Notes
Article history
The research reported in this issue of the journal was funded by PGfAR as project number RP-PG-0407-10384. The contractual start date was in July 2008. The final report began editorial review in July 2016 and was accepted for publication in April 2017. As the funder, the PGfAR programme agreed the research questions and study designs in advance with the investigators. The authors have been wholly responsible for all data collection, analysis and interpretation, and for writing up their work. The PGfAR editors and production house have tried to ensure the accuracy of the authors’ report and would like to thank the reviewers for their constructive comments on the final report document. However, they do not accept liability for damages or losses arising from material published in this report.
Declared competing interests of authors
Gavin J Murphy reports grants from the National Institute for Health Research (NIHR), the British Heart Foundation and Zimmer Biomet during the conduct of the study. He also received consultancy fees from AbbVie and Thrasos Inc. with respect to the conduct of trials of organ protection interventions in cardiac surgery. Andrew D Mumford reports grants from the NIHR and the British Heart Foundation during the conduct of the study. Chris A Rogers reports grants from the NIHR and the British Heart Foundation during the conduct of the study. Sarah Wordsworth reports grants from the NIHR during the conduct of the study. Elizabeth A Stokes reports grants from the NIHR during the conduct of the study. Tracy Kumar reports grants from Zimmer Biomet during the conduct of the study. Marcin Wozniak reports grants from the British Heart Foundation and Zimmer Biomet during the conduct of the study. Jonathan A Sterne reports grants from the NIHR during the conduct of the study. Gianni D Angelini reports grants from the NIHR and the British Heart Foundation during the conduct of the study. Barnaby C Reeves reports grants from the NIHR during the conduct of the study.
Permissions
Copyright statement
© Queen’s Printer and Controller of HMSO 2017. This work was produced by Murphy et al. under the terms of a commissioning contract issued by the Secretary of State for Health. This issue may be freely reproduced for the purposes of private research and study and extracts (or indeed, the full report) may be included in professional journals provided that suitable acknowledgement is made and the reproduction is not associated with any form of advertising. Applications for commercial reproduction should be addressed to: NIHR Journals Library, National Institute for Health Research, Evaluation, Trials and Studies Coordinating Centre, Alpha House, University of Southampton Science Park, Southampton SO16 7NS, UK.
Chapter 1 Introduction
The clinical problem
Organ failure in cardiac surgery
Cardiac surgery with cardiopulmonary bypass (CPB) results in a complex inflammatory response attributable to haematological activation (coagulation and protease cascades, platelets, leucocytes) by the extracorporeal circuit. 1 This often results in activation of vascular endothelium, tissue hypoxia and organ injury, particularly in those with pre-existing organ dysfunction. 2 In addition, cardioplegic arrest results in a myocardial-specific ischaemia–reperfusion injury. This can result in low cardiac output post surgery that compounds the tissue hypoxia, inflammation and organ injury attributable to CPB. 3 Organ injury is an important cause of morbidity and mortality and results in an increased use of hospital resources. In a study by Murphy et al. ,4 clinically significant kidney, lung and myocardial injury occurred in 34%, 16% and 11% of patients respectively, contributing to 41%, 36% and 24% of all deaths respectively. Every year, cardiac surgery is performed in > 35,000 UK patients and > 1 million patients worldwide. The number of elderly patients with pre-existing organ dysfunction referred for cardiac surgery increases year on year. 5 Reducing perioperative organ failure therefore presents an ever-increasing challenge for clinicians and health services.
Blood management
The safe and effective management of perioperative anaemia and coagulopathic bleeding is a key determinant of outcome following cardiac surgery. 6 Anaemia and coagulopathic bleeding are common, often require multiple blood management interventions and are associated with increases in the rates of organ failure, sepsis and death. However, there is clinical uncertainty as to how these conditions should be managed clinically because of our limited understanding of the underlying mechanisms and the lack of clinical efficacy for most blood management interventions that have been evaluated in clinical trials. 7 This has led to significant variability in care.
Coagulopathic bleeding is a potential complication of every cardiac operation. Death as a direct consequence of bleeding is rare; in the Blood Conservation Using Antifibrinolytics in a Randomised Trial (BART) study, 23 of 2330 patients (1%) died of uncontrolled blood loss. 8 However, emergency sternotomy for life-threatening bleeding and large-volume blood transfusion (LVBT; > 4 units of red cells) are common and are associated with significant increased risks of organ failure, sepsis and death. LVBT occurs in 22% of UK cardiac surgery patients. 9 This has been associated with an eightfold increase in the risk of death. 10 Emergency re-sternotomy for life-threatening bleeding or tamponade occurs in 4% of UK cardiac surgery patients and increases the risk of death two- to fivefold. 11–13
The causes of coagulopathy are complex and poorly understood but they relate to the activation of platelets and serum proteases by the extracorporeal bypass circuit. These are discussed in detail in Chapter 2. Summaries of interventions in common clinical use to prevent or reverse coagulopathy are described in Table 1. The most effective of these is tranexamic acid, a lysine analogue that inhibits the serum protease plasmin, thereby reducing fibrinolysis and promoting clot stability. Tranexamic acid reduces blood loss, transfusion rates and, most importantly, death in cardiac surgery patients. 16–18 Other interventions to prevent or reverse coagulopathic haemorrhage reduce blood loss and transfusion but lack the clinical efficacy of tranexamic acid (see Table 1) and their use has declined. 22 Transfusion of non-red cell blood components [pooled platelets, fresh-frozen plasma (FFP) and cryoprecipitate] remains the primary treatment for coagulopathic haemorrhage. However, their use is empirical, their risks and benefits are poorly defined and the frequency of their use is highly variable (described in more detail in Chapter 2).
Intervention | Reference | n studies/n participants | Effect on transfusion, risk ratio (95% CI) | Effect on clinical outcome, risk ratio (95% CI) |
---|---|---|---|---|
Minimise loss of autologous red cells | ||||
Preoperative autologous donation | Henry et al.14 | 14/1506 | 0.32 (0.22 to 0.47) | Infection: 0.70 (0.34 to 1.43) |
Thrombosis: 0.82 (0.21 to 3.13) | ||||
Any transfusion: 1.24 (1.02 to 1.51) | ||||
Acute normovolaemic haemodilution | Davies et al.15 | 11/1423 | 0.36 (0.25 to 0.51) | Infection: 0.70 (0.34 to 1.43) |
Thrombosis: 0.82 (0.21 to 3.13) | ||||
Mechanical cell salvage (high-risk patients) | Murphy et al.16 | 4/223 | 0.74 (0.58 to 0.93) | Death: 0.97 (0.64 to 1.47) |
Thrombosis: not estimable | ||||
Infection: 0.4 (0.18 to 0.87) | ||||
Mechanical cell salvage (medium-risk patients) | Murphy et al.16 | 3/384 | 0.74 (0.5 to 1.12) | Death, thrombosis and infection: not reported |
Stimulate erythropoiesis | ||||
Intravenous iron vs. placebo or no intravenous iron (surgery) | Murphy et al.16 | 5/467 | 0.77 (0.59 to 0.99) | Death: 1.1 (0.49 to 2.47) |
Thrombosis: – | ||||
Infection: 1.23 (0.63 to 2.42) | ||||
Oral iron vs. intravenous iron | Murphy et al.16 | 6/699 | 1.2 (0.56 to 2.61) | Death: 1.22 (0.58 to 2.56) |
Thrombosis: – | ||||
Infection: – | ||||
Erythropoietin vs. placebo (surgery) | Murphy et al.16 | 12/1663 | 0.59 (0.53 to 0.67) | Death: 1.55 (0.79 to 3.07) |
Thrombosis: 1.37 (0.73 to 2.56) | ||||
Infection: not estimable | ||||
Erythropoietin plus intravenous iron vs. placebo | Murphy et al.16 | 2/283 | 0.51 (0.39 to 0.67) | Death: 0.33 (0.01 to 7.93) |
Thrombosis: not estimable | ||||
Infection: not estimable | ||||
Erythropoietin plus oral iron vs. oral iron | Murphy et al.16 | 2/880 | 0.06 (0.02 to 0.25) | Death: 0.88 (0.39 to 1.96) |
Thrombosis: 1.71 (0.68 to 4.3) | ||||
Infection: 0.5 (0.05 to 4.98) | ||||
Reverse coagulopathy | ||||
Tranexamic acid vs. placebo (high-risk surgery) | Murphy et al.16 | 38/4105 | 0.71 (0.63 to 0.81) | Death: 0.73 (0.15 to 3.66) |
Thrombosis: 0.69 (0.44 to 1.07) | ||||
Infection: 0.93 (0.22 to 3.93) | ||||
Tranexamic acid vs. placebo (moderate-risk surgery) | Murphy et al.16 | 25/4577 | 0.45 (0.38 to 0.52) | Death: 0.73 (0.15 to 3.66) |
Thrombosis: 0.69 (0.44 to 1.07) | ||||
Infection: 0.93 (0.22 to 3.93) | ||||
Tranexamic acid high dose vs. tranexamic acid low dose (surgery) | Ker et al.17 | 129/10,488 | 0.62 (0.58 to 0.65) | Death: 0.61 (0.38 to 0.98) |
Myocardial infarction: 0.68 (0.43 to 1.09) | ||||
Pulmonary embolism: 1.14 (0.65 to 2.00) | ||||
Tranexamic acid vs. placebo (trauma) | Ker et al.18 | 2/20,367 | 0.98 (0.96 to 1.01) | Death: 0.90 (0.85 to 0.96) |
Myocardial infarction: 0.61 (0.40 to 0.92) | ||||
Tranexamic acid plus cell salvage vs. cell salvage (high-risk surgical patients) | Murphy et al.16 | 5/514 | 0.71 (0.6 to 0.85) | Death: 1.04 (0.07 to 16.41) |
Tranexamic acid plus cell salvage vs. tranexamic acid (high-risk surgical patients) | Murphy et al.16 | 1/63 | 0.79 (0.43 to 1.45) | Death: 7.71 (0.43 to 137.53) |
Aprotinin vs. placebo | Henry et al.19 | 108/1172 | 0.66 (0.60 to 0.72) | Death: 0.81 (0.63 to 1.06) |
Myocardial infarction: 0.87 (0.69 to 1.11) | ||||
Renal failure: 1.10 (0.79 to 1.54) | ||||
Aprotinin vs. tranexamic acid | Henry et al.19 | 21/4185 | 0.90 (0.81 to 1.01) | Death: 1.35 (0.94 to 1.93) |
Myocardial infarction: 1.00 (0.71 to 1.42) | ||||
Renal failure: 1.02 (0.79 to 1.31) | ||||
Desmopressin | Carless et al.20 | 19/1387 | 0.96 (0.87 to 1.06) | Death: 1.72 (0.68 to 4.33) |
Thrombosis: 1.46 (0.64 to 3.35) | ||||
Hypotension: 2.81 (1.50 to 5.27) | ||||
Recombinant activated factor VII | Simpson et al.21 | 8/868 | 0.85 (0.72 to 1.01) | Death: 1.04 (0.55 to 1.97) |
Arterial thromboembolic events: 1.45 (1.02 to 2.05) |
Identifying those most likely to bleed may benefit patients by allowing preoperative optimisation or the use of targeted blood management interventions such as the reversal of the effects of antiplatelet agents or the reversal of specific coagulation defects identified by laboratory tests or preferably point-of-care (POC) diagnostic tests for coagulopathy. Current guidance recommends that patients undergo careful risk assessment prior to surgery, in combination with POC haemostasis testing to direct therapy in bleeding patients. 23,24 Existing bleeding risk scores have significant limitations, however (reviewed in Chapter 3), and are not widely used. Moreover, the evidence to support the use of POC tests is of low quality. 25,26 These limitations are reflected by wide variability in the assessment and management of bleeding patients. In a UK audit, 12% of units reported that they did not use POC tests for coagulopathy, 24% reported that they were used in < 25% of cases, LVBT rates ranged from 8% to 35% and emergency re-sternotomy rates ranged from 0.3% to 12%. 9 To better define the roles of preoperative clinical assessment and near-patient platelet and viscoelastometry testing for the prediction of severe bleeding, we undertook the COagulation and Platelet laboratory Testing in Cardiac surgery (COPTIC) study in a large cohort of adult cardiac surgery patients (see Chapter 2). We hypothesised that the use of near-patient testing would improve the prediction of severe bleeding beyond the use of clinical risk factors alone. We also critically reviewed the limitations of previously published clinical risk scores and developed two new risk scores for preoperative risk assessment (see Chapter 3).
Anaemia, defined as a blood haemoglobin concentration of < 12 g/dl, can be identified in approximately 30% of cardiac surgery patients presenting for surgery in the UK. 9 A significant number also develop intraoperative or postoperative anaemia. The most common cause of anaemia is chronic disease (45%), with relatively small proportions of patients presenting with diagnosable and reversible causes of anaemia such as iron deficiency (7%) or vitamin B12 deficiency (11%). 27 Additional aetiological factors for intra- and postoperative anaemia are haemodilution, haemorrhage or impaired erythropoiesis. It is hypothesised that anaemia contributes to organ failure and death by reducing tissue oxygen delivery during CPB, resulting in hypoxic cellular injury and organ dysfunction. 28 Organs with high metabolic demands are considered more susceptible to injury in the presence of anaemia and there are strong associations between perioperative anaemia and kidney, myocardial and brain injury. 29,30
Preoperative interventions for the treatment or reversal of anaemia have limited clinical benefit (see Table 1) and the preferred treatment for acute perioperative anaemia is red cell transfusion. The severity of anaemia, or haemoglobin threshold, that triggers a red cell transfusion differs between clinicians and units. As a result, red cell transfusion rates vary widely. In the UK, red cell transfusion rates range from 32% to 75% in different centres. 9 In the USA, rates range from 45% to 92%. 31 This is potentially important as red cell transfusion is strongly associated with increased rates of postoperative organ failure and death (see Table 1). Transfusion is also associated with an increased frequency of sepsis. 32 It has been hypothesised that these complications are the result of pathological changes that occur in red cells during storage, termed ‘the storage lesion’, which result in inflammation and organ injury in recipients. 33
Differentiating the cause and effect of anaemia on adverse outcomes from those of transfusion is not possible from existing epidemiological analyses because these variables are so strongly linked. Furthermore, in complete contrast to observational studies, randomised controlled trials (RCTs) comparing restrictive haemoglobin transfusion thresholds (6.5–8 g/dl) with more liberal thresholds (8–10 g/dl) suggest that the reversal of anaemia with red cell transfusion may benefit patients. The Transfusion Indication Threshold Reduction (TITRe2) trial4 demonstrated higher mortality in patients randomised to more restrictive thresholds, that is, with more severe anaemia. These observations were supported by the results of a systematic review of this and four similar trials,32 with more liberal transfusion thresholds found to reduce mortality [risk ratio (RR) 0.7, 95% confidence interval (CI) 0.49 to 1.02]. In trials that included only patients with severe symptomatic cardiovascular disease, the reduction in mortality was statistically significant (RR 0.67, 95% CI 0.47 to 0.95; Table 2). This suggests that conditions predisposing to anaemia are the principal contributors to organ failure and death observed in epidemiological analyses, rather than red cell transfusion.
Outcome | Observational analyses | RCTs of liberal vs. restrictive thresholds in cardiac surgery | RCTs of liberal vs. restrictive thresholds in symptomatic cardiovascular disease | |||
---|---|---|---|---|---|---|
n studies/n participants | Odds ratio (95% CI) | n studies/n participants | RR (95% CI) | n studies/n participants | RR (95% CI) | |
Death | 19/138,357 | 1.48 (1.42 to 1.53) | 5/3304 | 0.70 (0.49 to 1.02) | 7/3439 | 0.67 (0.47 to 0.95) |
Myocardial infarction | 8/35,763 | 1.55 (1.48 to 1.62) | 1/2003 | 1.34 (0.30 to 6.02) | – | – (–) |
Stroke | 7/43,649 | 1.41 (1.34 to 1.48) | 1/2003 | 1.14 (0.57 to 2.30) | – | – (–) |
Acute kidney injury | 14/59,003 | 1.73 (1.65 to 1.83) | 5/3304 | 0.86 (0.68 to 1.09) | – | – (–) |
Pulmonary injury | 7/43,431 | 1.68 (1.63 to 1.74) | 6/3357 | 0.94 (0.76 to 1.17) | – | – (–) |
Infection | 11/88,025 | 1.81 (1.73 to 1.89) | 4/2802 | 0.97 (0.79 to 1.19) | – | – (–) |
The evidence from randomised trials notwithstanding, clinical uncertainty as to the indications for red cell transfusion in anaemic patients remains. Transfusion guidelines published by the UK National Institute for Health and Care Excellence (NICE)16 recommend more liberal transfusion thresholds in patients with severe cardiovascular disease; however, many other guidelines recommend restrictive thresholds, including those published by the American Association of Blood Banks,34 the Society of Thoracic Surgeons24 and the American College of Critical Care Medicine (ACCM). 35 In addition, a limitation of existing ‘transfusion trigger’ trials is that they compare protocolised transfusion thresholds. It has been hypothesised that the critical haemoglobin level, that is, the threshold below which oxygen delivery becomes impaired, is different both between patients and in individual patients during their perioperative course. 36,37 Changes in tissue oxygen requirements are not reflected by protocolised transfusion thresholds and recent commentaries have stressed the importance of more personalised measures of the need for transfusion. 38 We evaluate a personalised red cell transfusion algorithm in the PAtient-SPecific Oxygen monitoring to Reduce blood Transfusion during heart surgery (PASPORT) trial in Chapter 4.
Safer red cells may move the balance of risks and benefits to favour the more aggressive treatment of anaemia. The Red Cell Storage Duration Study (RECESS)39 randomised participants to red cells that had been stored for longer (> 21 days) or shorter (< 10 days) periods of time to test the hypothesis that the storage lesion directly contributed to organ injury. There was no difference between the groups in this trial for the primary outcome of multiple organ dysfunction [95% CI for the mean difference (MD) –0.6 to 0.3; p = 0.44]. This study was limited in that the difference in severity of the storage lesion between day 10 and day 21 red cells is small. Furthermore, epidemiological analysis that compared organ injury and death in many thousands of patients receiving younger red cells or older red cells did not suggest important differences between red cells stored for these different time periods. 40 The clinical importance of the storage lesion in transfused patients remains uncertain. In Chapter 5 we consider the effects of an intervention to modify the storage lesion in the REDWASH trial.
Medical devices
A medical device may be defined as any instrument, apparatus, appliance, software, material or other article used specifically for diagnostic and/or therapeutic purposes. CPB circuits, prosthetic heart valves and other implants, monitoring equipment and web-based mortality risk scores are examples of devices used in cardiac surgery. The most common devices in clinical use as blood management adjuncts are cell salvage devices that collect shed mediastinal blood for autotransfusion. These have been used in cardiac surgery for decades. However, despite their widespread use, the evidence of clinical benefit to patients from these devices is limited. Cell salvage devices reduce red cell transfusion rates and infections when used in isolation in patients at high risk for bleeding and red cell transfusion. 16 This benefit is not observed when patients are also administered tranexamic acid (see Table 1). This apparent lack of clinical benefit has emerged only in recent analyses,16 prompted by the evidence of effectiveness for tranexamic acid from a high-quality RCT in trauma41 and a subsequent systematic review in surgical patients. 17 This reflects a common criticism of the levels of evidence required for approval of medical devices compared with that required to introduce a medicinal product into clinical care. 42 Unlike pharmacological interventions, medical devices do not require evidence of efficacy from RCTs. Rather, in Europe, manufacturers are required to submit a dossier of supporting evidence to a notified body. Notified bodies are private companies that specialise in evaluating many products, including medical devices, for European Conformity (CE) marks. Notified bodies are designated by a national competent authority, such as the Medicines and Healthcare products Regulatory Agency in the UK, to cover certain types of devices. If the notified body deems that a device meets the standards for performance and reliability testing linked to the risks of its intended use, it verifies a Certificate of Conformity and the device may enter clinical use. This process has been criticised for lack of transparency, and variable quality, as well as the fact that CE marks may be obtained in the absence of evidence of clinical efficacy. 43 On the one hand the flexibility of the process promotes innovation, but on the other it may lead to widespread use of ineffective or even harmful medical devices.
We identified multiple devices in common use as blood management adjuncts in our own clinical practice, for which there was uncertainty as to their clinical efficacy. Principal among these were POC haemostasis tests for the management of coagulopathic bleeding. These devices are of uncertain utility as existing studies show only limited predictive accuracy for coagulopathic bleeding and clinical efficacy, in terms of the benefit to patients from their use. 25,26 We also identified a knowledge gap with respect to clinical risk assessment. Importantly, there was no available published risk calculator available for clinical use. Near-infrared spectroscopy (NIRS), a device for measuring regional tissue oxygenation in the forebrain, is also widely used in cardiac surgery. It has been suggested that these devices may be used to personalise transfusion decisions by identifying the critical haematocrit below which tissues become hypoxic. 44,45 Finally, we noted that mechanical cell salvage devices are used in some clinical situations to wash allogenic red cells prior to transfusion, with the intention of removing harmful metabolites of red cell storage and attenuating inflammation and organ injury in recipients. 46,47
Structure of this report
The overarching aim of this programme was to provide high-quality evidence on the efficacy and cost-effectiveness of devices in common clinical use as blood management adjuncts. A common theme of all of the workstreams was to design trials that would address the bias and limitations that were identified in our reviews of existing data.
Our work was divided into three workstreams, summarised in the following sections.
Workstream 1: diagnosis of coagulopathy and assessment of bleeding and transfusion risk
Our objectives were to:
-
evaluate the predictive accuracy of clinical risk assessment using data available at baseline for bleeding, organ injury, sepsis and death following cardiac surgery
-
evaluate the additional clinical value of routine POC tests of platelet function, coagulation and fibrinolysis
-
evaluate the additional clinical value of pre- and postoperative laboratory reference tests (LRTs) of coagulation
-
evaluate the cost-effectiveness of the routine introduction of POC tests into clinical practice
-
review the evidence supporting the clinical use of POC haemostasis tests in a systematic review and meta-analysis of RCTs
-
develop two new clinical risk prediction scores for red cell transfusion and bleeding.
Our plan of investigation to address these objectives was as follows.
-
A systematic review and meta-analysis of RCTs comparing POC haemostasis test-based algorithms for the prevention of post-cardiac surgery bleeding and organ injury (reported in Chapter 2).
-
A prospective diagnostic accuracy study evaluating the benefits of pre- and postoperative POC tests for the prediction of bleeding and adverse outcome over those achieved using routinely measured baseline factors (the COPTIC study; reported in Chapter 2).
-
A prospective diagnostic accuracy study evaluating the benefits of pre- and post-LRTs for the prediction of bleeding and adverse outcome over those achieved using routinely measured baseline factors (the COPTIC study; reported in Chapter 2).
-
A health economic analysis to assess the cost-effectiveness of introducing POC tests into routine clinical care (reported in Chapter 2).
-
A narrative review of existing risk prediction scores for bleeding and transfusion in cardiac surgery (reported in Chapter 3).
-
The development and validation of novel web-based risk scores for preoperative clinical risk assessment (reported in Chapter 3).
Workstream 2: the use of near-infrared spectroscopy as a patient-specific indicator of regional tissue oxygenation and the need for red cell transfusion in cardiac surgery
Our objectives were to:
-
evaluate the efficacy of a personalised NIRS-based algorithm that incorporated a restrictive red cell transfusion threshold compared with standard care during CPB in a multicentre RCT
-
estimate the cost-effectiveness of introducing into clinical practice a NIRS-based algorithm to guide the conduct of CPB and red cell transfusion
-
review the evidence to support the clinical use of cerebral NIRS monitors and NIRS-based algorithms during CPB in a systematic review and meta-analysis of the PASPORT trial and other similar trials.
Our plan of investigation to address these objectives was as follows.
-
A multicentre randomised controlled efficacy trial comparing a patient-specific NIRS-based algorithm with standard care (the PASPORT trial; reported in Chapter 4).
-
A health economic analysis of the cost-effectiveness of introducing a NIRS-based algorithm into routine clinical care (reported in Chapter 4).
-
A systematic review of RCTs that have assessed the effectiveness of NIRS-based algorithms for the reduction of red cell transfusion and organ injury in cardiac surgery (reported in Chapter 4).
Workstream 3: the use of mechanical red cell washing devices to remove the red cell supernatant from stored red cells, thereby reducing inflammation and organ injury attributable to the storage lesion
Our objectives were to:
-
compare clinical outcomes including inflammation, organ injury and sepsis in participants randomised to receive mechanically washed allogenic red cells compared with standard care
-
evaluate the effects of mechanical washing on the structure, function and inflammatory properties of allogenic red cells in vitro and in participants in vivo
-
evaluate the cost-effectiveness of introducing red cell washing into clinical care.
Our plan of investigation to address these objectives was as follows.
-
A multicentre RCT to assess the efficacy of allogenic red cell washing compared with standard care for the prevention of past cardiac surgery inflammation and organ injury (reported in Chapter 5).
-
A substudy to explore the mechanisms underlying the results of our clinical trial (reported in Chapter 5).
Trial registration
Studies in the programme were prospectively registered as follows:
-
ISRCTN20778544 – coagulation and platelet laboratory testing in cardiac surgery (the COPTIC study)
-
PROSPERO CRD42016033831 – the routine use of point-of-care tests for the diagnosis and treatment of coagulopathic bleeding in cardiac surgery: a systematic review
-
ISRCTN 23557269 – a randomised controlled trial of patient-specific oxygen monitoring to reduce blood transfusion during heart surgery (the PASPORT trial)
-
PROSPERO CRD42015027696 – efficacy of near-infrared spectroscopy on the outcome of patients undergoing cardiac surgery: a systematic review
-
ISRCTN 27076315 – a randomised controlled trial of red cell washing for the attenuation of transfusion associated organ injury in cardiac surgery (the REDWASH trial).
Changes to the original programme of work described in the grant application
The original programme of work was completed in its entirety, with the exception of the REDWASH study, which was terminated prematurely because of poor recruitment. For this reason no cost-effectiveness analysis was performed in the REDWASH trial as originally planned. In addition, two systematic reviews are included (see Chapters 2 and 4) and a mechanisms substudy was performed alongside the REDWASH trial (see Chapter 5).
Patient and public involvement
Patient and public involvement (PPI) groups in Bristol and Leicester were involved in the development, governance, conduct and dissemination of this programme. The groups were composed of cardiac surgery patients, some of whom had participated in clinical trials, and members of the public, many of whom had PPI clinical research experience in local and national organisations. Of these, the Leicester Cardiac Surgery PPI group was the most developed, with specific subgroups dealing with consultation in respect of research protocols and materials, research activity including the development of recruitment strategies, trial governance and the enhanced patient visitor role, raising awareness about research in the public forum and disseminating research findings in partnership with the research team. The activities of this group have ensured that patient needs have remained at the centre of the research programme throughout.
Transparency declaration
The lead author (GJM) affirms that the manuscript is an honest, accurate and transparent account of the studies being reported; that no important aspects of the study have been omitted; and that any discrepancies from the study as planned (and, if relevant, registered) have been explained.
Chapter 2 Point-of-care coagulation and platelet laboratory testing in cardiac surgery: a predictive accuracy study with linked health economic analysis and parallel systematic review of efficacy trials of viscoelastometry
Abstract
Background: Coagulopathic bleeding is a common and severe complication of cardiac surgery.
Objective: To assess the benefits of introducing routine POC tests or an expanded range of laboratory diagnostic tests for coagulopathy compared with the current standard of care (clinical risk assessment) for the management of cardiac surgery patients with severe bleeding.
Methods: A diagnostic accuracy study and linked cost-effectiveness analysis compared POC platforms in current use for the prediction of post-surgery haemorrhage [rotational thromboelastometry (ROTEM) (ROTEM® Delta; TEM International GmbH, Munich, Germany), thromboelastography (TEG) (TEG® 5000 Thromboelastograph® Haemostasis Analyzer; Haemonetics Corporation, Niles, IL, USA) and Multiplate® analyzer (Roche, Rotkreuz, Switzerland)], as well as alternative LRTs of coagulopathy, with standard care. The clinical effectiveness of POC testing in cardiac surgery was evaluated in a systematic review of RCTs.
Results: In the predictive accuracy study 2541 participants were recruited between March 2010 and September 2012. Perioperative POC and LRT results did not result in clinically important improvements in predictive accuracy beyond that achieved using clinical risk prediction alone. The cost-effectiveness analysis demonstrated very little difference between any of the POC tests and current practice in either costs or life-years saved. The systematic review demonstrated that the routine use of POC tests did not improve clinical outcomes.
Conclusions: Existing diagnostic tests for coagulopathy have limited additional value for the diagnosis and treatment of coagulopathic bleeding in cardiac surgery beyond the current standard of care.
Study registration: Current Controlled Trials ISRCTN20778544 and PROSPERO CRD42016033831.
Background
The clinical problem
Coagulopathic bleeding is a common and severe complication of cardiac surgery. In the UK up to 5% of all patients require emergency re-exploration for bleeding in the immediate postoperative period. 9 This is associated with a fourfold increase in mortality and resource use (Figure 1). Adverse events associated with severe bleeding may be attributable to the severity of the underlying illness and the complexity of surgery, which contribute to coagulopathy, as well as the consequences of significant haemorrhage and shock. The adverse effects of coagulopathic bleeding may also be attributable in part to the side effects of treatment. Large-volume red cell transfusions or the administration of pro-haemostatic therapies such as platelet and FFP transfusion and recombinant activated factor VII (rFVIIa) have well-documented risks. 7 These risks, although offset by the risks of ongoing bleeding in coagulopathic patients, may be clinically significant in those without coagulopathy or when administered to those who are not actively bleeding.
FIGURE 1.
Forest plots summarising effect estimates from observational studies that have considered the effects of emergency reternotomy for bleeding on mortality. M–H, Mantel–Haenszel.

A range of medical devices are in common use as POC diagnostic tests for the prediction of severe bleeding in patients and its treatment; however, the evidence to support their use is inconsistent and this is reflected by wide variation in their use. 9 This chapter will establish whether commonly used POC testing devices have good predictive accuracy for coagulopathic bleeding when used routinely and consider whether the introduction of POC testing algorithms for the diagnosis and management of bleeding is cost-effective. It will also consider whether the introduction of additional diagnostic tests improves predictive accuracy and effectiveness.
Coagulopathic bleeding
Coagulopathic haemorrhage is poorly defined. The current consensus definition of coagulopathy is a prolonged prothrombin time (PT) of > 1.2 times normal. 51 However, prolonged PT and abnormalities in other standard laboratory tests (SLTs) have not been found to have predictive accuracy for coagulopathic haemorrhage in cardiac or non-cardiac surgery. 52,53 Many patients with abnormal standard laboratory coagulation tests do not develop bleeding and coagulopathic bleeding may occur when these tests are normal. This reflects the limitations of the tests and the complex aetiology of coagulopathy. This means, however, that it is often difficult to distinguish coagulopathic bleeding from non-coagulopathic bleeding. Defining excessive bleeding is also problematic in situations in which bleeding occurs to some degree in all patients. The efficacy of haemostatic interventions is also unclear. The lack of a clear definition of coagulopathic bleeding complicates epidemiological analyses and the development of accurate diagnostic tests and treatments. Excessive bleeding in cardiac surgery may be defined clinically as:
-
Emergency re-sternotomy for life-threatening haemorrhage or cardiac tamponade within hours of surgery. This definition includes patients with very severe coagulopathic haemorrhage in which conventional pro-haemostatic therapies have failed to control bleeding. This has limitations as a definition because emergency re-sternotomy may be required for ‘surgical’ bleeding in the absence of coagulopathy. There are also important institutional differences in how patients with haemorrhage are managed and how readily patients are re-explored when tamponade is suspected. This is reflected by emergency re-sternotomy rates that range from 0.3% to 12% between UK units. 9 This definition may therefore underestimate the proportion of patients with coagulopathic haemorrhage.
-
Large-volume red cell transfusion. Massive blood transfusion (MBT) defined as ≥ 10 units of red cells within 24 hours, or 4 units within 1 hour, is rare in cardiac surgery. Large-volume red cell transfusion (≥ 4 units of red cells) is common, however. In the UK 22% of patients receive a LVBT perioperatively. 9 This equates to approximately 1000 ml of packed red cells or 20% of the circulating blood volume of a 70-kg adult. Transfusion of ≥ 4 units has been shown to be associated with significant increases in perioperative morbidity and mortality in cardiac surgery. 10 A limitation of this definition is that red cells are also commonly administered for the treatment of anaemia and this may therefore overestimate the frequency of coagulopathic haemorrhage. Late transfusions, for example after 24 hours postoperatively, are also unlikely to represent treatment for bleeding.
-
Transfusion of allogenic non-red cell components to promote haemostasis. In the UK up to 25% of cardiac surgery patients receive FFP or platelets to arrest or prevent coagulopathic bleeding. 9 The limitation of this definition is that currently non-red cell transfusions are often administered empirically based on subjective assessments by clinical staff. As a consequence there is variation in the frequency of non-red cell component transfusion across UK units. 9 This reflects differences in case mix and institutional practices; however, it also suggests that many patients receive unnecessary transfusions. This definition would therefore overestimate the frequency of coagulopathic bleeding.
-
Excessive blood loss. The volume of blood loss in the drains is routinely measured. Excessive bleeding has been defined as > 10 ml/kg/hour. 54 This has limitations as an indicator of coagulopathic bleeding because (1) patients with coagulopathic bleeding who receive effective pro-haemostatic treatment may not have excessive blood loss, (2) excessive blood loss may be masked by blocked chest drains and this may present as tamponade or late pleural and pericardial collections, (3) excessive blood loss may have a surgical as opposed to a coagulopathic cause and (4) drain losses may not represent active bleeding and may consist of pleural or pericardial collections and effusions.
In summary, existing consensus definitions of coagulopathy or coagulopathic haemorrhage in cardiac surgery have important limitations. Clinical studies must anticipate these limitations by, for example, excluding patients with clear ‘surgical’ causes of bleeding; by careful assessment of adherence to blood management protocols for transfusion and the use of therapeutic adjuncts such as cell salvage or antifibrinolytic use; and by using definitions of drain losses that are most likely to reflect active bleeding. Assessment of predictive accuracy should also consider alternative definitions of coagulopathic haemorrhage within analyses to obtain a balanced assessment of utility. These are features of the COPTIC study.
Clinical risk factors for coagulopathic bleeding
Careful clinical assessment can be used to identify patients at risk of coagulopathic haemorrhage and this is recommended as part of standard care. 24 Diagnostic tests for coagulopathy should be interpreted, and pro-haemostatic treatments should be administered, only in the context of known clinical characteristics.
Preoperative factors
Heritable coagulopathy
Mild heritable coagulation factor defects are prevalent in the general population and are associated with increased bleeding during surgery. 55 These may be detected by routine screening SLTs or by clinical assessment and a history of bleeding after surgical procedures. These require further detailed investigation by a haematologist.
Antiplatelet agents
Most patients with symptomatic cardiovascular disease receive aspirin. In addition, patients with acute coronary syndromes or those who have received coronary stents receive dual antiplatelet therapy (DAPT) with the addition of the P2Y12 receptor antagonists clopidogrel, prasugrel (Efient®; Eli Lilly and Co., Indianapolis, IN, USA) or ticagrelor (Brilique®; AstraZeneca, Cambridge, UK), because of the proven benefit of DAPT in these settings. 56–58 Platelet inhibition increases blood loss during surgery,59 but, although desirable, early withdrawal of antiplatelet therapy increases the risk of preoperative acute cardiovascular events and death in those at greatest risk. Current guidelines recommend that aspirin be continued until the time of surgery as RCTs have indicated that, although this increases blood loss marginally, there are no serious adverse events attributable to this blood loss and there are clear benefits in terms of fewer adverse cardiovascular events prior to surgery. 60 Observational analyses demonstrate more severe bleeding and adverse events attributable to platelet inhibition with DAPT relative to aspirin alone61,62 and current guidelines (class I recommendation, evidence level B) recommend that P2Y12 receptor antagonists be stopped 3–5 days prior to surgery. 24 This empirical approach is not without risk; many patients may be left at increased risk of adverse cardiovascular events prior to surgery and this is not detected by observational studies that commonly analyse events from surgery, a form of lead time bias. Conversely, other patients may have significant residual platelet inhibition at operation. This variability in responsiveness is more commonly observed with clopidogrel than with the newer agents such as ticagrelor and prasugrel, for which pharmacokinetics are more predictable.
Preoperative morbidity
Clinical risk prediction scores have been developed that allow objective assessment of bleeding risk. These identify age, female sex, low body mass index (BMI), preoperative anaemia, chronic kidney disease, poor left ventricular function and recent myocardial ischaemia as predictive of excessive bleeding or MBT. 63,64 The mechanism by which these factors contribute to bleeding is poorly understood. Existing scores are not widely used because of the limited generalisability of risk models developed in single centres or subpopulations of patients. This is considered in detail in Chapter 3.
Intra- and postoperative factors
Protease activation/clotting factor consumption/fibrinolysis
Direct activation of the contact system of proteases (kallikrein, kininogen) by the negatively charged surface of the CPB circuit results in the generation of factor XIIa and activation of the intrinsic clotting cascade (Figure 2). In addition, release of tissue factor by surgical trauma and the activation of inflammatory cells will activate the extrinsic coagulation pathway. Both result in activation of the common coagulation pathway and the uncontrolled generation of thrombin during CPB. Thrombin and activated contact proteases also promote fibrinolysis through the activation of tissue plasminogen activator. High levels of thrombin formation and fibrinolysis ultimately result in factor depletion and deranged haemostasis, which is most evident after prolonged CPB duration. 66 This is further impaired by haemodilution with circuit prime, platelet dysfunction and depletion.
FIGURE 2.
Intrinsic, extrinsic and common coagulation protease cascades. TFPI, tissue factor pathway inhibitor. Reproduced from Wikimedia Commons. 65 This material is distributed in accordance with the terms of the Creative Commons Attribution-ShareAlike 3.0 Unported (CC BY-SA 3.0) licence, which permits others to distribute, remix, adapt and build upon this work, provided the original work is properly cited. See: https://creativecommons.org/licenses/by-sa/3.0/.

Platelet dysfunction
The circulation of blood through the CPB circuit directly activates circulating platelets, as do thrombin and contact proteases, which then adhere to the circuit or activated endothelium and leucocytes. This may lead to depletion of platelets, whose number may be further reduced by haemodilution. Non-adherent activated platelets become desensitised to further stimuli and the degree of platelet dysfunction correlates well with CPB duration. 67 Post-cardiac surgery platelet dysfunction is common and is enhanced by the administration of antiplatelet agents such as aspirin or P2Y12 receptor antagonists preoperatively. Administration of more potent antiplatelet agents such as P2Y12 receptor antagonists up to the time of surgery may be associated with severe bleeding. 61
Acidosis, hypothermia and haemodilution
These factors serve to reduce coagulation factor and platelet activity through direct suppression of enzyme activity. They also promote inflammation, endothelial dysfunction and organ injury. 68
Inflammation and organ dysfunction
Protease and platelet activation during CPB lead to oxidative stress, leucocyte and endothelial activation, leucocyte extravasation, endothelial dysfunction, refractory tissue hypoxia and ultimately organ injury. 2 The contribution of these factors to coagulopathy is poorly understood; however, there are strong associations between coagulopathy and post-cardiac surgery organ failure in observational studies. 69
Heparin
Heparin is used in almost every patient undergoing cardiac surgery to provide systemic anticoagulation and prevent fibrin formation and thrombosis within the patient or activated CPB circuit. Heparin is reversed by the administration of protamine once the patient has been weaned from bypass successfully. Heparin is a small highly sulfated glycosaminoglycan and can accumulate in the extravascular compartment during CPB, where protamine may be less effective at reversal. Subsequent re-entry of heparin to the intravascular space may lead to residual heparin activity or ‘heparin rebound’ and contribute to bleeding. 70
Blood management adjuncts
Evidence from RCTs indicates that the administration of antifibrinolytics such as tranexamic acid reduces bleeding and improves survival in cardiac surgery. 17 Concomitant use of blood management adjuncts is an important consideration when determining the effectiveness of blood management interventions being evaluated in clinical trials.
Diagnosis of coagulopathy
Standard laboratory tests
Prothrombin time is a measure of the extrinsic pathway of coagulation. The PT is the time that plasma takes to clot after the addition of tissue factor. PT is often expressed as the international normalised ratio, which is the ratio of a patient’s PT to a normal (control sample) referenced against the international sensitivity index value for the analytical system used and corrects for differences in assay responses to different sources of tissue factor.
The activated partial thromboplastin time (aPTT) measures the ‘intrinsic’ or contact activation pathway and the common coagulation pathway. An activated matrix (e.g. silica, celite, kaolin) and calcium are mixed into the plasma sample and the time that the sample takes to clot is measured.
Fibrinogen (factor I) is a soluble plasma protein that is cleaved by thrombin to generate insoluble fibrin polymers, a principal component of the primary haemostatic response. Fibrinogen levels are commonly measured using the Clauss assay, with the time taken for plasma to clot in the presence of high thrombin concentrations compared with that of reference plasma with a known level of fibrinogen calibrated against a known international standard. Low preoperative fibrinogen levels or depletion of fibrinogen during prolonged CPB have been associated with excessive blood loss and adverse clinical events in observational studies. 71,72
Cross-linked fibrin is cleaved in the presence of plasma into fibrin degradation products, the largest of which is the D-dimer. Levels of the D-dimer are therefore considered as markers of thrombus (fibrin) formation and lysis.
Standard laboratory tests are considered to have limited utility in the setting of acute haemorrhage and coagulopathy. These limitations include the following:
-
There is a slow turnaround time for results of 40 minutes on average, which precludes their use in unstable bleeding patients.
-
These tests (aPTT, PT, fibrinogen) evaluate time to clot formation in platelet-poor plasma at 37 °C in standardised conditions and were developed to screen for clotting factor deficiencies or to monitor anticoagulant therapy. They were not designed to diagnose coagulopathy in the acutely bleeding surgical patient. 73 Moreover, the effects of important factors such as the patient’s temperature, acidosis or platelet function are not reflected in these tests.
-
The tests lack specificity with regard to the specific defect in the coagulation pathway responsible for prolonged PT or aPTT values and do not reflect platelet dysfunction.
-
Platelet counts in whole blood do not account for alterations in platelet function or clinical status (antiplatelet therapy), which limits their utility in cardiac surgery.
As a consequence of these limitations, SLTs have poor predictive and prognostic utility in cardiac surgery. 52,53 Despite this, they are routinely performed pre- and postoperatively in all patients.
Laboratory reference tests
Reference assays have important advantages compared with SLTs. They can measure specific components of the coagulation pathway, as well as provide quantitative measures of pathway activity. They have important disadvantages, however, in that they are expensive and time-consuming to perform and this limits clinical utility. However, they may be used to accurately define specific phenotypes of coagulopathy that other tests may be compared against and have a valuable role as reference tests in studies of coagulopathy.
Factor XIII or fibrin-stabilising factor is a circulating transglutaminase that is cleaved by thrombin to form activated factor XIII. This acts on fibrin to form cross-links between fibrin molecules to form an insoluble clot. Factor XIII becomes depleted during CPB and has been implicated in post-cardiac surgery coagulopathy. 74 Factor XIII activity can be assessed using the Berichrom® XIII assay (Sysmex, Kobe, Japan), which measures ammonia release (transglutaminase activity) by activated factor XIII in soluble fibrin.
Incomplete reversal of heparin leads to anticoagulation and bleeding. There is no accurate SLT for incomplete reversal or ‘heparin rebound’ and it is common for this to be evaluated using the activated clotting/coagulation time (ACT), a measure of global clotting activity in whole blood. However, the ACT may be affected by non-heparin factors and can be normal in the presence of residual heparin activity or coagulopathy. 75 Heparin acts by binding to antithrombin (see Figure 2). This inhibits both thrombin (factor II) and factor Xa. Anti-Xa activity is less sensitive to the effects of non-heparin factors than ACT or aPTT and is considered a more accurate measure of residual heparin activity. 76 Anti-Xa activity may be measured accurately in automated laboratory analysers using a commercial assay (anti-Xa kit; HYPHEN BioMed, Neuville-sur-Oise, France).
The endogenous thrombin potential (ETP) is a test of global coagulation pathway function, similar to the PT and aPTT, performed using a thrombinoscope. Unlike SLTs, the output of this assay is presented as a thrombin generation curve, rather than simply the time to clot formation. This curve reflects all three phases of coagulation: initiation, which corresponds to the PT or aPTT depending on the activator, followed by propagation and termination, which are quantitative measures of thrombin activity. It has been hypothesised that this is a measure of the thrombin burst, a functional, rather than a static, measure of common coagulation pathway activity. The propagation and termination phases of the curve can be quantified as the area under the curve (AUC), which is termed the ETP. In one study the ETP was shown to discriminate between cardiac surgery patients who developed severe blood loss and those who did not. 77
Von Willebrand factor (vWF) is a multimeric adhesive glycoprotein that mediates the adhesion of platelets to injured subendothelium (collagen) and to the platelet surface receptor GPIb. It is required for the formation of the initial platelet plug that achieves primary haemostasis following vascular injury. It also serves as the specific carrier protein for coagulation factor VIII in plasma, preventing proteolytic degradation. vWF, as a large multimeric protein, is subject to shear and fragmentation during CPB. Depletion of multimeric vWF has been implicated in post-CPB bleeding. 78 vWF activity can be measured using the collagen binding assay, which detects the ability of multimeric forms of vWF to bind collagen.
In addition to data from specific LRTs, routinely recorded data obtained from automated cell counters used as part of standard clinical care may be able to provide information that will discriminate between patients who are likely to bleed and those who are not. Automated cell counters that measure the standard platelet count can detect thrombocytopenia, a risk factor for bleeding. In addition, they also measure the immature platelet count. This is a measure of thrombopoiesis that reflects the accelerated reduction of immature platelets in the setting of accelerated platelet consumption, as seen, for example, following prolonged CPB. High levels of immature platelets would implicate low platelet function as a cause of associated coagulopathy. Conversely, an elevated mean platelet volume (MPV) is associated with increased platelet activation and thrombosis. A low platelet volume may reflect reduced platelet activity. As no additional tests are performed beyond existing care, these data are available at no additional cost as they are obtained routinely as part of usual care. The predictive accuracy of these measures for bleeding has not previously been reported.
Point-of-care tests
Near-patient tests allow clinical teams to undertake rapid, reproducible and repeated assays of coagulation with short turnaround times at the POC. These offer more personalised and effective management in bleeding patients, whose clinical status may be unstable. The two most common POC testing platforms in clinical use are the viscoelastic tests ROTEM and TEG.
These devices have limited ability to discriminate between platelet dysfunction and defects in fibrin generation and are often complemented by platforms that can detect specific defects in platelet function. 79 A commonly used platelet function testing platform in the UK is the Multiplate analyzer.
Rotational thromboelastometry
Previously known as rotational TEG, the ROTEM assays are based on the oscillation of a disposable sensor pin suspended within a citrated whole blood sample (340 µl) placed within a disposable cuvette (sample cup). Coagulation is activated by a range of assays and as the clot forms the resistance to the rotation of the pin is increased to provide a measure of clot firmness. This is detected by an optical sensor and translated into the ROTEM output (Figure 3). By using different activators discrete components of clot formation can be assessed within a single integrated platform. The individual assays are as follows:
-
INTEM. Uses an activator of the contact (kallikrein) proteases and measures the intrinsic clotting pathway.
-
EXTEM. Uses tissue factor as an activator and is a measure of the extrinsic pathway. This assay is not affected by heparin.
-
HEPTEM. Uses an activator of the contact proteases along with heparinise (effectively the INTEM assay in the presence of heparinase) and indicates the presence of residual heparin activity.
-
FIBTEM. Uses tissue factor as an activator along with an inhibitor of platelet activation (effectively the EXTEM pathway in the absence of platelet function). The output of this assay denotes fibrin formation and is considered a measure of fibrinogen concentration.
FIGURE 3.
Diagrammatic representation of ROTEM output. Reproduced with permission from ROTEM® (TEM International GmbH, Munich, Germany; www.rotem.de). A5, amplitude 5 minutes after CT; CFT, clot formation time; CT, clotting time; MCF, maximum clot firmness; LI30, lysis index at 30 minutes (percentage decrease in MCF 30 minutes after maximum amplitude is reached).

Each test generates multiple values that reflect dynamic clot formation and lysis. A separate graphical output of results is produced for each assay. A representative output is displayed in Figure 3. Output definitions are listed in Box 1.
Clotting time (CT). Time from activation until initial fibrin formation; represents activation of the intrinsic and common (INTEM) and extrinsic and common (EXTEM) pathways respectively. Prolongation of the CT in INTEM and EXTEM assays denotes coagulation factor deficiencies or residual heparin activity (INTEM only). Prolongation of the INTEM CT relative to the HEPTEM CT specifically denotes residual heparin activity.
Clot formation time (CFT). Time from the start of fibrin formation until a clot firmness of 20 mm has been achieved. These values reflect primarily platelet function and fibrinogen concentration, along with other clotting factors.
Amplitude 10 minutes after CT (A10). A predictive indicator of maximum clot firmness (MCF) at 10 minutes, a global measure of clotting factor and platelet function. This result is available earlier than MCF. Initial results for coagulation factor activity, platelet function and fibrin formation are therefore available within 10 minutes of assay commencement.
Maximum clot firmness. The greatest vertical amplitude of the ROTEM trace. Low MCF values denote deficiencies in platelet function, fibrinogen levels or clot stabilisation (factor XIII). The difference between the MCF for EXTEM and FIBTEM denotes the contribution of platelets to clot formation. The absolute MCF value for FIBTEM reflects fibrinogen levels.
Lysis index at 30 minutes (LY30). The percentage decrease in MCF at 30 minutes post clot activation. This is a measure of clot lysis. The time taken to obtain this result means that is has limited clinical utility in the setting of severe haemorrhage.
Thromboelastography
The TEG system is also based on a viscoelastic test, with some differences between this system and ROTEM. In this system 340 µl of citrated whole blood is placed in a cuvette. A disposable pin is then lowered into the fluid blood and the cuvette is rotated. As the first fibrin strands are formed the pin becomes tethered to the cup and starts to follow its motion. When maximum clot firmness (MCF) is achieved, the cup and pin move in unison. The motion of the pin is detected by a torsion wire linked to a transducer that is interpreted as a graphical trace by computer software. As with ROTEM, different activators may be used to evaluate different components of clot formation:
-
kaolin is used as an activator of the intrinsic pathway – analogous to the INTEM assay
-
heparinase is used along with kaolin – analogous to the HEPTEM assay.
Other activators and assays have been described, including a functional fibrinogen assay and platelet mapping; however, these are not in common use and are associated with additional and significant costs relative to the two principal assays. As with ROTEM, the graphical output provides visual information related to dynamic clot formation and also provides information that reflect specific stages of clot formation (Figure 4). Definitions of TEG outputs are listed in Box 2.
FIGURE 4.
Diagrammatic representation of TEG output. (TEG® Haemostasis Analyzer tracing images presented by permission of Haemonetics Corporation, Niles, IL, USA; www.haemonetics.com. TEG® and Thromboelastograph® are registered trademarks of Haemonetics Corporation in the US, other countries or both.) α, α-angle; EPL, estimated per cent lysis (estimated rate of change in amplitude after the MA is reached); K, K time; LY30, lysis index at 30 minutes (percentage decrease in MCF 30 minutes after maximum amplitude is reached); MA, maximum amplitude; R, R time.

R time. Time from assay activation until fibrin formation. Prolongation of this value denotes deficiency in coagulation factor concentrations from the intrinsic and common pathways or residual heparin activity. Prolongation of the R time from the kaolin assay relative to the R time from the kaolin and heparinase assay specifically denotes residual heparin activity.
K time or α-angle. The K time is the time from fibrin/clot formation until clot amplitude reaches 20 mm. The α-angle is the angle of the slope between the R time and the point on the TEG output that corresponds to time K. These are measures of fibrin clot formation. Prolongation of the K time or the α-angle, or reduction of the maximum amplitude (MA), reflects fibrinogen concentrations, platelet function or clotting factor activity (factor XIII).
Maximum amplitude. A measure of the maximum strength of the clot. Low MA values indicate deficiencies in fibrinogen concentrations, platelet function and clotting factor activity (factor XIII).
Lysis index at 30 minutes (LY30). Percentage decrease in MCF 30 minutes after MA is reached. This is a measure of clot lysis.
Multiplate
Platelets are activated by a wide array of stimuli that include thrombin, tissue factor, adenosine diphosphate (ADP) and arachidonic acid metabolites such as thromboxane. Activation is accompanied by aggregation and adhesion to other platelets via fibrin strands and to exposed extravascular matrix through interaction with vWF. Aggregation can be measured in small volumes of whole blood (1.2 ml) by multiple electrode aggregometry (MEA) using the Multiplate device. The instrument performs the analysis by employing two independent sensor units for every test cell, representing an internal control of the reaction. Each unit consists of two silver-coated, highly conductive copper wires on which the platelets adhere on specific activation. The aggregation function is measured in terms of increasing electrical impedance between the metallic electrodes of the sensor as platelets aggregate on the electrodes in the presence of an activator. The results are displayed graphically (Figure 5) within minutes. Up to four activators may be assessed in a single assay. The cyclo-oxygenase pathway is activated with 0.5 mM of arachidonic acid (ASPI) (ASPItest®), the P2Y12 receptor pathway with 6.5 µM of ADP (ADPtest®) and the thrombin receptor with a thrombin receptor-activating peptide (TRAP) (TRAPtest®). By comparing these results with those for maximal platelet activation using high adrenaline (epinephrine) concentrations (20 mg/ml) (ADRENtest®), the degree of inhibition of specific activation pathways can be assessed. Outcomes are expressed as the AUC [in aggregation units (AUs)*min] or the maximum amplitude (in AUs). The test results can be assessed more rapidly using the test velocity, expressed as dAU/dt, within minutes of activation (see Figure 5). A limitation of the Multiplate is that platelet counts must ideally be > 100 × 109/l to obtain reproducible results.
FIGURE 5.
Representative MEA graphs for (a) ADPtest; (b) ASPItest; (c) TRAPtest; and (d) ADRENtest. Outputs may be expressed as the AUC (AU*min), maximum amplitude (AU) or test velocity (dAU/dt). This result in a post CPB patient demonstrates diminished platelet responsiveness in response to ADP, TRAP and adrenaline (epinephrine), but not arachidonic acid.
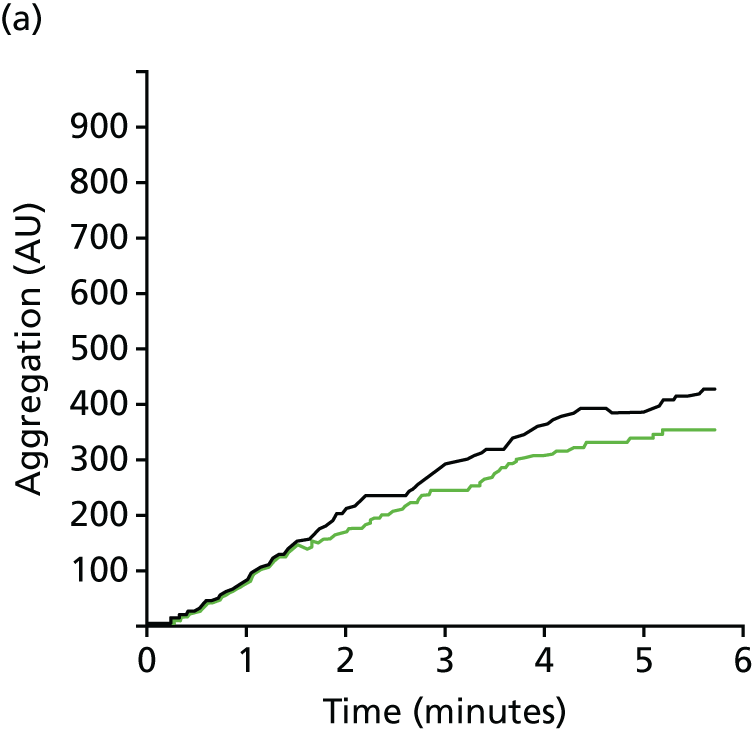

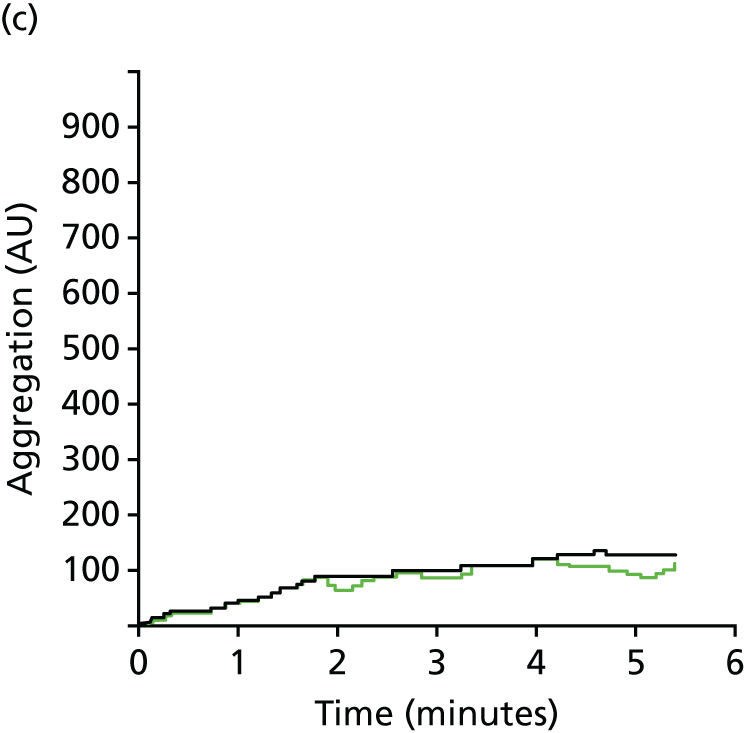
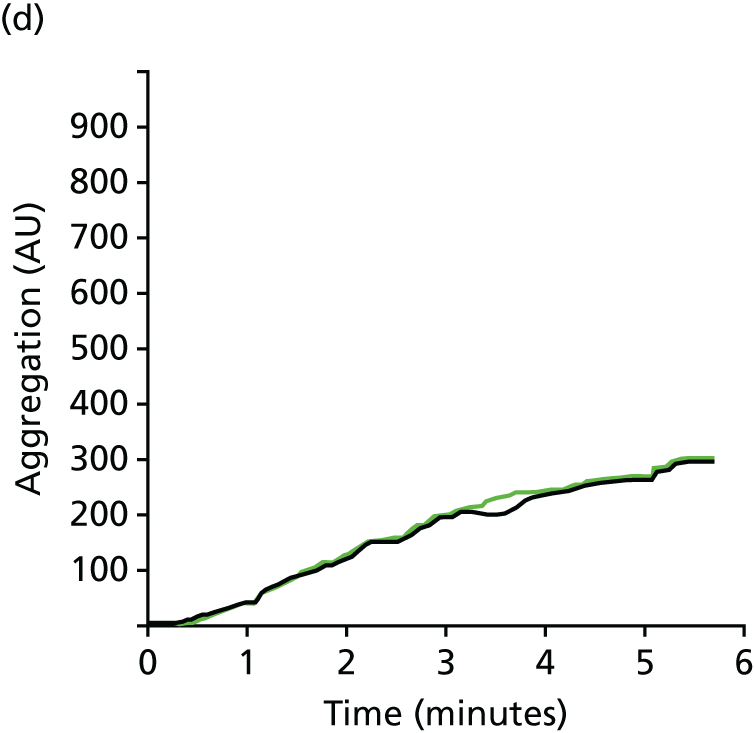
Treatment
Without accurate diagnostic tests to identify specific defects in the coagulation pathway, which if treated or reversed can improve clinical outcomes, the management of coagulopathy is often empirical, non-specific and based on the assumption that reversal of coagulopathy is beneficial.
Fresh-frozen plasma
One unit of FFP is derived from a single unit of donated red cells and contains variable concentrations of the coagulation factors I (fibrinogen), II, V, VII, VIII, IX, X and XI. It also contains anticoagulants such as protein C, protein S and antithrombin along with a large number of proteins such as immunoglobulins, albumin and acute-phase proteins. A standard dose of FFP is 2 units and there is uncertainty whether this significantly increases coagulation factor concentrations in recipients. Uncertainty over the risks and benefits of FFP80,81 is reflected by the variability in its use, with use ranging from 9% to 59% of patients between centres (mean 25%). 9 Cardiac surgery uses up to 12% of all FFP produced by NHS Blood and Transplant. 82
Platelets
In the UK a standard unit of platelets contains the pooled platelet component of up to six donors. There is very little evidence to guide platelet transfusion in the setting of coagulopathic haemorrhage. A recent RCT reported that empirical transfusion of higher platelet ratios, in combination with higher plasma ratios, improves haemostasis and leads to fewer deaths from bleeding at 24 hours in trauma patients. 83 Evidence on the risks and benefits of platelet transfusion in cardiac surgery comes from observational analyses and shows conflicting results. 84,85 The rate of platelet transfusion in cardiac surgery is variable, ranging from 10% to 56% (mean 28%) of patients between UK cardiac surgery units. Cardiac surgery is one of the largest single consumers of platelets (17%) produced by NHS Blood and Transplant. 82
Fibrinogen
UK guidelines recommend that fibrinogen is replaced during major blood loss or as part of the management of disseminated intravascular coagulation once the Clauss fibrinogen value falls below 1.5 g/l, although this recommendation is based on weak evidence. 86 Cryoprecipitate is the first-line treatment in the UK for acquired hypofibrinogenaemia and a standard adult dose (two pools) raises the plasma fibrinogen level by 1 g/l. A Cochrane review of predominantly cardiac surgical RCTs found that fibrinogen reduced transfusion requirements (very low-quality evidence), but there was insufficient evidence to evaluate differences in important clinical end points, including mortality. 87
Recombinant activated factor VII
Recombinant activated factor VII is a potent pharmacological pro-haemostatic agent licensed for use in patients with haemophilia. This has led to the off-label use of rFVIIa for the treatment of severe coagulopathic bleeding in trauma and surgical settings as an adjunct to conventional non-red cell blood components.
Prothrombin complex concentrates
Prothrombin complex concentrates are plasma-derived coagulation factor concentrates that contain three or four vitamin K-dependent factors at high concentration, typically factors II, VII, IX and X, as well as variable amounts of anticoagulants and heparin. These are increasingly used off-licence in cardiac surgery in the setting of coagulopathic haemorrhage; however, this is not supported by evidence.
Current compared with proposed new standards of care for the diagnosis and treatment of coagulopathy
As both preoperative and operative variables may contribute to coagulopathy and bleeding in cardiac surgery, we considered how improved testing of coagulopathy both before and after surgery would benefit patients when used in conjunction with careful clinical assessment.
Preoperative testing
Current standard of care
Patients undergoing cardiac surgery undergo preoperative screening with SLTs including tests of PT and aPTT. In some centres preoperative fibrinogen is also measured routinely. Platelet counts are measured on standard haematology analysers. Abnormal screening tests or a clinical history of excessive blood loss following previous dental extractions or minor surgical procedures may lead to further haematological investigations on an individual basis. In addition to standard care, current blood management guidelines recommend (class 1, level of evidence C24) that patients undergo a formal assessment and documentation of bleeding risk. Risk scores that may accurately predict the risk of bleeding and red cell transfusion exist;63,64,88,89 however, these are not widely used. The predictive accuracy of any new diagnostic test should therefore complement appropriate clinical assessment and the benefits of additional testing should be assessed beyond those attributable to clinical assessment combined with standard testing.
Proposed new standard of care
Our proposed new standard of care includes routine preoperative platelet function testing in addition to current standard care. We suggest that this may have clinical benefits by allowing targeted interventions to be given to patients with pre-existing platelet dysfunction that will reduce the risk of severe bleeding and its complications, particularly in patients receiving antiplatelet therapy preoperatively. Currently, it is recommended that aspirin be continued until surgery but that P2Y12 receptor antagonists be discontinued 3–5 days prior to surgery. 24 This reflects the reversal characteristics of current P2Y12 receptor antagonists and clinical outcomes in observational studies in which patients on recent DAPT have undergone cardiac surgery. There is marked variation in the implementation of these guidelines. Specifically, increases in the numbers of non-elective surgeries for patients with unstable cardiovascular disease or recent coronary stenting in the setting of myocardial infarction (MI) mean that patients frequently present to surgery within 3–5 days of taking DAPT or require DAPT because of the risk of acute stent thrombosis. 90 In these patients preoperative platelet function testing may allow more informed decision-making as to the risks of surgery and bleeding and the need for pre-emptive platelet transfusion or deferment of surgery.
What evidence supports this change in the current standard of care for preoperative testing?
Corredor et al. 25 recently reviewed clinical studies that have evaluated the predictive accuracy of POC platelet function tests to detect platelet dysfunction and predict postoperative bleeding in cardiac surgery (Table 3). They identified 16 studies in which platelet function tests had been used in isolation to predict postoperative haemorrhage. The studies included in the systematic review were at high risk of bias and only five of 15 studies reported test discrimination for bleeding or transfusion. Overall, the Multiplate was evaluated in the largest number of patients and showed modest discrimination. Test results were more likely to accurately predict bleeding in patients receiving P2Y12 receptor antagonists prior to surgery. The authors concluded that there was inconsistent evidence that routine preoperative platelet function testing had predictive accuracy for bleeding, particularly in patients receiving DAPT. This uncertainty is reflected in current treatment guidelines. The Society of Thoracic Surgeons clinical practice guidelines (updated in 2012) state that ‘For patients on dual antiplatelet therapy, it is reasonable to make decisions about surgical delay based on tests of platelet inhibition rather than arbitrary use of a specified period of surgical delay (Class Iia, Level of Evidence B)’. 91 Conversely, a recent position statement has recommended routine Multiplate use in patients receiving P2Y12 receptor antagonists. 92 To address this uncertainty we assessed the predictive accuracy of routine preoperative platelet function tests in the COPTIC A study.
Study | Patient population | Number of patients | POC assay | Timing of POC test | SIGNa | Summary of findings |
---|---|---|---|---|---|---|
Platelet function testing | ||||||
Lasne et al. (20) | Coronary artery bypass grafting | 146 | PFA-100 | Preoperatively and 5 minutes and 5 hours post protamine | + | No correlation between blood loss and pre- or postoperative PFA-100 values |
Slaughter et al. (21) | Coronary artery bypass grafting | 76 | PFA-100 | Preoperatively, intraoperatively and postoperatively | + | Low positive predictive value (18%) for post-bypass collagen/ADP closure times. Negative predictive value 96% |
Forestier et al. (22) | Cardiac surgery with bypass | 45 | PFA-100/haemostatus | Postoperatively | + | No correlation between POC testing and chest drain output |
Fattorutto et al. (24) | Cardiac surgery with bypass | 70 | PFA-100 | Pre and post bypass | + | Weak correlation between pre-bypass collagen/adrenaline (epinephrine) closure time and 2-hour mediastinal blood loss (r = 0.34; p = 0.01) |
Chen et al. (27) | Coronary artery bypass grafting | 90 | PFA-100 | Preoperatively | + | ADP aggregometry was a better predictor of blood loss and platelet and/or red cell transfusion than PFA-100 |
Gerrah et al. (28) | Off-pump coronary artery bypass grafting | 18 | Cone and platelet analyser | Preoperatively and perioperatively | + | Preoperative average size and surface coverage values correlated with postoperative bleeding (r = 0.7; p = 0.01) |
Dalen et al. (41) | Coronary artery bypass grafting, receiving DAPT | 50 | Plateletworks | Preoperatively | + | Correlation between ADP-induced aggregation and postoperative blood loss (r = 0.83; p < 0.01) |
Orlov et al. (45) | Cardiac surgery with bypass | 100 | Plateletworks | Pre bypass and postoperatively | ++ | Functional platelet count increase associated with high blood loss. Rewarming RR 0.89 (95% CI 0.82 to 0.97; p = 0.006). Post-protamine RR 0.87 (95% CI 0.78 to 0.98; p = 0.02) |
Lennon et al. (26) | Cardiac surgery with bypass | 50 | Plateletworks | Preoperatively | + | Poor correlation with postoperative blood loss (r = 0.14; p = 0.34) |
Ranucci et al. (40) | Cardiac surgery, exposed to thienopyridines | 87 | Multiplate | Preoperatively | + | Multiplate ADP (cut-off value 31 U) predicts postoperative bleeding risk. Sensitivity 72%, specificity 66% |
Rahe-Meyer et al. (34) | Cardiac surgery with bypass | 60 | Multiplate | Preoperatively and postoperatively | + | Pre- and postoperative ADP test predicts risk of platelet transfusion (AUC 0.74; p = 0.001). No relationship between decreased platelet aggregation and postoperative blood loss |
Velik-Salchner et al. (35) | Coronary artery bypass grafting | 70 | Multiplate, LTA | Preoperatively and 15 minutes and 3 hours post protamine | ++ | Multiplate and LTA detect bypass-induced platelet dysfunction but do not predict blood loss |
Reece et al. (39) | Coronary artery bypass grafting | 44 | Multiplate | Pre and post bypass | ++ | Patients requiring blood transfusion had significantly reduced platelet aggregation compared with non-transfused patients. ADP: 18 U vs. 29 U (p = 0.01); thrombin receptor agonist peptide-6: 65 U vs. 88 U (p = 0.01) |
Schimmer et al. (43) | Mixed cardiac surgery with bypass | 223 | Multiplate | Preoperatively and postoperatively | + | Abnormal ADP test and thrombin receptor agonist peptide test significantly predict postoperative blood transfusion requirements |
Petricevic et al. (44) | Coronary artery bypass grafting | 211 | Multiplate | Preoperatively, post bypass and post protamine | + | Multiple values correlated with 24-hour chest tube output. Arachidonic acid < 20 AU and ADP < 73 AU were ‘bleeder’ determinants |
Ranucci et al. (46) | Cardiac surgery, exposed to P2Y12 receptor inhibitors | 361 | Multiplate | Preoperatively | ++ | ADP test and thrombin receptor agonist peptide-6 test significantly associated with postoperative bleeding (p = 0.001) |
Combined platelet function testing and viscoelastic tests | ||||||
Wahba et al. (18) | Cardiac surgery with bypass | 40 | Hepcon Haemostasis Management System, PFA-100 | Preoperatively | + | Significant correlation between preoperative PFA-100 and blood loss (r = 0.41; p = 0.022). No correlation with Hepcon Haemostasis Management System |
Dietrich et al. (19) | Cardiac surgery with bypass | 16 | TEG, impedence aggregometry, PFA-100 | Preoperatively, intraoperatively and postoperatively | + | None of the methods predicted postoperative blood loss |
Cammerer et al. (25) | Cardiac surgery with bypass | 255 | TEG, PFA-100 | Pre, during and post bypass | + | High negative predictive value for bleeding post bypass. ROTEG α-angle 82%, PFA-100-ADP 76% |
Ostrowsky et al. (29) | Cardiac surgery with bypass | 35 | Plateletworks/TEG | Preoperatively, post protamine and 24 hours postoperatively | + | Plateletworks collagen tubes correlated with postoperative bleeding (r = 0.324; p = 0.048). No correlation with TEG |
Kotake et al. (30) | Cardiac surgery with bypass | 26 | Whole-blood aggregometry, Sonoclot | Pre and post bypass | + | No correlation between postoperative blood loss and reduced platelet aggregation |
Hertfelder et al. (31) | Coronary artery bypass grafting | 49 | PFA-100, impedence aggregometry, TEG | Pre and post bypass | + | PFA-100 and impedence aggregometry do not predict postoperative blood loss |
Alstrom et al. (36) | Coronary artery bypass grafting, receiving DAPT | 60 | VerifyNow/TEG 5000, platelet mapping | Preoperatively and postoperatively | + | Weak correlation between preoperative platelet inhibition measured by VerifyNow and postoperative blood loss (r = 0.29; p = 0.03). No significant correlation observed with TEG 5000 |
Kwak et al. (37) | Off-pump coronary artery bypass grafting, receiving clopidogrel | 100 | TEG, platelet mapping | Preoperatively | + | Association between 70% platelet inhibition and postoperative transfusion requirements (AUC = 0.77, 95% CI 0.67 to 0.87; p < 0.001) |
Preisman et al. (38) | Coronary artery bypass grafting, receiving DAPT | 59 | TEG, platelet mapping | Preoperatively | ++ | Only maximum amplitude ADP predicts excessive postoperative blood loss. Sensitivity 78%, specificity 84% |
Viscoelastic tests | ||||||
Dorman et al. (17) | Coronary artery bypass grafting | 60 | TEG | Preoperatively | + | TEG failed to predict blood loss (r < 0.25; p = 0.78) |
Lee et al. (42) | Cardiac surgery with bypass | 321 | ROTEM | Preoperatively and postoperatively | + | ROTEM did not improve performance of statistical model predicting blood loss |
Ti et al. (23) | Coronary artery bypass grafting | 40 | TEG | 10 and 60 minutes post protamine | + | Limited predictive ability. Positive predictive value 58% at 10 minutes and 55% at 60 minutes |
Reinhofer et al. (32) | Cardiac surgery with bypass | 150 | ROTEM | Pre and post bypass | + | Positive predictive value and specificity for > 600-ml blood loss: EXTEM assay clot formation time 71%/94%; FIBTEM assay MCF 73%/95% |
Davidson et al. (33) | Coronary artery bypass surgery | 58 | ROTEM | Preoperatively and postoperatively 1–3 hours | + | Poor positive predictive value of 14.8% for predicting postoperative bleeding |
The COPTIC A study
The objective of this study was to examine the relationship between preoperative abnormalities in blood coagulation and coagulopathic bleeding after cardiac surgery. Although numerous abnormalities in both clotting factors and platelets have been reported in patients before cardiac surgery, the most prevalent and clinically important abnormalities are likely to be in platelets. This is because antiplatelet drugs such as aspirin and the P2Y12 blockers clopidogrel, ticagrelor or prasugrel are prescribed widely to patients before cardiac surgery and markedly reduce platelet function.
Approximately 50% of cardiac surgery patients receive aspirin alone up to the day of surgery and approximately 25% receive DAPT. For the dual-therapy subgroup, current practice guidelines suggest withdrawal of the P2Y12 blocker approximately 3–5 days before cardiac surgery but continuation of aspirin up to the day of surgery. 24,60 In practice, the duration of withdrawal varies between 0 and 7 days before surgery because of clinical operational constraints. We hypothesised that exposure to aspirin alone or exposure to aspirin plus a P2Y12 blocker would be associated with more clinical concern about bleeding (CCB) than no exposure to antiplatelet drugs. We also hypothesised that, in the DAPT subgroup, a short time of withdrawal of the P2Y12 blocker would be associated with more coagulopathic bleeding than a long time of withdrawal.
It is already known that antiplatelet drugs cause abnormal results in some laboratory tests of platelet function. Some other preoperative factors may also influence platelet function tests. It is unknown whether any laboratory test or combination of tests of platelet function can be used to predict CCB. The overall aim of the COPTIC A study was to estimate the association between the results from tests of platelet function and platelet count performed before cardiac surgery and CCB in all patients. As antiplatelet drugs are known to influence Multiplate results, we also estimated the association between test results and coagulopathic bleeding in patient subgroups (no antiplatelet drugs vs. aspirin alone vs. aspirin plus P2Y12 blockers). We also estimated the association between test results and duration of withdrawal of P2Y12 blockers and the relationship between duration of withdrawal of P2Y12 blockers and coagulopathic bleeding. Other preoperative, intraoperative and postoperative factors were considered as potential confounders of the association between platelet test results and coagulopathic bleeding. Finally, estimates of association were used to generate a parsimonious predictive model of the probability of coagulopathic bleeding based on preoperative platelet test results and baseline clinical factors.
Postoperative laboratory testing
Current standard of care
A recent audit of UK cardiac centres9 demonstrated wide variation in the diagnosis and management of postoperative bleeding across UK centres. The majority of centres use routine SLTs in combination with ad hoc POC tests in patients who are bleeding or in those considered as being at high risk. In the 2011 UK audit of blood transfusion in adult cardiac surgery,9 of the 17 UK centres that replied to a questionnaire on the use of blood management adjuncts, two reported that they did not use POC testing, four reported that POC testing was used in 1–25% of patients and six reported the use of POC tests in 75–100% of patients.
Proposed new standard of care
The routine use of pre- and postoperative POC tests in all patients, including TEG, ROTEM and Multiplate, would have important clinical benefits beyond those attributable to the current standard of care by enabling early and accurate diagnosis of coagulopathy and the early targeted treatment of patients who are likely to develop coagulopathic haemorrhage.
What evidence supports a change in the current standard of care for postoperative testing?
Corredor et al. 25 summarised the results of existing clinical studies that have considered the predictive accuracy of TEG/ROTEM and platelet function tests in cardiac surgery in a systematic review (see Table 3). All of these studies were of poor methodological quality. Most were small single-centre studies that recruited non-consecutive cohorts and included only small numbers of patients with coagulopathic bleeding, limiting study power and precision. In addition, clinicians in most studies were aware of the test results and this is likely to have influenced the study outcomes. The definition of bleeding was not standardised between studies and in some cases outcome definitions identified only those with the most severe coagulopathic bleeding, inflating estimates of diagnostic accuracy and reducing the likelihood that the findings would translate to unselected populations. The main limitation was that most studies reported associations between test results and bleeding and not measures of diagnostic accuracy, limiting interpretation of the likely clinical utility of the tests. The authors of the review concluded that platelet function tests in combination with TEG/ROTEM had greater discriminatory power than the assessment of platelet function by TEG/ROTEM alone. They also concluded that there was uncertainty as to the most appropriate tests for the management of postoperative bleeding in cardiac surgery patients.
The results of RCTs in cardiac surgery patients that have evaluated the clinical effectiveness of POC test-based treatment algorithms for coagulopathic bleeding have been summarised in a systematic review undertaken by Whiting et al. 26 This formed the basis for recent NICE guidelines that recommended the use of TEG/ROTEM devices for the management of coagulopathic haemorrhage in cardiac surgery. 23 The authors identified 11 RCTs that had evaluated the use of POC testing algorithms in 1089 patients. The included trials evaluated either ROTEM or TEG, with (n = 2) or without (n = 9) additional platelet function testing. Two trials evaluated ad hoc testing in bleeding patients and nine evaluated routine testing. Eight trials undertook serial pre- and postoperative tests. Quantitative meta-analysis that included all trials concluded that the intervention, POC testing algorithms, reduced the rate of red cell transfusion (RR 0.88, 95% CI 0.80 to 0.96). There was no difference between intervention and control groups for important clinical outcomes including death (RR 0.87, 95% CI 0.35 to 2.18) and reoperation for bleeding (RR 0.72, 95% CI 0.41 to 1.26) or for intensive care or hospital length of stay (LOS).
Whiting et al. 26 also undertook a cost–utility analysis of the use of POC tests in cardiac surgery. No formal cost-effectiveness analysis of the use of POC tests has been performed to date and therefore clinical events, costs and utilities [quality-adjusted life-years (QALYs)] were estimated from published data. This analysis concluded that TEG/ROTEM dominated SLTs, being cost saving and more effective. This analysis was limited, however, in that it was based largely on a previous study conducted for the Scottish NHS in 2006 and published in 2008. 93 The authors used several assumptions from the 2006 analysis that recent clinical studies have shown to be incorrect. The principal limitation of this analysis is that the economic model was based on the difference in transfusion rates between groups allocated to POC testing algorithms and groups allocated to standard care identified in the systematic review. 26 The economic model in this analysis presupposed that red cell transfusion causes harm, an assumption based on a previous observational analysis showing significant associations between red cell transfusion and adverse clinical outcomes. 94 However, the association between transfusion and harm is most likely the result of confounding. 32 Other limitations include the omission from the economic model of the assessment of clinical outcomes measured in the trials and that the model used estimates of utilities (QALYs) from 199695 and not measured values. All of these limitations will have biased the analyses towards the use of POC testing.
In summary, analyses of trial evidence have concluded that the addition of routine POC tests to standard care will reduce transfusion rates and be cost-effective. The evidence from predictive accuracy studies suggests that these tests may discriminate between patients who will develop severe bleeding and those who will not. There is considerable uncertainty around these findings, however, because of variable quality of the evidence. This uncertainty is reflected in the wide variation in the use of POC tests in UK cardiac surgery centres. To address this uncertainty and to provide high-quality evidence to inform treatment decisions we conducted the COPTIC B study.
Rationale for the COPTIC B study
We hypothesised that the routine use of POC tests in all patients, including TEG, ROTEM and the Multiplate test, would have important clinical benefits beyond those attributable to the current standard of care plus appropriate preoperative risk assessment. We also hypothesised that clinical benefits would be achieved by enabling early and accurate diagnosis of coagulopathy and the early targeted treatment of patients who are likely to develop coagulopathic haemorrhage.
The COPTIC B study considered whether routine pre- and postoperative POC tests would have predictive accuracy for the development of coagulopathic haemorrhage and whether the routine use of these tests would be cost-effective if introduced alongside standard care.
The COPTIC B study evaluated both TEG and ROTEM. We hypothesised that ROTEM would have better predictive accuracy than TEG because it has greater specificity for some common coagulopathies in cardiac surgery, such as fibrinogen deficiency. TEG and ROTEM have not previously been compared directly in cardiac surgery. We also evaluated the additional benefits of using the Multiplate test in isolation or in combination with ROTEM and TEG. We hypothesised that this would further improve discrimination as suggested by the systematic review of predictive accuracy studies. 25 We hypothesised that any additional benefit would be attributable to the added value of a test that can detect the effects of antiplatelet drugs and discriminate between platelet dysfunction and fibrin deficiency. 79
Finally, if coagulopathic haemorrhage has a causal relationship with adverse clinical outcomes, it is sensible to suggest that POC tests that improve clinical prediction for bleeding may also have the potential to predict adverse clinical outcomes. This has not previously been investigated. We tested this hypothesis in an exploratory analysis within the COPTIC B study.
Reference test analysis
In addition to our evaluation of POC tests we also considered whether the predictive accuracy of POC testing would be improved by the use of additional LRTs to identify coagulopathies that are not detected or which are misclassified by the TEG and ROTEM assays. Assay systems that measure fibrinogen, D-dimer, vWF and factor XIII concentrations as well as assays of anti-Xa activity and thrombin generation are now available commercially. Additional data on platelet size and maturity are routinely measured using automated cell counters as part of standard care and may also have clinical utility. Introduction of these tests, or the development of rapid testing for these assays, may permit detailed investigation of specific coagulopathies in cardiac surgery and improve the predictive accuracy of current tests. However, there is no high-quality evidence available on which additional assays should be performed and whether the potential increase in patient benefit from performing additional assays justifies testing. To address this issue we therefore assessed the potential benefits of introducing a range of LRTs of coagulopathy alongside the current standard of care.
If our analyses identified significant improvements in predictive accuracy with the use of either POC or LRTs it was our intention to assess the relationship between diagnostic error and clinical outcomes, including bleeding, and use these data to generate alternative management algorithms that consider the value of additional reference tests. The potential clinical and economic benefits of these refined algorithms would then be estimated by modelling using the clinical and resource use data collected in the study combined with NHS costs obtained from reference sources.
Systematic review
We identified important limitations in the evidence used by NICE when recommending that viscoelastic POC devices enter routine use in adult cardiac surgery. 23,26 To address the limitations of the evidence used to support the current NICE guidance, and to place the results of the COPTIC study in context, we undertook a systematic review of RCTs that have assessed the clinical efficacy of viscoelastic tests in cardiac surgery. We assessed important clinical outcomes included kidney injury, stroke and prolonged respiratory support and we summarised the evidence using the GRADE (Grades of Recommendation, Assessment, Development and Evaluation) approach (GRADEpro version 3.6 for Windows; Jan Brozek, Andrew Oxman and Holger Schünemann, McMaster University, 2008).
COPTIC study aim and objectives
Study aim
The aim of the COPTIC study was to estimate patient benefit from the measurement of coagulation factors and platelet function using POC testing platforms in common use or a range of LRTs in patients undergoing cardiac surgery.
Study objectives
The specific study objectives common to each analysis were as follows:
-
determine the clinical characteristics of participants who develop the reference outcome CCB, a composite outcome of objective measures of clinically important bleeding, as defined in Definition of study end points, compared with the clinical characteristics of those who do not develop CCB
-
characterise the association between prespecified clinical characteristics and CCB, with specific consideration of the effects of DAPT administration in close proximity to surgery
-
characterise the distributions of a range of index tests from POC devices in common clinical use or a range of LRTs in participants with and without CCB
-
develop a clinical risk prediction model for CCB using baseline clinical data and quantify its predictive accuracy for CCB
-
evaluate the benefits of introducing the results from separate platforms to the clinical prediction model, either as single tests or as combinations of different testing platforms
-
develop a clinical risk prediction score for a range of secondary clinical outcomes using baseline clinical data and quantify its predictive accuracy
-
compare these results with the predictive accuracy of the clinical risk prediction score plus the results of index tests, either as single tests or as combinations of different testing platforms.
Methods
Overview
We carried out a prospective, single-centre observational cohort study to test the predictive accuracy of a range of diagnostic tests for coagulopathic bleeding in adult cardiac surgery. A schematic of the timings of the (index) test samples and the determination of reference outcomes is shown in Figure 6. We collected venous blood samples pre- and postoperatively (times T0 and T3 respectively) and conducted a range of POC and laboratory tests on these samples. The primary (reference) outcome of the study was an estimate of the frequency of coagulopathic haemorrhage, termed CCB, which is defined in Definition of study end points. Our analyses considered the importance of key preoperative predictors of bleeding that could be used to estimate the risk of CCB without the need for diagnostic tests. We then estimated the additional value of the index test results for the prediction of CCB. We considered all participants as well as the subgroup of participants receiving P2Y12 receptor blockers preoperatively, those receiving aspirin and those receiving no antiplatelet therapy. The study included three distinct analyses:
-
COPTIC A. This analysis considered the benefits of the measurement of preoperative platelet function using Multiplate aggregometry.
-
COPTIC B. This analysis considered the benefits of postoperative POC tests including TEG and ROTEM with or without pre- and postoperative measurement of platelet function using the Multiplate test.
-
LRTs. This analysis considered the benefits of:
-
preoperative measurements of red cell mass and platelet morphology derived from automated cell counters (LRT analysis 1)
-
preoperative and postoperative data on red cell mass and platelet morphology derived from automated cell counters and LRTs of coagulation (LRT analysis 2).
-
FIGURE 6.
Timeline indicating research procedures undertaken in the COPTIC study. CICU, cardiac intensive care unit.

The LRT analyses were initially intended to complement the COPTIC B analysis; however, this did not occur because of the poor predictive accuracy of the LRTs.
The study was conducted in accordance with the International Conference for Harmonisation of Good Clinical Practice guidelines, the Research Governance Framework for Health and Social Care, the European Union Directive 2001/20/EC on clinical trials, the Data Protection Act 199896 and the Human Tissue Act 2004. 97 The research was conducted as a partnership between the Bristol Heart Institute and the University of Bristol, UK. The study was sponsored by the University Hospitals Bristol NHS Foundation Trust and authorised by a UK NHS research ethics committee (REC) (reference 09/H0104/53) and was registered prospectively (ISRCTN20778544). The study protocol is available along with this report online as Report Supplementary Material 1. Changes to the study design after commencement of the study are listed in Appendix 1. The study is reported in accordance with the STARD (Standards for Reporting Diagnostic Accuracy) statement98 (see Appendix 1).
Study population
Study setting
The study was conducted in the Bristol Heart Institute, University Hospitals Bristol NHS Foundation Trust, a tertiary cardiac surgery centre in the UK.
Study population
Consecutive adult patients undergoing cardiac surgery that necessitated opening the pericardium were assessed for eligibility according to the following criteria:
-
inclusion criteria:
-
aged > 18 years
-
undergoing cardiac surgery at Bristol Heart Institute
-
-
exclusion criteria:
-
prisoners
-
patients unable to give prospective or retrospective consent through mental incapacity.
-
Characteristics of non-study patients
To evaluate the representativeness of the study participants, demographic and clinical data were compared between the following groups:
-
ineligible patients
-
eligible patients who were not approached
-
eligible patients who were approached but who did not consent
-
consented patients who did not provide a postoperative blood sample
-
consented patients who did provide a postoperative blood sample for which at least one laboratory test result was available.
The reasons why eligible patients were not approached, why eligible patients refused consent and why samples were not taken from consenting patients were documented. Formal statistical comparisons between the final analysis group and those eligible and not included in the analyses were undertaken using t-tests, Mann–Whitney tests and chi-squared tests as appropriate. Comparisons between all other groups were descriptive.
Withdrawals
Participants (or clinicians on their behalf) could withdraw from the study at any time post consent (including prior to surgery). The data available for analysis depended on the timing of withdrawal (see Figure 6). Data collected up to the point of withdrawal were retained for analysis.
Protocol deviations
The protocol stated that all research samples should be collected after the return of cell saver and/or pump blood and after all ‘routine’ protamine doses had been administered. All samples that were recorded as not being collected at this specific time were reviewed and excluded if necessary. The overlap with the return of pump blood was of particular concern as this is known to alter coagulation.
Analysis populations
The analysis population was defined as all eligible, consenting participants who provided a pre- and/or postoperative blood sample for testing. The final data sets were restricted to complete cases, those with laboratory assay results from the research blood samples and case report form and clinical data extracted from the hospital information systems – the cardiac surgery Patient Analysis and Tracking System® (Dendrite Clinical Systems, Henley-on-Thames, UK) and the intensive care Innovian® data management system (Drager Medical Inc., Telford, PA, USA) – to define the primary outcome. Consenting participants who had a research blood sample collected but who did not undergo surgery for medical or other reasons were excluded from the analysis population. The risk of bias was examined by comparing the characteristics of participants retained and excluded from the proposed analysis population. Effect sizes in the form of standardised mean differences (SMDs) were calculated. These can be interpreted as follows: SMDs of 0.20 are ‘small’ in magnitude, those around 0.50 are ‘medium’ and those ≥ 0.80 are ‘large’. 99 Involvement in any other Bristol Clinical Trials and Evaluation Unit studies was also reviewed and any interventions deemed to have an impact were reported.
Definition of study end points
Primary outcome (reference outcome)
As discussed in Coagulopathic bleeding there is no consensus definition of coagulopathic haemorrhage that could serve as the reference outcome. Anticipating the limitations of existing outcomes we used a novel composite definition of postoperative CCB, that is, bleeding that was considered likely to cause direct patient harm or indirect patient harm by precipitating a non-routine pro-haemostatic treatment or reoperation for bleeding for which no direct surgical cause was identified. A participant was classified as having experienced the primary outcome of postoperative CCB if any of the three circumstances below was documented within the designated time interval:
-
Postoperative blood loss (volume collected through the chest drain) of > 600 ml at 6 hours post protamine reversal of heparin (between T3 and T5; interval 2a in Figure 6). It was considered that drain losses after this time may not represent active bleeding.
-
Intervention with a non-routine early pro-haemostatic treatment, defined as additional protamine after heparin reversal (this did not include additional protamine administered routinely after re-transfusion of pump blood), FFP, cryoprecipitate, platelets, antifibrinolytic drugs, rFVII, prothrombin complex concentrate or fibrinogen concentrate during interval 2b (T3–T6; see Figure 6).
-
Reoperation for bleeding recorded during the hospital stay and failure to demonstrate a surgical cause for bleeding, as recorded in the operation record.
For the COPTIC A study a modification of this definition was used. As a preoperative test could potentially influence pro-haemostatic treatments administered at any time during the course of the operation, it would be more correct to consider any such treatment administered after the start of the procedure (intervals 1 and 2, between T0 and T6; see Figure 6) in addition to treatment as in the postoperative CCB definition. For the purposes of clarity this is referred to in the analyses as any CCB.
Secondary clinical outcomes
Secondary clinical outcomes and the data sources used to identify these are listed in Table 4. Red cell transfusion of > 4 units has been used as a definition of coagulopathic bleeding in previous studies;10 however, because red cell transfusion is also likely to reflect the treatment of anaemia in addition to the treatment of bleeding, it was recorded as a secondary outcome in the COPTIC study.
Secondary outcome | Definition | Data source |
---|---|---|
Red cell transfusion | Any red cell transfusion between the time of the postoperative blood sample and discharge from the CICU (T3–T7; see Figure 6) | Hospital transfusion laboratory, Innovian data management system |
Red cell transfusion of > 4 units | Any red cell transfusion of > 4 units during interval 2 (T3–T7; see Figure 6) | Innovian data management system |
Death | Death in the operating theatre or during hospital admission | Patient Analysis and Tracking System database |
MI | Any ST elevation or non-ST elevation MI occurring before discharge or death from MI in hospital | Innovian data management system and Patient Analysis and Tracking System database |
Stroke | Any postoperative stroke, transient ischaemic attack, hemiplegia, paralysis, paraplegia, dysphasia, cerebral infarct or embolus | Innovian data management system and Patient Analysis and Tracking System database |
Acute kidney injury | Serum creatinine increased by ≥ 26.5 µmol/l from the preoperative value within 48 hours of surgery or serum creatinine increased by ≥ 1.5 times the preoperative value within 7 days of surgery | Innovian data management system and hospital laboratory database |
Infection during hospital admission | Any chest infection, wound infection, mediastinitis, graft site infection, sternal wound infection, urinary tract infection, sepsis or bloodborne infection | Innovian data management system and Patient Analysis and Tracking System database |
Test predictors
Predictors from index POC tests are listed in Table 5, predictors from index differential cell counter tests are listed in Table 6 and predictors from index SLTs and LRTs are listed in Table 7.
Test group | Test | Units | Likely predictor of CCB |
---|---|---|---|
Preoperative MEA | ADPtest AUC | AU | Low test result |
ASPItest AUC | AU | Low test result | |
TRAPtest AUC | AU | Low test result | |
ADRENtest AUC | AU | Low test result | |
Postoperative MEA | ADPtest AUC | AU | Low test result |
ASPItest AUC | AU | Low test result | |
TRAPtest AUC | AU | Low test result | |
ADRENtest AUC | AU | Low test result | |
Postoperative ROTEM | INTEM CT | Minutes | High test result |
INTEM α-angle | Degrees | Low test result | |
INTEM MCF | mm | Low test result | |
INTEM ML | % reduction in MCF | High test result | |
INTEM Vmax | mm/second | Low test result | |
INTEM tVmax | Seconds | High test result | |
EXTEM CT | Minutes | High test result | |
EXTEM α-angle | Degrees | Low test result | |
EXTEM MCF | mm | Low test result | |
EXTEM ML | % reduction in MCF | High test result | |
EXTEM Vmax | mm/second | Low test result | |
EXTEM tVmax | Seconds | High test result | |
FIBTEM MCF | mm | Low test result | |
EXTEM MCF – FIBTEM MCF | mm | Low test result | |
INTEM CT – HEPTEM CT | Minutes | High test result | |
Postoperative TEG | TEG CK R | Minutes | High test result |
TEG CK α-angle | Degrees | Low test result | |
TEG CK MA | mm | Low test result | |
TEG CK LY60 | % of MA | High test result | |
TEG CK R – CKH R | Minutes | High test result |
Test result | Test (platform) | Units | Likely predictor of CCB |
---|---|---|---|
Haemoglobin concentration | FBC (XE-2100™ Automated Hematology System; Sysmex, Milton Keynes, UK) | g/dl | Low test result |
Haematocrit | FBC (XE-2100 Automated Hematology System) | % | Low test result |
White blood cell count | FBC (XE-2100 Automated Hematology System) | ×109/l | Low test result |
Absolute neutrophil count | FBC (XE-2100 Automated Hematology System) | ×109/l | Low test result |
Absolute platelet count | FBC (XE-2100 Automated Hematology System) | ×109/l | Low test result |
MPV | FBC (XE-2100 Automated Hematology System) | fl | High or low test result |
MPV × platelet count | FBC (XE-2100 Automated Hematology System) | fl × 109/l | Low test result |
Immature platelet fraction | FBC (XE-2100 Automated Hematology System) | % | High or low test result |
Absolute reticulocytes | FBC (XE-2100 Automated Hematology System) | ×109/l | High or low test result |
Test result | Test (platform) | Units | Likely predictor of CCB |
---|---|---|---|
PT | Innovin® (CS-2100i analyser; Sysmex, Kobe, Japan) | Seconds | High test result |
aPTT | Actin FS (CS-2100i analyser) | Seconds | High test result |
Clauss fibrinogen activity | Thrombin reagent (CS-2100i analyser) | g/l | Low test result |
D-dimer | INNOVANCE® (CS-2100i analyser) | ng/ml | High test result |
Anti-Xa heparin activity | Anti-Xa kit (HYPHEN BioMed) | IU/ml | High test result |
Factor XIII activity | Berichrom® XIII (CS-2100i analyser) | IU/dl | Low test result |
vWF | Ristocetin cofactor activity (BC RiCof assay; Sysmex) | IU/dl | Low test result |
ETP | FluCa-kit (Stago, Asnières sur Seine, France) (Fluoroskan Ascent™; ThermoFisher Scientific, Paisley, UK) | nM/minute | Low test result |
Timing of test results
We evaluated the potential advantages of using early test results for each assay when these were available (Table 8). The early time frame tests have never been incorporated into POC testing algorithms developed empirically by others in cardiac surgery. However, they may be more useful clinically by virtue of providing informative test results more quickly than ‘standard’ tests. Models were fitted first for the standard time frame. Then, models were fitted for the early time frame, testing whether this resulted in a ‘significant’ deterioration in prediction. Comparing early and standard time frames is interesting because there may be a trade-off between the potential benefits of earlier decision-making (e.g. allows definitive treatment to be provided more quickly, thereby preventing blood loss) and the potential harms (e.g. somewhat less good prediction). For each platform, the test results were classified as being relevant to an early or ‘standard’ time frame, for instance:
-
Multiplate:
-
early = velocity
-
standard = AUC, amplitude
-
-
ROTEM:
-
early = A10 or A5 [amplitude 10 or 5 minutes after clotting time (CT)]
-
standard = MCF variables (measurements 20 minutes after starting the analysis)
-
-
TEG:
-
early = no immediately available measurements comparable with those for ROTEM (but available in principle)
-
standard = MCF variables (measurements 20 minutes after starting the analysis).
-
Standard test result | Early alternative |
---|---|
Multiplate ADPtest AUC | ADP test velocity |
Multiplate AAtest AUC (Multiplate ASPItest) | AA test velocity (ASPI velocity) |
Multiplate TRAPtest AUC | TRAP test velocity |
Multiplate ADRtest AUC (Multiplate ADRENtest) | ADR test velocity (ADREN velocity) |
ROTEM INTEM CT | NA |
ROTEM INTEM α-angle | NA |
ROTEM INTEM MCF | INTEM A5 or A10 |
ROTEM INTEM ML | NA |
ROTEM INTEM Vmax | NA |
ROTEM INTEM tVmax | NA |
ROTEM EXTEM CT | NA |
ROTEM EXTEM α-angle | NA |
ROTEM EXTEM MCF | EXTEM A5 or A10 |
ROTEM EXTEM ML | NA |
ROTEM EXTEM Vmax | NA |
ROTEM EXTEM tVmax | NA |
ROTEM FIBTEM MCF | FIBTEM A5 or A10 |
ROTEM EXTEM MCF – FIBTEM MCF | EXTEM A5 – FIBTEM A5; EXTEM A10 – FIBTEM A10 |
ROTEM INTEM CT – HEPTEM CT | NA |
TEG CK R | NA |
TEG CK α-angle | NA |
TEG CK MA | CK A30 |
TEG CK LY60 | NA |
TEG CK R – CKH R | NA |
Predictor (index) tests and reference outcomes in the COPTIC analyses
The primary predictor (index) tests and reference outcomes of interest differed by analysis (Table 9).
Analysis | Reference outcome | Predictor (index) tests |
---|---|---|
COPTIC A | Any CCB | Preoperative Multiplate aggregometry |
Postoperative CCB | Preoperative Multiplate aggregometry | |
COPTIC B | Postoperative CCB | Preoperative Multiplate aggregometry |
Postoperative Multiplate aggregometry | ||
Postoperative ROTEM | ||
Postoperative TEG | ||
Combinations of the above | ||
Secondary clinical outcomes | Preoperative Multiplate aggregometry | |
Postoperative Multiplate aggregometry | ||
Postoperative ROTEM | ||
Postoperative TEG | ||
Combinations of the above | ||
LRT analysis 1 | Any CCB | Preoperative measures of red cell mass and platelet morphology derived from automated cell counters |
LRT analysis 2 | Postoperative CCB | Preoperative and postoperative data on red cell mass and platelet morphology derived from automated cell counters and LRT of coagulation |
Combinations of the above |
Research procedures
Patient recruitment
Consecutive patients undergoing cardiac surgery at the Bristol Heart Institute during the recruitment period were screened for eligibility and approached for consent when research staff were available. Some patients were not approached because admission occurred outside normal working hours or because staff members were on leave. Participants provided informed consent on admission to hospital. In the rare event of a patient with diminished capacity being unable to physically complete the consent form, verbal consent was accepted and this was documented on the trial-specific consent form and in the medical notes. The person taking consent and the participant (or someone in a qualifying relationship) were required to sign the request for participation. This was agreed with the REC and the sponsor, as it was recognised that critically ill patients undergoing emergency surgery are among those most likely to benefit from improved diagnosis and treatment of coagulopathic bleeding. All recruited participants ultimately provided informed consent for enrolment in the study.
Blood sampling
For the research, two 22.5-ml blood samples (45 ml in total) were obtained in the operating theatre at the following time points (see Figure 6):
-
immediately before induction of anaesthesia (T0)
-
on reversal of heparin anticoagulation (T3).
All blood samples were taken from existing arterial lines inserted as part of standard clinical care. Blood samples for MEA platelet function testing were collected into 3-ml vacuum collection tubes containing hirudin (final concentration 15 µg/ml). Blood samples for TEG, ROTEM and SLTs and LRTs were collected into 4.5-ml vacuum collection tubes containing trisodium citrate (final concentration 0.105 M). The MEA, ROTEM and TEG tests were analysed in a research laboratory specifically staffed and equipped for the COPTIC study using whole blood within 120 minutes of blood collection. For the reference tests, platelet-poor plasma was prepared by centrifugation of the blood samples at 2240 g for 10 minutes. The plasma was then decanted into a new specimen tube before recentrifugation using the same conditions. Plasma samples were then stored in aliquots at –70 °C for analysis in batches.
Rotational thromboelastometry
For the ROTEM tests, 300 µl of anticoagulated blood was recalcified in the reaction cup with 20 µl of star-TEM® reagent and was then activated with the following reagents in accordance with the manufacturer’s instructions: (1) EXTEM reagent, (2) FIBTEM reagent, (3) INTEM reagent and (4) HEPTEM reagent (all reagents manufactured by ROTEM, TEM International GmbH, Munich, Germany). Changes in clot viscoelastic strength were measured for 60 minutes. Analyses were performed on two ROTEM Delta devices.
Thromboelastography
For the TEM tests, 20 µl of 0.2 M CaCl2 was added to each TEG cup. In accordance with the manufacturer’s instructions, 1 ml of anticoagulated blood was added to kaolin reagent TEG bottle and mixed gently. For each test, 340 µl of this mixture was then added to either the plain or the heparinase-coated TEG cups. Changes in clot viscoelastic strength were measured for 60 minutes. Analyses were performed on two TEG® 5000 Thromboelastograph® Haemostasis Analyzer Systems (Haemonetics Corporation, Niles, IL, USA).
For both the ROTEM and the TEG analyses, traces that were identified as being of poor quality during the initial analyses were immediately repeated using spare blood samples. After completion of the assays, numerical parameters derived from the TEG curves by the device automated algorithms were inspected manually. For traces in which the derived parameters had not been correctly recorded, correct estimates were obtained manually by inspection of the x,y co-ordinates of the raw viscoelastometry traces. ROTEM and TEG parameters that were absent or incorrect and which could not be resolved by this method were excluded from the analyses.
Multiplate
The hirudinised blood was first mixed 1 : 1 with 0.9% NaCl preheated to 37 °C in standard test cells on a Multiplate multiple electrode aggregometer according to the manufacturer’s instructions. After 3 minutes’ incubation, platelet activation was initiated by the addition of the following agonists: (1) ADPtest reagent (ADP final concentration 6.5 µM), (2) ASPItest reagent (arachidonic acid final concentration 0.5 mM), (3) TRAPtest reagent (TRAP final concentration 32 µM) and (4) ADRENtest [adrenaline (epinephrine) final concentration 20 mg/ml]. The increase in electrical impedance from the two electrode pairs within each test cell was recorded for 6 minutes and was expressed as a mean of the AUC measurements in arbitrary units of AU*min, converted to U (where 1 U = 10 AU*min) to enable comparison with other clinical studies. Internal MEA quality control software generates an error flag if the difference in AUC measurements between the two electrode pairs exceeds 20% of the mean of the AUC measurements. In this circumstance, the assay was immediately repeated using the same blood sample. Mean AUC measurements were excluded from the analyses if repeat testing also failed to generate a result within this quality control threshold.
Laboratory reference tests
The reference tests for soluble coagulation factor activities and clotting times were all performed in batches on thawed plasma aliquots using standard manufacturer’s protocols on a Sysmex CS2100i analyser. Differential cell counts were performed on a Sysmex XE-2100 automated haematology analyser. The following test reagents were used: PT – Innovin®; aPTT – Actin FS; Clauss fibrinogen activity – thrombin reagent; vWF – ristocetin cofactor activity (BC RiCof assay); factor XIII activity – Berichrom XIII; and D-dimer concentration – INNOVANCE® (all from Sysmex, Kobe, Japan). The anti-Xa assay was performed using the HYPHEN BioMed anti-Xa kit, using an unfractionated heparin standard. Thrombin generation was measured using a Fluoroskan Ascent™ device (ThermoFisher Scientific, Paisley, UK) and the FluCa-kit (Stago, Asnières sur Seine, France), according to the manufacturer’s instructions.
Measurement of clinical outcomes
Clinical outcomes as described in Definition of study end points were measured as follows. Blood loss at 6 hours, non-routine administration of protamine and administration of non-routine pro-haemostatic treatments during surgery (T0–T3, interval 1; see Figure 6) were recorded on case report forms. Reopening for bleeding, MI, stroke, acute kidney injury (AKI) requiring dialysis, wound infection and death were recorded on the hospital’s Patient Analysis and Tracking System. Baseline participant demographics, comorbidity and operative details were also recorded on the Patient Analysis and Tracking System. Non-routine early pro-haemostatic treatment, reopening for bleeding, MI, stroke, AKI requiring dialysis and sepsis were recorded on the intensive care Innovian data management system. Serum creatinine data required for the estimation of AKI were obtained from the hospital’s clinical biochemistry database. Details of data extraction and coding are recorded in the statistical analysis plans (available online in Report Supplementary Materials 2 and 3).
Duration of follow-up
The duration of follow-up was until hospital discharge or death.
Clinical management of study subjects
Participants underwent standard preoperative, anaesthetic, surgical and postoperative care according to existing unit protocols. Decisions about intra- and postoperative haemostasis and transfusion treatment were guided by ad hoc TEG analyses and standard laboratory investigations (PT, aPTT, ACT, fibrinogen, platelet count), performed at the discretion of the responsible clinician in accordance with routine institutional practice. These decisions were not influenced by participation in the study.
Measures taken to avoid bias
Selection bias was minimised by selecting consecutive participants who satisfied the prespecified inclusion criteria. There were few exclusion criteria and this was intended to select a population that was as representative as possible of the reference cardiac surgery population. Selection bias was also minimised by the development of a dedicated COPTIC laboratory, to ensure that all consented participants were recruited and samples analysed. We tested for selection bias by comparing the characteristics of patients who were recruited with the characteristics of those who were not recruited.
Index tests included POC tests, SLTs in common use for the management of coagulopathic bleeding in cardiac surgery and LRTs that can diagnose common acquired coagulopathies in cardiac surgery. The timing of the tests corresponded to critical stages in the patient journey when these tests are commonly performed. Interventions based on pre- and postoperative POC and coagulation tests have been shown to reduce coagulopathic bleeding in cardiac surgery patients. All tests were performed in a laboratory that was separate from the site of clinical care of the study subjects and almost all tests were carried out by staff who were blinded to the clinical course of the patients. Quality assurance steps were taken on an ongoing basis to ensure adequate data quality. Laboratory test results were unavailable to the clinicians responsible for direct patient care and therefore did not influence the clinical management of participants or the reference outcome.
We used a novel definition of the primary (reference) outcome CCB. We considered that this had important advantages over definitions of coagulopathic bleeding used in previous studies. We also evaluated the robustness of our primary analysis by considering alternative definitions of CCB in sensitivity analyses.
Data collection and linkage
Almost all data were collected from routine sources. Data required to characterise (1) participants preoperatively, (2) operations and (3) postoperative complications were collected through the audit infrastructure currently in place for all patients undergoing cardiac surgery (Patient Administration and Tracking System) and the intensive care unit (ICU) Innovian data management system. All SLT and reference test data and TEG, Multiplate and ROTEM data were stored in electronic laboratory databases until linkage. Data describing transfusions (red cells, platelets, FFP) were obtained from the hospital blood bank. Creatinine measurements for classification of AKI were obtained from laboratory data collected for routine clinical care. A minimal number of data not available from the above sources (e.g. blood products in theatre given prior to sample collection and blood loss at 6 and 12 hours post admission to intensive care) were collected from medical records or postoperative care charts and recorded on case report forms. Information on treatment taken (aspirin, clopidogrel, prasugrel or a combination) and the time that treatment was stopped was recorded using the Patient Analysis and Tracking System. The data were linked for analysis on the basis of a pseudonymised participant identifier, that is, hospital number, in addition to the date of operation (Figure 7). Further details of the data linkage procedures are listed in the statistical analysis plans (available online in Report Supplementary Materials 2 and 3). Data were collected and retained in accordance with the UK Data Protection Act 1998. 96
FIGURE 7.
Sequence of linkage of data sources. BRI, Bristol Royal Infirmary; CICU, cardiac intensive care unit; CRF, case report from; FBC, full blood count.
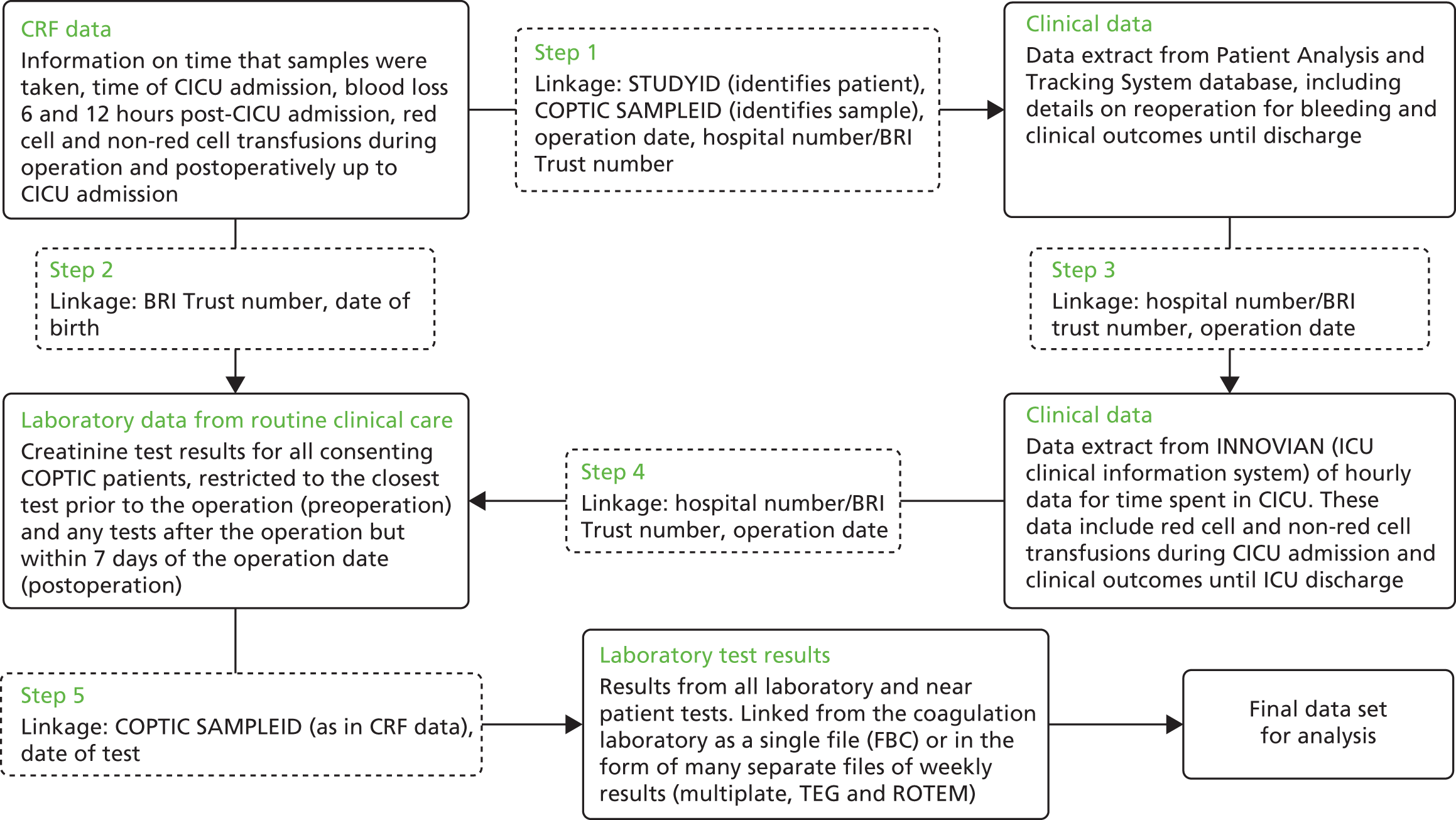
Storage of clinical material
To enable retrospective analysis of confounding variables and for laboratory quality control and assay validation purposes, blood samples not used in the initial analyses were stored at –80°C in a secure storage facility in the hospital laboratory for the duration of the study.
Sample size considerations
The study sample size detailed in the protocol (version 6, 21 March 2012) was 2400, which corresponds to the entire predicted patient throughput at Bristol Heart Institute for the 2-year study period, allowing for up to 10% of patients to opt out. We estimated that a sample size of 2400 would have > 95% power to detect significant associations between continuous exposures and outcomes at a two-tailed significance level of 0.01. As an example, assuming that (1) the prevalence of clopidogrel withdrawal < 5 days from surgery is 50% and (2) the overall risk of excess bleeding is 15% (historical Patient Analysis and Tracking System data for Bristol), the study would have about 90% power to detect a RR of developing the primary outcome of about 1.7 (11% vs. 19%) at a two-tailed significance level of 0.05 in the subgroup of participants (approximately 800) taking clopidogrel prior to surgery.
More recent data extraction from the Patient Analysis and Tracking System led to a review of this estimate. This demonstrated that the proportion of patients on clopidogrel in a more contemporary cohort was 29% (estimated as 33% in the previous example), the proportion of patients who have clopidogrel withdrawn within 4 days of surgery was 38.6% (estimated as 50% in the previous example) and the excess bleeding rate was 13.1% (estimated as 15% in the previous example). Using these figures, we estimated that the COPTIC study would now have 84% power to detect a 1.9-fold increase in the risk of excess bleeding between those who had clopidogrel withdrawn and those who did not (incidence of excess bleeding 9.03% vs. 17.17% respectively) at a two-tailed significance level of 0.05. This revised estimate did not indicate that a change in the sample size was required to meet the study objectives.
Plan of analysis
Overview
The objective of the COPTIC analyses was to estimate how well the results of POC blood tests, or LRTs, assist in the prediction of CCB. This was achieved by developing predictive models for the reference outcome CCB, initially using variables related to existing participant characteristics. Index (predictor) POC or LRT variables were then included in refined models and the predictive value compared with that of models using participant characteristics alone. Differences in predictive accuracy were expressed as differences in the AUC or the number of participants reclassified as having the reference outcome. Finally, we fitted separate regression models to estimate associations between the results of POC tests and secondary outcomes and developed models that would be informative in predicting the risk of each secondary outcome according to POC test results. Statistical analyses and reporting were undertaken in accordance with the STARD guidelines,98 as described in Appendix 1.
Clinical prediction model
To develop a clinical prediction model for CCB that would complement the current standard of care we prespecified a priori expected relationships between a series of baseline participant characteristics and an increased likelihood of CCB. This included whether or not participants were receiving DAPT, aspirin alone or no antiplatelet treatment. The DAPT subgroup was also further subdivided into those participants who had stopped their P2Y12 receptor blocker > 7 days before surgery, 6–7 days before surgery, 3–5 days before surgery or 0–2 days before surgery. This was stratified by the type of cardiac surgery. Cardiac procedures are recorded and categorised in the Patient Analysis and Tracking System as CABG (coronary artery bypass graft) only, CABG and valve replacement surgery, CABG and other, CABG and valve replacement surgery plus other, valve replacement surgery alone, valve replacement surgery plus other and other procedure. These three factors (DAPT, timing of withdrawal and type of operation) co-vary with one another in that the treatments of interest (DAPT and timing of withdrawal) are not necessarily relevant to all cardiac procedures. Therefore, we considered these factors by making a categorical variable that coded clinically relevant combinations. The nature of the ‘other’ cardiac procedures was heterogeneous and required additional characterisation. Any combination that included an ‘other’ procedure was reviewed and classified according to whether or not the ‘other’ procedure was considered to be the primary determinant of the risk of bleeding (from the point of view of the operation being carried out). Participants undergoing an ‘other’ procedure classified as being the determinant of bleeding became a new subset; all participants with an ‘other’ procedure were reclassified according to their main procedure, for example ‘CABG and other’ was reclassified as ‘CABG only’. If the only procedure was an ‘other’ procedure judged to have a high bleeding risk, it was classified with the new ‘other’ subset; if it was judged to have a low bleeding risk, for example atrial fibrillation radiofrequency ablation, the participant was excluded from the analysis population. The ‘other’ procedures identified as having a high bleeding risk were K08 ‘Repair of double outlet ventricle (Clean)’, K33 ‘Aortic root replacement’ and L19 ‘Other replacement of aneurysmal segment of aorta’. The new categories combining the treatment group, time since withdrawal of antiplatelet therapy and cardiac procedure are as follows [reducing a three-dimensional matrix of four cells (cardiac procedure) × three cells (treatment group) × three cells (time since withdrawal)], as shown in Box 3.
-
Age.
-
Sex.
-
Diabetes.
-
Procedure and treatment group:
-
CABG, no antiplatelet treatment
-
CABG + valve, no antiplatelet treatment
-
valve, no antiplatelet treatment
-
CABG, aspirin, no P2Y12 blocker or P2Y12 blocker stopped > 7 days before surgery
-
CABG + valve, aspirin, no P2Y12 blocker or P2Y12 blocker stopped > 7 days before surgery
-
valve, aspirin, no P2Y12 blocker or P2Y12 blocker stopped > 7 days before surgery
-
CABG, aspirin, P2Y12 blocker stopped 0–2 days before surgery
-
CABG, aspirin, P2Y12 blocker stopped 3–5 days before surgery
-
CABG, aspirin, P2Y12 blocker stopped 6–7 days before surgery
-
CABG + valve, aspirin, P2Y12 blocker stopped ≤ 7 days before surgery
-
valve, aspirin, P2Y12 blocker stopped ≤ 7 days before surgery
-
other high bleeding risk procedure, any or no antiplatelet treatment.
-
-
Surgical priority:
-
elective
-
urgent.
-
-
Estimated glomerular filtration rate (ml/minute/1.73m2).
-
Preoperative haematocrit (%).
-
Preoperative platelet count (×109/l).
-
BMI (kg/m2).
Valve, valve replacement surgery.
Specific analysis procedures
We evaluated the benefits of introducing the results from separate platforms to the clinical prediction model [Multiplate (preoperative), ROTEM, TEG, Multiplate (postoperative)]. Platforms were added individually in the first instance. We then evaluated whether the inclusion of additional platforms would improve the prediction of CCB. The reason for considering groups of variables by platform (rather than simply choosing the ‘best’ subset of variables) was that there is no cost of using extra variables from one platform, but there is an extra cost of carrying out a second test.
The specific steps undertaken were as follows. In step 1 we fitted regression models to estimate associations between the results of laboratory tests and CCB. For all continuous terms the linearity of any potential associations was investigated using multivariable fractional polynomial (FP) models. If the model fit improved by transformation of the term, this was performed. The prediction achieved using participant characteristics was determined and an optimal model based only on ‘baseline’ covariates was defined. Separate models were developed for any CCB (intraoperative or postoperative) for the COPTIC A study and the evaluation of the LRTs and for postoperative CCB for the COPTIC A and B studies and the evaluation of the LRTs.
In step 2 we reviewed the predictive accuracy of the POC test results for ROTEM, TEG and Multiplate to obtain the best-performing model for each (highest AUC), including the baseline covariates. First, the contributions of all of these baseline characteristics to the prediction of CCB were incorporated into a single score, which was forced into models of laboratory predictors with a coefficient of 1.00 to enable comparisons between different models based on the various laboratory test platforms. Next, all of the test results from each device were included for each model, with multivariable FP techniques used to investigate the linearity of terms. All transformations and centring found to improve the fit of the models were implemented. Backward elimination was undertaken to select the final models, with a threshold of inclusion of 0.05 and a threshold of exclusion of 0.10. Initial models considered only the standard test results (see Tables 6 and 7); subsequently, the ‘early-phase’ alternatives were considered for any terms that significantly contributed to the model. Goodness of fit for models of baseline characteristics and best-performing models was assessed using the Hosmer–Lemeshow test and by calculating Brier scores.
The following models were constructed (Figure 8):
-
model 2A – preoperative Multiplate platform in relation to any CCB
-
model 2B – preoperative Multiplate platform in relation to postoperative CCB
-
model 2C – postoperative Multiplate platform in relation to postoperative CCB
-
model 2D – postoperative ROTEM in relation to postoperative CCB
-
model 2E – postoperative TEG in relation to postoperative CCB.
FIGURE 8.
Summary of analyses of preoperative and postoperative tests.
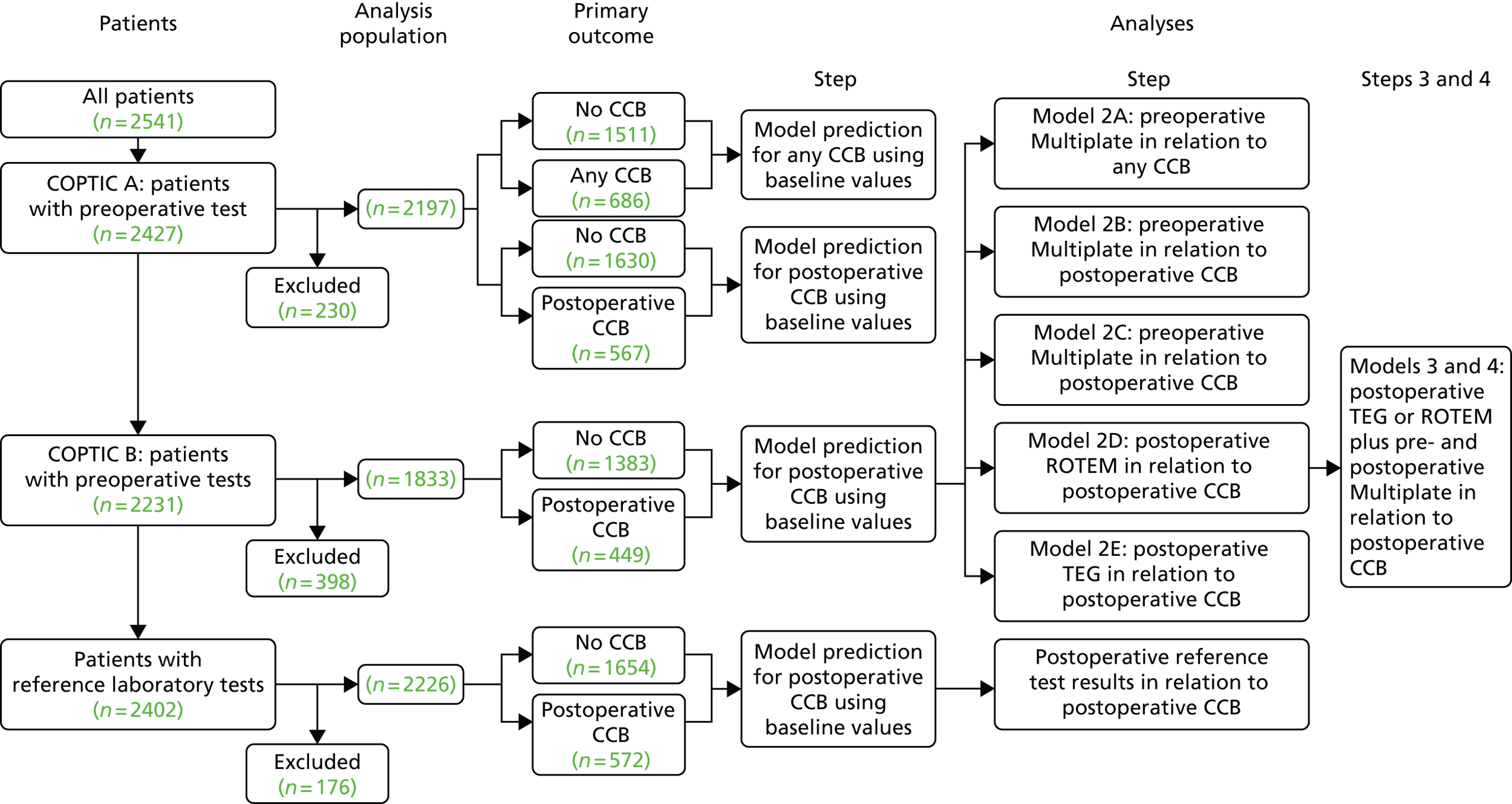
Each model was then compared with a baseline model containing only the baseline characteristics to evaluate the contribution of the selected laboratory test results to the prediction of CCB. These analyses are presented as receiver operating characteristic (ROC) curves. We hypothesised that each of these models would consider all variables for the test/time point, that is, consider the test/time point as a ‘package’. The first model stands alone for any CCB. For postoperative CCB, this step will identify which of the other four models is the best platform for predicting CCB, that is, provides the best prediction. We hypothesised that the best platform would one of the postoperative platforms because the information from these is more ‘proximal’ to the outcome for these models, that is, postoperative CCB. For the best test/time point we also investigated whether a model using test variables that are available early (for the test identified as best) performs as well as a model using the standard test variables available at ‘test completion’.
In step 3 we tested whether the addition of one of the other (postoperative) platforms would improve the best-performing model for postoperative CCB (highest AUC) identified in step 2. We expected, on the basis of existing data, that this would be a test of whether or not addition of the postoperative Multiplate platform improves prediction compared with either ROTEM or TEG alone (or the addition of ROTEM or TEG to the postoperative Multiplate platform, as ROTEM and TEG assess similar mechanisms and the Multiplate platform assesses different mechanisms). The combination of ROTEM and TEG together was not considered. For the test/time point added at step 3, we also investigated whether or not a model using test variables that are available early (for the test/time point added at step 3) performed as well as a model using the standard test variables available at ‘test completion’.
In step 4 we tested whether or not the addition of the preoperative Multiplate platform would improve the model identified in step 3. If the preoperative Multiplate platform was added at step 4, we also planned to consider whether or not a model using test variables that are available early for the preoperative Multiplate platform performs as well as a model using the standard test variables available at ‘test completion’.
Finally, for the best-performing predictive model, the predictive value was expressed as the proportion of participants correctly classified as having CCB or no CCB, with those with a fitted probability of CCB of ≥ 0.5 classified as having CCB. The predictive value was also described by the change in post-test probability conditional on the test results in the model, as a function of pre-test probability. 100 The net reclassification improvement (NRI) was calculated. This index quantifies how well the best-performing predictive model reclassifies subjects – either appropriately or inappropriately – compared with the baseline characteristics model.
All analyses were undertaken using Stata® version 14 (StataCorp LP, College Station, TX, USA).
Sensitivity analyses
We undertook sensitivity analyses to address uncertainty in relation to the reference outcome CCB. These sensitivity analyses were specified in the context of there being a large proportion of participants classified as having CCB because they received one or more pro-haemostatic treatments but who did not experience excessive bleeding (chest drain < 600 ml at 6 hours post operation) or did not require a reoperation. Anecdotal evidence suggests that it is common for one pro-haemostatic agent (1 unit of pooled platelets or 2 units of FFP) to be administered to participants considered to be at high risk of bleeding in the absence of evidence of bleeding (i.e. a priori concern about bleeding). Therefore, the sensitivity analyses were intended to explore whether discrimination on the basis of platelet function might be improved by excluding this group that contained an unknown (and unknowable) proportion of participants in whom pro-haemostatic treatment had been administered without evidence of bleeding or evidence to cause concern about bleeding. Two sensitivity analyses were prespecified:
-
Sensitivity analysis 1 (SA1). Those defined as having received early pro-haemostatic treatment but who did not experience excessive bleeding (chest drain < 600 ml at 6 hours post operation) or require reoperation for bleeding were excluded from the analyses and the models and ROC curves detailed in Specific analysis procedures were repeated.
-
Sensitivity analysis 2 (SA2). Those defined as having received early pro-haemostatic treatment but who did not experience excessive bleeding (chest drain < 600 ml at 6 hours post operation) or require reoperation for bleeding were included in the analyses but were reclassified as being free from CCB.
In addition, we performed an additional post hoc sensitivity analysis:
-
Sensitivity analysis 3 (SA3). Those defined as having received only a single treatment of early pro-haemostatic treatment defined as 1 pooled unit of platelets and 2 units of FFP were included in the analysis but reclassified as free from CCB. The resulting CCB definition was considered to have reflected more severe coagulopathy i.e. not responsive to initial early pro-haemostatic treatment.
Internal validation
The AUC statistics from the primary outcome models were internally validated by bootstrapping with 1000 replicates and cross-validated by removing one observation and then using the remaining study population to create models that generated the predicted probability of CCB for that one observation. After repeating for each observation, the predicted probabilities were used to build ROC curves and to calculate AUC statistics.
External validation
The baseline-only model was validated in a separate cohort of adult cardiac surgery participants (n = 1611) recruited to the multicentre TITRe2 trial between July 2009 and February 2013,4 excluding participants at the Bristol Heart Institute. The data for this cohort did not exactly match those collected for the COPTIC cohort in the following ways: (1) the preoperative platelet count was not measured in the TITRe2 trial; (2) blood loss was not measured at 6 hours post operation and so was interpolated from measurements at 4 and 12 hours; (3) procedure type and antiplatelet medication were not recorded in identical ways and (4) non-standard care pro-haemostatic treatments were recorded for the hospital admission in the TITRe2 trial, which was longer than the observation interval in the COPTIC study. To accommodate these differences, small changes were made in the baseline-only model to enable comparison between the cohorts.
Secondary outcomes
Coagulopathic bleeding is strongly associated with adverse outcomes. To assess whether or not prediction of bleeding risk may also translate into accurate prediction of a range of adverse clinical outcomes we developed baseline clinical predictive models for our prespecified secondary outcomes. Steps 1–4, as described earlier, were then repeated to assess whether or not the addition of the postoperative POC test results evaluated in the COPTIC B study would improve the discrimination of these models.
Analyses of laboratory reference tests
Using the COPTIC B data set we performed two separate analyses for the LRTs.
In LRT analysis 1 we developed a baseline clinical prediction score for the reference outcome any CCB, as described in Clinical prediction model. Next, we repeated steps 1 and 2, described earlier, to identify the final model. In LRT analysis 2 we included the results of multiple tests and multiple testing platforms. In this analysis we repeated steps 1–4, as described earlier. Sensitivity analyses (SA1–3) were also performed to test uncertainty in relation to the reference outcome, as described earlier.
Statistical analysis plans
The statistical analysis plans were written prior to the analyses being carried out. Separate statistical analysis plans were written for the preoperative, postoperative and reference test analyses (available online in Report Supplementary Materials 2, 3 and 4).
Results
The results of the predictive accuracy study are considered in two parts. The analyses of the COPTIC A and B studies are reported together. The analysis of the reference tests was originally intended to complement the COPTIC B analysis to assess whether or not the addition of specific reference tests would improve the predictive accuracy of the best-performing model developed using the POC tests (highest AUC). This analysis was not performed because of the poor predictive accuracy of all of the models and therefore the results of the reference tests are reported separately.
Study and analysis cohorts
A total of 3638 participants were identified as being eligible for the study between 30 March 2010 and 31 August 2012, of whom 2541 (69.8% of those who were eligible) provided consent to participate (Figure 9). Among the 1097 participants who did not consent to participate, the proportion undergoing other (9.2% vs. 2.5%; SMD 0.32) or urgent (46.7% vs. 35.7%; SMD 0.23) procedures was higher. Other differences were considered small (SMD < 0.2) (Table 10).
FIGURE 9.
Standards for Reporting Diagnostic Accuracy (STARD) flow chart for the COPTIC study. PATS, Patient Analysis and Tracking System; UHBristol, University Hospitals Bristol NHS Foundation Trust. a, Defined as eligible: surgery, not weekend, not emergency, not bank holiday, not Easter/Christmas study closure dates, aged ≥ 18 years; b, using the appropriate final model with the highest AUC.
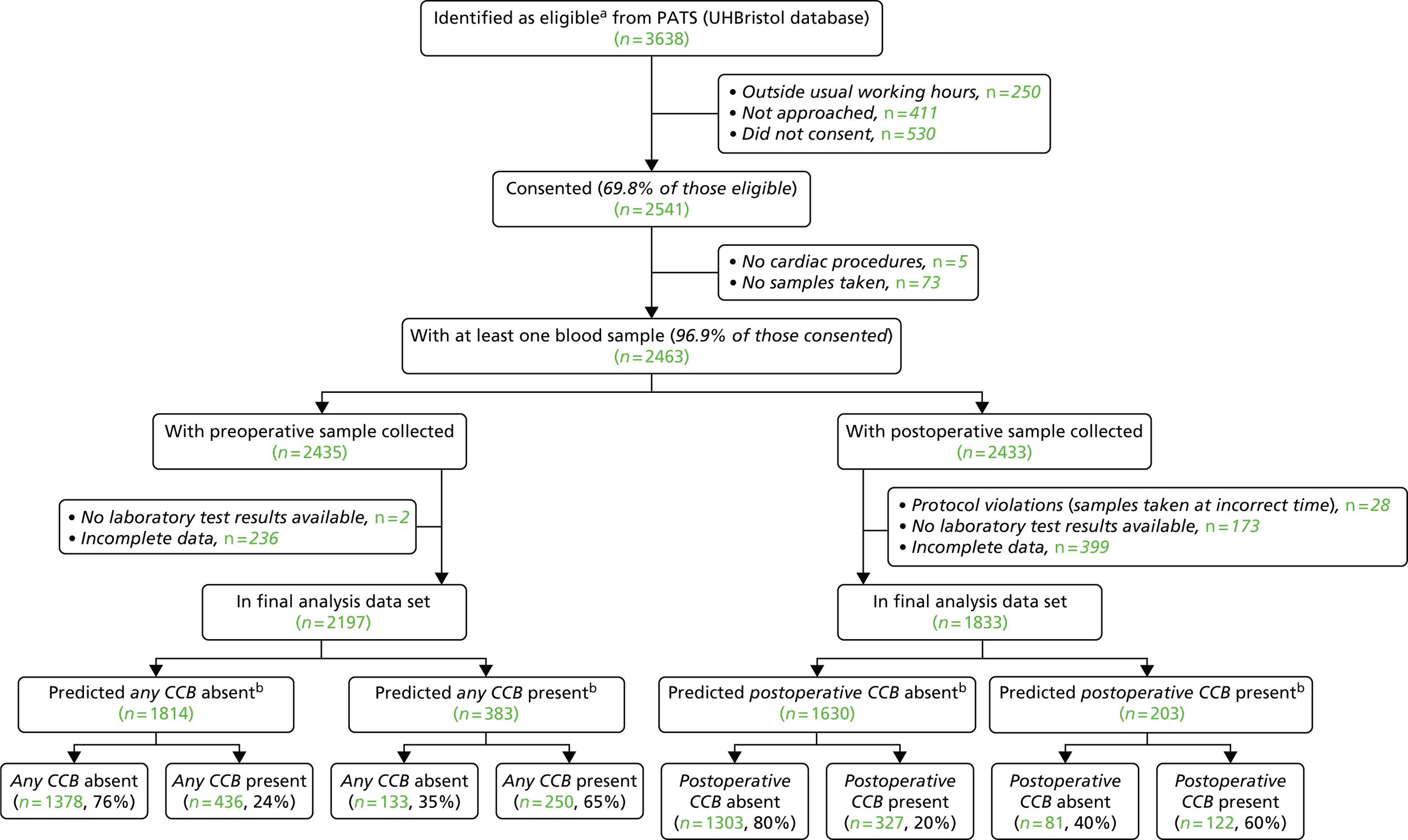
Characteristic | Not consented (N = 1097) | Consented (N = 2541) | SMD |
---|---|---|---|
Age (years),a mean (SD) | 65.76 (14.7) | 67.17 (11.9) | 0.11 |
Sex, n/N (%) | |||
Female | 348/1081 (32.2) | 645/2541 (25.4) | 0.15 |
Male | 733/1081 (67.8) | 1896/2541 (74.6) | |
Diabetes, n/N (%) | 209/1073 (19.5) | 499/2493 (20.0) | 0.01 |
Cardiac procedure, n/N (%) | |||
CABG only | 529/1074 (49.3) | 1405/2490 (56.4) | 0.14 |
CABG + valve | 102/1074 (9.5) | 278/2490 (11.2) | 0.05 |
CABG + valve + other | 13/1074 (1.2) | 30/2490 (1.2) | 0.001 |
CABG + other | 17/1074 (1.6) | 33/2490 (1.3) | 0.02 |
Valve alone | 225/1074 (20.9) | 555/2490 (22.3) | 0.03 |
Valve + other | 89/1074 (8.3) | 126/2490 (5.1) | 0.14 |
Other | 99/1074 (9.2) | 63/2490 (2.5) | 0.32 |
Operative priority, n/N (%) | |||
Elective | 563/1076 (52.3) | 1633/2541 (64.3) | 0.25 |
Urgent | 502/1076 (46.7) | 908/2541 (35.7) | 0.23 |
Emergency | 3/1076 (0.3) | 0/2541 (0.0) | 0.10 |
Salvage | 8/1076 (0.7) | 0/2541 (0.0) | 0.16 |
BMI (kg/m2),b mean (SD) | 27.33 (5.1) | 27.95 (4.7) | 0.13 |
In the COPTIC A analysis of preoperative test results 2435 participants had a preoperative sample collected, of whom 2427 had both the sample analysed and the primary outcome recorded. In total, 344 participants were not included in the analysis because of incomplete baseline or laboratory data, leaving an analysis population of 2197 participants. The analysis population had a median age of 67.3 years [interquartile range (IQR) 61.7–75.7 years] and included 1674 males (76.2%). Most of the analysis population underwent CABG with aspirin treatment (38.4%) (Table 11). Among the 344 participants who were excluded from further analysis because of incomplete data, the proportion of men was lower (67.2%; SMD 0.21). The proportion of participants undergoing CABG and valve replacement surgery with aspirin treatment was higher among the participants not included in the analysis (9.9% vs. 7.2%; SMD 0.10), as was the proportion of participants undergoing CABG only who had been taking DAPT within 6–7 days of surgery (7.0% vs. 4.7%; SMD 0.11) and the proportion of patients in the ‘other’ (high-risk) group (7.6% vs. 5.1%; SMD 0.11). Otherwise, the baseline characteristics of these groups were similar. Given that the differences between the groups were small (defined as a SMD of < 0.2), with the exception of male sex (SMD 0.21), we concluded that the analysis population was representative of the entire study population (see Table 11).
Characteristic | Analysis data set (N = 2197) | Consented participants not in analysis data set (N = 344) | SMD |
---|---|---|---|
Age (years), mean (SD) | 67.33 (11.6) | 66.39 (13.6) | 0.12 |
Age (years), median (IQR) | 68.9 (61.7–75.7) | 69.3 (58.9–76.2) | – |
Sex male, n/N (%) | 1674/2197 (76.2) | 231/344 (67.2) | 0.21 |
Diabetes, n/N (%) | 440/2197 (20.0) | 59/295 (20.0) | 0.00 |
Procedure and treatment group, n/N (%) | |||
CABG only, no APT | 74/2197 (3.4) | 5/172 (2.9) | 0.03 |
CABG + valve, no APT | 81/2197 (3.7) | 5/172 (2.9) | 0.04 |
Valve only, no APT | 377/2197 (17.2) | 23/172 (13.4) | 0.10 |
CABG only, ASP | 843/2197 (38.4) | 64/172 (37.2) | 0.02 |
CABG + valve, ASP | 159/2197 (7.2) | 17/172 (9.9) | 0.10 |
Valve only, ASP | 176/2197 (8.0) | 12/172 (7.0) | 0.04 |
CABG only, DAPT (0–2 days) | 119/2197 (5.4) | 9/172 (5.2) | 0.01 |
CABG only, DAPT (3–5 days) | 124/2197 (5.6) | 12/172 (7.0) | 0.06 |
CABG only, DAPT (6–7 days) | 103/2197 (4.7) | 12/172 (7.0) | 0.11 |
CABG + valve, DAPT (≤ 7 days) | 22/2197 (1.0) | 0/172 (0.0) | 0.10 |
Valve only, DAPT (≤ 7 days) | 7/2197 (0.3) | 0/172 (0.0) | 0.06 |
Other, high bleeding risk procedure | 112/2197 (5.1) | 13/172 (7.6) | 0.11 |
Operative priority, n/N (%) | |||
Elective | 1442/2197 (65.6) | 216/344 (62.8) | 0.06 |
Urgent | 755/2197 (34.4) | 128/344 (37.2) | 0.06 |
eGFR (ml/minute/1.73m2), mean (SD)a | 77.12 (29.2) | 77.48 (30.1) | 0.01 |
eGFR (ml/minute/1.73m2), median (IQR)a | 73.8 (57.1–93.5) | 73.2 (55.0–97.5) | – |
Preoperative haematocrit (%), mean (SD)b | 36.01 (4.4) | 35.72 (4.5) | 0.07 |
Preoperative haematocrit (%), median (IQR)b | 36.0 (33.0–39.0) | 36.0 (33.0–39.0) | – |
Preoperative platelet count (×109/l), mean (SD)c | 210.03 (61.9) | 216.10 (71.2) | 0.10 |
Preoperative platelet count (×109/l), median (IQR)c | 204.0 (168.0–241.0) | 208.0 (170.5–252.0) | – |
BMI (kg/m2), mean (SD) | 27.98 (4.7) | 27.69 (5.1) | 0.06 |
BMI (kg/m2), median (IQR) | 27.5 (24.7–30.8) | 27.1 (23.9–30.8) | – |
In the COPTIC B analysis, of the 2433 participants for whom a postoperative sample was collected, 202 were excluded because of incomplete POC test data or components of the primary outcome, leaving 2231 participants for whom both the sample was analysed and the primary outcome was reported. For the comparison of a predictive model that incorporated the baseline characteristics of the participants and alternative models that also included all preoperative and postoperative test results, a further 399 participants were excluded for incompleteness of baseline data, outcome data or preoperative or postoperative test data (see Figure 9). The primary analysis was restricted to the 1833 participants (72.1% of those consented) with a complete set of baseline characteristics and predictor test results. The analysis population had a median age of 68.9 years (IQR 61.9–75.7) and included 1389 males (75.8%). Most of the analysis population underwent CABG with aspirin treatment (40.2%), valve replacement without antiplatelet drugs (16.4%) or valve replacement with aspirin treatment (7.3%; Table 12). Among the 708 participants who were excluded from further analysis because of incomplete data, the proportion undergoing CABG with aspirin treatment was lower than in the analysis population (31.7%; SMD 0.18) and the proportion undergoing valve replacement with aspirin treatment was higher than in the analysis population (10.3%; SMD 0.11). Otherwise, the baseline characteristics of the groups were similar, indicating that the analysis population was representative of the entire study population (see Table 12).
Characteristic | Analysis data set (N = 1833) | Consented participants not in analysis data set (N = 708) | SMD |
---|---|---|---|
Age (years), median (IQR) | 68.9 (61.9–75.7) | 69.1 (59.9–75.9) | 0.09a |
Sex male, n/N (%) | 1389/1833 (75.8) | 516/708 (72.9) | 0.07 |
Diabetes, n/N (%) | 380/1833 (20.7) | 119/659 (18.1) | 0.07 |
Procedure and treatment group, n/N (%) | |||
CABG only, no APT | 61/1833 (3.3) | 18/536 (3.4) | 0.00 |
CABG + valve, no APT | 66/1833 (3.6) | 20/536 (3.7) | 0.01 |
Valve only, no APT | 301/1833 (16.4) | 99/536 (18.5) | 0.05 |
CABG only, ASP | 737/1833 (40.2) | 170/536 (31.7) | 0.18 |
CABG + valve, ASP | 127/1833 (6.9) | 49/536 (9.1) | 0.08 |
Valve only, ASP | 133/1833 (7.3) | 55/536 (10.3) | 0.11 |
CABG only, DAPT (0–2 days) | 98/1833 (5.3) | 30/536 (5.6) | 0.01 |
CABG only, DAPT (3–5 days) | 114/1833 (6.2) | 22/536 (4.1) | 0.09 |
CABG only, DAPT (6–7 days) | 88/1833 (4.8) | 27/536 (5.0) | 0.01 |
CABG + valve, DAPT (≤ 7 days) | 14/1833 (0.8) | 8/536 (1.5) | 0.08 |
Valve only, DAPT (≤ 7 days) | 5/1833 (0.3) | 2/536 (0.4) | 0.02 |
Other high bleeding risk procedure | 89/1833 (4.9) | 36/536 (6.7) | 0.08 |
Priority, n/N (%) | |||
Elective | 1204/1833 (65.7) | 454/708 (64.1) | 0.03 |
Urgent | 629/1833 (34.3) | 254/708 (35.9) | 0.03 |
eGFR (ml/minute/1.73m2), median (IQR)b | 73.7 (56.9–93.7) | 73.8 (56.5–93.6) | 0.04a |
Preoperative haematocrit (%), mean (SD)c | 35.94 (4.4) | 36.11 (4.4) | 0.04 |
Preoperative platelet count (×109/l), median (IQR)d | 205.0 (170.0–242.0) | 201.0 (165.0–241.0) | 0.04a |
BMI (kg/m2), median (IQR) | 27.4 (24.8–30.8) | 27.5 (24.3–30.8) | 0.03a |
Clinical outcomes
The primary outcome of any CCB was recorded in 686 out of 2197 (31%) of the COPTIC A study cohort (Figure 10). The largest single group included those receiving non-routine pro-haemostatic treatments (n = 622; 28%). Drain losses of > 600 ml at 6 hours occurred in 334 participants (15%) and reoperations for bleeding occurred in 119 participants (4%). Postoperative CCB occurred in 449 out of 1833 (24%) of the COPTIC B study cohort (Figure 11). The proportion of participants receiving non-routine pro-haemostatic treatments was less (20%) than the proportion receiving non-routine pro-haemostatic treatments using the any CCB definition, whereas the proportions with excessive drain loss (14%) and reoperation for bleeding (3%) were similar. This was expected as the difference in the any CCB and postoperative CCB definitions was the intraoperative administration of pro-haemostatic treatments.
FIGURE 10.
Primary outcome in the COPTIC A study (preoperative samples): any CCB (686/2197; 31.0%) (participants with missing data for one component of the primary outcome are not included in the Venn diagram).

FIGURE 11.
Primary outcome in the COPTIC B study (postoperative samples): postoperative CCB (449/1833; 24.5%) (participants with missing data for one component of the primary outcome are not included in the Venn diagram).

