Notes
Article history
The research reported in this issue of the journal was funded by the PHR programme as project number 11/3004/02. The contractual start date was in January 2013. The final report began editorial review in January 2015 and was accepted for publication in May 2015. The authors have been wholly responsible for all data collection, analysis and interpretation, and for writing up their work. The PHR editors and production house have tried to ensure the accuracy of the authors’ report and would like to thank the reviewers for their constructive comments on the final report document. However, they do not accept liability for damages or losses arising from material published in this report.
Declared competing interests of authors
none
Permissions
Copyright statement
© Queen’s Printer and Controller of HMSO 2015. This work was produced by Perkins et al. under the terms of a commissioning contract issued by the Secretary of State for Health. This issue may be freely reproduced for the purposes of private research and study and extracts (or indeed, the full report) may be included in professional journals provided that suitable acknowledgement is made and the reproduction is not associated with any form of advertising. Applications for commercial reproduction should be addressed to: NIHR Journals Library, National Institute for Health Research, Evaluation, Trials and Studies Coordinating Centre, Alpha House, University of Southampton Science Park, Southampton SO16 7NS, UK.
Chapter 1 Background and aims
Background to the project
In April 2011 the National Institute for Health Research (NIHR) Public Health Research (PHR) programme invited research proposals to assess the impact of street lighting reduction schemes on public health. The background to the commissioning brief was as follows:
The UK has about 7.5 million street lights which cost up to £500 million per year. The cost of running and maintenance has risen significantly over the last five years. A number of local authorities are changing the provision of street lighting for financial reasons, often between midnight and 5am. Very little research has explored the public health impact of street lighting. The little research available was conducted in the UK and the USA predominantly in the 1970s. Conclusions on the public health impact of street lighting are uncertain at best. In this call, the programme is interested in comparatively short studies. 1
A research proposal from a team comprising academics from the Transport and Health Group, London School of Hygiene & Tropical Medicine, and the Department of Security and Crime Science, University College London, was commissioned, and the research project started in January 2013.
Aims of the project
The aims of the project were:
-
to conduct a nationwide analysis of street-level data for all local authorities in which street lighting reduction schemes had been introduced by 2012 and to quantify (with adequate power and precision) the impact of these schemes on the incidence of road traffic injuries and crime
-
to use a rapid appraisal to identify articulated public views about street lighting reduction schemes and their unforeseen consequences or benefits, the role that these views have played in local decision-making and less explicit concerns evidenced in accounts of the impact of reduced street lighting
-
to develop an inventory of all quantified costs and benefits of street lighting reduction schemes and to examine variation in the costs and benefits of schemes
-
to convene a workshop with representatives of key stakeholder groups in order to maximise knowledge transfer.
This report presents the rapid appraisal first (see Chapter 2), followed by the impacts of street lighting reduction on crime and road traffic injuries (see Chapter 3) and then the study of costs (see Chapter 4). Finding from the stakeholder workshop are integrated in the final discussion (see Chapter 5).
Stakeholder collaboration
There are a number of key stakeholders with an interest in street lighting, including the local authorities and other lighting authorities that provide it, and a range of ‘publics’ who might benefit from its provision or reduction. To obtain good-quality data, we partnered with the Institution of Lighting Professionals (ILP), the main professional organising of local authority lighting engineers, and local authorities across England and Wales. It is difficult, however, to identify a sensible ‘representative’ for public groups, as there are a number of organisations with vested interests (e.g. astronomy groups or environmental non-governmental organisations), but no obvious ‘population’ representatives. The rapid appraisal was designed specifically to engage the public and to identify which ‘publics’ might have an interest in the topic, so it would have been difficult to identify a ‘representative’ of the public before the project was complete. We did, however, include a range of stakeholders in the project advisory group, including representatives from lighting professionals and local government.
The project was, therefore, called the LANTERNS (Local Authority collaborators’ National Evaluation of Reduced Night-time Streetlight) project.
Chapter 2 Public views and private concerns: a rapid appraisal of the impact of reduced street lighting at night on well-being in England and Wales
Introduction
Electric street lighting has been a feature of urban and suburban settlement since the end of the nineteenth century. 2 Indeed, the electrification of lighting has in many ways defined the modern city, in extending the visibility of its public spaces, inhabitants and itinerants beyond the hours of natural daylight. 3,4 As an essential, if somewhat underappreciated, feature of modern places, abundant electric light has come to provide the mundane backdrop to contemporary life in the cities and towns of high-income countries and mark what Augé calls the ‘non-places’ of supermodernity, such as brightly lit service stations, motorways and airports, in which artificial light erodes the temporal markers of day and night. 5
However, in many areas of England and Wales, as in other countries, the taken-for-granted assumption that streets and public spaces will be lit at night has been disrupted in recent years. Many local authorities responsible for street lighting across England and Wales have reduced, or are considering reducing, some street lighting at night, in part to reduce costs, but also with considerations of contributing towards climate change mitigation and reducing environmental light pollution. 6 A rapid growth of technological innovations over the past 20 years has enabled greater control over the colour, intensity and switching on schedules of public lighting stock,7 enabling local lighting authorities to reduce street lighting at night using a range of interventions. These include removing or switching off lanterns in street light columns; reducing the number of hours that they are switched on (part-night lighting), replacing sodium lanterns with white light-emitting diodes (LEDs); and dimming lanterns through centrally managed systems. These changes, particularly switch-off in urban areas, have attracted considerable public and media concern, centring on crime, perceptions of safety, and road safety. 8,9 However, potential positive well-being impacts of reduced lighting have also been noted, in particular for amateur astronomy. 10 Reductions in light at night might, in theory, also mitigate the negative health impacts some have claimed result from a growth in, and changing frequencies of, artificial light in the environment. 11,12 Although the evidence base to date is weak,13 a growing concern with light pollution as a potential hazard to health draws on studies of animals14 and shift workers to identify disruptions in circadian rhythms and endocrine processes, which can affect sleep15 and, theoretically, health outcomes such anxiety, depression and even cancer incidence. 16,17 The amount, and quality, of light at night has thus become a public health as well as political issue.
There is, however, little research on public concerns about the potential detrimental or positive health impacts of street lighting reductions. To date, studies on fear of crime and perceptions of safety have focused largely on improvements to lighting, with findings identifying mixed results on perceptions of crime, personal security and actual travel behaviour after lighting improvements. 18,19 Reductions in street lighting have received little attention in research and it is not known how far the concerns voiced in media coverage are shared by the wider public. Specifically, there is no literature on how the public view the relationships between reductions in night-time street lighting and well-being. As a first step in studying the impact of reduced street lighting on crime and road injury, therefore, we conducted a rapid appraisal in eight areas of England and Wales to explore public views of the broader potential well-being impacts of reduced street lighting.
Methods
Given the limited evidence to date on how street lighting does impact on health outcomes, and the diverse interventions being implemented across England and Wales, this component was exploratory rather than hypothesis testing. The aims were to map public views on the effects that street lighting reductions have on health and well-being to identify areas for further research and to provide context for the impact evaluation. We therefore needed to include settings with a range of specific interventions and diverse localities (rural, suburban, urban) and to document ‘public views’ across different forums to identify key domains of interest to the public, strength of feeling and implications of these views for well-being. A rapid appraisal design enabled us to include a broad range of settings and of sources of data. Originally developed for rural development projects, rapid appraisal is characterised by the production of results in a shorter time frame than traditional ethnographic methods. There has been some interest in its use in public health research in high-income countries, particularly in conjunction with epidemiological methods. 20 Beebe21 identified three key elements to the approach: a system perspective, the triangulation of different data sources and an iterative approach, generally utilised by teams drawing on more than one discipline. We adopted this approach, with a small multidisciplinary team of researchers conducting focused ethnographic visits (2–3 days) to the study areas to generate a range of data. The eight areas (Table 1) were chosen to reflect the range of lighting authorities and interventions across England, Wales and different geographical settings across the two countries.
Local authority area | Street lighting intervention | Fieldwork settings |
---|---|---|
Hertfordshire County Council | PNL approved, trialled and implemented from November 2010 with about 70% of lights operating on this basis; also trimming and white light | Rural/suburban towns and villages in London’s commuter belt |
Buckinghamshire County Council | Switch-off selected street lights at 12 a.m. from August 2007; extended to more areas in April 2008 | Suburban towns and villages |
Shropshire Council | PNL scheme to convert 70% of lights in progress since 2012; also trialled dimming and white light | Shrewsbury and town and parish councils in surrounding towns and villages |
Wakefield Council | Trials of dimming and some white light | City centre and suburbs |
Swansea Council | Around 1000 lights switched off; also dimming and white light | City centre, suburbs and surrounding rural areas |
London Borough of Hackney | White light | Inner London borough |
London Borough of Southwark | White light, trimming | Outer London borough |
City of Westminster | White light-only policy since 2004 | London borough with large numbers of visitors |
These areas were included to provide a maximum variation sample in terms of local populations and interventions, rather than to provide comparative cases. We focused fieldwork in those areas with part-night lighting or switch-off.
Ethnographic data
Within each area, we interviewed key stakeholders (local authority lighting professionals and councillors); collected documentary evidence (including local authority plans, blogs, e-mails and letters to residents’ associations, local newspapers and local authorities); reviewed local authority consultations (if available); and conducted focused ethnographic visits. The data from these visits included field notes from ‘walk-arounds’ of areas with street lighting reductions, including informal intercept interviews and in-depth individual and group interviews (which were recorded and transcribed) with a mix of residents, visitors and workers. A topic guide for the formal interviews included prompts on whether or not changes had been noticed; what impact they had on mobility, sleep, security and feelings about their neighbourhood; what they did differently after lights had changed; and whether or not they had taken part in any formal consultations. Participants were asked to also complete a brief questionnaire covering demographic information [age range, gender, (last) occupation and ethnicity]. Group interviews were all with natural groups of participants who knew each other already. Intercept interviewees were told we were researching street lighting, and asked if they had noticed changes in their area and what they thought about those changes, if noticed. Fieldwork was conducted between April 2013 and December 2014.
Sampling and recruitment for the rapid appraisal
For the formal in-depth individual and group interviews, we used a purposive sampling strategy to identify a range of participants. To do this, we first identified contacts for community groups in the selected areas using web searches. These included sports clubs, residents’ associations, choirs and youth organisations. We also approached key informants likely to be knowledgeable about the impact of reduced street lighting, such as residents’ association chairs and police officers. These contacts were sent information about the project and invited to take part. From those who responded, we selected a range of participants (by age, location and employment) and snowballed the sample by inviting them to pass the invite on to colleagues or neighbours. Sampling was iterative, in that ongoing analysis suggested new lines of enquiry or individuals to include. For instance, young people were identified as particularly affected by reductions, so we recruited through a youth mentoring scheme. Once formal interviews were scheduled, we undertook field visits which included walk-arounds in daytime and after dark in locations affected by part-night or switch-off across the eight areas. Given that those volunteering for formal interviews may be more likely to have strong views about the topic, intercept interviews were an opportunity to gauge other perspectives. Intercept interviews were opportunistic, in that the research team approached those using cafes, pubs, public transport and shops during walk-arounds between and after scheduled formal interviews. We deliberately included workers likely to be using the streets early in the mornings or at night, such as police officers, hospitality workers and transport workers. The aim was not to get a statistically representative sample of any wider population, but to ensure that we included a large range of people in different contexts, such as group discussions and more private settings. This sample has the limitations of any volunteer (for the formal interviews) and convenience (for the intercept interviews) sample, in that the views elicited may not be representative of the whole population. However, the sample was appropriate for our aim for the ethnographic component of the rapid appraisal, which was to map the content and range of views, in a relatively efficient way, in a range of contexts. It is unlikely that any more systematic sampling strategy would have identified additional content domains. In total, the data set comprised formal individual or group interviews with 59 residents or workers and 16 stakeholders (local authority lighting professionals, lighting providers); 63 informal intercept interviews; 112 documents; and field notes from locations across the case study areas.
Given the limitations of a convenience sample for assessing the extent or representativeness of views, we also included a household survey in one area after initial fieldwork had generated the main domains of interest to the public.
Household survey
The aim of the household survey was to estimate the prevalence of reported negative and positive well-being impacts of reduced street lighting and identify whether or not reduced street lighting did have an impact on well-being in core domains identified in ethnographic work. We identified one area in Shropshire where lighting reductions at night had been introduced in selected streets and were scheduled for other streets. Using data provided by the local authority on implemented and planned lighting changes, the roads were divided into 12 strata based on whether or not lighting reductions had been introduced (yes/no), tertile (low/medium/high) of deprivation of the census lower super output areas (LSOAs) in which the roads were located, and proportion of postcodes within the LSOAs that were pubs, restaurants, shops or other businesses (higher/lower than 2%, the median proportion). We then randomly selected roads within each of the 12 strata and obtained the addresses for every residence on the selected roads to achieve a total sample of 500 houses in streets where lighting had been reduced at night and 500 in streets unaffected to date. Even if only half of households responded to the survey, the study would have 90% power to detect a 10% absolute difference between affected and unaffected streets in prevalence of well-being impacts at the 5% significance level. A self-completed questionnaire was designed to assess feelings about neighbourhood and local authorities, safety, fear of crime, impact of street lighting on sleep and the importance of seeing the night sky (see Appendix 1). After piloting, this was mailed to residents, with a choice of return by enclosed stamped addressed envelope or online, followed up by one reminder. A total 483 responses (476 postal and 7 online) were received, comprising 250 (i.e. 50%) responses from the part-night lighting group and 233 (47%) from the unaffected streets. There were no significant differences in the distributions of respondents from the affected and unaffected streets according to gender, age group or the number of adults in the household.
Analysis
Qualitative data (interviews transcripts, field notes, documentary data and open comments on the questionnaire) were analysed using thematic content analysis,22 with a coding frame drawing on both the literature and inductive coding of early data. The project team met to open code an initial transcript and generate a draft coding frame (see Appendix 2). This was applied to early data, with emerging analysis used to identify further sampling.
Data from the survey questionnaire were entered into a database and each record was then checked for accuracy. We created binary variables for each questionnaire item using the extreme of the response options (e.g. when asking about personal safety we dichotomised using the response ‘very safe’; when asking ‘how often do you walk alone in your neighbourhood at night’ we dichotomised using the response ‘at least once a week’, etc.). The difference in the prevalence of each negative or positive well-being impact between respondents in affected and unaffected streets was estimated using odds ratios with 95% confidence intervals (CIs). The significance of any differences in prevalence was tested using a chi-squared test. Results were compared with national surveys using similar indicators to estimate representativeness. Data were analysed using Stata 13 statistical software (StataCorp, College Station, TX, USA).
Ethics
In quoted extracts, we have removed identifying place and other information, with tags indicating local authority area (except for stakeholder interviews, where this might breach confidentiality) and source of data: in-depth individual or pair interview (I), group interview (G), stakeholder interview (S), document (D) or field notes, including informal intercept interviews (F). Written consent was obtained for transcribed in-depth, group and stakeholder interviews; intercept interviewees were told that we were ‘conducting research on street lighting’. Approval for the study was provided by the London School of Hygiene & Tropical Medicine Ethics Committee (number 6341).
Findings
Public views: strong and polarised
Unsurprisingly, views expressed about switch-off or part-night lighting in public domains such as local authority consultations and newspaper letters pages tended to be strong and polarised, with more people expressing strong views on the negative impacts on well-being than giving positive views of street light reductions. Key domains of concern on the potential or experienced negative impacts were impact on personal security when walking at night, fear of crime and potential for increased road traffic injuries. Letters to one local newspaper in Hertfordshire, a county that had introduced part-night lighting, are typical of both the polarisation and the strength of the individual views expressed in public domains:
. . . this is great for the criminal fraternity that prowl our streets . . .
Letter to The Comet, 16 August 2012, p. 19, D923
I am a serving police office and work long shifts . . . I am not impressed why, after I pay my taxes, that I now have to walk home in pitch black.
Letter to The Comet, 24 May 2012, p. 18, D1424
The great switch-off has begun [. . .] Never have I felt so frightened to walk back to my house from the road after a night out.
Letter to The Comet, 17 May 2012, p. 19, D2325
Am I alone in thinking it’s a fantastic idea? . . . the money saved . . . can be ploughed back into schools and lollipop ladies . . . Sleeping in the pitch black will have amazing health benefits for the people of this town. Not to mention the impact on the environment.
Letter to The Comet, 10 May 2012, p. 18, D2726
Local authorities that had engaged in consultations over planned reductions solicited comments from both representative groups (parish councils, residents associations), and individual members of the public. One phase of Buckingham County Council’s consultation over ‘switching off’ lighting, for instance, attracted 34 comments from individual residents, of whom 24 protested the decision, and eight supported it, with two making other or mixed comments. In addition to noting details of particular roads or junctions of concern, negative comments also questioned likely cost or carbon emission savings and focused on safety and the competence of the authority to make decisions:
This is a regressive plan that will make roads more dangerous. Endangering lives to save money is gross mismanagement.
Anonymous comment, public consultation, Buckinghamshire D104
Positive impacts reported or expected in public comments were benefits in sleep with less light pollution, impact on environment through reductions in carbon emissions and improved ability to see the night sky. Both the polarisation of views and the preponderance of negative views were reflected in open comments on the household survey. Around half of all respondents added comments: a higher number of these expressed negative views of street light reductions than gave positive views (see Appendix 3).
Negotiating views: deliberative settings
If views expressed in public domains suggest strongly held public opinion, a somewhat different tenor was evident in more deliberative settings. When asked about street lighting reductions in contexts such as group interviews, participants were typically more equivocal or hesitant in offering their assessments and more willing to consider potential benefits as well as risks. For instance, despite some in this group of neighbours being concerned about the dark streets at night, they all went on to agree on the benefits of being able to see the night sky:
I really notice being able to see the stars.
Yes, yes, I have, too.
And one night I even . . .
It’s lovely.
I woke up because we had our curtains open, and I woke up and it was really bright outside . . . It was just so beautiful.
G3 Hertfordshire
In groups, participants were also more likely to explicitly note that there might be trade-offs between different outcomes likely to accrue from reductions in street lighting and that these would impact differently across their communities. Here, for instance, after rehearsing the negative impacts, particularly (they felt) for older residents, these members of a residents’ association then went on to discuss potential benefits of changes:
Uh, it’s, there’s a balance to strike, isn’t there? . . .
[indicates agreement]
I’m a reasonable man but I know if there was no cost to it I, I’d probably rather have more lights than less. And I find it quite hard. I probably haven’t got very good night vision . . .
I do feel slightly better for thinking, well, we’re doing our bit towards cutting down carbon.
G5 Buckinghamshire
Similarly, this group of teachers in Wakefield debated the likely impact of reductions in street lighting in their neighbourhood, drawing on news coverage, their own experience and anecdotes from others to offer and test out rather more tentative views than those expressed in public consultations:
And they’re, they are actually energy saving . . . there were a big piece on [local TV news programme] or something about them . . .
You wouldn’t want them to just totally switch things off. I guess if they did it at a certain time, you know, when the majority of people aren’t on the roads?
I don’t know, because it’s, on estates, because I know what [colleague] was saying, since she got burgled, because it’s so well lit where she is. She says, the, the policeman said if, if it hadn’t been able as well lit and they hadn’t been able to see into your garden, your house, your garage.
