Notes
Article history
The research reported in this issue of the journal was funded by PGfAR as project number RP-PG-1210-12003. The contractual start date was in January 2014. The final report began editorial review in September 2019 and was accepted for publication in September 2020. As the funder, the PGfAR programme agreed the research questions and study designs in advance with the investigators. The authors have been wholly responsible for all data collection, analysis and interpretation, and for writing up their work. The PGfAR editors and production house have tried to ensure the accuracy of the authors’ report and would like to thank the reviewers for their constructive comments on the final report document. However, they do not accept liability for damages or losses arising from material published in this report.
Permissions
Copyright statement
© Queen’s Printer and Controller of HMSO 2021. This work was produced by Perera et al. under the terms of a commissioning contract issued by the Secretary of State for Health and Social Care. This issue may be freely reproduced for the purposes of private research and study and extracts (or indeed, the full report) may be included in professional journals provided that suitable acknowledgement is made and the reproduction is not associated with any form of advertising. Applications for commercial reproduction should be addressed to: NIHR Journals Library, National Institute for Health Research, Evaluation, Trials and Studies Coordinating Centre, Alpha House, University of Southampton Science Park, Southampton SO16 7NS, UK.
2021 Queen’s Printer and Controller of HMSO
SYNOPSIS
Background
Advances in health promotion and better prevention, detection and treatment of diseases have led to important demographic changes in the UK, with consistent increases in healthy life expectancy. Paradoxically, this also means that a greater number of people are now living longer with one or more long-term conditions, such as diabetes, kidney disease or coronary heart disease. 1,2 In the UK, the management of these conditions has shifted from secondary care to primary care, partly because of the continuity of care that primary care provides, but also because of the generalist approach used in primary care to deal with multiple, not necessarily related, conditions. 3 A major aspect of the good management of these conditions is long-term monitoring. However, despite the considerable economic cost for most of these conditions, there is no or poor evidence on the monitoring strategies used, for example how, what and when to monitor. 4
In particular, the frequency of monitoring has received little attention. A higher frequency of monitoring does not necessarily lead to better management. 5 Besides the financial costs, there are considerable downsides to overfrequent measurement. There are personal costs, such as the time spent, the need for invasive tests, the extra levels of anxiety and sometimes unnecessary hospital visits. More directly relevant, a higher frequency of monitoring increases the potential for inadequate management based on test results showing, incorrectly, deterioration when, in reality, the observed change is due to measurement variability only. 5,6 The overall aim of this programme was to develop a better understanding, extend the methods, increase the evidence base and improve the practice of clinical monitoring in UK primary care.
As background for this programme, our group, and others, had already developed evidence in the areas of cardiovascular disease (CVD) monitoring (cholesterol and blood pressure), warfarin monitoring and the monitoring of diabetic nephropathy. 5,7–10 This programme extended this work to other important clinical areas in primary care that represent two extremes in the way monitoring is carried out in primary care: renal monitoring in chronic kidney disease (CKD) and monitoring people with chronic heart failure (CHF). In the renal monitoring workstream (WS), we emphasised the non-diabetic population with CKD, to complement our previous report on diabetic nephropathy. 7
Diagnosis and management of CKD is based around estimated glomerular filtration rate (eGFR) measurements (creatinine and cystatin C based). Measurement of eGFR-creatinine (i.e. eGFR based on a creatinine measure) is recommended whenever a request for serum creatinine is made;11 as laboratory testing in primary care has seen a 9% annual increase in the previous two decades,12 the majority of those classified with CKD will have mild/moderate disease [G1 to G3b, based on eGFR-creatinine, and A1/A2 based on albumin–creatinine ratio (ACR)]. Therefore, most of the management/monitoring for CKD carried out in primary care will be in this group, with the final stages of disease (renal failure needing dialysis or transplantation) occurring rarely or only after many years. Current National Institute for Health and Care Excellence (NICE) guidance for the management of CKD11 recommends that monitoring should be based around measurements of eGFR-creatinine and ACR, with the frequency determined jointly by the patient and the health practitioner, but suggests at least one test per year for those with mild or moderate reduction in kidney function (G3a or higher) or for those with moderately increased ACR (A2).
For CHF, on the other hand, diagnosis is carried out by a heart failure multidisciplinary team based around symptoms, signs and investigations. N-terminal prohormone of B-type natriuretic peptide (NT-proBNP) is currently the only recommended test used as part of this diagnostic process, but there are high levels of uncertainty regarding the threshold used for this. 13 Although some improvement in the management of this condition has been made, the outlook after diagnosis is still poor; therefore, the majority of monitoring, even when carried out in primary care, is conducted in the later stages of disease. 14 There is, however, strong evidence of the prognostic association between NT-proBNP level and survival,15 as well as evidence that treatment of heart failure can improve patient quality of life, in terms of both physical and emotional well-being. 16 With regard to monitoring, NICE recommends that this should be based around clinical reviews and recommends the use of NT-proBNP as part of treatment optimisation only in a specialist care setting for people with CHF aged < 75 years who have a reduced ejection fraction and who do not have CKD of G3a or higher. 13
The research programme
The overall aim of this programme is to improve the management of long-term conditions in primary care by optimising the utility and frequency of tests used for monitoring. To achieve this, we carried out a series of inter-related projects on two specific areas, arranged as two WSs: WS1 – CKD and WS2 – CHF. Each WS was designed to (1) provide a summary of current practice and current evidence in this area, (2) generate new quantitative and qualitative evidence for monitoring these conditions and (3) provide a cost-effectiveness framework (or full model) to integrate this evidence.
The programme was integrated by a series of studies using different methodological approaches: systematic reviews, analysis of electronic health records, cohort and feasibility studies, interviews and focus groups, and statistical and health economic modelling. These studies were carried out to answer the following research questions:
-
Does the choice of biomarker for the monitoring of CKD in a primary care population better predict renal function decline and is this choice cost-effective?
-
Can the number of tests that are used to monitor individuals with stage G2 and G3 CKD be reduced, and hence the associated costs?
-
Is it useful to monitor CHF in primary care using natriuretic peptide (NP) or weight as markers?
-
Is it feasible and affordable to use point-of-care (POC) NP measurement as part of a monitoring strategy in primary care?
-
What is the acceptability and impact of alternative monitoring regimes among individuals experiencing these conditions?
The research was carried out between January 2013 and May 2019.
The links between the different WSs and the different projects in each WS are summarised in Figure 1. There was shared methodology in both WSs and a single stakeholder group, which provided guidance and direction throughout.
FIGURE 1.
Overview of the programme. BNP, B-type natriuretic peptide.

Workstream 1: chronic kidney disease
Prevalence rates from the Quality and Outcomes Framework (QOF) England returns indicate that > 1.8 million adults are classified as having moderate to severe CKD (defined as persistent proteinuria or eGFR from serum creatinine), representing 4.09% of those aged ≥ 18 years. 17 CKD (see Appendix 1 for the five main stages) is associated with increased CVD risk (predominantly stroke, ischaemic heart disease and heart failure)2,18–21 and increased all-cause mortality. 2,19,21
Given that prevalence of CKD rises exponentially with age, published estimates vary according to the populations used to derive them. Less severe stages of early CKD are likely to be more prevalent, as suggested by US data that estimated that approximately 14% of the population could be classified as having stage G1, 10% as having stage G2, 6.7% as having stage G3 and 0.5% as having stages G4 and G5. Nevertheless, the majority of studies focus only on moderate or severe renal impairment, at the level of CKD stage G3+, which means that there is a lack of evidence for the management of the majority of those with CKD.
Most patients with CKD are managed in primary care (98%) using a multifactorial approach of repeated monitoring, maintenance of blood pressure below agreed guideline limits (140/90 mmHg, or < 130/80 mmHg in those with diabetes or raised proteinuria), treatment of hypertension with angiotensin-converting enzyme (ACE) inhibitors or angiotensin receptor blockers (ARBs), and encouragement to lead a healthy lifestyle. 11 Current NICE guidance for the management of CKD11 recommends that monitoring should be based around measurements of eGFR-creatinine and ACR, with the frequency determined jointly by the patient and the health practitioner and tailored to take into account several factors, but suggests at least one test per year in those with mild or moderate reduction in kidney function (stage G3a or higher) or those with moderately increased ACR (stage A2). One of the reasons given for monitoring people with CKD is to be able to identify accelerated progression, which is currently defined as a sustained decrease in glomerular filtration rate (GFR) of ≥ 25% and change in GFR category within 12 months, or a sustained decrease in GFR of 15 ml/minute/1.73 m2 in ≥ 1 year. Accelerated CKD progression is one of several potential criteria for hospital referral. 11
What questions are being addressed?
We studied current practice in monitoring CKD to establish a point of reference for our subsequent research, and we reviewed the evidence for treatment options in CKD, because the value of monitoring depends, in large part, on the availability of actions that can be taken when disease has progressed. The value of monitoring also depends on the strength of the signal (true change) compared with the noise (apparent change due to imprecision in laboratory tests). We therefore studied the signal and noise of the current monitoring test, eGFR-creatinine, using different equations, and used this to compare the value of longer versus shorter intervals between laboratory tests. Furthermore, we established a cohort study in which to compare the laboratory test currently used to calculate eGFR-creatinine with a promising alternative using eGFR-cystatin C (i.e. eGFR based on a cystatin C measure). We accompanied these quantitative studies of the overall properties of CKD monitoring with detailed investigation of the experience of CKD monitoring in practice from patients and health practitioners. This WS addressed the following questions:
-
What is current practice in monitoring kidney disease in primary care?
-
Is there evidence for preferring the Modification of Diet in Renal Disease (MDRD) or Chronic Kidney Disease Epidemiology Collaboration (CKD-EPI) equation when estimating eGFR-creatinine?
-
What interventions are known to slow the progression of early-stage CKD?
-
What are the properties of CKD monitoring based on eGFR-creatinine, in particular the rate of change and the accuracy of diagnosed CKD stages?
-
Does the use of serum cystatin C (instead of serum creatinine) to estimate GFR improve the predictive value of CKD monitoring?
-
What are the views from patients and health practitioners on how monitoring CKD is carried out in primary care and the acceptability of any changes from current practice?
-
What is the cost-effectiveness of different monitoring strategies for CKD in primary care?
Current practice
Serum creatinine testing 1993–2013 in Oxfordshire (Oke et al.22)
To determine if the frequency of kidney function testing has changed over time, we looked at who is tested and how frequently these tests are conducted (see Appendix 2). We obtained details of serum creatinine tests sent to the Oxford University Hospitals NHS Foundation Trust Clinical Biochemistry laboratories from both primary and secondary care in Oxfordshire (> 1.2 million people) over a 20-year period (1993–2013). To determine the frequency of monitoring, we used Poisson regression models to adjust for the initial level of kidney function, glycated haemoglobin (HbA1c) testing, evidence of albuminuria or proteinuria, sex and age.
We found that the number of serum creatinine tests ordered from primary and secondary care steadily increased over the 20-year period (reaching > 220,000 in 2013 in primary care alone). Increased rates of testing were attributed, in part, to the expansion of the area that the laboratory serves and the ageing population, but trends did not seem to be affected by guideline changes or incentive payments. Older people with higher HbA1c levels and people with reduced kidney function were tested most frequently.
This analysis did not include data on patient history, prescriptions or reasons for test ordering, and so was unable to determine whether the tests were for diagnosis or monitoring or whether or not they were ordered with appropriate frequency. As the data are from a single region of the UK, we were unable to comment on whether or not these represent nationwide trends.
Therefore, we looked at national data to examine kidney function testing using data from > 600 general practices across the UK.
UK primary care kidney function testing 2005–2013 (Feakins et al.23)
To comment on regional variation in kidney function testing rates and how these differ by chronic diseases and prescriptions, we used routinely collected data from 4,573,275 patients from 630 UK general practices contributing to the Clinical Practice Research Datalink (CPRD) between 2005 and 2013 (see Appendices 3 and 4). The analyses were based on serum creatinine and urinary protein measures as markers of kidney function, and codes indicating the presence of chronic conditions, as well as drug prescriptions for which kidney monitoring is recommended.
We found that the rate of serum creatinine testing increased linearly across all age groups year on year, and the rate of proteinuria testing increased sharply in the 2009–10 financial year, but only for patients aged ≥ 60 years. For patients with established CKD, creatinine testing increased rapidly in 2006–7 and 2007–8, and urinary protein measurement increased rapidly in 2009–10, aligning with the introduction of respective QOF indicators. In adjusted analyses, the presence of a Read code for CKD was associated with up to a twofold increase in the rate of serum creatinine testing, whereas the presence of other chronic conditions and the prescription of potentially nephrotoxic drugs were associated with up to a sixfold increase. Regional variation in the rate of serum creatinine testing predominantly reflected country boundaries; in particular, Northern Ireland had higher rates of testing than other UK regions.
These findings suggest that the significant increases in the number of patients having kidney function tests annually and the frequency of testing are driven by changes in the recommended management of CKD in primary care, namely the QOF. Future studies should address whether or not increased testing has led to better outcomes.
Current evidence
Comparison of the bias and accuracy of the Modification of Diet in Renal Disease and Chronic Kidney Disease Epidemiology Collaboration Equations (McFadden et al.24)
Measuring eGFR is important in primary care as it determines the stage of CKD, referral decisions and changes to doses of commonly prescribed medicines. However, there is uncertainty over the optimal eGFR-creatinine equation to be used in community-based populations because the existing equations for eGFR-creatinine are derived mainly from younger patients with renal disease, whereas, in community populations, the patients are older and have a lower prevalence of established renal disease. We set out to examine how eGFR from existing equations, the CKD-EPI and MDRD, differ from measured glomerular filtration rate (mGFR) in populations that are equivalent to a primary care population (see Appendix 5). We undertook a systematic review and meta-analysis of studies (up to June 2017) that recruited patients similar to a primary care population, extracting data on the difference between estimated and measured GFR for CKD-EPI and MDRD equations. We also developed methods for meta-analysis to combine data on differences into summary statistics.
We found that the MDRD equation underestimates true renal function by 4.7 ml/minute/m2 [95% confidence interval (CI) 0.8 to 8.7 ml/minute/m2], and the CKD-EPI equation is more accurate in community-based populations. At higher levels of mGFR, which reflect community populations, the MDRD equation was less accurate (by 4.6%, 95% CI 2.9% to 6.2%) and more biased (by 3.2 ml/minute/1.73 m2, 95% CI 1.6 to 4.8 ml/minute/1.73 m2) than the CKD-EPI equation. Our data were limited in that the quality rating was not deemed high in many studies that were single centre, and the recruitment methods were not always clearly stated. This work allowed us to have a baseline of bias and accuracy for creatinine-based estimates of GFR in primary care.
Effects of medication on the progression of stages G3 and G4 chronic kidney disease (Taylor et al.25)
Treatment of people with CKD aims to prevent or reduce disease progression, prevent complications and minimise the risk of CVD (see Appendix 6). We conducted a systematic review and meta-analysis of randomised controlled trials (RCTs) (March 1999 to July 2018) to examine and compare the effects on the progression of CKD of four classes of drugs: antihypertensives, lipid-modifying drugs, glycaemic-control medications in patients with diabetes, and sodium bicarbonate. Our focus was on patients managed in primary care or by shared care with specialist nephrology services. The review summarised 35 studies including > 51,000 patients: 12 studies of antihypertensive drugs, 14 of lipid-modifying drugs, one study of an antihypertensive drug and a lipid-modifying drug, six studies of glycaemic-control drugs, and two of sodium bicarbonate. Nineteen studies provided summary data based on populations with CKD of stages G3 and G4 only. None of these studies provided data on sodium bicarbonate medication. Pooled estimates from these showed that, for antihypertensive drugs, there were no significant differences in renal function, and that eGFR was 4% higher in those taking lipid-modifying drugs (ratio of means 1.04, 95% CI 1.00 to 1.08) and 6% higher (ratio of means 1.06, 95% CI 1.02 to 1.10) in those taking glycaemic-control drugs (no studies provided data on sodium bicarbonate medication). Furthermore, and as expected, treatment with lipid-modifying drugs led to a significant reduction in the risk of CVD [risk ratio (RR) 0.64, 95% CI 0.52 to 0.80] and all-cause mortality (RR 0.74, 95% CI 0.56 to 0.98). There were no significant differences in cardiovascular events or mortality in studies of antihypertensive and glycaemic-control drugs. We found some evidence that glycaemic-control and lipid-modifying drugs slow the progression of CKD, and no evidence of renal benefit or harm from antihypertensive drugs.
New quantitative evidence
Modelling deterioration of kidney function (Oke et al.26)
This project (see Appendix 7) aimed to estimate the rates of progression of renal function stratified by urine albumin status, age and sex in a general population of people attending primary care.
We used the CPRD and constructed four cohorts based on their urine albumin status at baseline. The statistical method specified in the protocol (based on linear models6,27) proved unsuccessful; instead, a method for categorical data (i.e. a hidden Markov model27) was used to estimate the true underlying kidney function while accounting for measurement error and within-person variability due to eGFR-creatinine. Models were adjusted for age, sex, heart failure and previous diagnosis of cancer, and stratified by albuminuria status (< 3 mg/mmol vs. 3–30 mg/mmol vs. > 30 mg/mmol) at baseline.
The estimated rate of true transition from one CKD stage to the next, per year, was approximately 2% for people with normal urine albumin, between 3% and 5% for people with microalbuminuria (urine albumin of 3–30 mg/mmol) and between 3% and 12% for people with macroalbuminuria (> 30 mg/mmol). Misclassification of CKD stage due to measurement variability in serum creatinine, and hence eGFR-creatinine, is estimated to occur in between 12% and 15% of all tests in primary care. Progression of kidney function becomes faster with increasing age, and is faster among men, among people with elevated urine albumin and among patients with heart failure or with a previous diagnosis of cancer.
The true rate of progression of CKD is relatively slow, and eGFR-creatinine is an imperfect measurement that can lead to misclassification of disease stage every time it is used. This suggests that, when annual monitoring detects an apparent change of disease stage, this is more likely to be attributable to the imperfections of the measurement than to a true change.
Limitations of this analysis include missing data, which arise from the use of routinely collected electronic health records data rather than a clinical study. See the following section for a further study of the progression of CKD.
Prospective cohort study of monitoring chronic kidney disease
Cross-sectional evidence suggests that cystatin C provides better estimates of current kidney function than serum creatinine. We designed a study [Frequency Of Renal Monitoring – Creatinine and Cystatin C (FORM-2C)] to investigate whether or not cystatin C also provides better prognostic information than serum creatinine for future change in renal function. Because direct (radiological) measurement of GFR is invasive and expensive, making large studies impractical, we used change in eGFR (respectively eGFR-creatinine and eGFR-cystatin C) as the study outcome: the study report in Appendix 8 gives more details.
A cohort of 747 patients with an eGFR-creatinine of 30–89 ml/minute/1.73 m2 was recruited and followed up for 2 years, with blood samples taken at recruitment, 2 weeks and 12 weeks to establish baseline eGFR (both measures), and at 6, 12, 18 and 24 months after recruitment to measure the change in eGFR (both measures) over time. There were sufficient data from 629 patients to carry out analysis. When using serum creatinine to estimate GFR, baseline eGFR-creatinine had a concordance statistic (c-statistic) (also known as concordance index) of 0.495 (95% CI 0.471 to 0.521) for predicting future change in eGFR-creatinine. When using cystatin C to estimate GFR, baseline eGFR-cystatin C had a c-statistic of 0.500 (95% CI 0.474 to 0.525) for predicting future change in eGFR-cystatin C. Similar results were seen in sensitivity analyses, as described in Appendix 8. Either method of estimating baseline eGFR was predictive of the 2-year value [c-statistic for eGFR-creatinine 0.833 (95% CI 0.811 to 0.851) and for eGFR-cystatin C 0.889 (95% CI 0.877 to 0.898)], because there was, on average, little change over 2 years (see Appendix 8, Table 9).
Regardless of the method of estimation, eGFR (both measures) does not appear to usefully predict future change in eGFR (both measures). This is relevant, as change in eGFR has been shown to be significantly associated with all-cause mortality, end-stage renal disease (ESRD), and cardiovascular events beyond that observed for mGFR. 28,29 The study is limited by the use of surrogate measurements of renal function in the outcome, as well as in the index tests. One study using radiological measurement of kidney function is due to report in June 2021. If confirmed, our preliminary results suggest that the rate of change of renal status does not depend strongly on current state: there is no ‘acceleration’ of renal decline, at least across CKD stages G2 and G3a, which dominate our cohort.
New qualitative evidence
Qualitative evaluation for chronic kidney disease
To understand the acceptability and impact of current monitoring regimes among individuals with CKD, we used a mixture of patient interviews and focus groups with health professionals30 (see Appendices 9 and 10). For the patient interviews, we sought to recruit people who were having regular checks of their kidney function because they had stage G1–3 CKD. For the focus groups with health professionals, we used a variety of methods to recruit participants, mainly drawn from practices in Oxfordshire and the wider Thames Valley area. Participant numbers for the focus groups were small, so the views expressed may not necessarily be regarded as representative of these professions.
Forty-six people were recruited for the patient interviews; one withdrew after interview. Stage of CKD was unknown for seven participants, 35 had stage G3, two had stage G2 and two had been recently referred to specialist care because of more advanced kidney disease. The main findings were published in 2015 on https://healthtalk.org/ (accessed 5 May 2020) as ‘topic summaries’. 31 Sixteen people participated in four health professionals’ focus groups.
A gap was revealed between what health professionals seek to explain about CKD and what patients may understand. Primary care professionals often avoided using the term ‘CKD’ when talking to patients with early-stage kidney impairment in an attempt to avoid causing unnecessary anxiety. Patients’ accounts of receiving information about their kidney health echoed the phrases described by health professionals. However, patient interpretations showed some of these phrases to be unhelpful, raising further questions and adding to, rather than diminishing, initial concerns. The use of phrases such as ‘kidney damage’ or ‘kidney failure’ could be frightening, and the term ‘chronic’ was sometimes misinterpreted as meaning serious, whereas a description of the decrease in kidney function as a percentage or stage seemed less alarming. However, the exact meaning of the test results was often unclear, and those who were told their CKD stage did not always understand what this meant. Some patients found it difficult to understand that they had been diagnosed with CKD when they did not experience symptoms. Attempting to reassure patients that their kidney impairment was nothing to worry about, without providing explanatory information about the condition, could leave patients concerned and wanting to know more about possible causes, the meaning of test results and whether or not they could do anything to prevent further decline.
The GPs all confessed to being unfamiliar with the latest updates to NICE guidelines for either CHF or CKD or both, arguing that work pressures did not allow time to read these for all conditions. NICE’s website was considered confusing and difficult to navigate, and GPs were more likely to learn about patient management recommendations from other sources, such as the Clinical Commissioning Group or QOF alerts. On the current CKD guidelines,11 specifically, there was confusion about when ACRs should be requested from the laboratory and how the results should be interpreted and acted on.
Our research led to a mixed-methods study that calls for a rethink in how doctors talk to patients with reduced kidney health, replacing the term ‘CKD’ with different bands of kidney age (see Appendix 11).
Economic models
Review of economic models
We asked the following questions: what is known, from existing models, about the cost-effectiveness of monitoring of CKD? What can be inferred about the cost-effectiveness of CKD monitoring from the results of our CKD WS project (described above)? To what extent has the cost-effectiveness of CKD monitoring been studied, and, in particular, what modes of CKD monitoring have been studied? What modes of CKD monitoring should be prioritised for study (see Appendix 12)?
We reviewed the literature to identify existing cost-effectiveness models in CKD that could be suitable for such an assessment (up to January 2019). Although there are many model-based evaluations of interventions in CKD, we included only models that could be useful to evaluate the cost-effectiveness of CKD monitoring in the context of current clinical guidelines. Therefore, a lifetime cost-effectiveness model was reviewed if (1) CKD stages were defined using eGFR-creatinine (i.e. not exclusively based on proteinuria status), (2) at least two distinct states prior to renal replacement therapy (RRT) (i.e. renal dialysis or renal transplant) were included (i.e. the model states were not simply ‘pre RRT’ and ‘RRT’) and (3) the incidence of important cardiovascular events was modelled.
A pearl-growing search strategy was used to identify eligible cost-effective models. First, four suitable key studies (‘pearls’) were identified32–36 by the authors using their previous experience, including the development of one of these models. 36 The reference lists and citations of each included study were reviewed. One additional study was included37 and classified as a new pearl, and the search was repeated for another iteration, as a result of which no relevant papers were identified. To ensure that no important manuscripts were missed, a quick scoping search was performed in Google Scholar (Google Inc., Mountain View, CA, USA) (using the search line ‘cost-effectiveness “chronic kidney disease” progression’), followed by a review of the references included in an in-house review of models of CKD progression (Iryna Schlackow, University of Oxford, 2018, personal communication). These reviews did not yield further eligible studies.
Thus, five long-term cost-effectiveness models potentially useful to assess CKD monitoring were reviewed. Detailed descriptions of the models included in our review are presented in Appendix 12. Of the five models, two were developed to assess the cost-effectiveness of CVD prevention interventions (e.g. statins) in CKD,32,36 two were developed in the context of screening for CKD/proteinuria in general population33,37 and one aimed to accommodate both CKD identification and treatment. 35 Parameters of the models were mostly collated from published literature and/or derived from different data sources,32,33,35,37 with only one model based largely on individual patient-level data. 36 Only two models separate the ESRD state into dialysis and renal transplant states, which are associated with different outcomes and costs. 35,36 All models included validation of their performance, but this validation was not in the context of a general CKD population. Only the screening models included early CKD stages (e.g. CKD stage G2, which constitutes the bulk of the CKD population in a primary care setting) in their target populations. 33,35,37 The CVD prevention models considered only CKD stage G3 and beyond. 32,36 In the screening models, effects of ACE inhibitors and ARB treatments were implemented, but in only one model were these treatments assumed to affect CVD risks;37 in the other two models, these treatments were assumed to affect only CKD progression and mortality. 33,35 Only one model considered heart failure as an end point,35 but it was unclear how the treatment effect on heart failure was implemented, and what the impact of this treatment on other transitional probabilities was. None of the identified studies assessed effects of monitoring eGFR.
In summary, none of the identified models included the elements required for the assessment of cost-effectiveness of monitoring strategies in UK primary care. Therefore, it was decided to develop a new cost-effectiveness model to support the evaluation of CKD monitoring.
Cost-effectiveness of monitoring kidney function in UK primary care (Schlackow et al.38 and Appendix 14)
We sought to incorporate the findings from the programme into a model of the cost-effectiveness of monitoring CKD in primary care. We assessed the following frequencies of GFR monitoring: no monitoring, monitoring every 5, 4, 3 and 2 years and annual monitoring. Based on the systematic review of interventions (see Appendix 6),25 other published meta-analyses39–41 and discussions with clinicians and stakeholders, it was agreed that a key objective of eGFR monitoring in primary care is to guide treatments to reduce CVD risk among people with reduced kidney function. To project health outcomes, we supplemented the model of variability and progression (see Appendix 7)26 with additional modelling that included CVD risk equations with a term for stage of eGFR. Full details of the model, including estimated cost and quality-of-life impact of cardiovascular outcomes, are given in Schlackow et al. 38
Appendix 14 gives details of the application of the model to GFR monitoring, including the assumptions made about monitoring costs and prescription costs, and assumptions made about treatment. Current clinical guidelines recommend that CVD preventative treatments (i.e. statin, antihypertensive or antiplatelet drugs) be considered for patients with reduced kidney function and, therefore, in the cost-effectiveness analysis we studied the impact of GFR monitoring on their use. Based on the systematic review of interventions (see Appendix 6),25 we assumed statin treatment to be the most relevant.
We could not justify monitoring in people with CKD to guide cardiovascular prevention as changes in eGFR did not trigger changes in CVD treatment recommendations (see Appendix 14). Briefly, this arises because, for people with clinical CKD [i.e. meeting the Kidney Disease: Improving Global Outcomes (KDIGO)42 definition], statin treatment is recommended;43 many of them are also indicated for antihypertensive treatment44 and, if they have a history of CVD, for antiplatelet therapy. 44 These therapies are also widely recommended in people with CVD. 43 Therefore, under current guidelines,11 monitoring the eGFR among patients with clinical CKD or with a history of CVD would largely not change indicated cardiovascular therapies.
We also considered eGFR monitoring in people with impaired eGFR (i.e. an eGFR of < 90 ml/minute/1.73 m2) but no CKD (defined as macro/microalbuminuria or an eGFR of < 60 ml/minute/1.73 m2). For some of these people, monitoring eGFR to detect CKD is potentially beneficial, compared with no monitoring, but the model does not currently separate potential harms of overdiagnosis. The optimal (i.e. most cost-effective) interval of eGFR monitoring in this group depends on a patient’s age and our analyses indicate that, at £20,000 per QALY cost-effectiveness threshold, that is about every 3 years for people aged < 60 years, every 4 years for people aged 60 to 69 years, and no monitoring among those aged ≥ 70 years.
Conclusions
This WS focused on management/monitoring of CKD in primary care, researching current practice and providing new evidence to guide this management. In particular, we concluded that:
-
Over the last decade, renal function testing has increased in volume at the national level. Specifically, for those with CKD, this increase is driven by national guidance for reporting monitoring.
-
At higher levels of mGFR, which reflects primary care populations, the MDRD equation was less accurate and more biased than the CKD-EPI equation.
-
Lipid-modifying and glycaemic-control medications are possibly beneficial to slow the progression of early-stage CKD. However, evidence of glycaemic control exists only in diabetic populations.
-
The rate of change in eGFR is slow, and misclassification is more common than real change.
-
The rate of change in eGFR is slow, regardless of the baseline eGFR, in patients in primary care. Cystatin C is not a better predictor of change than creatinine.
-
The terminology around ‘chronic’ and ‘disease’ is problematic for patients and GPs when communicating about early stage, or small decreases in renal function.
-
Before this programme, there were no models available that were able to assess the cost-effectiveness of monitoring strategies in UK primary care, to our knowledge.
-
A new cost-effectiveness model to assess frequency of eGFR modelling was developed in this programme; people with an eGFR of between 60 and 90 ml/minute/1.73 m2 were identified as a group for whom monitoring could potentially be beneficial.
-
Application of our cost-effectiveness model to those with an eGFR of between 60 and 90 ml/minute/1.73 m2 suggests that monitoring eGFR in people aged < 70 years and without cardiovascular disease every 3–4 years to guide cardiovascular prevention may be cost-effective.
Research recommendations
-
Estimates of the bias, and accuracy, of eGFR-creatinine equations varied greatly (statistical heterogeneity) between studies in our systematic review. It would benefit the NHS to determine when (in what settings) and how (with what protocol) the most accurate results can be obtained and which protocol should be recommended nationwide.
-
High variability in eGFR-creatinine, and hence inaccuracy in CKD staging, suggest a probable benefit to combining eGFR measurements (several creatinine and/or several cystatin C), rather than simply repeating them and acting on the most recent; this potential route to better management should be researched.
-
Cystatin C appears to have some advantages over serum creatinine for estimating GFR, for example in predicting rapid progression; these findings, from secondary analyses only, should be confirmed.
-
At least one alternative to the term ‘CKD’ has now been proposed, in response to our qualitative work and patient and public involvement (PPI), and its potential to improve communication should be investigated (see Patient and public involvement).
-
Closing the gap (identified in the systematic review of interventions) of evidenced interventions that specifically protect renal function would have the greatest potential to improve the cost-effectiveness of CKD monitoring.
Overall, these can be summarised as three key questions:
-
When and how can the most accurate estimates of GFR be obtained?
-
What is the longer-term relationship between current eGFR (either measure) and future progression of CKD?
-
How can the progression of CKD be prevented or delayed in patients at risk?
Implications for practice
-
Laboratories could improve the accuracy of eGFR testing by switching to the CKD-EPI equation for its calculation, as recommended by NICE,11 if they have not done so already.
-
Potential treatments that might positively affect kidney function are lipid-modifying treatment and glycaemic-control medication.
-
The rate of change of kidney function in a primary care population is slow, and most apparent changes will be due to measurement noise (error) and not real change.
-
This rate of change is slow regardless of the initial CKD stage (in a primary care population).
-
The terms ‘chronic’ and ‘disease’ act as barriers in the communication between health practitioners and patients. A potential solution is the use of alternative terms, such as ‘kidney age’. 45
-
Monitoring individuals with CKD is difficult to justify by the usual logic of treatment initiation or titration. Alternative ways of quantifying its benefit might be required.
Reflections
We find that CKD monitoring, using blood tests in particular, but also urine tests, has been a huge growth area in the NHS. However, our research highlighted a substantial gap in the evidence base for this monitoring. The usual rationale for monitoring a clinical condition includes an action, typically a treatment change, that can be taken in response to the results of monitoring tests. In a large systematic review, we found no evidence that antihypertensive treatment or administration of sodium bicarbonate are useful actions to slow progression of kidney disease, and the treatments that might slow progression, in particular lipid-modifying treatments, are likely to be in use already in patients with CKD, particularly those with stage G3 disease onwards. Following these findings through to a full model of cost-effectiveness emphasised that monitoring of CKD in primary care, especially as frequently (i.e. annually) as currently recommended, cannot be rationalised as monitoring to guide treatment. This complements our previous finding that monitoring diabetic nephropathy as frequently as annually is unlikely to be cost-effective. 7
It would, however, be premature to abandon all monitoring of CKD. Arguments may be made that monitoring CKD (e.g. in stage G3) could encourage lifestyle changes, promote adherence to already indicated medications, help avoid nephrotoxic treatments such as ibuprofen in primary care and help avoid sudden descent from apparent full kidney health into late-stage kidney disease in secondary care. These benefits will be harder to quantify than an obvious treatment for CKD, but such a research effort is needed to provide a sound rationale for monitoring and to design a cost-effective monitoring schedule. Current practice, that is annual monitoring, has become a growing cost to the NHS, yet lacks a clear evidence base.
The other major finding to emerge from this work was the problematic terminology ‘CKD’, which is misunderstood by patients and avoided or used with reluctance by GPs. This emerged from our formal qualitative research, but it emerged also, and sooner, from the reactions of patients approached to take part in our studies, and from the patient and public members of our stakeholder group. We return to this in Patient and public involvement.
Workstream 2: chronic heart failure
A 2002 report suggested that ≈ 900,000 people in the UK had CHF. 46 Since then, the prevalence of heart failure in the UK has been increasing, probably as a result of demographic changes and improvement in the management of CHF, with an observed increase of 23% between 2002 and 2014. 47 In a prospective community screening study,48 definitive CHF, according to the European Society of Cardiology (ESC) criteria,49 was present in 2.3% of the population, of whom the proportion with a left ventricular ejection fraction (LVEF) of < 40% was 41%; the prevalence of definitive CHF rose to 8% in those aged > 75 years. 50
The incidence in the UK is less clear, but the crude rate has been estimated to be 1.3 cases per 1000 population per year for those aged ≥ 25 years, reaching 7.4 cases per 1000 population per year for those aged 75–84 years and 11.6 cases per 1000 per year for those aged ≥ 85 years. 48 The absolute incidence of CHF in the UK has also increased considerably since 2002 (12% between 2002 and 2014), even though the age-standardised incidence is actually decreasing. 47 Overall, a GP with a patient population of 2000 will care for approximately 40 to 50 patients with heart failure and see two or three new cases each year.
Early diagnosis is seen as critical because early-stage heart failure may be reversible. However, the diagnosis and management of CHF remains challenging. For example, although CHF is frequently diagnosed by GPs, it is confirmed by echocardiography in only approximately one-third of cases. 51 NICE13 recommends that a heart failure multidisciplinary team carry out the CHF diagnosis using a combination of symptoms, signs and investigations. The use of a NT-proBNP measure is the only biomarker recommended as part of this diagnostic process, but with recognition of the uncertainty regarding the absolute threshold used. 13 Despite recent improvements, patients diagnosed with heart failure have a poor prognosis: approximately 40% of patients diagnosed die within the first year. After that, the mortality rate decreases to approximately 10% per year. 52–54 Survival rates are worse than those of cancer of the breast or prostate. 55
People diagnosed with heart failure have high levels of use of health-care resources not only in terms of GP consultations and community-based drug therapies, but also because of referrals to outpatient clinics and inpatient bed-days. Heart failure accounts for 5% of all emergency medical admissions; it is estimated that the total annual cost of heart failure to the NHS is approximately 2% of the total budget, with approximately 70% of this total attributable to the costs of hospitalisation. 56,57 Moreover, re-admissions are common, with approximately one in four patients re-admitted within 3 months. 58,59 Patients also report a dramatic decrease in their quality of life, with a consequent impact not only on agencies such as social services and the benefits system, but also on their families and caregivers. 58 There is, however, considerable evidence58,60,61 that pharmacological treatments can improve the prognosis of heart failure. Both pharmacological and non-pharmacological treatments can improve patient quality of life, in terms of both physical functioning and well-being. 58
With regard to monitoring, NICE13 recommends that this should be based around clinical reviews, and recommends the use of NT-proBNP as part of treatment optimisation only in a specialist care setting for people with CHF aged < 75 years who have reduced ejection fraction and who do not have CKD of stage G3a or higher. 13 Increased levels of B-type natriuretic peptide (BNP) and NT-proBNP in patients with heart failure have been demonstrated, in some studies,62,63 to have, not only diagnostic, but also prognostic, utility.
Previous work has identified BNP as having large clinical variation in non-severe populations, thereby probably reducing its utility for monitoring CHF. 64 POC NP testing has been suggested as an alternative method to reduce this variability. 65 At the same time, POC NP testing would enable GPs to rapidly refer the appropriate patients or, if CHF can be excluded, investigate alternative causes of clinical symptoms (e.g. dyspnoea).
What questions are being addressed?
We reviewed the evidence for NP-guided treatment in CHF, including how a potential intervention using this approach could be implemented, because this has been the most promising area for which monitoring of this group could be effective. For NP monitoring to be of use, the noise (i.e. within-person variability) should be relatively small compared with the signal (true change). We therefore studied the noise of NP measures based on a secondary analysis of a clinical trial. As an alternative strategy, to minimise NP variability, we studied the accuracy of available POC NP devices, as well as testing the feasibility of their use in a general practice. To understand where monitoring should take place, we summarised the evidence relating to strategies for remote monitoring (RM) (from home) in this population. As for the CKD WS, we carried out a detailed investigation of the experience of CHF monitoring in practice from the perspectives of patients and health practitioners. Finally, we summarised the evidence available regarding health economic models to evaluate monitoring strategies in CHF and provided a framework to carry out this assessment. This WS addressed the following questions:
-
Is there evidence to suggest that NP-guided treatment improves outcomes in patients with CHF?
-
What would be the relevant components of an intervention to implement NP-guided treatment?
-
What are the properties of NP measures used when monitoring CHF, in particular, the within-person coefficient of variation (CV)?
-
Are POC NP measures accurate and, of the devices available for measuring POC NP, which would be best to use?
-
Is it feasible to implement a POC NP test/device in a primary care practice?
-
What is the evidence regarding the efficacy of RM in CHF?
-
What are the views from patients and health practitioners of how monitoring CHF is carried out in primary care and the acceptability of any changes to current practice?
-
What health economic models have been used, and what issues need to be addressed, to study the cost-effectiveness of monitoring CHF in UK primary care?
Current practice
Although the age-adjusted heart failure incidence in the UK is decreasing, the absolute incidence and prevalence have seen a substantial increase over the previous decade, potentially due to demographic changes and improvement in management. 47 Recommended management of CHF patients is based around a multidisciplinary team working in collaboration with primary care, with the primary care team taking over routine management once the patient has stabilised. 13 Measurement of patient NT-proBNP is also recommended as part of the diagnostic pathway and as part of a monitoring strategy to optimise treatment, but only in a specialist setting for people aged < 75 years with heart failure, with reduced ejection fraction and with an eGFR of > 60 ml/minute/1.73 m2.
An analysis of current management of CHF patients in primary care66 identified that, at least until 2013, only a small proportion of those with a current diagnosis of CHF have ever had NP measured in a primary care setting (4.85%, 95% CI 4.71% to 4.99%); that most of these measurements are probably used as part of a diagnostic strategy (74% of individuals with a single NP measurement); and that the type of NP most commonly used in primary care since 2007 is NT-proBNP. This analysis66 confirmed the strong association observed between high levels of NPs and all-cause mortality in this group. It also suggests that measurements of NP are underused in primary care as part of the diagnostic pathway (not consistent with NICE guidance13) and not used as part of a monitoring strategy (consistent with NICE guidance13). 66
Current evidence
Natriuretic peptide-guided management of heart failure (McLellan et al.67,68)
We carried out a systematic review and meta-analysis (see Appendices 15 and 16) to assess whether or not treatment guided by serial NP monitoring improves outcomes, compared with treatment guided by clinical assessment alone (up to November 2017). In 2016, we found inconclusive evidence for a reduction in all-cause mortality (13%) and heart failure mortality (16%). Heart failure admission was reduced 30% by NP-guided treatment, but the evidence was inconclusive for all-cause admission. Six studies reported on adverse events; however, the results could not be pooled. Only four studies provided results for cost of treatment: three of these studies reported a lower cost for NP-guided treatment, whereas one reported a higher cost. The evidence showed uncertainty for quality-of-life data. Heterogeneity was low for all outcomes bar heart failure admission, which was substantial.
In 2017, the addition of the Guiding Evidence Based Therapy Using Biomarker Intensified Treatment in Heart Failure (GUIDE-IT) study69 increased the total number of participants by 24% and substantially increased the precision of the estimates. This altered the findings of the Cochrane systematic review,67 and the evidence now indicated that NP-guided treatment could improve all-cause mortality by 13%. The effect on heart failure admission was a relative reduction of 20%, whereas the findings for all other outcomes were similar to those from the Cochrane systematic review.
We concluded that the current pooled evidence indicates a beneficial effect of NP-guided therapy on all-cause mortality and heart failure admissions. However, despite the publication of the GUIDE-IT study,69 the largest in this field, the effectiveness estimated from the meta-analysis is marginal and the conclusions are not yet robust, with a high chance that these will change as new evidence emerges.
Effectiveness of remote monitoring for heart failure
Remote monitoring, a collective term for telemonitoring (TM) and structured telephone support (STS), of heart failure is increasingly becoming an option for patients, as a result of the ongoing advancement in technology and familiarity with its use (see Appendix 17). RM aims to collect and transmit physiological data through devices in the patient’s own home that could potentially increase early detection of clinical deterioration, improve patients’ quality of life and decrease health-care delivery costs.
Despite the lack of NICE-recommended use of RM (201070 and 201813 guidelines), the NHS Technology Enabled Care Services Resource for Commissioners,71 in response to the NHS Five Year Forward View,72 recognised that RM technology would be important in the future.
This systematic review and meta-analysis (up to February 2019) aimed to evaluate the clinical effectiveness of home TM and/or STS interventions, compared with standard care, among adults with heart failure for all-cause mortality, all-cause hospital admission, heart failure hospital admission, length of stay, health-related quality of life, adherence, acceptability, heart failure knowledge and self-care. This was an update of a previous review by Inglis et al. 73
We identified 12 new RCTs, including the second- and fourth-largest studies to date, to our knowledge, to add to the existing 41 RCTs previously identified by Inglis et al. 73 in 2015. Screening and data extraction were carried out by two co-authors. For binary outcomes, we used fixed-effects meta-analyses to estimate the RR; for continuous outcomes, the standardised mean difference (SMD) was used.
The pooled results suggest that the rates of all-cause mortality and heart failure hospital admissions were reduced and quality of life was improved with the use of RM (all-cause mortality: STS – RR 0.85, 95% CI 0.76 to 0.96; I2 = 0%; TM – RR 0.82, 95% CI 0.73 to 0.92; I2 = 20%; heart failure admission: STS – RR 0.85, 95% CI 0.77 to 0.93; I2 = 27%; TM – RR 0.74, 95% CI 0.64 to 0.84; I2 = 17%; quality of life: STS – SMD 0.13, 95% CI 0.09 to 0.18; I2 = 86%; TM – SMD 0.24, 95% CI 0.20 to 0.28; I2 = 98%). However, although there was a suggestion that the rate of all-cause hospital admissions may be reduced by RM, this was not statistically significant. It was not possible to pool data for length of stay, adherence, acceptability, heart failure knowledge or self-care. For the outcomes of all-cause mortality and heart failure hospital admission, the heterogeneity was low and, although moderate for all-cause hospital admission, no particular explanation for the higher heterogeneity was revealed by subgroup analysis or meta-regression. The findings for quality of life should be viewed with caution as the heterogeneity was very high and testing of the robustness of the effect estimate by using a random-effects model resulted in the TM finding no longer being statistically significant.
This is a rapidly changing area of research, and a further 24 studies were recorded as ongoing; therefore, the evidence will continue to need updating as these are published. Furthermore, the technology continues to advance, meaning further updates will be required. Many RM interventions are complex, and further investigation of these using component analysis would be beneficial.
Diagnostic accuracy of point-of-care natriuretic peptide tests for chronic heart failure (Taylor et al.74)
To assess the diagnostic accuracy of POC tests compared with echocardiography, clinical examination or combinations of these, we conducted a systematic review and meta-analysis (up to March 2017) of all POC NP diagnostic accuracy studies (see Appendix 18). We included only studies that were carried out in primary care or other ambulatory care settings, as this is where the test would be used in practice.
We identified 42 publications of 39 individual studies and included 37 studies in the analysis. These studies assessed the diagnostic accuracy of two different natriuretic tests (BNP and NT-proBNP); only five studies had been undertaken in primary care. The studies varied considerably in the types of patients they included, the health-care settings and the thresholds used. There was a wide range of sensitivity and specificity at different thresholds reported in the various studies. For the BNP test, the pooled sensitivity and specificity were 0.95 (95% CI 0.91 to 0.97) and 0.57 (95% CI 0.43 to 0.70), respectively, whereas, for NT-proBNP, pooled sensitivity and specificity were 0.99 (95% CI 0.57 to 1.00) and 0.60 (95% CI 0.44 to 0.74), respectively. However, it was important to note that the sensitivity was more variable in the studies conducted in primary care.
Our review showed that NP tests have variable ability to exclude CHF in patients in ambulatory care. A positive test would need to be confirmed with cardiac imaging and also appropriate follow-up. We also highlighted that certain thresholds recommended by guidelines might be relevant, but further research is needed to confirm which thresholds are the most appropriate in an ambulatory care setting and whether or not implementing them can improve patient care.
Limitations of the analysis were that very few data were available in primary care settings and only a small number of studies were conducted using NT-proBNP. Several included studies were also at unclear risk of bias, which may potentially lead to an overestimate of the accuracy measures. The heterogeneity across the studies may also affect the generalisability of the results.
Given the lack of studies in primary care and the methodological limitations we identified in the studies, high-quality evidence in the form of randomised trials is needed to better guide the use of POC NP tests in the primary care and ambulatory care setting. Such studies should also clarify the appropriate thresholds to improve outcomes in patients with CHF. As part of this programme grant, a study in primary care of the accuracy of POC NT-proBNP tests was undertaken to address this research gap.
New quantitative evidence
Essential components in natriuretic peptide-guided management of heart failure (Oke et al.75)
This work aimed to identify the key components of NP-guided treatment interventions that reduced rates of hospitalisation in patients with heart failure (see Appendix 19). We extracted detailed information on the components of interventions from studies of NP-guided treatment of heart failure identified in a systematic review67,68 (see Appendices 15 and 16).
Detailed information on the interventions used in the studies was extracted from the papers in the review. We looked at univariate associations between components of the interventions and the strength of the reduction in the rate of heart failure hospitalisations and all-cause mortality. We compared intervention options in studies with significant and non-significant results to find components common to the more effective forms of the intervention.
We identified eight components across 10 studies that reported heart failure hospitalisation rates. The common-components analysis identified four components: a predefined treatment protocol, the locale of the heart failure clinic, setting a stringent NP target and incorporating a relative target as potential key components to reducing heart failure hospitalisations using NP-guided therapy.
The extent of what we could say about the effective components was limited by the number of studies that reported heart failure hospitalisations. Being unable to control for patient-level differences across studies may have masked the active components. Future studies should be conducted using individual patient data for their analyses.
Estimation of the variability of B-type natriuretic peptide and weight
To investigate variability in BNP concentrations and weight in patients with heart failure, we analysed data from the control arm of a RCT among patients with stable New York Heart Association (NYHA) class II or III CHF (see Appendix 20). The data were collected over 13 weeks of follow-up. Thirty patients in the control arm reported weight measurements weekly. BNP was measured every 6 weeks at 1, 7 and 13 weeks. The influence of age and obesity was investigated.
The between-person CV of weight was < 26% and of BNP was 137%. Between-person variation in BNP was higher for younger patients than for older patients with heart failure (the CV was 170% for age < 55 years and 88% for age ≥ 55 years), but did not appear to be related to obesity. Within-person variation was also substantial, but was smaller than between-person variation (the CV was 46% for BNP and 1.2% for weight).
The results suggest that monitoring over 3 months is unlikely to detect true BNP change against background noise in this small, chronically stable, heart failure population.
Feasibility study of the use of point-of-care natriuretic peptide testing in primary care
It has been postulated that routine monitoring of NP could assist in improving the care of patients with heart failure in the community. 70 Recent advances in technology provide the possibility of POC NP testing, but there is currently no evidence to support the use of such devices as part of routine care of patients with heart failure in primary care.
The primary aim of this study was to determine the variability in NP measurements made using POC NP technology in patients with heart failure in primary care settings, including both between- and within-person variability. Secondary outcomes assessing feasibility included the proportion of planned tests for which results were available (see Appendix 21).
We recruited adults with a recorded confirmed diagnosis of heart failure from three general practices in Oxfordshire between 20 February and 28 March 2018; follow-up was to 29 March 2019. Participants attended three scheduled visits at 0, 6 and 12 months from baseline. At all three visits, three venous blood samples were taken: one for POC NT-proBNP measurement (cobas® h 232 POC system; Roche Diagnostics, Rotkreuz, Switzerland), and two to be sent to the local laboratory for NT-proBNP and renal function testing.
Of 27 recruited participants, all attended visit 1, 23 attended visit 2 and 24 attended visit 3 (see Appendix 21). POC NT-proBNP measurements were successfully obtained at all visits attended by participants.
Within-person variability in POC NT-proBNP over 12 months was 881 pg/ml (95% CI 380 to 1382 pg/ml). Between-person variability was calculated using data from all 27 participants. Between-person variability in POC NT-proBNP over 12 months was 1972 pg/ml (95% CI 1525 to 2791 pg/ml).
We found that it was feasible to carry out POC NT-proBNP testing in primary care, with testing successfully carried out in 100% of planned study visits. However, no tests were carried out during routine primary care visits by the study participants. Between-person variability in POC NT-proBNP was around twice as large as within-person variability over 12 months. This would indicate that deviations from individual set points for NT-proBNP are likely to be more helpful than population-level thresholds when considering a monitoring strategy.
New qualitative evidence
Qualitative evaluation for chronic heart failure
To understand the acceptability and impact of current monitoring regimes in individuals with CHF, we used a mixture of patients’ interviews and focus groups with health professionals (see Appendix 9). We used existing interviews conducted between 2003 and 2013, as well as new interviews focusing on monitoring. Participants for the focus groups with health professionals were recruited using a variety of methods, but were mainly drawn from practices from Oxfordshire and the wider Thames Valley area. Participant numbers for the focus groups were small, so the views expressed cannot be regarded as being representative of these professions.
Forty-three previously existing interviews and 16 new ones were analysed. The analysis aimed to focus on patients’ attitudes to both the content and frequency of monitoring, adherence to and understanding of medications, and triggers of consultation. The analyses of the original and new interviews were published in (respectively) 2014 and 2016. 76 Sixteen health professionals participated in four focus groups: two consisting of community heart failure nurses (CHFNs), one of GPs and one of practice nurses.
A range of strategies was reported, with some people monitoring their weight and blood pressure at home, and some using TM. Monitoring could take place in either primary or secondary care (or both); care by specialist nurses was particularly valued. Frequency of check-ups ranged from yearly to weekly. Being monitored provided people with reassurance. A few expressed criticisms, which were minor, about their current regime, although, for some, titrating medication to optimal levels took too long. Advice about when to seek help did not seem to have been given other than for emergency situations. Approval for hypothetical future changes to monitoring regimens would be forthcoming, provided the rationale was explained. Increased reliance on blood testing in future would be acceptable, and POC testing in GPs’ surgeries would be appreciated by people who would become anxious waiting days or weeks for test results.
As expected, CHF nurses were fully cognisant of the NICE guidelines for managing people with CHF, and used them daily to guide their care, with adjustment based on patient complexity (comorbidities). GPs and practice nurses expressed unfamiliarity with the latest updates to NICE guidelines. NICE’s website was considered confusing and difficult to navigate, and both GPs and practice nurses were more likely to learn about patient management recommendations from other sources, such as the Clinical Commissioning Group or QOF alerts. Given this, CHF nurses mentioned that they usually lead on treatment plans.
Chronic heart failure patients are regularly seen by practice nurses, but only as part of their management for other long-term conditions (driven by the QOF). GPs were unsure what tests they should be doing, and when and how to interpret and act on the results. Although they would welcome specific guidance from specialists about how to manage individual patients discharged from secondary care, they felt criticised by cardiologists for up-titrating a patient’s medicines too slowly, when, in reality, this process could be challenging as a result of patient factors beyond their control.