Of the 535 (29.2%) participants with any CCB in the COPTIC B analysis population, 449 (24.5% of the analysis population) were recorded as having postoperative CCB (Table 13). Eighty-six participants (4.7% of the analysis population) had CCB reported both during and after surgery. All participants in the analysis population with recorded CCB during surgery also had recorded CCB after surgery. The frequency of the secondary outcomes is also reported in Table 13. Overall, the red cell transfusion rate was 32% in the COPTIC B analysis population, with 3.3% of participants requiring > 4 units in intensive care. The mortality rate as well as the rates of major morbidities were typical of reported event rates for a UK cardiac surgery population, with the exception of AKI, which occurred in 45% of participants.
Outcome | n/N (%) |
---|---|
Primary outcome: any CCB | 535/1833 (29.2) |
Postoperative blood loss > 600 ml after 6 hours | 244/1799 (13.6) |
Non-routine medical treatment of bleeding after 12 hoursa | 457/1801 (25.4) |
Reoperation for bleeding during hospital staya | 57/1802 (3.2) |
Primary outcome: postoperative CCB | 449/1833 (24.5) |
Postoperative blood loss > 600 ml after 6 hours | 244/1799 (13.6) |
Non-routine medical treatment of bleeding after the postoperative sample and prior to 12 hours after CICU admissiona | 362/1801 (20.1) |
Reoperation for bleeding during hospital staya | 57/1802 (3.2) |
Secondary outcomes | |
Any red cell transfusiona | 581/1795 (32.4) |
Red cell transfusion of ≥ 5 unitsa | 59/1802 (3.3) |
Mortality | 34/1833 (1.9) |
MI | 19/1828 (1.0) |
Stroke | 19/1828 (1.0) |
AKI | 819/1833 (44.7) |
Sepsis | 104/1826 (5.7) |
Baseline characteristics and clinical concern about bleeding
The frequencies of expected baseline risk factors in participants with any CCB in the COPTIC A cohort and participants with postoperative CCB in the COPTIC B cohort are shown in Tables 14 and 15 respectively. No formal comparison of these values in the CCB and non-CCB groups was performed.
Characteristic | No CCB (N = 1511) | Any CCB (N = 686) | Total (N = 2197) |
---|---|---|---|
Age (years), mean (SD) | 66.65 (11.4) | 68.84 (11.9) | 67.33 (11.6) |
Age (years), median (IQR) | 68.0 (61.2–74.8) | 71.2 (63.3–77.7) | 68.9 (61.7–75.7) |
Sex male, n/N (%) | 1135/1511 (75.1) | 539/686 (78.6) | 1674/2197 (76.2) |
Diabetes, n/N (%) | 344/1511 (22.8) | 96/686 (14.0) | 440/2197 (20.0) |
Procedure and treatment group, n/N (%) | |||
CABG only, no APT | 59/1511 (3.9) | 15/686 (2.2) | 74/2197 (3.4) |
CABG + valve, no APT | 47/1511 (3.1) | 34/686 (5.0) | 81/2197 (3.7) |
Valve only, no APT | 267/1511 (17.7) | 110/686 (16.0) | 377/2197 (17.2) |
CABG only, ASP | 690/1511 (45.7) | 153/686 (22.3) | 843/2197 (38.4) |
CABG + valve, ASP | 69/1511 (4.6) | 90/686 (13.1) | 159/2197 (7.2) |
Valve only, ASP | 111/1511 (7.3) | 65/686 (9.5) | 176/2197 (8.0) |
CABG only, DAPT (0–2 days) | 64/1511 (4.2) | 55/686 (8.0) | 119/2197 (5.4) |
CABG only, DAPT (3–5 days) | 78/1511 (5.2) | 46/686 (6.7) | 124/2197 (5.6) |
CABG only, DAPT (6–7 days) | 80/1511 (5.3) | 23/686 (3.4) | 103/2197 (4.7) |
CABG + valve, DAPT (≤ 7 days) | 4/1511 (0.3) | 18/686 (2.6) | 22/2197 (1.0) |
Valve only, DAPT (≤ 7 days) | 2/1511 (0.1) | 5/686 (0.7) | 7/2197 (0.3) |
Other high bleeding risk procedure | 40/1511 (2.6) | 72/686 (10.5) | 112/2197 (5.1) |
Operative priority, n/N (%) | |||
Elective | 1011/1511 (66.9) | 431/686 (62.8) | 1442/2197 (65.6) |
Urgent | 500/1511 (33.1) | 255/686 (37.2) | 755/2197 (34.4) |
eGFR (ml/minute/1.73m2), mean (SD)a | 79.57 (29.0) | 71.72 (29.0) | 77.12 (29.2) |
eGFR (ml/minute/1.73m2), median (IQR)a | 76.4 (59.3–96.8) | 69.4 (51.3–6.2) | 73.8 (57.1–93.5) |
Preoperative haematocrit (%), mean (SD)b | 36.28 (4.1) | 35.41 (4.8) | 36.01 (4.4) |
Preoperative haematocrit (%), median (IQR)b | 37.0 (34.0–39.0) | 36.0 (32.0–39.0) | 36.0 (33.0–39.0) |
Preoperative platelet count (×109/l), mean (SD)c | 214.55 (60.7) | 200.06 (63.6) | 210.03 (61.9) |
Preoperative platelet count (×109/l), median (IQR)c | 208.0 (173.0–245.0) | 193.0 (157.0–230.0) | 204.0 (168.0–241.0) |
BMI (kg/m2), mean (SD) | 28.38 (4.7) | 27.11 (4.6) | 27.98 (4.7) |
BMI (kg/m2), median (IQR) | 27.8 (25.1–31.2) | 26.7 (24.0–29.6) | 27.5 (24.7–30.8) |
Characteristic | No CCB (N = 1384) | Postoperative CCB (N = 449) | Total (N = 1833) |
---|---|---|---|
Age (years), mean (SD) | 66.74 (11.5) | 69.60 (11.2) | 67.44 (11.5) |
Age (years), median | 68.1 (61.3–75.1) | 71.3 (64.1–77.7) | 68.9 (61.9–75.7) |
Sex male, n/N (%) | 1029/1384 (74.3) | 360/449 (80.2) | 1389/1833 (75.8) |
Diabetes, n/N (%) | 314/1384 (22.7) | 66/449 (14.7) | 380/1833 (20.7) |
Procedure and treatment group, n/N (%) | |||
CABG only, no APT | 50/1384 (3.6) | 10/449 (2.2) | 61/1833 (3.3) |
CABG + valve, no APT | 47/1384 (3.4) | 17/449 (3.8) | 66/1833 (3.6) |
Valve only, no APT | 239/1384 (17.3) | 61/449 (13.6) | 301/1833 (16.4) |
CABG only, ASP | 619/1384 (44.7) | 119/449 (26.5) | 737/1833 (40.2) |
CABG + valve, ASP | 68/1384 (4.9) | 61/449 (13.6) | 127/1833 (6.9) |
Valve only, ASP | 90/1384 (6.5) | 44/449 (9.8) | 133/1833 (7.3) |
CABG only, DAPT (0–2 days) | 58/1384 (4.2) | 40/449 (8.9) | 98/1833 (5.3) |
CABG only, DAPT (3–5 days) | 76/1384 (5.5) | 38/449 (8.5) | 114/1833 (6.2) |
CABG only, DAPT (6–7 days) | 72/1384 (5.2) | 16/449 (3.6) | 88/1833 (4.8) |
CABG + valve, DAPT (≤ 7 days) | 4/1384 (0.3) | 10/449 (2.2) | 14/1833 (0.8) |
Valve only, DAPT (≤ 7 days) | 2/1384 (0.1) | 3/449 (0.7) | 5/1833 (0.3) |
Other high bleeding risk procedure | 59/1384 (4.3) | 30/449 (6.7) | 89/1833 (4.9) |
Operative priority, n/N (%) | |||
Elective | 928/1384 (67.1) | 276/449 (61.5) | 1204/1833 (65.7) |
Urgent | 456/1384 (32.9) | 173/449 (38.5) | 629/1833 (34.3) |
eGFR (ml/minute/1.73m2), mean (SD)a | 78.84 (29.2) | 70.68 (27.7) | 76.84 (29.0) |
eGFR (ml/minute/1.73m2), median (IQR)a | 75.7 (58.7–96.2) | 68.4 (51.2–86.1) | 73.7 (56.9–93.7) |
Preoperative haematocrit (%), mean (SD)b | 36.12 (4.2) | 35.37 (4.8) | 35.94 (4.4) |
Preoperative haematocrit (%), median (IQR)b | 36.0 (34.0–39.0) | 36.0 (32.0–39.0) | 36.0 (33.0–39.0) |
Preoperative platelet count (×109/l), mean (SD)c | 214.67 (61.7) | 131.13 (47.3) | 211.24 (62.2) |
Preoperative platelet count (×109/l), median (IQR)c | 207.5 (173.0–245.0) | 125.0 (97.0–156.0) | 205.0 (170.0–242.0) |
BMI (kg/m2), mean (SD) | 28.32 (4.6) | 26.98 (4.6) | 27.99 (4.7) |
BMI (kg/m2), median (IQR) | 27.7 (25.0–31.1) | 26.6 (24.0–29.4) | 27.4 (24.8–30.8) |
Point-of-care tests and clinical concern about bleeding
The results of the preoperative MEA test results and the postoperative MEA, ROTEM and TEG results are shown subdivided according to analysis population and occurrence of CCB in Table 16.
Test | No CCB | CCB | All participants |
---|---|---|---|
COPTIC A population | N = 1511 | N = 686 | N = 2197 |
Preoperative ADPtest AUC (U), median (IQR) | 77.1 (57.4–94.6) | 65.6 (46.7–87.5) | 73.4 (54.0–92.4) |
Preoperative ASPItest AUC (U), median (IQR) | 27.9 (16.9–69.5) | 24.5 (15.0–60.4) | 26.9 (16.3–66.8) |
Preoperative TRAPtest AUC (U), mean (IQR) | 121.1 (101.4–137.0) | 114.4 (94.3–133.3) | 118.7 (99.0–136.1) |
Preoperative ADRtest AUC (U), mean (SD) | 25.3 (13.1) | 24.7 (13.6) | 25.1 (13.3) |
COPTIC B population | N = 1384 | N = 449 | N = 1833 |
Preoperative ADPtest AUC (AU*min), mean (SD) | 76.1 (27.1) | 67.0 (27.7) | 73.8 (27.6) |
Preoperative ASPItest AUC (AU*min), median (IQR) | 27.5 (16.4–71.1) | 22.5 (14.1–47.8) | 25.9 (15.7–65.8) |
Preoperative TRAPtest AUC (AU*min), median (IQR) | 120.8 (101.1–136.9) | 113.2 (95.2–133.1) | 118.7 (99.5–136.3) |
Preoperative ADRtest AUC (AU*min), mean (SD) | 25.1 (13.2) | 24.7 (13.6) | 25.0 (13.3) |
Multiplate ADPtest AUC (AU*min), median (IQR) | 53.8 (37.0–76.5) | 38.6 (26.1–59.9) | 49.8 (33.7–73.1) |
Multiplate ADRtest AUC (AU*min), median (IQR) | 20.5 (13.3–35.7) | 17.3 (11.0–26.9) | 19.7 (12.6–33.4) |
Multiplate AAtest AUC (AU*min), median (IQR) | 119.6 (92.4–147.6) | 105.6 (78.7–133.6) | 115.9 (87.9–144.5) |
Multiplate TRAPtest AUC (AU*min), mean (SD) | 24.9 (14.1) | 23.8 (15.1) | 24.6 (14.4) |
ROTEM INTEM CT (seconds), median (IQR) | 166.0 (151.0–182.5) | 167.0 (154.0–184.0) | 166.0 (152.0–183.0) |
ROTEM INTEM α-angle (°), median (IQR) | 75.0 (72.0–77.0) | 73.0 (69.0–76.0) | 74.0 (71.0–77.0) |
ROTEM INTEM MCF (mm), median (IQR) | 62.0 (58.0–66.0) | 60.0 (55.0–64.0) | 62.0 (57.0–65.0) |
ROTEM INTEM ML (%), median (IQR) | 5.0 (2.0–7.0) | 4.0 (2.0–7.0) | 4.0 (2.0–7.0) |
ROTEM INTEM Vmax (mm/second), mean (SD) | 17.3 (5.3) | 15.4 (5.2) | 16.9 (5.3) |
ROTEM INTEM tVmax (seconds), mean (SD) | 200.7 (35.4) | 207.3 (40.1) | 202.3 (36.7) |
ROTEM EXTEM CT (seconds), median (IQR) | 56.0 (51.0–62.0) | 57.0 (52.0–65.0) | 56.0 (51.0–63.0) |
ROTEM EXTEM α-angle (°), median (IQR) | 74.0 (70.0–77.0) | 72.0 (68.0–75.0) | 74.0 (70.0–77.0) |
ROTEM EXTEM MCF (mm), median (IQR) | 64.0 (60.0–67.0) | 61.0 (56.0–65.0) | 63.0 (59.0–67.0) |
ROTEM EXTEM ML (%), median (IQR) | 5.0 (2.0–8.0) | 4.0 (2.0–7.0) | 4.0 (2.0–8.0) |
ROTEM EXTEM Vmax (mm/second), median (IQR) | 16.0 (13.0–20.0) | 15.0 (12.0–18.0) | 16.0 (13.0–19.0) |
ROTEM EXTEM tVmax (seconds), median (IQR) | 101.0 (77.0–117.0) | 106.0 (82.0–124.0) | 102.0 (79.0–119.0) |
ROTEM FIBTEM MCF (mm), median (IQR) | 13.0 (11.0–17.0) | 12.0 (9.0–15.0) | 13.0 (10.0–17.0) |
ROTEM EXTEM MCF – FIBTEM MCF (mm), median (IQR) | 49.0 (47.0–51.0) | 48.0 (46.0–51.0) | 49.0 (47.0–51.0) |
ROTEM INTEM CT – HEPTEM CT (seconds), median (IQR) | 0.0 (–10.0 to 9.0) | –1.0 (–11.0 to 9.0) | 0.0 (–10.0 to 9.0) |
TEG CK R (minutes), median (IQR) | 6.0 (5.1–7.1) | 5.8 (5.0–6.8) | 5.9 (5.1–7.0) |
TEG CK α-angle (°), median (IQR) | 63.5 (59.0–67.5) | 62.9 (57.7–66.8) | 63.4 (58.7–67.4) |
TEG CK MA (mm), mean (SD) | 59.6 (6.1) | 56.7 (7.0) | 58.9 (6.5) |
TEG CK LY60 (%), median (IQR) | –0.2 (–1.2 to 0.6) | –0.1 (–0.8 to 1.3) | –0.1 (–1.1 to 0.8) |
TEG CK R – CKH R (minutes), median (IQR) | 0.1 (–0.1 to 0.4) | 0.1 (–0.1 to 0.4) | 0.1 (–0.1 to 0.4) |
Results of specific analysis procedures
Step 1: modelling for baseline characteristics
We developed a clinical prediction model for any CCB in the COPTIC A analysis population and a predictive model for postoperative CCB in the COPTIC B analysis population using regression analyses. The baseline characteristics of male sex, absence of diabetes, procedure and antiplatelet treatment group, low preoperative haematocrit, low preoperative platelet count and low BMI explained most of the variation in any CCB and postoperative CCB (Tables 17 and 18). There were a number of similarities between the models: any CCB and postoperative CCB were less evident among female, diabetic and elective patients. Increasing the preoperative haematocrit and increasing BMI were associated with reduced odds of CCB. A reduced preoperative platelet count was also associated with reduced odds of CCB. The odds ratios (ORs) for preoperative platelet count are not comparable between the preoperative analysis and the postoperative analysis as these data were transformed for the any CCB model. In terms of the procedure and treatment categories, compared with the CABG with no treatment group, the participants with the highest odds of CCB were those undergoing CABG and valve replacement on DAPT, although this was a very small group (n = 22 in the preoperative analysis; n = 14 in the postoperative analysis). Increased odds of CCB in relation to the CABG with no treatment group were also observed for CABG and valve replacement patients on aspirin, CABG-only patients on DAPT with withdrawal at 0–2 days before surgery and patients undergoing high-risk other procedures, for any CCB only. Participating in another study also appeared to be related to the odds of CCB; in particular, those participants also enrolled in the TITRe2 RCT4 had increased odds of CCB.
Variable | Any CCB (preoperative analysis) | Postoperative CCB (postoperative analysis) |
---|---|---|
Increased age | Observed, additionally shown using quintiles (not shown here) | Observed, additionally shown using quintiles (not shown here) |
Female | Not observed | Not observed |
Diabetes | Not observed | Not observed |
Other > CABG + valve > valve > CABG only | Observed | CABG + valve > other > valve alone > CABG only |
Operative priority urgent > elective | Observed | Observed |
Decreased eGFR | Observed, additionally shown using quintiles (not shown here) | Observed, additionally shown using quintiles (not shown here) |
Decreased preoperative haematocrit | Observed, additionally shown using quintiles (not shown here) | Not clear |
Low preoperative platelet count (fitted continuously) | Observed, additionally shown using quintiles (not shown here) | Not clear |
Aspirin + P2Y12 blocker (clopidogrel or prasugrel) > aspirin > no drugs | Observed | Observed |
Decreased time from P2Y12 blocker withdrawal | Observed | Observed |
Reduced BMI | Observed, additionally shown using quintiles (not shown here) | Observed, additionally shown using quintiles (not shown here) |
Characteristic | Preoperative analysis (any CCB) | Postoperative analysis (postoperative CCB) | ||
---|---|---|---|---|
OR (95% CI) | p-value | OR (95% CI) | p-value | |
Age | 1.00 (0.99 to 1.02) | 0.52 | 1.01 (1.00 to 1.02) | 0.14 |
Sex, female | 0.62 (0.47 to 0.80) | < 0.001 | 0.57 (0.42 to 0.78) | < 0.001 |
Diabetic | 0.56 (0.42 to 0.75) | < 0.001 | 0.57 (0.41 to 0.79) | 0.001 |
CABG only, no APT | 1.00 | 1.00 | ||
CABG + valve, no APT | 2.15 (1.02 to 4.54) | < 0.001 | 1.46 (0.61 to 3.48) | < 0.001 |
Valve only, no APT | 1.36 (0.72 to 2.58) | 1.06 (0.51 to 2.22) | ||
CABG only, ASP | 0.81 (0.44 to 1.49) | 0.84 (0.42 to 1.69) | ||
CABG + valve, ASP | 4.39 (2.24 to 8.58) | 3.35 (1.56 to 7.20) | ||
Valve only, ASP | 1.94 (0.99 to 3.81) | 1.82 (0.84 to 3.96) | ||
CABG only, DAPT (0–2 days) | 3.63 (1.79 to 7.34) | 3.53 (1.59 to 7.85) | ||
CABG only, DAPT (3–5 days) | 2.04 (1.01 to 4.13) | 2.13 (0.97 to 4.69) | ||
CABG only, DAPT (6–7 days) | 1.13 (0.53 to 2.41) | 1.09 (0.46 to 2.60) | ||
CABG + valve, DAPT (≤ 7 days) | 13.71 (3.91 to 48.10) | 8.59 (2.18 to 33.88) | ||
Valve only, DAPT (≤ 7 days) | 7.32 (1.21 to 44.30) | 4.97 (0.70 to 35.18) | ||
Other high-risk bleeding procedure | 6.31 (3.07 to 12.98) | 1.94 (0.85 to 4.41) | ||
Operative priority elective | 1.00 | 1.00 | ||
Operative priority urgent | 1.28 (1.02 to 1.61) | 0.03 | 1.26 (0.97 to 1.64) | 0.09 |
Preoperative eGFR | 0.99 (0.99 to 1.00) | 0.04 | 1.00 (0.99 to 1.00) | 0.20 |
Preoperative haematocrit | 0.96 (0.94 to 0.99) | 0.002 | 0.97 (0.94 to 0.99) | 0.01 |
BMI | 0.97 (0.94 to 0.99) | 0.01 | 0.96 (0.93 to 0.99) | 0.004 |
Preoperative platelet counta | 14.63 (5.60 to 38.23) | < 0.001 | 1.00 (0.99 to 1.00) | < 0.001 |
Participated in another study | ||||
None | 1.00 | 1.00 | ||
TITRe2 (liberal)4 | 2.03 (1.35 to 3.07) | 0.01 | 2.25 (1.43 to 3.52) | 0.01 |
TITRe2 (restrictive)4 | 1.56 (1.01 to 2.40) | 1.83 (1.12 to 2.97) | ||
PASPORT (control) | 0.71 (0.29 to 1.74) | 0.73 (0.25 to 2.14) | ||
PASPORT (intervention) | 1.34 (0.58 to 3.12) | 1.39 (0.43 to 4.53) | ||
Other (HArVeST/ProMPT/VeRDiCT/Protectionb) | 0.98 (0.67 to 1.44) | 1.19 (0.77 to 1.85) | ||
Constant | 0.53 (0.10 to 2.76) | 0.45 | 4.40 (0.69 to 28.24) | 0.12 |
Predictive models incorporating the baseline characteristics generated AUCs of 0.74 (95% CI 0.72 to 0.76) for any CCB (COPTIC A population) and 0.72 (95% CI 0.69 to 0.75) for postoperative CCB (COPTIC B population). The Hosmer–Lemeshow test demonstrated a satisfactory model fit (p = 0.28 and p = 0.59 for the COPTIC A and B baseline models respectively) and the Brier statistics were close to zero (0.18 for the COPTIC A model and 0.16 for the COPTIC B model). Bootstrapping the estimates of the baseline characteristics-only model gave AUCs of 0.74 (95% CI 0.72 to 0.76) for the COPTIC A population and 0.72 (95% CI 0.69 to 0.75) for the COPTIC B population and internal cross-validation gave AUCs of 0.74 (95% CI 0.72 to 0.76) for the COPTIC A population and 0.72 (95% CI 0.69 to 0.75) for the COPTIC B population. This model correctly classified 76.8% of participants in the COPTIC B analysis population as having CCB or having no CCB. The adapted baseline characteristics model for predicting any CCB in the COPTIC study, allowing for differences between the COPTIC population and the test data set, as described in Clinical prediction model, had an AUC of 0.70 (95% CI 0.67 to 0.73). The predictive model was externally validated in a cohort of 1611 participant recruited to the TITRe2 trial at 16 UK cardiac surgery centres (excluding Bristol) between July 2009 and February 2013. 4 The adapted model is reported in Table 19. The AUC for any CCB in the TITRe2 cohort was 0.64 (95% CI 0.60 to 0.67).
Characteristic | OR (95% CI) | p-value |
---|---|---|
Age | 1.01 (1.00 to 1.03) | 0.04 |
Sex, female | 0.52 (0.39 to 0.70) | < 0.001 |
Diabetic | 0.58 (0.42 to 0.80) | 0.001 |
CABG only, no APT | 1.00 | < 0.001 |
CABG + valve, no APT | 1.64 (0.69 to 3.89) | |
Valve only, no APT | 1.25 (0.61 to 2.60) | |
CABG only, ASP | 0.86 (0.43 to 1.71) | |
CABG + valve, ASP | 3.79 (1.78 to 8.05) | |
Valve only, ASP | 2.21 (1.03 to 4.76) | |
CABG only, DAPT (0–5 days) | 2.57 (1.23 to 5.36) | |
CABG only, DAPT (6–7 days) | 1.08 (0.45 to 2.58) | |
CABG + valve, DAPT (≤ 7 days) | 9.88 (2.55 to 38.31) | |
Valve only, DAPT (≤ 7 days) | 4.4 (0.64 to 30.35) | |
Other high-risk bleeding procedure | 2.51 (1.12 to 5.66) | |
Operative priority elective | 1.00 | 0.29 |
Operative priority urgent | 1.15 (0.89 to 1.49) | |
Preoperative eGFR | 1.00 (0.99 to 1.00) | 0.24 |
Preoperative haematocrit | 0.97 (0.94 to 1.00) | 0.03 |
BMI | 0.96 (0.93 to 0.99) | 0.01 |
Constant | 1.29 (0.22 to 7.41) | 0.78 |
Step 2: modelling for test predictors
Sequential addition of postoperative MEA, postoperative ROTEM and postoperative TEG test results to the baseline characteristics model caused a progressive increase in the AUC for prediction of postoperative CCB.
Model 2A: predictive accuracy of preoperative Multiplate (multiple electrode aggregometry) values for any clinical concern about bleeding
Of the preoperative MEA test predictors, only the ASPItest AUC was significantly associated with any CCB after taking the baseline characteristics into account; increasing the ASPItest AUC was associated with reduced odds of CCB (Table 20). The difference between the ROC curves illustrated in Figure 12 was not significant (0.74 vs. 0.74; p = 0.274), which is clearly demonstrated by the overlapping curves for the baseline model and the baseline model plus Multiplate preoperative measurements. To examine early test results, ASPI velocity was included as a potential determinant of CCB along with the ASPItest AUC. The selection process identified the ASPItest AUC as having a stronger effect. After bootstrapping the estimates, the model had an AUC of 0.74 (95% CI 0.72 to 0.76); cross-validation resulted in an AUC of 0.74 (95% CI 0.72 to 0.76). The model had an adequate fit (Hosmer–Lemeshow p-value = 0.28; Brier score = 0.18).
Test predictor | OR (95% CI) | p-value |
---|---|---|
ASPItest AUC ÷ 10a | 0.97 (0.94 to 1.00) | 0.02 |
Constant | 1.00 (0.91 to 1.10) | 0.99 |
preop_score | 1.00 |
FIGURE 12.
Preoperative Multiplate (MEA) measurements in relation to any CCB (model 2A).
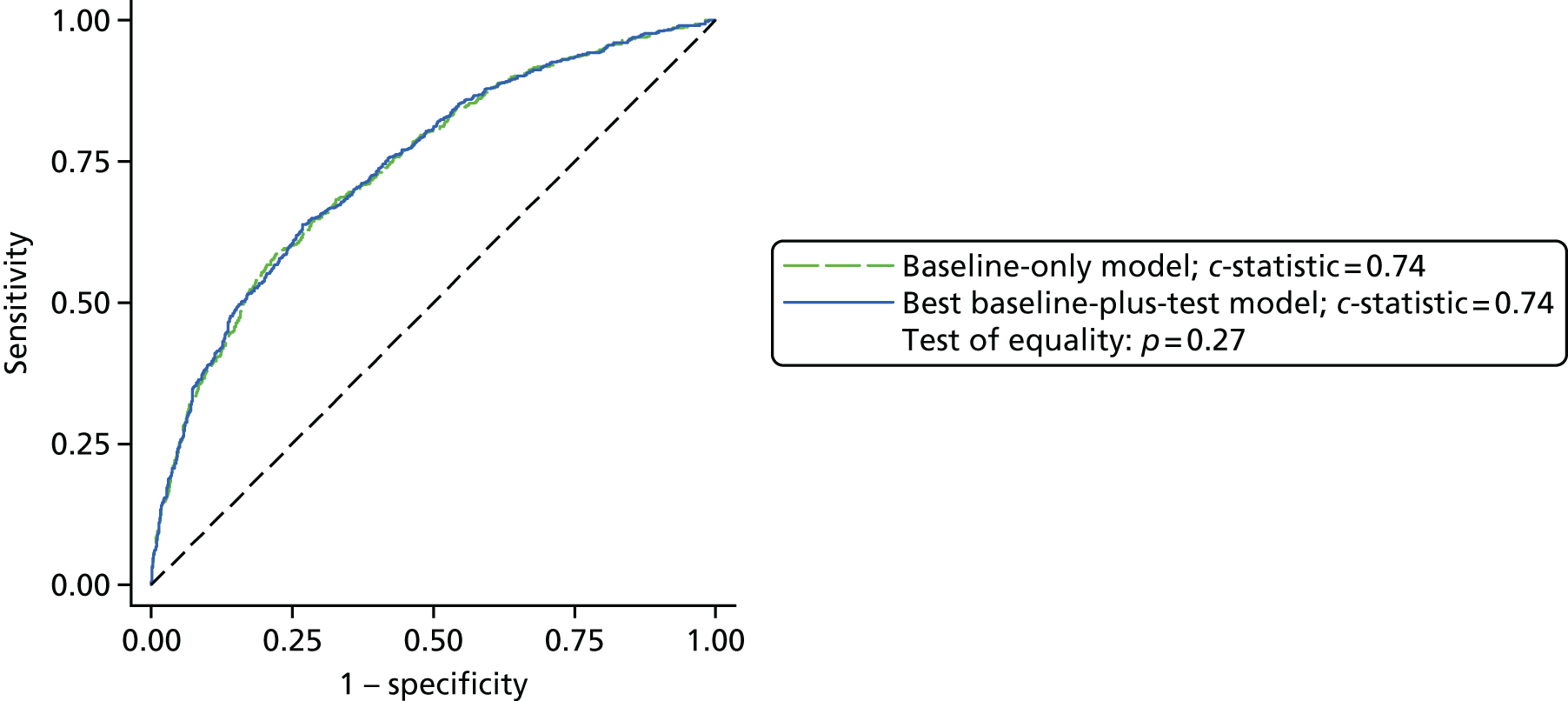
Model 2B: predictive accuracy of preoperative Multiplate (multiple electrode aggregometry) values for postoperative clinical concern about bleeding
Only the preoperative ASPItest AUC was significantly associated with postoperative CCB after taking the baseline characteristics into account (Table 21). Increasing the preoperative ASPItest AUC was associated with reduced odds of CCB. The difference between the ROC curves was not significant (0.72 vs. 0.72; p = 0.3603; Figure 13). To examine early test results, the ASPI velocity and ASPItest AUC were both included as potential determinants of CCB. The selection process identified the ASPI velocity as having a stronger effect (ROC 0.72); however, this was still not significantly better than the clinical risk model (Table 22).
Test predictor | OR (95% CI) | p-value |
---|---|---|
ASPItest AUC ÷ 10a | 0.96 (0.93 to 1.00) | 0.03 |
Constant | 1.00 (0.88 to 1.11) | 0.87 |
postop_score | 1.00 |
FIGURE 13.
Preoperative Multiplate (MEA) measurements in relation to postoperative CCB (model 2B).

Test predictor | OR (95% CI) | p-value |
---|---|---|
ASPI velocity | 0.98 (0.97 to 1.00) | 0.03 |
Constant | 0.99 (0.88 to 1.11) | 0.86 |
postop_score | 1.00 |
Model 2C: predictive accuracy of postoperative Multiplate (multiple electrode aggregometry) values for postoperative clinical concern about bleeding (COPTIC B study)
The natural log of the postoperative ADPtest AUC and the postoperative ADRENtest AUC were significantly associated with postoperative CCB after taking the baseline characteristics into account (Table 23); increasing the log of the postoperative ADPtest AUC was associated with reduced odds of CCB and increasing the postoperative ADRENtest AUC was associated with increased odds of CCB. The difference between the ROC curves was statistically significant (best baseline characteristics plus test 0.74 vs. baseline characteristics-only model 0.72; p = 0.001; Figure 14). To examine early test results, ADPtest velocity and ADRENtest velocity were both included as potential determinants of CCB alongside the ADPtest AUC and the ADRENtest AUC. The selection process identified the model detailed in Table 23 as the best model (ROC 0.74).
Test predictor | OR (95% CI) | p-value |
---|---|---|
Loge (ADPtest AUC ÷ 100) | 0.43 (0.32 to 0.57) | < 0.001 |
ADRENtest AUC ÷ 10 | 1.18 (1.06 to 1.31) | 0.002 |
Constant | 0.82 (0.72 to 1.11) | 0.94 |
postop_score | 1.00 |
FIGURE 14.
Postoperative Multiplate (MEA) measurements in relation to postoperative CCB (model 2C).

Model 2D: predictive accuracy of postoperative rotational thromboelastometry for postoperative clinical concern about bleeding
Of the postoperative ROTEM predictors, only INTEM MCF was significantly associated with CCB after taking baseline characteristics into account. ROTEM FIBTEM MCF was dropped from the model because of colinearity. Increasing the INTEM MCF was associated with reduced odds of CCB (OR 0.95, 95% CI 0.94 to 0.97; Table 24). The difference between the ROC curves was statistically significant (best baseline characteristics plus test model 0.73 vs. baseline characteristics-only model 0.72; p = 0.0148; Figure 15). To examine early test results, INTEM A5 and INTEM A10 (measurements 5 and 10 minutes after starting the analysis) were both included as potential determinants of CCB alongside INTEM MCF. The selection process identified the standard tests model, that is, the model with results available at test completion, as the best model (ROC 0.73).
Test predictor | OR (95% CI) | p-value |
---|---|---|
ROTEM INTEM MCF | 0.95 (0.94 to 0.97) | < 0.001 |
Constant | 0.96 (0.85 to 1.08) | 0.48 |
postop_score | 1.00 |
FIGURE 15.
Postoperative ROTEM measurements in relation to postoperative CCB (model 2D).

Model 2E: predictive accuracy of postoperative thromboelastography for postoperative clinical concern about bleeding
Of the postoperative TEG values, celite kaolin (CK) α-angle and CK maximum amplitude (MA) were both significantly associated with CCB after taking baseline characteristics into account; increasing the CK α-angle was associated with increased odds of CCB (OR 1.02, 95% CI 1.00 to 1.04) and increasing the CK MA was associated with reduced odds of CCB (OR 0.93, 95% CI 0.91 to 0.96; Table 25). The difference between the ROC curves was statistically significant (best baseline characteristics plus test model 0.74 vs. baseline characteristics-only model 0.72; p = 0.006; Figure 16). To examine early test results, CK A30 (amplitude 30 minutes after clotting time) was included as a potential determinant of CCB alongside the CK α-angle and CK MA. The selection process selected CK A30 as a stronger predictor than the combination of CK α-angle and CK MA (see Table 25). The ROC for this model was 0.73, higher than that for the baseline model (0.72; p = 0.0167). However, the ROC for the initial model that did not use rapid rest results (Table 26) was marginally higher (0.74) and so may be considered preferable.
FIGURE 16.
Postoperative TEG measurements in relation to postoperative CCB (model 2E).

Test predictor | OR (95% CI) | p-value |
---|---|---|
CK α-angle | 1.02 (1.00 to 1.04) | 0.09 |
CK MA | 0.93 (0.91 to 0.96) | < 0.001 |
Constant | 0.94 (0.84 to 1.06) | 0.35 |
postop_score | 1.00 |
Test predictor | OR (95% CI) | p-value |
---|---|---|
CK A30 | 0.95 (0.93 to 0.97) | < 0.001 |
Constant | 0.96 (0.85 to 1.07) | 0.45 |
postop_score | 1.00 |
Step 3: modelling for the predictive accuracy of combinations of postoperative thromboelastography/rotational thromboelastometry and Multiplate parameters
Model 2C (postoperative Multiplate parameters) and model 2E (TEG parameters) appear to demonstrate marginally better prediction of CCB than model 2D (ROTEM parameters). Step 3 considered whether the combination of postoperative measurements from the viscoelastic and Multiplate platforms could improve the model further.
All measurements from each of these models were considered for inclusion in the model. The test results for CK α-angle, CK MA, CK R – celite kaolin heparinase (CKH) R (TEG parameters) and ADRENtest AUC (Multiplate) were centred; the ADPtest AUC was log-transformed and centred. The final model from this stage is shown in Figure 17 and Table 27. The difference between the ROC curves was statistically significant (best baseline characteristics plus test model 0.75 vs. baseline characteristics-only model 0.72; p < 0.001). To test whether the addition of the postoperative Multiplate measurements significantly improved the model based on TEG parameters alone, the ROC curve for the TEG model was compared with the ROC curve for the model based on TEG and postoperative Multiplate parameters (0.75 vs. 0.74; p = 0.003). Additionally, the inclusion of the early-phase alternative parameters demonstrated that TEG CK A30 was a marginally inferior predictor than TEG CK MA, with a ROC curve of 0.75. After bootstrapping the estimates, the model had an AUC of 0.74 (95% CI 0.72 to 0.77); cross-validation resulted in an AUC of 0.74 (95% CI 0.72 to 0.77). The model had an adequate fit (Hosmer–Lemeshow p-value = 0.15; Brier score = 0.16).
FIGURE 17.
Postoperative TEG and postoperative Multiplate measurements in relation to postoperative CCB (model 3).
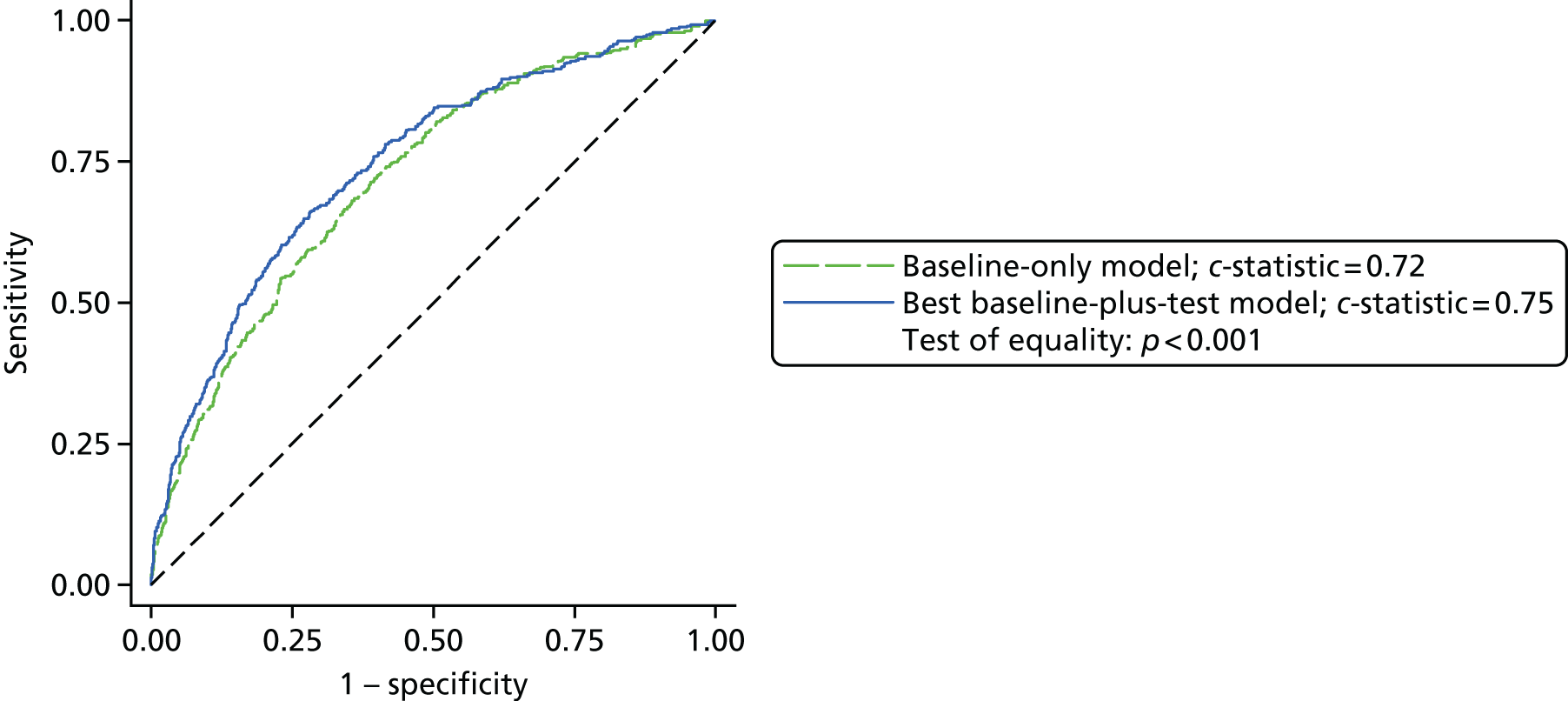
Test predictor | OR (95% CI) | p-value |
---|---|---|
CK MA | 0.96 (0.94 to 0.98) | < 0.001 |
Loge (ADPtest AUC ÷ 100) | 0.50 (0.37 to 0.67) | < 0.001 |
ADRENtest AUC ÷ 10 | 1.19 (1.07 to 1.32) | 0.001 |
Constant | 0.82 (0.71 to 0.94) | 0.004 |
postop_score | 1.00 |
As the ROC curve for the model based on TEG parameters (model 2E) was only marginally better than that for the model based on ROTEM parameters (model 2D), this approach was repeated for the ROTEM parameters, that is, the postoperative Multiplate parameters were also considered for inclusion in the model (Figure 18 and Table 28). Increasing the ROTEM INTEM α-angle and log-transformed ADPtest AUC were associated with reduced odds of CCB. Increasing the ADRENtest AUC was associated with increased odds of CCB. The ROC curve for the model based on the ROTEM and postoperative Multiplate parameters was significantly better than that for the baseline model (0.74 vs. 0.72; p < 0.001). The difference in ROC curves between the ROTEM model and the model with both ROTEM and postoperative Multiplate parameters was significant (0.74 vs. 0.73; p = 0.01), suggesting that the addition of the postoperative Multiplate parameters improved the predictive ability of the model. Inclusion of the early-phase tests as alternatives to the standard test results did not change this final model.
FIGURE 18.
Postoperative ROTEM and postoperative Multiplate measurements in relation to postoperative CCB (model 3).

Test predictor | OR (95% CI) | p-value |
---|---|---|
ROTEM INTEM α-angle | 0.96 (0.93 to 0.98) | < 0.001 |
Loge (ADPtest AUC ÷ 100) | 0.49 (0.36 to 0.66) | < 0.001 |
ADRENtest AUC | 1.18 (1.06 to 1.31) | 0.002 |
Constant | 0.82 (0.71 to 0.94) | 0.01 |
postop_score | 1.00 |
Step 4: modelling for preoperative and postoperative test predictors
Model 3 with the TEG and postoperative Multiplate parameters appears to be marginally better than the model based on TEG parameters alone. Step 4 considered whether the inclusion of the preoperative Multiplate measurements could improve this model further. No terms were selected and so the model reported in step 3 remains the best-performing predictive model. The increase in AUC attributable to the inclusion of the postoperative TEG and Multiplate results corresponded to an increase in the correct classification of only 0.98% of participants compared with the baseline model. From the baseline model to the best-performing predictive model, 63 participants were correctly reclassified as having CCB and 45 were incorrectly reclassified, a net improvement of 18 participants (NRI = 0.078; p < 0.001).
Sensitivity analyses of point-of-care test results
We used sensitivity analyses to address uncertainty around the definition of our reference outcome. As stated previously, all components of the CCB definition have limitations. The use of an early pro-haemostatic treatment may overestimate the frequency of coagulopathic bleeding, whereas outcomes such as drain losses or reoperation for bleeding may underestimate the frequency of coagulopathic haemorrhage. We therefore tested the predictive accuracy of the models developed in the base-case analysis using revised definitions of the primary outcome. We repeated the processes for model 1 and models 2A–E, with new models fitted for each sensitivity analysis. The parameters for each model were reselected for each step. The AUCs for these sensitivity analyses are reported in Table 29. The revised CCB definitions were as follows:
-
In SA1, those defined as having received early pro-haemostatic treatment but who did not experience excessive bleeding (chest drain < 600 ml at 6 hours post operation) or require reoperation for bleeding were excluded from the analyses. This changed the frequency of the primary outcome as follows: any CCB, 351 out of 1862 (18.9%) participants; postoperative CCB, 268 out of 1652 (16.2%) participants. This revised definition resulted in only marginal improvements in predictive accuracy relative to the base-case analysis (see Table 29). Furthermore, it resulted in a reduction in the best-performing model (model 3).
-
In SA2, those defined as having received early pro-haemostatic treatment but who did not experience excessive bleeding (chest drain < 600 ml at 6 hours post operation) or require reoperation for bleeding were included in the analyses but were reclassified as being free from CCB. This changed the frequency of the primary outcome as follows: any CCB, 351 out of 2197 (16.0%) participants; postoperative CCB, 268 out of 1833 (14.6%) participants. The discrimination of all of the models was markedly reduced using this definition (see Table 29).
-
In SA3, after reviewing the results of our base-case analysis and the sensitivity analyses, we considered whether a definition of ‘more severe’ CCB would improve discrimination. We hypothesised that any pro-haemostatic therapy beyond the ‘first line’ treatment of bleeding, which in the Bristol Heart Institute consists of 1 unit of pooled platelets and 2 units of FFP, would denote more severe coagulopathic haemorrhage. We also hypothesised that a definition used in previous analyses of > 4 units of red cells transfused within 12 hours of surgery would also denote severe coagulopathy. Ultimately, this latter criterion was not included in the definition. Of the 13 COPTIC participants who received > 4 units of red cells in this period, nine were already classified as having CCB. Three were classified as not having CCB because their reoperation was defined as having a surgical cause. Consequently, one additional participant would now be classified as having CCB; this participant did not have a chest drain loss of > 600 ml at 6 hours, did not have a reoperation for bleeding and did not receive any products other than the > 4 units of red cells. This revised definition of CCB resulted in the following estimates of CCB: any CCB, 396 out of 1832 (21.6%) participants; postoperative CCB, 349 out of 1832 (19.1%) participants. Using this revised definition in a post hoc analysis did not improve the discrimination of any of the models and marginally reduced the discrimination of the best-performing model (model 3; see Table 29).
Model | Original analysis | SA1 | SA2 | SA3 |
---|---|---|---|---|
Baseline characteristics (preoperative) | 0.74 (0.71 to 0.76) | 0.75 (0.72 to 0.78) | 0.70 (0.67 to 0.74) | 0.73 (0.71 to 0.76) |
Model 2A: preoperative Multiplate parameters vs. any CCB | 0.74 (0.72 to 0.77) | 0.75 (0.72 to 0.78) | 0.71 (0.67 to 0.74) | 0.74 (0.71 to 0.77) |
Baseline characteristics (postoperative) | 0.72 (0.69 to 0.75) | 0.72 (0.69 to 0.75) | 0.70 (0.67 to 0.74) | 0.71 (0.68 to 0.74) |
Model 2B: preoperative Multiplate parameters vs. postoperative CCB | 0.72 (0.70 to 0.75) | 0.73 (0.69 to 0.76) | 0.71 (0.67 to 0.74) | 0.72 (0.69 to 0.75) |
Model 2C: postoperative Multiplate parameters vs. postoperative CCB | 0.74 (0.71 to 0.76) | 0.74 (0.70 to 0.77) | 0.72 (0.69 to 0.75) | 0.73 (0.70 to 0.76) |
Model 2D: postoperative ROTEM parameters vs. postoperative CCB | 0.73 (0.71 to 0.76) | 0.74 (0.71 to 0.77) | 0.72 (0.69 to 0.75) | 0.73 (0.70 to 0.76) |
Model 2E: postoperative TEG parameters vs. postoperative CCB | 0.74 (0.71 to 0.76) | 0.74 (0.71 to 0.77) | 0.72 (0.689 to 0.75) | 0.73 (0.70 to 0.76) |
Model 3: postoperative TEG plus postoperative Multiplate parameters vs. postoperative CCB | 0.75 (0.72 to 0.77) | 0.72 (0.69 to 0.76) | 0.70 (0.66 to 0.73) | 0.74 (0.71 to 0.77) |
Model 3: postoperative ROTEM plus postoperative Multiplate parameters vs. postoperative CCB | 0.74 (0.72 to 0.77) | 0.73 (0.69 to 0.76) | 0.69 (0.66 to 0.72) | 0.74 (0.71 to 0.77) |
Secondary outcomes
The preoperative Multiplate results and the postoperative Multiplate, ROTEM and TEG results are shown according to the analysis population and occurrence of the secondary outcomes in Appendix 1 (see Tables 103–109). Inclusion of near-patient test results improved the model prediction for any postoperative red cell transfusion (AUC 0.85, 95% CI 0.83 to 0.87 for the baseline model plus postoperative TEG results vs. AUC 0.83, 95% CI 0.81 to 0.85 for the baseline model alone; p < 0.001) and sepsis (AUC 0.74, 95% CI 0.70 to 0.79 for baseline model plus postoperative ROTEM results vs. AUC 0.70, 95% CI 0.65 to 0.75 for the baseline model alone; p = 0.01; Table 30). Inclusion of near-patient test results did not increase the predictive value of the baseline model for the other secondary outcomes (see Table 30).
Secondary outcome | Baseline model AUC (95% CI) | Best predictive modelb AUC (95% CI) | p-value |
---|---|---|---|
Red cells intraoperatively/postoperativelyc (0 vs. ≥ 1 units) | 0.85 (0.83 to 0.87) | 0.85 (0.83 to 0.87) | 0.58 |
Red cells intraoperatively/postoperativelyc (≤ 4 vs. > 4 units) | 0.85 (0.81 to 0.89) | No change | – |
Red cells postoperatively (0 vs. ≥ 1 units) | 0.83 (0.81 to 0.85) | 0.85 (0.83 to 0.87) | < 0.001 |
Red cells postoperatively (≤ 4 vs. > 4 units) | 0.83 (0.78 to 0.88) | 0.84 (0.78 to 0.89) | 0.44 |
Death | 0.84 (0.77 to 0.91) | No change | – |
MI | 0.68 (0.55 to 0.82) | No change | – |
Stroke | 0.81 (0.72 to 0.90) | No change | – |
AKI | 0.76 (0.74 to 0.78) | 0.76 (0.74 to 0.79) | 0.08 |
Sepsis | 0.70 (0.65 to 0.75) | 0.74 (0.70 to 0.79) | 0.01 |
Analysis of the predictive accuracy of laboratory reference tests
Overview
Two questions were considered in this analysis:
-
LRT analysis 1 – can the predictive accuracy of a risk model for any CCB derived from baseline clinical factors be improved by the addition of data from automated cell counters used on blood samples obtained preoperatively? This analysis tested the hypothesis that measures of the differential platelet count, immature platelet count and MPV, along with other data obtained from automated cell counters preoperatively, such as the haematocrit, may be used to predict CCB, allowing earlier and more effective intervention.
-
LRT analysis 2 – can the predictive accuracy of a risk model for postoperative CCB derived from baseline clinical factors be improved by the addition of all pre- and postoperative test results from automated cell counters and specific LRTs of coagulation using blood samples obtained at the end of surgery? This analysis tested the hypothesis that the use of pre- and postoperative data from automated cell counters as well as postoperative measures of specific components of the coagulation pathway, or pathway activity, may be used to more accurately predict those patients likely to bleed and allow targeted prevention strategies.
Study and analysis cohorts
As with the analyses of the POC tests, the final analysis data set was defined as those with complete baseline characteristics data, primary outcome data and at least one of the reference tests considered [PT, aPTT, D-dimer, fibrinogen, anti-Xa, vWF, ETP, factor XIII, platelet count and haematocrit]. As all reference tests were considered together and not as a comparison across platforms, any participant who had at least one test result was included in the analysis data set.
Table 31 describes the population included in the analysis data set (n = 2226) and those who consented but who were excluded (n = 315) (Figure 19). The mean age of the analysis population was 67 years. The relative proportions of the groups categorised by antiplatelet treatment or by type of surgical procedure were similar to those described in the COPTIC B analysis. There were some differences between the analysis population and the population excluded from the analysis (see Table 31). The proportion of males was lower in the excluded group (68.9% vs. 75.8%; SMD 0.16) and the median age was younger among those excluded (median 67.7 vs. 69.0 years; SMD 0.18). The proportion of patients undergoing valve replacement surgery and receiving aspirin was higher in the excluded group than in the analysis data set (14.0% vs. 7.5%; SMD 0.24). These differences were considered small. There were no other differences between the analysed population and the excluded population and we concluded that the two groups were similar.
Characteristic | Analysis data set (N = 2226) | Consented participants not in analysis data set (N = 315) | SMD |
---|---|---|---|
Age (years), mean (SD) | 67.39 (11.6) | 65.86 (13.6) | 0.18 |
Age (years), median (IQR) | 69.0 (61.8–75.7) | 67.7 (58.3–76.3) | – |
Sex male, n/N (%) | 1688/2226 (75.8) | 217/315 (68.9) | 0.16 |
Diabetes, n/N (%) | 450/2226 (20.2) | 49/266 (18.4) | 0.04 |
Procedure and treatment group, n/N (%) | |||
CABG only, no APT | 77/2226 (3.5) | 2/143 (1.4) | 0.11 |
CABG + valve, no APT | 82/2226 (3.7) | 4/143 (2.8) | 0.05 |
Valve only, no APT | 377/2226 (16.9) | 23/143 (16.1) | 0.02 |
CABG only, ASP | 863/2226 (38.8) | 44/143 (30.8) | 0.16 |
CABG + valve, ASP | 163/2226 (7.3) | 13/143 (9.1) | 0.07 |
Valve only, ASP | 168/2226 (7.5) | 20/143 (14.0) | 0.24 |
CABG only, DAPT (0–2 days) | 118/2226 (5.3) | 10/143 (7.0) | 0.07 |
CABG only, DAPT (3–5 days) | 128/2226 (5.8) | 8/143 (5.6) | 0.01 |
CABG only, DAPT (6–7 days) | 105/2226 (4.7) | 10/143 (7.0) | 0.11 |
CABG + valve, DAPT (≤ 7 days) | 21/2226 (0.9) | 1/143 (0.7) | 0.03 |
Valve only, DAPT (≤ 7 days) | 7/2226 (0.3) | 0/143 (0.0) | 0.06 |
Other high-risk bleeding procedure | 117/2226 (5.3) | 8/143 (5.6) | 0.02 |
Operative priority, n/N (%) | |||
Elective | 1467/2226 (65.9) | 191/315 (60.6) | 0.11 |
Urgent | 759/2226 (34.1) | 124/315 (39.4) | 0.11 |
eGFR (ml/minute/1.73m2), mean (SD) | 77.11 (29.2) | 77.64 (29.7) | 0.02 |
eGFR (ml/minute/1.73m2), median (IQR) | 73.9 (57.0–93.5) | 72.1 (55.5–97.5) | – |
BMI (kg/m2), mean (SD) | 28.00 (4.7) | 27.51 (4.8) | 0.10 |
BMI (kg/m2), median (IQR) | 27.4 (24.7–30.8) | 27.0 (23.9–30.5) | – |
FIGURE 19.
Flow chart for the COPTIC study: LRTs. a, Defined as eligible: surgery, not weekend, not emergency, not bank holiday, not Easter/Christmas study closure dates, aged ≥ 18 years.

Clinical outcomes
The primary outcome of any CCB was recorded in 684 out of 2200 (31.1%) of the reference test cohort for the analysis of preoperative automated cell counter data (LRT analysis 1) (Figure 20).
FIGURE 20.
Overlap between components of the primary outcome of any CCB in the reference test analysis population (LRT analysis 1) (participants with missing data for one component of the primary outcome are not included in the Venn diagram).

The primary outcome of postoperative CCB was recorded in 572 out of 2226 (25.7%) of the reference test cohort for the analysis of postoperative automated cell counter data and LRTs of coagulation (LRT analysis 2) (Figure 21).
FIGURE 21.
Overlap between components of the primary outcome of postoperative CCB in the reference test analysis population (LRT analysis 2) (participants with missing data for one component of the primary outcome are not included in the Venn diagram).
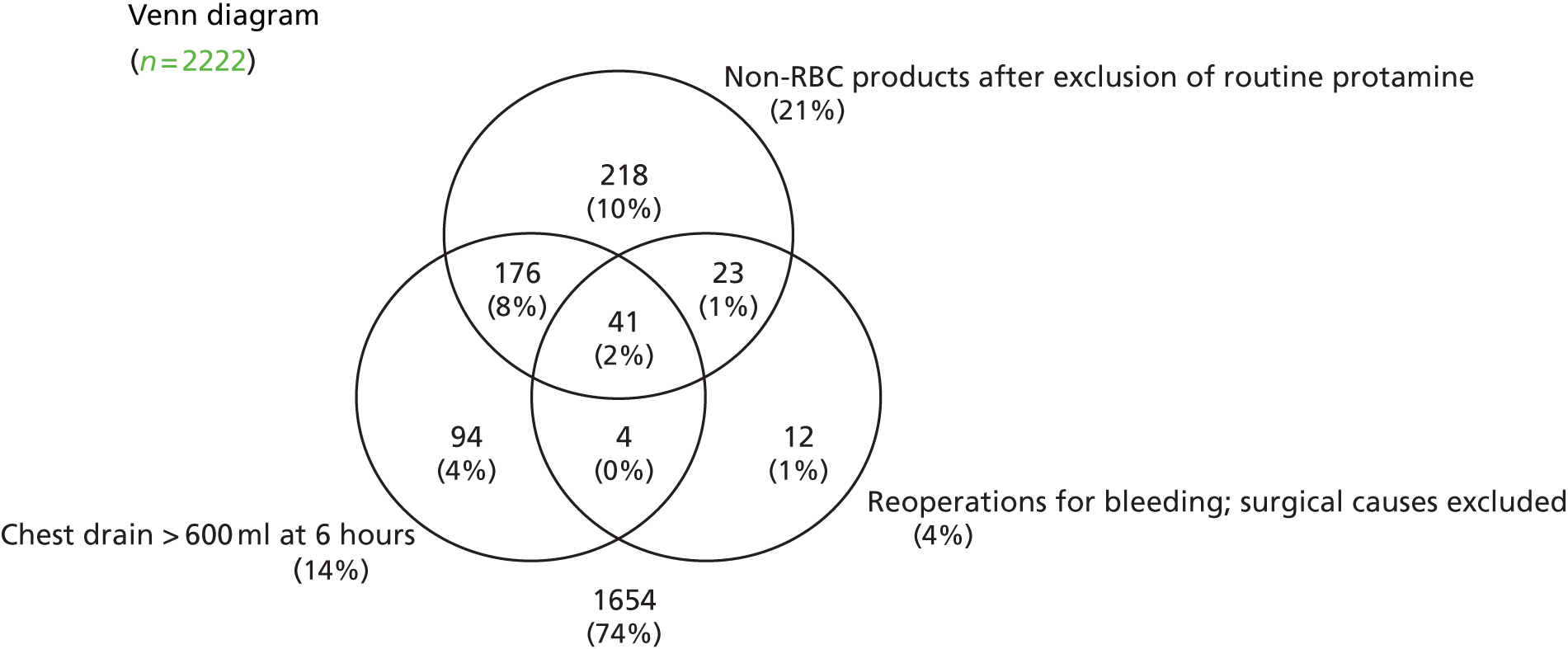
The proportions of the different components of the primary outcomes were as for the COPTIC B analysis population, as were the secondary outcomes (see Figures 20 and 21 and Table 32).
Outcome | Analysis data set (N = 2226), n/N (%) |
---|---|
Primary outcome: any CCB (LRT analysis 1) | 690/2206 (31.3) |
Postoperative blood loss of > 600 ml after 6 hoursa | 316/2184 (14.5) |
Intervention with a non-routine early pro-haemostatic treatmenta | 590/2160 (27.3) |
Reoperation for bleeding recorded during hospital stay, no surgical cause | 82/2187 (3.8) |
Primary outcome: postoperative CCB (LRT analysis 2) | 572/2226 (25.7) |
Postoperative blood loss of > 600 ml after 6 hoursa | 316/2184 (14.5) |
Intervention with a non-routine early pro-haemostatic treatmenta | 460/2186 (21.0) |
Reoperation for bleeding recorded during hospital stay, no surgical cause | 82/2187 (3.8) |
Secondary outcomes collected prior to discharge | |
Red cell transfusion given intraoperatively or postoperativelya | 812/2154 (37.76) |
≥ 5 units of red cells transfused intraoperatively or postoperativelya | 125/2152 (5.8) |
Red cell transfusion given postoperatively (surgical causes excluded) | 719/2177 (33.0) |
> 4 units of red cells transfused postoperatively (surgical causes excluded) | 77/2186 (3.5) |
Intervention with a non-routine early pro-haemostatic treatmenta | 460/2186 (21.0) |
Mortality | 41/2226 (1.8) |
MI | 26/2219 (1.2) |
Any stroke | 29/2219 (1.3) |
AKI | 988/2226 (44.4) |
Stage 1 | 987/2226 (44.3) |
Stage 2 | 257/2226 (11.6) |
Stage 3 | 151/2226 (6.8) |
Infective complication | 133/2215 (6.0) |
Baseline characteristics and clinical concern about bleeding
The frequencies of expected baseline risk factors in participants who did or did not develop any CCB (LRT analysis 1) are provided in Table 33. The frequencies of expected baseline risk factors in participants who did or did not develop postoperative CCB (LRT analysis 2) are provided in Table 34.
Characteristic | No CCB (N = 1516) | Any CCB (N = 690) | Adjusted ORa (95% CI) | p-value |
---|---|---|---|---|
Age (years), median (IQR) | 68.1 (61.3–74.9) | 71.2 (63.3–77.5) | 1.01 (1.00 to 1.02) | 0.11 |
Sex male, n (%) | 1139 (75.1) | 538 (78.0) | 0.54 (0.42 to 0.69) | < 0.001 |
Diabetes, n (%) | 345 (22.8) | 100 (14.5) | 0.60 (0.45 to 0.79) | 0.002 |
Procedure and treatment group, n (%) | ||||
CABG only, no APT | 61 (4.0) | 16 (2.3) | 1.00 | < 0.001 |
CABG + valve, no APT | 48 (3.2) | 34 (4.9) | 2.21 (1.07 to 4.56) | |
Valve only, no APT | 266 (17.5) | 109 (15.8) | 1.43 (0.77 to 2.66) | |
CABG only, ASP | 699 (46.1) | 155 (22.5) | 0.78 (0.43 to 1.40) | |
CABG + valve, ASP | 72 (4.7) | 90 (13.0) | 3.99 (2.08 to 7.65) | |
Valve only, ASP | 101 (6.7) | 65 (9.4) | 2.19 (1.14 to 4.22) | |
CABG only, DAPT (0–2 days) | 63 (4.2) | 55 (8.0) | 3.24 (1.63 to 6.44) | |
CABG only, DAPT (3–5 days) | 79 (5.2) | 47 (6.8) | 2.01 (1.01 to 3.99) | |
CABG only, DAPT (6–7 days) | 81 (5.3) | 23 (3.3) | 0.99 (0.47 to 2.09) | |
CABG + valve, DAPT (≤ 7 days) | 3 (0.2) | 18 (2.6) | 17.86 (4.57 to 69.83) | |
Valve only, DAPT (≤ 7 days) | 2 (0.1) | 5 (0.7) | 7.04 (1.21 to 41.08) | |
Other high-risk bleeding procedure | 41 (2.7) | 73 (10.6) | 6.49 (3.23 to 13.06) | |
Operative priority, n (%) | ||||
Elective | 1014 (66.9) | 440 (63.8) | 1.00 | 0.11 |
Urgent | 502 (33.1) | 250 (36.2) | 1.15 (0.92 to 1.45) | |
eGFR (ml/minute/1.73m2), median (IQR) | 76.4 (59.2–96.7) | 69.6 (51.8–86.6) | 1.00 (0.99 to 1.00) | 0.08 |
BMI (kg/m2), mean (SD) | 28.37 (4.6) | 27.12 (4.7) | 51.01 (4.48 to 581.40) | < 0.001 |
Characteristic | No CCB (N = 1654) | Postoperative CCB (N = 572) | Adjusted ORa (95% CI) | p-value |
---|---|---|---|---|
Age (years), median (IQR) | 68.2 (61.2–75.0) | 69.26 (64.1–77.7) | 1.01 (1.00 to 1.02) | 0.05 |
Sex male, n (%) | 1228 (74.2) | 435 (80.3) | 0.53 (0.41 to 0.69) | < 0.001 |
Diabetes, n (%) | 365 (22.1) | 77 (14.2) | 0.65 (0.49 to 0.86) | 0.002 |
Procedure and treatment group, n (%) | ||||
CABG only, no APT | 61 (3.7) | 16 (2.8) | 1.00 | < 0.001 |
CABG + valve, no APT | 56 (3.4) | 26 (4.5) | 1.38 (0.66 to 2.89) | |
Valve only, no APT | 293 (17.7) | 84 (14.7) | 1.03 (0.55 to 1.92) | |
CABG only, ASP | 721 (43.6) | 142 (24.8) | 0.38 (0.43 to 1.23) | |
CABG + valve, ASP | 84 (5.1) | 79 (13.8) | 2.92 (1.53 to 5.58) | |
Valve only, ASP | 112 (6.8) | 56 (9.80) | 1.72 (0.89 to 3.32) | |
CABG only, DAPT (0–2 days) | 70 (4.2) | 48 (8.4) | 2.52 (1.27 to 5.02) | |
CABG only, DAPT (3–5 days) | 88 (5.3) | 40 (7.0) | 1.56 (0.78 to 3.13) | |
CABG only, DAPT (6–7 days) | 83 (5.0) | 22 (3.8) | 0.97 (0.46 to 2.04) | |
CABG + valve, DAPT (≤ 7 days) | 8 (0.5) | 13 (2.3) | 4.31 (1.48 to 12.57) | |
Valve only, DAPT (≤ 7 days) | 4 (0.2) | 3 (0.5) | 2.03 (0.40 to 10.34) | |
Other high-risk bleeding procedure | 74 (4.5) | 43 (7.5) | 2.07 (1.04 to 4.15) | |
Operative priority, n (%) | ||||
Elective | 1107 (66.9) | 360 (62.9) | 1.00 | 0.14 |
Urgent | 547 (33.1) | 212 (37.1) | 1.19 (0.94 to 1.50) | |
eGFR (ml/minute/1.73m2), median (IQR) | 76.0 (58.7–96.4) | 69.2 (51.8–86.0) | 1.00 (0.99 to 1.00) | 0.08 |
BMI (kg/m2), mean (SD) | 28.34 (4.7) | 27.00 (4.5) | 0.95 (0.93 to 0.98) | 0.001 |
Reference test results and clinical concern about bleeding
Reference test results in participants with and without any CCB (LRT analysis 1) or postoperative CCB (LRT analysis 2) are summarised in Table 35. No formal comparison of these values was performed.
Predictor | No CCB, median (IQR) | CCB, median (IQR) |
---|---|---|
Preoperative results: any CCB (LRT analysis 1) | N = 1516 | N = 684 |
Preoperative haemoglobin (g/dl) | 12.9 (11.8–13.8) | 12.6 (11.3–13.7) |
Preoperative haematocrit (ratio) | 37.0 (34.0–39.0) | 36.0 (32.0–39.0) |
Preoperative WBC (×109/l) | 6.7 (5.6–8.0) | 6.4 (5.3–7.7) |
Preoperative neutrophil count (×109/l) | 4.1 (3.2–5.1) | 4.1 (3.1–5.1) |
Preoperative platelet count (×109/l) | 207.0 (173.0–244.0) | 193.0 (157.0–230.5) |
Preoperative MPV (fl/l) | 10.6 (10.0–11.3) | 10.5 (9.9–11.2) |
Preoperative immature platelet fraction (%) | 2.7 (1.8–4.0) | 2.8 (1.9–4.1) |
Preoperative absolute reticulocyte count (×109/l) | 45.5 (35.5–57.6) | 44.5 (34.4–56.5) |
Preoperative MPV × platelet count (fl/l) | 2190.9 (1864.4–2580.0) | 2041.7 (1705.6–2400.0) |
Preoperative results: postoperative CCB (LRT analysis 2) | N = 1654 | N = 572 |
Preoperative haemoglobin (g/dl) | 12.8 (11.7–13.8) | 12.7 (11.3–13.7) |
Preoperative haematocrit (ratio) | 37.0 (34.0–39.0) | 36.0 (32.0–39.0) |
Preoperative WBC (×109/l) | 6.7 (5.6–7.9) | 6.4 (5.3–7.8) |
Preoperative neutrophil count (×109/l) | 4.1 (3.2–5.1) | 4.0 (3.2–5.2) |
Preoperative platelet count (×109/l) | 206.0 (172.0–244.0) | 193.0 (157.0–231.0) |
Preoperative MPV (fl/l) | 10.6 (10.0–11.3) | 10.6 (9.9–11.3) |
Preoperative immature platelet fraction (%) | 2.7 (1.8–4.0) | 2.7 (1.9–4.1) |
Preoperative absolute reticulocyte count (×109/l) | 45.7 (35.5–57.7) | 44.1 (34.0–56.5) |
Preoperative MPV × platelet count (fl/l) | 2183.8 (1851.3–2568.0) | 2042.2 (1706.3–2401.2) |
Postoperative results: postoperative CCB (LRT analysis 2) | N = 1654 | N = 572 |
Preoperative haemoglobin (g/dl) | 10.0 (8.9–11.3) | 9.2 (8.2–10.1) |
Preoperative haematocrit (ratio) | 28.0 (25.0–32.0) | 26.0 (23.0–29.0) |
Preoperative WBC (×109/l) | 8.6 (6.6–11.0) | 8.2 (6.4–10.4) |
Postoperative neutrophil count (×109/l) | 6.7 (4.9–9.0) | 6.6 (5.1–8.6) |
Postoperative platelet count (×109/l) | 144.0 (117.0–177.0) | 124.0 (97.0–154.0) |
Preoperative MPV (fl/l) | 10.4 (9.7–11.0) | 10.2 (9.7–11.0) |
Preoperative immature platelet fraction (%) | 3.0 (2.0–4.4) | 3.0 (2.0–4.9) |
Preoperative absolute reticulocyte count (×109/l) | 39.0 (30.9–49.8) | 36.7 (29.0–45.8) |
Postoperative MPV × platelet count (fl/l) | 1498.5 (1222.1–1832.6) | 1260.0 (1009.8–1604.8) |
PT (seconds) | 12.2 (11.7–13.0) | 12.5 (11.9–13.4) |
aPTT (seconds) | 31.2 (28.3–34.5) | 32.6 (29.6–36.5) |
Clauss fibrinogen (g/l) | 2.1 (1.7–2.7) | 1.8 (1.5–2.3) |
Anti-Xa (IU/dl) | 0.2 (0.1–0.3) | 0.2 (0.1–0.3) |
D-dimer (ng/ml) | 396.0 (256.6–763.2) | 447.3 (268.1–913.0) |
Factor XIII (IU/dl) | 81.1 (68.4–94.0) | 72.2 (61.3–84.1) |
vWF (IU/dl) | 149.6 (115.6–192.6) | 155.1 (116.1–196.9) |
ETP (nM/minute) | 1055.0 (699.0–1376.5) | 1066.5 (687.0–1352.1) |
Results of specific analyses of laboratory-based test predictors
Laboratory reference test analysis 1 results
As with the analyses of the early tests, the predictive models were built by first considering the contributions to the prediction of any CCB of the baseline characteristics. The best model for all reference tests considered, after adjusting for baseline characteristics, was then found using a stepwise approach. Non-linearity of the reference test results was considered. Preoperative haematocrit and MPV were all associated with any CCB. Lower haematocrit levels and lower platelet count × MPV increased the likelihood of CCB (Table 36). The final best model developed with these predictors is shown in Figure 22. The AUC for the baseline characteristics model was 0.72 (95% CI 0.70 to 0.74) and for the full model was 0.74 (95% CI 0.71 to 0.76). This improvement in the AUC was small but statistically significant (p = 0.001). The percentage of participants correctly classified in the final model was 74.32% and in the baseline characteristics model was 72.60%.
Test predictor | OR (95% CI) | p-value |
---|---|---|
Preoperative HCT | 0.97 (0.95 to 0.99) | 0.97 |
(1000Preoperative PLT×MPV)2 | 5.79 (3.15 to 10.63) | < 0.001 |
Constant | 0.88 (0.79 to 0.98) |
FIGURE 22.
Predictive accuracy of LRTs for any CCB (LRT analysis 1). AUC baseline = 0.72, AUC baseline plus laboratory tests = 0.74 (p = 0.001).

Laboratory reference test analysis 1 sensitivity analyses
As in the analyses in the COPTIC A and COPTIC B studies, we conducted sensitivity analyses to address uncertainty around the reference outcome, CCB. Using the definition of CCB in SA1, we identified 348 out of 1864 (18.7%) participants in the LRT analysis 1 population who developed any CCB. The coefficients for the reference test predictors from the final model are shown in Table 36. Only the preoperative haematocrit and platelet count × MPV terms were predictors in this model. The AUC for the baseline model was 0.71 (95% CI 0.68 to 0.74) and for the full model including baseline characteristics plus the LRT results was 0.73 (95% CI 0.70 to 0.76). This difference was statistically significant (p = 0.004). ROC curves are shown in Figure 23.
FIGURE 23.
Laboratory reference tests in relation to any CCB (LRT analysis 1, SA1).
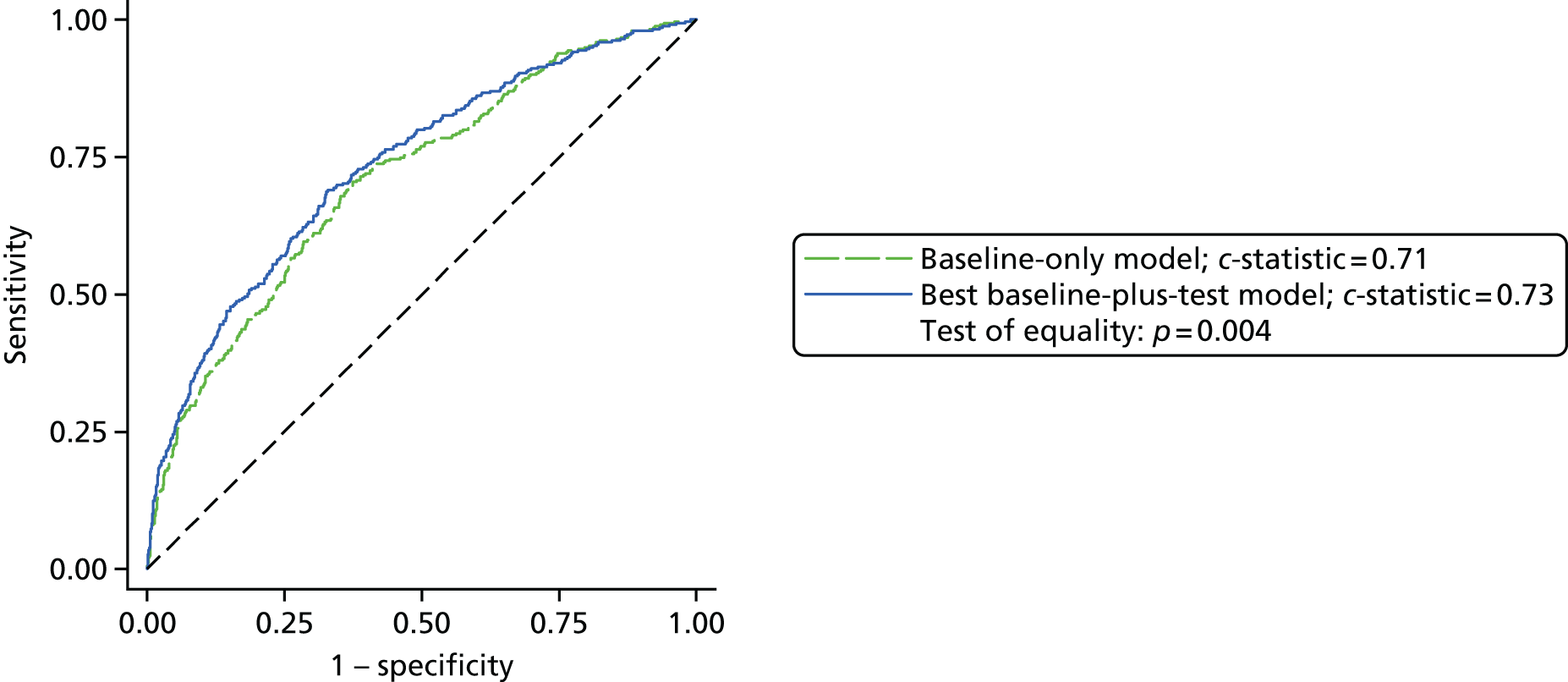
For the second sensitivity analysis we used the definition of CCB as described in SA2. This identified 334 out of 2200 (15.2%) participants in the LRT analysis 1 population as having any CCB. In this analysis, preoperative haematocrit and platelet count × MPV remained significant predictors of CCB. The AUC for the baseline model was 0.68 (95% CI 0.65 to 0.71) and for the full model including baseline characteristics plus LRT results was 0.6946 (95% CI 0.66 to 0.73). This difference was statistically significant (p = 0.02). ROC curves are shown in Figure 24.
FIGURE 24.
Laboratory reference tests in relation to any CCB (LRT analysis 1, SA2).

Laboratory reference test analysis 2 results
The baseline and full models were developed as previously described. Fibrinogen concentration, D-dimer concentration, haematocrit and factor XIII concentration were all associated with postoperative CCB. Lower preoperative haematocrit, lower postoperative fibrinogen levels and lower factor XIII levels increased the likelihood of CCB. Higher D-dimer levels increased the likelihood of CCB (Table 37). The final best model developed with these predictors is shown in Figure 25. The AUC for the baseline characteristics model was 0.6976 (95% CI 0.67 to 0.72) and for the full model was 0.73 (95% CI 0.71 to 0.76). This improvement in the AUC was small but statistically significant (p < 0.001). The percentage of participants correctly classified in the final model was 76.91% and in the baseline characteristics model was 76.28%.
Test predictor | OR (95% CI) | p-value |
---|---|---|
Preoperative haematocrit | 0.96 (0.94 to 0.99) | 0.004 |
Clauss fibrinogen | 0.68 (0.56 to 0.82) | < 0.001 |
D−dimer1000 | 1.18 (1.06 to 1.30) | 0.002 |
(100FXIII)2 | 1.59 (1.24 to 2.05) | < 0.001 |
(100FXIII)2 × ln (100FXIII)2 | 1.26 (1.08 to 1.47) | < 0.001 |
Constant | 0.83 (0.74 to 0.94) | 0.003 |
FIGURE 25.
Predictive accuracy of LRTs for postoperative CCB (LRT analysis 2). AUC baseline = 0.70, AUC baseline plus laboratory tests = 0.73 (p < 0.001).

Laboratory reference test analysis 2 sensitivity analyses
We conducted sensitivity analyses to address uncertainty around the reference outcome, postoperative CCB. Using the definition of CCB in SA1 we identified 354 out of 2008 (17.6%) participants in the LRT analysis 2 population who developed postoperative CCB. Only the preoperative haematocrit, fibrinogen level and D-dimer concentration were predictors in this model. The AUC for the baseline model was 0.69 (95% CI 0.66 to 0.73) and for the full model including baseline characteristics plus LRTs was 0.7437 (95% CI 0.71 to 0.77). This difference was statistically significant (p-value < 0.001) ROC curves are shown in Figure 26.
FIGURE 26.
Laboratory reference tests in relation to postoperative CCB (LRT analysis 2, SA1).

For the second sensitivity analysis we used the definition of CCB as described in SA2. This identified 354 out of 2226 (15.9%) participants in the LRT analysis 2 population as having CCB. In this analysis the preoperative haematocrit and neutrophil count and postoperative fibrinogen level and D-dimer concentration remained significant predictors for postoperative CCB. The AUC for the baseline model was 0.67 (95% CI 0.64 to 0.71) and for the full model including baseline characteristics plus the LRT results was 0.73 (95% CI 0.69 to 0.76). This difference was statistically significant (p < 0.001). ROC curves are shown in Figure 27.
FIGURE 27.
Laboratory reference tests in relation to postoperative CCB (LRT analysis 2, SA2).
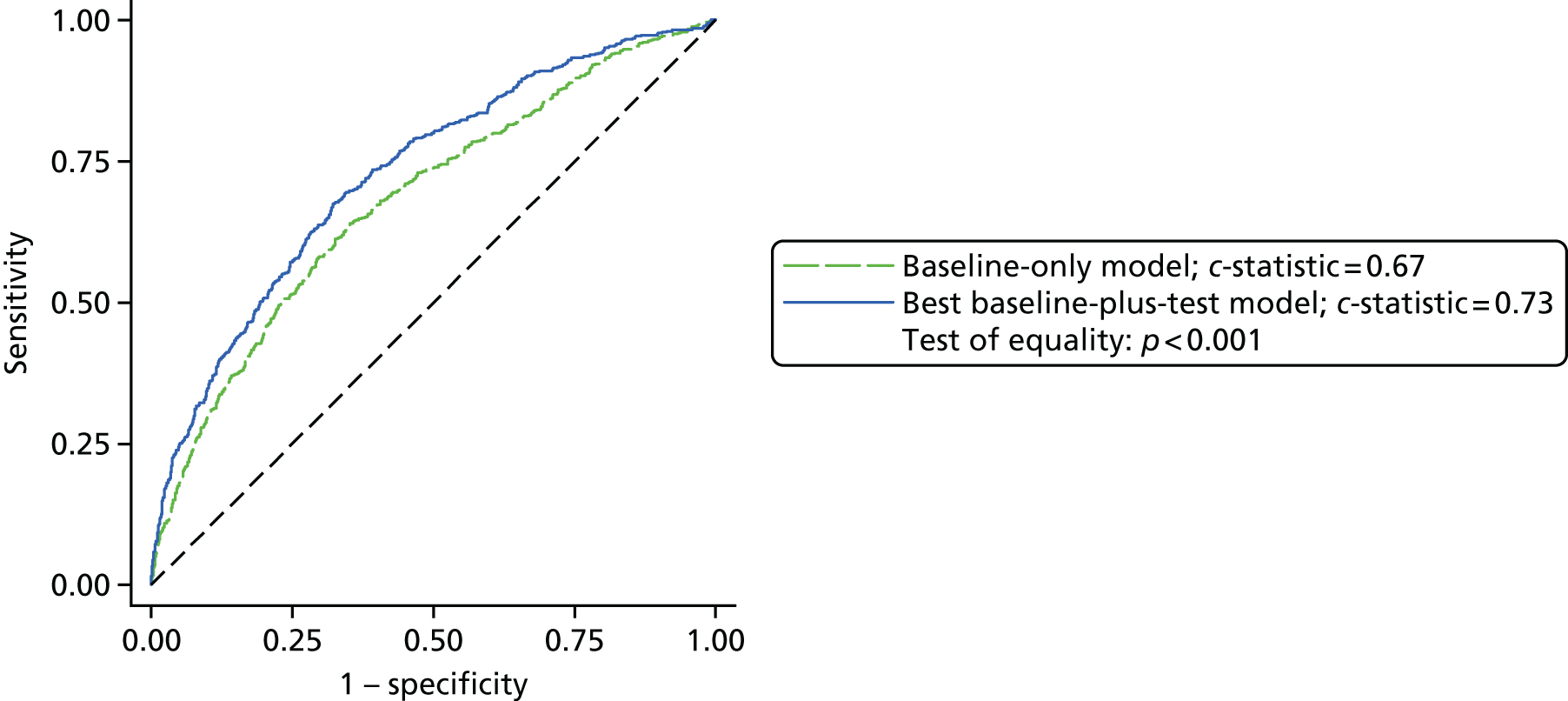
Summary of the main findings of the COPTIC predictive accuracy studies
COPTIC A study
We conducted a prospective observational cohort study to examine the predictive accuracy of the addition to standard care of routine preoperative platelet function testing using the Multiplate device. We enrolled a large representative consecutive sample of all adult patients undergoing cardiac surgery at a single UK cardiac centre. Complete data were available for all demographic, baseline, clinical, laboratory and Multiplate parameters for 2197 (91%) participants, of whom 686 (31%) were suspected of having CCB. There was no evidence that the participants excluded from the analysis population had been selected in a non-random manner. We developed a clinical risk prediction model based on baseline factors that had good predictive accuracy for bleeding. We found that participants receiving DAPT were at increased risk of bleeding and this risk was greatest in those participants undergoing CABG or CABG and valve replacement procedures and taking DAPT within 2 days of surgery. After introduction to the model of the preoperative Multiplate results as predictors, only the ASPItest was significantly associated with bleeding; however, the addition of this value to the predictive accuracy model did not significantly improve discrimination. The early ASPItest result (velocity) showed a stronger association with bleeding than the ASPItest AUC value; however, the addition of this to the predictive accuracy model also failed to improve discrimination. On the basis of these results we conclude that preoperative Multiplate testing has low or no predictive accuracy for post-cardiac surgery coagulopathic bleeding beyond that achieved using clinical risk factors alone.
COPTIC B study
In this study the analysis population included 1833 participants, with complete data available for all demographic, baseline, clinical, laboratory and TEG, ROTEM and Multiplate parameters for 71% of participants. Of these, 535 (29%) developed any CCB and 449 (24%) developed postoperative CCB. The clinical prediction models for CCB showed good discrimination, with similarities in the principal clinical determinants of bleeding, as observed in the COPTIC A model. The addition of preoperative Multiplate test predictors did not improve discrimination for any CCB. However, the addition of postoperative test predictors for the Multiplate, ROTEM or TEG resulted in a progressive increase in predictive accuracy for postoperative CCB. The best-performing model for predicting bleeding was the combination of TEG and postoperative Multiplate parameters (AUC 0.75, 95% CI 0.72 to 0.77). Addition of preoperative Multiplate parameters did not show additional benefit. Sensitivity analyses that tested uncertainty around the definition of the reference outcome CCB by excluding components of the outcome that would either over- or underestimate the frequency of coagulopathic bleeding did not improve predictive accuracy. On the basis of these results we conclude that the routine use of postoperative TEG and Multiplate tests alongside a clinical risk prediction score would have greater discrimination than is observed with the clinical score alone. The magnitude of the difference was small, however, and the clinical importance was uncertain.
Laboratory reference test analyses
The analysis population included 2226 participants. For LRT analysis 1, 684 out of 2200 (31.1%) participants were classified as having any CCB. For LRT analysis 2, 572 out of 2226 (25.7%) participants were classified as having postoperative CCB. LRT analysis 1 considered whether the use of data from automated cell counters not otherwise used in standard care may be used to improve the predictive accuracy of baseline (preoperative) clinical prediction models. This demonstrated that the preoperative haematocrit as well as the product of the platelet count and MPV were predictors for bleeding. The inclusion of these variables in the predictive model showed greater discrimination for any CCB than the clinical risk prediction score alone; however, the magnitude of the difference appeared small. In LRT analysis 2, when pre- and postoperative automated cell counter results were combined with LRTs of coagulation, preoperative haematocrit and postoperative fibrinogen, D-dimer and factor XIII activity were found to be predictors for postoperative CCB. As with the other analyses the effect of adding these laboratory test results to the clinical prediction model was small. Sensitivity analyses confirmed that the definition of CCB was not the main explanation for this relatively poor ability of these tests to improve the prediction of CCB.
Health economic analysis
Aim of the cost-effectiveness analysis
The previous section described the clinical analyses of pre- and postoperative POC testing in cardiac surgery patients. The results showed that the addition of a combination of postoperative TEG and Multiplate test values to a clinical risk prediction model offered the greatest predictive accuracy for the prediction of postoperative bleeding. However, the magnitude of the difference between the final model and the clinical risk model alone was small and of uncertain clinical significance. This section considers whether introducing POC tests routinely into clinical practice would be cost-effective in a UK NHS setting. Good practice guidelines on the conduct of economic evaluations were followed101,102 and the analysis is described in accordance with the Consolidated Health Economic Evaluation Reporting Standards (CHEERS) statement. 103
Our original intention was to use the clinical analyses to identify the most effective test (or combination of tests) and to perform a cost-effectiveness analysis of the test(s). As the preoperative Multiplate test showed little ability to predict CCB, we have focused on the postoperative tests and postoperative CCB. However, as the predictive accuracy results were similar for the different postoperative tests, we have evaluated the cost-effectiveness of all of the tests. As the Multiplate test indicates the need for platelets only, rather than indicating the need for a range of blood products, it was deemed most clinically plausible to consider its introduction into routine practice in combination with either TEG or ROTEM, rather than on its own. The test options evaluated were therefore TEG and ROTEM used separately and each in combination with the Multiplate test.
For each of these four test options the aim was to evaluate the routine use of POC testing in all patients. Although current practice did not include the routine use of these tests (at the time of the study), the care of some participants may have been guided by the ad hoc use of a POC test; specifically, the TEG test was in clinical use at the Bristol Heart Institute during the study. The comparison was therefore between current practice (which may have included the ad hoc use of a TEG test) and routine postoperative POC testing in all patients undergoing cardiac surgery. The research question was therefore whether or not it is it cost-effective to introduce routine postoperative POC testing in all patients undergoing cardiac surgery.
The predictive accuracy analyses evaluated the performance of the POC tests in predicting CCB, rather than evaluating how they would be used in practice and their effect on treatments given to patients. To evaluate the cost-effectiveness of the tests, it is necessary to consider how they would impact on a patient’s care pathway, rather than on an intermediary outcome such as predicting CCB, and how they would be used in practice: what test result cut-offs would indicate a positive test result and what treatments this could result in. In the cost-effectiveness analyses, POC tests were considered to ‘rule in’ treatments for patients but not to ‘rule out’ treatments. If a POC test result was positive (or an ad hoc TEG test was positive), this was assumed to lead to prophylactic treatment with protamine, platelets, FFP and/or cryoprecipitate. If a test result was negative this was assumed to have no impact on a patient’s care pathway.
Although introducing a test for all patients adds a cost to everyone in the short term, the hypothesis was that this would provide better treatment guidance and could reduce overall costs in the longer term. The aim of routine testing is to prevent severe blood loss, reopening of the chest for bleeding, large-volume transfusion, further pro-haemostatic treatments (second-line FFP, platelets, cryoprecipitate or rFVIIa) and shock and major morbidity (MI, AKI, stroke or sepsis) by prophylactic treatment. 104 Patients with a positive test result would receive timely and appropriate prophylactic treatment to prevent the development of CCB and reduce the frequency of adverse events attributable to coagulopathic bleeding. This reduction in adverse events could lead to a reduction in total health-care costs and improve outcomes.
Economic decision model methods
To address the question of whether it is cost-effective to introduce routine postoperative POC testing in all patients undergoing cardiac surgery, an economic decision model was built to simulate the costs and effects of introducing a POC test for all patients compared with current practice. As uncertainty surrounds the performance of the POC tests in practice, a flexible framework was required to facilitate the assessment of uncertainty around parameter estimates. Models provide a simplification of the real world and will never capture every last possible scenario associated with an intervention, but the key consequences need to be included when models are conceptualised and structured. 105 In this analysis the model was developed in consultation with clinical experts on the COPTIC study team. Figure 28 highlights the model scenarios. The initial model scenarios are based on the test results and are slightly different for current practice and postoperative POC testing for all. Thereafter, the model scenarios are the same for each arm of the model.
FIGURE 28.
Cost-effectiveness model scenarios. a, Treatment in the form of protamine, platelets, clotting factors, reoperation for bleeding or > 4 units of red cells; b, complications defined as MI, stroke, sepsis or AKI. POC test results were used to estimate the likely results of the ad hoc TEG tests.

Cost-effectiveness model structure
The model combines the use of decision trees and Markov models and was developed in TreeAge Pro 2013 (TreeAge Software, Inc., Williamstown, MA, USA). All participants start in the ‘year 1’ health state of the Markov model and move through a decision tree, which is used to model the patient pathway after leaving the operating theatre up to 1 year post surgery. At the end of the decision tree, participants move to one of three further health states in the Markov model (alive having had no complications during the index hospital admission, alive having had complications in hospital and dead), which was then used to assess longer-term consequences on an annual basis. The model structure was split into two main arms (branches), with the top branch representing current practice and the bottom branch representing postoperative POC testing for all.
Current practice arm of the model to 1 year
Figure 29 shows the current practice arm of the model for the first year. The decision tree subdivides the starting cohort of participants undergoing cardiac surgery into two, depending on whether participants have an ad hoc TEG test or not. Some participants in the current practice arm had a TEG test and it was assumed that the results were acted on; it is therefore important to account for this in the current practice pathway.
FIGURE 29.
Current practice arm of the model to 1 year. RBC, red cell units.

In the current practice arm, participants who have an ad hoc TEG test are then divided into those whose test is positive and those whose test is negative. The next branches in the tree divide participants into those whose POC test (whichever of the four options is being considered) is positive and those whose POC test is negative. Although this information is not used clinically in current practice, it has been incorporated within the structure of the current practice arm to facilitate the population of the model using the COPTIC study data. Participants with a positive ad hoc TEG result will receive first-line prophylactic treatment on the basis of the test result; this is likely to be protamine, platelets and/or FFP. This prophylactic treatment may be successful and CCB may be prevented or CCB may develop despite the prophylactic treatment. Participants with a negative ad hoc TEG test, and therefore no prophylactic treatment, may also go on to develop CCB or not. Participants are divided into those with and without CCB.
In the current practice arm, participants who do not have an ad hoc TEG test are divided into those whose POC test is positive and those whose POC test is negative. As for those with an ad hoc TEG test, this is for ease when populating the tree with COPTIC study data. The next branches in the tree divide these participants into those with CCB and those without CCB.
Regardless of whether participants have an ad hoc test or not (and whether this was positive or negative), the remaining branches in the tree are identical. After CCB or no CCB, participants are divided into those who receive treatment for clotting abnormalities or bleeding in the form of protamine, platelets or clotting factors in the first 12 hours after surgery, a reoperation for bleeding or > 4 units of red cells and those who do not. By definition, the only treatment that participants without CCB would receive is > 4 units of red cells. For participants with a negative ad hoc test or no ad hoc test, this includes any protamine, platelets or clotting factors received; for participants who receive prophylactic treatment, the protamine, platelets and/or clotting factors are in addition to those received as prophylaxis. As red cells may be given for reasons other than bleeding, for example 1 or 2 units of red cells may be given in response to a low haematocrit level or anaemia, it was considered more appropriate to regard large-volume transfusions (> 4 units of red cells) rather than any red cell transfusions as reliably reflecting clinically important bleeding,10 hence the criterion set here.
The remaining branches in the tree consider subsequent key events for participants: whether they develop any of four key complications in hospital (MI, stroke, sepsis or AKI), whether they survive to hospital discharge and whether they are alive at 1 year.
Postoperative point-of-care testing for all
The lower half of the model is shown in Figure 30 and illustrates the intervention arm and the routine use of a postoperative POC test for all participants. The structure of the model is the same regardless of whether the test introduced is TEG or ROTEM or either test in combination with the Multiplate test. The postoperative POC test is conducted and the cohort is split into two groups depending on whether the test result is positive or negative.
FIGURE 30.
Postoperative POC test arm of the model to 1 year. RBC, red cell units.

Regardless of whether the POC test is positive or negative, participants are then divided into whether they had an ad hoc TEG test or not under current practice. Those with an ad hoc test are further divided into whether this is positive or negative. Participants are divided into these additional groups for the purposes of populating the model, as described in Model probabilities to 1 year: test-all arm.
If the POC test result is positive, then prophylactic treatment will be given to try to prevent the development of CCB. For participants with a positive POC test result, the following branches then indicate whether this prophylactic treatment is successful or not in preventing CCB. The following branches then indicate whether participants receive further pro-haemostatic treatments, > 4 units of red cells or a reopening for bleeding and, as in the current practice arm, whether they develop complications, their survival status at hospital discharge and 1 year and then the three health states in the Markov model, as described above.
If the POC test result is negative, this has no impact on a patient’s care pathway compared with current practice. The following branches indicate whether participants develop CCB or not and, as above, whether they receive further treatments or not, whether they develop complications and their survival status at hospital discharge and 1 year.
Both arms of the model beyond 1 year
Figure 31 shows the structure of the model beyond 1 year. In each arm, all participants start in ‘year 1’ of the Markov model and move through the decision tree, which models the participant pathway to 1 year, as shown in Figures 29 and 30. From 1 year, the structure of the model is the same in both arms. At 1 year, participants move into one of three health states in the Markov model: alive having had complications in their index hospital admission; alive having not had complications in their index hospital admission; and dead (see Figure 31). In each of the annual cycles thereafter, participants in the alive with complications health state can remain in that health state or die. Similarly, participants in the alive without complications health state can remain in that health state or die. The death state is an absorbing state (i.e. participants remain there). The analysis used a 5-year time horizon as it was anticipated that all major resource use (and outcomes) would occur within this time frame.
FIGURE 31.
Model structure for both arms beyond 1 year.
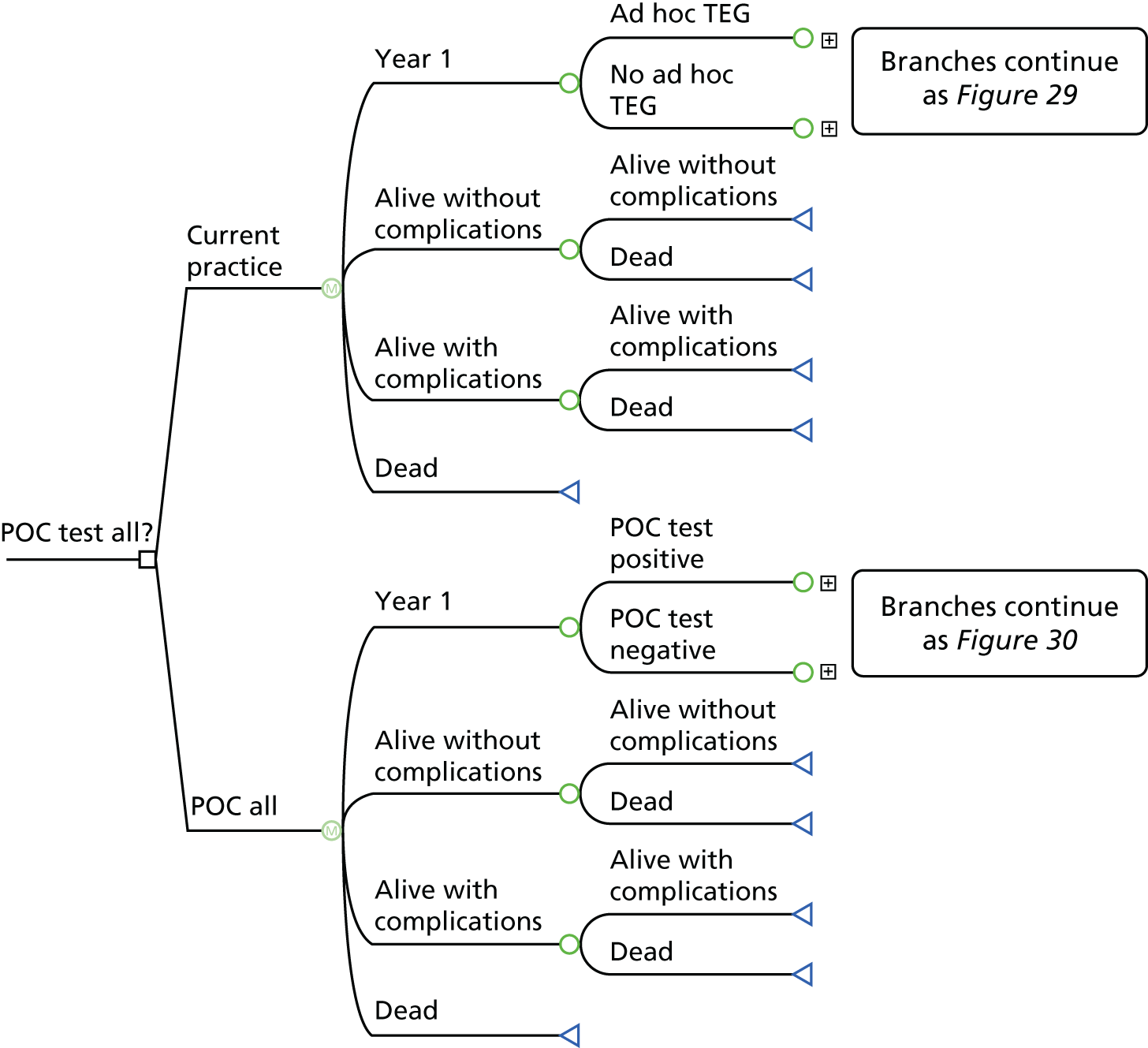
Data for the model
The majority of the parameters in the model were populated with estimates derived from patient-level data from the COPTIC study conducted at Bristol Heart Institute as part of this programme of work, which incorporated the collection of key health economic variables. Analyses of these data (including the POC test results and resource use data) were conducted in Stata version 12. Of the 1833 participants included in the COPTIC B analysis population, 31 were excluded from the cost-effectiveness analyses. These 31 participants had a reoperation with a surgical cause of their bleeding; they were not classed as having CCB but, unlike other participants without CCB, they may have received non-red cell products in the first 12 hours after surgery or have had postoperative blood loss of > 600 ml at 6 hours collected in the chest drain, but these were assumed to be related to the surgical cause of bleeding. The cohort for the cost-effectiveness analyses therefore included 1802 participants. Participants had a mean age of 67 years and 76% were male. Most participants underwent CABG (59%) or valve replacement surgery (22%) and 25% of participants were classed as having postoperative CCB.
The following sections describe how the model transition probabilities, costs and outcomes were estimated to 1 year, largely based on the observational data. The final section describes how model inputs were estimated beyond 1 year. Tables 110–120 in Appendix 1 list the probabilities, costs and outcome estimates used to populate the base-case models. The analysis was conducted from a NHS and personal social services perspective,106 with costs expressed in 2013/14 UK pounds and outcomes as life-years. Costs and effects were not discounted in the first year and thereafter a discount rate of 3.5% per year was applied. 107
Model probabilities to 1 year: current practice arm
The probabilities for each branch of the model to 1 year were generated using the patient-level data from the COPTIC study. Of the starting cohort of participants, 16% had an ad hoc TEG test (284/1802). The result of the ad hoc TEG test was not captured in the COPTIC study and so the results from the POC TEG test were used to determine the results. The TEG test produces a number of results, which have published normal ranges. 108 In consultation with clinical experts on the study team, and based on 100% of the normal ranges, if any of the criteria in Box 4 was met a participant was considered to have a positive ad hoc TEG test result.
-
CK R : CKH R > 1.5 and if CK R > 8 minutes.
-
CK R > 8 minutes.
-
CK α-angle < 47°.
-
CK MA < 55 mm.
-
CK LY60 > 15%.
α-angle, measures the speed of fibrin build-up; LY60, per cent lysis 60 minutes after MA (percentage decrease in clot amplitude); R, reaction time (minutes) to initial fibrin formation.
Participants are then divided according to whether their POC test is positive or negative. Details of the criteria used to determine whether a POC test (or test combination) is considered positive or negative are provided in the following section (Model probabilities to 1 year: test-all arm); these criteria were also used to divide participants into POC test positive or negative in the current practice arm.
Participants were classed as having CCB or not based on the outcomes reported in the COPTIC study, with one adjustment for participants with a positive ad hoc TEG test. Any participant with a positive ad hoc TEG test and who received quantities of protamine, FFP or platelets that could be considered only first-line prophylactic treatment, and who had no other element of the CCB outcome, was reclassified from CCB to no CCB. The protamine, FFP or platelets given that caused the participant to be classified as CCB were considered in this case to be prophylactic and were deemed to have prevented CCB. It was not known what treatments were given to participants specifically as a result of the ad hoc test, but in-hospital treatment data were available. It was assumed that if participants received additional protamine only, or up to 1 unit of platelets and 2 units of FFP but no additional protamine in the first 12 hours after surgery, and no cryoprecipitate in the first 12 hours, and did not have CCB as a result of blood in the chest drain or a reoperation for bleeding, then they had had successful prophylactic treatment. In the base-case analysis, 16 participants were reclassified to ‘no CCB’ on this basis.
The subsequent probabilities of whether participants received treatment in the form of protamine, platelets, clotting factors (FFP, cryoprecipitate or rFVIIa), > 4 units of red cells and/or a reoperation for bleeding and whether they developed complications (MI, stroke, sepsis or AKI) were straightforward to obtain from the COPTIC study. Only complications occurring in the index hospital admission before discharge were captured; it is not known whether additional events occurred after discharge.
It was known from the Patient Analysis and Tracking System database whether participants were discharged from hospital or died in hospital and survival status at 1 year was available for all 1802 participants from the NHS Strategic Tracing Service (NSTS). The probabilities of being discharged from hospital and being alive at 1 year were therefore obtained from the COPTIC study. There were 73 deaths by 1 year, 31 in the index hospital admission and 42 between hospital discharge and 1 year.
Model probabilities to 1 year: test-all arm
The TEG, ROTEM and Multiplate POC tests all produce a number of results, many of which have published normal ranges. 108–111 In consultation with clinical experts on the study team, and informed by the normal ranges and a published algorithm for ROTEM,112 criteria were set to define positive test results and what treatments would be given as a result of positive tests. For TEG and ROTEM these values were approximately 80% of the normal ranges.
If any of the criteria in Box 5 was met a participant was considered to have a positive TEG test result in the base-case analysis. Criterion 1 was considered first and, if this indicated that a participant required protamine, it was assumed that he or she was given 50 mg of protamine and that this resolved the problem and was all that was needed. If the test did not suggest that a participant required protamine, then criteria 2–5 were considered and all treatments indicated were given.
-
If CK R : CKH R > 1.5 and if CK R > 10 minutes, give protamine.
-
If CK R > 10 minutes, give FFP.
-
If CK α-angle < 37.6°, give FFP and platelets.
-
If CK MA < 44, give FFP and platelets.
-
If CK LY60 > 18.75%, give tranexamic acid.
The TEG results from the COPTIC study classified 3.6% (65/1802) of the cohort as having a positive test result. The criteria for determining positive ad hoc TEG test results and positive POC test results under a ‘test-all’ strategy were different. An ad hoc TEG test is likely to have been given to participants who clinicians were concerned about and clinical opinion in the study research team was that these test results would be interpreted less conservatively than routine tests for all participants, many of whom give clinicians no cause for concern.
Box 6 describes the criteria for a positive ROTEM test result in the base-case analysis, together with the treatments indicated. As for TEG, if protamine was indicated then it was assumed that no further treatment was required. If protamine was not required, then the other ROTEM criteria (2–5) were considered in their entirety and any treatments indicated were given.
-
If INTEM CT > 300 seconds and HEPTEM CT : INTEM CT < 0.64, give protamine.
-
If EXTEM CT > 100 seconds or HEPTEM CT > 300 seconds, give FFP.
-
If EXTEM A10 ≤ 32 mm and FIBTEM A10 < 8 mm, give cryoprecipitate.
-
If EXTEM A10 ≤ 32 mm and FIBTEM A10 ≥ 8 mm, give FFP and platelets.
-
If FIBTEM A10 < 5.6 mm or FIBTEM MCF < 7.2 mm, give cryoprecipitate.
Note that although TEG and ROTEM are being considered as alternatives, and EXTEM A10 is in some ways comparable to the α-angle or MA in TEG, ROTEM has the ability to discriminate between giving FFP and platelets and giving cryoprecipitate using the FIBTEM values; there is not an equivalent to this available in TEG. The ROTEM results from the COPTIC study classified 3.4% (61/1802) of the cohort as having a positive test result.
Box 7 describes the criteria for a positive Multiplate test result. If any of the criteria was met then platelets were indicated. In the event of a positive Multiplate test result, pooled platelets are transfused; however, the Multiplate test result was not considered on its own.
-
AUC TRAPtest < 50.
-
AUC ASPItest < 30.
-
AUC ADPtest < 30.
If any of the TEG/ROTEM or Multiplate criteria were met then the combined test result was considered positive. In terms of treatment, if the Multiplate test was introduced alongside TEG or ROTEM it was assumed that, if the TEG or ROTEM test indicated the need for protamine, then that was all that was required and the Multiplate test result was not used. If the TEG or ROTEM test did not indicate the need for protamine, then the Multiplate criteria were considered alongside the other TEG/ROTEM criteria.
If the Multiplate test indicated the need for platelets (even if TEG/ROTEM did not), platelets would be given alongside any other treatments indicated by TEG/ROTEM, as the Multiplate test would be considered more sensitive for platelet defects. If the reverse were true and TEG/ROTEM indicated the need for platelets but the Multiplate test did not, it was assumed that platelets were not given. This is really the added benefit of the Multiplate test in practice; if abnormal TEG/ROTEM parameters indicated the need for FFP and platelets but the Multiplate test result was normal, it was assumed that a participant would be given only FFP and that unnecessary treatment would be avoided.
Test results from the COPTIC study classified 5.0% (91/1802) of the cohort as having a positive test result according to the TEG and Multiplate tests combined and 4.9% (89/1802) as having a positive test result according to the ROTEM and Multiplate tests combined.
For participants who had a positive test result (from TEG or ROTEM or either TEG/ROTEM in combination with the Multiplate test) and who were given prophylactic treatment, an estimate of the probability that the prophylactic treatment was successful and that they would not develop CCB was needed. This is one of the key parameters in the model, but also a major unknown. Although it was not possible to observe this probability, the observational data were used to estimate it. The proportion of participants with CCB who received more than first-line treatment and hence for whom it was assumed that first-line treatment had failed was used as an estimate of the failure rate of prophylactic treatment. Primary treatment was assumed to have failed if a participant received > 1 unit of platelets or > 2 units of FFP, any cryoprecipitate, rFVIIa or a reoperation for bleeding. In total, according to this definition, treatment failed in 154 out of 449 (34.3%) participants with CCB. The base-case probability of prophylactic treatment failing was therefore 0.34 (with the complement of 0.66 signifying success). Alternative failure rates were explored in a sensitivity analysis.
Once participants had been classified according to whether their POC test was positive or negative based on the criteria described above, it was then straightforward to use the COPTIC study data to divide them into those who had an ad hoc TEG test and those who did not. For those who had an ad hoc TEG test, the POC TEG results were used to classify the ad hoc test as positive or negative, using the same criteria as in the current practice arm (described in Model probabilities to 1 year: current practice arm).
As the POC tests conducted during the observational study were not used to guide treatment, it is not possible to directly observe the impact of the test results on patients’ care pathways and on their treatments, costs and outcomes from this point forward in the model. However, at this point in the model, participants are classified into the same 12 groups based on their POC test result, whether they had an ad hoc test and its result (if applicable) as in the current practice arm. This was the reason for structuring the model by dividing participants in the current practice arm according to whether their POC test was positive or negative and dividing participants in the test-all arm according to whether they had an ad hoc test and its result. Participants in both arms of the model are therefore divided into the same 12 groups, but in a different order, as can be seen in Figure 32. In the figure, branches labelled with the same number in each arm include the same categories of participants. For example, participants in group 1 in both arms of the model had a positive ad hoc test and a positive POC test. The probabilities going forward from the branches numbered 1–12 in the test-all arm can then be considered to be as in the current practice arm, but with some adjustments, which are described next. Costs and life-years, which are described later, were also based on these 12 groups, again with some adjustments.
FIGURE 32.
Current practice groups of participants used to populate the test-all arm: (a) current practice; and (b) test all.
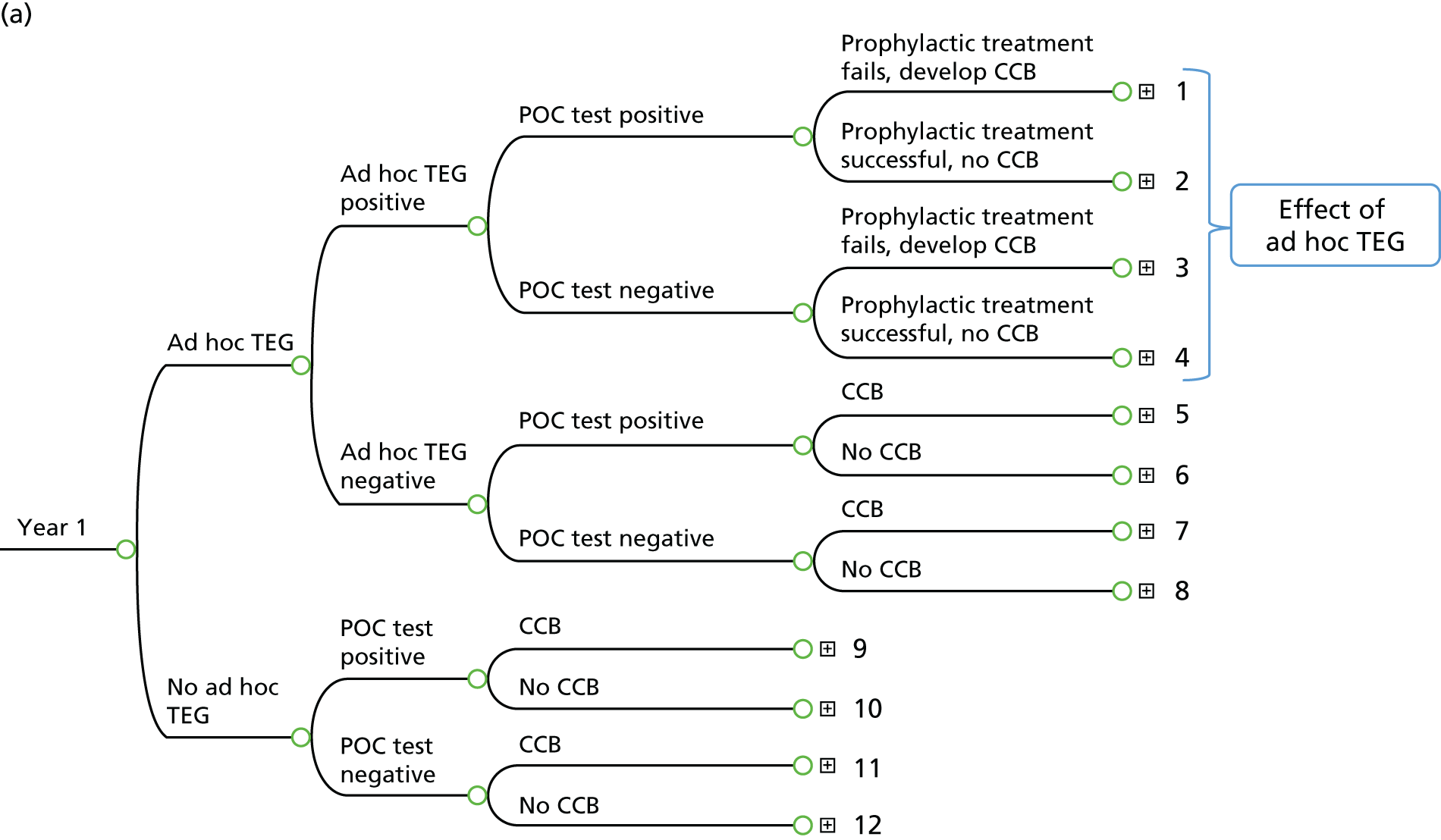

Looking at the POC test-all arm, participants in groups 1 and 2, 5 and 6 and 9 and 10 all have a positive POC test. Under a test-all strategy, these participants would receive prophylactic treatment, with the aim of preventing CCB. Participants in groups 1 and 2 had a positive ad hoc test and it is therefore assumed that these participants had prophylactic treatment as a result of that test, which is already incorporated into the current practice arm. Therefore, for groups 1 and 2, no additional effects of the POC test are incorporated into the test-all arm.
Participants in groups 5 and 6 had a negative ad hoc test and participants in groups 9 and 10 had no ad hoc test and so none of these participants would have received prophylactic treatment as a result of the ad hoc test. The effect of the POC test therefore needs to be added here. The existing probabilities of CCB in groups 5 and 9 need to be multiplied by the probability that prophylactic treatment fails, thus increasing the probability that participants are in groups 6 and 10, rather than 5 and 9, that is, increasing the probability that they do not have CCB (compared with those groups under current practice).
In reality, there were no participants in group 6 in any of the base-case models and therefore it was not possible to switch participants from group 5 to group 6. However, there were fewer than four participants in group 5 in each of the models and so this will not have had a significant impact on the results. Participants in groups 5 and 6 had a negative ad hoc TEG test but a positive POC test; as less stringent criteria were used to determine whether the ad hoc TEG was positive than the more stringent criteria used to determine whether the POC test was positive, there are very few participants in these groups. In some of the sensitivity analyses of less stringent criteria for a positive POC test result it was possible to switch participants from group 5 to group 6. In the base-case models there were 15–20 participants in group 9 under current practice; these are the patients for whom the POC test could have a positive impact.
A limitation of not having observational data from which to estimate parameters in the test-all arm of the model is that it was not possible to ‘undo’ the effect of the ad hoc tests that are used in current practice. It was not possible to ‘take out’ the effect of the ad hoc TEG test in groups 1 and 2 and add back the effect of the POC test result; the assumption is that care would be the same under both scenarios (a reasonable assumption if the POC test is a TEG test like the ad hoc test, but less realistic if it is a different POC test). Additionally, for participants in groups 3 and 4 in the test-all arm who have a negative POC test but in reality who have had the effect of a positive ad hoc test incorporated into their care, it was not possible to take out the effect of any treatment as a result of the ad hoc test. There were between 95 and 107 participants in groups 3 and 4 in each of the base-case models to whom this applied.
Cost data to 1 year
In the current practice arm, the costs included were health-care costs to hospital discharge, health-care costs from hospital discharge to 1 year and the cost of the ad hoc TEG test (if applicable). In the test-all arm, the costs included were health-care costs to hospital discharge, health-care costs from hospital discharge to 1 year, the cost of the POC test and the cost of prophylactic treatment indicated by a positive test (for participants with positive results). Each of these cost categories are described next. The methods used for costing resource use for participants in the COPTIC study are summarised first.
Costs from the end of surgery to hospital discharge were estimated for each participant in the COPTIC study using the resource use data collected. Although a small number of data were collected on the study case report forms, the majority of the resource use data were obtained from two routine data sets: the Patient Analysis and Tracking Systems database and Innovian, the clinical information system that records hourly data for time spent in the cardiac intensive care unit (CICU). A considerable amount of additional work was undertaken by the team at Bristol to address known limitations of these data sets. For example, because of the number of missing data in the Patient Analysis and Tracking System for serious postoperative complications such as sepsis and AKI, Innovian data were also used to identify these complications, either by the event recorded in the Innovian database or by review of five free-text fields (see Figure 7). Data were available on the number of units of red cells, platelets, FFP and cryoprecipitate given during the CICU stay, any additional doses of protamine given after initial heparin reversal, the time spent in critical care and on a ward and the time of extubation, any complications and the use of rFVIIa. The time of extubation was used to differentiate between the time spent in critical care that should be considered as intensive care (CICU) and the time spent in a high-dependency unit (HDU). Complications captured and costed were stroke, MI, sepsis and AKI (a cost was attached to stage 3 AKI only). Any reoperations and use of an intra-aortic balloon pump (IABP) were also recorded and costed. The Patient Analysis and Tracking System database included variables for gastrointestinal and pulmonary complications, haemodynamic support and arrhythmias; however, > 60% of these data were missing. These complications were not secondary clinical outcomes and therefore additional work was not carried out to address these missing data. The decision was taken not to use these variables in the costings.
For the variables that were used, missing resource use data were assumed to be missing at random and were handled by applying multiple imputation using a series of chained regression equations. 113 Twenty values were predicted for each missing data cell, essentially creating 20 different data sets. A method called Rubin’s rule (which acknowledges variability between as well as within imputed data sets) was used to summarise data across the 20 data sets. 114 Overall, < 1% of resource use data were missing.
Table 38 summarises the observed resource use to hospital discharge for participants in the cohort. The 16 participants who had a positive ad hoc TEG test and who were deemed to have had successful prophylactic treatment are classified as having no CCB here. Tables 121 and 122 in Appendix 1 show the unit costs (and their sources) used to value this resource use; the resultant expected cost estimates for all participants and according to CCB status are shown in Table 39. The costs of any ad hoc TEG tests used are not included in these data as these costs were added into the model separately.
Resource/complication | All participants (N = 1802) | Participants with CCB (N = 433) | Participants with no CCB (N = 1369) | Participants with CCB vs. participants with no CCB, % or mean (SE) difference |
---|---|---|---|---|
Blood products (units), mean (SE) | ||||
Red blood cells | 0.73 (0.04) | 1.70 (0.08) | 0.42 (0.05) | 1.28 (0.10) |
Platelets | 0.26 (0.16) | 1.02 (0.02) | 0.02 (0.01) | 1.00 (0.03) |
FFP | 0.22 (0.02) | 0.86 (0.03) | 0.01 (0.02) | 0.85 (0.04) |
Cryoprecipitate | 0.06 (0.01) | 0.24 (0.02) | 0.00 (0.01) | 0.24 (0.02) |
LOS (days), mean (SE) | ||||
Time on CICU | 0.69 (0.03) | 0.95 (0.07) | 0.60 (0.04) | 0.35 (0.08) |
Time on HDU | 3.82 (0.12) | 4.80 (0.24) | 3.52 (0.13) | 1.28 (0.27) |
Time on ward | 5.05 (0.12) | 5.41 (0.24) | 4.94 (0.13) | 0.47 (0.27) |
Complications/additional treatments, n (%) | ||||
Protamine | 121 (7) | 118 (27) | 3 (0) | 27 |
rFVIIa | 5 (0) | 5 (1) | 0 (0) | 1 |
Reoperation | 86 (5) | 65 (15) | 21 (2) | 13 |
AKI stage 3 | 122 (7) | 49 (11) | 73 (5) | 6 |
MI | 18 (1) | 4 (1) | 14 (1) | 0 |
Stroke | 18 (1) | 8 (2) | 10 (1) | 1 |
Sepsis | 100 (6) | 34 (8) | 66 (5) | 3 |
IABP | 5 (0) | 4 (1) | 1 (0) | 1 |
Cost component | Cost (£), mean (SE) | Participants with CCB vs. participants with no CCB, mean (SE) difference (£) | ||
---|---|---|---|---|
All participants (N = 1802) | Participants with CCB (N = 433) | Participants with no CCB (N = 1369) | ||
Red blood cellsa | 98 (6) | 226 (11) | 57 (6) | 169 (12) |
Platelets | 54 (3) | 213 (5) | 4 (3) | 209 (6) |
FFP | 6 (0) | 24 (1) | 0 (0) | 24 (1) |
Cryoprecipitate | 11 (2) | 46 (3) | 0 (2) | 46 (3) |
Protamine | 0 (0) | 1 (0) | 0 (0) | 1 (0) |
rFVIIa | 7 (3) | 29 (6) | 0 (4) | 29 (7) |
Time on CICU | 825 (41) | 1147 (83) | 723 (47) | 424 (96) |
Time on HDU | 2393 (73) | 3003 (149) | 2201 (84) | 803 (171) |
Time on ward | 2001 (46) | 2142 (93) | 1956 (53) | 186 (107) |
Reoperations | 403 (42) | 1268 (84) | 129 (47) | 1139 (96) |
AKI stage 3 | 98 (9) | 165 (17) | 78 (10) | 87 (20) |
Other complicationsb | 32 (6) | 52 (12) | 25 (7) | 27 (14) |
Total costs | 5928 (127) | 8315 (252) | 5173 (141) | 3143 (289) |
Populating the model with observed health-care costs to hospital discharge
Health-care costs to hospital discharge for each of the different pathways from the end of surgery to discharge could have been estimated by simply averaging the costs to hospital discharge for participants in that pathway. There are many individual pathways, however, and therefore a large number of cost estimates would have been required (in excess of 35), the majority of which would have been based on only a few participants (< 10). Cost estimates based on only a few participants may not be very accurate or reliable and could be heavily influenced by any outliers occurring by chance. Regression analysis was therefore used to pool participants whose costs were not statistically significantly different, across individual pathways, to increase the sample size for cost estimates; this also reduced the number of cost inputs required.
In Figure 32, 12 groups of participants were identified, based on their ad hoc test and POC test results, six with CCB and six without CCB. These 12 groups of participants were then divided into whether they received treatment in the form of protamine, platelets, clotting factors, > 4 units of red cells or a reoperation, whether they developed complications and whether they were alive at hospital discharge. For each of the eight different combinations of treatment/no treatment, complications/no complications and alive at discharge/died in hospital, regression was used to determine whether the costs to discharge were similar across some or all of the 12 groups of patients and for which groups costs could be pooled.
Initially, ordinary least squares (OLS) regression was conducted, with health-care costs as the dependent variable and dummy variables included for 11 of the 12 groups of participants (see Figure 32). Group 12, patients with no ad hoc test and a negative POC test, was taken as the base group as this was the largest group of patients. Any of groups 1–11 was considered suitable to be pooled with group 12 if its coefficient was not statistically significantly different from that for group 12. If several groups were statistically significantly different from group 12, Wald tests (which test the significance of particular explanatory variables, in this case dummy variables for groups) were conducted to determine whether these groups could be combined.
As the cost data were skewed, alternatives to OLS regression (and the normal distribution) were considered. A gamma distribution is positively skewed and often fits cost data well; a generalised linear model (GLM) with a gamma family and a log link was also conducted. As the results from the OLS and GLM models were not always identical, a modified Parks test was conducted for each regression to determine the most appropriate family115 and a Box-Cox test was performed to determine the most appropriate link function. 116 Results from the GLM with the most appropriate family and link function were used to determine which groups could be combined. Generally, many groups could be combined and only one or two pooled cost estimates were required for each of the eight different combinations of treatment, complications and outcome at discharge, thus successfully increasing the sample size for each cost estimate. All cost estimates used in the models are reported in Tables 114–119 in Appendix 1.
Populating the model with observed health-care costs from hospital discharge to 1 year
As resource use data were not collected beyond hospital discharge in the COPTIC study, estimates for health-care costs beyond hospital discharge were sought from secondary sources. All participants who were discharged from hospital were assumed to incur health-care costs between hospital discharge and 1 year. Participants who had complications during their index admission were assumed to incur higher health-care costs during this time period.
Health-care costs from hospital discharge to 1 year were based on the Office of Health Economics estimate of the annual cost per person of NHS care,117 inflated to 2013/14 prices using the Hospital and Community Health Services inflation index,118 multiplied by the proportion of the first year that was not part of the index admission (based on the average LOS of participants alive at 1 year). All participants who survived to 1 year after their surgery were assumed to incur health-care costs of £2116 between hospital discharge and 1 year. Participants who died in the first year were assumed to incur health-care costs of £922 after hospital discharge, based on the same annual cost and the average proportion of 1 year for which they were alive and which was not part of the index admission.
For participants who had complications during their index admission, an additional cost was assigned between hospital discharge and 1 year. MI and stroke were assumed to be associated with continuing care costs. These annual costs were taken from Greenhalgh et al. 119 and inflated to 2013/14 prices. Separate costs were provided for disabling and non-disabling stroke; according to Davies et al. ,15 58% of strokes are disabling and this percentage was used to weight the two continuing care costs for stroke. An annual cost associated with complications was calculated by weighting estimates of the annual costs of MI and stroke by the number of participants alive at hospital discharge with these complications divided by the number of participants alive at discharge with any complications. Costs associated with complications between hospital discharge and 1 year were then calculated separately for participants alive at 1 year and for those who had died by 1 year, based on the proportion of the year for which they were alive and not in hospital for the index admission. Continuing care costs across all participants with complications were estimated to be £92 for participants alive at 1 year and £35 for participants who had died by 1 year.
Costs of the point-of-care and ad hoc thromboelastography tests
Pricing information was obtained from a published source for the TEG and ROTEM tests23 and from the manufacturer (Roche) for the Multiplate test. The price of capital equipment was converted into an equivalent annual cost to take account of depreciation and discounting, using a lifespan of 7 years for equipment and a 3.5% discount rate. It was assumed that, for any test introduced into routine practice at the Bristol Heart Institute, two analysers would be required. In terms of throughput for the analysers, information was taken from the National Adult Cardiac Surgery Audit (NACSA). According to this audit, 1449 cardiac surgery operations were conducted in 2012/13 at the Bristol Heart Institute. 120 It was therefore assumed that each analyser would be used for 725 patients per year. Including both the capital and consumable elements, the cost of a TEG test per patient was estimated to be £37, the cost of a ROTEM test per patient was estimated to be £32 and the cost of a Multiplate test per patient was estimated to be £11.
The cost of an ad hoc TEG test under current practice was estimated to be higher than the cost of a TEG test if it were introduced for all patients. Although the consumable costs were the same as for the POC TEG test, the equipment costs were higher as the throughput for the analysers was lower. Two TEG analysers were in use at the time of the study and, as only 16% of participants received an ad hoc TEG test, each analyser was assumed to be used for 114 patients per year. The cost of the ad hoc TEG test under current practice was estimated to be £64.
Cost of prophylactic treatment indicated by positive point-of-care test results
When blood products were indicated, the following numbers of units were assumed to be given: platelets, 1 unit; FFP, 2 units; and cryoprecipitate, 2 units. A dose of protamine was assumed to be 50 mg.
For each participant in the COPTIC study classified as having a positive POC test result, the cost of the prophylactic treatment indicated by the test result was calculated. For example, 65 participants had a positive TEG test, of whom nine needed protamine, 29 FFP only and 27 platelets and FFP. The average cost [standard error (SE)] of prophylactic treatment for participants with a positive TEG test was £135 (£14). Similarly, the average cost (SE) of prophylactic treatment was £309 (£19) for participants with a positive ROTEM test, £96 (£8) for participants with a positive TEG/Multiplate test and £277 (£14) for participants with a positive ROTEM/Multiplate test.
Life-years to 1 year
Outcomes for the cost-effectiveness model were measured in life-years, as EuroQol-5 Dimensions (EQ-5D) data were not collected and therefore it was not possible to present the results using QALYs. Life-years to 1 year were calculated for each participant in the COPTIC study and participants alive at 1 year accrued 1 life-year in that time. For participants who died by 1 year, either in hospital or after discharge, the date of death was captured and therefore it was possible to calculate the number of days to death from the operation date and divide by 365 days to calculate the proportion of a life-year accrued. To populate the model, life-years were pooled in a similar way to costs to hospital discharge. OLS regression was used to determine whether there were any differences in the life-years accrued by the 12 groups of participants identified in Figure 32, for each combination of treatment/no treatment, complications/no complications and died in hospital/died after discharge but by 1 year. Frequently, life-year estimates could be pooled across all of the groups.
Populating the model beyond 1 year
No data were available from the COPTIC study beyond 1 year (follow-up was until hospital discharge or death and survival data were captured from the NSTS at 1 year) and so secondary sources of information were sought to populate the model. For each annual cycle beyond 1 year, estimates of survival and annual health-care costs were required.
Survival beyond 1 year
At 1 year, participants are split into three health states: alive having had no complications during the index hospital admission, alive having had complications in hospital and dead. In terms of survival, age- and sex-adjusted population life tables were used as the starting point,121 but these needed to be adjusted to account for the increased risk of death in participants who have had cardiac surgery compared with the general population. A further adjustment was required for participants who experienced complications, as these participants are known to be at an increased risk of death compared with participants without complications.
Adjusting for increased mortality after cardiac surgery
Data on the proportion of patients surviving each year after cardiac surgery from the NACSA were used to adjust for the increased risk of mortality after cardiac surgery compared with that in the general population. 120 Using the separate survival curves for CABG, mitral valve surgery and aortic valve surgery available on the audit website, the proportion of patients surviving each year after surgery (up to 12 years) was read off the graphs. A weighted average of the proportion of patients surviving each year after surgery was calculated for all cardiac surgery, based on the proportions of the different types of surgery included in the audit.
To estimate the additional risk of mortality associated with having had cardiac surgery, the odds of dying each year after surgery were calculated for the NACSA population and for an age- and sex-matched population from life tables. The OR of dying in the cardiac surgery population compared with the general population was then calculated for each subsequent year after surgery. The OR was estimated to be 3.0 in the second year after surgery and it gradually reduced to 2.2 by 5 years after surgery (the OR for the first year after surgery was not required as survival to 1 year was available for participants in the observational study). These ORs were used to adjust the life table estimates for the increased risk of mortality after cardiac surgery for all participants in the model; life table probabilities were converted to odds to be adjusted and were then converted back to probabilities.
It is recognised that this will likely underestimate survival slightly for patients without complications, as the NACSA data include patients with and without complications. However, this underestimation will affect both the current practice arm of the model and the test-all arm and so should not affect the differences between treatment options.
Adjusting for increased mortality in the presence of complications after cardiac surgery
A second adjustment to survival was required for participants who experienced complications. Of the 777 participants alive at 1 year who experienced complications during their index admission, 749 had AKI (of whom 543 had stage 1, 113 had stage 2 and 93 had stage 3 AKI according to Acute Kidney Injury Network criteria for AKI122), 83 had sepsis, 17 had a stroke and 15 had a MI (these are not mutually exclusive events). As the data are dominated by AKI, rather than adjusting mortality for a number of different complications, mortality was adjusted for AKI only.
As individual patient data were available for survival to 1 year, it was important that estimates of increased mortality in participants with AKI (compared with those without AKI) from other sources did not include mortality in the first year after surgery as well as longer-term mortality, otherwise mortality in the first year would be double counted. In a review of long-term outcomes after AKI, Sawhney et al. 123 identified two studies that compared mortality with and without AKI and looked at the timing of outcomes. Of the two studies reported, one was not specific to cardiac surgery124 but the other reported hazard ratios (HRs) for mortality at 12, 24, 36 and 60 months after cardiac surgery with and without AKI. 125 HRs for group III (50–99% creatinine increase from baseline) in the study by Ishani et al. 125 corresponded to participants with stage 1 AKI here. This slightly underestimated mortality for COPTIC participants with AKI, as they have a mix of stage 1–3 AKI. A sensitivity analysis was therefore conducted using HRs for group IV (> 100% creatinine increase from baseline) from the study by Ishani et al. 125 HRs were used to adjust survival for participants who experienced complications (compared with those who did not); survival probabilities were converted to rates and adjusted using the HRs and were then converted back to probabilities.
Health-care costs beyond 1 year
Health-care costs beyond 1 year costs were calculated using the unit costs used to estimate health-care costs between hospital discharge and 1 year (described earlier). Annual health-care costs for participants alive in a subsequent year were estimated to be £2173; an additional annual cost of £99 was assigned to participants alive in a subsequent year who experienced a complication during their index admission.
Model analysis
For each model, once the structure had been set up in TreeAge and all probabilities, costs and outcomes entered for current practice, the expected costs and life-years associated with this arm were obtained for the first year, along with the probabilities of being in each of the three Markov states at the end of the first year. These costs, life-years and probabilities were calculated separately in Stata and therefore provided a robust check that all of the inputs had been entered correctly into TreeAge. Once the current practice arm of the model was set up, this was used to set up the test-all arm of the model. Separate calculations were conducted in Stata and Microsoft Excel® 2013 (Microsoft Corporation, Redmond, WA, USA) to estimate the differences between the strategies at 1 year, again as a check that all inputs in TreeAge were correct.
Costs and life-years beyond 1 year were discounted at a rate of 3.5%107 and a half-cycle correction was applied (which adjusts costs and deaths to occur half-way through each annual cycle, rather than at the beginning of the year). 126 Cost-effectiveness analyses were run and expected costs and life-years associated with current practice and the test-all arm were calculated. The incremental cost-effectiveness ratio (ICER) was derived from the differences in costs and life-years between the test-all arm and current practice. The ICER is the cost per life-year gained of introducing a POC test compared with current practice. Analyses were conducted separately for TEG, ROTEM and each of TEG and ROTEM in combination with the Multiplate test.
Sensitivity analyses
Both one-way sensitivity analyses and probabilistic sensitivity analyses were conducted.
One-way sensitivity analyses
One-way sensitivity analyses were used to assess the sensitivity of the base-case results to key uncertainties in the model. Two key uncertainties were the probability of a positive POC test result and the probability that prophylactic treatment indicated by a positive test result was successful and prevented the development of CCB. Table 40 summarises the sensitivity analyses conducted.
SA | Parameter varied | Base case | Alternative strategies in sensitivity analysis |
---|---|---|---|
1 | Probability of a positive test result (which influences the cost of prophylactic treatment) | Approximately 80% of normal ranges | Approximately 100% of normal ranges. This also changes the average cost of prophylactic treatment |
2 | Probability that prophylactic treatment is not successful in preventing CCB | 0.343 | Varied by ± 25% |
3 | Probability that prophylactic treatment is not successful in preventing CCB | 0.343 | Change to 0 |
4 | Probability of CCB for participants in the test-all arm with a positive ad hoc TEG test and a negative POC test | 0.61–0.62 depending on the POC test | Multiply the probability of no CCB by the probability that prophylactic treatment fails |
5 | Cost of the ad hoc TEG | £64 | Varied by ± 25% |
6 | Cost of the POC test | £32–48 (see Table 119) | Varied by ± 25% |
7 | Cost of prophylactic treatment | £135–309 (see Table 119) | Varied by ± 25% |
8 | Unit cost for bed-days | CICU £1203, HDU £626, ward £396 | Varied by ± 25% |
9 | Unit cost for red cells | £122.09 | Varied by ± 25% |
10 | Unit cost for platelets | £208.09 | Varied by ± 25% |
11 | Increased risk of death for participants who experienced complications compared with those who did not | HRs for participants with a 50–99% increase in serum creatinine level since baseline from Ishani et al.125 | HRs for participants with a ≥ 100% increase in serum creatinine since baseline from Ishani et al.125 |
12 | Time horizon | 5 years | 10 years |
The rationale for the sensitivity analyses is described in the following sections.
For the POC tests there is uncertainty around the thresholds at which test parameters indicate a positive test and the need for prophylactic treatment. These thresholds still need to be validated. Less conservative thresholds for treatment were therefore considered in sensitivity analyses. The base-case thresholds used were approximately 80% of the normal ranges; 100% of the normal ranges were used to consider less conservative thresholds for treatment.
For TEG, the less conservative criteria in Box 8 were used to indicate positive test results (these are the same criteria used to identify positive ad hoc test results).
-
If CK R : CKH R > 1.5 and if CK R > 8 minutes, give protamine.
-
If CK R > 8 minutes, give FFP.
-
If CK α-angle < 47°, give FFP and platelets.
-
If CK MA < 55, give FFP and platelets.
-
If CK LY60 > 15%, give tranexamic acid.
α-angle, measures the speed of fibrin build-up; LY60, per cent lysis 60 minutes after MA (percentage decrease in clot amplitude); R, reaction time (minutes) to initial fibrin formation.
Based on these criteria, 32.8% (591/1802) of participants were classified as having a positive TEG test result and the average (SE) cost of their prophylactic treatment indicated was £222 (£3).
For ROTEM, the less conservative criteria in Box 9 were used to indicate positive test results.
-
If INTEM CT > 240 seconds and HEPTEM CT : INTEM CT < 0.8, give protamine.
-
If EXTEM CT > 80 seconds or HEPTEM CT > 240 seconds, give FFP.
-
If EXTEM A10 ≤ 40 mm and FIBTEM A10 < 10 mm, give cryoprecipitate.
-
If EXTEM A10 ≤ 40 mm and FIBTEM A10 ≥ 10 mm, give FFP and platelets.
-
If FIBTEM A10 < 7 mm or FIBTEM MCF < 9 mm, give cryoprecipitate.
Based on these criteria, 10.6% (191/1802) of participants were classified as having a positive ROTEM test result and the average (SE) cost of their prophylactic treatment indicated was £262 (£12).
For the Multiplate test, the base-case criteria were increased by 25% to give the less conservative criteria in Box 10 for determining positive test results.
-
AUC TRAPtest < 62.5.
-
AUC ASPItest < 37.5.
-
AUC ADPtest < 37.5.
Based on these less conservative criteria, 34.1% (615/1802) of participants were classified as having a positive TEG/Multiplate test result and the average (SE) cost of their prophylactic treatment indicated was £67 (£2); 12.5% (225/1802) of participants were classified as having a positive ROTEM/Multiplate test result with an average (SE) cost of prophylactic treatment of £263 (£11).
There is considerable uncertainty around the probability that the prophylactic treatment indicated by a positive test result is successful and prevents the development of CCB; this is a key parameter in the model. The base-case failure rate of 34.3% was varied by ± 25% in sensitivity analyses.
In the test-all arm it was not possible to ‘take out’ the effect of a positive ad hoc TEG test for participants who had a negative POC test. This was crudely attempted in a sensitivity analysis by shifting some of these participants from no CCB to CCB on the basis that they would not have received prophylactic treatment as a result of a positive ad hoc test and more of them may have developed CCB. Specifically, the probability of being in the no CCB group for participants with a positive ad hoc test but a negative POC test (group 4 in Figure 32) was multiplied by the probability that the prophylactic treatment failed.
To quantify the uncertainty around these one-way sensitivity analyses, parameters were assigned distributions (rather than fixed values) so that SEs and 95% CIs could be estimated; this was carried out in the same way as for the probabilistic sensitivity analyses that were conducted.
Probabilistic sensitivity analyses
As the majority of parameters in the model were estimated from the COPTIC study rather than the whole population of patients undergoing cardiac surgery, uncertainty exists around these estimates. Parameter uncertainty was investigated using probabilistic sensitivity analysis, which enabled the joint impact on the cost-effectiveness results of all of the uncertain parameters in the model to be assessed simultaneously. 126–128 Rather than taking fixed values, model parameters were assigned probability distributions to describe the range of plausible values that they could take based on the precision of the estimates available. The type of distribution assigned was matched to the nature of the parameter, for example a beta distribution lies between 0 and 1 and is therefore appropriate for parameterising the probability of two mutually exclusive events and a gamma distribution is positive and continuous and was used to parameterise costs. The type of distribution assigned to each parameter is shown in Table 110 in Appendix 1.
Randomly selected values from each distribution for each model parameter were simultaneously generated using Monte Carlo simulation; this was repeated 1000 times and the results were calculated for each run of the model, generating a distribution of costs and effects that was used to calculate 95% CIs around the cost and life-year differences between strategies, enabling the effect of the parameter uncertainty on conclusions to be investigated. The results are presented on the cost-effectiveness plane and are also expressed in terms of a cost-effectiveness acceptability curve, which indicates the likelihood that a POC test is cost-effective for different amounts that health-care decision-makers are willing to pay for an additional life-year. 102
Results of the economic evaluation
Base-case results
The expected costs and life-years gained under current practice and for each of the POC test options are shown in Table 41, together with the cost-effectiveness results for each POC test compared with current practice. For each of the POC test options, the MDs in costs and life-years compared with current practice are small and not statistically significant; the largest difference in costs is £33, approximately 0.2% of the total costs, and the largest difference in life-years is 0.0043, equivalent to 1.6 days.
Strategy | Costs (£), mean (SE) | Life-years, mean (SE) | Difference in costs from current practice (£), mean (95% CI) | Difference in life-years from current practice, mean (95% CI) | ICER (£) compared with current practice |
---|---|---|---|---|---|
Current practice | 16,057 (133) | 4.5917 (0.0241) | |||
TEG | 16,081 (134) | 4.5917 (0.0233) | 24 (–34 to 85) | –0.00002 (–0.0050 to 0.0041) | Current practice dominant (–1,122,030) |
ROTEM | 16,066 (132) | 4.5960 (0.0240) | 9 (–56 to 76) | 0.0043 (–0.0032 to 0.0137) | 2050 |
TEG and Multiplate | 16,090 (134) | 4.5911 (0.0252) | 33 (–28 to 98) | –0.0006 (–0.0084 to 0.0065) | Current practice dominant (–55,934) |
ROTEM and Multiplate | 16,075 (134) | 4.5955 (0.0255) | 18 (–49 to 87) | 0.0038 (–0.0047 to 0.0142) | 4727 |
Based on the point estimates of the ICER, TEG and TEG plus the Multiplate test are dominated by current practice as they are both more costly and less effective, but ROTEM and ROTEM plus the Multiplate test are likely to be considered cost-effective. However, there is great uncertainty around all of these results, as shown in plots of the 1000 simulated cost and life-year differences from the probabilistic sensitivity analysis for each POC test (Figure 33). In each figure a large number of points are in two or three quadrants of the cost-effectiveness plane. The black dot is the point estimate of the cost and life-year difference and in each figure is close to the origin.
FIGURE 33.
Plots of 1000 simulated cost and life-year differences from the probabilistic sensitivity analysis for test all vs. current practice: (a) TEG; (b) ROTEM; (c) TEG and Multiplate; and (d) ROTEM and Multiplate.
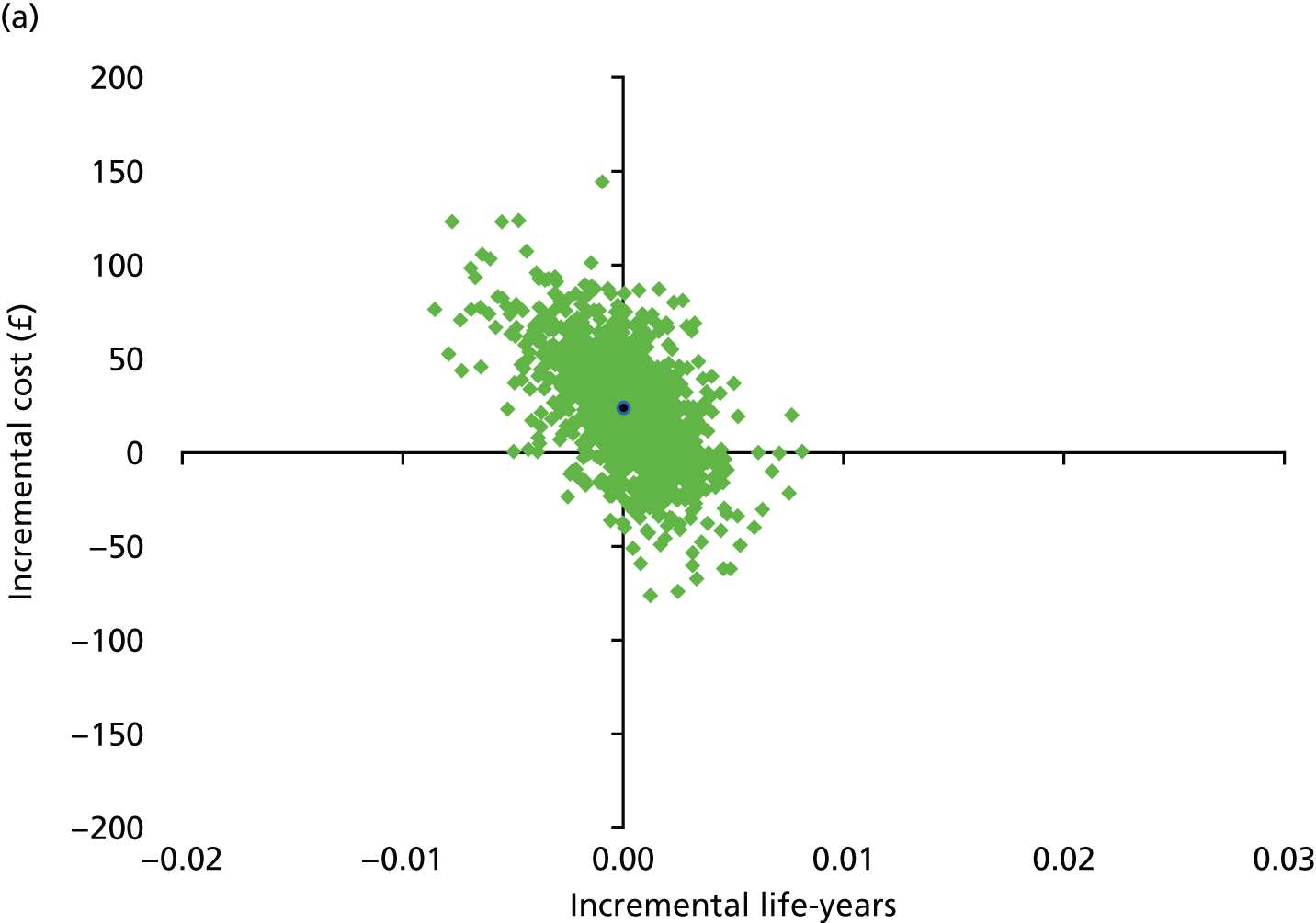
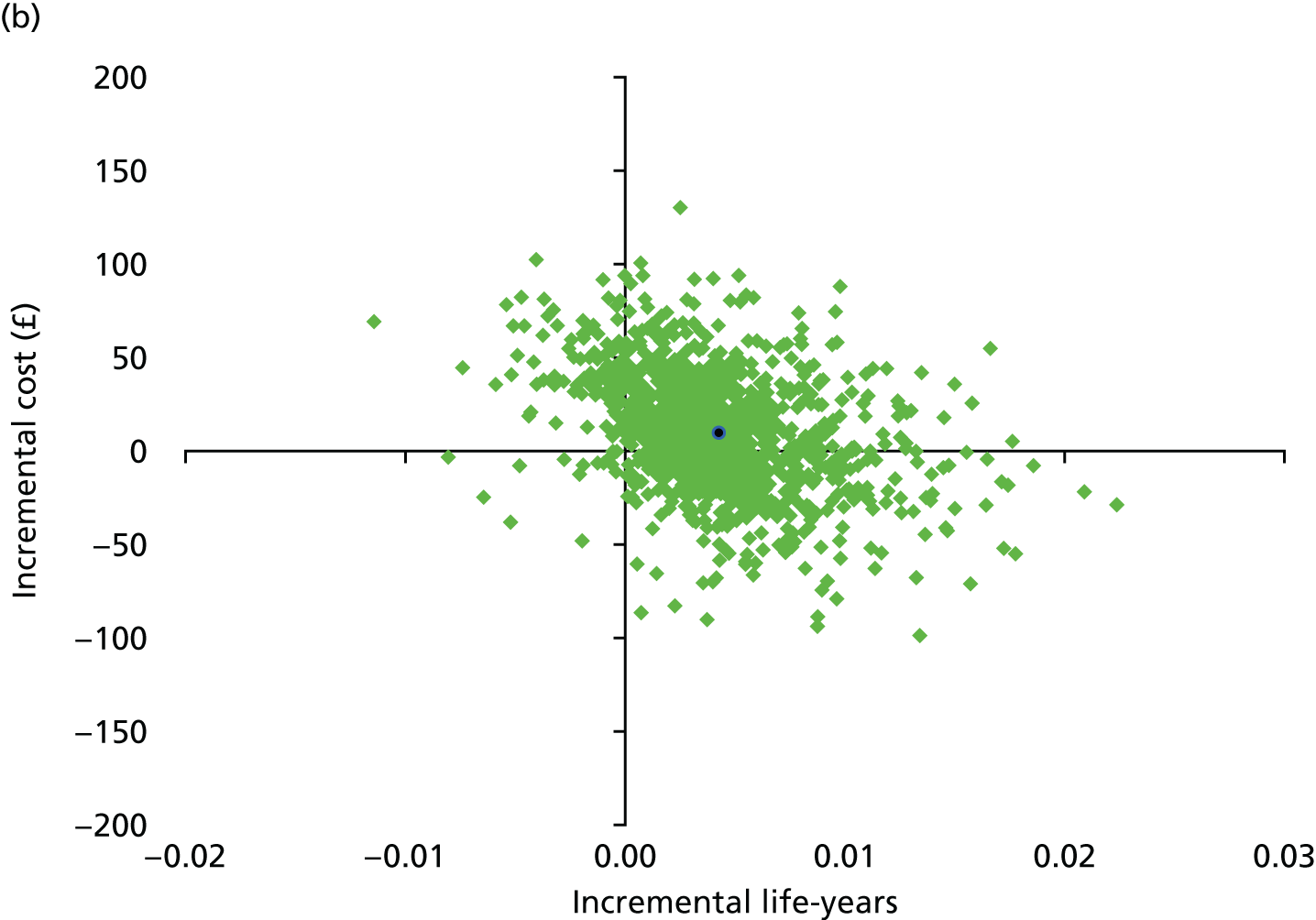
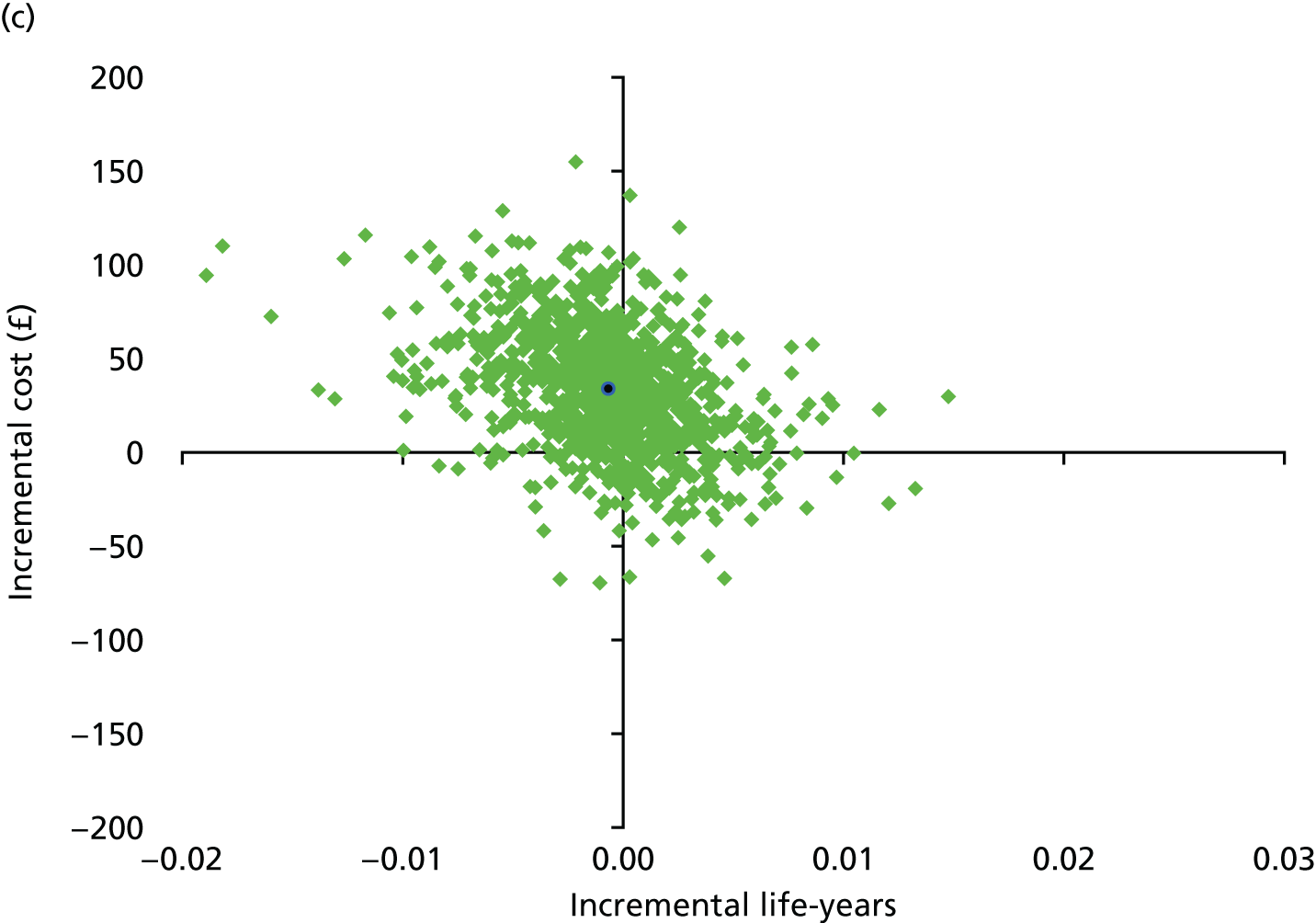