I suppose you’re never going to please everybody are you?
G9 Wakefield
Despite one group interview participant suggesting ‘I think people seem to be either very for lighting or very against lighting’ (G4 Buckinghamshire), in deliberative settings, the tenor of most discussion was that there were likely to be both positive and negative health and well-being outcomes and that the balance of these would depend on individual circumstances.
Accounting for well-being consequences: social attributes
In accounting for who would be most affected by the potential consequences of street lighting reductions on safety and mobility, participants drew on normative assumptions about how social attributes such as age and gender affected mobility, safety and risk in public spaces after dark. Older adults, for instance, often cited their age as a reason for not noticing or being affected by street lighting, as they reported rarely going out after dark. Younger adults, even when negative about reduced street lighting, couched their concerns in terms not of impacts on their own mobility, but the potential for harm to older neighbours. One couple in their thirties, for instance, recalled moving from an urban area to a commuter town which had part-night lighting and their initial surprise and discomfort on realising that their local streets were dark by the time they returned from a night out:
. . . we might be coming home at gone midnight . . . once you’ve turned off [main road] you would yeah, literally around 12 o’clock, you would watch lights going out in front of you as you walked down the road . . . it would be pretty pitch black.
. . . no street lights at all!
I7 Hertfordshire
However, despite reportedly having to take care not to trip over unseen hazards and having to walk in the road to avoid bumping into cars parked on the pavement in the narrow streets late at night, they deflected any suggestion that the 12 a.m. switch-off had constrained their activities or particularly affected their well-being. Indeed, despite a long list of inconveniences and, at times, dangerous situations (including nearly being run over on an early morning walk), they cited their (young) age to deflect any question of significant impact on their routines:
Does the lack of street lighting affect what you choose to do?
I wouldn’t say it bothers me . . . if I want to go out, I’ll go out . . . but I’m not a 70-year-old person . . .
. . . it doesn’t impact on anything as in we’d not do something because of the lighting.
I7 Hertfordshire
Symmetrically, older participants disavowed any impact on their own behaviour, in their case because they were at less risk than younger people, being less likely to be out and about later, but also because they described themselves as more likely to be adequately prepared to cope with navigating dark streets:
I think it needs to be said that, um, someone spoke to me about their teenage children coming back from London, nights out – And they were concerned [about] picking up the, the children coming home from the station and it was dark. Now this has been said to me.
Well, I’ve walked up from the bottom in the dark when it’s been the blackout and I felt perfectly safe . . . I just walk in the middle of the road . . . I found it quite surprising the first time I was coming back in the dark, but actually I’ve, oh, because I’m, I’m fairly sober and not wearing silly shoes.
G3 Hertfordshire
Thus, in public, participants were often careful to claim that, even where lighting reductions were unwelcome, that they themselves could ‘cope’ and that their own competence would offset any impacts on well-being. In a group interview with a group of neighbours who were all deeply opposed to the local switch-off at 12 a.m., one woman, for instance, describes how she has ‘taught’ younger workers how to cope with darkness:
I gave two of my apprentices torches . . . Because they were complaining about coming home drunk from [town] and couldn’t walk and find their way. So I gave them torches [brief laugh] . . . and I gave them a torch and was like put that in your handbag because it’s, they’re really light . . . I mean they thought it was quite funny because they’re about 18 and 19, but at the same time they were like we really can’t see.
G2 Hertfordshire
Similarly, this young man describes in detail the importance of street lighting for security in his neighbourhood (‘if it’s more, it’s more dim, it’s more dark, it’s easier for crime to take place’) but again claims competence in the form of both maturity (‘I’m getting to the stage now where that sort of thing shouldn’t bother me’) and local familiarity; others, in contrast, he suggests, might be more vulnerable:
Personally I don’t feel unsafe around here because this is my neighbourhood to be honest, I’m quite familiar around everyone and the surroundings, however that doesn’t mean everyone who lives here or lives around here or comes here feels safe . . . street lighting . . . it’s a mental thing but I just think it gives people a kind of a reassurance that . . . nobody wouldn’t try anything because they’ve got light.
I64 Hackney
Concerns were, then, typically rationalised as concerns for others: of different age groups or those lacking local familiarity. The exception was female gender, which was on occasion used in discussion to justify concern participants’ own concerns. Many women of all ages reported that personal security was an issue for them, or at least that they could flag up concerns ‘as women’ about security. Some did report avoiding travelling after dark on their own. However, the role of street lighting per se, rather than street lighting as an indicator of time of day, was ambiguous. Two railway workers, for instance, first suggested lack of street lighting early in the morning when they travel to work as an issue, particularly for women, but then went on to suggest that the problem is the time of night, rather than the darkness itself, being inherently dangerous:
I take a taxi. As a young woman I wouldn’t want to be walking out at that time in the morning.
Does it make a difference, no street lights?
No, I’d get a taxi anyway. It does put you off, not knowing who’s around, especially as it’s dark. But I’d come by taxi anyway – 10 years ago, would have been different – you don’t think about it when you’re young do you? But you know, as a woman, you don’t want to be out on your own walking around.
No, not as a woman. Mind you, same for a lot of men – wouldn’t want to be walking, you never know who’s out and about.
F3 Shropshire
Direct reports of reduced lighting affecting mobility were rare and reported impacts on well-being were largely marginal. They included reports from a few people who had changed their behaviour to avoid walking, running or cycling in the dark:
What it has changed for me is, because I’m a runner, I run, I can’t run early in the mornings like I used to. Or not in the winter anyway. It’s too dangerous, with all the narrow roads and that. I have to drive somewhere now to go for a run.
F33 Swansea
However, as for this woman, the impacts of lighting reductions had, in general, entailed manageable changes to routines rather than the abandonment of activities that would have positive impacts on well-being. Strategies included taking a torch when walking the dog at night, or using main roads for as long as possible when walking home rather than taking short cuts through unlit side roads.
Lack of opinion
Expressed public views were, then, strong and polarised, and those in more deliberative settings were more considered. However, this suggests a level of engagement with the topic of street lighting that was not evident in the walk-arounds of affected areas. Most intercept interviews during fieldwork suggested that, for the majority in affected areas, changes in street lighting had had little impact or had not been noticed. Changes such as white light and dimming had gone largely unnoticed, and even residents in streets with lights switched off typically said they did not know if there had been any changes, or had not noticed, or that it would not affect them as they were rarely out after 12 a.m. A group of customers and barman in a local pub in a town in Shropshire, for instance, were typical in that although they reported going home after 12 a.m. (the time of local switch-off), they were unsure if their streets were affected, or, if aware, were unconcerned about the implications for their own mobility or safety:
I don’t notice to be honest.
That’s because you’re pissed! [All laugh]
They’re all off in my area – I think they go off at 2 or something.
People are leaving about 2, 2:30 – we kick them out then and, to be honest, most of them are getting taxis home anyways. It’s just one of them things, street lights, doesn’t really make much difference . . . for most people, no one’s going to notice – in my street, it’s only really me coming back past midnight, no one else.
F4 Shropshire
Indeed, the most common response to general enquiries such as ‘have you noticed any changes in street lighting locally?’ was ‘To be honest, I haven’t’. For those who had noticed change, this was often a vague ‘the colour may be different’, and few had concerns about the impact on their own well-being:
I don’t notice really, as I don’t go out after dark.
F22 Swansea
[T]hey’ve changed the orange lights – they’re not as bright. To be honest I haven’t got an opinion, you just get used to it.
F22 Swansea
It’s not an issue, most of us drive, or get a taxi to [town], or someone’s always driving that way and you can get a lift.
F34 Hertfordshire
Few intercept interviewees mentioned negative well-being impacts and, where street lighting reductions had been noticed, the most common responses were spontaneous comments related to seeing the night sky. Although this was not often an issue in more suburban areas (where light pollution from nearby towns and cities was too great to see stars anyway), it was more frequently raised in the more rural neighbourhoods. One young man, who had moved to a rural area relatively recently, described walking home at 12 a.m. the night before: ‘there was no sun, no moon – but I could still see the way; no torch – just starlight – you get amazing skies here’ (F31 Swansea). Another in the same area had noticed changes: ‘They’ve changed them to what do you call it, low density lights – good for the stargazing – now you can see the sky, see whole . . . you’ve got the whole world in front of you!’ (F23 Swansea). In urban areas with white light interventions, if people had noticed this or had a view about it, this was more likely to relate to light pollution and the impact on sleep from existing lights:
Have Southwark reduced street lighting?
It’s definitely dimmer down the other end of [X] road.
. . . maybe only the quieter roads. Is it only on minor roads? This one outside [light in front of house] is still really bright – we worried about that when we moved in.
Well yes, but it has been OK, we haven’t really noticed it have we?
F1 Southwark
The lack of concern reported in intercept interviews was echoed in responses to invitations to take part in more in-depth interviews, with many expressing surprise that this was a topic for research. Even those who went on to make thoughtful and considered comments about the possible impacts of street light reductions on well-being were often initially unsure if they would have any views to share. One young woman who went on to provide a detailed account of how lighting in her neighbourhood affected how, when and who walked around it commented: ‘I’m not going to lie, when you first suggested it to me, I was like, eh, street lights? That’s a random topic’ (I60 Hackney). It was also reflected by some local authority consultations; one, over a proposed introduction of a white light scheme, was described by the lighting engineer as having gone ‘down like a lead balloon – we didn’t get any response at all’ (S2). It may, then, be reasonable to assume that, for the majority of those in affected areas, there was little discernible impact on well-being and few concerns about potential effects on well-being from changes made.
We deliberately included those likely to be out after dark or before sunrise, including workers such as taxi drivers, hospitality workers and police officers. In general, even those who did report working in or leaving and returning home in dark streets did not report significant impacts from street lighting reduction: largely, they reported using cars to get to and from work and the only impacts of dark streets were marginal, in that taxi drivers, for instance, reported that it could be difficult to see house numbers. The exception was police officers, some of whom did report very strong negative views about the impact of street lighting switch off on their work. Three police officers included in the fieldwork all agreed that switching off street lights had an impact on public perceptions of fear of crime, even if there was no evidence that it had an impact on crime levels. Two had particularly strong views that lights should not be switched off, particularly on housing estates, as dark streets both fostered crime and made it more difficult for them to respond appropriately, given the added time it could take to find addresses in the dark and also to see the perpetrators of low-level crime: ‘we go out and we literally can’t see them – we can hear them making a noise, but you can’t see who it is’ (S10).
Private concerns
The typical lack of engagement encountered in intercept interviews might suggest that characterising public concern by the expressed views of those who do write into local authority consultation risks exaggerating the strength and negativity of views. However, there were also some deeply held concerns that tended not to be expressed in public. In public, accounts of the impact of street lighting reductions on personal security or mobility were circumscribed by age and gender appropriate presentations of the self, with participants reluctant to admit that fear of the dark, for instance, would curtail their activities. Well-being impacts of reduced street lighting were, therefore, largely presented as rational concerns about road safety or crime and its impact on more vulnerable others. In more private settings, though, a rather different tone was sometimes apparent, with interviewees expressing more deep-seated concerns about impacts on well-being of both darkness itself and, more specifically, the ‘switching off’ of lights. These more private concerns were elicited in a number of contexts: typically, at the end of group discussions when participants asked to speak privately to the team, and in in-depth individual interviews. Here, participants were more likely to reflect on the meaning of dark nights and express more uncertainty and anxiety about the implications of reduced street lighting, not just for their own individual well-being, but also for the social well-being of their neighbourhoods:
[after the public meeting] a couple of women are keen to talk more privately [. . .] One says when she first got here ‘I thought there had been a power cut! I feel quite vulnerable – I won’t wait for a bus, because I don’t like not being seen. In winter, it does stop me going out – I struggle even to put the bins out’ [. . .] Another woman then tells a story of a lively village visited on holiday and [concludes] [. . .] ‘ Here, it is as dead as a doornail, because no one ventures out’.
Field notes, Hertfordshire, December 2013
Three themes characterised these more private views: fear of the dark, wonder at the lights going out and concern about ‘going backwards’. Fear of the dark is perhaps difficult to admit to in public, given the need to present oneself as a mature, coping adult, but in more private settings participants were more willing to speak about how darkness affected their mobility, often prefaced by an apologetic ‘I don’t know if this is just me. . .’. This young woman, for instance, talked about visiting a family member who lived in the suburbs, where ‘come 8 o’clock all the street lights are knocked off’, which affected her willingness to walk there:
It’s quiet, like there’s no shops around there. Like come I think 10 o’clock it’s just dead, you need a car basically to move around there. So yeah, and it’s really scary, like you have to take, when I’m going there, say if I go there late at night I have to take a cab there, I wouldn’t walk there.
I60 Hackney
Women in particular described why they feel unsafe walking at night in unlit streets:
I don’t like to walk in the dark. I could carry a torch – but that shows you up. If there’s a street light, people can say [if something happened] ‘I saw a lady walk past us’. If I’m carrying a torch, all they can see is the torch.
I17 Hertfordshire
It [street lighting] matters hugely – you obviously don’t feel so safe when its dark, you can’t see who is there, and it is probably imagination, but you imagine someone there in the shadows.
F20 Westminster
I worry as a dog walker – I do go out at night. Your eyes don’t get their night vision, because there are these pools of light, and the pavements are very uneven and dangerous – and that’s all apart from the worry about personal safety. My mother won’t go out after dark, she’s afraid of falling.
I29 Buckinghamshire
One woman, who described a recent emergency at night that necessitated driving, discussed how she now preferred living in a relatively well-lit town:
I used to live out in [small village], it’s very rural there, it’s completely different. When I was there I didn’t go out as much at night, I didn’t feel as secure. Here I go out to the theatre, cinema, I feel safe . . . in [village] the lighting wasn’t very good – it was well spaced out, and they didn’t light the whole lane . . . you feel much safer in the town. You do think about that more as you get older.
I20 Wakefield
If the dark was, for some, inherently disturbing and a factor that might shape preferences for more urban settings, for others it was the switch-off itself which was unsettling in some way. First, the instant change from lit streets to dark ones evoked a range of emotions in those living in areas where local authorities had instigated part-night lighting. This included wonder, again something participants often flagged as perhaps an individual, or idiosyncratic, reaction:
I know this sounds weird, but I got quite obsessed by it when they first started turning them off at midnight, I started to stay up to watch them going out – just to see how dark it was. It was so strange watching the lights going out – an odd thing to happen.
I37 Hertfordshire
For others, the anomaly of lights going out at a certain time was simply strange, and was often described as a largely humorous disruption in terms of expectations one might have of either modernity or what a walk home should entail:
They go off at midnight – it’s hilarious, it’s like going back to the Dark Ages!
F34 Hertfordshire
And literally it was quite funny, because there were some people walking ahead of us, and the light was literally turning off as they got to it . . . and we thought, ha, ha, that’s quite funny!
I7 Hertfordshire
The concern, it is implied, is not the dark per se, but the surprise of the dark, something which, in urban areas, was out of time and out of place. If some described this in humorous terms, others were more disturbed. Private accounts included some reflection on the meaning of this switch-off and the symbolic breach in expectations of modernity that it heralded:
The streetlight thing seemed to me a big step forward in quality of life . . . the thought of actually going backwards seems to be quite appalling. I’ve never lived in a non-suburban or non-town area my entire life. Um, and I think, and I would choose not, I, you know, I would actively choose not to live in the country . . . I would prefer to be somewhere where there are lights.
I2 Buckinghamshire
As this response suggests, location shaped the meaning of light at night. If those who were out and about at night in built-up residential neighbourhoods were made anxious by the switch-off, those in more rural areas presented themselves as ‘hardier’ to the removal of artificial light; for many, living in a rural area meant taking a pride in coping with the absence of such indicators of modernity as street lighting:
It wouldn’t bother me to go out at night. Since I was young I’ve done that, I’ve grown up in the country.
G5 Hertfordshire
Indeed, lighting was an important marker of the division between ‘town’ and ‘country’. First, ‘bright lights’ signify ‘the city’:
I’m always relieved when I’ve been driving along country roads to get back to the bright lights of the city.
F19 Buckinghamshire
Second, lighting operates as a marker of normative expectations attributed to residents of town and country and, thus, as the second quote suggests, operates as a potential political faultline in localities which bridge the urban and rural in commuter villages:
If you’re used to being in [city] with loads of streetlights, it’s kind of weird getting here and finding it in darkness.
I7 Hertfordshire
The city people want street lights – there is a new-build estate, and its second generation people coming from London, with different expectations – they want them. It is a divide – people do talk about it as something people come to the country and then expect it to be like the city.
F38 Buckinghamshire
Light demarcates time and place
Key stakeholder interviews with lighting professionals also focused on place and time. Lighting was explicitly used to signal appropriate use of public space in time, and reductions in hours of street lighting were often justified in terms of normative accounts of the ‘proper’ hours for doing things, as suggested by this parish councillor’s discussion of a plan for 12 a.m. switch-off, which he described as having been pushed through despite some residents’ disapproval:
Between the hours of midnight and 5.30 what is anyone doing anyway? Because people are asleep at that time. The only time I’ve been out at that time is Christmas Eve, coming back from church. And that was about 12.30 and I did think, oh, it’s dark!
S9
Outside urban centres, being out and about after dark still risks raising questions about propriety. Deliberately leaving places dark could, therefore, demarcate places where people were not ‘meant’ to be: a reading understood by both lighting professionals and residents:
We do switch down park lights . . . because we don’t want to encourage people into the parks . . .
S2
We’re going into a park or something, a park after dark like to just go and mess about and chill and whatever. That’s the only time we use our phones as torches because there’s no lighting in parks, people assume that nobody’s in the park at that time, so there’s obviously going to be no lighting . . .
I59 Hackney
Lighting engineers discussed in detail how ‘the right light, in the right place, at the right time’ (S1) – a mantra of their professional aims, repeated during the fieldwork many times – was essential not only to the security of the communities they served, but also to creating appropriate lighting, with cues to the proper use of space as well as time. In the city centres, this often implied a welcoming light, which encouraged residents and visitors to use services and facilities. As the introduction to Westminster’s guidance on lighting puts it: ‘Light changes everything: it illuminates our way and makes spaces inviting to enter’ (City of Westminster, undated, D45). For many, lighting was not just about making places more visible, but marking them as identifiable locales which enhanced community well-being, through selecting the right quality, intensity, direction and colour of public lighting. Commercial interests play a large role in this, with a rising number of companies now vying for local authority contracts to provide lighting. In Wakefield, for instance, in an advertising brochure Philips Lighting describe their brief as not only to help the council to save money but to ‘provide a welcoming environment’ through the use of LEDs. The use of light to create visual coherence has been to extent ‘corporatised’ in many high-income countries (see e.g. Jones27 on London’s South Bank and lighting), but for many local authorities in England and Wales, there was a still a keen sense that good lighting was a duty of local government, part of the obligation of public servants to the well-being of local communities. One lighting professional is particularly eloquent on the wide-ranging impacts that good-quality lighting can have across the range of environmental, economic and social aspects of localities:
A lot, a lot safer to do it, you know, even, even simple things as you, yourself and a neighbour going to play a game of badminton twice a week . . . and stopping for a glass of wine and a slice of, or a chicken salad on the way home . . . And it’s going to serve local people and feed back into the local economy [and on] inclusion, civic pride . . . We deliver a lighting installation but which can contribute towards our Transport Department’s drive for sustainable forms of transportation, on our education sector who are looking for safer routes to school to encourage parents to walk . . .
