Notes
Article history
The research reported in this issue of the journal was funded by PGfAR as project number RP-PG-0609-10195. The contractual start date was in June 2011. The final report began editorial review in November 2017 and was accepted for publication in November 2018. As the funder, the PGfAR programme agreed the research questions and study designs in advance with the investigators. The authors have been wholly responsible for all data collection, analysis and interpretation, and for writing up their work. The PGfAR editors and production house have tried to ensure the accuracy of the authors’ report and would like to thank the reviewers for their constructive comments on the final report document. However, they do not accept liability for damages or losses arising from material published in this report.
Declared competing interests of authors
Anne Spaight reports grants from East Midlands Ambulance Service NHS Trust during the conduct of the study. Steve Goodacre is a member of the Health Technology Assessment (HTA) Clinical Trials Board, HTA Funding Boards Policy Group and HTA IP Methods Group. Helen Snooks is a member of National Institute for Health Research HTA and Efficacy and Mechanism Evaluation Editorial Board.
Permissions
Copyright statement
© Queen’s Printer and Controller of HMSO 2019. This work was produced by Turner et al. under the terms of a commissioning contract issued by the Secretary of State for Health and Social Care. This issue may be freely reproduced for the purposes of private research and study and extracts (or indeed, the full report) may be included in professional journals provided that suitable acknowledgement is made and the reproduction is not associated with any form of advertising. Applications for commercial reproduction should be addressed to: NIHR Journals Library, National Institute for Health Research, Evaluation, Trials and Studies Coordinating Centre, Alpha House, University of Southampton Science Park, Southampton SO16 7NS, UK.
2019 Queen’s Printer and Controller of HMSO
SYNOPSIS
Background
The NHS provides emergency care for a diverse population of patients who need medical care for a wide range of conditions and have different levels of urgency. For many patients, the first point of contact with the NHS is when they request help from the ambulance service.
Demand for ambulance services has been steadily increasing for many years. In 1974, ambulance services in England responded to 1.5 million emergency (‘999’) calls per year. By 2016/17 this had increased to > 6 million responses to almost 10 million calls. 1 In the past, the main purpose of the ambulance service was to respond to serious emergencies and transport patients to the nearest hospital emergency department (ED). As the number of calls has grown, so has the type of health problems people call 999 for.
Ambulance services now provide care for conditions that range from life-threatening emergencies, such as heart attacks, stroke and serious injury, a wide range of illnesses and problems associated with chronic disease and long-term conditions, to relatively minor illness or injury. In response to these changes, ambulance services have also adapted and developed.
In 2005, the Department of Health and Social Care policy document Taking Healthcare to the Patient: Transforming NHS Ambulance Services2 recognised that patients who call 999 should receive care that not only is timely but also best meets their clinical needs. Patients with serious medical emergencies and injury still need a fast response, early treatment and good clinical care at the scene, followed by transport to hospital. However, research evidence also showed that, for some patients with less urgent problems, a trip to the ED was not always necessary and their needs could be better met by providing the care at home or in a community service. 3
As a result of the 2005 report, and subsequent broader policy initiatives focusing on delivering care closer to home for urgent problems and developing expert centres for specialist care such as stroke units and major trauma centres for emergencies,4 there have been a variety of innovations in types of response provided by the ambulance service. These types of response now fall into three main categories:
-
More detailed clinical telephone assessment of some 999 calls by nurses or paramedics so that patients who do not need an emergency ambulance can be provided with self-care advice or referred to the right service – ‘hear and treat’.
-
Development of paramedic skills including advanced paramedic practitioners so they can treat minor illness and injury at home and, when needed, refer to other services for follow-up care, for example community falls services – ‘see and treat’.
-
Improving prehospital assessment and care pathways so that patients who need specialist care can be taken straight to the best facility (e.g. a stroke or heart attack centre) and other patients taken to the nearest ED – ‘see and convey’.
The changes in the way that ambulance services provide care have contributed to broader efforts to improve emergency and urgent care by developing a more ‘joined up’ system approach in which ambulance, accident and emergency (A&E), and community services work in partnership to ensure that patients can access and receive care in the right place and at the right time. 4
These changes also need to reflect the NHS principles set out in the Next Stage Review,5 which are to provide high-quality services through improving effectiveness, safety and patient experience. If these aims are to be achieved, then the assessment and monitoring of the quality of services and the wider system in which they operate become important tasks. 6 This means that we need ways of measuring how well ambulance services are performing, in terms of both the services they are delivering and the impact that these services have on the patients that they care for.
Quality assessment and quality improvement can be achieved only if we can find ways of routinely and consistently measuring these important aspects of patient care so that we can identify where care is good and where it needs to be improved. Measuring performance and quality of care is never easy but is particularly difficult for ambulance services, as they provide care to such a diverse group of patients and those patients will be in their care for a relatively short period of time only.
For many years, the quality of ambulance service care has been mainly assessed by measuring how quickly they respond to 999 calls – response time performance. This is not unique to the UK and has been the predominant performance or quality measure for Emergency Medical Services (EMS) internationally. 7 Response time has been used as a proxy measure because of the relationship between speed of response and survival following out-of-hospital cardiac arrest – the faster the response, the more likely a patient is to survive. 8 However, cardiac arrest accounts for a very small proportion of 999 calls only, currently 0.6% in England,9 and research evidence shows that, for other calls, the speed of response has little impact on survival or outcomes. 10–12 Response time also tells us nothing about what clinical care was given or the impact of that care on patient outcomes so, overall, it is a poor measure of service quality for the vast majority of patients who request an ambulance.
The need to develop better ways of measuring ambulance service performance and quality of care, particularly the effect on patient outcomes that are relevant to all people who use the service, not just a few, has been recognised for some time. This need was a key recommendation of Taking Healthcare to the Patient: Transforming NHS Ambulance Services2 and also a high priority in a UK Delphi study assessing priorities for prehospital care research. 13 Despite this recognition, a review of outcome measurement in prehospital care found few research studies investigating this area and found that most published literature focused on discussion of the need to develop measures rather than any solution to the problem. 14
In England, some progress has been made with the introduction of a set of 11 ambulance service quality indicators adopted in 2011. 15 Having a set of indicators, rather than just a single measure of response time performance, that had relevance to all calls was a big step forward, as was the inclusion of a small number of clinical indicators designed to measure the care provided for some key conditions like cardiac arrest, stroke and heart attack. 16 However, the focus remained on processes [what the ambulance service did in terms of response times, type of service (e.g. how many calls are managed using ‘hear and treat’) and providing treatment for a small number of conditions] rather than how care affected patients. It is also fair to say that these indicators or measures have mainly been developed by health professionals and academics so may not include aspects of care that are important to patients.
There has been a major problem holding back the development of better ways of measuring how well ambulance services provide care to the population who call 999 and that was the lack of information available to them about what happens to patients and their outcome once they had left ambulance care. If the only information they have is their own data about how they respond and what they do then, it is not surprising that these are the only things that were measured. These data are also incident rather than patient based, which makes it difficult to monitor related calls for the same patient. However, if ambulance service information about patients could be linked to, for example, hospital information, so that they have a more complete picture of what happens to patients and their outcomes, then a better assessment could be made of the impact of care and the benefits it produced. Creating linked data sets can also potentially add value by creating comprehensive information sources that can be used to provide feedback on outcomes and improve performance and practice, but this was not something that was being done routinely.
This programme was designed to try and resolve these problems by bringing together the two key themes of:
-
developing more meaningful ambulance service performance and quality measures that reflect the key principles of quality, safety and effectiveness of care
-
creating linked data sets that can combine ambulance service, hospital and mortality data to provide the information needed to support better performance and quality measurement.
Even with a linked data set that includes patient outcomes, measuring impact is problematic. Outcomes can be influenced by a whole range of factors in addition to ambulance care received, such as a patient’s age, illness severity or hospital care. One way of overcoming this is to develop performance measures that can take account of these intrinsic and extrinsic factors by using risk or case-mix adjustment models. These have been successfully developed and used for research and audit in settings such as trauma17 and intensive care18 and have shown they can make an important difference to improving processes and outcomes of care.
Aims and objectives
The aim of this programme was to develop new ways of measuring the impact of care provided by ambulance services. This could support quality improvement by providing information to monitor and audit service performance and assess the impact of change through service evaluation. The programme was called PhOEBE (Prehospital Outcomes for Evidence Based Evaluation).
Its objectives were to:
-
review and synthesise the research literature on prehospital care outcome measures and use consensus methods to identify a small set of measures relevant to the NHS and patients for further development
-
create a data set linking routinely collected prehospital (ambulance service) data, hospital data and mortality data to provide outcome information
-
use the linked data to develop the measures identified in objective 1 by building case-mix adjustment models that could potentially be used to assess ambulance service performance and detect change over time with repeated measurement
-
explore the practical use of the linked data set and the case-mix adjustment models to measure the effectiveness and quality of ambulance service care and assess how they can be best used to support quality improvement strategies.
Programme design
The programme was designed to be conducted in four linked stages or workstreams (Figure 1).
-
Workstream 1: a review and synthesis of the evidence on ambulance service-related performance and quality measures to produce a list of potential measures. Then use consensus methods to assess and prioritise these measures and to identify a small number that are relevant to different stakeholders, including patients and NHS staff, research academics and patient representatives for further development.
-
Workstream 2: linking ambulance service call information and patient information from the care record completed by ambulance clinicians with routine ED and hospital information [Hospital Episode Statistics (HES)] and national records of patient deaths. This creates a single data set that follows what happens to each patient who makes a 999 call.
-
Workstream 3: explore and develop case-mix-adjusted models for processes and outcomes in patients attended by the ambulance service using the linked data. This allows us to assess whether or not case-mix adjustment is needed to improve the usefulness of process or outcome measures, and their potential as indicators to measure quality and performance between services or within services over time.
-
Workstream 4: testing the risk adjustment models to assess if they can be used to measure effectiveness and quality. This looks at how they might be used in practice and explores with users (patients and staff) how useful they are and how they might be best implemented in the NHS. In addition, the linked data can be used to estimate the costs of different types of ambulance response.
FIGURE 1.
The PhOEBE programme overview. APC, admitted patient care; CAD, computer-aided dispatch; ePRF, electronic patient report form; ONS, Office for National Statistics.

Figures 1 and 2 provide a summary of the four workstreams and how they are linked together.
FIGURE 2.
Workstream 1. PPI, patient and public involvement.
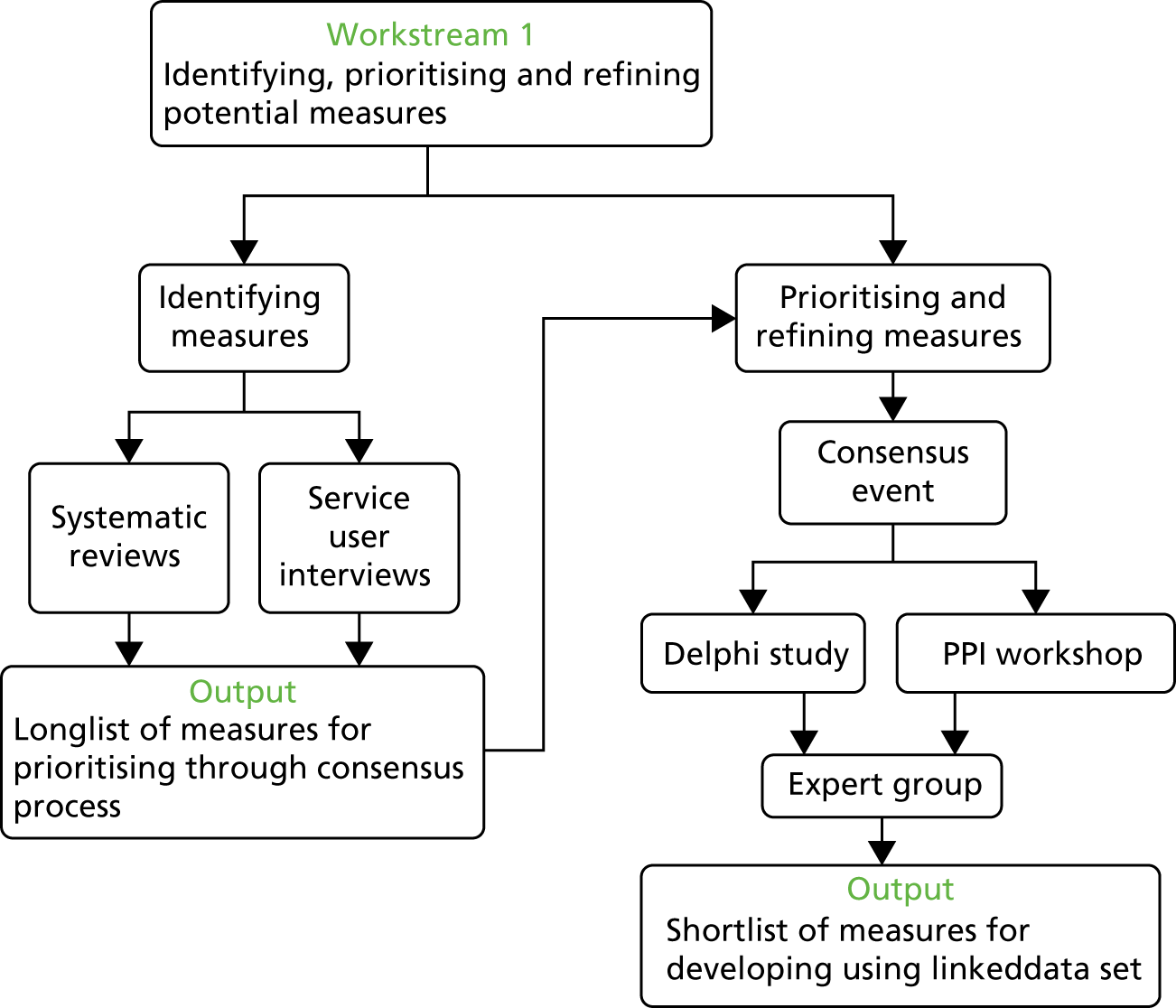
The programme was overseen and supported by a project management group that included all of the programme collaborators, the research team, representatives from the two ambulance services taking part and a public involvement member. We also had a project steering committee comprising key members of the management group and external advisors representing ambulance services, the College of Paramedics, NHS commissioners and emergency care research. The programme was carried out over the 6-year period of 2011–17.
Public involvement
Public involvement made an important contribution to the PhOEBE programme. At the outset we formed a public involvement reference group of three members who provided substantial support and input to each stage of the programme, both individually and by providing links to other relevant external groups. Our public involvement reference group not only contributed advice but also co-produced some of the programme work and outputs. We describe the patient involvement work in more detail under Patient and public involvement below.
Changes from the original proposal
During the course of the programme we encountered a major problem during workstream 2. The data linkage component was contracted to the then Health and Social Care Information Centre (HSCIC) (now NHS Digital). NHS Digital held the central HES data and also provided a trusted data linkage service. We planned to use this service to link the ambulance service, hospital and Office for National Statistics (ONS) mortality data. The plan was to obtain the first set of linked data by the end of year 2 and a second set in year 3 (2013–14).
During 2013, major data security issues arose at NHS Digital and, as a result, there was a major review and restructuring of the organisation. This meant that no data were released for (any) research use for almost 2 years. These issues are described in more detail in Creating a linked data set but the overall impact was that, because of these external delays, we did not receive a linked data set until October 2016 – 4 months after the original expected end date of the programme. We were fortunate to obtain a 1-year extension to the programme, which enabled us to complete the workstream 3 work to develop the risk-adjusted measures, albeit within a much reduced time frame.
The delays meant that we were unable to conduct much of the planned work for workstream 4, principally because the second linked data set that we had intended to use to test and validate the measures developed in workstream 3 arrived too late and we had no time left to complete this. The implications of these delays and the difficulties in obtaining and managing the linked data are discussed in more detail in Discussion and conclusions.
Identifying potential measures to assess ambulance service performance and quality of care
The overall aim of this first workstream (see Figure 2) was to explore, as broadly as possible, the range of potential measures that might be used to assess ambulance service performance and quality of care and then, through a consensus process, reduce this down to those suitable for further development as risk-adjusted measures. This was achieved using a stepwise process of five different activities:
-
Two systematic searches and syntheses of the relevant literature to identify candidate measures.
-
A qualitative study with recent users of the ambulance service to identify which aspects of ambulance service care were important to patients and carers.
-
A consensus event at which we presented the outputs from steps 1 and 2 to a group of people representing different interests in ambulance service care and asked participants to rate the importance of the potential measures.
-
The highest-scoring measures from the consensus event were then developed into more detailed measures and a Delphi survey and a patient and public involvement (PPI) event were conducted to further rate and prioritise them.
-
A review and assessment of the results from step 4 by the programme steering group to identify the final small set of measures for development in workstream 3.
Systematic searches and review of related research evidence
We conducted two systematic searches to review, assess and synthesise the research literature for existing and potential process and patient outcome measures for prehospital care. These were not conventional systematic reviews in that we were not appraising evidence of the effects of prehospital care. The aim was to identify all measures that had been used to assess the impact, quality and safety of prehospital care as well as potential and as yet untested measures, using systematic searching and evidence synthesis strategies. We conducted two reviews so that we could examine both policy literature and primary research evidence.
Review 1: documentary analysis of policy documents
The first review was designed to identify actual and aspirational quality and performance measures of ambulance and prehospital care. We used a comprehensive search strategy to search four electronic databases: MEDLINE, Scirus, Scopus and Google Scholar (see Appendix 1, Table 15). We also searched relevant websites such as the Department of Health and Social Care,19 National Association of Emergency Medical Services Physicians20 and NHS Confederation. 21 We supplemented the searches with our own extensive archive from previous related research studies. Any policy documents produced by national, regional or professional organisations or agencies were included, but these were limited to those in the English language published between 2000 and 2011 to ensure relevance. Searches were conducted in August 2011. The results of the searches are given in Figure 3.
FIGURE 3.
The Preferred Reporting Items for Systematic Reviews and Meta-Analyses (PRISMA) flow chart for review 1. Reproduced from Moher et al. 22 © 2009 Moher et al. This is an open-access article distributed under the terms of the Creative Commons Attribution License, which permits unrestricted use, distribution, and reproduction in any medium, provided the original author and source are credited.
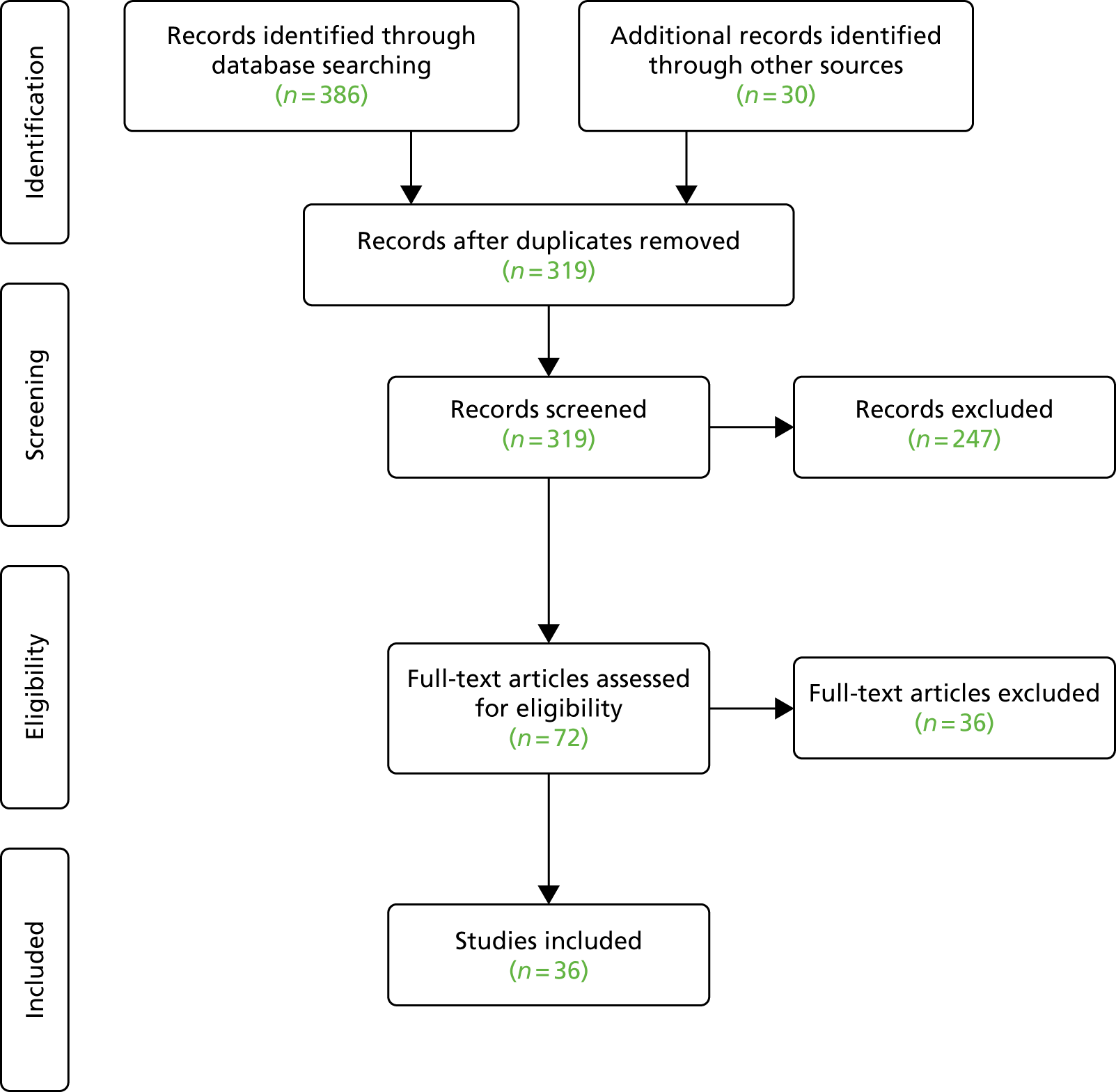
References were screened by six members of the research team. We screened 319 potential references, assessed 72 full-text papers and included 36 documents.
Double data extraction of included references was carried out by the same six researchers and the measures identified were classified using established frameworks of health-care quality (structure, process and outcome23 and timeliness; efficiency; effectiveness; safety; patient centredness and equity24).
Of the included references, the majority were discussion documents. Some were specific to ambulance services (also known as EMS), setting out the case for the inadequacies of using response times as a performance measure and the need to find alternatives, but stopping short of providing specific alternatives. Others were strategy documents for the management of specific conditions (primarily stroke, coronary heart disease or major trauma) that contained a section on prehospital management with suggestions for potential quality measures, for example time from a call to arriving at a specialist unit for stroke patients.
Of the documents describing performance measures in use or suggestions for measures, these documents were, unsurprisingly, dominated by time measures. After time measures, the most common measures were also process related, mainly recording what ambulance clinicians did to either assess patients or provide treatments. Service measures included types of response (e.g. if a paramedic was sent), how accurately a clinical problem was identified at the time of the emergency call and how calls were managed (e.g. the proportion managed at home and taken to hospital). There were few examples of patient outcome measures and this category was dominated by survival from cardiac arrest. Several documents supported the need to measure patient experience and satisfaction and some identified relief of symptoms, such as pain as important, but there were no examples of methods to do this routinely through quality measures.
Review 2: systematic literature search and synthesis of primary research studies
For the second review, we conducted a systematic literature search and synthesis of longitudinal studies, audits and evaluations of ambulance services (or EMS) performed at a local, regional or national level. The aim was to identify potential performance and quality measures that may have been used to assess differences between, or change in, the delivery of ambulance service care in primary research projects. Some of these may not have been considered as routine measures but could potentially be developed and adapted for this purpose.
We conducted a systematic search of five electronic databases: MEDLINE, EMBASE, CINAHL (Cumulative Index to Nursing and Allied Health Literature), ISI Web of Science and The Cochrane Library (see Appendix 1, Table 16). This was supplemented with references identified in review 1, hand-searching of included studies and articles from our own relevant archive. Any relevant research study that had investigated ambulance service delivery and care from a service perspective and incorporated some measurement of change was included.
We were aware that there was an enormous amount of related research literature for specific patient groups, particularly cardiac arrest and trauma, which could potentially overwhelm our search. Much of this research is about the clinical management of patients and its effectiveness. We therefore excluded studies for which the primary aim was to assess a specific clinical intervention or if it was a descriptive study. The key focus here was comparative research and the measurement of change. Included studies were limited to those in the English language and published between 2000 and 2011. Searches were conducted in October 2011.