Generally, both GPs and CHF nurses recognise NP as a useful diagnostic test for CHF, but CHF nurses could see no benefit of measuring NP as part of routine monitoring, instead suggesting that any changes in severity would be reflected in patients’ symptoms. All groups believed that the cost of the test (both financial and in terms of professionals’ time and extra blood samples required from the patient) would outweigh any potential benefits.
Economic issues
We aimed to identify health economic models used to address research questions related to monitoring heart failure in primary care, and hence to identify key issues. We reviewed the research questions addressed, the model structures and the data sources used to populate the models (see Appendix 22). A previous review by Goehler et al. 77 (including studies published be June 2010) was updated to August 2018 to identify analytical frameworks developed to evaluate heart failure interventions and management programmes in primary care. Studies were excluded if the research question was based fully within secondary care or if they reported local non-UK adaptations of already-included models.
Forty studies were identified: three focused on diagnosing heart failure, 11 focused on management strategies and 26 focused on drug interventions. Thirty-one studies used Markov model specifications with several different model structures. There was limited use of individual patient characteristics in the models. The data used to populate the parameters of the economic models were sourced predominantly from RCTs (undertaken several years ago) among patients with CHF recruited in hospitals.
Different models have been used to evaluate primary care interventions for patients with CHF. Despite the small number of studies that took into account patient characteristics, this appears to be increasing over time. Data from RCTs are widely used for estimating disease risks, quality-adjusted life-years (QALYs) and costs. Participants in these trials, frequently completed some years previously, were unlikely to be representative of the general CHF population in primary care. Future work should consider individual patient risk factors and include more recent data from CHF patients in primary care.
Three key issues were identified. First, the model structures were not generally sufficiently detailed to allow assessment of subtle improvements in disease monitoring. Second, a very small number of models accounted for individual patient characteristics and risks; however, this problem appears to be increasingly tackled in more recent models. Third, data to parameterise the economic models almost exclusively came from RCTs, many of which were completed some years previously and recruited patients exclusively in hospitals. Therefore, these data are unlikely to be representative of the target CHF population in primary care in the UK. Future work should consider individual patient risk factors and source more recent data from primary care.
Conclusions
This WS focused on management/monitoring of CHF in primary care, researching current practice and providing new evidence to guide this management. In particular, we concluded that:
-
NP tests appear to be underused in primary care as part of diagnostic pathways (at least until 2013), which is not consistent with NICE guidance,13 and are not used as part of a monitoring strategy (consistent with NICE guidance).
-
NP-guided treatment might be beneficial in this population in reducing all-cause mortality and the rate of heart failure hospital admissions, although some uncertainty remains with regard to the size and robustness of this effect.
-
We identified three components (plus being located in a specialist setting) that may be essential for NP-guided therapy in reducing the rate of heart failure hospitalisations – (1) using a predefined treatment protocol, (2) setting a stringent target and (3) the use of patient-specific targets.
-
TM and STS in this population reduce the rate of all-cause mortality and hospital admission. Quality of life might also be improved by RM, although large levels of heterogeneity reduce our confidence in this beneficial effect.
-
Within-person variability of BNP and weight is large, thereby reducing the chances of identifying true change in the condition using these measures.
-
POC NP tests have variable ability to exclude CHF in patients in ambulatory care at low thresholds, but the ESC threshold for non-acute care for NT-proBNP might be an appropriate cut-off point for POC testing in this setting.
-
It is feasible to carry out POC NT-proBNP testing in primary care, with testing successfully carried out in 100% of planned study visits. However, no opportunistic tests were conducted.
-
Between-person variability in POC NT-proBNP is around twice as large as within-person variability over 12 months.
-
Professionals consider the use of NP tests for monitoring expensive, of limited benefit and, potentially, a waste of scarce resources.
-
Health economic studies increasingly consider individual patient factors, but are limited by a lack of data from CHF patients typically seen in primary care.
Research recommendations
-
Further studies evaluating the efficacy of NP-guided treatment in CHF are needed. Based on a 13% relative reduction from a 15% mortality (baseline), an excess of 10,000 participants will be required to have a sufficiently powered (≥ 80%) study. Current systematic reviews/meta-analyses include just over 4000 participants.
-
Any such studies should collect resource data on length of hospital stay and re-admission rates, which are critical for the health service and will facilitate assessment of cost-effectiveness.
-
The potential mechanisms for an effect on mortality, such as increased patient and physician adherence to treatment regimens, should be explored.
-
New and ongoing studies of RM need to be incorporated promptly to systematic reviews; these should consider changes in the technologies used for this purpose.
-
Determining the appropriate threshold for the use of POC NP testing is needed if this technology is to be incorporated as part of a diagnostic or monitoring pathway.
-
Exploring the barriers to the routine use of NP and POC NP testing is needed to increase health practitioners’ use of these tests.
-
More detailed data on sociodemographic and clinical characteristics, including disease progression and health-care use, from CHF patient cohorts in primary care are required to inform assessments of monitoring strategies.
Implications for practice
-
If NP tests are not currently used as part a diagnostic pathway in primary care, which goes against the NICE recommendation, their use could be incentivised.
-
RM, using either TM or STS, in the care of CHF should be considered, as it is likely to reduce the rates of all-cause mortality and heart failure hospital admissions, and may improve quality of life.
-
NP tests have variable ability to exclude CHF in patients in ambulatory care at low thresholds, but the ESC threshold for non-acute care for NT-proBNP might be an appropriate cut-off point for POC testing in this setting.
-
As between-person variability in POC NT-proBNP was around twice as large as within-person variability over 12 months, this indicates that deviations from individual targets for NT-proBNP are likely to be more helpful than population-level thresholds when considering a monitoring strategy.
-
Both NP and weight are highly variable measures; therefore, any change observed should be interpreted with caution.
-
Use of POC tests to measure NP in general practice is feasible, but does not lead to reductions in observed variability.
-
There are substantial barriers to the implementation of NP-guided treatment in primary care. In particular, the perception from health practitioners, both nurses and clinicians, is that the use of NP measures may not be beneficial.
Reflections
Pharmacological treatments for heart failure in primary care are well established, but must be managed (titrated) with great care and may temporarily worsen patient quality of life. This may contribute to the major underuse of evidence-based therapies in routine practice, which may, in turn, increase the likelihood of urgent admissions with worsening heart failure. Identifying ways of detecting heart failure deterioration is therefore likely to be important for patients and health-care systems. There is, therefore, strong prima facie evidence for a role for monitoring, but we find that the candidate monitoring measures – NP, and also weight – have relatively high within-measurement variability. In this context, it is perhaps not surprising that we find encouraging, but inconsistent, evidence from trials that monitoring to guide treatment, by clinicians or at home, is potentially life-saving. Future research should investigate the settings and methods in which monitoring has the most benefit, and we suggest that refining the reliability of measurements, whether through new biochemical methods or through averaging several measurements to reduce noise, is a promising direction in which to start.
Patient and public involvement
Background
The target audiences for this programme of research were patients/the public and health practitioners, so a stakeholder group was formed comprising these two groups. We planned to include PPI representatives (6/12), nurse practitioners (3/12), pharmacists (1/12) and clinicians (2/12). The group’s tasks included providing views on the plans for design, implementation and evaluation of monitoring strategies; participating in developing the plans for understanding patient and GP factors associated with monitoring; contributing to plans to explore the views of patients and practitioners of potential changes to the type of test used, frequency of testing and setting of testing; guiding and advising on the dissemination of individual research projects in the programme; and providing representation on the steering group. Two members joined the steering group: one PPI member and one clinician. PPI members were offered remuneration for their time and travel expenses. However, not all PPI members claimed any or all of the offered expenses. Training and support for PPI members were available and were provided by the Nuffield Department of Primary Care Health Sciences. The group met regularly, every 6 months, throughout the duration of the programme, with the principal investigator (PI), deputy PIs and the programme managers. Agendas were prepared and sent in advance, together with relevant paperwork, via e-mail. Any member of the stakeholder group could suggest changes and additions to the agenda. The PI chaired these meetings, while the programme managers took minutes, which were circulated to all members, usually within 1 month of the meeting. Views and opinions from the group were collected during the meetings, but were also accepted via e-mail, particularly when members were not able to attend in person. The terms of reference for this group are in Appendix 23.
Processes and contributions
We held seven face-to-face meetings and one teleconference. For all face-to-face meetings, teleconference facilities were available, although they were used infrequently. All meetings lasted for between 1 and 2 hours. Once per year (on four occasions), the stakeholder group was invited to also attend the senior management meetings and research presentations to help with the dissemination of the findings. Finally, in April 2019 we held a dissemination day for the stakeholder group that lasted 5.5 hours (including lunch).
As the programme lasted for > 5 years, the membership of the stakeholder group changed, but some members (notably four of the PPI contributors) remained throughout the grant. Overall, during the term of the programme, a total of 15 members contributed: seven patients or lay representatives; two primary care nurses, four GPs, one pharmacist and one heart failure nurse from secondary care.
Working practices between the stakeholder group and the research team were a collaborative undertaking. Communications were established and maintained to ensure that the stakeholder group was fully informed of the research projects, at all stages. In accordance with the terms of reference, the stakeholder group assisted the research team in five main areas: (1) designing and conducting the primary quantitative research projects (FORM-2C and feasibility of POC BNP testing in monitoring), (2) planning qualitative research in current monitoring, (3) planning qualitative research on potential changes in monitoring regimes, (4) guiding and advising on the dissemination of research and (5) representing the stakeholders on the steering group for the programme.
The stakeholders were instrumental in helping the research team design the quantitative primary research studies and were involved in the very earliest stages of development, before seeking ethics approval. Examples of the influence that the group (principally the patient and lay members) had on the design and execution of the research are as follows: for FORM-2C, the group designed a schedule of visits that it felt could be incorporated into regular visits to the doctor’s surgery and the development of the patient information materials. In the POC feasibility study, it again advised us about the development of the protocol.
For the qualitative components of the programme, the stakeholder group commented on the draft interview guides for CHF projects and specifically considered whether or not it covered all relevant aspects, whether or not the language was appropriate, and how onerous the interview might be for people with heart failure (is it overambitious?). The group was also asked to suggest proposed sampling categories. One member helped with recruitment. The group also commented on the published https://healthtalk.org/ (accessed 5 May 2020) site, particularly concerning the CKD interviews.
The research team developed a few short audio/Microsoft PowerPoint® (Microsoft Corporation, Redmond, WA, USA) presentations to show during the focus groups with health professionals, to trigger discussion. The stakeholder group commented on these presentations, which were then modified accordingly. They also provided feedback on the study results.
As the programme progressed, we discussed the methodological approaches taken, and the stakeholder group provided comments. At one point, it queried whether or not we were analysing the most appropriate data in a project,23 as we were not analysing rates of renal testing using cystatin C. The group was familiar with cystatin C as a serum marker of kidney function, as it is the alternative marker used in the FORM-2C project. We were able to clarify that, at that time, cystatin C was not routinely used in clinical practice. However, had this been an oversight, this would have been identified.
A recurrent theme during the programme was feedback regarding terminology, such as the use of the label CKD, with the term ‘disease’ being considered as frightening. The interviews conducted as part of the qualitative evaluation for CKD alerted some stakeholder members to the reluctance of GPs to tell patients about CKD, and patients’ confusions over the terminology of CKD ultimately led to a spin-off project, Kidney Age45 (see Appendix 11). This, in turn, has been the foundation of small, additionally funded, projects to develop patient and health professional communication resources to enhance discussions about kidney health and ageing. The stakeholder group was very influential in this work, discussing the terminology that is currently used, developing a patient-facing information sheet and helping devise the methodological approach to evaluate the potential usefulness of such a resource. It felt that this research was vital to provide signposting and communication for patients, as providing clear information can relieve anxiety.
Once individual projects started to produce outputs, the stakeholder group provided editorial and content comments on lay summaries for the paper by Taylor et al. 74 (see Appendix 18) and the plain language summary for the paper by McLellan et al. 67 (see Appendix 15), and gave comments on drafted manuscripts for most projects.
Potential mechanisms to disseminate the findings of the programme were discussed. Suggestions included via The Kidney Alliance, the Nuffield Department of Primary Care Health Sciences PPI newsletter, the GP magazine Pulse and the knowledge summaries on the NICE website.
Stakeholder group’s reflections on the findings and implications for NHS and funders
Towards the end of the period of funding, we held an internal dissemination meeting attended by five members of the stakeholder group. A senior qualitative researcher with a particular interest in PPI facilitated this meeting.
The stakeholder members were supportive of the research approaches that we had taken and reported that they found the results interesting and congruent with their experiences, views and beliefs. Overall, they felt that the generalisability of the research was good, and saw it as delivering benefit to research and patients.
They felt that the results serve to add to the case for NHS England to continue to support and encourage evidence-based practices throughout the NHS, especially in the light of the benefits to patients and the potential for funding to be saved. The results also suggest that consistency in the use of evidence-based treatments across all 44 NHS integrated care systems would benefit patients across England driving down inequalities in health care provision. Regarding research funding, it was felt that the results emphasised the importance of continuing to fund ongoing research into wide-ranging aspects of current clinical care practices, for both patient benefit and achieving value for money.
Lessons learnt on the role and impact of patient and public involvement: reflections from members of the stakeholder group
Members of the stakeholder group reflected on their experiences of participating in this programme, and felt that it had been well organised, with good administration, communication and paperwork. They felt that PPI contributors were treated with every consideration and that their needs were very well catered for in all respects. They felt appreciated, valued, accepted and included as part of the team. One member commented that they liked that the senior staff always attended the meetings, not just more junior researchers in the team. Members reported that their experience in this programme was better than in previous PPI groups, with one participant saying that they felt that, when PPI was first introduced, it was actually held in contempt, even more so because participants tended to be older, and older people were held in contempt too. However, the whole concept of PPI has been moving forward over the years and the participants who attended the dissemination event uniformly had an enjoyable and beneficial experience that exceeded their expectations.
One participant reported that they would have valued greater clarity on what to expect at the beginning, such as the time taken and demands. They were told you ‘can do as much as you want’, but, as a numbers person, they felt that this did not help. They would have appreciated a ‘job description’. Partly in response to this comment, and incorporating suggestions from this participant, the Nuffield Department of Primary Care Health Sciences PPI co-ordinator has subsequently produced guidance for PPI members on future projects. Another member commented that the concurrent training programme for PPI contributors was also a helpful adjunct to those involved in the programme. As a result, the experience of being a PPI contributor to this programme was very positive, especially feeling that, as a layperson, and in a relatively small way, they had been able to contribute to this important piece of work.
Regarding the practicalities of attending meetings, all the attendees preferred the face-to-face meetings; in fact, they would have complained or not participated if many of the meetings had been carried out by teleconference. Covering travelling costs and having lunches were seen as important to ongoing engagement.
Lessons learnt: reflections of the research team
The research team found it easier to recruit to the CKD streams than to the heart failure stream. The patient and lay participants who withdrew during the term of the programme did so because of deteriorating health, although, at times, they contributed via telephone or e-mail, as that was manageable with their health condition. Initially, at the start of the programme, the balance of the stakeholder group was more towards the health professionals than the public, although, over time, several of the health professionals dropped out because of work commitments. During the second half of the programme, the stakeholder group principally constituted patient and lay members.
Regarding how best to incorporate PPI, we feel that, although, for some projects (specifically FORM-2C), PPI was initiated at the design stage, a clearer strategy for when PPI could be included could have been developed. A projected overview of what, when and how PPI would be needed for each project would have made the process run even smoother. At times, it felt that we were looking for places to include PPI. However, perhaps this ebbing and flowing of the need for involvement of any type of participant (PPI, statistician, clinician) is an inherent part of the research process, in that it differs according to the stage of the research. This is a reflection too on the nature of the projects in the WSs: much of our research has been systematic reviews and methodological work, and maybe it is easier to see a role for PPI in primary research that has direct participant involvement than in research using routine or previously collected data.
Overall conclusions
Management of chronic disease requires monitoring, almost by definition. CKD is an example of a condition for which monitoring measures the progression of disease to manage long-term risk, whereas CHF is an example of a condition for which monitoring has been suggested as being important for guiding treatment.
In some chronic conditions, randomised trials have been conducted of monitoring either as an intervention in its own right or as a component of a management strategy. For example, multiple randomised trials of monitoring have been conducted in hypertension78 and diabetes,79 but these trials usually use surrogate outcome measures such as blood pressure or HbA1c, instead of hard outcomes such as CVD. Evaluation of medical testing against hard outcomes usually requires modelling, rather than randomised trials. 80
For each of the two exemplar conditions, we began by reviewing current practice. For CKD, monitoring based on blood tests and urine testing is widespread, accounting for millions of tests in the NHS each year. For CHF, blood tests such as NP are available, but are currently little used.
Mant81 suggested that the following minimum criteria should be in place for a monitoring strategy to be justifiable. First, it is necessary that ‘clinically significant changes in the condition . . . occur over time’. 81 For CKD and CHF, the time scales differ, but changes in condition can be highly clinically significant.
Second, there must be ‘an available monitoring test that reliably detects clinically significant changes when they occur’. 81 We studied the properties of blood tests for CKD, and of BNP and weight as monitoring tests for CHF. For CKD, we found that different blood tests and different equations are similarly useful for estimating GFR. For CHF, we found a range of devices available for testing NP at the POC, but no clear evidence on which device may be the best for use in practice. For both CKD and CHF, we found that blood tests (serum creatinine and NP, respectively) have non-trivial measurement error, that is that the within-person or within-measurement variation is not small, compared with the between-person variation and rate of change. This implies that any monitoring strategy that is sensitive to changes may not be specific, and, in the case of CKD, we quantified this, showing that most of the observed changes in CKD stage are due to misclassification. This is consistent with previous studies of monitoring conditions such as hypertension, dyslipidaemia, diabetes and diabetic kidney disease in primary care. 7,9,10,82 In all these conditions, monitoring programmes are likely to detect many apparent changes in condition due to measurement variability, and this problem is exacerbated by frequency of monitoring. 6
Third, ‘cost-effective action can be taken on the basis of the test result’. 81 We conducted systematic reviews of available actions in both chronic conditions. For CKD, we found evidence that some glucose-lowering and lipid-modifying interventions, in particular, may be beneficial to slow the progress of CKD, as well as reduce the risk of other conditions. For CHF, we found gaps in the current evidence for monitoring and management interventions as a whole, but there are established pharmacological therapies, including ACE inhibitors, ARBs, beta blockers, mineralocorticoid receptor antagonists, diuretics or aldosterone antagonists, for reducing risk and improving life expectancy16 in people living with CHF.
For both CKD and CHF, whether or not the available actions are cost-effective is more difficult to determine. Our cost-effectiveness model of CKD found minimal evidence to support monitoring in the early stages of CKD, with most patients already eligible for the treatments that may be indicated, making it difficult to justify monitoring in the scheme proposed by Mant. 81 For heart failure, cost-effectiveness of the treatments is established,77 but cost-effectiveness of monitoring as a whole is harder to establish because of other evidence gaps, for primary care populations in particular, as most evidence available does not include this population.
Thus, for both conditions, although our qualitative work and feasibility study demonstrate the acceptability and potential usefulness of monitoring, there are substantial gaps in the evidence to demonstrate that monitoring is cost-effective. In CKD, the main evidence gap is for an action to be taken, in response to worsening CKD, which would not already be in place for most patients anyway. In CHF, evidence-based treatments are established but there is no proven reliable test for monitoring (e.g. large variability of NPs and weight).
Limitations
We did not attempt to produce RCT evidence, which has both challenges and limitations for monitoring strategies,80 but we did systematically review existing trials of CHF monitoring. For CKD, we systematically reviewed and synthesised RCT evidence for treatments because, as discussed previously, one of the minimum criterion for monitoring to be beneficial is the existence of an action that can usefully be taken when monitoring detects change. Limitations of individual projects are discussed elsewhere in this document [Oke et al. 22 (see Appendix 2); Feakins et al. 23 (see Appendix 3); McFadden et al. 24 (see Appendix 5); Taylor et al. 25 (see Appendix 6); Oke et al. 26 (see Appendix 7); prospective cohort study of monitoring CKD (FORM-2C) (see Appendix 8); qualitative evaluation for CKD30 (see Appendices 9 and 10); review of economic models (see Appendix 12); cost-effectiveness of monitoring kidney function in UK primary care38 (see Appendix 14); McLellan et al. 67,68 (see Appendices 15 and 16); remote monitoring, telemonitoring and structured telephone support to monitor heart failure (see Appendix 17); Taylor et al. 74 (see Appendix 18); Oke et al. 75 (see Appendix 19); estimation of the variability of BNP and weight (see Appendix 20); feasibility study of use of POC NP tests in primary care (see Appendix 21); qualitative evaluation for CHF (see Appendix 9); and economic issues (see Appendix 22)]. Here, we call attention in particular to the limitation of our CKD WS: that the benefits of (e.g. annual) monitoring are difficult to quantify in the absence of a quantifiable, evidence-based, pathway to follow when monitoring detects deterioration. We have advanced knowledge about the reliability of NP and weight in the management of CHF. Given the size of the variability of each of these measures, the only situations in which either would prove useful would be if the signal detected was substantially larger or the noise could be minimised. This programme has not evaluated whether or not such clinical situations or measurement strategies could exist.
All publications from this programme of research are listed in Publications.
Acknowledgements
We would like to thank the following colleagues, clinicians, patients and public for their help and support in this research programme:
-
Philip Alderson, Research synthesis, NICE (Steering Committee).
-
Julie Barker, Research and Development Manager, Oxfordshire Clinical Commissioning Group.
-
Kristina Bennert, Research Associate (contributed to qualitative interviews).
-
John Burden (PPI).
-
Alison Clements, Senior Qualitative Researcher (contributed to essential components in NP-guided management of heart failure).
-
Kiren Collison, Clinical Chair of the Oxfordshire Clinical Commissioning Group (stakeholder group).
-
Di Croft (stakeholder).
-
Robert Cummings (PPI, Steering Committee).
-
Andrew Farmer, Professor of General Practice, Nuffield Department of Primary Care Health Sciences (contributed to application of the programme grant).
-
Robin Ferner, Professor of Clinical Pharmacology (Steering Committee).
-
Natasha Francis (PPI).
-
Alice Fuller, Research Officer (data management).
-
Laura Hill (stakeholder group).
-
Elizabeth Holloway (PPI).
-
Jade Howard, Research Assistant for the Health Experiences Research Group (contributed to qualitative focus groups).
-
Jeremy Horwood, Senior Research Fellow in Ethnography/Qualitative Social Science (contributed to qualitative interviews and analysis).
-
Jennifer A Hirst, Senior Primary Care Researcher (contributed to systematic review of GFR equation comparability).
-
Tim James, Laboratory Manager (laboratory tests).
-
Marion Judd (PPI).
-
Peter Kirby (PPI).
-
Emily C McFadden, Senior Statistical Epidemiologist (data manager and systematic review of GFR equation comparability).
-
Joanne Noble, Community Heart Failure Nurse (contributed to feasibility study of POC NP monitoring in patients with heart failure in primary care).
-
Mercia Page (Steering Committee).
-
Frank Palma (PPI).
-
Rachael Patel (PPI).
-
Christopher Price, Visiting Professor in Clinical Biochemistry (contributed to diagnostic accuracy POC NP testing for CHF in ambulatory care).
-
Kristy Ravenhall, Administration Assistant (data entry).
-
Oliver Rivero-Arias, Professor in Health Economics (contributed to the application of the programme grant).
-
Mary Selwood, Research Nurse/Study Manager (former lead to the observational cohort study for primary care patients with reduced eGFR).
-
Debs Smith (PPI).
-
Matthew Thompson, Professor of Family Medicine (contributed to the application of the programme grant).
-
David Timmins, FORM-2C Project Manager (contributed to the observational cohort study for primary care patients with reduced eGFR).
-
Sula Wiltshire, Associate Director of Quality at Oxfordshire Clinical Commissioning Group.
The observational cohort study for primary care patients with reduced eGFR and the feasibility study of the use of POC NP monitoring in patients with heart failure in primary care would not have been possible without the help of the participating patients. We would like to thank the following general practices and their practice nurses for their participation and co-operation with this study: Bicester Health Centre (Emily Ackland and Claire Giles), Didcot Health Centre (Ruth Atkinson and Sarah Hyner), Observatory Medical Practice (Kathryn Balmford and Antonia Michalikova), Summertown Health Centre (Mandy Beckett, Wendy Cubiss and Sam Towers), Botley Medical Centre (Liz Burquest), Westongrove Research Centre (Paula Caldwell), Gosford Hill Medical Centre (Sam Davies), Islip Medical Practice (Debbie Denton), Woodlands Medical Centre (Angela Fountain), The Boathouse Surgery (Wioletta Kowalczyk-Williams), Woodlands Medical Centre (Sue Paton and Jackie Peck), Eynsham Medical Group (Leanda Rankin and Janice Williams), Broadshires Health Centre (Sarah Sanders), White Horse Medical Practice (Debbie Warwick) and Berinsfield Health Centre (Sarah Williams). In addition, we would like to acknowledge the support of the National Institute for Health Research Clinical Research Network.
Contributions of authors
Professor Rafael Perera (https://orcid.org/0000-0003-2418-2091) (PI, Director of Medical Statistics) designed, interpreted data for and prepared for publication the study of local variation of practice (Oxford) data;22 conducted all aspects of the study of national variations in practice – CPRD data; designed and prepared for publication the systematic review of interventions for CKD stages G3 and G4; designed, interpreted data for and prepared for publication the estimation of variability of eGFR; designed, analysed, interpreted data for and prepared for publication the prospective cohort study of monitoring CKD (FORM-2C); conducted all aspects of the evaluation of intervention components; conducted the systematic review of BNP-guided treatment for heart failure; prepared for publication the systematic review of RM of CHF; designed and prepared for publication the systematic review of the diagnostic accuracy of POC devices; conducted all aspects of the estimation of variability of BNP and weight; and designed and prepared for publication the feasibility study of use of POC BNP testing in primary care.
Professor Richard Stevens (https://orcid.org/0000-0002-9258-4060) (Deputy PI, Deputy Director of Medical Statistics) interpreted data for and prepared for publication the study of local variation of practice (Oxford) data; conducted all aspects of the study of national variations in practice – CPRD data; designed, analysed, interpreted data for and prepared for publication the systematic review of GFR equation comparability; prepared for publication the systematic review of economic models of CKD; prepared for publication the systematic review of interventions for CKD stages G3 and G4; designed and prepared for publication the estimation of variability of eGFR; designed, interpreted data for and prepared for publication the prospective cohort study of monitoring CKD (FORM-2C); prepared for publication the qualitative evaluation of monitoring CKD; prepared for publication the cost-effectiveness model of strategies for CKD; prepared for publication the estimation of variability of BNP and weight; prepared for publication the feasibility study of use of POC BNP testing in primary care; and prepared for publication the systematic review of economic models of CHF.
Professor Jeffrey K Aronson (https://orcid.org/0000-0003-1139-655X) (Consultant Physician and Clinical Pharmacologist, pharmacology) designed, interpreted data for and prepared for publication the study of national variations in practice – CPRD data;23 and designed, interpreted data for and prepared for publication the systematic review of interventions for CKD stages G3 and G4.
Professor Amitava Banerjee (https://orcid.org/0000-0001-8741-3411) (Senior Lecturer in Clinical Data Science and Honorary Consultant in Cardiology, heart failure service, cardiac services) designed, analysed and prepared for publication the study of the evaluation of intervention components; designed and prepared for publication the estimation of variability of BNP and weight; designed and prepared for publication the feasibility study of use of POC BNP testing in primary care; designed the qualitative evaluation of monitoring CHF; and designed, interpreted data for and prepared for publication the systematic review of economic models of CHF.
Dr Julie Evans (Senior Researcher) conducted all aspects of the qualitative evaluation of monitoring CKD and conducted the qualitative evaluation of monitoring CHF.
Dr Benjamin G Feakins (https://orcid.org/0000-0002-3928-6750) (Statistician, Epidemiologist) conducted all aspects of the study for national variations in practice – CPRD data.
Dr Susannah Fleming (https://orcid.org/0000-0001-7205-2051) (Senior Qualitative Researcher, monitoring and diagnosis) analysed, interpreted data for and prepared for publication the systematic review of interventions for CKD stages G3 and G4; conducted all aspects of the prospective cohort study of monitoring CKD (FORM-2C); and conducted the feasibility study of use of POC BNP testing in primary care.
Professor Paul Glasziou (https://orcid.org/0000-0001-7564-073X) (Director of the Institute for Evidence Based Healthcare, evidence-based medicine) designed, analysed and interpreted data for the evaluation of intervention components, and conducted the systematic review of BNP-guided treatment for heart failure.
Professor Carl Heneghan (https://orcid.org/0000-0002-1009-1992) (Director of the Centre for Evidence-Based Medicine and Programs in Evidence-Based Health Care, epidemiologist) designed, analysed and prepared for publication the systematic review of BNP-guided treatment for heart failure; designed the estimation of variability of BNP and weight; and designed the systematic review of economic models of CHF.
Professor FD Richard Hobbs (https://orcid.org/0000-0001-7976-7172) (Head of Nuffield Department of Primary Care Health Sciences, Senior Researcher in cardiovascular epidemiology and clinical trials) designed, interpreted data for and prepared for publication the systematic review of GFR equation comparability; designed, interpreted data for and prepared for publication the systematic review of interventions for CKD stages G3 and G4; interpreted data for and prepared for publication the study of estimation of variability of eGFR; designed and prepared for publication the prospective cohort study of monitoring CKD (FORM-2C); designed and prepared for publication the study of estimation of variability of BNP and weight; and designed and prepared for publication the feasibility study of use of POC BNP testing in primary care.
Dr Louise Jones (https://orcid.org/0000-0002-0519-2334) (Clinical Trials Manager, health-care research) conducted the prospective cohort study of monitoring CKD (FORM-2C).
Dr Milena Kurtinecz (https://orcid.org/0000-0001-6869-5628) (Clinical Researcher, evidence-based medicine) conducted all aspects of the study for estimation of variability of BNP and weight.
Professor Daniel S Lasserson (https://orcid.org/0000-0001-8274-5580) (Professor of Ambulatory Care, acute medical care) designed, analysed and prepared for publication the study of local variation of practice (Oxford) data; conducted all aspects of the systematic review of GFR equation comparability; designed, interpreted data for and prepared for publication the systematic review of economic models of CKD; designed, analysed and prepared for publication the systematic review of interventions for CKD stages G3 and G4; designed the work for the estimation of variability of eGFR; designed and prepared for publication the prospective cohort study of monitoring CKD (FORM-2C); and designed and prepared for publication the cost-effectiveness model of strategies for CKD.
Professor Louise Locock (https://orcid.org/0000-0002-8109-1930) (Professor in Health Services Research Unit, Qualitative Social Science Researcher) conducted all aspects of the qualitative evaluation of monitoring CKD and conducted the qualitative evaluation of monitoring CHF.
Mrs Julie McLellan (https://orcid.org/0000-0002-2868-8631) (Programme Manager and Research Officer, systematic reviews) analysed, interpreted data for and prepared for publication the systematic review of GFR equation comparability; conducted all aspects of the systematic review of interventions for CKD stages G3 and G4; analysed, interpreted data for and prepared for publication the evaluation of intervention components; analysed, interpreted data for and prepared for publication the systematic review of BNP-guided treatment for heart failure; and conducted all aspects of the systematic review of RM of CHF.
Professor Borislava Mihaylova (https://orcid.org/0000-0002-0951-1304) (Senior Health Economist, economic aspects of chronic disease) designed, analysed and prepared for publication the systematic review of economic models of CKD; interpreted data for the systematic review of interventions for CKD stages G3 and G4; designed and prepared for publication estimation of variability of eGFR; conducted all aspects of the cost-effectiveness model of strategies for CKD; and conducted all aspects of the systematic review of economic models of CHF.
Professor Christopher A O’Callaghan (https://orcid.org/0000-0001-9962-3248) (Professor of Medicine, bioinformatics, statistics and computational biology), designed and prepared for publication the study of local variation of practice (Oxford) data; prepared for publication the study of national variations in practice – CPRD data; designed and prepared for publication the systematic review of GFR equation comparability; designed and prepared for publication the systematic review of interventions for CKD stages G3 and G4; designed and prepared for publication the estimation of variability of eGFR; designed and prepared for publication the prospective cohort study of monitoring CKD (FORM-2C); and designed and prepared for publication the cost-effectiveness model of strategies for CKD.
Dr Jason L Oke (https://orcid.org/0000-0003-3467-6677) (Senior Statistician, overdiagnosis and overtreatment), analysed, interpreted data and prepared for publication the study of local variation of practice (Oxford) data; analysed, interpreted data and prepared for publication the study of national variations in practice – CPRD data; analysed, interpreted data for and prepared for publication the estimation of variability of eGFR; and analysed, interpreted data for and prepared for publication the evaluation of intervention components.
Ms Nicola Pidduck (https://orcid.org/0000-0002-6491-1159) (Programme Manager and Researcher, systematic reviews) interpreted data for and prepared for publication the systematic review of interventions for CKD stages G3 and G4; interpreted data for and prepared for publication the systematic review of BNP-guided treatment for heart failure; and designed, interpreted data for and prepared for publication the systematic review of RM for CHF.
Dr Annette Plüddemann (https://orcid.org/0000-0003-2101-0390) (Course Director of the MSc in Evidence-Based Health Care and Senior Research Fellow, evidence-based medicine) conducted all aspects of the systematic review of the diagnostic accuracy of POC devices.
Ms Nia Roberts (https://orcid.org/0000-0002-1142-6440) (Librarian, research and clinical care) designed and prepared for publication the systematic review of interventions for CKD stages G3 and G4, and designed and prepared for publication the systematic review of BNP-guided treatment for heart failure. She also completed the updated publication search for the systematic review, remote monitoring, telemonitoring and structured telephone support to monitor heart failure.
Dr Iryna Schlackow (https://orcid.org/0000-0002-4154-1431) (Senior Researcher, economics in CKD and CVD) conducted all aspects of the systematic review of economic models of CKD; analysed, interpreted data for and prepared for publication estimation of variability of eGFR; and conducted all aspects of the cost-effectiveness model of strategies for CKD.
Dr Brian Shine (https://orcid.org/0000-0002-1016-8638) (Consultant Chemical Pathologist, endocrinology and diabetes) conducted all aspects of the study of local variation of practice (Oxford) data, and designed and prepared for publication the feasibility study of use of POC BNP testing in primary care.
Dr Claire L Simons (https://orcid.org/0000-0003-0822-7261) (Senior Researcher), interpreted data and reviewed content for estimation of variability of eGFR; analysed, interpreted data for and prepared for publication the cost-effectiveness model of strategies for CKD; and conducted all aspects of the systematic review of economic models of CHF.
Dr Clare J Taylor (https://orcid.org/0000-0001-8926-2581) (National Institute for Health Research Academic Clinical Lecturer, heart failure) interpreted data for and prepared for publication the study of national variations in practice (Oxford) data; interpreted data for and prepared for publication the evaluation of intervention components; interpreted data for and prepared for publication the systematic review of home-monitoring for CHF; conducted all aspects of the feasibility study of use of POC BNP testing in primary care; and interpreted data for and prepared for publication the systematic review of economic models of CHF.
Dr Kathryn S Taylor (https://orcid.org/0000-0001-6589-5456) (Medical Statistician, systematic reviews and meta-analysis) conducted all aspects of the systematic review of economic models of CHF; analysed, interpreted data for and prepared for publication the prospective cohort study of monitoring CKD (FORM-2C); analysed, interpreted data for and prepared for publication the systematic review of home-monitoring for CHF; and interpreted data for the systematic review of the diagnostic accuracy of POC devices.
Dr Jan Y Verbakel (https://orcid.org/0000-0002-7166-7211) (Clinical Researcher, diagnosis of serious diseases in primary care) designed, interpreted data for and prepared for publication the systematic review of interventions for CKD stages G3 and G4, and interpreted data for and prepared for publication the systematic review of the diagnostic accuracy of POC devices.
Professor Clare Bankhead (https://orcid.org/0000-0003-1588-3849) (Deputy PI, Senior Researcher) designed the study of national variations in practice (Oxford) data; designed and analysed the systematic review of GFR equation comparability; designed, analysed, interpreted data for and prepared for publication the systematic review of interventions for CKD stages G3 and G4; conducted all aspects of the prospective cohort study of monitoring CKD (FORM-2C); designed the qualitative evaluation of monitoring CKD; conducted the evaluation of intervention components; conducted all aspects of the systematic review of BNP-guided treatment for heart failure; conducted the systematic review of the diagnostic accuracy of POC devices; designed and analysed the feasibility study of use of POC BNP testing in primary care; and designed the qualitative evaluation of monitoring CHF.
Publications
Horwood J, Evans J The Language of Chronic Kidney Disease Diagnosis: A Qualitative Study of Patient Experiences and Primary Care Professionals’ Views. Society for Academic Primary Care; 2015. URL: https://sapc.ac.uk/conference/2015/abstract/language-of-chronic-kidney-disease-diagnosis-qualitative-study-of-patient (accessed 5 May 2020).
Oke J. Trends in Kidney Function Assessments in Oxfordshire Between 1985–2013, Too Much Testing? Society for Academic Primary Care; 2015. URL: https://sapc.conferenceservices.net/reports/template/onetextabstract.xml?xsl=template/onetextabstract.xsl%26conferenceID=3890%26abstractID=855104 (accessed 2015).
Oke J, Shine B, McFadden E, Stevens R, Lasserson D, Perera R. Trends in serum creatinine testing in Oxfordshire, UK, 1993–2013: a population-based cohort study. BMJ Open 2015;5:e009459. https://doi.org/10.1136/bmjopen-2015-009459
Selwood M, Perera-Salazar R, Stevens R, Bankhead C. Frequency of Renal Monitoring with Cystatin C and Creatinine: The FORM-2C Study. Society for Academic Primary Care; 2015. URL: https://sapc.conferenceservices.net/reports/template/onetextabstract.xml?xsl=template/onetextabstract.xsl%26conferenceID=3890%26abstractID=854942 (accessed 5 May 2020).
Selwood M, Perera-Salazar R, Bankhead C. Adaptable, Pragmatic Approach to Facilitate Research in Practice: The FORM-2C Strategy. Society for Academic Primary Care; 2015. URL: https://sapc.conferenceservices.net/reports/template/onetextabstract.xml?xsl=template/onetextabstract.xsl%26conferenceID=3890%26abstractID=847614 (accessed 5 May 2020).
Healthtalk.org. Heart Failure: Overview. 2016. URL: https://healthtalk.org/heart-failure/overview (accessed 5 May 2020).
McLellan J, Heneghan CJ, Perera R, Clements AM, Glasziou PP, Kearley KE, et al. B-type natriuretic peptide-guided treatment for heart failure. Cochrane Database Syst Rev 2016;12:CD008966. https://doi.org/10.1002/14651858.CD008966.pub2
Simmonds R, Evans J, Feder G, Blakeman T, Lasserson D, Murray E, et al. Understanding tensions and identifying clinician agreement on improvements to early-stage chronic kidney disease monitoring in primary care: a qualitative study. BMJ Open 2016;6:e010337. https://doi.org/10.1136/bmjopen-2015-010337
Fleming S. Discordant Baseline CKD Staging using Creatinine and Cystatin C in Participants Enrolled in FORM-2C, an Observational Study of Primary Care Patients with Reduced eGFR. Society for Academic Primary Care – South West Regional Conference 2017, Oxford, UK, 23–24 March 2017.
Fleming S. Baseline Characteristics of Participants Enrolled in FORM-2C, an Observational Study of Primary Care Patients with Reduced eGFR. Society for Academic Primary Care; 2017. URL: https://sapc.ac.uk/conference/2017/abstract/baseline-characteristics-of-participants-enrolled-form-2c-observational (accessed 5 May 2020).
Healthtalk.org. Kidney Health: Overview. 2017. URL: https://healthtalk.org/kidney-health/overview (accessed 5 May 2020).
Kurtinecz M. B-type and N-terminal Pro-B-type Natriuretic Peptides in Heart Failure. PhD thesis. Oxford: University of Oxford; 2018.
McFadden EC, Hirst JA, Verbakel JY, McLellan JH, Hobbs FDR, Stevens RJ, et al. Systematic review and meta-analysis comparing the bias and accuracy of the modification of diet in renal disease and chronic kidney disease epidemiology collaboration equations in community-based populations. Clin Chem 2018;64:475–85. https://doi.org/10.1373/clinchem.2017.276683
McLellan J, Bankhead C. Natriuretic peptide guided therapy for management of patients with heart failure does not show improvement compared with usual medical therapy alone. BMJ Evid Based Med 2018;23:74–5. https://doi.org/10.1136/bmjebm-2017-110880
Oke J, Clements A, McLellan J, Bankhead C, Taylor CJ, Spence G, et al. Essential components in natriuretic peptide-guided management of heart failure: an intervention synthesis. Open Heart 2018;5:e000826. https://doi.org/10.1136/openhrt-2018-000826
Oxford Mail. Oxford Researchers Call for Doctors to Say ‘Kidney Age’ Not ‘Kidney Disease’. 2018. URL: www.oxfordmail.co.uk/news/news_bites/16133132.oxford-researchers-call-doctors-say-kidney-age-not-kidney-disease/ (accessed 5 May 2020).
Plüddemann A. Rapid POCT for Diagnosing HF. Centre for Evidence-Based Medicine; 2018. URL: www.cebm.net/2018/06/rapid-natriuretic-peptide-tests-for-diagnosing-heart-failure/ (accessed 5 May 2020).
Maddocks L, Stevens R, Holloway E. Scientific and Lay Authors: Writing Together. PPI Pulse, 2018, pp. 12–14. URL: www.clahrc-oxford.nihr.ac.uk/files/get-involved/ppi-pulse_sprin-2018.pdf (accessed 5 May 2020).
Stevens RJ, Evans J, Oke J, Smart B, Hobbs FDR, Holloway E, et al. Kidney age, not kidney disease. Can Med Assoc J 2018;190:E389–93. https://doi.org/10.1503/cmaj.170674
Stevens R. Kidney Age, Not Kidney Disease. International Health Congress 2018, Oxford, UK, 28–30 June 2018.
Taylor KS, Verbakel JY, Feakins BG, Price CP, Perera R, Bankhead C, et al. Diagnostic accuracy of point-of-care natriuretic peptide testing for chronic heart failure in ambulatory care: systematic review and meta-analysis. BMJ 2018;361:k1450. https://doi.org/10.1136/bmj.k1450
Bankhead C. Comorbidities in the FORM-2C Cohort of Patients with Reduced eGFR. South West regional meeting of the Society for Academic Primary Care, Southampton, UK, 13–14 March 2019.
Feakins B, Oke J, McFadden E, Aronson J, Lasserson D, O’Callaghan C, et al. Trends in kidney function testing in UK primary care since the introduction of the quality and outcomes framework: a retrospective cohort study using CPRD. BMJ Open 2019;9:e028062. https://doi.org/10.1136/bmjopen-2018-028062
Fleming S. Does eGFR Calculated by Creatinine or Cystatin C Predict Future Decline in Kidney Function? Results of the FORM-2C Observational Study of Primary Care Patients with Reduced eGFR. Society for Academic Primary Care; 2019. URL: https://sapc.ac.uk/conference/2019/abstract/does-egfr-calculated-creatinine-or-cystatin-c-predict-future-decline-kidney (accessed 5 May 2020).
Oke J. Kidney Disease Progression and the Factors Influencing Progressive Loss of Kidney Function in a Primary Care Population. A Retrospective Database Analysis. Society for Academic Primary Care; 2019. URL: https://sapc.ac.uk/sites/default/files/sapc_asm_2019_exeter_abstracts.pdf (accessed 5 May 2020).
Oke J, Feakins B, Schlackow I, Mihaylova B, Simons C, O’Callaghan C, et al. Statistical models for the deterioration of kidney function in a primary care population: a retrospective database analysis [version 1; peer review: 2 approved with reservations]. F1000Research 2019;8:1618. https://doi.org/10.12688/f1000research.20229.1
Schlackow I, Simons C, Oke J, Feakins B, Lasserson D, Stevens R, Perera-Salazar R, Mihaylova B. Model of Cardiovascular Disease in People with Reduced Kidney Function using Routine Healthcare Data. Society for Academic Primary Care; 2019. URL: https://sapc.ac.uk/sites/default/files/sapc_asm_2019_exeter_abstracts.pdf (accessed 5 May 2020).
Schlackow I. Model of Cardiovascular Disease in People with Reduced Kidney Function Developed using Routine Healthcare Data. The Health Economists’ Study Group; 2019. URL: https://hesg.org.uk/wp-content/uploads/2019/04/Abstract-Booklet.pdf (accessed 5 May 2020).
Taylor KS, McLellan J, Verbakel JY, Aronson JK, Lasserson DS, Pidduck N, et al. Effects of antihypertensives, lipid-modifying drugs, glycaemic control drugs and sodium bicarbonate on the progression of stages 3 and 4 chronic kidney disease in adults: a systematic review and meta-analysis. BMJ Open 2019;9:e030596. https://doi.org/10.1136/bmjopen-2019-030596
McLellan J, Bankhead CR, Oke JL, Hobbs FDR, Taylor CJ, Perera R. Natriuretic peptide-guided treatment for heart failure: a systematic review and meta-analysis. BMJ Evid Based Med 2020;25:33–7. https://doi.org/10.1136/bmjebm-2019-111208
Schlackow I, Simons C, Oke J, Feakins B, O’Callaghan CA, Hobbs FDR, et al. Long-term health outcomes of people with reduced kidney function in the UK: a modelling study using population health data. PLOS Med 2020;17:e1003478. https://doi.org/10.1371/journal.pmed.1003478
Data-sharing statement
All data requests should be submitted to the corresponding author for consideration. Access to anonymised data may be granted following review.
Patient data
This work uses data provided by patients and collected by the NHS as part of their care and support. Using patient data is vital to improve health and care for everyone. There is huge potential to make better use of information from people’s patient records, to understand more about disease, develop new treatments, monitor safety, and plan NHS services. Patient data should be kept safe and secure, to protect everyone’s privacy, and it’s important that there are safeguards to make sure that it is stored and used responsibly. Everyone should be able to find out about how patient data are used. #datasaveslives You can find out more about the background to this citation here: https://understandingpatientdata.org.uk/data-citation.
Disclaimers
This report presents independent research funded by the National Institute for Health Research (NIHR). The views and opinions expressed by authors in this publication are those of the authors and do not necessarily reflect those of the NHS, the NIHR, CCF, NETSCC, PGfAR or the Department of Health and Social Care. If there are verbatim quotations included in this publication the views and opinions expressed by the interviewees are those of the interviewees and do not necessarily reflect those of the authors, those of the NHS, the NIHR, NETSCC, the PGfAR programme or the Department of Health and Social Care.
References
- Tran J, Norton R, Conrad N, Rahimian F, Canoy D, Nazarzadeh M, et al. Patterns and temporal trends of comorbidity among adult patients with incident cardiovascular disease in the UK between 2000 and 2014: a population-based cohort study. PLOS Med 2018;15. https://doi.org/10.1371/journal.pmed.1002513.
- Wen CP, Cheng TY, Tsai MK, Chang YC, Chan HT, Tsai SP, et al. All-cause mortality attributable to chronic kidney disease: a prospective cohort study based on 462 293 adults in Taiwan. Lancet 2008;371:2173-82. https://doi.org/10.1016/S0140-6736(08)60952-6.
- Charles A, Ewbank L, McKenna H, Wenzel L. The NHS Long-Term Plan Explained n.d. www.kingsfund.org.uk/publications/nhs–long–term–plan–explained?gclid=CjwKCAjw8–LnBRAyEiwA6eUMGu–u6SXLamiAbcZvNiQAj9mEDE–PYAtP57Bq1YKZyD5kpEYqvZ–n4xoCoYgQAvD_BwE (accessed 11 May 2020).
- Selby PJ, Banks RE, Gregory W, Hewison J, Rosenberg W, Altman DG, et al. Methods for the evaluation of biomarkers in patients with kidney and liver diseases: multicentre research programme including ELUCIDATE RCT. Programme Grants Appl Res 2018;6. https://doi.org/10.3310/pgfar06030.
- Glasziou PP, Irwig L, Heritier S, Simes RJ, Tonkin A. LIPID Study Investigators . Monitoring cholesterol levels: measurement error or true change?. Ann Intern Med 2008;148:656-61. https://doi.org/10.7326/0003-4819-148-9-200805060-00005.
- Stevens RJ, Oke J, Perera R. Statistical models for the control phase of clinical monitoring. Stat Methods Med Res 2010;19:394-41. https://doi.org/10.1177/0962280209359886.
- Farmer AJ, Stevens R, Hirst J, Lung T, Oke J, Clarke P, et al. Optimal strategies for identifying kidney disease in diabetes: properties of screening tests, progression of renal dysfunction and impact of treatment – systematic review and modelling of progression and cost-effectiveness. Health Technol Assess 2014;18. https://doi.org/10.3310/hta18140.
- Heneghan C, Ward A, Perera R, Bankhead C, Fuller A, Stevens R, et al. Self-monitoring of oral anticoagulation: systematic review and meta-analysis of individual patient data. Lancet 2012;379:322-34. https://doi.org/10.1016/S0140-6736(11)61294-4.
- Keenan K, Hayen A, Neal BC, Irwig L. Long term monitoring in patients receiving treatment to lower blood pressure: analysis of data from placebo controlled randomised controlled trial. BMJ 2009;338. https://doi.org/10.1136/bmj.b1492.
- Perera R, McFadden E, McLellan J, Lung T, Clarke P, Pérez T, et al. Optimal strategies for monitoring lipid levels in patients at risk or with cardiovascular disease: a systematic review with statistical and cost-effectiveness modelling. Health Technol Assess 2015;19. https://doi.org/10.3310/hta191000.
- National Institute for Health and Care Excellence (NICE) . Chronic Kidney Disease: Early Identification and Management of Chronic Kidney Disease in Adults in Primary and Secondary Care 2014.
- O’Sullivan JW, Stevens S, Hobbs FDR, Salisbury C, Little P, Goldacre B, et al. Temporal trends in use of tests in UK primary care, 2000–15: retrospective analysis of 250 million tests. BMJ 2018;363. https://doi.org/10.1136/bmj.k4666.
- National Institute for Health and Care Excellence . Chronic Heart Failure in Adults: Diagnosis and Management [NG106] n.d. www.nice.org.uk/guidance/ng106/resources/chronic-heart-failure-in-adults-diagnosis-and-management-pdf-66141541311685 (accessed 28 January 2021).
- Conrad N, Judge A, Canoy D, Tran J, O’Donnell J, Nazarzadeh M, et al. Diagnostic tests, drug prescriptions, and follow-up patterns after incident heart failure: a cohort study of 93,000 UK patients. PLOS Med 2019;16. https://doi.org/10.1371/journal.pmed.1002805.
- Bettencourt P, Azevedo A, Pimenta J, Friões F, Ferreira S, Ferreira A. N-terminal-pro-brain natriuretic peptide predicts outcome after hospital discharge in heart failure patients. Circulation 2004;110:2168-74. https://doi.org/10.1161/01.CIR.0000144310.04433.BE.
- Rossignol P, Hernandez AF, Solomon SD, Zannad F. Heart failure drug treatment. Lancet 2019;393:1034-44. https://doi.org/10.1016/S0140-6736(18)31808-7.
- Quality and Outcomes Framework . Achievement, Prevalence and Exceptions Data 2018–19 n.d. https://digital.nhs.uk/data–and–information/publications/statistical/quality–and–outcomes–framework–achievement–prevalence–and–exceptions–data/2018–19–pas (accessed 12 May 2020).
- James MT, Hemmelgarn BR, Tonelli M. Early recognition and prevention of chronic kidney disease. Lancet 2010;375:1296-309. https://doi.org/10.1016/S0140-6736(09)62004-3.
- Go AS, Chertow GM, Fan D, McCulloch CE, Hsu CY. Chronic kidney disease and the risks of death, cardiovascular events, and hospitalization. N Engl J Med 2004;351:1296-305. https://doi.org/10.1056/NEJMoa041031.
- O’Hare AM, Bertenthal D, Covinsky KE, Landefeld CS, Sen S, Mehta K, et al. Mortality risk stratification in chronic kidney disease: one size for all ages?. J Am Soc Nephrol 2006;17:846-53. https://doi.org/10.1681/ASN.2005090986.
- Rifkin DE, Shlipak MG, Katz R, Fried LF, Siscovick D, Chonchol M, et al. Rapid kidney function decline and mortality risk in older adults. Arch Intern Med 2008;168:2212-18. https://doi.org/10.1001/archinte.168.20.2212.
- Oke J, Shine B, McFadden E, Stevens R, Lasserson D, Perera R. Trends in serum creatinine testing in Oxfordshire, UK, 1993–2013: a population-based cohort study. BMJ Open 2015;5. https://doi.org/10.1136/bmjopen-2015-009459.
- Feakins B, Oke J, McFadden E, Aronson J, Lasserson D, O’Callaghan C, et al. Trends in kidney function testing in UK primary care since the introduction of the quality and outcomes framework: a retrospective cohort study using CPRD. BMJ Open 2019;9. https://doi.org/10.1136/bmjopen-2018-028062.
- McFadden EC, Hirst JA, Verbakel JY, McLellan JH, Hobbs FDR, Stevens RJ, et al. Systematic review and metaanalysis comparing the bias and accuracy of the Modification of Diet in Renal Disease and Chronic Kidney Disease Epidemiology Collaboration equations in community-based populations. Clin Chem 2018;64:475-85. https://doi.org/10.1373/clinchem.2017.276683.