The cost-effectiveness acceptability curves in Figure 34 show the probability that each POC test is cost-effective for a range of willingness-to-pay thresholds. If a decision-maker is willing to pay £20,000 for an additional life-year, then the probability of the test-all strategy being cost-effective is 0.38, 0.83, 0.32 and 0.74 for TEG, ROTEM, TEG plus the Multiplate test and ROTEM plus the Multiplate test respectively. Across willingness-to-pay thresholds from £0 to £100,000, TEG and TEG plus the Multiplate test are less likely to be cost-effective than current practice. As the willingness-to-pay threshold increases, ROTEM and ROTEM and Multiplate are more likely to be cost-effective than current practice. There is, however, much uncertainty around these findings. The dotted lines at 0.1 and 0.9 indicate the 80% confidence limits for the probability that a POC test is cost-effective. As these horizontal lines do not cut the curve at any point on any of the graphs, the 80% confidence limits on cost-effectiveness do not exist.
FIGURE 34.
Cost-effectiveness acceptability curves for test all vs. current practice: (a) TEG; (b) ROTEM; (c) TEG and Multiplate; and (d) ROTEM and Multiplate.
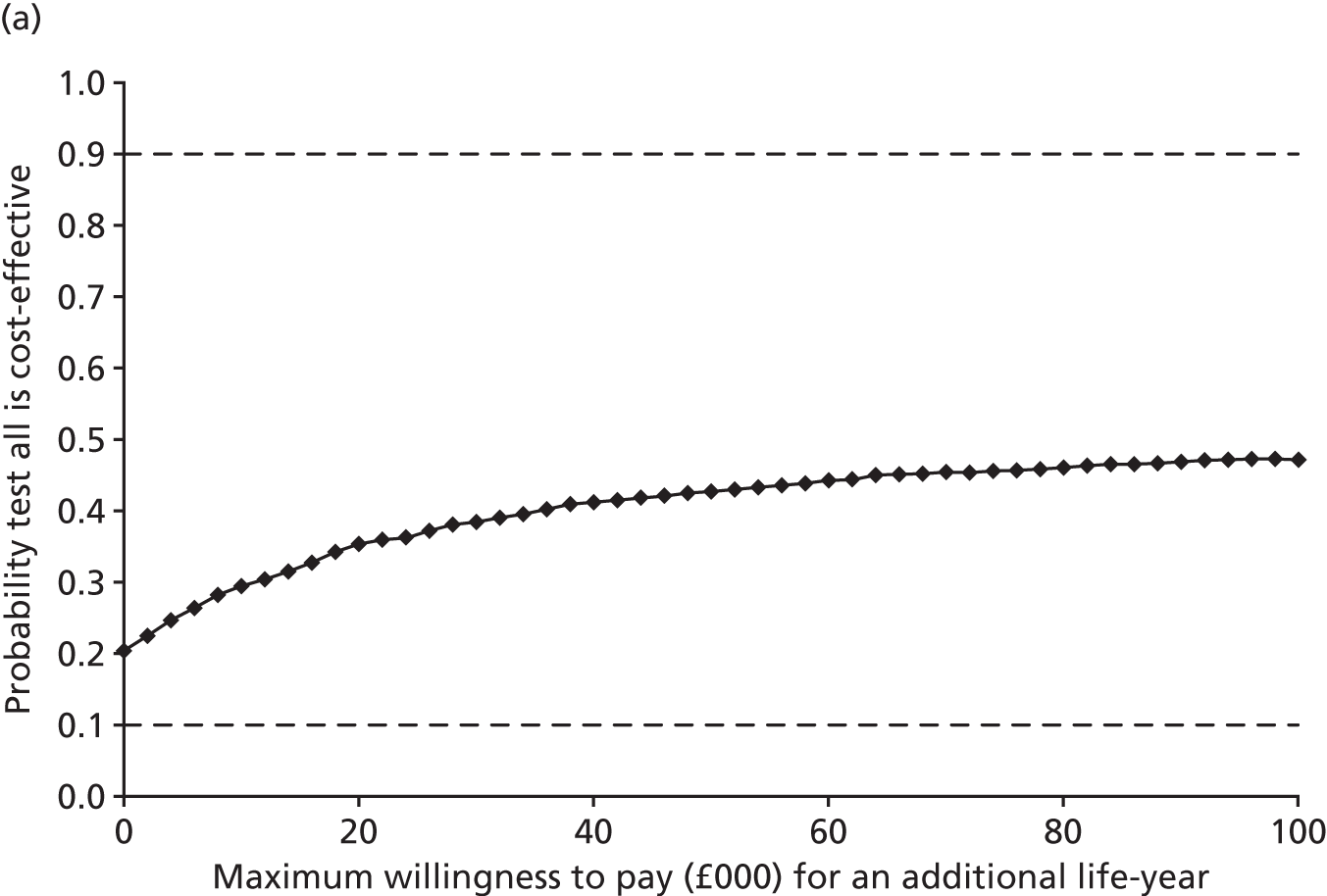
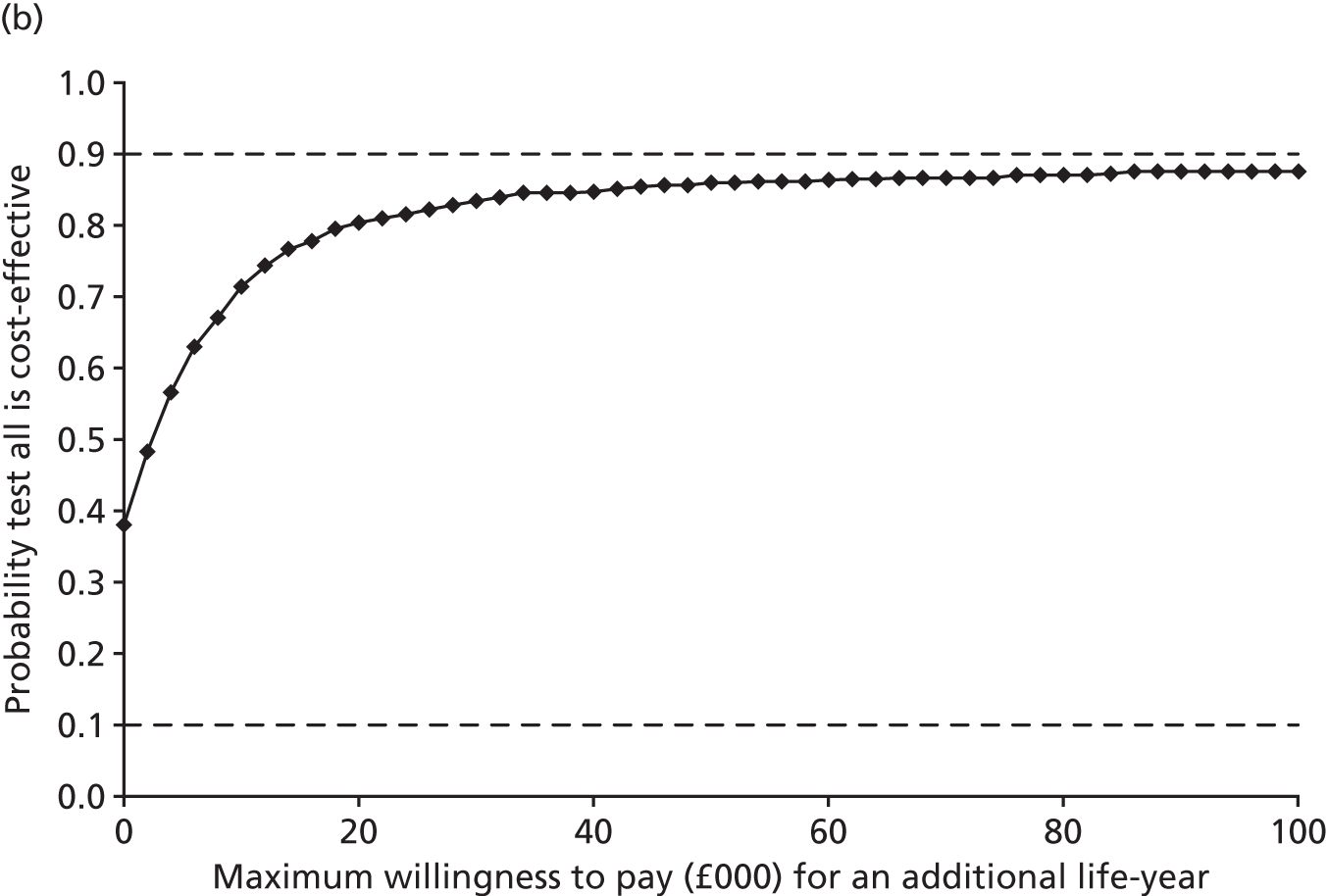
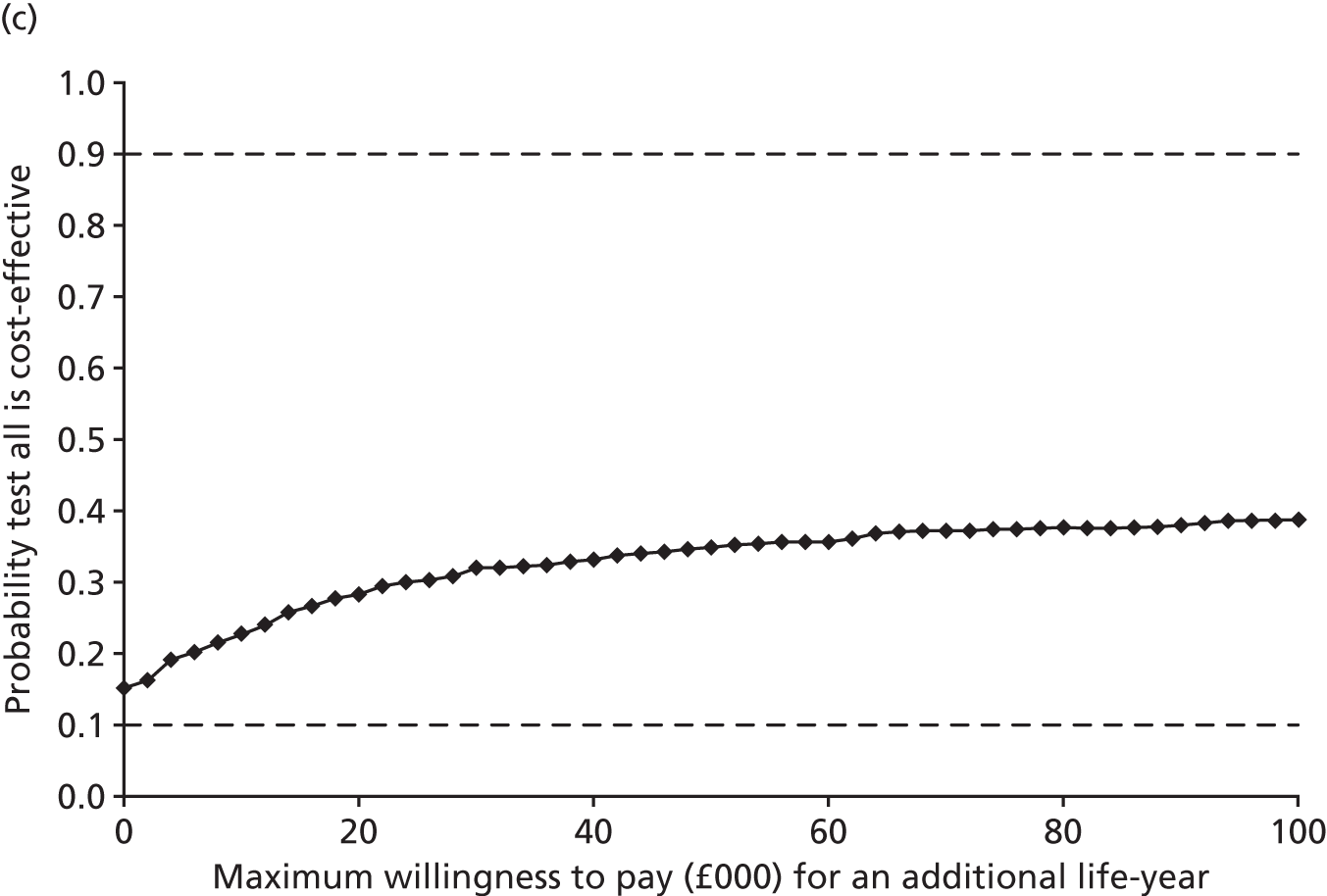

Sensitivity analyses
The results of the sensitivity analyses are shown in Table 42. In three of the sensitivity analyses (SA1, 3 and 4) interpretation of the point estimates of cost-effectiveness was different from that in the base case for one or more of the POC tests. In all cases, however, differences in costs and life-years were very small and the uncertainty around the results remained.
SA | Strategy | Costs (£), mean (SE) | Life-years, mean (SE) | Difference in costs from current practice (£), mean (95% CI) | Difference in life-years from current practice, mean (95% CI) | ICER (£) compared with current practice |
---|---|---|---|---|---|---|
Base case | Current practice | 16,057 (133) | 4.5917 (0.0241) | |||
TEG | 16,081 (134) | 4.5917 (0.0233) | 24 (–34 to 85) | –0.00002 (–0.0050 to 0.0041) | Current practice dominant (–1,122,030) | |
ROTEM | 16,066 (132) | 4.5960 (0.0240) | 9 (–56 to 76) | 0.0043 (–0.0031 to 0.0137) | 2050 | |
TEG and Multiplate | 16,090 (134) | 4.5911 (0.0252) | 33 (–28 to 98) | –0.0006 (–0.0084 to 0.0065) | Current practice dominant (–55,934) | |
ROTEM and Multiplate | 16,075 (134) | 4.5955 (0.0255) | 18 (–49 to 87) | 0.0038 (–0.0047 to 0.0142) | 4727 | |
SA1 | TEG | 16,097 (128) | 4.5990 (0.0232) | 41 (–19 to 102) | 0.0073 (–0.0014 to 0.0182) | 5606 |
ROTEM | 16,060 (126) | 4.5931 (0.0240) | 3 (–69 to 73) | 0.0033 (–0.0039 to 0.0115) | 989 | |
TEG and Multiplate | 16,074 (127) | 4.5986 (0.0237) | 17 (–55 to 83) | 0.0069 (–0.0027 to 0.0185) | 2537 | |
ROTEM and Multiplate | 16,063 (127) | 4.5964 (0.0242) | 7 (–69 to 80) | 0.0047 (–0.0031 to 0.0134) | 1425 | |
SA2: –25% | TEG | 16,080 (134) | 4.5917 (0.0233) | 23 (–35 to 85) | –0.00002 (–0.0050 to 0.0041) | Current practice dominant (–957,420) |
ROTEM | 16,063 (132) | 4.5966 (0.0240) | 6 (–59 to 74) | 0.0049 (–0.0031 to 0.0149) | 1281 | |
TEG and Multiplate | 16,088 (134) | 4.5910 (0.0252) | 31 (–30 to 97) | –0.0007 (–0.0084 to 0.0065) | Current practice dominant (–46,665) | |
ROTEM and Multiplate | 16,071 (134) | 4.5960 (0.0255) | 14 (–54 to 84) | 0.0042 (–0.0044 to 0.0151) | 3242 | |
SA2: +25% | TEG | 16,082 (134) | 4.5917 (0.0233) | 25 (–33 to 85) | –0.00002 (–0.0049 to 0.0041) | Current practice dominant (–1,336,055) |
ROTEM | 16,068 (133) | 4.5955 (0.0240) | 11 (–53 to 78) | 0.0037 (–0.0032 to 0.0124) | 3049 | |
TEG and Multiplate | 16,092 (134) | 4.5912 (0.0252) | 35 (–26 to 100) | –0.0005 (–0.0083 to 0.0065) | Current practice dominant (–67,985) | |
ROTEM and Multiplate | 16,079 (134) | 4.5950 (0.0256) | 22 (–44 to 91) | 0.0033 (–0.0047 to 0.0132) | 6658 | |
SA3 | TEG | 16,078 (134) | 4.5917 (0.0233) | 21 (–39 to 83) | –0.00003 (–0.0049 to 0.0041) | Current practice dominant (–632,966) |
ROTEM | 16,055 (132) | 4.5983 (0.0241) | –2 (–69 to 66) | 0.0065 (–0.0029 to 0.0190) | ROTEM dominant (–234) | |
TEG and Multiplate | 16,082 (134) | 4.5908 (0.0252) | 26 (–38 to 93) | –0.0009 (–0.0086 to 0.0063) | Current practice dominant (–28,397) | |
ROTEM and Multiplate | 16,059 (133) | 4.5974 (0.0254) | 2 (–71 to 75) | 0.0057 (–0.0043 to 0.0189) | 316 | |
SA4 | TEG | 16,129 (137) | 4.5871 (0.0240) | 72 (4 to 140) | –0.0047 (–0.0139 to 0.0026) | Current practice dominant (–15,448) |
ROTEM | 16,131 (139) | 4.5926 (0.0245) | 74 (–1 to 153) | 0.0009 (–0.0092 to 0.0155) | 80,351 | |
TEG and Multiplate | 16,132 (137) | 4.5864 (0.0258) | 76 (9 to 149) | –0.0053 (–0.0153 to 0.0045) | Current practice dominant (–14,344) | |
ROTEM and Multiplate | 16,133 (140) | 4.5922 (0.0259) | 76 (–3 to 164) | 0.0005 (–0.0100 to 0.0113) | 167,948 | |
SA5: –25% | Current practice | 16,054 (133) | 4.5917 (0.0241) | |||
TEG | 16,081 (134) | 4.5917 (0.0233) | 27 (–31 to 88) | –0.00002 (–0.0050 to 0.0041) | Current practice dominant (–1,238,736) | |
ROTEM | 16,066 (132) | 4.5960 (0.0240) | 11 (–53 to 78) | 0.0043 (–0.0031 to 0.0137) | 2637 | |
TEG and Multiplate | 16,090 (134) | 4.5911 (0.0252) | 36 (–25 to 101) | –0.0006 (–0.0084 to 0.0065) | Current practice dominant (–60,169) | |
ROTEM and Multiplate | 16,075 (134) | 4.5955 (0.0255) | 20 (–46 to 90) | 0.0038 (–0.0047 to 0.0142) | 5399 | |
SA5: +25% | Current practice | 16,059 (133) | 4.5917 (0.0241) | |||
TEG | 16,081 (134) | 4.5917 (0.0233) | 22 (–37 to 83) | –0.00002 (–0.0050 to 0.0041) | Current practice dominant (–1,005,323) | |
ROTEM | 16,066 (132) | 4.5960 (0.0240) | 6 (–59 to 73) | 0.0043 (–0.0031 to 0.0137) | 1463 | |
TEG and Multiplate | 16,090 (134) | 4.5911 (0.0252) | 31 (–31 to 96) | –0.0006 (–0.0084 to 0.0065) | Current practice dominant (–51,699) | |
ROTEM and Multiplate | 16,075 (134) | 4.5955 (0.0255) | 15 (–51 to 85) | 0.0038 (–0.0047 to 0.0142) | 4056 | |
SA6: –25% | TEG | 16,072 (134) | 4.5917 (0.0233) | 15 (–43 to 76) | –0.00002 (–0.0050 to 0.0041) | Current practice dominant (–693,921) |
ROTEM | 16,058 (132) | 4.5960 (0.0240) | 1 (–64 to 68) | 0.0043 (–0.0031 to 0.0137) | 187 | |
TEG and Multiplate | 16,076 (134) | 4.5911 (0.0252) | 20 (–42 to 85) | –0.0006 (–0.0084 to 0.0065) | Current practice dominant (–33,260) | |
ROTEM and Multiplate | 16,062 (134) | 4.5955 (0.0255) | 6 (–60 to 75) | 0.0038 (–0.0047 to 0.0142) | 1464 | |
SA6: +25% | TEG | 16,090 (134) | 4.5917 (0.0233) | 33 (–25 to 95) | –0.00002 (–0.0050 to 0.0041) | Current practice dominant (–1,550,138) |
ROTEM | 16,074 (132) | 4.5960 (0.0240) | 17 (–48 to 84) | 0.0043 (–0.0031 to 0.0137) | 3912 | |
TEG and Multiplate | 16,103 (134) | 4.5911 (0.0252) | 47 (–15 to 112) | –0.0006 (–0.0084 to 0.0065) | Current practice dominant (–78,608) | |
ROTEM and Multiplate | 16,087 (134) | 4.5955 (0.0255) | 30 (–36 to 100) | 0.0038 (–0.0047 to 0.0142) | 7990 | |
SA7: –25% | TEG | 16,080 (134) | 4.5917 (0.0233) | 23 (–35 to 85) | –0.00002 (–0.0050 to 0.0041) | Current practice dominant (–1,076,955) |
ROTEM | 16,064 (132) | 4.5960 (0.0240) | 7 (–57 to 74) | 0.0043 (–0.0031 to 0.0137) | 1661 | |
TEG and Multiplate | 16,089 (134) | 4.5911 (0.0252) | 32 (–29 to 97) | –0.0006 (–0.0084 to 0.0065) | Current practice dominant (–54,301) | |
ROTEM and Multiplate | 16,072 (134) | 4.5955 (0.0255) | 15 (–51 to 85) | 0.0038 (–0.0047 to 0.0142) | 4103 | |
SA7: +25% | TEG | 16,082 (133) | 4.5917 (0.0233) | 25 (–33 to 86) | –0.00002 (–0.0050 to 0.0041) | Current practice dominant (–1,167,105) |
ROTEM | 16,067 (132) | 4.5960 (0.0240) | 10 (–54 to 77) | 0.0043 (–0.0031 to 0.0137) | 2439 | |
TEG and Multiplate | 16,091 (134) | 4.5911 (0.0252) | 34 (–27 to 99) | –0.0006 (–0.0084 to 0.0065) | Current practice dominant (–57,567) | |
ROTEM and Multiplate | 16,077 (134) | 4.5955 (0.0255) | 20 (–46 to 90) | 0.0038 (–0.0047 to 0.0142) | 5352 | |
SA8: –25% | Current practice | 14,752 (112) | 4.5917 (0.0241) | |||
TEG | 14,776 (112) | 4.5917 (0.0233) | 25 (–25 to 77) | –0.00002 (–0.0050 to 0.0041) | Current practice dominant (–1,136,243) | |
ROTEM | 14,765 (112) | 4.5960 (0.0240) | 13 (–43 to 72) | 0.0043 (–0.0031 to 0.0137) | 3036 | |
TEG and Multiplate | 14,786 (112) | 4.5911 (0.0252) | 34 (–19 to 90) | –0.0006 (–0.0084 to 0.0065) | Current practice dominant (–57,849) | |
ROTEM and Multiplate | 14,775 (113) | 4.5955 (0.0255) | 23 (–35 to 85) | 0.0038 (–0.0047 to 0.0142) | 6209 | |
SA8: +25% | Current practice | 17,362 (153) | 4.5917 (0.0241) | |||
TEG | 17,385 (157) | 4.5917 (0.0233) | 24 (–43 to 93) | –0.00002 (–0.0050 to 0.0041) | Current practice dominant (–1,107,976) | |
ROTEM | 17,366 (153) | 4.5960 (0.0240) | 5 (–69 to 80) | 0.0043 (–0.0031 to 0.0137) | 1064 | |
TEG and Multiplate | 17,394 (157) | 4.5911 (0.0252) | 32 (–38 to 108) | –0.0006 (–0.0084 to 0.0065) | Current practice dominant (–54,024) | |
ROTEM and Multiplate | 17,374 (157) | 4.5955 (0.0255) | 12 (–63 to 93) | 0.0038 (–0.0047 to 0.0142) | 3246 | |
SA9: –25% | Current practice | 16,034 (131) | 4.5917 (0.0241) | |||
TEG | 16,059 (133) | 4.5917 (0.0233) | 24 (–34 to 85) | –0.00002 (–0.0050 to 0.0041) | Current practice dominant (–1,126,825) | |
ROTEM | 16,044 (131) | 4.5960 (0.0240) | 9 (–55 to 75) | 0.0043 (–0.0031 to 0.0137) | 2135 | |
TEG and Multiplate | 16,068 (133) | 4.5911 (0.0252) | 33 (–27 to 98) | –0.0006 (–0.0084 to 0.0065) | Current practice dominant (–56,189) | |
ROTEM and Multiplate | 16,053 (134) | 4.5955 (0.0255) | 18 (–47 to 87) | 0.0038 (–0.0047 to 0.0142) | 4843 | |
SA9: +25% | Current practice | 16,079 (133) | 4.5917 (0.0241) | |||
TEG | 16,103 (135) | 4.5917 (0.0233) | 24 (–34 to 86) | –0.00002 (–0.0050 to 0.0041) | Current practice dominant (–1,117,185) | |
ROTEM | 16,087 (133) | 4.5960 (0.0240) | 8 (–57 to 77) | 0.0043 (–0.0031 to 0.0137) | 1965 | |
TEG and Multiplate | 16,112 (135) | 4.5911 (0.0252) | 33 (–29 to 99) | –0.0006 (–0.0084 to 0.0065) | Current practice dominant (–55,673) | |
ROTEM and Multiplate | 16,096 (135) | 4.5955 (0.0255) | 17 (–49 to 88) | 0.0038 (–0.0047 to 0.0142) | 4611 | |
SA10: –25% | Current practice | 16,043 (132) | 4.5917 (0.0241) | |||
TEG | 16,067 (134) | 4.5917 (0.0233) | 24 (–34 to 84) | –0.00002 (–0.0050 to 0.0041) | Current practice dominant (–1,105,324) | |
ROTEM | 16,052 (132) | 4.5960 (0.0240) | 9 (–55 to 75) | 0.0043 (–0.0031 to 0.0137) | 2119 | |
TEG and Multiplate | 16,073 (134) | 4.5911 (0.0252) | 33 (–28 to 97) | –0.0006 (–0.0084 to 0.0065) | Current practice dominant (–55,505) | |
ROTEM and Multiplate | 16,061 (134) | 4.5955 (0.0255) | 18 (–48 to 86) | 0.0038 (–0.0047 to 0.0142) | 4680 | |
SA10: +25% | Current practice | 16,070 (133) | 4.5917 (0.0241) | |||
TEG | 16,095 (134) | 4.5917 (0.0233) | 25 (–34 to 86) | –0.00002 (–0.0050 to 0.0041) | Current practice dominant (–1,138,535) | |
ROTEM | 16,079 (133) | 4.5960 (0.0240) | 9 (–57 to 78) | 0.0043 (–0.0031 to 0.0137) | 1981 | |
TEG and Multiplate | 16,104 (134) | 4.5911 (0.0252) | 34 (–28 to 99) | –0.0006 (–0.0084 to 0.0065) | Current practice dominant (–56,420) | |
ROTEM and Multiplate | 16,088 (135) | 4.5955 (0.0255) | 18 (–49 to 89) | 0.0038 (–0.0047 to 0.0142) | 4783 | |
SA11 | Current practice | 15,966 (137) | 4.5519 (0.0287) | |||
TEG | 15,990 (137) | 4.5519 (0.0277) | 24 (–33 to 84) | –0.00004 (–0.0050 to 0.0043) | Current practice dominant (–545,627) | |
ROTEM | 15,975 (137) | 4.5563 (0.0286) | 9 (–55 to 76) | 0.0044 (–0.0030 to 0.0136) | 2054 | |
TEG and Multiplate | 16,000 (138) | 4.5514 (0.0294) | 33 (–27 to 99) | –0.0005 (–0.0083 to 0.0068) | Current practice dominant (–62,647) | |
ROTEM and Multiplate | 15,985 (139) | 4.5559 (0.0299) | 18 (–47 to 88) | 0.0039 (–0.0045 to 0.0144) | 4618 | |
SA12 | Current practice | 21,951 (157) | 7.2527 (0.0479) | |||
TEG | 21,975 (154) | 7.2526 (0.0458) | 24 (–31 to 82) | –0.00009 (–0.0084 to 0.0072) | Current practice dominant (–269,602) | |
ROTEM | 21,966 (159) | 7.2598 (0.0477) | 15 (–47 to 76) | 0.0070 (–0.0051 to 0.0221) | 2066 | |
TEG and Multiplate | 21,984 (160) | 7.2519 (0.0492) | 33 (–23 to 94) | –0.0008 (–0.0134 to 0.0109) | Current practice dominant (–40,334) | |
ROTEM and Multiplate | 21,974 (160) | 7.2591 (0.0489) | 23 (–40 to 90) | 0.0064 (–0.0071 to 0.0230) | 3592 |
Sensitivity analysis 1, which considered less stringent criteria for determining whether a POC test result was positive, had the greatest impact on the results for TEG and TEG plus the Multiplate test. Using the less stringent criteria, the point estimates suggest that these tests are more costly than current practice, as in the base case, but are now more effective; the ICERs are < £6000 per life-year, suggesting that the tests would be considered cost-effective. Neither the difference in costs nor the difference in life-years is statistically significant and the uncertainty around the results remains.
Under an extreme sensitivity analysis (SA3), assuming that prophylactic treatment is always successful, ROTEM became marginally less costly than current practice (by £2) and the point estimate of the ICER suggests that ROTEM dominates current practice, but there is huge uncertainty around this result, as demonstrated by the CIs around the cost and life-year differences compared with current practice.
When an attempt was made to ‘take out’ the effect of a positive ad hoc test result for participants in the test-all arm with a negative POC test result (SA4), the costs under each of the POC strategies increased, life-years decreased and ROTEM and ROTEM plus the Multiplate test were no longer likely to be considered cost-effective.
All other sensitivity analyses had very little impact on the results. In most cases there was little impact on the average costs and life-years in each group and therefore little impact on the differences between groups and the ICERs. Altering bed-day costs by ± 25% did alter average costs by approximately £1300, but this effect was seen under all strategies and therefore there was virtually no change in the MD in costs between the POC tests and current practice. Based on the point estimates, TEG and TEG plus the Multiplate test remain dominated by current practice, whereas ROTEM and ROTEM plus the Multiplate test are likely to be cost-effective (all ICERs are < £8000 per life-year except for SA4); however, as in the base case, differences are very small and the results are very uncertain.
Summary of the health economic analysis
There was very little difference between any of the POC tests and current practice in terms of either costs or life-years and there was great uncertainty around the cost-effectiveness results. Mean life-years to 5 years were 4.6 for all strategies considered; the greatest difference in life-years of 0.0043 is equivalent to 1.6 days. Costs were approximately £16,100 under each strategy and the largest MD compared with current practice was £33. Point estimates suggested that TEG and TEG plus the Multiplate test were more costly and less effective than current practice and therefore dominated by it and that ROTEM and ROTEM plus the Multiplate test were more costly and more effective than current practice; if a decision-maker was willing to pay £8000 for an additional life-year, then they would be considered cost-effective, according to the base case and all point estimates in the sensitivity analyses (except for the extreme case in SA4). However, given the small differences in costs and life-years, there was much uncertainty around these results. There was no clear difference in the cost-effectiveness of any of the POC test options compared with current practice.
Systematic review
Aims and objectives
Existing NICE guidance23 supports the routine use of POC tests for the management of bleeding patients in cardiac surgery. However, the results of the COPTIC study suggest that viscoelastic tests have limited or no benefits for the management of these patients. We have also identified limitations in the Health Technology Assessment (HTA) study26 that was used to inform the NICE guidance. Clinical uncertainty as to the utility of viscoelastic devices is reflected by variability in their use; the 2011 UK audit of blood transfusion in adult cardiac surgery concluded that these devices were not in common use in as many as 25% of units sampled. 9 To address this uncertainty, and the limitations of the evidence used to support the current NICE guidance, we undertook a systematic review of RCTs assessing the clinical efficacy of viscoelastic tests in cardiac surgery. Unlike the HTA assessment,26 we included the results of a pragmatic multicentre trial that enrolled 7402 participants in 12 Canadian hospitals and which was published in 2016,129 we assessed important clinical outcomes including mortality, kidney injury, stroke and prolonged respiratory support and we summarised the evidence using the GRADE approach.
Methods
Search strategy and selection criteria
Search methods, data extraction, assessment and presentation were performed as recommended by the Cochrane Handbook for Systematic Reviews of Interventions (version 5.1.0). 130 The analysis was specified in advance and registered on the PROSPERO international prospective register of systematic reviews (reference number CRD42016033831) on 31 January 2016. This review is reported in accordance with the Preferred Reporting Items for Systematic Reviews and Meta-Analyses (PRISMA) statement (see Appendix 1). 131
Eligibility criteria
Randomised controlled trials, irrespective of blinding, language, publication status, date of publication and sample size, were considered eligible for this study. Participants of any age undergoing cardiac surgery for acquired or congenital disease or aortovascular disease, with or without CPB, were considered eligible. No age restriction was applied. There were no exclusion criteria.
Type of intervention
Eligible trials were those evaluating the risks and benefits of viscoelastic POC testing devices for coagulopathy [ROTEM, TEG or the Sonoclot® Coagulation & Platelet Function Analyzer (Sienco Inc., CO, USA)], alone or combined with platelet function testing.
Comparator
The comparator was represented by a combination of clinical judgement and/or SLTs including PT, aPTT, ACT and plasma fibrinogen levels. We did not distinguish between these comparators for the purpose of this review.
Types of outcome measures
The primary outcome was 30-day or hospital all-cause mortality. The secondary outcomes were adverse events including reoperation for bleeding, red cell, platelet and FFP transfusion, AKI, cerebrovascular accident (CVA), MI, ventilation time and ICU and hospital LOS.
Information sources
Potentially eligible trials were identified by searching the Cochrane Central Register of Controlled Trials (CENTRAL) (Internet), MEDLINE (PubMed, 1946 to present) and EMBASE (Ovid, 1975 to present) and the Cumulative Index to Nursing and Allied Health Literature (CINAHL) Plus (1979 to present) using a combination of subject headings and text words to identify relevant trials. The following MEDLINE search strategy was adapted as appropriate for other databases: ((Cardiopulmonary Bypass) OR (Cardiac Surgery) OR (Extra Corporeal Circulation) OR (Perioperative Morbidity) OR (Bleeding) OR (Coagulopathy) OR (Coagulopathic bleeding)) AND ((Thrombelastography) OR (Thromboelastometry) OR (Transfusion) OR (Viscoelastic testing devices)). The last search was run on 3 December 2016. In addition to searching databases we searched trial registries and checked reference lists of included studies.
Risk of bias assessment
The quality of the studies was assessed using the Cochrane Collaboration’s tool for assessing risk of bias. 132 The items assessed were (1) sequence generation, (2) allocation concealment, (3) blinding of outcome assessor, (4) incomplete outcome data and (5) selective outcome reporting. The risk of bias was graded as unclear, high or low.
Study selection and data abstraction
Two authors (GJM, GFS) independently screened the search output to identify records of potentially eligible trials examining the outcomes, the full texts of which were retrieved and assessed for inclusion. Excluded studies and the reasons for exclusion were recorded.
A standardised form was used to extract data from the included studies for assessment of study quality and evidence synthesis. Extracted information included:
-
year and language of publication
-
country of participant recruitment
-
year of conduct of the trial
-
study setting: university teaching hospital, non-university teaching hospital
-
study population and inclusion and exclusion criteria
-
sample size
-
participant demographics
-
baseline characteristics
-
type of surgery
-
outcomes and times of measurement
-
information for assessment of the risk of bias.
Data extraction forms were completed by one author (GFS) and checked by a second author (GJM). Similarly, quality assessment was carried out by one author (GFS) and checked by a second (GJM).
Assessment of reporting bias
When ≥ 10 studies were identified for each outcome, publication was assessed by the visual assessment of funnel plots and Egger’s test. 133
Measures of treatment effect
For dichotomous variables we calculated the RR with 95% CI. For continuous variables such as hospital stay we calculated the MD with 95% CI; for quality of life (when different scales were used) we calculated the SMD with 95% CI.
Missing data
We performed an intention-to-treat analysis when possible. For dichotomous data presented only as percentages we estimated frequencies using reported sample sizes for these outcomes. For continuous outcomes, if the mean and the standard deviation (SD) were not available from the trial report, we sought this information from the trial authors. When this information was still not available, we calculated the mean and SD from the median and IQR using the software available in Review Manager version 5 (The Cochrane Collaboration, The Nordic Cochrane Centre, Copenhagen, Denmark).
Assessment of heterogeneity
We anticipated that major sources of clinical heterogeneity would be associated with different patient groups, the use of different goal-directed algorithms, the use of co-interventions such as restrictive transfusion thresholds and differences in the methodology used to assess coagulative dysfunction. We explored heterogeneity within each meta-analysis using a chi-squared test, with significance set at a p-value of 0.10, and we expressed the percentage of heterogeneity due to variation rather than chance as the I2 value. 134 We defined heterogeneity as follows:
-
I2 0–40%: no or mild heterogeneity
-
I2 40–80%: moderate heterogeneity
-
I2 > 80%: severe heterogeneity.
In the presence of severe heterogeneity, meta-analysis was not performed.
Data synthesis
Meta-analyses were performed using the software package Review Manager version 5.2 and in accordance with the recommendations of the Cochrane Handbook for Systematic Reviews of Intervention. 130 For the primary analysis we compared the results of a random-effects model with those of a fixed-effects model to assess the effects of small studies. For continuous outcomes we pooled MDs or SMDs using the inverse variance method.
Subgroup analyses
Subgroup analyses were performed for different viscoelastic POC testing devices and by participant group: CABG compared with non-CABG. Tests for subgroup differences were carried out using Review Manager, with a p-value of < 0.05 considered statistically significant.
Sensitivity analyses
Sensitivity analyses were carried out excluding trials with a high risk of bias for random sequence generation, allocation concealment, blinding of participants, health-care providers or outcome assessors, incomplete outcome data or attrition, or with other sources of bias, including source of funding.
Summary of findings
The main results of the review are presented in a summary of findings table. We included the following outcomes:
-
risk of mortality
-
risk of reoperation and bleeding
-
risk of red cell, FFP and platelet transfusion
-
resource use: ICU and hospital LOS.
We used GRADEpro software to prepare the summary of findings table. We judged the overall quality of the evidence for each outcome as ‘high’, ‘moderate’, ‘low’ or ‘very low’ using the GRADE approach. We considered the following:
-
impact of the risk of bias of individual trials
-
precision of the pooled estimate
-
inconsistency or heterogeneity (clinical, methodological and statistical)
-
indirectness of the evidence
-
impact of selective reporting and publication bias on the effect estimate.
Results
A total of 5125 abstracts were retrieved from the searches (Figure 35), of which 4570 articles were excluded as they were duplicate publications, were studies carried out in animals or were reviews and 533 articles were excluded on the basis of title and abstract. In total, therefore, 22 potentially relevant publications were retrieved for further assessment. Fifteen trials that randomised a total of 8737 participants met the inclusion criteria and were included in the analysis. 112,129,135–147 Two of the included studies were published in abstract form. 139,144 The two review authors (GJM, GFS) were in agreement on the selection of included studies. The key characteristics of the individual studies are described in Table 43.
FIGURE 35.
Preferred Reporting Items for Systematic Reviews and Meta-Analyses (PRISMA) flow diagram.

Study | Number of participants | Mean (SD) age (years) | Inclusion criteria | Intervention test | Control test | Outcomes |
---|---|---|---|---|---|---|
Ak et al., 2009135 | 228 | 64 (20) | CABG | TEG | Clinician decision and SLT | Transfusion, bleeding, reoperation, death, ICU LOS, hospital LOS |
Avidan et al., 2004136 | 102 | 64 | CABG | TEG, platelet function test and ACT | SLT | Transfusion, bleeding and reoperation |
Cui et al., 2010137 | 100 | 30.9 (25.8) months | Cardiac surgery for cyanotic disease | TEG | Clinician decision | Transfusion of blood products, drainage, ICU LOS, hospital LOS, ventilation time |
Girdauskas et al., 2010138 | 56 | 62 (16) | Aortic surgery | ROTEM | Clinician decision and SLT | Transfusion, bleeding, reoperation, death, AKI, stroke, re-intubation, ICU LOS, hospital LOS |
Karkouti et al., 2016129 | 7402 | Median (IQR) 67 (59–75) | Mixed cardiac surgery | ROTEM, Plateletworks® (Helena Laboratories, Beaumont, TX, USA | SLT | Transfusion of blood products, major bleeding, major complications |
Kempfert 2011139 | 104 | 67.2 (0.4) | Patients with excessive bleeding after cardiac surgery | ROTEM | SLT | Blood transfusion, drainage loss |
Kultufan Turan et al., 2006140 | 40 | 53 | Mixed cardiac surgery | ROTEG | Clinician decision and SLT | Transfusion |
Nakayama et al., 2015141 | 100 | Median (IQR) 12 (4–24) months | Cardiac surgery for paediatric disease | ROTEM | SLT | Transfusion of blood products |
Nuttal et al., 2001142 | 92 | 63 | Mixed cardiac surgery | TEG, PT, aPTT, platelet function test and FIB | Clinician decision and SLT | Transfusion |
Paniagua et al., 2011143 | 22 | NR | Mixed cardiac surgery | ROTEM | SLT | Transfusion |
Rauter et al., 2007144 | 213 | NR | Mixed cardiac surgery | ROTEM, clinical signs | Clinician decision and SLT | Transfusion |
Royston and von Kier, 2001145 | 60 | NR | Mixed cardiac surgery | TEG | Clinician decision and SLT | Transfusion, bleeding, reoperation and death |
Shore-Lesserson et al., 1999146 | 107 | 66 (15) | Mixed cardiac surgery | TEG, platelet function test and FIB | Clinician decision and SLT | Transfusion, bleeding, reoperation and death |
Weber et al., 2012112 | 100 | 71 (8) | Mixed cardiac surgery | ROTEM, platelet function tests | SLT | Transfusion, bleeding, reoperation, AKI, sepsis, death, ICU LOS, hospital LOS |
Westbrook et al., 2009147 | 60 | 64 (20) | Mixed cardiac surgery | TEG | Clinician decision and SLT | Transfusion, bleeding, reoperation, ICU LOS, hospital LOS |
Included studies
Of the 15 trials identified in our search, 13 enrolled adults undergoing CABG (n = 2),135,136 mixed cardiac surgery (n = 10),112,129,139,140,142–147 or surgery on the thoracic aorta (n = 1)138 and two enrolled children undergoing surgery for congenital disease. 137,141 The sample size ranged from 22143 to 7402129 participants. The largest trial,129 which enrolled more participants (n = 7402) than all of the other trials, used a multicentre stepped-wedge cluster RCT design. To adjust for the stepped-wedge cluster design we recalculated the effective sample size for this trial as per the recommendations in the Cochrane Handbook for Systematic Reviews of Interventions130 using the intracluster coefficient calculation of 0.095 stated in the trial methods. 129
All of the trials evaluated the efficacy of blood management algorithms based on viscoelastic test results. One trial140 evaluated the ROTEG device, seven trials evaluated ROTEM,112,129,138,139,141,143,144 and seven trials evaluated TEG. 135–137,142,145–147 Blood management in control groups was at the clinicians’ discretion in combination with SLTs in eight trials,135,138,140,142,144–147 according to SLTs alone in six trials 112,129,136,139,141,143 and according to clinical judgement alone in one trial. 137 Ten trials provided data on the length of follow-up,112,129,136,138,140,141,143,144,146,147 which ranged from 24 hours to 3 years.
Excluded studies
Seven trials that met our inclusion criteria were excluded after review of the full manuscript (Table 44). Four studies were excluded because of insufficient data to judge the study design. 149–152 In the trial by Agarwal et al. ,148 viscoelastic testing algorithms were applied in both the treatment group and the control group. Traverso et al. 153 performed the randomisation in abdominal aortic aneurysm. Two trials (NCT00772239 and NCT01218074) were published only as protocols, with no data available.
Study | Type | Country | Sample | Viscoelastic device | Comments |
---|---|---|---|---|---|
NCT00772239 | RCT | France | 100 (estimated) | ROTEM | Trial was terminated; no data available |
NCT01218074 | RCT | Italy | 400 (estimated) | TEG | Ongoing study; not TEG/ROTEM vs. other treatment |
Agarwal et al., 2015148 | RCT | UK | 249 | MEA, TEG | Kaolin heparinase TEG performed in the control arm after completion of the administration of protamine |
Thai et al., 2011149 | Unclear | USA | 24 | TEG | – |
Forestier et al., 2001150 | Unclear | France | 41 | TEG, Sonoclot | – |
Dietrich et al., 1998151 | Unclear | Germany | 16 | Unclear | – |
Miyaschita and Kuro, 1998152 | Unclear | Japan | 15 | Sonoclot | Diagnostic test accuracy study |
Risk of bias in included studies
The overall quality of the studies was evaluated based on the major sources of bias (domains), as described earlier. None of the parallel-group randomised trials could be classified as being at low risk of bias. The various bias domains are presented in Figure 36.
FIGURE 36.
Risk of bias summary: (a) all studies; and (b) individual studies. +, low risk of bias; –, high risk of bias; ?, unclear risk of bias.


Risk of bias in the stepped-wedge cluster randomised controlled trial
The trial by Karkouti et al. 129 was judged to be at low risk of bias for the domains of recruitment bias, baseline imbalance, loss of clusters and incorrect analysis.
Sequence generation
Random sequence generation was adequate in four trials112,138,141,146 and unclear in eight trials. 135–137,139,140,143,144,147 Two trials had a high risk of bias for this domain. 142,145
Allocation concealment
Allocation concealment was unclear in 13 trials. 112,135–141,143–147 One trial had a high risk of bias for this domain. 142
Blinding of participants and personnel
There was evidence of blinding of participants and clinical staff caring for participants in two trials,135,146 unclear evidence in five trials139–141,143,145 and evidence of lack of blinding in seven trials. 112,136–138,142,144,147 There was evidence of blinding of outcome assessors in two trials,136,146 unclear evidence of blinding of outcome assessors in seven trials,135,139–141,143,145,147 and a high risk of detection bias in five trials. 112,137,138,142,144
Incomplete outcome data
Nine trials reported completeness of follow-up for the primary outcome. 112,135,136,138,141–143,146,147 All of them reported < 10% loss to follow-up. Three trials that failed to report completeness of follow-up were considered to be at high risk of attrition bias. 137,144,145 Insufficient reporting of attrition/exclusion to permit a judgement was detected in two trials. 139,140
Selective reporting
Three trials were published only in abstract form;139,143,144 in these trials some outcomes were not reported or treatment effects were not reported and thus they were considered to be at high risk of bias for selective reporting. All of the others trials but one129 were considered to be at unclear risk of bias because we were unable to retrieve the original protocols of the trials or evidence of publication in a trial registry.
Other potential sources of bias
Nine trials disclosed the funding source. 112,129,136,137,140,141,143,144,146 Of these, three trials were at risk of funder bias. 112,129,141 For the remaining studies, the funding source was defined as unknown. Sample size calculations were reported in eight trials. 112,129,135,136,138,142,145,146
Effects of interventions
A summary of the findings for the main comparison between TEG or ROTEM and usual care for patients undergoing cardiac surgery is reported in Table 45.
Study | Outcome | |||||||||||||||||||
---|---|---|---|---|---|---|---|---|---|---|---|---|---|---|---|---|---|---|---|---|
Mortality, n (%) | Red blood cells transfused, n (%) | FFP transfused, n (%) | Platelets transfused, n (%) | Reoperation for bleeding, n (%) | AKI, n (%) | Cerebrovascular accident, n (%) | Ventilation time (hours), mean (SD) | ICU LOS (hours), mean (SD) | Hospital LOS (days), mean (SD) | |||||||||||
VE | C | VE | C | VE | C | VE | C | VE | C | VE | C | VE | C | VE | C | VE | C | VE | C | |
Ak et al., 2009135 | 3 (2.6) | 2 (1.8) | 52 (45.6) | 60 (54.5) | 19 (16.7) | 31 (28.2) | 17 (14.9) | 29 (26.4) | 6 (5.26) | 5 (4.54) | 7 (6.1) | 9 (8.2) | – | – | 8.2 (2.1) | 7.9 (4.7) | 23.3 (5.7) | 25.3 (11.1) | 6.2 (1.1) | 6.3 (1.4) |
Avidan et al., 2004136 | – | – | 34 (66.6) | 35 (68.6) | 2 (3.9) | 0 | 2 (3.9) | 1 (2) | 1 (2) | 1 (2) | – | – | – | – | – | – | – | – | – | – |
Cui et al., 2010137 | – | – | 3 (17.6) | 5 (35.7) | – | – | 5 (29.4) | 5 (35.7) | – | – | – | – | – | – | – | – | – | – | – | – |
Girdauskas et al., 2010138 | 4 (18.5) | 5 (13.8) | 24 (88.9) | 27 (93.1) | 9 (33.3) | 25 (86.2) | 14 (51.8) | 23 (79.3) | 5 (18.5) | 7 (24.1) | 5 (18.5) | 7 (24.1) | 4 (14.8) | 3 (10.3) | 144 (139) | 137 (172) | 175 (218) | 194 (201) | 16.6 (16.4) | 17 (14.8) |
Karkouti et al., 2016129 | – | – | 58 (45.7) | 52 (44.1) | 30 (23.6) | 24 (20.3) | 31 (24.4) | 31 (26.3) | – | – | – | – | – | – | – | – | – | – | – | – |
Kempfert 2011139 | – | – | – | – | – | – | – | – | 15 (28.8) | 13 (25) | – | – | – | – | – | – | – | – | – | – |
Kultufan Turan et al., 2006140 | – | – | 7 (35) | 12 (60) | – | – | 1 (5) | 0 | – | – | – | – | – | – | – | – | – | – | – | – |
Nakayama et al., 2015141 | 0 | 0 | 42 (84) | 45 (90) | 42 (84) | 43 (86) | 22 (44) | 22 (44) | – | – | – | – | – | – | – | – | – | – | – | – |
Nuttal et al., 2001142 | – | – | – | – | – | – | – | – | 0 | 6 (11.8) | – | – | – | – | – | – | – | – | – | – |
Paniagua et al., 2011143 | 3 (11.5) | 4 (22.2) | 23 (88.5) | 16 (88.9) | 12 (46.1) | 8 (44.4) | 10 (38.5) | 10 (55.5) | 3 (11.5) | 5 (27.8) | 8 (30.8) | 13 (72.2) | – | – | 15.6 (12.3) | 32 (59) | 132 (120) | 236 (168) | 13.6 (7.1) | 25.8 (19.2) |
Rauter et al., 2007144,a | – | – | Mean 0.8 | Mean 1.3 | 0 | 4 | 0 | 0 | – | – | – | – | – | – | – | – | – | – | – | – |
Royston and von Kier, 2001145 | 0 | 0 | – | – | – | – | – | – | 1 (3.3) | 1 (3.3) | – | – | – | – | – | – | – | – | – | – |
Shore-Lesserson et al., 1999146 | 0 | 2 (3.8) | 22 (41.5) | 31 (41.5) | 4 (7.54) | 16 (30.8) | 7 (13.2) | 15 (28.8) | 0 | 2 (3.8) | – | – | 1 (1.9) | 0 | – | – | – | – | – | – |
Weber et al., 2012112 | 2 (4) | 10 (20) | 42 (84) | 49 (98) | 20 (40) | 40 (80) | 28 (56) | 50 (66) | 5 (10) | 8 (16) | 3 (6) | 10 (20) | – | – | – | – | – | – | – | – |
Westbrook et al., 2009147 | – | – | 14 (43.7) | 33 (89.2) | – | – | – | – | – | – | – | – | – | – | – | – | – | – | – | – |
Summary effect estimate, RR (95% CI) | 0.55 (0.28 to 1.10) | 0.88 (0.79 to 0.97) | 0.68 (0.46 to 1.00) | 0.78 (0.66 to 0.93) | 0.82 (0.55 to 1.23) | 0.42 (0.20 to 0.86) | 1.61 (0.45 to 5.81) | 0.29 (–0.66 to 1.23) | –31.76 (–94.7 to 31.2) | –3.11 (–9.57 to 3.34) |
Primary outcome
Hospital mortality was reported in seven trials. 112,135,138,141,143,145,146 Mortality was lower in participants treated with TEG- or ROTEM-guided algorithms (12/350) than in participants receiving usual care (23/339); however, this was not statistically significant (RR 0.55, 95% CI 0.28 to 1.10; I2 = 1%, chi-squared test for heterogeneity p = 0.40, random effect) (Figure 37).
FIGURE 37.
Mortality. df, degrees of freedom; M–H, Mantel–Haenszel.
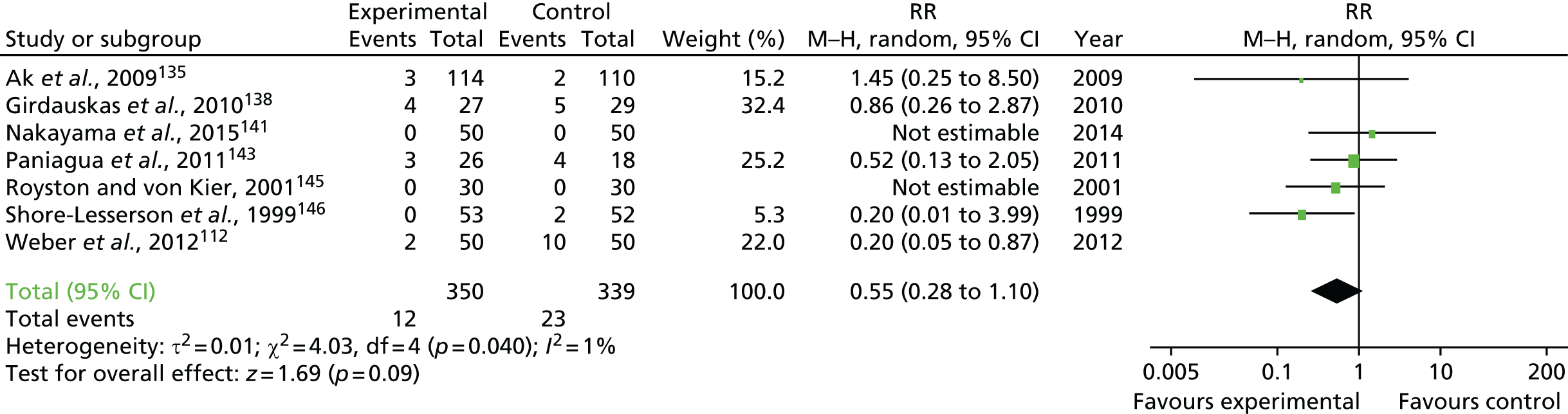
Secondary outcomes
Eleven RCTs reported data on the number of participants transfused with allogenic red cells. 112,129,135–138,140,141,143,146,147 Red cell transfusion was reduced in participants treated with TEG- or ROTEM-guided algorithms, with some heterogeneity (RR 0.88, 95% CI 0.79 to 0.97; I2 = 43%; chi-squared test for heterogeneity p = 0.06, random effect) (Figure 38). Exclusion of the trial by Karkouti et al. 129 did not alter the summary effect estimate or the heterogeneity (RR 0.86, 95% CI 0.76 to 0.96; I2 = 52%; chi-squared test for heterogeneity p = 0.03, random effect).
FIGURE 38.
Red cell transfusion. df, degrees of freedom; M–H, Mantel–Haenszel.
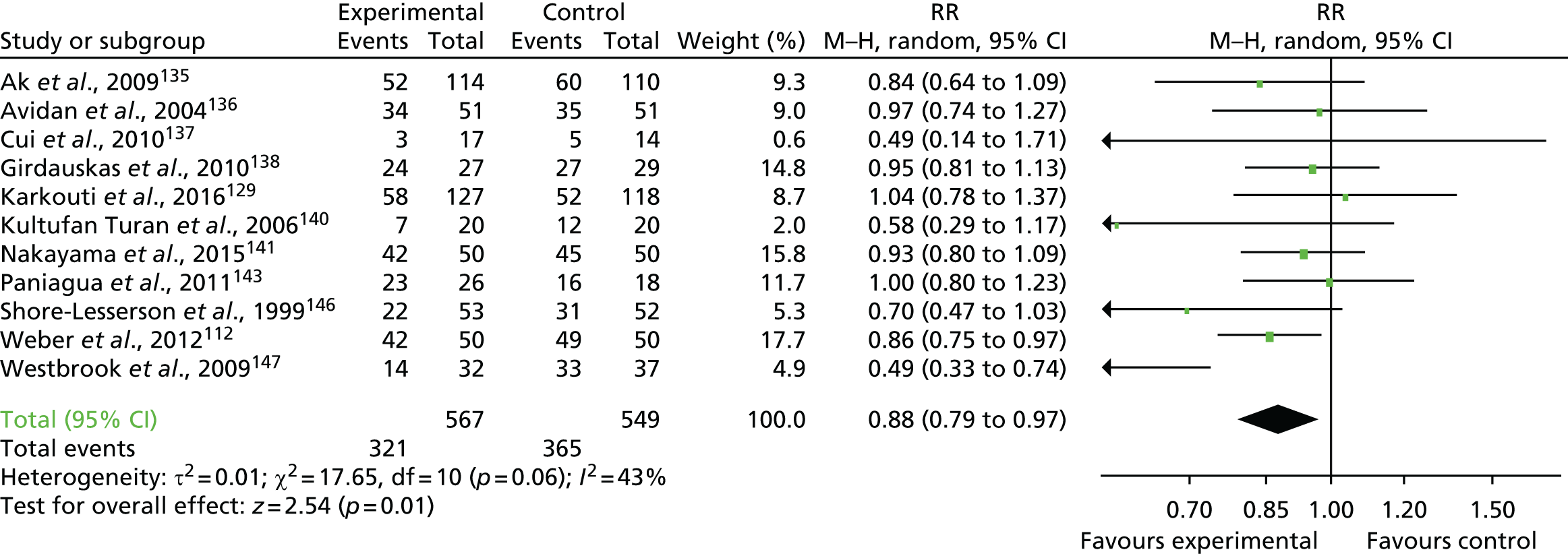
Eight RCTs reported data on the number of participants receiving FFP. 112,129,135,136,138,141,143,146 The summary effect estimate for TEG- or ROTEM-guided algorithms compared with controls was a RR of 0.68 (95% CI 0.46 to 1; I2 = 79%; chi-squared test for heterogeneity p = 0.0001, random effect) (Figure 39). Exclusion of the trial by Karkouti et al. 129 did not significantly alter the summary effect estimate or reduce the heterogeneity (RR 0.62, 95% CI 0.38 to 0.99; I2 = 84%; chi-squared test for heterogeneity p = 0.05, random effect).
FIGURE 39.
Fresh-frozen plasma transfusion. df, degrees of freedom; M–H, Mantel–Haenszel.

Ten RCTs reported data on the number of participants receiving platelet transfusions. 112,129,135–138,140,141,143,146 The use of viscoelastic tests resulted in a significant reduction in the number of participants receiving platelet transfusions (RR 0.78, 95% CI 0.66 to 0.93; I2 = 0%; chi-squared test for heterogeneity p = 0.60, random effect) (Figure 40). Exclusion of the trial by Karkouti et al. 129 did not alter these findings (RR 0.76, 95% CI 0.63 to 0.91; I2 = 0%; chi-squared test for heterogeneity p = 0.003, random effect).
FIGURE 40.
Platelet transfusion. df, degrees of freedom; M–H, Mantel–Haenszel.
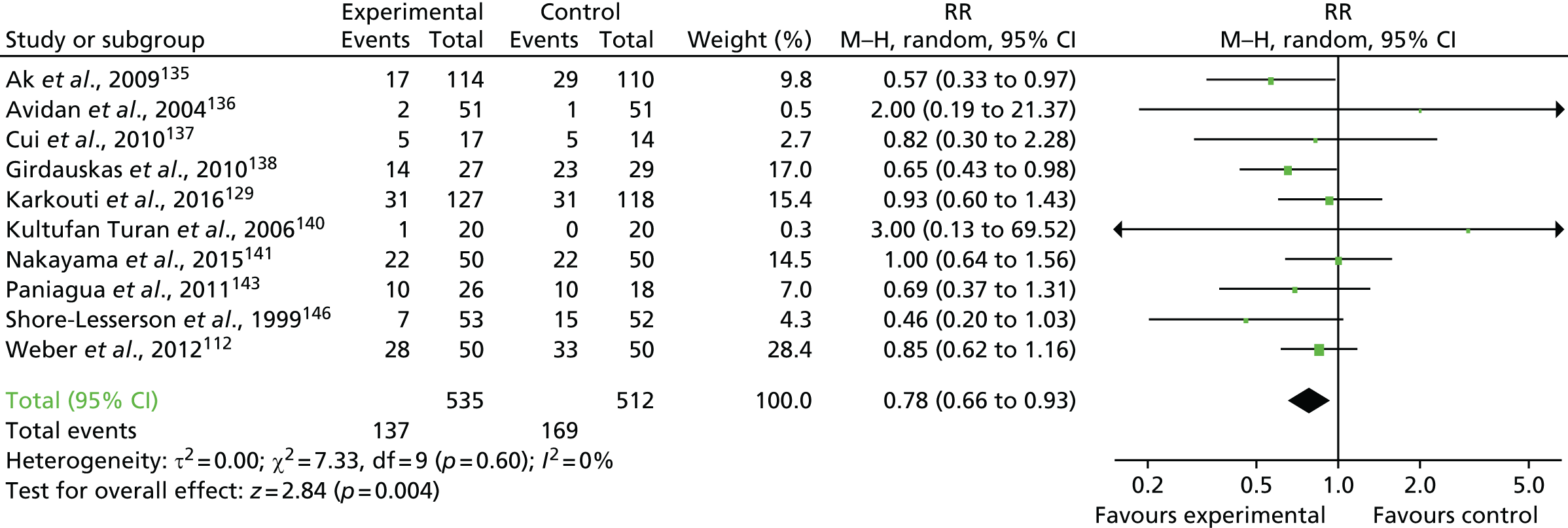
Nine trials reported the number of participants who required reoperation for bleeding. 112,135,136,138,139,142,143,145,146 The use of viscoelastic tests resulted in a reduction in the of number of reoperations for bleeding; however, this effect did not reach statistical significance (RR 0.82, 95% CI 0.55 to 1.23; I2 = 0%; chi-squared test for heterogeneity p = 0.63, random effect) (Figure 41).
FIGURE 41.
Reoperation for bleeding. df, degrees of freedom; M–H, Mantel–Haenszel.
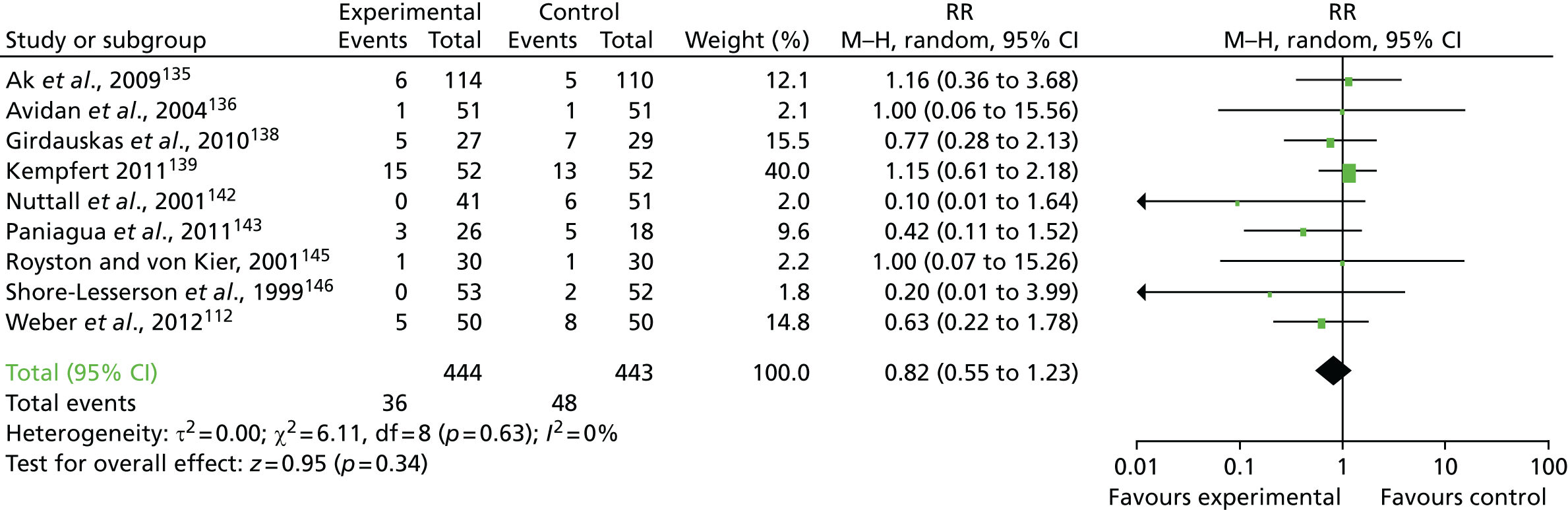
Four trials reported the frequency of severe AKI. 112,135,138,143 The use of viscoelastic tests reduced the frequency of severe AKI compared with usual care, with moderate heterogeneity (RR 0.42, 95% CI 0.20 to 0.86; I2 = 26%; chi-squared test for heterogeneity p = 0.02, random effect) (Figure 42).
FIGURE 42.
Acute kidney injury. df, degrees of freedom; M–H, Mantel–Haenszel.

Two trials138,146 evaluated the number of participants with CVA. There was no difference between the viscoelastic test group and the control group with respect to the frequency of stroke (RR 1.61, 95% CI 0.45 to 5.81; I2 = 0%; chi-squared test for heterogeneity p = 0.47, random effect) (Figure 43).
FIGURE 43.
Cerebrovascular accident. df, degrees of freedom; M–H, Mantel–Haenszel.

Three trials135,138,143 provided continuous data on the ventilation time in each group. Meta-analysis demonstrated a shorter ventilation time in the viscoelastic test group; however, this was not statistically significant (MD 0.28, 95% CI –0.66 to 1.23; I2 = 0%; chi-squared test for heterogeneity p = 0.56, random effect) (Figure 44).
FIGURE 44.
Ventilation time (hours). df, degrees of freedom; IV, inverse variance.

This outcome was reported in three studies. 135,138,143 The ICU LOS was shorter in participants randomised to TEG- or ROTEM-guided algorithms than in those receiving usual care; however, this difference was not statistically significant (MD –31.76 hours, 95% CI –94.68 to 31.17 hours; I2 = 59%; chi-squared test for heterogeneity p = 0.32, random effect) (Figure 45).
FIGURE 45.
Intensive care unit LOS (hours). df, degrees of freedom; IV, inverse variance; VE, viscoelastic test group.

This outcome was reported in three studies. 135,138,143 Hospital LOS was shorter in participants randomised to TEG- or ROTEM-guided algorithms than in those receiving usual care; however, this difference was not statistically significant and there was moderate heterogeneity (MD –3.11 days, 95% CI –9.57 to 3.34 days, I2 = 69%; chi-squared test for heterogeneity p = 0.34, random effect) (Figure 46).
FIGURE 46.
Hospital LOS (days). df, degrees of freedom; IV, inverse variance; VE, viscoelastic test group.

Publication bias
For two outcomes (red cell transfusion and FFP transfusion) a sufficient number of trials provided data (≥ 10 studies identified for each outcome) for the evaluation of publication bias. The funnel plot of the SE compared with the RR for red cell transfusion showed an asymmetrical distribution and a regression test for the funnel plot asymmetry model [z = –2.21; p < 0.026 (random-effects version of Egger’s test)] that indicated likely publication bias (Figure 47a). The funnel plot of the SE compared with the RR for platelet transfusion showed a symmetrical distribution and a regression test for the funnel plot asymmetry model [z = 0.22; p = 0.818 (random-effects version of Egger’s test)] that indicated no publication bias (see Figure 47b). Exclusion of the trial by Karkouti et al. 129 did not alter these findings (p = 0.015 for red cell transfusion and 0.724 for platelet transfusion).
FIGURE 47.
Funnel plots: (a) red cell transfusion; and (b) platelet transfusion.
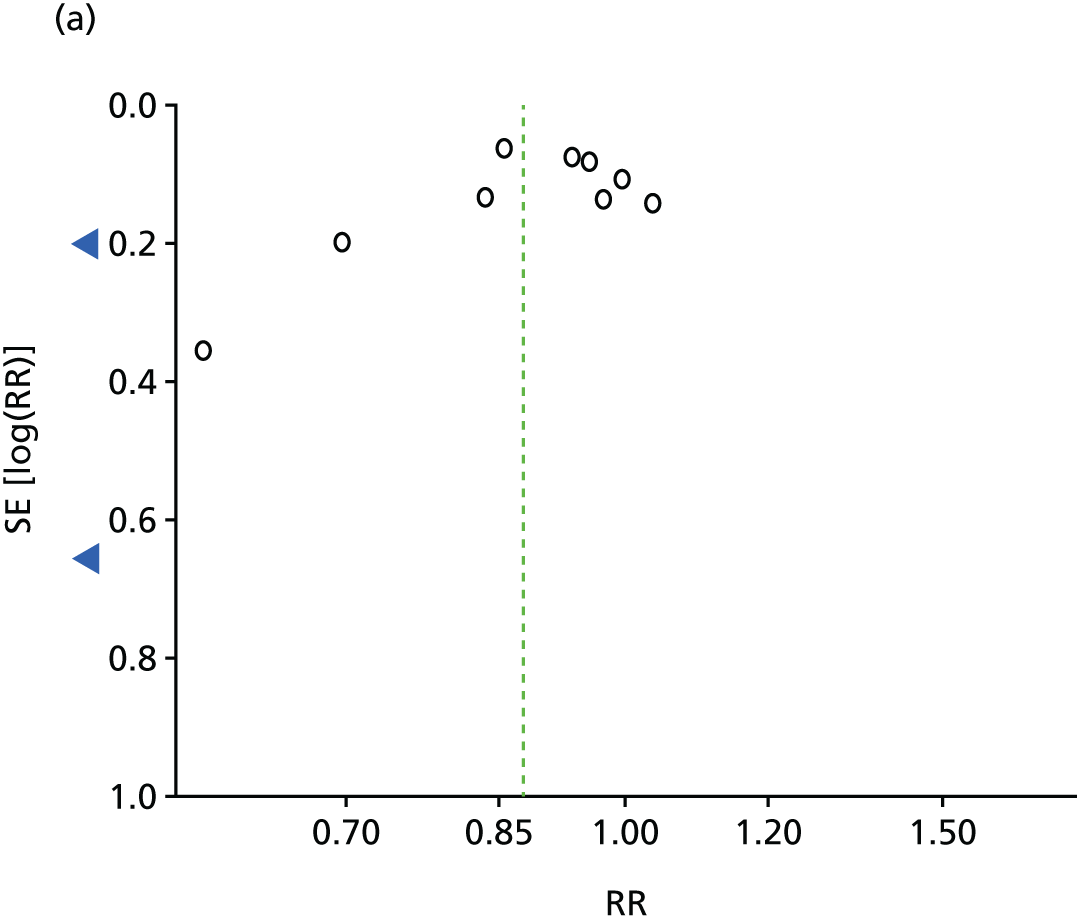

Sensitivity analyses
Sensitivity analysis was not conducted because none of the included trials was considered to be at low risk of bias.
Subgroup analyses
Results of the subgroup analyses for TEG algorithms compared with ROTEM algorithms, TEG algorithms compared with other algorithms and CABG compared with non-CABG are shown in Table 46.
Subgroup | CABG | Non-CABG | Test for subgroup | TEG + other devices | ROTEM/ROTEG | TEG only | Test for subgroup differences |
---|---|---|---|---|---|---|---|
Mortality, RR (95% CI) | 1.45 (0.25 to 8.50), 1 trial, n = 224, I2 = NA | 0.46 (0.22 to 0.98), 6 trials, n = 465, I2 = 0% | χ2 = 1.35, df = 1 (p = 0.25), I2 = 26% | 0.19 (0.01 to 4.03), 1 trial, n = 105, I2 = NA | 0.42 (0.16 to 1.07), 4 trials, n = 300, I2 = 11% | 1.46 (0.24 to 8.91), 2 trials, n = 284, I2 = NA | χ2 = 1.88, df = 2 (p = 0.39), I2 = 0% |
AKI, RR (95% CI) | 0.73 (0.26 to 2.05), 1 trial, n = 224, I2 = NA | 0.32 (0.14 to 0.74), 3 trials, n = 200, I2 = 18% | χ2 = 1.51, df = 1 (p = 0.22), I2 = 33.6% | No trials | 0.32 (0.14 to 0.74), 3 trials, n = 200, I2 = 18% | 0.73 (0.26 to 2.05), 1 trial, n = 224 I2 = NA | χ2 = 1.51, df = 1 (p = 0.22), I2 = 33.6% |
Reoperation, RR (95% CI) | 1.14 (0.37 to 3.48), 2 trials, n = 326, I2 = 0% | 0.70 (0.40 to 1.20), 7 trials, n = 561, I2 = 0% | χ2 = 0.60, df = 1 (p = 0.44), I2 = 0% | 0.28 (0.05 to 1.45), 3 trials, n = 299, I2 = 0% | 0.84 (0.54 to 1.32), 4 trials, n = 304, I2 = 0% | 1.13 (0.39 to 3.29), 2 trials, n = 284, I2 = 0% | χ2 = 2.02, df = 2 (p = 0.36), I2 = 0.8% |
Red cell transfusion, RR (95% CI) | 0.75 (0.48 to 1.18), 2 trials, n = 326. I2 = 0% | 0.44 (0.25 to 0.80), 9 trials, n = 790, I2 = 70% | χ2 = 1.98, df = 1 (p = 0.16), I2 = 49.4% | 0.39 (0.16 to 0.96), 4 trials, n = 307, I2 = 66% | 0.66 (0.37 to 1.17), 6 trials, n = 585, I2 = 25% | 0.70 (0.41 to 1.18), 1 trial, n = 224, I2 = NA | χ2 = 1.26, df = 2 (p = 0.53), I2 = 0% |
FFP transfusion, RR (95% CI) | 1.02 (0.16 to 6.39), 2 trials, n = 326, I2 = 53% | 0.67 (0.43 to 1.04), 6 trials, n = 650,I2 = 84% | χ2 = 0.19, df = 1 (p = 0.67), I2 = 0% | 0.79 (0.04 to 14.57), 2 trials, n = 207, I2 = 72% | 0.84 (0.55 to 1.28), 4 trials, n = 445, I2 = 74% | 0.59 (0.36 to 0.98), 1 trial, n = 224, I2 = NA | χ2 = 1.09, df = 2 (p = 0.58), I2 = 0% |
Platelet transfusion, RR (95% CI) | 0.62 (0.33 to 1.15), 2 trials, n = 326, I2 = 19% | 0.81 (0.67 to 0.97), 7 trials, n = 690, I2 = 0% | χ2 = 0.67, df = 1 (p = 0.41), I2 = 0% | 0.63 (0.34 to 1.15), 3 trials, n = 238, I2 = 0% | 0.83 (0.69 to 1.00), 6 trials, n = 585, I2 = 0% | 0.57 (0.33 to 0.97), 1 trial, n = 224, I2 = NA | χ2 = 2.32, df = 2 (p = 0.31), I2 = 13.6% |
ICU LOS, MD (95% CI) | –2.00 (–4.34 to 0.34), 1 trial, n = 224, I2 NA | –67.77 (–149.99 to –14.45), 2 trials, n = 100, I2 = 87% | χ2 = 2.46 df = 1 (p = 0.12), I2 = 59.3% | No trials | –47.99 (–130.95 to 34.97), 2 trials, n = 144, I2 = 72% | 8.00 (–14.45 to –1.55), 1 trial, n = 69, I2 = NA | χ2 = 0.89 df = 1 (p = 0.35), I2 = 0% |
Hospital LOS, MD (95% CI) | –0.10 (–0.43 to 0.23), 1 trial, n = 224. I2 = NA | –42.04 (–141.60 to 57.51), 2 trials, n = 100, I2 = 80% | χ2 = 0.68, df = 1 (p = 0.41), I2 = 0% | No trials | –42.04 (–141.60 to 57.51), 2 trials, n = 100, I2 = 80% | –0.10 (–0.43 to 0.23), 1 trial, n = 224, I2 = NA | χ2 = 0.68, df = 1 (p = 0.41), I2 = 84% |
GRADE summary
The results of the GRADE summary of the evidence are provided in Table 47. There was no difference between POC testing and usual care for mortality, red cell transfusion, bleeding and major morbidity, with the exception of severe AKI. The quality of the evidence was low or very low for all of the outcomes assessed, implying a high level of uncertainty with respect to these results.
Outcomes | Anticipated absolute effectsb (95% CI) | Relative effect (95% CI) | Number of participants (RCTs) | Quality of the evidence (GRADE)c | |
---|---|---|---|---|---|
Risk with control | Risk with VE tests | ||||
Mortality | 68 per 1000 | 37 per 1000 (19 to 75 per 1000) | RR 0.55 (0.28 to 1.10) | 689 (7) | ⊕⊕○○ Lowd |
Red cell transfusion | 391 per 1000 | 585 per 1000 (388 to 564 per 1000) | RR 0.88 (0.79 to 0.97) | 1116 (11) | ⊕○○○ Very lowd,e |
Platelet transfusion | 330 per 1000 | 257 per 1000 (218 to 307 per 1000) | RR 0.78 (0.66 to 0.93) | 1116 (11) | ⊕⊕○○ Lowd |
Severe AKI | 188 per 1000 | 89 per 1000 (44 to 166 per 1000) | RR 0.42 (0.20 to 0.86) | 1047 (10) | ⊕○○○ Very lowd |
Reoperation for bleeding | 108 per 1000 | 89 per 1000 (60 to 133 per 1000) | RR 0.82 (0.55 to 1.23) | 744 (4) | ⊕○○○ Very lowe,f |
ICU LOS | The mean ICU LOS in the intervention group was 31.8 hours lower (–94.7 to 31.1 hours) | ⊕○○○ Very lowg |
|||
Hospital LOS | The mean hospital LOS was 3.1 days lower (–9.6 to 3.3 days) | ⊕⊕○○ Lowh |
Discussion
Main findings
Predictive accuracy study
We recruited a large cohort of consecutive adult patients undergoing cardiac surgery, of whom approximately 30% developed CCB, a composite of objective criteria indicative of bleeding that is likely to lead to patient harm. We found that clinical risk factors were able to accurately predict CCB and that the clinical risk prediction model was dominated by the complexity of the operative procedure and treatment with P2Y12 receptor blockers close to the time of surgery. We demonstrated that the addition of preoperative platelet function tests to this model did not improve predictive accuracy. In contrast, postoperative POC tests, including the results of the Multiplate, TEG and ROTEM tests, improved predictive accuracy. The best predictive model was achieved by the combination of postoperative TEG and Multiplate values. Although statistically significant, the magnitude of the incremental increase in predictive accuracy was small and of uncertain clinical utility.
Health economic analysis
We evaluated the cost-effectiveness of the introduction of POC tests for coagulopathy into routine clinical care using decision-analytic modelling. For each of the POC test options considered, the MDs in costs and life-years compared with current practice were small and not statistically significant; the largest difference in costs was £33, approximately 0.2% of the total costs, and the largest difference in life-years was 0.0043, equivalent to 1.6 days. Based on the point estimates of the ICER, TEG and TEG/Multiplate test were dominated by current practice as they were both more costly and less effective. ROTEM and ROTEM/Multiplate test were more costly and more effective than current practice and would be considered cost-effective if a decision-maker was willing to pay £8000 for an additional life-year. However, there was great uncertainty around all of these results because of the very small differences in costs and life-years.
Systematic review
A systematic review of trials of viscoelastic POC tests in cardiac surgery identified 15 RCTs, of which none was judged to be free from potential bias. Pooled effect estimates from the trials showed that a TEG- or ROTEM-guided algorithm for the management of coagulopathic haemorrhage reduced the number of patients requiring transfusion, but had no effect on mortality, stroke, prolonged intubation and emergency reopening for bleeding or on ICU and hospital LOS. There was a significant reduction in the frequency of severe AKI in four trials in which this was reported. The GRADE assessment concluded that the quality of the evidence was low or very low for all of the outcomes.
Strengths and limitations
Predictive accuracy study
The COPTIC study has several important strengths. The study design reduced the likelihood of bias observed in previous studies that have considered the predictive accuracy of POC tests in cardiac surgery. 25 It evaluated POC tests in an unselected consecutive cohort of patients in whom these devices are in common clinical use and recruited 70% of all eligible patients undergoing surgery at a single large cardiac centre over a 2.5-year period. Data on demographics, baseline clinical characteristics and laboratory and POC test results were missing in a proportion of participants; however, our analysis suggested that ‘missingness’ occurred at random and was unlikely to have introduced bias. The index tests were performed in a dedicated laboratory by trained staff, with careful quality controls and standard operating procedures in place to ensure data quality. The use of the tests was timed to coincide with their common use in the patient pathway and test predictors were evaluated that are used in clinical practice, increasing the likelihood that the results of our analyses are relevant to the clinical setting. The predictor tests were also conducted independently of the clinical setting and did not influence clinical care or the reference outcome. We used a reference outcome that was a composite of objective clinical measures of bleeding that on balance we considered to accurately reflect bleeding likely to cause harm. We tested uncertainty over the accuracy of this reference outcome in a series of sensitivity analyses by using alternative definitions of CCB that we considered would either underestimate or overestimate the frequency of severe bleeding or identify only very severe bleeding; however, this did not alter the results. A final strength is that the COPTIC study is the largest ever study to consider the predictive accuracy of POC tests in bleeding patients. To date, it recruited more participants than all of the previous predictive accuracy studies evaluating Multiplate or viscoelastic tests, including TEG and ROTEM, combined.
The limitations of the COPTIC study are important considerations when interpreting the results. First, the COPTIC study was a single-centre study and therefore our findings may not be generalisable to other centres. Our clinical risk prediction score, for example, had only moderate discrimination when externally validated in a multicentre cohort of participants from the TITRe2 trial. 4 This is a limitation of almost all centre-specific risk prediction scores for bleeding (see Chapter 3). It also suggests that blood management in Bristol may differ from that in other centres. This was also suggested by the findings of the 2011 UK audit of blood transfusion in adult cardiac surgery,9 in which the Bristol Heart Institute had rates of red cell (44%, UK mean 53%), platelet (22%, UK mean 28%) and FFP (15%, UK mean 24%) transfusion and a reopening rate (4.6%, UK mean 4.9%) below the national averages. In the national transfusion audit,9 differences in red cell transfusion and reopening rates persisted after adjustment for baseline clinical characteristics (platelet and FFP transfusion were not analysed after risk adjustment). This implies that existing blood management protocols are applied effectively at the Bristol Heart Institute, which is supported by the use of ad hoc POC tests in 15% of participants in this study. This could reduce the likely cost-effectiveness of POC testing as an intervention. In contrast, the implementation of effective blood management protocols would also tend to increase the predictive accuracy of POC tests as the unnecessary use of non-routine pro-haemostatic treatments would be less frequent and the objective measures of CCB used in the study would be more likely to accurately represent coagulopathic bleeding.
Second, the number of missing data in the COPTIC B analysis is a limitation. We have shown that ‘missingness’ occurred at random; however, our analysis population included 1833 (72%) of the total cohort. This is a reflection of the complexity of the study and the limitations of the POC platforms, which can be unreliable and often provide incomplete panels of results. Because of the size and overall costs of the study we elected to perform the POC tests for each sample in singlicate. All results were then immediately reviewed by the laboratory technician. When the panel of results was incomplete the tests were repeated; however, reproducibility was limited by the maximum interval allowed between sampling and testing, which we specified as 120 minutes for ROTEM and TEG and 240 minutes for the Multiplate test. No previously published predictive accuracy study of POC tests has reported the degree of incomplete test results and we are therefore unable to compare the degree of missing data in this study with that found in other studies. This is less likely to limit the use of these devices in clinical practice because of the redundancy of multiple measures of similar processes (e.g. α-angle = K time = MA).
Third, there is no consensus definition of coagulopathic bleeding and the outcome measure of CCB was developed by the clinical team to capture the different ways in which bleeding might be apparent. The resulting definition, which effectively defined coagulopathic haemorrhage by its treatment, is imprecise and may lead to over- or underestimation of the disease prevalence. In other screening programmes, for example for breast cancer, a patient with a positive screen would have a biopsy taken and a definitive diagnosis would be obtained. Here, there is no gold standard test for coagulopathy. Moreover, coagulopathy manifests clinically as a heterogeneous condition, in which multiple defects in platelet function and coagulation may co-exist. In the COPTIC B study we attempted to address this complexity using a series of LRTs of coagulation factors and coagulation pathway function. Fibrinogen concentration and D-dimer levels were, as expected, predictors of coagulopathic haemorrhage; however, the effect size of these measures was modest, implying that other unmeasured factors may also be important. Until the pathogenesis of coagulopathy is better defined we must accept that the predictive accuracy of the POC tests is unclear.
Health economic analysis
Our health economic analysis had important strengths. This was the first cost-effectiveness analysis of POC tests in cardiac surgery for which the majority of the model inputs (probabilities, costs and life-years) were estimated directly from an observational cohort of patients, rather than from published secondary sources. In contrast, the health economic analyses of POC tests in cardiac surgery26 used to inform current NICE guidance23 were based on estimates of resource use and outcomes from published sources. The health economic analysis in the COPTIC study also benefited from the insights gained by the researchers in related economic evaluations undertaken for the TITRe24 and PASPORT154 trials. The cost-effectiveness analysis evaluated predictive test thresholds that are in current clinical use. 112 The robustness of these thresholds was tested in a series of sensitivity analyses and the likelihood of cost-effectiveness was investigated in probabilistic sensitivity analyses.
There were also limitations of the health economic analysis. First, the limitations of the predictive accuracy study are also limitations of the cost-effectiveness analysis. Specifically, the definition of the reference outcome by its treatment prevented determination of whether transfusion of FFP or platelets was an effective intervention to prevent CCB or whether it occurred as a consequence of CCB. Sensitivity analysis in the predictive accuracy study used alternative definitions of CCB, including a definition in which only participants who received > 2 units of FFP, > 1 unit of platelets or > 2 units of cryoprecipitate were adjudged to meet the CCB criteria or definition in which CCB was defined solely by blood loss and emergency re-sternotomy. These alternative definitions did not materially alter our findings. It is therefore unlikely that definitions of more severe CCB would alter the results of the cost-effectiveness analysis. The lack of a gold standard definition of the primary outcome is also a limitation. Typically, the sensitivity and specificity of diagnostic tests would be incorporated into the decision model; however, as stated above, the true discrimination of these tests is unclear. In sensitivity analyses we modelled the effect of using different treatment thresholds for each of the POC tests evaluated; again, this did not materially alter our results.
Second, there were a number of participants in the COPTIC cohort who underwent an ad hoc TEG test and whose care pathway was assumed to have been influenced by a positive result. This may have biased the results in favour of the POC tests in the POC test arm of the model as it was not possible to ‘take out’ the effect of the ad hoc test; the tests were much less favourable in a sensitivity analysis that crudely attempted to take out the effect of a positive ad hoc TEG test. Although data on the ad hoc use of TEG in theatre and in the CICU were collected in the COPTIC study, the results of these TEG tests were not recorded and it is not known how or whether or not results were acted on. With hindsight it would have been helpful to capture further information here to more accurately reflect what happened to these participants.
Third, there were relatively few positive POC test results (compared with the number of participants with CCB in the COPTIC study) and therefore relatively few participants for whom the positive POC test could impact positively on their pathway (between 15 and 20 participants). Even if the POC tests had a significant impact for these few participants, it is perhaps not surprising that, across a cohort of 1802 participants, the differences in resulting costs and life-years between strategies were small. Recognising that there are only small differences between the strategies also explains why the majority of the sensitivity analyses had very little impact on the cost and life-year differences between the POC tests and current practice; to a very large extent the same changes were made to each strategy.
Fourth, ideally quality of life as well as quantity of life would have been considered and QALYs used as the outcome measure. QALYs can be compared across a broad range of treatments, interventions and clinical areas. However, it was not possible to collect information on quality of life as part of the observational study and so life-years were used as the outcome measure. It is therefore more difficult to draw conclusions about the value for money of the POC tests, particularly in comparison to other treatments and interventions in health care, although comparisons can be made with other studies that report life-years.
Fifth, if a POC test were introduced in practice, it is likely that any patients who continued to bleed after a positive test result and initial prophylactic treatment would be retested to identify whether or not further treatment was required. Retesting patients was not taken into account in this model.
Sixth, there were limitations around the complications data available for participants in the COPTIC study. Although Innovian data were used to supplement the Patient Analysis and Tracking System data for postoperative complications that were secondary clinical outcomes in the study to reduce the number of missing data, there were additional complications (such as pulmonary and gastrointestinal complications) that were not included as secondary outcomes in the clinical analyses but which ideally would have been costed. Data from the Patient Analysis and Tracking System for these complications were missing for > 60% of participants and data on these complications were deemed not useable, although we consider it likely that the impact of these complications will have been partially captured by other measures of resource use, for example ICU stay. 155
Seventh, although information was available on survival status at 1 year, resource use was captured only until hospital discharge. Although secondary sources were used to make assumptions about resource use beyond hospital discharge, it would have been beneficial to have individual patient data on, for example, hospital readmissions or further health-care resource use beyond hospital discharge up to 1 year (and even beyond).
A final limitation is that our cost-effectiveness analysis was based on observational data. The cost-effectiveness of a clinical intervention is best estimated from measures of resource use and outcomes obtained from appropriately designed RCTs.
Systematic review
The principal limitation of the systematic review is that the findings and interpretations are constrained by the quality and quantity of the available evidence. We judged that all of the 14 parallel-group trials had significant limitations in terms of methodological quality. The risk of procedural bias was high in these trials as there was little or no allocation concealment or blinding of clinical personnel. The potential to produce summary effect estimates was limited by the wide variety of outcomes reported and a lack of standardisation of the way in which these were measured. The assessment of heterogeneity was limited by the relatively small number of studies that contributed to each meta-analysis. Our assessment of likely bias differed from that in a recent Cochrane review. 156 For example, in the Cochrane review the use of sealed envelopes was considered to represent a low risk of bias for allocation concealment. However, Cochrane guidance132 states that only sealed opaque envelopes may be considered as evidence of allocation concealment. When the term opaque was omitted we therefore classified the risk of bias as unclear rather than low. Similarly, the Cochrane reviewers assessed several studies as having a low risk of reporting bias, despite the absence of prospective trial registration or the availability of trial protocols for reference. 156 We judged that these trials should be categorised as having unclear evidence of reporting bias; hence, we found no trial to be at low risk of bias in our analysis. These limitations notwithstanding, the current meta-analysis provides the most comprehensive evaluation of viscoelastic POC tests in cardiac surgery to date. It considered all of the trials identified in two recent meta-analysis,26,156 along with the results of a recent large cluster randomised trial not included in these previous analyses. 129 This trial by Karkouti et al. 129 enrolled 7402 participants, almost five times the total number of participants enrolled in the previous RCTs identified in our search. We evaluated the effects of including/excluding this study from our primary analysis in terms of its influence on the effect estimates. This analysis demonstrated that the inclusion of this trial did not substantially alter the summary effect estimates for those outcomes for which data were available, specifically, that viscoelastic tests reduced the numbers of participants receiving red cell and platelet transfusions.
Clinical importance
Clinical risk prediction for clinical concern about bleeding
A principal finding of our study was that preoperative clinical risk assessment has good predictive accuracy for coagulopathic bleeding. Key preoperative risk factors included the use of P2Y12 receptor blockers shortly before surgery and planned longer complex procedures. Interestingly, a low preoperative haematocrit was found to be a risk factor for bleeding. This suggests a link between chronic inflammatory diseases, the main cause of preoperative anaemia in cardiac surgery,27 and bleeding that is not well recognised. This may be an area for future study. Clinical risk prediction scores are not widely used despite class I recommendations that they are included in standard care. 24 This is because existing scores have been developed in small single-centre cohorts and, when they are externally validated, they are often found to lack discrimination or calibration. Scores developed in multiple centres in multiple countries are more likely to have generalisability. This is the subject of Chapter 3.
Point-of-care tests
The COPTIC study addressed uncertainty over the predictive accuracy of platelet function testing in cardiac surgery. We showed that preoperative Multiplate testing did not improve discrimination beyond clinical risk assessment. This contrasts with the recommendations of established blood management guidelines in cardiac surgery24 and a recent consensus statement from UK researchers recommending routine preoperative platelet function testing. 92 Corredor et al. 25 reviewed studies that had considered the predictive accuracy of preoperative platelet function testing in cardiac surgery. The evidence for preoperative testing was inconsistent and positive studies considered only patients receiving P2Y12 receptor blockers. In the largest of these studies, Ranucci et al. 157 evaluated the predictive accuracy of preoperative Multiplate values for subsequent bleeding in 352 participants receiving DAPT within 7 days of surgery. They found that ADPtest AUC values of < 22 U had a negative predictive value for bleeding of 94% and a positive predictive value of 20%. The ROC AUC was 0.6. When combined with a TRAPtest cut-off of < 75 U, these tests identified seven of the 27 participants in the study who experienced severe bleeding. 157 These thresholds were very low, however, as the normal range for the ADPtest AUC is 51–75 U. This study showed that preoperative platelet function testing had only moderate predictive accuracy, even in selected participants receiving P2Y12 receptor antagonists. Together with the results of the COPTIC study we conclude that clinical risk prediction alone, that is, knowing how recently P2Y12 receptor blockers have been stopped, in combination with other determinants of bleeding, can provide good predictive accuracy and that preoperative platelet function testing has no added benefit.
The COPTIC analyses identified only small incremental increases in predictive accuracy with the use of postoperative POC viscoelastic tests. The limitations of the COPTIC analyses notwithstanding, it is difficult to reconcile evidence of limited predictive accuracy with limited trial evidence of efficacy as shown by the systematic review and the widespread use of these devices in clinical care. In a recent RCT by Weber et al. ,112 the use of an integrated ROTEM and Multiplate algorithm resulted in a reduction in mortality compared with a treatment algorithm based on SLTs. This study was noteworthy for several reasons. First, the POC-based management algorithms were applied to bleeding patients, thereby increasing their potential clinical effectiveness. This is different from the COPTIC analyses, which considered the routine use of POC devices in all patients, in whom their clinical effectiveness would be expected to be less. Second, the bleeding algorithm was complex and required clinical insight and knowledge of the limitations of the testing platforms. We modelled our health economic analysis algorithm for the ROTEM and Multiplate tests on the published Weber et al. 112 algorithm, but this may not have adequately captured the clinical decision-making process in complex bleeding patients. Third, the Weber et al. 112 study allowed repeat testing in bleeding patients; this was not a feature of the COPTIC analysis, although, for a significant number of patients in the COPTIC analyses, ad hoc TEG tests were used to manage active bleeding. The difficulties in evaluating the effects of these interventions in the COPTIC analyses have been described earlier (see Economic decision model methods). Fourth, the Weber et al. 112 study enrolled only 100 participants and was stopped prematurely because of the difference in mortality between the groups, limiting the precision of the estimates in the trial. A final comment, however, is that the COPTIC health economic analysis, discussed in more detail below, identified ROTEM, as well as the combination of ROTEM and the Multiplate test, as being potentially cost-effective, although the effect was small and of uncertain significance. These points serve to highlight the uncertainty around the clinical effectiveness and cost-effectiveness of POC testing.
Given the evidence of poor predictive accuracy and limited clinical efficacy demonstrated in the COPTIC study and other recent analyses it is interesting to speculate why these devices remain in widespread use. The main reason is that there are no alternatives; there have been no significant developments in this technology for several decades. Moreover, if these devices are licensed for use and are supported by treatment guidelines there are few financial incentives for the development of new devices. This also reflects the limitations of our understanding of coagulopathy. The COPTIC trial enrolled 2542 participants and was the largest predictive accuracy study of cardiac surgery coagulopathy ever conducted. All previous predictive accuracy studies that have evaluated POC tests in cardiac surgery have enrolled a total of 1338 participants. As discussed earlier, many of these studies were at high risk of bias, but they have been used to support the widespread use of these devices, for example the preoperative use of platelet aggregometry, for which we have found no evidence. The scale of this research effort is also relatively modest; bleeding potentially affects every patient undergoing cardiac surgery, with > 1 million procedures performed worldwide per year. Research into the pathogenesis of coagulopathy and the development of new technology and diagnostic tests are clearly warranted.
Laboratory reference tests
We evaluated the predictive accuracy of a range of LRTs that may be used to diagnose common acquired coagulopathies. Although none of these tests is available currently as a POC test, evidence of utility would be a driver towards the development of such a test. Our findings confirmed that SLTs, PT and aPTT did not increase predictive accuracy beyond that of our clinical risk score. Reference tests for vWF, factor Xa activity and ETP also failed to improve discrimination. These results address conflicting evidence from previous small studies that have considered the interaction between these measures and bleeding,77,158,159 although all of these studies were at high risk of bias. A low recombinant factor XIII level has also been shown to be associated with coagulopathic bleeding in previous studies. 160,161 This was associated with bleeding in some of our models but not others. A recent RCT has shown that recombinant factor XIII administration does not reduce bleeding or transfusion rates. 162 A consistent finding in all studies is the association between low fibrinogen concentrations and bleeding. In a study in 4202 cardiac surgery patients, Karkouti et al. 163 observed a fibrinogen threshold of 2 g/dl below which blood loss increased. Several small RCTs have also shown that fibrinogen administration reduces bleeding in cardiac surgery164,165 and a Cochrane review of cardiac and non-cardiac surgery trials reported a similar finding. 87 No previous study has evaluated the predictive accuracy of low fibrinogen levels. In the COPTIC study we confirmed that this added to the discrimination of the model; however, the effect was small. FIBTEM values were also predictive; however, this was omitted from the ROTEM model because of collinearity with the MA value, which can also reflect fibrin deficiency. The dominance of low fibrinogen levels in these analyses is of interest as it provides an alternative explanation for the apparently conflicting results of the COPTIC study and NICE analyses23 in that tests that do not have predictive accuracy have clinical efficacy when evaluated in clinical trials. This explanation is that it is the treatment algorithm and not the diagnostic test that provides the clinical benefit. In the RCT by Weber et al. ,112 in which a ROTEM/Multiplate-based algorithm resulted in reductions in bleeding, transfusion and mortality, the treatment algorithm was characterised by early fibrinogen administration. Similar clinical benefits were not observed in trials in which platelets were administered along with FFP as initial treatment. 26 We suggest that low fibrinogen levels may contribute to bleeding in a significant proportion of patients and that this group may derive a benefit from prompt fibrinogen administration. Future work will consider whether a hierarchical clustering analysis of the COPTIC data may permit early identification of this group of patients.
Health economic analysis
To inform recent NICE guidelines23 a HTA study26 considered the introduction of POC tests in several clinical areas, including cardiac surgery. In the literature review of cost-effectiveness analyses of POC tests, only one study was identified, a model conducted for the Scottish NHS. 93 This study was largely based on a previous decision tree used to assess methods for minimising perioperative allogeneic blood transfusion and which considered the relationship between transfusion and subsequent complications and adverse events. 15 Whiting et al. 26 also used a decision tree to assess the cost-effectiveness of POC tests in cardiac surgery, based on these previous studies but updating many of the parameter estimates with data from their clinical effectiveness review. Whiting et al. 26 compared TEG and ROTEM with standard laboratory testing and their cost-effectiveness results indicated that POC testing was dominant; each POC test was less costly and more effective than standard laboratory testing. 26 Their conclusions were robust to various sensitivity analyses conducted. Across a broad range of willingness-to-pay thresholds, the probability of the POC tests being cost-effective was approximately 0.80.
The results of the Whiting et al. 26 analyses differ from the results of the COPTIC cost-effectiveness analyses. This may be explained by differences between the studies and by their limitations. Whiting et al. 26 concluded that there was evidence of efficacy of POC test-based algorithms based on evidence from RCTs, which constitutes a higher level of evidence than the COPTIC study. The main outcome measure in the Whiting et al. 26 study was the proportion of patients receiving a red cell transfusion under a POC testing strategy compared with standardised laboratory testing. However, the RCTs in the Whiting et al. 26 analyses were considered to be at high risk of bias and we have assessed the quality of this evidence as being low or very low using the GRADE approach. Moreover, the reduction in red cell transfusion demonstrated by Whiting et al. 26 is particularly susceptible to performance bias in unblinded trials. Furthermore, our own analysis has indicated that the reporting of this outcome is likely to be subject to publication bias. The interpretation of the economic analysis was further limited by the extrapolation of these results to calculated differences in clinical outcomes based on the estimated risks of transfusion obtained from older published observational studies94 that are now known to be attributable to bias. 32 For example, the probabilities of adverse events attributed to red cell transfusion were based on an analysis that demonstrated that the HR of death for transfused compared with non-transfused patients was 6.69 (95% CI 3.66 to 15.1). This estimate was based on our earlier work. 94 A more recent meta-analysis of RCTs has shown that more liberal transfusion strategies that increase the risk of transfusion (OR 1.7) also reduce the risk of death (HR 0.61) in patients with cardiovascular disease. 32 However, all of the differences in clinical outcomes in the Whiting et al. 26 analysis were based on the transfusion rates; patients were assumed not to have complications such as MI, stroke and renal dysfunction if they did not receive a transfusion. In contrast, in the COPTIC analysis, in which clinical outcomes were measured rather than estimated, we observed that in many cases COPTIC participants who did not have a red cell transfusion experienced complications. Furthermore, we observed no difference between POC testing and standard care in a meta-analysis of trial data with respect to death, major morbidity or resource use. Although in the meta-analysis there was a reduction in severe AKI associated with viscoelastic tests, this was based on an analysis of 62 events in four trials, all of which were at high risk of bias. There was also a reduction in major bleeding attributable to viscoelastic testing in the Karkouti et al. trial;129 however, this was a composite end point and the frequencies of individual components of the endpoint were not available, despite a direct request to the authors, limiting our ability to evaluate these results. The trial by Karkouti et al. 129 also failed to demonstrate any difference in overall complication rates, although again the exact complications and their frequencies were not specified. Overall, the results of this trial mirrored the findings of the 2016 Cochrane review,156 notably that the use of viscoelastic tests reduced transfusion rates but had no benefit with respect to objective clinically important end points.
The COPTIC study was observational and therefore the analyses cannot prove cause and effect. However, the COPTIC study was at low risk of bias for many of the domains considered in the quality assessment of predictive accuracy studies. 166 It also included more participants than the combined number of participants in all of the trials that were included in the systematic review by Whiting et al. 26 and considered measured differences in the frequencies of important clinical end points rather than estimates derived from published risks attributable to blood transfusion. The results of the COPTIC study along with the results of our systematic reviews of RCTs evaluating the efficacy of POC devices do not support the current NICE recommendations. 23,26
Summary and conclusions
What is known on this topic?
Severe coagulopathic bleeding is a common and severe complication of cardiac surgery. A recent technology evaluation by NICE23 concluded that the use of POC tests for the diagnosis and treatment of coagulopathic bleeding reduced the rate of transfusions and was cost-effective in cardiac surgery, resulting in a recommendation for their use. However, systematic reviews of the evidence supporting this guidance have shown inconsistencies with regard to whether these tests have predictive accuracy for bleeding or whether their use results in important clinical benefits. Importantly, the existing NICE guidance was based on estimates of resource use and outcomes from studies that have subsequently been superseded by new evidence.
What does this study add?
We performed a prospective observational cohort study that evaluated the predictive accuracy of POC tests that are in common use for the management of coagulopathic bleeding in cardiac surgery. We also evaluated a range of LRTs to establish whether the addition of these tests would increase predictive accuracy beyond that of existing POC tests. Our results showed that preoperative Multiplate testing did not improve predictive accuracy beyond the use of a clinical risk prediction score. Postoperative POC tests, including combinations of ROTEM or TEG plus Multiplate testing resulted in small incremental improvements in discrimination, as did measures of fibrinogen concentration or fibrinolysis. Although statistically significant, these changes were of uncertain clinical significance. We addressed this by undertaking a cost-effectiveness analysis of the introduction of these tests into routine clinical care using direct measures of resource use and survival from the COPTIC cohort and secondary sources to extend the time horizon to 5 years. We concluded that there was no clear difference in the cost-effectiveness of any of the POC test options compared with current practice. In a systematic review of RCTs that have evaluated the clinical effectiveness of POC test-based algorithms we concluded that there was no evidence of clinical benefit beyond reductions in transfusion. These findings are not congruent with the conclusions of a recent HTA of POC tests in cardiac surgery26 and the NICE guidelines based on these conclusions.
Future research
Ongoing and future RCTs comparing POC testing with the current standard of care in cardiac surgery are required to reconcile prevailing uncertainty over the clinical efficacy and cost-effectiveness of POC devices in current clinical use.
Chapter 3 A study to develop and validate a clinical risk prediction score for red cell transfusion and large-volume red cell transfusion in adult cardiac surgery
Abstract
Background: The aim of this study was to develop two novel clinical risk prediction scores for transfusion and bleeding risk assessment in cardiac surgery.
Methods: Clinical data prospectively collected from 27 cardiac surgical centres in the UK and Europe were used to develop two risk prediction models, one for any red cell transfusion and the other for LVBT (≥ 4 units), an index of severe blood loss. Complete case data were available for 24,749 participants. Multiple imputation was used for missing covariate data, with the imputed data set containing 39,970 participants. Risk models were developed in the complete case data set, with internal validation using leave-one-centre-out cross-validation (LOCOCV). The final selected models were fitted to the imputed data set. The final risk scores were compared with the performance of three existing risk scores: the Transfusion Risk and Clinical Knowledge (TRACK) score, the Transfusion Risk Understanding Scoring Tool (TRUST) and the Papworth Bleeding Risk Score (BRiSc).
Results: The AUC was 0.77 (95% CI 0.77 to 0.77) and 0.80 (95% CI 0.79 to 0.80) for the any transfusion and LVBT scores, respectively, in the imputed data set. The LVBT model also showed good calibration (Hosmer–Lemeshow p = 0.32). In the imputed data set the AUCs for the TRACK and TRUST scores for any transfusion were 0.71 and 0.71, respectively, and the AUC for the BRiSc score for LVBT was 0.69.
Conclusion: Two new risk scores for any transfusion and LVBT among cardiac surgery patients have excellent discrimination and could inform clinical decision-making.
Background
The clinical problem
There are strong arguments for reducing the exposure of cardiac surgery patients to allogenic transfusion. First, cardiac surgery is a major consumer of blood; up to 35,000 adults undergo complex cardiac surgery in the UK per year, using as much as 5% of all allogenic red cell transfusions. 9,82,120 Second, there is circumstantial evidence suggesting that bleeding and LVBT may be harmful, with observational clinical studies indicating strong associations between transfusion and bleeding and postoperative complications, short and long-term mortality and increased hospitalisation costs. 32,94,167 Third, there is a wealth of evidence suggesting that appropriately targeted blood management strategies may reduce exposure to allogenic blood components. 7,16,24 Finally, there are strong economic benefits of more efficient use of blood, in addition to the direct reduction in transfusion costs; lower transfusion rates post cardiac surgery are associated with reduced hospitalisation costs, attributable to fewer adverse clinical events. Economic arguments may become more prominent in the future. The combination of an ageing population undergoing ever more complex surgical procedures with a diminishing blood supply from younger donors is predicted to lead to blood shortages in the near to mid-term. 168
Despite these drivers to improve blood management in cardiac surgery, wide regional and inter-institutional variability persists. In the USA, transfusion rates range from 7.8% to 92.8% for red cells, from 0% to 97.5% for FFP and from 0.4% to 90.4% for platelets. 169 In the UK, transfusion rates range from 25% to 74% for red cell transfusion, from 9% to 59% for FFP transfusion and from 10% to 56% for platelet transfusion. Emergency re-sternotomy rates for bleeding in the UK range from 0.3% to 12%. 9 Although this variability undoubtedly reflects differences in case mix and uncertainty over the appropriate indications for many blood management interventions, it also reflects variability in the preoperative assessment of bleeding risk and the utilisation of existing interventions. For example, in the 2011 UK audit of blood transfusion in adult cardiac surgery,9 one of 17 units that responded to a blood management questionnaire reported that tranexamic acid, an antifibrinolytic agent that reduces transfusions, blood loss and mortality in cardiac surgery,17 was administered to between 1% and 25% of patients. Two of 17 units reported its use in 50–75% of patients and 14 units reported its use in 76–100% of patients. Blood management strategies are also more likely to improve outcomes and to be cost-effective in patients at greatest risk for bleeding or massive transfusion. Preoperative assessment of bleeding risk is considered a class I recommendation (level of evidence C) in the Society of Thoracic Surgeons blood conservation guideline,24 but is rarely implemented or documented. Accurate and accessible risk prediction tools may therefore promote the wider and more uniform implementation of existing interventions. Transfusion or bleeding risk scores have other uses. They enable comparisons of transfusion rates between units, with appropriate adjustment for differences in case mix, and can demonstrate changes in practice as part of quality improvement initiatives by comparing observed with expected transfusion rates. They may also be used to select patients at increased risk for enrolment in trials of blood management interventions.
Transfusion risk prediction
Risk stratification may be achieved using clinical risk prediction scores that assess the likelihood of developing one of three determinants of transfusion (Table 48):
-
baseline anaemia: patients’ total preoperative haemoglobin level, a product of their circulating volume, haematocrit and red cell haemoglobin concentration
-
haemodilution: the likelihood of significant haemodilution during surgery, a product of the total volume of pump prime or other fluids administered during the course of the surgery, the patients’ preoperative circulating volume and the total preoperative haemoglobin level
-
the likelihood of significant blood loss: a product of congenital or acquired preoperative bleeding tendency, complexity and duration of the procedure, surgical attention to haemostasis, postoperative coagulopathy and the individual and institutional management protocols for the treatment of severe blood loss.
Variable | Reference | Risk factor for | ||
---|---|---|---|---|
Low preoperative haemoglobin | Haemodilution | Excessive blood loss | ||
Increased age | 88,170–177 | ✓ | ✓ | |
Female sex | 88 | ✓ | ✓ | |
Lower preoperative haemoglobin level or similara | 88,170–179 | ✓ | ✓ | ✓ |
Type of operationa | 88,179 | ✓ | ✓ | |
Operative priority – emergency | 88,170,172,175,176,179 | ✓ | ||
Previous cardiac surgery | 88,170,174,175,177 | ✓ | ||
Increased number of diseased coronary vessels | 179 | |||
Catheterisation during same admissiona or complications resulting from catheterisation | 175,177 | ✓ | ✓ | |
Lower body surface area or BMI or weight | 88,170–173,175,176 | ✓ | ✓ | ✓ |
Poor left ventricular ejection fraction | 170,174,175 | ✓ | ✓ | |
Peripheral vascular disease | 172,175 | |||
Insulin-dependent diabetes | 175,177 | ✓ | ||
High serum creatinine level or renal failure | 88,170,175,176,179 | ✓ | ✓ | |
Albumin < 4 g/dl | 175 | ✓ | ✓ | |
PT | 175 | ✓ | ||
Coagulation defects | 177 | ✓ | ||
Cardiogenic shock | 175 | ✓ | ||
Current smoker | 177 | |||
Recent Q wave MI | 177 | ✓ | ||
Increased time on CPB | 171,175,176,179 | ✓ | ✓ |
Importantly, blood management interventions may be directed at any or all of these factors.
In Chapter 2 we demonstrated that a clinical risk score had good predictive accuracy for clinically important bleeding. However, this risk score performed poorly in an external data set from the TITRe2 trial. 4 This is a common feature of existing predictive models for blood transfusion or bleeding, all of which have been developed in single-centre cardiac surgery populations. 88,170–179 Other limitations include heterogeneity in the definition of the reference outcome (any transfusion, red cell transfusion, bleeding), restriction to a particular type of cardiac surgery (e.g. isolated CABG170–173) or small sample sizes176,179 or presentation of regression model beta-coefficients rather than a useable risk score. As a consequence no score is widely used, preoperative bleeding risk assessment is often subjective and there is wide variability in bleeding and transfusion rates.
The essential characteristics of a clinical risk prediction score are that it:
-
has high diagnostic accuracy
-
is transferable between patient populations
-
is practical and easy to use.
In this chapter we report the development of two new risk scores for bleeding and transfusion that incorporate the strengths, but minimise the limitations, of existing risk scores. These existing risk scores are described in the following sections.
Transfusion Risk Understanding Scoring Tool
The TRUST score88 was developed using data on 11,113 patients operated on at Toronto General Hospital, Toronto, Canada, between 1999 and 2004, who were split into development and validation cohorts. The reference outcome was any blood transfusion occurring within 24 hours of surgery, which occurred in 3858 out of 7448 patients in the development cohort. Key predictors identified using logistic regression (Table 49) were assigned a value of 1 to provide an additive score, with a minimum value of 0 and a maximum value of 8. Different scores equated to the following probabilities of transfusion: 0 = baseline risk, with a probability of transfusion of 0.00–0.19; 1 = low risk, with a probability of transfusion of 0.20–0.39; 2 = intermediate risk, with a probability of transfusion of 0.40–0.59; 3 = high risk, with a probability of transfusion of 0.60–0.79; 4–8 = very high risk, with a probability of transfusion of 0.80–1.00. The AUC for the score in the validation cohort was 0.78. External validation in a cohort of 5316 patients from a second Toronto cardiac surgery centre, Sunnybrook and Women’s College Health Sciences Centre, demonstrated an AUC of 0.81. 88 More recently, a TRUST score of ≥ 3 was used to select patients at increased risk of red cell transfusion in the multicentre RECESS trial,39 in which the positive predictive value was 74%.
Variable | Score |
---|---|
Haemoglobin level < 13.5 g/dl | 1 |
Weight < 77 kg | 1 |
Female sex | 1 |
Aged > 65 years | 1 |
Non-elective surgery | 1 |
Serum creatinine level > 120 µmol/l | 1 |
Previous cardiac surgery | 1 |
Non-isolated surgery | 1 |
The Reducing Bleeding in Cardiac Surgery research group risk prediction score
The Reducing Bleeding in Cardiac Surgery (RBC) research group risk prediction score was developed in a cohort of 10,667 patients who had undergone cardiac surgery at a single large-volume Canadian centre (Toronto General Hospital) between 2000 and 2005. 63 The score was designed to identify patients at risk of MBT, defined as transfusion of > 4 units of red cells within 48 hours of surgery, which is considered an index of severe bleeding. This was based on a previous study that had demonstrated significant increases in mortality with transfusions of greater volumes of red cells than this volume,10 with the rationale that identifying and reducing transfusion in these patients was more likely to improve clinical outcomes. The authors developed a prediction rule for MBT in a training subset (60%) of patients, with independent risk factors for transfusion identified using logistic regression. The prediction rule was shown to have a high degree of accuracy in the training data set (AUC 0.88). The prediction rule was then simplified to generate an additive risk score (range 0–14) based on the beta-coefficients of the independent variables identified in the regression analysis (Table 50). Using the simplified score, cut-offs were then identified for classifying patients as being at low, moderate or high risk for MBT and the predictive accuracy of the model was assessed in a validation subset (40%) of patients. This accurately classified patients who were at low or high risk for MBT but was less discriminatory for medium-risk patients. The RBC research group went on to validate the simplified risk score in an external multicentre data set from seven cardiac surgery centres. 180 The prediction rule performed well at most sites. Its pooled positive predictive value for excessive blood loss (defined as MBT or re-sternotomy for bleeding) was 71% (range 63%–89%) and its negative predictive value for excessive blood loss was 90% (range 87%–93%). The score performed less well for prediction of any blood transfusion or MBT alone, however. This reflects a major limitation of any score developed within a single institutional database. Interinstitutional variability in transfusion practice has as much influence on transfusion practice as almost any other risk factor. 9,169
Variable | Scorea |
---|---|
Aged ≥ 80 years | 1.0 |
Aged 70–80 years | 0.5 |
Body surface area ≤ 1.5 m2 | 1.0 |
Body surface area 1.5–1.9 m2 | 0.5 |
Preoperative shock | 1.0 |
Preoperative platelet count < 100 × 109/l | 2.0 |
Preoperative platelet count 100–150 × 109/l | 0.5 |
Preoperative haemoglobin ≤ 11 g/dl | 1.0 |
Preoperative haemoglobin 11–13 g/dl | 0.5 |
Complex procedure (procedures other than isolated CABG or single-valve surgery) | 0.5 |
High blood loss surgeon (LVBT rate greater than the median institutional rate) | 0.5 |
Redo surgery | 1.0 |
Non-elective surgery | 0.5 |
Circulatory arrest ≥ 30 minutes | 2.0 |
Circulatory arrest 0–30 minutes | 0.5 |
CPB duration ≥ 180 minutes | 2.5 |
CPB duration 120–180 minutes | 1.0 |
Nadir CPB haematocrit ≤ 18% | 1.0 |
Nadir CPB haematocrit 18%–22% | 0.5 |
Transfusion Risk and Clinical Knowledge score
The TRACK score developed by Ranucci et al. 89 uses preoperative variables and determines the risk of requiring any red cell transfusion. The score was developed in a series of 8989 consecutive adult patients undergoing cardiac surgery at the IRCCS Policlinico San Donato, Milan, Italy. Independent predictors of allogenic blood transfusion were identified using logistic regression. In an attempt to simplify the score and increase its utility, a panel selected the five most clinically relevant predictors of transfusion from these variables. A logistic regression model was created using these five predictors, with the prediction score derived from the value of the beta-coefficients of the logistic regression equation (regression coefficient for each variable multiplied by 10 and rounded to the next integer) (Table 51). The TRACK score performed well in the development data set as well as in a validation series of 2371 patients who had undergone a cardiac operation at another institution. 89 The score also performed favourably compared with other risk scores. One limitation of this score was that it performed less well in isolated CABG patients than in complex cardiac surgery patients. The latter contributes potentially 7 out of 32 points or 22% of the maximum possible score, which does not contribute to risk prediction in the CABG patients.
Variable | Score (range 0–32) |
---|---|
Age > 67 years | 6 |
Weight < 60 kg (female) or < 85 kg (male) | 2 |
Female sex | 4 |
Complex surgery | 7 |
Haematocrit (continuous) | 1 point for each value below 40% (max. 13 points) |
The Papworth Bleeding Risk Score
The BRiSc score was developed in a cohort of 11,592 patients operated on in a single UK centre over an 8-year period. 64 The index outcome for this study was defined as blood loss in drains exceeding 2 ml/kg/hour over the first 3 hours following admission to the ICU post surgery. This occurred in 8% of all patients. The data were split into a development data set (60%) and a validation data set. Logistic regression was used to select risk factors for an additive score, which was further simplified by combining some factors with similar bleeding outcomes (Table 52). In the validation data set 3% of patients with a minimum score of zero developed the index outcome. Conversely, for patients with the maximum score of 5, 27% developed the primary outcome. Conventional measures of predictive accuracy were not presented, limiting interpretation. The discrimination of the score has also not been evaluated in any external data set. However, this score is noteworthy in that it attempted to identify patients with excessive bleeding rather than red cell transfusion and is the only contemporary risk score that has been developed in a UK population.
Risk factor | Value = 0 | Value = 1 |
---|---|---|
Surgery priority | Elective | Urgent or emergency |
Surgery type | CABG or single-valve surgery | All other surgery types |
Aortic valve disease | None | Stenosis, regurgitation or both |
BMI | BMI ≥ 25 kg/m2 | BMI < 25 kg/m2 |
Age | > 75 years | < 75 years |
Desirable characteristics for a transfusion risk score
Key considerations for any risk score are its ability to target therapy, prevent transfusion and above all contribute to improved patient outcome. A desirable risk score would therefore be composed solely of preoperative variables so that effective blood management could be started preoperatively or at the beginning of an operation. Preoperative risk models fail to address important intraoperative variables such as CPB duration or hypothermic circulatory arrest, however, reducing their predictive accuracy. Conversely, models that introduce intraoperative factors may improve accuracy but have a lesser effect on clinical behaviour, as the decision to use most blood management interventions, such as cell salvage or tranexamic acid, is made prior to the end of the surgical procedure. A risk score that was able to guide therapy towards any of the three main contributory factors to transfusion would also be desirable. Ideally, the score would also indicate different strata of risk and therefore indicate the use of a greater number of, or more potent, blood management therapies. Scores that employ a small number of predictor variables are simple to use at the patient’s bedside but may lack precision, particularly in external data sets. Conversely, increasing score complexity, or a large number of contributory variables, may limit the widespread adoption of a risk score in clinical practice, although this has not happened in the case of the internet-based Society of Thoracic Surgeons risk score [http://riskcalc.sts.org/stswebriskcalc/#/ (accessed 7 May 2017)] or the European System for Cardiac Operative Risk Evaluation (euroScore) calculator [www.euroscore.org/ (accessed 7 May 2017)] because of their availability as e-calculators.
A major limitation of all risk models is the inability to adjust for unmeasured confounders such as the local management of bleeding patients in individual units. The use of blood management interventions varies according to centre, surgeon and patient and this will have significant effects on transfusion risk, but the effects of these variables are not reflected in contemporary transfusion risk scores. Similarly, ease of access to platelets and plasma for transfusion will also affect transfusion rates, but these are rarely measured in observational databases. It is of interest that in the multicentre validation of the RBC risk score the interinstitutional variability was large for receiving any perioperative blood transfusion, but much less for MBT or re-exploration. 180 This suggests that local factors are a more important contributor to the former outcome whereas measurable differences in patient and operative characteristics are more important, and with respect to risk score development more accurate, determinants of the latter outcome. A desirable risk score must therefore predict a clinically useful outcome with prognostic accuracy in multiple institutions and in multiple countries (Table 53).
Risk score | TRUST score88 | RBC score63 | TRACK score89 | BRisC64 |
---|---|---|---|---|
Reference outcome | Any red cell transfusion at < 24 hours | LVBT (> 4 red cell units) | Any red cell transfusion | Severe postoperative bleeding at < 3 hours |
Derivation time period | 1999–2004 | 2000–2 | 2001–7 | 2000–8 |
Centres included | Single centre | Single centre | Single centre | Single centre |
Derivation sample size | 7446 | 6651 | 8989 | 6906 |
Frequency of outcome | 51.5% | 8.7% | 55.4% | 7.5% |
Number of variables | 8 | 12 | 5 | 5 |
Discrimination/calibration | AUC 0.79 | AUC 0.88 | AUC 0.73 | PPV 20–25% |
External validation | Yes | Yes | Yes | No |
Discrimination in external validation | Single centre: AUCROC = 0.81 | 7 Canadian centres: PPV 65% (range 35–81%) | Single centre: AUCROC = 0.71 |
Aims and objectives
Aims
The aims of this study were to develop two novel transfusion and bleeding risk prediction scores for cardiac surgical patients that would have good predictive accuracy, generalisability and widespread utility for clinical practice, quality assurance and research.
Objectives
-
To pool data from four different data sets, reconciling differences in definitions and coding of predictor variables and assessing the degree of missing data to generate a complete case data set for the development of risk prediction models.
-
To generate an imputed data set for validation of each risk score, anticipating significant amounts of missing data.
-
To develop two novel risk scores for bleeding and assess their discrimination and calibration in the complete case data set, each individual data set and the imputed data set.
-
To compare the discrimination of the final risk models with that of existing risk scores.
-
To assess the possible utility of these scores presented as e-calculators for personalised medicine, quality assurance and cohort enrichment in clinical trials.
Plan of investigation
Study design
This study developed risk prediction scores for any (red cell) transfusion and LVBT using prospectively collected data from 27 UK and one Italian cardiac centre. The study was undertaken by the School of Social and Community Medicine, University of Bristol, UK, in collaboration with clinical investigators at the Bristol Heart Institute, Bristol, UK, the IRCCS Policlinico San Donato, Milan, Italy, and New Cross Hospital, Wolverhampton, UK. The study also used data from a national transfusion audit carried out by the NHS Blood and Transplant National Audit Programme, based at NHS Blood and Transplant, Oxford, UK. 9 The study was sponsored by University Hospitals Bristol NHS Foundation Trust and had received ethical approval (REC reference number 11/SW/0075). Changes to the study design after commencement of the study are listed in Appendix 2. The study has been reported in accordance with the Strengthening the Reporting of Observational Studies in Epidemiology (STROBE) statement. 181
Study population
The study used data from three institutional cardiac audit data sets – University Hospitals Bristol NHS Foundation Trust, Bristol, UK (April 1996–December 2010), New Cross Hospital, Wolverhampton, UK (April 2004–March 2010) and IRCCS Policlinico San Donato, Milan, Italy (2002–10) – plus data from the UK Cardiac Surgery National Transfusion Audit, which contains data from 26 UK hospitals collected between April and June 2010 that were extracted from data routinely submitted as part of the UK National Audit for Adult Cardiac Surgery. 9 This audit undergoes internal and external quality control procedures and is considered to be of high quality. The Italian data set was obtained from a prospectively collected research data set that has contributed to multiple research studies, including the development of the TRACK score. 89 Transfusion data were recorded prospectively as part of the Milan and Wolverhampton data collection process. Transfusion data for the Bristol data set were obtained by linkage with the institutional blood bank database, as previously described. 94 Transfusion data were collected for the UK Cardiac Surgery National Transfusion Audit as described previously. 9 Researchers from Toronto (RBC, TRUST) and Papworth (BRisC) who had been involved in the development of existing risk scores were approached but declined to participate in the study.
Inclusion criteria
Participants could enter the study if they were aged > 18 years and were undergoing elective or urgent cardiovascular surgery.
Exclusion criteria
Participants could not enter the study if any of the following applied:
-
they were undergoing an emergency or a salvage procedure
-
they were undergoing heart or lung transplantation
-
they were undergoing a ventricular assist device procedure
-
there was an inability to link clinical and transfusion data.
Outcomes
Reference outcomes
‘Any transfusion’ was defined as the administration of red cells within the index hospitalisation (in theatre, in the critical care unit or on the ward). ‘LVBT’ was defined as transfusion of ≥ 4 units of red cells within the index hospitalisation. This is comparable to previous definitions of MBT in cardiac surgery, equates to approximately 1000 ml of allogenic red cells transfused and is considered an index of severe blood loss. 10,63
Predictor variables
The UK Society for Cardiothoracic Surgery in Great Britain and Ireland national cardiac surgery audit definitions for perioperative variables were used. 120 Operations were classified by procedure(s), namely CABG, valve or ‘other cardiac procedures’ or any combination of the three. A subgroup of the ‘other cardiac procedures’ category, all procedures involving the aorta, was additionally categorised as major aortic procedures. CPB used at any point during operation was treated as a preoperative variable, as it is usually known in advance of the operation. Operative priority was defined as elective (admission from home), urgent (surgery within the current hospital admission), emergency (cardiac compromise, no delay in surgery) and salvage (cardiopulmonary resuscitation pre anaesthetic induction). International definitions of urgent differed. The term ‘urgent’ in the Milan data set was equivalent to the term ‘emergency’ in UK terminology. Preoperative haemoglobin levels were measured in g/dl, with haemoglobin levels in the Milan data set obtained by transforming haematocrit readings. Preoperative serum creatinine levels were measured in µmol/l.
Cardiac-related indicators included cardiogenic shock, ejection fraction [categorised as good (≥ 50%), moderate (30–49%) and poor (< 30%)], previous cardiac surgery and an indicator for whether the patient had had a MI < 30 days prior to operation. Preoperative cardiac-related interventions included failed percutaneous transluminal coronary angioplasty (PTCA), IABP used preoperatively and the administration of intravenous heparin or nitrates until operation.
Comorbidities included chronic obstructive pulmonary disease (COPD), including emphysema, previous neurological disease [transient ischaemic attack (TIA), reversible ischaemic neurological deficit, CVA or other significant neurological disease], peripheral vascular disease, chronic dialysis (onset > 6 weeks prior to cardiac surgery) and diabetic on medication (oral therapy or insulin). Age, sex, height and weight measurements were also extracted. Data on congestive heart failure and active endocarditis were present only in a subset of the data sets so could not be used for model development. The coding of candidate predictors was driven by the format of the source data, which generally took the form of a categorical or binary response. Continuous variables were global mean centred using the following means: age 65.1 years, weight 77.86 kg, height 169.48 cm, preoperative serum creatinine level 105.1 µmol/l and preoperative haemoglobin level 13.41 g/dl.
Data sources
Data fields and definitions for each of the sources are described in detail in the study protocol. 182 They are summarised in the following sections.
The Bristol data set
The Bristol Royal Infirmary established a database of adult cardiac surgery patients in April 1996 (Patient Analysis and Tracking System). A standard set of perioperative and postoperative data is collected prospectively by the anaesthetist, surgeon and ICU, HDU and ward nurses. All adult patients (aged > 16 years) in this database up to 31 December 2010 whose data could be linked to the hospital blood bank database of blood products issued and used (n = 17,869) were to be included.
The Milan data set
Data on > 16,000 consecutive patients have been prospectively collected in the institutional database of the IRCCS Policlinico San Donato, Milan, Italy, since 2002.
The Wolverhampton data set
New Cross Hospital in the Royal Wolverhampton NHS Trust has high-quality records obtained prospectively on 4900 consecutive patients who underwent cardiac surgery from 2004. These data have been matched with data on exposure to allogeneic blood components from the blood bank, as well as haematological and biochemistry data, such as perioperative laboratory test results for haemoglobin and creatinine.
The UK audit of blood transfusion in adult cardiac surgery data set
In 2010, NHS Blood and Transplant initiated a national audit of UK cardiac surgical transfusion practice to inform the research agenda. 9 This audit assessed the frequency of blood component transfusion in all patients undergoing cardiac surgery in UK hospitals over a 3-month period. The audit also assessed contemporary patient blood management in UK cardiac surgery using a questionnaire. The aim of the audit was to assess the variability in UK cardiac surgery transfusion practice that can be ascribed to institutional practice, as opposed to differences in case mix and patient populations. We used the anonymous data set generated through this audit to develop our bleeding and transfusion risk scores. The audit data set included all patients who underwent adult cardiac surgery in the UK between 1 April and 30 June 2010 whose risk factors, operation details and in-hospital outcomes were recorded in the Patient Analysis and Tracking System or institution equivalent for submission to the Central Cardiac Audit Database as part of the ongoing national cardiac audit. The clinical data represented a single 3-month download for each institution. Patients were included in the study analyses if their Patient Analysis and Tracking System/database record had been successfully linked to the institution transfusion databases that record the blood products issued and used. The units approached to take part in the national audit of blood transfusion in adult cardiac surgery included all those routinely contributing data to the Central Cardiac Audit Database hosted by the Care Quality Commission; these units are listed in Table 123 in Appendix 2.
Analyses
Data considerations
The four data sets were cleaned to remove implausible entries and duplicate observations and were then merged to allow variables to be matched across the data sets. All patients aged ≥ 18 years undergoing elective or urgent cardiac surgery were included. Patients undergoing emergency or salvage procedures or for whom it was not possible to link serum creatinine and transfusion data or for whom sex or type of cardiac operation was missing were excluded. In the pooled data set data were missing for some variables (item-wise missing data < 5% for most variables), with patterns of missing data varying in magnitude and nature by source data set. A ‘complete case’ data set was obtained by including all cases with complete data for the following set of preoperative, intraoperative and postoperative variables:
-
preoperative: female, age, height, weight, ejection fraction category, MI status, cardiogenic shock, preoperative IABP, failed PTCA, heparin/nitrates until operation, peripheral vascular disease, chronic dialysis, COPD, previous neurological accident, diabetic on medication, preoperative serum creatinine, preoperative haemoglobin level, previous cardiac surgery, operation type, major aortic procedure, CPB
-
intraoperative: CPB time, cardioplegia, type of cardioplegia, receipt of FFP, receipt of platelets
-
postoperative outcome: IABP postoperatively, surgical revision required.
Model development in the complete case data set
Candidate predictor variables were selected on the basis of data availability, consistency of measurement across the four data sets and existing literature on predictors of transfusion identified in our narrative review. Crude associations between each candidate and the outcome measures were assessed using logistic regression, with variables selected for inclusion in multivariable models based on a liberal p-value threshold of 0.2. For each outcome, a main-effects model was developed by retaining variables based on a p-value of 0.1, using backwards stepwise selection. Pairwise first-order interactions were retained if their p-values were ≤ 0.001 and < 0.1 for the any transfusion and LVBT models, respectively, and if they were clinically plausible. All retained interactions were added to the main-effects model and the relative contribution of each interaction to the model was assessed by dropping one interaction term in turn and observing the fit. A set of candidate models was developed by adding the interaction terms cumulatively to the main-effects model in order of decreasing significance.
We checked for non-linear effects of continuous variables in our main-effects model using univariable FP regressions run separately for men and women within each data set, FP progressions run separately for men and women within each data set with the top and bottom 2.5% of observations removed and, lastly, separate FP regressions by sex on the complete case data set (pooled across all four cohorts). In each case we treated the linear model as our default and selected the best FP models of order 1 and 2 models using the deviance criterion of Sauerbrei and Royston183 for each sex in each cohort. Our final decision on whether or not to adopt a linear model was based on three decision rules: (1) if indicated in three or more cohorts for each variable–sex combination, based on a p-value of > 0.1, or else (2) if indicated in the FP analyses or else (3) if the best FP models selected in different data sets differ widely, then adopt a linear specification to avoid different specifications by data sets and sex.
Leave-one-centre-out cross-validation184 was used to identify the most stable model across the four cardiac data sets. For each cohort and candidate model we calculated the difference between the deviances when the model was fitted in the pooled data excluding that cohort and when the model was fitted using that cohort’s data alone. The deviance difference was then averaged across cohorts and the model with the smallest mean deviance difference was selected for further refinement. First, FP analyses were employed to determine whether or not a linear relationship between each continuous variable and the outcome measure could be assumed and alternative specifications were tested when appropriate. As a further check for potential non-linearity, the effect of adding quadratic terms for age, height, weight, preoperative serum creatinine level and preoperative haemoglobin level to the main-effects model was also examined. LOCOCV was then repeated for a further set of candidate models containing main effects, interaction terms and increasing numbers of quadratic terms (added in order of statistical significance). The model with the smallest mean deviance difference was selected as the final model.
Multiple imputation
The robustness of the complete case model results were tested by fitting the final complete case model in multiply imputed data. Thirty imputed data sets were constructed using multiple imputation and chained equations. Multiple imputation was carried out separately by sex and data set (Bristol, Milan, Wolverhampton and audit data sets) using the Stata command –mi ice185 and 20 cycles. For each variable with missing data the predictor variables for the imputation process were selected on three grounds: first, all variables featuring in the complete case any transfusion model were included; second, variables known or suspected to influence completeness, such as the version of Patient Analysis and Tracking System used or hospital, were added; third, variables that explained a sizable component of the variance of the variable with missing data were included. 186 Augmented logistic regression was applied when separation occurred and predictive mean matching was used for all of the continuous variables apart from preoperative haemoglobin level. 113 For each sex/data set combination 30 imputed data sets were generated and the eight resulting sets of imputed data sets were combined to produce 30 cross-centre imputed data sets.
The degree of heterogeneity between parameter estimates across the cohorts in the imputed data sets was assessed using the DerSimonian and Laird estimate187,188 of the between-data set variance, tau2. The any transfusion and LVBT risk scores were based on coefficients from the models fitted to imputed data, to avoid selection bias and to utilise the larger sample size. To best reflect contemporaneous surgical practice, the final models were fitted to data excluding observations prior to 2002 from the Bristol data set.
Model discrimination
Model discrimination was assessed by calculating the AUCs for the final any transfusion and LVBT models in the imputed data sets. Following Vergouwe et al. ,189 Rubin’s rules were used to obtain the AUCs for the imputed data sets. 190
Comparison with existing risk scores
The discriminative performance of the two scores was compared with that of three existing risk scores for transfusion associated with cardiac surgery: the TRACK score,89 the TRUST score88 and the BRiSc. 64 We were unable to replicate the RBC research group score63 in our data as it required surgeon-specific data and information on preoperative platelet count, which were not recorded in the four data sets. All analyses were carried out using Stata version 11.
Utility
To promote dissemination of the risk scores they were made available as web-based calculators. Estimates of positive predictive values and negative predictive values were also provided to inform clinical decision-making and to assist with the design of research studies. Finally, the risk score was used to risk adjust observed transfusion rates from the UK national audit to better assess the distribution across UK units with adjustment for baseline risk.
Study cohort
Analysis data sets
The data sets used for the development and validation of the any transfusion and LVBT models are summarised in Figure 48. Once duplicates had been removed, 43,081 cases were considered for inclusion in the analysis sample. Of these, 17 participants aged < 18 years, 1528 participants undergoing emergency or salvage procedures, 1190 participants with missing transfusion data and 13 participants with missing sex or operation type data were excluded from the sample. Two hospitals that submitted data to the national audit were dropped: Hammersmith Hospital, London, as it did not return transfusion data, and Bristol Royal Infirmary, as the records duplicated those in the larger Bristol data set. The analysis data set comprised 39,970 consecutive operations: 17,457 from Bristol, 12,617 from Milan, 4651 from Wolverhampton and 5245 from the audit data set. Data completeness was generally good, with item-wise missing data typically totalling < 5% per data set, but with some exceptions. The Milan data set did not record the number of units of red cells transfused and so LVBT analyses are based on the remaining three data sources.
FIGURE 48.
Schematic showing participant cohorts used for the any transfusion and LVBT model development in the complete case and imputed data sets.