As in review 1, references were screened by six members of the research team. For included studies, data extraction was completed using a two-stage process. As review 2 yielded a larger number of studies than review 1, double data extraction was carried out for a 10% random sample of references. For the first stage, six reviewers extracted descriptive information on study aim, population, setting and the main process or outcome measures used. For the second stage, three reviewers carried out a more detailed data extraction on each measure identified including the type of measure, how it was measured and how it was reported.
We screened 5088 references by title and abstract, reviewed 257 full-text references and included 139 references. We identified 136 different measures that were recorded 483 times in the included studies. The results of the searches are given in Figure 4.
FIGURE 4.
The Preferred Reporting Items for Systematic Reviews and Meta-Analyses (PRISMA) flow chart for review 2. Reproduced from Moher et al. 22 © 2009 Moher et al. This is an open-access article distributed under the terms of the Creative Commons Attribution License, which permits unrestricted use, distribution, and reproduction in any medium, provided the original author and source are credited.

We classified measures into three broad groups: service (operational) measures, patient management measures and patient outcomes (see Appendix 1, Boxes 5–7). The largest group was service measures (41%), which mainly included a large number of time-interval measurements. This group also included call handling, skill level of response and type of response (e.g. transported or not transported). The patient management group accounted for 29% of measures and these were mainly concerned with clinical procedures and interventions, such as assessing symptoms and condition and treatment provided (e.g. drugs given, splinting, defibrillation and oxygen therapy).
It also included decisions about where to take patients (i.e. to the nearest ED or to a specialist hospital) and subsequent hospital measures, such as length of stay or where a patient was discharged to. The third group of patient outcomes included 30% of the identified measures but this was dominated by the single measure of survival (or mortality), which accounted for more than half of the measures. The reason for this is that there were many different end points for measuring survival ranging from < 1 day up to 5 years. A small number of functional measures were recorded, which included quality-of-life measures, physical disability and cognitive (brain) function. Some examples of the types of measures included in each category are provided in Table 1.
Category | Measure |
---|---|
Clinical management | Accuracy of call-taker identification of different conditions (e.g. cardiac arrest, heart attack, stroke, low-urgency calls suitable for nurse advice) |
Proportion of people with diabetes mellitus treated at home | |
Accuracy of paramedic diagnosis, for example agreement of on-scene and final hospital diagnosis | |
Compliance with protocols and guidelines (e.g. triage or transport protocols) | |
Whole system | Completeness and accuracy of patient records |
Frequency with which ambulance staff administer treatments (e.g. inserting breathing tubes, heart monitoring, oxygen therapy, defibrillation) | |
Proportion of all calls that receive an ambulance response with patients who are not conveyed to hospital/other health facility | |
Volume and nature of complaints | |
Patient outcomes | Survival at different time points after the event. For example, in hospital, 24 hours, 7 days, 30 days, 90 days, 1 year |
Health/quality-of-life status | |
Proportion of patients left at home who have a contact with any emergency/urgent health service within 72 hours | |
Pain measurement and symptom relief |
Example search strategies for each review and a table of the categorisation of the 136 included measures are provided in Appendix 1. Further details on the findings and individual measures identified in the two reviews and the final list of combined measures identified from both reviews is provided in a supplementary file available at www.sheffield.ac.uk/scharr/sections/hsr/mcru/phoebe/reports. 25
An update of the searches in 2016 did not reveal any new measures that need to be considered. In our original proposal we said that we would conduct a third systematic review of any tools or instruments that we identified that had been specifically constructed to measure performance for prehospital care. However, we found no relevant instruments and the only composite measures were already validated general tools to assess, for example, quality of life [EuroQol-5 Dimensions (EQ-5D)] or functional outcome (Glasgow Outcome Score); therefore, this review was not conducted. The results of both reviews were pooled and a list of potential measures was constructed using the three broad groups described above. This list provided the focus for the next stage of workstream 1 – the consensus event to begin identifying and prioritising measures for further development.
Consensus event
The next step in workstream 1 was to begin to reduce the number of potential measures identified in the evidence reviews by making an assessment of their importance and beginning the process of prioritising which of these might be suitable for further development.
Performance and quality measures can serve different purposes for different groups of people. For services, this can be how well they are providing a timely and appropriate response to people who request their help and whether or not they are providing the best clinical care determined by current best practice. For commissioners, these measures may help to judge whether or not a local service is performing well and where improvement is needed, which may require additional support and investment. For patients, these measures should provide some indication of what type of service they are likely to receive and whether or not this meets their expectations. For policy-makers and government, these measures should provide an overview of the current level of service provision, whether or not this is consistent with expected standards and to what extent there is variation in different parts of the country. A good set of performance and quality measures should then, as far as possible, be relevant to different groups that have a legitimate interest in how well ambulance services are being delivered. This means that we had to try to find a set of measures that were agreed as important and relevant by the different groups that would potentially be the end users of these measures.
Our first step was to hold a consensus event that brought together different groups of people (i.e. ambulance operational and clinical staff, commissioners, patients and the public, emergency care clinicians, policy-makers and academics) to discuss, assess and rate the potential measures identified by the reviews. We also wanted to include measures that were important to patients who may not have been identified by the reviews. We conducted a separate interview study with recent users of the ambulance service (see Patient and carer views of ambulance service care) at around the same time and, although this was still in progress, there were some emerging themes that we were able to include in the consensus event. To supplement this, we also conducted a small focus group with 10 patient and public participants immediately before the consensus event. Participants in this focus group described their experiences and expectations of ambulance service care and identified a small number of important factors that were added to the list of measures for discussion.
We held the consensus event over 1 day in July 2012. The event was attended by 42 people (excluding the research team) who represented ambulance services, emergency medicine clinicians, patient and public representatives, commissioners, policy-makers and academics. Participants were mainly from the UK, but there were three international attendees.
The reviews identified a large number of potential measures. To make the process of prioritising more manageable for a 1-day event we did two things:
-
We reduced some measures to a single principle rather than all of the possible options. An example was ‘survival’, which we kept as a single measure rather than providing all of the different time cut-off points identified in the review. Overall, 42 measures (excluding time measures) were presented.
-
There were a large number of time measures and we did not want the discussions to be dominated by discussions of these to the detriment of other potential measures. We did recognise that time is an important factor, not just in terms of outcome for a small group of patients but more generally as a patient expectation. We therefore conducted a separate exercise where all 28 time measures were listed in a spreadsheet and participants asked to rate how important they thought each was by e-mail. The results of this exercise were subsequently combined with the ratings of the other measures discussed at the consensus event.
On the day we assessed the potential measures in two ways:
-
Participants were randomly allocated to small groups. Using a nominal group method each group was provided with a list of potential measures with explanatory notes and allowed to discuss and share their opinions about the measures presented. There was also the opportunity for participants to add their own ideas. Each group was facilitated by a member of the research team and participants could add notes to the list.
-
After the discussions, each measure was presented to the whole group as a Microsoft PowerPoint® (Microsoft Corporation, Redmond, WA, USA) slide (including any new measures identified) and participants were asked to vote on whether they thought the measure was essential, desirable or irrelevant using a live electronic voting system. This meant that all participants could contribute to discussions but also cast their votes independently and anonymously.
This two-step process was repeated three times, once for each of the main categories of measures (service, patient management and patient outcome). After the event the results of the voting were analysed and the measures ranked according to the proportion voting essential, desirable or irrelevant. This was carried out for each of the three groups and for all measures combined. A full description of the results of the voting for all of the measures considered is provided on the PhOEBE programme’s website. 25
Table 2 shows an illustration of the voting using the top-10 measures ranked according to the proportion voted essential.
Rank | Measure | Voting, n (%) | Total, n | ||
---|---|---|---|---|---|
Essential | Desirable | Irrelevant | |||
1 | Accuracy of dispatch decisions | 36 (86) | 6 (14) | 0 (0) | 42 |
2 | Completeness and accuracy of patient records | 35 (85) | 5 (12) | 1 (2) | 41 |
3 | Accuracy of call-taker identification of different conditions or needs (e.g. heart attack, stroke, suitable for nurse advice) | 33 (79) | 7 (17) | 2 (5) | 42 |
4 | Pain measurement and symptom relief | 33 (79) | 7 (17) | 2 (5) | 42 |
5 | Patient experience | 31 (78) | 9 (23) | 0 (0) | 40 |
6 | Measuring patient safety | 32 (76) | 9 (23) | 1 (2) | 42 |
7 | Overtriage and undertriage rates | 31 (76) | 9 (22) | 1 (2) | 41 |
8 | Compliance with end-of-life care plans | 31 (76) | 7 (17) | 3 (2) | 41 |
9 | Proportion of calls treated by most appropriate service (whole 999 population) | 30 (75) | 9 (23) | 1 (3) | 40 |
10 | Compliance with protocols and guidelines | 29 (69) | 12 (29) | 1 (2) | 42 |
Delphi survey
The consensus event allowed us to begin to prioritise the large number of candidate measures and reject some measures that were agreed as not important. For the next stage, we used another consensus method – a Delphi survey to further rate and prioritise measures. At this point we considered not just what could be measured but also how this might be done.
A total of 67 measures were included and these were categorised into the same three groups: patient outcomes (n = 25), whole service measures (n = 32) and clinical management measures (n = 10). The number of items was larger than those considered in the consensus event because, at this stage, we included time measures and began to develop more explicit, discrete descriptions of potential indicators. For example, where a broad principle such as accuracy of dispatch decisions was used for the consensus work, this was refined into multiple descriptions for specific conditions or call types. Potential measures were presented in a survey that enabled responses to be completed and returned electronically. Participants were asked to consider each measure and score their level of agreement on a scale of 1 to 9 (strongly disagree to strongly agree) using the statement:
This measure (either on its own or within a set of measures) is a good reflection of the quality of care provided by ambulance services and is likely to be a good indicator of the quality of the 999 ambulance service care pathway.
Participants were able to suggest additional indicators for inclusion. Responses to round 1 were recorded and the median score was calculated for each measure. This was followed by a second round during which revisions to measure descriptions were made following suggestions from the first round. Participants were provided with their own and the group median score and asked to score the measures again on the same 1–9 scale.
There were 23 participants who completed the round 1 form and 20 completed round 2 with an overall response rate of 74%. As in the consensus event, the participants represented a wide range of service provider and professional viewpoints, and most UK ambulance trusts. Some participants had also participated in the consensus event. Scores from round 2 were recorded and median scores calculated. A large number of measures scored highly, so a median score of 8 was used to discriminate between measures, with 20 (67%) participants ranked as high scoring.
We intended to include patient and public participants in the Delphi survey but our PPI reference group thought that the level of technical detail would make meaningful participation difficult. Instead, we held a separate event for PPI participants so that the concepts and measures could be explained and discussed in a face-to-face format.
Using a similar format to the previous consensus event, measures were presented for each of the three categories and small group discussions held. Participants then used an electronic voting system to rank each measure. Eighteen PPI representatives attended the PPI workshop and represented a range of people, including young people and vulnerable groups. The results of the PPI event were added to the results of the Delphi survey for the final stage of this workstream.
Two published papers26,27 are freely available and describe in more detail the methods and results of the consensus event and Delphi survey,26 and the co-produced event created with our PPI reference group to complement the Delphi study. 27
Patient and carer views of ambulance service care
At the outset of the PhOEBE programme we were aware that little research had been done to investigate the aspects of emergency ambulance service care that are valued by people who use the service. This includes patients but also their carers, who may be the person who makes a 999 call asking for help. To address this we conducted a qualitative study in which we interviewed people who had recently used the ambulance service in one of our study services. Ethics approval for the study was sought and gained from the National Research Ethics Service Committee East Midlands – Northampton (Research Ethics Committee reference 12/EM/0022) on 23 February 2012. During 2012, we talked to 22 patients and eight of their spouses (n = 30) using a semistructured face-to-face (n = 18) or telephone (n = 14) interview. We felt that it was important to explore the processes and outcomes of care that were important to ambulance users and we wanted to ensure that we captured issues that were relevant to the range of ambulance users, not just those with a life-threatening condition. We therefore included patients and carers who had called for serious problems requiring transport to hospital, those who had an ambulance crew attending but who were managed at home, and those managed by telephone advice. In the first part of the interview, we explored positive and negative aspects of their ambulance service experience and this was followed with questions about what they valued about the service and how performance might be measured. Interviews were recorded, transcribed and then analysed using framework analysis. An initial thematic framework was developed and then interviews coded to these themes, adding new ones as they emerged. A thematic map was constructed related to issues participants valued.
Participants in our study, regardless of clinical condition or level of ambulance service response received, valued similar aspects of their prehospital care experience. Users were often extremely anxious about their health and the outcome they valued was reassurance provided by ambulance service staff to alleviate the anxiety, fear or panic that they experienced at the time of calling an ambulance. They also valued reassurance that they were receiving appropriate advice, treatment and care, and this was enhanced by the professional behaviour of staff, which instilled confidence in their care, communication, waiting times for help (i.e. a short wait), and continuity during transfers. These features are themselves a consequence of the ability of call-takers and ambulance clinicians to competently recognise what the problem is and deliver appropriate advice and care and so implicitly reflect good-quality care. A timely response was valued in terms of allaying anxiety quickly. Participants valued the experience that they had, not just with ambulance crews who attended them but also the call-takers when they made their 999 call.
The interviews with users highlighted very clearly that, regardless of the actual clinical problem, the ability of the emergency ambulance service to allay the high levels of fear and anxiety felt by patients and their carers was crucial to the delivery of a high-quality service. Measures developed to assess and monitor the performance of emergency ambulance services have predominantly focused on actions such as response times or treatments provided. However, it was the more human interactions with the service that users recalled and described, and which could be included in the development of ambulance service patient experience measures. We used the findings from this study to add context to the description of ‘patient experience’ as a potential measure within the consensus work. Although it was recognised as important, it was acknowledged that measurement of patient experience is a longer-term objective outside the scope of the programme.
The qualitative study has been published as an open-access peer-reviewed journal article. 28
Final selection of measures for further development
The reviews and consensus work allowed us to consider a large number of potential ambulance service performance and quality measures, and to determine which were considered important to a range of end users. The final stage was to select from this list a small set of measures that could reflect the range of perspectives (service measures, patient management and patient outcomes) and take account of the broad population of people calling 999, not just a few with specific conditions.
The final set was selected using an expert panel drawn from our programme management and steering groups. The panel comprised 13 members and included representatives of the research team (reflecting research, statistics, ambulance service clinicians, PPI, emergency medicine) and external expertise from a further emergency medicine consultant, consultant paramedic and commissioner. We assessed all measures considered in the consensus work26 to avoid missing potentially important measures that did not feature highly in the rating exercises. Each measure was rated using a set of criteria that considered, for example, how highly it ranked in the consensus meetings and Delphi survey, the population it applied to, feasibility and availability of data, relevance to ambulance care, importance, meaningfulness and whether or not an item was already being measured. A score was derived for each potential measure using these criteria and the final set selected using these scores and expert judgements so that the set as a whole provided a balanced assessment of the different aspects of ambulance care considered to be important. The full set of criteria used and 56 measures assessed is available in Appendix 2 (see Table 17).
For two measures, survival from an emergency condition and accuracy of call identification, we had to identify a set of relevant conditions, as not all 999 calls were appropriate. We had previously conducted some consensus work as part of a study to develop emergency care system indicators and in this work identified a set of 16 emergency conditions [with relevant International Classification of Diseases, Tenth Edition (ICD-10),29 codes] that were considered appropriate to include in the indicators. We therefore used this same set of validated conditions for this work, including only patients with this diagnosis at discharge from hospital or as a cause of death. 30 The 16 emergency conditions are listed in Box 1.
Acute heart failure.
Acute myocardial infarction.
Anaphylaxis.
Asphyxiation.
Asthma.
Cardiac arrest.
Falls in patients aged < 75 years.
Fractured neck of femur.
Meningitis.
Pregnancy and birth related.
Road traffic collision.
Ruptured aortic aneurysm.
Self-harm.
Septic shock.
Serious head injury.
Stroke.
The final set of six measures selected for further development, included in workstream 3, is shown in Table 3. We initially included two further measures in this list. First, the compliance of ambulance clinicians with protocols and guidelines for specific conditions. The current ambulance service Clinical Quality Indicators31 for England already include a measure of compliance with expected care bundles for a small number of conditions. The purpose of this measure was to explore whether or not the availability of linked data and better information on patient outcome could be used to improve this indicator. However, the problems in obtaining the linked data and reduced time available to develop the performance measures meant that we had to exclude at least one intended measure and, as this measure already exists at least in part, we decided to concentrate on new measures. Second, we included a measure of mortality in patients with urgent problems, that is, those who have a low risk of dying. However, the lack of information on final diagnosis for patients not admitted to hospital made it impossible to identify all relevant patients. Instead, we took a different approach with this measure and explored the use of a structured judgement review process to identify potentially avoidable deaths.
Measure description | Aim |
---|---|
Change in pain score (mean/median) | To calculate the change in pain score for patients who received an ambulance response and had more than one pain score recorded |
Accuracy and appropriateness of call ID | To identify the proportion of patients with serious emergency conditions whose condition is appropriately categorised by the ambulance service |
Response time | To calculate a range of mean and percentile ambulance response times to explore alternative ways of displaying performance for an ambulance service |
Proportion of decisions to leave a patient at scene (‘hear and treat’ and ‘see and treat’) that resulted in re-contacts and/or death (within 3 days) | To identify the frequency of potentially inappropriate non-conveyance decisions |
Proportion of ambulance patients with a serious emergency condition who survive to admission, and to 7 days post admission | To identify the proportion of people with a serious emergency condition who survive to admission (within 7 days of ambulance contact) and, of those, the proportion who survive to 7 days post admission |
Proportion of patients transported to ED by 999 emergency ambulance who were discharged to usual place of residence or care of GP, without treatment or investigation(s) that needed hospital facilities | To identify the frequency of potentially inappropriate conveyance decisions |
Summary
Workstream 1 encompassed a number of related activities. The evidence reviewed revealed a large number of potential measures although many were variations on a single theme, such as time. The consensus work allowed us to consider this broad range of measures from a number of different perspectives. In particular, there was strong patient and public input including use of a novel approach to meaningful participation in the consensus process. The final set of measures for further development represented the potential to provide a broader and more balanced view of ambulance service care. These were relevant to all people who used the service rather than the current focus on single processes, such as response time or smaller populations with important but more specific conditions (e.g. cardiac arrest). The qualitative study produced new and important primary research evidence in an area that has not been well studied and revealed important insights into patient perceptions that were poorly understood. We found that:
-
Previous quality measures and performance indicators were dominated by time measures and accounted for over one-third of identified measures.
-
Outcome measures were dominated by varying durations of survival or mortality, spanning the range from admission to hospital to up to 5 years post admission, in a small number of longitudinal primary research studies.
-
Measures of accuracy were most frequently voted as essential, followed by measures (including pain) that reflected patient experience.
-
Patients felt that addressing anxiety and providing reassurance were important. This applied to the call process as well as face-to-face interaction with ambulance clinicians.
Workstream 1 produced a set of candidate measures potentially suitable for further development as indicators of ambulance service quality and performance. Development required an information source that brought together details of what happened to patients at the time of the incident and after their ambulance service contact. This was the focus of the next workstream.
Creating a linked data set
Introduction
Health-care information relating to a single person is often held by different services and is usually unconnected. In addition, different systems may be event rather than person based, so the same person can have multiple and different unconnected event records. The purpose of linking data is to match data from different information systems, bringing them together to create a single record of events for an individual person.
Having access to linked health data provides a real advantage for assessing health-care quality and performance. Patient pathways often involve multiple service providers or service contacts. If we base our assessments of how good care is on information from a single health provider or service, this provides only a ‘partial view’ of quality and performance and does not capture the range of services or complex care pathways available in today’s health care. 32
This is important for the ambulance service as, although they are a key service providing immediate help to people with an emergency or urgent health-care problem, very often this is a relatively short component, being only the first step in a longer set of contacts with different parts of the health-care system. In most cases, the impact of ambulance service care may not be obvious until further along the episode of care and this is particularly true of patient outcome information.
The availability of linked patient information enables important outcomes to be measured. In addition, making better use of the routine information collected along an episode or pathway of care for a population of patients, such as those who call 999, means that it becomes possible to monitor and compare processes and outcomes of care over time. Although linking information from different parts of the health service into a single patient record might seem obvious in the digital age, this is still not routinely available.
There have been previous attempts to link ambulance service data with ED data in the UK33 and Australia. 34 In both of these previous studies, data linkage was achieved but only after problems with data quality, finding suitable patient identifiers (IDs) and developing statistical matching processes had been overcome. Within the PhOEBE programme, our aim for workstream 2 was to revisit this problem and attempt to create a data set that linked routinely collected health service and national mortality information for individuals who used the 999 emergency ambulance service. The objectives were to:
-
develop data linkage processes that are acceptable to patients, data processors and data controllers, and comply with information legislation
-
obtain the necessary research and data approvals
-
link routinely collected ambulance service information about the 999 call and the clinical care given to patients with routinely collected hospital information and national mortality information, using a third-party data processor (NHS Digital)
-
create a new information source that provides a single record of the emergency care pathway for each patient contacting the 999 ambulance service.
Types and sources of information included in the linked data
We used five different types of information from three sources to create the linked data set. A full list of data and variables included in the linked data set can be found in a supplementary file at www.sheffield.ac.uk/scharr/sections/hsr/mcru/phoebe/reports. 25
Ambulance information
We used two different types of ambulance service data.
-
Computer-aided dispatch (CAD) data: this is the information that is recorded in the ambulance control room for every 999 call they receive. It contains items relating to call management and triage (e.g. the assessment of what the health problem is and how urgent it is). Items include timings (e.g. call received, ambulance sent, arrival on scene), location, reason for the call, urgency category, resources sent, disposition and patient demographic information.
-
Electronic patient report form (ePRF): a comprehensive record of clinical care provided to patients at the incident scene for those patients who are sent an ambulance response. It includes descriptions of condition, results of assessment and any treatment provided and is recorded directly to a hand-held computer.
Hospital information
We used two types of information on hospital events relating to ED care and hospital admission. The source of this information was HES data. This is a centrally managed data warehouse containing details of all admissions to NHS hospitals in England. HES information is stored as a large collection of separate records, one for each period of care, in a secure data warehouse. Each HES record contains a wide range of information about an individual patient admitted to a NHS hospital. For example:
-
clinical information about diagnoses and operations
-
information about the patient, such as age group, sex and ethnic category
-
administrative information, such as time waited, date of admission and discharge destination
-
geographical information on where the patient was treated and the area in which they lived.
Within HES data, we obtained information from two subsets of data.
-
HES A&E: these are individual records for all ED attendances occurring in England and contains information on patient details, dates and times, health problem or condition, investigations and treatments.
-
HES admitted patient care (APC): these are individual records for all patients admitted to hospital in England and contain information on diagnosis, treatment, length of stay, ward or facility type and medical specialty (e.g. cardiology, orthopaedics).
The advantage of national HES data is that all episodes of care for an individual patient are recorded. This means that we could identify not only any hospital care associated with the initial 999 call but also any subsequent related ED attendances or hospital admissions within a defined period of time.
National mortality data
Mortality data were obtained from the ONS. 35 The ONS collects information on the date and cause of death from the death certificate when the death of an individual is registered. The death certificate also records a list of other conditions or diseases that the patient had at the time of death.
Some people die in hospital and this is recorded in the HES data. However, other people die outside hospital. Adding ONS mortality information to our linked data provides a better and more accurate picture as it gives more detailed information on the cause of death and allows us to identify this important outcome for people who may have died without being admitted to hospital or after they have been discharged from hospital.
Study services and planned data collection periods
Two ambulance services in England took part in the programme: East Midlands Ambulance Service NHS Trust (EMAS) and Yorkshire Ambulance Service NHS Trust (YAS). When the PhOEBE programme started in 2011, the use of the ePRF was not widespread among ambulance services. EMAS had ePRF coverage for > 80% of the population who it served and YAS for one small operational area (about 15% of the service population). In our original plan we intended to create two linked data sets for each service (four data sets in total) for two time periods in year 2: July –December 2012 and January–June 2013. Delays in obtaining the right permissions meant that this moved by 6 months so the final data linkage periods were January–June 2013 and July–December 2013. Two separate time periods were used as the intention was to use the first data sets to construct the performance and quality measures in workstream 3 and the second sets to test the measures in workstream 4.
