Notes
Article history
The research reported in this issue of the journal was funded by PGfAR as project number RP-PG-0407-10064. The contractual start date was in September 2008. The final report began editorial review in August 2015 and was accepted for publication in March 2016. As the funder, the PGfAR programme agreed the research questions and study designs in advance with the investigators. The authors have been wholly responsible for all data collection, analysis and interpretation, and for writing up their work. The PGfAR editors and production house have tried to ensure the accuracy of the authors’ report and would like to thank the reviewers for their constructive comments on the final report document. However, they do not accept liability for damages or losses arising from material published in this report.
Declared competing interests of authors
Nigel Arden reports personal fees from Bioventus, Flexion, Merck Sharp & Dohme, Regeneron, Smith & Nephew, Freshfields Bruckhaus Deringer, and grants from Bioibérica and Novartis outside the submitted work. Daniel Prieto-Alhambra reports grants from Servier Laboratories, AMGEN and Bioibérica S.A., and advisory board fees from AMGEN outside the submitted work. Andrew Judge reports personal fees from Anthera Pharmaceuticals, Inc., Servier, the UK Renal Registry, Blood Journal and Freshfields Bruckhaus Deringer, and grants and personal fees from the Oxford Craniofacial Unit, and grants from Roche-Chugai outside the submitted work. Jeremy Latham reports personal fees from Zimmer Biomet, Lima Corporate, MatOrtho and DePuy Synthes outside the submitted work. Rafael Pinedo-Villanueva reports personal fees from Freshfields Bruckhaus Deringer outside the submitted work. David Murray reports grants and personal fees from Zimmer Biomet and grants from Stryker and Health Technology Assessment (HTA) outside the submitted work. James Raftery reports that he is an editor on the National Institute for Health Research HTA and EME editorial board. In addition, James Raftery was previously a Director of the Wessex Institute and Head of the National Institute for Health Research (NIHR) Evaluation, Trials and Studies Coordinating Centre (NETSCC).
Permissions
Copyright statement
© Queen’s Printer and Controller of HMSO 2017. This work was produced by Arden et al. under the terms of a commissioning contract issued by the Secretary of State for Health. This issue may be freely reproduced for the purposes of private research and study and extracts (or indeed, the full report) may be included in professional journals provided that suitable acknowledgement is made and the reproduction is not associated with any form of advertising. Applications for commercial reproduction should be addressed to: NIHR Journals Library, National Institute for Health Research, Evaluation, Trials and Studies Coordinating Centre, Alpha House, University of Southampton Science Park, Southampton SO16 7NS, UK.
Chapter 1 Introduction
Background
Osteoarthritis (OA) is one of the most common musculoskeletal conditions worldwide and accounts for > 90% of total knee replacement (TKR) and total hip replacement (THR) procedures in the UK. 1 There is currently no acceptable medical therapy for reducing the onset or progression of OA and the current treatments are aimed at symptom relief and increasing mobility. 2,3 The only successful treatment for patients with OA of the lower limbs is arthroplasty.
A wide range of high-quality support measures and treatments are needed for individuals suffering from musculoskeletal conditions. Choice of treatment is based on type of condition, severity of symptoms and access to health-care services and professionals. An estimated 30% of general practitioner (GP) consultations4 and 40% of visits to NHS walk-in centres are for musculoskeletal-related conditions. 5,6
Currently, lower limb arthroplasty is the most common and most successful elective orthopaedic procedure undertaken in the UK in patients with OA affecting the hip and knee joints. 7,8 It provides substantial relief from pain and improves physical function,2,8–10 and is considered the most successful and cost-effective operation for end-stage disease. 2,9,11,12 It is therefore not unexpected that the workload of trauma and orthopaedic surgical services has intensified.
Musculoskeletal conditions are a major cause of ill health, pain and disability, placing a significant burden on the NHS. 13 Evidence suggests that this burden will only increase as a result of a growing elderly population and an increase in obesity. 14 A combination of these factors, in association with related comorbidities,15 highlights an urgent need for accurate and reliable data to ensure effective long-term planning and equitable resource allocation across all regions in the UK. It is therefore essential that the current needs for surgery are accurately described and future trends estimated for effective planning of health-care services.
Surgical trends
It has been estimated that a total of 52,048 THRs and 44,645 TKRs were performed in 2002, and the number of elective procedures increased markedly in the period 1989–2004. 16 These surgical interventions remain the top elective procedures performed by orthopaedic surgeons in the NHS and, in the 12 months to April 2012, a total of 75,366 hip replacements and 76,497 knee replacements were performed17 in the NHS.
The reasons for the increase in demand are widely debated and suggested explanations include improved instrumentation and prostheses survival rates, but also increasing numbers of patients with OA (related or unrelated to comorbid chronic conditions such as obesity) and the significant increase in total joint replacements (TJRs) among individuals aged > 55 years. 18
Patient choice is important to understand and may be a factor affecting demand, as suggested by a Canadian study assessing OA patients’ perception of total joint arthroplasty as an intervention, which found that the willingness to undergo surgery was inversely associated with misperceptions about its appropriateness. 19 The same team also found low rates of willingness among those with disabling arthritis. 20 Women seemed to be less willing than men to undergo surgery21 and less willing to undergo TKR than THR22 as a suitable intervention. Jüni et al. 23 previously reported that 32% of patients considered for TKR, for a variety of reasons, did not consider surgery an option. The group conducted a population-based study of TKR in the south-west of England, using an assessment of need based on the New Zealand Score,24 and found that differences in the perception of disease severity may account for some of the underprovision reported by the study team.
Some estimates have been produced from historical rates of arthroplasty but up-to-date information is essential to estimate future rates. Historically, published findings were often based on small data sets unrepresentative of a general population and administrative codes lacked specificity. The potential of these codes in providing accurate and clinical descriptions was recognised, and revision codes were updated in October 2005. 25 Furthermore, published results have used simplistic models to predict changes in future rates and have produced unrealistic information. 22
Predictors of surgery outcomes and prediction tool
Although the majority of patients improve after hip and knee arthroplasty, an important group of patients continue to experience some pain and functional disability after THRs and TKRs, and some experience no improvement or get worse. 26–35 This especially applies to TKR surgeries, as a number of studies have identified that a small minority of patients are not satisfied with their knee replacements. 26,34,36
Arthroplasties are successful interventions for end-stage disease and have been known to provide pain relief and improved physical function. 8 However, for years, the approach has tended to focus on revision as an outcome, with few data on patient-reported outcomes. In more recent years, the government has accepted the importance of patient-reported outcomes for this operation and introduced the patient-reported outcome measures (PROMs) for monitoring the outcomes of such patients.
There is consistent evidence in the joint arthroplasty literature that up to 30% of patients are dissatisfied with their outcomes. 37,38 It still not entirely clear from the available evidence what factors contribute to dissatisfaction. For example, Gandhi et al. 38 and Hawker et al. 26 found that preoperative pain and function were not associated with patient satisfaction and, yet, Kim et al. 39 and Scott et al. 40 demonstrated that less preoperative pain is suggestive of increased satisfaction.
As a result of advances in arthroplasty devices and improvements in technical surgical skills and expertise, a successful long-term outcome is now achieved in the majority of patients undergoing hip or knee replacements. It has, however, also become evident that prosthesis survival may not be an accurate or true measure of success when patient satisfaction is taken into account and, by this criterion, a small but important group of patients do not improve or even get worse. 27 Following this understanding, the focus has moved away from implant survival to patient-reported outcomes that concentrate on the patient’s experience and level of satisfaction with the operation. 41 The difficulty lies with identifying the determinants of outcome, as well as using the most appropriate and accurate method for collecting and interpreting the patient-reported outcomes. A successful joint replacement should result in pain relief, function improvement and patient satisfaction. 42
Total hip replacement is successful in the majority of patients. However, there is growing evidence that a small, but important, minority of patients show no improvement or get worse. 27,32,33,35 PROMs are now commonly used to determine the result of knee and hip surgical interventions. However, little work has been done to establish the predictors of good or bad patient-reported outcomes after THR. 43
Determinants of outcomes for THR have been widely researched and include baseline levels of pain and function,32,44–47 severity of clinical disease,45 age,45,47,48 sex,45,46,49 radiographic grade,45,50 education,32,44,49,50 obesity,46,48 comorbidities,32,46 living alone,46,51 mental health47 and patients’ expectation of surgery. 52 The results in literature are conflicting. For example, some studies have found that age, sex, body mass index (BMI) and comorbidities are not predictive of outcome,26,37–40 whereas others have found that a lower level of education and higher BMI are associated with dissatisfaction,26 and Noble et al. 53 found an association between age and satisfaction. 53
Validation of some key issues about age, sex, rates and indication for surgery is needed for a better understanding of these surgical interventions in order to effectively target treatment. Of the patient factors for poor outcome, there is ongoing controversy as to the role of obesity. 54 Although some have found no difference in clinical outcome after THR6 or TKR,55 others have demonstrated that obesity is a recognised risk factor for poor outcome after TKR. 6,56
Surgical technique and implant type are important factors in the outcome of hip and knee arthroplasty. However, in a recent systematic review, Kynaston-Pearson et al. 57 reported that there is no clinical evidence of effectiveness in one-quarter of available hip prostheses in the UK. The relationships between implant users, manufacturers and suppliers have been in development over a number of years, as implant costs contribute appreciably to the overall cost of surgery. Lack of implant regulation became an area of focused concentration recently as a result of the adverse outcomes in metal-on-metal resurfacings and large-bearing-surface implants. 58
It is only recently that regulatory frameworks have started to focus on the safety regulations around implants, and much more needs to be done to ensure that tested prosthetics are both safe and clinically effective. 3 Other technical factors include case volume, technique and choice of prosthesis. Technical factors in performing surgery, such as component alignment,59 influence both the short- and long-term success rates. The technical ability of the surgeon also plays a vital role in the successful outcome of hip and knee replacements, and continues to drive the ongoing development and refinement of implants, surgical techniques, skills and training.
Historically, most research has focused on implant failure as the main outcome. There are three main causes of implant failure: aseptic osteolysis, infection and inflammation. Osteolysis results, in part, from resin wear, leading to local inflammation and accelerated bone resorption. 60 Differences between implant design, resin storage and type affect resin wear and osteolysis. 61,62 Currently, radiographic assessment has poor sensitivity for detecting osteolysis, requiring at least 50% of demineralisation to occur before osteolysis can be detected. However, osteolysis may be better detected using structural measurements, such as fractal analysis, than changes in density. 63,64 Although initially considered a purely degenerative disease, there is increased inflammation in both synovial fluid and cell membranes of osteoarthritic joints, and this may play a key role in arthroplasty failure. 65
Other common complications of arthroplasties include infection, vascular or thrombotic compromise, dislocation, instability and fracture. Infection is a common cause of early failure and can lead to significantly poorer clinical outcomes, such as amputation or revision surgery. Deep-seated infections may be the leading cause of implant failure over the next 20 years. 66 The diagnosis of surgical site infection following TJR requires a balance between quality and practicality. Revision after septic failures has a higher failure rate than revision after aseptic failures, highlighting the importance of accurate identification of sepsis. 60
Extensive research into the diseases commonly associated with degenerative changes in joints has been conducted, as an understanding of the underlying causes could assist with predicting the outcome of surgery. One of the most common musculoskeletal conditions, OA, accounted for > 90% of the total knee and hip arthroplasties (153,000 procedures) in the UK up to 2010. 1 OA is the major cause of health, pain and disability and increased mortality. Two main risk factors for OA are age and obesity, both of which are increasing in the population in the West. 67 For this reason, it is almost inevitable that the prevalence of OA will increase substantially in the next 20 years. 68
Osteoarthritis has been recognised as a global burden and is the most frequent primary indication for total hip and knee replacements in the UK,69 accounting for 93% of hip replacements and 97% of knee replacements in England and Wales. 70 In the UK, 550,000 people have moderate to severe knee OA and 210,000 moderate to severe hip OA. Each year approximately 2 million people consult their GP for OA and 115,000 are admitted to hospital. The prevalence of hip and knee OA is particularly high in the population aged > 60 years. 71–74 The lifetime risk of hip OA has been calculated at 25%75 and of knee OA has been calculated at 45%. 14,71,76 Changes in the reported prevalence of OA, such as the overall increase and increased prevalence in younger patients found by Kim,14 have to be substantiated and validated to inform new treatment algorithms for local services.
Patient selection,3,77 implant design and surgical technique are all key factors that could affect the durability of a prosthetic implant. 78 Historically, outcome studies used continuous variables at population levels to identify statistically significant predictors; however, their clinical relevance is less clearly understood, especially by patients. Understanding and identifying patients at risk of poor patient-reported outcomes and presenting these in a clinically meaningful way to an individual patient will enable clinicians to evaluate the risks and benefits of surgery on an individual level.
The lack of information led to well-publicised decisions by primary care trusts (PCTs) in Suffolk to temporarily withhold hip and knee arthroplasty from obese subjects. 79 This decision was overturned because of a lack of supporting evidence. We urgently need data to identify patients at a high risk of poor outcome both before surgery, in order to minimise risk factors, and in the early postoperative period, to initiate urgent interventions to improve outcomes and prioritise resources.
A number of individual determinants of implant failure have been described in the literature; however, the majority of patients exhibit more than one cause of failure,59 and the benefit of combining risk factors are not known. The current literature describes a wide range of risk factors in a prognostic model,80,81 including age, sex, education, obesity, mental health status, preoperative level of pain and function, indication for surgery, coexisting conditions, radiographic variables (radiographic grade) and surgery-related risk factors (i.e. femoral component offset). In this programme we aimed to develop similar prognostic models for the knee and hip, and to include a wider range of risk factors to predict pain and function outcomes.
As personalised medicine becomes increasingly common, it is essential that the correct patients are chosen to undergo hip and knee arthroplasties, which are important but complex procedures. This emphasises the importance of understanding the predictors of patient-reported outcomes of satisfaction and pain or function scores. This programme aims to address these issues. Previous work on outcomes focused very heavily on prosthesis and little attention was paid to surgical- and patient-related factors that predict outcomes. It is important to look at all three components and their interactions to predict surgery outcomes accurately. The information then can be used to identify patients with good or poor outcomes and form the clinical decision-making tool to allow stratification of patients for surgeries with patient-informed consent.
With increasing restrictions on funding in the NHS, it is critical to have accurate and reliable data from practice, alongside current and future population-based estimates, for a better understanding of these surgical interventions. This would aid our understanding of the clinical effectiveness and cost-effectiveness of lower limb arthroplasty and help to target resources more efficiently.
Cost-effectiveness of implementation of the tools
Lower limb arthroplasties are a considerable burden on NHS resources. Estimations by Jenkins et al. 82 suggest the cost per procedure to be in excess of £7000. In the USA, TJR is a cost-saving or cost-effective procedure in those with significant functional limitation as it avoids high care costs resulting from the disability of OA. 83 Early improvements in the management of patients, such as decreasing length of stay, resulted in overall reduced costs. 84,85 Although the overall costs of primary TJR have decreased, the procedural costs of revision surgery continue to increase. 86 In the UK implant survival data are impressive, with a 5-year revision rate of 4.5% for THR and 5.1% for TKR. 7 Yet, although it may be a technically successful replacement, up to 20% of knee replacement patients still have a poor outcome and a small, but important, proportion of patients who have had hip replacements do not achieve a clinically meaningful symptomatic improvement or their symptoms get worse. 27,87
Accurate cost-effectiveness data are essential for the appropriate evaluation within the NHS of the incremental cost-effectiveness ratio (ICER) of using more expensive prosthetic components that may improve implant survival. 88,89 As well as validated predictors of poor outcome following TJR outcome, cost implications are important for informing patient expectations. 90
In addition to optimising the outcome of patients undergoing arthroplasty, it is important for NHS commissioners to have accurate data on the cost-effectiveness and cost–utility of these operations. Current health economic data are limited for several reasons. The main limitations of the data on utility gains post surgery are that they are from small cohorts, they do not differentiate between different patient profiles, they are limited to outcomes at 10 years, with limited data on short-term gains, and, importantly, they use revision surgery and not ongoing health-care utilisation as a result poor functional outcomes.
In this programme we aimed to design a clinical tool to predict patients who will experience poor outcomes following THR and TKR. Taking into account the fact that these procedures are costly and exert a significant burden on the NHS, we need to ascertain if the additional cost of the implementing tools would be worthwhile in terms of benefits to an already overstretched current health-care system; that is, if the tools would be a cost-effective use of resources in the UK health-care system. With this in mind, we aim to provide an economic evaluation of the implementation of the tool in the health-care setting. The availability of predictive tools, and detailed cost-effectiveness and cost–utility data will help to produce a coherent strategy for the provision of a clinically effective and cost-effective strategy for the provision of lower limb arthroplasty in the NHS. The information collected and analysed in the development of a predictive tool for hip and knee replacement will also support the development of patient-based, informed decision-making programmes. 91
External validation of the tool
As part of the programme we aimed to test the productiveness, practicality and cost-effectiveness of the developed tool in the pragmatic cohort of NHS setting. This required us to recruit a cohort of patients undergoing hip and knee arthroplasties in which the productiveness, practicality and cost-effectiveness of the tools would be tested.
Aims of the programme grant
We aimed to inform the policy-makers of the current health-care system in the UK about predicting the outcomes and failure of lower limb arthroplasty, and give advice on the cost-effectiveness of implementation of predictive tools. We set out to achieve this through four work packages (1–4), as described in the subsequent chapters (see Chapters 2–5) of this report.
In Chapter 2 (work package 1) we describe the current and future needs for primary and revision lower limb joint replacement surgeries in the UK using a national longitudinal prospective database.
In Chapter 3 (work package 2) we look at the predictors of poor outcome following lower limb arthroplasties using extant databases. We report on combining these databases to produce predictive tools separately for knee and hip for patient-reported outcomes at 12 months.
In Chapter 4 (work package 3) we describe the detailed body of work looking at the cost-effectiveness of implementing the tool to predict the outcomes following knee and hip arthroplasties using the extant databases, nationally available routine data and our prospective cohort of patients who were recruited in work package 4.
In Chapter 5 (work package 4) we describe our prospective new cohort and the steps performed in this prospective pragmatic cohort to detail the external validation of the tools developed in work package 2.
Chapter 2 Work package 1: current and future rates of lower limb arthroplasties
This chapter describes the current and future needs for primary and revision lower limb joint replacement surgeries in the UK using a national prospective database.
The chapter contains information covered in work package 1. The objectives in this work package were to:
-
describe and estimate the rates of THR, TKR and unicompartmental knee replacement (UKR) in the UK
-
describe regional and national variation in hip and knee replacement surgery in the UK
-
describe the mechanics of revision for hip and knee arthroplasty and quantify the rates in the UK
-
predict future trends in hip and knee surgery in the UK, accounting for projected changes in age and obesity.
Design and setting
In order to quantify the rates of lower limb arthroplasty in the UK we used a prospective cohort from the Clinical Practice Research Datalink (CPRD), formerly known as the General Practice Research Database (GPRD). The CPRD is a recognised and frequently utilised database in epidemiological studies,92 and is validated. 69,93 It is a computerised medical records system that is representative of the UK population and has been validated for a wide range of medical conditions. 94 The CPRD database has data from over 6 million patients across more than 600 practices and has been collecting data since 1987. The data set has been validated and audited, and only practices providing good-quality data are admitted. Every patient is registered with one general practice. GPs are responsible for providing primary care and referral services to their patients and keep comprehensive records that contain prescription data, clinical events, specialist referrals, hospital admissions and their major outcomes. These systems are commonly used in general practice for the classification of diseases. Personal details are encoded and all patients are provided with clinic identifiers to ensure confidentiality. The database is administered by the Medicines and Healthcare products Regulatory Agency. The CPRD database provides a unique resource to examine the outcome of joint arthroplasty.
The CPRD database is accepted as being broadly representative of the UK population with respect to age, sex, socioeconomic circumstances and region; the data and Read codes for diseases (see Appendix 1), which are cross-referenced to the International Classification of Diseases, Ninth Edition,94–96 are stored in the Oxford Medical Information System (OXMIS).
The CPRD data were used to answer several research questions within different outputs, which contributed to the results described in this chapter. The design details of the bespoke computer programs written to manipulate and post-process the raw CPRD data are not provided. The methods used in the published articles,67,97–99 produced as part of work package 1, are described in general terms.
The CRPD data are routinely gathered for contributing practices and are not explicitly censored, other than when patients die or when they leave a general practice. The data delivered to our research team were truncated at 31 December 2006 for practical purposes, as the data ‘cut’ were taken from the main CPRD database shortly thereafter. No minimum contribution time was imposed, but the CPRD does impose a practice-level requirement that the data delivered to the database by each practice should be ‘up to standard’ according to the CPRD’s definitions, which in effect means that each practice submits data for up to 6 months before the data were confirmed as being up to standard. Other than this exclusion criterion, which is applied to all CPRD studies, we applied no further inclusion/exclusion criteria other than those reported in the individual research outputs written. Consequently, studies using CPRD data are ‘real world’, in the sense that the data recorded in general practice are used as research data, and therefore constitute a sensible sample from which to make population-level inferences about the UK population of GP-registered patients.
Sets of Read codes (see Appendix 1) (including remapped OXMIS codes, which have been phased out) were used for all of the data selection from the CPRD. Two or more clinicians validated the code lists for replacement procedures and clinical consensus was reached. Regarding the potential miscoding of primary THR/TKR, we took the first code match as the date of primary replacement.
A small number of subjects were found to have more than two primary operations, which is not strictly possible according to the usual definition, and without linkage to register data it is impossible to know which are genuine and which are not. Similarly, sidedness (left or right) of the procedure is not identifiable from Read codes. We took a pragmatic but consistent approach by identifying the first primary encountered as the one to use. In addition, we acknowledge that, without detail on sidedness, we cannot be sure that, for example, a left-sided revision was matched with a left-sided primary, but register data suggest that the effect of these potential mismatches on estimated incidence rates, lifetime risks and hazard ratios (HRs) would be small.
The first phase of using the data set for this work package involved the construction and validation of the GPRD data set with internal checks for consistency. During the analyses we also compared the summary data set with other available and appropriate data sets such as the National Joint Registry (NJR) for England and Wales, Hospital Episode Statistics (HES) and Health Survey for England (HSE), and population forecast data from the Office for National Statistics (ONS) in order to establish external validity.
Main exposure
The main exposure is primary arthroplasty. The selected cohort contains all of the patients with a code for primary or revision hip or knee replacement surgery and meets the criteria for each particular analysis.
Outcome measures
This work package centres on describing the epidemiology of hip and knee replacements. The relevant outcome measures are incidence rates, estimates of revision risk and future projections of procedure counts.
Rates of total hip replacement, total knee replacement and unicompartmental knee replacement in the UK
Temporal trends
Temporal trends in hip and knee replacement in the UK67
Total joint arthroplasty is a successful surgical intervention and is considered reliable and effective for pain relief and improved function and quality of life, with 90% prosthesis survival at 10 years. 3,8 In total, 160,000 hip and knee replacements were carried out in England and Wales in the 12 months prior to April 2010. 70 This number is expected to rise, and studies from the USA predict an increase in hip replacements (174%) and knee replacements (673%) to nearly 3.5 million per annum by 2030. 66 More than 650,000 knee replacements alone were carried out in the USA in 2008,100 and almost 80,000 in the UK in 2009. 17 The USA saw the number of knee surgeries increase from 31.2 per 100,000 person-years [95% confidence interval (CI) 25.3 to 37.1 per 100,000 person-years] in 1971–1976, to 220.9 per 100,000 person-years (95% CI 206.7 to 235.0 per 100,000 person-years) in 2008. 101
For the analysis we selected the patient data with a medical diagnosis code for THR (n = 27,113) or TKR (n = 23,843) between 1991 and the end of 2006. Patients were included if they were aged > 18 years at the time of operation. Evidence suggests that 20% of THR/TKRs in England are carried out in private institutions102 and the impact of this on arthroplasty provision needs further investigation. However, as the CPRD database had not been validated at this time, private practice codes were not included in the analysis. The NHS rates were validated using the HES (2005–6)103 and NJR. 104
Directly age- and sex-standardised replacement rates for calendar years were calculated using 10-year age groups with the mid-year population estimates for 2003 as the reference standard, as published by the ONS,105,106 the General Register Office for Scotland and the Northern Ireland Statistics and Research Agency. The 95% CI was computed using the Poisson model appropriate for directly standardised rates. The mean age at total replacement was calculated for the hip and knee for each calendar year and 95% CIs computed. To investigate patterns over time we calculated age distribution at operation by sex for three consecutive 5-year periods for the hip and knee.
The estimated age-standardised rate of THR increased from 60.3 per 100,000 person-years (95% CI 53.7 to 67.0 per 100,000 person-years) to 144.6 per 100,000 person-years (95% CI 138.1 to 151.1 per 100,000 person-years) for women (Figure 1a), and from 35.8 per 100,000 person-years (95% CI 30.4 to 41.3 per 100,000 person-years) to 88.6 per 100,000 person-years (95% CI 83.4 to 93.7 per 100,000 person-years) for men (Figure 1b). The increase in rates over time for THRs were steady between 1993 and 2005. The rate of TKR increased from 42.5 per 100,000 person-years (95% CI 37.0 to 48.0 per 100,000 person-years) to 138.7 per 100,000 person-years (95% CI 132.3 to 145.0 per 100,000 person-years) for women (see Figure 1a), and from 28.7 per 100,000 person-years (95% CI 23.9 to 33.6 per 100,000 person-years) to 99.4 per 100,000 person-years (95% CI 93.9 to 104.8 per 100,000 person-years) for men (see Figure 1b). The temporal trend for knees showed a marked plateau from the mid-1990s, followed by a sharp rise from 2000.
FIGURE 1.
Trends in primary THR and TKR rates in (a) women; and (b) men, based on data from Culliford et al. 67

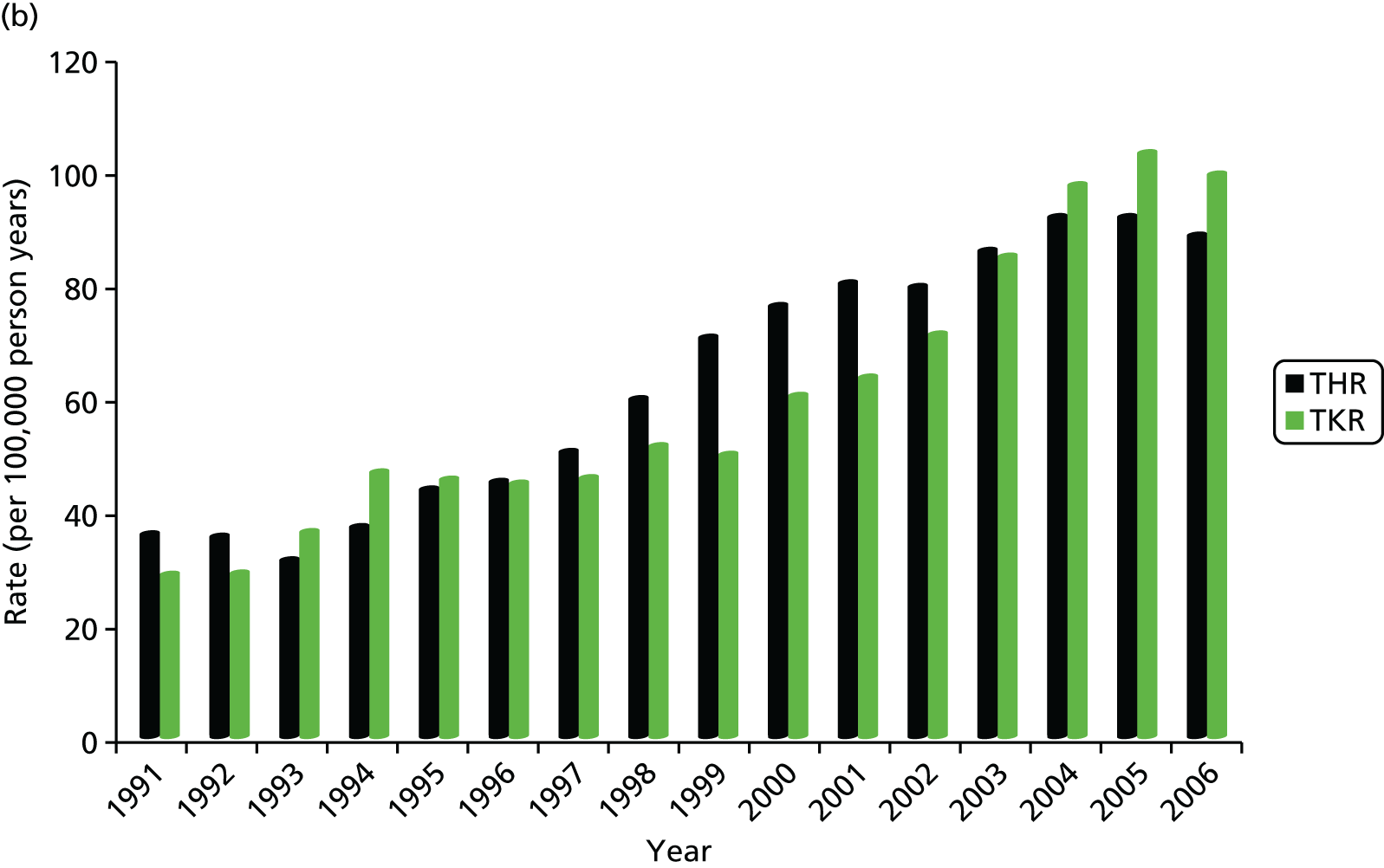
The mean age at operation was significantly higher for women than for men for all years after 1991: the mean age at THR was 70.3 years (95% CI 69.8 to 70.8 years) for women and 67.6 years (95% CI 66.9 to 68.2 years) for men; and the mean age at TKR was 70.1 years (95% CI 69.6 to 70.5 years) for women and 69.2 years (95% CI 68.6 to 69.7 years) for men. The highest rates of THRs and TKRs were for women aged between 70 and 79 years, with a mean rate of THR of 541.8 per 100,000 person-years (95% CI 501.0 to 582.5 per 100,000 person-years) and of TKR of 555.3 per 100,000 person-years (95% CI 514.1 to 596 per 100,000 person-years).
The final results showed that the rates of hip and knee arthroplasty continued to increase, but that the rise was more marked for knees than for hips. Women were 67% more likely than men to undergo THR, and 45% were more likely to undergo TKR, but sex ratios have been consistent over time, as demonstrated in Figure 2.
FIGURE 2.
The sex ratio for the number of hip and knee replacements vs. year of procedure based on data from Culliford et al. 67
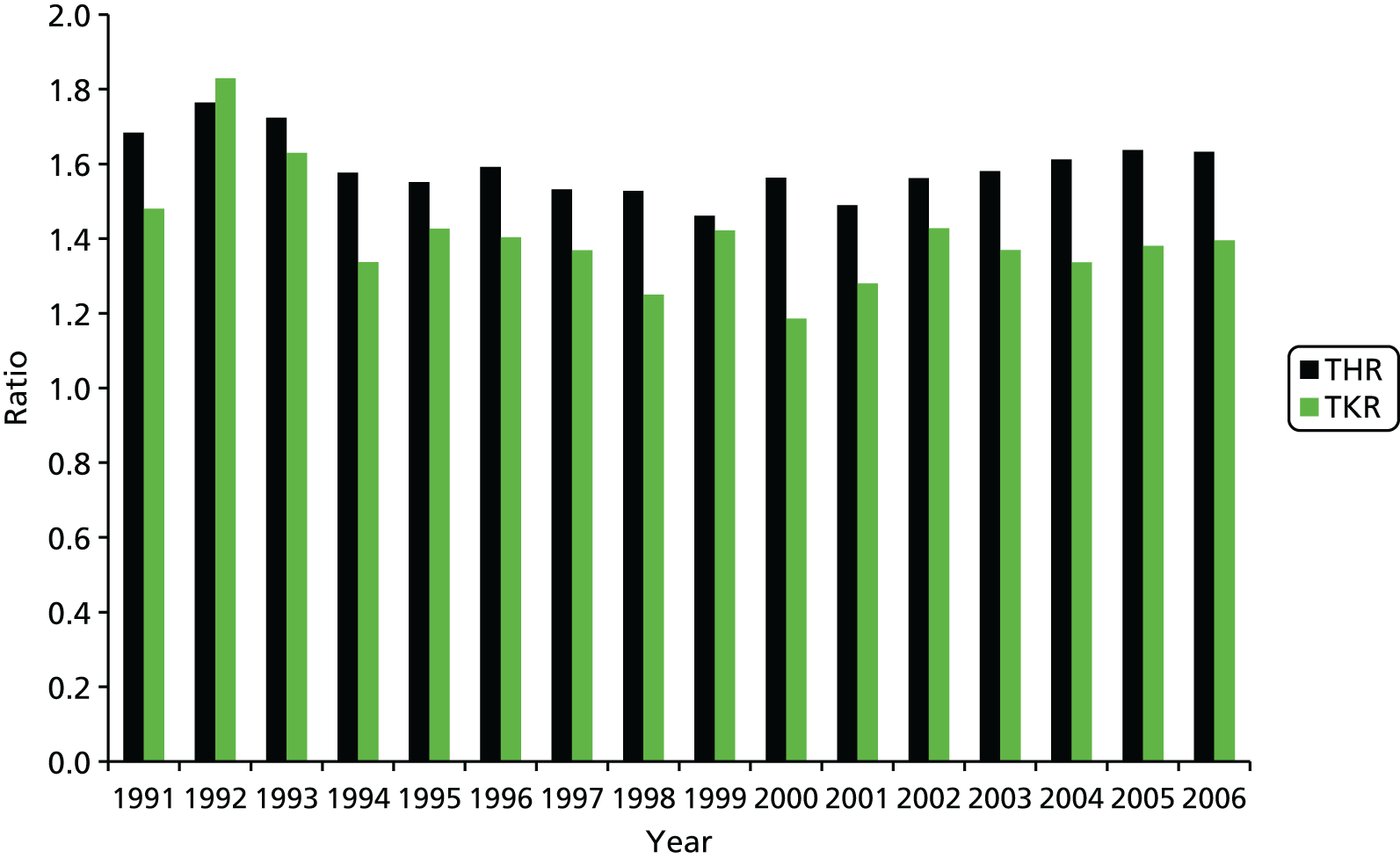
Women were, on average, 3 years older than men at THR, but the age difference between men and women undergoing knee replacements was only half as great. BMI was significantly higher for patients undergoing TKR than for those undergoing THR (p < 0.0001) and was higher for women than for men. There was little sex difference in the number of replacements carried out in patients between the ages of 60 and 79 years, who made up almost two-thirds of the total number of patients undergoing arthroplasty during 1991–2006.
Unicompartmental knee replacements are also becoming more popular, and the rates have increased over the last decade. We, again, used CPRD data and Read/OXMIS codes to identify all patients who underwent primary TKR or UKR between 1986 and 2006. However, the final analysis was restricted to the use of data between 1999 and 2006, as very few UKRs were carried out before this time.
The results of the statistical analysis give the number of TKRs and UKRs performed in each year; the mean age [and standard deviation (SD)] of patients of each sex and undergoing each type of operation was calculated to explore the profile for each intervention. The total numbers of TKRs and UKRs performed in the UK in 2006 were estimated by applying the CPRD rates to the population of the UK in that year.
There were substantially more TKRs (n = 18,450) than UKRs (n = 266) in 2006. The rate of TKRs increased from 55.4 per 100,000 person-years in 1999 to 123.5 per 100,000 person-years in 2006. The rate of UKRs increased from 0.25 per 100,000 person-years in 1999 to 3.0 per 100,000 person-years in 2006. Both men and women undergoing UKR were, on average, younger than those undergoing TKR (p < 0.0001). Men who underwent TKR were, on average, younger than women undergoing TKR (p < 0.0001). There was no statistically significant difference between the mean age of men and women undergoing UKRs (p = 0.74). TKR was performed more often in women (n = 10,836) than in men (n = 7614), but UKR was performed less often in women (n = 126) than in men (n = 140). The ratio of TKRs to UKRs fell from 250 : 1 in 1999 to 40 : 1 in 2006. The estimated numbers of operations performed in 2006 were 74,800 TKRs and 1800 UKRs.
The results showed a 12-fold increase in UKRs since 1999, and that this was still significantly less than TKRs, and UKRs are performed on a younger age group than TKRs.
Regional and national variation for hip and knee replacement surgery in the UK
Geographical and sociodemographic variations play an important role in the provision of, and access to, health care. Estimates of the mismatch of need and provision have been published by Judge et al. 107 and were found to be greater for TKR than for THR. There seems to be a wide variation in intervention rates for revision surgery across PCTs and the reasons need to be understood more clearly.
We looked at regional variations in the UK using CPRD data for 1986–2006 and found inter-regional differences in joint replacement rates. Using Read/OXMIS codes we identified 28,068 THRs and 24,364 TKRs.
Incidence was calculated by dividing the number of replacement operations by the number of person-years in the GPRD population. The rates were directly age and sex standardised, and computed by region, using a reference population (mid-2003 ONS population estimates). A 95% CI was calculated using a Poisson model.
Marked temporal changes were observed within and between certain regions. The reason for these differences is not clear, but factors such as medical indications and contraindications, personal and social perceptions of surgery as well as the availability of orthopaedic services should be considered. Figure 3 shows the example of regional differences in hip replacement rates between south-west England and London, standardised by age.
The GPRD data showed significant inter-regional differences in joint replacement rates in the UK in the period 1991–2006. Marked temporal changes were observed within and between certain regions, and the differences are larger for hips than for knees. This is supported by other studies also using national databases and registries within the UK. Inequities and inequalities currently exist within the UK health-care system,109 but the reasons need further investigation.
Describing the revision rates for hip and knee arthroplasty, and quantifying the rates in the UK
Total joint replacements are very successful operations, but a number of patients continue to have problems or are not satisfied with the outcome. Hawker27 estimated that up to 30% do not have symptomatic improvement after surgery. A further 20% of patients report unfavourable long-term pain. 87 There are many types of implants, and they are likely to be revised within the lifetime of a patient; for example, it is expected that a metal-on-polyethylene implant will need to be replaced after 20 years because of wear or prosthetic loosening. This is one of the reasons why THR and TKRs were indicated for mainly older patients. Even more modern prosthetics, making use of the latest technological advances, are not routinely recommended for younger patients. A UK population-based survey of patients after TKR found that 20% were not satisfied. 36 TJRs have, on average, a prosthesis survival rate of 90% at 10 years,110 and none has an indefinite lifespan. 17,111 They are considered economical because of the low failure rate,78,112,113 but surgical intervention is recommended when they do fail. Revision surgery is a high-risk procedure with a significantly higher mortality and morbidity than primary joint replacement, and is more costly than primary replacements. 114,115
The revision rate is expected to increase as the population that requires hip and knee surgery increases because of an increase in lifespan in developed countries and changing demographics. Dixon et al. 116 examined the trend in primary and revision TJRs in England and found a rapid increase in the proportion of hip surgeries requiring subsequent revision between 1991 and 2000, from 1 in 12 to 1 in 5; the number of knee revisions tripled over the same period, from 1 in 33 to 1 in 11. 116 The increase in revision rates is expected to continue in parallel with the steady increase of primary joint replacements. 117 Kurtz et al. 66 predicted an increase of 137% in hip revisions and of 601% in knee revisions in the USA by 2030 than 2005. Evidence from the Scandinavian National Joint Registries118 further demonstrates that the mean age for joint replacement is also decreasing.
An understanding of the reasons for failure, and success, of arthroplasty surgery is essential for guidance with implant design and clinical decision-making. Revision surgery is primarily indicated by implant loosening, instability through implant wear, or osteolysis and complications.
National and international registry data have been used extensively to estimate time to revision119 and to model prosthesis survival time in order to assess which specific demographic, clinical and prosthetic-specific factors are associated with time to failure. 120,121 Appropriate commissioning of services will reduce waiting times by matching demand with capacity and improved health-care delivery. Compared with primary TJR, revision TJR is more costly and more technically difficult, and results in only a 65% improvement in symptoms, although it remains a cost-effective method for improving function, pain relief and quality of life. 117 Revision rates continue to increase despite advances in surgical technique and implant design and the reasons for this remain unclear. Without this understanding it is difficult to address implant survival and long-term patient outcomes. 122
To determine the revision rates for the UK, we obtained data between 1991 and 2006 from the CPRD database. We used Read/OXMIS codes to identify all revisions to hip and knee replacements. Patients aged > 18 years at the time of operation were included in the analysis. Private practices were excluded because of lack of validation within the CPRD at this time.
For the analysis we calculated directly age- and sex-standardised rates for the incidence of revision for each calendar year. We used 10-year age groups, with 2003 mid-year population estimates as the reference standard. These rates have been constructed to represent the incidence of revision in the overall UK population and do not reflect the risk of revision for those already having undergone hip or knee replacement. The population estimates used for standardisation were as published by the ONS,106 the General Register Office for Scotland and the Northern Ireland Statistics and Research Agency. We computed 95% CIs using a Poisson model appropriate for directly standardised rates.
Mean age at revision was calculated for hips and knees for each calendar year and 95% CIs computed. The distribution of age at revision was calculated for three consecutive 5-year periods, separately for hip and knee by sex, to investigate patterns over time.
A total of 1689 hip revisions and 634 knee revisions were identified in the CPRD database. During the period 1991–2006, women underwent 59% more hip revisions and 6% more knee revisions than men. Women were, on average, > 3 years older than men at hip revision and approximately 2.5 years older in the case of knee revisions. Since 1994, the female-to-male ratio among patients undergoing revision surgery has remained reasonably stable, with ratios for hips varying around 2 : 1 and for knees 1.4 : 1, with further variation by age group, showing higher ratios for 70- to 79-year-olds (Figures 4 and 5).
FIGURE 4.
Revision rates for (a) hip; and (b) knee. Age profile of replacement rates by sex.


FIGURE 5.
Trends in revision rates with 95% CIs 1991 to 2006: (a) hip; and (b) knee.

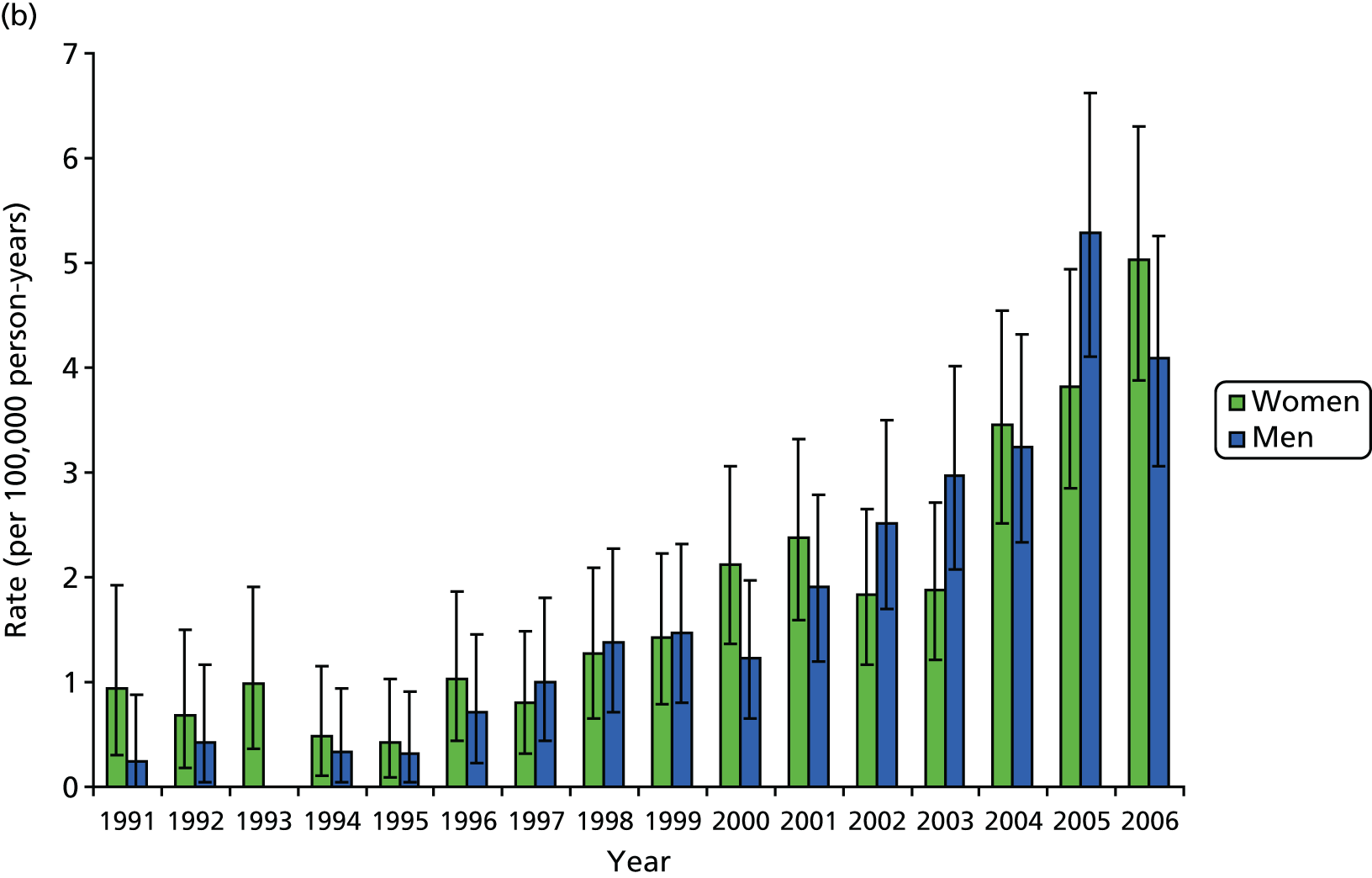
Patients undergoing TKR had a significantly higher BMI than those undergoing THR (p < 0.0001) and the difference in BMI was greater for women than for men (Table 1).
Variable | Surgery | |||
---|---|---|---|---|
THR | TKR | |||
Female (n = 17,560) | Male (n = 10,508) | Female (n = 14,462) | Male (n = 9902) | |
Age (years), mean (range) | 70.4 (18–103) | 67.5 (19–100) | 70.9 (18–99) | 69.4 (19–98) |
BMI (kg/m2), mean (IQR) | 27.0 (23.3–30.1) | 27.5 (24.6–29.9) | 29.1 (25.2–32.5) | 28.4 (25.4–30.9) |
Smoker (%) | 11.3 | 14.4 | 8.1 | 12.3 |
Deprivation (% from practices in the most deprived quintile) | 17.3 | 17.1 | 18.8 | 19.8 |
Between 1991 and 2006, the estimated age-standardised rates of hip revision arthroplasty increased from 2.3 per 100,000 person-years (95% CI 1.2 to 3.8 per 100,000 person-years) to 7.7 per 100,000 person-years (95% CI 6.2 to 9.2 per 100,000 person-years) for women and from 1.3 per 100,000 person-years (95% CI 0.5 to 2.5 per 100,000 person-years) to 6.3 per 100,000 person-years (95% CI 5.0 to 7.8 per 100,000 person-years) for men. The majority of the increase occurred between 1991 and 1994, with rates stabilising between 1994 and 2006. When the rates of revision hip replacement in 2006 were applied to the mid-2006 population estimates for the UK, we obtained an estimated total number of primary THRs (excluding private practice) of 1887 (95% CI 1538 to 2270) for women and 1447 (95% CI 1148 to 1780) for men.
Over the same period, the estimated age-standardised rates of knee revision arthroplasty increased from 0.9 per 100,000 person-years (95% CI 0.3 to 1.9 per 100,000 person-years) to 5.0 per 100,000 person-years (95% CI 3.9 to 6.3 per 100,000 person-years) for women and from 0.2 per 100,000 person-years (95% CI 0.0 to 3.1 per 100,000 person-years) to 4.1 per 100,000 person-years (95% CI 3.1 to 5.3 per 100,000 person-years) for men. The temporal trend in rates of knee revision shows a marked increase, with a steep rise after 1995. Estimated rates for women increased almost fivefold between 1996 and 2006. When we apply the 2006 rates for knee replacement to the mid-2006 UK population estimates, we obtain an estimated total number of revision TKRs (excluding private practice) of 1225 (95% CI 946 to 1540) for women and 942 (95% CI 706 to 1211) for men.
In 2006, the mean age at operation for hip revisions was 72.7 years (95% CI 70.3 to 75.0 years) for women and 69.5 years (95% CI 67.3 to 71.7 years) for men, and for knee revisions it was 71.0 years (95% CI 68.8 to 73.2 years) for women and 67.8 years (95% CI 65.3 to 70.3 years) for men. Among women, the highest incidence rate of hip revision is in the 80–89 years age group and of knee revision is in the 70–79 years age group. The rates in these groups are 35.1 (95% CI 22.1 to 48.1) for hips and 19.9 (95% CI 12.1 to 27.8) for knees. The number of replacements for those aged 60–79 years comprises almost two-thirds of the total for knees (64.7%) and a similar proportion for hips (61.0%), with men having a higher proportion than women in this age group for both hips and knees.
The mean age at hip revision was higher in women than in men for almost all years after 1991 (Figure 6a), but the difference was statistically significant in only 2 of the years. For knee revision (Figure 6b), the sex difference in mean age at operation is much narrower than for hip revision. Since 1999, the sex-specific mean ages at knee revision have been very similar, with women slightly older than men, but by 2006 there is virtually no discernible difference between the sexes.
FIGURE 6.
Mean age at revision, with 95% CIs, in 1991–2006: (a) hip; and (b) knee.
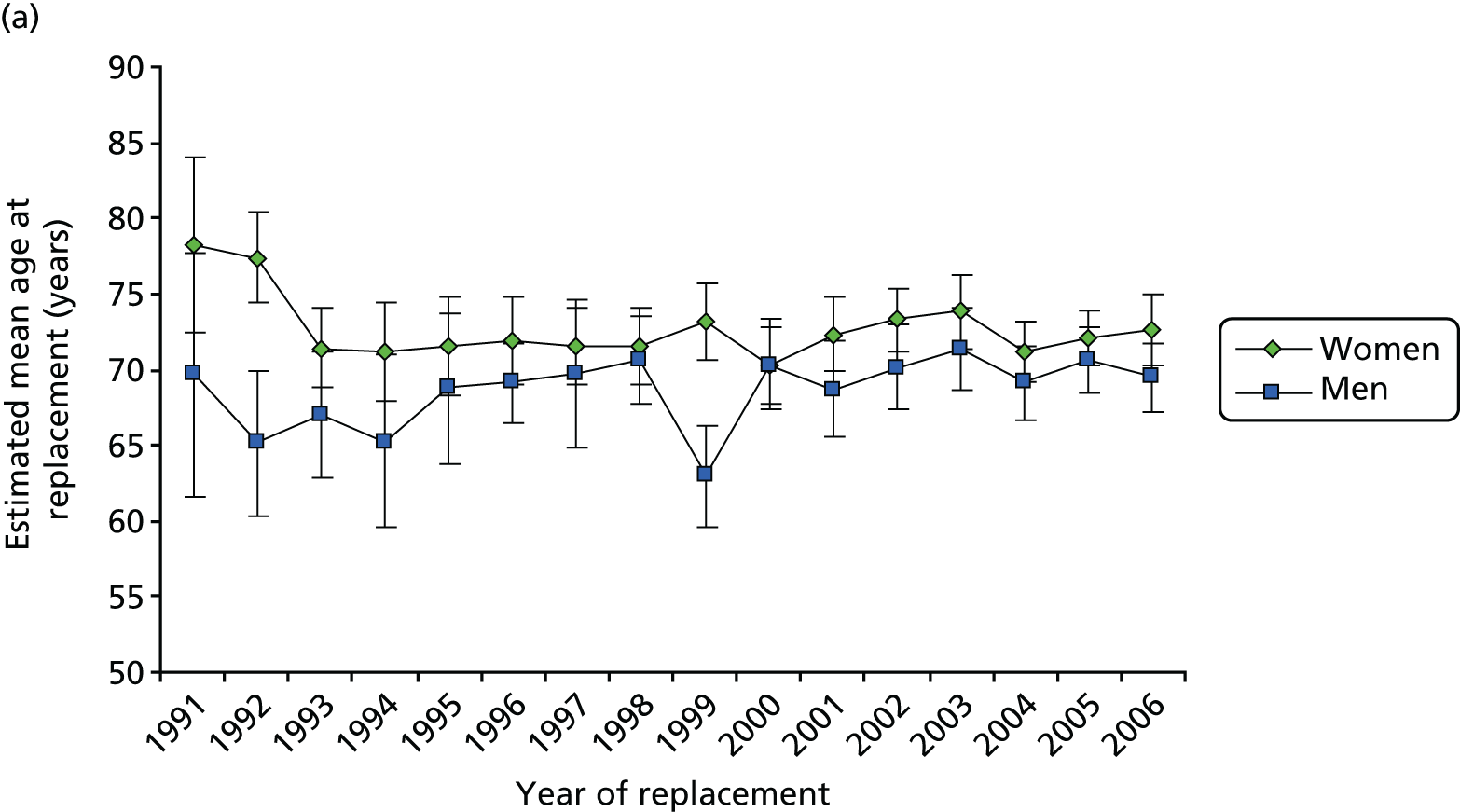

To explore the possibility that there had been a change in the distribution in age of people undergoing revision surgery, we examined the distribution of age in 10-year age bands over three time periods: 1991–5, 1996–2000 and 2001–5. For the two earlier periods the counts of revision operations were generally too low to enable an effective comparison between age distributions over time. However, in the period 2001–5 it was observed that the distributions were similar between the sexes and also between hip and knee revisions. During the same period, the 10-year age group at which most revisions were carried out was 70–79 years (between 37% and 39% of revisions, whether for hips or knees for either sex).
The ratio of knee-to-hip revision incidence rates (Figure 7) was low for both men and women during the mid-1990s, at around 0.15 : 1. This ratio then began to rise steeply in both sexes in 1996 such that, by 2006, the incidence of knee revision was two-thirds of that of hip revision.
FIGURE 7.
Ratio of incidence rates for knee to hip revisions for men and women between 1991 and 2006.

When we compared our estimated revision incidence rates for 2006 with the corresponding rates for primary operations using the same GPRD data set, we found that the ratio of primary operations to revisions was approximately 17 : 1 for hips and 25 : 1 for knees.
Analysis of the UK national database over a 10-year period revealed that knee revision rates increased more than fivefold over this period in both men and women. The rate of THR revision has remained relatively static, with no significant increase identified between 1994 and 2006.
The fivefold increase in knee revision rates may be multifactorial and a reflection of the increased number of primary replacements over this 10-year period as well as rapid advances in implant technology alongside improved surgical experience. 66 Clinicians may be much more likely to intervene at an earlier stage, especially in complex cases that in the past would have been considered beyond salvage. Another contributing factor may be the increased usage of unicompartmental knee arthroplasty, which has been associated with higher revision rates.
The picture looks different for hip replacements, as the results showed a marginal increase in revision rates. Technological advances, such as improved bearing surfaces and fixation methods, should have decreased the need for revision but may not have had the predicted impact on primary total hip arthroplasty (THA) longevity. Registry data from Scandinavia120,121 demonstrate that the longevity of more conventional cemented implants is superior to that of modern cementless or resurfacing designs. In 1996 there was good evidence to support THA in < 30% of primary cases in the UK; in 2010 there was good evidence for their use in < 40% of cases. This is an important observation, as newer implants tend to be more expensive and may in fact be adding to the revision burden if they are not introduced in a co-ordinated manner. Significant demographical differences were found, with women 59% more likely to require hip revision than men.
In comparison with our findings, Kurtz et al. 66 have previously reported a 79% increase in revision THA and a 200% increase in revision total knee arthroplasty (TKA) in the USA between 1990 and 2002. 66 This group also looked at future projections between 2006 and 2030 and estimated that revision THA would increase by 137% and revision TKA by 601% by 2030. These findings confirm the predicted trends in revision arthroplasty in the UK, with dramatic increases in knee revisions and a smaller, but still significant, increase in hip revisions. The cost implications for this increase would be significant, and accurate modelling of revision THA and TKA demand is therefore required for adequate and appropriate long-term health planning.
We have further investigated the role of a risk factor, particularly the role of BMI, on the time of revision for hip and knee arthroplasties. We used methods from survival analysis to present population-based estimates for the risk of revision following TJR of the hip and knee. We described these associations and published the results. 97
Association of body mass index with time of revision
A population-based survival analysis describing the association of body mass index on time to revision for total hip and knee replacements: results from the UK General Practice Research Database97
For this task we selected 63,162 THR and 54,276 TKRs from the CPRD database. The average age at replacement was similar in both groups and the proportion for women in both procedures was greater (Table 2).
Characteristic | Surgery | |||
---|---|---|---|---|
THR (N = 63,162) | TKR (N = 54,276) | |||
Female (n = 39,292) | Male (n = 23,870) | Female (n = 31,682) | Male (n = 22,594) | |
Age (years), mean (SD) | 70.5 (11.1) | 67.7 (11.0) | 70.7 (9.6) | 69.4 (9.4) |
Sex (%) | 62.2 | 37.8 | 58.3 | 41.6 |
BMI (kg/m2), mean (SD) | 27.2 (5.1) | 27.7 (4.3) | 29.6 (5.6) | 28.8 (4.4) |
Missing BMI (%) | 19.1 | 19.3 | 13.8 | 14.0 |
Revisions, n (%) | 1000 (2.55) | 811 (3.40) | 572 (1.8) | 614 (2.7) |
Deaths pre revision, n (%) | 6615 (16.8) | 4201 (17.6) | 4110 (13.0) | 3349 (14.8) |
Number of comorbid conditions (%) | ||||
0 | 42.8 | 48.1 | 37.5 | 43.7 |
1 | 34.2 | 31.0 | 37.4 | 35.8 |
≥ 2 | 23.0 | 20.9 | 25.2 | 20.6 |
Table 2 also describes the baseline characteristics of the cohort, including summary statistics and missing data percentages for all explanatory variables for which complete data were not observed.
Eighty per cent of preoperative BMI values used were recorded within 5 years of the primary operation; among those with a recorded BMI, the proportion of obese patients (BMI ≥ 30 kg/m2) was 26.2% for THR and 39.8% for TKR, and of morbidly obese patients (BMI ≥ 40 kg/m2) was 1.6% for THR and 3.6% for TKR.
In a single-predictor (univariable) survival model allowing for the competing risk of death over the entire period of follow-up, we estimated that THR participants had a 3% increase in the subhazard of revision [subhazard ratio (SHR) 1.030, 95% CI 1.020 to 1.041; p < 0.001] for each extra unit (kg/m2) of BMI, with TKR participants showing a 2.6% increase per unit (SHR 1.026, 95% CI 1.013 to 1.038; p < 0.001]. The SHR was significantly greater for men than for women for both THR (SHR 1.35, 95% CI 1.23 to 1.48; p < 0.001) and TKR 2 (SHR 1.54, 95% CI 1.37 to 1.72; p < 0.001).
Age at TJR was also a significant univariable predictor of both hip and knee revision surgery, with THR participants estimated to have a 3% reduction (SHR 0.970, 95% CI 0.967 to 0.973; p < 0.001) for each extra year of age, and TKR participants showing a 4.3% reduction (SHR 0.957, 95% CI 0.952 to 0.961; p < 0.001). The effects for all three variables (sex, age and BMI) were then estimated in multivariable competing risks regression models after adjusting for smoking status, drinking status and the number of comorbid conditions over the entire period of follow-up. For age, the estimates were almost exactly the same as those from the univariable model for both hip and knee, but for sex (SHR 1.23 for hip and 1.51 for knee) and BMI (SHR 1.020 for hip and 1.015 for knee) the estimates were smaller. Nevertheless, all three variables remained statistically significant for both hip and knee in the presence of adjustment.
For a 5-kg/m2 and 10-kg/m2 increase in BMI, this represents an increase in THR revision risk of 10.4% and 21.9%, respectively (7.7% and 16.1% for TKR). Testing for two-way interactions between age, sex and BMI did not produce any significant effects. All subhazard estimates (with 95% CI and p-values) from the univariable and multivariable models are given in Tables 3 and 4.
Variable | Analysis | |||||
---|---|---|---|---|---|---|
Univariable | Adjusteda | |||||
HR | 95% CI | p-value | HR | 95% CI | p-value | |
BMI (kg/m2) (per additional unit)b | 1.030 | 1.020 to 1.041 | < 0.001 | 1.020 | 1.009 to 1.032 | < 0.001 |
Sex | ||||||
Female (reference) | 1.00 | 1.00 | ||||
Male | 1.35 | 1.23 to 1.48 | < 0.001 | 1.23 | 1.10 to 1.38 | < 0.001 |
Age (years at THR) (per additional year) | 0.970 | 0.967 to 0.973 | < 0.001 | 0.971 | 0.966 to 0.975 | < 0.001 |
Variable | Analysis | |||||
---|---|---|---|---|---|---|
Univariable | Adjusteda | |||||
HR | 95% CI | p-value | HR | 95% CI | p-value | |
BMI (kg/m2) (per additional unit)b | 1.026 | 1.013 to 1.038 | < 0.001 | 1.015 | 1.002 to 1.028 | 0.023 |
Sex | ||||||
Female (reference) | 1.00 | 1.00 | ||||
Male | 1.54 | 1.37 to 1.72 | < 0.001 | 1.51 | 1.32 to 1.73 | < 0.001 |
Age (years at THR) (per additional year) | 0.957 | 0.952 to 0.961 | < 0.001 | 0.957 | 0.951 to 0.962 | < 0.001 |
To further explore the effect of estimates for BMI, we ran the same adjusted age–sex–BMI model described but used categorical rather than continuous BMI. For morbidly obese TKR participants (BMI > 40 kg/m2) there was a 43.9% increase (95% CI 2.6% to 103.9%; p = 0.040) in the rate than those with a normal BMI (18.5–25 kg/m2), but the effect for THR was larger (an increase of 65.5%) and stronger (95% CI 15.4% to 137.3%; p = 0.006).
The effect sizes were similar to those obtained when using the adjusted SHR estimate of continuous BMI for a participant with a BMI of 45 kg/m2 relative to one with a BMI of 22 kg/m2 (an increase of 57.7% for THR and 40.8% for TKR). For obese patients in the range 30–40 kg/m2 compared with those with a normal BMI, the estimated SHR for revision was weakly significant for THR (15.7% increase, 95% CI 0.2% to 33.7%; p = 0.048) but not for TKR (17.9% increase, 95% CI –1.9% to 41.6%; p = 0.079). As a sensitivity analysis, we also performed standard Cox regressions with revision surgery as the event of interest, and when no distinction was made between death and other censoring events. Univariable models for age, sex and BMI gave very similar results to the competing risks analysis, as did the multivariable models that adjusted for the same factors as in the competing risks regression. Results from the Cox regression models are given in Tables 5 and 6.
Variable | Analysis | |||||
---|---|---|---|---|---|---|
Univariable | Adjusteda | |||||
HR | 95% CI | p-value | HR | 95% CI | p-value | |
BMI (kg/m2) (per additional unit)b | 1.029 | 1.017 to 1.040 | < 0.001 | 1.019 | 1.008 to 1.031 | 0.001 |
Sex | ||||||
Female (reference) | 1.00 | 1.00 | ||||
Male | 1.36 | 1.24 to 1.49 | < 0.001 | 1.26 | 1.13 to 1.41 | < 0.001 |
Age (years at THR) (per additional year) | 0.978 | 0.974 to 0.983 | < 0.001 | 0.977 | 0.972 to 0.982 | < 0.001 |
Variable | Analysis | |||||
---|---|---|---|---|---|---|
Univariable | Adjusteda | |||||
HR | 95% CI | p-value | HR | 95% CI | p-value | |
BMI (kg/m2) (per additional unit)b | 1.024 | 1.012 to 1.037 | < 0.001 | 1.015 | 1.003 to 1.028 | 0.019 |
Sex | ||||||
Female (reference) | 1.00 | 1.00 | < 0.001 | |||
Male | 1.58 | 1.41 to 1.77 | < 0.001 | 1.55 | 1.36 to 1.77 | |
Age (years at THR) (per additional year) | 0.962 | 0.956 to 0.967 | < 0.001 | 0.961 | 0.955 to 0.968 | < 0.001 |
In addition, we also calculated that it would take 175 patients with TKR to reduce their baseline BMI from obese to normal in order to prevent one revision operation after 5 years. For patients with THR this number reduces to 152.
Finally, we assessed whether or not the higher incidence of hip revision surgery during the first year following THR might compromise the proportionality assumption and, therefore, suggest the inclusion of time-dependent effects. Separate univariable piecewise competing risk models for hip revision were fitted for sex, age (≤ 65 years vs. > 65 years) and BMI (> 40 kg/m2 vs. ≤ 40 kg/m2). A single change point at 1 year was used to simultaneously estimate two SHRs for revision (before and after 1 year following THR).
The only model that provided some evidence for a different SHR during the first year was with BMI (> 40 kg/m2 vs. ≤ 40 kg/m2) as the predictor (SHR 2.619, 95% CI 1.502 to 4.560; p = 0.001), but this was not matched with a statistically significant estimate for revision after the first year (SHR 0.575, 95% CI 0.238 to 1.170; p = 0.130).
Cumulative incidence rates of revision were higher for men than for women and higher for hips than for knees. Age, sex and BMI were estimated to be significant predictors of time to revision in an adjusted model allowing for the competing risk of death. Severely obese patients undergoing THR had a higher risk of revision surgery during the first year following replacement, but the same effect was not observed for knee replacement.
Projected future trends for total hip replacement/total knee replacement accounting for projected changes in age and obesity
Estimating the lifetime risk of total knee and hip arthroplasties
The lifetime risk of total hip and knee arthroplasty: results from the UK General Practice Research Database99
Lifetime risk is a patient-centred measure of risk for the onset of disease or the occurrence of specific events. The concept is easily understood by clinicians and policy-makers, and can be made even more informative by also calculating interval risks (e.g. 10 years) at different ages to establish the periods of greatest lifetime risk. Population-based estimates are needed for effective and efficient health-care planning and resource allocation. No lifetime risk estimates were available in the literature for patients who were undergoing these surgical procedures, but published incidence rates existed for hip and knee replacement in the UK67,116 and internationally. 119,123,124
The primary aim of this analysis was to use the CPRD database combined with the ONS mortality data to provide estimates for the lifetime risk of undergoing a primary THR or TKR in the UK. OXMIS/Read codes were used to identify THRs and TKRs for the period 1991–2006 in the CPRD database. Patients were included if aged ≥ 50 years at the time of replacement. Sex-specific all-cause mortality data from the ONS were obtained for the same period. 125
The analysis was done with CPRD data that were aggregated into single-year age intervals, with the age label defined as age at last birthday at the end of a calendar year, starting at the age of 50 years. We used data for the time period 1991–2006 and identified 49,105 patients who had undergone a THR (n = 25,845) or TKR (n = 23,260). Consistent definitions were applied to death data and exposure to risk. Incidence rates for joint replacement were computed by dividing the count of primary THRs and TKRs in the CPRD data by the corresponding amount of person-time spent by those in the entire CPRD population who matched the age band, sex and time interval of interest. This was achieved by a life table method similar to that described by Kim et al. 39 CIs at the 95% level were estimated under a Poisson model. 126 Risks were estimated separately for sex and hip/knee. This was repeated with 60, 70 and 80 years of age as the starting point for the risk of replacement. We further computed 10-year risk percentages from age 50 years up to the age of 80 years. All estimates for single calendar years used mortality data matched to the same calendar years, but for the estimates based on the entire study period we used 2006 mortality rates with a sensitivity analysis. Lifetime risks of THR and TKR, stratified by sex for individual calendar years, were estimated in order to compare temporal trends.
The results, using rates from 2005, showed that the estimated mortality-adjusted lifetime risk of THR at age 50 years was 11.6% for women and 7.1% for men. For the aggregated data over the period 1991–2006, the mortality-adjusted lifetime risk of THR at age 50 years was estimated at 8.3% for women and 5.2% for men. The lifetime risk of THR at age 50 years rose from 4.0% (95% CI 3.0% to 5.0%) to 11.1% (95% CI 9.9% to 12.2%) for women and from 2.2% (95% CI 1.4% to 3.0%) to 6.6% (95% CI 5.7% to 7.5%) for men. Therefore, our findings estimated that, between 1991 and 2006, the lifetime risk of THR at age 50 years rose from 4.0% (95% CI 3.0% to 5.0%) to 11.1% (95% CI 9.9% to 12.2%) for women and from 2.2% (95% CI 1.4% to 3.0%) to 6.6% (95% CI 5.7% to 7.5%) for men.
Again, using the rates from 2005, we estimated that the mortality-adjusted lifetime risk of TKR at age 50 years was 10.8% for women and 8.1% for men. The aggregated data for the period 1991–2006 estimated the mortality-adjusted lifetime risk for TKR at age 50 years at 7.0% for women and at 5.2% for men. The same time period for TKR saw an increased risk for women from 2.9% (95% CI 2.1% to 3.8%) to 10.6% (95% CI 9.5% to 11.7%) and for men from 1.8% (95% CI 1.1% to 2.6%) to 7.7% (95% CI 6.8% to 8.7%). As with hips, TKR estimates of risk also increased, for women from 2.9% (95% CI 2.1% to 3.8%) to 10.6% (95% CI 9.5% to 11.7%) and for men from 1.8% (95% CI 1.1% to 2.6%) to 7.7% (95% CI 6.8% to 8.7%).
As a sensitivity analysis these estimates were recalculated using 1991 mortality data, but this resulted in only small reductions in the lifetime risk estimates of between 0.6 and 0.8 percentage points at age 50 years and of 0.2 and 0.3 percentage points at age 80 years. These reductions were seen for both THR and TKR, and for men and women.
The lifetime risk decreases with increasing age for both THR and TKR in men and women. At age 80 years, the sex gap in risk of THR reduced to 40% higher for women than for men (22% higher for TKR). Estimated risk percentages at ages 50, 60, 70 and 80 years are presented in Table 7.
Current age (years) | Risk of primary TKR, % (95% CI) | Risk of primary THR, % (95% CI) | ||
---|---|---|---|---|
Female | Male | Female | Male | |
50 | 10.8 (9.7 to 11.9) | 8.1 (7.1 to 9.1) | 11.6 (10.4 to 12.7) | 7.1 (6.2 to 8.0) |
60 | 10.1 (9.0 to 11.2) | 7.9 (6.9 to 8.9) | 10.8 (9.7 to 12.0) | 6.7 (5.8 to 7.7) |
70 | 7.8 (6.7 to 8.8) | 6.2 (5.2 to 7.2) | 8.1 (7.1 to 9.2) | 5.3 (4.3 to 6.2) |
80 | 3.3 (2.6 to 4.1) | 2.7 (1.8 to 3.6) | 3.8 (3.0 to 4.7) | 2.7 (1.8 to 3.6) |
The sex gaps in the estimates obtained for the whole study period were similar to those for the 2005 estimates.
Our results showed that between 1991 and 2006 the lifetime risk of THR at age 50 years increased from 4.0% (95% CI 3.0% to 5.0%) to 11.1% (95% CI 9.9% to 12.2%) for women and from 2.2% (95% CI 1.4% to 3.0%) to 6.6% (95% CI 5.7% to 7.5%) for men. For TKR, the risk increased for women from 2.9% (95% CI 2.1% to 3.8%) to 10.6% (95% CI 9.5% to 11.7%) and for men from 1.8% (95% CI 1.1% to 2.6%) to 7.7% (95% CI 6.8% to 8.7%) (Figure 8).
FIGURE 8.
Estimated lifetime risk at age 50 years of undergoing (a) THR; or (b) TKR based on age- and sex-specific incidence adjusted for mortality. Data from the GPRD. 99


