.org/10.1016/j.amjcard.2010.03.026.
- Bursi F, Vassallo R, Weston SA, Killian JM, Roger VL. Chronic obstructive pulmonary disease after myocardial infarction in the community. Am Heart J 2010;160:95-101. https://doi.org/10.1016/j.ahj.2010.05.004.
- Salisbury AC, Reid KJ, Spertus JA. Impact of chronic obstructive pulmonary disease on post-myocardial infarction outcomes. Am J Cardiol 2007;99:636-41. https://doi.org/10.1016/j.amjcard.2006.09.112.
- Huiart L, Ernst P, Ranouil X, Suissa S. Oral corticosteroid use and the risk of acute myocardial infarction in chronic obstructive pulmonary disease. Can Respir J 2006;13:134-8. https://doi.org/10.1155/2006/935718.
- Albouaini K, Andron M, Alahmar A, Egred M. Beta-blockers use in patients with chronic obstructive pulmonary disease and concomitant cardiovascular conditions. Int J Chron Obstruct Pulmon Dis 2007;2:535-40.
- Hurst JR, Vestbo J, Anzueto A, Locantore N, Müllerova H, Tal-Singer R, et al. Susceptibility to exacerbation in chronic obstructive pulmonary disease. N Engl J Med 2010;363:1128-38. https://doi.org/10.1056/NEJMoa0909883.
- Gottlieb SS, McCarter RJ, Vogel RA. Effect of beta-blockade on mortality among high-risk and low-risk patients after myocardial infarction. N Engl J Med 1998;339:489-97. https://doi.org/10.1056/NEJM199808203390801.
- Dransfield MT, Rowe SM, Johnson JE, Bailey WC, Gerald LB. Use of beta blockers and the risk of death in hospitalised patients with acute exacerbations of COPD. Thorax 2008;63:301-5. https://doi.org/10.1136/thx.2007.081893.
- Short PM, Lipworth SI, Elder DH, Schembri S, Lipworth BJ. Effect of beta blockers in treatment of chronic obstructive pulmonary disease: a retrospective cohort study. BMJ 2011;342. https://doi.org/10.1136/bmj.d2549.
- Whitaker HJ, Farrington CP, Spiessens B, Musonda P. Tutorial in biostatistics: the self-controlled case series method. Stat Med 2006;25:1768-97. https://doi.org/10.1002/sim.2302.
- Ho PM, Tsai TT, Maddox TM, Powers JD, Carroll NM, Jackevicius C, et al. Delays in filling clopidogrel prescription after hospital discharge and adverse outcomes after drug-eluting stent implantation: implications for transitions of care. Circ Cardiovasc Qual Outcomes 2010;3:261-6. https://doi.org/10.1161/CIRCOUTCOMES.109.902031.
- Unstable Angina and NSTEMI: The Early Management of Unstable Angina and Non-ST-Segment-Elevation Myocardial Infarction. London: NICE; 2010.
- Eisenstein EL, Anstrom KJ, Kong DF, Shaw LK, Tuttle RH, Mark DB, et al. Clopidogrel use and long-term clinical outcomes after drug-eluting stent implantation. JAMA 2007;297:159-68. https://doi.org/10.1001/jama.297.2.joc60179.
- Wiederkehr D, Ogbonnaya A, Casciano R, Makenbaeva D, Mozaffari E, Corbelli J. Clinical impact of early clopidogrel discontinuation following acute myocardial infarction hospitalization or stent implantation: analysis in a nationally representative managed-care population. Curr Med Res Opin 2009;25:2327-34. https://doi.org/10.1185/03007990903156087.
- Butler MJ, Eccleston D, Clark DJ, Ajani AE, Andrianopoulos N, Brennan A, et al. The effect of intended duration of clopidogrel use on early and late mortality and major adverse cardiac events in patients with drug-eluting stents. Am Heart J 2009;157:899-907. https://doi.org/10.1016/j.ahj.2009.02.018.
- LaFleur J, Nelson RE, Sauer BC, Nebeker JR. Overestimation of the effects of adherence on outcomes: a case study in healthy user bias and hypertension. Heart 2011;97:1862-9. https://doi.org/10.1136/hrt.2011.223289.
- Rosenthal GE, Shah A, Way LE, Harper DL. Variations in standardized hospital mortality rates for six common medical diagnoses: implications for profiling hospital quality. Med Care 1998;36:955-64. https://doi.org/10.1097/00005650-199807000-00003.
- Jarman B, Gault S, Alves B, Hider A, Dolan S, Cook A, et al. Explaining differences in English hospital death rates using routinely collected data. BMJ 1999;318:1515-20. https://doi.org/10.1136/bmj.318.7197.1515.
- Dr Foster Intelligence . My Hospital Guide 2013 n.d. http://myhospitalguide.drfosterintelligence.co.uk (accessed 4 December 2016).
- Krumholz HM, Chen J, Wang Y, Radford MJ, Chen YT, Marciniak TA. Comparing AMI mortality among hospitals in patients 65 years of age and older: evaluating methods of risk adjustment. Circulation 1999;99:2986-92. https://doi.org/10.1161/01.CIR.99.23.2986.
- Peterson ED, Roe MT, Mulgund JF, DeLong ER, Lytle BL, Brindis RG, et al. Association between hospital process performance and outcomes among patients with acute coronary syndromes. JAMA 2006;295:1912-20. https://doi.org/10.1001/jama.295.16.1912.
- Bradley EH, Herrin J, Elbel B, McNamara RL, Magid DJ, Nallamothu BK, et al. Hospital quality for acute myocardial infarction: correlation among process measures and relationship with short-term mortality. JAMA 2006;296:72-8. https://doi.org/10.1001/jama.296.1.72.
- Patel MR, Chen AY, Roe MT, Ohman EM, Newby LK, Harrington RA, et al. A comparison of acute coronary syndrome care at academic and nonacademic hospitals. Am J Med 2007;120:40-6. https://doi.org/10.1016/j.amjmed.2006.10.008.
- Bernheim SM, Grady JN, Lin Z, Wang YF, Savage SV, Bhat K, et al. National patterns of risk-standardized mortality and readmission for acute myocardial infarction and heart failure. Update on publicly reported outcomes measures based on the 2010 release. Circ Cardiovasc Qual Outcomes 2010;3:459-67. https://doi.org/10.1161/CIRCOUTCOMES.110.957613.
- Menon V, Rumsfeld JS, Roe MT, Cohen MG, Peterson ED, Brindis RG, et al. Regional outcomes after admission for high-risk non-ST-segment elevation acute coronary syndromes. Am J Med 2006;119:584-90. https://doi.org/10.1016/j.amjmed.2006.01.018.
- Murphy NF, MacIntyre K, Stewart S, Capewell S, McMurray JJ. Reduced between-hospital variation in short term survival after acute myocardial infarction: the result of improved cardiac care?. Heart 2005;91:726-30. https://doi.org/10.1136/hrt.2004.042929.
- Alter DA, Austin PC, Tu JV. Canadian Cardiovascular Outcomes Research Team . Community factors, hospital characteristics and inter-regional outcome variations following acute myocardial infarction in Canada. Can J Cardiol 2005;21:247-55.
- Leyland AH, Boddy FA. League tables and acute myocardial infarction. Lancet 1998;351:555-8. https://doi.org/10.1016/S0140-6736(97)09362-8.
- Rasmussen S, Zwisler AD, Abildstrom SZ, Madsen JK, Madsen M. Hospital variation in mortality after first acute myocardial infarction in Denmark from 1995 to 2002: lower short-term and 1-year mortality in high-volume and specialized hospitals. Med Care 2005;43:970-8. https://doi.org/10.1097/01.mlr.0000178195.07110.d3.
- Bradley EH, Herrin J, Curry L, Cherlin EJ, Wang Y, Webster TR, et al. Variation in hospital mortality rates for patients with acute myocardial infarction. Am J Cardiol 2010;106:1108-12. https://doi.org/10.1016/j.amjcard.2010.06.014.
- Alpert JS, Thygesen K, Antman E, Bassand JP. Myocardial infarction redefined – a consensus document of The Joint European Society of Cardiology/American College of Cardiology Committee for the redefinition of myocardial infarction. J Am Coll Cardiol 2000;3:959-69.
- Wiviott SD, Morrow DA, Frederick PD, Antman EM, Braunwald E. National Registry of Myocardial Infarction . Application of the thrombolysis in myocardial infarction risk index in non-ST-segment elevation myocardial infarction: evaluation of patients in the National Registry of Myocardial Infarction. J Am Coll Cardiol 2006;47:1553-8. https://doi.org/10.1016/j.jacc.2005.11.075.