Data permissions
Linking different sources of health data together must be done in a way which is ethical and secure, acceptable to patients and service users and meets the requirements of the Data Protection Act36,37 and other relevant legislation. A central principle of data-linking studies is that individual concerns about the use of personal information must be balanced against the research benefits for the general population, so measures to manage risk and safeguard personal health information must be in place. 38 We therefore had to obtain a number of relevant permissions and put in place the required information governance and secure data management processes before we could request and obtain our linked data. Patient identifiable data were needed to enable the processes that linked ambulance service, hospital and mortality data. However, no identifiable data were transferred or processed outside the NHS and, therefore, no patient identifiable data were retained in the final linked data set we used for our research.
We obtained the following permissions:
-
NHS research ethics approval – as elements of the process required patient identifiable data, approval was sought and gained on 12 July 2012 from the NHS health research authority through the National Research Ethics Service Committee East Midlands – Derby (Research Ethics Committee reference 12/EM/0251, Integrated Research Application System project number 84751).
-
Confidentiality Advice Group – approval is required from this group (previously the National Information Governance Board) where research studies wish to use personal-identifiable patient information without consent, for purposes other than the direct care of patients and where it is not possible to use anonymised data or to seek patient consent. In this project, seeking individual consent was not feasible as the number of patients was very large (the individual ambulance services respond to > 400,000 999 calls per year) and we anticipated that some patients would have died. Approval was confirmed on 17 August 2012.
-
NHS Digital Data Access Request Service – permission is required from the NHS Digital Data Access Advisory Group to process and receive data. As part of this approval process, the legal basis for accessing the data, information governance and security arrangements, including data storage systems, and whether or not the project has a purpose beneficial to the health system is assessed.
The process of applying for data permissions and approvals proved to be very challenging and time-consuming. When we started this process, the HES data were held by NHS Digital. This organisation was also engaged to provide the data linkage service. We initially obtained the necessary (at that time) approvals in 2012 and began the process for obtaining the first set of ambulance data for linkage in January 2013. However, shortly after this a number of serious internal problems at HSCIC meant that there was a major reorganisation into what is now NHS Digital. During this period no data were released from NHS Digital. The reorganisation also meant that new approvals processes were put in place and the data permissions process had to begin again. This was completed in May 2015 and it was then a further 4 months before we received any NHS Digital data. Additional work and data approvals were then required owing to poor match rates for some patient groups, meaning it was not until October 2016 that we received the first adequate data set required for the workstream 3 work. Figure 5 provides a summary of the timelines and processes for the data linkage work.
FIGURE 5.
Timetable of data permissions and processes for obtaining linked data. CAG, Confidentiality Advice Group; DoB, date of birth; DSA, data sharing agreement; IG, Information Governance; NIGB, National Information Governance Board; R&D, research and development; UoS, University of Sheffield.
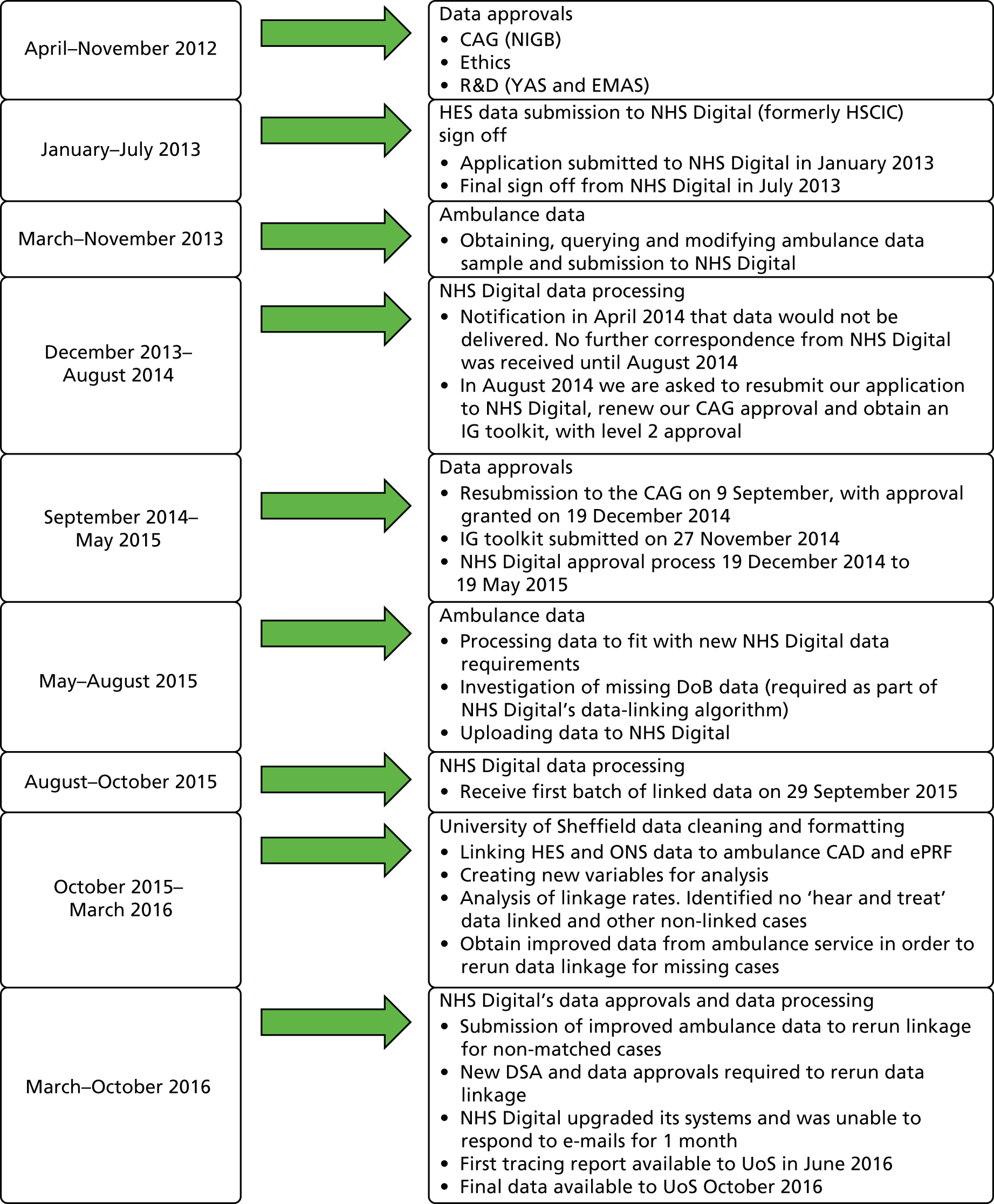
Creating the linked data sets
To create the linked data that we needed for the programme, a number of steps were needed to bring the different types of information together. Within NHS Digital, processes are already in place to link HES records and ONS mortality data. The new task for this project was to link ambulance service electronic records with these subsequent health records.
The first step was to retrieve the relevant information from ambulance service CAD and ePRF records. The starting point was all 999 calls received in the relevant time frame. Some calls were excluded at this point such as attendances with no ePRF, interhospital transfers, calls passed to other ambulance services and duplicate calls for the same incident. The exception was ‘hear and treat’ calls, defined as those calls that received input from a clinician (nurse or paramedic) but which have no ePRF record as no ambulance is sent.
The following stepwise process was then followed:
-
Yorkshire Ambulance Service and EMAS selected and extracted the study data sample, based on all included ambulance service contacts within the specified time period.
-
The study ambulance services linked the CAD and ePRF data (except ‘hear and treat’ calls) for all selected ambulance service contacts and produced a linked data set in Microsoft Excel® (Microsoft Corporation, Redmond, WA, USA). These data contain a large number of variables recording details of the patient, call processes, response provided, clinical assessment and treatment.
-
The ambulance services assigned a unique ID code to each individual patient record.
-
The ambulance services created a version of the data set that contained only the clinical data from the ePRF, non-identifiable emergency call and dispatch information from CAD and the unique ID number. This anonymised file, in the form of a password-protected Excel spreadsheet, was sent via secure encrypted e-mail to the research team at the University of Sheffield.
-
The ambulance services created a second version of the data set that contained only the variables required for data linking including patient identifiable data. These included, for example, date, time and location of incident, patient name, date of birth, address, hospital attended, the unique ID number, and (when available) NHS number. For cases for which there was no NHS number available, these were traced by NHS Digital. This data set was sent to NHS Digital as a password-protected Excel spreadsheet via NHS Digital’s secure electronic file transfer system.
The next step was to link the ambulance service data with HES and ONS mortality data. This was undertaken by NHS Digital using its data-linking algorithm. This was a deterministic linkage of NHS number, sex, date of birth and postcode using a series of progressive steps39 to match the same information in one data set with that in another. When the NHS number was unavailable, we used NHS Digital’s NHS number-tracing service to look up NHS numbers using date of birth and patient name. NHS digital linked ambulance data with a large number of variables from the HES A&E, HES patient admission and ONS death records so we could identify all patients who subsequently attended an ED, were admitted to hospital or died. The unique patient ID provided by the ambulance service was retained in this linked data set. After all possible records were linked, NHS Digital removed identifiable data and, when necessary, replaced it into a pseudonymised variable, for example date of birth was transformed into age. The de-identified data were returned to the research team using the same secure transfer processes.
The final step was for the research team to re-link the clinical and CAD data provided by the ambulance services with the HES and ONS data provided by NHS Digital, using the unique ID number contained in each data set to produce our final linked data set. Figure 6 shows the data flow processes used for workstream 2.
FIGURE 6.
Data flow processes.

Because of the delays in obtaining linked data, we were unable to obtain the intended four complete data sets in our original plan. The first best-quality data received was that created for EMAS data for the period January–June 2013 in October 2016. We did subsequently obtain linked data for YAS for the same period and also the linked data for both EMAS and YAS for the second period of July–December 2013. However, given the time needed to then process these data sets into formats needed for the programme research, we were unable to use them within the time available. These data will be available for further research but the description below of data processing and the number of cases included in the linked data used in this programme was confined to the first EMAS data set we were able to fully utilise.
Processing the linked data
Data were housed on a secure virtual machine and read for processing into R (The R Foundation for Statistical Computing, Vienna, Austria), which is an open source programming language and data management software programme for statistical computing. The processing involved data cleaning and standardisation to create variables required for the study, for example calculating time intervals.
A full list of the variables included in the data sets from each information source, a detailed description of the processes for requesting and returning data, the technical specification of the linkage algorithm and a description of how each data package was created are provided in the supplementary file at www.sheffield.ac.uk/scharr/sections/hsr/mcru/phoebe/reports. 25
Data complexity
Ambulance service data are complex and services hold data about patients in multiple data sets. For example, call data are stored within CAD, clinical patient data are stored in ePRF and process data about resources sent to incidents are stored in a separate resources data set. We also obtained another data file containing lower super output area to provide information about geographical area and deprivation index for each incident. This was used to calculate variables such as rural and urban incidents. The process of linking the ambulance data sets together was very complex. It is possible for multiple vehicles to be sent to the same incident. The first attending resource may not be the resource that takes the patient to hospital; therefore, calculating time on scene or total prehospital time involves multiple rows of data in multiple data sets. There can also be more than one patient for the same incident, meaning that one row of CAD data are linked with multiple ePRF files. Added to this complexity is that patients may re-contact the ambulance service many times within the 6-month data sample. For some of the PhOEBE programme indicators we were required to link together all ambulance contacts for the same person within a specific time period (e.g. 3 or 7 days).
Analytical decisions
Calls can be analysed for individual patients or individual calls. We decided to count decisions, not patients, because we were interested in the performance of the ambulance service at each point of contact, rather than for each individual; multiple 999 calls still present multiple opportunities for a service to make an appropriate/inappropriate decision on each occasion, even if the calls all relate to the same patient. Counting decisions allows us to recognise this in a way that simply counting the overall experience of each patient (once) would not [i.e. if the same patient phoned three times in 3 days, was left at scene each time and then died (still within 3 days of the first call), that would be three care decisions even though they relate to a single patient].
Additional linkages
Poor initial matching results for one service and for specific types of patients within another service meant that investigation of the data quality for the linkages was required. Ambulance services were subsequently able to provide better-quality linking information by either accessing alternative data sources or obtaining missing patient data from previous contacts and attaching it to the study data.
Cases included in the final data set
In our first complete EMAS data set, 83% (154,927/187,426) of patients in the sample were successfully traced and their records linked. Unsuccessful traces were due to missing or incomplete patient ID data from the CAD or ePRF records, so these could not be linked to subsequent health-care information or deaths. However, subsequent re-contacts with the ambulance service were identifiable using a unique HES ID generated for each patient. Figure 7 shows the numbers of cases and proportions of cases included and traced and Table 4 shows the numbers and proportions of calls traced at each tracing step.
FIGURE 7.
Numbers of cases traced and included in the linked data set.

Criterion | Traced call numbers | |||||
---|---|---|---|---|---|---|
All EMAS calls (including non-ePRF attendances) | Calls in raw sample (‘hear and treat’, and attended with ePRF) | Calls in cleaned sample 1 | Traced first attempt | Calls in cleaned sample 2 | Traced second attempt | |
‘Hear and treat’ | 10,648 | 0 | 10,634 | 2521 | ||
Conveyed to A&E | 122,882 | 105,618 | 122,797 | 106,822 | ||
Discharged at scene or conveyed to other destinations (not A&E) | 53,882 | 44,419 | 53,856 | 45,584 | ||
Total | 362,714 | 188,414 | 187,412 | 150,037 | 187,287 | 154,927 |
% of total calls | 51.95 | 51.67 | 41.37 | 51.63 | 42.71 | |
% of sample in use at the time | 80.06 | 82.72 |
Linkage of data for patients with an ePRF (‘see and treat’ and ‘see and convey’) was high, leading to a high overall (> 82%) match rate for the PhOEBE programme data sample (all calls attended with an ePRF and ‘hear and treat’). However, data-linking success for different patient groups was variable because of differences in the quality of data recorded for different types of patients. In particular, linkage rates for ‘hear and treat’ patients were very low. This is because at the time that the data were recorded, it was not standard practice for date of birth to be recorded on the CAD system. Date of birth was a key part of the NHS Digital data-linking algorithm and without this information the algorithm produced a non-match. As the CAD data system was the only data source available for ‘hear and treat’ patients, this resulted in an initial match rate of zero. A final linkage rate of 23.7% was achieved through searching subsequent and previous ambulance attendance data for additional linking information for ‘hear and treat’ patients. This potentially introduced a bias into the sample as the ‘hear and treat’ patients who were matched within our sample were those that had previously contacted and been seen by the ambulance service. These patients were more likely to be sicker than the ‘hear and treat’ patients in our sample where linkage was not possible. We assessed whether or not there were differences between patient characteristics for those with linked or unlinked data and found little evidence of differences for those discharged at scene or conveyed to ED. We did, however, find differences for ‘hear and treat’ patients; for example, linked ‘hear and treat’ patients were older than patients with non-linked data. This was most likely because older people were more likely to have had other contacts with the ambulance service.
Summary
We were able to develop data linkage processes that were acceptable to patients and the public and to data controllers, met with data legislation and were technically possible.
Although it was technically possible to link the data within the context of a research project, the complexity and time-consuming nature of data approvals, obtaining linked data and processing that data means that this is not feasible for individual ambulance services to undertake this routinely at present.
We found the following:
-
For cases involving patient contact with ambulance staff it was possible to link ambulance, hospital and mortality data for > 85% of ambulance calls.
-
Much lower rates of linkage were possible with ‘hear and treat’ calls, resulting in a potentially biased sample. This also made it more difficult to accurately establish consequent events such as re-contacts with other parts of the urgent-care system.
-
Recording date of birth was essential for linking data sets and ambulance services could improve this in future for data processing.
-
We were able to define the steps and processes required to link ambulance, hospital and mortality data for future research studies, assuming that the regulatory requirements remain unchanged. Future data linkage for evaluation could be achieved more efficiently through data-sharing agreements between ambulance services and hospitals with linkage performed at an NHS organisation if there were sufficient resources and expertise to do this.
The completion of the data linkage work allowed us to proceed to the next activity: exploring the use of case mix and building statistical models to measure the six indicators identified in workstream 1.
Developing case-mix-adjusted performance indicators
Introduction
The objective of this workstream was to use the linked data created in workstream 2 to explore the development of the performance and quality indicators identified in workstream 1. For each indicator, we could simply measure the related process or outcome (e.g. discharge from hospital with no treatment or survival from an emergency condition). However, there could have been factors other than receiving ambulance service care that influenced the processes or outcomes. Some of these are intrinsic to patients, for example age or type and seriousness of the health problem they call for. Others will be related to extrinsic or other NHS factors, such as when the call is made (day or night), incident location or hospital attended. Only considering the crude values of a measure means that inaccurate and unhelpful judgements might be made about the performance or quality of ambulance service care. For example, we could calculate the survival rate for all patients receiving an ambulance response in two ambulance services. In service 1, the rate is 95% and in service 2 it is 85%. We might then judge that service 2 is providing poorer care. However, the patients attended by service 2 may be much older or the proportion of calls for serious emergencies (rather than minor urgent problems) may be higher and, hence, the risk of dying will also be higher in these patients. The lower survival rate may therefore be a consequence of these case-mix differences and not ambulance service care.
For this reason we considered the effect of intrinsic and extrinsic factors by building case-mix adjustment models for some indicators. This allowed us to assess whether or not an indicator needed case-mix adjustment, if it did what characteristics or variables were important and needed to be taken into account, and if it was possible to build models that are statistically robust enough to be useful using currently available information. Developing each indicator using statistical techniques to include case-mix adjustment that allows patient and other differences to be taken into account may provide more accurate and realistic measurement so that differences between services or within services over time can be detected.
Indicator development methods
We used a complex, stepwise statistical approach to the development of each indicator. These are described in detail along with the comprehensive results of the analyses for each indicator in a supplementary file available at www.sheffield.ac.uk/scharr/sections/hsr/mcru/phoebe/reports. 25
For each indicator, the basic approach was to develop a ‘model’ that predicted the outcome (or process) from characteristics of the incident and the patient. For simplicity, only the outcome is used in the following description.
Step 1
For indicators where the outcome is binary (e.g. a simple yes or no measure) we used logistic regression to examine the association with patient and incident characteristics and prehospital measures of the patient condition. The strength of the relationship between each characteristic and the outcome was calculated using statistical testing. For indicators where the outcome is continuous (e.g. a pain score where there are many different recorded values) multiple linear regression was used to assess association between characteristics and the outcome.
Step 2
Here we conducted multivariable model building using a stepwise strategy. All single variables identified in step 1 that were significantly related to the outcome were entered into the multivariable model building analysis. To begin, the subset of terms independently predicting the outcome were selected. Then, other variables that were not important on their own were added to the model one at a time to check if they had become important in the presence of others. The next stage was to check for statistically significant interactions between the retained predictor variables. This is important because, for example, the rates of survival within conditions may change with age. Essentially, step 2 examines different combinations of characteristics and finds the one that provides the best ‘fit’ for predicting the outcome being considered. As an example, for the indicator ‘survival from an emergency condition’, we know from our linked data whether or not each patient with one of our specified conditions survived or died. The statistical modelling allowed us to work from these known outcomes and find the best combination of characteristics (e.g. age, sex, condition) that can predict whether a patient will survive or die.
Step 3
In this step we did further statistical testing to assess how well the final model for each indicator could discriminate between patients who experienced the event being tested or did not experience it and tested the relationship between the predicted probability and estimated actual probability for the outcome. This additional testing allowed us to check that the model was as ‘true’ as we could make it (i.e. that it was accurately measuring the outcome and not systematically over- or underestimating the predicted outcome). We also at this stage calculated standardised rates for subgroups. For example, different geographical areas will have patients and incidents with different characteristics; standardisation allows for these differences so that fair comparison can be made. Finally, we used funnel plots to observe the standardised rates for different geographical areas to see if there were any outliers.
The end product for each indicator is a case-mix-adjusted model that allows us to calculate a standardised rate for the outcome that takes into account patient and event factors. Using our survival example, this means that we might measure a crude survival rate of 95% but using the case-mix-adjusted model this may change to 94.6%. If we then measure this rate again at different time points it will account for any differences in the population and so changes are much more likely to be a consequence of changing ambulance care.
For each indicator we assessed what it might be useful for depending on the characteristics included in the final model, such as if it could measure differences between ambulance services or if it was only accurate for changes over time at an individual service level.
Summary of indicators developed
Tables 5–11 provide a summary of each indicator and how they were constructed. Table 12 compares response times for urban and rural calls. The abbreviations used for data sources and case identification are described in Box 2.