S8
For most lighting professionals, providing high-quality lighting for residents was, then, framed in terms of their obligations to provide a good ‘service’ and a responsive one which made communities feel that the local authority cared about them. Residents who discussed negative well-being implications of reduced light at night typically referenced a perceived failure in this regard. As one resident said: ‘What does disturb me is they are starting to turn lights off to save money. Because you never know when you’re going to be out at that time of night’ (I20 Wakefield). A feeling that security was being compromised by cost saving contributed to a sense of neglect and lack of trust that the local authority was acting in ‘good faith’:
Why do local authorities think it’s OK to light up the shopping areas – they seem more concerned at shops and businesses than they do the local residents and people’s own homes. We’re a bit of an island – when the lights are off, on this road, we’re cut off.
G2 Hertfordshire
For some, it was not, then, necessarily the darkness in and of itself that was disturbing, but rather the loss of a public good that had been enjoyed.
How much difference does part-night lighting make to well-being?
The household survey in one area of Shropshire provided some insight into both how typical the views outlined thus far were, and whether or not reductions in street lighting did make a difference to feelings about the local area, walking and driving after dark, fear of crime, sleep disturbance from street lights and seeing the night sky (Table 2).
Survey item | PNL (N = 250), n (%) | Non-PNL (N = 233), n (%) | Odds ratio (95% CI) | p-value |
---|---|---|---|---|
Thinking about your neighbourhood in general, would you say that people can rely on each other for help? | ||||
Never | 9 (3.6) | 4 (1.7) | 2.14 (0.59 to 9.62) | 0.20 |
Do you trust your local council to do its best for your neighbourhood? | ||||
Never | 14 (5.6) | 15 (6.4) | 0.86 (0.38 to 1.97) | 0.70 |
Thinking about the spring and autumn periods, how often do you usually walk alone in your neighbourhood after dark? | ||||
At least once a week | 73 (29.2) | 79 (33.9) | 0.80 (0.54 to 1.20) | 0.27 |
Thinking about the spring and autumn periods, how safe do you feel walking alone in your neighbourhood after dark? | ||||
Very safe | 39 (15.6) | 61 (26.2) | 0.52 (0.32 to 0.84) | 0.004 |
Thinking about the spring and autumn periods, how safe do you feel driving home after dark? | ||||
Very safe | 115 (46.0) | 117 (50.2) | 0.84 (0.58 to 1.23) | 0.35 |
How worried are you about having your car stolen or broken into after dark in your neighbourhood? | ||||
Very worried | 7 (2.8) | 7 (3.0) | 0.93 (0.27 to 3.16) | 0.89 |
Please indicate how much you agree with the following statements | ||||
(a) ‘There is enough street lighting to see clearly at night in my street’ | ||||
Strongly disagree | 69 (27.6) | 63 (27.0) | 1.03 (0.68 to 1.57) | 0.89 |
(b) ‘Street lights outside my home keep me awake at night’ | ||||
Strongly disagree | 186 (74.4) | 162 (69.5) | 1.27 (0.84 to 1.94) | 0.23 |
(c) ‘On a clear night, I can see the stars if I stand outside in my street’ | ||||
Strongly agree | 141 (56.4) | 135 (57.9) | 0.94 (0.64 to 1.37) | 0.73 |
(d) ‘It’s important to me to be able to see the stars at night’ | ||||
Strongly agree | 105 (42.0) | 116 (49.8) | 0.73 (0.50 to 1.06) | 0.09 |
Thinking about your house or flat, have you or the owner done any of the following within the last 2 years? | ||||
(a) Installed any lights at the front or garden, to improve visibility or security? | ||||
Yes | 79 (31.6) | 74 (31.8) | 0.99 (0.66 to 1.48) | 0.97 |
(b) Installed any lights at the back or garden, to improve visibility or security? | ||||
Yes | 84 (33.6) | 75 (32.2) | 1.07 (0.72 to 1.59) | 0.74 |
(c) Installed a burglar alarm? | ||||
Yes | 24 (9.6) | 24 (10.3) | 0.92 (0.49 to 1.76) | 0.80 |
(d) Made other improvements to the visibility of your front entrance? | ||||
Yes | 33 (13.2) | 34 (14.6) | 0.89 (0.51 to 1.54) | 0.66 |
Has the number or brightness of street lights in your neighbourhood been reduced within the last few years? | ||||
Yes in my street | 126 (50.4) | 56 (24.0) | 3.21 (2.14 to 4.84) | < 0.001 |
Do you carry a torch with you when you go out at night? | ||||
Always | 33 (13.2) | 28 (12.0) | 1.11 (0.63 to 1.99) | 0.70 |
The survey included a population that are generally in line with national views on how far they trust their local authority, with around 6% of people in streets affected and unaffected by part-night lighting reported that they ‘never’ trust their local council to do its best for their neighbourhood, compared with the national Citizenship Survey 2009–10, where the proportion was 7.9%. 28 Although respondents were significantly more likely to report that the number or brightness of street lights had been reduced within the last few years in the affected streets, only half had noticed the change. The only item where those in streets affected by part-night lighting made significantly different responses was ‘Thinking about the spring and autumn periods, how safe do you feel walking alone in your neighbourhood after dark?’, with fewer of those in affected streets more likely to report feeling ‘very safe’ (15.6% vs. 26.2%; p = 0.004). In the national Citizenship Survey 2009–10, the proportion reporting feeling ‘very safe’ was 31.8%. 28 There were no significant differences, however, in reporting walking alone after dark (29% vs. 34%; p = 0.27). In the Survey of English Housing 2004–5, the proportion reporting ever walking alone at night was 46.4%. 29
A small proportion (3%) of people reported being ‘very worried’ about having their car stolen or broken into after dark and this was similar in the affected and unaffected streets. Compared with national data, this is low: in the British Crime Survey 2007–8,30 10.7% reported being very worried about having their car stolen and 9.1% reported being very worried about having things stolen from their car.
In general, then, in a random sample of the population in affected and non-affected streets, there was little evidence that the introduction of part-night lighting had made significant differences to well-being, except with regard to residents’ feelings of personal security.
Pathways linking lighting reductions to well-being
We took the findings from the rapid appraisal, in the light of the literature on the impact of artificial light on health, to map out the possible pathways through which lighting reductions might theoretically impact on the public health. In summary, reductions in street lighting at night can affect well-being through a number of pathways. First, there are pathways from the direct effects of darker streets at night, which have both positive effects (improved sleep, existential capital from being able to see the night sky) and negative effects (anxiety from fear of crime, constraints on mobility at night). Although negative ones are emphasised in public accounts, deliberative public views balance these implications for personal well-being against broader determinants of health, such as carbon emission reduction. There are also pathways which are mediated by feelings about place and the governance of place. These relate to expectations that urban settings will be well lit, to the meaning of street lighting reductions in terms of trust in local government to make decisions in the interests of the neighbourhood and also more wide-ranging concerns about the implications of financial imperatives to ‘switch off’ lights. Finally, claims about well-being impacts are shaped by social attributes (such as age and gender) which potentially change the meaning of dark streets (in, for instance, making it more or less likely that one would be in them), but also the possibilities of expressing concern about them. Although highlighted in the scientific literature, concerns about the potential impacts of increases in LEDs on cancer or other physical health outcomes were not raised in any settings, public or private. These pathways are illustrated in Figure 1, with those of concern to the public in shaded boxes.
FIGURE 1.
A model of potential pathways linking reductions in street lighting to health and well-being. Health outcomes are on the right, with those raised in the rapid appraisal in shaded boxes. GHG, greenhouse gases.

Discussion
A strength of this pragmatic rapid appraisal design was the mix of different accounts that was generated on the issue of street lighting and well-being. Rather than use these for triangulation,21 we have highlighted the variations in thematic content and tone generated across these methods. It is not surprising, perhaps, that expressed public views (such as those elicited through local authority consultations) were often strong and polarised, given that only those who did have strong feelings were likely to volunteer their time to respond. Informal interviews with members of the public in ethnographic encounters were less forceful; indeed, for the majority of the population, reductions in street lighting (even in areas with part-night lighting or switch-off) had little impact on their well-being and largely went unnoticed. When engaged in more deliberative discussions, such as group interviews, many were also often willing to change their opinion and were reflective on the mix of risks and benefits likely to accrue from interventions such as part-night lighting or dimming. A second advantage is that drawing on these diverse sources of evidence also sheds light on the social as well as individual impacts of street lighting reductions, in that key informants such as local authority lighting engineers and residents couched contributions in terms of locality, and how this mediates the meaning and impact of lighting and its reduction.
Broadly, then, the direct impacts on individual well-being from reductions in street lighting at night were reported as minimal. In a few cases, people reported getting more sleep or being afraid to go out after dark, but data from the survey in one area suggested that street lighting reductions, at a population-health level, are likely to have few significant effects, in that reductions were not significantly associated with levels of fear of crime, willingness to go out or lack of sleep due to street lighting. However, this survey was carried out in an area reporting representative levels of trust in the local authority, compared with national data. In other case study areas, in which residents may have had lower trust in local authorities, reported effects (particularly of switch-off) could be significant for a minority, and had potentially far-reaching effects on residents’ views of their neighbourhoods as places that could be ‘ignored’ by their local authorities. Further, some more private views suggest rather deeper anxieties about the meaning of street lighting for a sense of well-being, one that is linked to understandings of locality, neighbourhood and governance. ‘Bright lights’, it seems, have a cultural significance beyond the immediate concerns of crime and road safety. Permanent and abundant lighting first signifies the ‘modern’, and electric lighting has long marked ‘progress’. 4 Abundant lighting is symbolically associated with a developed, modern infrastructure, marking a contrast with the Victorian city or the low-income country. 3 For those in urban and suburban localities, the sense of ‘going backwards’ generated a measure of ontological insecurity, even where this was expressed humorously. In a context of financial constraints, with the ‘politics of austerity’ framing some contributions to this debate, reductions in service not only disrupted taken-for-granted assumptions about the abundance of resources available in a developed economy, but also potentially undermined local residents’ faith in good governance. In this respect, the decisions of local authorities to reduce street lighting may have been as important for how they reflected a lack of engagement with residents’ views. As others have noted in relation to problems such as dog faeces,31 which also causes considerable concern despite the limited evidence on its public health impacts, ‘switching off’ lights may have more meaning as a perceived mark of disrespect than for its direct impacts on mobility, safety or sleep.
As Shaw7 has noted, if an ability to ‘keep the lights on’ becomes a symbolic indicator of the administration’s ability to maintain order, the ‘lights going out’ makes very visible a failure in governance. A telling detail of the coverage of the US city of Detroit’s bankruptcy in 2013 was the way in which the decline of the city was described in news coverage, with most newspapers using the same three indicators of a city where governance has failed:
As it stands, 40% of Detroit street lights are broken. It takes, on average, an hour for police or ambulance services to respond . . . Gang murders are carried out in vacant buildings . . .
Observer, 20 July 201332
That the fact that street lights are broken can be used as an indicator on a par with gang murders is a notable reminder that permanently well-lit streets, for urban and suburban citizens, evoke more than a safer place to walk or drive: they indicate good governance, affluence and a taken-for-granted location in the ‘modern’. Although few participants in this study explicitly voiced extreme concerns about reductions in lighting at night, the more private views touched on both fears of the phenomenological meaning of darkness33 and a sense of insecurity in any easy assumptions about continued affluence or progress. In rural areas, by contrast, accounts emphasised the ‘natural’, in terms of being able to appreciate dark skies at night, and the ‘hardiness’ of rural citizens who cited reliance on artificial lighting as symptomatic of the dependencies of urban residents.
The impact of street light reductions on well-being is, then, meditated by place. First, it is mediated directly, in that identification as a rural or urban resident, for instance, changes the meanings of darkness and artificial light, and the perceived role of lighting in safeguarding well-being. Second, place mediates the well-being implications of street lighting in terms of how perceptions of neighbourhoods and their governance shape the likely impact of an intervention. Here, trust in local lighting authorities to do the right thing and act in good faith is crucial. Given that views were, in deliberative settings, malleable, this does suggest that well-conducted consultations about street lighting reductions might have a role in mitigating negative public health effects, through the pathways linking feelings about neighbourhood and well-being.
The various pathways theoretically linking street lighting to public health are illustrated in Figure 1.
Empirical research is needed on whether reductions in street lighting do achieve financial or carbon emission reductions which would have a positive impact on the public health, and whether any negative impacts on the public health through changes in injury, mobility or sleep accrue from different interventions (dimming, part-night lighting and switch-off). However, these direct health impacts are not the only ones of concern. This study has suggested that to achieve gains in these areas without compromising other well-being outcomes for affected residents and workers, attention should be paid to the more symbolic effects of street light reductions as well as the direct health impacts. Given the malleability and reflexivity of public views, negative well-being effects of reductions in street lighting may be mitigated by wider consultation with local communities.
In conclusion, for the majority of residents in areas where street lighting had been reduced, the impacts on individual well-being were likely to be minimal, in that only a minority reported deteriorations in personal security, fear of crime, mobility or other negative effects. Similarly, few reported significant positive effects, such as better sleep or enjoyment of the night sky. However, at a social level, reduced street lighting may have significant effects in urban and suburban settings, where residents associate well-lit streets with competent and trustworthy governance. The well-being impacts of reduced street lighting at night may reflect, then, not darker streets per se, but the fact that lights which were once provided are no longer.
Chapter 3 The effect of reduced street lighting on crime and road traffic injuries at night in England and Wales: a controlled interrupted time series analysis
This chapter follows the STROBE (Strengthening of the Reporting of Observational Studies in Epidemiology) reporting guidelines for observational study designs (www.strobe-statement.org). 34
Introduction
Background/rationale
As described in Chapter 2, many UK local authorities responsible for street lighting have reduced, or are planning to reduce, some street lighting at night in order to reduce costs and greenhouse gas emissions. Local authorities are able to reduce street lighting at night using a range of interventions, including switching off lights permanently (switch-off), reducing the number of hours that lights are switched on (part-night lighting), replacing sodium lamps with white lights or LEDs and dimming lamps through centrally managed systems. Reductions to street lighting at night have attracted considerable public and media concern, centred on crime and road safety.
A previous systematic review35 of the effects of increasing street lighting on crime found a reduction in crime for those studies that examined changes over the course of the entire day (i.e. during hours of both daylight and darkness). However, for those that examined crime that occurred during the night-time alone (four studies), there was no evidence of an impact of changes to street lighting on crime. A major limitation of this research was the use of inadequate control areas (only two studies used multiple control areas, and in some studies the control area was adjacent to the treatment area) in the primary studies on which the review was based.
Previous research into the effects of the introduction of street lighting on road traffic injury found some evidence for improved road safety with increased street lighting [rate ratio (RR) 0.78, 95% CI 0.63 to 0.97]. 36 This systematic review included 17 controlled before-and-after studies; seven used a designated control site, while the other 10 studies collected data at one site only and used daytime data as the control. The pooled results of studies that used daytime data as the control also provided evidence for a stronger protective effect (RR 0.68, 95% CI 0.61 to 0.77). However, the methodological quality of the included studies was considered to be poor, with a high risk of bias.
We identified no previous research into effects of reduced street lighting on crime or road safety. By working together in collaboration with the local authorities of England and Wales, the LANTERNS project aimed to answer the question of whether or not reducing night-time street light for environmental and energy reasons has any impact on road traffic injuries and crime.
Objectives
The objectives of this component of the study were to collate information from local authorities in England and Wales on street light reduction and energy-saving schemes and to examine whether or not these schemes were associated with any changes to the rates of road traffic injuries or crime.
Methods
Study design
The study was a controlled interrupted time series analysis. It was based on national data on changes made to street lighting provision at night. Intervention data included the nature of changes to lighting made (e.g. switch-off, part-night lighting, dimming and white light), plus the dates of change and geographic location of street light columns.
To control for all of the important sources of confounding, we analysed street-level and area-level data as a ‘panel’ study, such that any factors that were constant over time in an area (e.g. road design) contributed no information to the analysis. The analysis was, therefore, of any association between changes to street lighting provision within streets/areas over time and any changes in counts of crime and traffic injuries.
Setting
We began in 2013 by establishing a clear project identity and framework for collaborating with the local authorities of England and Wales. This included a study acronym, ‘LANTERNS’, and a website (http://lanterns.lshtm.ac.uk/) that emphasised the collaborative nature of our project working with local authorities. We aimed to invite all local authorities that had made changes to street light provision at night to participate in the project. We developed a project database containing contact details of chief executives and street lighting managers, and summaries of lighting reduction schemes in the public domain (populated through searches of the web and other resources). In addition to local authority web pages, useful sources of information identified include previous freedom of information requests (e.g. www.whatdotheyknow.com), public sector database (www.public-sector.co.uk), street lighting Private Finance Initiative (PFI) websites and websites of interested organisations (e.g. www.stargazerslounge.com). The database was populated by April 2013 and, with NIHR approval for the website, project launch e-mails were sent to all contacts in the database on 10 April 2013.
Local authorities who responded to the first e-mail, or who registered interest in the project via the project website, were contacted by telephone. This personalised approach was intended to encourage participation in the project, to ascertain whether or not street lighting reduction schemes had been implemented, to discuss data extraction, to identify individuals who might offer expert lighting advice and to identify local authorities for the rapid appraisal. Further e-mails were sent in mid-April 2013 to follow up non-responding local authorities and to confirm the contact details of responders. A personalised letter was also sent from the principal investigator to all chief executive officers of England and Wales at the end of April 2013 to ask for their support of the project. In May 2013 the LANTERNS project was invited to partner with the ILP and the London Lighting Engineers Group. These organisations provided technical support and assisted in making further contact with non-responding local authorities.
A data request was discussed with the ILP and piloted with several local authorities. In June 2013 a formal letter and data request was sent to the 75 local authorities within which a contact person had been nominated, or with whom the LANTERNS project team had made telephone contact. In November 2013 a project newsletter was sent to all chief executives and primary street lighting contacts in the local authorities and made available on the LANTERNS website. This generated further interest and responses from the local authorities. By May 2014 we had direct contact with 140 (80%) of the 174 local authorities in England and Wales, resulting in useable data sets from 62 local authorities. Two local authorities additionally provided data via their unmetered supply operators (energy suppliers).
Participants
The study participants were, effectively, all people injured in road traffic collisions and all victims of crime who reported offences to the police in the local authorities in which street lighting reduction had been implemented and for which data were available (as described above). We used two routine data sources (described below), covering all local authorities and police forces in England and Wales, for the ascertainment of cases of road traffic injury and crime.
Variables
The exposure (intervention) and outcome variables used in the analysis were as follows.
Exposure (intervention) variables
These comprised street lighting reduction or energy-saving schemes, including switching off street lights permanently; switching street lights off for part of the night; dimming the lighting level (brightness) in the evening and early hours of morning; change from yellow/orange light to white light/LEDs; trimming the period where lights are switched on; and any combinations of the above.