The lifetime risks of hip and knee replacements are estimated to be between 5% and 10%, which is substantially below the estimated lifetime risk of hip and knee OA. Our estimates based on UK GPRD data from 2005 suggest a lifetime risk of THR and TKR for women or men aged 50 years living in the UK of 10–11% and 6–7%, respectively.
Future projections of total hip and knee arthroplasties
Future projections of total hip and knee arthroplasty in the UK: results from the UK Clinical Practice Research Datalink98
Future predictions for lower limb arthroplasty in the UK are limited,116,123 and international predictions are mainly concentrated around the USA and Europe. 34,68,127,128 With the steady increase in rates of hip and knee surgery, up-to-date future predicted rates are necessary as part of our understanding of this treatment intervention. The most recent published future projections of the UK covered England only,116 were based on a 10-year period of HES data and did not account for BMI changes or other important risk factors for arthroplasty.
Deciding on the correct method for forecasting is important and dependent on high-quality research data. More sophisticated modelling approaches require at least one population-based cohort or survey data set with long-term follow-up. 129
We produced and published the national future projections for THR and TKR. 98 We used three national data sets that were representative of age, sex and BMI in the UK population. Using the CPRD database, in combination with national population forecasts from the ONS, we aimed to calculate age- and sex-specific forecasts for the number of THR and TKR operations per year in the UK between 2010 and 2035. Secondary analysis aimed to produce forecasts that reflect the changing distribution of BMI during the same period. To project estimated THR and TKR rates, HSE data were used. We constructed a denominator to estimate BMI-specific rates and obtained sex-specific population projections from the ONS for the period 2011–35. 106 The methods of Kurtz et al. 66 were further extended to incorporate the inclusion of BMI, in addition to age and sex.
Analysis: estimation
The CPRD data (1991–2010) were used to estimate annual incidence rates for THR/TKRs, and standard log-linear regression models were used to produce calendar year-, age- and sex-specific rates, but were extended to include BMI-specific rates. Unweighted aggregated data from the HSE, for the same period, were used as a proxy for the change in the distribution of BMI in the UK population. The CPRD data were remodelled by calendar year, age, sex and BMI. Age and BMI were grouped in categories, and rates for hips and knees were estimated separately. The calendar year-/age-/sex-specific values of BMI in the HSE were used to partition the calendar year-/age-/sex-specific denominator values in the CPRD to further break them down by BMI. Regarding the numerator for the rate (i.e. the counts of TJRs), the counts were weighted by BMI for those TJR patients with an observed preoperative BMI in their record. This was the case for approximately 80%. We made the decision not to use missing data methods (such as multiple imputation) because of a high rate of observed BMI. If preoperative BMI had been available in, for example, 50–60% of cases, we could have reconsidered and use multiple imputation methods. Our BMI-specific projections were in categorical bandings; therefore, fewer concerns were raised about sensitivity to missingness.
The ONS data were split into age- and sex-specific forecasts, by BMI group, prior to applying the estimated incidence rates obtained from the HSE. Two methods were used: BMI proportions fixed at 2010 levels and BMI proportions increasing linearly based on ordinary least squares (OLS) regression estimates derived from the HSE BMI data from 1991 to 2010. A hyperbolic tangent function, similar to the method described in the Foresight report, was used to smooth the proportions over the forecasting time frame. 130
Analysis projection
Two different projections methods were used on each of the two future UK population scenarios. Hips and knees were analysed separately. The first method used THR/TKR incidence rate estimates held at 2010 levels, applied to the two population scenarios. The second used an exponential extrapolation directly from the log-linear model-estimated rates for THR/TKR. The two population forecast data sets76 contained exactly the same population growth estimates by age and sex over time, as forecast by ONS, with a difference that one population data set assumed a static BMI distribution (held fixed at 2010), whereas the other reflected HSE- and CPRD-based estimates of forecast BMI distribution change in the UK.
The results from analysis of the CPRD database contained 50,000 THRs and 45,609 TKRs between 1991 and 2010, and all sets included age, sex and BMI. The average age at time of operation was similar for THR and TKR, and the proportion of women was greater for both TKR and THR (Table 8).
Variable | Surgery | |||
---|---|---|---|---|
TKR (N = 45,609) | TKR (N = 50,000) | |||
Female (n = 26,623) | Male (n = 18,986) | Female (n = 31,148) | Male (n = 18,852) | |
Sex (%) | 58.4 | 41.6 | 62.2 | 37.8 |
Age (years), mean (SD) | 70.3 (9.5) | 69.4 (9.2) | 69.9 (10.9) | 67.8 (10.7) |
BMI (kg/m2), mean (SD) | 29.6 (5.4) | 28.8 (4.4) | 27.2 (5.1) | 27.7 (4.2) |
Preoperative BMI was slightly higher for TKR than for THR. There was little sex-specific difference in counts when comparing fixed or varying future estimates of BMI category distribution in hips. Knee estimates, however, suggested a 9% higher rate when using the varying BMI distribution.
Hospital Episode Statistics data (1991–2010) were used to estimate future BMI distribution and contained 186,174 subjects with measured BMI. The breakdown of this distribution by age, sex and BMI values is depicted in Tables 9 and 10.
BMI group (kg/m2) | Total number of subjects (N = 186,174) | |||
---|---|---|---|---|
Female [n = 100,576 (54.0%)] | Male [n = 85,598 (46.0%)] | |||
n | % | n | % | |
< 20 | 6117 | 6.1 | 2933 | 3.4 |
20 to 25 | 39,261 | 39.0 | 27,347 | 31.9 |
25 to 29 | 33,361 | 33.2 | 38,681 | 45.2 |
30 to 39 | 19,688 | 19.6 | 16,216 | 18.9 |
≥ 40 | 2149 | 2.1 | 421 | 0.5 |
Age group (years) | Total number of subjects (N = 186,174) | |||
---|---|---|---|---|
Female [n = 100,576 (54.0%)] | Male [n = 85,598 (46.0%)] | |||
n | % | n | % | |
18–39 | 37,664 | 37.4 | 32,527 | 38.0 |
40–49 | 18,503 | 18.4 | 15,704 | 18.3 |
50–59 | 15,620 | 15.5 | 13,640 | 15.9 |
60–69 | 13,813 | 13.7 | 12,433 | 14.5 |
70–79 | 10,430 | 10.4 | 8504 | 9.9 |
≥ 80 | 4546 | 4.5 | 2790 | 3.3 |
The static rate projection method, with BMI distribution held fixed at levels estimated for 2010, forecasts an annual number of THRs of up to 97,516 and of TKRs of up to 110,306 by 2035. Using the same projection method, but with changing BMI distribution, the estimated rates are expected to grow by up to 95,877 for hips and 118,666 for knees.
Using the log-linear projection method, with BMI distribution held fixed at levels estimated for 2010, the annual number forecast for hips is up to 437,708 and for knees is up to 1,071,790 by 2035. Using the same method, but with changing BMI distribution, the rates are exponential and increase to 439,097 for hip and 1,219,362 for knees by 2035. Five-yearly projections for all four scenarios up to 2035 are shown in Table 11.
Year | Projection | |||||||
---|---|---|---|---|---|---|---|---|
THR incidence rates | TKR incidence rates | |||||||
Estimated rates fixed at the 2010 level | Estimated rates increasing log-linearly | Estimated rates fixed at the 2010 level | Estimated rates increasing log-linearly | |||||
BMI category proportions fixed at 2010 estimates | BMI category proportions changing over time | BMI category proportions fixed at 2010 estimates | BMI category proportions changing over time | BMI category proportions fixed at 2010 estimates | BMI category proportions changing over time | BMI category proportions fixed at 2010 estimates | BMI category proportions changing over time | |
2015 | 72,762 | 72,418 | 96,314 | 95,945 | 82,610 | 85,019 | 128,944 | 133,063 |
2020 | 79,716 | 79,048 | 141,626 | 140,945 | 90,555 | 94,783 | 221,653 | 234,244 |
2025 | 85,988 | 85,026 | 205,464 | 204,793 | 97,780 | 103,657 | 376,384 | 407,400 |
2030 | 91,496 | 90,202 | 296,354 | 296,106 | 103,810 | 111,015 | 632,257 | 701,052 |
2035 | 97,516 | 95,877 | 437,708 | 439,097 | 110,306 | 118,666 | 1,071,790 | 1,219,362 |
The results that follow present counts split by sex, BMI and age, all of which are estimated using the static projection method (see Table 11).
Hip and knee projected counts by sex are shown in Table 12. There is little sex difference in counts at 2035 when we compare projections, with fixed or varying future estimates, of BMI category distribution for hips. Knees results are different, however, especially for women, whose TKR count at 2035 is estimated to be 9% higher when using varying BMI distribution as opposed to fixed.
Year | Surgery | |||||||
---|---|---|---|---|---|---|---|---|
THR | TKR | |||||||
Women | Men | Women | Men | |||||
BMI category proportions fixed at 2010 estimates | BMI category proportions changing over time | BMI category proportions fixed at 2010 estimates | BMI category proportions changing over time | BMI category proportions fixed at 2010 estimates | BMI category proportions changing over time | BMI category proportions fixed at 2010 estimates | BMI category proportions changing over time | |
2015 | 45,143 | 44,905 | 27,618 | 27,513 | 47,703 | 49,207 | 34,908 | 35,812 |
2020 | 49,207 | 48,752 | 30,509 | 30,296 | 51,931 | 54,638 | 38,624 | 40,145 |
2025 | 52,949 | 52,307 | 33,039 | 32,719 | 55,785 | 59,604 | 41,995 | 44,054 |
2030 | 56,255 | 55,426 | 35,241 | 34,776 | 58,919 | 63,665 | 44,891 | 47,350 |
2035 | 59,909 | 58,850 | 37,607 | 37,026 | 62,493 | 68,082 | 47,813 | 50,584 |
Discussion
The increasing trends in THR and TKR up to the year 2000 have continued and are more marked in knees than in hips. Although there is a marked increase in the number of knee replacements being carried out per year, the number of TKRs are similar to those for THR. The increase in knee surgery may be because the burden of OA of the knee is more easily identified in radiographs. 131 The number of TKRs per year is similar to the number of THRs, despite the much higher prevalence of OA of the knee. 75,76 It is possible that the level of provision of THR is appropriate to the burden of OA of the hip, whereas the level for the TKR is still below that required by surgeons operating on patients with lower levels of pain and disability.
The reason for the mismatch between lifetime risk of OA75,76 and the established intervention (THR/TKR) is not clear, but could be in part a result of the lack of consensus on the type or severity of symptoms. 132 The mismatch may also be a result of the difference between the need for and provision of hip and knee arthroplasty in the UK, as described by Judge et al. 107,133 Attempts have been made to understand the geographical and sociodemographic characteristics of different countries,30 and UK-based studies from the late 1990s22,23,134 found little mismatch in THR provision but a large mismatch between the estimated need for TKR and provision. Data from the NJR70 show that, for England and Wales, growth in provision was much slower over the period 2007–10 than in 1995–2005,67 suggesting that the perceived gap between need and provision is unlikely to have been substantially narrowed in the time since the end of our analysis in 2006. This would depend on there being no change in the risk of developing OA over the same period.
Surgical thresholds are also important and hold their own implications for access and provision of care. There have been a number of strategies to cope with this increase in demand, for example national waiting list initiatives, but without accurate and reliable information long-term planning is difficult. It is therefore essential to have up-to-date and accurate disease-specific information available to estimate the future burden of the interventions, as well as the underlying diseases that are indications for hip and knee arthroplasty. This information also needs to be substantiated and validated for an accurate consensual agreement of the changing trends within the surgical community. Estimates from the USA are useful but not always consistent with epidemiological findings from the UK.
Accurate and reliable evidence of the demand and need for hip and knee arthroplasty is necessary for future planning. However, estimating rates for forecasts is difficult because surgical capacity is influenced and limited by governmental planning. Nevertheless, in the absence of supply-side forecasts, future projections based on the extrapolation of observed data98 suggest that modest increases in the number of arthroplasties are to be expected in the UK, and that the dramatic increases forecast for the USA up to 203066 are unlikely to be matched in this country.
We estimated the high rates of THR and TKR using the log-linear model. We think that simply using a straight log-linear model (which projects exponentially) to estimate future projections is not helpful as a substantive analysis, particularly over such a long projection period. Given the rise in the temporal trends in the incidence rates (1991–2010) on which the projections are anchored, it is not surprising that the curvature on a log-linear scale will eventually (by 2035) produce a very large number. Therefore, we did not major on this simple log-linear extrapolation but took a more refined and considered approach.
From the CPRD database we considered the first primary replacement for each subject to be the one of interest, and for the survival study we ignored subsequent primaries, even though the second primary may be a genuine contralateral primary. Likewise, we took the first revision encountered as the one that matches up with the primary. This approach, although arbitrary, is consistent, and is not likely to be biased with respect to any other important factors of interest in our studies (e.g. age, sex and BMI). For survival analyses, taking the first revision recorded is less of an issue, given that multiple revisions on the same-side joint are not only possible but quite common, over and above those revision procedures that are designed to be performed in two separate stages. We found the distributions of certain event coding to be good, considering that the CPRD is derived from routinely recorded data; however, the coding is not always perfect.
The main limitation of the work performed in this work package was the lack of individual validation of events in the data of the CPRD. However, several studies have shown the data to be accurate and complete for the clinical codes corresponding to OA, fracture and other crucial variables. The diagnosis of OA in general practice is often based on clinical symptoms without radiological support. This is because current general practice guidelines do not recommend radiographs to make a diagnosis of uncomplicated OA. Furthermore, we have examined a random sample of patients from general practice with a clinical diagnosis of knee OA to validate the diagnosis. We found that > 75% of GP diagnoses were confirmed using validated criteria. Moreover, there is an increasing need to study the epidemiology and management of the more clinically relevant diagnosis of clinically diagnosed hip OA.
The provisional number of patients identified in the GPRD is consistent with the expected number of patients for hip and knee joint replacements, which are less likely to be a result of misclassification or under-reporting.
The CPRD data are routinely gathered for all contributing practices and are not explicitly censored when requested, including left-censoring. The CPRD has a practice-level requirement that the data delivered to the database by each practice should be ‘up to standard’, but this does not affect patient-level data. Similar to most users of CPRD data, we used only data from practices after the point at which the CPRD deemed them to be ‘up to standard’. Although a subject may have had a primary THR/TKR event after his/her practice began submission of data but before that practice was deemed to be ‘up to standard’, we used only the up-to-standard data. Therefore, if a subject satisfying the study selection criteria is registered with a valid CPRD practice for any length of registration period, he/she will be in the data set so long as that period coincides with the time during which the practice is supplying up-to-standard data.
Another limitation of our work was that it was possible for us to encounter revisions whereby the matching primary was carried out before the up-to-standard date, before the practice began submission or even before the patient registered with that practice. Once again, our consistent and conservative approach was not to use revision events in our survival or lifetime risk analyses unless we had a valid preceding primary replacement event for the same subject.
Conclusion
In conclusion, rates of hip and knee replacement rose between 1991 and 2006. Projections of future growth in the number of procedures to 2035 suggest a slower increase than that observed since the early 1990s. The long-term risk of revision for hip and knee replacements is slightly higher for subjects with higher BMI, but the effect is small.
Chapter 3 Work package 2: designing the statistical tool to predict surgery outcome
This chapter describes the important risk factors for poor outcome and combine them to produce a clinically relevant instrument (tool) to predict poor outcome and replacement failure.
The chapter contains information from publications that were based on work package 2.
Our objectives for work package 2 were to:
-
describe the risk factors for primary and revision surgery using the data from existing national and hospital prospective arthroplasty cohort studies
-
combine these risk factors to produce a clinically meaningful panel of predictors for poor outcome.
Predictors of outcome
Identification of operational, clinical and biological predictors of poor outcome after TJR is urgently required for a balanced provision of services and avoidance of unjustified use of resources when there is a high risk of implant failure. Identification of risk factors for poor outcome will guide translational research in terms both of the specific interventions and specific patient group selection. In addition, this will provide vital information to clinicians, patients and their carers, as those with a high risk of revision may wish to forgo surgery whereas those with a lower risk would be reassured.
Baker et al. 36 used a NJR to determine the role of pain and function in postoperative patient satisfaction and found that pain was a significant factor in patients not being satisfied with their operation.
Aims and objectives
In this work package we identify the important operational, clinical, biological and other important risk factors for poor outcome for lower limb joint replacements. We then combine previously described risk factors in order to develop a statistical tool for identification of patients with poor outcomes following THRs and TKRs. To achieve this goal, the work has been done to:
-
initially define the good and bad PROMs
-
identify the role of univariable as well as multivariable risk factors in patient-reported outcomes
-
develop a statistical tool to predict poor outcomes following THR and TKR surgeries.
We have completed further work to confirm the role of individual predictors for hip and knee replacement surgeries, particularly BMI, age and sex, patient’s preoperative expectation, premorbidities (such as OA) and the type of implant. We have summarised our findings on the potential risk factors of good or bad patient-reported outcomes after THR and TKR, and revision results from the GPRD, currently known as the CPRD, in a number of publications. 135,136
In this chapter we discuss each individual risk factor and its association with surgery outcomes, as described in published papers. At the end of each subsection for risk factors we will summarise the publications when these associations were reported.
Design and setting
Cohorts and databases
The list of databases and cohorts we used in work package 2 is outlined in this section.
European Collaborative Database of Cost and Practice Patterns of Total Hip Replacement137,138
The European Collaborative Database of Cost and Practice Patterns of Total Hip Replacement (EUROHIP) study consists of 327 patients receiving THR treatment across 20 orthopaedic centres in Europe. Patients completed self-administered questionnaires on demographic variables and baseline of pain, stiffness, mobility and quality of life. In addition, they were asked about their expectations of surgery 1 year after the operation. We also collected preoperative radiographs and data on operative procedure, including the prosthesis type used. Patients undergoing primary hip replacement in whom the indication for surgery was OA were included, but those with hip disease other than OA, severe mental conditions and/or dementia were excluded.
Exeter Primary Outcomes Study139
In the Exeter Primary Outcomes Study (EPOS), participants were recruited between January 1999 and January 2002 from seven centres in England and Scotland. Patients received primary THR using a cemented Exeter femoral stem component (Stryker Howmedica Osteonics, Mahwah, NJ, USA). 140 A variety of cemented and uncemented acetabular components were used in included patients. Ethics approval was obtained from the North Western Multiple Centre Research Ethics Committee and the local research ethics committees. The cohort was representative of a wider orthopaedic cohort, as the participating hospitals (both teaching and district general hospitals) covered a wide geographical area including urban and rural locations; thus, it covered both affluent and somewhat deprived inner-city suburban areas with an overall population of 1 million.
Elective Orthopaedic Centre141
The Elective Orthopaedic Centre (EOC), also known as the South West London Elective Orthopaedic Centre (SWLEOC), is a purpose-built orthopaedic treatment centre that was opened in 2004. The centre serves a population of 1.5 million people in south-west London and it performs TKR surgeries across four acute NHS trusts: Kingston, St George’s, Mayday, and Epsom and St Helier. In this work package we included patients who received either primary UKR or TKR.
Knee Arthroplasty Trial142
Participants in the Knee Arthroplasty Trial (KAT) received primary TKR across 34 centres in the UK between July 1999 and January 2003. The primary outcome was the Oxford Knee Score (OKS) at 12 months after surgery. For this work package we used a wide range of preoperative predictors such as patient characteristics, as well as clinical and surgical factors.
Portsmouth and North Staffordshire143
We used the data on patients undergoing THR from two districts in England, Portsmouth and North Staffordshire, with a population of approximately 1 million. The districts were selected for our work because, first, they were specialised in the assessment and treatment of hip OA; second, there was much support from the local orthopaedic surgeons; and, third, the district had a diverse socioeconomic profile. The orthopaedic surgeons recorded all men and women aged > 45 years who were listed for primary THA between 1993 and 1995. For our work we excluded the patients who had sustained a hip fracture within the past year, with a diagnosis of rheumatoid arthritis (RA) or ankylosing spondylitis, and those with secondary OA.
Clinical Practice Research Datalink77,94
The CPRD, formerly known as the GPRD, is an English NHS observational data and interventional research service. It is jointly funded by the NHS National Institute for Health Research (NIHR) and the Medicines and Healthcare products Regulatory Agency. The database is designed to facilitate the linkage of anonymised patients’ clinical data, thus enabling a number of epidemiological studies which would subsequently be beneficial for improved health-care services. The CPRD has become a gold standard in utilising data by observational researches. Currently over 890 clinical reviews and publications have benefited from the service. More information about the database has been previously detailed in Chapter 2.
A summary of the cohorts and data sets used in work package 2 is provided in Table 13.
Cohort/database | Year of inception | Arthroplasty | Number of patients | Longest duration of observation (years) | Pain outcome | Joint failure |
---|---|---|---|---|---|---|
CPRD | 1987 | Hip | 27,155 | 18 | N | Y |
Knee | 23,536 | |||||
EPOS | 1999 | Hip | 1411 | 5 | Y | Y |
KAT | 2000 | Knee | 2000 | 5 | Y | Y |
Portsmouth and North Staffordshire | 1993 | Hip | 643 | 8 | Y | Y |
St Helier | 1995 | Hip | 4089 | 12 | Y | Y |
EOC | 2004 | Hip and knee | Still recruiting – > 10,000 | 3 | Y | Y |
Outcome measures
We used two scoring systems as a measure of surgery outcome:
-
PROMs, which are a condition-specific instrument jointly made up of the condition-specific Oxford score, a generic instrument EuroQol-5 Dimensions (EQ-5D) and general patient-specific questions144
-
the Western Ontario and McMaster Universities Osteoarthritis Index (WOMAC).
Oxford Hip Score/Oxford Knee Score
Two of the most commonly used and nationally recommended scores are the Oxford Hip Score (OHS) and OKS,145 incorporated into PROMs as described but also used as stand-alone questionnaires. They were originally designed as joint-specific scores for use in clinical trials to measure population-based changes,146 and widely assessed for reliability and validity145–147 for this intended purpose. The OHS and OKS consist of 12 questions asking patients about their joint-specific pain and function in the preceding 4 weeks. Questions are scored on a Likert scale from 0 to 4, with the results added up to give a total score. The overall score maximum is 48 units, with 0 the worst possible score, indicating poor function and/or severe pain; and 48 representing the best score, suggesting no adverse symptoms and excellent joint function. Overall satisfaction of Oxford scores is measured by a visual analogue scale (VAS) with scores from 0 to 100 units.
EuroQol-5 Dimensions
The EQ-5D is joint non-specific questionnaire asking patients about their general health state, mobility, self-care, usual activities, pain and anxiety/depression. 148
Satisfaction
In a number of cohorts, patients were asked about their satisfaction with surgery. In many cohorts, the measure of satisfaction is split between satisfaction with the procedure and satisfaction with the service. Satisfaction in some cohorts is a dichotomous variable, whereas in others it measured on a VAS.
Western Ontario and McMaster Universities Osteoarthritis Index
The WOMAC consists of 24 items with three domains: pain, stiffness and physical function. The scores range from 0 (no symptoms) to 100 (symptoms with extreme severity). The total score is created by adding the scores from each domain, multiplying by 100 and then dividing by the maximum score. Combination of these three domains adds up to a total score of 96, which is then converted into a normalised score. In order to classify whether or not patients improved 1 year after their surgery, we used the Outcome Measures in Rheumatology Clinical Trials/Osteoarthritis Research Society International (OARSI) responder criteria. For statistical analysis we used logistic regression to describe association between preoperative expectations and response to surgery.
Short Form questionnaire-12 items
The Short Form questionnaire-12 items (SF-12) consists of 12 questions and is the shorter version of the Short Form questionnaire-36 items (SF-36) health survey. The questionnaire is designed to collect the data on patients’ mental and physical functioning, and also overall quality of life related to health. SF-12 is not age or disease specific, but is a general measure of health. The physical and mental component summary scores are combined and range from 0 to 100, in which 0 is indicative of the lowest possible status of health and 100 of the best possible status of health.
Main exposure
Primary and revision hip and knee replacement surgery.
Sample size
For a diagnostic (predictive) model to be of use in predicting outcomes it needs to have a sensitivity of at least 90% and a specificity of at least 75%. The power calculations are based conservatively on having data for 32,500 hip arthroplasties and 27,300 knee arthroplasties. It is assumed that we have complete data for only 80% of the patients and that 16% will experience arthroplasty failure, as defined by poor functional outcome at 2 years. This would result in there being 5200 patients with whom to estimate sensitivity and 27,300 patients with whom to estimate specificity for THR. With 5200 patients, a true sensitivity of 90% can be estimated to within 1% (95% CI 89% to 91%), and a true specificity of 75% can be estimated to within 0.5% (95% CI 74.5% to 75.5%). For TKR, there would be 4370 patients with whom to estimate sensitivity and 22,930 patients with whom to estimate specificity for knee arthroplasty, giving similar precision for the estimated sensitivity of 90% (95% CI 89% to 91%) and specificity of 75% (95% CI 74.4% to 75.6%).
Variables
A detailed literature search was conducted for an up-to-date list of potential risk factors for poor outcome. Our a priori list of factors can be grouped into three main groups: (1) technical factors, including type of prosthesis and cemented compared with uncemented; (2) other non-patient-related factors, such as the hospital where the surgery took place (size, throughput and expertise) and year of surgery; and (3) patient-related factors, such as age, sex, obesity, underlying arthritic condition, comorbid medical problems, radiographic parameters, intraoperative findings, postoperative complications and medication, such as non-steroidal anti-inflammatory drugs, bisphosphonates, hormone replacement therapy, statins and corticosteroids.
Preoperative radiographs were graded for severity using the Kellgren and Lawrence (K/L)149 grading system for OA and the Sharpe score for RA. Grading of radiographs was done by trained research assistants and in cases of disagreement a formal consensus reading was performed. Radiographs were digitally scanned for a more detailed assessment of bone quality and shape.
The early postoperative radiographs (approximately 6 weeks post surgery) were assessed for joint alignment and the quality of subchondral bone as early predictors of joint failure.
Statistical methods
For identifying and combining predictors we have used various statistical methods. The list of such methods is detailed for each work/publication in Appendix 2. Statistical methods for designing predictive tools are detailed in Developing a predictive model.
Defining good and bad patient-reported outcome measures
Patient-reported outcome measures have been in development over a number of years for different reasons, and often for use as outcomes in clinical trials. They are now increasingly being used to assess a patient’s satisfaction with hip or knee replacements. 150,151 They are also increasingly considered as a potential tool to prioritise patients for surgery. This has raised some methodological concerns as these scoring systems traditionally accounted for the mean improvement of population group by using pre- and postsurgical scores as a continuous variable, with little validation for use as an outcome measure in individuals.
Some of the most commonly used PROMs include OHSs146 and OKSs,147 all validated to assess pain, stiffness and function. However, the scores have historically been used mostly as continuous outcome measures.
The government has introduced the PROM, as the recognised PROM for all patients having a hip or knee replacement,152 and attempts have been made to use PROMs to prioritise patients for surgery, limiting those with lower (worse) scores. The intention is that all data collected as part of the PROM programme are published and used towards the patient choice agenda. This kind of access to data provides patients and health-care professionals with the opportunity to discuss the information available regarding their care options, and results in shared informed decision-making. 153
Initially, the routine collection of PROMs has been brought in to clinical trials and national audits followed by the government initiative in 2009 to introduce PROMs throughout the NHS as a measure of improvement of clinical quality within the health-care system. 150 OHSs and OKSs, which form part of PROMs, were not, however, designed to be utilised in this way. Moreover, little work has been done to suggest that Oxford scores can actually predict surgery outcome. We therefore investigated how preoperative Oxford scores can be used for the definition of patient-reported outcomes, a prerequisite for prioritising patients to access surgery.
What is a good patient-reported outcome after total hip replacement?154
To assess the suitability of the Oxford scores, we investigated the possibility of defining a postoperative OHS threshold anchored on patient satisfaction, as described in Arden et al. 154 As OHS is a continuous variable ranging from 0 units, as the worst possible score, to 48 units, as the best possible score, it is unclear which score on this continuous scale would define a cut-off point indicating patient satisfaction with surgery. To explore this, we used the St Helier Hospital Outcome Programme data for defining a postoperative OHS threshold anchored on patient satisfaction. To investigate patients’ satisfaction at 12 and 24 months after the surgery, patients were asked ‘are you satisfied with the result of your hip replacement?’, to which patients would answer yes or no.
From baseline characteristics, we included BMI, sex, age, OHS and duration of pain. Table 14 shows the median values of these characteristics for the full cohort (799 patients).
Baseline characteristics | Median (full cohort, n = 799) | Non-respondents | |
---|---|---|---|
12-month follow-up (n = 180) | 24-month follow-up (n = 160) | ||
BMI (kg/m2) | |||
All, median (IQR) | 27 (24–30), n = 487 | 27 (24–30), n = 106 | 27 (23–30), n = 93 |
Low tertile, median (range) | 23 (15–25), n = 197 | 23 (15–25), n = 42 | 22 (15–24), n = 32 |
Medium tertile, median (range) | 27 (26–29), n = 143 | 27 (26–29), n = 33 | 27 (25–29), n = 34 |
High tertile, median (range) | 32 (30–43), n = 147 | 33 (30–42), n = 31 | 33 (30–39), n = 27 |
Sex | |||
Female, frequency (%) | 480 (60.1) | 107 (59.4) | 96 (60.0) |
Male, frequency (%) | 319 (39.9) | 73 (40.6) | 64 (40.0) |
Age (years) | |||
All, median (IQR) | 68 (58–76), n = 797 | 65 (54–74), n = 179 | 67.5 (56–77), n = 158 |
Low tertile, median (range) | 54 (20–62), n = 269 | 51 (20–60), n = 65 | 53 (20–60), n = 54 |
Medium tertile, median (range) | 68 (63–73), n = 275 | 65 (63–71), n = 56 | 68 (61–74), n = 52 |
High tertile, median (range) | 79 (74–100), n = 253 | 78.5 (72–100), n = 58 | 80 (75–100), n = 52 |
OHS (units) | |||
All, median (IQR) | 17 (11–23), n = 799 | 17 (12–24), n = 180 | 17 (12–23), n = 160 |
Low tertile, median (range) | 9 (0–13), n = 278 | 10 (1–15), n = 71 | 10 (1–14), n = 57 |
Medium tertile, median (range) | 17 (14–21), n = 277 | 18 (16–21), n = 51 | 17 (15–20), n = 50 |
High tertile, median (range) | 27 (22–41), n = 244 | 26.5 (24–30), n = 58 | 25 (21–36), n = 53 |
Duration of pain, median (IQR) | 1–3 years (1–3 years, 3–5 years), n = 630 | 1–3 years (1–3 years, 3–5 years), n = 83 | No observations |
A total of 799 patients who had THR were eligible in the period 1986–2007. Of those, 77.5% (n = 619) completed the 12-month follow-up questionnaires and 80.0% (n = 639) completed the 24-month follow-up questionnaires. The underlying diagnosis was OA in 95.4% of selected patients. Outcome measures included age, height, weight, sex, expectation and satisfaction questions, and OHS.
Indication for surgery was available for only 239 patients, which was a limitation of this data set. Within this group, 95.4% of operations were carried out because of OA/coxarthrosis. The remaining 4.6% of cases were a result of avascular necrosis (n = 4), failed postfracture fixation (n = 3), acetabular erosion secondary to hemiarthroplasty (n = 1), unspecified arthritis (n = 1), hip dysplasia (n = 1) and joint pain (n = 1). Only 487 patients had a baseline BMI measurement and, among these patients, the median BMI was 27 kg/m2 [interquartile range (IQR) 24–30 kg/m2]. The duration of pain measurement was available in 630 patients, and sex and baseline OHS were present in all.
Two different statistical methods were used to identify the cut-off points, which were anchored on patient satisfaction: one was the receiver operating characteristic (ROC) curve technique, which was used to identify the thresholds to maximise sensitivity and specificity; and the other was the 75th percentile approach. The OHS, which is quoted to patient satisfaction, at 12 months was ≥ 38 units using the ROC curve technique and ≥ 38 units by the 75th percentile approach. At 24 months, the figures were 33 units and 40 units, respectively (Figure 9). Using changing OHS as the outcome of choice, the ROC curve revealed that the value of satisfaction was 15 at 12 months and 14 at 24 months.
FIGURE 9.
Patient satisfaction at 2 years after surgery: (a) ROC plot; and (b) 75th percentile. The 75th percentile shows the cut-off point in which positive responses are within the 75% data. Reprinted from Osteoarthritis and Cartilage, vol. 19, Arden NK, Kirna A, Judge A, Biant LC, Javaid MK, Murray DW, et al. , What is a good patient-reported outcome after total hip replacement? pp. 155–162, 2011, with permission from Elsevier. 154

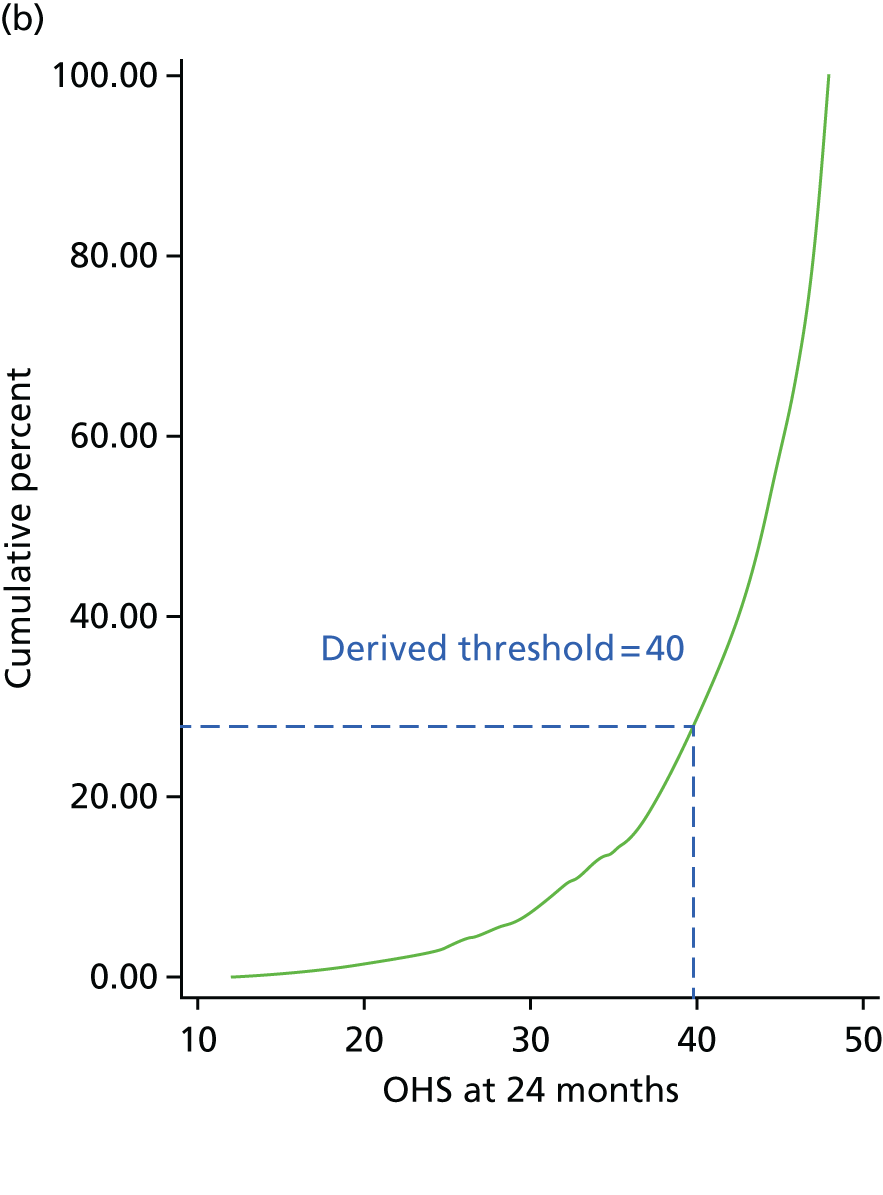
We took the 75th percentile from the top end of the OHS curve, leaving the cut-off point at 25%. At follow-up, 91.9% were satisfied at 12 months and 92.8% at 24 months, whereas 24 patients were unsatisfied at 24 months.
To assess whether or not these cut-off points varied according to important baseline characteristics, we performed a stratified analysis. The characteristics were sex, age (tertiles), BMI (tertiles), baseline OHS (tertiles), preoperative expectation of pain (‘not at all painful’ vs. ‘any pain’) and expectations for function (‘not limited at all’ vs. ‘any limitation’).
We demonstrated that the cut-off points, when using the change in OHS between the baseline and 24 months, have greater variation across patients than when using only 24-month OHSs (Figure 10). The value associated with satisfaction was greater in women than men. There were also greater variations accounting for BMI and patients’ preoperative expectations. The patients who had the highest preoperative OHS required the lowest change in the score in order to be satisfied.
FIGURE 10.
The ROC curve cut-off points for satisfaction at 24 months using 24-month OHSs, stratified by baseline clinical variables. 154 Reprinted from Osteoarthritis and Cartilage, vol. 19, Arden NK, Kirna A, Judge A, Biant LC, Javaid MK, Murray DW, et al. , What is a good patient-reported outcome after total hip replacement? pp. 155–162, 2011, with permission from Elsevier. 154

Using the 12-month data, we demonstrated the heterogeneity in the cut-off points, particularly when stratified for BMI and age. The greatest discrepancy was seen for change and baseline OHS, but with the lesser changes seen in percentage for potential improvement.
This study below confirms that we could identify the cut-off point for outcome of hip replacement surgery, which could be used for our research. This was based on the patient-accepted symptom state (PASS) methodology. In view of the heterogeneity discovered on stratification, however, this was not acceptable for clinical decision-making as a single outcome measure and more work would be required to stratify outcome measures if this was to be the case.
Interpretation of patient-reported outcomes for hip and knee replacement surgery155
Having identified a cut-off score for outcome of hip arthroplasty at 12 months, we then used a second cohort, the EOC, to produce a PASS score for the OHS but also to validate the OHS. This purpose-built EOC performs hip and knee replacement surgeries for four acute NHS trusts (Mayday, Kingston, St George’s, and Epsom and St Helier) serving 1.5 million people. The database routinely collected OHS and OKS preoperatively and 6 months after surgery.
We obtained baseline and 6-month postoperative Oxford scores from 1523 patients undergoing hip replacement and 1784 patients undergoing knee replacement. Six months after the surgery, patients were asked to complete their overall satisfaction with surgery using a VAS. On a VAS, 0 depicts no satisfaction and 100 shows a complete satisfaction (very satisfied). We identified a threshold value of ≥ 50. This cut-off point was observed with 93% patients who had hip arthroplasty and with 89% who had knee arthroplasty.
A ROC curve was used to identify PASS score thresholds for absolute changes in OHS and OKS. For OHS this cut-off point was 14 when 97.6% patients declared satisfaction with surgery, and for OKS the cut-off point was 11 when 95.4% said that they were satisfied with surgery.
Table 15 shows these results at the baseline and follow-up at 6 months scores in tabular format.
Time point | Surgery | |
---|---|---|
OKS | OHS | |
Preoperative | ||
Mean (SD) | 19.9 (8.0) | 19.7 (8.8) |
Median (IQR) | 20 (14–25) | 19 (13–26) |
6 months | ||
Mean (SD) | 34.5 (9.1) | 38.8 (8.7) |
Median (IQR) | 36 (29–42) | 41 (34–46) |
Patients satisfied with surgery, n (%) | 1591 (89.2) | 1415 (92.9) |
The mean improvement (the absolute change) in OHS was 19 units (10.5 units) and in OKS was 14.5 units (9.8 units). Of interest, 80 patients undergoing THR had no change or worsening of OHS at 6 months, of whom 56.3% still declared themselves satisfied with surgery. Of the 143 patients whose OKS remained unchanged or deteriorated, 54.6% reported that they were satisfied with surgery. Of those whose pain score improved, 94.9% of hip replacement patients and 92.2% of knee replacement patients were satisfied. Using ROCs, the OHS associated with satisfaction at 6 months was 35 units (95% CI 32.9 to 37.1 units) and, of the patients achieving this score, 98% were satisfied with their surgery compared with 78.6% of those not meeting the threshold. The same figures for OKS were 96.7% and 70.1%, respectively. Figure 11 shows the thresholds according to baseline Oxford scores. Overall, it can be seen that patients starting with a higher baseline score, not surprisingly, have higher thresholds for satisfaction. Patients with worst pain/function baseline scores require higher change in Oxford scores to achieve the highest level of satisfaction, in contrast to patients with the better preoperative scores. However, patients with low preoperative scores (severe symptoms) require lower 6 months’ postoperative Oxford scores to achieve a higher state of satisfaction.
FIGURE 11.
Bar charts illustrating the cut-off points of (a) OHS; and (b) OKS in relation to satisfaction 6 months post surgery. Reproduced with permission and copyright © of the British Editorial Society of Bone and Joint Surgery. 155


A PROMs score on its own does not translate into a clinically meaningful outcome for either clinicians or patients. We aimed to describe how absolute changes in Oxford scores can relate to patients’ satisfaction with surgery. We used PASS156 to identify the cut-off points for the difference between the scores at baseline and 6 months after surgery. PASS depicts the value of OHS/OKS beyond which a patient’s consideration of his or her own health status is good. These cut-off points for the change, as well as 6-month scores, are useful for both patients and clinicians as they improve the understanding of representation of a ‘very good outcome’ as opposed to a ‘good outcome’ and of the expectations of the operation.
Overall, this study demonstrated that thresholds for satisfaction could be identified for use in this research programme. The value obtained for the knee was not dissimilar from the previous study and a new value for the OKS was identified. This was substantially, and significantly, lower than the hip score.
Novel methodological approach for measuring symptomatic change following total joint arthroplasty157
There has been confusion in the orthopaedic literature owing to the different methods used to define patient-reported outcomes. Some papers have used the score as the main outcome with or without adjustments for baseline score. Others have used the changes in score. This has often caused discrepant results in predictors of outcome, most notably when using baseline functional score and pain score as predictors. Harmonising the outcome measure is needed in current research. Both of these functional and pain scores have had limitations. We propose a new score – percentage of potential change (PoPC). PoPC is computed as the actual change divided by the potential improvement multiplied by 100. Thus, PoPC is a measure to express relativity of an actual change in PROMs in relation to a potential change, that is what could have been attained (Figure 12).
FIGURE 12.
Percentage of potential changes. Reprinted from The Journal of Arthroplasty, vol. 29, Kiran A, Hunter DJ, Judge A, Field RE, Javaid MK, Cooper C, Arden NK. A novel methodological approach for measuring symptomatic change following total joint arthroplasty, pp. 2140–5, 2014, with permission from Elsevier. 157

We have used the data from the EOC of patients who underwent THA and TKA between 2004 and 2009. Patients had completed OHS and OKS questionnaires both preoperatively and 6 months postoperatively. For the analysis, 1523 OHS and 1784 OKS completed questionnaires were used. In addition to Oxford scores, patients were also asked to complete a short questionnaire about their satisfaction with surgery on a VAS. A threshold of ≥ 50 units was used to generate a binary variable to identify whether or not patients were satisfied with surgery. Patients with potential improvement have a PoPC of > 0 units, with a potential worsening PoPC of < 0 units and patients with no actual change have a PoPC value of 0 units. PoPC allows the expression of how much a patient’s symptoms have improved or worsened.
Kiran et al. ,157 again, demonstrated excellent improvements following knee and hip replacement surgeries. Correlation (Spearman’s rank-order correlation) of patient satisfaction score with each of the outcome measures was greatest for PoPC and lowest for the relative change (Table 16).
Surgery | ||
---|---|---|
OHS | OKS | |
Follow-up score | 0.49 (0.45 to 0.53) | 0.57 (0.54 to 0.60) |
Actual change | 0.43 (0.40 to 0.47) | 0.49 (0.46 to 0.53) |
Relative change | 0.30 (0.25 to 0.34) | 0.34 (0.30 to 0.38) |
PoPC | 0.52 (0.49 to 0.56) | 0.58 (0.55 to 0.61) |
The results showed that the ROC analysis, anchored on satisfaction as the outcome area under the curve (AUC) for hip replacement were follow-up score of 0.86 units, PoPC of 0.86 units, actual change of 0.83 units, relative change of 0.75 units, and for knee replacement were 0.85, 0.85, 0.8 and 0.72 units, respectively.
Kiran et al. 157 have demonstrated the importance of defining outcome measures. Different outcome measures identified different numbers of patients as being responders and non-responders, which has major implications in terms of health economics and health-care planning. It also demonstrates that outcomes for individual subjects differ depending on the outcome measures. This is critically important when trying to define outcome in an individual patient, as in this programme.
In summary, so far we have demonstrated that the OHSs and OKSs can be used to define patient-reported outcome following hip and knee replacement surgery. We have defined cut-off points for the OHSs and OKSs that equate to patient satisfaction with the procedure for use in the following sections to identify predictors of outcome.
Value-added publication
Assessing patients for joint replacement: can preoperative Oxford Hip and Knee Scores be used to predict patient satisfaction following joint replacement surgery and to guide patient selection?50
In response to increasing moves to use the Oxford scores to ration access to lower limb joint replacement, we investigated the predictive nature of preoperative OHS and OKS in determining patient satisfaction postoperatively. We used the database from the SWLEOC, in which OHS or OKS were routinely collected with the addition of a satisfaction questionnaire both preoperatively and 6 months postoperatively. A total of 1523 THR patients and 1784 TKR patients were selected. Patients were asked routinely to complete the questionnaires with OHSs and OKSs at baseline, preoperatively and 6 months after their surgery. They were also asked to measure their overall satisfaction with surgery using a VAS, with scores from 0 to 100.
We used scatterplots to identify, and describe, the associations between participants’ preoperative Oxford scores and satisfaction 6 months postoperatively. We found no such association, as shown in the scatterplots (Figure 13).
FIGURE 13.
Scatterplots showing relationship between (a) baseline OKS; and (b) baseline OHS compared with satisfaction at 6 months after surgery. Reproduced with permission and copyright © of the British Editorial Society of Bone and Joint Surgery. 50

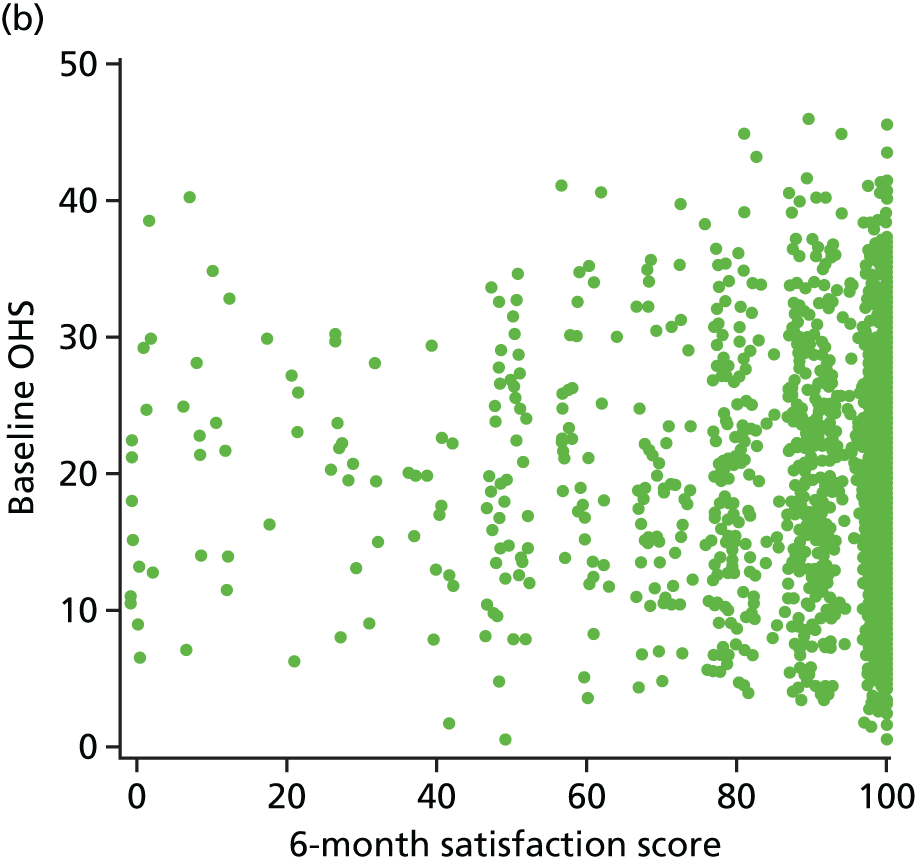
Spearman’s rank-correlation coefficients between Oxford scores and satisfaction at 6 months postoperatively were –0.04 units for the OHS (95% CI –0.09 to 0.01 units) and 0.04 for the OKS (95% CI –0.01 to 0.08 units). We also found no differences in median satisfaction scores by baseline OHS (Table 17) or OKS (Table 18) with the Kruskal–Wallis test. Interestingly, TKR results suggested that TKR patients with the lowest preoperative scores were most dissatisfied with their surgery at 6 months after their operation; conversely, scores were not predictive in patients undergoing THR.
OHS | Number of patients | Number of satisfied patients at 6 months after surgery (IQR) | Kruskal–Wallis p-value | Patients satisfied at 6 months after surgery (%) | Chi-squared p-value |
---|---|---|---|---|---|
Total | 1523 | 100 (90–100) | 1415 (92.9) | ||
Baseline OHS of ≤ 26 units | 1170 | 100 (90–100) | 0.45 | 1085 (92.7) | 0.63 |
Baseline OHS of > 26 units | 353 | 100 (90–100) | 330 (93.5) | ||
Baseline OHS of 0–15 units (low) | 533 | 100 (90–100) | 0.36 | 496 (93.1) | 0.97 |
Baseline OHS of 16–24 units (medium) | 546 | 100 (90–100) | 506 (92.7) | ||
Baseline OHS of 25–46 units (high) | 444 | 100 (90–100) | 413 (93.0) |
OKS | Number of patients | Number of satisfied patients at 6 months after surgery (IQR) | Kruskal–Wallis p-value | Patients satisfied at 6 months after surgery (%) | Chi-squared p-value |
---|---|---|---|---|---|
Total | 1784 | 90 (80–100) | 1591 (89.2) | ||
Baseline OKS of ≤ 20 units | 954 | 90 (75–100) | 0.079 | 834 (87.4) | 0.01 |
Baseline OKS of > 20 units | 830 | 90 (80–100) | 757 (91.2) | ||
Baseline OKS of 0–16 units (low) | 623 | 90 (75–100) | 0.36 | 540 (86.7) | 0.037 |
Baseline OKS of 17–23 units (medium) | 568 | 90 (80–100) | 511 (90.0) | ||
Baseline OKS of 24–47 units (high) | 593 | 90 (80–100) | 540 (91.1) |
This study suggests that, based on 6-month outcomes, preoperative Oxford scores should not be used to predict patient satisfaction after surgery. We conclude that it is unlikely that PROMs can be used on their own to predict patient satisfaction, but it is likely that a combination of multifactorial elements would play an important factor. Such a multidimensional instrument would incorporate a wide range of risk factors in the assessment of pain, function, satisfaction and health-related quality of life (HRQoL).
Identifying the role of univariable as well as multivariable risk factors in patient-reported outcomes
In this work package we report on the identification of the operational, clinical, biological and other important risk factors for poor outcome, and on the combining of risk factors, with the aim of producing a clinically relevant instrument to predict poor outcome. We have summarised our findings on the potential risk factors of good or bad patient-reported outcomes after THR and TKR, and revision results from GPRD and the new CPRD. 135,136
Data from a number of cohorts were used to inform the analysis. In particular, four data sets, which have been reported in detail elsewhere, were used extensively:
In this section, we will discuss each individual risk factors and its association with outcomes, as described in published studies.
Preoperative Oxford Hip Score and Oxford Knee Score
Introduction
In the UK, within the NHS, PROMs have been routinely used as the outcome measure. PROMs comprise the OHS, the OKS, the EQ-5D and several questions asking patients about their satisfaction with surgery, services and expectations. The OHS was developed in 1996 mainly for use in clinical trials. 146 The OHS and OKS are joint-specific measures,145,158 whereas EQ-5D is non-specific, asking patients about their general health state, mobility, self-care, usual activities, pain and anxiety/depression. 148
As previously described, the OHS and OKR score patients’ satisfaction between 0 and 48 units, with 0 describing the worst possible pain and function, and 48 indicating the best possible outcomes. These scales have been used to identify patients with surgery failure; however, scores alone are not sufficient to inform clinicians and patients of the outcome. For this reason, we introduced a dichotomous variable, the PASS score. 156 The PASS score is a necessary measure to translate the continuous Oxford score variable into a binary variable, that is, to calculate a cut-off point as an indicative score for surgery satisfaction or dissatisfaction. It is a useful variable that provides clinicians and patients with more meaningful information about outcomes of surgery. In addition to PROMs, we have also used the WOMAC, which assesses pain, stiffness and function. 159
Findings
Knee
We investigated the baseline OKS from the EOC database. We included patients undergoing total and UKR surgeries, but excluded those with previous knee surgeries and bilateral operations. Judge et al. 138 investigated the OKS at baseline and 6 months after surgery, as well as the absolute change in OKS. 138 The histogram shows a relatively normal distribution of the baseline OKS (Figure 14a). The OKS distribution after 6 months is skewed to the right, indicating that pain and function improved in the majority of patients (Figure 14b).
FIGURE 14.
Distributions of OKS at (a) baseline; and (b) 6 months after surgery. Reproduced from Judge A, Arden NK, Cooper C, Kassim Javaid M, Carr AJ, Field RE, Dieppe PA. Predictors of outcomes of total knee replacement surgery. Rheumatology, 2012, vol. 51, pp. 1804–13, with permission of Oxford University Press. 141

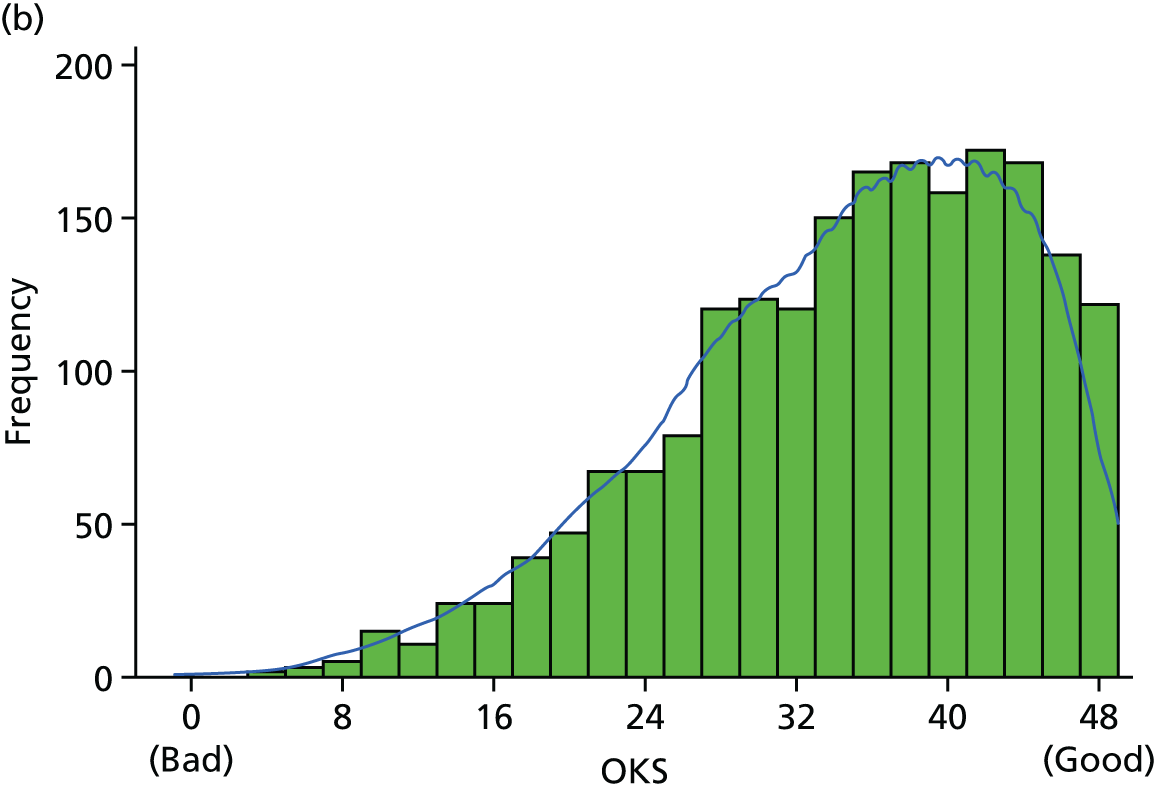
We established a PASS score using the ROC curve in order to produce the outcome for this study. A score of 30 units was used as a PASS score, which identified 71.7% of the patients (OKS ≥ 30 units) as satisfied at 6 months postoperatively. A higher baseline OKS predicts better outcome, defined by the PASS score, at the 6-month follow-up [odds ratio (OR) 1.52, 95% CI 1.40 to 1.66]. It also predicted a higher follow-up OKS (β = 1.70, 95% CI 1.43 to 1.96).
In another study, Sánchez-Santos et al. 142 used the data from 1967 patients from the KAT across 34 UK centres in the UK. 142 The results showed a baseline OKS mean of 18.2 units (SD 7.5 units) among patients who completed both pre- and postoperative questionnaires. The study found an association between the OKS at baseline and the OKS 12 months after surgery; patients with a better preoperative OKS achieved better pain and functional outcome following TKR (β = 0.35, 95% CI 0.29 to 0.42).
Hip
Our work confirmed that a higher PROMs score was associated with a better outcome. 139,160 The mean baseline OHS was 16.5 units (SD 7.6 units) in patients from the EPOS cohort139 and 16.49 units (SD 7.7 units), 15.67 units (SD 8.61 units), 19.51 units (SD 8.77 units) and 17.52 units (SD 8.30 units) in a meta-analysis combining responders at 12 months of the four cohorts. 160 Baseline SF-36 physical function score, collected from responders of two England health districts, was 20 units (SD 5.35 units).
Primary hip replacement for OA was assessed by using the EPOS prospective cohort. 139 The study showed that better preoperative pain/function was associated with a higher postoperative score. Figure 15a shows the left-skewed histogram of baseline OHS. Figure 15b depicts the 1-year OKS distribution, indicating that the majority of patients exhibited better outcomes at 1–5 years using a repeated measures linear regression model (baseline OHS 10 units; β = 2.68, 95% CI 2.16 to 3.21).
FIGURE 15.
The distribution of OHSs at (a) baseline; and (b) 1 year postoperatively. Reproduced from Judge et al. 139 This is an open-access article distributed under the terms of the Creative Commons Attribution Noncommercial license, which permits use, distribution, and reproduction in any medium, provided the original work is properly cited, the use is non commercial and is otherwise in compliance with the licence.
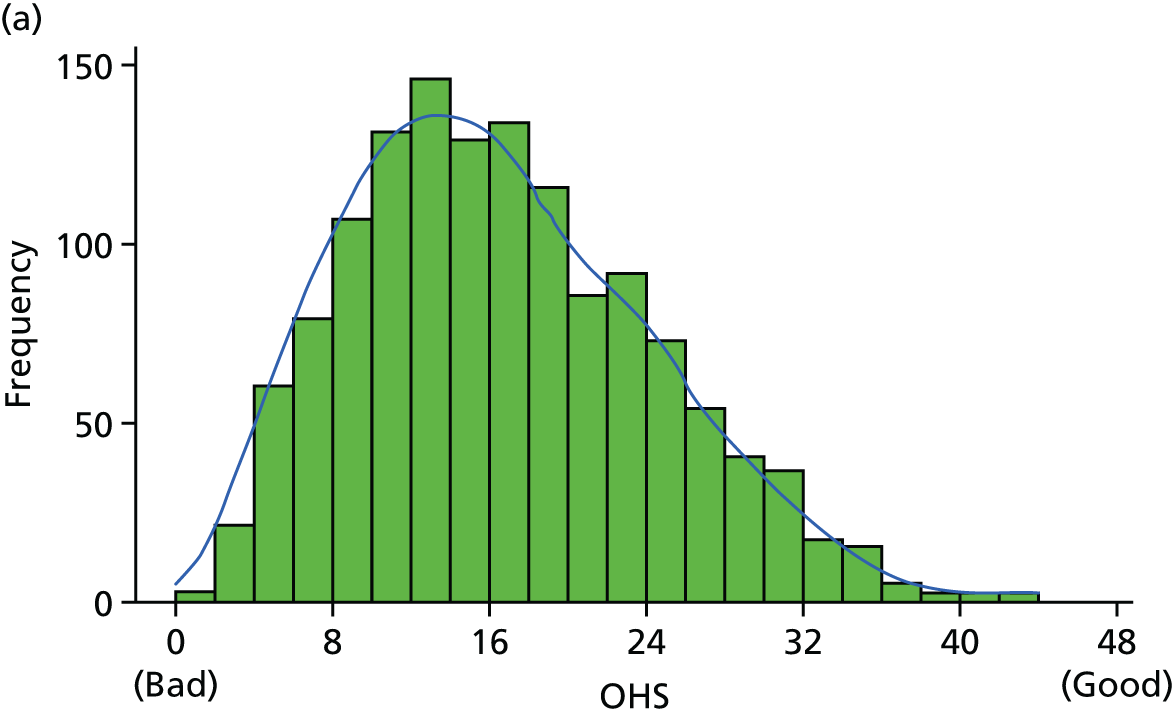

More importantly, this study found that, regardless of the preoperative OHS, participants attained a statistically significant improvement in pain and function after THR. 139 We observed that the patients with the worst baseline scores attained the greatest improvements in pain and function (patients with a preoperative OHS of < 5 achieved a 28.8-point change in the score). However, the patients with the best baseline scores still attained a substantial improvement (patients with a baseline OHS of > 30 achieved a 10.6-point change) (Figure 16).
FIGURE 16.
Change in OHS over time, stratified by baseline score, from Judge et al. 139 This is an open-access article distributed under the terms of the Creative Commons Attribution Noncommercial license, which permits use, distribution, and reproduction in any medium, provided the original work is properly cited, the use is non commercial and is otherwise in compliance with the licence.
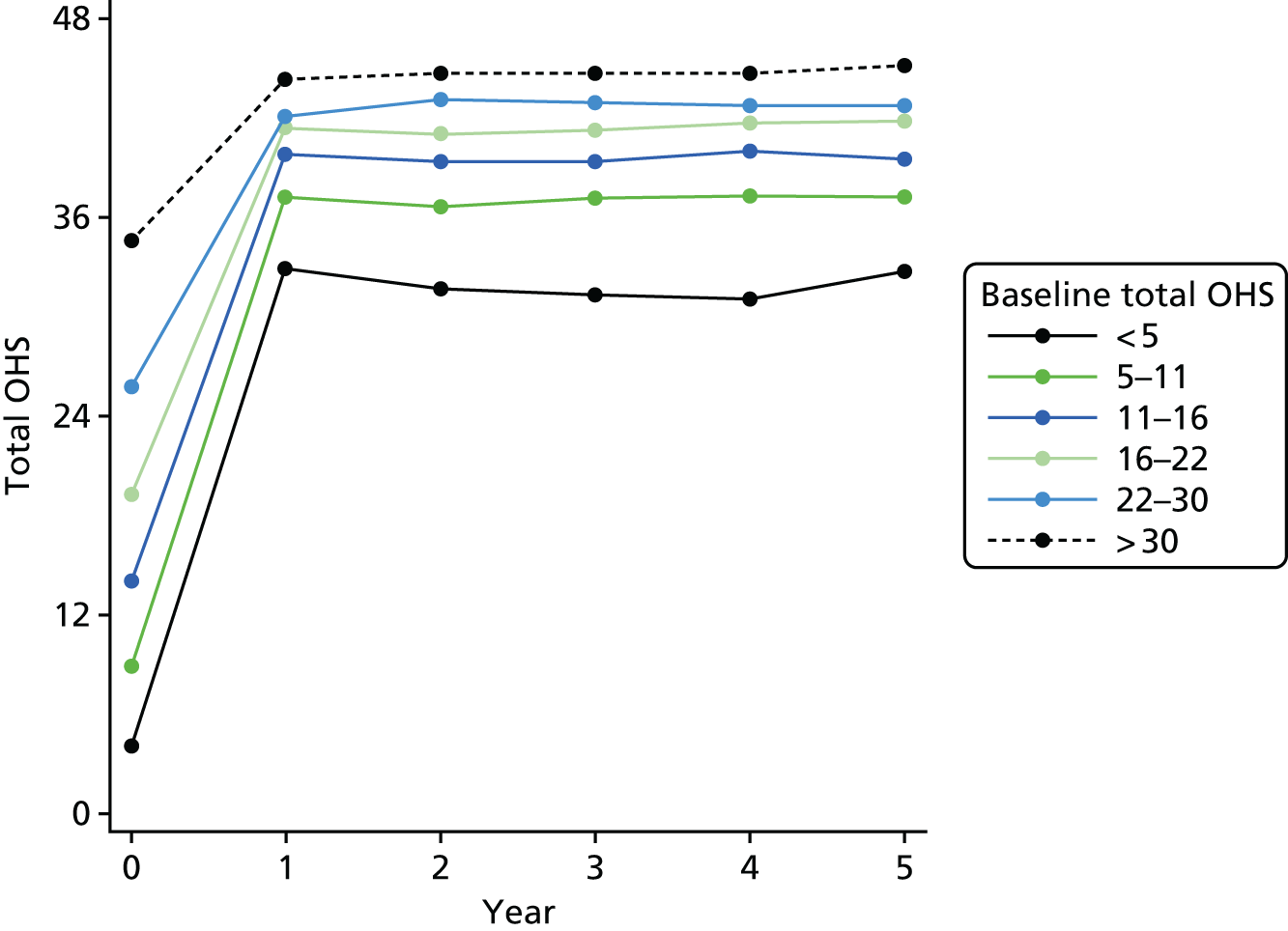
We have investigated the association of the baseline OHS and BMI. 160 We observed that the baseline OHS decreased as BMI increased; that is, patients of normal weight (BMI 18.5–25 kg/m2) had an OHS of 17.02 units (IQR 15.69–18.34 units), whereas patients with the highest weight (BMI ≥ 40 kg/m2) had an OHS of 12.25 units (IQR 9.02–15.49 units). Underweight patients (< 17.02 kg/m2) also had a low OHS (14.01 units, IQR 9.54–18.48 units). A higher baseline OHS was associated with a better outcome.
Interestingly, a further study investigating patients from two England public health districts (Portsmouth and North Staffordshire districts) demonstrated that patients reporting better baseline physical function were at higher risk of a bad postoperative outcome. 143 This can be explained by the fact that the study used a different measure from the OHS, that is, the SF-36. Functional improvement was classified as change in SF-36 physical function score in the upper quartile. The cut-off point for improved outcome on the SF-36 was defined as ≥ 30 units, which falls in the upper quartile. Patients with better preoperative scores had also improved outcomes, although they achieved a lesser change between pre- and postoperative scores. Finally, we observed that the increased baseline OHS was associated with a greater OHS. 138 This study emphasises the importance of classifying outcome similarly across all studies.
Conclusion
Preoperative pain/function was one of the strongest predictors of outcome. A high baseline preoperative score was related to a better postoperative score. It has been established that patients with lower preoperative pain and better preoperative function attain the highest postoperative pain and function, whereas patients with the worst baseline scores achieve the highest change between baseline and follow-up. 32,44–47 We also need to consider floor and ceiling effects when we estimate change because the level of satisfaction attained differs according to the baseline degree of functional disability. It is also known that patients with the lower preoperative scores never obtain the original functional and pain levels, although this outcome is possible in patients with better preoperative scores. Table 19 summarises our findings.
Cohort | Hip/knee | PROMs/revision | Outcome | Association found | For example | Reference |
---|---|---|---|---|---|---|
EOC | Knee | PROMs | 6-month PASS score | High OS predicts better outcome | Adjusted ORtotal OKS: 1.52 (95% CI 1.40 to 1.66)a | Judge et al.141 |
EOC | Knee | PROMs | 6-month OKS | High OS predicts better outcome | Linear model coefficienttotal OKS: 1.70 (95% CI 1.43 to 1.96)a | Judge et al.141 |
KAT | Knee | PROMs | 12-month OKS | High OS predicts better outcome | Linear model coefficientlog total OKS: 5.6 (95% CI 4.4 to 6.7)b | Sánchez-Santos et al.142 |
EPOS | Hip | PROMs | 1- to 5-year OHS | High OS predicts better outcome | Δ linear model coefficientOHS (10 units): 2.68 (95% CI 2.16 to 3.21)c | Judge et al.139 |
EPOS, EUROHIP, EOC and St Helier | Hip | PROMs | 12-month OHS | High OS predicts better outcome | Linear model coefficientOHS (10 units): 1.48 (95% CI 0.62 to 2.34)d | Judge et al.160 |
Portsmouth and North Staffordshire | Hip | PROMs | 6 months, with ≥ 30 points in the SF-36 | High OS predicts lower risk of good functional outcome | Adjusted OROHS (10 units): 0.73 (95% CI 0.60 to 0.89)e | Judge et al.143 |
EPOS and EUROHIP | Hip | PROMs | 12-month OHS and WOMAC score | High OS predicts better outcome | Linear model coefficientOHS (10 units): 2.23 (95% CI 1.68 to 2.79)f | Judge et al.161 |
Age
Introduction
Previous research on the association of age with the outcome of TJR has been heterogeneous. Some authors have found an association between age and outcome, but others have found no such evidence. 44–49,51,162 Literature reviews suggest that age is not a strong predictor of outcome. 43
Findings
Knee
Data from the EOC database showed no association with age overall but a minor effect on OKS function, with younger patients having a better outcome. 141 However, despite the statistical significance, the effect size observed was small. We further explored this association using the KAT data and found that patients aged < 60 years and ≥ 80 years presented a worse pain and functional status at 12 months after knee surgery. 142 We observed that younger women (aged < 60 years) had a better outcome than men, whereas in the oldest age group (aged ≥ 80 years) women had a worse outcome than men.
Age at TJR was also a significant predictor of revision for knee. Importantly, Culliford et al. 97 found that the patients undergoing TKR had a 4.3% reduction in revision rates for each extra year of age. 97 Figure 17 illustrates that patients aged between 55 and 65 years had up to 10% revision rates at 15 years after primary knee replacement; patients aged > 85 years had a < 2% revision rate; interestingly, the youngest group of patients had the highest revision rate.
FIGURE 17.
Cumulative incidence estimate for revision of TKR by age. Reproduced from Culliford et al. 97 This is an Open Access article distributed in accordance with the Creative Commons Attribution-Noncommercial (CC BY-NC 3.0) license, which permits others to distribute, remix, adapt, build upon this work non-commercially, and license their derivative works on different terms, provided the original work is properly cited and the use is non-commercial.
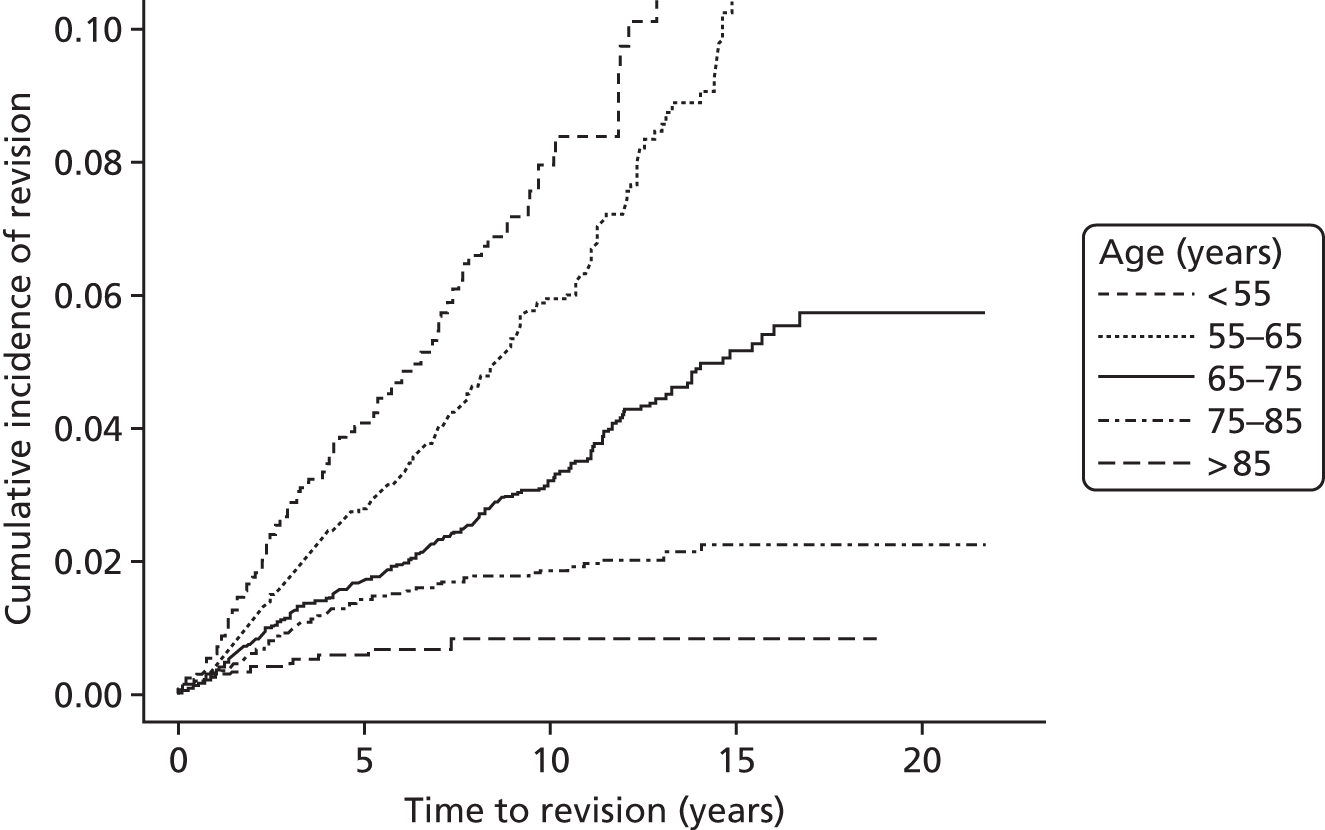
Hip
Judge et al. 30 investigated the association between age and patient outcomes following THR surgery in the EUROHIP cohort. Age was grouped at baseline as < 50, 50–69 or ≥ 70 years. The results showed that there were no statistically significant differences across the age groups, although the youngest responders presented better outcomes. 30 In another study, Judge et al. 143 collected data from 282 patients from two England health districts: Portsmouth and North Staffordshire. The primary outcome was the long-term functional improvement after THR. Patients included in the study were aged ≥ 45 years and were listed for THR for primary OA. To identify the risk factors to predict functional improvement in the long term (≈8 years), we used the logistic regression modelling. The results showed that older patients were less likely to have an improvement in physical function; however, the association found in the study was weak. 143 Furthermore, data analysis from EPOS and EUROHIP cohorts revealed a non-linear association between age and outcome in patients undergoing THR. 138 In those patients aged ≥ 75 years, increasing age was associated with worse outcomes. In addition, a worse outcome was found in those patients aged 50–60 years than in those who are younger (aged < 50 years) and older (aged > 60 years),139 although there was a small, statistically significant change in achieved postoperative outcome associated with patient age (Figure 18).
FIGURE 18.
The change in OHS over time, layered by age, from Judge et al. 139 This is an open-access article distributed under the terms of the Creative Commons Attribution-Noncommercial license, which permits use, distribution, and reproduction in any medium, provided the original work is properly cited and the use is non-commercial and is otherwise in compliance with the licence.

Among patients aged > 65 years, revision rates 15 years after the primary hip replacement were up to 10%97 (Figure 19). Among THR patients, the revision rate fell by 3% for each extra year of age, after adjusting for the competing risk of death.
FIGURE 19.
Cumulative incidence estimate for revision of THR by age. Reproduced from Culliford et al. 97 This is an Open Access article distributed in accordance with the Creative Commons Attribution-Noncommercial (CC BY-NC 3.0) license, which permits others to distribute, remix, adapt, build upon this work non-commercially, and license their derivative works on different terms, provided the original work is properly cited and the use is non-commercial.

Conclusion
We have demonstrated that increasing age reduces the risk of revision surgery. 97 Different, and heterogeneous, results were seen when using PROMs as the primary outcome. Some of our work showed that the effect of age on joint replacement surgery outcome is not significant,30,141,160 whereas other work showed the effect of age to be non-linear, with the youngest and oldest patients having the worst outcomes. 138,139,142 Age was associated with functional outcome following TKR and THR, although size effect of the association was small.
Overall, the small statistically significant differences relating to patient age at the time of surgery were greatly outweighed by the substantial change in PROMs achieved by these patients. 139 Table 20 summarises associations found between age and outcomes.
Cohort | Hip/knee | PROMs/revision | Outcome | Association found | For example | Reference |
---|---|---|---|---|---|---|
EOC | Knee | PROMs | 6-month PASS score | NS pain effect. Weak function effect: younger | Adjusted ORpain: 0.98 (95% CI 0.92 to 1.05) | Judge et al.141 |
Higher likelihood of improvement | Adjusted ORfunction: 0.93 (95% CI 0.87 to 0.99) | |||||
EOC | Knee | PROMs | 6-month OKS | NS pain effect. Weak function effect: younger | Linear model coefficientpain: 0.01 (95% CI –0.10 to 0.12) | Judge et al.141 |
Higher likelihood of improvement | Linear model coefficientfunction: –0.21 (95% CI –0.3 to –0.08) | |||||
GPRD | Knee | Revision | 15 years | Increasing age reduces risk | SHR: 0.957 (95% CI 0.951 to 0.962)a | Culliford et al.97 |
KAT | Knee | PROMs | 12-month OKS | Younger (< 60 years) and older (> 80 years) worst outcome | Linear model coefficient(> 80): –2.8 (95% CI –5.6 to 0.1) | Sánchez-Santos et al.142 |
EPOS | Hip | PROMs | 1- to 5-year OHS | Very old (> 80 years) worst outcome, but NS worse for young | Δ linear model coefficient(> 80): –3.81 (95% CI –5.29 to –2.33) Δ linear model coefficient(50–60): –1.87 (95% CI –3.22 to –0.53) |
Judge et al.139 |
GPRD | Hip | Revision | 15 years | Increasing age reduces risk | Adjusted OR(1 year): 0.971 (95% CI 0.966 to 0.975) | Culliford et al.97 |
EPOS, EUROHIP, EOC and St Helier | Hip | PROMs | 12-month OHS | NS | Linear model coefficient: –0.28 (95% CI –1.12 to 0.57) | Judge et al.160 |
EUROHIP | Hip | PROMs | 12-month WOMAC score | NS effect, but young did better | ORreturn to normal (< 50): 1.7 (95% CI 0.6 to 4.6) | Judge et al.30 |
Portsmouth and North Staffordshire | Hip | PROMs | 6 months, with ≥ 30 points in the SF-36 | Weak significant effect, younger age higher likelihood of improvement | Adjusted OR(10 unit): 0.94 (95% CI 0.90 to 0.98) | Judge et al.143 |
EPOS and EUROHIP | Hip | PROMs | 12-month OHS and WOMAC score | Increasing age. When aged > 75 years worst outcome | Linear model coefficient(≥ 75): –2.00 (95% CI –3.55 to –0.45) | Judge et al.161 |
Sex
Introduction
The work carried out in work package 1 showed that the lifetime risk of THR in the UK is higher among women than among men. 98,99 It was observed that, at the age of 50 years in 2005, the risk of THR was 11.6% (95% CI 11.1% to 12.1%) for women and 7.1% (95% CI 6.7% to 7.5%) for men. Similarly, the risk of TKR was also greater among women (10.8%, 95% CI 10.3% to 11.3%) than among men (8.1%, 95% CI 7.6% to 8.5%). 99 In work package 2 we aimed to confirm sex, as previously described in the literature, as a predictor of surgery outcome following THR and TKR.
Findings
Knee
Judge et al. 141 found that, among the EOC cohort patients undergoing TKA, functional outcomes were worse in women. The attained 6-month OKS was 0.88 units lower in women than in men (95% CI 0.08 to 1.68 units). In the KAT cohort, the responders showed strong evidence of an interaction between age and sex: younger women (aged < 60 years) and older men (aged ≥ 80 years) had better outcomes. 142 This difference of sex was not found on OKS outcome in the middle age groups (60–80 years).
Conversely, Culliford et al. 97 showed that the revision risks were significantly higher among men than among women following TKR. The adjusted overall SHR was greater in men than in women in the adjusted competing risk analysis (SHR 1.51, 95% CI 1.32 to 1.73).
Hip
In hip arthroplasty patients we did not find an association between sex and surgery outcomes. 30,139,143,160 Slight improvements were observed in the EUROHIP and Portsmouth and North Staffordshire Health districts’ cohorts, although this difference was found to be non-significant. 30,143 On the other hand, Culliford et al. 97 described a significantly higher risk of revision THR in men than in women: men had a 23% higher risk of revision arthroplasty than women (1.23, 95% CI 1.10 to 1.38). Although women and men did not present differences in the OHS, WOMAC and SF-36 scores at 12 months, 1–5 years and close to 8 years after surgery,30,139,143,160 it was found that men had a greater risk of revision THR. 97
Conclusion
The results from the knee and hip studies show that sex may have small effects in knee arthroplasty patients with little effect on hip arthroplasty when using PROMs as the outcome. In general, women had a worse PROMs outcome (of minor clinical significance),141,142 whereas men were at a higher risk for prosthesis failure resulting in the revision arthroplasties. 97 Our results are summarised in Table 21.
Cohort | Hip/knee | PROMs/revision | Outcome | Association found | For example | Reference |
---|---|---|---|---|---|---|
EOC | Knee | PROMs | 6-month PASS score | NS | Adjusted ORfemale: 0.92 (95% CI 0.72 to 1.17) | Judge et al.141 |
EOC | Knee | PROMs | 6-month OKS | Female worse | Linear model coefficientfemale: –0.88 (95% CI –1.68 to –0.08) | Judge et al.141 |
GPRD | Knee | Revision | 15 years | Higher risk in men | Adjusted ORmale: 1.54 (95% CI 1.37 to 1.72) | Culliford et al.97 |
KAT | Knee | PROMs | 12-month OKS | High predicts better outcome. Younger women and older men had a better outcome | Linear model coefficientmale: –4.6 (95% CI –7.7 to –1.4) | Sánchez-Santos et al.142 |
EPOS | Hip | PROMs | 1- to 5-year OHS | NS | – | Judge et al.139 |
GPRD | Hip | Revision | 15 years | Higher risk in men | Adjusted ORmale: 1.35 (95% CI 1.23 to 1.48) | Culliford et al.97 |
EPOS, EUROHIP, EOC and St Helier | Hip | PROMs | 12-month OHS | NS | Linear model coefficient: –0.88 (95% CI –0.67 to 2.43) | Judge et al.160 |
EUROHIP | Hip | PROMs | 12-month WOMAC SCORE | No significant difference, but women had a slightly better improvement | Adjusted OROMERACT/OARSI (female): 1.6 (95% CI 0.9 to 2.8) | Judge et al.30 |
Portsmouth and North Staffordshire | Hip | PROMs | 6 months, with ≥ 30 points in the SF-36 | NS | Adjusted OR(female): 0.37 (95% CI 0.19 to 0.72) | Judge et al.143 |
Body mass index
Introduction
The World Health Organization recommends that BMI is classified into four categories: underweight (< 18.5 kg/m2), normal (between 18 and 25 kg/m2), overweight (> 25 to 30 kg/m2) and obese (class I, > 30 to 35 kg/m2; class II, > 35 to 40 kg/m2; and class III, > 40 kg/m2). BMI is widely recognised as an important predictor for many conditions, including OA, and, as such, a number of patients referred for lower limb arthroplasty will have a raised BMI. Although some studies identified a positive relationship between increased BMI and susceptibility to knee and hip OA, and the need for replacement surgeries,163–165 there is increasing concern that obesity could be seen as an obstacle to accessing replacement surgeries. We aimed to confirm an association between BMI and patient-reported outcomes of THR surgeries. A number of detailed studies investigating the BMI effects on surgery outcomes have been carried out. This section will detail the results from several publications.
Knee
We used the GPRD data to describe the association of BMI with revision rates. 97 The data were collected from patients who had undergone hip and knee replacement surgeries between 1998 and 2011. From this cohort we then identified those with subsequent revision surgeries. We investigated the effects of BMI on the time of revision surgery. We estimated cumulative incidences of TKR revisions at 1, 5, 10 and 15 years. The results showed that at 5 years the estimated cumulative revision rate for TKR was 1.9% (95% CI 1.8% to 2.1%). The cumulative incidences across all BMI groups are shown in Figure 20. For each increased unit of BMI the estimated risk of TKR revision increased by 1.015 (95% CI 1.002 to 1.028).
FIGURE 20.
Estimated cumulative incidence for TKRs by BMI. Reproduced from Culliford et al. 97 This is an Open Access article distributed in accordance with the Creative Commons Attribution-Noncommercial (CC BY-NC 3.0) license, which permits others to distribute, remix, adapt, build upon this work non-commercially, and license their derivative works on different terms, provided the original work is properly cited and the use is non-commercial.
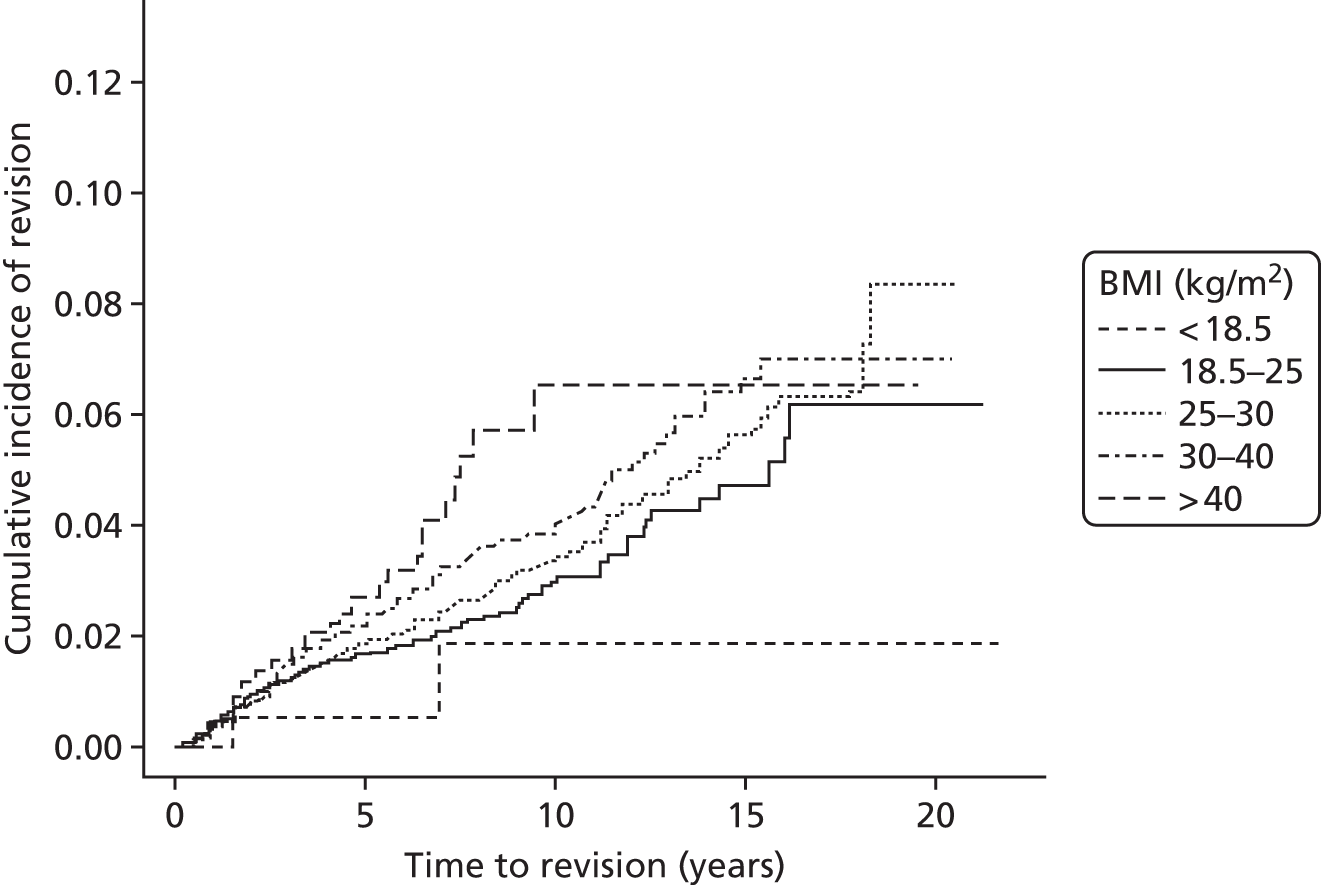
Our results suggested that BMI appears to be a significant risk factor of time to revision of TKR. The risk of revision for morbidly obese TKR patients was found as high as 6% after 10 years following surgery. Up to approximately 7 years there was a more even distribution across all BMI categories; however, there was a much higher risk for the morbidly obese patients between 7 and 10 years.
In Judge et al. ,141 a high BMI was related to a worse outcome at 6 months after TKR (coefficient total OKS –0.44 units, 95% CI –0.86 to –0.01 units), although BMI was not found to be a clinically important predictor of outcome taking into account the PASS score binary variable (OR total OKS 0.90 units, 95% CI 0.80 to 1.01 units) (Table 22). Patients who had a higher BMI showed worse functional outcomes (coefficient function OKS –0.33 units, 95% CI –0.57 to –0.09 units). Cohort analysis by Sánchez-Santos et al. 142 showed that a high BMI was a determinant factor associated with a decreased OKS at 12 months’ follow-up. Although statistically significant, the effect sizes are small and below the minimal clinically important difference of the OKS.
Cohort | Hip/knee | PROMs/revision | Outcome | Association found | For example | Reference |
---|---|---|---|---|---|---|
EOC | Knee | PROMs | 6-month PASS score | NS | Adjusted ORtotal OKS: 0.90 (95% CI 0.80 to 1.01) | Judge et al.141 |
EOC | Knee | PROMs | 6-month OKS | High BMI, worse outcome | Linear model coefficienttotal OKS: –0.44 (95% CI –0.86 to –0.01) | Judge et al.141 |
KAT | Knee | PROMs | 12-month OKS | High BMI, worse outcome | Linear model coefficientBMI (10 units): –1.5 (95% CI –2.4 to –0.6) | Sánchez-Santos et al.142 |
GPRD | Knee | Revision | 15 years | Increasing BMI increases risk (small) | Adjusted ORTKR: 1.015 (95% CI 1.002 to 1.028) | Culliford et al.97 |
CPRD | Knee | PROMs | DVT/PE 6 months after surgery | Increasing BMI increases risk | Adjusted ORTKR (obese): 1.59 (95% CI 1.26 to 1.99) | Wallace et al.77 |
CPRD | Knee | PROMs | Anaemia 6 months after surgery | Obesity decreases TKR risk | Adjusted ORTKR (obese): 0.74 (95% CI 0.58 to 0.94) | Wallace et al.77 |
CPRD | Knee | PROMs | A wound infection 6 months after surgery | Increasing BMI increases risk | Adjusted ORTKR (obese): 1.23 (95% CI 1.01 to 1.50) | Wallace et al.77 |
CPRD | Knee | PROMs | A UTI 6 months after surgery | TKR NS | Adjusted ORTKR (obese): 0.93 (95% CI 0.74 to 1.17) | Wallace et al.77 |
CPRD | Knee | PROMs | Death 6 months after surgery | Underweight increases risk | Adjusted ORTKR (underweight): 4.61 (95% CI 1.64 to 13.01) | Wallace et al.77 |
Wallace et al. 77 further investigated the association between BMI and the risks of complications 6 months following TKR. We used the CPRD to collect baseline BMI measurements, as recorded by GP practices, on patients who had undergone primary TKR between 1995 and 2011. We selected 32,485 TKR patients (including those who died within 6 months of their surgery) and, of those, < 1% were underweight, 17% were of normal weight, 38% were overweight, 29% were obese class I, 12% were obese class II and 4% were obese class III. The following outcomes were recorded following their surgeries: myocardial infarction, stroke, deep-vein thrombosis (DVT) or pulmonary embolism (PE), respiratory infection, anaemia, urinary tract infection, wound infection and death.
The study analysis showed that a higher BMI was associated with a significantly higher risk of developing wound infections, up from 3% to 4.1% (adjusted p < 0.05), in TKR patients. An association was also found between increased BMI and DVT/PE risk, up from 2.0% to 3.3% (adjusted p < 0.01), in TKR patients. Interestingly, no association was found between BMI and other confounders, particularly myocardial infarction, stroke and mortality.
Hip
We have published several studies investigating associations between BMI and THR outcomes. 97,160,166 Table 23 shows these associations. The results of the study by Culliford et al. 97 show that at 5 years the estimated cumulative rate of revision surgery for THR was 2% (95% CI 1.8% to 2.1%). Figure 21 depicts cumulative incidences across all BMI groups for patients undergoing THR. BMI was a significant predictor of revision. Severely obese THR patients seem to have an increased risk of revision surgery in the first year. For each additional unit of BMI, the estimated risk of THR revision rises by 1.02 units (95% CI 1.009 to 1.032 units).
Cohort | Hip/knee | PROMs/revision | Outcome | Association found | For example | Reference |
---|---|---|---|---|---|---|
GPRD | Hip | Revision | 15 years | Increasing BMI increases risk (small) | Adjusted ORTHR: 1.020 (95% CI 1.009 to 1.032) | Culliford et al.97 |
EPOS | Hip | PROMs | 1- to 5-year OHS | High BMI worse outcome but small effect | Δ linear model coefficientBMI (10 units): –1.54 (95% CI –2.45 to –0.64) | Judge et al.139 |
EPOS, EUROHIP, EOC and St Helier | Hip | PROMs | 12-month OHS | Higher BMI worse outcome | Linear model coefficientBMI (5 units): –0.81 (95% CI –1.08 to –0.54) | Batra et al.166 |
EPOS, EUROHIP, EOC and St Helier | Hip | PROMs | 12-month OHS | Higher BMI worse outcome but not clinically significant | Linear model coefficientBMI (5 units): –0.78 (95% CI –1.28 to –0.27) | Judge et al.160 |
EUROHIP | Hip | PROMs | 12-month WOMAC score | The obese improved less than the non-obese | ORreturn to normal (obese): 0.8 (95% CI 0.5 to 1.3) | Judge et al.30 |
Portsmouth and North Staffordshire | Hip | PROMs | 6 months, with ≥ 30 points in the SF-36 | NS. No influence of BMI on functional outcome | Crude OR: 1.00 (95% CI 0.93 to 1.07) | Judge et al.143 |
EPOS and EUROHIP | Hip | PROMs | 12-month OHS and WOMAC score | High BMI, worse outcome | Linear model coefficientBMI (5 units): –0.66 (95% CI –1.10 to –0.22) | Judge et al.161 |
CPRD | Hip | PROMs | DVT/PE 6 months after surgery | Increasing BMI increases risk | ORTHR (obese): 1.64 (95% CI 1.34 to 2.00) | Wallace et al.77 |
CPRD | Hip | PROMs | Anaemia 6 months after surgery | THR NS | ORTHR (obese): 1.03 (95% CI 0.83 to 1.28) | Wallace et al.77 |
CPRD | Hip | PROMs | A wound infection 6 months after surgery | Increasing BMI increases risk | ORTHR (obese): 1.52 (95% CI 1.21 to 1.90) | Wallace et al.77 |
CPRD | Hip | PROMs | A UTI 6 months after surgery | Obesity increases THR risk | ORTHR (obese): 1.25 (95% CI 1.02 to 1.55) | Wallace et al.77 |
CPRD | Hip | PROMs | Death 6 months after surgery | Underweight increases risk | ORTHR (underweight): 2.71 (95% CI 1.67 to 4.39) | Wallace et al.77 |
FIGURE 21.
Figure showing estimated cumulative incidence for THRs by BMI. Reproduced from Culliford et al. 97 This is an Open Access article distributed in accordance with the Creative Commons Attribution-Noncommercial (CC BY-NC 3.0) license, which permits others to distribute, remix, adapt, build upon this work non-commercially, and license their derivative works on different terms, provided the original work is properly cited and the use is non-commercial.