Criterion | Descriptor |
---|---|
Aim | To calculate the mean change in pain score for patients who were sent an ambulance response and had more than one pain score recorded, using a predictive model |
Rationale | The focus of this measure is on the management and relief of pain. Measuring a change in subsequent pain scores is a direct way of measuring the effect of care provided by the ambulance service |
Data sources | CAD, ePRF |
Data provider | Ambulance service |
Population | Ambulance service users who are attended by an ambulance-dispatched response. This measure is not restricted by ICD-10 codes. Only patients with more than one pain score can be included in the predictive model but this restriction does not apply to the contextual measures |
Measurement details | Adults and children (aged ≥ 2years) verbal/converted Faces Pain Scale score 0–10. 0 = no pain at all and 10 = worst imaginable pain |
Construction | Identify population with more than one pain score. Categorises as pain expected or not expected (based on AMPDS condition or incident type) |
Calculate the difference between the pain scores. Where patients have more than two pain scores, use the first and last recorded pain score | |
Calculate the mean difference in pain scores | |
Case-mix adjustment variables | No important variables identified |
Exclusion criteria | Exclude the following:
|
Cases included |
165,853 cases for contextual descriptive analysis (excluding dead/unconscious, ‘hear and treat’, children aged < 2 years) 35,824 after excluding no score, no pain and only one score included in the case-mix analyses |
Contextual measures results |
Of the 165,853 cases 27.5% had no pain score recorded, 72.5% had at least one pain score and 48.8% two or more pain scores. 42.4% had a pain score of 0 (58.5% excluding cases with no score). 76.3% of cases categorised as pain not expected had a pain score of 0 compared with 23.0% categorised as pain expected 21.4% had a pain score of ≥ 5 and of these 25.6% did not have a second pain score recorded |
Criterion | Descriptor |
---|---|
Aim | To identify the proportion of patients with a serious emergency conditions whose condition is correctly categorised by the ambulance service |
Rationale | Serious emergency conditions require prompt and specialist treatment. Identification of these conditions by the ambulance service means that patients get access to the right care in the right place from the start of their care episode. This may lead to improved patient outcomes, health-care cost savings and improved patient experience. Accuracy of call identification is measured at the point of call triage and is not concerned with the abilities of crews |
Data sources | CAD, ePRF, HES APC, ONS mortality |
Data provider | Ambulance service, NHS Digital |
Population | Patients identified as having one of 16 prespecified serious emergency conditions (from HES APC or ONS mortality ICD-10 codes) |
Measurement details |
AMPDS code assigned at ambulance dispatch (CAD data set) Clinical impression (ePRF) Final diagnosis within 24 hours (e.g. ICD-10 diagnosis or cause of death) |
Construction |
|
Case-mix adjustment variables | Age, sex, condition, IMD |
Inclusion/exclusion criteria |
Include: patients with one of 16 serious emergency conditions who are admitted to hospital or die, on day 0 or day 1 post call Exclude: calls with no AMPDS codes [e.g. 111, GP, police calls, calls with no ICD-10 code (from admission or death) within 1 day of call] |
Cases included | 9314 cases with ICD-10 code for 16 conditions and an AMPDS code |
Descriptive accuracy by condition (in all cases/% correct) | Acute heart failure (737/59.8%); acute myocardial infarction (1176/69.0%); anaphylaxis (39/74.4%); asphyxiation (54/61.1%); asthma (382/84.0%); cardiac arrest (68/47.1%); falls in those aged < 75 years (1615/54.6%); fractured neck of femur (905/31.0%); meningitis (14/35.7%); pregnancy and birth related (6/0%); road traffic crash (137/73.0%); ruptured aortic aneurysm (139/10.1%); self-harm (1726/85.5%); septic shock (433/25.2%); serious head injury (594/58.6%); stroke (1289/70.0%) |
Criterion | Descriptor |
---|---|
Aim | To calculate the average ambulance response time |
Rationale | Response time is a commonly used process measure but it is usually measured against a time threshold, such as 75% within 8 minutes. This can incentivise operational behaviours that are concerned with chasing targets rather than clinical care and may produce long waits for some patients. Using an indicator based on the average response time ensures that all response times are included and is more representative of whole service performance |
Data sources | CAD, ePRF |
Data provider | Ambulance service |
Population | All service users who call 999 and where a response is sent |
Construction | Use the ‘clock start’ variable and the time the first ambulance service dispatched response arrived on scene variable |
Calculate the difference | |
Calculate measures of location (e.g. mean, median, trimmed mean) | |
Calculate measures of variability (e.g. standard deviation, interquartile range) | |
Case-mix adjustment | None: this is an operational process controlled by the ambulance service |
Inclusion/exclusion criteria |
Include: all patients where an ambulance is sent Exclude: ‘hear and treat’ calls, negative response times |
Cases included | 176,515 face-to-face responses to 999 calls |
Criterion | Descriptor |
---|---|
Aim | To identify the frequency of potentially inappropriate non-conveyance decisions (‘hear and treat’ and ‘see and treat’) |
Rationale | Not all patients who contact the ambulance service are taken to an ED. Some are treated and left at scene and others receive advice over the telephone. By identifying subsequent re-contacts and mortality outcomes for non-conveyed patients we may be able to assess whether or not the decision to leave at scene was appropriate |
Data sources | CAD, ePRF, HES APC, ONS mortality |
Data provider | Ambulance service, NHS Digital |
Population | ‘Hear and treat’, and ‘see and treat’ traced calls |
Construction |
Identify decisions not to convey a patient to hospital (denominator) – the same patient may have multiple contacts with the ambulance service so each separate contact (decision) is used rather than patient Identify which non-conveyed patients had re-contacts (hospital admission) or died |
Case-mix adjustment variables | Age, sex, reason for the call, IMD quintile (deprivation index) |
Inclusion/exclusion criteria |
Deaths and hospital admissions can be from any cause Exclude: end-of-life care cases (identified by clinical review of cause of death) and patients who have no re-contacts (death or hospital admission) within 3 days of the call |
Cases included | 45,310 traced cases. Additional 15,899 non-conveyed cases that were not traced (so no re-contacts or deaths identified) were included in the sensitivity analysis |
Criterion | Descriptor | ||||||||||||||||
---|---|---|---|---|---|---|---|---|---|---|---|---|---|---|---|---|---|
Aim | To identify the frequency of potentially unnecessary conveyance decisions | ||||||||||||||||
Rationale | Current NHS England policy highlights the importance of the right care in the right place at the right time. This measure identifies the proportion of all patients taken to ED by ambulance who could potentially be managed by other services and who are less likely to benefit from hospital resources | ||||||||||||||||
Data sources | CAD, ePRF, HES A&E | ||||||||||||||||
Data provider | Ambulance service, NHS Digital | ||||||||||||||||
Population | All patients attending ED brought in by ambulance | ||||||||||||||||
Construction | Identify all patients taken to ED (type 1 or 2) by ambulance (exclude self-presentations) | ||||||||||||||||
Identify patients who only had the following investigations or treatments and disposition at ED: InvestigationTreatmentDispositionNonePrescriptionsDischarged – following treatment to be provided by GPUrinalysisGuidance/advice onlyDischarged – did not require any follow-up treatmentPregnancy testRecording vital signsLeft department before being treatedDental investigationDental treatmentPrescriptionNone |
Investigation | Treatment | Disposition | None | Prescriptions | Discharged – following treatment to be provided by GP | Urinalysis | Guidance/advice only | Discharged – did not require any follow-up treatment | Pregnancy test | Recording vital signs | Left department before being treated | Dental investigation | Dental treatment | Prescription | None | |
Investigation | Treatment | Disposition | |||||||||||||||
None | Prescriptions | Discharged – following treatment to be provided by GP | |||||||||||||||
Urinalysis | Guidance/advice only | Discharged – did not require any follow-up treatment | |||||||||||||||
Pregnancy test | Recording vital signs | Left department before being treated | |||||||||||||||
Dental investigation | Dental treatment | ||||||||||||||||
Prescription | |||||||||||||||||
None | |||||||||||||||||
These criteria were derived for a previous study40 and a modified version used in a similar study41 being conducted by the Yorkshire and Humber Collaboration for Leadership in Applied Health Research and Care Calculate how many patients had no other investigations or treatments than those identified in the criteria and were discharged as per the codes above. This is the total number of patients who could have been treated elsewhere |
|||||||||||||||||
Case-mix adjustment variables | Age, hospital (different hospitals may do different rates of investigations), deprivation, sex, call type | ||||||||||||||||
Inclusion/exclusion criteria |
All patients aged ≥ 2 years taken to ED by emergency ambulance Exclude: all infants and babies aged < 2 years (ambulance service policy is to take all under 2s to hospital) Patients attending walk-in centres and minor injury units managed by the ED |
||||||||||||||||
Cases included | 100,435 | ||||||||||||||||
Contextual analyses | We conducted subgroup analyses to look at the possible effects on this outcome of different factors such as time of call and the specific condition types, alcohol intoxication and mental health problems to identify examples of patient groups where the rate of inappropriate attendance may vary |
Criterion | Descriptor |
---|---|
Aim | To identify the proportion of people with a serious emergency condition who survive to admission (within 7 days of ambulance contact) and, of those, the proportion who survive to 7 days post admission |
Rationale | This indicator identifies early deaths following an ambulance contact, through HES APC data or ONS mortality data. It is a system indicator that relates to improving the number of people with a serious emergency condition who survive. Survival may improve through taking the right patients to the right specialist care. It can be used to assess trends over time |
Data sources | CAD, ePRF, HES APC, ONS mortality |
Data provider | Ambulance service, NHS Digital |
Population | Ambulance service users with an ICD-10 code indicating one of 16 serious emergency conditions |
Construction | Identify 16 serious emergency conditions from the diagnosis on admission (for all admitted patients) and ICD-10 cause of death (only for those patients who died without being admitted) |
There are two parts to indicator 6 (denoted 6a and 6b):
|
|
Case-mix adjustment variables | Age, condition, IMD quintile (deprivation), hospital (by trust: for 6b only) |
Inclusion/exclusion criteria | Include patients with (hospital) ICD-10 codes for the 16 emergency conditions who were admitted to hospital or died |
Cases included | 6a, 11,750; 6b, 11,154 (excluding patients who died before admission) |
Indicator | Case-mix factors in risk adjustment model | Importance of risk adjustment |
---|---|---|
Mean change in pain score | First pain score, age, sex and total prehospital time | Not important |
Proportion of serious emergency conditions correctly identified | Age, sex, condition and IMD | Limited importance |
Response time | None | N/A |
Proportion of decisions to leave a patient at scene which were potentially inappropriate | Age, sex, reason for the call and IMD | Limited importance |
Proportion of patients transported to ED by 999 emergency ambulance, but who were discharged without treatment or investigation(s) | Age, hospital, deprivation, sex and call type | Important |
Proportion of ambulance patients with a serious emergency condition who survive to admission, and to 7 days post admission | Age, condition, IMD and hospital | Important |
Response time (minutes:seconds) | ||
---|---|---|
Urban (n = 140,853) | Rural (n = 35,550) | |
Mean | 17:29 | 21:42 |
Standard deviation | 29:06 | 32:24 |
Median | 07:46 | 12:32 |
Interquartile range | 04:49–17:20 | 07:36–21:44 |
90th percentile | 41:07 | 45:37 |
95th percentile | 64:08 | 70:59 |
99th percentile | 147:08 | 171:16 |
Trimmed mean (90th) | 10:11 | 13:41 |
Trimmed mean (95th) | 12:19 | 15:55 |
Trimmed mean (99th) | 15:30 | 19:27 |
Winsorised mean (90th) | 13:16 | 16:53 |
Winsorised mean (95th) | 14:55 | 18:40 |
Winsorised mean (99th) | 16:49 | 20:58 |
Geometric mean | 08:43 | 11:45 |
CAD: the ambulance service computer system that records all the details of 999 calls, such as times, incident type, patient details and location.
ePRF: a mobile (laptop, tablet) patient record completed by ambulance clinicians at the scene of an incident to record patient details, presenting problem and treatment given.
HES A&E: HES ED record of times, clinical findings, treatments and referrals.
HES APC: record of details of diagnosis; dates, times and place of admission; length of stay; place discharged to or if died in hospital.
ONS mortality: records from the register of deaths including date and cause.
AMPDS: a computer triage system used by ambulance services to assess 999 calls. Produces a code that describes the problem (a symptom, e.g. chest pain, or an incident type, e.g. fall).
ICD-10: a dictionary of codes providing a detailed description of the diagnosed problem for each admission or cause of death.
IMD: a value indicating deprivation and poverty in geographical areas. Deprivation is known to affect health outcomes.
AMPDS, Advanced Medical Priority Dispatch System; IMD, Index of Multiple Deprivation.
Indicator 1: mean change in pain score
What did we find?
We examined the relationship between mean change in pain score (see Table 5) for a number of variables and found (1) a negative correlation between first pain score and change in pain score indicating that overall pain was reduced during the prehospital phase; (2) a weak negative correlation between total prehospital time and change in pain score suggesting that pain decreases as total prehospital time increases; and (3) a very weak negative correlation between age and change in pain score suggesting that pain decreases as age at incident increases. First pain score recorded was the only variable to capture any meaningful variation in change in pain score. The final multivariable model incorporating all of these variables showed that a case-mix-adjusted model was able to explain only about 21% of the total variation of mean change in pain score and so has poor predictive ability. The results suggest that this model is not suitable for case-mix adjustment and if mean change in pain score is used as a care quality indicator the crude values are as useful as a case-mix-adjusted statistical model using currently available information.
The assumptions used for this indicator were that, first, pain score is given before treatment; it is possible for subsequent pain scores to increase as well as decrease, and, in some cases, it may be appropriate for only one pain score to be present. For example, if the patient reported no pain or short transit times, this meant that there was not time to do multiple pain scores.
It is not possible to identify the time point at which pain scores were carried out, the time that the medication was given or the impact of short journey times on patient care. Therefore, total prehospital time was used in the predictive model. It is possible that if more accurate information on these variables were to be recorded in the future then a case-mix-adjusted model may be more useful.
Indicator 2: proportion of serious emergency conditions correctly identified at the time of the 999 call
What did we find?
There was considerable variation in the percentage of calls accurately identified for each condition (see Table 6). There is a linear relationship between age and the accurate identification of condition, with accuracy decreasing as age increases. This most probably reflects the increasing complexity of health problems as people age and may make it more difficult to differentiate between multiple symptoms or problems reported at the time of the call. Condition type was also associated with accuracy of identification and so the final multivariable model included age and condition. The predictive ability of this model is lower than would ideally be expected for a case-mix adjustment model but was still considered better than using the crude accuracy rates as an indicator of performance.
We also calculated standardised rates per 100 calls by Clinical Commissioning Group (CCG), time of call (weekday or weekend, in or out of hours) and ambulance operation centre. This showed that variation was within limits that will allow this model to be used to compare different geographical areas and different times of the day or week as well as monitoring performance over time. For example, the standardised rate of call accuracy for the 16 emergency conditions calculated for 22 CCGs varied from 57.4 to 65.2 per 100 calls.
This measure can include only patients who were admitted to hospital or who died, and works by looking backwards from the ICD-10 data to assess the ambulance dispatch decision. However, the 999-call triage system is not a diagnostic tool and while some conditions (e.g. stroke) may have clear symptoms, others (e.g. sepsis or meningitis) may not and could be coded in a number of ways. In addition, some conditions (e.g. falls) may be coded to a different Advanced Medical Priority Dispatch System (AMPDS) category if an obvious injury becomes apparent during the call assessment. AMPDS codes were mapped to ICD-10 codes, bearing in mind that there could be multiple correct codes, but there may be appropriate codes for a condition we have not included. Further analysis of all of the AMPDS codes assigned to each condition may reveal additional relevant codes, which could improve precision.
Indicator 3: response time
What did we find?
For this indicator we looked at different ways of displaying response time performance by calculating percentile response times and different variations on mean time (see Table 7). Mean values include all cases but can be skewed by small numbers of very high or low values. We looked at alternatives such as trimmed and Winsorised means, which treat extreme values differently and may provide a more useful indicator. We calculated response times for different types of call to illustrate how this could be used for within- and between-service comparisons, for example urban versus rural times using lower super output area data and different categories of urgency. These are presented in detail in Appendix 2. An example is given in Table 12.
The tables have shown that displaying mean and percentile response times can provide a useful indicator of response performance that can highlight differences in service provision; for example, it can be used to compare performance in urban and rural areas or at different times. These are important when considering resource management and provision of an equitable service. It also provides a picture of variation across the whole response time spectrum, not just a proportion of calls. Displaying percentiles adds transparency so that longer as well as shorter response times are visible.
The different approaches to calculating mean and percentile response times by displaying standard mean (using all values) and alternative methods for managing extreme values (trimmed and Winsorised means) present different pictures. In particular, the differences are more marked for call categories with longer expected response time standards (e.g. 30 minutes) than those with short expected standards (e.g. 8 minutes). The larger mean values for the standard mean reflect an important issue in ambulance service delivery in that these take into account the very long waits that some patients experience. It is also possible that some of the very long response times reported may be the consequence of data inaccuracies rather than a real extended response time and, therefore, present a distorted picture that could lead to erroneous assumptions about performance. Trimmed or Winsorised means allow these potential data problems to be accounted for. All of the methods for calculating means, and the corresponding percentiles, have their own advantages and disadvantages and the choice of which to use will be as much a feature of what they will be used for and who by. For example, if reflecting long waits is important then standard means may tell a better story, but for frequent monitoring of overall patterns or trends in performance, use of an alternative may provide a more consistent approach that smooths out the effects of short-term variation, such as call demand spikes or bad weather, which inevitably affect response performance.
We presented the alternative ways of describing response times to our PPI reference group. From a user perspective, they found the median and 90th percentile measures most useful as these could be interpreted as, respectively, half of calls or 9 out of 10 calls being responded to within a given time. They thought that presenting actual times was more informative and transparent than percentages within a specified time, as the latter option obscured what was happening to calls outside the target time.
Indicator 4: proportion of decisions to leave a patient at scene (‘hear and treat’ and ‘see and treat’), which were potentially inappropriate
What did we find?
For this indicator (see Table 8), we examined four variables and included three in the final multivariable model. There was a non-linear relationship between re-contacts or admission and age with the probability of re-contact increasing with age up to the 70–80 years age group and then the probability decreased as age increased further. Sex and reason for the call were also associated with re-contact. Reason for the call was characterised by 10 broad groups: abdominal pain, breathing problems, cardiovascular, falls, fitting, injury, psychiatric, sick person, unconscious and other conditions. There was a small proportion of cases where no problem was recorded (e.g. transfers from NHS111).
We also calculated standardised rates per 100 calls by CCG and time of call to test if the model could be used to assess performance between areas and at different times. Although the model did not meet the statistical threshold for a good case-mix adjustment model, the results suggested that case-mix adjustment improved the usefulness of the indicator by controlling for factors that could make interpretation of crude re-contact or admission rates for non-conveyed patients inaccurate. Overall, the model could be used to measure standardised rates between areas and over time and case-mix adjustment provided a potentially more sensitive measure. The re-contact or admission rate for non-conveyed patients ranged from 5 to 10.2 per 100 calls across 22 CCGs. This means that between 90% and 95% of decisions to not convey a patient to hospital were appropriate.
Sensitivity analysis showed that standardised rates were smaller if untraced calls were included. Better information enabling more calls to be traced would improve the model. A limitation is that we cannot know what proportion of untraced calls had a re-contact or died and how many were untraced simply because their problem was resolved by the ambulance service and they had no further contact with the health service within the time frames of our linked data. This is a particular issue with ‘hear and treat’ calls. Some re-contacts may be justified as the condition has worsened but the original decision was appropriate but the indicator is useful as we would expect the standardised rate to remain stable or improve over time. If it becomes worse, or there are substantially different rates between services, this would indicate a problem.
This indicator is linked to the next indicator (5 – potentially unnecessary conveyance).
Indicator 5: proportion of patients transported to emergency department by 999 emergency ambulance, but who were discharged to usual place of residence or care of general practitioner without treatment or investigation(s) that needed hospital facilities
What did we find?
For this indicator (see Table 9), the final multivariable model included age, which was a non-linear association, deprivation index and hospital as all these variables were important. The model was more complex as each deprivation category (1–5) and each hospital were treated as individual variables. The statistical analysis showed that this model had good predictive ability and thus that case-mix adjustment improves the usefulness of the measure as a means of identifying change in performance.
We also calculated standardised rates per 100 calls and compared a small number of contextual factors. The standardised rate of unnecessary transports to ED was small but variable across CCGs ranging from 2.4 to 8.0 per 100 calls. This probably reflects the differences in hospitals that the model showed had an effect, as they were associated with CCG areas. We found the highest rate of unnecessary ED attendances were out of hours at weekends and that the rate was much higher for patients with alcohol intoxication and with mental health problems than those without. For those with mental health problems, this may be because of our definition (which was based on treatments and investigations), which may not be relevant to this patient group. In the case-mix-adjusted model, we found that hospital had a substantial effect on the outcome being measured. This means that if this indicator is used for comparing ambulance services areas, this could be confounded by the effect of the hospital. However, it could be used within areas to measure change over time, where the effect of hospital is constant. It may also be used as a system indicator (i.e. to measure the performance of an ambulance service and hospital together).
Indicator 6: proportion of ambulance patients with a serious emergency condition who survive to admission, and to 7 days post-admission
What did we find?
This indicator, ‘Proportion of ambulance patients with a serious emergency condition who survive to admission, and to 7 days post admission’, had two parts (see Table 10): survival to admission (6a) and to 7 days post admission (6b). For the first part (6a – survival to admission for the combined 16 emergency conditions), the final multivariable model included age (which had a linear relationship, with probability of survival decreasing as age increased) and condition. The statistical analysis showed this case-mix-adjusted model was suitable for prediction and, therefore, measurement of change that took into account the effects that age and condition had on the outcome. The standardised survival rate ranged from 90.5 to 97.3 per 100 calls across the 222 CCGs. There was little variation in the standardised survival rates by time of call. This illustrated that a large proportion (> 90%) of the 999 population were calls for problems that were not life-threatening emergencies. The results suggest that this indicator can be used to make comparisons between different geographical or administrative areas, different times of the day/week, or to monitor performance over time as it was not confounded by hospital influences.
For the second part (6b – survival to 7 days post admission) the final multivariable model included age (which had a linear relationship as in 6a), condition and hospital. The statistical analysis also showed that this case-mix-adjusted model was suitable for prediction and measurement of change, taking into account the effects that age and condition had on the outcome, but in this model hospital was also an important factor associated with outcome. Standardised rates for the model including hospital suggest strong confounding between CCG and hospital of admission. This meant that the first model, which excluded hospital effects, could potentially be used to measure differences between regions or services, or monitor performance over time in the same service. The second model was not suitable for measuring between-service differences. Hospital care also had a strong influence on outcome but it could be used to monitor within-service changes over time. It could also potentially be used as a system measure rather than an ambulance measure where the standardised rates could then be presented at a hospital level.
For this indicator, there were some problems identifying patients with cardiac arrest as they may have been coded under other conditions when admitted to hospital. There were also a large number of prehospital deaths that were not coded as cardiac arrest as the primary cause of death.
Summary of the indicator development
We have examined the case-mix factors that influence the outcome of the six performance indicators that were derived in earlier workstreams as being important and potentially useful in assessing the performance of ambulance services in England. The results of these analyses, summarised in Table 11, suggest that most of the indicators do need to be adjusted for case mix before being used to make ‘fair’ comparisons. The indicator for mean change in pain score was not improved by risk adjustment. The indicators for accuracy of correct identification of emergency conditions and re-contact rates for patients left at home or managed by telephone did not quite reach the statistical significance for a good predictive model, but nevertheless appeared to be useful for monitoring changes over time and could be improved with some further work to improve precision. The best indicators, in terms of their predictive ability, were those for transporting potentially unnecessary attendances to ED and survival from a set of emergency conditions.
The advantage of this set of indicators is that it provided a more complete picture of ambulance service care than the current focus on a single process (response time) and clinical outcomes from a small set of acute conditions (cardiac arrest, stroke and heart attack) that comprise only a small proportion of ambulance service workload. The PhOEBE programme indicators are more inclusive as they represent patients with both emergency and urgent conditions, consider patient- as well as process-related outcomes and have expanded the measurement of survival to a broader group of patients than just cardiac arrest.
The models fitted as part of the development of case-mix-adjusted indicators are an indication of what models may need to be used to enable ‘fair’ comparisons to be made between services, areas or time periods. As the models were fitted using data from one ambulance service, they may not be generalisable to all ambulance services in England. Therefore, before the indictors could be used to compare and monitor performance within and between all ambulance services, the models would need re-fitting using national data.
We identified one other potential indicator at the end of workstream 1 – survival rates for patients who call for urgent conditions – as we would expect the risk of dying to be low in this patient group and it could tell us something about unexpected deaths, which are an important aspect of patient safety. We did construct a case-mix-adjusted model for this indicator. However, identification of patients with one of the specified urgent conditions relied on having an ICD-10 code that was available only for patients who were admitted to hospital or died. After discussion with the programme steering group we abandoned this indicator as it was recognised that including only admitted patients or those who died, and who were therefore more likely to be sick, introduced a serious bias. A substantial number of patients with urgent conditions will be managed by ‘hear and treat’ or at scene and these cases were not included in the model as we could not determine if they had one of the urgent conditions. Instead, we conducted a separate piece of work that allowed us to look at potential unexpected deaths by reviewing all deaths based on calculated risk of dying from our linked data. This is described in the next section.
Patient safety in prehospital ambulance care: exploratory structured judgement case record review study
Introduction
Retrospective case record reviews have been widely used within hospitals to assess quality of care and are supported by policy initiatives advocating the review of patient records for care quality and safety to identify shortfalls in care and inform improvement. 42 In-hospital mortality reviews have focused on ascertaining whether or not deaths were caused by problems in care and studies have reported preventable death rates ranging from 3.4% to 6%. 43,44
There is limited evidence of using retrospective case reviews in prehospital ambulance settings but it is possible that prehospital mortality may be higher and, therefore, more easily linked to failure to provide good care. Problems with safety may be caused by deficiencies in the initial assessment, care at the scene, poor record keeping or failure to transport patients to hospital for further assessment and treatment. Structured judgement case note review (SJR) is a retrospective case note review method for assessing quality of care, including safety issues following death of a patient, and may be applicable to the prehospital setting. 45
We aimed to assess the feasibility and usefulness of undertaking patient safety reviews within a prehospital setting by using SJR to assess records of patients with a low risk of death who died within 3 days of an ambulance contact.
Methods
We used SJR, which is a standardised clinical judgement-based case note review method. Reviewers provide quality and safety judgements about each phase of care, resulting in explicit written comments about care for each phase, scoring care for each phase and overall. 45,46
We used our linked data set to identify and select patients who died within 3 days of the initial ambulance call. Cases were stratified according to age group (aged 0–2, 3–10, 11–20, 21–30, 31–40, 41–50, 51–60, 61–70, 71–80, 81–90 and 91–120 years), dispatch codes and urgency (Red1–Green4) to ensure maximum variation. The number of calls within each group and the number of deaths (within 3 days of a call) within each group was calculated. The death rate for each group (based on dispatch code, urgency and age) was determined and groups were sorted by this rate, randomly selecting three in-hours and three out-of-hours patients from each group.
The review process
Five reviewers [nurse, paramedic, general practitioner (GP), community physician and hospital physician/medically qualified health service manager] each reviewed cases using SJR to assess quality, safety and potential preventability of mortality events. One reviewer attended training on structured judgement review and provided cascade training to the other four. Each reviewer had access to current guidelines for ambulance care. 47 Only information on prehospital care was provided.
Reviewers made judgement statements about the quality of care provided by the emergency ambulance service, taking into consideration the following phases of care: initial assessment, care on scene, care en route to hospital, quality of the records and overall quality of care, as well as rating the quality of each phase of care. Overall quality of care was also rated using a scale (Box 3).
-
Very poor care: may have led to severe harm(s) or even death.
-
Poor care: may have caused moderate or minor harm(s) or led to patient/family distress.
-
Adequate care.
-
Good care.
-
Excellent care.
Reviewers also assessed whether or not there were potential shortfalls in care that may have contributed to a patient’s death, to inform a judgement on whether or not death might have been prevented. Of course, not all deaths are the result of poor practice. For example, patients with terminal conditions, or who have complications after appropriate management, may die from causes that are not avoidable.
Avoidability of death was rated using a validated 6-point Likert scale. 48 Deaths were judged avoidable if reviewers felt that there was a > 50% chance that the death was avoidable, and this included all deaths that scored ≤ 3.