- Gale CP, Manda SO, Weston CF, Birkhead JS, Batin PD, Hall AS. Evaluation of risk scores for risk stratification of acute coronary syndromes in the Myocardial Infarction National Audit Project (MINAP) database. Heart 2009;95:221-7. https://doi.org/10.1136/hrt.2008.144022.
- Spiegelhalter DJ. Funnel plots for comparing institutional performance. Stat Med 2005;24:1185-202. https://doi.org/10.1002/sim.1970.
- Moons KG, Donders RA, Stijnen T, Harrell FE. Using the outcome for imputation of missing predictor values was preferred. J Clin Epidemiol 2006;59:1092-101. https://doi.org/10.1016/j.jclinepi.2006.01.009.
- Knorr-Held L. Analysis of incomplete multivariate data. Statist Med 2000;19:1006-8. https://doi.org/10.1002/(SICI)1097-0258(20000415)19:7<1006::AID-SIM384>3.0.CO;2-T.
- Lilford R, Mohammed MA, Spiegelhalter D, Thomson R. Use and misuse of process and outcome data in managing performance of acute medical care: avoiding institutional stigma. Lancet 2004;363:1147-54. https://doi.org/10.1016/S0140-6736(04)15901-1.
- Mohammed MA, Deeks JJ, Girling A, Rudge G, Carmalt M, Stevens AJ, et al. Evidence of methodological bias in hospital standardised mortality ratios: retrospective database study of English hospitals. BMJ 2009;338. https://doi.org/10.1136/bmj.b780.
- Mant J, Hicks N. Detecting differences in quality of care: the sensitivity of measures of process and outcome in treating acute myocardial infarction. BMJ 1995;311:793-6. https://doi.org/10.1136/bmj.311.7008.793.
- Adab P, Rouse AM, Mohammed MA, Marshall T. Performance league tables: the NHS deserves better. BMJ 2002;324:95-8. https://doi.org/10.1136/bmj.324.7329.95.
- Rose G. Rose’s Strategy of Preventive Medicine. Oxford: Oxford University Press; 2008.
- Gale CP, Cattle BA, Moore JF, Dawe HF, Greenwood DC, West RM. Impact of missing data on standardised mortality ratios for acute myocardial infarction: evidence from the Myocardial Ischaemia National Audit Project (MINAP) 2004–7. Heart 2011;97:1926-31. https://doi.org/10.1136/hrt.2010.204883.
- Myocardial Infarction with ST-Segment Elevation: Acute Management. London: NICE; 2013.
- Rothwell PM. Limitations of the usual blood-pressure hypothesis and importance of variability, instability, and episodic hypertension. Lancet 2010;375:938-48. https://doi.org/10.1016/S0140-6736(10)60309-1.
- Mancia G, Fagard R, Narkiewicz K, Redon J, Zanchetti A, Bohm M, et al. 2013 ESH/ESC guidelines for the management of arterial hypertension: the Task Force for the Management of Arterial Hypertension of the European Society of Hypertension (ESH) and of the European Society of Cardiology (ESC). Eur Heart J 2013;34:2159-219. https://doi.org/10.1093/eurheartj/eht151.
- Thygesen K, Alpert JS, White HD. Joint ESC/ACCF/AHA/WHF Task Force for the Redefinition of Myocardial Infarction . Universal definition of myocardial infarction. Eur Heart J 2007;28:2525-38. https://doi.org/10.1093/eurheartj/ehm355.
- Van de Werf F, Bax J, Betriu A, Blomstrom-Lundqvist C, Crea F, Falk V, et al. Management of acute myocardial infarction in patients presenting with persistent ST-segment elevation: the Task Force on the Management of ST-Segment Elevation Acute Myocardial Infarction of the European Society of Cardiology. Eur Heart J 2008;29:2909-45. https://doi.org/10.1093/eurheartj/ehn416.
- Kushner FG, Hand M, Smith SCJ, King SB, Anderson JL, Antman EM, et al. 2009 focused updates: ACC/AHA guidelines for the management of patients with ST-elevation myocardial infarction (updating the 2004 guideline and 2007 focused update) and ACC/AHA/SCAI guidelines on percutaneous coronary intervention (updating the 2005 guideline and 2007 focused update) a report of the American College of Cardiology Foundation/American Heart Association Task Force on Practice Guidelines. J Am Coll Cardiol 2009;54:2205-41. https://doi.org/10.1016/j.jacc.2009.10.015.
- Hamm CW, Bassand JP, Agewall S, Bax J, Boersma E, Bueno H, et al. ESC Guidelines for the management of acute coronary syndromes in patients presenting without persistent ST-segment elevation: The Task Force for the management of acute coronary syndromes (ACS) in patients presenting without persistent ST-segment elevation of the European Society of Cardiology (ESC). Eur Heart J 2011;32:2999-3054. https://doi.org/10.1093/eurheartj/ehr236.
- Anderson JL, Adams CD, Antman EM, Bridges CR, Califf RM, Casey DE, et al. 2012 ACCF/AHA focused update incorporated into the ACCF/AHA 2007 guidelines for the management of patients with unstable angina/non-ST-elevation myocardial infarction: a report of the American College of Cardiology Foundation/American Heart Association Task Force on Practice Guidelines. J Am Coll Cardiol 2013;61:e179-e347. https://doi.org/10.1016/j.jacc.2013.01.014.
- Birkmeyer JD, Dimick JB. Understanding and reducing variation in surgical mortality. Annu Rev Med 2009;60:405-15. https://doi.org/10.1146/annurev.med.60.062107.101214.
- Ross JS, Normand SL, Wang Y, Ko DT, Chen J, Drye EE, et al. Hospital volume and 30-day mortality for three common medical conditions. N Engl J Med 2010;362:1110-18. https://doi.org/10.1056/NEJMsa0907130.
- Bradley EH, Curry LA, Spatz E, Herrin J, Cherlin EJ, Curtis J, et al. Hospital strategies for reducing risk-standardized mortality rates in acute myocardial infarction. Ann Intern Med 2012;156:618-26. https://doi.org/10.7326/0003-4819-156-9-201205010-00003.
- Popescu I, Werner RM, Vaughan-Sarrazin M, Cram P. Characteristics and outcomes of America’s lowest-performing hospitals: an analysis of acute myocardial infarction hospital care in the United States. Circ Cardiovasc Qual Outcomes 2009;2:221-7. https://doi.org/10.1161/CIRCOUTCOMES.108.813790.
- Limb M. Unexplained variations in hospital admissions must be tackled, report says. BMJ 2011;342. https://doi.org/10.1136/bmj.d2380.
- Packer C, Simpson S, Stevens A. EuroScan: the European Information Network on New and Changing Health Technologies . International diffusion of new health technologies: a ten-country analysis of six health technologies. Int J Technol Assess Health Care 2006;22:419-28. https://doi.org/10.1017/S0266462306051336.
- Widimsky P, Wijns W, Fajadet J, de Belder M, Knot J, Aaberge L, et al. Reperfusion therapy for ST elevation acute myocardial infarction in Europe: description of the current situation in 30 countries. Eur Heart J 2010;31:943-57. https://doi.org/10.1093/eurheartj/ehp492.
- Carlsson P. Health technology assessment and priority setting for health policy in Sweden. Int J Technol Assess Health Care 2004;20:44-5. https://doi.org/10.1017/S0266462304000777.
- Li J, Li X, Wang Q, Hu S, Wang Y, Masoudi FA, et al. ST-segment elevation myocardial infarction in China from 2001 to 2011 (the China PEACE-Retrospective Acute Myocardial Infarction Study): a retrospective analysis of hospital data. Lancet 2015;385:441-51. https://doi.org/10.1016/S0140-6736(14)60921-1.
- Bannink L, Wells S, Broad J, Riddell T, Jackson R. Web-based assessment of cardiovascular disease risk in routine primary care practice in New Zealand: the first 18,000 patients (PREDICT CVD-1). N Z Med J 2006;119.
- Aragam KG, Tamhane UU, Kline-Rogers E, Li J, Fox KA, Goodman SG, et al. Does simplicity compromise accuracy in ACS risk prediction? A retrospective analysis of the TIMI and GRACE risk scores. PLOS ONE 2009;4. https://doi.org/10.1371/journal.pone.0007947.
- D’Ascenzo F, Biondi-Zoccai G, Moretti C, Bollati M, Omedè P, Sciuto F, et al. TIMI, GRACE and alternative risk scores in Acute Coronary Syndromes: a meta-analysis of 40 derivation studies on 216,552 patients and of 42 validation studies on 31,625 patients. Contemp Clin Trials 2012;33:507-14. https://doi.org/10.1016/j.cct.2012.01.001.
- Mehta RH, Newby LK, Patel Y, Hoekstra JW, Miller CD, Chen AY, et al. The impact of emergency department structure and care processes in delivering care for non-ST-segment elevation acute coronary syndromes. Am Heart J 2006;152:648-60. https://doi.org/10.1016/j.ahj.2006.04.015.