Batra et al. 166 used the analysis from four prospective cohort studies of patients who had undergone THR for OA: EPOS, SWLEOC, St Helier and EUROHIP. We determined the relationship between BMI and OHS at 1 year following THR. This was adjusted for the baseline OHS. We used a meta-analysis to combine the results from separately built models in all four cohorts. All models were adjusted for common variables such as sex and age. The analysis showed that, for every 5-unit rise in BMI, the 1-year OHS fell by 0.81 units (95% CI 0.55 to 1.08 units)166 (Figure 22).
FIGURE 22.
Fixed-effects meta-analysis from Batra et al. 166 Osteoarthritis and Cartilage, vol. 20, Batra RN, Judge A, Javaid MK, Thomas GE, Beard D, Murray D, et al. Pre-operative BMI as a predictor of patient reported outcomes of primary hip replacement surgery: a combined analysis of 4 prospective cohort study, pp. S152–3, 2012, with permission from Elsevier.
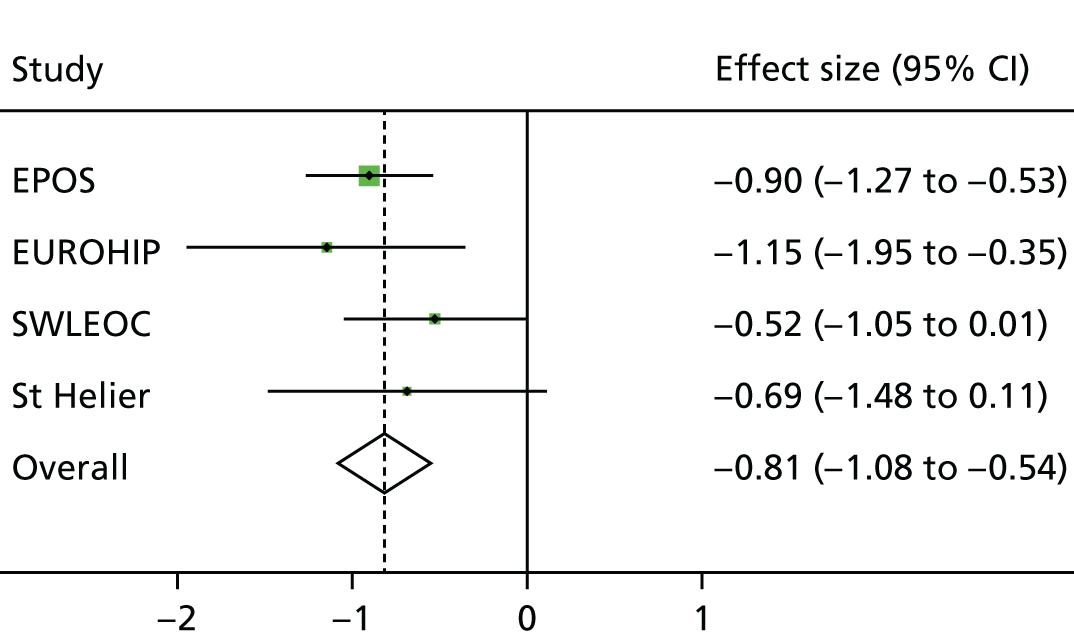
We then combined the data from all cohorts and used multiple imputations for missing data. With this analysis, when the sex and age variables were adjusted, every 5-unit rise in BMI was associated with a 1-year fall in OHS of 0.72 units (95% CI 0.46 to 0.99 units); when adjusting for all potential confounders, this reduction was decreased to 0.51 units (95% CI 0.09 to 0.92 units). The results suggest that, for each 5-unit increase in BMI, the difference in 1-year OHS becomes more significant.
Following these preliminary results, Judge et al. 160 further expanded the investigation and used the same cohorts, exposure, primary outcome OHS and confounding variables to reinvestigate whether or not BMI is a clinically significant predictor of patient-reported outcomes in patients with THR. Tables 24 and 25 show that patients achieved significant improvement in their OHS, regardless of their baseline BMI value.
BMI categories (kg/m2) | OHS, mean (95% CI) | |
---|---|---|
Baseline | 12 months | |
Underweight (< 18.5) | 14.01 (9.54 to 18.48) | 39.31 (34.93 to 43.68) |
Normal (18.5–25) | 17.02 (15.69 to 18.34) | 40.04 (38.72 to 41.36) |
Overweight (25–30) | 16.65 (15.38 to 17.91) | 39.01 (37.75 to 40.28) |
Obese class I (30–35) | 14.23 (12.81 to 15.64) | 36.95 (35.54 to 38.37) |
Obese class II (35–40) | 13.69 (11.82 to 15.57) | 35.90 (34.01 to 37.79) |
Obese class III (> 40) | 12.25 (9.02 to 15.49) | 36.43 (33.10 to 39.76) |
BMI categories (kg/m2) | OHS, mean (95% CI) | |
---|---|---|
Baseline | 12 months | |
Underweight (< 18.5) | 14.04 (9.56 to 18.52) | 39.34 (34.97 to 43.71) |
Normal (18.5–25) | 16.83 (15.25 to 18.40) | 39.85 (38.25 to 41.45) |
Overweight (25–30) | 16.79 (15.22 to 18.36) | 39.15 (37.56 to 40.75) |
Obese class I (30–35) | 14.93 (13.13 to 16.72) | 37.66 (35.93 to 39.39) |
Obese class II (35–40) | 14.71 (12.51 to 16.91) | 36.92 (34.72 to 39.11) |
Obese class III (> 40) | 13.66 (10.24 to 17.07) | 37.83 (34.25 to 41.41) |
The results confirmed the following associations between BMI and OHS: for each 5-kg/m2 increase in BMI, the OHS at 1 year decreased by 0.78 units (95% CI 0.27 to 1.28 units; p < 0.001). Obese class II patients would have a 1-year OHS 2.34 units lower than that of people with a normal BMI.
Our results confirmed that the effect of BMI on 1-year postoperative OHS is statistically significant, although the degree of significance is low: patients who are obese class II would have an OHS 2.34 units lower than patients with a normal BMI. In addition, patients who are rated as obese class II achieved a 22.2-unit change in their OHS following THR. As suggested by Murray et al. ,145 the smallest change in OHS that can be regarded as clinically important is approximately 5 units. Therefore, although a difference of 2.34 units is statistically significant, a difference of this magnitude in OHS at 1 year across all categories of BMI will have clinical significance only in patients who are rated as obese class II or III. This effect is greatly outweighed by a significant change in OHS in obese class II people (22.2-unit change), indicating substantial improvement in outcomes in this patients over the year. Thus, we conclude that BMI should not be indicative to deny patients access to hip replacement surgery.
Judge et al. 138 analysed the data from the EPOS cohort preoperatively and every year up to 5 years after the patients’ THR surgery. The analysis showed that a 10-kg/m2 increase in BMI was associated with decrease in OHS of 1.54 units (95% CI 0.64 to 2.45 units) averaged between the 1- and 5-year follow-ups, although these differences were small compared with the overall benefit of the operation (Figure 23). 139 Similarly, patients from the EUROHIP cohort presented similar results at 1 year after their THR [OR return to normal (obese) 0.8 units, 95% CI 0.5 to 1.3 units]. 30 In contrast, there was no association found between BMI and functional outcomes in THA patients from Portsmouth and North Staffordshire districts. 143
FIGURE 23.
Change in OHS over time, stratified by BMI from Judge et al. 139 This is an open-access article distributed under the terms of the Creative Commons Attribution-Noncommercial license, which permits use, distribution, and reproduction in any medium, provided the original work is properly cited, the use is non commercial and is otherwise in compliance with the licence.

Wallace et al. 77 investigated the association between BMI and the risks of complications 6 months after THR surgery using the CPRD. 77 We collected the baseline BMI measurements from the CPRD for THR patients between 1995 and 2011. For THR we selected 31,817 patients. From this cohort, BMI distribution was as follows: 1.5% underweight, 28% normal weight, 40% overweight, 21% obese class I, 7% obese class II and 2% obese class III. The results showed that in THR patients increased BMI was associated with a significantly higher risk of wound infections, ranging from 1.6% to 3.5% (adjusted p < 0.01). The association between increased BMI and DVT/PE risk was significant, with increased BMI from 2.2% to 3.3% (adjusted p < 0.01) in THR patients.
Conclusion
We found weak associations between increasing BMI and worse PROMs outcome. In accordance with other studies,167 however, the effect sizes were small and often below the minimal clinically important difference for the Oxford scores. BMI was found not to be a strong predictor of functional outcomes. Therefore, high preoperative BMI should not be a deterrent to knee or hip replacement surgeries. 26,43
Obese patients have a high risk of developing of DVT, PE, wound infection and urinary tract infection following knee and hip replacement surgeries.
We cannot advocate selecting patients for joint replacement surgeries without consideration of BMI, but we do suggest that denial of surgery based on high BMI is unwarranted.
Deprivation
Introduction
Historically, deprivation was measured using the Index of Multiple Deprivation (IMD) 2004. 53 This index was extracted from the residence area where the patients lived at the time of surgery. The index is a compound of seven deprivation indices, employing the indicated weightings: income (22.5%), employment (22.5%), health deprivation and disability (13.5%), education, skills and training (13.5%), barriers to housing and services (9.3%), crime (9.3%) and living environment (9.3%). Poorer areas attract a higher deprivation score and more prosperous areas have a lower score. In addition, educational level was considered in some of our studies as a proxy of socioeconomic,30,161 as well as employment, status. 30 Table 26 shows postoperative scores in relation to deprivation indices.
Cohort | Hip/knee | PROMs/revision | Outcome | Association found | For example | Reference |
---|---|---|---|---|---|---|
EOC | Knee | PROMs | 6-month PASS score | Deprived worse outcome | Adjusted ORtotal OKS: 0.73 (95% CI 0.62 to 0.87) | Judge et al.141 |
EOC | Knee | PROMs | 6-month OKS | Deprived worse outcome | Linear model coefficienttotal OKS: –1.40 (95% CI –1.96 to –0.85) | Judge et al.141 |
KAT | Knee | PROMs | 12-month OKS | Deprived worse outcome | Linear model coefficient: –0.5 (95% CI –0.9 to –0.1) | Sánchez-Santos et al.142 |
EPOS, EUROHIP, EOC and St Helier | Hip | PROMs | 12-month OHS | College/university better outcome | Linear model coefficient: 3.39 (95% CI 0.12 to 6.67) | Judge et al.160 |
EPOS and EUROHIP | Hip | PROMs | 12-month OHS and WOMAC score | College/university better outcome | Linear model coefficient: 2.08 (95% CI 0.59 to 3.57) | Judge et al.161 |
EUROHIP | Hip | PROMs | 12-month WOMAC score | Employment no significant effect, but employed not significantly better | Adjusted ORreturn to normal (employed): 0.8 (95% CI 0.5 to 1.5) | Judge et al.30 |
EUROHIP | Hip | PROMs | 12-month WOMAC score | Education better for dichotomous outcomes but not continuous | Adjusted ORreturn to normal (university degree): 2.9 (95% CI 1.4 to 5.9) | Judge et al.30 |
Findings
Knee
The data analysed from the EOC and KAT cohorts showed that deprivation is one of the main predictors of worse outcome in knee patients. 141,142 The IMD 200453 score in the KAT cohort showed that, for each 10-unit increase in deprivation index, the 12-month OKS was reduced by 0.5 units (95% CI 0.1 to 0.9 units), whereas increased deprivation was associated with a lower OR of achieving a 6-month PASS score (OR 0.73, 95% CI 0.62 to 0.87) (see Table 26).
Hip
We did not identify any THR cohorts for which IMD data were available,53 and so we used data on educational level and occupation as imperfect surrogates. The data showed that patients who had higher levels of education had better outcomes. 30,160,161 There was no significant difference between employed and retired patients. 30
Conclusion
Higher levels of deprivation were associated with worse patient outcomes following TKR. A higher attained educational level was associated with better postoperative reported outcomes following THR.
Indication for surgery
Introduction
The most frequent indication for THR and TKR in the UK is OA, which is the most common type of arthritis in developed countries and for which TJR is the only effective therapy in severe cases. 2 The total number of hip procedures reported in the UK in 2013 was 89,945. Of these, 80,194 were primary procedures and 9751 were revision replacements. 168 The indication for primary hip replacement was OA in 91% of cases. The total number of knee procedures reported in the UK in 2013 was 91,703. Of these, 85,920 were primary procedures and 5783 were revision replacements. The indication for primary knee replacement was OA in 97% of cases.
Another important disease for THR and TKR indication is RA. RA is a chronic autoimmune disease affecting joints. Severe RA also requires surgical intervention. RA indication for THR and TKR in 2013 was 1%. 168 Since the licensing of biological agents for the treatment of RA, the number of arthroplasties in patients with RA is declining. Association between the indication for lower limb joint replacement (OA or RA) and postoperative score is shown in Table 27.
Cohort | Hip/knee | PROMs/revision | Outcome | Association found | For example | Reference |
---|---|---|---|---|---|---|
EOC | Knee | PROMs | 6-month PASS score | RA better outcome | Adjusted ORtotal OKS: 2.17 (95% CI 1.02 to 4.60) | Judge et al.141 |
EOC | Knee | PROMs | 6-month OKS | Not significantly (RA better for pain) | Linear model coefficienttotal OKS pain: 1.75 (95% CI 0.61 to 2.89) | Judge et al.141 |
KAT | Knee | PROMs | 12-month OKS | No model differences in OA vs. OA + RA analysis | – | Sánchez-Santos et al.142 |
EPOS, EUROHIP, EOC and St Helier | Hip | PROMs | 12-month OHS | More joints with OA, worse improvement | Linear model coefficient(number of joints with OA): –1.24 (95% CI –1.71 to –0.77) | Judge et al.160 |
Portsmouth and North Staffordshire | Hip | PROMs | 6 months with ≥ 30 points in the SF-36 | OA worse preoperative radiograph grade, higher improvement | Adjusted OR: 2.15 (95% CI 1.17 to 3.93) | Judge et al.143 |
EPOS and EUROHIP | Hip | PROMs | 12-month OHS and WOMAC score | OA, superomedial, medial or concentric disease, had worse outcomes | Linear model coefficient(superomedial, medial or concentric): –1.44 (95% CI –2.79 to –0.09) | Judge et al.161 |
EPOS and EUROHIP | Hip | PROMs | 12-month OHS and WOMAC score | More joints with OA, worse improvement | Linear model coefficient: –1.11 (95% CI –1.48 to –0.74) | Judge et al.161 |
EPOS and EUROHIP | Hip | PROMs | 12-month OHS and WOMAC score | More joints with surgery, worse improvement | Linear model coefficient: –0.78 (95% CI –1.50 to –0.06) | Judge et al.161 |
Findings
Knee
The data analysis from EOC showed that patients diagnosed with RA had a better outcome than those diagnosed with OA. 141 Clinical outcomes in patients with RA were more than twice as better than in those with primary OA, as indicated by the 6-month PASS score (OR 2.17, 95% CI 1.02 to 4.60), and patients with RA had a better PASS pain score than those with OA (OR 2.33, 95% CI 1.03 to 5.29). There was no difference in PASS function scores between patients with RA and OA (OR 1.56, 95% CI 0.73 to 3.31). However, the result using the continuous OKS was not statistically significant (see Table 27).
Hip
We collected data on the number of joints affected by OA, apart from the hip. 160 The adjusted multivariable analysis showed that, for each additional joint affected by OA, the 12-month OHS fell by 1.24 units (95% CI 0.77 to 1.71 units) (see Table 27).
Baseline data on preoperative radiographic severity were collected from OA patients in two health districts in England (Portsmouth and North Staffordshire). 143 Radiographic grades (Croft grading system) of hip for surgery were grouped as 0–3, 4 and 5. Patients with worse preoperative radiographic grades had greater improvement (OR 2.15, 95% CI 1.17 to 3.93). In another study we collected data from preoperative anteroposterior radiographs of the pelvis and its intra-articular pattern of disease distribution (superolateral, superomedial, medial or concentric). 161 The pattern of OA was strongly associated with the outcome. Patients with superomedial, medial or concentric disease presented with a lower improvement than those with a superolateral pattern of OA or no reduction in joint space. Furthermore, the number of additional joints affected by arthritis and the number of joint replacements was also related to worse outcomes. Therefore, for each extra joint affected by OA, the 12-month OHS was reduced by 1.11 units (95% CI 0.74 to 1.48 units), and for each extra joint with surgery the OHS was reduced by 0.78 units (95% CI 0.06 to 1.50 units).
Conclusion
Most of the patients undergoing lower limb joint replacements have been diagnosed with OA. In only one study did patients diagnosed with RA have better outcomes than those diagnosed with OA. 141 We adjusted the model by the confounding factor age, as the patient population diagnosed with RA is younger than that diagnosed with OA141,169 Similar results were also observed for OKS pain score. The other studies were related to OA patterns. These studies unveiled better TKR outcomes in patients with a superolateral pattern of OA or no reduction in joint space, any or a low number of other joints affected by OA, as well as any or a low number of previous interventions on other joints. In summary, fewer baseline OA complications were related to better outcomes.
American Society of Anesthesiologists/comorbidities
Introduction
The American Society of Anesthesiologists (ASA) status classification system is a standard measure of fitness for surgery, scored from 1 (normal, healthy) to 6 (brain-dead patients whose organs are being removed for donor purposes). Our studies included patients up to ASA 4 (severe systemic disease that is a constant threat to life).
In addition, we considered some coexisting diseases that could affect surgery outcomes. We take into account DVT and PE, urinary tract infection, other musculoskeletal diseases and neurological, respiratory, cardiovascular, renal or hepatic disease. ASA status and number of comorbidities were analysed in several cohorts, listed in Table 28. Some of these coexistent diseases were also related to BMI (see Tables 18 and 20).
Cohort | Hip/knee | PROMs/revision | Outcome | Association found | For example | Reference |
---|---|---|---|---|---|---|
EOC | Knee | PROMs | 6-month PASS score | ASA NS | Adjusted ORtotal OKS (ASA 1 vs. 2): 1.30 (95% CI 0.81 to 2.08) | Judge et al.141 |
EOC | Knee | PROMs | 6-month OKS | ASA NS | Linear model coefficienttotal OKS (ASA 1): 1.00 (95% CI –0.29 to 2.29) | Judge et al.141 |
KAT | Knee | PROMs | 12-month OKS | Worse ASA grade worse outcome | Linear model coefficient(ASA 3/4 vs. ASA 1): –2.6 (95% CI –4.1 to –1.1) | Sánchez-Santos et al.142 |
EPOS | Hip | PROMs | 1- to 5-year OHS | Increasing number of comorbidities associated with worse outcome | Δ linear model coefficient(number of comorbidities): –0.90 (95% CI –1.27 to –0.54) | Judge et al.139 |
EPOS, EUROHIP, EOC and St Helier | Hip | PROMs | 12-month OHS | ASA NS | Linear model coefficient(number of joints with OA): –0.56 (95% CI –3.24 to 2.12) | Judge et al.160 |
EUROHIP | Hip | PROMs | 12-month WOMAC score | Continuous no effect | p = 0.13 | Judge et al.30 |
Findings
Knee
The data analysis in EOC cohort study showed that the ASA grade was not statistically significant when normal healthy patients (ASA 1) or those with severe systemic disease (ASA 3) were compared with patients with mild systemic disease (ASA 2). 141 On the other hand, the KAT cohort study142 showed that a worse ASA grade was linked to a worse OKS outcome (see Table 28). 142
Hip
In the EUROHIP cohort, there was no statically significant association between ASA grade and change in WOMAC score at 12 months: the median OHS for ASA 1 patients was 40.6 (95% CI 35.2 to 46.0), for ASA 2 patients was 35.4 (95% CI 32.8 to 38.1), for ASA 3 patients was 33.3 (95% CI 27.8 to 38.9) and for ASA 4 patients was 38.5 (95% CI 30.9 to 46.2) (p = 0.13). 30 We did not find differences between preoperative ASA grades and the OHS 12 months after hip surgery. 160
In EPOS, the number of coexistent diseases was statistically significantly associated with outcome, that is, for each additional preoperative disease the OHS between 1 year and 5 years was reduced by 0.90 units (95% CI 0.54 to 1.27 units)139 (Figure 24).
FIGURE 24.
Change in OHS over time, stratified by number of coexisting diseases, from Judge et al. 139 This is an open-access article distributed under the terms of the Creative Commons Attribution Noncommercial license, which permits use, distribution, and reproduction in any medium, provided the original work is properly cited, the use is non commercial and is otherwise in compliance with the licence.
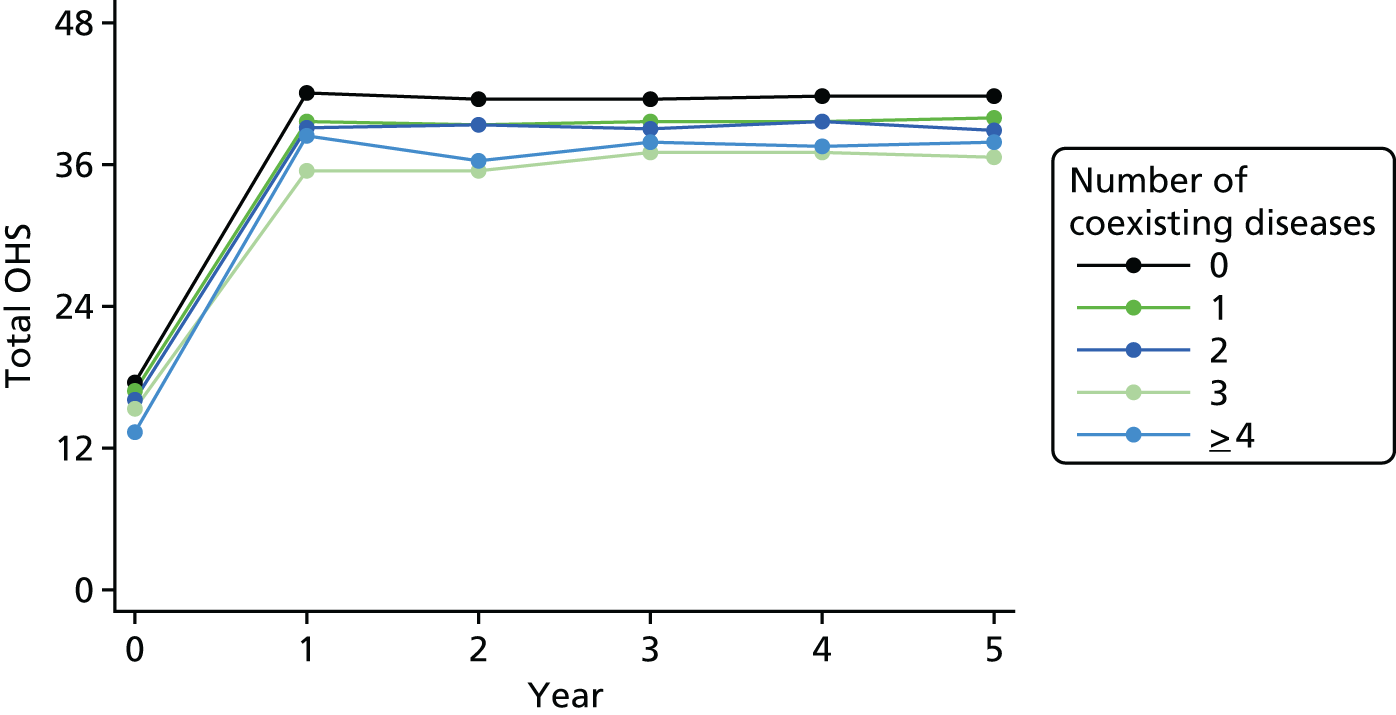
Conclusion
We can conclude that ASA grade is not an important predictor of outcomes, although healthier preoperative conditions could relate to better outcome. On the other hand, the number of other coexisting diseases is a predictor of worse outcome, although the effect size was small and it would be necessary to identify the weight for each illness in the final outcome.
Anxiety/depression
Introduction
We employed several scoring systems to assess mental health. For instance, patients from EUROHIP, the EOC, St Helier and EPOS cohorts completed a SF-36 questionnaire. This tool measures quality of life through eight health concepts, from which we selected mental health (psychological distress and psychological well-being). 170,171 In the KAT cohort, quality of life was assessed using a subset of those in the SF-36 (SF-12). Both questionnaires score from 0 (the worst possible health) to 100 (best possible health). In addition, we estimated anxiety and depression among EOC participants using the EQ-5D. 148
Findings
Knee
In the EOC cohort, those patients with moderate or extreme anxiety and/or depression presented a worse TKR outcome, that is, worse EQ-5D scores were related to worse outcome using the continuous OKS as outcome. 141 We observed lower OKS in anxious or depressed patients, with moderate anxiety or depression being associated with a reduction of 0.85 units (95% CI 0.03 to 1.68 units), and extreme anxiety or depression a reduction of 2.21 units (95% CI 0.09 to 4.34 units), compared with non-anxious or depressed patients. In the KAT cohort, the SF-12 questionnaire was used and demonstrated that worse mental health is found in those with poor outcomes. 142 These results highlight the clinical relevance of mental health in relation to outcome.
Hip
In the EPOS cohort, lower preoperative SF-36 mental health scores were associated with reduced postoperative improvement in OHS between 1 and 5 years (Table 29). 139 The differences in achieved postoperative OHS among categorised mental health levels were not substantial, although they were statistically significant when we followed up between 1 and 5 years (Figure 25). We observed the same results when we followed up patients at 12 months and we used the same questionnaire (coefficient 0.76 units, 95% CI 0.18 to 1.33 units). 160 Finally, we obtained a similar increase in the OHS outcome (coefficient 0.59 units, 95% CI 0.24 to 0.94 units) in patients from the EPOS and EUROHIP cohorts followed up at 12 months. 161
Cohort | Hip/knee | PROMs/revision | Outcome | Association found | For example | Reference |
---|---|---|---|---|---|---|
EOC | Knee | PROMs | 6-month PASS score | NS. Anxiety/depression worse outcome | Adjusted ORtotal OKS (extremely anxious/depressed): 0.70 (95% CI 0.42 to 1.18) | Judge et al.141 |
EOC | Knee | PROMs | 6-month OKS | Anxiety/depression worse outcome | Linear model coefficienttotal OKS (extremely anxious/depressed): –2.21 (95% CI –4.34 to –0.09) | Judge et al.141 |
KAT | Knee | PROMs | 12-month OKS | Worse mental health score associated with poor outcome | Linear model coefficientSF-12 (10 units): 0.9 (95% CI 0.4 to 1.3) | Sánchez-Santos et al.142 |
EPOS | Hip | PROMs | 1- to 5-year OHS | Lower SF-36 mental health score associated with poor outcome | Δ linear model coefficientSF-36 (10 units): 0.76 (95% CI 0.46 to 1.07) | Judge et al.139 |
EPOS, EUROHIP, EOC and St Helier | Hip | PROMs | 12-month OHS | Lower SF-36 mental health score associated with poor outcome | Linear model coefficientSF-36 (10 units): 0.76 (95% CI 0.18 to 1.33) | Judge et al.160 |
EPOS and EUROHIP | Hip | PROMs | 12-month OHS and WOMAC score | Lower SF-36 mental health score associated with poor outcome | Linear model coefficientSF-36 (10 units): 0.59 (95% CI 0.24 to 0.94) | Judge et al.161 |
FIGURE 25.
Change in OHS over time, stratified by SF-36 mental health categories from Judge et al. 139 This is an open-access article distributed under the terms of the Creative Commons Attribution Noncommercial license, which permits use, distribution, and reproduction in any medium, provided the original work is properly cited, the use is non commercial and is otherwise in compliance with the licence.

Conclusion
Our results show an association between preoperative mental health and the PROM. Therefore, patients having worse preoperative mental health were more likely to have worse postoperative outcome scores. This is concordant with other studies in the literature which used detailed measures of mental health28,29,172,173 or more specific ones, for example the Beck Depression Inventory. 174 However, there were no clinical important differences among mental health categories and the outcome found after surgery.
Clinical, surgical and drug predictors
Introduction
Surgical variables are not usually included in models assessing lower limb joint replacement. We considered clinical examination findings, that is, the presence of fixed flexion deformity, joint deformity (valgus, varus, no deformity) and presence of anterior cruciate ligaments (ACLs) and posterior cruciate ligaments. We also considered variables extrinsic to the patient, that is grade of operating surgeon (consultant, associate specialist staff, registrar and senior house officer) and the grade of senior surgeon present at the operation (consultant, associate specialist staff and registrar). 142 Furthermore, information about the surgical approach (anterolateral and posterior) and patient position (supine and lateral) was also collected. 139
Findings
Knee
Clinical variables were included in a general linear model to predict OKS patient outcome. 142 We found better outcomes in patients with fixed flexion deformity than in those with no deformity (linear model coefficient 1.5, 95% CI 0.6 to 2.4). In addition, we found better outcomes in patients with varus deformity than in those with no deformity and those with an absent preoperative ACL than in those with an intact ACL (linear model coefficient 1.5, 95% CI 0.0 to 3.0).
Hip
In EUROHIP, we observed increasing differences between the 12-month follow-up and baseline scores from K/L grade 2 to K/L grade 4, that is, the median WOMAC score for K/L grade 2 was 29.7 units (95% CI 22.6 to 36.8 units), K/L grade 3 was 34.4 units (95% CI 31.3 to 37.4 units) and K/L grade 4 was 38.5 units (95% CI 35.4 to 41.7 units); differences among groups were statistically significant (p = 0.03). Although this effect seems to increase also from grade 2 to grade 0, the absolute numbers of patients in groups 0 and 1 (six and five patients, respectively) did not let us affirm that K/L grade was a U-shaped curve, with group 2 being the worst. We also analysed K/L grade in patients from four cohorts, being K/L grades 1 and 2 versus K/L grade 4, and found this not to be statistically significant, that is 0.43 units (95% CI –2.75 to 3.62 units) and 0.86 units (95% CI –4.73 to 6.44 units), respectively (Table 30). We observed better outcomes in hip replacement associated with a larger offset size (offset of ≥ 44 mm).
Cohort | Hip/knee | PROMs/revision | Outcome | Association found | For example | Reference |
---|---|---|---|---|---|---|
KAT | Knee | PROMs | 12-month OKS | Fixed flexion deformity, varus deformity, absent preoperative ACL were associated with better outcome | Linear model coefficientfixed flexion deformity: 1.5 (95% CI 0.6 to 2.4) | Sánchez-Santos et al.142 |
Linear model coefficientno valgus/varus deformity: –1.6 (95% CI –3.0 to –0.3) | ||||||
Linear model coefficientpreoperative ACL absent: 1.5 (95% CI 0.0 to 3.0) | ||||||
EUROHIP | Hip | PROMs | 12-month WOMAC score | K/L grade change increases from 2 to 4 | K/L grade 2 worse outcome; p = 0.03 | Judge et al.30 |
EPOS, EUROHIP, EOC and St Helier | Hip | PROMs | 12-month OHS | NS K/L grade | Linear model coefficient(K/L 1): 0.43 (95% CI to 2.75 to 3.62) | Judge et al.160 |
Linear model coefficient(K/L 2): 0.86 (95% CI –4.73 to 6.44) | ||||||
EPOS | Hip | PROMs | 1- to 5-year OHS | Femoral offset size larger = better outcome | Δ linear model coefficient(offset): 0.17 (95% CI 0.06 to 0.28) | Judge et al.139 |
EPOS and EUROHIP | Hip | PROMs | 12-month OHS and WOMAC score | Femoral offset size larger = better outcome | Linear model coefficient(offset): 0.18 (95% CI 0.03 to 0.32) | Judge et al.161 |
EPOS | Hip | PROMs | 12-month OHS | Posterior approach = better outcome | Linear model coefficient: 2.2 (95% CI 1.1 to 3.3) | Judge et al.139 |
EPOS and EUROHIP | Hip | PROMs | 12-month OHS and WOMAC score | Posterior approach = better outcome | Linear model coefficient: 2.42 (95% CI 0.44 to 4.39) | Judge et al.161 |
GPRD | Hip and knee | Revision | 10-year implant survival | HRT reduces revision rates | HRHRT ≥ 6 months: 0.62 (95% CI 0.41 to 0.94) | Prieto-Alhambra et al.175 |
Change among femoral offset size categories had a small statistically significant difference in the postoperative OHS achieved (Figure 26). We found a significant interaction between offset size and sex, with the effect limited to women. 139
FIGURE 26.
Change in OHS over time, layered by femoral component offset (mm offset) categories, from Judge et al. 139 This is an open-access article distributed under the terms of the Creative Commons Attribution Noncommercial license, which permits use, distribution, and reproduction in any medium, provided the original work is properly cited, the use is non commercial and is otherwise in compliance with the licence.

We also identified the effect of surgical approach as significant, with the posterior approach having better outcomes than anterolateral; that is, there is a difference in the OHS at 12 months of 2.2 units (95% CI 1.1 to 3.30 units). 139 This result was very similar to those presented by Judge et al. ;161 that is, the difference at 12 months for the OHS was 2.42 units (95% CI 0.44 to 4.39 units).
Finally, we found that patients from GPRD receiving hormone replacement therapy had a lower risk of joint (hip and knee replacements) revision surgery after 6 months (adjusted HR 0.62, 95% CI 0.41 to 0.94) and after 12 months (adjusted HR 0.48, 95% CI 0.29 to 0.78)175 (Figure 27 and see Table 30).
FIGURE 27.
Kaplan–Meier estimates of probability of revision surgery according to hormone replacement therapy use. Reproduced from Annals of the Rheumatic Diseases, Hormone replacement therapy and mid-term implant survival following knee or hip arthroplasty for osteoarthritis: a population-based cohort study, Prieto-Alhambra D, Javaid MK, Judge A, Maskell J, Cooper C, Arden NK, COASt Study Group. vol. 74, pp. 557–63, 2015, with permission from BMJ Publishing Group Ltd. 175 HRT, hormone replacement therapy.

Conclusion
Clinical factors have demonstrated their importance in TKR as predictors of OKS outcomes. 142 Among them, we identified fixed flexion deformity, preoperative valgus/varus deformity and preoperative damaged ACL. We unveiled an association between femoral offset size and THR, with femoral offsets > 44 mm related to better outcomes,139,161 although this finding may happen only in women. 139 In addition, we discovered that a posterior approach may have better outcomes than an anterolateral approach. 139,161 Furthermore, K/L status was not a good predictor of the outcome. 30,160
Conclusion
We have identified a number of predictors of outcome for both THR and TKR. With the exception of baseline Oxford score, the majority of the predictors have statistically significant, but clinically small, effects in isolation. We have identified that the predictors of PROMs may be different from the predictors of revision and complications of surgery, this was best evidenced by the data relating to age and sex. We found that increasing age was associated with a lower risk of knee and hip revision,97 whereas in knee studies younger patients had a higher likelihood of improvement and the older patients a worse outcome. 142 Furthermore, men had a higher risk of TKR revision,97 whereas women had worse PROMs after TKR. 141 Therefore, in order to understand the risks and benefits of lower limb arthroplasty, results from PROMs outcomes would complement those obtained from revision studies.
When comparing predictors of THR and TKR, we found that the results were broadly similar for the majority of the risk factors assessed. We therefore surmise that the features of patients affecting TKR and THR outcomes would be similar.
Having identified a number of predictors of outcome, it is now important to combine them into a model to assess their independence and to search for interactions. This will be described in the next section.
Developing a predictive model
Statistical tool for predicting poor outcomes following total hip or knee replacement surgeries
The final work of work package 2 was to combine the identified risk factors to develop a prognostic tool to predict poor outcome following TKR and THR surgeries. We published a number of studies and combined them into the statistical tools (for both hip and knee) for each surgery category. The papers are currently under internal review and waiting for external validation in the pragmatic cohort study Clinical Outcomes in Arthroplasty Study (COASt). Although the previous research provides information on different type of predictors, it is important to understand how the interplay of these predictors can play a role in the development of poor functional and pain outcome after THR/TKR.
We aimed to combine risk factors into clinically meaningful tools for stratifying patients for THR and TKR surgeries. For this purpose we collected and used the data from EPOS, EUROHIP and KAT.
Hip predictive tool
The work for the development of the prognostic tool to predict the outcome following THR was done and summarised in the article by Judge et al. ,138 as described in the next section.
Patient-reported outcomes following primary hip replacement surgery: development and internal validation of a prognostic tool138
Following identification and confirmation of risk factors within this work package we aimed to develop a clinical risk prediction tool for both hip and knee arthroplasty patients. Such tools will be beneficial for clinical decision-making and patients’ expectation of their surgery.
The recent literature describes combining of data from multiple risk factors and, thus, including the broad range of predictors in a prognostic model. 80,81 This includes patient-related risk factors such as age, sex, education, obesity and mental health status; clinical predictors such as preoperative level of pain and function, indication for surgery, coexisting conditions and radiographic (grade) variables; and surgery-related risk factors, such as femoral component offset. In this work we aimed to develop a similar prognostic model allowing inclusion of a number of risk factors to predict pain and function following hip replacement surgery.
We collected data from prospective cohorts of patients receiving primary THR for OA: EUROHIP and EPOS. From EUROHIP we used the data from 845 patients and from EPOS we used the data from 1247 patients. By combining the data from these large cohorts we took into account a comprehensive range of both already-investigated risk factors and novel predictors.
As OHS was one of the predictors included for the analysis, we collected OHS questionnaires preoperatively and 1 year postoperatively. OHS was used as a primary outcome measure. In the EUROHIP cohort, outcome measures were predominantly collected via WOMAC, in contrast to EPOS, which collected OHS. To derive OHSs from WOMAC we used the truncated regression model for 110 patients in whom both OHS and WOMAC data are complete. Using this model we could predict WOMAC score at baseline (R2 = 75.5%) and at 1 year (R2 = 63.4%).
Predictor variables we included in this work are represented in Table 31.
Variable | Cohort, data available (%) | |
---|---|---|
EUROHIP (n = 845) | EPOS (n = 1247) | |
Patient variables | ||
Preoperative and 12-month follow-up OHSa | 100 | 100 |
Age (years) | 99 | 99 |
Sex | 95 | 100 |
BMI (kg/m2) | 92 | 95 |
Employment/occupation | 98 | 100 |
Education | 88 | 0 |
Mental healthb | 98 | 68 |
ASA grade | 87 | 0 |
Years of hip pain | 99 | 0 |
Care for someone else | 99 | 0 |
Fixed flexion | 0 | 92 |
Preoperative expectations | 100 | 0 |
Preoperative comorbidity | 0 | 69 |
Preoperative medication use | 100 | 0 |
Analgesic/NSAIDs | 99 | 92 |
Radiographic variables | ||
K/L grade | 93 | 0 |
Pattern of OA | 87 | 0 |
Prosthesis type | 97 | 0 |
Number of joints OA | 100 | 0 |
Number of joints surgery | 100 | 0 |
Number of sites osteophytes | 85 | 0 |
Surgical variables | ||
Grade of operator | 0 | 100 |
Surgical approach | 0 | 73 |
Patient’s position | 0 | 100 |
Femoral component size (mm offset) | 0 | 100 |
Femoral head | 0 | 100 |
Head size | 0 | 100 |
Duration of operation | 0 | 95 |
In the EPOS study, patients completed the SF-36. The SF-36 is an instrument that measures quality of life in eight domains. One of these domains is the mental health component, which we have selected for this work. In SF-36 the lowest score, 0, is indicative of the worst possible health and the highest score, 100, of the best possible health. We obtained the fixed flexion range of motion variable in degrees from the Charnley Modification of D’Aubigné–Postel Grade questionnaire. 176 From comorbidities we collected information on coexistent diseases, such as DVT, PE, urinary tract infection, other musculoskeletal disorders, neurological, renal, cardiovascular, respiratory or hepatic disease or treatment for other medical conditions. We have also collected detailed intraoperative information: grade of operating surgeon and patient position. From the implant information we obtained and used data such as implant material (stainless steel or ceramic), femoral head size in millimetres and the femoral component size (offset).
From the EUROHIP cohort we obtained data on home circumstances, employment, education, duration of pain in the affected hip, the number of preoperative expectations of surgery162 and other joints affected by OA, as well as other surgeries in other joints. Medications (prior to surgery) that were considered relevant to the study included analgesic/non-steroidal anti-inflammatory drugs (NSAIDs), bisphosphonates, medications for heart disease, anticoagulants, antidepressants, bronchodilators and antidiabetic drugs. We obtained EQ-5D scores for the patient’s health state today, mobility, self-care, usual activities and pain and anxiety. We obtained the data collected from baseline radiographs of the pelvis taken in the anteroposterior view. Radiographic variables included the standard K/L grade (0–4)162,176–179 and the intra-articular pattern of disease distribution (superolateral, superomedial, medial or concentric). Osteophyte size in the superior–femoral, superior–acetabular, inferior–femoral and inferior–acetabular regions was recorded. For osteophytes with moderate sizes, we created an ordinal variable of the number of sites. From intraoperative data we also obtained records from surgical teams on patients’ height and weight, prosthesis type and ASA status, the last being a standard measure of fitness for surgery, scored from 1 (normal, healthy) to 4 (severe systemic disease that is a constant threat to life).
Methodology for combining variables
Because cohorts contained a different set of confounders, combining the two studies resulted in a high proportion of missing data. Appendix 3 shows the variables available in all cohorts.
Previously methodology has been developed to combine data from multiple sources. 80,81,178,179 For instance, Heymans et al. 178 described the method multivariate imputation by chained equations (MICE), based on a Bayesian approach,176 in which data from three controlled trials were combined to identify risk factors of chronic low back pain. Jackson et al. 80 used the multiple imputation methods within Bayesian graphical modelling and described the associations between low birthweight and air pollution, thus incorporating adjustments for crucial confounders that were not available within the individual data set. These two approaches have been further compared in both simulation180 and cases studies. 181 It was concluded that, even when the proportion of missing data is high, the performance of both approaches is similar. Moreover, they both correct most of the bias with a non-hierarchical (simple) data structure.
In our work we used MICE to combine the data sources as we were more familiar with this methodology, it is easier to implement in standard statistical software and it takes less time to run the models.
Use of the multiple imputation method allows the assumption that the data are missing at random. This is plausible, as the reason behind the missing data is that not all covariates were collected in each study. 178 At first, we created 25 copies of the data set. In each copy missing values were replaced by imputed values,176,182,183 with 20 cycles of regression switching. Imputations were made by drawing from the posterior predictive distribution of each variable that required imputation. We included all of the covariates together with the outcome variable (12-month postoperative OHS) in the imputation model, as this carries information about the missing values of predictors. 176 Prior to imputation we transformed continuous variables so that they were approximately normally distributed. For the imputation of continuous variables we used the linear regression method, whereas for categorical variables we used logistic, ordinal and multinomial regression. At the second stage we fitted a statistical model to each of the imputed data sets separately. The results were then averaged to obtain a single estimate of the association. We calculated standard errors using Rubin’s rule, as it accounts for the variability between results of imputed data sets and reflects the uncertainty associated with imputing missing data. 176
Although the methodology we used has been tested and used before and proved to be transparent, and it is relatively easy to implement within the standard statistical software, its use required us to make the assumption that data were missing at random. This was plausible in the context of this study because the reason for the missing data was that the variables were not collected. This was further supported by inclusion of a wide range of covariates to ensure that sufficient predictors were included to recover missing data for missing information. We are aware, however, that there were too many missed data in this study. Even if the missing at random assumption is valid, with increased proportion of missing variables the reliability of the regression coefficient may diminish. 182 However, we are reassured by the previous simulations and case studies in which the methods have been shown to be effective, even with extreme numbers (> 90%) of missing data. 180,181
Statistical methods used
For statistical analysis we used Stata version 12.1 (StataCorp LP, College Station, TX, USA). We used analysis of covariance (ANCOVA) to identify predictors of the 12-month follow-up OHS, adjusting for preoperative OHS. In order to model non-linear relationships for continuous variables we used linear splines. We use MICE in order to combine data from two studies and adjust for a wider range of variables. 80,81 In the imputation model we included all of the listed covariates together with the outcome variable (12-month postoperative OHS). Before imputation we log-transformed continuous variables so that they were approximately normally distributed. We created 25 imputed data sets for missing data by using MICE in Stata. The final regression model included all predictor variables and was fitted to each imputed data set. This was then averaged for overall estimated associations. We applied the automatic backward selection per 200 bootstrap samples of imputed data sets. The variables retained were those consistently selected for at least 70% of the time.
We assessed the performance of the tool by using the calibration and discrimination methods. 184,185 By calibration we could judge how close the predicted OHS was to the observed OHS for each tenth of predicted score in 10 equally sized groups. By discrimination we assessed the measure of variation and for this we used R2. In total, 200 bootstrap samples with replacement and combined with multiple imputations were used to estimate overoptimism in the predictive ability of the model, and obtain bias-corrected estimates of R2. We compared R2 from models developed in 200 bootstrap samples with R2 in the same models applied to the original sample. As previously described in this chapter, a change in OHS by fewer than 5 units is described as clinically not significant. We used this knowledge to finally test the ability of the predictive tool to identify patients with the worst possible scores after their THR (i.e. identify the patients with < 5-unit changes in OHS). We then used a logistic regression model and assessed the discriminatory ability of the tool by calculating the area under the ROC curve.
Results
From the selected patients, only 63.7% in EUROHIP and 87.1% in EPOS had completed both a baseline and 1-year follow-up OHS. We observed discrepancies between the patients who did not answer the 1-year follow-up questionnaire and those who responded (Table 32). In the EUROHIP cohort, responders had better baseline pain and function and EQ-5D mental health scores, lower educational level and higher levels of obesity as well as greater ASA scores. In the EPOS study we found differences in younger responders and also in those employed and with a better preoperative SF-36 score.
Variables | Cohort | |||
---|---|---|---|---|
EUROHIP | EPOS | |||
Responders (n = 908) | Non-responders (n = 419) | Responders (n = 987) | Non-responders (n = 437) | |
ASA grade, n (%) | ||||
Fit and healthy | 123 (15.5) | 86 (22.5) | ||
Asymptomatic no restriction | 505 (63.7) | 214 (56.0) | ||
Symptomatic minimal | 160 (20.2) | 77 (20.2) | ||
Severe restriction | 5 (0.6) | 5 (1.3) | ||
K/L grade, n (%) | ||||
0 | 6 (0.7) | 0 (–) | ||
1 | 5 (0.6) | 1 (0.4) | ||
2 | 26 (3.2) | 6 (2.4) | ||
3 | 394 (49.2) | 116 (46.4) | ||
4 | 370 (46.2) | 127 (50.8) | ||
Number of coexisting diseases, n (%) | ||||
0 | 136 (31) | 295 (30) | ||
1 | 147 (33) | 351 (35) | ||
2 | 99 (22) | 216 (22) | ||
3 | 43 (10) | 97 (10) | ||
4 | 16 (4) | 31 (3) | ||
WOMAC score | OHS | |||
Pain score, mean (SD) | 54.5 (17.6) | 57.7 (18.1) | 16.1 (8.2) | 16.5 (7.6) |
The mean age of patients in EUROHIP was 65.7 years (SD 10.6 years) and 68 years (SD 10.7 years) in EPOS. There were 55.2% and 62.8% women in EUROHIP and EPOS, respectively. BMI was similar across the two cohorts, with a mean BMI of 27.8 kg/m2 (SD 4.4 kg/m2) in EUROHIP and 27.3 kg/m2 (SD 4.9 kg/m2) in EPOS.
Table 33 displays the predictors that have been found statistically significant.
Variable | % included | Coefficient (95%) | p-value |
---|---|---|---|
Patient variables | |||
Baseline total OHS (10 units) | 100.00 | 2.23 (1.68 to 2.79) | < 0.001 |
Age < 75 years (per 10 years) | 75.00 | 0.26 (–0.19 to 0.71) | 0.252 |
Age ≥ 75 years (per 10 years) | 82.50 | –2.00 (–3.55 to –0.45) | 0.011 |
BMI (kg/m2) (5 units) | 90.00 | –0.66 (–1.10 to –0.22) | 0.003 |
Education | |||
None | 0 | ||
College/university | 100.00 | 2.08 (0.59 to 3.57) | 0.006 |
SF-36 mental component summary score preoperative (10 units) | 100.00 | 0.59 (0.24 to 0.94) | 0.001 |
Radiographic variables | |||
Pattern of OA | |||
Superolateral | 0 | ||
Superomedial/medial/concentric | 89.00 | –1.44 (–2.79 to –0.09) | 0.037 |
No reduction | 2.50 | –1.72 (–4.80 to 1.35) | 0.272 |
Number of joints with OA | 100.00 | –1.11 (–1.48 to –0.74) | < 0.001 |
Number of joints with surgery | 85.00 | –0.78 (–1.50 to –0.06) | 0.034 |
Surgical variables | |||
Surgical approach | |||
Anterolateral | 0 | ||
Posterior | 100.00 | 2.42 (0.44 to 4.39) | 0.017 |
Femoral component size (mm offset) | 82.50 | 0.18 (0.03 to 0.32) | 0.016 |
For example, higher baseline OHS (better pain and function) was associated with an increased follow-up OHS, which is indicative of better pain and function outcomes. The effect of age was non-linear and there was a threshold effect. Patients aged > 75 years had worse outcomes. Worse outcomes were also observed in patients with an increased BMI, lower levels of education and lower baseline SF-36 scores. The pattern of OA was found to be an important predictor of outcome as a radiographic variable. Patients with superomedial, medial or concentric OA had worse outcomes than those with a superolateral pattern of disease or with no reduction in joint space. Worse outcomes were also linked with having had previous surgeries in other joints and having had arthritis in other joints. A posterior surgical approach and femoral component offset of ≥ 44 mm were associated with significantly better outcomes.
Internal validation and model performance
To validate the model internally we used bootstrapping to the imputed data sets. To ensure that the risk factors are replicated in other external validation studies we ensured that all predictors identified were those consistently selected across the 200 bootstrap resamples at least 70% of the time. The performance of the model was assessed by calibration and discrimination (see Table 34). Calibration of the predicted 12-month postoperative OHS was good, except in the lowest deciles of OHS, in which case the model overestimated the predicted score. Calibration of this predicted change in OHS was very good across all deciles of change in OHS. The model showed a discriminatory ability with a bias-corrected R2 of 23.1%. We assessed the performance of the model and found the calibration of the predicted 12-month OHS to be good. The exception was the lowest deciles of OHS, where we found that the developed model overestimated the predicted score. The performance of the model is described in Table 34.
Calibration | OHS | |||||
---|---|---|---|---|---|---|
12 months | Change | |||||
Observed | Predicted | Ratio | Observed | Predicted | Ratio | |
Deciles | ||||||
1 | 26.78 | 31.79 | 0.84 | 10.53 | 11.49 | 0.92 |
2 | 33.88 | 34.90 | 0.97 | 15.42 | 16.36 | 0.94 |
3 | 35.85 | 36.30 | 0.99 | 18.25 | 19.08 | 0.96 |
4 | 38.19 | 37.50 | 1.02 | 20.74 | 20.95 | 0.99 |
5 | 39.55 | 38.49 | 1.03 | 22.77 | 22.51 | 1.01 |
6 | 41.02 | 39.41 | 1.04 | 23.00 | 24.02 | 0.96 |
7 | 42.14 | 40.36 | 1.04 | 26.16 | 25.54 | 1.02 |
8 | 42.74 | 41.53 | 1.03 | 27.98 | 27.20 | 1.03 |
9 | 43.67 | 42.90 | 1.02 | 29.42 | 29.00 | 1.01 |
10 | 44.57 | 45.22 | 0.99 | 33.79 | 31.97 | 1.06 |
Discrimination (%) | ||||||
R 2 | 24.00 | |||||
Optimism | 0.80 | |||||
Corrected R2 | 23.10 |
We also calculated the predicted the absolute change in OHS by subtracting the predicted 12-month score from the observed preoperative score. It was observed that the calibration of this absolute predicted change was very good. Importantly, the model also showed good discriminatory ability, with a bias-corrected R2 of 23.1%.
Development of a risk prediction tool
We have developed a web-based tool that can be used to inform patients of the likely outcomes following their surgery. This web-based tool (Figure 28) uses the predictive equation:
FIGURE 28.
Example of a web-based calculator displaying the predictive tool based on the patient’s individual preoperative characteristics.
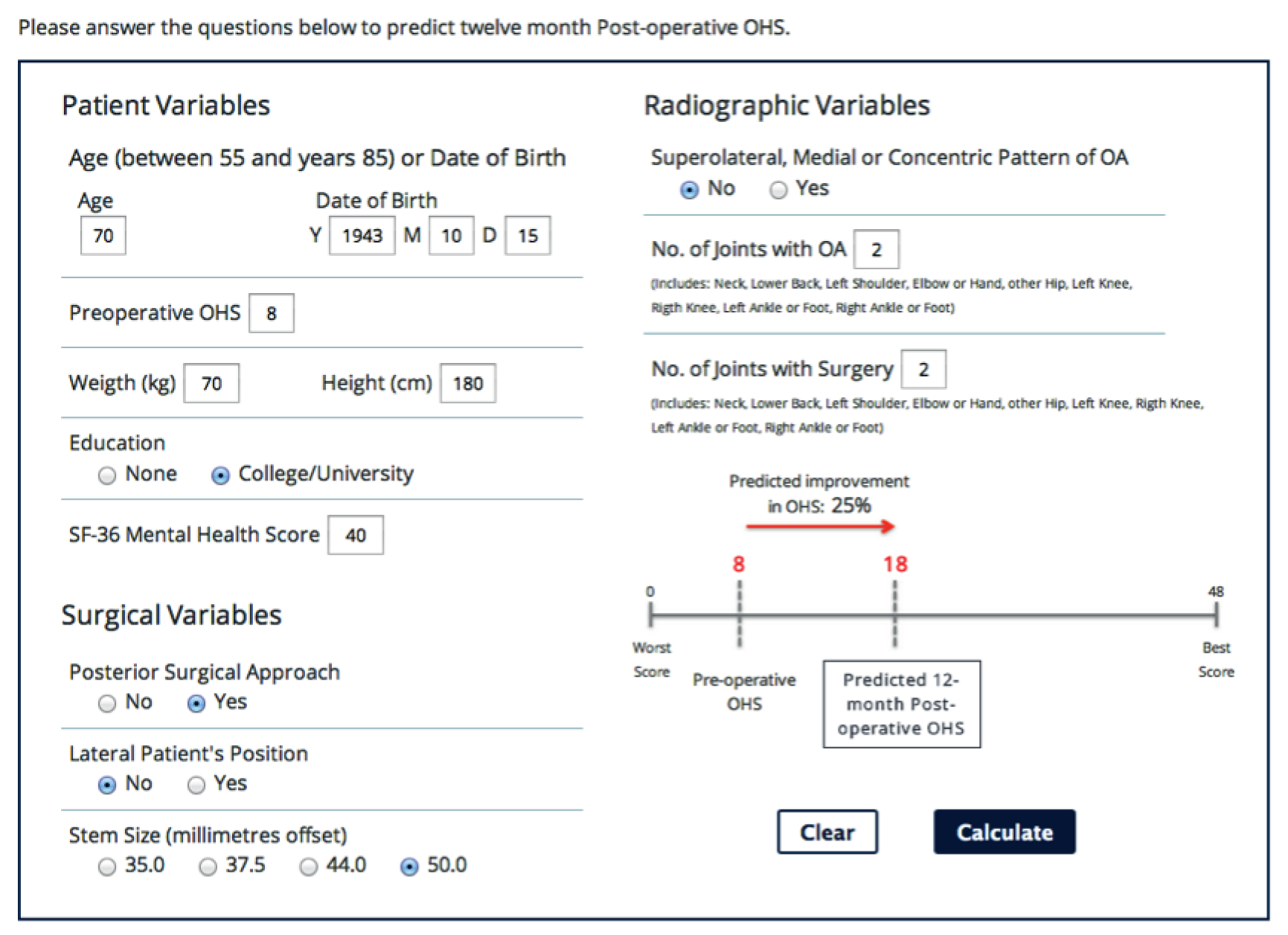
We tested the ability of the tool to identify the patients with the worst possible outcomes of surgery. The worst outcomes are defined as those THR patients whose preoperative score does not improve for at least 5 units over the 12 months. For 89 patients who met the criteria of the worst possible outcome, the discriminatory ability of the tool was good, with an area under the ROC curve of 0.78 (95% CI 0.73 to 0.82) (Figure 29). The tool had a sensitivity of 72% and a specificity of 73%. The calibration was also reasonable, as indicated by the Hosmer–Lemeshow p-value of 0.13.
FIGURE 29.
The ROC curve showing discriminatory ability of the model to identify those patients with the worst outcome (change in OHS of 5 units or fewer).
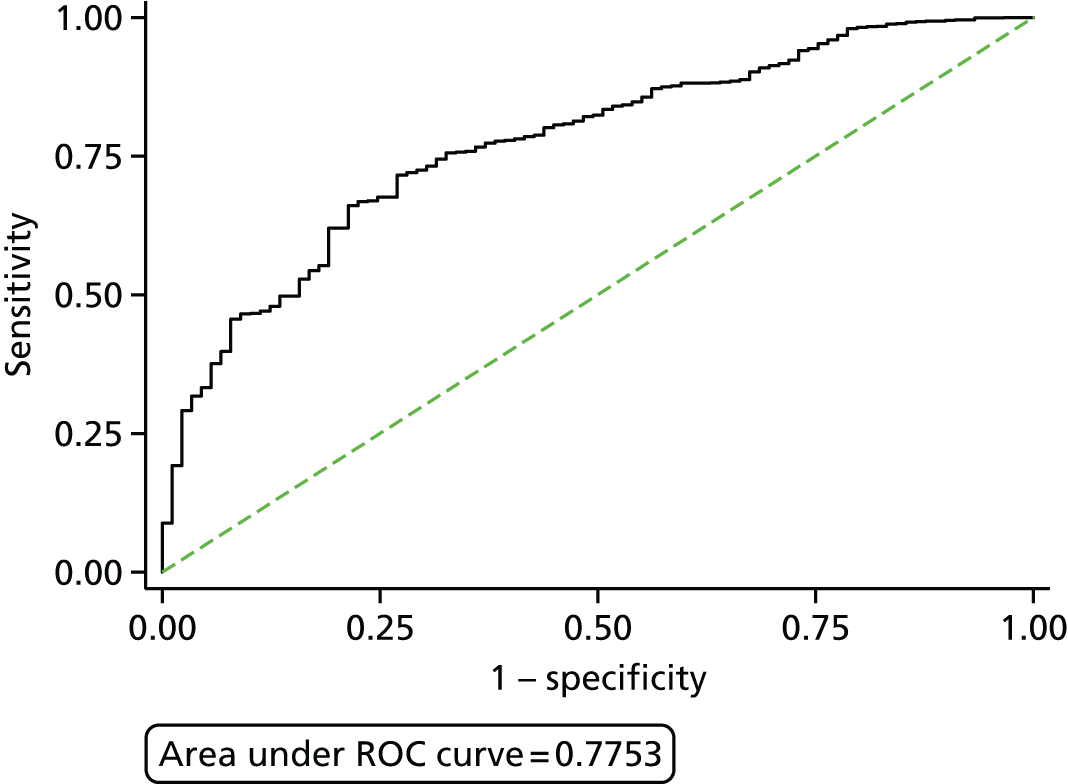
Main findings
Our study found that predictors associated with worse outcomes were old age, worse preoperative mental health score, higher BMI and the lower education attainment. Better preoperative pain and function were associated with better postoperative pain and function, and vice versa, patients with worse preoperative pain and function were observed to obtain the greatest symptomatic improvement (greatest change) between baseline and follow-ups described in previous literature. 52,186 As for the surgical factors, much less is described previously in the literature of their association with patient-reported outcomes of THR. In this work we confirmed that the larger femoral component was associated with a better THR outcome.
A new finding was observed with radiographic variables. Specifically, the pattern of joint space narrowing was found to be a strong predictor: patients with a medial, superomedial or concentric pattern of OA, as identified on a radiograph, had worse outcomes, as opposed to those with a superolateral pattern or with no intra-articular space. This observation applies only to patients with OA, as patients with other types of arthritis (such as RA) were not included in the analysis. The results can be explained by the likelihood that the superolateral pattern of disease developed as a result of subtle local mechanical factors such as abnormalities of acetabulum or the femoral head, that is acetabular dysplasia and cam-type femoroacetabular impingement. 33 It was previously suggested that these types of mechanical risk factors are associated with superolateral disease. 27,48 Superomedial, medial and concentric patterns of OA are more commonly observed in women, with the presence of Heberden’s nodes47 and are generally bilateral. 49 Therefore, the observed relationship between the pattern of OA and the outcome may be because of patients with mechanical disease having better pain and functional outcomes.
No associations were found between the radiographic severity of disease and the surgery outcome using K/L grade. This may be explained by the existing of other confounding factors in the model, that is, other markers of radiographic severity of disease.
We conclude that the tool developed within this work package to predict THR outcomes had calibration and discriminatory ability. The tool has promising potential to inform patients and their clinicians of the outcomes and help them with making the decision regarding whether or not to select patients for THR. The tool predicts the outcomes 12 months postoperatively and provides the absolute change in OHS expressed as a percentage. The performance of the tool to identify patients with the worst outcome following THR was also good. We tested the model for internal validation but future work will be done to provide external validation in the prospective cohort of pragmatic setting of NHS patients.
Knee predictive tool
The work for the development of the prognostic tool to predict the outcome following TKR was done and summarised in the manuscript by Sanchez-Santos et al. ,142 as described in the next section.
A clinical tool for the prediction of patient-reported outcomes after knee replacement surgery: a prospective cohort study142
To combine previously described risk factors into a prognostic tool to predict outcomes 12 months after TKA we used the data from the KAT cohort. 187,188 KAT is a pragmatic, partial factorial, unblinded randomised controlled trial (RCT). In total, 2352 participants were recruited through a random sample to KAT between July 1999 and January 2003, and then stratified by surgeon by sex, age and site of disease. The trial contains information on patients receiving primary TKR surgeries across 34 centres in the UK. One hundred patients who received UKR withdrew from the trial before the procedure or died were excluded from the analysis. From the remaining 2252 patients with primary TKR, 1649 agreed to complete both baseline and postoperative questionnaires. All TKR types were included whether or not a metal-backed tibial component, patellar resurfacing and a mobile bearing were used. Patients had completed questionnaires pre- and postoperatively (at 3 months, 1 year and annually after their surgery). The primary outcome measure for our analysis was 12-month postoperative OKS. Predictor variables collected for this work are summarised in Table 35.
Variable | Additional information |
---|---|
Patients’ characteristic | |
Age | From 32 to 93 years |
Sex | Male/female |
Marital status |
|
BMI | Weight/height2 (kg/m2) |
Preoperative OKS | 0 (worst) to 48 (best) |
Preoperative SF-12 mental component summary score | Maximum score of 75.3 |
IMD 200453 | Score ranged from 2.1 (least deprived) to 79.3 (most deprived) |
Clinical factors | |
Disease type | OA or RA |
Disease side |
|
ASA grade | Grades 1 to 3–4 (grades 3 and 4 were collapsed) |
Previous knee surgery | Yes or no |
Previous contralateral TKR | Yes or no |
Other condition affecting mobility | Yes or no |
Surgical factors | |
Fixed flexion deformity | Yes or no |
Knee deformity |
|
State of ACL |
|
State of posterior cruciate ligament |
|
Grade of operator |
|
Grade of senior surgeon |
|
Statistical methods used
For statistical analysis we used Stata version 12.1. We compared patient characteristics in responders and non-responders with preoperative and 12-month postoperative questionnaires. This helped with determining selection (response) bias. We assessed the relationship between the potential predictors on two outcomes: continuous 12-month postoperative OKS and change between pre- and postoperative OKS.
For continuous 12-month postoperative OKS we used general linear models to identify risk factors. We assessed the linearity of continuous variables with the outcome by the fractional polynomials and the collinearity between variables by variance inflation factor (VIF). Owing to the heteroscedasticity of the variance of residuals, robust standard errors were used to estimate the sandwich variance estimator. We used the chained equations to generate 40 imputed data sets to investigate the effects of missing data. We used all potential predictors (including outcome) and combined estimated parameters by using Rubin’s rule. Afterwards we selected 200 bootstrap samples with replacement from these 40 imputed data sets. We applied automatic backward selection within each bootstrap sample (significance level of 0.157). Age and sex were force entered into all models. All variables appearing for > 70% of the time were then used in the final regression model.
For change in the OKS, for identified predictors we used the repeated measures linear regression in which pre- and postoperative OKSs were included as an outcome. In order to describe the change in the outcome, we included the interaction term between each potential risk factor and time point.
Results
Descriptive statistics
Descriptive statistics and comparison of responders (patients who completed both pre- and postoperative questionnaires) compared with non-responders is summarised in Table 36.
Variables | Responders (N = 1649) | Non-responders (N = 603) | p-value |
---|---|---|---|
Patients’ characteristic | |||
Preoperative OKS (units), mean (SD) | 18.3 (7.5) | 17.0 (7.7) | < 0.001 |
Age (years), mean (SD) | 70.4 (8.1) | 71.1 (8.9) | 0.053 |
Sex, n (%) | |||
Female | 921 (55.9) | 350 (58.0) | 0.353 |
Male | 728 (44.2) | 253 (42.0) | |
Marital status, n (%) | |||
Married | 1082 (66.0) | 376 (65.4) | 0.348 |
Single | 65 (4.0) | 31 (5.4) | |
Widowed/divorced | 492 (30.0) | 168 (29.2) | |
BMI (kg/m2), mean (SD) | 29.7 (5.4) | 29.6 (5.3) | 0.925 |
Preoperative SF-12, mean (SD) | 50.2 (11.5) | 48.6 (11.7) | < 0.01 |
IMD 200453 score, medium (IQR) | 15.6 (9.6–25.5) | 14.5 (8.9–24.9) | 0.273 |
Clinical factors | |||
Disease type, n (%) | |||
OA | 1561 (95.3) | 541 (94.6) | 0.492 |
RA | 77 (4.7) | 31 (5.4) | |
Disease side, n (%) | |||
One knee | 432 (26.4) | 137 (24.0) | 0.521 |
Both knees | 642 (39.2) | 232 (40.6) | |
General | 564 (34.4) | 203 (35.5) | |
ASA grade, n (%) | |||
Fit and healthy | 277 (17.5) | 89 (15.8) | < 0.05 |
Asymptomatic no restriction | 991 (62.8) | 329 (58.5) | |
Symptomatic minimal/severe restriction | 311 (19.7) | 144 (25.6) | |
Previous knee surgery, n (%) | |||
No | 1039 (63.4) | 381 (66.6) | 0.172 |
Yes | 599 (36.6) | 191 (33.4) | |
Previous contralateral TKR, n (%) | |||
No | 1422 (86.8) | 494 (86.4) | 0.785 |
Yes | 216 (13.2) | 78 (13.6) | |
Other condition affecting mobility, n (%) | |||
No | 1408 (86.1) | 474 (83.5) | 0.121 |
Yes | 227 (13.9) | 94 (16.6) | |
Surgical factors | |||
Preoperative fixed flexion deformity, n (%) | |||
No | 690 (42.6) | 257 (45.3) | 0.272 |
Yes | 930 (57.4) | 311 (54.8) | |
Preoperative valgus/varus deformity, n (%) | |||
Varus | 1006 (63.0) | 334 (60.7) | 0.434 |
Valgus | 325 (20.3) | 111 (20.2) | |
No deformity | 267 (16.7) | 105 (19.1) | |
Preoperative ACL, n (%) | |||
Intact | 1054 (65.4) | 362 (64.3) | 0.694 |
Damaged | 379 (23.5) | 131 (23.3) | |
Absent | 179 (11.1) | 70 (12.4) | |
Preoperative posterior cruciate ligament, n (%) | |||
Intact | 1302 (81.1) | 433 (77.3) | < 0.05 |
Recessed/damaged | 144 (9.0) | 72 (12.9) | |
Divided | 160 (10.0) | 55 (9.8) | |
Grade of operator, n (%) | |||
Consultant | 968 (59.1) | 357 (61.9) | 0.376 |
Associate specialist staff | 216 (13.2) | 80 (13.9) | |
Specialist registrar | 432 (26.4) | 131 (22.7) | |
Senior house officer | 22 (1.3) | 9 (1.6) | |
Grade of senior surgeon, n (%) | |||
Consultant | 829 (72.0) | 289 (71.4) | 0.647 |
Associate specialist staff | 185 (16.1) | 72 (17.8) | |
Specialist registrar | 138 (12.0) | 44 (10.9) |
As Table 36 shows, responders have a better (higher) baseline OKS and SF-12 mental health score than non-responders. Responders seem to have a higher preoperative OKS and better preoperative SF-12 mental health score than non-responders. Responders also tend to have a higher ASA grade and had a less damaged preoperative posterior cruciate ligament than non-responders. The percentage of missing data for the majority of predictors was < 10%. A high percentage of missing data (> 30%) was mainly observed for socioeconomic status and grade of senior surgeon.
From the initial 19 variables we had used in the backward regression model, we identified 12 variables as predictors of 12-month postoperative OKS (Table 37).
Predictor variables (reference category) | Overall (n = 1649) | ||
---|---|---|---|
% retained in final model | Coefficient (95% CI) | p-value | |
Age (years) | |||
(< 60) | |||
60–69 | 100 | 0.7 (–1.5 to 3.0) | 0.524 |
70–79 | 100 | 1.1 (–1.2 to 3.3) | 0.354 |
≥ 80 | 100 | –2.8 (–5.6 to 0.1) | 0.059 |
Sex | |||
(Female) | |||
Male | 100 | –4.6 (–7.7 to –1.4) | 0.004 |
Age × sex | 78.0% (61.5% to 98.5%) | 0.001 | |
Log-preoperative total OKS | 100 | 5.6 (4.4 to 6.7) | 0.000 |
IMD 200453 score (10 units) | 96 | –0.5 (–0.9 to –0.1) | 0.009 |
BMI (kg/m2) (10 units) | 97 | –1.5 (–2.4 to –0.6) | 0.001 |
SF-12 mental component summary score (10 units) | 98 | 0.9 (0.4 to 1.3) | 0.000 |
ASA | |||
(Fit and healthy) | |||
Asymptomatic no restriction | 52.5 | –1.0 (–2.2 to 0.2) | 0.097 |
Symptomatic minimal/severe restriction | 96.5 | –2.6 (–4.1 to –1.1) | 0.001 |
Other condition affecting mobility | |||
(No) | |||
Yes | 99.5 | –2.9 (–4.3 to –1.6) | 0.000 |
Previous knee surgery | |||
(No) | |||
Yes | 100 | –1.7 (–2.6 to –0.7) | 0.000 |
Fixed flexion deformity | |||
(No) | |||
Yes | 96.5 | 1.5 (0.6 to 2.4) | 0.002 |
Preoperative valgus/varus deformity | |||
(Varus) | |||
No deformity | 85.5 | –1.6 (–3.0 to –0.3) | 0.014 |
Valgus | 12.0 | –0.1 (–1.3 to 1.1) | 0.865 |
Preoperative ACL | |||
(Intact) | |||
Damaged | 77.0 | 0.8 (–0.2 to 1.8) | 0.108 |
Absent | 82.5 | 1.5 (0.0 to 3.0) | 0.047 |
R 2 | 20.9% | ||
Optimism | 0.67 | ||
Bias-corrected R2 | 20.2% |
The worst functional and pain outcomes were identified in association with the following predictors: worse preoperative OKS, worse SF-12 mental health status, ASA grade 3 or 4, presence of other conditions affecting patient’s mobility and having had previous knee surgery. From surgical factors, the presence of a fixed flexion deformity as well as preoperative varus deformity and absence of ACL as opposed to intact ACL were found to be strongly associated with a better postoperative outcome. We found a significant interaction between age and sex (p < 0.001) and included this in the model. From the age variable we found that patients aged < 60 and > 80 years had a worse pain and function outcome. The effect of age also varied by sex: women aged < 60 years had a better outcome than men, and women aged > 80 years had a worse outcome than men. We found no difference across sex on the outcome in the age group between 60 and 80 years.
Internal validation and model performance
For the internal validation we used 200 bootstrap samples with replacement in the combination of multiple imputations and assessed predictive ability for bias-corrected estimates. We examined the discrimination (R2-statistic) and calibration to assess predictive ability. In the final predictive model the R2 was 20.2%. In total, 14.3% of the variability of outcome was explained by age, sex and preoperative risk factors. With the inclusion of other patient characteristics the variability was 16.6%, and with the inclusion of clinical and surgical variables the variance reached 18.9% and 20.2%, respectively. We found that the model calibration was good, with close agreement between predicted and observed values of 12-month postoperative OKS.
Main findings
Although the association between outcome of TKR and several potential determinants has been previously established,189,190 we aimed to identify surgical variables as novel predictors of pain and functional outcome at 12 months postoperatively following knee replacement surgery. From surgical factors it was found that the presence of fixed flexion deformity and preoperative varus deformity and absence of ACL were associated with better postoperative outcome. Preoperative deformity of fixed flexion leads to significant changes in the biomechanics of the knee, subsequently leading to a debilitating condition. The surgery aims to correct the knee malalignment and fixed flexion deformity, thus improving biomechanics. Hence, this may be the cause of the improvement in pain and function. Severe deformity and absence of the ACL may also result in more severe joint damage and may manifest as severe symptoms of OA. The discriminatory ability of the model was also improved by inclusion of these surgical predictors in the model compared with the model with only patient characteristics. From patient risk factors such as age and sex, we identified that younger women had a better outcome than men, but in the older age group men performed better. The worst outcomes were associated with the lowest baseline OKS, low socioeconomic determinants, high BMI, worse mental health (as indicated by SF-12 score), worse ASA grade, history of previous knee surgery and the presence of other conditions impacting on mobility. From radiographic variables, patients with more severe disease achieve a greater degree of improvement after the knee replacement.
This work offers clinicians and patients a tool to predict outcomes 12 months after TKA. The tool can be used to decide whether or not patients should be selected for surgery. Potentially modifiable risk factors (such as BMI and mental health) can be improved before surgery, whereas factors such as age and sex will simply help to inform the decision of whether or not an operation is sensible. We have validated the tool internally and the next step would be to develop a web-based calculator, similar to the hip predictive tool, which would enable clinicians and patients to predict outcome after their knee surgery. The external validation of the tool is also required. This would be carried out in the prospective cohort of patients in the pragmatic NHS setting.
Chapter 4 Work package 3: economic evaluation
This chapter will evaluate the cost-effectiveness of implementation of the predictive tools designed in work package 2.
Introduction
Hip and knee arthroplasties (THRs and TKRs, respectively) are regarded as one of the most successful interventions in orthopaedics because they have been able to reduce pain, and improve joint function and patient quality of life. 191,192 Moreover, these benefits are achieved at a cost that makes the intervention largely worthwhile. In fact, THRs are considered highly cost-effective,134 not only in the UK but also in many other countries. 193,194 According to an article published in The Lancet in 2007, THR has been given the title of ‘operation of the century’. 195
Despite the clinical success and established value for money of THRs and TKRs, the National Institute for Health and Care Excellence (NICE) continues to explore the clinical effectiveness and cost-effectiveness of these interventions. In particular, additional collection and analysis of long-term outcomes following arthroplasties has been motivated. 196 Given the large and growing number of procedures being performed, the important portion of the health budget that they consume and the increasing number of data available to assess their clinical effectiveness and cost-effectiveness, it is expected that economic evaluations of these procedures will continue to be performed. Future assessments can be expected to explore cost-effectiveness at higher levels of detail, such as the impact of different elements of the surgical process (e.g. fixation type, prosthesis brand and model) or cost-effectiveness stratified by patient subgroups (e.g. according to sex, age, BMI and comorbidities).
In addition, although procedures have become increasingly advanced,195 there is evidence showing that a number of patients undergoing THRs achieve little or no improvement in terms of mobility or are not satisfied with the results. 197–199 In a study based on 1100 randomly selected THR patients from five different regions in the UK, dating back to 2002, 11% of patients were found to be dissatisfied with the procedure at 1 year, whereas only 2.6% had had a revision replacement by then,35 indicating that the need for revision is not an indicator of patient satisfaction.
If potential poor or unsatisfactory outcomes following THR could be predicted, then these patients could be treated in some other way that benefited them the most, without the health-care system having to incur costs that could otherwise serve those same patients or others more efficiently. There has been work linking age, sex, marital status, comorbidity and the physical status ASA score to THR outcome,197 whereas anxiety/depression198 and socioeconomic factors such as education and employment199 have also been found to be associated with patient outcomes and satisfaction.
An outcome prediction tool has been developed under work package 2 of this programme to identify, preoperatively, patients with poor outcome after THR and TKR. It is important to ascertain whether or not the implementation of the tools would be worth it in terms of its additional costs and benefits. It is possible that the tool could effectively identify patients who would not have a satisfactory or very good outcome after surgery, yet its potential higher benefits may be lower than the health benefits displaced elsewhere in the system by directing resources to implement it. In short, it needs to be considered whether or not the outcome prediction tool would be a cost-effective use of resources for the UK health-care system. This work package provides such an economic evaluation of the tool.
To that aim, we developed a lifetime Markov model featuring two unique elements: it starts at the orthopaedic surgeon’s assessment and it distinguishes between two outcome categories after primary and revision procedures. To facilitate populating this and other economic models with health utility estimates, we compared the performance of several econometric models mapping OHS on to the EQ-5D index. All models reported high predictive power. Transition probabilities for the model were obtained from expert elicitation, the NHS PROMs initiative and the EPOS and KAT studies. The NHS PROMs, EPOS and KAT were also used to estimate health utilities. Procedure costs and primary care costs were obtained from NHS and CPRD data, respectively. An important contribution of this research was the estimation of a model predicting surgery outcome category based on resource use.
Data sources about the outcomes of THRs and TKRs for UK patients (such as the PROMs), together with other large patient-level data sets (such as the CPRD) made available by this programme grant, offered our research a unique opportunity to estimate the majority of the model’s input parameters from patient-level data. Most economic evaluations have to rely on data from small RCTs or observational cohorts, and more often than not some input parameters are also obtained from previously published sources. We strived to make this economic evaluation one populated mainly with data obtained from large representative patient-level routinely collected data sets about the current practice of THRs and TKRs in the UK. Therefore, results benefit from the highest levels of confidence, as well as extraordinary validity for UK decision-makers, health-care professionals and patients.
Much is known about the cost-effectiveness of THRs and TKRs, but little about predicting unsatisfactory outcomes. This knowledge gap is substantially reduced by the findings from this research, although ample scope remains for future research. There are many implications of our results, both for current policy and for future research.
Methods for total hip replacement
Economic model
Most economic evaluations of THRs have been performed based on Markov models, very appropriate given the chronic nature of the disease and the ability to represent disease progression within OA using discrete health states. In fact, one specific model structure, shown in Appendix 4, has been previously used in six studies. 200–205
Although a patient-level simulation model would have provided greater power to identify variability between patients, and allowed for analysis at a more detailed level that would require patient-level data on both costs and outcomes, that is often not available. As will be explained in detail in the section on model inputs, we had access to nationally representative routinely collected patient-level data on primary care resource use on the one hand, and secondary care together with outcomes on the other. However, these two data sets were not linked and, therefore, a patient-level simulation could not be populated with the required data.
We therefore developed an extended Markov model, which, as shown in Figure 30, begins at a stage of surgical assessment as opposed to the primary THR, as most other models do. From surgical assessment, patients may be referred to either the waiting list for a primary THR or to any of two non-surgical health states: risk factor modification or long-term medical management. Patients referred for a consultation with an orthopaedic surgeon would be likely candidates for arthroplasty because they would have been checked and perhaps even treated by other health-care professionals before they were seen by the surgeon. Some of those patients consulting with the surgeon would incidentally present conditions that could compromise the outcome of the replacement surgery. When any of these or other relevant risk factors are present, they would need to be dealt with before the patient could be put on the waiting list for THR. These patients would therefore be referred to the appropriate risk factor modification programme, where they would remain until they were found to be fit for surgery at a later reassessment. Meanwhile, they would also have their hip pain treated, commonly with painkillers and/or physiotherapy.
FIGURE 30.
Markov model for the cost-effectiveness of an outcome prediction tool for THR. a, Transitions from each health state to the absorbing state of death.