Outcome variables
STATS19 (the official data set of personal injury road collisions and casualties that occur on the public highway in the UK) road traffic injury data were obtained from the Department for Transport. 37 These data include all road traffic collisions that result in personal injuries and are reported to the police. The outcome variable used was the number (count) of collisions on each road. Crime data were obtained from the Police.uk website. 38 These data include crimes reported to each police force. Detailed crime data were also obtained from one police force (including specific locations and times of offences) to use in a methodological substudy to assess the optimum use of the Police.uk data for this study39 and for sensitivity analyses.
Data sources/measurement
As described above, every local authority in England and Wales was approached in 2013 with a request for the specific locations of all street light columns where changes to street lighting either had been implemented or were planned, together with the month and year that changes were introduced.
For road traffic injuries, STATS19 data were obtained for the period 2000 to 2013. These data include the date, time of day, location (easting and northing of the location of the road traffic collision), severity (slight injury, serious injury or fatal injury) and type of casualty (pedestrian, cyclist, car occupant or powered two-wheeler) for all reported road collisions.
For crime, data from the Police.uk website were obtained from December 2010 (the earliest date for which the data are available) to December 2013. This large data set was downloaded and stored on a server to be interrogated as required. These data comprise the month, the name of roads where incidents occurred, the approximate geographic co-ordinates and the type of crime. One disadvantage of this publicly available data set is that time of day is not included. Another is that the spatial accuracy of the data is unknown as the data are obfuscated to preserve the anonymity of victims. However, as part of this project we assessed the reliability of these data by comparing them with detailed crime data (including the time and location of offences) from one police force. 39
Using a geographic information system (GIS) the data on crime and road traffic injuries were linked to a road segment database that includes the characteristics of all classified and unclassified roads. The road network used to link the data sets was the current version (at the time of analysis) of the Ordnance Survey Mastermap Integrated Transport Network. 40 We initially linked data to roads for two local authorities to test the concept (using Camden borough as an urban area and Norfolk as a rural location).
Street lighting data from the local authorities were formatted and imported into the GIS. Each road segment was classified according to the type of street lighting reduction scheme implemented (e.g. part-night lighting, dimming, part-night lighting and dimming, etc.) and by the census LSOA within which it was located. LSOAs contain around 670 households and represent the smallest census areas for which some data used in the analysis (indices of deprivation) are available.
From the combined data set, counts of crime and road traffic collisions for each road segment were generated by month and by year.
Bias
For optimal control of biases our analysis compared changes in counts of crimes and traffic collisions on roads before and after street lighting was changed, relative to trends on other roads. The estimated effect is, therefore, specific to roads with decreased street lighting compared with other roads.
We examined potential bias due to ‘regression to the mean’. This bias might arise if, for example, unusually low numbers of collisions or crime were factors influencing local decisions to reduce lighting in some areas. An increase in collisions or crime in those areas may then have been expected as a result of random variation over time, rather than owing to the lighting intervention. To test for this bias, we repeated our analysis excluding data for periods of time prior to the introduction of street lighting changes.
To examine whether or not potential under-reporting of events to police might bias the estimates of effect, we analysed separately the most reliably reported events (i.e. killed or seriously injured casualties, and crime for which insurance claims require a crime report, e.g. burglary of dwelling or theft of vehicle).
Study size
Sample size (power) calculations assumed that street lighting changes had been implemented on streets for which only 1% of pre-intervention traffic injuries and crime occurred.
For road traffic injuries, statistical power was maximised by using data for a period of up to 10 years before lighting changes were implemented. Assuming that 150 night-time injuries per year might be expected on intervention roads, this was expected to give 1500 night-time injuries on intervention roads during the 10 years before lighting reduction was implemented and 150 injuries 1 year after the reduction was implemented, providing 90% power to detect an increase of 32% above pre-intervention injury levels. This magnitude of effect is consistent with the reduction in injuries estimated in a systematic review of the introduction of street lighting. 36
For crime, based on data from the crime survey of England and Wales30 and police-recorded crime data, we assumed that around 20,000 daytime and night-time offences would be expected per year on intervention roads, such that the study would have 90% power to detect a 5% increase in crime above pre-intervention levels (a much smaller increase than the estimated decrease of 22% associated with increased lighting reported in Welsh and Farrington35), and for major crime subcategories (e.g. violence against the person) it would have 90% power to detect increases of about 10% in crime.
Quantitative variables
We aimed to estimate the association between changes to street lighting provision over time and changes in counts of collisions at night, and counts of criminal offences that commonly occur at night: burglary, theft of (or from) a vehicle, robbery, antisocial behaviour, criminal damage and violence including sexual assault. We defined ‘night-time’ as 1 hour after sunset and 1 hour before sunrise. For the analysis of part-night lighting, ‘night-time’ was defined as 12 a.m. to 6 a.m., a common period used by local authorities when switching lights off for part of the night.
Road traffic injuries
We used the STATS19 road traffic collisions data, combining all transport modes and all injury severities in order to maximise statistical power to detect associations. We estimated the association between changes to street lighting provision and changes in counts of collisions at street level.
Crime
In a methodological study conducted as part of this project, we quantified the extent to which counts of crime generated using the Police.uk data at different census (and postcode) area levels agree with counts of crime using detailed police-recorded crime data. 39 The conclusion of this methodological study was that the open Police.uk data are reliable when used at middle super output area (MSOA) level (census areas which contain around 3200 homes) or at LSOA level (which contain around 670 homes), but not reliable if used either at output area level (which contain around 130 homes) or at postcode level (which contain around 15 homes). For crime outcomes we therefore estimated the association between changes to street light provision at MSOA (and LSOA) level and changes in counts of crime in MSOAs (and LSOAs). We used the proportion of total km of road in each MSOA (and LSOA) that had the street lighting intervention implemented as the explanatory variable in the analysis. The crime categories analysed were burglary, vehicle (theft of, or from), robbery, violence and the aggregate count of these offences. Antisocial behaviour and criminal damage were excluded from analysis because of incomplete data on these offences by some police forces.
Statistical methods
To control for all of the important sources of confounding we analysed street-level and area-level data using a panel study design with fixed effects. This approach uses data within (not between) the streets/areas (panels) to estimate any association between lighting reduction and changes in crime/traffic injuries. Any factors that are constant in a street/area over time (e.g. road design, presence of trees and buildings, etc.), therefore, contribute no information and cannot confound the primary association of interest. Other types of analyses (e.g. random effects for street/area) draw some information from how interventions correlate with overall outcome rates across streets/areas, but at the cost of potential confounding by other things that vary over space (but not time).
Road traffic injuries
For each region of England and Wales, we estimated how much on average rates of collisions had changed in each road segment after lighting was changed adjusting for possible biases by using a conditional Poisson regression model. 41 This approach is broadly similar to that used previously to study changes after introduction of 20 mph speed limit zones. 42 Seasonal variations and temporal trends were adjusted for by using step functions (indicators) for calendar month and year. This approach was chosen after exploratory analyses showed more parsimonious models fitted the data less well by Akaike’s information criterion. All street lighting interventions (switch-off, part-night lighting, dimming and white light) were entered into the same model to avoid mutual confounding.
Because changes in street lighting would be expected to have a direct impact only at night, we restricted attention to night-time collisions. However, to guard against bias due to changes concurrent with lighting interventions that might impact on the overall (i.e. day and night) collision rates, our model also estimated the change in daytime collisions rates associated with interventions and, as a refined measure of change in outcome rates following each intervention, the ratio between the night-time and daytime changes. We consider this ‘daytime collision rate corrected’ measure to be the most robust estimate of the change in collision rates following the changes to street lighting.
The final model was thus [expression (3) from Armstrong et al. 41]:
where i = 1 . . . I is the study month (months elapsed since the start of the study in 2000); s = 1 . . . S is the road segment; Yi,s is the number of collisions in month i on road segment s; and night-time and daytime collisions are included for each month, distinguished by an indicator variable.
The vector χi of explanatory variables has the following components.
Potentially confounding variables
-
Indicator variables for calendar month (1–12) to control for seasonal patterns and month duration.
-
Indicator variables for calendar year (2000 to 2013) to control for time trends.
To allow adjustment for patterns of daytime collisions
-
An indicator variable for night-time collisions (1 for night-time, 0 for daytime).
-
Interaction of the night-time indicator with each potentially confounding variable (above) to allow different seasonal patterns and time trends for night-time and day-time collisions.
Variables of interest
-
Indicator variables for each lighting intervention: switch-off, part-night lighting, dimming and white light (0 before intervention, 1 after intervention).
-
Interaction of the intervention indicators with the night-time indicator; the coefficients (βadj_change) of these variables estimate change in the night-time collision rate following the intervention adjusting for any changes observed in the daytime collision rate.
This model was fitted to each region of England and Wales. The mean over regions of the coefficients of interest for each intervention was estimated using a standard meta-analysis model. Because we found no evidence for heterogeneity, fixed effect models were used.
Crime
The model for crime rates had the same basic form as that for collisions, but with the following differences, reflecting different geographical resolution (i.e. counts at MSOA level rather than road segment level): the data origins (i.e. data collected at police force level), the larger average counts and complex background temporal patterns.
Instead of indicator variables for pre-intervention and post-intervention months, intervention variables comprised, for each year-month, the proportion (0–1) of total km of road length in a MSOA subject to the intervention; thus, 0 would represent no roads with the intervention and 1 would represent all roads with the intervention.
Because time of day of crime is not available from Police.uk data, total crime numbers were included and no night-time interaction variables were needed; the coefficients of interest for each intervention were the ‘main effects’ of the intervention variables.
We fitted models to each police force (of 31 police forces included in the data) separately, in view of their different data collection systems and evidence for different background time patterns across them.
Given evidence for more complex temporal patterns than allowed for by month and year indicators, we fitted indicator variables for the number of months (1–36) elapsed from the start of the study data series (i.e. a step function for elapsed month from December 2010).
Residual variance greater than that expected (i.e. ‘overdispersion’) in the Poisson statistical distribution was allowed for in standard errors using a scale factor estimated from the Pearson chi-squared statistic divided by the degrees of freedom of the residuals. (There was no evidence of overdispersion in the sparser collision analyses.)
The models were implemented in Stata statistical software. See Appendix 4 for the code implemented in Stata.
Subgroups
We explored the moderating effect of area-level deprivation on the associations between changes to street lighting and road traffic collisions and crime. Road segments and MSOAs were stratified using thirds (high, medium and low levels) of deprivation based on the Index of Multiple Deprivation (IMD) 2010. 43 For road segments deprivation was based on the LSOA in which segments were located. If road segments crossed multiple LSOAs we selected one LSOA at random. This exploratory stratified analysis excluded Wales, as the IMD covers English local authorities only.
Model checking and sensitivity analysis
We investigated evidence for zero inflation in our models. For road traffic collisions, where the models were fitted at regional level, we evaluated zero inflation by comparing the marginal fraction of counts that were zero in each region with the theoretical probabilities calculated using the Poisson mean. For crime, zero inflation was evaluated using a negative binomial model (implemented in Stata using ‘zinb’). We also conducted several sensitivity analyses by assuming a negative binomial rather than quasi-Poisson model, by allowing for zero inflation in this model and by fitting models dropping autoregression terms.
Missing data
The analysis was of routinely available data sets that are known to be incomplete owing to the under-reporting of some crime and road traffic injuries. To examine the sensitivity of results to the potential under-reporting of events, we analysed separately the most reliably reported events (i.e. killed/seriously injured casualties; crime for which insurance claims require a crime report, e.g. burglary, criminal damage to dwelling, theft of vehicle). Any missing data in the records of reported crime/traffic injuries were not imputed. Data on street lighting reduction were not available from all local authorities of England and Wales, and so the analysis was conducted only for those local authorities that provided data. Missing data in the provided data sets on street lighting interventions (e.g. type of lighting reduction) were not imputed. We used all available data on crime/traffic injuries for analysis and so ‘loss to follow-up’ is not applicable in this study.
Regression to the mean
As described above, had we found evidence for an association between street lighting reduction and road traffic injuries or crime we planned to assess whether or not this association may be partly due to bias from ‘regression to the mean’. We planned to exclude data from the analysis of road traffic injuries for periods of 1, 2 and 3 years before the street lighting reduction and rerun the analysis; for the analysis of crime we planned to exclude data for a period of 3 months before the street lighting reduction owing to the crime data being available only from December 2010.
Results
Participants
Local authorities in England and Wales were generally very positive about participating in the LANTERNS project. Although some of the local authorities were keen to participate, they were unable to provide the data required within the time scale. Figure 2 shows the flow of local authorities participating in the project and Figure 3 shows which local authorities were included in the analysis. Eight local authorities were unable to extract the required data from their asset management systems; three authorities confirmed that they had made no relevant changes to street lighting in their areas. In the case of 32 local authorities, despite an initial interest in the project and several follow-up e-mails and telephone calls, we could obtain no further response. Agreement to participate was obtained from 82 local authorities, of which 71 were able to provide data within the time scale. Of the 71 data sets received, 62 spanned sufficient periods of time to contribute to the time series analysis (see Figure 2).
FIGURE 2.
Flow of local authority participants in the LANTERNS project.

FIGURE 3.
Local authorities included in LANTERNS analysis. Contains National Statistics data © Crown copyright and database right 2013; contains Ordnance Survey data © Crown copyright and database right 2013.

Descriptive data
The street lighting interventions of primary interest were switch-off, part-night lighting, dimming, trimming and white light. However, many local authorities had introduced these interventions in combination with others, for example changing lamps to white light and using part-night lighting. Before proceeding with the analysis, we specified which combinations would be considered in addition to the single interventions. Trimming is where the time period during which lights are switched on is slightly reduced. This is usually achieved using photo cells which are set to switch lights on/off when it is darker (e.g. at 35 lx rather than 70 lx). Thus, the period of time whereby a trimming intervention can have an effect on crime or road safety is very marginal compared with the other light-reduction interventions, which last for several hours. For the purposes of analysis we therefore merged all combinations of lighting interventions that included trimming with the same combinations that excluded trimming. The street lighting interventions analysed were, therefore, switch-off, part-night lighting (including part-night lighting with trimming), white light (including white light with trimming) and dimming (including dimming with trimming).
The introduction of street lighting adaptation strategies increased steadily from 2009 (Figure 4). By December 2013, the local authorities participating in this study had implemented white light on a total of 15,833 km of road (7% of total road km in the 62 participating local authorities); part-night lighting on 12,101 km of road (5%); dimming on 10,519 km of road (4%); and switch-off on 946 km of road (0.4%). The proportions of total km of road in each MSOA that had lights switched off ranged from 0% to 60% [median 0.03%, interquartile range (IQR) 0.01–0.05%]. For part-night lighting the proportions ranged from 0% to 84% (median 0.2%, IQR 0.1–18%); for dimming the proportions ranged from 0% to 93% (median 0.14%, IQR 0.07–5%); and for white light the proportions ranged from 0% to 81% (median 1%, IQR 0.15–11%).
FIGURE 4.
Road km with lighting adaptation strategies implemented. PNL, part-night lighting.

Outcome data
Road traffic injuries
There were 859,935 collisions in the 62 local authorities included in the analysis; of these, 161,049 (19%) were night-time collisions and 153,442 (18%) collisions had resulted in a serious injury or fatality. Of the night-time collisions, 1202 (0.7%) occurred on roads along which switch-off had been introduced by December 2013; 5670 (4%) were on roads with part-night lighting; 11,634 (7%) were on roads with dimming; and 12,423 (8%) were on roads with white light. Total numbers of road traffic casualties from STATS19 data included in the analysis from 2000 to 2013 are shown by local authority in the appendices (see Appendix 5, Tables 14 and 15).
Crime
Total numbers of offences from Police.uk included in the analysis from December 2010 to December 2013 are shown by local authority in the appendices (see Appendix 5, Table 16). In the local authorities participating in this study, during the period December 2010 to December 2013, there were 581,837 burglaries, 475,657 vehicle thefts, 67,470 robberies, 2,882,758 offences of antisocial behaviour, 486,367 offences of criminal damage and 730,280 offences of violence.
Main results
Road traffic injuries
We found little evidence for any associations between street lighting adaptation strategies and day-adjusted night-time collision rates (Figure 5).
FIGURE 5.
Associations between street lighting adaptation strategies and night-time road traffic collisions. (a) Switch-off; (b) part-night lighting; (c) dimming; and (d) white light. PNL, part-night lighting.
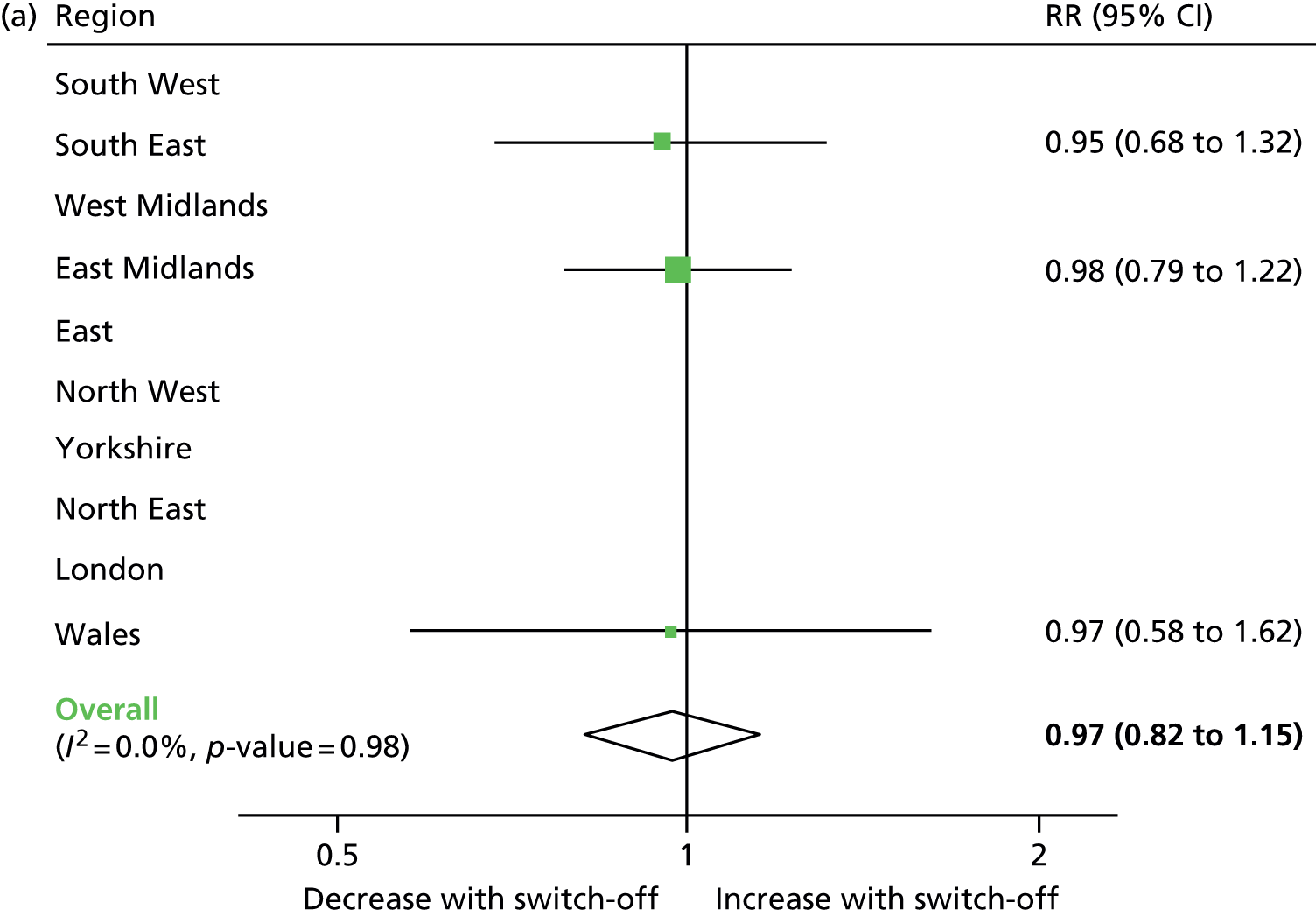



There was no evidence from any of the regional models or the overall estimates for an association between switch-off (RR 0.97, 95% CI 0.82 to 1.15), part-night lighting (RR 0.95, 95% CI 0.84 to 1.07) or dimming (RR 1.00, 95% CI 0.91 to 1.10).