- Tierney S, Cook G, Mamas M, Fath-Ordoubadi F, Iles-Smith H, Deaton C. Nurses’ role in the acute management of patients with non-ST-segment elevation acute coronary syndromes: an integrative review. Eur J Cardiovasc Nurs 2013;12:293-301. https://doi.org/10.1177/1474515112451555.
- Lee CH, Tan MF, Yan AT, Yan R, Fitchett D, Grima EA, et al. Use of cardiac catheterization for non-ST-segment elevation acute coronary syndromes according to initial risk: reasons why physicians choose not to refer their patients. Arch Intern Med 2008;168:291-6. https://doi.org/10.1001/archinternmed.2007.78.
- Thiele H, Rach J, Klein N, Pfeiffer D, Hartmann A, Hambrecht R, et al. Optimal timing of invasive angiography in stable non-ST-elevation myocardial infarction: the Leipzig Immediate versus early and late PercutaneouS coronary Intervention triAl in NSTEMI (LIPSIA-NSTEMI Trial). Eur Heart J 2012;33:2035-43. https://doi.org/10.1093/eurheartj/ehr418.
- Buber J, Goldenberg I, Kimron L, Guetta V. One-year outcome following coronary angiography in elderly patients with non-ST elevation myocardial infarction: real-world data from the Acute Coronary Syndromes Israeli Survey (ACSIS). Coron Artery Dis 2013;24:102-9. https://doi.org/10.1097/MCA.0b013e32835c8f53.
- Mehta SR, Granger CB, Boden WE, Steg PG, Bassand JP, Faxon DP, et al. Early versus delayed invasive intervention in acute coronary syndromes. N Engl J Med 2009;360:2165-75. https://doi.org/10.1056/NEJMoa0807986.
- Gyenes GT, Yan AT, Tan M, Welsh RC, Fox KA, Grondin FR, et al. Use and timing of coronary angiography and associated in-hospital outcomes in Canadian non-ST-segment elevation myocardial infarction patients: insights from the Canadian Global Registry of Acute Coronary Events. Can J Cardiol 2013;29:1429-35. https://doi.org/10.1016/j.cjca.2013.04.035.
- Anderson JL, Adams CD, Antman EM, Bridges CR, Califf RM, Casey DE, et al. 2012 ACCF/AHA focused update incorporated into the ACCF/AHA 2007 guidelines for the management of patients with unstable angina/non-ST-elevation myocardial infarction: a report of the American College of Cardiology Foundation/American Heart Association Task Force on Practice Guidelines. Circulation 2013;127:e663-828. https://doi.org/10.1161/CIR.0b013e31828478ac.
- Jabara R, Gadesam R, Pendyala L, Chronos N, Crisco LV, King SB, et al. Ambulatory discharge after transradial coronary intervention: preliminary US single-center experience (Same-day TransRadial Intervention and Discharge Evaluation, the STRIDE Study). Am Heart J 2008;156:1141-6. https://doi.org/10.1016/j.ahj.2008.07.018.
- Birkhead JS, Weston C, Lowe D. Impact of specialty of admitting physician and type of hospital on care and outcome for myocardial infarction in England and Wales during 2004–5: observational study. BMJ 2006;332:1306-11. https://doi.org/10.1136/bmj.38849.440914.AE.
- Savage J. Ethnography and health care. BMJ 2000;321:1400-2. https://doi.org/10.1136/bmj.321.7273.1400.
- Pope C, Mays N. Critical reflections on the rise of qualitative research. BMJ 2009;339. https://doi.org/10.1136/bmj.b3425.
- Dunckley M, Quinn T, Dickson R, Jayram R, Wright C, McDonald R. Acute coronary syndrome nurses: perceptions of other members of the health care team. Accid Emerg Nurs 2006;14:204-9. https://doi.org/10.1016/j.aaen.2006.07.005.
- Smallwood A. Cardiac assessment teams: a focused ethnography of nurses roles. Br J Cardiac Nurs 2009;4:132-9. https://doi.org/10.12968/bjca.2009.4.3.40050.
- Stukel TA, Alter DA, Schull MJ, Ko DT, Li P. Association between hospital cardiac management and outcomes for acute myocardial infarction patients. Med Care 2010;48:157-65. https://doi.org/10.1097/MLR.0b013e3181bd4da7.
- Dunham M, Challen K, Walter D. Risk stratification of patients with acute chest pain without a rise in troponin: current practice in England. Emerg Med J 2010;27:461-4. https://doi.org/10.1136/emj.2008.068163.
- de Belder MA. Interventional management of acute coronary syndromes: applying the lessons of ST-elevation services to non-ST-elevation myocardial infarction. Heart 2012;98:1407-11. https://doi.org/10.1136/heartjnl-2012-302459.
- Sampson F, O’Cathain A, Goodacre S. Feeling fixed and its contribution to patient satisfaction with primary angioplasty: a qualitative study. Eur J Cardiovasc Nurs 2009;8:85-90. https://doi.org/10.1016/j.ejcnurse.2008.07.003.
- Radcliffe EL, Harding G, Rothman MT, Feder GS. ’It got right to the spot’ the patient experience of primary angioplasty: a qualitative study. Eur J Cardiovasc Nurs 2009;8:216-22. https://doi.org/10.1016/j.ejcnurse.2009.02.001.
- Dullaghan L, Lusk LF, McGeough MF, Donnelly PF, Herity NF, Fitzsimons D. ‘I am still a bit unsure how much of a heart attack it really was!’ Patients presenting with non ST elevation myocardial infarction lack understanding about their illness and have less motivation for secondary prevention. Eur J Cardiovasc Nurs 2014;13:270-6. https://doi.org/10.1177/1474515113491649.
- Reeves S, Lewin S. Interprofessional collaboration in the hospital: strategies and meanings. J Health Serv Res Policy 2004;9:218-25. https://doi.org/10.1258/1355819042250140.
- Landman AB, Spatz ES, Cherlin EJ, Krumholz HM, Bradley EH, Curry LA. Hospital collaboration with emergency medical services in the care of patients with acute myocardial infarction: perspectives from key hospital staff. Ann Emerg Med 2013;61:185-95. https://doi.org/10.1016/j.annemergmed.2012.10.009.
- Keogh B. Review into the Quality of Care and Treatment Provided by 14 Hospital Trusts in England: Overview Report 2013.
- Strategy for UK Life Sciences One Year On. London: Department for Business, Innovation and Skills; 2012.
- Strengthening the UK’s Capability in Health Informatics Research Conference Report 2013. http://masoninstitute.org/wp-content/uploads/2013/08/Conference-Report-HIRC-launch-May-2013.pdf (accessed 27 February 2016).
- Realising the Potential of Stratified Medicine. London: Academy of Medical Sciences; 2013.
- Data in Safe Havens. London: Academy of Medical Sciences; 2014.
- Big Data Road Map. London: ABPI; 2013.
- Information and Technology for Better Care 2015–20 Draft Report for Consultation. Leeds: Health and Social Care Information Centre; 2015.
- Health and Social Care Information Centre . Data Release Review 2014.
- Caulfield M, Davies DK, Fowler T, Mahon-Pearson J, Turnbull C, Woods K. Genomics England Clinical Interpretation Partnership (GeCIP): Guidance for Expressions of Interest to Form Domains within GeCIP. London: Genomics England; 2014.
- Best Care at Lower Cost: The Path to Continuously Learning Health Care in America. Washington, DC: The National Academies Press; 2012.
- Huynh T, Birkhead J, Huber K, O’Loughlin J, Stenestrand U, Weston C, et al. The pre-hospital fibrinolysis experience in Europe and North America and implications for wider dissemination. JACC Cardiovasc Interv 2011;4:877-83. https://doi.org/10.1016/j.jcin.2011.05.013.
- International Organization for Standardisation 2013. www.iso.org/obp/ui/#iso:std:iso-iec:27001:ed-2:v1:en (accessed 4 December 2016).
- Sekhri N, Feder GS, Junghans C, Eldridge S, Umaipalan A, Madhu R, et al. Incremental prognostic value of the exercise electrocardiogram in the initial assessment of patients with suspected angina: cohort study. BMJ 2008;337. https://doi.org/10.1136/bmj.a2240.
- Haq IU, Skinner JS, Adams PC. 63 Implications of a likelihood based approach to diagnostic testing in coronary artery disease: impact of the new NICE guidelines. Heart 2011;97. https://doi.org/10.1136/heartjnl-2011-300198.63.
- Sheppard C, Edmund J, Frawley K, Dubey G, Baron J, Burn S, et al. 128 more discharges, less follow-up and similar rates of coronary angiography: initial ‘real-world’experience of NICE guidance on assessment of chest pain of recent onset in the rapid access chest pain clinic. Heart 2013;99. https://doi.org/10.1136/heartjnl-2013-304019.128.