- Taylor KS, Mclellan J, Verbakel JY, Aronson JK, Lasserson DS, Pidduck N, et al. Effects of antihypertensives, lipid-modifying drugs, glycaemic control drugs and sodium bicarbonate on the progression of stages 3 and 4 chronic kidney disease in adults: a systematic review and meta-analysis. BMJ Open 2019;9. https://doi.org/10.1136/bmjopen-2019-030596.
- Oke J, Feakins B, Schlackow I, Mihaylova B, Simons C, O’Callaghan C, et al. Statistical models for the deterioration of kidney function in a primary care population: a retrospective database analysis [version 1; peer review: 1 approved with reservations]. F1000Research 2019;8. https://doi.org/10.12688/f1000research.20229.1.
- Oke JL. Statistical Methods for Studying the Frequency of Monitoring Chronic Conditions. Oxford: Oxford University; 2014.
- Ku E, Xie D, Shlipak M, Hyre Anderson A, Chen J, Go AS, et al. Change in measured GFR versus eGFR and CKD outcomes. J Am Soc Nephrol 2016;27:2196-204. https://doi.org/10.1681/ASN.2015040341.
- Turin TC, Coresh J, Tonelli M, Stevens PE, de Jong PE, Farmer CK, et al. Change in the estimated glomerular filtration rate over time and risk of all-cause mortality. Kidney Int 2013;83:684-91. https://doi.org/10.1038/ki.2012.443.
- Simmonds R, Evans J, Feder G, Blakeman T, Lasserson D, Murray E, et al. Understanding tensions and identifying clinician agreement on improvements to early-stage chronic kidney disease monitoring in primary care: a qualitative study. BMJ Open 2016;6. https://doi.org/10.1136/bmjopen-2015-010337.
- Healthtalk.org . Kidney Health: Overview n.d. https://healthtalk.org/kidney-health/overview (accessed 5 May 2020).
- Erickson KF, Japa S, Owens DK, Chertow GM, Garber AM, Goldhaber-Fiebert JD. Cost-effectiveness of statins for primary cardiovascular prevention in chronic kidney disease. J Am Coll Cardiol 2013;61:1250-8. https://doi.org/10.1016/j.jacc.2012.12.034.
- Hoerger TJ, Wittenborn JS, Segel JE, Burrows NR, Imai K, Eggers P, et al. A health policy model of CKD: 1. Model construction, assumptions, and validation of health consequences. Am J Kidney Dis 2010;55:452-62. https://doi.org/10.1053/j.ajkd.2009.11.016.
- Hoerger TJ, Wittenborn JS, Segel JE, Burrows NR, Imai K, Eggers P, et al. A health policy model of CKD: 2. The cost-effectiveness of microalbuminuria screening. Am J Kidney Dis 2010;55:463-73. https://doi.org/10.1053/j.ajkd.2009.11.017.
- Orlando LA, Belasco EJ, Patel UD, Matchar DB. The chronic kidney disease model: a general purpose model of disease progression and treatment. BMC Med Inform Decis Mak 2011;11. https://doi.org/10.1186/1472-6947-11-41.
- Schlackow I, Kent S, Herrington W, Emberson J, Haynes R, Reith C, et al. A policy model of cardiovascular disease in moderate-to-advanced chronic kidney disease. Heart 2017;103:1880-90. https://doi.org/10.1136/heartjnl-2016-310970.
- Go DS, Kim SH, Park J, Ryu DR, Lee HJ, Jo MW. Cost-utility analysis of the National Health Screening Program for chronic kidney disease in Korea. Nephrology 2019;24:56-64. https://doi.org/10.1111/nep.13203.
- Schlackow I, Simons C, Oke J, Feakins B, O’Callaghan CA, Hobbs FDR, et al. Long-term health outcomes of people with reduced kidney function in the UK: a modelling study using population health data. PLOS Med 2020;17. https://doi.org/10.1371/journal.pmed.1003478.
- Ninomiya T, Perkovic V, Turnbull F, Neal B, Barzi F, Cass A, et al. Blood pressure lowering and major cardiovascular events in people with and without chronic kidney disease: meta-analysis of randomised controlled trials. BMJ 2013;347. https://doi.org/10.1136/bmj.f5680.
- Palmer SC, Di Micco L, Razavian M, Craig JC, Perkovic V, Pellegrini F, et al. Antiplatelet agents for chronic kidney disease. Cochrane Database Syst Rev 2013;2. https://doi.org/10.1002/14651858.CD008834.pub2.
- Herrington WG, Emberson J, Mihaylova B, Blackwell L, Reith C, Solbu MD, et al. Impact of renal function on the effects of LDL cholesterol lowering with statin-based regimens: a meta-analysis of individual participant data from 28 randomised trials. Lancet Diabetes Endocrinol 2016;4:829-39. https://doi.org/10.1016/S2213-8587(16)30156-5.
- Kidney Disease Improving Global Outcomes (KDIGO) CKD Work Group . KDIGO 2012 Clinical practice guideline for the evaluation and management of chronic kidney disease. Kidney Int Suppl 2013;3:1-150.
- National Institute for Health and Care Excellence (NICE) . Lipid Modification: Cardiovascular Risk Assessment and the Modification of Blood Lipids for the Primary and Secondary Prevention of Cardiovascular Disease. NICE Clinical Guideline, No. 181 2014.
- National Institute for Health and Care Excellence (NICE) . Chronic Kidney Disease in Adults: Assessment and Management. NICE Clinical Guideline, No. 182 2014.
- Stevens RJ, Evans J, Oke J, Smart B, Hobbs FDR, Holloway E, et al. Kidney age, not kidney disease. Can Med Assoc J 2018;190:E389-E93. https://doi.org/10.1503/cmaj.170674.
- Petersen S, Rayner M, Wolstenholme J. Coronary Heart Disease Statistics: Heart Failure Supplement. Claygate: British Heart Foundation; 2002.
- Conrad N, Judge A, Tran J, Mohseni H, Hedgecott D, Crespillo AP, et al. Temporal trends and patterns in heart failure incidence: a population-based study of 4 million individuals. Lancet 2018;391:572-80. https://doi.org/10.1016/S0140-6736(17)32520-5.
- Cowie MR, Wood DA, Coats AJ, Thompson SG, Poole-Wilson PA, Suresh V, et al. Incidence and aetiology of heart failure; a population-based study. Eur Heart J 1999;20:421-8. https://doi.org/10.1053/euhj.1998.1280.
- The Task Force on Heart Failure of the European Society of Cardiology . Guidelines for the diagnosis of heart failure. Eur Heart J 1995;16:741-51.
- Davies M, Hobbs F, Davis R, Kenkre J, Roalfe AK, Hare R, et al. Prevalence of left-ventricular systolic dysfunction and heart failure in the Echocardiographic Heart of England Screening study: a population based study. Lancet 2001;358:439-44. https://doi.org/10.1016/S0140-6736(01)05620-3.
- Zaphiriou A, Robb S, Murray-Thomas T, Mendez G, Fox K, McDonagh T, et al. The diagnostic accuracy of plasma BNP and NTproBNP in patients referred from primary care with suspected heart failure: results of the UK natriuretic peptide study. Eur J Heart Fail 2005;7:537-41. https://doi.org/10.1016/j.ejheart.2005.01.022.
- Cowie MR, Wood DA, Coats AJ, Thompson SG, Suresh V, Poole-Wilson PA, et al. Survival of patients with a new diagnosis of heart failure: a population based study. Heart 2000;83:505-10. https://doi.org/10.1136/heart.83.5.505.
- Hobbs FD, Roalfe AK, Davis RC, Davies MK, Hare R. Midlands Research Practices Consortium (MidReC) . Prognosis of all-cause heart failure and borderline left ventricular systolic dysfunction: 5 year mortality follow-up of the Echocardiographic Heart of England Screening Study (ECHOES). Eur Heart J 2007;28:1128-34. https://doi.org/10.1093/eurheartj/ehm102.
- Kalogeropoulos A, Georgiopoulou V, Kritchevsky SB, Psaty BM, Smith NL, Newman AB, et al. Epidemiology of incident heart failure in a contemporary elderly cohort: the health, aging, and body composition study. Arch Intern Med 2009;169:708-15. https://doi.org/10.1001/archinternmed.2009.40.
- Stewart S, MacIntyre K, Hole DJ, Capewell S, McMurray JJ. More ‘malignant’ than cancer? Five-year survival following a first admission for heart failure. Eur J Heart Fail 2001;3:315-22. https://doi.org/10.1016/S1388-9842(00)00141-0.
- Berry C, Murdoch DR, McMurray JJ. Economics of chronic heart failure. Eur J Heart Fail 2001;3:283-91. https://doi.org/10.1016/S1388-9842(01)00123-4.
- Stewart S, Jenkins A, Buchan S, McGuire A, Capewell S, McMurray JJ. The current cost of heart failure to the National Health Service in the UK. Eur J Heart Fail 2002;4:361-71. https://doi.org/10.1016/S1388-9842(01)00198-2.
- Hobbs FD, Kenkre JE, Roalfe AK, Davis RC, Hare R, Davies MK. Impact of heart failure and left ventricular systolic dysfunction on quality of life: a cross-sectional study comparing common chronic cardiac and medical disorders and a representative adult population. Eur Heart J 2002;23:1867-76. https://doi.org/10.1053/euhj.2002.3255.
- Ross JS, Chen J, Lin Z, Bueno H, Curtis JP, Keenan PS, et al. Recent national trends in readmission rates after heart failure hospitalization. Circ Heart Fail 2010;3:97-103. https://doi.org/10.1161/CIRCHEARTFAILURE.109.885210.
- National Collaborating Centre for Chronic Conditions . Chronic Heart Failure: National Clinical Guideline for Diagnosis and Management in Primary and Secondary Care 2003.
- Scottish Intercollegiate Guidelines Network (SIGN) . Management of Chronic Heart Failure 2016. www.sign.ac.uk/sign–147–management–of–chronic–heart–failure (accessed 14 May 2020).
- Clerico A, Fontana M, Zyw L, Passino C, Emdin M. Comparison of the diagnostic accuracy of brain natriuretic peptide (BNP) and the N-terminal part of the propeptide of BNP immunoassays in chronic and acute heart failure: a systematic review. Clin Chem 2007;53:813-22. https://doi.org/10.1373/clinchem.2006.075713.
- Doust JA, Pietrzak E, Dobson A, Glasziou P. How well does B-type natriuretic peptide predict death and cardiac events in patients with heart failure: systematic review. BMJ 2005;330. https://doi.org/10.1136/bmj.330.7492.625.
- Mant D, Hobbs FR, Glasziou P, Wright L, Hare R, Perera R, et al. Identification and guided treatment of ventricular dysfunction in general practice using blood B-type natriuretic peptide. Br J Gen Pract 2008;58:393-9. https://doi.org/10.3399/bjgp08X299209.
- Maisel AS, Koon J, Krishnaswamy P, Kazenegra R, Clopton P, Gardetto N, et al. Utility of B-natriuretic peptide as a rapid, point-of-care test for screening patients undergoing echocardiography to determine left ventricular dysfunction. Am Heart J 2001;141:367-74. https://doi.org/10.1067/mhj.2001.113215.
- Kurtinecz M. B-Type and N-Terminal Pro-B-Type Natriuretic Peptides in Heart Failure 2018.
- McLellan J, Heneghan CJ, Perera R, Clements AM, Glasziou PP, Kearley KE, et al. B-type natriuretic peptide-guided treatment for heart failure. Cochrane Database Syst Rev 2016;12. https://doi.org/10.1002/14651858.CD008966.pub2.
- McLellan J, Bankhead CR, Oke JL, Hobbs FDR, Taylor CJ, Perera R. Natriuretic peptide-guided treatment for heart failure: a systematic review and meta-analysis. BMJ Evid Based Med 2020;25:33-7. https://doi.org/10.1136/bmjebm-2019-111208.
- Felker GM, Anstrom KJ, Adams KF, Ezekowitz JA, Fiuzat M, Houston-Miller N, et al. Effect of natriuretic peptide-guided therapy on hospitalization or cardiovascular mortality in high-risk patients with heart failure and reduced ejection fraction: a randomized clinical trial. JAMA 2017;318:713-20. https://doi.org/10.1001/jama.2017.10565.
- National Institute for Health and Care Excellence . Chronic Heart Failure in Adults: Management. Clinical Guideline [CG108] n.d. www.nice.org.uk/guidance/cg108 (accessed 24 June 2014).
- NHS Commissioning Assembly . Technology Enabled Care Services: Resource for Commissioners 2015. www.england.nhs.uk/wp–content/uploads/2014/12/TECS_FinalDraft_0901.pdf (accessed 11 April 2019).
- NHS England . Five Year Forward View 2014. www.england.nhs.uk/publication/nhs-five-year-forward-view/ (accessed 11 November 2020).
- Inglis SC, Clark RA, Dierckx R, Prieto-Merino D, Cleland JG. Structured telephone support or non-invasive telemonitoring for patients with heart failure. Cochrane Database Syst Rev 2015;10. https://doi.org/10.1002/14651858.CD007228.pub3.
- Taylor KS, Verbakel JY, Feakins BG, Price CP, Perera R, Bankhead C, et al. Diagnostic accuracy of point-of-care natriuretic peptide testing for chronic heart failure in ambulatory care: systematic review and meta-analysis. BMJ 2018;361. https://doi.org/10.1136/bmj.k1450.
- Oke J, Clements A, McLellan J, Bankhead C, Taylor CJ, Spence G, et al. Essential components in natriuretic peptide-guided management of heart failure: an intervention synthesis. Open Heart 2018;5. https://doi.org/10.1136/openhrt-2018-000826.
- Healthtalk.org . Heart Failure 2016. https://healthtalk.org/heart-failure/overview (accessed 5 May 2020).
- Goehler A, Geisler BP, Manne JM, Jahn B, Conrads-Frank A, Schnell-Inderst P, et al. Decision-analytic models to simulate health outcomes and costs in heart failure: a systematic review. PharmacoEconomics 2011;29:753-69. https://doi.org/10.2165/11585990-000000000-00000.
- Tucker KL, Sheppard JP, Stevens R, Bosworth HB, Bove A, Bray EP, et al. Individual patient data meta-analysis of self-monitoring of blood pressure (BP-SMART): a protocol. BMJ Open 2015;5. https://doi.org/10.1136/bmjopen-2015-008532.
- Farmer A, Gibson OJ, Tarassenko L, Neil A. A systematic review of telemedicine interventions to support blood glucose self-monitoring in diabetes. Diabet Med 2005;22:1372-8. https://doi.org/10.1111/j.1464-5491.2005.01627.x.
- Bossuyt PMM, Glasziou PP, Irwig L, Aronson JK. Evidence-based Medical Monitoring: From Principles to Practice. Malden, MA: Blackwell Publishing, Inc.; 2008.
- Mant D, Glasziou PP, Irwig L, Aronson JK. Evidence-based Medical Monitoring: From Principles to Practice. Malden, MA: Blackwell Publishing, Inc.; 2008.
- Oke JL, Stevens RJ, Gaitskell K, Farmer AJ. Establishing an evidence base for frequency of monitoring glycated haemoglobin levels in patients with type 2 diabetes: projections of effectiveness from a regression model. Diabet Med 2012;29:266-71. https://doi.org/10.1111/j.1464-5491.2011.03412.x.
- Levey AS, de Jong PE, Coresh J, El Nahas M, Astor BC, Matsushita K, et al. The definition, classification, and prognosis of chronic kidney disease: a KDIGO Controversies Conference report. Kidney Int 2011;80:17-28. https://doi.org/10.1038/ki.2010.483.
- National Institute for Health and Care Excellence (NICE) . Chronic Kidney Disease: Assessment and Management (Update) n.d. www.nice.org.uk/guidance/indevelopment/gid-ng10118 (accessed 3 December 2020).
- National Institute for Health and Care Excellence (NICE) . Chronic Kidney Disease: Early Identification and Management of Chronic Kidney Disease in Adults in Primary and Secondary Care 2008.
- Fleming S, Perera-Salazar R, Taylor K, Jones L, Hobbs FR, James T, et al. FORM-2C (Frequency of Renal Monitoring – Creatinine and Cystatin C): an observational cohort study of primary care patients with reduced eGFR [published online ahead of print May 27 2021]. Br J Gen Pract 2021. https://doi.org/10.3399/BJGP.2020.0940.
- Coyne IT. Sampling in qualitative research. Purposeful and theoretical sampling; merging or clear boundaries?. J Adv Nurs 1997;26:623-30. https://doi.org/10.1046/j.1365-2648.1997.t01-25-00999.x.
- Heaton J. Secondary analysis of qualitative data: an overview. Hist Soc Res 2008;33:33-45.
- Nuffield Department of Primary Care Health Sciences . OxRen n.d. www.phc.ox.ac.uk/research/kidney-disease/studies/oxren (accessed 25 January 2021).
- National Institute for Health and Care Excellence (NICE) . Hypertension in Adults: Diagnosis and Management 2011.
- Ramer SL. Site-ation pearl growing: methods and librarianship history and theory. J Med Libr Assoc 2005;93:397-400.
- Tangri N, Kitsios GD, Inker LA, Griffith J, Naimark DM, Walker S, et al. Risk prediction models for patients with chronic kidney disease: a systematic review. Ann Intern Med 2013;158:596-603. https://doi.org/10.7326/0003-4819-158-8-201304160-00004.
- Echouffo-Tcheugui JB, Kengne AP. Risk models to predict chronic kidney disease and its progression: a systematic review. PLOS Med 2012;9. https://doi.org/10.1371/journal.pmed.1001344.
- Schlackow I, Kent S, Herrington W, Emberson J, Haynes R, Reith C, et al. Cost-effectiveness of lipid lowering with statins and ezetimibe in chronic kidney disease. Kidney Int 2019;96:170-9. https://doi.org/10.1016/j.kint.2019.01.028.
- Mihaylova B, Briggs A, Armitage J, Parish S, Gray A, Collins R. Heart Protection Study Collaborative Group . Cost-effectiveness of simvastatin in people at different levels of vascular disease risk: economic analysis of a randomised trial in 20,536 individuals. Lancet 2005;365:1779-85. https://doi.org/10.1016/S0140-6736(05)63014-0.
- Mihaylova B, Briggs A, Armitage J, Parish S, Gray A, Collins R. Heart Protection Study Collaborative . Lifetime cost effectiveness of simvastatin in a range of risk groups and age groups derived from a randomised trial of 20,536 people. BMJ 2006;333. https://doi.org/10.1136/bmj.38993.731725.BE.
- Matsushita K, van der Velde M, Astor BC, Woodward M, Levey AS, . Chronic Kidney Disease Prognosis Consortium . Association of estimated glomerular filtration rate and albuminuria with all-cause and cardiovascular mortality in general population cohorts: a collaborative meta-analysis. Lancet 2010;375:2073-81. https://doi.org/10.1016/S0140-6736(10)60674-5.
- Matsushita K, Coresh J, Sang Y, Chalmers J, Fox C, Guallar E, et al. Estimated glomerular filtration rate and albuminuria for prediction of cardiovascular outcomes: a collaborative meta-analysis of individual participant data. Lancet Diabetes Endocrinol 2015;3:514-25. https://doi.org/10.1016/S2213-8587(15)00040-6.
- National Institute for Health and Care Excellence (NICE) . Lipid Modification: Cardiovascular Risk Assessment and the Modification of Blood Lipids for the Primary and Secondary Prevention of Cardiovascular Disease. Clinical Guideline [CG181] 2014.
- National Institute for Health and Care Excellence (NICE) . Guide to the Methods of Technology Appraisal 2013 2013.
- Herrett E, Gallagher AM, Bhaskaran K, Forbes H, Mathur R, van Staa T, et al. Data resource profile: Clinical Practice Research Datalink (CPRD). Int J Epidemiol 2015;44:827-36. https://doi.org/10.1093/ije/dyv098.
- Hippisley-Cox J, Coupland C, Brindle P. Development and validation of QRISK3 risk prediction algorithms to estimate future risk of cardiovascular disease: prospective cohort study. BMJ 2017;357. https://doi.org/10.1136/bmj.j2099.
- NHS Digital Prescribing & Medicines Team . Prescription Cost Analysis England 2017 2018 n.d. https://digital.nhs.uk/data-and-information/publications/statistical/prescription-cost-analysis/prescription-cost-analysis-england-2017 (accessed 5 February 2019).
- Ballantyne CM, Abate N, Yuan Z, King TR, Palmisano J. Dose-comparison study of the combination of ezetimibe and simvastatin (Vytorin) versus atorvastatin in patients with hypercholesterolemia: the Vytorin Versus Atorvastatin (VYVA) study. Am Heart J 2005;149:363-73. https://doi.org/10.1016/j.ahj.2004.11.023.
- Law M, Rudnicka AR. Statin safety: a systematic review. Am J Cardiol 2006;97:52C-60C. https://doi.org/10.1016/j.amjcard.2005.12.010.
- Mansfield KE, Nitsch D, Smeeth L, Bhaskaran K, Tomlinson LA. Prescription of renin-angiotensin system blockers and risk of acute kidney injury: a population-based cohort study. BMJ Open 2016;6. https://doi.org/10.1136/bmjopen-2016-012690.
- Pletcher MJ, Lazar L, Bibbins-Domingo K, Moran A, Rodondi N, Coxson P, et al. Comparing impact and cost-effectiveness of primary prevention strategies for lipid-lowering. Ann Intern Med 2009;150:243-54. https://doi.org/10.7326/0003-4819-150-4-200902170-00005.
- NHS England . National Schedule of NHS Costs 2018 19 2021. www.england.nhs.uk/publication/2018-19-national-cost-collection-data-publication/ (accessed 1 May 2021).
- Sattar N, Preiss D, Murray HM, Welsh P, Buckley BM, de Craen AJ, et al. Statins and risk of incident diabetes: a collaborative meta-analysis of randomised statin trials. Lancet 2010;375:735-42. https://doi.org/10.1016/S0140-6736(09)61965-6.
- Preiss D, Seshasai SR, Welsh P, Murphy SA, Ho JE, Waters DD, et al. Risk of incident diabetes with intensive-dose compared with moderate-dose statin therapy. JAMA 2011;305:2556-64. https://doi.org/10.1001/jama.2011.860.
- Park JI, Baek H, Jung HH. CKD and health-related quality of life: the Korea National Health and Nutrition Examination Survey. Am J Kidney Dis 2016;67:851-60. https://doi.org/10.1053/j.ajkd.2015.11.005.
- Kent S, Haynes R, Hopewell JC, Parish S, Grey A, Landray MJ, et al. Effects of vascular and nonvascular adverse events and of extended-release niacin with laropiprant on health and healthcare costs. Circ Cardiovasc Qual Outcomes 2016;9:348-54. https://doi.org/10.1161/CIRCOUTCOMES.115.002592.
- Kent S, Schlackow I, Lozano-Kuhne J, Reith C, Emberson J, Haynes R, et al. What is the impact of chronic kidney disease stage and cardiovascular disease on the annual cost of hospital care in moderate-to-severe kidney disease?. BMC Nephrol 2015;16. https://doi.org/10.1186/s12882-015-0054-0.
- NHS Prescription Services . NHS Electronic Drug Tariff 2019. www.nhsbsa.nhs.uk/sites/default/files/2019–05/Drug%20Tariff%20June%202019.pdf (accessed 26 July 2019).
- National Institute for Health and Care Excellence (NICE) . Hypertension in Adults: Diagnosis and Management [NG136] 2019.
- National Cost Collection for the NHS . 2018/2019/National/Cost/Collection/Data n.d. https://improvement.nhs.uk/resources/national-cost-collection/#ncc1819 (accessed 1 May 2020).
- Curtis L, Burns A. Unit Costs of Health and Social Care 2019. Canterbury: PSSRU, University of Kent; 2019.
- Kemp CD, Conte JV. The pathophysiology of heart failure. Cardiovasc Pathol 2012;21:365-71. https://doi.org/10.1016/j.carpath.2011.11.007.
- Townsend N, Wickramasinghe K, Bhatnagar P, Smolina K, Nichols M, Leal J, et al. Coronary Heart Disease Statistics: A Compendium of Health Statistics. 2012 Edition. London: British Heart Foundation; 2012.
- Azad N, Lemay G. Management of chronic heart failure in the older population. J Geriatr Cardiol 2014;11:329-37. https://doi.org/10.11909/j.issn.1671-5411.2014.04.008.
- Zannad F. Pharmacotherapy in heart failure with reduced ejection fraction during the last 20 years, and the way ahead for precision medicine. Eur Heart J Cardiovasc Pharmacother 2015;1:10-2. https://doi.org/10.1093/ehjcvp/pvu006.
- Polsinelli VB, Shah SJ. Advances in the pharmacotherapy of chronic heart failure with preserved ejection fraction: an ideal opportunity for precision medicine. Expert Opin Pharmacother 2017;18:399-40. https://doi.org/10.1080/14656566.2017.1288717.
- Maggioni AP, Anker SD, Dahlstrom U, Filippatos G, Ponikowski P, Zannad F, et al. Are hospitalized or ambulatory patients with heart failure treated in accordance with European Society of Cardiology guidelines? Evidence from 12,440 patients of the ESC Heart Failure Long-term Registry. Eur J Heart Fail 2013;15:1173-84. https://doi.org/10.1093/eurjhf/hft134.
- Glasziou PP, Irwig L, Aronson JK. Evidence-based Medical Monitoring: From Principles to Practice. Malden, MA: Blackwell Publishing, Inc.; 2008.
- Aronow WS, Shamliyan TA. Comparative effectiveness of disease management with information communication technology for preventing hospitalization and readmission in adults with chronic congestive heart failure. J Am Med Dir Assoc 2018;19:472-9. https://doi.org/10.1016/j.jamda.2018.03.012.
- Carbo A, Gupta M, Tamariz L, Palacio A, Levis S, Nemeth Z, et al. Mobile technologies for managing heart failure: a systematic review and meta-analysis. Telemed J E Health 2018;24:958-68. https://doi.org/10.1089/tmj.2017.0269.
- Pekmezaris R, Tortez L, Williams M, Patel V, Makaryus A, Zeltser R, et al. Home telemonitoring in heart failure: a systematic review and meta-analysis. Health Aff 2018;37:1983-9. https://doi.org/10.1377/hlthaff.2018.05087.
- Yun JE, Park JE, Park HY, Lee HY, Park DA. Comparative effectiveness of telemonitoring versus usual care for heart failure: a systematic review and meta-analysis. J Card Fail 2018;24:19-28. https://doi.org/10.1016/j.cardfail.2017.09.006.
- Koehler F, Koehler K, Deckwart O, Prescher S, Wegscheider K, Kirwan BA, et al. Efficacy of Telemedical Interventional Management in patients with Heart Failure (TIM-HF2): a randomised, controlled, parallel-group, unmasked trial. Lancet 2018;392:1047-57. https://doi.org/10.1016/S0140-6736(18)31880-4.
- Koehler F, Koehler K, Deckwart O, Prescher S, Wegscheider K, Winkler S, et al. Telemedical Interventional Management in Heart Failure II (TIM-HF2), a randomised, controlled trial investigating the impact of telemedicine on unplanned cardiovascular hospitalisations and mortality in heart failure patients: study design and description of the intervention. Eur J Heart Fail 2018;20:1485-93. https://doi.org/10.1002/ejhf.1300.
- Kotooka N, Asaka M, Sato Y, Kinugasa Y, Nochioka K, Mizuno A, et al. Home telemonitoring study for Japanese patients with heart failure (HOMES-HF): protocol for a multicentre randomised controlled trial. BMJ Open 2013;3. https://doi.org/10.1136/bmjopen-2013-002972.
- Kotooka N, Kitakaze M, Nagashima K, Asaka M, Kinugasa Y, Nochioka K, et al. The first multicenter, randomized, controlled trial of home telemonitoring for Japanese patients with heart failure: home telemonitoring study for patients with heart failure (HOMES-HF). Heart Vessels 2018;33:866-76. https://doi.org/10.1007/s00380-018-1133-5.
- Olivari Z, Giacomelli S, Gubian L, Mancin S, Visentin E, Di Francesco V, et al. The effectiveness of remote monitoring of elderly patients after hospitalisation for heart failure: the renewing health European project. Int J Cardiol 2018;257:137-42. https://doi.org/10.1016/j.ijcard.2017.10.099.
- Smeets CJ, Storms V, Vandervoort PM, Dreesen P, Vranken J, Houbrechts M, et al. A novel intelligent two-way communication system for remote heart failure medication uptitration (the CardioCoach Study): randomized controlled feasibility trial. JMIR Cardio 2018;2. https://doi.org/10.2196/cardio.9153.
- Wagenaar KP, Broekhuizen BD, Dickstein K, Jaarsma T, Hoes AW, Rutten FH. Effectiveness of an interactive platform, and the ESC/HFA heartfailurematters.org website in patients with heart failure: design of the multicentre randomized e-Vita heart failure trial. Eur J Heart Fail 2015;17:1310-16. https://doi.org/10.1002/ejhf.413.
- Wagenaar KP, Broekhuizen BDL, Jaarsma T, Kok I, Mosterd A, Willems FF, et al. Effectiveness of the European Society of Cardiology/Heart Failure Association website ‘heartfailurematters.org’ and an e–health adjusted care pathway in patients with stable heart failure: results of the ‘e–Vita HF’ randomized controlled trial. Eur J Heart Fail 2019;21:238-46. https://doi.org/10.1002/ejhf.1354.
- Pandor A, Thokala P, Gomersall T, Baalbaki H, Stevens JW, Wang J, et al. Home telemonitoring or structured telephone support programmes after recent discharge in patients with heart failure: systematic review and economic evaluation. Health Technol Assess 2013;17. https://doi.org/10.3310/hta17320.
- Ong MK, Romano PS, Edgington S, Aronow HU, Auerbach AD, Black JT, et al. Effectiveness of remote patient monitoring after discharge of hospitalized patients with heart failure: the Better Effectiveness After Transition – Heart Failure (BEAT-HF) randomized clinical trial. JAMA Intern Med 2016;176:310-18. https://doi.org/10.1001/jamainternmed.2015.7712.
- Black JT, Romano PS, Sadeghi B, Auerbach AD, Ganiats TG, Greenfield S, et al. A remote monitoring and telephone nurse coaching intervention to reduce readmissions among patients with heart failure: study protocol for the Better Effectiveness After Transition – Heart Failure (BEAT-HF) randomized controlled trial. Trials 2014;15. https://doi.org/10.1186/1745-6215-15-124.
- Hutton B, Salanti G, Caldwell DM, Chaimani A, Schmid CH, Cameron C, et al. The PRISMA extension statement for reporting of systematic reviews incorporating network meta-analyses of health care interventions: checklist and explanations. Ann Intern Med 2015;162:777-84. https://doi.org/10.7326/M14-2385.
- Higgins JP, Altman DG, Gotzsche PC, Juni P, Moher D, Oxman AD, et al. The Cochrane Collaboration’s tool for assessing risk of bias in randomised trials. BMJ 2011;343. https://doi.org/10.1136/bmj.d5928.
- Higgins JPT, Green S. Cochrane Handbook for Sytematic Reviews of Interventions Version 5.1.1 [updated March 2011]. London: The Cochrane Collaboration; 2011.
- Follmann D, Elliott P, Suh I, Cutler J. Variance imputation for overviews of clinical trials with continuous response. J Clin Epidemiol 1992;45:769-73. https://doi.org/10.1016/0895-4356(92)90054-Q.
- Bekelman DB, Plomondon ME, Carey EP, Sullivan MD, Nelson KM, Hattler B, et al. Primary results of the Patient-Centered Disease Management (PCDM) for heart failure study: a randomized clinical trial. JAMA Intern Med 2015;175:725-32. https://doi.org/10.1001/jamainternmed.2015.0315.
- Dang S, Karanam C, Gómez-Marín O. Outcomes of a mobile phone intervention for heart failure in a minority county hospital population. Telemed J E Health 2017;23:473-84. https://doi.org/10.1089/tmj.2016.0211.
- Dang S, Karanam C, Gómez-Orozco C, Gómez-Marín O. Mobile phone intervention for heart failure in a minority urban county hospital population: usability and patient perspectives. Telemed J E Health 2017;23:544-54. https://doi.org/10.1089/tmj.2016.0224.
- Ritchie C, Richman J, Sobko H, Bodner E, Phillips B, Houston T. The E-coach transition support computer telephony implementation study: protocol of a randomized trial. Contemp Clin Trials 2012;33:1172-9. https://doi.org/10.1016/j.cct.2012.08.007.
- Ritchie CS, Houston TK, Richman JS, Sobko HJ, Berner ES, Taylor BB, et al. The E-Coach technology-assisted care transition system: a pragmatic randomized trial. Transl Behav Med 2016;6:428-37. https://doi.org/10.1007/s13142-016-0422-8.
- Karhula T, Vuorinen AL, Rääpysjärvi K, Pakanen M, Itkonen P, Tepponen M, et al. Telemonitoring and mobile phone-based health coaching among finnish diabetic and heart disease patients: randomized controlled trial. J Med Internet Res 2015;17. https://doi.org/10.2196/jmir.4059.
- Pedone C, Rossi FF, Cecere A, Costanzo L, Antonelli Incalzi R. Efficacy of a Physician-led multiparametric telemonitoring system in very old adults with heart failure. J Am Geriatr Soc 2015;63:1175-80. https://doi.org/10.1111/jgs.13432.
- Pérez-Rodríguez G, Brito-Zurita OR, Sistos-Navarro E, Benítez-Aréchiga ZM, Sarmiento-Salazar GL, Vargas-Lizárraga JF. Telemetric monitoring reduces visits to the emergency room and cost of care in patients with chronic heart failure. Cir Cir 2015;83:279-85. https://doi.org/10.1016/j.circir.2015.02.001.
- Dendale P, De Keulenaer G, Troisfontaines P, Weytjens C, Mullens W, Elegeert I, et al. Effect of a telemonitoring-facilitated collaboration between general practitioner and heart failure clinic on mortality and rehospitalization rates in severe heart failure: the TEMA-HF 1 (TElemonitoring in the MAnagement of Heart Failure) study. Eur J Heart Fail 2012;14:333-40. https://doi.org/10.1093/eurjhf/hfr144.
- GESICA Investigators . Randomised trial of telephone intervention in chronic heart failure: DIAL trial. BMJ 2005;331. https://doi.org/10.1136/bmj.38516.398067.E0.
- Koehler F, Winkler S, Schieber M, Sechtem U, Stangl K, Böhm M, et al. Impact of remote telemedical management on mortality and hospitalizations in ambulatory patients with chronic heart failure: the telemedical interventional monitoring in heart failure study. Circulation 2011;123:1873-80. https://doi.org/10.1161/CIRCULATIONAHA.111.018473.
- Sisk JE, Hebert PL, Horowitz CR, McLaughlin MA, Wang JJ, Chassin MR. Effects of nurse management on the quality of heart failure care in minority communities: a randomized trial. Ann Intern Med 2006;145:273-83. https://doi.org/10.7326/0003-4819-145-4-200608150-00007.
- Angermann CE, Störk S, Gelbrich G, Faller H, Jahns R, Frantz S, et al. Mode of action and effects of standardized collaborative disease management on mortality and morbidity in patients with systolic heart failure: the Interdisciplinary Network for Heart Failure (INH) study. Circ Heart Fail 2012;5:25-3. https://doi.org/10.1161/CIRCHEARTFAILURE.111.962969.
- Baker DW, Dewalt DA, Schillinger D, Hawk V, Ruo B, Bibbins-Domingo K, et al. The effect of progressive, reinforcing telephone education and counseling versus brief educational intervention on knowledge, self-care behaviors and heart failure symptoms. J Card Fail 2011;17:789-96. https://doi.org/10.1016/j.cardfail.2011.06.374.
- Barth V. A nurse-managed discharge program for congestive heart failure patients: outcomes and costs. Home Health Care Manag Pract 2001;13:436-43. https://doi.org/10.1177/108482230101300604.
- Bento VF, Brofman PR. Impact of the nursing consultation on the frequency of hospitalizations in patients with heart failure in Curitiba, Parana State. Arq Bras Cardiol 2009;92. https://doi.org/10.1590/s0066-782x2009000600013.
- Capomolla S, Pinna G, La Rovere MT, Maestri R, Ceresa M, Ferrari M, et al. Heart failure case disease management program: a pilot study of home telemonitoring versus usual care. Eur Heart J 2004;6:F91-8. https://doi.org/10.1016/j.ehjsup.2004.09.011.
- Chaudhry SI, Mattera JA, Curtis JP, Spertus JA, Herrin J, Lin Z, et al. Telemonitoring in patients with heart failure. N Engl J Med 2010;363:2301-9. https://doi.org/10.1056/NEJMoa1010029.
- Cleland JGF, Louis AA, Rigby AS, Janssen U, Balk AHMM. the Trans-European Network-Home-Care Management System (TENS-HMS) Study . Noninvasive home telemonitoring for patients with heart failure at high risk of recurrent admission and death. J Am Coll Cardiol 2005;45:1654-64. https://doi.org/10.1016/j.jacc.2005.01.050.
- DeBusk RF, Miller NH, Parker KM, Bandura A, Kraemer HC, Cher DJ, et al. Care management for low-risk patients with heart failure: a randomised, controlled trial. Ann Intern Med 2004;141:606-13. https://doi.org/10.7326/0003-4819-141-8-200410190-00008.
- DeWalt DA, Malone RM, Bryant ME, Kosnar MC, Corr KE, Rothman RL, et al. A heart failure self-management program for patients of all literacy levels: a randomised, controlled trial. BMC Health Serv Res 2006;13. https://doi.org/10.1186/1472-6963-6-30.
- Domingues FB, Clausell N, Aliti GB, Dominguez DR, Rabelo ER. Education and telephone monitoring by nurses of patients with heart failure: randomized clinical trial. Arq Bras Cardiol 2011;96:233-9. https://doi.org/10.1590/S0066-782X2011005000014.
- Galbreath AD, Krasuski RA, Smith B, Stajduhar KC, Kwan MD, Ellis R, et al. Long-term healthcare and cost outcomes of disease management in a large, randomized, community-based population with heart failure. Circulation 2004;110:3518-26. https://doi.org/10.1161/01.CIR.0000148957.62328.89.
- Gattis WA, Hasselblad V, Whellan DJ, O’Connor CM. Reduction in heart failure events by the addition of a clinical pharmacist to the heart failure management team. Arch Intern Med 1999;159:1939-45. https://doi.org/10.1001/archinte.159.16.1939.
- Krum H, Forbes A, Yallop J, Driscoll A, Croucher J, Chan B, et al. Telephone support to rural and remote patients with heart failure: the chronic heart failure assessment by telephone (CHAT) study. Cardiovasc Ther 2013;31:230-7. https://doi.org/10.1111/1755-5922.12009.
- Laramee AS, Levinsky SK, Sargent J, Ross R, Callas P. Case management in a heterogenous congestive heart failure population: a randomised controlled trial. Arch Intern Med 2003;163:809-17. https://doi.org/10.1001/archinte.163.7.809.
- Mortara A, Pinna GD, Johnson P, Maestri R, Capomolla S, LaRovere MT, et al. Home telemonitoring in heart failure patients: the HHH study (Home of Hospital in Heart Failure). Eur J Heart Fail 2009;11:312-18. https://doi.org/10.1093/eurjhf/hfp022.
- Rainville EC. Impact of pharmacist intervention on hospital readmissions for heart failure. Am J Health-Syst Pharm 1999;56:1339-42. https://doi.org/10.1093/ajhp/56.13.1339.
- Riegel B, Carlson B, Kopp Z, LePetri B, Glaser D, Unger A. Effect of a standardized nurse case-management telephone intervention on resource use in patients with chronic heart failure. Arch Intern Med 2002;162:705-12. https://doi.org/10.1001/archinte.162.6.705.
- Riegel B, Carlson B, Glaser D, Romero T. Randomized controlled trial of telephone case management in Hispanics of Mexican origin with heart failure. J Card Fail 2006;12:211-19. https://doi.org/10.1016/j.cardfail.2006.01.005.
- Tsuyuki RT, Fradette M, Johnson JA, Bungard TJ, Eurich DT, Ashton T, et al. A multicenter disease management program for hospitalised patients with heart failure. J Cardiac Fail 2004;10:473-80. https://doi.org/10.1016/j.cardfail.2004.02.005.
- Wakefield BJ, Ward MM, Holman JE, Ray A, Scherubel M, Burns TL, et al. Evaluation of home telehealth following hospitalization for heart failure: a randomized trial. Telemed J E Health 2008;14:753-61. https://doi.org/10.1089/tmj.2007.0131.
- Antonicelli R, Testarmata P, Spazzafumo L, Gagliardi C, Bilo G, Valentini M, et al. Impact of telemonitoring at home on the management of elderly patients with congestive heart failure. J Telemed Telecare 2008;14:300-5. https://doi.org/10.1258/jtt.2008.071213.
- Balk AHMM, Davidse W, Van Dommelen P, Klaassen E, Caliskan K, Van der Burg P, et al. Tele-guidance of chronic heart failure patients enhances knowledge about the disease. Eur J Heart Fail 2008;10:1136-42. https://doi.org/10.1016/j.ejheart.2008.08.003.
- Biannic C, Coutance G, Calus J, Belin A, Loiselet P, Michel L, et al. Educational home follow-up by telemedicine in cases of cardiac insufficiency. Randomised, multicentric study from the Basse-Normandie region. Preliminary results. Eur Res Telemed 2012;1:40-8. https://doi.org/10.1016/j.eurtel.2012.02.002.
- Blum K, Gottlieb SS. The effect of a randomized trial of home telemonitoring on medical costs, 30-day readmissions, mortality, and health-related quality of life in a cohort of community-dwelling heart failure patients. J Card Fail 2014;20:513-21. https://doi.org/10.1016/j.cardfail.2014.04.016.
- de Lusignan S, Wells S, Johnson P, Meredith K, Leatham E. Compliance and effectiveness of 1 year’s home telemonitoring. The report of a pilot study of patients with chronic heart failure. Eur J Heart Fail 2001;3:723-30. https://doi.org/10.1016/S1388-9842(01)00190-8.
- Giordano A, Scalvini S, Zanelli E, Corrà U, Longobardi GL, Ricci VA, et al. Multicentre randomised trial on home-based telemanagement to prevent hospital readmission of patients with chronic heart failure. Int J Cardiol 2009;131:192-9. https://doi.org/10.1016/j.ijcard.2007.10.027.
- Goldberg LR, Piette JD, Walsh MN, Frank TA, Jaski BE, Smith AL, et al. Randomized trial of a daily electronic home monitoring system in patients with advanced heart failure: the Weight Monitoring in Heart Failure (WHARF) trial. Am Heart J 2003;146:705-12. https://doi.org/10.1016/S0002-8703(03)00393-4.
- Lyngå P, Persson H, Hägg-Martinell A, Hägglund E, Hagerman I, Langius-Eklof A, et al. Weight monitoring in patients with severe heart failure (WISH). A randomized controlled trial. Eur J Heart Fail 2012;14:438-44. https://doi.org/10.1093/eurjhf/hfs023.
- Seto E, Leonard KJ, Cafazzo JA, Barnsley J, Masino C, Ross HJ. Mobile phone-based telemonitoring for heart failure management: a randomized controlled trial. J Med Internet Res 2012;14. https://doi.org/10.2196/jmir.1909.
- Soran OZ, Piña IL, Lamas GA, Kelsey SF, Selzer F, Pilotte J, et al. A randomised clinical trial of the clinical effects of enhanced heart failure monitoring using a computer-based telephonic monitoring system in older minorities and women. J Cardiac Fail 2008;114:711-7. https://doi.org/10.1016/j.cardfail.2008.06.448.
- Villani A, Malfatto G, Compare A, Della Rosa F, Bellardita L, Branzi G, et al. Clinical and psychological telemontoring and telecare of high risk heart failure patients. J Telemed Telecare 2014;20:468-75. https://doi.org/10.1177/1357633X14555644.
- Vuorinen AL, Leppänen J, Kaijanranta H, Kuliju M, Heliö T, Van Gils M, et al. Use of home telemonitoring to support multidisciplinary care of heart failure patients in Finland: randomised controlled trial. J Med Internet Res 2014;16. https://doi.org/10.2196/jmir.3651.
- Woodend KA, Sherrard H, Fraser M, Stuewe L, Cheung T, Struthers C. Telehome monitoring in patients with cardiac disease who are at high risk of readmission. Heart Lung 2008;37:36-45. https://doi.org/10.1016/j.hrtlng.2007.04.004.
- Ramachandran K, Husain N, Maikhuri R, Seth S, Vij A, Kumar M, et al. Impact of a comprehensive telephone-based disease management programme on quality-of-life in patients with heart failure. Natl Med J India 2007;20:67-73.
- Scherr D, Kastner P, Kollmann A, Hallas A, Auer J, Krappinger H, et al. Effect of home-based telemonitoring using mobile phone technology on the outcome of heart failure patients. J Med Internet Res 2009;11. https://doi.org/10.2196/jmir.1252.
- Brandon AF, Schuessler JB, Ellison KJ, Lazenby RB. The effects of an advanced practice nurse led telephone intervention on outcomes of patients with heart failure. Appl Nurs Res 2009;22:e1-7. https://doi.org/10.1016/j.apnr.2009.02.003.
- Flodgren G, Rachas A, Farmer AJ, Inzitari M, Shepperd S. Interactive telemedicine: effects on professional practice and health care outcomes. Cochrane Database Syst Rev 2015;9. https://doi.org/10.1002/14651858.CD002098.pub2.
- Knox L, Rahman RJ, Beedie C. Quality of life in patients receiving telemedicine enhanced chronic heart failure disease management: a meta-analysis. J Telemed Telecare 2017;23:639-49. https://doi.org/10.1177/1357633X16660418.
- ClinicalTrials.gov . Integrated Tele-Monitoring and Patient-Centric Health Coaching Strategy in Patients Hospitalized With Heart Failure (Tele-HC) n.d. https://clinicaltrials.gov/ct2/show/NCT02391987 (accessed 7 December 2020).
- ClinicalTrials.gov . Daily Ambulatory Remote Monitoring System For Post-Dischage Management Of ADHF (DAVID-HF) n.d. https://clinicaltrials.gov/ct2/show/NCT03072693 (accessed 7 December 2020).
- ClinicalTrials.gov . Future Patient – Telerehabilitation of Heart Failure Patients n.d. https://clinicaltrials.gov/ct2/show/NCT03388918 (accessed 7 December 2020).
- ClinicalTrials.gov . Post-Discharge Monitoring of Patients With Heart Failure (CARDIOENF) n.d. https://clinicaltrials.gov/ct2/show/NCT03512782 (accessed 7 December 2020).
- ClinicalTrials.gov . Heart Failure Events Reduction With Remote Monitoring and EHealth Support Investigator Initiated Trial (HERMeS) n.d. https://clinicaltrials.gov/ct2/show/NCT03663907 (accessed 7 December 2020).
- ClinicalTrials.gov . Patient-Centered Mobile Health Intervention to Improve Self-Care in Patients With Chronic Heart Failure (iCardia4HF) n.d. https://clinicaltrials.gov/ct2/show/NCT03642275 (accessed 7 December 2020).
- ClinicalTrials.gov . A Self–care Mobile Health App in Individuals With Heart Failure n.d. https://clinicaltrials.gov/ct2/show/NCT03509506 (accessed 7 December 2020).
- Schulte P, Olson L, Bruce C. Remote TELEmonitoring and Health Coaching for patients with acute heart failure: results of the TELE-HC randomized trial. Eur Heart J 2020;41. https://doi.org/10.1093/ehjci/ehaa946.1242.
- ClinicalTrials.gov . A Multi-Center, Placebo-Controlled Study to Evaluate the Safety of GSK716155 and Its Effects on Myocardial Metabolism, Myocardial Function, and Exercise Capacity in Patients With NYHA Class II III Congestive Heart Failure n.d. https://clinicaltrials.gov/ct2/show/NCT01357850 (accessed 16 December 2020).
- Lepore JJ, Olson E, Demopoulos L, Haws T, Fang Z, Barbour AM, et al. Effects of the novel long-acting GLP-1 agonist, albiglutide, on cardiac function, cardiac metabolism, and exercise capacity in patients with chronic heart failure and reduced ejection fraction. JACC Heart Fail 2016;4:559-66. https://doi.org/10.1016/j.jchf.2016.01.008.
- American Heart Association, Inc . Classes of Heart Failure n.d. www.heart.org/en/health-topics/heart-failure/what-is-heart-failure/classes-of-heart-failure (accessed 8 December 2020).
- Laird NM, Ware JH. Random-effects models for longitudinal data. Biometrics 1982;38:963-74. https://doi.org/10.2307/2529876.
- Fraser CG. Biological Variation: From Principles to Practice. Washington, DC: American Association for Clinical Chemistry; 2001.
- Iglesias N, Petersen PH, Ricós C. Power function of the reference change value in relation to cut-off points, reference intervals and index of individuality. Clin Chem Lab Med 2005;43:441-8. https://doi.org/10.1515/CCLM.2005.078.
- Frankenstein L, Wu AH, Hallermayer K, Wians FH, Giannitsis E, Katus HA. Biological variation and reference change value of high-sensitivity troponin T in healthy individuals during short and intermediate follow-up periods. Clin Chem 2011;57:1068-71. https://doi.org/10.1373/clinchem.2010.158964.
- The Renal Association . CKD Stages n.d. https://renal.org/health-professionals/information-resources/uk-eckd-guide/ckd-stages (accessed 8 December 2020).
- Troughton RW, Richards AM. Outpatient monitoring and treatment of chronic heart failure guided by amino-terminal proB-type natriuretic peptide measurement. Am J Cardiol 2008;101:72-5. https://doi.org/10.1016/j.amjcard.2007.11.027.
- Meijers WC, van der Velde AR, Muller Kobold AC, Dijck-Brouwer J, Wu AH, Jaffe A, et al. Variability of biomarkers in patients with chronic heart failure and healthy controls. Eur J Heart Fail 2017;19:357-65. https://doi.org/10.1002/ejhf.669.
- Thygesen K, Mair J, Mueller C, Huber K, Weber M, Plebani M, et al. Recommendations for the use of natriuretic peptides in acute cardiac care: a position statement from the Study Group on Biomarkers in Cardiology of the ESC Working Group on Acute Cardiac Care. Eur Heart J 2012;33:2001-6. https://doi.org/10.1093/eurheartj/ehq509.
- Peng Q, Hu W, Su H, Yang Q, Cheng X. Levels of B-type natriuretic peptide in chronic heart failure patients with and without diabetes mellitus. Exp Ther Med 2013;5:229-32. https://doi.org/10.3892/etm.2012.760.
- Wu AH. Serial testing of B-type natriuretic peptide and NTpro-BNP for monitoring therapy of heart failure: the role of biologic variation in the interpretation of results. Am Heart J 2006;152:828-34. https://doi.org/10.1016/j.ahj.2006.08.021.
- Piper S, deCourcey J, Sherwood R, Amin-Youssef G, McDonagh T. Biologic variability of soluble ST2 in patients with stable chronic heart failure and implications for monitoring. Am J Cardiol 2016;118:95-8. https://doi.org/10.1016/j.amjcard.2016.04.017.
- National Clinical Guideline Centre . Chronic Heart Failure: The Management of Chronic Heart Failure in Adults in Primary and Secondary Care 2010.
- Denny N, Lasserson D, Price C, Heneghan C, Thompson M, Plüddemann A. Diagnostic Technology: Point of Care B-Type Natriuretic Peptide Testing. Horizon Scan Report 0019 2011.
- Bruins S, Fokkema MR, Romer JW, Dejongste MJ, van der Dijs FP, van den Ouweland JM, et al. High intraindividual variation of B-type natriuretic peptide (BNP) and amino-terminal proBNP in patients with stable chronic heart failure. Clin Chem 2004;50:2052-8. https://doi.org/10.1373/clinchem.2004.038752.
- Frankenstein L, Remppis A, Frankenstein J, Hess G, Zdunek D, Slottje K, et al. Variability of N-terminal probrain natriuretic peptide in stable chronic heart failure and its relation to changes in clinical variables. Clin Chem 2009;55:923-9. https://doi.org/10.1373/clinchem.2008.112052.
- Nordenskjold AM, Ahlstrom H, Eggers KM, Frobert O, Venge P, Lindahl B. Short- and long-term individual variation in NT-proBNP levels in patients with stable coronary artery disease. Clin Chim Acta 2013;422:15-20. https://doi.org/10.1016/j.cca.2013.03.025.
- Schindler EI, Szymanski JJ, Hock KG, Geltman EM, Scott MG. Short- and long-term biologic variability of galectin-3 and other cardiac biomarkers in patients with stable heart failure and healthy adults. Clin Chem 2016;62:360-6. https://doi.org/10.1373/clinchem.2015.246553.
- Takeda Y, Takeda Y, Suzuki S, Kimura G. Within-person variation of the plasma concentration of B-type natriuretic peptide: safety range in stable patients with heart failure. Am Heart J 2009;157:97-101. https://doi.org/10.1016/j.ahj.2008.09.002.
- Hex C, Smeets M, Penders J, Van Hoof V, Verbakel J, Buntinx F, et al. Accuracy, user-friendliness and usefulness of the Cobas h232 point-of-care test for NT-proBNP in primary care. J Clin Pathol 2018;71:539-45. https://doi.org/10.1136/jclinpath-2017-204746.
