Notes
Article history
The research reported in this issue of the journal was funded by the PHR programme as project number 17/92/31. The contractual start date was in June 2019. The final report began editorial review in April 2022 and was accepted for publication in January 2023. The authors have been wholly responsible for all data collection, analysis and interpretation, and for writing up their work. The PHR editors and production house have tried to ensure the accuracy of the authors’ manuscript and would like to thank the reviewers for their constructive comments on the final manuscript document. However, they do not accept liability for damages or losses arising from material published in this manuscript.
Permissions
Copyright statement
Copyright © 2024 Naughton et al. This work was produced by Naughton et al. under the terms of a commissioning contract issued by the Secretary of State for Health and Social Care. This is an Open Access publication distributed under the terms of the Creative Commons Attribution CC BY 4.0 licence, which permits unrestricted use, distribution, reproduction and adaptation in any medium and for any purpose provided that it is properly attributed. See: https://creativecommons.org/licenses/by/4.0/. For attribution the title, original author(s), the publication source – NIHR Journals Library, and the DOI of the publication must be cited.
2024 Naughton et al.
Chapter 1 Introduction
Tobacco use remains a threat to public health worldwide, accounting for more than 8 million deaths every year. 1 While smoking rates are decreasing in more economically developed countries including the UK, the total number of smokers worldwide is increasing2 and so the delivery of effective cessation support remains crucial. In England, tobacco smoking is the leading cause of preventable illness and premature death and is the largest single contributor to the UK disease burden. 3 It is estimated that 22% of annual hospital admissions for respiratory diseases, 15% of admissions for circulatory diseases and 9% of admissions for cancers are attributable to smoking. 4 While quitting smoking reduces the risk of smoking-related health problems and reduces the burden on healthcare services, the success rate of those attempting to quit remains low. Of the 3 million UK smokers who attempt to quit each year, over 80% relapse within 1 year of their attempt. 5
Lapses and cues to smoke
Any smoking early on in a quit attempt (a ‘lapse’) is highly predictive of longer-term return to smoking (‘relapse’). 6,7 For example, one large prospective study found that 22% of smokers who lapsed early, a mean of 10 days after they started their quit attempt, were abstinent at 6 months compared to 71% who did not lapse early on in their quit attempt. 7 This relationship also exists when a lapse is experimentally induced. 8 Evidence, therefore, indicates that maintaining abstinence during the first few weeks of a quit attempt seems to be particularly crucial for long-term success.
Almost half of all lapses are estimated to be induced by cues to smoke from the environment or setting,9 through the generation of a type of craving referred to as ‘cue-induced craving’. Cues have been categorised as ‘proximal’, such as seeing unlit cigarettes or other people smoke, and ‘distal’, that is the actual environments that an individual smokes in or has smoked in. 10,11 Distal cues can be generic, such as a bar, or specific, such as someone’s back garden. Studies have shown that smokers will experience cravings after viewing generic images of environments associated with smoking selected by an experimenter, such as a bus stop, and that this effect is magnified when using images taken by participants of their own specific smoking locations. 12,13 Given that people attempting to quit smoking will very likely experience cravings driven by the exposure to both proximal and distal cues in their daily life, with a higher magnitude of cravings when both types are present simultaneously,11 the effective management of cues could prevent early lapses and increase successful smoking cessation.
However, there is a lack of effective support available to help smokers manage cue-induced cravings. The most used medications for smoking cessation do not address cue-induced cravings. This includes steady-state medications such as bupropion, varenicline and nicotine patches. 14–17 While fast-acting nicotine replacement therapy (NRT) can help reduce these cravings,16 only a minority of NRT users use this type18 and the proportion of people attempting to quit in England who use NRT has been steadily decreasing, currently standing at 11% in 2022. 19 Cognitive or behavioural lapse prevention strategies, such as using self-talk or avoiding other smokers, can help smokers avoid or manage cue-induced cravings,16,20,21 though the strategies smokers are most likely to use are those least effective or with the least strong evidence base. 22 An additional challenge with targeting cue-induced cravings with a behavioural response is that the window of opportunity for intervening is short. One of the only studies of its kind found half of lapses brought about by acute craving episodes occurred on average within 11 minutes after craving onset. 9 Support to address cue-induced cravings therefore needs to be rapid, easily accessible in different locations and deliver evidence-based strategies.
Digital cessation interventions
While each year many hundreds of smoking cessation apps become publicly available, the vast majority demonstrate poor adherence to clinical guidance and evidence-based approaches23 and even fewer have been evaluated. 24 Among those where evaluation research has been undertaken, there is a lack of clinical trials. 25 A 2019 Cochrane Review pooled five RCTs of cessation apps identified and found there was no evidence of effectiveness for cessation apps compared to a less intensive cessation intervention comparator. A more recent review (2021) identified 11 RCTs, 4 of which showed evidence of effectiveness. 26 However, smartphone apps are primarily delivery mechanisms for delivering support and so different therapeutic approaches delivered by apps are likely to have different success rates for cessation. RCTs have provided evidence that acceptance and commitment therapy can be effectively delivered through an app to promote cessation27 but that mindfulness training for smoking cessation delivered via an app was not effective when compared to a monitoring-only intervention. 28 Furthermore, trials randomising smokers recruited after installing an app from an app store provide evidence that adding a chatbot feature to a cessation app increases short-term effectiveness29 but that a multiple-features cessation app including craving feedback, counselling messages and NRT and e-cigarette advice was no more effective than a basic scaled-down cessation app. 30
Smartphone apps may be a potentially effective way of addressing cue-induced cravings. Unlike traditional in-person delivered cessation interventions, digital interventions, such as smartphone apps, have the advantage of portability and so are much more likely to be on hand when needed. Some mobile phone-based interventions have features that specifically aim to deliver support to reduce lapse risk in response to need identified by randomly triggered assessment prompts [via Ecological Momentary Assessment (EMA)]31 or on-demand requests for craving support. 32 However, given the rapid time to lapse after the onset of a craving identified above, and evidence that some user-initiated on-demand craving tools are seldom used beyond a first try,33–35 the efficacy of such features in addressing cue-induced craving may be limited.
Despite the potential of apps to deliver effective cessation support at the time of need, very few to date have been developed to achieve this and, among those that have,31 there have been a lack of RCTs to provide a better estimate of their impact. 36 Based on a recent systematic review,31 only one RCT has been undertaken testing a just-in-time support system for smoking cessation. 37 This pilot RCT (N = 81) tested an app (Smart-T2) where lapse risk was identified by randomly sent EMAs, which then triggered ‘in the moment’ support, and found that support could be feasibly delivered, although the study was underpowered to identify its impact on smoking and did not assess potential mechanisms of action.
The idea of developing cessation apps that passively ‘sense’ high-risk situations for smokers and respond in real time have been proposed for almost a decade,38,39 with the expectation that they could reduce reliance and burden on the user to identify and respond to high-risk or high craving situations. Despite modern smartphones having the technological capability to support such apps, other than Quit Sense (see below), there have been no apps developed or evaluated that sense and adapt cessation support, so it is relevant to real-time context.
Quit Sense
Quit Sense was developed to address this important gap in digital quit smoking support and is a theory-guided ‘context aware’ smartphone app, also referred to as a Just-In-Time Adaptive Intervention (JITAI). 39 Quit Sense is trained by the individual smoker before their quit attempt starts in order to learn about their smoking habits, including the locations where they smoke and the smoking cues they are exposed to within these locations that precede their smoking. Then, once their quit attempt starts, Quit Sense delivers behavioural support triggered by and tailored to those smoking locations and the main cues within them. Alongside this, it provides additional components of behavioural support to prevent lapse and relapse that are not tailored to real time context.
Previous research has demonstrated that Quit Sense succeeds in providing ‘in the moment’ support to smokers, including the provision of lapse prevention strategies, and that it is both engaged with and found to be acceptable by users (N = 15). 10 Logging a smoking episode, the primary method of training the app, took on average 13 seconds, including the identification of key smoking cues and location. There was an average of 70 sessions of app use per participant, the majority of which were to report smoking, complete a survey or view a support message, for the duration of use (up to 4 weeks). However, qualitative interviews identified barriers to logging smoking episodes due primarily to forgetting and environmental constraints, such as when driving or in the presence of others. These barriers led to under-reporting of smoking behaviour on approximately half of days pre-quit attempt. Participants overall were positive about having access to real-time support and had no privacy concerns with the collection of location data. Findings from this formative work were used to guide app optimisation. Optimisation included improved prompting and feedback to promote fuller recording of smoking episodes, the removal of the requirement for an internet connection to receive location-triggered tailored support, improved power efficiency of location sensors and enhancements to the app’s user interface. Changes were also made due to the COVID-19 pandemic, including ensuring support messages did not give recommendations that might contradict COVID guidance and ensuring the app was adapted to higher rates of working from home. A feature to record audio reports of users’ views of the app and how it could be improved was also added to the feasibility trial (‘auto-process evaluation’) to explore novel methods of evaluation.
Aims and objectives
The overarching aim of the proposed research was to establish the feasibility of a future RCT of the Quit Sense app.
Objectives
Objectives were to estimate:
-
completion rates for the anticipated primary outcome for a full trial (6-month self-reported abstinence with biochemical validation, based on the Russell standard);
-
usual care arm cessation rate;
-
cost of recruitment using online advertising;
-
rates of app installation, use and acceptability;
-
completion of smoking cessation-related resource use and quality-of-life (QoL) data;
-
intervention effect on anticipated primary outcome;
-
intervention effect on hypothesised mechanisms of action of app at 6 weeks post enrolment;
-
participant views of the app, as part of a qualitative process evaluation.
Chapter 2 Methods
Some text in this chapter has been reproduced from Naughton et al. 40 This is an open access article distributed in accordance with the terms of the Creative Commons Attribution (CC BY 4.0) licence, which permits others to distribute, remix, adapt and build upon this work, for commercial use, provided the original work is properly cited. See: https://creativecommons.org/licenses/by/4.0/. The text below includes minor additions and formatting changes to the original text.
Study design
This was a two-arm parallel-group randomised controlled feasibility trial, allocating smokers recruited online to a ‘usual care’ arm (link to NHS SmokeFree website) or an intervention arm who received ‘usual care’ plus access to the Quit Sense app (link with a code to app via Google Play app store).
The study also had a nested qualitative process evaluation with a subsample of participants to assess participant experiences of the intervention and how these experiences might help explain the causal pathway towards smoking behaviour change. Usual care participants were also asked about their experience of participating in the study, informing an assessment of the feasibility of randomised study design for a definitive trial.
Before initiation of the trial, the protocol (Naughton et al.,40 all informed consent forms and any material given to the prospective participants were approved by the Wales REC7 NHS Research Ethics Committee (reference 19/WA/0361). A Trial Steering Committee (TSC) with a majority of independent members oversaw the conduct of the trial.
Participants
Inclusion criteria for participation were: a current smoker; age 16 years and above; smoked at least 7 cigarettes per week; willing to make a quit attempt within 14 days of enrolling in the study; own an Android smartphone (version 5.0 or above); a resident in England; and able and willing to provide informed consent using the web-based form. Exclusion criteria were that participants must not have previously participated in the trial.
Setting
The setting was online and the intervention was delivered online via the participants’ smartphones. This matches the ‘real-world’ setting after implementation to maximise validity. Participants saw online recruitment adverts and completed screening, consent, baseline and follow-up measures online.
Online recruitment
Recruitment took place through paid-for online adverts with Facebook (which includes Instagram) and Google search, limited to England-based IP addresses and targeted at Android devices. The period of recruitment was specifically planned to be over 6 weeks. Online adverts were managed by a partner company called Nativve who specialise in digital marketing for recruiting to research studies.
In line with our patient and public involvement (PPI) panel’s recommendations, Nativve, on our behalf, initially tested different advert wording and images to assess which led to the highest recruitment rate using imbedded Google analytics in our study website (using REDCap, Research Electronic Data Capture, a web-based software platform designed to support research studies, with built-in audit trails and data export functions41,42) (see Appendix 1 for advert examples). They then prioritised the best-performing adverts primarily based on the lowest cost per recruit.
The distribution of socioeconomic status (SES) was monitored throughout the recruitment via the study website, using the National Statistics Socio-Economic Classification (NSSEC). 43 This was undertaken to monitor whether the sampling strategy was promoting major socioeconomic inequalities of access. It was pre-planned that if after 3 months of recruitment or when 35% of the target sample was reached, < 45% of our sample were categorised as low SES, then oversampling in favour of low SES participants would be undertaken to increase low SES representation. Low SES was defined as individuals who have a semiroutine or routine and manual occupation, class 5 in the NSSEC, or who have never worked or are long term unemployed. This action primarily included using Facebook advert targeting, which allows the targeting of users according to their education, job titles, workplace, etc. We also used Facebook advert targeting to attempt to increase the ethnic diversity of the sample.
Procedure
Enrolment and engagement
Once an individual had clicked on a study advert, they were directed to the study website where they were given information on the study including the downloadable participant information sheet. If, after reading information about participating, they were interested in taking part, they completed a screening survey to ensure that they met the study inclusion criteria. If eligible, they were asked to provide consent to participate by providing an e-signature. Participants were then asked to complete an online baseline questionnaire and afterwards they were randomly allocated to either the usual care or the Quit Sense app arm (see Table 1 for study assessments).
Study period | ||||
---|---|---|---|---|
Enrolment (online) | Allocation (online) | Post allocation (online or telephone) | Close-out (online or telephone) | |
TIME POINT | 0 | 0 | 6 weeks FU (from enrolment) a | 6 months FU (from enrolment) b |
ENROLMENT: | ||||
Eligibility screen | ✗ | |||
Informed consent | ✗ | |||
Allocation | ✗ | |||
INTERVENTIONS: | ||||
Usual care (link to www.smokefree.nhs.uk) | ✗ | |||
Quit Sense app + usual care | ✗ | |||
ASSESSMENTS: | ||||
Demographics | ✗ | |||
Use of smartphone and apps | ✗ | ✗ | ||
Smoking behaviour and dependence | ✗ | ✗ | ||
Smoking beliefs | ✗ | ✗ | ||
Cessation self-efficacy | ✗ | ✗ | ✗ | |
Strength and frequency of urges to smoke | ✗ | ✗ | ✗ | |
Automaticity and associative processes subscales (WISDM-37) | ✗ | ✗ | ||
EQ-5D-5L | ✗ | ✗ | ✗ | |
Smoking lapse incidence | ✗ | |||
Smoking abstinence outcomes | ✗ | ✗ | ||
Use of lapse prevention strategies | ✗ | |||
Smoking cessation resource use | ✗ | |||
Views on the app (Quit Sense arm only) | ✗ | |||
Assessment of tobacco exposure (postal saliva sample) | ✗ |
To promote continued engagement in the study, 5 days after enrolment all participants were sent a study text message thanking them for their continued involvement in the study. After 12 weeks, participants were sent a postcard (in an envelope for privacy) with study information. The PPI panel recommended that we should provide summary information on the characteristics of participants in the study, such as age groups, and provide recruitment rates and follow-up rates to promote continued engagement, particularly among those in the usual care arm (see Appendix 2).
Interventions
Usual care arm
After randomisation, both arms received an automated text message to their phone providing them with a link to the NHS SmokeFree website (www.smokefree.nhs.uk), and, if requested, also by e-mail. At the time the trial was run, this website provided access and signposting to digital, telephone and in-person cessation support in England. The support offered on the SmokeFree website included information and links to six main types of cessation support: in-person (via a Stop Smoking Service), the SmokeFree National telephone helpline, an online chat facility with an adviser, an e-mail cessation programme, a SMS text message programme and the NHS SmokeFree smartphone app. The main difference between the NHS app and Quit Sense is that the NHS app does not require users to log their smoking behaviour, it does not proactively deliver real-time support to help smokers manage urges, and in addition it is not tailored to the user and most of the tips and support it provides are user- rather than phone-triggered.
Quit Sense app arm
In addition to usual care, Quit Sense arm participants received an automated text message providing them with a link to the Quit Sense app on the Google Play store, along with a unique app activation code. If participants in the Quit Sense arm had not installed the app after 3 days, a reminder text message was sent to encourage participants to install the app. If the participant still had not installed the app 5 days later, a further text message was sent enquiring why they had not installed the app and inviting a reply by text message. They were asked to respond with one of the following reasons for not having installed the app: (1) for no internet; (2) for not enough storage; (3) for forgot; (4) for don’t want app; (5) for problem installing; and (6) for other. If participants replied ‘5’ or indicated a problem installing the app, the lead researcher contacted them manually (by text or phone) to offer help with installation.
Quit Sense is a ‘context aware’ smartphone app, also known as a JITAI, designed for smokers willing to make a quit attempt. It is informed by learning theory (LT) and two theory-guided SMS text message systems,35,44 which are in turn informed by social cognitive theory (SCT). 45 Quit Sense targets three key determinants that map on to LT and six that map on to SCT: learned associations between smoking and an individual’s physical environment (LT); learned associations between smoking and mood triggered by the environment (LT); the presence of other smokers (LT, SCT); awareness of smoking triggers (antecedents) (SCT); outcome expectancies of a lapse (SCT); goal/intention for complete abstinence (SCT); self-efficacy in resisting urges to smoke (SCT); and knowledge of and self-efficacy for lapse prevention strategy use (SCT). Twenty-one corresponding behaviour change techniques (BCTs)46,47 are used to target these determinants. See Appendix 3 for a ‘logic model’ for Quit Sense and connecting evidence.
The central feature is ‘geofence-triggered support’ (GTS), which is orientated around three stages within the app (see Appendix 4 for screenshots):
Stage 1 (‘train the app’): The user sets a quit date (default suggestion is 7 days’ time) and trains the app to learn about their smoking behaviour via the app’s smoking reporting tool. This requires the user to report in the app each smoking episode and the situational context when they ‘light up’ in real time [stress, mood,48 urge strength,49 setting description (home, work, working from home, socialising, other), presence of other smokers], while the app records geolocation using location sensors. If a user reports smoking more than once in the same location (as defined by the app), the app creates a geofence (a circular virtual perimeter) around that location. Each geofence zone represents a high-risk area for smoking for that user, with users typically having multiple geofence zones in different locations.
Stage 2 (‘commit to quit’ – a 28-day abstinence challenge): After their quit date has passed, the app monitors the user’s location. If they enter (and remain in for at least 5 minutes) a geofence, the app will then determine whether to trigger a GTS message. This decision is informed by the user’s smoking reporting history, including time of day, for that location. Messages are individually tailored using the context information collected in stage 1 and provide lapse prevention support (strategies, motivation enhancement, encouragement, etc.) to help users avoid or cope with triggers they are likely to experience in that location that cue urges to smoke. Further decisions about whether to trigger messages are made after each 3-hour interval of remaining in that location (default between 8 a.m. and 9.30 p.m. or defined by the user). New geofences are created in this stage if smoking is reported more than once in the same location, as with stage 1.
Stage 3 (maintaining abstinence – ‘maintain the change’): The app continues to deliver GTS for 2 further months but reduces the frequency by one-half every month (i.e. one in every two scheduled messages sent for first month, then one in every four scheduled messages sent). After 3 months post quit date, the GTS support stops, unless the user opts to restart their quit attempt, which they can do at any time (see feature description below).
Quit Sense has six additional features (relevant to all stages, unless specified otherwise):
-
After each smoking report is submitted, tailored feedback is provided on screen. This feedback in stage 1 includes messages focused on preparing to quit, boosting motivation and self-efficacy. These messages are tailored to nine characteristics collected during app initiation (hardest situation to avoid smoking, number of cigarettes smoked, living with a smoker, longest quit attempt to date, primary reason for quitting, perceived primary downside to quitting, self-efficacy, gender and pregnancy status). In stage 2, the feedback after a smoking report is submitted is orientated around lapse and relapse prevention.
-
An ‘End of Day’ (EoD) survey that users are invited to complete each day, recording the number of cigarettes smoked that day, craving48 and self-efficacy44,45 (stages 1 and 2 only). After the EoD survey is completed, the app provides a feedback message. If the number of cigarettes reported in real time during the day is different from the number reported in the EoD survey, the feedback message promotes adherence to logging smoking behaviour.
-
A ‘My Profile’ section including number of days quit, money saved (based on smoking rate), a calendar showing emoji feedback with use of a smiley, neutral and sad emoji to reflect value ranges for smoking, cravings and self-efficacy for each day. In addition, smoking pattern feedback is provided using graphical and written summaries for smoking triggers based on the user’s historical reporting of smoking behaviour.
-
A ‘Library’ of quitting advice split into six categories: ‘getting ready’, ‘boost your motivation’, ‘effects of smoking and quitting’, ‘early days of quitting’, ‘staying quit’, ‘smoking after your quit date’. It includes an option for users to write and submit their own support messages.
-
Scheduled non-tailored daily support messages delivered each morning orientated around the quit date (stages 1 and 2 only) – targeting outcome expectancies, motivation, preparation, self-efficacy (three sets of different messages to prevent message duplication in successive attempts).
-
The option for the user to reset their quit date in stage 1, if they are not feeling ready, or stage 2 if they relapse. Relapse is defined as more than one smoking episode reported each day over 2 consecutive days during a quit attempt (either through smoking episode logs or the EoD survey). If this occurs, users are invited to either recommit to their quit attempt and continue or reset their quit date, and they then go back to stage 1.
As described earlier, Quit Sense users are also able to use an audio-record feature embedded in the app where they can provide feedback on their views and experiences of using Quit Sense, as part of the process evaluation. They receive two prompts and two reminders to provide such feedback at key time points: 2 days before quit date, with a reminder 1 day later if no feedback provided, and 10 days after quit date, with a reminder 1 day later if no feedback provided.
Follow-up
One day before the 6-week questionnaire was due to be completed, participants received a text message letting them know that they would receive the 6-week questionnaire link the next day, which they were sent the following day and informed that they would receive a £5 Amazon voucher for completing it. If participants had not completed this after 4 days, they were sent a reminder text message. If it was still not completed a further 3 days later, they were informed by text message that a researcher would get in contact to collect the information over the phone, and they were also given the questionnaire link again in the text message. Shortly after this last message was sent, if the questionnaire had still not been completed, the researcher contacted them by telephone. Up to five contact attempt episodes were made.
Included in the 6-week questionnaire was a question asking about interest in participating in a qualitative interview by telephone to investigate experiences of participation in the trial and, from Quit Sense arm participants, to gain feedback on the app. We planned to interview 20 participants (15 in the Quit Sense arm, 5 in the usual care arm). Purposive sampling was used to identify participants with varied characteristics. For further information, see Qualitative process evaluation.
Other approaches to increase response rates were also used. For example, participants were issued with a £5 voucher following completion of the 6-week follow-up questionnaire and interview participants were given a £20 gift voucher.
Six and a half months after enrolment (referred to as the 6-month follow-up), all participants were sent a text message inviting them to complete the final questionnaire. As with the 6-week follow-up procedure, a reminder text message was sent and a message about contacting them by telephone instead to complete the questionnaire at 4 and 7 days after the initial text message, respectively. As part of the SWAT nested within the trial, participants were randomly allocated to be offered either £10 or £20 to complete the 6-month follow-up questionnaire (see SWAT for more details). If manual follow-up by telephone was unsuccessful, participants were sent a text message inviting them to respond to the primary smoking outcome question. Participants who reported a 7-day abstinence in the 6-month questionnaire were informed at the end of the questionnaire that they would be sent a saliva test kit in the post and that they would receive a further £5 for returning it. This information was also sent by text message.
Measures
Feasibility outcomes
Feasibility outcomes used to estimate key parameters to inform a fuure trial50 include:
-
completeness of the anticipated primary outcome for a future definitive trial (6-month self-reported abstinence with biochemical validation; see Smoking and related outcomes);
-
abstinence rate of usual care arm, using the anticipated primary outcome for a future definitive trial;
-
cost per recruit, based on advertising costs;
-
rates of app installation and use and acceptability [recommend Quit Sense to a friend and ease of use (five-point scale)];
-
completion of smoking cessation-related resource use, including usual care use, and QoL (EQ-5D-5L)51 data;
-
hypothesised mechanisms of action of Quit Sense;
-
participant experiences and feedback on app usage.
Smoking and related outcomes
The primary abstinence outcome for which we monitored completeness of ascertainment was based on the Russell standard. 52 Abstinence was defined as: self-reported abstinence in the previous 6 months allowing for no more than five cigarettes and not smoking in the previous week, biochemically validated by a saliva cotinine concentration of < 10 ng/ml,52,53 and for those using nicotine substitution (e.g. NRT, or e-cigarettes), an anabasine concentration of < 0.2 ng/ml. 53,54 Given evidence that some e-liquid, believed to be that made outside of the UK, can contain anabasine53 we monitored the proportion of e-cigarette users who reported abstinence from smoking but had an anabasine level above the chosen threshold.
Given recommendations to include additional smoking outcomes based on different time periods of abstinence and time points,55 we also measured 7-day point prevalence abstinence at 6-month follow-up (self-report and biochemically verified) and 7-day point prevalence abstinence at 6-week follow-up (self-report).
We collected the following hypothesised mechanisms of action at 6-week follow-up:
-
Lapse incidence (any smoking, even a puff) in the first 2 weeks since the initial quit attempt or since enrolment,9 which is highly predictive of relapse. 6,77
-
Lapse prevention strategy use,22 which is associated with lapse prevention. 16 This was a mean score of the frequency of use [4 categories: not at all (1), 1–5 times (2), 6–10 times (3), more than 10 times (4)] for 7 strategies for avoiding smoking and 13 for coping with the desire to smoke.
-
Smoking cessation self-efficacy,44,45 which prospectively predicts lapse and relapse to smoking. 56 This self-efficacy measure includes four items which are scored on a five-point scale from not at all (1) to extremely (5) and then averaged; self-efficacy to quit smoking for good (adapted from original) and self-efficacy relating to avoiding smoking during habitual (after eating), social (when with other smokers) and emotional (when anxious or stressed) situations. This measure demonstrates good internal consistency (α = 0.81). 44
-
The strength of urges to smoke (SUTS) measure,48 which prospectively predicts abstinence and is superior to other urge-measures in doing so,49 and frequency of urges to smoke (FUTS). 48
-
Automaticity and associative processes subscales from the Wisconsin Inventory of Smoking Dependence Motives (WISDM-37). 57 WISDM items are scored on a seven-point scale from ‘not true of me at all’ (1) to ‘extremely true of me’ (7). The automaticity subscale is derived from four items and the associative processes subscale is from three items.
Sample size
As this was a feasibility study, sample size was determined as that likely to provide reasonably accurate estimates for key ‘full trial’ parameters, rather than to detect a difference in smoking outcome between the two arms.
In line with recommendations that feasibility studies with binary outcome measures recruit at least 60 participants per group (minimum of 120) and a maximum of 100 per group (maximum of 200),58 the proposed sample size for this feasibility trial was 200 smokers (100 per arm). Originally, we planned to recruit 160 participants but during recruitment we found that the cost per participant was much lower than anticipated and the speed of recruitment could be controlled by increasing the daily spend on adverts. Therefore, with approval from the Trial Steering Group, sponsor and National Institute for Health and Care Research, and having notified the REC, we increased the planned sample size to 200. This sample size would enable key parameters to inform a future definitive trial to be estimable within the following precision [defined as the 95% condifence interval (CI) half-width]:
-
Primary outcome completion – we estimated a follow-up rate for self-reported smoking status at 6 months of 80%, with precision of ± 6%. This was based on smoking cessation evaluation trials with online recruitment that were most similar to the proposed trial, namely trials of a cessation smartphone app59 (2-month follow-up = 84%) and a cessation website60 (7-month follow-up = 72%). A Cochrane Review of web-based cessation trials showed that few report retention rates higher than 80%. 61 For biochemical validation, we estimated that 75% of participants reporting abstinence would return a saliva sample by post, with precision of ± 22%. This was based on estimates from a cessation website trial with a 6-month follow-up60 (75%) and a SMS text message cessation intervention trial with a 3-month follow-up33 (80%).
-
Cessation rate in usual care arm – based on the control arm abstinence rate in a Cochrane Review of mobile phone-based cessation interventions,62 we estimated an abstinence rate of 5% at 6-month follow-up, providing precision of ± 5%
-
App installation and initiation – we estimated that 85% of intervention participants would install the app, with precision of ± 7%. This was based on installation rates (92%) provided by a study examining engagement with a cessation app among an online population of smokers63 – we assumed slightly lower installation rates as, unlike this other study, we did not use financial incentives to promote initial engagement.
-
App engagement – in our acceptability study, 71% used the app for more than 1 week (a time frame deemed meaningful by our PPI panel). With a similar rate in this trial, precision would be ± 9%. This is in line with engagement rates reported in other studies identified when this study was designed. 63
Randomisation
Sequence generation, allocation and blinding
Randomisation was stratified by smoking rate (< 16 vs. ≥ 16 cigarettes/day; based on mean smoking rates from trials recruiting smokers online)59,64 and SES (low vs. high), based on the NSSEC self-coded method. 43 Participants were randomised to the usual care or Quit Sense arms in a 1 : 1 ratio. Allocation sequences were generated by a computer program (using REDCap) with random permuted blocks (varying block sizes). Randomisation was integrated into the enrolment process on the study website. As allocation was integrated into the study website, the allocation sequence was concealed from participants until assignment and concealed from members of the trial team, other than the statistician, developers of the study database and the lead researcher who needed to be unblinded for potentially providing app installation support and selecting participants to interview as part of the qualitative evaluation.
Statistical methods
The feasibility and acceptability of all measures were assessed by their level of completeness and via interviews with a subsample of participants.
Outcomes
Completeness of the anticipated primary outcome for a future trial (feasibility outcome i.) and the abstinence rate using this anticipated primary outcome for the usual care arm (outcome ii.) are described as proportions with 95% CIs and translated into interpretable probabilities using the Bayesian approach relevant in preliminary trials65 with the objective of powering the definitive trial. This method produces the estimates of the probability that the underlying odds ratio (OR) is 1.7 or higher, or at other values relevant to providing plausibility to the effect size used to power a subsequent trial such as 1.5 or higher, or 2.0 or higher. It is based on treating the CI for the log OR as a normal distribution. Due to the small number of events in the feasibility trial,65 a method involving a continuity correction was used for the log OR and its standard error. 66
Cost per recruit (outcome iii.), rates of app installation (outcome iv.) and completion of smoking cessation-related resource use and QoL (EQ-5D-5L) (outcome v.) are also described using summary statistics with 95% CIs.
For the primary outcome measure, the intervention effect on abstinence at 6 months (self-reported, with abstinence validated by a saliva test) was estimated using multiple logistic regression, providing ORs with 95% CIs, while adjusting for stratification variables (smoking rate and SES at baseline), prognostic covariates (heaviness index at baseline) and treatment group, defined by the Statistical Analysis and Health Economics Plan (SHEAP; https://osf.io/mt6s5/). For the primary outcome measure, we assumed that those participants withdrawn, non-responders or missing were not abstinent,52 as a conservative model. Analysis results using exact inference are also presented, as a more appropriate approach, due to the relatively small numbers of abstinent participants.
Between-group analyses of mechanisms of action variables at 6 weeks were undertaken for lapse incidence (2 weeks, 1 month), lapse prevention average scores and subscale scores, WISDM subscale scores, strength of urge to smoke, frequency of urge to smoke and self-efficacy average scores. An appropriate parametric/non-parametric statistical test was used to test for a difference between treatment groups, with the two-sample t- test for continuous variables reasonably satisfying the normality assumption, and the chi-squared or Mann–Whitney test otherwise, or for categorical variables.
Sensitivity analyses of abstinence at 6 months were conducted using an analogous logistic model as described for the primary outcome, excluding withdrawals, as well as a complete case analysis. Secondary analyses, again using an analogous logistic model, were carried out for 7-day abstinence at 6 months with biochemical validation by saliva test and self-reported 7-day abstinence at 6 weeks and 6 months. A missing data analysis using the full information maximum likelihood method was originally planned; however, for a binary outcome, it was unfortunately not currently technically possible in commonly available statistical software.