Some other patients may have been referred for the surgical consultation only for the surgeon to diagnose that their hip problem, for example, was not related to the hip (problems with the spine, for instance, are known to cause pain in the hip region) or was not an orthopaedic problem. These, as well as those patients who are found by the orthopaedic surgeon not to be candidates for a THR for any other reason, and the patients who despite being candidates decide that they are not willing to go through surgery, would be referred back to primary or secondary care for long-term medical management of their condition. After being reassessed, some of these patients may eventually be found fit and willing to receive a THR.
As the second defining feature of this Markov model is the distinction between surgery outcomes, the states that patients may find themselves in after a primary THR are categorised according to a combination of a measure of their postoperative pain and mobility functions together with their satisfaction. After a primary THR in our model, patients may be in a state of good outcome (in which they would be mostly free from pain and satisfied with surgery results) or in a state of poor outcome (in which pain and functional limitations persist on patients generally dissatisfied with the results of the operation). Making such a distinction also allows for different consumption levels of health-care resources by the two outcome categories.
The distinction between good and poor outcomes after surgery is not exclusive to the primary operation. THR patients who require a revision face the same possible outcomes (i.e. some will do better than others): some will be satisfied whereas others will not, and those who feel better because pain was reduced and mobility increased are more likely to be satisfied. The same distinction between outcome categories used after primary THR was therefore applied in the Markov model following the revision operation, such that a (potentially different) threshold in the OHS can be used to differentiate good from poor outcomes anchored in post-revision satisfaction. Figure 30 illustrates the model schema in a slightly simplified form by combining under ‘primary THR’ and ‘revision THR’ the first year after the operation, which the economic model actually treated as two separate health states, one for each outcome category. The model showing all health states and transition probabilities is shown in Appendix 5.
The model was conceived to operate with yearly cycles and for as long as patients remain alive.
Patient subgroups
The economic model was populated with data corresponding to different patient subgroups. Patient cohorts were selected according to sex and age. The impact of these factors on THR revision rates, as well as their proven effect on the likelihood of achieving a clinically significant physical functioning improvement after arthroplasty,143 justifies exploring, separately, cost-effectiveness of the prediction tool by these subgroups. Using a combination of sex and four different starting ages (45, 60, 70 and 80 years) produced a total of eight groups. The starting age of 45 years was selected because, even though THRs and TKRs are sometimes performed on younger patients, it is only after 45 years of age that sufficient numbers of patients are found from which to draw reliable data inputs to populate the model. A cohort entering the economic model would be, for example, 45-year-old women, and then a separate analysis would be performed for 60-year-old women. This would also be the case for the remaining two subgroups of women and the four equivalent male subgroups. Model input parameters were estimated from data about patients aged 45–60 years for the model cohorts with a starting age of 45 years, about patients aged 60–70 years for the model cohorts with a starting age of 60 years, and so on. For the purpose of populating the model, nevertheless, whenever data for an input parameter were not available for a specific subgroup, a common value was applied to several or all subgroups.
We were also interested in performing the analysis controlling for BMI. BMI thresholds have been applied by some PCTs for patients’ assessment and eligibility for joint replacement surgery. 206–209 However, it has been found that BMI does not influence the ability of patients to benefit from THR. 143 This contradiction between the policy being implemented in some parts of England and the evidence already available provides grounds for the inclusion of BMI in this evaluation. BMI was not available, however, in the main sources of data used to populate the model and, therefore, its impact on the cost-effectiveness of the prediction tool for THR was not analysed here.
Model inputs for total hip replacements
Transition probabilities for total hip replacement
Preoperative transitions
The first section of the model covering the states and transitions between the surgical consultation and primary THR rendered this model not only novel but also contingent on information not systematically collected before. This is because no data were found in the published literature that described referral decisions by orthopaedic surgeons. In order to obtain estimates for these probabilities, we conducted a systematic expert elicitation exercise to estimate mean referral rates as well as uncertainty around those values.
We used an individual, direct method of expert judgement elicitation, which was a mathematical approach that revealed experts’ answers as distributions. We presented experts with a set of questions about their referral decisions of hypothetical THR patients. The histogram technique was employed, whereby a frequency chart showing intervals for the range of answers were presented to experts, who were asked to specify their relative subjective probabilities for each interval by placing a finite number of crosses throughout the grid. We conducted a pilot exercise and then decided to run individual guided interviews with the seven selected expert surgeons from hospitals in Southampton, Bournemouth, Oxford and Portsmouth. Responses from these surgeons were analysed, with their responses aggregated. Appendix 6 details the individual responses.
The deterministic model was populated with the mean values obtained from the responses provided in the expert elicitation. For the probabilistic sensitivity analysis (PSA), we assigned the corresponding beta distribution if two conditions were met: (1) the resulting probability density function had to appropriately fit the corresponding pooled probability distribution from experts’ responses; and (2) there was no significant difference between the observed mean value and that generated by the inverse of the cumulative density function evaluated at 0.5 (a difference > 0.05 was considered excessive). Table 38 shows the mean and SD, as well as the distribution and its parameters, if applicable, used to populate preoperative transition probabilities for the deterministic and probabilistic economic model.
Transition probability | Mean | SD | Distribution | Alpha | Beta |
---|---|---|---|---|---|
Surgical assessment to risk factor modification | 0.136 | 0.093 | Beta | 1.714 | 10.916 |
Surgical assessment to long-term medical management | 0.167 | 0.208 | Empirical | ||
Risk factor modification to reassessment | 0.679 | 0.285 | Empirical | ||
Reassessment after risk factor modification to THR | 0.840 | 0.111 | Beta | 8.287 | 1.581 |
Long-term medical management to reassessment | 0.106 | 0.066 | Beta | 2.208 | 18.598 |
Reassessment after long-term medical management to THR | 0.315 | 0.300 | Empirical |
Postoperative transitions
Data for the breakdown of good and poor outcomes after primary THR were obtained from the NHS PROMs initiative. The COASt project obtained, from the Health and Social Care Information Centre, the non-identifiable HES and PROMs records of all patients who had a hip replacement operation and who consented to participate in the PROMs initiative. Of a total of 171,881 PROMs records for the three fiscal years between 2009 and 2012, only 128,084 were linkable with HES. After dropping records for other hip replacement procedures, removing records from patients aged < 45 years and keeping only those with non-missing postoperative OHS so that their outcome category could be determined, the data set of primary THR by age and sex group comprised 68,156 interventions.
The outcomes of operations could all be classified as good or poor based on the set criteria. Thus, an OHS below 38 units at 1 year after the primary surgery was considered a poor outcome. The percentage of patients in each outcome category after primary THR, based on postoperative OHS reported in the HES PROMs data from 2009 to 2012, allowed for an estimate of the probability of poor outcome by patient group. As the split between good and poor outcome can naturally be considered binomial data, we fitted a beta distribution to the probability of poor outcome immediately after a primary THR based on the counts of good and poor outcomes within each patient subgroup, as reported in Table 39. Given the large number of observations, uncertainty around these parameter values was quite low.
Transition probability/patient subgroup (sex and age) | Mean | Distribution | Alpha | Beta |
---|---|---|---|---|
Men | ||||
45–59 years | 0.298 | Beta | 1431 | 3370 |
60–69 years | 0.262 | Beta | 2647 | 7546 |
70–79 years | 0.310 | Beta | 3128 | 6974 |
≥ 80 years | 0.398 | Beta | 1112 | 1682 |
Women | ||||
45–59 years | 0.359 | Beta | 2253 | 4014 |
60–69 years | 0.329 | Beta | 4399 | 8956 |
70–79 years | 0.410 | Beta | 6099 | 8768 |
≥ 80 years | 0.514 | Beta | 3015 | 2852 |
We used data collected preoperatively and annually during 5 years after a primary THR by the EPOS group to estimate the probabilities of moving from each of the outcome categories in the first year to each outcome category in year 2, and of moving between health states representing outcome categories during the second and subsequent years after the primary. The EPOS data available to us on primary THR patients included OHSs and other demographic information from a total of 1589 patients.
Table 40 reports the transition probabilities estimated from the sample for each patient subgroup as well as the distribution parameters. Only one transition from each outcome category is reported as the other will result from calculating one minus the probability of death, minus the probability of revision (reported in the next section) and minus the probability reported in Table 40.
Transition probability/patient subgroup (age, sex) | Mean | Distribution | Alpha | Beta |
---|---|---|---|---|
Good outcome year 1 to good outcome year 2 | ||||
Men | ||||
45–69 years | 0.929 | Beta | 131 | 10 |
≥ 70 years | 0.987 | Beta | 156 | 2 |
Women | ||||
45–69 years | 0.966 | Beta | 196 | 7 |
≥ 70 years | 0.920 | Beta | 230 | 20 |
Poor outcome year 1 to poor outcome year 2 | ||||
Men | ||||
45–69 years | 0.444 | Beta | 24 | 30 |
≥ 70 years | 0.472 | Beta | 17 | 19 |
Women | ||||
45–69 years | 0.578 | Beta | 52 | 38 |
≥ 70 years | 0.505 | Beta | 56 | 55 |
Using data from the EPOS trial, we extrapolated proportions of good and poor outcomes up to 10 years after the primary THR by calculating the mean transition probability for remaining in each outcome category between years 2 and 5 and applying it to the transitions between years 5 and 10. Table 41 shows those mean probabilities and the parameters of the beta distributions calculated based on average counts. Finally, yearly mortality rates from both model states were assumed to be the same all-cause sex- and age-specific death rates used preoperatively. 125
Transition probability/patient subgroup (age, sex) | Mean | Distribution | Alpha | Beta |
---|---|---|---|---|
Good outcome to good outcome | ||||
Men | ||||
45–69 years | 0.958 | Beta | 157.3 | 7.0 |
≥ 70 years | 0.919 | Beta | 144.0 | 12.7 |
Women | ||||
45–69 years | 0.945 | Beta | 223.7 | 13.0 |
≥ 70 years | 0.899 | Beta | 239.7 | 27.0 |
Poor outcome to poor outcome | ||||
Men | ||||
45–69 years | 0.682 | Beta | 18.3 | 8.7 |
≥ 70 years | 0.492 | Beta | 8.3 | 8.7 |
Women | ||||
45–69 years | 0.728 | Beta | 38.0 | 14.0 |
≥ 70 years | 0.666 | Beta | 50.0 | 25.0 |
Revision rates were estimated for our two categories of outcome after THR. These rates were based on the rates proposed by Kalairajah et al. 210 for four groups, using 27, 33 and 41 as cut-off points on postoperative OHS at 6 months after primary surgery based on a sample of over 15,000 THRs from the New Zealand Joint Registry. Based on the overall proportion of patients classified as having poor and good outcomes in the HES PROMs data set (45% and 55%, respectively), we chose to consider the three Kalairajah et al. ’s groups scoring up to 41 units as poor (42%) and those above 41 units as good (58%). We estimated instantaneous revision rates (assuming the rate was constant over the 2 years) and then probabilities of revision at 1 year for the two outcome categories. This is described in detail in Appendix 7. Table 42 shows the distribution parameters of each revision rate for the PSA, which will be applied to all patient subgroups as the data were not reported by sex and age groups.
Transition probability | Mean | Distribution | Alpha | Beta |
---|---|---|---|---|
Good outcome first year to revision THR | 0.0026 | Beta | 22.2 | 8609.3 |
Poor outcome first year to revision THR | 0.0130 | Beta | 93.4 | 7098.2 |
Good outcome year 2 and onwards to revision THR | 0.0077 | Beta | 66.5 | 8565.0 |
Poor outcome year 2 and onwards to revision THR | 0.0477 | Beta | 343.4 | 6848.2 |
The probabilities of good and poor outcome following a THR revision were estimated using HES PROMs data, analogously to the approach taken for the probabilities following primary THRs. As the study identifying cut-off points for outcome categories used data from primary THRs only,154 and it has not been replicated on revision operations, we used the threshold identified for the second year (OHS 33) to classify our HES PROMs patient records into good or poor outcomes. We chose the lower second year cut-off point as opposed to that for the first year after the operation because patients undergoing a revision THR would have had problems with their primary prosthesis and are less likely to perform well than the broader spectrum of patients undergoing a THR for the first time. The resulting transition probabilities are shown in Table 43.
Transition probability/patient subgroup (sex and age) | Mean | Distribution | Alpha | Beta |
---|---|---|---|---|
Men | ||||
45–59 years | 0.455 | Beta | 160 | 192 |
60–69 years | 0.447 | Beta | 314 | 388 |
70–79 years | 0.391 | Beta | 383 | 596 |
≥ 80 years | 0.474 | Beta | 157 | 174 |
Women | ||||
45–59 years | 0.510 | Beta | 255 | 245 |
60–69 years | 0.476 | Beta | 390 | 429 |
70–79 years | 0.450 | Beta | 492 | 601 |
≥ 80 years | 0.500 | Beta | 272 | 272 |
As in the case of primary THRs, our economic model required two sets of transition probabilities as the cohort moves through the Markov model following a revision procedure. First, after their first year in good or poor outcome immediately following the revision, patients who do not die would transit into good or poor outcome at year 2, which are modelled as separate health states; and, second, patients in either outcome category at year 2 or onwards may remain in the health state they are in or move to the other one at each iteration.
Given that no other data were available with yearly follow-ups of revision THR patients, we used the same data from EPOS primary THR records to produce estimates for the transition probabilities between outcome categories after a revision, using 33 as the cut-off point for outcome classification at 1 year after revision THR. Table 44 shows the estimated probabilities of the transition between good or poor outcomes during the first year following the revision to the same outcome category the second year after the procedure. Table 45 shows the mean transition probabilities and distribution parameters entered in the model for the transition of patients between outcome categories after the second year following the revision procedure.
Transition probability/patient subgroup (sex and age) | Mean | Distribution | Alpha | Beta |
---|---|---|---|---|
Good outcome year 1 to good outcome year 2 | ||||
Men | ||||
45–69 years | 0.902 | Beta | 148 | 16 |
≥ 70 years | 0.954 | Beta | 167 | 8 |
Women | ||||
45–69 years | 0.913 | Beta | 219 | 21 |
≥ 70 years | 0.878 | Beta | 259 | 36 |
Poor outcome year 1 to poor outcome year 2 | ||||
Men | ||||
45–69 years | 0.581 | Beta | 18 | 13 |
≥ 70 years | 0.579 | Beta | 11 | 8 |
Women | ||||
45–69 years | 0.717 | Beta | 38 | 15 |
≥ 70 years | 0.606 | Beta | 40 | 26 |
Transition probability/patient subgroup | Mean | Distribution | Alpha | Beta |
---|---|---|---|---|
Good outcome to good outcome | ||||
Men | ||||
45–69 years | 0.958 | Beta | 157.3 | 7.0 |
≥ 70 years | 0.919 | Beta | 144.0 | 12.7 |
Women | ||||
45–69 years | 0.945 | Beta | 223.7 | 13.0 |
≥ 70 years | 0.899 | Beta | 239.7 | 27.0 |
Poor outcome to poor outcome | ||||
Men | ||||
45–69 years | 0.682 | Beta | 18.3 | 8.7 |
≥ 70 years | 0.492 | Beta | 8.3 | 8.7 |
Women | ||||
45–69 years | 0.728 | Beta | 38.0 | 14.0 |
≥ 70 years | 0.666 | Beta | 50.0 | 25.0 |
Costs for total hip replacements
Resource use was obtained primarily from the CPRD, a database containing primary care data on approximately 4.8 million patients from about 600 GP practices in the UK. The extract of the CPRD data set employed for our analysis identified controls by matching sex, GP practice and age (±5 years) to each case. Clinical factors, however, were expected to vary between cases and controls. We relied on the large number of observations to balance out the differences in comorbidities, so that the effect of controls with more comorbidities and, hence, greater resource use than cases would be offset by the effect of those with fewer comorbidities and, therefore, less use of resources. We calculated the mean quantity of each resource used by sets of controls and subtracted this from the level reported by corresponding cases. We interpreted this difference as the amount of resources used by each hip pain patient in excess of what their controls, on average, demanded from the health-care system. The overall mean of these differences was then an estimate of the resource use attributable to the hip problem. This approach also allowed us to obtain an estimate of variability from the observed resource use attributable to hip pain.
As the analysis was performed from the perspective of the NHS, data on prices are those reported in the Department of Health’s Publication of 2010–11 Reference Costs211 for inpatient events, the Personal Social Services Research Unit’s Unit Costs of Health and Social Care 2011212 for primary care, and the British National Formulary (BNF)213 for drug prices. The NHS reference costs correspond to the period 2011–12,214 Personal Social Services Research Unit’s unit costs are based on the period 2010–11 and the online version of the BNF used was last updated in November 2011. All unit costs are therefore in 2011 GBP.
Preoperative costs
For the costs associated with all health states previous to a THR, records about both consultations and prescriptions were considered. Consultation and medication costs were then added together and, thus, the progression of estimated total costs attributable to hip pain used by patients during the 15 years prior to their THR was produced, showing an expected upwards trend, especially in the 2 years prior to the primary surgery. This growing estimated costs confirmed the increasing burden generated by unresolved hip pain and problems experienced by patients who are referred for a THR. We used the estimates for the year immediately prior to a THR to populate all preoperative states. Deterministic analysis was based on the above mean values for costs attributable to hip problems. For PSA, a normal distribution was used to model the uncertainty around the difference in resource use between the two groups. The process for estimating these preoperative costs is discussed in more detail in Appendix 8. Table 46 shows the estimated cost of all preoperative model health states by patient subgroup.
State/patient subgroup (sex and age) | Mean cost (£) | SD (£) | Distribution |
---|---|---|---|
Men | |||
45–59 years | 98.00 | 200.35 | Normal |
60–69 years | 98.20 | 209.08 | Normal |
70–79 years | 87.60 | 219.69 | Normal |
≥ 80 years | 101.10 | 222.50 | Normal |
Women | |||
45–59 years | 121.70 | 216.32 | Normal |
60–69 years | 118.90 | 237.33 | Normal |
70–79 years | 97.40 | 240.31 | Normal |
≥ 80 years | 92.00 | 257.74 | Normal |
Primary total hip replacement costs
The cost of a primary THR was estimated separately for each patient subgroup based on Healthcare Resource Group (HRG) assignment. In order to do this, we obtained the table of relative frequencies of HRGs assigned by the payment by results system in the NHS to each THR reported in HES and eligible for PROMs for fiscal year 2011–12 (N Gutacker, Centre for Health Economics, University of York, 2013, personal communication). Based on these frequencies and the NHS reference costs by HRGs for the same year,214 we calculated the mean cost for a primary THR by patient subgroup (Table 47).
Patient subgroup (sex and age) | Number of patients | Mean cost (£) | SD (£) |
---|---|---|---|
Men | |||
45–59 years | 4696 | 6069 | 249.40 |
60–69 years | 7632 | 6102 | 233.50 |
70–79 years | 7948 | 6186 | 182.00 |
≥ 80 years | 2578 | 6352 | 143.00 |
Women | |||
45–59 years | 5121 | 6063 | 262.40 |
60–69 years | 10,164 | 6083 | 208.60 |
70–79 years | 12,838 | 6139 | 111.70 |
≥ 80 years | 6043 | 6307 | 104.90 |
Postoperative costs
To estimate postoperative primary health-care costs, we followed the same process used for preoperative costs. However, the resulting costs pool together the costs associated with good and poor outcomes. To produce separate estimates for the latter, we developed a model to predict outcome categories after surgery based on health-care resource use during the first year after the primary surgery. This was done using data from the COASt cohort, described in Chapter 5, and Appendix 9 explains in detail the estimation and use of the model. This model proved to be a unique and a valuable instrument, as the COASt cohort appears to be the only data set with both postoperative outcome and resource use data available. The connection between these was then used to estimate the outcome category of patients reported in the CPRD who have a wealth of resource use data but no outcome measure available. The classification of patients by outcome group using the model led to an estimated mean of £280 for likely poor-outcome patients and £24 for likely good-outcome patients during the first year after the primary. The mean values and distribution of costs for the first year after the operation added to the mean costs of surgery to produce the total costs associated to the model states combining the primary THR and the first postoperative year in good or poor outcome. These are shown in Table 48 with parameter distributions set to normal. As THR costs were estimated regardless of surgery outcome, the slightly higher overall mean costs of poor outcomes are explained by their higher primary care costs.
State/patient subgroup (sex and age) | Mean cost (£) | SD (£) | Distribution |
---|---|---|---|
Primary THR and first year in good outcome | |||
Men | |||
45–59 years | 6070 | 123.50 | Normal |
60–69 years | 6082 | 120.40 | Normal |
70–79 years | 6143 | 134.90 | Normal |
≥ 80 years | 6320 | 149.30 | Normal |
Women | |||
45–59 years | 6049 | 125.60 | Normal |
60–69 years | 6054 | 140.10 | Normal |
70–79 years | 6096 | 139.60 | Normal |
≥ 80 years | 6250 | 153.30 | Normal |
Primary THR and first year in poor outcome | |||
Men | |||
45–59 years | 6352 | 215.20 | Normal |
60–69 years | 6379 | 227.30 | Normal |
70–79 years | 6469 | 255.50 | Normal |
≥ 80 years | 6637 | 222.60 | Normal |
Women | |||
45–59 years | 6376 | 242.50 | Normal |
60–69 years | 6362 | 223.00 | Normal |
70–79 years | 6421 | 228.80 | Normal |
≥ 80 years | 6570 | 284.80 | Normal |
Costs for the second and subsequent years in either outcome category were estimated based on CPRD records of resource use, as above, but with the application of an adjusted surgery outcome prediction model. Once CPRD records were labelled as likely poor and likely good outcomes, records for years 2 through 10 after the primary surgery were pooled together, and mean values and distributions estimated to represent the yearly cost of primary care for patients 2 years and onwards after a THR. These values are reported in Table 49 by patient subgroup.
State/patient subgroup (sex and age) | Mean cost (£) | SD (£) | Distribution |
---|---|---|---|
Second and subsequent year in good outcome | |||
Men | |||
45–59 years | 21 | 183.30 | Normal |
60–69 years | 19 | 191.50 | Normal |
70–79 years | 5 | 213.80 | Normal |
≥ 80 years | 34 | 250.60 | Normal |
Women | |||
45–59 years | 33 | 206.60 | Normal |
60–69 years | 17 | 224.80 | Normal |
70–79 years | 9 | 225.70 | Normal |
≥ 80 years | –1 | 239.10 | Normal |
Second and subsequent year in poor outcome | |||
Men | |||
45–59 years | 316 | 304.50 | Normal |
60–69 years | 237 | 264.30 | Normal |
70–79 years | 241 | 245.00 | Normal |
≥ 80 years | 298 | 363.20 | Normal |
Women | |||
45–59 years | 314 | 295.70 | Normal |
60–69 years | 285 | 285.60 | Normal |
70–79 years | 255 | 296.80 | Normal |
≥ 80 years | 253 | 355.90 | Normal |
Revision total hip replacement and postoperative costs
Parameters for the four revision and post-revision model states were estimated following the same process as that of post-primary health states. Table 50 reports the mean and SDs of the costs associated to the revision THR operation by patient subgroup.
Patient subgroup (sex and age) | Number of patients | Mean (£) | SD (£) |
---|---|---|---|
Men | |||
45–59 years | 499 | 7899 | 885.30 |
60–69 years | 835 | 8096 | 697.50 |
70–79 years | 1247 | 8145 | 479.60 |
≥ 80 years | 488 | 8191 | 941.60 |
Women | |||
45–59 years | 636 | 7733 | 777.50 |
60–69 years | 1080 | 7910 | 664.60 |
70–79 years | 1537 | 7996 | 458.90 |
≥ 80 years | 885 | 8001 | 574.70 |
For the costs of primary care provided to revision THR patients we used CPRD data. In order to distinguish between primary care costs provided to good and poor outcome patients after a revision THR, we employed the predictive model described previously using OHS 33 units as a threshold for outcome categories and resource use data from primary surgery. Given the low number of revision cases further divided after fitting the predictive model to the data, results for the primary care cost component could not be presented for each of the eight patient subgroups and were instead produced in aggregate form for all patients. Table 51 shows the mean cost associated to the model states combining group-specific revision THR and common primary care costs for the first postoperative year in which the differences by outcome categories are explained entirely by primary care costs associated to each group.
State/patient subgroup (sex and age) | Mean (£) | SD (£) | Distribution |
---|---|---|---|
Primary THR and first year in good outcome | |||
Men | |||
45–59 years | 7937 | 38.00 | Normal |
60–69 years | 8134 | 38.00 | Normal |
70–79 years | 8183 | 38.00 | Normal |
≥ 80 years | 8229 | 38.00 | Normal |
Women | |||
45–59 years | 7771 | 38.00 | Normal |
60–69 years | 7948 | 38.00 | Normal |
70–79 years | 8034 | 38.00 | Normal |
≥ 80 years | 8039 | 38.00 | Normal |
Primary THR and first year in poor outcome | |||
Men | |||
45–59 years | 8264 | 337.90 | Normal |
60–69 years | 8460 | 337.90 | Normal |
70–79 years | 8510 | 337.90 | Normal |
≥ 80 years | 8555 | 337.90 | Normal |
Women | |||
45–59 years | 8098 | 337.90 | Normal |
60–69 years | 8275 | 337.90 | Normal |
70–79 years | 8361 | 337.90 | Normal |
≥ 80 years | 8365 | 337.90 | Normal |
For the model states representing each outcome category after a revision THR, Table 52 indicates the mean and SD of primary care costs that, given the low number of CPRD observations, were applied to all patient subgroups as well. These were estimated by pooling together all CPRD records for the years 2 through to 8.
State/patient subgroup | Mean cost (£) | SD (£) | Distribution |
---|---|---|---|
Second and subsequent year in good outcome | |||
All patient subgroups | 43 | 229.60 | Normal |
Second and subsequent year in poor outcome | |||
All patient subgroups | 261 | 242.20 | Normal |
Quality-adjusted life-years for total hip replacements
Mapping Oxford Hip Score to EuroQol-5 Dimensions
Since April 2009, NHS providers performing unilateral hip replacements have been required to collect both EQ-5D scores and the OHS, a condition-specific outcome measure. 144 Before this, however, EQ-5D was not routinely collected from THR patients, whereas the OHS questionnaire was commonly collected and regarded as an important indicator of the success of THR. 145 The OHS consists of 12 patient-completed statements covering pain, mobility and ability to carry out regular tasks. The current scoring system assigns values between 0 and 4 to each item, higher scores corresponding to better outcomes. Individual scores are summed, giving a total score ranging from 0 (worst) to 48 (best). 145 The EQ-5D is a widely used generic measure of health outcomes. It produces a summary index for each of the 243 descriptive health states by applying a preference-based valuation derived from a sample of the general population. 215
The ability to estimate EQ-5D scores based on the OHS would enable estimation of utility data for older data sets for which OHSs were collected but EQ-5D scores were not. Older data sets are of key importance given the need for long-term follow-up of hip replacement patients whose prostheses, in most cases, last for many years without the need for replacement.
In order to estimate the summary EQ-5D index from OHS responses, we employed two known conversion algorithm techniques: transfer-to-utility (TTU) regression and response mapping. The TTU regression approach uses regression equations to predict the values of one outcome measure, using scores from a second measure as regressor(s). 216 We used three different TTU regression methods in this modelling exercise: two are variations of the linear regression model, and the third is a two-part model combining a binary outcome and a linear regression model. The first model regressed total OHSs on the EQ-5D summary index using OLS. The second method employed responses to all 12 questions of the OHS questionnaire as categorical regressors. The third was a two-part logit OLS model, whereby a first logit model would estimate whether or not OHS responses are associated to an EQ-5D score of 1 unit, and if not a second OLS model would predict the value (< 1 units). Meanwhile, response mapping seeks to predict the responses to each of the five individual EQ-5D questions instead of predicting the summary score directly. 217 A multinomial logistic model was applied to do this. Each of these models is described in more detail in Appendix 10.
Data were obtained from the SWLEOC database. The full data comprised 3504 hip replacements, each with preoperative and/or 6-month postoperative responses to the OHS and EQ-5D questionnaires, plus basic demographic, socioeconomic and clinical information. All except two operations were performed between 2006 and 2008. All models were estimated on 1759 operations for which we had data on both pre- and postoperative OHS and EQ-5D scores, sex, age and deprivation. As we were interested in cross-sectional mapping, we pooled pre- and postoperative records together, providing 3518 outcome observations.
The simplest model, continuous OLS with total OHS as the only regressor, was statistically significant with residuals approximately normally distributed. The categorical version of the linear regression model including all OHS separate questions also reported residuals nearly normally distributed. However, it produced some coefficients that were both statistically non-significant and inconsistent with the positive relationship between OHS and EQ-5D; that is, they were either negative or did not follow an increasing progression within the same question. This was fixed by pooling response levels together. For the two-part approach, we estimated the first (logistic) part of the model and found that only 13 of the 48 regressors were statistically significant. For the second part (categorical OLS) we estimated the model on observed EQ-5D scores lower than 1 and included all OHS questions, as none could be excluded based on repetitive likelihood ratio tests. We collapsed response levels using the same methods as with the categorical OLS model. Again, we found that residuals were approximately normally distributed but with a high peak at zero from perfectly fitted cases of observed EQ-5D equal to 1. For the response mapping approach we found that all five multinomial models (one for each EQ-5D question) were statistically significant (p < 0.001); however, many of the individual regressors were not. These results are also detailed further in Appendix 10.
All selected variations of each model were internally validated and predictive power was highest for both OLS models. In addition to assessing the models’ ability to predict the observed mean EQ-5D score, we also calibrated them by recording prediction errors through the range of values of the dependent variable. All models reported a high predictive power of the aggregate mean, although the level of precision was not uniform across the full range of scores. Overall performance of the four models was within the range of other reported mapping studies based on their root-mean-square errors of around 0.20. 218
Preoperative quality-adjusted life-years
The HES PROMs data used to estimate the probabilities of good and poor outcome after a primary THR were the most appropriate to estimate the HRQoL of patients before they undergo the operation given its large number of observations and national representativeness. In order to fit a distribution to these health utilities, which range from –0.5 to 1 quality-adjusted life-years (QALYs), disutilities (the distance in QALYs between the recorded utility and its maximum value of 1) were calculated so that the range becomes positive and bound by zero. As disutilities, a gamma distribution was fitted using the mean and SDs to produce distribution parameters. Table 53 shows mean values and distribution parameters for disutilities associated to all preoperative model states for each patient subgroup.
State/patient subgroup (age, sex) | Mean | Distribution | Alpha | Beta |
---|---|---|---|---|
Men | ||||
45–59 years | 0.615 | Gamma | 3.82 | 0.161 |
60–69 years | 0.592 | Gamma | 3.68 | 0.161 |
70–79 years | 0.597 | Gamma | 3.74 | 0.160 |
≥ 80 years | 0.656 | Gamma | 4.33 | 0.151 |
Women | ||||
45–59 years | 0.694 | Gamma | 4.61 | 0.151 |
60–69 years | 0.653 | Gamma | 4.16 | 0.157 |
70–79 years | 0.666 | Gamma | 4.32 | 0.154 |
≥ 80 years | 0.724 | Gamma | 5.05 | 0.143 |
Quality-adjusted life-years after primary total hip replacement
Patients undergoing a primary THR accrued health utilities depending on their outcome category. First, for health utilities associated to model states including the operation, we considered the EQ-5D postoperative summary scores by patient subgroups reported in the HES PROMs data set. We produced QALYs from the latter by considering the progression of scores following a primary THR observed in EPOS because the latter reported a measure at 3 months that helps better understand patients’ rate of improvement during the first year after the primary surgery. Figure 31 shows estimated EQ-5D scores before, 3 months and 1 year after the operation mapped from the summary OHSs using the mapping method reported above. As evidenced in this graph, poor outcomes not only improve less in the first 3 months than good outcomes, but they also do not improve any more after that, on average, whereas good outcomes still improve a bit more in the last 9 months of the first year after the THR. This progression was used to estimate QALYs during the first year after the primary surgery using the HES PROMs data.
FIGURE 31.
Mean EQ-5D summary scores mapped from OHSs reported by EPOS patients.
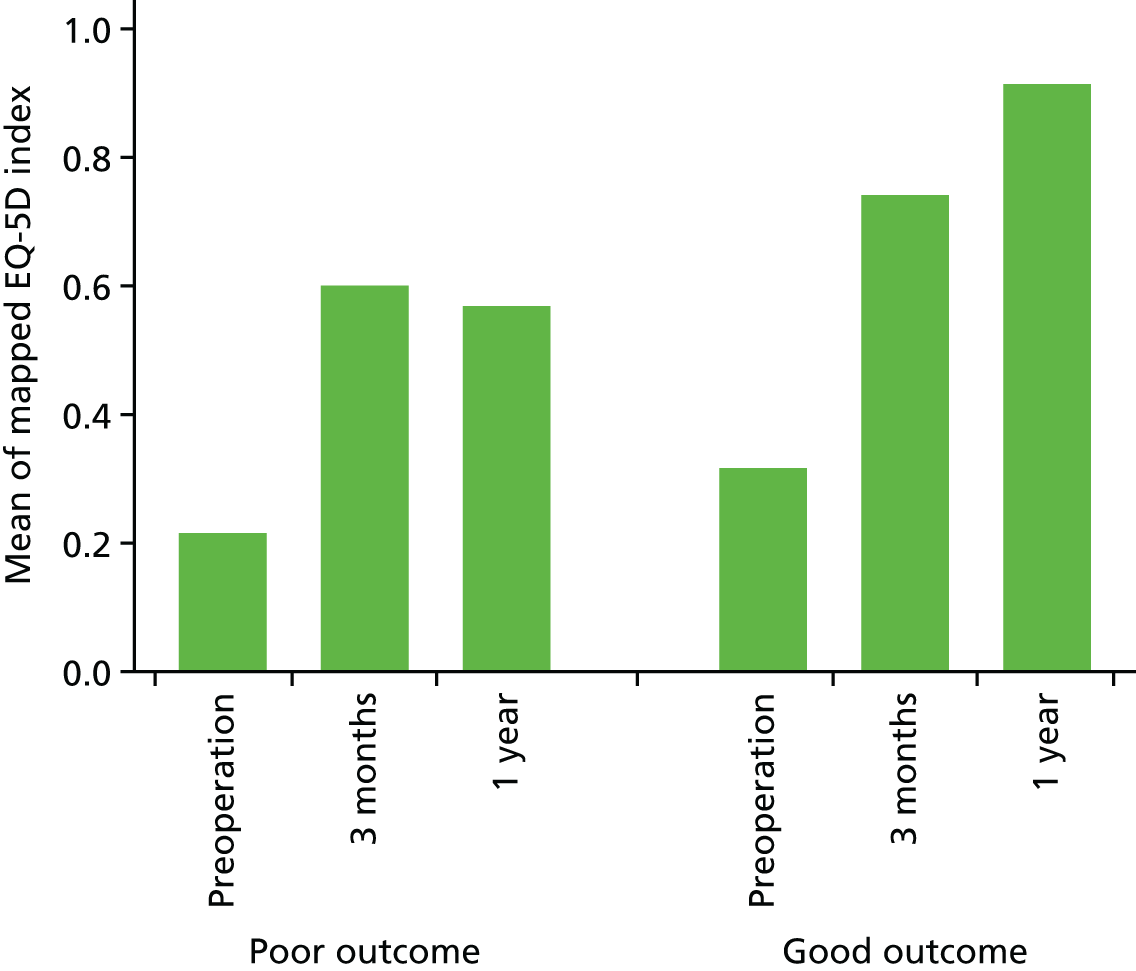
We applied the progression patterns to the data on pre- and postoperative EQ-5D summary scores reported in the HES PROMs data set, to estimate QALYs associated to the first year after primary THR for each patient subgroup by outcome category. We calculated disutilities and estimated the parameters for the corresponding gamma distributions. Table 54 shows the mean value, as well as the parameters for the gamma distributions, describing uncertainty around the mean value of the QALYs associated to the first year after a primary THR for both good and poor outcome patients.
State/patient subgroup (sex and age) | Mean | Distribution | Alpha | Beta |
---|---|---|---|---|
Primary THR and first year in poor outcome | ||||
Men | ||||
45–59 years | 0.540 | Gamma | 4.20 | 0.129 |
60–69 years | 0.485 | Gamma | 4.29 | 0.113 |
70–79 years | 0.450 | Gamma | 4.37 | 0.103 |
≥ 80 years | 0.457 | Gamma | 4.87 | 0.094 |
Women | ||||
45–59 years | 0.536 | Gamma | 4.24 | 0.126 |
60–69 years | 0.478 | Gamma | 4.22 | 0.113 |
70–79 years | 0.454 | Gamma | 4.60 | 0.099 |
≥ 80 years | 0.462 | Gamma | 4.40 | 0.105 |
Primary THR and first year in good outcome | ||||
Men | ||||
45–59 years | 0.217 | Gamma | 2.14 | 0.101 |
60–69 years | 0.212 | Gamma | 2.09 | 0.101 |
70–79 years | 0.220 | Gamma | 2.39 | 0.092 |
≥ 80 years | 0.247 | Gamma | 2.72 | 0.091 |
Women | ||||
45–59 years | 0.248 | Gamma | 2.39 | 0.104 |
60–69 years | 0.239 | Gamma | 2.45 | 0.098 |
70–79 years | 0.245 | Gamma | 2.68 | 0.091 |
≥ 80 years | 0.270 | Gamma | 2.98 | 0.091 |
For the health states representing the second and subsequent years after the primary surgery in either outcome category, we also benefited from the longer follow-up performed under EPOS and the representativeness in the HES PROMs data when estimating the required QALY values. We estimated EQ-5D scores among patients in the second and subsequent years after surgery based on the data in the EPOS and HES PROMs by combining the yearly relationships found in the former with the point estimates, variability and representativeness of the latter. Table 55 shows the mean values and distribution parameters for disutilities during the second and subsequent years after the primary THR for each outcome category and by patient subgroup.
State/patient subgroup (sex and age) | Mean | Distribution | Alpha | Beta |
---|---|---|---|---|
Second and subsequent years after primary THR in poor outcome | ||||
Men | ||||
45–59 years | 0.427 | Gamma | 10.42 | 0.041 |
60–69 years | 0.398 | Gamma | 10.23 | 0.039 |
70–79 years | 0.473 | Gamma | 15.95 | 0.030 |
≥ 80 years | 0.471 | Gamma | 20.02 | 0.024 |
Women | ||||
45–59 years | 0.489 | Gamma | 14.48 | 0.034 |
60–69 years | 0.459 | Gamma | 13.92 | 0.033 |
70–79 years | 0.405 | Gamma | 23.18 | 0.017 |
≥ 80 years | 0.414 | Gamma | 29.39 | 0.014 |
Second and subsequent years after primary THR good outcome | ||||
Men | ||||
45–59 years | 0.134 | Gamma | 1.97 | 0.068 |
60–69 years | 0.128 | Gamma | 2.19 | 0.058 |
70–79 years | 0.139 | Gamma | 2.12 | 0.065 |
≥ 80 years | 0.160 | Gamma | 2.47 | 0.065 |
Women | ||||
45–59 years | 0.139 | Gamma | 1.75 | 0.080 |
60–69 years | 0.135 | Gamma | 1.81 | 0.074 |
70–79 years | 0.185 | Gamma | 2.97 | 0.062 |
≥ 80 years | 0.215 | Gamma | 4.13 | 0.052 |
Quality-adjusted life-years after revision total hip replacement
Health utility estimates were obtained following an analogous procedure as that used for after a primary THR. For the health states, including the revision procedure and the first year in either of the outcome categories, QALYs were estimated based on pre- and postoperative EQ-5D summary scores from patients undergoing a revision THR in HES PROMs combined with the estimated EQ-5D progression by EPOS primary THR patients used in the previous section. We used the progression of scores after a primary procedure because no data set was available containing follow-up HRQoL measures for revision THR patients before and 1 year after the operation, as well as at a third point in between. Table 56 shows the means and SDs of the EQ-5D summary scores of revision THR patients extracted from the HES PROMs data set.
Patient subgroup (sex and age) | Health utility estimates | |||||
---|---|---|---|---|---|---|
Preoperative | Postoperative | |||||
Poor outcome | Good outcome | |||||
Mean | SD | Mean | SD | Mean | SD | |
Men | ||||||
45–59 years | 0.338 | 0.353 | 0.367 | 0.284 | 0.811 | 0.194 |
60–69 years | 0.355 | 0.349 | 0.395 | 0.290 | 0.840 | 0.169 |
70–79 years | 0.406 | 0.329 | 0.459 | 0.276 | 0.824 | 0.173 |
≥ 80 years | 0.358 | 0.319 | 0.496 | 0.241 | 0.790 | 0.193 |
Women | ||||||
45–59 years | 0.341 | 0.347 | 0.404 | 0.309 | 0.798 | 0.197 |
60–69 years | 0.365 | 0.343 | 0.431 | 0.286 | 0.819 | 0.191 |
70–79 years | 0.364 | 0.329 | 0.479 | 0.266 | 0.804 | 0.188 |
≥ 80 years | 0.312 | 0.331 | 0.490 | 0.246 | 0.789 | 0.185 |
In order to estimate the QALYs associated to this first year after the revision THR, we connected the start and end points reported in Table 56 using the differential progression by outcome category found for primary patients. We estimated QALYs based on this progression, converted them into disutilities and produced the mean values and gamma distribution parameters shown in Table 57.
State/patient subgroup (sex and age) | Mean | Distribution | Alpha | Beta |
---|---|---|---|---|
Revision THR and first year in poor outcome | ||||
Men | ||||
45–59 years | 0.655 | Gamma | 6.24 | 0.105 |
60–69 years | 0.627 | Gamma | 5.35 | 0.117 |
70–79 years | 0.565 | Gamma | 4.90 | 0.115 |
≥ 80 years | 0.535 | Gamma | 5.81 | 0.092 |
Women | ||||
45–59 years | 0.616 | Gamma | 4.65 | 0.133 |
60–69 years | 0.592 | Gamma | 5.06 | 0.117 |
70–79 years | 0.549 | Gamma | 4.97 | 0.110 |
≥ 80 years | 0.543 | Gamma | 5.75 | 0.094 |
Revision THR and first year in good outcome | ||||
Men | ||||
45–59 years | 0.280 | Gamma | 2.41 | 0.116 |
60–69 years | 0.259 | Gamma | 2.54 | 0.102 |
70–79 years | 0.262 | Gamma | 2.50 | 0.105 |
≥ 80 years | 0.297 | Gamma | 2.77 | 0.107 |
Women | ||||
45–59 years | 0.294 | Gamma | 2.51 | 0.117 |
60–69 years | 0.272 | Gamma | 2.22 | 0.122 |
70–79 years | 0.288 | Gamma | 2.68 | 0.108 |
≥ 80 years | 0.313 | Gamma | 3.01 | 0.104 |
The lack of follow-up data on sufficient revision THR patients meant that, for the model states representing the second and subsequent years after their revision operation, we used the patterns of progression observed in patients who underwent a primary surgery. Estimated EQ-5D summary scores, assumed to remain constant over the year, were converted into disutilities, and their mean values and gamma distribution parameters estimated. These values are shown in Table 58.
State/patient subgroup (sex and age) | Mean | Distribution | Alpha | Beta |
---|---|---|---|---|
Second and subsequent years after revision THR in poor outcome | ||||
Men | ||||
45–59 years | 0.531 | Gamma | 23.38 | 0.023 |
60–69 years | 0.517 | Gamma | 23.20 | 0.022 |
70–79 years | 0.541 | Gamma | 15.96 | 0.034 |
≥ 80 years | 0.529 | Gamma | 19.80 | 0.027 |
Women | ||||
45–59 years | 0.551 | Gamma | 20.05 | 0.027 |
60–69 years | 0.529 | Gamma | 19.53 | 0.027 |
70–79 years | 0.462 | Gamma | 45.08 | 0.010 |
≥ 80 years | 0.454 | Gamma | 49.52 | 0.009 |
Second and subsequent years after revision THR good outcome | ||||
Men | ||||
45–59 years | 0.217 | Gamma | 3.17 | 0.069 |
60–69 years | 0.209 | Gamma | 3.17 | 0.066 |
70–79 years | 0.190 | Gamma | 2.40 | 0.079 |
≥ 80 years | 0.214 | Gamma | 3.16 | 0.068 |
Women | ||||
45–59 years | 0.209 | Gamma | 2.49 | 0.084 |
60–69 years | 0.196 | Gamma | 2.50 | 0.079 |
70–79 years | 0.238 | Gamma | 4.06 | 0.059 |
≥ 80 years | 0.255 | Gamma | 5.33 | 0.048 |
Parameter values when using the prediction tool
As the economic evaluation compared current practice as described in the values and transition probabilities above with the hypothetical scenario of implementing the outcome prediction tool estimated in Chapter 3, a new set of model parameter values had to be estimated to populate the latter. Most model inputs remained the same, but a few key ones that were directly associated with the implementation of the tool as a guide for patient referral before the operation had to be adjusted. The probability of being referred for a THR would be directly affected by the implementation of the tool, as would the probability of being referred to risk factor modification because patients who are referred to that path are, in principle, considered suitable for a THR. The probability of a patient going into long-term medical management will necessarily be adjusted to compensate for the changes made to referrals for the previous two surgical pathways.
Data from 2092 patients were used to estimate the linear model predicting OHS at 1 year. We used the estimating data set to explore the effects of using the prediction tool as the definitive guide to refer patients to a THR (including risk factor modification) or to the long-term medical management state. Although not applying the tool in fact led to all patients in the data set being referred for surgery, fitting the model to those same patients indicated that, if the threshold had been an OHS of 38 units, only 60% of patients currently receiving a THR would have been referred for the operation (or risk factor modification) and the remaining 40% would have been sent for long-term medical management instead.
Under current practice and according to our expert elicitation, 17% of patients are referred for long-term medical management and 83% are considered suitable for a THR. These figures would change considerably if the outcome prediction tool were to be applied. Table 59 shows the transition probabilities with the tool being used.
Transition probability | Mean | SD | Distribution | Alpha | Beta |
---|---|---|---|---|---|
Surgical assessment to risk factor modification | 0.082 | 0.093 | Empirical | ||
Surgical assessment to long-term medical management | 0.495 | 0.208 | Beta | 2.365 | 2.413 |
The different probabilities of good and poor outcomes are the main reason why the tool is considered in the first place; they will also be affected by its implementation, by definition leading to a smaller proportion of poor outcomes. Table 60 shows the probability of poor outcome if the tool had been in place using a threshold of an OHS of 38 units to refer patients.
Transition probability/patient subgroup (sex and age) | Mean | Distribution | Alpha | Beta |
---|---|---|---|---|
Men | ||||
45–59 years | 0.168 | Beta | 22 | 109 |
60–69 years | 0.165 | Beta | 38 | 192 |
70–79 years | 0.133 | Beta | 23 | 150 |
≥ 80 years | 0.237 | Beta | 9 | 29 |
Women | ||||
45–59 years | 0.167 | Beta | 19 | 95 |
60–69 years | 0.141 | Beta | 32 | 195 |
70–79 years | 0.178 | Beta | 43 | 199 |
≥ 80 years | 0.234 | Beta | 15 | 49 |
Given that those patients having the operation are expected to perform well, and that there exists an association between outcome and preoperative OHS as well as between OHS and EQ-5D, the QALYs associated with all preoperative states but the surgical assessment would also be affected by the shuffling of patients at this stage. Table 61 shows the mean disutilities and distribution parameters separately for the two preoperative branches of the economic model.
State/patient subgroup (sex and age) | Mean | Distribution | Alpha | Beta |
---|---|---|---|---|
Risk factor modification, and reassessment after risk factor modification | ||||
Men | ||||
45–59 years | 0.497 | Gamma | 3.89 | 0.128 |
60–69 years | 0.484 | Gamma | 3.76 | 0.129 |
70–79 years | 0.490 | Gamma | 3.81 | 0.128 |
≥ 80 years | 0.518 | Gamma | 4.01 | 0.129 |
Women | ||||
45–59 years | 0.536 | Gamma | 3.84 | 0.139 |
60–69 years | 0.511 | Gamma | 3.76 | 0.136 |
70–79 years | 0.522 | Gamma | 3.87 | 0.135 |
≥ 80 years | 0.539 | Gamma | 4.07 | 0.132 |
Long-term medical management and reassessment after long-term medical management | ||||
Men | ||||
45–59 years | 0.954 | Gamma | 19.55 | 0.049 |
60–69 years | 0.942 | Gamma | 20.99 | 0.045 |
70–79 years | 0.933 | Gamma | 18.17 | 0.051 |
≥ 80 years | 0.948 | Gamma | 21.10 | 0.045 |
Women | ||||
45–59 years | 0.951 | Gamma | 19.39 | 0.049 |
60–69 years | 0.946 | Gamma | 20.60 | 0.046 |
70–79 years | 0.943 | Gamma | 18.44 | 0.051 |
≥ 80 years | 0.957 | Gamma | 19.51 | 0.049 |
Assumptions
Modelling assumptions
As with all models, the one presented here attempts to reflect the true care pathway of patients as they are assessed for a THR, which most undergo, but it necessarily simplifies what in reality is a more complex process. Our model, as any other, simplifies reality so that we can produce estimates for the cost-effectiveness of the outcome prediction tool. This simplification is achieved by making a number of assumptions that can make the model feasible.
First, this model assumes that the outcome prediction tool is capable of identifying potential poor surgical outcomes before patients have the operation. In particular, we are assuming that the information in the EPOS and EUROHIP data sets is representative of the equivalent characteristics and outcomes in the wider population eligible to undergo THR in the UK. Second, we are assuming that all, or most, patients with an OHS of ≥ 38 units the first year after their primary surgery are free from pain and major mobility limitations as well as satisfied with the operation, and that the opposite is true for those who have a OHS of < 38 units. Third, we assume that all patients found to be candidates for surgery, but presenting a risk factor that should be dealt with before the operation, whether it is excessive weight, diabetes, high blood pressure or something else, can all be grouped together into the risk factor modification state (in which most patients would be expected to stay for only a short period). Similarly, we group a diverse set of patients into the health state of long-term medical management. Fourth, we assume that probabilities of good and poor outcomes are the same in the model whether the patient comes from the risk factor modification section or from that of long-term medical management. Fifth, we do not allow for multiple revisions. And, sixth, we assume that the tool would be used by orthopaedic surgeons.
Parameter assumptions
During the process of identifying parameter values to populate the economic model, a number of assumptions were made, whether because of the simplification forced by the fact that we were modelling a complex reality or because of limitations in the data available.
For transitions, first, although preoperative transition probabilities may vary between patient subgroups, the values extracted from the expert elicitation exercise were assumed to apply to all patients regardless of age or sex. Second, transitions between good and poor outcomes after year 2 post operation were estimated based on follow-up questionnaires only up to year 5. Third, we assume that surgery outcome after the primary has no bearing on surgery outcome after revision (as patients can move between outcome categories after a primary). Fourth, we used the cut-off point derived for primary THRs to categorise outcomes after revisions. Transition probabilities between outcome categories were also assumed to be equivalent after primaries and after revisions when those calculated for the former were applied to the latter. Fifth, and finally, we applied all-cause mortality rates from the general population.
For HRQoL values, first we assumed that the pattern of progression by outcome category during the first year after the operation in EPOS is generalisable to the wider population. We also assumed that the connection between OHSs in the first and second years is representative of the changes all or most patients would experience. Second, we did not consider a utility decrement when assigning health utility estimates to the good and poor outcome states where most patients would remain for long periods of time, until death in many cases. Third, we assumed that the progression of health utility estimated from primary THR patients in EPOS was also applicable to revision THR patients. Fourth, and finally, we did not consider any uncertainty around the time trade-off weights reported in the literature for the EQ-5D. 215
Regarding assumptions about cost parameters, the first significant assumption was that there was no cost to the risk factor modification state. Second, surgery costs were assumed to be the same regardless of outcome category 1 year after the operation. Third, the costs of complications were not explicitly included. And, fourth, in using preliminary results from the COASt cohort for sections of the cost estimation exercise, we assumed that the cohort is representative of clinical practice and, more generally, of patients in the UK.
The implications of these modelling and parameter assumptions, as well as related limitations, are discussed at great length in Appendix 11.
Methods for total knee replacements
The economic evaluation of implementing the outcome prediction tool for TKRs followed the same methodology as that applied to the economic analysis for the THR tool. Variations with regard to data sources and methods specifically applied to the cost-effectiveness analysis for TKRs are detailed below.
Economic model for total knee replacements
The cost-effectiveness analysis was conducted following the same exact Markov model employed for hips as the surgeons involved in consultation about the model structure agreed that it largely applied to both THR and TKR. Figure 30 illustrates the simpler schema of the model for TKRs, whereas the full model structure including the split of ‘primary TKR’ and ‘revision TKR’ each into two health states representing the first year in a good or poor outcome would be exactly equivalent to that shown in Appendix 5 for THRs.
Model inputs
Transition probabilities for total knee replacements
For preoperative transitions, a similar expert elicitation exercise to that conducted for THRs was performed for TKRs. Five expert orthopaedic surgeons, from three different hospitals, participated in the elicitation exercise. An overwhelming agreement on distribution of probabilities of referral to risk modification state was observed, whereas for long-term medical management and TKR expert opinion was very heterogeneous, probably reflecting the different groups surgeons work with or the many factors that influence the decision to place patients on the waiting list for a TKR (Figure 32). For the PSA, exactly as was done with THRs, some probability distributions were used to estimate beta distribution parameters and in some other cases the empirical distribution elicited from experts was used.
FIGURE 32.
Expert elicitation exercise: opinions on distribution of probabilities of referral to risk modification state.

Before probabilities of good and poor outcomes after a primary or revision TKR for each patient subgroup could be estimated from the HES PROMs data, a cut-off point for outcome categories had to be identified. We chose a method to split outcome into categories based on patient satisfaction. This was extensively discussed in the case of THRs, all of which applies to TKRs as well. The study by Judge et al. 155 suggested a cut-off point of an OKS of 30 units at 6 months as optimal as it maximised the sensitivity (77.8%) and specificity (78.2%), identified via the 45-degree line on the ROC curve (AUC 0.85). However, this cut-off point was estimated based on data from patients following a primary TKR, from a sample of 1784 patients and using a threshold of 50 units out of a total possible score of 100 units in a VAS satisfaction question answered by patients 6 months after their operation.
As we needed a cut-off point for outcome categories not only for primary but also for revision TKRs, and as satisfaction after the latter is known to be significantly lower than after the former, we replicated the method followed by Judge et al. 155 on the HES PROMs data. This allowed us to estimate more robust cut-off points derived from a much larger and representative sample, using satisfaction at 1 year following surgery (which is the cycle length in our model) as the anchor, and identifying patients as ‘satisfied’ when their answer to the question ‘How would you describe the results of your operation?’ was ‘excellent’, ‘very good’ or ‘good’, leaving as dissatisfied those who answered ‘fair’ or ‘poor’. Data from 95,349 patients undergoing a primary TKR were used to estimate a cut-off point after primaries, whereas data from 3068 patients who underwent a revision TKR were used to estimate a separate cut-off point following revisions. The cut-off point in the OKS anchored in satisfaction 1 year after surgery was estimated to be 30 units for primaries (sensitivity 80.7%, specificity 82.0%, AUC 0.89) and 24 units for revisions (sensitivity 77.9%, specificity 78.6%, AUC 0.87) (Figure 33).
FIGURE 33.
Receiver operating characteristic curve: primaries.
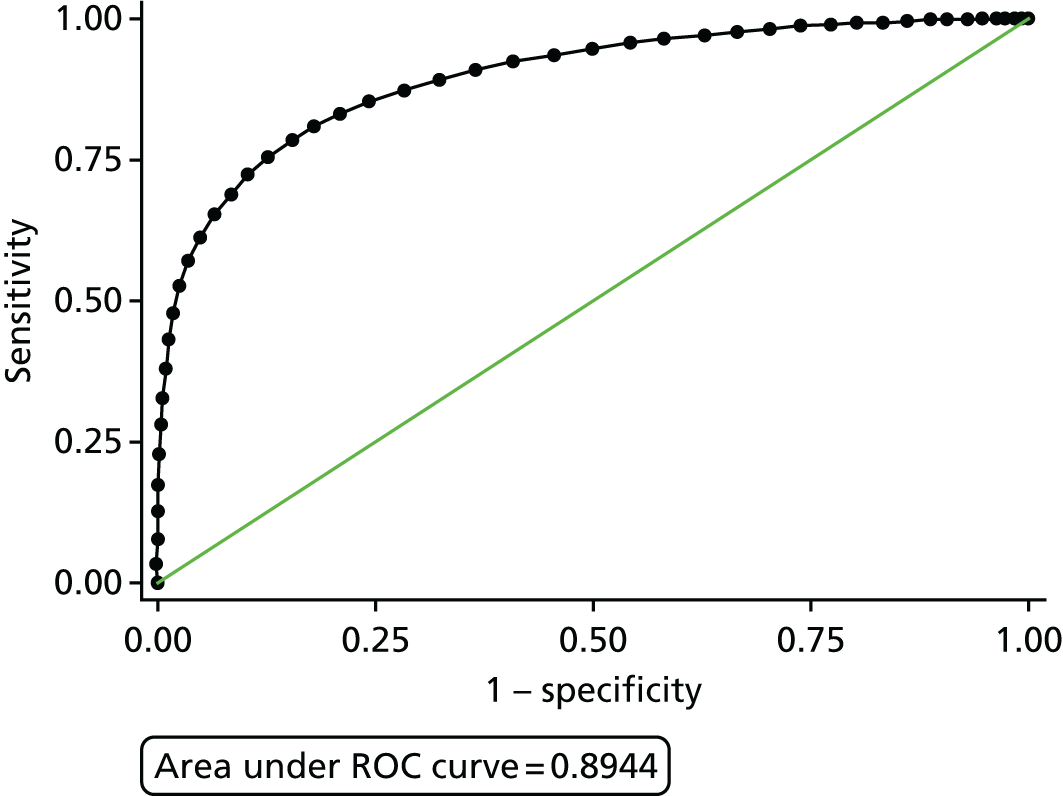
Based on the above cut-off points for outcome categorisation, the probabilities of good and poor outcomes following primary and revision surgeries based on HES PROMs data are shown in Table 62 and illustrated in Figure 34.
Patient subgroup (sex and age) | Surgery | |
---|---|---|
Primary | Revision | |
Men | ||
45–59 years | 0.377 | 0.609 |
60–69 years | 0.268 | 0.441 |
70–79 years | 0.229 | 0.341 |
≥ 80 years | 0.244 | 0.316 |
Women | ||
45–59 years | 0.416 | 0.534 |
60–69 years | 0.312 | 0.400 |
70–79 years | 0.306 | 0.418 |
≥ 80 years | 0.337 | 0.395 |
FIGURE 34.
Percentage of good and poor outcome following primary TKR, by outcome groups. W, women; M, men.

The probabilities of a revision surgery after patients moved to either outcome category health state during the first year after the primary or at ≥ 2 years were estimated based on the weighted average of annual revision rates reported by the NJR219 for cemented, cementless and hybrid for all patients. For revisions at ≥ 2 years, the annual reported revision rates for a first revision at years 2 through to 10 were averaged into one common rate. The overall NJR rates were combined with the proportion of good and poor outcomes after TKR found in the HES PROMs data set and the relative rates of revision between outcome groups reported by Rothwell et al. 220 for THRs because no similar data have been found for TKRs. From Rothwell et al. ‘s220 rates by outcome groups, we considered poor outcomes those reporting a postoperative OHS of < 33 units, as that was the closer cut-off point to the 30 units on the OKS identified in the HES PROMs data set. Estimated revision rates by outcome groups for the first and subsequent years are shown in Tables 63 and 64.
Outcome | Revised | Not revised | Total | Revision rates (%) |
---|---|---|---|---|
Poor | 0.286 | 29.634 | 29.920 | 0.96 |
Good | 0.108 | 69.972 | 70.080 | 0.15 |
Overall revision rates | 0.394 | 99.606 | 100 | 0.39 |
Outcome | Revised | Not revised | Total | Revision rates (%) |
---|---|---|---|---|
Poor | 1.710 | 28.210 | 29.920 | 5.71 |
Good | 0.646 | 69.434 | 70.080 | 0.92 |
Overall revision rates | 2.356 | 97.644 | 100 | 2.36 |
Mortality rates during the first year after the primary were obtained from the NJR219 for each patient group. For the transition probabilities between outcome groups, whether in the first or following years and after primary or revision, we used the same values identified for THRs.
Costs for total knee replacements
For the model’s preoperative states leading to a primary TKR we used primary care costs from the CPRD, for health states involving a surgical procedure we combined secondary care costs derived from HRGs and primary care costs during the first year following the primary or revision operation, and for postoperative costs we derived primary care costs from the CPRD, again from the second year after the operation and beyond.
For the primary care costs derived from the CPRD, as with THRs, we estimated the excess resource use to patients’ joint pain problems and arthroplasty by comparing cases with controls. Cases were patients with a CPRD record of having undergone a TKR, whereas controls were those with no record of OA, knee pain or arthroplasty ever in the system and of the same sex, similar age (±5 years) and attending the same GP practice. We kept sets only with cases and at least one control, so that cases with no controls as well as controls with no cases were excluded from our analysis. When data for more than one control were available, resource use for controls was averaged and subtracted from the resource use by the case to obtain an estimate of the excess resource use associated to OA, knee pain and TKR. We considered data for up to 10 years before and 10 years after the first recorded primary TKR, and included years for which patients were reported to be active in the GP practice for at least 6 months; otherwise records were dropped. Consultations for the same health-care staff roles and as well as prescriptions for the same drugs as those considered for THRs were included in the resource use analysis, with the addition of neuropathic drugs [gabapentin (Neurontin, Pfizer Ltd) and pregabalin] that were considered common prescriptions for TKR patients. The costs associated to consultations were obtained from the Personal Social Services Research Unit report for 2014,221 whereas for prescriptions we applied the weighted average cost of daily treatment to the excess number of treatment days per drug. The weighted average was calculated based on the items dispensed by Pharmacy and Appliance Contractors in England during January 2015,222 current unit prices for the NHS for those dispensed prescriptions223 and defined daily doses established by the World Health Organization224 (or the BNF225 when information for a specific prescription did not appear in the World Health Organization reference).
Primary care costs leading to the first primary TKR increased, on average, for all patient groups pooled together, from nearly £60 excess costs per annum 10 years before the primary to slightly above £250 during the 365 days preceding the operation. As patients are assumed to enter the model during that year before the operation, the values obtained per patient subgroup were applied to all preoperative health states prior to the primary TKR. Table 65 reports the mean and CIs of preoperative excess costs for each patient subgroup.
Patient subgroup (sex and age) | Observations | Mean cost (£) | Standard error (£) | 95% CI (£) |
---|---|---|---|---|
Men | ||||
45–59 years | 952 | 247.61 | 13.13 | 221.85 to 273.36 |
60–69 years | 3164 | 231.84 | 7.40 | 217.33 to 246.34 |
70–79 years | 3672 | 178.07 | 7.28 | 163.80 to 192.35 |
≥ 80 years | 1274 | 182.46 | 12.75 | 157.44 to 207.48 |
Women | ||||
45–59 years | 1222 | 332.65 | 13.77 | 305.63 to 359.68 |
60–69 years | 3576 | 251.85 | 7.75 | 236.65 to 267.05 |
70–79 years | 5192 | 209.27 | 6.24 | 197.03 to 221.51 |
≥ 80 years | 2254 | 192.66 | 9.50 | 174.03 to 211.30 |
The model states involving a primary or revision TKR were combined with a first year either in good or in poor outcome following surgery based on the postoperative OKS of 30 units for primaries and of 24 units for revisions (as explained in the previous section). The secondary care component of the costs associated with these health states were obtained from the HRG associated with TKR. For primaries, we used the mean elective inpatient unit cost for HRG codes relating to ‘major knee procedures for trauma’ and ‘intermediate knee procedures for trauma’, both categories 1 and 2 and with or without comorbidities and complications. For revisions, we averaged the ‘major’ procedures only, in all cases as reported in the NHS reference costs tables. The mean total cost of primaries was set to £5165, whereas that for revisions was estimated to be £6022, and these costs were applied to all patient subgroups as the patient-level estimation carried out for THRs showed no significant differences between costs for different patient subgroups based on age, sex or outcome group following surgery.
For the primary care costs of patients following primary or revision surgery, we used the estimated excess use of consultations and the CPRD-reported prescriptions to predict the outcome group. This was done based on a model estimated from the COASt cohort of 483 patients, in which both OKSs and resource use following primary knee replacement were available. A logit model was estimated for poor outcomes after primary surgery in which patients scoring < 30 units at the 1-year follow-up were considered to have a poor outcome. Sex, age, consultations with GPs, nurses or physiotherapists and prescriptions for all drug groups included in the CPRD analysis were entered in the model. A manual backward stepwise selection method was used to exclude variables that were not statistically significant, arriving at a model for poor outcome after primary with GP consultations and prescription of opioid drugs as significant predictors. This model, with 0.5 as the probability cut-off point, achieved a sensitivity of 12% and a specificity of 98%, with 80% correctly classified and an AUC of 68%, as illustrated in Figure 35.
FIGURE 35.
The ROC curve for the COASt-derived model predicting poor outcome based on resource use.

The same method was applied to predict outcome after revision. The same estimating data set was used but applying 24 units as the cut-off postoperative OKS for outcome groups. The resulting model included not only GP visits and prescription of opioids but also sex as well as prescription of antidepressants and neuropathic drugs. The model for poor outcomes after revision achieved a sensitivity of 13% and a specificity of 99%, with 90% correctly predicted and an AUC of 78%.
The above models were applied to the CPRD data allowing the identification of patients as likely to have good or poor outcomes, and their data extracted as input parameters for the corresponding model states. The distribution of costs for the first year after a primary TKR by outcome group is shown in Figure 36. As with THRs, there is a circular relationship because resource use is the main predictor of outcome category; hence, costs will follow the pattern of high resource use equals poor outcome equals high cost. However, only selected resource use variables are employed and, as Figure 36 shows, there is a clear overlap in the curves, indicating that many patients likely to experience a poor outcome incur lower primary care costs than those likely to experience a good outcome, thus breaking to a certain extent the circularity. Mean primary care costs during the first year after primary TKR for each patient subgroup are reported in Table 66.
FIGURE 36.
Distribution of costs for the first year following primary TKR.

Patient subgroup (sex and age) | Likely poor outcomes (£) | Likely good outcomes (£) |
---|---|---|
Men | ||
45–59 years | 615.23 | 115.64 |
60–69 years | 599.81 | 119.91 |
70–79 years | 602.01 | 74.401 |
≥ 80 years | 647.60 | 80.07 |
Women | ||
45–59 years | 757.77 | 148.75 |
60–69 years | 653.98 | 108.32 |
70–79 years | 603.89 | 79.94 |
≥ 80 years | 555.03 | 75.71 |
The mean values for revisions are reported in Appendix 12 (see Table 122). For both primaries and revisions, the connection between resource use and outcome category was maintained for the years following the first after surgery and, accordingly, the same model applied. This was based on the fact that, as will be shown in Quality-adjusted life-years for total knee replacements, no significant change was observed in health utility between the first year and the next four, regardless of outcome category, after primary TKR. This, together with the broad stability of total resource use observed in the CPRD from the second year onwards, allowed us to obtain estimates of primary care costs for the good and poor outcome states ≥ 2 years following primaries and revisions by averaging the costs incurred in the years between the primary and the first revision for the former, and between the first and second revision for the latter. These values are reported in Appendix 12.
Quality-adjusted life-years for total knee replacements
Health utilities for all health states before primary TKR were derived from preoperative EQ-5D scores reported in the HES PROMs data set. For this and all other health utilities, as was done with THRs, a PSA was performed by modelling the gamma distribution corresponding to the disutilities (the inverse of QALYs), subsequently reconverted into QALYs. Regardless of outcome group after the primary operation, mean health utility increased significantly with the operation, as shown in Figure 37.
FIGURE 37.
Mean health utility accounted by sex and age. M, men; W, women.

For the first year following the primary surgery, we found that poor outcomes achieved the entirety of their 1-year change by the third month after their primary surgery, whereas good outcomes continue improving after that time, achieving the first 85% of the total change in the first 3 months and the remainder in the following 9 months. This progression was observed in 1500 patients from the KAT, with available EQ-5D summary scores preoperatively and at 3 months and 1 year postoperatively. Figure 38 shows these findings from the KAT data set. Based on the latter, the overall mean QALYs experienced by patients experiencing a good or a poor outcome during the first year following the primary TKR were 0.742 and 0.449, respectively, leading to a QALY gain of 0.281 for good outcomes and of 0.170 for poor outcomes.
FIGURE 38.
The mean EQ-5D score observed in KAT patients before and after TKR.

Data from the KAT also show that, for good outcomes, the mean EQ-5D summary score remains at the same level during years 2 through to 5, whereas for poor outcomes there is a reduction of approximately 10% when pooling together those 4 years compared with the first after the primary surgery. Figure 39 shows this progression. Based on this, we maintained the health utility level achieved by good outcomes after the first year for the health state comprising all subsequent unrevised years as a good outcome, whereas for poor outcomes we reduced the level attained at year 1 by 10%, keeping the same SD for uncertainty purposes. Given the lack of follow-up data on patients after a revision, we assumed this same progression for the post-revision states of the model.
FIGURE 39.
Progression of health utility, based on the KAT. First group of five bars correspond to ‘good outcomes’ and the second group of five bars correspond to ‘poor outcomes’.