Complete case data sets
The complete case data set for the any transfusion model included 24,749 (61%) participants and that for the LVBT model included 13,636 participants (after exclusion of the Milan data). The characteristics of the participants by data set are shown in Table 54. The Milan data set had a higher proportion of female participants than the other three data sets. A preoperative haemoglobin level of < 10 g/dl was proportionally more common in the audit data set than in the other data sets. The vast majority of operations across all cardiac centres were carried out using CPB, although the rate of CPB in the Bristol data set was significantly lower than the rates in the Milan, Wolverhampton and audit data sets (66.5%, 98.2%, 91.1% and 90.3% respectively).
Characteristic | Bristol (N = 17,457), n (%) | Milan (N = 12,617), n (%) | Wolverhampton (N = 4651), n (%) | Audit (N = 5245), n (%) |
---|---|---|---|---|
Sex | ||||
Male | 12,988 (74.4) | 8621 (68.3) | 3557 (76.5) | 3786 (72.2) |
Female | 4469 (25.6) | 3996 (31.7) | 1094 (23.5) | 1459 (27.8) |
Age (years) | ||||
< 65 | 7477 (42.8) | 5195 (41.2) | 1855 (39.9) | 1859 (38.8) |
65–74 | 6627 (38) | 4617 (36.6) | 1811 (38.9) | 1599 (33.4) |
≥ 75 | 3353 (19.2) | 2805 (22.2) | 985 (21.2) | 1332 (27.8) |
Height (cm) | ||||
< 150 | 215 (1.2) | 107 (0.8) | 50 (1.1) | 125 (2.4) |
150–159 | 1894 (10.8) | 1653 (13.1) | 571 (12.3) | 747 (14.2) |
160–169 | 4737 (27.1) | 5062 (40.1) | 1360 (29.2) | 1580 (30.1) |
170–179 | 7337 (42) | 4646 (36.8) | 1963 (42.2) | 1992 (38) |
180–189 | 2741 (15.7) | 992 (7.9) | 663 (14.3) | 638 (12.2) |
≥ 190 | 533 (3.1) | 157 (1.2) | 44 (0.9) | 163 (3.1) |
Weight (kg) | ||||
< 50 | 299 (1.8) | 287 (2.3) | 40 (0.9) | 79 (1.5) |
50–59 | 1135 (6.7) | 1394 (11.2) | 263 (5.7) | 319 (6.2) |
60–69 | 2777 (16.3) | 3126 (25) | 734 (15.8) | 846 (16.5) |
70–79 | 4520 (26.6) | 3873 (31) | 1183 (25.5) | 1267 (24.7) |
80–89 | 4562 (26.8) | 2390 (19.1) | 1147 (24.7) | 1249 (24.3) |
90–99 | 2183 (12.8) | 1012 (8.1) | 736 (15.9) | 755 (14.7) |
≥ 100 | 1515 (8.9) | 410 (3.3) | 535 (11.5) | 620 (12.1) |
Preoperative haemoglobin level (g/dl) | ||||
< 10 | 333 (2) | 310 (2.5) | 80 (1.7) | 234 (4.8) |
10–11.9 | 2040 (12.5) | 2121 (16.8) | 532 (11.5) | 866 (17.9) |
≥ 12 | 13,898 (85.4) | 10,186 (80.7) | 4021 (86.8) | 3747 (77.3) |
Preoperative serum creatinine level (µmol/l) | ||||
< 50 | 3 (0) | 92 (0.7) | 16 (0.3) | 31 (0.6) |
50–110 | 10,664 (61.9) | 8959 (71.4) | 3723 (80.3) | 4211 (81.2) |
> 110 | 6573 (38.1) | 3505 (27.9) | 895 (19.3) | 946 (18.2) |
Congestive heart failure | ||||
No | 10,323 (93.6) | 12,078 (95.7) | 4352 (93.6) | – |
Yes | 711 (6.4) | 539 (4.3) | 299 (6.4) | – |
Cardiogenic shock | ||||
No | 17,351 (99.7) | 12,600 (99.9) | 4645 (99.9) | 5194 (99.5) |
Yes | 51 (0.3) | 17 (0.1) | 6 (0.1) | 24 (0.5) |
IABP used preoperatively | ||||
No | 17,236 (99.6) | 12,593 (99.8) | 4601 (98.9) | 4869 (98) |
Yes | 68 (0.4) | 24 (0.2) | 50 (1.1) | 98 (2) |
Active endocarditis | ||||
No | 6772 (98.8) | 12,549 (99.5) | – | – |
Yes | 79 (1.2) | 68 (0.5) | – | – |
Failed PTCA (< 24 hours) | ||||
No | 10,356 (99.9) | 12,601 (99.9) | 4649 (100) | 5074 (99.5) |
Yes | 11 (0.1) | 16 (0.1) | 2 (0) | 23 (0.5) |
MI during the operation or within 30 days of the operation | ||||
No | 15,838 (91) | 10,610 (84.1) | 3938 (84.7) | 4222 (86.8) |
Yes | 1575 (9) | 2007 (15.9) | 713 (15.3) | 641 (13.2) |
Ejection fraction (category) | ||||
Good (> 50%) | 12,570 (73.6) | 7931 (69.8) | 3065 (65.9) | 3520 (71.2) |
Fair (30–49%) | 3605 (21.1) | 2894 (25.5) | 1210 (26) | 1156 (23.4) |
Poor (< 30%) | 910 (5.3) | 543 (4.8) | 376 (8.1) | 265 (5.4) |
Heparin or nitrates until operation | ||||
No | 16,567 (95.5) | 12,007 (95.2) | 4448 (95.6) | 4986 (95.5) |
Yes | 784 (4.5) | 610 (4.8) | 203 (4.4) | 235 (4.5) |
Peripheral vascular disease | ||||
No | 16,062 (92.3) | 12,121 (96.1) | 3811 (81.9) | 4608 (88.5) |
Yes | 1335 (7.7) | 496 (3.9) | 840 (18.1) | 601 (11.5) |
Chronic dialysis | ||||
No | 17,340 (99.6) | 12,527 (99.3) | 4632 (99.6) | 5193 (99.7) |
Yes | 63 (0.4) | 90 (0.7) | 18 (0.4) | 16 (0.3) |
COPD (or emphysema) | ||||
No | 16,476 (94.7) | 11,776 (93.3) | 4308 (92.6) | 4782 (91.6) |
Yes | 920 (5.3) | 841 (6.7) | 343 (7.4) | 440 (8.4) |
Previous neurological accident | ||||
No | 16,096 (92.5) | 12,047 (95.5) | 4242 (91.2) | 4823 (92.7) |
Yes | 1305 (7.5) | 570 (4.5) | 409 (8.8) | 382 (7.3) |
Diabetic on medication | ||||
No | 15,030 (86.4) | 11,014 (87.3) | 3683 (79.2) | 4100 (82.2) |
Yes | 2358 (13.6) | 1603 (12.7) | 968 (20.8) | 888 (17.8) |
Urgent operation | ||||
No | 10,622 (60.9) | – | 3330 (71.6) | 3716 (71.1) |
Yes | 6815 (39.1) | – | 1321 (28.4) | 1512 (28.9) |
Previous cardiac surgery | ||||
No | 16,464 (94.4) | 11,864 (94) | 4523 (97.2) | 4895 (93.7) |
Yes | 980 (5.6) | 753 (6) | 128 (2.8) | 328 (6.3) |
Operation type | ||||
CABG only | 11,346 (65) | 6127 (48.6) | 3068 (66) | 2781 (53) |
Valve only | 2946 (16.9) | 2912 (23.1) | 685 (14.7) | 1136 (21.7) |
Other only | 503 (2.9) | 727 (5.8) | 17 (0.4) | 155 (3) |
CABG + valve | 1588 (9.1) | 1241 (9.8) | 567 (12.2) | 705 (13.4) |
CABG + other | 251 (1.4) | 537 (4.3) | 98 (2.1) | 104 (2) |
Valve + other | 680 (3.9) | 803 (6.4) | 147 (3.2) | 294 (5.6) |
CABG + valve + other | 143 (0.8) | 270 (2.1) | 69 (1.5) | 70 (1.3) |
Major aortic procedure | ||||
No | 16,895 (96.8) | 12,119 (96.1) | 4556 (98) | 4854 (96.2) |
Yes | 562 (3.2) | 498 (3.9) | 95 (2) | 193 (3.8) |
CPB | ||||
No | 5849 (33.5) | 233 (1.8) | 416 (8.9) | 506 (9.7) |
Yes | 11,595 (66.5) | 12,384 (98.2) | 4233 (91.1) | 4733 (90.3) |
Multiple imputation data sets
The imputed data set contained 39,970 participants for the any transfusion analysis and 27,353 participants for the LVBT analysis. The prevalence of transfusion and LVBT in each data set is shown in Table 55. The characteristics of the transfused and non-transfused participants in the complete case and imputed data sets are shown in Table 56. In the complete case sample, 41.7% received a red cell transfusion and 13.5% received a LVBT. In the imputed sample, 45.1% of participants received a red cell transfusion and 15.0% received a LVBT. Rates of red cell transfusion (LVBT) varied from 36.2% (11.4%) in the Wolverhampton data set to 51.8% (20.2%) in the audit data set. Within the audit data set, any transfusion rates ranged from 24–78%, with LVBT rates ranging from 8%–35%.
Data set | Red cell transfusion | LVBT | ||||||
---|---|---|---|---|---|---|---|---|
Complete case | Imputed | Complete case | Imputed | |||||
N | Any transfusion, n (%) | N | Any transfusion, n (%) | N | LVBT, n (%) | N | LVBT, n (%) | |
Bristol | 6594 | 2755 (41.8) | 17,457 | 8344 (47.8) | 6594 | 810 (12.3) | 17,457 | 2523 (14.5) |
Milan | 11,113 | 4590 (41.3) | 12,617 | 5274 (41.8) | ||||
Wolverhampton | 4246 | 1585 (37.3) | 4651 | 1684 (36.2) | 4246 | 506 (11.9) | 4651 | 532 (11.4) |
Audit | 2796 | 1392 (49.8) | 5245 | 2715 (51.8) | 2796 | 527 (18.8) | 5245 | 1057 (20.2) |
Total | 24,749 | 10,322 (41.7) | 39,970 | 18,017 (45.1) | 13,636 | 1843 (13.5) | 27,353 | 4112 (15.0) |
Characteristic | Receiving any red cell transfusion | Receiving LVBT | ||
---|---|---|---|---|
Complete case | Imputed | Complete case | Imputed | |
n (%) | n (%) | n (%) | n (%) | |
Female participant | ||||
No | 17,730 (36.0) | 28,952 (39.0) | 10,110 (11.2) | 20,331 (12.8) |
Yes | 7019 (56.1) | 11,018 (61.0) | 3526 (20.1) | 7022 (21.5) |
Age (years) | ||||
< 65 | 9736 (29.9) | 16,557 (34.4) | 5226 (7.9) | 11,361 (9.8) |
65–74 | 9106 (43.5) | 14,804 (47.6) | 4993 (13.9) | 10,187 (15.8) |
≥ 75 | 5907 (58.4) | 8609 (61.2) | 3417 (21.5) | 5805 (24.0) |
Height (cm) | ||||
< 150 | 268 (67.5) | 505 (73.3) | 189 (24.9) | 398 (27.1) |
150–159 | 3134 (58.9) | 4933 (63.6) | 1678 (21.6) | 3266 (23.5) |
160–169 | 8500 (46.1) | 12,906 (49.5) | 3982 (15.2) | 7799 (16.3) |
170–179 | 9829 (35.7) | 16,147 (39.2) | 5683 (11.0) | 11,463 (12.6) |
180–189 | 2830 (28.8) | 5107 (32.7) | 1959 (9.4) | 4106 (11.7) |
≥ 190 | 188 (27.1) | 373 (33.4) | 145 (11.0) | 321 (13.9) |
Weight (kg) | ||||
< 50 | 397 (66.0) | 736 (73.7) | 151 (31.8) | 441 (36.0) |
50–59 | 2056 (61.8) | 3203 (65.8) | 831 (25.9) | 1784 (27.6) |
60–69 | 4991 (51.9) | 7637 (56.2) | 2203 (19.3) | 4477 (21.0) |
70–79 | 6886 (42.2) | 11,014 (46.3) | 3444 (14.9) | 7111 (15.5) |
80–89 | 5479 (35.0) | 9489 (38.3) | 3350 (10.0) | 7082 (11.3) |
90–99 | 2991 (29.8) | 4757 (32.0) | 2084 (9.1) | 3739 (10.5) |
≥ 100 | 1949 (24.7) | 3134 (25.9) | 1573 (7.6) | 2720 (8.3) |
Preoperative haemoglobin (g/dl) | ||||
< 10 | 653 (80.7) | 1007 (83.7) | 394 (53.0) | 698 (54.5) |
10–11.9 | 3822 (69.1) | 5816 (73.9) | 1968 (29.3) | 3695 (32.4) |
≥ 12 | 20,274 (35.3) | 33,147 (38.8) | 11,274 (9.4) | 22,960 (11.0) |
Preoperative serum creatinine (µmol/l) | ||||
< 50 | 105 (44.8) | 143 (47.7) | 30 (23.3) | 51 (27.8) |
50–110 | 17,942 (38.0) | 27,795 (40.9) | 10,126 (10.8) | 18,793 (11.9) |
> 110 | 6702 (51.6) | 12,032 (54.7) | 3480 (21.4) | 8509 (21.8) |
Cardiogenic shock | ||||
No | 24,689 (41.6) | 39,870 (45.0) | 13,593 (13.4) | 27,270 (14.9) |
Yes | 60 (83.3) | 100 (83.5) | 43 (48.8) | 83 (56.6) |
IABP used preoperatively | ||||
No | 24,559 (41.5) | 39,721 (44.9) | 13,469 (13.3) | 27,127 (14.9) |
Yes | 190 (67.4) | 249 (68.4) | 167 (32.3) | 226 (35.8) |
MI during the operation or within 30 days of the operation | ||||
No | 21,016 (40.5) | 34,969 (44.3) | 11,640 (12.8) | 24,359 (14.7) |
Yes | 3733 (48.5) | 5001 (50.7) | 1996 (17.5) | 2994 (18.0) |
Ejection fraction (category) | ||||
Good (> 50%) | 17,477 (39.1) | 28,494 (42.4) | 9724 (11.7) | 19,658 (13.1) |
Fair (30–49%) | 5870 (45.9) | 9295 (50.0) | 3043 (17.4) | 6110 (19.1) |
Poor (< 30%) | 1402 (56.6) | 2181 (58.9) | 869 (20.5) | 1585 (22.9) |
Heparin or nitrates until operation | ||||
No | 23,670 (41.3) | 38,131 (44.7) | 13,107 (13.2) | 26,124 (14.7) |
Yes | 1079 (50.3) | 1839 (53.7) | 529 (21.6) | 1229 (21.9) |
Peripheral vascular disease | ||||
No | 22,653 (41.2) | 36,689 (44.6) | 11,994 (13.0) | 24,568 (14.7) |
Yes | 2096 (47.4) | 3281 (50.6) | 1642 (17.5) | 2785 (18.4) |
Chronic dialysis | ||||
No | 24,622 (41.6) | 39,780 (45.0) | 13,588 (13.4) | 27,253 (14.9) |
Yes | 127 (68.5) | 190 (71.2) | 48 (41.7) | 100 (46.5) |
COPD (or emphysema) | ||||
No | 23,083 (41.3) | 37,420 (44.6) | 12,729 (13.3) | 25,644 (14.8) |
Yes | 1666 (47.7) | 2550 (51.7) | 907 (17.1) | 1709 (19.0) |
Previous neurological accident | ||||
No | 23,086 (41.0) | 37,296 (44.4) | 12,484 (13.0) | 25,249 (14.6) |
Yes | 1663 (51.1) | 2674 (54.3) | 1152 (18.9) | 2104 (20.7) |
Diabetic on medication | ||||
No | 20,954 (40.6) | 34,000 (44.3) | 11,318 (12.9) | 22,986 (14.6) |
Yes | 3795 (47.8) | 5970 (49.4) | 2318 (16.6) | 4367 (17.2) |
Previous cardiac surgery | ||||
No | 23,420 (40.9) | 37,777 (44.3) | 12,951 (12.7) | 25,913 (14.2) |
Yes | 1329 (55.2) | 2193 (58.1) | 685 (29.1) | 1440 (30.4) |
Operation type | ||||
CABG only | 13,577 (35.1) | 23,322 (39.2) | 8099 (8.1) | 17,195 (9.9) |
Valve only | 5083 (42.5) | 7679 (47.4) | 2525 (14.7) | 4767 (17.8) |
Other only | 807 (37.4) | 1402 (38.9) | 265 (17.7) | 675 (18.8) |
CABG + valve | 2720 (64.4) | 4101 (67.6) | 1627 (31.8) | 2860 (33.5) |
CABG + other | 754 (54.0) | 990 (56.3) | 262 (18.7) | 453 (24.5) |
Valve + other | 1372 (46.9) | 1924 (51.2) | 670 (21.0) | 1121 (24.1) |
CABG + valve + other | 436 (66.3) | 552 (67.2) | 188 (33.0) | 282 (36.2) |
Major aortic procedure | ||||
No | 23,822 (41.2) | 38,611 (44.6) | 13,159 (12.9) | 26,491 (14.4) |
Yes | 927 (53.5) | 1359 (58.0) | 477 (29.8) | 862 (35.5) |
CPB used at any point | ||||
No | 3419 (23.7) | 7015 (25.5) | 3228 (4.6) | 6781 (5.0) |
Yes | 21,330 (44.6) | 32,955 (49.2) | 10,408 (16.3) | 20,572 (18.4) |
Urgent operation | ||||
No | 8937 (37.4) | 17,693 (41.5) | 8937 (10.8) | 17,693 (11.9) |
Yes | 4699 (50.8) | 9660 (56.0) | 4699 (18.7) | 9660 (20.7) |
Missing | 11,113 (41.3) | 12,617 (41.8) | 0 (0.0) | 0 (0.0) |
Failed PTCA (< 24 hours) | ||||
No | 24,713 (41.7) | 32,823 (42.3) | 13,616 (13.5) | 20,222 (13.9) |
Yes | 36 (55.6) | 57 (58.1) | 20 (25.0) | 41 (25.9) |
Missing | 0 (0.0) | 7090 (57.9) | 0 (0.0) | 7090 (18.2) |
Congestive heart failure | ||||
No | 15,505 (39.2) | 26,753 (43.3) | 4896 (11.0) | 14,675 (13.2) |
Yes | 851 (61.1) | 1549 (66.8) | 347 (28.0) | 1010 (31.7) |
Missing | 8393 (44.4) | 11,668 (46.4) | 8393 (14.4) | 11,668 (15.9) |
Results of the modelling analyses
Model development in the complete case data set
Any red cell transfusion
Univariable regression analyses in the complete case data set produced a set of 17 candidate covariates for inclusion in the any transfusion model (Table 57).
Risk factors | OR (95% CI) | |
---|---|---|
Any red cell transfusion | LVBT | |
Female vs. male | 2.28 (2.15 to 2.41) | 2.00 (1.80 to 2.21) |
Age per 10 years | 1.51 (1.48 to 1.55) | 1.51 (1.43 to 1.59) |
Height per 10 cm | 0.63 (0.61 to 0.65) | 0.69 (0.66 to 0.73) |
Weight per 10 kg | 0.73 (0.72 to 0.75) | 0.74 (0.71 to 0.76) |
Ejection fraction category | ||
Good (> 50%) | 1 | 1 |
Fine (30–49%) | 1.32 (1.24 to 1.40) | 1.60 (1.43 to 1.79) |
Poor (< 30%) | 2.03 (1.82 to 2.26) | 1.95 (1.64 to 2.33) |
MI during the operation or within 30 days of the operation | 1.38 (1.29 to 1.48) | 1.45 (1.27 to 1.64) |
Cardiogenic shock | 7.02 (3.56 to 13.84) | 6.17 (3.39 to 11.24) |
IABP used preoperatively | 2.91 (2.15 to 3.94) | 3.12 (2.25 to 4.33) |
Failed PTCA | 1.75 (0.91 to 3.38) | 2.14 (0.78 to 5.88) |
Heparin or nitrates until operation | 1.44 (1.27 to 1.63) | 1.81 (1.46 to 2.24) |
Peripheral vascular disease | 1.29 (1.18 to 1.41) | 1.43 (1.24 to 1.64) |
Chronic dialysis | 3.06 (2.1 to 4.45) | 4.61 (2.59 to 8.20) |
COPD | 1.30 (1.17 to 1.43) | 1.35 (1.13 to 1.62) |
Previous neurological accident | 1.50 (1.36 to 1.66) | 1.56 (1.33 to 1.82) |
Diabetic on medication | 1.34 (1.25 to 1.44) | 1.34 (1.19 to 1.52) |
(Preoperative serum creatinine level in µmol/l)/100 | 1.74 (1.62 to 1.86) | 2.30 (2.05 to 2.58) |
Preoperative haemoglobin level in g/dl | 0.62 (0.61 to 0.63) | 0.61 (0.60 to 0.63) |
Previous cardiac surgery | 1.77 (1.59 to 1.98) | 2.82 (2.37 to 3.35) |
Operation type | ||
CABG only | 1 | 1 |
Valve only | 1.37 (1.28 to 1.46) | 1.95 (1.70 to 2.23) |
Other only | 1.11 (0.95 to 1.28) | 2.44 (1.76 to 3.38) |
CABG + valve | 3.34 (3.06 to 3.64) | 5.28 (4.63 to 6.02) |
CABG + other | 2.17 (1.87 to 2.51) | 2.61 (1.89 to 3.59) |
Valve + other | 1.64 (1.46 to 1.83) | 3.02 (2.47 to 3.70) |
CABG + valve + other | 3.63 (2.97 to 4.45) | 5.57 (4.07 to 7.63) |
Major aortic procedure | 1.64 (1.44 to 1.87) | 2.86 (2.33 to 3.50) |
CPB | 2.58 (2.38 to 2.81) | 4.05 (3.41 to 4.81) |
Urgent operation | – | 1.91 (1.73 to 2.11) |
n | 24,749 | 13,636 |
Candidate pair-wise interaction terms that were individually significant (p ≤ 0.001) were CPB*preoperative haemoglobin level, weight*preoperative haemoglobin level, CPB*height, CPB*female, chronic dialysis*preoperative serum creatinine level, diabetic on medication*preoperative haemoglobin level, major aortic procedure*height, female*weight, female*operation type, age*operation type and height*operation type. The terms CPB*female, female*weight, diabetic on medication*preoperative haemoglobin level, age*operation type and height*operation type no longer met the criterion for inclusion when all 11 interaction terms were added to the model together and so were dropped from further models:
-
model 1: main effects + (weight*preoperative haemoglobin level)
-
model 2: model 1 + (female*operation type)
-
model 3: model 2 + (CPB*preoperative haemoglobin level)
-
model 4: model 3 + (age*operation type)
-
model 5: model 4 + (chronic dialysis*preoperative serum creatinine level)
-
model 6: model 5 + (CPB*height)
The six interaction terms all appeared to make sense clinically. Cross-validation of the main-effects model (model 0) and models 1–6 (main effects plus interaction terms) showed that, of these seven candidate models, model 3 (main effects + three interactions) had the smallest mean deviance difference (Table 58). FP analyses of the relationship between each continuous variable and receipt of red cell transfusion yielded results that varied by data set and by sex. Using decision rule 1 a linear specification was indicated for weight and height for men and women and for age and preoperative haemoglobin level for women. Decision rule 2 suggested that preoperative serum creatinine level should be treated as linear for women. There was little agreement across the four data sets for the choice of specification of the relationship between transfusion for male participants and three variables: age, preoperative haemoglobin level and preoperative serum creatinine level. Therefore, the effect of adding quadratic terms to our model was explored.
Model | Description of terms included in the model | Mean deviance difference | Deviance difference | |||
---|---|---|---|---|---|---|
Bristol | Milan | Wolverhampton | Audit | |||
0 | Main effects only | 665.39 | 689.40 | 1694.20 | 111.25 | 166.70 |
1 | Main effects + 1 interaction | 661.24 | 662.63 | 1696.72 | 117.61 | 168.01 |
2 | Main effects + 2 interactions | 662.11 | 669.52 | 1691.10 | 118.65 | 169.18 |
3 | Main effects + 3 interactions | 639.89 | 625.34 | 1642.91 | 120.89 | 170.43 |
4 | Main effects + 4 interactions | 655.53 | 631.66 | 1664.07 | 135.38 | 190.99 |
5 | Main effects + 5 interactions | 652.21 | 627.37 | 1655.54 | 132.73 | 193.19 |
6 | Main effects + 6 interactions | 647.43 | 622.12 | 1639.35 | 134.39 | 193.85 |
0 | Main effects + 3 interactions | 639.89 | 625.34 | 1642.91 | 120.89 | 170.43 |
1 | Main effects + 3 interactions + 1 quadratic | 611.75 | 599.17 | 1557.06 | 110.02 | 180.75 |
2 | Main effects + 3 interactions + 2 quadratics | 610.69 | 597.70 | 1553.33 | 113.77 | 177.95 |
3 | Main effects + 3 interactions + 3 quadratics | 631.27 | 603.92 | 1620.28 | 119.09 | 181.79 |
4 | Main effects + 3 interactions + 4 quadratics | 653.57 | 619.39 | 1690.39 | 124.82 | 179.69 |
5 | Main effects + 3 interactions + 5 quadratics | 651.40 | 620.23 | 1679.67 | 128.45 | 177.25 |
6 | Main effectsa + 3 interactions | 620.23 | 621.62 | 1579.87 | 114.09 | 165.34 |
7 | Main effectsa + 3 interactions + 1 quadratic | 585.08 | 597.31 | 1460.28 | 104.13 | 178.61 |
8 | Main effectsa + 3 interactions + 2 quadratics | 583.79 | 595.86 | 1455.80 | 107.87 | 175.65 |
9 | Main effectsa + 3 interactions + 3 quadratics | 605.32 | 602.16 | 1526.39 | 113.34 | 179.41 |
10 | Main effectsa + 3 interactions + 4 quadratics | 627.22 | 617.50 | 1595.24 | 118.53 | 177.60 |
11 | Main effectsa + 3 interactions + 5 quadratics | 625.60 | 618.40 | 1586.88 | 121.99 | 175.14 |
All five quadratic terms were highly significant and so five further candidate models were created by adding the five quadratic terms incrementally to the candidate model with the smallest mean deviance difference in the initial cross-validation exercise (model 3). The results of the cross-validation of these candidate models are provided in Table 58. All candidate models performed best in the Wolverhampton data set and the audit data set.
The 11 candidate models all performed considerably less well in the Milan data set than in other data sets in terms of deviance difference. We attempted to identify the reasons for the poorer performance in the Milan data set by comparing the coefficients produced in the main-effects model fitted on each of the four data sets in turn and by comparing descriptive statistics for the four estimation samples. Although there were some differences in terms of ORs and the significance of some coefficients, these were generally small. We also compared the coefficients from the best-performing candidate model estimated in the complete case data set and the coefficients from the best-performing candidate model estimated in the complete case data set once the Milan data set had been removed. We then carried out cross-validation of the candidate models estimated without the use of the Milan data set. The main effects-only model performed best in terms of minimal deviance difference. We concluded that we had insufficient evidence to support dropping the Milan data set from the model development sample, especially as doing so would reduce the sample size considerably. The final any transfusion model (Table 59) included participant sex, height, weight, cardiogenic shock, preoperative IABP, previous neurological accident, diabetic on medication, previous cardiac surgery, MI within < 30 days of surgery, major aortic procedure, ejection fraction, use of CPB, three interaction terms (weight*preoperative haemoglobin level, female*operation type, CPB*preoperative haemoglobin level) and two linear and quadratic terms: age and preoperative creatinine level.
Variable | OR (95% CI) |
---|---|
Age per 10 years | 1.29 (1.25 to 1.32) |
Age per 10 years2 | 1.03 (1.02 to 1.04) |
Height per 10 cm | 0.96 (0.92 to 1.00) |
Weight per 10 kg | 0.85 (0.83 to 0.87) |
Cardiogenic shock (yes vs. no) | 2.38 (1.26 to 4.49) |
IABP used preoperatively (yes vs. no) | 1.82 (1.31 to 2.54) |
Previous neurological accident (yes vs. no) | 1.28 (1.16 to 1.41) |
Diabetic on medication (yes vs. no) | 1.13 (1.05 to 1.21) |
(Preoperative serum creatinine level in µmol/l)/100 | 2.12 (1.94 to 2.31) |
[(Preoperative serum creatinine level in µmol/l)/100]2 | 0.89 (0.88 to 0.91) |
Previous cardiac surgery (yes vs. no) | 1.74 (1.56 to 1.95) |
MI within 30 days of operation (yes vs. no) | 1.37 (1.28 to 1.48) |
Major aortic procedure (yes vs. no) | 2.02 (1.73 to 2.36) |
Ejection fraction category | |
Good (> 50%) | 1 |
Fine (30–49%) | 1.09 (1.02 to 1.15) |
Poor (< 30%) | 1.40 (1.25 to 1.57) |
CPB used at any point (yes vs. no) | 2.33 (2.15 to 2.52) |
Preoperative haemoglobin level by CPB status | |
Preoperative haemoglobin level in g/dl if CPB | 0.71 (0.70 to 0.73) |
Preoperative haemoglobin level in g/dl if no CPB | 0.60 (0.57 to 0.63) |
Female vs. male | 1.76 (1.60 to 1.94) |
Operation type by sex | |
Female and CABG only | 1 |
Female and valve only | 0.85 (0.76 to 0.95) |
Female and other only | 0.59 (0.48 to 0.72) |
Female and CABG + valve | 1.66 (1.41 to 1.95) |
Female and CABG + other | 1.05 (0.74 to 1.49) |
Female and valve + other | 0.83 (0.70 to 0.99) |
Female and CABG + valve + other | 1.16 (0.80 to 1.68) |
Male and CABG only | 1 |
Male and valve only | 1.05 (0.97 to 1.15) |
Male and other only | 1.14 (0.93 to 1.40) |
Male and CABG + valve | 2.38 (2.16 to 2.62) |
Male and CABG + other | 1.70 (1.44 to 2.00) |
Male and valve + other | 1.22 (1.03 to 1.44) |
Male and CABG + valve + other | 2.16 (1.70 to 2.75) |
Constant | 0.22 (0.20 to 0.23) |
Large volume blood transfusion
Candidate variables for LVBT derived from the complete case data are listed in Table 60. The following interaction terms were found to be significant at p = 0.1 or better when added individually to the main-effects model: urgent*status, previous neurological accident*preoperative serum creatinine level, previous cardiac surgery*preoperative haemoglobin level, MI status*preoperative haemoglobin level, female*preoperative serum creatinine level, age*preoperative haemoglobin level, urgent*age, chronic dialysis*previous neurological accident, MI status*weight, female*IABP used preoperatively, urgent*preoperative haemoglobin level, IABP used preoperatively*preoperative haemoglobin level, diabetic on medication*MI status, previous cardiac surgery*MI status and CPB*operation type.
Model | Description of terms included in the model | Mean deviance difference | Deviance difference | ||
---|---|---|---|---|---|
Bristol | Wolverhampton | Audit | |||
0 | Main effects only | 236.67 | 344.11 | 266.98 | 98.92 |
1 | Main effects + 1 interaction | 235.45 | 342.40 | 265.50 | 98.45 |
2 | Main effects + 2 interactions | 238.85 | 350.72 | 263.77 | 102.06 |
3 | Main effects + 3 interactions | 240.31 | 353.04 | 264.44 | 103.47 |
0 | Main effects + 1 interaction | 235.45 | 235.45 | 265.50 | 98.45 |
1 | Main effects + 1 interaction + 1 quadratic | 239.37 | 349.90 | 265.68 | 102.52 |
2 | Main effects + 1 interaction + 2 quadratics | 236.72 | 343.39 | 262.38 | 104.38 |
3 | Main effects + 1 interaction + 3 quadratics | 237.58 | 343.47 | 263.83 | 105.45 |
4 | Main effects + 1 interaction + 4 quadratics | 241.23 | 351.01 | 264.02 | 108.65 |
Three candidate models containing one, two and three interaction terms, respectively, were generated by adding the interaction terms listed above to the main-effects model in a cumulative manner, starting with the most significant interaction. LOCOCV across the three cohorts of the complete case data set (Bristol, Wolverhampton and audit) showed that the deviance difference was smallest for the model containing main effects and one interaction term: urgent*MI status (see upper part of Table 60).
Quadratic terms for age, weight, preoperative serum creatinine level and preoperative haemoglobin level were added in turn to the best-performing interaction model. LOCOCV was then applied to candidate models containing the main effects, one interaction term and from zero to four quadratic terms. The model with the lowest mean deviance, model 1, included the main effects, one interaction term and one quadratic term (see lower part of Table 60). The final LVBT model (Table 61) included age, sex, preoperative IABP, dialysis, diabetes, serum creatinine level, haemoglobin level, previous cardiac surgery, major aortic surgery, ejection fraction, use of CPB and the interaction term urgent*MI status.
Variable | OR (95% CI) |
---|---|
Female vs. male | 1.12 (1.00 to 1.24) |
Age per 10 years | 1.22 (1.17 to 1.28) |
Weight per 10 kg | 0.86 (0.83 to 0.89) |
IABP used preoperatively (yes vs. no) | 1.94 (1.37 to 2.74) |
Chronic dialysis (yes vs. no) | 0.29 (0.15 to 0.59) |
Previous neurological accident (yes vs. no) | 1.13 (0.98 to 1.31) |
Diabetic on medication (yes vs. no) | 1.22 (1.09 to 1.37) |
(Preoperative serum creatinine level in µmol/l)/100 | 1.97 (1.74 to 2.23) |
Preoperative haemoglobin level in g/dl | 0.71 (0.69 to 0.73) |
Previous cardiac surgery (yes vs. no) | 2.25 (1.91 to 2.65) |
Major aortic procedure (yes vs. no) | 2.61 (2.05 to 3.33) |
CPB used at any point (yes vs. no) | 3.37 (2.90 to 3.93) |
Ejection fraction category | |
Good (> 50%) | 1 |
Fine (30–49%) | 1.18 (1.06 to 1.31) |
Poor (< 30%) | 1.05 (0.89 to 1.25) |
Operation type | |
CABG only | 1 |
Valve only | 1.17 (1.03 to 1.34) |
Other only | 1.66 (1.25 to 2.20) |
CABG + valve | 2.73 (2.41 to 3.09) |
CABG + other | 2.07 (1.57 to 2.72) |
Valve + other | 1.52 (1.21 to 1.92) |
CABG + valve + other | 2.43 (1.75 to 3.38) |
Urgency status*MI within 30 of operation | |
No MI and not urgent operation | 1 |
MI and urgent operation | 1.84 (1.59 to 2.12) |
MI and not urgent operation | 1.90 (1.34 to 2.70) |
No MI and urgent operation | 1.69 (1.53 to 1.87) |
Constant | 0.02 (0.02 to 0.03) |
Model discrimination in the imputed data set
Meta-analysis comparing the stability of the coefficients across all four cohorts in the imputed data set (excluding Bristol cases before 2002) for the any transfusion model (Table 62) and for the three UK cohorts for LVBT (Table 63) revealed only minimal differences. The AUC for both risk scores in each of the cardiac data sets is shown in Table 64. The AUC in the any transfusion model for the imputed data set excluding Bristol cases before 2002 was 0.78 (asymptotic 95% CI 0.77 to 0.78). The any transfusion model fitted in the contemporaneous imputed data had good discriminatory ability in the Wolverhampton data set (AUC 0.76, 95% CI 0.75 to 0.78) and Milan data set (AUC 0.71, 95% CI 0.70 to 0.72) and very good discriminatory ability in the Bristol data set (AUC 0.83, 95% CI 0.82 to 0.83) and audit data set (AUC 0.80, 95% CI 0.78 to 0.81). Likewise, the final LVBT model had good discriminatory ability in the contemporary imputed data set as a whole (AUC 0.81, 95% CI 0.80 to 0.82) and the Bristol data set (AUC 0.83, 95% CI 0.82 to 0.84), but performed slightly less well in the Wolverhampton and audit data sets (AUC 0.78, 95% CI 0.76 to 0.80, and AUC 0.78, 95% CI 0.76 to 0.79 respectively). The loss of fit stemming from fitting the model in the imputed data set rather than the relevant sample (e.g. the sample under consideration) can be assessed by comparing the two AUCs. In all samples the loss of fit was small, not exceeding 2 percentage points (see Table 64).
Variables | Bristol excluding early cases: 1 | Milan: 2 | Wolverhampton: 3 | Audit: 4 | Tau2 comparing 1–4 | Bristol including early cases |
---|---|---|---|---|---|---|
Age per 10 years | 1.29 (1.22 to 1.36) | 1.31 (1.26 to 1.37) | 1.08 (1.00 to 1.17) | 1.25 (1.16 to 1.34) | 0.008 | 1.17 (1.12 to 1.22) |
Age per 10 years2 | 1.00 (0.98 to 1.03) | 1.04 (1.02 to 1.06) | 0.99 (0.95 to 1.04) | 1.04 (1.00 to 1.07) | 0.000 | 0.99 (0.97 to 1.00) |
Height per 10 cm | 0.93 (0.86 to 1.00) | 0.98 (0.92 to 1.05) | 0.97 (0.86 to 1.09) | 0.89 (0.81 to 0.98) | 0.000 | 0.92 (0.87 to 0.97) |
Weight per 10 kg | 0.76 (0.73 to 0.79) | 0.86 (0.83 to 0.89) | 0.77 (0.72 to 0.81) | 0.80 (0.76 to 0.84) | 0.002 | 0.74 (0.72 to 0.76) |
Cardiogenic shock | 2.23 (0.66 to 7.52) | 1.07 (0.34 to 3.37) | 2.90 (0.27 to 30.56) | 2.76 (0.80 to 9.48) | 0.000 | 2.63 (0.90 to 7.64) |
IABP used preoperatively (yes vs. no) | 2.63 (1.20 to 5.78) | 2.03 (0.77 to 5.35) | 1.03 (0.53 to 2.00) | 1.46 (0.84 to 2.52) | 0.000 | 2.16 (1.03 to 4.53) |
Previous neurological accident (yes vs. no) | 1.20 (1.01 to 1.43) | 1.14 (0.95 to 1.37) | 1.28 (1.01 to 1.62) | 1.21 (0.94 to 1.56) | 0.000 | 1.09 (0.95 to 1.26) |
Diabetic on medication (yes vs. no) | 1.20 (1.04 to 1.38) | 1.11 (0.99 to 1.25) | 1.17 (0.98 to 1.39) | 1.00 (0.84 to 1.19) | 0.000 | 1.11 (0.99 to 1.24) |
(Preoperative serum creatinine in µmol/l)/100 | 3.27 (2.63 to 4.07) | 1.73 (1.54 to 1.95) | 2.66 (1.96 to 3.61) | 2.26 (1.71 to 2.98) | 0.454 | 3.93 (3.30 to 4.69) |
[(Preoperative serum creatinine in µmol/l)/100]2 | 0.84 (0.78 to 0.89) | 0.93 (0.91 to 0.95) | 0.87 (0.77 to 0.98) | 0.89 (0.83 to 0.96) | 0.002 | 0.83 (0.80 to 0.87) |
Previous cardiac surgery (yes vs. no) | 1.30 (1.05 to 1.61) | 1.96 (1.66 to 2.31) | 1.78 (1.18 to 2.70) | 1.80 (1.34 to 2.42) | 0.099 | 1.27 (1.07 to 1.51) |
MI during the operation or within 30 days of the operation | 1.34 (1.15 to 1.55) | 1.44 (1.29 to 1.60) | 1.30 (1.08 to 1.58) | 1.65 (1.34 to 2.03) | 0.001 | 1.07 (0.93 to 1.22) |
Major aortic procedure (yes vs. no) | 3.08 (2.23 to 4.26) | 1.38 (1.10 to 1.72) | 3.38 (1.85 to 6.16) | 1.41 (0.92 to 2.18) | 0.444 | 2.81 (2.11 to 3.75) |
Ejection fraction category | ||||||
Good (> 50%) | 1 | 1 | 1 | 1 | – | 1 |
Fine (30–49%) | 1.32 (1.17 to 1.49) | 1.11 (1.01 to 1.22) | 0.90 (0.76 to 1.05) | 1.03 (0.88 to 1.21) | 0.022 | 1.35 (1.23 to 1.48) |
Poor (< 30%) | 1.43 (1.15 to 1.79) | 1.71 (1.40 to 2.08) | 1.05 (0.81 to 1.36) | 1.01 (0.74 to 1.37) | 0.079 | 1.44 (1.20 to 1.71) |
CPB used at any point (yes vs. no) | 4.13 (3.65 to 4.68) | 1.94 (1.37 to 2.75) | 2.89 (2.14 to 3.90) | 2.58 (2.01 to 3.30) | 0.989 | 5.48 (4.98 to 6.02) |
Preoperative haemoglobin by CPB status | ||||||
Preoperative haemoglobin in g/dl if CPB | 0.56 (0.53 to 0.58) | 0.80 (0.78 to 0.82) | 0.67 (0.63 to 0.71) | 0.65 (0.61 to 0.68) | 0.014 | 0.58 (0.56 to 0.60) |
Preoperative haemoglobin in g/dl if no CPB | 0.61 (0.57 to 0.64) | 0.67 (0.55 to 0.83) | 0.66 (0.55 to 0.80) | 0.60 (0.51 to 0.69) | 0.000 | 0.6 (0.57 to 0.63) |
Female vs. male | 2.15 (1.79 to 2.57) | 1.43 (1.24 to 1.66) | 1.68 (1.29 to 2.19) | 1.86 (1.42 to 2.44) | 0.093 | 2.02 (1.75 to 2.34) |
Operation type by sex | ||||||
Female participant and CABG only | 1 | 1 | 1 | 1 | – | 1 |
Female participant and valve only | 0.73 (0.58 to 0.92) | 0.90 (0.77 to 1.07) | 0.68 (0.50 to 0.93) | 0.58 (0.43 to 0.79) | 0.013 | 0.59 (0.49 to 0.70) |
Female participant and other only | 0.44 (0.30 to 0.66) | 0.69 (0.52 to 0.91) | 0.60 (0.14 to 2.56) | 0.80 (0.45 to 1.42) | 0.010 | 0.31 (0.22 to 0.42) |
Female participant and CABG + valve | 2.54 (1.68 to 3.85) | 1.27 (1.00 to 1.62) | 1.33 (0.88 to 2.01) | 1.34 (0.86 to 2.09) | 0.055 | 1.93 (1.33 to 2.80) |
Female participant and CABG + other | 1.81 (0.59 to 5.54) | 1.21 (0.79 to 1.84) | 0.89 (0.28 to 2.85) | 2.65 (0.56 to 12.64) | 0.000 | 1.11 (0.47 to 2.63) |
Female participant and valve + other | 0.71 (0.48 to 1.07) | 0.92 (0.73 to 1.16) | 0.98 (0.52 to 1.86) | 0.82 (0.51 to 1.32) | 0.000 | 0.53 (0.37 to 0.76) |
Female participant and CABG + valve + other | 1.88 (0.53 to 6.70) | 1.27 (0.80 to 2.01) | 1.54 (0.55 to 4.32) | 1.09 (0.34 to 3.50) | 0.000 | 1.73 (0.50 to 5.97) |
Male and CABG only | 1 | 1 | 1 | 1 | – | 1 |
Male and valve only | 0.86 (0.72 to 1.02) | 1.05 (0.92 to 1.20) | 1.02 (0.77 to 1.34) | 0.80 (0.65 to 0.99) | 0.008 | 0.63 (0.55 to 0.72) |
Male and other only | 0.61 (0.39 to 0.97) | 1.51 (1.16 to 1.96) | 1.34 (0.23 to 7.89) | 1.40 (0.79 to 2.49) | 0.270 | 0.5 (0.35 to 0.72) |
Male and CABG + valve | 2.16 (1.78 to 2.61) | 2.22 (1.89 to 2.59) | 2.26 (1.78 to 2.88) | 2.22 (1.75 to 2.83) | 0.000 | 1.67 (1.42 to 1.95) |
Male and CABG + other | 1.57 (1.07 to 2.30) | 2.04 (1.64 to 2.53) | 1.26 (0.75 to 2.12) | 1.46 (0.87 to 2.44) | 0.048 | 1.32 (0.94 to 1.84) |
Male and valve + other | 0.80 (0.57 to 1.12) | 1.41 (1.10 to 1.82) | 1.50 (0.86 to 2.62) | 1.31 (0.85 to 2.03) | 0.100 | 0.51 (0.38 to 0.68) |
Male and CABG + valve + other | 2.30 (1.30 to 4.09) | 2.46 (1.76 to 3.45) | 2.40 (1.23 to 4.68) | 1.13 (0.60 to 2.14) | 0.349 | 1.65 (0.98 to 2.78) |
Constant | 0.20 (0.18 to 0.23) | 0.21 (0.14 to 0.29) | 0.19 (0.14 to 0.26) | 0.34 (0.26 to 0.43) | 0.001 | 0.26 (0.24 to 0.29) |
Variable | Bristol (excluding early cases): 1 | Wolverhampton: 2 | Audit: 3 | Tau2 comparing 1–3 | Bristol including early cases |
---|---|---|---|---|---|
Female vs. male | 1.16 (1.00 to 1.35) | 1.33 (1.04 to 1.71) | 1.02 (0.85 to 1.23) | 0.005 | 1.11 (0.99 to 1.24) |
Age per 10 years | 1.27 (1.19 to 1.35) | 1.23 (1.10 to 1.37) | 1.12 (1.04 to 1.21) | 0.005 | 1.23 (1.17 to 1.29) |
Weight per 10 kg | 0.85 (0.81 to 0.89) | 0.85 (0.79 to 0.92) | 0.85 (0.80 to 0.90) | 0.000 | 0.84 (0.81 to 0.87) |
IABP used preoperatively (yes vs. no) | 1.91 (1.00 to 3.65) | 1.49 (0.70 to 3.19) | 1.89 (1.13 to 3.17) | 0.000 | 1.95 (1.09 to 3.51) |
Chronic dialysis (yes vs. no) | 0.44 (0.18 to 1.10) | 0.11 (0.02 to 0.52) | 0.12 (0.02 to 0.57) | 0.002 | 0.31 (0.15 to 0.67) |
Previous neurological accident (yes vs. no) | 1.04 (0.84 to 1.28) | 1.61 (1.20 to 2.16) | 1.05 (0.80 to 1.38) | 0.034 | 1.13 (0.96 to 1.33) |
Diabetic on medication (yes vs. no) | 1.32 (1.10 to 1.57) | 1.14 (0.88 to 1.46) | 1.16 (0.95 to 1.41) | 0.000 | 1.16 (1.01 to 1.33) |
(Preoperative serum creatinine in µmol/l)/100 | 1.95 (1.64 to 2.33) | 2.67 (1.98 to 3.61) | 2.07 (1.63 to 2.63) | 0.020 | 2.10 (1.83 to 2.42) |
Preoperative haemoglobin in g/dl | 0.69 (0.66 to 0.72) | 0.80 (0.75 to 0.86) | 0.73 (0.69 to 0.76) | 0.002 | 0.71 (0.69 to 0.74) |
Previous cardiac surgery (yes vs. no) | 2.03 (1.61 to 2.56) | 3.18 (2.06 to 4.90) | 2.27 (1.70 to 3.03) | 0.032 | 1.81 (1.52 to 2.17) |
Major aortic procedure (yes vs. no) | 3.15 (2.23 to 4.45) | 4.31 (2.26 to 8.21) | 1.78 (1.16 to 2.73) | 0.811 | 3.16 (2.36 to 4.24) |
CPB used at any point (yes vs. no) | 3.70 (3.03 to 4.50) | 2.86 (1.52 to 5.36) | 2.08 (1.48 to 2.91) | 0.858 | 3.90 (3.36 to 4.52) |
Ejection fraction category | |||||
Good (> 50%) | 1 | 1 | 1 | – | 1 |
Fine (30–49%) | 1.32 (1.13 to 1.54) | 1.05 (0.84 to 1.33) | 1.09 (0.91 to 1.31) | 0.010 | 1.34 (1.19 to 1.50) |
Poor (< 30%) | 1.38 (1.07 to 1.77) | 1.02 (0.72 to 1.45) | 0.78 (0.55 to 1.11) | 0.067 | 1.41 (1.17 to 1.70) |
Operation type | |||||
CABG only | 1 | 1 | 1 | – | 1 |
Valve only | 1.23 (1.01 to 1.50) | 1.51 (1.11 to 2.04) | 0.91 (0.73 to 1.15) | 0.053 | 1.10 (0.96 to 1.26) |
Other only | 1.23 (0.83 to 1.84) | 0.73 (0.15 to 3.65) | 2.12 (1.35 to 3.34) | 0.166 | 1.04 (0.76 to 1.42) |
CABG + valve | 2.60 (2.14 to 3.16) | 3.18 (2.45 to 4.13) | 2.73 (2.20 to 3.39) | 0.000 | 2.32 (2.01 to 2.68) |
CABG + other | 2.50 (1.69 to 3.72) | 1.31 (0.67 to 2.55) | 1.96 (1.19 to 3.21) | 0.139 | 1.95 (1.40 to 2.72) |
Valve + other | 1.15 (0.81 to 1.65) | 2.31 (1.32 to 4.06) | 1.64 (1.12 to 2.39) | 0.105 | 0.97 (0.73 to 1.31) |
CABG + valve + other | 2.56 (1.55 to 4.24) | 2.19 (1.10 to 4.35) | 2.10 (1.16 to 3.79) | 0.000 | 2.31 (1.52 to 3.50) |
Urgency status*MI during the operation or within 30 days of the operation | |||||
No MI and not urgent operation | 1 | 1 | 1 | – | 1 |
MI and urgent operation | 1.76 (1.41 to 2.21) | 1.61 (1.20 to 2.16) | 2.41 (1.89 to 3.08) | 0.081 | 1.41 (1.17 to 1.71) |
MI and not urgent operation | 1.72 (0.98 to 3.04) | 2.07 (0.88 to 4.83) | 1.85 (1.09 to 3.13) | 0.000 | 1.08 (0.63 to 1.84) |
No MI and urgent operation | 1.59 (1.38 to 1.84) | 1.73 (1.34 to 2.24) | 1.94 (1.60 to 2.34) | 0.007 | 1.58 (1.42 to 1.76) |
Constant | 0.02 (0.02 to 0.02) | 0.02 (0.01 to 0.04) | 0.06 (0.04 to 0.08) | 0.000 | 0.03 (0.02 to 0.03) |
Sample | Any transfusion risk score | LVBT risk score | ||||
---|---|---|---|---|---|---|
N | AUC (95% CI) | N | AUC (95% CI) | |||
Imputed data (excluding early Bristol cases) | Relevant sample | Imputed data (excluding early Bristol cases) | Relevant sample | |||
All imputed data excluding early Bristol cases | 33,960 | 0.78 (0.77 to 0.78) | – | 21,343 | 0.81 (0.80 to 0.82) | – |
All imputed data | 39,970 | 0.77 (0.77 to 0.77) | 0.77 (0.77 to 0.78) | 27,353 | 0.80 (0.79 to 0.80) | 0.80 (0.79 to 0.81) |
Bristol, including early cases | 17,457 | 0.83 (0.82 to 0.83) | 0.84 (0.84 to 0.85) | 17,457 | 0.81 (0.80 to 0.81) | 0.81 (0.80 to 0.82) |
Bristol, excluding early cases | 11,447 | 0.85 (0.84 to 0.85) | 0.85 (0.84 to 0.86) | 11,447 | 0.83 (0.82 to 0.84) | 0.83 (0.82 to 0.84) |
Milan | 12,617 | 0.71 (0.70 to 0.72) | 0.71 (0.70 to 0.72) | – | – | – |
Wolverhampton | 4651 | 0.76 (0.75 to 0.78) | 0.77 (0.76 to 0.79) | 4651 | 0.78 (0.76 to 0.80) | 0.80 (0.78 to 0.82) |
Audit | 5245 | 0.80 (0.78 to 0.81) | 0.80 (0.79 to 0.81) | 5245 | 0.78 (0.76 to 0.79) | 0.78 (0.77 to 0.80) |
Comparison with existing risk scores
The results of fitting existing risk scores to the data sets used for model development are shown in Table 65. The AUCs for the TRUST, TRACK and BRiSc scores in the imputed data set were 0.71 (95% CI 0.71 to 0.72), 0.71 (95% CI 0.71 to 0.72) and 0.69 (95% CI 0.68 to 0.70) respectively. Separate results are shown for participants whose operation involved CPB as the TRUST and TRACK scores were developed for this subset of participants. In all four cardiac data sets the any transfusion risk score had a greater AUC than the TRUST and TRACK scores by around 5 percentage points. The LVBT risk score had a greater AUC by 10 percentage points relative to the BriSc score.
Sample | Any blood transfusion | LVBT | TRUST | TRACK | BriSca | |||||
---|---|---|---|---|---|---|---|---|---|---|
N | AUC (95% CI) | N | AUC (95% CI) | N | AUC (95% CI) | N | AUC (95% CI) | N | AUC (95% CI) | |
All available imputed data | 39,970 | 0.77 (0.77 to 0.77) | 21,343 | 0.81 (0.80 to 0.82) | 39,970 | 0.71 (0.71 to 0.72) | 39,970 | 0.71 (0.71 to 0.72) | 22,702 | 0.69 (0.68 to 0.70) |
Excluding those participants with no CPB or missing data with regard to CPB | ||||||||||
All available data | 32,945 | 0.75 (0.74 to 0.75) | 20,561 | 0.77 (0.77 to 0.78) | 32,945 | 0.70 (0.69 to 0.70) | 32,945 | 0.70 (0.69 to 0.70) | 16,328 | 0.65 (0.64 to 0.66) |
Bristol | 11,595 | 0.80 (0.79 to 0.81) | 11,595 | 0.77 (0.76 to 0.78) | 11,595 | 0.75 (0.74 to 0.76) | 11,595 | 0.72 (0.71 to 0.73) | 11,595 | 0.64 (0.63 to 0.65) |
Milan | 12,384 | 0.71 (0.70 to 0.72) | – | – | 12,384 | 0.67 (0.66 to 0.68) | 12,384 | 0.67 (0.66 to 0.68) | – | – |
Wolverhampton | 4233 | 0.76 (0.74 to 0.77) | 4233 | 0.77 (0.75 to 0.80) | 4233 | 0.72 (0.71 to 0.74) | 4233 | 0.71 (0.70 to 0.73) | – | – |
Audit | 4733 | 0.79 (0.78 to 0.80) | 4733 | 0.77 (0.75 to 0.79) | 4733 | 0.75 (0.74 to 0.76) | 4733 | 0.75 (0.74 to 0.76) | 4733 | 0.68 (0.66 to 0.69) |
Clinical utility
The potential of the any transfusion and LVBT risk scores to assess risk-adjusted institutional variation in transfusion rates associated with cardiac surgery is illustrated in Figure 49. The funnel plots show risk-adjusted LVBT rates for 24 hospitals submitting data to the audit and used in our model development process. The units circled in green indicate those that have become outliers (99% CI) following risk adjustment. Low sample sizes in some of the hospitals mean that the results should be viewed with caution. To promote dissemination the scores were also made available as online calculators that may be used on any phone, computer or tablet [www.cardiacsurgeryleicester.com/large-volume-blood-transfusion-calculator/ and www.cardiacsurgeryleicester.com/our-research/blood-transfusion/ (accessed 19 May 2017)]. To assist interpretation we also estimated the predictive accuracy of different cut-off scores for any transfusion and LVBT (Table 66). We also used the LVBT risk score in the REDWASH trial as a cohort enrichment tool for the selection of participants at increased risk of LVBT, which is described in the following chapter.
FIGURE 49.
Funnel plots showing the frequency of LVBT in 26 UK cardiac surgery centres in 2010: (a) unadjusted frequency of LVBT; and (b) effect of risk adjustment based on the LVBT risk score on the number of centres with a LVBT rate exceeding the 99% CI. Green circles denote centres identified as outliers following risk adjustment.
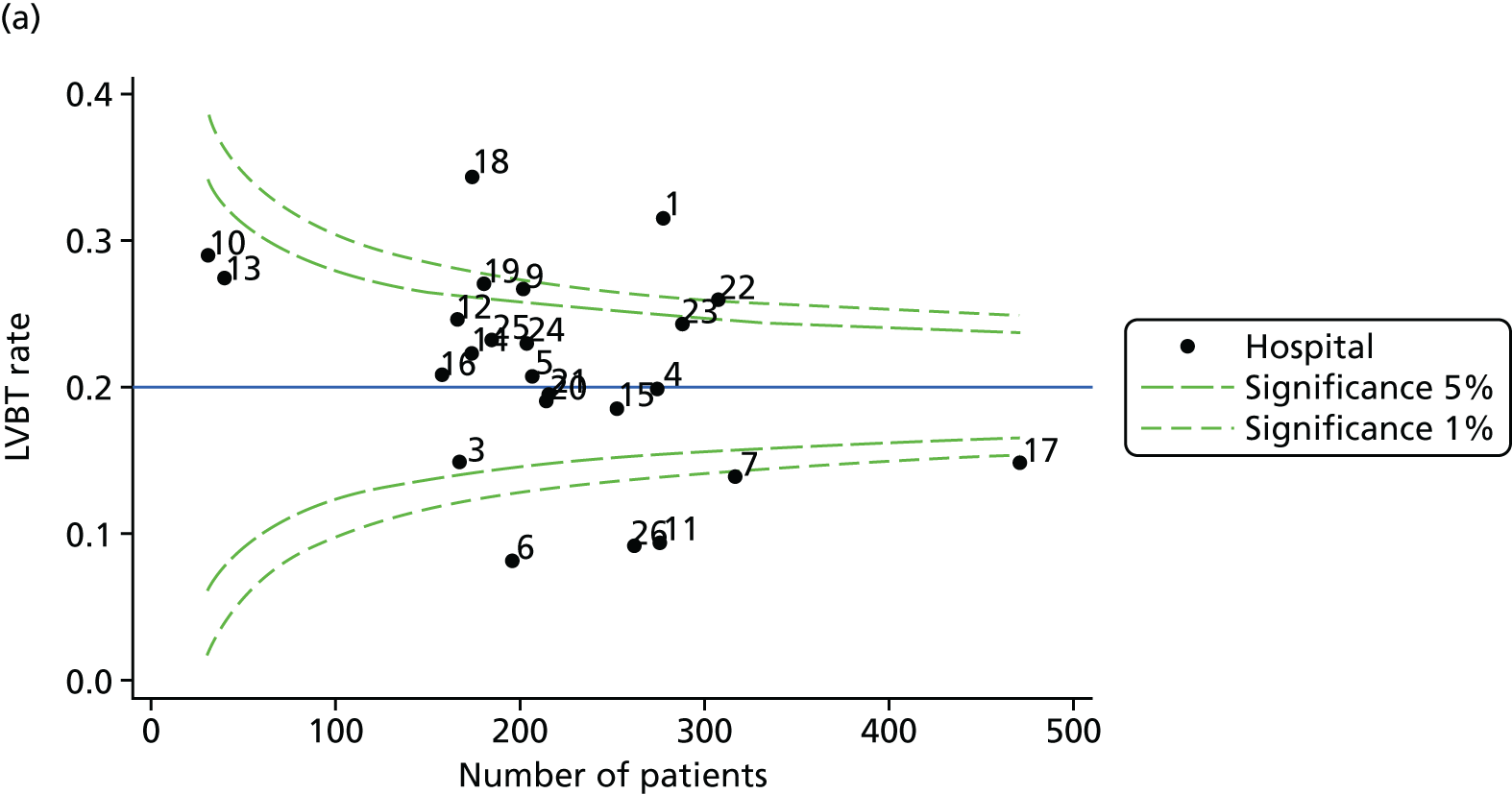

Score | Discrimination | ||||
---|---|---|---|---|---|
Cut-point | Sensitivity | Specificity | PPV | NPV | |
Any transfusion | 0.2 | 100.0 | 0.0 | 52.7 | – |
0.3 | 94.6 | 17.5 | 56.1 | 74.4 | |
0.4 | 81.5 | 45.9 | 62.6 | 69.0 | |
0.5 | 66.1 | 67.2 | 69.1 | 64.0 | |
0.6 | 49.1 | 81.5 | 74.7 | 59.0 | |
0.7 | 32.0 | 91.0 | 79.8 | 54.6 | |
0.8 | 15.9 | 96.5 | 83.6 | 50.8 | |
0.9 | 3.8 | 99.4 | 88.4 | 48.2 | |
LVBT | 0.2 | 100.0 | 0.0 | 42.2 | – |
0.3 | 85.4 | 30.2 | 47.2 | 74.0 | |
0.4 | 59.5 | 65.4 | 55.7 | 68.8 | |
0.5 | 39.5 | 84.0 | 64.3 | 65.5 | |
0.6 | 24.6 | 92.8 | 71.5 | 62.8 | |
0.7 | 12.5 | 97.5 | 78.5 | 60.4 | |
0.8 | 5.2 | 99.1 | 81.0 | 58.9 | |
0.9 | 1.1 | 99.8 | 78.3 | 58.0 | |
0.9 | 1.1 | 99.8 | 78.3 | 58.0 |
Discussion
Main findings
In this study we developed two novel risk scores using a large multicentre cohort of cardiac surgery patients from two countries. Our any transfusion risk score demonstrated good discrimination, whereas the LVBT risk score demonstrated both excellent discrimination and calibration. Both scores had better discrimination than published risk prediction scores for bleeding or transfusion in cardiac surgery.
Strengths and limitations
Our study has six main strengths. First, it uses clinical factors that are well defined and routinely collected in cardiac surgery centres, increasing its potential utility. Second, by using a large multicentre international cohort our risk scores were designed to overcome the main limitation of existing scores, specifically the wide interinstitutional variation in blood management practice, which has limited the utility of existing scores beyond the centre in which they were developed. This study utilised patient data from the majority of units and surgeons in the UK, reducing the likelihood that non-random selection of low or high transfusion risk units or surgeons will have introduced bias. We therefore suggest that the accurate representation of interinstitutional variability in the data used to develop the models will increase the generalisability and utility of the risk scores across centres. Third, model development within the complete case data set used a LOCOCV technique in preference to the traditional development–validation approach, in which loss of fit is measured in a single, arbitrarily selected data set that is not used for model development. Here, we take account of the cohort structure to make the process symmetrical by fitting the model on all but one cohort and looking at the loss of fit in the omitted cohort and rotating this around all cohorts. We can then select the model for which the loss of fit is least, on average, that is, the one that best generalises across all cohorts. Fourth, we compared models developed using data collected over different time periods, that is, including and excluding both older data (pre 2002) and more recent data (the national audit data collected in 2010). Model coefficients and discrimination were not significantly altered across these analyses, suggesting that the major clinical risk factors for transfusion and LVBT have not changed over time, despite changes in blood management practice. Fifth, we assessed the stability of the coefficients when the models were estimated in different samples, quantifying the between-centre/between-data set variance in ORs for each predictor. This permitted objective identification of predictors that were stronger/weaker predictors in a particular cohort (perhaps because of the different case mix). This highlighted differing roles for CPB, major aortic procedures and preoperative serum creatinine level in the models fitted in different data sets, reflecting different prevalence rates across the data sets. However, it also demonstrated that there were similar effect sizes when the models were fitted in a range of different samples. Sixth, the risk scores are freely available to clinicians or other researchers as web-based calculators. These take < 1 minute to complete and can be performed at the bedside on any phone or tablet, increasing their utility.
The study has several limitations. First, many of the participants included in the study will have been exposed to a range of blood management interventions. These may act as confounders in our analyses. However, our study suggests that these variables may be less important than the preoperative factors used in model development. For example, aprotinin, a serine protease inhibitor that reduces transfusion and coagulopathic bleeding beyond that achieved with alternatives,6 was administered only to participants in the Bristol and Wolverhampton data sets. However, the risk estimates for individual variables across the data sets as well as the overall discrimination of the scores did not differ significantly between data sets in which aprotinin had been administered and data sets in which aprotinin had not been delivered. Second, the timing of cessation of aspirin and/or clopidogrel therapy was not recorded consistently in all of the data sets and these variables were not assessed in the models. In Chapter 2 we demonstrated that administration of DAPT within 5 days of surgery was an important predictor of subsequent bleeding. However, in the COPTIC study this occurred in approximately 11% of participants and we may consider that our any transfusion and LVBT risk scores are applicable to the great majority of cardiac surgery patients. Third, intraoperative events not included in the risk scores, such as prolonged CPB time, are also potential confounders. It was our intention, however, for the risk scores to have greatest utility in terms of cohort enrichment for research or personalised medicine, by developing them using only data that were available preoperatively. Thus, the scores will inform the use of the most common blood management interventions that are principally administered at the beginning of surgery (antifibrinolytics, low-volume prime) or during surgery (cell salvage). Fourth, although multiple imputation is now widely used, diagnostic tests for the imputation process are less well developed, so some bias may result from the assumptions used in the imputation process. However, the small number of missing data, and thus imputed values, suggests that any such bias is likely to be minimal. Fifth, the discrimination of the any transfusion risk score was lowest in the Italian data set. This may reflect differences in clinical definitions of routinely collected data between countries; the variable urgent operation was not included in the any transfusion model for this reason. It may also reflect demographic differences; the participant population in the Italian data set was different in some respects from the UK population. These considerations notwithstanding, we would caution against widespread utilisation of the score in a non-UK population without further validation. A final limitation is that we were unable to completely match the variables in all of the comparison risk scores to those in our data. The end points specified in these scores were also different to the end point that we used. We stress that the utility of the scores that we have described will be determined only by independent external validation.
Clinical utility
Cohort enrichment is an important consideration in RCTs of blood management interventions. For example, enrolment of a patient cohort at mixed risk of bleeding and transfusion in two recent multicentre cardiac surgery RCTs, one evaluating antifibrinolytics and another recombinant factor XII, is considered to have significantly influenced the results of these trials. 8,162,191 The LVBT risk score described in the current study provides a simple transparent technique for cohort enrichment in clinical trials in cardiac surgery. Our risk score, which is available as an e-calculator, may also be used by clinicians to accurately risk assess individual patients, a class I recommendation in recent cardiac surgery blood management guidelines. 24 Although careful preoperative assessment and effective blood management is practised in many centres, the 2011 UK audit of blood transfusion in adult cardiac surgery has demonstrated wide variability in transfusion rates and blood management between units. 9 Similar variation has also been reported in other countries. 169 The risk scores described in the current study may enable more consistent application of perioperative blood management interventions by identifying objectively those patients at increased risk. Finally, we have shown that the risk scores may be used to allow risk adjustment and comparison of blood management strategies across centres.
Summary and conclusions
We have reported the development of two novel transfusion risk scores in a multicentre cardiac surgery patient cohort that may be used to inform individual treatment decisions, compare blood management between institutions and enrich cohorts for clinical research. The utility of these scores requires further independent external validation.
Chapter 4 Effectiveness of patient-specific near-infrared spectroscopy-based algorithms for the optimisation of cerebral tissue oxygenation during cardiac surgery: randomised trial with cost-effectiveness analysis and parallel systematic review
Abstract
Background: We assessed whether or not a NIRS-based algorithm in common use for the personalised optimisation of cerebral oxygenation during CPB combined with a restrictive red cell transfusion threshold would reduce perioperative organ injury and be cost-effective.
Methods: In a RCT participants in three UK centres were allocated to a NIRS-based ‘patient-specific’ algorithm that included a restrictive red cell transfusion threshold (haematocrit 18%) or to a ‘generic’ non-NIRS-based algorithm (standard care). The primary outcome for the trial was cognitive function measured up to 3 months post surgery. Secondary outcomes, including biomarkers of brain, kidney and myocardial injury and adverse clinical events, and cost-effectiveness were compared between groups. A parallel systematic review of this and similar trials up to November 2016 was also undertaken.
Results: Of the 204 participants who entered the trial between December 2009 and January 2014, 175 with complete follow-up data for the primary end point formed the analysis cohort. Cognitive testing scores were higher in the verbal fluency domain for the patient-specific group (MD 3.73, 95% CI 1.50 to 5.96); however, there was no difference between the groups for other domains and this did not meet our prespecified definition of an improvement in overall cognitive function. There was no difference between the groups with respect to secondary outcomes or cost-effectiveness. The systematic review showed similar results.
Conclusions: Near-infrared spectroscopy-based algorithms for the management of CPB do not have clinical benefits in adult cardiac surgery.
Study registration: Current Controlled Trials ISRCTN23557269 and PROSPERO CRD42015027696.
Background
The clinical problem
Brain injury is a common and severe complication of cardiac surgery with CPB, affecting up to 40% of patients and contributing to morbidity, mortality and the increased use of hospital resources. 192 The pathophysiology of CPB-associated brain injury is multifactorial2 but is thought to involve regional hypoperfusion and tissue hypoxia,193,194 often within vascular beds that are already abnormal because of advanced age or comorbidities such as diabetes. 195,196 Adequate cerebral tissue oxygen delivery during CPB is achieved by optimisation of several parameters including CPB pump flows, perfusion pressure, haematocrit and the oxygen saturation of arterial blood. Currently, the adequacy of perfusion is determined by the use of global measures of oxygen utilisation, such as mixed venous oxygen saturation (SvO2), or evidence of tissue hypoxia, as implied by indicators of metabolic acidosis or elevated serum lactate, a marker of cell anaerobic metabolism. However, global measures may be normal in the presence of regional hypoxia197,198 and it has been suggested that direct measures of cerebral oxygenation may have greater clinical utility for the development of goal-directed organ protection interventions during CPB. 44
Haemoglobin concentration is a key determinant of oxygen delivery during CPB. A low haemoglobin concentration or low haematocrit reduces tissue oxygen delivery and is associated with tissue hypoxia28 and injury affecting the brain, heart and kidneys. 29,199–201 In an apparent paradox, however, reversal of anaemia during CPB with red cell transfusion is associated with a further increase in the risk of developing organ failure. 29 It is unclear whether this additional risk is attributable to transfusion or the effects of unmeasured confounders. Trials in non-cardiac surgery patients indicate that more liberal (higher) haemoglobin thresholds for red cell transfusion increase transfusion rates and mortality. 32 These findings are reflected by recommendations that restrictive transfusion thresholds are used in patients with cardiovascular disease in contemporary transfusion guidelines. 34 In contrast, in a recent RCT, adult cardiac surgery patients randomised to liberal haemoglobin thresholds had lower mortality. 4 However, this trial considered only postoperative transfusion thresholds. It is well recognised that tissue oxygen utilisation is different during and after surgery. 37 Transfusion thresholds during CPB (haematocrits of 18–23%) are also lower than those used after surgery (haematocrits of 23–29%). Thus far, only two small trials have compared restrictive and liberal transfusion protocols during CPB, yielding inconclusive results. 202,203 Furthermore, protocolised (fixed) transfusion thresholds have distinct disadvantages in that they do not reflect temporal and patient-specific differences in oxygen utilisation. 36,37 It has been suggested that a more appropriate transfusion indicator would be personalised (patient specific), and would assess the adequacy of tissue oxygenation, other important determinants of tissue oxygen utilisation including age and comorbidity or differences in oxygen utilisation over time. 38 No RCT has thus far attempted to evaluate the clinical effectiveness of a patient-specific transfusion threshold in cardiac surgery.
The intervention
Direct measures of regional tissue oxygen levels such as gastric tonometry, laser Doppler flowmetry of the intestinal mucosa or measurement of cerebral venous oxygen saturation using a jugular bulb catheter have been used to optimise tissue oxygenation in clinical trials, but these modalities are invasive, which limits their use. 204 In contrast, NIRS can be used to monitor cerebral oxygenation non-invasively. 204 NIRS is based on the principle that light in the infrared spectrum can travel through bone and tissue for up to several centimetres. Different chromophores, for example oxygenated and deoxygenated haemoglobin, absorb near-infrared light at different wavelengths. The INVOS™ 5100 device manufactured by Covidian (Medtronic, Minneapolis, MN, USA) is a NIRS device used in many cardiac surgery centres in the UK. It operates by projecting near-infrared light into the skull from a light-emitting diode placed on the forehead. The non-absorbed light is detected by a proximal or distal detector applied several centimetres from the emitter. These allow subtraction of the NIRS absorbance in the skin and skull and provide spatial resolution of NIRS absorbance in the forebrain. Measurement of NIRS absorbance at 730 and 810 nm allows assessment of the relative absorbance of oxyhemoglobin compared with total haemoglobin within the cerebral arterioles and venules of the forebrain. 205 The result is a scale of oxygenation expressed as a percentage, which is expressed as a serial measure in real time. As the total volume of blood in the forebrain is dominated by the venous compartment, this measure is considered to accurately reflect cerebral venous oxygen saturation. NIRS vales have been shown to correlate closely with other direct and invasive measures of cerebral venous oxygen saturation. 204
Cerebral desaturation is associated with adverse clinical outcomes206,207 and early clinical studies demonstrated that the use of NIRS-based goal-directed algorithms that aimed to optimise cerebral oxygenation were associated with reductions in brain injury. 208 This led to the hypothesis that the use of NIRS-based interventions would lead to reductions in brain injury. It was also hypothesised that the brain would act as an ‘index’ organ and that optimisation of cerebral NIRS values would result in improved regional oxygenation and reduced organ injury in other tissues such as the kidney or intestine. 44 Finally, it was hypothesised that cerebral NIRS values might be used to direct red cell transfusion by identifying the critical haematocrit, which is thought to be patient and time specific,36,37 and provide an indicator for red cell transfusion. 209 This would theoretically reduce the risks of under- and overtransfusion that have been attributed to protocolised transfusion thresholds. 38 However, in the absence of high-quality evidence to support these hypotheses there is clinical uncertainty over the clinical utility or cost-effectiveness of NIRS-based algorithms. 210 This is reflected by the wide variation in their use. 211
How the intervention might work
Existing protocols to optimise tissue oxygenation during CPB deploy manipulations of CPB (according to levels of blood markers such as lactate or pH) and the transfusion of red cells to maintain the haematocrit above a prespecified threshold. Patient-specific NIRS-based algorithms differ from standard care in two key ways. First, they are ‘goal-directed’ in that they are specifically targeted to maintain cerebral oxygen delivery during CPB (monitored by NIRS), a measure of regional rather than global tissue hypoxia. Second, they allow the manipulation of multiple variables to optimise regional oxygenation for individual patients, allowing personalised interventions. The most widely cited patient-specific algorithm, developed by Denault et al. ,212 aims to optimise the cerebral oxygen supply/demand balance during CPB by (1) increasing oxygen supply using hyperoxygenation, increased pump flow or perfusion pressure or hypercapnic cerebral vasodilation, (2) increasing oxygen offloading by the use of nitrates or (3) reducing oxygen demand by deepening anaesthesia. Cerebral oxygen saturation approaching a low threshold in the presence of anaemia (haematocrit of between 18% and 23%) and despite optimisation of other parameters suggests that the cerebral critical haematocrit is about to be reached and transfusion is indicated. Therefore, this algorithm is patient and time specific and goal directed to optimise a validated objective measure of tissue oxygenation. This should potentially reduce health-care costs associated with complications attributable to tissue hypoxia during CPB or unnecessary allogeneic red cell transfusions.
What knowledge gap is being addressed by this study
Central venous oxygen desaturation is common following cardiac surgery (30%),193 as are neurocognitive decline (40%),213 AKI (25–30%), major adverse cardiovascular events (25–30%) and red cell transfusion (32–75%). 4,214 Existing studies that have demonstrated benefits from the use of NIRS-based algorithms to optimise cerebral oxygenation have been predominantly observational in nature and no systematic review has attempted to summarise the available evidence from RCTs. The use of this technology is variable and there is evidence of equipoise with regard to its effectiveness. 211 To address this uncertainty we undertook a multicentre RCT to assess the clinical utility and cost-effectiveness of patient-specific goal-directed NIRS-based algorithms in cardiac surgery. We also undertook a systematic review and meta-analysis of the available evidence from RCTs that have assessed the clinical utility of this technology, to identify knowledge gaps in the existing evidence and provide recommendations for further research.
Aims and objectives
We aimed to compare generic and patient-specific algorithms for optimising tissue oxygenation during CPB in adult cardiac surgery patients in a RCT. Compared with the generic algorithm (including a haematocrit transfusion threshold of 23%), we hypothesised that the patient-specific, goal-directed algorithm (based on optimising regional cerebral oxygen saturation), combined with a prespecified ‘restrictive’ haematocrit transfusion threshold of 18%, would result in fewer red cell transfusions and would reduce complications arising from low oxygen levels during CPB.
The specific objectives of this multicentre RCT were to:
-
compare the effects of the patient-specific algorithm with those of the generic algorithm in terms of cognitive function
-
compare the effects of the patient-specific algorithm with those of the generic algorithm with respect to a range of secondary outcomes
-
estimate the incremental cost and incremental cost-effectiveness of the patient-specific algorithm compared with the generic algorithm and describe this in terms of a cost-effectiveness acceptability curve.
To better understand the importance of our trial findings, we also undertook a systematic review and meta-analysis of this and other similar trials to establish whether the use of perioperative goal-directed interventions that aim to optimise cerebral NIRS readings result in reductions in measures of cerebral injury (neurocognitive function, serum biomarkers, stroke), other major morbidity (heart, kidney), bleeding, red cell transfusion rates, mortality and resource use.
PASPORT trial methods
Trial design
This trial was a multicentre RCT. Participants were randomised to either a generic algorithm for optimising tissue oxygenation during CPB that included a fixed transfusion threshold or a patient-specific algorithm that incorporated cerebral NIRS monitoring and a restrictive red cell transfusion threshold. The trial is registered as ISRCTN23557269. Participants provided written informed consent preoperatively but became eligible for randomisation only if they scored ≥ 24 on the Mini Mental State Examination (indicating no cognitive impairment). A UK NHS REC approved the study (reference number 09/H0102/13). The trial protocol has been published. 215 Changes to the study design and trial outcomes after commencement of the study are listed in Appendix 3. The trial has been reported in accordance with the 2010 updated Consolidated Standards of Reporting Trials (CONSORT) statement (see Appendix 3). 216
Participants
Study population
-
The study population consisted of adults of either sex undergoing valve surgery or combined CABG and valve surgery.
Inclusion criteria
-
Age ≥ 16 years at recruitment.
-
Gave informed consent to participate and suitable for allocation to either transfusion protocol.
Exclusion criteria
-
Patients undergoing emergency cardiac surgery.
-
Patients prevented from receiving blood and blood products according to a system of beliefs.
-
Patients with higher perioperative haemoglobin requirements or critical limb ischaemia.
-
Patients with congenital or acquired red cell, platelet or clotting factor disorders.
-
Patients with a neurological disorder.
-
Patients with a diagnosed psychiatric disorder or drug or alcohol addiction.
-
Patients with an already-identified cognitive impairment, as defined by psychometric assessment or a preoperative Mini Mental State Examination score of < 24.
-
Patients who had previously sustained a stroke or intracerebral haemorrhage or who had an acquired brain injury.
-
Patients with a pre-existing inflammatory state.
-
Patients with end-stage renal failure or patients who had undergone renal transplantation.
-
Patients unable to complete the cognitive assessments required for the trial, for example because of language difficulties or visual or hearing impairment.
-
Patients unable to give full informed consent for the study.
-
Patients already participating in another clinical (interventional) study.
Settings
Participants were recruited in three specialist cardiac surgery centres in UK NHS hospitals: the Bristol Heart Institute at the Bristol Royal Infirmary, the Department of Cardiothoracic Surgery at Castle Hill Hospital (Hull) and the Department of Cardiothoracic Surgery at Glenfield Hospital (Leicester).
Interventions
The trial compared two algorithms for optimising tissue oxygenation during CPB: generic and patient specific. The interventions were defined as follows:
-
Generic algorithm (including a standard transfusion threshold) – a generic algorithm for optimising tissue oxygenation based on global measures of oxygen utilisation and including a predefined intraoperative haematocrit transfusion threshold of 23%.
-
Patient-specific algorithm (including a restrictive transfusion threshold) – a patient-specific, goal-directed algorithm based on the monitoring and optimisation of regional cerebral oxygen saturation combined with a predefined ‘restrictive’ intraoperative haematocrit transfusion threshold of 18%.
For all participants a NIRS machine was set on arrival into the anaesthetic room and baseline readings were taken pre anaesthetic induction.
For participants allocated to the patient-specific algorithm, optimisation of cerebral oxygenation used a protocol modified from that of Denault et al. 212 Target regional oxygen saturation values were specified as no less than 70% of preinduction values or an absolute value of 50%. If regional oxygen saturation did not fall below the specified target values, but the haematocrit fell to 18%, then red cell transfusion was indicated. This represented a measure to increase safety and protect participants from possible risks associated with the experimental intervention. The steps in Table 67 were taken to maintain these targets.
Equipment check | Bypass pump, oximeter sensors, head and aortic cannula position |
---|---|
CPP < 60 mmHg (CPP = MABP – CVP) | Raise CPP to > 60 mmHg: administer 0.5 mg of metaraminol |
If no effect and CPP < 80 mmHg | Raise CPP to > 80 mmHg: administer 0.5 mg of metaraminol |
PaCO2 < 35 | Raise PaCO2 to 40–45: reduce gas flows |
FiO2 < 0.6 | Raise FiO2 |
If no effect and FiO2 < 1.0 | Raise FiO2 to 1.0 |
Decrease cerebral metabolic rate | Increase depth of anaesthesia: increase propofol (Diprivan®, AstraZeneca, Cambridge, UK) infusion rate |
Increase pump flow to maximum tolerated by venous reservoir | |
Target not met and haematocrit 18–23% | Transfuse 1 unit of red cells |
Although participants in the generic algorithm group had their oxygen saturation levels recorded throughout their operation, the NIRS machine data were concealed so that the perfusionist would not be influenced by these. The duration of intervention in the trial was the duration of a participant’s time on CPB. Clinicians were allowed to transfuse, or refuse to transfuse, in contravention of the allocated treatment arm but were required to document their reason for doing this. Furthermore, a clinician could decide that it was in the best interests of a participant to permanently discontinue the allocated treatment; this did not constitute a withdrawal and the participant was followed up as normal.
Outcomes
Primary outcome
The primary outcome was cognitive function 3 months after surgery. This was measured to determine whether the patient-specific algorithm (designed to optimise cerebral oxygenation and restrict transfusion) improved cognitive outcomes compared with current practice (in which cerebral oxygenation monitoring is not implemented and a generic transfusion threshold is used). Cognitive function was assessed by a qualified examiner blinded to treatment allocation preoperatively, between 4 and 7 days postoperatively and again at 3 months. We tested all key domains of cognitive function recommended by a consensus statement. 217 Key domains assessed and specific tests used218 were as follows:
-
attention – sustained and divided attention: Trail Making Test parts A and B114,219
-
verbal memory – Rey Auditory Verbal Learning Test (RAVLT)7
-
visuospatial – the Block Design Test from the Wechsler Adult Intelligence Scale (WAIS)220
-
psychomotor speed – Digit Symbol Test from the WAIS220
-
executive function/verbal fluency – Controlled Oral Word Association Test (COWAT)221
-
motor co-ordination – Grooved Pegboard Test, dominant and non-dominant hand. 219
In addition to these tests, to help interpret the cognitive function data, the following assessments related to cognitive testing were carried out for all participants:
-
the Wechsler Test of Adult Reading (WTAR)220 provided a measure of intellectual ability, preoperatively only
-
documentation of medications known to interfere with neuropsychological functions (including hypnotics, sedatives, neuroleptics, anxiolytics, antidepressants and beta-blockers) preoperatively, 4–7 days postoperatively and 3 months postoperatively
-
assessment of participants’ current mental health using the General Health Questionnaire (GHQ-30) and Hospital Anxiety and Depression Scale (HADS) preoperatively, 4–7 days postoperatively and 3 months postoperatively, to take into account the potential interaction between postoperative cognition and mood.
Secondary outcomes
All secondary outcomes were collected in the time between randomisation and 3 months’ follow-up, unless otherwise stated:
-
Units of red cells and other blood components transfused – the number of units of red cells and other blood components transfused during the operative period and postoperative hospital stay were recorded.
-
Cerebral oxygenation during the operative period – NIRS readings were recorded for both groups for comparison. Monitoring started before preoxygenation and anaesthetic induction and continued until participants left theatre.
-
Oxygen delivery and utilisation during CPB – serial measurements of oxygen delivery and utilisation were collected from the clinical perfusion record.
-
Quality of life, measured using the EuroQol-5 Dimensions three-level version (EQ-5D-3L),222 was assessed preoperatively and at 6 weeks and 3 months post randomisation.
-
Duration of ICU or HDU stay, calculated as the total time between randomisation and discharge from the cardiac unit that the participant was in (the CICU, HDU or general ICU ward), including any periods of readmission to that area.
-
Duration of hospital stay, calculated as the time between randomisation and discharge from the cardiac unit.
-
Clinical outcomes, defined as:
-
Infectious complications, that is sepsis [defined antibiotic treatment for suspected infection and the presence of systemic inflammatory response syndrome (SIRS) within 24 hours of the start of antibiotic treatment] or wound infection [ASEPSIS score223 > 20, sternum, leg and arm (if applicable) wounds were assessed at least once during a participant’s hospital stay; a questionnaire was administered at 3 months to identify wound infections arising after discharge]. Sepsis occurring post discharge contributed only if the event resulted in admission to hospital or death. [SIRS is central to the diagnosis of infective complications. It was defined as two or more of the following conditions: temperature > 38 °C or < 36 °C; heart rate > 90 beats/minute; respiratory rate > 20 breaths/minute or partial pressure of carbon dioxide in arterial blood (PaCO2) < 32 mmHg; white blood cell count > 12,000/mm3 or < 4000/mm3. Blood test results and temperature were classified using standard reference ranges.]
-
Stroke (validated by computerised tomography scanning): blinded assessment of brain imaging (computerised tomography or magnetic resonance imaging), in association with new-onset focal or generalised neurological deficit (defined as deficit in motor, sensory or co-ordination functions).
-
ST elevation myocardial infarction (STEMI) accompanied by a troponin level of > 5 ng/ml.
-
Postoperative AKI criteria122 stage 1, 2 or 3.
-
Respiratory complications, that is, re-intubation, ventilation of > 48 hours, tracheostomy or adult respiratory distress syndrome.
-
-
Health and personal social services resource use and costs.
-
All-cause mortality within 30 days of surgery.
-
Biochemical markers of organ injury: the following were measured from venous blood samples taken preoperatively, on return to the ICU/HDU/CICU and at 6, 24, 48 and 96 hours postoperatively:
-
S100/S100B (brain)
-
troponin I or T (heart)
-
creatinine clearance (derived from serum creatinine, urine creatinine and urine volume) (kidney)
-
interleukin (IL)-6 and IL-8 (systemic inflammation).
-
-
We assessed a range of urine biomarkers that are considered to reflect different mechanisms underlying post-cardiac surgery AKI, specifically inflammation, altered haem metabolism and glomerular and tubular injury. The following were measured from urine collected for over a 3-hour period (one sample taken preoperatively and three samples taken over the first 2 postoperative days):
-
urinary microalbumin, a marker of glomerular and tubular damage
-
neutrophil gelatinase-associated lipocalin (NGAL), a marker of renal inflammation/altered haem metabolism
-
renal liver-type fatty acid-binding protein (LFABP), a marker of renal inflammation/altered haem metabolism
-
IL-18, a marker of renal tubular inflammation
-
kidney injury molecule-1 (KIM-1), a marker of renal tubular epithelial mesenchymal transition
-
alpha- and pi-glutathione S-transferase, markers of renal tubular injury.
-
Adverse events
Expected adverse events were specified in the study protocol and captured via the study case report forms, both for the postoperative in-hospital period (serious and non-serious) and at the 3-month follow-up (serious only). All other events were considered unexpected; any such events satisfying one or more criteria for classification as serious were recorded in detail on purpose-designed serious adverse event forms.
Sample size
The sample size for the study was 150 participants. The basis for this choice was as follows. The co-primary outcomes (see Appendix 3 for discussion of subsequent removal of one of the co-primary outcomes) are continuously scaled and so 150 participants (75 per group) would be sufficient to detect a small to moderate standardised difference. Specifically, standardised differences of 0.33 for cognitive function (adjusting for baseline), 0.46 for the infectious outcome and costs and 0.4 for the biochemical markers (adjusting for baseline and using four repeated measures) could be detected with 80% power and 5% significance (two-tailed). Correlations between repeated measures were assumed to be 0.7. For time to ICU/hospital discharge, the sample size allowed a HR of 1.65 to be detected.
At the request of the funder, the sample size was reviewed after 100 participants had been recruited. The correlation between repeated post-intervention measures was as anticipated (i.e. approximately 0.7) but the correlation between baseline and post-intervention cognitive function was lower than expected (closer to 0.5 than 0.7). Also, there was a higher than anticipated dropout rate early on in the trial (see Appendix 3). To account for this lower correlation and to allow for a 12% dropout rate across the trial, the target sample size was increased from 150 to 200 participants. The target standardised differences for the primary outcomes remained unchanged and no comparison between groups was made. This increase in study size increased the power to detect differences in the biochemical markers and the length of hospital stay. It also provided 72% (80%) power to detect a standardised difference of 0.33 (0.36) in cognitive function at the 2.5% significance level (reduced to allow for multiple cognitive function domains). This amendment was approved by the REC on 7 August 2013.
Interim analyses
There were no planned interim analyses.
Randomisation
Participants were randomly allocated to either the generic algorithm or the patient-specific algorithm for optimising tissue oxygenation during CPB in a 1 : 1 ratio, stratified by centre and surgical procedure (valve surgery only or combined CABG and valve surgery). Allocations, blocked with varying block sizes, were generated by computer and concealed. A secure password-protected internet-based randomisation system was developed by the Clinical Trials and Evaluation Unit. Staff in participating centres were required to enter information to identify a participant uniquely and to confirm eligibility before the randomised allocation was revealed. Randomisation occurred preoperatively after written informed consent was given and eligibility confirmed and as close to the scheduled surgery time as possible. If the surgery was unexpectedly rescheduled, participants retained their study number and allocation.
Blinding
It was not possible to blind surgical, anaesthetic and perfusion staff in theatre to the randomised allocation. However, every effort was made to ensure that the treatment allocation was concealed from participants, ICU and other ward care staff and outcome assessors. Particular importance was placed on the blinding of staff involved in the administration and scoring of the neurocognitive assessments. To further minimise bias, outcome measures were defined, as far as possible, on the basis of objective criteria. The success of participant blinding was checked by asking participants if they knew their allocation at the time of their 3-month post-surgery follow-up.
Data collection
In-hospital data collection included the following elements:
-
screening data for all non-emergency participants undergoing cardiac surgery:
-
distinguishing patient information but without recording identifiable data electronically
-
whether or not a patient information leaflet was sent
-
whether or not a patient was approached for the trial
-
planned operation
-
assessment of eligibility and, if ineligible, reasons for ineligibility
-
whether or not a patient was asked to give written informed consent for the trial
-
-
for all randomised participants:
-
preoperative characteristics
-
operative details
-
details of random allocation, including whether this was adhered to
-
observations required for the primary and secondary outcomes, including dates and times of relevant events
-
scores on the neurocognitive tests preoperatively and at days 4–7 postoperatively and information about relevant concomitant medications
-
EQ-5D, HADS and GHQ-30 questionnaires completed preoperatively and HADS and GHQ-30 questionnaires completed between day 4 and day 7 postoperatively
-
other key resource use data
-
any adverse events.
-
Research staff in participating centres collected data on the trial screening log (custom-designed access database retained locally) and preprinted case report forms. These data were transferred promptly to a secure computerised database maintained on a NHS server, allowing data to be checked centrally. Queries about specific data items were listed on the database and were immediately apparent to centre staff when they logged on.
Data collection after hospital discharge consisted of the following elements:
-
Six weeks after the operation – the EQ-5D was posted to participants.
-
Three months after the operation – the neurocognitive assessments were administered at a follow-up visit along with the EQ-5D, HADS and GHQ-30 questionnaires and information about relevant concomitant medications was recorded. A 3-month follow-up questionnaire was also administered. The questionnaire was composed of items eliciting information about:
-
adverse events occurring after discharge, with further details of any event suspected to contribute to the primary outcome or meet the definition of an serious adverse event sought from either the admitting hospital or the participant’s general practitioner (GP)
-
surgical wound infections occurring after discharge (ASEPSIS post-discharge surveillance questionnaire)
-
resource use after discharge from hospital
-
a participant’s awareness of his or her random allocation.
-
-
Occasionally, data collection was delayed beyond the planned follow-up times. When this occurred the following rule was used to determine whether or not data should be included in the analyses:
-
Three-month questionnaire: questionnaire items were phrased specifically in relation to the 3-month postoperative period and staff completing the questionnaires were trained to record information relating to this period only; therefore, data from all questionnaires were used.
-
Data collection is summarised in Table 68.
Data collection | Pre surgery | Intraoperatively | In CICU/ward | At discharge | Follow-up (post randomisation) | ||
---|---|---|---|---|---|---|---|
Generic protocol | Patient-specific protocol | 6 weeks | 3 months | ||||
Eligibility | ✓ | ||||||
Written consent | ✓ | ||||||
Demographics, medical history, comorbidity, type of operation | ✓ | ||||||
Operative details | ✓ | ✓ | |||||
Haematocrit | ✓ | ✓ | ✓ | ✓ | |||
Renal functiona | ✓ | ✓ | |||||
Mini Mental State Examination and WTAR | ✓ | ||||||
Cognitive function testsb | ✓ | ✓c | ✓ | ||||
GHQ-30 and HADS questionnaires | ✓ | ✓c | ✓ | ||||
EQ-5D questionnaire | ✓ | ✓ | ✓ | ||||
Concomitant medicationsd | ✓ | ✓c | ✓ | ||||
Laboratory serum analysis | ✓e | ✓e | |||||
Laboratory urine analysis | ✓f | ✓f | |||||
Randomised allocation | ✓ | ||||||
NIRS values | ✓ | ✓ | ✓ | ||||
NIRS algorithmg | ✓ | ✓ | |||||
CPB managementh | ✓ | ✓ | |||||
Transfusioni | ✓ | ✓ | ✓ | ||||
ASEPSIS assessment of wound infection | ✓ | ||||||
ASEPSIS post-discharge surveillance | ✓ | ||||||
Surgical complications and adverse events | ✓ | ✓ | ✓ | ✓ | ✓ | ||
ICU, HDU and hospital stay | ✓ | ||||||
Resource use data | ✓ | ✓ |
Laboratory analyses
Serum troponin, calculated creatinine clearance and NGAL were key biomarker-based outcomes of interest and were measured in all available samples. Assessment of S100B, IL-6, IL-8, IL-18, LFABP and KIM-1 was carried out only in subgroups of consecutive participants, because of budget limitations.
After collection serum samples were left to clot for at least 30 minutes and were then centrifuged at 3500 rpm for 5 minutes. Remaining serum was aliquoted into cryovials and frozen at –80 °C. Urine samples were centrifuged at 1500 g for 15 minutes, aliquoted into cryovials and frozen at –80 °C. Frozen urine and serum samples were shipped in batches to the central laboratory at University Hospitals of Leicester NHS Trust for analysis. From 2010, serum troponin T was measured in the University Hospitals Bristol NHS Foundation Trust biochemistry laboratory on fresh samples using established standard operating procedures, using the Enzo® Troponin I (human) enzyme-linked immunosorbent assay (ELISA) kit (Enzo Life Sciences, Inc., Ann Arbor, MI, USA). Prior to this, serum troponin I was measured at the University Hospitals Bristol NHS Foundation Trust laboratory. Serum creatinine, urine creatinine and urine microalbumin were measured using the ADVIA® 2400 Clinical Chemistry System (Siemens, Frimley, UK). S100B (S100B Human ELISA, BioVendor Research and Diagnostic Products, Oxford, UK), IL-6 (EASIA kit; Invitrogen, Waltham, MA, USA), IL-8 (ELISA kit; Bender MedSystems GmbH, Vienna, Austria) NGAL, LFABP, IL-18 (all EIA kit; EKF Diagnostics, Barleben, Germany) and KIM-1 (ELISA assay kit; BioAssay, US Biological Life Sciences, Salem, MA, USA) were measured on the DS2® two-plate ELISA Processing System (Dynex Technologies, Chantilly, VA, USA).
Strategies to optimise recruitment
When the study opened to recruitment in Bristol in 2009, potential participants were identified from operating lists and were then sent study information by post/fax in advance of them coming to the hospital for their operation. Approach and consent took place once participants attended hospital for their operation. The short time period between receiving the study information and the operation led to a number of patients declining the study. In 2011, a ‘day of surgery admission’ policy was introduced to maximise bed space. Day of surgery admission patients attend the hospital for a short visit the day before surgery to give consent for their procedure and undertake routine clinical tests. They then return for their surgery the following day. Around 50% of patients became day of surgery admission cases after this policy was implemented, which significantly limited the time available to enrol patients into the study, conduct neurocognitive assessments and take trial samples. To help overcome this problem, ethics approval was obtained in February 2012 to provide patients with information at the outpatient clinic and then approach and consent them at the pre-assessment clinic. When time permitted, the neurocognitive tests were also carried out at the pre-assessment clinic. If this was not possible, patients were asked to come in an hour earlier on the day of their admission to complete the baseline assessments. Patients were also give a sample pot so that they could collect a 3-hour urine sample on the morning of their admission and bring it with them.
Adherence
Non-adherence to the randomisation protocol
Non-adherence to the randomisation protocol was defined as either of the following:
-
Participant did not meet one or more of the pre-consent study eligibility criteria but was consented and treated in the study.
-
Participant consented and met the inclusion criteria but was not randomised. Such participants were excluded from the analysis population.
Non-adherence to the algorithm for optimising tissue oxygenation
Two types of non-adherence to the algorithm for optimising tissue oxygenation were defined: (1) when the optimisation of cerebral oxygenation using the Murkin algorithm was used outside the protocol and (2) when the optimisation of cerebral oxygenation using the Murkin algorithm was not used in accordance with the protocol and should have been. Adherence was assessed for the duration of CPB.
Non-adherence to the transfusion protocol
Two types of non-adherence to the transfusion protocol were defined: (1) a participant received a red cell transfusion outside the protocol (‘extra’ transfusion) and (2) a participant was not given a red cell transfusion that, according to the protocol, should have been given (‘withheld’ transfusion). Adherence was assessed for the duration of CPB.
Statistical analysis
The analysis population included all randomised participants, excluding participants who died or who withdrew after randomisation but before surgery. The safety population consisted of the analysis population plus any participants who died after randomisation but before surgery.
Study data and outcomes, which include safety outcomes, are reported by intention to treat and were directed by a prespecified statistical analysis plan (available online in Report Supplementary Material 5. Continuous variables are summarised using the mean and SD (or median and IQR if the distribution is skewed) and categorical data are summarised as a number and percentage. NIRS data are summarised as AUCs; minimum values are also described. For comparability each participant’s AUC was first standardised according to the duration of the operation and then calibrated relative to the largest standardised AUC observed (i.e. the largest standardised AUC was set to 100, with all other participant AUCs < 100).
Primary and secondary outcomes were compared using linear mixed model (continuous variables measured at multiple time points), multiple (continuous variables summarised as a single measurement), logistic (binary variables) or Cox proportional hazards (time-to-event variables) regression, with the generic algorithm as the reference group. Model validity was checked using standard methods; if a model fitted poorly, transformations/alternative models were explored. Outcomes analysed on a logarithmic scale were transformed back to the original scale after analysis and results were presented as geometric mean ratios (GMRs). Binary outcomes were compared formally only if at least 10 participants in total experienced the outcome (with at least one event in each treatment group).
All analyses were adjusted for the stratification variables, centre and surgical procedure. Neurocognitive outcomes were also adjusted for reading ability at recruitment (i.e. WTAR), medication (any vs. no medication) and preoperative GHQ-30 score (≥ 72 vs. < 72), HADS anxiety score (≥ 8 vs. < 8) and HADS depression score (≥ 8 vs. < 8). GHQ-30, HADS and medication data were also collected at 4–7 days and 3 months postoperatively and the analyses were carried out with and without the inclusion of these ‘updated’ covariates in the model as a sensitivity analysis. For continuous outcomes that were measured preoperatively as well as postoperatively, preoperative and postoperative values were modelled jointly to avoid the necessity of excluding or imputing missing preoperative values.
For outcomes measured at multiple time points, if the time*treatment interaction term was statistically significant at the 10% level then separate treatment effects are presented for each time point; otherwise, an overall treatment effect is reported. For LOS outcomes, deaths prior to discharge were treated as censored observations. Low concentrations of biomarkers cannot be detected reliably in biomarker assays (concentrations below the lower limit of detection). Values that were lower than the lower limit of detection were treated as ‘not detectable’ and not as genuine readings. Many participants had undetectable troponin concentrations at baseline. To account for this, the baseline troponin concentration was categorised into (1) not detectable, (2) detectable but less than the median concentration observed and (3) concentration at or greater than the median. All other biomarkers were detectable at all time points. The trial was not powered to detect differences in clinical outcomes and their frequencies are tabulated descriptively.
Likelihood ratio tests were used to determine statistical significance and two-tailed p-values of < 0.05 were considered statistically significant. No formal adjustment was made for multiple testing but, in interpreting the results, consideration was given to the number of statistical tests performed and the consistency, magnitude and direction of treatment estimates for different outcomes. Additionally, for the primary outcome, which was measured across six dimensions, it was specified in the statistical analysis plan that four out of six outcomes significant at the 5% level would provide evidence to support the study hypotheses.
All analyses were performed in SAS version 9.3 (SAS Institute Inc., Cary, NC, USA) and Stata version 13.0.
PASPORT trial study cohort
Study centres
The study opened to recruitment in Bristol in December 2009. The study was initially designed as a single-centre study but was subsequently extended to two further centres to increase recruitment. The centres in Hull and Leicester were opened for recruitment in January 2012 and February 2013 respectively.
Patients screened
From study opening in December 2009 until the study closed to recruitment in January 2014, a total of 764 patients were screened for inclusion in the study. Of the 764 patients screened, 135 (17.7%) were ineligible and 185 (24.2%) were eligible but were not asked to participate (Figure 50).
FIGURE 50.
PASPORT trial CONSORT diagram. Hb, haemoglobin; MMSE, Mini Mental State Examination.

The main reasons for ineligibility were either clinical (elevated preoperative haemoglobin requirement; active inflammatory state or sepsis; red cell, platelet or clotting factor disorder) or neurological (previous stroke, intracerebral haemorrhage, acquired brain injury, neurological disorder or disability that prevented the patient completing the neurological assessments). The main reasons why eligible patients were not approached were changes in operating schedules (e.g. cancellations or patients moved to a surgeon not participating in the study) or because the surgeon did not feel that it was in a patient’s best interests to take part.
Of the remaining 444 patients invited to join the study, 227 (51.1%) declined, mainly because of not wanting to be part of research or because of anxiety (see Figure 50). Of the 217 who consented to participate, nine were withdrawn before randomisation, four because they failed the post-consent neurocognitive screening test (Mini Mental State Examination score of < 24) and five because they withdrew their consent.
Participants recruited
Between 13 December 2009 and 8 January 2014 a total of 208 participants were therefore recruited and randomised; 188 in Bristol, six in Hull and 14 in Leicester. The Hull participants were randomised in the year from 8 June 2012 to 17 June 2013 and the Leicester centre randomised its first and last participants on 20 March 2013 and 12 November 2013 respectively. Follow-up of all study participants was completed on 29 April 2014. Of the 208 participants randomised, 106 were allocated to the generic algorithm and 102 to the patient-specific algorithm.
Participant withdrawals
There was one death and three withdrawals post randomisation but before surgery in the patient-specific group; one participant decided not to go ahead with the surgery, one was identified as ineligible and the third was withdrawn because of an emergency change to the operating schedule. The analysis population therefore included 204 participants, 106 of whom were allocated to the generic algorithm and 98 of whom were allocated to the patient-specific algorithm.
A further seven participants withdrew after their surgery, but all were willing for data and samples collected prior to withdrawal to be used. The reasons for withdrawal included not wanting to participate in follow-up and personal reasons (Table 69).
Variable | Randomised to generic algorithm (N = 106), n/N (%) | Randomised to patient-specific algorithm (N = 102), n/N (%) | Overall (n = 208), n/N (%) |
---|---|---|---|
Any withdrawala | 5/106 (5) | 5/102 (5) | 10/208 (5) |
Of those who withdrew: | |||
Time of withdrawal | |||
After randomisation but before intervention | 0/5 (0) | 3/5 (60) | 3/10 (30) |
After intervention | 5/5 (100) | 2/5 (40) | 7/10 (70) |
Decision for withdrawal | |||
Participant withdrawal | 5/5 (100) | 3/5 (60) | 8/10 (80) |
Clinician withdrawal | 0/5 (0) | 2/5 (40) | 2/10 (20) |
Reason for withdrawal | |||
Treatment allocation | 0/5 (0) | 0/5 (0) | 0/10 (0) |
Participant found to be ineligible after randomisation | 0/5 (0) | 1/5 (20) | 1/10 (10) |
Participant did not give reason | 1/5 (20) | 0/5 (0) | 1/10 (10) |
Surgery no longer required | 0/5 (0) | 0/5 (0) | 0/10 (0) |
Otherb | 4/5 (80) | 4/5 (80) | 8/10 (80) |
Protocol deviations
There were 30 protocol deviations, 11 in the generic algorithm group and 19 in the patient-specific algorithm group. In the generic algorithm group, eight of the 11 deviations were transfusion related: either a transfusion was given when the haematocrit was > 23% or a transfusion was withheld when the haematocrit fell below 23% (Table 70). In the patient-specific algorithm group, there were 18 instances in which the Murkin algorithm was not followed and two cases of transfusion-related non-adherence. Further details are provided in Table 124 in Appendix 3.
Variable | Randomised to generic algorithm (N = 106), n/N (%) | Randomised to patient-specific algorithm (N = 98), n/N (%) | Overall (n = 204), n/N (%) |
---|---|---|---|
Any protocol deviation | 11/106 (10) | 19/98 (19) | 30/204 (15) |
Allocated treatment adherence | |||
Ineligible but treated | 1/106 (1) | 0/98 (0) | 1/204 (0) |
Did not receive an intervention | 1/106 (1) | 0/98 (0) | 1/204 (0) |
Transfusion threshold adherence | |||
Transfused when haematocrit > 23% | 3/106 (3) | 0/98 (0) | 3/204 (1) |
Transfused when haematocrit > 18% without Murkin algorithm being followed | 0/106 (0) | 2/98 (2) | 2/204 (1) |
Did not receive a transfusion when haematocrit dropped below 23% | 5/106 (5) | 0/98 (0) | 5/204 (2) |
Other non-adherence | |||
Murkin algorithm not followed when NIRS reading dropped below 70% of baseline or to an absolute value of 50% | 0/106 (0) | 18/98 (18) | 18/204 (9) |
Murkin algorithm followed for participant in generic algorithm group, i.e. crossover between treatment groups | 1/106 (1) | 0/98 (0) | 1/204 (0) |
Participant follow-up
Of the 204 randomised participants who underwent surgery, 194 were eligible for follow-up at 3 months. Seven withdrew (see Table 69) and three died following surgery. Of these 194 participants, 175 completed the 3-month questionnaire and attended for the neurocognitive assessment. The remaining 19 participants (generic algorithm group, n = 12; patient-specific algorithm group, n = 7) were contacted but declined to participate further (see Figure 50).
Participant demography and past history
Participant characteristics and past history are summarised in Table 71. Overall, the mean age of participants was 68 years (SD 11 years) and 31% were female. Generally, there was a good balance between the groups; the majority of participants had good left ventricular function (86%) and 30% had evidence of coronary disease. The median logistic euroScore was 5 in both groups. By chance, there were slightly more diabetics in the patient-specific algorithm group (12% vs. 6%) and fewer past or current smokers in the generic algorithm group (47% vs. 54%). Beta-blockers were also more commonly prescribed in the generic algorithm group (49% vs. 38%) and a higher proportion in the generic algorithm group were taking aspirin in the 5 days prior to surgery (52% vs. 39%). Overall, 89% of participants underwent elective surgery and 11% were considered urgent.
Characteristic | Randomised to generic algorithm (N = 106) | Randomised to patient-specific algorithm (N = 98) | Overall (N = 204) |
---|---|---|---|
Demographics | |||
Female sex, n/N (%) | 32/106 (30) | 32/98 (33) | 64/204 (31) |
Age (years), mean (range) | 70.0 (29.5–88.7) | 65.9 (18.5–86.6) | 68.0 (18.5–88.7) |
BMI (kg/m2), mean (SD) | 27.5 (4.6) | 27.8 (5.6) | 27.6 (5.1) |
NYHA class, n/N (%) | |||
I | 25/106 (24) | 20/98 (20) | 45/204 (22) |
II | 50/106 (47) | 43/98 (44) | 93/204 (46) |
III | 30/106 (28) | 33/98 (34) | 63/204 (31) |
IV | 1/106 (1) | 2/98 (2) | 3/204 (1) |
CSS class, n/N (%) | |||
Asymptomatic | 49/106 (46) | 53/98 (54) | 102/204 (50) |
I | 18/106 (17) | 21/98 (21) | 39/204 (19) |
II | 21/106 (20) | 15/98 (15) | 36/204 (18) |
III | 15/106 (14) | 9/98 (9) | 24/204 (12) |
IV | 3/106 (3) | 0/98 (0) | 3/204 (1) |
Angiography/echocardiography results | |||
Left ventricular function, n/N (%) | |||
Good (> 50%) | 90/106 (85) | 85/98 (87) | 175/204 (86) |
Moderate (30–50%) | 16/106 (15) | 13/98 (13) | 29/204 (14) |
50% disease in left main stem | 2/106 (2) | 0/98 (0) | 2/204 (1) |
Coronary disease: number of vessels,a n/N (%) | |||
None | 71/106 (67) | 70/97 (72) | 141/203 (69) |
Single | 15/106 (14) | 11/97 (11) | 26/203 (13) |
Double | 11/106 (10) | 9/97 (9) | 20/203 (10) |
Triple | 9/106 (8) | 4/97 (4) | 13/203 (6) |
Not investigated | 0/106 (0) | 3/97 (3) | 3/203 (1) |
Blood and urine results | |||
Haemoglobin level (g/dl), median (IQR) | 14.2 (13.1–15.5) | 14.7 (13.3–112.0) | 14.4 (13.2–16.2) |
Haematocrit (%), median (IQR)b | 40.0 (37.7–42.0) | 41.0 (38.0–43.0) | 41.0 (38.0–43.0) |
Platelets (×109/l), median (IQR)c | 220.0 (192.0–258.0) | 212.0 (186.0–252.0) | 217.0 (186.5–255.5) |
Creatinine level (µmol/l), median (IQR) | 92.0 (77.0–105.0) | 87.5 (77.0–104.0) | 90.0 (77.0–105.0) |
Urine output over 3 hours (ml), median (IQR)d | 221.0 (144.0–303.0) | 250.0 (157.0–370.5) | 230.0 (152.0–342.0) |
Medical history | |||
Diabetic, n/N (%) | |||
No | 100/106 (94) | 86/98 (88) | 186/204 (91) |
Diet | 2/106 (2) | 4/98 (4) | 6/204 (3) |
Oral | 4/106 (4) | 6/98 (6) | 10/204 (5) |
Insulin | 0/106 (0) | 2/98 (2) | 2/204 (1) |
Pacemaker, n/N (%) | |||
No | 101/106 (95) | 96/98 (98) | 197/204 (97) |
Temporary | 1/106 (1) | 0/98 (0) | 1/204 (1) |
Permanent | 4/106 (4) | 2/98 (2) | 6/204 (3) |
Heart rhythm, n/N (%) | |||
Sinus | 82/106 (77) | 76/98 (78) | 158/204 (77) |
Atrial fibrillation | 21/106 (20) | 19/98 (19) | 40/204 (20) |
Block | 1/106 (1) | 1/98 (1) | 2/204 (1) |
Paced | 2/106 (2) | 2/98 (2) | 4/204 (2) |
CVA or TIA | 5/106 (5) | 11/98 (11) | 16/204 (8) |
Smoking status, n/N (%) | |||
No | 56/106 (53) | 45/98 (46) | 101/204 (50) |
Ex-smoker (> 1 month) | 43/106 (41) | 47/98 (48) | 90/204 (44) |
Yes | 7/106 (7) | 6/98 (6) | 13/204 (6) |
Previous cardiac surgery, n/N (%) | 6/106 (6) | 5/98 (5) | 11/204 (5) |
MI, n/N (%) | 12/106 (11) | 8/98 (8) | 20/204 (10) |
Operative priority, n/N (%) | |||
Elective | 94/106 (89) | 88/98 (90) | 182/204 (89) |
Urgent | 12/106 (11) | 10/98 (10) | 22/204 (11) |
Additive euroScore, median (IQR) | 5.0 (4–7) | 5.0 (3–6) | 5.0 (4–7) |
Medications | |||
Heparin, n/N (%) | 2/106 (2) | 0/98 (0) | 2/204 (1) |
Nitrates until theatre, n/N (%) | 1/106 (1) | 0/98 (0) | 1/204 (1) |
Clexane® (enoxaparin; Sanofi-Aventis, Guildford, UK) within 12 hours preoperatively, n/N (%) | 1/106 (1) | 1/98 (1) | 2/204 (1) |
Aspirin within 5 days preoperatively, n/N (%) | 55/106 (52) | 38/98 (39) | 93/204 (46) |
Clopidogrel within 5 days preoperatively, n/N (%) | 3/106 (3) | 3/98 (3) | 6/204 (3) |
Beta-blockers, n/N (%) | 52/106 (49) | 37/98 (38) | 89/204 (44) |
Psychotic medications, n/N (%) | |||
Hypnotics | 4/106 (4) | 1/98 (1) | 5/204 (2) |
Sedatives | 4/106 (4) | 1/98 (1) | 5/204 (2) |
Anxiolytics | 4/106 (4) | 0/98 (0) | 4/204 (2) |
Antidepressants | 9/106 (8) | 6/98 (6) | 15/204 (7) |
Any psychotic drug | 17/106 (16) | 8/98 (8) | 25/204 (12) |
WTAR | |||
WTAR standard score, median (IQR)e | 118.5 (108.0–123.0) | 117.0 (108.0–122.0) | 118.0 (108.0–123.0) |
Operative characteristics
Operative characteristics are described in Table 72. Overall, 157 (77%) participants were listed for valve surgery and 47 (23%) were listed for combined CABG and valve surgery. The majority of value procedures involved the repair or replacement of an aortic (65%) or a mitral (34%) valve. By chance, there were proportionally more aortic value procedures in the generic algorithm group (72% vs. 58%) and proportionally more mitral value procedures in the patient-specific algorithm group (28% vs. 40%). Most aortic procedures involved a replacement of the valve (97% of those with data recorded, not specified for 29%). The majority of mitral valves were replaced (75% of those with data recorded, not specified for 26%). The duration of bypass and cross-clamp and the overall duration of the surgery were similar in the two groups; in all but five participants blood cardioplegia was used. For the majority of participants (85%) a Quadrox oxygenator was used. All participants were alive at the end of the surgery.
Characteristic | Randomised to generic algorithm (N = 106) | Randomised to patient-specific algorithm (N = 98) |
---|---|---|
Type of surgery,a n/N (%) | ||
Valve | 81/106 (76) | 76/98 (78) |
CABG and valve | 25/106 (24) | 22/98 (22) |
Duration of operation (hours), mean (SD)b | 3.7 (0.8) | 3.6 (0.9) |
Bypass duration (minutes), mean (SD)c | 107.7 (29.0) | 109.9 (32.2) |
Cross-clamp duration (minutes), mean (SD)d | 78.6 (22.5) | 81.2 (25.1) |
Myocardial protection,e n/N (%) | ||
Blood | 98/103 (95) | 98/98 (100) |
Crystalloid | 5/103 (5) | 0/98 (0) |
Coated circuit,f n/N (%) | 11/104 (11) | 6/98 (6) |
Oxygenator,f n/N (%) | ||
D903 (Sorin, Gloucester, UK) | 2/104 (2) | 0/98 (0) |
EOS (Sorin) | 7/104 (7) | 3/98 (3) |
Quadrox (Maquet, Sunderland, UK) | 86/104 (83) | 87/98 (89) |
Other | 9/104 (9) | 8/98 (8) |
Prime volumes in bypass circuit (ml) | ||
Crystalloid, median (IQR)f | 1000.0 (1000.0–1000.0) | 1000.0 (1000.0–1000.0) |
Colloid, median (IQR)f | 500.0 (500.0–500.0) | 500.0 (500.0–500.0) |
Mannitol, median (IQR)g | 200.0 (150.0–223.5) | 198.0 (150.0–240.0) |
Blood used,g,h n/N (%) | 3/104 (3) | 0/97 (0) |
Valve surgery, n/N (%) | ||
Aortic valve replacement/repair | 76/106 (72) | 57/98 (58) |
Mitral valve replacement/repair | 30/106 (28) | 39/98 (40) |
Tricuspid valve replacement/repair | 3/106 (3) | 4/98 (4) |
Pulmonary valve replacement/repairi | 0/103 (0) | 3/94 (3) |
Primary outcome
The neurocognitive outcomes are shown in Table 73. As might be expected, participants’ attention deteriorated after surgery (average score at 5 days was higher than at baseline) but improved thereafter. In both groups, the average attention scores were lower at 3 months than at recruitment. The difference between the groups was small (average 1% lower in the patient-specific algorithm group; GMR 0.99, 95% CI 0.93 to 1.07) and this was unchanged when adjusting for medication, anxiety and depression and overall health status during follow-up (see Tables 125–129, Appendix 3, for medication and mental health data). A similar pattern was observed for verbal memory, visuospatial skills, psychometer speed and visual motor co-ordination, which all declined after surgery and then improved by 3 months to levels that were similar to or better than those observed at baseline. These measures were also all similar between the two groups when adjusting for baseline medication and mental health and when accounting for medication and mental health during follow-up (see Table 73).
Assessment | Time point | Randomised to generic algorithm (N = 106) | Randomised to patient-specific algorithm (N = 98) | Adjusted for baseline covariates | Adjusted for time-specific covariates | ||
---|---|---|---|---|---|---|---|
Effect (95% CI) | p-value | Effect (95% CI) | p-value | ||||
1. Attention: Trail Making Test (lower score is better) | |||||||
Trail B completion (seconds), median (IQR) | Baselinea | 98.5 (74.0–140.0) | 86.0 (62.0–111.0) | ||||
5 days postoperativelyb | 104.5 (80.0–159.0) | 102.0 (76.0–142.0) | |||||
3 months postoperativelyc | 87.0 (64.0–126.0) | 76.0 (60.0–98.0) | |||||
Treatment*time interaction | 0.329 | 0.166 | |||||
Overall | GMR 0.99 (0.93 to 1.07) | 0.882 | GMR 0.99 (0.91 to 1.06) | 0.709 | |||
2. Verbal memory: RAVLT (higher score is better) | |||||||
Trial VI, having adjusted for age, mean (SD) | Baselined | 90.8 (17.5) | 96.2 (17.0) | ||||
5 days postoperativelye | 80.9 (16.0) | 81.2 (14.9) | |||||
3 months postoperativelyf | 92.6 (17.1) | 93.9 (14.8) | |||||
Treatment*time interaction | 0.534 | 0.832 | |||||
Overall | MD –1.75 (–5.22 to 1.72) | 0.326 | MD –0.96 (–4.65 to 2.74) | 0.614 | |||
3. Visuospatial skills: WAIS III (Wechsler) (higher score is better) | |||||||
Block design, having adjusted for age, mean (SD) | Baselineg | 11.3 (3.6) | 11.2 (2.6) | ||||
5 days postoperativelyh | 10.9 (3.6) | 10.5 (2.8) | |||||
3 months postoperativelyf | 12.0 (3.5) | 11.6 (3.0) | |||||
Treatment*time interaction | 0.815 | 0.961 | |||||
Overall | MD –0.25 (–0.77 to 0.28) | 0.364 | MD –0.001 (–0.57 to 0.57) | 0.153 | |||
4. Psychomotor speed: WAIS III (Wechsler) (higher score is better) | |||||||
Digital symbol coding, having adjusted for age, mean (SD) | Baselinei | 9.3 (2.6) | 9.3 (2.6) | ||||
5 days postoperativelyj | 8.1 (2.6) | 8.4 (2.5) | |||||
3 months postoperativelyk | 10.4 (2.8) | 10.4 (2.7) | |||||
Treatment*time interaction | 0.624 | 0.961 | |||||
Overall | MD 0.08 (–0.37 to 0.52) | 0.733 | MD 0.33 (–0.12 to 0.79) | 0.153 | |||
5. Executive function/verbal fluency: multilingual aphasia (COWAT) (higher score is better) | |||||||
Word score, having adjusted for age and years of education, mean (SD) | Baselinel | 42.2 (12.3) | 44.2 (11.2) | ||||
5 days postoperativelym | 37.1 (12.9) | 42.6 (13.7) | |||||
3 months postoperativelyn | 41.6 (10.5) | 45.6 (14.1) | |||||
Treatment*time interaction | 0.428 | 0.141 | |||||
Overall | MD 2.72 (0.64 to 4.80) | 0.01 | MD 3.73 (1.50 to 5.96) | 0.0011 | |||
6. Visual motor co-ordination: Grooved Pegboard Test (lower score is better) | |||||||
Pegboard completion (seconds), median (IQR) | Baselineo | 83.0 (71.0–104.0) | 77.0 (69.0–102.0) | ||||
5 days postoperativelye | 97.0 (77.0–120.0) | 94.0 (78.0–123.0) | |||||
3 months postoperativelyp | 83.5 (72.0–100.0) | 80.0 (70.0–97.0) | |||||
Treatment*time interaction | 0.412 | 0.608 | |||||
Overall | GMR 1.01 (0.97 to 1.07) | 0.533 | GMR 0.99 (0.94 to 1.04) | 0.736 |
The one measure of cognitive function to show a difference between the groups was executive function/verbal fluency, which was significantly better for participants allocated to the patient-specific algorithm group (MD 2.72, 95% CI 0.64 to 4.80), with the difference increasing when adjusting for medication and mental health, both at baseline and during follow-up (MD 3.73, 95% CI 1.50 to 5.96). Although this difference was statistically significant (p ≤ 0.01), it was the only measure to show a difference. We prespecified in the statistical analysis plan that ‘when interpreting the primary outcome results against the study hypotheses, if either all six dimensions or four out of six are statistically significant at the 5% level we would suggest this gives evidence to support the study hypotheses. Any other combinations will be considered insufficient evidence for the study hypotheses’. On this basis we must infer that cognitive outcome is similar for the two strategies.
Secondary outcomes
The results for the secondary outcomes (excluding biomarkers and quality of life outcomes) are shown in Table 74.
Outcome | Randomised to generic algorithm (N = 106) | Randomised to patient-specific algorithm (N = 98) | Overall (N = 204) | Effect (95% CI) | p-value |
---|---|---|---|---|---|
Transfusions, n/N (%) | |||||
Total red cell units transfused | |||||
0 | 62/106 (58) | 61/98 (62) | 123/204 (60) | ||
1 | 16/106 (15) | 11/98 (11) | 27/204 (13) | ||
2 | 10/106 (9) | 11/98 (11) | 21/204 (10) | ||
3 | 8/106 (8) | 9/98 (9) | 17/204 (8) | ||
> 3 | 10/106 (9) | 6/98 (6) | 16/204 (8) | ||
Any red cell units | 44/106 (42) | 37/98 (38) | 81/204 (40) | RR 0.88 (0.63 to 1.23) | 0.47 |
FFP | 17/106 (16) | 14/98 (14) | 31/204 (15) | ||
Platelets | 16/106 (15) | 20/98 (20) | 36/204 (18) | ||
Cryoprecipitate | 5/106 (5) | 4/98 (4) | 9/204 (4) | ||
rFVIIa | 0/106 (0) | 0/98 (0) | 0/204 (0) | ||
Cerebral oxygenation during the operative period (%), mean (SD) | |||||
Minimum cerebral oxygenation | 33.8 (8.9) | 34.1 (10.4) | 34.0 (9.6) | ||
AUCb | 75.7 (8.6) | 77.0 (8.8) | 76.3 (8.7) | MD 0.65 (–1.49 to 2.79) | 0.544 |
Oxygen delivery during CPB (ml/minute), mean (SD) | |||||
Start of CPBc | 678.5 (147.1) | 704.2 (169.4) | 690.6 (158.1) | ||
20 minutes after the start of CPBd | 676.4 (146.8) | 706.9 (149.2) | 691.0 (148.4) | ||
40 minutes after the start of CPBe | 686.2 (150.0) | 713.0 (152.3) | 699.0 (151.3) | ||
Pre-warmf | 681.6 (151.1) | 716.6 (157.6) | 698.0 (154.8) | ||
Pre-weang | 703.8 (145.8) | 723.8 (150.6) | 713.5 (148.1) | ||
Time*treatment interaction | 0.715 | ||||
Overall treatment effect | MD 6.13 (–11.84 to 24.10) | 0.495 | |||
Oxygen utilisation during CPB (ml/minute), mean (SD) | |||||
Start of CPBh | 173.2 (56.8) | 181.8 (62.2) | 177.2 (59.4) | ||
20 minutes after the start of CPBi | 158.8 (44.9) | 167.0 (45.0) | 162.7 (45.0) | ||
40 minutes after the start of CPB | 156.9 (49.4) | 166.5 (51.4) | 161.4 (50.4) | ||
Pre-warmj | 164.9 (48.5) | 176.8 (53.1) | 170.5 (50.9) | ||
Pre-weank | 210.6 (57.4) | 215.1 (59.1) | 212.8 (58.1) | ||
Time*treatment interaction | 0.844 | ||||
Overall treatment effect | MD 0.24 (–7.51 to 7.99) | 0.950 | |||
LOS, median (IQR) | |||||
CICU LOS (hours)l | 89.9 (53.6–119.5) | 73.6 (50.9–118.0) | 79.3 (51.3–119.0) | HR 1.15 (0.87 to 1.52) | 0.32 |
Hospital LOS (days) | 8.0 (6.0–10.0) | 7.0 (6.0–10.0) | 7.0 (6.0–10.0) | HR 1.10 (0.83 to 1.45) | 0.51 |
Clinical outcomes, n/N (%) | |||||
Infectionm | 28/92 (30) | 22/86 (26) | 50/178 (28) | ||
Stroken | 0/89 (0) | 1/89 (1) | 1/178 (1) | ||
STEMIn | 2/89 (2) | 1/89 (1) | 3/178 (2) | ||
AKIo | 18/92 (20) | 14/91 (15) | 32/183 (17) | ||
Respiratory complicationsp | 11/91 (12) | 3/89 (3) | 14/180 (8) | ||
Any clinical outcomeq | 45/94 (48) | 30/88 (34) | 75/182 (41) | RR 0.73 (0.51 to 1.05) | 0.08 |
Death within 30 days of surgeryn | 2/89 (2) | 1/89 (1) | 3/178 (2) | HR 0.52 (0.05 to 5.77) | 0.60 |
Postoperative characteristics not listed as outcomes are provided in Table 130 (see Appendix 3).
Blood transfusions
In total, 44 (42%) participants in the generic algorithm group received a red cell transfusion, compared with 37 (38%) in the patient-specific algorithm group (RR 0.88, 95% CI 0.63 to 1.23). In most cases either 1 or 2 units of red cells were given (Figure 51). Use of FFP, platelets and cryoprecipitate was similar in both groups. No participants were given activated factor VII.
FIGURE 51.
Percentage of participants receiving a red cell transfusion by group: (a) generic algorithm group; and (b) patient-specific algorithm group.

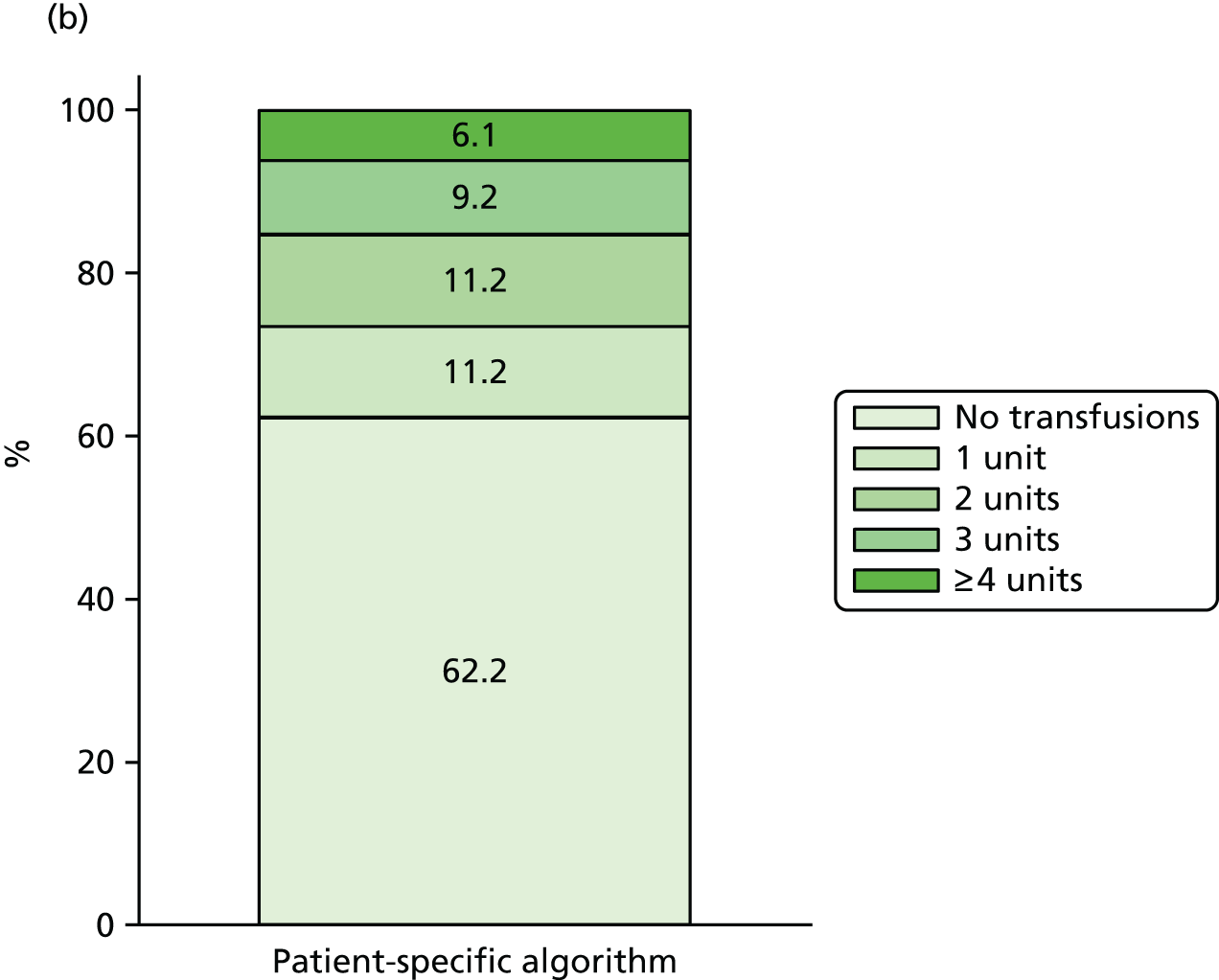
Cerebral oxygenation during the operative period
The minimum cerebral oxygen level was similar in the generic algorithm group (33.8%) and the patient-specific algorithm group (34.1%). The mean AUC was 75.7 (SD 8.6) in the generic algorithm group and 77.0 (SD 8.8) in the patient-specific algorithm group (MD after adjustment +0.65, 95% CI –1.49 to 2.79), suggesting higher oxygen levels in the patient-specific algorithm group. These data are summarised further in Figure 52.
FIGURE 52.
Cerebral oxygenation during the operative period (NIRS): (a) generic algorithm group; and (b) patient-specific algorithm group.

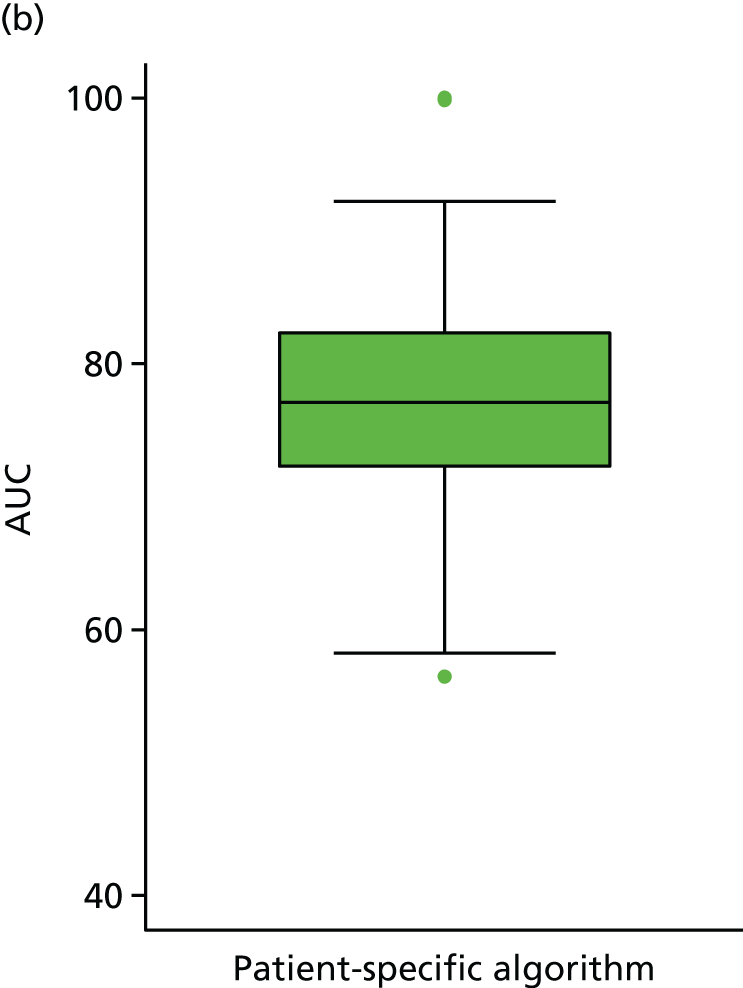
Oxygen delivery and utilisation during cardiopulmonary bypass
Oxygen delivery and utilisation were measured at five time points, from the start of CPB to weaning off CPB. At all five time points mean levels were slightly higher in the patient-specific algorithm group, but not significantly so. There was no evidence to suggest that the MD between the groups changed over time (p = 0.72 and p = 0.84 for oxygen delivery and utilisation respectively). Overall, oxygen delivery was on average 6.13 ml/minute (95% CI –11.84 to 24.10 ml/minute) higher in the patient-specific algorithm group, where oxygen utilisation was similar (p = 0.95). The profile of oxygen delivery and utilisation over time is shown is Figure 53.
FIGURE 53.
(a) Oxygen delivery and (b) oxygen utilisation during CPB. a, Generic algorithm group. b, Patient-specific algorithm group.
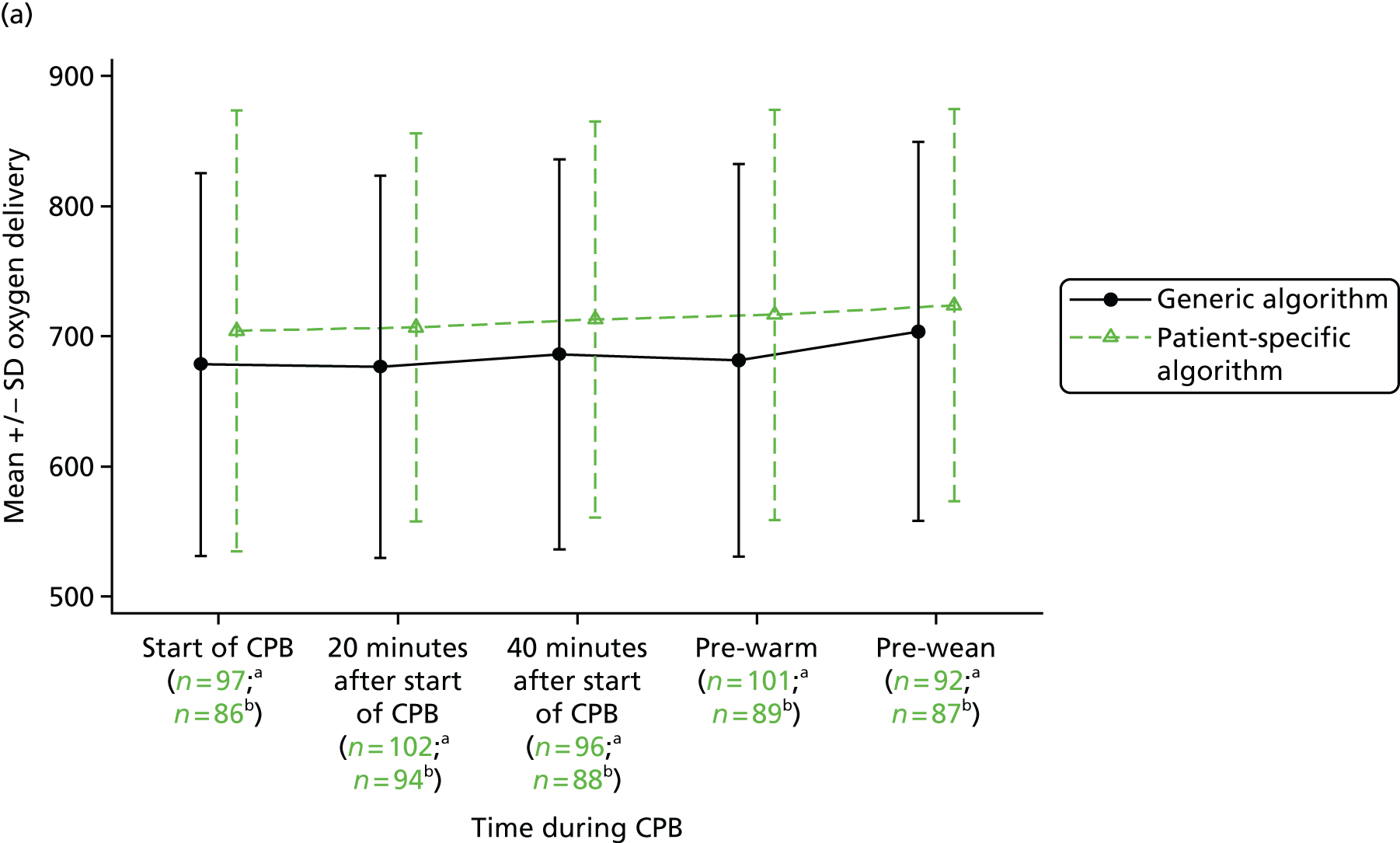
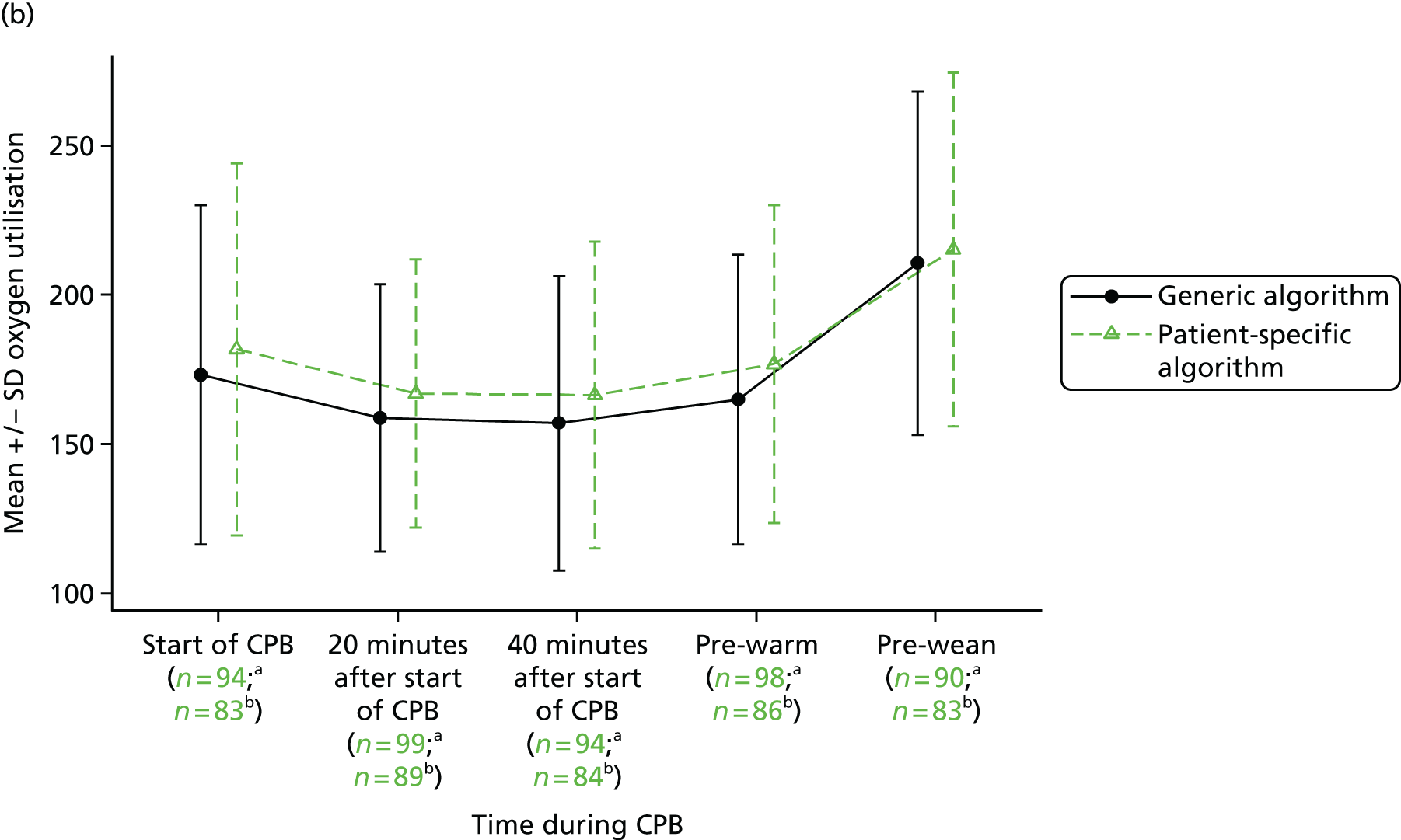
Clinical outcomes
Clinical outcomes included infection, stroke, STEMI, AKI and respiratory complications. Of these five outcomes, infection was the most frequent (30% in the generic algorithm group, 26% in the patient-specific algorithm group), followed by AKI (20% in the generic algorithm group, 15% in the patient-specific algorithm group) and respiratory complications (12% in the generic algorithm group, 3% in the patient-specific algorithm group). Overall, 48% of participants in the generic algorithm group had at least one of these complications compared with 34% in the patient-specific algorithm group (RR 0.73, 95% CI 0.51 to 1.05; p = 0.08). There were three deaths within 30 days of the surgery: two in the generic algorithm group and one in the patient-specific algorithm group.
Length of cardiac intensive care unit and hospital stay
On average, participants in the generic algorithm group spent 90 hours in the CICU whereas those in the patient-specific algorithm group spent 79 hours in the CICU. After adjustment, the ‘risk’ of discharge from the CICU was 15% greater for the patient-specific algorithm group (HR 1.15, 95% CI 0.87 to 1.52; p = 0.32). A similar difference was observed for the total post-surgery hospital stay (median 8 days vs. 7 days), but again the difference was not statistically significant (HR 1.10, 95% CI 0.83 to 1.45; p = 0.51). Time to discharge from the CICU and from hospital is shown in Figure 54.
FIGURE 54.
Time to discharge post surgery: (a) discharge from the CICU; and (b) discharge from hospital. Note that one participant randomised to the generic algorithm group had a CICU LOS of 299 hours and one participant randomised to the patient-specific algorithm had a CICU LOS of 812 hours. Three participants randomised to the generic algorithm group had hospital lengths of stay of 23, 25 and 35 days and one participant randomised to the patient-specific algorithm group had a hospital LOS of 34 days. These patients were omitted from the graphs.
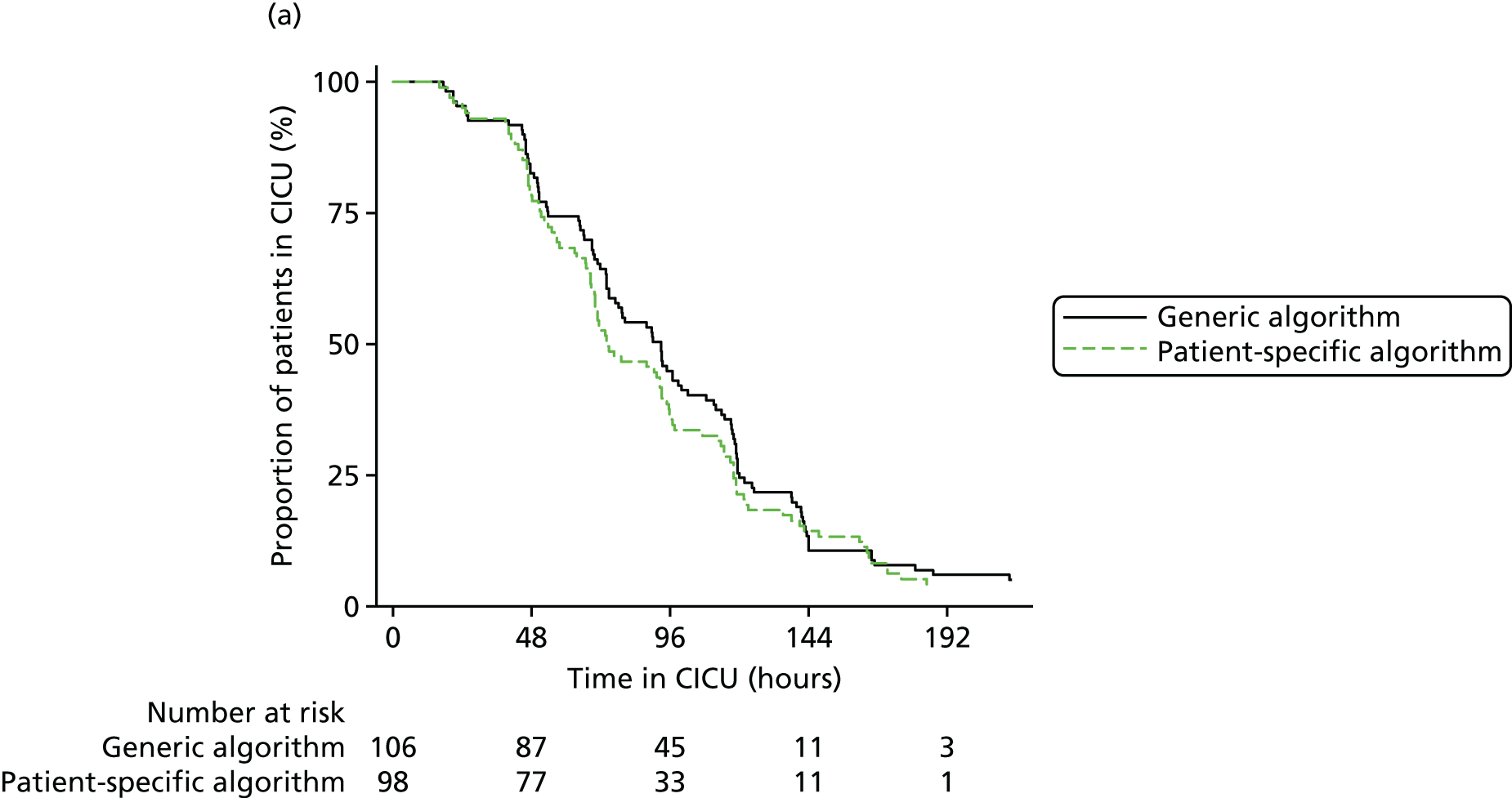

Quality of life
Quality of life, measured using the EQ-5D, is summarised in Table 75. In both groups the summary index scores declined after surgery (suggesting lower quality of life at 6 weeks) but at 3 months the median scores had increased to levels similar to those observed at baseline. In contrast, the median visual analogue scale scores were higher at both 6 weeks and 3 months than at baseline. The odds of having less than perfect health after surgery (summary index score of < 1) was higher for the patient-specific algorithm group, but not significantly so (OR 1.75, 95% CI 0.88 to 3.50; p = 0.11). Category responses to the EQ-5D questionnaire are provided in Table 131 (see Appendix 3).
Outcomes | Randomised to generic algorithm (N = 106), median (IQR) | Randomised to patient-specific algorithm (N = 98), median (IQR) | Effect (95% CI) | p-value |
---|---|---|---|---|
EQ-5D single summary index | ||||
Preoperativelya | 0.80 (0.66–1.00) | 0.85 (0.69–1.00) | ||
6 weeks postoperativelyb | 0.76 (0.69–0.88) | 0.76 (0.69–0.85) | ||
3 months postoperativelyc | 0.88 (0.76–1.00) | 0.80 (0.73–1.00) | ||
Time*treatment interaction | 0.594 | |||
Overall treatment effect | OR 1.75 (0.88 to 3.50) | 0.106 | ||
EQ-5D visual analogue scale | ||||
Preoperativelyd | 68.0 (50.0–80.0) | 70.0 (51.0–80.0) | ||
6 weeks postoperativelye | 73.0 (62.5–85.0) | 75.0 (65.0–83.5) | ||
3 months postoperativelyf | 80.0 (70.0–90.0) | 80.0 (70.0–90.0) | ||
Time*treatment interaction | 0.616 | |||
Overall treatment effect | GMR –0.06 (–3.70 to 3.59) | 0.976 |
Biomarkers of organ injury
Serum S100
The biomarker S100 was measured in 98 study participants, 52 in the generic algorithm group and 46 in the patient-specific algorithm group. The results for this biomarker are summarised in Figure 55a and Table 76. The difference in S100 concentration between the groups was similar at all postoperative time points (p = 0.83). On average, the S100 concentration was 6% higher in the patient-specific algorithm group than in the generic algorithm group (GMR 1.06, 95% CI 0.95 to 1.19; p = 0.29).
FIGURE 55.
Serum biomarkers of inflammation and organ injury: (a) S100 concentration over time; (b) IL-6 concentration over time; (c) IL-8 concentration over time; and (d) serum creatinine concentration over time. a, Generic algorithm group. b, Patient-specific algorithm group.


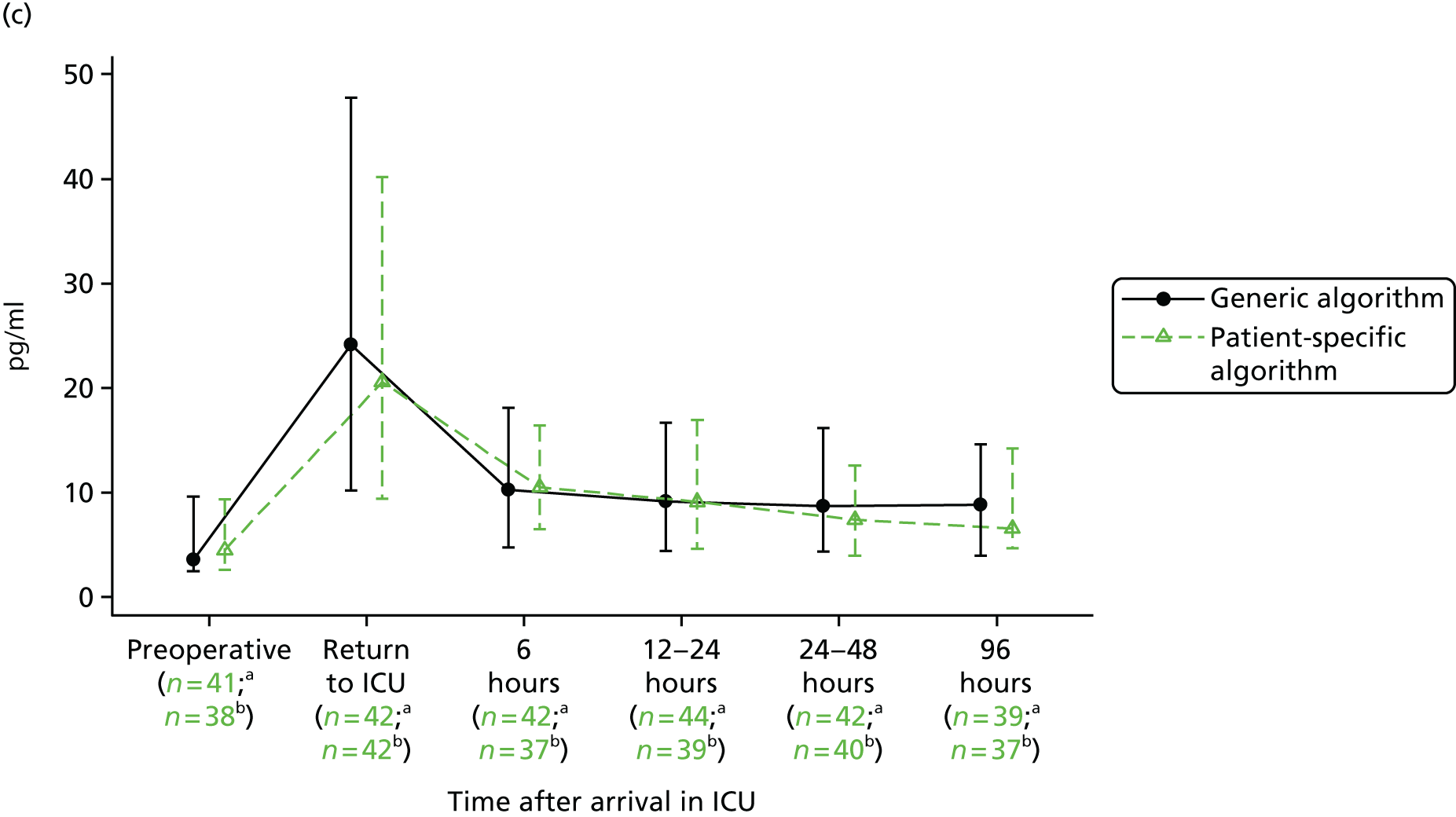

Serum biomarker | Randomised to generic algorithm (N = 106) | Randomised to patient-specific algorithm (N = 98) | Overall (N = 204) | Effect (95% CI) | p-value |
---|---|---|---|---|---|
S100 level (ng/ml) | |||||
Subgroup analysis, n/N (%) | 52/106 (49) | 46/98 (47) | 98/204 (48) | ||
Preoperatively,a median (IQR) | 27.7 (21.5–37.1) | 24.1 (20.8–32.4) | 27.0 (21.5–35.1) | ||
Return from ICU,b median (IQR) | 139.0 (104.8–203.1) | 137.9 (86.2–221.9) | 139.0 (94.4–211.9) | ||
6 hours,c median (IQR) | 54.5 (46.1–72.7) | 57.2 (39.6–81.2) | 55.3 (45.1–80.5) | ||
12–24 hours,d median (IQR) | 49.7 (41.7–60.8) | 50.2 (37.6–70.4) | 49.8 (39.2–63.1) | ||
24–48 hours,e median (IQR) | 44.0 (38.0–53.9) | 45.8 (38.0–54.9) | 44.2 (38.0–54.9) | ||
96 hours,f median (IQR) | 34.8 (27.8–41.1) | 33.3 (26.4–38.9) | 34.1 (26.8–40.1) | ||
Time*treatment interaction | 0.831 | ||||
Overall treatment effect | GMR 1.06 (0.95 to 1.19) | 0.288 | |||
IL-6 level (pg/ml) | |||||
Subgroup analysis, n/N (%) | 43/106 (41) | 43/98 (44) | 86/204 (42) | ||
Preoperatively,g median (IQR) | 14.7 (1.4–16.5) | 5.7 (1.3–14.9) | 13.6 (1.3–16.1) | ||
Return from ICU,h median (IQR) | 207.0 (64.0–411.2) | 165.4 (93.6–331.5) | 181.1 (84.7–370.6) | ||
6 hours,i median (IQR) | 164.4 (94.4–313.9) | 148.8 (64.2–208.4) | 157.0 (70.5–261.1) | ||
12–24 hours,j median (IQR) | 171.6 (94.7–233.6) | 125.8 (64.2–238.7) | 143.7 (85.7–234.4) | ||
24–48 hours,k median (IQR) | 194.8 (130.3–298.7) | 171.5 (97.2–267.6) | 172.9 (106.4–282.1) | ||
96 hours,l median (IQR) | 38.4 (17.2–51.4) | 33.4 (12.8–45.7) | 35.7 (12.8–49.1) | ||
Time*treatment interaction | 0.868 | ||||
Overall treatment effect | GMR 0.90 (0.66 to 1.21) | 0.444 | |||
IL-8 level (pg/ml) | |||||
Subgroup analysis, n/N (%) | 56/106 (53) | 53/98 (54) | 109/204 (53) | ||
Preoperatively,m median (IQR) | 3.6 (2.5–9.6) | 4.3 (2.7–9.4) | 4.1 (2.7–9.4) | ||
Return from ICU,n median (IQR) | 22.4 (10.7–45.8) | 21.9 (9.7–41.5) | 22.2 (10.1–42.3) | ||
6 hours,o median (IQR) | 10.5 (4.7–18.1) | 10.5 (6.5–16.4) | 10.5 (5.6–17.8) | ||
12–24 hours,p median (IQR) | 9.1 (4.3–16.9) | 9.1 (4.6–15.5) | 9.1 (4.5–16.9) | ||
24–48 hours,q median (IQR) | 8.7 (4.3–16.1) | 6.9 (3.9–12.4) | 7.4 (4.1–14.5) | ||
96 hours,r median (IQR) | 9.0 (4.1–14.3) | 6.4 (4.4–14.5) | 8.0 (4.1–14.4) | ||
Time*treatment interaction | 0.293 | ||||
Overall treatment effect | GMR 0.99 (0.79 to 1.23) | 0.902 | |||
Troponin T level (heart) (ng/ml)s | |||||
Subgroup analysis, n/N | 91/106 (86) | 98/106 (92) | 189/204 (93) | ||
Preoperatively,t median (IQR) | 14.0 (14.0–16.0) | 14.0 (14.0–17.0) | 14.0 (14.0–16.0) | ||
Return from ICU,u median (IQR) | 476.0 (291.0–676.0) | 423.0 (310.0–665.0) | 427.0 (300.5–670.5) | ||
12–24 hours,v median (IQR) | 448.0 (325.0–615.0) | 469.5 (334.0–678.0) | 457.0 (334.0–633.0) | ||
24–48 hours,w median (IQR) | 333.5 (244.0–518.5) | 384.0 (271.0–515.0) | 356.0 (258.0–515.0) | ||
Time*treatment interaction | 0.571 | ||||
Overall treatment effect | GMR 1.03 (0.89 to 1.20) | 0.685 | |||
Serum creatinine level (µmol/l) | |||||
Preoperatively, median (IQR) | 88.0 (76.0–105.0) | 87.5 (77.0–103.0) | 88.0 (76.5–104.0) | ||
6 hours,π median (IQR) | 87.0 (74.0–97.0) | 86.0 (71.0–96.0) | 86.0 (72.0–96.0) | ||
12 hours, median (IQR) | 92.5 (76.0–115.0) | 89.0 (79.0–104.0) | 91.0 (77.0–106.5) | ||
24–48 hours, median (IQR) | 95.5 (76.0–122.0) | 90.0 (75.0–114.0) | 92.5 (75.0–116.5) | ||
96 hours,ππ median (IQR) | 83.0 (68.0–102.0) | 81.0 (68.0–100.0) | 83.0 (68.0–101.0) | ||
Time*treatment interaction | 0.253 | ||||
Overall treatment effect | GMR 0.99 (0.96 to 1.03) | 0.189 | |||
Creatinine clearance (ml/minute) | |||||
Preoperatively,θ median (IQR) | 145.5 (106.8–192.3) | 177.4 (100.7–237.3) | 154.2 (105.2–222.3) | ||
24–48 hours,θθ median (IQR) | 132.4 (82.4–224.4) | 177.0 (112.1–249.4) | 149.9 (92.2–237.9) | ||
Overall treatment effect | GMR 1.14 (0.94 to 1.40) | 0.189 |
Serum interleukin-6
The biomarker IL-6 was measured in 86 study participants, 43 in each group. The results for this biomarker are summarised in Figure 55b and Table 76. The difference in IL-6 concentration between the groups was similar at all postoperative time points (p = 0.87). On average, the IL-6 concentration was 10% lower in the patient-specific algorithm group than in the generic algorithm group (GMR 0.90, 95% CI 0.66 to 1.21; p = 0.44).
Serum interleukin-8
The biomarker IL-8 was measured in 109 study participants, 56 in the generic algorithm group and 53 in the patient-specific algorithm group. The results for this biomarker are summarised in Figure 55c and Table 76. The difference in IL-8 concentration between the groups was similar at all postoperative time points (p = 0.29). On average, the IL-8 concentration was just 1% lower in the patient-specific algorithm group than in the generic algorithm group (GMR 0.99, 95% CI 0.79 to 1.23; p = 0.90).
Serum troponin T
During the trial, troponin I assays were replaced by highly sensitive troponin T assays as measures of myocardial injury. The biomarker troponin T was measured in 176 study participants, 91 in the generic algorithm group and 85 in the patient-specific algorithm group. Troponin I was measured in the remaining 28 participants (data not shown). The troponin T data are summarised in Table 76. The troponin T concentration was similar between the patient-specific algorithm group and the generic algorithm group (GMR 1.03, 95% CI 0.89 to 1.20; p = 0.69) and did not differ across the postoperative period (test for treatment*time interaction p = 0.57).
Serum creatinine
The serum creatinine results are summarised in Figure 55d and Table 76. Serum creatinine concentration in the first 96 hours after surgery was similar between the patient-specific algorithm group and the generic algorithm group (GMR 0.99, 95% CI 0.96 to 1.03; p = 0.19) and did not differ across the postoperative period (test for treatment*time interaction p = 0.25).
Calculated creatinine clearance
Creatinine clearance was measured at baseline and once postoperatively (see Table 76). Creatinine clearance was, on average, 14% higher in the patient-specific algorithm group than in the generic algorithm group, but this difference was not significant (GMR 1.14, 95% CI 0.94 to 1.40; p = 0.19).
Urinary neutrophil gelatinase-associated lipocalin: renal inflammation and haem metabolism
The biomarker NGAL was measured in 170 participants, 88 in the generic algorithm group and 82 in the patient-specific algorithm group. The results for this biomarker are summarised in Figure 56a and Table 77. In contrast to the biomarkers described above, the difference in NGAL concentration between the groups varied across the postoperative time points (p = 0.0129). Twelve hours after surgery, the NGAL concentration was significantly lower in the patient-specific algorithm group (GMR 0.69, 95% CI 0.51 to 0.93; p = 0.0135), whereas at the other postoperative times the concentrations were similar.
FIGURE 56.
Urinary biomarkers of AKI: (a) NGAL concentration over time; (b) LFABP concentration over time; (c) IL-18 concentration over time; (d) KIM-1 concentration over time; and (e) urinary microalbumin concentration over time. a, Generic algorithm group. b, Patient-specific algorithm group.