Economic evaluation
The methods used to estimate the costs and benefits have been described in detail in the SHEAP. Briefly, they are as follows: we aimed to collect baseline and follow-up data that would enable us to inform the decision as to how cost (based on resource use data) and benefit (in terms QoL) data are estimated in any future more definitive study. Completion rates were thereby estimated.
In terms of costs, a societal cost perspective was adopted, where we estimated the costs associated with the intervention (e.g. app maintenance) and smoking-related costs to both the individual (e.g. NRT) and the NHS [e.g. NHS stop smoking services/general practitioner (GP) visits]. Appropriate unit costs67 were attached to all reported items of resource use (at 2020–21 price levels) in order to identify major cost drivers.
In line with guidance at the analysis stage,68 QoL was measured via the EQ-5D-5L51 [as this enables the calculation of quality-adjusted life-year (QALY) scores] and scored via the crosswalk mapping function. 69
Additionally, as outlined in the SHEAP, a preliminary cost-effectiveness analysis was conducted, the results of which need to be treated with caution due to the small sample size. A complete case analysis was conducted, where only those with complete cost (based on the 6-month resource use questionnaire) and outcome (EQ-5D-5L data at baseline, 6 weeks and 6 months) were included. Seemingly unrelated regression analysis70 was undertaken to estimate the mean incremental cost and mean QALY gain associated with Quit Sense compared to usual care. In the absence of dominance (where one intervention is both more costly and less effective), the incremental cost-effectiveness ratio (incremental cost/QALY gain) was estimated and assessed in relation to a range of cost-effectiveness thresholds (e.g. £20,000–30,000 per QALY)71 to provide a preliminary assessment of whether Quit Sense constitutes value for money. The associated level of uncertainty was also estimated.
SWAT assessing the effect of incentives on follow-up rates
SWAT objective
The objective of the SWAT was to determine whether the offer of a higher financial incentive for questionnaire completion improved response rates at 6-month follow-up. Findings will be used to inform a future definitive trial.
SWAT methods
Participants were randomly allocated to either receive an incentive worth £10 (the rate advertised to them originally) or £20 (the increased incentive) for completing the ‘primary outcome’ question at 6-month follow-up.
All participants were sent automated notifications by SMS 1 week before the 6-month questionnaire was due (pre-questionnaire notification as planned), at the 6-month time point with a link to the questionnaire and 7 days after the questionnaire due date with a reminder message. Two weeks after the questionnaire due date, the Research Associate attempted manual follow-up to collect the data by telephone (as previously planned and this still entitled the participant to the incentive) and a final text message primary outcome question was sent if there was no success from the manual follow-up. The automated notifications reminded the participant of the follow-up incentive, at the previous or increased rate, depending on SWAT randomisation group. The SWAT protocol has been accepted on to the Medical Research Council (MRC)-funded SWAT store (SWAT #164; www.qub.ac.uk/sites/TheNorthernIrelandNetworkforTrialsMethodologyResearch/SWATSWARInformation/Repositories/SWATStore/).
SWAT outcome measures
Primary: Questionnaire response rate. This included those responding by all methods (text, online or by telephone follow-up) within the allowable window for the primary outcome question (smoking status at 6 months).
Secondary:
-
proportion of participants requiring manual follow-up by the lead researcher (i.e. cost implications of staff time);
-
time taken for participants to respond to the questionnaire;
-
completeness of responses (counted as the number of missing items from all items asked, including secondary outcomes) and comparison to the response rate for the primary outcome (smoking status at 6 months).
SWAT analysis plan
Sample characteristics at baseline (by incentive arm), response rates at 6-month follow-up and other related response data, including completeness of responses, were summarised. For the primary analysis, a logistic regression model was run, using Wald CIs for the OR, including stratification variables as covariates in the model and any participant characteristics that appeared to be imbalanced between the different incentive groups, with response (binary) as the dependent variable. For secondary analyses, further logistic regression models were run using the same approach, and covariates for assessing the effect of incentives on manual follow-up rates and Cox proportional hazards analysis were undertaken with the log-rank test to assess the effects of incentives on response time.
Qualitative process evaluation
An embedded qualitative study was undertaken to assess participant perspectives. The aims of the qualitative process evaluation were:
-
to understand participant experiences of participating in the study;
-
to gather user views on app usage to inform further optimisation.
A purposively selected subsample of participants was invited to take part in a qualitative interview. The process evaluation was guided by the MRC guidance on process evaluation of complex interventions. 72 Part of this was to use interview data to better understand participant experiences of the intervention and how these experiences might help explain the causal pathway towards smoking behaviour change where it was observed. Qualitative interview topic guides (see Appendix 5) were used to probe for in-depth descriptions of experiences (e.g. of cravings experienced and how the app delivered support that may have helped ‘in the moment’, or not). Detailed information gathered qualitatively allowed for a richer understanding of situations and experiences than would be possible to gather through the app or quantitatively. In addition to using interview data, to meet the second aim of the process evaluation study, the Quit Sense app included an audio recording feature (‘auto-process evaluation’) so that users could leave feedback on their views and experiences of using the app. The study therefore also assessed the feasibility of this user-initiated process evaluation approach where data can be collected in a highly ecologically valid context. Any relevant data submitted were explored alongside the interview data, to potentially provide a broader range of views from a more user-controlled perspective.
At the 6-week follow-up, participants, including those in the Quit Sense and usual care arms, were invited to participate in a telephone qualitative interview as part of the process evaluation (aiming for a recruitment ratio of 3 : 1 from the Quit Sense and usual care arms, respectively). We used purposive sampling for intervention participants, where possible, by aiming to recruit individuals with high and low SES (as per study stratification), varied rates of app engagement and to include both abstainers and continuing smokers. For control participants, a mixture of high and low SES individuals, including smokers and abstainers, were purposively sampled. During the interview, intervention participants were asked about their experience and views of self-monitoring smoking using the app, including any environmental context factors/triggers missing from the smoking report survey, probing for detail and specific examples in order that causal pathways to behaviour change could potentially be identified. The interview topic guide was employed flexibly, allowing participants to discuss the study and app engagement in a manner that was comfortable for them. According to the topic guide, interviewees were also asked about the ease of using the app; types of messages they liked most and least; their views on the timing of messages when entering or dwelling in a smoking zone (geofence); their views on the ‘My Profile’ and ‘Library’ sections of the app; their views on features they would like that are not currently provided; and how personalisation of the app could be improved. Control participants were asked about their experience of participating in the study, informing an assessment of the feasibility of randomised study design for a definitive trial. Both groups of participants were asked about their use or interest in the use of other smoking cessation aids and different types of cessation support available including e-cigarettes; use of other smoking cessation apps; other health and well-being apps; and NHS-orientated smoking cessation support options.
Interviews were audio-recorded by the interviewer (AH) and transcribed to intelligent verbatim standard by an administrator. Interview transcripts were checked for accuracy, and all were anonymised (removing names, places and other distinguishing features). NVivo was used to support the qualitative analysis.
Analysis of process evaluation data
An inductive thematic analysis of the first three to four interview transcripts was initially undertaken (by AH). 73 AH and CN then met to discuss coding and to develop a coding framework. This was agreed by consensus. AH then continued to code the remaining data according to the framework. Coding consistency was checked through the independent coding of 10% of the data (conducted by CN). At verification meetings, AH and CN agreed codes by consensus and were satisfied that coding was consistent. Refinement of themes continued until the themes were agreed. CN and AH met regularly to discuss the coding process and ultimately agreed on the key themes arising from data analysis for reporting purposes.
Analysis of process evaluation data focused on identifying key themes associated with using the app and views and experiences of using other relevant apps, supplemented with a descriptive analysis of relevant experiences to begin to hypothesise intervention causal pathways towards behaviour change. A second stage of analysis worked with descriptions of experiences of lapse or lapse avoidance in the context of the app and considering unique participant contexts. These are reported as vignettes and used to explore participant experiences and to identify mechanisms of action and casual pathways towards behaviour change. There were not many audio-recordings submitted via Quit Sense’s auto-process evaluation feature and so, as pre-planned, we did not code these data for thematic analysis but instead provided a basic summary of the content.
Chapter 3 Results
Study advertisements were promoted on Facebook (including Instagram) and Google AdWords in two phases – the first ran from 27 November to 12 December 2020 and the second from 5 January to 25 January 2021. Participant identification and recruitment is summarised in the study Consolidated Standards of Reporting Trials flow (Figure 1) and the advertisement engagement flow diagrams (Figure 2). The total number of ‘impressions’ (when the advert is shown on a web page or app) were 30,835 and 98,767 for Facebook for phases 1 and 2, respectively (total of 129,602), and 24,143 and 630 for Google across phases 1 and 2 (total of 24,773). This led to 560 and 858 advert ‘link clicks’ (total of 1418) for Facebook and 407 and 18 for Google (total of 425) for phases 1 and 2, respectively. There was a total of 1275 landings on the study website, of which 323 (25%) people completed the eligibility assessment, 299 (23%) were eligible, 267 (21%) provided informed consent to participate and 209 (16%) continued to randomisation (117 in phase 1 and 92 in phase 2). Of those assessed for eligibility, 93% were eligible, and of those eligible, 70% consented and were randomised. One individual was randomised but had been enrolled by their partner and so was removed from the randomised sample as they were not eligible as they had not provided informed consent.
FIGURE 1.
Trial flow.
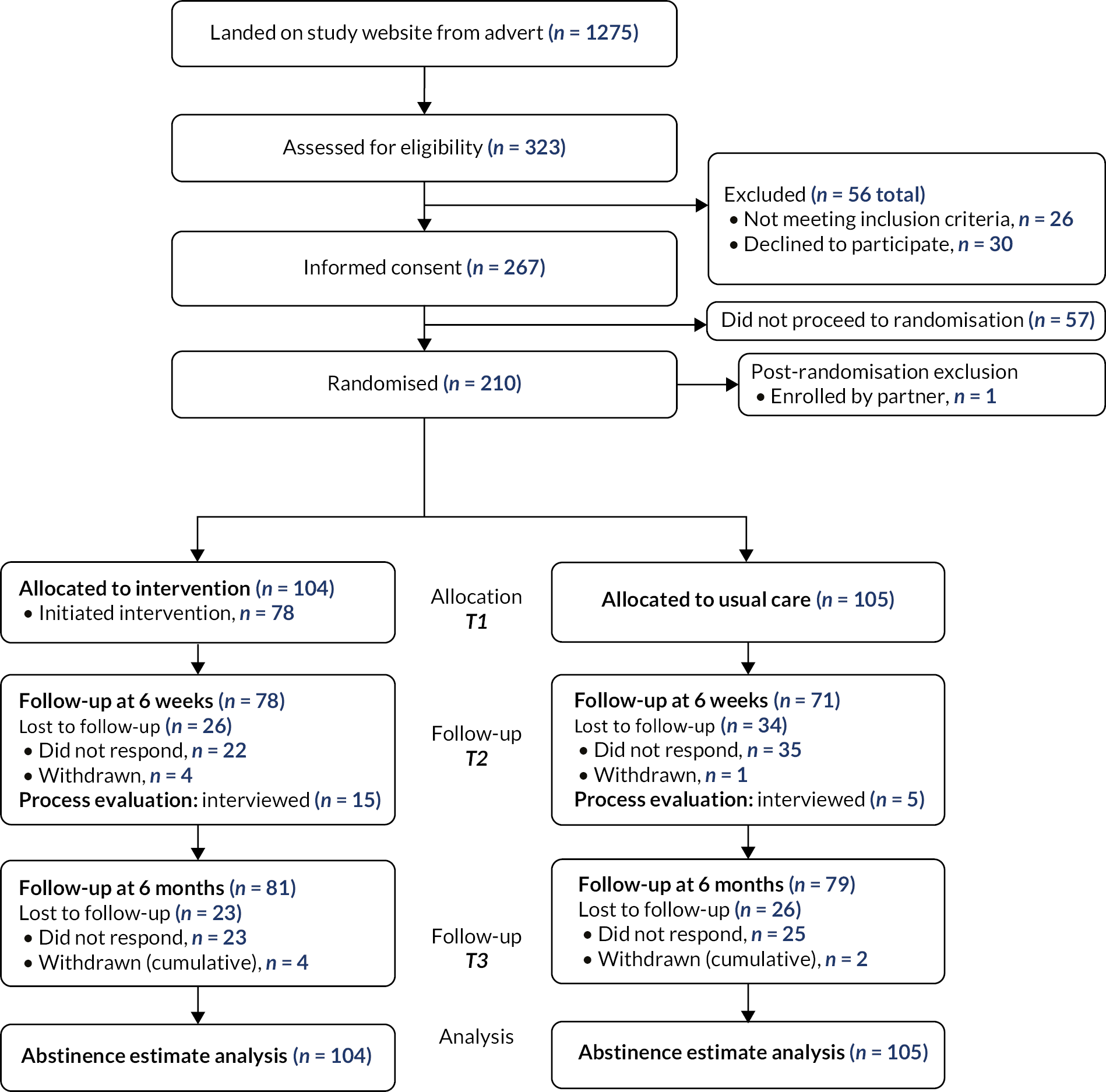
FIGURE 2.
Flow diagram of advert reach, engagement and enrolment rates and costs.
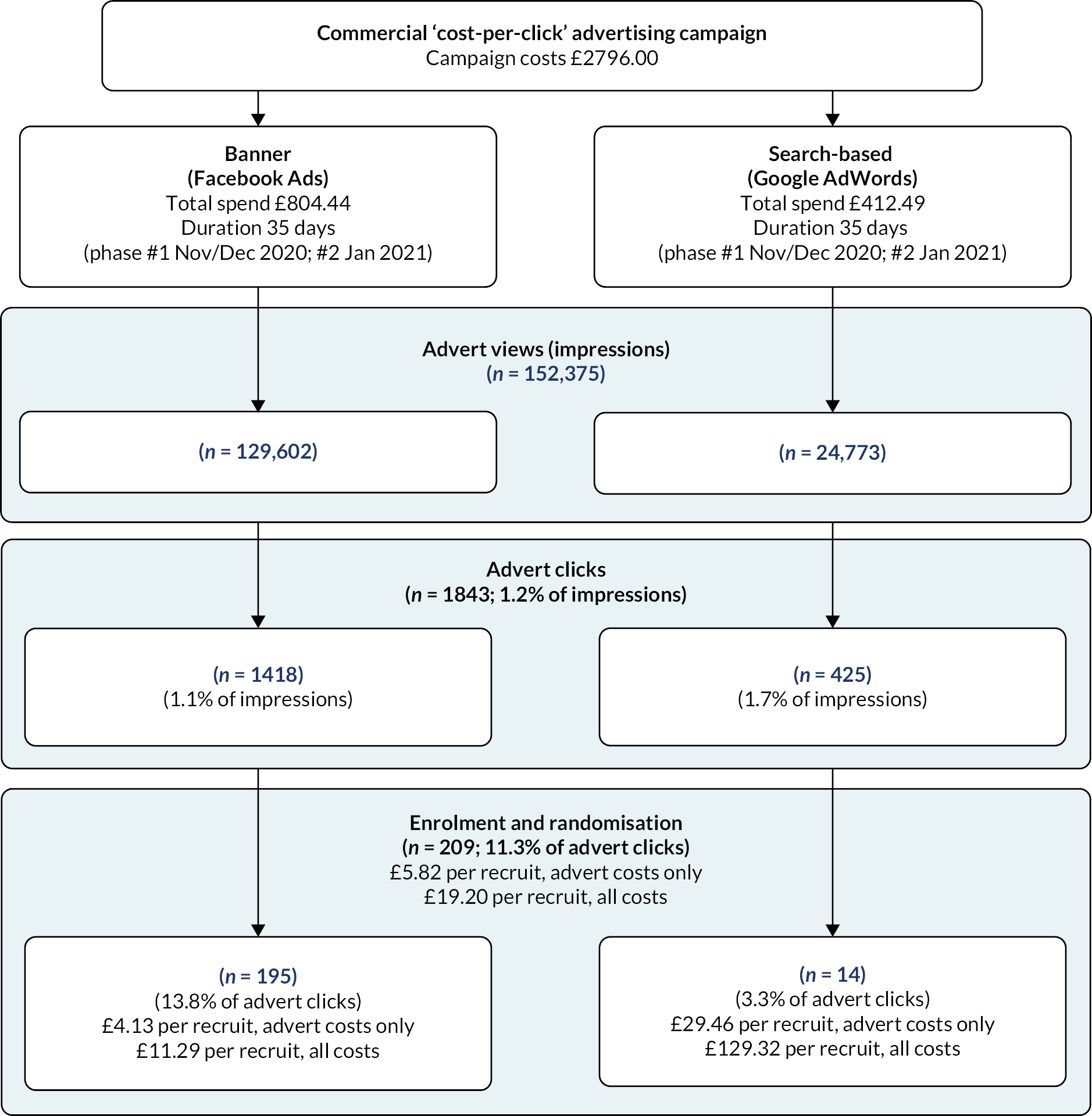
Removing the one participant randomised but enrolled by their partner, 209 remaining individuals were randomised, 104 allocated to the Quit Sense arm and 105 to the usual care arm. At 6 weeks, 149 (71%; 95% CI 65% to 77%) were followed up and at 6 months this was 160 (77%; 95% CI 71% to 82%). There were six withdrawals, four from the Quit Sense arm and two from the usual care arm.
Table 2 provides baseline sample characteristics. The sample had a mean age of 41 years (range 18–61), 56% were female, 29% classified as low SES and 9% were of non-white ethnicity. Mean baseline smoking rate was 15 cigarettes per day and 31%, 59% and 10% were classed as low, moderate and high heaviness of smoking, respectively.
Quit Sense (n = 104) |
Usual care (n = 105) |
Overall (n = 209) |
|
---|---|---|---|
Age at consent: mean (SD) | 39.6 (10.0) | 42.6 (10.0) | 41.1 (10.0) |
Gender: n (%) | |||
Male | 45 (43.3) | 48 (45.7) | 93 (44.5) |
Female | 59 (56.7) | 57 (54.3) | 116 (55.5) |
Number of cigarettes smoked per day: n (%) | |||
< 16 | 64 (61.5) | 63 (60.0) | 127 (60.8) |
16 or more | 40 (38.5) | 42 (40.0) | 82 (39.2) |
SES: n (%) | |||
Low | 30 (28.9) | 31 (29.5) | 61 (29.2) |
High | 74 (71.2) | 74 (70.5) | 148 (70.8) |
Socioeconomic grade: n (%) | |||
1 (highest) | 48 (57.1) | 46 (65.7) | 94 (61.0) |
2 | 8 (9.5) | 5 (7.1) | 13 (8.4) |
3 | 4 (4.8) | 7 (10.0) | 11 (7.1) |
4 | 13 (15.5) | 8 (11.4) | 21 (13.6) |
5 (lowest) | 11 (13.1) | 4 (5.7) | 15 (9.7) |
Missing | 20 | 35 | 55 |
Ethnicity: n (%) | |||
White | 95 (91.4) | 96 (91.4) | 191 (91.4) |
Indian | 0 (0.0) | 1 (1.0) | 1 (0.5) |
Pakistani | 2 (1.9) | 0 (0.0) | 2 (1.0) |
Bangladeshi | 1 (1.0) | 2 (1.9) | 3 (1.4) |
Black African | 1 (1.0) | 1 (1.0) | 2 (1.0) |
Black (other) | 1 (1.0) | 0 (0.0) | 1 (0.5) |
Asian | 2 (1.9) | 1 (1.0) | 3 (1.4) |
Mixed race | 1 (1.0) | 2 (1.9) | 3 (1.4) |
Not given | 1 (1.0) | 2 (1.9) | 3 (1.4) |
Number of cigarettes usually smoked a day: mean (SD) | 15.4 (7.6) | 15.5 (6.5) | 15.4 (7.1) |
Employment status: n (%) | |||
In work during last 12 months | 83 (79.8) | 70 (66.7) | 153 (73.2) |
Out of work for more than 12 months | 19 (18.3) | 27 (25.7) | 46 (22.0) |
Retired | 1 (1.0) | 0 (0.0) | 1 (0.5) |
Full-time student | 1 (1.0) | 8 (7.6) | 9 (4.3) |
Occupation: n (%) | |||
Modern professional | 22 (26.2) | 17 (24.3) | 39 (25.3) |
Clerical | 9 (10.7) | 9 (12.9) | 18 (11.7) |
Senior manager/administration | 11 (13.1) | 11 (15.7) | 22 (14.3) |
Technical | 7 (8.3) | 8 (11.4) | 15 (9.7) |
Semiroutine manual/service | 13 (15.5) | 6 (8.6) | 19 (12.3) |
Routine manual/service | 7 (8.3) | 2 (2.9) | 9 (5.8) |
Middle/junior manager | 6 (7.1) | 10 (14.3) | 16 (10.4) |
Traditional professional | 9 (10.7) | 7 (10.0) | 16 (10.4) |
Missing | 20 | 35 | 55 |
Highest qualification: n (%) | |||
No formal | 6 (5.8) | 7 (6.7) | 13 (6.2) |
General Certificate of Secondary Education or similar | 22 (21.2) | 20 (19.1) | 42 (20.1) |
A/Advanced Subsidiary Level or similar | 24 (23.1) | 28 (26.7) | 52 (24.9) |
Degree or similar | 46 (44.2) | 44 (41.9) | 90 (43.1) |
Other | 6 (5.8) | 6 (5.7) | 12 (5.7) |
Heaviness of Smoking Index: n (%) | |||
Low | 36 (34.6) | 29 (27.6) | 65 (31.1) |
Moderate | 57 (54.8) | 67 (63.8) | 124 (59.3) |
High | 11 (10.6) | 9 (8.6) | 20 (9.6) |
FUTS: n (%) | |||
A little of the time | 12 (11.5) | 5 (4.8) | 17 (8.1) |
Some of the time | 29 (27.9) | 39 (37.1) | 68 (32.5) |
A lot of the time | 39 (37.5) | 47 (44.8) | 86 (41.2) |
Almost all the time | 17 (16.4) | 6 (5.7) | 23 (11.0) |
All the time | 7 (6.7) | 8 (7.6) | 15 (7.2) |
SUTS: n (%) | |||
No urges | 1 (1.0) | 0 (0.0) | 1 (0.5) |
Slight urges | 5 (4.8) | 6 (5.7) | 11 (5.3) |
Moderate urges | 36 (34.6) | 49 (46.7) | 85 (40.7) |
Strong urges | 38 (36.5) | 31 (29.5) | 69 (33.0) |
Very strong urges | 20 (19.2) | 14 (13.3) | 34 (16.3) |
Extremely strong urges | 4 (3.9) | 5 (4.8) | 9 (4.3) |
EQ-5D-5L utility score: mean (SD) | 0.79 (0.22) | 0.77 (0.21) | 0.78 (0.22) |
Missing | 1 | 0 | 1 |
WISDM Automaticity subscale score: mean (SD) | 4.71 (1.87) | 4.79 (1.62) | 4.75 (1.75) |
WISDM Associative processes subscale score: mean (SD) | 4.70 (1.34) | 4.50 (1.34) | 4.60 (1.34) |
Self-efficacy score: mean (SD) | 1.69 (0.92) | 1.65 (0.81) | 1.67 (0.87) |
Measurement, recruitment, intervention engagement and smoking outcomes
Completion rates for the anticipated primary outcome for a full trial
Completion of self-reported abstinence for the primary outcome at 6 months was 77% (160/209; 95% CI 71% to 82%) (Table 3). By arm, response rates were 78% and 75% for the Quit Sense and usual care arms, respectively. The return of a viable saliva sample for biochemical validation of those self-reporting abstinence for the primary outcome was 39% (16/41; 95% CI 24% to 54%), and by arm 52% (13/25) and 19% (3/16) for the Quit Sense and usual care arms, respectively. In addition, one participant (in the Quit Sense arm) returned a sample which had insufficient saliva in order to assess cotinine/anabasine levels. More detailed outcome data at 6 weeks and 6 months can be found in Appendix 6, Tables 19 and 20.
Outcome (denominator) | Count/N | Proportion (%) | 95% Wald CI |
---|---|---|---|
Of those landing on the website, proportion then checked for eligibility | 323/1275 | 25 | 23% to 28% |
Of those checked for eligibility, proportion eligible | 299/323 | 93 | 90% to 95% |
Of those eligible, proportion then randomised into the trial | 209/299 | 70 | 65% to 75% |
Trial participants with self-reported smoking status at 6 weeksa | 149/209 | 71 | 65% to 77% |
Trial participants with self-reported smoking status at 6 months | 160/209 | 77 | 71% to 82% |
Self-reported abstinent participants at 6 months that returned a saliva test | 16/41 | 39 | 24% to 54% |
Trial participants with EQ-5D-5L at 6 weeksa | 148/209 | 71 | 65% to 77% |
Trial participants with EQ-5D-5L at 6 months | 147/209 | 70 | 64% to 77% |
Usual care arm cessation rate
Three participants in the usual care arm were classified as abstinent according to the primary outcome, a rate of 2.9% (95% CI 0.0% to 6.0%).
Cost of recruitment using online advertising
Advertising costs were split into running costs for the campaigns, managed by the commercial partner organisation Nativve, and the costs of the adverts on Facebook (which includes Instagram) and Google (all costs inclusive of value-added tax) (see Figure 2). Total advertising running costs were £2796, broken down into a set-up fee (£1068) and 6-week campaigns for both Facebook and Google (£864 each). Total advert costs were £804.44 for Facebook and £412.49 for Google. Total cost per recruit was £19.20, which included running costs (£13.38) and advert costs (£5.82). One hundred and ninety-five participants were recruited via Facebook and 14 from Google. The advert cost per recruit was lower for Facebook (£4.13) than for Google (£29.46). In phase 2, Facebook adverts were purposively targeted towards non-white and low SES groups by Nativve using filters including for language and interests to attempt to increase the proportion of participants from these groups. While the proportion of non-white (including missing) participants was higher in phase 2 (13.8%) relative to phase 1 (5.2%), there were no meaningful differences in the proportion classed as low SES (phase 1 30.4% vs. phase 2 29.9%). The estimated cost per recruit was higher in phase 2 (calculated range £2.27–8.11) compared with phase 1 (calculated range £1.16–4.61), although the data comes from web analytics and so is not fully reliable.
Rates of app installation and use and acceptability
The installation rate of the Quit Sense app, defined as the submission of the unique code provided to intervention participants as recorded by the server hosting the app, was 75% (95% CI 67% to 83%; 78/104) (Table 4). All but one participant (99%; 77/78) who installed the app did so before the installation text message reminder was sent. Among the 28 participants sent a reminder, one installed the app on the day of the reminder and three replied to the subsequent text message inviting a reason for not installing, where two indicated they had had problems installing (‘option 5’) and one that had had no internet (‘option 1’). The three responding to the text message were contacted by the lead researcher but none of them installed the app. A small number of participants who installed the app (n = 9) did not have engagement data uploaded to the server due to a technical issue. In a few of these cases, missing engagement data for some engagement variables were determined from data recorded in follow-up questionnaires or during process evaluation interviews. Among those who installed the app, 100% (95% CI 95% to 100%; 70/70) set a quit date in the app and 51% (95% CI 39% to 63%; 38/74) engaged with the app for more than 7 days, and 23% for more than 30 days (95% CI 13% to 33%), with a median duration of use of 10 days [interquartile range (IQR) 30)]. The median number of days between the date when the quit date was set and the actual quit date (‘quit date delay’) was 9 (IQR 8). Among those who engaged with the app up until their quit date (36/74; 49%), the total median duration of app engagement was 27 days (IQR 91) and duration of app engagement after their quit date had passed was 22 days (IQR 99), excluding two participants whose app engagement period could not be accurately calculated due to a technical issue.
Quit Sense arm | |
---|---|
Data uploaded to server from Quit Sense app | |
Participants that installed the app n (%) | 78 (75.0) |
Median number of days into future quit date set for: median (q25,q75) | 9 (8) |
Duration of app use (days): median (q25,q75); minimum, maximum (N = 71)a | 10 (1, 31); 0, 261 |
Number of active engagements: median (q25,q75), maximum, minimum (N = 69)a |
11 (2, 39); 0, 271 |
Participants that engaged with the app for more than 7 days (N = 74)a (%) | 38 (51.4) |
Participants that engaged with the app for more than 30 days (N = 74)a (%) | 17 (23.0) |
Participants that set a quit date in the app (N = 70)a (%) | 70 (100) |
Number of app quit dates per participant: median (q25,q75), maximum, minimum (N = 74)a | 1 (1, 1); 0, 8 |
Participants that engaged with the app up until their quit date (of those that set a quit date; N = 74)a (%) | 36 (48.6) |
Self-reported data at follow-up | |
Participants reporting that they would recommend Quit Sense to a friend trying to quit: n (%) (N = 43)a,b | |
Unsure | 0 (0.0) |
No | 1 (2.3) |
Maybe | 13 (30.2) |
Yes | 29 (67.4) |
Missing | 31 |
Participants reporting that they found the app easy to use (4 or 5 on 5-point scale) (N = 44)a,b: n (%) | 38 (86.4) |
Among intervention participants who installed the app and were followed up at 6 weeks, 67% (29/46) said they would recommend Quit Sense to a friend trying to quit, with 30% (13/46) saying ‘maybe’ and 2% (1/46) saying they would not recommend it. The majority of participants either strongly agreed (55%; 24/44) or agreed (32%; 14/44) that Quit Sense was easy to use, with 11% (5/44) neither agreeing nor disagreeing and 2% (1/44) disagreeing.
Completion of smoking cessation-related resource use and quality-of-life data
At 6-month follow-up, the response rate for both resource use and QoL data was 147/209 (70%; 95% CI 64% to 77%).
Intervention effect on anticipated primary outcome and secondary abstinence outcomes
Analysis of the primary outcome, self-reported abstinence as smoking no more than five cigarettes within the 6-month study period, biochemically verified by saliva test, found a higher abstinence rate in the Quit Sense arm (11.5%; 12/104) compared to the usual care arm (2.9%; 3/105) (unadjusted OR 4.44, 95% CI 1.21 to 16.21; p = 0.024) (Table 5). This shows a positive association, with moderate evidence to suggest that the odds of abstinence for the Quit Sense group is 4.4 times that of the usual care group. When adjusting for stratification variables and prognostic factors, results and conclusions in terms of direction, size, precision and statistical significance of the effect remain consistent (adjusted OR 4.46, 95% CI 1.19 to 16.69; p = 0.023) (see also Appendix 6, Table 21). This analysis adjusted for heaviness of smoking index as a key prognostic variable, which appeared potentially imbalanced between arms at baseline, but due to relatively small numbers of abstinent participants, the model fit was potentially problematic. A more appropriate approach of exact inference (using SAS 9.4 statistical software) was therefore used, which produced more conservative (wider CIs), but overall consistent results and conclusions (adjusted OR 4.36, 95% CI 1.10 to 25.22; p = 0.033) (see Appendix 6, Table 22). Sensitivity analyses were undertaken where (1) withdrawals were excluded and (2) complete cases only included. Other than changing the abstinence proportions, results and conclusions of effect size, direction, uncertainty and statistical significance also remain consistent (see Appendix 6, Tables 23–27).
Outcome | App group n (%) N = 104 |
Usual care n (%) N = 105 |
Difference % (95% CI) | Unadjusted ORa,b (95% CI) |
p-value | Adjusted OR model 1c (95% CI) |
Adjusted OR model 2c,d (95% CI) |
---|---|---|---|---|---|---|---|
Primary (6-month prolonged abstinence validated by saliva test) | 12 (11.5) | 3 (2.9) | 8.7% (1.6% to 16.5%) | 4.44 (1.21 to 16.21) | 0.024 | 4.46e (1.19 to 16.69) | 4.36 (1.10 to 25.22) |
Secondary (7-day point prevalence abstinence at 6 months validated by saliva test) | 16 (15.4) | 5 (4.8) | 10.6% (2.4% to 19.2%) | 3.64 (1.28 to 10.33) | 0.015 | 3.67e (1.27 to 10.60) | 3.59 (1.18 to 13.18) |
Secondary (7-day point prevalence abstinence at 6 months, self-report) | 28 (26.9) | 20 (19.1) | 7.9% (−3.6% to 19.1%) | 1.57 (0.82 to 3.01) | 0.178 | 1.54 (0.80 to 2.97) | 1.53 (0.76 to 3.11) |
Secondary (7-day point prevalence abstinence at 6 weeks, self-report) | 20 (19.2) | 21 (20.0) | −0.8% (−11.5% to 10.0%) | 0.95 (0.48 to 1.89) | 0.889 | 0.91 (0.45 to 1.83) | 0.91 (0.43 to 1.93) |
By using the Bayesian approach relevant in preliminary trials, it is estimated that there is 90% probability that the underlying OR favouring the intervention is 1.7 or higher, 93% that it is 1.5 or higher and 85% that it is 2.0 or higher, indicating good support for a subsequent trial in which this range of effect sizes is considered.