We used a thematic approach to analyse textual comments about the quality of care provided and related these to phase and overall care scores. We then related these themes to the avoidability of mortality judgement statements.
Results
From a total cohort of 150,003 linked records, we sampled 153 cases in which patients died within 3 days representing patients from different age, condition and urgency groups with the lowest risk of death. Almost one in five patients (19%; 29/153) were not transported to hospital at the initial attendance. Only these 29 patients were reviewed in detail as we could not distinguish hospital from prehospital effects in those taken to hospital.
There was variation in scoring between the five raters: the intraclass correlation coefficient (ICC) was calculated to determine the degree of consistency between rates. The single measures ICC, an index for the reliability of the ratings for one, typical, single rater was 0.51 [95% confidence interval (CI) 0.35 to 0.67]. The average measures ICC, representing a measure of the reliability of different raters averaged together, was 0.84 (95% CI 0.73 to 0.92), representing satisfactory inter-rater reliability in scoring.
Overall, 8 cases out of 29 (27.6%) scored between 2.4 and 2.8 (1 = definitely avoidable, 2 = strong evidence of avoidability), 8 cases (27.6%) scored between 3.0 and 4.6 (3 = probably avoidable, 4 = possibly avoidable) and the remaining 13 cases (44.8%) scored between 4.0 and 5.8 (5 = slightly avoidable, 6 = definitely not avoidable).
Common themes among cases determined to have strong evidence of avoidability were symptoms or physical findings indicating a potentially serious condition and refusal by patients or their carers to be transported to hospital (Table 13).
Case number | Sex | Age range (years) | Clinical impression (ambulance) | Description | Mean avoidability score | Final diagnosis of death | Days to death (1–3) |
---|---|---|---|---|---|---|---|
1 | Male | 61–70 | None recorded | Patient vomiting, with slurred speech and fall, found on bedroom floor by next of kin. Patient due for renal dialysis next day. On arrival lying on the floor, alert, with a GCS score of 15, good colour, pulse 88 beats per minute, BP 170/96 mmHg, FAST negative, no injuries apparent and 12-lead ECG normal. Patient did not wish to be transported to hospital | 2.4 | Coronary atherosclerosis | 2 |
2 | Female | 51–60 | Depression | Patient with depression on medication has become more depressed. Lives with sister who has been asking her to see her GP but she has declined. Sister reports patient becoming more lethargic. Patient has full capacity. Stated she will go to see her GP next morning | 2.6 | Hypertensive heart disease without (congestive) heart failure | 2 |
3 | Female | 51–60 | Chest infection | Patient with epilepsy and a current chest infection had a seizure for 10–12 minutes and given midazolam (Hypnovel®, Roche) by her family. After the fit she had hiccoughs and was twitching for several hours. It was decided by her parents to monitor at home | 2.6 | Chest infection | 1 |
4 | Male | 51–60 | Acute abdomen | Patient with abdominal pain, nausea and productive cough that began during the night. Cough caused him to retch with right-sided abdominal pain just below ribs. He has had abdominal pains for several weeks but has not been to see GP. History of previous heart attacks. On arrival of crew, patient not coughing but pointed to where it hurt when he coughed. Normal ECG. Took paracetamol and ibuprofen for his pain. Discussed with patient that it would be more beneficial to seek advice from GP – so partner to ring for an emergency appointment | 2.6 | Small bowel ischaemia | 2 |
5 | Male | 51–60 | Patient with diabetes mellitus found with speech problems by relative. Blood glucose level of 2.2 mmol/l. Patient given sugar and jam by sister. In addition, dizzy, diarrhoea, generally unwell, persistent cough for 4 weeks, poor appetite and lower leg swelling for 7 days. Took normal medications, except missed evening dosage. On arrival patient alert and orientated, blood glucose level of 5.6 mmol/l, pale, clammy, hypothermic and bradycardic and breathless on exertion with expiratory wheeze, reduced oxygen saturation (83%) and lower limb oedema. Appointment made to attend GP surgery at 11.50 | 2.6 | Mitral valve disease | 1 |
Discussion
We found that it was possible to use SJR to assess quality and safety of care in people who died. However, variability in the results from the five reviewers limited the usefulness of mortality reviews. Although standardised training may help to offset some of this variation, it may be more useful to focus on common themes and lessons for improving patient safety.
Although we used a sampling strategy to identify those at least risk of dying, we considered only patients who were not transported to hospital because we would not be able to identify preventable factors in patients admitted to hospital. We have reviewed only patients attended by one ambulance service and so the results will not be generalisable to all services. We also relied on linked data that is not currently routinely available to ambulance services. We did not include an ED physician as a reviewer, which was a limitation. However, the linked data did provide a valuable way of identifying patients who have died and calculating mortality rates for different groups of patients. This allowed selection of individual patients for review for whom the likelihood of dying was low and, therefore, may be potentially avoidable. Reviewing case notes to learn from examples of good- and poor-quality care is an important method for improving the quality and safety of patient care and service delivery. The findings of this type of periodic review are directly useful for informing efforts to improve quality of care and prevention of future deaths relating to care provided by ambulance service.
A more detailed description of the mortality review and the results is provided in a supplementary file at www.sheffield.ac.uk/scharr/sections/hsr/mcru/phoebe/reports. 25
Patient and public involvement
Introduction
Patient and public involvement is recognised as an important component of high-quality health services research in the UK and internationally,49 and was an integral part of our programme:
The long-running PhOEBE project has had PPI at its heart from the beginning.
Maggie Marsh, PhOEBE programme PPI reference group member
Patients offer unique insights and knowledge of a clinical condition or experience of care that researchers may not possess. Our PPI reference group helped the research team to focus on meaningful and relevant issues, improving the overall quality and credibility of the programme. As the PhOEBE study was a publicly funded programme that was focused on improving ambulance care, it was crucial that patients and the public were involved, helping to improve the NHS and people’s health-care outcomes and experiences, moving from being ‘mere users and choosers to being makers and shapers of health services’. 50
Reference group
A PPI reference group was created at the outset of the programme to independently consider the PPI issues and perspectives relevant to the programme and advise the research team. We aimed to optimise PPI input by identifying innovative ways to involve patients and the public.
The PhOEBE programme PPI reference group included three lay members: Maggie Marsh and Dan Fall from the Sheffield Emergency Care Forum (which is a PPI group representing patients and the public in prehospital emergency care research in Sheffield and across the UK) and Andrea Broadway-Parkinson, a freelance Disability Consultant, patient and public perspective ‘champion’ and ‘expert patient/advisor’ working with YAS NHS Trust, focusing on patient safety, experience, patient voice and involvement strategies. Details are provided in Box 4.
Andrea has experience of working in the voluntary, public and private sectors nationally and regionally and has focused recently on ‘expert patient’ and patient experience/quality-focused work. Key roles currently include Expert Patient/Advisor and Patient Research Ambassador with YAS, member of Pressure Ulcer Research Service User Network (PURSUN), member of the Yorkshire and Humber Research Design Service Public Involvement Forum and other freelance work as a PPI/lay representative.
Maggie MarshMaggie is a retired primary teacher. Her father was a GP. She became interested in medical research when her late husband developed heart problems and she was invited to take part in various research projects. She has become more interested in patient care and experience as she has taken part in further research projects on emergency care. She is a member of Sheffield Emergency Care Forum.
Dan FallDan previously worked as a researcher on ambulance studies as well as a wide range of odd jobs including coach driving. He participated in the PPI reference group for the PhOEBE programme to provide a view from the middle-aged working man and generally to be involved in the research process from a public perspective. He is a member of Sheffield Emergency Care Forum.
These three PPI members had previous experience of involvement in ambulance-related research or ambulance service patient involvement. This was necessary given the complexity of programme. However, an important part of their role was to ensure that there was wider PPI involvement and participation at key points during the work by drawing on much wider networks, including hard-to-reach and vulnerable patient groups.
Recognition of the role of public representatives also grew in the PhOEBE programme. Initially, PPI representatives were acknowledged in reports or included in group authorship as members of a steering or advisory committee. Increasingly they were recognised as co-authors of publications and co-presenters at conferences. Dan, Andrea and Maggie’s work on the PhOEBE programme represented a broad spectrum of involvement activities from consultative to event co-design, co-presenting and as co-authors on research outputs.
The PPI reference group benefited from having a dedicated member of the research team act as PPI co-ordinator to support its activities across the increasingly complex and multiple workstreams within the programme.
Co-ordination and activities
Key components of the PPI co-ordinator’s role were to:
-
provide an accessible single point of contact
-
build and sustain relationships across the PPI reference group and research team
-
act as an advocate for the needs and wishes of the PPI reference group
-
translate the research and research team’s wishes back to the PPI reference group
-
respond to PPI reference group lay members’ input and ideas to maximise co-production and adoption of general collaborative principles and practice
-
learn more about PPI-related issues in order to transfer learning to other research projects and other external research partners/stakeholders.
The PPI reference group developed its own Gantt chart to clarify the PPI tasks and related timetable, roles and responsibilities. This guided planning of PPI reference group meetings and agendas, and enhanced integration of PPI into management meetings and vice versa, which created a sense of a proactive rather than reactive PPI function:
The contributors’ roles and requirements must be spelt out from day 1 to ensure everyone knows their place in the structure.
Dan Fall
A terms of reference document was co-written with reference group members, setting out the purpose of the group, responsibilities of members, general approach to working, accountability of members and co-ordinator as well as a mission statement.
The specific responsibilities of the group are outlined in the terms of reference document:
-
To review all patient-facing aspects of the planned project including writing lay summaries in the initial funding application and ethics applications from a patient and public perspective, and to suggest any changes that might be made.
-
[One member] to sit on the project steering and management committees, attend all committee meetings, feedback PPI-related activity to the committee (with support from the PPI co-ordinator from the research team when this was required) and report back to the PPI reference group.
-
To help review summaries of research findings for a lay audience.
-
To plan for and help disseminate research findings and wider PPI/engagement activities.
-
To develop and deliver key PPI-specific activities (e.g. consensus events, focus groups, workshops and conference events).
-
To maintain communication with INVOLVE and other panels or conferences relating to lay involvement in research.
-
To work with the PPI co-ordinator in any other PPI-related tasks as appropriate.
The PPI reference group meetings lasted 2–3 hours and were held at The University of Sheffield School of Health and Related Research approximately every 3 months with communication by e-mail in between. The meetings were attended by at least two PPI reference group lay members (MM, DF or AB-P) and the PPI co-ordinator, project administrator and members of the research team as required.
One PPI member (DF) acted as a link and attended the Project Management Group and Study Steering Committee when possible. This mechanism helped to ensure a lay perspective on significant decisions within the project was considered:
My role in PhOEBE has been to participate within both the PPI reference group and also to be the PPI connection into the management and steering group of the project. This has been a 5-year project where I have been intended to have a day per month for input, contribute to the PPI element of the project and also to be the PPI presence within the core of the research team.
Dan Fall
The PPI reference group and wider patient and public contributors played an essential role in the PhOEBE programme, helping to steer and define measures for further development reflecting both service provider and public perspectives. The group contributed to the qualitative study in workstream 1 by commenting on the study protocol, patient information, consent forms and interview schedules results and interpretation.
The PPI reference group members and members of the Sheffield Emergency Care Forum were active participants in the initial interview study and subsequent consensus workshop and PPI event. The members also provided invaluable help in recruiting members of the public via local PPI networks as well as personal and professional contacts. Their most innovative work came with the co-production of a PPI workshop to support the Delphi study and ensure a patient and public lay input into the final choice of measures for development in workstream 3:
I had the inspiration to increase [PPI] to a manageable number, perhaps 20, of lay people to deliberate, choose and vote on their preferences of the measures in a new consensus day, closely working with the research team to bring this to fruition.
Maggie Marsh
The broad aim was to develop a more interactive way to listen to those who used and cared about ambulance services beyond a mere ‘tick-box’ exercise, and also to meet the requirements of the PhOEBE research programme:
It seems that within the concept of involving the public and patient representatives within academic research there is first an idea that the subject matter must be understandable by lay people. This needs to be a priority issue at the outset of any project. If genuine lay people are being used there is a high chance that the subject might be very alien.
Dan Fall
This resulted in a paper, co-written by PPI reference group members, which was published in Health Expectations,27 after editorial advice to ensure that the ‘voice’ of the PPI reference group was clearer in the paper. This was a real success for the PPI voice in the PhOEBE programme. The PPI reference group has also presented posters51,52 about its work on the PhOEBE programme at both an INVOLVE and a 999 EMS Research Forum conference, with other conference and public event attendances pending.
As the PhOEBE programme drew to a close, the PPI reference group was instrumental in the creation of another ‘first’ for a research programme with the development of an animation video to support dissemination of the programme findings. Group members have invested a huge amount of time, effort and enthusiasm in this activity and their experiences will be shared at the INVOLVE conference in November 2017. The animation video can be viewed online. 53
There is no doubt that the PhOEBE programme has presented some real challenges and difficult concepts that have tested our PPI reference group. It has risen to this challenge and found innovative and new ways of ensuring that the views and needs of those who are the users of our ambulance services have been truly embedded in the programme. Its work has not only benefited this programme but has more broadly generated new knowledge and methods for meaningful PPI in research that will hopefully influence future research studies.
A more detailed narrative about the PPI contribution to the programme, co-produced by our reference group, is provided in a supplementary file at www.sheffield.ac.uk/scharr/sections/hsr/mcru/phoebe/ppi.
How useful could the indicators be in the future and what are the costs associated with different clinical management options?
Introduction
In our original programme proposal and plan the primary intention of this final workstream (workstream 4) was to conduct further analytic work to test and validate the case-mix-adjusted indicators developed in workstream 3. However, as we have described earlier in this report, the substantial delays that occurred in obtaining and creating the linked data set meant that we were unable to conduct this work even with the extension period we had been granted. Instead, we have considered the conceptual issues related to the indicators we have developed, their value as ‘good’ indicators of performance or quality and their potential advantage over existing measures.
In our proposal, we also set out an objective to use the linked data to assess the costs associated with different types of ambulance response. We have been able to conduct this work and this is reported here.
What is a good performance indicator?
As part of the PhOEBE programme, we developed candidate indicators to assess the performance of emergency ambulance services. The process of development has included wide consultation with patients, ambulance service, commissioners, policy-makers and researchers. We have also calculated the indicators and examined their statistical reliability and whether or not case-mix adjustment makes them a potentially more reliable way of assessing performance or quality. To decide whether or not to recommend these indicators for inclusion in a national assessment framework, we have also considered wider questions about what characteristics a good indicator should exhibit.
There is a wide literature on performance indicators, some of which is focused on what makes a good indicator and how to choose and develop them. We have examined some of the publications issued by UK organisations, as well as a comprehensive review, to try to identify a ‘checklist’ of the characteristics of good indicators. This literature is not comprehensive or based on a systematic search as we are only trying to achieve ‘saturation’ of the ideas about the criteria that good indicators should possess.
Sets of indicators
Because services have many components, and many objectives, assessment of the quality of services will ideally be based on sets of, rather than individual, indicators. Before looking at the quality of individual indicators it is worth asking what properties this set should have, but there is little literature on this question. Based on previous research undertaken by PhOEBE programme study investigators,40 we consider that the set should ideally be:
-
Inclusive – the set of indicators should ensure that service or system performance is relevant to all patient groups that might use the service. If some patients are excluded, then there is the possibility of distorting the service or system to focus on only those groups included. This is related to equity.
-
Comprehensive – addressing all the important dimensions of performance quality, such as effectiveness of services and care, efficiency, cost, equity and safety. A comprehensive indicator set should also address outcomes, processes and structures.
-
Co-ordinated – indicators should work independently or with each other, not against each other. For example, including both the proportion of traumatic brain injury patients treated in a neurosurgery centre and the total number of transfers could cause conflict.
-
Parsimonious – equally, a good set of indicators should avoid unnecessary duplication (indicators that are measuring or indicating performance in the same area). An over-riding principle identified by the Royal Statistical Society working group on indicators is the need for parsimony. 54
Types of indicator
Indicators or measures
One issue to consider in selecting types of indicator is the question of whether they are ‘indicators’ or ‘measures’. Indicators do just that, as they are said to ‘resonate’ with performance and quality but are not direct measures of it. For example, in emergency and urgent care, one indicator could be based on the number of attendances at an ED on a Monday morning compared with the average number on other weekday mornings. There is known to be a Monday morning blip, which in itself does not matter, nor is it a measure of anything; however, when it is high it might indicate poor access to the emergency and urgent-care system over the weekend. Therefore, this could be a measure that resonates with the quality and performance of the emergency and urgent-care system over the weekend.
We take the view that measures are generally preferable and that the set of indicators we choose for assessing ambulance service performance should, as far as possible, actually measure aspects of the service performance that are important. Individually, if these measures change it could indicate a better performance overall and, taken together as a set, are an indicator of the quality of the service. Nevertheless, we have also considered some service-specific measures that were considered to be indicators of performance.
Characteristics of good performance indicators
Several lists of the attributes of a good performance indicator have been published. We have synthesised the lists published by Pringle et al. 54 (12 items), The Audit Commission55 (13 items), the Royal Statistical Society56 (14 items), the Institute for Innovation and Improvement (11 items relevant to individual indicators and two items focused on the set of indicators)57 and the literature review and expert assessment carried out by Jones et al. ,58 which although focused on health care generally has considered services, organisations and policy as well as frontline ‘care’ (15 items). There are many other published lists but a review of this sort seeks saturation rather than completeness and we have found that adding other lists is simply repetitive.
Broadly speaking, these checklists have identified six key criteria for good indicators. Criteria should:
-
be important to users
-
be valid and evidence based
-
use reliable data
-
be statistically robust
-
be simple to understand
-
be remediable.
We have taken the view that the ambulance service indicators should be chosen principally with either performance monitoring within a system in mind, to answer the question ‘are things getting better?’, or performance assessment to determine whether or not a change in the system has improved performance. Between-service comparisons and the construction of league tables are fraught with difficulties and should not be a priority in choosing indicator sets for ambulance services, but some of our indicators would make this possible. This was illustrated in two of the indicators that we developed, for which the effects of individual hospitals on outcome was very apparent. With this in mind, we have placed emphasis not on issues such as comparability or consistency between places, nor on the question of whether or not they are ‘context free’, but rather on their value as signals of change in individual services or the ambulance service component of an emergency and urgent-care system.
We have used these six main criteria to assess all our candidate indicators to ensure that we have made an all-round assessment of their quality. Within each of the six main criteria there are several characteristics and those that have been explicitly identified in the lists we have examined are presented in the results. We have used these characteristics as a guideline of the issues to consider in making the assessment of each criterion.
Assessment of measures for the PhOEBE programme
Each of the measures developed for the PhOEBE programme were assessed against the six key criteria for good indicators by a small group of five ‘experts’, drawn from the programme steering committee, who assessed the indicators from the perspectives of health-care commissioners, clinical academics, ambulance providers and statisticians.
Each of the six key criteria for good indicators was broken down into several subcomponents (based on those identified in the literature). The PhOEBE programme’s indicators were assessed against each of the subcomponents by our expert group and the results were collated (Table 14). The PhOEBE programme’s ‘toolkit’, which set out the definitions and construction of each indicator, was provided to each of the panel to facilitate the assessment.
Attribute | Attribute components | Pain | Accuracy of call ID | Response time | Re-contacts | Unnecessary ED attendance | Survival from emergency conditions | ||||||||||||||||||||||||
---|---|---|---|---|---|---|---|---|---|---|---|---|---|---|---|---|---|---|---|---|---|---|---|---|---|---|---|---|---|---|---|
Important | Relevant to people | ||||||||||||||||||||||||||||||
Valuable | |||||||||||||||||||||||||||||||
Avoid perverse incentives | |||||||||||||||||||||||||||||||
Valid | Well defined | ||||||||||||||||||||||||||||||
Evidence based | |||||||||||||||||||||||||||||||
Effective | |||||||||||||||||||||||||||||||
Timely | |||||||||||||||||||||||||||||||
Cost-effective | |||||||||||||||||||||||||||||||
Use reliable data | Consistent | ||||||||||||||||||||||||||||||
Reliable | |||||||||||||||||||||||||||||||
Statistically robust | Statistically reliable | ||||||||||||||||||||||||||||||
Responsive | |||||||||||||||||||||||||||||||
Simple to understand | Interpretable | ||||||||||||||||||||||||||||||
Communicable | |||||||||||||||||||||||||||||||
Remediable | Attributable | ||||||||||||||||||||||||||||||
Deconstructable | |||||||||||||||||||||||||||||||
Remediable |
On the whole, all the measures met or partly met most of the criteria. This means that the measures are potentially good indicators of ambulance service quality and performance.
One of the panel members frequently felt that they required more information than was available to assess the measures, as shown by the high number of times they used the ‘do not know/unable to assess’ option. Our expert panel agreed that all the PhOEBE programme measures are relevant to people. This was also identified through the large amount of consensus work undertaken by the study in workstream 1. There were only 5 cases out of 510 ratings that were rated as ‘does not meet’. Four of these cases related to the survival from emergency conditions measure and there was some uncertainty about whether this measure is remediable or deconstructable. There was also some uncertainty about the reliability of the pain measure, which may be because the pain scales used to assess pain are subjective.
Summary
Overall, the set of indicators created appeared to provide an inclusive picture of different aspects of ambulance service care. They have relevance to different patient groups – for example, response time includes all calls, pain to a substantial proportion, accuracy of call problem identification and survival to the most urgent cases – and separate but complementary measures for patients who are and are not taken to hospital encompass all service users. Combined, they also fulfil the different domains of quality set out by the Institute of Medicine framework23 and some are relevant to more than one dimension. For example, response time relates to timeliness and efficiency; re-contact rates and unnecessary attendances relate to effectiveness and patient centredness (providing the most appropriate clinical response for patient need) and also safety; and change in pain score and survival are patient-centred outcomes that are relevant to a much larger proportion of the 999-call population than existing measures (which have focused on small and specific groups, such as cardiac arrest). There is no direct measure of equity but the ability of the indicators to measure potential differences across different geographical areas or populations can address this dimension.
In this respect, the set of measures or indicators that we have explored has achieved our objective of developing measures that can tell us something useful and valuable about the service and care delivered to all patients who contact the ambulance service and that consider both processes and outcomes that are important to a range of stakeholders with different perspectives.
Exploring the use of case-mix adjustment has revealed that, for some measures, there are patient and environmental factors that exert effects on processes and outcomes that are independent of the response and care provided by the ambulance service. Adding case-mix adjustment to performance or quality indicators takes these factors into account and allows a more meaningful and precise assessment of the impact of ambulance care that crude unadjusted measures, as currently used, cannot convey. This is particularly important if comparisons were to be made between different services or areas within services. It is also important for single-service comparisons over time. If there are changes in the 999 population, for example as a result of shifting demographics with an increasing proportion of elderly patients, and the effect that this has on processes and outcomes is not controlled for, there is a real risk that apparent changes in performance or quality will be erroneously attributed to poor or deteriorating ambulance service provision when this may not be true.
The PhOEBE programme has created a potential set of ambulance service performance and quality indicators that fulfil the requirements of good indicators and the dimensions considered important to good-quality health care. How they might be used in future is explored in more detail in Discussion and conclusions, Implications for practice.
Estimating the cost impact of alternative types of ambulance response
Introduction
As we have seen earlier, there have been innovations within ambulance services that have changed the way they respond to people who call 999, including advice over the telephone and treatment at home as well as hospital attendance. These changes have come about both to provide more appropriate clinical care for patients that is responsive to their needs, and to help improve efficiency and make best use of ambulance and hospital resources.
The response received by the patient will depend on the assessment by the ambulance service. However, similar conditions may potentially be assessed and treated differently and there is potential for inappropriate decisions to be made, which could waste ambulance service resources, lead to deaths or lead to future hospital admissions. The performance and quality indicators developed in workstream 3 have encompassed these elements by examining different processes and outcomes. Another important element is the impact that decisions have on costs to both the ambulance service and the wider health system. The creation of the linked data in workstream 2 and the development of indicators to identify potentially incorrect decisions provided a unique opportunity to assess the impact of different types of response on NHS costs. For this piece of work we have examined the economic effects of different types of response in two ways. First, by comparing the average costs of treatment associated with each response type and, second, by estimating the average costs associated with incorrect decisions to either leave patients who need to go to hospital or transport patients when this is unnecessary. This allows us to show current costs and the potential cost reductions associated with a change in the type of response.