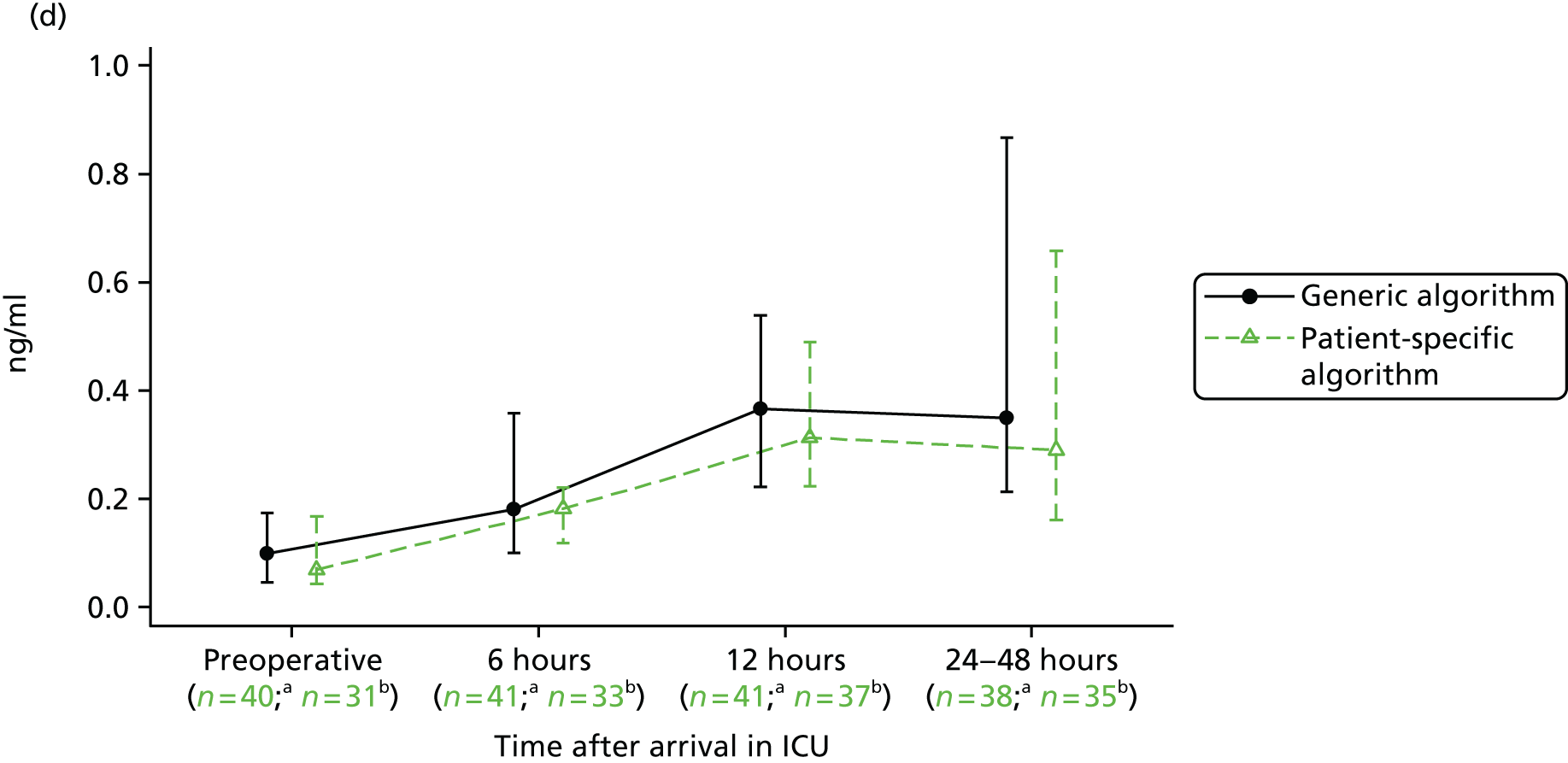

Urine biomarker | Randomised to generic algorithm (N = 106) | Randomised to patient-specific algorithm (N = 98) | Overall (N = 204) | Effect (95% CI) | p-value |
---|---|---|---|---|---|
NGAL level (ng/ml) | |||||
Subgroup analysis, n/N (%) | 88/106 (83) | 82/98 (84) | 170/204 (83) | ||
Preoperatively,a median (IQR) | 18.8 (11.4–37.3) | 20.0 (13.5–35.2) | 19.5 (12.1–35.2) | ||
6 hours,b median (IQR) | 204.4 (118.0–411.8) | 194.0 (103.8–344.9) | 199.9 (106.0–385.8) | GMR 0.89 (0.67 to 1.19) | 0.424 |
12 hours,c median (IQR) | 151.4 (68.7–254.9) | 93.8 (48.4–207.8) | 114.3 (56.2–242.1) | GMR 0.69 (0.51 to 0.93) | 0.0135 |
24–48 hours,d median (IQR) | 58.7 (37.5–109.2) | 68.4 (36.2–113.8) | 61.5 (37.3–113.4) | GMR 1.01 (0.78 to 1.30) | 0.949 |
Time*treatment interaction | 0.0129 | ||||
LFABP level (ng/ml) | |||||
Subgroup analysis, n/N (%) | 56/106 (53) | 52/98 (53) | 108/204 (53) | ||
Preoperatively,i median (IQR) | 3.4 (1.7–5.5) | 4.9 (2.1–10.9) | 3.5 (1.8–7.5) | ||
6 hours,j median (IQR) | 27.3 (14.4–45.7) | 25.7 (16.0–50.3) | 26.4 (15.6–45.7) | ||
12 hours,k median (IQR) | 23.9 (14.8–37.3) | 20.0 (13.0–34.4) | 22.3 (13.6–36.6) | ||
24–48 hours,a median (IQR) | 15.6 (8.3–24.0) | 10.7 (6.5–32.2) | 14.1 (7.9–29.0) | ||
Time*treatment interaction | 0.536 | ||||
Overall treatment effect | GMR 0.85 (0.62 to 1.17) | 0.315 | |||
IL-18 level (pg/ml) | |||||
Subgroup analysis, n/N (%) | 55/106 (52) | 52/98 (53) | 107/204 (52) | ||
Preoperatively,e median (IQR) | 43.3 (29.9–61.7) | 46.5 (21.0–54.0) | 44.9 (28.4–59.8) | ||
6 hours,f median (IQR) | 71.1 (45.6–98.7) | 76.4 (46.8–115.3) | 72.6 (46.2–107.4) | ||
12 hours,g median (IQR) | 85.3 (53.3–124.1) | 108.0 (55.9–174.9) | 95.9 (55.5–147.2) | ||
24–48 hours,h median (IQR) | 62.2 (46.6–95.5) | 64.1 (42.7–195.3) | 63.7 (43.2–128.0) | ||
Time*treatment interaction | 0.914 | ||||
Overall treatment effect | GMR 1.22 (1.01 to 1.47) | 0.033 | |||
KIM-1 level (ng/ml) | |||||
Subgroup analysis, n/N (%) | 45/106 (42) | 43/98 (44) | 88/204 (43) | ||
Preoperatively,l median (IQR) | 0.1 (0.0–0.2) | 0.1 (0.0–0.2) | 0.1 (0.0–0.2) | ||
6 hours,m median (IQR) | 0.2 (0.1–0.4) | 0.2 (0.1–0.2) | 0.2 (0.1–0.3) | ||
12 hours,n median (IQR) | 0.4 (0.2–0.5) | 0.3 (0.2–0.5) | 0.3 (0.2–0.5) | ||
24–48 hours,o median (IQR) | 0.3 (0.2–1.0) | 0.3 (0.2–0.7) | 0.3 (0.2–0.8) | ||
Time*treatment interaction | 0.264 | ||||
Overall treatment effect | GMR 1.05 (0.79 to 1.38) | 0.744 | |||
Urinary microalbumin level (g/l) | |||||
Subgroup analysis, n/N | 106/106 | 98/98 | 204/204 | ||
Preoperatively,p mean (SD) | 40.2 (3.3) | 40.7 (3.7) | 40.4 (3.5) | ||
6 hours,q mean (SD) | 23.7 (4.1) | 23.8 (4.3) | 23.8 (4.2) | ||
12 hours, mean (SD) | 26.9 (3.7) | 27.1 (4.0) | 27.0 (3.8) | ||
24–48 hours,r mean (SD) | 26.9 (2.8) | 26.8 (3.5) | 26.8 (3.1) | ||
96 hours,s mean (SD) | 26.8 (3.3) | 27.2 (3.6) | 27.0 (3.5) | ||
Time*treatment interaction | 0.253 | ||||
Overall treatment effect | MD –0.04 (–0.77 to 0.70) | 0.911 |
Urinary liver-type fatty acid-binding protein: renal inflammation and haem metabolism
The biomarker LFABP was measured in 108 study participants: 56 in the generic algorithm group and 52 in the patient-specific algorithm group. The results for this biomarker are summarised in Figure 56b and Table 77. The difference in LFABP concentration between the groups was similar at all postoperative time points (p = 0.54). On average, the LFABP concentration was 15% lower in the patient-specific algorithm group than in the generic algorithm group (GMR 0.85, 95% CI 0.62 to 1.17; p = 0.32).
Urinary interleukin-18: renal tubular inflammation
The biomarker IL-18 was measured in 107 study participants: 55 in the generic algorithm group and 52 in the patient-specific algorithm group. The results for this biomarker are summarised in Figure 56c and Table 77. The difference in IL-18 concentration between the groups was similar at all postoperative time points (p = 0.91). On average, the IL-18 concentration was 22% higher in the patient-specific algorithm group than in the generic algorithm group (GMR 1.22, 95% CI 1.01 to 1.47; p = 0.033).
Urinary kidney injury molecule-1: renal tubular epithelial mesenchymal transition
The biomarker KIM-1 was measured in 88 study participants, 45 in the generic algorithm group and 43 in the patient-specific algorithm group. The results for this biomarker are summarised in Figure 56d and Table 77. The difference in KIM-1 concentration between the groups was similar at all postoperative time points (p = 0.26). On average, the KIM-1 concentration was 5% lower in the patient-specific algorithm group than in the generic algorithm group (GMR 1.05, 95% CI 0.79 to 1.38; p = 0.744).
Urinary microalbumin
The urinary microalbumin results are summarised in Figure 56e and Table 77. The urinary microalbumin concentration in the first 96 hours after surgery was similar between the patient-specific algorithm group and the generic algorithm group (MD –0.04 g/l, 95% CI –0.77 to 0.70 g/l; p = 0.911) and did not differ across the postoperative period (test for treatment*time interaction p = 0.25).
Postoperative complications
In addition to the secondary clinical outcomes, data were collected on all postoperative complications that might be expected after cardiac surgery and any unexpected complications that met the definition of ‘serious’. Details of the complications experienced by study participants in the period from recruitment to 3 months are provided in Table 78. For completeness, all adverse events are reported, including those that formed part of the secondary outcomes. Most complications were rare and occurred with similar frequency in the two groups. There were 15 unexpected serious adverse events in the generic algorithm group and 10 in the patient-specific algorithm group.
Adverse event | Randomised to generic algorithm (N = 106) | Randomised to patient-specific algorithm (N = 98) | ||||||
---|---|---|---|---|---|---|---|---|
Adverse events | Serious adverse events | Adverse events | Serious adverse events | |||||
Events/participants | % | Events/participants | % | Events/participants | % | Events/participants | % | |
Expected events (i.e. listed in the study protocol) | ||||||||
STEMIa | 2/2 | 2 | 1/1 | 1 | 1/1 | 1 | 0/0 | 0 |
Cardiac arresta | 3/3 | 3 | 3/3 | 3 | 0/0 | 0 | 0/0 | 0 |
SVT/AFb | 49/48 | 45 | 8/8 | 8 | 43/43 | 44 | 6/6 | 6 |
VF/VTa | 2/2 | 2 | 1/1 | 1 | 0/0 | 0 | 0/0 | 0 |
New pacinga | 50/50 | 47 | 10/10 | 9 | 37/37 | 38 | 8/8 | 8 |
Singlec | 27/27 | 25 | 5/5 | 5 | 20/20 | 20 | 4/4 | 4 |
Doubled | 22/22 | 21 | 0/0 | 0 | 16/16 | 16 | 0/0 | 0 |
Permanenta | 3/3 | 3 | 2/2 | 2 | 3/3 | 3 | 3/3 | 3 |
Use of inotropesa | 56/55 | 52 | 10/10 | 9 | 54/54 | 55 | 12/12 | 12 |
Use of IABPa | 0/0 | 0 | 0/0 | 0 | 1/1 | 1 | 1/1 | 1 |
Use of pulmonary artery cathetere | 1/1 | 1 | 0/0 | 0 | 2/2 | 2 | 0/0 | 0 |
Use of vasodilatore | 20/20 | 19 | 0/0 | 0 | 21/21 | 21 | 0/0 | 0 |
Low cardiac outputa | 5/5 | 5 | 0/0 | 0 | 3/3 | 3 | 1/1 | 1 |
Tracheostomya | 0/0 | 0 | 0/0 | 0 | 1/1 | 1 | 1/1 | 1 |
Mask CPAPa | 8/8 | 8 | 2/2 | 2 | 2/2 | 2 | 0/0 | 0 |
Pneumothorax or effusion requiring drainingf | 8/8 | 8 | 5/5 | 5 | 8/8 | 8 | 3/3 | 3 |
AKIa | 20/20 | 19 | 4/4 | 4 | 15/15 | 15 | 2/2 | 2 |
Stage 1 | 10/10 | 9 | 0/0 | 0 | 10/10 | 10 | 1/1 | 1 |
Stage 2 | 5/5 | 5 | 1/1 | 1 | 3/3 | 3 | 0/0 | 0 |
Stage 3 | 5/5 | 5 | 3/3 | 3 | 2/2 | 2 | 1/1 | 1 |
Haemofiltration/dialysis since heart operationa | 4/4 | 4 | 2/2 | 2 | 1/1 | 1 | 1/1 | 1 |
Peptic ulcer/GI bleed/perforationg | 1/1 | 1 | 0/0 | 0 | 3/3 | 3 | 1/1 | 1 |
Other GIa | 1/1 | 1 | 1/1 | 1 | 1/1 | 1 | 1/1 | 1 |
Permanent strokea | 1/1 | 1 | 1/1 | 1 | 2/2 | 2 | 2/2 | 2 |
TIAh | 0/0 | 0 | 0/0 | 0 | 1/1 | 1 | 0/0 | 0 |
Excessive bleeding not requiring reoperationa | 1/1 | 1 | 0/0 | 0 | 1/1 | 1 | 1/1 | 1 |
Wound dehiscencea | 2/2 | 2 | 2/2 | 2 | 1/1 | 1 | 1/1 | 1 |
Reoperationi | 4/4 | 4 | 1/1 | 1 | 6/6 | 6 | 0/0 | 0 |
Unexpected serious adverse events (i.e. not listed in the study protocol) | ||||||||
Cardiac failure | 2/2 | 2 | 1/1 | 1 | ||||
Cardiac pseudoaneurysm | 1/1 | 1 | 0/0 | 0 | ||||
Cardiac tamponade | 1/1 | 1 | 0/0 | 0 | ||||
Cardiomegaly | 1/1 | 1 | 0/0 | 0 | ||||
Cholelithiasis | 0/0 | 0 | 2/2 | 2 | ||||
Confused state | 2/2 | 2 | 0/0 | 0 | ||||
Convulsion | 2/2 | 2 | 1/1 | 1 | ||||
Duodenal ulcer haemorrhage | 0/0 | 0 | 2/2 | 2 | ||||
Electrocardiogram T-wave inversion | 1/1 | 1 | 0/0 | 0 | ||||
Endocarditis | 1/1 | 1 | 0/0 | 0 | ||||
Fluid retention | 1/1 | 1 | 0/0 | 0 | ||||
Gastritis | 0/0 | 0 | 1/1 | 1 | ||||
Hyponatraemia | 1/1 | 1 | 1/1 | 1 | ||||
Mental disorder | 1/1 | 1 | 0/0 | 0 | ||||
Multiorgan failure | 1/1 | 1 | 1/1 | 1 | ||||
Oedema | 0/0 | 0 | 1/1 | 1 | ||||
Panic attack | 0/0 | 0 | 1/1 | 1 | ||||
Pericardial effusion | 4/4 | 4 | 3/ 3 | 3 | ||||
Renal failure acute | 1/1 | 1 | 0/0 | 0 | ||||
Right ventricular failure | 1/1 | 1 | 0/0 | 0 |
Health economics
Aims and objectives
The economic evaluation part of the study aimed to estimate the incremental cost and the incremental cost-effectiveness of the patient-specific algorithm compared with the generic algorithm for optimising tissue oxygenation during CPB in adult cardiac surgery patients. In line with the main trial analysis, we paid particular attention to any resources associated with cognitive function issues and postoperative infectious complications.
Economic evaluation methods overview
A cost–utility analysis was conducted, with outcomes measured using the EQ-5D-3L (the PASPORT trial began before the introduction of the five-level version of the EQ-5D). The patient-specific algorithm was considered to be cost-effective if the ICER fell below £20,000 per QALY, which is generally considered as the threshold at which an intervention is considered cost-effective by NICE. 106 Good practice guidelines on the conduct of economic evaluations were followed for the economic evaluation. 101,102 The analysis is described in accordance with the CHEERS statement. 103 Table 79 summarises the methods used in the economic evaluation, with further details provided in the accompanying text.
Aspect of methodology | Strategy used in the base-case analysis |
---|---|
Form of economic evaluation | Cost–utility analysis for comparison of the patient-specific algorithm with the generic algorithm |
Perspective | NHS and Personal Social Services |
Time horizon | A within-trial analysis, taking a 3-month time horizon (up to the primary clinical time point) |
Population | All randomised participants were included, except those randomised in error and those who withdrew consent for their data to be used |
Costs included in the analysis | Surgery, blood products, blood-saving techniques (e.g. cell saver, tranexamic acid), LOS by ward type (including ICU and HDU), medications, reoperations, investigations and treatments relating to complications, such as the use of inotropes, serious adverse events such as SVT/AF requiring treatment, post-discharge costs [readmissions to hospital, other hospital accident and emergency and outpatient visits (e.g. warfarin clinic) and community health and social care contacts] |
Utility measurement (primary economic outcome) | EQ-5D-3L (administered preoperatively and at 6 weeks and 3 months postoperatively) |
QALY calculations | Assumed that participants’ utility changes linearly between utility measurements |
Adjustment for baseline utility | Regression used to adjust QALY calculations for differences in baseline utility |
Missing data | Mean imputation and multiple imputation |
Form of analysis and primary outcome measure
The primary outcome measure for the economic evaluation was QALYs, as advocated by NICE. 106 This outcome combines quantity and quality of life into a single measure. The difference in mean costs between the two trial arms was divided by the difference in mean QALYs between the two groups to calculate an ICER and, specifically, the incremental cost per QALY gained by switching from a generic algorithm to a patient-specific algorithm.
Perspective and time horizon
The primary perspective of the evaluation was that of the UK NHS and personal social services, as recommended by NICE. 106 The perspective for outcomes included the patients undergoing treatment. A within-trial analysis, from the day of surgery, taking a 3-month time horizon was conducted. It was anticipated that all major resource use would occur within this time frame and would therefore be captured.
Resource use and costs
Resource use data were collected for all significant health service resource inputs for the trial participants up to the point of the 3-month follow-up. Aside from the cost of the intervention, data were collected from the trial case report forms on the type of initial cardiac surgery performed and any reoperations, blood products transfused, inpatient days by ward type, blood-saving techniques used, complications and serious adverse events and regular medications. At 3 months postoperatively, a bespoke resource use questionnaire was used to obtain estimates of health-care resources used since hospital discharge, for example readmissions to hospital and further contact with health professionals in primary or secondary care. The costs of unrelated care post discharge were excluded. For example, our analysis included the cost of outpatient visits for cardiology and gastroenterology, but excluded the cost of outpatient visits to a breast clinic. Costing decisions (such as resource use assumed for complications) were made without knowledge of the allocation of participants to trial groups.
The cost of the intervention was calculated to incorporate the cost of both the capital equipment and the sensors required for each participant. The price of the NIRS machine was converted into an equivalent annual cost to take account of depreciation and discounting, using a life span of 6 years for the machine and assuming a 3.5% discount rate. This figure was then divided by the number of patients estimated to be using the machine per annum [based on the number of cardiac surgery operations performed at the Glenfield Hospital in 2012/13 (n = 264) according to the Blue Book Online120 and assuming that a machine was used for 50% of operations and that there were two machines]. The cost of the intervention was estimated to be £218.08; the vast majority of this cost was accounted for by the sensors (£212.34) rather than the cost of the equipment (£5.75).
Unit costs used to value hospital and community health-care resource use were largely obtained from national sources, for example NHS Blood and Transplant price lists for blood products,224 the National Schedule of Reference Costs for ICU, HDU and cardiac ward costs, magnetic resonance imaging and computerised tomography scans and complications225,226 and Unit Costs of Health and Social Care for community costs. 118,227 The costs of drugs provided in hospital were taken from the Electronic Marketing Information Tool228 when possible, which provides the reduced prices paid for generic drugs in hospital. Drug costs not available from this source were taken from the British National Formulary (BNF). 229 When possible we drew on the unit costs that we had sourced in a previous economic evaluation for the TITRe2 trial. 230 Costs were inflated to 2013–14 prices using the Hospital and Community Health Services inflation index. 118 Details of all unit costs and their sources are provided in Tables 132–139 in Appendix 3.
Health-related quality of life and quality-adjusted life-years
Health-related quality of life was estimated using the EQ-5D-3L,222 administered to participants preoperatively and at 6 weeks and 3 months postoperatively. Respondents were assigned valuations derived from published UK population tariffs. 231 The number of QALYs accrued by each participant was calculated assuming that a participant’s utility changed linearly between each of the time points. Participants who died were allocated a value of zero for quality of life to represent death.
Statistical methods
Our base-case analysis included all participants randomised into the trial except those randomised in error and those who withdrew consent for their data to be used, which is consistent with the main clinical analyses. Analyses were performed on an intention-to-treat basis. Missing resource use and EQ-5D data were imputed by multiple imputation using a series of chained regression equations. 113 Twenty values were predicted for each missing data cell and a method called Rubin’s rule was used to summarise data across the 20 data sets. 114 When resource use data were partially missing, for example for linked questions in which only the first part was answered, mean imputation was used. For example, if a participant reported visiting his or her GP but not the number of visits, the mean number of GP visits from other participants was assigned. Overall, 5% of resource use data were completely missing and 13% of EQ-5D scores were missing across the three time points (pre surgery, 6 weeks and 3 months).
Given that baseline utility directly contributes to QALY calculations, it is important to control for any potential imbalances in baseline utility in the estimation of the MD in QALYs between treatment groups, to avoid introducing bias. 232 QALYs were therefore adjusted for baseline EQ-5D scores. Costs and effects were not discounted as the time horizon was < 12 months. The ICER was derived from the average costs and QALYs gained in each trial group, producing an incremental cost per QALY gained from implementing a patient-specific algorithm in place of a generic algorithm. Non-parametric bootstrapping of costs and QALYs was then used to quantify the degree of uncertainty around the ICER. In total, 1000 bootstrap samples were drawn for each of the 20 imputed data sets. 233
Presentation of results
The mean costs and QALYs gained in each trial arm (with SEs and 95% CIs) are presented as well as the ICER. Uncertainty around the ICER is represented graphically on the cost-effectiveness plane by the bootstrap replicates of the MDs in costs and QALYs between the groups. The bootstrap replicates are also used to express the results in terms of a cost-effectiveness acceptability curve, which indicates the likelihood that the patient-specific algorithm is cost-effective for different levels that health-care decision-makers are willing to pay for health gain. The patient-specific algorithm would be considered cost-effective if the ICER falls below £20,000 per QALY, the level below which NICE generally recommends interventions to the NHS; however, the ICERs and cost-effectiveness acceptability curves presented allow decision-makers to assess cost-effectiveness at a willingness-to-pay threshold of their choice.
Sensitivity analyses
Deterministic sensitivity analyses were used to investigate the impact on the results of varying key parameters or major cost drivers and also to investigate the impact of uncertainty on the cost-effectiveness results. Key unit costs that were varied included the cost of the intervention, ward costs and the costs of complications, as shown in Table 80. The costs associated with any outliers in the trial were also investigated (e.g. any participants with an especially long length of hospital stay).
SA | Resource/complication | Unit costs used in the base-case analysis (£) | Alternative strategy for sensitivity analysis |
---|---|---|---|
1 | NIRS | 218.08 | ± 25% |
2 | Bed-days in first admission | CICU 1203, HDU 626, cardiac ward 418, another unit/hospital ward 268 | Alter all bed-day costs in first admission by ± 25% |
3 | Bed-days in readmissions | ICU 1181, ward 268 | Alter readmission ICU/ward costs by ± 25% |
4 | Pacing | 3107 | ± 25% |
5 | Chest drain | 4224 | ± 25% |
6 | Reoperations | 8233 | ± 25% |
Results of the economic evaluation
Resource use and costs
Table 81 presents the resource use information for the two trial groups. Participants in the patient-specific algorithm group were transfused slightly fewer red blood cells than those in the generic algorithm group, but the difference was small. Those in the patient-specific algorithm group spent 1 day less, on average, as an inpatient following their surgery than those following the generic algorithm. Participants in the intervention group were also slightly less likely to be given antibiotics postoperatively or to require pacing and fewer in the intervention group had their pump blood processed after CPB. Other resource use and the occurrence of complications were very similar between the trial groups. Tables 132–138 (see Appendix 3) provide information on the unit costs that were attached to the resource use information in Table 81.
Resource use component | Patient-specific algorithm (N = 98) | Generic algorithm (N = 106) | Patient-specific vs. generic algorithm, % or mean (SE) difference |
---|---|---|---|
Cardiac procedure, n (%) | |||
CABG and valve surgery | 22 (22) | 25 (24) | –2 |
Valve surgery – single | 71 (72) | 77 (73) | –1 |
Valve surgery – more than one | 5 (5) | 4 (4) | 1 |
Blood products, number of units per participant, mean (SE) | |||
Red blood cells | 0.96 (0.17) | 1.10 (0.18) | –0.14 (0.24) |
FFP | 0.40 (0.10) | 0.41 (0.10) | –0.01 (0.14) |
Platelets | 0.35 (0.11) | 0.21 (0.05) | 0.14 (0.12) |
Cryoprecipitate | 0.07 (0.04) | 0.09 (0.04) | –0.02 (0.06) |
Inpatient complications, n (%) | |||
Antibiotics | 26 (27) | 36 (34) | –7 |
Reoperation | 6 (6) | 3 (3) | 3 |
Re-intubation | 1 (1) | 3 (3) | –2 |
Suspected MI | 1 (1) | 2a (2) | –1 |
Cardiac arrest | 0 (0) | 3a (3) | –3 |
SVT/AF requiring treatment | 42 (43) | 46a (44) | –1 |
VF/VT requiring intervention | 0 (0) | 2a (2) | –2 |
Pacing | 37 (38) | 49a (47) | –9 |
Inotropes | 54 (55) | 55a (52) | 3 |
IABP inserted | 1 (1) | 0a (0) | 1 |
Pulmonary artery catheter | 2 (2) | 1a (1) | 1 |
Vasodilator | 21 (21) | 20a (19) | 2 |
Low cardiac output | 3 (3) | 5a (5) | –2 |
Tracheostomy | 1 (1) | 0a (0) | 1 |
Mask CPAP | 2 (2) | 8a (8) | –6 |
Adult respiratory distress syndrome | 0 (0) | 0a (0) | 0 |
Pneumothorax or pleural effusion requiring drainage | 6 (6) | 5b (5) | 1 |
Haemofiltration/renal dialysis | 1 (1) | 4a (4) | –3 |
Gut infarction | 0 (0) | 0b (0) | 0 |
Peptic ulcer/GI bleed/perforation | 1b (1) | 0c (0) | 1 |
Pancreatitis | 0b (0) | 0c (0) | 0 |
Other GI | 1b (1) | 1d (1) | 0 |
Permanent stroke | 2 (2) | 1a (1) | 1 |
TIA | 0e (0) | 0f (0) | 0 |
Deep-vein thrombosis | 0 (0) | 0a (0) | 0 |
Pulmonary embolus | 0 (0) | 0a (0) | 0 |
Excess bleeding | 0 (0) | 1b (1) | –1 |
Wound dehiscence | 1 (1) | 2a (2) | –1 |
Inpatient LOS, days per participant, mean (SE) | |||
CICU | 0.62 (0.28) | 0.46 (0.07) | 0.16 (0.29) |
HDU | 3.23 (0.17) | 3.44 (0.18) | –0.21 (0.25) |
Ward | 4.69 (0.35) | 5.07 (0.41) | –0.38 (0.54) |
Another unit/hospital | 1.02 (0.61) | 1.55c (0.69) | –0.53 (0.93) |
Blood-saving techniques, n (%) | |||
Post-CPB processing of pump blood | 57 (58) | 77a (73) | –15 |
Tranexamic acid | 66a (68) | 72a (69) | –1 |
Aprotinin | 2 (2) | 0b (0) | 2 |
Cell saver | 23 (23) | 28a (27) | –4 |
Readmissions to hospital,g days per participant (mean) (SE) | 1.57 (0.57) | 2.15 (0.77) | –0.58 (0.96) |
A&E attendances,g number per participant (mean) (SE) | 0.11 (0.03) | 0.06 (0.02) | 0.06 (0.04) |
Outpatient appointments,g number per participant, mean (SE) | |||
Cardiac surgery outpatient visits | 1.04 (0.07) | 0.93 (0.06) | 0.11 (0.09) |
Cardiology outpatient visits | 0.21 (0.06) | 0.09 (0.03) | 0.12 (0.07) |
Other outpatient visits | 0.11 (0.04) | 0.06 (0.03) | 0.06 (0.05) |
Other health-care contacts,g number per participant, mean (SE) | |||
GP at surgery | 2.31 (0.22) | 1.81 (0.18) | 0.50 (0.28) |
GP at home | 0.30 (0.10) | 0.21 (0.05) | 0.09 (0.11) |
Practice nurse | 3.68 (0.50) | 2.72 (0.38) | 0.96 (0.63) |
District nurse | 1.68 (0.52) | 0.96 (0.17) | 0.73 (0.55) |
A breakdown of the total costs (resource use multiplied by unit costs) of care from surgery to 3 months is shown in Table 82. The key drivers of total costs were the surgery, the intervention, complications and LOS. Most, but not all, of the difference in cost of surgery between the groups could be explained by the cost of the intervention (£218 per participant). However, those in the patient-specific algorithm group had, on average, lower complication and serious adverse event costs and lower LOS costs during the inpatient episode than those in the generic algorithm group.
Cost component | Cost (£), mean (SE) | Patient-specific vs. generic algorithm, mean (SE) difference (£) | |
---|---|---|---|
Patient-specific algorithm (N = 98) | Generic algorithm (N = 106) | ||
Inpatient episode | |||
Initial cardiac surgery | 7554 (88) | 7282 (79) | 272 (118) |
Red blood cells | 127 (22) | 145 (23) | –18 (32) |
Other blood products | 97 (25) | 77 (19) | 20 (32) |
Complications and serious adverse events | 2202 (348) | 2347 (289) | –145 (449) |
LOSa | 5005 (506) | 5241 (339) | –236 (600) |
Blood-saving techniques | 80 (13) | 105 (15) | –25 (20) |
Regular medications | 2 (0) | 2 (0) | 0 (1) |
Total | 15,067 (722) | 15,200 (512) | –133 (875) |
Post discharge | |||
Readmissions | 853 (322) | 1242 (406) | –389 (523) |
A&E visits | 23 (8) | 10 (5) | 13 (9) |
Outpatient appointments | 360 (20) | 301 (17) | 58 (26) |
Other medical/social care | 287 (30) | 235 (18) | 52 (34) |
Regular medications | 1 (0) | 3 (1) | –2 (1) |
Total | 1524 (326) | 1791 (410) | –267 (529) |
Total costs | 16,591 (799) | 16,992 (656) | –400 (1027) |
The patient-specific algorithm group had a lower cost for readmissions and slightly higher costs for outpatient visits and other visits than the generic algorithm group. The difference in readmission costs is unexpected and worth closer inspection. The readmission costs consist of LOS costs, the costs of complications and serious adverse events, the cost of admission through accident and emergency (A&E) or a GP and the cost of travel by ambulance. The difference in readmission costs between the two groups is the result of two factors. First, the generic algorithm group had, on average, an additional 0.6 readmission days compared with the patient-specific algorithm group, which made their readmission LOS costs approximately £200 higher than in the patient-specific algorithm group. Second, costs associated with complications and serious adverse events were approximately £160 higher in the generic algorithm group; this was largely attributable to one participant undergoing temporary pacing during a readmission and this being converted to permanent pacing. Overall, though, the costs are similar between the two treatment groups. The differences in costs between the groups are small, although there is substantial uncertainty around these differences (as is evident from the large SEs in the final column of the table). In terms of the distribution of costs across the trial groups, the histograms presented in Figure 57 show that the cost data are skewed for both groups, which is a common finding in health economic evaluations. There is one clear outlier in the patient-specific algorithm group.
FIGURE 57.
Mean total health-care cost per participant: (a) patient-specific algorithm group; and (b) generic algorithm group.
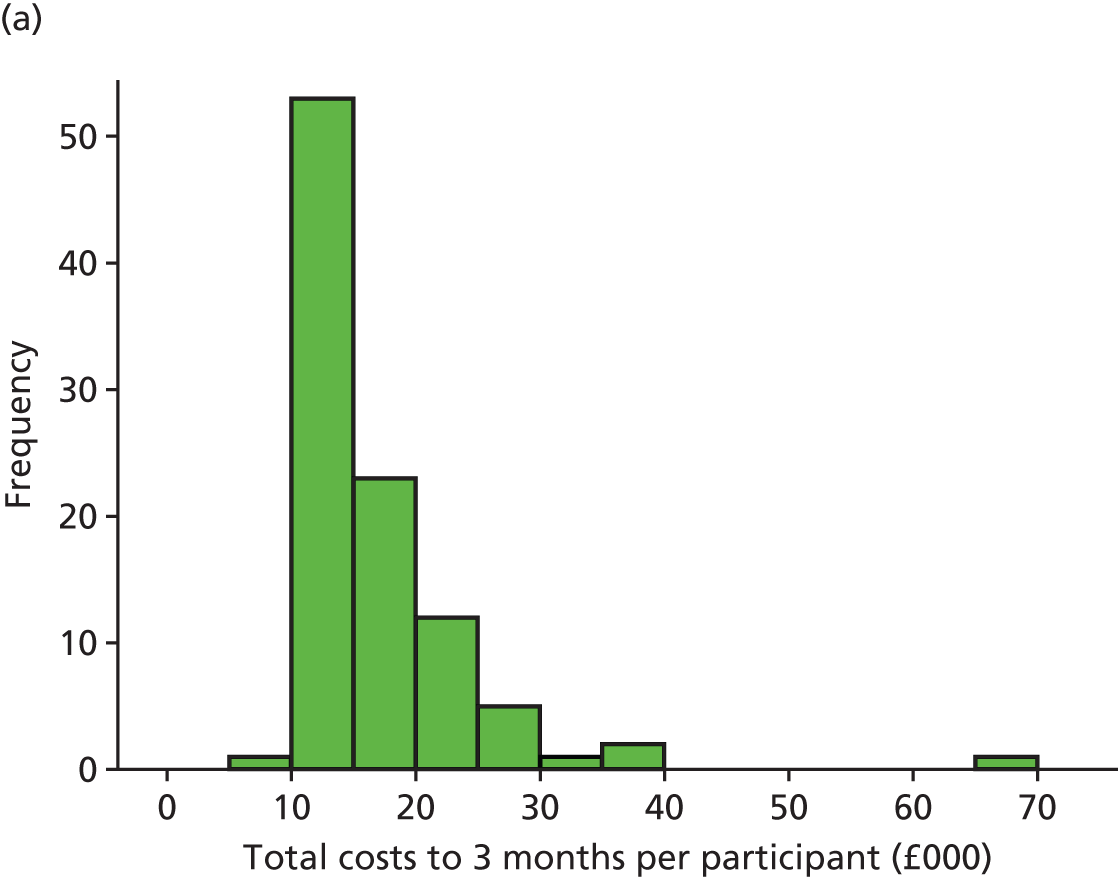

Health-related quality of life and quality-adjusted life-years
Three participants died during the trial, with all three deaths occurring in hospital during the inpatient stay following surgery. Two participants in the control group died 3 days and 9 days after surgery, respectively, and one participant in the intervention group died 4 days after surgery. For the cost analysis we included information on costs up to the point of death (therefore, we did not exclude the costs of these participants) and for quality of life we allocated a value of zero to these participants and included them in the analysis.
Mean EQ-5D scores at each of the three time points are shown in Table 83. Participants who died were included at 6 weeks and 3 months using a value of zero and missing data were imputed. On average, the EQ-5D score did not return to its preoperative level by 3 months for participants following the patient-specific algorithm, but did so for participants in the generic algorithm group. Table 83 also presents QALYs to 3 months, which are based on imputed data and adjusted for baseline EQ-5D scores. The table shows that QALYs to 3 months were 0.1840 in the patient-specific algorithm group and 0.1917 in the generic algorithm group, with a MD of only –0.0078 (SE 0.0062), equivalent to 2.8 quality-adjusted days.
Variable | Patient-specific algorithm (N = 98), mean (SE) | Generic algorithm (N = 106), mean (SE) | Patient-specific vs. generic algorithm, MD (SE) |
---|---|---|---|
EQ-5D scorea | |||
Baseline | 0.799 (0.020) | 0.775 (0.021) | 0.024 (0.029) |
6 weeks | 0.693 (0.028) | 0.729 (0.025) | –0.035 (0.038) |
3 months | 0.793 (0.025) | 0.818 (0.024) | –0.025 (0.035) |
QALYs to 3 months (adjusted for baseline EQ-5D score) | 0.1840 (0.0044) | 0.1917 (0.0043) | –0.0078 (0.0062) |
QALYs to 3 months (unadjusted, for comparison) | 0.1857 (0.0053) | 0.1901 (0.0050) | –0.0045 (0.0072) |
Within each treatment group, and at each time point, those with missing EQ-5D data had a longer total inpatient LOS than those who were not missing EQ-5D data. It seems reasonable, therefore, that the average scores with imputation at 6 weeks and 3 months are slightly lower than the average scores without imputation (data not shown).
Base-case cost-effectiveness results
The ICER for the patient-specific algorithm group compared with the generic algorithm group is shown in Table 84. This analysis generated a trade-off between costs and effectiveness: the patient-specific algorithm group was less costly but also less effective than the generic algorithm group. Neither the difference in costs nor the difference in QALYs was statistically significant between the groups. The ICER of £51,616 is the incremental saving associated with the loss of 1 QALY by adopting a patient-specific algorithm rather than a generic algorithm. Based on the point estimate of the ICER, if a decision-maker’s willingness to accept compensation for the loss of 1 QALY were £20,000, then a patient-specific algorithm would be considered cost-effective. However, the uncertainty around this result is shown on the cost-effectiveness plane in Figure 58. The black dot is the point estimate of the cost and QALY difference. The bootstrap replicates are mainly found in three out of four of the quadrants, indicating a lot of uncertainty around the result.
Total costs (95% CI) (£) | QALYs (95% CI) (adjusted for baseline EQ-5D scores) | ICER, cost per QALY (£) | Probability (%) patient-specific algorithm is | Probability (%) patient-specific algorithm is cost-effective at a ceiling ratio of | Probability (%) patient-specific algorithm is | ||||||||
---|---|---|---|---|---|---|---|---|---|---|---|---|---|
Patient-specific algorithm (N = 98) | Generic algorithm (N = 106) | Difference | Patient-specific algorithm (N = 98) | Generic algorithm (N = 106) | Difference | Dominant | Dominated | £20,000 per QALY | £50,000 per QALY | £100,000 per QALY | More effective | Less costly | |
16,591 (15,012 to 18,171) | 16,992 (15,732 to 18,251) | –400 (–2423 to 1623) | 0.1840 (0.1754 to 0.1925) | 0.1917 (0.1833 to 0.2002) | –0.0078 (–0.0198 to 0.0043) | 51,616 | 10 | 34 | 60 | 51 | 41 | 11 | 65 |
FIGURE 58.
Cost-effectiveness plane (so that points are visible, only the first 50 bootstrap replicates for each of the 20 imputations are plotted).

The cost-effectiveness acceptability curve in Figure 59 shows the probability that the patient-specific algorithm is cost-effective for a range of willingness-to-pay thresholds (or ceiling ratios). As the patient-specific algorithm is both less costly and less effective than the generic algorithm (rather than more costly and more effective), these ceiling ratios are in fact the thresholds at which a decision-maker is willing to accept compensation for the loss of 1 QALY (rather than the amount that they are willing to pay for an additional QALY). If a decision-maker is willing to accept compensation of £20,000 for the loss of 1 QALY, then the probability that the patient-specific algorithm is cost-effective is 60%. At accepted ceiling ratios (£20,000–30,000 per QALY), the patient-specific algorithm is more likely to be cost-effective than the generic algorithm, but there is much uncertainty around this. The dotted lines at 0.1 and 0.9 indicate 80% confidence limits for the probability that the patient-specific algorithm is cost-effective. As these horizontal lines do not cut the curve at any point, the 80% confidence limits on cost-effectiveness do not exist; indeed, across ceiling ratios from £0 to £100,000 it is not possible to define even 50% limits on cost-effectiveness.
FIGURE 59.
Cost-effectiveness acceptability curve.
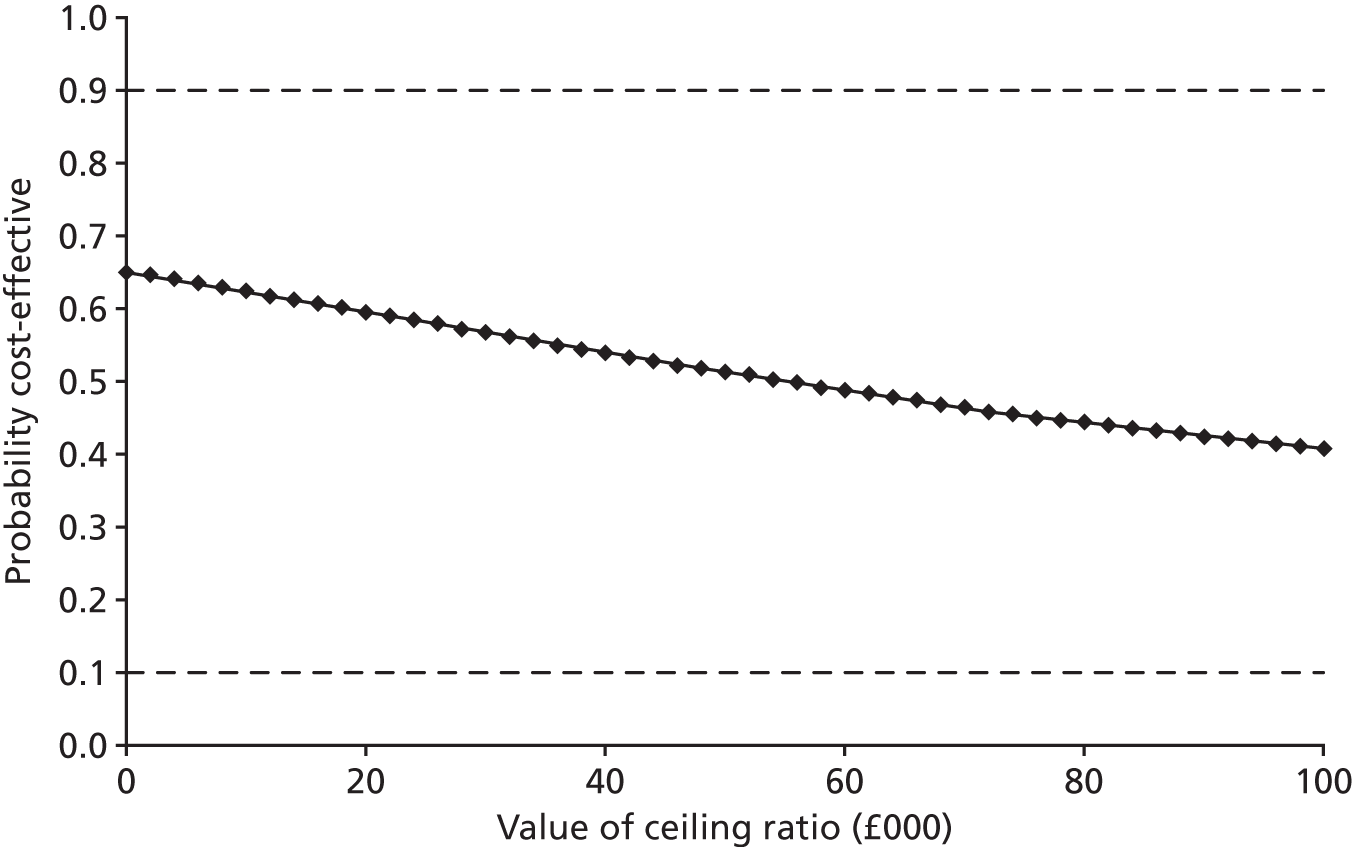
Sensitivity analyses
The results of the sensitivity analyses around unit costs are shown in Table 85. Although sensitivity analyses 1–4 had an impact on the cost difference between the groups, in none of the sensitivity analyses did this cause the conclusions to change. In each case the patient-specific algorithm group was less costly than the generic algorithm group.
Sensitivity analysis | Patient-specific algorithm (N = 98), mean (SE) cost (£) | Generic algorithm (N = 106), mean (SE) cost (£) | Patient-specific vs. generic algorithm, mean (SE) cost difference (£) |
---|---|---|---|
Base case | 16,591 (799) | 16,992 (656) | –400 (1027) |
SA1 (NIRS) | |||
+25% | 16,646 (799) | 16,992 (656) | –346 (1027) |
–25% | 16,537 (799) | 16,992 (656) | –455 (1027) |
SA2 (bed-days, first admission) | |||
+25% | 17,843 (902) | 18,302 (711) | –459 (1140) |
–25% | 15,340 (703) | 15,681 (607) | –341 (925) |
SA3 (bed-days, readmissions) | |||
+25% | 16,726 (824) | 17,177 (693) | –451 (1071) |
–25% | 16,456 (777) | 16,806 (623) | –349 (989) |
SA4 (pacing) | |||
+25% | 16,885 (811) | 17,365 (672) | –480 (1047) |
–25% | 16,298 (789) | 16,619 (641) | –321 (1010) |
SA5 (chest drain) | |||
+25% | 16,678 (806) | 17,062 (662) | –384 (1037) |
–25% | 16,505 (793) | 16,921 (650) | –416 (1019) |
SA6 (reoperations) | |||
+25% | 16,717 (819) | 17,050 (664) | –333 (1047) |
–25% | 16,465 (781) | 16,933 (649) | –468 (1010) |
A final sensitivity analysis was conducted to assess the impact on findings of the cost outlier in the patient-specific algorithm group. This participant had a stroke and stayed in hospital with a high level of care for some time; there are no grounds for excluding this participant but it is instructive to investigate the impact on the cost and cost-effectiveness results as this outlier could easily have arisen by chance. If this participant was excluded, the mean (SE) costs in the patient-specific algorithm group fell to £16,044 (3588) and the mean (SE) cost difference between the groups was –£948 (£887). The mean (SE) QALYs gained in the patient-specific algorithm group increased to 0.1850 (0.0044) and the resulting point estimate of the ICER was £143,772. This participant clearly had a significant impact on the cost and cost-effectiveness results.
Summary of the findings
There was very little difference between the patient-specific algorithm group and the generic algorithm group in terms of either costs or effects, and uncertainty around the cost-effectiveness results. The mean cost in the patient-specific algorithm group was £16,591 (SE £799) and in the generic algorithm group was £16,992 (SE £656), a difference of –£400 (SE £1027). QALYs to 3 months were very slightly lower in the patient-specific algorithm group than in the generic algorithm group, with a MD of only –0.0078 (SE 0.0062), equivalent to 2.8 quality-adjusted days. On average, therefore, the patient-specific algorithm group was less costly but gained fewer QALYs than the generic algorithm group, with an incremental saving associated with the loss of 1 QALY by adopting a patient-specific algorithm rather than a generic algorithm of £51,616. If a decision-maker’s willingness to accept compensation for the loss of 1 QALY were £20,000, then based on the point estimate of the ICER the patient-specific algorithm would be considered cost-effective. However, there was considerable uncertainty around this finding, largely because of the very small differences in costs and effects.
Systematic review and meta-analysis
Overview
The results of the PASPORT trial did not support our primary hypothesis. We demonstrated that the use of the NIRS-based algorithm did not reduce neurocognitive impairment or injury to other organs, including the kidneys, heart and brain, or reduce transfusion rates. Our health economic analysis demonstrated no difference in cost-effectiveness between the NIRS-based algorithm and standard care. However, previous trials have reported benefits from the use of NIRS-based algorithms. 235 To reconcile these disparate findings, and to address equipoise with regard to effectiveness and variations in use,211 we undertook a systematic review and meta-analysis of the available evidence from RCTs to assess the clinical utility of this technology, identify knowledge gaps in the existing evidence and provide recommendations for further research. The review protocol was registered (PROSPERO CRD42015027696) and the review is reported in accordance with the PRISMA statement131 (see Appendix 1).
Objectives
The objective of this review was to establish whether or not the use of perioperative goal-directed interventions that aim to optimise cerebral NIRS readings result in reductions in measures of cerebral injury (neurocognitive function, serum biomarkers, stroke), other major morbidity (heart, kidney), bleeding, red cell transfusion rates, mortality and resource use.
Methods
Types of studies
Randomised controlled trials irrespective of blinding, language, publication status, date of publication and sample size were included in the review.
Types of participants
Patients undergoing cardiac surgery for acquired or congenital disease or aortovascular disease with or without CPB were included in the review. No age restriction was applied and there were no exclusion criteria.
Types of interventions
-
Intervention: goal-directed NIRS algorithm.
-
Comparator/control: an untreated group or alternative (non-NIRS based) goal-directed therapy.
Types of outcome measure
Primary outcome
-
Mortality: 30-day or hospital all-cause mortality.
Secondary outcomes
-
Acute brain injury: stroke and TIA, as defined by study authors.
-
Low cardiac output, as defined by study authors.
-
MI, as defined by study authors.
-
Acute kidney Injury stage 3 or requiring haemofiltration, as defined by study authors.
-
Neurocognitive function; group means, as described by neurocognitive tests. Tests recommended by a consensus statement217 for testing all key domains of cognitive function are indicated with an asterisk. Studies were categorised as ‘yes/no’ according to whether or not they assessed the key domains described in the consensus statement. Key domains assessed218 and examples of likely tests were:
-
attention – sustained and divided attention: consensus statement217 recommends the Trail Making Test parts A* and B*219,236
-
verbal memory – consensus statement217 recommends the RAVLT*236
-
visuospatial – the Block Design Test from the WAIS220
-
psychomotor speed – consensus statement217 recommends tests such as the Digit Symbol Test from the WAIS220
-
executive function/verbal fluency – consensus statement217 recommends tests such as the COWAT221
-
motor co-ordination – consensus statement217 recommends tests such as the Grooved Pegboard Test*, dominant and non-dominant hand219
-
frontal lobe – saccadic and antisaccadic eye movements
-
assessment of important covariates (Mini Mental State Examination, Anxiety and depression, intellectual ability, and concomitant medication) in these analyses were documented.
-
-
Neurocognitive dysfunction, as reported by the study authors. The consensus definition is a change in a single test of > 1 SD. This may be defined as change in a group mean (adjusted for baseline) or change for individual patients. 237 Studies were categorised as defining cognitive dysfunction using a consensus or a non-consensus definition.
-
Risk of receiving a blood transfusion, as defined by study authors.
-
Reoperation for bleeding, as defined by study authors.
-
Resource use: ICU and hospital LOS, as defined by study authors.
-
S100B levels (a marker of brain injury), as reported by study authors.
Search methods for identification of studies
Potentially eligible trials were identified by searching the CENTRAL, MEDLINE, EMBASE and CINAHL Plus databases, using a combination of subject headings and text words to identify relevant trials from inception until November 2016. The following MEDLINE search strategy was adapted as appropriate for other databases:
((Cardiopulmonary Bypass) OR (Cardiac Surgery) OR (Coronary Artery Bypass) OR (Extra Corporeal Circulation) OR (Perioperative Morbidity)) AND ((Near Infra-Red Spectroscopy) OR (Oximetry) OR (Brain/Metabolism) OR (Cerebral Desaturation) OR (Cerebral Perfusion) OR (Cerebral Ischaemia) OR (Cerebral Oximetry) OR (Cerebral Saturation) OR (Near Infrared Oximetry) OR (Cognitive))
To identify ongoing or unpublished trials we searched ClinicalTrials.gov using the following search terms:
Search terms: Randomised
Study type: Interventional Studies
Conditions: Cardiac surgery OR Cardiopulmonary bypass
Interventions: Near Infra-Red Spectroscopy OR Near Infrared Oximetry OR Cerebral Desaturation
We also examined the reference lists of eligible trials and reviews. Searches were not restricted by language or publication status.
Data collection and analysis
The review was performed in accordance with the Cochrane Handbook for Systematic Reviews of Interventions. 238
Selection of studies
Two reviewers (GJM, GFS) identified trials for inclusion independently. Excluded studies and the reasons for exclusion were recorded.
Data extraction (selection and coding)
Two authors independently screened the search output to identify records of potentially eligible trials examining the outcomes, the full texts of which were retrieved and assessed for inclusion.
A standardised form was used to extract data from the included studies for assessment of study quality and evidence synthesis. Extracted information included:
-
year and language of publication
-
country of participant recruitment
-
year of conduct of the trial
-
study setting: university teaching hospital, non-university teaching hospital
-
study population: inclusion and exclusion criteria
-
sample size
-
participant demographics
-
baseline characteristics
-
type of surgery
-
details of the NIRS algorithm (Murkin, non-Murkin212) and cointerventions (restrictive vs. non-restrictive red cell transfusion thresholds)
-
details of the comparator: non-NIRS goal-directed therapy, standard care (protocolised care)
-
outcomes and times of measurement
-
information for assessment of the risk of bias.
Two review authors extracted data independently and discrepancies were identified and resolved through discussion (with a third author when necessary). Missing data were requested from study authors. If there was doubt whether trials shared participants completely or partially (with common authors and centres), we attempted to contact the study authors to ascertain whether the study report had been duplicated.
Risk of bias
The following risk of bias domains were assessed as being low, uncertain or high based on instructions given in the Cochrane Handbook for Systematic Reviews of Interventions:132
-
sequence generation
-
allocation concealment
-
blinding of participants and personnel
-
blinding of outcome assessors
-
incomplete outcome data
-
selective outcome reporting
-
source of funding bias.
Trials were classified as having a low risk of bias if they were graded as being at low risk of bias for all of these domains. Two review authors independently assessed the risk of bias in all of the studies. Discrepancies were resolved by discussion with a third author.
Assessment of reporting bias
When ≥ 10 studies were identified for each outcome publication bias was assessed by the visual assessment of funnel plots and using Egger’s test. 133
Measurement of treatment effect
For dichotomous variables we calculated the RR with 95% CI. For continuous variables we calculated the MD with 95% CI for outcomes such as hospital stay and the SMD with 95% CI for quality of life (when different scales were used).
Dealing with missing data
We performed an intention-to-treat analysis when possible. For dichotomous data presented only as percentages we estimated frequencies using reported sample sizes. For continuous outcomes, if the mean and SD were not available from the trial report, we sought this information from the trial authors. If this information was still not available, we calculated the mean and SD from the median and IQR using the software available in Review Manager version 5.
Assessment of heterogeneity
We anticipated that major sources of clinical heterogeneity would be associated with different patient groups (adults, children, congenital vs. acquired disease), the use of different goal-directed NIRS algorithms, the use of cointerventions such as restrictive transfusion thresholds and differences in the methodology used to assess neurocognitive dysfunction. We explored heterogeneity within each meta-analysis using a chi-squared test, with significance set at a p-value of 0.10, and we expressed the percentage of heterogeneity due to variation rather than chance as the I2 value. 134 We defined heterogeneity as follows:
-
I2 0–40% – no or mild heterogeneity
-
I2 40–80% – moderate heterogeneity
-
I2 > 80% – severe heterogeneity.
In the presence of severe heterogeneity meta-analysis was not performed.
Data synthesis
Meta-analyses were performed using the software package Review Manager version 5.2 and in accordance with the recommendations of the Cochrane Handbook for Systematic Reviews of Intervention. 130 For the primary analysis we compared the results of a random-effects model with those of a fixed-effects model to assess the effects of small studies. For continuous outcomes we pooled MDs or SMDs by using the inverse variance method.
Subgroup analyses
Subgroup analysis were performed in trials in which the Murkin algorithm212 was used to guide goal-directed therapy compared with trials in which the Murkin algorithm was not used. Subgroup analyses were also performed by participant group: CABG compared with non-CABG, adults compared with children and assessment of neurocognitive function that incorporated tests described in the previous consensus statement217 compared with assessment that did not incorporate tests described in the previous consensus statement. Tests for subgroup differences were performed using Review Manager, with a p-value of < 0.05 considered statistically significant.
Sensitivity analyses
Sensitivity analysis excluded trials with a high risk of bias for any of the following: random sequence generation, allocation concealment, blinding of participants, health-care providers or outcome assessors, incomplete outcome data, attrition and other sources of bias, including source of funder.
Summary of findings
The main results of the review are presented in a summary of findings table. We included the following outcomes:
-
risk of mortality
-
risk of stroke, MI or severe AKI
-
risk of red cell transfusion
-
neurocognitive impairment
-
resource use: ICU and hospital LOS.
We used GRADEpro software to prepare the summary of findings table. We judged the overall quality of the evidence for each outcome as ‘high’, ‘moderate’, ‘low’ or ‘very low’ according to the GRADE approach. 239 We considered the following:
-
impact of risk of bias of individual trials
-
precision of the pooled estimate
-
inconsistency or heterogeneity (clinical, methodological and statistical)
-
indirectness of the evidence
-
impact of selective reporting and publication bias on effect estimates.
Systematic review results
Description of studies
Search results
A total of 17,792 references were identified through electronic searches of CENTRAL (n = 1347), MEDLINE (n = 9924), EMBASE (n = 6159) and CINAHL Plus (n = 362). From ClinicalTrials.gov we identified 18 trials that potentially met the inclusion criteria. The study flow is provided in Figure 60. We excluded 7909 duplicate references and 9820 clearly irrelevant references after reading titles and abstracts. No additional references were identified by reference searching. Of 79 study reports retrieved in full, we excluded 69 for the reasons listed in Figure 60. In total, 11 publications45,235,240–248 describing nine RCTs fulfilled the inclusion criteria and provided quantitative data for this review. In addition, we included the results of the PASPORT trial,154 resulting in a total of 10 trials for inclusion (Table 86).
FIGURE 60.
Preferred Reporting Items for Systematic Reviews and Meta-Analyses (PRISMA) flow chart of included studies.
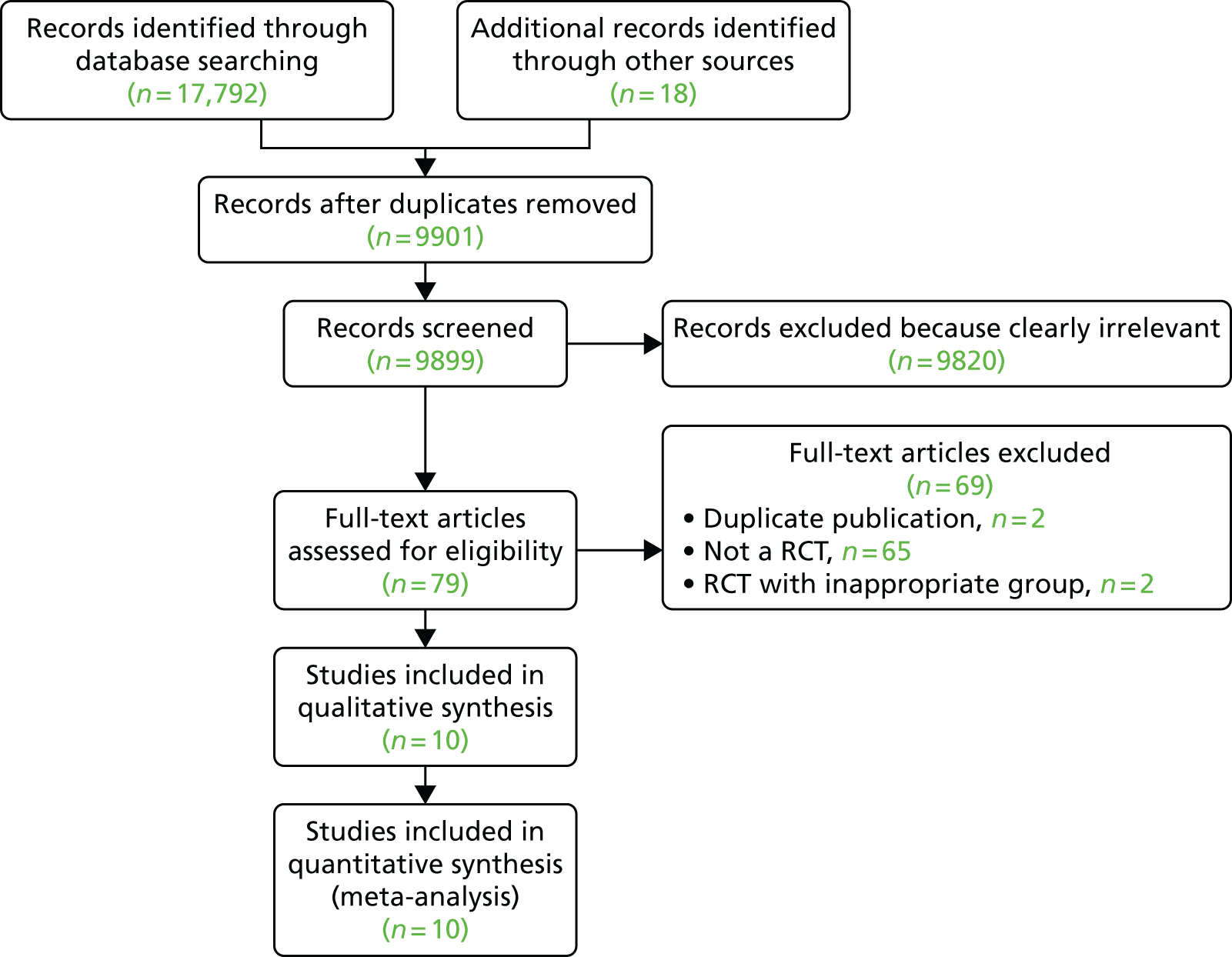
Study | Location and funding | Sample size | Time period | Inclusion and exclusion criteria | Mean age (years) (intervention group/control group) | Female (%) (intervention group/control group) | NIRS device | Use of Murkin algorithm | Control | Outcomes |
---|---|---|---|---|---|---|---|---|---|---|
Deschamps et al., 2016241 | Multicentre, Canada; regional funders | 201 | 2012–13 | Inclusion: high-risk cardiac surgery. Exclusion: off-pump coronary artery bypass surgery, emergency surgery, planned deep hypothermic circulatory arrest, acute endocarditis and presence of active delirium or encephalopathy | 69 ± 12.6/72 ± 9.4 | 27.4/28.3 | FORE-SIGHT, EQUANOX Classic 7600, INVOS 5100C-PB | SC | Primary outcome: success rate of reversing cerebral desaturations below 10% relative to baseline in the intervention group. Secondary outcomes: cerebral desaturation load, 30-day follow-up for adverse events | |
Kara et al., 2015244 | Single centre, Sakarya, Turkey | 79 | 2013–15 | Inclusion: CABG. Exclusion: other procedures, high degree of aortic atherosclerosis | 59.1 ± 9.4/61.2 ± 10.3 | 23.3/19.4 | INVOS | SC | Primary: cognitive impairment (Montreal Cognitive Assessment test score). Secondary: ICU and hospital LOS | |
PASPORT, 2017154 | Three UK centres; NIHR funded | 204 | 2009–14 | Inclusion: CABG and non-CABG. Exclusion: patients undergoing emergency cardiac surgery, patients prevented from receiving blood and blood products because of their system of beliefs | 70 ± 10/65.9 ± 12.3 | 30/33 | INVOS | Yes | SC | Primary: cognitive function. Secondary: units of red cells transfused, cerebral oxygenation, oxygen delivery and utilisation during CPB, quality of life, infectious complications, stroke, MI, ICU and hospital LOS, postoperative AKI, respiratory complications, health and personal social services resource use and costs, all-cause mortality within 30 days of surgery, biochemical markers of organ injury |
Colak et al., 2015240 | Single centre, Zagreb, Croatia; institutional support grant | 200 | 2009–10 | Inclusion: CABG. Exclusion: carotid artery stenosis, previous stroke or head injury, seizure, psychiatric illness (NYHA class III/IV), ejection fraction < 25%, emergency off-pump CABG and severely impaired renal and liver function | 61.9 ± 7.1/63.4 ± 8.8 | 20/24 | INVOS | Yes | SC | Primary: cognitive impairment, Mini Mental State Examination, Color Trail Test 1, Grooved Pegboard Test. Secondary: coma, stupor, TIA or stroke, neuropsychological deficits |
Deschamps et al., 2013242 | Single centre, Quebec, Canada; institutional support grant | 49 | NS | Inclusion: CABG and non-CABG. Exclusion: emergency surgery, first-time CABG surgery, single-valve surgery | 70.2 ± 9.2/71.1 ± 7.9 | 15/15 | INVOS | Yes | SC | Primary: rSO2. Secondary: ICU and hospital LOS |
Vretzakis et al., 201345 | Single centre, Greece; institutional support grant | 50 | 16-month period | Inclusion: CABG and non-CABG. Exclusion: emergency, redo operations, combined cardiac and carotid surgery, circulatory arrest, haematological disease, coagulation abnormality, advanced cirrhosis and renal dysfunction | 67.3 ± 8.5/65.9 ± 9.5 | 12/15 | INVOS | SC | Primary: red cell transfusion, ICU and hospital LOS, death. Secondary: major complications | |
Mohandas et al., 2013245 | Single centre, Bangalore, India | 100 | NS | Inclusion: on-pump cardiac surgery. Exclusion: pre-existing neuropsychiatric disorders, inability to correctly perform the neurocognitive tests and Mini Mental State Examination score of < 23 | 38.05 ± 15.81/34.60 ± 16.28 | 44/44 | NONIN EQUANOX | Yes | SC | Primary: cognitive decline (Mini Mental State Examination, anti-saccadic eye movements). Secondary: ICU LOS |
Harilall et al., 2014243 | Single centre, South Africa | 40 | NS | Inclusion: CABG. Exclusion: pregnancy, history of stroke or persistent neurological residue, history of TIA and stenosis of carotid artery | 55.3 ± 9.7 (all) | 30 (all) | INVOS | Yes | SC | Primary: rSO2. Secondary: S100B |
Slater et al., 2009246 | Single centre, NJ, USA | 265 | 2004–6 | Inclusion: CABG. Exclusion: pre-existing neuropsychiatric disorders, inability to correctly perform the neurocognitive tests and Mini Mental State Examination score of ≤ 23 | 64.8 ± 10.1/64.78 ± 9.9 | 31 (all) | INVOS | Yes | SC | Primary: cognitive impairment. Secondary: ICU LOS |
Murkin et al., 2007235 | Single centre, Ontario, Canada; industry support | 200 | 2002–4 | Inclusion: aged > 18 years, CABG | 61.8 ± 10.3/61.8 ± 9.3 | 12.5 (all) | INVOS | Yes | SC | Primary: 30-day death, major organ morbidity or mortality. Secondary: LOS |
Description of excluded studies
Four references that met our inclusion criteria were excluded after review of the full manuscripts (Table 87). Two were reports – an interim analysis247 and a post hoc analysis248 – of a trial that was also reported in full235 (see Table 86). Another was a report of a trial comparing NIRS values in on-pump and of-pump CABG patients. 249 A fourth trial by Dullnekopf et al. 250 was reported in the abstract to be a RCT; however, randomisation involved placing the NIRS sensors on either the right or the left forehead and there was no clinical comparison of a NIRS-based intervention compared with a control group.
Study | Study type and location | Sample size | Time period | Inclusion and exclusion criteria | Mean age (years) | Female (%) | NIRS device | Murkin algorithm | Control | Outcomes |
---|---|---|---|---|---|---|---|---|---|---|
Dullenkpof et al., 2007250 | Prospective RCT, single centre, Zurich, Switzerland; no intervention | 35 | NS | Inclusion: elective cardiac surgery with insertion of pulmonary artery catheter. Exclusion: cerebral perfusion disturbance, CAD, intracardiac shunt | 65.5 ± 10.9 | 74.2 | INVOS | Primary: rSO2 | ||
Kok et al., 2014249 | Single centre, Groningen, the Netherlands | 59 | 2011–12 | Inclusion: CABG. Exclusion: other procedures, difficulty completing cognitive tests, difficulty with Dutch language, impaired function of the dominant arm or hand, history of head trauma, stroke or neurosurgery, severe CAD | 62.8 ± 9.4 | 10 | INVOS 5100C, FORE-SIGHT | On-pump vs. off-pump surgery | Primary: cerebral desaturation. Secondary: postoperative cognitive dysfunction, major complications, ICU and hospital LOS |
Description of included studies
Participants
Overall, 1388 participants took part in the 10 trials included in this systematic review. 45,154,235,240–246 The average age of participants ranged from 34.6 ± 16.3 to 71.1 ± 11.2 years. 45,154,235,240–246 The proportion of females ranged from 12.5% to 44% in the trials that provided this information. 45,154,235,240,242–246 The proportion of post-randomisation withdrawals ranged from 0% to 4.11%. After withdrawal, 1452 participants were included in the analysis cohort in this systematic review and meta-analysis. Five trials were conducted in participants undergoing CABG only,235,240,243,244,246 and five trials were conducted in participants undergoing valve surgery or CABG or valve surgery or other cardiac surgical procedures. 45,154,241,242,245 Further details of the participating trials are provided in Table 86.
Intervention
Cerebral NIRS values were measured with the INVOS™ 5000 (Covidian, Medtronic, Minneapolis, MN, USA), or a modification of this device, in nine trials. In one trial241 three different devices were used: INVOS, FORE-SIGHT (CAS Medical Systems Inc., USA) and EQUANOX Classic 7600 (Nonin Medical Inc., USA). In two trials the target NIRS value was > 75% of baseline. 235,243 In three trials the target value was > 80%. 242,244,246 In one trial the target NIRS value was greater than an absolute NIRS value of 60% or > 20% compared with the mean value during pulmonary artery catheter insertion. 45 In two trials the target NIRS value was a combination of > 80% of baseline or an absolute measure > 50%. 240,245 In one trial the target regional oxygen saturation value was specified as > 70% of the pre-induction value or an absolute value of > 50%. 154 In one trial the intervention threshold was specified as a 10% decrease in the regional cerebral oxygen saturation (rSO2) value relative to baseline for a duration exceeding 15 seconds. 241 Seven trials used a version of the Murkin algorithm to optimise NIRS values. 154,235,240,242,243,245,246 Three studies used non-Murkin algorithms. 45,241,244
Control
All trials used standard (protocolised) care in the control group. In seven trials NIRS values were measured in the control group, although this was hidden from the clinical personnel. 154,235,241–243,245,246 No trial considered an alternative patient-specific goal-directed algorithm.
Cointerventions
In two trials the Murkin algorithm was combined with a restrictive transfusion trigger. 45,154 In these studies a prespecified objective was to determine whether NIRS could be utilised as part of a patient-specific red cell transfusion indicator.
Risk of bias in included studies
Risk of bias in the individual trials is shown in Figure 61 and the proportions of trials with a low risk, an unclear risk and a high risk of bias in each of the domains are shown in Figure 62. Clinical personnel were not blinded in any of the trials; however, two trials were considered to be at low risk of bias in every domain. 45,154
FIGURE 61.
Risk of bias summary for individual studies. +, low risk of bias; –, high risk of bias; ?, unclear risk of bias.

FIGURE 62.
Risk of bias summary: all studies.
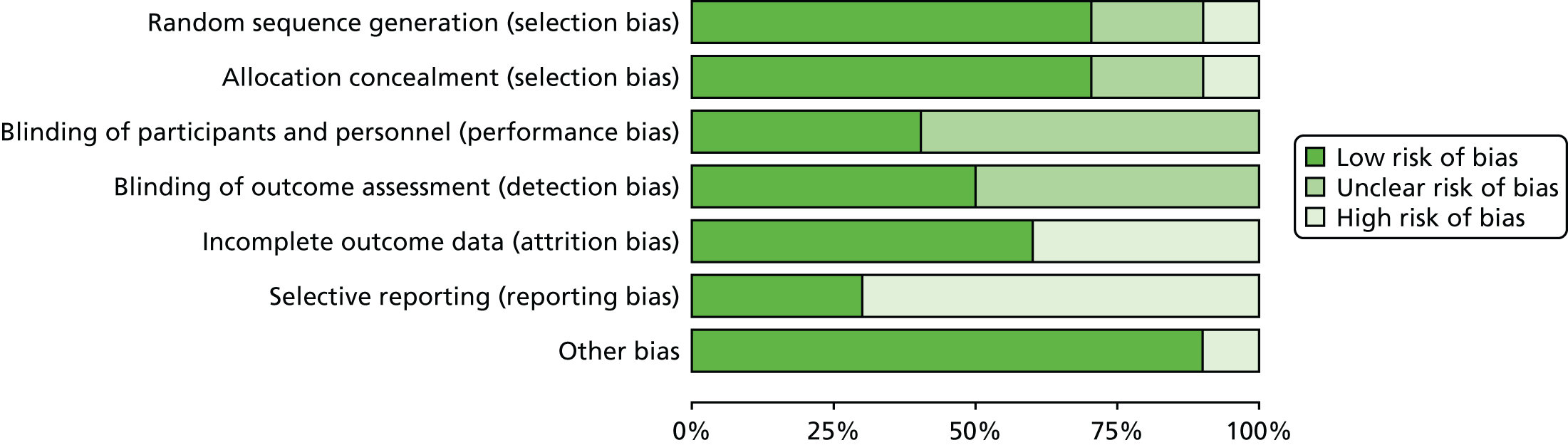
Sequence generation
Random sequence generation was adequate in seven trials,45,154,235,240,241,243,246 and unclear in two trials. 242,245 There was a high risk of bias for random sequence generation in one trial. 244
Allocation
Allocation concealment was adequate in seven trials45,154,235,240,241,243,246 and unclear in two trials. 242,245 There was a high risk of bias for random sequence generation in one trial. 244
Blinding
Theatre staff were unblinded in all of the studies. There was evidence of blinding of participants and clinical staff caring for participants postoperatively in four trials,45,154,242,244 with unclear evidence in six trials. 235,240,241,243,245,246 Three trials reported the frequency of protocol compliance. 45,154,241 In seven trials non-compliance was not monitored or not specified. There was evidence of blinding of outcome assessors in five trials45,154,235,240,244 and unclear evidence of blinding of outcome assessors in five trials. 241–243,245,246
Incomplete outcome data
Six trials reported completeness of follow-up for the primary outcome. 45,154,235,241,243,244 Of these, five trials reported < 10% loss to follow-up154,235,241,243,244 and one trial reported > 10% loss to follow-up. 45 Four trials that failed to report completeness of follow-up were considered to be at high risk of attrition bias. 240,242,245,246
Selective reporting
Only one of the 10 trials included in this review had a published trial protocol. 154 Another five trials reported details of primary and secondary outcomes in trial registries. 45,240–242,246 Of these, three trials failed to report all of the prespecified outcomes. 240,242,246 Of the remaining four trials,235,243–245 none was registered or had a published protocol and so these trials were considered to be at high risk of selective outcome reporting bias.
Source of funding bias
Sources of funding were reported in six trials. 45,154,235,240–242 In one trial the study was supported in part by the NIRS device manufacturer and was therefore considered to be at high risk of funding bias. 235 Four studies that failed to report the source of funding were considered at uncertain risk of funding bias. 243–246
Effects of interventions
A summary of the findings for all primary and secondary end points is provided in Table 88 and Figures 63–70.
Study | Outcome | |||||||||||||||
---|---|---|---|---|---|---|---|---|---|---|---|---|---|---|---|---|
Mortality, n (%) | MI, n (%) | Stroke, n (%) | AKI, n (%) | Reoperation for bleeding, n (%) | Transfusion, n (%) | ICU LOS (days), mean ± SD | Hospital LOS (days), mean ± SD | |||||||||
NIRS | Control | NIRS | Control | NIRS | Control | NIRS | Control | NIRS | Control | NIRS | Control | NIRS | Control | NIRS | Control | |
Deschamps et al., 2016241 | 4 (11.7) | 6 (13.0) | 0 | 0 | 1 (2.9) | 2 (4.3) | 12 (35.2) | 17 (47.2) | – | – | – | – | 2.65 ± 2.42 | 3.35 ± 3.61 | 11 ± 7.2 | 9.9 ± 5.8 |
Kara et al., 2015244 | – | – | – | – | – | – | – | – | – | – | – | – | 1.74 ± 0.81 | 2.12 ± 1.05 | 7.15 ± 1.39 | 7.67 ± 1.14 |
PASPORT, 2017154 | 1 (1) | 2 (1.88) | 1 (1) | 2 (1.88) | 1 (1) | 0 | 2 (2) | 5 (4.71) | 6 (6.1) | 4 (3.77) | 37 (37.7) | 44 (45.8) | 3.3 ± 0.81 | 3.67 ± 0.79 | 8.5 ± 4.2 | 8.9 ± 4.6 |
Colak et al., 2015240 | – | – | 8 (8.5) | 7 (7.29) | 4 (4.25) | 1 (1) | 0 | 1 (1) | 0 | 1 (1) | 77 (81) | 73 (76) | 2.7 ± 6.2 | 1.9 ± 0.9 | – | – |
Deschamps et al., 2013242 | – | – | – | – | – | – | – | – | – | – | – | – | 2.99 ± 2.26 | 0.39 ± 2.05 | 7.6 ± 5.4 | 7.9 ± 3.2 |
Vretzakis et al., 201345 | 1 (1.33) | 1 (1.33) | 2 (2.66) | 2 (2.66) | 1 (1.33) | 0 | 1 (1.33) | 0 | 1 (1.33) | 1 (1.33) | 51 (68) | 63 (84) | 2.7 ± 3.8 | 2.7 ± 3.6 | 10.9 ± 3.6 | 10.2 ± 10.7 |
Mohandas et al., 2013245 | – | – | – | – | 0 | 2 (4) | – | – | – | – | – | – | 1.49 ± 0.38 | 1.7 ± 0.49 | – | – |
Harilall et al., 2014243 | – | – | – | – | – | – | – | – | – | – | – | – | – | |||
Slater et al., 2009246 | – | – | 1 (0.8) | 1 (0.86) | 1 (0.8) | 0 | 1 (0.8) | 1 (0.86) | – | – | – | – | – | – | – | |
Murkin et al., 2007235 | 0 | 1 (1) | 1 (1) | 3 (3) | 1 (1) | 4 (4) | 0 | 0 | 1 (1) | 1 (1) | 8 (8) | 10 (10) | 1.25 ± 0.84 | 1.87 ± 2.67 | 6.1 ± 4.4 | 6.9 ± 5.5 |
Summary effect estimate, RR (95% CI) | 0.76 (0.30 to 1.96) | 0.90 (0.43 to 1.89) | 1.08 (0.40 to 2.91) | 0.88 (0.52 to 1.49) | 1.23 (0.46 to 3.32) | 0.93 (0.77 to 1.12) | MD –0.13 (–0.47 to 0.22) | MD –0.45 (–0.90 to –0.01) |
FIGURE 63.
Forest plot of effect estimates for mortality. df, degrees of freedom; M–H, Mantel–Haenszel.

FIGURE 64.
Forest plot of effect estimates for stroke. df, degrees of freedom; M–H, Mantel–Haenszel.
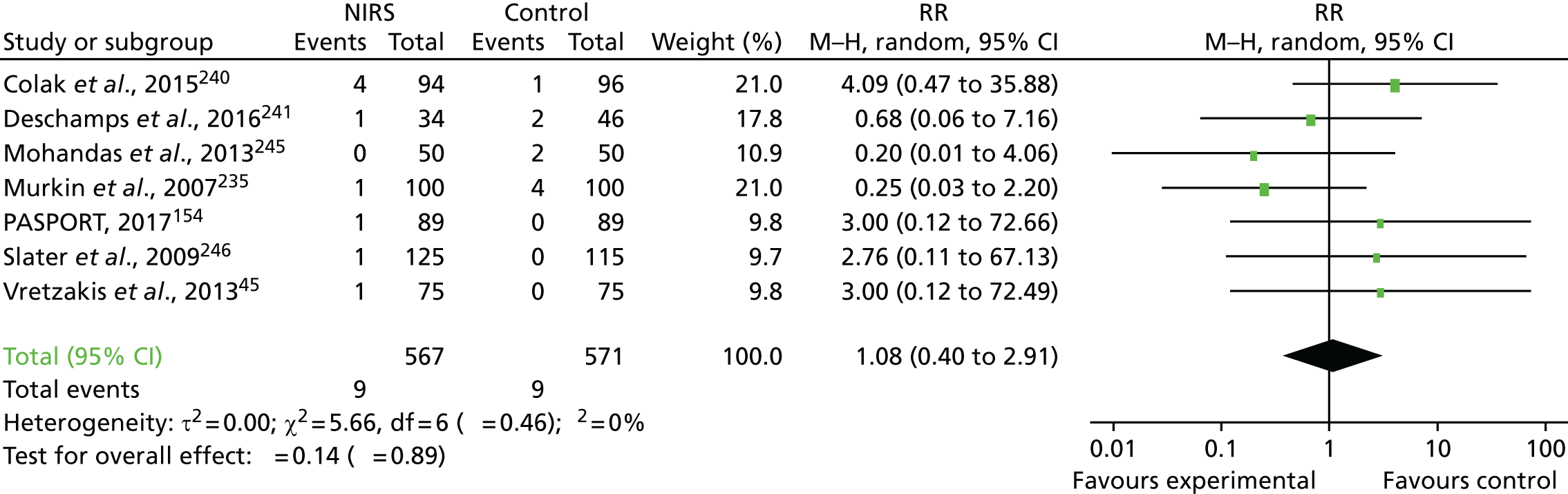
FIGURE 65.
Forest plot of effect estimates for MI. df, degrees of freedom; M–H, Mantel–Haenszel.

FIGURE 66.
Forest plot of effect estimates for severe AKI. df, degrees of freedom; M–H, Mantel–Haenszel.
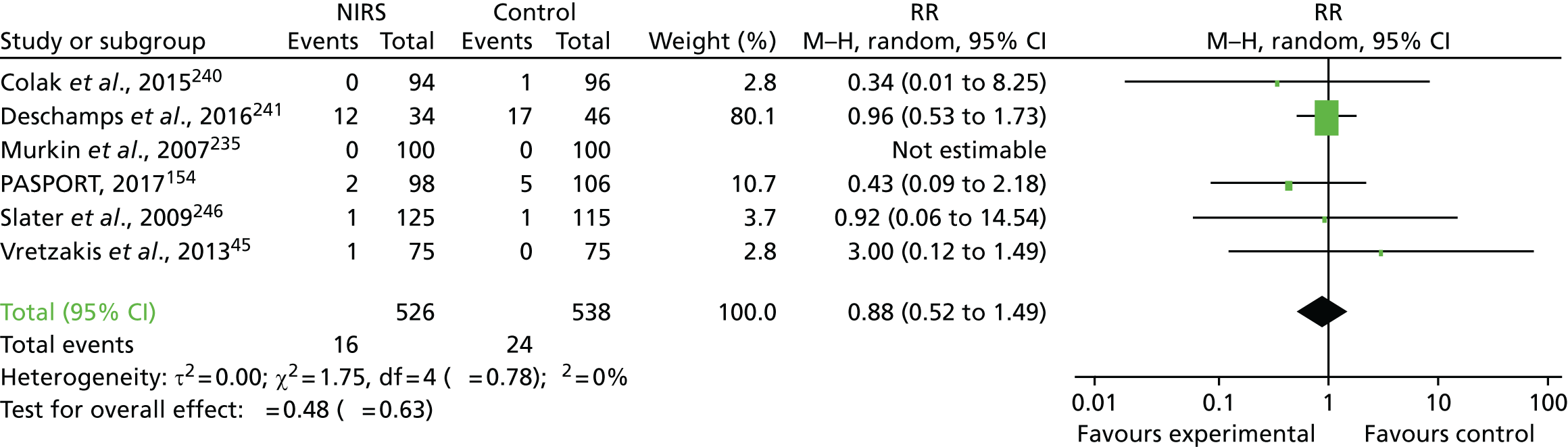
FIGURE 67.
Forest plot of effect estimates for red blood cell transfusion. df, degrees of freedom; M–H, Mantel–Haenszel.
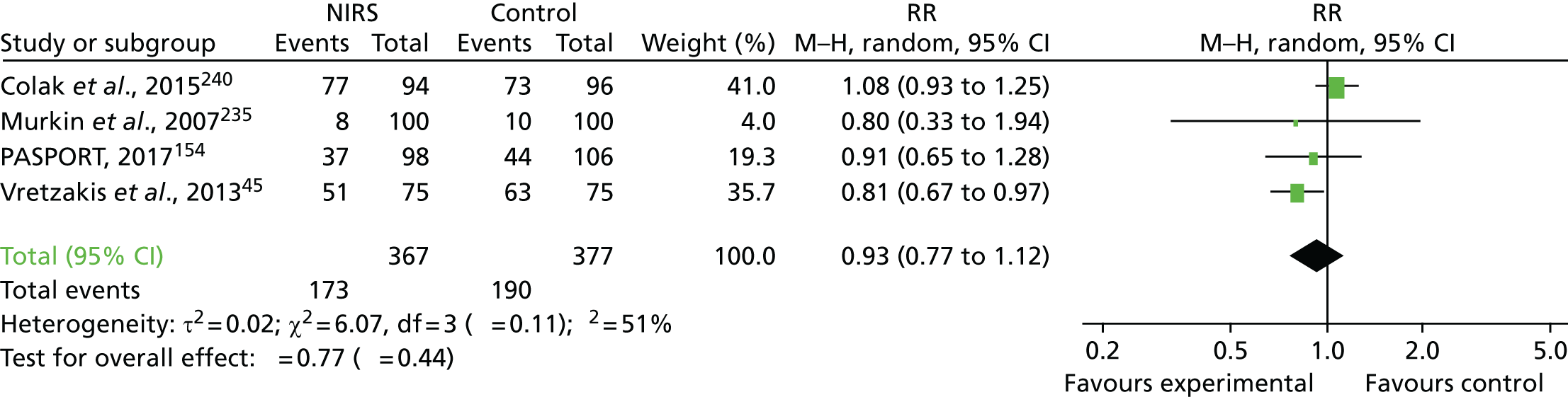
FIGURE 68.
Forest plot of effect estimates for reoperation for bleeding. df, degrees of freedom; M–H, Mantel–Haenszel.
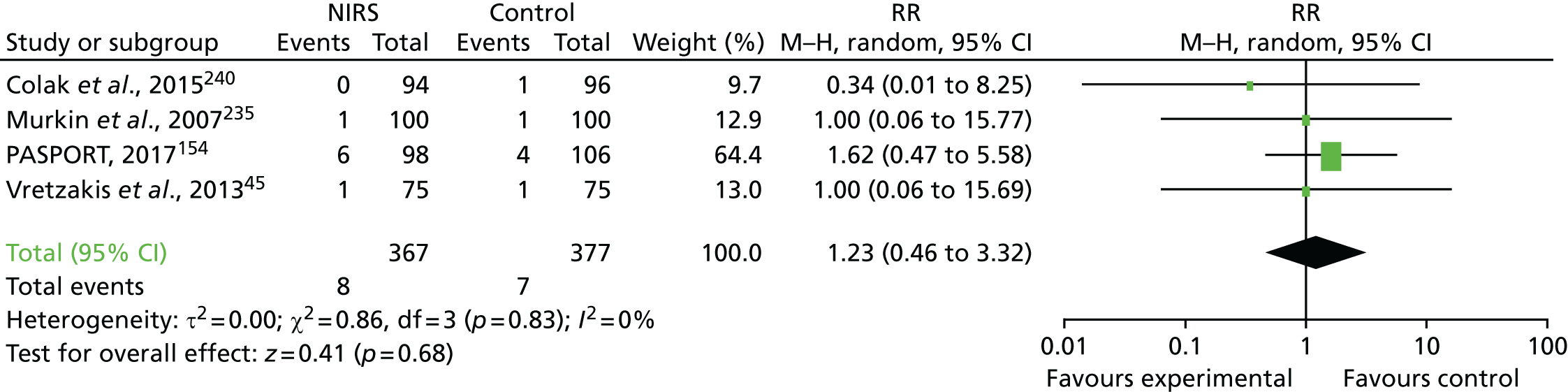
FIGURE 69.
Forest plot of effect estimates for ICU LOS. df, degrees of freedom; IV, inverse variance.

FIGURE 70.
Forest plot of effect estimates for hospital LOS. df, degrees of freedom; IV, inverse variance.
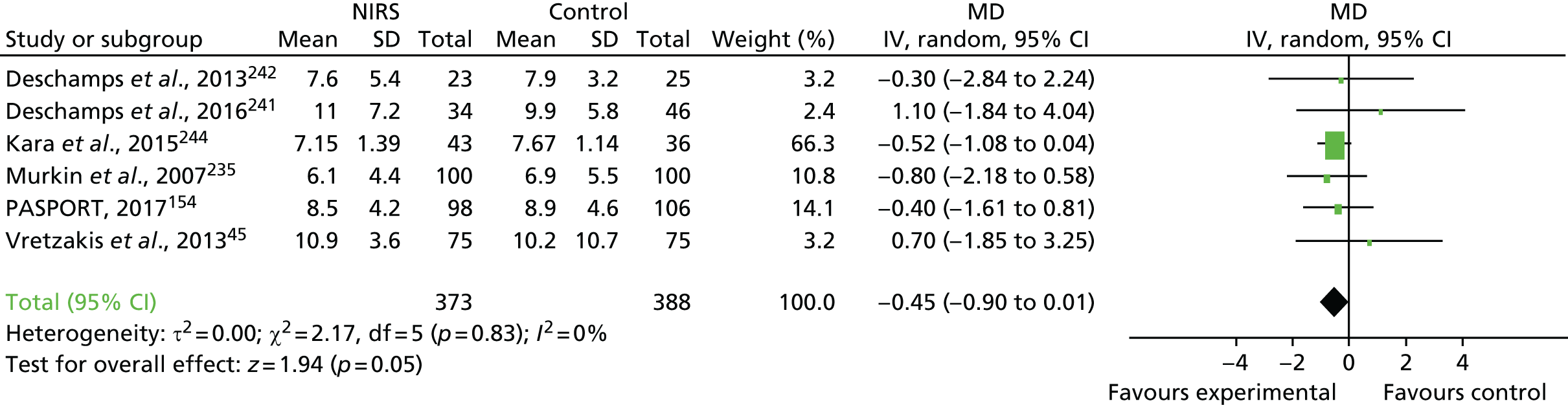
Mortality
Four trials with 608 participants reported this outcome. 45,154,235,241 There was no statistically significant difference in mortality between the NIRS group and the control group (random-effects RR 0.76, 95% CI 0.30 to 1.96; I2 = 0%; chi-squared test for heterogeneity p = 0.92; see Figure 63). A fixed-effect model resulted in similar estimates.
Stroke
Seven trials with 1138 participants reported this outcome. 45,154,235,240,241,245,246 There was no statistically significant difference in the frequency of stroke between the NIRS group and the control group (RR 1.08, 95% CI 0.40 to 2.91; I2 = 0%; chi-squared test for heterogeneity p = 0.46; see Figure 64). A fixed-effect model resulted in similar estimates.
Myocardial infarction
Six trials with 1038 participants reported this outcome. 45,154,235,240,241,246 There was no statistically significant difference in the frequency of MI between the NIRS group and the control group (RR 0.90, 95% CI 0.43 to 1.89; I2 = 0%; chi-squared test for heterogeneity p = 0.86; see Figure 65). A fixed-effect model resulted in similar estimates.
Severe acute kidney injury
Six trials with 1064 participants reported this outcome. 45,154,235,240,241,246 There was no statistically significant difference in the frequency of severe AKI between the NIRS group and the control group (RR 0.88, 95% CI 0.52 to 1.49; I2 = 0%; chi-squared test for heterogeneity p = 0.78; see Figure 66). A fixed-effect model resulted in similar estimates.
Red cell transfusion
Four trials with 744 participants reported this. 45,154,235,240 There was no statistically significant difference in the frequency of red cell transfusion between the NIRS group and the control group (RR 0.93, 95% CI 0.77 to 1.12; I2 = 51%; chi-squared test for heterogeneity p = 0.11; see Figure 67). A fixed-effect model resulted in similar estimates.
Reoperation for bleeding
Four trials with 744 participants reported this outcome. 45,154,235,240 There was no difference in the frequency of reoperation for bleeding between the NIRS group and the control group (RR 1.23, 95% CI 0.46 to 3.32; I2 = 0%; chi-squared test for heterogeneity p = 0.83; see Figure 68). A fixed-effect model resulted in similar estimates.
Neurocognitive testing and measuring neurocognitive dysfunction
Five trials that recruited 813 participants reported this outcome. 154,240,244–246 Details of these trials are provided in Table 89. The consensus statement for the assessment of neurocognitive function in cardiac surgery217 recommends that the following core tests are performed at baseline and up to 3 months post surgery to assess the neurocognitive domains of attention, verbal memory and motor co-ordination: the RAVLT, the Trail Making Test parts A and B and the Grooved Pegboard Test. The consensus statement defines cognitive decline as a difference for the individual of > 1 SD from baseline or a difference of > 1 SD between group means, with adjustment for baseline, for at least one test. There was significant heterogeneity between the trials in this review for this outcome. Only two trials154,246 measured cognitive function as recommended by the consensus statement. Both trials reported no difference in neurocognitive function between the groups. In one trial246 no test data were presented to support this conclusion. In the other trial154 there was a significant difference between the groups for the COWAT, which assesses the domain of executive function/verbal fluency; however, this test is not included in the consensus statement. The three remaining trials used non-consensus testing protocols and non-consensus definitions of neurocognitive decline,240,244,245 with only one testing participants at 3 months post surgery. 245 Because of the degree of heterogeneity we did not perform meta-analyses of these outcome. Overall, four out of five trials detected differences in neurocognitive test scores between the NIRS group and the control group.
Domain | Test protocol | Study | ||||
---|---|---|---|---|---|---|
Kara et al., 2015244 | Colak et al., 2015240 | PASPORT, 2017154 | Mohandas et al., 2013245 | Slater et al., 2009246 | ||
Timing | Baseline and pre discharge | Baseline and 7 days | Baseline, 7 days and 3 months | Baseline, 7 days and 3 months | Baseline, pre discharge and 3 months | |
Attention | Trail Making Test parts A and B or equivalent | ✗ (CTT 1) | ✗ | ✗ (SCW) | ||
Verbal memory | RAVLT or Hopkins Verbal Learning Test | ✗ | ✗ | |||
Visuospatial | Block Design Test from the WAIS | ✗ | ||||
Psychomotor speed | Digit Symbol Test from the WAIS | ✗ | ||||
Executive function/verbal fluency | COWAT | ✗ | ||||
Motor co-ordination | Grooved Pegboard Test | ✗ | ✗ | ✗ | ||
Other | Saccadic and antisaccadic eye movements | ✗ | ✗ | ✗ | ||
Montreal Cognitive Assessment | ✗ | |||||
Measurement of confounders | MMSE | ✗ | ✗ | ✗ | ✗ | |
Anxiety and depression | ✗ | ✗ | ||||
Adult Reading Test | ✗ | |||||
Medication | ✗ | |||||
Delirium Rating Scale | ✗ | |||||
Presented test data | ✗ | ✗ | ✗ | |||
Definition of cognitive decline | Maximum score on Montreal Cognitive Assessment test is 30; > 25 = normal, 19–25 = mild cognitive impairment, < 19 = serious cognitive impairment | Decrease in MMSE score of ≥ 3 points from the baseline value and decrease of ≥ 1 SD in performance on the CTT 1 and Grooved Pegboard Test | Difference in group mean (p < 0.05) in more than three of six domains | Postoperative MMSE impairment was defined as a decrease in score of > 20% of the preoperative value. Postoperative antisaccadic eye movement impairment was defined as a decrease in score of > 30% of the preoperative value | A decline of ≥ 1 SD in performance on one or more of the neuropsychological tests | |
Reported difference in cognitive function | ✗ | ✗ | ✗ | |||
Estimate of difference | Mild cognitive impairment: NIRS 7/43, control 16/36 (p = 0.01); severe cognitive impairment: NIRS 0/42, control 3/36 (p = 0.09) | Incidence of cognitive decline: NIRS 28%, control 52% (p = 0.0002) | Significant difference in COWAT score: MD 3.73 (95% CI 1.5 to 5.96) (p = 0.0011) | Mean (SD) antisaccadic eye movement score at 3 months: NIRS 17.68 (1.79), control 15.69 (3.99) (p < 0.001) | Incidence of cognitive decline: NIRS 58%, control 61% |
Intensive care unit length of stay
Eight trials with 1051 participants reported this outcome. 45,154,235,240–242,244,245 There was no statistically significant difference in the duration of ICU stay between the NIRS group and the control group, with moderate heterogeneity (MD –0.13 days, 95% CI –0.47 to 0.22 days; I2 = 75%; chi-squared test for heterogeneity p = 0.0003; see Figure 69). A fixed-effects model also showed no difference in ICU stay between the groups (MD –0.23 days, 95% CI –0.38 to –0.14 days; I2 = 75%; chi-squared test for heterogeneity p = 0.0003).
Hospital length of stay
Six trials with 761 participants reported this outcome. 45,154,235,241,242,244 There a reduction in the duration of hospital stay in the NIRS group compared with the control group, with no heterogeneity (MD –0.45 days, 95% CI –0.90 to 0.01 days; I2 = 0%; chi-squared test for heterogeneity p = 0.82; see Figure 70). This did not reach conventional statistical significance (p = 0.05). A fixed-effect model resulted in similar estimates.
S100B
Two trials reported this outcome in 138 participants. 154,243 One trial with 40 participants243 measured S100B pre- and postoperatively and reported a significant reduction in S100B concentration in NIRS participants (MD –99.87 pg/ml, 95% CI –105.18 to –94.56 pg/ml). The time of S100B measurement post surgery was not reported. Another trial with 98 participants154 measured S100B preoperatively and at five post-surgery time points: on return to the ICU and at 6, 12–24, 24–48 and 96 hours. There was no difference between the groups (GMR 1.06, 95% CI 0.95 to 1.19; p = 0.29). No meta-analysis was performed because of the heterogeneity for this outcome.
Subgroup analyses
The results of the subgroup analyses for Murkin compared with non-Murkin algorithms, CABG compared with non CABG, consensus neurocognitive assessment compared with non-consensus neurocognitive assessment and restrictive red cell transfusion trigger compared with no restrictive red cell transfusion trigger are provided in Table 90.
Outcome | Subgroup | |||||||||||
---|---|---|---|---|---|---|---|---|---|---|---|---|
CABG | Non-CABG | Test for subgroup differences | Murkin algorithm | Non-Murkin algorithm | Test for subgroup differences | Consensus neurocognitive assessment | Non-consensus neurocognitive assessment | Test for subgroup differences | Restrictive red cell transfusion trigger | No restrictive red cell transfusion trigger | Test for subgroup differences | |
Mortality, RR (95% CI) | 0.33 (0.01 to 8.09), 1 trial, n = 200, I2 = NA | 0.81 (0.30 to 2.18), 3 trials, n = 408, I2 = 0% | χ2 = 0.50, df = 3 (p = 0.92), I2 = 0% | 0.77 (0.26 to 2.32), 2 trials, n = 289, I2 = NA | 0.67 (0.11 to 3.94), 2 trials, n = 328, I2 = 0% | χ2 = 0.50, df = 3 (p = 0.92), I2 = 0% | 0.50 (0.05 to 5.42), 1 trial, n = 178, I2 = NA | 0.94 (0.39 to 2.26), 3 trials, n = 430, I2 = 4% | χ2 = 0.23, df = 3 (p = 0.97), I2 = 0% | 0.67 (0.11 to 3.94), 2 trials, n = 328, I2 = 0% | 0.77 (0.26 to 2.32), 2 trials, n = 280, I2 = NA | χ2 = 0.50, df = 3 (p = 0.92), I2 = 0% |
Stroke, RR (95% CI) | 1.18 (0.38 to 3.63), 3 trials, n = 630, I2 = 43% | 0.89 (0.26 to 3.10), 4 trials, n = 508, I2 = 1% | χ2 = 5.66, df = 6 (p = 0.46), I2 = 0% | 0.84 (0.33 to 2.11), 5 trials, n = 810, I2 = 14% | 3.00 (0.32 to 28.54), 3 trials, n = 328, I2 = 0% | χ2 = 5.66, df = 6 (p = 0.46), I2 = 0% | 3.00 (0.12 to 72.66), 1 trial, n = 178, I2 = NA | 1.00 (0.39 to 2.56), 6 trials, n = 960, I2 = 22% | χ2 = 5.66, df = 6 (p = 0.46), I2 = 0% | 3.00 (0.32 to 28.54), 2 trials, n = 328, I2 = 0% | 0.84 (0.33 to 2.11), 5 trials, n = 810, I2 = 14% | χ2 = 5.66, df = 6 (p = 0.46), I2 = 0% |
MI, RR (95% CI) | 0.92 (0.40 to 2.10), 3 trials, n = 630, I2 = 0% | 0.75 (0.17 to 3.30), 3 trials, n = 408, I2 = 0% | χ2 = 0.05, df = 1 (p = 0.82), I2 = 0% | 0.92 (0.40 to 2.10), 4 trials, n = 710, I2 = 0% | 0.75 (0.17 to 3.30), 2 trials, n = 328, I2 = 0% | χ2 = 0.05, df = 1 (p = 0.82), I2 = 0% | 0.50 (0.05 to 5.42), 1 trial, n = 178, I2 = NA | 0.93 (0.43 to 1.99), 5 trials, n = 860, I2 = 0% | χ2 = 0.24, df = 1 (p = 0.63), I2 = 0% | 0.75 (0.17 to 3.30), 2 trials, n = 328, I2 = 0% | 0.92 (0.40 to 2.10), 4 trials, n = 710, I2 = 0% | χ2 = 0.05, df = 1 (p = 0.82), I2 = 0% |
Severe AKI, RR (95% CI) | 0.58 (0.08 to 4.41), 3 trials, n = 630, I2 = 0% | 0.88 (0.51 to 1.12), 4 trials, n = 434, I2 = 0% | χ2 = 0.15, df = 1 (p = 0.70), I2 = 0% | 0.90 (0.51 to 0.19), 4 trials, n = 710, I2 = 0% | 0.67 (0.18 to 2.56), 2 trials, n = 354, I2 = 12% | χ2 = 0.15, df = 1 (p = 0.70), I2 = 0% | 3.00 (0.12 to 72.49), 1 trial, n = 150, I2 = NA | 0.96 (0.55 to 1.68), 3 trials, n = 780, I2 = 0% | χ2 = 0.48, df = 1 (p = 0.49), I2 = 0% | 0.67 (0.18 to 2.56), 2 trials, n = 354, I2 = 12% | 0.90 (0.51 to 1.19), 2 trials, n = 630, I2 = 0% | χ2 = 0.15, df = 1 (p = 0.70), I2 = 0% |
Transfusion, RR (95% CI) | 1.04 (0.89 to 1.23), 2 trials, n = 390, I2 = 0% | 0.85 (0.71 to 1.01), 2 trials, n = 354, I2 = 0% | χ2 = 2.81, df = 1 (p = 0.09), I2 = 64.4% | 1.04 (0.89 to 1.23), 2 trials, n = 390, I2 = 0% | 0.85 (0.71 to 1.01), 2 trials, n = 354, I2 = 0% | χ2 = 2.81, df = 1 (p = 0.09), I2 = 64.4% | 0.91 (0.65 to 1.28), 1 trial, n = 150, I2 = NA | 0.94 (0.83 to 1.06), 5 trials, n = 860, I2 = 66% | χ2 = 0.04, df = 1 (p = 0.85), I2 = 0% | 0.85 (0.71 to 1.01), 2 trials, n = 354, I2 = 0% | 1.04 (0.89 to 1.23), 4 trials, n = 710, I2 = 0% | χ2 = 2.81, df = 1 (p = 0.09), I2 = 64.4% |
ICU LOS (days), MD (95% CI) | –0.39 (–0.71 to –0.06), 3 trials, n = 469, I2 = 51% | –0.24 (–0.37 to –0.10), 5 trials, n = 582, I2 = 82% | χ2 = 0.70, df = 1 (p = 0.4), I2 = 0% | –0.19 (–0.35 to –0.03), 5 trials, n = 618, I2 = 84% | –0.36 (–0.55 to –0.17), 3 trials, n = 433, I2 = 0% | χ2 = 1.87, df = 1 (p = 0.17), I2 = 46.5% | –0.37 (–0.59 to –0.15), 1 trial, n = 204, I2 = NA | –0.21 (–0.50 to 0.09), 6 trials, n = 747, I2 = 81% | χ2 = 0.77, df = 1 (p = 0.38), I2 = 0% | –0.36 (–0.57 to –0.14), 2 trials, n = 354, I2 = 0% | –0.21 (–0.36 to –0.06), 6 trials, n = 697, I2 = 81% | χ2 = 1.18, df = 1 (p = 0.28), I2 = 14.9% |
Hospital LOS (days), MD (95% CI) | –056 (–1.08 to –0.04), 2 trials, n = 279, I2 = 0% | –0.46 (–0.77 to –0.15), 4 trials, n = 482, I2 = 0% | χ2 = 0.1, df = 1 (p = 0.75), I2 = 0% | –0.43 (–1.55 to 0.69), 3 trials, n = 328, I2 = 0% | –0.49 (–0.77 to –0.22), 3 trials, n = 433, I2 = 0% | χ2 = 0.01, df = 1 (p = 0.91), I2 = 0% | –0.50 (–0.82 to –0.18), 1 trial, n = 204, I2 = NA | –0.46 (0.05 to 0.93), 5 trials, n = 557, I2 = 0% | χ2 = 0.02, df = 1 (p = 0.89), I2 = 0% | –0.48 (–0.80 to –0.17), 2 trials, n = 354, I2 = 0% | –0.50 (–1.00 to –0.00), 4 trials, n = 407, I2 = 0% | χ2 = 0.00, df = 1 (p = 0.95), I2 = 0% |
Publication bias
Funnel plots were not generated as there were < 10 trials reporting results for any of the specified end points in the review.
Sensitivity analyses
We conducted a sensitivity analyses in two trials identified as being at low risk of bias. 45,154 These trials reported outcomes in 328 participants. Both trials incorporated restrictive red cell transfusion thresholds within the NIRS algorithm. Both trials reported no difference between the NIRS group and the control group for mortality (RR 0.67, 95% CI 0.11 to 24.08; I2 = 0%; chi-squared test for heterogeneity p = 0.14), stroke (RR 3.0, 95% CI –0.32 to 28.54; I2 = 0%; chi-squared test for heterogeneity p = 0.34), MI (RR 0.76, 95% CI 0.17 to 3.41; I2 = 0%; chi-squared test for heterogeneity p = 0.72), severe AKI (RR 0.69, 95% CI 0.14 to 3.49; I2 = 0%; chi-squared test for heterogeneity p = 0.65) and reoperation for bleeding (RR 1.50, 95% CI 0.48 to 4.62; I2 = 0%; chi-squared test for heterogeneity p = 0.75). Analyses of the data from the two trials suggested that the use of a NIRS-based algorithm resulted in reductions in red cell transfusion (RR 0.83, 95% CI 0.71 to 0.98; I2 = 0%; chi-squared test for heterogeneity p = 0.52), ICU LOS (RR –0.36, 95% CI –0.57 to –0.14; I2 = 0%; chi-squared test for heterogeneity p = 0.55) and hospital LOS (RR –0.48, 95% CI –0.80 to –0.17; I2 = 0%; chi-squared test for heterogeneity p = 0.36). One of the trials, the PASPORT trial,154 reported the results of neurocognitive assessments and concluded that there was no difference between the NIRS group and the control group.
Summary of the main findings
The main findings are summarised in Table 91. In this systematic review we examined the utility of NIRS-based patient-specific goal-directed algorithms for the management of cardiac surgery patients. All of the trials were conducted in adult patients and NIRS-based patient-specific goal-directed algorithms did not reduce mortality or severe morbidity, red cell transfusion or bleeding or resource use. A qualitative review demonstrated inconsistent evidence that the use of NIRS-based patient-specific goal-directed algorithms resulted in improvements in neurocognitive function. A post hoc sensitivity analysis that considered two trials with a low risk of bias showed similar results to those of the primary analysis. Both trials combined NIRS with a restrictive red cell transfusion threshold and meta-analysis demonstrated a reduction in red cell transfusion with this approach, with no difference between the NIRS group and the control group with respect to mortality, morbidity and ICU or hospital stay. Overall, the GRADE quality of the evidence was low or very low for all of the outcomes measured.
Outcomes | Anticipated absolute effectsa (95% CI) | Relative effect (95% CI) | Number of participants (RCTs) | Quality of the evidence (GRADE)b | |
---|---|---|---|---|---|
Risk with control | Risk with NIRS | ||||
Mortality | 32 per 1000 | 25 per 1000 (10 to 63 per 1000) | RR 0.76 (0.30 to 1.96) | 608 (4) | ⊕⊕○○ Low |
Red cell transfusion | 504 per 1000 | 469 per 1000 (388 to 564 per 1000) | RR 0.93 (0.77 to 1.12) | 744 (4) | ⊕⊕○○ Low |
Stroke | 16 per 1000 | 17 per 1000 (6 to 46 per 1000) | RR 1.08 (0.40 to 2.91) | 1138 (7) | ⊕○○○ Very low |
MI | 29 per 1000 | 26 per 1000 (12 to 54 per 1000) | RR 0.90 (0.43 to 1.89) | 1038 (6) | ⊕○○○ Very low |
Renal failure | 71 per 1000 | 62 per 1000 (41 to 95 per 1000) | RR 0.88 (0.58 to 1.34) | 1043 (6) | ⊕○○○ Very low |
Reoperation for bleeding | 19 per 1000 | 21 per 1000 (8 to 56 per 1000) | RR 1.11 (0.41 to 3.04) | 744 (4) | ⊕○○○ Very low |
ICU LOS | The mean ICU LOS in the intervention group was 0.13 days lower (0.47 days lower to 0.22 days higher) | ⊕○○○ Very low |
|||
Hospital LOS | The mean hospital LOS was 0.45 days lower (0.9 days lower to 0.01 days higher) | ⊕⊕○○ Low |
Discussion
The PASPORT trial
Main findings
The PASPORT trial tested the hypothesis that a NIRS-based patient-specific algorithm for the management of CPB would result in reductions in organ injury (brain, kidney, heart) and red cell transfusion and would be cost-effective. Our findings did not support this hypothesis; we observed no difference between the groups in the primary outcome, cognitive dysfunction, and in a range of secondary outcomes including transfusion, adverse events, quality of life and costs. There was also no difference between the groups in levels of biomarkers of inflammation or in brain, kidney or myocardial injury. There was no clear difference between the cost-effectiveness of the NIRS-based algorithm the cost-effectiveness of standard care.
Strengths and limitations
The PASPORT trial had several strengths. It compared a widely used patient-specific NIRS algorithm with standard care in three UK centres where this technology is in common use for the optimisation of tissue oxygenation during CPB. It used detailed neurocognitive assessments and complementary clinical measures and biomarkers of injury and dysfunction in multiple organ systems. The principal limitation of the trial was the lack of blinding of health-care personnel. We addressed this by documenting protocol compliance. This demonstrated differences in the nature of protocol non-adherence by group and also that the proportion of participants with non-adherence to the Murkin algorithm in the intervention arm was also high (18%). This occurred despite all of the members of the clinical team at each of the recruiting centres being familiar with the technology prior to conducting the trial. However, high levels of protocol non-adherence have been reported in other trials45 and we suggest that our results may reflect the normal application of this technology, given the complexity and variability of the operating theatre environment.
In the PASPORT trial detailed neurocognitive assessments were conducted at three time points. The sample size was increased to adjust for higher than anticipated attrition and the resulting sample size was adequate to test the primary hypothesis of the trial. There were no differences between the groups with respect to any of the core domains as defined by the consensus statement for the assessment of neurocognitive function in cardiac surgery. 217 We detected a difference in the domain of executive function/verbal fluency that favoured the NIRS group; however, this is not a core domain and did not meet the prespecified definition of neurocognitive dysfunction in our statistical analysis plan. We therefore concluded that this was not sufficient to demonstrate efficacy. This conclusion was supported by there being no difference between the groups for the brain injury biomarker S100B. NIRS levels were similar in both groups, with relatively few participants experiencing cerebral desaturation. We suggest that this may have contributed to the clinical findings; low frequencies of cerebral desaturation reduce the likelihood that NIRS-based algorithms will have clinical utility. This is perhaps attributable to the supranormal levels of oxygen delivery during CPB measured in the study; almost no participant had a level approaching critical oxygen delivery (< 500 ml/minute). The potential for NIRS to contribute to cerebral protection will also have been influenced by the presence or absence of other factors such as cerebral emboli or pre-existing white matter changes, which may be more frequent and significant contributors to post-cardiac surgery cognitive decline. 251 There were no differences between the groups in injury to other organs, specifically the heart and kidney, further supporting the result of our primary analysis. These findings do not support the hypothesis that a patient-specific algorithm that aims to optimise cerebral NIRS values may have additional important benefits for these organs. 44
Economic evaluation
There was very little difference between the patient-specific algorithm group and the generic algorithm group in terms of either costs or effects, and uncertainty around the cost-effectiveness results. The differences seen in costs and QALYs between the groups were not statistically significant. On average, the patient-specific algorithm group was less costly but also gained fewer QALYs than the generic algorithm group. The incremental saving associated with the loss of 1 QALY by adopting a patient-specific algorithm rather than a generic algorithm was £51,616. If a decision-maker’s willingness to accept compensation for the loss of 1 QALY were £20,000, then based on the point estimate of the ICER a patient-specific algorithm would be considered cost-effective. However, there is uncertainty around this finding, largely because of the very small differences in costs and effects.
With respect to the budget impact of the cost reduction observed with the intervention, a difference of approximately £400 between the groups is a modest cost difference. However, as 14,535 procedures (either valve surgery or valve surgery plus CABG) were undertaken in the UK in 2013/14,120 this cost reduction would have resulted in savings of £5.8M for the NHS per annum. In terms of making recommendations for clinical decision-making based on these cost-effectiveness results, decision-makers need to decide if they are willing to make a trade-off between costs and effects, that is, if they are willing to accept a slightly less effective option for patients (one that provides marginally fewer QALYs) to have a slightly cheaper option.
Systematic review
Main findings
In the systematic review we examined the clinical effectiveness of NIRS-based patient-specific goal-directed algorithms for the prevention of organ failure, sepsis and death in cardiac surgery patients. All of the trials included in the review were conducted in adult patients and the NIRS-based patient-specific goal-directed algorithms did not reduce mortality or severe morbidity, red cell transfusion, bleeding or resource use. A qualitative review of studies that had assessed the effect of NIRS on neurocognitive function did not resolve uncertainty over the benefits of NIRS with respect to this outcome. A post hoc sensitivity analysis that considered two trials with a low risk of bias showed similar results to those of the primary analysis. Both trials combined NIRS with a restrictive red cell transfusion threshold and meta-analysis demonstrated a reduction in red cell transfusion with this approach, in addition to reductions in the ICU and hospital stay. Overall, the GRADE quality of the evidence was very low for all of the outcomes measured.
Strengths and limitations
Overall completeness and applicability of the evidence
This review is applicable to adults undergoing cardiac surgery. No paediatric trials were identified although this is a common clinical setting for the use of NIRS. This review has therefore identified a clear knowledge gap with respect to the utility of this intervention in paediatric cardiac surgery.
Quality of the evidence
Overall, the quality of the evidence was very low for the measured outcomes, most often because of the high risk of bias of individual trials. In addition, many trials did not report important clinical outcomes such as death, although it is highly likely that this outcome was measured. Furthermore, in many cases, although the trial report stated that important assessments had been carried out, particularly with respect to the testing of cognitive function, the results of these assessments were not reported. We approached the authors of the studies to request access to the missing data, of whom three responded. This resulted in a high risk of selective reporting bias.
Potential bias in the review process
This is the most comprehensive evaluation of NIRS-based patient-specific goal-directed algorithms in cardiac surgery to date. It used comprehensive search strategies in a wide range of registries and data sources, had access to the full texts of all identified trials, used contemporary risk of bias assessments (GRADE) and assessed a wide range of outcomes after cardiac surgery. The main limitation of the review is that we did not have access to all of the source data. We realise that consistent analyses of all of the studies can be carried out only when data on individual participants are combined. In addition, the review identified important limitations of existing data. All nine of the published RCTs had limitations in terms of methodological quality. The risk of procedural bias was high in these trials as there was no blinding of clinical personnel. Furthermore, only two of nine trials attempted to define the likelihood of procedural bias by describing the degree of protocol adherence. The reporting of outcomes was also heterogeneous between the trials, limiting the number of studies that could be included for each outcome. This was most evident for the outcome of cognitive function. A final limitation is that we included the unpublished results of the PASPORT trial in the systematic review. Our assumptions are therefore potentially based on an incomplete data set, as the exclusion of any other trials awaiting publication may have influenced our findings. This limitation will be addressed by an updated systematic review once the results of the PASPORT trial have been published. However, despite these sources of bias and heterogeneity, the results were remarkably consistent, with low or no heterogeneity for all but one of the analyses of prespecified primary and secondary end points, all of which indicated a lack of efficacy of NIRS-based algorithms.
Clinical importance
Monitoring technology has the potential to enable more personalised, goal-directed medical management. NIRS monitoring meets many of these specifications: it is non-invasive and tissue specific and provides data that correlate closely with jugular venous saturations, a measure of cerebral oxygen extraction. 204 This has led to its use in multiple settings in addition to adult cardiac surgery, including the assessment of visceral perfusion in paediatric cardiac surgery198 and cerebral oxygenation in neonatal intensive care. 252 It is important, therefore, to consider the negative findings that we have presented. First, we must consider that our results are attributable to chance. Our GRADE assessment was very low for all of the prespecified outcomes, indicating a high likelihood that these conclusions may be altered by subsequent trials. There was almost no heterogeneity for these outcomes, however, and these findings were consistent with the results of the PASPORT trial, which was at low risk of bias for all of the domains assessed. An alternative hypothesis is that NIRS has limited or no efficacy. This is not implausible: cerebral venous oxygen saturation, as measured by NIRS, may be influenced by multiple factors that result in high values in the presence of tissue hypoxia. Supranormal oxygen delivery or alternatively diminished tissue oxygen utilisation in states in which cerebral autoregulation is impaired, as is frequently observed during CPB,253,254 may result in spuriously high values. Our observation that, despite the low frequency of cerebral desaturation, 41% of participants had dysfunction of at least one organ system supports this hypothesis. Alternative non-invasive monitors of cellular oxygenation exist. On the basis of our current findings we suggest that further research must evaluate technologies that focus on tissue oxygen utilisation, rather than mixed venous oxygenation, as measures of therapeutic effectiveness.
Summary and conclusions
What this study shows
-
We conducted a multicentre RCT to evaluate the utility and cost-effectiveness of a NIRS-based patient-specific goal-directed algorithm compared with standard care in adult cardiac surgery.
-
In this RCT NIRS was not found to reduce neurocognitive impairment, inflammation or organ injury. There was no clear difference between the cost-effectiveness of the NIRS-based intervention and that of standard care.
-
To assess the implications of these findings in the context of existing evidence we conducted a systematic review of this and other similar trials to test the hypothesis that the use of NIRS would have clinical benefits, reduce resource use or assist with personalised red cell transfusion.
-
On the basis of very low-quality evidence we have determined that the use of NIRS-based patient-specific goal-directed algorithms may reduce the duration of hospitalisation for adults undergoing cardiac surgery. There was no reduction in mortality or major morbidity or transfusion rates.
-
The combination of a NIRS-based algorithm and a restrictive red cell transfusion trigger may result in reduced red cell transfusion rates, although this did not result in other important clinical benefits.
Further research
-
The use of a GRADE approach to judge the overall quality of the evidence suggests that there is a high likelihood that further well-conducted trials will alter the effect estimates that have been reported in this review.
-
There is a need for further RCTs at low risk of bias to assess the clinical utility of NIRS in both adult and paediatric cardiac surgery.
-
Future studies should be powered to assess the effect of NIRS on important clinical outcomes. The cost-effectiveness of this technology has also been assessed only in a single trial to date.
Chapter 5 A randomised controlled trial of red cell washing for the attenuation of transfusion-associated organ injury in cardiac surgery: the REDWASH trial
Abstract
Background: Experimental studies suggest that mechanical cell washing to remove pro-inflammatory materials that accumulate in the supernatant of stored donor red cells may reduce inflammation and organ injury in transfused patients.
Methods: Cardiac surgery patients at increased risk of LVBT were eligible. Participants were randomised to receive either mechanically washed allogenic red cells or standard care red cells. The primary outcome was serum IL-8 concentration, measured at baseline and at four post-surgery time points. A mechanism substudy evaluated the effects of washing on stored red cells in vitro, as well as on markers of platelet, leucocyte and endothelial activation in trial participants.
Results: Sixty adult cardiac surgery patients at three UK cardiac centres were enrolled between September 2013 and March 2015. Participants received a median of 3.5 units (IQR 2–5.5 units) of red cells, stored for a mean of 21 days (SD 5.2 days), within 48 hours of surgery. Mechanical washing reduced levels of red cell-derived microvesicles but increased cell-free haemoglobin levels in red cell supernatant relative to standard care red cells. There was no difference between the trial groups with respect to perioperative serum IL8 levels (adjusted MD 0.239 pg/ml, 95% CI –0.231 to 0.709 pg/ml; p = 0.318) or levels of plasma red cell microvesicles, platelet and leucocyte activation, plasma cell-free haemoglobin levels, endothelial activation or biomarkers of myocardial, lung or kidney injury.
Conclusions: These results do not support a hypothesis that allogenic red cell washing has clinical benefits in cardiac surgery.
Trial registration: Current Controlled Trials ISRCTN27076315.
Background
The clinical problem
Organ failure and sepsis are common and severe complications of cardiac surgery that contribute to the majority of postoperative deaths. In a recent trial, sepsis and acute kidney, lung and myocardial injury occurred in 23%, 34%, 16% and 11% of participants, respectively, and preceded 39%, 41%, 36% and 24% of all deaths respectively. 4 Organ injury and immunomodulation associated with red cell transfusion have been attributed to the ‘storage lesion’, changes in red cell properties and accumulation of inflammatory particles in the supernatant of red cell units during storage. 33 Experimental studies have shown that these changes cause inflammatory organ injury through complex mechanisms including platelet and monocyte activation, endothelial injury and oxidative stress and the loss of microcirculatory autoregulation. 255–258 This results in paradoxical tissue hypoxia, despite apparently adequate oxygen delivery, tissue inflammation and organ dysfunction. The most important change that occurs in stored red cells over time is the depletion of high-energy phosphates. 33 This leads to the loss of autoregulatory function, erythrocyte deformability and changes in erythrocyte morphology, which are associated with abnormal gas transfer and microcirculatory flow. A significant aspect of these changes is the overexpression of phosphatidylserine on the red cell surface, attributable to diminished function of the ATP-dependent membrane-bound flippase enzyme, which acts to maintain membrane asymmetry by transporting phosphatidylserine to the interior of the membrane and phosphatidylcholine to the exterior. 259 High levels of phosphatidylserine expression are associated with rapid uptake of donor red cells by recipient myeloid tissue and hence diminished donor cell survival. They are also associated with the release of membrane vesicles from erythrocytes during storage. 260 These microvesicles express high levels of phosphatidylserine, which has potent inflammatory effects. 261 Microvesicle levels increase progressively from the onset of storage and may be removed by mechanical cell washing. On the basis of these results we hypothesised that the release of microvesicles by erythrocytes during storage is associated with inflammatory organ injury in cardiac surgery patients receiving blood transfusion and that this may be prevented by red cell washing prior to transfusion. We tested this hypothesis in the REDWASH trial.
Red cell washing
Red cell washing devices are ubiquitous in cardiac surgery. The salvage, washing and transfusion of autologous blood lost from the operative field is part of standard care and has been shown to improve clinical outcomes, perhaps by reducing allogenic red cell exposure. 16 Washing of allogenic red cells was also until recently widely used in paediatric cardiac surgery. Low-birthweight neonates and small infants are susceptible to the high ion (free haemoglobin, potassium and calcium) concentrations that are present in older red cell units and it is standard practice in many centres to wash these red cells using cell salvage devices prior to administration. 47 However, a RCT of red cell washing in paediatric cardiac surgery patients failed to show a clinical benefit, although there was a reduction in a measure of inflammation (IL-6 : IL-10 ratio). 46 Importantly, no adverse effects of this technique were reported in recipients. Standard cell saver devices use low-speed centrifugation with resuspension in normalised saline without apparent detriment to human autologous or allogenic red cells. 31,262 Our own studies (unpublished) have also documented significant homology between the effect of mechanical red cell washing using low-speed centrifugation with the Fresenius Continuous AutoTransfusion System (CATS®, Fresenius AG, Bad Homburg, Germany) and the washed red cells produced by the UK National Blood Service for patients at increased risk of hypersensitivity reactions. We considered that the pre-transfusion washing of stored donor cells at the bedside using commonly used cell salvage devices offered a simple and practical blood safety intervention.
Data from experimental studies of red cell washing
Effects of washing on red cell-derived microparticles, monocyte and leucocyte activation and organ injury
In previous work we have shown that microvesicles positive for CD235a and annexin V accumulate throughout human red cell storage. 263 Mechanical washing of red cells significantly reduced concentrations of CD235a/annexin V microvesicles as well as total microvesicle concentrations at day 41 and day 21, the median storage time of red cells transfused in the REDWASH trial. In further (unpublished) work we have shown that oxidised lipids on the surface of microvesicles directly activate monocytes and phosphatidylserine on the surface of microvesicles directly activates platelets. In a porcine model (unpublished observations) we observed that transfusion of 14-day stored red cells (D14) resulted in alveolar protein leak, histological lung injury, platelet activation (as indicated by a blunted aggregation response using Multiplate aggregometry), IL-6 release and leucocyte (CD16 and CD14) invasion of the lungs and kidney. Red cell washing (D14W) attenuated all of these changes. On the basis of these experimental observations we hypothesised that red cell-derived microvesicles activate platelets and leucocytes and that this contributes to inflammation and lung injury in transfusion recipients. We tested this hypothesis in a prespecified mechanism substudy conducted in the first 60 participants recruited to the REDWASH trial.
Effects of washing on cell-free haemoglobin concentrations, endothelial injury and organ injury
In previous work we have demonstrated that cell-free haemoglobin levels increase progressively in the supernatant of human red cell units during storage. 263 Mechanical washing did not reduce levels of free haemoglobin in 35-day stored human red cells and doubled free haemoglobin levels in 21-day stored red cells. In human umbilical vein endothelial cell static culture experiments we demonstrated that free haemoglobin (1 mg/ml) directly increased endothelial permeability, whereas microvesicles, or phosphate-buffered saline (PBS) (negative control), did not. In further static culture experiments we demonstrated that free haemoglobin did not increase expression of endothelial E-selectin expression. Conversely, free haemoglobin increased expression of activated integrin-β1 and connecting segment-1 (CS-1) fibronectin on the surface of human umbilical vein endothelial cells, indicating an alternative activation pathway for haemoglobin in endothelial cells. 263 In the porcine model we observed that recipients of washed 14-day stored red cells developed significantly higher plasma free haemoglobin levels than other groups. Transfusion of washed 14-day stored cells also resulted in increased pulmonary levels of tumour necrosis factor-α (TNF-α), pulmonary vascular endothelial dysfunction and increased haemoxygenase-1 expression in porcine lungs, renal vascular endothelial dysfunction, an acute increase in serum creatinine levels and increased haemoxygenase-1 expression in kidney. These changes were not attributable to differences in haematocrit, red cell survival, tissue hypoxia-inducible factor-1α expression, nitric oxide bioavailability or plasma iron levels. 263 On the basis of these results we concluded that mechanical red cell washing resulted in the accelerated release of cell-free haemoglobin and that this causes endothelial activation, dysfunction and pulmonary and kidney injury in experimental models in vivo. We considered whether there was evidence of similar haemoglobin-mediated endothelial injury in a post hoc exploratory analysis of the first 60 participants recruited to the REDWASH trial.
Aims and objectives
The REDWASH trial proposed to test the hypothesis that the severity of the postoperative inflammatory response would be less and postoperative recovery would be faster if patients undergoing cardiac surgery with CPB who are at risk of massive transfusion received stored allogenic red cells that have been washed prior to transfusion rather than receiving standard care, in which stored red cells are administered without washing. A secondary hypothesis was that the adverse effects of transfusion are mediated by platelet and monocyte activation by microvesicles within the storage supernatant and that by removing the supernatant this would be attenuated.
The specific objectives of this trial were to:
-
estimate the MDs in biochemical markers of the systemic inflammatory response between participants allocated to receive washed red cells and participants allocated to receive unwashed red cells
-
estimate the MD in hospital LOS between participants allocated to receive washed red cells and participants allocated to receive unwashed red cells
-
estimate the MD in the frequency of inflammatory organ injury or death between participants allocated to receive washed red cells and participants allocated to receive unwashed red cells
-
estimate the cost-effectiveness of washed compared with unwashed red cells
-
establish whether red cell washing attenuates postoperative platelet and monocyte activation in a mechanism substudy.
Trial methods
Study design
This study was a multicentre, single-blinded, parallel-group RCT of allogenic red cell washing prior to transfusion compared with standard care (no washing). The South West Leicestershire REC approved the trial protocol on the 15 May 2013 (reference number 12/EM/0475). The University of Leicester was the trial sponsor and the trial was registered as ISRCTN27076315. A trial protocol has been published. 264 Changes to the study design after commencement of the study are listed in Appendix 4. The trial has been reported in accordance with the updated CONSORT statement216 (see Appendix 4).
Participants
Setting
The study was carried out at three tertiary cardiac surgery centres in the UK: the University Hospitals of Leicester NHS Trust, the Blackpool Victoria Hospital and the University Hospitals Coventry and Warwickshire NHS Trust. These units perform > 3500 major cardiac procedures per year, of which > 875 were at risk of LVBT. 9,120
Study population
We considered it more likely that the clinical benefits of red cell washing and the impact on resource use would be most apparent in patients receiving a LVBT. Patients at risk of a LVBT were therefore identified preoperatively using a risk score developed and validated by us in a multicentre population,182 as described in Chapter 3.
Inclusion criteria
Participants could enter the study if all of the following applied:
-
Adult cardiac surgery patient (aged ≥ 16 years) undergoing cardiac surgery with blood cardioplegia.
-
Identified as representing a high-risk group for LVBT using a modified risk score. The score for inclusion was ≥ 25. This has a 55% positive predictive value for LVBT. 182
The exclusion criteria for the study are listed in Box 11.
Participants could not enter study if any of the following applied:
-
emergency or salvage procedure
-
ejection fraction < 20%, i.e. very poor left ventricular function
-
end-stage renal failure defined as an estimated glomerular filtration rate of < 15 ml/minute/1.72m2, calculated from the Modification of Diet in Renal Disease equation,265 or on long-term dialysis or have undergone renal transplantation
-
prevented from having blood and blood products according to a system of beliefs (e.g. Jehovah’s Witness)
-
congenital or acquired red cell, platelet or clotting factor disorder (excluding those receiving antiplatelet therapy, warfarin or other systemic oral anticoagulants)
-
in a critical preoperative state [Kidney Disease: Improving Global Outcomes (KDIGO) stage 3 AKI266 or requiring inotropes, ventilation or an IABP]
-
pregnancy
-
participating in another interventional clinical study.
Intervention being investigated
Treatment regimens
Patients were screened by the investigators to assess their eligibility for entry into the study. Eligible patients undergoing cardiac surgery with CPB who consented to participate were randomly allocated in a 1 : 1 ratio to:
-
group A – unwashed allogenic red cells (standard care)
-
group B – washed allogenic red cells.
Red cell transfusion
Allogenic red cells, harvested in citrate–phosphate–dextrose, buffy coat removed, leucocyte depleted, saline–adenine–glucose–mannitol-suspended red cell units, issued by NHS Blood and Transplant as per standard practice, were used. For the intervention each unit of red cells was added to the CATS, washed using a centrifugal method as per the device instructions and resuspended in 0.9% normal saline. The washed red cells were then immediately administered to participants as per standard practice.
The haematocrit threshold for transfusion was 23%. Clinician discretion was allowed in certain situations, that is, during bleeding, when use of haematocrit thresholds is impractical. The clinical indication and timing of every transfusion was recorded. This was a single-blinded study; it was impossible to blind all clinical staff and participants to the intervention as red cell washing occurred by the participants’ bedside. However, the investigators, including those responsible for the collection of postoperative data and laboratory and statistical analyses, were blinded when possible.
Administration of study treatment
Red cell units (washed or unwashed) were administered via the appropriate giving set, preferably 1 unit at a time. Clinician discretion was necessary, however, in certain clinical situations, such as bleeding, when > 1 unit might need to be administered. Before transfusion of washed or unwashed cells, the anaesthetist or ICU staff member carried out standard blood transfusion checks to ensure that the blood bag had the correct participant identification number. Because the washed red cells contained no additive solution, it was necessary to administer these as soon as possible after preparation. For cell washing and administration in the theatre and ICU, safety protocols were adopted as for the washing and autotransfusion of mediastinal blood, that is, after appropriate identification checks the cells were washed at the participants’ bedside and administered immediately. Once washed, red cell units were not stored for future use. Shed mediastinal blood not returned directly to the CPB machine using sump suction was washed alongside allogenic red cells. No mediastinal blood shed after chest closure in theatre or in the ICU was washed and autotransfused in the study.
Protocol adherence
Participants who underwent randomisation but who did not undergo surgery were not considered in the analysis. A major protocol violation was defined as receipt of only unwashed blood for participants randomised to receive washed red cells or the receipt of only unwashed blood for those in the standard care arm. Receipt of some units of unwashed blood in a participant randomised to receive washed blood, and who received at least 1 unit of washed blood, was considered a minor protocol violation. Participants not followed up to the end of the trial (3 months) were considered to be minor protocol violations. Participants with missing data for laboratory and biochemical values were also considered to be minor protocol violations. In the event of any deviation from the trial protocol, the deviation was documented and participants continued to be treated according to the randomised allocation for all subsequent transfusions.
Duration of the intervention
The intervention was transfusion of any allogenic blood product between the commencement of surgery and 48 hours postoperatively. The duration of the trial for individual participants was from the commencement of surgery to the completion of the 3-month questionnaire.
Outcomes
Primary outcome
The primary outcome for the trial was the severity of the systemic inflammatory response as indicated by serum levels of the cytokine IL-8. These were measured from venous blood samples taken preoperatively, on return to the ICU and at 6, 24 and 48 hours postoperatively. Our previous research indicated that blood transfusion is associated with increases in the levels of this pro-inflammatory cytokine that are maximal between 4 and 24 hours postoperatively. 267
Secondary outcomes
The secondary outcome measures are described in Table 92.
Outcome | Definition/method of verification |
---|---|
Inflammatory organ injury, sepsis or death | Sepsis, defined as antibiotic treatment for suspected infection and the presence of SIRS within 24 hours of the start of antibiotic treatment, with SIRS defined as two or more of the following conditions: temperature > 38 °C or < 36 °C, heart rate > 90 beats/minute, respiratory rate > 20 breaths/minute or PaCO2 < 32 mmHg, white blood cell count > 12,000/mm3 or < 4000/mm3 or antibiotic treatment for wound infection |
AKI, defined as KDIGO stage 1, 2 or 3266 | |
Acute lung injury, defined as a PaO2/FiO2 ratio of < 300 mmHg or a requirement for respiratory support, invasive ventilation for > 48 hours, non-invasive ventilation for > 4 hours, re-intubation, tracheostomy or adult acute respiratory distress syndrome268 | |
Low cardiac output, defined as new intra- or postoperative IABP insertion or a cardiac index of < 2.2 l/minute/m2 refractory to appropriate intravascular volume expansion after correction or attempted correction of any dysrhythmias or the administration of inotropes including dobutamine, enoximone, milrinone, levosimendan and adrenaline (epinephrine) | |
Death | |
Differences in Multiple Organ Dysfunction Score269 at days 1, 2, 3 and 5 | |
Bleeding and transfusion | Blood loss at 6 hours postoperatively |
The number of units of red cells and other blood components transfused during the operative period and the postoperative hospital stay was recorded | |
Transfusion reactions | Febrile transfusion reactions |
Non-haemolytic transfusion reactions | |
Haemolytic transfusion reactions | |
Other clinical outcomes | Stroke, diagnosed by brain imaging (computerised tomography or magnetic resonance imaging) in association with new-onset focal or generalised neurological deficit (defined as a deficit in motor, sensory or co-ordination functions) |
STEMI accompanied by a troponin I level of > 5000 pg/ml | |
Hospital stay and cumulative resource use | ICU, HDU and hospital LOS were determined by assessment of care level |
Compliance with the washing protocol | Data were collected for all participants during surgery to characterise compliance with the randomly assigned washing protocol |
Additional markers of inflammation and organ injury | Urinary LFABP270 and NGAL271 at baseline and 6, 12 and 24 hours for a subgroup of participants (n = 40 per group) |
Serum troponin I level at baseline and 24 and 48 hours | |
Platelet aggregation (using Multiplate aggregometry) in the first 48 hours for a subgroup of participants (participants at Glenfield Hospital only) | |
Age of each unit of red cells transfused | |
Transfused red cell characteristics (washed and unwashed): ATP levels, 2,3-diphosphoglycerate (2,3DPG) levels, deformability, osmotic fragility, cytokine levels | |
Serum levels of granulocyte macrophage colony-stimulating factor, interferon-γ, IL-1β, IL-2, IL-4, IL-5, IL-6, IL-10 and TNF-α at the same time points as for the primary end point | |
Platelet and monocyte activation as determined by flow cytometry for a subgroup of participants (participants at Glenfield Hospital only) | |
Endothelial injury as determined by quantification of endothelial-derived microparticles by flow cytometry (participants at Glenfield Hospital only) | |
Effect of blood harvested from recipients on platelet and monocyte activation within a microfluidics system |
Adverse events
Serious and other adverse events were recorded and reported in accordance with the International Conference for Harmonisation of Good Clinical Practice guidelines and the sponsor’s (University of Leicester) research-related adverse event reporting policy. The Blackpool Victoria Hospital and the University Hospitals Coventry and Warwickshire NHS Trust also notified the trial team of all serious adverse events. Data on adverse events were collected from the time of surgery for the duration of each participant’s postoperative hospital stay and for the 3-month follow-up period.
Clinical management of study subjects
Standard anaesthetic and CPB protocols were used. These have been described in detail elsewhere. 264 Non-red cell components were administered according to standard unit protocols, with the indication, volume and timing of their administration recorded. Intraoperative cell salvage was used in every participant, with washing, resuspension in normal saline and autotransfusion carried out as per standard care. A single cell salvage device was used for allogenic and autologous red cell washing and transfusion. Cardiotomy suction was returned directly to the extracorporeal circuity without washing. Postoperative salvage of mediastinal fluid was not performed. Tranexamic acid was administered to every participant. In participants refractory to two standard doses of non-red cell blood components (a standard dose was 1 pooled unit of adult platelets and 2–4 units of FFP and 2 units of cryoprecipitate), rFVIIa or prothrombin complex concentrate could be administered at the discretion of the attending clinician.
Research procedures
Screening and eligibility assessment
Potential trial participants were identified from surgical theatre lists and sent study information by post/fax. Patients’ risk score was calculated at the preoperative assessment clinic or from standardised inpatient referral protocols that include detailed clinical and demographic information. Elective patients were approached on admission for surgery. After written consent was obtained participants were randomised by a member of the research team prior to surgery. Confirmation of a participant’s identity and eligibility needed to be entered into a database before the randomised allocation was generated. Details of all patients approached for the trial and reason(s) for non-participation (e.g. reason for being ineligible: patient or clinician preference or patient refusal) were documented. Participants were consented at admission for surgery or earlier for those undergoing urgent surgery (40% of all patients).
Randomisation and code breaking
Participants were randomly assigned in a 1 : 1 ratio using an internet-based randomisation system (Sealed Envelope Ltd, London, UK, a Medicines and Healthcare products Regulatory Agency-recognised facility). Randomisation was stratified by (1) study site and (2) type of procedure: CABG, valve surgery, CABG and valve surgery or other. Staff in participating centres were required to enter information to enable a participant to be identified uniquely and to confirm eligibility before the randomised allocation was revealed. Randomisation occurred preoperatively after written informed consent was given and eligibility confirmed and as close to the scheduled surgery time as possible. If the surgery was unexpectedly rescheduled, participants retained their study number and allocation.
Trial-specific tests and procedures
Urine samples were collected on the day before surgery, on the day of surgery (6 and 12 hours post surgery) and on day 1 (24 hours post surgery) for the measurement of AKI biomarkers and the calculation of creatinine clearance. Urine concentrations of NGAL were determined using a commercially available assay (human NGAL EIA kit; EKF Diagnostics), according to the manufacturer’s instructions.
Blood samples were collected from all participants in the trial at the following time points: preoperatively, postoperatively, on return to the ICU, 6–12 hours postoperatively, 24 hours postoperatively, 48 hours postoperatively, 72 hours postoperatively and 96 hours postoperatively or at hospital discharge, whichever was the earliest. Inflammatory markers in serum [IL-6, IL-8, TNF-α, monocyte chemotactic protein-1 (MCP-1) and macrophage inflammatory protein-1 (MIP-1)] were measured on the MAGPIX® multiplex platform (Luminex Corporation, Austin, TX, USA) using the ProcartaPlex® Immunoassay kit (Affymetrix eBioscience, San Diego, CA), according to the manufacturers’ instructions.
Clinical outcomes post discharge and quality of life were assessed using the EQ-5D at baseline, 6 weeks (routine clinic visit) and 3 months after surgery (postal questionnaire) and a health resource usage questionnaire conducted at 6 weeks (routine clinic visit) and 3 months after surgery (postal or telephone questionnaire).
Data collection
Before surgery, the research nurse/trial co-ordinator collected data on preprinted case report forms from patients who were approached about the trial (e.g. eligibility criteria, key demographic data, serum creatinine, ejection fraction, willingness to participate and, if not willing, main reason for refusal). These data were entered promptly into a computerised database to allow the randomised allocation codes to be generated for study participants. Other preoperative data likely to influence the speed of postoperative recovery, operative details (procedure, bypass time, cross-clamp time), postoperative morbidity, duration of ICU and hospital stay and other non-biochemical secondary outcomes were collected prospectively on preprinted forms, including dates and times of relevant outcomes. These data were entered into a database immediately after discharge. Follow-up data (collected at the routine 4- to 6-week follow-up visit and by telephone or postal questionnaire at 3 months) included the results of the resource use and EQ-5D questionnaires. Key data collection points are described in Table 93.
Data collection | Preoperatively | Day of operation | Day 1 | Day 2 | Day 3 | Day 4 | Discharge | 6 weeks | 3 months |
---|---|---|---|---|---|---|---|---|---|
Eligibility | ✓ | ||||||||
Written consent | ✓ | ||||||||
Randomisation | ✓ | ||||||||
EQ-5D questionnaire | ✓ | ✓ | ✓ | ✓a | |||||
Bloods: serum biochemistry (creatinine and troponin T/I) | ✓b | ✓b (CICU and 6–12 hours) | ✓b (24 hours) | ✓b (48 hours) | ✓b (72 hours) | ✓c (96 hours) | ✓c | ||
Bloods: serum inflammatory biomarkers | ✓b | ✓b (CICU and 6–12 hours) | ✓b (24 hours) | ✓b (48 hours) | ✓b (72 hours) | ✓c (96 hours) | ✓c | ||
Bloods: full blood count | ✓ | ✓ (CICU and 6–12 hours) | ✓ (24 hours) | ✓ (48 hours) | ✓ (72 hours) | ✓c (96 hours) | ✓c | ||
Bloods: plasma sample for microparticle analysis and monocyte activation | ✓ | ✓ (CICU) | ✓ (24 hours) | ✓ (48 hours) | |||||
Urine sample and volume: NGAL, urea and electrolytes | ✓ | ✓ (6 and 12 hours) | ✓ (24 hours) | ✓b,d (48 hours) | |||||
Operative details | ✓b | ||||||||
Clinical outcomes | ✓ | ✓ | ✓e | ||||||
Serious adverse event monitoring | ✓ | ✓ | ✓ | ✓ | ✓ | ✓ | ✓e | ||
Resource use data | ✓ | ✓e | ✓a | ||||||
Bloods: platelet response | ✓f | ✓f (CICU and 6–12 hours) | ✓f (24 hours) | ✓f (48 hours) |
Strategies to optimise recruitment
The cohort enrichment tool used to identify high-risk cardiac surgery patients identified one in every 12 cardiac surgery patients as being eligible. It was therefore planned from the outset that multiple UK sites would be required to recruit the required numbers of participants. Initially, four units – University Hospitals Leicester NHS Trust, University Hospitals Coventry and Warwickshire NHS Trust, Plymouth Hospitals NHS Trust and University Hospitals Southampton NHS Foundation Trust – were evaluated as recruiting centres. These had been leading recruiters in a previous cardiac surgery transfusion trial. 4 The unit in Coventry recruited participants early in the trial; however, planned restructuring of the Clinical Research Networks in 2014 led to staff vacancies in the research team, such that all recruitment stopped after two participants had been recruited. By the end of the trial no research nurses were available to undertake any of the research procedures. Similar restrictions in research nurse numbers also occurred in Plymouth. These issues were not resolved despite multiple sites visits and applications to the Clinical Research Networks for further resources. A particular problem in Southampton was the availability of suitably trained clinical staff who could undertake the washing procedure at the participants’ bedside on an on-call basis at nights and weekends, as determined by patient recruitment. This was necessary because of the requirement for safe transfusion practice, which was required even in clinical settings, where participants could be bleeding or haemodynamically unstable. This remained a key problem despite the addition of a new site, Blackpool Teaching Hospitals NHS Foundation Trust, which was able to show evidence that it had the staff resources to recruit patients to the trial. In September 2014 the funder agreed to provide additional funds to meet the costs of on-call staff who could undertake the washing procedure. By January 2015, however, it was clear that the recruitment timeline for the study sample size could not be met within a reasonable time period and the study was terminated by the funder.
Measures taken to avoid bias
All necessary steps were taken to reduce the risk of bias. 132,272 The trial data were analysed on an intention-to-treat basis, that is, outcomes were analysed according to treatment allocation, irrespective of future management and events, and every effort was made to include all randomised participants. Selection bias was minimised by concealed randomised allocation. As the intervention took place at the participants’ bedside it was impossible to blind clinical staff and participants to the intervention. Detection bias was minimised, however, by blinding of laboratory staff analysing cytokines, biomarkers and inflammatory processes. Specifically, urine and serum samples obtained exclusively for the trial were identified only by a trial acronym, the participants’ study identification number, initials and date of birth and the time at which the samples were taken, ensuring that laboratory staff performing the analyses were blinded. Detection bias for the clinical outcomes was also minimised by the use of objective outcome criteria, as defined in Outcomes. Blood and other urine samples, which were obtained as part of routine care, were analysed routinely in NHS laboratories by personnel who were unaware that the participants were taking part in a trial. Decisions about discharge from the ICU, the HDU and hospital were made by clinical staff on the basis of existing institutional protocols. ICU/HDU transition was defined as transition from level 3 (nursing ratio of 1 : 1) to level 2 (nursing ratio of 1 : 2). HDU/ward transition was defined as time of arrival on the ward. To minimise attrition bias we aimed to include data for all randomised participants in the data analyses.
Mechanism analyses
Detection and quantification of microparticles
Microparticles were analysed in platelet-poor plasma collected from trial participants. To prepare platelet-poor plasma, 4.3 ml of blood anticoagulated with sodium citrate was centrifuged twice at 1550 g for 15 minutes. Aliquots of platelet-poor plasma were taken from each sample and immediately frozen at –80 °C for further analysis. Microparticles were labelled using annexin V–phycoerythrin (PE) (BD Pharmingen, Oxford, UK) and antibodies against CD235a-FITC (fluorescein isothiocyanate) and CD14-FITC (both eBioscience, Abingdon, UK). Labelled microparticles were analysed using flow cytometry (CyAn™ ADP driven by Summit V4.3.02 Build 2451 software; Beckman Coulter, Inc., Brea, CA, USA). The size distribution and concentration of microparticles was estimated using nanoparticle tracking analysis (NTA) (NanoSight NS500; Malvern Instruments Ltd, Malvern, UK). Microparticle samples were diluted 1 : 50 in PBS and three 60-second videos were acquired followed by analysis with dedicated software.
Assessment of plasma microparticle concentrations and platelet and leucocyte activation
Preoperatively, on return to the CICU and at 24 and 48 hours postoperatively, additional blood was collected for analysis of microparticle levels and platelet and monocyte activation using flow cytometry and Multiplate aggregometry, as per our experimental protocols. Platelets were labelled with CD62P-FITC (Abcam, Cambridge, UK), CD41-PE (Affymetrix, Santa Clara, CA, USA) and platelet-activating complex-1 (PAC-1)-FITC (BD Biosciences, Abingdon, UK) antibodies. Leucocyte activation was assessed in citrated whole blood with specific antibodies against CD64 (FITC conjugated), CD163 (PE conjugated), CD11 [allophycocyanin (APC) conjugated] and CD14 (FITC conjugated) from eBioscience and against CD16 (APC conjugated) from BD Biosciences followed by lysis by with FACS™ lysing solution (BD Biosciences). Microparticle analysis was carried out in citrated plasma samples spun twice at 1500 g and red cell bag supernatant spun at 1850 g after a 1 : 1 dilution with PBS. Concentration and size distribution were estimated using the NanoSight NS500 nanoparticle tracking device. Derivation of microparticle and phosphatidylserine exposure was determined with FITC-coupled CD235a, CD14 and CD284 antibodies and annexin V (PE coupled) (Affymetrix).
Exploratory analysis: assessment of haem metabolism and oxidative stress
Cell-free haemoglobin was measured in plasma. Absorbance at 415, 450 and 700 nm was measured using the EnSpire® Multimode Plate Reader (PerkinElmer, Waltham, MA, USA). Nitric oxide in plasma was measured using a colorimetric R&D Systems (Abingdon, UK) kit. Tissue haem and iron metabolism were assessed indirectly by measuring serum bilirubin on the ADVIA 2400 Clinical Chemistry System, serum non-transferrin-bound iron and changes in levels of the primary iron regulatory hormone hepcidin (ELISA kit; Abbexa, Cambridge, UK). Levels of serum intercellular adhesion molecule-1 (ICAM-1) (ProcartaPlex Immunoassay kit) and endothelial-derived microparticles expressing integrin-β1, a component of very late antigen 5 (VLA5), were measured as indicators of endothelial activation.
Statistical analyses
Sample size considerations
The primary outcome, serum IL-8 level, was continuously scaled and so the target differences were specified as ‘standardised differences’ (0.2 = small, 0.5 = moderate, 0.8 = large). On the assumption that there would be a moderate correlation of 0.7 between pre- and post-intervention measures and between repeated post-intervention measures, as observed in previous work,267 and on the basis that there would be one baseline and five postoperative measures, we estimated that a sample size of 150 patients would enable us to detect a small to moderate target difference between groups of 0.4, with 90% power and 5% significance (two-tailed). We aimed to recruit 170 participants (85 per group), assuming an attrition rate of between 10% and 15% for incomplete sampling, participant death and withdrawal. In addition, a substudy to explore the mechanism that would underlie the effects of the intervention was planned. The study was exploratory and the variance of the key outcomes, monocyte and platelet activation, as determined by flow cytometry, was unknown. We therefore estimated that a sample size of 60 participants would be able to provide insight into the process underlying measured differences in the primary or other clinical outcomes, as was our experience previously. 267
Plan of analysis
The REDWASH trial was terminated by the funder because of slow recruitment. This report includes the results of a prespecified mechanistic substudy planned for the first 60 participants recruited in the trial. 264 The analysis was performed on an intention-to-treat basis on all randomised participants who entered the trial (underwent surgery) and who had the primary outcome measured at least one time point (including baseline). Means for continuous outcomes for clinical and experimental data (transformed logarithmically if required) were compared using mixed-effects models, adjusting for baseline values when available. The findings are reported as effect sizes with 95% CIs.
For some trial variables we evaluated the Box–Cox power transformation of each physiological and immunological variable and, if required, identified the appropriate transformation function to account for the increased variability of the variable with the corresponding mean. Each of the transformed (or untransformed) physiological and immunological variables were analysed using a linear mixed model incorporating available baseline variables and group as fixed effects. The model included a random intercept for each participant and, if necessary, a random time-specific slope for each participant. In addition, we explored different variance structures to deal with the residual heterogeneity in the data. For the binary data on COPD, adult respiratory distress syndrome, non-acute lung injury and acute lung injury–non-adult respiratory distress syndrome we fitted a logistic mixed model with a logit link function assuming the Bernoulli distribution of the individual variable. The model included group and time as fixed effects and participant as a random effect. We fitted a cumulative link mixed model on the ordinal data for the Berlin acute respiratory distress syndrome score and AKI; the model incorporated group as a fixed effect and patient as a random effect. All statistical analyses were carried out in the R software environment (2015; The R Foundation for Statistical Computing, Vienna, Austria) using appropriate packages (nlme, lme4, ordinal). A statistical analysis plan providing full details of the statistical analysis was written prior to database lock (available online in Report Supplementary Material 6). The analysis was performed using SAS version 9.4. Because of the early termination of the trial no health economic analysis was performed.
Study cohort
Study centres
The study opened for recruitment in Leicester in May 2013. The trial recruited participants in Leicester, Coventry and Blackpool.
Patients screened
From opening in May 2013 until the study closed to recruitment in March 2015, a total of 1448 patients were screened for inclusion in the study. The main reasons for ineligibility were a LVBT score of < 23. The main reasons why eligible patients were not approached were unavailability of research staff to undertake red cell washing at the bedside or to take postoperative blood and urine samples at the serial time points required by the study protocol. In total, 123 patients were invited to join the study, of whom 63 declined, mainly because of not wanting to be part of a research study or because of anxiety (Figure 71).
FIGURE 71.
REDWASH trial CONSORT diagram.
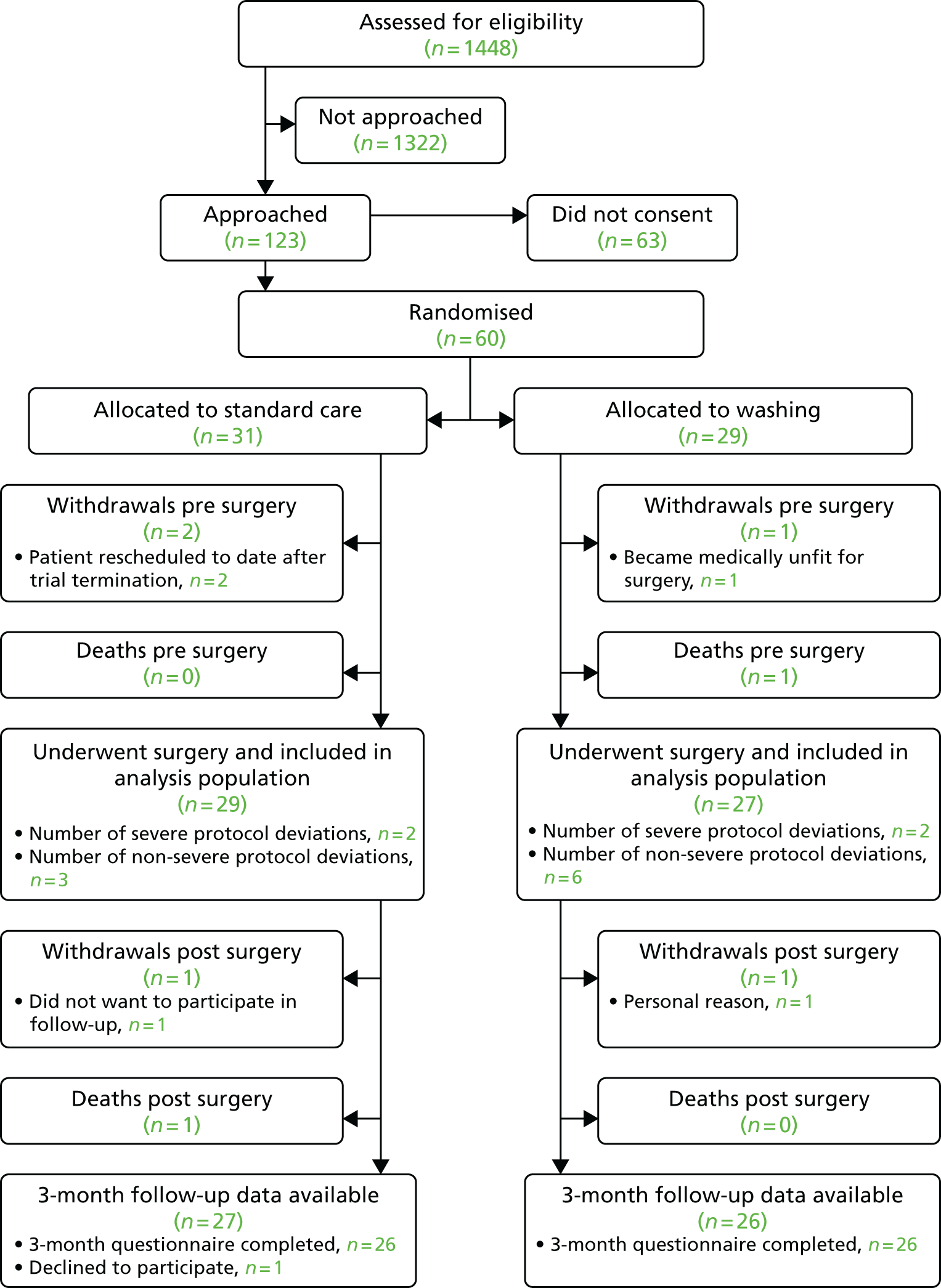
Participants recruited
Between 16 June 2013 and 13 March 2015 a total of 60 participants were recruited and randomised; 54 were randomised in Leicester, two were randomised in Coventry and four were randomised in Blackpool. The Coventry participants were randomised between 6 January 2014 and 25 March 2014 and the Blackpool participants were randomised between 12 December 2014 and 4 March 2015. The last participant was recruited in Leicester on 13 March 2015. Follow-up of all study participants was completed on 24 June 2015. Of the 60 participants randomised, 31 were allocated to the standard care arm and 29 were allocated to the red cell washing arm.
Participant withdrawals
Of the 60 participants who were randomised, four were withdrawn prior to surgery (Table 94). Of these, one died, one suffered a preoperative stroke and two had their surgery rescheduled to a date post study termination. The analysis population therefore consisted of 56 participants, of whom 29 were allocated to the standard care arm and 27 were allocated to the red cell washing arm. Following surgery one participant died and two participants withdrew. Both withdrawals were willing for data and samples collected prior to withdrawal to be used. These participants withdrew because they did not want to participate in follow-up (n = 1) and for personal reasons (n = 1).
Withdrawals | Randomised participants, n (%) | ||
---|---|---|---|
Standard care (N = 31) | Red cell washing (N = 29) | Overall (N = 60) | |
Any withdrawal | 4 (12.9) | 3 (10.3) | 7 (11.7) |
Of those who withdrew | |||
Time of withdrawal | |||
Before surgery | 2 (50.0) | 2 (66.7) | 4 (57.1) |
After surgery | 2 (50.0) | 1 (33.3) | 3 (42.9) |
Reason for withdrawal | |||
Died | 1 (25.0) | 1 (33.3) | 2 (28.6) |
Participant unfit for surgery | 1 (33.3) | 1 (14.3) | |
Surgery rescheduled | 2 (50.0) | 2 (28.6) | |
Surgery no longer required | |||
Unwilling to continue | 1 (25.0) | 1 (14.3) | |
Personal reasons | 1 (33.3) | 1 (14.3) |
Protocol deviations
There were four severe protocol deviations, two in the standard care group and two in the red cell washing group (Table 95). In the standard care group two participants received washed red cells at the request of the attending physician. In the red cell washing group two participants received only unwashed red cells. In one case this was because of oversight and in one case this was because of the need to rapidly transfuse the participant during severe haemorrhage.
Deviation | Randomised participants, n (%) | ||
---|---|---|---|
Standard care (N = 29) | Red cell washing (N = 27) | Overall (N = 56) | |
Any protocol deviation | 5 (17.2) | 8 (29.6) | 13 (23.3) |
Severe protocol deviation | 2 (6.9) | 2 (7.4) | 4 (7.1) |
Receipt of only unwashed cells in washing group | 2 (7.4) | 2 (3.6) | |
Receipt of only washed cells in standard care group | 2 (6.9) | 2 (3.6) | |
Non-severe protocol deviation | 3 (10.3) | 6 (22.2) | 9 (16.1) |
Washed group receiving unwashed cells | 5 (18.5) | 5 (8.9) | |
Standard care group receiving any washed cells | |||
No red cell transfusion | 3 (10.3) | 1 (3.7) | 4 (7.1) |
Missing data primary end point |
Participant follow-up
Of the 56 randomised participants who underwent surgery, 53 were eligible for follow-up at 3 months. Of these, 52 completed the 3-month follow-up questionnaire. The remaining participant was contacted but declined to participate (see Figure 71).
Participant demography and past history
Participant characteristics and past history are summarised in Table 96. Overall, the mean age of participants was 74 years (SD 9.0) and 50.0% (n = 28) were female. Generally, there was good balance between the groups and the average LVBT score was similar. By chance, there was a lower baseline estimated glomerular filtration rate (eGFR) in the red cell washing group than in the standard care group [68.5 (SD 24.5) ml/minute/1.73m2 vs. 88.5 (SD 29.7) ml/minute/1.73m2].
Characteristic | Randomised participants | ||
---|---|---|---|
Standard care (N = 29) | Red cell washing (N = 27) | Overall (N = 56) | |
Demographics | |||
Female sex, n (%) | 15 (51.7) | 13 (48.1) | 28 (50.0) |
Age (years), mean (SD) | 74.6 (8.9) | 74.1 (9.2) | 74.4 (9.0) |
BMI (kg/m2), mean (SD) | 28.9 (4.8) | 29.2 (6.1) | 29.1 (5.4) |
NYHA class, n (%) | |||
I | 5 (17.2) | 4 (14.8) | 9 (16.1) |
II | 17 (58.6) | 11 (40.7) | 28 (50.0) |
III | 6 (20.7) | 12 (44.4) | 18 (32.1) |
IV | 1 (3.4) | 0 (0.0) | 1 (1.8) |
CSS class, n (%) | |||
Asymptomatic | 14 (48.3) | 13 (48.1) | 27 (48.2) |
I | 7 (24.1) | 8 (29.6) | 15 (26.8) |
II | 6 (20.7) | 2 (7.4) | 8 (14.3) |
III | 2 (6.9) | 3 (11.1) | 5 (8.9) |
IV | 0 (0.0) | 1 (3.7) | 1 (1.8) |
Haemoglobin (g/dl), mean (SD) | 11.1 (1.5) | 11.2 (1.4) | 11.2 (1.4) |
Haematocrit (%), mean (SD) | 33.0 (4.6) | 33.5 (3.6) | 33.2 (4.1) |
Platelets (×109/l), mean (SD) | 263.7 (123.6) | 245.5 (67.6) | 254.6 (99.1) |
Creatinine (mmol/l), mean (SD) | 6.8 (3.9) | 6.8 (4.3) | 6.8 (4.1) |
eGFR (ml/minute/1.73m2), mean (SD) | 88.5 (29.7) | 68.5 (24.5) | 78.9 (28.9) |
Diabetic, n (%) | |||
No | 14 (48.3) | 17 (63.0) | 31 (55.4) |
Diet | 3 (10.3) | 2 (7.4) | 5 (8.9) |
Oral | 9 (31.0) | 4 (14.8) | 13 (23.2) |
Insulin | 3 (10.3) | 4 (14.8) | 7 (12.5) |
Pacemaker, n (%) | |||
No | 26 (89.7) | 25 (92.6) | 51 (91.1) |
Temporary | 0 (0.0) | 0 (0.0) | 0 (0.0) |
Permanent | 2 (6.9) | 2 (7.4) | 4 (7.1) |
CVA or TIA, n (%) | 3 (10.3) | 4 (14.8) | 7 (12.5) |
Smoking status, n (%) | |||
No | 13 (44.8) | 4 (14.8) | 17 (30.4) |
Ex (> 1 month) | 13 (44.8) | 20 (74.1) | 33 (58.9) |
Yes | 1 (3.4) | 0 (0.0) | 1 (1.8) |
Previous cardiac surgery, n (%) | 3 (10.3) | 5 (18.5) | 8 (14.3) |
MI, n (%) | 4 (13.8) | 4 (14.8) | 8 (14.3) |
Operative priority, n (%) | |||
Elective | 15 (51.7) | 13 (48.1) | 28 (50.0) |
Urgent | 14 (48.3) | 14 (51.9) | 28 (50.0) |
Logistic euroScore, median (IQR) | 9.6 (7.1–19.1) | 10.7 (6.0–23.5) | 10.0 (6.6–19.3) |
LVBT score, median (IQR) | 33.1 (30.0–39.2) | 34.6 (31.2–41) | 34.0 (28.8–40.0) |
Medications | |||
Heparin, n (%) | 0 (0.0) | 0 (0.0) | 0 (0.0) |
Clexane® (enoxaparin; Sanofi-Aventis, Guildford, UK) within 12 hours preoperatively, n (%) | 1 (3.4) | 0 (0.0) | 1 (1.8) |
Aspirin within 5 days preoperatively, n (%) | 15 (51.7) | 16 (59.3) | 31 (55.4) |
Clopidogrel within 5 days preoperatively, n (%) | 3 (10.3) | 3 (11.1) | 6 (10.7) |
Operative characteristics
Operative characteristics are described in Table 97. The different types of procedure were well matched between groups. Bypass and cross-clamp times were also similar. Cold blood cardioplegia was used in all participants. All participants were alive at the end of surgery. Monitoring during CPB demonstrated an increase in serum lactate concentrations in the red cell washing group that was not present in the standard care group.
Characteristic | Randomised participants | ||
---|---|---|---|
Standard care (N = 29) | Red cell washing (N = 27) | Overall (N = 56) | |
Type of surgery, n (%) | |||
CABG | 4 (13.8) | 6 (22.2) | 10 (17.9) |
Valve | 10 (34.5) | 9 (33.3) | 19 (33.9) |
CABG and valve | 10 (34.5) | 9 (33.3) | 19 (33.9) |
Other | 5 (17.2) | 3 (11.1) | 8 (14.3) |
Conduct of CPB, mean (SD) | |||
Bypass duration (hours) | 2.1 (0.9) | 1.9 (0.8) | 2.0 (0.9) |
Cross-clamp duration (hours) | 1.3 (0.6) | 1.3 (0.7) | 1.3 (0.6) |
Myocardial protection, n (%) | |||
Blood | 29 (100.0) | 27 (100.0) | 56 (100.0) |
Crystalloid | 0 (0.0) | 0 (0.0) | 0 (0.0) |
Baseline values, mean (SD) | |||
CPB start | |||
MABP (mmHg) | 60.1 (10.8) | 57.6 (13.3) | 58.9 (12.0) |
Haematocrit (%) | 24.1 (5.2) | 23.4 (4.7) | 23.7 (4.9) |
Lactate (mmol/l) | 1.5 (1.3) | 1.5 (2.0) | 1.5 (1.6) |
20-minute CPB time | |||
MABP (mmHg) | 58.3 (9.3) | 60.6 (6.5) | 59.4 (8.1) |
Haematocrit (%) | 23.8 (2.7) | 22.6 (3.8) | 23.2 (3.3) |
Lactate (mmol/l) | 1.8 (1.2) | 1.7 (2.1) | 1.7 (1.7) |
40-minute CPB time | |||
MABP (mmHg) | 60.8 (8.8) | 62.7 (8.4) | 61.7 (8.6) |
Haematocrit (%) | 25.2 (2.9) | 24.1 (3.4) | 24.7 (3.1) |
Lactate (mmol/l) | 1.9 (1.4) | 2.0 (2.7) | 2.0 (2.1) |
Pre-CPB rewarming | |||
MABP (mmHg) | 61.6 (10.8) | 62.4 (9.0) | 62.0 (10.0) |
Haematocrit (%) | 25.4 (2.9) | 24.2 (3.2) | 24.8 (3.1) |
Lactate (mmol/l) | 2.0 (1.5) | 2.1 (2.9) | 2.1 (2.2) |
Pre-CPB weaning | |||
MABP (mmHg) | 60.9 (9.5) | 64.1 (11.5) | 62.5 (10.6) |
Haematocrit (%) | 26.4 (2.2) | 25.3 (2.7) | 25.8 (2.5) |
Lactate (mmol/l) | 2.2 (1.5) | 2.5 (2.8) | 2.3 (2.3) |
Values on return to ICU | |||
MABP (mmHg) | 76.4 (11.9) | 71.1 (16.2) | 73.9 (14.2) |
Haematocrit (%) | 27.7 (4.3) | 28.8 (3.9) | 28.2 (4.2) |
Lactate (mmol/l) | 2.6 (1.7) | 3.7 (3.7) | 3.1 (2.9) |
Temperature (°C) | 35.8 (0.9) | 36.1 (0.7) | 35.9 (0.8) |
PaO2 (kPa) | 17.3 (6.1) | 16.4 (5.5) | 16.9 (5.8) |
PaCO2 (kPa) | 5.4 (0.7) | 5.7 (0.7) | 5.5 (0.7) |
Red cell transfusion
Red cell transfusion characteristics are provided in Table 98. A total of 221 units of allogenic red cells were transfused in the study. Of the 29 participants randomised to the standard care arm, three did not receive any transfusion and two received washed red cells. The median (IQR) number of units transfused in the standard care arm was 3 (2–5). In the red cell washing arm, one participant did not receive any red cells and two received only unwashed red cells. The median (IQR) number of red cells transfused was greater in the red cell washing arm [4 (2–6); p = 0.04]. The distribution of the timings (intra- vs. postoperative) of the red cell transfusions was similar between the groups. The mean (SD) storage age of the red cells was 19.7 (4.1) days and 21.7 (5.6) days in the standard care and red cell washing arms respectively (p = 0.078). There was no difference between the groups with respect to blood loss (4 and 12 hours) and serial haemoglobin concentrations and haematocrits (Figure 72). The exposure to non-red cell components was equal between the groups. No transfusion reactions occurred in the study.
Variable | Randomised participants | Effect (95% CI) | p-value | ||
---|---|---|---|---|---|
Standard care (N = 29) | Red cell washing (N = 27) | Overall (N = 56) | |||
Units transfused, n (%) | |||||
0 | 3 (10.3) | 1 (3.7) | 4 (7.1) | ||
1 | 3 (10.3) | 1 (3.7) | 4 (7.1) | ||
2 | 5 (17.2) | 5 (18.5) | 10 (17.9) | ||
3 | 6 (20.7) | 4 (14.8) | 10 (17.9) | ||
4 | 4 (13.8) | 4 (14.8) | 8 (14.3) | ||
> 4 | 8 (27.6) | 12 (44.4) | 20 (35.7) | ||
Total units transfused, n | 98 | 123 | 221 | ||
By setting | |||||
Theatre, median (IQR) | 1 (0–2) | 2 (0–3) | 2 (0–2) | ||
ICU, median (IQR) | 2 (1–3) | 3 (1–4) | 2 (1–4) | ||
Total units, median (IQR) | 3 (2–5) | 4 (2–6) | 3.5 (2–5.5) | 1.32a (1.01 to 1.73) | 0.040 |
Storage age (days), mean (SD) | 19.7 (4.08) | 21.7 (5.6) | 21.0 (5.2) | 0.078 | |
Blood loss (ml), mean (SD) | |||||
4 hours | 228.3 (144.5) | 251.9 (174.5) | 239.7 (158.6) | 28.08b (–109.02 to 52.86) | 0.489 |
12 hours | 470.1 (258.2) | 497.1 (253.3) | 483.1 (253.9) | 27.71b (–154.67 to 99.26) | 0.663 |
Non-red cell components, median (IQR) | 0.5 (0–4) | 2 (0–5) | 1 (0–5) | 1.01a (0.71 to 1.44) | 0.939 |
Transfusion reactions, n | 0 | 0 | 0 |
FIGURE 72.
Trial results: (a) haemoglobin; (b) serum IL-8; (c) Multiple Organ Dysfunction Score (MODS); (d) partial pressure of oxygen in arterial blood (PaO2)/inspired oxygen concentration (FiO2) ratio; (e) serum troponin; (f) urine NGAL; and (g) serum creatinine. Values are mean (SD). The findings were no different in prespecified per-protocol and safety analyses.