Secondary smoking outcomes (see Table 5 and Appendix 6, Tables 27–34) collected at 6 months also favoured Quit Sense over usual care, although this was only statistically significant for the biochemically validated outcome: biochemically validated 7-day point prevalence abstinence (Quit Sense 15.4%; usual care 4.8%, OR; 3.64, 95% CI 1.28 to 10.33; p = 0.015) and self-reported 7-day point prevalence abstinence (Quit Sense 26.9%; usual care 19.1%, OR 1.57, 95% CI 0.82 to 3.01; p = 0.18). Self-reported 7-day point prevalence abstinence at 6 weeks did not favour Quit Sense over usual care (Quit Sense 19.2%; usual care 20.0%, OR 0.95, 95% CI 0.48 to 1.89; p = 0.89). Due to there being potential between-arm differences in saliva sample return rates, we undertook an additional post hoc sensitivity analysis for the primary outcome analysis but using self-reported prolonged abstinence only. The findings favoured the Quit Sense arm, though the between-arm difference was not statistically significant (Quit Sense 24.0%; usual care 15.2%, OR 1.76, 95% CI 0.88 to 3.53; p = 0.11).
Intervention effect on hypothesised mechanisms of action of app at 6 weeks post enrolment
At 6-week follow-up, the proportion of participants who reported smoking in the first 2 weeks of a quit attempt (or since enrolment if no quit date set) was 70.4% in the Quit Sense arm and 80.8% in the usual care arm, which was not a statistically significant difference [X2, 1 (degrees of freedom), N = 149] = (X2 statistic = 2.17), p = 0.14] (Table 6). Use of lapse prevention strategies varied, with ‘contacting a friend/family member for support or distraction’ as the least commonly used strategy (47% participants used) to ‘focusing on other tasks’ as the most commonly used (91%) (Table 7). There was no evidence of a difference between arms on average lapse prevention strategy use [MD −0.07, 95% CI −0.26 to 0.12, T-statistic: −0.75; p = 0.46] or when broken down into avoidance (MD −0.10, 95% CI −0.33 to 0.13, T-statistic: −0.58; p = 0.39) or coping strategies (MD −0.06, 95% CI −0.26 to 0.14, T-statistic: −0.58; p = 0.56) (Table 8).
Categorical/continuous variable at 6 weeks | App (n = 71) | Standard (n = 78) | Statistical test | p-value |
---|---|---|---|---|
Did not smoke tobacco in the first 2 weeks of quit attempt (or first 2 weeks of study) (lapse incidence) (%) | 21 (29.6) | 15 (19.2) | Chi-squared | 0.141 |
Did not smoke any tobacco in the first month of quit attempt (or first month of study) (lapse incidence) (%) | 11 (15.5) | 7 (9.0) | Chi-squared | 0.223 |
SUTS (%)a | Mann–Whitney | 0.228 | ||
No urges | 12 (16.9) | 3 (3.9) | ||
Slight urges | 9 (12.7) | 20 (25.6) | ||
Moderate urges | 29 (40.9) | 22 (28.2) | ||
Strong urges | 7 (9.9) | 16 (20.5) | ||
Very strong urges | 8 (11.3) | 9 (11.5) | ||
Extremely strong urges | 6 (8.5) | 8 (10.3) | ||
FUTS (%)a | Mann–Whitney | 0.825 | ||
Not at all | 10 (14.1) | 6 (7.7) | ||
A little of the time | 15 (21.1) | 21 (26.9) | ||
Some of the time | 17 (23.9) | 17 (21.8) | ||
A lot of the time | 12 (16.9) | 18 (23.1) | ||
Almost all the time | 9 (12.7) | 9 (11.5) | ||
All the time | 8 (11.3) | 7 (9.0) | ||
Self-efficacy average score (five-point scale) | 1.89 (1.26) | 1.71 (1.11) | Mann–Whitney | 0.391 |
WISDM Automaticity subscale score (seven-point scale) | 3.56 (2.26) | 3.83 (2.30) | Mann–Whitney | 0.512 |
Lapse prevention strategy at 6 weeks | App (n = 71) (%) |
Standard (n = 78) (%) |
Overall (n = 149) (%) |
---|---|---|---|
Focusing on other tasks | 87 | 94 | 91 |
Avoiding spending time with other smokers | 45 | 58 | 52 |
Changing my routine | 77 | 74 | 76 |
Avoiding places where I would usually smoke | 55 | 56 | 56 |
Avoiding stressful situations | 68 | 60 | 64 |
Avoiding places where I can buy cigarettes | 48 | 51 | 50 |
Trying not to think about smoking | 87 | 85 | 86 |
Going for a walk or doing some exercise | 79 | 78 | 79 |
Keeping my mouth busy, for example, chewing mints | 63 | 72 | 68 |
Contacting a friend/family member for support or distraction | 49 | 45 | 47 |
Replacing smoking with something else, for example, food | 76 | 77 | 77 |
Using self-talk, for example, ‘I can do it’ | 73 | 81 | 77 |
Delaying smoking | 80 | 83 | 82 |
Using deep breathing | 59 | 51 | 55 |
Reminding myself how bad I will feel if I smoke | 83 | 83 | 83 |
Thinking about the harmful effects of smoking | 83 | 79 | 81 |
Thinking about the benefits of quitting | 94 | 94 | 94 |
Thinking about rewards I could have for not smoking | 76 | 77 | 77 |
Doing things that help me relax | 77 | 77 | 77 |
Thinking about saving money | 73 | 79 | 77 |
Continuous variable at 6 weeks | Mean difference | 95% CI | t- test p-value |
---|---|---|---|
Lapse prevention average scorea | −0.07 | (−0.26 to 0.12) | 0.455 |
Lapse prevention subscale 7 average scorea | −0.10 | (−0.33 to 0.13) | 0.394 |
Lapse prevention subscale 13 average scorea | −0.06 | (−0.26 to 0.14) | 0.560 |
WISDM cue exposure/associative processes subscale score | 0.14 | (−0.36 to 0.64) | 0.577 |
There were no evidence of differences between arms on self-efficacy (Mann–Whitney U = 5550.50, p = 0.39) and strength of urges to quit (Mann–Whitney U = 5016.00, p = 0.23), frequency of urges to quit (Mann–Whitney U = 5267.50, p = 0.83) and WISDM automaticity (Mann–Whitney U = 5153.50, p = 0.51) and associative processes (MD 0.14, 95% CI −0.36 to 0.64, T-statistic: 0.56; p = 0.58) subscale scores at 6 weeks (see Tables 6 and 8).
Process evaluation findings
Participants who made use of the ‘auto-process evaluation’ feature did so primarily to provide positive suggestions for app improvement, which mainly focused on logging unique contexts when smoking, but also to raise some issues and problems with the app. These are summarised in Table 9. However, this feature was not well-used. Six participants out of those who installed the app (6/78; 7.7%) chose to submit an audio message. Two of these participants also took part in a qualitative interview. One participant submitted an audio message on two occasions (once on commencement of the app and another having used it for some time).
ID | Theme(s) and summary notes | Evidence |
---|---|---|
ID017 | Did not engage with app training (thus app did not send correctly targeted messages) | ‘The reason I got the app was because I searched “quit smoking” and so at that point I needed something that was like, quit now, in that moment because that’s when people are ready to quit. When they are searching the terms, not like, a week later.’ |
ID014 | Technical issues Reporting smoking as diary impacts behaviour Notifications act as smoking reminders |
‘There’s time where, when I’ve closed the app down, I try to open a different app and this app reopens. Which is a bit annoying.’ ‘I’ve actually noticed while tracking my smoking, that I’ve actually reduced how much I smoke as well. Which will hopefully make tomorrow easier.’ ‘Too many notifications, after you’ve gone past the first week of quitting. I’m getting notifications when I’ve not been thinking about smoking.’ |
ID010 | Capture link between smoking and eating (activity context) | ‘When I am um, informing you that I am smoking, it could be really good if I could say that I have just eaten or what the actual activity was, rather than if I’m just at home or working and such like. That would be really good information to share for my habits around smoking. Especially around eating.’ |
ID011 | Capture link between smoking and driving (activity context) | ‘It would be good if there was an option in there to recognise when you were driving, or at least have the option. I know one of the places, you can select where you are and when you’re smoking and it does obviously, have an option for work. But driving would be a big one, so if there was a way it could recognise when I’m driving and respond to that, that would be great.’ |
ID017 | Capture link between smoking and driving (activity context) Changing context (active) |
‘Most of the time when I do smoke, it’s when I’m on my own driving or when I’m going somewhere.’ |
ID015 | Capture routine habit | ‘It doesn’t have an option for just a habit. You know, when you just pick a cigarette up, light it, great. Then you realise you’ve been smoking a cigarette because it’s part of a habit. It would be nice to have that as part of this app, if at all possible.’ |
Participants of the qualitative interview study (N = 20) were purposefully sampled, with 15 recruited from the intervention group, to inform aspects of app engagement and trial procedures, and 5 from the usual care group, to capture experiences of participating in that group. We specifically chose participants from those consenting to a qualitative interview who had both achieved smoking abstinence and continued to smoke, or had relapsed to smoking (Table 10)
Smoking status | Intervention (n = 15) | Usual care (n = 5) |
---|---|---|
Abstinent | 9 | 1 |
Smoking | 6 | 4 |
The qualitative sample were broadly representative of the total trial sample with two exceptions – higher rates of highest qualification as degree and higher rates of those from lower SES groups in the interview sample, with the latter due to deliberate oversampling to ensure a broader range of views were captured (Table 11).
Interview sample | Trial sample | |
---|---|---|
Age: mean (range) | 39 (28–51) years | 41 (18–61) years |
Gender: n (%) | ||
Male | 10 (50.0) | 93 (44.5) |
Female | 10 (50.0) | 116 (55.5) |
Ethnicity: n (%) | ||
White | 15 (75.0) | 191 (91.4) |
Indian | 0 (0.0) | 1 (0.5) |
Pakistani | 0 (0.0) | 2 (1.0) |
Bangladeshi | 2 (10.0) | 3 (1.4) |
Black African | 0 (0.0) | 2 (1.0) |
Black (other) | 1 (5.0) | 1 (0.5) |
Asian | 0 (0.0) | 3 (1.4) |
Mixed race | 1 (5.0) | 3 (1.4) |
Not given | 1 (5.0) | 3 (1.4) |
SES: n (%) | ||
Low | 10 (50.0) | 61 (29.2) |
High | 10 (50.0) | 148 (70.8) |
Highest qualification: n (%) | ||
No formal | 1 (5.0) | 13 (6.2) |
General Certificate of Secondary Education or similar | 2 (10.0) | 42 (20.1) |
A/Advanced Subsidiary Level or similar | 2 (10.0) | 52 (24.9) |
Degree or similar | 14 (70.0) | 90 (43.1) |
Other | 1 (5.0) | 12 (5.7) |
Number of cigarettes usually smoke a day: mean (range) | 14.7 (5–30) | 15.4 (1–40) |
Self-reported as not smoking at 6 weeks (of those followed up): n (%) | 10 (50.0) | 49 (33.1) |
Five main themes were identified from the qualitative analysis: ‘engaging’, ‘disconnecting’, ‘suggestions for app improvement’, ‘pathways to change’ and ‘time and context’.
‘Engaging’
Finding out about the study and reasons for taking part
Participants did not have concerns about being recruited remotely online and providing personal details such as their names, addresses and telephone numbers as part of registering for the study. They felt that the study advertisements appeared genuine. Participants commented that they were reassured by the associations the study had with both a university and the NHS. Participants tended to report that they signed up immediately after landing on the study website and based only on reading the basic participant information sheet rather than downloading and reading the longer participant information sheet.
I’ve seen it’s also in relation with the NHS. So […] I enrolled straight away […].
Female, usual care arm, ID110
Everything was kind of straight forward and […] informative […] So, I didn’t download the detailed information sheet to be honest [laughs]. […] no concerns whatsoever.
Male, usual care arm, ID116
The main reason given for participation was a desire to quit smoking. Related motivations included the desire to be held accountable in making a quit attempt, seeking extra support and a sense of belonging (i.e. being part of a group with others who had similar motivations):
It was the accountability almost; just to be able to, you know, put a bit of pressure on myself rather that than just say, ‘I’m going to finish’ and inevitably, I never do.
Male, usual care arm, ID116
I thought, I’ll have a stab at it and give something a go. Just sort of as many aids as we can get really.
Male, Quit Sense arm, ID115
I found it on Facebook [ … ] fed to me on the feed. It felt good to be a part because it’s certainly something that I’m interested in or at any rate, something that I need [ … ] and it also felt good to be a part of a research group [ … ] like you were part of something.
Male, Quit Sense arm, ID116
‘Disconnecting’
Those participants who were retained in the study reported disconnecting with the study and intervention app due to a variety of reasons connected to either the use of the technology or changes in their own motivations and behaviours.
Technical issues
Several participants reported having technical issues with the app resulting in feelings of frustration and annoyance. Issues included the following: unwanted and repeated battery optimisation messages, crashing other applications, the app opening when it was not wanted, an inability to complete surveys and inaccurate recording of cigarettes reported.
Not being able to use it at the beginning of the quitting smoking process, when it was like the most important [ … ] I found it quite frustrating [ … ].
Female, Quit Sense arm, ID106
Change in smoking behaviour
Some participants had uninstalled Quit Sense from their phones. Reasons included not quitting and lapsing:
No, I used it for about a month. Of course, when I started smoking again after that, I felt bad and embarrassed, so I didn’t use it much after that [ … ] Just disengaged by that point.
Female, Quit Sense arm, ID210
Other participants disengaged because they felt that they no longer needed app support, such as because they had quit cigarettes and/or transitioned to vaping. Interestingly, one participant who thought the app was very useful in the preparation stage ceased actively engaging with it once quit day arrived because they associated it with smoking:
So, quit day came and although I checked back in on the app, I did find that I really didn’t use the app at all. Partially because when I quit, I wanted to be, ‘I’ve quit’. When you quit you throw your cigarettes and lighters away. One of the top tips [from the app] is to throw anything away that reminds you of your life when you were smoking. In my mind, that was also to get rid of the stop smoking app as well because you’ve stopped smoking. The messages did still pop up.
Male, Quit Sense arm, ID216
Some participants disengaged with the app and/or study more broadly because they either did not make a quit attempt or lapsed:
I haven’t felt like I’ve been particularly involved in a study [ … ] me enrolling on the stop smoking and the timing of me stopping smoking [ … ] I hadn’t stopped smoking.
Female, usual care arm, ID108
Not meeting motivational needs
Some participants had been interested to try an app to assist their quit attempt but had found that this form of support (at least alone) did not meet their needs:
I removed it after a few days. I thought yeah, it’s not going to work for me [ … ] I just realised it’s all, shall we say, psychological support. [ … ] To stop smoking it has to be at least a week or two of something physical [ … ] Supervised or patches, pills, I don’t know.
Female, usual care arm, ID110
Suggestions for app improvement
Participants felt that alcohol, daily routine and boredom were important triggers for smoking that were missing from the app:
I think it could have been a bit more specific. As someone that smokes and likes a bit of a drink every now and again, I felt that just clicking ‘socialising’ didn’t quite cover it. I don’t know, I felt like it might have been beneficial for your data to say, ‘okay, so alcohol was involved’.
Male, Quit Sense arm, ID115
Sometimes it can be as simple as after a meal.
Female, Quit Sense arm, ID109
Boredom is probably a lot of people’s triggers for smoking. But for me, quite often, I didn’t fit into a category. I didn’t have one because I felt a certain way, I just had one because I felt like I should have one now. There’s no real reason or purpose. [ … ] It was having one for the sake of it, for the routine of it.
Male, Quit Sense arm, ID216
Some participants wanted to be able to record that they had smoked while en route to other places, for example, while walking or driving:
I like to smoke whilst I’m walking. So in between actual locations and venues; it doesn’t give you the option to say that.
Male, Quit Sense arm, ID116
How I personally see how it could be slightly improved for future use, would be the ability to tell the app that you are driving, or for the app to recognise that. [ … ] the roads where I would smoke the most [ … ] trigger roads.
Male, Quit Sense arm, ID216
Related to the desire to record smoking while being out and about was the suggestion that the app should have a ‘quick report’ button or allow users to add details later after the smoking event:
When you’re travelling around, the process of logging that you’ve had a cigarette can be quite a long process. Which isn’t a problem at all, if you have a job working behind a desk or if you physically stop what you’re doing to go and have a cigarette break, you’ve got time to log it and go through your phone etc, etc.
Male, Quit Sense arm, ID216
Yeah, so when there’s like a questionnaire at the end of the day that say’s how many cigarettes you’d had that day, you could put it in there but you couldn’t log the time of day and what the triggers were. So, you have to sort of like put a ‘I smoked 10 in that day’ but if you didn’t log it at the time, you couldn’t go through the options of what triggered that craving etc.
Female, Quit Sense arm, ID111
One participant liked the idea of being able to make notes in the app, for example, how she was feeling on quit date that she could look back on as an additional aid to monitoring progress:
Yeah, I mean, maybe just have a section that would explain more of how we felt that day. I think sometimes just getting it off your chest. I know I ticked a box, ‘did you find it easy’, maybe you could write or type something, as it’s on your phone [ … ] you could look back on it and see how you’ve improved.
Female, Quit Sense arm, ID210
Several participants thought that the app could further boost motivation by having a reward system such as points for not smoking or badges:
Every time you read a message maybe it could get something like a star or a tick or whatever. [ … ] Just to get people a bit more engaged with the app. With a points system, maybe they could have like free books to read or whatever.
Female, Quit Sense arm, ID215
Yeah, it just gives you a little more of a boost that you’re doing something good. I might not notice it, but it is doing something. You also get little, you know, badges – ‘Yay, one week smoke free!’ that you can then share and things like that, which then gives you a sense of achievement; especially if you’ve told family and friends. It’s things you can share and then go – look how well I’m doing. Which I think we all … it’s really tough to quit smoking so I think that little pat on the back is really nice [laughs].
Female, Quit Sense arm, ID109
One participant who found that messages about smoking occasionally prompted him to think about smoking suggested that some could be reworded to congratulate users on not smoking in locations where they previously had reported:
I think, and again, I don’t know how possible this even is, but I always found it interesting to think back about ‘how many cigarettes have I not smoked?’ So, if for example, the app knew that you were driving and then said afterwards that I would usually have five cigarettes on that journey, it could be that when you were driving it would say, ‘well done, that’s five cigarettes you didn’t have.’ So, it’s still recognising that it is somewhere where you usually would have smoked but instead of reminding you and then having a cigarette, it would be like, ‘oh great, a little milestone or achievement that I haven’t had any cigarettes on that journey.
Male, Quit Sense arm, ID216
Some participants felt that when they were in the same location for prolonged periods of time, such as due to COVID-19 lockdown restrictions, messages could be better tailored by arriving at the times that they reported smoking, so based less on location but more on reported times of smoking (e.g. after meals):
I was working from home, so I was pretty much sat in the same place all of the time [ … ] either I was at the dining room table, or I was on the sofa. They were the only two places.
Female, Quit Sense arm, ID114
They just sort of came at times that I felt was a bit random. I didn’t feel that they came at specific times, like tea breaks and stuff because that’s the key periods really when you’re more likely to go and have a smoke.
Male, Quit Sense arm, ID115
I liked the idea of the application training itself to my pattern and what I’m doing. But then I expected at the time that the application would identify the time that I was more likely to be longing for a cigarette and send me a message. So, I’m not sure if that is in the app or not but I would find that helpful.
Female, Quit Sense arm, ID208
‘Pathways to change’
Three main mechanisms by which the app supported behaviour change were identified. A further mechanism (‘disrupting’) which was associated more with contextual factors is presented under ‘Time and Context’. Participant vignettes which expand on this theme can be found in Appendix 6, Table 35.
Committing, reporting and preparing for quit day
The preparation phase (stage 1) of the app was valued as an opportunity to make a formal commitment to quitting, including setting a fixed goal by establishing a quit date. This stage was also seen as something novel and not offered by other quit smoking apps:
All the other smoking apps don’t do that. On those ones, you’ve quit now. So, that was a nice one to be like, right, this is the day I’m going to quit, and it was that countdown [ … ] it gave you that focus.
Female, Quit Sense arm, ID109
It made me make a commitment [ … ]. It was something a bit more concrete and an actual set date and it really did keep me on track.
Female, Quit Sense arm, ID315
Some participants attributed a reduction in their cigarette intake during stage 1 to the act of reporting smoking via the app. One reason provided was that logging smoking required effort and, in some cases, it appeared easier to forgo the cigarette than to answer the questions about the smoking event. An alternative explanation of this point could be that participants felt more accountable for their smoking behaviour when asked to report it, so became more aware of it, and that in itself prompted behaviour change. Participants also said that repeatedly reporting smoking made smoking a conscious decision again, which in turn prompted them to question whether they really wanted a cigarette and raised their self-awareness about triggers:
When I did need to go for a cigarette, I would do it without thinking about it. Using that app made me think about it a lot. Do I really need one? Should I really go for one right now? Using that app, it just helped us to cut down after the first four days to be fair. Now, the first four days, they were a bit stressful in my life, but the app did help. Every time going for one, I had to write it up. [ … ] The training was very good. Every time I was writing in one of those little boxes, when I was going for a smoke. The app would come up and be like, ‘Do you really need to do this right now?’ Little subliminal messages in my head just stuck there. Every time I was pulling one out of the box, it just kept going over in my head – ‘Do you really need to have one right now?’ [ … ] Every time I would have to go for one, I’d have to report it. So, in the end I was just thinking, I’m not going to go for one because I’d have to report it. That would then take my mind of wanting one to be fair.
Male, Quit Sense arm, ID206
So, I found on the lead up to my quit date, it firstly made me realise how many I smoked. [ … ] When you’re on the app and you see how many cigarettes you’ve had. Moreover, I would have a cigarette and then 10 minutes later, I would have another one. Why? Why did I have that cigarette that I didn’t need anyway and then half an hour later, I’ll have another one? I found it useful, looking at that to see my own reasons for validating my own smoking. There was no hiding behind the ‘Oh, well, I’ve only had a few cigarettes’ [ … ] it’s in black and white and actually, no I haven’t.
Male, Quit Sense arm, ID216
I very much attribute my quitting to the app because it helped me subtract the patterns of me smoking. It allowed me to see when I was smoking more, and it then allowed me to unpick why I was smoking more. That was the key to the lock for me. It was helping me see that was why I was smoking more, when the only solution I could draw was because I felt I should.
Male, Quit Sense arm, ID311
One participant discussed how the combination of reporting and looking at the progress calendar in ‘My Profile’ helped to motivate her to reduce her cigarette intake:
To be honest, I really thought I was going to fail at quitting, but it actually works. [ … ] I think it was that because if you smoked more than five in a day, you got a little sad face as well. So, I noticed as I was getting towards the quit date, I was actually thinking, do I need to really have this smoke or not? I was cutting down before which I think helps for when I did quit. I hadn’t been smoking as much just before then.
Female, Quit Sense arm, ID114
Interestingly, another participant used the report smoking feature to understand and then to avoid locations, which for him triggered cravings to smoke, like a personal diary:
It didn’t last very long because I set a very short quitting date, but I like the idea of reporting where and when. I did that for a couple of days and then I purposely went to different routes; when I was on [a] walk and that in the mornings, I would go on different routes to avoid that place. It became a routine that I would light a cigarette at that place, so I went a different way.
Male, Quit Sense arm, ID211
‘Validating’: the role of app messages
App messages were largely deemed to be encouraging, supportive and motivational, offering validation of efforts to quit and helping to re-enforce the goal of quitting:
What they do is they sort of they just bolster your defences a little bit. The odd fact here, the odd figure there. [ … ] a lot of the messages are very good. They give that validation that you’re looking for. They give that little reason to smile about why you’re doing it.
Male, Quit Sense arm, ID216
Yeah, they were very motivational, without being lecturing, if you know what I mean? They were just on the right level, for me I felt.
Female, Quit Sense arm, ID210
Having given up smoking, it kind of suggested that you’d be very proud. You’d be able to say, ‘I’ve given up’. [ … ] I found that very useful. [ … ] I think they’re very, very good. Very supportive.
Male, Quit Sense arm, ID211
I guess the main effect for me is in terms of it inspiring me; the tips that you would … you know, every time I recorded that I’d had a cigarette, it would say something. Some of those ideas were new [ … ] that encouragement was needed and definitely had an effect.
Male, Quit Sense arm, ID116
Some participants described the app as a ‘positive voice’ and one which some participants felt able to internalise:
Yes, it’s definitely like a positive voice in your head; that’s how I view the app. Having the encouragement coming from inside you. Sometimes you are experiencing a negative mood, so I would just be like, ignore it and let me have my cigarette. Having the [app] is like another positive sound in your head. It’s something that’s constantly encouraging you.
Female, Quit Sense arm, ID208
All in all, the app is really great. Little messages really, really help. Especially when you know in the back of your head that you don’t need to go for one just because you’re bored. With the little messages there, for extra support, just telling you what your mind knows, that you don’t need to go for one. An app knows that you don’t need to go for one, so why are you going for one?
Male, Quit Sense arm, ID206
While the information presented in the messages was not necessarily new to participants, they reported finding the content provided a helpful reminder:
I think it was mostly stuff that I already knew but it’s always helpful to be reminded and was more like of a prompt to think about stuff that I already knew. If that makes sense, because sometimes there’s stuff that you know about but you kind of need to be reminded of it.
Female, Quit Sense arm, ID106
‘Equipping’
When asked, several participants recalled strategies suggested by the app that they had attempted including breathing exercises, delaying smoking for a few minutes, removing visual cues to smoke and using distraction techniques:
I think the ones that were just sort of saying, you know, 'make sure you’ve cleared everything away beforehand.' So, I would make sure that the night before, I would have my last smoke and then make sure that the tobacco I had then went away, out of sight. Got rid of the ashtrays [ … ] Like I said, in the first week, I found it the most useful. I think I’d gone and brought a pack of Mint Imperials, so if I’m sat bored in a meeting, at least I can have one of those, to keep my hands and mouth busy for a few minutes.
Female, Quit Sense arm, ID114
It was about changing up something about when you had a trigger.
Female, Quit Sense arm, ID210
I did the exercise ones, and I spent a lot more time indoors with my daughter. That was the strategy that said, 'be indoors with people who don’t smoke.'
Female, Quit Sense arm, ID315
I used the ones that said, try and preoccupy yourself with something else. I found that if I happened to catch it at that point when I was having a craving then I did tend to find it helpful.
Male, Quit Sense arm, ID115
‘Time and context’
COVID-19
As interviews were collected during a national COVID-19 lockdown in England, participants were asked how their smoking habits had been impacted by the pandemic, if at all.
Several participants felt that due to lockdown they had more free time, especially at home and they attributed these circumstances to an increase in boredom and consequently of increased smoking:
If anything, I’m smoking more because I’ve got more time on my hands.
Male, Quit Sense arm, ID308
I think it’s probably true that I actually smoke more now because I’m indoors, at home, in a situation that’s quite comfortable, to be quite truthful. In that, I’m at home and doing my own thing, so I can do as I please. So, I probably smoke more than if I were going to work or going out and about, I would probably reduce intake. If I was in public or in a building where smoking wasn’t allowed etc., then I’d be smoking less.
Male, Quit Sense arm, ID116
I think it kind of confirmed. I knew as soon as I was working from home that I was getting bored. So, I’d be sitting there working, particularly in a meeting. When you’re sat in a meeting for 2 hours; there’s nothing much else you can do, so I’d think, oh, I’ll just have a smoke [laughs].
Female, Quit Sense arm, ID114
Well, unfortunately, we started smoking more at the beginning of it because of nothing to do; sheer boredom.
Female, Quit Sense arm, ID315
Other participants had found lockdown had helped to reduce their smoking and had been useful in making a quit attempt. One reason for this was the lack of social contact including with other smokers and not going to locations where they would ordinarily have smoked:
The only time I will have been out is when we’ve been shopping, as of recently. Or we’ll have been food shopping, or I’ll have gone to work when I’ve needed to. But apart from that, I haven’t really seen anyone who would influence me on my smoking. If that makes sense?
Male, Quit Sense arm, ID206
To be fair, it probably helped in a strange way, just because of the lack of socialising, which was kind of my key issue. My days in the office are a lot lower, so again, the smoke breaks, things like that; the temptations go. It has made it easier with, I’m not going say, for dinner or for a drink and things like that. Where normally my previous attempts have ended, let’s say.
Male, usual care arm, ID116
Some participants had come under increasing financial pressures due to the pandemic which motivated a reduction in smoking:
I don’t think [Covid] had a massive impact, apart from being a slight motivator. Mostly, to be honest, my motivation to stop smoking was financial. I think I, like a lot of people, got made redundant towards the start of the pandemic. Again, financially, it was that I can’t continue to spend money that I don’t have on smoking. And you know, the knowledge too that if I continue to smoke, it improves my chances of bad health if I were to get Covid. I also think the fact that I’ve not been around other people that smoke.
Female, Quit Sense arm, ID106
There were additional references to health as a motivator, including the possibility of quitting providing improved resilience to COVID. These were from a participant who was still smoking at the time of interview. However, for this participant concerns about smoking and COVID had disappeared over time and were no longer a primary motivator.
It’s been scary at times hasn’t it. I mean, really, when it first came out a year ago, it made me think that I need to stop smoking because if I catch this, I’m going to have a better chance of getting over it if I don’t smoke. To now, when we’re all just so depressed aren’t we? How long is this going to go on, you know [laughs]? I think alcohol and cigarettes … everyone’s behaviour. You’re not going to the gym, you’re not …
Female Quit Sense arm, ID210
For some other participants who continued to work as usual throughout lockdown, there were no significant changes reported:
Exactly the same. Yeah, there’s no difference in lockdown for me. I’m still working. It’s the same.
Female, Quit Sense arm, ID208
Feasting and fasting
Recruitment for the study took a break between mid-December 2020 and early January 2021 to avoid the main festivities of Christmas and New Year. Nonetheless, a small number of participants commented that their attempts at quitting had been negatively impacted by Christmas and New Year festivities:
Christmas and New Year’s intruded within the time period that I’d set myself. I think I’d started using the app around the beginning of November, possibly late December. It came to Christmas and New Year and I found myself smoking and reaching for cigarettes even more rapidly than I had been, certainly more so than the early stages of me using the app. The app had a direct effect on reducing the number of cigarettes at first, probably for the first two or three weeks, so not really so long. Then towards Christmas, it would be too easy to pick up … and when I realised that I’d failed; I’d crossed the line, and I hadn’t given up. It just picked up again.
Male, Quit Sense arm, ID120
Some interviews coincided with preparations for Ramadan and Ramadan itself, with Muslim participants discussing how this was a positive factor – helping to motivate and enforce their quit attempt:
Something good that is going to happen – I’m Muslim, so Ramadan is going to begin in less than two weeks. When you’re fasting, you cannot eat, drink or smoke as well. So, I always look forward to Ramadan because it does help with stopping smoking. Because you’re fasting, here in the UK, you’ll be fasting for more like 10 hours a day.