There was weak evidence in London for an association between the introduction of white light and increased night-time collisions (RR 1.30, 95% CI 1.03 to 1.65); however, the overall national estimate provides no evidence for an association (RR 1.01, 95% CI 0.93 to 1.09).
When models were restricted to collisions where casualties had suffered a serious injury or were killed, the estimated associations with street lighting adaptation strategies were unchanged and estimates were less precise (Figure 6).
FIGURE 6.
Associations between street lighting adaptation strategies and night-time collisions (casualties killed or seriously injured). (a) Switch-off; (b) part-night lighting; (c) dimming; and (d) white light. PNL, part-night lighting.
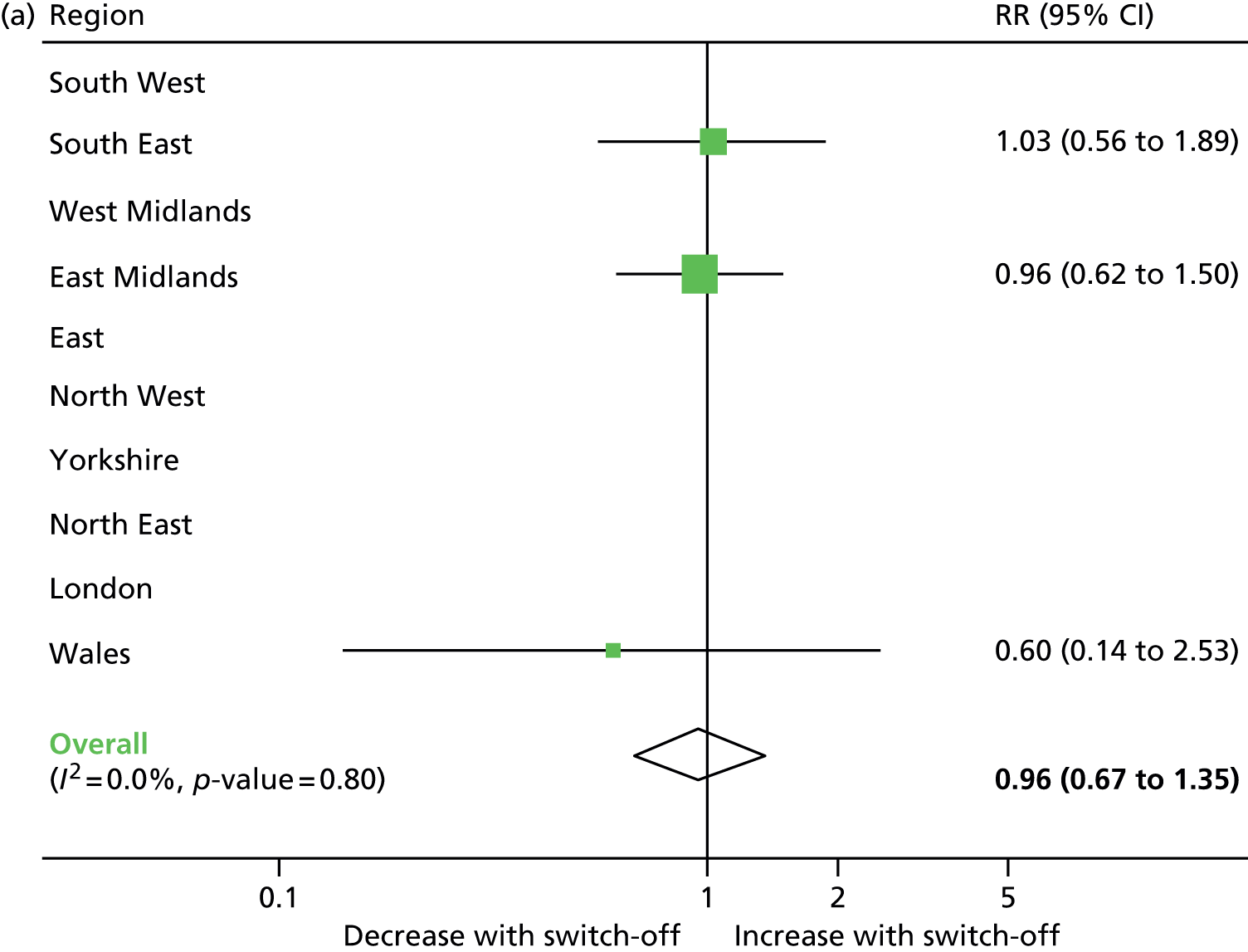

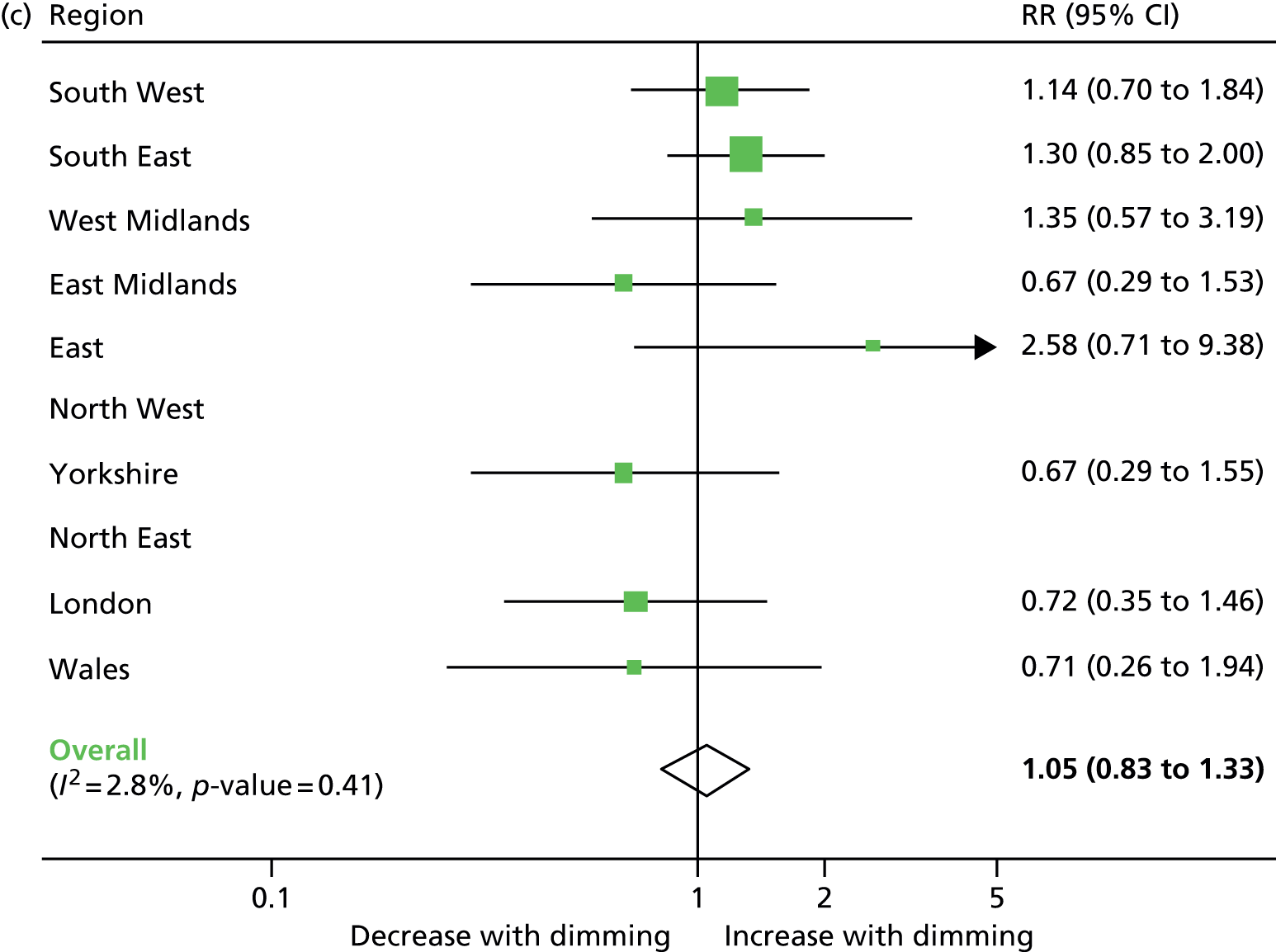

Crime
Figure 7 shows the estimated mean associations between changes in the proportion of total km of road in each MSOA that had introduced each street lighting adaptation intervention and counts of each offence across England and Wales. The RRs indicate the expected change in crime if 100% of total km of road in an area were to receive the lighting intervention.
FIGURE 7.
Associations between street lighting adaptation strategies and crime. (a) Switch-off; (b) part-night lighting; (c) dimming; and (d) white light. PNL, part-night lighting.


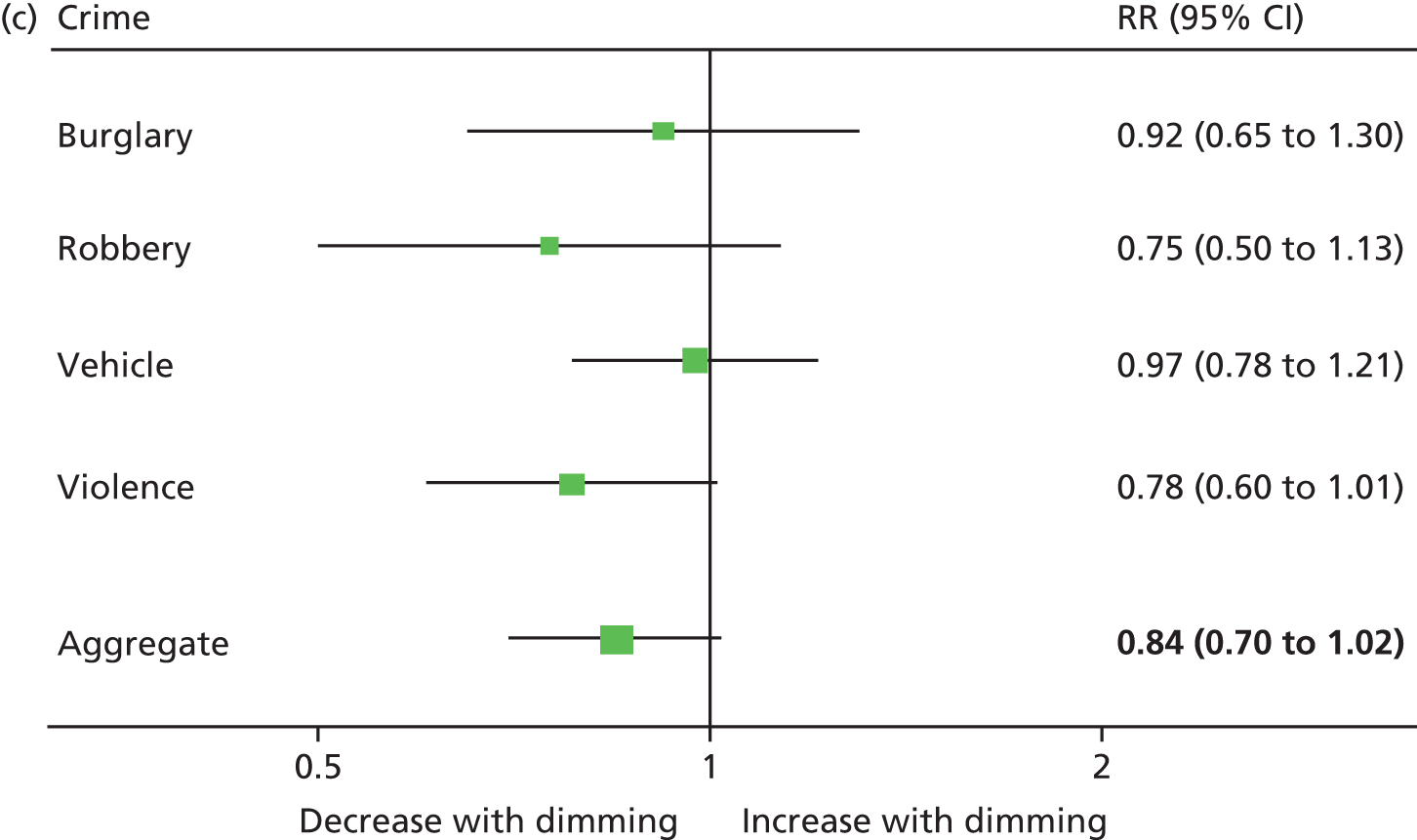

There was no evidence from the overall estimates for an association between the aggregate count of crime and switch off (RR 0.11, 95% CI 0.01 to 2.75) or part-night lighting (RR 0.96, 95% CI 0.86 to 1.06).
There was weak evidence for a reduction in the aggregate count of crime and dimming (RR 0.84, 95% CI 0.70 to 1.02) and white light (RR 0.89, 95% CI 0.77 to 1.03).
These RRs estimate change in outcomes associated with 100% implementation of the street lighting intervention in an area. To assist with interpretation, if dimming was applied to street lights on 10% of total road km in an area, the estimated change in crime in the area would be equivalent to 0.840.10 = 0.98 (i.e. a 2% decrease in crime) with a 95% CI from 0.700.10 = 0.96 to 1.020.10 = 1.00 (i.e. between a 4% decrease and no change in crime). If applied to street lights on 50% of roads, the change would be 0.840.50 = 0.92 (i.e. an 8% decrease in crime) with a 95% CI from 0.84 to 1.01 (i.e. between a 6% decrease and a 1% increase in crime).
We found significant heterogeneity between the estimates at police force level (see Appendix 6). There was strong evidence for an association between part-night lighting, dimming and white light and a decrease in crimes in some police forces, and strong evidence for an association between part-night lighting, dimming and white light and an increase in crimes in others.
When specific crime offences were considered, the estimates provide suggestive evidence that part-night lighting may be associated with an increase in robbery (RR 1.48, 95% CI 0.99 to 2.21) and that dimming may be associated with a decrease in violence (RR 0.78, 95% CI 0.60 to 1.01).
We found consistency in the direction of the estimated associations between crime and dimming, with all point estimates indicating reductions in crime. There was similar consistency in the estimated associations with white light, all suggesting reductions in crime.
Other analyses
Area deprivation (England only)
When the analysis was repeated in each of three strata of areas based on the IMD,43 the stratum-specific estimates were not materially different from the overall estimates, and each was imprecise (Table 3). There were insufficient data for switch-off.
Adaptation | Overall RR (95% CI) | IMD1 (least deprived) | IMD2 | IMD3 (most deprived) |
---|---|---|---|---|
Switch-off | 0.11 (0.01 to 2.75) | Insufficient data | Insufficient data | Insufficient data |
Part-night lighting | 0.96 (0.86 to 1.06) | 0.90 (0.79 to 1.02) | 0.92 (0.78 to 1.08) | 0.99 (0.79 to 1.24) |
Dimming | 0.84 (0.70 to 1.02) | 0.86 (0.58 to 1.27) | 0.99 (0.79 to 1.24) | 0.95 (0.77 to 1.16) |
White light | 0.89 (0.77 to 1.03) | 1.01 (0.76 to 1.33) | 0.91 (0.71 to 1.16) | 0.90 (0.75 to 1.08) |
There was no strong evidence for any associations between any street lighting changes and road traffic injuries within any IMD strata (Table 4).
Adaptation | Overall RR (95% CI) | IMD1 (least deprived) | IMD2 | IMD3 (most deprived) |
---|---|---|---|---|
Switch-off | 0.97 (0.82 to 1.15) | 0.88 (0.68 to 1.14) | 1.02 (0.71 to 1.45) | 1.23 (0.83 to 1.82) |
Part-night lighting | 0.95 (0.84 to 1.07) | 1.02 (0.85 to 1.23) | 0.81 (0.66 to 0.99) | 1.15 (0.84 to 1.59) |
Dimming | 1.00 (0.91 to 1.10) | 1.05 (0.88 to 1.25) | 0.95 (0.80 to 1.14) | 1.01 (0.84 to 1.21) |
White light | 1.01 (0.93 to 1.09) | 0.93 (0.80 to 1.09) | 0.95 (0.82 to 1.09) | 1.14 (1.01 to 1.29) |
Regression to the mean
Had we observed any evidence for an increase in collisions or crime following reduction of street lighting we had planned to assess whether or not the associations may be, in part, due to bias from regression to the mean. We found no evidence for increases in either outcome and so this analysis was not conducted. In addition, there were insufficient months of crime data available prior to street lighting interventions being implemented for an assessment of potential bias due to regression to the mean to be meaningful.
Model checking and sensitivity analysis
We found no evidence for overdispersion in the road collisions analysis. There was evidence for overdispersion in the crime analysis. Table 5 presents a summary of the residual scale overdispersion found in the crime data over crimes and police forces. The average overdispersion for the aggregate count of crime was 1.69 (i.e. compared with 1.0, which would indicate a Poisson distribution with no overdispersion).
Crime | Mean | Minimum | Maximum |
---|---|---|---|
Burglary | 1.66 | 1.34 | 2.04 |
Robbery | 1.12 | 0.56 | 1.52 |
Vehicle crime | 1.69 | 1.30 | 2.13 |
Violence | 1.35 | 1.16 | 1.99 |
Aggregate count | 1.69 | 1.31 | 2.24 |
We investigated evidence for zero-inflation in our models for collisions at regional level. The marginal fraction of zero counts in each region was very similar to theoretical probabilities based on a Poisson mean (i.e. within 0.001) and we concluded no zero-inflation. For crime models zero-inflation was evaluated in a negative binomial model and no evidence was found. The results allowing for zero inflation are presented in Table 6 with the results of the other sensitivity analyses. Although small differences were apparent, they did not alter the interpretation based on the main results.