- van Hout BA, Wielink G, Bonsel GJ, Rutten FF. Effects of ACE inhibitors on heart failure in the Netherlands. PharmacoEconomics 1993;3:387-97. https://doi.org/10.2165/00019053-199303050-00006.
- Paul SD, Kuntz KM, Eagle KA, Weinstein MC. Costs and effectiveness of angiotensin converting enzyme inhibition in patients with congestive heart failure. Arch Intern Med 1994;154:1143-9. https://doi.org/10.1001/archinte.1994.00420100129016.
- Glick HA, Cook J, Kinosian B, Pitt B, Bourassa MG, Pouleur H. Costs and effects of enalapril therapy in patients with symptomatic heart failure: an economic analysis of the Studies of Left Ventricular Dysfunction (SOLVD) treatment trial. J Cardiac Fail 1995;1:371-80. https://doi.org/10.1016/S1071-9164(05)80006-5.
- Delea TE, Vera-Llonch M, Richner RE, Fowler MB, Oster G. Cost effectiveness of carvedilol for heart failure. Am J Cardiol 1999;83:890-6. https://doi.org/10.1016/S0002-9149(98)01066-2.
- Levy AR, Briggs AH, Demers C, O’Brien BJ. Cost-effectiveness of beta-blocker therapy with metoprolol or with carvedilol for treatment of heart failure in Canada. Am Heart J 2001;142:537-43. https://doi.org/10.1067/mhj.2001.116479.
- Varney S. A cost-effectiveness analysis of bisoprolol for heart failure. Eur J Heart Fail 2001;3:365-71. https://doi.org/10.1016/S1388-9842(01)00132-5.
- Gregory D, Udelson JE, Konstam MA. economic impact of beta blockade in heart failure. Am J Med 2001;110:74S-80S. https://doi.org/10.1016/S0002-9343(98)00387-8.
- Barry M. Cost effectiveness of beta blocker therapy for patients with chronic severe heart failure in Ireland. Ir Med J 2002;95:174-7.
- Tilson L, McGowan B, Ryan M, Barry M. Cost-effectiveness of spironolactone in patients with severe heart failure. Ir J Med Sci 2003;172:70-2. https://doi.org/10.1007/BF02915250.
- Morimoto T, Hayashino Y, Shimbo T, Izumi T, Fukui T. Is B-type natriuretic peptide-guided heart failure management cost-effective?. Int J Cardiol 2004;96:177-81. https://doi.org/10.1016/j.ijcard.2003.05.036.
- Heidenreich PA, Gubens MA, Fonarow GC, Konstam MA, Stevenson LW, Shekelle PG. Cost-effectiveness of screening with B-type natriuretic peptide to identify patients with reduced left ventricular ejection fraction. J Am Coll Cardiol 2004;43:1019-26. https://doi.org/10.1016/j.jacc.2003.10.043.
- Cowper PA, DeLong ER, Whellan DJ, LaPointe NMA, Califf RM. Economic effects of beta-blocker therapy in patients with heart failure. Am J Med 2004;116:104-11. https://doi.org/10.1016/j.amjmed.2003.07.016.
- Inomata T, Izumi T, Kobayashi M. Cost-effectiveness analysis of carvedilol for the treatment of chronic heart failure in Japan. Circ J 2004;68:35-40. https://doi.org/10.1253/circj.68.35.
- Caro JJ, Migliaccio-Walle K, O’Brien JA, Nova W, Kim J, Hauch O. Economic implications of extended-release metoprolol succinate for heart failure in the MERIT-HF trial: a US perspective of the MERIT-HF trial. J Card Fail 2005;11:647-56. https://doi.org/10.1016/j.cardfail.2005.06.433.
- Göhler A, Geisler BP, Manne JM, Kosiborod M, Zhang Z, Weintraub WS. Utility estimates for decision-analytic modeling in chronic heart failure — health states based on New York Heart Association classes and number of rehospitalizations. Value Health 2009;12:185-7. https://doi.org/10.1111/j.1524-4733.2008.00425.x.
- Chan DC, Heidenreich PA, Weinstein MC, Fonarow GC. Heart failure disease management programs: a cost-effectiveness analysis. Am Heart J 2008;155:332-8. https://doi.org/10.1016/j.ahj.2007.10.001.
- Miller G, Randolph S, Forkner E, Smith B, Galbreath AD. Long-term cost-effectiveness of disease management in systolic heart failure. Med Decis Making 2009;29:325-33. https://doi.org/10.1177/0272989X08327494.
- Rosen VM, Taylor DC, Parekh H, Pandya A, Thompson D, Kuznik A. Cost effectiveness of intensive lipid-lowering treatment for patients with congestive heart failure and coronary heart disease in the US. PharmacoEconomics 2010;28:47-60. https://doi.org/10.2165/11531440-000000000-00000.
- Cowie MR, Cure S, Bianic F, McGuire A, Goodall G, Tavazzi L. Cost-effectiveness of highly purified omega-3 polyunsaturated fatty acid ethyl esters in the treatment of chronic heart failure: Results of Markov modelling in a UK setting. Eur J Heart Fail 2011;13:681-9. https://doi.org/10.1093/eurjhf/hfr023.
- Moertl D, Steiner S, Coyle D, Berger R. Cost–utility analysis of nt-probnp-guided multidisciplinary care in chronic heart failure. Int J Technol Assess Health Care 2013;29:3-11. https://doi.org/10.1017/S0266462312000712.
- Ford E, Adams J, Graves N. Development of an economic model to assess the cost-effectiveness of hawthorn extract as an adjunct treatment for heart failure in Australia. BMJ Open 2012;2. https://doi.org/10.1136/bmjopen-2012-001094.
- Thokala P, Baalbaki H, Brennan A, Pandor A, Stevens JW, Gomersall T. Telemonitoring after discharge from hospital with heart failure: cost-effectiveness modelling of alternative service designs. BMJ Open 2013;3. https://doi.org/10.1136/bmjopen-2013-003250.
- Banka G, Heidenreich PA, Fonarow GC. Incremental cost-effectiveness of guideline-directed medical therapies for heart failure. J Am Coll Cardiol 2013;61:1440-6. https://doi.org/10.1016/j.jacc.2012.12.022.
- Ademi Z, Pasupathi K, Krum H, Liew D. Cost effectiveness of eplerenone in patients with chronic heart failure. Am J Cardiovasc Drugs 2014;14:209-16. https://doi.org/10.1007/s40256-014-0066-3.
- Griffiths A, Paracha N, Davies A, Branscombe N, Cowie MR, Sculpher M. The cost effectiveness of ivabradine in the treatment of chronic heart failure from the UK National Health Service perspective. Heart 2014;100:1031-6. https://doi.org/10.1136/heartjnl-2013-304598.
- Lee CH, Li YH, Cheng CL, Chen JH, Yang YHK. The Incidence and Predicting Factors of Heart Failure After First Acute Myocardial Infarction: A Population-Based Study in Taiwan n.d.
- Reed SD, Neilson MP, Gardner M, Li Y, Briggs AH, Polsky DE. Tools for economic analysis of patient management interventions in heart failure cost-effectiveness model: a web-based program designed to evaluate the cost-effectiveness of disease management programs in heart failure. Am Heart J 2015;170:951-60. https://doi.org/10.1016/j.ahj.2015.08.015.
- Kansal AR, Cowie MR, Kielhorn A, Krotneva S, Tafazzoli A, Zheng Y. Cost-effectiveness of ivabradine for heart failure in the United States. J Am Heart Assoc 2016;5. https://doi.org/10.1161/JAHA.116.003221.
- Thanh NX, Ezekowitz JA, Tran DT, Kaul P. Cost effectiveness of eplerenone for the treatment of systolic heart failure with mild symptoms in Alberta, Canada. Am J Cardiovasc Drugs 2016;16:365-76. https://doi.org/10.1007/s40256-016-0177-0.
- Sandhu AT, Ollendorf DA, Chapman RH, Pearson SD, Heidenreich PA. Cost-effectiveness of sacubitril-valsartan in patients with heart failure with reduced ejection fraction. Ann Intern Med 2016;165:681-9. https://doi.org/10.7326/M16-0057.
- King JB, Shah RU, Bress AP, Nelson RE, Bellows BK. Cost-effectiveness of sacubitril-valsartan combination therapy compared with enalapril for the treatment of heart failure with reduced ejection fraction. JACC Heart Fail 2016;4:392-40. https://doi.org/10.1016/j.jchf.2016.02.007.
- Gaziano TA, Fonarow GC, Claggett B, Chan WW, Deschaseaux-Voinet C, Turner SJ. Cost-effectiveness analysis of sacubitril/valsartan vs enalapril in patients with heart failure and reduced ejection fraction. JAMA Cardiol 2016;1:666-72. https://doi.org/10.1001/jamacardio.2016.1747.
- Dang W, Yi A, Jhamnani S, Wang SY. Cost-effectiveness of multidisciplinary management program and exercise training program in heart failure. Am J Cardiol 2017;120:1338-43. https://doi.org/10.1016/j.amjcard.2017.06.071.
- Monahan M, Barton P, Taylor CJ, Roalfe AK, Hobbs FDR. REFER Investigators . MICE or NICE? An economic evaluation of clinical decision rules in the diagnosis of heart failure in primary care. Int J Cardiol 2017;241:255-61. https://doi.org/10.1016/j.ijcard.2017.02.149.
- Liang L, Bin-Chia Wu D, Aziz MIA, Wong R, Sim D, Leong KTG. Cost-effectiveness of sacubitril/valsartan versus enalapril in patients with heart failure and reduced ejection fraction. J Med Econ 2018;21:174-81. https://doi.org/10.1080/13696998.2017.1387119.
- van der Pol S, Degener F, Postma MJ, Vemer P. An economic evaluation of sacubitril/valsartan for heart failure patients in the Netherlands. Value Health 2017;20:388-96. https://doi.org/10.1016/j.jval.2016.10.015.
- Maru S, Byrnes JM, Carrington MJ, Stewart S, Scuffham PA. Long-term cost-effectiveness of home versus clinic-based management of chronic heart failure: the WHICH? study. J Med Econ 2017;20:318-27. https://doi.org/10.1080/13696998.2016.1261031.
- Grustam AS, Severens JL, De Massari D, Buyukkaramikli N, Koymans R, Vrijhoef HJM. Cost-effectiveness analysis in telehealth: a comparison between home telemonitoring, nurse telephone support, and usual care in chronic heart failure management. Value Health 2018;21:772-82. https://doi.org/10.1016/j.jval.2017.11.011.
- Mohiuddin S, Reeves B, Pufulete M, Maishman R, Dayer M, Macleod J. Model-based cost-effectiveness analysis of B-type natriuretic peptide-guided care in patients with heart failure. BMJ Open 2016;6. https://doi.org/10.1136/bmjopen-2016-014010.
- Pufulete M, Maishman R, Dabner L, Mohiuddin S, Hollingworth W, Rogers CA. Effectiveness and cost-effectiveness of serum B-type natriuretic peptide testing and monitoring in patients with heart failure in primary and secondary care: an evidence synthesis, cohort study and cost-effectiveness model. Health Technol Assess 2017;21. https://doi.org/10.3310/hta21400.
- Troughton RW, Frampton CM, Yandle TG, Espiner EA, Nicholls MG, Richards AM. Treatment of heart failure guided by plasma aminoterminal brain natriuretic peptide (N-BNP) concentrations. Lancet 2000;355:1126-30. https://doi.org/10.1016/S0140-6736(00)02060-2.
- Sullivan PW, Lawrence WF, Ghushchyan V. A national catalog of preference-based scores for chronic conditions in the United States. Med Care 2005;43:736-49. https://doi.org/10.1097/01.mlr.0000172050.67085.4f.
- Zannad F, McMurray JJV, Krum H, van Veldhuisen DJ, Swedberg K, Shi H, et al. Eplerenone in patients with systolic heart failure and mild symptoms. N Engl J Med 2011;364:11-2. https://doi.org/10.1056/NEJMoa1009492.
- Yao G, Freemantle N, Calvert MJ, Bryan S, Daubert JC, Cleland JG. The long-term cost-effectiveness of cardiac resynchronization therapy with or without an implantable cardioverter-defibrillator. Eur Heart J 2007;28:42-51. https://doi.org/10.1093/eurheartj/ehl382.
- Swedberg K, Komajda M, Böhm M, Borer J, Robertson M, Tavazzi L, et al. Effects on outcomes of heart rate reduction by ivabradine in patients with congestive heart failure: is there an influence of beta-blocker dose?: findings from the SHIFT study. J Am Coll Cardiol 2012;59:1938-45. https://doi.org/10.1016/j.jacc.2012.01.020.
- Yusuf S, Pitt B, Davis CE, Hood WB, Cohn JN. SOLVD Investigators . Effect of enalapril on survival in patients with reduced left ventricular ejection fractions and congestive heart failure. N Engl J Med 1991;325:293-302. https://doi.org/10.1056/NEJM199108013250501.
- Freudenberger RS, Kim J, Tawfik I, Sonnenberg FA. Optimal medical therapy is superior to transplantation for the treatment of class I, II, and III heart failure: a decision analytic approach. Circulation 2006;114:I62-6. https://doi.org/10.1161/CIRCULATIONAHA.105.001412.
Appendix 1 Chronic kidney disease by glomerular filtration rate and albuminuria stages
FIGURE 2.
Prognosis of CKD by GFR and albuminuria category. Albuminuria = proteinuria. Light blue: low risk (if no other markers of disease, no CKD); purple: moderately increased risk; mid-blue: high risk; orange: very high risk. Reprinted from Kidney International, Vol. 80, Levey AS, de Jong PE, Coresh J, Nahas MEI, Astor BC, Kunihiro Matsushita K, et al. 83 The definition, classification, and prognosis of chronic kidney disease: a KDIGO Controversies Conference report, Copyright 2011, with permission from Elsevier.

Based on NICE guidance11 from 2014 (updated in January 2015 and checked by NICE in April 2017; due to be updated by 30 June 202184), the following monitoring schedule is recommended:
-
≤ 1 per year: G1/A1 and G2/A1
-
1 per year: G1/A2, G2/A2, G3a/A1 and G3a/A2
-
≥ 1 per year: G1/A3 and G2/A3
-
≤ 2 per year: G3b/A1
-
2 per year: G3a/A3, G3b/A2, G4/A1, and G4/A2
-
≥ 2 per year: G3b/A3
-
3 per year: G4/A3
-
4 per year: G5/A1
-
≥ 4 per year: G5/A2 and G5/A3.
Appendix 2 Trends in serum creatinine testing in Oxfordshire, UK, 1993–2013: a population-based cohort study
Oke et al. 22 https://doi.org/10.1136/bmjopen-2015-009459
Appendix 3 Trends in kidney function testing in UK primary care since the introduction of the quality and outcomes framework: a retrospective cohort study using the Clinical Practice Research Datalink
Feakins et al. 23 https://doi.org/10.1136/bmjopen-2018-028062
Appendix 4 Protocols for research using the Clinical Practice Research Datalink
This appendix contains a quotation from NICE. 85 © NICE 2008 Chronic Kidney Disease: Early Identification and Management of Chronic Kidney Disease in Adults in Primary and Secondary Care. Available from www.nice.org.uk/guidance/CG73 All rights reserved. Subject to Notice of rights. The NICE guidance is prepared for the NHS in England. All NICE guidance is subject to regular review and may be updated or withdrawn. NICE accepts no responsibility for the use of its content in this product/publication.
Figure 1 is reprinted from Kidney International, Vol. 80, Levey AS, de Jong PE, Coresh J, Nahas MEI, Astor BC, Kunihiro Matsushita K, et al. 83 The definition, classification, and prognosis of chronic kidney disease: a KDIGO Controversies Conference report, Copyright 2011, with permission from Elsevier.
Appendix 5 Systematic review and meta-analysis comparing the bias and accuracy of the Modification of Diet in Renal Disease and Chronic Kidney Disease Epidemiology Collaboration Equations in community-based populations
McFadden et al. 24 https://doi.org/10.1373/clinchem.2017.276683
Appendix 6 Effects of antihypertensives, lipid-modifying drugs, glycaemic control drugs and sodium bicarbonate on the progression of stages G3 and G4 chronic kidney disease in adults: a systematic review and meta-analysis
Taylor et al. 25 https://doi.org/10.1136/bmjopen-2019-030596
Appendix 7 Statistical models for the deterioration of kidney function in a primary care population: a retrospective database analysis
Oke et al. 26 https://doi.org/10.12688/f1000research.20229.1
Appendix 8 An observational cohort study of primary care patients with reduced estimated glomerular filtration rate
Flemming et al. 86 https://doi.org/10.3399/BJGP.2020.0940
Appendix 9 Qualitative evaluation of monitoring chronic kidney disease and chronic heart failure with patients and health practitioners
Overview
Aim
These WSs aimed to use qualitative methods to explore patients’ and health professionals’ experiences of, and views about, monitoring for CKD and CHF. This was achieved through analysis of individual patient interviews and focus group interviews with GPs, practice nurses and CHFNs. Qualitative research methods are widely regarded as the most appropriate means of collecting, analysing and understanding patients’ experiences.
Alterations to the original study design
The original design for the patient interview studies had been to use the same methodology for both conditions. This comprised a secondary analysis of existing data sets from the Nuffield Department of Primary Care Health Sciences (NDPCHS) Health Experiences Research Group’s (HERG’s) archive of studies published on the health information website https://healthtalk.org/ (accessed 5 May 2020), followed by new interviews to explore, in more depth, issues raised by the secondary analyses. However, owing to unforeseen delays, a collaborative research project between the University of Bristol, University of Manchester, University College London, University of Southampton and University of Oxford to produce the CKD data set had not been started, so a different approach was taken for the CKD patient study.
The work to produce the CKD patient interview collection took place in collaboration with the current work programme, with additional funds from the National Institute for Health Research School for Primary Care Research (project reference number NSPCR ID FR4.120). This enabled the incorporation of questions about patients’ experiences of being monitored in the primary study. One-third of the interviews were conducted by the Oxford-based researcher from the current work programme (JE), the remainder by a researcher in Bristol. The researchers collaborated closely, using the same methodology and interview guide, and jointly analysed the data. All interviews were conducted using existing HERG ethics approval and were copyrighted to the University of Oxford, with permission for sharing with other researchers.
For the focus group study, rather than convening separate groups to discuss CKD and CHF, we decided to discuss both conditions in each group except for those involving just CHFNs. Focus groups were conducted with each professional group separately.
Methodology used for all patient interviews
The methodology used for all HERG studies leading to publication on https://healthtalk.org/ (accessed 5 May 2020) has been tried and tested since 2000 (see https://healthtalk.org/uploads/files/HERGresearch.pdf); accessed 25 January 2021, approved by the NHS National Research Ethics Service Committee South Central – Berkshire (reference number 12/SC/0495) and recommended by the NHS National Knowledge Service as the ‘gold standard’ for research into patient experiences. All patient interviews included in the current work programme were collected and analysed using the HERG methodology and ethics approval.
For all HERG/healthtalk studies, 40–50 interviewees were sought from a range of backgrounds, age groups, geographical areas and experiences of health care, with the aim of achieving a ‘maximum variation sample’. 87 Interviews took place in a person’s home or another place of their choice. Participants were asked to tell the story of their illness or health condition, without interruption from the researcher, after which questions were asked to elicit more detail or raise issues not mentioned by the participant. Interviews typically lasted between 40 and 120 minutes. Interviewing continued until data saturation was reached on key categories, such as experiences of diagnosis and treatments, and information and support needs, and no new themes emerged. Interviews were recorded and transcribed verbatim and returned to the participant for optional review. Transcripts were anonymised and coded using qualitative data indexing software (NVivo; QSR International, Warrington, UK). The data in each coding report were then thematically analysed in detail. Analyses were written with the aim of explaining ‘what is going on in the data’ while taking account of all the issues raised, not just the most common ones.
The chronic heart failure patient study
Method
The existing CHF collection consisted of 43 patient interviews conducted between 2003 and 2013. For the current work programme, 16 new patient interviews in 2015 focused on monitoring, which had received little attention in the original collection. Interview guides for the original and new interviews are presented in Tables 1 and 2. Table 3 presents sample characteristics. The secondary analysis of the existing interview collection was conducted first so that the findings could inform the new interviews. Using Heaton’s categories of secondary analysis,88 our approach was that of ‘supra-analysis’ (examining new empirical, theoretical or methodological questions that were not the focus of the primary study). The analysis aimed to focus on patients’ attitudes to both the content and frequency of monitoring, adherence to and understanding of medications, and triggers to consultation.
Questions | Prompts |
---|---|
Could you tell me how you first discovered that you had heart failure? How did it start? What made you seek help/medical advice? |
|
When you were diagnosed with heart failure, what were you told about the illness? Who told you that you had heart failure and how did s/he go about it? How did you feel about it at that time? What were you told about heart failure? |
|
What do you think caused you to have heart failure? | None |
What did you know about heart failure before you found out that you had it? | Do you know anyone else who has heart failure? |
What do you understand heart failure to mean? | None |
Have you wanted to find out more about heart failure since your first diagnosis? |
|
Have you had any other medical problems and can you tell me about them? | How have they affected your heart failure? And medication for them? |
(Given that you may have had lots of treatment for heart failure) Can you tell me now about the treatment you have had so far? |
|
Are you having any home visits from nurses now and what do they do? | None |
Other people will be interested in the kind of medicine you’re taking, so can you tell me which drugs you’re taking at the moment and what they do to you? | None |
What do they make you feel like? (e.g. diuretics can cause leg cramps, need to go to loo often and urgently.) Are you aware of any side effects now or in the past? |
|
(You mentioned breathlessness/tiredness/not coping) . . . can you describe what it feels like when you have an episode of this? | How do you usually cope with your breathlessness/tiredness/not coping? |
How has heart failure affected you physically? |
|
What about your usual way of life; can you describe how heart failure has affected that? |
|
What kind of effect has heart failure had on you and your family? |
|
What about the mental and emotional side of living with heart failure? How have you found that? |
|
What are your thoughts about the future and do you talk to anyone about your thoughts? |
|
Are there any messages that you would like to give to doctors and nurses about the treatment of heart failure? Are there still questions about heart failure that you would like answered? | None |
What kinds of advice, based on your own experience of living with heart failure, would you like to pass onto others with the same condition? | Living with it, partnerships, changing your lifestyle |
Is there anything else I’ve not mentioned about living and coping with heart failure that you would like to tell me? |
Topic | Questions |
---|---|
Part 1: initial open-ended question to elicit narrative | |
As you know, my study is focusing on how people’s heart failure is monitored or managed, but, in order to get some background about your situation, please could you spend a few minutes telling me about the following three things:
|
|
Part 2: ask any specific questions to seek clarification about anything in part 1 | |
Then cover the following topics as required to elicit further information (time allowing) in any order, but with an emphasis on monitoring | |
Understanding heart failure |
|
Other health conditions | Do you have any other health problems/medical conditions?
|
Heart treatments past and present |
|
Monitoring |
|
Self-management and lifestyle behaviours |
|
Impacts of heart failure | Please tell me how your heart condition impacts on your life
|
What are your thoughts about the future? |
|
Satisfaction with health professionals | How do you feel about the health professionals that are looking after you?
|
And finally . . . |
|
Characteristic | CKD | CHF | ||
---|---|---|---|---|
n | % | n | % | |
Sex | ||||
Men | 25 | 55.6 | 38 | 64.4 |
Women | 20 | 44.4 | 21 | 35.6 |
Age (years) at time of interview | ||||
< 40 | 1 | 2.2 | 3 | 5.1 |
40–49 | 1 | 2.2 | 5 | 8.5 |
50–59 | 7 | 15.6 | 11 | 18.6 |
60–69 | 5 | 11.1 | 17 | 28.8 |
70–79 | 26 | 57.8 | 13 | 22.0 |
80–89 | 4 | 8.89 | 10 | 16.9 |
90–99 | 1 | 2.2 | ||
Recruited via | ||||
GP | 36 | 80.0 | 17 | 28.8 |
Support group/charity | 5 | 11.1 | 17 | 28.8 |
Nurse | 9 | 15.3 | ||
Consultant | 4 | 6.8 | ||
Hospice | 1 | 1.7 | ||
Snowballing | 1 | 2.2 | 1 | 1.7 |
Other study | 2 | 4.44 | 1 | 1.7 |
Social media | 1 | 2.2 | ||
Unknown | 8 | 13.6 |
Questioning in the new interviews focused on patients’ attitudes to monitoring: what they felt about the timing of visits, what they gained from the visit, what they understood about the purpose of monitoring and how they would feel about any changes in how monitoring is organised. We also explored attitudes to medication and decision thresholds as to when to seek medical attention. The new interviews were initially analysed without reference to the secondary analysis, to allow the coding structure to emerge from the data, rather than coding to predetermined codes. Codes concerning managing/monitoring of the condition and medication were examined in greater detail and coded more finely (Table 4). Each of the subcodes was then thematically analysed. Written analyses were prepared separately for the original and new data sets, before being combined.
Secondary analysis | Primary analysis of new interviews |
---|---|
Heart events or symptoms | Background to their heart condition and its treatment |
Diagnostic tests and procedures | |
Surgical procedures and angioplasty | |
Learning and understanding the heart failure diagnosis | |
Causes of heart problems | Causes |
Information about heart failure | Information |
Medication | Medications |
What drugs | |
Level of understanding | |
Drug regimen and dose changes | |
Adherence and self-management | |
Side effects and interactions | |
Complementary medicines or therapies | Complementary/alternative medicines and over-the-counter medicines and recreational drugs |
Other | Oxygen treatment |
Monitoring and access to professionals | Management of the condition |
Health professional appointments and tests | Tests and check-ups with a health professional |
Access between appointments and triggers to consult | Unplanned access to health professionals |
Self-monitoring | Self-monitoring |
Psychological impacts of monitoring | Attitudes towards |
Weight | |
Blood pressure | |
Oxygen saturation | |
International normalised ratio | |
Remote monitoring of implanted cardiac devices | Telemonitoring |
Other | |
Satisfaction with care | |
Views about changes to monitoring | |
Healthy lifestyle behaviours | |
Physical activity and exercise | |
Diet and weight | |
Stress avoidance | |
Smoking | |
Alcohol | |
Coping strategies or attitudes | |
Living with heart failure | Impacts of heart failure |
The future | |
Satisfaction with health professionals or the NHS | Support (from professionals, family, friends, peer support groups) |
Other health conditions | Other medical conditions |
Results
The original CHF interviews contained little material about monitoring the condition, as the study had pre-dated NICE guidelines on this topic. Our combined analyses of the original and new interviews were published online in 201676 as a major update and restructuring of the existing site, which included new topic summaries on the following: heart failure monitoring – check-ups with health professionals; access to health professionals between appointments; heart failure monitoring at home; and attitudes to heart failure monitoring. Key findings related to monitoring are summarised in the following paragraphs.
Monitoring took place in primary or secondary care or a combination; care delivered by specialist nurses was particularly valued. Intervals between check-ups with a health professional ranged from 1 year to 1 week. Some people monitored their weight and blood pressure at home. A few interviewed in 2015 had experience of automated systems of monitoring such as telephone calls or texts; one had taken part in a telemonitoring trial. People with implanted cardiac devices and some of those with pacemakers had a machine at home for sending data downloads automatically to the hospital.
Having their CHF monitored provided people with reassurance that someone was keeping an eye on them and that test results were satisfactory. Current monitoring regimens were largely acceptable; a few expressed minor criticisms. Although many people had been told to contact a health professional if they had any concerns, and had done so, advice about when to seek help did not seem to have been given, other than for emergency situations. It had often taken a long time for the optimum level of medication to be achieved and unwelcome side effects were common. People said they disliked having to take medicines, but accepted that it was necessary.
Responses to questions about hypothetical future changes to monitoring regimens indicated that approval would be forthcoming provided the rationale was explained, in particular for changes to frequency for reasons other than a change in disease severity or stability. However, systemic changes of the professionals involved in heart failure monitoring could be problematic if patients had developed an especially trusting relationship with a particular professional. Self-monitoring would not be acceptable to all, but some people who were not already doing it would be prepared to do so, provided measurements were not required more frequently than their disease severity appeared to warrant. Equipment would need to be provided for some patients. Those with experience of telemonitoring of weight and blood pressure measurements found it acceptable. Home monitoring of implanted cardiac devices was valued by all those who had it. An increased reliance on blood testing in future would be acceptable, and POC testing in GPs’ surgeries would be appreciated by people who become anxious waiting days or weeks for test results.
The chronic kidney disease patient study
Method
The sampling categories and interview guide were developed with help from an expert advisory panel (Table 5). We sought to recruit people who were having regular checks of their kidney function because they had stage G1–3 CKD. The Bristol-based researcher recruited participants entirely through GPs in the Bristol area, aided by service support costs. The Oxford-based researcher recruited nationwide using the usual range of methods for healthtalk studies (GPs, hospital consultants, advisory panel members, support organisations/charities, social media and snowballing through personal contacts) and also via the OxRen cohort study being conducted within the Oxford NDPCHS. 89 Forty-six people were recruited; one withdrew after interview. The stage of CKD was unknown for seven participants, 35 had stage G3, two had stage G2, and two had been recently referred to specialist care because of more advanced kidney disease, but could reflect back on their experiences of being monitored in primary care. See Table 3 for sample characteristics.
Name | Position |
---|---|
Dr Jeremy Horwood | PI, School of Social and Community Medicine, University of Bristol (chairperson) |
Dr Kristina Bennert | Qualitative Researcher, School of Social and Community Medicine, University of Bristol |
Dr Tom Blakeman | Co-applicant, National Institute for Health Research Clinical Lecturer in Primary Care |
Dr Fergus Caskey | Renal Specialist, North Bristol NHS Trust |
Ms Di Croft | Specialist Diabetes Nurse in Primary Care, Oxford |
Dr Julie Evans | Senior Qualitative Researcher, HERG, University of Oxford |
Professor Gene Feder | Co-applicant, Professor of Primary Care, School of Social and Community Medicine, University of Bristol |
Dr Kathryn Griffith | Royal College of General Practitioners Clinical Champion for Chronic Kidney Disease, University of York |
Ms Sarah Griffith | Diabetes and Hypertension Nurse in Primary Care, NHS Bristol Clinical Commissioning Group |
Dr Daniel S Lasserson | GP and Senior Clinical Researcher, University of Oxford |
Dr Louise Locock | Co-applicant, Deputy Research Director, HERG, University of Oxford |
Ms Fiona Loud | Patient Representative, Director of the Kidney Alliance |
Mr Andy Williamson | Patient Representative, Kidney Patient Guide Forum Moderator |
To avoid participants learning about their diagnosis through taking part in the study, we had stipulated that health professionals should recruit only participants who had been told either that they had CKD or that their kidney function was being monitored. Given disagreement about the meaning of the term CKD, we described the topic of our research in recruitment literature as ‘kidney health’. However, despite health professionals’ assurances that they had told participants the reason why they were approached, it became apparent during interviews that some lacked awareness that their kidney function was impaired. To prevent these participants from learning of their CKD from the researcher, certain questions in the interview schedule were omitted or reformulated, and exploration of experience tended to focus to a greater extent on other comorbidities for this subgroup. The interview guide is presented in Table 6.
Topic | Questions |
---|---|
Narrative section | |
Opening question: as you know, we are interested in hearing about your experiences of having regular checks of your kidney function, but, in order to provide some context for that, perhaps you could start by telling me about your health in general, any conditions you have been diagnosed with and how they affect you, any treatments you are having, and how the kidney function checks fit into the overall picture? | |
Supplementary question | |
Thank you. Now please could you tell me about your kidney checks. The sorts of things I’d like to hear about are how the subject of your kidney function first came up, how often you are checked and by whom, what the checks consist of, what you are told about the results, and how you feel about having these checks. Don’t worry if you forget to talk about some of these things: when you have finished, I will ask you questions to fill in any gaps. | |
Semistructured questioning as necessary | |
General health |
|
Introducing kidney function |
|
Information |
|
Initial knowledge |
|
Kidney checks |
|
Impacts |
|
Support |
|
Future |
|
Messages to others |
|
Final questions |
|
Results
The main findings were published online in 201531 as ‘topic summaries’, illustrated with extracts from the interviews. Topic summaries about monitoring (check-ups in general practice and hospital; diagnosing problems with kidney health; tests used to monitor kidney health; receiving and making sense of test results; and attitudes towards monitoring kidney health) were complemented by summaries about the diagnosis and its disclosure, information and support needs, and looking after kidney health. Key findings are summarised in the following paragraph.
A gap was revealed between what health professionals seek to explain about CKD and what patients may understand. Primary care professionals often avoided using the term ‘CKD’ when talking to patients with early-stage kidney impairment in an attempt to avoid causing unnecessary anxiety. Patients’ accounts of receiving information about their kidney health echoed the phrases described by health professionals. However, patient interpretations showed some of these phrases to be unhelpful, raising further questions and adding to, rather than diminishing, initial concerns. The use of phrases such as ‘kidney damage’ or ‘kidney failure’ could be frightening, and the term ‘chronic’ was sometimes misinterpreted as meaning serious, whereas a description of the decrease in kidney function as a percentage or stage seemed less alarming. However, the exact meaning of the test results was often unclear, and those who were told their CKD stage did not always understand what this meant. For some patients, it could be difficult to understand that they had been diagnosed with CKD when they did not experience symptoms. Attempting to reassure patients that their kidney impairment was nothing to worry about, without providing explanatory information about the condition, could leave patients concerned and wanting to know more about possible causes, the meaning of test results and whether or not they could do anything to prevent further decline.
The focus group study with health professionals
Method
With approval from the University of Oxford’s Central University Research Ethics Committee (reference number R49627/RE001), we recruited individual GPs and practice nurses from Oxfordshire, and CHFN teams from the wider Thames Valley area (because the numbers were small). CHFN team leads were contacted and invited to forward the study information to their members. GPs and practice nurses known to us through other studies run by the NDPCHS were invited to participate. GP colleagues from the NDPCHS were invited and asked to forward details to their practice nurses. Members of the Oxfordshire practice nurse forum were also invited.
Recruitment proved difficult, resulting in few participants of all professional types, exacerbated by people dropping out at short notice because of unforeseen circumstances. Sixteen people participated in four focus groups. One was held with each of two teams of CHFNs as part of their regular team meeting, another with GPs, held at the NDPCHS, with light refreshments provided. We succeeded in recruiting enough practice nurses for a fourth group only by offering a complimentary meal at an Oxford college. Table 7 presents the participant characteristics.
Characteristic | CHFNs | GPs | Practice nurses | Total |
---|---|---|---|---|
Groups held (n) | 2 | 1 | 1 | 4 |
Participants (n) | 7 | 5 | 4 | 16 |
Men (n) | 0 | 1 | 0 | 1 |
Age range (years) | 41–49 | 46–57 | 53–72 | |
Minority ethnic participants (n) | 3 | 0 | 0 | 3 |
Years in practice (range) | < 1–10 | 18–23 | 3–38 |
Before each session, the participants signed their consent, including to be video-recorded. As an ‘ice-breaker’, practice nurses were asked to explain the ways in which they were involved in managing patients with CKD or CHF; CHFNs and GPs were asked to complete the following sentence: ‘In managing patients with CKD or CHF, NICE (National Institute for Health and Care Excellence) guidelines are helpful because . . .’. Further questions and prompts followed to elicit how NICE guidelines affected real-life clinical management and any difficulties in applying them, before discussing how the monitoring of these conditions might be improved. Short pre-recorded video presentations stimulated debate on potential impacts of future developments in monitoring arising from the wider research programme. These concerned the possible use of the term ‘kidney age’ when talking to patients about CKD (GPs and practice nurses only), monitoring CHF patients’ NPs to guide treatment, and use of a POC device for measuring NP. Each session was designed to last 1 hour and closed with participants feeding back what, if anything, they had learned.
Focus groups were audio- and video-recorded and the research assistant’s notes were expanded while listening to the recording to form a rough anonymised transcript. A thematic framework analysis was structured around the main areas of questioning and any emergent themes. Selected quotations were fully transcribed for presentation; hesitations and overlapping speech were removed to aid readability. Data collection and analysis proceeded in parallel so that early findings could inform questioning in the subsequent focus groups. Video-recordings were used to examine non-verbal cues and interactions between focus group members and how these affected the content.
Results
National Institute for Health and Care Excellence guidelines
Community heart failure nurses were fully cognisant of the NICE guidelines for managing people with CHF and used them on a daily basis to guide their care. However, not all patients could be treated strictly according to the guidelines, either because of competing treatment targets for comorbidities or because patients could not tolerate certain medicines. CHFNs therefore used their clinical knowledge, skills and close links with cardiology consultants to adapt the recommended treatments to suit individual patients.
Community heart failure nurses sometimes found it difficult working with GPs, who were not always up to date with NICE guidance and appeared to use different thresholds for blood pressure or kidney function when altering medication. When communicating with GPs about specific patients, CHFNs said they had taken to explaining that their proposed treatment plan accorded with NICE guidelines, a strategy that usually resulted in the GP’s agreement:
Sometimes GPs are not that up to date on certain things, but usually when you say, ‘These are the NICE guidelines’, they usually follow suit. But sometimes that can be a bit challenging.
CHFN 07
The GPs all confessed to being unfamiliar with the latest updates to NICE guidelines for either CHF or CKD, or both, arguing that work pressures did not allow time to read these for all conditions. The NICE website was considered confusing and difficult to navigate, and GPs were more likely to learn about patient management recommendations from other sources, such as the Clinical Commissioning Group or QOF alerts.
Some aspects of NICE guidelines that GPs were familiar with were perceived to be impractical and did not reflect real life in general practice, although one participant pointed out that such aspirational guidelines could be used to lever change at commissioning level. On the current CKD guidelines,11 specifically, there was confusion about when albumin–creatinine ratios should be requested from the laboratory and how the results should be interpreted and acted on.
One practice nurse said she liked to read NICE guidelines, but agreed with the others that, although they would like to understand the evidence base for management strategies, most practice nurses do not have time to read NICE guidelines and they tend to work according to QOF targets and local protocols unquestioningly, rather than to national guidelines. However, one practice nurse said she occasionally consulted NICE guidelines for specific treatment advice.
Monitoring patients with chronic heart failure
Although there was no structured recall for CHF patients via the QOF, and practice nurses did not see patients specifically about their heart failure, both GPs and practice nurses saw many patients with CHF in clinics for other long-term conditions. The practice nurses primarily saw patients about their diabetes, hypertension or respiratory disease and were not expert in CHF. Practice nurses suggested that management of CHF could possibly be improved by greater provision of education to patients about lifestyle to try to prevent the condition from developing in the first place, or to optimise their health once they had it, and about what to do when things go wrong.
The GPs’ discussion on this topic centred on their uncertainties about how they should manage CHF patients. GPs were unsure what tests they should be doing and when, and how, to interpret and act on the results. They did not know how to identify opportunities for intervention that might benefit the patient, such as cardiac resynchronisation. They would welcome specific guidance from specialists about how to manage individual patients discharged from secondary care:
So an interesting primary care question is: how do we understand what the threshold or the point where we could, would or should be referring these people back for more echoes [echocardiography] or at what point might they benefit? Because if the burden of managing the vast majority of people with heart failure is with us and there is an intervention that’s effective, but we don’t know how to identify when they need to go back, that strikes me as quite important.
GP01
General practitioners sometimes felt criticised by cardiologists for up-titrating a patient’s medicines too slowly, when, in reality, this process could be challenging because of patient factors beyond their control. They also struggled to manage patients who had CHF with preserved ejection fraction, who are not seen in Oxfordshire by CHFNs. By contrast, the CHFNs in one group said they accepted patients with all types of CHF. One GP mentioned developments in automated RM of CHF patients, but believed that few of their own patients would take to using such technology. However, CHFNs who used these technologies found that their more elderly patients got on well with this method of monitoring and felt reassured that someone was keeping an eye on them.
Community heart failure nurses in one group felt that wider use of telemonitoring was one way in which heart failure monitoring could be improved. They would also welcome Skype™ (Microsoft Corporation, Redmond, WA, USA) clinics that would enable patients to remain at home, and joint clinics with respiratory and renal specialists and pharmacists. They would also like communication between professionals to be improved by means of shared information technology systems across administrative boundaries, instead of the current fragmented arrangements, and more coherent ways of working among CHFNs nationally:
Having a system that we could all just write on, one system, would be lovely. I mean it’s like we have a system, and yes that talks to community matrons, district nurses and mental health, but it doesn’t talk to the COPD [chronic obstructive pulmonary disease] team or the GP.
CHFN 07
Community heart failure nurses in the other group said their work could be made easier if they had more staff with which to share their case load, especially as they typically became involved in dealing with non-CHF issues, being the only professional visiting the patients at home.
Potential future use of natriuretic peptide measurements in chronic heart failure monitoring
Only one of the practice nurses was familiar with NP tests and had used them for diagnostic purposes; the others had never heard of them and queried how using NP measurements for monitoring might change patient management. One GP was attracted by the idea of a measure that could potentially show that they had optimised a particular patient’s treatment. Other GPs could not see where monitoring a patient’s NP would fit into their management:
So the question is where would the [BNP] test sit? How often would it need to be done? What would it let us do that we’re not doing now? What could it help us do better from a patient point of view?
GP01
Both GPs and CHFNs, and one of the practice nurses, recognised NP as a useful diagnostic test for CHF and to rule out other causes of breathlessness such as chronic obstructive pulmonary disease. However, the CHFNs could see no benefit of measuring NP as part of routine monitoring. Changes in NP were believed to reflect the severity of symptoms in most patients and CHFNs believed in managing their patients according to symptoms, not numbers. It was unclear how knowing the NP would alter their management in most cases, and telling a patient that their NP was rising could negatively affect a patient’s well-being:
I’m not convinced it’s going to change your treatment, whatever the reading is going to be. Not like a renal function or a haemoglobin or, you know, those kind of things would change your management maybe. But pro-BNP I don’t think would change that.
CHFN 06
But with people that you know have got no other comorbidities, have got major heart failure anyway, what are numbers going to tell us? I mean, our consultants are quite anti-numbers, aren’t they, anyway. You know, they’ll say ‘Go on symptoms, don’t go on that their blood pressure’s 80 systolic or their renal function’s 300; go on how they’re feeling and what we need to do’. [. . .] Actually, I think we get very fixated on numbers, and it might be more detrimental to patients. ‘Yes, you know, your BNP was 4000 last week, oh damn, it’s 8000 this week, oh it’s getting worse.’ What’s that going to tell them, really? I mean, it tells us their heart failure is getting worse but we can probably see that by looking at them.
CHFN 07
Furthermore, all groups believed that the cost of the test (both financial and in terms of professionals’ time and extra blood samples required from the patient) would outweigh any potential benefits. One CHFN suggested that the money could be better spent on providing cardiac rehabilitation for people with CHF, which had proven benefits. The practice nurses debated the relative merits of educating patients to self-monitor their condition, such as by regular weight measurements, and to seek help if it changed.
One GP raised the issue of inaccurate NP measurements resulting from blood samples taken from surgeries that were far from the laboratory, which led to a suggestion that a POC device to measure NP could be a useful alternative for GPs. A CHFN pointed out that use of a NP POC device in general practice could speed up diagnosis because their laboratory ran the NP assay only once per week. A practice nurse suggested that using a POC device for monitoring NP could prevent a patient being ‘over-diuresed’ while waiting for a laboratory test result to arrive. However, CHFNs would be reluctant to add a POC device to the already large collection of equipment that they have to carry when visiting patients, because they see no value in its routine use. One added that they would also like to know a patient’s renal function at the same time as their NP before changing any medicines.
Talking to patients about chronic kidney disease
In both the GP and practice nurse groups, the problem of terminology about kidney function was raised spontaneously before the researcher showed the presentation about the suggested alternative term ‘kidney age’. Both groups said that CKD needed a new name, and the nurses mentioned that kidney function naturally declines with increasing age:
And it’s difficult because I hate the term ‘chronic kidney disease’. I’ve found it difficult to navigate a way through those conversations constructively with patients.
GP01
After watching the presentation, the practice nurses said that they liked the suggested term ‘kidney age’ because it demedicalised CKD, thereby making it easier to discuss with patients, and they drew parallels with the existing concept of lung age. The opportunity to demedicalise kidney impairment through embracing the term ‘kidney age’ served to reinforce the view of one practice nurse that people should not have a part of the normal ageing process monitored unless it could be reversed:
I’d much rather have that terminology of saying that your kidney age . . . It’s like the lung age, isn’t it, the same sort of conversation. Which isn’t too upsetting because you’re not telling them they’ve got a disease, you’re telling them that their kidneys are older than they should be. I think that’s it. Reasonable discussion with the patient. And then what can we do, if anything, about your lifestyle that would facilitate it lessening the progression, or making things better, or looking at your drugs?
Practice nurse 02
The nurses debated what lifestyle and other interventions might help slow or reverse kidney impairment, such as optimising treatment for diabetes, CHF and hypertension; avoiding prescribing medicines that can damage kidney function; quitting smoking; losing weight; and reducing dietary intake of alcohol or salt. However, none was sure if any of this would make a difference. Some practice nurses felt that monitoring kidney function could help to identify factors that could precipitate a rapid decline to genuine kidney disease. One drew parallels with her work on respiratory disease.
Although all disliked the term CKD and wanted a workable alternative, the GPs were not convinced that ‘kidney age’ was the answer to the current communication problem. ‘Kidney age’ was potentially a helpful concept for doctors to understand declining kidney function, and it might mean that they intervened less as a result, but they were not sure that they would want to use it when explaining the condition to patients. It was suggested that, unlike ‘lung age’, whereby patients can be motivated to quit smoking to improve their lung function, there was nothing equivalent that the patient could do to improve their kidney function, except perhaps helping to optimise their blood pressure control. Therefore, telling a patient that their kidneys are considerably older than their biological age could cause unnecessary anxiety:
And I think that use of ‘kidney age’ reminds me of ‘lung age’, which is possibly what it’s based on. But at least, with ‘lung age’, on the whole you are using it as a motivational factor, aren’t you, for smoking cessation, and it is pretty powerful; it does shock some people in a way that seems surprising to a medical mind when you think ‘I can tell you all this time this and that and this and that, and none of it made any impact till I told you you had a lung age of 75 when you’re 50’. But it just goes to show the terrible assumptions we make and how you have to explain things in terms that people understand. But there’s a point to that, you’re telling . . . Whereas, in this situation, you’re saying, ‘Your kidneys have aged darling’, and . . .
Your lungs can look 10 years younger just as your face does when you . . . Yeah, all those . . . But actually, your kidneys, there’s something much more frightening. [. . .] So we think it needs a different name but not that one.
When asked if they could suggest alternatives to ‘kidney age’, the practice nurses suggested that kidney function could be measured on a scale of ‘kidney health’ as opposed to ‘kidney disease’, so as to adopt a more positive emphasis. One GP said they liked to use the term ‘kidney reserve’ because it gave a sense of what you could do with a certain level of kidney function, and it seemed less emotive than ‘kidney age’.
Interactions between participants and non-verbal cues
In both CHFN groups, an element of peer pressure to agree to the session being video-recorded was apparent, and one nurse was teased by her team leader for being uncharacteristically quiet during the discussion. In both groups, it was difficult to keep the discussion flowing, the team lead spoke the most and there was a tendency for the participants to agree with each other. Most of the conversation was directed at the researcher rather than to each other.
The GPs and practice nurses appeared happy to agree to the video-recording. Four of the five GPs were also academics and knew each other. Two of the practice nurses worked in the same practice. Discussion in the GP and practice nurse groups was more animated than in the CHFN groups, proceeded with ease and lasted longer; the researcher interjected only to change the topic. There were intermittent bouts of laughter and multiple interruptions of each other’s speech. However, when one particular GP interrupted, all the other participants stopped talking and paid attention. This GP appeared to be considered more expert because of their involvement in commissioning. Discussion in the practice nurse group was dominated by one particular nurse, although it did not appear to prevent others speaking. Apart from the opposing opinions among the practice nurses about the relative merits of monitoring kidney function, consensus among participants in each group was high. All the participants sat in a relaxed manner throughout and there were few instances of body language that could suggest unease, dissent or lack of engagement.
Limitations
Recruitment took place across just two counties and participant numbers were small, so the views expressed cannot be regarded as representative of these professions as a whole. The focus groups in which both CHF and CKD were discussed (GPs and practice nurses) over-ran the time allowed, which was insufficient for in-depth discussion of all the relevant issues.
Conclusions
The organisation of heart failure services currently varies across administrative boundaries, even within counties. In one area, the CHFN team dealt with patients with all types of CHF; in the other areas, CHFNs saw patients with left ventricular systolic dysfunction only. Information technology systems are fragmented, so professionals cannot access information about patients when they are treated in a different area. More uniform service provision and coherent information technology systems could increase the efficiency of patient management.
Community heart failure nurses use NICE guidelines as an essential tool in managing their patients and in eliciting support from individual patients’ GPs for proposed treatment plans. GPs are uncertain how to manage patients with CHF, yet they do not have time to read updated NICE guidelines, which could provide some of the information they lack. GPs would like to receive specific guidance from specialists about how to manage individual patients. CHFNs who offer this kind of advice say it is well received by GPs. Practice nurses do not routinely read NICE guidelines, their work being driven by QOF targets and local protocols. Because there is no structured recall in the QOF for CHF patients, GPs and practice nurses tend to see them for medication reviews and in clinics for other long-term conditions, rather than specifically about their CHF. So although these patients receive relatively intensive monitoring, it is not specifically for their CHF, and GPs do not think about monitoring for this condition in isolation. Primary care staff need to be better educated about how to manage CHF patients.
Patients often felt frustrated at the time it took to up-titrate their CHF medicines, and GPs felt pressured by specialists to do it more quickly than was possible in practice.
Patients with CHF are amenable to possible future changes in monitoring regimens, provided the rationale for change is explained to them. Although all groups of professionals recognised that a NP POC device could be useful in general practice to speed up diagnosis of CHF and to produce more accurate test results, measuring NP as a regular part of patient monitoring was not seen as adding anything useful to what the professionals already did in managing their patients. Furthermore, CHFNs would be reluctant to alter patient management on the basis of a NP measurement without also knowing a patient’s renal function. Professionals also considered the NP test expensive and potentially a waste of scarce resources. Further evaluation of the evidence around NP monitoring is needed to establish what changes in routine management could lead to patient benefits.
Although CHF patients are well aware of their condition and commonly felt reassured by having it monitored by professionals, improvements are needed in the ways in which GPs communicate with patients about CKD, which is a much less readily understandable ‘diagnosis’. This finding contributed to a mixed-methods article that calls for a rethink in how doctors talk to some patients with reduced kidney health, replacing the term ‘CKD with different bands of kidney age. 45
General practitioners and practice nurses were keen for CKD to be given a different name. Practice nurses felt that using ‘kidney age’ would facilitate conversations with patients because it demedicalised the condition, and they would be keen to discuss lifestyle issues that might slow the decline. By contrast, GPs were not keen on the proposed term ‘kidney age’ because of concerns that it could still cause unnecessary anxiety if a patient’s kidney function had declined beyond what was normal for their age, believing that there was little, if anything, that could be done to slow or reverse the decline.
Appendix 10 Understanding tensions and identifying clinician agreement on improvements to early-stage chronic kidney disease monitoring in primary care: a qualitative study
Simmonds et al. 30 https://doi.org/10.1136/bmjopen-2015-010337
Appendix 11 Kidney age, not kidney disease
Stevens et al. 45 https://doi.org/10.1503/cmaj.170674
Appendix 12 Review of economic models of chronic kidney disease
Introduction
Monitoring and management of CKD aims to reduce the incidence of cardiovascular complications and slow the progression of kidney disease. 11 To fully assess the benefits and costs of CKD monitoring, a long-term model of the disease over the duration of a patient’s lifetime is required. We reviewed the literature to identify existing cost-effectiveness models among patients with CKD that could be suitable for such an assessment.
Methods
The review focused on the models that could potentially be used specifically to evaluate cost-effectiveness of eGFR monitoring in the context of current clinical guidelines. 11,43,90 Therefore, a lifetime cost-effectiveness model was included only if (1) the target population was relatively non-specific (e.g. models aimed at CKD in type 1 diabetes or patients with secondary hyperparathyroidism were excluded), (2) CKD stages were defined using eGFR (i.e. not exclusively based on proteinuria status), (3) at least two distinct pre-RRT states were included (e.g. models with states ‘pre-RRT’ and ‘RRT’ were excluded) and (4) incidence of important cardiovascular events was modelled, and could support the implementation of cardio-protective treatments (e.g. statins, blood pressure-lowering medications, antiplatelets/anticoagulants).
A pearl-growing strategy91 was chosen instead of a standard systematic review approach to more efficiently complete the review. The decision was based on a previous experience of an in-house review of models of CKD progression (unpublished), which resulted in tens of thousands of citations, the vast majority of which were not relevant. First, a set of key studies (‘pearls’) was identified by the authors using their previous experience and two published systematic reviews. 92,93 The reference lists and citations of each included study were reviewed, with relevant papers included, classified as new pearls, and the search repeated for another iteration, until no more relevant papers were identified. To ensure that no important manuscripts were missed, a scoping search was performed in Google Scholar (using the search line “cost-effectiveness ‘chronic kidney disease’ progression”), followed by a review of references (including those listed as excluded and original cost–utility analyses) in the relevant (health) economics sections of the NICE clinical guidelines for CKD. 11 All eligible published studies were included, with no restrictions on search databases or year of publication. The search was performed in January 2016 and updated in January 2019. Because the aim of the review was to identify all key studies, rather than to be exhaustive, a formal protocol registration was not sought.