- Management of Stable Angina. London: NICE; 2011.
- Griffin SC, Barber JA, Manca A, Sculpher MJ, Thompson SG, Buxton MJ, et al. Cost effectiveness of clinically appropriate decisions on alternative treatments for angina pectoris: prospective observational study. BMJ 2007;334. https://doi.org/10.1136/bmj.39129.442164.55.
- Management of Stable Angina: Evidence Update September 2012. Evidence Update 23: A Summary of Selected New Evidence Relevant to NICE Clinical Guideline 126 ‘Management of stable angina’ (2011). Manchester: NICE; 2012.
- Sutaria S, Philipson P, Fitzpatrick NK, Abrams K, Moreno SG, Timmis A, et al. Translational phases of evidence in a prognostic biomarker: a systematic review and meta-analysis of natriuretic peptides and the prognosis of stable coronary disease. Heart 2012;98:615-22. https://doi.org/10.1136/heartjnl-2011-300862.
- Ticagrelor for the Treatment of Acute Coronary Syndromes. 2011.
- Timmis A, Rapsomaniki E, Chung S-C, Pujades-Rodriguez M, Moayyeri A, Stogiannis D, et al. Prologed dual antiplatelet therapy in stable coronary disease: comparative observational study of benefits and harms in unselected versus trial populations. BMJ 2016;353.
- Pasea L, Chung S-C, Pujades-Rodriguez M, Moayyeri A, Denaxas S, Fox KAA, et al. Personalising the decision for prolonged dual antiplatelet therapy: development, validation and potential impact of prognostic models for cardiovascular events and bleeding in myocardial infarction survivors. Eur Heart J 2017.
- Mowatt G, Cook JA, Hillis GS, Walker S, Fraser C, Jia X, et al. 64-Slice computed tomography angiography in the diagnosis and assessment of coronary artery disease: systematic review and meta-analysis. Heart 2008;94:1386-93. https://doi.org/10.1136/hrt.2008.145292.
- Pontone G, Andreini D, Bartorelli AL, Bertella E, Cortinovis S, Mushtaq S, et al. A long-term prognostic value of CT angiography and exercise ECG in patients with suspected CAD. JACC Cardiovasc Imaging 2013;6:641-50. https://doi.org/10.1016/j.jcmg.2013.01.015.
- Hemingway H, McCallum A, Shipley M, Manderbacka K, Martikainen P, Keskimäki I. Incidence and prognostic implications of stable angina pectoris among women and men. JAMA 2006;295:1404-11. https://doi.org/10.1001/jama.295.12.1404.
- PLOS Medicine Editors . Observational studies: getting clear about transparency. PLOS Med 2014;11. https://doi.org/10.1371/journal.pmed.1001711.
- Sheikh A, McLean S, Cresswell K, Pagliari C, Pappas Y, Car J, et al. The Impact of EHealth on the Quality and Safety of Healthcare: An Updated Systematic Overview and Synthesis of the Literature 2011.
Appendix 1 Summary of published papers relating to individual studies in the programme
Study number | Authors | Title | Journal | Year of publication |
---|---|---|---|---|
1 | Denaxas SC, George J, Herrett E, Shah AD, Kalra D, Hingorani AD, et al.15 | Data resource profile: cardiovascular disease research using linked bespoke studies and electronic health records (CALIBER) | International Journal of Epidemiology | 2012 |
2 | Herrett E,a Shah AD,a Boggon R, Denaxas S, Smeeth L, van Staa T, et al.28 | Completeness and diagnostic validity of recording acute myocardial infarction events in primary care, hospital care, disease registry, and national mortality records: cohort study | BMJ | 2013 |
3 | Hemingway H, Croft P, Perel P, Hayden JA, Abrams K, Timmis A, et al.29 | Prognosis Research Strategy (PROGRESS) 1: a framework for researching clinical outcomes | BMJ | 2013 |
3 | Riley RD, Hayden JA, Steyerberg EW, Moons KG, Abrams K, Kyzas PA, et al.31 | Prognosis Research Strategy (PROGRESS) 2: prognostic factor research | PLOS Medicine | 2013 |
3 | Steyerberg EW, Moons KG, van der Windt DA, Hayden JA, Perel P, Schroter S, et al.32 | Prognosis Research Strategy (PROGRESS) 3: prognostic model research | PLOS Medicine | 2013 |
3 | Hingorani AD, Windt DA, Riley RD, Abrams K, Moons KG, Steyerberg EW, et al.30 | Prognosis Research Strategy (PROGRESS) 4: stratified medicine research | BMJ | 2013 |
3 | Peat G, Riley RD, Croft P, Morley KI, Kyzas PA, Moons KG, et al.33 | Improving the transparency of prognosis research: the role of reporting, data sharing, registration, and protocols | PLOS Medicine | 2014 |
4 | Pujades-Rodriguez M, Timmis A, Stogiannis D, Rapsomaniki E, Denaxas S, Shah A, et al.34 | Socioeconomic deprivation and the incidence of 12 cardiovascular diseases in 1.9 million women and men: implications for risk prediction and prevention | PLOS ONE | 2014 |
4 | George J,a Rapsomaniki E,a Pujades-Rodriguez M, Shah AD, Denaxas S, Herrett E, et al.35 | How Does Cardiovascular disease first present in women and men? Incidence of 12 cardiovascular diseases in a contemporary cohort of 1,937,360 people | Circulation | 2015 |
5 | Zaman MJ, Philipson P, Chen R, Farag A, Shipley M, Marmot MG, et al.36 | South Asians and coronary disease: is there discordance between effects on incidence and prognosis? | Heart | 2013 |
6 | Shah AD, Langenberg C, Rapsomaniki E, Denaxas S, Pujades-Rodriguez M, Gale CP, et al.37 | Type 2 diabetes and incidence of cardiovascular diseases: a cohort study in 1.9 million people | The Lancet Diabetes & Endocrinology | 2015 |
7 | Rapsomaniki E, Timmis A, George J, Pujades-Rodriguez M, Shah AD, Denaxas S, et al.38 | Blood pressure and incidence of twelve cardiovascular diseases: lifetime risks, healthy life-years lost, and age-specific associations in 1.25 million people | The Lancet | 2014 |
10 | Herrett E, George J, Denaxas S, Bhaskaran K, Timmis A, Hemingway H, Smeeth L41 | Type and timing of heralding in ST-elevation and non-ST-elevation myocardial infarction: an analysis of prospectively collected electronic healthcare records linked to the national registry of acute coronary syndromes | European Heart Journal: Acute Cardiovascular Care | 2013 |
11 | Shah AD, Martinez C, Hemingway H42 | The freetext matching algorithm: a computer program to extract diagnoses and causes of death from unstructured text in electronic health records | BMC Medical Informatics and Decision Making | 2012 |
12 | Wang Z, Shah AD, Tate AR, Denaxas S, Shawe-Taylor J, Hemingway H43 | Extracting diagnoses and investigation results from unstructured text in electronic health records by semi-supervised machine learning | PLOS ONE | 2012 |
13 | Cramer H, Evans M, Featherstone K, Johnson R, Zaman MJ, Timmis AD, et al.44 | Treading carefully: a qualitative ethnographic study of the clinical, social and educational uses of exercise ECG in evaluating stable chest pain | BMJ Open | 2012 |
13 | Johnson R, Evans M, Cramer H, Bennert K, Morris R, Eldridge S, et al.45 | Feasibility and impact of a computerised clinical decision support system on investigation and initial management of new onset chest pain: a mixed methods study | BMC Medical Informatics and Decision Making | 2015 |
15 | Shah AD, Nicholas O, Timmis AD, Feder G, Abrams KR, Chen R, et al.47 | Threshold haemoglobin levels and the prognosis of stable coronary disease: two new cohorts and a systematic review and meta-analysis | PLOS Medicine | 2011 |
17 | Hemingway H, Philipson P, Chen R, Fitzpatrick NK, Damant J, Shipley M, et al.49 | Evaluating the quality of research into a single prognostic biomarker: a systematic review and meta-analysis of 83 studies of C-reactive protein in stable coronary artery disease | PLOS Medicine | 2010 |
18 | Patel RS, Asselbergs FW, Quyyumi AA, Palmer TM, Finan CI, Tragante V, et al.50 | Genetic variants at chromosome 9p21 and risk of first versus subsequent coronary heart disease events: a systematic review and meta-analysis | Journal of the American College of Cardiology | 2014 |
20 | Rapsomaniki E, Shah A, Perel P, Denaxas S, George J, Nicholas O, et al.52 | Prognostic models for stable coronary artery disease based on electronic health record cohort of 102 023 patients | European Heart Journal | 2014 |
21 | Asaria M, Walker S, Palmer S, Gale CP, Shah AD, Abrams KR, et al.53 | Using electronic health records to predict costs and outcomes in stable coronary artery disease | Heart | 2016 |
21 | Walker S, Asaria M, Manca A, Palmer S, Gale CP, Shah AD, et al.54 | Long-term healthcare use and costs in patients with stable coronary artery disease: a population-based cohort using linked electronic health records (CALIBER) | European Heart Journal – Quality of Care and Clinical Outcomes | 2016 |
22 | Chung SC, Gedeborg R, Nicholas O, James S, Jeppsson A, Wolfe C, et al.55 | Acute myocardial infarction: a comparison of short-term survival in national outcome registries in Sweden and the UK | The Lancet | 2014 |