Female, Quit Sense arm, ID208
To be fair, yeah, it’s been okay. It’s kind of gave me a bit of a push to cut down a lot more. I don’t know if you’re aware but it’s Ramadan at the minute as well and I’m fasting. So, I’m hoping that by the end of the 30 days I will have eliminated it altogether, but we’ll just have to wait and see.
Male, Usual care arm, ID209
Economic evaluation
From the reported levels of smoking cessation aid resource use over the 6-month follow-up period (Table 12) we can see that these are generally at relatively low levels, and that e-cigarettes/vaporisers (which are not routinely funded by the NHS) seem to be the most frequently used. This coupled with the finding that 80% of NRT users reported paying for NRT themselves (see Table 12) demonstrates that the recommended NHS and Personal Social Services (PSS) cost perspective (National Institute for Health and Care Excellence, 2013) would miss much of the costs associated with smoking cessation aids. The importance of taking a more societal cost perspective can also be seen when the levels of resource use are combined with estimated unit costs (Table 13) to give total costs (in Table 14 it can be seen that e-cigarettes/vaporisers and NRT account for more than 70% of the total cost when excluding intervention costs).
Resource use, mean value (SD) (n) | App (n = 104) | Standard care (n = 105) | Overall (n = 209) |
---|---|---|---|
NHS stop smoking support, mean number of sessions | 0.46 (1.36) (n = 74) | 0.70 (1.87) (n = 73) | 0.58 (1.63) (n = 147) |
NRT, mean number of weeks | 0.96 (3.38) (n = 74) | 1.74 (3.09) (n = 73) | 1.35 (3.25) (n = 147) |
Number who reported they paid for NRT | 10 (n = 14) (1 missing) | 23 (n = 27) | 33 (n = 41) (1 missing) |
Varenicline/Champix, mean number of weeks | 0.18 (0.91) (n = 74) | 0.30 (1.62) (n = 73) | 0.24 (1.31) (n = 147) |
Bupropion/Zyban, mean number of weeks | 0.14 (0.83) (n = 74) | 0.00 (0.00) (n = 73) | 0.07 (0.59) (n = 147) |
E-cigarettes/vaporisers, mean number of weeks | 3.77 (7.27) (n = 74) | 3.26 (5.00) (n = 73) | 3.52 (6.23) (n = 147) |
Telephone quitline, mean number of calls | 1.09 (5.65) (n = 74) | 0.10 (0.48) (n = 73) | 0.90 (4.69) (n = 147) |
GP, mean number of visits (for any reason) | 1.00 (1.66) (n = 74) | 1.06 (2.18) (n = 72) | 1.03 (1.93) (n = 147) |
Resource (source) | Unit cost (£) |
---|---|
NHS stop smoking support session | 7.33a |
NRT (e.g. nicotine gum), cost per week | 22.22 |
Varenicline/Champix, cost per week | 21.38 |
Bupropion/Zyban, cost per week | 19.34 |
E-cigarettes/vaporisers, cost per week | 7.32b |
Telephone quitline, cost per call | 7.33 |
GP visit | 34.45c |
Cost component, mean (SD) | App (n = 104) |
Standard care (n = 105) |
Overall (n = 209) |
---|---|---|---|
Advertising/recruitment costs | £14.09 (£0.00) (n = 104) | £14.09 (£0.00) (n = 105) | £14.09 (£0.00) (n = 209) |
Maintaining the app | £14.42(£0.00) (n = 104) | £0.00 (£0.00) (n = 105) | N/A |
Total cost of the intervention | £28.51 (£0.00) (n = 104) | N/A | N/A |
NHS stop smoking support sessions | £3.37 (£9.94) (n = 74) | £5.12 (£13.69) (n = 73) | £4.24 (£11.94) (n = 147) |
NRT | £21.32 (£75.14) (n = 74) | £38.66 (£68.69) (n = 73) | £28.71 (£69.74) (n = 147) |
Varenicline/Champix | £3.76 (£19.50) (n = 74) | £6.44 (£34.67) (n = 73) | £5.09 (£28.01) (n = 147) |
Bupropion/Zyban | £2.61 (£16.11) (n = 74) | £0.00 (£0.00) (n = 73) | £1.32 (£11.46) (n = 147) |
E-cigarettes/vaporisers | £107.69 (£264.36) (n = 74) | £81.19 (£120.63) (n = 73) | £94.53 (£205.66) (n = 147) |
Telephone quitline | £1.09 (£5.65) (n = 74) | £0.70 (£3.49) (n = 73) | £0.90 (£4.69) (n = 147) |
GP visits | £34.45 (£57.02) (n = 74) | £36.37 (£75.14) (n = 72) | £35.40 (£66.35) (n = 147) |
Total non-intervention cost (societal) | £174.28 (£282.03) (n = 74) | £167.55 (£185.21) (n = 73) | £170.96 (£238.44) (n = 147) |
Total cost (societal) | £202.80 (£282.03) (n = 74) | £167.55 (£185.21) (n = 73) | £185.14 (£239.07) (n = 147) |
In terms of the cost of the intervention, above it was reported that the mean advertising cost per participant recruited was estimated to be £14.09 (across all participants). We assumed that this was our best estimate of what the recruitment costs would be if the intervention were to be provided again. Additionally, it was assumed that the cost of maintaining the Quit Sense app would be £1500 per annum. When apportioned across the 104 participants in the intervention arm, this equates to £14.42 per participant, and this was assumed to be our best estimate of what the Quit Sense app maintenance costs would be if the intervention were to be provided again. Together this gave a total intervention cost (for future provision) of £28.51 per participant. When added to the total non-intervention cost (for those with data on this variable), the mean per participant total cost can be seen to be approximately £35 higher in the intervention group (without adjustment for any differences there might be between groups) (see Table 14).
The completion rates for the QoL measure (EQ-5D-5L) at the baseline, 6-week and 6-month follow-up points were 100% (208/209), 71% (148/209) and 70% (147/209), respectively. Also, in both groups, compared to the baseline EQ-5D-5L score (see Table 2), the mean follow-up scores for both those who completed the EQ-5D-5L at baseline and 6 weeks, and at baseline and 6 months, were lower than that at baseline (see Appendix 6, Tables 19 and 20). The total QALY scores are also reported in Appendix 6, Table 20; however, as the intervention group had a higher mean EQ-5D-5L score at baseline these need to be treated with caution (adjustment for this is made in the below regression analysis).
Acknowledging that the results need to be treated with caution, for example due to the small sample size, regression analysis was conducted in order to estimate the mean difference in cost between treatment groups (incremental cost of the intervention) and mean difference in effect between treatment groups (incremental QALY gain associated with the intervention). Based on the total costs, which included the estimated total intervention cost (for future provision), the results show that the incremental cost of the intervention was estimated to be £6.68, with an incremental QALY gain of −0.006 (Table 15). However, it should be noted that there was no significant difference in cost or effect on QoL between the two groups and that such differences could therefore have arisen due to chance. Nonetheless, with these mean estimates, as the intervention is estimated to be both more costly and less effective it is referred to as dominated by standard care, and the intervention would not be estimated to be cost-effective. In keeping with this, the cost-effectiveness acceptability curve (CEAC) estimated that there was a 32.4% probability that the intervention was cost-effective at a threshold of £20,000 per QALY (a cost/QALY below £20,000/QALY has been deemed to be cost-effective). 71
Cost perspective (Nc,Ni) | Incremental cost (95% CI) | Incremental effect (95% CI) | Net benefit (£) | CEACa (%) |
---|---|---|---|---|
Base case (64,64) | £6.68 (£72.58 to −£59.23) | −0.006 (0.017 to −0.029) | −128.58 | 32.4 |
Sensitivity analysis (64,64) | −£21.84 (£44.07 to −£87.74) | −0.006 (0.017 to −0.029) | −100.63 | 38.1 |
Finally, a sensitivity analysis was conducted using different assumptions about the total intervention cost for future provision. This was not specified in the health economics analysis plan but was justified on the basis that we sought to assess whether the above results might change when more favourable assumptions about the intervention cost were used. We assumed that, in terms of recruitment costs, the difference in cost between the intervention and standard care would be zero as in this trial standard care also had the same estimated recruitment costs. Additionally, the cost of maintaining the app was assumed to be negligible as this might be an appropriate assumption if the app were adopted at scale by a large number of people. The results of this sensitivity analysis (see Table 15) show that when the intervention is assumed to have a zero cost, the incremental cost is estimated to be −£21.84 (the estimated incremental QALY gain was unchanged). It has been argued that the incremental cost-effectiveness ratio values can be misleading with negative cost/effect estimates. 74 Accordingly, we estimated the net benefit74 at the threshold value of £20,000 per QALY. In this sensitivity analysis, the net benefit was estimated to be negative (see Table 15), which denotes that the intervention is again not estimated to be cost-effective at the £20,000/QALY threshold. Additionally, the intervention was estimated to have a 38.1% probability of being cost-effective according to the CEAC (see Table 15).
When estimating the treatment effect, estimates have been adjusted for baseline EQ-5D-5L score (QALYs only), age, gender, baseline smoking rate, baseline SES and baseline heaviness index; 95% CI = 95% CI; Nc (Ni) = number of intervention (app) and standard care (control) participants who were included in the analysis; a, estimated probability of the intervention being cost-effective on the CEAC at the threshold (λ) of £20,000 per QALY.
SWAT assessing effect of incentives for increasing follow-up
Baseline characteristics for SWAT groups can be found in Appendix 6, Table 36. At 6-month follow-up, there was no evidence of a difference in overall response rate among participants offered a £20 voucher incentive compared with a £10 incentive (79% vs. 74%, unadjusted OR = 1.35, 95% CI 0.71 to 2.60; p = 0.36; fully adjusted OR = 1.29, 95% CI 0.66 to 2.54; p = 0.46) (Table 16). However, the likelihood of requiring manual follow-up differed between incentive groups; 46% of those in the £20 incentive group required manual follow-up (by text, phone or e-mail) compared with 62% of the £10 incentive group, representing a 49% reduction for the £20 incentive (unadjusted OR = 0.51, 95% CI 0.29 to 0.89; p = 0.018; adjusted OR = 0.53, 95% CI 0.29 to 0.95; p = 0.032).
Modela N = 204 |
£20 incentive n (%) N = 101 |
£10 incentive n (%) N = 103 |
ORb | 95% Wald CIc | p-valued |
---|---|---|---|---|---|
Overall response rate | |||||
Incentive group only (unadjusted) | 80 (79.2) | 76 (73.8) | 1.35 | (0.71 to 2.60) | 0.362 |
Adjusted model, including incentive group and stratification variables | 80 (79.2) | 76 (73.8) | 1.38 | (0.71 to 2.69) | 0.337 |
Adjusted model, including incentive group, stratification and prognostic variables | 80 (79.2) | 76 (73.8) | 1.29 | (0.66 to 2.54) | 0.462 |
Rate of manual follow-up | |||||
Incentive group only (unadjusted) | 46 (45.5) | 64 (62.1) | 0.51 | (0.29 to 0.89) | 0.018 |
Adjusted model, including incentive group and stratification variables | 46 (45.5) | 64 (62.1) | 0.53 | (0.30 to 0.94) | 0.030 |
Adjusted model, including incentive group, stratification and prognostic variables | 46 (45.5) | 64 (62.1) | 0.53 | (0.29 to 0.95) | 0.032 |
The log-rank test, which compares expected and observed questionnaire completions over time by incentive group, shows moderate evidence that there was a difference in survival probability between incentive groups, with the £20 incentive group showing on average a lower completion time (median: 7.0 days, IQR 0.08, 18.13) than the £10 incentive group (median: 14.9 days, IQR 0.15, 24.55), which was statistically significant (unadjusted p = 0.008) (Table 17). This is confirmed by a Cox proportional hazards analysis showing that at any particular point in time, the £20 incentive group were 55% more likely (1.55 times) to respond to the 6-month questionnaire, compared to those participants in the £10 incentive group (unadjusted HR = 1.55, 95% CI 1.12, 2.15, p = 0.008). The failure plot (Figure 3) suggested that failure probability increased over time, with a higher probability of completing the 6-month questionnaire when close to 0 day. Adjusting for stratification and prognostic variables for the above analyses did not alter the findings meaningfully.
Modela | £20 incentive group N = 101 |
£10 incentive group N = 103 |
Hazard ratiob | 95% CIc | p-valued |
---|---|---|---|---|---|
Incentive group only | 7.00 (0.08, 18.13)e | 14.85 (0.15, 24.55)e | 1.55 | (1.12, 2.15) | 0.008 |
Adjusted model, including incentive group and stratification variables | 7.00 (0.08, 18.13)e | 14.85 (0.15, 24.55)e | 1.53 | (1.10, 2.12) | 0.012 |
Adjusted model, including incentive group, stratification and prognostic variables | 7.00 (0.08, 18.13)e | 14.85 (0.15, 24.55)e | 1.53 | (1.10, 2.13) | 0.012 |
FIGURE 3.
Failure plot (estimate of 1 minus the survival function) for time taken to complete the 6-month follow-up questionnaire by incentive arm.
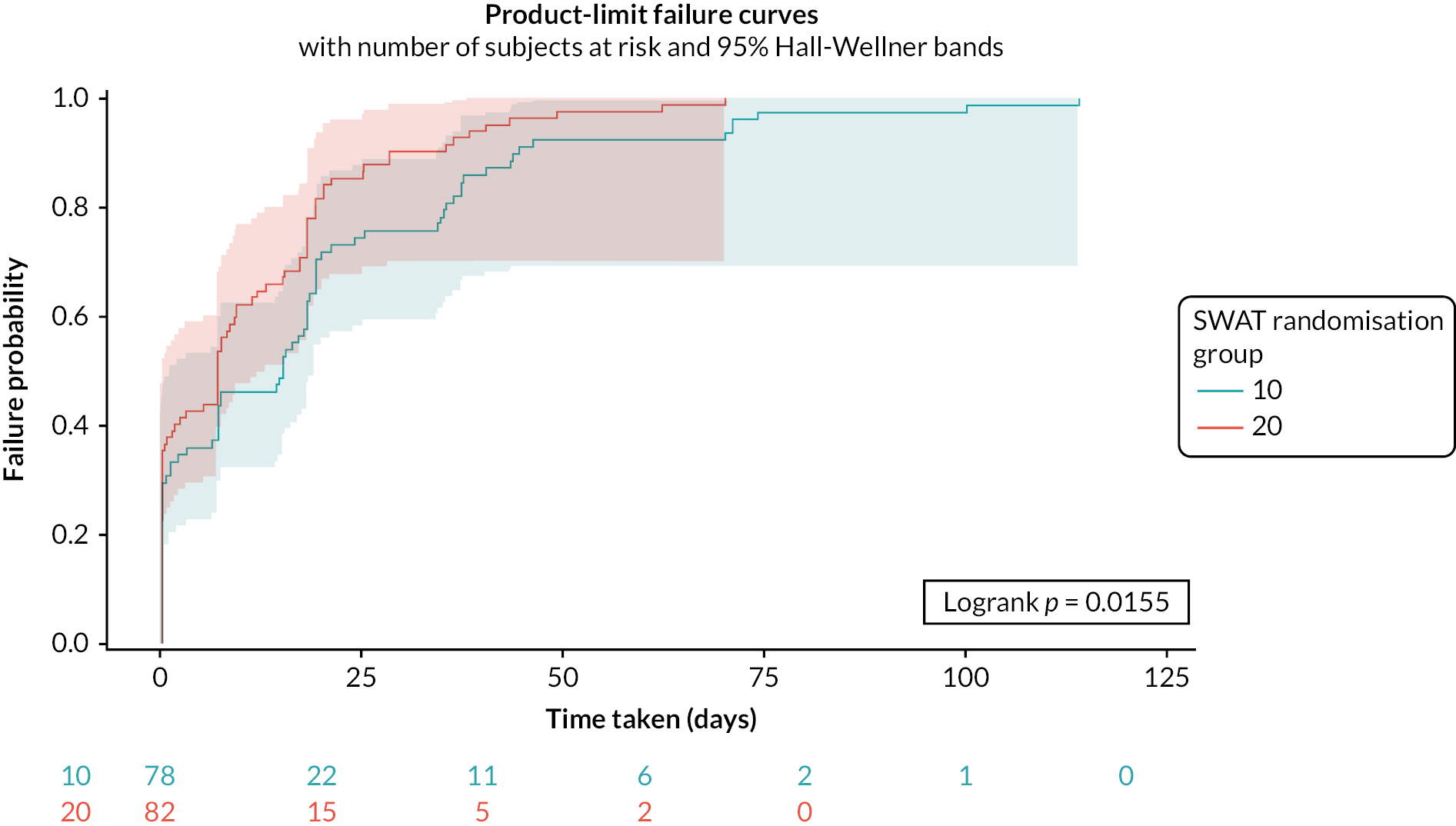
Response rates to questionnaire items other than the primary outcome were also higher in the £20 incentive group compared to the £10 group (see Appendix 6, Table 37). For example, 78% versus 68% of participants reported urge items data and 78% versus 66% completed EQ-5D-5L questions in the £20 and £10 incentive groups, respectively.
Chapter 4 Discussion
This is the first RCT undertaken which evaluated a JITAI smartphone app for smoking cessation that uses mobile sensing to trigger support. In addition to assessing the feasibility of key trial parameters and estimating intervention effects, a qualitative process evaluation was undertaken as well as a SWAT to investigate incentives for improving follow-up rates.
The innovative online trial design employed was feasible and enabled the sample size target to be reached within the planned period of recruitment and for a lower cost than originally planned (£19 vs. £50 per participant). Importantly, given that the sample was exclusively identified online via adverts, the follow-up rate estimate was close to anticipated levels and is towards the higher end of the rates achieved in other web-based cessation trials with comparable samples in terms of mean age, gender and education. 61 The SWAT findings suggested that higher incentives (i.e. £20 compared to £10) would reduce the proportion of people requiring manual follow-up, which is time-consuming and takes up study resources, and speed up the completion of follow-up. However, rates of response to the request to return a saliva sample were lower than anticipated. It is possible that this was affected by the COVID-19 pandemic, potentially due to more limited access to postal services due to changes in movement and time spent outside of the home or hesitation to provide a sample. Given the positive influence of a higher incentive on follow-up identified by the SWAT, it is likely also though the relatively low incentive of £5 for a returned sample may have also been a factor. This may have also contributed to the imbalance in return rates between arms.
The trial also demonstrated that three-quarters of smokers assigned to the Quit Sense app will install it on their phones and engage with it at least to the point of setting a quit date. There have been very few studies reporting the rates of uptake for cessation apps and those that do have either offered incentives for installation63 or required participants to install an app for study inclusion. 75 Rates of engagement in the app were slightly lower than anticipated, with around half of those who installed it continuing to use the app for 1 week or more. Compared to the largest cessation app evaluation undertaken to date, for the acceptance and commitment therapy-based app ‘iCanQuit’,27 Quit Sense participants had a higher median number of days use (10 days; server recorded, vs. 6 days; self-report). 27 Few other app evaluations provide engagement duration data although these can vary considerably across apps and countries, as demonstrated by a cessation app evaluation in Japan showing that after 6 months 88% of the intervention arm were continuing to engage with the ‘CureApp Smoking Cessation’ system. 75
The trial findings provided moderate evidence that Quit Sense increased verified cessation at 6-month follow-up compared to usual care, having found a larger effect size than originally anticipated, though estimated with considerable imprecision in line with a feasibility trial. Self-reported outcomes showed smaller non-significant effects favouring Quit Sense. No effects of Quit Sense were observed at 6-week follow-up, suggesting that any benefit from Quit Sense, relative to usual care, was more likely due to maintaining abstinence in the longer term among those attempting to quit rather than from increasing the proportion of participants initiating a quit attempt. However, there was no quantitative evidence that Quit Sense affected hypothesised mechanisms of action at 6-week follow-up relative to usual care, including use of lapse prevention strategies, urge strength and frequency, self-efficacy or the extent to which smoking is automatic and associated with environmental cues, although these analyses may have been underpowered. Smoking rates early on in the study, potentially reflecting lapse rates among those attempting to quit, were lower among Quit Sense participants, which could indicate one potential pathway towards cessation, although this difference was not statistically significant. The qualitative process evaluation, however, provided greater depth into the potential pathways to abstinence from using the app. One unexpected pathway, although supported from a Quit Sense pilot study,10 was the participants’ description of benefiting in particular from the training phase of using the app (stage 1). They reported how this self-monitoring had helped them better understand and reflect on the drivers of their smoking behaviour and reinforce their commitment to quit. Self-monitoring is not a commonly employed BCT in smoking cessation interventions and few, if any, prompt the systematic logging of cues and triggers for smoking with feedback. Self-monitoring of experiences during a quit attempt has been found to reduce the intensity of cravings and other emotional triggers for smoking76 and may be an under-used approach to support cessation. Participants also indicated valuing the support delivered once they embarked on their quit attempt and that this ‘bolstered’ their defences against urges to smoke and helped keep them motivated and committed to quitting. Though other participants found they disengaged from the app because they did not feel they needed or wanted the support or because they relapsed back to smoking.
The health economics analyses indicated that the mean total costs for the Quit Sense arm were £35 higher than for usual care, though there were no differences in QoL at 6 months between arms. However, most benefits from stopping smoking will be realised beyond the 6-month follow-up time point77 and the cost-effectiveness analysis did not model long-term health outcomes, for example due to cessation differences between arms. As such, an economic model would be required in any future more definitive study. A further potential limitation of the health economic analysis is that an NHS/PSS cost perspective was not included as a further sensitivity analysis. That said, we did include a sensitivity analysis based on more favourable cost assumptions and in that the intervention was still not estimated to be cost-effective. Were an NHS/PSS cost perspective to be adopted then, based on results reported here (see Table 15), this would likely give the same result, as the estimated cost of the intervention is not estimated to be offset by any differences in NHS costs (e.g. NHS stop smoking services/GP visits). Finally, one cost that is challenging to estimate is the maintenance costs of the app. While during the trial these were estimated based on programmer time, if rolled out and Quit Sense was made available and used by far higher numbers of people, the annual maintenance costs would likely be much greater, though when spread across a larger number of people the per participant costs would likely be lower.
In the original grant proposal for this project four key feasibility criteria were outlined to help guide whether to proceed to a definitive trial of Quit Sense. As specified in Table 18, for each criterion, thresholds are considered to have been met if they are included in or are lower than the 95% CI around the estimate value (i.e. the upper 95% CI is higher than the threshold).
Progression criterion | Thresholda | Estimate (95% CI) |
Threshold met |
---|---|---|---|
Completion of self-reported abstinence for primary outcome | Upper 95% CI of estimate is ≥ 70% | 77% (71% to 82%) | Yes |
Return rate of saliva samples for those reporting abstinence, for primary outcome | Upper 95% CI of estimate is ≥ 70% | 39% (24% to 54%) | No |
Rate of Quit Sense app installation in intervention arm | Upper 95% CI of estimate is ≥ 70% | 75% (67% to 83%) | Yes |
Actively engaged in using Quit Sense app for more than 1 week (of those installing Quit Sense) | Upper 95% CI of estimate is ≥ 60% | 51% (39% to 63%) | Yes |
All but the saliva sample return rate progression criterion reached a priori thresholds. As already indicated, several factors were believed to have contributed to the low return rate of saliva samples, though the relatively low financial incentive was considered to be the primary factor. Other online cessation trials using procedures that this trial was informed by but with higher incentives for returning saliva samples experienced higher response rates. For example, the StopAdvisor trial59 provided a £20 incentive for returning a sample and obtained a 75% response rate (personal communication). Other trials of digital cessation interventions with hard-to-reach populations (pregnant smokers) have provided £30 incentives and attained a 70% return rate. 78 Therefore, it is expected that increasing the incentive in a future trial should substantially increase the response rate.
Duration of engagement with Quit Sense was slightly lower than anticipated, with half of those installing Quit Sense using the app for more than 1 week. Engagement with digital interventions is challenging to assess and device-derived data are considered limited for doing so, particularly as it fails to differentiate between effective and routine engagement and misses active engagement with the process of behaviour change outside of the intervention. 79 This was demonstrated in part in our qualitative findings. Some participants reported feeling that a key benefit from the app was gained from the initial training stage and some individuals actively avoided using the app once their quit attempt started in order to avoid smoking-related objects. Participants also reported stopping using the app when they relapsed, and relapse is most likely to occur in the first week or so of a quit attempt. Therefore, it is difficult to determine what an ideal engagement rate is given most participants of any cessation intervention will fail to quit and most of these will do so early on in their quit attempt. Having participants actively engage in the preparation of quitting and gain benefit from the app through effective engagement79 may be a more important and realistic goal. This was supported by evidence indicating that all those installing the app set a quit date, with the 10-day (median) period spent using the app reflecting engagement in the process of training the app and self-monitoring smoking behaviour.
Strengths and limitations
A key strength and novelty of this trial was that most procedures were fully automated, from recruitment to follow-up, and so the resources required to run the trial were relatively low. In terms of manual follow-up, for those receiving a £20 incentive for completing the 6-month questionnaire, only a minority required contacting by telephone, further lowering the required resources for this and any future definitive trial. The trial also tested a novel ‘auto-process evaluation’ feature where Quit Sense participants could orally describe their views of the app in a high ecologically valid context, further investigating novel approaches for efficient trial design. In terms of quality, the trial used gold standard assessments of smoking abstinence,52 applied robust randomisation and intervention delivery fidelity and published protocols (trial and SWAT) and made the statistical and health economic analysis plan publicly accessible prior to analysis on the open science framework, embracing the principles of open science. 80
Reinforcing the value of undertaking a feasibility trial, there were limitations identified which, if rectified, would improve the quality of a future definitive trial. A key limitation, as identified above, was the poor return rate of saliva samples and the potential bias created from these being higher in the Quit Sense arm compared to usual care. A further limitation was that the oldest participant in the trial was 61 years old and so our sample did not include those in older age groups. This likely reflects the online advertising approach adopted. Trials undertaken in the USA of digital cessation apps have found Facebook and Google adverts resulted in 11% and 4% of participants aged over 65, respectively,81 which, although still relatively low, was higher than found in this trial. Interestingly, the mean age of participants in the present trial aligns closely with trials of digital cessation interventions where smokers were recruited offline,61 and so may reflect a narrow age distribution of participants rather than one shifted towards younger age groups. However, users of cessation apps from app stores, more closely representing the ‘real world’, have a lower mean age than that found in online cessation trials, around 30 years [standard deviation (SD) 10],82 and those participating in app store-based cessation app trials also have a similar lower mean age. 83 Nevertheless, working on approaches to increase the age diversity of research participants would be valuable in future work.
A further limitation was that this trial was undertaken during an unprecedented time of changed habits and routines due to the COVID-19 pandemic. Participants reported reduced movement due to lockdown and similar measures to reduce movement outside of the home. It is likely this affected the exposure and time spent in different smoking locations and consequently the app’s ability to deliver context-specific support. It is not possible to say whether COVID-related changes made quitting easier or harder for trial participants, but evidence from a nationally representative survey indicates that on average smoking cessation activity increased during the 2020 lockdown, relative to the same time in 2018–9, though smoking prevalence in younger age groups (18–34) also increased. 84 The extent to which increased cessation activity continued into 2021 and beyond, however, is unclear.
Implications and future research
This feasibility trial supports progress to a definitive trial, with some minor amendments relating to trial procedures and minor intervention optimisation.
The project generated evidence that incentives were likely important for reducing trial workload related to follow-up, speeding up follow-up responses and potentially increasing the proportion of data completed in the final follow-up questionnaire. Although speculative, there is also an expectation that increased incentives for returning a saliva sample would increase response rates. These changes to incentives are warranted based on the findings and are also highly relevant for informing online automated trials in general.
The trial also found that Facebook advertising was much less expensive than Google advertising and that when Facebook adverts dominated in the second phase of recruitment, they led to higher rates of non-white ethnicity participants. Such findings are important beyond this project given the growing interest in online recruitment.
In terms of the intervention, some participants mentioned experiencing some technical issues during the qualitative interviews, although it was not possible to determine for how many across the whole Quit Sense arm. In addition, a small proportion of participants (9%) seemed to be able to use the app normally but without their data being uploaded to the server. Minor technical issues are common with smartphone apps, particularly those with relatively low development budgets compared to commercially funded apps. Further ‘bug fixing’ combined with extensive testing as well as updating the app to the latest Android operating system is required prior to a definitive trial to reduce the risk of technical issues affecting the user experience, potentially leading to disengagement. Ultimately an iOS (Apple iPhone) version will need to be developed should the app be ‘rolled out’, although given that there are few differences found between Android and iOS users of cessation apps,85 there is no strong rationale for doing this prior to a definitive trial.
The qualitative findings indicate a few areas where the app could be enhanced to improve usability, satisfaction and potential efficacy. These include increasing the options for reporting smoking in stage 1 of the app, such as increasing the smoking trigger and situation options, adding gamification elements such as rewards or ‘badges’, and improving the tailoring of the timing for support delivery when users dwell in the same location for long periods of time, such as at home. There were no suggestions or indications that the overall structure of the app or its user interface should be changed and, given the vast majority reported that the app was easy to use, only very minor modifications appear to be required.
Finding ways of enhancing engagement with apps remains a challenge in the field of digital health. While the engagement rates with Quit Sense were positive, they were slightly lower than those anticipated, and those rates found in the feasibility study. 10 Opportunities for enhancing engagement with Quit Sense, based on review and qualitative evidence,86,87 could include greater use of statistical progress information, coping games, community networking and social interaction, personification of the app and use of rewards (e.g. badges/trophies).
The efficacy estimate findings, combined with the qualitative findings, suggest that there is potential for using an app to help both the individual and a system to learn about smoking patterns and triggers to inform extrinsic (app) and intrinsic (individual) support during a quit attempt. Greater emphasis on the potential benefit of engagement with this pre-quit learning phase could help users gain greater insight into their own smoking behaviour to help them more successfully maintain abstinence long term. Other smoking cessation apps may also benefit from providing this feature. The moderate efficacy estimate evidence generated in this trial suggests that this uncommon approach for promoting cessation should be more widely investigated. Additionally, it would be useful to better understand the mechanisms with which Quit Sense might lead to increased abstinence relative to usual care.
Further research investigating the mechanism of action of Quit Sense would help improve our understanding of how apps might support people to quit smoking and could be used to optimise Quit Sense support. Furthermore, exploration into additional methods of passively identifying high-risk situations for smoking, such as through the use of Bluetooth to identify other individuals the app user smokes with (co-location) or geographic information system data, may help improve the accuracy of JITAIs like Quit Sense.
Patient and public involvement
A PPI panel contributed throughout all stages of the project. This included input at the pre-submission stage of the original grant application to advise on the project plan (four panel members who were smokers or ex-smokers), during the study to advise on design, procedures and intervention content and delivery (two panel members who were smokers/ex-smokers) and anticipated input for dissemination activities (two panel members who were smokers/ex-smokers). PPI input was achieved through dedicated PPI-based in-person and online meetings, from written feedback via e-mail, involvement in quarterly team meetings and through a PPI-based independent member of the TSC. During the project we attempted to increase the number of PPI members in the panel by advertising formally and approaching individuals informally though without success.
In addition to active participation in study meetings, to help with study preparation PPI members provided feedback on study design, the participant journey and promotion of study engagement, participant-facing materials and study information including the full and basic participant information sheet, the screening process, consent form and baseline measures, and they helped to choose the Quit Sense logo. PPI members also reviewed the intervention messages delivered by the Quit Sense app, reviewed wording of study procedural SMS messages and provided more general feedback on the Quit Sense app overall including aesthetics and functionality. Furthermore, PPI members participated in meetings specifically to discuss and help validate qualitative findings.