A set of criteria was developed to help gauge model suitability for the research question, namely assessment of cost-effectiveness of CKD monitoring in the UK general primary care population. The criteria, based on authors’ previous experiences in disease modelling,36,94–96 included questions on generalisability of the model target population to that in UK primary care (i.e. whether or not patients with an eGFR of 60–90 ml/minute/1.73 m2 were included), alignment of the modelled cardiovascular outcomes and treatment pathways with those in the clinical guidelines [i.e. whether or not the model enables implementation of the effect of antihypertensive, low-density lipoprotein- (LDL-) lowering and antiplatelet interventions], separation of RRT modalities to allow for their different health-care outcomes and costs (i.e. dialysis and renal transplantation), and model validation. For each model, information on each criterion, as well as model identifier, data sources used for populating the model and brief description of the model structure, was extracted (IS) and summarised in a table.
Results
Four suitable key studies (‘pearls’) were identified at the first iteration32–36 in 2016–17; the review of reference lists and citations of the included studies, a scoping search in Google Scholar and review of the NICE clinical guideline did not yield further eligible studies. When the searches were repeated in January 2019, one additional newly published study was identified,37 and no further studies were identified by iterating the search. Therefore, five long-term cost-effectiveness models, potentially useful to assess CKD monitoring, were included in the review.
Detailed characteristics of the included models are presented in Table 8. Of the five models, two were developed to assess the cost-effectiveness of CVD prevention interventions (e.g. statins) in CKD,32,36 two were developed in the context of screening for CKD/proteinuria in the general population33,34,37 and one aimed to accommodate both types of interventions. 35 Only one model targeted patients with mildly reduced renal function (eGFR of ≥ 60 ml/minute/1.73 m2), which constitutes the bulk of the population with renal impairment in a primary care setting,37 although patients with an eGFR of 60–90 ml/minute/1.73 m2 were not separated from those with an eGFR of ≥ 90 ml/minute/1.73 m2.
Model identifier | Target population | Modelled interventions, cardiovascular end points and treatment pathways | Modelled CKD stages | Modelled costs and quality-of-life utilities | Data sources | Structure | Validation |
---|---|---|---|---|---|---|---|
Erickson et al.,32 2013. Cost-effectiveness of statins for primary cardiovascular prevention in chronic kidney disease | Patients with CKD stage G3a, G3b or G4 with no previous cardiovascular event | Statin therapy. The intervention is assumed to affect directly the incidence of cardiovascular events (myocardial infarction, stroke). In a sensitivity analysis, the intervention is also assumed to affect CKD progression (and hence, indirectly, incidence of cardiovascular events) | CKD stage G3a, CKD stage G3b, CKD stage G4, CKD stage G5 (includes RRT) |
|
Estimates of all parameters were based on published data (Framingham equations; studies in CKD; USRDS) | Lifetime Markov model with a 3-month transition cycle applied to simulated patients with predefined sets of baseline characteristics. Patients progress through modelled CKD stages using constant annual GFR decline. Probability of cardiovascular events and death depend on patient’s CKD stage. Cost and quality depend on patient’s CKD stage and CVD history. Potential adverse effects of statins are also implemented | Yes: model results were compared with US life tables by age, sex, CKD status and hypertension. Alternative assumptions (e.g. different speed of GFR decline, further potential adverse effects of statins) were explored, and all model inputs were varied in sensitivity analyses |
Go et al.,37 2017. Cost–utility analysis of the National Health Screening Program for chronic kidney disease in Korea | General population; results also stratified by person’s hypertension and diabetes status | CKD screening. The intervention is assumed to affect prescription of ACE inhibitors, intensive glycaemic and/or blood pressure control. This, in turn, directly affects incidence of cardiovascular events (myocardial infarction, stroke) and CKD progression and, indirectly, incidence of cardiovascular events | eGFR of ≥ 60 ml/minute/1.73 m2 (including no CKD, CKD stages G1 and G2), CKD stage G3, CKD stage G4, CKD stage G5 kidney transplant, haemodialysis and peritoneal dialysis |
|
All model parameters were derived from published data from large cohort studies in the Korean population, claims data or literature from other countries | Lifetime Markov cohort model with an annual transition cycle applied to a simulated cohort. Patients’ progress through CKD stages with transition probabilities depending on patient’s starting CKD stage, hypertension and diabetes status. Probability of cardiovascular events depends on patient’s CKD stage, and complications such as hypertension and diabetes | Yes: model’s prevalence of each health state was compared with national statistics |
Hoerger et al.,33 2010. A health policy model of CKD: 1. Model construction, assumptions, and validation of health consequences | General population; results also stratified by person’s hypertension and diabetes status | Screening for microalbuminuria. The intervention is assumed to affect prescription of ACE inhibitors or ARB treatments. This, in turn, is assumed to affect micro- to macroalbuminuria transition, mortality, CKD progression, and, indirectly, incidence of cardiovascular events (stroke, coronary heart disease) | No CKD, CKD stage G1, CKD stage G2, CKD stage G3, CKD stage G4, CKD stage G5, ESRD (all modalities combined) |
Costs: yes, health-care costs of each disease state, CKD complications and risk factors Utilities: quality-of-life utilities for each disease state; quality-of-life decrements for CKD complications |
Estimates of all model parameters were taken from the literature (e.g. Framingham equation), CKD expert panel or derived using data from NHANES and Medicare claims | Discrete-state microsimulation with annual transitions applied to a simulated cohort of patients. Patients progress forward through CKD stages according to their eGFR decrease. It is also possible to progress from micro- to macroalbuminuria. Probability of cardiovascular events depend on patient’s risk factors and CKD stage | Yes: for each CKD stage, model-predicted CKD prevalence was compared with that reported in external studies (NHANES, USRDS) |
Hoerger et al.,34 2010. A health policy model of CKD: 2. The cost-effectiveness of microalbuminuria screening | |||||||
Orlando et al.,35 2011. The chronic kidney disease model: a general purpose model of disease progression and treatment | General population | According to the authors, the model can accommodate treatment with ACE inhibitors, ARB, statin, management by a nephrologist, control of diabetes and hypertension, calcium and phosphorus management; CKD screening programme. However, only one hypothetical treatment is presented (20% relative reduction in vascular disease). It is unclear how the treatment pathway is implemented, given the many inter-relations between model states. Cardiovascular complications modelled include myocardial infarction, stroke and congestive heart failure | No CKD, CKD stage G1, CKD stage G2, CKD stage G3, CKD stage G4, CKD stage G5, kidney transplantation, haemodialysis |
|
Estimates of all model parameters were derived from literature, based on experts’ opinion or from an analysis of an external data set | Lifetime Markov Monte Carlo simulation with a monthly transition cycle. In each cycle, patient’s CKD state and complications are determined. Transition probabilities between CKD stages and to cardiovascular conditions depend on patient’s current CKD stage. In addition, at each stage, the patients could develop comorbid conditions (e.g. diabetes, hypertension or calcium/phosphorus abnormalities) | Yes: model outputs for myocardial infarction, mortality rates and GFR changes were compared with those reported in literature |
Schlackow et al.,36 2017. A policy model of cardiovascular disease in moderate-to-advanced chronic kidney disease | Patients with CKD stage G3b or more advanced, including those with a kidney transplant or on dialysis | Lipid-lowering or other treatments directly affecting risk of cardiovascular end points (major atherosclerotic or vascular event). Incidence of cardiovascular events is assumed to be affected directly by the treatment, as well as indirectly as contemporaneous CVD history affects future cardiovascular events and CKD progression in the model | CKD stage G3b, CKD stage G4, CKD stage G5, kidney transplantation, dialysis |
|
Model parameters were derived from the individual patient-level data in the SHARP randomised control trial. External literature/data sets were used for non-vascular disease risks and statin adverse effects | Lifetime Markov cohort simulation with an annual transition cycle. In each cycle, a patient’s CKD stage and cardiovascular complications were modelled. All transition probabilities were derived from parametric risk equations and depend on patient’s baseline characteristics, and time-updated CKD state and CVD history | Yes: model-predicted rates of disease events were compared with those observed in the SHARP trial and three external cohorts. Predicted 5-year risks of major events were compared with observed rates in SHARP and those predicted by other models widely used in CVD or CKD |
Parameters of the models were mostly collated from published literature and/or derived from different data sources, with only one model36 based largely on individual patient-level data. Three models separated the ESRD state into dialysis and renal transplant states,35–37 which are associated with different outcomes and costs. All models included validation of their performances. Two models enabled implementation of lipid-lowering medications, such as statins;32,36 and four models enabled implementation of blood pressure-lowering medications, such as ACE inhibitors or ARBs33,36,37 (although, in one of these, the medications were assumed to affect CKD progression only, rather than incidence of cardiovascular events directly33,34 and implementation of treatment pathway was unclear in another model35). Only one model considered heart failure as an end point, but it was unclear how the treatment effect on heart failure, as well as the impact of this treatment on other transition probabilities, were implemented. 35 None of the models satisfied all suitability criteria (Table 9).
Study/model | Suitability criteria | |||
---|---|---|---|---|
Does the target population include a separate category of patients with an eGFR of 60–90 ml/minute/1.73 m2? | Are major cardiovascular events (e.g. myocardial infarction, stroke, heart failure) included in the model and do these allow implementing effects of treatments? | Were dialysis and renal transplantation modelled separately? | Was any model validation done? | |
Erickson et al.32 | No | Yes, excluding heart failure | No | Yes |
Go et al.37 | No | Yes, excluding heart failure | Yes | Yes |
Hoerger et al.33,34 | No | No | No | Yes |
Orlando et al.35 | No | Yes, but unclear how | Yes | Yes |
Schlackow et al.36 | No | Yes, excluding heart failure | Yes | Yes |
Discussion
None of the identified models included the key elements required for the assessment of cost-effectiveness of monitoring strategies in UK primary care in the context of current clinical guidelines. Therefore, it was decided to develop a new cost-effectiveness model to support the evaluation of CKD monitoring. A possible limitation of the review is employing the pearl-growing strategy instead of performing a comprehensive systematic search. However, given the checks performed and our concurrent involvement in CKD modelling research over a number of years and awareness of available relevant data, we are confident that we have not missed relevant studies.
Appendix 13 Cost-effectiveness model in people with reduced kidney function
Schlackow et al. 38 https://doi.org/10.1371/journal.pmed.1003478
Appendix 14 Cost-effectiveness of monitoring kidney function in UK primary care
Introduction
The association between increasing kidney function impairment and increasing cardiovascular and other adverse events is well recognised. 97,98 Current guidelines for the management of CKD underline the need to monitor kidney function and reduce CVD risks accordingly. 11,42 In the UK, NICE recommends kidney function monitoring in people with, or at risk of, CKD using eGFR with the frequency of monitoring determined by the degree of kidney function impairment and the presence of proteinuria measured by the urinary ACR. 11 The evidence base for the recommended frequencies of GFR monitoring, however, is unclear. Current guidelines recommend that cardiovascular preventative treatments (i.e. statin, antihypertensive or antiplatelet) are considered for patients with reduced kidney function. For people with clinical CKD (i.e. meeting the CKD definition in the KDIGO guideline42 adopted by NICE11) statin treatment is recommended, many of whom are also indicated for antihypertensive treatment and, if they have had a prior CVD diagnosis, for antiplatelet therapy. These therapies are also widely recommended for people with CVD. 11,99 Therefore, under current guidelines, monitoring eGFR among patients with clinical CKD or with a history of CVD is not expected to modify the indication for cardiovascular therapies.
The category of patients who could derive benefit from eGFR monitoring are those with mildly impaired kidney function (eGFR of 60–90 ml/minute/1.73 m2), not yet with clinical CKD and without previous CVD, and this is the category of patients for whom we studied the cost-effectiveness of different frequencies of eGFR monitoring.
We used the CPRD CKD–CVD model, a long-term cost-effectiveness model of declining kidney function, and evolving CVD and mortality,38 to assess the long-term health outcomes and health-care costs, under kidney function monitoring regimens of different frequency in UK primary care. The comparative cost-effectiveness of different regimens in categories of patients with reduced kidney function is also summarised.
Methods
The study was developed from the perspective of the UK health service and took a lifetime patient perspective. The health benefits were measured in incremental QALYs. We report incremental costs per QALY with future costs and QALYs discounted at 3.5% per year, as recommended in the NICE reference case. 100
Study population
We assessed the value of eGFR monitoring of different frequency among people with evidence of declining kidney function (eGFR < 90 ml/minute/1.73 m2) in UK primary care. 101 From the overall open cohort of patients with eGFR 60–90 ml/minute/1.73 m2 38 and without a history of CVD in CPRD, we randomly sampled 5000 men and 5000 women while maintaining the age distribution in each group.
Kidney function monitoring regimens assessed
We assessed the following frequencies of eGFR monitoring: ‘no monitoring’, and monitoring every 1, 2, 3, 4 and 5 years. In discussions with members of the Programme Management Group and stakeholders, it was agreed that the key objective of eGFR monitoring in primary care is to guide treatments to reduce cardiovascular risk among people with reduced kidney function. The latest NICE clinical guideline for management of adults with CKD11 and adults with eGFR 60–90 ml/minute/1.73 m2 but no CKD99 was used to derive a schematic of the use of the three classes of guideline-indicated cardiovascular prevention pharmacotherapies (i.e. statins, antihypertensive and antiplatelet) to implement in the CPRD CKD–CVD model (Table 10). We used the QRISK3 risk score to annually calculate the CVD risk among people without previous CVD. 102 Atorvastatin 20 mg daily, the statin treatment recommended for people without a history of CVD but with clinical CKD, and atorvastatin 80 mg/daily, the statin treatment recommended for all other patients indicated for statin treatment, were used. We used ramipril 10 mg capsule daily, the most widely used antihypertensive in England,103 to simulate the cost of antihypertensive treatment (relative risk reductions across ACE inhibitors were used), and aspirin 75 mg daily for the use of antiplatelet treatment. The treatment effects of these three classes of treatments were informed from recent meta-analyses of randomised evidence for the therapies39–41 (Table 11).
Pharmacotherapy | Without history of CVD | With history of CVD |
---|---|---|
Statin therapy | Atorvastatin 20 mg per day for people:
|
Atorvastatin 80 mg/day |
Antihypertensive therapy | Antihypertensive treatment (ramipril 10 mg per day) for people: | |
Antiplatelet therapy | None | Aspirin 75 mg per day |
Treatment and dose (mg per day) | CKD stage, rate ratio (95% CI) | |||||
---|---|---|---|---|---|---|
G2 | G3a | G3b | G4 | G5 | Dialysis | |
Atorvastatin, 20 mga,b | ||||||
Vascular death | 0.78 (0.73 to 0.85) | 0.88 (0.80 to 0.97) | 0.90 (0.79 to 1.02) | 0.81 (0.69 to 0.95) | 0.82 (0.70 to 0.96) | 1.00 (0.87 to 1.15) |
MI | 0.65 (0.60 to 0.69) | 0.64 (0.57 to 0.73) | 0.74 (0.61 to 0.91) | 0.82 (0.62 to 1.09) | 0.83 (0.63 to 1.09) | 0.85 (0.66 to 1.10) |
Stroke | 0.77 (0.70 to 0.85) | 0.75 (0.65 to 0.87) | 0.89 (0.72 to 1.10) | 0.80 (0.62 to 1.03) | 0.81 (0.63 to 1.09) | 1.11 (0.86 to 1.42) |
Atorvastatin, 80 mga,b | ||||||
Vascular death | 0.75 (0.68 to 0.82) | 0.86 (0.76 to 0.97) | 0.88 (0.76 to 0.97) | 0.77 (0.63 to 0.94) | 0.78 (0.65 to 0.95) | 1.00 (0.84 to 1.19) |
MI | 0.59 (0.54 to 0.64) | 0.58 (0.50 to 0.68) | 0.70 (0.55 to 0.89) | 0.79 (0.56 to 1.11) | 0.80 (0.57 to 1.11) | 0.82 (0.60 to 1.13) |
Stroke | 0.73 (0.65 to 0.83) | 0.71 (0.59 to 0.85) | 0.87 (0.67 to 1.12) | 0.76 (0.56 to 1.04) | 0.77 (0.57 to 1.04) | 1.13 (0.84 to 1.53) |
ACEIc,d | ||||||
Vascular death | 0.87 (0.78 to 0.98) | 0.80 (0.69 to 0.93) | 0.80 (0.69 to 0.93) | 0.80 (0.69 to 0.93) | 0.80 (0.69 to 0.93) | 0.80 (0.69 to 0.93) |
MI | 0.79 (0.75 to 0.87) | 0.81 (0.71 to 0.93) | 0.81 (0.71 to 0.93) | 0.81 (0.71 to 0.93) | 0.81 (0.71 to 0.93) | 0.81 (0.71 to 0.93) |
Stroke | 0.85 (0.71 to 1.03) | 0.81 (0.68 to 0.96) | 0.81 (0.68 to 0.96) | 0.81 (0.68 to 0.96) | 0.81 (0.68 to 0.96) | 0.81 (0.68 to 0.96) |
Heart failure hospitalisation | 0.90 (0.74 to 1.09) | 0.75 (0.63 to 0.91) | 0.75 (0.63 to 0.91) | 0.75 (0.63 to 0.91) | 0.75 (0.63 to 0.91) | 0.75 (0.63 to 0.91) |
Aspirin 75 mgd,e | ||||||
Vascular death | 0.87 (0.65 to 1.15) | 0.87 (0.65 to 1.15) | 0.87 (0.65 to 1.15) | 0.87 (0.65 to 1.15) | 0.87 (0.65 to 1.15) | 0.87 (0.65 to 1.15) |
MI | 0.68 (0.46 to 0.99) | 0.68 (0.46 to 0.99) | 0.68 (0.46 to 0.99) | 0.68 (0.46 to 0.99) | 0.68 (0.46 to 0.99) | 0.68 (0.46 to 0.99) |
Stroke | 1.00 (0.58 to 1.72) | 1.00 (0.58 to 1.72) | 1.00 (0.58 to 1.72) | 1.00 (0.58 to 1.72) | 1.00 (0.58 to 1.72) | 1.00 (0.58 to 1.72) |
The impact of kidney function monitoring on an individual’s health is driven by the actions taken (e.g. treatments initiated) to improve the patient’s health using the acquired new information about the patient’s kidney function from monitoring. For example, statin therapy is not recommended for patients with mildly reduced kidney function (eGFR > 60 ml/minutes/1.73m2) if they are otherwise at low cardiovascular risk (i.e. without albuminuria or other cardiovascular risk factors), but is recommended for the same patients if their kidney function declines below 60 ml/minute/1.73 m2. 11,99 We assessed the value of different frequencies of GFR monitoring among people with reduced kidney function assuming that the NICE clinical guidelines for the management of CKD11 and cardiovascular risk99 were fully implemented. In our assessment, all patients indicated (because of a new cardiovascular event or increased cardiovascular risk) or, in the course of GFR monitoring (i.e. decline of kidney function), becoming indicated for particular cardiovascular therapies (i.e. statin, antihypertensive or antiplatelet) and receiving the respective guideline-indicated therapies. Statins are associated with small increases in the risk of incident diabetes, myopathy and rhabdomyolysis. 105 Declining kidney function and use of ACE inhibitors are both associated with increased risk of acute kidney injury. 106 Increased rates of these and associated health-care costs and quality-of-life effects were implemented in the monitoring scenarios simulated (Table 12).
Adverse event | Incidence per 100,000 treated (per year) | Quality-of-life decrement (per case) | Health-care cost (per case) (£, 2018) |
---|---|---|---|
Statin (excess risks related to treatment)a | |||
Myopathy | 11 | 0.0014b | 38.95b |
Rhabdomyolysis | 3.4 | 2.67%b 10% risk of death | 3827c |
Diabetes |
98 (atorvastatin 20 mg/day)d |
||
Acute kidney injury (not on ACEI)f risk due to CKD | |||
G3a | 5.5 | 0.07825 | 1995g |
G3b | 17.9 | 0.07825 | 1995 |
G4 | 46.1 | 0.07825 | 1995 |
Acute kidney injury (on ACEI)f excess risk due to ACEI | |||
G3a | 0.9 | 0.07825 | 1995 |
G3b | 3.2 | 0.07825 | 1995 |
G4 | 8.3 | 0.07825 | 1995 |
The analytical approach
The CPRD CKD–CVD model was developed using a large, open cohort of patients with reduced kidney function (eGFR < 90 ml/minute/1.73 m2). The model was derived from the UK CPRD database of routine health-care data from 388 general practices in England with the CPRD data linked to UK Hospital Episode Statistics mortality data from the Office for National Statistics and the Townsend multiple deprivation quintile. It was used to simulate the long-term health outcomes and health-care costs of patients under different monitoring regimens. The model, described in detail in Schlackow et al. ,38 uses kidney function decline and cardiovascular risk estimates, derived from the CPRD cohort and quality-of-life36,99,111 and UK-specific costs112,113 for each model state informed from published sources.
The underlying trajectories of eGFR were modelled using the statistical models for the deterioration of kidney function, outlined in Oke et al. 26 The calculated ‘observed’ eGFR categories from these models were used, rather than the calculated ‘true’ eGFR categories, to reflect the use of observed eGFR in the modelling of CVD risks, in the randomised trials for cardiovascular treatments, and during monitoring of eGFR in primary care where observed measure of kidney function informs disease management decisions.
The impact of eGFR monitoring was implemented in the models via updating of the eGFR category in the annual period in which the kidney function was ‘measured’ given the particular monitoring frequency. This updated, observed eGFR category informs the review of treatments. For example, in the scenarios of ‘no monitoring’, patients’ eGFR categories remain fixed at their entry into the model levels throughout the duration of the simulation for the purpose of eGFR-driven treatment initiation, while in the scenarios with annual monitoring, patients’ eGFR category is updated annually for the same purpose. Independently of eGFR monitoring frequency, however, patients’ underlying kidney function is updated annually in the model to drive projection of cardiovascular risk and survival.
Cost of estimated glomerular filtration rate monitoring and related cardiovascular therapies
Each eGFR monitoring cycle in simulations involves a creatinine test and a nurse visit (Table 13). The costs of atorvastatin of 20 and 80 mg per day, ramipril of 10 mg per day and aspirin of 75 mg per day were informed by the NHS drug tariff. 114 NICE guidelines informed the resources for monitoring statin treatment (i.e. an annual medication review with a nurse including cholesterol test) and antihypertensive (e.g. annual clinic blood pressure measurement with nurse appointment). 11,99,115 It was assumed that, if indicated, a single nurse appointment in a year (replaced by a GP appointment once every 5 years) would be sufficient to monitor CKD progression and all three classes of cardiovascular medications.
Category of resources | Costs (£, 2018/19) | Source and further notes |
---|---|---|
Health-care resources involved in monitoring eGFR | ||
Creatinine test; total cholesterol test | 1.10 | 2018/2019 National Cost Collection Data116 for a clinical biochemistry test |
Appointment with a nurse | 11.88 | Unit Costs of Health and Social Care 2019;117 cost of a 20-minute nurse (band 6) appointment |
Pharmacotherapies | ||
Statin (per 28 days) | 0.81 (atorvastatin 20 mg/day) | NHS Electronic Drug Tariff 114 |
1.65 (atorvastatin 80 mg/day) | ||
Antihypertensive (per 28 days) | 1.08 (ramipril 10 mg) | NHS Electronic Drug Tariff.114 Note that the Prescription Cost Analysis England 2017/2018103 identifies ramipril 10-mg capsules as the most commonly used ACEI and, therefore, ramipril 10-mg capsules have been used to cost ACEI in the scenarios |
Antiplatelet (per 28 days) | 0.71 (aspirin 75 mg) | NHS Electronic Drug Tariff 114 |
Extra annual monitoring with or without pharmacotherapies | ||
Statin | 12.98 or 34.10 (every 5 years) | Unit Costs of Health and Social Care 2019117 and 2018/2019 National Cost Collection Data;116 an appointment with a nurse, or (every 5 years) a GP, plus a non-HDL cholesterol test |
Antihypertensive | 11.88 or 33.00 (every 5 years) | Unit Costs of Health and Social Care 2019;117 an appointment with a nurse (including blood pressure test), or (every 5 years) a GP; only if statin is not used concomitantly (otherwise in same visit with statin annual check) |
Antiplatelet/anticoagulant | – | Administered at the same time with statin or antihypertensive; no extra monitoring costs |
CKD progression | – | Administered at the same time with statin, as all patients with CKD need to be prescribed statins |
Results
The baseline characteristics of the 10,000 sampled cohort of patients with reduced kidney function, used to simulate results of eGFR monitoring strategies, are presented in Table 14, overall and by age at start of monitoring.
Characteristic | 18–59 years | 60–69 years | 70–79 years | ≥ 80 years | All with eGFR 60–90 ml/minute/1.73 m2 |
---|---|---|---|---|---|
n | 5404 | 2645 | 1500 | 451 | 10,000 |
Age (years) | 50 (8) | 65 (3) | 75 (3) | 85 (4) | 59 (13) |
Male | 2581 (48%) | 1450 (55%) | 771 (51%) | 198 (44%) | 5000 (50%) |
Never smoked | 3002 (56%) | 1329 (50%) | 793 (53%) | 267 (59%) | 5391 (54%) |
Current smoker | 1178 (22%) | 450 (17%) | 204 (14%) | 45 (10%) | 1877 (19%) |
Previous smoker | 1224 (23%) | 866 (33%) | 503 (34%) | 139 (31%) | 2732 (27%) |
Body mass index | 28 (6) | 28 (7) | 27 (5) | 25 (4) | 28 (6) |
Systolic blood pressure | 132 (17) | 139 (17) | 142 (18) | 143 (18) | 136 (18) |
Diastolic blood pressure | 81 (10) | 81 (10) | 79 (10) | 77 (10) | 80 (10) |
HDL cholesterol | 1.4 (0.4) | 1.5 (0.4) | 1.5 (0.4) | 1.6 (0.5) | 1.5 (0.4) |
LDL cholesterol | 3.3 (1.0) | 3.3 (1.0) | 3.1 (1.0) | 3.1 (1.0) | 3.3 (1) |
Total cholesterol | 5.4 (1.1) | 5.3 (1.1) | 5.2 (1.1) | 5.4 (1.1) | 5.4 (1.1) |
Hypertension (Read code) | 1362 (25%) | 1083 (41%) | 697 (46%) | 191 (42%) | 3333 (33%) |
Rheumatoid arthritis | 75 (1%) | 37 (1%) | 31 (2%) | 4 (1%) | 147 (1%) |
Family history of coronary heart disease | 1110 (21%) | 470 (18%) | 225 (15%) | 29 (6%) | 1834 (18%) |
Diabetes, type 1 | 24 (0%) | 0 (0%) | 1 (0%) | 0 (0%) | 25 (0%) |
Diabetes, type 2 | 392 (7%) | 319 (12%) | 195 (13%) | 36 (8%) | 942 (9%) |
Cancer | 176 (3%) | 217 (8%) | 186 (12%) | 68 (15%) | 647 (6%) |
Albuminuria, not measured | 3249 (60%) | 1477 (56%) | 790 (53%) | 237 (53%) | 5753 (58%) |
Albuminuria, measured and normal | 2089 (39%) | 1114 (42%) | 684 (46%) | 205 (45%) | 4092 (41%) |
Indicated statins at baselinea | 1362 (25%) | 2162 (82%) | 1498 (100%) | 451 (100%) | 5473 (55%) |
Indicated antihypertensives at baselinea | 1072 (20%) | 1254 (47%) | 873 (58%) | 281 (62%) | 3480 (35%) |
Stage 1 hypertensionb and no diabetes (i.e. would be indicated antihypertensives if eGFR declines to < 60 ml/minute/1.73 m2) | 941 (17%) | 141 (5%) | 0 (0%) | 0 (0%) | 1082 (11%) |
The results from our scenarios of frequency of eGFR monitoring regimens in people with reduced kidney function, without a history of CVD, followed by guideline-recommended cardiovascular pharmacotherapies, indicate that, on average, across this target population and eGFR levels, a monitoring regimen every 4 years may be cost-effective at a threshold of £20,000 per QALY (Table 15). However, the patient’s age at the start of monitoring has an important effect on the cost-effective frequency of monitoring. At a threshold of £20,000K per QALY, the cost-effective frequencies of eGFR monitoring were every 3 years among people aged < 60 years, every 4 years among people aged 60–69 years, and monitoring was not cost-effective among people aged ≥ 70 years. Compared with no eGFR monitoring strategies, these regimens of monitoring would produce 0.2732 extra QALYs per person monitored among those aged < 60 years and 0.0524 extra QALYs among those aged 60–69 years. The components of long-term costs with monitoring strategies of different frequencies (Table 16) indicate that the direct eGFR monitoring and cardiovascular treatment costs contribute similarly to the excess costs related to different strategies.
Patient category | Total cost (£) | Life-years | QALYs | Incremental cost (vs. next less frequent monitoring) | Incremental QALYs (vs. next less frequent monitoring) | Incremental cost per QALYa (vs. next less frequent monitoring) |
---|---|---|---|---|---|---|
All | ||||||
No monitoring | 24,430 (22,160 to 26,758) | 29.2712 (28.8275 to 29.6388) | 21.858 (21.2676 to 22.3747) | – | – | – |
5-yearly monitoring | 24,705 (22,399 to 27,045) | 29.3548 (28.9076 to 29.7223) | 22.0147 (21.4174 to 22.5395) | 274 (211 to 345) | 0.1567 (0.1278 to 0.1823) | 3173 (2526 to 4221) |
4-yearly monitoring | 24,726 (22,420 to 27,066) | 29.3559 (28.9087 to 29.7236) | 22.0172 (21.4196 to 22.5423) | 22 (21 to 22) | 0.0025 (0.0021 to 0.0028) | 12,185 (10,507 to 14,298) |
3-yearly monitoring | 24,765 (22,459 to 27,106) | 29.3571 (28.9098 to 29.725) | 22.0199 (21.4218 to 22.5452) | 39 (39 to 40) | 0.0026 (0.0023 to 0.003) | 21,333 (18,476 to 25,007) |
2-yearly monitoring | 24,836 (22,528 to 27,177) | 29.3581 (28.9109 to 29.7261) | 22.0222 (21.4239 to 22.5476) | 70 (69 to 71) | 0.0024 (0.002 to 0.0027) | 41,226 (35,771 to 48,505) |
Annual monitoring | 25,033 (22,725 to 27,375) | 29.3592 (28.9119 to 29.7269) | 22.0246 (21.4259 to 22.5499) | 197 (194 to 200) | 0.0024 (0.0021 to 0.0028) | 110,451 (95,716 to 129,435) |
Age < 60 years | ||||||
No monitoring | 31,400 (28,327 to 34,559) | 38.4411 (37.8221 to 38.9387) | 28.9296 (28.1554 to 29.6081) | – | – | – |
5-yearly monitoring | 31,821 (28,701 to 34,955) | 38.5778 (37.969 to 39.0887) | 29.1945 (28.406 to 29.879) | 422 (314 to 543) | 0.2649 (0.2154 to 0.3082) | 2865 (2240 to 3860) |
4-yearly monitoring | 31,851 (28,730 to 34,984) | 38.5795 (37.9705 to 39.0903) | 29.1985 (28.41 to 29.8828) | 29 (28 to 30) | 0.004 (0.0034 to 0.0046) | 10,188 (8761 to 12,039) |
3-yearly monitoring | 31,904 (28,783 to 35,038) | 38.5813 (37.9724 to 39.0921) | 29.2028 (28.4143 to 29.8869) | 54 (53 to 55) | 0.0043 (0.0037 to 0.0049) | 17,786 (15,405 to 20,904) |
2-yearly monitoring | 31,999 (28,876 to 35,132) | 38.5829 (37.9743 to 39.0936) | 29.2066 (28.4181 to 29.8906) | 94 (92 to 96) | 0.0038 (0.0033 to 0.0044) | 33,726 (29,292 to 39,630) |
Annual monitoring | 32,260 (29,133 to 35,395) | 38.5845 (37.9761 to 39.0952) | 29.2105 (28.422 to 29.8943) | 261 (257 to 265) | 0.0039 (0.0033 to 0.0045) | 88,473 (76,709 to 103,846) |
Age 60–69 years | ||||||
No monitoring | 19,572 (17,845 to 21,294) | 22.5154 (22.1987 to 22.7779) | 16.5549 (16.0866 to 16.9694) | – | – | – |
5-yearly monitoring | 19,717 (18,000 to 21,430) | 22.5521 (22.2358 to 22.8127) | 16.606 (16.1303 to 17.0198) | 145 (125 to 167) | 0.0512 (0.0421 to 0.0591) | 4256 (3540 to 5418) |
4-yearly monitoring | 19,732 (18,016 to 21,445) | 22.5529 (22.2365 to 22.8134) | 16.6073 (16.1315 to 17.021) | 15 (15 to 16) | 0.0012 (0.0011 to 0.0014) | 14,751 (12,748 to 17,284) |
3-yearly monitoring | 19,761 (18,045 to 21,473) | 22.5536 (22.2372 to 22.8141) | 16.6085 (16.1327 to 17.0221) | 29 (28 to 29) | 0.0012 (0.001 to 0.0014) | 27,957 (24,079 to 32,892) |
2-yearly monitoring | 19,813 (18,097 to 21,525) | 22.5543 (22.2378 to 22.8147) | 16.6096 (16.1338 to 17.0232) | 52 (51 to 53) | 0.0011 (0.001 to 0.0013) | 53,329 (45,887 to 62,706) |
Annual monitoring | 19,962 (18,246 to 21,675) | 22.5549 (22.2384 to 22.8153) | 16.6108 (16.1349 to 17.0243) | 150 (148 to 151) | 0.0012 (0.001 to 0.0013) | 146,044 (125,294 to 172,860) |
Age 70–79 years | ||||||
No monitoring | 12,959 (11,826 to 14,009) | 14.5129 (14.3403 to 14.6449) | 10.5539 (10.2507 to 10.8139) | – | – | – |
5-yearly monitoring | 13,003 (11,870 to 14,054) | 14.5131 (14.3406 to 14.6451) | 10.5541 (10.2509 to 10.8142) | 45 (44 to 45) | 0.0002 (0.0002 to 0.0003) | 273,239 (231,518 to 337,069) |
4-yearly monitoring | 13,013 (11,880 to 14,063) | 14.5131 (14.3406 to 14.6451) | 10.5542 (10.2509 to 10.8142) | 9 (9 to 9) | < 0.0001 | 694,078 (590,086 to 820,561) |
3-yearly monitoring | 13,028 (11,895 to 14,079) | 14.5131 (14.3406 to 14.6451) | 10.5542 (10.2509 to 10.8142) | 16 (16 to 16) | < 0.0001 | 1,141,711 (969,606 to 1,358,290) |
2-yearly monitoring | 13,060 (11,926 to 14,110) | 14.5131 (14.3406 to 14.6452) | 10.5542 (10.251 to 10.8142) | 31 (31 to 32) | < 0.0001 | 2,240,790 (1,902,092 to 2,693,706) |
Annual monitoring | 13,153 (12,020 to 14,204) | 14.5131 (14.3406 to 14.6452) | 10.5542 (10.251 to 10.8142) | 94 (93 to 95) | < 0.0001 | 11,647,270 (9,879,952 to 14,013,001) |
Age ≥ 80 years | ||||||
No monitoring | 7572 (6945 to 8176) | 8.1008 (8.0015 to 8.1775) | 5.8227 (5.6568 to 5.9727) | – | – | – |
5-yearly monitoring | 7600 (6973 to 8204) | 8.1008 (8.0015 to 8.1775) | 5.8227 (5.6568 to 5.9727) | 28 (27 to 28) | 0 | N/A |
4-yearly monitoring | 7605 (6978 to 8209) | 8.1008 (8.0015 to 8.1775) | 5.8227 (5.6568 to 5.9727) | 5 (5 to 5) | 0 | N/A |
3-yearly monitoring | 7614 (6987 to 8218) | 8.1008 (8.0015 to 8.1775) | 5.8227 (5.6568 to 5.9727) | 9 (8 to 9) | 0 | N/A |
2-yearly monitoring | 7631 (7004 to 8235) | 8.1008 (8.0015 to 8.1775) | 5.8227 (5.6568 to 5.9727) | 17 (17 to 18) | 0 | N/A |
Annual monitoring | 7683 (7056 to 8287) | 8.1008 (8.0015 to 8.1775) | 5.8227 (5.6568 to 5.9727) | 52 (51 to 52) | 0 | N/A |
Patient category | Total cost (£) | eGFR monitoring cost (£) | Cost of CVD treatments, monitoring and adverse effects (£) | Hospital care costs (£) |
---|---|---|---|---|
All | ||||
No monitoring | 24,430 (22,135 to 26,760) | – | 807 (787 to 824) | 23,623 (21,344 to 25,958) |
5-yearly monitoring | 24,705 (22,396 to 27,053) | 83 (81 to 83) | 1193 (1170 to 1213) | 23,429 (21,123 to 25,775) |
4-yearly monitoring | 24,726 (22,417 to 27,075) | 102 (100 to 103) | 1197 (1174 to 1217) | 23,427 (21,120 to 25,773) |
3-yearly monitoring | 24,765 (22,455 to 27,114) | 133 (131 to 135) | 1207 (1184 to 1227) | 23,425 (21,117 to 25,771) |
2-yearly monitoring | 24,836 (22,523 to 27,183) | 197 (194 to 199) | 1216 (1193 to 1236) | 23,423 (21,114 to 25,769) |
Annual monitoring | 25,033 (22,716 to 27,380) | 387 (381 to 391) | 1225 (1202 to 1245) | 23,421 (21,112 to 25,766) |
Age < 60 years | ||||
No monitoring | 31,400 (28,239 to 34,570) | – | 764 (737 to 787) | 30,636 (27,511 to 33,802) |
5-yearly monitoring | 31,821 (28,650 to 34,975) | 106 (105 to 108) | 1413 (1381 to 1441) | 30,302 (27,160 to 33,433) |
4-yearly monitoring | 31,851 (28,678 to 35,004) | 131 (129 to 133) | 1420 (1388 to 1449) | 30,299 (27,157 to 33,429) |
3-yearly monitoring | 31,904 (28,730 to 35,057) | 173 (170 to 175) | 1436 (1404 to 1464) | 30,295 (27,153 to 33,424) |
2-yearly monitoring | 31,999 (28,821 to 35,151) | 256 (252 to 259) | 1450 (1418 to 1479) | 30,292 (27,150 to 33,419) |
Annual monitoring | 32,260 (29,076 to 35,412) | 506 (498 to 512) | 1465 (1433 to 1493) | 30,289 (27,146 to 33,415) |
Age 60–69 years | ||||
No monitoring | 19,572 (17,837 to 21,294) | – | 980 (964 to 995) | 18,591 (16,854 to 20,321) |
5-yearly monitoring | 19,717 (17,991 to 21,442) | 65 (64 to 66) | 1113 (1095 to 1128) | 18,539 (16,811 to 20,254) |
4-yearly monitoring | 19,732 (18,006 to 21,458) | 79 (78 to 80) | 1115 (1097 to 1129) | 18,538 (16,810 to 20,254) |
3-yearly monitoring | 19,761 (18,035 to 21,487) | 104 (102 to 105) | 1120 (1102 to 1135) | 18,537 (16,809 to 20,253) |
2-yearly monitoring | 19,813 (18,087 to 21,539) | 152 (150 to 154) | 1124 (1106 to 1139) | 18,536 (16,808 to 20,252) |
Annual monitoring | 19,962 (18,237 to 21,689) | 298 (294 to 302) | 1128 (1111 to 1143) | 18,535 (16,808 to 20,252) |
Age 70–79 years | ||||
No monitoring | 12,959 (11,813 to 14,009) | – | 766 (756 to 774) | 12,193 (11,048 to 13,241) |
5-yearly monitoring | 13,003 (11,857 to 14,054) | 44 (44 to 44) | 766 (757 to 774) | 12,193 (11,048 to 13,241) |
4-yearly monitoring | 13,013 (11,866 to 14,063) | 53 (53 to 54) | 766 (757 to 774) | 12,193 (11,048 to 13,241) |
3-yearly monitoring | 13,028 (11,882 to 14,079) | 69 (68 to 70) | 767 (757 to 774) | 12,193 (11,048 to 13,241) |
2-yearly monitoring | 13,060 (11,913 to 14,110) | 100 (99 to 101) | 767 (757 to 774) | 12,193 (11,048 to 13,241) |
Annual monitoring | 13,153 (12,007 to 14,204) | 194 (192 to 196) | 767 (757 to 774) | 12,193 (11,048 to 13,241) |
Age ≥ 80 years | ||||
No monitoring | 7572 (6942 to 8168) | – | 444 (439 to 448) | 7128 (6498 to 7723) |
5-yearly monitoring | 7600 (6970 to 8196) | 28 (27 to 28) | 444 (439 to 448) | 7128 (6498 to 7723) |
4-yearly monitoring | 7605 (6975 to 8201) | 33 (33 to 33) | 444 (439 to 448) | 7128 (6498 to 7723) |
3-yearly monitoring | 7614 (6984 to 8210) | 41 (41 to 42) | 444 (439 to 448) | 7128 (6498 to 7723) |
2-yearly monitoring | 7631 (7001 to 8227) | 59 (58 to 59) | 444 (439 to 448) | 7128 (6498 to 7723) |
Annual monitoring | 7683 (7053 to 8279) | 111 (110 to 112) | 444 (439 to 448) | 7128 (6498 to 7723) |
Discussion
In the present study, the cost-effectiveness of different intervals of eGFR monitoring among people with eGFR 60–90 ml/minute/1.73 m2 but without a history of CVD, and without albuminuria, were evaluated. Among these patients, the cost-effective interval of monitoring ranged from every 3 years among patients aged < 60 years to no monitoring among those aged ≥ 70 years and produced from 0.27 to 0.05 extra QALYs per person monitored across the age categories < 60 years and 60–69 years. There was a gradual decrease in the indicated cost-effective frequency of monitoring with increasing age and monitoring was not cost-effective above the age of 70 years.
Current NICE guidance suggests that less than annual monitoring of kidney function is sufficient among patients with eGFR 60–90 ml/minute/1.73 m2 but does not recommend a particular frequency of monitoring. 11 Although our results are concordant with this guidance, they go further to suggest that the optimal interval of monitoring would depend on the patient’s age, with older patients unlikely to benefit cost-effectively from eGFR monitoring.
In the present study, we focused on people with only mildly reduced kidney function and without a history of CVD. This was motivated by the observation that people with eGFR< 60 ml/minute/1.73 m2 or a history of CVD are already indicated for the cardiovascular prevention therapies used with monitoring. 11 Monitoring eGFR among these patients for the purpose of initiating cardiovascular treatments, therefore, is unlikely to bring any further benefits under the assumption of full uptake and persistence with the indicated statin, antihypertensive and antiplatelet treatments.
In the present study, the effect of monitoring for albuminuria was not investigated because of a lack of sufficient ACR test data in primary care to allow models of trajectories of ACR to be developed. Annual screening for albuminuria, however, has been previously reported to be cost-effective in people with a history of diabetes. 7 We also did not model effects of lipid-lowering and glycaemic control interventions on CKD progression as no reliable evidence was identified to suggest that these treatments modified progression through CKD stages. Nevertheless, treatments that protect renal function have the potential to substantially improve the cost-effectiveness of CKD monitoring in primary care.
In the cost-effectiveness study, the observed eGFR during monitoring is used to inform treatment decisions during monitoring, and probabilities of misclassification due to test variability are explicitly modelled as recommended in economic evaluations of frequency of disease monitoring to penalise inappropriate actions because of this misclassification. In the context of the present study, however, there are no clear penalties for misclassification as the cardiovascular treatments used will be reducing cardiovascular risk even if misclassification of eGFR category has occurred (and the scenarios already include the safety issues with these medications). Furthermore, CVD risks in the present study, as well as treatment effectiveness of cardiovascular therapies in the randomised studies, were evaluated using observed measures of kidney function in the respective patients under rates of misclassification that were likely to be similar. Nevertheless, future studies could investigate the net effects of monitoring under an assumption for further dis-benefit from ‘earlier’ initiation of cardiovascular therapies.
A large, UK primary care data set and a detailed individual patient data-driven cost-effectiveness model informed the present analysis of frequency of eGFR monitoring in primary care. However, a number of potential limitations need to be acknowledged. First, we did not explicitly include screening for eGFR < 90 ml/minute/1.73 m2 in our model, opting instead to study kidney function monitoring once patients were observed to have eGFR < 90 ml/minute/1.73 m2. Although the vast majority of such patients in CPRD did not have a corresponding kidney function diagnosis code, their identification with automatic review of electronic records in primary care is likely to be straightforward. Second, although all patients in our target patient cohort did not have evidence of albuminuria at baseline (thus defined as without clinical CKD), we did not model their albuminuria status over time, nor did we investigate the effects of ACR monitoring as we did not have data on which to base such analyses. Similarly, blood pressure changes over time have also not been modelled. Future model development including modelling time trajectories of ACR and blood pressure in this population will be informative. Third, the present analyses focused exclusively on monitoring eGFR at earlier stages of kidney function decline to optimise cardiovascular risk reduction. Monitoring kidney function to guide early identification of people requiring dialysis/transplant or other interventions (e.g. vaccinations recommended at different CKD stages) was beyond the scope of this work. Finally, the present analyses are importantly based on the available patient data in routine primary care records. These data are influenced by indication-based measurement of disease and other clinical parameters (e.g. blood pressure is measured more often among people with higher blood pressure) with missing data probably not missing at random. Such data issues might have transcended in the disease model and, therefore, further validation of the model in different cohorts are required to confirm its validity.
In conclusion, the present analyses indicate that eGFR monitoring is indicated among people with mildly reduced kidney function and without CVD history up to the age of 70 years. In such patients, the optimal frequency of monitoring depends on the patient’s age with patients aged < 60 years indicated for eGFR monitoring every 3 years, whereas those aged between 60 and 69 years are indicated for eGFR monitoring every 4 years. Monitoring eGFR among people aged ≥ 70 years, without CVD history and with only mildly reduced kidney function is not indicated.
Appendix 15 B-type natriuretic peptide-guided treatment for heart failure
McLellan et al. 67 https://doi.org/10.1002/14651858.CD008966.pub2
Appendix 16 Natriuretic peptide-guided treatment for heart failure: a systematic review and meta-analysis
McLellan et al. 68 https://doi.org/10.1136/bmjebm-2019-111208
Appendix 17 Remote monitoring, telemonitoring and structured telephone support to monitor heart failure: systematic review and meta-analysis
This project was conducted by Julie McLellan, Nicola Pidduck, Clare J Taylor, Kathryn S Taylor and Rafael Perera.
Introduction
Description of the condition
Heart failure is a condition in which the heart is unable to pump enough blood to supply sufficient oxygen to the rest of the body, leading to breathlessness, fatigue and oedema. 118 In the UK, an estimated 920,000 people have heart failure. Incidence increases with age and is highest in adults aged > 75 years. With an ageing population and improved survival of patients with heart disease, it is predicted that there will be an increasing prevalence of heart failure in the future. 119
The goals of treatment in patients with established heart failure are to relieve symptoms, prevent hospital admission and, when possible, improve survival. 13,120 A range of drugs, in addition to devices and rehabilitation, have been shown to have a role in improving outcomes. 121 Diuretic treatment is effective in reducing fluid overload in all types of heart failure. 13 ACE inhibitors, beta-adrenoceptor blocking agents and mineralocorticoid receptor antagonists have shown prognostic benefit in patients with heart failure with reduced ejection fraction, but, to date, the same survival gains have not been shown in trials of heart failure with preserved ejection fraction. 122 Recommended therapies are often underutilised and only a small proportion of heart failure patients receive optimal treatment. 123
An important aspect of good management is long-term monitoring. Monitoring has the potential to optimise treatment for heart failure by identifying patients who do and patients who do not need further drug treatment and can improve patient quality of life, both in terms of physical and emotional well-being. 124
Description of the intervention and why it is important to do this review
Remote monitoring, a collective term for TM and STS, of heart failure is increasingly becoming an option for patients as a result of advancing technology and increasing familiarity with its use. RM aims to collect and transmit physiological data through devices in the patient’s own home that could potentially increase early detection of clinical deterioration, improve patient’s quality of life and decrease health-care delivery costs.
There have been a number of systematic reviews and meta-analyses on the effectiveness of RM. In the previous 5 years, there have been 15 meta-analyses and four in 2018 alone. 125–128 Findings from previous reviews have been inconsistent, although most favour using RM to reduce all-cause hospital admission. Primary research continues to be undertaken; in the preceding year, a further five studies have been published in this field. 129–136 Two meta-analyses have been prominent in this research area. The first by Pandor et al. 137 was published in 2013 as part of a Health Technology Assessment programme, and updated earlier reviews. The second, in 2015, by Inglis et al. ,73 was a Cochrane review, which also updated earlier reviews, and was selected as the main source of evidence for the 2018 NICE CHF guideline update. 13 These two systematic reviews examined broadly the same studies, although Inglis et al. 73 included only RCTs. Both reviews reported trends towards RM reducing all-cause mortality and all-cause hospital admission, although, for Pandor et al. ,137 it was inconclusive. They varied in their findings for heart failure-related hospital admission. Since the publication of Inglis et al. ,73 two further large RM studies have reported findings: the Telemedical Interventional Management in Heart Failure II (TIM-HF2)129,130 trial, with the second-largest number of participants to date, and the Better Effectiveness After Transition – Heart Failure (BEAT-HF)138,139 trial, with the fourth-largest number of participants to date.
The 2010 NICE guideline70 did not recommend the use of RM for management of CHF because it was unclear whether any of the reported benefits were due specifically to RM or to additional monitoring in general. However, NICE did recommend further research into the effectiveness of home monitoring for patients with CHF. Despite a large number of studies since 2010, the latest NICE guideline update in 201813 also did not recommend the use of RM for management of CHF because of lack of evidence of effectiveness. A further research recommendation for this area was not included in the 2018 guideline. NICE explain that this was because of the rapidly advancing nature of technology in the field, and the lack of any plateau in terms of the technology available to clinicians, which makes it difficult to future-proof any further research recommendations. Other factors preventing NICE from granting approval are the lack of consensus between manufacturers about the appropriate interface and between clinicians about what physiological parameters should be measured.
However, the NICE CHF guideline of 2018 does acknowledge that developments in information technology and in the use of telephone-based and direct TM technologies have the potential to improve further the delivery of health care. The NHS Technology Enabled Care Services: Resource for Commissioners,71 in meeting the NHS Five Year Forward View,72 highlights RM of vital health signs as a key enabler in detecting deterioration in a patient’s health.
Therefore, despite the lack of NICE recommendations for the use of RM, the potential for RM technology is recognised. Widespread use of smart technology and further developments over time will mean that the question of whether or not RM for CHF is effective will continue to be clinically relevant.
Objective
The objective of this study was to evaluate the clinical effectiveness of RM (home TM and/or STS) interventions, compared with standard care, in adults with heart failure for all-cause mortality, all-cause hospital admission, heart failure-related hospital admission, length of stay, health-related quality of life, adherence, acceptability, heart failure knowledge and self-care in RCTs. This will update the existing systematic review by Inglis et al. 73
Methods
This systematic review was reported in line with the Preferred Reporting Items for Systematic Reviews and Meta-Analyses (PRISMA) statement. 140 The protocol was registered with PROSPERO (CRD42019134922).
Search strategy
The search strategy was based on the search strategies published in Inglis et al. 73 Searches were conducted in the Cochrane Central Register of Controlled Trials, Ovid® (Wolters Kluwer, Alphen aan den Rijn, the Netherlands) MEDLINE® ePub Ahead of Print, MEDLINE In-Process & Other Non-Indexed Citations, Ovid MEDLINE Daily and Ovid MEDLINE, EMBASE™ (Elsevier, Amsterdam, the Netherlands), Cumulative Index to Nursing and Allied Health Literature, Science Citation Index Expanded (Clarivate Analytics, Philadelphia, PA, USA), Conference Proceedings Citation Index – Science (Clarivate Analytics), Allied and Complementary Medicine Database, and the Institute of Electrical and Electronic Engineers’ digital library (IEEE Xplore®, Institute of Electrical and Electronic Engineers, Piscataway, NJ, USA). No restriction was made for language or publication type. All publications from January 2015 to February 2019 were considered for inclusion.
Study selection
Two reviewers (JM and NP) screened the title and abstract of publications independently, to identify potentially relevant studies. Considering the full text of these publications, two reviewers (JM and NP) selected studies independently to be included in the review by using predetermined inclusion criteria. When a relevant study was identified from a conference abstract, the study was included provided a full-text peer-reviewed publication was available, even if it was published after February 2019. In all cases, disagreements about study inclusion were resolved by consensus; a third reviewer was consulted if disagreements persisted.
All studies included by Inglis et al. 73 were included in the review, in addition to new studies meeting the same inclusion criteria, as outlined in the following section.
Inclusion criteria
Study design
All RCTs that evaluated interventions of non-invasive TM or STS compared with usual post-discharge care.
Participants
-
Adults (aged ≥ 18 years) with a definite diagnosis of heart failure living in the community [including a relative’s home, but excluding nursing homes or convalescence (rehabilitation) homes].
-
Participants may be recently discharged from an acute setting or already managed in the community.
-
No restriction on sex or ethnicity.
-
Studies with participants who had general cardiac disorders were excluded.