Parameter values when using the tool
Again, as with the model for THRs, the present model is assessing the cost-effectiveness of implementing the TKR outcome prediction tool compared with current practice. Current practice has been described by the model input parameters detailed above, whereas the hypothetical scenario of the prediction tool being implemented would change some key parameters.
First, the prediction tool is assumed to be the ultimate guide for patient referral to TKR. Patients expected to have a good outcome, that is to have an OKS of ≥ 30 units 1 year after their primary TKR in our base case, would be considered candidates for the operation (TKR or risk factor management in the model), whereas the rest would be placed in long-term medical management. After validating the outcome prediction tool in the COASt cohort, it was shown that the tool would have instead kept 7% of those primary knee replacement patients in a long-term medical management state, allowing the remaining 93% to be candidates for surgery. Table 67 shows how these figures would change if the cut-off value for categorisation of outcomes is an OKS as low as 24 units or as high as 34 units.
OKS cut-off point for prediction tool (units) | Candidate for TKR (%) | No TKR (%) |
---|---|---|
24 | 99.67 | 0.33 |
26 | 99.17 | 0.83 |
28 | 97.84 | 2.16 |
30 | 93.37 | 6.63 |
32 | 87.56 | 12.44 |
34 | 75.79 | 24.21 |
Implementing the outcome prediction tool would therefore change all transition probabilities originating from the consultation with the orthopaedic surgeon. Both TKR and risk factor management were adjusted by the percentage drop reported above, and transition to long-term medical management adjusted accordingly. Table 68 shows mean values for these transition probabilities as well as the distribution parameters for the PSA. Under these circumstances, moreover, the probabilities of good and poor outcome after surgery are also affected, the former upwards and the latter downwards, in accordance with the prediction tool’s sensitivity and specificity. At an OKS of < 30 units, and based on the application of the tool to the COASt cohort, the probabilities of poor outcome decreased to the levels shown in Table 69 for each patient subgroup.
Transition probability | Mean | SD | Distribution | Alpha | Beta |
---|---|---|---|---|---|
Surgical assessment to risk factor modification | 0.116 | Empirical | |||
Surgical assessment to long-term medical management | 0.393 | 0.208 | Beta | 2.766 | 2.579 |
Patient subgroup (sex and age) | Mean | Distribution | Alpha | Beta |
---|---|---|---|---|
Men | ||||
45–59 years | 0.1494 | Beta | 13 | 74 |
60–69 years | 0.1429 | Beta | 29 | 174 |
70–79 years | 0.1891 | Beta | 38 | 163 |
≥ 80 years | 0.1111 | Beta | 8 | 64 |
Women | ||||
45–59 years | 0.1494 | Beta | 13 | 74 |
60–69 years | 0.1429 | Beta | 29 | 174 |
70–79 years | 0.1891 | Beta | 38 | 163 |
≥ 80 years | 0.1111 | Beta | 8 | 64 |
Finally, preoperative QALYs would also be affected inasmuch as the health utilities of those patients kept from a TKR referral would tend to be lower than the mean of all preoperative records. For those considered candidates for a TKR, health utilities observed in the COASt cohort experienced only a non-significant increase compared with the overall mean (equivalent to not using the tool). This can be explained by the fact that only 6.6% of the sample would be removed; hence, we maintained the same values used for current practice and obtained from the larger HES PROMs data set. For those 6.6% of patients who would be held back and referred for long-term medical management, health utilities were significantly lower than what was applied to current practice. Again, this can be explained by the fact that those patients expected to have a postoperative OKS of < 30 units are likely to have a very low baseline OKS, as this was the main predictor of the former. A mean disutility of 0.915 was used for all patient subgroups in this health state, as this was estimated from the COASt cohort and its sample size was only 38 patients in total. However, given this low and potentially unrealistic level of QALYs, this input parameter was chosen for one-way sensitivity analysis to explore its effect on final results.
Assumptions
The assumptions made for this TKR model are exactly the same as those reported for the THR model.
Results
Total hip replacements
Expected (mean) results
Expected costs and QALYs over the lifetime of the cohorts entering the model were calculated for current practice and the hypothetical scenario of implementing the outcome prediction tool with a predicted OHS of 38 units as the threshold to direct patients to THR (> 38 units) or to long-term medical management (< 38 units). The results for each patient subgroup, including the corresponding incremental costs and QALYs (prediction tool minus current practice) and the corresponding ICERs are shown in Table 70.
Patient subgroup (sex and age) | Current practice | Prediction tool | Incremental | ICER (£ per QALY lost) | |||
---|---|---|---|---|---|---|---|
Costs (£) | QALY | Costs (£) | QALY | Costs (£) | QALY | ||
Women | |||||||
45 years | 11,562 | 14.52 | 10,437 | 10.87 | –1125 | –3.66 | 308 |
60 years | 9282 | 11.08 | 7853 | 7.97 | –1429 | –3.11 | 460 |
70 years | 7891 | 7.93 | 6199 | 5.56 | –1692 | –2.37 | 714 |
80 years | 6520 | 4.75 | 4676 | 3.26 | –1844 | –1.49 | 1240 |
Men | |||||||
45 years | 10,086 | 14.61 | 9055 | 10.70 | –1031 | –3.92 | 263 |
60 years | 8196 | 10.81 | 6890 | 7.64 | –1306 | –3.17 | 412 |
70 years | 7062 | 7.64 | 5495 | 5.30 | –1567 | –2.34 | 669 |
80 years | 5954 | 4.48 | 4367 | 3.07 | –1587 | –1.40 | 1130 |
As Table 71 shows, implementation of the outcome prediction tool is associated with lower costs as well as lower QALY gains than current practice for all patient subgroups. As current lifetime costs for the average patient assessed for a surgical intervention are between £6000 and £11,500 higher than lifetime costs incurred by patients without a hip condition, and this for a gain of 4.5–14.5 QALYs, with values mainly depending on age, implementing the outcome prediction tool would reduce such costs by £1000–1500 but also reduce QALY gain by as much as 4 years in full health, or its equivalent.
Mean health utility estimate | Current practice | Prediction tool | Incremental | |||
---|---|---|---|---|---|---|
Costs (£) | QALY | Costs (£) | QALY | Costs (£) | QALY | |
0.057 (base case) | 7891 | 7.93 | 6199 | 5.56 | –1692 | –2.37 |
0.112 | 7891 | 7.93 | 6199 | 5.73 | –1692 | –2.20 |
0.167 | 7891 | 7.93 | 6199 | 5.91 | –1692 | –2.02 |
0.223 | 7891 | 7.93 | 6199 | 6.08 | –1692 | –1.85 |
0.278 | 7891 | 7.93 | 6199 | 6.26 | –1692 | –1.67 |
0.334 (current practice) | 7891 | 7.93 | 6199 | 6.43 | –1692 | –1.50 |
As a result, ICERs were estimated to be around £250–300 per QALY forgone for men or women assessed at 45 years of age, up to £1100–1200 per QALY lost for 80-year-old men or women considered for a THR. As the prediction tool would reduce costs at the expense of QALYs gained, thus placing the cost-effectiveness ratio in the south-west quadrant, only ICERs above £30,000 per QALY forgone might be considered cost-effective under the assumption that the health-care system would be willing to reduce costs at the expense of length and quality of life at the same rate that it is willing to adopt technologies that increase QALYs at a positive cost. Hence, the above deterministic results suggest that the outcome prediction tool would only be cost-effective if the health-care system was willing to exchange reduction in costs for reduction in length and quality of life at a rate lower than the reported ICERs.
Scenario sensitivity analyses
We conducted one-way sensitivity analyses on the discount rate and on the health utility estimate applied to long-term medical management, as this is the parameter value for which there were no highly representative data and, therefore, the one subject to the largest uncertainty. We also performed a sensitivity analysis on the cut-off point assumed by the outcome prediction tool to direct patients to THR or to long-term medical management as the non-surgical alternative. The analyses were performed only on women entering the model at 70 years of age to illustrate effects, because of the eight subgroups considered this is the largest one receiving THRs in the UK.
The average health utility estimate for long-term medical management, when the prediction tool is assumed to be in place, played an important part in the tool producing fewer QALYs than current practice. Although its low value (around 0.05) was justified by the tool’s discriminatory raison d’être based primarily on preoperative OHS, we performed sensitivity analysis on the QALY estimate associated to this state in order to ascertain whether or not it affected results in any significant manner. As Table 71 shows, varying the mean value of health utility assigned to this health state in five equal steps from the low 0.05 to the same value applied to the simulation for current practice (0.334), increases the QALY gain when using the tool, but not enough to reach the levels attained by current practice, ceteris paribus. The difference in QALY gain is driven by the higher proportion of good outcomes in current practice and which, if the prediction tool were implemented, would have been kept from surgery in long-term medical management because of the tool’s imperfect specificity.
As is customary in economic evaluations and suggested in NICE’s Guide to the Methods of Technology Appraisal,226 we performed sensitivity analysis by dropping the discount rate for both costs and benefits from 3.5% to 1.5%. The results obtained when applying this lower rate did not affect results in any significant way. Both incremental costs and QALYs were again in the negative range, with costs savings slightly larger (£1712 instead of £1692) and QALYs lost increasing marginally, from 2.37 to 2.78. In neither of the above two cases would the expected effects of changing original mean values be enough to change the decision of not adopting the tool, unless the health service were willing to forgo QALYs for savings at a rate of only £1600–1700 saved per QALY lost.
Arguably the parameter associated with the greatest uncertainty is the application of the outcome prediction tool itself, as all results modelled here are, although based on the patient-level data used to estimate the statistical tool, hypothetical. We therefore conducted a sensitivity analysis on the cut-off point at which the outcome prediction tool would be used to direct patients into surgery, or not, to explore potential effects on final results. By changing this cut-off point, five probabilities and a number of health utility estimates would all change: the probabilities of being referred for a THR, for risk factor modification, for long-term medical management, the probabilities of good and poor outcome, and the QALY estimate for all preoperative states with the exception of surgical assessment. As the base-case analysis used an OHS of 38 units as the reference cut-off point for the prediction tool, we adjusted the above model input parameters accordingly for scenarios in which the tool would direct patients based on cut-off points of an OHS of 32, 34, 36, 40 or 42 units. Table 72 shows the resulting total costs and QALYs of each alternative, as well as the corresponding incremental differences and ICERs.
Cut-off point (OHS) | Current practice | Prediction tool | Incremental | ICER (£) | |||
---|---|---|---|---|---|---|---|
Costs (£) | QALY | Costs (£) | QALY | Costs (£) | QALY | ||
32 units | 7891 | 7.93 | 7630 | 7.02 | –261 | –0.91 | 288.72 |
34 units | 7891 | 7.93 | 7394 | 6.67 | –497 | –1.26 | 394.74 |
36 units | 7891 | 7.93 | 6896 | 6.18 | –995 | –1.75 | 569.83 |
38 units (base case) | 7891 | 7.93 | 6199 | 5.56 | –1692 | –2.37 | 714.19 |
40 units | 7891 | 7.93 | 5337 | 4.95 | –2554 | –2.97 | 858.80 |
42 units | 7891 | 7.93 | 4648 | 4.58 | –3243 | –3.35 | 967.34 |
As expected, with changes to the parameters of the simulation under application of the prediction tool, total costs and QALYs for current practice considering the cohort of 70-year-old women did not change, but those for the tool did. As the tool becomes more lenient and directs patients with a predicted postoperative OHS of < 38 units for a THR, savings with respect to current practice are reduced because more patients ultimately have their hips replaced. Application of the tool thus calibrated would also mean that the QALYs generated would approach those attained by current practice because more potential good outcomes wrongly held back before in long-term medical management would now be put forward for a THR, hence attaining the higher QALYs that the operation achieves on most patients. The opposite effect was obtained when parameters were adjusted to a prediction tool that applied cut-off points of > 38 units to decide which patients should receive a THR or not: more money would be saved, but more QALYs would be forgone.
Probabilistic sensitivity analysis
We conducted a full PSA by allowing all parameter values to change stochastically and independently based on their distribution. Figure 40 shows the results of running 1000 Monte Carlo simulations and placing the corresponding incremental costs and QALYs on the cost-effectiveness plane for women and men entering the model at 70 years of age. The vast majority of the simulations placed incremental cost-effectiveness results on the south-west quadrant. More specifically, in 87% of cases for women and 88% of cases for men aged 70 years, implementing the tool was expected to cost less but also produce fewer QALYs than current practice.
FIGURE 40.
Results of Monte Carlo simulations on the cost-effectiveness plane. (a) Women aged 70 years; and (b) men aged 70 years.
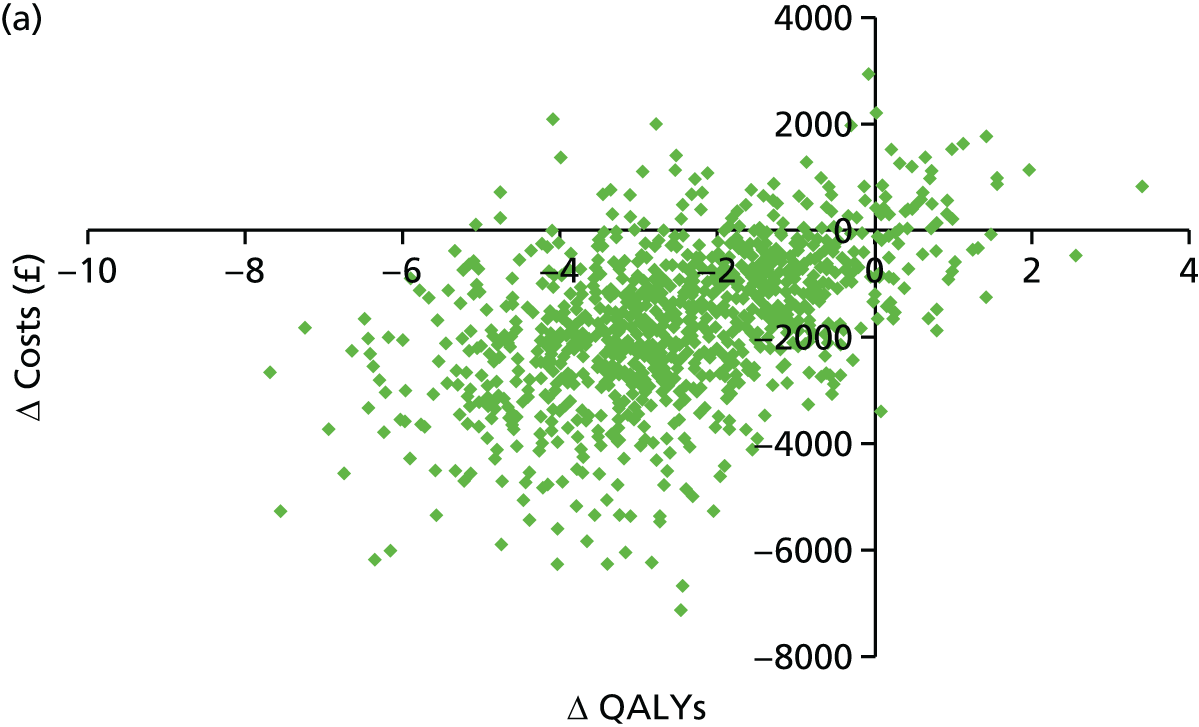
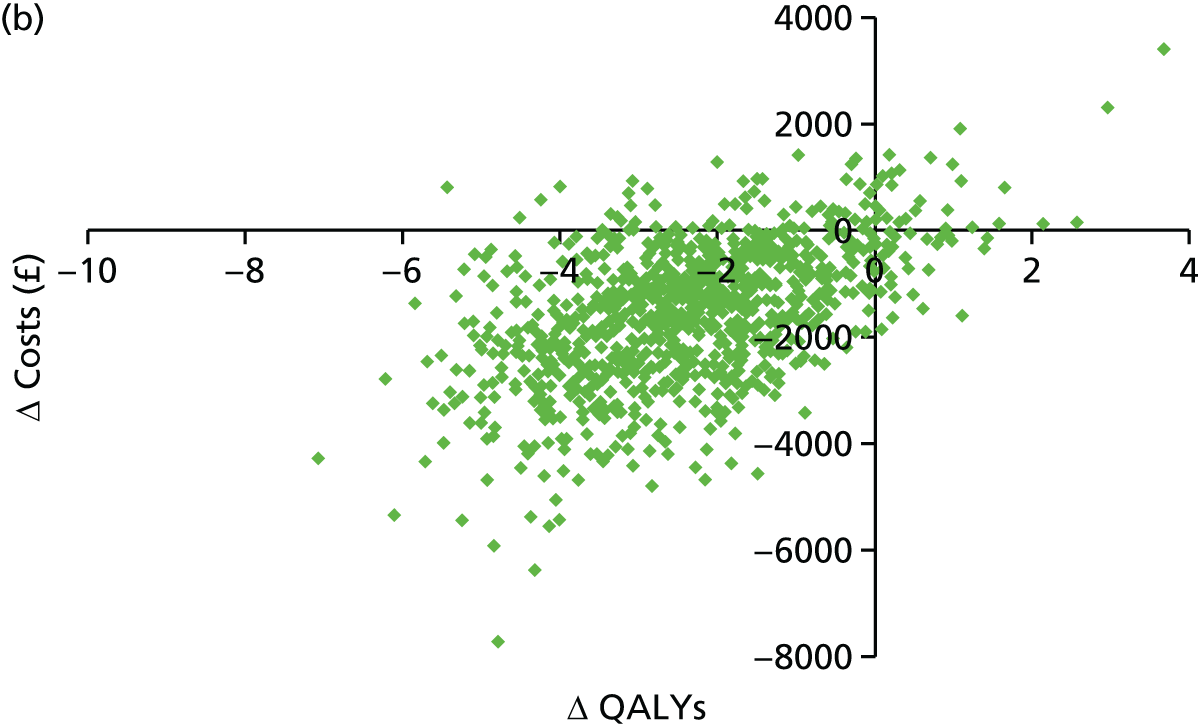
These Monte Carlo simulations produced a cost-effectiveness acceptability curve (CEAC), which is shown in Figure 35 only for women entering the model at 70 years of age (there is no significant difference in results by sex). The curves representing the probability that either current practice or the tool would be deemed cost-effective at the various thresholds represented in the x-axis crossed at a point between £700 and £800 per QALY. This is consistent with the reported expected ICER of £714. This is shown in Figure 41 by the decreasing probability of the outcome prediction tool being cost-effective as the threshold increases, with this probability falling under that for current practice at a point exactly or near the ICER for women entering the model at 70 years of age.
FIGURE 41.
Cost-effectiveness acceptability curve.
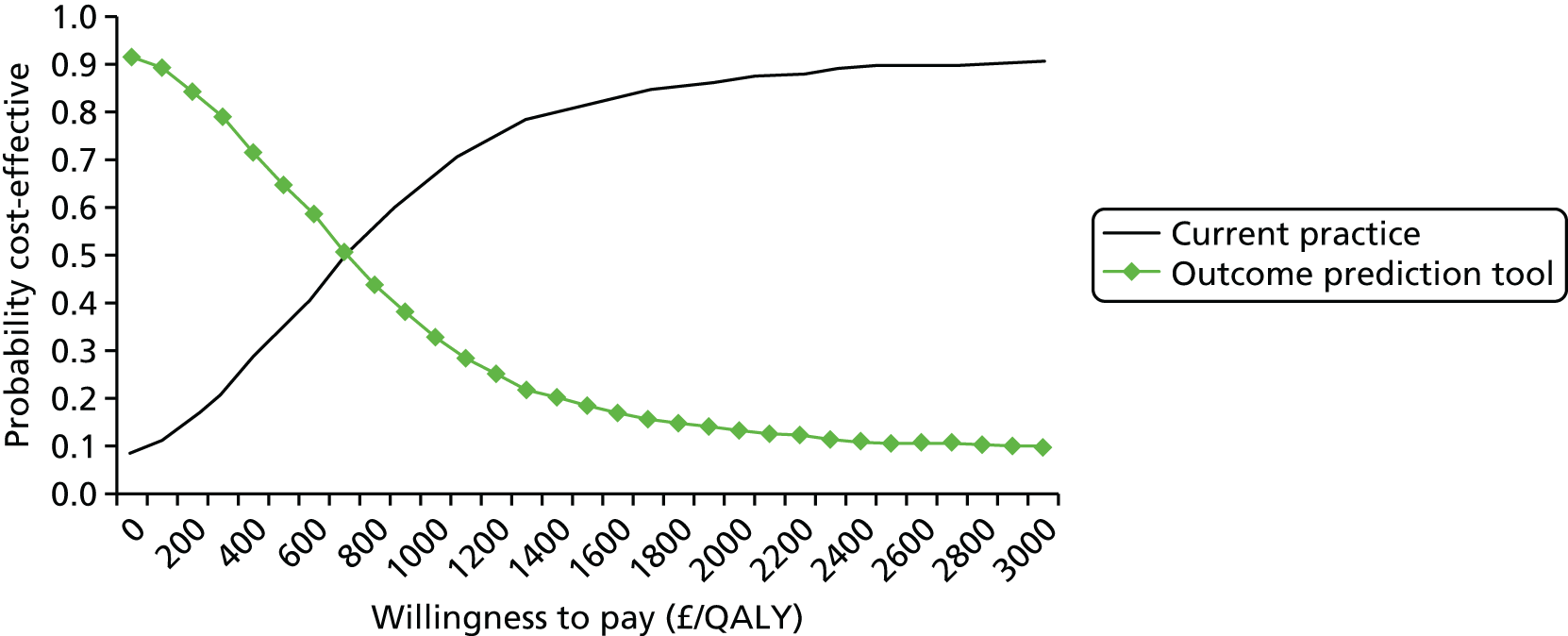
It is important to stress, however, that the range of willingness-to-pay thresholds within which implementing the outcome prediction tool would be cost-effective refers actually to scenarios of cost reduction and fewer QALYs generated with respect to current practice. This is effectively the range of willingness-to-pay thresholds at which both alternatives produce net monetary losses, with the outcome prediction tool generating lower net losses than current practice. It is, ultimately, a range of ‘willingness to save’ resources at the expense of QALYs forgone. This is also shown for all patient subgroups, for which we found no significant differences between sexes and a slight trend depending on age. The range of willingness-to-pay thresholds at which the outcome prediction tool remains cost-effective increases as the age of patients gets higher. Figure 41 shows this for female patient subgroups. In other words, if the willingness to save resources at the expense of QALYs forgone progressively drops from £30,000 per QALY, for example, implementing the outcome prediction tool would become cost-effective for older patients first, and then gradually for younger cohorts.
Total knee replacements
Expected (mean) results
Similar to our findings with the tool for THRs, our cost-effectiveness analysis of the hypothetical scenario of implementing the outcome prediction tool for TKRs would reduce costs to the NHS, but would also decrease the QALYs that the TKRs achieve.
Table 73 shows current patients receiving a TKR cost the health-care system between £4000 and £14,000 over the course of their lives starting the year before they receive their primary TKR. The amount varies slightly with sex, with women being somewhat more expensive, but greatly with age. This is to be expected because we used a lifetime model, meaning that the younger patients are, the more likely they are to receive an expensive revision joint replacement and the longer they live, hence using primary care resources for longer time. For similar reasons, the mean number of QALYs associated with TKRs currently is only marginally different between sexes but significantly so, depending on the age patients entered the model. Although 45-year-old patients accrued, on average, between 12 and 13 QALYs after discounting following their primary TKR, patients aged > 80 years would only accumulate about 4.5 QALYs.
Patient subgroup (sex and age) | Current practice | Prediction tool | Incremental | ICER (£) | |||
---|---|---|---|---|---|---|---|
Costs (£) | QALY | Costs (£) | QALY | Costs (£) | QALY | ||
Men | |||||||
45 years | 11,597 | 12.86 | 11,285 | 11.73 | –312 | –1.13 | 275 |
60 years | 8083 | 9.94 | 7890 | 8.66 | –193 | –1.27 | 151 |
70 years | 5914 | 7.30 | 5782 | 6.16 | –132 | –1.14 | 116 |
80 years | 4270 | 4.49 | 4075 | 3.74 | –195 | –0.75 | 260 |
Women | |||||||
45 years | 14,972 | 12.56 | 14,460 | 11.76 | –512 | –0.80 | 637 |
60 years | 10,147 | 10.03 | 9807 | 9.01 | –340 | –1.02 | 335 |
70 years | 7479 | 7.43 | 7237 | 6.49 | –242 | –0.94 | 258 |
80 years | 5152 | 4.57 | 4842 | 3.97 | –310 | –0.60 | 521 |
Implementing the outcome prediction tool for TKRs developed as part of this project would keep a small percentage of patients from being placed in the waiting list for surgery and keep them under long-term non-surgical management. Our model suggests that this would reduce lifetime costs by £100–500, while at the same time reducing the cumulative QALYs by 0.7 to 1.3. The impact of implementing the tool does not vary significantly between sexes or with patients’ age. These potentially forgone results are consistent with our observations about the improvement in pain and function that those who we have defined as having ‘poor’ outcomes achieve despite not reaching the cut-off point of 30 units in their postoperative OKS. This improvement, paired with their QALY gain, is forgone because of the application of a tool that keeps the latter patients from surgery and, hence, from increasing their quality of life, even if by a lower magnitude than those expected to reach the 30-unit cut-off point.
The above results place the deterministic cost-effectiveness ratio in the south-west quadrant of the cost-effectiveness plane, as it did with THRs. Interpretation of the ICER in these circumstances, estimated at between £100 and £700 per QALY, must be conducted with care, as it reflects not the additional cost per additional QALY gained with the assessed intervention, but rather the costs saved by each additional QALY forgone as a result of implementing the tool. The threshold band of £20,000–30,000 commonly applied by NICE when recommending health-care interventions to be implemented by the NHS normally applies to results in the north-east quadrant; therefore, the ICERs presented here must not be compared with this threshold, as they are instead a measure of the impacts of disinvestment in the quality of life of patients considered for TKR.
Scenario sensitivity analyses
As with our analysis of the THR tool, the single most uncertain model input parameter populating our economic model is likely to be the quality of life associated to patients in long-term medical management and subsequent reassessment health states of the model when the outcome prediction tool is modelled. Although extracted from the validation of the tool on the COASt cohort and based on observed preoperative health utility scores reported by patients, this value was only based on 38 observations and we found it to be significantly low. We therefore conducted a one-way sensitivity analysis on this specific parameter, allowing it to vary in equal steps from the value used in our base case (mean disutility 0.915 or 0.085 of a QALY) to the value applied under current practice (disutility 0.620 or 0.380 of a QALY) for women entering the model at 70 years of age, as was done with the analysis for THRs. Table 74 shows how, with costs unaffected, increasing the health utility accrued by patients in long-term medical management and its reassessment up to the same level used for current practice would reduce the QALYs forgone from approximately 0.9 to 0.3, still keeping deterministic results in the south-west quadrant.
Mean health utility estimate | Current practice | Prediction tool | Incremental | |||
---|---|---|---|---|---|---|
Costs (£) | QALY | Costs (£) | QALY | Costs (£) | QALY | |
0.085 (base case) | 7479 | 7.43 | 7237 | 6.49 | –242 | –0.94 |
0.144 | 7479 | 7.43 | 7237 | 6.63 | –242 | –0.80 |
0.203 | 7479 | 7.43 | 7237 | 6.76 | –242 | –0.67 |
0.262 | 7479 | 7.43 | 7237 | 6.90 | –242 | –0.53 |
0.321 | 7479 | 7.43 | 7237 | 7.04 | –242 | –0.40 |
0.380 (current practice) | 7479 | 7.43 | 7237 | 7.17 | –242 | –0.26 |
As the intervention being assessed in this analysis is the outcome prediction tool, a relevant sensitivity analysis would be to vary the cut-off point at which the tool would ‘decide’ to allow patients to be referred for the waiting list for TKR or keep them under long-term medical management. We performed this sensitivity analysis based on the proportion of patients identified in the COASt cohort as being expected to be candidates for TKR (expected to score above the predictive tool cut-off point) or not (expected to score less than the cut-off level). As with THRs, however, the allocation of patients was the only parameter of the model changed while all remaining transition probabilities, costs and QALYs per health state remained constant. Again, for women entering the model at 70 years of age, Table 75 shows full deterministic results for all patient subgroups at different cut-off levels of the expected postoperative OKS for the application of the tool.
Cut-off point (OKS) | Current practice | Prediction tool | Incremental | ICER (£ per QALY lost) | |||
---|---|---|---|---|---|---|---|
Costs (£) | QALY | Cost (£) | QALY | Costs (£) | QALY | ||
24 units | 7479 | 7.43 | 7328 | 6.69 | –151 | –0.74 | 202 |
26 units | 7479 | 7.43 | 7321 | 6.67 | –158 | –0.76 | 208 |
28 units | 7479 | 7.43 | 7302 | 6.63 | –177 | –0.80 | 221 |
30 units (base case) | 7479 | 7.43 | 7237 | 6.49 | –242 | –0.94 | 258 |
32 units | 7479 | 7.43 | 7153 | 6.32 | –326 | –1.12 | 291 |
34 units | 7479 | 7.43 | 6983 | 5.95 | –496 | –1.48 | 335 |
As Table 75 shows, the higher the cut-off point, that is the more stringent the tool guiding the referral of patients for TKR, the lower the number of patients getting a replacement, leading to lower costs but also fewer QALYs were the tool to be implemented.
Probabilistic sensitivity analysis
We conducted a full PSA by running a Monte Carlo simulation of 1000 iterations, where input values for each parameter feeding the economic model were independently drawn from their distributions based on the observed heterogeneity of our patient-level data or the probability distributions obtained from the expert elicitation exercise.
Figure 42 shows, for women entering the model at 70 years of age, how the small savings and levels of QALY forgone estimated in the deterministic analysis become a cloud of possible results covering all four quadrants of the cost-effectiveness plane, although concentrated mostly in the south-west quadrant (52% of iterations). The spread of the cloud was largely similar for all patient subgroups, in all cases therefore suggesting that, accounting for the uncertainty and heterogeneity of all model input parameters, an outcome prediction tool such as the one assessed in this analysis is capable of saving funds for the health-care system, although it may also prove more expensive in some cases, and that the tool can limit the QALYs produced by TKRs but in some circumstances it can also increase them.
FIGURE 42.
Results of the probabilistic sensitivity analysis in the cost-effectiveness plane for women aged 70 years.
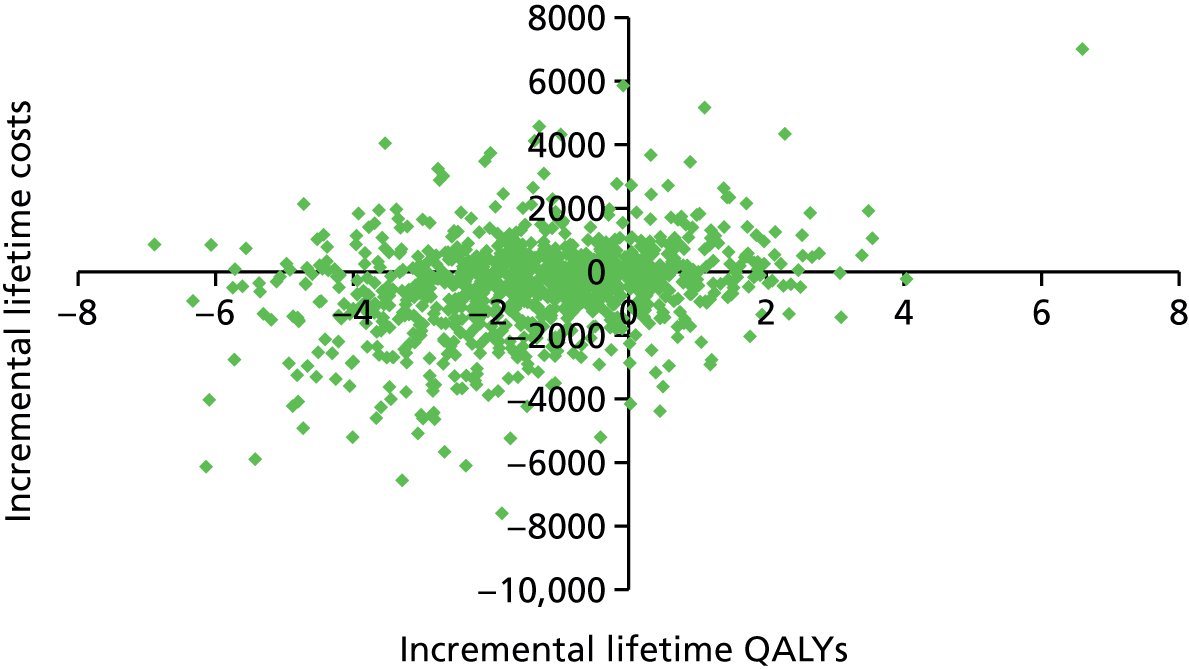
A PSA is often accompanied by a CEAC showing the likelihood of the intervention being cost-effective at various thresholds, as was done with results for THRs. However, given that, again, results are mostly set in the south-west quadrant of the cost-effectiveness plane, where, as we indicated above, the context becomes one of disinvestment and where a cost-effectiveness threshold does not directly apply as it is common employed, we do not show corresponding CEACs to avoid misleading messages.
Discussion
Main findings
No ground for rationing
The outcome prediction tool for THRs and TKRs developed under COASt would, as intended, reduce the number and proportion of unsatisfactory and poor outcomes after the operation, saving NHS resources in the process. However, the tool would do so at the cost of keeping a number of patients from surgery who would have otherwise improved significantly in their OHS and HRQoL, meaning that the tool would also produce fewer QALYs than current practice.
The highest savings per QALY forgone were reported by the oldest patient subgroups (men and women aged ≥ 80 years) with an ICER around £1200 per QALY for THRs. We believe that this is probably an overestimate of the cost-effectiveness of the tool based on having simulated its implementation through an internal validation on patient-level data and no input from a surgeon. As a result, applied in reality, this tool would probably ration joint replacements but to a lesser extent than our simulation did, thereby potentially closing the gap of savings and of QALY losses. Nevertheless, even at the above possibly overestimated levels, these results are unlikely to be deemed cost-effective for the NHS in England even assuming that the £30,000 per QALY threshold currently used for more costly interventions that produce more QALYs were applicable in a disinvestment scenario in which money is saved but QALYs are forgone. Keeping patients from surgery, therefore, appears unlikely to be cost-effective for any tool applied to such a highly successful operation, unless the tool is extremely sensitive and specific, to a level that the one assessed here appears not to reach.
In this context, it seems highly unlikely that simple preoperative Oxford scores could direct patients more efficiently than current practice, or even compared with the prediction tools assessed here. Nonetheless, documents such as the 2010/11 South West London Effective Commissioning Initiative227 suggest that a primary THR should be provided to patients as long as they have a preoperative OHS of < 26 units, or if other criteria involving pain and functional limitation are met. Justification for this specific threshold is not provided, other than a reference about patients with preoperative OHS of < 20 units achieving the greatest benefit from THR,158 although this does not appear clearly stated in the publication and neither does the publication address cost-effectiveness considerations behind the definition of a cut-off point to consider THR. The same criterion was applied by the former Cheshire and Merseyside PCT,228 whereas Derby City and Derbyshire specified a cut-off point of ≥ 30 units to fund a primary THR,229 again with no indication of evidence to justify the specific OHS threshold and furthermore pointing in the opposite direction from the South West London document, that is that THRs should be performed on patients who are not at their worst in pain and mobility. The outcome prediction tools assessed in this evaluation considered not only preoperative Oxford scores but also age, BMI and a number of environmental and surgical variables to predict scores at 1 year after surgery. These prediction models are more comprehensive and appropriate than using merely preoperative Oxford scores to guide the decision about performing a THR/TKR or not, and it did not prove cost-effective. Using only preoperative scores would most likely be associated with even higher net benefit losses than those found for the outcome prediction tool, which suggests that the rationing policy based on Oxford scores should be stopped.
New tool or new definition of outcome categories?
The prediction tools are simply not sensitive and specific enough, or, in other words, THRs and TKRs are just remarkably effective interventions producing notable increases in the disease-specific outcome measure as well as in a generic HRQoL one, even for patients labelled as having poor outcomes based on a combination of satisfaction and Oxford scores.
One way forward is to work on improving the statistical tool. Other potential predictors of outcome (such as the volume of operations performed in the hospital or the experience of the particular surgeon performing the operation) have previously been found to be associated with outcome not only for hip procedures,158,230 but also for arthroplasties of the knee,231 and could be included. More complete data not requiring as much imputation of missing values could also be employed in the estimating sample to produce a more accurate tool.
Improving the predictive power of the tool seems necessary for it to achieve better QALY results by keeping from surgery only the small proportion of patients who would not improve, or would do so only slightly, while sending all others achieving significant QALY gains through to surgery. The sensitivity analysis conducted around the Oxford scores cut-off point at which the tools would direct patients to THR/TKR or to long-term medical management showed that, regardless of the cut-off point, the prediction tools, as developed, would not be able to achieve better QALY results than current practice. It is, therefore, not a matter of calibrating the current prediction tool. A second approach to improve performance of the tool could involve the adjustment of all model input parameters associated to what we termed good and poor outcomes based on the OHS and OKS thresholds of 38 and 30 units, respectively, to reflect the various thresholds identified by Arden et al. 154 for specific patient subgroups based on sex, age, baseline OHS, BMI and expectations.
Given the significant clinical effectiveness and cost-effectiveness of THRs and TKRs as they are performed in the UK, we believe that a new description of the outcome group intended to be prevented is the optimal way forward. Furthermore, we believe that this outcome group should be limited to those patients who do not improve in their Oxford scores or EQ-5D, or who do so only very slightly. Using the postoperative OHS and OKS thresholds of 38 and 30 units, respectively, to distinguish between two outcome categories and employing an outcome prediction tool to prevent patients from falling into the lower scoring group is a waste of potential significant improvements in HRQoL. The basis for this Oxford scores thresholds was that they were found to be the level that best distinguishes between satisfied and unsatisfied total replacement patients. Satisfaction does not, however, seem to be a valid proxy for HRQoL gain. The notable improvement in EQ-5D summary score of those labelled as poor, and hence likely unsatisfied outcomes, confirms this. If a new definition of the ‘poor’ outcome category could be identified such that it grouped patients who do not or only hardly improve after the operation, and a prediction tool capable of accurately identifying them can be developed, then THRs and TKRs could lead to better outcomes and lower costs.
The sooner, the better?
The model presented here incorporated a long-term medical management arm that essentially worked as a surgery delay mechanism, which for a certain proportion of patients meant that they would not get a TJR before they died. This was particularly important because, if the outcome prediction tools were to be implemented, they would identify patients who are likely to perform poorly and those patients would be kept from surgery precisely by placing them in this medical management state. The PROMs showed, however, that waiting until the disease affects patients more severely tends to reduce their improvement.
For both good and poor outcomes the mean EQ-5D summary score increased significantly after surgery, and it also showed that poor outcomes started at a lower EQ-5D score than good outcomes (0.18 vs. 0.35 for the lowest scoring patient subgroups in the case of THRs) and achieved a smaller improvement (0.25 vs. 0.44). Assuming that the disease progresses with time and, therefore, that the longer patients remain without a replacement, the lower their Oxford scores and EQ-5D scores, would be a delay mechanism, such as the one implicitly put into place by the outcome prediction tools, which appears to potentially reduce the ability of patients to improve. Field et al. 158 have already suggested that delaying surgery could make it more difficult for patients to achieve the best possible improvement. At least one economic evaluation comparing THR against watchful waiting was structured assuming the exact opposite, that is that patients were to remain in watchful waiting until their quality of life dropped to very low levels. 202 Based on the above evidence, it would be important to perform similar assessments using as comparator a watchful waiting alternative in which patients in need of a THR or TKR do not wait so long, perhaps until their pain, mobility and quality of life began to decrease in a sustained manner but not beyond that point.
These findings must be handled with care, as they may be viewed as an indication for THR/TKR for all OA patients early in their disease stage when it is also a fact that an important number of patients do perform poorly after surgery. The complex prediction tools assessed in this study included a measure of disease progression by incorporating preoperative Oxford scores as one of the predictors, and yet it lacked the necessary accuracy to identify poor outcomes with sufficient sensitivity and specificity to make them cost-effective interventions. Having a THR/TKR when patients are not at their worst may increase the average improvement obtained, but that does not guarantee that poor outcomes will be reduced.
The improvement reported above and shared, albeit in different magnitudes, by the outcome category groups we have called good and poor outcomes suggests that the term ‘poor’ lacks accuracy. A more appropriate label for these groups would be better and worse outcomes. The timing of arthroplasty, nonetheless, remains a complex and extremely policy-relevant question; but a question also that this study did not attempt to answer, although it would hopefully contribute to future research.
Differentiated rates of revision
Our findings in this study support distinguishing between outcome categories when performing economic evaluations for which the clearly different outcome groups are relevant. This is supported even further by the revision rates reported by Rothwell et al. 220 for the four different outcome groups suggested by Kalairajah et al. ,210 as the 2-year revision rate in patients with a postoperative OHS score < 27 units was reported to be 7.6%, compared with 0.5% for those patients with a postoperative OHS score of > 41 units. Although equivalent revision rates have not been calculated for the UK, it is sensible to expect a similar pattern whereby worse outcomes have their replacements revised at a significantly higher rate than better outcomes. Given the high cost of revision surgeries, this is yet another good reason to continue working on the development of an outcome prediction tool because by accurately preventing worse outcomes after a primary replacement, it would not only be preventing the higher primary costs during the lifetime of the primary prosthesis, but it would also be preventing the much higher costs of a revision THR or TKR.
A large prosthesis market
In the case of THRs, over 100 different brands of acetabular cups and more than 140 brands of femoral components were used in the UK during 2011. 17 Equivalent figures for TKRs are similarly high. Furthermore, these components of a TJRs can be fixed with cement, without cement or with a combination thereof (hybrid), with an additional classification by head size (varying between 22 and 60 mm) and bearing surface (with different combinations of metal, ceramic and polyethylene) in the case of THRs. As a result, to speak of a THR or a TKR in general terms, as we have done for this assessment, means that we did not make any distinction between the significant number of combinations of components and types of each of these interventions, all of which are associated with different prosthesis survival rates. 17 We intended to incorporate specific revision rates by fixation type that are reported to the NJR, but regrettably our request for the data was denied.
Nevertheless, having access to these data is essential not only to refine economic evaluations such as this one, but also to explore the effects that they may have on outcome after surgery. Again, for THRs, for example, in its eighth annual report, the NJR reported that 935 different combinations of acetabular cups and femoral components had been used in the 7-year period during which the registry had been collecting data. Of those, at least 20 had been used on 2500 patients or more, reporting 5-year revision rates as far apart as 0.58% (95% CI 0.42% to 0.79%) for the Exeter V40 (Stryker UK Limited, Newbury, UK) with Elite Plus Ogee (Depuy Synthes UK, Leeds, UK) (13,000 patients) and 3.6% (95% CI 2.72% to 4.76%) for the SL-Plus Cementless Stem (Smith & Nephew, Watford, UK) with Exceed (Biomet UK Limited, Bridgend, UK) (3500 patients). 1 As NICE’s technology appraisal guidance 304 issued in 2014 recommends the use of prostheses for primary THR as long as prostheses have rates (or projected rates) of revision of ≤ 5% at 10 years, research on the comparative performance of prosthesis brands is of paramount importance. 233 The above evidence on differential survival of the prostheses and the significant difference in prosthesis costs232 support further research specific by prosthesis type, something Pennington et al. 234 have recently started to address with a cost-effectiveness analysis of THR by fixation type in 2012.
Long-term follow-up
Finally, as joint replacements are interventions that impact patients for a long time and revision surgeries have been found to be important drivers of cost-effectiveness,205 access to long-term follow-up data on THR and TKR patients is essential. The Swedish Hip Arthroplasty Register has been following up patients since the late 1970s and has also been collecting HRQoL data since 2002. 235 This is a good example for the UK to follow; the main commitment must be maintaining the collection of data over time regarding not only the failure of prosthesis but also patient-reported outcomes, prosthesis types and brands, details about the hospital where the procedure was performed as well as the surgeons involved and, crucially, sociodemographic information about the patients.
Important additions to the information collected would be all likely determinants of outcome such as stage of disease progression, diagnoses, coexistent conditions and previous treatment received. In terms of health-care use, it would be important to achieve high degrees of effective linkage between the clinical follow-up and hospital as well as primary care records before and after the operation. In the case of the UK, this would mean building and maintaining links between an extended version of the PROMs initiative with the records being collected by the NJR, the NHS hospital episode records, the NHS outpatient data and the CPRD. Given that between 20% and 25% of THRs are performed privately, of which about half are privately funded,1 links from the NJR data to the corresponding records in the private sector will contribute to building a most complete database of relevant information about joint replacements in the country.
Efforts made by the UK in the direction of improving the data collected to evaluate THRs and TKRs are noteworthy. The establishment of the NJR in 2002 was a major first step, followed by including hip and knee replacements as two of the four interventions for which PROMs are systematically collected as a measure of treatment outcome and quality of care in the NHS. Although the national initiative only involves one preoperative and one postoperative measure 6 months after surgery, the NJR has begun a project extending the follow-up period for hip and knee replacement patients by sending PROM forms to 35,000 patients in England at 1, 3 and 5 years after surgery. 236 These initiatives, combined with the measures described above, will make an important contribution to building a solid body of data that, available to researchers, will help shape policy on THR and joint replacement surgery for the benefit of patients and the efficiency of the health-care system.
Strengths and limitations
In general, this research benefited from using the best available sources of data to populate a cost-effectiveness model. First, the only source of data not based on patient-level records was the expert elicitation exercise, which is comprehensively reported in the section dedicated to preoperative transition probabilities as inputs for the hip and knee models. When expert opinion has been used in similar previous assessments, the details about how the elicitation was conducted were not reported. 83,237 For our economic evaluation, every step of the process of collecting and synthesising experts’ judgement was described.
Apart from the expert judgements, all other sources of data consisted of patient-level data sets with the most appropriate, representative and up-to-date information on the probabilities, health utility and resource use associated with THRs in the UK, both before and after the operation. The HES PROMs data set, the CPRD, EPOS, KAT and the COASt cohort provide the best data on hip arthroplasty in the UK, and the only model parameter estimated based on data from elsewhere was revision rates by outcome category, which were published on data from New Zealand but ultimately adjusted to match the UK’s overall revision rate and relative sizes of the outcome groups.
The level of detail provided by the above data sources allowed estimating model parameter values for patient subgroups by age and sex. This not only made it possible to present results separately by these subgroups, but critically it also allowed for adjusting all parameter values in the model so that not only death rates but almost all other parameters changed in the simulation as patients became older. If results are only as good as the data feeding the model, then those produced by this research are results in which we can have great confidence.
However, no research is exempt from shortcomings. First of all, the interventions that were assessed with our economic evaluations have not been implemented yet. Although the final work package of the COASt research programme involves a validation of the prediction tool on the cohort of patients recruited from Oxford and Southampton hospitals, our study was performed assuming that the results of the tool would be those of its internal validation in the case of THRs and the actual validation for TKRs. Although an internal validation would generally be associated with better results than external ones, the prediction tool was estimated after merging large data sets and performing a substantial imputation of values that were missing or simply not collected.
As reported in the section detailing the assumptions made surrounding the model’s structure and parameterisation, there are a number of limitations that, although clinically feasible, constrain results. Although the expert elicitation was conducted with a sound methodology and the frequency of convergent results speak of understanding of the process by and agreement among surgeons, a validation of those values at a national level would improve the model’s robustness. Revision rates by outcome groups were adjusted from those reported on patients from New Zealand, while equivalent values can now be produced for the UK thanks to the PROMs initiative. The lack of long-term follow-up of patients who do not receive a THR and even of those who do have their hips replaced for both primary and revision operations forced us to make a number of assumptions that, if replaced by evidence, would improve reliability of results. Further research can focus on these limitations.
Research recommendations
Some of the former PCTs in England were using BMI thresholds for THR referrals, up until PCTs ceased to exist with the introduction of the new structure of the health-care system in England in April of 2013. 238 BMI thresholds of 25 kg/m2,206,207 30 kg/m2207 and 35 kg/m2208,209 were defined as a basis to encourage weight reduction before referral for THR. It is not clear whether or not the newly formed clinical commissioning groups will continue applying these criteria to ration THRs but, as with OHS thresholds, they lack appropriate economic evaluations. We originally intended to include BMI as one of the defining criteria for the patient subgroups in our analysis, but were not able to do so because height and weight were available in only about 40% of CPRD records. BMI was also unavailable in the HES PROMs data set, a limitation that disappears if records are linked to the NJR as they do collect height and weight measures from hospitals performing THRs. Although our economic evaluation focused on the application of an outcome prediction tool and did not include BMI groups in the analysis, it did show that current practice of THRs in the UK is remarkably cost-effective and, therefore, suggests that any rationing such as that possibly still in place based on BMI must be carefully reviewed, as it may be denying a significant improvement in health to patients and an opportunity to invest health-care resources in a very cost-effective manner.
Chapter 5 Work package 4: external validation of the tool
Work package 4 has been designed to test and externally validate the predictive tools, developed in work package 2, in a pragmatic NHS setting of a prospective cohort of lower limb arthroplasty patients (observational study COASt). We have obtained ethics approval to recruit 3200 hip and knee arthroplasty patients to COASt across two NHS hospitals, and collected the data longitudinally. The study assesses a strategy for predicting patients at risk of poor functional outcome following lower limb joint arthroplasty.
Aim
The aims of this work package were to:
-
evaluate the practicality and effectiveness of the prediction tool generated in work package 2 in a large pragmatic cohort study of hip and knee replacement surgeries
-
advise on improved design of future interventional clinical trials, which would aid improvement of the outcomes following lower limb arthroplasties
-
further assess the ability of the tools developed in work package 2 to predict patients’ outcomes at an early postoperative stage.
Background
Our work suggests that the postoperative patient-reported outcomes are dependent on preoperative Oxford scores. 139,141,155,160 Although routinely used in the selection of patients for joint replacement surgeries, there is an increasing trend to utilise PROMs in a more meaningful way for patients and surgeons, which would aid the selection process of patients for joint replacement surgeries. For patients, knowing only by how many points surgery would change their score is not informative. A more meaningful way would be to associate this score with a classification of poor or good outcomes. Within this programme, in work package 2, we have carried out work to introduce cut-off points to identify patient satisfaction, which would serve for a better understanding during clinical decision-making rather than a score on a continuous scale. 154,155 However, clinical applications of the thresholds are limited because of the combination of preoperative predictors affecting the outcome of surgery.
Previously methodology has been developed to combine a wide range of predictors that can be included in a statistical model to predict outcomes. In work package 2 we have included and investigated the effects of the interplay of a wide range of risk factors as described in Chapter 3, using the Oxford scores as a primary outcome. Not only we have confirmed previously described risk factors, but we have also identified the interplay between these individual predictors, which have an important role in poor outcomes following hip or knee replacement surgeries. 138,142 Similarly, as part of this programme, we aimed to develop a prognostic tool to predict outcomes following THR and TKR surgeries. Currently, there are two drafts describing hip and knee predictive models. The knee model has been validated successfully using the COASt data set. Indeed, external validation predicts 21% of the variance of the outcome. The knee manuscript is under internal review before its submission to a peer-reviewed journal. The hip predictive model is currently waiting for external validation with COASt. 138,142 For work package 4 we have obtained ethics approval to recruit and obtain the data from a new prospective observational study from patients receiving total hip and knee replacement surgeries at two participating hospitals: the Nuffield Orthopaedic Centre (NOC) and Southampton General Hospital.
In this work package we set out to validate the predictive tool developed in work package 2, which used preoperative information including technical, patient and medical factors through the ROC curve analysis to predict 12-month postoperative PROMs. We also aimed to collect the data to populate a cost-effectiveness analysis of the implementation of the model in the current health-care system and thus complete work package 3.
Description of the study
Study design
The Clinical Outcomes in Arthroplasty Study is a prospective, dual-centre longitudinal cohort study of patients listed for hip and knee arthroplasties across two hospitals [the University Hospitals Southampton NHS Foundation Trust (UHS) and NOC, which is the part of the Oxford University Hospitals NHS Trust (OUH)]. The study collects baseline, intraoperative and follow-up information for up to 5 years after surgery. It also collects patients’ preoperative, intraoperative and 1- and/or 2-year postoperative samples. The COASt grant application was made to cover the costs for a 2-year follow-up. However, we believed that the longitudinal collection of patients’ data would add great scientific value to the analysis in terms of long-term prosthesis survival. Therefore, we obtained ethics approval to follow-up study participants for 5 years.
Important changes to the design of the study
The initial grant application for the programme was submitted to recruit 1600 patients across both categories, hip and knee arthroplasties, as described in Sample size. However, the work carried out in work package 2 demonstrated that patients undergoing TKR have different outcomes from those undergoing THR, that is, outcome following TKR is poorer than that of THR. 141,143 This resulted in a change to our original plan and, hence, the subsequent increase in the recruitment up to 1600 patients in each category (in total 3200 participants). We believed that this would enable us to have sufficient statistical power to test the productivity of the predictive tools for hip and knee replacement surgeries separately. We have, therefore, been granted an extension from NIHR to enable us to carry out this important work.
Owing to the number of the data collected during the study and the need to integrate them with the existing information technology (IT) systems, we encountered unforeseen difficulties in the database development and data extraction. There was an unexpected change in the core staff, specifically a high-grade database manager. In addition, we experienced problems with incompatibilities and inconsistences within the NHS systems from which we needed to obtain a substantial number of source data. We, thus, have been granted a second extension of 9 months. Since we identified these problems, we had employed a number of staff including a higher-grade database manager, study co-ordinator and several data assistants. We have made substantial changes to the database design and have successfully overcome incompatibility issues.
Participating centres
Two hospitals have been participating in the study: Southampton General Hospital and NOC. There are three subcohorts within the study: North COASt study (Oxford) (NCOASt), South COASt study (Southampton) and Oxford Musculoskeletal Biobank (OMB). Patients recruited at the NOC under COASt ethics (Oxford) constituted the NCOASt part of the study, and the patients recruited at the Southampton General Hospital (SGH) constituted the South COASt part of the study. COASt also utilises the data and samples collected by the OMB, under OMB ethics, which has also recruited patients listed for hip or knee arthroplasties at the NOC.
At the beginning of work package 4, COASt had made a successful application to the OMB to access the data and samples of patients who were listed for hip or knee arthroplasties at the NOC, signed the written consent form and had met the COASt eligibility criteria. The OMB is a research tissue and data bank that has been reviewed and approved by Oxford Research Ethics Committee C (reference number 09/H0606/11, 3 March 2009) and is regulated and licensed by the Human Tissue Authority (HTA) (licence number 12217). As all questions in the pre- and postoperative questionnaires are mostly identical, the data collected from OMB and COASt are comparable. In addition to data, OMB also provides the study with long-term storage sample capacity, as approved and governed by the HTA.
For a variety of trial management considerations, a subgroup of patients who were already recruited under the OMB ethics was reconsented (converted) under COASt ethics and allocated to NCOASt. The breakdown of patients groups is shown in the pie chart (Figure 43).
FIGURE 43.
Pie chart showing the breakdown of COASt eligible patients cohorts. SCOASt, South COASt Study.

The majority of COASt eligible patients have been recruited under OMB ethics (2675 participants in Oxford). In Oxford, the study recruited 295 NCOASt patients, of whom 156 were initially consented by the OMB but were then reconsented into COASt at a later stage. In Southampton, where recruitment still continues, the study has recruited 741 patients to date.
Clinical Outcomes in Arthroplasty Study team
Overall responsibility for the study falls to chief investigator who, in turn, delegates various responsibilities to the team members. The principal investigator of the Oxford site is delegated to take up the responsibilities to run the study in Oxford.
Recruitment and research visits are carried out by registered research nurses or physiotherapists. The study manager and co-ordinator oversee overall monitoring of the recruitment, data collection and study milestones, ensuring that the protocol is conducted in accordance with ethical and regulatory standards at all times. The study has also been supported by the team of statisticians, a health economist, epidemiologist, database manager, imaging research assistant and clerical and data entry assistants. Sample collection and management have been delegated to a laboratory research assistant in Southampton, whereas in Oxford this has been carried out by the OMB members.
Signature and delegation logs are regularly updated for each site, Oxford and Southampton. Although patient recruitment and sample and data collection takes place at both centres, management, co-ordination and data entry/validation are handled from Oxford.
The study milestones and the dissemination policy is supported by two committees: the Steering Committee and the Data and Sample Access Committee (DSAC). Figure 44 shows the allocation of personnel at each site of the study.
FIGURE 44.
Showing personnel allocated to the study in Oxford and Southampton.

Study population
Patient inclusion and exclusion criteria
The broad inclusion criteria of COASt have allowed maximising the recruitment rate. All patients who have been listed for hip and knee arthroplasties have been considered for the study. Inclusion and exclusion criteria are shown in Table 76.
Inclusion criteria | Exclusion criteria |
---|---|
Aged > 18 years | Unwilling or unable to give consent |
On waiting list for hip or knee arthroplasty | Charcot’s arthropathy or other severe neurological disorders |
Consent competent and willing to consent | Other severe neurological disorders |
We have identified 10 procedures on the database to represent the preoperative assessments for eligibility for COASt (Table 77). Actual surgery types performed within the COASt cohort are listed in Table 78.
Preoperative procedure knee | Preoperative procedure hip |
---|---|
Hemiarthroplasty | Hip resurfacing arthroplasty |
Knee patellofemoral resurfacing | THA |
TKA | THA revision |
Knee patellofemoral resurfacing/TKA | |
TKA revision | |
Unicompartmental knee arthroplasty | |
Unicompartmental knee arthroplasty/TKA |
Knee procedure | Hip procedure |
---|---|
Patellofemoral resurfacing | Hip resurfacing arthroplasty |
Primary TKA (primary TKA) | Primary THA (primary THA) |
Revision TKA (revision TKA) | Revision THA (revision THA) |
Unicompartmental knee arthroplasty | Hip – other |
Knee arthroscopy | |
Knee – other |
Patient pathway
Consent and patient recruitment
All participants who are listed for hip and knee replacement surgeries are potentially eligible for inclusion in the study. Once potential participants are identified, they are sent a recruitment pack, which includes the patient information sheet (PIS), sample consent form and recruitment letter. Participant involvement in this study does not normally provide direct personal benefits but such involvement facilitates future research in this particular field and in the orthopaedic community.
Owing to their own time constraints, work and family commitments or any other reasons, some participants have been unable to fully commit themselves to the study. However, they have supported the research by allowing the study members to collect and use their data with their minimal participation. By adopting a minimally intrusive method we have accommodated a number of participants: we excluded their research visits from the consent form; however, we retained consent on collecting data relevant to their surgeries from medical records and, thus, enhanced the generalisability of the study results. In such cases we used the specific consent form of minimum level of participation that permits team members to access and gather information from participants’ medical records without the patients’ active participation.
Potential participants are normally identified by the orthopaedic team. They can be any patients who are listed for knee or hip replacement surgeries at the NOC or SGH. The orthopaedic team identifies if patients meet other inclusion criteria and then provides them with a recruitment pack containing the PIS, a sample consent form and a recruitment letter. Depending on time and resources, the recruitment packs are either given to participants on the day of their preassessment appointments or posted to them after their clinics.
After approximately 2 weeks, ensuring that the patient has had sufficient time for consideration, a member of the COASt team contacts them to discuss the study in more detail, as specified in the PIS. The COASt member takes a verbal consent during the telephone discussion. The verbal consent includes an agreement that the participant is happy to receive the patient self-assessment booklet and the second morning urine sample instruction sheet with a sample pot, when applicable. Written consent is taken at the research appointment date, which is preliminarily agreed between the study member and the patient over the telephone.
At the NOC, the majority of the patients listed for hip or knee replacement already complete the patient self-assessment questionnaire (which is essentially similar to the questionnaire designed specifically for COASt) for scheduled surgery as part of their routine care. This process ensures that the patients are not overburdened and completing the questionnaire is not repeated specifically for the research. In this instance, data are accessed either through the OMB or by the NCOASt directly from the NOC medical records.
Once consent is taken, participants are assigned a site-specific screening number, which is then entered into the screening log. In some cases, participants are listed for surgery for different joints (hip vs. knee and left vs. right) at different time points. When the participant is willing for the research to collect data relating to the second operation, second consent is taken. In this case a new study number is allocated. This ensures the high quality of data collection and management. The screening log contains the details of all participants, whether or not they have enrolled to the study. Patients who choose to opt out from the research are flagged on the screening log.
Preoperative visits
After patients decide that they would like to take part in the study, they are contacted by a member of the research team. At the research appointment, patients bring along a completed patient self-assessment for inpatient surgery form. During the research appointment the patient signs the consent form, as described in the section on consent. The following additional tests are undertaken: whole-body density [via dual-energy X-ray absorptiometry (DEXA)], a physical assessment and blood and urine sample collection.
Inpatient data and sample collection
The COASt collects inpatient data and intraoperative samples with patients’ explicit consent.
Postoperative visits
Patients may also be invited to 1- or 2-year follow-up research appointments at their choice. These visits may include a physical assessment, a DEXA scan, and blood and urine sample collection.
Follow-up
With patients’ consent, study personnel post follow-up questionnaires at 6 weeks and then annually for up to 5 years postoperatively. The participants complete the questionnaires and return them in the prepaid envelope provided.
Patient pathways at University Hospitals Southampton NHS Foundation Trust and Nuffield Orthopaedic Centre
The patient pathway fundamentally differs between the two hospitals, which subsequently affected recruitment rates across the two centres.
At the NOC, the study worked closely with the trust to combine the research documentation with the extant NHS documentation, which was then adopted by the trust and subsequently used in patients’ routine care. The Oxford site then used the documentation for the collection of preoperative and inpatient data. In particular, this includes preoperative booklets such as patient self-assessment for inpatient surgery, procedure-specific physical assessment and admission, and inpatient and discharge information forms. As the preoperative booklets have been incorporated into patients’ routine care, it significantly reduced the overburdening of patients, leading to a very high rate of recruitment, with > 90% of patients approached consenting to take part in the study; in contrast, in Southampton, a more conservative recruitment strategy result in 40% of patients approached being recruited.
There are also slight differences in terms of the study milestones across the two centres. This has been reflected in the recruitment process; as recruitment has proved to be very successful in Oxford, recruitment has ceased at the NOC but it still continues at SGH. In addition to preoperative patients’ visits, in Southampton patients are also invited in at 1 or 2 years after their surgery. These visits include a procedure-specific physical assessment, blood and urine sample collection and a DEXA scan (Figure 45).
FIGURE 45.
Recruitment process in SGH. The flow chart shows that the patient is either consented fully to the study (right column) or with limited participation (left column of the flow chart). The right-hand side light-green boxes depict the phase of data collection and follow-up visits.

As previously described, the recruitment process has been more complex in Oxford. At the beginning of the project, COASt had recruited patients under OMB ethics. A subset of OMB patients has been reconsented to be followed up by COASt. This is because the OMB is restricted by its licence and generic ethics, which does not currently permit research-specific procedures. We have amended the internal application to OMB to request permission to follow up those patients recruited as part of the COASt–OMB collaboration.
Figure 46 shows that some patients have remained under the OMB ethics (green box) whereas some of the OMB patients (dark-green box under the green box, dotted line) have been reconsented into NCOASt at the NOC; we had only invited patients for a preoperative physical assessment, preoperative sample collection and a DEXA scan. Follow-up visits at the NOC were not carried out.
FIGURE 46.
Recruitment flow chart at the NOC. Three types of consents were taken: patients in the left-hand column consent to limited participation to the study; patients in the right-hand column are fully consented to participate in the study and are allocated to NCOASt; and patients in the middle column are recruited under OMB ethics. The collection of baseline data and samples, and postoperative follow-up data for OMB happens similarly to the NCOASt procedure; at some stage, some OMB patients are reconsented and followed up by NCOASt, and this may happen at any stage. Hence, the flow is indicated by the dashed arrows. Light-green boxes indicate the stage of the data collection and preoperative visit.
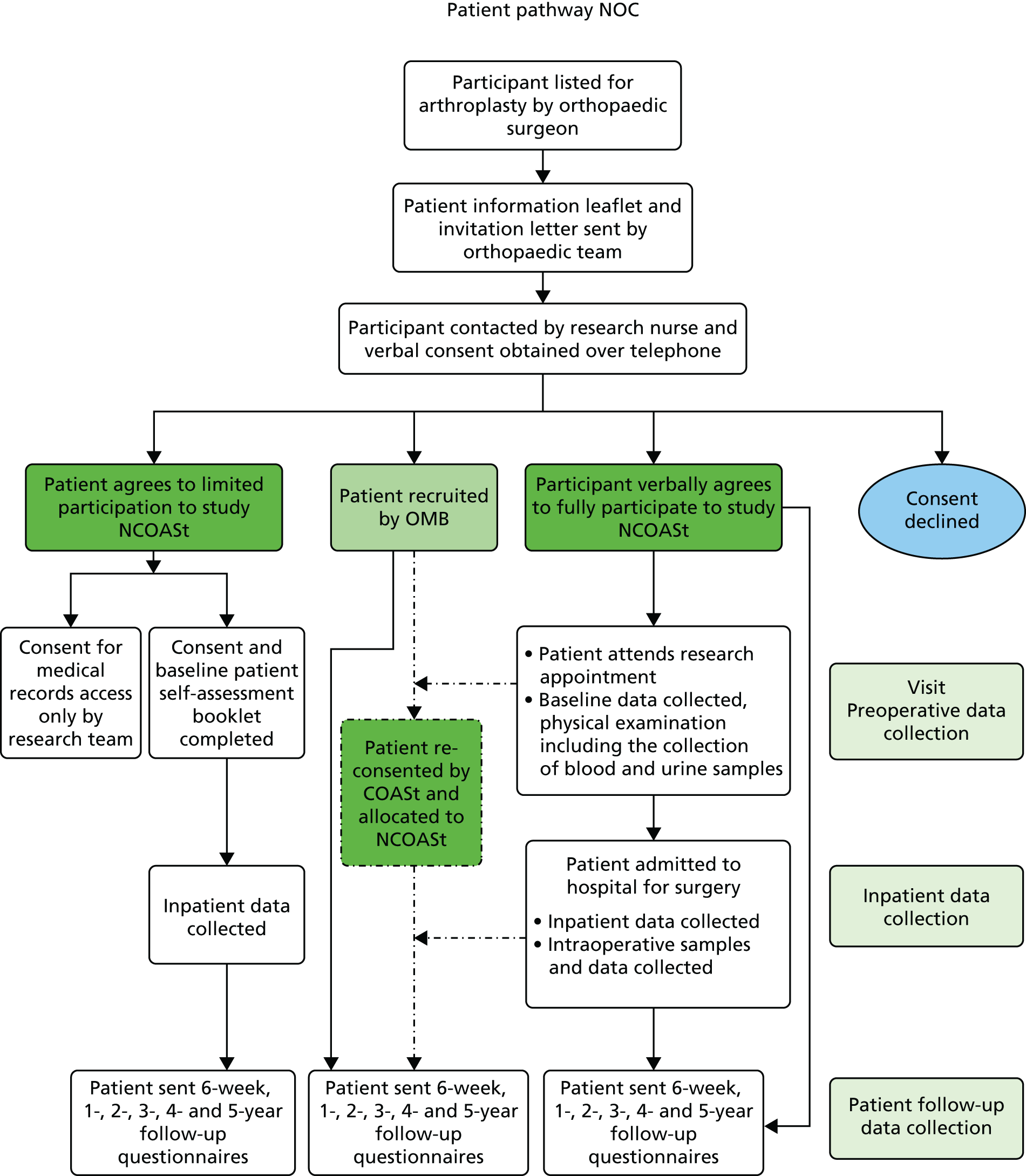
Ethics
The study has been approved by the Oxford Research Ethics Committee A (reference number 10/H0604/91). The sponsoring organisation of the study is the UHS. Although UHS is the sponsor for the study, the majority of the co-ordination has been done in Oxford, where the study chief investigator is now based. Oxford has considerable experience in setting up and running multicentre studies, is cognisant of the issues on research governance and other frameworks, which are essential for conducting and maintaining clinical studies. COASt has been conducted and maintained in accordance with International Conference of Harmonisation Good Clinical Practice guidelines and is in compliance of other regulatory requirements and governing bodies.
Recruitment figures
Proposed sample size
The initial population sample size was calculated to test the predictive tool developed in work package 2. This would have a sensitivity of at least 90% and a specificity of at least 75%. The power calculation was based on having data for 1400 TJRs, which would result in there being 224 patients with which to estimate sensitivity and 1176 patients with which to estimate specificity for hip arthroplasty patients. With 224 patients, a true sensitivity of 90% can be estimated to within 4% (95% CI 86% to 94%) and a true sensitivity of 75% can be estimated to within 2.5% (95% CI 72.5% to 77.5%). The sample size doubled in the course of the programme, as described in Important changes to the design of the study.
Actual recruitment figures
We have had an excellent rate of recruitment and went over the target by 15.96% from the proposed sample size of 3200. Over-recruitment allowed us to maximise data collection, despite the loss of patients during the long-term follow-up. The number of patients included in the study across both categories, hip and knee, is illustrated in Figure 47.
FIGURE 47.
Number of patients included in COASt.
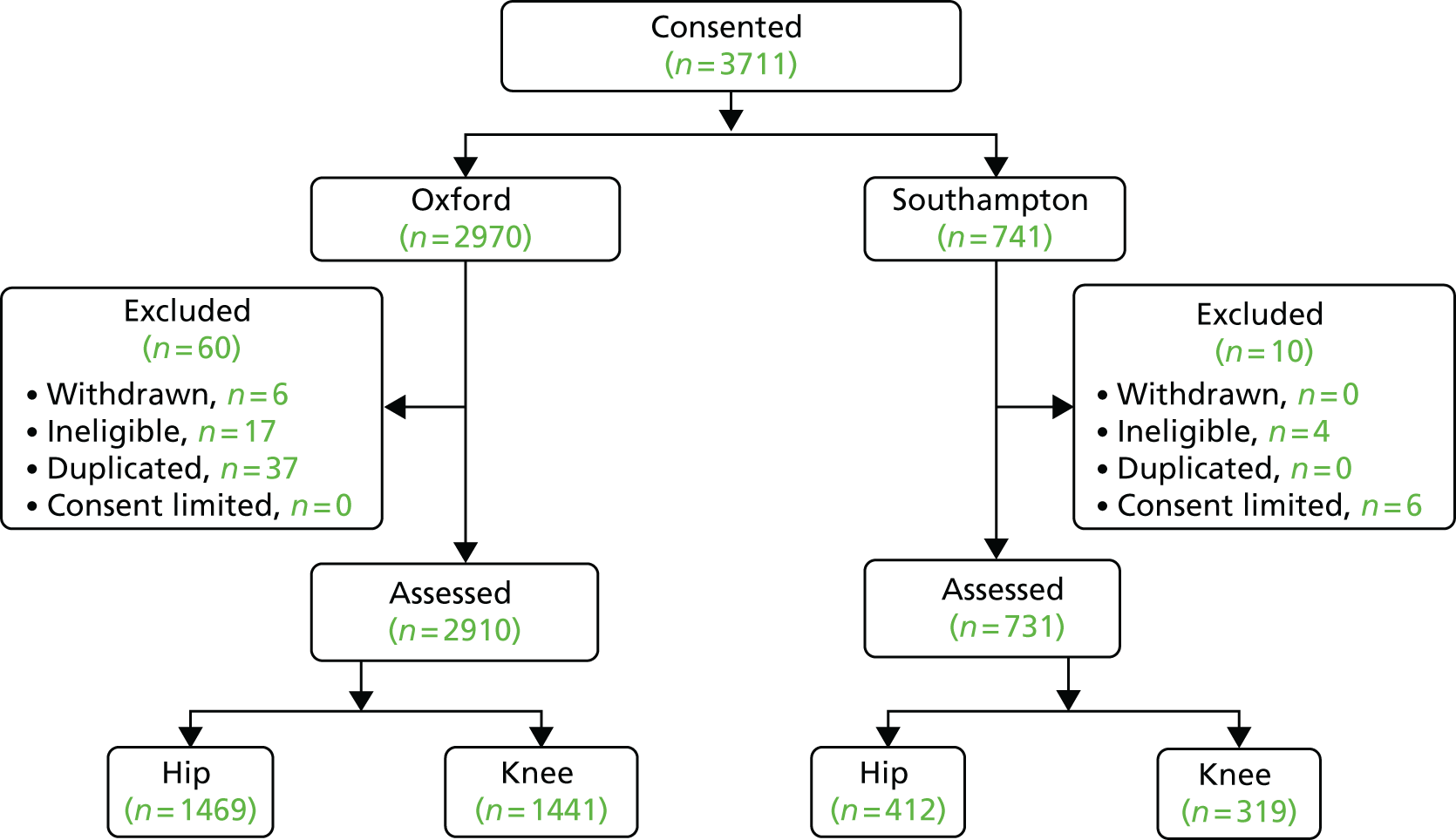
As Figure 47 shows, the overall number of consented patients across the two centres is 3711 (2970 in Oxford and 741 in Southampton).
A subset of patients was excluded during the course of the trial for the variety of reasons. For instance, during the study some patients with progressive degenerative diseases, such as Parkinson’s disease and multiple sclerosis, were recruited. Although not exhibiting severe symptoms at the time of the recruitment, because of the longitudinal nature of the study, it was expected that in these patients symptoms were likely to deteriorate over time. Therefore, considering ethical implications, these participants have been excluded from the study and flagged as ‘ineligible for COASt’. During the data cleaning and auditing, a small number of patients in Oxford have been identified as duplicates. The errors have been rectified by flagging these cases and removing them from the analysis. Only six patients have withdrawn their consent from the study in Oxford. The minimum data set of withdrawn patients have been kept intact on the database for linkage and auditing purposes. Six patients in Southampton have been recruited with an optional level of participation (see Consent and patient recruitment) and marked as ‘consent limited’ on the database.
To summarise, 60 patients have been excluded from the study in Oxford either because they withdrew from the study (six participants) or because they were ineligible (17 participants were diagnosed with severe neurological disorders), or had been allocated duplicate study identification numbers (37 participants). Ten patients were excluded from COASt because of the ineligibility criteria (four participants) and/or because only limited consent was obtained (six patients). This left 2910 participants in Oxford and 731 participants in Southampton in whom data were collected pre- and postoperatively.
Retention has also been good and reached a rate of 87% at 1 year after surgery. The number of recruited patients who returned 1-year follow-up booklets is shown in Tables 79 and 80. The tables list the categories of actual surgeries that were used by COASt.
Main group | Subgroup | Oxford (n) | Southampton (n) | Total (n) | ||||||
---|---|---|---|---|---|---|---|---|---|---|
Yes | Subgroup | No | Yes | Subgroup | No | Yes | Subgroup | No | ||
Patients assessed | 1469 | 412 | 1881 | |||||||
Died before surgery | 5 | 1 | 6 | |||||||
Had any surgeries vs. waiting | 1417 | 47 | 395 | 16 | 1812 | 63 | ||||
Hip: othera | 1 | 0 | 1 | |||||||
Hip: HRA | 11 | 5 | 16 | |||||||
Hip: THA | 1229 | 316 | 1545 | |||||||
Hip: THA revision | 176 | 73 | 249 | |||||||
Knee: TKAb | 0 | 1 | 1 | |||||||
Had COASt surgery (either hip or knee) | 1416 | 1 | 395 | 0 | 1811 | 1 | ||||
Had COASt surgery on assessed joint | 1416 | 0 | 394 | 1 | 1810 | 1 | ||||
Died without returning 6-week follow-up questionnaire | 16 | 3 | 19 | |||||||
Returned 6-week follow-up questionnaire | 590 | 790 | 355 | 37 | 945 | 827 | ||||
Died without returning 1-year follow-up questionnaire | 5 | 1 | 6 | |||||||
Returned 1-year follow-up forms (including by telephone)c | 1010 | 385 | 317 | 74 | 1327 | 459 |
Main group | Subgroup | Oxford (n) | Southampton (n) | Total (n) | ||||||
---|---|---|---|---|---|---|---|---|---|---|
Yes | Subgroup | No | Yes | Subgroup | No | Yes | Subgroup | No | ||
Patients assessed | 1441 | 319 | 1760 | |||||||
Died before surgery | 2 | 2 | 4 | |||||||
Had any surgeries vs. waiting | 1358 | 81 | 303 | 14 | 1661 | 95 | ||||
Hip: THAa | 2 | 2 | 4 | |||||||
Knee: arthroscopy | 18 | 0 | 18 | |||||||
Knee: otherb | 1 | 1 | 2 | |||||||
Knee: patellofemoral resurfacing | 13 | 3 | 16 | |||||||
Knee: TKA | 635 | 224 | 859 | |||||||
Knee: TKA revision | 99 | 11 | 110 | |||||||
Knee: unicompartmental knee arthroplasty | 590 | 62 | 652 | |||||||
Had COASt surgery (hip or knee) | 1339 | 19 | 302 | 1 | 1641 | 20 | ||||
Had COASt surgery on assessed joint | 1337 | 2 | 300 | 2 | 1637 | 4 | ||||
Died without returning 6-week follow-up questionnaire | 14 | 1 | 15 | |||||||
Returned 6-week follow-up questionnaire | 560 | 751 | 277 | 24 | 837 | 775 | ||||
Died without returning 1-year follow-up questionnaire | 5 | 3 | 8 | |||||||
Returned 1-year follow-up questionnaire (including by telephone)c | 916 | 404 | 231 | 67 | 1147 | 471 |
Table 79 shows that 1881 hip patients in total (SGH and NOC) were included in the study. Of this cohort, six patients died and 63 patients did not undergo surgery. Overall, 19 patients died in the 6 weeks after surgery and six patients died before the end of the first year after their surgery. One patient in Southampton was initially assessed for hip replacement surgery but actually underwent knee replacement surgery. Therefore, in total, COASt followed up only 1327 patients at 1 year. This, however, does not reflect the true retention rate because the number excludes those who had surgeries recently and who have not reached the 1-year postoperative time point. Therefore, these patients have not yet been sent the 1-year follow-up booklets.
For the knee cohort, all patients who were listed for knee replacement surgery and were aged > 18 years, consent competent and had not had severe neurological disorder were selected for the study (1760 patients in total). Table 80 shows that 1760 patients have been assessed for knee replacement surgery. Of these, four died before their surgery, 15 died in the 6 weeks after surgery and eight died before the end of the first year after their surgery. In total, 95 patients have not had their surgery yet. Interestingly, four patients were initially assessed for knee replacement but actually underwent primary total hip arthroscopy. Therefore, only 1637 patients were selected to be sent the 1-year follow-up booklet. Overall, 1147 patients have returned the 1-year follow-up booklet or supplied the data over the telephone. This number, however, is not a true reflection for the rate of 1-year follow-up success, as it excludes the patients who have not reached the 1-year postoperative time point.
Data, samples and radiographic variables
Data
The data have been collected in accordance with Good Clinical Practice and the Data Protection Act 1998. 239 COASt data collection has gone though the appropriate approval processes. The data have been collected either from participants’ hospital records or directly from participants. This includes:
-
Baseline data – patient self-assessment for inpatient surgery and PROMs, both of which are completed by patients, and procedure-specific physical assessment carried out by a qualified research nurse, physiotherapist or podiatrist.
-
Inpatient data – from participants’ medical notes various perioperative clinical information have been extracted including radiographic variables and other clinical information relevant to the study, such as intraoperative variables.
-
Postoperative data – questionnaires completed by patients at 6 weeks and 1, 2, 3, 4 and 5 years after their surgery. Follow-up questionnaires are posted to study participants. The completed booklet is then returned in a stamped addressed envelope.
Radiographic variables
Radiographic variables such as radiographs and DEXA scans are collected pre- and postoperatively (DEXA scans are carried out for the study at 1 and/or 2 years after surgery, as specified in COASt protocol).
Samples
The study team collected samples donated by participants. Participants’ specimens are allocated a unique specimen number alongside their unique study number. All collected samples are processed and stored in the OMB. This includes urine, blood and intraoperative biomaterial.
Urine samples are collected for future storage and deoxypyridinoline cross-link analysis. At visits a second urine sample is collected. Blood samples are collected for storage and the serum is analysed for high-sensitivity C-reactive protein (hsCRP) concentration. For this report we analysed blood samples for hsCRP to use as a predictor for TJR outcome.
The COASt also collects intraoperative biomaterial such as femoral condyle, tibial plateau, meniscus, ligament, synovium, synovial fluid, fat, femoral head, acetabular bone/cartilage, labrum, ligamentum teres, synovium and synovial fluid.
Collection of samples will enable tests to be conducted and more extensive profiling to take place in the future. It will also contribute significantly to clinical research in orthopaedics, rheumatology and musculoskeletal sciences.
Donated blood and urine speciments are centrifuged and aliquoted in several cryovials. This takes place immediately after the collection. Samples that are not immediately analysed for deoxypyridinoline and C-reactive protein are sent to the OMB for storage. Samples are analysed blind by the laboratory at SGH, that is laboratory staff are not provided with sensitive patient information but only the study identifications. Blood and urine sample types, number of aliquotes and tests that are carried out for the study are shown in Table 81.
Test | Sample type | Number of tubes |
---|---|---|
C-reactive protein | Blood | 1 × 5 ml |
DpD | Urine | 1 × 10 ml |
For samples for storage: extra blood maximum of 50 ml allowed | ||
C-reactive protein | Blood for serum (gold) | 1 × 6 ml |
C-reactive protein | Blood for serum (red) | 1 × 6 ml |
C-reactive protein | Blood for plasma | 1 × 6 ml |
DNA | Blood | 1 × 5 ml |
For samples on admission | ||
DpD | Urine | 1 × 10 ml |
All biological samples are collected and stored in accordance with the local trust guidelines and HTA. Biomaterial for long storage is snap frozen and kept in the OMB, for testing, at –80 °C in a securely monitored freezer.
If the results arising from sample testing generate any clinical concerns or queries, the case will be referred to the principal investigator or chief investigator, who may in turn contact the patient’s GP/consultant to inform them of the results.
Radiographic variables
Dual-energy X-ray absorptiometry scan
This is an additional test carried out by the study and was not included in the NIHR grant application. In SGH and NOC we used densitometers Hologic Discovery QDR (Hologic, Bedford, MA, USA) and Hologic Discovery A (Hologic, Bedford, MA, USA), respectively, for DEXA scans to measure bone mineral density (BMD). The majority of DEXA scans are carried out at SGH and a relatively small number of successive patients at the NOC. In SGH the scan is done preoperatively and at 1 or 2 years post surgery, whereas at the NOC the scan was performed only before the patient’s surgery. BMD of the index joint will be used to assess the quality of the bone into which the prosthesis is going to be implanted and will be used in future research for assessing the risk of a poor PROMs outcome, postoperative osteoporosis fracture and the risk of revision surgery. BMD measurements are made at the lumbar spine (L1–L4), hip, whole body and proximal tibia/subchondral region, and an instant vertebral assessment has also been collected.
Radiographs
Patients’ preoperative radiographs of the index joint are obtained as a part of routine care by using a picture archiving and communication system (PACS). Baseline radiographs are taken within the routine care in the trusts for the majority of patients. These have been obtained by the study with patients’ consent. A small minority of radiographs are taken out of the trust area and, therefore, are unavailable. Radiographic variables have been taken mainly from the Digital Imaging and Communications in Medicine (DICOM) format. DICOM format retains maximum image quality and accurate image size in order to be used for assessing semiquantitative and quantitative morphology and OA variables for research purposes. When DICOM was not available, Joint Photographic Experts Group (JPEG) images were used for baseline variables.
The radiographs used were clinical images taken during routine patient care and surgery preparation, and were not standardised specifically for COASt. Therefore, the anteroposterior weight-bearing images that were required for grading were not available for all patients. Radiographs were anonymised at the point they were downloaded from the PACS, with all patient-identifiable information removed. Images were saved in DICOM (.dcm) format in order to retain the best-quality imaging data.
Data storage and database
Database
All COASt data have been stored on servers located at the University of Oxford and managed by the Medical Sciences Division IT. The patient-identifiable data have been collected and stored in two monitoring databases (one for Oxford’s patients and one for Southampton’s patients) using Microsoft Access® databases (2010, Microsoft Corporation, Redmond, WA, USA) located in high-compliance systems (HCSs). The other, non-sensitive, data have been collected via a web interface developed in PHP and JavaScript accessible only within the Nuffield Department of Orthopaedics, Rheumatology and Musculoskeletal Sciences (NDORMS), and stored in a password-protected MySQL database (version 5.6.12; Cupertino, CA, USA).
Data security and confidentiality
Patient-identifiable data have been collected and stored in a password-protected HCS provided by the Information Management Services Unit (IMSU) at NDORMS in Oxford to ensure patient data protection and confidentiality. Only the NIHR Biomedical Research Unit IT manager, the database manager and approved members from the study team have password-protected access to the patient-identifiable information, for the purposes of patient follow-up, data validation and error checking. No sensitive data have gone outside the HCSs. Each participant has been given a unique study-specific identifier used to identify them safely and anonymously across the COASt databases. Password-protected access to the non-sensitive information has been given to the database manager and approved study members for data checking and data entry purposes, respectively.
Error checking and validation
A number of layers of data checking and validation have been implemented to allow only sensible data to be entered into the databases. The NHS algorithm has been used to identify possible typing mistakes in the NHS numbers. Lower and upper limits have been set in the database and interface for numeric fields in order to accept only a range of possible answers according to the question being requested. When applicable, and appropriate, for the question, a set of answers has been presented in the interface to facilitate data entry and minimise human mistakes. Daily automated reviews of the data have been run overnight to identify any outliers and, when present, automatic e-mails have been sent to the COASt team for further checking and corrections.
Audit trail
The databases have recorded a full history of user access and user actions (i.e. data insertions, updates and deletion). Audit tables in the databases have stored an original copy of any information that has been entered, updated or deleted.
Backups
Backups of the databases have been performed regularly. The HCS Microsoft Access databases have been backed up daily by the IMSU. The MySQL database has been dumped and stored daily on a dedicated IMSU server at NDORMS. Only the NIHR Biomedical Research Unit IT manager and the COASt database manager have authorised password-protected access to these data backups.
Safety reporting
Study-related risk assessments have been carried out and participants are informed of such risks, however small, in PISs or in discussions, when necessary. Study safety reports are generated annually to Research Ethics Committee and reported, as specified in the protocol.
Patients and scientific community
Data sharing
Results deriving from work package 4 will be presented as publications in high-calibre journals, national and international research meetings. We have a very strong track record of informing health policy nationally and internationally, through organisations such as the European League Against Rheumatism, OARSI, World Health Organization and the Institute of Fundraising. The intellectual property arising from the programme is owned by the UHS. However, the trust had discussed the possibility of a future collaboration between Southampton and Oxford around some potentially synergistic intellectual property, which is currently being developed in Oxford and the outputs of the COASt.
We believe in the benefits of actively sharing the achievements of research with the public and the wider scientific community. The results will be shared with the participants by posting newsletters detailing our latest achievements and conclusions we have made so far. The templates of newsletters and any communication will vary according to the phase of the study and the interpretations available at the time. We are planning to design an external website for promoting use of COASt data and samples in order to maximise the study potential and encourage collaborations with other scientific groups for the benefits of the public. For this purpose we established the DSAC.
The DSAC facilitates the provision of access to the data set and samples collected. The DSAC operates under its terms of reference and the protocol. The committee consists of several members of the local research group, an independent chairperson and the patient representative from the patient and public involvement (PPI). The committee ensures that the common principles on data and tissue policy are adhered to, allows appropriate sharing of the study samples/data for scientific research with the participants’ privacy and well-being protected within the scope of their informed consent, and ensures compliance with regulatory requirements.
In order to access the COASt data and samples, a researcher sends a completed application form to the DSAC. The applicants must be employees of a recognised academic institution, health service organisation or commercial research organisation with experience in medical research with sufficient scientific merit. Transfer of the data and samples outside the UK, within or outside the European Economic Area, requires a full protocol that ensures conformity to UK legal, safety and ethical standards.
Patient and public involvement
We are committed to deliver credible data and results for the benefits of general public. At the same time we ensure that the rights, safety and confidentiality of participants are respected and protected. Team members involved in this research have been appropriately qualified by education, training and their experience. In parallel, as described in our grant application, we have appointed the patient and public representatives (PPRs) to represent patients’ rights from each participating centres.
At the beginning of work package 4 a PPR was appointed to the steering committee. At a later stage PPR was involved in the dissemination strategy as a DSAC committee member, in which they supported the study dissemination policy and ensured that the participants’ anonymity is observed while sharing the data with other scientific groups.
The COASt PPR is a member of general public, works on voluntary basis and has interest in the topic we research. The PPR’s contribution in the study involved:
-
discussion on substantial amendments of the study
-
data sharing and dissemination policy
-
consultation on lay summaries.
During the meetings, discussing COASt substantial amendments, the PPI had the opportunity to emphasise a number of issues and, most importantly, effectively design the patient information leaflets. The leaflets were designed to be well presented, informative and easy to understand, which certainly enhanced recruitment success rates. There was a concern in the group that patients should not be overburdened with different clinical studies that take place in local trusts. The PPR felt that, as a patient themselves, it is important that patients are offered the opportunity to participate in as many observational studies as they wish as long as they are happy to do so.
The PPR had more involvement in later stage of the study, especially during the dissemination phase, as the DSAC member PPI had opportunity to review DSAC original documentation and share any suggestions they had. Although previous meetings have happened face to face, meetings for the DSAC have been carrying out remotely via teleconferences. The PPR has also been involved in consultation for lay summaries such as designing newsletters and writing a lay summary for this report.
Although our PPR had involvement on a number of occasions, we fully understand that this involvement could be expanded further. We would take the ideas forward and expand our relationships with the public. In particular, the accent will be made on more active involvement at the initial stages, before grant application, in which the ideas would be closely discussed with focus groups. Patients who have similar interests in the study would have a clear vision of what patients would expect from the research and whether or not the research would benefit patients. In the Research Ethics Committee application stage, the focus groups would discuss the detailed patient pathway whether or not the research would affect patients routine pathway, whether or not research would undertake suitable pathway to recruit optimum number of patients, whether or not patients would have sufficient time for reading patient information leaflets and other related documents and if the information provided in the leaflets and consent form is easy to understand and sufficiently comprehensive. In the active phase, PPRs could review and comment on proposed patient booklets and questionnaires, as well as data collection methods, potentially contribute to the implementation of the results, and advise on different techniques and avenues for dissemination of the results.
Statistical analysis (methods) and end points
This report describes the external validation of two prediction models for THR138 and TKR,142 using the prospective COASt cohort. We also present results of the evaluation of the incremental value of additional variables (radiographs and the K/L grade) on the work package 2 COASt knee and hip models.
Total knee replacement: Clinical Outcomes in Arthroplasty Study knee model
We developed a clinical risk prediction tool to predict the OKS at 12 months after undergoing TKR surgery. 142 We used the data from 1649 patients enrolled in the KAT across 34 centres in the UK between July 1999 and January 2003. The presence of a fixed flexion deformity or other deformities (compared with no deformity) and the absence of a preoperative ACL (rather than an intact ACL) were associated with a better outcome. The clinical factors associated with a worse outcome were a worse ASA grade, the presence of other conditions affecting mobility and previous knee surgery. A lower preoperative OKS, living in a poor area, a high BMI and a worse SF-12 mental component summary score were also associated with a worse outcome. The discrimination and calibration statistics were good. Patient characteristics explained 16.6% of the outcome variability. The addition of clinical variables, then surgical variables increased the explained outcome variance to 18.9% and 20.2%, respectively.
Total hip replacement: Clinical Outcomes in Arthroplasty Study hip model
We developed a clinical tool to predict patient-reported outcomes following primary hip replacement surgery for OA using data from the Exeter Primary Outcome Study and EUROHIP. 138 The OA pattern, one of the radiographic variables, was an important determinant of outcome (i.e. patients with superolateral disease had a better outcome). Arthritis and previous surgery on other joints led to a worse outcome. Among the surgical variables, a posterior surgical approach and a larger femoral component offset had a better outcome. The patient factors of worse preoperative pain/function, increased age once > 75 years, increased BMI, a lower educational attainment level and a worse preoperative SF-36 mental health score also gave a worse outcome. These factors were operationalised into a clinical risk prediction tool. The tool was well calibrated and discriminated well (R2 = 23.1%). The tool performed well in identifying the patients with the worst outcomes (c-statistic = 0.77).
Assessment of Clinical Outcomes in Arthroplasty Study hip radiographs
Radiograph scoring methods
Two validated semiquantitative scoring methods were used to assess common features of OA visible on a radiograph. The K/L score is a global grading system consisting of a combination of radiographic features (osteophytes and joint space narrowing) in both the medial and lateral compartments. 177,240 This score ranges from 0, indicating no evidence of radiographic OA, to grade 4, which indicates severe radiographic OA. The atlas developed by the OARSI was also utilised in order to score individual features and compartments of radiographic OA. 241 Osteophytes were scored based on size in four locations: inferior acetabular, inferior femoral, superior acetabular and superior femoral. Joint space narrowing was graded in two locations: superior and medial. The presence or absence of subchondral cysts and subchondral sclerosis was also recorded.
Femoral head migration has been previously identified as an important predictor of outcome after hip replacement surgery. 137 As there is no single method for assessing this score, information from several references were combined to replicate this measure and create a standardised, reproducible method of assessment. 242–246 Categories were selected to match the research by Dieppe et al. :137 superolateral, superomedial, medial or concentric.
The following classifications were used to describe femoral head migration on the COASt images:
-
superolateral – definite loss of joint space in the superolateral region of the joint leading to the migration of the femoral head towards the lateral sourcil
-
superomedial – definite loss of joint space in the superomedial region of the joint leading to the migration of the femoral head towards the medial sourcil
-
medial – inferomedial migration of the femoral head with associated medial and inferior joint space loss
-
concentric – joint space loss, which resulted in a relatively even width of the intra-articular joint space between the femoral head and acetabulum superolaterally to medially
-
none – no definite joint space loss, across a regional area, between the femoral head and acetabulum.
Radiograph scoring
Two readers with expertise in radiographic assessment of OA and joint morphology graded all COASt hip radiographs. Preparation consisted of several weeks of intensive training, in which test sets of hip radiographs were graded independently by each reader and then compared. Additional training and adjudication of discrepancies identified from grading the test data set were provided by a consultant rheumatologist with expertise in radiographic hip OA. Official reproducibility was evaluated using 50 radiographs randomly selected from a population-based cohort (the Chingford study247) reflecting the full range of radiographic disease (as assessed by K/L grade). Each reader graded the full set of 50 radiographs twice, approximately 2 weeks apart, in order to calculate both intra- and interobserver reproducibility.
Methods
We present a numerical description of the variables in the hip and the knee COASt data sets. For the hip data set, we separately considered data from the patients who completed and returned all forms (550 patients, forming the complete data set) and those who completed and returned, at minimum, the 1-year follow-up form (1098 patients, forming the incomplete data set). The two data sets were summarised to examine any pattern of missing data. Graphical methods were used to compare the outcome and baseline OHS. The knee data set was also split into data from patients who completed and returned all forms (608 patients) and data from those who completed and returned, at minimum, the 1-year follow-up form (1025 records). However, the knee data set suffered from a substantial number of missing data. For example, 608 patients returned all the forms, but only 182 patients provided complete information on all the variables that are required for validation of the knee model. Both the 608 and 1025 record data sets were also summarised to examine the missing data patterns in the data sets.
The coefficient of determination (R2) was used to quantify the goodness of fit of both models. R2 is computed by comparing the sum of squares from the regression line with the sum of squares from a model defined by the null hypothesis. For external validation, the R2 of prediction is given as:
where Y¯ is the arithmetic mean of the dependent variable in the data used to develop the model, Yi is the predicted value and Y^i is the observed value from the validation data set. An approximate approach is to calculate the multiple R2 of Yi and Y^i. We used this approximate approach as Y¯ may not be readily available in practice.
In addition to R2, the calibration of the hip and knee models on the COASt data sets was assessed at the tenths of the predicted values, obtained from the developed models. The ratio of the mean of the observed and predicted scores was compared within each tenth. A ratio close to 1 signified that the observed and predicted scores were in agreement. We also plotted the observed and predicted scores. The calibration slope was used to assess the degree of agreement between the observed outcomes and predicted values (from the prediction models).
Although the hip and knee COASt data sets included complete data for most of the baseline variables, some important variables had missing values. We used predictive mean matching to impute missing values in the COASt data sets. This method is similar to regression, except, for each missing value, it randomly imputes a value from a set of observed values whose predicted values are closest to the predicted value for the missing value from the simulated regression model. We used single imputation to validate the predictive mean matching. In single imputation, a single-imputed data set is taken from a multiply imputed data set. We used the first imputed data set created from 10 multiply imputed data sets. Although single imputation is not the most efficient method for variable selection in the presence of missing data, it maintains the convenience of dealing with a single data set. It also avoids the inefficiency of the complete-case approach.
We assessed whether or not the hip and knee models could be improved by adding additional variables. We evaluated the improvement after adding each new variable singly and jointly. We used bootstrapping for internal validation, as it assesses and quantifies overfitting. We used 300 bootstrap samples to quantify possible overfitting in the improved hip and knee models.
Results
Part 1: Clinical Outcomes in Arthroplasty Study hip model
Numerical description of the hip Clinical Outcomes in Arthroplasty Study data
Table 82 shows the characteristics of the COASt participants for all the variables that were required for validating the COASt hip model. The data set included 550 complete records and 1098 incomplete records. Patient factors included the baseline and outcome OHS values, age, BMI, the level of education attained (education) and a surrogate for the SF-36 mental health score. Three radiographic variables were included: the OA pattern (superomedial), the presence of arthritis in other joints (arthritis) and surgery in other joints (surgery). Two surgical variables were included: which posterior surgical approach was used (posterior) and stem size. Model improvements were assessed by adding variables measuring the level of C-reactive protein and the K/L grade.
Variables and outcome | Data set | % missing | |
---|---|---|---|
Complete (N = 550) | Entire (N = 1098) | ||
Mean OHS (SD) at 12 months | 41.06 (8.96) | 41.20 (8.56) | 5.7 |
Predictors | |||
Mean baseline OHS (SD) | 18.63 (8.05) | 19.35 (6.70) | 12.0 |
Mean age in year (SD) | 68.23 (10.36) | 67.97 (10.93) | 0 |
Mean BMI (SD) | 27.97 (3.88) | 28.13 (5.00) | 0 |
Education attainment, n (%) | 24.4 | ||
None | 192 (34.9) | 320 (38.6) | |
O level | 44 (8.0) | 64 (7.7) | |
A level | 38 (6.9) | 26 (3.1) | |
Further education | 117 (21.3) | 161 (19.4) | |
Higher education | 159 (28.9) | 259 (31.2) | |
SF-36 (surrogate for mental health), n (%) | 21.0 | ||
Yes | 125 (22.7) | 416 (48.0) | |
No | 425 (77.3) | 451 (52.0) | |
Superomedial, n (%) | 48.9 | ||
None | 49 (8.9) | 50 (8.9) | |
Concentric | 184 (33.5) | 189 (33.7) | |
Medial | 131 (23.8) | 132 (23.5) | |
Superomedial | 48 (8.7) | 49 (8.7) | |
Superolateral | 138 (25.1) | 141 (25.1) | |
Arthritis, n (%) | 23.0 | ||
Yes | 396 (72.0) | 603 (71.3) | |
No | 154 (28.8) | 243 (28.7) | |
Mean surgery (SD) | 0.07 (0.26) | 0.07 (0.26) | 24.4 |
Posterior, n (%) | 11.5 | ||
Hardinge | 109 (19.8) | 192 (19.8) | |
Posterior | 440 (80.0) | 779 (80.1) | |
Other | 1 (0.2) | 1 (0.1) | |
Mean stem size (SD) | 40.25 (4.15) | 40.25 (4.15) | 11.6 |
New variables | |||
Mean C-reactive protein (SD) | 5.21 (7.77) | 5.21 (7.77) | 40.7 |
K/L grade, n (%) | 48.9 | ||
0 | 3 (0.9) | 3 (0.5) | |
1 | 5 (1.4) | 5 (0.9) | |
2 | 28 (8.0) | 29 (5.2) | |
3 | 135 (38.6) | 136 (24.2) | |
4 | 179 (51.0 | 388 (69.2) |
The complete and incomplete data sets in Table 83 have similar distributions, as they had similar ranges, means and SDs for each variable. The number of missing data in the radiographic variable superomedial was high (48.91%). The two additional variables, C-reactive protein and K/L grade, also had a high number of missing data (40.71% and 48.91%, respectively). It is therefore not surprising that these variables had identical distributions in the complete and incomplete data sets.
Variable | Data set, n (%) | % missing | |
---|---|---|---|
Complete (N = 550) | Entire (N = 1098) | ||
Sex | 0 | ||
Male | 219 (39.8) | 445 (40.5) | |
Female | 331 (60.2) | 653 (59.5) | |
Smoking | 20.6 | ||
Yes | 42 (7.6) | 68 (6.2) | |
No | 508 (92.4) | 804 (73.2) | |
Alcoholism | 20.8 | ||
Yes | 7 (1.3) | 12 (1.1) | |
No | 543 (98.7) | 858 (78.1) | |
Anxiety | 21.7 | ||
Yes | 68 (12.4) | 101 (9.2) | |
No | 482 (87.6) | 759 (69.1) | |
Depression | 21.04 | ||
Yes | 97 (17.6) | 153 (13.9) | |
No | 453 (82.4) | 714 (65.0) | |
Fractures | 23.0 | ||
Yes | 234 (42.6) | 368 (33.5) | 21.1 |
No | 316 (57.5) | 498 (45.4) | |
Back pain | 22.0 | ||
Yes | 219 (39.8) | 367 (33.4) | |
No | 331 (60.2) | 489 (44.5) | |
OA | 23.0 | ||
Yes | 396 (72.0) | 603 (54.9) | |
No | 154 (28.0) | 243 (22.1) | |
RA | 22.4 | ||
Yes | 38 (6.9) | 55 (5.0) | |
No | 512 (93.1) | 797 (72.6) | |
Diabetes | 20.8 | ||
Yes | 38 (6.9) | 66 (6.0) | |
No | 512 (93.1) | 804 (73.2) | |
Hypertension | 21.9 | ||
Yes | 239 (43.5) | 367 (33.4) | |
No | 311 (56.6) | 491 (44.7) | |
Gout | 21.7 | ||
Yes | 36 (6.6) | 58 (5.3) | |
No | 514 (93.5) | 802 (73.0) | |
Osteoporosis | 23.5 | ||
Yes | 47 (8.6) | 73 (6.7) | |
No | 503 (91.5) | 767 (69.9) | |
High cholesterol | 22.0 | ||
Yes | 154 (28.0) | 251 (22.9) | |
No | 386 (70.2) | 606 (55.2) | |
Bowel | 21.6 | ||
Yes | 147 (26.7) | 251 (22.9) | |
No | 403 (73.3) | 610 (55.6) | |
Renal | 21.1 | ||
Yes | 31 (5.6) | 45 (4.1) | |
No | 519 (94.4) | 821 (74.8) | |
Ethnicity | 18.4 | ||
British | 526 (95.6) | 836 (76.1) | |
Irish | 9 (1.6) | 11 (1.0) | |
Any other white | 10 (1.8) | 12 (1.1) | |
White and black Caribbean | 2 (0.2) | ||
White and black African | 14 (1.3) | ||
White and Asian | 1 (0.2) | 13 (1.2) | |
Any other mixed background | 2 (0.4) | 5 (0.5) | |
Indian | 2 (0.2) | ||
Pakistani | 1 (0.2) | 1 (0.1) |
We also compared additional general patient factors between the complete and incomplete data sets (see Table 83). Apart from sex, the number of missing data was similar across all of the factors examined. The numerical exploration of the arthritis and examination variables, questionnaire scores and 12-month follow-up measures in the hip data set are presented in Appendix 13, Tables 105 and 106.
We imputed the incomplete data (1098 records) using MICE, as described in Methods. Figure 48 shows the correlation matrix for the outcome variable and some of the continuous variables in the hip data set. The correlations between the outcome and the surgery, stem size and C-reactive protein variables were not significant (p = 0.313, 0.072 and 0.317, respectively) in the model using the complete data set; however, these variables were significant in the model using the imputed data set. In the case of stem size, this was explained by increasing statistical power, whereas for the other two variables, there were also increases in the correlation coefficient suggesting an actual change in the association measured.
FIGURE 48.
Correlation matrix plot for (a) the complete; and (b) imputed hip data sets.