Methods
We used the linked data to create a new data set with additional cost data added. For each incident we extracted the relevant data fields: age, sex, call type, urgency, response, admission and discharge details, treatment type, ICD-10 primary diagnosis code, primary operation code, episode start and end date. For inpatient hospital stays we allocated a Healthcare Resource Group code (based on diagnostic code) to assign inpatient costs and used these to calculate the cost per inpatient spell. For ED attendances we assigned a cost per attendance and for ambulance costs a cost per response type (‘hear and treat’, ‘see and treat’ and ‘see and convey’). All costs were assigned using National Reference Costs. 59 For individual patients, not all hospital attendances may be related to the ambulance incident, so we made some assumptions (e.g. excluding elective inpatient episodes and using only the first ED attendance within 3 days of the call) to account for this.
We calculated mean costs per call for ‘hear and treat’, ‘see and treat’ and ‘see and convey’ responses and compared categories. However, we know that there will be differences in the characteristics of calls within each type of response. To control for this we also created matched groups of calls for patients with the same characteristics. We applied exact matching on call code and condition, and Mahalanobis distance matching on age and sex. 60 Exact matching was used for the categorical variables to ensure that a ‘hear and treat’ caller with a given call code and condition was matched to a ‘see and treat’ or ‘convey’ caller with the same call code and condition. Mahalanobis, matching is used to minimise the distance in terms of age and sex for the matched observations, for instance a caller is matched to another caller, within the same call code and condition category, that is closest in terms of age and sex. We created matched samples to compare costs for:
-
‘hear and treat’ with ‘see and treat’
-
‘hear and treat’ with ‘see and convey’
-
‘see and treat’ with ‘see and convey’.
Incorrect decisions were identified for calls where ‘hear and treat’ or ‘see and treat’ patients attend ED or are admitted to hospital within 3 days, or patients are taken to hospital and discharged from ED using the same criteria set out in indicator 5 (workstream 3). Each incorrect decision was matched with an equivalent case that had the correct decision. We then compared the costs of an ‘incorrect’ decision with a ‘correct decision’ for each response type to enable us to estimate the costs associated with less than ideal decision-making.
Results
A total of 182,566 cases were included in the analysis, and 10,151 (5.6%) calls received a ‘hear and treat’ response, 51,223 (28.0%) calls received a ‘see and treat’ response and 121,192 (66.4%) calls resulted in the patient being taken to hospital. Using the results of the matched case analysis, as these are more precise, we found that the total mean cost of a ‘hear and treat’ call was £125, ‘see and treat’ was £415 and ‘see and convey’ was £1745. The main reasons for these differences are the differences in inpatient costs. For the calls that initially received a ‘hear and treat’ response, the mean length of stay was 0.24 days, compared with 0.68 days in the ‘see and treat’ group, and 4.46 days in the ‘see and convey’ group. The majority of patients have 0 days of inpatient stay with only 2.35% of ‘hear and treat’ cases, 5.44% of the ‘see and treat’ cases and 46.73% of ‘see and convey’ cases having an inpatient stay.
For the analysis comparing costs of correct and incorrect decisions we found that the mean total cost of a correct conveyance decision is £3728.99 and the mean total cost of an incorrect non-conveyance decision is £4042.38; therefore, the additional cost to the emergency services of making an inappropriate decision of this type is £313.39. This difference is the result of higher call and inpatients costs (relating to, approximately, 1 day in hospital). The mean total cost of an appropriate non-conveyance decision is £109 and the mean total cost of an incorrect conveyance decision is £346, resulting from higher call and ED costs (relating to, approximately, the cost of one ambulance call out and one A&E attendance).
Summary
The cost analysis has allowed us, for the first time, to estimate the actual costs of different types of ambulance response using real data on all NHS contacts for each type of response. The matched analysis suggests that, for many conditions, alternative responses are possible with lower costs, but imperfect matching and the lack of patient outcome information means that these results should be treated with caution. However, this type of information is helpful to support planning and commissioning of services and where efficiencies might be achieved. Our analyses of two different types of incorrect decision are more robust and show that these decisions are associated with higher costs. The development of processes to identify the rates of incorrect decisions through the workstream 3 indicator and ability to now assign a cost to these decisions provides a way of estimating what costs could be saved if efforts are put in place to reduce the number of incorrect decisions.
A more detailed description of the methods, results and limitations of the cost analysis can be accessed as a supplementary file at www.sheffield.ac.uk/scharr/sections/hsr/mcru/phoebe/reports. 25
Discussion and conclusions
Summary of programme achievements
We set out an ambitious programme to explore and test the potential use of case-mix-adjusted measures to assess ambulance service performance and quality. We have achieved most of the objectives we set out in our original proposal. To fulfil the first objective of identifying potential indicators for development we used a systematic approach to the identification of potential measures and indicators by conducting and triangulating different evidence sources. This included collating and summarising the existing evidence on process and outcome measures from both policy and primary evaluation research and new primary research on patient views of ambulance service care. Consensus methods allowed us to rate and prioritise potential measures using an inclusive, interdisciplinary approach ensuring that a range of views and perspectives were considered in the final choice of measures or indicators for further development. The final set of measures identified for development reflected a range of important processes and outcomes that, together, provide the potential to make a balanced assessment of ambulance performance and quality that encompass all users of the emergency ambulance service. This could be achieved with a relatively small set of just six indicators that have relevance to patients, providers and commissioners. The six indicators were reduction in pain, accuracy of 999-call triage, response times, inappropriate non-conveyance to hospital, unnecessary transport to hospital, and survival for 16 emergency conditions.
The second objective was the development of a linked data set that could capture processes and patient outcomes for the whole episode of care, including hospital care, related to each individual call, not just the prehospital component. Despite considerable difficulties and delays we have, for the first time in England (to our knowledge), created a comprehensive data set that provides information on ambulance service response and patient care, hospital events and mortality outcome for > 150,000 individual 999 calls. For the third objective on indicator development, the primary purpose was to assess whether or not case-mix adjustment could improve the measurement of processes and outcomes, whether or not this was feasible and what characteristics might be important. We found that for four of the indicators (i.e. triage accuracy, inappropriate non-conveyance, unnecessary conveyance and survival) case-mix adjustment did identify patient and environmental factors that influenced the process or outcome being measured. This confirmed the value of taking this approach if measures are to be useful and meaningful in detecting changes that are a consequence of ambulance service care rather than other influences that are outside the control of a service. In particular, the significant effect that individual hospitals have on some outcomes was confirmed and highlights the difficulties of separating the impact of ambulance service care from that delivered by other parts of the health-care system. This means that the utility of the indicators and what they can usefully be used to compare varies. For the outcomes that are solely within the control of the ambulance service (i.e. triage accuracy, decisions not to convey to hospital and survival to arrival at hospital), the indicator could be used to make both within- and between-service comparisons. When other system factors (e.g. the hospital) have an influence, the indicator would be most useful for measuring within-service changes over time. The indicators also revealed that rates for some of the indicators were relatively low; for example, the rate of inappropriate decisions to leave patients at home was between 5% and 10% across CCGs. Survival to admission to hospital was 90.5–97.3% for 16 emergency conditions, which confirmed that a very small proportion of 999 calls are for immediately life-threatening conditions. This does bring in to question the value of using survival (or mortality) rates as an indicator of ambulance service quality.
The fourth objective was to test the indicators on a separate data set and further assess the validity and utility, but delays in obtaining the data meant that we were unable to meet this objective.
The creation of the linked data also allowed us to make a comprehensive assessment of the costs associated with different types of ambulance response and provide a method, using the indicators, for estimating the potential cost savings that could be made if decision-making about conveyance to hospital could be improved. This is the first time that calculation of costs for the complete episode of care, including contacts and re-contacts with the wider health system, has been possible.
The programme has been underpinned by a comprehensive component of PPI despite some of the complex concepts involved. Most people understand what the ambulance service do; understanding performance measurement, why it is needed and how it should be done is more difficult. By embedding PPI within our project, we were able to prioritise a potential set of ambulance outcome and performance measures, which have considered the preferences of professionals involved in prehospital ambulance care and also factors that are important to patient and public representatives. The animation developed with our PPI representatives will help disseminate key messages from this study around the way ambulance services have evolved and how their performance is measured to the general public.
Challenges and limitations
Clearly the biggest challenge to the programme has been the delays encountered in workstream 2 and the excessive time taken to obtain the linked data. Although we recognise that it was unfortunate that our programme coincided with a particularly difficult period for NHS Digital and that we had no control over these events, there have been substantial consequences. The related issues took so long to resolve that we had not received any data by the time the programme was due to end in May 2016 – 5 years after the programme had started. We were mindful that the National Institute for Health Research had invested considerable funds in this programme, so to have finished at this stage would have been wasteful of the money already invested. With careful management of the budget and allocating staff to other projects during the periods when we could make no progress, we were able to ask for, and we were granted, a 1-year no-cost extension to the programme. This means that we were able to complete the work planned in workstream 3, but this put considerable pressure on the team as we condensed > 1 year of planned work into 8 months. The delays do mean that we were unable to complete some of the work that we had planned in order to further test and validate the case-mix-adjusted indicators. We were also unable to re-assess their usefulness in a ‘real-world’ setting, in terms of both their relevance to the ambulance service and wider emergency and urgent-care system. It has also meant that of the four planned data sets, we have been able to process and use only one.
An important consideration of linked data sets is completeness. In this programme, although the overall linkage rate was high (> 82%), this was variable across different patient groups and was very low for the ‘hear and treat’ group because of the quality of recorded data about this patient group. This potentially introduces bias into our sample and our analysis. We assessed the patient characteristics of those with linked and those with unlinked data for all of the study patient groups and found very little evidence of difference for those that were conveyed to ED or seen and treated at the scene. However, the ‘hear and treat’ patients with linked data were older than patients with non-linked data and this limits the generalisability of these findings across the whole ‘hear and treat’ population.
There are other broader challenges and limitations that have arisen from our experiences in obtaining the linked data that have implications for the future use of the set of indicators that we have developed. First, the process of creating the linked CAD and ePRF data within the study ambulance services into a format that could maximise the number of calls traced at NHS Digital proved to be more complex and time-consuming than we had envisaged. Having worked through the process, lessons have been learned that would make this easier in the future but it does require a substantial investment of time to complete this work. Ambulance service information systems that enable linkage within and across services are still variable in terms of implementation, adoption and capability and trying to improve this is a work in progress. Second, even assuming that the NHS Digital data linkage service is operating normally, it takes time for tracing, matching and processing. There are also natural delays because NHS Digital’s own processes for cleaning and checking HES data means that this is not available for matching for several months. This means that using this type of approach is unsuitable for performance measurement that is near to ‘real time’. Data will always be several months old before it is useable. Third, once the linked data were obtained, the processing required to transform it into a format suitable for conducting the indicator model development was also complex and time consuming. Again, having done this and refined the processes needed, this would be less onerous to replicate but the resource needed to carry out this work cannot be underestimated. These challenges are not unique to England. Recent work in Australia linking health data across different jurisdictions found the same problems, that is, the processes remain costly and time-consuming. 61 However, the challenges are not insurmountable. In Scotland, an urgent-care data set linking NHS 24, ambulance service, primary care out of hours, ED, hospital and mortality data has been developed and contains complete patient pathway records from 2011. 62
We were fortunate to have research funds to support this work. We also requested a very large number of variables for the linked data from both the ambulance service and NHS Digital. Having constructed the case-mix-adjusted indicators and identified which variables are needed, a much smaller data set would be needed in future to replicate these measures, which would simplify the process. Nevertheless, without considerable investment or better data sources that are more easily linked, it will not be feasible for ambulance services to routinely create linked data for performance and quality measurement in the short term.
The construction of the case-mix-adjusted indicators was a complex process requiring multiple iterations to identify the most suitable predictive model. For some indicators, there were issues with missing data that limited the value of the model. This was particularly true for cases that could not be matched, which then reduced the number of incidents that were included in a model and potentially introduced bias into the measure. We also had to make some assumptions that need to be taken into account when interpreting the measures. For example, when measuring re-contacts it may be that some subsequent hospital attendances or admissions are justifiable and the consequence of a worsening problem and the original decision to not take a patient to hospital was correct at the time it was made. The indicator cannot tell us which individual decisions are ‘right’ or ‘wrong’, and we cannot assume that all re-contacts are the result of an incorrect decision – there will be some natural variance. The value of the case-mix-adjusted indicators is that they calculate a rate and it is variation in this rate that potentially provides a signal that the decision-making is changing over time, or varies between areas in the same service. However, there are limitations. The models fitted as part of the development of case-mix-adjusted indicators are an indication of what models may need to be used to enable ‘fair’ comparisons to be made between services, areas or time periods. We have demonstrated that direct risk standardised rates can be calculated to make comparisons between performance in different geographical areas within the ambulance service and how funnel plots can be used to identify outliers. As the models were fitted using data from one ambulance service, they may not be generalisable to all ambulance services in England. Before the indictors could be used to compare and monitor performance within and between all ambulance services, the models would need re-fitting using national data and this may lead to changes in the structure of models as well as the model coefficients. Furthermore, we have examined only the influence of case-mix factors that have been measured and that were available to us. There may be other factors that we have not explored that matter, and so before these indicators are used it would be important for policy-makers and other stakeholders to consider whether or not they believe that the case-mix-adjusted indicators do give a fair reflection of an ambulance service’s performance.
We have been unable to test the ability of the case-mix-adjusted measures to detect changes or differences, as was our original intention, which limits the usefulness at present. Further work needs to be conducted to establish if the models can be replicated with different data sets and to test whether or not they are sensitive enough to measure important changes or differences.
Implications for practice
The development of more useful and outcome-based performance and quality measures has been an issue of international interest and importance for a long period of time. Despite this, little progress has been made beyond the measurement of simple process measures such as response time in most countries. 7 The most commonly measured outcome has been survival from out-of-hospital cardiac arrest, which is relevant only to a very small proportion of calls (in England, this is currently 0.6% of 999 calls). 9 The UK has used a combination of system and clinical indicators for a number of years, but the limitations of predominantly process measures and an overemphasis on response time performance has been acknowledged. 16
A recent Delphi study identified potential measures from existing literature that could be used as key performance indicators for prehospital care and captured many of the same items that we found in our own reviews63 but the prioritised measures comprised single items. Little attention has been paid to case-mix adjustment with only one US study addressing this issue,64 although no outputs in terms of validated indicators have been identified. The advantage of the set of indicators that we have developed is that they are composite measures, for example including 16 emergency conditions in a single indicator, and incorporate case-mix adjustment where we have identified that this is important. Although they need further testing, there are real potential advantages in measuring a small but inclusive and comprehensive set of indicators. However, as we have shown, most of these indicators require linked data and the issues encountered in this programme suggest that it is not feasible to create the necessary data sets in a routine and timely way using current systems.
The problems associated with the creation of linked data sets and their use in research are a consequence of the much broader and more difficult policy issues concerned with use of technology and data. In England, the Five Year Forward View65 acknowledges the shortcomings of previous attempts to streamline and connect information across the NHS and there is a clear intention to address this issue with the formation of the National Information Board. Within this is an explicit statement of the need to bring together different data sources to support quality improvement and research. 65 The NHS Digital data and information strategy also sets out ambitious objectives to create better data and access to data that can support service planning and delivery and measurement of quality and performance. 66,67 Access to data is particularly challenging issue. Internationally, the opportunities that the use of ‘big data’ can potentially bring to improving and transforming health care are recognised but critical issues remain around how data are collected, stored and used. 61,68 In particular, important factors around regulation, privacy and the need to reassure the public that their data are used legitimately and safely still need to be overcome. A policy analysis by Heitmueller et al. 68 highlights how policy and legislation may help or hinder change in digital information gathering. We have described our experiences within the context of one programme of research but the solutions to overcoming the problems we encountered will need changes that require revisiting these broader issues at a national level.
We have considered the short- and long-term implications for practice and how the indicators we have developed might be used.
In the short term:
-
Two of the indicators require ambulance data only and so could be implemented. The indicator that explored alternative ways of displaying response time performance has already had an impact. In 2015, NHS England embarked on a programme of work to improve emergency ambulance response performance – the Ambulance Response Programme (ARP). 9 Members of the PhOEBE programme research team have also evaluated this programme. The main changes tested in the ARP were allowing additional time for call assessment and a revision of the 999-call categories to better reflect urgency and response needed. As part of this programme, there was also a revision of the current ambulance system and clinical quality indicators. The PhOEBE programme helped this work in two ways. First, we utilised the outputs of workstream 1 to support a workshop that reviewed the current quality indicators. Second, the work on the response time indicator has been adopted and the 75% within 8 minutes response time target has been replaced by reporting mean and 90th percentile response times for each of the new call categories. 69 The ARP changes were approved by the Secretary of State for Health in July 2017 and implemented in all ambulance services in England by November 2017.
-
The indicator measuring mean change in pain score also requires ambulance data only. In the ARP indicator review, pain management was identified as an important outcome. The national ambulance clinical indicators are currently being reviewed and there is now scope to include the pain measure within this revision for some specific condition types.
In the long term, progress will be dependent on resolving the broader issues around provision of and access to data. Current ambulance quality indicators use aggregated data. A basic principle for the indicators that we have developed is that they require individual call data to provide the information, such as demographics, condition and outcomes, needed to construct the indicator. Should data linkage become a simpler process, there would still be a burden on individual services to support the process by providing call-level data. One potential solution would be to design and support a standardised way of collecting individual call- or patient-level data that could be warehoused centrally. This could be a similar process to the recently developed Emergency Care Data Set that now provides a standard data repository for EDs. A national data set could potentially be a more efficient way of handling the individual records needed for data linkage. It would also provide an important resource for research that could also reduce pressure on individual ambulance services as they are increasingly asked to provide data for research studies but have limited capacity to support these requests.
If a national ambulance data set were created that could be linked easily to the Emergency Care Data Set, then it would be possible to use the indicator developed to measure the rate of unnecessary ED attendance in conveyed patients as this does not require inpatient or death data.
The three indicators measuring accuracy of call assessment, re-contacts and admissions for patients who were not transported, and survival do all need complete linked data. It will not be feasible to measure these indicators routinely until simpler, more efficient and timelier processes for linking data can be found. A national ambulance data set could in part facilitate this. It would also enable indicators to be measured centrally rather than separately by individual services, which would improve efficiency. If better and more meaningful performance and quality indicators are to be implemented then it also needs to be recognised that these are also more complex to measure and will need the resources necessary to support data collection and management, and analysis.
The mortality review provided a useful exercise and is a potential method for reviewing patient safety. Using the linked data to identify deaths and assess risk of death provided a structured and systematic way of sampling cases for further review. The process is resource intensive and not suitable as a regular monitoring tool but could be used on a periodic basis to add another dimension to quality improvement.
The case-mix-adjusted indicators may also have value as outcome measures for new research projects in which, for example, change in service delivery is being evaluated. If an indicator is relevant then the data collection requirements and methods for construction of the indicator are already specified or could be modified. A future national, patient-level ambulance service data set that has interoperability with related data sets in other parts of the urgent-care system, similar to that provided in Scotland, could enable the development of more sophisticated measures of performance and quality, based on those described here.
Recommendations for research
The different studies conducted as part of the PhOEBE programme open up a wide range of potential future research:
-
The measures prioritised through the consensus studies should be further developed, validated and examined to investigate their importance, validity, feasibility, relevance and sensitivity to differences in services, service changes and quality improvement efforts in practice.
-
New measures, such as patient-related experience measures, should be developed based on our understanding of what is important to patients using ambulance services.
-
Further work could investigate how to use the indicator to monitor performance over time and the frequency with which the indictors could be calculated statistically and feasibly.
-
The existing and future data sets linking ambulance, hospital and mortality data could be used to investigate the effects, safety and costs of different pathways and processes for a variety of clinical conditions and patient outcomes.
Conclusions
We identified and prioritised, through systematic reviews of the literature followed by a series of formal consensus processes with a wide range of stakeholders, a set of potential ambulance service quality measures that reflect the preferences of both services and users. We also created a comprehensive linked data set providing information for individual calls that extends beyond the prehospital component of care, although this proved to be a complex and time-consuming process. Six candidate indicators were developed using case-mix adjustment and, of these, four were found to need adjustment to make fair comparisons. Hospital was found to have a substantial effect on the process or outcome for two indicators, which means that these are suitable only for use at an individual service or system level. Other indicators could be used to make comparisons between regions or services. The complexities of both creating linked data and constructing the indicators means that, at present, they are of limited value as it would not be possible to measure them routinely. This indicator set, or subsets of indicators, could potentially be used to compare ambulance services or regions or measure performance over time after further testing to establish their utility when used in practice. Substantial improvements will be needed in both the collection and management of ambulance data and the mechanisms for linking data across services if more complex and comprehensive outcome-based measures are to be adopted for routine monitoring and quality improvement.
Acknowledgements
We thank the patients, service users and public representatives, including members of the PhOEBE programme’s PPI Reference Group, Sheffield Emergency Care Forum, and Healthier Aging Patient and Public Involvement (HAPPI) Group, that supported and contributed to the programme; ambulance service staff and academics who contributed to the consensus and Delphi studies; Joseph Akanuwe [Community and Health Research Unit (CaHRU)], Greg Whitley (EMAS), Rod Johnson (EMAS), Dr Nadeeka K Chandraratne (CaHRU) and Dr KGRV Pathirathna (CaHRU) who assisted with the mortality review; Darren Cox (EMAS) and Russell Danby (YAS) for support with ambulance data provision; members of the PhOEBE programme’s management and steering groups including Fiona Lecky, Daniel Mason, Andy Newton and John Brazier for their advice and support; and, finally, Marc Chattle for administering the programme including its management and steering groups.
Contributions of authors
Janette Turner (programme co-lead, co-applicant) co-led the design of the programme and was substantially involved with all aspects of the programme, and led the drafting of the final report and synopsis and the assimilation of report components and appendices.
A Niroshan Siriwardena (programme co-lead, chief investigator, corresponding author) made substantial contributions to the conception, design and analysis and interpretation of the data.
Joanne Coster made substantial contributions to the conception, design, and acquisition, analysis and interpretation of the data, including leading the consensus studies and workstream 2, gaining approval for data linkage and accessing data, contributing to the systematic reviews and drafting these sections of the report and the sections on what makes a good indicator and the assessment of measures.
Richard Jacques made a substantial contribution to the analysis and interpretation of data through conducting the statistical analysis of data and developing the risk adjustment models.
Andy Irving made a substantial contribution to the acquisition, analysis and interpretation of the data through facilitating PPI in the study, undertaking the Delphi study and leading the writing of the PPI section of the report.
Annabel Crum made substantial contributions to the acquisition, analysis and interpretation and provided data management support.
Helen Bell Gorrod made a substantial contribution to the analysis and interpretation of data by leading on the economic analysis and drafted this section of the report.
Jon Nicholl made a substantial contribution to the conception, design and interpretation of the data, and advised on all aspects of the programme, particularly the development of statistical models and good indicators.
Viet-Hai Phung made a substantial contribution to the systematic reviews and the qualitative research through acquisition, analysis and interpretation of the data.
Fiona Togher made a substantial contribution to the acquisition, analysis and interpretation of the qualitative data through undertaking fieldwork, analysing data and writing a publication.
Richard Wilson made a substantial contribution to the design acquisition and analysis of data, particularly for the systematic reviews.
Alicia O’Cathain made a substantial contribution to the design, acquisition, interpretation and analysis of data by leading the qualitative interview study and participating in the consensus events.
Andrew Booth made a substantial contribution to the design, acquisition, analysis and interpretation of the systematic reviews by designing and leading this aspect of work.
Daniel Bradbury made a substantial contribution to the acquisition, analysis and interpretation of data for the systematic reviews and was involved in the consensus work.
Steve Goodacre, Ronan Lyons, Helen Snooks and Mike Campbell made substantial contributions to the conception and design of the study, provided methodological advice and contributed to the analysis and interpretation of data and the development of the indicators.
Anne Spaight, Jane Shewan and Richard Pilbery made substantial contributions to the analysis and interpretation of the data and the development of the indicators and also helped access to ambulance service sites and data.
Daniel Fall, Maggie Marsh and Andrea Broadway-Parkinson made substantial contributions to the design, analysis and interpretation of data through their role as PPI representatives for the study. They also took part in the consensus research and contributed to writing the PPI sections of the report.
Mike Campbell made a substantial contribution to the design of the study and to the analysis of data and the risk-adjusted models for the indicators.
All of the authors were involved with drafting and/or revising the report, approved the final version and agree to be accountable for all aspects of the work in ensuring that questions related to the accuracy or integrity of any part of the work are appropriately investigated and resolved.