Intervention
-
Telemonitoring (non-invasive): using patient-initiated external electronic devices remotely, with transfer of physiological data from the patient to the health-care provider by digital/broadband/satellite/wireless or Bluetooth® (Bluetooth Special Interest Group, Kirkland, WA, USA) transmission.
-
Structured telephone support: monitoring or self-monitoring of telephone contact between patients and health-care providers using simple telephone technology, although data may be collected by and stored on computers. Data reported to be symptoms and/or physiological data. Studies of STS to include patients with access to touch-tone telephones.
-
Intervention to be delivered as the only aftercare.
-
Intervention to be targeted towards patients, rather than caregivers.
-
Studies with interventions that included home visits by specialised heart failure health-care professionals or study personnel for the purpose of education or clinical assessment, other than an initial visit to set up equipment, were excluded.
Control/comparator
Usual care was defined as standard post-discharge care without intensified attendance at cardiology clinics or clinic-based heart failure disease management programme, or home visits by specialised heart failure health-care professionals or study personnel for the purpose of education or clinical assessment, other than an initial visit to set up equipment.
Studies with any previous exposure to TM or STS for the intervention or usual-care arms prior to the start of the study were excluded.
Outcome(s)
-
Primary outcomes: all-cause mortality, all-cause hospital admission (at least once during the follow-up period), heart failure-related hospital admission (at least once during the follow-up period).
-
Secondary outcomes: length of stay (days in hospital), health-related quality of life (assessed by a validated questionnaire), adherence (compliance), acceptability (satisfaction and usability), heart failure knowledge and self-care.
Studies that did not report any data in an extractable format for the above outcomes were excluded.
Data extraction and quality assessment
Data extraction and methodological quality assessment were conducted by one reviewer and independently checked by a second reviewer for accuracy. A data extraction form was used to pilot an initial sample of 10 studies, before proceeding to extract data for all studies. Extracted data included participant details (age, sex, ethnicity, autonomy, comorbidities), study details (design type, country, intervention and control details, follow-up period, control group, source of funding) and outcome data.
The methodological quality of studies was assessed using the same criteria as Inglis et al. ,73 which used the Cochrane Risk of Bias tool. 141 This considers potential biases in studies owing to selection bias (random sequence generation, allocation concealment), blinding of outcome assessors, incomplete outcome data and selective reporting. Blinding of participants is not feasible for this type of intervention; therefore, only blinding of outcome assessment was assessed. Methodological quality was assessed for the primary outcomes only because of the lack of reporting for secondary outcomes. Publication bias (small-study effect) was explored using funnel plots. 142
Disagreements about extracted data and assessments of methodological quality were resolved by discussion or referral to a third reviewer.
Data analysis
For the primary outcomes (all-cause mortality, all-cause hospital admission and heart failure-related hospital admission), the number of people who had an event at the end of the study follow-up in each arm were pooled in a meta-analysis using a fixed-effects model based on the Mantel–Haenszel method and specifying a RR effect measure, with 95% CIs. 142
A fixed-effects model, based on the inverse variance method, was used to pool quality-of-life data at the end of the trial using the SMD as effect measure. When required, data were inverted to ensure that all questionnaire scores had the same direction of effect. The standard error (SE) of the SMD was estimated from the data using methods derived from the Cochrane handbook. 142 The correlation coefficient was assumed to be 0.5. 143 When it was not possible to calculate the SE, it was imputed using the average of the SEs from studies using similar quality-of-life questionnaires.
Owing to the variation in data reported for length of stay, adherence, acceptability, heart failure knowledge and self-care, it was not possible to pool these data, so they were reported narratively, as in Inglis et al. 73
Statistical heterogeneity was measured using the I2 statistic. 142
Subgroup analysis
In line with Inglis et al. ,73 we investigated potential causes of heterogeneity using subgroup analysis:
-
Technology – comparison of:
-
telephone calls
-
videophone
-
interactive voice response – a manual input of data using a telephone keypad in response to questions from a computerised interactive voice response system and computer-assisted telephone interviewing
-
complex/clinical TM – automatic transmission of physiological data from a measuring device to a central server via telephonic, satellite or broadband capabilities for interpretation by the health-care team
-
mobile phones or personal digital assistant (PDA) – automatic transmission of physiological data from a measuring device to a mobile phone or PDA.
-
-
Telemonitoring intensity – office hours (i.e. Monday–Friday, 09.00–17.00), compared with 24 hours per day, 7 days per week.
-
Structured telephone support focus – clinical monitoring of physiological data with clinical support, compared with self-management.
-
Publication date – comparisons between pre 2000, 2000–7, 2008–9 and post 2010. The year 2000 marks significant developments in heart failure treatments. The NICE CHF guideline was updated in 2007 and a new guideline was issued in 2010. The latest update of the NICE heart failure guidelines in 2018 will not have had sufficient time to show any effect. 13
-
Mean/median age – < 70 years, compared with ≥ 70 years.
Meta-regression was conducted for the primary outcomes to investigate whether or not the effect size depended on the average age of patients in the study or publication date (variables on a continuous scale).
Owing to the considerable heterogeneity found in the quality-of-life data, the robustness of the pooled results was tested using a random-effects model as a post hoc analysis.
Sensitivity analysis
Prespecified sensitivity analyses were performed to assess if the effect estimates were sensitive to the length of follow-up. Analyses were restricted to studies with follow-up periods of > 6 months.
Post hoc sensitivity analyses were conducted for the quality-of-life outcome to ensure that our effect estimates were not sensitive to our choice of questionnaire, our choices of included data and imputations. Analyses were rerun using an alternative imputation of the SE, based on all other included studies, rather than just those based on the same quality-of-life questionnaire, and, in a separate analysis, excluding studies for which imputed data had been used.
All analyses were carried out using Stata® version 14.236 (StataCorp LP, College Station, TX, USA) and/or RevMan version 5.337 (The Cochrane Collaboration, The Nordic Cochrane Centre, Copenhagen, Denmark).
Results
Search results
The search identified 3013 records, from which 12 new studies129–136,138,139,144–151 met the inclusion criteria and could be added to the existing 41 studies identified by Inglis et al. 73 (Figure 3).
FIGURE 3.
Flow chart of study selection.

Study characteristics
The characteristics of the 12 new included studies are provided in Tables 17 and 18. Of the 12 new included studies, three studies examined STS144–148 and nine studies examined TM. 129–136,138,139,149–151 The characteristics of the 41 existing studies are provided in Inglis et al. 73 In STS studies, the total number of patients increased to 10,296, and in TM studies, the total number of patients increased to 8329, compared with 9332 and 3860, respectively, in Inglis et al. 73 Study sample sizes ranged from 34 to 1653 participants in STS studies, and from 20 to 1571 participants in TM studies.
Study (first author and year of publication) | Study name | RM type | Country | Total number of patients | RM intervention description | Comparator | Follow-up duration (months) | Study primary outcome | Study secondary outcome |
---|---|---|---|---|---|---|---|---|---|
Bekelman 2015144 | PCDM for Heart Failure | STS | USA | 392 |
|
Usual care | 12 |
|
|
Dang 2017145,146 | – | STS | USA | 61 |
|
Usual care | 3 |
|
– |
Karhula 2015149 | Renewing Health Finland | TM | Finland | 269 |
|
Usual care | 12 |
|
|
Koehler 2018129,130 | TIM-HF2 | TM | Germany | 1571 |
|
Usual care | 12 | Days (%) lost because of unplanned cardiovascular hospital admission or all-cause mortality |
|
Kotooka 2018131,132 | HOMES-HF | TM | Japan | 183 | Electronic scale with a body composition metre, a sphygmomanometer and a device called a ‘receiver’, which received acquired physiological data wirelessly and transmitted the data to the central web server via the internet | Usual care | 15 |
|
|
Olivari 2018133 | Renewing Health Italy | TM | Italy | 399 | Personal Health System, composed of a wearable Wrist Clinic device and a digital weight scale for clinical data collection | Usual care | 12 | All-cause mortality or at least one hospitalisation for HF |
|
Ong 2016138,139 | BEAT-HF | TM | USA | 1437 | Three components:
|
Usual care | 6 | All-cause re-admission rate (180 day) |
|
Pedone 2015150 | – | TM | Italy | 96 |
|
Standard care | 6 | All-cause hospital admission or all-cause mortality (180 days) | – |
Pérez-Rodríguez 2015151 | – | TM | Mexico | 40 |
|
Traditional | 3 |
|
– |
Ritchie 2016147,148 | E Coach | STS | USA | 511 |
|
Usual care | 1 | All-cause rehospitalisation (30 days) |
|
Smeets 2018134 | Cardio-Coach | TM | Belgium | 24 | Two-way communication platform connected to RM devices. All vital signs measurements were transmitted automatically to the CardioCoach application, without manual patient input | Usual care | 6 |
|
– |
Wagenaar 2019135,136 | e-Vita HF | TM | The Netherlands | 450 |
|
Usual care | 12 | Self-care |
|
Study (first author and year of publication) | Study name | RM type | Age (years)a | Sex (male %) | NYHA class (%) | Acute or stable | Inclusion criteria |
---|---|---|---|---|---|---|---|
Bekelman 2015144 | PCDM | STS | 68 | 96.60 | NR | Mixed acute and stable |
|
Dang 2017145,146 | – | STS | 55.3 ± 9.8 | 39 | Class I: 47.5, class II: 37.7, class III or IV: 14.8 | Stable |
|
Karhula 2015149 | Renewing Health Finland | TM | 69.1 ± 9.1 | 66.2 | NR | Stable |
|
Koehler 2018129,130 | TIM-HF2 | TM | 70 | 70 | Class I: 1, class II: 52, class III: 47 | Discharged in previous 12 months |
|
Kotooka 2018131,132 | HOMES-HF | TM | 66 | 60 | Class II: 78, class III: 22 | Acute |
|
Olivari 2018133 | Renewing Health Italy | TM | 79.9 | 64 | Class II: 48, class III: 47, class IV: 5 | Acute |
|
Ong 2016138,139 | BEAT-HF | TM | 73 (median) | 53.8 | Class III or IV: 61.2b | Acute |
|
Pedone 2015150 | – | TM | 80 ± 7 | 39 | Class II: 32, class III: 57, class IV: 11 | Mixed acute and stable |
|
Pérez-Rodríguez 2015151 | – | TM | 68.5 | 65 | NR | Stable |
|
Ritchie 2016147,148 | E Coach | TM | 63 ± 12 | 51.4 | NR | Acute |
|
Smeets 2018134 | CardioCoach | TM | 61.75 | 62.3 | Class II: 41.8, class III: 45.9 | Acute |
|
Wagenaar 2019135,136 | e-Vita HF | TM | 66.8 ± 11.0 | 74.2 | Class I: 44, class II: 36, class III: 14, class IV: 6c | Stable |
|
Risk of bias
We considered the methodological quality of the 12 new included studies (Figure 4). Two studies131,132,147,148 were assessed as being of high quality, as they were deemed to be at ‘low’ risk of bias across all domains. The majority of studies were assessed as being at low risk of bias for random sequence generation, allocation concealment and selective reporting. However, all studies, bar two, were assessed as having an unclear or high risk of bias for blinding of outcome assessment. Inglis et al. 73 assessed four studies as being at low risk of bias across all domains. 152–155
FIGURE 4.
Risk-of-bias summary (12 new included studies).

Combining the risk-of-bias assessments for the 12 new included studies with the 39 existing studies from Inglis et al. 73 in a risk-of-bias graph (Figure 5) resulted in little variation from the previous graph reported by Inglis et al. 73 Overall, for the majority of risk-of-bias domains, studies were split between having a low or unclear risk of bias, although, for selective reporting, > 80% of studies were considered to be at low risk of bias. Few studies were considered to be at high of risk of bias in any domain, except for attrition bias, for which approximately 25% of studies were considered to be at high risk of bias.
FIGURE 5.
Risk-of-bias graph (all 53 studies).

We considered publication bias for all 53 studies based on the primary outcomes and, similar to Inglis et al. ,73 found evidence of strong publication bias (Figure 6).
FIGURE 6.
Funnel plots. (a) Funnel plot comparison for all-cause mortality for STS, (b) funnel plot comparison for all-cause mortality for TM, (c) funnel plot comparison for all-cause hospital admission for STS, (d) funnel plot comparison for all-cause hospital admission for TM, (e) funnel plot comparison for heart failure hospital admission for STS and (f) funnel plot comparison for heart failure hospital admission for TM.

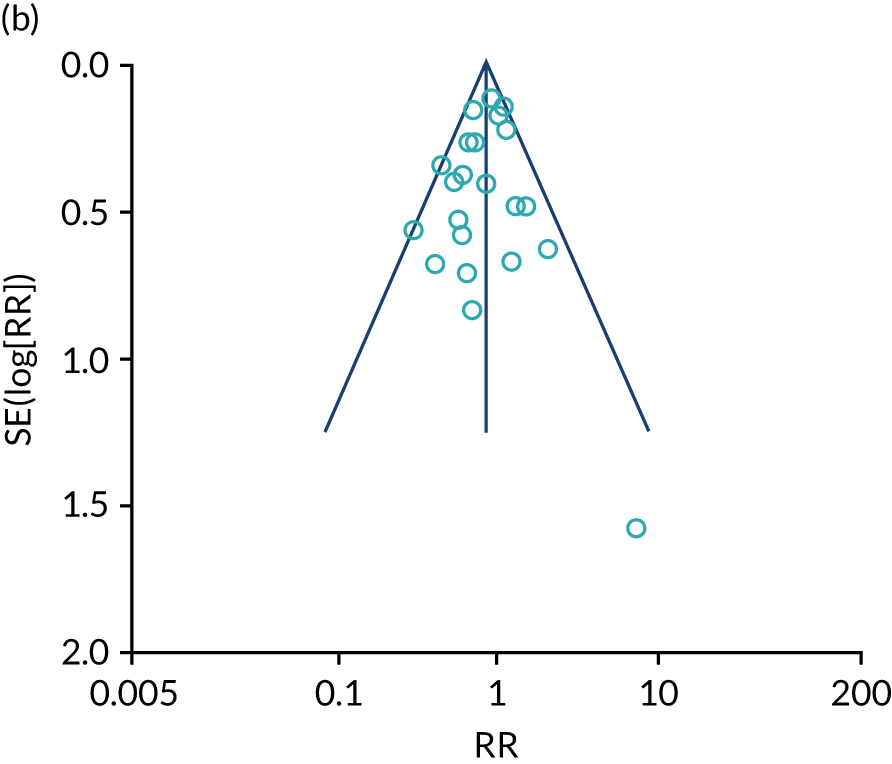
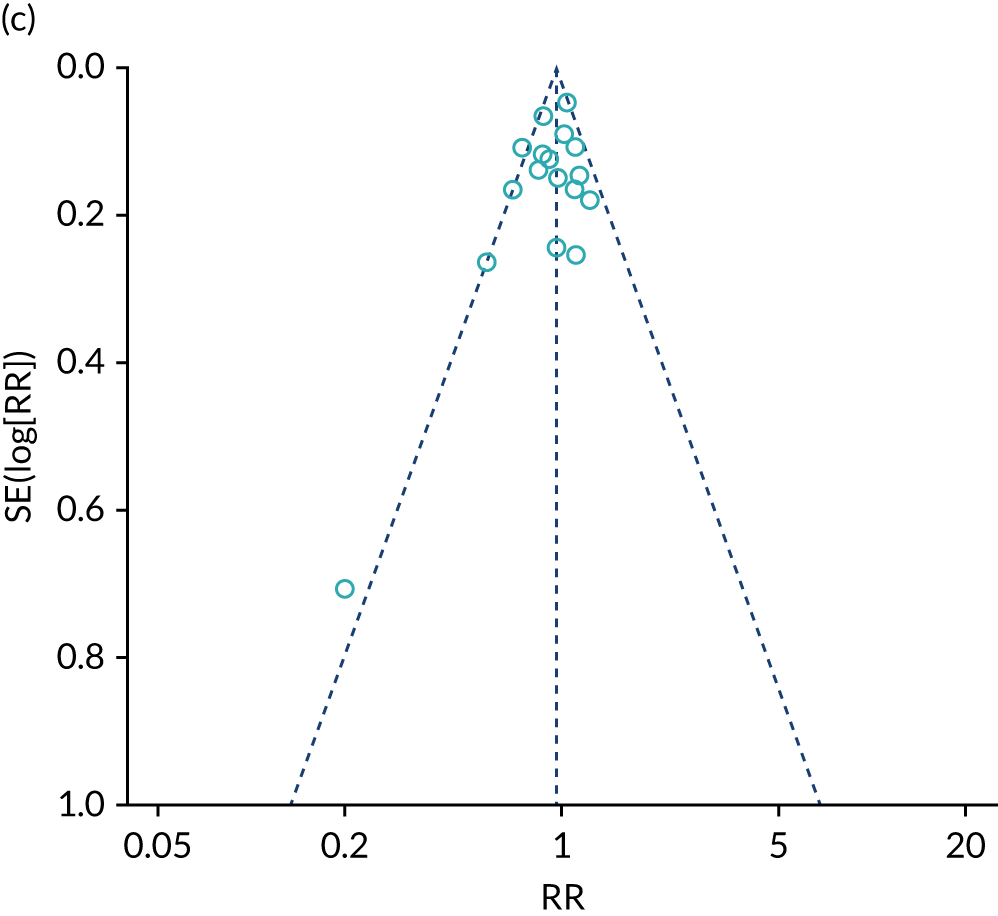
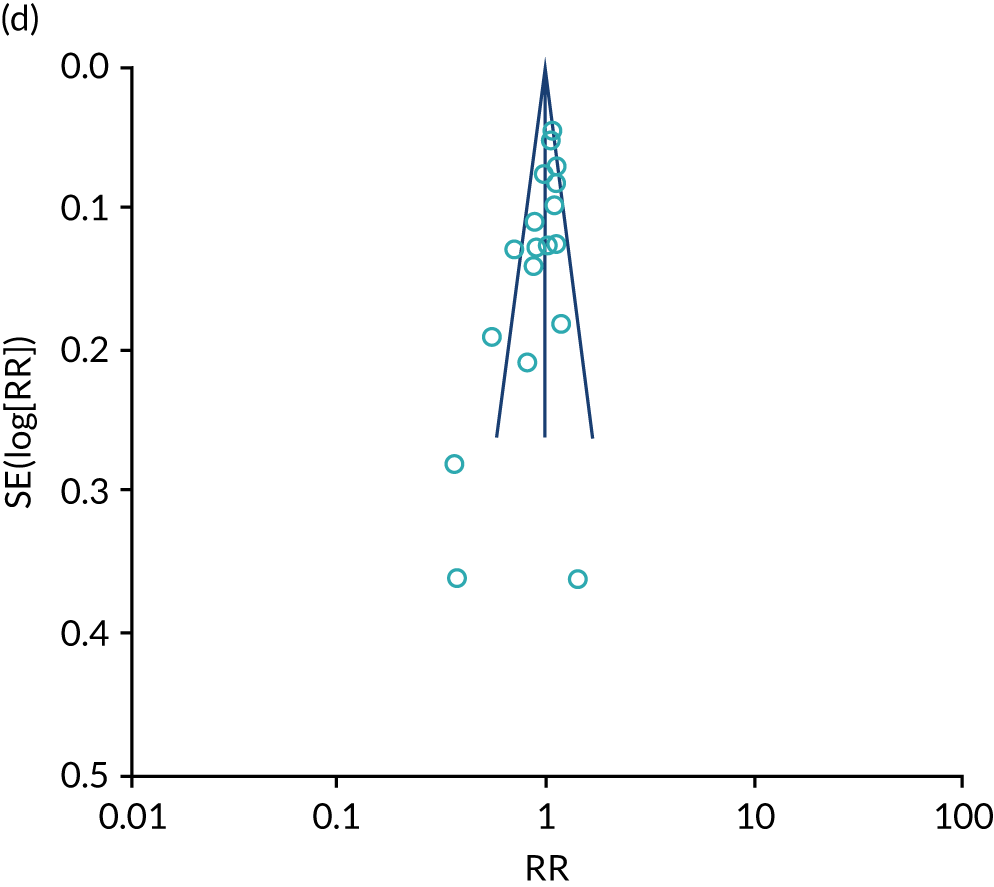

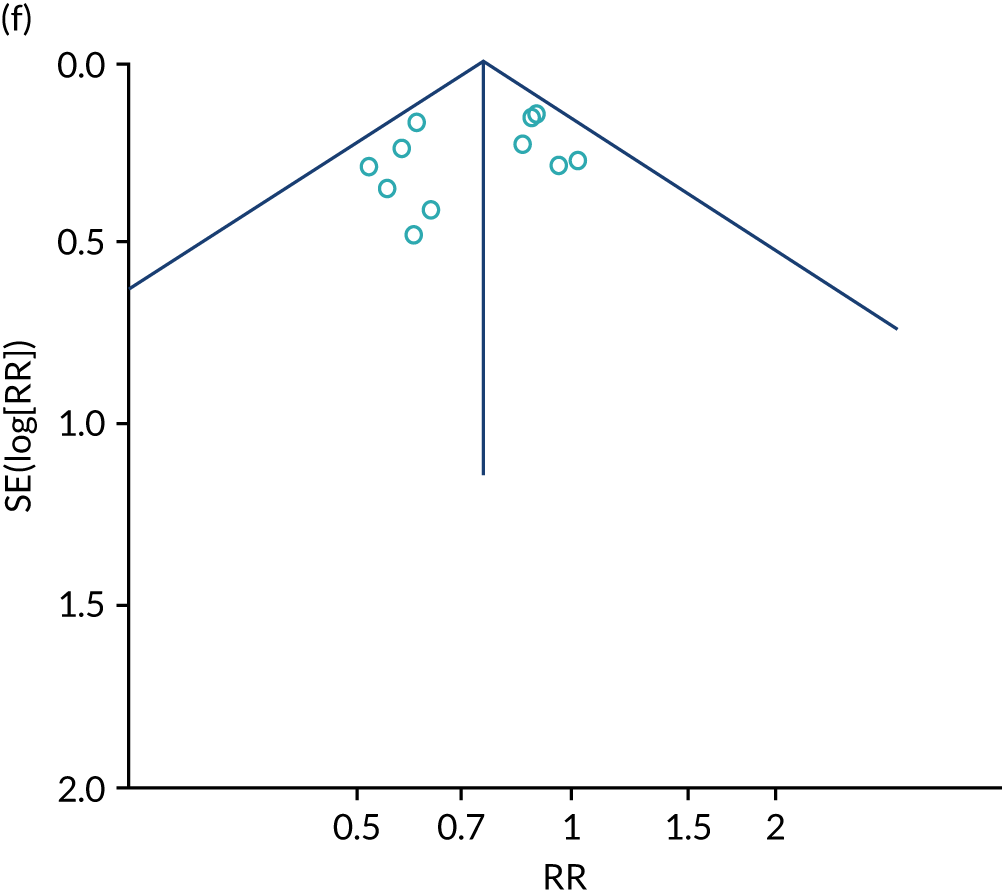
All-cause mortality
Nine of the 12 new included studies reported data for all-cause mortality. 129–133,135,136,138,139,144,147,148,150,151 The data from these studies were pooled with data from the existing 39 studies in Inglis et al. 73 (Figure 7). Based on all 51 studies, when compared with usual care, RM reduced mortality among patients with heart failure (STS: RR 0.85, 95% CI 0.76 to 0.96; I2 = 0%; TM: RR 0.82, 95% CI 0.73 to 0.92; I2 = 20%).
FIGURE 7.
Forest plots to show RM vs. usual care on all-cause mortality. (a) STS; and (b) TM. M–H, Mantel–Haenszel.
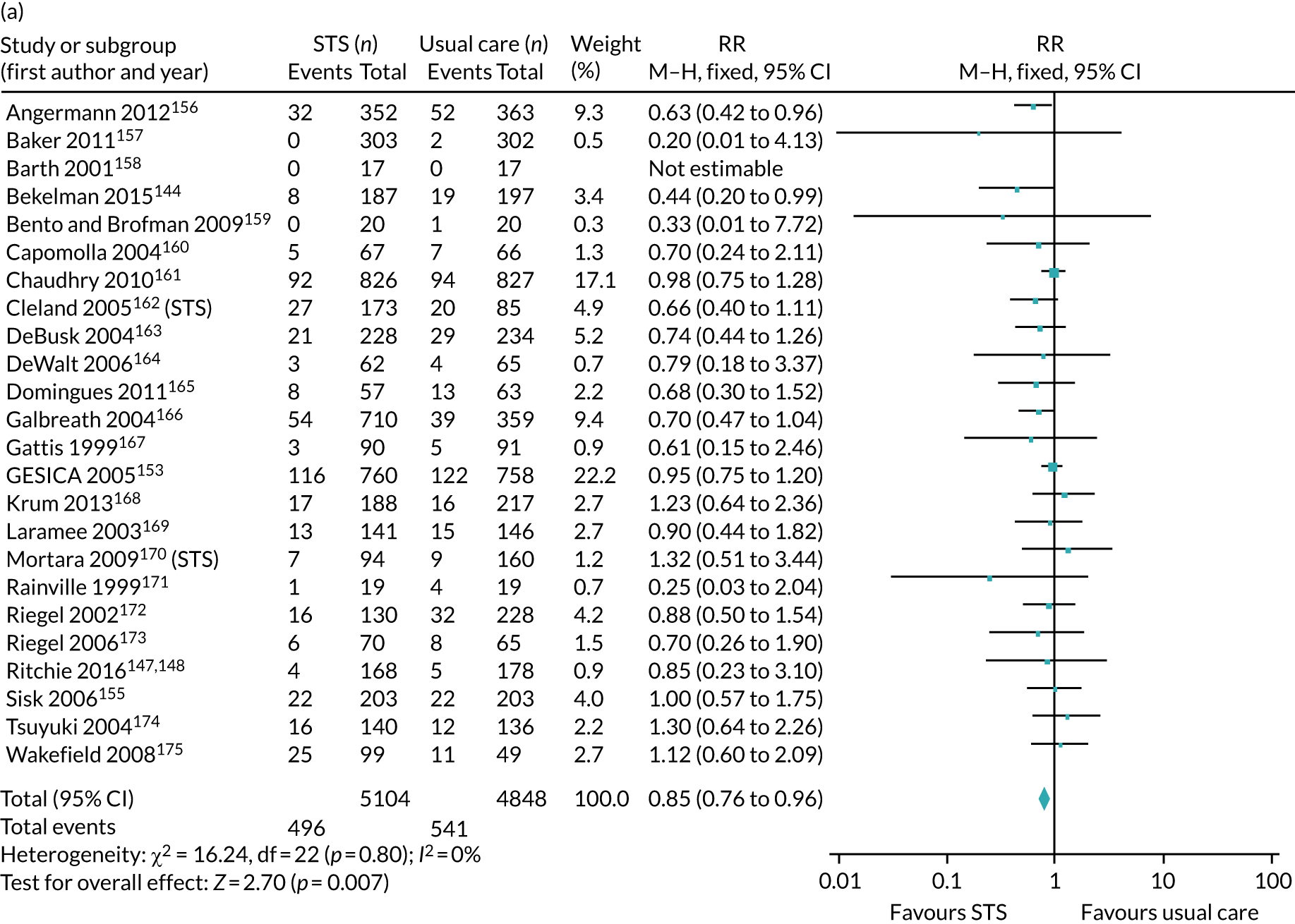
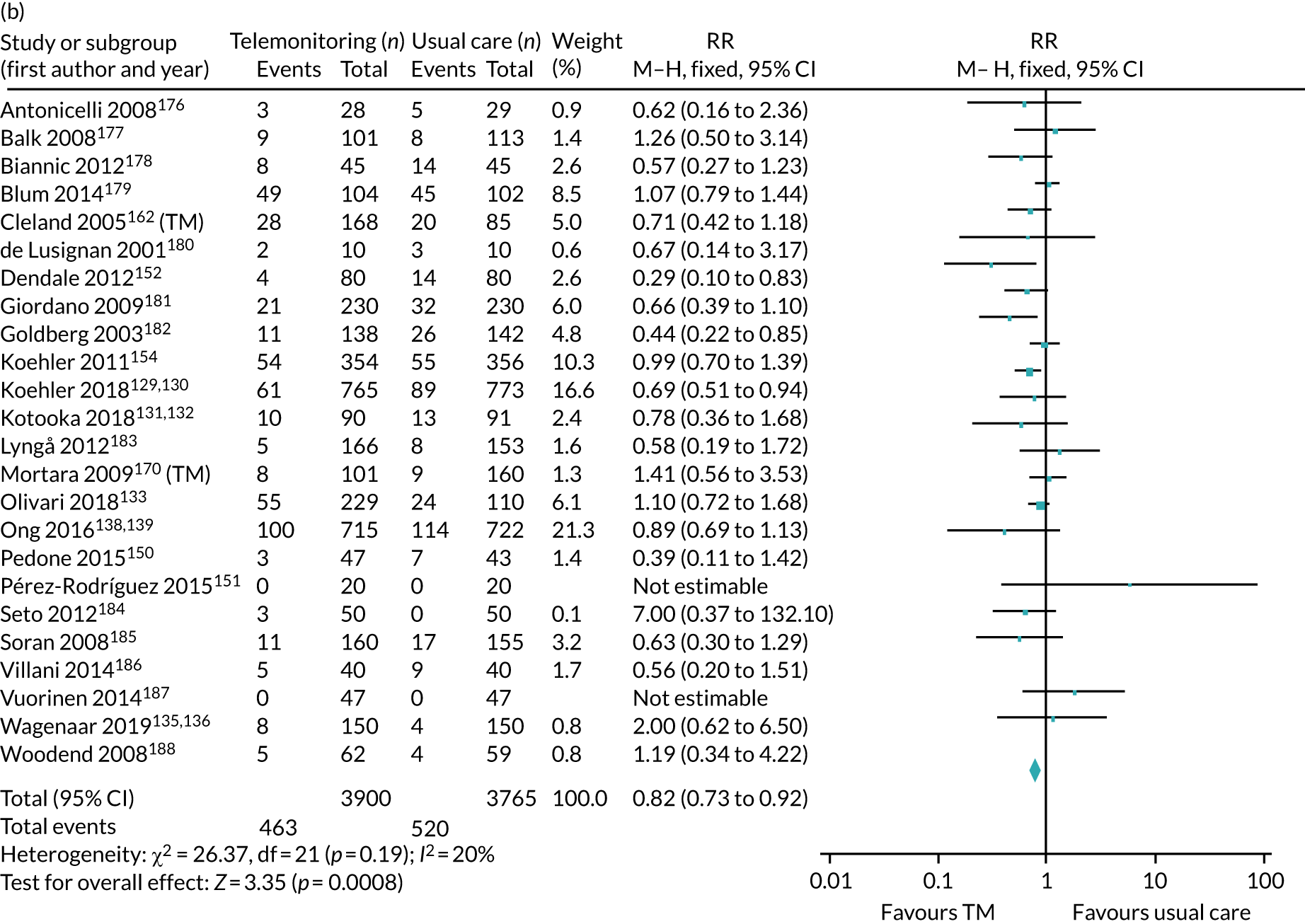
Subgroup analyses to compare between technologies used or by the focus of the monitoring did not show any significant difference between groups. For these analyses and subgroup analysis by year of publication, most of the studies fell into one subgroup; therefore, this subgroup was similar to the overall findings for STS and TM. Subgroup analysis comparing the average age of patients (< 70 vs. ≥ 70 years) found that, for nine STS studies with patients aged ≥ 70 years, the pooled result was no longer significant, although the difference between groups was not significant (p = 0.82). The opposite effect was seen in 12 TM studies with patients < 70 years, where for this group, the effect was no longer significant, although again the difference between groups was not significant (p = 0.56). Sensitivity analyses excluding studies with < 6 months of follow-up did not change the overall findings for STS, and, for TM, the RR was similar, but no longer significant (RR 0.87, 95% CI 0.75 to 1.00) (Table 19).
Analysis | Effect estimate (95% CI) | Number of studies | I2 (%) | Test for subgroup differences (p-value) |
---|---|---|---|---|
All-cause mortality (RR, < 1 favours RM) | ||||
STS vs. usual care | ||||
Fixed effects, Mantel–Haenszel method | 0.85 (0.76 to 0.96)a | 24 | 0 | NA |
Subgroup by technology: telephone call | 0.81 (0.71 to 0.93)a | 17 | 0 | p = 0.39 |
Subgroup by technology: videophone | 1.12 (0.60 to 2.09) | 1b | NA | |
Subgroup by technology: interactive voice response | 0.93 (0.77 to 1.17) | 6 | 0 | |
Subgroup by focus: clinical support | 0.87 (0.96 to 0.98)a | 19 | 0 | p = 0.42 |
Subgroup by focus: self-management education | 0.73 (0.48 to 1.10) | 5 | 16 | |
Subgroup by publication year: pre 2000 | 0.45 (0.14 to 1.40) | 2b | 0 | p = 0.18 |
Subgroup by publication year: 2000–7 | 0.86 (0.74 to 0.99)a | 13 | 0 | |
Subgroup by publication year: 2008–10 | 1.01 (0.79 to 1.28) | 4 | 0 | |
Subgroup by publication year: post 2010 | 0.70 (0.53 to 0.94)a | 5 | 0 | |
Subgroup by mean/median age: < 70 years | 0.96 (0.75 to 0.99)a | 15 | 0 | p = 0.83 |
Subgroup by mean/median age: ≥ 70 years | 0.84 (0.67 to 1.04) | 9 | 0 | |
Sensitivity analysis: excluding studies with < 6 months of follow-up | 0.85 (0.74 to 0.99)a | 12 | 0 | NA |
TM vs. usual care | ||||
Fixed effects, Mantel–Haenszel method | 0.82 (0.73 to 0.92)a | 24 | 20 | NA |
Subgroup by technology: videophone | 1.19 (0.34 to 4.22) | 1b | NA | p = 0.69 |
Subgroup by technology: complex/clinical TM | 0.83 (0.73 to 0.93)a | 19 | 25 | |
Subgroup by technology: mobile phone/PDA | 0.71 (0.46 to 1.11) | 4 | 25 | |
Subgroup by intensity: office hours | 0.70 (0.53 to 0.92)a | 11 | 0 | p = 0.36 |
Subgroup by focus: 24 hours per day/7 days per week | 0.81 (0.69 to 0.95)a | 10 | 43 | |
Subgroup by publication year: 2000–7 | 0.58 (039 to 0.86)a | 3b | 0 | p = 0.18 |
Subgroup by publication year: 2008–10 | 0.81 (0.59 to 1.12) | 6 | 0 | |
Subgroup by publication year: post 2010 | 0.86 (0.75 to 0.97)a | 15 | 33 | |
Subgroup by mean/median age: < 70 years | 0.86 (0.70 to 1.05) | 12 | 27 | p = 0.56 |
Subgroup by mean/median age: ≥ 70 years | 0.80 (0.70 to 0.92)a | 12 | 23 | |
Sensitivity analysis: excluding studies with < 6 months of follow-up | 0.87 (0.75 to 1.00) | 14 | 0 | NA |
All-cause hospitalisation (RR, < 1 favours RM) | ||||
STS vs. usual care | ||||
Fixed effects, Mantel–Haenszel method | 0.95 (0.90 to 1.00) | 17 | 44 | NA |
Subgroup by technology: telephone call | 0.93 (0.86 to 0.99)a | 12 | 51 | p = 0.08 |
Subgroup by technology: videophone | 0.70 (0.50 to 0.97)a | 1b | NA | |
Subgroup by technology: interactive voice response | 1.00 (0.91 to 1.09) | 4 | 65 | |
Subgroup by focus: clinical support | 0.94 (0.89 to 1.00) | 15 | 49 | p = 0.32 |
Subgroup by focus: self-management education | 1.08 (0.84 to 1.38) | 2b | 0 | |
Subgroup by publication year: pre 2000 | 0.57 (0.34 to 0.95)a | 1b | NA | p = 0.14 |
Subgroup by publication year: 2000–7 | 0.93 (0.86 to 1.01) | 8 | 0 | |
Subgroup by publication year: 2008–10 | 1.01 (0.92 to 1.10) | 4 | 73 | |
Subgroup by publication year: post 2010 | 0.95 (0.82 to 1.09) | 4 | 55 | |
Subgroup by mean/median age: < 70 years | 0.95 (0.89 to 1.02) | 11 | 52 | p = 0.83 |
Subgroup by mean/median age: ≥ 70 years | 0.94 (0.85 to 1.04) | 6 | 36 | |
Sensitivity analysis: excluding studies with < 6 months of follow-up | 0.89 (0.82 to 0.96)a | 7 | 37 | NA |
TM vs. usual care | ||||
Fixed effects, Mantel–Haenszel method | 0.96 (0.91 to 1.01) | 18 | 67 | NA |
Subgroup by technology: videophone | 1.06 (0.97 to 1.16) | 1b | NA | p = 0.02a |
Subgroup by technology: complex/clinical TM | 0.98 (0.93 to 1.03) | 14 | 67 | |
Subgroup by technology: mobile phone/PDA | 0.77 (0.63 to 0.95)a | 3b | 40 | |
Subgroup by intensity: office hours | 0.84 (0.76 to 0.94)a | 7 | 72 | p = 0.03a |
Subgroup by focus: 24 hours per day/7 days per week | 0.97 (0.90 to 1.05) | 9 | 66 | |
Subgroup by publication year: 2000–7 | 0.94 (0.79 to 1.12) | 2b | 0 | p = 0.37 |
Subgroup by publication year: 2008–10 | 0.89 (0.79 to 1.01) | 5 | 88 | |
Subgroup by publication year: post 2010 | 0.98 (0.93 to 1.04) | 11 | 61 | |
Subgroup by mean/median age: < 70 years | 0.96 (0.88 to 1.04) | 9 | 54 | p = 0.90 |
Subgroup by mean/median age: ≥ 70 years | 0.96 (0.90 to 1.03) | 9 | 77 | |
Sensitivity analysis: excluding studies with < 6 months of follow-up | 0.94 (0.88 to 1.01) | 11 | 71 | NA |
Heart failure hospitalisation (RR, < 1 favours RM) | ||||
STS vs. usual care | ||||
Fixed effects, Mantel–Haenszel method | 0.85 (0.77 to 0.93)a | 16 | 27 | NA |
Subgroup by technology: telephone call | 0.76 (0.67 to 0.86)a | 13 | 2 | p = 0.007a |
Subgroup by technology: interactive voice response | 0.99 (0.86 to 1.14) | 3b | 0 | |
Subgroup by focus: clinical support | 0.84 (0.76 to 0.93)a | 15 | 31 | p = 0.56 |
Subgroup by focus: self-management education | 0.95 (0.64 to 1.39) | 1b | NA | |
Subgroup by publication year: pre 2000 | 0.24 (0.10 to 0.58)a | 2b | 48 | p = 0.002a |
Subgroup by publication year: 2000–7 | 0.78 (0.69 to 0.89)a | 10 | 0 | |
Subgroup by publication year: 2008–10 | 1.02 (0.88 to 1.19) | 2b | 0 | |
Subgroup by publication year: post 2010 | 0.79 (0.57 to 1.08) | 2b | 0 | |
Subgroup by mean/median age: < 70 years | 0.86 (0.77 to 0.96)a | 8 | 51 | p = 0.53 |
Subgroup by mean/median age: ≥ 70 years | 0.81 (0.67 to 0.96)a | 8 | 27 | |
Sensitivity analysis: excluding studies < 6 months of follow-up | 0.76 (0.66 to 0.88)a | 7 | 0 | NA |
TM vs. usual care | ||||
Fixed effects, Mantel–Haenszel method | 0.74 (0.64 to 0.84)a | 12 | 17 | NA |
Subgroup by technology: complex/clinical telemonitoring | 0.79 (0.68 to 0.92)a | 9 | 14 | p = 0.05 |
Subgroup by technology: mobile phone/PDA | 0.58 (0.44 to 0.77)a | 3b | 0 | |
Subgroup by intensity: office hours | 0.72 (0.58 to 0.89)a | 7 | 22 | p = 0.83 |
Subgroup by focus: 24 hours/7 days per week | 0.70 (0.57 to 0.86)a | 4 | 14 | |
Subgroup by publication year: 2000–7 | 0.84 (0.55 to 1.30) | 1b | NA | p = 0.56 |
Subgroup by publication year: 2008–10 | 0.66 (0.51 to 0.86)a | 3b | 41 | |
Subgroup by publication year: post 2010 | 0.76 (0.64 to 0.89)a | 8 | 19 | |
Subgroup by mean/median age: < 70 years | 0.75 (0.64 to 0.88)a | 9 | 7 | p = 0.71 |
Subgroup by publication year: ≥ 70 years | 0.71 (0.57 to 0.89)a | 3b | 57 | |
Sensitivity analysis: excluding studies < 6 months of follow-up | 0.80 (0.69 to 0.93)a | 7 | 0 | NA |
Quality of life (SMD, > 1 favours RM) | ||||
STS vs. usual care | ||||
Fixed effects, inverse variance method | 0.13 (0.09 to 0.18)a | 12 | 86 | NA |
Subgroup by technology: telephone call | 0.14 (0.09 to 0.18)a | 9 | 90 | p = 0.78 |
Subgroup by technology: videophone | 0.10 (–0.14 to 0.34) | 1b | NA | |
Subgroup by technology: interactive voice response | 0.07 (–0.14 to 0.27) | 2b | 0 | |
Subgroup by focus: clinical support | 0.16 (0.11 to 0.22)a | 8 | 89 | p = 0.10 |
Subgroup by focus: self-management education | 0.08 (0.01 to 0.16)a | 3b | 85 | |
Subgroup by publication year: 2000–7 | 0.09 (0.02 to 0.16)a | 5 | 91 | p = 0.003a |
Subgroup by publication year: 2008–10 | 0.40 (0.23 to 0.56)a | 3b | 86 | |
Subgroup by publication year: post 2010 | 0.13 (0.07 to 0.19)a | 4 | 75 | |
Subgroup by mean/median age: < 70 years | 0.20 (0.15 to 0.25)a | 10 | 83 | p = < 0.0001a |
Subgroup by mean/median age: ≥ 70 years | –0.06 (–0.15 to 0.03) | 2b | 63 | |
Sensitivity analysis: excluding studies < 6 months of follow-up | 0.10 (0.03 to 0.17) | 6 | 89 | NA |
Sensitivity analysis: using alternative EQ-5D data for Riegel et al.173 | 0.14 (0.10 to 0.18)a | 12 | 86 | NA |
Sensitivity analysis: using alternative EQ-5D (single item) data for Riegel et al.173 | 0.13 (0.09 to 0.17)a | 12 | 87 | NA |
Sensitivity analysis: using alternative SF-36 (mental component) data for Galbreath et al.166 | 0.17 (0.13 to 0.22)a | 12 | 81 | NA |
Sensitivity analysis: using alternative imputation of SE based on mean from all studies | 0.18 (0.13 to 0.22)a | 12 | 82 | NA |
Sensitivity analysis: excluding studies with imputed data | 0.19 (0.14 to 0.24)a | 9 | 85 | NA |
Sensitivity analysis: using random effects model | 0.24 (0.10 to 0.37)a | 12 | 86 | NA |
TM vs. usual care | ||||
Fixed effects, inverse variance method | 0.24 (0.20 to 0.28)a | 11 | 98 | NA |
Subgroup by technology: complex/clinical telemonitoring | 0.26 (0.22 to 0.31)a | 9 | 98 | p = 0.003a |
Subgroup by technology: mobile phone/PDA | 0.08 (–0.04 to 0.19) | 2b | 68 | |
Subgroup by intensity: office hours | 0.08 (–0.08 to 0..23) | 3b | 0 | p = 0.01a |
Subgroup by focus: 24 hours/7 days per week | 0.29 (0.23 to 0.36)a | 5 | 99 | |
Subgroup by publication year: 2000–7 | 0.06 (–0.07 to 0.20) | 2b | 0 | p = 0.004a |
Subgroup by publication year: 2008–10 | 0.00 (–0.27 to 0.27) | 1b | NA | |
Subgroup by publication year: post 2010 | 0.27 (0.22 to 0.31)a | 8 | 98 | |
Subgroup by mean/median age: < 70 years | 0.33 (0.27 to 0.39)a | 6 | 99 | p = < 0.0001a |
Subgroup by publication year: ≥ 70 years | 0.15 (0.09 to 0.21)a | 5 | 95 | |
Sensitivity analysis: excluding studies < 6 months of follow-up | No change | NA | ||
Sensitivity analysis: using alternative SF-36 (physical component) data for Blum and Gottlieb179 | 0.24 (0.19 to 0.28)a | 11 | 98 | NA |
Sensitivity analysis: using alternative SF-36 (mental component) data for Blum and Gottlieb179 | 0.26 (0.22 to 0.31)a | 11 | 98 | NA |
Sensitivity analysis: using alternative SF-36 (mental component) data for Antonicelli et al.176 | 0.25 (0.21 to 0.29)a | 11 | 98 | NA |
Sensitivity analysis: using alternative SF-36 v.2 (mental component) data for Olivari et al.133 | 0.25 (0.21 to 0.29)a | 11 | 98 | NA |
Sensitivity analysis: using alternative SF-36 (mental component) data for Karhula et al.149 | 0.23 (0.19 to 0.27)a | 11 | 98 | NA |
Sensitivity analysis: using alternative imputation of SE based on mean from all studies | 0.25 (0.20 to 0.29)a | 11 | 98 | NA |
Sensitivity analysis: excluding studies with imputed data | 0.26 (0.21 to 0.30)a | 10 | 98 | NA |
Sensitivity analysis: using random effects model | 0.25 (–0.05 to 0.55) | 11 | 98 | NA |
Meta-regression investigating whether or not the effect estimates depended on the average age of study participants or the year of publication did not find any association for either STS (publication date: p = 0.63, age: p = 0.3) or TM (publication date: p = 0.23; age: p = 0.69).
All-cause hospital admission
Six new included studies reported data for all-cause hospital admission. 131–133,135,136,138,139,147,148,150 Figure 8 shows the pooled data from these six studies, along with data from 29 studies identified by Inglis et al. 73 Based on 35 studies comparing RM with usual care, the findings are inconclusive for all-cause hospital admission among patients with heart failure (STS: RR 0.95, 95% CI 0.90 to 1.0; TM: RR 0.96, 95% CI 0.91 to 1.01). Heterogeneity was moderate to substantial (STS: 44%; TM: 67%).
FIGURE 8.
Forest plots to show RM vs. usual care on all-cause hospital admissions. (a) STS; and (b) TM. M–H, Mantel–Haenszel.
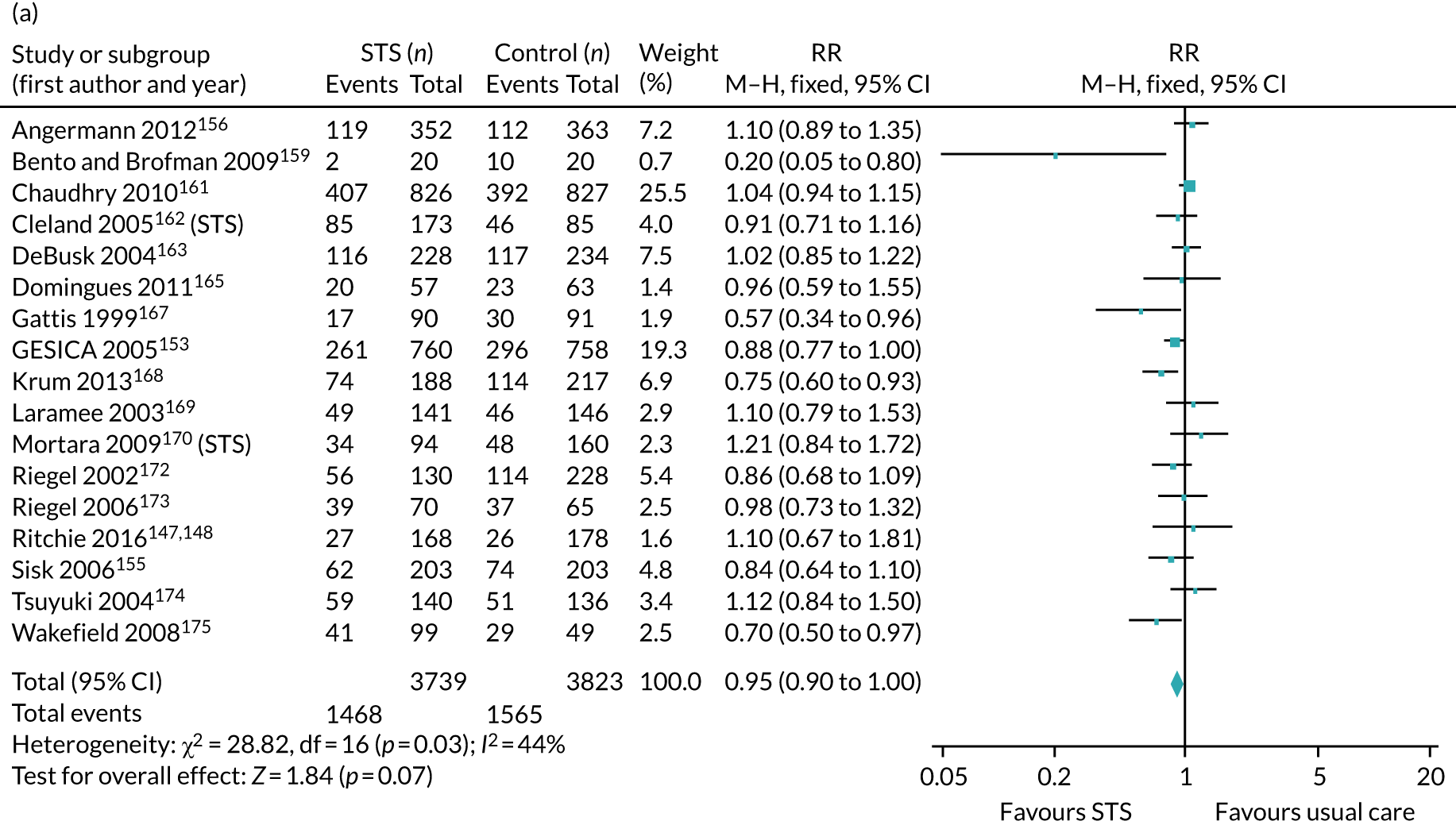
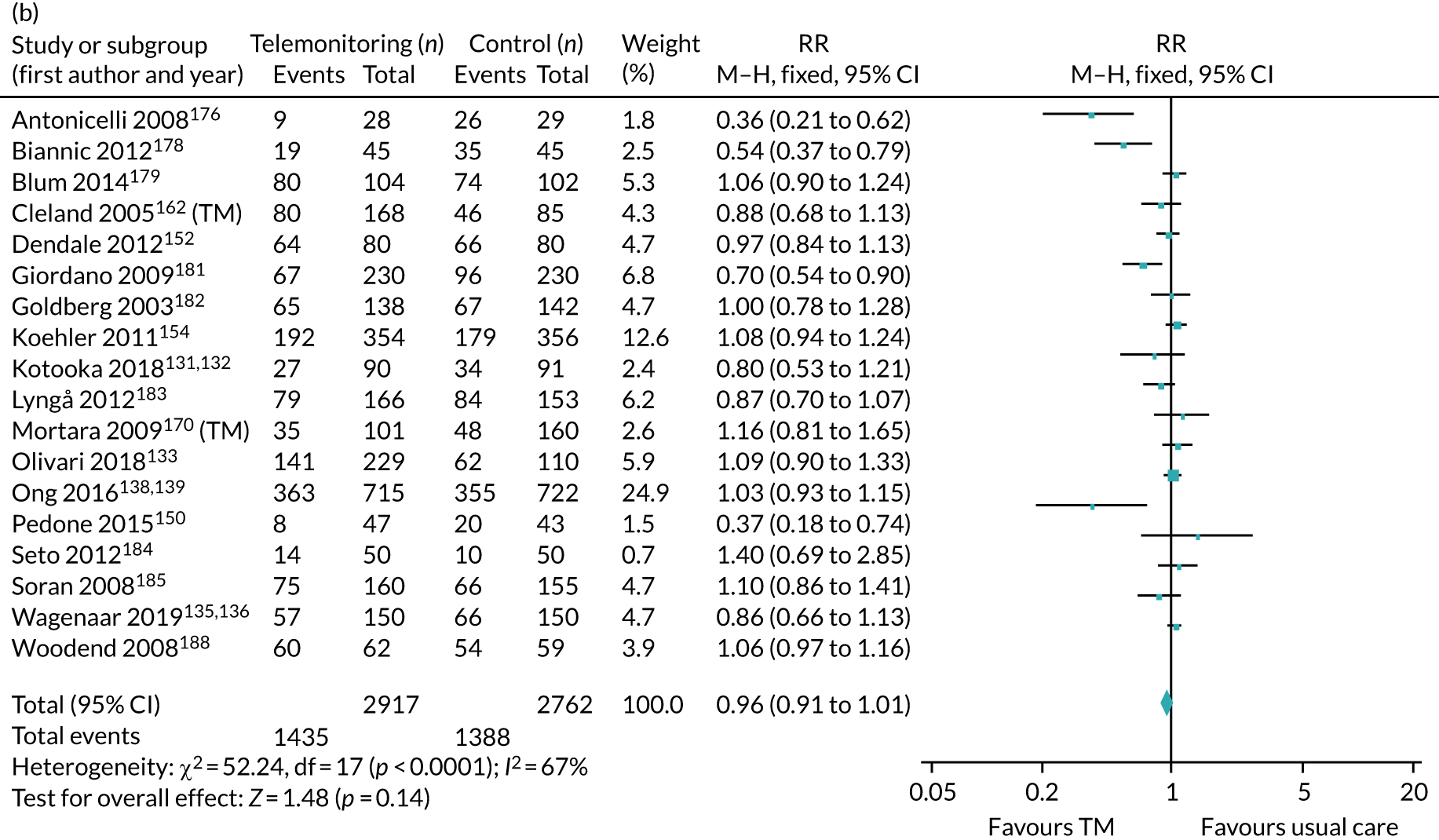
Subgroup analyses of STS versus usual care by technology type suggest that telephone calls as a technology used may favour STS, as this subgroup, 12 of 17 total studies, showed a significant pooled effect (RR 0.93, 95% CI 0.86 to 0.99), although the test for subgroup difference was not significant (p = 0.08). All other subgroups analysed showed no difference to the overall findings in terms of direction of effect and statistical significance. A sensitivity analysis excluding studies with < 6 months of follow-up reported a statistically significant result, with reduced heterogeneity (RR 0.89, 95% CI 0.82 to 0.96; I2 = 37%).