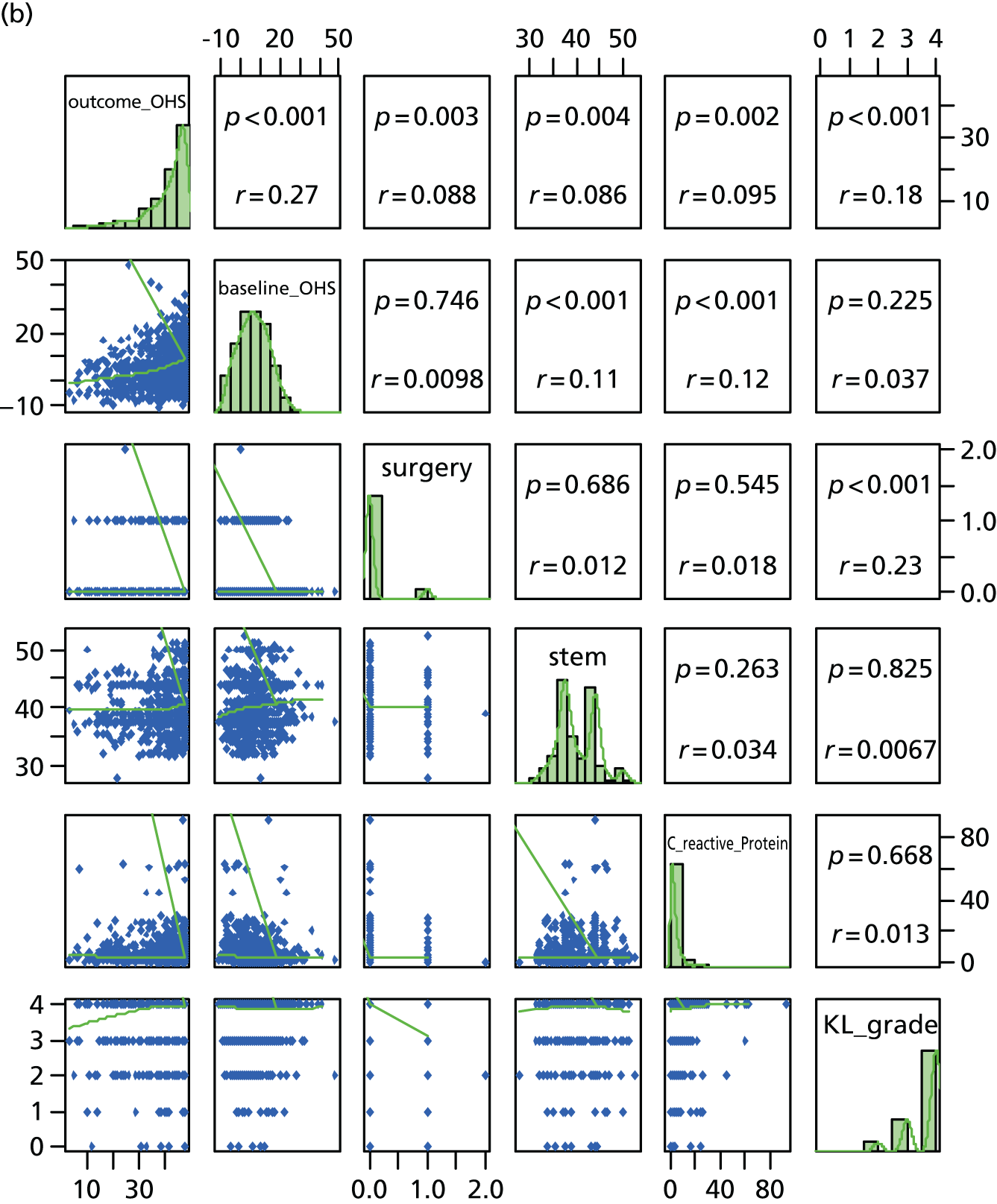
Statistical analysis of hip replacement surgery
We carried out model validation using both the complete and incomplete data sets. We used multiple imputation to ‘fill in’ the missing observations in the incomplete data set, as discussed in Methods. The rationale for using multiple imputation to fill in the missing data is not to gain power from having increased sample size but to be able to show if there are systematic differences in the predictive performances of the models validated using the complete and the completed data sets. We will expect that if the assumption underlying the missing data is ‘true’, then the imputed data set should outperform the complete data set in terms of predictive accuracy. Otherwise, the missing data have a distribution different from the observed data.
The model to predict the OHS at 12 months is defined as:
where
These variables were identified in the validation data set. The baseline OHS was computed after rescaling the score to the range from 0 to 48. The outcome was computed using the same method. The SF-36 questionnaire was used to measure mental health in the model development data set. However, the COASt did not collect this score. We used a surrogate score; a positive value was assigned if the patient reported being treated for anxiety or depression, or if they scored 2 or 3 units on the mental health component of the EQ-5D.
We assessed the performance of the model by evaluating calibration and discrimination (Table 84), as described in the Methods section.
Tenths of the predicted OHS | OHS | ||
---|---|---|---|
Observed | Predicted | Observed : predicted | |
14.2–19.6 | 37.62 | 17.90 | 2.10 |
19.6–21.8 | 39.07 | 20.66 | 1.89 |
21.8–23.6 | 38.31 | 22.73 | 1.69 |
23.6–25.5 | 40.58 | 24.62 | 1.65 |
25.5–27 | 42.09 | 26.21 | 1.61 |
27–28.7 | 42.21 | 27.79 | 1.52 |
28.7–30.1 | 42.53 | 29.44 | 1.45 |
30.1–31.7 | 41.43 | 30.86 | 1.34 |
31.7–34.4 | 44.38 | 32.91 | 1.35 |
34.4–44.7 | 42.46 | 36.70 | 1.16 |
R 2 | 0.042 |
The distribution of the observed scores is not identical to the predicted scores, as can easily be seen in Figure 49, which shows density plots of the observed and predicted scores using the complete records.
FIGURE 49.
Density plot of the observed and predicted scores for the hip data using the complete data set. (a) Observed OHS; and (b) predicted OHS.


Figure 50 shows the baseline OHS and absolute change in OHS. We have computed the change in score as a difference between observed and the baseline scores.
FIGURE 50.
Histograms of the outcome using (a) baseline; and (b) absolute change in the OHS, using the complete data set (550 records).
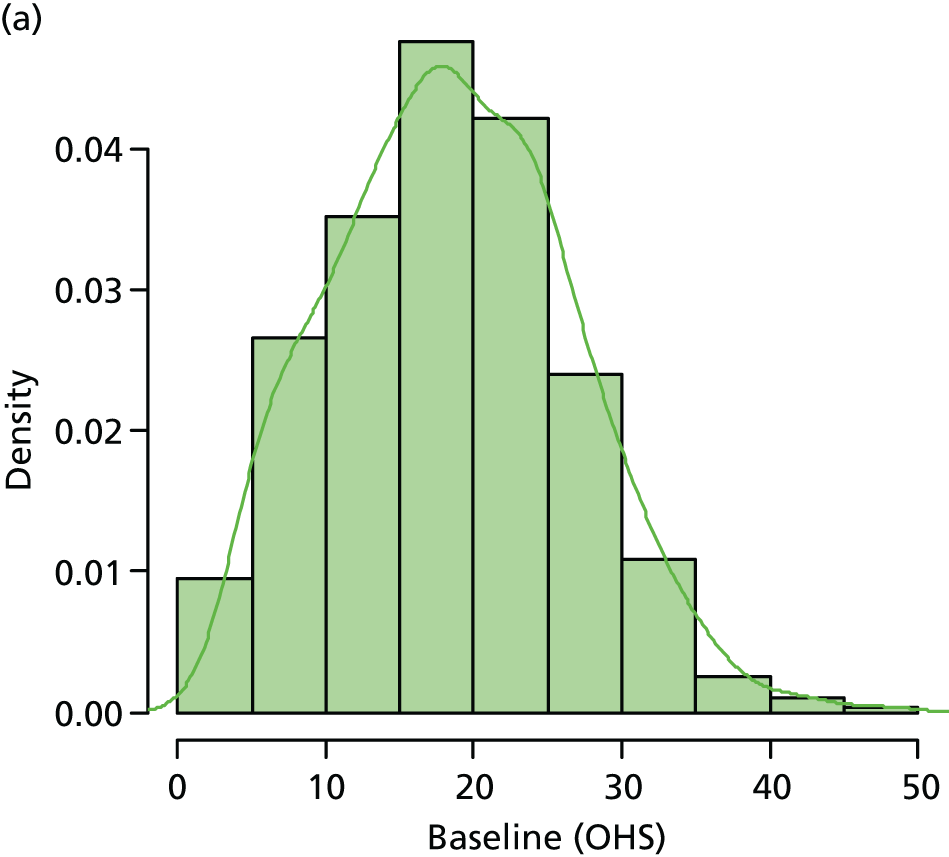

The model also had poor discriminatory ability (R2 = 4.2%), which was substantially less than that achieved in the original model from work package 2. Model calibration was also generally unacceptable (see Table 84 and Figure 43), with the model generally predicting a worse outcome than seen in the observed data. This was particularly true for the lowest deciles of outcome, for which the predicted scores were very substantially less. These are the exact patients that we would want to model to predict accurately.
The calibration plot in Figure 51 graphically illustrates poor agreement between the observed OHS at 12 months and the predicted 12-month OHS from the prediction model.
FIGURE 51.
Calibration plot of the validated hip model using the complete data set.
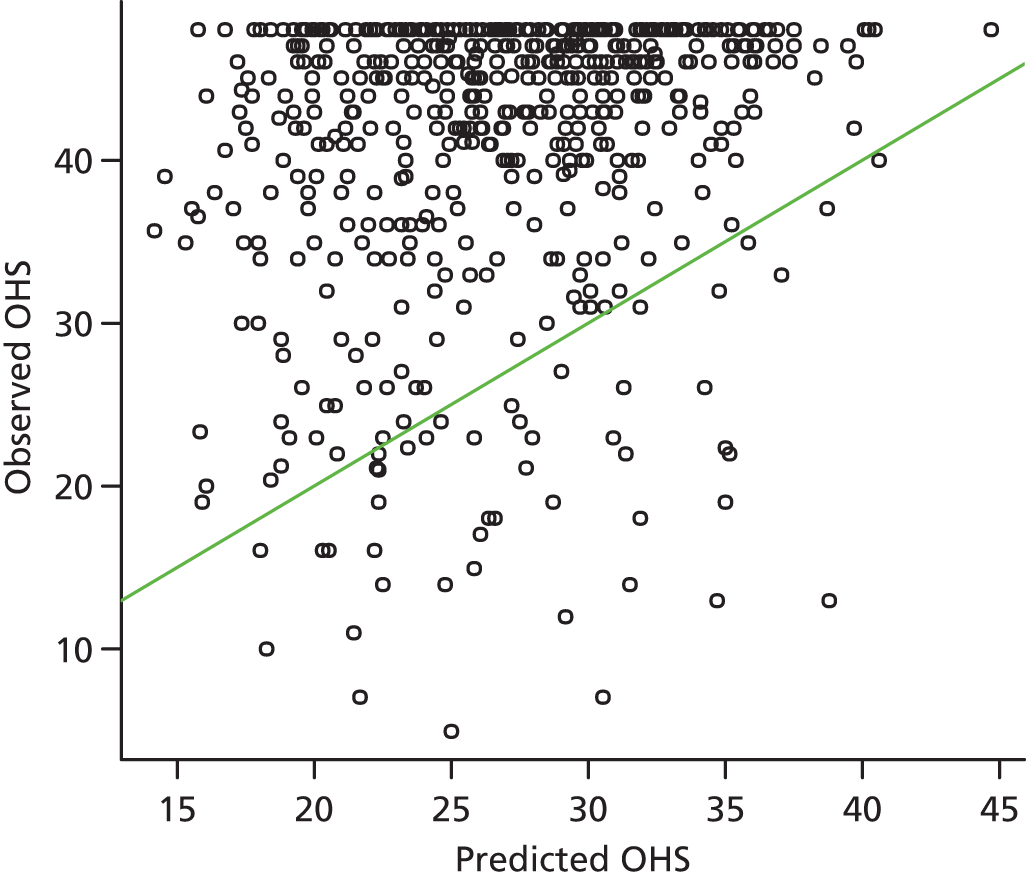
Figure 52 shows the density plots of the observed and predicted scores using the complete records. The distribution of the observed scores is bimodal, whereas the distribution of the predicted scores is unimodal.
FIGURE 52.
Density plot of the (a) observed; and (b) predicted scores for the hip data using the complete data set.


We also examined the model performance using imputed data (1098 records). The discrimination and calibration of the predicted model was worse than that using the complete data set. Table 85 shows the observed and predicted OHSs at 12 months by tenths of predicted OHS. The R2 value of the model using imputed data was 3.4%, which was smaller than that using the complete data set (4.2%). This result is supported by the calibration plot in Figure 53.
Tenths of the predicted OHS | OHS | ||
---|---|---|---|
Observed | Predicted | Observed : predicted | |
9.04–16.6 | 38.04 | 14.53 | 2.62 |
16.6–18.8 | 39.79 | 17.71 | 2.25 |
18.8–20.6 | 39.03 | 19.72 | 1.98 |
20.6–22.5 | 40.32 | 21.55 | 1.87 |
22.5–24.4 | 41.44 | 23.40 | 1.77 |
24.4–26.2 | 41.63 | 25.22 | 1.65 |
26.2–27.9 | 41.97 | 27.04 | 1.55 |
27.9–29.7 | 41.58 | 28.79 | 1.44 |
29.7–32.2 | 43.28 | 30.90 | 1.40 |
32.2–42.0 | 43.59 | 34.26 | 1.27 |
R 2 | 0.034 |
FIGURE 53.
Calibration plot of the validated hip model using the imputed data.

Statistical analysis of the incremental value of the hip model predictors
We examined the performance of the hip prediction model by evaluating the improvement in its predictive ability when additional predictors (listed below) that were not initially considered for inclusion in the model were added:
-
C-reactive protein (mg/l) [bi_preop_crp_mg_lt]
-
K/L grades to assess OA severity [xr_preop_kellgren_and_lawrence].
We used the model based on the complete data set, as its validation performance was better than the model based on the imputed data set.
An OLS regression was predicted to the COASt data set using variables identified as appropriate surrogates for the variables in the original data. The C-reactive protein and K/L grades variables were added singly and jointly to see if they would improve the model. Table 86 shows the uncorrected and corrected multiple R2 values. The corrected R2 was based on 300 bootstrap samples used to estimate optimism because of possible overfitting.
R 2 | Original variables | C-reactive protein added | K/L grades added | C-reactive protein and K/L grades added |
---|---|---|---|---|
Uncorrected | 0.144 | 0.144 | 0.164 | 0.164 |
Corrected | 0.110 | 0.108 | 0.125 | 0.125 |
As noted from Figure 48a, the correlation between the C-reactive protein variable and the outcome was not significant (r = 0.043, p = 0.317). It added noise and did not improve the model (corrected R2 = 0.108). The K/L grades variable was significantly associated with the outcome variable and improved the model’s predictive performance from 0.110 to 0.125. Adding both new variables did not improve the model’s predictive ability further.
We therefore formally assessed a model that included the additional K/L grades variable:
where:
Table 87 shows the model calibration and discrimination. The R2 value (12.5%) showed a significant improvement over the R2 from the validation process (4.2%), but was lower than the original R2 (23.1%). In terms of calibration, the ratio of the observed and predicted scores within the tenth of the predicted OHSs was close to 1 within each of the tenths, showing good agreement between the scores across all tenths of predicted OHS. In general, this model did not improve the performance of the original model developed by developed by Judge et al. 138 Figure 54 shows the density plot of the predicted ‘improved’ hip model. The distribution of the predicted scores behaved better than the predicted scores in Figure 52.
Tenths of the predicted OHS | OHS | ||
---|---|---|---|
Observed | Predicted | Observed : predicted | |
31.2–36.3 | 34.16 | 34.54 | 0.99 |
36.3–38 | 35.92 | 37.20 | 0.97 |
38–39.1 | 38.23 | 38.51 | 0.99 |
39.1–40.3 | 40.95 | 39.71 | 1.03 |
40.3–41.2 | 40.89 | 40.77 | 1.00 |
41.2–42.2 | 43.01 | 41.65 | 1.03 |
42.2–43.2 | 43.40 | 42.68 | 1.02 |
43.2–44.2 | 42.67 | 43.67 | 0.98 |
44.2–45.5 | 44.42 | 44.80 | 0.99 |
45.5–49.7 | 46.66 | 46.97 | 0.99 |
R 2 | 0.125 |
FIGURE 54.
Density plot of the predicted scores for the improved model using the hip data.

In terms of calibration, the ratio of the observed and predicted scores within the tenth of the predicted OHSs was close to 1 within each of the tenths, showing good agreement between the scores across all tenths of predicted OHS. In general, this model did not improve the performance of the original model developed by Judge et al. 138 Figure 54 shows the density plot of the predicted ‘improved’ hip model. The distribution of the predicted scores behaved better than the predicted scores (see Figure 52).
Table 88 shows the associations between the outcome variable and the variables in the improved OHS COASt model. The variables education attainment, superomedial, arthritis in other joints, stem size and posterior surgical approach did not have significant effects at 0.157. Backward selection with a p-value threshold of 0.157 was used to choose variables for this model.
Predictor variables (reference category) | Overall | Imputed |
---|---|---|
Complete case (n = 550) (standard error) | Hip data (n = 1098) (standard error) | |
Intercept | 28.79 | 36.31 |
Baseline (OHS) | 0.28 (0.05)* | 0.23 (0.03) * |
Age (years) | 0.01 (0.04) | –0.04 (0.02)** |
BMI (kg/m2) | –0.25 (0.08)* | –0.20 (0.05)* |
Education | 0.42 (0.22) | 0.20 (0.15) |
SF-36 mental health score | –1.43 (0.87)** | –1.67 (0.50)* |
Superomediala | 0.22 (0.29) | 0.23 (0.19) |
Arthritis in other joints | 0.27 (0.80) | –0.57 (0.54) |
Surgery in other joints | –0.59 (1.39) | –1.61(0.91)** |
Posterior | 1.10 (0.88) | 0.44 (0.61) |
Stem size (mm offset) | 0.09 (0.09) | 0.11 (0.06) |
K/L grade | 2.04 (0.57)* | 1.89 (0.37)* |
Part 2: Clinical Outcomes in Arthroplasty Study knee model
Numerical description of the knee Clinical Outcomes in Arthroplasty Study data
Table 89 shows the variables that were required for validating the COASt knee model. The two incomplete data sets contained 608 and 1025 records, and both suffered from a large number of missing data. The patient factors included the baseline and outcome OKSs, age, sex, IMD 200453 score, BMI and a surrogate for the SF-12 mental health score. Three clinical factors were included: (1) the presence of other conditions affecting mobility; (2) a binary variable measuring whether or not knee surgery had previously occurred; and (3) the ASA grade, which categorises patients as fit and healthy, asymptomatic on restriction or symptomatic with minimal/severe restriction. The presence of other conditions affecting mobility was collected as a binary variable that measured whether or not a patient suffered from RA, gout, inflammatory joint disease, avascular necrosis, Paget’s disease, childhood conditions, multiple sclerosis, Parkinson’s, neurological problems, back pain, sciatica or joint contracture. Three surgical factors were also included in the model, measuring the presence of a fixed flexion deformity, preoperative deformity (derived from the American Knee Society Score Left Knee alignment and classified as normal, valgus or varus) and the state of the ACL (intact, damaged or absent). The C-reactive protein variable was, again, used to assess possible model improvements.
Variable | Data set | |||
---|---|---|---|---|
Incomplete (N = 608) | Entire (N = 1025) | |||
Outcome | Incomplete data set | % missing | Entire data set | % missing |
Mean OKS (SD) at 12 months | 37.46 (9.74) | 6.1 | 37.49 (9.64) | 6.6 |
Predictors | ||||
Mean baseline OKS (SD) | 20.31 (7.69) | 3.1 | 9.33 (6.68) | 7.8 |
Mean age in year (SD) | 68.71 (9.12) | 0 | 68.78 (9.36) | 0 |
Sex, n (%) | 0 | 0 | ||
Male | 274 (45.1) | 456 (44.5) | ||
Female | 334 (54.9) | 569 (55.5) | ||
Mean IMD 200453 score (SD) | 11.62 (8.24) | 0 | 11.88 (8.63) | 0.3 |
Mean BMI (kg/m2) (SD) | 30.52 (5.43) | 0 | 30.34 (5.27) | 0 |
SF-12 (surrogate for mental health), n (%) | 2.3 | 11.6 | ||
Yes | 141 (23.2) | 381 (37.2) | ||
No | 453 (74.5) | 525 (51.2) | ||
ASA grade, n (%) | 0.8 | 11.9 | ||
Fit | 94 (15.5) | 131 (12.8) | ||
Asymptomatic | 421 (69.2) | 633 (61.8) | ||
Symptomatic | 88 (14.5) | 88 (8.6) | ||
Factors affecting mobility, n (%) | 6.4 | 6.5 | ||
Yes | 340 (55.9) | 537 (52.4) | ||
No | 229 (37.7) | 421 (41.1) | ||
Previous knee surgery, n (%) | 2.8 | 12.4 | ||
Yes | 85 (14.0) | 122 (11.9) | ||
No | 506 (83.2) | 776 (75.7) | ||
Fixed flexion deformity, n (%) | 48.9 | 34.9 | ||
Yes | 176 (29.0) | 489 (47.7) | ||
No | 135 (22.2) | 178 (17.4) | ||
Preoperative deformity, n (%) | 51.6 | 36.5 | ||
Normal | 75 (12.3) | 102 (10.0) | ||
Valgus | 93 (15.3) | 105 (10.2) | ||
Varus | 126 (20.7) | 444 (43.3) | ||
ACL, n (%) | 8.9 | 34.7 | ||
Intact | 419 (68.9) | 513 (50.1) | ||
Damage | 123 (20.2) | 141 (13.8) | ||
Absent | 12 (2.0) | 15 (1.5) | ||
New variable, n (%) | ||||
Mean C-reactive protein (mg/l) (SD) | 6.13 (12.37) | 25.7 | 5.90 (11.27) | 37.6 |
We also explored additional general patient factors, shown in Table 90. Again, the COASt knee data set with 1025 records had more missing data than the 608-record data set. Our numerical exploration of the arthritis and examination variables, questionnaire scores and 12-month follow-up measures in the knee data are presented in Appendix 13, Tables 107 and 108.
Variable | Data set, n (%) | |||
---|---|---|---|---|
Incomplete (N = 608) | Entire (N = 1025) | |||
Value | % missing | Value | % missing | |
Smoking | 0.2 | 9.3 | ||
Yes | 38 (6.3) | 59 (5.8) | ||
No | 569 (93.6) | 871 (85.0) | ||
Alcoholism | 1.0 | 9.8 | ||
Yes | 8 (1.3) | 14 (1.4) | ||
No | 594 (97.7) | 911 (88.9) | ||
Anxiety | 1.0 | 10.1 | ||
Yes | 64 (10.5) | 104 (10.2) | ||
No | 538 (88.5) | 818 (79.8) | ||
Depression | 1.3 | 10.2 | ||
Yes | 124 (20.4) | 191 (18.6) | ||
No | 476 (78.3) | 730 (71.2) | ||
Fractures | 1.2 | 10.0 | ||
Yes | 264 (43.4) | 392 (38.2) | ||
No | 337 (55.4) | 531 (51.8) | ||
Back pain | 1.7 | 10.4 | ||
Yes | 189 (31.1) | 307 (30.0) | ||
No | 409 (67.3) | 611 (59.6) | ||
OA | 4.6 | 13.1 | ||
Yes | 413 (67.9) | 644 (62.8) | ||
No | 167 (27.5) | 247 (24.1) | ||
RA | 3.3 | 11.6 | ||
Yes | 61 (10.0) | 93 (9.1) | ||
No | 527 (86.7) | 813 (79.3) | ||
Diabetes | 0.5 | 9.6 | ||
Yes | 61 (10.0) | 99 (9.7) | ||
No | 544 (89.5) | 828 (80.8) | ||
Hypertension | 1.0 | 10.5 | ||
Yes | 316 (52.0) | 482 (47.0) | ||
No | 286 (47.0) | 435 (42.4) | ||
Gout | 3.0 | 11.9 | ||
Yes | 34 (5.6) | 63 (6.2) | ||
No | 556 (91.5) | 840 (82.0) | ||
Osteoporosis | 3.6 | 12.2 | ||
Yes | 47 (78.3) | 78 (7.6) | ||
No | 539 (88.7) | 822 (80.2) | ||
High cholesterol | 2.6 | 11.5 | ||
Yes | 189 (31.1) | 292 (28.5) | ||
No | 403 (66.3) | 615 (60) | ||
Bowel | 3.5 | 12.7 | ||
Yes | 159 (26.2) | 260 (25.4) | ||
No | 428 (70.4) | 635 (62.0) | ||
Renal | 1.3 | 10.0 | ||
Yes | 32 (5.3) | 54 (5.3) | ||
No | 568 (93.4) | 568 (55.4) | ||
Ethnicity | 2.1 | 8.0 | ||
British | 581 (97.7) | 895 (87.3) | ||
Irish | 5 (0.8) | 6 (0.6) | ||
Any other white | 1 (0.2) | 8 (0.8) | ||
White and black Caribbean | 1 (0.1) | |||
White and black African | 1 (0.1) | |||
White and Asian | 1 (0.2) | 1 (0.1) | ||
Any other mixed background | 2 (0.3) | 7 (0.7) | ||
Indian | 4 (0.7) | 11 (1.1) | ||
Pakistani | 1 (0.1) | |||
Bangladeshi | 2 (0.2) | |||
Any other Asian background | 1 (0.2) | 6 (0.6) | ||
Caribbean | 2 (0.2) | |||
Chinese | – | 1 (0.1) | ||
Any other ethnic group | – | 1 (0.1) |
We imputed the two data sets using MICE, as described in Methods. Figure 55 shows the correlation matrix for the outcome variable and some of the continuous variables in the knee data set. The correlation between the outcome and C-reactive protein variables was not significant using the 608-record data set, but was significant using the 1025-record data set. This difference may have been caused by the high degree of missingness in these variables. The correlations between the baseline OKS and IMD 200453 score and C-reactive protein variables were also not significant using the 608-record data set, but were significant using the 1025-record data set. This difference may have been caused by the degree of missing data in the variables and, more importantly, by the distribution of the baseline OKS variable in the two data sets (the baseline OKS variable was 20.31 units and 9.33 units for the 608- and 1025-record data sets, respectively; see Tables 84 and 85).
FIGURE 55.
Correlation matrix plot for the incomplete knee data sets with (a) 608 records; and (b) 1025 records.
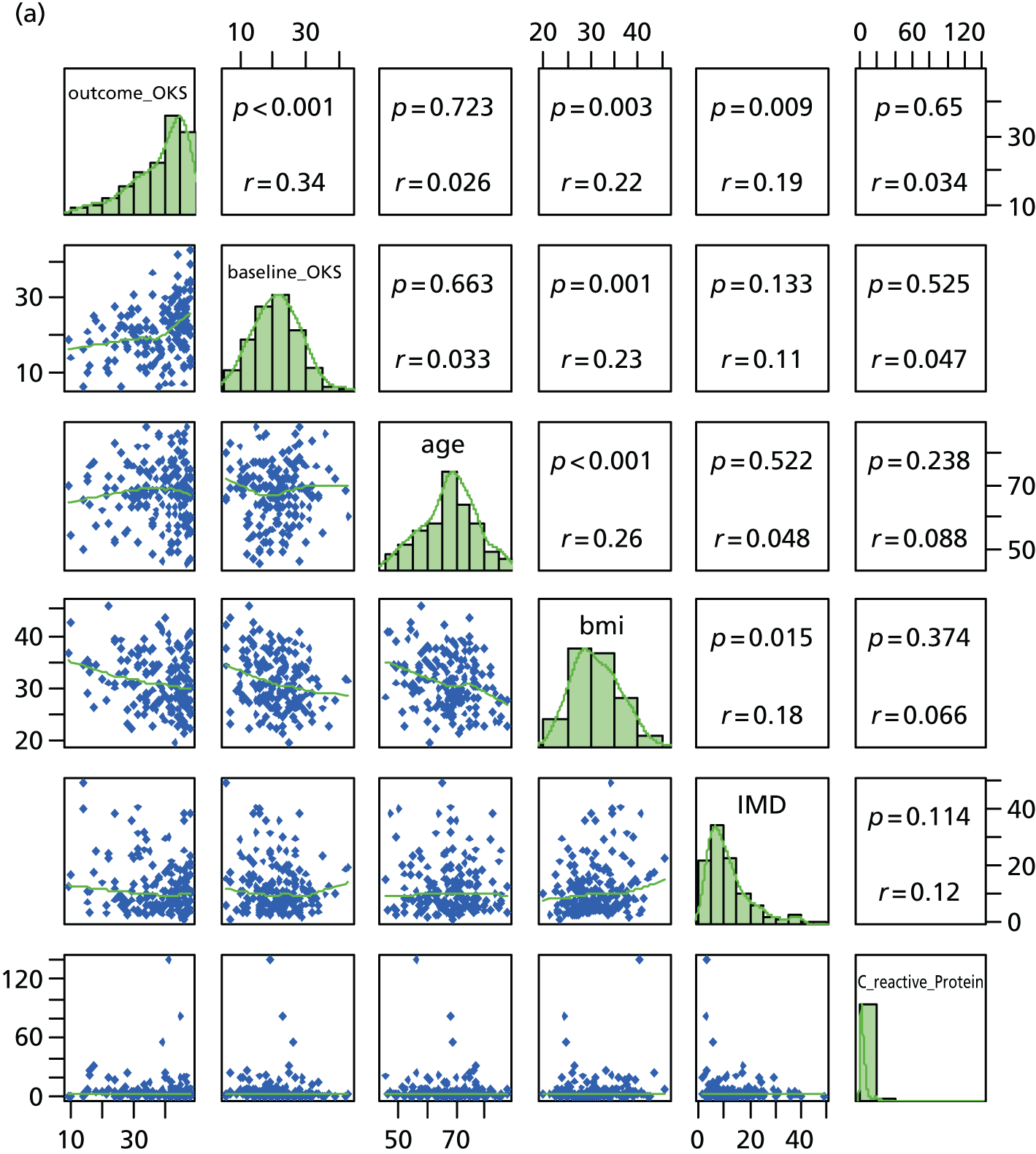
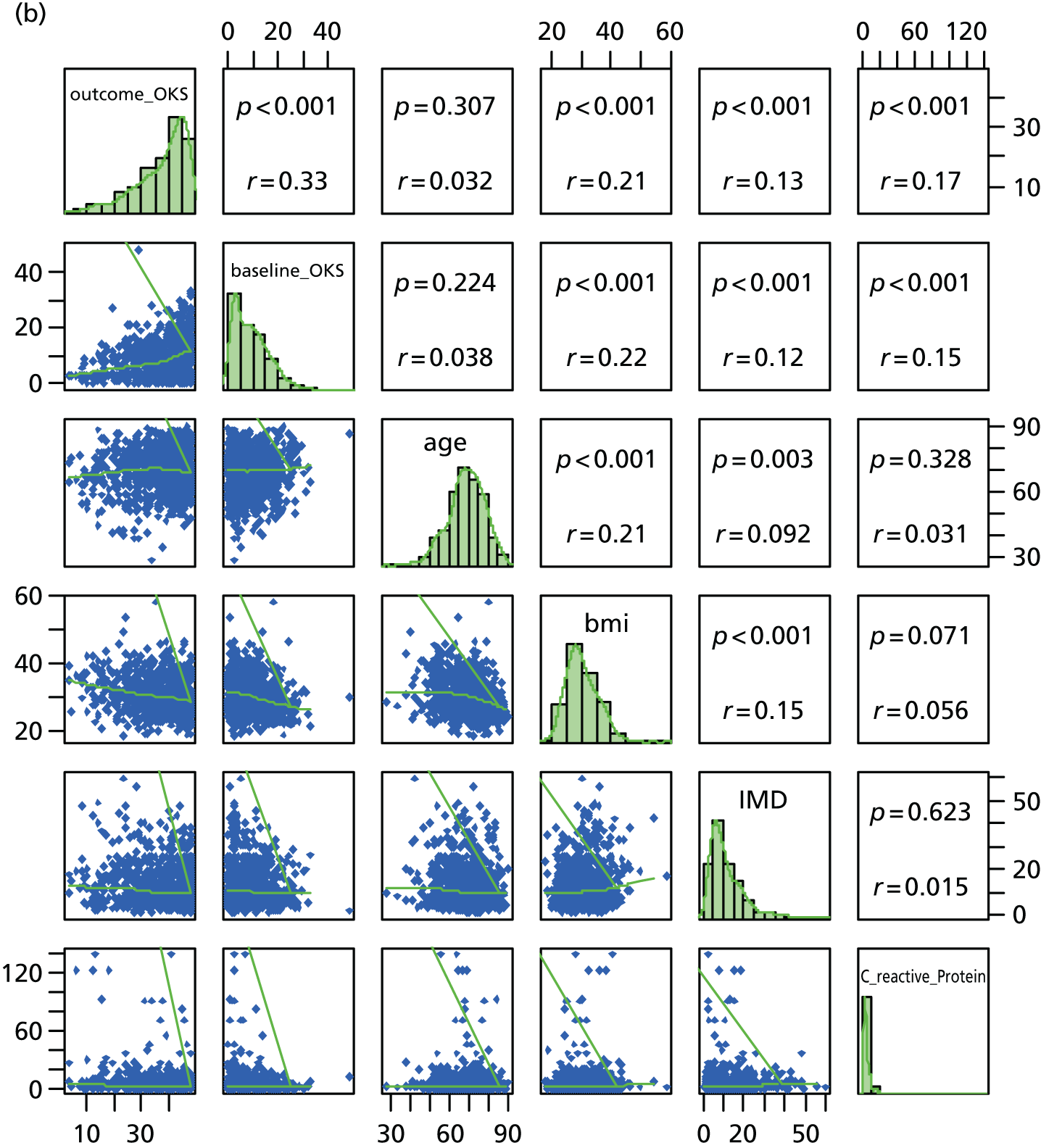
Statistical analysis of knee replacement surgery
We validated the model using both incomplete knee data sets. To improve the statistical power of the validation, we imputed the missing values using MICE and predictive mean matching.
The predicted 12-month OKS is:
where:
Details of the model, including the variable interpretations, can be found in Sánchez-Santos et al. 142
These variables were identified in the validation data set. We computed the baseline OKS using the self-assessment questions sa_OKS_1 to sa_OKS_12. The model development data set had value ranges of 0 to 4 for these variables, whereas the COASt data set had value ranges of 1–5. We therefore rescaled the composite score as 60 – (sa_OKS_1 +sa_OKS2 +. . .+ sa_OKS_12), allowing the score to lie in the range 0–48. We formed appropriate subvariables for the age category in the original model. The categorical variable groups in the model were formed using the ‘factor’ function in the R statistical software package (The R Foundation for Statistical Computing, Vienna, Austria) and included in the R base function ‘formula’. We gave the variable categories not included in the model zero coefficients during validation.
The SF-12 questionnaire score was used to measure mental health in the model development data set. However, this score was not collected in COASt. We used a surrogate score that assigned a positive value if a patient reported being treated for anxiety or depression, or scored 2 or 3 on the mental health component of the EQ-5D.
The model performance was assessed using calibration and discrimination (Table 91), as described in the methodology. Model calibration was generally poor and was worse in the lower deciles of the OKS. The model had good discriminatory ability (R2 = 14.1%) as the original model had a discriminatory ability of 20.2%.
Tenths of the predicted OKS | OKS | ||
---|---|---|---|
Observed | Predicted | Observed : predicted | |
10.8–20.9 | 29.63 | 18.39 | 1.61 |
20.9–23.6 | 35.89 | 22.51 | 1.59 |
23.6–26.3 | 34.67 | 25.04 | 1.39 |
26.3–28.9 | 35.89 | 27.48 | 1.31 |
28.9–31 | 38.14 | 30.08 | 1.27 |
31–32.8 | 37.42 | 31.94 | 1.17 |
32.8–34.7 | 37.92 | 33.77 | 1.12 |
34.7–36.9 | 41.11 | 35.79 | 1.15 |
36.9–39.6 | 40.40 | 38.20 | 1.06 |
39.6–51 | 44.10 | 42.39 | 1.04 |
R 2 | 0.141 |
We also examined the model performance using the 1025-record data set (Table 92). The calibration and the discrimination measures were poorer than the data set with 608 records.
Tenths of the predicted OKS | OKS | ||
---|---|---|---|
Observed | Predicted | Observed : predicted | |
6.17–15 | 32.60 | 12.29 | 2.65 |
15–17.8 | 33.65 | 16.55 | 2.03 |
17.8–19.8 | 35.15 | 18.73 | 1.88 |
19.8–21.5 | 35.73 | 20.70 | 1.73 |
21.5–23 | 36.99 | 22.23 | 1.66 |
23–24.7 | 39.58 | 23.86 | 1.66 |
24.7–26.2 | 37.94 | 25.41 | 1.49 |
26.2–28.3 | 39.97 | 27.29 | 1.47 |
28.3–31.2 | 40.79 | 29.63 | 1.38 |
31.2–52.3 | 43.01 | 34.49 | 1.25 |
R 2 | 0.103 |
The calibration plot in Figure 56 shows that the model performed better at the upper deciles. The model had good discriminatory ability (R2 = 14.1%); however, this is still less than the original model (R2 = 20.2%). As with the hip model, model calibration was also generally unacceptable, with the model generally predicting a worse outcome than seen in the observed data. This was particularly true for the lowest deciles of outcome, for which the predicted scores were very substantially less, and this is the area of greatest interest.
FIGURE 56.
Calibration plot of the validated knee model using the 608-record data set.
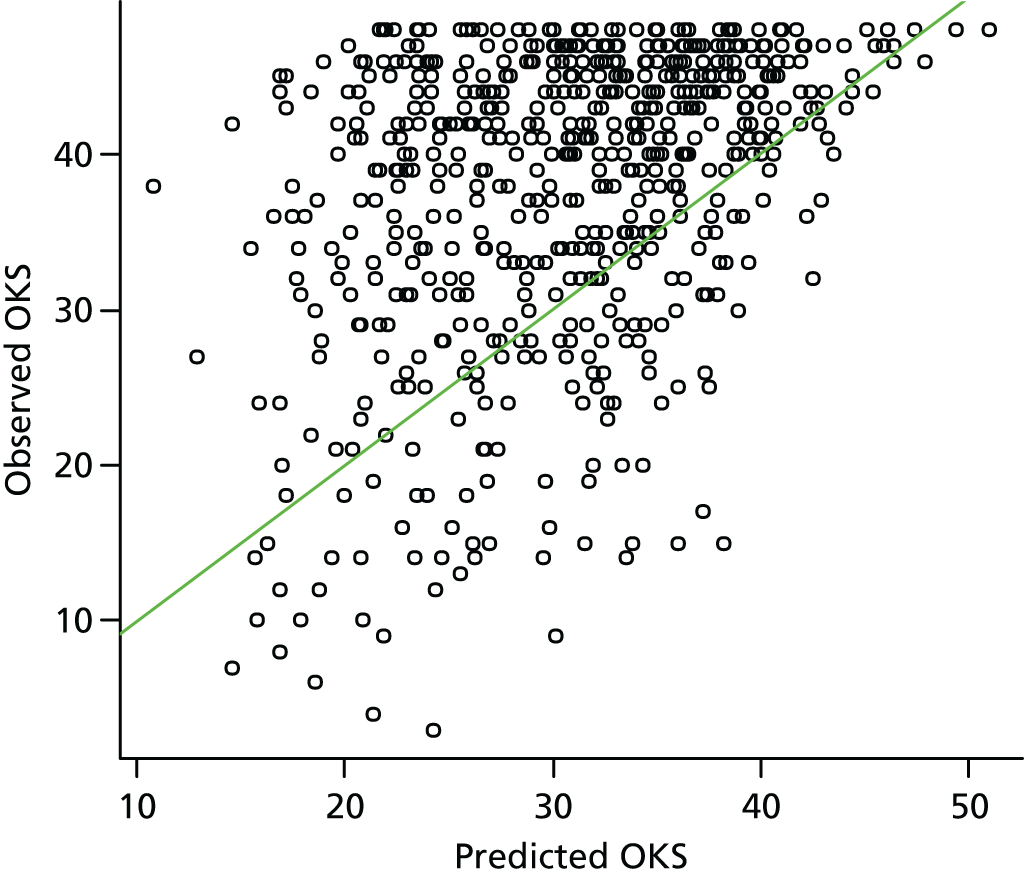
Figure 57 shows the density plots of the observed and predicted knee data scores, which form a mixture of two distributions with different variances.
FIGURE 57.
Density plot of the (a) observed; and (b) predicted OKS using the 608-record data set.
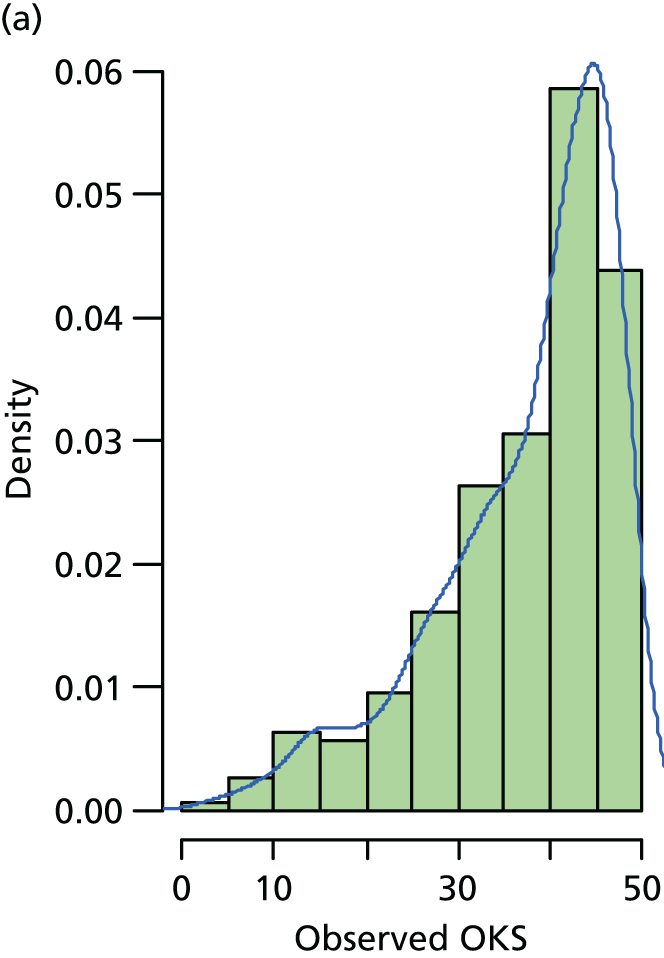
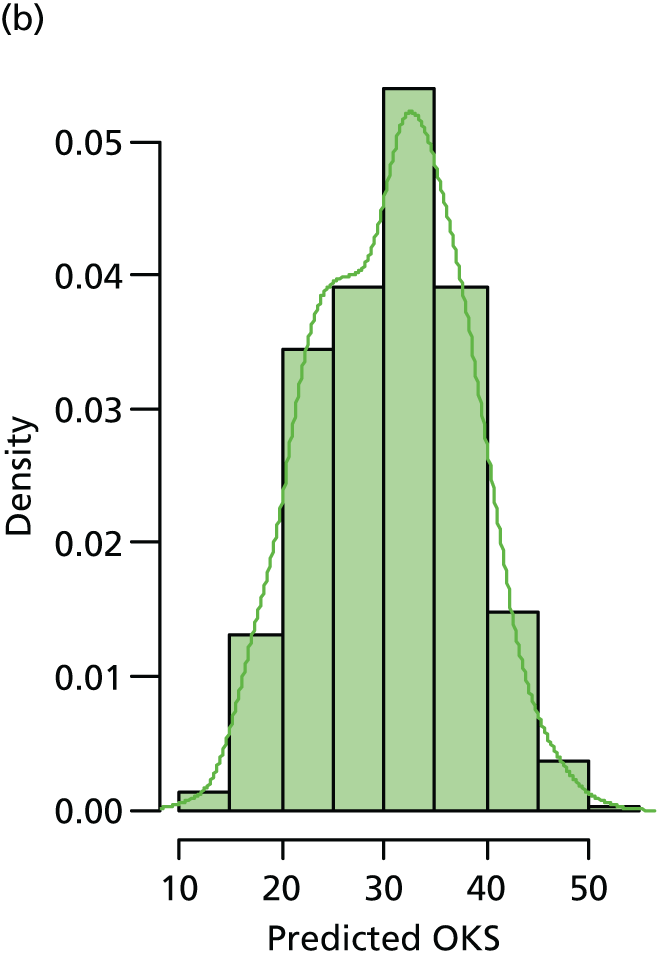
Figure 58 shows the baseline OKS and absolute change in OKS. We computed the change in score as a difference between the observed and baseline scores.
FIGURE 58.
Histograms of the (a) baseline; and (b) absolute change in the OKS, using the 608-record data set with imputed data.