Publications
Coster J, Turner J, Siriwardena AN, Wilson R, Phung VH, O’Cathain A, et al. Prioritising Outcomes Measures for Ambulance Service Care: A Three Stage Consensus Study. In Society for Social Medicine 57th Annual Scientific Meeting, 11–13 September 2013, University of Sussex, Brighton, UK.
Coster J, Turner J, Siriwardena AN, Wilson R, Phung VH. Prioritising outcomes measures for ambulance service care: a three stage consensus study. J Epidemiol Comm Health 2013;67(Suppl. 1):A33–4.
Coster J, Turner J, Wilson R, Phung VH, Siriwardena AN. Prioritising Prehospital Outcome Measures with A Multi-stakeholder Group: A Consensus Methods Study. In International Forum on Quality & Safety in Health Care, 16–19 April 2013, ICC Excel, London, UK.
Phung VH, Booth A, Coster J, Turner J, Wilson R, Siriwardena AN. Prehospital Outcomes for Ambulance Service Care: Systematic Review. In Making an Impact: what is new in emergency prehospital care research? 27 February 2013, Novotel, Cardiff, UK.
Phung VH, Coster J, Wilson R, Booth A, Turner J, Bradbury D, et al. Prehospital Outcomes for Evidence-Based Evaluation (PhOEBE): A Systematic Review. In World Congress on Disasters and Emergency Medicine, 28–30 May 2013, Manchester Central Convention, Manchester, UK.
Phung VH, Coster, J, Wilson R, Turner J, Booth A, Siriwardena AN. Systematic review of prehospital outcomes for evidence-based evaluation of ambulance service care. Prehosp and Disaster Med 2013;28(Suppl. 1):S102.
Turner J, Coster J, Wilson R, Phung VH, Siriwardena AN. What outcome measures should be developed for prehospital care? Results of a consensus event. Prehosp and Disaster Med 2013;28(Supp. 1):S89.
Coster J, Irving A, Turner J, Wilson R, Phung VH, Siriwardena AN. How Should we Measure Ambulance Service Quality and Performance? Results from a Delphi Study. In 8th European Congress on Emergency Medicine, 28 September to 1 October 2014, Westergasfabriek BV, Amsterdam, Netherlands.
Coster J, Turner J, Irving A, Wilson R, Siriwardena AN, Phung VH. How Should We Measure Ambulance Service Quality and Performance? In International Conference on Emergency Medicine, 11–14 June 2014, Hong Kong Convention and Exhibition Centre, Hong Kong.
Coster J, Turner J, Irving A, Wilson R, Siriwardena AN, Phung VH. Moving on from Response Rates: Linking Patient-level Ambulance Data to ED, Hospital and Survival Data to Assess Quality and Performance. In International Conference on Emergency Medicine, 11–14 June 2014, Hong Kong Convention and Exhibition Centre, Hong Kong.
Turner J, Coster J, Wilson R, Siriwardena AN, Phung VH. Developing New Ways of Measuring the Impact of Ambulance Service Care. In 8th European Congress on Emergency Medicine, 28 September to 1 October 2014, Westergasfabriek BV, Amsterdam, Netherlands.
Togher F, O’Cathain A, Phung V-H, Turner J, Siriwardena A. Reassurance as a key outcome valued by emergency ambulance service users: a qualitative interview study. Health Expect 2015;18:2951–61.
Coster J, Jacques R, Turner J, Crum A, Nicholl J, Siriwardena AN. New indicators for measuring patient survival following ambulance service care. Emerg Med J 2017;34:e4.
Coster J, Siriwardena AN, Turner J, Jacques R, Crum A, Nicholl J. Multi-method development of new ambulance service quality and performance measures. Emerg Med J 2017;34:e2.
Crum A, Coster J, Turner J, Siriwardena AN. Creating a linked dataset to explore patient outcomes after leaving ambulance care. Emerg Med J 2017;34:e6.
Coster J, Irving AD, Turner JK, Phung VH, Siriwardena AN. Prioritizing novel and existing ambulance performance measures through expert and lay consensus: a three-stage multimethod consensus study. Health Expect 2018;21:249–60.
Irving A, Turner J, Marsh M, Broadway-Parkinson A, Fall D, Coster J, Siriwardena AN. A coproduced patient and public event: an approach to developing and prioritizing ambulance performance measures. Health Expect 2018;21:230–8.
Siriwardena AN, Akanuwe J, Crum A, Coster J, Jacques R, Turner J. Preventable mortality in patients at low risk of death requiring prehospital ambulance care: retrospective case record review study. BMJ Open 2018;8.
Turner J, Jacques R, Coster J, Nicholl J, Crum A, Siriwardena N. Development of risk adjusted indicators of ems performance and quality (PhOEBE programme). BMJ Open 2018;8.
Data-sharing statement
Owing to the conditions attached to original ethics agreements, there are no data available for wider use. All queries should be submitted to the corresponding author in the first instance.
Patient data
This work uses data provided by patients and collected by the NHS as part of their care and support. Using patient data is vital to improve health and care for everyone. There is huge potential to make better use of information from people’s patient records, to understand more about disease, develop new treatments, monitor safety, and plan NHS services. Patient data should be kept safe and secure, to protect everyone’s privacy, and it’s important that there are safeguards to make sure that it is stored and used responsibly. Everyone should be able to find out about how patient data are used. #datasaveslives You can find out more about the background to this citation here: https://understandingpatientdata.org.uk/data-citation.
Disclaimers
This report presents independent research funded by the National Institute for Health Research (NIHR). The views and opinions expressed by authors in this publication are those of the authors and do not necessarily reflect those of the NHS, the NIHR, CCF, NETSCC, PGfAR or the Department of Health and Social Care. If there are verbatim quotations included in this publication the views and opinions expressed by the interviewees are those of the interviewees and do not necessarily reflect those of the authors, those of the NHS, the NIHR, NETSCC, the PGfAR programme or the Department of Health and Social Care.
References
- Ambulance Quality Indicators 2016/17. Leeds: NHS England; 2017.
- Taking Healthcare to the Patient: Transforming NHS Ambulance Services. London: The Stationery Office; 2005.
- Turner J, Nicholl J, Webber L, Cleary K. A preliminary analysis of the nature and management of category C calls (abstract). Pre-Hospital Immediate Care 1999;3.
- High Quality Care for All, Now and for Future Generations: Transforming Urgent and Emergency Care Services in England – Urgent and Emergency Care Review End of Phase 1 Report. Leeds: NHS England; 2013.
- High Quality Care for All – NHS Next Stage Review Final Report. London: Department of Health and Social Care; 2008.
- Office of Public Sector Information . Health Act 2009 (C21). Quality &Amp; Delivery of NHS Services in England. Chapter 2 Quality Accounts 2009. www.opsi.gov.uk/acts/acts2009/ukpga_20090021_en_1 (accessed 30 July 2018).
- Emergency Services Review – A Comparative Review of Ambulance Service International Best Practice. London: Department of Health and Social Care; 2009.
- O’Keeffe C, Nicholl J, Turner J, Goodacre S. Role of ambulance response times in the survival of patients with out-of-hospital cardiac arrest. Emerg Med J 2011;28:703-6. https://doi.org/10.1136/emj.2009.086363.
- Turner J, Jacques R, Crum A, Coster J, Stone T, Nicholl J. Ambulance Response Programme: Evaluation of Phase 1 and Phase 2. Sheffield: Centre for Urgent and Emergency Care Research, University of Sheffield; 2017.
- Turner J, O’Keeffe C, Dixon S, Warren K, Nicholl J. The Costs and Benefits of Changing Ambulance Service Response Time Performance Standards. Sheffield: Medical Care Research Unit, University of Sheffield; 2006.
- Pons PT, Haukoos JS, Bludworth W, Cribley T, Pons KA, Markovchick VJ. Paramedic response time: does it affect patient survival?. Acad Emerg Med 2005;12:594-600. https://doi.org/10.1197/j.aem.2005.02.013.
- Blackwell TH, Kline JA, Willis JJ, Hicks GM. Lack of association between pre-hospital response times and patient outcomes. Prehosp Emeg Care 2009;13:444-50. https://doi.org/10.1080/10903120902935363.
- Snooks H, Evans A, Wells B, Peconi J, Thomas M, Woollard M, et al. What are the highest priorities for research in emergency prehospital care?. Emerg Med J 2009;26:549-50. https://doi.org/10.1136/emj.2008.065862.
- Turner J. Building the Evidence Base in Pre-Hospital Emergency and Urgent Care: A Review of Research Evidence and Priorities for Future Research 2010. https://assets.publishing.service.gov.uk/government/uploads/system/uploads/attachment_data/file/216064/dh_117198.pdf (accessed 7 March 2019).
- Measuring Patient Outcomes: Clinical Quality Indicators. London: Association of Ambulance Chief Executives; n.d.
- Siriwardena AN, Shaw D, Donohoe R, Black S, Stephenson J. National Ambulance Clinical Audit Steering Group. Development and pilot of clinical performance indicators for English ambulance services. Emerg Med J 2010;27:327-31. https://doi.org/10.1136/emj.2009.072397.
- Champion HR, Sacco WJ, Copes WS, Gann DS, Gennarelli TA, Flanagan ME. A revision of the Trauma Score. J Trauma 1989;29:623-9. https://doi.org/10.1097/00005373-198905000-00017.
- Harrison DA, Brady AR, Parry GJ, Carpenter JR, Rowan K. Recalibration of risk prediction models in a large multicentre cohort of admissions to adult, general critical care units in the United Kingdom. Crit Care Med 2006;34:1378-88. https://doi.org/10.1097/01.CCM.0000216702.94014.75.
- Department of Health and Social Care n.d. www.gov.uk/government/organisations/department-of-health-and-social-care (accessed 7 March 2019).
- National Association of Emergency Medical Services Physicians (NAEMSP) n.d. https://naemsp.org/ (accessed 7 March 2019).
- NHS Confederation n.d. www.nhsconfed.org/ (accessed 7 March 2019).
- Moher D, Liberati A, Tetzlaff J, Altman DG. The PRISMA Group . Preferred Reporting Items for Systematic Reviews and Meta-Analyses: the PRISMA statement. PLOS Med 2009;6. https://doi.org/10.1371/journal.pmed.1000097.
- Crossing the Quality Chasm. A New Health System for the 21st Century. Washington, DC: National Academies Press; 2001.
- Donabedian A. Evaluating the quality of medical care. Milbank Mem Fund Q 1966;44:166-20. https://doi.org/10.2307/3348969.
- Turner J, Coster J, Phung V-H, Booth A, Wilson R, Siriwardena N. Systematic Searches and Review of Related Research Evidence on Ambulance Service Performance and Quality Measurement. Supplemental File to PhOEBE Programme. Sheffield: University of Sheffield; 2018.
- Coster JE, Irving AD, Turner JK, Phung VH, Siriwardena AN. Prioritizing novel and existing ambulance performance measures through expert and lay consensus: a three-stage multimethod consensus study. Health Expect 2018;21:249-60. https://doi.org/10.1111/hex.12610.
- Irving A, Turner J, Marsh M, Broadway-Parkinson A, Fall D, Coster J, et al. A coproduced patient and public event: an approach to developing and prioritizing ambulance performance measures. Health Expect 2018;21:230-8. https://doi.org/10.1111/hex.12606.
- Togher FJ, O’Cathain A, Phung VH, Turner J, Siriwardena AN. Reassurance as a key outcome valued by emergency ambulance service users: a qualitative interview study. Health Expect 2014;18:2951-61. https://doi.org/10.1111/hex.12279.
- International Classification of Diseases (ICD-10) Online Versions. Geneva: World Health Organization; n.d.
- Coleman P, Nicholl J. Consensus methods to identify a set of potential performance indicators for systems of emergency and urgent care. J Health Serv Res Policy 2010;15:12-8. https://doi.org/10.1258/jhsrp.2009.009096.
- NHS England . Ambulance Quality Indicators n.d. www.england.nhs.uk/statistics/statistical-work-areas/ambulance-quality-indicators/ (accessed 7 March 2018).
- Data Matters: Linking Data to Unlock Information. The Use of Linked Data in Healthcare Performance Assessment. Chatswood, NSW: Bureau of Health Information; 2015.
- Downing A, Wilson R, Cooke M. Linkage of ambulance service and accident and emergency department data: a study of assault patients in the west midlands region of the UK. Injury 2005;36:738-44. https://doi.org/10.1016/j.injury.2004.12.045.
- Crilly JL, O’Dwyer JA, O’Dwyer MA, Lind JF, Peters JA, Tippett VC, et al. Linking ambulance, emergency department and hospital admissions data: understanding the emergency journey. Med J Aust 2011;194:S34-7.
- Office for National Statistics n.d. www.ons.gov.uk/ (accessed 7 March 2019).
- Principles of Advice. Bristol: Health Research Authority; 2015.
- Data Protection Act 1998. London: The Stationery Office; 1998.
- Oderkirk J, Ronchi E, Klazinga N. International comparisons of health system performance among OECD countries: opportunities and data privacy protection challenges. Health Policy 2013;112:9-18. https://doi.org/10.1016/j.healthpol.2013.06.006.
- Dusetzina SB, Tyree S, Meyer AM, Meyer A, Green L, Carpenter WR. Linking Data for Health Services Research: A Framework and Instructional Guide. Rockville, MD: Agency for Healthcare Research and Quality (US); 2014.
- Nicholl J, Coleman P, Jenkins J, Knowles E, O’Cathain A, Turner J. The Emergency and Urgent Care System. Final report to the Department of Health and Social Care. Sheffield: University of Sheffield, Medical Care Research Unit; 2011.
- O’Keeffe C, Mason S, Jacques R, Nicholl J. Characterising non-urgent users of the emergency department (ED): a retrospective analysis of routine ED data. PLOS ONE 2018;13. https://doi.org/10.1371/journal.pone.0192855.
- Makary MA, Daniel M. Medical error-the third leading cause of death in the US. BMJ 2016;353. https://doi.org/10.1136/bmj.i2139.
- Hayward RA, Hofer TP. Estimating hospital deaths due to medical errors: preventability is in the eye of the reviewer. JAMA 2001;286:415-20. https://doi.org/10.1001/jama.286.4.415.
- Briant R, Buchanan J, Lay-Yee R, Davis P. Representative case series from New Zealand public hospital admissions in 1998 – III: adverse events and death. N Z Med J 2006;119.
- Shojania KG, Dixon-Woods M. Estimating deaths due to medical error: the ongoing controversy and why it matters. BMJ Qual Saf 2017;26:423-8. https://doi.org/10.1136/bmjqs-2016-006144.
- National Mortality Case Record Review Programme Resources. London: Royal College of Physicians; n.d.
- Hogan H, Healey F, Neale G, Thomson R, Black N, Vincent C. Learning from preventable deaths: exploring case record reviewers’ narratives using change analysis. J R Soc Med 2014;107:365-75. https://doi.org/10.1177/0141076814532394.
- Hutchinson A. National Mortality Case Record Review Programme. London: Royal College of Physicians; 2016.
- Why Research Matters. Southampton: National Institute for Health Research; n.d.
- Cornwall A, Gaventa J. From users and choosers to makers and shapers: repositioning participation in social policy. IDS Bulletin 2000;31:50-61. https://doi.org/10.1111/j.1759-5436.2000.mp31004006.x.
- Irving A, Broadway-Parkinson A, Marsh M, Fall D. Who Cares about Ambulance Performance measures?! The Role of PPI in Developing an Animation for Dissemination of the PhOEBE Programme n.d.
- Irving A, Broadway-Parkinson A, Marsh M, Fall D. Revolution in PPI or revolt? A meeting of minds or a clash of opinions. Emerg Med J 2015;32.
- University of Sheffield . Ambulance Research: The PhOEBE Project n.d. www.youtube.com/watch?v=g2saLhBv9-U&feature=youtu.be (accessed 6 April 2019).
- Pringle M, Wilson T, Grol R. Measuring ‘goodness’ in individuals and healthcare systems. BMJ 2002;325:704-7. https://doi.org/10.1136/bmj.325.7366.704.
- Target: The Practice of Performance Indicators. London: Audit Commission; 2000.
- Bird SM, Cox Sir D, Farwell VT, Goldstein H, Holt T, Smith PC. Performance indicators: good, bad, and ugly. J R Statist Soc 2005;168:1-27.
- Pencheon D. The Good Indicators Guide: Understanding How to Use and Choose Indicators n.d. www.england.nhs.uk/improvement-hub/wp-content/uploads/sites/44/2017/11/The-Good-Indicators-Guide.pdf (accessed 7 March 2019).
- Jones P, Shepherd M, Wells S, Le Fevre J, Ameratunga S. Review article: what makes a good healthcare quality indicator? A systematic review and validation study. Emerg Med Australas 2014;26:113-24. https://doi.org/10.1111/1742-6723.12195.
- NHS Reference Costs 2015–16. London: DHSC; 2016.
- Mahalanobis PC. Analysis of race mixture in Bengal. J Asiatic Soc Bengal 1927;23:301-33.
- Langton JM, Goldsbury D, Srasuebkul P, Ingham JM, O’Connell DL, Pearson SA. Insights from linking routinely collected data across Australian health jurisdictions: a case study of end-of-life health service use. Public Health Res Pract 2018;28. https://doi.org/10.17061/phrp2811806.
- NHS National Services Scotland . Unscheduled Care Data Mart. Information Services Division n.d. www.isdscotland.org/Health-Topics/Emergency-Care/Patient-Pathways/UrgentCareDataMartBackgroundPaper_20171002.pdf (accessed 27 July 2018).
- Murphy A, Wakai A, Walsh C, Cummins F, O’Sullivan R. Development of key performance indicators for prehospital emergency care. Emerg Med J 2016;33:286-92. https://doi.org/10.1136/emermed-2015-204793.
- Spaite DW, Maio R, Garrison HG, Desmond JS, Gregor MA, Stiell IG, et al. Emergency Medical Services Outcomes Project (EMSOP) II: developing the foundation and conceptual models for out-of-hospital outcomes research. Ann Emerg Med 2001;37:657-63. https://doi.org/10.1067/mem.2001.115215.
- Five Year Forward View. London: NHS England; 2014.
- NHS Digital Data and Information Strategy. Leeds: NHS Digital; 2016.
- Ray D, Roebuck C, Smith O. Delivering Linked Datasets to Support Health and Care Delivery and Research. Leeds: NHS Digital; 2018.
- Heitmueller A, Henderson S, Warburton W, Elmagarmid A, Pentland AS, Darzi A. Developing public policy to advance the use of big data in health care. Health Aff 2014;33:1523-30. https://doi.org/10.1377/hlthaff.2014.0771.
- Ambulance Quality Indicators Data 2018/19. London: NHS England; n.d.
- Transforming urgent and emergency care services in England. Urgent and emergency care review. End of phase 1 report. Leeds: NHS England; 2013.
Appendix 1 Systematic searches and summary of identified measures
Database search results | Number of papers |
---|---|
MEDLINE | 190 |
Scirus | 23 |
Scopus | 60 |
Google Scholar | 101 |
Total | 374 |
Total after removal of duplicates | 319 |
MEDLINE search strategy | |
1 performance {Including Related Terms} (24,050) 2 Cost-Benefit Analysis/or cost effective.mp. (79,285) 3 Efficiency/or efficiency.mp. (214,001) 4 utilis$.mp. (26,792) 5 Benchmarking/or best practice.mp. (11,982) 6 benchmark$.mp. (18,516) 7 economic$.mp. or Economics/(173,384) 8 manag$.mp. (774,167) 9 clinical quality.mp. (738) 10 patient pathway$.mp. (138) 11 outcome indicator$.mp. (721) 12 or/1-11 (1,224,729) |
Performance terms |
13 *Ambulances/(2586) | Ambulance |
14 limit 13 to (english language and yr = “2000 -Current”) (826) 15 12 and 14 (190) |
limits |
Scirus and Scopus search strategy | |
ambulance | Ambulance |
(performance OR “cost effective” OR efficient OR utilisation OR benchmarking OR economics OR management OR “clinical quality” OR “patient pathway” OR outcome) | Performance terms |
Additional search for “Emergency Medical Services Outcomes Project” or EMSOP | |
Google Scholar | 19 |
Database search results | Number of papers |
---|---|
CINAHL | 434 |
Cochrane | 570 |
MEDLINE | 2726 |
EMBASE | 2259 |
Web of Science | 78 |
Total | 6067 |
Total after removal of duplicates | 5088 |
MEDLINE and EMBASE search strategy | |
1 age factors/ (339,379) 2 sex factors/ (190,416) 3 risk factors/ (466,520) 4 socioeconomic factors/ (94,879) 5 1 or 2 or 3 or 4 (931,516) |
Factors effecting performance |
6 Emergency Medical Service Communication Systems/ (1385) 7 emergency medical services/ (27,586) 8 emergency medical technicians/ (4414) 9 ambulances/ (4418) 10 transportation of patients/ (7475) 11 emergency service, hospital/ (35,248) 12 trauma centers/ (5254) 13 first aid/ (6667) 14 air ambulances/ (1586) 15 emergency medicine/ (8598) 16 intubation, intratracheal/ (26,814) 17 resuscitation/ (20,109) 18 infusions, intravenous/ (44,377) 19 critical care/ (22,332) 20 emergency treatment/ (7111) 21 or/6-20 (197,189) |
Emergency services terms |
22 quality of health care/ (49,639) 23 quality assurance, health care/ (44,252) 24 Prognosis/ (314,870) 25 treatment outcome/ (496,656) 26 time factors/ (908,764) 27 injury severity score/ (8750) 28 Trauma Severity Indices/ (4625) 29 survival rate/ (106,582) 30 survival analysis/ (85,469) 31 length of stay/ (48,560) 32 severity of illness index/ (136,335) 33 “Outcome and Process Assessment (Health Care)”/ (19,308) 34 “Outcome Assessment (Health Care)”/ (40,411) 35 hospitalization/ (61,536) 36 forecasting/ (65,384) 37 Glasgow Coma Scale/ (5589) 38 hospital mortality/ (17,030) 39 mortality/ (31,411) 40 or/22-39 (2,072,503) |
Quality-of-care terms |
41 5 and 21 and 40 (4901) | |
42 medical audit/ (13,307) 43 prospective studies/ (306,088) 44 retrospective studies/ (395,821) 45 evaluation studies as topic/ (119,831) 46 practice guidelines as topic/ (64,559) 47 health care surveys/ (19,579) 48 program evaluation/ (39,204) 49 cross-sectional studies/ (131,582) 50 follow-up studies/ (433,177) 51 cohort studies/ (127,326) 52 Cost-benefit analysis/ (52,318) 53 Chi-Square Distribution/ (47,593) 54 Pilot Projects/ (68,129) 55 research/ (163,768) 56 Randomized Controlled Trials as Topic/ (76,653) 57 “costs and cost analysis”/ (39,208) 58 or/42-57 (1,788,497) |
Limit by study type |
59 5 and 21 and 40 and 58 (2726) | |
Web of Science strategy | |
TS = (“age factor*” or “sex factor*” or “risk factor *” or “socioeconomic factor*”) | Factors effecting performance |
ts = (“emergency Medical Service Communication System*” or “emergency medical service” or “emergency medical technician” or ambulance* or “transportation of patient*” or “hospital emergency service” or “trauma center” or “first aid” or “emergency medicine” or “intratracheal intubation” or resuscitation or “intravenous intubation” or “critical care” or “emergency treatment”) | Emergency services terms |
ts = (“quality of health care” or “health care quality assurance” or prognosis or “treatment outcome” or “time factor*” or “injury severity score” or “trauma severity indices” or “survival rate” or “survival analysis” or “length of stay” or “severity of illness index” or “outcome and process assessment” or “outcome assessment” or hospitalization or forecasting or “glasgow coma scale” or “hospital mortality” or “mortality”) | Quality of care terms |
ts = (“medical audit” or “prospective stud*” or “evaluation stud*” or “practice guideline*” or “health care survey*” or “program evaluation” or “cross?sectional stud*” or “follow?up stud*” or “cohort stud*” or “cost?benefit analysis” or “chi-square distribution” or “pilot project*” or research or “randomized control trial*” or “costs and cost analysis”) | Limit by study type |
#1 and #2 and #3 and #4 |
Calls sent for telephone nurse advice that are returned for an ambulance response.
Accuracy of call-taker identification of different conditions (e.g. cardiac arrest, heart attack, stroke, serious illness, low-urgency calls suitable for nurse advice) or needs. Includes:
-
measures of call assessment accuracy, such as sensitivity
-
appropriateness of triage decision
-
risk of undertriage
-
risk of overtriage.