Subgroup analyses of TM versus usual care suggest a significant subgroup difference for technologies used (p = 0.02), with mobile phones and PDAs now suggesting a statistically significant reduction in all-cause hospital admission (RR 0.77, 95% CI 0.63 to 0.95), whereas videophone and complex/clinical TM remained inconclusive. Subgroup analyses for the intensity of monitoring suggest that monitoring during office hours, compared with 24 hours per day, 7 days per week, is statistically significant (RR 0.84, 95% CI 0.76 to 0.94); the test for subgroup difference was significant (p = 0.03). A sensitivity analysis excluding studies with shorter follow-up duration did not change the finding (see Table 19).
Meta-regression testing for any association between the effect estimates and the average age of study patients or year of publication found no evidence for either STS (publication date: p = 0.68; age: p = 0.87) or TM (publication date: p = 0.6; age: p = 0.33).
Heart failure hospital admission
Only four new included studies, all TM studies, reported data for all-cause hospital admission. 131–133,135,136,151 The data from these four studies were pooled with data from 24 studies identified by Inglis et al. 73 (Figure 9). RM compared with usual care (28 studies) reduces the rate of heart failure hospital admissions among patients with heart failure (STS: RR 0.85, 95% CI 0.77 to 0.93; I2 = 27%; TM: RR 0.74, 95% CI 0.64 to 0.84; I2 = 17%).
FIGURE 9.
Forest plots to show RM vs. usual care on heart failure hospital admissions. (a) STS; and (b) TM. M–H, Mantel–Haenszel.
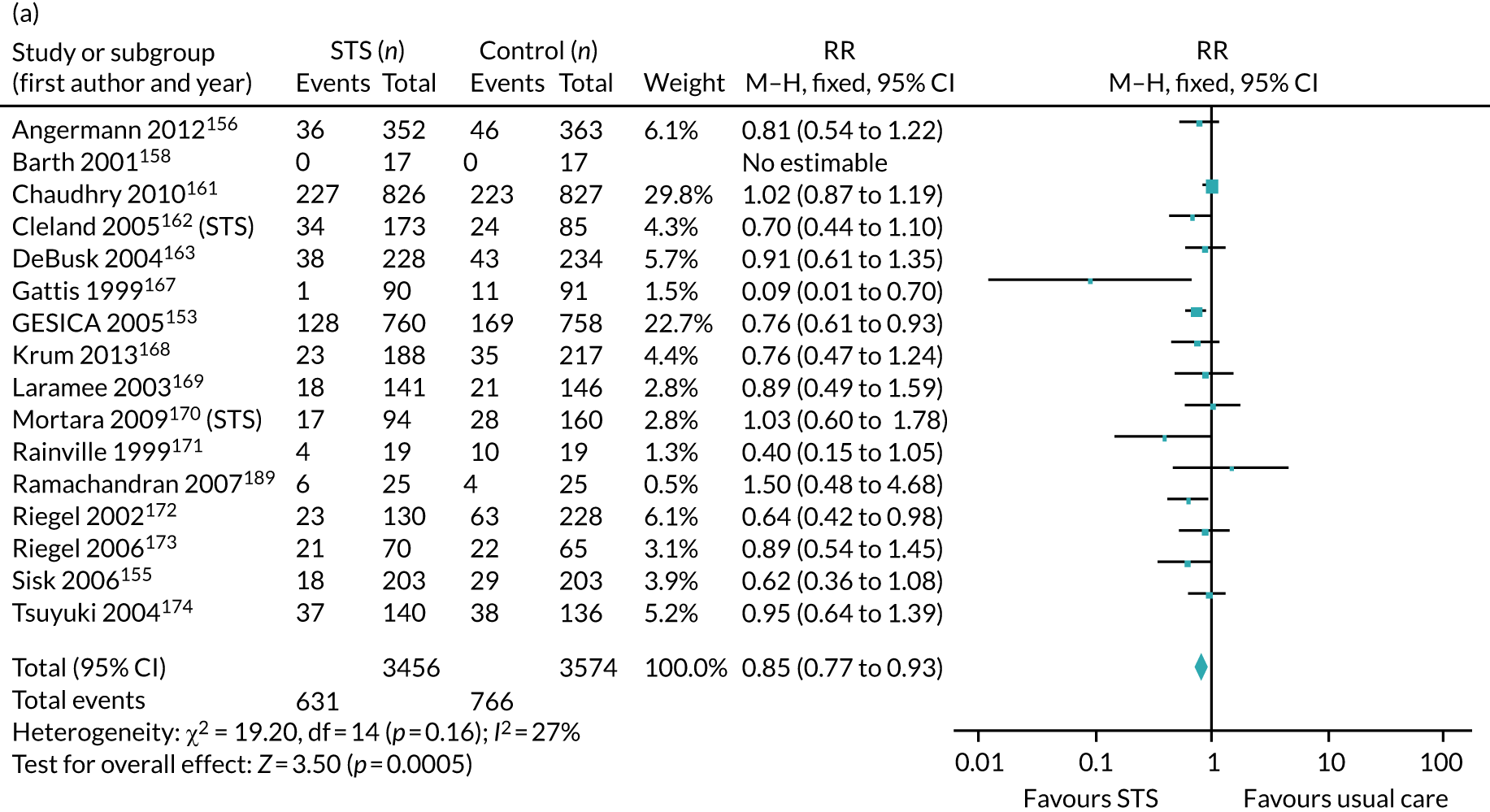

Subgroup and sensitivity analyses for STS and TM were similar. All subgroup analyses and sensitivity analyses excluding studies with < 6 months of follow-up showed the same direction of effect and statistical significance as the overall finding. Testing for subgroup difference was significant for STS only by technology used (p = 0.007) and year of publication (p = 0.002) (see Table 19).
Similar to the other two primary outcomes, meta-regression testing for any association between the effect estimates and the average age of study patients or year of publication found no evidence for either STS (publication date: p = 0.13; age: p = 0.42) or TM (publication date: p = 0.6, age: p = 0.82).
Quality of life
Nine of the 12 new included studies reported data for quality of life,129–133,135,136,138,139,144–149 although one of these could not be pooled. 129,130 Inglis et al. 73 did not report a pooled result for quality of life; however, 15 studies153–157,166,173,175,176,179,180,182,184,189,191 did report these data in a format that could be pooled with the newly acquired data. Based on 27 studies, RM, compared with usual care, improved quality of life among patients with heart failure (STS: SMD 0.13, 95% CI 0.09 to 0.18; TM: SMD 0.24, 95% CI 0.20 to 0.28; Figure 10). However, these results should be viewed with caution as the heterogeneity was considerable (STS: I2 = 86%; TM: I2 = 98%). Using a random-effects model, the TM effect was found to no longer be significant (STS: SMD 0.24, 95% CI 0.1 to 0.37; TM: SMD 0.25, 95% CI –0.05 to 0.55; see Table 19).
FIGURE 10.
Forest plots to show RM vs. usual care on quality of life. (a) STS; and (b) TM. IV, inverse variance.
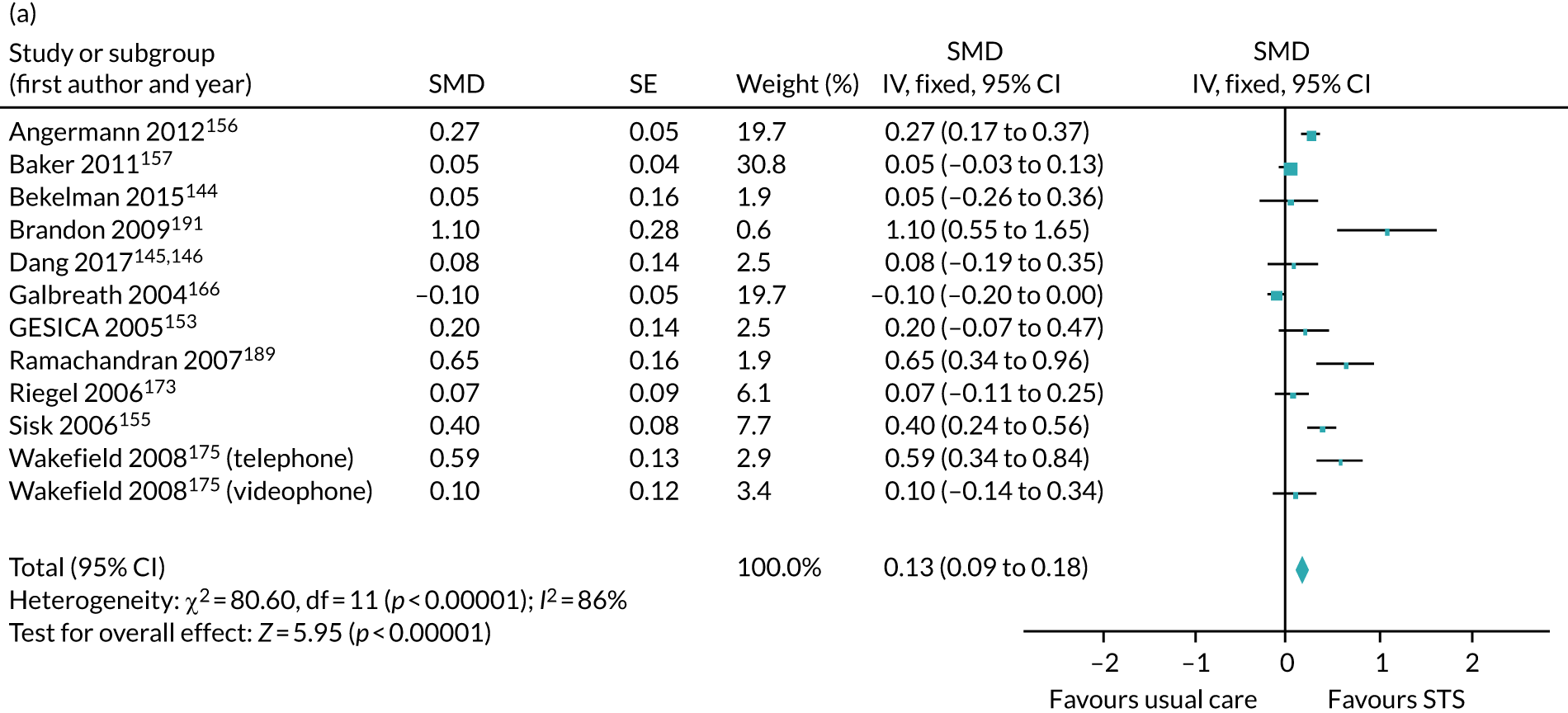
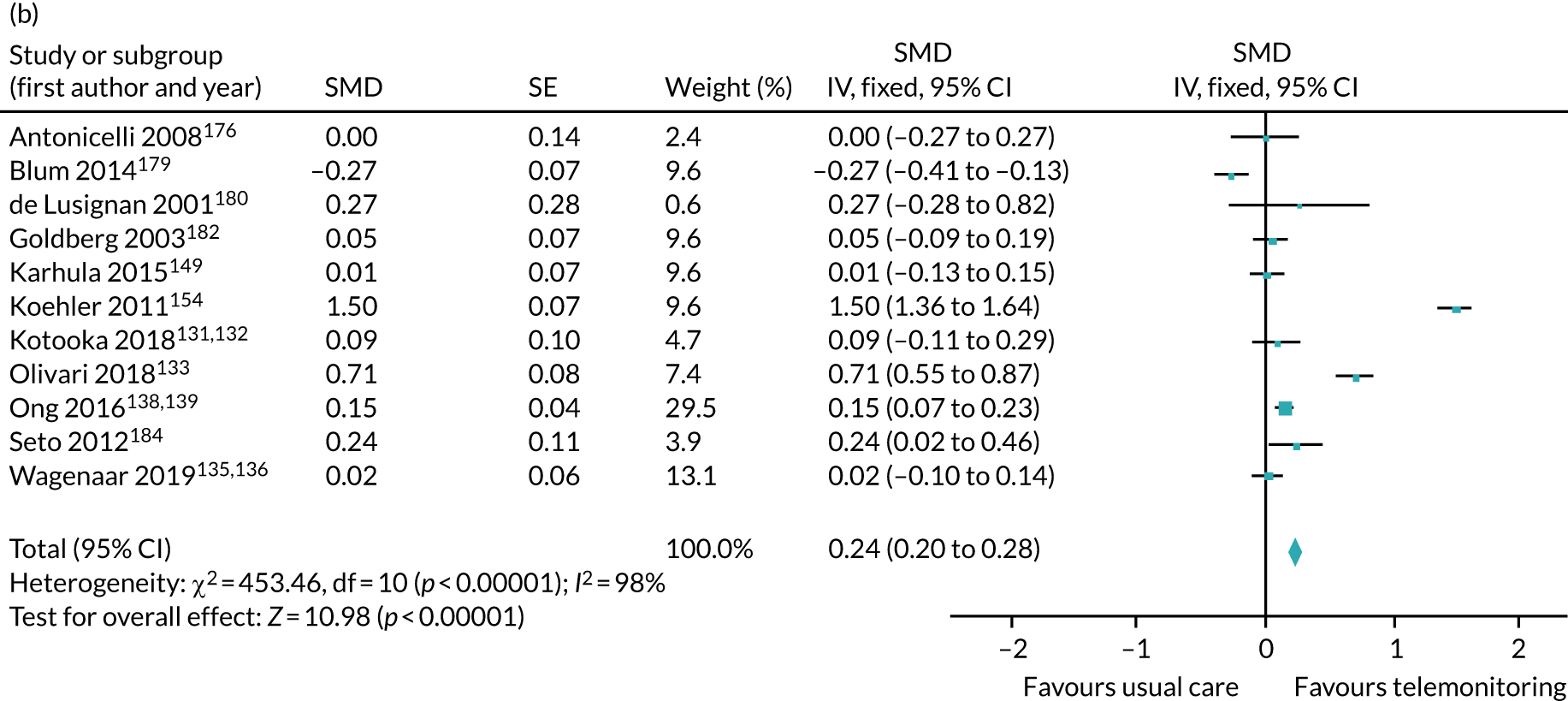
Subgroup analyses for STS versus usual care showed significant results for a test for difference between groups for year of publication (p = 0.003) and average age (p ≤ 0.0001); however, heterogeneity remained considerable for all groups. For all subgroup analyses of TM versus usual care, the test for group differences were significant; however, heterogeneity remained substantial for all pooled results; when it was reduced, it was for groups that pooled only one to three studies. Excluding studies with shorter follow-up durations and post hoc analyses using alternative quality-of-life surveys, imputed SE values or exclusion of studies with imputed data showed no difference to the main finding in the direction of effect, statistical significance or heterogeneity. For all analyses, see Table 19.
Length of stay, adherence, acceptability, heart failure knowledge and self-care
Data were reported for these secondary outcomes, but, similar to Inglis et al. ,73 data were limited and sufficiently varied in how they were measured and reported such that it was not possible to pool the evidence.
Of the 12 new included studies, two reported outcome data for length of stay in hospital: one for STS147,148 and one for TM. 133 Neither study reported any difference between the RM and usual-care groups (p = 0.76 and p = 0.21, respectively). This was consistent with the findings in Inglis et al. ,73 in which the majority of studies for both STS and TM reported no significant difference in the length of stay for hospital admission.
Six new included studies, all TM versus usual care, reported adherence data. 129–132,134,138,139,145,146,149 Each study measured this differently, so comparison was not possible. For each study, compliance data were reported without comment, or for Kotooka et al. 131,132 and Smeets et al. 134 as ‘sufficiently high’ and ‘excellent’, respectively. All adherence rates for the eight studies, both STS and TM, in Inglis et al. 73 were reported as being > 55%.
Only two studies,134,145,146 both newly included TM versus usual-care studies, considered acceptability (usability); both reported high satisfaction by patients for all aspects of the intervention. Furthermore, patients in Smeets et al. 134 ‘experienced an extra sense of security’. Inglis et al. 73 stated narratively that six of the 41 studies reported satisfaction of > 75% with RM interventions and that two, which were videophone studies, had low satisfaction rates.
Dang et al. 145,146 and Wagenaar et al. 135,136 reported data for heart failure knowledge using the Dutch Heart Failure Knowledge Scale. Both these TM studies reported no difference between the TM and usual-care groups: Dang et al. 145,146 reported a non-significant mean difference at 3 months (p = 0.09) and Wagenaar et al. 135,136 reported no ‘clear difference’ at 12 months. Only one newly included study reported data on heart failure self-care: Wagenaar et al. 135,136 They reported no effect difference between the two groups (unadjusted and adjusted for self-care at baseline: p = 0.082 and p = 0.184, respectively). This varied from Inglis et al. ,73 in which five of the six studies evaluating heart failure knowledge and five studies evaluating heart failure self-care reported improvements in patients.
Discussion
Main findings
The evidence we pooled in this systematic review suggests that all-cause mortality and heart failure hospital admission rates reduced and that quality of life improved with the use of RM (both TM and STS). There was little difference between STS and TM in terms of the strength of effect or the degree of uncertainty surrounding it. Although there was some suggestion that RM may reduce all-cause hospital admission, this was not statistically significant. It was not possible to pool data for length of stay, adherence, acceptability, heart failure knowledge or self-care.
For the outcomes of all-cause mortality and heart failure hospitalisation, the heterogeneity was low. Sensitivity analyses restricting the evidence to studies with longer follow-up durations suggest that the findings were not robust for TM, as, for both outcomes, it was no longer a statistically significant result. Heterogeneity was moderate for all-cause hospitalisation; this was not explained by subgroup analysis or meta-regression. The findings for quality of life should be viewed with caution as the heterogeneity was considerable, and testing of the robustness of the effect estimate by using a random-effects model resulted in the TM finding no longer being statistically significant.
Although it was not possible to pool data for secondary outcomes other than quality of life, it was possible to draw some narrative indications of the effectiveness of RM. There appeared to be no effect on the length of hospital stay for patients. In general, adherence and satisfaction with RM was good. In contrast to the Inglis et al. 73 review in 2015, the two more recent (2015–19) studies135,136,145,146 reporting on heart failure knowledge and self-care found no improvement when using RM, compared with previously reported improvements.
Comparison with other reviews
This review is comparable with a number of previous reviews. The results are consistent with the findings of all four reviews published in 2018125–128 in terms of the direction of effect, except for Carbo et al. ,126 who reported a trend towards the control group for all-cause hospital admission, although this was based on only five studies (compared with 24 studies in this review). Consistent with our review, all pooled results from previous systematic reviews are inconclusive for all-cause hospital admission. There is still variation between previous pooled results for all-cause mortality and heart failure-related hospital admission over whether or not the effect is statistically significant.
This systematic review was an update of the review by Inglis et al. ,73 and added 12 studies published since January 2015. The pooled results from this review are consistent with those of Inglis et al. ,73 reporting similar conclusions for the outcomes of all-cause mortality, all-cause hospital admission and heart failure-related hospital admission, and, for the majority, with marginally increased confidence in the findings. For this update, we were able to pool data for the secondary outcome of quality of life, which was reported only narratively in Inglis et al. 73
Two previous systematic reviews have pooled data for quality of life. Our findings suggest a statistically significant improvement in quality of life using RM, is consistent with both Flodgren et al. 192 and Knox et al. 193
Strengths and limitations
To our knowledge, this systematic review is the most comprehensive to date. There was no restriction on language or publication type in the study selection, although a study found in abstract form was included only if a full-text publication was available. Nevertheless, no cases of this were found in this update. However, it is possible that we missed relevant studies. Furthermore, we believe that this systematic review is the first to include evidence from the largest TM study to date. 129,130 Our ability to assess the methodological quality of studies was limited because of the number of studies assessed as being at unclear risk of bias in a domain; in particular, > 50% of studies were judged to have an unclear risk of bias for allocation concealment and blinding of outcome assessment. Further bias may be present as there was a strong suggestion of publication bias. We pooled data even in cases when there were few studies or high statistical heterogeneity, to give an overall indication of intervention effectiveness. We used reported average ages for each study to explore its association with the outcomes of interest, as done in the Inglis et al. 73 review that we used as the basis for this one. This is not recommended as it masks different population distributions found across the included studies. To have an adequate exploration of the impact that age has on these outcomes, individual patient data arising from these studies would be required.
Implication for clinical practice and research
In response to the 2010 NICE guideline,70 there has been an increase in the number of studies investigating the clinical effectiveness of RM. This review includes 12 new studies published since 2015 and classifies 24 studies as ongoing. Seven studies are registered with www.clinicaltrials.gov. 194–200 One study has now presented their findings at conference,194,201 one study is due to start in January 2021,195 and five studies are completed or estimated to complete by August 2021, but have not yet published findings. 196–200 To consider whether or not sufficient evidence has been accumulated to date for STS studies, a simple sample size calculation (using ‘power two proportions’ in Stata) indicates that, to show an effect size as presented in this review (15% reduction in all-cause mortality), a study would need 10,120 patients to be sufficiently powered (baseline risk 11.4%,161 two-sided alpha of 5% with a power of 80%). This review includes 9952 patients for this outcome. A similar calculation for TM studies indicates that, for an effect size of 18% reduction in all-cause mortality, a study would need 6544 patients to be sufficiently powered (baseline risk 12%129,130). Our review includes 7665 patients. The pooled evidence in this review indicates that, for the outcome of all-cause mortality, the threshold has now been reached for the comparison of TM with usual care. It is short of the threshold for STS versus usual care, although by < 500 patients. Therefore, there can be some level of confidence in the pooled results for all-cause mortality. As a result, we do not perceive the need for any new studies until the ongoing studies have reported their results, although this decision can be reviewed then in respect of other outcomes.
The 2018 NICE guideline13 did not recommend the use of RM because of lack of evidence of effectiveness; our review would question this. Furthermore, although a reticence to recommend RM because of a lack of plateau in terms of technology advancement is understandable, we would argue that the pace of change and development of functionality is unlikely to stop, so recommendations should be made in spite of potential changes. However, it is true that RM interventions are complex and feature a number of interconnected factors. A key method to move these research findings into clinical practice would be to identify the beneficial components of the RM interventions that are associated with a clinical benefit to patients. Intervention synthesis75 could be used to tease out the effect of components, such as different physiological measurements, frequency and duration of monitoring, incorporation of educational or motivational training, rural or urban settings, treatment protocols and severity of disease.
Conclusions
This review confirms the evidence base to date that all-cause mortality and heart failure hospital admission rates were reduced with the use of RM (both TM and STS). In addition, the review suggests that quality of life would be improved using RM; however, this result should be viewed with caution as the heterogeneity was considerable. The review does not provide evidence of an effect of RM on the rate of all-cause hospital admission.
Appendix 18 Diagnostic accuracy of point-of-care natriuretic peptide testing for chronic heart failure in ambulatory care: systematic review and meta-analysis
Taylor et al. 74 https://doi.org/10.1136/bmj.k1450
Appendix 19 Essential components in natriuretic peptide-guided management of heart failure: an intervention synthesis
Oke et al. 75 https://doi.org/10.1136/openhrt-2018-000826
Appendix 20 Estimation of variability of B-type natriuretic peptide and weight in the monitoring of chronic heart failure
Parts of this appendix have been reproduced from chapter 6 of Milena Kurtinecz’s PhD thesis66 with permission from Milena Kurtinecz (University of Oxford, 2021, personal communication).
Aim
The aim was to investigate the effects of factors that determine the BNP concentration and to estimate variability.
Proposed methodology
Secondary analyses of previously collected data from routinely collected data (CPRD) and pilot studies will be used to model BNP and weight to (1) identify biological variability and measurement error and determine coefficients of variation and (2) calculate rates of change by age, stratified by CHF severity.
Modifications
Evaluation of CPRD data identified that BNP measures are not commonly used. A small proportion (approximately 6%) of patients with CHF would have a BNP measure recorded, with most of these being a single measure. Instead, we obtained data from a RCT that collected repeated BNP measures on a small number of individuals with CHF. We determined that these would provide a better estimate for the variability of BNP in this population.
Objectives
-
To estimate the within-subject short-term variability of BNP in non-diabetic, stable mild and moderate heart failure subjects (NYHA class II or III).
-
To explore the impact of clinical, cardiac and other factors on the BNP variability.
Clinical factors: heart rate, systolic and diastolic blood pressure, and renal dysfunction.
Cardiac factors: LVEF, cardiac output, left ventricular end-diastolic and end-systolic volumes obtained from an echocardiography.
Other factors: age, sex and body mass index.
Methods
The objectives were addressed based on data from a phase 2 clinical trial in stable CHF patients in a post hoc analysis. This clinical trial is registered on www.clinicaltrials.gov with the clinical identifier number NCT01357850. 202
The information provided in the register includes study description, condition/disease, study design, study start and completion dates, eligibility criteria, primary and secondary outcomes, participating sites and the primary publication. 203 Access to additional study documents, such as the statistical analysis plan, individual participant data sets, clinical study report, annotated case report forms, data specification, informed consent and study protocol, can be requested through www.clinicaltrials.gov. 202 A clinical trial setting provides the ideal opportunity to investigate the BNP variability because patients are assessed similarly and at the same time intervals throughout the study duration. 124
Description of trial design
The study was a multicentre, randomised, placebo-controlled clinical trial that evaluated the safety of a new therapeutic agent in stable NYHA class II or III heart failure subjects. The investigational drug was in development for improved myocardial efficiency and increased exercise ability in heart failure patients. The clinical researchers and subjects were blinded to the study drug. The study design was a parallel group with four arms: placebo and three increasing dose levels of the new therapeutic agent. The 30 patients included in this appendix were not exposed to any dose of a new investigational medicine. Therefore, the BNP variability studied here cannot be attributed to such potential confounding. The study duration was 13 weeks, followed by an additional visit approximately 1 month after the study was completed. The clinical trial was initiated in September 2010 and ended in August 2012.
The BNP, cardiac output, LVEF, left ventricular end-diastolic volume, and left ventricular end-systolic volume was measured every 6 weeks, whereas the weight, heart rate, diastolic and systolic blood pressure measurements were collected weekly. The serum creatinine level was measured every other week, as described in Table 20.
Measurement | Week | ||||||||||||
---|---|---|---|---|---|---|---|---|---|---|---|---|---|
1 | 2 | 3 | 4 | 5 | 6 | 7 | 8 | 9 | 10 | 11 | 12 | 13 | |
BNP | ✗ | ✗ | ✗ | ||||||||||
Cardiac output | ✗ | ✗ | ✗ | ||||||||||
LVEF | ✗ | ✗ | ✗ | ||||||||||
LVEDV | ✗ | ✗ | ✗ | ||||||||||
LVESV | ✗ | ✗ | ✗ | ||||||||||
Heart rate | ✗ | ✗ | ✗ | ✗ | ✗ | ✗ | ✗ | ✗ | ✗ | ✗ | ✗ | ✗ | ✗ |
Blood pressure | ✗ | ✗ | ✗ | ✗ | ✗ | ✗ | ✗ | ✗ | ✗ | ✗ | ✗ | ✗ | ✗ |
Weight | ✗ | ✗ | ✗ | ✗ | ✗ | ✗ | ✗ | ✗ | ✗ | ✗ | ✗ | ✗ | ✗ |
Serum creatinine | ✗ | ✗ | ✗ | ✗ | ✗ | ✗ | ✗ |
Study participants
The clinical trial included clinically stable CHF patients on optimal therapies for at least 3 months before the baseline visit. The aetiology of CHF was ischaemic or non-ischaemic cardiomyopathy. Patients were in NYHA class II or III204 for at least 6 months before enrolment in the study with a reduced LVEF (e.g. LVEF of < 40%) at any time during the previous 24 months from the study start. Both males and females were between 21 and 75 years of age.
Patients with any of the following current or recent conditions were excluded: active ischaemia, diabetes mellitus, thyroid disease, genetic disorders of skeletal muscle, clinically significant pericardial diseases, renal dysfunction, history of coronary revascularisation, history of alcohol or drug abuse, history of deep-vein thrombosis or pancreatitis and other medical conditions with a life expectancy of < 1 year. Diabetes mellitus was assessed to be present based on a fasting glucose level of > 140 mg/dl or HbA1c of > 7%. The renal dysfunction was determined based on an eGFR of < 40 ml/minute/1.73 m2 at screening. Patients with a positive hepatitis B or C or human immunodeficiency virus test in the previous 3 months were excluded. Other reasons for exclusions at screening were calcitonin levels of > 100 pg/ml, triglycerides levels of > 850 mg/dl, resting low (< 85 mmHg) or high (> 170 mmHg) systolic blood pressure or high diastolic blood pressure (> 110 mmHg). Mentally incapacitated patients and pregnant women were also excluded from the study.
Outcomes
The primary outcome of this analysis was BNP. The unit of reporting was ng/l, which is the same as pg/ml. Although the assay type was not collected, all blood tests were processed at a central laboratory. The within-subject BNP variability was estimated over a 3-month period.
The between-subject BNP variability was described in subgroups of patients based on demographics, clinical characteristics, medical history and previous heart failure medication exposure. The changes in BNP levels up to 3 months were described.
Sample size and randomisation
The sample size of 30 participants includes only those that were randomised into the placebo arm of the trial and, therefore, did not receive any investigational medicine. The original study included a total of 82 participants with the other 50 participants allocated to one of three doses of the investigational medicine (with different allocation ratios). These 50 participants are not included in the present analysis.
Blinding
The clinical investigators and patients were blinded to the treatment received.
Statistical methods
The approach was exploratory and descriptive; therefore, no formal statistical hypothesis was tested. Summary statistics (e.g. n, mean, 95% CI for the mean, geometric mean, 95% CI for the geometric mean, standard deviation, SE, between-subject CV, median, range, interquartile range) were generated for BNP, weight, heart rate, serum creatinine level and systolic and diastolic blood pressure over time. A similar approach was adopted for the absolute changes from baseline and per cent changes from baseline in BNP over time.
Repeated-measures mixed models were employed to estimate the within-subject variability. Unlike the generalised linear models, mixed models incorporate random and fixed effects, allowing for correlations among measurements, as well as heterogeneous variance, while the normality assumption of the observations remains. Random factors were included to account for sources of variation, and their values were assumed to be a random sample from a population of interest whose values are normally and independently distributed. Consequently, some values could be higher or lower than the overall average effect. The model fit was evaluated by examining the marginal residuals, conditional residuals, covariance parameter diagnostics and influence diagnostics.
Using matrix notations, the general form of the mixed models is Y = Xβ + Zγ + ε,205 where X is the known design matrix for the fixed effects, β is the vector of fixed effects, Z is the known design matrix for the random effects, γ is the unknown parameter vector of random effects and ε is the normally distributed random error. When variability is of interest, the focus is on the random part of the mixed models. Random intercept models with the intercept as a random effect and week as a fixed effect were fitted, as well as random coefficient models, where the subject was a random effect and week was a fixed effect. The natural logarithm of the BNP baseline value was also modelled as a covariate.
B-type natriuretic peptide measurements taken over time in the same subject are expected to be correlated. Usually, the further apart the measurements, the lesser the correlation. The following covariance structures were considered: variance components, unstructured, compound symmetry, heterogeneous autoregressive and heterogeneous Toeplitz.
In the statistical analysis, BNP was transformed using natural logarithm because of the right skewness of its distribution, but the summary statistics are presented in the original scale.
The within-subject CV was calculated using the following formula:
where σ is the within-subject mean-square error from the mixed-model analysis of the BNP log-transformed levels. 205
The reference change values (RCVs) were calculated using a log-normal approach based on the following derivations:206–208
The statistical analyses of weight, heart rate, serum creatinine level, and systolic and diastolic blood pressure were conducted on the original scale, without any data transformation. The within-subject CV was estimated as the square root of the residual variance from the intercept model divided by the overall mean. As each subject had several measurements taken, a subject’s means were calculated for weight, heart rate, serum creatinine level, and systolic and diastolic blood pressure. In addition, the within-subject CV from a subject’s mean was computed for each subject. An average within-subject CV was obtained for the clinical variable of interest.
The between-subject CV [CVb(%)] was calculated according to the following formula:
Patients’ characteristics were summarised using means and standard deviations for the continuous variables and counts and percentages for the categorical variables.
The BNP measurement for week 1 was considered as baseline. Changes in BNP over time were calculated as follows:
-
Change in BNP to week 7 = BNPweek7 – BNPbaseline.
-
Change in BNP to week 13 = BNPweek13 – BNPbaseline.
-
Per cent change in BNP to week 7 (%) = 100 × (BNPweek7 – BNPbaseline)/BNPbaseline.
-
Per cent change in BNP to week 13 (%) = 100 × (BNPweek13 – BNPbaseline)/BNPbaseline.
The body mass index was categorised into the following subgroups: underweight (< 18.5 kg/m2), normal (18.5–24.99 kg/m2), overweight (25–29.99 kg/m2) and obese (≥ 30 kg/m2). Kidney function was categorised into CKD stages G1–5 based on their GFR. 209 All statistical analyses were performed with SAS® version 9.3 (SAS Institute Inc., Cary, NC, USA).
Results
Participants’ characteristics
Thirty subjects were included in this analysis. One subject was withdrawn as a result of not entering the follow-up period and one subject had a missing BNP value at week 13. These two missing BNP values at week 13 were not imputed. Data for these two subjects were available for weeks 1 and 7. Therefore, 30 subjects were included in the analyses at week 1 and week 7, and 28 subjects were included in the analysis at week 13.
The study population was relatively young for a CHF group, with a mean age of 55.6 years. Most participants were male (70%) and of white ethnicity (86.7%), with almost half of the cohort categorised as obese (46.6%). The mean serum cholesterol level was 4.8 mmol/l, of which the average LDL cholesterol level was 2.7 mmol/l. The triglyceride levels were mostly in the normal upper range, with a mean of 1.7 mmol/l. Less than one-quarter (23.3%) were categorised as CKD stage G3, and approximately one-third (30%) had normal kidney function (CKD stage G1). The mean creatinine levels were 89.6 µmol/l, and urea levels were slightly higher than average. The study excluded diabetes patients, and the average blood glucose levels were slightly higher than normal. The platelets, calcium, potassium and sodium levels were normal. The patient characteristics of the cohort are presented in Table 21.
Patient characteristics | Summary statistics |
---|---|
Age (years), mean (SD) | 55.6 (9.6) |
0–44, n (%) | 4 (13.3) |
45–54, n (%) | 9 (30) |
55–64, n (%) | 13 (43.4) |
65–74, n (%) | 4 (13.3) |
Female, n (%) | 9 (30) |
Male, n (%) | 21 (70) |
Body mass index (kg/m2), mean (SD) | 31.3 (6.4) |
Normal weight, n (%) | 5 (16.7) |
Overweight, n (%) | 11 (36.7) |
Obese, n (%) | 14 (46.6) |
Ethnicity, n (%) | |
African/American | 3 (10) |
Native Hawaiian/Pacific Islander | 1 (33) |
White | 26 (86.7) |
Cholesterol (mmol/l), mean (SD) | 4.8 (1.1) |
LDL cholesterol (mmol/l), mean (SD) | 2.7 (1) |
Triglycerides (mmol/l), mean (SD) | 1.7 (0.8) |
GFR (ml/m/1.73 m2), mean (SD) | 77.3 (21.8) |
CKD stage, n (%) | |
G1 | 9 (30) |
G2 | 14 (46.7) |
G3 | 7 (23.3) |
Creatinine (µmol/l), mean (SD) | 89.6 (22.8) |
Urea (mmol/l), mean (SD) | 7.2 (2.3) |
HbA1c (%), mean (SD) | 5.8 (0.4) |
Platelet (GI/l), mean (SD) | 221.7 (50.2) |
Calcium (mmol/l), mean (SD) | 2.4 (0.1) |
Potassium (mmol/l), mean (SD) | 4.5 (0.4) |
Sodium (mmol/l), mean (SD) | 139.1 (2.5) |
Approximately half (53.3%) of the participants reported hypertension as part of their medical history; however, a little over one-third (36.7%) of the cohort were hypertensive at baseline. The aetiology of the heart failure was mostly non-ischaemic. Eighty per cent of these CHF patients had left ventricular systolic dysfunction, and 56.7% had a history of arrhythmia. As expected, most of the patients had taken beta blockers, ACE inhibitors, diuretics and aldosterone antagonists. The cardiovascular medical history and past exposure to the cardiovascular medication are summarised in Table 22.
Cardiovascular medical history | Participants (N = 30), n (%) |
---|---|
Angina | 4 (13.3) |
Arrhythmia | 17 (56.7) |
Hypertension | 16 (53.3) |
Ischaemic cardiomyopathy | 11 (36.7) |
Non-ischaemic cardiomyopathy | 19 (63.3) |
Left ventricular systolic dysfunction | 24 (80) |
Left ventricular diastolic dysfunction | 8 (26.7) |
Myocardial infarction | 5 (16.7) |
Valvular heart disease | 7 (23.3) |
Cardiovascular medication exposure history | |
ACE inhibitor | 22 (73.3) |
Aldosterone antagonist | 20 (66.7) |
Angiotensin II antagonist | 8 (26.7) |
Beta blocker | 27 (90) |
Diuretics | 21 (70) |
The average weight was close to 95 kg. On average, the mean heart rate was normal, centred around 70 beats per minute. Although a little over half of the cohort had reported a diagnosis of hypertension in the past, most of the subjects were exposed to blood pressure-lowering medication before entering the study. During the study period, the blood pressure for the cohort was normal, on average. The mean diastolic blood pressure for the cohort was < 70 mmHg during most of the weeks. On average, the serum creatinine level was in the low 90s (of µmol/l), with a standard deviation varying from 21.6 µmol/l (week 13) to 23.9 µmol/l (week 3).
Consistent with a stable, relatively young, CHF population, there were relatively few heart failure medication changes over the course of the clinical trial. One patient was prescribed losartan (100 mg once daily) on the 10th day into the trial and then added amlodipine (5 mg) on the 29th day into the clinical trial to control his hypertension. Another patient had a dose increase in carvedilol [i.e. from 3.125 mg bis in die (b.i.d.) on day 76 to 6.25 mg b.i.d. on day 77]. One patient had a dose reduction in lisinopril (i.e. from 30 mg once daily starting on day 6 to 20 mg once daily beginning on day 29) while taking furosemide (30 mg b.i.d.). Another patient was given an ACE inhibitor starting on day 45 (i.e. lisinopril, 2.5 mg once daily), adding a diuretic (i.e. furosemide, 40 mg once daily) and a potassium replacement on day 49. Another patient had a decrease in diuretics during the trial (i.e. furosemide, 40 mg b.i.d. from day 49 to 40 mg once daily starting from day 52).
B-type natriuretic peptide measures
The baseline BNP levels ranged from 5 ng/l to 605 ng/l at baseline. The BNP levels were < 100 ng/l for 60% of the patients (n = 18) and were between 100 and 199.9 ng/l for eight subjects. There were only four placebo subjects with a baseline BNP value of > 200 ng/l, and, for two of them, such values were > 400 ng/l at baseline. Counts and frequency of patients based on their BNP levels at baseline are presented in Table 23.
BNP category (ng/l) | Counts | Per cent of patients |
---|---|---|
< 100 | 18 | 60 |
100–199.9 | 8 | 26.6 |
200–400 | 2 | 6.7 |
> 400 | 2 | 6.7 |
Total | 30 | 100 |
The means and standard deviations over time for weight, heart rate, systolic and diastolic blood pressure, serum creatinine level and BNP are presented in Table 24.
Week | Weight (kg) | Heart rate (bpm) | Systolic blood pressure (mmHg) | Diastolic blood pressure (mmHg) | Creatininea (µmol/l) | BNPb (ng/l) |
---|---|---|---|---|---|---|
1 | 93.4 ± 23.2 | 71.4 ± 12.7 | 114.8 ± 14.6 | 69 ± 8.4 | 93.9 ± 22.8 | 114.8 ± 136.8 |
2 | 93.6 ± 23 | 68.9 ± 10.1 | 116.2 ± 21.9 | 66.9 ± 10.6 | – | – |
3 | 94.7 ± 23.4 | 67.9 ± 10.8 | 115.6 ± 17.2 | 68.5 ± 8.1 | 90.2 ± 23.8 | – |
4 | 95.1 ± 22.8 | 69.1 ± 12.8 | 115.6 ± 16.7 | 69.7 ± 8.4 | – | – |
5 | 94 ± 23.1 | 71.9 ± 11.4 | 115.6 ± 17.1 | 69.9 ± 8.8 | 94.1 ± 23.5 | – |
6 | 94.3 ± 23.6 | 69.7 ± 13.6 | 112.5 ± 17.3 | 66.7 ± 7.6 | – | – |
7 | 94 ± 23 | 66.8 ± 11.2 | 115.2 ± 15.6 | 67.7 ± 9 | 91.1 ± 23.4 | 112.9 ± 121.6 |
8 | 93.7 ± 23.8 | 66.8 ± 10.2 | 112.7 ± 17 | 67.9 ± 10.3 | – | – |
9 | 95.5 ± 22.9 | 68.9 ± 11.2 | 114.6 ± 18.4 | 68.2 ± 10.9 | 90.1 ± 20.9 | – |
10 | 94.8 ± 24 | 69.2 ± 10.7 | 114.2 ± 16.5 | 68.6 ± 9.1 | – | – |
11 | 94.5 ± 23.7 | 68.2 ± 11.8 | 111 ± 18.7 | 68.5 ± 11.6 | 92.7 ± 22.8 | – |
12 | 94.3 ± 23.8 | 69.4 ± 10.6 | 113.7 ± 17.1 | 68.3 ± 9.1 | – | – |
13 | 93.8 ± 24 | 69.6 ± 11.7 | 115.6 ± 16.7 | 70.7 ± 10.8 | 92.9 ± 21.6 | 103.5 ± 141.6 |
Between-subject B-type natriuretic peptide variability
The ranges of BNP values were 5 ng/l to 605 ng/l, 6 ng/l to 601 ng/l and 3 ng/l to 721 ng/l at week 1, week 7 and week 13, respectively. Two subjects did not have BNP measurements at week 13 either because of withdrawal or missing values. The individual BNP profiles (Figure 11) reveal no trends of BNP levels over time. One subject had BNP values of > 600 ng/l at all time points: 605 ng/ml at week 1, 601 ng/ml at week 7 and 721 ng/l at week 13, from the study start. This subject was a 50-year-old white male, weighing 84.7 kg. His medical history included left ventricular systolic dysfunction and dilated cardiomyopathy, as well as pulmonary embolic disease, which explains the high BNP values. Prior medication exposure included ramipril (5 mg once daily), bisoprolol (1.25 mg once daily), spironolactone (25 mg once daily), furosemide (80 mg once daily) and warfarin (7 mg once daily). Current medication during the study period included furosemide (40 mg once daily) and erythromycin (500 mg) taken during the seventh week in the trial for an upper tract respiratory infection.
FIGURE 11.
Individual profiles of BNP throughout the clinical trial duration.
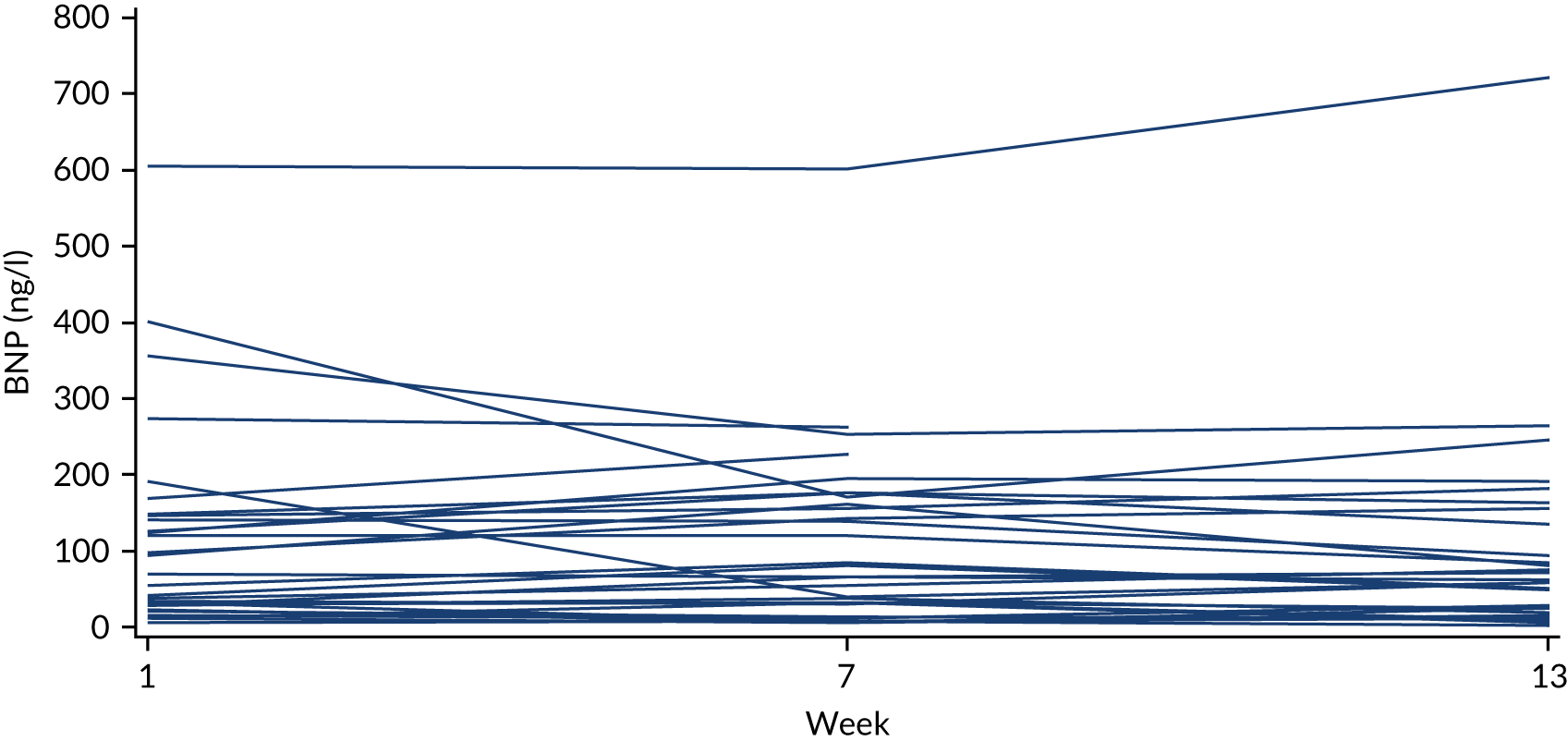
As illustrated in Figure 12, average BNP levels decreased over time. The mean BNP levels were 114.8 ng/l at week 1, 112.9 ng/l at week 7 and 103.5 ng/l at week 13. Compared with baseline, between-patient BNP variation decreases in week 7, and then increases at week 13. The between-subject CV was 119.2%, 107.7% and 136.8% at weeks 1, 7 and 13, respectively. This shows the extent of BNP variability about the mean of the population. Summary statistics for BNP values are presented in Table 25.
FIGURE 12.
Mean/SE plot for BNP levels over time. (a) Original; and (b) logarithmic scales.


Summary measure | Week 1 | Week 7 | Week 13 |
---|---|---|---|
Number of participants included | 30 | 30 | 28 |
Arithmetic mean (95% CI) | 114.8 (63.7 to 165.8) | 112.9 (67.5 to 158.3) | 103.5 (48.6 to 158.4) |
Standard deviation | 136.8 | 121.6 | 141.6 |
SE | 25 | 22.2 | 26.8 |
Geometric mean (95% CI) | 60.3 (40.4 to 99.5) | 60.3 (40.4 to 99.5) | 49.4 (30 to 81.5) |
Standard deviation on natural logarithmic scale | 1.2 | 1.3 | 1.3 |
Between-subject CV (CVb%) | 119.2% | 107.7% | 136.8% |
Median | 62 | 73 | 61 |
Minimum, maximum | 5, 605 | 6, 601 | 3, 721 |
Lower quartile, upper quartile (Q1, Q3) | 28, 146 | 32, 170 | 21.5, 145.5 |
Interquartile range (Q3 – Q1) | 118 | 138 | 124 |
On average, there were no significant changes in BNP from baseline to week 7 or week 13. All summary statistics generated for the change from baseline in BNP are presented in Table 26, and in Table 27 for the per cent changes from baseline.
Summary measure | Change from baseline to week 7 | Change from baseline to week 13 |
---|---|---|
Number of participants included | 30 | 28 |
Arithmetic mean (95% CI) | –1.8 (–25 to 21.3) | –3.7 (–25.1 to 17.7) |
Standard deviation | 62 | 55.2 |
SE | 11.3 | 10.4 |
Geometric mean (95% CI) | 1 (0.8 to 1.3) | 0.9 (0.7 to 1.1) |
Standard deviation on natural logarithmic scale | 0.7 | 0.5 |
CV (CVb%) | –3381 | –1500 |
Median (range) | 3 (302) | –3 (271) |
Minimum, maximum | –231, 71 | –155, 116 |
Lower quartile, upper quartile (Q1, Q3) | –5, 30 | –12.5, 30 |
Interquartile range (Q3–Q1) | 35 | 42.5 |
Summary measure | Per cent change from baseline to week 7 | Per cent change from baseline to week 13 |
---|---|---|
Number of participants included | 30 | 28 |
Mean | 21.5% | 7% |
Standard deviation | 66.8% | 56.2% |
Median (range) | 8.1% (270%) | –11% (196.9%) |
Minimum, maximum | –79.1%, 190.9% | –69.6%, 127% |
Between-subject B-type natriuretic peptide variability in subgroups of patients
Heart failure is a heterogeneous disease. The between-subject variability was generated by subgroups defined by age, sex, body mass, ethnicity and clinical characteristics. The BNP between-subject CV varied over time and was higher for those aged < 55 years. The cohort included only five subjects with a normal body mass index. Therefore, in the present appendix, weight was categorised as normal/overweight versus obese to allow meaningful comparisons between subgroups. For both normal/overweight and obese subgroups, the BNP between-subject CVs decreased from baseline to week 7 and then peaked at week 13. The between-subject CV was lower among obese subjects than among normal or overweight subjects (Table 28).
Parameter | Subgroup | CVb (%); number of participants (n) | ||
---|---|---|---|---|
Week 1 | Week 7 | Week 13 | ||
Age (years) | < 55 | 149.3; n = 13 | 145; n = 13 | 169.9; n = 13 |
≥ 55 | 100; n = 17 | 74.2; n = 17 | 88.4; n = 15 | |
Body mass index | Normal/overweight | 129.7; n = 16 | 112.6; n = 16 | 133.5; n = 16 |
Obese | 104.9; n = 14 | 97.6; n = 14 | 120.4; n = 12 |
The BNP between-subject CVs for various subgroups defined by a history of hypertension, arrhythmia and ischaemic and non-ischaemic cardiomyopathy are presented in Table 29. The between-subject variability in BNP was also described in subgroups of subjects defined by prior exposure to medication commonly prescribed to heart failure patients: beta blockers (e.g. carvedilol, carvedilol phosphate, metoprolol, metoprolol succinate, nebivolol hydrochloride and bisoprolol), ACE inhibitors (e.g. captopril, lisinopril, enalapril, ramipril or quinapril), aldosterone antagonists (e.g. spironolactone or eplerenone), angiotensin II antagonists (e.g. losartan, losartan potassium, valsartan, candesartan or candesartan cilexetil) and diuretics (e.g. torasemide, furosemide, metolazone, hydrochlorothiazide or bumetanide). As, often, heart failure patients have multiple comorbidities and are prescribed multiple medications, the table should be read in the context of each line without comparing the subgroups or multiple lines at a time. The between-subject CV was high in those with a history of non-ischaemic cardiomyopathy and those previously exposed to aldosterone antagonists, ACE inhibitors, beta blockers or diuretics.