Intervention | Switch-off RR (95% CI) | PNL RR (95% CI) | Dimming RR (95% CI) | White light RR (95% CI) |
---|---|---|---|---|
Main result | 0.11 (0.01 to 2.75) | 0.96 (0.86 to 1.06) | 0.84 (0.70 to 1.02) | 0.89 (0.77 to 1.03) |
Negative binomial model | 0.16 (0.01 to 2.13) | 0.96 (0.87 to 1.06) | 0.85 (0.71 to 1.01) | 0.88 (0.76 to 1.02) |
Zero-inflated negative binomial model | 0.16 (0.01 to 2.13) | 0.98 (0.88 to 1.09) | 0.85 (0.71 to 1.01) | 0.88 (0.76 to 1.02) |
No auto-regression terms | 0.18 (0.01 to 2.49) | 0.99 (0.90 to 1.10) | 0.83 (0.70 to 1.00) | 0.86 (0.74 to 1.01) |
Discussion
Key results
This study has provided detailed evidence on the impact of street lighting energy-saving schemes on two important public health outcomes, namely crime and road traffic injury. We obtained data from 62 local authorities in England and Wales, which collectively account for a total of over 30,000 road km affected by lighting changes by 2013.
In summary, the key results are:
-
Switch-off (permanently turning street lights off) was not associated with an increase in night-time traffic collisions or crime; however, the results are imprecise because of the small number of areas in which switch-off was implemented, and so should be treated with caution.
-
Part-night lighting (e.g. street lights are switched off between 12 a.m. and 6 a.m.) was not associated with an increase in night-time traffic collisions or crime.
-
Replacing conventional yellow lighting with white light was not associated with an increase in night-time traffic collisions and was associated with a reduction in crime, though estimates were imprecise.
-
Dimming of conventional yellow light or white light was not associated with an increase in night-time traffic collisions and was associated with a reduction in crime, though estimates were imprecise.
These results were not materially changed by the sensitivity analyses undertaken.
Limitations
The study used routine data sources on road traffic injuries (police STATS19 data) and crime (Police.uk data). These data sets have several limitations; in particular, they may be incomplete owing to under-reporting. However, for under-reporting to affect the results of our analysis, there would have had to have been differential changes over time in the recording of crime and road traffic injuries in the streets where lighting has been changed, compared with streets without changes to street lighting. This seems unlikely.
Before making crime data publicly available, the spatial locations of reported crimes in the Police.uk data are altered to protect the anonymity of victims. This introduces the potential for misclassifying crime to roads adjacent to those on which they occurred, which could lead to them being incorrectly allocated to roads on which lighting reduction interventions have been introduced (or vice versa). To address this limitation, we conducted a reliability study that compared these data with detailed police-recorded crime data, and to identify the spatial level at which counts of crime using both sources are in good agreement. Analyses were then conducted at this level of spatial resolution. Thus, while we are reasonably confident in the direction of the associations between street lighting reductions and crime, we must remain cautious about their relative magnitudes as the effects are measured at the area, rather than street segment, level.
To address the potential for under-reporting to bias estimates of effect on collisions, we analysed separately those collisions where casualties were fatally or seriously injured, as these collisions are more likely to be reported to the police. These analyses found no evidence for associations between street lighting changes and night-time collisions. We also analysed crimes that are more likely to be reported to the police (i.e. burglary and vehicle theft reports are required for insurance claims). The estimated effects were not consistently larger for these two types of crime. Our analysis did not, however, include any measures of fear of crime, which might be expected to have increased in those areas with reduced lighting.
We could not take into account the potential impact of other road safety or crime prevention initiatives, such as improved road markings, policing interventions or CCTV, for example. If such measures were introduced more often in streets where lighting has been changed than elsewhere, it is possible that some of the associated changes in crime in areas where lighting has been changed may be attributable to these other measures. It is likely that such confounding will account for part of the observed associations with crime within the areas where street lighting was changed, compared with other areas.
We found no convincing evidence for associations between street lighting reductions and road traffic injuries. In designing the study we had estimated the hypothesised effect of reducing street lighting on casualties (a 32% increase) by using the inverse of the relative risk estimated in the Cochrane systematic review. 36 We had assumed there would be 1500 night-time injuries on intervention roads during 10 years before lighting reduction and 150 injuries 1 year after, to achieve 90% power to detect this increase of 32% above pre-intervention injury levels. For our analysis of switch-off, we were able to include over 1780 night-time casualties on roads before street lighting was reduced (i.e. during 2000–10) and 298 night-time casualties after street lighting was reduced (i.e. during 2011–13). It is possible that we did not have sufficient statistical power to detect smaller, plausible associations. However, for other street lighting interventions, such as part-night lighting and dimming, the numbers of night-time collisions included in the analysis were much larger.
It is also possible that the numbers of pedestrians, cyclists, motorcyclists and car drivers who use and travel within the streets and areas where street lighting was reduced declined at the same time as the lighting was reduced, resulting in fewer road casualties in those streets and areas. If so, any increase in hazard to pedestrians and cyclists due to lower lighting conditions might have been obscured by a reduction in numbers of people exposed to road injury risk at night.
Interpretation
In the context of reported concern about street lights going out at night, this study found little evidence of harmful effects of switch-off, part-night lighting, dimming or changes to white light/LEDs on road collisions or crime in England and Wales. Given previous evidence that introducing street lighting is associated with a reduction in road traffic collisions, it is notable that we found no evidence for an increase in collisions where street lighting was reduced at night. We did not, however, have direct measures of exposure, and so we do not know whether or not there were changes in cars or pedestrians using the affected areas. Neither do we have measures of risk compensation, which might shed light on whether or not road users take more care in darker streets. Certainly, the mechanisms linking the reduction of street lighting to impacts on crime and road safety may be different from those linking improvements to those impacts. First, given potential pathways that operate through feelings about neighbourhood or trust in local authority, removal of a public good may have very different effects from maintaining or improving it. There are, then, potential explanations that relate to both the motivations for the intervention on the part of local authorities and the ways in which those interventions are understood by those living in, and using, affected streets. As Chapter 2 outlined, concerns about dark streets are overlaid to some extent by concerns about the fact that a local authority has removed a public good: this may play out differently in different areas of the country. Indeed, we found significant evidence for heterogeneity in the impacts estimated at police force level, indicating the importance of context. In some forces there were statistically significant reductions in crime, whereas in others there were significant increases.
Permanently switching lights off in an area may signal decline and a lack of governance. 35 If switch-off is taken as a sign of disengagement with a community, mechanisms such as that described by the ‘broken window’ thesis, whereby disorder generates further disorder,44,45 might operate to escalate crime incidence. Dark streets might also encourage some forms of criminal activity. For instance, thieves may feel less conspicuous and less concerned about being seen or identified by either passers-by or CCTV operators. Further research is necessary to uncover the mechanism(s) through which changes (increases or decreases) to street lighting might bring about their effects on crime. To date, though, it appears that the decisions made by those local authorities that have shared data with us have not increased the risks of road traffic injuries or crime to their residents.
Generalisability
This study used data from a range of settings in the UK, including urban, rural, deprived and affluent areas, and has shown that the effect of changes to street lighting on crime (but not road traffic injuries) appears to vary across contexts. Our stratified analyses must be treated with a degree of caution, but they suggest that switch-off was associated with an increase in burglary and vehicle crime in more deprived areas and city/town centres, whereas part-night lighting was associated with decreases in burglary and vehicle crime in less deprived areas. The extent to which these results may be applied to a single area or street remains a matter for further enquiry.
Chapter 4 Cost–benefit analysis: methodological challenges of evaluating large-scale public health interventions and a worked example of the costs and benefits of part-night lighting
Introduction
Public health research is increasingly concerned with the assessment of value for money of large-scale public policies and interventions which impact on public health. Some of these large-scale public policies can incorporate characteristics of what economists call ‘public goods’. Public goods are goods that are available to everyone without exclusion, that can be enjoyed by all simultaneously and where one person’s use does not reduce the availability of the goods to others. Examples of public health interventions with characteristics of public goods include the introduction of bicycle lanes to encourage more active travel and the building of flood defences to protect homes and businesses against extreme weather. As recent articles have pointed out, the methods used by researchers to determine whether or not large-scale public policies offer value for money are currently inadequate,46–48 and when these policies involve characteristics of public goods, evaluations face even greater challenges. 49
In this final chapter we highlight some of the broad methodological issues of undertaking an economic evaluation of large-scale public health interventions, and use the reduction of street lighting by local authorities in England and Wales as a case study to explore the challenges facing evaluations of interventions involving characteristics of public goods. Finally, we use methodological recommendations from the literature and results from Chapter 3 of this report to structure a cost–benefit analysis (CBA) of switch-off regimes.
Types of economic evaluation
There are four main types of economic evaluation currently used in public health: cost-effectiveness analysis (CEA), cost–utility analysis (CUA), cost–consequence analysis (CCA) and CBA. CEA, which calculates a cost per unit of health outcome for each intervention, tends to be the most common form of economic evaluation in public health. CEAs allow policy-makers to compare different interventions to achieve what economists call ‘technical efficiency’: using the minimum amount of inputs to produce a given output. For example, a recent CEA compared two obesity prevention strategies (‘traffic-light’ nutrition labelling and ‘junk-food’ tax) by calculating the cost of each strategy per body mass index unit saved. 50
Cost–utility analyses are similar to CEAs, except that instead of measuring the effect of an intervention in units of health outcomes, CUAs measure the impact of an intervention in terms of a preference-weighted, health-related quality of life measure, such as a quality-adjusted life-year (QALY). Using a quality of life measure approach allows very different health outcomes potentially to be rendered (more) comparable, which helps to facilitate comparisons. In practice, if the cost per unit of QALY of the intervention is below a certain threshold (£20,000–30,000 in the UK) then the intervention is considered to offer acceptable value for money. These thresholds help policy-makers to improve what economists call ‘allocative efficiency’, in other words an optimal distribution of services. However, while very useful for evaluating health technologies, the focus of CEAs on a single health-related outcome and of CUAs on QALYs is usually inadequate for large-scale public health interventions, which often have multiple outcomes and broader objectives than just health gain. 51
Cost–consequence analyses solve some of the shortcomings of CEAs and CUAs for evaluations of large-scale public health interventions by measuring the impact of an intervention on a number of related outcomes. Policy-makers can then compare the costs of the intervention with multiple outcomes. However, CCAs have their own challenges: they do not synthesise benefits and costs and, therefore, oblige policy-makers to apply a (usually) implicit weighting to outcomes based on their own set of preferences. Judging the worth of an intervention based on an implicit set of preferences may not be particularly helpful for policy-makers, as it lacks transparency and makes it difficult to take decisions on a consistent basis. Although CCAs provide a systematic technique for assessing interventions,52 they cannot be used to rank interventions. For instance, Trueman and Anokye53 evaluated the costs and consequences of exercise referral schemes. Costs were estimated at £22M to the health-care provider and £12M to participants. Benefits were estimated at an additional 3900 people becoming active, 51 avoided cases of coronary heart disease, 16 avoided cases of stroke and 86 avoided cases of diabetes. Policy-makers must decide how exercise referral schemes compare with other physical activity interventions, which may have different health outcomes.
Unlike CCAs, CBAs synthesise all costs and benefits of an intervention by valuing all costs and benefits in monetary units. In principle, this approach is more attractive, as all outcomes and inputs are valued in the same units, making comparisons more straightforward. When the calculation of all of the values of costs and benefits are carefully justified and made explicit, CBAs can lead to more transparent decision-making. Academics have, therefore, argued that more attention should be placed on the CBA framework when evaluating large-scale public policy interventions. 52 Although CBAs in public health are a relatively recent phenomenon, CBAs have been used to evaluate a number of road danger reduction strategies such as roundabouts,54 speed cameras55 and 20 mph zones. 56
Although the CBA framework offers potential to be a useful decision-making tool, recent critiques have pointed out some conceptual and methodological challenges of these frameworks in general47 and when applied to evaluations of large-scale public health interventions in particular. 46,48 Conceptually, determining the scope of economic evaluations, that is which costs and benefits, outcomes and effects to include in the analysis, can be contentious. Often, in practice, the included costs and benefits are limited to those that are measurable, which has potential to bias decision-making. Choosing the time frame of an economic evaluation can also be challenging. Large-scale public policies which address some of the more structural or upstream determinants of health may take many years to achieve benefits and some critics have suggested that too much focus is often placed on short-term outcomes. As a general framework, economic evaluations have also been criticised for failing to consider issues around equity. In addition to these general critiques of economic evaluations, critiques specific to evaluations of large-scale policies and interventions have focused on three main areas: (1) determining the total costs of intervention, (2) determining the ‘wider’ health effects attributable to the intervention and (3) putting a monetary value on the effects of the intervention.
Costs of interventions
Although determining the costs of interventions is necessary in any economic evaluation, costing large-scale public health interventions can have particular challenges. Costs of large-scale public health interventions may be incurred by a range of agencies, making estimates of total costs tricky. For example, the costs of introducing free bus travel for young people in London, an intervention with many potential public health implications,57,58 were incurred by two different agencies. The costs of manufacturing and posting bus passes to young people were incurred by Transport for London (i.e. administrative costs of the scheme). The costs of providing increased bus capacity were incurred by the bus network operators within London (i.e. operating costs of the scheme). The marginal cost of an additional concessionary journey can be estimated using a variety of methods, which can lead to different results, further complicating cost estimation. In addition to having costs shared by multiple agencies, large-scale public health interventions may also shift costs (for instance, from public service authorities to individual households) making total cost calculations even more problematic.
‘Wider’ effects of policies
Traditionally, public health research has focused on the direct impacts of interventions on the health of individuals. This ‘micro-level’ focus often excludes important outcomes that indirectly effect health, such as social capital, community empowerment, etc. 48 Most research argues that these types of outcomes should ideally be included in evaluations of large-scale policies and interventions. Large-scale interventions can also affect non-health outcomes47 and conceptual decisions need to be made about whether or not these outcomes are included in the scope of the analyses. Identifying the wider health effects (and non-health effects if they are to be included) of large-scale policies is not straightforward. Causal pathways are complex and often unknown. Complicating matters is the fact that large-scale public policies are often implemented within what are called ‘complex systems’ with their own emergent properties that can have important health effects. 46
Attribution of health and wider effects to large-scale interventions is also problematic. Large-scale interventions can occur at the same time as other changes, making it difficult to assess which specific changes in health outcomes result from the intervention. In complex environments there are often no obvious comparison or ‘control’ areas to help contextualise changes. Randomised controlled trial (RCT) designs are often not feasible (or necessarily appropriate) in evaluations of large-scale policies and so evaluations may need to rely on robust observational methods to attribute health and wider effects of policies.
Monetising the effects of interventions
Valuing large-scale public health interventions also begets a number of methodological concerns. There are two main ways of valuing the benefits of interventions: revealed preference techniques and stated preference techniques. Revealed preference techniques use market information to place a monetary value on particular benefits. For example, economists can use the difference in compensation for different types of jobs to monetise the ‘risk’ of different types of workplace injuries. However, there are a number of fundamental challenges in identifying the trade-offs that individuals make (particularly the difficulty of controlling for other influences on decisions). Revealed preference techniques are very rarely used in monetising interventions of public goods: the non-excludable nature of public goods ensures that there are fewer opportunities for trade-offs to be identifiable. Therefore, research aiming to monetise benefits of large-scale public health interventions tends to concentrate on stated preference techniques. Stated preference techniques ask individuals to place a value on changes in their health and welfare, usually through a process called contingent valuation. Broadly, contingent valuation processes ask individuals hypothetically about the maximum amount of money they would be willing to pay for the benefits associated with the introduction of a service, or about the minimum amount of money they would be willing to accept as compensation for the removal of a service.
Although contingent valuation methods are the dominant valuation techniques used in public health, they involve a number of methodological choices which may impact on their validity and reliability. Briefly, these issues include how best to construct the hypothetical ‘scenario’ presented to individuals, the choice of ‘payment vehicle’ (the means by which payment will hypothetically be made), the time period for valuation and whether individuals are valuing the intervention under conditions of certainty or risk. 59 The choice of methods may have implications for the extent of hypothetical and strategic bias present in the valuation. Large-scale interventions that involve characteristics of public goods present even greater challenges for stated preference valuations. Evidence for ‘free rider’ hypotheses suggests that public goods are often undervalued and principles of ‘loss aversion’, the ‘endowment effect’ and the well-known willingness-to-pay/willingness-to-accept gap suggest that it is particularly difficult to value removal of a public good.
We use street lighting as a case study to illustrate some of the complexities of CBA of large-scale public health interventions with public good characteristics.
Case study: street lighting
Street lighting is an intervention with essential characteristics of a public good. Street lighting can be experienced by all residents and visitors of a particular area simultaneously and without exclusion. Once street lighting is provided, it is impossible to exclude others from benefiting, and one person’s use of street lighting does not reduce its availability to anyone else.
Many local authorities in England and Wales are considering reducing, or have reduced, some street lighting provision at night with the aim of reducing costs, but also with considerations of contributing towards climate change mitigation and reducing environmental light pollution. 6 However, the reduction of street lighting at night may have other unintended consequences (see Figure 1). Public concerns about these proposals have centred on crime, public perceptions of safety, and road safety. Potential positive impacts of reduced lighting have also been noted, in particular for amateur astronomy,10 and reductions might, in theory, mitigate the negative health impacts some have claimed result from ‘light at night’, such as disrupted sleep. 15
Although some work has been done on evaluating the costs and benefits of improving street lighting,60,61 to our knowledge no studies have assessed the costs and benefits of reducing street lighting. Reduced street lighting presents a helpful case study for exploring some of conceptual and methodological issues of CBA frameworks because the intervention has (deceptively) complicated costs, the intervention has many ‘wider’ effects and it presents valuation difficulties.
Costs of intervention
In England and Wales, street lighting is delivered by different agencies depending on the location of the street. The Highways Authority is responsible for providing street lighting on motorways. For the most part, local authorities deliver street lighting on roads within their boundaries. However, in major metropolitan areas, street lighting on ‘trunk roads’ (usually major roads that span different local authorities) is provided by metropolitan agencies.
Interventions to reduce street lighting have taken a number of forms. Some local authorities have introduced part-night lighting, where street lights are turned off between certain hours of the night (typically between 12 a.m. and 6 a.m., although this can vary). Other local authorities have introduced dimming, where lights stay on all night but emit a reduced amount of light. Trimming, where street lights are switched on slightly later and switched off slightly earlier, is another popular intervention. Finally, local authorities have also switched to different (usually more energy efficient) types of lighting. Many local authorities introduce multiple interventions involving the same street light.
If we take a societal perspective in the CBA framework, calculating the costs of these different interventions to different agencies poses a number of problems. Local authorities incur four major costs associated with street lighting reduction interventions, energy costs, maintenance costs, lighting adaptation costs and consultation costs, each of which may be difficult to tease out. Not all local authorities consult residents about changes to street lighting provision; among those that do, consultations on reduced street lighting can take place within a broader discussion of local issues, which means making some assumptions when attributing costs to the street lighting intervention in particular. In terms of energy costs, energy tariffs differ not only by company but by time of day, and energy companies have sometimes changed their prices in response to reduced street lighting. Maintenance costs are also problematic. Reduced street lighting is often part of a larger programme of works to update street lighting generally, so, again, some assumptions need to be made to attribute part of these costs to the street lighting intervention.