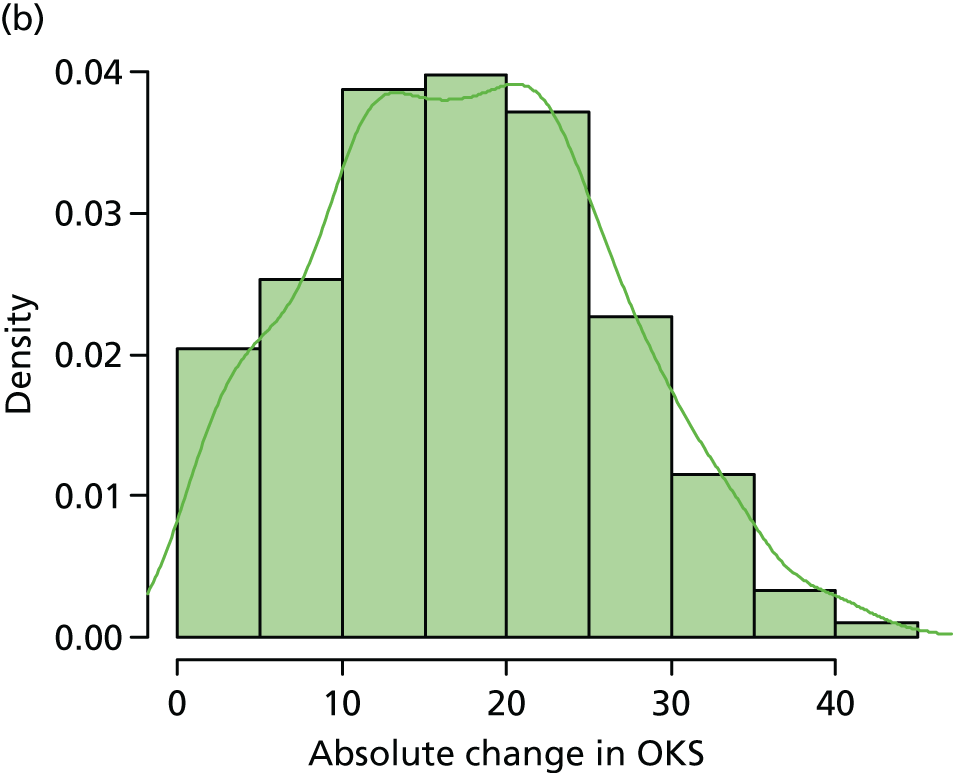
Statistical analysis of the incremental value of a knee data set predictor
As the model performed better with the 608-record data set than with the 102-record data set, we used the smaller data set to assess the possible benefits of adding an additional variable to the original knee model. We examined the model performance by evaluating the improvement in model predictions after adding the C-reactive protein variable (mg/l) [bi_preop_crp_mg_lt] as an additional predictor.
We predicted an OLS regression to the COASt knee data using the original variables and the C-reactive protein variable. We calculated the corrected R2 value using 300 bootstrap samples estimating optimism because of possible overfitting. Table 93, showing the corrected and uncorrected multiple R2 values, shows that the addition of the C-reactive protein variable improved the model’s discrimination ability from 21.6% to 23%. Both discrimination values were higher than those calculated for the original development data set. Table 94 shows that the model was well calibrated, as the ratio of the observed and predicted scores was close to 1 for all of the deciles. Figure 59 shows the density plot of the predicted scores for the improved knee model.
R 2 | Original variable | C-reactive protein variable added |
---|---|---|
Uncorrected | 0.259 | 0.277 |
Corrected | 0.216 | 0.230 |
Tenths of the predicted values | Mean score | ||
---|---|---|---|
Observed | Predicted | Observed : predicted | |
17.6–31 | 26.71 | 28.51 | 0.94 |
31–33.3 | 33.02 | 32.29 | 1.02 |
33.3–34.9 | 33.14 | 34.12 | 0.97 |
34.9–36.5 | 36.85 | 35.89 | 1.03 |
36.5–37.6 | 37.69 | 36.98 | 1.02 |
37.6–39.1 | 39.03 | 38.33 | 1.02 |
39.1–40.6 | 41.43 | 39.71 | 1.04 |
40.6–41.9 | 41.52 | 41.29 | 1.01 |
41.9–43.7 | 41.75 | 42.83 | 0.98 |
43.7–52.4 | 44.43 | 45.62 | 0.97 |
R 2 | 0.230 |
FIGURE 59.
Density plot of the predicted scores for the improved knee model.
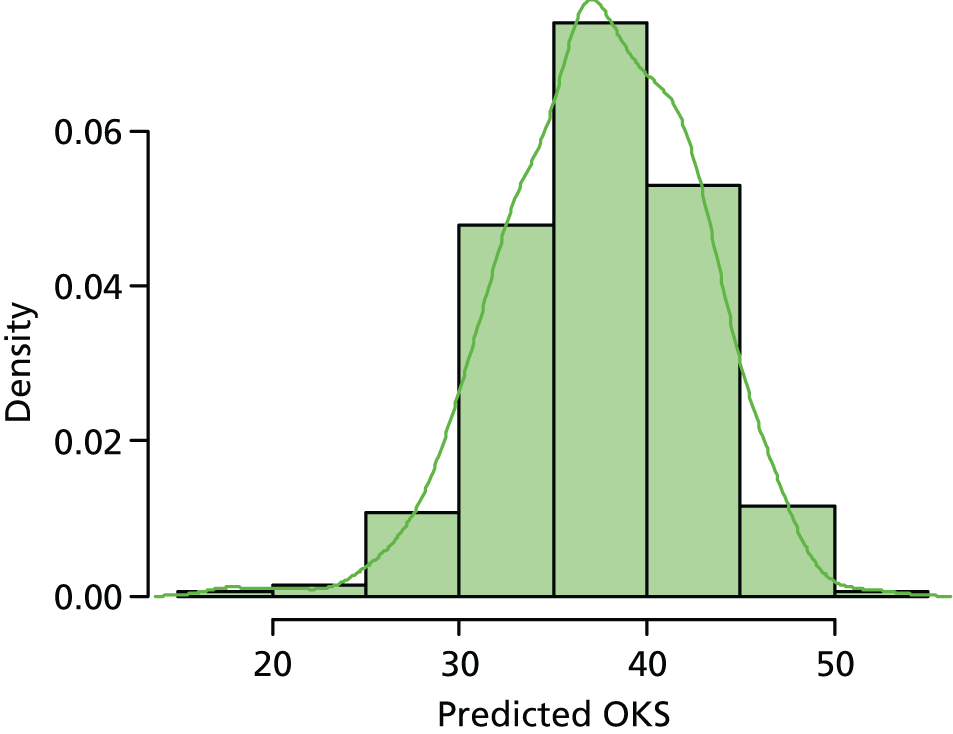
The improved predicted OKS model is:
where:
Appendix 13, Table 120, shows the association between the variables in the improved OKS COASt model and the outcome. Sex and the IMD 200453 score did not have a significant effect at 0.157. Backward selection with a p–value threshold of 0.157 was used to select the variables retained in the knee model.
Absolute differences between baseline and the 12-month follow-up Oxford scores
Table 95 shows the (absolute) difference between the mean score at baseline and at the 12-month follow-up for both the hip and the knee data sets. The average score showed improvement in the outcome of the patients in the complete record data sets and the entire data sets. The baseline average score for the entire knee data set is 9.33 units, which is far smaller than its counterparts in the complete-record data set (20.31 units).
OHS/OKS | Mean score | Absolute difference | |
---|---|---|---|
Baseline | 12-month follow-up | ||
Complete OHS data with 550 records and OKS with 608 records | |||
OHS | 18.63 | 41.06 | 22.43 |
OKS | 20.31 | 37.46 | 17.15 |
Entire data sets | |||
OHS | 19.35 | 41.20 | 21.85 |
OKS | 9.33 | 37.49 | 28.16 |
Patient expectation and outcome
Table 96 shows patients’ expectations and outcomes. For both the complete and the entire data sets, and for the hip and the knee scores, patients were very satisfied with their outcomes. The percentage of dissatisfied patients ranges between 1.8% and 2.0%.
Joint | Data set, n (%) | |
---|---|---|
Complete | Entire | |
Hip |
|
|
Knee |
|
|
Discussion
This work package evaluated the performance of models produced in work package 2, designed to predict PROMs following THR and TKR at 12 months. In order to do this, we recruited a large cohort of patients from two hospitals with a wide variety of data and samples collected. This is the most comprehensive cohort of arthroplasty patients collected to date. Neither model performed well in the validation cohort. However, when the variables from the models were used to produce a new model within the validation cohort, the performance improved, especially for the knee model. However, the models still did not reach the same levels of performance as the development models. Both models performed poorly in the lower deciles of outcome, which is unfortunately the area in which, for clinical utility, they would need to perform well.
There are many reasons why the models did not perform well in the validation cohort, which include the difference in patient characteristics, the different surgical techniques and the implants used in the development and the validation cohorts, the degree of imputation used in both the development and validation cohort, and the exact definition of the variables used in the development and validation cohorts.
When accessing the performance of the predictive model in a new cohort, it is extremely important to evaluate the comparability of the two cohorts in question. The model can fail to perform well in a new cohort because of limitations of the model itself or, more commonly, because of significant differences in the new population. If the population is identical, then a poor performance would imply a failure of validation of the model; however, if the population is significantly different, this could reflect a failure of transportation. There are several important differences between the development and the validation cohorts in the study. In the case of the hip replacement model, the developmental cohorts used were EUROHIP and EPOS; EUROHIP is a pan-European cohort and EPOS was a RCT, both of which will introduce potential issues related to case mix. Furthermore, the development cohorts were collected between 10 and 20 years ago, when surgical implants and techniques were very different than those used today and will vary across the contributing European sites in the EUROHIP cohort. In addition, patient selection has also changed, with increasing provision of THRs to the older population with more comorbidities and also to younger, more active patients. This is demonstrated by the validation cohort having a higher BMI, being older than the EUROHIP cohort but younger than the EPOS cohort and having a higher proportion of patients with severe radiographic OA (K/L grade 4). These factors would certainly mean that the development and validation cohorts were very different in nature, which may explain some of the reduced performance of the model.
To produce the knee predictive model, we used the data from KAT, which was a RCT of knee replacement and would have involved a different population from those attending the two hospitals through the NHS system. This is demonstrated by the validation cohort being younger and including a lower proportion of patients with a valgus deformity, a lower proportion of patients with an ASA grade of symptomatic and a lower proportion of patients having had previous knee surgery. Furthermore, because of the Oxford site’s expertise in UKR, a large proportion of patients there underwent UKR, an operation often performed on younger male patients with more mild OA. The same considerations of temporal changes, patient selection and implant technology apply as they did with the THR.
Significant degrees of imputation were required for both the development and validation cohorts because of missing data. This may have affected the performance of the model, as the imputation process may have produced inaccurate distributions of missing variables. There were substantially more missing data in the development cohorts than in the validation cohort. The most important issue to consider is the reason for missing data. If data were missing completely at random or missing at random, then the imputation would be relatively unbiased, but may still reduce the statistical power of the model. If, however, the absence was related to any of the explanatory variables in the model or the outcome, the imputed data could significantly affect the performance of the model. Although there were substantial missing data in the EPOS and EUROHIP cohorts, this was generally because the variables were not collected and was therefore these data were likely to be missing completely at random. However, in the case of some of the main variables, up to 10% of missing data may have been missing not at random, which may have been more problematic. Furthermore, in the validation cohort, the hip model performed less well than the knee model and the development cohorts for the hip model were more reliant on imputation than the knee model, supporting the potential role of imputation in explaining the poor performance of the model.
It was interesting to note that, in the validation cohort, the performance of the model was worse when using the entire data set, which included substantial imputation, than when using the complete cohort set, which relied less on imputed variables. Furthermore, slightly more variables were missing for the hip cohort than for the knee cohort. Both of these facts support the role of imputation in the poor performance of the models. We feel that the imputation in the developmental cohorts is probably the main factor in the poor performance of the models.
Another potential issue was the use of surrogate variables in the validation cohort. In designing the questionnaire for the validation cohort, we were mindful to use the most recent validated questionnaires to measure the traits of interest. Unfortunately, many of the development cohorts used outdated or non-validated questions and questionnaires and, as such, the two were not directly comparable between the two cohorts. An example was the mental health score: the hip validation tool used the mental component summary score of the SF-36 questionnaire, the knee validation tool used the SF-12 questionnaire and the validation cohort used a surrogate variable using self-reported depression, anxiety or use of medications for anxiety and depression. For the clinical variables identified in the development cohorts, there were few data available on the method of collection and definitions used, meaning that there was significant potential for misclassification between the development and validation cohorts.
It was somewhat disappointing to discover that the models in the validation cohorts performed particularly poorly in the lower deciles of outcome of both THR and TKR. This is the area in which the tools will be particularly useful if used to help patients and clinicians to make informed choices about the relative pros and cons of surgery. The exact reason for this is uncertain; however, because of the paucity of patients experiencing a poor outcome and the fact that some of these outcomes may be because of rare events (such as surgical mishap or very individualised specific factors, such as chronic pain syndrome), it is not surprising that they are difficult to predict. Hopefully when using the full data set of the validation cohort, we may be able to improve prediction within these lower deciles.
The addition of highly sensitive C-reactive protein did not add to the performance of the hip model. It did, however, add slightly to the performance of the knee model. This may reflect the greater range in inflammatory processes present in those patients undergoing knee replacement for OA, which is traditionally thought to have a higher inflammatory component than hip OA. The addition of radiographic severity, measured by the K/L scale, did add significantly to the performance of the hip replacement model. Both of these results are tantalising clues to the potential improvements in modelling that we may achieve in the future using the whole array of extra variables collected in the validation cohort. We are particularly interested in the role of vitamin D, urine collagen cross-links and BMD as markers of bone health and metabolism. We are currently performing these analyses and would hope to have provisional results in the near future. We will also run a complete new model using the whole validation cohort data with the hope of identifying novel predictive markers but also of improving the performance of the model. This would obviously require an external data set and we are in provisional discussions with the Geneva Arthroplasty Registry, which does collect very similar data to the validation cohort.
We also intend to follow the validation cohort going forward, to obtain annual follow-up scores for at least 5 and, hopefully, 10 years with a view to having long-term PROMs and also revision and complication rates. We feel this will significantly add to the current literature.
Conclusion
We demonstrated that the models produced in the development cohort did not perform well in the validation cohort and feel that a combination of different variable definitions, the degree of imputation used and the temporal changes in patient and implant selection would explain this. We hope that a new bespoke model produced in this cohort and validated in a contemporary Geneva Arthroplasty Registry will get around a number of these points and allow a model with much better performance to be produced.
Chapter 6 Summary of the programme
The overall aim of this programme was to design and implement a strategy for predicting patients at risk of poor functional outcome following lower limb joint arthroplasty, for use within the NHS.
The programme was divided into four work packages. Each work package had its own aims and designs, but all four were linked together to arrive to the final purpose of the programme. The schematic representation of the programme is illustrated in Figure 60.
FIGURE 60.
Summary of programme. WP, work programme.
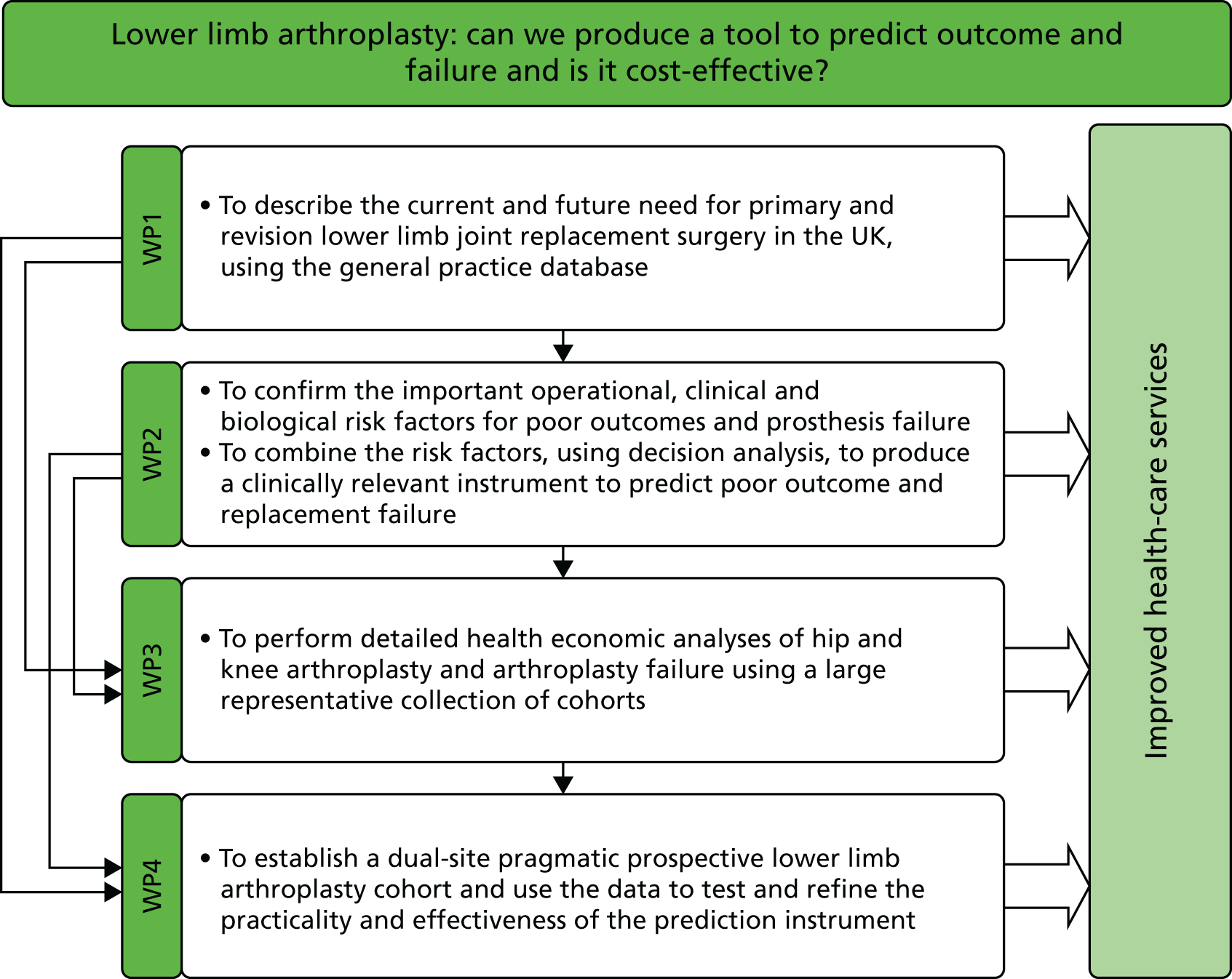
Work package 1
The aim of the work package was determined by the need to accurately quantify the rates of primary and revision lower limb arthroplasties in the UK in order to allocate health resources effectively. In addition to national data, it is important to explore data at a regional level. More important is the need to project the number of procedures likely to be required in the future. Previous attempts at projections had not accounted for the increase in age and levels of obesity in the population.
Our analysis using the CPRD database demonstrated that the rates for hip and knee arthroplasty have increased substantially over this period, but with different trends. The rates of THR have increased steadily, whereas the rates of TKR increased slowly initially but then rapidly since 2000, such that by 2006 the rates of both procedures were very similar. Women were 67% more likely to have THR and 45% more likely to have TKR performed than men. 67 Trends in UKR have also increased over the last decade, and the ratio of TKRs to UKRs fell from 250 : 1 in 1999 to 40 : 1 in 2006. The estimated numbers in 2006 were 74,800 for TKRs and 1800 for UKRs, with UKRs being performed on a younger age group than TKRs. We produced the lifetime risk of a TKR for 50-year-old men (8.1%) and women (10.8%), and THR for 50-year-old men (7.1%) and women (11.6%), which are substantially below the estimated lifetime risk for hip and knee OA. We feel that this is a valuable way of describing risk to patients.
Interrogating the CPRD data from 1991 to 2006, we found marked inter-regional differences in joint replacement rates in the UK. These were higher for hips than for knees and have not narrowed over time but, if anything, may have become more pronounced. The reason for these differences between the regions cannot be explained using this data set, but requires urgent investigation. Potential explanations include variation in medical indications and contraindications, personal and social perceptions of surgery, and the availability of orthopaedic services, both NHS and private.
We have demonstrated that the rates of revision surgery are low compared with those for primary operations but with very different temporal patterns. The rates of hip revision have remained essentially stable for the last 10 years, whereas for knee revision, the rates have increased substantially. This may partially reflect the recent increase in the rates of primary TKR but also the established techniques and prostheses for THR. We have demonstrated that increasing BMI is a risk factor for revision surgery for both the hip and knee. This is an important piece of information but it has to be considered along with the effect of BMI on PROMs and also of complications, for which the adverse effects of obesity on outcome are more obvious.
We have demonstrated that the numbers of THRs and TKRs performed are projected to increase dramatically over the next 20 years. The different methodologies used give very different estimates. For THR, we feel that the model using rates fixed at 2010 levels and varying BMI gives the most sensible estimate, and this suggests a figure of 95,877 THRs, a 34% increase in the number of procedures from 2015 to 2035. For TKR, we feel that the rates in 2010 do not represent a balance between need and provision, and that the rates will continue to rise. The real number required will therefore be greater than the fixed rates and varying BMI model (118,666), but less than the estimates produced using the log-linear model (1,219,362).
In this work package, we have estimated the current and future rates for lower limb arthroplasty, which should aid in the long-term planning of health-care resources for the UK. The number of procedures required will increase substantially over the next 20 years, especially those for revision arthroplasty. The increase in rates is driven predominantly by the increasing age and obesity levels in the population. These assumptions, particularly about rates of obesity, may be subject to change, particularly when considering the current NHS focus on obesity. The projected rates of TKR are less certain than those of THR because of the presumed need/provision gap.
In planning orthopaedic services, potentially one of the most cost-effective tools would be to stratify patients for knee and hip replacement surgeries in order to avoid operations in those who would have a poor outcome. This stratification necessitates the development of a statistical tool to predict poor outcomes for surgeries. Work package 2 aimed to develop the predictive tools for knee and hip separately.
Work package 2
In this work package we describe the predictors for poor patient-reported outcomes at 12 months for THR and TKR, and combine them into a statistical tool that could be used to identify patients with poor outcomes before lower limb replacement surgeries.
As there was no existing definition of poor outcome using the Oxford scores, we used existing cohorts from SWLEOC, with PROMs at 6, 12 and 24 months, to define values of the OHS and OKS that were associated with patient satisfaction with the operation at 12 months. We used two different statistical methods to identify the cut-off points: the ROC curve and the 75th percentile approach. The values were an OKS of 30 units for TKR and an OHS of 33 units for THR; however, a single score is not recommended as stratified analyses demonstrated varying values depending on age, sex and baseline score.
Using the above methodology, we identified a number of predictors of outcome for both THR and TKR, including preoperative Oxford scores, age, sex, BMI, deprivation index, indication for surgery, anxiety and depression, and radiographic variables. With the exception of the baseline Oxford score, the majority of the predictors were observed to have statistically significant, but clinically small, effects. Age had a variable association with PROMs, with worse outcomes in the youngest and oldest patients, whereas younger age was associated with a higher revision rate. Increasing BMI was associated with a higher rate of revision and, although it was associated with a poorer PROM, the effect size was very small, suggesting that it should not be a barrier to surgery. However, obese patients are at an increased risk of developing postoperative DVT, PE, wound infection and urinary tract infection, which needs to be considered when discussing the risks and benefits of surgery.
Preoperative pain/function was one of the strongest predictors of the outcome: better preoperative pain and function was associated with better outcomes, and vice versa. 32,44–47 Higher levels of deprivation were associated with worse patient outcomes following TKR. Higher attained educational level was associated with better postoperative reported outcomes following THR. Radiographic variables, specifically the pattern of joint space narrowing, were found to be a strong predictor of outcome for THR; patients with a superolateral pattern of joint space loss had better outcomes than those with medial, superomedial or concentric patterns. However, no associations were found between the radiographic severity of disease and the surgery outcome using K/L grade. Unfortunately, none of our extant TKR cohorts had routinely collected preoperative radiographic variables to analyse.
We have demonstrated, for the first time, that the use of bisphosphonates reduces the risk of revision knee and hip surgery by 46%. 248 We have now validated these findings in a Danish registry. Furthermore, hormone replacement therapy reduced the risk by 38% if used for at least 6 months postoperatively. 175,249 In addition, we have described an increased postoperative risk of fracture, which is prevented by bisphosphonate use.
Using our findings on predictors and previous literature reviews, we designed the predictive model for knee and hip separately. For these statistical tools we performed internal validation to test their calibration and discrimination ability. The hip predictive tool included age, sex, baseline OHS, BMI, education, SF-36, SF-36 mental component summary score, number of joints with OA, number of joints with surgery, radiographic pattern of OA and two surgical variables (femoral offset size and surgical approach). The model performed well with a corrected R2 of 23.1% and had good calibration with only slight overestimation of OHS in the lowest decile of outcome. We developed a tool to predict the outcomes of THR at 12 months after surgery. The tool provides the absolute change in OHS expressed as a percentage change.
The knee predictive tool included age, sex, baseline OKS, BMI, deprivation score, SF-12, SF-12 mental component summary score, ASA grade, other conditions affecting mobility, previous knee surgery, fixed flexion deformity, valgus/varus deformity at baseline and preoperative ACL state (intact yes/no). The model performed less well than the hip model with a corrected R2 of 20.2%; however, it had good calibration.
Having identified a number of predictors of outcome of both THR and TKR, we produced predictive models that were validated internally, but external validation of these predictive models is required in a NHS setting. This required us to establish a new prospective cohort with extensive baseline phenotyping of all patients (work package 4). We also need to assess the cost–utility of implementing this model in the NHS (work package 3).
Work package 3
Having developed an outcome prediction tool to preoperatively identify patients with poor outcomes after their THR and TKR, it is important to ascertain whether or not implementing the tool will be cost-effective in the health-care system and more importantly if it provides benefits to patients. In this work package we provided an economic evaluation of the predictive tools.
We developed a lifetime Markov model featuring two unique elements: it starts at the orthopaedic surgeon’s assessment and it distinguishes between two patient-reported outcome categories after primary and revision procedures. Although these features are not unique to other disease areas and interventions, they have not been combined in previous economic models of surgical interventions of OA patients. We highlight the preoperative starting point of the model and the split of postoperative health states because they allowed the analysis to focus on the hypothetical implementation of a prediction tool to guide the decision for surgery as well as the reduction of poor surgical outcomes. Transition probabilities for the model were obtained from expert elicitation, HES PROMs and the CPRD, EPOS, KAT and COASt cohorts (work package 4). For most cohorts available to us, EQ-5D data were missing, but Oxford scores were available. We therefore used several econometric models to map the OHS on to the EQ-5D index to enable health utilities to be obtained from all cohorts. Procedure costs, as well as primary care costs, were obtained from NHS and CPRD data, respectively. This research benefited from using the best available sources of data to populate a cost-effectiveness model. The only source of data not based on patient-level records was an expert elicitation exercise reported in a fully comprehensive manner. Apart from this, all other sources of data consisted of patient-level data sets with the most appropriate, representative and up-to-date information on the probabilities, health utility and resource use associated to THRs in the UK, both before and after the operation.
The level of detail provided by the above data sources allowed the development of model parameter values for patient subgroups by age and sex. This made it possible not only to present results separately by these subgroups but, critically, also to adjust all parameter values in the model so that not only death rates but almost all other parameters changed in the simulation as patients became older.
At 12 months post surgery, the EQ-5D scores increased substantially for both hip and knee replacement operations (0.44 and 0.32 units, respectively). Even patients defined as having poor outcomes, using the criteria defined in this programme, exhibited a substantial improvement in scores (0.28 and 0.19 units, respectively). Thus, with the cost of surgery being £4000 and £6000, respectively, the operations are, on average, clearly cost-effective interventions. This set a high hurdle for the predictive tool to be a cost-effective tool. The outcome prediction tool for THRs and TKRs developed did, as intended, reduce the number and proportion of unsatisfactory and poor outcomes after the operation, saving NHS resources in the process. However, the tool would do so at the cost of keeping from surgery a number of patients who would otherwise experience a significant improvement in OHS and HRQoL, meaning that the tool would also produce fewer QALYs than current practice.
The highest savings per QALY forgone were reported from the oldest patient subgroups (men and women aged ≥ 80 years), with a reported ICER around £1200 per QALY for THRs. This would probably not be a cost-effective alternative for the NHS. Keeping patients from surgery, therefore, appears unlikely to be cost-effective for any tool applied to such a highly successful operation, unless the tool is extremely sensitive and specific, to a level that the one assessed here appears not to reach.
Our economic evaluation demonstrated that both THR and TKR are highly effective operations associated with substantial health utility gain. Within the area of health care, these are achieved at a relatively low cost, making this a cost-effective procedure. The predictive tool was effective in reducing subjects experiencing a poor outcome, but as even these patients experienced health utility gain, it did so with an associated overall utility loss. The Markov model produced will now be extremely useful for assessing the impact of current local strategies aimed at restricting access to lower limb arthroplasty, including those based on BMI thresholds. It will also be essential for any improvements or novel predictive models produced in work package 4 or by other researchers.
Work package 4
Work package 4 has been designed to evaluate and possible improve the predictive tools, developed in work package 2, in a pragmatic NHS setting involving a prospective cohort of lower limb arthroplasty patients. To do this, we aimed to recruit 3200 hip and knee arthroplasty patients across two NHS hospitals, and extensively phenotype them preoperatively and follow them at 6 weeks and annually thereafter. We recruited 3711 patients who were listed for knee and hip replacement surgeries across two NHS trusts: OUH and UHS. This is the most comprehensive cohort of arthroplasty patients collected to date.
The cohort had good rates of recruitment and follow-up and confirmed the excellent patient-reported outcomes of each operation: a THR preoperative OHS of 18.63 units (SD 8.05 units) and postoperative OHS of 41.06 units (SD 8.96 units) with 92% satisfied, and a TKR preoperative OKS of 20.31 units (SD 7.69 units) and postoperative OKS of 37.46 units (SD 9.74 units) with 87% satisfied. It provided essential data on health resource use both pre- and postoperatively. The performance of the knee model was modest (R2 = 0.14) and that of the hip model poor (R2 = 0.04). However, when the same variables were used to produce a new model, the performance of the knee model improved (R2 = 0.216) compared with the development model (R2 = ±0.202). Both models performed better in predicting good, rather than poor, outcomes. The addition of radiographic OA severity improved the performance of the hip model (R2 = 0.125 vs. R2 = 0.110) and hsCRP improved the performance of the knee model (R2 = 0.230 vs. R2 = 0.216).
There are many reasons why the models did not perform well in the validation cohort, which include the difference in patient characteristics, the surgical techniques and the implants used in the development and the validation cohorts, the degree of imputation used in both the development and validation cohort and the exact definition of the variables used in the discovery and validation cohorts.
When assessing the performance of the predictive model in a new cohort, it is extremely important to evaluate the comparability of the two cohorts in question. The model can fail to perform well in a new cohort because of limitations of the model itself or more commonly because of significant differences in the new population. There are several important differences between the development and the validation cohorts in the study. In the case of the hip replacement model, the developmental cohorts used were EUROHIP and EPOS: EUROHIP is a pan-European cohort and EPOS was a RCT, both of which will introduce potential issues related to case mix. Furthermore, the development cohorts were collected between 10 and 20 years ago, when surgical implants and techniques were very different from those used today, and will vary across the contributing European sites in the EUROHIP cohort. In addition, patient selection has also changed, with increasing provision of THRs in the older population with more comorbidities and also in younger, more active, patients. This is demonstrated by the validation cohort having a higher BMI, being older than the EUROHIP cohort but younger than the EPOS cohort and having a higher proportion of patients with severe radiographic OA (K/L grade 4). These factors would certainly mean that the development and validation cohorts were very different in nature, which may explain some of the reduced performance of the model.
To produce the knee predictive model, we used the data from KAT, which was a RCT of knee replacement and involved a different population from those attending the two hospitals through the NHS system. This is demonstrated by the validation cohort being younger and including a lower proportion of patients with a valgus deformity, a lower proportion of patients with an ASA grade of symptomatic and a lower proportion of patients having had previous knee surgery. Furthermore, because of its expertise in UKR, a large proportion of patients at the Oxford site underwent UKR, an operation often performed on younger male patients with more mild OA. The same considerations of temporal changes, patient selection and implant technology applies as it did with the THR.
Significant degrees of imputation were required for both the development and validation cohorts because of missing data, especially in the development cohorts. Although there were a substantial number of missing data in the EPOS and EUROHIP cohorts, this was generally a result of variables not being collected and data were therefore likely to be missing completely at random. In the validation cohort, the hip model performed less well than the knee model and the development cohorts for the hip model were more reliant on imputation that the knee model, supporting the potential role of imputation in explaining the poor performance of the model.
Furthermore, in the validation cohort, the performance of the model was worse when using the entire data set, which included more imputation, than when using the complete cohort set, which relied less on imputed variables. Both of these support the role of imputation in the poor performance of the models. We feel that the imputation in the developmental cohorts is probably the main factor in the poor performance of the models.
The addition of hsCRP improved the performance of the knee model (R2 = 0.230 vs. R2 = 0.216), but not the hip model. This may reflect the greater range in inflammatory processes present in those patients undergoing knee replacement for OA, which is traditionally thought to have a higher inflammatory component than hip OA. The addition of radiographic severity, measured by the K/L scale, did add significantly to the performance of the hip replacement model (R2 = 0.125 vs. R2 = 0.110). Both of these results are tantalising clues to the potential improvements in modelling that we may achieve in the future using the whole array of extra variables collected in the validation cohort. We are particularly interested in the role of vitamin D, urine collagen crosslinks and BMD as markers of bone health and metabolism. We are currently performing these analyses and would hope to have provisional results in the near future. We will also run a completely new model using the whole validation cohort data with the hope of identifying novel predictive markers but also of improving the performance of the model. This would obviously require an external data set and we are in provisional discussions with the Geneva Arthroplasty Registry, which does collect very similar data to the validation cohort.
Research implications
There are several areas of future research that would build on the results of this programme to:
-
develop and test a potential postoperative prediction model as possibly useful in reducing risk of poor outcome
-
use the COASt cohort as an opportunistic resource to test policy
-
use prediction models to explore patients who have a good outcome after THR and TKR
-
produce explore novel potential predictors of PROMs following THR and TKR in the validation cohort (BMD, vitamin D, bone markers, better phenotyping of mental status, etc.)
-
produce a new bespoke model in the validation cohort with external validation in a contemporary Geneva Arthroplasty Registry
-
follow the validation cohort going forward to obtain annual follow-up scores for at least 5 and, hopefully, 10 years with a view to having long-term PROMs and also revision and complication rates
-
use the Markov model to explore the economic effects of new therapeutic (e.g. postoperative bisphosphonates) and care delivery interventions (e.g. BMI restriction criteria)
-
perform a RCT of postoperative bisphosphonate use to reduce fracture and revision rates.
Conclusions
This programme has described the number of hip and knee replacement operations performed and projected to be performed in the future, which will help in planning services. It has defined a poor outcome using PROMs and identified a number of important predictors of PROMs. Increased BMI is statistically significantly associated with a worse PROM; however, the effect size is small and almost certainly not clinically significant. It is, however, associated with an increased risk of revision surgery and postoperative complications, which need to be considered in making a decision to operate. We have demonstrated and validated that bisphosphonates reduce postoperative fractures and the need for revision surgery, which warrants further investigation.
Both hip and knee surgery are cost-effective procedures, and, although we have produced a predictive tool for outcome, it would not be cost-effective to implement in its current form. Further work is being performed to refine and improve the predictive tools using extensive and novel risk factors. They will also prove to be very useful as part of patient decision aids in the future. The Markov model produced will prove extremely useful for assessment of any future therapeutic or health-care delivery interventions.
Acknowledgements
The authors would like to acknowledge the significant contributions of the following people.
Carole Ball (Research Nurse, UHS), who contributed significantly to study conduct, recruitment and maintenance in Southampton.
Professor Christopher Edwards (Professor of Rheumatic Diseases, UHS), who acted as the principal investigator at the Southampton site and contributed to study set-up.
Phil Costello (Research Nurse, UHS) for his significant contribution to the setting up of the Southampton site.
Lindsey Goulston (Rheumatology Research Team Leader, UHS) for setting up and maintenance of the quality assurance system and expert authorisation of the patient physical assessment in Southampton.
Kirsten Leyland (Postdoctoral Epidemiologist, NDORMS, Oxford) for her significant contribution to interpretation, validation and grading of study radiographs and for her significant contribution to a quality assurance system for obtaining patients’ images from OUH PACS.
Katherine Edwards (Imaging Assistant, NDORMS, Oxford) for interpretation and grading of radiographs.
Kitty Chang (Laboratory Assistant, UHS) for significant administrative support and sample management in Southampton.
Jennifer Rowe (Physiotherapist, OUH), Nick Kenny (Physiotherapist, OUH), Adam Toner (Physiotherapist, OUH), Lucy Gates (Podiatrist, UHS), Paloma O’Dogherty (Administrative Assistant, NDORMS), Edmund Wyatt (Administrative Assistant, NDORMS), Simon Shayler (Data Assistant, NDORMS) and all the COASt team in Oxford and Southampton for the recruitment and patient follow-up, data/sample collection, data validation and entry, and database and administrative support.
Amit Kiran (Statistician, NDORMS) for contribution to work package 2.
Cesar Garriga-Fuentes (Epidemiologist, NDORMS) for contribution to write up of work package 2.
David Turner (currently at University of East Anglia, at the time of the project Principal Health Economist at University of Southampton) for important contributions to work package 3.
Professor Sion Glyn-Jones (Professor Orthopaedic Surgery and Honorary Consultant Orthopaedic Surgeon) and other orthopaedic surgeons for help with recruitment and for allowing access to their patients by the COASt team.
Finally, we would like to thank the patients that participated in the study.
Contributions of authors
Professor Nigel Arden (Chief Investigator), as a grant applicant, has been responsible for design, initiation and maintenance of the programme. He has acted as a chief investigator of the prospective cohort study, COASt.
Professor Doug Altman (Co-Director of Oxford Clinical Trials Research Unit) has contributed to the design and statistical analysis of work package 2, and the development of the predictive tools.
Professor David Beard (Co-Director of Surgical Trials Unit, Oxford), as a grant applicant, has contributed to the design and initiation of the programme.
Professor Andrew Carr (Head of NDORMS, Oxford), as a grant applicant, has contributed to the design and initiation of the programme.
Professor Nicholas Clarke (Consultant Orthopaedic Surgeon), as a grant applicant, has contributed to the design and initiation of the programme.
Dr Gary Collins (Associate Professor, Deputy Director of the Centre of Statistics, Centre for Statistics in Medicine, Oxford) has contributed to the design and statistical analysis of work package 2.
Professor Cyrus Cooper (Director of the Medical Research Council Lifecourse Epidemiology Unit), as a grant applicant, has contributed to the design and initiation of the programme.
Dr David Culliford (Senior Medical Statistician, Faculty of Health Sciences, University of Southampton) has been responsible for design, initiation and critical analysis of work package 1.
Dr Antonella Delmestri (Database Manager, NDORMS) has been responsible for data validation, maintaining vital databases and reporting of the data.
Stefanie Garden (COASt Manager, NDORMS) has contributed, as the COASt manager, to study design, overseeing of the study milestones and write up of this report.
Tinatin Griffin (COASt Co-ordinator, NDORMS) has contributed to the day-to-day maintenance of the study, reviewing study milestones, co-ordinating the recruitment centres and all study-associated tasks, and co-ordinating and writing up this report.
Dr Kassim Javaid (Associate Professor, Consultant in Metabolic Medicine, Oxford), as a grant applicant, contributed to the initiation and design of the programme.
Dr Andrew Judge (Associate Professor, Senior Statistician, NDORMS) has been responsible for initiation, design and critical analysis of work package 2, and the development of the predictive tools.
Jeremy Latham (Orthopaedic and Trauma Surgeon Consultant), as a grant applicant, has contributed to the initiation and design of the programme.
Mark Mullee (Associate Professor, Director of the NIHR Research Design Service South Central), as a grant applicant, has contributed to the initiation and design of the programme.
David Murray (Professor of Orthopaedic Surgery at the University of Oxford) has acted as the COASt principal investigator in Oxford and also, as a grant applicant, was responsible for design and initiation of the programme.
Emmanuel Ogundimu (Statistician, NDORMS) has been responsible for external validation of the predictive tools in the prospective cohort of COASt.
Dr Rafael Pinedo-Villanueva (Senior Research Associate in Health Economics, NDORMS) has been responsible for the design, initiation and critical analyses of work package 3, and also overseeing the data analysis of work package 4.
Professor Andrew Price (Consultant Orthopaedic Surgeon) has acted as COASt principal investigator in Oxford and also, as a grant applicant, was responsible for design and initiation of the programme.
Dr Daniel Prieto-Alhambra (Associate Professor and NIHR Clinician Scientist) has contributed to work package 2 and was responsible for investigation of bisphosphonate studies.
Professor James Raftery (Professor of Health Technology Assessment, Chair NIHR Evaluation, Trials and Studies Coordinating Centre, Director Wessex Institute), as a grant applicant, has contributed to the grant application of the programme.
The authors of this report would like to acknowledge the support from NIHR for funding this project; NIHR OMB Research Unit and its members (University of Oxford) for significant contributions to the study and productive collaboration.
Data sharing statement
As outlined in Chapter 5, research groups who require COASt data should apply for access as approved by the sponsoring organisation, UHS. Please contact the corresponding author for further details.
Disclaimers
This report presents independent research funded by the National Institute for Health Research (NIHR). The views and opinions expressed by authors in this publication are those of the authors and do not necessarily reflect those of the NHS, the NIHR, CCF, NETSCC, PGfAR or the Department of Health. If there are verbatim quotations included in this publication the views and opinions expressed by the interviewees are those of the interviewees and do not necessarily reflect those of the authors, those of the NHS, the NIHR, NETSCC, the PGfAR programme or the Department of Health.
References
- National Joint Registry for England and Wales: 8th Annual Report. Hemel Hempstead: NJR; 2011.
- Harris WH, Sledge CB. Total hip and total knee replacement (1). N Engl J Med 1990;323:725-31. http://dx.doi.org/10.1056/NEJM199009133231106.
- Carr AJ, Robertsson O, Graves S, Price AJ, Arden NK, Judge A, et al. Knee replacement. Lancet 2012;379:1331-40. http://dx.doi.org/10.1016/S0140-6736(11)60752-6.
- European Action towards Better Musculoskeletal Health: A Public Health Strategy to Reduce the Burden of Musculoskeletal Conditions. Cornwall: The Bone and Joint Decade; 2004.
- Arthritis: The Big Picture. Chesterfield: Arthritis Research Campaign; 2002.
- Ibrahim T, Hobson S, Beiri A, Esler CN. No influence of body mass index on early outcome following total hip arthroplasty. Int Orthop 2005;29:359-61. https://doi.org/10.1007/s00264-005-0012-8.
- Judge A, Chard J, Learmonth I, Dieppe P. The effects of surgical volumes and training centre status on outcomes following total joint replacement: analysis of the Hospital Episode Statistics for England. J Public Health 2006;28:116-24. http://dx.doi.org/10.1093/pubmed/fdl003.
- Ethgen O, Bruyère O, Richy F, Dardennes C, Reginster JY. Health-related quality of life in total hip and total knee arthroplasty. A qualitative and systematic review of the literature. J Bone Joint Surg Am 2004;86–A:963-74. https://doi.org/10.2106/00004623-200405000-00012.
- Harris WH, Sledge CB. Total hip and total knee replacement (2). N Engl J Med 1990;323:801-7. http://dx.doi.org/10.1056/NEJM199009203231206.
- Cooper C, Arden NK. Excess mortality in osteoarthritis. BMJ 2011;342. http://dx.doi.org/10.1136/bmj.d1407.
- Nwachukwu BU, Bozic KJ, Schairer WW, Bernstein JL, Jevsevar DS, Marx RG, et al. Current status of cost utility analyses in total joint arthroplasty: a systematic review. Clin Orthop Relat Res 2015;473:1815-27. http://dx.doi.org/10.1007/s11999-014-3964-4.
- Liang MH, Cullen KE, Larson MG, Thompson MS, Schwartz JA, Fossel AH, et al. Cost-effectiveness of total joint arthroplasty in osteoarthritis. Arthritis Rheum 1986;29:937-43. https://doi.org/10.1002/art.1780290801.
- Framework for Vocational Rehabilitation: A Plan for Getting People Back to Work if They are Ill or have Hurt Themselves. London: Department for Work and Pensions; 2004.
- Kim S. Changes in surgical loads and economic burden of hip and knee replacements in the US: 1997–2004. Arthritis Rheum 2008;59:481-8. http://dx.doi.org/10.1002/art.23525.
- Singh JA, Lewallen DG. Time trends in the characteristics of patients undergoing primary total knee arthroplasty. Arthritis Care Res 2014;66:897-906. http://dx.doi.org/10.1002/acr.22233.
- The Musculoskeletal Services Framework – A Joint Responsibility: Doing it Differently. London: Department of Health; 2006.
- National Joint Registry for England and Wales: 9th Annual Report. Hemel Hempstead: NJR; 2012.
- Mehrotra C, Remington PL, Naimi TS, Washington W, Miller R. Trends in total knee replacement surgeries and implications for public health, 1990–2000. Public Health Rep 2005;120:278-82.
- Hawker GA, Wright JG, Badley EM, Coyte PC. Perceptions of, and willingness to consider, total joint arthroplasty in a population-based cohort of individuals with disabling hip and knee arthritis. Arthritis Rheum 2004;51:635-41. http://dx.doi.org/10.1002/art.20524.
- Hawker GA, Wright JG, Coyte PC, Williams JI, Harvey B, Glazier R, et al. Determining the need for hip and knee arthroplasty: the role of clinical severity and patients’ preferences. Med Care 2001;39:206-16. https://doi.org/10.1097/00005650-200103000-00002.
- Jüni P, Low N, Reichenbach S, Villiger PM, Williams S, Dieppe PA. Gender inequity in the provision of care for hip disease: population-based cross-sectional study. Osteoarthr Cartil 2010;18:640-5. http://dx.doi.org/10.1016/j.joca.2009.12.010.
- Dieppe P, Dixon D, Horwood J, Pollard B, Johnston M. MOBILE research team . MOBILE and the provision of total joint replacement. J Health Serv Res Policy 2008;13:47-56. http://dx.doi.org/10.1258/jhsrp.2008.008018.
- Jüni P, Dieppe P, Donovan J, Peters T, Eachus J, Pearson N, et al. Population requirement for primary knee replacement surgery: a cross-sectional study. Rheumatology 2003;42:516-21. https://doi.org/10.1093/rheumatology/keg196.
- Hadorn DC, Holmes AC. The New Zealand priority criteria project. Part 1: Overview. BMJ 1997;314:131-4. https://doi.org/10.1136/bmj.314.7074.131.
- Bozic K. CMS changes ICD9 and DRG codes for revision TJA. AAOS Bulletin 2005;3:17-21.
- Hawker G, Wright J, Coyte P, Paul J, Dittus R, Croxford R, et al. Health-related quality of life after knee replacement. J Bone Joint Surg Am 1998;80:163-73. https://doi.org/10.2106/00004623-199802000-00003.
- Hawker GA. Who, when, and why total joint replacement surgery? The patient’s perspective. Curr Opin Rheumatol 2006;18:526-30.
- Heck DA, Robinson RL, Partridge CM, Lubitz RM, Freund DA. Patient outcomes after knee replacement. Clin Orthop Relat Res 1998;356:93-110. https://doi.org/10.1097/00003086-199811000-00015.
- Jones CA, Voaklander DC, Suarez-Alma ME. Determinants of function after total knee arthroplasty. Phys Ther 2003;83:696-70.
- Judge A, Cooper C, Williams S, Dreinhoefer K, Dieppe P. Patient-reported outcomes one year after primary hip replacement in a European Collaborative Cohort. Arthritis Care Res 2010;62:480-8. http://dx.doi.org/10.1002/acr.20038.
- Kennedy LG, Newman JH, Ackroyd CE, Dieppe PA. When should we do knee replacements?. Knee 2003;10:161-6. https://doi.org/10.1016/S0968-0160(02)00138-2.
- MacWilliam CH, Yood MU, Verner JJ, McCarthy BD, Ward RE. Patient-related risk factors that predict poor outcome after total hip replacement. Health Serv Res 1996;31:623-38.
- Nilsdotter AK, Petersson IF, Roos EM, Lohmander LS. Predictors of patient relevant outcome after total hip replacement for osteoarthritis: a prospective study. Ann Rheum Dis 2003;62:923-30. https://doi.org/10.1136/ard.62.10.923.
- Robertsson O, Dunbar M, Pehrsson T, Knutson K, Lidgren L. Patient satisfaction after knee arthroplasty: a report on 27,372 knees operated on between 1981 and 1995 in Sweden. Acta Orthop Scand 2000;71:262-7. http://dx.doi.org/10.1080/000164700317411852.
- Williams O, Fitzpatrick R, Hajat S, Reeves BC, Stimpson A, Morris RW, et al. Mortality, morbidity, and 1-year outcomes of primary elective total hip arthroplasty. J Arthroplasty 2002;17:165-71. https://doi.org/10.1054/arth.2002.29389.
- Baker PN, van der Meulen JH, Lewsey J, Gregg PJ. National Joint Registry for England and Wales . The role of pain and function in determining patient satisfaction after total knee replacement. Data from the National Joint Registry for England and Wales. J Bone Joint Surg Br 2007;89:893-900. http://dx.doi.org/10.1302/0301-620X.89B7.19091.
- Noble M, Wright G, Dibben C, Smith G, McLennan D, Anttila C, et al. Indices of Deprivation 2004. Report to the Office of the Deputy Prime Minister. London: Neighbourhood Renewal Unit; 2004.
- Gandhi R, Davey JR, Mahomed NN. Predicting patient dissatisfaction following joint replacement surgery. J Rheumatol 2008;35:2415-18. http://dx.doi.org/10.3899/jrheum.080295.
- Kim TK, Chang CB, Kang YG, Kim SJ, Seong SC. Causes and predictors of patient’s dissatisfaction after uncomplicated total knee arthroplasty. J Arthroplasty 2009;24:263-71. http://dx.doi.org/10.1016/j.arth.2007.11.005.
- Scott CE, Howie CR, MacDonald D, Biant LC. Predicting dissatisfaction following total knee replacement: a prospective study of 1217 patients. J Bone Joint Surg Br 2010;92:1253-8. http://dx.doi.org/10.1302/0301-620X.92B9.24394.
- Darzi AW. High Quality Care for All. NHS Next Stage Review Final Report – Summary. London: Department of Health; 2008.
- Dorr LD, Chao L. The emotional state of the patient after total hip and knee arthroplasty. Clin Orthop Relat Res 2007;463:7-12. https://doi.org/10.1097/blo.0b013e318149296c.
- Santaguida PL, Hawker GA, Hudak PL, Glazier R, Mahomed NN, Kreder HJ, et al. Patient characteristics affecting the prognosis of total hip and knee joint arthroplasty: a systematic review. Can J Surg 2008;51:428-36.
- Fortin PR, Clarke AE, Joseph L, Liang MH, Tanzer M, Ferland D, et al. Outcomes of total hip and knee replacement: preoperative functional status predicts outcomes at six months after surgery. Arthritis Rheum 1999;42:1722-8. http://dx.doi.org/10.1002/1529-0131(199908)42:8<1722::AID-ANR22>3.0.CO;2-R.
- Cushnaghan J, Coggon D, Reading I, Croft P, Byng P, Cox K, et al. Long-term outcome following total hip arthroplasty: a controlled longitudinal study. Arthritis Rheum 2007;57:1375-80. https://doi.org/10.1002/art.23101.
- Jones CA, Voaklander DC, Johnston DW, Suarez-Almazor ME. The effect of age on pain, function, and quality of life after total hip and knee arthroplasty. Arch Intern Med 2001;161:454-60. https://doi.org/10.1001/archinte.161.3.454.
- Quintana JM, Escobar A, Aguirre U, Lafuente I, Arenaza JC. Predictors of health-related quality-of-life change after total hip arthroplasty. Clin Orthop Relat Res 2009;467:2886-94. http://dx.doi.org/10.1007/s11999-009-0868-9.
- Braeken AM, Lochhaas-Gerlach JA, Gollish JD, Myles JD, Mackenzie TA. Determinants of 6-12 month postoperative functional status and pain after elective total hip replacement. Int J Qual Health Care 1997;9:413-18. https://doi.org/10.1093/intqhc/9.6.413.
- Rissanen P, Aro S, Sintonen H, Slätis P, Paavolainen P. Quality of life and functional ability in hip and knee replacements: a prospective study. Qual Life Res 1996;5:56-64. https://doi.org/10.1007/BF00435969.
- Judge A, Arden NK, Price A, Glyn-Jones S, Beard D, Carr AJ, et al. Assessing patients for joint replacement: can pre-operative Oxford hip and knee scores be used to predict patient satisfaction following joint replacement surgery and to guide patient selection?. J Bone Joint Surg Br 2011;93:1660-4. http://dx.doi.org/10.1302/0301-620X.93B12.27046.
- Hopman WM, Mantle M, Towheed TE, MacKenzie TA. Determinants of health-related quality of life following elective total hip replacement. Am J Med Qual 1999;14:110-16. https://doi.org/10.1177/106286069901400302.
- Gandhi R, Davey JR, Mahomed N. Patient expectations predict greater pain relief with joint arthroplasty. J Arthroplasty 2009;24:716-21. http://dx.doi.org/10.1016/j.arth.2008.05.016.
- Noble M, Wright G, Dibben C, Smith GAN, McLennan D, Anttila C, et al. Indices of Deprivation 2004. Report to the Office of the Deputy Prime Minister. London: Neighbourhood Renewal Unit; 2004.
- Gillespie GN, Porteous AJ. Obesity and knee arthroplasty. Knee 2007;14:81-6. http://dx.doi.org/10.1016/j.knee.2006.11.004.
- Stern SH, Insall JN. Total knee arthroplasty in obese patients. J Bone Joint Surg Am 1990;72:1400-4. https://doi.org/10.2106/00004623-199072090-00020.
- Berend KR, Lombardi AV, Mallory TH, Adams JB, Groseth KL. Early failure of minimally invasive unicompartmental knee arthroplasty is associated with obesity. Clin Orthop Relat Res 2005;440:60-6. https://doi.org/10.1097/01.blo.0000187062.65691.e3.
- Kynaston-Pearson F, Ashmore AM, Malak TT, Rombach I, Taylor A, Beard D, et al. Primary hip replacement prostheses and their evidence base: systematic review of literature. BMJ 2013;347. http://dx.doi.org/10.1136/bmj.f6956.
- Langton DJ, Jameson SS, Joyce TJ, Hallab NJ, Natu S, Nargol AV. Early failure of metal-on-metal bearings in hip resurfacing and large-diameter total hip replacement: a consequence of excess wear. J Bone Joint Surg Br 2010;92:38-46. http://dx.doi.org/10.1302/0301-620X.92B1.22770.
- Mulhall KJ, Ghomrawi HM, Scully S, Callaghan JJ, Saleh KJ. Current etiologies and modes of failure in total knee arthroplasty revision. Clin Orthop Relat Res 2006;446:45-50. https://doi.org/10.1097/01.blo.0000214421.21712.62.
- Chiew YF, Theis JC. Comparison of infection rate using different methods of assessment for surveillance of total hip replacement surgical site infections. ANZ J Surg 2007;77:535-9. http://dx.doi.org/10.1111/j.1445-2197.2007.04145.x.
- Engh GA, Lounici S, Rao AR, Collier MB. In vivo deterioration of tibial baseplate locking mechanisms in contemporary modular total knee components. J Bone Joint Surg Am 2001;83–A:1660-5. https://doi.org/10.2106/00004623-200111000-00007.
- Fehring TK, Murphy JA, Hayes TD, Roberts DW, Pomeroy DL, Griffin WL. Factors influencing wear and osteolysis in press-fit condylar modular total knee replacements. Clin Orthop Relat Res 2004;428:40-5. https://doi.org/10.1097/01.blo.0000148853.37270.67.
- Messent EA, Buckland-Wright JC, Blake GM. Fractal analysis of trabecular bone in knee osteoarthritis (OA) is a more sensitive marker of disease status than bone mineral density (BMD). Calcif Tissue Int 2005;76:419-25. https://doi.org/10.1007/s00223-004-0160-7.
- Messent EA, Ward RJ, Tonkin CJ, Buckland-Wright C. Differences in trabecular structure between knees with and without osteoarthritis quantified by macro and standard radiography, respectively. Osteoarthr Cartil 2006;14:1302-5. http://dx.doi.org/10.1016/j.joca.2006.07.012.
- Wilkinson JM, Wilson AG, Stockley I, Scott IR, Macdonald DA, Hamer AJ, et al. Variation in the TNF gene promoter and risk of osteolysis after total hip arthroplasty. J Bone Miner Res 2003;18:1995-2001. http://dx.doi.org/10.1359/jbmr.2003.18.11.1995.
- Kurtz S, Ong K, Lau E, Mowat F, Halpern M. Projections of primary and revision hip and knee arthroplasty in the United States from 2005 to 2030. J Bone Joint Surg Am 2007;89:780-5. https://doi.org/10.2106/00004623-200704000-00012.
- Culliford DJ, Maskell J, Beard DJ, Murray DW, Price AJ, Arden NK. Temporal trends in hip and knee replacement in the United Kingdom: 1991 to 2006. J Bone Joint Surg Br 2010;92:130-5. http://dx.doi.org/10.1302/0301-620X.92B1.22654.
- Otten R, van Roermund PM, Picavet HS. Trends in the number of knee and hip arthroplasties: considerably more knee and hip prostheses due to osteoarthritis in 2030. Ned Tijdschr Geneeskd 2010;154.
- van Staa TP, Dennison EM, Leufkens HG, Cooper C. Epidemiology of fractures in England and Wales. Bone 2001;29:517-22. https://doi.org/10.1016/S8756-3282(01)00614-7.
- National Joint Registry for England and Wales: 7th Annual Report. Hemel Hempstead: NJR; 2010.
- Felson DT, Naimark A, Anderson J, Kazis L, Castelli W, Meenan RF. The prevalence of knee osteoarthritis in the elderly. The Framingham Osteoarthritis Study. Arthritis Rheum 1987;30:914-18. https://doi.org/10.1002/art.1780300811.
- Davies AP, Vince AS, Shepstone L, Donell ST, Glasgow MM. The radiologic prevalence of patellofemoral osteoarthritis. Clin Orthop Relat Res 2002;402:206-12. https://doi.org/10.1097/00003086-200209000-00020.
- Dagenais S, Garbedian S, Wai EK. Systematic review of the prevalence of radiographic primary hip osteoarthritis. Clin Orthop Relat Res 2009;467:623-37. http://dx.doi.org/10.1007/s11999-008-0625-5.
- Quintana JM, Arostegui I, Escobar A, Azkarate J, Goenaga JI, Lafuente I. Prevalence of knee and hip osteoarthritis and the appropriateness of joint replacement in an older population. Arch Intern Med 2008;168:1576-84. http://dx.doi.org/10.1001/archinte.168.14.1576.
- Murphy LB, Helmick CG, Schwartz TA, Renner JB, Tudor G, Koch GG, et al. One in four people may develop symptomatic hip osteoarthritis in his or her lifetime. Osteoarthr Cartil 2010;18:1372-9. http://dx.doi.org/10.1016/j.joca.2010.08.005.
- Murphy L, Schwartz TA, Helmick CG, Renner JB, Tudor G, Koch G, et al. Lifetime risk of symptomatic knee osteoarthritis. Arthritis Rheum 2008;59:1207-13. http://dx.doi.org/10.1002/art.24021.
- Wallace G, Judge A, Prieto-Alhambra D, de Vries F, Arden NK, Cooper C. The effect of body mass index on the risk of post-operative complications during the 6 months following total hip replacement or total knee replacement surgery. Osteoarthr Cartil 2014;22:918-27. http://dx.doi.org/10.1016/j.joca.2014.04.013.
- Rand JA, Trousdale RT, Ilstrup DM, Harmsen WS. Factors affecting the durability of primary total knee prostheses. J Bone Joint Surg Am 2003;85–A:259-65. https://doi.org/10.2106/00004623-200302000-00012.
- Dehn T. Joint replacement in the overweight patient. Ann Royal Coll Surg Engl 2007;89. https://doi.org/10.1308/003588407X183247.
- Jackson CH, Best NG, Richardson S. Bayesian graphical models for regression on multiple data sets with different variables. Biostatistics 2009;10:335-51. http://dx.doi.org/10.1093/biostatistics/kxn041.
- Vergouw D, Heymans MW, Peat GM, Kuijpers T, Croft PR, de Vet HC, et al. The search for stable prognostic models in multiple imputed data sets. BMC Med Res Methodol 2010;10. http://dx.doi.org/10.1186/1471-2288-10-81.
- Jenkins PJ, Clement ND, Hamilton DF, Gaston P, Patton JT, Howie CR. Predicting the cost-effectiveness of total hip and knee replacement: a health economic analysis. Bone Joint J 2013;95–B:115-21. http://dx.doi.org/10.1302/0301-620X.95B1.29835.
- Chang RW, Pellisier JM, Hazen GB. A cost-effectiveness analysis of total hip arthroplasty for osteoarthritis of the hip. JAMA 1996;275:858-65. https://doi.org/10.1001/jama.1996.03530350040032.
- Healy WL, Finn D. The hospital cost and the cost of the implant for total knee arthroplasty. A comparison between 1983 and 1991 for one hospital. J Bone Joint Surg Am 1994;76:801-6. https://doi.org/10.2106/00004623-199406000-00002.
- Rorabeck CH, Murray P. The cost benefit of total knee arthroplasty. Orthopedics 1996;19:777-9.
- Ong KL, Mowat FS, Chan N, Lau E, Halpern MT, Kurtz SM. Economic burden of revision hip and knee arthroplasty in Medicare enrollees. Clin Orthop Relat Res 2006;446:22-8. https://doi.org/10.1097/01.blo.0000214439.95268.59.
- Beswick AD, Wylde V, Gooberman-Hill R, Blom A, Dieppe P. What proportion of patients report long-term pain after total hip or knee replacement for osteoarthritis? A systematic review of prospective studies in unselected patients. BMJ Open 2012;2. http://dx.doi.org/10.1136/bmjopen-2011-000435.
- Gillespie WJ, Pekarsky B, O’Connell DL. Evaluation of new technologies for total hip replacement. Economic modelling and clinical trials. J Bone Joint Surg Br 1995;77:528-33.
- Bozic KJ, Morshed S, Silverstein MD, Rubash HE, Kahn JG. Use of cost-effectiveness analysis to evaluate new technologies in orthopaedics. The case of alternative bearing surfaces in total hip arthroplasty. J Bone Joint Surg Am 2006;88:706-14. http://dx.doi.org/10.2106/JBJS.E.00614.
- Mahomed NN, Liang MH, Cook EF, Daltroy LH, Fortin PR, Fossel AH, et al. The importance of patient expectations in predicting functional outcomes after total joint arthroplasty. J Rheumatol 2002;29:1273-9.
- Adam JA, Khaw FM, Thomson RG, Gregg PJ, Llewellyn-Thomas HA. Patient decision aids in joint replacement surgery: a literature review and an opinion survey of consultant orthopaedic surgeons. Ann R Coll Surg Engl 2008;90:198-207. http://dx.doi.org/10.1308/003588408X285748.
- Herrett E, Thomas SL, Schoonen WM, Smeeth L, Hall AJ. Validation and validity of diagnoses in the General Practice Research Database: a systematic review. Br J Clin Pharmacol 2010;69:4-14. http://dx.doi.org/10.1111/j.1365-2125.2009.03537.x.
- Van Staa TP, Leufkens HG, Abenhaim L, Zhang B, Cooper C. Use of oral corticosteroids and risk of fractures. J Bone Miner Res 2000;15:993-1000. http://dx.doi.org/10.1359/jbmr.2000.15.6.993.
- Srikanth VK, Fryer JL, Zhai G, Winzenberg TM, Hosmer D, Jones G. A meta-analysis of sex differences prevalence, incidence and severity of osteoarthritis. Osteoarthr Cartil 2005;13:769-81. http://dx.doi.org/10.1016/j.joca.2005.04.014.
- Parkinson J, Davis S, van Staa T, Mann R, Andrews EB. Pharmacovigilance. Chichester: John Wiley & Sons Ltd; 2007.
- Hollowell J. The General Practice Research Database: quality of morbidity data. Popul Trends 1997;87:36-40.
- Culliford D, Maskell J, Judge A, Arden NK. COAST Study group . A population-based survival analysis describing the association of body mass index on time to revision for total hip and knee replacements: results from the UK general practice research database. BMJ Open 2013;3. http://dx.doi.org/10.1136/bmjopen-2013-003614.
- Culliford D, Maskell J, Judge A, Cooper C, Prieto-Alhambra D, Arden NK. COASt Study Group . Future projections of total hip and knee arthroplasty in the UK: results from the UK Clinical Practice Research Datalink. Osteoarthr Cartil 2015;23:594-600. http://dx.doi.org/10.1016/j.joca.2014.12.022.
- Culliford DJ, Maskell J, Kiran A, Judge A, Javaid MK, Cooper C, et al. The lifetime risk of total hip and knee arthroplasty: results from the UK general practice research database. Osteoarthr Cartil 2012;20:519-24. http://dx.doi.org/10.1016/j.joca.2012.02.636.
- Agency for Healthcare Research and Quality . HCUPnet: 2008 Outcomes by Patient and Hospital Characteristic for ICD-9-CM Principal Procedure Code n.d. http://hcupnet.ahrq.gov/HCUPnet.app/ (accessed 16 December 2011).
- Singh JA, Vessely MB, Harmsen WS, Schleck CD, Melton LJ, Kurland RL, et al. A population-based study of trends in the use of total hip and total knee arthroplasty, 1969–2008. Mayo Clin Proc 2010;85:898-904. http://dx.doi.org/10.4065/mcp.2010.0115.
- Williams B, Whatmough P, McGill J, Rushton L. Private funding of elective hospital treatment in England and Wales, 1997–8: national survey. BMJ 2000;320:904-5. https://doi.org/10.1136/bmj.320.7239.904.
- NHS Digital . Hospital Episode Statistics n.d. www.hesonline.nhs.uk (accessed 25 September 2008).
- National Joint Registry for England and Wales: 2nd Annual Report. Hemel Hempstead: NJR; 2005.
- National Joint Registry for England and Wales: 5th Annual Report. Hemel Hempstead: NJR; 2009.
- ONS . Key Population and Vital Statistics Series – No. 30 2003 Edition n.d. www.statistics.gov.uk/downloads/theme_population/KPVS30_2003?KPVS2003.pdf (accessed 30 November 2009).
- Judge A, Welton NJ, Sandhu J, Ben-Shlomo Y. Modeling the need for hip and knee replacement surgery. Part 1. A two-stage cross-cohort approach. Arthritis Rheum 2009;61:1657-66. http://dx.doi.org/10.1002/art.24892.
- Culliford DJ, Mullee MA, Arden NK. Annual Meeting of the British Society Rheumatology/Spring Meeting of the British Health Professional in Rheumatology, Liverpool, England. Rheumatology 2008:22-5.
- Dixon T, Shaw ME, Dieppe PA. Analysis of regional variation in hip and knee joint replacement rates in England using Hospital Episodes Statistics. Public Health 2006;120:83-90. http://dx.doi.org/10.1016/j.puhe.2005.06.003.
- Segal HE, Bellamy TN. The Joint Health Benefits Delivery Program: improving access and reducing costs – successes and pitfalls. Mil Med 1988;153:430-1.
- Kurtz S, Mowat F, Ong K, Chan N, Lau E, Halpern M. Prevalence of primary and revision total hip and knee arthroplasty in the United States from 1990 through 2002. J Bone Joint Surg Am 2005;87:1487-97. http://dx.doi.org/10.2106/JBJS.D.02441.
- Callaghan JJ, Albright JC, Goetz DD, Olejniczak JP, Johnston RC. Charnley total hip arthroplasty with cement. Minimum twenty-five-year follow-up. J Bone Joint Surg Am 2000;82:487-97. https://doi.org/10.2106/00004623-200004000-00004.
- Mullins MM, Norbury W, Dowell JK, Heywood-Waddington M. Thirty-year results of a prospective study of Charnley total hip arthroplasty by the posterior approach. J Arthroplasty 2007;22:833-9. http://dx.doi.org/10.1016/j.arth.2006.10.003.
- Maradit Kremers H, Visscher SL, Moriarty JP, Reinalda MS, Kremers WK, Naessens JM, et al. Determinants of direct medical costs in primary and revision total knee arthroplasty. Clin Orthop Relat Res 2013;471:206-14. http://dx.doi.org/10.1007/s11999-012-2508-z.
- Vanhegan IS, Malik AK, Jayakumar P, Islam SUI, Haddad FS. A financial analysis of revision hip arthroplasty: the economic burden in relation to the national tariff. J Bone Joint Surg Br 2012;94:619-23. http://dx.doi.org/10.1302/0301-620X.94B5.27073.
- Dixon T, Shaw M, Ebrahim S, Dieppe P. Trends in hip and knee joint replacement: socioeconomic inequalities and projections of need. Ann Rheum Dis 2004;63:825-30. http://dx.doi.org/10.1136/ard.2003.012724.
- Burns AW, Bourne RB, Chesworth BM, MacDonald SJ, Rorabeck CH. Cost effectiveness of revision total knee arthroplasty. Clin Orthop Relat Res 2006;446:29-33. https://doi.org/10.1097/01.blo.0000214420.14088.76.
- Grotle M, Hagen KB, Natvig B, Dahl FA, Kvien TK. Prevalence and burden of osteoarthritis: results from a population survey in Norway. J Rheumatol 2008;35:677-84.
- Kurtz SM, Ong KL, Lau E, Widmer M, Maravic M, Gómez-Barrena E, et al. International survey of primary and revision total knee replacement. Int Orthop 2011;35:1783-9. http://dx.doi.org/10.1007/s00264-011-1235-5.
- Jameson SS, Baker PN, Mason J, Porter ML, Deehan DJ, Reed MR. Independent predictors of revision following metal-on-metal hip resurfacing: a retrospective cohort study using National Joint Registry data. J Bone Joint Surg Br 2012;94:746-54. http://dx.doi.org/10.1302/0301-620X.94B6.29239.
- Havelin LI, Robertsson O, Fenstad AM, Overgaard S, Garellick G, Furnes O. A Scandinavian experience of register collaboration: the Nordic Arthroplasty Register Association (NARA). J Bone Joint Surg Am 2011;93:13-9. http://dx.doi.org/10.2106/JBJS.K.00951.
- Bozic KJ, Kurtz SM, Lau E, Ong K, Chiu V, Vail TP, et al. The epidemiology of revision total knee arthroplasty in the United States. Clin Orthop Relat Res 2010;468:45-51. http://dx.doi.org/10.1007/s11999-009-0945-0.
- Birrell F, Johnell O, Silman A. Projecting the need for hip replacement over the next three decades: influence of changing demography and threshold for surgery. Ann Rheum Dis 1999;58:569-72. https://doi.org/10.1136/ard.58.9.569.
- Merx H, Dreinhöfer K, Schräder P, Stürmer T, Puhl W, Günther KP, et al. International variation in hip replacement rates. Ann Rheum Dis 2003;62:222-6. https://doi.org/10.1136/ard.62.3.222.
- Interim Life Tables: United Kingdom, 1980–82 to 2007–09. London: ONS; 2010.
- Schouten LJ, Straatman H, Kiemeney LA, Verbeek AL. Cancer incidence: life table risk versus cumulative risk. J Epidemiol Community Health 1994;48:596-600. https://doi.org/10.1136/jech.48.6.596.
- Kurtz S, Lau E, Halpern M, Ong K. Trend shows growing orthopedic surgery case load. Will surgeons be able to keep up?. Mater Manag Health Care 2006;15:61-2.
- Pedersen AB, Johnsen SP, Overgaard S, Søballe K, Sørensen HT, Lucht U. Total hip arthroplasty in Denmark: incidence of primary operations and revisions during 1996-2002 and estimated future demands. Acta Orthop 2005;76:182-9. https://doi.org/10.1080/00016470510030553.
- Holt HL, Katz JN, Reichmann WM, Gerlovin H, Wright EA, Hunter DJ, et al. Forecasting the burden of advanced knee osteoarthritis over a 10-year period in a cohort of 60-64 year-old US adults. Osteoarthr Cartil 2011;19:44-50. http://dx.doi.org/10.1016/j.joca.2010.10.009.
- McPherson K, Marsh T, Brown M. Tackling Obesities: Future Choices – Modelling Future Trends in Obesity and Their Impact on Health. London: Department of Innovation Universities and Skills; 2008.
- Arden NK, Crozier S, Smith H, Anderson F, Edwards C, Raphael H, et al. Knee pain, knee osteoarthritis, and the risk of fracture. Arthritis Rheum 2006;55:610-15. https://doi.org/10.1002/art.22088.
- Gossec L, Paternotte S, Maillefert JF, Combescure C, Conaghan PG, Davis AM, et al. The role of pain and functional impairment in the decision to recommend total joint replacement in hip and knee osteoarthritis: an international cross-sectional study of 1909 patients. Report of the OARSI-OMERACT Task Force on total joint replacement. Osteoarthr Cartil 2011;19:147-54. http://dx.doi.org/10.1016/j.joca.2010.10.025.
- Judge A, Welton NJ, Sandhu J, Ben-Shlomo Y. Modeling the need for hip and knee replacement surgery. Part 2. Incorporating census data to provide small-area predictions for need with uncertainty bounds. Arthritis Rheum 2009;61:1667-73. http://dx.doi.org/10.1002/art.24732.
- Frankel S, Eachus J, Pearson N, Greenwood R, Chan P, Peters TJ, et al. Population requirement for primary hip-replacement surgery: a cross-sectional study. Lancet 1999;353:1304-9. https://doi.org/10.1016/S0140-6736(98)06451-4.
- Herrett E, Gallagher AM, Bhaskaran K, Forbes H, Mathur R, van Staa T, et al. Data Resource Profile: Clinical Practice Research Datalink (CPRD). Int J Epidemiol 2015;44:827-36. http://dx.doi.org/10.1093/ije/dyv098.
- Walley T, Mantgani A. The UK General Practice Research Database. Lancet 1997;350:1097-9. https://doi.org/10.1016/S0140-6736(97)04248-7.
- Dieppe P, Judge A, Williams S, Ikwueke I, Guenther KP, Floeren M, et al. Variations in the pre-operative status of patients coming to primary hip replacement for osteoarthritis in European orthopaedic centres. BMC Musculoskelet Disord 2009;10. http://dx.doi.org/10.1186/1471-2474-10-19.
- Judge A, Batra RN, Murray D, Dieppe PA, Sanchez-Santos MT, Thomas G, et al. Patient reported outcomes following primary hip replacement surgery: development and internal validation of a prognostic tool. Osteoarthritis Cartilage 2014;22. https://doi.org/10.1016/j.joca.2014.02.401.
- Judge A, Arden NK, Batra RN, Thomas G, Beard D, Javaid MK, et al. The association of patient characteristics and surgical variables on symptoms of pain and function over 5 years following primary hip-replacement surgery: a prospective cohort study. BMJ Open 2013;3. http://dx.doi.org/10.1136/bmjopen-2012-002453.
- Hossain M, Parfitt DJ, Beard DJ, Darrah C, Nolan J, Murray DW, et al. Does pre-operative psychological distress affect patient satisfaction after primary total hip arthroplasty?. BMC Musculoskelet Disord 2011;12. http://dx.doi.org/10.1186/1471-2474-12-122.
- Judge A, Arden NK, Cooper C, Kassim Javaid M, Carr AJ, Field RE, et al. Predictors of outcomes of total knee replacement surgery. Rheumatology 2012;51:1804-13. http://dx.doi.org/10.1093/rheumatology/kes075.
- Sánchez-Santos MT, Judge A, Batra RN, Murray D, Price A, Liddle AD, et al. A clinical tool for the prediction of patient-reported outcomes after knee replacement surgery: a prospective cohort study. Osteoarthritis Cartilage 2014;22. https://doi.org/10.1016/j.joca.2014.02.776.
- Judge A, Javaid MK, Arden NK, Cushnaghan J, Reading I, Croft P, et al. Clinical tool to identify patients who are most likely to achieve long-term improvement in physical function after total hip arthroplasty. Arthritis Care Res 2012;64:881-9. http://dx.doi.org/10.1002/acr.21594.
- Guidance on the Routine Collection of Patient Reported Outcome Measures (PROMs). London: Department of Health; 2008.
- Murray DW, Fitzpatrick R, Rogers K, Pandit H, Beard DJ, Carr AJ, et al. The use of the Oxford hip and knee scores. J Bone Joint Surg Br 2007;89:1010-14. http://dx.doi.org/10.1302/0301-620X.89B8.19424.
- Dawson J, Fitzpatrick R, Carr A, Murray D. Questionnaire on the perceptions of patients about total hip replacement. J Bone Joint Surg Br 1996;78:185-90.
- Dawson J, Fitzpatrick R, Murray D, Carr A. Questionnaire on the perceptions of patients about total knee replacement. J Bone Joint Surg Br 1998;80:63-9. https://doi.org/10.1302/0301-620X.80B1.7859.
- Dolan P. Modeling valuations for EuroQol health states. Med Care 1997;35:1095-108. https://doi.org/10.1097/00005650-199711000-00002.
- Kellgren JH, Lawrence JS. Osteo-arthrosis and disk degeneration in an urban population. Ann Rheum Dis 1958;17:388-97. https://doi.org/10.1136/ard.17.4.388.
- Dawson J, Doll H, Fitzpatrick R, Jenkinson C, Carr AJ. The routine use of patient reported outcome measures in healthcare settings. BMJ 2010;340. http://dx.doi.org/10.1136/bmj.c186.
- Wylde V, Dieppe P, Hewlett S, Learmonth ID. Total knee replacement: is it really an effective procedure for all?. Knee 2007;14:417-23. http://dx.doi.org/10.1016/j.knee.2007.06.001.
- Standard NHS Contract for Acute Services. London: Department of Health; 2007.
- Stacey D, Hawker G, Dervin G, Tomek I, Cochran N, Tugwell P, et al. Management of chronic pain: improving shared decision making in osteoarthritis. BMJ 2008;336:954-5. http://dx.doi.org/10.1136/bmj.39520.701748.94.
- Arden NK, Kiran A, Judge A, Biant LC, Javaid MK, Murray DW, et al. What is a good patient reported outcome after total hip replacement?. Osteoarthr Cartil 2011;19:155-62. http://dx.doi.org/10.1016/j.joca.2010.10.004.
- Judge A, Arden NK, Kiran A, Price A, Javaid MK, Beard D, et al. Interpretation of patient-reported outcomes for hip and knee replacement surgery: identification of thresholds associated with satisfaction with surgery. J Bone Joint Surg Br 2012;94:412-18. http://dx.doi.org/10.1302/0301-620X.94B3.27425.
- Tubach F, Ravaud P, Baron G, Falissard B, Logeart I, Bellamy N, et al. Evaluation of clinically relevant states in patient reported outcomes in knee and hip osteoarthritis: the patient acceptable symptom state. Ann Rheum Dis 2005;64:34-7. http://dx.doi.org/10.1136/ard.2004.023028.
- Kiran A, Hunter DJ, Judge A, Field RE, Javaid MK, Cooper C, et al. Oxford NDORMS Musculoskeletal Epidemiology Unit Writing Committee . A novel methodological approach for measuring symptomatic change following total joint arthroplasty. J Arthroplasty 2014;29:2140-5. http://dx.doi.org/10.1016/j.arth.2014.06.008.
- Field RE, Cronin MD, Singh PJ. The Oxford hip scores for primary and revision hip replacement. J Bone Joint Surg Br 2005;87:618-22. http://dx.doi.org/10.1302/0301-620X.87B5.15390.
- Bellamy N. WOMAC: a 20-year experiential review of a patient-centered self-reported health status questionnaire. J Rheumatol 2002;29:2473-6.
- Judge A, Batra RN, Thomas GE, Beard D, Javaid MK, Murray DW, et al. Body mass index is not a clinically meaningful predictor of patient reported outcomes of primary hip replacement surgery: prospective cohort study. Osteoarthr Cartil 2014;22:431-9. http://dx.doi.org/10.1016/j.joca.2013.12.018.
- Judge A, Batra RN, Murray D, Dieppe PA, Sanchez-Santos MT, Thomas G, et al. Patient Reported Outcomes Following Primary Hip Replacement Surgery: Development and Internal Validation of a Prognostic Tool. Oxford: Oxford NIHR Musculoskeletal Biomedical Research Unit Nuffield Department of Orthopaedics, Rheumatology and Musculoskeletal Sciences (NDORMS), Rheumatology and Musculoskeletal Sciences, University of Oxford; 2015.
- Judge A, Cooper C, Arden NK, Williams S, Hobbs N, Dixon D, et al. Pre-operative expectation predicts 12-month post-operative outcome among patients undergoing primary total hip replacement in European orthopaedic centres. Osteoarthr Cartil 2011;19:659-67. http://dx.doi.org/10.1016/j.joca.2011.03.009.
- Jiang L, Rong J, Wang Y, Hu F, Bao C, Li X, et al. The relationship between body mass index and hip osteoarthritis: a systematic review and meta-analysis. Joint Bone Spine 2011;78:150-5. http://dx.doi.org/10.1016/j.jbspin.2010.04.011.
- Jiang L, Tian W, Wang Y, Rong J, Bao C, Liu Y, et al. Body mass index and susceptibility to knee osteoarthritis: a systematic review and meta-analysis. Joint Bone Spine 2012;79:291-7. http://dx.doi.org/10.1016/j.jbspin.2011.05.015.
- Holliday KL, McWilliams DF, Maciewicz RA, Muir KR, Zhang W, Doherty M. Lifetime body mass index, other anthropometric measures of obesity and risk of knee or hip osteoarthritis in the GOAL case-control study. Osteoarthr Cartil 2011;19:37-43. http://dx.doi.org/10.1016/j.joca.2010.10.014.
- Batra RN, Judge A, Javaid MK, Thomas GE, Beard D, Murray D, et al. Pre-operative BMI as a predictor of patient reported outcomes of primary hip replacement surgery: a combined analysis of 4 prospective cohort study. Osteoarthr Cartil 2012;20:S54-296. https://doi.org/10.1016/j.joca.2012.02.224.
- Kane RL, Saleh KJ, Wilt TJ, Bershadsky B. The functional outcomes of total knee arthroplasty. J Bone Joint Surg Am 2005;87:1719-24. http://dx.doi.org/10.2106/JBJS.D.02714.
- National Joint Registry for England and Wales: 1st Annual Report. Hemel Hempstead: NJR; 2004.
- Salamon L, Morovic-Vergles J, Marasovic-Krstulovic D, Kehler T, Sakic D, Badovinac O, et al. Differences in the prevalence and characteristics of metabolic syndrome in rheumatoid arthritis and osteoarthritis: a multicentric study. Rheumatol Int 2015;35:2047-57. https://doi.org/10.1007/s00296-015-3307-0.
- Ware JE. How to Score the Revised MOS Short Form Health Scales. Boston, MA: The Health Institute, New England Medical Center Hospital; 1998.
- Stewart AL, Hays RD, Ware JE. The MOS short-form general health survey. Reliability and validity in a patient population. Med Care 1988;26:724-35. https://doi.org/10.1097/00005650-198807000-00007.
- Lingard EA, Katz JN, Wright EA, Sledge CB. Kinemax Outcomes Group. Predicting the outcome of total knee arthroplasty. J Bone Joint Surg Am 2004;86–A:2179-86. https://doi.org/10.2106/00004623-200410000-00008.
- Lingard EA, Riddle DL. Impact of psychological distress on pain and function following knee arthroplasty. J Bone Joint Surg Am 2007;89:1161-9. http://dx.doi.org/10.2106/JBJS.F.00914.
- Brander V, Gondek S, Martin E, Stulberg SD. Pain and depression influence outcome 5 years after knee replacement surgery. Clin Orthop Relat Res 2007;464:21-6.
- Prieto-Alhambra D, Javaid MK, Judge A, Maskell J, Cooper C, Arden NK. COASt Study Group . Hormone replacement therapy and mid-term implant survival following knee or hip arthroplasty for osteoarthritis: a population-based cohort study. Ann Rheum Dis 2015;74:557-63. http://dx.doi.org/10.1136/annrheumdis-2013-204043.
- Sterne JA, White IR, Carlin JB, Spratt M, Royston P, Kenward MG, et al. Multiple imputation for missing data in epidemiological and clinical research: potential and pitfalls. BMJ 2009;338. http://dx.doi.org/10.1136/bmj.b2393.
- Kellgren JH, Lawrence JS. Radiological assessment of osteo-arthrosis. Ann Rheum Dis 1957;16:494-502. https://doi.org/10.1136/ard.16.4.494.
- Heymans MW, van Buuren S, Knol DL, van Mechelen W, de Vet HC. Variable selection under multiple imputation using the bootstrap in a prognostic study. BMC Med Res Methodol 2007;7. http://dx.doi.org/10.1186/1471-2288-7-33.
- Molitor NT, Best N, Jackson C, Richardson S. Using Bayesian graphical models to model biases in observational studies and to combine multiple data sources: application to low birth-weight and water disinfection by-products. J R Stat Soc Series A 2008;172:615-37. https://doi.org/10.1111/j.1467-985X.2008.00582.x.
- Mason A, Richardson S, Best N. A Comparison of Fully Bayesian and Two-Stage ImputatIon Strategies for Missing Covariate Data 2012.
- Best N, Mason A, Richardson S, McCandless L. Bayesian Approaches for Combining Multiple Data Sources to Adjust for Missing Confounders n.d.
- Royston P. Multiple imputation of missing values. Stata J 2004;4:227-41.
- Marshall A, Altman DG, Holder RL, Royston P. Combining estimates of interest in prognostic modelling studies after multiple imputation: current practice and guidelines. BMC Med Res Methodol 2009;9. http://dx.doi.org/10.1186/1471-2288-9-57.
- Royston P. Multiple imputation of missing values: update of ice. Stata J 2005;5:527-36.
- Collins GS, Altman DG. An independent external validation and evaluation of QRISK cardiovascular risk prediction: a prospective open cohort study. BMJ 2009;339. http://dx.doi.org/10.1136/bmj.b2584.
- Fitzgerald JD, Orav EJ, Lee TH, Marcantonio ER, Poss R, Goldman L, et al. Patient quality of life during the 12 months following joint replacement surgery. Arthritis Rheum 2004;51:100-9. http://dx.doi.org/10.1002/art.20090.
- Dakin H, Gray A, Fitzpatrick R, Maclennan G, Murray D. KAT Trial Group . Rationing of total knee replacement: a cost-effectiveness analysis on a large trial data set. BMJ Open 2012;2. http://dx.doi.org/10.1136/bmjopen-2011-000332.
- Johnston L, MacLennan G, McCormack K, Ramsay C, Walker A. KAT Trial Group . The Knee Arthroplasty Trial (KAT) design features, baseline characteristics, and two-year functional outcomes after alternative approaches to knee replacement. J Bone Joint Surg Am 2009;91:134-41. http://dx.doi.org/10.2106/JBJS.G.01074.
- Baker PN, Deehan DJ, Lees D, Jameson S, Avery PJ, Gregg PJ, et al. The effect of surgical factors on early patient-reported outcome measures (PROMS) following total knee replacement. J Bone Joint Surg Br 2012;94:1058-66. http://dx.doi.org/10.1302/0301-620X.94B8.28786.
- Bjorgul K, Novicoff WM, Saleh KJ. Evaluating comorbidities in total hip and knee arthroplasty: available instruments. J Orthop Traumatol 2010;11:203-9. http://dx.doi.org/10.1007/s10195-010-0115-x.
- Shields RK, Enloe LJ, Leo KC. Health related quality of life in patients with total hip or knee replacement. Arch Phys Med Rehabil 1999;80:572-9. https://doi.org/10.1016/S0003-9993(99)90202-2.
- Bozic KJ, Saleh KJ, Rosenberg AG, Rubash HE. Economic evaluation in total hip arthroplasty: analysis and review of the literature. J Arthroplasty 2004;19:180-9. https://doi.org/10.1016/S0883-5403(03)00456-X.
- Maynard A. Developing the health care market. Econ J 1991;101:1277-86. https://doi.org/10.2307/2234443.
- Bourne RB, Rorabeck CH, Laupacis A, Feeny D, Wong C, Tugwell P, et al. A randomized clinical trial comparing cemented to cementless total hip replacement in 250 osteoarthritic patients: the impact on health related quality of life and cost effectiveness. Iowa Orthop J 1994;14:108-14.
- Learmonth ID, Young C, Rorabeck C. The operation of the century: total hip replacement. Lancet 2007;370:1508-19. http://dx.doi.org/10.1016/S0140-6736(07)60457-7.
- NICE . Health Technology Appraisal – Total Hip Replacement and Resurfacing Arthroplasty for the Treatment of Pain or Disability Resulting from End Stage Arthritis of the Hip (Review of Technology Appraisal Guidance 2 and 44): Final Scope 2012 n.d. www.nice.org.uk/nicemedia/live/13690/61348/61348.pdf (accessed 20 November 2012).
- Husted H, Holm G, Jacobsen S. Predictors of length of stay and patient satisfaction after hip and knee replacement surgery: fast-track experience in 712 patients. Acta Orthop 2008;79:168-73. http://dx.doi.org/10.1080/17453670710014941.
- Rolfson O, Dahlberg LE, Nilsson JA, Malchau H, Garellick G. Variables determining outcome in total hip replacement surgery. J Bone Joint Surg Br 2009;91:157-61. http://dx.doi.org/10.1302/0301-620X.91B2.20765.
- Schäfer T, Krummenauer F, Mettelsiefen J, Kirschner S, Günther KP. Social, educational, and occupational predictors of total hip replacement outcome. Osteoarthr Cartil 2010;18:1036-42. http://dx.doi.org/10.1016/j.joca.2010.05.003.
- Briggs A, Sculpher M, Britton A, Murray D, Fitzpatrick R. The costs and benefits of primary total hip replacement. How likely are new prostheses to be cost-effective?. Int J Technol Assess Health Care 1998;14:743-61. https://doi.org/10.1017/S0266462300012058.
- Fitzpatrick R, Shortall E, Sculpher M, Murray D, Morris R, Lodge M, et al. Primary total hip replacement surgery: a systematic review of outcomes and modelling of cost-effectiveness associated with different prostheses. Health Technol Assess 1998;2.
- Vale L, Wyness L, McCormack K, McKenzie L, Brazzelli M, Stearns SC. A systematic review of the effectiveness and cost-effectiveness of metal-on-metal hip resurfacing arthroplasty for treatment of hip disease. Health Technol Assess 2002;6. https://doi.org/10.3310/hta6150.
- Briggs A, Sculpher M, Dawson J, Fitzpatrick R, Murray D, Malchau H. Modelling the Cost-Effectiveness of Primary Hip Replacement: How Cost-Effective is the Spectron Compared to the Charnley Prosthesis?. York: Centre for Health Economics, The University of York; 2003.
- McKenzie L, Vale L, Stearns S, McCormack K. Metal on metal hip resurfacing arthroplasty. An economic analysis. Eur J Health Econ 2003;4:122-9. https://doi.org/10.1007/s10198-002-0158-x.
- Briggs A, Sculpher M, Dawson J, Fitzpatrick R, Murray D, Malchau H. The use of probabilistic decision models in technology assessment: the case of total hip replacement. Appl Health Econ Health Policy 2004;3:79-8. https://doi.org/10.2165/00148365-200403020-00004.
- NHS Hertfordshire . Referral Criteria for Patients from Primary Care Presenting With Hip Pain Due to Ostoarthritis, and Clinical Thresholds for Elective Primary Hip Replacement Surgery 2012. www.enhertsccg.nhs.uk/sites/default/files/documents/Mar2015/guidance_32-hip-referral-and-surgery-thresholds-updated-feb12.pdf (accessed 11 March 2013).
- NHS Warwickshire . Referral and Surgical Threshold Criteria for Elective Primary Hip Replacement Surgery 2011. www.coventry.nhs.uk (accessed 11 March 2013).
- Peninsula Commissioning Priorities Group . Commissioning Decision: Hip and Knee Replacement Surgery in Obese Patients (Those With a Body Mass Index of 30 or Greater) 2011. www.devonpct.nhs.uk/Library/Treatments_commissioning_policies (accessed 11 March 2013).
- NHS Yorkshire and Humber Public Health Observatory . Clinical Thresholds: Hip Replacement for the Treatment of Joint Symptoms and Functional Limitation 2010. www.yhpho.org.uk (accessed 11 March 2013).
- Kalairajah Y, Azurza K, Hulme C, Molloy S, Drabu KJ. Health outcome measures in the evaluation of total hip arthroplasties – a comparison between the Harris hip score and the Oxford hip score. J Arthroplasty 2005;20:1037-41. http://dx.doi.org/10.1016/j.arth.2005.04.017.
- Publication of 2010–11 Reference Costs. London: Department of Health; 2011.
- Curtis L. Unit Costs of Health and Social Care 2011. Canterbury: Personal Social Services Research Unit, University of Kent; 2011.
- British National Formulary. London: BMJ Group and Pharmaceutical Press; 2011.
- NHS Reference Costs: Financial Year 2011 to 2012. London: Department of Health; 2012.
- Dolan P, Gudex C, Kind P, Williams A. The time trade-off method: results from a general population study. Health Econ 1996;5:141-54. https://doi.org/10.1002/(SICI)1099-1050(199603)5:2%3C141::AID-HEC189%3D3.0.CO;2-N.
- Mortimer D, Segal L. Comparing the incomparable? A systematic review of competing techniques for converting descriptive measures of health status into QALY-weights. Med Decis Making 2008;28:66-89. http://dx.doi.org/10.1177/0272989X07309642.
- Gray AM, Rivero-Arias O, Clarke PM. Estimating the association between SF-12 responses and EQ-5D utility values by response mapping. Med Decis Making 2006;26:18-29. http://dx.doi.org/10.1177/0272989X05284108.
- Brazier JE, Yang Y, Tsuchiya A, Rowen DL. A review of studies mapping (or cross walking) non-preference based measures of health to generic preference-based measures. Eur J Health Econ 2010;11:215-25. http://dx.doi.org/10.1007/s10198-009-0168-z.
- National Joint Registry for England and Wales: 11th Annual Report. Hemel Hempstead: NJR; 2014.
- Rothwell AG, Hooper GJ, Hobbs A, Frampton CM. An analysis of the Oxford hip and knee scores and their relationship to early joint revision in the New Zealand Joint Registry. J Bone Joint Surg Br 2010;92:413-18. http://dx.doi.org/10.1302/0301-620X.92B3.22913.
- Curtis L. Unit Costs of Health and Social Care 2014. Canterbury: Personal Social Services Research Unit, University of Kent; 2014.
- NHS Prescription Services . Prescription Cost Analysis (PCA) Data 2015 n.d. www.nhsbsa.nhs.uk/PrescriptionServices/3494.aspx (accessed 4 June 2015).
- NHS Prescription Services . Drug Tariff 2015 n.d. www.nhsbsa.nhs.uk/PrescriptionServices/4940.aspx (accessed 4 June 2016).
- WHO Collaborating Centre for Drug Statistics Methodology . ATC/DDD/Index/2015 2015. www.whocc.no/atc_ddd_index/ (accessed 4 June 2015).
- British National Formulary. London: BMJ Group and Pharmaceutical Press; n.d.
- Guide to the Methods of Technology Appraisal 2013. London: NICE; 2013.
- South West London Public Health Network . 2010/11/South/West/London/Effective/Commissioning/Initiative 2010. www.kingstonpct.nhs.uk/Downloads/Board%20Papers/24%20May%202010/Att%20D%20-ECI%20%20MASTER%20VERSION%202010-11%20DRAFT%207.pdf (accessed 13 June 2011).
- Contract and Information Shared Services Unit . Cheshire &Amp; Merseyside Prior Approval Scheme: Incorporating Procedures of Lower Clinical Priority 2010. www.haltonandsthelenspct.nhs.uk/library/documents/HTSHcmfinalforcontractspapolicyfor2011122303114.pdf (accessed 17 April 2012).
- NHS Derby City and NHS Derbyshire County . Commissioning Policy for Procedures of Limited Clinical Value (PLCV) 2011. www.derbycitypct.nhs.uk/UserFiles/Documents/1101%20Policy%20for%20PLCV%202011.pdf (accessed 13 June 2011).
- Losina E, Walensky RP, Kessler CL, Emrani PS, Reichmann WM, Wright EA, et al. Cost-effectiveness of total knee arthroplasty in the United States: patient risk and hospital volume. Arch Intern Med 2009;169:1113-21. http://dx.doi.org/10.1001/archinternmed.2009.136.
- Slover JD, Tosteson AN, Bozic KJ, Rubash HE, Malchau H. Impact of hospital volume on the economic value of computer navigation for total knee replacement. J Bone Joint Surg Am 2008;90:1492-500. http://dx.doi.org/10.2106/JBJS.G.00888.
- Murray DW, Carr AJ, Bulstrode CJ. Which primary total hip replacement?. J Bone Joint Surg Br 1995;77:520-7.
- NICE . Total Hip Replacement and Resurfacing Arthroplasty for End-Stage Arthritis of the Hip, Technology Appraisal Guidance (TA304) n.d. www.nice.org.uk/guidance/ta304/chapter/1-Guidance (accessed May 2017).
- Pennington M, Grieve R, Sekhon JS, Gregg P, Black N, van der Meulen JH. Cemented, cementless, and hybrid prostheses for total hip replacement: cost effectiveness analysis. BMJ 2013;346. http://dx.doi.org/10.1136/bmj.f1026.
- Sahlgrenska University Hospital . Swedish Hip Arthroplasty Register: Annual Report 2008. Shortened Version 2009 n.d. www.jru.orthop.gu.se/ (accessed 28 March 2011).
- NJR . NJR Patient Reported Outcome Measures n.d. www.njrcentre.org.uk/njrcentre/Research/NJRPROMs/tabid/203/Default.aspx (accessed 3 July 2012).
- Saleh KJ, Wood KC, Gafni A, Gross AE. Immediate surgery versus waiting list policy in revision total hip arthroplasty. An economic evaluation. J Arthroplasty 1997;12:1-10. https://doi.org/10.1016/S0883-5403(97)90040-1.
- NHS . The NHS Structure Explained n.d. www.nhs.uk/NHSEngland/thenhs/about/Pages/nhsstructure.aspx (accessed 22 July 2013).
- Data Protection Act 1998. London: The Stationery Office; 1998.
- Lawrence JS. Rheumatism in Populations. London: William Heinemann Medical Books Ltd; 1977.
- Altman RD, Gold GE. Atlas of individual radiographic features in osteoarthritis, revised. Osteoarthr Cartil 2007;15:1-56. http://dx.doi.org/10.1016/j.joca.2006.11.009.
- Resnick D. Patterns of migration of the femoral head in osteoarthritis of the hip. Roentgenographic-pathologic correlation and comparison with rheumatoid arthritis. Am J Roentgenol Radium Ther Nucl Med 1975;124:62-74. https://doi.org/10.2214/ajr.124.1.62.
- Solomon L. Patterns of osteoarthritis of the hip. J Bone Joint Surg Br 1976;58:176-83.
- Ledingham J, Dawson S, Preston B, Milligan G, Doherty M. Radiographic patterns and associations of osteoarthritis of the hip. Ann Rheum Dis 1992;51:1111-16. https://doi.org/10.1136/ard.51.10.1111.
- Maillefert JF, Gueguen A, Nguyen M, Berdah L, Lequesne M, Mazières B, et al. A composite index for total hip arthroplasty in patients with hip osteoarthritis. J Rheumatol 2002;29:347-52.
- Maheu E, Cadet C, Marty M, Dougados M, Ghabri S, Kerloch I, et al. Reproducibility and sensitivity to change of various methods to measure joint space width in osteoarthritis of the hip: a double reading of three different radiographic views taken with a three-year interval. Arthritis Res Ther 2005;7:R1375-85. https://doi.org/10.1186/ar1831.
- Hart DJ, Mootoosamy I, Doyle DV, Spector TD. The relationship between osteoarthritis and osteoporosis in the general population: the Chingford Study. Ann Rheum Dis 1994;53:158-62. https://doi.org/10.1136/ard.53.3.158.
- Prieto-Alhambra D, Javaid MK, Judge A, Murray D, Carr A, Cooper C, et al. Association between bisphosphonate use and implant survival after primary total arthroplasty of the knee or hip: population based retrospective cohort study. BMJ 2011;343. http://dx.doi.org/10.1136/bmj.d7222.
- Prieto-Alhambra D, Lalmohamed A, Abrahamsen B, Arden NK, de Boer A, Vestergaard P, et al. Oral bisphosphonate use and total knee/hip implant survival: validation of results in an external population-based cohort. Arthritis Rheumatol 2014;66:3233-40. https://doi.org/10.1002/art.38789.
- Fine JP, Gray RJ. A proportional hazards model for the subdistribution of a competing risk. J Am Stat Assoc 1999;94:496-509. http://dx.doi.org/10.1080/01621459.1999.10474144.
- Prieto-Alhambra D, Javaid MK, Judge A, Maskell J, Kiran A, Cooper C, et al. Bisphosphonate use and risk of post-operative fracture among patients undergoing a total knee replacement for knee osteoarthritis: a propensity score analysis. Osteoporos Int 2011;22:1555-71. http://dx.doi.org/10.1007/s00198-010-1368-1.
- de Verteuil R, Imamura M, Zhu S, Glazener C, Fraser C, Munro N, et al. A systematic review of the clinical effectiveness and cost-effectiveness and economic modelling of minimal incision total hip replacement approaches in the management of arthritic disease of the hip. Health Technol Assess 2008;12. https://doi.org/10.3310/hta12260.
- Pinedo-Villanueva R. Total Hip Replacement in the UK: Cost-effectiveness of a Prediction Tool and Outcomes Mapping. Southampton: University of Southampton; 2013.
- Wooldridge J. Introductory Econometrics – A Modern Approach. Independence, KY: South-Western CENGAGE Learning; 2009.
- Neuburger J, Hutchings A, Allwood D, Black N, van der Meulen JH. Sociodemographic differences in the severity and duration of disease amongst patients undergoing hip or knee replacement surgery. J Public Health 2012;34:421-9. http://dx.doi.org/10.1093/pubmed/fdr119.
- Judge A, Welton NJ, Sandhu J, Ben-Shlomo Y. Equity in access to total joint replacement of the hip and knee in England: cross sectional study. BMJ 2010;341. http://dx.doi.org/10.1136/bmj.c4092.
- Lalmohamed A, Vestergaard P, Klop C, Grove EL, de Boer A, Leufkens HG, et al. Timing of acute myocardial infarction in patients undergoing total hip or knee replacement: a nationwide cohort study. Arch Intern Med 2012;172:1229-35. https://doi.org/10.1001/archinternmed.2012.2713.
- Lalmohamed A, Vestergaard P, Cooper C, de Boer A, Leufkens HG, van Staa TP, et al. Timing of stroke in patients undergoing total hip replacement and matched controls: a nationwide cohort study. Stroke 2012;43:3225-9. http://dx.doi.org/10.1161/STROKEAHA.112.668509.
- Charnley J. The long-term results of low-friction arthroplasty of the hip performed as a primary intervention. J Bone Joint Surg Br 1972;54-B:61-76.
Appendix 1 Read codes
General Practice Research Database medical codes
medcode | readcode | readterm |
---|---|---|
1104 | N053512 | Hip OA NOS |
2209 | N05z511 | Hip OA NOS |
6812 | N05zJ00 | OA NOS, of hip |
medcode | readcode | readterm |
---|---|---|
665 | N05z611 | Knee OA NOS |
2487 | N05zL00 | OA NOS, of knee |
medcode | readcode | readterm |
---|---|---|
394 | 7K22z00 | Total prosthetic replacement of hip joint NOS |
2224 | 7K20.1G | THR – total prosthetic replacement of hip joint using cement |
5481 | 7K20.00 | Total prosthetic replacement of hip joint using cement |
2734 | 7K22.12 | THR – other total prosthetic replacement of hip joint |
9762 | 7K22.00 | Other total prosthetic replacement of hip joint |
33439 | 7K22000 | Primary total prosthetic replacement of hip joint NEC |
589 | 7K21.17 | THR – total prosthetic replacement hip joint without cement |
18442 | 7K21.00 | Total prosthetic replacement of hip joint not using cement |
16671 | 7K20.13 | Charnley total replacement of hip joint using cement |
10856 | 7K20000 | Primary cemented THR |
28468 | 7K20.14 | Exeter total replacement of hip joint using cement |
17860 | 7K20.11 | Arthroplasty of hip joint using cement |
47483 | 7K21000 | Primary uncemented THR |
38001 | 7K20y00 | Total prosthetic replacement of hip joint using cement NOS |
10348 | 7K20300 | Primary hybrid total replacement of hip joint NEC |
47812 | 7K20z00 | Total prosthetic replacement of hip joint using cement NOS |
37631 | 7K22y00 | Other specified total prosthetic replacement of hip joint |
29977 | 7K20.1E | Stanmore total replacement of hip joint using cement |
38332 | 7K20.17 | Furlong total replacement of hip joint using cement |
38347 | 7K21z00 | Total prosthetic replacement hip joint not using cement NOS |
52714 | 7K20011 | Charnley cemented THR |
6013 | 7K20.1C | Muller total replacement of hip joint using cement |
36590 | 7K20.18 | Howse total replacement of hip joint using cement |
47735 | 7K21.12 | Furlong total replacement of hip joint not using cement |
48220 | 7K22100 | Conversion to total prosthetic replacement of hip joint NEC |
71351 | 7K20.12 | Aufranc total replacement of hip joint using cement |
31843 | 7K20100 | Conversion to cemented THR |
47715 | 7K20.1B | Monk total replacement of hip joint using cement |
34997 | 7K20.1A | McKee total replacement of hip joint using cement |
10341 | 7K21y00 | Total prosthetic replacement hip joint not using cement NOS |
62092 | 7K20.1F | Turner total replacement of hip joint using cement |
62133 | 7K20400 | Conversion to hybrid THR NEC |
52901 | 7K20.16 | Freeman total replacement of hip joint using cement |
50624 | 7K21100 | Conversion to uncemented THR |
67778 | 7K20x00 | Conversion from cemented THR |
56215 | 7K20.19 | Ilch total replacement of hip joint using cement |
66139 | 7K20.15 | Farrer total replacement of hip joint using cement |
73951 | 7K21.16 | Ring total replacement of hip joint not using cement |
51519 | 7K21.15 | Monk total replacement of hip joint not using cement |
53109 | 7K21.11 | Freeman total replacement of hip joint not using cement |
67306 | 7K20600 | Conversion from hybrid total prosthetic hip joint replacement NEC |
94273 | 7K20.1D | Pretoria total replacement of hip joint using cement |
96435 | 7K21.13 | Lord total replacement of hip joint not using cement |
2032 | 7K22200 | Revision of total prosthetic replacement of hip joint NEC |
8895 | 7K20200 | Revision cemented THR |
29101 | 7K21200 | Revision uncemented THR |
38942 | 7K23200 | Revision cemented hemiarthroplasty of hip |
41370 | 7K22300 | Attention to THR NEC |
41184 | 7K24200 | Revision uncemented hemiarthroplasty of hip |
36700 | 7K20x11 | Removal previous cemented total prosthetic replacement hip joint |
28784 | 7K69100 | Revision of resurfacing arthroplasty |
55662 | 7K20500 | Revision of hybrid THR NEC |
38791 | 7K22x00 | Conversion from previous total prosthetic replacement hip joint NEC |
45930 | 7K68300 | Conversion to excision arthroplasty |
66363 | 7K22211 | Revision of hybrid THR NEC |
97176 | 7K22112 | Conversion to hybrid THR NEC |
99651 | 7K22x12 | Conversion from hybrid total prosthetic hip joint replacement NEC |
medcode | readcode | readterm |
---|---|---|
5362 | 7K30.1V | TKR – total prosthetic replacement of knee joint using cement |
3414 | 7K30.00 | Total prosthetic replacement of knee joint using cement |
673 | 7K32z00 | Other total prosthetic replacement of knee joint NOS |
3973 | 7K32.12 | TKR – other total prosthetic replacement of knee joint |
8555 | 7K32.00 | Other total prosthetic replacement of knee joint |
28048 | 7K32000 | Primary TKR NEC |
11847 | 7K32200 | Revision of TKR NEC |
20746 | 7K30000 | Primary cemented TKR |
17471 | 7K31.00 | Total prosthetic replacement of knee joint not using cement |
9877 | 7K31.12 | TKR – total prosthetic replacement knee joint without cement |
10553 | 7K30200 | Revision cemented TKR |
11225 | 7K37.00 | Cemented UKR |
10372 | 7K30y00 | Total prosthetic replacement of knee joint using cement NOS |
8006 | 7K30z00 | Total prosthetic replacement of knee joint using cement NOS |
9817 | 7K38.00 | Uncemented UKR |
36343 | 7K37000 | Primary cemented UKR |
49053 | 7K31000 | Primary uncemented TKR |
54343 | 7K38000 | Primary uncemented UKR |
41545 | 7K31200 | Revision uncemented TKR |
37979 | 7K32y00 | Other total prosthetic replacement of knee joint NOS |
58612 | 7K31z00 | Total prosthetic replacement knee joint not using cement NOS |
55470 | 7K39.00 | Hybrid UKR |
54756 | 7K32400 | Attention to TKR NEC |
37950 | 7K39000 | Primary hybrid UKR |
46475 | 7K30.16 | Charnley total replacement of knee joint using cement |
61687 | 7K30.13 | Attenborough total replacement of knee joint using cement |
50829 | 7K31y00 | Total prosthetic replacement knee joint not using cement NOS |
48815 | 7K32x11 | Removal previous total prosthetic replacement knee joint NEC |
93344 | 7K30.11 | Anametric total replacement of knee joint using cement |
38740 | 7K30x11 | Removal previous cemented total prosthetic replacement knee |
44775 | 7K30.18 | Denham total replacement of knee joint using cement |
54860 | 7K30.19 | Freeman total replacement of knee joint using cement |
55991 | 7K30.1P | Sheehan total replacement of knee joint using cement |
42259 | 7K32211 | Revision hybrid TKR NEC |
62757 | 7K32100 | Conversion to TKR NEC |
66156 | 7k32200 | Revision of total knee replacement NEC |
44926 | 7K30.1R | Stanmore total replacement of knee joint using cement |
83544 | 7K32011 | Primary hybrid TKR NEC |
47301 | 7K30.1N | Polycentric total replacement of knee joint using cement |
49813 | 7K30.17 | Deane total replacement of knee joint using cement |
69999 | 7K30100 | Conversion to cemented TKR |
41820 | 7K32x00 | Conversion from TKR NEC |
63086 | 7K30.1T | Uci total replacement of knee joint using cement |
49716 | 7K30.1S | Swanson total replacement of knee joint using cement |
58980 | 7K37200 | Revision cemented UKR |
92246 | 7K30.1H | Liverpool total replacement of knee joint using cement |
38073 | 7K39200 | Revision hybrid UKR |
47223 | 7K32411 | Attention to hybrid TKR NEC |
61288 | 7K38200 | Revision uncemented UKR |
63802 | 7K30.1A | Geomedic total replacement of knee joint using cement |
66707 | 7K30.1E | Herbert total replacement of knee joint using cement |
70507 | 7K30.1Q | Shiers total replacement of knee joint using cement |
71456 | 7K30.15 | Cavendish total replacement of knee joint using cement |
73075 | 7K30x00 | Conversion from cemented TKR |
93435 | 7K32112 | Conversion to hybrid TKR NEC |
97341 | 7K31x00 | Conversion from uncemented TKR |
97400 | 7K37x00 | Conversion from cemented UKR |
99912 | 7K30.1I | Manchester total replacement of knee joint using cement |
28784 | 7K69100 | Revision of resurfacing arthroplasty |
45930 | 7K68300 | Conversion to excision arthroplasty |
medcode | readcode | readterm |
---|---|---|
286 | N094K12 | Hip pain |
1330 | N094512 | Hip joint pain |
33407 | N094K00 | Arthralgia of hip |
medcode | readcode | readterm |
---|---|---|
9517 | 1M10.00 | Knee pain |
554 | N094611 | Knee joint pain |
6044 | N094M00 | Arthralgia of knee |
6166 | N094W00 | Anterior knee pain |
10389 | 1M12.00 | Anterior knee pain |
medcode | readcode | readterm |
---|---|---|
7334 | N06z511 | Hip arthritis NOS |
17561 | N010511 | Hip pyogenic arthritis |
66483 | N01zH00 | Infective arthritis NOS, of hip |
medcode | readcode | readterm |
---|---|---|
2852 | N06z611 | Knee arthritis NOS |
62037 | N03xB00 | Arthritis associated with other disease, knee |
56895 | N01zK00 | Infective arthritis NOS, of knee |
medcode | readcode | readterm |
---|---|---|
17176 | N072100 | Degenerative lesion of articular cartilage of knee |
Appendix 2 Statistical methods
Predictors of outcomes of total knee replacement surgery141
Statistical methods: ANCOVA was used to identify predictors of the 6-month follow-up OKS, adjusting for preoperative OKS. A multivariable model was fitted including all predictor variables. Analyses were repeated for the total OKSs, pain scores and function scores separately. Regression diagnostics were checked to ensure that the assumptions underlying the linear regression model (ANCOVA) were met. As there was evidence of heteroscedasticity (variance of the residuals is non-constant), robust standard errors were used with the sandwich variance estimator. Performance of the predictive model was assessed in terms of calibration and discrimination.
The ROC curve analyses were used to identify cut-off points for the 6-month follow-up OKS associated with satisfaction with surgery. Logistic regression modelling was used to identify predictors of the 6-month PASS score. Calibration was assessed using a Hosmer–Lemeshow goodness-of-fit test. Discrimination was assessed by calculating the area under the ROC curve. Regression diagnostics were checked to ensure that the assumptions underlying the logistic regression model were met.
A population-based survival analysis describing the association of body mass index on time to revision for total hip and knee replacements: results from the UK general practice research database97
Statistical methods: we used competing risks regression methods of Fine and Gray. 250 Proportionality of hazards assumptions was assessed by examining complementary log–log plots of the cumulative incidence. As a sensitivity analysis we modelled the same data using a standard Cox regression analysis.
The association of patient characteristics and surgical variables on symptoms of pain and function over 5 years following primary hip replacement surgery: a prospective cohort study139
Statistical methods: a repeated measures linear regression model was fitted when the outcomes were the OHS. A generalised estimating equation was used to account for clustering within the data using an exchangeable correlation matrix. Fractional polynomial regression modelling was used to explore evidence of non-linear relationships for continuous variables.
Body mass index is not a clinically meaningful predictor of patient-reported outcomes of primary hip replacement surgery: prospective cohort study160
Statistical methods: linear regression modelling is used adjusting for the baseline OHS and confounding factors. A repeated measures linear regression model is fitted, in which the outcome is the pre- and postoperative OHS, and an interaction term fitted between BMI and time, to describe the change in OHS over time within BMI categories, adjusting for confounding factors.
Bisphosphonate use and risk of postoperative fracture among patients undergoing a total knee replacement for knee osteoarthritis: a propensity score analysis251
Statistical methods: univariable and multivariable Cox regression models were used. The proportional hazards assumption was checked using the Schoenfeld residuals formal test and the smoothing splines in time plots. The linearity of quantitative covariates was assessed by inspection of the Martingale’s residuals plots. Delta beta plots were used to look for possible influential cases.
Preoperative expectation predicts 12-month postoperative outcome among patients undergoing primary total hip replacement in European orthopaedic centres162
Statistical methods: ordered logistic regression modelling was used.
The effect of body mass index on the risk of postoperative complications during the 6 months following total hip replacement or total knee replacement surgery77
Statistical methods: for each operation (THR or TKR), logistic regression methods were used to assess whether or not the likelihood of experiencing each outcome varied by BMI category. Robust standard errors adjusting for clustering of patients within GP practices were used. Adjustment was made for potential confounding variables.
Association between bisphosphonate use and implant survival after primary total arthroplasty of the knee or hip: population-based retrospective cohort study248
Statistical methods: we used propensity score adjustment to reduce the effects of confounding by indication. The propensity score for bisphosphonate use represents the probability that a patient is prescribed bisphosphonate treatment, and was estimated for the whole study population by multivariate logistic regression modelling.
Patient-reported outcomes 1 year after primary hip replacement in a European Collaborative Cohort30
Statistical methods: a random-effects logistic regression model was fitted that controlled for evidence of clustering across countries. Multivariable analyses were then fitted to obtain adjusted ORs. Wald’s tests were used to explore linear trends.
Clinical tool to identify patients who are most likely to achieve long-term improvement in physical function after total hip arthroplasty143
Statistical methods: logistic regression modelling was used to identify predictors of functional improvement. Univariable models explored the association between each of the predictor variables and the outcome. Linearity was assessed using likelihood ratio tests comparing a model with a categorical variable to one with the variable as a score. To avoid overfitting the model, 10 times as many outcome events as predictor variables (more specifically, degrees of freedom) are required, restricting the regression model to six degrees of freedom. Regression diagnostics were checked to ensure that the assumptions underlying the logistic regression model were met.
A clinical tool for the prediction of patient-reported outcomes after knee replacement surgery: a prospective cohort study142
Statistical methods: general linear models were used to identify risk factors on postoperative OKS. Linearity of continuous variables with the outcome was assessed using fractional polynomials and collinearity between variables was assessed by the VIF. Because the variance of the residuals is non-constant (evidence of heteroscedasticity), robust standard errors were used with the sandwich variance estimator.
Patient-reported outcomes following primary hip replacement surgery: development and internal validation of a prognostic tool138
Statistical methods: ANCOVA was used to identify predictors of the 12-month follow-up OHS, adjusting for preoperative OHS. Linear splines were used to model non-linear relationships for continuous variables.
Appendix 3 Variable list
Variable | Available, n (%) | Categories (for binary/categorical) data | Cohort | |||
---|---|---|---|---|---|---|
EPOS (n = 1366) | EUROHIP (n = 888) | St Helier (n = 787) | SWLEOC (n = 1598) | |||
Patient ID | 4639 (100) | 100 | 100 | 100 | 100 | |
Study ID | 4639 (100) | EPOS (n = 1366), EUROHIP (n = 888), St Helier (n = 787) and SWLEOC (n = 1598) | 100 | 100 | 100 | 100 |
OHS preoperative | 4639 (100) | 100 | 100 | 100 | 100 | |
OHS 12 months | 4413 (95) | 93 | 96 | 89 | 100 | |
PASS score: quality of life | 4413 (95) | Not satisfied (score of < 36) (n = 1003) and satisfied (score of ≥ 36) (n = 3410) | 93 | 96 | 89 | 100 |
Age at operation | 4618 (100) | 99 | 99 | 100 | 100 | |
Sex: 1 = female, 0 = male | 4585 (99) | 0 (n = 1772) and 1 (n = 2813) | 100 | 95 | 100 | 100 |
BMI | 2892 (62) | 93 | 92 | 59 | 21 | |
Weight (kg) | 2495 (54) | 98 | 93 | 0 | 21 | |
Height (cm) | 2440 (53) | 94 | 92 | 0 | 21 | |
Living alone | 886 (19) | Spouse/partner (n = 619), somebody else (n = 50) and alone (n = 217) | 0 | 100 | 0 | 0 |
Caring for someone else | 875 (19) | No (n = 718) and yes (n = 157) | 0 | 99 | 0 | 0 |
Employment | 872 (19) | Employed (n = 216), retired (n = 507), unemployed (n = 25), retired early (n = 66) and housework (n = 58) | 0 | 98 | 0 | 0 |
Employed | 2781 (60) | Unemployed (n = 2208) and employed (n = 573) | 100 | 98 | 0 | 34 |
Occupation | 1366 (29) | Heavy manual (n = 40), light manual (n = 87), office/professional (n = 106), housewife (n = 175) and unemployed/retired (n = 958) | 100 | 0 | 0 | 0 |
EQ-5D anxiety/depression preoperative | 2418 (52) | None (n = 1327), moderate (n = 975) and extreme (n = 116) | 0 | 99 | 0 | 96 |
Hospital Anxiety and Depression Scale score | 118 (2.5) | 0 | 13 | |||
SF-36 mental component summary score preoperative | 916 (20) | 67 | 0 | 0 | 0 | |
ASA grade | 1151 (25) | Grade1 (n = 183), grade 2 (n = 763), grade 3 (n = 200) and grade 4 (n = 5) | 0 | 88 | 0 | 23 |
School education | 781 (17) | Postgraduate (n = 28), graduate (n = 91), college (n = 244) and none (n = 418) | 0 | 88 | 0 | 0 |
College education | 781 (17) | None (n = 418) and college/university (n = 363) | 0 | 88 | 0 | 0 |
Years of hip pain | 1044 (23) | < 1 (n = 121), 1–2 (n = 317), 3–5 (n = 305) and 6–8 (n = 301) | 0 | 99 | 21 | 0 |
Fixed flexion | 1254 (27) | 0 (n = 643), 0–9 (n = 131), 10–19 (n = 275), 20–29 (n = 127), 30–59 (n = 68) and ≥ 60 (n = 10) | 92 | 0 | 0 | 0 |
Preoperative expectation: care_self | 888 (19) | 0 (n = 831) and 1 (n = 57) | 0 | 100 | 0 | 0 |
Preoperative expectation: less_pain | 1050 (23) | No (n = 722) and yes (n = 328) | 0 | 100 | 21 | 0 |
Preoperative expectation: care_others | 888 (19) | 0 (n = 864) and 1 (n = 24) | 0 | 100 | 0 | 0 |
Preoperative expectation: housework | 888 (19) | No (n = 677) and yes (n = 211) | 0 | 100 | 0 | 0 |
Preoperative expectation: work | 888 (19) | 0 (n = 831) and 1 (n = 57) | 0 | 100 | 0 | 0 |
Preoperative expectation: shopping | 888 (19) | 0 (n = 804) and 1 (n = 84) | 0 | 100 | 0 | 0 |
Preoperative expectation: walk_normally | 888 (19) | No (n = 564) and yes (n = 324) | 0 | 100 | 0 | 0 |
Preoperative expectation: as_much_as_possible | 888 (19) | 0 (n = 832) and 1 (n = 56) | 0 | 100 | 0 | 0 |
Preoperative expectation: drive | 888 (19) | 0 (n = 847) and 1 (n = 41) | 0 | 100 | 0 | 0 |
Preoperative expectation: garden | 888 (19) | No (n = 727) and yes (n = 161) | 0 | 100 | 0 | 0 |
Preoperative expectation: holiday | 888 (19) | No (n = 866) and yes (n = 22) | 0 | 100 | 0 | 0 |
Preoperative expectation: Activities of Daily Living | 1049 (23) | No (n = 758) and yes (n = 291) | 0 | 100 | 20 | 0 |
Preoperative expectation: exercise_leisure | 888 (19) | No (n = 708) and yes (n = 180) | 0 | 100 | 0 | 0 |
Preoperative comorbidity: dvt | 947 (20) | No (n = 881) and yes (n = 66) | 69 | 0 | 0 | 0 |
Preoperative comorbidity: pulmo | 945 (20) | No (n = 915) and yes (n = 30) | 69 | 0 | 0 | 0 |
Preoperative comorbidity: uti | 948 (20) | No (n = 884) and yes (n = 64) | 69 | 0 | 0 | 0 |
Preoperative comorbidity: muscu | 948 (20) | No (n = 606) and yes (n = 342) | 69 | 0 | 0 | 0 |
Preoperative comorbidity: neuro | 945 (20) | No (n = 887) and yes (n = 58) | 69 | 0 | 0 | 0 |
Preoperative comorbidity: respi | 945 (20) | No (n = 828) and yes (n = 117) | 69 | 0 | 0 | 0 |
Preoperative comorbidity: cardi | 945 (20) | No (n = 559) and yes (n = 386) | 69 | 0 | 0 | 0 |
Preoperative comorbidity: renal | 945 (20) | No (n = 924) and yes (n = 21) | 69 | 0 | 0 | 0 |
Preoperative comorbidity: hepat | 943 (20) | No (n = 937) and yes (n = 6) | 69 | 0 | 0 | 0 |
Number of comorbidities | 948 (20) | 0 (n = 198), 1 (n = 489), 2 (n = 199), 3 (n = 47), 4 (n = 13) and 5 (n = 2) | 69 | 0 | 0 | 0 |
Medication use: bisphosphonates | 888 (19) | 0 (n = 873) and 1 (n = 15) | 0 | 100 | 0 | 0 |
Medication use: anticoagulant | 888 (19) | 0 (n = 831) and 1 (n = 57) | 0 | 100 | 0 | 0 |
Medication use: antidepressants | 888 (19) | No (n = 818) and yes (n = 70) | 0 | 100 | 0 | 0 |
Medication use: bronchodilators | 888 (19) | 0 (n = 849) and 1 (n = 39) | 0 | 100 | 0 | 0 |
Medication use: antidiabetic | 888 (19) | 0 (n = 833) and 1 (n = 55) | 0 | 100 | 0 | 0 |
Medication use: analgesic NSAIDs | 2079 (45) | No (n = 446) and yes (n = 1633) | 99 | 81 | 0 | 0 |
Medication use: heart | 720 (16) | No (n = 258) and yes (n = 462) | 0 | 81 | 0 | 0 |
K/L grade preoperative | 823 (18) | K/L grade 0–2 (n = 123), K/L grade 3 (n = 273) and K/L grade 4 (n = 427) | 0 | 93 | 0 | 0 |
Pattern of OA | 771 (17) | No reduction of joint space (n = 36), superolateral (n = 335) and superomedial/medial/concentric (n = 400) | 0 | 87 | 0 | 0 |
Hypertrophic | 779 (17) | None (n = 662), hyper (n = 45) and atrophic (n = 72) | 0 | 88 | 0 | 0 |
Prosthesis type | 2223 (48) | Hybrid (n = 354), cemented (n = 1556) and uncemented (n = 313) | 100 | 97 | 0 | 0 |
Superior acetabular osteophyte | 803 (17) | 0 (n = 49), 1 (n = 243), 2 (n = 319), 3 (n = 162), 7 (n = 8), 8 (n = 12) and 9 (n = 10) | 0 | 90 | 0 | 0 |
Superior femoral osteophyte | 803 (17) | 0 (n = 87), 1 (n = 217), 2 (n = 269), 3 (n = 193), 7 (n = 10), 8 (n = 17) and 9 (n = 10) | 0 | 90 | 0 | 0 |
Inferior acetabular osteophyte | 803 (17) | 0 (n = 106), 1 (n = 197), 2 (n = 264), 3 (n = 192), 7 (n = 12), 8 (n = 22) and 9 (n = 10) | 0 | 90 | 0 | 0 |
Inferior femoral osteophyte | 803 (17) | 0 (n = 122), 1 (n = 270), 2 (n = 232), 3 (n = 135), 7 (n = 11), 8 (n = 23) and 9 (n = 10) | 0 | 90 | 0 | 0 |
Acetabular sclerosis | 803 (17) | 0 (n = 319), 1 (n = 74), 2 (n = 376), 7 (n = 18), 8 (n = 6) and 9 (n = 10) | 0 | 90 | 0 | 0 |
Acetabular cysts | 803 (17) | 0 (n = 442), 1 (n = 332), 7 (n = 14), 8 (n = 5) and 9 (n = 10) | 0 | 90 | 0 | 0 |
Acetabular attrition | 802 (17) | 0 (n = 637), 1 (n = 140), 7 (n = 11), 8 (n = 4) and 9 (n = 10) | 0 | 90 | 0 | 0 |
Femoral sclerosis | 801 (17) | 0 (n = 409), 1 (n = 43), 2 (n = 313), 7 (n = 18), 8 (n = 8) and 9 (n = 10) | 0 | 90 | 0 | 0 |
Femoral cysts | 801 (17) | 0 (n = 336), 1 (n = 434), 7 (n = 14), 8 (n = 7) and 9 (n = 10) | 0 | 90 | 0 | 0 |
Femoral attrition | 800 (17) | 0 (n = 526), 1 (n = 248), 7 (n = 11), 8 (n = 5) and 9 (n = 10) | 0 | 90 | 0 | 0 |
Superior joint space | 801 (17) | 0 (n = 155), 1 (n = 82), 2 (n = 259), 3 (n = 282), 7 (n = 9), 8 (n = 4) and 9 (n = 10) | 0 | 90 | 0 | 0 |
Medial joint space | 800 (17) | 0 (n = 287), 1 (n = 155), 2 (n = 218), 3 (n = 115), 7 (n = 9), 8 (n = 6) and 9 (n = 10) | 0 | 90 | 0 | 0 |
Chondrocalcinosis | 799 (17) | 0 (n = 771), 1 (n = 6), 7 (n = 8), 8 (n = 4) and 9 (n = 10) | 0 | 90 | 0 | 0 |
Number of joints with OA | 884 (19) | 0 | 100 | 0 | 0 | |
Number of joints with surgery | 884 (19) | 0 (n = 494), 1 (n = 272), 2 (n = 88), 3 (n = 25) and 4 (n = 5) | 0 | 100 | 0 | 0 |
Lower back/foot/ankle OA | 881 (19) | 0 (n = 635) and 1 (n = 246) | 0 | 99 | 0 | 0 |
Lower back/foot/ankle surgery | 884 (19) | 0 (n = 797) and 1 (n = 87) | 0 | 100 | 0 | 0 |
Grade of operator | 1361 (29) | Consultant, locum consultant, associated specialist/staff (n = 865) and fellow, senior registrar, registrar, locum registrar (n = 496) | 100 | 0 | 0 | 0 |
Surgical approach | 985 (21) | Anterolateral (n = 641) and posterior (n = 344) | 72 | 0 | 0 | 0 |
Patient’s position | 1359 (29) | Supine (n = 225) and lateral (n = 1134) | 99 | 0 | 0 | 0 |
Lavage system (acetabular) | 1334 (29) | No (n = 47) and yes (n = 1287) | 98 | 0 | 0 | 0 |
Cement pressurisation (acetabular) | 1355 (29) | No (n = 265) and yes (n = 1090) | 99 | 0 | 0 | 0 |
Cement pressurisations (femur) | 1350 (29) | No (n = 13) and yes (n = 1337) | 99 | 0 | 0 | 0 |
Stem size (mm offset) | 1363 (29) | 31 (n = 64), 38 (n = 576), 44 (n = 695) and 50 (n = 28) | 100 | 0 | 0 | 0 |
Femoral head | 1362 (29) | Stainless steel (n = 1250) and ceramic – zirconia/alumina (n = 112) | 100 | 0 | 0 | 0 |
Head size | 1366 (29) | 22 (n = 243), 26 (n = 507) and 28 (n = 616) | 100 | 0 | 0 | 0 |
Duration of operation (minutes) | 1308 (28) | 96 | 0 | 0 | 0 | |
Type of polythene | 1363 (29) | UHMWPE (n = 809) and duration (n = 554) | 100 | 0 | 0 | 0 |
Acetabular cup inclination (degrees) | 923 (20) | 68 | 0 | 0 | 0 | |
Acetabular cup version (degrees) | 923 (20) | 68 | 0 | 0 | 0 | |
Hip dislocation | 923 (20) | No (n = 903) and yes (n = 20) | 68 | 0 | 0 | 0 |
IMD 200453 deprivation score | 1575 (34) | 0 | 0 | 0 | 99 | |
Marital status | 301 (6) | Divorced (n = 2), married (n = 288), single (n = 7) and widow (n = 4) | 0 | 0 | 0 | 19 |
Appendix 4 Hip replacement model by Briggs et al.200
This popular model shown in Figure 61 was first published by Briggs et al. 200 in 1998. One other study made a slight change, keeping the same structure shown in the figure but substituting ‘death’ for ‘non-operative management’, allowing transitions to death from all states. 252
FIGURE 61.
Hip replacement model used by six economic evaluations and first reported by Briggs A, Sculpher M, Britton A, Murray D, Fitzpatrick R. The costs and benefits of primary total hip replacement. How likely are new prostheses to be cost-effective? Int J Technol Assess Health Care vol. 14, issue 4, pp. 743–61200 reproduced with permission.
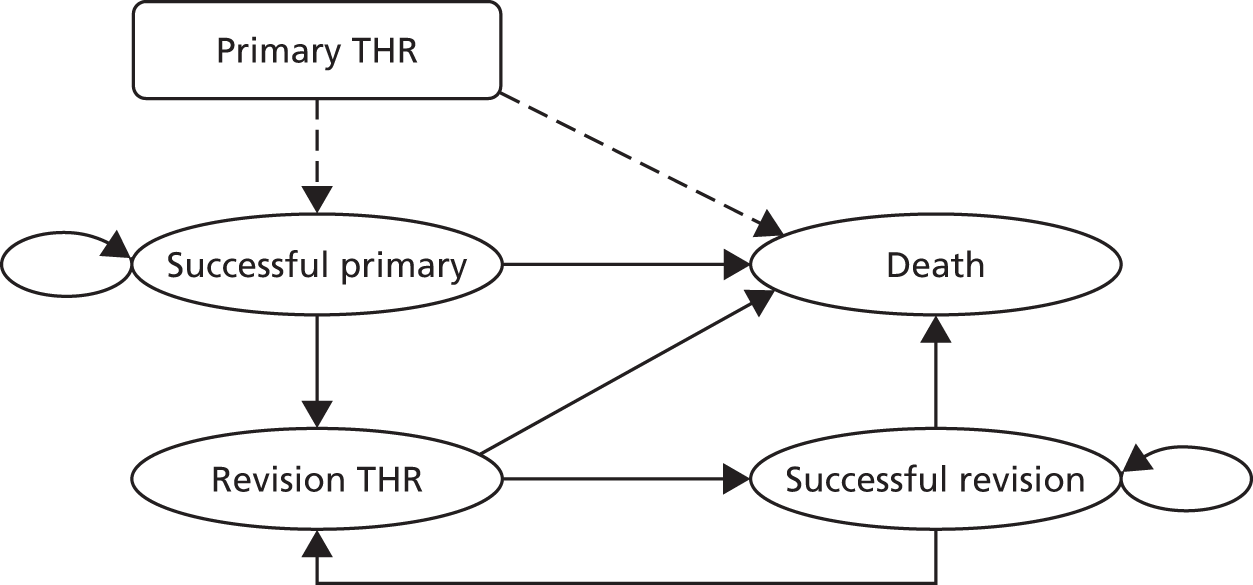
Appendix 5 Full total hip replacement economic model schema
FIGURE 62.
Full THR economic model schema. Boxes, hexagons and oval represent health states. Arrows represent possible transitions between health states. Death can occur following any other health state in the model. a, Transitions from each health state to the absorbing state of death. Reproduced with permission from Pinedo-Villanueva. 253