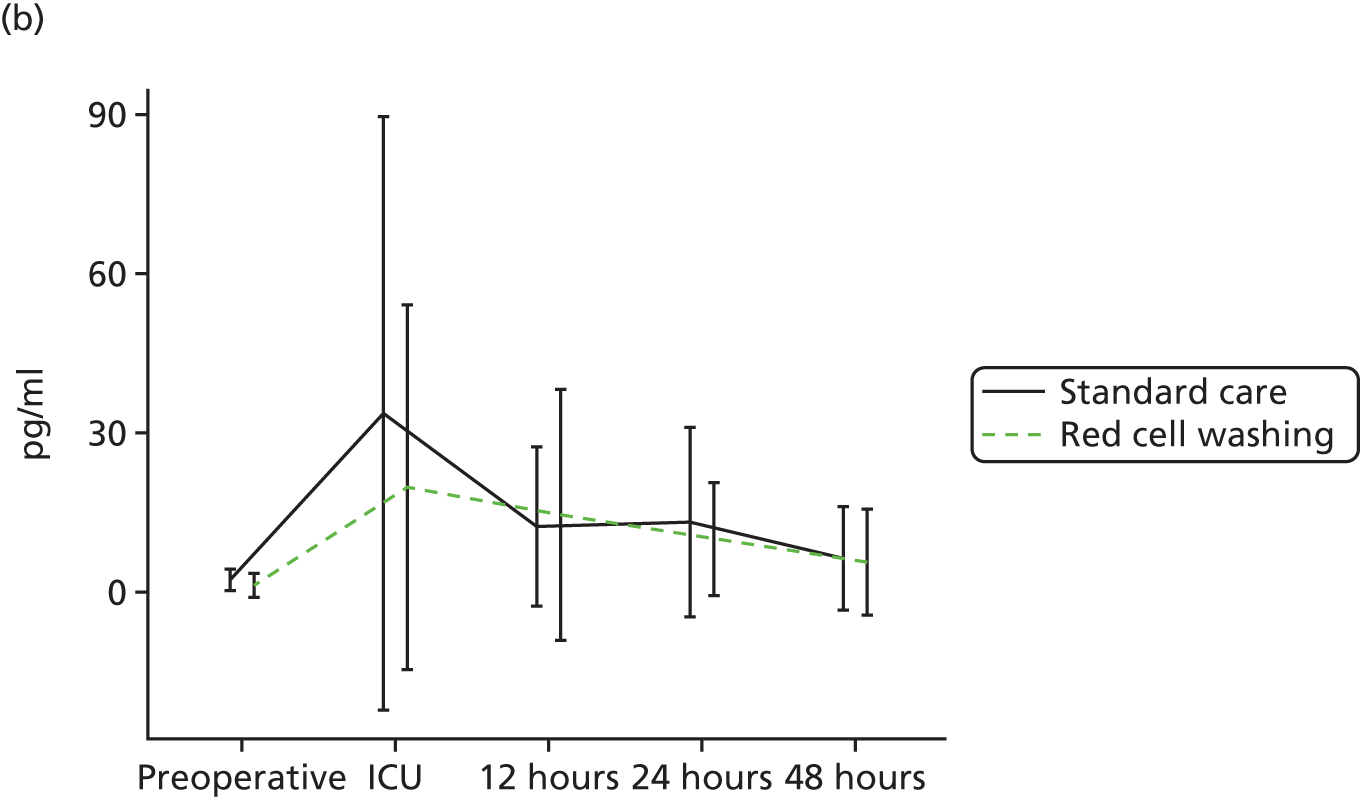
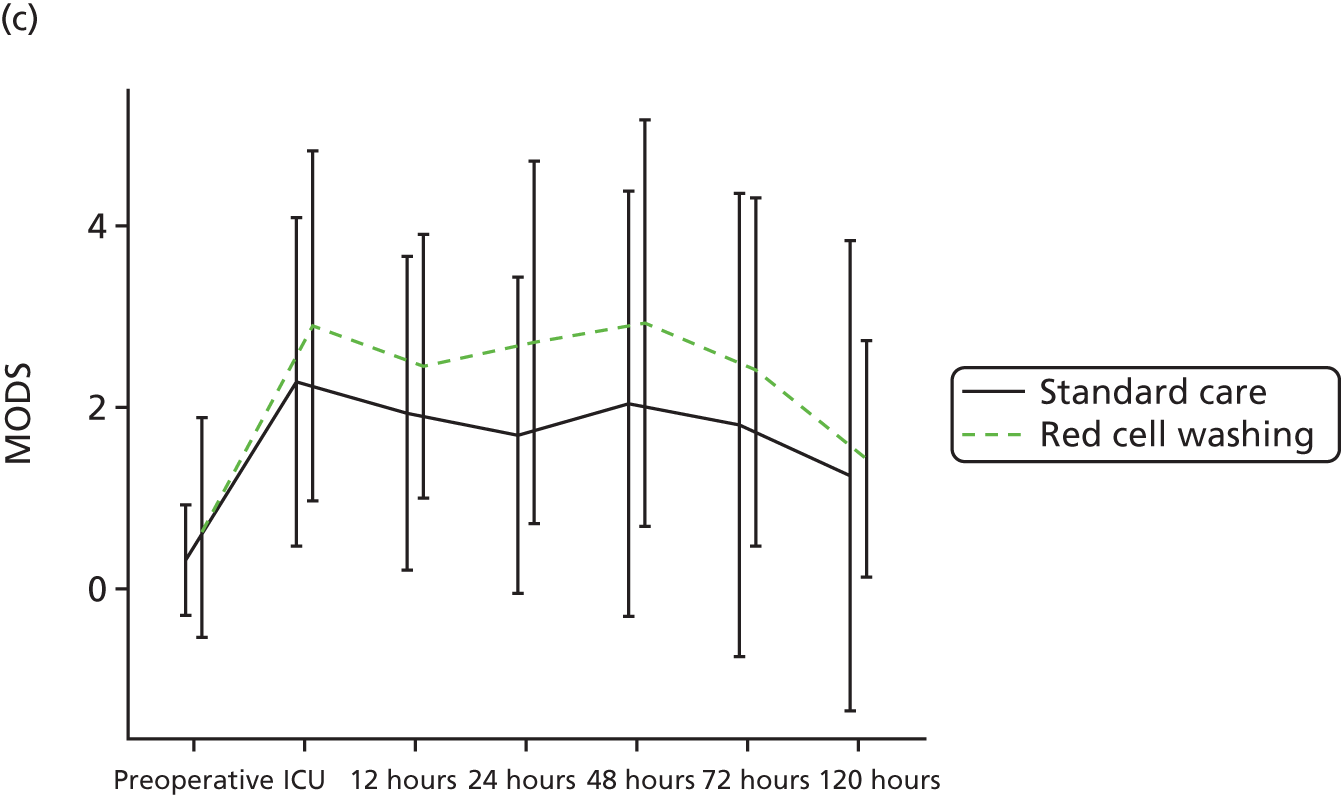



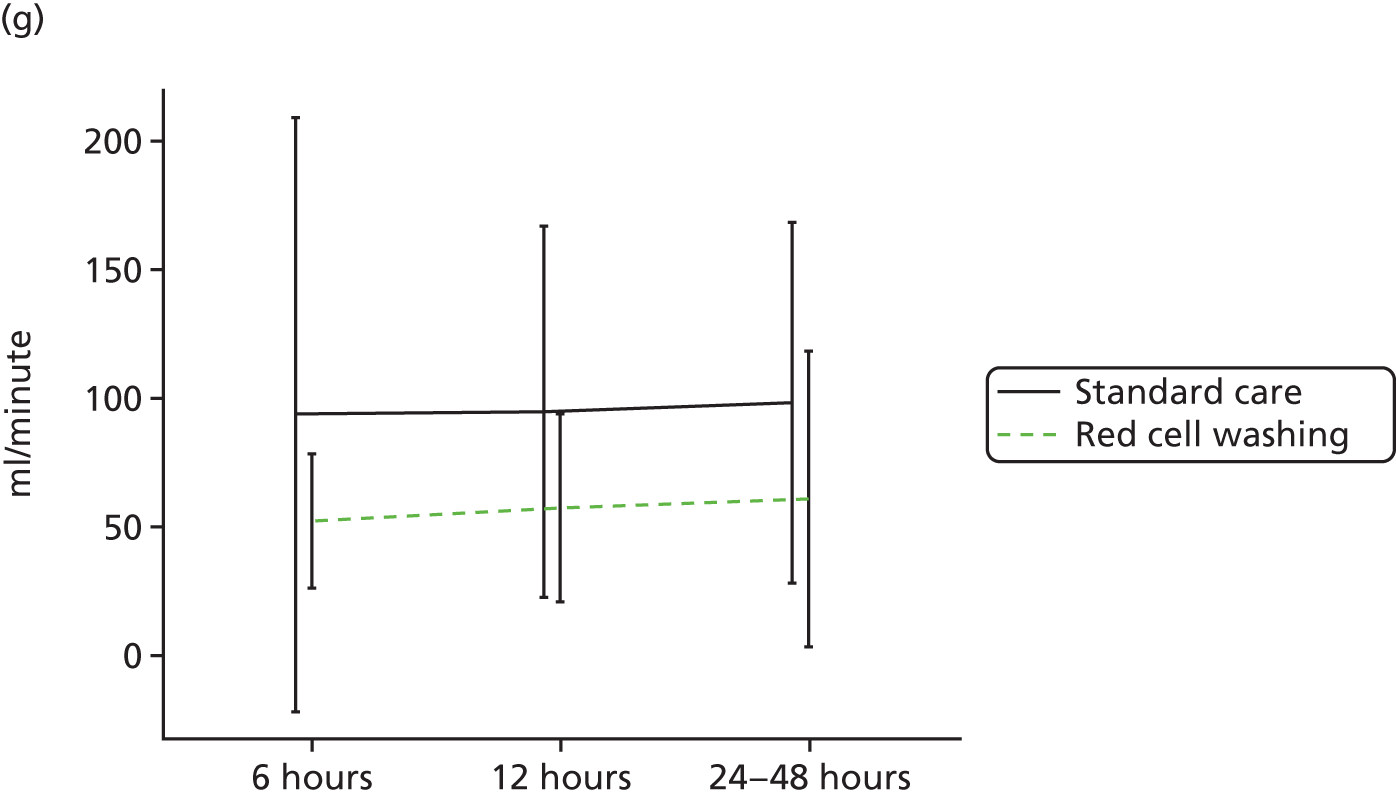
Results
Primary outcome
Serial IL-8 levels in the intervention and control arms are shown in Figure 72 and Table 99. In both groups the peak IL-8 level was observed immediately post surgery on return to the ICU. After adjustment for baseline values, IL-8 levels were higher in the standard care group than in the red cell washing group; however, this difference was small and not statistically significant. Sensitivity analysis of complete cases as well as a per-protocol analysis demonstrated similar findings (see Table 99).
Biomarker | Randomised participants, mean (SD) | Effect (95% CI) | p-value | |
---|---|---|---|---|
Standard care | Red cell washing | |||
Log(serum IL-8) | n = 29 | n = 26 | ||
Baseline | 0.659 (1.083) | –0.105 (1.243) | ||
Return to ICU | 2.409 (1.382) | 1.730 (1.543) | –0.193 (–0.894 to 0.509) | 0.591 |
6–12 hours | 1.942 (1.289) | 1.785 (1.205) | 0.367 (–0.202 to 0.936) | 0.207 |
24 hours | 1.906 (1.343) | 1.772 (1.163) | 0.361 (–0.224 to 0.945) | 0.226 |
48 hours | 1.228 (1.204) | 1.072 (1.147) | 0.393 (–0.166 to 0.952) | 0.166 |
Full analysis population with imputed values,a overall effect | 0.239 (–0.231 to 0.709) | 0.318 | ||
Full analysis population: complete cases, overall effect | 0.262 (–0.265 to 0.788) | 0.322 | ||
Per-protocol population,b overall effect | n = 26 | n = 23 | 0.182 (–0.318 to 0.683) | 0.475 |
Log(TNF-α) | n = 23 | n = 22 | ||
Baseline | –1.033 (1.718) | –1.324 (1.720) | ||
Return to ICU | –0.058 (1.734) | –0.915 (1.773) | –0.679 (–1.545 to 0.187) | 0.121 |
6–12 hours | –0.760 (1.581) | –1.281 (1.650) | –0.311 (–1.049 to 0.427) | 0.399 |
24 hours | –0.810 (1.912) | –0.932 (1.962) | 0.089 (–0.637 to 0.814) | 0.806 |
48 hours | –0.607 (1.789) | –1.354 (1.654) | –0.558 (–1.467 to 0.351) | 0.228 |
Overall effect | –0.362 (–1.022 to 0.299) | 0.283 | ||
Log(serum IL-6) | n = 23 | n = 22 | ||
Baseline | 2.309 (1.871) | 1.940 (1.860) | ||
Return to ICU | 5.401 (1.729) | 5.549 (1.603) | 0.323 (–0.512 to 1.158) | 0.448 |
6–12 hours | 5.266 (1.250) | 5.513 (1.223) | 0.400 (–0.197 to 0.998) | 0.189 |
24 hours | 5.035 (1.036) | 5.272 (0.995) | 0.355 (–0.148 to 0.857) | 0.167 |
48 hours | 4.702 (0.950) | 4.681 (1.142) | 0.108 (–0.389 to 0.606) | 0.670 |
Overall effect | 0.310 (–0.173 to 0.792) | 0.209 | ||
Log(MCP-1) | n = 23 | n = 22 | ||
Baseline | 4.309 (0.834) | 4.061 (1.507) | ||
Return to ICU | 5.772 (1.038) | 4.476 (2.845) | –0.933 (–1.977 to 0.110) | 0.078 |
6–12 hours | 4.955 (0.978) | 4.637 (1.804) | –0.339 (–1.239 to 0.561) | 0.451 |
24 hours | 4.679 (1.100) | 4.060 (2.132) | –0.640 (–1.685 to 0.404) | 0.222 |
48 hours | 4.380 (0.937) | 3.884 (2.403) | –0.458 (–1.567 to 0.650) | 0.407 |
Overall effect | –0.638 (–1.444 to 0.167) | 0.120 | ||
Log(MIP-1) | n = 23 | n = 22 | ||
Baseline | 2.241 (1.500) | 1.450 (2.149) | ||
Return to ICU | 3.085 (1.015) | 2.233 (1.640) | –0.658 (–1.473 to 0.156) | 0.110 |
6–12 hours | 2.797 (1.494) | 1.814 (1.906) | –0.678 (–1.701 to 0.345) | 0.188 |
24 hours | 2.357 (1.856) | 1.436 (2.098) | –0.256 (–1.040 to 0.529) | 0.514 |
48 hours | 2.281 (1.650) | 1.672 (2.098) | –0.118 (–1.022 to 0.786) | 0.798 |
Overall effect | –0.440 (–1.085 to 0.205) | 0.182 |
Secondary outcomes
Biomarkers of inflammation and organ injury
Biomarkers of inflammation (IL-6, TNF-α, MCP-1 and MIP-1) were measured in serum samples along with the primary outcome (see Table 99). There was no significant difference between the groups at baseline for any of these biomarkers and peak values were observed on return from theatre. Similar findings were reported for serum troponin, a biomarker of myocardial injury. Levels of urine NGAL, a biomarker of AKI, were higher in the red cell washing group (log-adjusted MD 0.435, 95% CI 0.022 to 0.849; p = 0.039; Table 100); however, after adjustment for baseline eGFR this difference was no longer significant. Calculated creatinine clearance at 6, 12 and 24 hours post surgery was statistically significantly lower in the red cell washing group; however, this effect was no longer statistically significant after adjustment for baseline eGFR (see Table 100)
Outcome | Randomised participants, mean (SD) | Effect (95% CI) | p-value | |
---|---|---|---|---|
Standard care (N = 29) | Red cell washing (N = 26) | |||
MODS total | ||||
Baseline | 0.32 (0.61) | 0.69 (1.23) | ||
Return to ICU | 2.32 (1.83) | 3.00 (1.88) | 0.52 (–0.47 to 1.51) | 0.293 |
6–12 hours | 1.96 (1.75) | 2.50 (1.45) | 0.42 (–0.43 to 1.27) | 0.325 |
24 hours | 1.71 (1.76) | 2.69 (2.04) | 1.02 (–0.01 to 2.04) | 0.051 |
48 hours | 2.07 (2.39) | 2.92 (2.24) | 0.67 (–0.50 to 1.84) | 0.254 |
72 hours | 1.82 (2.60) | 2.38 (1.92) | 0.57 (–0.63 to 1.78) | 0.341 |
120 hours | 1.25 (2.59) | 1.42 (1.30) | 0.11 (–1.02 to 1.24) | 0.843 |
Full analysis population, overall effect | 0.56 (–0.26 to 1.39) | 0.177 | ||
MODS maximum values | ||||
Worst-value MODS | ||||
Baseline | 0.31 (0.60) | 0.67 (1.21) | ||
Sum of worst values | 4.41 (3.13) | 5.37 (2.57) | 0.73 (–0.65 to 2.11) | 0.296 |
Log(serum troponin) | ||||
Baseline | 3.074 (0.843) | 3.474 (1.369) | ||
Return to ICU | 8.731 (1.029) | 8.520 (1.014) | –0.183 (–0.734 to 0.368) | 0.516 |
6–12 hours | 8.900 (1.156) | 8.737 (0.873) | –0.129 (–0.683 to 0.426) | 0.650 |
24 hours | 8.449 (1.285) | 8.251 (0.809) | –0.151 (–0.733 to 0.430) | 0.610 |
48 hours | 7.801 (1.479) | 7.659 (0.961) | –0.096 (–0.766 to 0.573) | 0.778 |
72 hours | 7.306 (1.481) | 7.065 (0.892) | –0.185 (–0.848 to 0.478) | 0.584 |
96 hours | 6.784 (1.429) | 6.614 (0.873) | –0.111 (–0.754 to 0.532) | 0.736 |
Overall effect | –0.175 (–0.705 to 0.354) | 0.516 | ||
Log(urine NGAL) | ||||
Baseline | 2.006 (1.240) | 1.433 (1.031) | ||
6 hours | 3.374 (0.699) | 3.446 (0.773) | 0.161 (–0.254 to 0.576) | 0.446 |
12 hours | 2.861 (1.227) | 3.346 (0.748) | 0.515 (–0.072 to 1.101) | 0.086 |
24 hours | 2.576 (0.928) | 3.119 (0.684) | 0.496 (–0.017 to 1.101) | 0.058 |
Overall effect | 0.435 (0.022 to 0.849) | 0.039 | ||
Overall effect adjusted for baseline eGFR | 0.217 (–0.202 to 0.636) | 0.311 | ||
Calculated log(creatinine clearance) | ||||
6 hours | 4.147 (0.778) | 3.850 (0.578) | –0.331 (–0.717 to 0.054) | 0.092 |
12 hours | 4.318 (0.770) | 3.829 (0.727) | –0.531 (–0.962 to –0.100) | 0.016 |
24–48 hours | 4.189 (1.063) | 3.750 (0.878) | –0.473 (–0.999 to 0.054) | 0.078 |
Overall effect | –0.445 (–0.793 to –0.097) | 0.012 | ||
Overall effect adjusted for baseline eGFR | –0.243 (–0.584 to 0.097) | 0.161 | ||
Log (serum creatinine) | ||||
Baseline | 4.31 (0.32) | 4.57 (0.34) | ||
Return to ICU | 4.39 (0.32) | 4.56 (0.45) | –0.05 (–0.18 to 0.08) | 0.474 |
6–12 hours | 4.52 (0.34) | 4.75 (0.37) | –0.03 (–0.13 to 0.08) | 0.611 |
24 hours | 4.57 (0.38) | 4.84 (0.41) | 0.04 (–0.09 to 0.18) | 0.537 |
48 hours | 4.61 (0.45) | 4.93 (0.55) | 0.02 (–0.16 to 0.20) | 0.862 |
72 hours | 4.48 (0.47) | 4.83 (0.59) | 0.07 (–0.14 to 0.28) | 0.503 |
120 hours | 4.45 (0.44) | 4.75 (0.56) | 0.02 (–0.19 to 0.22) | 0.873 |
Overall effect | –0.02 (–0.15 to 0.12) | 0.809 | ||
PaO2/FiO2 ratio | ||||
Baseline | 482.05 (108.31) | 484.27 (164.26) | ||
Return to ICU | 292.55 (98.67) | 277.06 (100.94) | –14.63 (–68.15 to 38.90) | 0.585 |
6–12 hours | 345.89 (111.73) | 340.84 (97.75) | –6.24 (–61.55 to 49.07) | 0.822 |
24 hours | 354.86 (122.56) | 323.04 (79.82) | –20.73 (–79.18 to 37.72) | 0.479 |
48 hours | 328.32 (115.74) | 325.91 (112.62) | –7.69 (–64.21 to 48.83) | 0.785 |
72 hours | 339.77 (104.53) | 358.10 (139.20) | 18.57 (–46.09 to 83.22) | 0.566 |
120 hours | 411.64 (139.19) | 384.05 (102.08) | –28.53 (–92.91 to 35.85) | 0.377 |
Overall effect | –9.95 (–50.30 to 30.40) | 0.622 |
Multiple Organ Dysfunction Score
The Multiple Organ Dysfunction Score was higher in the red cell washing arm than in the standard care arm, but this differences was not statistically significant (adjusted mean 0.56, 95% CI –0.26 to 1.39; p = 0.177; see Figure 72 and Table 100). Analysis of the results from the individual MODS domains demonstrated that serum creatinine values and pressure-adjusted heart rate (PAHR) were higher in the red cell washing group, but these differences were not significant (log serum creatinine adjusted mean –0.02, 95% CI –0.15 to 0.12, p = 0.809; PAHR adjusted mean 0.51, 95% CI –0.71 to 1.72; p = 0.405), with the difference in serum creatinine largely attributable to differences in baseline values. Analysis of worst MODS values, as described in a recent transfusion trial,39 also demonstrated no statistically significant differences between the groups (see Table 100).
Clinical outcomes
Overall, 85.7% of participants in the trial developed the composite outcome of sepsis, organ injury or death within 30 days of surgery. The frequency of the composite end point was higher in the red cell washing group than in the standard care group [92.6% (25/27) vs. 79.3% (23/29)], but this was not statistically significant (OR 3.26, 95% CI 0.58 to 18.21; p = 0.179). The frequencies of individual components of the composite clinical outcome are shown in Table 101.
Clinical outcome | Randomised participants | Effect (95% CI) | p-value | |
---|---|---|---|---|
Standard care (N = 29) | Red cell washing (N = 27) | |||
Organ injury, sepsis or death, n (%) | 23 (79.3) | 25 (92.6) | 3.26a (0.58 to 18.21) | 0.179 |
Sepsis, n (%) | 13 (44.8) | 14 (51.9) | 1.26a (0.43 to 3.70) | 0.677 |
Low cardiac output, n (%) | 2 (6.9) | 3 (11.1) | b | |
AKI (any), n (%) | 15 (51.7) | 20 (74.1) | 2.70a (0.83 to 8.85) | 0.100 |
Stage 1 | 11 (37.9) | 12 (44.4) | ||
Stage 2 | 2 (6.9) | 4 (14.8) | ||
Stage 3 | 2 (6.9) | 4 (14.8) | ||
Overall | 1.68a (1.01 to 2.82) | 0.048 | ||
Adjusted for baseline eGFR | 1.37a (0.80 to 2.36) | 0.254 | ||
Berlin adult respiratory distress syndrome (any), n (%) | 17 (58.6) | 18 (66.7) | 1.57a (0.48 to 5.14) | 0.456 |
Mild adult respiratory distress syndrome | 12 (41.4) | 12 (44.4) | ||
Moderate adult respiratory distress syndrome | 5 (17.2) | 4 (14.8) | ||
Severe adult respiratory distress syndrome | 0 (0.0) | 0 (0.0) | ||
Stroke, n (%) | 0 (0.0) | 1 (3.7) | b | |
STEMI, n (%) | 1 (3.4) | 0 (0.0) | b | |
Death within 30 days of surgery, n (%) | 1 (3.4) | 0 (0.0) | b | |
LOS, median (IQR) | ||||
Ventilation time (hours) | 10.3 (8.8–16.2) | 11.2 (8.6–27.7) | 1.50c (0.85 to 2.64) | 0.162 |
CICU LOS (hours) | 52.4 (24.9–144.8) | 115.1 (48.2–147.6) | 1.12c (0.65 to 1.93) | 0.696 |
Cardiac unit LOS (days) | 11.1 (9.0–24.2) | 14.2 (9.1–22.0) | 0.93c (0.53 to 1.61) | 0.783 |
EQ-5D VAS summary index, mean (SD) | ||||
Preoperative | 0.70 (0.21) | 0.81 (0.18) | ||
6–12 hours | 0.72 (0.23) | 0.75 (0.15) | –0.02 (–0.12 to 0.07) | 0.621 |
6 weeks postoperatively | 0.77 (0.20) | 0.77 (0.16) | 0.02 (–0.08 to 0.12) | 0.683 |
3 months postoperatively | 0.73 (0.20) | 0.76 (0.15) | 0.04 (–0.06 to 0.14) | 0.433 |
Overall treatment effect | 0.01d (–0.05 to 0.08) | 0.685 |
Hospital stay and resource use
There was no difference between the two groups with respect to ventilation times, ICU LOS or time to discharge from hospital (see Table 101).
Quality of life
There was no difference in quality of life between the groups at any time point as assessed by the EQ-5D (see Table 101).
Postoperative adverse events
In addition to the secondary clinical outcomes, data were collected on all postoperative complications that might be expected after cardiac surgery and any unexpected complications that met the definition of ‘serious’. Details of the complications experienced by study participants in the period from recruitment to 3 months are provided in Table 102. For completeness, all adverse events are reported, including those that formed part of the secondary outcomes. Most complications were rare and occurred with similar frequency in the two groups. There were five unexpected serious adverse events in the standard care group and four in the red cell washing group. For the presentation of safety results, participants were allocated to the treatment group ‘as treated’, that is, participants in the control group who received at least 1 unit of washed red cells were allocated to the washed group and participants in the washed group who received only unwashed blood were allocated to the control group. Participants who received no blood were omitted from the analysis.
Expected events (i.e. listed in the study protocol) | Received standard care (N = 26) | Received washed blood (N = 26) | ||||||
---|---|---|---|---|---|---|---|---|
Adverse events | Serious adverse events | Adverse events | Serious adverse events | |||||
Events/participants | % | Events/participants | % | Events/participants | % | Events/participants | % | |
STEMI | 1 | 3.8 | 0 | 0.0 | 0 | 0.0 | 0 | 0.0 |
Cardiac arrest | 0 | 0.0 | 0 | 0.0 | 0 | 0.0 | 0 | 0.0 |
SVT/AF | 14 | 53.8 | 0 | 0.0 | 9 | 34.6 | 1 | 3.8 |
VF/VT | 0 | 0.0 | 0 | 0.0 | 1 | 3.8 | 0 | 0.0 |
New pacing | 4 | 15.4 | 0 | 0.0 | 7 | 26.9 | 0 | 0.0 |
Use of inotropes | 24 | 92.3 | 1 | 3.8 | 25 | 96.2 | 2 | 7.7 |
Use of IABP | 1 | 3.8 | 0 | 0.0 | 0 | 0.0 | 0 | 0.0 |
Use of pulmonary artery catheter | 9 | 34.6 | 0 | 0.0 | 7 | 26.9 | 0 | 0.0 |
Use of vasodilator | 4 | 15.4 | 0 | 0.0 | 4 | 15.4 | 0 | 0.0 |
Low cardiac output | 2 | 7.7 | 0 | 0.0 | 3 | 11.5 | 0 | 0.0 |
Tracheostomy | 1 | 3.8 | 1 | 3.8 | 0 | 0.0 | 0 | 0.0 |
Mask CPAP | 1 | 3.8 | 0 | 0.0 | 5 | 19.2 | 1 | 3.8 |
Pneumothorax or effusion requiring draining | 3 | 11.5 | 1 | 3.8 | 7 | 26.9 | 1 | 3.8 |
AKI | 15 | 57.7 | 0 | 0.0 | 19 | 73.1 | 1 | 3.8 |
Stage 1 | 11 | 42.3 | 0 | 0.0 | 11 | 42.3 | 0 | 0.0 |
Stage 2 | 2 | 7.7 | 0 | 0.0 | 4 | 15.4 | 0 | 0.0 |
Stage 3 | 2 | 7.7 | 0 | 0.0 | 4 | 15.4 | 1 | 3.8 |
Haemofiltration/dialysis since heart operation | 1 | 3.8 | 0 | 0.0 | 3 | 11.5 | 1 | 3.8 |
Peptic ulcer/GI bleed/perforation | 1 | 3.8 | 1 | 3.8 | 1 | 3.8 | 1 | 3.8 |
Other GI | 0 | 0.0 | 0 | 0.0 | 1 | 3.8 | 0 | 0.0 |
Permanent stroke | 0 | 0.0 | 0 | 0.0 | 1 | 3.8 | 1 | 3.8 |
TIA | 0 | 0.0 | 0 | 0.0 | 0 | 0.0 | 0 | 0.0 |
Excessive bleeding not requiring reoperation | 0 | 0.0 | 0 | 0.0 | 0 | 0.0 | 0 | 0.0 |
Wound dehiscence | 2 | 7.7 | 1 | 3.8 | 0 | 0.0 | 0 | 0.0 |
Reoperation | 0 | 0.0 | 0 | 0.0 | 0 | 0.0 | 0 | 0.0 |
Mechanism analysis: the effects of red cell washing on plasma microparticle levels and platelet and leucocyte activation in vivo
In contrast to the experimental findings, in trial participants there was no difference between the groups with respect to serial measures of total, phosphatidylserine-positive or red cell-derived (CD235a-positive) microparticles in plasma (Figure 73). We also demonstrated no difference between the trial groups with respect to levels of CD64/163-positive leucocytes, activated (PAC-1-positive or CD40/CD62P-positive) platelets, as determined by flow cytometry, or platelet activation, as assessed by Multiplate aggregometry in serial blood samples (see Figure 73).
FIGURE 73.
Effects of red cell washing on microparticle concentrations and activation of platelets and leucocytes in trial participants: (a) total microparticle concentrations in citrated plasma samples; (b) fractional content of annexin V-positive microparticles; (c) fractional content of CD235a-positive microparticles; (d) activation of monocytes indicated by expression of CD64 on monocytes (CD163); (e) expression of integrin-αIIb/β3 (PAC-1) on platelets; (f) expression of CD62P on platelets (CD41); (g) results of the ADPtest assay on the Multiplate device; (h) results of the ASPItest assay on the Multiplate device; and (i) results of the TRAPtest assay on the Multiplate device.


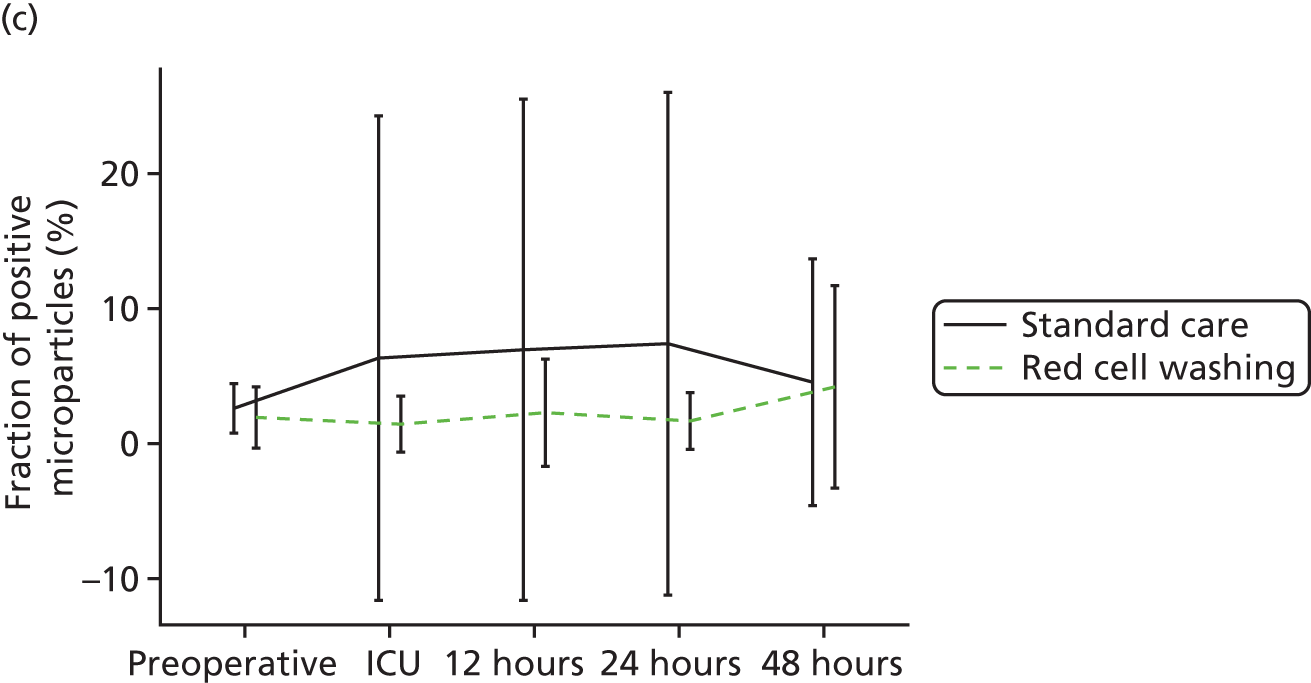
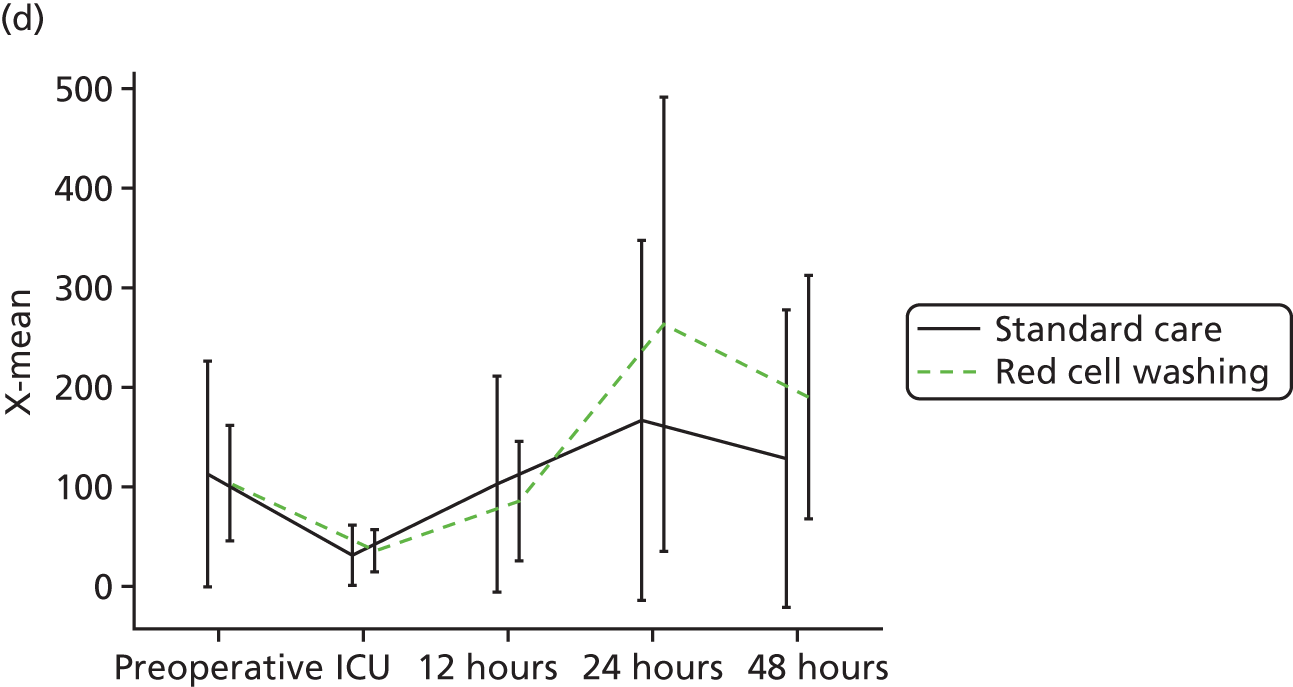
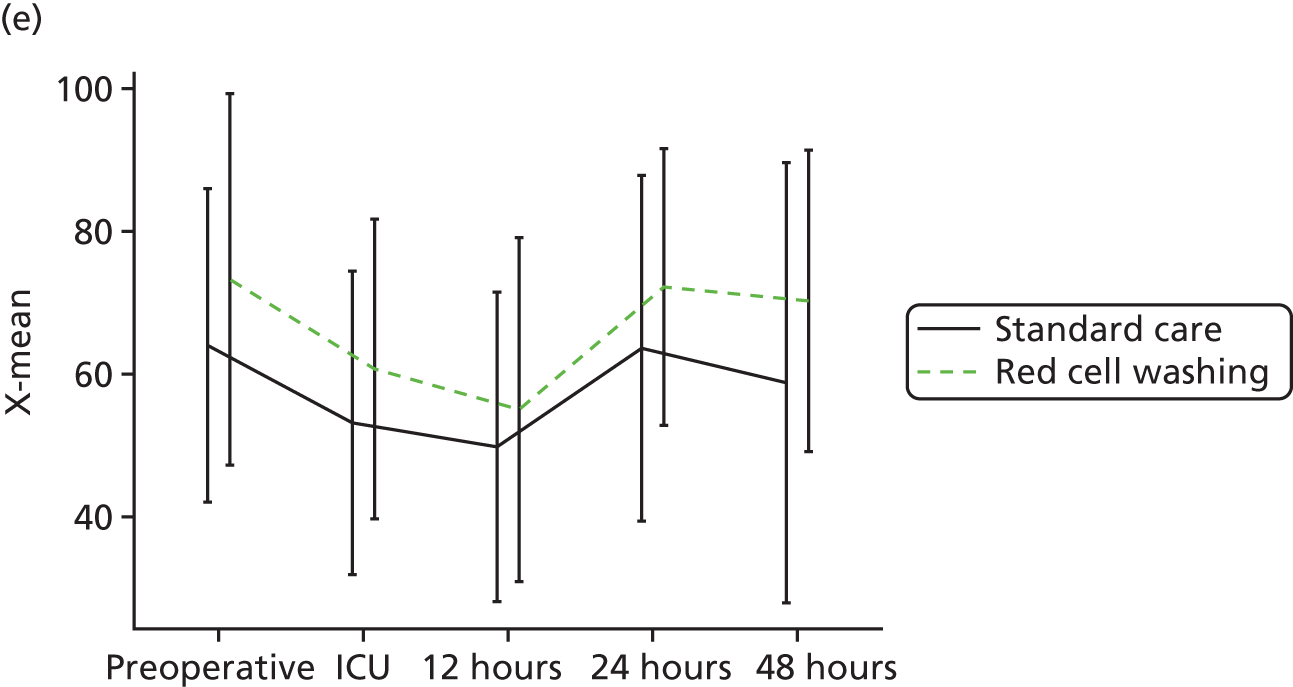


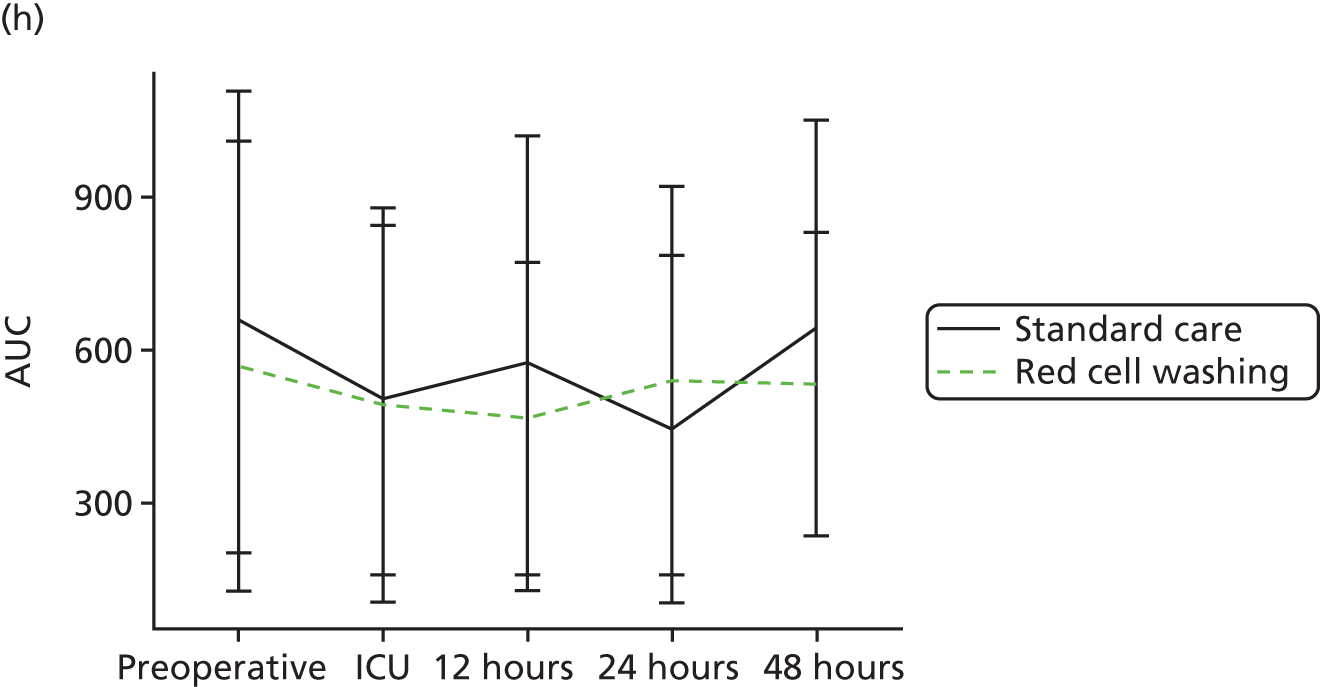
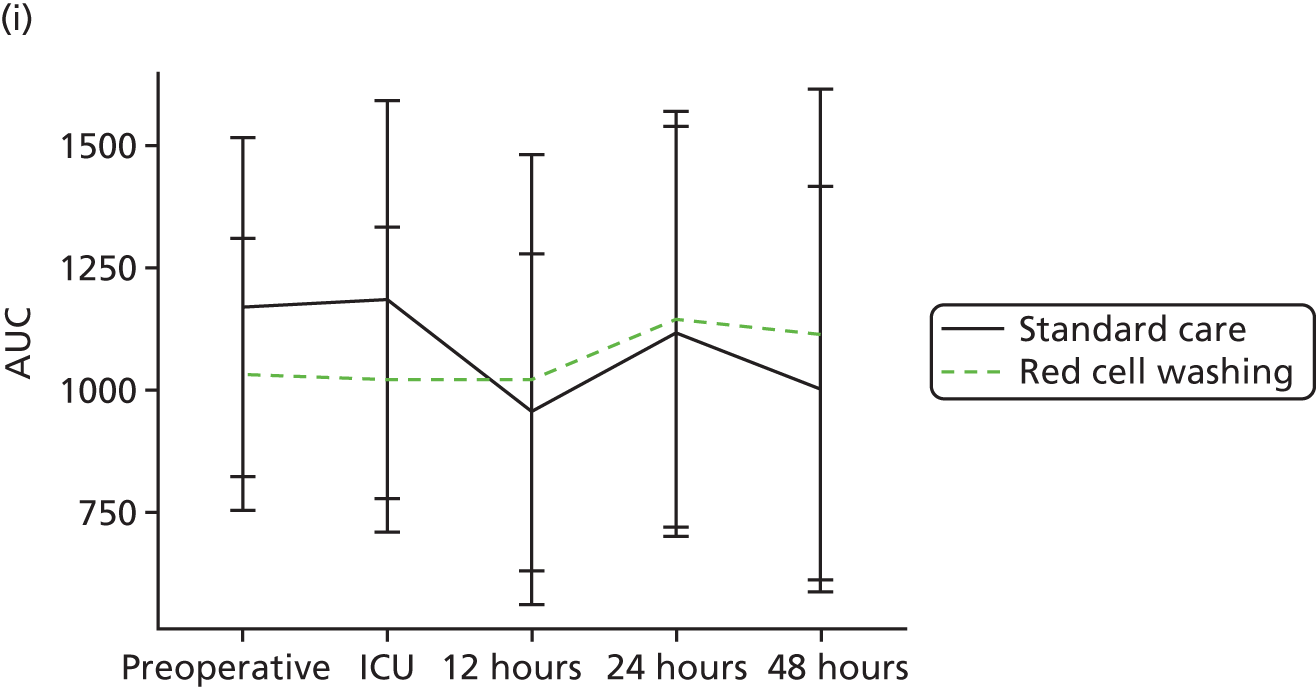
Exploratory analyses: assessment of the effects of red cell washing on cell-free haemoglobin, oxidative stress and endothelial injury
In trial participants cell-free haemoglobin levels were increased significantly in both treatment groups immediately post surgery (Figure 74a). However, there was no difference between the trial groups in cell-free haemoglobin, plasma iron, serum haematocrit, serum total bilirubin, non-transferrin-bound iron, hepcidin, nitric oxide bioavailability or serum ICAM-1- or CD144-positive microparticles, an indicator of endothelial activation (see Figure 74a–i). The endothelial-derived microparticles did not co-express integrin-β1 (see Figure 74i), a measure of VLA5 activation. This suggests that the greater part of the cell-free haemoglobin immediately post surgery was not altered by red cell washing but was most likely caused by lysis of recipient red cells by the CPB circuit. However, free haemoglobin released following washing of stored red cells is more reactive than that released by lysed fresh cells, as generated by the CPB circuit (see Figure 74j), implying that similar plasma free haemoglobin concentrations in trial groups may bely qualitative differences in biological activity. However we did not detect differences in measures of oxidative stress in serum, a putative mechanism by which free haemoglobin may damage cells, as measured by protein carbonylation or lipid peroxidation (see Figure 74k and l).
FIGURE 74.
Effects of washing and rejuvenation on cell-free haemoglobin, oxidative stress and endothelial injury: (a) plasma cell-free haemoglobin; (b) plasma total iron; (c) serial haematocrit; (d) total bilirubin; (e) non-transferrin-bound iron; (f) serum hepcidin; (g) nitric oxide bioavailability; (h) serum ICAM-1; (i) endothelial (CD144) microparticles expressing integrin-β1; (j) oxidative potential of cell-free haemoglobin derived from stored or fresh red cells; (k) serum protein carbonylation; and (l) serum thiobarbituric acid reactive substances (TBARS) (lipid peroxidation). Values are mean (SD). *p < 0.05 for fresh RBC lysate vs. HS sup, and for fresh RBC lysate vs. old RBC lysate. Hgb, haemoglobin; HS sup, supernatant of stored allogenic red cells fractionated by high-speed centrifugation; RBC, red cell units; ROS, reactive oxygen species.
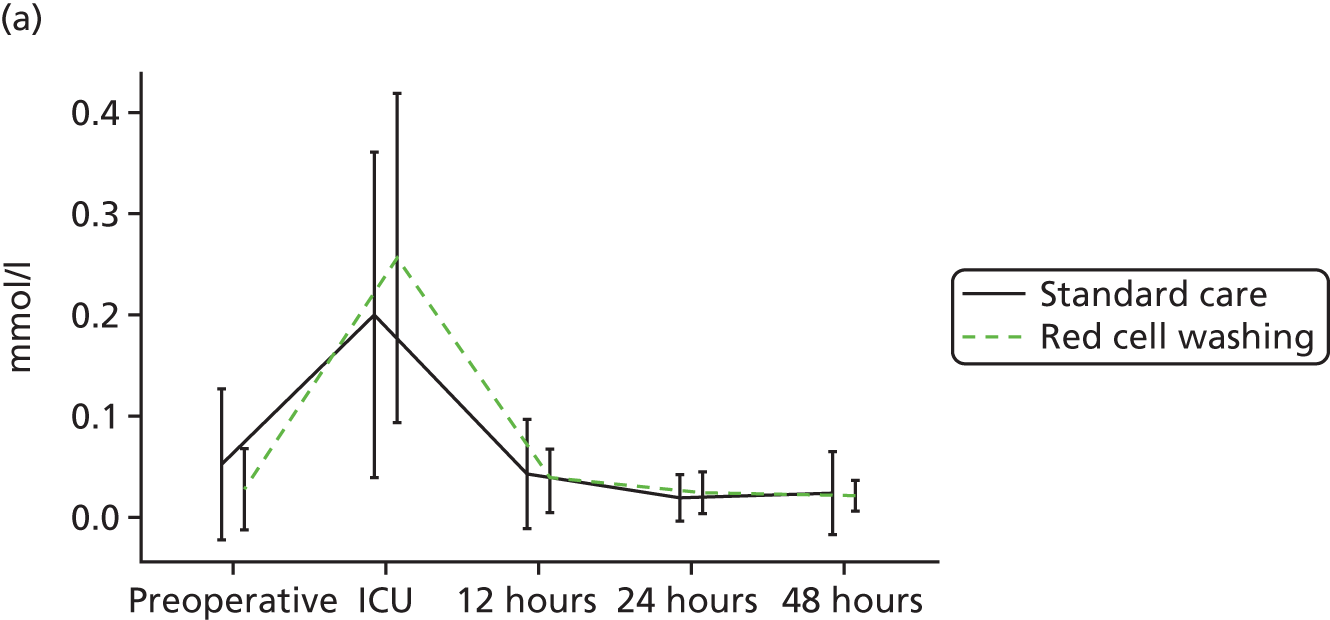
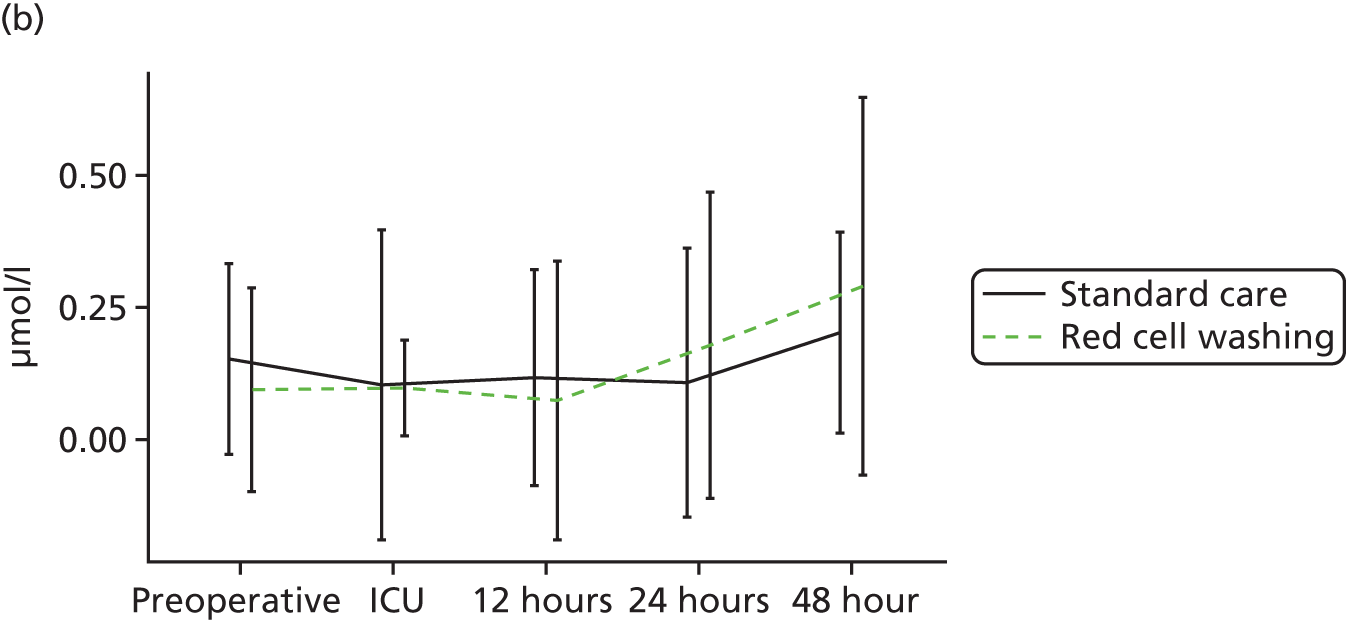
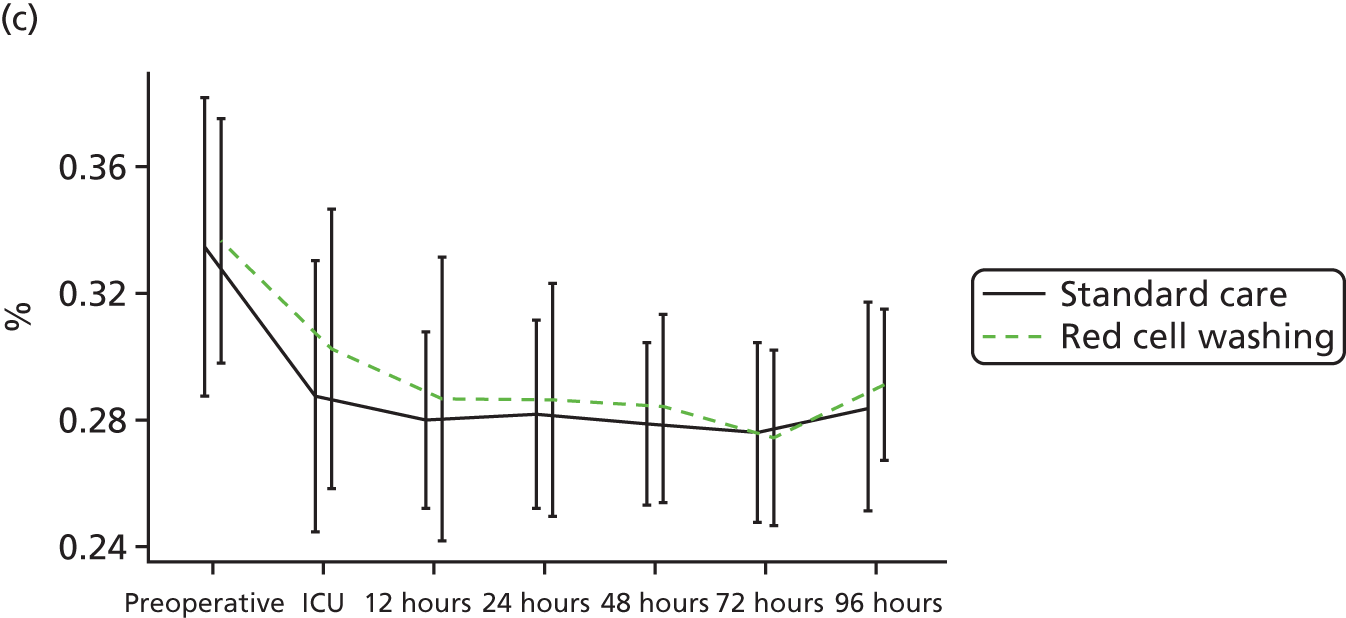


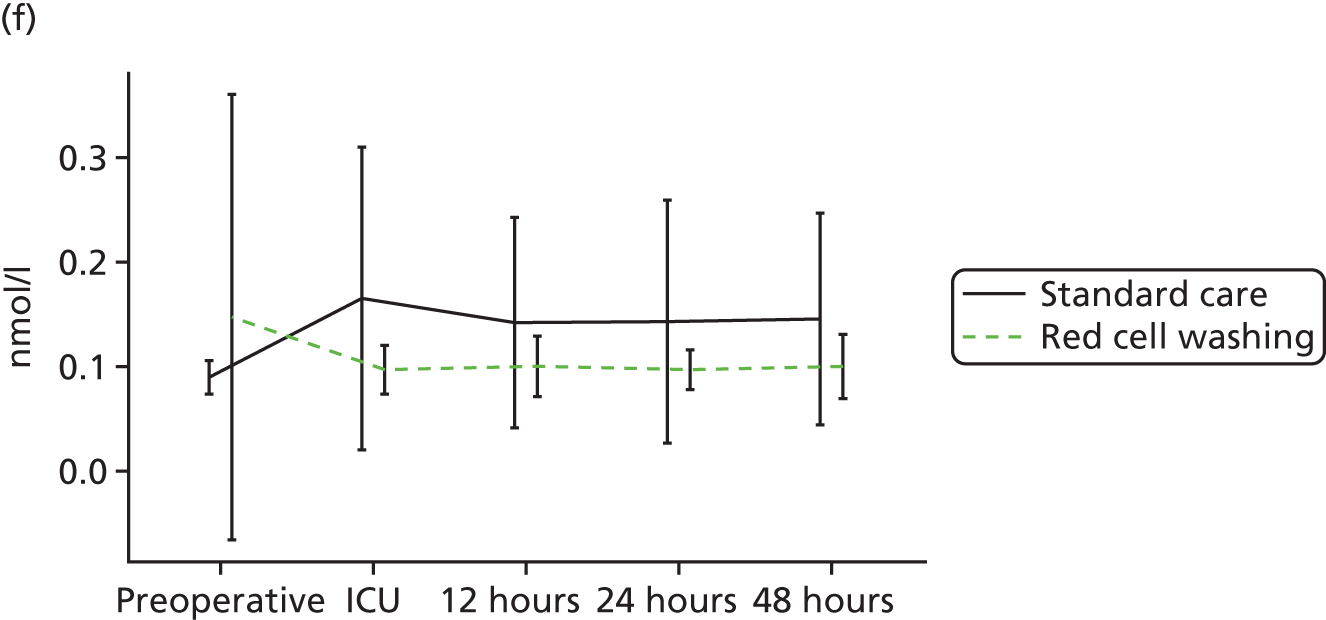
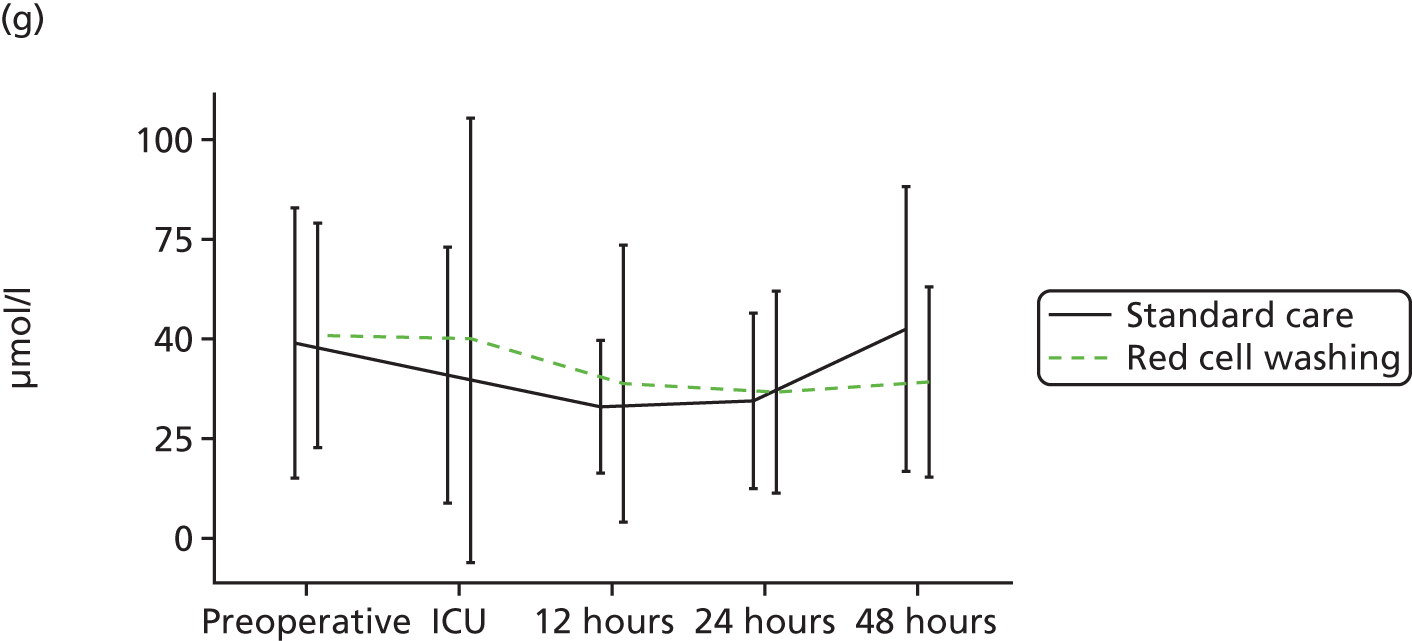
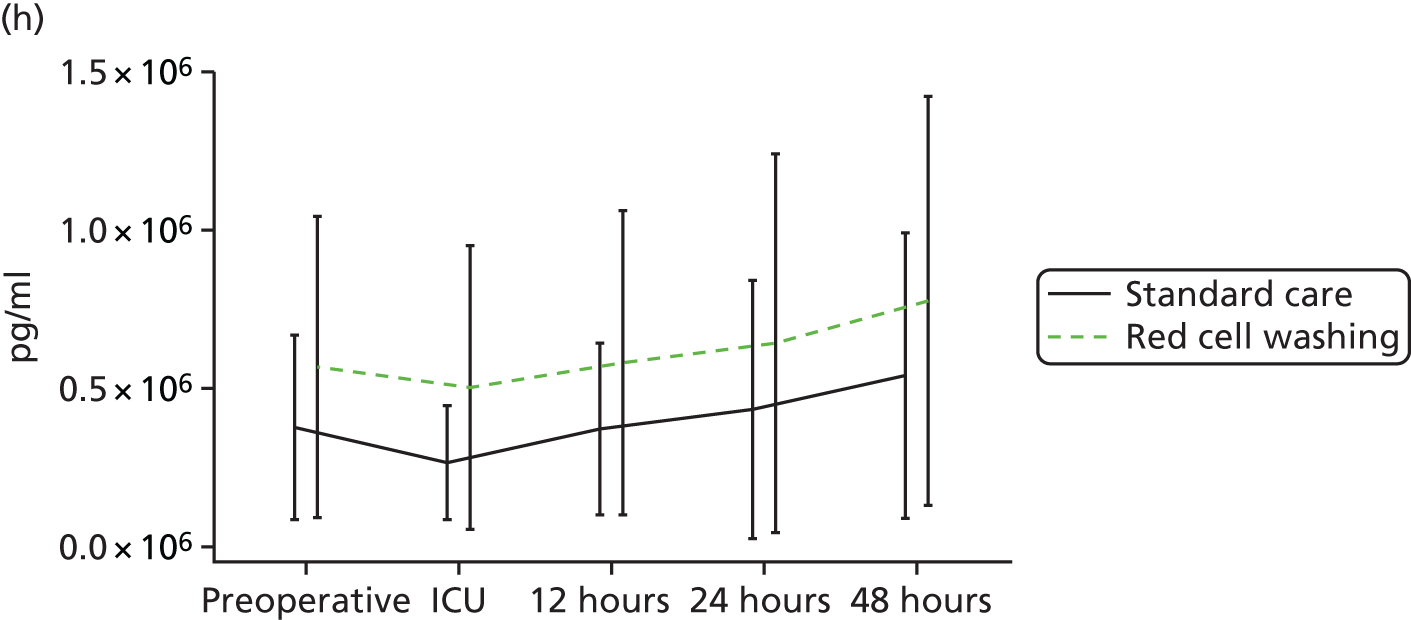