The input from the PPI panel has been impactful across numerous aspects of the study. One such aspect, made prior to the project starting, was the PPI recommendation that the design of the study should enable a reasonable estimate of the impact of the Quit Sense app. This led to a proposed sample size that was at the higher end of what would typically be recommended for a feasibility trial and added weight to the mid-study proposal to increase the sample size further due to lower than anticipated cost and speed of recruitment. This was felt to be important to increase the precision of the effect estimate of the app and for feasibility outcomes. A further example of impactful input related to the design and refinement of app content. This included PPI members reviewing and providing suggestions for intervention message content that needed to be updated to accommodate changes related to COVID such as new working from home messages and reviewing other messages to ensure that they were COVID appropriate and relevant. PPI members also provided highly valuable input by reading through and validating the summary of qualitative findings to help with interpretation relating to app refinement. Both PPI members felt the qualitative findings relating to the app resonated with their own experiences and highlighted the importance and novelty of the training stage (stage 1) of the app. Based on their own experiences, PPI members felt that participants’ suggestions for additional ways of creating positive feedback, such as the option for the user to indicate times when they had resisted smoking and adding gamification components such as badges and rewards, would be valuable additions to the app.
Patient and public involvement members have indicated that they are happy to continue involvement in project dissemination and in the proposed full trial application and subsequent project if funded. Their continued involvement in dissemination activities will likely include recommendations on dissemination channels, advising on dissemination content and headline findings and on approaches to communicate findings to the public via infographics and media-based press releases.
Equality, diversity and inclusion
As identified in Strengths and limitations, the diversity of age was limited, having excluded those in higher age groups. This is an area for improvement in a future trial.
While it was considered a strength that a target was set for the proportion of the sample who were from low SES backgrounds, the final sample fell short of the proposed target of 45%, with only 29% from low SES recruited. One factor influencing this was the decision to use a conservative definition of low SES – either in a semiroutine or routine and manual occupation, class 5 in the NSSEC, or having never worked or were long term unemployed. Including class 4 of the NSSEC may have been justifiable and would have resulted in a sample proportion of low SES very close to reaching the 45% target, although this would have diluted the low SES classification. While in the second recruitment period we attempted to increase the proportion of participants from low SES groups, and from non-white backgrounds, this did not appear to be effective for SES and coincided with increased advertising costs, though this was not formally evaluated. Improved targeting of low SES groups may help to increase the proportion from the lowest SES group. This might be achieved via a more systematic application of targeting criteria for the online adverts from the outset, consideration of approaching online forums or organisations well represented by key diverse groups or through more tailored adverts, supported by PPI input and co-design.
The diversity of the trial sample in terms of non-white ethnicity was also relatively low (9%) and was lower than the proportion in England and Wales (15%). 88 The proportion of non-white ethnicity participants recruited in the second recruitment period, including those missing, when this characteristic was purposively targeted for in the advertising filters, was higher (14%) than in the first period (5%). Improving ethnic diversity through advertising filters may, therefore, be feasible and effective, though this may still fall short of ensuring good representation from minority groups. For the qualitative process evaluation, we had a higher diversity of participants, including 25% of participants from non-white ethnicity and 50% classed as low SES.
Throughout the study and for all procedures, we sought input from our PPI panel, representing our target group, on wording and images used in participant-facing materials. This was considered to help identify potential issues with wording or images and help ensure that materials were accessible to a range of reading levels and backgrounds. While we attempted to identify further PPI members, particularly from diverse groups, we had no success. This will remain an objective for a future definitive trial.
Reflecting on the research team’s composition, there was a lack of diversity in team members’ characteristics, although there was even gender balance. There was, however, slightly higher ethnic diversity in the Trial Steering Group, who had oversight responsibility for the trial. Increasing the diversity of researchers and those involved in oversight as well as study samples remains an important goal for research projects. There was a mixture of career levels across the research team, although no one was below a PhD or equivalent level of experience, and earlier career researchers had significant leadership roles in the project.
Chapter 5 Conclusions (implications for health care, recommendations for research)
The automated trial design worked effectively and implemented procedures enabled trial delivery with satisfactory or above satisfactory rates in all key feasibility outcomes other than the saliva sample return rate. This included higher estimated outcome completion rates than expected, three out of four Quit Sense participants installing the app and half of these using it for longer than 1 week. Evidence generated regarding the benefit of increasing incentive payments from £10 to £20 for improving follow-up engagement would suggest that increased incentives would likely improve saliva sample return rates in future work. The trial also demonstrated the feasibility of recruiting exclusively via online advertising for a lower than anticipated cost, although further refinements in advert filtering or consideration of other platforms may be needed to help increase the diversity of the sample.
Based on the abstinence outcome, representing the anticipated primary outcome for a definitive trial, Quit Sense led to a higher rate of biochemically verified abstinence relative to usual care and all end-of-trial abstinence measures favoured the app and represented a clinically meaningful effect estimate, although return rates for saliva samples were imbalanced between arms. Nevertheless, this work adds to a growing body of evidence supporting the efficacy potential of smartphone apps for smoking cessation. How Quit Sense might bring about a change in smoking behaviour is still not well understood, although, for some, learning about their own smoking triggers through the app was felt to be key. This remains an important research question for future studies.
Progression to a definitive trial to assess the effectiveness of Quit Sense, with minor refinements to trial procedures and the app as discussed above, is warranted.
Additional information
Acknowledgements
The authors would like to express their heartfelt thanks to the following for their contribution, time and efforts in ensuring the success of this project:
-
The participants of the study and all those supporting their involvement.
-
The recruitment partner (Nativve) for their efficient, professional and effective management of recruitment advertising.
-
The Norwich Clinical Trials Unit for their management and support for trial delivery.
-
NHS Norfolk and Waveney Integrated Care Board.
-
Darren Leader Studio Ltd for designing the Quit Sense logo.
Author contributions
Felix Naughton (https://orcid.org/0000-0001-9790-2796) (Professor of Health Psychology) conceived the study project and obtained project funding, led on app design, led on protocol development and led on drafting the report.
Aimie Hope (https://orcid.org/0000-0002-1920-0876) (Senior Research Associate) led on protocol development, contributed to app design, led on data collection, led on the process evaluation and contributed to drafting the report.
Chloë Siegele-Brown (https://orcid.org/0000-0002-9229-3351) (Academic Clinical Fellow in Public Health) led on app development and contributed to drafting the report.
Kelly Grant (https://orcid.org/0000-0001-5319-8127) (CTU Trial Statistician) led on the statistical analysis and contributed to drafting the report.
Caitlin Notley (https://orcid.org/0000-0003-0876-3304) (Professor of Addiction Sciences) helped conceive the study project and obtain project funding, contributed to the qualitative process evaluation and contributed to drafting the report.
Antony Colles (https://orcid.org/0000-0002-2268-0903) (Senior Data Programmer) developed the study database and landing website and contributed to drafting the report.
Claire West (https://orcid.org/0000-0001-7649-9600) (Senior Data Programmer) developed the study database and landing website and contributed to drafting the report.
Cecilia Mascolo (https://orcid.org/0000-0001-9614-4380) (Professor of Mobile Systems) helped conceive the study project and obtain project funding, contributed to app design and contributed to drafting the report.
Tim Coleman (https://orcid.org/0000-0002-7303-4805) (Professor of Primary Care) helped conceive the study project and obtain project funding and contributed to drafting the report.
Garry Barton (https://orcid.org/0000-0001-9040-011X) (Professor of Health Economics) helped conceive the study project and obtain project funding, led on the health economics and contributed to drafting the report.
Lee Shepstone (Professor of Medical Statistics) led on the statistical analysis and contributed to drafting the report.
Toby Prevost (https://orcid.org/0000-0003-1723-0796) (Nightingale-Saunders Chair of Complex Clinical Trials and Statistics) helped conceive the study project and obtain project funding and contributed to drafting the report.
Stephen Sutton (https://orcid.org/0000-0003-1610-0404) (Professor of Behavioural Science) helped conceive the study project and obtain project funding and contributed to drafting the report.
David Crane (https://orcid.org/0000-0002-9755-0214) (Chief Executive Officer of Smoke Free) helped conceive the study project and obtain project funding and contributed to drafting the report.
Felix Greaves (https://orcid.org/0000-0001-9393-3122) (Clinical Senior Lecturer) helped conceive the study project and obtain project funding and contributed to drafting the report.
Juliet High (https://orcid.org/0000-0003-2555-2349) (Senior Trials Manager) contributed to regulatory and Clinical Trials Unit related content and contributed to drafting the report.
Disclosure of interests
Full disclosure of interests: Completed ICMJE forms for all authors, including all related interests, are available in the toolkit on the NIHR Journals Library report publication page at https://doi.org/10.3310/KQYT5412.
Primary conflicts of interest: David Crane is the Chief Executive Officer of Smoke Free, a smoking cessation app unrelated to this project, and owner of 23 Ltd. Garry Barton is a member of an NIHR-funded Clinical Trials Unit and receives income via employment by UEA. Toby Prevost has been a member of an NIHR Public Health Research Funding Board and NIHR Covid-19 Reviewing Committee. Felix Greaves has been a member of an NIHR Public Health Research Funding Board, NIHR Public Health Research Prioritisation Group and NIHR Public Health Research Programme Advisory Board. Felix Greaves is also employed by NICE. None of the other authors have competing interests to declare.
Data-sharing statement
All requests for data should be submitted to the corresponding author for consideration. Access to anonymised data, excluding location data, may be granted following review.
Ethical approval
Ethical approval was received from the Wales REC7 NHS Research Ethics Committee (reference 19/WA/0361) on 28 January 2020.
Department of Health and Social Care disclaimer
This publication presents independent research commissioned by the National Institute for Health and Care Research (NIHR). The views and opinions expressed by the interviewees in this publication are those of the interviewees and do not necessarily reflect those of the authors, those of the NHS, the NIHR, MRC, NIHR Coordinating Centre, the PHR programme or the Department of Health and Social Care.
This monograph was published based on current knowledge at the time and date of publication. NIHR is committed to being inclusive and will continually monitor best practice and guidance in relation to terminology and language to ensure that we remain relevant to our stakeholders.
Disclaimers
This manuscript presents independent research funded by the National Institute for Health and Care Research (NIHR). The views and opinions expressed by authors in this publication are those of the authors and do not necessarily reflect those of the NHS, the NIHR, the PHR programme or the Department of Health and Social Care. If there are verbatim quotations included in this publication the views and opinions expressed by the interviewees are those of the interviewees and do not necessarily reflect those of the authors, those of the NHS, the NIHR, the PHR programme or the Department of Health and Social Care.
References
- World Health Organization . Report on Global Tobacco Epidemic 2011.
- GBD 2019 . Tobacco collaborators. spatial, temporal, and demographic patterns in prevalence of smoking tobacco use and attributable disease burden in 204 countries and territories, 1990–2019: a systematic analysis from the Global Burden of Disease Study 2019. Lancet 2021;397:2337-60. https://doi.org/10.1016/S0140-6736(21)01169-7.
- Murray CJ, Richards MA, Newton JN, Fenton KA, Anderson HR, Atkinson C, et al. UK health performance: findings of the global burden of disease study 2010. Lancet 2013;381:997-1020. https://doi.org/10.1016/S0140-6736(13)60355-4.
- NHS Digital . Statistics on Smoking – England 2018: Statistics on Smoking. Part 1: Smoking-Related Ill Health and Mortality 2018. https://digital.nhs.uk/data-and-information/publications/statistical/statistics-on-smoking/statistics-on-smoking-england-2018/part-1-smoking-related-ill-health-and-mortality (accessed 27 April 2020).
- West R, Brown J. Smoking Toolkit Study (STS) 2017. www.smokinginengland.info/ (accessed 1 July 2017).
- Kenford SL, Fiore MC, Jorenby DE, Smith SS, Wetter D, Baker TB. Predicting smoking cessation: who will quit with and without the nicotine patch. JAMA 1994;271:589-94. https://doi.org/10.1001/jama.1994.03510320029025.
- Deiches JF, Baker TB, Lanza S, Piper ME. Early lapses in a cessation attempt: lapse contexts, cessation success, and predictors of early lapse. Nicotine Tob Res 2013;15:1883-91. https://doi.org/10.1093/ntr/ntt074.
- Shadel WG, Martino SC, Setodji C, Cervone D, Witkiewitz K, Beckjord EB, et al. Lapse-induced surges in craving influence relapse in adult smokers: an experimental investigation. Health Psychol 2011;30:588-96. https://doi.org/10.1037/a0023445.
- Shiffman S, Paty JA, Gnys M, Kassel JA, Hickcox M. First lapses to smoking: within-subjects analysis of real-time reports. J Consult Clin Psychol 1996;64:366-79. https://doi.org/10.1037/0022-006X.64.2.366.
- Naughton F, Hopewell S, Lathia N, Schalbroeck R, Brown C, Mascolo C, et al. A context-sensing mobile phone app (Q Sense) for smoking cessation: a mixed-methods study. JMIR Mhealth Uhealth 2016;4. https://doi.org/10.2196/mhealth.5787.
- Conklin CA, McClernon FJ, Vella EJ, Joyce CJ, Salkeld RP, Parzynski CS, et al. Combined smoking cues enhance reactivity and predict immediate subsequent smoking. Nicotine Tob Res 2019;21:241-8. https://doi.org/doi:10.1093/ntr/nty009.
- Conklin CA, Perkins KA, Robin N, McClernon FJ, Salkeld RP. Bringing the real world into the laboratory: personal smoking and nonsmoking environments. Drug Alcohol Depend 2010;111:58-63. https://doi.org/doi:10.1016/j.drugalcdep.2010.03.017.
- Ahnallen CG, Tidey JW. Personalized smoking environment cue reactivity in smokers with schizophrenia and controls: a pilot study. Psychiatry Res 2011;188:286-8. https://doi.org/10.1016/j.psychres.2011.04.005.
- Ferguson SG, Shiffman S. Effect of high-dose nicotine patch on craving and negative affect leading up to lapse episodes. Psychopharmacology (Berl) 2014;231:2595-602. https://doi.org/10.1007/s00213-013-3429-6.
- Ferguson SG, Shiffman S. Effect of high-dose nicotine patch on the characteristics of lapse episodes. Health Psychol 2010;29:358-66. https://doi.org/10.1037/a0019367.
- Ferguson SG, Shiffman S. The relevance and treatment of cue-induced cravings in tobacco dependence. J Subst Abuse Treat 2009;36:235-43. https://doi.org/10.1016/j.jsat.2008.06.005.
- Versace F, Stevens EM, Robinson JD, Cui Y, Deweese MM, Engelmann JM, et al. Brain responses to cigarette-related and emotional images in smokers during smoking cessation: no effect of varenicline or bupropion on the late positive potential. Nicotine Tob Res 2019;21:234-40. https://doi.org/10.1093/ntr/ntx264.
- Balmford J, Borland R, Hammond D, Cummings KM. Adherence to and reasons for premature discontinuation from stop-smoking medications: data from the ITC Four-Country Survey. Nicotine Tob Res 2011;13:94-102. https://doi.org/10.1093/ntr/ntq215.
- Buss V, West R, Kock L, Kale D, Brown J. Smoking in England 2022. https://smokinginengland.info/ (accessed 23 November 2023).
- Brodbeck J, Bachmann MS, Znoj H. Distinct coping strategies differentially predict urge levels and lapses in a smoking cessation attempt. Addict Behav 2013;38:2224-9. https://doi.org/10.1016/j.addbeh.2013.02.001.
- O’Connell KA, Hosein VL, Schwartz JE. Thinking and/or doing as strategies for resisting smoking. Res Nurs Health 2006;29:533-42. https://doi.org/10.1002/nur.20151.
- Naughton F, McEwen A, Sutton S. Use and effectiveness of lapse prevention strategies among pregnant smokers. J Health Psychol 2015;20:1427-33. https://doi.org/10.1177/1359105313512878.
- Abroms LC, Lee WJ, Bontemps-Jones J, Ramani R, Mellerson J. A content analysis of popular smartphone apps for smoking cessation. Am J Prev Med 2013;45:732-6. https://doi.org/10.1016/j.amepre.2013.07.008.
- Thornton L, Quinn C, Birrell L, Guillaumier A, Shaw B, Forbes E, et al. Free smoking cessation mobile apps available in Australia: a quality review and content analysis. Aust N Z J Public Health 2017;41:625-30. https://doi.org/10.1111/1753-6405.12688.
- Vilardaga R, Casellas-Pujol E, McClernon JF, Garrison KA. Mobile applications for the treatment of tobacco use and dependence. Curr Addict Rep 2019;6:86-97. https://doi.org/10.1007/s40429-019-00248-0.
- Chu KH, Matheny SJ, Escobar-Viera CG, Wessel C, Notier AE, Davis EM. Smartphone health apps for tobacco cessation: a systematic review. Addict Behav 2021;112. https://doi.org/10.1016/j.addbeh.2020.106616.
- Bricker JB, Watson NL, Mull KE, Sullivan BM, Heffner Jaimee L. Efficacy of smartphone applications for smoking cessation: a randomized clinical trial. JAMA Intern Med 2020;180. https://doi.org/10.1001/jamainternmed.2020.4055.
- Garrison KA, Pal P, O’Malley SS, Pittman BP, Gueorguieva R, Rojiani R, et al. Craving to quit: a randomized controlled trial of smartphone app-based mindfulness training for smoking cessation. Nicotine Tob Res 2020;22:324-31. https://doi.org/10.1093/ntr/nty126.
- Perski O, Crane D, Beard E, Brown J. Does the addition of a supportive chatbot promote user engagement with a smoking cessation app? An experimental study. Digit Health 2019;5. https://doi.org/10.1177/2055207619880676.
- Etter JF, Khazaal Y. The Stop-tabac smartphone application for smoking cessation: a randomized controlled trial. Addiction 2021;117:1406-15. https://doi.org/10.1111/add.15738.
- Perski O, Hebert ET, Naughton F, Hekler EB, Brown J, Businelle MS. Technology-mediated just-in-time adaptive interventions (JITAIs) to reduce harmful substance use: a systematic review. Addiction 2021;117:1220-41. https://doi.org/10.1111/add.15687.
- Free C, Knight R, Robertson S, Whittaker R, Edwards P, Zhou W, et al. Smoking cessation support delivered via mobile phone text messaging (txt2stop): a single-blind, randomised trial. Lancet 2011;378:49-55. https://doi.org/10.1016/S0140-6736(11)60701-0.
- Devries KM, Kenward MG, Free CJ. Preventing smoking relapse using text messages: analysis of data from the txt2stop trial. Nicotine Tob Res 2012;15:77-82. https://doi.org/10.1093/ntr/nts086.
- Naughton F, Cooper S, Foster K, Emery J, Leonardi-Bee J, Sutton S, et al. Large multi-centre pilot randomized controlled trial testing a low-cost, tailored, self-help smoking cessation text message intervention for pregnant smokers (MiQuit). Addiction 2017;112:1238-49. https://doi.org/10.1111/add.13802.
- Naughton F, Jamison J, Boase S, Sloan M, Gilbert H, Prevost AT, et al. Randomized controlled trial to assess the short-term effectiveness of tailored web- and text-based facilitation of smoking cessation in primary care (iQuit in Practice). Addiction 2014;109:1184-93. https://doi.org/10.1111/add.12556.
- Whittaker R, McRobbie H, Bullen C, Rodgers A, Gu Y, Dobson R. Mobile phone text messaging and app-based interventions for smoking cessation. Cochrane Database Syst Rev 2019;10. https://doi.org/10.1002/14651858.CD006611.pub5.
- Hebert ET, Ra CK, Alexander AC, Helt A, Moisiuc R, Kendzor DE, et al. A mobile just-in-time adaptive intervention for smoking cessation: pilot randomized controlled trial. J Med Internet Res 2020;22. https://doi.org/10.2196/16907.
- McClernon FJ, Roy CR. I am your smartphone and I know you are about to smoke: the application of mobile sensing and computing approaches to smoking research and treatment. Nicotine Tob Res 2013;15:1651-4. https://doi.org/10.1093/ntr/ntt054.
- Naughton F. Delivering ‘Just-In-Time’ smoking cessation support via mobile phones: current knowledge and future directions. Nicotine Tob Res 2016;19:379-83. https://doi.org/10.1093/ntr/ntw143.
- Naughton F, Brown C, High J, Notley C, Mascolo C, Coleman T, et al. Randomised controlled trial of a just-in-time adaptive intervention (JITAI) smoking cessation smartphone app: the Quit Sense feasibility trial protocol. BMJ Open 2021;11. https://doi.org/10.1136/bmjopen-2020-048204.
- Harris PA, Taylor R, Minor BL, Elliott V, Fernandez M, O’Neal L, et al. REDCap Consortium. The REDCap consortium: building an international community of software platform partners. J Biomed Inform 2019;95. https://doi.org/10.1016/j.jbi.2019.103208.
- Harris PA, Taylor R, Thielke R, Payne J, Gonzalez N, Conde JG. Research electronic data capture (REDCap) – a metadata-driven methodology and workflow process for providing translational research informatics support. J Biomed Inform 2009;42:377-81. https://doi.org/10.1016/j.jbi.2008.08.010.
- Office for National Statistics . The National Statistics Socio-Economic Classification User Manual 2005.
- Naughton F, Prevost AT, Gilbert H, Sutton S. Randomised controlled trial evaluation of a tailored leaflet and SMS text message self-help intervention for pregnant smokers (MiQuit). Nicotine Tob Res 2012;14:569-77. https://doi.org/10.1093/ntr/ntr254.
- Bandura A. Social Foundations of Thought and Action: A Social Cognitive Theory. Englewood Cliffs, NJ: Prentice-Hall; 1986.
- Michie S, Richardson M, Johnston M, Abraham C, Francis J, Hardeman W, et al. The behavior change technique taxonomy (v1) of 93 hierarchically clustered techniques: building an international consensus for the reporting of behavior change interventions. Ann Behav Med 2013;46:81-95. https://doi.org/10.1007/s12160-013-9486-6.
- Michie S, Hyder N, Walia A, West R. Development of a taxonomy of behaviour change techniques used in individual behavioural support for smoking cessation. Addict Behav 2011;36:315-9. https://doi.org/10.1016/j.addbeh.2010.11.016.
- West R, Hajek P. Evaluation of the mood and physical symptoms scale (MPSS) to assess cigarette withdrawal. Psychopharmacology (Berl) 2004;177:195-9. https://doi.org/10.1007/s00213-004-1923-6.
- Fidler JA, Shahab L, West R. Strength of urges to smoke as a measure of severity of cigarette dependence: comparison with the Fagerstrom Test for Nicotine Dependence and its components. Addiction 2011;106:631-8. https://doi.org/10.1111/j.1360-0443.2010.03226.x.
- Craig P, Dieppe P, Macintyre S, Michie S, Nazareth I, Petticrew M. Developing and evaluating complex interventions: the new Medical Research Council guidance. BMJ 2008;337. https://doi.org/10.1136/bmj.a1655.
- Herdman M, Gudex C, Lloyd A, Janssen M, Kind P, Parkin D, et al. Development and preliminary testing of the new five-level version of EQ-5D (EQ-5D-5L). Qual Life Res 2011;20:1727-36. https://doi.org/10.1007/s11136-011-9903-x.
- West R, Hajek P, Stead L, Stapleton J. Outcome criteria in smoking cessation trials: proposal for a common standard. Addiction 2005;100:299-303. https://doi.org/10.1111/j.1360-0443.2004.00995.x.
- Benowitz NL, Bernert JT, Foulds J, Hecht SS, Jacob P, Jarvis MJ, et al. Biochemical verification of tobacco use and abstinence: 2019 update. Nicotine Tob Res 2019;22:1086-97. https://doi.org/10.1093/ntr/ntz132.
- Jacob P, Hatsukami D, Severson H, Hall S, Yu L, Benowitz Neal L. Anabasine and anatabine as biomarkers for tobacco use during nicotine replacement therapy. Cancer Epidemiol Biomarkers Prev 2002;11:1668-73.
- Cheung KL, de Ruijter D, Hiligsmann M, Elfeddali I, Hoving C, Evers SMAA, et al. Exploring consensus on how to measure smoking cessation. A Delphi study. BMC Public Health 2017;17. https://doi.org/10.1186/s12889-017-4902-7.
- Gwaltney CJ, Shiffman S, Balabanis MH, Paty JA. Dynamic self-efficacy and outcome expectancies: prediction of smoking lapse and relapse. J Abnorm Psychol 2005;114:661-75. https://doi.org/10.1037/0021-843X.114.4.661.
- Smith SS, Piper ME, Bolt DM, Fiore MC, Wetter DW, Cinciripini PM, et al. Development of the brief Wisconsin Inventory of Smoking Dependence Motives. Nicotine Tob Res 2010;12:489-99. https://doi.org/10.1093/ntr/ntq032.
- Teare MD, Dimairo M, Shephard N, Hayman A, Whitehead A, Walters SJ. Sample size requirements to estimate key design parameters from external pilot randomised controlled trials: a simulation study. Trials 2014;15. https://doi.org/10.1186/1745-6215-15-264.
- Brown J, Michie S, Geraghty AW, Yardley L, Gardner B, Shahab L, et al. Internet-based intervention for smoking cessation (StopAdvisor) in people with low and high socioeconomic status: a randomised controlled trial. Lancet Respir Med 2014;2:997-1006. https://doi.org/10.1016/S2213-2600(14)70195-X.
- Bricker JB, Mull KE, Kientz JA, Vilardaga R, Mercer LD, Akioka KJ, et al. Randomized, controlled pilot trial of a smartphone app for smoking cessation using acceptance and commitment therapy. Drug Alcohol Depend 2014;143:87-94. https://doi.org/10.1016/j.drugalcdep.2014.07.006.
- Taylor GMJ, Dalili MN, Semwal M, Civljak M, Sheikh A, Car J. Internet-based interventions for smoking cessation. Cochrane Database Syst Rev 2017;9. https://doi.org/10.1002/14651858.CD007078.pub5.
- Whittaker R, McRobbie H, Bullen C, Rodgers A, Gu Y. Mobile phone-based interventions for smoking cessation. Cochrane Database Syst Rev 2016;4. https://doi.org/10.1002/14651858.CD006611.pub4.
- Iacoviello BM, Steinerman JR, Klein DB, Silver Theodore L, Berger AG, Luo SX, et al. Clickotine, a personalized smartphone app for smoking cessation: initial evaluation. JMIR Mhealth Uhealth 2017;5. https://doi.org/10.2196/mhealth.7226.
- Mason D, Gilbert H, Sutton S. Effectiveness of web-based tailored smoking cessation advice reports (iQuit): a randomized trial. Addiction 2012;107:2183-90. https://doi.org/10.1111/j.1360-0443.2012.03972.x.
- Burton PR, Gurrin LC, Campbell MJ. Clinical significance not statistical significance: a simple Bayesian alternative to p values. J Epidemiol Community Health 1998;52:318-23. https://doi.org/10.1136/jech.52.5.318.
- Fleiss JL. Statistical Methods for Rates and Proportions. New York: John Wiley; 1981.
- Jones KC, Burns A. Unit Costs of Health and Social Care 2021. Kent, UK: Personal Social Services Research Unit; 2021.
- National Institute for Health and Clinical Excellence (NICE) . Position Statement on Use of the EQ-5D-5L Valuation Set (updated October 2019) 2019. https://www.nice.org.uk/about/what-we-do/our-programmes/nice-guidance/technology-appraisal-guidance/eq-5d-5l (accessed 1 March 2024).
- van Hout B, Janssen MF, Feng YS, Kohlmann T, Busschbach J, Golicki D, et al. Interim scoring for the EQ-5D-5L: mapping the EQ-5D-5L to EQ-5D-3L value sets. Value Health 2012;15:708-15. https://doi.org/10.1016/j.jval.2012.02.008.
- Willan AR, Briggs AH, Hoch JS. Regression methods for covariate adjustment and subgroup analysis for non-censored cost-effectiveness data. Health Econ 2004;13:461-75. https://doi.org/10.1002/hec.843.
- National Institute for Health and Clinical Excellence (NICE) . Guide to the Methods of Technology Appraisal 2013 2013. https://www.nice.org.uk/process/pmg9/chapter/foreword (accessed 1 March 2024).
- Moore GF, Audrey S, Barker M, Bond L, Bonell C, Hardeman W, et al. Process evaluation of complex interventions: Medical Research Council guidance. BMJ 2015;350. https://doi.org/10.1136/bmj.h1258.
- Braun V, Clarke V. Using thematic analysis in psychology. Qual Res Psychol 2006;3:77-101. https://doi.org/10.1191/1478088706qp063oa.
- Stinnett AA, Mullahy J. Net health benefits: a new framework for the analysis of uncertainty in cost-effectiveness analysis. Med Decis Making 1998;18:S68-80. https://doi.org/10.1177/0272989X98018002S09.
- Masaki K, Tateno H, Nomura A, Muto T, Suzuki S, Satake K, et al. A randomized controlled trial of a smoking cessation smartphone application with a carbon monoxide checker. NPJ Digit Med 2020;3. https://doi.org/10.1038/s41746-020-0243-5.
- McCarthy DE, Minami H, Yeh VM, Bold KW. An experimental investigation of reactivity to ecological momentary assessment frequency among adults trying to quit smoking. Addiction 2015;110:1549-60. https://doi.org/10.1111/add.12996.
- Berg ML, Cheung KL, Hiligsmann M, Evers S, de Kinderen RJA, Kulchaitanaroaj P, et al. Model-based economic evaluations in smoking cessation and their transferability to new contexts: a systematic review. Addiction 2017;112:946-67. https://doi.org/10.1111/add.13748.
- Coleman T, Clark M, Welch C, Whitemore R, Leonardi-Bee J, Cooper S, et al. Effectiveness of offering tailored text message, self-help smoking cessation support to pregnant women who want information on stopping smoking: MiQuit3 randomised controlled trial and meta-analysis. Addiction 2022;117:1079-94. https://doi.org/10.1111/add.15715.
- Yardley L, Spring BJ, Riper H, Morrison LG, Crane DH, Curtis K, et al. Understanding and promoting effective engagement with digital behavior change interventions. Am J Prev Med 2016;51:833-42. https://doi.org/10.1016/j.amepre.2016.06.015.
- Munafo M. Open science and research reproducibility. Ecancermedicalscience 2016;10. https://doi.org/10.3332/ecancer.2016.ed56.
- Watson NL, Mull KE, Heffner JL, McClure JB, Bricker JB. Participant recruitment and retention in remote eHealth intervention trials: methods and lessons learned from a large randomized controlled trial of two web-based smoking interventions. J Med Internet Res 2018;20. https://doi.org/10.2196/10351.
- BinDhim NF, McGeechan K, Trevena L. Who uses smoking cessation apps? A feasibility study across three countries via smartphones. JMIR Mhealth Uhealth 2014;2. https://doi.org/10.2196/mhealth.2841.
- BinDhim NF, McGeechan K, Trevena L. Smartphone Smoking Cessation Application (SSC App) trial: a multicountry double-blind automated randomised controlled trial of a smoking cessation decision-aid ‘app’. BMJ Open 2018;8. https://doi.org/10.1136/bmjopen-2017-017105.
- Jackson SE, Beard E, Angus C, Field M, Brown J. Moderators of changes in smoking, drinking and quitting behaviour associated with the first COVID-19 lockdown in England. Addiction 2022;117:772-83. https://doi.org/10.1111/add.15656.
- Ubhi HK, Kotz D, Michie S, van Schayck OCP, West R. A comparison of the characteristics of iOS and Android users of a smoking cessation app. Transl Behav Med 2017;7:166-71. https://doi.org/10.1007/s13142-016-0455-z.
- Szinay D, Jones A, Chadborn T, Brown J, Naughton F. Influences on the uptake of and engagement with health and well-being smartphone apps: systematic review. J Med Internet Res 2020;22. https://doi.org/10.2196/17572.
- Szinay D, Perski O, Jones A, Chadborn T, Brown J, Naughton F. Perceptions of factors influencing engagement with health and well-being apps in the United Kingdom: qualitative interview study. JMIR Mhealth Uhealth 2021;9. https://doi.org/10.2196/29098.
- Office for National Statistics . Population Estimates by Ethnic Group and Religion, England and Wales: 2019 2019. www.ons.gov.uk/peoplepopulationandcommunity/populationandmigration/populationestimates/articles/populationestimatesbyethnicgroupandreligionenglandandwales/2019 (accessed 20 March 2022).