‘Wider effects’ of street lighting
There are many potential effects of reduced street lighting on public health and many of these may be mediated through related changes that increase and/or decrease the likelihood of particular outcomes (see Figure 1). For instance, one theory suggests that reduced street lighting will lead to poorer visibility on the streets at night. This decrease in visibility will, in turn, lead to an increase in road traffic collisions and particular types of crime. However, reduced visibility at night may also reduce residents’ mobility, if they are less likely to go out at night because of fear of crime or injury. Reduced mobility may lead to a decrease in the number of crimes and road traffic injuries that take place in affected areas.
In addition, there are a number of non-health related outcomes that may be affected by street light interventions. For example, if reduced visibility leads individuals to travel more slowly or to avoid particular areas then this has implications for the cost of travel. A reduction in mobility due to the reduced street lighting schemes may also have implications for the night-time economy near affected areas. Conceptual decisions need to be made about whether or not to include these non-health outcomes.
In a real-world context, it may be difficult to attribute any change in health outcomes to the street lighting intervention. Local authorities choose to reduce street lighting in particular areas, for different reasons, but many choose to reduce street lighting in what they consider to be ‘low-risk’ areas. It may be difficult to identify matching comparison areas, there are a number of potential confounders, and other changes in the streets may have occurred around the same time (e.g. increased police presence; traffic-calming measures), making attribution of causation difficult. For instance, in response to concerns about crime, policing policies may have changed to increase presence of police in those areas affected by the interventions. Additionally, companies or individuals may have invested privately in their own lighting in response to the intervention, which may mitigate any effects of the intervention. Depending on the scope of an economic evaluation these private investments may need to be considered in the costs of the intervention.
Difficult valuation issues
Street lighting reduction offers a complex case for stated preference approaches to valuation for a number of reasons. Research into the value of introducing street lighting has identified a few technical challenges. First, in a context where there is a lack of transparency in local government spending,62 the public may lack trust in the agency responsible for street lighting provision. This presents challenges in choosing a method of eliciting willingness to pay/willingness to accept. Using repeated valuations may anger and surprise the respondent as a result of expectations about the first valuation representing actual cost of the intervention; respondents may wish to send a message about keeping prices low to the untrusted authority; and values may be contingent on other changes in local taxes that have occurred recently. 61 Second, individuals are likely to base their preferences for reduced street lighting on the direct effects of the intervention (such as crime, fear of crime and road safety concerns) and are unlikely to consider other more minor implications such as light pollution. 60
Overcoming challenges
A number of recent articles have made recommendations for the conduct of CBA in the face of these challenges. Broadly, these include questionnaires or consultations with key stakeholders to inform cost calculations, conceptual modelling and quasi-experimental methods to elucidate the wider benefits of an intervention, and utilising guidance and literature from a range of disciplines on valuation.
Costs of interventions
There are a number of different approaches to collecting costs of interventions. Macro-costing (or ‘top-down’ costing), often used to cost health-care episodes, looks at the total cost incurred by a government agency and then applies an appropriate proportion. Macro-costing has a number of disadvantages for large-scale public policy interventions: costs tend to be shared across multiple agencies and macro-costing methods typically fail to quantify indirect costs, such as those to individuals. Alternatively, micro-costing (or ‘bottom-up’ or ingredient) approaches sum the cost of each individual feature of the intervention. For instance, in the case of reduced street lighting ingredients would include public consultations, any changes to lighting infrastructure and energy costs. Micro-costing methods are able to include indirect costs and costs to multiple agencies, though some work is required in identifying these types of costs. Recently, a number of studies have created costs assessment tools,63 which ask different centres/institutions delivering the intervention about costs of particular ingredients. Alternatively, studies have relied on more informal consultations with key stakeholders to help to estimate costs of different ingredients.
‘Wider’ effects
Assessment of the ‘wider’ effects of large-scale public policies involves both conceptual challenges in determining the range of outcomes that may have been affected and methodological challenges in attributing any changes in outcome to the intervention under study.
Logic models are widely used in public health to present theoretical understandings of relationships between interventions and health outcomes. Developing a logic model linking large-scale interventions with wider outcomes that is able to include complexities such as feedback loops and emergent properties requires a systematic and iterative approach. Recent guidance has suggested embarking on conceptual modelling as a first step in understanding the ways that policies can shape the health of the population. 64 Conceptual models are based on the premise that causal pathways connect the wider determinants of health to both individual health outcomes and to patterns of population-level health. Guidance suggests assessing the intervention of interest at a variety of different analytic levels, including economic, social, political, and physical and biological factors. Once an initial model has been drafted, researchers can review the literature across a range of related disciplines to further explore elements of the model and refine and develop understandings of relationships and mechanisms. 65
Once all of the potential health and wider effects of large-scale interventions have been identified, any changes in these outcomes need to be attributed to the intervention. RCTs are the gold-standard method for attribution of effects and should be adopted for large-scale policies whenever possible and appropriate. 66 However, given that RCTs are often not feasible (and may in some circumstances miss crucial aspects of large-scale policies), a substantial literature has emerged on methods to attribute health effects to interventions in the absence of RCT evidence. Studies have used a number of quasi-experimental designs to attribute outcomes to large-scale policies. For instance, controlled interrupted time series methods have been used to quantify the effects of large-scale policies such as speed zones42 and policies that limit the availability of alcohol. 67 Natural experiment methods have been used to evaluate a wide range of policies from free bus travel for young people57 to large-scale food retailing. 68 Craig et al. have produced useful guidelines on methods to employ natural experiments to evaluate large-scale interventions. 69
Valuing outcomes
As discussed earlier, estimating willingness-to-pay/willingness-to-accept values for large-scale interventions as a whole presents methodological difficulties and has time and cost implications. An alternative method of valuation involves summing up stated preference estimates of the individual and wider benefits attributable to the intervention. The advantage of this method is that there are often valuations of particular benefits available in the economics (or related discipline) literature. In the reduced street lighting example, for instance, there are well-used willingness-to-pay valuations for the prevention of crimes70 and road traffic injuries. 71 There are even some valuations for some of the ‘wider’ benefits of public health interventions. In the street lighting example, there is some work estimating willingness to pay to reduce fear of crime and for improvements to the night sky. Although promising, this method faces some methodological challenges. The complex casual pathways linking large-scale interventions to health and wider outcomes are likely to include inter-related mechanisms and feedback loops, which could lead to the double counting of particular benefits. Further, the well-reported part–whole bias suggests that the sum of valuations placed on individual benefits may exceed the value of the intervention as a whole. Interventions with externalities or other market failures (which large-scale interventions are likely to have) are particularly vulnerable to part–whole bias.
Although interest in economic evaluations of large-scale interventions within public health disciplines is relatively recent, there is a longer tradition of valuation in environmental, agricultural and resource economics. Policies with characteristics of public goods are common within these disciplines, and economists have produced good-practice guidelines on, for example, how to value wetlands72 and forest goods and services. 73 Perhaps public health can learn from these disciplines to overcome some of the challenges of valuing large-scale policies.
Cost–benefit analysis of part-night lighting
The previous sections have outlined some of the challenges of conducting a CBA of reduced street lighting and provided some suggestion of methods to overcome these challenges. This section uses some of these methodological suggestions to compare the costs of one street light intervention, part-night lighting, with the public health benefits in a simple model.
In this CBA, we have based our assumption about numbers of street lights, crime rates and road traffic collisions for a typical local authority and we compare two scenarios:
-
part-night lighting scenario – a proportion (e.g. 5%) of lights are adapted to turn off between 12 a.m. and 6 a.m.
-
‘do nothing’ scenario – street lighting continues to be provided without adaptation.
We take a societal perspective and include all costs and benefits to society where we have estimates of an association with part-night lighting regimes. This necessarily excludes outcomes that are more difficult to measure, or outcomes that are difficult to attribute to the intervention. This analysis should, therefore, not be viewed as a comprehensive CBA of part-night lighting regimes; instead, we present it as a starting point for use by local authorities to assist with local discussions on whether or not part-night lighting regimes will offer value for money in their localities.
Methods
In our cost–benefit model, we have simulated forward-looking estimates of the costs and benefits of adapting a proportion of street lights in a typical local authority area over 5-, 10- and 15-year time horizons. All prices are reported in 2015 GBP.
(Dis)benefits
Using estimates presented in Chapter 3 of this report, this CBA considers night-time road traffic collisions and four types of crime, burglary, vehicle crime, robbery and violence, as the (dis)benefits of part-night lighting regimes. The model includes reductions in greenhouse gas emissions (CO2) from reduced energy use at night as a benefit.
Collisions
The estimates in Chapter 3 suggest that night-time road traffic collision rates decrease by 5% after the implementation of part-night lighting on roads (RR 0.95, 95% CI 0.84 to 1.07). It is important to note that this effect estimate is from a model fitted at road segment level and so applies at the road segment level, whereas this CBA considers costs and benefits of part-night lighting regimes at a local authority level. For the RR of 0.95 to apply to the whole local authority, it would require area-wide implementation of part-night lighting (i.e. 100% of all road segments). If, as in this CBA, a local authority is considering introducing part-night lighting on a fraction (e.g. 5%) of its roads, the reduction in traffic collisions expected is estimated by raising the RR to the power of the fraction of roads (i.e. 0.9 ^ 0.05).
Our CBA model assumes that, independent of changes to street lighting, night-time collision rates are changing over time: we derived the annual trends in night-time collisions using a local authority-level data set, by modelling yearly and monthly trends. The results suggest an average annual background decrease in night-time collisions of 1.6% (RR 0.984, 95% CI 0.983 to 0.985).
To estimate the monetary costs to society of night-time road collisions, we used valuations from the Department for Transport,74 which include loss of output due to injury, ambulance costs and costs of hospital treatments, human costs of casualties, costs of damage to vehicles and property, police costs and administrative costs of insurance. The estimated average value of preventing a road traffic collision is £79,174 (valuations converted to 2015 prices using the Retail Prices Index75).
Crime
The estimates in Chapter 3 suggest that if all street lights in an area were adapted to part-night lighting, the number of burglaries would decrease by 8% (RR 0.92, 95% CI 0.74 to 1.13), vehicle crimes would decrease by 9% (RR 0.91, 95% CI 0.76 to 1.09), robberies would increase by 48% (RR 1.48, 95% CI 0.99 to 2.21) and violent crimes would increase by 1% (RR 1.01, 95% CI 0.92 to 1.11).
To estimate background trends in crimes occurring independently of changes in street lighting, we modelled yearly and monthly trends in crimes in England and Wales at the area level (MSOA). Poisson models were fitted for each of the four crime types as the dependent variables, with a linear term for year and categorical terms for months. These models estimated an average annual decline of 8% in burglary (RR 0.915, 95% CI 0.912 to 0.918), a 5% decline in vehicle crime (RR 0.95, 95% CI 0.945 to 0.952), a 13% decline in robbery (RR 0.87, 95% CI 0.859 to 0.976), and an 8% decline in violent crime (RR 0.92, 95% CI 0.917 to 0.923).
To estimate the monetary cost of changes in burglary, vehicle crime, robbery and violent crime arising from adaptations to part-night lighting, we used valuations from the Home Office70,76 on the economic and social costs of crime. These valuations include the costs of anticipating a crime, costs of the consequences of a crime and costs of responding to a crime.
The categories used in Police.uk data for our models, however, do not map perfectly onto the Home Office categories (see Appendix 7).
Burglary in Police.uk data includes burglary in and outside a dwelling. According to Home Office reports,76 both types of burglary have similar social and economic costs associated with them: £3565 for burglary in a dwelling and £4185 for burglary outside a dwelling (valuations converted to 2015 prices using the Retail Prices Index75). An update of the Home Office valuations of the social and economic costs of crime70 reported that burglary in a dwelling should be valued at £4750. We therefore used £4750 as the value of the costs associated with burglary in our CBA model.
Vehicle crime in Police.uk data includes a number of Home Office crime categories with different valuations: theft of a vehicle (£6014), theft from a vehicle (£1247) and attempted vehicle theft (£741). To estimate an overall value for vehicle crime for the CBA, we used a weighted average value (£1759), based on the total annual numbers of each offence. 70
We used Home Office valuations of £10,585 for robberies and £15,127 for violent crimes. 70
Carbon emissions
The reduction in energy used by part-night lighting regimes would result in fewer CO2 emissions. To estimate the reduction in CO2 emissions for the CBA, we multiplied the kWh of energy saved by adaptations to street lighting by a greenhouse gas conversion factor for UK electricity. 77 To estimate the monetary value of these CO2 savings, we multiplied the amount saved (in kg) by short-term traded carbon values for UK public policy. 78
Costs
We used a micro-costing approach to estimate costs of a ‘do-nothing’ (no changes to street light provision) and part-night lighting scenario. Costs were largely determined through informal consultations with a number of local authorities (see Chapter 2), guidance issued by the ILP and Power Data Associates and case studies from PFI providers. We include three categories of cost in our cost estimations: energy costs, maintenance costs and capital costs. Consultation costs (of part-night lighting) are excluded as interviews with local stakeholders suggested that public consultations do not always occur and, when they do, the costs are minimal. Any potential benefits from the Carbon Reduction Commitment Energy Efficiency Scheme are also excluded from the model.
Energy costs
We assume that energy is currently supplied at an average cost of 9.5 pence/kWh. 79 Consultations with stakeholders also suggested that energy prices may change in direct response to street lighting adaptation measures. 79 We have assumed in our part-night lighting scenario, therefore, that the energy price rises to an average of 9.6 pence/kWh as a result of these lighting adaptations.
Maintenance costs
Maintenance costs of street lighting include lamp replacement, repair of lighting columns, cleaning and monitoring. In our model, we estimated these costs to be £30 per lantern per year (data from stakeholder interviews).
Capital costs
Most local authorities that have implemented part-night lighting have done so through a central management system (CMS). In this CBA, we assume that there is a capital cost of switching to a CMS of £100 per column (data from stakeholder interviews).
Local authority lighting, collisions and crime
Our cost–benefit model is calculated for a typical local authority, which we have assumed provides street lighting using 30,000 70-W sodium-vapour (SON) lamps. We assume that these lamps come on and turn off at 70 lx, resulting in 4151 hours of lighting provision each year. 79 We have assumed that in the local authority there were 2594 burglaries and 2366 vehicle crimes, 228 robberies, 3180 violent crimes and 159 night-time road collisions in the previous year, which are the median numbers of these outcomes in 2012 based on the local authorities that participated in the LANTERNS project.
Model
Our CBA model allows a proportion of street lighting in the local authority to be changed to part-night lighting. We simulate forward-looking estimates, over 5-, 10- and 15-year horizons, of the costs and benefits of adapting a proportion of street lights to part-night lighting.
In our ‘do-nothing’ scenario, where all lights remain lit at night, we calculate the total cost of street lighting provision each year as energy costs (number of lamps lit × wattage × hours of lighting provision × price of energy in kWh) + maintenance costs (number of lamps lit × maintenance cost per lamp).
The maintenance cost per lamp is assumed to rise each year at the rate of inflation, which is assumed to be 2.5%. 80 In line with current guidance,81 the price of energy is assumed to rise each year at 1.5% above the rate of inflation. There is some debate about by how much energy prices will rise, so alternative estimates of the rate of energy price increase are considered in sensitivity analyses. 82
In terms of benefits, our model assumes that night-time collisions and crimes decline at an average annual rate (as explained above). The model multiplies the numbers of collisions and crimes in an area by the appropriate valuation to estimate the economic and social costs of these outcomes. Benefits are discounted at a rate of 3.5% in accordance with HM Treasury guidance. 83
In our part-night lighting scenario, we assume that 5% of street lights in the local authority are switched off between 12 a.m. and 6 a.m. The total costs of street lighting under this scenario are identical to those in the ‘do-nothing’ scenario, with two important exceptions. First, energy prices in the part-night lighting scenario increase by 0.1 pence/kWh in the first year (an assumed increase in energy price by energy suppliers as a response to the lighting adaptations); in subsequent years, the energy price is assumed to rise each year by 1.5% above the rate of inflation. Second, in the first year of the part-night lighting scenario, the local authority incurs a one-time capital cost of £100 per lighting column to implement a CMS to control street lights remotely.
Benefits in the part-night lighting scenario are calculated in a similar way as the ‘do-nothing’ scenario, with two exceptions. First, benefits associated with carbon reduction are included. Second, in the first year of the part-night lighting scenario, collisions and crimes are assumed to change as a result of lighting adaptation. In subsequent years the model assumes that collisions and crimes will decline at the average background trend rate. In other words, the model assumes that there is a step-change in numbers of collisions and crime at the point where lights are first switched off between 12 a.m. and 6 a.m.
Results are presented in terms of net present values (NPVs) using 5-, 10- and 15-year time horizons, which compare the sum of the costs and benefits of the ‘do-nothing’ scenario with those of the part-night lighting scenario, where 5% of street lights in an area are switched off between 12 a.m. and 6 a.m. We present a range of values to account for uncertainty in the estimates around the effect of part-night lighting on collisions and crimes. Sensitivity analyses are used to examine NPVs over different time horizons and under different energy price assumptions.
Results
Results from the initial model suggest that after 5 years the cost of street lighting provision in the part-night lighting scenario are greater than in the ‘do-nothing’ scenario by £74,754 (Table 7). Over a 5-year time horizon, therefore, a reduction in energy consumption in the part-night lighting scenario is outweighed by the capital costs of implementing part-night lighting through a CMS. The benefits of the part-night lighting scenario, however, are also greater than the benefits of the ‘do-nothing’ scenario by £2,029,519 (range –£6,596,018 to £10,117,131). In other words, it appears that the disbenefits of an estimated increase in robbery and violence associated with the introduction of part-night lighting are outweighed by the benefits of the estimated decrease in collisions, burglary and vehicle crime and carbon reductions. It is important to note that the estimates of effect of part-night lighting on collisions and crimes are imprecise and so lead to big differences in estimates of benefits.
Time horizon | Difference in street lighting provision costs | Difference in (dis)benefits: collisions, crime and carbon emissions (range) | NPV (benefits – cost) (range) |
---|---|---|---|
5 years | 74,754 | 2,029,519 (–6,596,018 to 10,117,131) | 1,954,765 (–6,670,772 to 10,042,377) |
10 years | –16,794 | 3,640,780 (–11,293,68 to 17,618,549) | 3,657,574 (–11,276,887 to 17,635,343) |
15 years | –128,176 | 4,911,520 (–14,715,109 to 23,255,609) | 5,039,696 (–14,586,933 to 23,383,786) |
If we use the upper bounds from the CIs for estimated associations with part-night lighting, collisions and all crime types are estimated to increase with part-night lighting. In this case, the model indicates that after 5 years the disbenefits of estimated increases in collisions and crimes would outweigh the benefits from reductions in carbon emissions by £6,596,018.
Alternatively, if we use the lower bound of CIs around estimates of association between part-night lighting and collisions and crimes, all crimes and collisions would decrease after the implementation of part-night lighting. In this case the benefits of reductions in collisions and crimes in addition to benefits from a reduction in carbon emissions would total £10,117,131. Comparing the benefits to the costs of the part-night lighting scenario with the ‘do-nothing’ scenario results in a NPV of £1,954,765 (range –£6,670,772 to £10,042,377). Implementing part-night lighting on 5% of roads in this hypothetical local authority might, therefore, result in a net benefit to society of nearly £2M after 5 years.