23 | Simms AD, Weston CF, West RM, Hall AS, Batin PD, Timmis A, et al.56 | Mortality and missed opportunities along the pathway of care for ST-elevation myocardial infarction: a national cohort study | European Heart Journal: Acute Cardiovascular Care | 2015 |
24 | McNamara RL, Chung SC, Jernberg T, Holmes D, Roe M, Timmis A, et al.57 | International comparisons of the management of patients with non-ST segment elevation acute myocardial infarction in the United Kingdom, Sweden, and the United States: The MINAP/NICOR, SWEDEHEART/RIKS-HIA, and ACTION Registry-GWTG/NCDR registries | International Journal of Cardiology | 2014 |
25 | Boggon R, Timmis A, Hemingway H, Raju S, Malvestiti FM, Van Staa TP58 | Smoking cessation interventions following acute coronary syndrome: a missed opportunity? | European Journal of Preventive Cardiology | 2014 |
27 | Quint JK, Herrett E, Bhaskaran K, Timmis A, Hemingway H, Wedzicha JA, Smeeth L59 | Effect of β blockers on mortality after myocardial infarction in adults with COPD: population based cohort study of UK electronic healthcare records | BMJ | 2013 |
28 | Douglas IJ, Evans SJ, Hingorani AD, Grosso AM, Timmis A, Hemingway H, Smeeth L60 | Clopidogrel and interaction with proton pump inhibitors: comparison between cohort and within person study designs | BMJ | 2012 |
29 | Boggon R, van Staa TP, Timmis A, Hemingway H, Ray KK, Begg A, et al.61 | Clopidogrel discontinuation after acute coronary syndromes: frequency, predictors and associations with death and myocardial infarction – a hospital registry-primary care linked cohort (MINAP-GPRD) | European Heart Journal | 2011 |
32 | Chung SC, Sundström J, Gale CP, James S, Deanfield J, Wallentin L, et al.62 | Comparison of hospital variation in acute myocardial infarction care and outcome between Sweden and United Kingdom: population based cohort study using nationwide clinical registries | BMJ | 2015 |
Appendix 2 Transcript from clinician interview on what role can computerised decision support play in clinical decision-making in the Optimising the Management of Angina study?
Yeah but sometimes you need, you need to make thing, make sense of yourself, you don’t totally rely on technology to, to make your own decision or change your own decision. But that depends on experience and, and err other factors. [. . .] You need to guide the technology not the technology guide you.
There will always be outside cases [. . .] and I think it would be wrong to try and make a tool that [. . .] does everything because then it would be, because it would lose meaning, you know.
I think the whole nature of it, it would have to err on the side of caution wouldn’t it . . . because that’s the way these tool kits work because you haven’t got the whole experience aspect behind it to be able to make a judgement, that’s what the tool can’t do, is to do that judgement side of it.
If you’ve got a full validated tool [. . .] and you’ve got good studies that support it and all the rest of it then you probably would trust it, but I think initially, well like I do with this, I would use it to compare my decision with what . . . I mean it’s difficult because subsequently the tool represents a guideline doesn’t it! That’s how it is in my mind, and you’re still thinking ‘OK this is what I think I need to do’ and I know what the guidelines written on a piece of paper say, and maybe I should be doing that, but my gut feeling tells me ‘before you do that maybe you ought to do something else’.
It works like I said, it does work 85% of the time, I mean there are patients who will never fit into the protocol, that always happens but that needs to be discussed anyway I think, so OMA actually sort of, in a way screens patients who fit into the straight part of it and the good thing is for the people fitting into the straight part of it, it gives you a decision so that it could be used by the juniors. Now if they don’t fit into the straight part where it is good, the juniors need to discuss with the seniors.
Appendix 3 Transcript from clinician interview on clinicians’ perceptions of possible uses of the Optimising the Management of Angina clinical decision support system in clinical practice
Reassurance
I think part of it does because you kind of sometimes you’ve got that niggle at the back of your mind . . . are you making the right decision or not . . . and most of the time it is clear cut one way or another but you will always have that kind of borderline patients and there is something in the back of your mind that thinks wow, I’m not 100% and as a reassuring tool maybe or not or partially yes steer your decision, I think yes, it will be useful.
INT-VAL
Training for junior doctors
I think it would be useful for less experienced professionals, you know that’s the most important thing . . . because it gives you a suggestion. N and myself we are both are doctors and we can decided on our own. J is very experienced but in many other areas you find many juniors doing it or even not doctors doing the chest pain clinic.
INT-SUJ
Helping to implement National Institute for Health and Care Excellence guidance
That was what stuck in my mind, because that was the first question. What does the tool say? So I think where, certainly a couple of the cardiologists that are fully on board with this, I think where they see it fitting in is helping to imbed what NICE requires us to do.
INT-JT
Appendix 4 Participant consent form used in the Clinical Cohorts in Coronary disease Collaboration study
Appendix 5 Clinical Cohorts in Coronary disease Collaboration blood resource: sample collection and processing
FIGURE 80.
Clinical Cohorts in Coronary disease Collaboration blood resource: sample collection and processing. EDTA, ethylenediaminetetraacetic acid; PST, plasma separator tube; RNA, ribonucleic acid; SST, serum separator tube.

Appendix 6 Clinical Cohorts in Coronary disease Collaboration participant baseline health questionnaire
Appendix 7 Clinical Cohorts in Coronary disease Collaboration case record form for extraction of clinical data from the electronic health record
Appendix 8 Postgraduate qualifications and career development
Master of Science dissertations/Bachelor of Medicine, Bachelor of Surgery
Abell B. The Feasibility, Validity and Reproducibility of Novel Clinical Measurement Devices in the Assessment of Coronary Heart Disease: A Pilot Study in 22 Participants. MSc in Clinical and Experimental Medicine. London: University College London; 2010.
Crowther M. Improving the Quality of Care of Patients with Angina and Heart Attack. MSc in Medical Statistics. NIHR Research Methods Fellowship Programme. Leicester: University of Leicester; 2010.
Mole G. Gender Differences in Coronary Disease Investigation and Outcomes. MBBS. Brighton: Brighton and Sussex Medical School; 2011.
Kim H. The Impact of Drug Choice and Combinations on the Mortality and Morbidity among Stable Angina Patients in England: Prospective Approach using CALIBER Database. MSc in Health and Society: Social Epidemiology. London: University College London; 2011.
Zhang J. Smoking Effects on Different Manifestations of Coronary Heart Disease. MSc in Health and Society: Social Epidemiology. London: University College London; 2011.
Furr J. Internal Validation of the Accuracy of Diagnostic Categorizations in Coronary Heart Disease: Descriptive, Outcome and Temporal Analysis in a Linked Database. MSc in Health and Society: Social Epidemiology. London: University College London; 2011.
Choi M. Aetiological Effects of Depression on Different Phenotypes of Coronary Heart Disease: The Roles of Age, Sex and Region. MSc in Health and Society: Social Epidemiology. London: University College London; 2011.
Zhao M. The Association of Exercise ECG Variable beyond ST-segment with Prognosis in Patients with Suspected or Confirmed Coronary Disease: A Systematic Review and Meta-Analysis. MSc in Preventative Cardiology. London: Imperial College London; 2011.
Shah A. Comparison of Methods for Handling Missing Data in the CALIBER Dataset. MSc in Medical Statistics. London: London School of Hygiene and Tropical Medicine; 2012.
Fidler M. Hypertension Management, Social Deprivation, and Coronary-Specific Endpoints in 1,349,867 Patients: A CALIBER Study. MSc Health and Society: Social Epidemiology. London: University College London; 2012.
Root A. The Effect of Visit-to-Visit Blood Pressure Variability on Cardiovascular Events in a Hypertensive Primary Care Cohort. MSc Epidemiology. London: London School of Hygiene and Tropical Medicine; 2013.
Chen Y. Primary Care Utilisation and Acute Myocardial Infarction Hazards: An Open Cohort CALIBER Study. MSc in Health and Society: Social Epidemiology. London: University College London; 2013.