Appendix 1 Examples of recruitment adverts on Facebook and Google AdWords for both desktops and mobiles
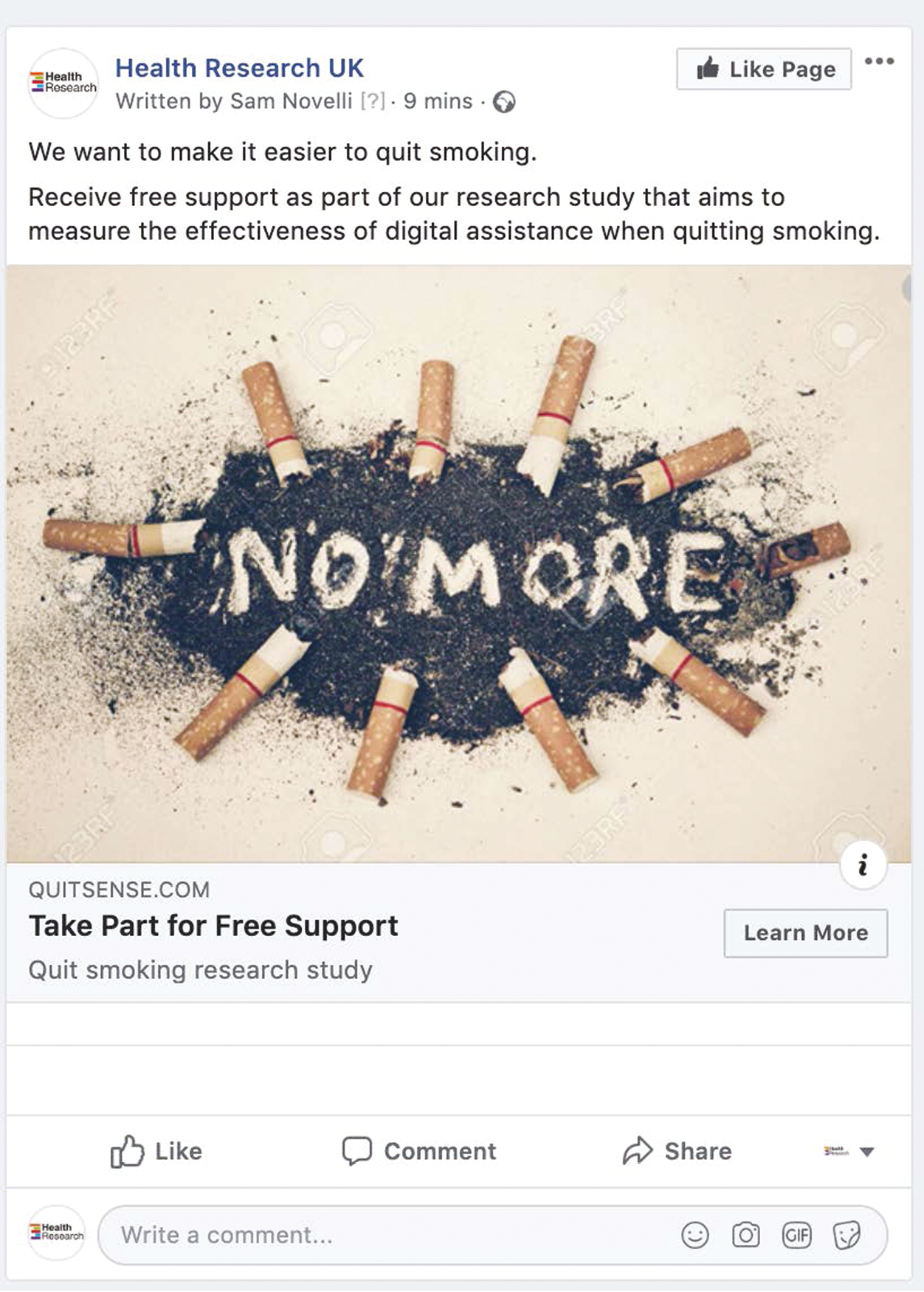
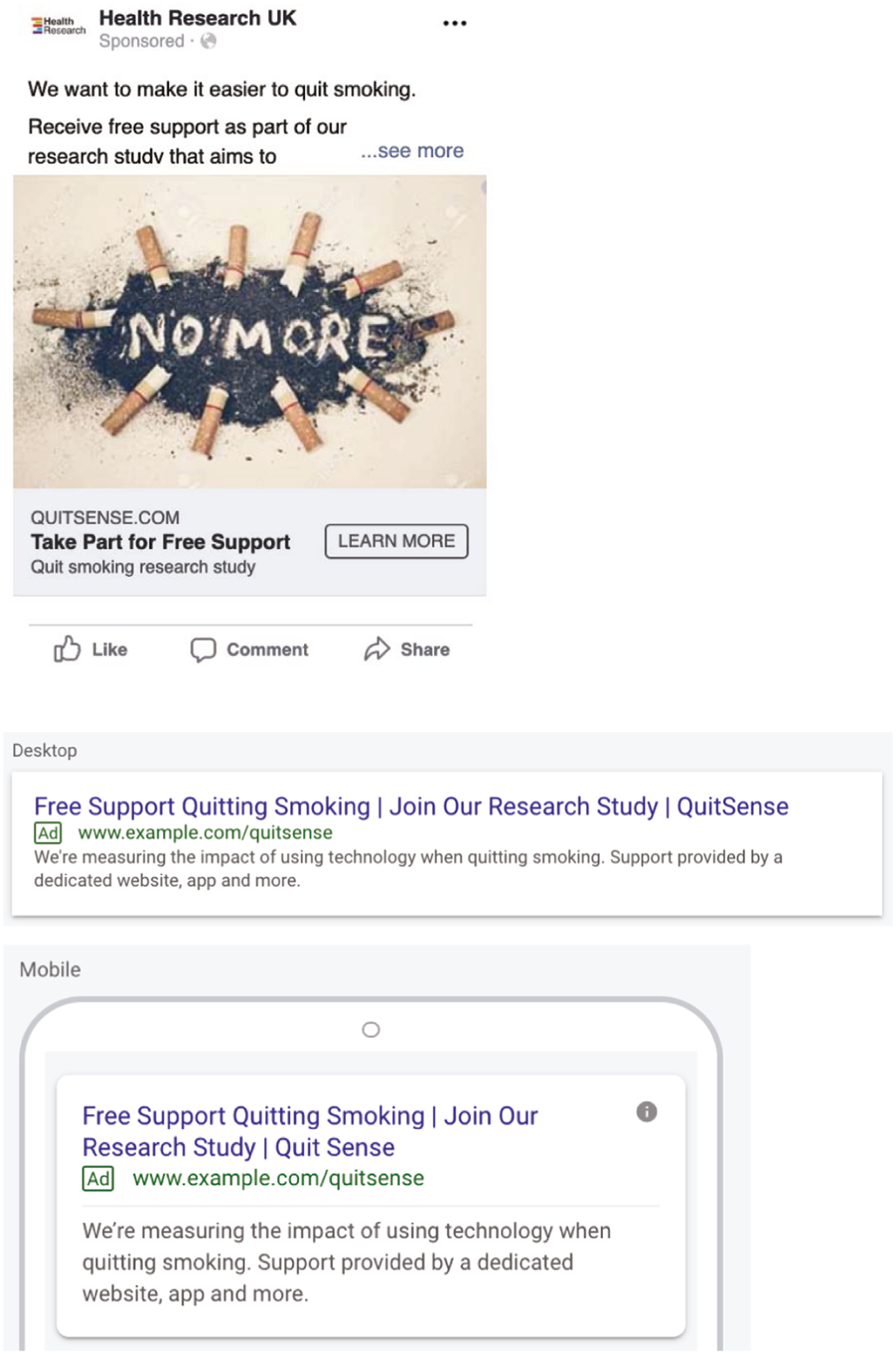
Appendix 2 Two-sided ‘postcard’ sent (in envelope) to all participants to encourage continued engagement with follow-up
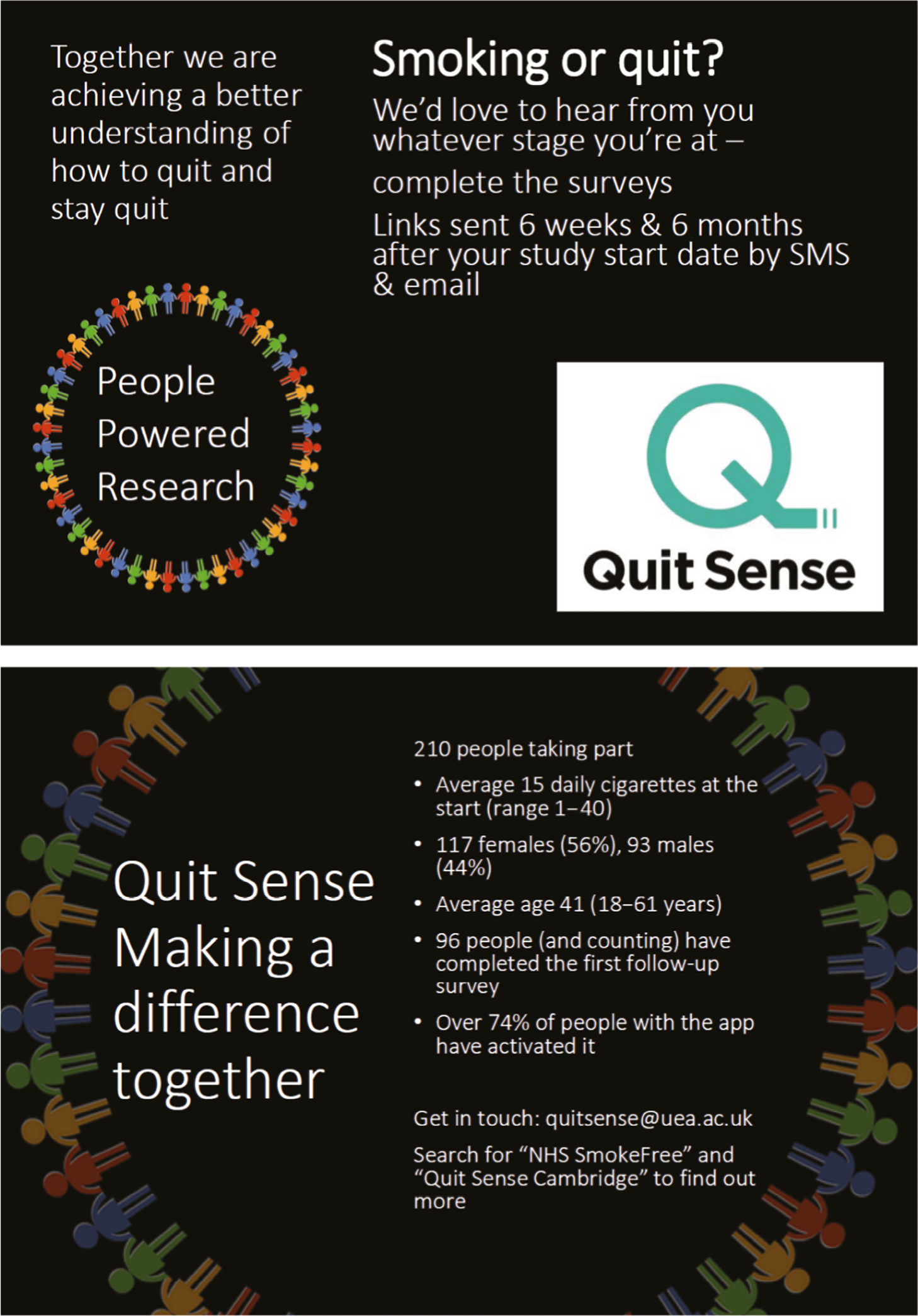
Appendix 3 Quit Sense logic model and supporting evidence
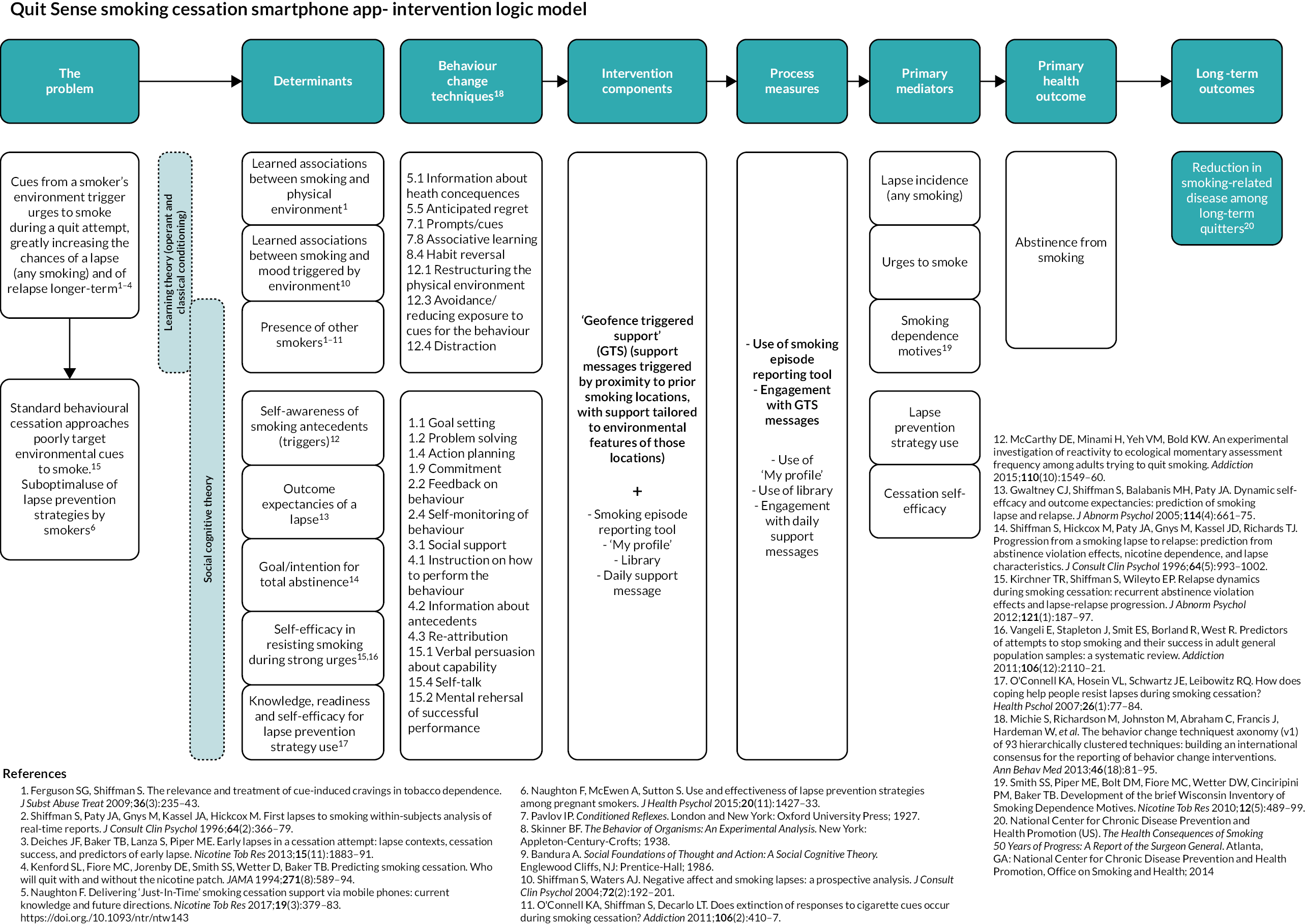
Appendix 4 Quit Sense app screenshots
Screenshot 1: Home screen (stage 1, pre-quit date)
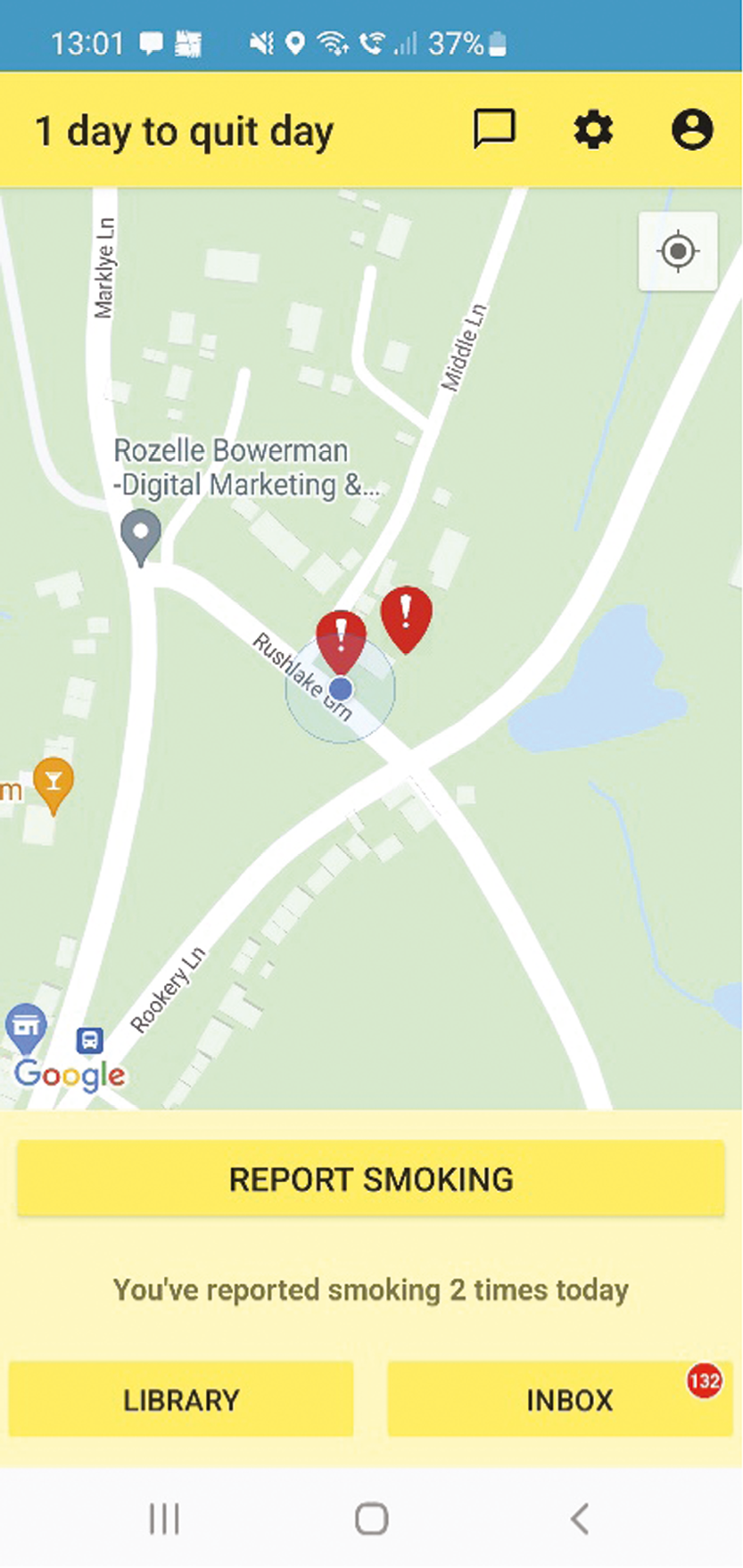
Screenshot 2: Library (three example screens; stage 1, pre-quit date)
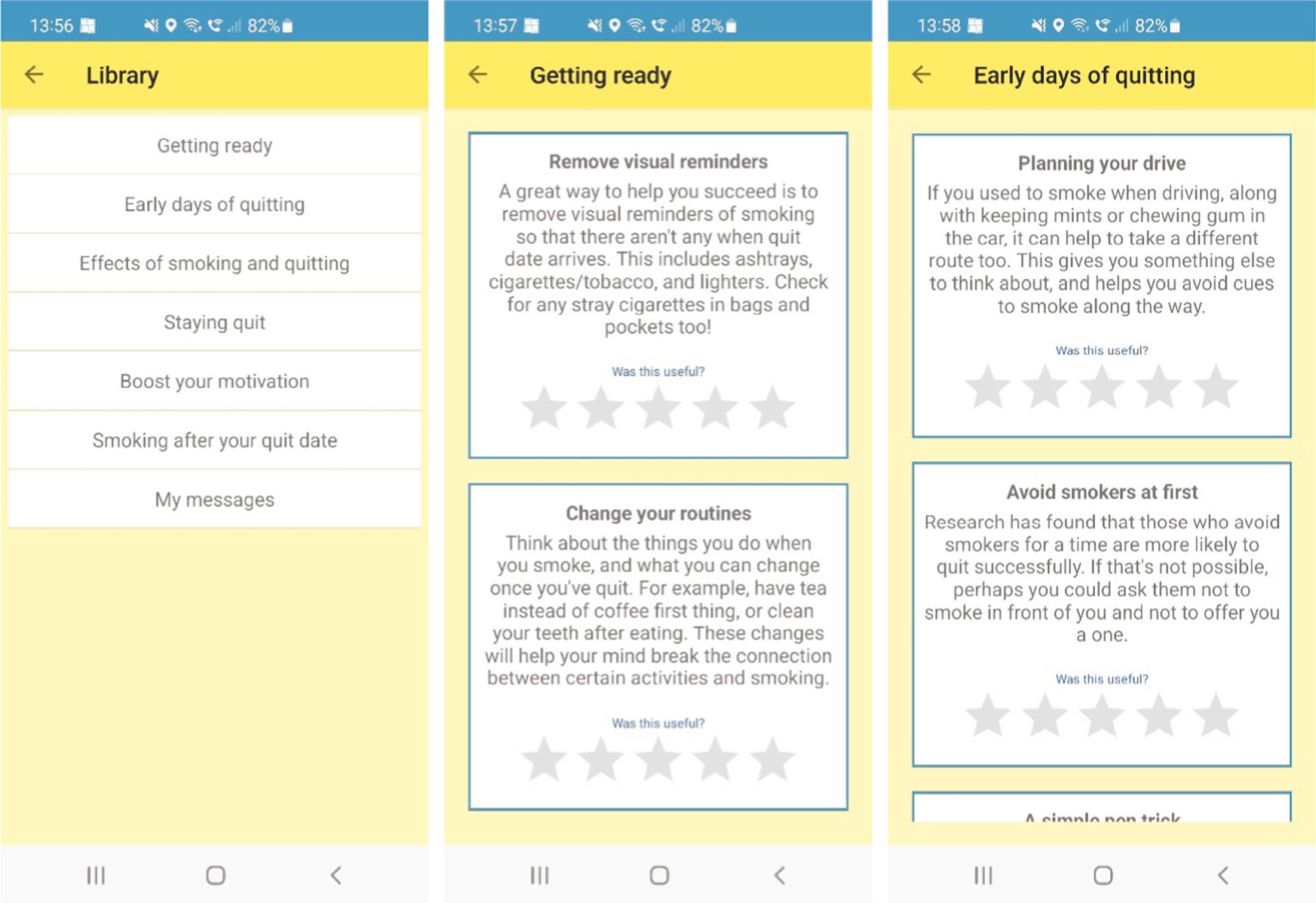
Screenshot 3: Reporting smoking (six screens; stage 1, pre-quit)
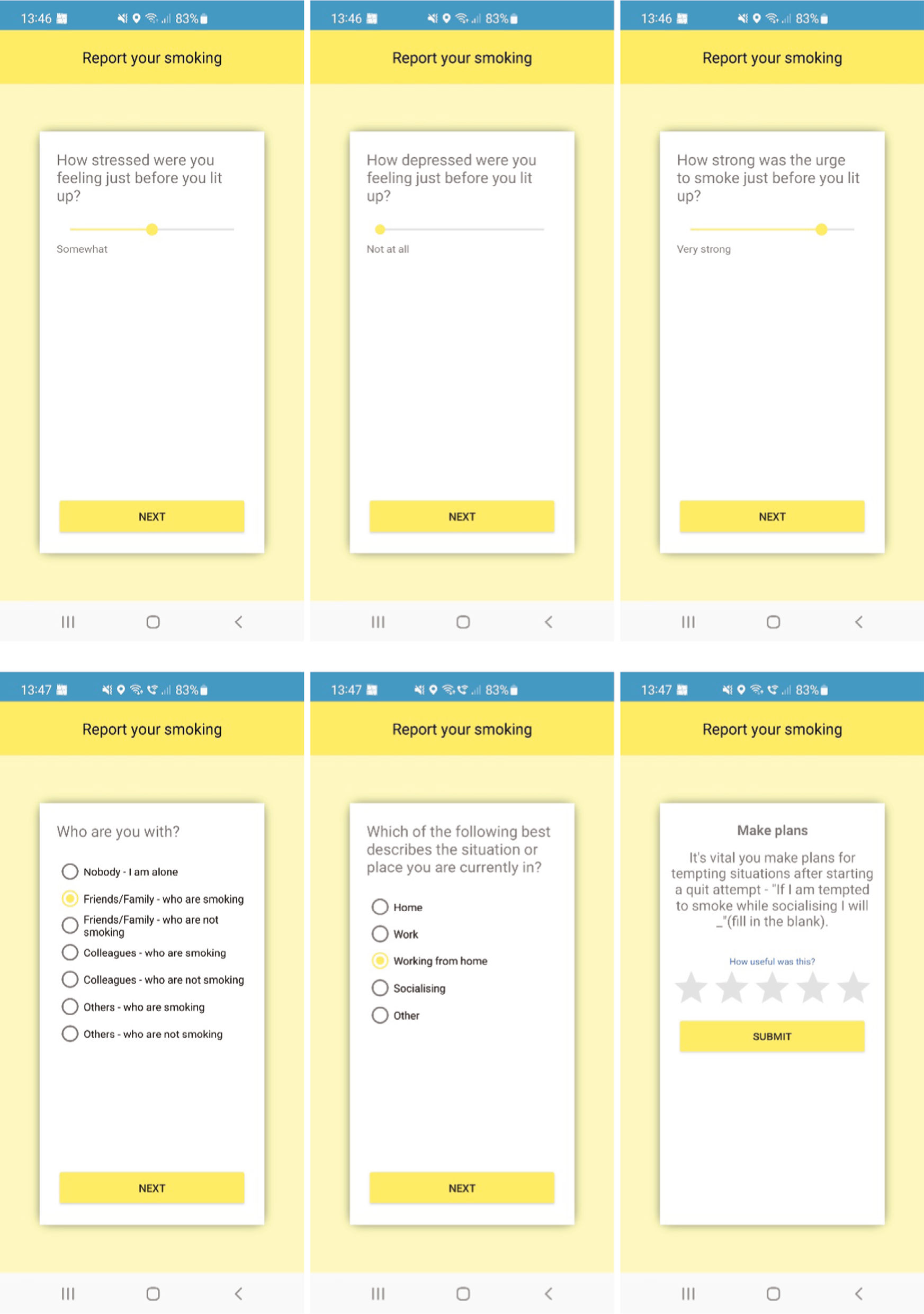
Screenshot 4: My smoking patterns (six screens; stage 1, pre-quit)
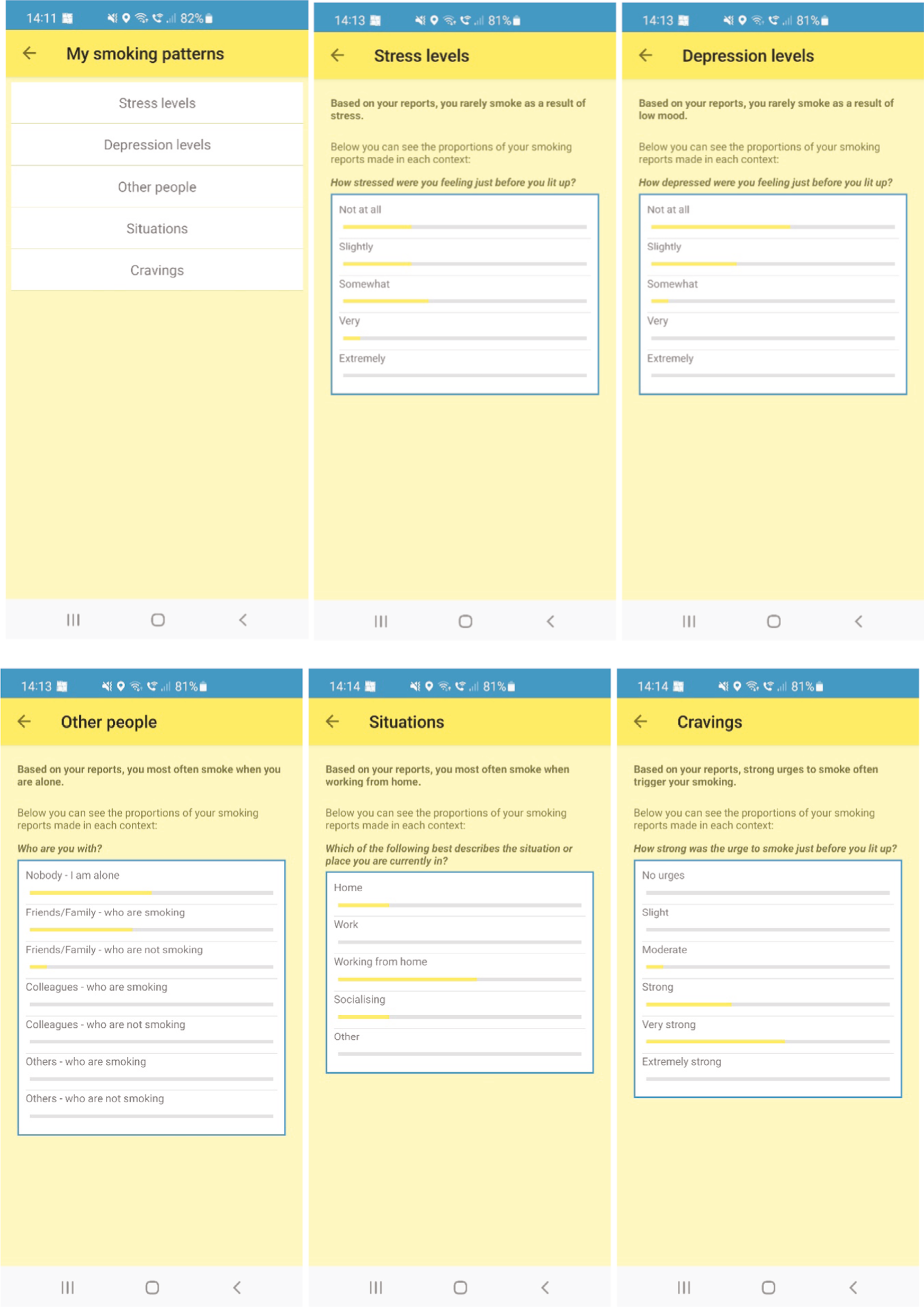
Screenshot 5: Inbox (stage 1, pre-quit)
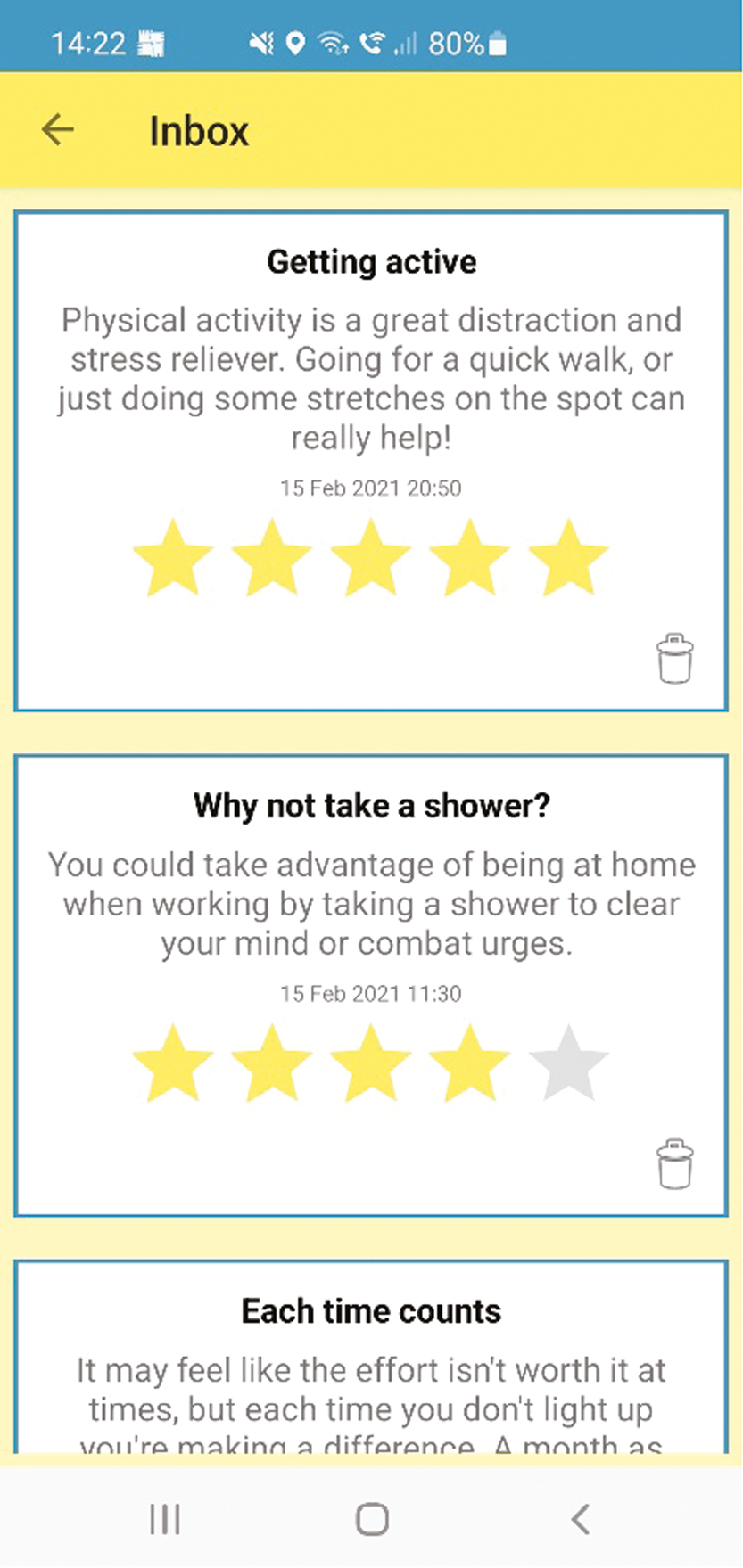
Screenshot 6: Daily support message (stage 2, 28-day quitting challenge)
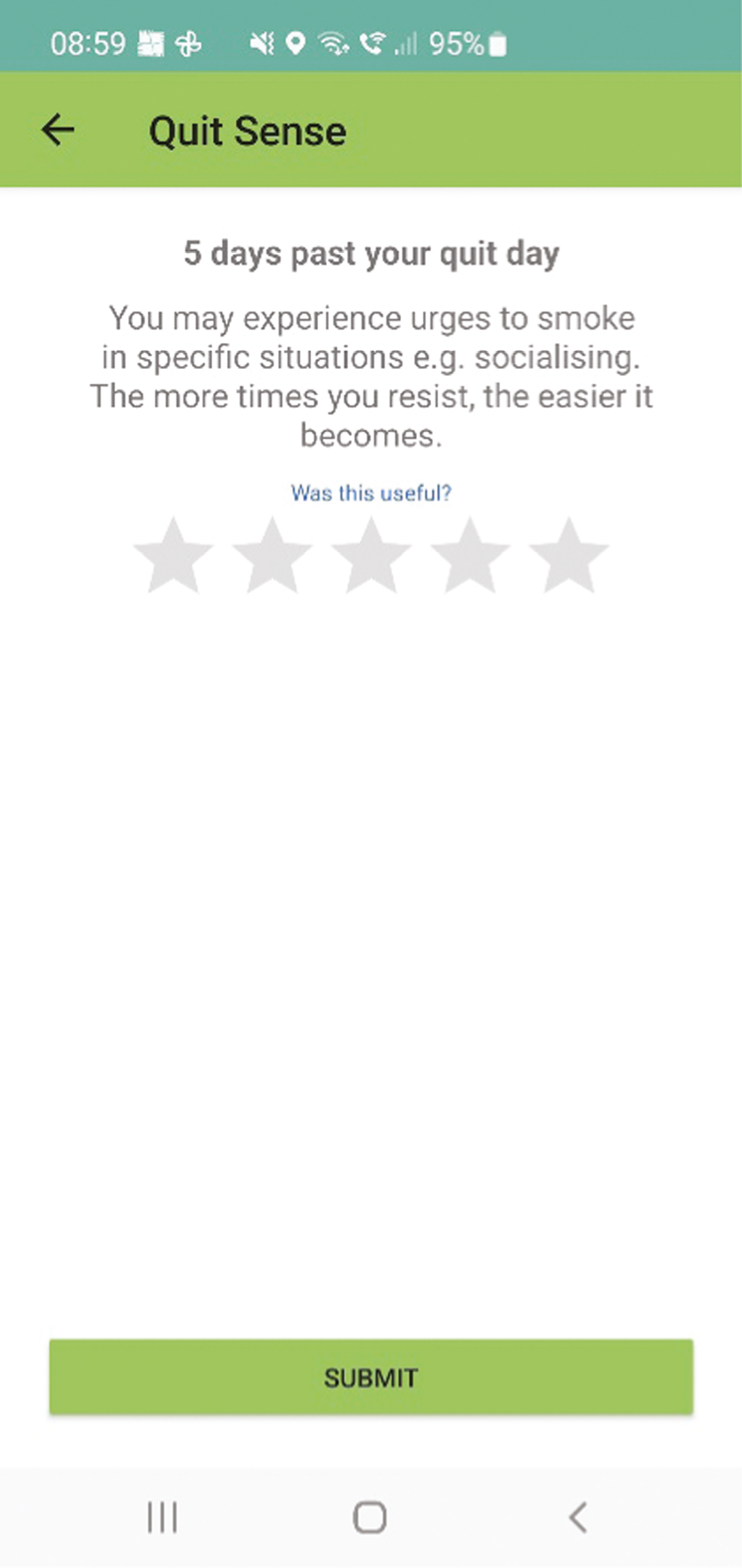
Screenshot 7: Geofence-triggered support messages (home and working from home geofences; stage 2, 28-day quitting challenge)
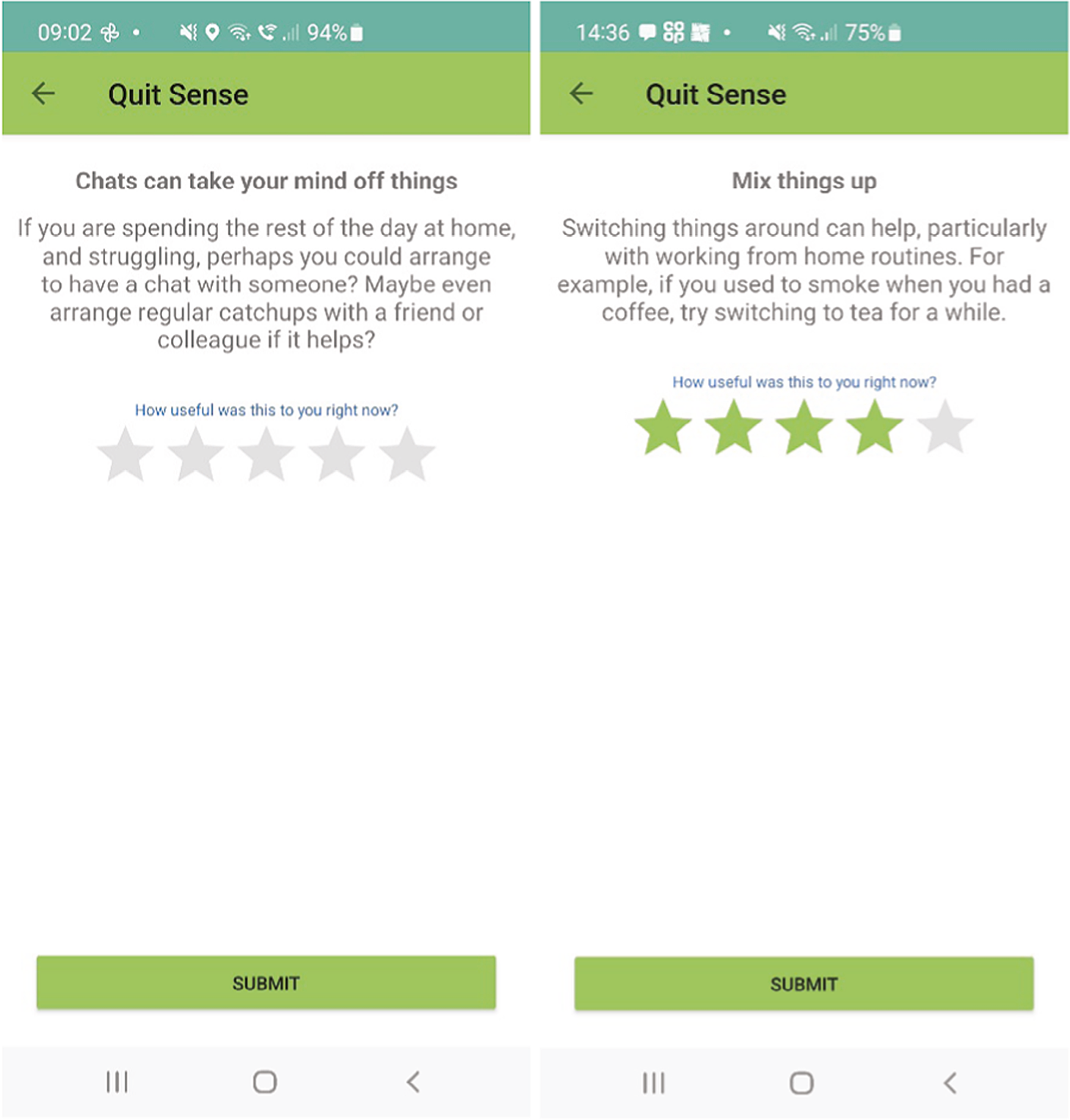
Screenshot 8: My Profile (stage 2, 28-day quitting challenge)
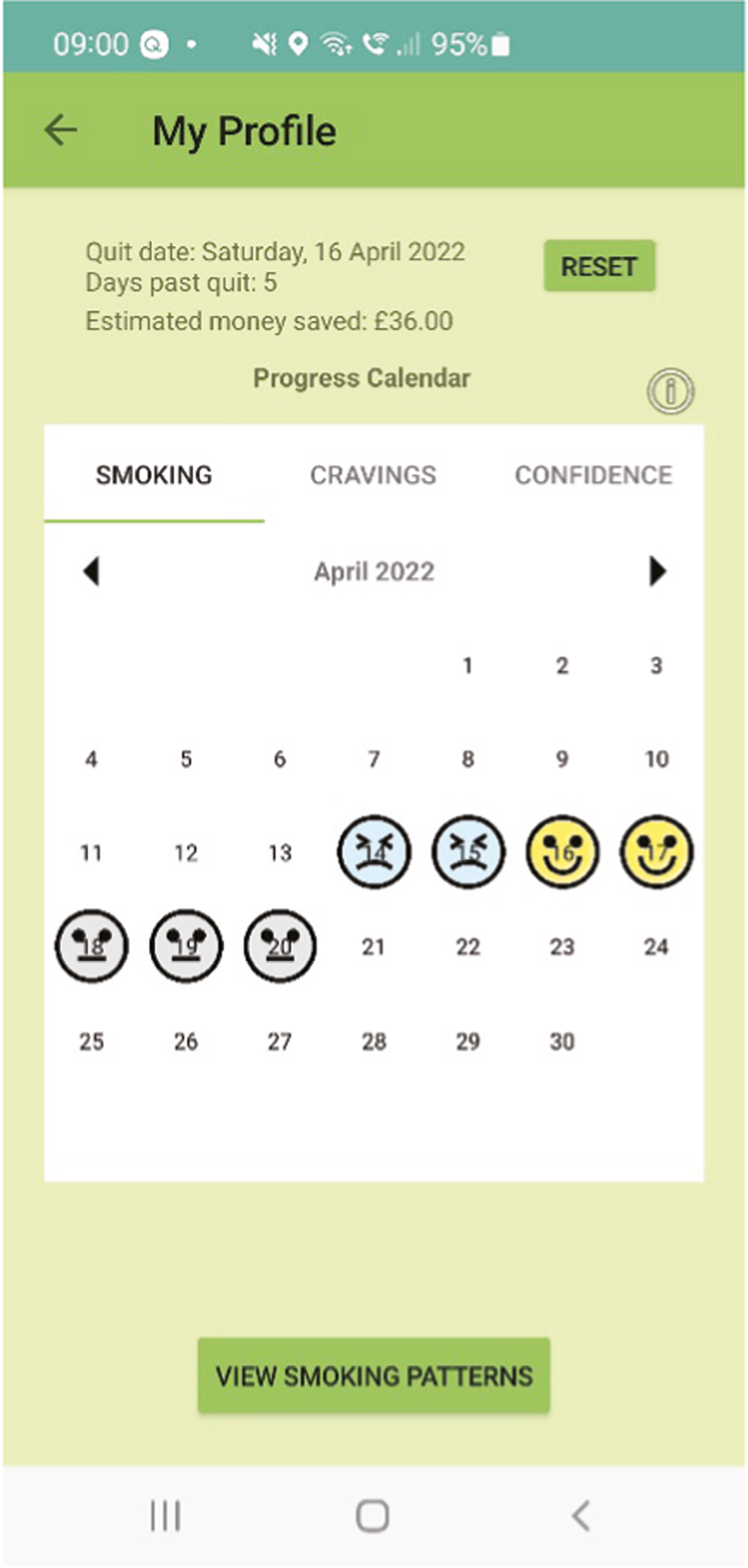
Screenshot 8: Settings and auto-process evaluation (stage 1, pre-quit)
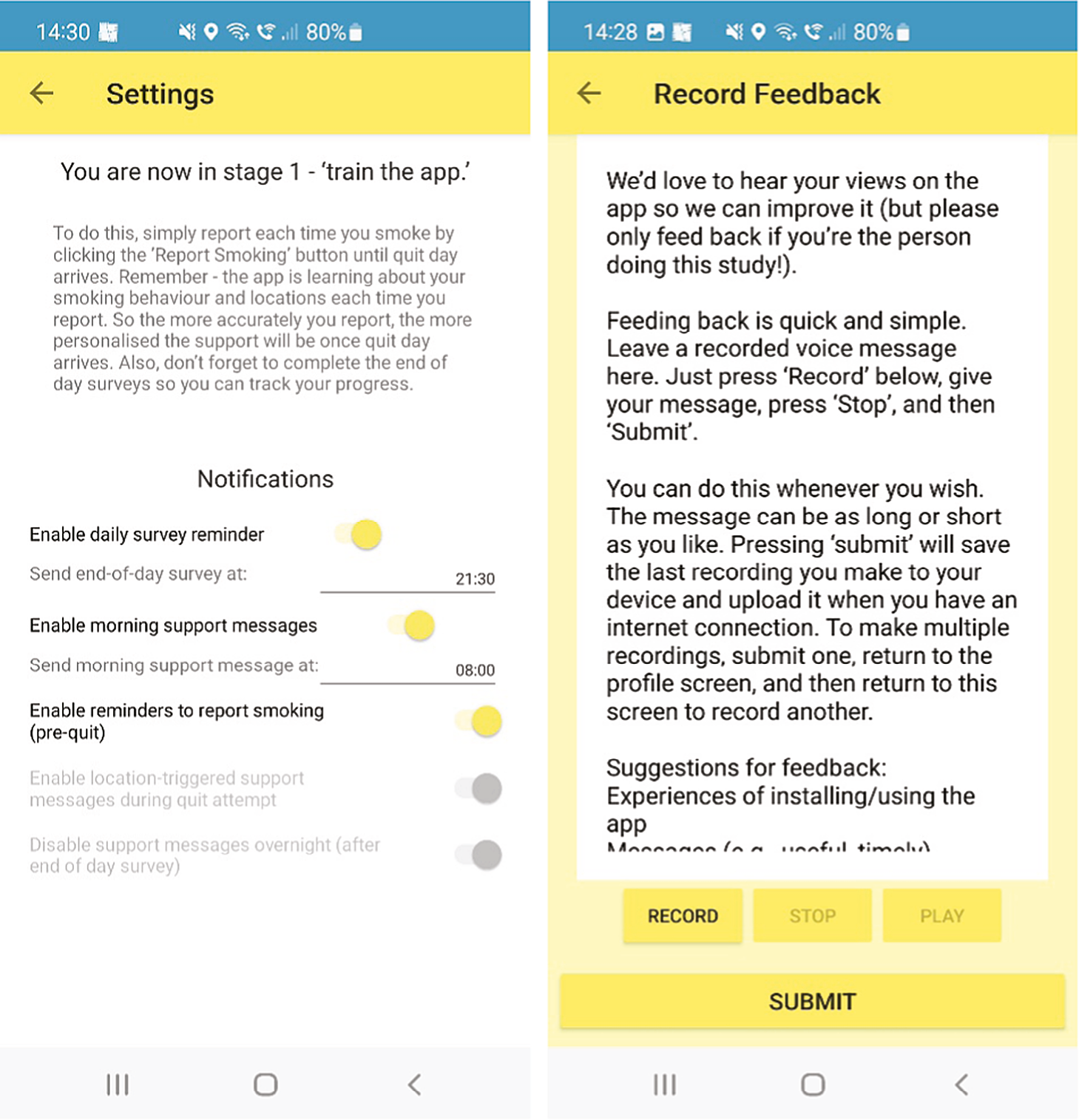
Appendix 5 Interview topic guide
Introduction/preamble to interviews
-
Introduce myself
-
Calling to get feedback on Quit Sense study. We really want to know about people’s experiences of this research so that this can be factored in when we design and run a much larger trial in future. Stress that there are no right or wrong answers and how important their feedback is
-
Check aware that call will be recorded > that it will be written up, that personally identifying details (e.g. names, places) will be removed from the written version and the recording will then be deleted
-
The call around half an hour but can be stopped at any point
-
Reaffirm verbal consent.
SEMISTRUCTURED INTERVIEW TOPIC GUIDE
*Prompts are in blue.
INTERVENTION ARM (Quit Sense + SmokeFree website)
-
Overall, how have you found using the Quit Sense app? *Has it met your expectations? Have you used the app as much as you thought you would? Why? Has that changed since you first installed it? [Probe for specific examples, e.g. if not met expectations, can you say how? If a feature is liked, which feature, and can you give an example of how you used it or what you liked about it? Also probe for technical issues (installing, running, updates, etc).]
REPORTING SMOKING
-
How have you found reporting smoking using the app? *Did you use this as a tool for yourself? Were you honest? Did you feel like you were being checked up on? Is it easier to report in some places or at some times than at others? When reporting smoking are there any situations that are missing/hard to categorise?
MESSAGES
-
What are your thoughts on the messages the app sends you? *Do you feel the messages delivered during your quit attempt (if you’ve made one) have been appropriate for where you have been spending your time? Ask for an example. Are there any situations where messages have been particularly helpful/unhelpful? Ask for example. Why is that? Any differences pre-quit + post quit. * Did you rate the messages? If no, why not?
USE OF STRATERGIES AND AIDS
-
Which strategies suggested by the app (if any) did you use? *Were there any app strategies which you found particularly helpful/unhelpful with managing cravings to smoke?
-
Have you used any quit smoking aids or other support since you started this study? In previous quit attempts? *If no – are you interested in using any? What have you used/tried? Examples – e-cigarettes, other cessation apps, other health and well-being apps and the NHS website
END OF DAY SURVEYS
-
Did you complete the end of day surveys? What did you think of these? *For example, in terms of timing and convenience. Any situations missing?
MY (PROFILE relevant IF they did the end of day surveys)