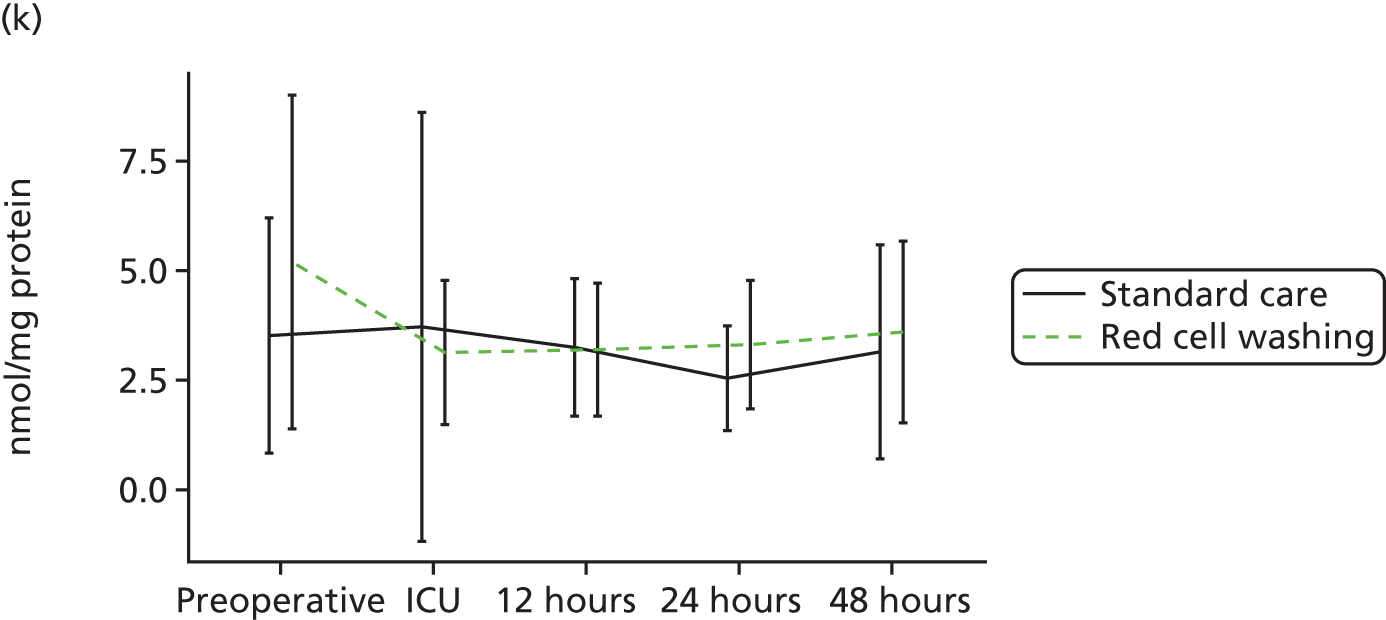
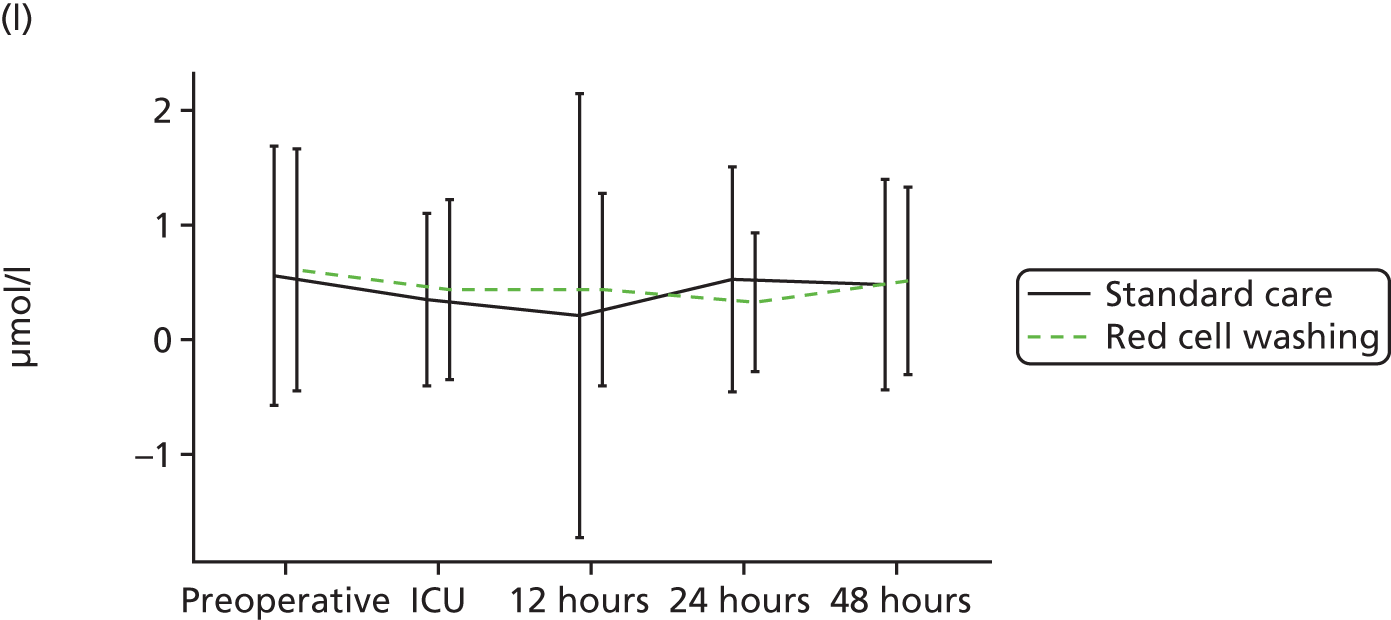
Discussion
Main findings
In the mechanism substudy of the REDWASH trial we did not find evidence to support our hypothesis that mechanical red cell washing would result in reductions in inflammation or organ injury in cardiac surgery patients at risk of LVBT. Complementary experimental models (as discussed in Red cell washing) indicate that washing reduces microparticle levels in stored red cell units and attenuates platelet and monocyte activation post transfusion. In experimental studies washing also results in accelerated release of cell-free haemoglobin, which results in oxidative stress, endothelial activation and kidney and lung injury in transfusion recipients. However, in cardiac surgery patients in the REDWASH trial, transfusion of washed red cells did not reduce platelet or leucocyte activation compared with standard red cells. Moreover, despite increased release of free haemoglobin from human red cells post washing, this was not reflected by differences between the treatment groups in plasma-free haemoglobin levels, markers of haem/iron metabolism or endothelial activation.
Strengths and limitations
The REDWASH trial had several strengths. This is the first randomised trial to have evaluated the risks and benefits of red cell washing in adults. We recruited a high-risk population of cardiac surgery patients who should, in principle, have benefited from safer red cell transfusion. Participants in both groups received LVBTs within 48 hours of surgery and experienced significant morbidity; 86% of participants experienced our composite clinical outcome of death, sepsis or organ failure. Participants in the red cell washing group received more red cell units despite similar levels of blood loss and serial serum haemoglobin concentrations perioperatively. This is consistent with previous clinical and experimental studies that have reported the loss of 10–25% of red cells during mechanical washing. 31,273 The chief limitations of the study were the limited power of our observations and the early termination of the main trial and for these reasons we can neither accept nor refute our primary hypothesis. This limitation notwithstanding the randomisation did generate two groups of participants who received significant volumes of washed or unwashed red cells and demonstrated no evidence of benefit for any measured biomarker of inflammation or organ injury. Even significant differences in inflammatory biomarkers often translate to marginal clinical effects. 267 On balance, these findings argue against washing having important clinical benefits.
Our mechanism substudy is strengthened by complementary experimental analyses that document both the risks and the benefits of red cell washing (see Red cell washing). We have demonstrated novel pathways by which components of the storage lesion contribute to experimental inflammation and organ injury. Our experimental studies using human cells in vitro and porcine cells in vivo were remarkably consistent, indicating that microparticles and free haemoglobin act through distinct mechanisms in platelets/monocytes and endothelial cells respectively. Our experimental findings did not support either the iron hypothesis255 or the nitric oxide hypothesis. 274 We demonstrated important pathological differences between experimental groups that were attributable to significant differences in microparticles and free haemoglobin, but measures of iron metabolism and nitric oxide bioavailability were similar. Importantly, we demonstrated that cell-free haemoglobin activates endothelial cells via a pathway previously described only in human aortic endothelial cells in the presence of minimally modified low-density lipoprotein. 275 Here, an alternative cyclic adenosine monophosphate (cAMP)/R-Ras/phosphatidylinositol-3-kinase (PI3K)-dependent pathway results in VLA5 (integrin-α5/β1) expression, retention of alternatively spliced CS-1 fibronectin on the surface of endothelial cells and endothelial–monocyte interaction. 276 We suggest that the activation is most likely mediated by lipids oxidised by increased levels of free haemoglobin,277 which happens either in the blood bags or after transfusion. 278
Clinical importance
Our experimental results were not replicated in the REDWASH trial. This may be attributable to differences in the time period over which red cells were transfused between the porcine experiments and the REDWASH trial; 1000 ml were transfused over 2 hours in the porcine experiments whereas a similar volume, on average, was transfused over 2 days in the clinical trial. The porcine experiments were also conducted without CPB. These factors may have diminished the clinical effects of washing in trial participants; neither microparticle depletion nor the release of free haemoglobin observed following washing were reflected by differences in plasma microparticle levels or cell-free haemoglobin in participants or by differences in inflammation and organ injury. Moreover, the peak mean IL-6 level in the porcine D14 group (transfusion of 14-day stored red cells) was 10 pg/ml whereas that in the standard care participants in the REDWASH trial was 221 pg/ml. This suggests that the effects of washing were superseded by the greater platelet and leucocyte activation, free haemoglobin release and endothelial injury, attributable to surgery and CPB. 2 This is consistent with the results of the RECESS trial. 39 Alternatively, we have demonstrated both potential harms and potential benefits of red cell washing that could have resulted in no overall difference between the trial groups. In our experimental studies red cell rejuvenation maximised the benefits by preventing microparticle production post washing and minimised the risks by preventing red cell damage and free haemoglobin release and resulted in significant attenuation of transfusion-mediated organ injury. We suggest that a RCT with rejuvenated red cells would resolve this uncertainty.
In summary, the results of the mechanism substudy of the REDWASH trial did not support our hypothesis that mechanical washing would attenuate platelet and leucocyte activation and organ injury. Complementary experimental studies have identified potential risks as well as potential benefits of mechanical red cell washing that could explain our negative findings; however, our results were also consistent with the findings of other recent trials that have failed to show any causal relationship between the red cell storage lesion and adverse outcomes in cardiac surgery.
Summary and conclusions
What this study shows
-
The LVBT risk score developed in Chapter 4 was used to select a cohort of cardiac surgery patients with large transfusion requirements and a very high frequency of sepsis or organ failure.
-
The REDWASH trial was terminated by the funder because of slow recruitment and therefore we were unable to adequately test our hypothesis.
-
The results of the trial, and in particular the results of the mechanistic substudy, did not support our hypothesis: there was no difference between washed red cells and standard care.
-
We demonstrated that red cell washing has both risk and benefits and this is an alternative explanation for the apparently negative findings.
-
The results are also consistent with the findings of other recent trials that have failed to show any causal relationship between transfusion of stored red cells and adverse outcomes.
Further research
-
The clinical effects of red cell washing need to be evaluated in an adequately powered RCT.
-
We suggest that a trial of rejuvenated red cells in high-risk cardiac surgery patients will address the remaining uncertainty over the risks and benefits of red cell transfusion in cardiac surgery.
Chapter 6 Summary statement
What this programme showed
This programme considered the clinical effectiveness and cost-effectiveness of medical devices in common clinical use as blood management adjuncts in cardiac surgery. We demonstrated that risk assessment using baseline clinical factors accurately predicts which patients are likely to develop severe bleeding post surgery. We developed two new risk scores that have greater predictive accuracy than existing scores. These are freely available to any clinician or researcher as e-calculators. We did not demonstrate any additional benefit from routine POC haemostasis testing or from the use of an expanded range of LRTs for the prediction of bleeding. The introduction of POC tests into routine clinical use was not estimated to be cost-effective. A review of existing trial data did not suggest that POC-based algorithms are clinically effective. We failed to demonstrate either clinical effectiveness or cost-effectiveness of NIRS-based algorithms for the optimisation of tissue oxygenation or to guide red cell transfusion. Finally, we demonstrated that mechanical washing of stored red cells results in accelerated pathological changes that may contribute to inflammation and organ injury in recipients. Overall, our findings suggest that the use of medical devices as diagnostic and therapeutic tools for safer blood management in cardiac surgery is not supported by existing evidence. These results have direct implications for practice; for example, on the basis that 36,000 cardiac surgery procedures are performed per year in the UK and that the additional per-patient costs of consumables alone are £48 for a POC test, £218 for NIRS and £150 per unit of washed red cells (with an average of 2 units of red cells used per patient), the annual cost to the NHS of using these tests routinely would be £14,256,000. These results also question the quality of current systems for approving the introduction of medical devices into clinical practice and underpin the value to patients and the NHS of careful clinical evaluation of novel technology prior to use.
Strengths and weaknesses
The programme used a range of research designs to address specific areas of clinical uncertainty with respect to a range of medical devices in common use as blood management adjuncts. Patients and the public were involved in all aspects of the programme, including consultation on study design, governance and dissemination and, latterly in the REDWASH trial, participation. Blood safety is an important area of concern for patients and the public and their input maintained this as the focus of the programme. Appropriate methodology was rigorously applied for each component of the programme, with full and transparent reporting in accordance with established guidelines for observational studies (STROBE),181 diagnostic accuracy studies (STARD),98 systematic reviews (PRISMA),131 RCTs (CONSORT)216 and health economic analyses (CHEERS). 103 The use of systematic reviews performed according to Cochrane guidelines130 to place the evaluation of devices within the appropriate clinical context was also a strength. We used these reviews to highlight areas of uncertainty, gaps in knowledge and the limitations of existing data. The subsequent prospective trials addressed these limitations.
In workstream 1 we described a recently published review of existing studies that had evaluated the predicative accuracy of POC tests. 25 These studies enrolled small cohorts of patients and were of poor methodological quality. We then addressed this in the COPTIC study. Here, the individual cohorts evaluated for each platform were larger than the number of participants included in all of the previous studies combined. Moreover, and unlike all of the existing studies, the COPTIC study design mitigated important sources of bias in diagnostic test accuracy studies. 166,279 These considerations increase our confidence in the veracity of our findings, notably that existing POC tests of coagulopathy do not accurately predict clinically important bleeding. It is perhaps unsurprising, therefore, that RCTs of POC test-based management algorithms for bleeding have failed to result in important clinical benefits. This absence of efficacy was reinforced by the health economic analysis, which concluded that the routine introduction of these tests would not significantly reduce costs or improve outcomes. It may be argued that a prospective RCT of POC test-based algorithms of a similar scale to that of the COPTIC study would have yielded stronger evidence for decision-makers with respect to recommendations for the routine use of these devices. However, such a trial assumes a priori that these tests can accurately diagnose the target condition. A recent large stepped-wedge cluster RCT of Multiplate- and ROTEM-based algorithms enrolling 7402 patients failed to demonstrate any clinical benefit compared with standard care (no POC tests) beyond a reduction in a composite outcome of LVBT and bleeding. 129 These results are not dissimilar to those of our own systematic review. The results of the COPTIC trial provide important insights into these effectiveness trials and question whether any future large trial of POC tests is justified. That every rigorous evaluation of POC tests in cardiac surgery thus far has failed to demonstrate clinical benefits should have direct implications for practice (see Implications for practice and future research).
In workstream 2 we employed a similar approach. In a systematic review we identified important limitations in previous studies that had evaluated NIRS-based devices. We then addressed these limitations in a multicentre trial, at low risk of bias. It has been suggested that tissue oximetry may have a role in the development of personalised transfusion thresholds. 38 An important strength of our trial was the use of an absolute restrictive transfusion threshold, with the ability to transfuse above this threshold in the presence of cerebral hypoxia refractory to other interventions, a personalised transfusion indicator. We demonstrated that this approach resulted in a modest reduction in transfusion (approximately 12%); however, there was no clinical benefit and, importantly, this approach was not cost-effective. A limitation of this workstream is that the PASPORT trial enrolled a relatively small cohort of patients such that it was not possible to assess the impact of NIRS on important clinical outcomes. Moreover, when the PASPORT trial data were added to our systematic review, the GRADE level of evidence remained low for all outcomes assessed. For this reason we cannot be certain that these findings would be altered by a well-conducted effectiveness trial.
In workstream 3 we performed a trial of an intervention widely believed to have clinical benefits, with a linked evaluation of disease mechanisms believed to underlie organ failure in cardiac surgery. To our knowledge this was the first evaluation of mechanical red cell washing as a blood safety intervention to have been performed in adults. The clinical trial was terminated prematurely and this limits our ability to determine the clinical impact of this intervention. However, the mechanism substudy did not demonstrate differences between the groups for any of the measures of inflammation, oxidative stress and platelet, leucocyte and endothelial cell activation. We therefore consider it unlikely that this will translate into clinical benefits. Our in vitro and in vivo experimental studies have identified both risks and benefits of mechanical washing (see Chapter 5, Red cell washing). Washing removed inflammatory microvesicles caused accelerated release of cell-free haemoglobin, which in experimental models causes endothelial injury, microvascular dysfunction and organ injury. The results of a similar trial (NCT02094118) evaluating the clinical efficacy of red cell washing that has recently started recruiting patients will provide greater insights into these results.
Implications for practice and future research
The essentially negative findings of the research reflect the overall paucity of evidence that is used to support transfusion practice in cardiac surgery and other settings. Our interpretation of the research will benefit from input from other disciplines. Moving forward we propose to work with collaborators undertaking similar research in critical care, trauma and blood management to more fully consider the impact of the results on current practice and further explore what additional research should be conducted. Our immediate conclusions are presented in the following sections.
Workstream 1
Our analysis of POC tests does not support current NICE guidance on the use of POC tests in cardiac surgery. 23 Our results showed that the routine use of these tests was not predictive of bleeding and was not clinically effective or cost-effective. Existing NICE guidance is based on a recent HTA review. 26 The findings of this systematic review of trial evidence, on which the NICE guidelines are based, were similar to our own, notably that trials were at high risk of bias and showed efficacy only for those end points at greatest risk of bias. The NICE recommendation that POC tests be used routinely23 was largely driven by a health economic analysis which suggested that the reduction in red cell transfusion identified in the systematic review would translate into clinical effectiveness and cost-effectiveness. This was based on an early study showing an association between transfusion and adverse clinical outcomes that has subsequently been shown to be attributable to confounding,4,32 as well as estimates of costs and utility from previously published studies. Conversely, a cost-effectiveness analysis of the COPTIC study showed no benefit. These results are not consistent with current NICE guidelines. Furthermore, as part of the COPTIC study we conducted a further analysis to evaluate the clinical value of a range of expanded reference tests that could potentially be developed as novel POC tests. We did not identify a single test that had clinical value beyond the predictive accuracy of clinical risk scores alone. This highlights our poor understanding of the links between coagulopathy, bleeding and outcome and identifies a knowledge gap that must be addressed by further research.
On the basis of these findings we suggest that future research should include:
-
Investigation of the causes and pathogenesis of coagulopathy. There is no clear definition of coagulopathy. It is highly likely that coagulopathy is both heterogeneous and dynamic, that is, that it is a rapidly evolving multifactorial process. It remains poorly characterised. In the COPTIC study, the largest study of post-cardiac surgery coagulopathy performed to date, existing tests of coagulation system and platelet dysfunction had only limited predictive accuracy for coagulopathic bleeding. Diagnostic tests for coagulopathy were also not predictive for adverse clinical outcomes, at odds with clinical evidence suggesting that these processes are strongly linked. This highlights important knowledge gaps with respect to the pathogenesis of coagulopathy. For example, there is very little research on the role of the systemic vasculature and vascular endothelium in coagulopathic bleeding, despite this being acknowledged for at least a century as a key determinant of haemostasis. We suggest that this should be the focus of future research into coagulopathy, bleeding and organ injury.
-
Investment into new diagnostic tests of coagulopathy. Existing POC platforms are relatively expensive tools in the care pathway of patients, with costs running into tens of thousands of pounds. In contrast, the evidence presented here suggests that these platforms have limited or no benefits. It is unlikely that current devices would be licensed based on the evidence presented here. Along with better understanding of the pathogenesis of coagulopathy these findings should promote the development of new platforms and diagnostic tests for coagulopathy. Evidence of efficacy should be mandatory prior to licensing of these devices for clinical use.
Workstream 2
Near-infrared spectroscopy devices are widely used in adult cardiac surgery. In a Canadian study it was estimated that they are used in 40–60% of patients. 211 We have conducted the most comprehensive evaluation of the clinical effectiveness and cost-effectiveness of this technology to date. Our results do not support the routine use of NIRS devices. The existing evidence consisted, in the most part, of trials at high risk of bias. The GRADE level of evidence was very low, indicating that further trials are required to address the remaining uncertainty. We conducted a trial that was at low risk of bias, the PASPORT trial, which used sensitive indicators of organ injury – detailed neurocognitive assessment and assessment of biomarkers of inflammation and cellular injury to the brain, heart and kidneys; however, this trial showed no benefit of NIRS. A cost–utility analysis also showed no benefit. We argue that this is an example of a medical device that has been CE marked for clinical use in the absence of evidence of efficacy. We hope that the wider dissemination of this evidence will allow physicians and health-care organisations to make better-informed decisions about the purchase of NIRS devices and their consumables in the face of strong ongoing marketing campaigns from manufacturers.
On the basis of these findings we suggest that future research should include:
-
Investigation into the role of tissue hypoxia in acute organ injury. Tissue oxygen saturations measured by NIRS during CPB were not found to be a determinant of brain injury. This suggests that brain tissue mixed venous oxygenation is not a determinant of cellular injury. The PASPORT trial results also indicated that brain mixed venous desaturation was uncommon during CPB. As indicated in our discussion, we have concluded that tissue oxygen saturation levels in all probability do not reflect cellular oxygen utilisation. We suggest that further research should focus on the development of new tests and technologies for the assessment of tissue oxygen utilisation and mitochondrial function on the basis that these are more likely to reflect critical oxygen delivery. Alternatively, future research should focus on changes in organs at baseline, as these are key determinants of organ injury. The baseline eGFR, for example, is the most important determinant of post-cardiac surgery AKI. 155 Pre-surgery white matter change is emerging as a key determinant of post-surgery brain injury. 251 How these factors interact with the inflammation caused by CPB and/or tissue hypoxia during CPB is poorly understood and should be the subject of ongoing studies.
-
Assessment of new personalised indicators of red cell transfusion. As hypothesised by the PASPORT trial, markers of tissue hypoxia are likely to represent personalised indicators for red cell transfusion in severely anaemic or bleeding patients. Current evidence suggests that higher haematocrits have benefits in patients undergoing CPB4,32 and highlights the harms attributable to severe anaemia in patients with severe symptomatic cardiovascular disease. Cardiac surgery remains a significant user of red cells in the UK and elsewhere. We suggest that a better understanding of the interaction between microvascular function in diseased organs, inflammation and hypoxia will lead to the development of new devices that can identify critical haematocrits and act as personalised indicators of the need for transfusion. One such example is the COMET device (Photonics Healthcare BV, Utrecht, the Netherlands), a non-invasive sensor of cutaneous mitochondrial oxygen tension. These devices require evaluation in clinical trials prior to clinical use.
Workstream 3
Red cell washing is used widely in paediatric cardiac surgery, particularly where blood stored for less than seven days is not routinely available. This has led to speculation that this may represent a blood safety strategy in adults. Our results did not support this; however, our trial was terminated prematurely. There is remaining uncertainty whether this intervention is safe and effective and further trials of this intervention are required.
On the basis of these findings we suggest that future research should include:
-
A randomised trial of rejuvenated red cells in patients undergoing cardiac surgery with CPB. Our experimental work suggests that red cell rejuvenation prior to washing may preserve the benefits and remove the harmful effects observed following washing alone. Rejuvenated red cells do not release free haemoglobin at an accelerated rate. Rejuvenation may have other benefits: restoration of red cell 2,3-diphosphoglycerate (2,3DPG) levels restores the P50 of haemoglobin to normal levels, which may have additional clinical benefits. A clinical trial from 1975 demonstrated that participants receiving rejuvenated red cells had higher post-surgery P50 values and improved cardiac function following cardiac surgery with CPB. 280 However, the safety and efficacy of rejuvenated red cells has not been evaluated since. A trial of rejuvenated red cells will establish whether improved red cell function will have clinical benefits and address residual uncertainty over the clinical importance of the storage lesion in transfused red cells in cardiac surgery. 39
-
An evaluation of the risks and benefits of red cell washing for other indications. Mechanically washed red cells are routinely produced by blood component manufacturers, albeit in small numbers, for patients with high antibody titres, at risk of non-haemolytic transfusion reactions. These cells normally have a 7-day shelf life post washing. Our mechanism substudy of the REDWASH trial suggested that this may result in red cell components with high concentrations of inflammatory microvesicles and free haem, as well as red cells that have undergone mechanical trauma. Although our results do not identify clinical harms attributable to these changes, our results may lead to further evaluation of current red cell washing protocols and evaluation of the efficacy of these red cells once transfused.
Conclusion
An 8-year National Institute for Health Research (NIHR)-funded programme of applied research evaluated the available evidence employed to support the use of a range of devices in common use as blood management adjuncts. These included POC diagnostic tests for coagulopathy and platelet dysfunction, NIRS and mechanical red cell washing devices. Areas of uncertainty and knowledge gaps were then addressed using appropriate prospective clinical studies. The programme did not find evidence to support the clinical use of these devices in cardiac surgery. We suggest that future research should focus on improving our understanding of the relationship between bleeding, anaemia, transfusion and adverse outcomes and on the development of new devices that can accurately measure processes that are causal to organ injury and subsequent adverse clinical outcomes and that may be used to more effectively stratify patients and enable targeted treatments.
Acknowledgements
This report presents independent research commissioned by the NIHR. The views and opinions expressed by the authors in this publication are those of the authors and do not necessarily reflect those of the NHS, the NIHR, the NIHR Evaluation, Trials and Studies Coordinating Centre (NETSCC), the Programme Grants for Applied Research programme or the Department of Health.
Funding
The trial was funded by the NIHR Programme Grants for Applied Research programme (reference number RP-PG-0407–10384). Investigators in the programme were funded by other agencies. Chris Rogers, Gianni Angelini, Gavin Murphy, Marcin Wozniak and Tracy Kumar were funded by the British Heart Foundation. Barnaby Reeves was funded by the Bristol Cardiovascular Biomedical Research Unit. Additional funding was acquired from the following sources to complete the programme: the COPTIC trial was supported by additional funds from the Bristol Cardiovascular Biomedical Research Unit (research nurse time); the PASPORT trial was supported by additional funds from the Bristol and Leicester Cardiovascular Biomedical Research Units (research nurse time); and the mechanism substudy of the REDWASH trial was supported by British Heart Foundation (grants RG/13/6/29947, CH/12/1/29419 and PG/11/95/29173) (research staff time and laboratory consumables) and the Leicester Cardiovascular Biomedical Research Unit (statistical analysis and laboratory consumables).
Contributions of authors
All of the listed authors can attest to the integrity of the components of the programme for which they were personally responsible, contributed to the writing of this report and approved the final version for submission. All authors attest to the contributions of their co-authors in the report. In addition, specific contributions are as follows:
Gavin J Murphy (Professor of Cardiac Surgery) was the lead applicant and chief investigator with overall responsibility for the programme, conceived and designed the studies in the programme and supervised the governance, co-ordination and analysis of the studies and their interpretation. He is the first author and corresponding author.
Andrew D Mumford (Reader in Haematology) was a co-applicant and was responsible for the design, supervision, analysis and interpretation of the COPTIC study.
Chris A Rogers (Reader in Statistics) was a co-applicant, designed and supervised the co-ordination, statistical analysis planning and implementation and interpretation of the COPTIC study and PASPORT trial.
Sarah Wordsworth (Associate Professor of Health Economics) was a co-applicant and designed and supervised the health economic analyses for the COPTIC study and PASPORT trial.
Elizabeth A Stokes (Postgraduate Student in Health Economics) undertook the health economic analyses for the COPTIC study and PASPORT trial.
Veerle Verheyden managed all aspects of the governance and co-ordination of the programme from January 2010 to January 2013.
Tracy Kumar managed all aspects of the governance and co-ordination of the programme from July 2013 to April 2016.
Jessica Harris (Medical Statistician) drafted the statistical analysis plan and undertook the statistical analysis in the COPTIC study.
Gemma Clayton (Medical Statistician) drafted the statistical analysis plan and undertook the statistical analysis in the PASPORT trial.
Lucy Ellis was the trial co-ordinator for the PASPORT trial and was responsible for the acquisition of trial data.
Zoe Plummer was the trial co-ordinator for the COPTIC study and was responsible for the acquisition of study data.
William Dott was the trial co-ordinator for the REDWASH trial and was responsible for the acquisition of study data.
Filiberto Serraino (Clinical Research Fellow) was responsible for the design, supervision, data acquisition and analysis for the systematic reviews of near-patient haemostasis testing and NIRS.
Marcin Wozniak (Research Fellow in Cardiovascular Sciences) designed and performed the mechanism substudy in the REDWASH trial.
Tom Morris (Medical Statistician) wrote the statistical analysis plan and undertook the statistical analysis in the REDWASH trial.
Mintu Nath (Medical Statistician) undertook the statistical analysis for the mechanism substudy of the REDWASH trial.
Jonathan A Sterne (Professor of Medical Statistics) was responsible for the design, development and implementation of the transfusion risk score.
Gianni D Angelini (Professor of Cardiac Surgery) was a co-applicant and was responsible for the supervision of the COPTIC study and PASPORT trial.
Barnaby C Reeves (Professor of Health Services Research) was a co-applicant and was responsible for the design, supervision, analysis and interpretation of the PASPORT trial and COPTIC study. Professor Reeves is the last author.
Contributions of others
The authors are extremely grateful to the patients who participated in the programme. They are also grateful to the cardiac surgery and cardiac surgery intensive care clinical teams at the Bristol Heart Institute; Glenfield Hospital; Leicester, Blackpool Victoria Hospital; Castle Hill Hospital, Hull; and University Hospitals Coventry and Warwickshire NHS Trust, without whom the studies would not have been possible.
The research teams for individual work packages were as follows.
The COPTIC study
Investigators: Dr Andrew Mumford (chief investigator, COPTIC study) and Professor Gavin Murphy (chief investigator, programme).
Trial co-ordinator: Dr Zoe Plummer.
Research nurses: Kim Wright (lead nurse), Maria Wahab, Emma Heron, Emma Hopkins, Rachel Wyatt, Kate Rajakaruna, Penny Lambert and Jenny Wilcox.
Laboratory technician: Curtis Lee.
Statistician: Jessica Harris.
Database: David Hutton.
Transfusion risk score
The authors are grateful to the committee members of the National Audit of Blood Transfusion in Adult Cardiac Surgery for providing the audit data: Professor Michael Murphy (University of Oxford), Dr Ravi Gill and Dr Mike Herbertson (both University Hospital Southampton NHS Foundation Trust), Dr Shubha Allard (Barts and The London NHS Trust) and Mr John Grant-Casey (NHS Blood and Transplant). Mr Mo Bhabra provided the Wolverhampton audit data. Dr Marco Ranucci provided the Milan data. Thanks also to Drs Jessica Harris and Chris Rogers (University of Bristol) for matching clinical and laboratory data in the Bristol data set and to Dr Rachael Hughes, Dr Margaret May, Professor Kate Tilling and Michael Lawton who provided advice on the analyses (all at the University of Bristol).
The PASPORT trial
Trial Steering Committee: Dr Edwin Massey, Mr Ian Millar, Mr Patrick Magee (deceased), Professor John Pepper, Dr Duncan Young, Dr Edwin Massey, Dr Gordon Taylor and Hon. Karin Smyth MP.
Data Monitoring and Safety Committee: Professor Gordon Murray (chairperson), Professor Tim Walsh and Professor Domenico Pagano
Trial co-ordinator: Teresa Henstridge and Alice Miles (until November 2010)/Lucy Ellis from (November 2010; prior to this Lucy Ellis was the trainee co-ordinator on the study).
Assistant co-ordinator: Rachael Heys.
Back-up trial co-ordinators: Lucy Dreyer and Kate Ashton (prior to going on maternity leave).
Statistician: Gemma Clayton (previously Kate Bayliss).
Database: David Hutton.
Health economic analyses: Danielle Bargo and Jilles Fermont.
Design and monitoring of the intervention: Richard Downes and Eamon Nicholson.
Bristol Heart Institute
Investigators: Gavin Murphy (until August 2012)/Serban Stoica (from August 2012).
Research nurses: Kim Wright (lead nurse), Maria Wahab, Emma Heron, Emma Hopkins, Rachel Wyatt, Kate Rajakaruna, Penny Lambert and Jenny Wilcox.
Castle Hill Hospital, Hull
Investigator: Sean Bennett.
Lead research nurse: Neil Smith.
Glenfield Hospital, Leicester
Investigator: Gavin Murphy.
Trial co-ordinator: Pia Nielsen (until September 2014)/ William Dott (from September 2014).
Sample analyses: Pia Nielsen and Ade Abidoye.
The REDWASH trial
Trial co-ordinator: William Dott (previously Pia Nielsen).
Trial manager: Tracy Kumar.
Science lead: Marcin Wozniak and Nikol Sullo.
Trial Steering Committee: Mr Anthony Locke, Mr Alan Phillips, Dr Karl Herbert, Professor Alison Goodall, Professor Nigel Brunskill and Dr Rakesh Vaja.
Professor Tim Walsh had accepted the role of chairperson of the Data Monitoring and Ethics Committee for the trial; however, as the trial was stopped by the funder, the Data Monitoring and Ethics Committee had not convened prior to the cessation of the trial.
Glenfield Hospital, Leicester
Investigator: Gavin Murphy.
Research nurse: Latisha Joel-Davies.
University Hospitals Coventry and Warwickshire NHS Trust
Investigator: Sunil Bhudia.
Blackpool Victoria Hospital
Investigator: Nidal Bittar.
Publications
Ellis L, Murphy GJ, Culliford L, Dreyer L, Clayton G, Downes R, et al. The effects of patient-specific cerebral oxygenation monitoring as part of an algorithm on post-operative cognitive function and infectious complications in patients having heart valve surgery using cardiopulmonary bypass: PASPORT, a multi-centre, randomised controlled trial. JMIR Res Protoc 2015;4:e137.
Goudie R, Sterne JAC, Verheyden V, Bhabra M, Ranucci M, Murphy GJ. Risk scores to facilitate pre-operative prediction of transfusion and large volume blood transfusion associated with adult cardiac surgery. Br J Anaesthesia 2015;114:757–66.
Norman JE, Lee K, Walker ME, Murden SL, Harris J, Mundell S, et al. Low multiple electrode aggregometry platelet responses are not associated with loss-of-function variants in G-protein coupled receptor genes. Thomb Haemostas 2015;136:818–24.
Murphy GJ, Verheyden V, Wozniak M, Sullo N, Dott W, Bhudia S, et al. Trial protocol for a randomised controlled trial of red cell washing for the attenuation of transfusion associated organ injury in cardiac surgery: the REDWASH trial. Open Heart 2016;3:e000344.
Serraino GF, Murphy GJ. Routine use of viscoelastic blood tests for diagnosis and treatment of coagulopathic bleeding in cardiac surgery: updated systematic review and meta-analysis. Br J Anaesthesia 2017;118:823–33.
Woźniak MJ, Sullo N, Qureshi S, Dott W, Cardigan R, Wiltshire M, et al. Randomized trial of red cell washing for the prevention of transfusion-associated organ injury in cardiac surgery. Br J Anaesthesia 2017;118:689–98.
Mumford AD, Harris J, Plummer Z, Lee K, Verheyden V, Reeves BC, et al. Prediction of bleeding after cardiac surgery with near-patient haemostasis tests. Res Pract Thromb Haemostas 2017. In press.
Rogers CA, Stoica S, Ellis L, Stokes EA, Wordsworth S, Dabner L, et al. A randomised trial of near infra-red spectroscopy for the personalised optimisation of cerebral tissue oxygenation during cardiac surgery. Br J Anaesth 2017. In press.
Serraino GF, Murphy GJ. Effects of cerebral near-infrared spectroscopy on the outcome of patients undergoing cardiac surgery: a systematic review of randomised trials. BMJ Open 2017. In press.
Data sharing statement
Patient-level data, a technical appendix and statistical code are available with open access from the corresponding author. Consent was not obtained for the sharing of patient-identifiable data but the anonymised data will be made available to researchers when appropriate governance approvals are in place.
Disclaimers
This report presents independent research funded by the National Institute for Health Research (NIHR). The views and opinions expressed by authors in this publication are those of the authors and do not necessarily reflect those of the NHS, the NIHR, CCF, NETSCC, PGfAR or the Department of Health. If there are verbatim quotations included in this publication the views and opinions expressed by the interviewees are those of the interviewees and do not necessarily reflect those of the authors, those of the NHS, the NIHR, NETSCC, the PGfAR programme or the Department of Health.
References
- Murphy GJ, Ascione R, Angelini GD. Coronary artery bypass grafting on the beating heart: surgical revascularization for the next decade?. Eur Heart J 2004;25:2077-85. https://doi.org/10.1016/j.ehj.2004.09.022.
- Murphy GJ, Angelini GD. Side effects of cardiopulmonary bypass: what is the reality?. J Card Surg 2004;19:481-8. https://doi.org/10.1111/j.0886-0440.2004.04101.x.
- Hausenloy DJ, Boston-Griffiths E, Yellon DM. Cardioprotection during cardiac surgery. Cardiovasc Res 2012;94:253-65. https://doi.org/10.1093/cvr/cvs131.
- Murphy GJ, Pike K, Rogers CA, Wordsworth S, Stokes EA, Angelini GD, et al. Liberal or restrictive transfusion after cardiac surgery. N Engl J Med 2015;372:997-1008. https://doi.org/10.1056/NEJMoa1403612.
- Hickey GL, Grant SW, Murphy GJ, Bhabra M, Pagano D, McAllister K, et al. Dynamic trends in cardiac surgery: why the logistic EuroSCORE is no longer suitable for contemporary cardiac surgery and implications for future risk models. Eur J Cardiothorac Surg 2013;43:1146-52. https://doi.org/10.1093/ejcts/ezs584.
- Menkis AH, Martin J, Cheng DC, Fitzgerald DC, Freedman JJ, Gao C, et al. Drug, devices, technologies, and techniques for blood management in minimally invasive and conventional cardiothoracic surgery: a consensus statement from the International Society for Minimally Invasive Cardiothoracic Surgery (ISMICS) 2011. Innovations 2012;7:229-41. https://doi.org/10.1097/IMI.0b013e3182747699.
- Ranucci M, Aronson S, Dietrich W, Dyke CM, Hofmann A, Karkouti K, et al. Patient blood management during cardiac surgery: do we have enough evidence for clinical practice?. J Thorac Cardiovasc Surg 2011;142.
- Fergusson DA, Hébert PC, Mazer CD, Fremes S, MacAdams C, Murkin JM, et al. A comparison of aprotinin and lysine analogues in high-risk cardiac surgery. N Engl J Med 2008;358:2319-31. https://doi.org/10.1056/NEJMoa0802395.
- Murphy MF, Murphy GJ, Gill R, Allard S, Herbertson M. National Comparative Audit of Blood Transfusion: 2011 Audit of Blood Transfusion in Adult Cardiac Surgery. Oxford: NHS Blood and Transplant; 2013.
- Karkouti K, Wijeysundera DN, Yau TM, Beattie WS, Abdelnaem E, McCluskey SA, et al. The independent association of massive blood loss with mortality in cardiac surgery. Transfusion 2004;44:1453-62. https://doi.org/10.1111/j.1537-2995.2004.04144.x.
- Karthik S, Grayson AD, McCarron EE, Pullan DM, Desmond MJ. Reexploration for bleeding after coronary artery bypass surgery: risk factors, outcomes, and the effect of time delay. Ann Thorac Surg 2004;78:527-34. https://doi.org/10.1016/j.athoracsur.2004.02.088.
- Moulton MJ, Creswell LL, Mackey ME, Cox JL, Rosenbloom M. Reexploration for bleeding is a risk factor for adverse outcomes after cardiac operations. J Thorac Cardiovasc Surg 1996;111:1037-46. https://doi.org/10.1016/S0022-5223(96)70380-X.
- Unsworth-White MJ, Herriot A, Valencia O, Poloniecki J, Smith EE, Murday AJ, et al. Resternotomy for bleeding after cardiac operation: a marker for increased morbidity and mortality. Ann Thorac Surg 1995;59:664-7. https://doi.org/10.1016/0003-4975(94)00995-3.
- Henry DA, Carless PA, Moxey AJ, O’Connell D, Forgie MA, Wells PS, et al. Pre-operative autologous donation for minimising perioperative allogeneic blood transfusion. Cochrane Database Syst Rev 2002;2.
- Davies L, Brown TJ, Haynes S, Payne K, Elliott RA, McCollum C. Cost-effectiveness of cell salvage and alternative methods of minimising perioperative allogeneic blood transfusion: a systematic review and economic model. Health Technol Assess 2006;10. https://doi.org/10.3310/hta10440.
- Murphy MF, Allard SA, Blackwell D, Donald G, Halligan K, Madgwick K, et al. Blood Transfusion 2015. www.nice.org.uk/guidance/ng24 (accessed 21 July 2017).
- Ker K, Edwards P, Perel P, Shakur H, Roberts I. Effect of tranexamic acid on surgical bleeding: systematic review and cumulative meta-analysis. BMJ 2012;344. https://doi.org/10.1136/bmj.e3054.
- Ker K, Roberts I, Shakur H, Coats TJ. Antifibrinolytic drugs for acute traumatic injury. Cochrane Database Syst Rev 2015;5. https://doi.org/10.1002/14651858.CD004896.pub4.
- Henry DA, Carless PA, Moxey AJ, O’Connell D, Stokes BJ, Fergusson DA, et al. Anti-fibrinolytic use for minimising perioperative allogeneic blood transfusion. Cochrane Database Syst Rev 2011;3. https://doi.org/10.1002/14651858.CD001886.pub4.
- Carless PA, Henry DA, Moxey AJ, O’Connell D, McClelland B, Henderson KM, et al. Desmopressin for minimising perioperative allogeneic blood transfusion. Cochrane Database Syst Rev 2004;1. https://doi.org/10.1002/14651858.CD001884.pub2.
- Simpson E, Lin Y, Stanworth S, Birchall J, Doree C, Hyde C. Recombinant factor VIIa for the prevention and treatment of bleeding in patients without haemophilia. Cochrane Database Syst Rev 2012;3. https://doi.org/10.1002/14651858.CD005011.pub4.
- Walkden GJ, Verheyden V, Goudie R, Murphy GJ. Increased perioperative mortality following aprotinin withdrawal: a real-world analysis of blood management strategies in adult cardiac surgery. Intensive Care Med 2013;39:1808-17. https://doi.org/10.1007/s00134-013-3020-y.
- Detecting, Managing and Monitoring Haemostasis: Viscoelastometric Point-of-Care Testing (ROTEM, TEG and Sonoclot Systems). London: NICE; 2014.
- Ferraris VA, Brown JR, Despotis GJ, Hammon JW, Reece TB, . Society of Thoracic Surgeons Blood Conservation Guideline Task Force . 2011 update to the Society of Thoracic Surgeons and the Society of Cardiovascular Anesthesiologists blood conservation clinical practice guidelines. Ann Thorac Surg 2011;91:944-82. https://doi.org/10.1016/j.athoracsur.2010.11.078.
- Corredor C, Wasowicz M, Karkouti K, Sharma V. The role of point-of-care platelet function testing in predicting postoperative bleeding following cardiac surgery: a systematic review and meta-analysis. Anaesthesia 2015;70:715-31. https://doi.org/10.1111/anae.13083.
- Whiting P, Al M, Westwood M, Ramos IC, Ryder S, Armstrong N, et al. Viscoelastic point-of-care testing to assist with the diagnosis, management and monitoring of haemostasis: a systematic review and cost-effectiveness analysis. Health Technol Assess 2015;19. https://doi.org/10.3310/hta19580.
- Hung M, Ortmann E, Besser M, Martin-Cabrera P, Richards T, Ghosh M, et al. A prospective observational cohort study to identify the causes of anaemia and association with outcome in cardiac surgical patients. Heart 2015;101:107-12. https://doi.org/10.1136/heartjnl-2014-305856.
- Ranucci M, Carboni G, Cotza M, Bianchi P, Di Dedda U, Aloisio T. Surgical and Clinical Outcome Research (SCORE) Group . Hemodilution on cardiopulmonary bypass as a determinant of early postoperative hyperlactatemia. PLOS ONE 2015;10. https://doi.org/10.1371/journal.pone.0126939.
- Habib RH, Zacharias A, Schwann TA, Riordan CJ, Engoren M, Durham SJ, et al. Role of hemodilutional anemia and transfusion during cardiopulmonary bypass in renal injury after coronary revascularization: implications on operative outcome. Crit Care Med 2005;33:1749-56. https://doi.org/10.1097/01.CCM.0000171531.06133.B0.
- Karkouti K, Wijeysundera DN, Beattie WS. Reducing Bleeding in Cardiac Surgery (RBC) Investigators . Risk associated with preoperative anemia in cardiac surgery: a multicenter cohort study. Circulation 2008;117:478-84. https://doi.org/10.1161/CIRCULATIONAHA.107.718353.
- Bennett-Guerrero E, Kirby BS, Zhu H, Herman AE, Bandarenko N, McMahon TJ. Randomized study of washing 40- to 42-day-stored red blood cells. Transfusion 2014;54:2544-52. https://doi.org/10.1111/trf.12660.
- Patel NN, Avlonitis VS, Jones HE, Reeves BC, Sterne JA, Murphy GJ. Indications for red blood cell transfusion in cardiac surgery: a systematic review and meta-analysis. Lancet Haematol 2015;2:e543-53. https://doi.org/10.1016/S2352-3026(15)00198-2.
- Tinmouth A, Fergusson D, Yee IC, Hébert PC. ABLE Investigators . Clinical consequences of red cell storage in the critically ill. Transfusion 2006;46:2014-27. https://doi.org/10.1111/j.1537-2995.2006.01026.x.
- Carson JL, Grossman BJ, Kleinman S, Tinmouth AT, Marques MB, Fung MK, et al. Red blood cell transfusion: a clinical practice guideline from the AABB. Ann Intern Med 2012;157:49-58. https://doi.org/10.7326/0003-4819-157-1-201206190-00429.
- Napolitano LM, Kurek S, Luchette FA, Corwin HL, Barie PS, Tisherman SA, et al. Clinical practice guideline: red blood cell transfusion in adult trauma and critical care. Crit Care Med 2009;37:3124-57. https://doi.org/10.1097/CCM.0b013e3181b39f1b.
- Casutt M, Seifert B, Pasch T, Schmid ER, Turina MI, Spahn DR. Factors influencing the individual effects of blood transfusions on oxygen delivery and oxygen consumption. Crit Care Med 1999;27:2194-200. https://doi.org/10.1097/00003246-199910000-00021.
- Utoh J, Moriyama S, Okamoto K, Kunitomo R, Hara M, Kitamura N. The effects of cardiopulmonary bypass on postoperative oxygen metabolism. Surg Today 1999;29:28-33. https://doi.org/10.1007/BF02482966.
- Klein HG, Flegel WA, Natanson C. Red blood cell transfusion: precision vs. imprecision medicine. JAMA 2015;314:1557-8. https://doi.org/10.1001/jama.2015.10890.
- Steiner ME, Ness PM, Assmann SF, Triulzi DJ, Sloan SR, Delaney M, et al. Effects of red-cell storage duration on patients undergoing cardiac surgery. N Engl J Med 2015;372:1419-29. https://doi.org/10.1056/NEJMoa1414219.
- Koch CG, Li L, Sessler DI, Figueroa P, Hoeltge GA, Mihaljevic T, et al. Duration of red-cell storage and complications after cardiac surgery. N Engl J Med 2008;358:1229-39. https://doi.org/10.1056/NEJMoa070403.
- Shakur H, Roberts I, Bautista R, Caballero J, Coats T, . CRASH-2 trial collaborators . Effects of tranexamic acid on death, vascular occlusive events, and blood transfusion in trauma patients with significant haemorrhage (CRASH-2): a randomised, placebo-controlled trial. Lancet 2010;376:23-32. https://doi.org/10.1016/S0140-6736(10)60835-5.
- Fraser AG, Daubert JC, Van de Werf F, Estes NA, Smith SC, Krucoff MW, et al. Clinical evaluation of cardiovascular devices: principles, problems, and proposals for European regulatory reform. Report of a policy conference of the European Society of Cardiology. Eur Heart J 2011;32:1673-86. https://doi.org/10.1093/eurheartj/ehr171.
- Lewi H, Frame JD. The scandal of device regulation in the UK. Lancet 2012;379. https://doi.org/10.1016/S0140-6736(12)60760-0.
- Murkin JM. Cerebral oximetry: monitoring the brain as the index organ. Anesthesiology 2011;114:12-3. https://doi.org/10.1097/ALN.0b013e3181fef5d2.
- Vretzakis G, Georgopoulou S, Stamoulis K, Tassoudis V, Mikroulis D, Giannoukas A, et al. Monitoring of brain oxygen saturation (INVOS) in a protocol to direct blood transfusions during cardiac surgery: a prospective randomized clinical trial. J Cardiothorac Surg 2013;8. https://doi.org/10.1186/1749-8090-8-145.
- Cholette JM, Henrichs KF, Alfieris GM, Powers KS, Phipps R, Spinelli SL, et al. Washing red blood cells and platelets transfused in cardiac surgery reduces postoperative inflammation and number of transfusions: results of a prospective, randomized, controlled clinical trial. Pediatr Crit Care Med 2012;13:290-9. https://doi.org/10.1097/PCC.0b013e31822f173c.
- Swindell CG, Barker TA, McGuirk SP, Jones TJ, Barron DJ, Brawn WJ, et al. Washing of irradiated red blood cells prevents hyperkalaemia during cardiopulmonary bypass in neonates and infants undergoing surgery for complex congenital heart disease. Eur J Cardiothorac Surg 2007;31:659-64. https://doi.org/10.1016/j.ejcts.2007.01.014.
- Dacey LJ, Munoz JJ, Baribeau YR, Johnson ER, Lahey SJ, Leavitt BJ, et al. Reexploration for hemorrhage following coronary artery bypass grafting: incidence and risk factors. Northern New England Cardiovascular Disease Study Group. Arch Surg 1998;133:442-7. https://doi.org/10.1001/archsurg.133.4.442.
- Ranucci M, Bozzetti G, Ditta A, Cotza M, Carboni G, Ballotta A. Surgical reexploration after cardiac operations: why a worse outcome?. Ann Thorac Surg 2008;86:1557-62. https://doi.org/10.1016/j.athoracsur.2008.07.114.
- Choong CK, Gerrard C, Goldsmith KA, Dunningham H, Vuylsteke A. Delayed re-exploration for bleeding after coronary artery bypass surgery results in adverse outcomes. Eur J Cardiothorac Surg 2007;31:834-8. https://doi.org/10.1016/j.ejcts.2007.02.001.
- Davenport R, Manson J, De’Ath H, Platton S, Coates A, Allard S, et al. Functional definition and characterization of acute traumatic coagulopathy. Crit Care Med 2011;39:2652-8. https://doi.org/10.1097/CCM.0b013e3182281af5.
- Gelb AB, Roth RI, Levin J, London MJ, Noall RA, Hauck WW, et al. Changes in blood coagulation during and following cardiopulmonary bypass: lack of correlation with clinical bleeding. Am J Clin Pathol 1996;106:87-99. https://doi.org/10.1093/ajcp/106.1.87.
- Prohaska W, Zittermann A, Inoue K, Tenderich G, Lüth JU, Köster-Eiserfunke W, et al. Preoperative haemostasis testing does not predict requirement of blood products in cardiac surgery. Eur J Med Res 2008;13:525-30.
- Dyke C, Aronson S, Dietrich W, Hofmann A, Karkouti K, Levi M, et al. Universal definition of perioperative bleeding in adult cardiac surgery. J Thorac Cardiovasc Surg 2014;147:1458-63.e1. https://doi.org/10.1016/j.jtcvs.2013.10.070.
- Tosetto A, Rodeghiero F, Castaman G, Bernardi M, Bertoncello K, Goodeve A, et al. Impact of plasma von Willebrand factor levels in the diagnosis of type 1 von Willebrand disease: results from a multicenter European study (MCMDM-1VWD). J Thromb Haemost 2007;5:715-21. https://doi.org/10.1111/j.1538-7836.2007.02444.x.
- Yusuf S, Zhao F, Mehta SR, Chrolavicius S, Tognoni G, Fox KK. Clopidogrel in Unstable Angina to Prevent Recurrent Events Trial I: effects of clopidogrel in addition to aspirin in patients with acute coronary syndromes without ST-segment elevation. N Engl J Med 2001;345:494-502. https://doi.org/10.1056/NEJMoa010746.
- Wiviott SD, Braunwald E, McCabe CH, Montalescot G, Ruzyllo W, Gottlieb S, et al. Prasugrel versus clopidogrel in patients with acute coronary syndromes. N Engl J Med 2007;357:2001-15. https://doi.org/10.1056/NEJMoa0706482.
- Wallentin L, Becker RC, Budaj A, Cannon CP, Emanuelsson H, Held C, et al. Ticagrelor versus clopidogrel in patients with acute coronary syndromes. N Engl J Med 2009;361:1045-57. https://doi.org/10.1056/NEJMoa0904327.
- Hansson EC, Jideus L, Aberg B, Bjursten H, Dreifaldt M, Holmgren A, et al. Coronary artery bypass grafting-related bleeding complications in patients treated with ticagrelor or clopidogrel: a nationwide study. Eur Heart J 2016;37:189-97. https://doi.org/10.1093/eurheartj/ehv381.
- Ferraris VA, Ferraris SP, Moliterno DJ, Camp P, Walenga JM, Messmore HL, et al. The Society of Thoracic Surgeons practice guideline series: aspirin and other antiplatelet agents during operative coronary revascularization (executive summary). Ann Thorac Surg 2005;79:1454-61. https://doi.org/10.1016/j.athoracsur.2005.01.008.
- Chen L, Bracey AW, Radovancevic R, Cooper JR, Collard CD, Vaughn WK, et al. Clopidogrel and bleeding in patients undergoing elective coronary artery bypass grafting. J Thorac Cardiovasc Surg 2004;128:425-31. https://doi.org/10.1016/j.jtcvs.2004.02.019.
- Maltais S, Perrault LP, Do QB. Effect of clopidogrel on bleeding and transfusions after off-pump coronary artery bypass graft surgery: impact of discontinuation prior to surgery. Eur J Cardiothorac Surg 2008;34:127-31. https://doi.org/10.1016/j.ejcts.2008.03.052.
- Karkouti K, O’Farrell R, Yau TM, Beattie WS. Reducing Bleeding in Cardiac Surgery Research Group . Prediction of massive blood transfusion in cardiac surgery. Can J Anaesth 2006;53:781-94. https://doi.org/10.1007/BF03022795.
- Vuylsteke A, Pagel C, Gerrard C, Reddy B, Nashef S, Aldam P, et al. The Papworth Bleeding Risk Score: a stratification scheme for identifying cardiac surgery patients at risk of excessive early postoperative bleeding. Eur J Cardiothorac Surg 2011;39:924-30. https://doi.org/10.1016/j.ejcts.2010.10.003.
- Coagulation. 2017.
- Despotis GJ, Avidan MS, Hogue CW. Mechanisms and attenuation of hemostatic activation during extracorporeal circulation. Ann Thorac Surg 2001;72:S1821-31. https://doi.org/10.1016/S0003-4975(01)03211-8.
- Weerasinghe A, Taylor KM. The platelet in cardiopulmonary bypass. Ann Thorac Surg 1998;66:2145-52. https://doi.org/10.1016/S0003-4975(98)00749-8.
- Hardy JF, de Moerloose P, Samama CM. The coagulopathy of massive transfusion. Vox Sang 2005;89:123-7. https://doi.org/10.1111/j.1423-0410.2005.00678.x.
- Karkouti K, Yau TM, Rensburg Av, McCluskey SA, Callum J, Wijeysundera DN, et al. The effects of a treatment protocol for cardiac surgical patients with excessive blood loss on clinical outcomes. Vox Sang 2006;91:148-56. https://doi.org/10.1111/j.1423-0410.2006.00813.x.
- Teoh KH, Young E, Blackall MH, Roberts RS, Hirsh J. Can extra protamine eliminate heparin rebound following cardiopulmonary bypass surgery?. J Thorac Cardiovasc Surg 2004;128:211-19. https://doi.org/10.1016/j.jtcvs.2003.12.023.
- Karlsson M, Ternström L, Hyllner M, Baghaei F, Nilsson S, Jeppsson A. Plasma fibrinogen level, bleeding, and transfusion after on-pump coronary artery bypass grafting surgery: a prospective observational study. Transfusion 2008;48:2152-8. https://doi.org/10.1111/j.1537-2995.2008.01827.x.
- Yang L, Vuylsteke A, Gerrard C, Besser M, Baglin T. Postoperative fibrinogen level is associated with postoperative bleeding following cardiothoracic surgery and the effect of fibrinogen replacement therapy remains uncertain. J Thromb Haemost 2013;11:1519-26. https://doi.org/10.1111/jth.12304.
- Dzik WH. Predicting hemorrhage using preoperative coagulation screening assays. Curr Hematol Rep 2004;3:324-30.
- Shainoff JR, Estafanous FG, Yared JP, DiBello PM, Kottke-Marchant K, Loop FD. Low factor XIIIA levels are associated with increased blood loss after coronary artery bypass grafting. J Thorac Cardiovasc Surg 1994;108:437-45.
- Galeone A, Rotunno C, Guida P, Bisceglie A, Rubino G, Schinosa Lde L, et al. Monitoring incomplete heparin reversal and heparin rebound after cardiac surgery. J Cardiothorac Vasc Anesth 2013;27:853-8. https://doi.org/10.1053/j.jvca.2012.10.020.
- Liveris A, Bello RA, Friedmann P, Duffy MA, Manwani D, Killinger JS, et al. Anti-factor Xa assay is a superior correlate of heparin dose than activated partial thromboplastin time or activated clotting time in pediatric extracorporeal membrane oxygenation. Pediatr Crit Care Med 2014;15:e72-9. https://doi.org/10.1097/PCC.0000000000000028.
- Karkouti K, McCluskey SA, Syed S, Pazaratz C, Poonawala H, Crowther MA. The influence of perioperative coagulation status on postoperative blood loss in complex cardiac surgery: a prospective observational study. Anesth Analg 2010;110:1533-40. https://doi.org/10.1213/ANE.0b013e3181db7991.
- Bolliger D, Dell-Kuster S, Seeberger MD, Tanaka KA, Gregor M, Zenklusen U, et al. Impact of loss of high-molecular-weight von Willebrand factor multimers on blood loss after aortic valve replacement. Br J Anaesth 2012;108:754-62. https://doi.org/10.1093/bja/aer512.
- Mallett SV, Armstrong M. Point-of-care monitoring of haemostasis. Anaesthesia 2015;70:73-7. https://doi.org/10.1111/anae.12909.
- Desborough M, Sandu R, Brunskill SJ, Doree C, Trivella M, Montedori A, et al. Fresh frozen plasma for cardiovascular surgery. Cochrane Database Syst Rev 2015;7. https://doi.org/10.1002/14651858.CD007614.pub2.
- Thomas D, Bolton-Maggs P, Watt A, Poles D, Davies A, Ball J, et al. Annual SHOT Report 2014 n.d. www.shotuk.org/wp-content/uploads/report-2014.pdf (accessed 21 July 2017).
- Wells AW, Llewelyn CA, Casbard A, Johnson AJ, Amin M, Ballard S, et al. The EASTR Study: indications for transfusion and estimates of transfusion recipient numbers in hospitals supplied by the National Blood Service. Transfus Med 2009;19:315-28. https://doi.org/10.1111/j.1365-3148.2009.00933.x.
- Holcomb JB, Tilley BC, Baraniuk S, Fox EE, Wade CE, Podbielski JM, et al. Transfusion of plasma, platelets, and red blood cells in a 1 : 1 : 1 vs. a 1 : 1 : 2 ratio and mortality in patients with severe trauma: the PROPPR randomized clinical trial. JAMA 2015;313:471-82. https://doi.org/10.1001/jama.2015.12.
- Karkouti K, Wijeysundera DN, Beattie WS. Platelet transfusions as a risk factor in cardiac surgery. Transfusion 2007;47:1739-40. https://doi.org/10.1111/j.1537-2995.2007.01356.x.
- Spiess BD, Royston D, Levy JH, Fitch J, Dietrich W, Body S, et al. Platelet transfusions during coronary artery bypass graft surgery are associated with serious adverse outcomes. Transfusion 2004;44:1143-8. https://doi.org/10.1111/j.1537-2995.2004.03322.x.
- Hunt BJ, Allard S, Keeling D, Norfolk D, Stanworth SJ, Pendry K. British Committee for Standards in Haematology . A practical guideline for the haematological management of major haemorrhage. Br J Haematol 2015;170:788-803. https://doi.org/10.1111/bjh.13580.
- Wikkelsø A, Lunde J, Johansen M, Stensballe J, Wetterslev J, Møller AM, et al. Fibrinogen concentrate in bleeding patients. Cochrane Database Syst Rev 2013;8. https://doi.org/10.1002/14651858.CD008864.pub2.
- Alghamdi AA, Davis A, Brister S, Corey P, Logan A. Development and validation of Transfusion Risk Understanding Scoring Tool (TRUST) to stratify cardiac surgery patients according to their blood transfusion needs. Transfusion 2006;46:1120-9. https://doi.org/10.1111/j.1537-2995.2006.00860.x.
- Ranucci M, Castelvecchio S, Frigiola A, Scolletta S, Giomarelli P, Biagioli B. Predicting transfusions in cardiac surgery: the easier, the better: the Transfusion Risk and Clinical Knowledge score. Vox Sang 2009;96:324-32. https://doi.org/10.1111/j.1423-0410.2009.01160.x.
- Mehta RH, Roe MT, Mulgund J, Ohman EM, Cannon CP, Gibler WB, et al. Acute clopidogrel use and outcomes in patients with non-ST-segment elevation acute coronary syndromes undergoing coronary artery bypass surgery. J Am Coll Cardiol 2006;48:281-6. https://doi.org/10.1016/j.jacc.2006.04.029.
- Ferraris VA, Saha SP, Oestreich JH, Song HK, Rosengart T, Reece TB, et al. 2012 update to the Society of Thoracic Surgeons guideline on use of antiplatelet drugs in patients having cardiac and noncardiac operations. Ann Thorac Surg 2012;94:1761-81. https://doi.org/10.1016/j.athoracsur.2012.07.086.
- Kong R, Trimmings A, Hutchinson N, Gill R, Agarwal S, Davidson S, et al. Consensus recommendations for using the Multiplate® for platelet function monitoring before cardiac surgery. Int J Lab Hematol 2015;37:143-7. https://doi.org/10.1111/ijlh.12279.
- Craig J, Aguiar-Ibanez R, Bhattacharya S, Downie S, Duffy S, Kohli H, et al. The Clinical and Cost Effectiveness of Thromboelastography/Thromboelastometry. Glasgow: NHS Health Improvement Scotland; 2008.
- Murphy GJ, Reeves BC, Rogers CA, Rizvi SI, Culliford L, Angelini GD. Increased mortality, postoperative morbidity, and cost after red blood cell transfusion in patients having cardiac surgery. Circulation 2007;116:2544-52. https://doi.org/10.1161/CIRCULATIONAHA.107.698977.
- Joint Health Surveys Unit of Social and Community Planning Research and University College London . Health Survey for England, 1996 n.d. http://doi.org/10.5255/UKDA-SN-3886-1 (accessed 21 July 2017).
- Data Protection Act 1998. London: The Stationery Office; 1998.
- Human Tissue Act 2004. London: The Stationery Office; 2004.
- Bossuyt PM, Reitsma JB, Bruns DE, Gatsonis CA, Glasziou PP, Irwig L, et al. STARD 2015: an updated list of essential items for reporting diagnostic accuracy studies. BMJ 2015;351. https://doi.org/10.1136/bmj.h5527.
- Cohen J. Statistical Power Analysis for the Behavioral Sciences. Mahwah, NJ: Lawrence Erlbaum; 1988.
- Pencina MJ, D’Agostino RB, D’Agostino RB, Vasan RS. Evaluating the added predictive ability of a new marker: from area under the ROC curve to reclassification and beyond. Stat Med 2008;27:157-72. https://doi.org/10.1002/sim.2929.
- Drummond MF, Sculpher MJ, Claxton K, Stoddart GL, Torrance GW. Methods for the Economic Evaluation of Health Care Programmes. New York, NY: Oxford University Press; 2015.
- Gray A, Clarke P, Wolstenholme J, Wordsworth S. Applied Methods of Cost Effectiveness Analysis in Health Care. Oxford: Oxford University Press; 2010.
- Husereau D, Drummond M, Petrou S, Carswell C, Moher D, Greenberg D, et al. Consolidated Health Economic Evaluation Reporting Standards (CHEERS) statement. BMJ 2013;346. https://doi.org/10.1136/bmj.f1049.
- Meybohm P, Zacharowski K, Weber CF. Point-of-care coagulation management in intensive care medicine. Crit Care 2013;17. https://doi.org/10.1186/cc12527.
- Roberts M, Russell LB, Paltiel AD, Chambers M, McEwan P, Krahn M, et al. Conceptualizing a model: a report of the ISPOR-SMDM Modeling Good Research Practices Task Force – 2. Value Health 2012;15:804-11. https://doi.org/10.1016/j.jval.2012.06.016.
- Guide to the Methods of Technology Appraisal 2013. London: NICE; 2013.
- The Green Book: Appraisal and Evaluation in Central Government. London: The Stationery Office; 2015.
- Walsh M, Thomas SG, Howard JC, Evans E, Guyer K, Medvecz A, et al. Blood component therapy in trauma guided with the utilization of the perfusionist and thromboelastography. J Extra Corpor Technol 2011;43:162-7.
- Calatzis A, Leitner M, Panzer S. Monitoring anticoagulation of primary haemostasis – estimation of platelet function in whole blood assays. Hamostaseologie 2009;29:279-84.
- Multiplate® Analyzer. Cut-off-Values ADPtest and ASPItest. Rotkreuz: Roche Diagnostics International Ltd; 2013.
- Reference Ranges for Multiplate® Analysis, Version 1.0. Rotkreuz: Roche Diagnostics International Ltd; 2013.
- Weber CF, Görlinger K, Meininger D, Herrmann E, Bingold T, Moritz A, et al. Point-of-care testing: a prospective, randomized clinical trial of efficacy in coagulopathic cardiac surgery patients. Anesthesiology 2012;117:531-47. https://doi.org/10.1097/ALN.0b013e318264c644.
- White IR, Royston P, Wood AM. Multiple imputation using chained equations: issues and guidance for practice. Stat Med 2011;30:377-99. https://doi.org/10.1002/sim.4067.
- Rubin D. Multiple Imputation for Non-response in Surveys. New York: John Wiley; 1987.
- Glick HA, Doshi JA, Sonnad SS, Polsky D. Economic Evaluation in Clinical Trials. Oxford: Oxford University Press; 2007.
- Deb P, Manning WG, Norton EC. Modeling Health Care Costs and Counts n.d. http://stagingharris.uchicago.edu/sites/default/files/iHEA_Sydney_minicourse.pdf (accessed 26 May 2017).
- Hawe E, Cockcroft L. OHE Guide to UK Health and Health Care Statistics. London: Office of Health Economics; 2013.
- Curtis L. Unit Costs of Health and Social Care 2014. Canterbury: Personal Social Services Research Unit, University of Kent; 2014.
- Greenhalgh J, Bagust A, Boland A, Martin Saborido C, Oyee J, Blundell M, et al. Clopidogrel and modified-release dipyridamole for the prevention of occlusive vascular events (review of technology appraisal no. 90): a systematic review and economic analysis. Health Technol Assess 2011;15. https://doi.org/10.3310/hta15310.
- Bridgewater B, Keogh B, Kinsman R, Walton P. Blue Book Online 2013. http://bluebook.scts.org (accessed 7 June 2017).
- National Life Tables, United Kingdom, 1980–82 to 2012–14. London: Office for National Statistics; 2015.
- Mehta RL, Kellum JA, Shah SV, Molitoris BA, Ronco C, Warnock DG, et al. Acute Kidney Injury Network: report of an initiative to improve outcomes in acute kidney injury. Crit Care 2007;11. https://doi.org/10.1186/cc5713.
- Sawhney S, Mitchell M, Marks A, Fluck N, Black C. Long-term prognosis after acute kidney injury (AKI): what is the role of baseline kidney function and recovery? A systematic review. BMJ Open 2015;5. https://doi.org/10.1136/bmjopen-2014-006497.
- Lafrance JP, Miller DR. Acute kidney injury associates with increased long-term mortality. J Am Soc Nephrol 2010;21:345-52. https://doi.org/10.1681/ASN.2009060636.
- Ishani A, Nelson D, Clothier B, Schult T, Nugent S, Greer N, et al. The magnitude of acute serum creatinine increase after cardiac surgery and the risk of chronic kidney disease, progression of kidney disease, and death. Arch Intern Med 2011;171:226-33. https://doi.org/10.1001/archinternmed.2010.514.
- Briggs A, Sculpher MJ, Claxton K. Decision Modelling for Health Economic Evaluation. Oxford: Oxford University Press; 2006.
- Briggs AH, Goeree R, Blackhouse G, O’Brien BJ. Probabilistic analysis of cost-effectiveness models: choosing between treatment strategies for gastroesophageal reflux disease. Med Decis Making 2002;22:290-308. https://doi.org/10.1177/027298902400448867.
- Claxton K, Sculpher M, McCabe C, Briggs A, Akehurst R, Buxton M, et al. Probabilistic sensitivity analysis for NICE technology assessment: not an optional extra. Health Econ 2005;14:339-47. https://doi.org/10.1002/hec.985.
- Karkouti K, Callum J, Wijeysundera DN, Rao V, Crowther M, Grocott HP, et al. Point-of-care hemostatic testing in cardiac surgery: a stepped-wedge clustered randomized controlled trial. Circulation 2016;134:1152-62. https://doi.org/10.1161/CIRCULATIONAHA.116.023956.
- Higgins JPT, Green S. Cochrane Handbook for Systematic Reviews of Interventions. The Cochrane Collaboration; 2011.
- Liberati A, Altman DG, Tetzlaff J, Mulrow C, Gotzsche PC, Ioannidis JP, et al. The PRISMA statement for reporting systematic reviews and meta-analyses of studies that evaluate healthcare interventions: explanation and elaboration. BMJ 2009;339. https://doi.org/10.1136/bmj.b2700.
- Higgins JPT, Sterne JAC, Higgins JPT, Green S. Cochrane Handbook for Systematic Reviews of Interventions. The Cochrane Collaboration; 2011.
- Sterne JAC, Egger M, Moher D, Higgins JPT, Green S. Cochrane Handbook for Systematic Reviews of Interventions. The Cochrane Collaboration; 2011.
- Higgins JP, Thompson SG. Quantifying heterogeneity in a meta-analysis. Stat Med 2002;21:1539-58. https://doi.org/10.1002/sim.1186.
- Ak K, Isbir CS, Tetik S, Atalan N, Tekeli A, Aljodi M, et al. Thromboelastography-based transfusion algorithm reduces blood product use after elective CABG: a prospective randomized study. J Card Surg 2009;24:404-10. https://doi.org/10.1111/j.1540-8191.2009.00840.x.
- Avidan MS, Alcock EL, Da Fonseca J, Ponte J, Desai JB, Despotis GJ, et al. Comparison of structured use of routine laboratory tests or near-patient assessment with clinical judgement in the management of bleeding after cardiac surgery. Br J Anaesth 2004;92:178-86. https://doi.org/10.1093/bja/aeh037.
- Cui Y, Hei F, Long C, Feng Z, Zhao J, Yan F, et al. Perioperative monitoring of thromboelastograph on blood protection and recovery for severely cyanotic patients undergoing complex cardiac surgery. Artif Organs 2010;34:955-60. https://doi.org/10.1111/j.1525-1594.2010.01148.x.
- Girdauskas E, Kempfert J, Kuntze T, Borger MA, Enders J, Fassl J, et al. Thromboelastometrically guided transfusion protocol during aortic surgery with circulatory arrest: a prospective, randomized trial. J Thorac Cardiovasc Surg 2010;140:1117-24.e2. https://doi.org/10.1016/j.jtcvs.2010.04.043.
- Kempfert J. Thromboelastography-guided blood component therapy after cardiac surgery: a randomised study. Interact Cardiovasc Thorac Surg 2011;13:S106-7.
- Kultufan Turan S, Aydinli B, Ayik I, Yagar S, Kazanci D, Karadeniz U. The role of rotational thromboelastography on decision of blood transfusion in open heart surgery. Gögüs-Kalp-Damar Anestezi Ve Yogun Bakim Dernegi Dergisi 2006;12:154-9.
- Nakayama Y, Nakajima Y, Tanaka KA, Sessler DI, Maeda S, Iida J, et al. Thromboelastometry-guided intraoperative haemostatic management reduces bleeding and red cell transfusion after paediatric cardiac surgery. Br J Anaesth 2015;114:91-102. https://doi.org/10.1093/bja/aeu339.
- Nuttall GA, Oliver WC, Santrach PJ, Bryant S, Dearani JA, Schaff HV, et al. Efficacy of a simple intraoperative transfusion algorithm for nonerythrocyte component utilization after cardiopulmonary bypass. Anesthesiology 2001;94:773-81.
- Paniagua P, Koller T, Requena T, Gil JM, Campos JM, Galan J. Randomized controlled trial to evaluate postoperative coagulation management with bed-side thromboelastometry (Rotem) compared with a transfusion protocol based on laboratory measurements in bleeding patients after cardiac surgery: preliminary data. Eur J Anaesthesiol 2011;28. https://doi.org/10.1097/00003643-201106001-00301.
- Rauter M, Kastenbauer T, Schwarz S, Fitzgerald R. Reduced number of red blood cell transfusions in cardiac surgery due to perioperative coagulation testing by thromboelastometry. Acta Anaesthesiol Scand 2007;51.
- Royston D, von Kier S. Reduced haemostatic factor transfusion using heparinase-modified thromboelastography during cardiopulmonary bypass. Br J Anaesth 2001;86:575-8. https://doi.org/10.1093/bja/86.4.575.
- Shore-Lesserson L, Manspeizer HE, DePerio M, Francis S, Vela-Cantos F, Ergin MA. Thromboelastography-guided transfusion algorithm reduces transfusions in complex cardiac surgery. Anesth Analg 1999;88:312-9. https://doi.org/10.1213/00000539-199902000-00016.
- Westbrook AJ, Olsen J, Bailey M, Bates J, Scully M, Salamonsen RF. Protocol based on thromboelastograph (TEG) out-performs physician preference using laboratory coagulation tests to guide blood replacement during and after cardiac surgery: a pilot study. Heart Lung Circ 2009;18:277-88. https://doi.org/10.1016/j.hlc.2008.08.016.
- Agarwal S, Johnson RI, Shaw M. Preoperative point-of-care platelet function testing in cardiac surgery. J Cardiothorac Vasc Anesth 2015;29:333-41. https://doi.org/10.1053/j.jvca.2014.06.025.
- Thai J, Reynolds EJ, Natalia N, Cornelissen C, Lemmens HJ, Hill CC, et al. Comparison between RapidTEG(R) and conventional thromboelastography in cardiac surgery patients. Br J Anaesth 2011;106:605-6. https://doi.org/10.1093/bja/aer054.
- Forestier F, Bélisle S, Contant C, Harel F, Janvier G, Hardy JF. Reproducibility and interchangeability of the Thromboelastograph, Sonoclot and Hemochron activated coagulation time in cardiac surgery. Can J Anaesth 2001;48:902-10. https://doi.org/10.1007/BF03017358.
- Dietrich W, Schöpf K, Spannagl M, Jochum M, Braun SL, Meisner H. Influence of high- and low-dose aprotinin on activation of hemostasis in open heart operations. Ann Thorac Surg 1998;65:70-7. https://doi.org/10.1016/S0003-4975(97)01123-5.
- Miyashita T, Kuro M. Evaluation of platelet function by Sonoclot analysis compared with other hemostatic variables in cardiac surgery. Anesth Analg 1998;87:1228-33. https://doi.org/10.1213/00000539-199812000-00002.
- Traverso CI, Arcelus JI, Gomez E, Luna D, Lopez-Cantareno M, Garcia J. Prospective assessment of the risk of deep vein thrombosis in elective abdominal surgery: predictive role of thromboelastography. Thromb Haemarrhagic Disord 1993;71:9-15.
- Rogers CA, Stoica S, Ellis L, Stokes EA, Wordsworth S, Dabner L, et al. A randomised trial of near infra-red spectroscopy for the personalised optimisation of cerebral tissue oxygenation during cardiac surgery. Br J Anaesth 2017. https://doi.org/10.1093/bja/aex182.
- Birnie K, Verheyden V, Pagano D, Bhabra M, Tilling K, Sterne JA, et al. Predictive models for kidney disease: improving global outcomes (KDIGO) defined acute kidney injury in UK cardiac surgery. Crit Care 2014;18. https://doi.org/10.1186/s13054-014-0606-x.
- Wikkelsø A, Wetterslev J, Møller AM, Afshari A. Thromboelastography (TEG) or thromboelastometry (ROTEM) to monitor haemostatic treatment versus usual care in adults or children with bleeding. Cochrane Database Syst Rev 2016;8. https://doi.org/10.1002/14651858.CD007871.pub3.
- Ranucci M, Colella D, Baryshnikova E, Di Dedda U. Surgical Clinical Outcome Research Group . Effect of preoperative P2Y12 and thrombin platelet receptor inhibition on bleeding after cardiac surgery. Br J Anaesth 2014;113:970-6. https://doi.org/10.1093/bja/aeu315.
- Radulovic V, Hyllner M, Ternstrom L, Karlsson M, Bylock A, Hansson KM, et al. Sustained heparin effect contributes to reduced plasma thrombin generation capacity early after cardiac surgery. Thromb Res 2012;130:769-74. https://doi.org/10.1016/j.thromres.2011.12.025.
- Solomon C, Rahe-Meyer N, Sorensen B. Fibrin formation is more impaired than thrombin generation and platelets immediately following cardiac surgery. Thromb Res 2011;128:277-82. https://doi.org/10.1016/j.thromres.2011.02.022.
- Blome M, Isgro F, Kiessling AH, Skuras J, Haubelt H, Hellstern P, et al. Relationship between factor XIII activity, fibrinogen, haemostasis screening tests and postoperative bleeding in cardiopulmonary bypass surgery. Thromb Haemost 2005;93:1101-7. https://doi.org/10.1160/TH04-12-0799.
- Ternstrom L, Radulovic V, Karlsson M, Baghaei F, Hyllner M, Bylock A, et al. Plasma activity of individual coagulation factors, hemodilution and blood loss after cardiac surgery: a prospective observational study. Thromb Res 2010;126:e128-33. https://doi.org/10.1016/j.thromres.2010.05.028.
- Karkouti K, von Heymann C, Jespersen CM, Korte W, Levy JH, Ranucci M, et al. Efficacy and safety of recombinant factor XIII on reducing blood transfusions in cardiac surgery: a randomized, placebo-controlled, multicenter clinical trial. J Thorac Cardiovasc Surg 2013;146:927-39. https://doi.org/10.1016/j.jtcvs.2013.04.044.
- Karkouti K, Callum J, Crowther MA, McCluskey SA, Pendergrast J, Tait G, et al. The relationship between fibrinogen levels after cardiopulmonary bypass and large volume red cell transfusion in cardiac surgery: an observational study. Anesth Analg 2013;117:14-22. https://doi.org/10.1213/ANE.0b013e318292efa4.
- Rahe-Meyer N, Hanke A, Schmidt DS, Hagl C, Pichlmaier M. Fibrinogen concentrate reduces intraoperative bleeding when used as first-line hemostatic therapy during major aortic replacement surgery: results from a randomized, placebo-controlled trial. J Thorac Cardiovasc Surg 2013;145:S178-85. https://doi.org/10.1016/j.jtcvs.2012.12.083.
- Ranucci M, Baryshnikova E, Crapelli GB, Rahe-Meyer N, Menicanti L, Frigiola A, et al. Randomized, double-blinded, placebo-controlled trial of fibrinogen concentrate supplementation after complex cardiac surgery. J Am Heart Assoc 2015;4. https://doi.org/10.1161/JAHA.115.002066.
- Whiting PF, Rutjes AW, Westwood ME, Mallett S, Deeks JJ, Reitsma JB, et al. QUADAS-2: a revised tool for the quality assessment of diagnostic accuracy studies. Ann Intern Med 2011;155:529-36. https://doi.org/10.7326/0003-4819-155-8-201110180-00009.
- Koch CG, Li L, Duncan AI, Mihaljevic T, Cosgrove DM, Loop FD, et al. Morbidity and mortality risk associated with red blood cell and blood-component transfusion in isolated coronary artery bypass grafting. Crit Care Med 2006;34:1608-16. https://doi.org/10.1097/01.CCM.0000217920.48559.D8.
- Greinacher A, Fendrich K, Brzenska R, Kiefel V, Hoffmann W. Implications of demographics on future blood supply: a population-based cross-sectional study. Transfusion 2011;51:702-9. https://doi.org/10.1111/j.1537-2995.2010.02882.x.
- Bennett-Guerrero E, Zhao Y, O’Brien SM, Ferguson TB, Peterson ED, Gammie JS, et al. Variation in use of blood transfusion in coronary artery bypass graft surgery. JAMA 2010;304:1568-75. https://doi.org/10.1001/jama.2010.1406.
- Arora RC, Legare JF, Buth KJ, Sullivan JA, Hirsch GM. Identifying patients at risk of intraoperative and postoperative transfusion in isolated CABG: toward selective conservation strategies. Ann Thorac Surg 2004;78:1547-54. https://doi.org/10.1016/j.athoracsur.2004.04.083.
- Dial S, Delabays E, Albert M, Gonzalez A, Camarda J, Law A, et al. Hemodilution and surgical hemostasis contribute significantly to transfusion requirements in patients undergoing coronary artery bypass. J Thorac Cardiovasc Surg 2005;130:654-61. https://doi.org/10.1016/j.jtcvs.2005.02.025.
- Isomatsu Y, Tsukui H, Hoshino S, Nishiya Y. Predicting blood transfusion factors in coronary artery bypass surgery. Jpn J Thorac Cardiovasc Surg 2001;49:438-42. https://doi.org/10.1007/BF02913909.
- Karkouti K, Cohen MM, McCluskey SA, Sher GD. A multivariable model for predicting the need for blood transfusion in patients undergoing first-time elective coronary bypass graft surgery. Transfusion 2001;41:1193-203. https://doi.org/10.1046/j.1537-2995.2001.41101193.x.
- Litmathe J, Boeken U, Feindt P, Gams E. Predictors of homologous blood transfusion for patients undergoing open heart surgery. Thorac Cardiovasc Surg 2003;51:17-21. https://doi.org/10.1055/s-2003-37281.
- Magovern JA, Sakert T, Benckart DH, Burkholder JA, Liebler GA, Magovern GJ, et al. A model for predicting transfusion after coronary artery bypass grafting. Ann Thorac Surg 1996;61:27-32. https://doi.org/10.1016/0003-4975(95)00808-X.
- Parr KG, Patel MA, Dekker R, Levin R, Glynn R, Avorn J, et al. Multivariate predictors of blood product use in cardiac surgery. J Cardiothorac Vasc Anesth 2003;17:176-81. https://doi.org/10.1053/jcan.2003.44.
- Surgenor DM, Churchill WH, Wallace EL, Rizzo RJ, Chapman RH, McGurk S, et al. Determinants of red cell, platelet, plasma, and cryoprecipitate transfusions during coronary artery bypass graft surgery: the Collaborative Hospital Transfusion Study. Transfusion 1996;36:521-32. https://doi.org/10.1046/j.1537-2995.1996.36696269511.x.
- McDonald MB, McMillan J. Predicting blood usage in cardiac surgery – the transfusion predictor product. J Extra Corpor Technol 2005;37:157-60.
- Moskowitz DM, Klein JJ, Shander A, Cousineau KM, Goldweit RS, Bodian C, et al. Predictors of transfusion requirements for cardiac surgical procedures at a blood conservation center. Ann Thorac Surg 2004;77:626-34. https://doi.org/10.1016/S0003-4975(03)01345-6.
- Karkouti K, Wijeysundera DN, Beattie WS, Callum JL, Cheng D, Dupuis JY, et al. Variability and predictability of large-volume red blood cell transfusion in cardiac surgery: a multicenter study. Transfusion 2007;47:2081-8. https://doi.org/10.1111/j.1537-2995.2007.01432.x.
- von Elm E, Altman DG, Egger M, Pocock SJ, Gotzsche PC, Vandenbroucke JP. Strengthening the Reporting of Observational Studies in Epidemiology (STROBE) statement: guidelines for reporting observational studies. BMJ 2007;335:806-8. https://doi.org/10.1136/bmj.39335.541782.AD.
- Goudie R, Sterne JA, Verheyden V, Bhabra M, Ranucci M, Murphy GJ. Risk scores to facilitate preoperative prediction of transfusion and large volume blood transfusion associated with adult cardiac surgery. Br J Anaesth 2015;114:757-66. https://doi.org/10.1093/bja/aeu483.
- Sauerbrei W, Royston P. Building multivariable prognostic and diagnostic models: transformation of the predictors by using fractional polynomials. J R Stat Soc Ser A (Stat Soc) 1999;162:71-94. https://doi.org/10.1111/1467-985X.00122.
- Royston P, Parmar MK, Sylvester R. Construction and validation of a prognostic model across several studies, with an application in superficial bladder cancer. Stat Med 2004;23:907-26. https://doi.org/10.1002/sim.1691.
- Royston P. Multiple imputation of missing values: update of ice. Stata J 2005;5:527-36.
- van Buuren S, Boshuizen HC, Knook DL. Multiple imputation of missing blood pressure covariates in survival analysis. Stat Med 1999;18:681-94. https://doi.org/10.1002/(SICI)1097-0258(19990330)18:6<681::AID-SIM71>3.0.CO;2-R.
- Bradburn MJ, Deeks JJ, Altman DG. Metan – a command for meta-analysis in Stata. Stata Tech Bull 1998;44:4-15.
- Sterne JAC. Meta-analysis in Stata: an Updated Collection from the Stata Journal. College Station, TX: Stata Press; 2009.
- Vergouwe Y, Royston P, Moons KG, Altman DG. Development and validation of a prediction model with missing predictor data: a practical approach. J Clin Epidemiol 2010;63:205-14. https://doi.org/10.1016/j.jclinepi.2009.03.017.
- Marshall A, Altman DG, Holder RL, Royston P. Combining estimates of interest in prognostic modelling studies after multiple imputation: current practice and guidelines. BMC Med Res Methodol 2009;9. https://doi.org/10.1186/1471-2288-9-57.
- Song HK, von Heymann C, Jespersen CM, Karkouti K, Korte W, Levy JH, et al. Safe application of a restrictive transfusion protocol in moderate-risk patients undergoing cardiac operations. Ann Thorac Surg 2014;97:1630-5. https://doi.org/10.1016/j.athoracsur.2013.12.025.
- Selnes OA, Gottesman RF, Grega MA, Baumgartner WA, Zeger SL, McKhann GM. Cognitive and neurologic outcomes after coronary-artery bypass surgery. N Engl J Med 2012;366:250-7. https://doi.org/10.1056/NEJMra1100109.
- Colak Z, Borojevic M, Ivancan V, Gabelica R, Biocina B, Majeric-Kogler V. The relationship between prolonged cerebral oxygen desaturation and postoperative outcome in patients undergoing coronary artery bypass grafting. Coll Antropol 2012;36:381-8.
- Yao FS, Tseng CC, Ho CY, Levin SK, Illner P. Cerebral oxygen desaturation is associated with early postoperative neuropsychological dysfunction in patients undergoing cardiac surgery. J Cardiothorac Vasc Anesth 2004;18:552-8. https://doi.org/10.1053/j.jvca.2004.07.007.
- Croughwell N, Lyth M, Quill TJ, Newman M, Greeley WJ, Smith LR, et al. Diabetic patients have abnormal cerebral autoregulation during cardiopulmonary bypass. Circulation 1990;82:IV407-12.
- Miyoshi S, Morita T, Kadoi Y, Goto F. Analysis of the factors related to a decrease in jugular venous oxygen saturation in patients with diabetes mellitus during normothermic cardiopulmonary bypass. Surg Today 2005;35:530-4. https://doi.org/10.1007/s00595-004-2977-0.
- Haydin S, Onan B, Onan IS, Ozturk E, Iyigun M, Yeniterzi M, et al. Cerebral perfusion during cardiopulmonary bypass in children: correlations between near-infrared spectroscopy, temperature, lactate, pump flow, and blood pressure. Artif Organs 2013;37:87-91. https://doi.org/10.1111/j.1525-1594.2012.01554.x.
- Moreno GE, Pilan ML, Manara C, Magliola R, Vassallo JC, Balestrini M, et al. Regional venous oxygen saturation versus mixed venous saturation after paediatric cardiac surgery. Acta Anaesthesiol Scand 2013;57:373-9. https://doi.org/10.1111/aas.12016.
- DeFoe GR, Ross CS, Olmstead EM, Surgenor SD, Fillinger MP, Groom RC, et al. Lowest hematocrit on bypass and adverse outcomes associated with coronary artery bypass grafting. Northern New England Cardiovascular Disease Study Group. Ann Thorac Surg 2001;71:769-76. https://doi.org/10.1016/S0003-4975(00)02393-6.
- Ganushchak YM, Fransen EJ, Visser C, De Jong DS, Maessen JG. Neurological complications after coronary artery bypass grafting related to the performance of cardiopulmonary bypass. Chest 2004;125:2196-205. https://doi.org/10.1378/chest.125.6.2196.
- Ranucci M, Romitti F, Isgro G, Cotza M, Brozzi S, Boncilli A, et al. Oxygen delivery during cardiopulmonary bypass and acute renal failure after coronary operations. Ann Thorac Surg 2005;80:2213-20. https://doi.org/10.1016/j.athoracsur.2005.05.069.
- Berger K, Sander M, Spies CD, Weymann L, Buhner S, Lochs H, et al. Profound haemodilution during normothermic cardiopulmonary bypass influences neither gastrointestinal permeability nor cytokine release in coronary artery bypass graft surgery. Br J Anaesth 2009;103:511-17. https://doi.org/10.1093/bja/aep201.
- von Heymann C, Sander M, Foer A, Heinemann A, Spiess B, Braun J, et al. The impact of an hematocrit of 20% during normothermic cardiopulmonary bypass for elective low risk coronary artery bypass graft surgery on oxygen delivery and clinical outcome – a randomized controlled study [ISRCTN35655335]. Crit Care 2006;10. https://doi.org/10.1186/cc4891.
- Vretzakis G, Georgopoulou S, Stamoulis K, Stamatiou G, Tsakiridis K, Zarogoulidis P, et al. Cerebral oximetry in cardiac anesthesia. J Thorac Dis 2014;6:60-9. https://doi.org/10.3978/j.issn.2072-1439.2013.10.22.
- Scheeren TW, Schober P, Schwarte LA. Monitoring tissue oxygenation by near infrared spectroscopy (NIRS): background and current applications. J Clin Monit Comput 2012;26:279-87. https://doi.org/10.1007/s10877-012-9348-y.
- Kim MB, Ward DS, Cartwright CR, Kolano J, Chlebowski S, Henson LC. Estimation of jugular venous O2 saturation from cerebral oximetry or arterial O2 saturation during isocapnic hypoxia. J Clin Monit Comput 2000;16:191-9. https://doi.org/10.1023/A:1009940031063.
- Sapire KJ, Gopinath SP, Farhat G, Thakar DR, Gabrielli A, Jones JW, et al. Cerebral oxygenation during warming after cardiopulmonary bypass. Crit Care Med 1997;25:1655-62. https://doi.org/10.1097/00003246-199710000-00014.
- Goldman S, Sutter F, Ferdinand F, Trace C. Optimizing intraoperative cerebral oxygen delivery using noninvasive cerebral oximetry decreases the incidence of stroke for cardiac surgical patients. Heart Surg Forum 2004;7:E376-81. https://doi.org/10.1532/HSF98.20041062.
- Creteur J, Neves AP, Vincent JL. Near-infrared spectroscopy technique to evaluate the effects of red blood cell transfusion on tissue oxygenation. Crit Care 2009;13. https://doi.org/10.1186/cc8009.
- Kane JM, Steinhorn DM. Lack of irrefutable validation does not negate clinical utility of near-infrared spectroscopy monitoring: learning to trust new technology. J Crit Care 2009;24:472.e1-7. https://doi.org/10.1016/j.jcrc.2008.05.004.
- Zacharias DG, Lilly K, Shaw CL, Pirundini P, Rizzo RJ, Body SC, et al. Survey of the clinical assessment and utility of near-infrared cerebral oximetry in cardiac surgery. J Cardiothorac Vasc Anesth 2014;28:308-16. https://doi.org/10.1053/j.jvca.2013.06.003.
- Denault A, Deschamps A, Murkin JM. A proposed algorithm for the intraoperative use of cerebral near-infrared spectroscopy. Semin Cardiothorac Vasc Anesth 2007;11:274-81. https://doi.org/10.1177/1089253207311685.
- Newman MF, Kirchner JL, Phillips-Bute B, Gaver V, Grocott H, Jones RH, et al. Longitudinal assessment of neurocognitive function after coronary-artery bypass surgery. N Engl J Med 2001;344:395-402. https://doi.org/10.1056/NEJM200102083440601.
- Hausenloy DJ, Candilio L, Evans R, Ariti C, Jenkins DP, Kolvekar S, et al. Remote ischemic preconditioning and outcomes of cardiac surgery. N Engl J Med 2015;373:1408-17. https://doi.org/10.1056/NEJMoa1413534.
- Ellis L, Murphy GJ, Culliford L, Dreyer L, Clayton G, Downes R, et al. The effect of patient-specific cerebral oxygenation monitoring on postoperative cognitive function: a multicenter randomized controlled trial. JMIR Res Protoc 2015;4. https://doi.org/10.2196/resprot.4562.
- Schulz KF, Altman DG, Moher D. CONSORT Group . CONSORT 2010 statement: updated guidelines for reporting parallel group randomised trials. BMJ 2010;340. https://doi.org/10.1136/bmj.c332.
- Murkin JM, Newman SP, Stump DA, Blumenthal JA. Statement of consensus on assessment of neurobehavioral outcomes after cardiac surgery. Ann Thorac Surg 1995;59:1289-95. https://doi.org/10.1016/0003-4975(95)00106-U.
- Mahanna EP, Blumenthal JA, White WD, Croughwell ND, Clancy CP, Smith LR, et al. Defining neuropsychological dysfunction after coronary artery bypass grafting. Ann Thorac Surg 1996;61:1342-7. https://doi.org/10.1016/0003-4975(95)01095-5.
- Newman SP. Analysis and interpretation of neuropsychologic tests in cardiac surgery. Ann Thorac Surg 1995;59:1351-5. https://doi.org/10.1016/0003-4975(95)00215-7.
- Weschler D. Manual of the Wechsler Test of Adult Reading (WTAR-UK). New York, NY: Psychological Corporation (Iglesias); 2001.
- Benton AHR. Multilingual Aphasia Examination. Iowa City, IA: University of Iowa; 1976.
- EuroQol Group . EuroQol – a new facility for the measurement of health-related quality of life. Health Policy 1990;16:199-208. https://doi.org/10.1016/0168-8510(90)90421-9.
- Wilson AP, Gibbons C, Reeves BC, Hodgson B, Liu M, Plummer D, et al. Surgical wound infection as a performance indicator: agreement of common definitions of wound infection in 4773 patients. BMJ 2004;329. https://doi.org/10.1136/bmj.38232.646227.DE.
- NHS Blood and Transplant Price List 2013–2014. Oxford: NHS Blood and Transplant; 2014.
- National Schedule of Reference Costs 2012–13. London: Department of Health; 2013.
- National Schedule of Reference Costs 2013–14. London: Department of Health; 2014.
- Curtis L. Unit Costs of Health and Social Care 2013. Canterbury: Personal Social Services Research Unit, University of Kent; 2013.
- Electronic Marketing Information Tool (eMIT). London: Department of Health; 2013.
- British National Formulary. London: BMJ Group and Pharmaceutical Press; 2013.
- Stokes EA, Wordsworth S, Bargo D, Pike K, Rogers CA, Brierley RC, et al. Are lower levels of red blood cell transfusion more cost-effective than liberal levels after cardiac surgery? Findings from the TITRe2 randomised controlled trial. BMJ Open 2016;6. https://doi.org/10.1136/bmjopen-2016-011311.
- Dolan P, Gudex C, Kind P, Williams A. A Social Tariff for Euroqol. Results from a UK General Population Survey. York: University of York; 1995.
- Manca A, Hawkins N, Sculpher MJ. Estimating mean QALYs in trial-based cost-effectiveness analysis: the importance of controlling for baseline utility. Health Econ 2005;14:487-96. https://doi.org/10.1002/hec.944.
- Briggs AH, Wonderling DE, Mooney CZ. Pulling cost-effectiveness analysis up by its bootstraps: a non-parametric approach to confidence interval estimation. Health Econ 1997;6:327-40. https://doi.org/10.1002/(SICI)1099-1050(199707)6:4<327::AID-HEC282>3.0.CO;2-W.
- Briggs A, Clark T, Wolstenholme J, Clarke P. Missing . . . presumed at random: cost-analysis of incomplete data. Health Econ 2003;12:377-92. https://doi.org/10.1002/hec.766.
- Murkin JM, Adams SJ, Novick RJ, Quantz M, Bainbridge D, Iglesias I, et al. Monitoring brain oxygen saturation during coronary bypass surgery: a randomized, prospective study. Anesth Analg 2007;104:51-8. https://doi.org/10.1213/01.ane.0000246814.29362.f4.
- Spreen OSE. A Compendium of Neuropsychological Tests. New York, NY: Oxford University Press; 1998.
- Murkin JM, Stump DA, Blumenthal JA, McKhann G. Defining dysfunction: group means versus incidence analysis – a statement of consensus. Ann Thorac Surg 1997;64:904-5. https://doi.org/10.1016/S0003-4975(97)00743-1.
- Higgins JPT, Higgins JPT, Green S. Cochrane Handbook for Systematic Reviews of Interventions. The Cochrane Collaboration; 2011.
- Schünemann HJ, Vist GE, Higgins JPT, Deeks JJ, Glasziou P, Higgins JPT, et al. Cochrane Handbook for Systematic Reviews of Interventions. The Cochrane Collaboration; 2011.
- Colak Z, Borojevic M, Bogovic A, Ivancan V, Biocina B, Majeric-Kogler V. Influence of intraoperative cerebral oximetry monitoring on neurocognitive function after coronary artery bypass surgery: a randomized, prospective study. Eur J Cardiothorac Surg 2015;47:447-54. https://doi.org/10.1093/ejcts/ezu193.
- Deschamps A, Hall R, Grocott H, Mazer CD, Choi PT, Turgeon AF, et al. Cerebral oximetry monitoring to maintain normal cerebral oxygen saturation during high-risk cardiac surgery: a randomized controlled feasibility trial. Anesthesiology 2016;124:826-36. https://doi.org/10.1097/ALN.0000000000001029.
- Deschamps A, Lambert J, Couture P, Rochon A, Lebon JS, Ayoub C, et al. Reversal of decreases in cerebral saturation in high-risk cardiac surgery. J Cardiothorac Vasc Anesth 2013;27:1260-6. https://doi.org/10.1053/j.jvca.2013.01.019.
- Harilall Y, Adam JK, Biccard BM, Reddi A. The effect of optimising cerebral tissue oxygen saturation on markers of neurological injury during coronary artery bypass graft surgery. Heart Lung Circ 2014;23:68-74. https://doi.org/10.1016/j.hlc.2013.07.002.
- Kara I, Erkin A, Sacli H, Demirtas M, Percin B, Diler MS, et al. The effects of near-infrared spectroscopy on the neurocognitive functions in the patients undergoing coronary artery bypass grafting with asymptomatic carotid artery disease: a randomized prospective study. Ann Thorac Cardiovasc Surg 2015;21:544-50. https://doi.org/10.5761/atcs.oa.15-00118.
- Mohandas BS, Jagadeesh AM, Vikram SB. Impact of monitoring cerebral oxygen saturation on the outcome of patients undergoing open heart surgery. Ann Card Anaesth 2013;16:102-6. https://doi.org/10.4103/0971-9784.109740.
- Slater JP, Guarino T, Stack J, Vinod K, Bustami RT, Brown JM, et al. Cerebral oxygen desaturation predicts cognitive decline and longer hospital stay after cardiac surgery. Ann Thorac Surg 2009;87:36-44. https://doi.org/10.1016/j.athoracsur.2008.08.070.
- Murkin JM, Adams S, Schaefer B. Monitoring cerebral oxygen saturation significantly decreases major organ morbidity in CABG patients: a randomized blinded study. Heart Surg Forum 2004;7.
- Murkin JM, Adams SJ, Pardy E, Quantz M, McKenzie FN, Guo L. Monitoring brain oxygen saturation during coronary bypass surgery improves outcomes in diabetic patients: a post hoc analysis. Heart Surg Forum 2011;14:E1-6. https://doi.org/10.1532/HSF98.20101065.
- Kok WF, van Harten AE, Koene BM, Mariani MA, Koerts J, Tucha O, et al. A pilot study of cerebral tissue oxygenation and postoperative cognitive dysfunction among patients undergoing coronary artery bypass grafting randomised to surgery with or without cardiopulmonary bypass. Anaesthesia 2014;69:613-22. https://doi.org/10.1111/anae.12634.
- Dullenkopf A, Baulig W, Weiss M, Schmid ER. Cerebral near-infrared spectroscopy in adult patients after cardiac surgery is not useful for monitoring absolute values but may reflect trends in venous oxygenation under clinical conditions. J Cardiothorac Vasc Anesth 2007;21:535-9. https://doi.org/10.1053/j.jvca.2006.09.010.
- Wang P, Acker MA, Bilello M, Melhem ER, Stambrook E, Ratcliffe SJ, et al. DENOVO (Determining Neurologic Outcomes from Valve Operations) Investigators . Sex, aging, and preexisting cerebral ischemic disease in patients with aortic stenosis. Ann Thorac Surg 2010;90:1230-5. https://doi.org/10.1016/j.athoracsur.2010.04.016.
- Hyttel-Sorensen S, Pellicer A, Alderliesten T, Austin T, van Bel F, Benders M, et al. Cerebral near infrared spectroscopy oximetry in extremely preterm infants: phase II randomised clinical trial. BMJ 2015;350. https://doi.org/10.1136/bmj.g7635.
- Balzer F, Sander M, Simon M, Spies C, Habicher M, Treskatsch S, et al. High central venous saturation after cardiac surgery is associated with increased organ failure and long-term mortality: an observational cross-sectional study. Crit Care 2015;19. https://doi.org/10.1186/s13054-015-0889-6.
- Habicher M, von Heymann C, Spies CD, Wernecke KD, Sander M. Central venous-arterial pCO2 difference identifies microcirculatory hypoperfusion in cardiac surgical patients with normal central venous oxygen saturation: a retrospective analysis. J Cardiothorac Vasc Anesth 2015;29:646-55. https://doi.org/10.1053/j.jvca.2014.09.006.
- Hod EA, Zhang N, Sokol SA, Wojczyk BS, Francis RO, Ansaldi D, et al. Transfusion of red blood cells after prolonged storage produces harmful effects that are mediated by iron and inflammation. Blood 2010;115:4284-92. https://doi.org/10.1182/blood-2009-10-245001.
- Looney MR, Nguyen JX, Hu Y, Van Ziffle JA, Lowell CA, Matthay MA. Platelet depletion and aspirin treatment protect mice in a two-event model of transfusion-related acute lung injury. J Clin Invest 2009;119:3450-61. https://doi.org/10.1172/JCI38432.
- Patel NN, Lin H, Jones C, Walkden G, Ray P, Sleeman PA, et al. Interactions of cardiopulmonary bypass and erythrocyte transfusion in the pathogenesis of pulmonary dysfunction in swine. Anesthesiology 2013;119:365-78. https://doi.org/10.1097/ALN.0b013e31829419d3.
- Patel NN, Lin H, Toth T, Welsh GI, Jones C, Ray P, et al. Reversal of anemia with allogenic RBC transfusion prevents post-cardiopulmonary bypass acute kidney injury in swine. Am J Physiol Renal Physiol 2011;301:F605-14. https://doi.org/10.1152/ajprenal.00145.2011.
- Verhoeven AJ, Hilarius PM, Dekkers DW, Lagerberg JW, de Korte D. Prolonged storage of red blood cells affects aminophospholipid translocase activity. Vox Sang 2006;91:244-51. https://doi.org/10.1111/j.1423-0410.2006.00822.x.
- Simak J, Gelderman MP. Cell membrane microparticles in blood and blood products: potentially pathogenic agents and diagnostic markers. Transfus Med Rev 2006;20:1-26. https://doi.org/10.1016/j.tmrv.2005.08.001.
- Mause SF, Weber C. Microparticles: protagonists of a novel communication network for intercellular information exchange. Circ Res 2010;107:1047-57. https://doi.org/10.1161/CIRCRESAHA.110.226456.
- Walpoth BH, Eggensperger N, Walpoth-Aslan BN, Neidhart P, Lanz M, Zehnder R, et al. Qualitative assessment of blood washing with the continuous autologous transfusion system (CATS). Int J Artif Organs 1997;20:234-9.
- Woźniak MJ, Sullo N, Qureshi S, Dott W, Cardigan R, Wiltshire M, et al. Randomized trial of red cell washing for the prevention of transfusion-associated organ injury in cardiac surgery. Br J Anaesth 2017;118:689-98. https://doi.org/10.1093/bja/aex083.
- Murphy GJ, Verheyden V, Wozniak M, Sullo N, Dott W, Bhudia S, et al. Trial protocol for a randomised controlled trial of red cell washing for the attenuation of transfusion-associated organ injury in cardiac surgery: the REDWASH trial. Open Heart 2016;3. https://doi.org/10.1136/openhrt-2015-000344.
- Levey AS, Bosch JP, Lewis JB, Greene T, Rogers N, Roth D. A more accurate method to estimate glomerular filtration rate from serum creatinine: a new prediction equation. Modification of Diet in Renal Disease Study Group. Ann Intern Med 1999;130:461-70. https://doi.org/10.7326/0003-4819-130-6-199903160-00002.
- AKI Working Group . KDIGO clinical practice guideline for acute kidney injury. Kidney Int Suppl 2012;2:1-138.
- Rogers CA, Pike K, Angelini GD, Reeves BC, Glauber M, Ferrarini M, et al. An open randomized controlled trial of median sternotomy versus anterolateral left thoracotomy on morbidity and health care resource use in patients having off-pump coronary artery bypass surgery: the Sternotomy Versus Thoracotomy (STET) trial. J Thorac Cardiovasc Surg 2013;146:306-16.e1.
- Ranieri VM, Rubenfeld GD, Thompson BT, Ferguson ND, Caldwell E, Fan E, et al. Acute respiratory distress syndrome: the Berlin definition. JAMA 2012;307:2526-33. https://doi.org/10.1001/jama.2012.5669.
- Marshall JC, Cook DJ, Christou NV, Bernard GR, Sprung CL, Sibbald WJ. Multiple organ dysfunction score: a reliable descriptor of a complex clinical outcome. Crit Care Med 1995;23:1638-52. https://doi.org/10.1097/00003246-199510000-00007.
- Portilla D, Dent C, Sugaya T, Nagothu KK, Kundi I, Moore P, et al. Liver fatty acid-binding protein as a biomarker of acute kidney injury after cardiac surgery. Kidney Int 2008;73:465-72. https://doi.org/10.1038/sj.ki.5002721.
- Haase M, Bellomo R, Devarajan P, Schlattmann P, Haase-Fielitz A, NGAL Meta-analysis Investigator Group. Accuracy of neutrophil gelatinase-associated lipocalin (NGAL) in diagnosis and prognosis in acute kidney injury: a systematic review and meta-analysis. Am J Kidney Dis 2009;54:1012-24. https://doi.org/10.1053/j.ajkd.2009.07.020.
- Moher D, Schulz KF, Altman DG. The CONSORT statement: revised recommendations for improving the quality of reports of parallel-group randomised trials. Lancet 2001;357:1191-4. https://doi.org/10.1016/S0140-6736(00)04337-3.
- Cortes-Puch I, Wang D, Sun J, Solomon SB, Remy KE, Fernandez M, et al. Washing older blood units before transfusion reduces plasma iron and improves outcomes in experimental canine pneumonia. Blood 2014;123:1403-11. https://doi.org/10.1182/blood-2013-11-539353.
- Baek JH, D’Agnillo F, Vallelian F, Pereira CP, Williams MC, Jia Y, et al. Hemoglobin-driven pathophysiology is an in vivo consequence of the red blood cell storage lesion that can be attenuated in guinea pigs by haptoglobin therapy. J Clin Invest 2012;122:1444-58. https://doi.org/10.1172/JCI59770.
- Shih PT, Elices MJ, Fang ZT, Ugarova TP, Strahl D, Territo MC, et al. Minimally modified low-density lipoprotein induces monocyte adhesion to endothelial connecting segment-1 by activating beta1 integrin. J Clin Invest 1999;103:613-25. https://doi.org/10.1172/JCI5710.
- Cole AL, Subbanagounder G, Mukhopadhyay S, Berliner JA, Vora DK. Oxidized phospholipid-induced endothelial cell/monocyte interaction is mediated by a cAMP-dependent R-Ras/PI3-kinase pathway. Arterioscler Thromb Vasc Biol 2003;23:1384-90. https://doi.org/10.1161/01.ATV.0000081215.45714.71.
- Rifkind JM, Mohanty JG, Nagababu E. The pathophysiology of extracellular hemoglobin associated with enhanced oxidative reactions. Front Physiol 2014;5. https://doi.org/10.3389/fphys.2014.00500.
- Huber J, Vales A, Mitulovic G, Blumer M, Schmid R, Witztum JL, et al. Oxidized membrane vesicles and blebs from apoptotic cells contain biologically active oxidized phospholipids that induce monocyte-endothelial interactions. Arterioscler Thromb Vasc Biol 2002;22:101-7. https://doi.org/10.1161/hq0102.101525.
- Leeflang MM, Deeks JJ, Takwoingi Y, Macaskill P. Cochrane diagnostic test accuracy reviews. Syst Rev 2013;2. https://doi.org/10.1186/2046-4053-2-82.
- Dennis RC, Vito L, Weisel RD, Valeri CR, Berger RL, Hechtman HB. Improved myocardial performance following high 2-3 diphosphoglycerate red cell transfusions. Surgery 1975;77:741-7.
- Stanworth SJ, Estcourt LJ, Powter G, Kahan BC, Dyer C, Choo L, et al. A no-prophylaxis platelet-transfusion strategy for hematologic cancers. N Engl J Med 2013;368:1771-80. https://doi.org/10.1056/NEJMoa1212772.
- The VeriQ System for Assessing Graft Flow During Coronary Artery Bypass Graft Surgery. London: NICE; 2011.
- NHS Reference Costs 2011–12. London: Department of Health; 2012.
Appendix 1 The COPTIC study
Changes to the protocol after study commencement
Before the start of formal analyses the protocol was reviewed and a detailed analysis plan was drawn up. Specific questions detailed in the protocol were discussed and an approach was decided on that not only aimed to address the original intentions as detailed in the protocol but also was informed by observations around the data collected, such as the combinations of the cardiac procedure and antiplatelet therapy. The decision to review each of the rapid test platforms in turn and assess the contributions of these tests to predicting CCB was agreed on and documented in the statistical analysis plans (available as an online supplement).
Supplementary tables
These tables report near-patient test results in participants with and without the clinical outcomes of interest in the COPTIC predictive accuracy study.
Measurement | Red cell transfusion intraoperatively or postoperatively: 0 units (N = 1137) | Red cell transfusion intraoperatively or postoperatively: ≥ 1 unit (N = 660) | Total (N = 1797) |
---|---|---|---|
Preoperative ADPtest AUC (U), mean (SD) | 740.0 (264.0) | 742.9 (292.2) | 741.1 (274.6) |
Preoperative ASPItest AUC (U), median (range) | 270.0 (15.0–1546.0) | 251.5 (8.0–1573.0) | 261.0 (8.0–1573.0) |
Preoperative TRAPtest AUC (U), median (range) | 1199.0 (51.0–2139.0) | 1176.0 (215.0–2185.0) | 1187.0 (51.0–2185.0) |
Preoperative ADRtest AUC (U), mean (SD) | 250.3 (130.3) | 252.3 (138.9) | 251.0 (133.5) |
Red cell transfusion postoperatively: 0 units (N = 1214) | Red cell transfusion postoperatively: ≥ 1 unit (N = 581) | Total (N = 1795) | |
Preoperative ADPtest AUC (U), mean (SD) | 271.0 (15.0–1573.0) | 246.0 (8.0–1567.0) | 261.0 (8.0–1573.0) |
Preoperative ASPItest AUC (U), median (range) | 1190.0 (51.0–2139.0) | 1174.0 (215.0–2185.0) | 1187.0 (51.0–2185.0) |
Preoperative TRAPtest AUC (U), mean (SD) | 248.5 (129.9) | 255.5 (139.7) | 250.8 (133.2) |
Preoperative ADRtest AUC (U), median (SD) | 542.5 (32.0–1746.0) | 424.0 (25.0–1607.0) | 501.0 (25.0–1746.0) |
Postoperative ADPtest AUC (U), median (range) | 208.0 (8.0–1609.0) | 176.0 (12.0–1849.0) | 197.0 (8.0–1849.0) |
Postoperative ASPItest AUC (U), median (range) | 1210.0 (131.0–2777.0) | 1055.0 (213.0–2395.0) | 1159.0 (131.0–2777.0) |
Postoperative TRAPtest AUC (U), median (range) | 251.0 (139.6) | 236.9 (150.2) | 246.4 (143.2) |
Postoperative ADRtest AUC (U), mean (SD) | 271.0 (15.0–1573.0) | 246.0 (8.0–1567.0) | 261.0 (8.0–1573.0) |
INTEM CT (seconds), median (range) | 166.0 (106.0–401.0) | 166.0 (104.0–327.0) | 166.0 (104.0–401.0) |
INTEM α-angle (°), median (range) | 75.0 (51.0–83.0) | 74.0 (44.0–83.0) | 74.0 (44.0–83.0) |
INTEM MCF (mm), median (range) | 62.0 (40.0–78.0) | 61.0 (36.0–79.0) | 62.0 (36.0–79.0) |
INTEM ML (%), median (range) | 5.0 (0.0–20.0) | 4.0 (0.0–20.0) | 4.0 (0.0–20.0) |
INTEM Vmax (mm/second), median (range) | 16.0 (6.0–39.0) | 15.0 (4.0–40.0) | 16.0 (4.0–40.0) |
INTEM tVmax (seconds), mean (SD) | 201.9 (35.3) | 202.6 (39.3) | 202.1 (36.6) |
EXTEM CT (seconds), median (range) | 56.0 (14.0–120.0) | 57.0 (20.0–145.0) | 56.0 (14.0–145.0) |
EXTEM α-angle (°), median (range) | 74.0 (50.0–84.0) | 73.0 (48.0–84.0) | 74.0 (48.0–84.0) |
EXTEM MCF (mm), median (range) | 63.0 (30.0–82.0) | 63.0 (37.0–79.0) | 63.0 (30.0–82.0) |
EXTEM ML (%), median (range) | 5.0 (0.0–31.0) | 4.0 (0.0–26.0) | 4.0 (0.0–31.0) |
EXTEM Vmax (mm/second), median (range) | 16.0 (6.0–52.0) | 15.0 (5.0–58.0) | 16.0 (5.0–58.0) |
EXTEM tVmax (seconds), median (range) | 101.0 (20.0–254.0) | 103.0 (40.0–292.0) | 103.0 (20.0–292.0) |
FIBTEM MCF (mm), median (range) | 13.0 (3.0–40.0) | 13.0 (3.0–42.0) | 13.0 (3.0–42.0) |
EXTEM MCF – FIBTEM MCF (mm), median (range) | 49.0 (14.0–62.0) | 49.0 (32.0–61.0) | 49.0 (14.0–62.0) |
INTEM CT – HEPTEM CT (seconds), median (range) | 0.0 (–92.0 to 158.0) | 0.0 (–89.0 to 91.0) | 0.0 (–92.0 to 158.0) |
CK R (minutes), median (range) | 6.2 (2.7–15.7) | 5.7 (2.5–18.4) | 5.9 (2.5–18.4) |
CK α-angle (°), median (range) | 62.8 (31.3–76.7) | 65.1 (25.1–78.7) | 63.4 (25.1–78.7) |
CK MA (mm), mean (SD) | 59.1 (5.9) | 58.5 (7.4) | 58.9 (6.5) |
CK LY 60 (%), median (range) | –0.2 (–11.6 to 12.9) | –0.1 (–11.1 to 7.7) | –0.1 (–11.6 to 12.9) |
CK R – CKH R (minutes), median (range) | 0.2 (–2.4 to 8.3) | 0.1 (–8.0 to 13.2) | 0.1 (–8.0 to 13.2) |
Measurement | Red cell transfusion intraoperatively or postoperatively: ≤ 4 units (N = 1700) | Red cell transfusion intraoperatively or postoperatively: > 4 units (N = 96) | Total (N = 1796) |
---|---|---|---|
Preoperative ADPtest AUC (U), mean (SD) | 743.5 (272.7) | 697.2 (305.9) | 741.1 (274.7) |
Preoperative ASPItest AUC (U), median (range) | 261.0 (14.0–1573.0) | 252.5 (8.0–1508.0) | 260.5 (8.0–1573.0) |
Preoperative TRAPtest AUC (U), median (range) | 1188.0 (51.0–2139.0) | 1135.0 (215.0–2185.0) | 1187.0 (51.0–2185.0) |
Preoperative ADRtest AUC (U), mean (SD) | 250.4 (133.5) | 261.9 (134.3) | 251.0 (133.5) |
Red cell transfusion intraoperatively or postoperatively: ≤ 4 units (N = 1743) | Red cell transfusion intraoperatively or postoperatively: > 4 units (N = 59) | Total (N = 1802) | |
Preoperative ADPtest AUC (U), mean (SD) | 741.9 (275.1) | 715.0 (251.5) | 741.0 (274.3) |
Preoperative ASPItest AUC (U), median (range) | 260.0 (8.0–1573.0) | 299.0 (33.0–1318.0) | 260.5 (8.0–1573.0) |
Preoperative TRAPtest AUC (U), mean (IQR) | 1187.0 (51.0–2185.0) | 1177.0 (527.0–1728.0) | 1187.0 (51.0–2185.0) |
Preoperative ADRtest AUC (U), median (SD) | 250.0 (132.9) | 271.8 (147.7) | 250.7 (133.4) |
Postoperative ADPtest AUC (U), median (range) | 502.0 (25.0–1746.0) | 441.0 (192.0–1297.0) | 500.5 (25.0–1746.0) |
Postoperative ASPItest AUC (U), median (range) | 197.0 (8.0–1849.0) | 200.0 (6.0–1314.0) | 197.0 (6.0–1849.0) |
Postoperative TRAPtest AUC (U), median (range) | 1163.0 (131.0–2777.0) | 1027.0 (311.0–1904.0) | 1160.0 (131.0–2777.0) |
Postoperative ADRtest AUC (U), mean (SD) | 245.8 (141.8) | 266.8 (189.3) | 246.5 (143.5) |
INTEM CT (seconds), median (range) | 166.0 (104.0–401.0) | 164.0 (128.0–269.0) | 166.0 (104.0–401.0) |
INTEM α-angle (°), median (range) | 74.0 (44.0–83.0) | 74.0 (48.0–83.0) | 74.0 (44.0–83.0) |
INTEM MCF (mm), median (range) | 62.0 (36.0–79.0) | 62.0 (41.0–77.0) | 62.0 (36.0–79.0) |
INTEM ML (%), median (range) | 5.0 (0.0–20.0) | 3.0 (0.0–12.0) | 4.0 (0.0–20.0) |
INTEM Vmax (mm/second), median (range) | 16.0 (4.0–40.0) | 16.0 (6.0–36.0) | 16.0 (4.0–40.0) |
INTEM tVmax (seconds), mean (SD) | 202.2 (36.6) | 200.7 (36.5) | 202.1 (36.6) |
EXTEM CT (seconds), median (range) | 56.0 (14.0–145.0) | 55.0 (34.0–81.0) | 56.0 (14.0–145.0) |
EXTEM α-angle (°), median (range) | 74.0 (48.0–84.0) | 73.0 (57.0–84.0) | 74.0 (48.0–84.0) |
EXTEM MCF (mm), median (range) | 63.0 (30.0–82.0) | 63.0 (44.0–79.0) | 63.0 (30.0–82.0) |
EXTEM ML (%), median (range) | 5.0 (0.0–31.0) | 3.0 (0.0–13.0) | 4.0 (0.0–31.0) |
EXTEM Vmax (mm/second), median (range) | 16.0 (5.0–58.0) | 15.0 (8.0–42.0) | 16.0 (5.0–58.0) |
EXTEM tVmax (seconds), median (range) | 103.0 (20.0–254.0) | 95.0 (44.0–292.0) | 103.0 (20.0–292.0) |
FIBTEM MCF (mm), median (range) | 13.0 (3.0–40.0) | 14.0 (6.0–42.0) | 13.0 (3.0–42.0) |
EXTEM MCF – FIBTEM MCF (mm), median (range) | 49.0 (14.0–62.0) | 48.0 (34.0–56.0) | 49.0 (14.0–62.0) |
INTEM CT – HEPTEM CT (seconds), median (range) | 0.0 (–92.0 to 158.0) | –2.0 (–57.0 to 91.0) | 0.0 (–92.0 to 158.0) |
CK R (minutes), median (range) | 5.9 (2.5–18.4) | 5.8 (3.3–14.1) | 5.9 (2.5–18.4) |
CK α-angle (°), median (range) | 63.4 (25.1–78.7) | 65.8 (34.4–76.0) | 63.4 (25.1–78.7) |
CK MA (mm), mean (SD) | 58.9 (6.4) | 59.2 (8.1) | 58.9 (6.5) |
CK LY 60 (%), median (range) | –0.1 (–11.6 to 12.9) | –0.1 (–6.9 to 3.9) | –0.1 (–11.6 to 12.9) |
CK R – CKH R (minutes), median (range) | 0.1 (–8.0 to 13.2) | 0.1 (–0.7 to 4.8) | 0.1 (–8.0 to 13.2) |
Measurement | No death (N = 1799) | Death (N = 34) | Total (N = 1833) |
---|---|---|---|
Preoperative ADPtest AUC (U), mean (SD) | 739.0 (274.6) | 710.3 (325.6) | 738.5 (275.5) |
Preoperative ASPItest AUC (U), median (range) | 259.0 (8.0–1573.0) | 291.0 (46.0–1422.0) | 259.0 (8.0–1573.0) |
Preoperative TRAPtest AUC (U), mean (IQR) | 1187.0 (24.0–2139.0) | 1161.5 (352.0–2185.0) | 1187.0 (24.0–2185.0) |
Preoperative ADRtest AUC (U), median (SD) | 249.9 (133.1) | 264.1 (137.6) | 250.1 (133.2) |
Postoperative ADPtest AUC (U), median (range) | 501.0 (25.0–1746.0) | 342.0 (129.0–1039.0) | 498.0 (25.0–1746.0) |
Postoperative ASPItest AUC (U), median (range) | 197.0 (6.0, 1849.0) | 170.0 (25.0, 1350.0) | 197.0 (6.0–1849.0) |
Postoperative TRAPtest AUC (U), median (range) | 1161.0 (131.0–2777.0) | 963.5 (277.0–1794.0) | 1159.0 (131.0–2777.0) |
Postoperative ADRtest AUC (U), mean (SD) | 246.8 (143.4) | 223.2 (155.8) | 246.4 (143.6) |
INTEM CT (seconds), median (range) | 166.0 (104.0–401.0) | 162.0 (132.0–216.0) | 166.0 (104.0–401.0) |
INTEM α-angle (°), median (range) | 74.0 (44.0–83.0) | 76.0 (58.0–82.0) | 74.0 (44.0–83.0) |
INTEM MCF (mm), median (range) | 61.0 (36.0–79.0) | 62.5 (49.0–74.0) | 62.0 (36.0–79.0) |
INTEM ML (%), median (range) | 5.0 (0.0–20.0) | 2.0 (0.0–14.0) | 4.0 (0.0–20.0) |
INTEM Vmax (mm/second), median (range) | 16.0 (4.0–40.0) | 18.0 (7.0–31.0) | 16.0 (4.0–40.0) |
INTEM tVmax (seconds), mean (SD) | 202.4 (36.8) | 196.6 (31.8) | 202.3 (36.7) |
EXTEM CT (seconds), median (range) | 56.0 (14.0–145.0) | 57.0 (24.0–93.0) | 56.0 (14.0–145.0) |
EXTEM α-angle (°), median (range) | 74.0 (48.0–84.0) | 74.0 (57.0–81.0) | 74.0 (48.0–84.0) |
EXTEM MCF (mm), median (range) | 63.0 (30.0–82.0) | 63.5 (51.0–76.0) | 63.0 (30.0–82.0) |
EXTEM ML (%), median (range) | 4.0 (0.0–31.0) | 3.0 (0.0–14.0) | 4.0 (0.0–31.0) |
EXTEM Vmax (mm/second), median (range) | 16.0 (5.0–58.0) | 16.0 (8.0–27.0) | 16.0 (5.0–58.0) |
EXTEM tVmax (seconds), median (range) | 103.0 (20.0–292.0) | 99.0 (52.0–157.0) | 102.0 (20.0–292.0) |
FIBTEM MCF (mm), median (range) | 13.0 (3.0–42.0) | 13.5 (4.0–24.0) | 13.0 (3.0–42.0) |
EXTEM MCF – FIBTEM MCF (mm), median (range) | 49.0 (14.0–62.0) | 49.5 (41.0–57.0) | 49.0 (14.0, 62.0) |
INTEM CT – HEPTEM CT (seconds), median (range) | 0.0 (–92.0 to 158.0) | 5.5 (–49.0 to 41.0) | 0.0 (–92.0 to 158.0) |
CK R (minutes), median (range) | 5.9 (2.5–18.4) | 5.8 (3.3–9.6) | 5.9 (2.5–18.4) |
CK α-angle (°), median (range) | 63.3 (25.1–78.7) | 66.3 (45.8–74.0) | 63.4 (25.1–78.7) |
CK MA (mm), mean (SD) | 58.9 (6.4) | 59.2 (7.8) | 58.9 (6.5) |
CK LY 60 (%), median (range) | –0.1 (–11.6 to 12.9) | –0.1 (–1.8 to 6.1) | –0.1 (–11.6 to 12.9) |
CK R – CKH R (minutes), median (range) | 0.1 (–8.0 to 13.2) | 0.1 (–1.3 to 2.7) | 0.1 (–8.0 to 13.2) |
Measurement | No MI (N = 1809) | MI (N = 19) | Total (N = 1828) |
---|---|---|---|
Preoperative ADPtest AUC (U), mean (SD) | 738.5 (275.5) | 746.1 (315.9) | 738.6 (275.8) |
Preoperative ASPItest AUC (U), median (range) | 259.0 (8.0–1573.0) | 266.0 (67.0–1147.0) | 259.0 (8.0–1573.0) |
Preoperative TRAPtest AUC (U), mean (IQR) | 1187.0 (24.0–2185.0) | 1166.0 (598.0–1783.0) | 1185.5 (24.0–2185.0) |
Preoperative ADRtest AUC (U), median (SD) | 250.4 (133.2) | 252.9 (131.2) | 250.4 (133.2) |
Postoperative ADPtest AUC (U), median (range) | 499.0 (25.0–1746.0) | 466.0 (75.0–1103.0) | 498.0 (25.0–1746.0) |
Postoperative ASPItest AUC (U), median (range) | 197.0 (8.0–1849.0) | 188.0 (25.0–545.0) | 197.0 (8.0–1849.0) |
Postoperative TRAPtest AUC (U), median (range) | 1157.0 (131.0–2777.0) | 1338.0 (568.0–1722.0) | 1158.5 (131.0–2777.0) |
Postoperative ADRtest AUC (U), mean (SD) | 246.3 (143.7) | 269.0 (135.0) | 246.5 (143.6) |
INTEM CT (seconds), median (range) | 166.0 (104.0–401.0) | 157.0 (133.0–228.0) | 166.0 (104.0–401.0) |
INTEM α-angle (°), median (range) | 74.0 (44.0–83.0) | 74.0 (67.0–81.0) | 74.0 (44.0–83.0) |
INTEM MCF (mm), median (range) | 62.0 (36.0–79.0) | 60.0 (54.0–73.0) | 62.0 (36.0–79.0) |
INTEM ML (%), median (range) | 4.0 (0.0–20.0) | 5.0 (1.0–14.0) | 4.0 (0.0–20.0) |
INTEM Vmax (mm/second), median (range) | 16.0 (4.0–40.0) | 16.0 (11.0–28.0) | 16.0 (4.0–40.0) |
INTEM tVmax (seconds), mean (SD) | 202.3 (36.8) | 196.5 (29.4) | 202.2 (36.7) |
EXTEM CT (seconds), median (range) | 56.0 (14.0–145.0) | 50.0 (35.0–85.0) | 56.0 (14.0–145.0) |
EXTEM α-angle (°), median (range) | 74.0 (48.0–84.0) | 74.0 (67.0–81.0) | 74.0 (48.0–84.0) |
EXTEM MCF (mm), median (range) | 63.0 (30.0–82.0) | 63.0 (54.0–73.0) | 63.0 (30.0–82.0) |
EXTEM ML (%), median (range) | 4.0 (0.0–31.0) | 5.0 (0.0–15.0) | 4.0 (0.0–31.0) |
EXTEM Vmax (mm/second), median (range) | 16.0 (5.0–58.0) | 16.0 (12.0–25.0) | 16.0 (5.0–58.0) |
EXTEM tVmax (seconds), median (range) | 103.0 (20.0–292.0) | 100.0 (55.0–162.0) | 102.5 (20.0–292.0) |
FIBTEM MCF (mm), median (range) | 13.0 (3.0–42.0) | 13.0 (5.0–27.0) | 13.0 (3.0–42.0) |
EXTEM MCF – FIBTEM MCF (mm), median (range) | 49.0 (14.0–62.0) | 50.0 (45.0–55.0) | 49.0 (14.0–62.0) |
INTEM CT – HEPTEM CT (seconds), median (range) | 0.0 (–92.0 to 158.0) | 0.0 (–20.0 to 41.0) | 0.0 (–92.0 to 158.0) |
CK R (minutes), median (range) | 5.9 (2.5–18.4) | 5.5 (4.2–8.2) | 5.9 (2.5–18.4) |
CK α-angle (°), median (range) | 63.4 (25.1–78.7) | 65.1 (49.1–73.9) | 63.4 (25.1–78.7) |
CK MA (mm), mean (SD) | 58.9 (6.5) | 60.1 (5.7) | 58.9 (6.5) |
CK LY 60 (%), median (range) | –0.1 (–11.6 to 12.9) | –0.1 (–3.9 to 5.7) | –0.1 (–11.6 to 12.9) |
CK R – CKH R (minutes), median (range) | 0.1 (–8.0 to 13.2) | 0.1 (–1.3 to 1.8) | 0.1 (–8.0 to 13.2) |
Measurement | No stroke (N = 1809) | Stroke (N = 19) | Total (N = 1828) |
---|---|---|---|
Preoperative ADPtest AUC (U), mean (SD) | 736.7 (276.0) | 913.0 (203.3) | 738.6 (275.8) |
Preoperative ASPItest AUC (U), median (range) | 258.0 (8.0–1573.0) | 636.0 (22.0–1147.0) | 259.0 (8.0–1573.0) |
Preoperative TRAPtest AUC (U), mean (IQR) | 1184.0 (24.0–2185.0) | 1319.0 (850.0–1728.0) | 1185.5 (24.0–2185.0) |
Preoperative ADRtest AUC (U), median (SD) | 250.3 (133.3) | 259.3 (122.2) | 250.4 (133.2) |
Postoperative ADPtest AUC (U), median (range) | 500.0 (25.0–1746.0) | 386.0 (159.0–996.0) | 498.0 (25.0–1746.0) |
Postoperative ASPItest AUC (U), median (range) | 197.0 (8.0–1849.0) | 191.0 (85.0–727.0) | 197.0 (8.0–1849.0) |
Postoperative TRAPtest AUC (U), median (range) | 1159.0 (131.0–2777.0) | 1027.0 (466.0–1781.0) | 1158.5 (131.0–2777.0) |
Postoperative ADRtest AUC (U), mean (SD) | 246.7 (143.7) | 231.5 (135.0) | 246.5 (143.6) |
INTEM CT (seconds), median (range) | 166.0 (104.0–401.0) | 164.0 (133.0–185.0) | 166.0 (104.0–401.0) |
INTEM α-angle (°), median (range) | 74.0 (44.0–83.0) | 74.0 (66.0–81.0) | 74.0 (44.0–83.0) |
INTEM MCF (mm), median (range) | 62.0 (36.0–79.0) | 61.0 (51.0–71.0) | 62.0 (36.0–79.0) |
INTEM ML (%), median (range) | 4.0 (0.0–20.0) | 3.0 (0.0–10.0) | 4.0 (0.0–20.0) |
INTEM Vmax (mm/second), median (range) | 16.0 (4.0–40.0) | 15.0 (10.0–26.0) | 16.0 (4.0–40.0) |
INTEM tVmax (seconds), mean (SD) | 202.3 (36.8) | 196.8 (24.0) | 202.2 (36.7) |
EXTEM CT (seconds), median (range) | 56.0 (14.0–145.0) | 53.0 (34.0–118.0) | 56.0 (14.0–145.0) |
EXTEM α-angle (°), median (range) | 74.0 (48.0–84.0) | 73.0 (63.0–82.0) | 74.0 (48.0–84.0) |
EXTEM MCF (mm), median (range) | 63.0 (30.0–82.0) | 65.0 (53.0–72.0) | 63.0 (30.0–82.0) |
EXTEM ML (%), median (range) | 4.0 (0.0–31.0) | 3.0 (0.0–9.0) | 4.0 (0.0–31.0) |
EXTEM Vmax (mm/second), median (range) | 16.0 (5.0–58.0) | 16.0 (9.0–30.0) | 16.0 (5.0–58.0) |
EXTEM tVmax (seconds), median (range) | 103.0 (20.0–292.0) | 86.0 (40.0–148.0) | 102.5 (20.0–292.0) |
FIBTEM MCF (mm), median (range) | 13.0 (3.0–42.0) | 12.0 (7.0–29.0) | 13.0 (3.0–42.0) |
EXTEM MCF – FIBTEM MCF (mm), median (range) | 49.0 (14.0–62.0) | 49.0 (43.0–55.0) | 49.0 (14.0–62.0) |
INTEM CT – HEPTEM CT (seconds), median (range) | 0.0 (–92.0 to 158.0) | 0.0 (–30.0 to 14.0) | 0.0 (–92.0 to 158.0) |
CK R (minutes), median (range) | 5.9 (2.5–18.4) | 5.4 (3.5–9.5) | 5.9 (2.5–18.4) |
CK α-angle (°), median (range) | 63.4 (25.1–78.7) | 66.8 (55.1–76.3) | 63.4 (25.1–78.7) |
CK MA (mm), mean (SD) | 58.9 (6.5) | 60.1 (5.8) | 58.9 (6.5) |
CK LY 60 (%), median (range) | –0.1 (–11.6 to 12.9) | –0.1 (–3.4 to 3.9) | –0.1 (–11.6 to 12.9) |
CK R – CKH R (minutes), median (range) | 0.1 (–8.0 to 13.2) | 0.0 (–0.6 to 0.9) | 0.1 (–8.0 to 13.2) |
Measurements | No AKI (N = 1014) | AKI (N = 819) | Total (N = 1833) |
---|---|---|---|
Preoperative ADPtest AUC (U), mean (SD) | 735.5 (264.7) | 742.2 (288.5) | 738.5 (275.5) |
Preoperative ASPItest AUC (U), median (range) | 267.5 (8.0–1573.0) | 253.0 (19.0–1553.0) | 259.0 (8.0–1573.0) |
Preoperative TRAPtest AUC (U), mean (IQR) | 1194.0 (51.0–2139.0) | 1174.0 (24.0–2185.0) | 1187.0 (24.0–2185.0) |
Preoperative ADRtest AUC (U), median (SD) | 246.3 (130.5) | 254.9 (136.3) | 250.1 (133.2) |
Postoperative ADPtest AUC (U), median (range) | 503.0 (32.0–1746.0) | 491.0 (25.0–1669.0) | 498.0 (25.0–1746.0) |
Postoperative ASPItest AUC (U), median (range) | 199.0 (8.0–1849.0) | 193.0 (6.0–1457.0) | 197.0 (6.0–1849.0) |
Postoperative TRAPtest AUC (U), median (range) | 1178.5 (131.0–2395.0) | 1138.0 (213.0–2777.0) | 1159.0 (131.0–2777.0) |
Postoperative ADRtest AUC (U), mean (SD) | 244.5 (146.5) | 248.8 (140.0) | 246.4 (143.6) |
INTEM CT (seconds), median (range) | 166.0 (104.0–327.0) | 166.0 (111.0–401.0) | 166.0 (104.0–401.0) |
INTEM α-angle (°), median (range) | 74.0 (48.0–83.0) | 74.0 (44.0–83.0) | 74.0 (44.0–83.0) |
INTEM MCF (mm), median (range) | 61.0 (37.0–79.0) | 62.0 (36.0–79.0) | 62.0 (36.0–79.0) |
INTEM ML (%), median (range) | 5.0 (0.0–20.0) | 4.0 (0.0–20.0) | 4.0 (0.0–20.0) |
INTEM Vmax (mm/second), median (range) | 16.0 (6.0–39.0) | 16.0 (4.0–40.0) | 16.0 (4.0–40.0) |
INTEM tVmax (seconds), mean (SD) | 201.4 (35.8) | 203.3 (37.9) | 202.3 (36.7) |
EXTEM CT (seconds), median (range) | 56.0 (16.0–145.0) | 56.0 (14.0–140.0) | 56.0 (14.0–145.0) |
EXTEM α-angle (°), median (range) | 73.0 (50.0–84.0) | 74.0 (48.0–84.0) | 74.0 (48.0–84.0) |
EXTEM MCF (mm), median (range) | 63.0 (30.0–80.0) | 64.0 (38.0–82.0) | 63.0 (30.0–82.0) |
EXTEM ML (%), median (range) | 5.0 (0.0–21.0) | 4.0 (0.0–31.0) | 4.0 (0.0–31.0) |
EXTEM Vmax (mm/second), median (range) | 16.0 (6.0–58.0) | 16.0 (5.0–44.0) | 16.0 (5.0–58.0) |
EXTEM tVmax (seconds), median (range) | 103.0 (20.0–254.0) | 101.0 (40.0, 292.0) | 102.0 (20.0–292.0) |
FIBTEM MCF (mm), median (range) | 13.0 (3.0–38.0) | 13.0 (3.0–42.0) | 13.0 (3.0–42.0) |
EXTEM MCF – FIBTEM MCF (mm), median (range) | 49.0 (14.0–59.0) | 49.0 (31.0–62.0) | 49.0 (14.0–62.0) |
INTEM CT – HEPTEM CT (seconds), median (range) | 0.0 (–92.0 to 158.0) | –1.0 (–73.0 to 99.0) | 0.0 (–92.0 to 158.0) |
CK R (minutes), median (range) | 6.0 (2.5–14.7) | 5.9 (2.9–18.4) | 5.9 (2.5–18.4) |
CK α-angle (°), median (range) | 62.6 (31.3–77.1) | 64.4 (25.1–78.7) | 63.4 (25.1–78.7) |
CK MA (mm), mean (SD) | 58.4 (6.1) | 59.5 (6.8) | 58.9 (6.5) |
CK LY 60 (%), median (range) | –0.2 (–11.6 to 12.9) | –0.1 (–11.1 to 12.8) | –0.1 (–11.6 to 12.9) |
CK R – CKH R (minutes), median (range) | 0.1 (–8.0 to 7.1) | 0.1 (–2.4 to 13.2) | 0.1 (–8.0 to 13.2) |
Measurement | No sepsis (N = 1722) | Sepsis (N = 104) | Total (N = 1826) |
---|---|---|---|
Preoperative ADPtest AUC (U), mean (SD) | 739.0 (275.6) | 735.0 (280.6) | 738.8 (275.8) |
Preoperative ASPItest AUC (U), median (range) | 258.0 (8.0–1573.0) | 279.5 (26.0–1104.0) | 259.0 (8.0–1573.0) |
Preoperative TRAPtest AUC (U), mean (IQR) | 1188.0 (24.0–2185.0) | 1168.0 (215.0–1844.0) | 1187.0 (24.0–2185.0) |
Preoperative ADRtest AUC (U), median (SD) | 249.6 (132.8) | 263.4 (141.4) | 250.4 (133.3) |
Postoperative ADPtest AUC (U), median (range) | 500.0 (25.0–1746.0) | 477.0 (169.0–1561.0) | 498.5 (25.0–1746.0) |
Postoperative ASPItest AUC (U), median (range) | 197.0 (8.0–1849.0) | 195.0 (28.0–1350.0) | 197.0 (8.0–1849.0) |
Postoperative TRAPtest AUC (U), median (range) | 1164.0 (131.0–2777.0) | 1068.0 (306.0–2340.0) | 1158.5 (131.0–2777.0) |
Postoperative ADRtest AUC (U), mean (SD) | 246.5 (144.6) | 246.7 (128.3) | 246.5 (143.6) |
INTEM CT (seconds), median (range) | 166.0 (104.0–401.0) | 167.0 (131.0–267.0) | 166.0 (104.0–401.0) |
INTEM α-angle (°), median (range) | 74.0 (48.0–83.0) | 75.0 (44.0–83.0) | 74.0 (44.0–83.0) |
INTEM MCF (mm), median (range) | 61.0 (37.0–79.0) | 63.5 (36.0–78.0) | 62.0 (36.0–79.0) |
INTEM ML (%), median (range) | 5.0 (0.0–20.0) | 3.0 (0.0–14.0) | 4.0 (0.0–20.0) |
INTEM Vmax (mm/second), median (range) | 16.0 (5.0–40.0) | 18.0 (4.0–38.0) | 16.0 (4.0–40.0) |
INTEM tVmax (seconds), mean (SD) | 202.1 (36.8) | 204.3 (36.0) | 202.2 (36.7) |
EXTEM CT (seconds), median (range) | 56.0 (14.0–145.0) | 56.0 (24.0–110.0) | 56.0 (14.0–145.0) |
EXTEM α-angle (°), median (range) | 74.0 (50.0–84.0) | 75.0 (48.0–84.0) | 74.0 (48.0–84.0) |
EXTEM MCF (mm), median (range) | 63.0 (30.0–81.0) | 65.0 (38.0–82.0) | 63.0 (30.0–82.0) |
EXTEM ML (%), median (range) | 4.5 (0.0–26.0) | 3.5 (0.0–31.0) | 4.0 (0.0–31.0) |
EXTEM Vmax (mm/second), median (range) | 16.0 (6.0–58.0) | 17.0 (5.0–42.0) | 16.0 (5.0–58.0) |
EXTEM tVmax (seconds), median (range) | 101.0 (20.0–292.0) | 103.0 (50.0–196.0) | 102.0 (20.0–292.0) |
FIBTEM MCF (mm), median (range) | 13.0 (3.0–42.0) | 15.0 (3.0–36.0) | 13.0 (3.0–42.0) |
EXTEM MCF – FIBTEM MCF (mm), median (range) | 49.0 (14.0–59.0) | 49.0 (35.0–62.0) | 49.0 (14.0–62.0) |
INTEM CT – HEPTEM CT (seconds), median (range) | 0.0 (–92.0 to 158.0) | 0.0 (–57.0 to 69.0) | 0.0 (–92.0 to 158.0) |
CK R (minutes), median (range) | 6.0 (2.5–18.4) | 5.8 (2.8–11.4) | 5.9 (2.5–18.4) |
CK α-angle (°), median (range) | 63.2 (25.1–78.7) | 65.8 (38.9–76.7) | 63.4 (25.1–78.7) |
CK MA (mm), mean (SD) | 58.8 (6.4) | 60.4 (7.3) | 58.9 (6.5) |
CK LY 60 (%), median (range) | –0.1 (–11.6–12.9) | –0.1 (–4.4–7.0) | –0.1 (–11.6–12.9) |
CK R – CKH R (minutes), median (range) | 0.1 (–8.0 to 13.2) | 0.1 (–2.3 to 1.5) | 0.1 (–8.0 to 13.2) |
Supplementary figure
FIGURE 75.
Prediction of CCB and non-routine pro-haemostatic treatment during surgery. ORs with 95% CIs for CCB and non-routine pro-haemostatic treatment during surgery. For the categorical variables, ORs are presented for male sex relative to female sex, presence of diabetes relative to no diabetes, surgical procedure and antiplatelet treatment relative to CABG with no antiplatelet treatment and urgent surgery relative to elective surgery. ORs are adjusted for all other factors in the figure and for whether participants were included in an interventional study at our centre. APT, any antiplatelet treatment; ASP, aspirin alone or aspirin with P2Y12 blocker stopped > 7 days before surgery; DAPT, aspirin + P2Y12 blocker received ≤ 7 days before surgery shown with duration of omission of P2Y12 blocker before surgery; HCT, preoperative haematocrit; valve, valve replacement.
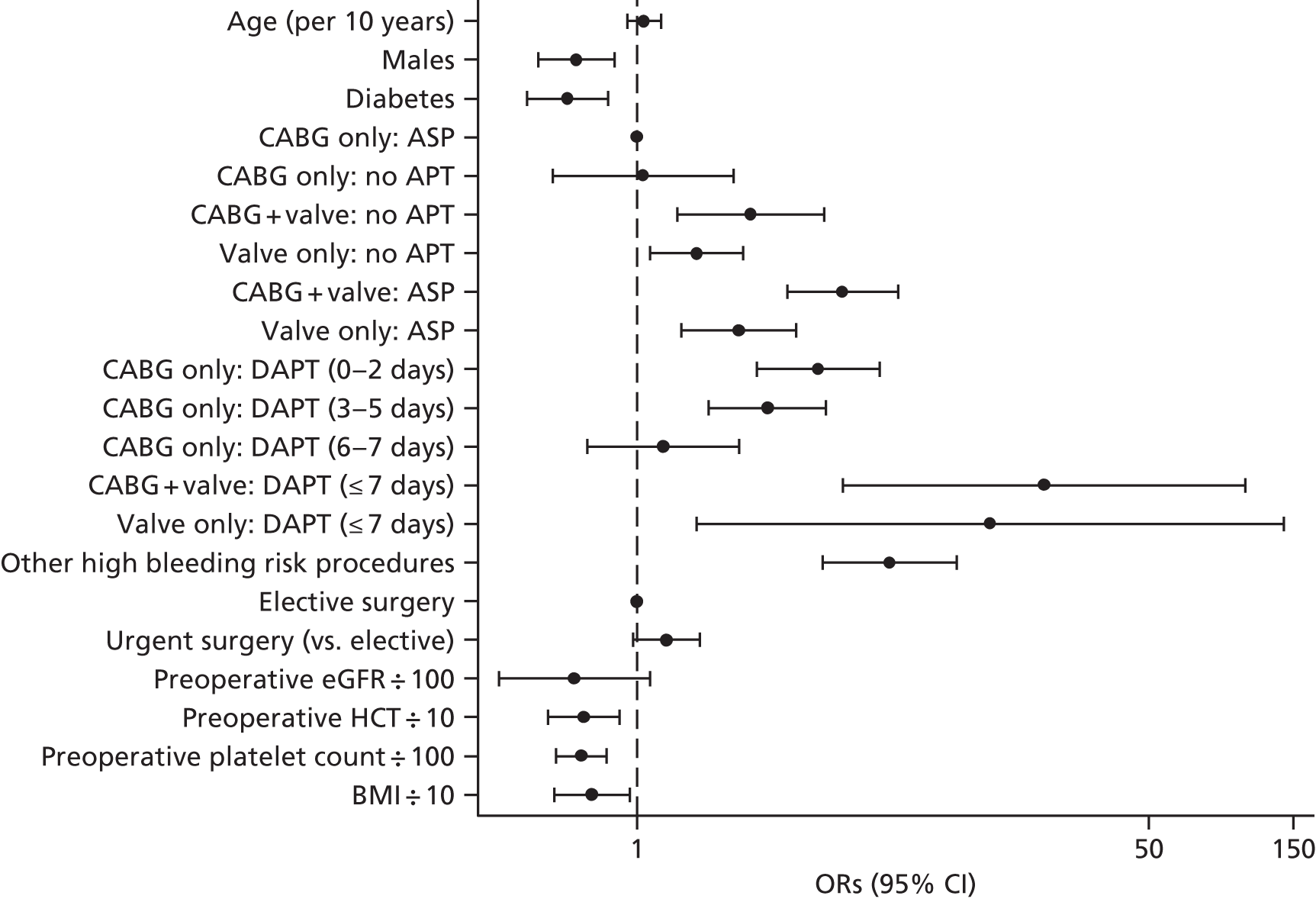
Supplementary results from the health economic analyses
Tables 110–119 list the parameter estimates (probabilities and costs) used to populate the four base-case COPTIC models. In all tables:
-
‘treatment’ refers to protamine, platelets, clotting factors, > 4 units of red cells and/or a reoperation for bleeding.
-
‘complications’ refers to MI, stroke, sepsis or AKI during the index hospital admission for cardiac surgery.
-
‘group’ refers to the 12 groups of participants identified in Figure 32.
Variable | Base-case value: positive/total | Distribution for probabilistic sensitivity analysis | Source |
---|---|---|---|
Current practice | |||
Probability of ad hoc TEG | 284/1802 | Beta | Observational study |
Current practice, ad hoc TEG | |||
Probability ad hoc TEG positive | 120/284 | Beta | Observational study |
Current practice, ad hoc TEG positive | |||
Probability POC test positive | 13/120 | Beta | Observational study |
Probability of CCB: POC test positive | 10/13 | Beta | Observational study |
Probability of treatment: CCB | 9/10 | Beta | Observational study |
Probability of MI, stroke, sepsis or AKI: treatment | 6/9 | Beta | Observational study |
Probability discharged: MI, stroke, sepsis or AKI | 6/6 | Beta | Observational study |
Probability alive at 1 year: discharged | 6/6 | Beta | Observational study |
Probability discharged: no MI, stroke, sepsis or AKI | 3/3 | Beta | Observational study |
Probability alive at 1 year: discharged, no MI, stroke, sepsis or AKI | 3/3 | Beta | Observational study |
Probability of MI, stroke, sepsis or AKI: no treatment | 1/1 | Beta | Observational study |
Probability discharged: MI, stroke, sepsis or AKI, no treatment | 1/1 | Beta | Observational study |
Probability alive at 1 year: discharged, MI, stroke, sepsis or AKI, no treatment | 1/1 | Beta | Observational study |
Probability of treatment: no CCB | 0/3 | Beta | Observational study |
Probability of MI, stroke, sepsis or AKI: no treatment | 3/3 | Beta | Observational study |
Probability discharged: MI, stroke, sepsis or AKI, no treatment | 3/3 | Beta | Observational study |
Probability alive at 1 year: discharged, MI, stroke, sepsis or AKI, no treatment | 3/3 | Beta | Observational study |
Probability of CCB: POC test negative | 65/107 | Beta | Observational study |
Probability of treatment: CCB | 59/65 | Beta | Observational study |
Probability of MI, stroke, sepsis or AKI: treatment | 26/59 | Beta | Observational study |
Probability discharged: MI, stroke, sepsis or AKI | 25/26 | Beta | Observational study |
Probability alive at 1 year: discharged | 24/25 | Beta | Observational study |
Probability discharged: no MI, stroke, sepsis or AKI | 33/33 | Beta | Observational study |
Probability alive at 1 year: discharged, no MI, stroke, sepsis or AKI | 30/33 | Beta | Observational study |
Probability of MI, stroke, sepsis or AKI: no treatment | 4/6 | Beta | Observational study |
Probability discharged: MI, stroke, sepsis or AKI, no treatment | 3/4 | Beta | Observational study |
Probability alive at 1 year: discharged, MI, stroke, sepsis or AKI, no treatment | 3/3 | Beta | Observational study |
Probability discharged: no MI, stroke, sepsis or AKI, no treatment | 2/2 | Beta | Observational study |
Probability alive at 1 year: discharged, no MI, stroke, sepsis or AKI, no treatment | 2/2 | Beta | Observational study |
Probability of treatment: no CCB | 0/42 | Beta | Observational study |
Probability of MI, stroke, sepsis or AKI: no treatment | 13/42 | Beta | Observational study |
Probability discharged: MI, stroke, sepsis or AKI, no treatment | 12/13 | Beta | Observational study |
Probability alive at 1 year: discharged, MI, stroke, sepsis or AKI, no treatment | 12/12 | Beta | Observational study |
Probability discharged: no MI, stroke, sepsis or AKI, no treatment | 29/29 | Beta | Observational study |
Probability alive at 1 year: discharged, no MI, stroke, sepsis or AKI, no treatment | 29/29 | Beta | Observational study |
Current practice, ad hoc TEG negative | |||
Probability POC test positive | 0/164 | Beta | Observational study |
Probability of CCB: POC test negative | 89/164 | Beta | Observational study |
Probability of treatment: CCB | 82/89 | Beta | Observational study |
Probability of MI, stroke, sepsis or AKI: treatment | 54/82 | Beta | Observational study |
Probability discharged: MI, stroke, sepsis or AKI | 50/54 | Beta | Observational study |
Probability alive at 1 year: discharged | 47/50 | Beta | Observational study |
Probability discharged: no MI, stroke, sepsis or AKI | 28/28 | Beta | Observational study |
Probability alive at 1 year: discharged, no MI, stroke, sepsis or AKI | 27/28 | Beta | Observational study |
Probability of MI, stroke, sepsis or AKI: no treatment | 6/7 | Beta | Observational study |
Probability discharged: MI, stroke, sepsis or AKI, no treatment | 6/6 | Beta | Observational study |
Probability alive at 1 year: discharged, MI, stroke, sepsis or AKI, no treatment | 6/6 | Beta | Observational study |
Probability discharged: no MI, stroke, sepsis or AKI, no treatment | 1/1 | Beta | Observational study |
Probability alive at 1 year: discharged, no MI, stroke, sepsis or AKI, no treatment | 1/1 | Beta | Observational study |
Probability of treatment: no CCB | 2/75 | Beta | Observational study |
Probability of MI, stroke, sepsis or AKI: treatment | 0/2 | Beta | Observational study |
Probability discharged: no MI, stroke, sepsis or AKI | 2/2 | Beta | Observational study |
Probability alive at 1 year: discharged, no MI, stroke, sepsis or AKI | 2/2 | Beta | Observational study |
Probability of MI, stroke, sepsis or AKI: no treatment | 39/73 | Beta | Observational study |
Probability discharged: MI, stroke, sepsis or AKI, no treatment | 39/39 | Beta | Observational study |
Probability alive at 1 year: discharged, MI, stroke, sepsis or AKI, no treatment | 36/39 | Beta | Observational study |
Probability discharged: no MI, stroke, sepsis or AKI, no treatment | 34/34 | Beta | Observational study |
Probability alive at 1 year: discharged, no MI, stroke, sepsis or AKI, no treatment | 34/34 | Beta | Observational study |
Current practice, no ad hoc TEG | |||
Probability POC test positive | 52/1518 | Beta | Observational study |
Probability of CCB: POC test positive | 15/52 | Beta | Observational study |
Probability of treatment: CCB | 13/15 | Beta | Observational study |
Probability of MI, stroke, sepsis or AKI: treatment | 4/13 | Beta | Observational study |
Probability discharged: MI, stroke, sepsis or AKI | 4/4 | Beta | Observational study |
Probability alive at 1 year: discharged | 4/4 | Beta | Observational study |
Probability discharged: no MI, stroke, sepsis or AKI | 9/9 | Beta | Observational study |
Probability alive at 1 year: discharged, no MI, stroke, sepsis or AKI | 9/9 | Beta | Observational study |
Probability of MI, stroke, sepsis or AKI: no treatment | 1/2 | Beta | Observational study |
Probability discharged: MI, stroke, sepsis or AKI, no treatment | 1/1 | Beta | Observational study |
Probability alive at 1 year: discharged, MI, stroke, sepsis or AKI, no treatment | 1/1 | Beta | Observational study |
Probability discharged: no MI, stroke, sepsis or AKI, no treatment | 1/1 | Beta | Observational study |
Probability alive at 1 year: discharged, no MI, stroke, sepsis or AKI, no treatment | 1/1 | Beta | Observational study |
Probability of treatment: no CCB | 1/37 | Beta | Observational study |
Probability of MI, stroke, sepsis or AKI: treatment | 1/1 | Beta | Observational study |
Probability discharged: MI, stroke, sepsis or AKI | 1/1 | Beta | Observational study |
Probability alive at 1 year: discharged | 1/1 | Beta | Observational study |
Probability of MI, stroke, sepsis or AKI: no treatment | 13/36 | Beta | Observational study |
Probability discharged: MI, stroke, sepsis or AKI, no treatment | 13/13 | Beta | Observational study |
Probability alive at 1 year: discharged, MI, stroke, sepsis or AKI, no treatment | 13/13 | Beta | Observational study |
Probability discharged: no MI, stroke, sepsis or AKI, no treatment | 23/23 | Beta | Observational study |
Probability alive at 1 year: discharged, no MI, stroke, sepsis or AKI, no treatment | 23/23 | Beta | Observational study |
Probability of CCB: POC test negative | 254/1466 | Beta | Observational study |
Probability of treatment: CCB | 183/254 | Beta | Observational study |
Probability of MI, stroke, sepsis or AKI: treatment | 99/183 | Beta | Observational study |
Probability discharged: MI, stroke, sepsis or AKI | 90/99 | Beta | Observational study |
Probability alive at 1 year: discharged | 86/90 | Beta | Observational study |
Probability discharged: no MI, stroke, sepsis or AKI | 84/84 | Beta | Observational study |
Probability alive at 1 year: discharged, no MI, stroke, sepsis or AKI | 81/84 | Beta | Observational study |
Probability of MI, stroke, sepsis or AKI: no treatment | 34/71 | Beta | Observational study |
Probability discharged: MI, stroke, sepsis or AKI, no treatment | 32/34 | Beta | Observational study |
Probability alive at 1 year: discharged, MI, stroke, sepsis or AKI, no treatment | 32/32 | Beta | Observational study |
Probability discharged: no MI, stroke, sepsis or AKI, no treatment | 37/37 | Beta | Observational study |
Probability alive at 1 year: discharged, no MI, stroke, sepsis or AKI, no treatment | 37/37 | Beta | Observational study |
Probability of treatment: no CCB | 16/1212 | Beta | Observational study |
Probability of MI, stroke, sepsis or AKI: treatment | 16/16 | Beta | Observational study |
Probability discharged: MI, stroke, sepsis or AKI | 13/16 | Beta | Observational study |
Probability alive at 1 year: discharged | 10/13 | Beta | Observational study |
Probability of MI, stroke, sepsis or AKI: no treatment | 515/1196 | Beta | Observational study |
Probability discharged: MI, stroke, sepsis or AKI, no treatment | 508/515 | Beta | Observational study |
Probability alive at 1 year: discharged, MI, stroke, sepsis or AKI, no treatment | 492/508 | Beta | Observational study |
Probability discharged: no MI, stroke, sepsis or AKI, no treatment | 678/681 | Beta | Observational study |
Probability alive at 1 year: discharged, no MI, stroke, sepsis or AKI, no treatment | 673/678 | Beta | Observational study |
Test all with TEG | |||
Probability POC test positive | 65/1802 | Beta | Observational study |
Probability of ad hoc TEG: POC test positive | 13/65 | Beta | Observational study |
Probability ad hoc TEG positive: ad hoc TEG, POC test positive | 13/13 | Beta | Observational study |
Probability of ad hoc TEG: POC test negative | 271/1737 | Beta | Observational study |
Probability ad hoc TEG positive: ad hoc TEG, POC test negative | 107/271 | Beta | Observational study |
Probability prophylactic treatment fails | 154/449 | Beta | Observational study |
Variable | Base-case value: positive/total | Distribution for probabilistic sensitivity analysis | Source |
---|---|---|---|
Current practice | |||
Probability of ad hoc TEG | 284/1802 | Beta | Observational study |
Current practice, ad hoc TEG | |||
Probability ad hoc TEG positive | 120/284 | Beta | Observational study |
Current practice, ad hoc TEG positive | |||
Probability POC test positive | 21/120 | Beta | Observational study |
Probability of CCB: POC test positive | 14/21 | Beta | Observational study |
Probability of treatment: CCB | 13/14 | Beta | Observational study |
Probability of MI, stroke, sepsis or AKI: treatment | 8/13 | Beta | Observational study |
Probability discharged: MI, stroke, sepsis or AKI | 8/8 | Beta | Observational study |
Probability alive at 1 year: discharged | 8/8 | Beta | Observational study |
Probability discharged: no MI, stroke, sepsis or AKI | 5/5 | Beta | Observational study |
Probability alive at 1 year: discharged, no MI, stroke, sepsis or AKI | 4/5 | Beta | Observational study |
Probability of MI, stroke, sepsis or AKI: no treatment | 1/1 | Beta | Observational study |
Probability discharged: MI, stroke, sepsis or AKI, no treatment | 1/1 | Beta | Observational study |
Probability alive at 1 year: discharged, MI, stroke, sepsis or AKI, no treatment | 1/1 | Beta | Observational study |
Probability of treatment: no CCB | 0/7 | Beta | Observational study |
Probability of MI, stroke, sepsis or AKI: no treatment | 2/7 | Beta | Observational study |
Probability discharged: MI, stroke, sepsis or AKI, no treatment | 2/2 | Beta | Observational study |
Probability alive at 1 year: discharged, MI, stroke, sepsis or AKI, no treatment | 2/2 | Beta | Observational study |
Probability discharged: no MI, stroke, sepsis or AKI, no treatment | 5/5 | Beta | Observational study |
Probability alive at 1 year: discharged, no MI, stroke, sepsis or AKI, no treatment | 5/5 | Beta | Observational study |
Probability of CCB: POC test negative | 61/99 | Beta | Observational study |
Probability of treatment: CCB | 55/61 | Beta | Observational study |
Probability of MI, stroke, sepsis or AKI: treatment | 24/55 | Beta | Observational study |
Probability discharged: MI, stroke, sepsis or AKI | 23/24 | Beta | Observational study |
Probability alive at 1 year: discharged | 22/23 | Beta | Observational study |
Probability discharged: no MI, stroke, sepsis or AKI | 31/31 | Beta | Observational study |
Probability alive at 1 year: discharged, no MI, stroke, sepsis or AKI | 29/31 | Beta | Observational study |
Probability of MI, stroke, sepsis or AKI: no treatment | 4/6 | Beta | Observational study |
Probability discharged: MI, stroke, sepsis or AKI, no treatment | 3/4 | Beta | Observational study |
Probability alive at 1 year: discharged, MI, stroke, sepsis or AKI, no treatment | 3/3 | Beta | Observational study |
Probability discharged: no MI, stroke, sepsis or AKI, no treatment | 2/2 | Beta | Observational study |
Probability alive at 1 year: discharged, no MI, stroke, sepsis or AKI, no treatment | 2/2 | Beta | Observational study |
Probability of treatment: no CCB | 0/38 | Beta | Observational study |
Probability of MI, stroke, sepsis or AKI: no treatment | 14/38 | Beta | Observational study |
Probability discharged: MI, stroke, sepsis or AKI, no treatment | 13/14 | Beta | Observational study |
Probability alive at 1 year: discharged, MI, stroke, sepsis or AKI, no treatment | 13/13 | Beta | Observational study |
Probability discharged: no MI, stroke, sepsis or AKI, no treatment | 24/24 | Beta | Observational study |
Probability alive at 1 year: discharged, no MI, stroke, sepsis or AKI, no treatment | 24/24 | Beta | Observational study |
Current practice, ad hoc TEG negative | |||
Probability POC test positive | 1/164 | Beta | Observational study |
Probability of CCB: POC test positive | 1/1 | Beta | Observational study |
Probability of treatment: CCB | 1/1 | Beta | Observational study |
Probability of MI, stroke, sepsis or AKI: treatment | 0/1 | Beta | Observational study |
Probability discharged: no MI, stroke, sepsis or AKI | 1/1 | Beta | Observational study |
Probability alive at 1 year: discharged, no MI, stroke, sepsis or AKI | 1/1 | Beta | Observational study |
Probability of CCB: POC test negative | 88/163 | Beta | Observational study |
Probability of treatment: CCB | 81/88 | Beta | Observational study |
Probability of MI, stroke, sepsis or AKI: treatment | 54/81 | Beta | Observational study |
Probability discharged: MI, stroke, sepsis or AKI | 50/54 | Beta | Observational study |
Probability alive at 1 year: discharged | 47/50 | Beta | Observational study |
Probability discharged: no MI, stroke, sepsis or AKI | 27/27 | Beta | Observational study |
Probability alive at 1 year: discharged, no MI, stroke, sepsis or AKI | 26/27 | Beta | Observational study |
Probability of MI, stroke, sepsis or AKI: no treatment | 6/7 | Beta | Observational study |
Probability discharged: MI, stroke, sepsis or AKI, no treatment | 6/6 | Beta | Observational study |
Probability alive at 1 year: discharged, MI, stroke, sepsis or AKI, no treatment | 6/6 | Beta | Observational study |
Probability discharged: no MI, stroke, sepsis or AKI, no treatment | 1/1 | Beta | Observational study |
Probability alive at 1 year: discharged, no MI, stroke, sepsis or AKI, no treatment | 1/1 | Beta | Observational study |
Probability of treatment: no CCB | 2/75 | Beta | Observational study |
Probability of MI, stroke, sepsis or AKI: treatment | 0/2 | Beta | Observational study |
Probability discharged: no MI, stroke, sepsis or AKI | 2/2 | Beta | Observational study |
Probability alive at 1 year: discharged, no MI, stroke, sepsis or AKI | 2/2 | Beta | Observational study |
Probability of MI, stroke, sepsis or AKI: no treatment | 39/73 | Beta | Observational study |
Probability discharged: MI, stroke, sepsis or AKI, no treatment | 39/39 | Beta | Observational study |
Probability alive at 1 year: discharged, MI, stroke, sepsis or AKI, no treatment | 36/39 | Beta | Observational study |
Probability discharged: no MI, stroke, sepsis or AKI, no treatment | 34/34 | Beta | Observational study |
Probability alive at 1 year: discharged, no MI, stroke, sepsis or AKI, no treatment | 34/34 | Beta | Observational study |
Current practice, no ad hoc TEG | |||
Probability POC test positive | 39/1518 | Beta | Observational study |
Probability of CCB: POC test positive | 15/39 | Beta | Observational study |
Probability of treatment: CCB | 12/15 | Beta | Observational study |
Probability of MI, stroke, sepsis or AKI: treatment | 11/12 | Beta | Observational study |
Probability discharged: MI, stroke, sepsis or AKI | 8/11 | Beta | Observational study |
Probability alive at 1 year: discharged | 8/8 | Beta | Observational study |
Probability discharged: no MI, stroke, sepsis or AKI | 1/1 | Beta | Observational study |
Probability alive at 1 year: discharged, no MI, stroke, sepsis or AKI | 1/1 | Beta | Observational study |
Probability of MI, stroke, sepsis or AKI: no treatment | 0/3 | Beta | Observational study |
Probability discharged: no MI, stroke, sepsis or AKI, no treatment | 3/3 | Beta | Observational study |
Probability alive at 1 year: discharged, no MI, stroke, sepsis or AKI, no treatment | 3/3 | Beta | Observational study |
Probability of treatment: no CCB | 0/24 | Beta | Observational study |
Probability of MI, stroke, sepsis or AKI: no treatment | 9/24 | Beta | Observational study |
Probability discharged: MI, stroke, sepsis or AKI, no treatment | 9/9 | Beta | Observational study |
Probability alive at 1 year: discharged, MI, stroke, sepsis or AKI, no treatment | 9/9 | Beta | Observational study |
Probability discharged: no MI, stroke, sepsis or AKI, no treatment | 15/15 | Beta | Observational study |
Probability alive at 1 year: discharged, no MI, stroke, sepsis or AKI, no treatment | 14/15 | Beta | Observational study |
Probability of CCB: POC test negative | 254/1479 | Beta | Observational study |
Probability of treatment: CCB | 184/254 | Beta | Observational study |
Probability of MI, stroke, sepsis or AKI: treatment | 92/184 | Beta | Observational study |
Probability discharged: MI, stroke, sepsis or AKI | 86/92 | Beta | Observational study |
Probability alive at 1 year: discharged | 82/86 | Beta | Observational study |
Probability discharged: no MI, stroke, sepsis or AKI | 92/92 | Beta | Observational study |
Probability alive at 1 year: discharged, no MI, stroke, sepsis or AKI | 89/92 | Beta | Observational study |
Probability of MI, stroke, sepsis or AKI: no treatment | 35/70 | Beta | Observational study |
Probability discharged: MI, stroke, sepsis or AKI, no treatment | 33/35 | Beta | Observational study |
Probability alive at 1 year: discharged, MI, stroke, sepsis or AKI, no treatment | 33/33 | Beta | Observational study |
Probability discharged: no MI, stroke, sepsis or AKI, no treatment | 35/35 | Beta | Observational study |
Probability alive at 1 year: discharged, no MI, stroke, sepsis or AKI, no treatment | 35/35 | Beta | Observational study |
Probability of treatment: no CCB | 17/1225 | Beta | Observational study |
Probability of MI, stroke, sepsis or AKI: treatment | 17/17 | Beta | Observational study |
Probability discharged: MI, stroke, sepsis or AKI | 14/17 | Beta | Observational study |
Probability alive at 1 year: discharged | 11/14 | Beta | Observational study |
Probability of MI, stroke, sepsis or AKI: no treatment | 519/1208 | Beta | Observational study |
Probability discharged: MI, stroke, sepsis or AKI, no treatment | 512/519 | Beta | Observational study |
Probability alive at 1 year: discharged, MI, stroke, sepsis or AKI, no treatment | 496/512 | Beta | Observational study |
Probability discharged: no MI, stroke, sepsis or AKI, no treatment | 686/689 | Beta | Observational study |
Probability alive at 1 year: discharged, no MI, stroke, sepsis or AKI, no treatment | 682/686 | Beta | Observational study |
Test all with ROTEM | |||
Probability POC test positive | 61/1802 | Beta | Observational study |
Probability of ad hoc TEG: POC test positive | 22/61 | Beta | Observational study |
Probability ad hoc TEG positive: ad hoc TEG, POC test positive | 21/22 | Beta | Observational study |
Probability of ad hoc TEG: POC test negative | 262/1741 | Beta | Observational study |
Probability ad hoc TEG positive: ad hoc TEG, POC test negative | 99/262 | Beta | Observational study |
Probability prophylactic treatment fails | 154/449 | Beta | Observational study |
Variable | Base-case value: positive/total | Distribution for probabilistic sensitivity analysis | Source |
---|---|---|---|
Current practice | |||
Probability of ad hoc TEG | 284/1802 | Beta | Observational study |
Current practice, ad hoc TEG | |||
Probability ad hoc TEG positive | 120/284 | Beta | Observational study |
Current practice, ad hoc TEG positive | |||
Probability POC test positive | 16/120 | Beta | Observational study |
Probability of CCB: POC test positive | 12/16 | Beta | Observational study |
Probability of treatment: CCB | 11/12 | Beta | Observational study |
Probability of MI, stroke, sepsis or AKI: treatment | 7/11 | Beta | Observational study |
Probability discharged: MI, stroke, sepsis or AKI | 7/7 | Beta | Observational study |
Probability alive at 1 year: discharged | 7/7 | Beta | Observational study |
Probability discharged: no MI, stroke, sepsis or AKI | 4/4 | Beta | Observational study |
Probability alive at 1 year: discharged, no MI, stroke, sepsis or AKI | 4/4 | Beta | Observational study |
Probability of MI, stroke, sepsis or AKI: no treatment | 1/1 | Beta | Observational study |
Probability discharged: MI, stroke, sepsis or AKI, no treatment | 1/1 | Beta | Observational study |
Probability alive at 1 year: discharged, MI, stroke, sepsis or AKI, no treatment | 1/1 | Beta | Observational study |
Probability of treatment: no CCB | 0/4 | Beta | Observational study |
Probability of MI, stroke, sepsis or AKI: no treatment | 4/4 | Beta | Observational study |
Probability discharged: MI, stroke, sepsis or AKI, no treatment | 4/4 | Beta | Observational study |
Probability alive at 1 year: discharged, MI, stroke, sepsis or AKI, no treatment | 4/4 | Beta | Observational study |
Probability of CCB: POC test negative | 63/104 | Beta | Observational study |
Probability of treatment: CCB | 57/63 | Beta | Observational study |
Probability of MI, stroke, sepsis or AKI: treatment | 25/57 | Beta | Observational study |
Probability discharged: MI, stroke, sepsis or AKI | 24/25 | Beta | Observational study |
Probability alive at 1 year: discharged | 23/24 | Beta | Observational study |
Probability discharged: no MI, stroke, sepsis or AKI | 32/32 | Beta | Observational study |
Probability alive at 1 year: discharged, no MI, stroke, sepsis or AKI | 29/32 | Beta | Observational study |
Probability of MI, stroke, sepsis or AKI: no treatment | 4/6 | Beta | Observational study |
Probability discharged: MI, stroke, sepsis or AKI, no treatment | 3/4 | Beta | Observational study |
Probability alive at 1 year: discharged, MI, stroke, sepsis or AKI, no treatment | 3/3 | Beta | Observational study |
Probability discharged: no MI, stroke, sepsis or AKI, no treatment | 2/2 | Beta | Observational study |
Probability alive at 1 year: discharged, no MI, stroke, sepsis or AKI, no treatment | 2/2 | Beta | Observational study |
Probability of treatment: no CCB | 0/41 | Beta | Observational study |
Probability of MI, stroke, sepsis or AKI: no treatment | 12/41 | Beta | Observational study |
Probability discharged: MI, stroke, sepsis or AKI, no treatment | 11/12 | Beta | Observational study |
Probability alive at 1 year: discharged, MI, stroke, sepsis or AKI, no treatment | 11/11 | Beta | Observational study |
Probability discharged: no MI, stroke, sepsis or AKI, no treatment | 29/29 | Beta | Observational study |
Probability alive at 1 year: discharged, no MI, stroke, sepsis or AKI, no treatment | 29/29 | Beta | Observational study |
Current practice, ad hoc TEG negative | |||
Probability POC test positive | 2/164 | Beta | Observational study |
Probability of CCB: POC test positive | 2/2 | Beta | Observational study |
Probability of treatment: CCB | 2/2 | Beta | Observational study |
Probability of MI, stroke, sepsis or AKI: treatment | 1/2 | Beta | Observational study |
Probability discharged: MI, stroke, sepsis or AKI | 0/1 | Beta | Observational study |
Probability discharged: no MI, stroke, sepsis or AKI | 1/1 | Beta | Observational study |
Probability alive at 1 year: discharged, no MI, stroke, sepsis or AKI | 1/1 | Beta | Observational study |
Probability of CCB: POC negative | 87/162 | Beta | Observational study |
Probability of treatment: CCB | 80/87 | Beta | Observational study |
Probability of MI, stroke, sepsis or AKI: treatment | 53/80 | Beta | Observational study |
Probability discharged: MI, stroke, sepsis or AKI | 50/53 | Beta | Observational study |
Probability alive at 1 year: discharged | 47/50 | Beta | Observational study |
Probability discharged: no MI, stroke, sepsis or AKI | 27/27 | Beta | Observational study |
Probability alive at 1 year: discharged, no MI, stroke, sepsis or AKI | 26/27 | Beta | Observational study |
Probability of MI, stroke, sepsis or AKI: no treatment | 6/7 | Beta | Observational study |
Probability discharged: MI, stroke, sepsis or AKI, no treatment | 6/6 | Beta | Observational study |
Probability alive at 1 year: discharged, MI, stroke, sepsis or AKI, no treatment | 6/6 | Beta | Observational study |
Probability discharged: no MI, stroke, sepsis or AKI, no treatment | 1/1 | Beta | Observational study |
Probability alive at 1 year: discharged, no MI, stroke, sepsis or AKI, no treatment | 1/1 | Beta | Observational study |
Probability of treatment: no CCB | 2/75 | Beta | Observational study |
Probability of MI, stroke, sepsis or AKI: treatment | 0/2 | Beta | Observational study |
Probability discharged: no MI, stroke, sepsis or AKI | 2/2 | Beta | Observational study |
Probability alive at 1 year: discharged, no MI, stroke, sepsis or AKI | 2/2 | Beta | Observational study |
Probability of MI, stroke, sepsis or AKI: no treatment | 39/73 | Beta | Observational study |
Probability discharged: MI, stroke, sepsis or AKI, no treatment | 39/39 | Beta | Observational study |
Probability alive at 1 year: discharged, MI, stroke, sepsis or AKI, no treatment | 36/39 | Beta | Observational study |
Probability discharged: no MI, stroke, sepsis or AKI, no treatment | 34/34 | Beta | Observational study |
Probability alive at 1 year: discharged, no MI, stroke, sepsis or AKI, no treatment | 34/34 | Beta | Observational study |
Current practice, no ad hoc TEG | |||
Probability POC test positive | 73/1518 | Beta | Observational study |
Probability of CCB: POC test positive | 20/73 | Beta | Observational study |
Probability of treatment: CCB | 17/20 | Beta | Observational study |
Probability of MI, stroke, sepsis or AKI: treatment | 8/17 | Beta | Observational study |
Probability discharged: MI, stroke, sepsis or AKI | 8/8 | Beta | Observational study |
Probability alive at 1 year: discharged | 8/8 | Beta | Observational study |
Probability discharged: no MI, stroke, sepsis or AKI | 9/9 | Beta | Observational study |
Probability alive at 1 year: discharged, no MI, stroke, sepsis or AKI | 9/9 | Beta | Observational study |
Probability of MI, stroke, sepsis or AKI: no treatment | 1/3 | Beta | Observational study |
Probability discharged: MI, stroke, sepsis or AKI, no treatment | 1/1 | Beta | Observational study |
Probability alive at 1 year: discharged, MI, stroke, sepsis or AKI, no treatment | 1/1 | Beta | Observational study |
Probability discharged: no MI, stroke, sepsis or AKI, no treatment | 2/2 | Beta | Observational study |
Probability alive at 1 year: discharged, no MI, stroke, sepsis or AKI, no treatment | 2/2 | Beta | Observational study |
Probability of treatment: no CCB | 2/53 | Beta | Observational study |
Probability of MI, stroke, sepsis or AKI: treatment | 2/2 | Beta | Observational study |
Probability discharged: MI, stroke, sepsis or AKI | 1/2 | Beta | Observational study |
Probability alive at 1 year: discharged | 1/1 | Beta | Observational study |
Probability of MI, stroke, sepsis or AKI: no treatment | 18/51 | Beta | Observational study |
Probability discharged: MI, stroke, sepsis or AKI, no treatment | 18/18 | Beta | Observational study |
Probability alive at 1 year: discharged, MI, stroke, sepsis or AKI, no treatment | 18/18 | Beta | Observational study |
Probability discharged: no MI, stroke, sepsis or AKI, no treatment | 33/33 | Beta | Observational study |
Probability alive at 1 year: discharged, no MI, stroke, sepsis or AKI, no treatment | 33/33 | Beta | Observational study |
Probability of CCB: POC test negative | 249/1445 | Beta | Observational study |
Probability of treatment: CCB | 179/249 | Beta | Observational study |
Probability of MI, stroke, sepsis or AKI: treatment | 95/179 | Beta | Observational study |
Probability discharged: MI, stroke, sepsis or AKI | 86/95 | Beta | Observational study |
Probability alive at 1 year: discharged | 82/86 | Beta | Observational study |
Probability discharged: no MI, stroke, sepsis or AKI | 84/84 | Beta | Observational study |
Probability alive at 1 year: discharged, no MI, stroke, sepsis or AKI | 81/84 | Beta | Observational study |
Probability of MI, stroke, sepsis or AKI: no treatment | 34/70 | Beta | Observational study |
Probability discharged: MI, stroke, sepsis or AKI, no treatment | 32/34 | Beta | Observational study |
Probability alive at 1 year: discharged, MI, stroke, sepsis or AKI, no treatment | 32/32 | Beta | Observational study |
Probability discharged: no MI, stroke, sepsis or AKI, no treatment | 36/36 | Beta | Observational study |
Probability alive at 1 year: discharged, no MI, stroke, sepsis or AKI, no treatment | 36/36 | Beta | Observational study |
Probability of treatment: no CCB | 15/1196 | Beta | Observational study |
Probability of MI, stroke, sepsis or AKI: treatment | 15/15 | Beta | Observational study |
Probability discharged: MI, stroke, sepsis or AKI | 13/15 | Beta | Observational study |
Probability alive at 1 year: discharged | 10/13 | Beta | Observational study |
Probability of MI, stroke, sepsis or AKI: no treatment | 510/1181 | Beta | Observational study |
Probability discharged: MI, stroke, sepsis or AKI, no treatment | 503/510 | Beta | Observational study |
Probability alive at 1 year: discharged, MI, stroke, sepsis or AKI, no treatment | 487/503 | Beta | Observational study |
Probability discharged: no MI, stroke, sepsis or AKI, no treatment | 668/671 | Beta | Observational study |
Probability alive at 1 year: discharged, no MI, stroke, sepsis or AKI, no treatment | 663/668 | Beta | Observational study |
Test all with TEG/Multiplate test | |||
Probability POC test positive | 91/1802 | Beta | Observational study |
Probability of ad hoc TEG: POC test positive | 18/91 | Beta | Observational study |
Probability ad hoc TEG positive: ad hoc TEG, POC test positive | 16/18 | Beta | Observational study |
Probability of ad hoc TEG: POC test negative | 266/1711 | Beta | Observational study |
Probability ad hoc TEG positive: ad hoc TEG, POC test negative | 104/266 | Beta | Observational study |
Probability prophylactic treatment fails | 154/449 | Beta | Observational study |
Variable | Base-case value: positive/total | Distribution for probabilistic sensitivity analysis | Source |
---|---|---|---|
Current practice | |||
Probability of ad hoc TEG | 284/1802 | beta | Observational study |
Current practice, ad hoc TEG | |||
Probability ad hoc TEG positive | 120/284 | Beta | Observational study |
Current practice, ad hoc TEG positive | |||
Probability POC test positive | 25/120 | Beta | Observational study |
Probability of CCB: POC test positive | 16/25 | Beta | Observational study |
Probability of treatment: CCB | 15/16 | Beta | Observational study |
Probability of MI, stroke, sepsis or AKI: treatment | 9/15 | Beta | Observational study |
Probability discharged: MI, stroke, sepsis or AKI | 9/9 | Beta | Observational study |
Probability alive at 1 year: discharged | 9/9 | Beta | Observational study |
Probability discharged: no MI, stroke, sepsis or AKI | 6/6 | Beta | Observational study |
Probability alive at 1 year: discharged, no MI, stroke, sepsis or AKI | 5/6 | Beta | Observational study |