Ukandu M. Study of the Association between Cerebrovascular Disease and Socio-economic Deprivation in Hypertensive Patients: A CALIBER Study. MSc in Health and Society: Social Epidemiology. London: University College London; 2013.
Fellowships/Doctor of Philosophy studentships
Warren-Gash C. Medical Research Council. The Role of Influenza and Acute Respiratory Infections as Triggers for Acute Cardiovascular Events. PhD Thesis. London: London School of Hygiene and Tropical Medicine; 2012.
Herrett E. Medical Research Council. Myocardial Infarction as the First Manifestation of Atherosclerotic Disease. PhD Thesis. London: London School of Hygiene and Tropical Medicine; 2012.
Johnson R. Shared Decision Making for Cardiovascular Conditions. NIHR In-Practice Fellowship. Bristol: University of Bristol; 2012.
George J. Gender Differences in Outcomes for 30,000 Myocardial Infarction Patients: Longitudinal Study Before and After Hospitalisation. NIHR Doctoral Fellowship. London: University College London; 2013.
Crowther M. Dynamic Clinical Risk Predictions in Personalised Medicine: Development and Application of Joint Models for Repeatedly Measured Biomarkers and Time-to-Event Data in Biomedical Research. NIHR Doctoral Research Fellowship. Leicester: University of Leicester; 2015.
Shah A. Differential Leucocyte Counts and the Prognosis of Specific Coronary Phenotypes. Wellcome Research Training Fellowship. London: University College London; 2015.
Archangelidi O. Heart Rate and Prognosis of Stable Coronary Disease. Servier-funded studentship. London: University College London; 2015.
Patel R. NIHR Clinical Lectureship in Cardiology. NIHR Clinical Lectureship. London: University College London; 2015.
Pasea L. Antithrombotic Therapies in Cardiovascular Diseases. Industry partnership PhD studentship with AstraZenica. London: University College London; 2016.
Wallace J. Examining the Determinants in the Time Course of Atrial Fibrillation: Factors Affecting Risk and Prognosis. Part of work for PhD thesis. London: Medical Research Council and University College London; not completed.
Hafidha Hikmayani N. Quality of Care in Incident Type 2 Diabetes: Attainment over Time, Treatment and Cardiovascular Prognosis. PhD thesis (Islamic Development Bank–University College London Joint Scholarship). London: University College London; in progress (date of completion: 2018).
Johnson R. Shared Decision Making for Cardiovascular Conditions. NIHR Doctoral Fellowship. Bristol: University of Bristol; ongoing (date of fellowship end: 2019).
Medical trainees
Juttner K, NIHR Academic Clinical Fellow, was involved in investigating relationships between depression and anxiety and CHD outcomes.
Zhang X, academic Foundation Year 2 junior doctor, developed the 4C questionnaire and manual.
Appendix 9 Invited talks and conferences
2009
Hemingway H. Epidemiology of Specific Coronary Phenotypes. Paper presented at the Royal Society of Medicine, London, January 2009.
Hemingway H. Higher Resolution Enquiry: How Electronic Health Records might Contribute to Understanding Coronary Disease Inequalities. Paper presented at the Wellcome Trust Symposium on Electronic Health Records. London, April 2009.
Hemingway H. Higher Resolution Enquiry: How Electronic Health Records might Contribute to Understanding Coronary Disease Inequalities. Paper presented at the Royal Statistical Society, London, April 2009.
Hemingway H. Opportunities in Large Scale Electronic Health Record Linkages: CALIBER. Paper presented at the Yale–UCL Collaboration, Yale, CT, May 2009.
Hemingway H. Higher Treating Chronic Stable Angina: Who’s Winning, Who’s Losing and Why? Paper presented at the British Cardiovascular Society, London, June 2009.
Hemingway H. Evolution of Prognosis Research: 10 Steps Forward. Paper presented at the Deutsche Gesellschaft fuer Epidemiologie, Münster, September 2009.
Hemingway H. Genes and Environment in Specific Coronary Phenotypes. Paper presented at the World Health Organization Co-ordinating Centre, Münster, September 2009.
Hemingway H, Timmis A, Feder G, Denaxas S, Fitzpatrick N. Optimising the Management of Angina. Expert Panel Meeting on the Investigation and Treatment of Suspected Angina. London, December 2009.
Hemingway H. Prognosis Research Strategy (PROGRESS) International Workshops. Chair of Interdisciplinary, International Group, London, December 2009.
2010
Cramer H. Using Ethnography to Inform the Development of a Complex Intervention for the Diagnosis and Management of Angina. South West Society Academic Primary Care, Oxford, March 2010.
Timmis A. Chest Pain Guidelines – What’s New? British Cardiac Society, Manchester, June 2010.
Johnson R. Developing a Complex Intervention: Combining an Ethnographic Analysis and a Psychological Theoretical Framework. Paper presented at the Society of Academic Primary Care Annual Conference, Norwich, July 2010.
Denaxas S. Linking the GPRD and the Mycoardial Ischaemia National Audit Project. Paper presented at the Clinical Practice Research Datalink International User Group meeting, Brighton, August 2010.
Timmis A. Acute Coronary Syndrome – Lessons from the Myocardial Ishaemia National Audit Project Registry. Paper presented at the Royal College of Physicians Update, Nottingham, September 2010.
Hemingway H. Prognosis Research Strategy (PROGRESS) International Workshops. Chair of Interdisciplinary, International Group, London, December 2010.
2011
Cramer H. Treading Carefully: An Ethnography of the Clinical, Social and Educational Uses of Exercise Electrocardiograms in Evaluating Stable Chest Pain. Paper presented at the Annual Society Academic Primary Care, Bristol, March 2011.
Hemingway H. What do Hospitals do for Patient Outcomes? 30-day Mortality after Heart Attack. Paper presented at the Future of Healthcare in Europe, London, May 2011.
Timmis A. NICE Angina Guidelines: Implications and Implementation. Paper presented at the British Cardiac Society, Manchester, June 2011.
Crowther M. Flexible Joint Modelling of Longitudinal and Survival Data. Paper presented at the International Society for Clinical Biostatistics, Ottawa, ON, August 2011.
Crowther M. Flexible Parametric Joint Modelling of Longitudinal and Survival Data. Paper presented at the Stata UK Users’ Group Meeting, London, September 2011.
Timmis A. MINAP – Developing a National Research Programme. Paper presented at the Royal College of Physicians, London, September 2011.
Hemingway H. Amplifying the Research Potential through Linkage: A Longitudinal Dimension in Acute Coronary Syndrome Research. Paper presented at the Royal College of Physicians MINAP Symposium, London, September, 2011.
Hemingway H. Predicting Outcomes in Stable Coronary Artery Disease. Paper presented at the Cardiovascular Forum, Girona, September 2011.
Hemingway H. CALIBER ClinicAl Disease Research sing Linked Bespoke Studies and Electronic Records eHealth Symposium. Paper presented at University College London, London, November 2011.
Crowther M. Flexible Parametric Joint Modelling of Longitudinal and Survival Data. Paper presented at the Workshop on Methodological Developments and Applications of Flexible Parametric Survival Models, Karolinska Institute, Stockholm, November 2011.
2012
Hemingway H. Linking Phenotypic and Genomic Resources to Primary Care: CALIBER. Paper presented at the Primary Care Database Symposium, Wellcome Collection, London, January 2012.
Cramer H. Using Qualitative Methods to Develop a Complex Intervention and Evaluate Trial Feasibility: The OMA Pilot Study. Paper presented at the MRC ConDuCT Hub workshop, Social and Community Medicine short course: Qualitative Research in Randomised Trials, Bristol, March 2012.
Cramer H. Using Theory to Enhance Qualitative Health Research: Ethnography and the Variation in Coronary Care study. Paper presented at the Cardiovascular research and theory, Birmingham CLAHRC, Birmingham, March 2012.
Crowther M. Joint Modelling of Repeatedly Measured Biomarkers and Time-to-Event Data. Survival Analysis for Junior Researchers, University of Leicester, Leicester, April 2012.
Hemingway H. The CALIBER Research Platform ClinicAl Disease Research using LInked Bespoke Studies and Electronic Records International Data Linkage. Paper presented at the International Data Linkage Conference, Perth, WA, May 2012.
Hemingway H. Prognostic Models for People with Stable Coronary Artery Disease based on 115,500 Patients: a CALIBER Study. Paper presented at the European Society of Cardiology, Munich, August 2012.
Crowther M. Adjusting for Measurement Error in Baseline Prognostic Biomarkers: A Joint Modelling Approach. Paper presented at the International Society for Clinical Biostatistics, Bergen, August 2012.