-
Did you view the My Profile feature? What did you think of it? *When did you look at it and why? Anything that could be added or improved?
-
Have you viewed the My Smoking Patterns feature? What did you think of it? *Did this tell you anything you didn’t already know about the things that make you want to smoke (e.g. places, moods, people, whether being aware made a difference to quit attempt). Example? *Have you noticed any changes to your triggers for wanting to smoke (i.e. cravings changed during the study)?
LAPSES
-
Have you smoked at all (even a puff) during your quit attempt? If yes, what effect did it have on your quit attempt? *Did this affect your confidence in being able to quit, did you report this on the app and, if so, was the app helpful? (>ASK ONLY IF FULLY RELAPSED > If you make another quit attempt in future would you use Quit Sense again?)
OVERALL APP (time allowing)
-
What one aspect did you most like about the app?
-
If you had to change one aspect of the app, what would it be and why?
STUDY EXPERIENCE
-
How have you found being part of this study so far? *Is it what you expected? Anything you think could be changed to improve your experience?
*It is possible to provide study feedback using the audio-record function of the app. Have you seen/used this feature? How did you/would you feel about leaving recorded feedback?
CURRENT CONTEXT (time allowing)
-
Has your personal situation changed as a result of the coronavirus pandemic (probe, e.g. working from home)?
-
Do you feel that the pandemic situation has impacted your smoking behaviour? Probe: more/less/different contexts of smoking? Aware of media reports around smoking and C19?
-
How have you found using the app during the pandemic situation? Probe: more helpful than it might have been, or less helpful than it might have been, messages tailored to situation or less relevant?
USUAL CARE ARM (SmokeFree website only)
INTRO along lines of > We are planning on doing a larger study in the future and we would therefore find your feedback on this study very helpful.
-
How have you found being part of this study so far? *Is it what you expected? Any suggestions on ways to improve the experience of participants?
-
Do you recall which group are you in (the app or SmokeFree only)? *How did you feel about being allocated to that group? Probe for awareness and understanding of randomisation. (Ask about ways to improve experience if people unhappy about allocation.)
-
How did you decide whether to take part? *For example, did you talk to anyone about it, download the participant information sheet (PIS), did you enrol right away or return later?
-
What has helped you to remain motivated to stay involved in the study? *For example, follow-up calls, incentives, postcard. Have you felt as involved in the study as you would like to be? If not, is there anything we could do to make you feel more involved?
-
Am I right in thinking that you found about this study through seeing an online advertisement? What did you think about the ad? *Any concerns about following the link?
-
What did you think about the study website? *For example, was it easy to use and understand? Could you easily find all the information you wanted about taking part?
-
What did you think about the questionnaires? *Baseline and 6-week. Length, content, functionality on mobile/computer, time taken.
-
What about the text messages you’ve received about the study? Have communications been too frequent, too few or about right? *Or follow-up phone calls?
-
Since starting this study, have you tried out any strategies to help you to stop smoking?
-
Have you used any quit smoking support? For example, apps, medicines, self-help. *Have you looked at/used the NHS SmokeFree website?
-
Do you have any other comments or suggestions? Anything you would like to ask us?
CURRENT CONTEXT (time allowing)
-
Has your personal situation changed as a result of the coronavirus pandemic (probe, e.g. working from home)?
-
Do you feel that the pandemic situation has impacted your smoking behaviour? Probe: more/less/different contexts of smoking? Aware of media reports around smoking and C19?