Probability of MI, stroke, sepsis or AKI: no treatment | 1/1 | Beta | Observational study |
Probability discharged: MI, stroke, sepsis or AKI, no treatment | 1/1 | Beta | Observational study |
Probability alive at 1 year: discharged, MI, stroke, sepsis or AKI, no treatment | 1/1 | Beta | Observational study |
Probability of treatment: no CCB | 0/9 | Beta | Observational study |
Probability of MI, stroke, sepsis or AKI: no treatment | 4/9 | Beta | Observational study |
Probability discharged: MI, stroke, sepsis or AKI, no treatment | 4/4 | Beta | Observational study |
Probability alive at 1 year: discharged, MI, stroke, sepsis or AKI, no treatment | 4/4 | Beta | Observational study |
Probability discharged: no MI, stroke, sepsis or AKI, no treatment | 5/5 | Beta | Observational study |
Probability alive at 1 year: discharged, no MI, stroke, sepsis or AKI, no treatment | 5/5 | Beta | Observational study |
Probability of CCB: POC test negative | 59/95 | Beta | Observational study |
Probability of treatment: CCB | 53/59 | Beta | Observational study |
Probability of MI, stroke, sepsis or AKI: treatment | 23/53 | Beta | Observational study |
Probability discharged: MI, stroke, sepsis or AKI | 22/23 | Beta | Observational study |
Probability alive at 1 year: discharged | 21/22 | Beta | Observational study |
Probability discharged: no MI, stroke, sepsis or AKI | 30/30 | Beta | Observational study |
Probability alive at 1 year: discharged, no MI, stroke, sepsis or AKI | 28/30 | Beta | Observational study |
Probability of MI, stroke, sepsis or AKI: no treatment | 4/6 | Beta | Observational study |
Probability discharged: MI, stroke, sepsis or AKI, no treatment | 3/4 | Beta | Observational study |
Probability alive at 1 year: discharged, MI, stroke, sepsis or AKI, no treatment | 3/3 | Beta | Observational study |
Probability discharged: no MI, stroke, sepsis or AKI, no treatment | 2/2 | Beta | Observational study |
Probability alive at 1 year: discharged, no MI, stroke, sepsis or AKI, no treatment | 2/2 | Beta | Observational study |
Probability of treatment: no CCB | 0/36 | Beta | Observational study |
Probability of MI, stroke, sepsis or AKI: no treatment | 12/36 | Beta | Observational study |
Probability discharged: MI, stroke, sepsis or AKI, no treatment | 11/12 | Beta | Observational study |
Probability alive at 1 year: discharged, MI, stroke, sepsis or AKI, no treatment | 11/11 | Beta | Observational study |
Probability discharged: no MI, stroke, sepsis or AKI, no treatment | 24/24 | Beta | Observational study |
Probability alive at 1 year: discharged, no MI, stroke, sepsis or AKI, no treatment | 24/24 | Beta | Observational study |
Current practice, ad hoc TEG negative | |||
Probability POC test positive | 3/164 | Beta | Observational study |
Probability of CCB: POC test positive | 3/3 | Beta | Observational study |
Probability of treatment: CCB | 3/3 | Beta | Observational study |
Probability of MI, stroke, sepsis or AKI: treatment | 1/3 | Beta | Observational study |
Probability discharged: MI, stroke, sepsis or AKI | 0/1 | Beta | Observational study |
Probability discharged: no MI, stroke, sepsis or AKI | 2/2 | Beta | Observational study |
Probability alive at 1 year: discharged, no MI, stroke, sepsis or AKI | 2/2 | Beta | Observational study |
Probability of CCB: POC negative | 86/161 | Beta | Observational study |
Probability of treatment: CCB | 79/86 | Beta | Observational study |
Probability of MI, stroke, sepsis or AKI: treatment | 53/79 | Beta | Observational study |
Probability discharged: MI, stroke, sepsis or AKI | 50/53 | Beta | Observational study |
Probability alive at 1 year: discharged | 47/50 | Beta | Observational study |
Probability discharged: no MI, stroke, sepsis or AKI | 26/26 | Beta | Observational study |
Probability alive at 1 year: discharged, no MI, stroke, sepsis or AKI | 25/26 | Beta | Observational study |
Probability of MI, stroke, sepsis or AKI: no treatment | 6/7 | Beta | Observational study |
Probability discharged: MI, stroke, sepsis or AKI, no treatment | 6/6 | Beta | Observational study |
Probability alive at 1 year: discharged, MI, stroke, sepsis or AKI, no treatment | 6/6 | Beta | Observational study |
Probability discharged: no MI, stroke, sepsis or AKI, no treatment | 1/1 | Beta | Observational study |
Probability alive at 1 year: discharged, no MI, stroke, sepsis or AKI, no treatment | 1/1 | Beta | Observational study |
Probability of treatment: no CCB | 2/75 | Beta | Observational study |
Probability of MI, stroke, sepsis or AKI: treatment | 0/2 | Beta | Observational study |
Probability discharged: no MI, stroke, sepsis or AKI | 2/2 | Beta | Observational study |
Probability alive at 1 year: discharged, no MI, stroke, sepsis or AKI | 2/2 | Beta | Observational study |
Probability of MI, stroke, sepsis or AKI: no treatment | 39/73 | Beta | Observational study |
Probability discharged: MI, stroke, sepsis or AKI, no treatment | 39/39 | Beta | Observational study |
Probability alive at 1 year: discharged, MI, stroke, sepsis or AKI, no treatment | 36/39 | Beta | Observational study |
Probability discharged: no MI, stroke, sepsis or AKI, no treatment | 34/34 | Beta | Observational study |
Probability alive at 1 year: discharged, no MI, stroke, sepsis or AKI, no treatment | 34/34 | Beta | Observational study |
Current practice, no ad hoc TEG | |||
Probability POC test positive | 61/1518 | Beta | Observational study |
Probability of CCB: POC test positive | 20/61 | Beta | Observational study |
Probability of treatment: CCB | 16/20 | Beta | Observational study |
Probability of MI, stroke, sepsis or AKI: treatment | 15/16 | Beta | Observational study |
Probability discharged: MI, stroke, sepsis or AKI | 12/15 | Beta | Observational study |
Probability alive at 1 year: discharged | 12/12 | Beta | Observational study |
Probability discharged: no MI, stroke, sepsis or AKI | 1/1 | Beta | Observational study |
Probability alive at 1 year: discharged, no MI, stroke, sepsis or AKI | 1/1 | Beta | Observational study |
Probability of MI, stroke, sepsis or AKI: no treatment | 0/4 | Beta | Observational study |
Probability discharged: no MI, stroke, sepsis or AKI, no treatment | 4/4 | Beta | Observational study |
Probability alive at 1 year: discharged, no MI, stroke, sepsis or AKI, no treatment | 4/4 | Beta | Observational study |
Probability of treatment: no CCB | 1/41 | Beta | Observational study |
Probability of MI, stroke, sepsis or AKI: treatment | 1/1 | Beta | Observational study |
Probability discharged: MI, stroke, sepsis or AKI | 0/1 | Beta | Observational study |
Probability of MI, stroke, sepsis or AKI: no treatment | 14/40 | Beta | Observational study |
Probability discharged: MI, stroke, sepsis or AKI, no treatment | 14/14 | Beta | Observational study |
Probability alive at 1 year: discharged, MI, stroke, sepsis or AKI, no treatment | 14/14 | Beta | Observational study |
Probability discharged: no MI, stroke, sepsis or AKI, no treatment | 26/26 | Beta | Observational study |
Probability alive at 1 year: discharged, no MI, stroke, sepsis or AKI, no treatment | 25/26 | Beta | Observational study |
Probability of CCB: POC test negative | 249/1457 | Beta | Observational study |
Probability of treatment: CCB | 180/249 | Beta | Observational study |
Probability of MI, stroke, sepsis or AKI: treatment | 88/180 | Beta | Observational study |
Probability discharged: MI, stroke, sepsis or AKI | 82/88 | Beta | Observational study |
Probability alive at 1 year: discharged | 78/82 | Beta | Observational study |
Probability discharged: no MI, stroke, sepsis or AKI | 92/92 | Beta | Observational study |
Probability alive at 1 year: discharged, no MI, stroke, sepsis or AKI | 89/92 | Beta | Observational study |
Probability of MI, stroke, sepsis or AKI: no treatment | 35/69 | Beta | Observational study |
Probability discharged: MI, stroke, sepsis or AKI, no treatment | 33/35 | Beta | Observational study |
Probability alive at 1 year: discharged, MI, stroke, sepsis or AKI, no treatment | 33/33 | Beta | Observational study |
Probability discharged: no MI, stroke, sepsis or AKI, no treatment | 34/34 | Beta | Observational study |
Probability alive at 1 year: discharged, no MI, stroke, sepsis or AKI, no treatment | 34/34 | Beta | Observational study |
Probability of treatment: no CCB | 16/1208 | Beta | Observational study |
Probability of MI, stroke, sepsis or AKI: treatment | 16/16 | Beta | Observational study |
Probability discharged: MI, stroke, sepsis or AKI | 14/16 | Beta | Observational study |
Probability alive at 1 year: discharged | 11/14 | Beta | Observational study |
Probability of MI, stroke, sepsis or AKI: no treatment | 514/1192 | Beta | Observational study |
Probability discharged: MI, stroke, sepsis or AKI, no treatment | 507/514 | Beta | Observational study |
Probability alive at 1 year: discharged, MI, stroke, sepsis or AKI, no treatment | 491/507 | Beta | Observational study |
Probability discharged: no MI, stroke, sepsis or AKI, no treatment | 675/678 | Beta | Observational study |
Probability alive at 1 year: discharged, no MI, stroke, sepsis or AKI, no treatment | 671/675 | Beta | Observational study |
Test all with ROTEM/Multiplate test | |||
Probability POC test positive | 89/1802 | Beta | Observational study |
Probability of ad hoc TEG: POC test positive | 28/89 | Beta | Observational study |
Probability ad hoc TEG positive: ad hoc TEG, POC test positive | 25/28 | Beta | Observational study |
Probability of ad hoc TEG: POC test negative | 256/1713 | Beta | Observational study |
Probability ad hoc TEG positive: ad hoc TEG, POC test negative | 95/256 | Beta | Observational study |
Probability prophylactic treatment fails | 154/449 | Beta | Observational study |
Treatment | Complications | Died before discharge | n | Groups | Cost (£), mean (SE) | Distribution for probabilistic sensitivity analysis | Source |
---|---|---|---|---|---|---|---|
No | No | No | 805 | ||||
776 | 3, 7, 8, 9, 10, 11, 12 | 4047 (72) | Gamma | Observational study | |||
29 | 4 | 4858 (654) | Gamma | Observational study | |||
No | No | Yes | 3 | All (12) | 3362 (1348) | Gamma | Observational study |
No | Yes | No | 618 | ||||
579 | 1, 2, 3, 4, 7, 9, 10, 11, 12 | 5859 (169) | Gamma | Observational study | |||
39 | 8 | 8548 (1020) | Gamma | Observational study | |||
No | Yes | Yes | 11 | All (3, 4, 11, 12) | 6818 (1698) | Gamma | Observational study |
Yes | No | No | 159 | ||||
93 | 9, 11 | 5054 (262) | Gamma | Observational study | |||
66 | 1, 3, 7, 8 | 7915 (552) | Gamma | Observational study | |||
Yes | No | Yes | 0 | ||||
Yes | Yes | No | 189 | ||||
175 | 1, 3, 7, 9, 11 | 10,564 (607) | Gamma | Observational study | |||
14 | 10, 12 | 26,747 (4095) | Gamma | Observational study | |||
Yes | Yes | Yes | 17 | All (3, 7, 11, 12) | 22,038 (3758) | Gamma | Observational study |
Treatment | Complications | Died before discharge | n | Groups | Cost (£), mean (SE) | Distribution for probabilistic sensitivity analysis | Source |
---|---|---|---|---|---|---|---|
No | No | No | 805 | ||||
781 | 2, 3, 7, 8, 9, 10, 11, 12 | 4048 (71) | Gamma | Observational study | |||
24 | 4 | 5003 (779) | Gamma | Observational study | |||
No | No | Yes | 3 | All (12) | 3362 (1348) | Gamma | Observational study |
No | Yes | No | 618 | ||||
579 | 1, 2, 3, 4, 7, 10, 11, 12 | 5859 (169) | Gamma | Observational study | |||
39 | 8 | 8548 (1020) | Gamma | Observational study | |||
No | Yes | Yes | 11 | All (3, 4, 11, 12) | 6818 (1698) | Gamma | Observational study |
Yes | No | No | 159 | ||||
93 | 9, 11 | 5054 (262) | Gamma | Observational study | |||
66 | 1, 3, 5, 7, 8 | 7915 (552) | Gamma | Observational study | |||
Yes | No | Yes | 0 | ||||
Yes | Yes | No | 189 | ||||
152 | 1, 7, 9, 11 | 9863 (592) | Gamma | Observational study | |||
23 | 3 | 15,202 (2277) | Gamma | Observational study | |||
14 | 12 | 26,747 (4095) | Gamma | Observational study | |||
Yes | Yes | Yes | 17 | ||||
11 | 3, 7, 9, 12 | 17,912 (3265) | Gamma | Observational study | |||
6 | 11 | 29,601 (8444) | Gamma | Observational study |
Treatment | Complications | Died before discharge | n | Groups | Cost (£), mean (SE) | Distribution for probabilistic sensitivity analysis | Source |
---|---|---|---|---|---|---|---|
No | No | No | 805 | ||||
776 | 3, 7, 8, 9, 10, 11, 12 | 4047 (72) | Gamma | Observational study | |||
29 | 4 | 4858 (654) | Gamma | Observational study | |||
No | No | Yes | 3 | All (12) | 3362 (1348) | Gamma | Observational study |
No | Yes | No | 618 | ||||
579 | 1, 2, 3, 4, 7, 9, 10, 11, 12 | 5859 (169) | Gamma | Observational study | |||
39 | 8 | 8548 (1020) | Gamma | Observational study | |||
No | Yes | Yes | 11 | All (3, 4, 11, 12) | 6818 (1698) | Gamma | Observational study |
Yes | No | No | 159 | ||||
93 | 9, 11 | 5054 (262) | Gamma | Observational study | |||
59 | 3, 7 | 7416 (544) | Gamma | Observational study | |||
7 | 1, 5, 8 | 12,119 (1911) | Gamma | Observational study | |||
Yes | No | Yes | 0 | ||||
Yes | Yes | No | 189 | ||||
175 | 1, 3, 7, 9, 11 | 10,564 (607) | Gamma | Observational study | |||
14 | 10, 12 | 26,747 (4095) | Gamma | Observational study | |||
Yes | Yes | Yes | 17 | All (3, 5, 7, 10, 11, 12) | 22,038 (3758) | Gamma | Observational study |
Treatment | Complications | Died before discharge | n | Groups | Cost (£), mean (SE) | Distribution for probabilistic sensitivity analysis | Source |
---|---|---|---|---|---|---|---|
No | No | No | 805 | ||||
781 | 2, 3, 7, 8, 9, 10, 11, 12 | 4048 (71) | Gamma | Observational study | |||
24 | 4 | 5003 (779) | Gamma | Observational study | |||
No | No | Yes | 3 | All (12) | 3362 (1348) | Gamma | Observational study |
No | Yes | No | 618 | ||||
579 | 1, 2, 3, 4, 7, 10, 11, 12 | 5859 (169) | Gamma | Observational study | |||
39 | 8 | 8548 (1020) | Gamma | Observational study | |||
No | Yes | Yes | 11 | All (3, 4, 11, 12) | 6818 (1698) | Gamma | Observational study |
Yes | No | No | 159 | ||||
93 | 9, 11 | 5054 (262) | Gamma | Observational study | |||
56 | 3, 7 | 7156 (527) | Gamma | Observational study | |||
10 | 1, 5, 8 | 12,166 (1626) | Gamma | Observational study | |||
Yes | No | Yes | 0 | ||||
Yes | Yes | No | 189 | ||||
153 | 1, 7, 9, 11 | 9885 (588) | Gamma | Observational study | |||
22 | 3 | 15,291 (2382) | Gamma | Observational study | |||
14 | 12 | 26,747 (4095) | Gamma | Observational study | |||
Yes | Yes | Yes | 17 | All (3, 5, 7, 9, 10, 11, 12) | 22,038 (3758) | Gamma | Observational study |
Outcome | Cost (£) |
---|---|
Health-care costs after hospital discharge to 1 year | |
For all participants alive at 1 year | 2116 |
For all participants who died between hospital discharge and 1 year | 922 |
Additional cost for those with in-hospital complications and alive at 1 year | 92 |
Additional cost for those with in-hospital complications and died by 1 year | 35 |
Health-care costs after 1 year | |
Annual health-care cost per participant | 2173 |
Additional annual cost for participants with in-hospital complications | 99 |
Test | Cost (£) | Distribution for probabilistic sensitivity analysis | Source |
---|---|---|---|
Ad hoc TEG | 64 | Capital and consumable costs for TEG and ROTEM came from a published source;23 costs for Multiplate were provided by Roche | |
TEG | 37 | ||
ROTEM | 32 | ||
TEG/Multiplate test | 48 | ||
ROTEM/Multiplate test | 43 | ||
Cost (£) of prophylactic treatment if POC test positive, mean (SE) | |||
TEG | 135 (14) | Gamma | Observational study |
ROTEM | 309 (19) | Gamma | Observational study |
TEG/Multiplate test | 96 (8) | Gamma | Observational study |
ROTEM/Multiplate test | 277 (14) | Gamma | Observational study |
Life-years for the base-case models
All participants alive at 1 year (known in the observational study) accrue 1 life-year. For participants who die, Table 120 summarises the life-years used in the base-case models. Although participants may be in different groups in each of the models, in each case all groups could be combined for each combination of treatment/complication and timing of death and therefore the estimates used in each of the models are the same.
Treatment | Complications | Died before discharge | Died at 1 year | n | Groups | Life-years, mean (SE) | Distribution for probabilistic sensitivity analysis | Source |
---|---|---|---|---|---|---|---|---|
No | No | Yes | – | 3 | All | 0.016 (0.007) | Beta | Observational study |
No | Yes | Yes | – | 11 | All | 0.013 (0.005) | Beta | Observational study |
Yes | No | Yes | – | 0 | – | – | – | |
Yes | Yes | Yes | – | 17 | All | 0.060 (0.014) | Beta | Observational study |
No | No | No | Yes | 5 | All | 0.516 (0.172) | Beta | Observational study |
No | Yes | No | Yes | 19 | All | 0.382 (0.060) | Beta | Observational study |
Yes | No | No | Yes | 7 | All | 0.658 (0.082) | Beta | Observational study |
Yes | Yes | No | Yes | 11 | All | 0.488 (0.093) | Beta | Observational study |
Resource | Unit costa (£) | Source |
---|---|---|
Blood products | ||
Red blood cells | 122.09 | NHS Blood and Transplant224 |
Red blood cell administration cost, first unit | 22 | Primary data collection of the nursing time and consumables associated with requesting blood and administering transfusions, undertaken with collaborators on the Trial Of Prophylactic versus no prophylactic platelet transfusions (TOPPS) trial,281 funded by NHS Blood and Transplant. Preliminary analyses show that it takes 49 minutes of nursing time and £6 of consumables to request and administer the first unit of red cells |
Red blood cell administration cost, subsequent units | 5 | As above; analyses found that it took 15 minutes of nursing time to administer subsequent units (no additional consumables) |
FFP | 27.98 | NHS Blood and Transplant224 |
Platelets | 208.09 | NHS Blood and Transplant224 |
Cryoprecipitate | 193.53 | NHS Blood and Transplant224 |
Inpatient stay | ||
CICU day | 1203 | NHS reference costs 2012/13:225 Critical Care Services – Adult: Critical Care Unit (weighted average of XC01Z–XC06Z, one to six organs supported) |
HDU day | 626 | NHS reference costs 2012/13:225 Critical Care Services – Adult: Critical Care Unit (XC07Z, no organs supported) |
Cardiac ward day | 396 | NHS reference costs 2012/13:225 weighted average of elective inpatient excess bed-day costs for relevant HRGs (EA14, EA16, EA17, EA19, EA20, EA22, EA51, EA52, EC01, EC02 and EC03, excluding any service codes for paediatrics) |
Additional treatments | ||
Protamine (50 mg) | 2.38 | eMIT228 |
Activated factor VII (5 mg intravenously) | 2514.30 | Oxford University Hospitals NHS Foundation Trust, 2013 |
Reoperation | 8390 | NHS reference costs 2012/13:225 elective inpatients – HRG code EA20 for service codes 170 (cardiothoracic surgery) and 172 (cardiac surgery). For each code the costs associated with the average LOS reported were subtracted at a cost of £392 per day (cardiac ward day cost before inflation to 2013/14 prices) and £1421 was subtracted for blood products based on data from an audit of blood transfusion in cardiac surgery.9 An average cost for the codes was then generated, weighted by activity |
IABP | 2807 | NICE282 |
Complication | Treatment/action assumed | Costa (£) | Reference |
---|---|---|---|
Sepsis | Antibiotics [piperacillin/tazobactam (Tazocin®, Pfizer Ltd, Tadworth, UK), 4.5 g intravenously three times a day for 5 days] | 28.72 | eMIT228 |
Permanent stroke | Rehabilitation | 141 | NHS reference costs 2012/13:225 Elective Inpatients. DZ30Z – Chest Physiotherapy, code 340 (respiratory medicine) |
Computerised tomography scan | 63 | NHS reference costs 2012/13:225 Diagnostic Imaging – Direct Access. RA08A – Computerised Tomography Scan, one area, no contrast, 19 years and over, code 100 (general surgery) | |
Total | 204 | ||
Suspected MI | Emergency angiogram | 1713 | NHS reference costs 2012/13225 |
Transthoracic echocardiogram | 122 | NHS reference costs 2012/13:225 Diagnostic Imaging – Outpatients. RA60A – Simple Echocardiogram, 19 years and over, code 172 (cardiac surgery) | |
Electrocardiogram | 54 | NHS reference costs 2012/13:225 Directly Accessed Diagnostic Services. EA47Z – Electrocardiogram Monitoring and Stress Testing | |
Total | 1889 | ||
AKI, stage 3 only | Haemofiltration (treatment for 2 days) | 1454 | NHS reference costs 2012/13:225 Renal Dialysis at Base. LE01A – Haemodialysis for Acute Kidney Injury, 19 years and over |
Standards for Reporting Diagnostic Accuracy (STARD) checklist: COPTIC study
Section/topic | Number | Item | Reported on page number |
---|---|---|---|
Title or abstract | |||
1 | Identification as a study of diagnostic accuracy using at least one measure of accuracy (such as sensitivity, specificity, predictive values or AUCs) | 9 | |
Abstract | |||
2 | Structured summary of study design, methods, results and conclusions (for specific guidance see STARD for Abstracts) | 9 | |
Introduction | |||
3 | Scientific and clinical background, including the intended use and clinical role of the index test | 9 | |
4 | Study objectives and hypotheses | 27 | |
Methods | |||
Study design | 5 | Whether data collection was planned before the index test and reference standard were performed (prospective study) or after (retrospective study) | 28 |
Participants | 6 | Eligibility criteria | 29 |
7 | On what basis potentially eligible participants were identified (such as symptoms, results from previous tests, inclusion in registry) | 29 | |
8 | Where and when potentially eligible participants were identified (setting, location and dates) | 29 | |
9 | Whether participants formed a consecutive, random or convenience series | 29 | |
Test methods | 10a | Index test described in sufficient detail to allow replication | 32 |
10b | Reference standard described in sufficient detail to allow replication | 30 | |
11 | Rationale for choosing the reference standard (if alternatives exist) | 30 | |
12a | Definition of and rationale for test positivity cut-off values or result categories of the index test, distinguishing prespecified from exploratory | 32 | |
12b | Definition of and rationale for test positivity cut-off values or result categories of the reference standard, distinguishing prespecified from exploratory | 30 | |
13a | Whether clinical information and reference standard results were available to the performers/readers of the index test | 37 | |
13b | Whether clinical information and index test results were available to the assessors of the reference standard | 37 | |
Analysis | 14 | Methods for estimating or comparing measures of diagnostic accuracy | 38 |
15 | How indeterminate index test or reference standard results were handled | 38 | |
16 | How missing data on the index test and reference standard were handled | 38 | |
17 | Any analyses of variability in diagnostic accuracy, distinguishing prespecified from exploratory | 38 | |
18 | Intended sample size and how it was determined | 38 | |
Results | |||
Participants | 19 | Flow of participants, using a diagram | 42 |
20 | Baseline demographic and clinical characteristics of participants | 47 | |
21a | Distribution of severity of disease in those with the target condition | 50 | |
21b | Distribution of alternative diagnoses in those without the target condition | NA | |
22 | Time interval and any clinical interventions between the index test and the reference standard | 28 | |
Test results | 23 | Cross-tabulation of the index test results (or their distribution) by the results of the reference standard | 53 |
24 | Estimates of diagnostic accuracy and their precision (such as 95% confidence intervals) | 54 | |
25 | Any adverse events from performing the index test or the reference standard | NA | |
Discussion | |||
26 | Study limitations, including sources of potential bias, statistical uncertainty and generalisability | 123 | |
27 | Implications for practice, including the intended use and clinical role of the index test | 129 | |
Other information | |||
28 | Registration number and name of registry | 29 | |
29 | Where the full study protocol can be accessed | 29 | |
30 | Sources of funding and other support, role of funders | 289 |
Preferred Reporting Items for Systematic Reviews and Meta-Analyses (PRISMA) checklist: viscoelastic tests systematic review
Section/topic | Number | Item | Reported on page number |
---|---|---|---|
Title | |||
Title | 1 | Identify the report as a systematic review, meta-analysis or both | 9 |
Abstract | |||
Structured summary | 2 | Provide a structured summary including, as applicable, background; objectives; data sources; study eligibility criteria, participants and interventions; study appraisal and synthesis methods; results; limitations; conclusions and implications of key findings; systematic review registration number | 9 |
Introduction | |||
Rationale | 3 | Describe the rationale for the review in the context of what is already known | 9 |
Objectives | 4 | Provide an explicit statement of questions being addressed with reference to participants, interventions, comparisons, outcomes and study design (PICOS) | 105 |
Methods | |||
Protocol and registration | 5 | Indicate if a review protocol exists and if and where it can be accessed (e.g. web address) and, if available, provide registration information including registration number | 106 |
Eligibility criteria | 6 | Specify study characteristics (e.g. PICOS, length of follow-up) and report characteristics (e.g. years considered, language, publication status) used as criteria for eligibility, giving rationale | 106 |
Information sources | 7 | Describe all information sources (e.g. databases with dates of coverage, contact with study authors to identify additional studies) in the search and date last searched | 106 |
Search | 8 | Present full electronic search strategy for at least one database, including any limits used, such that it could be repeated | 106 |
Study selection | 9 | State the process for selecting studies (i.e. screening, eligibility, included in systematic review and, if applicable, included in the meta-analysis) | 107 |
Data collection process | 10 | Describe method of data extraction from reports (e.g. piloted forms, independently, in duplicate) and any processes for obtaining and confirming data from investigators | 107 |
Data items | 11 | List and define all variables for which data were sought (e.g. PICOS, funding sources) and any assumptions and simplifications made | 107 |
Risk of bias in individual studies | 12 | Describe methods used for assessing risk of bias of individual studies (including specification of whether this was carried out at the study or outcome level) and how this information is to be used in any data synthesis | 106 |
Summary measures | 13 | State the principal summary measures (e.g. risk ratio, difference in means) | 107 |
Synthesis of results | 14 | Describe the methods of handling data and combining results of studies, if done, including measures of consistency (e.g. I2) for each meta-analysis | 108 |
Risk of bias across studies | 15 | Specify any assessment of risk of bias that may affect the cumulative evidence (e.g. publication bias, selective reporting within studies) | 107 |
Additional analyses | 16 | Describe methods of additional analyses (e.g. sensitivity or subgroup analyses, meta-regression), if done, indicating which were prespecified | 109 |
Results | |||
Study selection | 17 | Give numbers of studies screened, assessed for eligibility and included in the review, with reasons for exclusions at each stage, ideally with a flow diagram | 108 |
Study characteristics | 18 | For each study, present characteristics for which data were extracted (e.g. study size, PICOS, follow-up period) and provide the citations | 108 |
Risk of bias within studies | 19 | Present data on the risk of bias of each study and, if available, any outcome-level assessment (see Item 12) | 109 |
Results of individual studies | 20 | For all outcomes considered (benefits or harms), present, for each study: (a) simple summary data for each intervention group and (b) effect estimates and confidence intervals, ideally with a forest plot | 111 |
Synthesis of results | 21 | Present results of each meta-analysis done, including confidence intervals and measures of consistency | 111 |
Risk of bias across studies | 22 | Present results of any assessment of risk of bias across studies (see Item 15) | 122 |
Additional analysis | 23 | Give results of additional analyses, if done (e.g. sensitivity or subgroup analyses, meta-regression (see Item 16) | 122 |
Discussion | |||
Summary of evidence | 24 | Summarise the main findings including the strength of evidence for each main outcome; consider their relevance to key groups (e.g. health-care providers, users and policy-makers) | 123 |
Limitations | 25 | Discuss limitations at the study and outcome level (e.g. risk of bias) and at the review level (e.g. incomplete retrieval of identified research, reporting bias) | 128 |
Conclusions | 26 | Provide a general interpretation of the results in the context of other evidence and implications for future research | 129 |
Funding | |||
Funding | 27 | Describe sources of funding for the systematic review and other support (e.g. supply of data); role of funders in the systematic review | 289 |
Consolidated Health Economic Evaluation Reporting Standards (CHEERS) checklist: COPTIC study
Section/item | Number | Recommendation | Reported on page number |
---|---|---|---|
Title and abstract | |||
Title | 1 | Identify the study as an economic evaluation or use more specific terms such as ‘cost-effectiveness analysis’ and describe the interventions compared | 9 |
Abstract | 2 | Provide a structured summary of the objectives, perspective, setting, methods (including study design and inputs), results (including base-case and uncertainty analyses) and conclusions | 9 |
Introduction | |||
Background and objectives | 3 | Provide an explicit statement of the broader context for the study | 9 |
Present the study question and its relevance for health policy or practice decisions | 76 | ||
Methods | |||
Target population and subgroups | 4 | Describe the characteristics of the base-case population and subgroups analysed, including why they were chosen | 76 |
Setting and location | 5 | State relevant aspects of the system(s) in which the decision(s) need(s) to be made | 76 |
Study perspective | 6 | Describe the perspective of the study and relate this to the costs being evaluated | 76 |
Comparators | 7 | Describe the interventions or strategies being compared and state why they were chosen | 76 |
Time horizon | 8 | State the time horizon(s) over which costs and consequences are being evaluated and say why they are appropriate | 80 |
Discount rate | 9 | Report the choice of discount rate(s) used for costs and outcomes and say why they are appropriate | 88 |
Choice of health outcomes | 10 | Describe what outcomes were used as the measure(s) of benefit in the evaluation and their relevance for the type of analysis performed | 82 |
Measurement of effectiveness | 11a | Single study-based estimates: Describe fully the design features of the single effectiveness study and why the single study was a sufficient source of clinical effectiveness data | 82 |
11b | Synthesis-based estimates: Describe fully the methods used for identification of included studies and synthesis of clinical effectiveness data | NA | |
Measurement and valuation of preference-based outcomes | 12 | If applicable, describe the population and methods used to elicit preferences for outcomes | NA |
Estimating resources and costs | 13a | Single study-based economic evaluation: Describe approaches used to estimate resource use associated with the alternative interventions. Describe primary or secondary research methods for valuing each resource item in terms of its unit cost. Describe any adjustments made to approximate to opportunity costs | 88 |
13b | Model-based economic evaluation: Describe approaches and data sources used to estimate resource use associated with model health states. Describe primary or secondary research methods for valuing each resource item in terms of its unit cost. Describe any adjustments made to approximate to opportunity costs | NA | |
Currency, price date and conversion | 14 | Report the dates of the estimated resource quantities and unit costs. Describe methods for adjusting estimated unit costs to the year of reported costs if necessary. Describe methods for converting costs into a common currency base and the exchange rate | 82 |
Choice of model | 15 | Describe and give reasons for the specific type of decision-analytical model used. Providing a figure to show the model structure is strongly recommended | 77 |
Assumptions | 16 | Describe all structural or other assumptions underpinning the decision-analytical model | 77 |
Analytical methods | 17 | Describe all analytical methods supporting the evaluation. This could include methods for dealing with skewed, missing or censored data; extrapolation methods; methods for pooling data; approaches to validate or make adjustments (such as half-cycle corrections) to a model; and methods for handling population heterogeneity and uncertainty | 77 |
Results | |||
Study parameters | 18 | Report the values, ranges, references and, if used, probability distributions for all parameters. Report reasons or sources for distributions used to represent uncertainty where appropriate. Providing a table to show the input values is strongly recommended | 323 |
Incremental costs and outcomes | 19 | For each intervention, report mean values for the main categories of estimated costs and outcomes of interest, as well as mean differences between the comparator groups. If applicable, report incremental cost-effectiveness ratios | 96 |
Characterising uncertainty | 20a | Single study-based economic evaluation: Describe the effects of sampling uncertainty for the estimated incremental cost and incremental effectiveness parameters, together with the impact of methodological assumptions (such as discount rate, study perspective) | 97 |
20b | Model-based economic evaluation: Describe the effects on the results of uncertainty for all input parameters and uncertainty related to the structure of the model and assumptions | NA | |
Characterising heterogeneity | 21 | If applicable, report differences in costs, outcomes or cost-effectiveness that can be explained by variations between subgroups of participants with different baseline characteristics or other observed variability in effects that are not reducible by more information | 98 |
Discussion | |||
Study findings, limitations, generalisability and current knowledge | 22 | Summarise key study findings and describe how they support the conclusions reached. Discuss limitations and the generalisability of the findings and how the findings fit with current knowledge | 123 |
Other | |||
Source of funding | 23 | Describe how the study was funded and the role of the funder in the identification, design, conduct and reporting of the analysis. Describe other non-monetary sources of support | 289 |
Conflicts of interest | 24 | Describe any potential for conflict of interest of study contributors in accordance with journal policy. In the absence of a journal policy, we recommend authors comply with International Committee of Medical Journal Editors recommendations | i |
Appendix 2 Transfusion risk scores
Amendments to the protocol after commencement of the study
Amendment number: not applicable.
Previous version: 1.0.
Previous date: 21 June 2011
New version: 2.0.
New date: 29 February 2012.
Brief summary of change: Change in collaborator and data source (withdrawal of Toronto and addition of Wolverhampton).
Date of ethical approval (or ‘not applicable’ if non-substantial): not applicable.
Supplemental information
Unit | Database manager |
---|---|
NHS | |
Aberdeen Royal Infirmary | Mrs L Lawman |
Barts and The London | Ms E Kuforiji, Mr A Wood |
Blackpool Victoria Hospital | Ms C Malpas |
Bristol Royal Infirmary | Mr C Gummer, Mr D Finch |
Castle Hill Hospital, Hull | Hull Mr N Richards |
Cork University Hospital | Ms M Delaney |
Derriford Hospital, Plymouth | Mr R Gardner |
Edinburgh Royal Infirmary | Ms J Howlett |
Essex Cardiothoracic Centre, Basildon | Mr D McCormack |
Freeman Hospital, Newcastle | Ms S Jamieson |
Glenfield Hospital, Leicester | Mr P Stafford |
Golden Jubilee National Hospital, Glasgow | Ms A Cunningham, Ms M Kinnaird |
Guy’s and St Thomas’ Hospital, London | Ms G Tinto |
Hammersmith Hospital, London | Ms S Krikler |
Harefield Hospital, London | Mr P Kimberley |
James Cook University Hospital, Middlesbrough | Mrs T Smailes |
John Radcliffe Hospital, Oxford | Mr C Evans |
King’s College Hospital, London | Mr J Omigie |
Leeds General Infirmary | Mr R Higginson, Mr J Peckover |
Liverpool Heart and Chest Hospital | Ms L Bond, Ms L Jones, Mr A Ward |
Manchester Heart Centre | Ms S Chaisty |
Mater Misericordiae University Hospital, Dublin | Ms G Aherne, Mr P Marsden |
Morriston Hospital, Swansea | Mr S Morris |
North Staffordshire Royal Infirmary, Stoke-on-Trent | Mr A Lea |
New Cross Hospital, Wolverhampton | Ms J Sear |
Northern General Hospital, Sheffield | Ms J Daly, Ms L Kirk |
Nottingham City Hospital | Ms S Hammond |
Papworth Hospital, Cambridge | Ms S Powell |
Queen Elizabeth Hospital, Birmingham | Ms V Barnett |
Royal Brompton Hospital, London | Mr P Kimberley |
Royal Sussex County Hospital, Brighton | Mrs J Gayfer |
Royal Victoria Hospital, Belfast | Ms B Scullion |
Southampton General Hospital | Ms A Green |
St George’s Hospital, London | Dr O Valencia |
St James’s Hospital, Dublin | Ms M Houlihan |
St Mary’s Hospital, London | Ms J Turnbull |
The Heart Hospital, London | Mr M Curtis, Ms M Taylor |
University Hospital of Wales, Cardiff | Ms D Evans, Ms C Bray |
Walsgrave Hospital, Coventry | Ms A Nugent |
Wythenshawe Hospital, Manchester | Mr S Bullough |
Basingstoke and North Hampshire Hospital | Mr L Rowell |
Independent | |
BMI Manchester | |
London Bridge Hospital | Ms L Katsumbe |
St Anthony’s Hospital, North Cheam | |
Spire Southampton Hospital |
Strengthening the Reporting of Observational Studies in Epidemiology (STROBE) checklist
Section/topic | Number | Recommendation | Reported on page number |
---|---|---|---|
Title and abstract | |||
1 | (a) Indicate the study’s design with a commonly used term in the title or the abstract | 133 | |
(b) Provide in the abstract an informative and balanced summary of what was done and what was found | 133 | ||
Introduction | |||
Background/rationale | 2 | Explain the scientific background and rationale for the investigation being reported | 133 |
Objectives | 3 | State specific objectives, including any prespecified hypotheses | 139 |
Methods | |||
Study design | 4 | Present key elements of the study design early in the paper | 140 |
Setting | 5 | Describe the setting, locations and relevant dates, including periods of recruitment, exposure, follow-up and data collection | 140 |
Participants | 6 | (a) Give the eligibility criteria and the sources and methods of selection of participants. Describe methods of follow-up | 140 |
(b) For matched studies, give matching criteria and numbers of exposed and unexposed | NA | ||
Variables | 7 | Clearly define all outcomes, exposures, predictors, potential confounders and effect modifiers. Give diagnostic criteria, if applicable | 140 |
Data sources/measurement | 8 | For each variable of interest, give sources of data and details of the methods of assessment (measurement). Describe the comparability of assessment methods if there is more than one group | 141 |
Bias | 9 | Describe any efforts to address potential sources of bias | 142 |
Study size | 10 | Explain how the study size was arrived at | 141 |
Quantitative variables | 11 | Explain how quantitative variables were handled in the analyses. If applicable, describe which groupings were chosen and why | 142 |
Statistical methods | 12 | (a) Describe all statistical methods, including those used to control for confounding | 142 |
(b) Describe any methods used to examine subgroups and interactions | 142 | ||
(c) Explain how missing data were addressed | 142 | ||
(d) If applicable, explain how loss to follow-up was addressed | 142 | ||
(e) Describe any sensitivity analyses | 142 | ||
Results | |||
Participants | 13 | (a) Report numbers of individuals at each stage of the study, e.g. numbers potentially eligible, examined for eligibility, confirmed to be eligible, included in the study, completing follow-up and analysed | 144 |
(b) Give reasons for non-participation at each stage | 144 | ||
(c) Consider use of a flow diagram | 145 | ||
Descriptive data | 14 | (a) Give characteristics of study participants (e.g. demographic, clinical, social) and information on exposures and potential confounders | 146 |
(b) Indicate the number of participants with missing data for each variable of interest | 146 | ||
(c) Summarise follow-up times (e.g. average and total amount) | NA | ||
Outcome data | 15 | Report numbers of outcome events or summary measures over time | 149 |
Main results | 16 | (a) Give unadjusted estimates and, if applicable, confounder-adjusted estimates and their precision (e.g. 95% confidence interval). Make clear which confounders were adjusted for and why they were included | 152 |
(b) Report category boundaries when continuous variables were categorised | 149 | ||
(c) If relevant, consider translating estimates of relative risk into absolute risk for a meaningful time period | NA | ||
Other analyses | 17 | Report other analyses carried out, e.g. analyses of subgroups and interactions and sensitivity analyses | 156 |
Discussion | |||
Key results | 18 | Summarise key results with reference to study objectives | 163 |
Limitations | |||
Interpretation | 20 | Give a cautious overall interpretation of the results considering objectives, limitations, multiplicity of analyses, results from similar studies and other relevant evidence | 164 |
Generalisability | 21 | Discuss the generalisability (external validity) of the study results | 165 |
Other information | |||
Funding | 22 | Give the source of funding and the role of the funders for the present study and, if applicable, for the original study on which the present article is based | 289 |
Appendix 3 The PASPORT trial
Changes to the PASPORT trial design after commencement of the study
Initially, the study was designed as a single-centre study; however, to increase the recruitment rate the study was subsequently opened in two further centres.
Changes to the PASPORT trial outcomes after commencement of the study
Primary outcome
In November 2009, before starting recruitment, some amendments were made to the battery of neurocognitive tests used in the trial. The Block Design Test from the WAIS was added to assess visual spatial functioning. We also substituted the National Adult Reading Test (NART) with the WTAR. The NART was no longer in print whereas the WTAR was in print and is highly correlated with the NART.
In July 2011, an amendment was made to allow calculation of the ASEPSIS score based on only one assessment. The ASEPSIS tool for scoring wound infections was designed for daily scoring in the first postoperative week, with the scores then combined to give an overall total. It was recognised that wounds may not be scored every day and a minimum of one assessment was needed for the calculation. We had aimed to assess the wounds on days 3, 5 and 8 postoperatively. Many participants were discharged before day 8 and therefore had only two assessments. In the original protocol215 we had stated that wounds would be assessed at least twice during a participant’s hospital stay. However, a number of participants were discharged having had their wounds assessed only once. In rare cases this was because participants had already been discharged before their day 5 assessment, but more commonly this occurred when an assessment was missed (often at a weekend).
In June 2015 an amendment was made to the primary outcome. The study was designed with co-primary outcomes of cognitive function and infectious complications, to measure both the hypothesised benefits of preventing regional (cerebral) tissue hypoxia and a reduction in unnecessary red cell transfusion, as the evidence available at that time suggested that red cell transfusion was associated with increased rates of infection. In light of the finding of the TITRe2 trial,4 of consistent rates of infection with both liberal and restrictive postoperative transfusion thresholds (92% vs. 53% transfused – 25% infection rate in both groups, with > 20% due to sepsis), infective complications were removed as a co-primary outcome and added as a secondary clinical outcome. Similarly, a cumulative infection score, which we had intended to develop with data from the TITRe2 trial by supplementing data on wound infections with data describing the severity of sepsis, was removed and replaced with the occurrence of infectious complications. These changes were agreed before the data were analysed and the groups compared.
Secondary outcomes
In November 2009, before starting recruitment, amendments were made to the biochemical markers of organ injury in the study. A blood sample at day 4 (or 96 hours postoperatively) was added to increase the likelihood of capturing important changes and differences between the groups and the urinary sample test N-acetyl-beta-glucosaminidase was removed and replaced with NGAL and IL-18.
In May 2011, an additional sampling time for urine collection after surgery was added to increase the likelihood of capturing important changes and differences between the groups. The biomarkers for early detection of glomerular and tubular renal injury were also changed because novel and more sensitive and specific biomarkers had been identified since the study was initially designed. Cystatin C (kidney) and complement activation (systemic inflammation) were removed and replaced by LFABP and KIM-1.
In May 2012, two additional novel urinary biomarkers were added: alpha-glutathione S-transferase and pi- glutathione S-transferase. Tubular enzymes, such as glutathione S-transferase, are significantly elevated in the urine of patients with kidney injury. Historically, glutathione S-transferase-based assays have performed poorly as diagnostic markers. However, during the recruitment period the assays were refined to detect the alpha and pi analogues of the molecule, using the ELISA method. The relatively low protein levels in urine improve the accuracy of ELISA-based assays. Therefore, alpha- and pi-glutathione S-transferase tests were included in the panel of laboratory investigations.
In June 2015 a final change was made to the biochemical markers of organ injury. Alpha- and pi-glutathione S-transferase, novel markers of tubular and glomerular renal injury, were removed from the panel of markers after preliminary laboratory tests revealed that the ELISA kits did not work and the results were meaningless. These findings were fed back to the manufacturer. Blood and urine samples for assessing these markers were collected and stored for analysis at the end of the trial and so these changes did not impact on the integrity of the trial.
Summary of the PASPORT trial amendments
Amendment 1 (approved prior to commencement of study, 13 November 2009)
-
We increased the number of neurocognitive tests from five to six. The Block Design Test from the WAIS was added to assess visual spatial functioning. We also substituted the NART with the WTAR as the NART was no longer in print and the WTAR was in print and is highly correlated with the NART.
-
We increased the number of times that we sampled patients’ blood by one. We decided to include an analysis at day 4 (or 96 hours postoperatively). The additional day was added to increase the probability of capturing significant changes in blood values.
-
We changed the urinary sample test N-acetyl-beta-glucosaminidase to NGAL and IL-18.
Amendment 2 (approved 21 January 2010)
-
Inclusion of a GP contact letter to notify practitioners if their patients scored highly on the HADS questionnaire at 3 months.
-
Inclusion of the 3-month follow-up questionnaire.
Amendment 3 (approved 4 March 2010)
Clarification of study documents: no changes relating to study design or conduct.
Amendment 4 (submitted 10 November 2011, not approved)
Extension of study end date (date of follow-up of last patient) from 30 March 2011 to 31 March 2013.
Request to amend the labelling of study samples to include patient initials and date of birth in addition to study identification number. This part of the amendment resulted in disapproval. The REC were unhappy with identifiable information being recorded on the samples.
Amendment 5 (approved 17 May 2011)
-
Offering patients home visits when they were unable/did not wish to come back to hospital for their 3-month follow-up appointment.
-
Including an additional sample to the urine sample collected routinely on the first 2 days after surgery. The additional sample was added to increase the probability of capturing significant changes in urine values.
-
Update to sample analyses. We proposed to carry out the following analyses:
-
blood – the blood samples will be analysed in the laboratory for levels of the following biochemical markers of organ injury, using a variety of laboratory assays: S100/100B (brain), troponin I (heart), serum creatinine (kidney), cystatin C (kidney), complement activation (systemic inflammation) and ILs (systemic inflammation)
-
urine – the urine samples will be analysed in the laboratory for levels of urinary creatinine and electrolytes and for the following biochemical markers of organ injury, using a variety of laboratory assays: urinary microalbumin (kidney), NGAL and IL-18.
However, since the study was initially designed, novel and more sensitive and specific biomarkers have been identified for early detection of glomerular and tubular renal injury. These are important developments in the detection of postoperative acute kidney and we have therefore decided to perform these analysis across all study patients. Therefore, we now propose to carry out the following analyses:
-
blood – S100/100B (brain), troponin I or T (heart), creatinine clearance (derived from serum creatinine) and ILs (systemic inflammation)
-
urine – urinary creatinine, electrolytes, urinary microalbumin, NGAL, IL-18, LFABP and KIM-1(markers of tubular and glomerular renal injury).
-
-
Study sample labels to include an encrypted value of the patient’s date of birth in addition to the study identification number.
-
In the original submission we stated that the following were excluded: patients with neuropsychological impairment as defined by previous psychiatric illness, stroke, TIAs, intracerebral haemorrhage, a preoperative Mini Mental State Examination score of < 24 and/or alcohol or drug addiction. To avoid any confusion in the screening process we have decided to separately define neurological, psychiatric and cognitive impairments that exclude patients. These are now defined as:
-
patients with a neurological disorder (e.g. epilepsy, Alzheimer’s disease, dementia and Parkinson’s disease)
-
patients with a diagnosed psychiatric disorder (e.g. schizophrenia, psychosis) or drug or alcohol addiction
-
patients with an already-identified cognitive impairment (e.g. memory and/or attentional deficits) as defined by psychometric assessment or a preoperative Mini Mental State Examination score of < 24
-
patients who have previously sustained a stroke, intracerebral haemorrhage or acquired brain injury.
-
-
The following sentence was added: ‘Sepsis occurring post-discharge will only contribute to the primary outcome if the event results in admission to hospital or death’.
-
Lower respiratory tract infection was added to the expected adverse events section (section 5.1). This has always been an exclusion criteria and was included in the protocol under the ‘umbrella’ of suspected infection. However, in our case report forms it is listed separately so we have decided to add it as a separate event in the protocol to keep these documents in line and therefore avoid any confusion.
-
New Q waves in two contiguous leads and new ST depression of > 2 mm in two leads have been removed from the list of expected adverse events to fit in with our unit’s protocol template. Electrocardiogram changes are very common following cardiac surgery and troponin-based diagnosis of MI is more reliable in the postoperative period.
-
Raised troponin T or I level. Both can be collected and reported at this site.
-
Only units of platelet and FFP transfusions will be recorded. Time data for these was proving difficult to collect so it was decided to alter the way that collection is carried out.
Amendment 6 (approved 27 July 2011)
-
Addition of Castle Hill Hospital (Hull) – local NHS permissions to start the study at this site were granted on 24 January 2012.
-
The ASEPSIS tool for scoring wound infections was designed for daily scoring in the first postoperative week, with the scores then combined to give an overall total. It was recognised that wounds may not be scored every day and a minimum of one assessment is needed for the calculation. We currently aim to assess the wounds on days 3, 5 and 8 postoperatively. Many patients are discharged before day 8 and will therefore have only two assessments. The current protocol215 states that wounds will be assessed at least twice during a participant’s hospital stay. However, a number of patients are discharged having had their wounds assessed only once. In rare cases this may be because the patients have already been discharged before their day 5 assessment, but more commonly this occurs when an assessment is missed (often at a weekend). The incidence of infectious complications during the first 3 months after surgery is one of the primary outcomes for this trial and incorporates the patients’ ASEPSIS assessments. Patients with only one assessment would have missing data for this primary outcome, based on the current protocol (requiring at least two wound assessments). We are proposing that the requirement for two assessments is removed to allow calculation of an ASEPSIS score based on only one assessment.
-
Based on experience and changes made to this questionnaire for a sister trial we have decided to simplify the 3-month follow-up questionnaire.
Amendment 7 (approved 7 February 2012)
-
Consenting patients and collecting trial samples/data the day before or on the day of surgery can often be difficult because of time constraints. We are therefore looking to approach and consent patients at an earlier stage in their patient pathway:
-
Patients given information at the outpatient clinic and approached for consent at the preassessment clinic when possible. When time permits we will carry out the neurocognitive tests at the preassessment clinic too. If not, patients asked to come in an hour earlier on the day of their admission to do these. Patients given a sample pot so that they can collect a 3-hour sample on the morning of their admission and bring it in with them.
-
Amendment 8 (approved 1 May 2012)
-
Currently, we evaluate four different urinary biomarkers: IL-18, NGAL, LFABP and KIM-1. We would like to analyse two additional novel biomarkers: alpha- and pi-glutathione S-transferase. The study intervention was designed to both better maintain oxygen levels and reduce the likelihood of a patient having an unnecessary blood transfusion during surgery. One of the organ systems highly susceptible to hypoxia–reperfusion injury is the kidneys. AKI is a common and severe complication of cardiac surgery, affecting up to 30% of patients. Urinary biomarkers of organ injury are therefore included as a secondary outcome for the study. The panel currently includes the traditional laboratory tests (creatinine clearance, electrolytes and osmolality), as well as an assessment of biomarkers such as KIM-1, LFABP, IL-18, NGAL and alpha- and pi-glutathione S-transferase, in the panel of laboratory investigations. Urine analysis is a traditional non-invasive method used to diagnose, characterise the course of and predict the clinical outcome of numerous renal diseases. A test that allows early diagnosis of kidney injury will contribute to optimisation of postoperative care in cardiac surgery.
Amendment 9 (approved 22 August 2012)
-
Addition of Glenfield Hospital (Leicester) – local NHS permissions to start the study at this site were granted on 21 February 2013.
-
Serban Stoica took over the principal investigator role in Bristol.
-
Because of recruitment for the study being slower than anticipated, the study has been extended. Funding is in place until April 2014. It is expected that the study will finish recruiting in October 2013, with the final follow-up visit for the last patient completed in January 2014.
-
Central biomarker analysis will now take place at Glenfield Hospital, where Professor Murphy’s equipment and biomedical scientists will be based from now on. Samples from the Bristol Heart Institute and Castle Hill Hospital will be shipped every 3 months to Glenfield Hospital for analysis.
Amendment 10 (approved 7 August 2013)
-
Increase in patient sample size from 150 to 200 patients to account for withdrawals and patients lost to follow-up. To meet the increase in recruitment target from 150 to 200 patients we will finish recruiting at the end of December 2013. The final follow-up visit for the last patient will be completed in April 2014.
-
End-of-trial definition changed from:
This trial consists of two phases: an interventional phase (the duration of the patient’s time in theatre) and a three month follow up phase (see 3.9 above). The end of the trial is defined as completion of the final follow up assessment three month follow up visit and return of EQ5D questionnaire) for the last patient followed up in the trial.
to:
This trial consists of two phases: an interventional phase (the duration of the patient’s time in theatre) and a three month follow up phase (see 3.9 above). The end of the trial for an individual participant is defined as completion of the 3 month follow up. The definition of the end of the trial as a whole is the date when all participants have completed the 3 month follow up or have been lost to follow up, all sample analyses have been completed, all data queries resolved and the study database has been locked.
-
A large number of patients at Bristol are now consented at the preassessment clinic 2–3 weeks prior to their operation date. In these cases patients are often asked to come in an hour earlier the day before their surgery so that trial-related tests can be performed and preoperative samples collected. We have created a document as a reminder/checklist for patients of what is required of them when they come back to the hospital the day before their surgery.
Amendment 11 (submitted to the sponsor for review May 2015)
-
Change to the primary outcome from co-primary outcomes of cognitive function 3 months after surgery and infectious complications during the first 3 months after surgery to cognitive function alone (because of findings from the TITRe2 trial).
-
Update to the plan of analysis.
-
Removal of alpha- and pi-glutathione S-transferase from the secondary outcomes as preliminary laboratory tests revealed that the laboratory ELISA kits did not work and the results were meaningless.
Supplemental results
Protocol deviation | Allocated treatment group | Centre | Further details (exact nature dependent on type of deviation) |
---|---|---|---|
Ineligible but treated | Conventional group | Bristol | Stratified to valve repair/replacement and CABG but received CABG only |
Did not receive intervention | Conventional group | Bristol | Stratified to valve repair/replacement but the operation was abandoned as the valve was too damaged and therefore did not receive the intervention |
Transfused above haematocrit of 23% (conventional group) | Conventional group | Bristol | Had first red cell transfusion between 0 and 20 minutes after CPB when haematocrit was 29.7% at CPB0 |
Conventional group | Bristol | Had first red cell transfusion between 20 and 40 minutes after CPB when haematocrit was 25.6% at CPB20 | |
Conventional group | Bristol | Had first red cell transfusion between 20 and 40 minutes after CPB when haematocrit was 24% at CPB20 | |
Transfused above haematocrit of 23% without Murkin algorithm being followed (patient-specific group) | Patient-specific group | Bristol | Minimum haematocrit level was 24% and still transfused |
Patient-specific group | Bristol | Minimum haematocrit level was 23.5% and still transfused | |
Did not receive a transfusion when haematocrit dropped below 23% (conventional group) | Conventional group | Bristol | Minimum haematocrit level was 22.3% and no transfusion was given |
Conventional group | Bristol | Minimum haematocrit level was 20.6% and no transfusion was given | |
Conventional group | Bristol | Minimum haematocrit level was 18.8% and no transfusion was given | |
Conventional group | Bristol | Minimum haematocrit level was 22.9% and no transfusion was given | |
Conventional group | Hull | Minimum haematocrit level was 22% and no transfusion was given | |
Murkin algorithm not followed when NIRS reading dropped below 70% of baseline or to an absolute value of 50% | Patient-specific group | Bristol | None of the Murkin protocol was actioned when the NIRS reading dropped below the threshold |
Murkin algorithm followed for participant in the conventional group, i.e. participant crossed over between treatment groups | Conventional group | Bristol | The Murkin algorithm was followed for a participant in the conventional group |
Psychotic medication | Randomised to generic algorithm (n = 106), n/N (%) | Randomised to patient-specific algorithm (n = 98), n/N (%) | Overall (n = 204), n/N (%) |
---|---|---|---|
Baseline | |||
Hypnotics | 4/106 (4) | 1/98 (1) | 5/204 (2) |
Sedatives | 4/106 (4) | 1/98 (1) | 5/204 (2) |
Neuroleptics | 0/106 (0) | 0/98 (0) | 0/204 (0) |
Anxiolytics | 4/106 (4) | 0/98 (0) | 4/204 (2) |
Antidepressants | 9/106 (8) | 6/98 (6) | 15/204 (7) |
Any psychotic drug | 17/106 (16) | 8/98 (8) | 25/204 (12) |
5 days postoperativelya | |||
Hypnotics | 4/101 (4) | 7/96 (7) | 11/197 (6) |
Sedatives | 9/101 (9) | 6/96 (6) | 15/197 (8) |
Neuroleptics | 1/101 (1) | 0/96 (0) | 1/197 (1) |
Anxiolytics | 2/101 (2) | 2/96 (2) | 4/197 (2) |
Antidepressants | 8/101 (8) | 3/96 (3) | 11/197 (6) |
Any psychotic drug | 17/101 (17) | 17/96 (1) | 34/197 (17) |
3 months postoperativelyb | |||
Hypnoticsc | 0/69 (0) | 0/68 (0) | 0/137 (0) |
Sedativesc | 3/69 (4) | 2/68 (3) | 5/137 (4) |
Neurolepticsc | 1/69 (1) | 0/68 (0) | 1/137 (1) |
Anxiolyticsc | 2/69 (3) | 0/68 (0) | 2/137 (1) |
Antidepressantsc | 4/69 (6) | 2/68 (3) | 6/137 (4) |
Any psychotic drugd | 7/69 (10) | 4/68 (6) | 11/137 (8) |
Mental health outcome | Randomised to generic algorithm (n = 106) | Randomised to patient-specific algorithm (n = 98) | Overall (n = 204) |
---|---|---|---|
WTAR, median (IQR) | |||
Baseline scorea | 118.5 (108.0–123.0) | 117.0 (108.0–122.0) | 118.0 (108.0–123.0) |
Medications, n/N (%) | |||
Baseline | 60/106 (57) | 41/98 (42) | 101/204 (50) |
5 days postoperativelyb | 72/100 (72) | 52/96 (54) | 124/196 (63) |
3 months postoperativelyc | 54/69 (78) | 42/67 (63) | 96/136 (71) |
HADS summary measure, median (IQR) | |||
Anxiety | |||
Baseline scored | 5.0 (3.0–8.0) | 6.0 (4.0–9.0) | 5.0 (4.0–8.0) |
5-day postoperative scoree | 5.0 (2.0–8.0) | 5.5 (3.0–9.0) | 5.0 (3.0–9.0) |
3-month postoperative scoref | 2.0 (1.0–6.0) | 3.0 (1.0–7.0) | 3.0 (1.0–6.0) |
Depression | |||
Baseline scoreg | 3.5 (2.0–6.0) | 3.5 (2.0–6.0) | 3.5 (2.0–6.0) |
5-day postoperative scoreh | 5.0 (2.0–9.0) | 5.0 (2.0–8.2) | 5.0 (2.0–9.0) |
3-month postoperative scorei | 2.0 (1.0–4.0) | 2.0 (1.0–4.0) | 2.0 (1.0–4.0) |
GHQ-30 summary measure, median (IQR) | |||
Baseline scorej | 25.0 (19.0–34.0) | 25.0 (21.0–33.0) | 25.0 (20.0–33.5) |
5-day postoperative scorek | 30.0 (22.0–40.0) | 29.0 (20.0–39.0) | 30.0 (21.0–39.0) |
3-month postoperative scorel | 19.0 (15.0–26.0) | 20.0 (15.0–30.0) | 20.0 (15.0–28.0) |
Mental health outcome | Randomised to generic algorithm (n = 106) | Randomised to patient-specific algorithm (n = 98) | Overall |
---|---|---|---|
HADS summary measure, n/N (%) | |||
Anxiety (≥ 8 vs. < 8) | |||
Baseline score | 28/99 (28) | 29/90 (32) | 57/189 (30) |
5-day postoperative score | 28/89 (31) | 26/80 (33) | 54/169 (32) |
3-month postoperative score | 9/82 (11) | 15/85 (18) | 24/167 (14) |
Depression (≥ 8 vs. < 8) | |||
Baseline score | 14/103 (14) | 12/92 (13) | 26/195 (13) |
5-day postoperative score | 34/89 (38) | 27/84 (32) | 61/173 (35) |
3-month postoperative score | 7/83 (8) | 10/85 (12) | 17/168 (10) |
GHQ-30 (≥ 72 vs. < 72), n/N (%) | |||
Baseline score | 0/103 (0) | 0/93 (0) | 0/196 (0) |
5-day postoperative score | 0/87 (0) | 2/83 (2) | 2/170 (1) |
3-month postoperative score | 0/79 (0) | 0/80 (0) | 0/159 (0) |
Outcome | Randomised to generic algorithm (n = 106) | Randomised to patient-specific algorithm (n = 98) | Overall (n = 204) |
---|---|---|---|
Anxiety, n (%) | |||
I feel tense or wound up | |||
Preoperativelya | |||
Most of the time | 22 (21.8) | 20 (21.5) | 42 (21.6) |
A lot of the time | 64 (63.4) | 61 (65.6) | 125 (64.4) |
From time to time | 10 (9.9) | 8 (8.6) | 18 (9.3) |
Not at all | 5 (5.0) | 4 (4.3) | 9 (4.6) |
5 days postoperativelyb | |||
Most of the time | 23 (25.6) | 23 (27.4) | 46 (26.4) |
A lot of the time | 46 (51.1) | 44 (52.4) | 90 (51.7) |
From time to time | 15 (16.7) | 14 (16.7) | 29 (16.7) |
Not at all | 6 (6.7) | 3 (3.6) | 9 (5.2) |
3 months postoperativelyc | |||
Most of the time | 40 (48.2) | 41 (48.2) | 81 (48.2) |
A lot of the time | 38 (45.8) | 34 (40.0) | 72 (42.9) |
From time to time | 3 (3.6) | 6 (7.1) | 9 (5.4) |
Not at all | 2 (2.4) | 4 (4.7) | 6 (3.6) |
I get a sort of frightened feeling as if something awful is about to happen | |||
Preoperativelya | |||
Very definitely and quite badly | 42 (40.8) | 30 (32.3) | 72 (36.7) |
Yes, but not too badly | 32 (31.1) | 35 (37.6) | 67 (34.2) |
Not at all | 24 (23.3) | 19 (20.4) | 43 (21.9) |
Hardly at all | 5 (4.9) | 9 (9.7) | 14 (7.1) |
5 days postoperativelyb | |||
Very definitely and quite badly | 37 (41.1) | 36 (43.4) | 73 (42.2) |
Yes, but not too badly | 26 (28.9) | 22 (26.5) | 48 (27.7) |
Not at all | 20 (22.2) | 20 (24.1) | 40 (23.1) |
Hardly at all | 7 (7.8) | 5 (6.0) | 12 (6.9) |
3 months postoperativelyc | |||
Very definitely and quite badly | 57 (68.7) | 49 (57.6) | 106 (63.1) |
Yes, but not too badly | 15 (18.1) | 22 (25.9) | 37 (22.0) |
Not at all | 6 (7.2) | 8 (9.4) | 14 (8.3) |
Hardly at all | 5 (6.0) | 6 (7.1) | 11 (6.5) |
Worrying thoughts go through my mind | |||
Preoperativelya | |||
A great deal of the time | 40 (38.8) | 28 (30.1) | 68 (34.7) |
Not quite as much now | 38 (36.9) | 45 (48.4) | 83 (42.3) |
Definitely not so much now | 18 (17.5) | 14 (15.1) | 32 (16.3) |
Not at all | 7 (6.8) | 6 (6.5) | 13 (6.6) |
5 days postoperativelyb | |||
A great deal of the time | 38 (42.2) | 30 (35.7) | 68 (39.1) |
Not quite as much now | 35 (38.9) | 40 (47.6) | 75 (43.1) |
Definitely not so much now | 12 (13.3) | 9 (10.7) | 21 (12.1) |
Not at all | 5 (5.6) | 5 (6.0) | 10 (5.7) |
3 months postoperativelyc | |||
A great deal of the time | 49 (59.0) | 45 (52.9) | 94 (56.0) |
Not quite as much now | 26 (31.3) | 26 (30.6) | 52 (31.0) |
Definitely not so much now | 3 (3.6) | 9 (10.6) | 12 (7.1) |
Not at all | 5 (6.0) | 5 (5.9) | 10 (6.0) |
I can sit at ease and feel relaxed | |||
Preoperativelya | |||
Definitely | 42 (40.8) | 37 (40.2) | 79 (40.5) |
Usually | 56 (54.4) | 45 (48.9) | 101 (51.8) |
Not often | 5 (4.9) | 9 (9.8) | 14 (7.2) |
Not at all | 0 (0.0) | 1 (1.1) | 1 (0.5) |
5 days postoperativelyb | |||
Definitely | 38 (42.2) | 30 (35.7) | 68 (39.1) |
Usually | 37 (41.1) | 38 (45.2) | 75 (43.1) |
Not often | 13 (14.4) | 13 (15.5) | 26 (14.9) |
Not at all | 2 (2.2) | 3 (3.6) | 5 (2.9) |
3 months postoperativelyc | |||
Definitely | 55 (66.3) | 46 (54.1) | 101 (60.1) |
Usually | 25 (30.1) | 33 (38.8) | 58 (34.5) |
Not often | 3 (3.6) | 4 (4.7) | 7 (4.2) |
Not at all | 0 (0.0) | 2 (2.4) | 2 (1.2) |
I get a sort of frightened feeling like butterflies in the stomach | |||
Preoperativelya | |||
Not at all | 46 (45.1) | 30 (32.3) | 76 (39.0) |
Occasionally | 50 (49.0) | 55 (59.1) | 105 (53.8) |
Quite often | 5 (4.9) | 7 (7.5) | 12 (6.2) |
Very often | 1 (1.0) | 1 (1.1) | 2 (1.0) |
5 days postoperativelyb | |||
Not at all | 50 (56.2) | 39 (46.4) | 89 (51.4) |
Occasionally | 30 (33.7) | 37 (44.0) | 67 (38.7) |
Quite often | 7 (7.9) | 7 (8.3) | 14 (8.1) |
Very often | 2 (2.2) | 1 (1.2) | 3 (1.7) |
3 months postoperativelyc | |||
Not at all | 65 (78.3) | 60 (70.6) | 125 (74.4) |
Occasionally | 16 (19.3) | 23 (27.1) | 39 (23.2) |
Quite often | 1 (1.2) | 2 (2.4) | 3 (1.8) |
Very often | 1 (1.2) | 0 (0.0) | 1 (0.6) |
I feel restless as if I have to be on the move | |||
Preoperativelya | |||
Very much indeed | 30 (29.4) | 18 (19.4) | 48 (24.6) |
Quite a lot | 37 (36.3) | 41 (44.1) | 78 (40.0) |
Not very much | 30 (29.4) | 29 (31.2) | 59 (30.3) |
Not at all | 5 (4.9) | 5 (5.4) | 10 (5.1) |
5 days postoperativelyb | |||
Very much indeed | 32 (35.6) | 26 (31.7) | 58 (33.7) |
Quite a lot | 37 (41.1) | 31 (37.8) | 68 (39.5) |
Not very much | 17 (18.9) | 24 (29.3) | 41 (23.8) |
Not at all | 4 (4.4) | 1 (1.2) | 5 (2.9) |
3 months postoperativelyc | |||
Very much indeed | 34 (41.0) | 30 (35.3) | 64 (38.1) |
Quite a lot | 31 (37.3) | 30 (35.3) | 61 (36.3) |
Not very much | 13 (15.7) | 18 (21.2) | 31 (18.5) |
Not at all | 5 (6.0) | 7 (8.2) | 12 (7.1) |
I get sudden feelings of panic | |||
Preoperativelya | |||
Very often indeed | 56 (54.4) | 35 (38.5) | 91 (46.9) |
Quite often | 35 (34.0) | 39 (42.9) | 74 (38.1) |
Not very often | 4 (3.9) | 11 (12.1) | 15 (7.7) |
Not at all | 8 (7.8) | 6 (6.6) | 14 (7.2) |
5 days postoperativelyb | |||
Very often indeed | 42 (46.7) | 34 (41.0) | 76 (43.9) |
Quite often | 29 (32.2) | 36 (43.4) | 65 (37.6) |
Not very often | 14 (15.6) | 10 (12.0) | 24 (13.9) |
Not at all | 5 (5.6) | 3 (3.6) | 8 (4.6) |
3 months postoperativelyc | |||
Very often indeed | 54 (65.9) | 51 (60.0) | 105 (62.9) |
Quite often | 22 (26.8) | 24 (28.2) | 46 (27.5) |
Not very often | 1 (1.2) | 5 (5.9) | 6 (3.6) |
Not at all | 5 (6.1) | 5 (5.9) | 10 (6.0) |
Depression, n (%) | |||
I still enjoy the things I used to enjoy | |||
Preoperativelyd | |||
Definitely as much | 48 (46.6) | 40 (43.0) | 88 (44.9) |
Not quite as much | 45 (43.7) | 43 (46.2) | 88 (44.9) |
Only a little | 6 (5.8) | 7 (7.5) | 13 (6.6) |
Hardly at all | 4 (3.9) | 3 (3.2) | 7 (3.6) |
5 days postoperativelye | |||
Definitely as much | 37 (43.0) | 34 (42.0) | 71 (42.5) |
Not quite as much | 30 (34.9) | 26 (32.1) | 56 (33.5) |
Only a little | 16 (18.6) | 13 (16.0) | 29 (17.4) |
Hardly at all | 3 (3.5) | 8 (9.9) | 11 (6.6) |
3 months postoperativelyf | |||
Definitely as much | 51 (61.4) | 59 (69.4) | 110 (65.5) |
Not quite as much | 30 (36.1) | 23 (27.1) | 53 (31.5) |
Only a little | 2 (2.4) | 3 (3.5) | 5 (3.0) |
I can laugh and see the funny side of things | |||
Preoperativelyd | |||
As much as I always could | 83 (81.4) | 74 (79.6) | 157 (80.5) |
Not quite as much now | 16 (15.7) | 16 (17.2) | 32 (16.4) |
Definitely not as much now | 3 (2.9) | 3 (3.2) | 6 (3.1) |
5 days postoperativelye | |||
As much as I always could | 61 (67.8) | 58 (69.9) | 119 (68.8) |
Not quite as much now | 24 (26.7) | 20 (24.1) | 44 (25.4) |
Definitely not as much now | 5 (5.6) | 4 (4.8) | 9 (5.2) |
Not at all | 0 (0.0) | 1 (1.2) | 1 (0.6) |
3 months postoperativelyf | |||
As much as I always could | 81 (97.6) | 74 (87.1) | 155 (92.3) |
Not quite as much now | 2 (2.4) | 9 (10.6) | 11 (6.5) |
Definitely not so much now | 0 (0.0) | 1 (1.2) | 1 (0.6) |
Not at all | 0 (0.0) | 1 (1.2) | 1 (0.6) |
I feel cheerful | |||
Preoperativelyd | |||
Not at all | 76 (74.5) | 61 (66.3) | 137 (70.6) |
Not often | 14 (13.7) | 22 (23.9) | 36 (18.6) |
Sometimes | 7 (6.9) | 6 (6.5) | 13 (6.7) |
Most of the time | 5 (4.9) | 3 (3.3) | 8 (4.1) |
5 days postoperativelye | |||
Not at all | 48 (54.5) | 41 (49.4) | 89 (52.0) |
Not often | 27 (30.7) | 30 (36.1) | 57 (33.3) |
Sometimes | 6 (6.8) | 9 (10.8) | 15 (8.8) |
Most of the time | 7 (8.0) | 3 (3.6) | 10 (5.8) |
3 months postoperativelyf | |||
Not at all | 60 (72.3) | 66 (77.6) | 126 (75.0) |
Not often | 15 (18.1) | 12 (14.1) | 27 (16.1) |
Sometimes | 2 (2.4) | 3 (3.5) | 5 (3.0) |
Most of the time | 6 (7.2) | 4 (4.7) | 10 (6.0) |
I feel as if I am slowed down | |||
Preoperativelyd | |||
Nearly all the time | 11 (10.7) | 3 (3.2) | 14 (7.1) |
Very often | 42 (40.8) | 45 (48.4) | 87 (44.4) |
Sometimes | 28 (27.2) | 27 (29.0) | 55 (28.1) |
Not at all | 22 (21.4) | 18 (19.4) | 40 (20.4) |
5 days postoperativelye | |||
Nearly all the time | 7 (7.8) | 6 (7.2) | 13 (7.5) |
Very often | 32 (35.6) | 33 (39.8) | 65 (37.6) |
Sometimes | 21 (23.3) | 20 (24.1) | 41 (23.7) |
Not at all | 30 (33.3) | 24 (28.9) | 54 (31.2) |
3 months postoperativelyf | |||
Nearly all the time | 20 (24.1) | 21 (24.7) | 41 (24.4) |
Very often | 39 (47.0) | 42 (49.4) | 81 (48.2) |
Sometimes | 19 (22.9) | 16 (18.8) | 35 (20.8) |
Not at all | 5 (6.0) | 6 (7.1) | 11 (6.5) |
I have lost interest in my appearance | |||
Preoperativelyd | |||
Definitely | 72 (69.9) | 63 (67.7) | 135 (68.9) |
I don’t take as much care as I should | 20 (19.4) | 15 (16.1) | 35 (17.9) |
I may not take quite as much care | 3 (2.9) | 10 (10.8) | 13 (6.6) |
I take just as much care as ever | 8 (7.8) | 5 (5.4) | 13 (6.6) |
5 days postoperativelye | |||
Definitely | 42 (47.2) | 42 (50.0) | 84 (48.6) |
I don’t take as much care as I should | 18 (20.2) | 16 (19.0) | 34 (19.7) |
I may not take quite as much care | 14 (15.7) | 19 (22.6) | 33 (19.1) |
I take just as much care as ever | 15 (16.9) | 7 (8.3) | 22 (12.7) |
3 months postoperativelyf | |||
Definitely | 63 (75.9) | 66 (77.6) | 129 (76.8) |
I don’t take as much care as I should | 11 (13.3) | 9 (10.6) | 20 (11.9) |
I may not take quite as much care | 4 (4.8) | 6 (7.1) | 10 (6.0) |
I take just as much care as ever | 5 (6.0) | 4 (4.7) | 9 (5.4) |
I look forward with enjoyment to things | |||
Preoperativelyd | |||
As much as I ever did | 68 (66.0) | 61 (66.3) | 129 (66.2) |
Rather less than I used to | 27 (26.2) | 25 (27.2) | 52 (26.7) |
Definitely less than I used to | 7 (6.8) | 6 (6.5) | 13 (6.7) |
Hardly at all | 1 (1.0) | 0 (0.0) | 1 (0.5) |
5 days postoperativelye | |||
As much as I ever did | 63 (70.0) | 53 (63.9) | 116 (67.1) |
Rather less than I used to | 17 (18.9) | 20 (24.1) | 37 (21.4) |
Definitely less than I used to | 8 (8.9) | 7 (8.4) | 15 (8.7) |
Hardly at all | 2 (2.2) | 3 (3.6) | 5 (2.9) |
3 months postoperativelyf | |||
As much as I ever did | 64 (77.1) | 69 (81.2) | 133 (79.2) |
Rather less than I used to | 16 (19.3) | 14 (16.5) | 30 (17.9) |
Definitely less than I used to | 2 (2.4) | 2 (2.4) | 4 (2.4) |
Hardly at all | 1 (1.2) | 0 (0.0) | 1 (0.6) |
I can enjoy a good book or radio or TV programme | |||
Preoperativelyd | |||
Often | 84 (81.6) | 81 (87.1) | 165 (84.2) |
Sometimes | 16 (15.5) | 8 (8.6) | 24 (12.2) |
Not often | 1 (1.0) | 3 (3.2) | 4 (2.0) |
Very seldom | 2 (1.9) | 1 (1.1) | 3 (1.5) |
5 days postoperativelye | |||
Often | 52 (57.8) | 56 (67.5) | 108 (62.4) |
Sometimes | 25 (27.8) | 12 (14.5) | 37 (21.4) |
Not often | 7 (7.8) | 5 (6.0) | 12 (6.9) |
Very seldom | 6 (6.7) | 10 (12.0) | 16 (9.2) |
3 months postoperativelyf | |||
Often | 80 (96.4) | 72 (84.7) | 152 (90.5) |
Sometimes | 3 (3.6) | 13 (15.3) | 16 (9.5) |
Outcome | Randomised to generic algorithm (n = 106) | Randomised to patient-specific algorithm (n = 98) | Overall (n = 204) |
---|---|---|---|
1. Have you been able to concentrate on whatever you’re doing?, n/N (%) | |||
Preoperativelya | |||
Same as usual | 67 (65.0) | 61 (65.6) | 128 (65.3) |
Less than usual | 33 (32.0) | 29 (31.2) | 62 (31.6) |
Much less than usual | 3 (2.9) | 3 (3.2) | 6 (3.1) |
5 days postoperativelyb | |||
Better than usual | 2 (2.3) | 5 (6.0) | 7 (4.1) |
Same as usual | 31 (35.6) | 21 (25.3) | 52 (30.6) |
Less than usual | 43 (49.4) | 43 (51.8) | 86 (50.6) |
Much less than usual | 11 (12.6) | 14 (16.9) | 25 (14.7) |
3 months postoperativelyc | |||
Better than usual | 7 (8.9) | 5 (6.3) | 12 (7.5) |
Same as usual | 59 (74.7) | 52 (65.0) | 111 (69.8) |
Less than usual | 11 (13.9) | 21 (26.3) | 32 (20.1) |
Much less than usual | 2 (2.5) | 2 (2.5) | 4 (2.5) |
2. Have you lost much sleep over worry?, n/N (%) | |||
Preoperativelya | |||
Not at all | 23 (22.3) | 17 (18.3) | 40 (20.4) |
No more than usual | 52 (50.5) | 43 (46.2) | 95 (48.5) |
Rather more than usual | 26 (25.2) | 29 (31.2) | 55 (28.1) |
Much more than usual | 2 (1.9) | 4 (4.3) | 6 (3.1) |
5 days postoperativelyb | |||
Not at all | 25 (28.7) | 24 (28.9) | 49 (28.8) |
No more than usual | 31 (35.6) | 27 (32.5) | 58 (34.1) |
Rather more than usual | 25 (28.7) | 20 (24.1) | 45 (26.5) |
Much more than usual | 6 (6.9) | 12 (14.5) | 18 (10.6) |
3 months postoperativelyc | |||
Not at all | 40 (50.6) | 31 (38.8) | 71 (44.7) |
No more than usual | 33 (41.8) | 37 (46.3) | 70 (44.0) |
Rather more than usual | 6 (7.6) | 10 (12.5) | 16 (10.1) |
Much more than usual | 0 (0.0) | 2 (2.5) | 2 (1.3) |
3. Have you been having restless, disturbed nights?, n/N (%) | |||
Preoperativelya | |||
Not at all | 17 (16.5) | 16 (17.2) | 33 (16.8) |
No more than usual | 48 (46.6) | 38 (40.9) | 86 (43.9) |
Rather more than usual | 30 (29.1) | 32 (34.4) | 62 (31.6) |
Much more than usual | 8 (7.8) | 7 (7.5) | 15 (7.7) |
5 days postoperativelyb | |||
Not at all | 9 (10.3) | 9 (10.8) | 18 (10.6) |
No more than usual | 20 (23.0) | 18 (21.7) | 38 (22.4) |
Rather more than usual | 34 (39.1) | 33 (39.8) | 67 (39.4) |
Much more than usual | 24 (27.6) | 23 (27.7) | 47 (27.6) |
3 months postoperativelyc | |||
Not at all | 23 (29.1) | 20 (25.0) | 43 (27.0) |
No more than usual | 40 (50.6) | 31 (38.8) | 71 (44.7) |
Rather more than usual | 15 (19.0) | 21 (26.3) | 36 (22.6) |
Much more than usual | 1 (1.3) | 8 (10.0) | 9 (5.7) |
4. Have you been managing to keep yourself busy and occupied?, n/N (%) | |||
Preoperativelya | |||
More so than usual | 9 (8.7) | 7 (7.5) | 16 (8.2) |
Same as usual | 75 (72.8) | 68 (73.1) | 143 (73.0) |
Rather less than usual | 16 (15.5) | 14 (15.1) | 30 (15.3) |
Much less than usual | 3 (2.9) | 4 (4.3) | 7 (3.6) |
5 days postoperativelyb | |||
More so than usual | 7 (8.0) | 5 (6.0) | 12 (7.1) |
Same as usual | 35 (40.2) | 26 (31.3) | 61 (35.9) |
Rather less than usual | 28 (32.2) | 33 (39.8) | 61 (35.9) |
Much less than usual | 17 (19.5) | 19 (22.9) | 36 (21.2) |
3 months postoperativelyc | |||
More so than usual | 8 (10.1) | 9 (11.3) | 17 (10.7) |
Same as usual | 59 (74.7) | 52 (65.0) | 111 (69.8) |
Rather less than usual | 11 (13.9) | 17 (21.3) | 28 (17.6) |
Much less than usual | 1 (1.3) | 2 (2.5) | 3 (1.9) |
5. Have you been getting out of the house as much as usual?, n/N (%) | |||
Preoperativelya | |||
More so than usual | 11 (10.7) | 5 (5.4) | 16 (8.2) |
Same as usual | 65 (63.1) | 55 (59.1) | 120 (61.2) |
Less than usual | 17 (16.5) | 27 (29.0) | 44 (22.4) |
Much less than usual | 10 (9.7) | 6 (6.5) | 16 (8.2) |
5 days postoperativelyb | |||
More so than usual | 44 (50.6) | 36 (43.4) | 80 (47.1) |
Same as usual | 19 (21.8) | 15 (18.1) | 34 (20.0) |
Less than usual | 10 (11.5) | 17 (20.5) | 27 (15.9) |
Much less than usual | 14 (16.1) | 15 (18.1) | 29 (17.1) |
3 months postoperativelyc | |||
More so than usual | 13 (16.5) | 14 (17.5) | 27 (17.0) |
Same as usual | 50 (63.3) | 47 (58.8) | 97 (61.0) |
Less than usual | 13 (16.5) | 17 (21.3) | 30 (18.9) |
Much less than usual | 3 (3.8) | 2 (2.5) | 5 (3.1) |
6. Have you been managing as well as most people would in your shoes?, n/N (%) | |||
Preoperativelya | |||
Better than most | 28 (27.2) | 30 (32.3) | 58 (29.6) |
About the same | 71 (68.9) | 60 (64.5) | 131 (66.8) |
Rather less well | 3 (2.9) | 3 (3.2) | 6 (3.1) |
Much less well | 1 (1.0) | 0 (0.0) | 1 (0.5) |
5 days postoperativelyb | |||
Better than most | 17 (19.5) | 28 (33.7) | 45 (26.5) |
About the same | 56 (64.4) | 49 (59.0) | 105 (61.8) |
Rather less well | 11 (12.6) | 4 (4.8) | 15 (8.8) |
Much less well | 3 (3.4) | 2 (2.4) | 5 (2.9) |
3 months postoperativelyc | |||
Better than most | 28 (35.4) | 28 (35.0) | 56 (35.2) |
About the same | 50 (63.3) | 50 (62.5) | 100 (62.9) |
Rather less well | 1 (1.3) | 1 (1.3) | 2 (1.3) |
Much less well | 0 (0.0) | 1 (1.3) | 1 (0.6) |
7. Have you felt on the whole you were doing things well?, n/N (%) | |||
Preoperativelya | |||
Better than usual | 6 (5.8) | 2 (2.2) | 8 (4.1) |
About the same | 67 (65.0) | 68 (73.1) | 135 (68.9) |
Less well than usual | 28 (27.2) | 23 (24.7) | 51 (26.0) |
Much less well | 2 (1.9) | 0 (0.0) | 2 (1.0) |
5 days postoperativelyb | |||
Better than usual | 8 (9.2) | 9 (10.8) | 17 (10.0) |
About the same | 55 (63.2) | 49 (59.0) | 104 (61.2) |
Less well than usual | 14 (16.1) | 19 (22.9) | 33 (19.4) |
Much less well | 10 (11.5) | 6 (7.2) | 16 (9.4) |
3 months postoperativelyc | |||
Better than usual | 11 (13.9) | 21 (26.3) | 32 (20.1) |
About the same | 54 (68.4) | 45 (56.3) | 99 (62.3) |
Less well than usual | 14 (17.7) | 13 (16.3) | 27 (17.0) |
Much less well | 0 (0.0) | 1 (1.3) | 1 (0.6) |
8. Have you been satisfied with the way you’ve carried out your task?, n/N (%) | |||
Preoperativelya | |||
More satisfied | 5 (4.9) | 7 (7.5) | 12 (6.1) |
About the same as usual | 71 (68.9) | 57 (61.3) | 128 (65.3) |
Less satisfied than usual | 26 (25.2) | 28 (30.1) | 54 (27.6) |
Much less satisfied | 1 (1.0) | 1 (1.1) | 2 (1.0) |
5 days postoperativelyb | |||
More satisfied | 11 (12.6) | 7 (8.4) | 18 (10.6) |
About the same as usual | 52 (59.8) | 47 (56.6) | 99 (58.2) |
Less satisfied than usual | 18 (20.7) | 22 (26.5) | 40 (23.5) |
Much less satisfied | 6 (6.9) | 7 (8.4) | 13 (7.6) |
3 months postoperativelyc | |||
More satisfied | 15 (19.0) | 15 (18.8) | 30 (18.9) |
About the same as usual | 53 (67.1) | 51 (63.8) | 104 (65.4) |
Less satisfied than usual | 9 (11.4) | 14 (17.5) | 23 (14.5) |
Much less satisfied | 2 (2.5) | 0 (0.0) | 2 (1.3) |
9. Have you been able to feel warmth and affection for those near to you?, n/N (%) | |||
Preoperativelya | |||
Better than usual | 24 (23.3) | 31 (33.3) | 55 (28.1) |
About the same | 73 (70.9) | 57 (61.3) | 130 (66.3) |
Less well than usual | 6 (5.8) | 5 (5.4) | 11 (5.6) |
5 days postoperativelyb | |||
Better than usual | 34 (39.1) | 26 (31.3) | 60 (35.3) |
About the same | 47 (54.0) | 51 (61.4) | 98 (57.6) |
Less well than usual | 5 (5.7) | 5 (6.0) | 10 (5.9) |
Much less well | 1 (1.1) | 1 (1.2) | 2 (1.2) |
3 months postoperativelyc | |||
Better than usual | 20 (25.3) | 18 (22.5) | 38 (23.9) |
About the same | 58 (73.4) | 58 (72.5) | 116 (73.0) |
Less well than usual | 1 (1.3) | 4 (5.0) | 5 (3.1) |
10. Have you been finding it easy to get on with other people?, n/N (%) | |||
Preoperativelya | |||
Better than usual | 5 (4.9) | 5 (5.4) | 10 (5.1) |
About the same | 86 (83.5) | 80 (86.0) | 166 (84.7) |
Less well than usual | 9 (8.7) | 8 (8.6) | 17 (8.7) |
Much less well | 3 (2.9) | 0 (0.0) | 3 (1.5) |
5 days postoperativelyb | |||
Better than usual | 12 (13.8) | 8 (9.6) | 20 (11.8) |
About the same | 69 (79.3) | 67 (80.7) | 136 (80.0) |
Less well than usual | 5 (5.7) | 8 (9.6) | 13 (7.6) |
Much less well | 1 (1.1) | 0 (0.0) | 1 (0.6) |
3 months postoperativelyc | |||
Better than usual | 8 (10.1) | 10 (12.5) | 18 (11.3) |
About the same | 70 (88.6) | 69 (86.3) | 139 (87.4) |
Less well than usual | 1 (1.3) | 0 (0.0) | 1 (0.6) |
Much less well | 0 (0.0) | 1 (1.3) | 1 (0.6) |
11. Have you spent much time chatting with people?, n/N (%) | |||
Preoperativelya | |||
More time than usual | 19 (18.4) | 13 (14.0) | 32 (16.3) |
About the same as usual | 74 (71.8) | 67 (72.0) | 141 (71.9) |
Less time than usual | 7 (6.8) | 13 (14.0) | 20 (10.2) |
Much less than usual | 3 (2.9) | 0 (0.0) | 3 (1.5) |
5 days postoperativelyb | |||
More time than usual | 15 (17.2) | 16 (19.3) | 31 (18.2) |
About the same as usual | 51 (58.6) | 40 (48.2) | 91 (53.5) |
Less time than usual | 18 (20.7) | 23 (27.7) | 41 (24.1) |
Much less than usual | 3 (3.4) | 4 (4.8) | 7 (4.1) |
3 months postoperativelyc | |||
More time than usual | 20 (25.3) | 19 (23.8) | 39 (24.5) |
About the same as usual | 52 (65.8) | 47 (58.8) | 99 (62.3) |
Less time than usual | 5 (6.3) | 14 (17.5) | 19 (11.9) |
Much less than usual | 2 (2.5) | 0 (0.0) | 2 (1.3) |
12. Have you felt that you are playing a useful part in things?, n/N (%) | |||
Preoperativelya | |||
More so than usual | 7 (6.8) | 3 (3.2) | 10 (5.1) |
Same as usual | 67 (65.0) | 64 (68.8) | 131 (66.8) |
Less useful than usual | 26 (25.2) | 21 (22.6) | 47 (24.0) |
Much less useful | 3 (2.9) | 5 (5.4) | 8 (4.1) |
5 days postoperativelyb | |||
More so than usual | 14 (16.1) | 12 (14.5) | 26 (15.3) |
Same as usual | 41 (47.1) | 38 (45.8) | 79 (46.5) |
Less useful than usual | 19 (21.8) | 18 (21.7) | 37 (21.8) |
Much less useful | 13 (14.9) | 15 (18.1) | 28 (16.5) |
3 months postoperativelyc | |||
More so than usual | 9 (11.4) | 4 (5.0) | 13 (8.2) |
Same as usual | 55 (69.6) | 57 (71.3) | 112 (70.4) |
Less useful than usual | 12 (15.2) | 17 (21.3) | 29 (18.2) |
Much less useful | 3 (3.8) | 2 (2.5) | 5 (3.1) |
13. Have you felt capable of making decisions about things?, n/N (%) | |||
Preoperativelya | |||
More so than usual | 5 (4.9) | 6 (6.5) | 11 (5.6) |
Same as usual | 83 (80.6) | 78 (83.9) | 161 (82.1) |
Less so than usual | 15 (14.6) | 9 (9.7) | 24 (12.2) |
5 days postoperativelyb | |||
More so than usual | 8 (9.2) | 4 (4.8) | 12 (7.1) |
Same as usual | 50 (57.5) | 44 (53.0) | 94 (55.3) |
Less so than usual | 18 (20.7) | 29 (34.9) | 47 (27.6) |
Much less capable | 11 (12.6) | 6 (7.2) | 17 (10.0) |
3 months postoperativelyc | |||
More so than usual | 7 (8.9) | 9 (11.3) | 16 (10.1) |
Same as usual | 66 (83.5) | 60 (75.0) | 126 (79.2) |
Less so than usual | 6 (7.6) | 11 (13.8) | 17 (10.7) |
14. Have you felt constantly under strain?, n/N (%) | |||
Preoperativelya | |||
Not at all | 24 (23.3) | 13 (14.0) | 37 (18.9) |
No more than usual | 50 (48.5) | 49 (52.7) | 99 (50.5) |
Rather more than usual | 25 (24.3) | 30 (32.3) | 55 (28.1) |
Much more than usual | 4 (3.9) | 1 (1.1) | 5 (2.6) |
5 days postoperativelyb | |||
Not at all | 17 (19.5) | 18 (21.7) | 35 (20.6) |
No more than usual | 40 (46.0) | 32 (38.6) | 72 (42.4) |
Rather more than usual | 26 (29.9) | 28 (33.7) | 54 (31.8) |
Much more than usual | 4 (4.6) | 5 (6.0) | 9 (5.3) |
3 months postoperativelyc | |||
Not at all | 42 (53.2) | 36 (45.0) | 78 (49.1) |
No more than usual | 31 (39.2) | 30 (37.5) | 61 (38.4) |
Rather more than usual | 6 (7.6) | 14 (17.5) | 20 (12.6) |
15. Have you felt you couldn’t overcome your difficulties?, n/N (%) | |||
Preoperativelya | |||
Not at all | 30 (29.1) | 23 (24.7) | 53 (27.0) |
No more than usual | 56 (54.4) | 52 (55.9) | 108 (55.1) |
Rather more than usual | 16 (15.5) | 16 (17.2) | 32 (16.3) |
Much more than usual | 1 (1.0) | 2 (2.2) | 3 (1.5) |
5 days postoperativelyb | |||
Not at all | 24 (27.6) | 17 (20.5) | 41 (24.1) |
No more than usual | 40 (46.0) | 44 (53.0) | 84 (49.4) |
Rather more than usual | 17 (19.5) | 18 (21.7) | 35 (20.6) |
Much more than usual | 6 (6.9) | 4 (4.8) | 10 (5.9) |
3 months postoperativelyc | |||
Not at all | 40 (50.6) | 37 (46.3) | 77 (48.4) |
No more than usual | 33 (41.8) | 31 (38.8) | 64 (40.3) |
Rather more than usual | 6 (7.6) | 10 (12.5) | 16 (10.1) |
Much more than usual | 0 (0.0) | 2 (2.5) | 2 (1.3) |
16. Have you been finding life a struggle all the time?, n/N (%) | |||
Preoperativelya | |||
Not at all | 32 (31.1) | 29 (31.2) | 61 (31.1) |
No more than usual | 50 (48.5) | 38 (40.9) | 88 (44.9) |
Rather more than usual | 17 (16.5) | 23 (24.7) | 40 (20.4) |
Much more than usual | 4 (3.9) | 3 (3.2) | 7 (3.6) |
5 days postoperativelyb | |||
Not at all | 20 (23.0) | 21 (25.3) | 41 (24.1) |
No more than usual | 36 (41.4) | 37 (44.6) | 73 (42.9) |
Rather more than usual | 24 (27.6) | 21 (25.3) | 45 (26.5) |
Much more than usual | 7 (8.0) | 4 (4.8) | 11 (6.5) |
3 months postoperativelyc | |||
Not at all | 47 (59.5) | 43 (53.8) | 90 (56.6) |
No more than usual | 26 (32.9) | 25 (31.3) | 51 (32.1) |
Rather more than usual | 6 (7.6) | 10 (12.5) | 16 (10.1) |
Much more than usual | 0 (0.0) | 2 (2.5) | 2 (1.3) |
17. Have you been able to enjoy your normal day-to-day activities?, n/N (%) | |||
Preoperativelya | |||
More so than usual | 6 (5.8) | 2 (2.2) | 8 (4.1) |
Same as usual | 54 (52.4) | 49 (52.7) | 103 (52.6) |
Less so than usual | 34 (33.0) | 38 (40.9) | 72 (36.7) |
Much less than usual | 9 (8.7) | 4 (4.3) | 13 (6.6) |
5 days postoperativelyb | |||
More so than usual | 8 (9.2) | 5 (6.0) | 13 (7.6) |
Same as usual | 25 (28.7) | 27 (32.5) | 52 (30.6) |
Less so than usual | 35 (40.2) | 29 (34.9) | 64 (37.6) |
Much less than usual | 19 (21.8) | 22 (26.5) | 41 (24.1) |
3 months postoperativelyc | |||
More so than usual | 7 (8.9) | 9 (11.3) | 16 (10.1) |
Same as usual | 53 (67.1) | 50 (62.5) | 103 (64.8) |
Less so than usual | 17 (21.5) | 18 (22.5) | 35 (22.0) |
Much less than usual | 2 (2.5) | 3 (3.8) | 5 (3.1) |
18. Have you been taking things hard?, n/N (%) | |||
Preoperativelya | |||
Not at all | 36 (35.0) | 28 (30.1) | 64 (32.7) |
No more than usual | 47 (45.6) | 48 (51.6) | 95 (48.5) |
Rather more than usual | 18 (17.5) | 15 (16.1) | 33 (16.8) |
Much more than usual | 2 (1.9) | 2 (2.2) | 4 (2.0) |
5 days postoperativelyb | |||
Not at all | 16 (18.4) | 33 (39.8) | 49 (28.8) |
No more than usual | 49 (56.3) | 27 (32.5) | 76 (44.7) |
Rather more than usual | 16 (18.4) | 20 (24.1) | 36 (21.2) |
Much more than usual | 6 (6.9) | 3 (3.6) | 9 (5.3) |
3 months postoperativelyc | |||
Not at all | 46 (58.2) | 40 (50.0) | 86 (54.1) |
No more than usual | 27 (34.2) | 31 (38.8) | 58 (36.5) |
Rather more than usual | 5 (6.3) | 9 (11.3) | 14 (8.8) |
Much more than usual | 1 (1.3) | 0 (0.0) | 1 (0.6) |
19. Have you been getting scared or panicky for no good reason?, n/N (%) | |||
Preoperativelya | |||
Not at all | 51 (49.5) | 40 (43.0) | 91 (46.4) |
No more than usual | 34 (33.0) | 32 (34.4) | 66 (33.7) |
Rather more than usual | 14 (13.6) | 18 (19.4) | 32 (16.3) |
Much more than usual | 4 (3.9) | 3 (3.2) | 7 (3.6) |
5 days postoperativelyb | |||
Not at all | 23 (26.4) | 34 (41.0) | 57 (33.5) |
No more than usual | 44 (50.6) | 24 (28.9) | 68 (40.0) |
Rather more than usual | 16 (18.4) | 19 (22.9) | 35 (20.6) |
Much more than usual | 4 (4.6) | 6 (7.2) | 10 (5.9) |
3 months postoperativelyc | |||
Not at all | 59 (74.7) | 52 (65.0) | 111 (69.8) |
No more than usual | 16 (20.3) | 17 (21.3) | 33 (20.8) |
Rather more than usual | 3 (3.8) | 10 (12.5) | 13 (8.2) |
Much more than usual | 1 (1.3) | 1 (1.3) | 2 (1.3) |
20. Have you been able to face up to your problems?, n/N (%) | |||
Preoperativelya | |||
More so than usual | 9 (8.7) | 7 (7.5) | 16 (8.2) |
Same as usual | 86 (83.5) | 77 (82.8) | 163 (83.2) |
Less able than usual | 7 (6.8) | 8 (8.6) | 15 (7.7) |
Much less able | 1 (1.0) | 1 (1.1) | 2 (1.0) |
5 days postoperativelyb | |||
More so than usual | 5 (5.7) | 2 (2.4) | 7 (4.1) |
Same as usual | 61 (70.1) | 60 (72.3) | 121 (71.2) |
Less able than usual | 17 (19.5) | 19 (22.9) | 36 (21.2) |
Much less able | 4 (4.6) | 2 (2.4) | 6 (3.5) |
3 months postoperativelyc | |||
More so than usual | 8 (10.1) | 5 (6.3) | 13 (8.2) |
Same as usual | 69 (87.3) | 66 (82.5) | 135 (84.9) |
Less able than usual | 2 (2.5) | 9 (11.3) | 11 (6.9) |
21. Have you found everything getting on top of you?, n/N (%) | |||
Preoperativelya | |||
Not at all | 37 (35.9) | 32 (34.4) | 69 (35.2) |
No more than usual | 49 (47.6) | 48 (51.6) | 97 (49.5) |
Rather more than usual | 15 (14.6) | 10 (10.8) | 25 (12.8) |
Much more than usual | 2 (1.9) | 3 (3.2) | 5 (2.6) |
5 days postoperativelyb | |||
Not at all | 24 (27.6) | 28 (33.7) | 52 (30.6) |
No more than usual | 43 (49.4) | 37 (44.6) | 80 (47.1) |
Rather more than usual | 16 (18.4) | 16 (19.3) | 32 (18.8) |
Much more than usual | 4 (4.6) | 2 (2.4) | 6 (3.5) |
3 months postoperativelyc | |||
Not at all | 46 (58.2) | 43 (53.8) | 89 (56.0) |
No more than usual | 28 (35.4) | 29 (36.3) | 57 (35.8) |
Rather more than usual | 5 (6.3) | 8 (10.0) | 13 (8.2) |
22. Have you been feeling unhappy and depressed?, n/N (%) | |||
Preoperativelya | |||
Not at all | 60 (58.3) | 50 (53.8) | 110 (56.1) |
No more than usual | 21 (20.4) | 24 (25.8) | 45 (23.0) |
Rather more than usual | 21 (20.4) | 19 (20.4) | 40 (20.4) |
Much more than usual | 1 (1.0) | 0 (0.0) | 1 (0.5) |
5 days postoperativelyb | |||
Not at all | 29 (33.3) | 28 (33.7) | 57 (33.5) |
No more than usual | 30 (34.5) | 27 (32.5) | 57 (33.5) |
Rather more than usual | 21 (24.1) | 23 (27.7) | 44 (25.9) |
Much more than usual | 7 (8.0) | 5 (6.0) | 12 (7.1) |
3 months postoperativelyc | |||
Not at all | 57 (72.2) | 51 (63.8) | 108 (67.9) |
No more than usual | 15 (19.0) | 16 (20.0) | 31 (19.5) |
Rather more than usual | 3 (3.8) | 13 (16.3) | 16 (10.1) |
Much more than usual | 4 (5.1) | 0 (0.0) | 4 (2.5) |
23. Have you been losing confidence in yourself?, n/N (%) | |||
Preoperativelya | |||
Not at all | 51 (49.5) | 47 (50.5) | 98 (50.0) |
No more than usual | 38 (36.9) | 26 (28.0) | 64 (32.7) |
Rather more than usual | 13 (12.6) | 20 (21.5) | 33 (16.8) |
Much more than usual | 1 (1.0) | 0 (0.0) | 1 (0.5) |
5 days postoperativelyb | |||
Not at all | 26 (29.9) | 36 (43.4) | 62 (36.5) |
No more than usual | 36 (41.4) | 23 (27.7) | 59 (34.7) |
Rather more than usual | 19 (21.8) | 20 (24.1) | 39 (22.9) |
Much more than usual | 6 (6.9) | 4 (4.8) | 10 (5.9) |
3 months postoperativelyc | |||
Not at all | 48 (60.8) | 50 (62.5) | 98 (61.6) |
No more than usual | 20 (25.3) | 17 (21.3) | 37 (23.3) |
Rather more than usual | 10 (12.7) | 11 (13.8) | 21 (13.2) |
Much more than usual | 1 (1.3) | 2 (2.5) | 3 (1.9) |
24. Have you been thinking of yourself as a worthless person?, n/N (%) | |||
Preoperativelya | |||
Not at all | 78 (75.7) | 66 (71.0) | 144 (73.5) |
No more than usual | 17 (16.5) | 23 (24.7) | 40 (20.4) |
Rather more than usual | 8 (7.8) | 2 (2.2) | 10 (5.1) |
Much more than usual | 0 (0.0) | 2 (2.2) | 2 (1.0) |
5 days postoperativelyb | |||
Not at all | 50 (57.5) | 54 (65.1) | 104 (61.2) |
No more than usual | 27 (31.0) | 21 (25.3) | 48 (28.2) |
Rather more than usual | 9 (10.3) | 7 (8.4) | 16 (9.4) |
Much more than usual | 1 (1.1) | 1 (1.2) | 2 (1.2) |
3 months postoperativelyc | |||
Not at all | 64 (81.0) | 63 (78.8) | 127 (79.9) |
No more than usual | 10 (12.7) | 12 (15.0) | 22 (13.8) |
Rather more than usual | 4 (5.1) | 4 (5.0) | 8 (5.0) |
Much more than usual | 1 (1.3) | 1 (1.3) | 2 (1.3) |
25. Have you felt that life is entirely hopeless?, n/N (%) | |||
Preoperativelya | |||
Not at all | 87 (84.5) | 77 (82.8) | 164 (83.7) |
No more than usual | 12 (11.7) | 13 (14.0) | 25 (12.8) |
Rather more than usual | 4 (3.9) | 3 (3.2) | 7 (3.6) |
5 days postoperativelyb | |||
Not at all | 59 (67.8) | 60 (72.3) | 119 (70.0) |
No more than usual | 23 (26.4) | 18 (21.7) | 41 (24.1) |
Rather more than usual | 4 (4.6) | 4 (4.8) | 8 (4.7) |
Much more than usual | 1 (1.1) | 1 (1.2) | 2 (1.2) |
3 months postoperativelyc | |||
Not at all | 71 (89.9) | 66 (82.5) | 137 (86.2) |
No more than usual | 3 (3.8) | 11 (13.8) | 14 (8.8) |
Rather more than usual | 5 (6.3) | 2 (2.5) | 7 (4.4) |
Much more than usual | 0 (0.0) | 1 (1.3) | 1 (0.6) |
26. Have you been feeling hopeful about your own future?, n/N (%) | |||
Preoperativelya | |||
More so than usual | 25 (24.3) | 26 (28.0) | 51 (26.0) |
About the same as usual | 69 (67.0) | 55 (59.1) | 124 (63.3) |
Less so than usual | 7 (6.8) | 9 (9.7) | 16 (8.2) |
Much less hopeful | 2 (1.9) | 3 (3.2) | 5 (2.6) |
5 days postoperativelyb | |||
More so than usual | 27 (31.0) | 36 (43.4) | 63 (37.1) |
About the same as usual | 48 (55.2) | 35 (42.2) | 83 (48.8) |
Less so than usual | 11 (12.6) | 11 (13.3) | 22 (12.9) |
Much less hopeful | 1 (1.1) | 1 (1.2) | 2 (1.2) |
3 months postoperativelyc | |||
More so than usual | 17 (21.5) | 17 (21.3) | 34 (21.4) |
About same as usual | 54 (68.4) | 55 (68.8) | 109 (68.6) |
Less so than usual | 8 (10.1) | 6 (7.5) | 14 (8.8) |
Much less hopeful | 0 (0.0) | 2 (2.5) | 2 (1.3) |
27. Have you been feeling reasonably happy, all things considered?, n/N (%) | |||
Preoperativelya | |||
More so than usual | 11 (10.7) | 6 (6.5) | 17 (8.7) |
About the same as usual | 86 (83.5) | 76 (81.7) | 162 (82.7) |
Less so than usual | 5 (4.9) | 10 (10.8) | 15 (7.7) |
Much less than usual | 1 (1.0) | 1 (1.1) | 2 (1.0) |
5 days postoperativelyb | |||
More so than usual | 18 (20.7) | 20 (24.1) | 38 (22.4) |
About the same as usual | 55 (63.2) | 52 (62.7) | 107 (62.9) |
Less so than usual | 8 (9.2) | 9 (10.8) | 17 (10.0) |
Much less than usual | 6 (6.9) | 2 (2.4) | 8 (4.7) |
3 months postoperativelyc | |||
More so than usual | 15 (19.0) | 15 (18.8) | 30 (18.9) |
About the same as usual | 58 (73.4) | 58 (72.5) | 116 (73.0) |
Less so than usual | 6 (7.6) | 5 (6.3) | 11 (6.9) |
Much less than usual | 0 (0.0) | 2 (2.5) | 2 (1.3) |
28. Have you been feeling nervous and strung up all the time?, n/N (%) | |||
Preoperativelya | |||
Not at all | 56 (54.4) | 39 (41.9) | 95 (48.5) |
No more than usual | 32 (31.1) | 34 (36.6) | 66 (33.7) |
Rather more than usual | 13 (12.6) | 19 (20.4) | 32 (16.3) |
Much more than usual | 2 (1.9) | 1 (1.1) | 3 (1.5) |
5 days postoperativelyb | |||
Not at all | 40 (46.0) | 35 (42.2) | 75 (44.1) |
No more than usual | 31 (35.6) | 30 (36.1) | 61 (35.9) |
Rather more than usual | 14 (16.1) | 15 (18.1) | 29 (17.1) |
Much more than usual | 2 (2.3) | 3 (3.6) | 5 (2.9) |
3 months postoperativelyc | |||
Not at all | 58 (73.4) | 52 (65.0) | 110 (69.2) |
No more than usual | 17 (21.5) | 20 (25.0) | 37 (23.3) |
Rather more than usual | 4 (5.1) | 8 (10.0) | 12 (7.5) |
29. Have you felt that life isn’t worth living?, n/N (%) | |||
Preoperativelya | |||
Not at all | 90 (87.4) | 85 (91.4) | 175 (89.3) |
No more than usual | 10 (9.7) | 8 (8.6) | 18 (9.2) |
Rather more than usual | 2 (1.9) | 0 (0.0) | 2 (1.0) |
Much more than usual | 1 (1.0) | 0 (0.0) | 1 (0.5) |
5 days postoperativelyb | |||
Not at all | 65 (74.7) | 68 (81.9) | 133 (78.2) |
No more than usual | 17 (19.5) | 11 (13.3) | 28 (16.5) |
Rather more than usual | 5 (5.7) | 4 (4.8) | 9 (5.3) |
3 months postoperativelyc | |||
Not at all | 69 (87.3) | 69 (86.3) | 138 (86.8) |
No more than usual | 7 (8.9) | 8 (10.0) | 15 (9.4) |
Rather more than usual | 3 (3.8) | 3 (3.8) | 6 (3.8) |
30. Have you found at times you couldn’t do anything because your nerves were too bad?, n/N (%) | |||
Preoperativelya | |||
Not at all | 81 (78.6) | 75 (80.6) | 156 (79.6) |
No more than usual | 19 (18.4) | 14 (15.1) | 33 (16.8) |
Rather more than usual | 3 (2.9) | 4 (4.3) | 7 (3.6) |
5 days postoperativelyb | |||
Not at all | 57 (65.5) | 60 (72.3) | 117 (68.8) |
No more than usual | 18 (20.7) | 13 (15.7) | 31 (18.2) |
Rather more than usual | 8 (9.2) | 8 (9.6) | 16 (9.4) |
Much more than usual | 4 (4.6) | 2 (2.4) | 6 (3.5) |
3 months postoperativelyc | |||
Not at all | 72 (91.1) | 64 (80.0) | 136 (85.5) |
No more than usual | 6 (7.6) | 13 (16.3) | 19 (11.9) |
Rather more than usual | 1 (1.3) | 1 (1.3) | 2 (1.3) |
Much more than usual | 0 (0.0) | 2 (2.5) | 2 (1.3) |
Characteristic | Randomised to generic algorithm (n = 106) | Randomised to patient-specific algorithm (n = 98) | Overall (n = 204) |
---|---|---|---|
On return from theatre | |||
Temperature (°C), mean (SD)a | 35.9 (0.6) | 35.9 (0.7) | 35.9 (0.6) |
Haematocrit (%), mean (SD)b | 31.1 (4.3) | 30.9 (4.9) | 31.0 (4.6) |
Lactate (mmol/l),c median (IQR) | 1.4 (1.0–1.8) | 1.3 (1.0–1.8) | 1.3 (1.0–1.8) |
MABP (mmHg), mean (SD) | 72.7 (11.0) | 71.2 (12.5) | 72.0 (11.7) |
PaO2 (mmHg), median (IQR)d | 127.0 (95.6–149.0) | 141.0 (95.8–172.0) | 133.0 (95.6–157.0) |
PaCO2 (mmHg), median (IQR)b | 39.0 (34.3–44.0) | 38.0 (33.0–42.0) | 38.2 (34.0–43.0) |
Blood results (first 24 hours) | |||
Lowest haemoglobin (g/dl), median (IQR)c | 9.5 (8.4–10.9) | 9.3 (8.5–11.2) | 9.5 (8.5–11.0) |
Lowest haematocrit (%), mean (SD)e | 28.3 (4.1) | 27.9 (4.4) | 28.1 (4.3) |
Lowest MABP (mmHg), mean (SD)f | 61.8 (8.6) | 60.5 (7.1) | 61.1 (7.9) |
Highest lactate (mmol/l), median (IQR)f | 1.9 (1.7–2.6) | 2.1 (1.5–2.4) | 2.0 (1.6–2.5) |
Blood loss and fluid balance | |||
Fluid balance at 12 hours, median (IQR)d | 741.5 (401.0–1279.0) | 707.0 (335.0–1220.0) | 729.0 (347.0–1222.0) |
Total test tube drainage at 4 hours, median (IQR)d | 237.5 (150.0–400.0) | 225.0 (150.0–400.0) | 225.0 (150.0–400.0) |
Total test tube drainage at 12 hours, median (IQR)g | 400.0 (250.0–600.0) | 400.0 (300.0–600.0) | 400.0 (290.0–600.0) |
Reoperations | |||
Reoperation, n/N (%) | 3/106 (3) | 6/98 (6) | 9/204 (4) |
Type of reoperation, n/N (%) | |||
Chest reopened | 1/3 (33) | 5/6 (83) | 6/9 (67) |
Other | 2/3 (67) | 2/5 (40) | 4/8 (50) |
Reason for chest reopening, n/N (%) | |||
Tamponade | 1/1 (100) | 0/5 (0) | 1/6 (17) |
Bleeding | 0/1 (0) | 4/5 (80) | 4/6 (67) |
Mediastinitis | 0/1 (0) | 0/5 (0) | 0/6 (0) |
Cardiac arrest | 0/1 (0) | 0/5 (0) | 0/6 (0) |
Low cardiac output | 0/1 (0) | 1/5 (20) | 1/6 (17) |
Other | 0/1 (0) | 0/5 (0) | 0/6 (0) |
Reintubated, n/N (%) | 3/106 (3) | 1/98 (1) | 4/204 (2) |
Outcome | Randomised to generic algorithm (n = 106) | Randomised to patient-specific algorithm (n = 98) | Overall (n = 204) |
---|---|---|---|
Mobility, n (%) | |||
Preoperativelya | |||
I have no problems walking about | 60 (58.3) | 58 (63.7) | 118 (60.8) |
I have some problems walking about | 42 (40.8) | 33 (36.3) | 75 (38.7) |
I am confined to bed | 1 (1.0) | 0 (0.0) | 1 (0.5) |
6 weeks postoperativelyb | |||
I have no problems walking about | 64 (72.7) | 54 (66.7) | 118 (69.8) |
I have some problems walking about | 23 (26.1) | 25 (30.9) | 48 (28.4) |
I am confined to bed | 1 (1.1) | 2 (2.5) | 3 (1.8) |
3 months postoperativelyc | |||
I have no problems walking about | 67 (77.0) | 59 (68.6) | 126 (72.8) |
I have some problems walking about | 20 (23.0) | 27 (31.4) | 47 (27.2) |
Self-care, n (%) | |||
Preoperatively | |||
I have no problems with self-care | 94 (91.3) | 84 (92.3) | 178 (91.8) |
I have some problems with self-care | 9 (8.7) | 7 (7.7) | 16 (8.2) |
6 weeks postoperatively | |||
I have no problems with self-care | 75 (85.2) | 72 (88.9) | 147 (87.0) |
I have some problems with self-care | 12 (13.6) | 8 (9.9) | 20 (11.8) |
I am unable to wash and dress myself | 1 (1.1) | 1 (1.2) | 2 (1.2) |
3 months postoperatively | |||
I have no problems with self-care | 82 (94.3) | 79 (91.9) | 161 (93.1) |
I have some problems with self-care | 5 (5.7) | 7 (8.1) | 12 (6.9) |
Usual activities, n (%) | |||
Preoperatively | |||
I have no problems with doing my usual activities | 56 (54.4) | 50 (54.9) | 106 (54.6) |
I have some problems with doing my usual activities | 44 (42.7) | 37 (40.7) | 81 (41.8) |
I am unable to perform my usual activities | 3 (2.9) | 4 (4.4) | 7 (3.6) |
6 weeks postoperatively | |||
I have no problems with doing my usual activities | 33 (37.9) | 21 (26.6) | 54 (32.5) |
I have some problems with doing my usual activities | 46 (52.9) | 52 (65.8) | 98 (59.0) |
I am unable to perform my usual activities | 8 (9.2) | 6 (7.6) | 14 (8.4) |
3 months postoperatively | |||
I have no problems with doing my usual activities | 51 (58.6) | 53 (61.6) | 104 (60.1) |
I have some problems with doing my usual activities | 36 (41.4) | 32 (37.2) | 68 (39.3) |
I am unable to perform my usual activities | 0 (0.0) | 1 (1.2) | 1 (0.6) |
Pain/discomfort, n (%) | |||
Preoperatively | |||
I have no pain or discomfort | 55 (53.4) | 55 (60.4) | 110 (56.7) |
I have moderate pain or discomfort | 46 (44.7) | 34 (37.4) | 80 (41.2) |
I have extreme pain or discomfort | 2 (1.9) | 2 (2.2) | 4 (2.1) |
6 weeks postoperatively | |||
I have no pain or discomfort | 34 (39.1) | 24 (29.6) | 58 (34.5) |
I have moderate pain or discomfort | 53 (60.9) | 54 (66.7) | 107 (63.7) |
I have extreme pain or discomfort | 0 (0.0) | 3 (3.7) | 3 (1.8) |
3 months postoperatively | |||
I have no pain or discomfort | 56 (64.4) | 47 (54.7) | 103 (59.5) |
I have moderate pain or discomfort | 28 (32.2) | 35 (40.7) | 63 (36.4) |
I have extreme pain or discomfort | 3 (3.4) | 4 (4.7) | 7 (4.0) |
Anxiety/depression, n (%) | |||
Preoperatively | |||
I am not anxious or depressed | 71 (68.9) | 66 (72.5) | 137 (70.6) |
I am moderately anxious or depressed | 29 (28.2) | 25 (27.5) | 54 (27.8) |
I am extremely anxious or depressed | 3 (2.9) | 0 (0.0) | 3 (1.5) |
6 weeks postoperativelyb | |||
I am not anxious or depressed | 70 (79.5) | 57 (71.3) | 127 (75.6) |
I am moderately anxious or depressed | 17 (19.3) | 21 (26.3) | 38 (22.6) |
I am extremely anxious or depressed | 1 (1.1) | 2 (2.5) | 3 (1.8) |
3 months postoperativelyc | |||
I am not anxious or depressed | 76 (88.4) | 70 (81.4) | 146 (84.9) |
I am moderately anxious or depressed | 10 (11.6) | 15 (17.4) | 25 (14.5) |
I am extremely anxious or depressed | 0 (0.0) | 1 (1.2) | 1 (0.6) |
Supplemental data: health economic analysis
Resource | Unit costa (£) | Source |
---|---|---|
Cardiac surgery and the intervention | ||
CABG and valve | 7982 | NHS reference costs 2012/13.225 Elective inpatients; HRG code EA51 for service codes 170 (cardiothoracic surgery) and 172 (cardiac surgery). For each code the costs associated with the average LOS reported were subtracted at a cost of £413 per day (see Cardiac ward day) and £1421 was subtracted for blood products based on data from an audit of blood transfusion in cardiac surgery.9 An average cost for the codes was then generated, weighted by activity |
Single valve | 6908 | NHS reference costs 2012/13.225 Elective inpatients; HRG code EA17 (single valve) for service codes 170 and 172. For each code the costs associated with the average LOS reported were subtracted at a cost of £413 per day and £659 was subtracted for blood products based on data from an audit of blood transfusion in cardiac surgery.9 An average cost for the codes was then generated, weighted by activity |
More than one valve | 10,523 | NHS reference costs 2012/13.225 Elective inpatients. HRG code EA52 (more than 1 valve) for service codes 170 and 172. For each code, the costs associated with the average LOS reported were subtracted at a cost of £413 per day, and £659 was subtracted for blood products.9 An average cost for the codes was then generated, weighted by activity |
No valves (no surgical procedure performed except sternotomy) | 5003 | As for ‘Single valve’ but the lower quartile unit cost was used rather than the mean cost |
NIRS machine and sensors | 218.08 | INVOS 5100CKIT Cerebral/ Somatic Oximeter (£7995), Nottingham University Hospital, 2013. Cost of the sensors from University of Leicester, 2013 (price paid during the trial). Cost calculations are described in the main report |
Blood products | ||
Red blood cells | 122.09 | NHS Blood and Transplant price list 2013/14224 |
Red cell administration cost, first unit | 22 | Primary data collection of the nursing time and consumables associated with requesting blood and administering transfusions, undertaken with collaborators on the Trial Of Prophylactic versus no prophylactic platelet transfusions (TOPPS) trial,281 funded by NHS Blood and Transplant. Preliminary analyses show that it takes 49 minutes of nursing time and £6 of consumables to request and administer the first unit of red cells |
Red cell administration cost, subsequent units | 5 | As above; analyses found that it took 15 minutes of nursing time to administer subsequent units (no additional consumables) |
FFP | 27.98 | NHS Blood and Transplant224 |
Platelets | 208.09 | NHS Blood and Transplant224 |
Cryoprecipitate | 193.53 | NHS Blood and Transplant224 |
Clotting factors (Beriplex®; CSL Behring, Haywards Heath, UK) | 425 | Transfusion laboratory at a district general hospital, South Central (personal communication, 2014). Assumed 1500 IU intravenously |
Blood-saving techniques | ||
Intraoperative/postoperative cell salvage | 178 | Using data from Davies et al.15 Costs were inflated using the Hospital and Community Health Services inflation index118 |
Filter for haemofiltration before, during or after CPB | 30 | University Hospitals of Leicester NHS Trust (GR Majithia-Beet, Senior Perfusionist, 3 June 2015, personal communication) |
Tranexamic acid | 15.67 | Assumed 5 g intravenously229 |
Aprotinin (Trasylol®; Nordic Pharma Ltd, Reading, UK) | 320.36 | Using data from the BNF;229 assumed 6 million KIU intravenously |
Inpatient stay | ||
CICU day | 1203 | NHS reference costs 2012/13:225 Critical Care Services – Adult: Critical Care Unit (weighted average of XC01Z–XC06Z, one to six organs supported) |
HDU day | 626 | NHS reference costs 2012/13:225 Critical Care Services – Adult: Critical Care Unit (XC07Z, no organs supported) |
Cardiac ward day | 418 | NHS reference costs 2012/13:225 weighted average of excess bed-day cost [CABG with valve replacement/repair (HRG code EA51), single cardiac valve procedures (EA17), repair/replacement of more than one heart valve (EA52)] |
Ward day for another unit in the hospital or at another hospital | 268 | NHS reference costs 2012/13:225 non-elective inpatient excess bed-day cost across all activities |
Recorded on case report form | Route | Assumed drug | Assumed dose/frequency per day | Daily cost (£) | Source |
---|---|---|---|---|---|
Hypnotics | Oral | Temazepam | 10 mg once a day | 0.14 | Department of Health228 |
Sedatives | IV | Lorazepam | 10 mg once a day | 0.35 | Department of Health228 |
Neuroleptics | IV | Haloperidol | 5 mg once a day | 0.42 | Department of Health228 |
Anxiolytics | Oral | Diazepam | 5 mg three times a day | 0.02 | Department of Health228 |
Antidepressants | Oral | Fluoxetine | 20 mg once a day | 0.01 | Department of Health228 |
Beta-blockers | Oral | Atenolol | 25 mg once a day | 0.01 | Department of Health228 |
Drug name | Route | Assumed dose/frequency per day | Daily cost (£) | Source |
---|---|---|---|---|
Amoxicillin | Oral | 500 mg four times a day | 0.10 | Department of Health228 |
Amoxicillin | IV | 500 mg four times a day | 1.48 | Department of Health228 |
Benzyl-penicillin | IV | 1.2 g four times a day | 7.64 | BNF229 |
Cefuroxime | IV | 750 mg three times a day | 1.39 | Department of Health228 |
Ciprofloxacin | Oral | 500 mg twice a day | 0.05 | Department of Health228 |
Ciprofloxacin | IV | 400 mg twice a day | 2.04 | Department of Health228 |
Clarithromycin | Oral | 250 mg twice a day | 0.13 | Department of Health228 |
Clarithromycin | IV | 500 mg twice a day | 5.30 | Department of Health228 |
Co-amoxiclav | Oral | 375 mg three times a day | 0.21 | Department of Health228 |
Co-amoxiclav | IV | 600 mg three times a day | 1.66 | Department of Health228 |
Doxycycline | Oral | 200 mg first day, 100 mg once a day subsequent days | 0.07 day 1, 0.03 thereafter | Department of Health228 |
Flucloxacillin | Oral | 250 mg four times a day | 0.11 | Department of Health228 |
Flucloxacillin | IV | 0.25 g four times a day | 1.70 | Department of Health228 |
Gentamicin | IV | 80 mg three times a day | 1.59 | Department of Health228 |
Meropenem | IV | 0.5 g three times a day | 7.95 | Department of Health228 |
Metronidazole | Oral | 400 mg three times a day | 0.05 | Department of Health228 |
Metronidazole | IV | 500 mg three times a day | 1.21 | Department of Health228 |
Penicillin V | Oral | 1 g four times a day | 0.25 | Department of Health228 |
Piperacillin/tazobactam | IV | 4.5 g three times a day | 5.74 | Department of Health228 |
Teicoplanin | IV | 400 mg twice a day for three doses, subsequently 400 mg once a day | 12.38 day 1, 6.19 thereafter | Department of Health228 |
Trimethoprim | Oral | 200 mg twice a day | 0.03 | Department of Health228 |
Vancomycin | IV | 0.5 g twice a day | 2.35 | Department of Health228 |
Complication | Treatment/action | Total cost (£) | Assumptions |
---|---|---|---|
Sepsis | No additional treatment | 0 | Antibiotics recorded separately and costed |
Wound infection | No additional treatment | 0 | Antibiotics recorded separately and costed |
Reoperation | 8233 (9670 if as a readmission) | ||
Re-intubation/ventilation | Transoesophageal echocardiogram, three chest radiographs | 399 | |
Suspected MI | Emergency angiography, transthoracic echocardiogram, electrocardiogram | 1889 | |
Cardiac arrest | Cardiopulmonary resuscitation | 1508 | |
SVT/AF requiring treatment | Amiodarone – 1.2 g IV, then 200 mg orally three times a day for 1 week and two times a day for 1 week | 4.84 | |
VF/VT requiring intervention | Transoesophageal echocardiogram, emergency coronary angiography, chest radiography | 2029 | Emergency reoperations and re-intubation captured elsewhere |
Pacing (temporary) | 3107 | ||
Permanent pacemaker implanted | Permanent pacemaker | 14,726 | |
Inotropes | Noradrenaline (norepinephrine) – 1 mg/hour for 5 days | 57.94 | |
IABP | 2807 | ||
PAC | 1577 | ||
Vasodilator | Glyceryl trinitrate – 25 mg IV for 3 days | 6.69 | |
Low cardiac output | Transoesophageal echocardiogram, chest radiography (and IABP if IABP/PAC not already recorded) | 316 (+2807) | |
Tracheostomy | Tracheostomy, chest radiography | 5414 | |
Mask CPAP | CPAP, chest radiography | 545 | |
Pneumothorax or effusion requiring drainage | Chest radiography, chest drain | 4265 | |
Haemofiltration (assume for 2 days) | 1454 | ||
Permanent stroke | Rehabilitation (plus scan) | 141 | |
Computerised tomography scan | 63 | ||
Magnetic resonance imaging | 251 | ||
Peptic ulcer/GI bleed/perforation | Endoscopy | 684 | |
Other (GI complications) – Ileus | Computerised tomography scan | 63 | |
Other (GI complications) – laparoscopy | Laparoscopy | 2723 | |
Deep-vein thrombosis | Duplex scan of leg veins, intraveneous heparin, warfarin | 206.05 | |
Excess bleeding | Chest radiography | 41 | |
Wound dehiscence requiring rewiring/treatment | Minor treatment | 163 | |
Drainage of pus under local anaesthesia | 431 |
Treatment/action | Unit cost (£) | Source |
---|---|---|
24-hour Holter monitor | 206 | NHS reference costs 2012/13:225 Day Cases – EA47Z Electrocardiogram Monitoring and Stress Testing, code 320 (cardiology), lower quartile cost |
Adrenaline (epinephrine; 0.5 mg IV, three injections) | 0.13 | Department of Health228 |
Antibiotics (piperacillin/tazobactam, 4.5 g IV three times a day for 5 days) | 28.72 | Department of Health228 |
Amiodarone (1.2 g IV, then 200 mg orally three times a day for 1 week and two times a day for 1 week) | 4.84 | Department of Health228 |
Chest drain | 4224 | NHS reference costs 2012/13225 |
Chest radiography | 41 | John Radcliffe Hospital, 2012. Costs have been inflated using the Hospital and Community Health Services inflation index118 |
Coronary angiography | 1713 | NHS reference costs 2012/13225 |
CPAP | 504 | Costs have been inflated using the Hospital and Community Health Services inflation index102 |
Cardiopulmonary resuscitation | 1508 | NHS reference costs 2012/13225 |
Computerised tomography scan | 63 | NHS reference costs 2012/13:225 Diagnostic Imaging – Direct Access. RA08A Computerised Tomography Scan, one area, no contrast, 19 years and over, code 100 (general surgery) |
Diazepam (10 mg IV once) | 0.38 | Department of Health228 |
Diuretics (furosemide, 40 mg orally for 5 days) | 0.15 | BNF229 |
Diuretics – higher dose (furosemide, 80 mg orally for 5 days) | 0.30 | BNF229 |
Drainage of pus under local anaesthesia | 431 | NHS reference costs 2012/13:225 Elective inpatients. JC43A Minor Skin Procedures, 13 years and over, code 320 (cardiology) |
Duplex scan of leg veins | 157 | NHS reference costs 2012/13:225 Diagnostic Imaging – Outpatients. RA10Z Computerised Tomography Scan, one area, pre and post contrast, code 172 (cardiac surgery) |
Electrocardiogram | 54 | NHS reference costs 2012/13:225 Directly Accessed Diagnostic Services. EA47Z Electrocardiogram Monitoring and Stress Testing |
Echocardiogram – transthoracic | 122 | NHS reference costs 2012/13:225 Diagnostic Imaging – Outpatients. RA60A Simple Echocardiogram, 19 years and over, code 172 (cardiac surgery) |
Echocardiogram – transoesophageal | 275 | NHS reference costs 2012/13:225 Day Cases. EA45Z Complex Echocardiogram, including Transoesophageal and Fetal Echocardiography, code 320 (cardiology), lower quartile cost |
Endoscopy | 684 | NHS reference costs 2012/13225 |
Glyceryl trinitrate (25 mg IV for 3 days) | 6.69 | Department of Health228 |
Haemofiltration (assume for 2 days) | 1454 | NHS reference costs 2012/13:225 Renal Dialysis at Base. LE01A Haemodialysis for Acute Kidney Injury, 19 years and over |
IABP | 2807 | NICE.282 Costs have been inflated using the Hospital and Community Health Services inflation index118 |
Inotropes | 57.94 | Department of Health228 |
Intravenous fluids (Gelofusine®, B Braun Medical Ltd, Sheffield, UK; 1500 ml) | 13.64 | BNF229 |
Intravenous heparin (initially 5000 units, then 15,000 units every 12 hours for 5 days) | 47.96 | BNF229 |
Omeprazole (40 mg IV for 3 days, then 40 mg orally daily for 5 days) | 12.82 | BNF,229 Department of Health228 |
Lansoprazole (30 mg daily) | 0.03 daily | Department of Health228 |
Laparoscopy | 2723 | As laparotomy |
Laparotomy | 2723 | NHS reference costs 2012/13225 |
Levetiracetam (1 g per day) | 0.26 daily | Department of Health228 |
Midazolam (10 mg IV once) | 0.20 | Department of Health228 |
Minor treatment for wound dehiscence | 163 | NHS reference costs 2012/13:225 Elective inpatients. JC43A Minor Skin Procedures, 13 years and over, code 320 (cardiology), with the costs associated with the average LOS reported subtracted at a cost of £265 per day |
Magnetic resonance imaging | 251 | NHS reference costs 2012/13:225 Diagnostic Imaging – Direct Access. RA07Z Magnetic Resonance Imaging Scan, requiring extensive patient repositioning and/or more than one contrast agent, code 320 (cardiology) |
Permanent pacemaker | 14,726 | NHS reference costs 2012/13225 |
Phenytoin (1000 mg IV, followed by 100 mg IV three times a day for 3 days) | 6.96 | Department of Health228 |
Physiotherapy/rehabilitation | 141 | NHS reference costs 2012/13:225 Elective inpatients. DZ30Z Chest Physiotherapy, code 340 (respiratory medicine) |
Pulmonary artery catheter | 1577 | NHS reference costs 2012/13:225 Elective inpatients. EA36H Catheter with CC Score 0–1, code 320 (cardiology) |
Reoperation | 8233 (9670 if as a readmission) | NHS reference costs 2012/13:225 Elective inpatients. HRG code EA20 for service codes 170 and 172. For each code the costs associated with the average LOS reported were subtracted at a cost of £413 per day and £1421 was subtracted for blood products.9 An average cost for the codes was then generated, weighted by activity. For reoperations in readmissions, the costs of blood products was not subtracted (as they were not captured during follow-up) |
Temporary pacemaker | 3107 | NHS reference costs 2012/13:225 Elective inpatients. EA39B Pacemaker Procedure without Generator Implant, including Re-siting and Removal of Cardiac Pacemaker System, with CC Score 2–4, code 320 (cardiology), with the costs associated with the average LOS reported subtracted at a cost of £265 per day |
Tracheostomy | 5372 | NHS reference costs 2012/13225 |
Ultrasound | 68 | NHS reference costs 2012/13:225 Diagnostic Imaging – Outpatients. Weighted average of RA25Z Ultrasound Mobile Scan or Intraoperative Procedures, less than 20 minutes; RA26Z Ultrasound Mobile Scan or Intraoperative Procedures, 20 to 40 minutes; and RA27Z Ultrasound Mobile Scan or Intraoperative Procedures, more than 40 minutes. Code 100 (general surgery) |
Warfarin (3 mg daily) | 0.03 daily | BNF229 |
Resource | Unit cost (£) | Reference |
---|---|---|
Ward day for readmissions | 268 | NHS reference costs 2012/13:225 Non-elective inpatient excess bed-day cost across all activities |
ICU day for readmissions | 1181 | NHS reference costs 2012/13:225 Critical Care Services – Adult: Critical Care Unit (weighted average of XC01Z–XC07Z, 0–6 organs supported) |
A&E attendance, leading to admission | 156 | NHS reference costs 2012/13:225 Accident and Emergency Services, Excluding Dental Care. Weighted average of all admitted codes |
A&E attendance, not leading to admission | 102 | NHS reference costs 2012/13:225 Accident and Emergency Services, Excluding Dental Care. Weighted average of all non-admitted codes |
Ambulance to hospital | 233 | NHS reference costs 2012/13:225 Ambulance Services. ASS02 See and Treat and Convey |
Specialty | Unit cost (£) | Service code | Reference |
---|---|---|---|
Anticoagulation service | 25 | 324 | These were all sourced from NHS reference costs 2012–13.225 All represent the average cost for each specialty (from the Total – Outpatient Attendances page, Total activity) |
Cardiac rehabilitation | 42 | 327 | |
Cardiac surgery | 302 | 172 | |
Cardiology | 132 | 320 | |
Clinical haematology | 153 | 303 | |
Geriatric medicine | 206 | 430 | |
Gastroenterology | 139 | 301 | |
Ophthalmology | 87 | 130 | |
Pain management | 140 | 191 | |
Physiotherapy | 42 | 650 | |
Rehabilitation | 91 | 314 | |
Respiratory medicine | 152 | 340 | |
Stroke clinic | 202 | 328 | |
Urology | 102 | 101 |
Resource | Unit cost (£) | Source |
---|---|---|
GP at surgery | 34 | Curtis:227 10.8b, GP – unit costs. Per-patient contact lasting 11.7 minutes, excluding qualification costs and direct-care staff costs |
GP at home | 86 | Curtis:227 10.8b, GP – unit costs. Per out of surgery visit lasting 23.4 minutes, excluding qualification costs and direct-care staff costs |
Out-of-hours GP centre | 34 | As GP at surgery |
NHS walk-in centre | 34 | As GP at surgery |
GP nurse (at surgery) | 11.50 | Curtis:227 10.6, Nurse (GP practice). £44 per hour of face-to-face contact, excluding qualification costs. Average contact 15.5 minutes |
District/community nurse (at home) | 39 | Curtis:227 10.1, Community nurse. Using data from NHS reference costs 2011/12,283 the mean average cost for a face-to-face contact in district nursing services for 2012/13 was £39, with an IQR of £33–46. Costs were uprated using the Hospital and Community Health Services pay and prices inflator118 |
Other NHS or social services | ||
Cardiac rehabilitation/exercise class | 42 | National Schedule of Reference Costs 2012–13:225 Total – Outpatient Attendances, code 327 (Cardiac Rehabilitation) |
Cardiac nurse | 71 | National Schedule of Reference Costs 2012–13:225 Community Health Services – Nursing. N11AF Specialist Nursing – Cardiac Nursing/Liaison, Adult, Face to Face |
Anticoagulation service | 10 | National Schedule of Reference Costs 2012–13:225 Non Consultant Led Outpatient Attendances; Non-Admitted Non-Face to Face Attendance, Follow-up, code 324 (Anticoagulation Service) |
Physiotherapist | 34 | Curtis:227 13.1, Hospital physiotherapist. Using data from NHS reference costs 2011/12,283 the mean average cost for a non-consultant-led (non-admitted) follow-up physiotherapy attendance was £34, with an IQR of £28–38. Costs were uprated using the Hospital and Community Health Services pay and prices inflator118 |
Social worker | 88.16 | Curtis:227 11.2, Social worker (adult services). £159 per hour of face-to-face contact, excluding qualification costs. Contact assumed to be for 32.9 minutesa |
Other NHS or social services at home | ||
Cardiac rehabilitation/nurse | 71 | National Schedule of Reference Costs 2012–13:225 Community Health Services – Nursing. N11AF Specialist Nursing – Cardiac Nursing/Liaison, Adult, Face to Face |
Carer | 8.59 | Curtis:227 11.6, Home care worker. The mean hourly cost of all home care including local authority-funded and independent provision was £17. Just over half of local authority-funded visits lasted 30 minutes; 16% of visits lasted for 15 minutes and 19% of a home care worker’s time was spent travelling. Visit assumed to last for 30 minutes |
Intensive support team | 69 | National Schedule of Reference Costs 2012–13:225 Community Health Services – Nursing. N06AF – Specialist Nursing – Active Case Management (Community Matrons), Adult, Face to Face |
Occupational therapist | 74 | Curtis:227 9.2, NHS community occupational therapist. Using data from NHS reference costs 2011/12,283 the mean average cost of one-to-one contact with occupational therapy services was £73, with an IQR of £50–86. Costs were uprated using the Hospital and Community Health Services pay and prices inflator118 |
Paramedic | 176 | National Schedule of Reference Costs 2012–13:225 Ambulance Services. ASS01 See and Treat or Refer |
Physiotherapist | 48 | Curtis:227 9.1, Community physiotherapist. Using data from NHS reference costs 2011/12, the mean average cost of one-to-one contact with physiotherapy services was £47, with an IQR of £37–52. Costs were uprated using the Hospital and Community Health Services pay and prices inflator |
Psychiatric nurse | 61 | National Schedule of Reference Costs 2012–13:225 Community Health Services – Nursing. N29AF Other Specialist Nursing, Adult, Face to Face |
Social worker | 88.16 | Curtis:227 11.2, Social worker (adult services). £159 per hour of face-to-face contact time, excluding qualification costs. Contact assumed to be for 32.9 minutesa |
Consolidated Standards of Reporting Trials (CONSORT) statement checklist: PASPORT trial
Section/topic | Number | Item | Reported on page number |
---|---|---|---|
Title and abstract | |||
1a | Identification as a randomised trial in the title | 167 | |
1b | Structured summary of trial design, methods, results and conclusions (for specific guidance see CONSORT for Abstracts) | 167 | |
Introduction | |||
Background and objectives | 2a | Scientific background and explanation of rationale | 167 |
2b | Specific objectives or hypotheses | 169 | |
Methods | |||
Trial design | 3a | Description of trial design (such as parallel, factorial) including allocation ratio | 170 |
3b | Important changes to methods after trial commencement (such as eligibility criteria), with reasons | 170 | |
Participants | 4a | Eligibility criteria for participants | 170 |
4b | Settings and locations where the data were collected | 171 | |
Interventions | 5 | The interventions for each group with sufficient details to allow replication, including how and when they were actually administered | 171 |
Outcomes | 6a | Completely defined prespecified primary and secondary outcome measures, including how and when they were assessed | 172 |
6b | Any changes to trial outcomes after the trial commenced, with reasons | 167 | |
Sample size | 7a | How sample size was determined | 173 |
7b | When applicable, explanation of any interim analyses and stopping guidelines | 174 | |
Randomisation | |||
Sequence generation | 8a | Method used to generate the random allocation sequence | 174 |
8b | Type of randomisation; details of any restriction (such as blocking and block size) | 174 | |
Allocation concealment mechanism | 9 | Mechanism used to implement the random allocation sequence (such as sequentially numbered containers), describing any steps taken to conceal the sequence until interventions were assigned | 174 |
Implementation | 10 | Who generated the random allocation sequence, who enrolled participants and who assigned participants to interventions | 174 |
Blinding | 11a | If done, who was blinded after assignment to interventions (e.g. participants, care providers, those assessing outcomes) and how | 174 |
11b | If relevant, description of the similarity of interventions | NA | |
Statistical methods | 12a | Statistical methods used to compare groups for primary and secondary outcomes | 178 |
12b | Methods for additional analyses, such as subgroup analyses and adjusted analyses | 178 | |
Results | |||
Participant flow (a diagram is strongly recommended) | 13a | For each group, the numbers of participants who were randomly assigned, received intended treatment and were analysed for the primary outcome | 179 |
13b | For each group, losses and exclusions after randomisation, together with reasons | 180 | |
Recruitment | 14a | Dates defining the periods of recruitment and follow-up | 179 |
14b | Why the trial ended or was stopped | NA | |
Baseline data | 15 | A table showing baseline demographic and clinical characteristics for each group | 182 |
Numbers analysed | 16 | For each group, the number of participants (denominator) included in each analysis and whether the analysis was by original assigned groups | 180 |
Outcomes and estimation | 17a | For each primary and secondary outcome, results for each group and the estimated effect size and its precision (such as 95% confidence interval) | 186 |
17b | For binary outcomes, presentation of both absolute and relative effect sizes is recommended | NA | |
Ancillary analyses | 18 | Results of any other analyses performed, including subgroup analyses and adjusted analyses, distinguishing prespecified from exploratory | 193 |
Harms | 19 | All important harms or unintended effects in each group (for specific guidance see CONSORT for Harms) | 204 |
Discussion | |||
Limitations | 20 | Trial limitations, addressing sources of potential bias, imprecision and, if relevant, multiplicity of analyses | 242 |
Generalisability | 21 | Generalisability (external validity, applicability) of the trial findings | 244 |
Interpretation | 22 | Interpretation consistent with results, balancing benefits and harms and considering other relevant evidence | 244 |
Other information | |||
Registration | 23 | Registration number and name of trial registry | 170 |
Protocol | 24 | Where the full trial protocol can be accessed, if available | 170 |
Funding | 25 | Sources of funding and other support (such as supply of drugs), role of funders | 289 |
Preferred Reporting Items for Systematic Reviews and Meta-Analyses (PRISMA) checklist: near-infrared spectroscopy systematic review
Section/topic | Number | Item | Reported on page number |
---|---|---|---|
Title | |||
Title | 1 | Identify the report as a systematic review, meta-analysis or both | 167 |
Abstract | |||
Structured summary | 2 | Provide a structured summary including, as applicable, background; objectives; data sources; study eligibility criteria, participants and interventions; study appraisal and synthesis methods; results; limitations; conclusions and implications of key findings; systematic review registration number | 167 |
Introduction | |||
Rationale | 3 | Describe the rationale for the review in the context of what is already known | 167 |
Objectives | 4 | Provide an explicit statement of questions being addressed with reference to participants, interventions, comparisons, outcomes and study design (PICOS) | 219 |
Methods | |||
Protocol and registration | 5 | Indicate if a review protocol exists and if and where it can be accessed (e.g. web address) and, if available, provide registration information including registration number | 219 |
Eligibility criteria | 6 | Specify study characteristics (e.g. PICOS, length of follow-up) and report characteristics (e.g. years considered, language, publication status) used as criteria for eligibility, giving rationale | 219 |
Information sources | 7 | Describe all information sources (e.g. databases with dates of coverage, contact with study authors to identify additional studies) in the search and date last searched | 220 |
Search | 8 | Present full electronic search strategy for at least one database, including any limits used, such that it could be repeated | 220 |
Study selection | 9 | State the process for selecting studies (i.e. screening, eligibility, included in systematic review and, if applicable, included in the meta-analysis) | 220 |
Data collection process | 10 | Describe method of data extraction from reports (e.g. piloted forms, independently, in duplicate) and any processes for obtaining and confirming data from investigators | 221 |
Data items | 11 | List and define all variables for which data were sought (e.g. PICOS, funding sources) and any assumptions and simplifications made | 219 |
Risk of bias in individual studies | 12 | Describe methods used for assessing risk of bias of individual studies (including specification of whether this was done at the study or outcome level) and how this information is to be used in any data synthesis | 221 |
Summary measures | 13 | State the principal summary measures (e.g. risk ratio, difference in means) | 222 |
Synthesis of results | 14 | Describe the methods of handling data and combining results of studies, if done, including measures of consistency (e.g. I2) for each meta-analysis | 222 |
Risk of bias across studies | 15 | Specify any assessment of risk of bias that may affect the cumulative evidence (e.g. publication bias, selective reporting within studies) | 222 |
Additional analyses | 16 | Describe methods of additional analyses (e.g. sensitivity or subgroup analyses, meta-regression), if done, indicating which were prespecified | 222 |
Results | |||
Study selection | 17 | Give numbers of studies screened, assessed for eligibility and included in the review, with reasons for exclusions at each stage, ideally with a flow diagram | 223 |
Study characteristics | 18 | For each study, present characteristics for which data were extracted (e.g. study size, PICOS, follow-up period) and provide the citations | 223 |
Risk of bias within studies | 19 | Present data on risk of bias of each study and, if available, any outcome-level assessment (see Item 12) | 229 |
Results of individual studies | 20 | For all outcomes considered (benefits or harms), present, for each study: (a) simple summary data for each intervention group and (b) effect estimates and confidence intervals, ideally with a forest plot | 231 |
Synthesis of results | 21 | Present results of each meta-analysis done, including confidence intervals and measures of consistency | 231 |
Risk of bias across studies | 22 | Present results of any assessment of risk of bias across studies (see Item 15) | 240 |
Additional analysis | 23 | Give results of additional analyses, if done [e.g. sensitivity or subgroup analyses, meta-regression (see Item 16)] | 240 |
Discussion | |||
Summary of evidence | 24 | Summarise the main findings including the strength of evidence for each main outcome; consider their relevance to key groups (e.g. health-care providers, users and policy-makers) | 240 |
Limitations | 25 | Discuss limitations at the study and outcome level (e.g. risk of bias) and at the review level (e.g. incomplete retrieval of identified research, reporting bias) | 244 |
Conclusions | 26 | Provide a general interpretation of the results in the context of other evidence and implications for future research | 244 |
Funding | |||
Funding | 27 | Describe sources of funding for the systematic review and other support (e.g. supply of data); role of funders for the systematic review | 289 |
Consolidated Health Economic Evaluation Reporting Standards (CHEERS) checklist: PASPORT trial
Section/item | Number | Recommendation | Reported on page number |
---|---|---|---|
Title and abstract | |||
Title | 1 | Identify the study as an economic evaluation or use more specific terms such as ‘cost-effectiveness analysis’ and describe the interventions compared | 167 |
Abstract | 2 | Provide a structured summary of objectives, perspective, setting, methods (including study design and inputs), results (including base-case and uncertainty analyses) and conclusions | 167 |
Introduction | |||
Background and objectives | 3 | Provide an explicit statement of the broader context for the study | 167 |
Present the study question and its relevance for health policy or practice decisions | |||
Methods | |||
Target population and subgroups | 4 | Describe characteristics of the base-case population and subgroups analysed, including why they were chosen | 208 |
Setting and location | 5 | State relevant aspects of the system(s) in which the decision(s) need(s) to be made | 208 |
Study perspective | 6 | Describe the perspective of the study and relate this to the costs being evaluated | 208 |
Comparators | 7 | Describe the interventions or strategies being compared and state why they were chosen | 208 |
Time horizon | 8 | State the time horizon(s) over which costs and consequences are being evaluated and say why appropriate | 208 |
Discount rate | 9 | Report the choice of discount rate(s) used for costs and outcomes and say why appropriate | 209 |
Choice of health outcomes | 10 | Describe what outcomes were used as the measure(s) of benefit in the evaluation and their relevance for the type of analysis performed | 209 |
Measurement of effectiveness | 11a | Single study-based estimates: Describe fully the design features of the single effectiveness study and why the single study was a sufficient source of clinical effectiveness data | 208 |
11b | Synthesis-based estimates: Describe fully the methods used for identification of included studies and synthesis of clinical effectiveness data | NA | |
Measurement and valuation of preference-based outcomes | 12 | If applicable, describe the population and methods used to elicit preferences for outcomes | NA |
Estimating resources and costs | 13a | Single study-based economic evaluation: Describe approaches used to estimate resource use associated with the alternative interventions. Describe primary or secondary research methods for valuing each resource item in terms of its unit cost. Describe any adjustments made to approximate to opportunity costs | 209 |
13b | Model-based economic evaluation: Describe approaches and data sources used to estimate resource use associated with model health states. Describe primary or secondary research methods for valuing each resource item in terms of its unit cost. Describe any adjustments made to approximate to opportunity costs | NA | |
Currency, price date and conversion | 14 | Report the dates of the estimated resource quantities and unit costs. Describe methods for adjusting estimated unit costs to the year of reported costs if necessary. Describe methods for converting costs into a common currency base and the exchange rate | 209 |
Choice of model | 15 | Describe and give reasons for the specific type of decision-analytical model used. Providing a figure to show model structure is strongly recommended | 210 |
Assumptions | 16 | Describe all structural or other assumptions underpinning the decision-analytical model | 210 |
Analytical methods | 17 | Describe all analytical methods supporting the evaluation. This could include methods for dealing with skewed, missing or censored data; extrapolation methods; methods for pooling data; approaches to validate or make adjustments (such as half-cycle corrections) to a model; and methods for handling population heterogeneity and uncertainty | 210 |
Results | |||
Study parameters | 18 | Report the values, ranges, references and, if used, probability distributions for all parameters. Report reasons or sources for distributions used to represent uncertainty where appropriate. Providing a table to show the input values is strongly recommended | 211 |
Incremental costs and outcomes | 19 | For each intervention, report mean values for the main categories of estimated costs and outcomes of interest, as well as mean differences between the comparator groups. If applicable, report incremental cost-effectiveness ratios | 211 |
Characterising uncertainty | 20a | Single study-based economic evaluation: Describe the effects of sampling uncertainty for the estimated incremental cost and incremental effectiveness parameters, together with the impact of methodological assumptions (such as discount rate, study perspective) | 215 |
20b | Model-based economic evaluation: Describe the effects on the results of uncertainty for all input parameters and uncertainty related to the structure of the model and assumptions | NA | |
Characterising heterogeneity | 21 | If applicable, report differences in costs, outcomes or cost-effectiveness that can be explained by variations between subgroups of participants with different baseline characteristics or other observed variability in effects that are not reducible by more information | 218 |
Discussion | |||
Study findings, limitations, generalisability and current knowledge | 22 | Summarise key study findings and describe how they support the conclusions reached. Discuss limitations and the generalisability of the findings and how the findings fit with current knowledge | 218 |
Other | |||
Source of funding | 23 | Describe how the study was funded and the role of the funder in the identification, design, conduct and reporting of the analysis. Describe other non-monetary sources of support | 289 |
Conflicts of interest | 24 | Describe any potential for conflict of interest of study contributors in accordance with journal policy. In the absence of a journal policy, we recommend authors comply with International Committee of Medical Journal Editors recommendations | i |
Appendix 4 The REDWASH trial
Changes to the REDWASH trial after trial commencement
Changes made to the protocol264 after study commencement are summarised in Table 140. The study was stopped prematurely by the funder because of slow recruitment after 60 participants had been consented, of whom 56 entered the study. The analysis was performed on these 56 consented participants. The primary outcome was confirmed as the serum cytokine IL-8 level, with repeated measures at four post-surgery time points: on return to the ICU and at 6–12, 24 and 48 hours postoperatively. The 96-hour time point described in the original protocol was omitted from the analysis. The definition of adult respiratory distress syndrome was changed from the acute lung injury definition to the Berlin definition:268 ratio of partial pressure of oxygen in arterial blood (PaO2) to inspired oxygen concentration (FiO2) of < 300 mmHg, with a continuous positive airway pressure or positive end-expiratory pressure of > 5 cmH2O. Blood loss was recorded at 4 and 12 hours rather than at 6 hours, as stated in the protocol. The study did not measure granulocyte macrophage colony-stimulating factor, interferon-γ, IL-1β, IL-2, IL-4, IL-5 and IL-10, as stated in the protocol. Instead, levels of IL-6 and TNF-α were recorded as planned and MIP-1 and MCP-1 were recorded in addition to reflect monocyte and endothelial cell activation, as per our proposed mechanism hypothesis.
Amendment number | Previous version | Previous date | New version | New date | Brief summary of change |
---|---|---|---|---|---|
1 | 1.0 | 26/10/2012 | 2.0 | 22/04/2013 |
|
2 | 2.0 | 22/04/2013 | 3.0 | 04/08/2013 |
|
3 | 3.0 | 04/08/2013 | 4.0 | 16/09/2013 |
|
Consolidated Standards of Reporting Trials (CONSORT) statement checklist: REDWASH trial
Section/topic | Item number | Item | Reported on page number |
---|---|---|---|
Title and abstract | |||
1a | Identification as a randomised trial in the title | 247 | |
1b | Structured summary of trial design, methods, results and conclusions (for specific guidance see CONSORT for Abstracts) | 247 | |
Introduction | |||
Background and objectives | 2a | Scientific background and explanation of rationale | 247 |
2b | Specific objectives or hypotheses | 249 | |
Methods | |||
Trial design | 3a | Description of trial design (such as parallel, factorial) including allocation ratio | 249 |
3b | Important changes to methods after trial commencement (such as eligibility criteria), with reasons | 249 | |
Participants | 4a | Eligibility criteria for participants | 250 |
4b | Settings and locations where the data were collected | 250 | |
Interventions | 5 | The interventions for each group with sufficient details to allow replication, including how and when they were actually administered | 250 |
Outcomes | 6a | Completely defined prespecified primary and secondary outcome measures, including how and when they were assessed | 251 |
6b | Any changes to trial outcomes after the trial commenced, with reasons | 257 | |
Sample size | 7a | How sample size was determined | 257 |
7b | When applicable, explanation of any interim analyses and stopping guidelines | NA | |
Randomisation | |||
Sequence generation | 8a | Method used to generate the random allocation sequence | 252 |
8b | Type of randomisation; details of any restriction (such as blocking and block size) | 252 | |
Allocation concealment mechanism | 9 | Mechanism used to implement the random allocation sequence (such as sequentially numbered containers), describing any steps taken to conceal the sequence until interventions were assigned | 252 |
Implementation | 10 | Who generated the random allocation sequence, who enrolled participants and who assigned participants to interventions | 253 |
Blinding | 11a | If carried out, who was blinded after assignment to interventions (e.g. participants, care providers, those assessing outcomes) and how | 254 |
11b | If relevant, description of the similarity of interventions | NA | |
Statistical methods | 12a | Statistical methods used to compare groups for primary and secondary outcomes | 257 |
12b | Methods for additional analyses, such as subgroup analyses and adjusted analyses | 257 | |
Results | |||
Participant flow (a diagram is strongly recommended) | 13a | For each group, the numbers of participants who were randomly assigned, received the intended treatment and were analysed for the primary outcome | 257 |
13b | For each group, losses and exclusions after randomisation, together with reasons | 258 | |
Recruitment | 14a | Dates defining the periods of recruitment and follow-up | 258 |
14b | Why the trial ended or was stopped | 257 | |
Baseline data | 15 | A table showing baseline demographic and clinical characteristics for each group | 260 |
Numbers analysed | 16 | For each group, the number of participants (denominator) included in each analysis and whether the analysis was by original assigned groups | 258 |
Outcomes and estimation | 17a | For each primary and secondary outcome, results for each group and the estimated effect size and its precision (such as 95% CI) | 263 |
17b | For binary outcomes, presentation of both absolute and relative effect sizes is recommended | NA | |
Ancillary analyses | 18 | Results of any other analyses performed, including subgroup analyses and adjusted analyses, distinguishing prespecified from exploratory | 270 |
Harms | 19 | All important harms or unintended effects in each group (for specific guidance see CONSORT for Harms) | 270 |
Discussion | |||
Limitations | 20 | Trial limitations, addressing sources of potential bias, imprecision and, if relevant, multiplicity of analyses | 280 |
Generalisability | 21 | Generalisability (external validity, applicability) of the trial findings | 281 |
Interpretation | 22 | Interpretation consistent with results, balancing benefits and harms and considering other relevant evidence | 281 |
Other information | |||
Registration | 23 | Registration number and name of trial registry | 249 |
Protocol | 24 | Where the full trial protocol can be accessed, if available | 249 |
Funding | 25 | Sources of funding and other support (such as supply of drugs); role of funders | 289 |
Glossary
- Acute kidney injury
- A rapid deterioration of renal function, resulting in an inability to maintain fluid, electrolyte and acid–base balance. It is detected and monitored primarily by serial serum creatinine readings, which rise acutely.
- Acute lung injury
- A syndrome diagnosed by hypoxaemia, attributable to lung inflammation.
- Anaemia
- A decrease in the amount of red blood cells or haemoglobin in the blood. It is often defined clinically as a blood haemoglobin concentration of < 12g/dl.
- Area under the curve
- Area under the receiver operating characteristic curve. A measure of predictive accuracy.
- Bias in randomised trials
- Factors in the selection, randomisation, blinding, implementation of the intervention or control, assessment of outcomes or reporting of randomised controlled trials that question the validity of the results.
- Calibration
- A measure of whether observed event rates match expected event rates in a test or validation population.
- Cardiopulmonary bypass
- A technique that temporarily takes over the function of the heart and lungs during surgery, maintaining the circulation of blood and the oxygen content of the body.
- Clinical effectiveness
- A measure of the degree of beneficial effect under ‘real-world’ clinical settings that reflect routine clinical practice, expressed by the effect on outcomes that are essential for clinical decision-making.
- Clinical efficacy
- A measure of whether or not an intervention or test produces the expected effect on a disease or measured clinical outcome under ideal circumstances.
- Cost-effectiveness analysis
- A form of economic evaluation in which the difference in costs between the alternatives (the incremental cost) is compared with the difference in outcomes (the incremental effectiveness), with outcomes measured in natural health-related units.
- Decision modelling
- This compares the expected costs and outcomes of alternative interventions by defining an explicit set of consequences for each intervention, their likelihood and associated costs and outcomes.
- Discrimination
- The ability of a test to discriminate between the target condition and full health. This discriminative potential can be quantified by measures of diagnostic accuracy such as sensitivity and specificity, predictive values, likelihood ratios, the area under the receiver operating characteristic curve and diagnostic odds ratio.
- Endothelial activation
- A pro-inflammatory and pro-coagulant state of the endothelial cells lining the lumen of blood vessels. It is most characterised by an increase in interactions with white blood cells (leucocytes).
- Incremental cost-effectiveness ratio
- A statistic used in cost-effectiveness analysis that presents the cost per unit of health outcome gained by using a new intervention compared with an existing intervention. It is calculated by dividing the difference in mean costs between the alternatives in the population of interest by the difference in mean outcomes in the population of interest.
- Index test
- The test that is being evaluated.
- Large-volume blood transfusion
- Transfusion of four or more units of allogenic red cells. This is a marker of severe bleeding and a predictor for adverse clinical outcomes including death.
- Life-years gained
- The number of years of life gained by using a new intervention compared with an existing intervention.
- Meta-analysis
- Statistical techniques used to combine the results of two or more studies and obtain a combined estimate of effect.
- Microvesicles
- Fragments of plasma membrane ranging from 100 nm to 1000 nm shed from almost all cell types.
- Mixed venous oxygen
- A direct measure of the oxygen saturation in venous blood. Used to calculate oxygen utilisation when used alongside measures of arterial oxygen content or as an index of oxygen extraction.
- Multiple Organ Dysfunction Score (MODS)
- An objective scale to measure the severity of the multiple organ dysfunction syndrome, constructed using simple physiological measures of dysfunction in six organ systems.
- Near-infrared spectroscopy
- The use of differential absorbance of near-infrared light by chromophores to measure changes in the ratio of chromophore concentrations. A common clinical application is the measurement of changes in the relative proportions of oxyhaemoglobin and deoxyhaemoglobin in a sample.
- Negative predictive value
- The probability that subjects with a negative screening test truly do not have the disease.
- Neurocognitive dysfunction
- A reduction or impairment of function in one of the neurocognitive domains that consider perception, defragmentation of concepts, memory embeddedness, association and recall, in both the thought process and behaviour.
- Oxidative stress
- An imbalance between the production of free radicals and the ability of the body to counteract or detoxify their harmful effects through neutralisation by antioxidants.
- Oxygen consumption/extraction
- A measure of the oxygen utilised by tissues, often expressed as a ratio or percentage of that delivered.
- Oxygen delivery
- A measure of the volume of oxygen carried in blood to the tissues/organs every minute.
- Patient Analysis and Tracking System
- Audit system designed by Dendrite Clinical Systems and widely used for data collection as part of the National Audit of Blood Transfusion in Adult Cardiac Surgery.
- Performance bias
- A bias in outcomes found in clinical trials without blinding and attributable to behavioural responses by subjects or researchers to the knowledge that a subject is in or is not in a control group.
- Phosphatidylserine
- A phospholipid found in cell membranes, often expressed on the external surface of microvesicles.
- Platelet activation
- A complex phenomenon characterised by the release of granules that amplify activation, inflammation and coagulation, the release of thromboxane 2, shape change and the expression of surface markers that facilitate aggregation, including von Willebrand factor receptors and glycoprotein IIb/IIIa.
- Positive predictive value
- The probability that subjects with a positive screening test truly have the disease.
- Prediction study
- Study that evaluates the ability of a variable to predict an outcome.
- Prospective observational cohort study
- A study that follows over time a group of similar individuals (cohorts) who differ with respect to certain factors under study, to determine how these factors affect the rate of a certain outcome.
- Randomised controlled trial
- A type of scientific (often medical) experiment in which the people being studied are randomly allocated to one or other of the different treatments under study.
- Receiver operating characteristic curve
- A graph that illustrates the trade-offs between sensitivity and specificity that result from varying the diagnostic threshold.
- Red cell transfusion
- One unit of red blood cells corresponds to approximately 250 ml of packed allogenic red cells with a haematocrit of approximately 60%. Transfusion raises the circulating haemoglobin level by approximately 1 g/dl.
- Reference outcome
- The best currently available definition of the disease or event against which the index test is compared.
- Sensitivity
- The proportion of people with the target disorder who have a positive test result.
- Specificity
- The proportion of people without the target disorder who have a negative test result.
- Systematic review
- A literature review focused on a research question that tries to identify, appraise, select and synthesise all of the high-quality research evidence relevant to that question.
- Viscoelastic test
- A test that uses a viscoelastic method, either thromboelastometry or thromboelastography, to test for haemostasis.
List of abbreviations
- A5
- amplitude 5 minutes after clotting time
- A10
- amplitude 10 minutes after clotting time
- A30
- amplitude 30 minutes after clotting time
- A&E
- accident and emergency
- ACT
- activated clotting/coagulation time
- ADP
- adenosine diphosphate
- AKI
- acute kidney injury
- APC
- allophycocyanin
- aPTT
- activated partial thromboplastin time
- ASPI
- arachidonic acid
- AU
- aggregation unit
- AUC
- area under the curve
- BMI
- body mass index
- BNF
- British National Formulary
- BRiSc
- Papworth Bleeding Risk Score
- CABG
- coronary artery bypass graft
- CATS
- Continuous AutoTransfusion System
- CCB
- clinical concern about bleeding
- CE
- European Conformity
- CENTRAL
- Cochrane Central Register of Controlled Trials
- CHEERS
- Consolidated Health Economic Evaluation Reporting Standards
- CI
- confidence interval
- CICU
- cardiac intensive care unit
- CINAHL
- Cumulative Index to Nursing and Allied Health Literature
- CK
- celite kaolin
- CKH
- celite kaolin heparinase
- CONSORT
- Consolidated Standards of Reporting Trials
- COPD
- chronic obstructive pulmonary disease
- COPTIC
- COagulation and Platelet laboratory Testing in Cardiac surgery
- COWAT
- Controlled Oral Word Association Test
- CPB
- cardiopulmonary bypass
- CS-1
- connecting segment-1
- CT
- clotting time
- CVA
- cerebrovascular accident
- DAPT
- dual antiplatelet therapy
- eGFR
- estimated glomerular filtration rate
- ELISA
- enzyme-linked immunosorbent assay
- EQ-5D
- EuroQol-5 Dimensions
- EQ-5D-3L
- EuroQol-5 Dimensions three-level version
- ETP
- endogenous thrombin potential
- FFP
- fresh-frozen plasma
- FITC
- fluorescein isothiocyanate
- FP
- fractional polynomial
- GHQ-30
- General Health Questionnaire
- GLM
- generalised linear model
- GMR
- geometric mean ratio
- GP
- general practitioner
- GRADE
- Grades of Recommendation, Assessment, Development and Evaluation
- HADS
- Hospital Anxiety and Depression Scale
- HDU
- high-dependency unit
- HR
- hazard ratio
- HTA
- Health Technology Assessment
- IABP
- intra-aortic balloon pump
- ICER
- incremental cost-effectiveness ratio
- ICU
- intensive care unit
- IL
- interleukin
- IQR
- interquartile range
- KDIGO
- Kidney Disease: Improving Global Outcomes
- KIM-1
- kidney injury molecule-1
- LFABP
- liver-type fatty acid-binding protein
- LOCOCV
- leave-one-centre-out cross-validation
- LOS
- length of stay
- LRT
- laboratory reference test
- LVBT
- large-volume blood transfusion
- MA
- maximum amplitude
- MBT
- massive blood transfusion
- MCF
- maximum clot firmness
- MCP-1
- monocyte chemotactic protein-1
- MD
- mean difference
- MEA
- multiple electrode aggregometry
- MI
- myocardial infarction
- MIP-1
- macrophage inflammatory protein-1
- MPV
- mean platelet volume
- NACSA
- National Adult Cardiac Surgery Audit
- NART
- National Adult Reading Test
- NGAL
- neutrophil gelatinase-associated lipocalin
- NICE
- National Institute for Health and Care Excellence
- NIHR
- National Institute for Health Research
- NIRS
- near-infrared spectroscopy
- NRI
- net reclassification improvement
- NSTS
- NHS Strategic Tracing Service
- OLS
- ordinary least squares
- OR
- odds ratio
- PAC-1
- platelet-activating complex-1
- PaCO2
- partial pressure of carbon dioxide in arterial blood
- PAHR
- pressure-adjusted heart rate
- PASPORT
- PAtient-SPecific Oxygen monitoring to Reduce blood Transfusion during heart surgery
- PBS
- phosphate-buffered saline
- PE
- phycoerythrin
- POC
- point of care
- PPI
- patient and public involvement
- PRISMA
- Preferred Reporting Items for Systematic Reviews and Meta-Analyses
- PT
- prothrombin time
- PTCA
- percutaneous transluminal coronary angioplasty
- QALY
- quality-adjusted life-year
- RAVLT
- Rey Auditory Verbal Learning Test
- RBC
- Reducing Bleeding in Cardiac Surgery
- RCT
- randomised controlled trial
- REC
- research ethics committee
- RECESS
- Red Cell Storage Duration Study
- rFVIIa
- recombinant activated factor VII
- ROC
- receiver operating characteristic
- ROTEM
- rotational thromboelastometry
- RR
- risk ratio
- SD
- standard deviation
- SE
- standard error
- SIRS
- systemic inflammatory response syndrome
- SLT
- standard laboratory test
- SMD
- standardised mean difference
- STARD
- Standards for Reporting Diagnostic Accuracy
- STEMI
- ST elevation myocardial infarction
- STROBE
- Strengthening the Reporting of Observational Studies in Epidemiology
- SvO2
- mixed venous oxygen saturation
- TEG
- thromboelastography
- TIA
- transient ischaemic attack
- TITRe2
- Transfusion Indication Threshold Reduction
- TNF-α
- tumour necrosis factor-α
- TRACK
- Transfusion Risk and Clinical Knowledge
- TRAP
- thrombin receptor-activating peptide
- TRUST
- Transfusion Risk Understanding Scoring Tool
- VLA5
- very late antigen 5
- vWF
- von Willebrand factor
- WAIS
- Wechsler Adult Intelligence Scale
- WTAR
- Wechsler Test of Adult Reading