Herrett E. Heralding of STEMI and NSTEMI: A CALIBER Study. Paper presented at the International Conference of Pharmaco-epidemiology and Drug Safety, Barcelona, August 2012.
Hemingway H. Initial Presentations of Cardiovascular Diseases. Paper presented at the Cardiovascular Disease Forum, Cambridge University, Cambridge, September 2012.
George J. Smoking and Initial Presentation of Cardiac Disease: Cohort Study using linked Electronic Health Records. Paper presented at the Society for Social Medicine Scientific Programme, London School of Hygiene and Tropical Medicine, London, September 2012.
Cramer H. An Ethnography Trying to Explain Outcome Variation in Cardiac Care: The Process and Findings of the VICC Study. Paper presented at the Medical Sociology annual conference, Leicester, September 2012.
Hughes J. Up Close and Personal – Doing Ethnography of Acute Interventional Cardiology. Paper presented at the Medical Sociology annual conference, Leicester, September 2012.
Hemingway H. Population-Based Approaches to Tackling Non-Communicable Disease – EHR and Translation. Paper presented at the Singapore 1st Public Health Symposium, Singapore, October 2012.
Hemingway H. Comparative Effectiveness of Acute Myocardial Infarction Care Delivered In Sweden and the United Kingdom Using National Outcome Registries. Paper presented at the American Heart Association, Los Angeles, CA, November 2012.
Crowther M. Development and Application of Joint Models for Repeatedly Measured Biomarkers and Time-to-Event Data in Biomedical Research. Paper presented at the Joint meeting of Royal Statistical Society West Midlands Local group and MRC Midland Hub for Trials Methodology, University of Birmingham, Birmingham, November 2012.
George J. Differential Effects of Smoking on Specific Cardiovascular Presentations in Men and Women: Prospective Cohort Study in 900,000 Patients Using CALIBER Linked Electronic Health Records. Paper presented at the American Heart Association, Los Angeles, CA, November 2012.
Timmis A. Clinical Translation of National Cardiovascular Registries. Paper presented at the Grand Round, Cleveland Clinic, USA, December 2012.
2013
Crowther M. An Introduction to Joint Models for Repeatedly Measured Biomarkers and Time-to-Event Data in Biomedical Research. Paper presented at the Centre for Health Economics seminar series, University of York, York, February 2013.
Cramer H. Using Qualitative Methods to Develop a Complex Intervention and Evaluate Trial Feasibility: The Optimising Management of Angina Pilot Study. Paper presented at the MRC ConDuCT Hub workshop, Social and Community Medicine short course: Qualitative Research in Randomised Trials, Bristol, March 2013.
Hemingway H. e-Health Records Research: Benefits for Patients and Populations. Paper presented at the Westminster Health Forum, London, April 2013.
Shah A. Using Free Text in Primary Care Research. Paper presented at the Department of Primary Care and Population Health, Royal Free Campus, University College London, London, April 2013.
Hemingway H. Linked Electronic Health Records and New Insights on Prevention: CALIBER. Paper presented at the British Cardiovascular Society, London, June 2013.
Hemingway H. CHAPTER Health Records Research: Meeting the National and International Expectations. Paper presented at the Computational Life and Medical Sciences Network (CLMS) Annual Symposium, London, June 2013.
Shah A. Extracting Information for Research from Free Text in Electronic Health Records. Paper presented at the BRC MH Bioinformatics seminar, King’s College London, London, June 2013.
Timmis A. Clinical Applications of Prognosis Research. Paper presented at the Prognosis Research course, Keele, June 2013.
Hemingway H. Prognosis Research: New Opportunities in Linked Electronic Health Records. Paper presented at the Methods for Evaluating Medical Tests and Biomarkers Third International Symposium, Birmingham, July 2013.
Hemingway H. The Farr Institute, London: The First 3 Months. Paper presented at the Scottish Health Informatics Programme (SHIP), Biannual Conference, St Andrew’s, August 2013.
Hemingway H. Linked Electronic Health Records: New Opportunities for Prospective Studies. Paper presented at the Erasmus Summer School, Rotterdam, August 2013.
Shah A. Extracting Information for Research from Free Text in Electronic Health Records. Scottish Health Informatics Programme conference, St Andrews, UK, August 2013.
Shah A. Automated Phenotyping using Freetext in Primary Care Electronic Health Records in Patients with Coronary Artery Disease: A CALIBER Study. Paper presented at the Scottish Health Informatics Programme conference, St Andrews, August 2013.
Cramer H. Working Across and Against Boundaries: An Ethnographic Study of Heart Attack Care in Ten UK Hospitals. Paper presented at the York Medical Sociology conference, York, September 2013.
Crowther M. Joint Modelling of Longitudinal and Survival Data. Paper presented at the Department of Medical Epidemiology and Biostatistics Seminar, Karolinska Institutet, Stockholm, November 2013.
Hemingway H. Investing in Skills to Manage Datasets and Analytics. Paper presented at the ABPI R&D Conference 360° of Health Data – Harnessing big data for better health, London, November 2013.
Patel R. Coronary Artery Disease Genomics. Paper presented at the Royal Society of Medicine, London, December 2013.
Hemingway H. Linking Data across Primary Care, Secondary Care, Disease Registries and Mortality: The CALIBER Programme. Paper presented at the NCVIN Stakeholder Event: Using data and information to improve the quality of care and outcomes of people with cardiovascular disease: a new National Cardiovascular Intelligence Network (NCVIN), London, December 2103.
2014
Hemingway H. Academic Perspective. Paper presented at the UCL Partners Informatics Stakeholder Event: mobilising information for patient, population research benefit, London, January 2014.
Crowther M. Joint Modelling of Longitudinal and Survival Data in Biomedical Research. Paper presented at the Department of Medical Statistics seminar series, London School of Hygiene and Tropical Medicine, London, February 2014.
Hemingway H. A Retrospective Cohort Study of Health Outcomes and mortality in Patients with Stable Coronary Artery Disease (CAD) with a History of prior Myocardial Infarction (MI) in a UK setting. Paper presented at the Apollo Steering Committee Meeting, London, February 2014.
Hemingway H. The Wider UK Context. Data in Safe Havens, Paper presented at the Academy of Medical Sciences and Wellcome Trust, London, March 2014.
Cramer H. Using Qualitative Methods to Develop a Complex Intervention and Evaluate Trial Feasibility: The Optimising Management of Angina Pilot Study. Paper presented at the MRC ConDuCT Hub workshop, Social and Community Medicine short course: Qualitative Research in Randomised Trials, Bristol, March 2014.
Hemingway H. Higher Resolution Approach to Cardiovascular Risk Factors. Paper presented at the British Heart Foundation Informatics Symposium, London, April 2014.
Timmis A. UK National Cardiovascular Registries. Paper presented at the British Heart Foundation, London, April 2014.
Denaxas S. Electronic Health Record Phenotyping Algorithms and Tools. British Heart Foundation Electronic Health Record workshop, London, April 2014.
Timmis A. Translating the Electronic Health Record for Clinical Research. Paper presented at the Farr research Forum, Edinburgh, May 2014.
Crowther M. Joint Modelling of Longitudinal and Survival Data in Biomedical Research. Paper presented at the Probability and Statistics Group seminar series, University of Manchester, Manchester, May 2014.
Hemingway H. Farr London. Paper presented at the First Farr Research Forum, Edinburgh, May 2014.
Hemingway H. Big Data and Cardiovascular Disease Research: Opportunities in the Farr Institute. The Henderson Lecture, Welsh Cardiovascular Society, Cardiff, May 2014.
Timmis A. Clinical Applications of Prognosis Research. Paper presented at the Prognosis Research course, Keele, June 2014.
George J. Association of Gender, Smoking and Blood Pressure on Specific Cardiovascular Disease Presentations. Paper presented at the Public Health Department, Surrey County Council, Kingston upon Thames, June 2014.
Hemingway H. Linking Health System Data for Research: Early Experience in the UK Farr Institute. Paper presented at the Geisinger Medical Centre, Danville, PA, June 2014.
Crowther M. Flexible Joint Modelling of Longitudinal and Survival Data: Implementation in Stata. Paper presented at the Statistical Analysis of Multi-Outcome Data Workshop, University of Cambridge, Cambridge, June–July 2014.
Cramer H. Reflections on the Meanings, Interpretations and Impact of Chest Pain. Paper presented at the European Association of Social Anthropologists, Tallinn, July 2014.
Crowther M. Bayesian Modelling of Multivariate Biomarker Data using Informative Prior Distributions in order to Predict Clinical Outcomes. Paper presented at the International Society for Bayesian Analysis World Meeting, Cancun, July 2014.
Crowther M. Joint Modelling of Longitudinal and Survival Data Incorporating Delayed Entry: Application to Repeatedly Measured Mammographic Breast Density and Breast Cancer Survival. Paper presented at the International Society for Clinical Biostatistics, Vienna, August 2014.