Appendix 6 Supplementary tables and figures
App (n = 71) |
Standard (n = 78) |
Overall (n = 149) |
|
---|---|---|---|
Are you currently smoking tobacco? (%) | |||
No | 23 (32.4) | 26 (33.3) | 49 (32.9) |
Yes | 48 (67.6) | 52 (66.7) | 100 (67.1) |
Did you smoke any tobacco in the first 2 weeks of your quit attempt (or first 2 weeks of the study)? (%) | |||
No | 21 (29.6) | 15 (19.2) | 36 (24.2) |
Yes | 50 (70.4) | 63 (80.8) | 113 (75.8) |
Did you smoke any tobacco in the first month of your quit attempt (or first month of the study)? (%) | |||
No | 11 (15.5) | 7 (9.0) | 18 (12.1) |
Yes | 60 (84.5) | 71 (91.0) | 131 (87.9) |
When did you last smoke a cigarette? (%) | |||
In the last 24 hours | 43 (60.6) | 44 (56.4) | 87 (58.4) |
1–6 days ago | 8 (11.3) | 13 (16.7) | 21 (14.1) |
7–14 days ago | 1 (1.4) | 6 (7.7) | 7 (4.7) |
More than 14 days ago | 19 (26.8) | 15 (19.2) | 34 (22.8) |
FUTS: n (%) | |||
Not at all | 10 (14.1) | 6 (7.7) | 16 (10.7) |
A little of the time | 15 (21.1) | 21 (26.9) | 36 (24.2) |
Some of the time | 17 (23.9) | 17 (21.8) | 34 (22.8) |
A lot of the time | 12 (16.9) | 18 (23.1) | 30 (20.1) |
Almost all the time | 9 (12.7) | 9 (11.5) | 18 (12.1) |
All the time | 8 (11.3) | 7 (9.0) | 15 (10.1) |
SUTS: n (%) | |||
No urges | 12 (16.9) | 3 (3.9) | 15 (10.1) |
Slight urges | 9 (12.7) | 20 (25.6) | 29 (19.5) |
Moderate urges | 29 (40.9) | 22 (28.2) | 51 (34.2) |
Strong urges | 7 (9.9) | 16 (20.5) | 23 (15.4) |
Very strong urges | 8 (11.3) | 9 (11.5) | 17 (11.4) |
Extremely strong urges | 6 (8.5) | 8 (10.3) | 14 (9.4) |
EQ-5D-5L utility score: mean (SD) | 0.751 (0.249) | 0.734 (0.256) | 0.742 (0.246) |
Missinga | 1 | 0 | 1 |
EQ-5D-5L utility score: mean change (SD) | −0.020 (0.232) | −0.044 (0.178) | −0.031 (0.208) |
Missinga | 1 | 0 | 1 |
WISDM Automaticity subscale score: mean (SD) | 3.56 (2.26) | 3.83 (2.30) | 3.70 (2.28) |
WISDM cue exposure/associative processes subscale score: mean (SD) | 4.38 (1.55) | 4.24 (1.52) | 4.30 (1.53) |
Self-efficacy average score: mean (SD) | 1.89 (1.26) | 1.71 (1.11) | 1.80 (1.18) |
Lapse prevention strategy total useb: mean (SD) | 1.38 (0.57) | 1.46 (0.62) | 1.42 (0.59) |
Lapse prevention avoidance strategiesb: mean (SD) | 1.23 (0.73) | 1.33 (0.68) | 1.28 (0.70) |
Lapse prevention coping strategiesb: mean (SD) | 1.47 (0.56) | 1.53 (0.67) | 1.50 (0.62) |
App (n = 79) |
Standard (n = 81) |
Overall (n = 160) |
|
---|---|---|---|
Are you currently smoking tobacco? (%) | |||
No | 32 (41.6) | 30 (37.0) | 62 (39.2) |
Yes | 45 (58.4) | 51 (63.0) | 96 (60.8) |
Missing | 2 | 0 | 2 |
Have you smoked any tobacco in the last 6 months? (%) | |||
No, not a puff | 11 (14.3) | 5 (6.3) | 16 (10.3) |
Yes, a few puffs | 5 (6.5) | 2 (2.5) | 7 (4.5) |
Yes, 1–5 cigarettes | 9 (11.7) | 9 (11.4) | 18 (11.5) |
Yes, 5+ cigarettes | 51 (66.2) | 63 (79.8) | 114 (73.1) |
Cannot remember | 1 (1.3) | 0 | 1 (0.6) |
Missing | 2 | 2 | 4 |
When did you last smoke a cigarette? (%) | |||
In the last 24 hours | 42 (56.8) | 46 (60.5) | 88 (58.7) |
1–6 days ago | 4 (5.4) | 10 (13.2) | 14 (9.3) |
7–14 days ago | 4 (5.4) | 1 (1.3) | 5 (3.3) |
2–4 weeks ago | 4 (5.4) | 8 (10.5) | 12 (8.0) |
1–3 months ago | 5 (6.8) | 6 (7.9) | 11 (7.3) |
3–6 months ago | 7 (9.5) | 3 (4.0) | 10 (6.7) |
More than 6 months ago | 8 (10.8) | 2 (2.6) | 10 (6.7) |
Missing | 5 | 5 | 10 |
FUTS: n (%) | |||
Not at all | 16 (21.6) | 10 (13.3) | 26 (17.5) |
A little of the time | 18 (24.3) | 21 (28.0) | 39 (26.2) |
Some of the time | 18 (24.3) | 16 (21.3) | 34 (22.8) |
A lot of the time | (20.3) | 17 (22.7) | 32 (21.5) |
Almost all the time | 5 (6.8) | 5 (6.7) | 10 (6.7) |
All the time | 2 (2.7) | 6 (8.0) | 8 (5.4) |
Missing | 5 | 6 | 11 |
SUTS: n (%) | |||
No urges | 19 (25.7) | 11 (14.7) | 30 (20.1) |
Slight urges | 18 (24.3) | 14 (18.7) | 32 (21.5) |
Moderate urges | 14 (18.9) | 27 (36.0) | 41 (27.5) |
Strong urges | 16 (21.6) | 11 (14.7) | 27 (18.1) |
Very strong urges | 2 (2.7) | 8 (10.7) | 10 (6.7) |
Extremely strong urges | 5 (6.8) | 4 (5.3) | 9 (6.0) |
Missing | 5 | 6 | 11 |
EQ-5D-5L utility score: mean (SD) | 0.739 (0.297) | 0.743 (0.242) | 0.741 (0.270) |
Missinga | 5 | 8 | 13 |
EQ-5D-5L utility score: mean change (SD) | −0.046 (0.184) | −0.018 (0.161) | −0.032 (0.173) |
Missinga | 15 | 16 | 31 |
6 m total QALY score: mean (SD) | 0.379 (0.119) | 0.370 (0.108) | 0.375 (0.113) |
Missinga | 15 | 16 | 31 |
Abstinence based on primary outcome: n (%) | |||
Yes | 12 (18.5) | 3 (4.6) | 15 (11.5) |
No | 53 (81.5) | 63 (95.5) | 116 (88.6) |
Missing | 14 | 15 | 29 |
Self-efficacy average score: mean (SD) | 2.23 (1.26) | 1.76 (1.21) | 1.99 (1.25) |
Modela N = 209 |
App group abstinence n (%) N = 104 |
Standard group abstinence n (%) N = 105 |
ORb | 95% Wald CIc | p-valued |
---|---|---|---|---|---|
Adjusted for treatment group only | 12 (11.5) | 3 (2.9) | 4.44 | (1.21 to 16.21) | 0.024 |
Adjusted model, including treatment group and stratification variables | 12 (11.5) | 3 (2.9) | 4.57 | (1.23 to 16.94) | 0.023 |
Adjusted model, including treatment group, stratification and prognostic variables | 12 (11.5) | 3 (2.9) | 4.46 | (1.19 to 16.69)e | 0.026 |
Modela N = 209 |
App group abstinence n (%) N = 104 |
Standard group abstinence n (%) N = 105 |
ORb | 95% exact CIc | p-valued |
---|---|---|---|---|---|
Adjusted for treatment group only | 12 (11.5) | 3 (2.9) | 4.41 | (1.14 to 25.09) | 0.028 |
Adjusted model, including treatment group and stratification variables | 12 (11.5) | 3 (2.9) | 4.49 | (1.15 to 25.88) | 0.027 |
Adjusted model, including treatment group, stratification and prognostic variables | 12 (11.5) | 3 (2.9) | 4.36 | (1.10 to 25.22) | 0.033 |
Modela N = 203 |
App group abstinence n (%) N = 100 |
Standard group abstinence n (%) N = 103 |
ORb | 95% Wald CIc | p-valued |
---|---|---|---|---|---|
Adjusted for treatment group only | 12 (12.0) | 3 (2.9) | 4.55 | (1.24 to 16.63) | 0.022 |
Adjusted model, including treatment group and stratification variables | 12 (12.0) | 3 (2.9) | 4.61 | (1.24 to 17.09) | 0.022 |
Adjusted model, including treatment group, stratification and prognostic variables | 12 (12.0) | 3 (2.9) | 4.56 | (1.22 to 17.06)e | 0.024 |
Modela N = 203 |
App group abstinence n (%) N = 100 |
Standard group abstinence n (%) N = 103 |
ORb | 95% exact CIc | p-valued |
---|---|---|---|---|---|
Adjusted for treatment group only | 12 (12.0) | 3 (2.9) | 4.52 | (1.17 to 25.73) | 0.025 |
Adjusted model, including treatment group and stratification variables | 12 (12.0) | 3 (2.9) | 4.53 | (1.15 to 26.09) | 0.026 |
Adjusted model, including treatment group, stratification and prognostic variables | 12 (12.0) | 3 (2.9) | 4.45 | (1.13 to 25.76) | 0.030 |
Modela N = 131 |
App group abstinence n (%) N = 65 |
Standard group abstinence n (%) N = 66 |
ORb | 95% Wald CIc | p-valued |
---|---|---|---|---|---|
Adjusted for treatment group only | 12 (18.5) | 3 (4.5) | 4.76 | (1.27 to 17.74) | 0.020 |
Adjusted model, including treatment group and stratification variables | 12 (18.5) | 3 (4.5) | 4.83 | (1.28 to 18.31) | 0.020 |
Adjusted model, including treatment group, stratification and prognostic variables | 12 (18.5) | 3 (4.5) | 5.11 | (1.32 to 19.78)e | 0.018 |
Missing | 39 | 39 |
Modela N = 131 |
App group abstinence n (%) N = 65 |
Standard group abstinence n (%) N = 66 |
ORb | 95% exact CIc | p-valued |
---|---|---|---|---|---|
Adjusted for treatment group only | 12 (18.5) | 3 (4.5) | 4.70 | (1.18 to 27.32) | 0.023 |
Adjusted model, including treatment group and stratification variables | 12 (18.5) | 3 (4.5) | 4.71 | (1.17 to 27.64) | 0.025 |
Adjusted model, including treatment group, stratification and prognostic variables | 12 (18.5) | 3 (4.5) | 4.88 | (1.19 to 29.03) | 0.024 |
Missing | 39 | 39 |
Modela N = 209 |
App group abstinence n (%) N = 104 |
Standard group abstinence n (%) N = 105 |
ORb | 95% Wald CIc | p-valued |
---|---|---|---|---|---|
Adjusted for treatment group only | 25 (24.0) | 16 (15.2) | 1.76 | (0.88 to 3.53) | 0.112 |
Adjusted model, including treatment group and stratification variables | 25 (24.0) | 16 (15.2) | 1.78 | (0.87 to 3.63) | 0.114 |
Adjusted model, including treatment group, stratification and prognostic variables | 25 (24.0) | 16 (15.2) | 1.75 | (0.85 to 3.57) | 0.128 |
Modela N = 209 |
App group abstinence n (%) N = 104 |
Standard group abstinence n (%) N = 105 |
ORb | 95% exact CIc | p-valued |
---|---|---|---|---|---|
Adjusted for treatment group only | 25 (24.0) | 16 (15.2) | 1.76 | (0.83 to 3.79) | 0.153 |
Adjusted model, including treatment group and stratification variables | 25 (24.0) | 16 (15.2) | 1.76 | (0.82 to 3.87) | 0.159 |
Adjusted model, including treatment group, stratification and prognostic variables | 25 (24.0) | 16 (15.2) | 1.72 | (0.80 to 3.78) | 0.179 |
Modela N = 209 |
App group abstinence n (%) N = 104 |
Standard group abstinence n (%) N = 105 |
ORb | 95% Wald CIc | p-valued |
---|---|---|---|---|---|
Adjusted for treatment group only | 20 (19.2) | 21 (20.0) | 0.95 | (0.48 to 1.89) | 0.889 |
Adjusted model, including treatment group and stratification variables | 20 (19.2) | 21 (20.0) | 0.94 | (0.47 to 1.88) | 0.865 |
Adjusted model, including treatment group, stratification and prognostic variables | 20 (19.2) | 21 (20.0) | 0.91 | (0.45 to 1.83) | 0.793 |
Modela N = 209 |
App group abstinence n (%) N = 104 |
Standard group abstinence n (%) N = 105 |
ORb | 95% exact CIc | p-valued |
---|---|---|---|---|---|
Adjusted for treatment group only | 20 (19.2) | 21 (20.0) | 0.95 | (0.45 to 2.00) | 1.000 |
Adjusted model, including treatment group and stratification variables | 20 (19.2) | 21 (20.0) | 0.94 | (0.45 to 1.99) | 1.000 |
Adjusted model, including treatment group, stratification and prognostic variables | 20 (19.2) | 21 (20.0) | 0.91 | (0.43 to 1.93) | 0.935 |
Modela N = 209 |
App group abstinence n (%) N = 104 |
Standard group abstinence n (%) N = 105 |
ORb | 95% Wald CIc | p-valued |
---|---|---|---|---|---|
Adjusted for treatment group only | 16 (15.4) | 5 (4.8) | 3.64 | (1.28 to 10.33) | 0.015 |
Adjusted model, including treatment group and stratification variables | 16 (15.4) | 5 (4.8) | 3.69 | (1.29 to 10.59) | 0.015 |
Adjusted model, including treatment group, stratification and prognostic variables | 16 (15.4) | 5 (4.8) | 3.67 | (1.27 to 10.60)e | 0.016 |
Modela N = 209 |
App group abstinence n (%) N = 104 (%) |
Standard group abstinence n (%) N = 105 (%) |
ORb | 95% exact CIc | p-valued |
---|---|---|---|---|---|
Adjusted for treatment group only | 16 (15.4) | 5 (4.8) | 3.62 | (1.20 to 13.14) | 0.018 |
Adjusted model, including treatment group and stratification variables | 16 (15.4) | 5 (4.8) | 3.64 | (1.20 to 13.31) | 0.019 |
Adjusted model, including treatment group, stratification and prognostic variables | 16 (15.4) | 5 (4.8) | 3.59 | (1.18 to 13.18) | 0.021 |
Modela N = 209 |
App group abstinence n (%) N = 104 (%) |
Standard group abstinence n (%) N = 105 (%) |
ORb | 95% Wald CIc | p-valued |
---|---|---|---|---|---|
Adjusted for treatment group only | 28 (26.9) | 20 (19.1) | 1.57 | (0.82 to 3.01) | 0.178 |
Adjusted model, including treatment group and stratification variables | 28 (26.9) | 20 (19.1) | 1.56 | (0.81 to 3.00) | 0.183 |
Adjusted model, including treatment group, stratification and prognostic variables | 28 (26.9) | 20 (19.1) | 1.54 | (0.80 to 2.97) | 0.197 |
Modela N = 209 |
App group abstinence n (%) N = 104 |
Standard group abstinence n (%) N = 105 |
ORb | 95% exact CIc | p-valued |
---|---|---|---|---|---|
Adjusted for treatment group only | 28 (26.9) | 20 (19.1) | 1.56 | (0.78 to 3.19) | 0.234 |
Adjusted model, including treatment group and stratification variables | 28 (26.9) | 20 (19.1) | 1.55 | (0.77 to 3.16) | 0.243 |
Adjusted model, including treatment group, stratification and prognostic variables | 28 (26.9) | 20 (19.1) | 1.53 | (0.76 to 3.11) | 0.263 |
Participant | Case summary | Examples of themes and codes applied to excerpts | Mechanisms | Excerpts from interview |
---|---|---|---|---|
Quitting smoking | ||||
315 | Used the app to help get through strong cravings and avoid lapses (e.g. by charting progress in My Profile and Smoking Patterns). Also employed strategies suggested by the app. | Themes Time and context Smoking Support Cessation as a process Preparation stage Drivers to engagement Codes Motivations and justifications Lapses including previous quit attempts Current context including pandemic |
Increased financial constraints, health concerns | I quit pretty quick, which I’ve not been able to do before. I’ve been smoking on and off since I was 15, and the last time I was able to quit was when I was pregnant with my 15-year-old daughter. |
‘Equipping’ > Use of strategies suggested by app | Interviewer: What do you think helped with that this time? Well, I think money, definitely. I lost my job in October, so that was definitely motivation to stop. [ … ] It’s an expensive habit. But also, my health in general was a huge motivation [ … ] Interviewer [ … ] did you try out any of the strategies that the app suggested? |
|||
Strategies and aids Tracking cravings My Profile and Smoking Patterns Boredom Quit successful Quit date |
Tracking progress, monitoring craving levels | I did the exercise ones, and I spent a lot more time indoors with my daughter. That was the strategy that said, ‘be indoors with people who don’t smoke’. We don’t smoke inside, well, we don’t smoke at all now, but we don’t smoke inside the house, so I spent a lot more time like, forcing myself not to go outside. I also changed up my morning routine because we used to sit outside, have a cup of coffee, smoke and then go to work. So, my partner and I started exercising in the morning together and then we’d have a … well, I’d have a decaff coffee and then go to work, kind of thing [ … ] [ … ] I was tracking my cravings and making sure that I was still on track. And noticing, okay, so I’ve only got three more days until you [the cravings] should start going away, sort of thing. You know, my cravings went from being really high to non-existent, but it took about a week to actually get there. [ … ] Just to remind myself that I did want to stop [laughs]. |
||
‘Committing’, ‘Preparing’ | Interviewer: So, from your survey, it seems that [ … ] you haven’t smoked in the first two weeks of the study – how are things going now? I haven’t smoked at all. I have no desire to. I haven’t smoked since Dec 12th. Interviewer: [ … ] Do you think the app had any influence in this attempt, what would you attribute to … ? |
|||
Well, I think the app gave me the kick up the bum. It made me make a commitment, ’cause I’ve been umming and ahhhing about it for ages and just going, ‘oh, I need to quit but you know, it’s the pandemic and I’m bored’. [Laughs] I think it gave me … when you guys contacted me about using … well, I contacted you about using the app and then it said that I could use it, I think that gave me the push. It was something a bit more concrete and an actual set date and it really did keep me on track. | ||||
114 | Actively engaged with the app. She attributed her success to the app, at least in part. Training the app helped her reduce smoking before making the quit attempt. Used strategies from the app to maintain the quit attempt and avoid lapse. App used most in stage 1 (preparation) and then need for the app decreased. | Themes Drivers to engagement Preparation Cessation as a process Support Disconnecting/barriers to engagement |
‘Reporting and preparing for quit day’ > reporting smoking leads to smoking reduction | To be honest, I really thought I was going to fail at quitting but it [the app] actually works. I found that before the quit, when I had to log each of the times I was smoking, actually made me smoke less. |
‘Equipping’ > use of strategies suggested by app | Yeah, I think it was that because if you smoked more than five in a day, you got a little sad face as well. So, I noticed as I was getting towards the quit date, I was actually thinking, do I need to really have this smoke or not? I was cutting down before which I think helps for when I did quit. I hadn’t been smoking as much just before then. | |||
Codes Reporting leads to reduction Quit date Strategies suggested by the app |
Used nicotine replacement lozenges | [ … ] Like I said, in the first week, I found it [the app] the most useful. I think I’d gone and brought a pack of Mint Imperials, so if I’m sat bored in a meeting, at least I can have one of those, to keep my hands and mouth busy for a few minutes. |
||
[ … ] I was really, actually surprised that it worked. I’ve tried to do it before, and I failed within a week or two. So, it’s been really good. [ … ] Interviewer: Okay, so you said that you used the app quite a bit in the run up to quitting, are you still sort of looking at it or has that dropped off now? I think I’ve gone on it once in the last like, month probably. Just to see how much money I’ve saved. I do look in my bank account and think, I do not see that money in there [laughs]. But, I have just moved house, so that’s why. |
||||
211 | Briefly used the app to identify where he smoked and then avoided those trigger locations. Attributed his ability to quit both to lockdown and the app. | Themes Drivers of engagement Disconnecting/barriers to engagement Time and context Preparation Smoking |
‘Committing, reporting and preparing for quit day’ > setting quit date Used app to map and avoid contextual cues |
I like the … it didn’t last very long because I set a very short quitting date, but I like the idea of reporting where and when. I did that for a couple of days and then I purposely went to different routes; when I was on a walk and that in the mornings, I would go on different routes to avoid that place. It became a routine that I would light a cigarette at that place, so I went a different way. [ … ] So, I thought that was quite clever, recording where you’d had a cigarette and all that kind of stuff. [ … ] |
Codes Quit date Reporting smoking with app Used reporting to change routine |
Social context supportive of quit attempt | I think lockdown has played a major part in it [cessation]. I’m not out and about in public or out socialising with my mates and all that kind of stuff. Because my wife doesn’t smoke and because I’m at home, it’s like, I just can’t be bothered with it. [ … ] | ||
Mentions of family and others Lapses/previous quit attempts |
I’m extremely happy about it. I think it’s brilliant. I’ve tried giving up at least, I’d say half a dozen times in my life and each time I’ve given up, unfortunately I’ve gone back for one reason or the other. Obviously, this time, I’m determined not to do. In previous attempts, I found it hard work. It’s not easy. All these people that say they found it a walk in the park … nonsense. In the past I’ve found it very, very difficult but this time, I’ve not struggled at all. | |||
311 | Used the app extensively in stage 1 in multiple locations. He found the app made him aware of his smoking patterns and triggers and attributes this to being able to change his behaviour. Felt that the app messages had bolstered his motivation to maintain the quit. He gives an anecdote of when he was about to lapse when a message from the app reminds him that he is no longer a smoker. Largely disengaged with the app after doing the preparation stage because he saw it as connected with his old life as a smoker. | Themes Drivers of engagement Smoking Cessation as a process Support Preparation Smoking |
‘Validating’ > bolstering quit attempt Uses app to understand smoking patterns and triggers |
I think sometimes doing it for yourself or for someone else, isn’t always enough. Especially if you’ve got a really bad craving and this time around … I think I told you before that this time I haven’t been craving them whatsoever. But I know that in previous quit attempts, and there have been many. I would sit there with the cold sweats and the twitchy fingers going, ‘it’s okay, I’m doing something good for myself’. I think you’re always after that extra bit of validation. |
Codes Inspiring me/validation Cravings Lapses/previous quit attempts Messages – thoughts on Strategies and aids Self-efficacy |
‘Reporting’ > enabled to see smoking patterns | I think a lot of the messages that are displayed through the app, they do just that. They’re not so much reminding you why you’re quitting. I think you know why you’re quitting and that’s why you’re quitting. What they do is they sort of they just bolster your defences a little bit. The odd fact here, the odd figure there. [ … ] |
||
Reporting with the app Identity Message not appropriate for context/situation |
App messages re-enforced identity as a non-smoker | When I started using the app, quitting for me was always something that I would like to do but it just always felt like it’s just that little bit out of reach. I just couldn’t quite grasp it. It was always something that I wanted to do but I couldn’t quite get there. Before the app, that was no different. I very much attribute my quitting to the app because it helped me subtract the patterns of me smoking. It allowed me to see when I was smoking more, and it then allowed me to unpick why I was smoking more. That was the key to the lock for me. It was helping me see that was why I was smoking more, when the only solution I could draw was because I felt I should. That really is a pathetic reason and it sort of broke down that barrier of not being able to quit. | ||
[ … ] I remember [ … ] I was having a really bad day. [ … ] I called into a services and I don’t know why because I’d never been there before in my life but I went and got a drink and I said, ‘I’ll take 20 [names cigarette brand] and a lighter,’ and that bloody app pops up with a little message saying, ‘have you thought about smoking today?’ And I immediately said, ‘do you know what, forget the cigarettes’. The timing was absolutely impeccable. It was completely random because I’d never been in that services before. |
||||
I have no idea why the message popped up, but it did. So as much as I say that I ignored the app after I quit – but perhaps I wasn’t thinking about smoking, I was just a bit stressed, I was a bit irritable. Then this message pops up. Even though it was completely nonsensical, it allowed me to take a deep breath and think, okay, so that message doesn’t make sense to my situation at the moment, but I was a smoker, I’m now not. I’m a lot better off for it. So, my app use definitely waned after I quit. In my mind, that was part of the design. On the lead up to quitting, the way it tracks how you smoke, when you smoke, where you smoke. That doing that by itself is the reason that I do not smoke anymore. |
||||
115 | Employed strategies suggested by the app to manage cravings and avoid lapsing. His engagement with the app was greatest in stage 1 – preparing for quit | Themes Support Preparation Disconnecting Codes Strategies and aids/suggested by the app |
‘Equipping’ with strategies | Interviewer: So, the app suggests various strategies that you can try whilst on a quit attempt, did you try any of those? I don’t think I used any specific ones. I used the ones that said, try and preoccupy yourself with something else. I found that if I happened to catch it at that point when I was having a craving then I did tend to find it helpful. |
Cravings Vaping – substitution Quit date |
Substitution of smoking roll-ups with vaping ‘Preparing’ > used app to prepare for quit attempt |
Interviewer: Have you tried any other quit smoking aids or support since you started the study? Yeah, I’ve been using my vape, so -but I haven’t been using any other apps or anything like that. [ … ] Yeah, but I’m trying to sort of ease up on it and ween myself down. Obviously, I’ve still got nicotine in the vape. [ … ] |
||
Disengaged with app as felt reduced need for support | I didn’t find it any less useful per se [in stage 2], but I did find in that build up, it was more useful then in that build up. To be like, trying to ween down in this period and getting to the 1st of February when I set mine, then like I just found it … the further I got along with my quit attempt, the easier it was becoming. Interviewer: Yeah, so you didn’t feel the need to engage with it so much? |
|||
Yeah, so I found I had less reliance in the app itself, but I wouldn’t say I found it any less useful. Interviewer: Okay, are you still looking at the app from time to time or has that stopped now? I haven’t looked at it for a few days to be fair. |
||||
Lapse | ||||
206 | Engaged with the app by reporting smoking. This made him aware of his smoking patterns and triggers (e.g. boredom). Made the transition to vaping and reduced the number of cigarettes smoked. | Themes Support Smoking Drivers of engagement Codes Boredom Reduction Vaping – substitution Messages – thoughts on |
‘Reporting’ > promoting self-awareness of triggers and patterns Substitution of cigarettes with vaping ‘Equipping’ > use of strategies suggested by app |
It made me monitor pretty much everything in my life as well. Every time I needed to for a cigarette, like I say, I wouldn’t even think about it, I would just go. I was on 20+ cigarettes a day. At this moment in time, I’m on something called a vape. That’s mainly because it’s something to do with my hands. Now I have a cigarette in the morning, and I have a cigarette after my meal still too before bed. So, I’ve cut down from about 20+ a day to around 4–5 a day [ … ] Normally it would be, oh, ‘I’m bored’ or if I’m watching TV – this is on an advert; I’ll just quickly nip out and have a smoke now but it didn’t pop in my head. [ … ] In the end, what I was doing was making a cup of tea or sandwiches or … just not thinking about going for a smoke. |
Self-monitoring/self-regulation boosted | ‘Validating’ app messages | Interviewer: Okay, so quite a big change. Yeah. It’s about keeping my hands busy as well, it’s very difficult when you’ve been doing it for so long. I’ve been smoking since I was 11 [ … ] I could probably stand with a smoker and not think about it. The smell might put us on the edge a little bit but like I say, I’ve always got my vape in my pocket. [ … ] |
||
All in all, the app is really great. Little messages really, really help. Especially when you know in the back of your head that you don’t need to go for one just because you’re bored. With the little messages there, for extra support, just telling you what your mind knows, that you don’t need to go for one. An app knows that you don’t need to go for one, so why are you going for one? | ||||
111 | Did not feel ready to quit when her quit date arrived and didn’t reset her quit date. Became demotivated and decided that the goal of quitting was not attainable within the time frame and disengaged. | Themes Preparation Time and context Disconnecting/disengagement Suggestions from participants Codes Quit date Christmas/social situation outweighs support |
Didn’t succeed in resetting quit date Stress, time of year/situation Still smoking, discontinued efforts to quit |
I set my quit date for as far in advance as I could because I wanted my quit date to be on like the 1st January, so it was like a New Year’s resolution. I don’t think it gave me the option to do that, so I had to put it on a few days before that, which was like just after Christmas. So, it would have been good to be able to do that. I think at the time of my quit date, it was when I was working over Christmas, so it was like a really stressful period. So, I was actually smoking more. So, I felt like a bit of a disappointed with myself and when I realised it wasn’t really possible in that time frame, so that’s when I kind of stopped using it. |
120 | Liked the app and reported smoking and set a quit date. In stage 2 he lapsed. This was over Christmas while socialising. He did report the lapses but largely disengaged from the app because he considered his attempt had failed. | Themes Drivers to engagement Disconnecting/barriers Time and context Support Smoking |
Social situation outweighs cessation support Saw lapse as a failure and discontinued efforts to quit |
[ … ] it was really useful and possibly even inspirational. Just the tips and the nature of it, you know, something there to aid you and encourage you to give up, it was a good thing. Also, that it had a real-world effect, it thought that it had a … yeah, it’s a good idea and it was a good experience. [ … ] |
Codes Christmas/social situation outweighs support Reduction Reporting encourages self-monitoring Strategies and aids/inc. suggested by the app Reporting smoking post quit date |
Attempted some app strategies Disengaged with app support messages after reporting smoking post quit date |
[I] did start to tail off using it; the main reason for that is … the most risky situation for me to start smoking is either when I’m on my own and at a loose end, so kind of pottering around. Another one is, and even more so perhaps, socialising. Being with people. Christmas and New Year’s intruded within the time period that I’d set myself. I [ … ] I found myself smoking and reaching for cigarettes even more rapidly than I had been, certainly more so than the early stages of me using the app. | ||
The app had a direct effect on reducing the number of cigarettes at first, probably for the first 2 or 3 weeks, so not really so long. Then towards Christmas, it would be too easy to pick up … and when I realised that I’d failed; I’d crossed the line, and I hadn’t given up. It just picked up again. [ … ] |
||||
Yeah, so I’d tried to go back to it [the app]. Like I say, sort of Christmas and New Year were what broke me away from it. I thought I’d go back to it for a few days or so and then it just began to tail off even more, so, yeah. It’s unfortunate that it was … for me, anyway, was around that time [ … ] But certainly, at the beginning, first 2 or 3 weeks, I was battling and every cig … it really does get me down because every cigarette that I have, I think about it and you want to just record the stress and thinking about what it’s doing to me and so on. | ||||
[ … ] Interviewer: Did you try any of the strategies that were suggested by the app? I know that was quite a while ago now. [ … ] Yeah, I remember sort of delaying for a few minutes; I did do that, and it encouraged me to do that; smoking a cigarette is something that is often, not every time but often, I’d hesitate before doing it but then I would end up doing it anyway. So, there’s always that kind of delay. |
||||
Interviewer: Yeah, I can see that. So, I can see that you’ve reported smoking post the quit date on the app. Do you have any memory of how helpful or otherwise you found those messages? When you get a sort of – you’ve lapsed message. By the time I got to that stage, I wasn’t really following the app, so I didn’t really take in those messages. I saw that it was giving messages, but I didn’t really read them to be honest. The main thing was that I was still smoking post the date. |
||||
210 | Was smoking at the time of interview. She disengaged with the app after lapsing and deleted it. | Themes Smoking Disconnecting/disengagement |
Negative emotions around lapsing | No, I used it for about a month. Of course, when I started smoking again after that, I felt bad and embarrassed, so I didn’t use it much after that but … |
Codes Embarrassed Reporting smoking post quit date |
Saw lapse as a failure and discontinued efforts to quit | Interviewer: Okay, did you still see messages and things still popping up and did you still report when you were smoking after the quit date? | ||
Lapses including previous quit attempts Messages – thoughts on |
Disengaged with app support messages after reporting smoking post quit date | I did, yeah, and then after a couple of days, I deleted the app when I saw that it was using … ’cause I didn’t see the point of using the data when I was back smoking. Interviewer: Okay and did you find any of the messages that it sent you when you’d started smoking again … did you find those helpful at all or had you disengaged by that point? |
||
Just disengaged by that point. Just more about, oh, another failed attempt. I’ll stop eventually [laughs]. Interviewer: If you don’t mind me asking, what sort of triggered you to restart smoking again? Funnily enough, just the worst time to stop smoking, shifts and things.’ Cause I work for an agency, I don’t always get enough shifts and things, so that stresses me out. I’m thinking, ‘oh my god, how am I going to pay my bills?’ You end up smoking more and you shouldn’t be spending money on those things [laughs]. |
||||
[ … ] Interviewer: [ … ] if you try again at some point, would you be interested in trying the app again? Yeah, I would. It’s easy when you live alone to have something giving you support and reminders because you haven’t got someone here giving you a cheer and a, ‘well done’. You know. It’s good to have an app [laughs]. |
£20 incentive (n = 101) |
£10 incentive (n = 103) |
Overall (n = 204) |
|
---|---|---|---|
Age at consent: mean (SD) | 42.0 (9.6) | 40.3 (10.6) | 41.1 (10.2) |
Gender: n (%) | |||
Male | 51 (50.5) | 40 (38.8) | 91 (44.6) |
Female | 50 (49.5) | 63 (61.1) | 113 (55.4) |
Number of cigarettes smoked per day: n (%) | |||
Less than 16 | 63 (62.4) | 62 (60.2) | 125 (61.3) |
16 or more | 38 (37.6) | 41 (39.8) | 79 (38.7) |
SES: n (%) | |||
Low | 33 (32.7) | 26 (25.2) | 59 (28.9) |
High | 68 (67.3) | 77 (74.8) | 145 (71.1) |
Socioeconomic grade: n (%) | |||
1 (highest) | 45 (60.8) | 47 (61.8) | 92 (61.3) |
2 | 5 (6.8) | 8 (10.5) | 13 (8.7) |
3 | 7 (9.5) | 4 (5.3) | 11 (7.3) |
4 | 10 (13.5) | 10 (13.2) | 20 (13.3) |
5 (lowest) | 7 (9.5) | 7 (9.2) | 14 (9.3) |
Missing | 27 | 27 | 54 |
Ethnicity: n (%) | |||
White | 91 (90.1) | 95 (92.2) | 186 (91.2) |
Indian | 1 (1.0) | 0 (0.0) | 1 (0.5) |
Pakistani | 0 (0.0) | 2 (1.9) | 2 (1.0) |
Bangladeshi | 2 (2.0) | 1 (1.0) | 3 (1.5) |
Black African | 0 (0.0) | 2 (1.9) | 2 (1.0) |
Black (other) | 1 (1.0) | 0 (0.0) | 1 (0.5) |
Asian | 3 (3.0) | 0 (0.0) | 3 (1.5) |
Mixed race | 2 (2.0) | 1 (1.0) | 3 (1.5) |
Not given | 1 (1.0) | 2 (1.9) | 3 (1.5) |
Number of cigarettes usually smoke a day: mean (SD) | 15.6 (7.1) | 15.3 (6.8) | 15.4 (6.9) |
Employment status: n (%) | |||
In work during last 12 months | 74 (73.3) | 75 (72.8) | 149 (73.0) |
Out of work for more than 12 months | 26 (25.7) | 19 (18.5) | 45 (22.1) |
Retired | 0 (0.0) | 1 (1.0) | 1 (0.5) |
Full-time student | 1 (1.0) | 8 (7.8) | 9 (4.4) |
Occupation: n (%) | |||
Modern professional | 20 (27.0) | 17 (22.4) | 37 (24.7) |
Clerical | 10 (13.5) | 8 (10.5) | 18 (12.0) |
Senior manager/administration | 11 (14.9) | 11 (14.5) | 22 (14.7) |
Technical | 10 (13.5) | 5 (6.6) | 15 (10.0) |
Semiroutine manual/service | 6 (8.1) | 11 (14.5) | 17 (11.3) |
Routine manual/service | 5 (6.8) | 4 (5.3) | 9 (6.0) |
Middle/junior manager | 8 (10.8) | 8 (10.5) | 16 (10.7) |
Traditional professional | 4 (5.4%) | 12 (15.8) | 16 (10.7) |
Missing | 27 | 27 | 54 |
Highest qualification: n (%) | |||
No formal | 9 (8.9) | 4 (3.9) | 13 (6.4) |
General Certificate of Secondary Education or similar | 24 (23.8) | 18 (17.5) | 42 (20.6) |
A/Advanced Subsidiary Level or similar | 28 (27.7) | 23 (22.3) | 51 (25.0) |
Degree or similar | 36 (35.6) | 50 (48.5) | 86 (42.2) |
Other | 4 (4.0) | 8 (7.8) | 12 (5.9) |
Heaviness of Smoking Index: n (%) | |||
Low | 26 (25.7) | 37 (35.9) | 63 (30.9) |
Moderate | 67 (66.3) | 55 (53.4) | 122 (59.8) |
High | 8 (7.9) | 11 (10.7) | 19 (9.3) |
FUTS: n (%) | |||
A little of the time | 10 (9.9) | 6 (5.8) | 16 (7.8) |
Some of the time | 28 (27.7) | 38 (36.9) | 66 (32.4) |
A lot of the time | 41 (40.6) | 43 (41.8) | 84 (41.2) |
Almost all the time | 14 (13.9) | 9 (8.7) | 23 (11.3) |
All the time | 8 (7.9) | 7 (6.8) | 15 (7.4) |
SUTS: n (%) | |||
No urges | 1 (1.0) | 0 (0.0) | 1 (0.5) |
Slight urges | 6 (5.9) | 5 (4.9) | 11 (5.4) |
Moderate urges | 43 (42.6) | 39 (37.9) | 82 (40.2) |
Strong urges | 29 (28.7) | 38 (36.9) | 67 (32.8) |
Very strong urges | 16 (15.8) | 18 (17.5) | 34 (16.7) |
Extremely strong urges | 6 (5.9) | 3 (2.9) | 6 (5.9) |
WISDM Automaticity subscale score: mean (SD) | 4.92 (1.75) | 4.59 (1.72) | 4.75 (1.74) |
WISDM cue exposure/associative processes subscale score: mean (SD) | 4.48 (1.27) | 4.74 (1.40) | 4.61 (1.34) |
Self-efficacy average score: mean (SD) | 1.73 (0.86) | 1.63 (0.88) | 1.69 (0.87) |
6-month question | £10 incentive proportion N = 103 (%) |
£20 incentive proportion N = 101 (%) |
Overall proportion N = 204 (%) |
---|---|---|---|
Have you smoked any tobacco in the last 6 months? | 74 | 79 | 76 |
Are you currently smoking tobacco? | 74 | 81 | 77 |
How many cigarettes do you currently smoke? (among those reporting currently smoking) | 89 | 96 | 93 |
When did you last smoke a cigarette? | 69 | 78 | 74 |
How many serious quit attempts have you made during the study? | 68 | 78 | 73 |
FUTS | 68 | 78 | 73 |
SUTS | 68 | 78 | 73 |
Smoking aid questions | 66 | 78 | 72 |
App questions (intervention group only) | 71 | 76 | 73 |
EQ-5D-5L questions | 66 | 78 | 72 |
Self-efficacy questions | 67 | 78 | 73 |
List of abbreviations
- BCT
- behaviour change technique
- CEAC
- cost-effectiveness acceptability curve
- EMA
- Ecological Momentary Assessment
- EoD
- End of Day
- EQ-5D-5L
- EuroQol-5 Dimensions, five-level version
- FUTS
- frequency of urges to smoke
- GTS
- geofence-triggered support
- JITAI
- Just-In-Time Adaptive Intervention
- LT
- learning theory
- NRT
- nicotine replacement therapy
- NSSEC
- National Statistics Socio-Economic Classification
- PPI
- patient and public involvement
- PSS
- Personal Social Services
- QALY
- quality-adjusted life-year
- QOL
- quality of life
- REC
- Research Ethics Committee
- RCT
- randomised controlled trial
- SCT
- social cognitive theory
- SES
- socioeconomic status
- SHEAP
- Statistical Analysis and Health Economics Plan
- SUTS
- strength of urges to smoke
- SWAT
- Study Within A Trial
- TSC
- Trial Steering Committee
- WISDM
- Wisconsin Inventory of Smoking Dependence Motives