Subgroup | CVb (%); number of participants (n) | ||
---|---|---|---|
Week 1 | Week 7 | Week 13 | |
History of hypertension | 101.9; n = 16 | 83.6; n = 16 | 97.5; n = 14 |
History of arrhythmia | 94.1; n = 17 | 63; n = 17 | 73.5; n = 16 |
History of ischaemic cardiomyopathy | 102; n = 11 | 71; n = 11 | 75.6; n = 10 |
History of non-ischaemic cardiomyopathy | 124.4; n = 19 | 119.7; n = 19 | 154.8; n = 19 |
Prior beta blocker | 122.5; n = 27 | 111.5; n = 27 | 147; n = 25 |
Prior ACE inhibitor | 130; n = 22 | 125.9; n = 22 | 169.5; n = 20 |
Prior aldosterone antagonist | 134.8; n = 20 | 126.4; n = 20 | 165.8; n = 18 |
Prior angiotensin II antagonist | 92.5; n = 8 | 63.6; n = 8 | 71.5; n = 8 |
Prior diuretic | 121.7; n = 21 | 117.6; n = 21 | 148.4; n = 21 |
Within-subject B-type natriuretic peptide variability
Two random intercept models were fitted to the log-transformed BNP repeated measures, one including baseline BNP and one excluding this measure. Both models showed the same effect on the average increase in BNP per week; therefore, the random intercept model with the BNP baseline as a covariate was dropped to avoid model overparameterisation. This model included week as a fixed effect in addition to the random intercept, and was fitted using restricted maximum likelihood estimation for variance components. Most of the subjects had three BNP measurements over time, accounting for the 88 observations used in the model. The estimated BNP within-subject CV was 46%, with the rest of the model estimates reported in Tables 30 and 31. The average BNP value at baseline on the naturally logarithmic scale was 4.1267, corresponding to a BNP geometric mean of 62 ng/l, with no evidence of an average increase in BNP per week.
Covariance parameter | Estimate |
---|---|
Intercept | 1.3802 |
Residual | 0.1909 |
Within-subject CV | 45.86% |
RCV+ (upwards) | 242% |
RCV– (downwards) | –70.7% |
Effect | Estimate | SE | Degrees of freedom | t-value | Pr > |t| |
---|---|---|---|---|---|
Intercept | 4.1267 | 0.2291 | 29 | 18.01 | 0.0001 |
Week | –0.0055 | 0.0096 | 57 | –0.57 | 0.5704 |
As suggested by the BNP individual profiles over time (see Figure 11), the results of the random intercept model showed no evidence of progression or deterioration over time. Sensitivity analyses were conducted when excluding the two subjects with missing BNP values at week 13. The results were robust to transformation and differences in model selection.
Within-subject variability for BNP was much higher than the variability for other clinical variables often measured in primary care practice, such as weight, heart rate, blood pressure or serum creatinine. The estimated within-subject CVs are displayed in Table 32.
Group of patients | n | Estimated CVw (%) from mixed modelsa | Average CVw (%)b |
---|---|---|---|
Weight | 30 | 1.2 | 1.1 |
Heart rate | 30 | 10.3 | 9.6 |
Systolic blood pressure | 30 | 9.7 | 8.7 |
Diastolic blood pressure | 30 | 10.7 | 10.3 |
Serum creatinine level | |||
All | 30 | 8.9 | 7.3 |
Females | 9 | 10.7 | 8.2 |
Males | 21 | 8.1 | 6.9 |
Discussion
We used data from the placebo arm of a clinical trial providing repeated measurements taken over time to estimate the between- and within-subject variability for BNP. The study participants were stable CHF patients of NYHA class II or III. Ninety per cent of the cohort had been exposed to cardiovascular drugs before the baseline; therefore, their NP levels were expected to be similarly affected. This could be one of the reasons why BNP was stable, and few medications were changed over time.
The BNP within-subject variability over a 3-month period was 46%, with large RCVs. The results suggest that monitoring over 3 months is unlikely to detect true BNP change against background noise in this small, chronically stable, heart failure population. For reference, a change in BNP would have been detected as statistically significant if there had been an increase in BNP of at least 235% or a decrease of at least 70.2% between two consecutive visits.
Troughton and Richards210 reported variability of 30% between serial measurements in NT-proBNP. Meijers et al. 211 reported log-normal RCVs upwards and downwards of 104.9 and –40.1, respectively, in 83 heart failure patients over a 6-week period. The Study Group on Biomarkers in Cardiology of the ESC acknowledged intraindividual biological variability of between 30% and 50% for all NPs. 212 CHF patients are typically treated using several drugs to deal with their condition and various existing comorbidities. Drug–drug interactions and poor adherence are likely to affect increasing within-subject variability.
Limitations
The main limitations of this analysis are the small sample size and short duration of follow-up. The placebo cohort included only 30 subjects. Therefore, the CIs were wide, suggesting high imprecision. Similarly, subgroup analyses were affected by the small sample size. The younger age and lower body mass index subgroups included few subjects, but this was not surprising given the heart failure epidemiology. Laboratory and cardiac imaging parameters were summarised on a continuous scale, thus avoiding subgrouping whenever possible. Although 30% of the subjects were females, given the small sample size, this corresponded to only nine women. The 3-month study duration was relatively short: about 30–40% of CHF patients die within the first year of being diagnosed with heart failure. 52,53
The results are not generalisable to people with diabetes because the study did not include patients with type 2 diabetes mellitus. BNP values are higher in diabetic CHF patients. 213 The results are not generalisable to patients with a history of renal failure, characterised by a GFR of < 40 ml/minute/1.73 m2.
The BNP reference ranges and analytical performance depend on the assay being used to measure them. 214 The analytical imprecision of commercially available BNP assays varies from 3.1 to 16.7. 214 The NT-proBNP intra-assay precision was 5.4% for the IMMULITE® 2000 assay (Siemens Healthineers AG, Erlangen, Germany). 215 The 30 subjects included in this appendix were enrolled at 11 different sites. Unfortunately, the BNP assay was not collected; therefore, the assay imprecision could not be evaluated. The extent of this measurement bias is unknown in this study.
The aetiology of the CHF for all participants was cardiomyopathy of an ischaemic or non-ischaemic origin. However, the CHF duration was not available, but, given that this is a trial, it is unlikely that considerable differences existed between participants. The study included CHF patients with NYHA class II or III at screening; however, this symptomatic classification was not collected throughout the clinical trial. Thus, a potential change in NYHA class could not be assessed.
Implications for research and clinical practice
Owing to the small sample size of only 30 research subjects, the results of this study need to be replicated in larger cohorts of CHF patients, potentially of varying degrees of severity. Clinical trial data from CHF patients being monitored for > 3 months may provide additional insight.
The high within-subject variability of the BNP levels poses severe limitations when monitoring CHF patients in the primary care setting. In stable chronic, relatively young, heart failure patients, monitoring BNP over a 3-month period is of limited value. Although other clinical biomarkers (e.g. heart rate, weight, blood pressure and serum creatinine level) have a much lower within-subject variability in this population, only BNP has been identified as having a clear prognostic association with severity (e.g. all-cause mortality) among patients with CHF. Relevant to this, weight profiles in this study were mostly flat during the follow-up period. The within-subject CV of weight was 1.2%. The slight weekly fluctuations in weight during the study period did not appear to be associated with high BNP values.
Conclusion
The BNP within-subject variability over a 3-month period was 46%. The BNP upwards and downwards values for the log-normal RCVs were 235% and –70.2%, respectively.
Appendix 21 Observational feasibility study of point-of-care NT-proBNP monitoring in patients with heart failure in primary care
Authors: Susannah Fleming, Clare J Taylor, Richard Stevens and Clare Bankhead.
Introduction
Heart failure is a chronic disease, which can result in significant morbidity and mortality. NP levels are currently used as part of the diagnostic pathway in heart failure, and have been shown to correlate with poor prognosis. 216 Patients with heart failure receive much of their care in the community, either in primary care or through specialist community heart failure nurses, and are referred to secondary or tertiary care as required if they experience acute illness or deterioration.
It has been postulated that routine monitoring of NP could assist in improving the care of patients with heart failure in the community. 216 Recent advances in technology provide the possibility of POC NP testing, but there is currently no evidence to support the use of such devices as part of routine care of patients with heart failure in primary care.
Both BNP and NT-proBNP appear to have similar predictive value in heart failure. 216,217 However, NT-proBNP appears to have less biological variation than BNP in patients with heart failure. 211,218–222
The primary aim of this study was to determine the variability in NP measurements made using POC NP technology in patients with heart failure in primary care settings, including both between- and within-person variability.
Methods
This is a feasibility study, comprising an observational cohort study of POC NP measurement. A previous horizon scan of POC NP devices suitable for use in the diagnosis of heart failure in primary care identified two potential POC NT-proBNP devices. 217 Of these, we chose to use the Roche Cobas h 232 device (Roche Diagnostics, Switzerland), as this was the device for which the most evidence is available, and is also the only one for which a UK distributor could be identified. It also carries a Conformité Européenne (CE) mark and is currently marketed in the UK as a POC assessment of NT-proBNP for diagnosis of heart failure.
We recruited patients from three GP practices in Oxfordshire. Prospective patients were identified by searching the practice register for adults with a recorded diagnosis of heart failure, and were checked by a clinician against the inclusion and exclusion criteria for the study (Table 33). Eligible patients were invited to take part by post or direct invitation by a member of the practice team. Recruitment to the study took place from 20 February 2018 to 28 March 2018, with follow-up to 29 March 2019.
Inclusion criteria | Exclusion criteria |
---|---|
|
|
Participants attended three scheduled visits at 0, 6 and 12 months from baseline. Visits were carried out at the participant’s usual GP surgery, with a practice nurse trained in the use of the POC device. At all three visits, three venous blood samples were taken: one for POC NT-proBNP measurement and two to be sent to the local laboratory for NT-proBNP and renal function testing.
We also requested that, where possible, additional POC NT-proBNP measurements should be taken when study participants attended the practice outside their scheduled visits for any reason related to their heart failure. However, owing to the potential additional waiting time that this may cause for patients, we did not require that these measurements must be taken.
Statistics and sample size
The sample size for this study was chosen based on practical judgement rather than a formal sample size calculation, as this is a feasibility study. We judged that a sample of 30 patients would be sufficient to test our primary outcome measure, and recruited three practices based on a predicted recruitment of 10 patients per practice.
Where results from the POC device were reported at the end of the range, we analysed them using the value closest to the range of the device (i.e. values reported as < 60 pg/ml were analysed as 59 pg/ml and those reported as > 9000 pg/ml were analysed as 9001 pg/ml).
Within-person variability was calculated as the mean of within-person standard deviations, and between-person variability was calculated as the standard deviation of within-person means.
Results
Descriptive statistics of population
We recruited 27 participants from three GP practices to take part in the study. Figure 13 shows participant flow through the study. The median follow-up time was 366 days, with a maximum of 396 days, and the total study follow-up time was 24.5 person-years.
FIGURE 13.
Flow of participants in the observational study of POC NT-proBNP. Patients noted as discontinued did not attend any further visits. The two participants who missed visit 2 but were not discontinued continued to attend at visit 3.

Three participants discontinued participation during the study, two of whom only attended visit 1, and one participant attended both visit 1 and visit 2. Two participants discontinued because of changes in circumstances and one participant died during the course of the study.
Two participants did not attend visit 2 during the study but were not discontinued as they attended for visit 3. POC NT-proBNP measurements were successfully obtained at all visits attended by participants. No patient in the study had POC NT-proBNP measured at a routine GP appointment.
Table 34 summarises the baseline characteristics of the study population. The most common comorbidities reported at baseline were hypertension (15 participants, 55.6%), CKD (10 participants, 37.0%), arthritis (nine participants, 33.3%), and cancer (six participants, 22.2%).
Characteristic | Summary, n (%) |
---|---|
Age in years (mean, SD) | 77.6 (9.1) |
Sex | |
Male | 10 (37.0) |
Female | 17 (63.0) |
Ethnicity | |
White British | 25 (92.6) |
White other | 1 (3.7) |
Indian | 1 (3.7) |
Type of heart failure | |
Heart failure with preserved ejection fraction | 10 (37.0) |
Heart failure with reduced ejection fraction | 13 (48.1) |
Unknown | 4 (14.8) |
Baseline NYHA functional classification | |
Class I | 8 (29.6) |
Class II | 14 (51.9) |
Class III | 4 (14.8) |
Class IV | 1 (3.7) |
Primary analysis
Within-person variability was calculated using data from 25 participants. The two participants who were discontinued after visit 1 had only one POC measurement, and so could not contribute to this analysis. Within-person variability in POC NT-proBNP over 12 months was 881 pg/ml (95% CI 380 to 1382 pg/ml). Between-person variability was calculated using data from all 27 participants. Between-person variability in POC NT-proBNP over 12 months was 1972 pg/ml (95% CI 1525 to 2791 pg/ml).
Discussion
Principal findings
We found that it was feasible to carry out POC NT-proBNP testing in primary care, with testing successfully carried out in 100% of planned study visits. However, no tests were carried out during routine primary care visits by the study participants.
Between-person variability in POC NT-proBNP was around twice as large as within-person variability over a 12-month period. This would indicate that deviations from individual set points for NT-proBNP are likely to be more helpful than population-level thresholds when considering a monitoring strategy.
Strengths and weaknesses
As this is a feasibility study, the main weakness is the limited sample size. However, despite this, we have been able to clearly determine that it is possible to carry out POC NT-proBNP testing in primary care. However, this is limited to the situation of a pre-booked appointment with a clinician trained and prepared to use the POC device, and with sufficient time within the appointment to carry out venepuncture and wait for the POC device to provide a result. In this study, we did not mandate attempting to carry out POC testing during routine or unplanned appointments, or reporting why it was not carried out, so we do not have evidence of the barriers that prevented testing.
Comparison to other studies
Most previous studies assessing POC BNP or NT-proBNP have done so in secondary care. One previous study in Belgium223 assessed the practicality and accuracy of POC NT-proBNP for diagnosis of heart failure in primary care, using the same device as was used in this study. They found that GPs were able to incorporate the device into routine practice and showed high levels of acceptability from the GPs. A notable difference between the two studies is that all GPs at the participating practices in the study by Hex et al. 223 were trained to use the POC device, whereas in our study, only one or two practice nurses were trained to do so. This may have had implications for the practicality of carrying out unplanned POC tests.
Implications for clinicians or policy-makers
This study shows that it is feasible to monitor NT-proBNP in patients with heart failure using POC testing in primary care. This has important implications for the implementation of guideline recommendations that include NP monitoring following recent hospitalisation to prevent readmission. The lack of POC testing in routine appointments seen in our study is likely to reflect the current pressures in primary care. If unscheduled NP testing is to be successfully used, other factors such as sufficient time, training and staff available to carry out testing may need to be considered.
Unanswered questions and plans for future research
Further secondary analysis of the data gathered in this study should allow us to assess the potential impact that POC NT-proBNP testing in primary care has on clinical decision-making and to further investigate how the POC device fitted into the primary care workflow. Further research is needed to identify if and how this type of POC testing could be incorporated into routine primary care and to assess the potential benefit of monitoring of NT-proBNP in patients with heart failure who are managed in primary care.
Further research to characterise the short-term variability of POC NT-proBNP (e.g. within 1 day or 1 week) would also be valuable, as would assessments of whether or not accuracy and variability was dependent on operator skill level (e.g. nurse, health-care assistant or lab technician).
Appendix 22 A systematic review of economic models of chronic heart failure and economic issues of monitoring chronic heart failure
Introduction
The prevalence of CHF is rising47 and there is a growing interest in efforts to optimise its management in primary care. Economic evaluations are required to assess competing strategies and inform policy decisions on the efficient use of resources. These require long-term CHF models. We aimed to review available models of CHF that were potentially suitable to inform such assessments with a focus on the model structure and data used. We discuss limitations of available CHF economic models to assess CHF interventions in a primary care setting and make recommendations for future research.
Methods
An earlier published systematic literature review of decision-analytic models to project health outcomes and costs in patients with heart failure by Goehler et al. 77 was updated. Unlike Goehler et al. ,77 who considered all models regardless of care setting for CHF, we explicitly focused on models that could be used to assess management and treatment strategies for CHF in a primary care setting. Briefly, the search strategy in Goehler et al. 77 was implemented in MEDLINE and EMBASE, and was used to search for eligible publications from 1 June 2010 to 31 August 2018. The study inclusion criteria required the study to include a mathematical model that evaluated costs and health effects of health technologies. Studies that were short-term economic evaluations alongside clinical trials were excluded, as were studies where a model was used only to illustrate a methodological concept or was a non-UK adaptation of a study already included in the review model. Only full-text English-language studies were included. Since the review focused on methodological approaches, a formal protocol registration was not sought.
Studies were reviewed and data were extracted using a set of six broad questions:
-
What were the study characteristics?
-
How was the model structured?
-
How was disease progression modelled?
-
How was the treatment effect of the intervention modelled?
-
Did the model take into account patient characteristics?
-
What data sources were used to inform model parameters (i.e. for the disease end points, quality of life and costs)?
The information to answer these questions were independently extracted into a predefined spreadsheet from each study by either CS or BM with queries resolved by discussion (CS and BM). As in Goehler et al. ,77 we did not formally assess the quality of the methods used or studies from which data were sourced as the aim was to summarise the model features.
Results
The updated systematic literature search yielded 555 references published between June 2010 and August 2018. Duplicate papers (n = 17), conference abstracts (n = 315) and short-term cost-effectiveness studies carried out alongside RCTs (n = 6) or alongside cohort studies (n = 13) that did not require a model were excluded. The full texts of 68 papers featuring decision models were reviewed, during which additional papers were excluded because the study interventions were carried out in a secondary care setting only (n = 33), they focused on specific subgroups of patients with CHF (e.g. diabetes or hypertension), they were a non-UK-specific adaptation of already included models (n = 14), or were a review of cost-effectiveness studies or methods (n = 5). A total of 20 manuscripts from the new search were included in the final selection. One additional study was identified through a search of references. In addition, 19 papers from the previous review by Goehler et al. 77 were also included. In total, 40 models were included in the review (Figure 14).
FIGURE 14.
The PRISMA diagram of study selection.

Included models (Table 35) assessed diagnostic procedures (n = 3),13,234,257 disease management interventions (n = 11)233,238–240,243,245,250,256,260–263 (one model was reported in two studies262,263) and drug interventions (n = 26). 224–232,235–237,241,242,244,246–249,251–255,258,259 Modelling approaches included Markov models (n = 31), decision trees (n = 1), Markov model alongside a decision tree (n = 2), discrete event simulation (n = 4) and structural mathematical equations (n = 2).
Study (first author and year), country | Type of intervention | Target population | Perspective | Model type | Time horizon | Model structure | Outcome of the model |
---|---|---|---|---|---|---|---|
van Hout224 (1993), the Netherlands | Drugs | Aged 35–85 years, II–IV NYHA class | Societal | Markov | 10 years | Five states: four NYHA states, one death state | LY, costs, ICER |
Paul et al.225 (1994), USA | Drugs | EF < 35%, NYHA class | Societal | Markov | 10 years | Six states: five hospitalisation states (0, 1, 2, 3, ≥ 4 hospitalisations), one death state | LY, costs, ICER |
Glick226 (1995), USA | Drugs | EF < 35%, NYHA class | Societal | Markov | Lifetime | Weibull model for annual survival probabilities; four NYHA classes for functional status and quality of life | LY, QALYs, costs, ICER |
Delea227 (1999), USA | Drugs | EF < 35%, mean age 60 years, II–IV NYHA class | Societal | Markov | 20 years | Six states: five hospitalisation states (0, 1, 2, 3, ≥ 4 hospitalisations), one death state | LY, costs, ICER |
Levy228 (2001), Canada | Drugs | II–III NYHA class | Third party payer | Markov | 20 years | Six states: five hospitalisation states (0, 1, 2, 3, ≥ 4 hospitalisations), one death state | LY, costs, ICER |
Varney229 (2001), UK | Drugs | Symptomatic HF | Third party payer | Mathematical equations | 5 years | Survival model using actuarial method to estimate survival probabilities; hospitalisation rate estimated using trial data (to account for costs) | LY, costs, ICER |
Gregory230 (2001), USA | Drugs | II–III NYHA class | Not indicated | Mathematical equations | Not indicated | Survival model (sex-stratified Weibull model); hospitalisation model (exponential probability distribution model between hospitalisations) to account for costs | LY, costs, ICER |
Barry231 (2002), Ireland | Drugs | III–IV NYHA class | Not indicated | Markov | 10 years | Two states: alive, dead; CHF hospitalisation state for cost only | ICER |
Tilson232 (2003), Ireland | Drugs | EF < 35%, III–IV NYHA class | Not indicated | Markov | 10 years | Three states: severe HF, hospitalisation with severe HF and death | ICER |
Morimoto233 (2004), Japan | Management | EF < 40%, aged 35–85 years, II–III NYHA class | Not indicated | Markov | 18 months | Six states: five hospitalisation states (0, 1, 2, 3, ≥ 4 hospitalisations), one death state | Costs, ICER |
Heidenreich234 (2004), USA | Diagnostic | Asymptomatic with EF < 40%, NYHA class | Societal | Markov | Lifetime | Four states: three HF stages [symptomatic, non-symptomatic (both with low EF) and asymptomatic normal EF], one death state. Event state of hospitalisation | LY, QALYs, costs, ICER |
Cowper235 (2004), USA | Drugs | I–IV NYHA class | Societal and third party payer | Markov | 5 years | Five states: four NYHA states, one death state (probability of hospitalisations included in the model) | LY, costs, ICER |
Inomata236 (2004), Japan | Drugs | II–III NYHA class | Third party payer | Markov | 5 years | Two states: alive and dead; a further ‘hospitalisation due to worsening CHF’ to inform costs only | LY, costs |
Caro237 (2005), USA | Drugs | Aged 41–80 years, II–IV NYHA class | Third party payer | DES | 2 years | Events: hospitalisation (HF, CVD other); death (CVD, other); hospitalisation (cost and time); management (NYHA state, hospital visits, drug costs) | LY, costs, ICER |
Göhler238 (2008), Germany | Management | III–IV NYHA class | Societal | Markov | Lifetime | Five states: four hospitalisation states (index hospitalisation; rehospitalisations 1, 2 and ≥ 3), one death state | LY, QALYs, costs, ICER |
Chan239 (2008), USA | Management | EF < 35%, II–III NYHA class | Health-care provider | Markov | 15 years | Six states: five hospitalisation states (0, 1, 2, 3 and ≥ 4 hospitalisations), one death state | LY, cost, ICER |
Miller240 (2009), USA | Management | Echocardiographic evidence of CHF, NYHA I–III | Health-care system | Markov | Lifetime | Four states: NYHA I, II, III/IV and death | LY, QALYs, costs, ICER |
Rosen241 (2010), USA | Drugs | Symptomatic HF | Third party payer | Markov | Lifetime | Six states with combinations of major events (e.g. MI, stroke) and minor events (e.g. TIA), and non-cardiovascular and all-cause mortalities from states | LY, QALYs, costs, ICER |
Cowie242 (2011), UK | Drugs | NYHA II–III | Third party payer | Markov | Lifetime | 10 states: cardiovascular events [complication-free HF; HF hospitalisation, MI (first or second) and stroke (first, second or third) and CVD and other death] | LY, cost, QALY, ICER |
Moertl243 (2012), Austria/Canada | Management | NYHA III–IV | Payer | Markov | 20 years | Five states: event free, one hospitalisation, two hospitalisations, three or more hospitalisations and death | LY, cost, QALY, ICER |
Ford244 (2012), Australia | Drugs | II NYHA class | Government-funded health system perspective | Markov and decision tree | 5 years | Four states: NYHA classes I–IV. A decision tree (for each NYHA class) for hospitalisation and death | LY, cost, QALY, ICER |
Thokala245 (2013), UK | Management | Within 28 days of HF hospital admission | Health-care system | Markov | 30 years | Two states: alive and dead | QALY, cost, ICER |
Banka246 (2013), US | Drugs | EF < 35%, NYHA II–III | Single-payer/vertically integrated health-care system providing full coverage | Markov | Lifetime | Two states: alive and dead | LY, cost, QALY, ICER |
Ademi247 (2014), Australia | Drugs | Aged ≥ 55 years, LVEF ≤ 30%; on hypertensive treatment, NYHA class II | Australian health-care system | Markov | 10 years | Two states: alive and dead and additional cardiovascular transition states (no HF hospitalisation, HF hospitalisation) | LY, cost, QALY |
Griffiths248 (2014), UK | Drugs | Heart rate > 70 b.p.m., prior to HF hospitalisation, NYHA class II–IV | NHS | Markov | Lifetime | HF hospitalisation; cardiovascular hospitalisation, death, NYHA class, hospital length of stay | LY, cost, QALY, ICER |
Lee249 (2014), UK/Spain | Drugs | II NYHA class | Not clear | DES | Lifetime | Eight event states (other cardiovascular hospitalisation; HF hospitalisation; atrial fibrillation; adverse event; treatment continuation; devices; cardiovascular mortality; non-cardiovascular mortality) | LY, cost, QALY, ICER |
Reed250 (2015), USA | Management | Any NYHA class | Any | Individual patient simulation | Lifetime | A modified version of the Seattle Heart Failure Model in which the risk of death and treatment effects of medications were revised using recent clinical trials’ data | LY, cost, QALY, ICER |
Kansal251 (2016), USA | Drugs | LVEF < 35%; resting heart rate > 70 b.p.m.; sinus rhythm, NYHA class II–IV | Third party payer | Markov | 10 years | 10 states: interactions of HF events (0 to ≥ 3), cardiovascular events (0 to ≥ 3) where HF ≤ cardiovascular event, and death | Life expectancy, cost, QALY, ICER |
Thanh252 (2016), Canada (AB) | Drugs | Mild symptoms aged > 55 years | Health service | DES | Lifetime | Eight event states (HF hospitalisation, other CVD hospitalisation, AE, AF, implant, CVD death, other death, end of time horizon) | Life expectancy, cost, QALY, ICER |
Sandhu253 (2016), USA | Drugs | LVEF < 40%, II–IV NYHA class | Societal | Markov | Lifetime | Six states: HF hospitalisation, non-HF hospitalisation, ED HF visit, treatment intolerance, CVD death and non-CVD death | Life expectancy, cost, QALY, ICER |
King254 (2016), USA | Drugs | Reduced EF (< 35%) | Third party payer | Markov | 40 years (lifetime) | Combination of four NYHA classes and clinical events [no event, HF hospitalisation (30-day re-admission, no re-admission), death] | Life expectancy, cost, QALY, ICER |
Gaziano255 (2016), USA | Drugs | LVEF < 40%, II–IV NYHA class | Third party payer | Markov | 30 years | Two states: alive (with HF) and dead with hospitalisation classed as an event | Life expectancy, cost, QALY, ICER |
Dang256 (2017), USA | Management | Societal | Markov | 10 years | Eight states: three hospitalisation states (1, 2 and 3), four alive (but not hospitalised) states taking into account the number of previous hospitalisations and one death state | Life expectancy, costs, ICER | |
Monahan257 (2017), UK | Diagnostic | Symptoms suggestive of HF | NHS/Personal Social Services | Decision tree | Lifetime | Taken from a previous HF Health Technology Assessment (to present the different diagnostic strategies for patients presenting with HF symptoms in primary care) | Life expectancy, cost, QALY, ICER |
Liang258 (2017), Singapore | Drugs | Health-care payer | Markov | 10 years | NYHA classes I–IV and deaths; interacting with no event; HF hospitalisation (no admission; re-admission); death from CVD or other cause | Life expectancy, cost, QALY, ICER | |
van der Pol259 (2017), the Netherlands | Drugs | Health-care payer | Markov | 30 years | Four states: HF (home care); ward hospitalisation; intensive care unit hospitalisation; dead | Life expectancy, cost, QALY, ICER | |
Maru260 (2017), Australia | Management | II–IV NYHA class | Government-funded health system | Markov | 15 years | Five states: event free, HF hospitalisations (1, 2 and ≥ 3) and death | Life expectancy, cost, QALY, ICER |
Grustam261 (2018), the Netherlands | Management | Aged ≥ 70 years and I–IV NYHA class | Health care | Markov | 20 years | Five states: four NYHA I–IV states and one death state; annual cycle | Life expectancy, costs, QALYs, ICER |
NICE HF guideline13 (2018), UK | Diagnostic | People with suspected or diagnosed HF | UK NHS/PSS | Decision tree/Markov model | Lifetime |
A decision tree: proportion in each disease cohort and test referral Markov model: hospitalisation and death |
QALY, cost, ICER |
Mohiuddin262 (2016) and Pufulete263 (2016/2017), UK | Management | Subgroups by age and LVEF status, NYHA class | Government-funded health system perspective | Markov | 30/15 years | Two states (alive and dead); probabilities of death and hospitalisation recorded as an event that has an impact on the quality of life and costs | Life expectancy, cost, QALY, ICER |
Modelling of disease progression
There were several recurring approaches of varying complexity of modelling CHF disease progression (see Table 35). Ten studies used the NYHA classes in the basis of model disease progression. Of these studies, eight used a Markov model,224,226,235,240,248,254,258,261 one used a Markov model alongside a decision tree244 and one used a discrete event simulation. 237
Ten studies,225,227,228,233,235,238,239,243,256,260 all of which used Markov models, used the number of hospitalisations for heart failure as the basis of model disease progression. A further two studies229,230 used mathematical equations to model hospitalisations, and another259 used types of hospitalisation (ward or intensive care unit) as model states. One study234 used alternative definitions of CHF (symptomatic and ejection fraction) as states in their Markov model.
A two-state Markov model using ‘alive’ and ‘dead’ states was used in six studies. 226,231,236,245–247 Five Markov models237,241,242,252,253 used CVD events, including decompensated heart failure, myocardial infarction, stroke/transient ischaemic attack and cardiovascular death in modelling CHF progression. Three studies used composite states consisting of the NYHA class and number of hospital admissions for heart failure to model progression. 249,254,258 Two further Markov models248,251 used hospitalisation for heart failure combined with CVD events to model disease progression.
More recent studies were more likely to have a more detailed model structure, with all four studies having at least 10 states published post 2011. 242,251,254,258
Patient characteristics
Eleven studies considered a range of patient characteristics at entry into the models. In six studies non-fatal heart failure hospitalisations at entry influence the probability of death. 245–247,255,262,263 One study245 considered the impact of the time since discharge from heart failure hospital admission on the probability of death. One study238 used an age and sex adjustment for non-CVD death and overall mortality. In one study,248 which used parametric risk equations to model composite NYHA and CVD end points in the model, a range of baseline sociodemographic characteristics, use of heart failure medications or other cardiac therapies, prior cardiovascular history and biological characteristics were used. A further study250 used the Seattle Heart Failure Score (and updated it in the model) to inform the cause and probability of death.
More recent studies were more likely to incorporate patient characteristics in their models. All studies that considered interaction states were published after 2012. 248,249,251,254,258 Similarly, all seven studies245–247,255,256,262,263 one used a Markov model alongside a decision tree244 that considered the impact that heart failure hospitalisation had on death rates were published post 2013. Models published earlier than 2013, were more likely to use constant transition probabilities over time.
Data sources used to populate the models
End points
Thirty-nine economic models13,224–227,229–263 used data from RCTs to model CHF and disease progression, with the remaining study228 using cohort and meta-analysis data (Table 36). Twenty-six studies used only RCT data. The remaining studies used RCT data together with data from cohort studies (n = 4),230–232,250 administrative data (n = 8)13,235,236,240,256,259,262,263 or some combination of these (n = 2). 224,244 A total of 22 different RCTs were used across the 43 studies. One RCT explicitly stated that it had recruited from primary care. 257
Study (first author and year), country | Data to inform disease end points in model | Individual patient characteristics in model | Data to inform costs in model | Data to inform quality of life in model |
---|---|---|---|---|
van Hout224 (1993), the Netherlands | Unclear | Unclear | Unclear | Not collected |
Paul225 (1994), USA | Transition probabilities estimated using available trial data | Transition probabilities depend on time | US administrative data used to estimate a cost for a hospitalisation. Drug costs taken from retail pharmacy survey | Not collected |
Glick226 (1995), USA | Trial data used to estimate Weibull model for survival and the transition probabilities between NYHA states. Post-trial probabilities adjusted to reflect an increased all-cause mortality. Administrative data used to estimate ambulatory care event rates | Unclear | US administrative data used to cost all events (including death) and therapy | The visual analog scale, the Ladder of Life questionnaire, data in the trial was used to estimate quality of life for each NYHA state |
Delea227 (1999), USA | Trial data (from two studies): published data used to ‘solve’ for the different state-specific probabilities of hospitalisation and costs | Unclear | Drug and event costs taken from various US generic sources used to estimate cost of events and baseline costs | Not collected |
Levy228 (2001), Canada | Cohort data for monthly transition probabilities of event. Random effect meta-analysis of placebo-controlled trials used for treatment effect | Age-specific (5-year age bands) transition probabilities and length of hospital stay | Author assumptions for quantity; daily costs from administrative sources used to estimate costs of hospitalisation and death | Not collected |
Varney229 (2001), UK | Survival was estimated using the actuarial method using aggregated trial data; rate of hospitalisation estimated using aggregated trial data | Unclear | Administrative data and trial data used to estimate cost of hospitalisation and multiplied by hospitalisation rate | Not collected |
Gregory230 (2001), USA | Trial data and Framingham Heart Equations used to estimate lifetime survival distributions. Published data for estimating hospitalisations | Gender-stratified Weibull models | Administrative data used to derive a cost model estimating the relationship between the admissions and hospitalisation/outpatient/professional costs | Not collected |
Barry231 (2002), Ireland | Cohort data (hospital study) for probabilities of death and hospitalisation. Treatment effect from trial data | None (fixed rates) | Previous micro-costing for cost of drugs and hospitalisation | Not collected |
Tilson232 (2003), Ireland | Cohort data from hospital clinic (Ireland) (12-month period) for probabilities of death and hospitalisation. Treatment effect from trial data | None | Previous micro-costing used for cost of hospitalisation (carried out in the same hospital) | Not collected |
Morimoto233 (2004), Japan | Delea et al.227 used for comparator probability of admission (trial data). Troughton et al.264 used to estimate effects of treatment | None | Delea et al.227 | Taken from external time trade-off study – one quality of life for all alive states |
Heidenreich234 (2004), USA | Framingham Heart Study used for specificity and sensitivity of test. Trial data used to estimate event rates | Sex-specific specificity and sensitivity of BNP test | Administrative data used for resource use and costs for states | Time trade-off data from a previous trial used to estimate weights for asymptomatic/symptomatic states |
Cowper235 (2004), USA | Trial data used for transition probabilities between NYHA states. Observational data used to estimate number of allowed hospitalisations. Trial data supplemented by observational studies used for estimating treatment effects | Administrative data for each NYHA state and inpatient/outpatient states | Not collected | |
Inomata236 (2004), Japan | Trial data and administrative data (non-CHF-related mortality) | Cohort data used to cost an outpatient schedule | Not collected | |
Caro237 (2005), USA | Event rates taken from trial results and assigned to relevant states | Age, sex, NYHA class and EF assigned to each patient | Administrative data and resource-use profiles generated by the authors | Not collected |
Göhler238 (2008), Germany | Weibull and logistic regression of trial data for transition probabilities. Meta-analysis for effectiveness | Mortality risk a function of age and sex | Trial data for cost of hospitalisation; survey of doctors for resource use; treatment costs from administrative data (German diagnosis-related group grouper) | Trial data by using multivariate regression for estimating utilities allocated to each state |
Chan239 (2008), USA | Trial data (from two studies): published data used to ‘solve’ for the different state-specific probabilities of hospitalisation and costs | Estimated based on a review of literature on associated costs | Not collected | |
Miller240 (2009), USA | Trial data used to infer transition rates. Administrative data used to adjust mortality rates for age | Age | Trial data used for resource use. Costs of resources from administrative data | Baseline study SF-36 results used to develop utility adjustment weights |
Rosen241 (2010), USA | Trial data used for a Kaplan–Meier time to first event analysis (5-year probabilities assumed to be constant to year 10), after which pooled hazard ratios across treatments were used. Second event rates pooled estimates from trial data | Drug and event costs from published external sources | The EuroQol-5 Dimensions index scores from Sullivan et al.265 | |
Cowie242 (2011), UK | Trial data (both arms) used to estimate transition probabilities for the trial duration. Extrapolation period used literature and expert opinion assuming no difference between trial arms | Expert opinion and external data sources used to estimate the cost of each station/transition | Trial data used to estimate baseline utility. Expert opinion and external study data used for the impact that events had on quality of life | |
Moertl243 (2012), Austria/Canada | Trial data used (and extrapolated) to estimate transition probabilities | Data stratified by beta-blocker use | Trial costs (hospitalisation) and administrative data (medication costs) | Derived from trial data (patient questionnaire) |
Ford244 (2012), Australia | Trial data informs NYHA transitions. Administrative and cohort data inform other transitions | NYHA I to IV classes through model states | Administrative costs used for event, hospitalisation. Trial data used for drug costs | Trial data (same as for end points) used to estimate quality of life. Fixed disutility for hospitalisations |
Thokala245 (2013), UK | Trial data used to estimate monthly probabilities of death | Non-fatal hospitalisation influences the probability of death. Length of time since hospital discharge influence the probability of death | UK Healthcare Resource Group cost of HF and other hospital admissions; The Trans-European Network-Home-Care Managements System (TEN-HMS)162 trial; clinical opinion (programme costs) | Literature search used to estimate mean quality of life: first and subsequent years post HF admission; quality of life disutility with hospital admission |
Banka246 (2013), USA | Mortality rates and probability of hospitalisation derived from trials of each treatment comparison | Non-fatal hospitalisations influence the probability of death | Trial data used for costs of care; costs of test and drugs was taken from US Medicare data | The same health utility was applied for all non-fatal health states |
Ademi247 (2014), Australia | The Eplerenone in Mild Patients Hospitalization and Survival Study in Heart Failure (EMPHASIS-HF)266 trial data for placebo arm's event rates and hazard ratios between trial arms | Non-fatal hospitalisations influence the probability of death | Data from Australia-specific sources used to estimate cost of events | Single quality of life applied to the alive state; used in Ford et al.244 (Australian Study) derived from CARE-HF trial quality of life presented in Yao et al.267 |
Griffiths248 (2014), UK | Trial data used to generate parametric equations for cardiovascular mortality, hospitalisation and NYHA class | Baseline sociodemographic characteristics; baseline use of HF medications; baseline use of other cardiac therapies; prior cardiovascular history; biological characteristics; NYHA classes through model states | UK-based administrative costs for hospitalisations; drugs and deaths | Multilevel model using the EuroQol-5 Dimensions results gained in the Systolic HF Treatment with the If Inhibitor Ivabradine Trial (SHIFT):268 prediction based on treatment allocation, baseline characteristics, NYHA class (time updated) and hospitalisation episode |
Lee249 (2014), UK/Spain | Trial data used to generate parametric risk equations for events | Patient characteristics used in parametric risk equations | Trial data used for treatment costs. Proportions experiencing events used to estimate cost difference between arms and types of events | Formula from Göhler et al.238 used with baseline trial data |
Reed250 (2015), USA | Trial data (four studies) and observational cohorts used to derive competing risk regression for cause of death; various other studies used for treatment effects | Seattle Heart Failure Score (time updated) has an impact on cause and probability of death | Trial data used to estimate impact of Seattle Heart Failure Model score on medical resource use | Trial data used to estimate impact of Seattle Heart Failure Model score on health utility |
Kansal251 (2016), USA | US claims data and trial data used to estimate event rates and adverse events data; post hoc analysis of trial data used for cardiovascular mortality rates | Interaction between cardiovascular events and HF events through model structure | Administration and physician fee and coding data | Regressions using trial data used to estimate the change in baseline EuroQol-5 Dimensions index score due to event |
Thanh252 (2016), Canada | Trial data used (via parametric risk equation) to estimate end points | Age randomly assigned to patients | Pharmaceutical report and administrative data | Formula from Göhler et al.238 applied using trial data |
Sandhu253 (2016), USA | Placebo arm of trial used (via parametric survival equations) to estimate end points | Administrative data (adjusted by age and NYHA class) | Trial data (two studies) for baseline utility, treatment and disutilities | |
King254 (2016), USA | Life tables and trial data (two trials) used to estimate event rates | Structural: interaction between cardiovascular events and NYHA classes | Study of electronic records used for hospitalisation; administrative data for drug costs | Trial data used to estimate quality of life for the NYHA classes. Fixed disutility for hospitalisations |
Gaziano255 (2016), USA | Data from a RCT used to estimate all transition probabilities and hazard ratios | Non-fatal hospitalisations influence the probability of death | Administrative data and wholesale costs used | Trial data used to calculate a mixed-effects model based on EuroQol-5 Dimensions at baseline, age, time, hospitalisation and treatment status |
Dang256 (2017), USA | Trial cohorts and administrative data used to model the transition rates | Non-fatal hospitalisations influence the probability of death | Costs taken from a literature review | Not collected |
Monahan257 (2017), UK | Individual participant data from a prospective observational study was used to estimate probability of decision tree branches – probability of patients taking each branch of the decision tree. Framingham equations used to estimate prognosis post HF diagnosis | UK-based administrative data used for unit costs | Trial data (from the group who completed the 6-month questionnaire) used to estimate QALYs gained | |
Liang258 (2017), Singapore | Transition probabilities for end points estimated using parametric risk equations using data from a RCT subpopulation (Asian) | Structural: interaction between HF events and NYHA classes | Administrative data used to estimate hospital costs | Trial data used to estimate quality of life for the NYHA classes. Fixed disutility for hospitalisations |
van der Pol259 (2017), the Netherlands | Trial data, other study data and administrative data used to estimate the transition probabilities and treatment effects | Trial data and general Dutch administrative data used to estimate costs | Trial EuroQol-5 Dimensions data (UK based) used to estimate quality of life using the NYHA distribution in a trial | |
Maru260 (2017), Australia | Weibull model used to calculate the time-dependent probability of death adjusting for baseline covariates. Hospital re-admissions estimated using study data and assumptions beyond trial duration | Study data used to estimate cost of hospitalisation and community-based care with assumptions for increase in costs beyond the trial | Trial data (using regression) used to estimate baseline utility and the disutility due to hospital re-admission | |
Grustam261 (2018), the Netherlands | Trial data used to estimate the transition probabilities | Dutch administrative data used for all costs | Trial data informed the estimate of the utility using Dutch weights | |
NICE HF guideline13 (2018), UK | RCT data used to estimate transition probabilities and diagnostic pathways. UK life tables were used for all-cause mortality. Meta-analysis was used for the treatment effects | Administrative costs and author assumptions for resource use | Trial data used to estimate utility for patients with CHF diagnosis | |
Mouhiuddin262 (2016) and Pufulete263 (2016/2017), UK | Administrative (CPRD–ONS) data used for mortality and hospitalisation; trial data used to estimate treatment effects | Non-fatal hospitalisations (and age) influence the probability of death | General UK-based sources used to estimate medication and hospitalisation costs | Trial data used to estimate quality of life utilities in model states |
There were seven UK-based models; of these, six used RCT data only or RCT data alongside external data. 13,242,245,248,249,262,263 There was one study262 that used data from CPRD linked with mortality data from the Office for National Statistics along with hospital rates based on published cohort data. One study242 used expert opinion to model disease rates after the end of the trial.
Treatment effects on disease end points were integrated through adjusting the probabilities of death and hospitalisation (n = 24), and through direct estimation of separate transition probabilities for each intervention group from the trial data (n = 16).
Cost
Most studies used administrative unit cost data such as UK-based reference costs and and US prices data (n = 29) (see Table 36). Two studies used micro-costing methods. 231,232 Twelve studies used some RCT data, of which seven used RCT data in conjunction with other sources such as unit costs from the German diagnosis-related group grouper, the UK Healthcare Resource Groups costs or US Medicare costs of tests and drugs. 238,245,246 There was one study that used cohort data to estimate costs. 262 Expert opinion to estimate resource use was used in two studies. 242,245
Of the eight UK-based studies, most used administrative data including the British National Formulary, NHS Reference costs, Healthcare Resource Groups costs, Personal Social Services Research Unit costs, Prescription Cost Analysis and Scottish National Tariffs (n = 5). One study used RCT data alongside administrative data and expert opinion,245 one used expert opinion on resource use alongside administrative data242 and one model used trial data. 249
Quality-of-life data
In total, 30 out of the 40 included models evaluated patients’ quality of life in the model (see Tables 35 and 36).
The majority of studies used data about patients’ quality of life from RCTs (n = 23), of which a total of 15 different RCTs were used. Of these, one study used the trial data in conjunction with expert opinion about the quality of life of patients with CHF after a myocardial infarction or stroke. 242 There were five studies that used previously published data regarding quality of life to populate their models. 233,234,241,245,246
Seven out of the eight UK-based models incorporated quality-of-life data. Six of these used quality-of-life data from RCTs242,248,249,257,262,263 and one used previously published data. 245
The majority of the studies that included information on quality of life evaluated the impact that treatments had on quality of life through the transition probabilities in the model [i.e. quality-of-life estimates were applied to the states in the Markov model and treatment affected progression between the states (n = 17)]. There were two studies that directly derived utility estimates for patients using equations based on a range of patient characteristics. 248,255 Five studies included a direct effect of treatment on quality-of-life utility. Four studies evaluated effect of treatment on quality of life solely through its effect on the disease progression between model states. 246,247,254,261
Discussion
This review identified 40 economic models used to evaluate interventions for CHF patients with potential relevance to the primary care setting. A number of different model structures were employed with model complexity, judged by the number of model states or links between states, increasing over time. Individual patient characteristics were not commonly considered with only 11 (28%) of the models including these, although their use has increased in more recent studies. There was only one study that explicitly used patient data from primary care. The majority of studies used data from CHF patients recruited from hospital settings.
All studies used data from RCTs to inform their model parameters, with source trials frequently completed some years previously and unlikely to be representative of the current CHF population and disease management. For example, one study published in 2008239 used data from a trial that ran from 1986 to 1999. 269 In addition, trials recruited mostly in secondary care and their participants were often much younger than typical CHF patients seen in primary care. Other common sources of data were administrative data sources, mainly for all-cause mortality rates (n = 7). Data from the Framingham Heart Study was used to estimate CVD event risks in three studies. 230,234,257 Further cohorts were used, including the COPERNICUS cohort and a hospital clinic-based cohort. 232,270
Over time, the CHF patients’ NYHA classes were frequently used to inform the structure of disease models. However, the limited duration of most studies, the slow progression between NYHA classes in the stable CHF patients typically studied and the limited measurements of NYHA class during the duration of studies make this disease measure of limited longitudinal value. Modelling the number of hospital admissions, an alternative approach to measuring disease progression, was another common approach. However, hospital admissions are affected by health services provision and quality of care and, therefore, not necessarily appropriate. The difficulties of identifying appropriate proxies for disease progression persist in developing CHF models. 77
A number of studies used patient characteristics in risk equations to take into consideration individual patient’s disease risks. Incorporating the information from previous heart failure hospitalisations (through either the number of hospitalisations or the time since hospitalisation) was the most common way of incorporating patients’ disease severity; however, being an indirect proxy measure, it has limited ability to inform treatment and disease management decisions for individual patients. Further work on markers of CHF severity and factors associated with them is required to improve patient risk stratification in CHF.
This review has found that data from well-characterised CHF patient cohorts in primary care are lacking. Data from clinical trials have filled the gap but their use jeopardises the generalisability of findings to the population seen in primary care. The ECHOES cohort,53 which includes > 6000 patients with CHF followed up for at least 15 years, is one such cohort that could be used to derive information on transitional probabilities and quality-of-life data for use in economic models. 50 Routine health-care data, such as data in primary care databases and Hospital Episode Statistics, could inform factors such as CHF hospitalisations and death rates but are limited with respect to data about NYHA class or other markers of disease severity and progression.
Data about patients’ quality of life and costs specific to the CHF population are also lacking. Studies often used quality-of-life estimates derived from trials, and, as mentioned earlier, the duration of these trials may not be sufficient for the long-term impacts that disease progression has on quality of life to have emerged. Data about health-care costs are routinely collected in administrative data sources but are unlikely to inform models at the required granularity of disease severity (e.g. by NYHA class or patient comorbidities). More detailed quality-of-life data, resource use and cost information could be collected as part of future CHF patient cohorts.
Two broad areas in need of further research are identified as highly informative for future assessment of monitoring strategies of CHF in primary care. First, the increased use of well-characterised CHF patient cohorts in primary care, including data regarding disease status, disease progression, quality of life and health-care use, should be encouraged. Second, a broad consensus on appropriate markers of CHF disease severity and progression over time in primary care could be helpful in guiding prospective data collection as well as informing models of disease that are a better fit to assess the increasing range of CHF interventions.
Limitations
In this review, we did not assess whether or not the approaches employed in modelling CHF and effects of interventions were appropriate but instead we summarised model features and discussed these with respect to their suitability in assessing the monitoring and management of CHF in primary care.
Conclusion
A number of decision-analytic models in CHF are currently available and could be considered in assessments of the monitoring and management of interventions for patients with CHF in primary care. The majority of these models, however, were informed using data from CHF patients seen in secondary care who were recruited to randomised clinical trials up to 20 years previously. The use of more detailed data on CHF patients typically seen in contemporary primary care, including patient characteristics and markers of progression of CHF, should be encouraged.
Appendix 23 Stakeholder group: terms of reference and membership
Date: 21 May 2014, version: 0.3.
The role of the group
The role of the stakeholder group is to represent the views of health practitioners and patients (PPI) in the research activities conducted as part of the Monitoring Long Term Conditions programme. The group has been established for the purpose of this programme and will be active for its duration (until 31 May 2019).
Tasks
-
Provide views on the plans for design, implementation and evaluation of applied interventions.
-
Participate in developing the plans for understanding patient and GP factors associated with monitoring in CKD and CHD.
-
Contribute to plans to explore the views of patients and practitioners of potential changes to type of test used, frequency of testing and setting of testing (i.e. self-monitoring).
-
Guide and advise on the dissemination of individual research projects in the programme.
-
Attend senior management meeting (optional).
-
Representation on advisory group.
Membership
-
One panel that includes practitioners and patients; 12 people (six patients or lay persons, three nurses – patient-facing, one pharmacist, two clinicians – at least one GP).
-
For the PPI element, aim to have representation for non-CKD, non-CHF, CKD, CHF and, if possible, at least one member with both conditions.
-
Aim to have group set up for October 2014.
-
Members would be expected to attend at least one of the biannual meetings and to provide periodic feedback on research outputs (in person or in writing).
Review
The membership and commitment to the group will be reviewed half-way through the programme (autumn 2016).
Working methods
-
Face-to-face meetings every 6 months.
-
A maximum of 2 hours will be allocated for the stakeholders’ meeting.
-
An agenda and relevant paperwork will be circulated via e-mail 1 week before the meeting. This agenda will be initially created by the research team, but can be modified according to the group’s views.
-
Chairing and minute-taking will be done by members of the research team.
-
During the meeting, an update to the projects in the programme will be presented.
-
Views and opinions will be collected during the meeting. For members unable to attend, views and opinions can be given via e-mail.
-
Minutes from these meetings will be circulated within 1 month after the meeting.
-
After the face-to-face meeting, members of the group will be welcomed (but not obliged) to attend the senior management meeting (approximately 2 hours).
-
In the first instance, all material will be circulated via e-mail. If necessary, alternative methods for sharing information will be considered.
Payment and support (patient and public involvement only)
-
Patient and public involvement members will be remunerated for their time at a rate of £150 per day.
-
Travel expenses will be paid, in advance when possible, so that members will not be out of pocket.
-
Training and support for PPI members will be provided, if required, by the NDPCHS.
Definition of terms
-
Chronic kidney disease: for the purposes of this programme, it is early stages, that is when no or minor symptoms exist.
-
Chronic heart failure: for the purposes of this programme, this is symptomatic, and therefore needs to be actively managed by a clinician.
List of abbreviations
- ACE
- angiotensin-converting enzyme
- ACR
- albumin–creatinine ratio
- ARB
- angiotensin receptor blocker
- b.i.d.
- bis in die
- BNP
- B-type natriuretic peptide
- CHF
- chronic heart failure
- CHFN
- community heart failure nurse
- CI
- confidence interval
- CKD
- chronic kidney disease
- CKD-EPI
- Chronic Kidney Disease Epidemiology Collaboration
- CPRD
- Clinical Practice Research Datalink
- CV
- coefficient of variation
- CVD
- cardiovascular disease
- eGFR
- estimated glomerular filtration rate
- ESC
- European Society of Cardiology
- ESRD
- end-stage renal disease
- FORM-2C
- Frequency Of Renal Monitoring – Creatinine and Cystatin C
- GFR
- glomerular filtration rate
- GP
- general practitioner
- GUIDE-IT
- Guiding Evidence Based Therapy Using Biomarker Intensified Treatment in Heart Failure
- HbA1c
- glycated haemoglobin
- HERG
- Health Experiences Research Group
- KDIGO
- Kidney Disease: Improving Global Outcomes
- LDL
- low-density lipoprotein
- LVEF
- left ventricular ejection fraction
- MDRD
- Modification of Diet in Renal Disease
- mGFR
- measured glomerular filtration rate
- NDPCHS
- Nuffield Department of Primary Care Health Sciences
- NICE
- National Institute for Health and Care Excellence
- NP
- natriuretic peptide
- NSAID
- non-steroidal anti-inflammatory drug
- NT-proBNP
- N-terminal prohormone of B-type natriuretic peptide
- NYHA
- New York Heart Association
- PDA
- personal digital assistant
- PI
- principal investigator
- POC
- point of care
- PPI
- patient and public involvement
- PRISMA
- Preferred Reporting Items for Systematic Reviews and Meta-Analyses
- QALY
- quality-adjusted life-year
- QOF
- Quality and Outcomes Framework
- RCT
- randomised controlled trial
- RCV
- reference change value
- RM
- remote monitoring
- RR
- risk ratio
- RRT
- renal replacement therapy
- SE
- standard error
- SMD
- standardised mean difference
- STS
- structured telephone support
- TM
- telemonitoring
- WS
- workstream