Accuracy of dispatch decisions – includes:
-
choice of response type dispatched (rapid response car, ambulance, helicopter)
-
appropriateness of referral to other agencies (e.g. GP services)
-
use of alternatives to ambulance dispatch (e.g. nurse advice or make own way)
-
relationship between priority category and response (right resource to right call).
Accuracy of paramedic diagnosis:
-
agreement of on-scene and final hospital diagnosis
-
other measures of paramedic diagnosis accuracy (e.g. for specific conditions, such as stroke, trauma).
Compliance with protocols and guidelines:
-
with triage protocols
-
transport protocols (e.g. leave at home, alternative to ED)
-
with care and treatment guidelines (fits and convulsions, heart attack, stroke).
Proportion of people with respiratory distress (breathing difficulties) receiving mechanically assisted breathing.
Proportion of people with diabetes mellitus treated at home.
Proportion of elderly people attended within scope of advanced paramedic practice (e.g. treat and leave at home).
Proportion of people receiving spinal immobilisation (splints and collars) for back/neck injuries.
Re-contact with ambulance service within 24 hours (e.g. for calls closed with advice or patients not transported).
Hospital attendance or admission (e.g. within 24 hours, 7 days, 28 days).
Re-admission within 30 days for complications (e.g. pneumonia, wound infections).
Measuring patient safety:
-
adverse incidents (e.g. not recognising heart attack symptoms or leaving someone at home who needed hospital treatment)
-
errors in diagnosis.
Length of stay in hospital.
Duration of life support (intubation or ventilation) in hospital.
Discharge destinations:
-
home
-
continuing care
-
discharged needing continuing therapy (e.g. nursing care, supplemental oxygen, tube feeding, assisted breathing)
-
proportion of patients living at home at 3 months.
Proportion of cases treated within time guidelines including:
-
STEMI (heart attack) guidelines (90 minutes)
-
thrombolysis (clot busting) (60 minutes)
-
proportion FAST positive (suspected stroke) arriving at a stroke centre within 60 minutes.
FAST, Facial drooping, Arm weakness, Speech difficulties and Time to call emergency services; STEMI, ST-elevation myocardial infarction.
Days lost from work following the emergency episode.
Complications arising from care/treatment:
-
pneumonia
-
wound infections
-
adverse drug effects (reactions).
Neurological (brain function) outcome at different time points (discharge, 1 month, 6 months, 1 year, etc.) using a variety of measures including:
-
Glasgow Coma Scale score (adult and children)
-
Glasgow Outcome Score
-
Cerebral Performance Category (adult and children)
-
dementia score.
Health/quality-of-life status:
-
quality of life (EQ-5D, SF-36)
-
function (Katz Index of Independence in Activities of Daily Living, Knauss class, McCabe Score, FIM)
-
post-traumatic stress disorder.
Survival at different time points after the event:
-
in hospital
-
30 days
-
90 days
-
6 months
-
1 year
-
4–5 years.
Patient experience:
-
access
-
acceptability
-
decisions (e.g. to leave at home)
-
satisfaction
-
professionalism
-
holistic care (e.g. physical, social, emotional needs).
Statistical methods for measuring survival.
Pain measurement and symptom relief:
-
pain
-
nausea
-
shortness of breath
-
discomfort.
Return of spontaneous circulation (return of pulse).
FIM, Functional Independence Measure; SF-36, Short Form questionnaire-36 items.
Call numbers and caller types:
-
demographic (e.g. age, sex) characteristics of service population
-
call volumes (numbers)
-
call volumes by incident types
-
geographical differences in use of emergency number.
Call management characteristics (numbers and proportions):
-
calls assigned to different urgency categories
-
calls directed for nurse advice
-
calls closed with nurse advice
-
calls receiving paramedic response
-
calls abandoned before answered
-
ambulances cancelled.
Utilisation (frequency of ambulance use):
-
utilisation by age groups/ethnic group/sex/poverty/incident types
-
utilisation per 1000 population
-
unit-hour utilisation (use of resources).
Number of patients transported to hospital:
-
transport rates for serious calls
-
transport rates for non-serious calls.
Proximity of services:
-
% of operational area reachable within a specified time (e.g. 10 minutes, 20 minutes, 30 minutes, 1 hour)
-
% of population who can reach a major trauma centre within 45–60 minutes
-
scene-to-hospital distances.
Proportion of calls treated by most appropriate service (whole 999 population).
Completeness and accuracy of patient records.
Frequency with which ambulance staff administer treatments (e.g. inserting breathing tubes, heart monitoring, oxygen therapy, defibrillation).
Service costs:
-
cost per urgent call
-
cost per non-urgent call
-
cost per patient
-
mean cost of treatment (whole episode).
Ambulance service workforce characteristics:
-
age – average and proportions by group
-
attrition (staff turnover)
-
compensation claims.
Types and numbers of patient transportations:
-
transport rates (numbers and proportions transported and not transported to hospital)
-
numbers and proportions transported to alternatives to ED (e.g. minor injury unit)
-
numbers and proportions to different destination types (whole 999 population).
Ambulance staff training:
-
disability equality training
-
communication skills.
Volume and nature of complaints.
Overtriage rates and undertriage rates:
-
by category of urgency
-
advice only
-
condition specific [e.g. major trauma, stroke, STEMI (heart attack)].
STEMI, ST-elevation myocardial infarction.
Appendix 2 Assessment criteria for identifying indicator set
For the purposes of reducing the existing list of measures to a smaller list that could be encompassed by workstream 3, each measure was considered against a set of criteria (listed below). Each criterion was either answerable directly or functioned as a broader heading within which subcriteria provided the assessment detail. At issue was the scope, ‘measurability’ and applicability of each of the outcome measures:
-
Primary measure category (time response, compliance, accuracy call ID, re-contact, survival, other, pain, triage appropriate/accurate, time definitive).
-
Pathway (yes, no):
-
on-scene
-
pathway post prehospital
-
pathway call handling
-
pathway transport.
-
-
Measurement type (whole system, clinical management, patient outcome).
-
Population (all, specific):
-
population-specific detail
-
population quantity.
-
-
Relationship (related, independent):
-
relationship detail (particular measure related to).
-
-
Primary purpose (performance, outcome).
-
Measurable (yes, no):
-
measure detail
-
already measured? (yes, no).
-
-
Relevance (yes, no, unsure):
-
patients
-
commissioners
-
services.
-
-
Delphi score.
-
Second round median.
-
PPI vote (%).
-
Important?
-
Risk adjustment:
-
do we need to risk adjust?
-
Can we risk adjust?
Each measure was entered into a Microsoft Excel spreadsheet and the assessment against each criterion entered enabled a composite score to be obtained. Figure 8 shows an illustrative example of the scoring sheet.
FIGURE 8.
Assessment of potential measures.

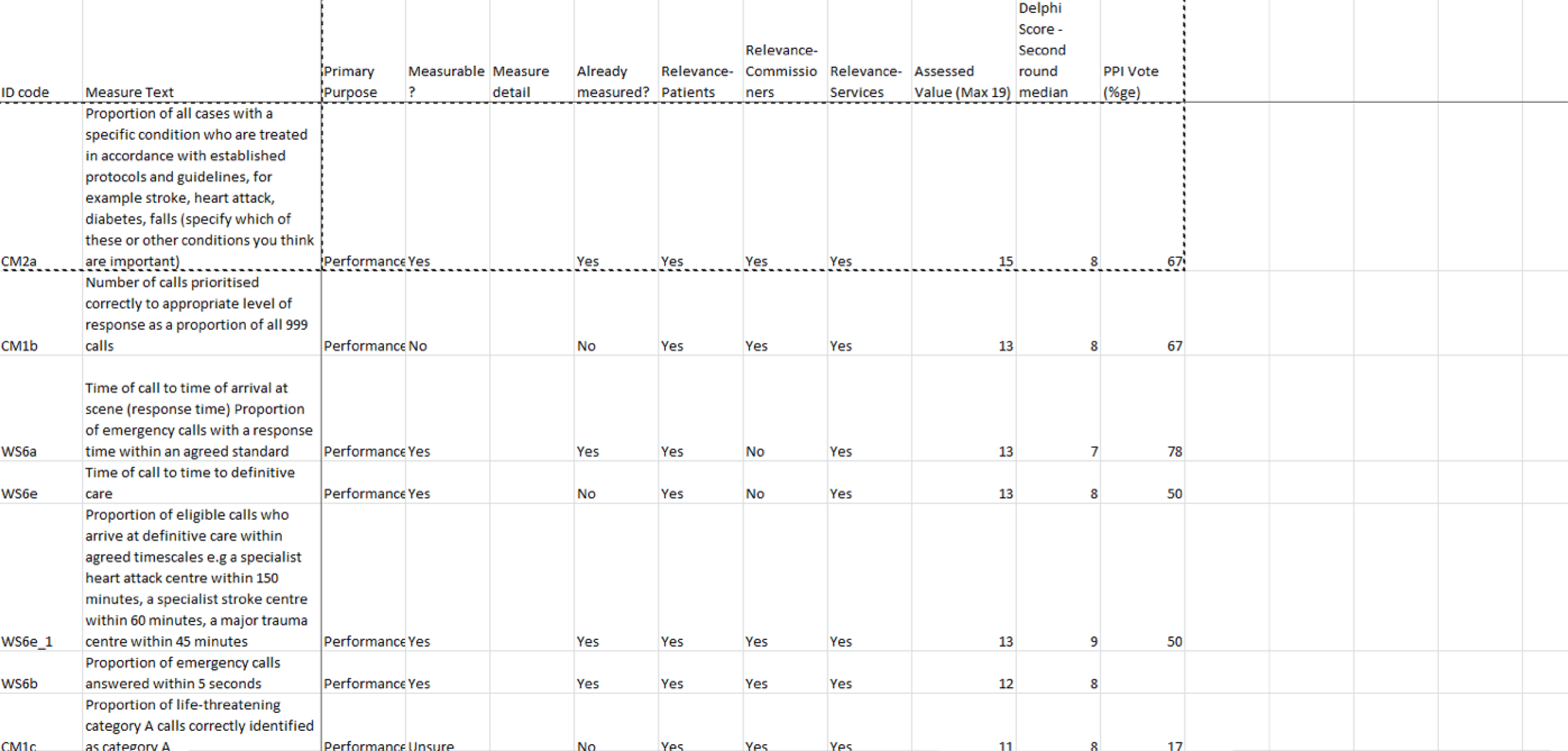
For the next stage, the results of the criteria assessment were considered and measures included or excluded using the following criteria.
Inclusion criteria
-
Is it an important outcome?
-
The raw data can be misleading.
-
Risk-adjusted data may be more useful.
-
The outcome says something about ambulance performance.
Exclusion criteria
-
The measure tells us something as it stands.
-
The measure cannot be risk adjusted.
Following this assessment the final set of indicators for further development was identified (Table 17).
ID code | Measure | Is it important? | Does it require risk adjustment? | Include/exclude |
---|---|---|---|---|
WS6a | Time of call to time of arrival at scene (response time). Proportion of emergency calls with a response time within an agreed standard | Response time is important but the arbitrary standard is not. Response time is important to patients, in terms of panic and anxiety. Response time is also related to outcome: shorter time = better outcomes, all things being equal. Overall, response time was judged important because of its relationship to the patient. However, if we have another measure in the list that is better, this will supersede this one | We would risk adjust to ensure fair comparisons, for example for an ambulance service which covers a large geographical area compared with a small area. Response times are already compared nationally without risk adjustment; therefore, there is no need to risk adjust here. We should use the mean or median response time. Mean of log or geometric mean | Include in workstream 4, no risk adjustment |
WS6ei | Proportion of eligible calls who arrive at definitive care within agreed timescales, for example a specialist heart attack centre within 150 minutes, a specialist stroke centre within 60 minutes, a major trauma centre within 45 minutes | This is important as time frame is related to a successful outcome. This could include the 8-minute response time for OHCA | A success is arriving in the right place (alive). It is not necessary to risk adjust if this measure is concerned with getting patients to the right place, but if it is for treatment we may want to case-mix adjust for the proportion that could benefit from treatment:
|
Include in workstream 4, no risk adjustment |
CM1b | Number of calls prioritised correctly to appropriate level of response as a proportion of all 999 calls |
Should this be the number of all calls prioritised correctly or do we want the number of serious calls that were prioritised correctly? Calls coded green that are left too long will be picked up in the mean response time measure Just take the serious emergency conditions (16 conditions) |
Superseded by CM1c – better measure | |
CM1c | Proportion of life-threatening category A calls correctly identified as category A | Change this to calls for serious emergency conditions and use 16 conditions. Clarify what the 16 conditions are |
We need to adjust for case mix. Some measures are difficult to categorise and others easy, for example ruptured aneurysm or OHCA We will adjust for case mix to level the playing field We will adjust for age |
Include in workstream 3, for risk adjustment |
CM2a | Proportion of all cases with a specific condition who are treated in accordance with established protocols and guidelines, for example stroke, heart attack, diabetes mellitus, falls (specify which of these or other conditions you think are important) | This is current CQI for five conditions and is important (asthma, STEMI, stroke, OHCA?) |
The CQIs are not risk adjusted The question here is whether or not patients with specific conditions were given the agreed best treatment. Therefore, there is no need to risk adjust Query – should we send a list of conditions that we are not risk adjusting for to ambulance services to ask them if they were performing badly on this measure, what would their excuse be? |
Include in workstream 4, no risk adjustment |
PO5c | Proportion of patients with a life-threatening condition who are discharged alive from hospital |
Use the 16 serious emergency conditions as the life-threatening conditions This is important to patients What about hospital effects? HSMR looks at the proportion of patients who die in hospital. Do we need to split this into prehospital and hospital components? If we have the HSMR we also need the prehospital SMR for all serious conditions. This is the proportion of deaths that occur before admission to a bed The HSMR is the proportion that die in hospital The system HSMR is the proportion that die before discharge |
HSMR is case-mix adjusted, so the PHSMR and the SHSMR will case-mix adjusted | Include in workstream 3, for risk adjustment |
PO1a | Proportion of all patients seen by an ambulance crew who have a pain assessment recorded | This is an explanatory variable for PO1c | Superseded by PO1c | |
PO1b | Proportion of patients who report pain who are given pain relief | This is an explanatory variable for PO1c | Superseded by PO1c | |
PO1c | Proportion of patients who have a reduction in pain score after analgesia treatment |
Should this be the mean reduction in pain (adjusted for time)? Remove after analgesia as other treatments, for example splints, can reduce pain |
Risk adjust for conditions, age and time We could start on this with just the ambulance data There may be high proportions of missing data as this measure requires two pain readings. We need to determine what to do with missing data We need a model with everything that is outside the ambulance control first |
Include in workstream 3, for risk adjustment |
PO1d | Proportion of patients reporting pain who have more than one pain score recorded | This is an explanatory variable for PO1c | Superseded by PO1c | |
WS6e | Time of call to time to definitive care | Too vague. Superseded by WS6ei | Superseded by WS6ei | |
PO6a | Proportion of all 999 calls re-contacting the ambulance service within 24 hours | The definition of re-contacts needs to be clearer. This should be re-contacts for all patients who were not conveyed to hospital. This is because patients who are conveyed to hospital may be discharged and re-contact the ambulance service | Superseded by PO6c | |
PO6c | Proportion of patients left at home who have a contact with any emergency/urgent health service within 24 hours |
This supersedes all other re-contact measures. Left at home = ’see and treat’ or ‘hear and treat’ Is a low rate good? This needs a reciprocal measure about patients who are taken to ED and not treated/admitted |
Risk adjust for age Time frame = 24 hours |
Include in workstream 3, for risk adjustment |
CM2c | Proportion of all cases with a specific condition who meet the established criteria for transfer, who are transported to an appropriate specialist facility, for example a heart attack, stroke or major trauma centre | This is covered by WS6e1 | Superseded by WS6e1 | |
PO5a_i | Proportion of 999 callers who die within: i. 0–48 hours of first call | We want to look at the proportion of 999 callers who die from specific causes within a specified time frame, where death was avoidable. We need to identify conditions where patients should not die. Look at the cause of death for patients in our sample and identify those that are preventable, for example hypothermia within 24 hours of call | Adjust for age | Include in workstream 3, for risk adjustment |
WS3b | Proportion of category A calls attended by a paramedic | Not important | Exclude | |
WS3c | Proportion of patients who are treated on scene or left at home who are referred to an appropriate pathway or primary care |
Is it the non-conveyance rate? Non-conveyance who are referred Not important |
Exclude | |
WS2a | Number of life-threatening (category A) calls not identified as category A as a proportion of all 999 calls | This is important but is the opposite of a measure that is already included (CM1c). Therefore this will be measured as part of CM1c | Superseded by CM1c | |
WS3f | Proportion of patients who potentially could be left at home who are successfully discharged at the scene | Better measures exist for this. It is difficult to know what the denominator is. We want to look at people who are transported and not having anything done. Superseded by WS3e | Superseded by WS3e | |
WS3e | Proportion of patients transported to ED by 999 emergency ambulance and discharged without treatment or investigation(s) that needed hospital facilities | This is important and supersedes other measures | ? Not sure | Include |
CM1a | Proportion of all calls referred for telephone advice returned for a 999 ambulance response | This relates to efficiency. This was highly scored in the Delphi as is easy to measure, but is not important | Exclude | |
PO3a | Proportion of patients with cardiac arrest where resuscitation is attempted at the incident scene who have a pulse on arrival at the ED |
ROSC is one of the CQIs There is scope for ROSC to be improved. Use in the 16 emergency conditions as a bundle but need to look at adjusted time to hospital discharge. This will give ROSC, survival to discharge and the system SMR What about non-transports? Can we pick these up? |
Needs to be adjusted for rhythm, witnessing, bystander CPR and adrenaline | |
PO6b | Proportion of all 999 calls referred for telephone advice only re-contacting the ambulance service within 24 hours | There are other re-contact measures that are better | Superseded by PO6c | |
PO6e | Proportion of patients left at home who are admitted to hospital within 72 hours | Left at home = ’hear and treat’ and ‘see and treat’ patients. We are interested in the proportion of patients who are not conveyed who are admitted to hospital. This could be part of a re-contacts set of measures. Include for the moment, but may be dropped | Risk adjust for age, condition type, avoidable emergency conditions. It is impossible to know where the hospital contact is for the same condition, or the extent to which the two are linked; therefore, we will assume that admissions within 3 days are related | Include in workstream 3, for risk adjustment |
R2_WS6a_2_3_ | Not important | Exclude | ||
WS6a_1 | Proportion of emergency calls with a response time within an agreed standard for calls for life-threatening conditions | This is dealt with by another measure | Exclude. Superseded by WS6a | |
WS2b | Number of calls that are not life-threatening identified as category A calls as a proportion of all 999 calls | We have another measure that looks at the opposite side of this (CM1c) | Exclude. Superseded by CM1c | |
CM1d | Proportion of calls for a specific condition correctly identified at the time of the call, e.g. cardiac arrest, stroke, heart attack | This is about accuracy and recognising conditions. The ambulance AMPDS code is not diagnostic and you would need to relate the AMPDS code to the admission code. This is of low importance and very difficult to verify, and can already be measured using similar measures that are already included. For example, with STEMIs sent to right place | Exclude. Superseded by WS6Ei and CM1c | |
CM2b | Proportion of cases that comply with end-of-life care plans when these are available | This is important but not currently measurable. ePRFs should record whether or not there is an end-of-life care plan | Exclude | |
WS6d | Time of call to CPR start time when CPR is required. Average time from call to start of CPR in cases of cardiac arrest | This is explanatory data for ROSC and survival and is a problem with bystander CPR. This is an explanatory process and not an outcome | Exclude | |
CM1e | Number of people attended within the scope of advanced paramedic practice (treat and leave at home) as a proportion of all people attended on scene | We cannot measure this as we do not know who the paramedics are. This measure is related to efficiency and is not important here. However, this links to policy because if the Keogh report70 becomes standard, more people will be left at home and ambulance services will need to have sufficient paramedics to deal with the demand | Exclude | |
CM3a | Number of ‘never events’ reported as a proportion of all requests for 999 ambulance care (never events applicable to the ambulance service include administration of drugs by the wrong route and failure to monitor and respond to reduced oxygen saturation) | Never events are already recorded. This does not come into the scope of the PhOEBE programme as not performance measures | Exclude | |
CM3b | Number of patient safety incidents reported as a proportion of all requests for 999 ambulance care | Same as CM3a | Exclude | |
PO2c | Proportion of patients who report that key aspects of care were delivered. (Examples of key aspects are timeliness of response, reassurance, professionalism, communication, smooth transition between different services or parts of the same service) | Not measured routinely. No patient satisfaction or experience measures | Exclude | |
PO2d | Proportion of patients who were satisfied with the overall service and separate components, for example the 999-call handling, attending ambulance crews | Not measured routinely. No patient satisfaction or experience measures | Exclude | |
PO5d | Proportion of patients with a specific clinical condition (e.g. stroke, heart attack, cardiac arrest) who are discharged alive from hospital | A mass measure would be for the 16 emergency conditions, but we may want to split this for specific conditions. This relates to PO5c and is part of a set | Adjust for case mix? | Include in workstream 3, for risk adjustment |
R2_WS6a_2-25 | Proportion of emergency calls for conditions that are not life-threatening with a response time of ≤ 25 minutes | This is not a measure | Exclude | |
R2_WS6a_4 | Proportion of emergency calls for life-threatening conditions with a response time of < 4 minutes (+ 4 minutes exact) | This applies only to cardiac arrest and we are looking at this in another measure | Exclude | |
R2_WS6a_4_8 | Proportion of emergency calls for life-threatening conditions with a response time of between 4 and 8 minutes | This applies only to cardiac arrest and we are looking at this in another measure | Exclude | |
R2_WS6E_3 | No | Exclude | ||
WS1a | Number of completed patient clinical records as a proportion of all cases attended by the ambulance service in accordance with minimum agreed data set | This is a data completeness measure | Exclude | |
WS3a | Number of calls transferred for telephone clinical advice assessment that are completed with self-care advice or referral to an appropriate service as a proportion of all calls transferred for clinical advice | This is an efficiency measure. This is superseded by other measures | Exclude | |
WS3d | Proportion of all calls who receive an ambulance response who are not conveyed to hospital or other health service facilities | This is the non-conveyance rate. This is being looked at by VAN | Exclude | |
WS4a | Proportion of staff who comply with mandatory training requirements for basic and advanced life support (BLS and ALS) | No. This is a service management measure | Exclude | |
WS4b | Proportion of operational staff trained as paramedics | No. This is a service management measure | Exclude | |
WS4c | Proportion of paramedics with advanced practitioner training | No. This is a service management measure | Exclude | |
WS5a | Unit-hour utilisation for the whole service (a unit-hour is a fully staffed ambulance for 1 hour). For any given time period, a service will have multiple unit-hours available. Unit-hour utilisation is how many of those hours are used within that time period |
Unit-hour utilisation works on the premise that calls are spread evenly and there are no spikes No. This is a service management measure |
Exclude | |
WS5b | Unit-hour utilisation for urban areas – compared with agreed utilisation | No. This is a service management measure | Exclude | |
WS5c | Unit-hour utilisation for rural areas – compared with agreed utilisation | No. This is a service management measure | Exclude | |
WS6a_2 | Proportion of emergency calls with a response time within an agreed standard for calls for non-life-threatening conditions | This will be covered by other measures (WS6a) | Exclude. Superseded by WS6a | |
WS6e_2 | Proportion of eligible calls who arrive at a specialist stroke centre within 60 minutes | This is covered by CM1c | Exclude. Superseded by CM1c |
List of abbreviations
- A&E
- accident and emergency
- AMPDS
- Advanced Medical Priority Dispatch System
- APC
- admitted patient care
- ARP
- Ambulance Response Programme
- CAD
- computer-aided dispatch
- CaHRU
- Community and Health Research Unit
- CCG
- Clinical Commissioning Group
- CI
- confidence interval
- CINAHL
- Cumulative Index to Nursing and Allied Health Literature
- ED
- emergency department
- EMAS
- East Midlands Ambulance Service NHS Trust
- EMS
- Emergency Medical Services
- ePRF
- electronic patient report form
- EQ-5D
- EuroQol-5 Dimensions
- GP
- general practitioner
- HES
- Hospital Episode Statistics
- ICC
- intraclass correlation coefficient
- ICD-10
- International Classification of Diseases, Tenth Edition
- ID
- identifier
- ONS
- Office for National Statistics
- PhOEBE
- Prehospital Outcomes for Evidence Based Evaluation
- PPI
- patient and public involvement
- SJR
- structured judgement case note review
- YAS
- Yorkshire Ambulance Service NHS Trust