Appendix 6 Expert elicitation
Estimates of seven probabilities were informed using expert elicitation. Table 107 shows the derived mean probabilities for the proportions of patients referred to primary THR, risk factor modification and long-term medical management. As transitions from the first health state in the model (surgical assessment) lead to the above three alternatives or to death (which we obtained from UK ONS life tables125), and all of these must add up to 1, we omitted the transition least agreed on, from surgical assessment to the waiting list for a THR, and let it instead be equal to the difference between one and the sum of all other probabilities.
Interviewed expert | Probability of referral from initial surgical assessment, mean (SD) | ||
---|---|---|---|
To waiting list for ‘THR’ | To ‘risk factor modification’ | To ‘long-term medical management’ | |
Expert 1 | 0.32 (0.088) | 0.04 (0.038) | 0.65 (0.060) |
Expert 2 | 0.31 (0.099) | 0.12 (0.055) | 0.20 (0.072) |
Expert 3 | 0.73 (0.034) | 0.15 (0.036) | 0.08 (0.025) |
Expert 4 | 0.84 (0.060) | 0.02 (0.025) | 0.08 (0.039) |
Expert 5 | 0.91 (0.033) | 0.06 (0.021) | 0.02 (0.024) |
Expert 6 | 0.69 (0.049) | 0.12 (0.043) | 0.04 (0.027) |
Expert 7 | 0.59 (0.066) | 0.31 (0.065) | 0.11 (0.059) |
Linear pool of experts | 0.63 (0.227) | 0.14 (0.093) | 0.17 (0.208) |
Table 108 shows the probability of referral from risk factor modification to reassessment and, specifically, for those reassessed, the probability of being referred to the waiting list for a primary THR. Table 109 shows the individual and pooled mean and SD of the derived transition probabilities between long-term medical management and reassessment, and from the latter to a primary THR.
Interviewed expert | Probability of referral from risk factor modification, mean (SD) | |
---|---|---|
To reassessment after 1 year | If reassessed, to THR | |
Expert 1 | 0.75 (0.063) | 0.97 (0.025) |
Expert 2 | 0.56 (0.110) | 0.85 (0.062) |
Expert 3 | 0.84 (0.037) | 0.88 (0.025) |
Expert 4 | 0.89 (0.055) | 0.87 (0.057) |
Expert 5 | 0.05 (0.038) | 0.63 (0.055) |
Expert 6 | 0.79 (0.047) | 0.79 (0.041) |
Expert 7 | 0.87 (0.067) | 0.90 (0.051) |
Linear pool of experts | 0.68 (0.286) | 0.84 (0.111) |
Interviewed expert | Probability of referral from medical management, mean (SD) | |
---|---|---|
To reassessment after 1 year | If reassessed, to THR | |
Expert 1 | 0.03 (0.036) | 0.50 (0.011) |
Expert 2 | 0.16 (0.076) | 0.54 (0.094) |
Expert 3 | 0.12 (0.029) | 0.15 (0.039) |
Expert 4 | 0.13 (0.062) | 0.07 (0.053) |
Expert 5 | 0.14 (0.035) | 0.85 (0.031) |
Expert 6 | 0.05 (0.042) | 0.05 (0.034) |
Expert 7 | 0.10 (0.475) | 0.05 (0.031) |
Linear pool of experts | 0.11 (0.066) | 0.31 (0.300) |
Appendix 7 Estimating 2-year revision rates by Kalairajah outcome classification using data from the New Zealand Joint Registry
Revision rates by categories of outcome based on postoperative OHS have been reported to date only by Rothwell et al. ,220 based on a sample of over 15,000 THRs from the New Zealand Joint Registry. The authors found that lower postoperative OHSs were strongly associated with higher revision rates at 2 years after the primary surgery. Revision rates were calculated for the four groups proposed by Kalairajah et al. 210 using 27, 33 and 41 units as cut-off points on postoperative OHS at 6 months after primary surgery. Table 110 reproduces the number of patients who had their primary surgeries revised within 2 years, the total number of patients by group and the corresponding 2-year revision rate, as reported by Rothwell et al. 220
Group (OHS) | Patients | Revised | Revision rate (%) |
---|---|---|---|
< 27 units | 944 | 72 | 7.6 |
27–33 units | 1452 | 32 | 2.2 |
34–41 units | 4170 | 50 | 1.2 |
> 41 units | 9257 | 44 | 0.5 |
We used the figures in Table 110 to produce estimated revision rates for our two categories of outcome after THR. As we are using a postoperative OHS of 38 units to classify patients into poor and good outcomes 1 year after the primary surgery, Kalairajah et al. ’s210 cut-off points of 34 or 41 units for the OHS could be used to recategorise the four groups into two. Based on the overall proportion of patients classified as having poor and good outcomes in the HES PROMs data set (45% and 55%, respectively), we chose to consider the two Kalairajah et al. ’s210 groups with scores up to 41 units as having a poor outcome (42%) and those above 41 units as having a good outcome (58%). Poor outcomes (OHS ≤ 41 units) were hence associated with a 2-year revision rate of 2.35% and good outcomes (OHS > 41 units) with 0.48%. The relative risk of revision thus indicates that patients with an OHS of ≤ 41 units at 6 months after their primary operation are 4.93 times more likely to have a revision in 2 years than patients scoring > 41 units.
In order to produce separate revision rates for good and poor outcomes during the first year after the primary surgery, we used the figures in Table 110 to produce instantaneous revision rates (assuming the rate was constant over the 2 years) and then probabilities of revision at 1 year for the two outcome categories. The 1-year probability of revision for the group scoring ≤ 41 units on the OHS was 1.18% and that of the patients scoring above 41 units was 0.24%. The relative risk of revision at 1 year was therefore 4.96%, all based on data from the sample of New Zealand THR patients used by Rothwell et al. 220
As a similar relative risk of revision by poor to good outcomes is not available for THR patients in the UK, we used this relative risk to produce revision rates stratified by outcome categories based on overall revision rates reported by the NJR and the proportion of good and poor outcomes found in the HES PROMs data set.
Appendix 8 Estimating primary care costs attributable to hip pain
As the CPRD stores a consultation as an event performed by a specific GP practice staff, we included only events performed by health-care professionals such as GPs, nurses, physiotherapists or alternative practitioners who would be directly involved in providing care to patients. To capture the use of medication, we searched the CPRD extract for 25 different drugs, identified and grouped into six categories: antidepressants, NSAIDs, opioid analgesics, non-opioid analgesics, laxatives and ulcer prevention medication. The mean number of consultations for each set of controls was calculated and subtracted from the number reported for their respective case. This was done for each health-care professional and type of event, and for each year. The difference was assumed to be an estimate of the number of consultations the case had with the specific health-care professional because of his or her hip problem.
Figure 63 shows the distribution of the number of day visits to GPs attributable to hip pain by the 21,572 cases included in the data set during the year prior to their operation. THR patients used, on average, an estimated 1.8 extra GP day visits during the year immediately before their operation compared with similar patients who did not have their hip replaced, suffer from OA or even suffer from hip pain. As Figure 63 shows, however, for many of these patients the number of extra visits to their GP was much higher, while for others it was considerably lower. This was a natural and expected result. Our assumption that comorbidities would balance between the two groups (cases and controls) refers to the aggregate level, given the matching process and the large number of observations. For individual sets of matched patients, however, no such balance was expected, as is confirmed by our results. The difference between cases and controls was not only because the former had OA and the latter did not, and other conditions must have been present in different rates between the two groups.
FIGURE 63.
Estimated GP day visits because of hip pain by THR patients during the year prior to surgery, patients aged > 45 years in the CPRD.

The use of medication associated with hip pain was estimated in the same manner as consultations. Mean prescriptions units were subsequently calculated for each set of controls and this value subtracted from that of the cases to obtain an estimate of the number of prescriptions associated with hip pain. For most drugs, the resulting difference was zero for the majority of patients in all preoperative years, whereas for paracetamol, for example, cases reported being prescribed 119 units more than their controls. As in the case of consultations, there were patients on the THR waiting list who were prescribed many more tablets than the mean of 119 units, and there were also many controls who, on average, were prescribed more tablets than the patients awaiting the operation. To estimate medication costs, the number of units attributable to hip pain was estimated as described above for the 272 different CPRD codes associated to the 25 included drugs and then multiplied by their unit cost, as reported in the BNF. 213
Consultation and medication costs were then added together and, thus, the progression of total costs attributable to hip pain incurred by patients during the 15 years before their THR could be estimated. The growing estimated costs confirmed the increasing burden generated by unresolved hip pain and problems experienced by patients who are referred for a THR, who markedly demand many more health-care resources during the year immediately prior to their operation. It is also notable that the relative weight of prescription as a portion of total costs increase much more rapidly than that of consultations as patients approach a THR, going from 20% to 27% to 36% at 8, 5 and 1 year before surgery, respectively. This indicates that patients are not able to successfully manage their pain through more consultations with health-care professionals and have to recourse to more medication until their hip is replaced.
We used the estimates for the year immediately prior to a THR to populate all preoperative states. Deterministic analysis was based on the above mean values for costs attributable to hip problems. For PSA, a normal distribution was used to model the uncertainty around the difference in resource use between the two groups.
Appendix 9 Logit model to predict surgery outcome
To produce separate estimates by outcome category, we developed a model to predict surgery outcome based on health-care resource use during the first year after the primary surgery, as collected from the COASt cohort. Table 111 shows how patients experiencing poor outcomes (i.e. those with a postoperative OHS < 38 units) reported more visits to health-care professionals and were more likely to be prescribed painkillers. Using these data, we estimated a logit model to predict poor outcome, as defined in this study. All resource use variables in the 1-year postoperative follow-up form used in the COASt cohort were originally included in the model, together with age and sex. Age and sex, as well as the number of nurse and physiotherapy visits, and drugs other than opioids and paracetamol were not statistically significant predictors of poor outcome. As Table 112 shows, a model explaining 24% of the variance of outcome category was estimated from a three-level categorical variable counting GP visits (zero being the base case), whether or not patients were taking paracetamol and the number of opioid drugs taken. As we lacked an external data set for validation, we fitted the model to the same estimation data set obtaining the ROC curve shown in Figure 64, which reported an AUC of 0.80. At certain cut-off points, the model was able to predict between 70% and 80% of both good and poor outcomes correctly.
Variable name | All patients | Outcome | Missing OHS | |
---|---|---|---|---|
Good | Poor | |||
Patients, n (%) | 329 (100) | 276 (84) | 38 (12) | 15 (5) |
Female, n (%) | 198 (60) | 166 (60) | 22 (58) | 10 (71) |
Age (years), mean (SD) | 68 (10.4) | 68 (10.3) | 71 (8.0) | 67 (15.8) |
BMI (kg/m2), mean (SD) | 28 (4.9) | 28 (4.9) | 30 (4.7) | 27 (4.7) |
OHS, mean (SD) | 42 (8.0) | 44 (4.1) | 24 (6.1) | – |
EQ-5D, mean (SD) | 0.82 (0.253) | 0.88 (0.185) | 0.40 (0.288) | 0.75 (0.238) |
Satisfied with outcome, n (%) | 298 (92) | 266 (96) | 19 (50) | 13 (87) |
Do not smoke, n (%) | 307 (94) | 258 (93) | 35 (92) | 14 (93) |
Visits to GP ≥ 2, n (%) | 46 (14) | 28 (10) | 16 (42) | 2 (13) |
Visits to NHS physiotherapist ≥ 1, n (%) | 89 (27) | 66 (24) | 17 (45) | 6 (40) |
Visits to NHS nurse ≥ 1, n (%) | 32 (10) | 24 (9) | 6 (16) | 2 (13) |
Taking any non-opioid drugs, n (%) | 50 (15) | 28 (10) | 17 (45) | 5 (33) |
Taking any NSAIDs, n (%) | 39 (12) | 28 (10) | 8 (21) | 3 (20) |
Taking any opioid drugs, n (%) | 59 (18) | 39 (14) | 15 (39) | 5 (33) |
Predictor | Coefficient | p-value | 95% CI |
---|---|---|---|
Number of GP visits, 1–4 | 2.120 | 0.000 | 1.446 to 2.794 |
Number of GP visits, ≥ 5 | 2.468 | 0.003 | 0.851 to 4.085 |
Paracetamol | 1.062 | 0.010 | 0.256 to 1.868 |
Number of opioid drugs | 1.113 | 0.002 | 0.421 to 1.804 |
Constant | –2.569 | 0.000 | –3.066 to –2.071 |
n = 314 | |||
Pseudo R2 = 0.241 |
FIGURE 64.
Receiver operating characteristic curve for the model predicting surgery outcome category.

As the predictors of poor surgery outcome were measures of resource use also available in the CPRD, we fitted the above model to CPRD’s postoperative data to predict the outcome category that patients would have most likely been classified into based on their patterns of resource use. We fitted the model to data from the first postoperative year after the primary because this, combined with the cost of surgery previously reported, produced overall costs associated with each model state covering primary THR and the first year in either outcome category.
Predictors in the CPRD were equivalent to those used to estimate the model in the COASt cohort. The model was estimated based on the number of visits to the GP specifically because of problems with the hip and, even though the CPRD collects the number of GP visits regardless of reason, we used the reported number of visits after subtracting the mean visits in controls as an approximation for visits because of hip problems. When fitting the model, we used the combined number of consultations, whether at surgery or night visits. Any presentation of paracetamol was regarded as valid for the binary predictor, and the number of opioid drugs was a straightforward count, also regardless of presentation, from any of the drugs included in the analysis and classified as opioid.
Those patients for whom the model estimated a probability > 0.4 of being classified as poor outcome were considered likely poor outcomes, and the rest were labelled as likely good outcomes.
Appendix 10 Mapping the Oxford Hip Score onto the EuroQol-5 Dimensions
Transfer to utility
The first model regressed total OHS onto the EQ-5D summary index using OLS. This model is described by Equation 19, where Ê is the expected EQ-5D summary score:
Although total OHS is an aggregation of twelve categorical responses, we treated it as a continuous variable under the assumption that it indicates levels of severity of hip arthritis. At www.orthopaedicscore.com (accessed 2015), ranges of OHSs are associated with different severity levels of the disease.
The second method employed responses to all 12 questions of the OHS questionnaire as categorical regressors and is shown in Equation 20:
where j is each of the 12 questions in the OHS questionnaire. One area of concern when including each of the 12 questions of the OHS as regressors is that some of them may be highly correlated, in which case there would be an effect over the variance of coefficients. 254 In order to explore the presence of multiple collinearity between OHS questions, Stata’s collin command was used on each pair of questions to assess their R2 value and the VIF between them. In both cases, the higher the values the greater the collinearity, with VIFs above 10 and R2 close to 1 being reasons of concern. The highest VIF reported was between the questions on description of pain and on pain interfering with work with a factor of 2.92, also showing the highest R2 at 0.66. Even though the collin command runs a simple correlation and ignores the fact that variables are categorical, running the correlation accounting for the categorical regressor hardly changes the value of the R2. Results suggest that none of the correlations between OHS questions are close enough to being nearly perfectly linear to cause concern when fitting a model that includes them all. The mean VIF when including all 12 OHS questions was 2.96.
The third TTU regression method used was a two-part logit OLS model. Many patients report having no problem in the five dimensions included in the EQ-5D after hip replacement, hence a high proportion of postoperative responses have scores of one (full health). As OLS would not predict a discrete score of one, we formulated this two-part model in order to be able to predict full-health states.
The first part employed a binary outcome logistic model to predict which patients would have an EQ-5D score of 1, as shown in Equation 21:
where y* is an unobserved latent variable indicating the log of odds of the EQ-5D score being equal to 1. We then converted this value into a probability using the exponential function, which determined if a score of 1 was to be recorded as the expected EQ-5D summary score for the selected observation. The second part used linear OLS regression to estimate EQ-5D values for those patients not predicted to score 1.
The underlying assumptions of the linear regression model were checked. Although there seemed to be evidence of heteroscedasticity, a linear association between OHS and EQ-5D was confirmed by a fractional polynomial plot and residuals were approximately normally distributed. For the categorical OLS and two-part models, different variations were estimated and compared by excluding some or all response categories of certain OHS questions. The best or more efficient variations of each class of model were assessed by internal and external validation.
Potential limitations with the TTU regression approach have been documented. 217 First, predicted values may fall outside the range of possible EQ-5D scores (–0.594 to 1 unit). Second, the actual values are unlikely to be matched by a linear regression. Third, regression methods have assumptions that need to hold for a model’s estimations to be efficient, or at least unbiased, and these may not always be met.
Response mapping approach
Response mapping seeks to predict the responses to each of the five individual EQ-5D questions instead of predicting the summary score directly. 217 A logistic regression model can then be used to estimate probabilities that each set of OHS responses would correspond to a response level of each EQ-5D question. The next step would be to use a Monte Carlo simulation to assign response levels to each EQ-5D question by comparing random numbers to these probabilities. In the original work by Gray et al. ,217 the authors rightly used the simulation procedure to generate a distribution and then assign the corresponding category, but reported only a single simulation because, given their large sample size, differences were very small. Based on our sample size, we also chose to assign health categories after one iteration only. The final index was then computed using the UK’s EQ-5D tariff. However, this comes at a cost, as assigning a wrong predicted response in just one of the EQ-5D dimensions would result in a significantly different fitted summary EQ-5D score. 216
Responses to EQ-5D questions are ordered, which intuitively implies that the ordered logistic model would be the most appropriate method to use. However, this requires the parallel regression assumption to hold. A likelihood ratio test was used to assess if this assumption held but it did not, therefore a multinomial logistic model was applied. Equation 22 was calculated for two of the three response categories of each EQ-5D dimension, and the third was the reference case against which these probabilities were calculated:
Here, pik is the probability that respondent i will be assigned response category k (1, 2 or 3) for the two non-reference categories (h). For the reference category, the numerator in Equation 22 becomes 1.
For all TTU regression and response mapping models we also ran variations that included additional regressors (sex, age, age2 and deprivation converted into a categorical variable). As none of these variations offered improved performance over the basic models, their results are not reported here.
Data
Data were obtained from the SWLEOC database. The centre performs hip and knee replacement surgeries for four acute NHS trusts in south-west London. The full data set comprised 3504 hip replacements each with preoperative and/or 6-month postoperative responses to the OHS and EQ-5D questionnaires, plus basic demographic, socioeconomic and clinical information. All except two operations were performed between 2006 and 2008. All models were estimated on 1759 operations, for which we had data on both pre- and postoperative OHSs and EQ-5D scores, sex, age and deprivation. As we were interested in cross-sectional mapping, we pooled pre- and postoperative records together, providing 3518 outcome observations.
We included primary and revision surgeries, as well as uni- and bilateral procedures. Multiple records for the same patient were allowed, as long as each record described a separate procedure. As we had at least two observations per patient (pre- and postoperative) our data set was clustered. We allowed for this using Stata’s robust cluster command during model estimation to show robust standard errors.
We treated the functional relationship between the OHS and the EQ-5D as being essentially the same regardless of circumstances and timing of data collection. Even though there could exist such a difference, we considered it would not significantly affect the estimation of the mean score of the group. The data were analysed using Stata/IC 11 statistical software.
Mapping results
The coefficients of the simplest model, continuous OLS with total OHS as the only regressor, are shown in Table 113.
Independent variables | Coefficient | Robust standard errorb | 95% CI |
---|---|---|---|
Total OHS | 0.0222 | 0.000 | 0.021 to 0.023 |
Constant | –0.0697 | 0.010 | –0.088 to –0.051 |
Table 114 shows coefficients for the categorical version of the linear regression and the two-part approaches.
OHS question: response level | OLS categorical | Two-part: logit (first stage) | Two-part: OLS (second stage) | |||
---|---|---|---|---|---|---|
Coefficient | p > |t| | Coefficient | p > |t| | Coefficient | p > |t| | |
Description of pain: 0 | Base case | Base case | Base case | |||
Description of pain: 1 | 0.171 | 0.000 | 0.339 | 0.764 | 0.171 | 0.000 |
Description of pain: 2 | 0.146 | 0.000 | 0.460 | 0.669 | 0.150 | 0.000 |
Description of pain: 3 | 0.174 | 0.000 | 1.866 | 0.072 | 0.158 | 0.000 |
Description of pain: 4 | 0.212 | 0.000 | 3.035 | 0.003 | 0.162 | 0.000 |
Night pain: 0 | Base case | Excluded | Base case | |||
Night pain: 1 | 0.036 | 0.012 | 0.038 | 0.008 | ||
Night pain: 2 | 0.037 | 0.009 | 0.039 | 0.005 | ||
Night pain: 3 | 0.037 | 0.028 | 0.040 | 0.017 | ||
Night pain: 4 | 0.047 | 0.002 | 0.049 | 0.001 | ||
Sudden pain: 0 | Base case | Excluded | Base case | |||
Sudden pain: 1 | 0.004 | 0.837 | 0.013 | 0.456 | ||
Sudden pain: 2 | 0.027 | 0.089 | 0.039 | 0.014 | ||
Sudden pain: 3 | 0.034 | 0.071 | 0.052 | 0.007 | ||
Sudden pain: 4 | 0.044 | 0.011 | 0.052 | 0.003 | ||
Limping: 0 | Base case | Base case | Base case | |||
Limping: 1 | 0.045 | 0.000 | ||||
Limping: 2 | 0.046 | 0.001 | 0.500 | 0.245 | 0.014 | 0.145 |
Limping: 3 | 0.045 | 0.001 | 0.929 | 0.003 | ||
Limping: 4 | 0.055 | 0.000 | 1.449 | 0.000 | ||
Walking duration: 0 | Base case | Excluded | Base case | |||
Walking duration: 1 | 0.006 | 0.738 | 0.009 | 0.624 | ||
Walking duration: 2 | 0.008 | 0.618 | 0.017 | 0.294 | ||
Walking duration: 3 | 0.031 | 0.050 | 0.048 | 0.004 | ||
Walking duration: 4 | 0.038 | 0.017 | 0.050 | 0.003 | ||
Climbing stairs: 0 | Base case | Base case | Base case | |||
Climbing stairs: 1 | 0.005 | 0.844 | ||||
Climbing stairs: 2 | 0.039 | 0.009 | 0.046 | 0.063 | ||
Climbing stairs: 3 | 0.058 | 0.001 | 0.738 | 0.006 | 0.073 | 0.006 |
Climbing stairs: 4 | 0.085 | 0.000 | 0.072 | 0.008 | ||
Socks and stockings: 0 | Base case | Base case | Base case | |||
Socks and stockings: 1 | 0.042 | 0.005 | ||||
Socks and stockings: 2 | 0.039 | 0.010 | 0.425 | 0.267 | 0.011 | 0.324 |
Socks and stockings: 3 | 0.055 | 0.001 | 0.946 | 0.005 | 0.018 | 0.143 |
Socks and stockings: 4 | 0.087 | 0.000 | 1.516 | 0.000 | 0.041 | 0.009 |
Pain from standing up from chair: 0 | Base case | Excluded | Base case | |||
Pain from standing up from chair: 1 | 0.072 | 0.004 | 0.076 | 0.001 | ||
Pain from standing up from chair: 2 | 0.101 | 0.000 | 0.107 | 0.000 | ||
Pain from standing up from chair: 3 | 0.117 | 0.000 | 0.128 | 0.000 | ||
Pain from standing up from chair: 4 | 0.118 | 0.000 | 0.127 | 0.000 | ||
Car and public transport: 0 | Base case | Base case | Base case | |||
Car and public transport: 1 | ||||||
Car and public transport: 2 | 0.034 | 0.018 | 0.037 | 0.011 | ||
Car and public transport: 3 | ||||||
Car and public transport: 4 | 0.044 | 0.014 | 0.934 | 0.000 | ||
Washing and drying: 0 | Base case | Base case | Base case | |||
Washing and drying: 1 | ||||||
Washing and drying: 2 | 0.018 | 0.256 | 0.019 | 0.223 | ||
Washing and drying: 3 | 0.049 | 0.005 | 1.105 | 0.036 | 0.051 | 0.003 |
Washing and drying: 4 | 0.063 | 0.001 | 0.059 | 0.001 | ||
House shopping: 0 | Base case | Base case | Base case | |||
House shopping: 1 | 0.001 | 0.967 | ||||
House shopping: 2 | 0.036 | 0.014 | 0.981 | 0.003 | 0.035 | 0.007 |
House shopping: 3 | 0.065 | 0.000 | 0.057 | 0.000 | ||
House shopping: 4 | 0.102 | 0.000 | 0.074 | 0.000 | ||
Pain interfering with work: 0 | Base case | Base case | Base case | |||
Pain interfering with work: 1 | 0.097 | 0.000 | 0.103 | 0.000 | ||
Pain interfering with work: 2 | 0.166 | 0.000 | 0.180 | 0.000 | ||
Pain interfering with work: 3 | 0.174 | 0.000 | 1.715 | 0.000 | 0.194 | 0.000 |
Pain interfering with work: 4 | 0.236 | 0.000 | 0.206 | 0.000 | ||
Constant | –0.165 | 0.000 | –9.816 | 0.000 | –0.154 | 0.000 |
Performance assessment and validation
All selected variations of each model were internally validated. Table 115 shows summary performance indicators for each model.
Model: regressors | Mean fitted EQ-5D | Difference of means (observed – fitted) | Range of fitted EQ-5D | Range of residuals | % within 0.10 utility | R2, EQ-5D observed vs. fitted | Root-mean-square error,b EQ-5D observed vs. fitted |
---|---|---|---|---|---|---|---|
Continuous OLS: total OHS | 0.5750 | 0.0000 | –0.070 to 0.995 | –0.91 to 0.76 | 41.6 | 0.67 | 0.20 |
Categorical OLS: all OHS questions | 0.5750 | 0.0000 | –0.165 to 0.967 | –0.91 to 0.78 | 52.0 | 0.72 | 0.19 |
Two-part logit OLSc | 0.5735 | 0.0015 | –0.154 to 1.000 | –1.11 to 0.82 | 51.5 | 0.70 | 0.19 |
Response mapping: all OHS questions | 0.5737 | 0.0013 | –0.484 to 1.000 | –0.98 to 1.03 | 49.0 | 0.57 | 0.23 |
Given the lack of other data sets recording both OHS and EQ-5D, we performed the external validation on 1616 observations from the subset of the original cohort of 3504 hip replacements that had not been selected for the estimation sample. The validation sample comprised records with OHS and EQ-5D responses for either the pre- or postoperative period, but not for both. Table 116 shows the performance of the four models when fitted to the validation sample.
Model: regressors | Mean fitted EQ-5D | Difference of means (observed – fitted) | Range of fitted EQ-5D | Range of residuals | % within 0.10 utility | R2, EQ-5D observed vs. fitted | Root-mean-square error,b EQ-5D observed vs. fitted |
---|---|---|---|---|---|---|---|
Continuous OLS: total OHS | 0.3805 | –0.0005 | –0.070 to 0.995 | –0.78 to 0.67 | 25.4 | 0.56 | 0.23 |
Categorical OLS: all OHS questions | 0.3845 | –0.0045 | –0.165 to 0.967 | –0.75 to 0.77 | 42.2 | 0.63 | 0.21 |
Two-part logit OLSc | 0.3820 | –0.0020 | –0.154 to 1.000 | –0.83 to 0.77 | 42.0 | 0.64 | 0.21 |
Response mapping: all OHS questions | 0.3758 | 0.0042 | –0.429 to 1.000 | –0.91 to 1.07 | 44.4 | 0.45 | 0.26 |
Discussion
The present work shows that models estimated here have a high predictive power when mapping OHS responses onto the summary EQ-5D score, and OHS changes onto EQ-5D change. Furthermore, it demonstrates that all models employed here for score mapping are able to estimate the mean EQ-5D index with a high level of precision. The simplest OLS continuous model achieved the closest estimation of the mean EQ-5D score, whereas response mapping proved to be the only approach capable of estimating individual scores well into the negative range and up to full health. An additional benefit of response mapping is that it allows for the estimation of mean EQ-5D scores using different valuation tariffs. For all models, predictive power varied considerably across the range of fitted EQ-5D scores with mean absolute error for predicted low EQ-5D scores doubling that of higher fitted values, a tendency also found in a previously published cross-walking study linking a condition-specific measure to a generic one. The OLS categorical model reported lower predictive errors across the range of scores than the other models. Overall performance of the four models was within range of other reported mapping studies, based on their root-mean-square errors of around 0.20.
Results of the continuous OLS model indicate that, based on the data used, 67% of the variation in hip patients’ EQ-5D scores is explained by their OHS. In other words, most of the variability in their HRQoL, as measured by EQ-5D, is associated with the impact their hip problem has on the pain and limitations they experience. We also found an association between severity of health problems and the models’ predictive power of individual scores so that, in general, better health leads to lower predictive errors of EQ-5D score mapping. This tendency, although explored by only a few authors in the past, has already been found in studies cross-walking from disease-specific and generic measures onto the EQ-5D.
The mapping exercise benefited from pooling together pre- and postoperative responses to the questionnaires, hence providing good power and the full range of scores for model estimation. We also found a number of similarities between the EQ-5D and the OHS; for example, both ask about pain, mobility and ability to perform tasks and functions. We felt that this was an extremely important factor in the good performance of the mapping algorithms. Similar mapping exercises are likely to be sensitive to similarities between instruments and it is very likely that mapping would perform poorly in cases where instruments are very different.
The mapping was performed using regression techniques that are very widely used and well understood, which facilitated analysis and interpretation of results. There are some limitations, however, that should be borne in mind when interpreting results. Although there is a substantial overlap between OHS and EQ-5D questions, there is one exception. One of the EQ-5D’s dimensions explores anxiety/depression, which is not covered in the OHS questionnaire; this limits the ability of the disease-specific measure to predict the scores of the generic one. In addition, we would ideally like to have used a completely different data set for external validation from that used for estimation. Our estimation and validation data sets are bound to have shared many characteristics; nevertheless, the large sample size and wide distribution of scores support the reliability of results. Although both the estimation and validation subsamples came from the same cohort, their method of selection made the validation process more robust than if they had been selected randomly. In most mapping studies, validation samples are built by randomly selecting cases from the same estimation data set. By doing so, the validation may simply confirm that the selection was truly random instead of actually testing whether or not results would vary on different data. Using a non-randomly selected validation sample, we were able to test the validity of the mapping methods while controlling for the equivalence effect of randomisation.
To conclude, the mapping methods tested here enable researchers, clinicians and policy-makers to obtain reliable estimates of mean EQ-5D scores and mean changes thereof after THR when these are not directly collected but responses to the OHS questionnaire are available. In Chapter 4, we report on the use of the above mapping methods to produce utility estimates based on OHS measures collected in the absence of EQ-5D data in order to populate our cost-effectiveness analysis. The models presented here report high predictive power. It is important to stress that, if mapped scores are to be used as part of economic evaluations, the uncertainty added by the mapping process must be properly incorporated into the analysis.
Appendix 11 Model assumptions and limitations
As with all models, the one presented here attempts to reflect the true care pathway of patients as they are assessed for a THR, which most undergo, but it necessarily simplifies what in reality is a more complex process: patients’ conditions may evolve in ways that have not been simulated in our model or health professionals or patients themselves may make decisions leading to a myriad of health states that are not specifically included in our schema. Modellers face the inevitable trade-off of attempting to capture the complexity of reality vis-à-vis building a manageable and parsimonious model that can be populated with good-quality data and produce results that aid the decision-making process. As long as these necessary simplifications do not contradict reality or produce misleading results, then the trade-off can only be expressed and the likely limitations of the simplified models made explicit. The model presented here captures the pathway of THR patients with greater detail and breadth than those used for previously published economic evaluations of THRs; nevertheless, assumptions have necessarily been made.
Our model, as any other, simplifies reality so that we can produce estimates for the cost-effectiveness of the outcome prediction tool. This simplification is achieved by making a number of assumptions that can make the model feasible. It is important to make these assumptions explicit and to consider their possible effects on final results.
First, this model assumes that the outcome prediction tool is capable of identifying potential poor surgical outcomes before patients have the operation. The methods employed to produce the tool are rigorous and appropriate, but they were applied to a set of patients that may or may not be representative of the entire population. We are therefore assuming that the information in the EPOS and EUROHIP data sets are representative of the equivalent characteristics and outcomes in the wider population susceptible of undergoing a THR in the UK. Based on their large number of participants and on the fact that EPOS is a UK-based study, and because EUROHIP is a multicentre study not only in the UK but also in other European countries, we believe that the prediction tool built on such data is applicable to the wider UK context.
Outcome categories are a key element in this study; hence, an important assumption we are making is that the way patients are classified in this model is valid and the most appropriate. We are assuming that all or most patients with an OHS of ≥ 38 units at 1 year after their primary surgery are all free from pain and major mobility limitations as well as satisfied with the operation, and that the opposite is true for those who score < 38 units. This may not necessarily be so. First, the method used to identify the cut-off point was anchored on satisfaction, which is a largely subjective concept. Second, satisfaction, and hence the cut-off point for good and poor outcomes, may also vary with sex, age, BMI, expectations or severity of disease, to name a few. In the study identifying this cut-off point on the postoperative OHS, the authors stratified their results by sex, age and BMI tertiles, and baseline OHS, but the differences were not statistically significant from the overall value. They also explored equivalent thresholds using the raw change in OHS after the operation and the percentage of potential improvement achieved as outcome, and in both cases stratifying by the above variables produced results whose difference to the overall values was not statistically significant. 154 We are, therefore, confident that an overall cut-off point is acceptable, as data appear to suggest that the connection between a postoperative OHS and satisfaction is stable across different groups of patients.
We also assume that all patients found to be candidates for surgery but presenting a risk factor that should be dealt with before the operation, whether it is excessive weight, diabetes, blood pressure or something else, can be grouped together and, therefore, the same costs, QALYs and transitions from the risk factor modification state can be applied. This is probably not the case in real life. However, we are using this health state essentially to introduce a delay into the path towards surgery, as attempts to modify risk factors were reported by surgeons to be common when assessing patients considered for an arthroplasty. The risk factor modification state (for which patients would be expected to stay for a short period in most cases) is not intended to reflect the specificities of the risk factor modification treatment. In fact, the costs of modification of risk factors are not included in the costs associated with this state. In addition, although HRQoL may differ depending on the type of risk factor patients have, we do not expect variations to be significant, as EQ-5D is largely sensitive to mobility, pain and limitation to usual activities, which all patients in the risk factor modification state would have in common as they have been found to be candidates for a THR. We therefore believe that the heterogeneity of patients diverted to risk factor modification state reflects clinical practice and that the variation in costs, QALYs and transition probabilities will be appropriately incorporated into results via PSA.
We are also grouping a diverse set of patients into the health state of long-term medical management. As above, we have given priority to what these patients have in common, namely their non-surgical treatment, as opposed to their potentially different costs, QALYs and transition probabilities based on what sets them apart. As health-care costs are expected to be driven by the non-surgical treatment of their problem, and this will be largely similar for all, bringing such diverse groups of patients together is warranted. QALYs, as explained above, are very much sensitive to hip pain and its consequences; hence, however diverse these patients, they are all likely to have similar HRQoL. Transition probabilities, however, may be different for patients in the long-term medical management state. One of the specific groups of patients that will transit into this state is that composed of potential candidates for a THR who are not willing to undergo the procedure. These patients, for example, are likely to be much more susceptible to the effects of an outcome prediction tool than patients whose problem is not orthopaedic or hip related, or simply those found unfit for surgery, all of whom will be in the long-term medical management state. Nevertheless, the distribution of the probability of transition from this health state will capture some of the variation within this group, which through PSA will allow results to incorporate this difference.
Another important assumption is that probabilities of good and poor outcomes are the same in the model whether the patient comes from the risk factor modification section or from that of long-term medical management. This is a clinically plausible assumption because long-term medical management patients, who are ultimately referred for a primary arthroplasty, are likely to be very similar to those referred for a THR from the risk factor modification state in all aspects relevant to surgery outcome.
The model presented here does not allow for multiple revisions. Although there are patients who undergo more than two THRs in their lifetime, not only are they a very small proportion of all patients who receive this operation but there are also no data available about the effect of surgical outcomes on a second or later revision of the prosthesis.
Finally, we are ultimately assuming that the tool will be used by orthopaedic surgeons, when in reality it would be very difficult to know whether or not the additional information it will provide will be taken into consideration by surgeons, or even patients. It would be unrealistic to think that if the tool predicts that a patient is likely to perform poorly, for example, that this information will supersede the surgeons’ criteria when they would otherwise refer the patient for the operation, or vice versa. We therefore perform the analysis comparing current practice against a hypothetical scenario in which the tool will dictate how patients are referred after the surgical assessment as an extreme case. The results will therefore show whether or not each unit of health benefit brought about by the strict use of this tool would require the NHS to assume additional costs at a rate lower or higher than the opportunity cost within the health system.
Parameter assumptions
During the process of identifying parameter values to populate the economic model, a number of assumptions were made, whether because of the simplification forced by the fact that we were modelling a complex reality or because of limitations in the data available. In this section we discuss the assumptions made on the various probabilities, costs and health utility estimates, their possible implications and general feasibility.
Although preoperative transition probabilities may vary between patient subgroups, the values extracted from the expert elicitation exercise were assumed to apply to all patients regardless of age or sex. The method of data collection posed an important limitation in this case. It would have been highly impractical to ask the same questions to all experts about each specific patient subgroup, and they may have not been able to provide different values for each group. Dividing the limited number of experts, to ask different surgeons about different subgroups, was not feasible either. A common estimate for the mean preoperative transition probabilities, therefore, may not capture the possible heterogeneity among groups. By including surgeons who specialise in a particular type of patient, however, the uncertainty represented in their answers was transferred to the pooled probability distributions, incorporating this heterogeneity into the analysis, ultimately reflected in PSA results.
Transitions between good and poor outcomes > 2 years post operation were estimated based on follow-up questionnaires only up to 5 years. Results from the EPOS records point to diminishing improvements over the first 5 years and the extrapolation of estimated probabilities took the levels of each outcome category to a plateau. It is possible, however, that, over time, and especially by 10 years after a primary THR, many of those good outcomes worsen and the proportion of poor outcomes increases. We do not have data to support this, yet it seems clinically plausible. We account for this with the distributions assigned to transition probabilities linked to the number of patients involved in EPOS from whom probabilities were estimated and that added some of this uncertainty into the results through PSA.
As patients transit the model from either outcome category after a primary THR to a revision THR and then to an outcome category immediately after this, we are assuming that surgery outcome after the primary surgery has no bearing on surgery outcome after revision. It may be possible that this is not the case, but no data are available to confirm either hypothesis. However, our assumption is clinically plausible inasmuch as this assumption implies that patients requiring a revision would be in a similar situation concerning their prosthesis regardless of their state of origin when they transitioned into the revision THR state. Such similarity would make them equally likely to perform well or poorly after the revision. In addition, although good- and poor-outcome patients after the primary would not generate similar levels of HRQoL or costs to the aggregate analysis, PSA did allow for variability such that these good- and poor-outcome patients after the primary would not be so different in these regards either.
An important assumption was made when we used the cut-off point derived for primary THRs to categorise outcomes after revisions. This was done because no similar cut-off point has been calculated for revision THR patients. The resulting probabilities of good and poor outcomes are, nonetheless, acceptable as they imply a slightly higher likelihood of performing poorly after a revision, which was consistently reported by surgeons in the various rounds of consultations. Transition probabilities between outcome categories were also assumed to be equivalent after primaries and after revisions when those calculated for the former were applied to the latter. This was done because there are no data sets available with long-term follow-up of revision THR patients. However, we considered it is very likely that these transition probabilities are indeed similar because they describe patients’ response > 1 year following major surgery, which primary and revision surgery both are. Assigning a distribution to these probabilities also reflects results accounting for the uncertainty around their true value.
Finally, we applied all-cause mortality rates from the general population to patients with OA or other conditions possibly leading to a THR assuming that such musculoskeletal problems do not affect their chance of dying. In addition, mortality rates applied to the first year after surgery, whether primary or revision, were those reported by the NJR, which describe only the risk of death without attempting to identify whether or not surgery itself had any effect. 17 We therefore assumed that those values were a true reflection of death rates of patients undergoing a THR regardless of the reason, which is what the model required. We further assumed that outcome at 1 year after surgery, again whether primary or revision, did not have any bearing on mortality rates during that period.
In regard to HRQoL values, it is important to note that both pre- and postoperative measures used for the economic model were taken at roughly the same time with respect to the operation but not necessarily at the same point in the progression of the disease. A recent study looking at the HES PROMs data from 2009–10, a subset of which we used to inform HRQoL parameters for our model, found that non-white and more deprived patients tend to have joint replacement operations at a point when their OHS is lower than their white and less deprived counterparts, suggesting that their disease had reached a more advanced stage. 255 We did not explore these inequalities here but, as the outcome prediction tool uses preoperative OHS as the main predictor variable, it is likely that the tool already takes account of such different disease stages regardless of patients’ race or deprivation level.
Regarding the use of the outcome prediction tool, inequalities in access to health services in general, to appropriate referrals and to surgery itself may also have an impact on the tool’s effects. These inequalities have already been identified in England based on sex, age, deprivation and ethnicity,256 but their possible effects on the application of the outcome prediction tool are outside the scope of this research.
When estimating QALYs for the model’s health states, we assumed that the pattern of progression by outcome category during the first year after the operation in EPOS is generalisable to the wider population. We also assumed that the connection between OHS in the first and second years is representative of the changes all or most patients would experience. Although this might not necessarily be strictly the case, these assumptions are highly plausible as EPOS is a multicentre study whose main limitation is that the prosthesis employed in the THR was of the Exeter brand. The most frequently used stem in cemented THRs in England and Wales, used in more than 60% of the interventions performed in 2011, is in fact the Exeter V40 (Stryker Corporation, Kalamazoo, MI, USA), with the second most common prosthesis being used in < 20% of arthroplasties;17 hence data from only THRs performed using Exeter prostheses are likely to be generalisable. In addition, although assumptions were made about the patterns of quality of life progression, these were applied to the HES PROMs data set, a highly representative source from which data were ultimately extracted.
The health states of good and poor outcome after primary or revision surgery are the states in which most patients would remain for long periods of time, until death in many cases. We did not consider a utility decrement when assigning health utility estimates to these states, which resulted in patients dying while still at high HRQoL levels, especially in the case of good outcomes. This is a potentially unfitting assumption, but it also becomes irrelevant in this analysis because results from the model employing the outcome prediction tool are compared with current practice. In addition, if a decrement was to be applied on the grounds of ageing, it would affect good and poor outcomes equally and for both comparators, and hence such effects would essentially cancel each other out. Therefore, final utility estimates from each separate model should not be considered an accurate estimation of health utilities obtained with or without the intervention, and should only be analysed with respect to one another.
Because of the lack of data sets with follow-up information on revision THR patients, we assumed that the progression of health utility estimated from primary THR patients in EPOS was also applicable to revision THR patients. Although primary and revision patients may evolve differently during the first few months after their operation, applying these patterns to observed pre- and postoperative scores reported by the highly representative HES PROMs meant that the estimation of the parameters would still be highly accurate. The reason for this is that the progression patterns applied only described how patients move from their preoperative to their postoperative scores and not the scores themselves. Health utility estimates for the model states describing the second and subsequent years after revision THR were also affected by our assumption that the connection between OHS at years 1 and 2 after primary is the same as that after a revision operation. Again, in the absence of data describing how revision patients evolve from years 1 to 2 after a revision, the best approximation available was those observed in primary patients, which is what we used to populate the model.
When estimating parameter distributions to characterise uncertainty around health utility estimates, we assumed the time trade-off weights reported in the literature for the EQ-5D215 without considering any uncertainty around such valuation. Although these values are commonly used when performing economic evaluations, it is important to acknowledge that other valuation methods exist that could ultimately produce different health utility estimates.
Regarding assumptions about cost parameters, the costs for the risk factor modification state considered only reported primary care consultations and prescriptions by patients before their THR. It did not include the cost of the risk factor modification programme itself because these vary according to the type of problem needing to be addressed (e.g. weight reduction or blood pressure) and, to date, we have no reliable data on the use of these programmes by THR patients before the operation. Moreover, the inclusion in the model of separate states for risk factor modification and long-term medical management was primarily justified by the intention to include a non-surgical treatment alternative as well as the reality of delayed primaries because of risk factor management. We did not expect costs of the risk factor modification programmes to have any significant effect on overall results.
Surgery costs, on the other hand, were explicitly included because they are the most resource-intensive state of the economic model and, furthermore, they were assumed to be the same regardless of outcome category 1 year after the operation. We had no reason to believe that there would be an association between the HRG assigned to the operation, whether primary or revision surgery, and surgical outcome 1 year later.
Costs of complications were not explicitly included but, in many cases, they were already part of the cost estimations. Perioperative complications were considered in HRG reference costs, and primary care resource use resulting from complications was also part of the CPRD data used to produce cost estimates. However, surgical complications such as DVT, PE, fracture and the more recently explored associations between THR and myocardial infarction257 or stroke258 were excluded from the analysis. As this economic evaluation assesses the implementation of an outcome prediction tool after THR, the effect of costs associated to complications would be relevant only inasmuch as the tool changes the proportion of patients going into surgery and these complications appear in statistically different rates between the outcome categories considered. As we lack data on the differential incidence of complications between good- and poor-outcome patients as defined here, and the rate of complications such as DVT and PE reported in other economic evaluations of THR is as low as 1%,252 these were not incorporated into the analysis.
In using preliminary results from the COASt cohort for sections of the cost estimation exercise, we assumed that the cohort is representative of clinical practice and, more generally, of patients in the UK. More specifically, we assumed that the list of medications used after a THR as well as the pattern of resource use and its relationship with surgery outcome observed in the COASt cohort is similar to the overall pattern and connection in the country as a whole.
We assumed that estimating the surgery outcome predictive model at an OHS threshold of 33 units on resource use data collected in COASt for the first year after a primary was a valid approximation of the coefficients and statistical significance that would have been obtained had the model been estimated from resource use collected during the second year. This was a necessary assumption, given the lack of data on resource use collected during the second postoperative year from THR patients with an available OHS. It is also a feasible assumption considering that, if resource use is associated with the level of pain and limitations as measured by the OHS, then the timing of the measure should be irrelevant and the resulting coefficients would represent the number of consultations and prescriptions associated with the groups scoring above or below the new threshold.
Regarding the application of the outcome prediction tool, we indicated that it would have the effect of lowering the probabilities of being referred for a THR, whether directly or through risk factor modification, and that the transition probability to long-term medical management would increase because patients not referred for a THR would be treated non-surgically. We assumed that the tool would not have any direct effect over the referral pattern of patients originally sent for long-term medical management because those patients had, by definition, not been considered for the operation because their problem would not be solved by the THR, they were unfit for surgery or they did not want to have it. None of these situations would feasibly be affected by the output of the outcome prediction tool.
Finally, we assumed that there was no correlation between model parameters within each model considering current practice or the application of the outcome prediction tool. The distinction is made because the difference between the two models is, in fact, that they are populated by a different set of probabilities and HRQoL measures that are associated with whether or not the tool is used. Any correlation among parameters beyond the changing patterns because of implementing the prediction tool was not considered in the economic model.
Appendix 12 Primary care costs for total knee replacements
Patient subgroup (sex and age) | ‘Likely poor’ outcomes (£) | ‘Likely good’ outcomes (£) |
---|---|---|
Men | ||
45–59 years | 919.38 | 170.66 |
60–69 years | 810.50 | 178.78 |
70–79 years | 730.61 | 142.98 |
≥ 80 years | 701.47 | 148.52 |
Women | ||
45–59 years | 919.38 | 177.52 |
60–69 years | 810.50 | 144.60 |
70–79 years | 730.61 | 113.33 |
≥ 80 years | 701.47 | 115.18 |
Patient subgroup (sex and age) | ‘Likely poor’ outcomes (£) | ‘Likely good’ outcomes (£) |
---|---|---|
Men | ||
45–59 years | 724.25 | 90.77 |
60–69 years | 701.86 | 69.09 |
70–79 years | 662.30 | 59.53 |
≥ 80 years | 672.77 | 53.03 |
Women | ||
45–59 years | 760.91 | 124.05 |
60–69 years | 718.00 | 91.40 |
70–79 years | 665.88 | 65.90 |
≥ 80 years | 646.93 | 59.42 |
Patient subgroup | ‘Likely poor’ outcomes (£) | ‘Likely good’ outcomes (£) |
---|---|---|
All subgroups | 923.23 | 101.94 |
Appendix 13 External validation of the model
Predictor variables (reference category) | Overall, complete case (n = 182) | Imputed knee data (n = 608) |
---|---|---|
Intercept | 41.25 | 40.95 |
Age (years) | ||
[< 60] | ||
60–69 | –4.80 (1.93)* | –1.90 (1.08)**** |
70 to 79 | –4.27 (2.02)* | –1.95 (1.11)**** |
≥ 80 | –4.03 (2.84)**** | –2.05 (1.43)**** |
Sex | ||
[Female] | ||
Male | 1.36 (1.35) | 0.40 (0.72) |
Preoperative total OKS | 0.33 (0.09)*** | 0.34 (0.05)*** |
IMD 200453 score | –0.09 (0.07) | –0.05 (0.04) |
BMI (kg/m2) | –0.20 (0.14) | –0.24 (0.07)*** |
SF-12 mental component summary score | –2.58 (1.55)**** | –1.71 (0.85)* |
ASA | ||
[Fit and healthy] | ||
Asymptomatic no restriction | 1.22 (1.76) | –0.67 (1.00) |
Symptomatic minimal/severe restriction | 0.17 (2.50) | –1.90 (1.33)**** |
Other condition affecting mobility | ||
[No] | ||
Yes | –2.11 (1.30)**** | –1.97 (0.72)** |
Previous knee surgery | ||
[No] | ||
Yes | –6.13 (1.89)** | –2.28 (1.04)* |
Fixed flexion deformity | ||
[No] | ||
Yes | –0.26 (1.32) | –1.11 (0.70)**** |
Preoperative valgus/varus deformity | ||
[Varus] | ||
No deformity | 2.10 (1.66) | 2.59 (0.91)** |
Valgus | 3.40 (1.57)* | 4.45 (0.88)*** |
Preoperative ACL | ||
[Intact] | ||
Damaged | 2.27 (1.71) | 0.77 (0.84) |
Absent | –7.68 (4.87)**** | –3.77 (2.35)**** |
C-reactive protein | –0.04 (0.05) | –0.09 (0.02)*** |
Variables (type) | Data set | ||
---|---|---|---|
Complete | Incomplete | ||
Frequency | % missing | Frequency | |
Arthritis variables: previous treatments | |||
Medication steroids (binary) | Yes, n = 840; no, n = 466 | 37.43 | Yes, n = 98; no, n = 589 |
Intra-articular steroid injection (binary) | Yes, n = 93); no, n = 457 | 22.59 | Yes, n = 151; no, n = 699 |
Intra-articular hyaluronic acid injection (binary) | Yes, n = 4; no, n = 546 | 23.77 | Yes, n = 6; no, n = 831 |
Soft tissue injection (binary) | Yes, n = 8; no, n = 542 | 51.28 | Yes, n = 8; no, n = 527 |
Infection treatment (binary) | Yes, n = 1; no, n = 549 | 22.59 | Yes, n = 7; no, n = 843 |
Arthritis variables: duration of suffering | |||
Suffered in days | 0 | 100 | |
Suffered in weeks | 0 | 100 | |
Suffered in months | Mean 6.79, SD 2.33; missing, 76.91% | 83.97 | Mean 6.90, SD 2.38 |
Suffered in years | Mean 5.13, SD 6.47; missing, 18.73% | 35.06 | Mean 5.96, SD 7.87 |
Procedure and operations | |||
Arthroscopy (binary) | Yes, n = 6; no, n = 544 | 23.22 | Yes, n = 10; no, n = 833 |
Radiography (binary) | Yes, n = 492; no, n = 58 | 22.68 | Yes, n = 751; no, n = 98 |
Examination | |||
‘Get up and go’ test (categorical) | Grade 1: 41 | 39.16 | Grade 1: 48 |
Grade 2: 242 | Grade 2: 295 | ||
Grade 3: 185 | Grade 3: 223 | ||
Grade 4: 51 | Grade 4: 63 | ||
Grade 5: 24 | Grade 5: 31 | ||
Grade 6: 7 | Grade 6: 8 | ||
‘Get up and go’ test in seconds (continuous) | Mean 16.42, SD 9.69 | 43.26 | Mean 15.85, SD 8.39 |
Handgrip strength: first right hand try (continuous) | Mean 27.97, SD 10.57 | 37.98 | Mean 27.91, SD 10.73 |
Handgrip strength: second right hand try (continuous) | Mean 27.26, SD 10.70 | 38.07 | Mean 27.25, SD 11.01 |
Handgrip strength: third right hand try (continuous) | Mean 27.07, SD 10.57 | 38.07 | Mean 27.19, SD 10.95 |
Handgrip strength: first left hand try (continuous) | Mean 26.36, SD 10.77 | 37.98 | Mean 26.29, SD 11.07 |
Handgrip strength: second left hand try (continuous) | Mean 25.92, SD 10.55 | 38.25 | Mean 26.03, SD 10.79 |
Handgrip strength: third left hand try (continuous) | Mean 25.60, SD 10.38 | 38.34 | Mean 25.81, SD 10.72 |
Right hip trochanteric bursitis (continuous) | Mean 0.32, SD 0.47 | 56.74 | Mean 0.30, SD 0.46 |
Left hip trochanteric bursitis (continuous) | Mean 0.34, SD 0.47 | 63.57 | Mean 0.28, SD 0.45 |
Right hip Trendelenburg sign (continuous) | Mean 0.50, SD 0.50 | 61.66 | Mean 0.41, SD 0.49 |
Left hip Trendelenburg sign (continuous) | Mean 0.54, SD 0.50 | 68.03 | Mean 0.43, SD 0.50 |
Charnley ABC categories259 |
|
36.16 |
|
Right hip internal rotation (continuous) | Mean 14.72, SD 7.97 | 52.28 | Mean 15.37, SD 7.98 |
Left hip internal rotation (continuous) | Mean 15.36, SD 8.91 | 63.12 | Mean 15.85, SD 8.43 |
Variables (type) | Data set | ||
---|---|---|---|
Complete, with missingness in some variables | Incomplete | ||
Frequency | % missing | Frequency | |
Health resources use | |||
Service GP | Yes, n = 495; no, n = 46; missing, 1.64% | 22.68 | Yes, n = 773; no, n = 76 |
Service GP NHS | Mean 3.57, SD 2.65; missing, 20.73% | 38.07 | Mean 3.57, SD 2.79 |
Service HDOC | Yes, n = 410; no, n = 106; missing, 6.18% | 26.41 | Yes, n = 657; no, n = 151 |
Service HDOC NHS | Mean 1.90, SD 1.44; missing, 33.82% | 47.18 | Mean 1.96, SD 1.40 |
Service physiotherapist | Yes, n = 220; no, n = 297; missing, 6.0% | 26.69 | Yes, n = 334; no, n = 471 |
Service physiotherapist NHS | Mean 3.27, SD 2.53; missing, 67.09% | 75.50 | Mean 3.44, SD 2.71 |
Service nurse | Yes, n = 106; no, n = 373; missing, 12.91% | 31.06 | Yes, n = 173; no, n = 576 |
Service nurse NHS | Mean 2.28, SD 2.22; missing, 84.55% | 88.07 | Mean 3.11, SD 5.56 |
Service alternative practitioner | Yes, n = 89; no, n = 440; missing, 3.82% | 23.77 | Yes, n = 129; no, n = 708 |
Service A&E | Yes, n = 58; no, n = 480; missing, 2.18% | 22.77 | Yes, n = 97; no, n = 751 |
Home care | Yes, n = 11; no, n = 530; missing, 1.64% | 22.31 | Yes, n = 18; no, n = 835 |
Questionnaire scores | |||
Pain detected: seven items used to form the scores – questions range from 0 to 5 | Mean 8.90, SD 6.27 | 31.24 | Mean 9.05, SD 6.39 |
Self-assessed EQ-5D | Mean 9.34, SD 1.40 | 12.02 | Mean 14.11, SD 1.97 |
Postoperative EQ-5D | Mean 6.49, SD 1.90 | 3.28 | Mean 6.50, SD 1.85 |
Intermittent and constant OA pain: five items used to form the scores. Questions range from 0 to 4 | Mean 1.56, SD 3.75 | 4.19 | Mean 1.31, SD 3.45 |
12-month follow-up | |||
Patient expectation and outcome |
|
|
Variables (type) | Data set | |||
---|---|---|---|---|
Incomplete with 608 records | Incomplete with 1025 records | |||
% missing | Frequency | % missing | Frequency | |
Arthritis variables: previous treatments | ||||
Medication steroids (binary) | 25.33 | Yes, n = 84; no, n = 370 | 31.22 | Yes, n = 128; no, n = 577 |
Intra-articular steroid injection (binary) | 1.81 | Yes, n = 142; no, n = 455 | 11.32 | Yes, n = 218; no, n = 691 |
Intra-articular hyaluronic acid injection (binary) | 4.28 | Yes, n = 7; no, n = 575 | 13.66 | Yes, n = 9; no, n = 876 |
Soft tissue injection (binary) | 4.11 | Yes, n = 14; no, n = 569 | 13.76 | Yes, n = 23; no, n = 861 |
Infection treatment (binary) | 2.63 | Yes, n = 7; no, n = 585 | 12.49 | Yes, n = 12; no, n = 885 |
Arthritis variables: duration of suffering | ||||
Suffered in days | 99.84 | – | 99.71 | – |
Suffered in weeks | 99.51 | – | 99.61 | – |
Suffered in months | 89.15 | Mean 6.82, SD 2.58 | 90.93 | Mean 6.67, SD 2.62 |
Suffered in years | 13.65 | Mean 8.45, SD 9.04 | 22.54 | Mean 8.44, SD 8.93 |
Procedure and operations | ||||
Arthroscopy (binary) | 2.80 | Yes, n = 85; no, n = 506 | 12.39 | Yes, n = 122; no, n = 776 |
Radiography (binary) | 2.30 | Yes, n = 516; no, n = 78 | 12.20 | Yes, n = 773; no, n = 127 |
Examination | ||||
‘Get up and go’ test (categorical) | 6.09 | Grade 1: 56 | 32.29 | Grade 1: 71 |
Grade 2: 286 | Grade 2: 354 | |||
Grade 3: 163 | Grade 3: 187 | |||
Grade 4: 44 | Grade 4: 56 | |||
Grade 5: 19 | Grade 5: 23 | |||
Grade 6: 3 | Grade 6: 3 | |||
‘Get up and go’ test in seconds (continuous) | 23.52 | Mean 14.17, SD 6.07 | 43.42 | Mean 14.21, SD 6.35 |
Handgrip strength: first right hand try (continuous) | 5.76 | Mean 28.33, SD 10.72 | 31.90 | Mean 28.08, SD 10.80 |
Handgrip strength: second right hand try (continuous) | 6.42 | Mean 27.61, SD 10.84 | 32.29 | Mean 27.44, SD 10.99 |
Handgrip strength: third right hand try (continuous) | 6.42 | Mean 27.17, SD 10.91 | 32.29 | Mean 27.09, SD 11.07 |
Handgrip strength: first left hand try (continuous) | 5.59 | Mean 26.49, SD 11.06 | 31.90 | Mean 26.16, SD 11.17 |
Handgrip strength: second left hand try (continuous) | 6.58 | Mean 26.31, SD 10.80 | 32.49 | Mean 26.03, SD 10.95 |
Handgrip strength: third left hand try (continuous) | 6.74 | Mean 25.95, SD 10.60 | 32.68 | Mean 25.74, SD 10.75 |
Knee effusion (binary) | 40.46 | Yes, n = 101; no, n = 261 | 57.37 | Yes, n = 124; no, n = 313 |
Knee fixed flexion deformity (continuous) | 48.85 | Mean 13.48, SD 64.99 | 62.63 | Mean 11.81, SD 58.71 |
Charnley ABC (continuous) | 50.66 | Mean 2.04, SD 0.71 | 63.51 | Mean 2.08, SD 0.72 |
Variables (type) | Data set | |||
---|---|---|---|---|
Incomplete with 608 records | Incomplete with 1025 records | |||
% missing | Frequency | % missing | Frequency | |
Health resources use | ||||
Service GP | 2.30 | Yes, n = 530; no, n = 64 | 12.20 | Yes, n = 806; no, n = 94 |
Service GP NHS | 23.19 | Mean 3.71, SD 2.88 | 31.81 | Mean 3.85, SD 3.30 |
Service HDOC | 6.58 | Yes, n = 472; no, n = 96 | 15.42 | Yes, n = 723; no, n = 144 |
Service HDOC NHS | 29.93 | Mean 2.30, SD 2.56 | 36.88 | Mean 2.27, SD 2.27 |
Service physiotherapist | 8.39 | Yes, n = 221; no, n = 336 | 18.05 | Yes, n = 336; no, n = 504 |
Service physiotherapist NHS | 69.74 | Mean 3.95, SD 4.43 | 72.59 | Mean 3.76, SD 4.21 |
Service nurse | 11.02 | Yes, n = 123; no, n = 418 | 21.56 | Yes, n = 183; no, n = 621 |
Service nurse NHS | 83.55 | Mean 3.30, SD 3.78 | 85.56 | Mean 3.23, SD 3.61 |
Service alternative practitioner | 4.93 | Yes, n = 69; no, n = 509 | 14.05 | Yes, n = 99; no, n = 782 |
Service A&E | 4.93 | Yes, n = 71; no, n = 507 | 14.34 | Yes, n = 99; no, n = 779 |
Home care | 3.95 | Yes, n = 16; no, n = 568 | 13.66 | Yes, n = 18; no, n = 867 |
Questionnaire scores | ||||
Pain detected: seven items used to form the scores – questions range from 0 to 5 | 14.15 | Mean 10.50, SD 6.33 | 24.20 | Mean 10.47, SD 6.39 |
Self-assessed EQ-5D | 3.29 | Mean 8.86, SD 1.37 | 7.81 | Mean 13.71, SD 1.67 |
Postoperative EQ-5D | 3.29 | Mean 6.85, SD 1.90 | 3.90 | Mean 6.91, SD 1.90 |
Intermittent and constant OA pain: five items used to form the scores – questions range from 0 to 4 | 4.11 | Mean 2.24, SD 4.18 | 4.00 | Mean 2.14, SD 4.06 |
12-month follow-up | ||||
Patient expectation and outcome | 12.67 |
|
12.78 |
|
List of abbreviations
- ACL
- anterior cruciate ligament
- ANCOVA
- analysis of covariance
- ASA
- American Society of Anesthesiologists
- AUC
- area under the curve
- BMD
- bone mineral density
- BMI
- body mass index
- BNF
- British National Formulary
- CEAC
- cost-effectiveness acceptability curve
- CI
- confidence interval
- COASt
- Clinical Outcomes in Arthroplasty Study
- CPRD
- Clinical Practice Research Datalink
- DEXA
- dual-energy X-ray absorptiometry
- DICOM
- Digital Imaging and Communications in Medicine
- DSAC
- Data and Sample Access Committee
- DVT
- deep-vein thrombosis
- EOC
- Elective Orthopaedic Centre
- EPOS
- Exeter Primary Outcomes Study
- EQ-5D
- EuroQol-5 Dimensions
- EUROHIP
- European Collaborative Database of Cost and Practice Patterns of Total Hip Replacement
- GP
- general practitioner
- GPRD
- General Practice Research Database
- HCS
- high-compliance system
- HES
- Hospital Episode Statistics
- HR
- hazard ratio
- HRG
- Healthcare Resource Group
- HRQoL
- health-related quality of life
- hsCRP
- high-sensitivity C-reactive protein
- HSE
- Health Survey for England
- HTA
- Human Tissue Authority
- ICER
- incremental cost-effectiveness ratio
- IMD
- Index of Multiple Deprivation
- IMSU
- Information Management Services Unit
- IQR
- interquartile range
- IT
- information technology
- K/L
- Kellgren and Lawrence grading system
- KAT
- Knee Arthroplasty Trial
- MICE
- multivariate imputation by chained equations
- NCOASt
- North COASt study (Oxford)
- NDORMS
- Nuffield Department of Orthopaedics, Rheumatology and Musculoskeletal Sciences
- NICE
- National Institute for Health and Care Excellence
- NIHR
- National Institute for Health Research
- NJR
- National Joint Registry
- NOC
- Nuffield Orthopaedic Centre
- NSAID
- non-steroidal anti-inflammatory drug
- OA
- osteoarthritis
- OARSI
- Osteoarthritis Research Society International
- OHS
- Oxford Hip Score
- OKS
- Oxford Knee Score
- OLS
- ordinary least squares
- OMB
- Oxford Musculoskeletal BioBank
- ONS
- Office for National Statistics
- OR
- odds ratio
- OUH
- Oxford University Hospitals NHS Trust
- OXMIS
- Oxford Medical Information System
- PACS
- picture archiving and communication system
- PASS
- patient-accepted symptom state
- PCT
- primary care trust
- PE
- pulmonary embolism
- PIS
- patient information sheet
- PoPC
- percentage of potential change
- PPI
- patient and public involvement
- PPR
- patient and public representative
- PROM
- patient-reported outcome measure
- PSA
- probabilistic sensitivity analysis
- QALY
- quality-adjusted life-year
- RA
- rheumatoid arthritis
- RCT
- randomised controlled trial
- ROC
- receiver operating characteristic
- SD
- standard deviation
- SF-12
- Short Form questionnaire-12 items
- SF-36
- Short Form questionnaire-36 items
- SGH
- Southampton General Hospital
- SHR
- subhazard ratio
- SWLEOC
- South West London Elective Orthopaedic Centre
- THA
- total hip arthroplasty
- THR
- total hip replacement
- TJR
- total joint replacement
- TKA
- total knee arthroplasty
- TKR
- total knee replacement
- TTU
- transfer to utility
- UHS
- University Hospitals Southampton NHS Foundation Trust
- UKR
- unicompartmental knee replacement
- VAS
- visual analogue scale
- VIF
- variance inflation factor
- WOMAC
- Western Ontario and McMaster Universities Osteoarthritis Index