Hemingway H. International Comparison of Outcomes Among 140,880 patients stable after Acute Myocardial Infarction; Real World Evidence from Electronic Health and Administrative Records. Paper presented at the Registry Hotline, European Society of Cardiology Congress, Barcelona, August 2014.
Rapsomaniki E, Stogiannis D, Emmas C, Chung S, Pasea L, Denaxas S, et al. Health Outcomes in Patients with Stable Coronary Artery Disease Following Myocardial Infarction; Construction of a PEGASUS-TIMI-54 Like Population in UK Linked Electronic Health Records. Paper presented at the European Society of Cardiology Congress, Barcelona, 31 August 2014.
Hemingway H. Session 3: Strategic Partners. Paper presented at the Francis Crick Institute and the Wellcome Trust Sanger Institute joint retreat, Oxford, September 2014.
Hemingway H. The Health Record Data (R)evolution: Early Experience of the Farr Institute. Paper presented at the Julius Seminar, Utrecht University Graduate School of Life Sciences, Utrecht, September 2014.
Hemingway H. Can Big Data Attack Heart Disease? Paper presented at the Life Sciences Seminar, Utrecht University Graduate School of Life Sciences (a monthly seminar for master’s students in various programmes, from molecular biology to drug innovation, from toxicology to epidemiology). Utrecht, September 2014.
Hemingway H. Big Health Data: Perspectives across the Patient Journey from Linking Multiple Record Sources. Paper presented at the Institute and Faculty of Actuaries’ International Mortality and Longevity Symposium, Birmingham, September 2014.
Herrett E. Clinical Presentations Before Myocardial Infarction: The Population Perspective. Paper presented at the UCL Special Grand Round, London, October 2014.
Hemingway H. Cardiovascular Risk in Patients with Atherosclerotic Disease: APOLLO Underlying Risk Scores. Paper presented at the Ticagrelor Forum, Amsterdam, October 2014.
2015
Hemingway H. Stress, Heart Attacks and Strokes – What can Big Data Tell Us? The Lancet Lecture, At the Limits: Cardiology, Diabetes and Nephrology, Cape Town, February 2015.
Hemingway H. Linked Electronic Health Records in the Prequel and Sequel to Trials. Paper presented at the EMBL European Bioinformatics workshop, London, April 2015.
Hemingway H. Pros and Cons of Disease Registries. Paper presented at the 17th International Vasculitis and ANCA Workshop, London, April 2015.
Hemingway H. eHealth Research – Value of UK Data Sources and Data Linkage Capabilities. Paper presented at the ABPI, The REAL WORLD Challenge: Are you maximising the effectiveness of RWE? London, May 2015.
Hemingway H. Big Data and Connected Challenges in Health: Examples in Cardiovascular Diseases. Paper presented at the Richard Doll Seminars in Public Health and Epidemiology, University of Oxford, Oxford, June 2015.
Hemingway H. Big Data and Connected Challenges in Cardiovascular Health. Annual Meeting of CANHEART (Cardiovascular Health In Ambulatory Care Research Team), Toronto, ON, June 2015.
Hemingway H. Big Data and Connected Challenges in Health: Opportunities in Cardiovascular Diseases. Paper presented at the Universal Healthcare: Investing in Wellbeing for All, Riga, June 2015.
Hemingway H. International Comparisons of Myocardial Infarction Care and Outcomes. Paper presented at the European Society of Cardiology Congress, London, September 2015.
Hemingway H. What are the Future Sources of Data? Paper presented at the Academy of Medical Science/Wellcome Trust ‘Evaluating evidence in health’ workshop, London, October 2015.
Hemingway H. Personalising the Decision for Prolonged Dual Antiplatelet Therapy. Paper presented at the AstraZeneca Risk Stratification Advisory Board, London, October 2015.
Hemingway H. Stratified Medicine: A Perspective from the Farr Institute. MRC Stratified Medicine Initiative: Data Management meeting, London, November 2015.
Hemingway H. Big Data: Novel Approaches to Strengthening English Burden of Disease Estimates on Morbidity using Electronic Health Records. Paper presented at the Findings of the Global Burden of Disease study England 2013: Changes in Health with Analysis by Region and Deprivation, London, December 2015.
Hemingway H. Data Science and Health Informatics. Paper presented at the NIHR University College London Hospitals Biomedical Research Centre. Independent Expert Panel Review, London, December 2015.
List of abbreviations
- 4C
- Clinical Cohorts in Coronary disease Collaboration
- ABPI
- Association of the British Pharmaceutical Industry
- ACCF
- American College of Cardiology Foundation
- ACEI
- angiotensin-converting enzyme inhibitor
- ACRE
- Appropriateness of Coronary REvascularisation
- ACS
- acute coronary syndrome
- ACTION
- Acute Coronary Treatment and Intervention Outcomes Network Registry
- AHA
- American Heart Association
- AMI
- acute myocardial infarction
- AR
- audio-recording
- ARB
- angiotensin receptor blocker
- BEAUTIFUL
- ivabradine for patients with stable coronary artery disease and left-ventricular systolic dysfunction
- BMI
- body mass index
- BMJ
- British Medical Journal
- BP
- blood pressure
- b.p.m.
- beats per minute
- CABG
- coronary artery bypass graft
- CAD
- coronary artery disease
- CALIBER
- ClinicAl disease research using LInked Bespoke studies and Electronic health Records
- CARDIoGRAM PlusC4D
- Coronary ARtery DIsease Genome wide Replication and Meta-analysis (CARDIoGRAM) plus The Coronary Artery Disease
- CCS
- Canadian Cardiovascular Society
- CDSS
- clinical decision support system
- CG
- Clinical Guideline (NICE)
- CHD
- coronary heart disease
- CI
- confidence interval
- CMOC
- cumulative missed opportunity for care
- COPD
- chronic obstructive pulmonary disease
- CPRD
- Clinical Practice Research Datalink
- CRP
- C-reactive protein
- CT
- computerised tomography
- CVD
- cardiovascular disease
- DBP
- diastolic blood pressure
- DNA
- deoxyribonucleic acid
- ECG
- electrocardiogram
- EHR
- electronic health record
- eMERGE
- Electronic Medical Records and Genomics
- EQ-5D
- EuroQol-5 Dimensions
- ESC
- European Society of Cardiology
- ETT
- exercise treadmill test
- FMA
- freetext matching algorithm
- FN
- field note
- GAD-7
- Generalized Anxiety Disorder 7-item scale
- GP
- general practitioner
- GPRD
- General Practice Research Database
- GRACE
- Global Registry of Acute Cardiac Events
- HbA1c
- glycated haemoglobin
- HDL
- high-density lipoprotein
- HES
- Hospital Episode Statistics
- HIC
- Health Informatics Collaborative
- HR
- hazard ratio
- HSMR
- Hospital Standardised Mortality Ratio
- ICD-10
- International Statistical Classification of Diseases and Health-Related Problems, Tenth Edition
- IHD
- ischaemic heart disease
- IMD
- Index of Multiple Deprivation
- INT
- interview (data analysis)
- IQR
- interquartile range
- IRR
- incident rate ratio
- ISIS-2
- Second International Study of Infarct Survival
- ISO
- International Organization for Standardization
- JNC 8
- Eighth Joint National Committee
- MINAP
- Myocardial Ischaemia National Audit Project
- MRC
- Medical Research Council
- NICE
- National Institute for Health and Care Excellence
- NICOR
- National Institute for Cardiovascular Outcomes Research
- NIHR
- National Institute for Health Research
- NSTEMI
- non-ST-segment elevation myocardial infarction
- OB
- observation
- OMA
- Optimising the Management of Angina
- ONS
- Office for National Statistics
- OPCS
- Office of Population, Censuses and Surveys
- OR
- odds ratio
- PAD
- peripheral arterial disease
- PCI
- (primary) percutaneous coronary intervention
- PHQ-9
- Patient Health Questionnaire-9
- PPI
- proton pump inhibitor
- PPV
- positive predictive value
- PROGRESS
- PROGnosis RESearch Strategy
- QALY
- quality-adjusted life-year
- RACPC
- rapid access chest pain clinic
- RCT
- randomised controlled trial
- S3CM
- Semi-supervised Set Covering Machine
- SBP
- systolic blood pressure
- SCAD
- stable coronary artery disease
- SCD
- sudden cardiac death
- SD
- standard deviation
- STEMI
- ST-segment elevation myocardial infarction
- SWEDEHEART/RIKS-HIA
- Swedish Web-system for Enhancement and Development of Evidence-based care in Heart disease Evaluated According to Recommended Therapies/Register of Information and Knowledge about Swedish Heart Intensive care Admissions
- TSVM
- transductive support vector machine