Looking at 10- and 15-year time horizons, the costs of street lighting provision under the part-night lighting scenario are lower than the costs under the ‘do-nothing’ scenario: that is, the reduction in spend on energy exceeds the capital cost of changing to a CMS over these longer time horizons. The benefits implementing part-night lighting driven by reductions in collisions, burglary, vehicle crime and carbon emissions remain large at £3,640,780 (range –£11,293,681 to £17,618,549) after 10 years and £4,911,520 (range –£14,715,109 to £23,255,609) after 15 years. Combined with cost savings these benefits drive a large overall benefit to society (NPVs) after 10 years of £3,657,574 (range –£11,276,887 to £17,635,343) and after 15 years of £5,039,696 (range –£14,586,933 to £23,383,786).
In the following sensitivity analyses we calculate the results under different assumptions about increases in energy prices. Table 8 presents results of the CBA model assuming energy prices rise with inflation only.
Time horizon | Difference in street lighting provision costs | Difference in (dis)benefits: collisions, crime and carbon emissions (range) | NPV (benefits – cost) (range) |
---|---|---|---|
5 years | 78,030 | 2,029,519 (–6,596,018 to 10,117,131) | 1,951,489 (–6,674,048 to 10,039,101) |
10 years | –3397 | 3,640,780 (–11,293,68 to 17,618,549) | 3,644,178 (–11,290,284 to 17,621,946) |
15 years | –95,525 | 4,911,520 (–14,715,109 to 23,255,609) | 5,007,045 (–14,619,584 to 23,351,134) |
Under this assumption, our CBA model estimates that implementing part-night lighting on 5% of road km in an area will result in marginally smaller benefits to society after 5, 10, and 15 years than with larger energy price rises.
Table 9 presents results of the CBA model using the assumption that energy prices will rise to 4% above inflation. Under this assumption, the costs savings from introducing part-night lighting are even greater than the initial model, contributing to an even larger net benefit to society from the intervention.
Time horizon | Difference in street lighting provision costs | Difference in (dis)benefits: crimes and carbon emissions (range) | NPV (range) |
---|---|---|---|
5 years | 69,000 | 2,029,519 (–6,596,018 to 10,117,131) | 1,960,519 (–6,665,018 to 10,048,131) |
10 years | –41,977 | 3,640,780 (–11,293,681 to 17,618,549) | 3,682,757 (–11,251,705 to 17,660,526) |
15 years | –194,025 | 4,911,520 (–14,715,109 to 23,255,609) | 5,105,544 (–14,521,084 to 23,449,634) |
Discussion
We conducted a CBA to compare the societal costs of street lighting reduction with its societal benefits. The scope of our CBA included infrastructure, maintenance and energy consumption costs, and any associated changes in crime and road traffic collisions (using estimates from Chapter 3 of this report). Our CBA framework considered two scenarios over different time horizons: one where street lighting is reduced and one where it is maintained. Specifically, we considered the economic argument for part-night lighting of a proportion of street lights in a typical local authority. We found that part-night lighting regimes are likely to represent a large net benefit to the local authority, although it is important to note the considerable uncertainty around the estimates.
Our results need to be interpreted with caution owing to a number of limitations. Our CBA model did not include other outcomes that may be hypothesised to be associated with part-night lighting regimes (see Figure 1). We might reasonably expect that most of these outcomes, such as the potential increase in fear of crime and decrease in mobility, if incorporated, would serve to reduce the net benefit to the local authority of part-night lighting regimes. This suggests that our CBA model is likely to have underestimated overall disbenefits from part-night lighting regimes. We cannot know whether or not these disbenefits, if incorporated, would outweigh benefits from reduced collisions, burglaries and vehicle crimes.
Another key limitation is the number of simplifying assumptions made within the model, such as those for energy prices, inflation rates and lighting maintenance costs. Our CBA was able to incorporate uncertainty around the estimates of the effect of part-night lighting on collisions and crime, leading to a wide range of estimates of NPVs of part-night lighting for local authorities. We did not consider uncertainty around other elements in the model, such as costs or time trends. If we were to incorporate these uncertainties, it is likely that the range of estimates of the NPV of part-night lighting regimes would widen. Furthermore, this CBA did not incorporate other public health outcomes, such as sleep patterns and neighbourhood morale, which might change when lighting reduction strategies are implemented. Reductions in energy use, CO2 emissions and crime, however, suggest that the economic case for street lighting reduction strategies may be promising.
Local authorities in England and Wales may wish to extend or adapt our CBA model to their localities, using energy costs, maintenance costs and lighting adaptation costs specific to their areas (the spreadsheet is available from the authors on request).
Chapter 5 Discussion
Reduced street lighting and public health outcomes
Local authority interventions to reduce street lighting at night are driven largely by financial and carbon reduction incentives, but they have potentially diverse impacts on the public health. Drawing on existing literature, discussions with stakeholders (see Appendix 7) and a rapid appraisal to explore public views, we mapped the key potential pathways linking street light reductions to health and well-being outcomes (see Figure 1). Despite rising interest in the public health effects of artificial light, particularly at night, on outcomes such as obesity84 and cancer,17 a key challenge in measuring impacts on many of these pathways is that there is, to date, little good-quality research evidence. 13 The pathways are also likely to be less linear in practice than this logic model suggests, given the possibilities of reinforcing loops between, for instance, fear of crime and restrictions on mobility, and the possibilities of emergent properties that may arise from, for instance, individual-level trust (or distrust) in the local authority. This study was designed to generate evidence on two specific pathways in this model: those linking reduced street lighting with crime and those linking reduced street lighting with road traffic injury. It was also designed to provide some insights into the broader context of well-being outcomes through a rapid appraisal and use a CBA to identify the economic implications of these interventions.
Our rapid appraisal concluded that public concern about street lighting reductions is perhaps less widespread, less strongly felt and less negative than media coverage might suggest. First, in deliberative settings, residents in areas with lighting reductions were, in general, adept at identifying both potential advantages and potential disadvantages of reduced lighting. Second, in intercept interviews in localities affected by street lighting reductions, most residents were unconcerned about changes: dimming and white light largely went unnoticed, and even switch-off and part-night lighting generated few reported negative impacts on well-being. Third, in a household survey, the only significant difference between those in streets with part-night lighting and those in streets with no intervention was that they were less likely to feel safe walking in the dark. The meaning of street lighting shifted dramatically across rural and urban environments. In more rural areas, residents saw dark streets as congruent with a rural life and reducing light pollution to enable better views of the night sky was reported as a benefit of reduced street lighting. This is not to conclude that there were not some profound concerns for a minority. Part-night lighting, in particular, was deeply unpopular with many residents in suburban housing estates and, for those using streets in the evening, street lighting was widely seen as a necessity for personal security, in line with existing research which identifies improved lighting as conducive to feelings of security,19 and indeed the limited psychological research which suggests that darkness in and of itself may foster dishonesty. 85
However, in practice, concerns about dark streets in urban and suburban areas were difficult to disentangle from concerns about the implications of a local authority removing a public good. In the context of austerity politics, social disquiet at the implications of switch-off relate to perceived neglect by local authorities and to wider concerns about modernity. The well-being implications of street lighting reduction are, then, likely to accrue through the pathways on our model linking confidence in local governance to feelings about neighbourhood, as well as directly from the effects of living and working in streets which are darker at night. Concerns about governance were mirrored by professionals in interviews and in our stakeholder workshop (see Appendix 8), who described the loss of professional lighting expertise within local authorities as budgets came under threat and noted a political context in which strategic lighting decisions may be driven largely by short-term cost implications rather than longer-term interests. In this context, outcomes with immediate cost implications, and ones that have direct costs (through potential legal liabilities) such as road traffic injuries, are more likely to be of concern to lighting authorities than to have distal health implications. As has been documented for other areas of public health,86,87 local authority officers have to juggle multiple responsibilities, to a variety of constituencies: neither health outcomes nor even lighting were likely to be considered in isolation or necessarily as priorities. However, to a large extent, the professionals interviewed in this study were working with a more holistic model of health than is customary in public health fields, in that providing a ‘good’ environment, conducive to social health, was a priority for many, despite the pressures to do this in a way which reduced costs as far as possible. The logic model, therefore, captured, for them, the key domains of interest, although there was debate at the stakeholder meeting about the relative priorities of various outcomes and the extent to which they, as local authorities, were responsible for some domains. All saw public streets as their responsibility, for instance, but some did not consider the visibility of private houses the concern of the local authority.
Context and methodological implications
The interventions summarised in the logic model as ‘reduced street lighting’ were, in practice, a mix of different technological innovations, ranging from completely switching off and removing lighting columns to minor trimming (which went largely unnoticed and, arguably, resulted not in darker, but in differently lit, streets). Since the rapid evolution of LED technology in the early twenty-first century, local authorities have been able to increasingly control lighting in the public realm through CMSs, which allow sophisticated regimes of switching off and dimming in response to environmental light levels, traffic flows or other needs.
A range of statutory and advisory guidance governs the levels at which different public roads should be lit,88 although these may be becoming increasingly outdated as technologies (such as road signs) evolve to function with lower levels of light and as concerns such as light pollution arise in addition to traditional ones around road injury. As Shaw7 has described, in this rapidly changing context, in which technologies are newly evolving, often untested over time, and in which (as above) there is little evidence on impacts on health or social practice, local authorities are having to make decisions with imperfect knowledge. Our study was designed to contribute by providing evidence on two outcomes that are of primary interest to both residents and professionals: crime and road injury. Given the complexity of both the interventions and the context, any evaluation faced challenges of providing evidence that would be both credible for policy-makers and defended against threats to internal and external validity. 89 As outlined in Chapter 3, our design for evaluating impacts on crime and road injury treated street lighting reductions as a natural experiment. 69 Strengths of our design were that it collated data from a large enough sample of local authorities to provide adequate statistical power, and that we compared counts in streets before and after changes had been adopted in order to control for potential confounders. We also incorporated a rapid appraisal to shed light on potential causal mechanisms for any changes identified in the quantitative evaluation. Strengths in terms of knowledge transfer are that we developed the logic model in tandem with policy-makers likely to be using our findings. However, this design has the same weaknesses of all study designs using non-random allocation of the intervention, with the inevitable difficulties in controlling for other sources of potential bias (such as changes in public use of streets or other changes to the road environment at the same time as street lighting is altered). Further, for crime outcomes, our analysis is at area level and thus we cannot be sure that implementation within LSOAs or MSOAs was not biased by, for instance, local knowledge of street segments with particular propensities to crime.
Implications of key findings
None of the street lighting interventions had a significant impact on counts of road traffic injury. As we have no direct measures of the number of trips taken or mode of travel before and after implementation of lighting interventions, we cannot know whether this reflects less mobility or changes in mode (e.g. from walking to car travel) in areas where either switch-off or part-night lighting had occurred. However, the rapid appraisal suggested that any changes in mobility in affected areas were likely to be marginal: even where residents were concerned about dark streets, they reported that their behaviour did not change. This was reflected in the household survey, in which no significant differences were identified in travel after dark between intervention and non-intervention streets.
Overall, there was no evidence for an increase in crime from any intervention at national level. However, we found significant evidence for heterogeneity in the estimated impacts on crime at police force level. It is plausible that lighting streets until 12 a.m. signals investment in communities, thus deterring crime. It is possible that streets that are unlit before 12 a.m. allow potential criminals to identify future targets at a times when they are less likely to attract the attention they would after 12 a.m. In the rapid appraisal, views were expressed by both police officers and residents which supported both lighting and lack of lighting being associated with crime: more research is needed on the mechanisms through which specific street lighting adaptation strategies affect crime in different contexts.
Overall, given that there were no significant impacts on road injury casualties or on crime outcomes, and few reported negative impacts on well-being, this suggests that, where street lighting is adapted by local authorities, the overall impact on the public health is likely to be negligible.
Given the complexities outlined in Chapter 4 of assessing the costs and benefits of street lighting interventions, any conclusion of the economic case for street lighting reductions must be made with caution. However, the case study presented suggests a promising economic case for part-night lighting. Although lighting professionals will have to make decisions about street lighting reductions in light of the needs of their own localities, the evidence presented in this report may help to inform these decisions by providing quantified estimates of the impact on two important public health outcomes, and by providing evidence from a rapid appraisal that public concerns may be less strong and polarised than suggested by media coverage. Careful consultation may offset the negative well-being outcomes associated with lighting reductions that signify lack of care.
Conclusions
Street lighting provision in England and Wales is a rapidly changing field. This study has mapped the key domains of concern to the public from reductions in street lighting, which relate primarily to personal security in urban areas, and has provided a robust evaluation of the impacts of different interventions on two key outcomes for policy-makers: crime and road injury. These suggest that there is no evidence that any of the street lighting reduction interventions have a detrimental effect on road casualties or crime. However, given public concerns about part-lighting and switch-off, alternative strategies utilising dimming and changes to white light/LEDs may be more acceptable to the public.
Further research
The links between lighting, crime and fear of crime are complex. We have identified some evidence for a decrease in crime with dimming and white light. More research is needed to understand how different lighting regimes affect opportunities for crime and crime prevention, and under what specific conditions any causal effects are most likely to be triggered. For example, are the effects more likely on certain types of roads (e.g. main roads vs. residential ones) or in combination with other interventions (e.g. CCTV)?
Public understanding of, and support for, initiatives which aim to reduce carbon emissions is complex. In this study, although respondents were supportive of efforts to reduce emissions, this support often did not outweigh other concerns, such as safety, and it also raised anxieties for some about the future of modernity. Given that future changes required to meet carbon reduction goals may be more dramatic, there is an urgent need to understand better what motivates, and inhibits, public support for changes such as reductions in street lighting.
Evidence for the impact of artificial light on human health is sparse, although there is a growing public health interest in the effects of duration of exposure, intensity and colour spectrum. As a first step to estimating the impact of reductions in street lighting through pathways leading to chronic disease and sleep disruption outcomes, a systematic review of the evidence for the effects of artificial light on health is needed.
Acknowledgements
We thank the members of the LANTERNS project advisory group, Ben Armstrong, Denise Kendrick, Emily Conner and Mark Norris, for providing valuable advice throughout the project.
We would also like to thank Richard Frost, Stuart Bulmer and Nigel Parry of the ILP, and James Everley of Power Data Associates, for their help and technical advice throughout the project; John Cairns at London School of Hygiene and Tropical Medicine for his guidance on the CBA; Christina Albertsen at London School of Hygiene and Tropical Medicine for her helpful administration of the project; and Caitlin Jarrett at London School of Hygiene and Tropical Medicine for assistance with data formatting for GIS.
We are grateful to all of the local authority street lighting managers who provided data for the project and those local authority officers, residents and workers in the eight case study areas who talked to us about their views of street lighting.
We thank Nicki Thorogood, Philip Corran, Jeni Gosling and Rebecca Green for their help with recruitment and fieldwork for the rapid appraisal.
The LANTERNS project was funded by the NIHR PHR programme. The NIHR is funded through the Department of Health to improve the health and wealth of the nation through research, with contributions from the Chief Scientist Office in Scotland, National Institute for Social Care and Health Research in Wales, and Health and Social Care Research and Development, Public Health Agency, Northern Ireland.
Material presented in Chapter 2 was previously published in Health Place 2015;34:171–80. Material presented in Chapter 3 was previously published in J Epidemiol Community Health 2015. doi: 10.1136/jech-2015-206012.
Contributions of authors
Chloe Perkins (research assistant in GIS) obtained, managed and collated street lighting data sets, assisted with data preparation in GIS, searched for public consultations, committee meeting minutes and local news stories, and contributed to the writing of the report.
Rebecca Steinbach (lecturer in GIS) obtained, managed and collated road traffic injury data sets, prepared the data in the GIS, conducted fieldwork interviews and analysis, conducted the CBA, conducted the statistical analyses and contributed to the writing of the report.
Lisa Tompson (Senior Research Associate in Security and Crime Science) obtained, managed and collated crime data from Police.uk and from the police forces and contributed to the writing of the report.
Judith Green (Professor of Sociology of Health) designed and supervised the rapid appraisal, designed the residents’ survey questionnaire, conducted fieldwork and analysis and contributed to the writing and editing of the report.
Shane Johnson (Professor of Security and Crime Science) provided expertise in crime data and its analysis, led on the methodological studies of the reliability of the open crime data and contributed to the writing and editing of the report.
Chris Grundy (lecturer in GIS) provided expert advice and input to data management in GIS.
Paul Wilkinson (Professor in Environmental Epidemiology) provided expert advice in the quantitative component and contributed to report writing.
Phil Edwards (senior lecturer in statistics) managed the project, supervised the statistical analysis, assisted with fieldwork interviews and design of the residents’ survey, and contributed to the writing and editing of the report.
Publication
Tompson L, Johnson S, Ashby M, Perkins C, Edwards P. UK open source crime data: accuracy and possibilities for research. Cartogr Geogr InformationSci 2014;42:97–111.
Green J, Perkins C, Steinbach R, Edwards P. Reduced street lighting at night and health: a rapid appraisal of public views in England and Wales. Health Place 2015;34:171–80.
Steinbach R, Perkins C, Tompson L, Johnson S, Armstrong B, Green J, et al. The effect of reduced street lighting on road casualties and crime in England and Wales: controlled interrupted time series analysis [published online ahead of print 28 July 2015]. J Epidemiol Community Health 2015. doi: 10.1136/jech-2015-206012.
Data sharing statement
The data sets on road traffic collisions and crime used in this study are publicly available. The data on changes to street lighting were obtained directly from local authorities, who provided data for the study on the understanding that they would remain anonymous. The street lighting data can, therefore, be obtained from the corresponding author in an anonymised format.
Disclaimers
This report presents independent research funded by the National Institute for Health Research (NIHR). The views and opinions expressed by authors in this publication are those of the authors and do not necessarily reflect those of the NHS, the NIHR, NETSCC, the PHR programme or the Department of Health. If there are verbatim quotations included in this publication the views and opinions expressed by the interviewees are those of the interviewees and do not necessarily reflect those of the authors, those of the NHS, the NIHR, NETSCC, the PHR programme or the Department of Health.
References
- Road Safety: The Public Health Impact of Decreasing the Amount of Street Lighting 11/3004. London: NIHR; 2011.
- Brox B. Brilliant: The Evolution of Artificial Light. London: Souvenir Press; 2010.
- Otter C. Making liberalism durable: vision and civility in the late Victorian city. Soc Hist 2002;27:1-15. http://dx.doi.org/10.1080/03071020110094174.
- Martland SJ. Progress illuminating the world: street lighting in Santiago, Valparaiso and La Plata, 1840–90. Urban History 2002;29:223-38. http://dx.doi.org/10.1017/S0963926802002043.
- Augé M. Non-Places: Introduction to an Anthropology of Supermodernity. London: Verso; 1995.
- Artificial Light in the Environment. London: The Stationery Office; 2009.
- Shaw R. Streetlighting in England and Wales: new technologies and uncertainty in the assemblage of streetlighting infrastructure. Env Plan A 2014;46:2228-42. http://dx.doi.org/10.1068/a130313p.
- Duffin C. Street lights turned off in their thousands to meet carbon emission targets. The Telegraph 2012. www.telegraph.co.uk/news/9637929/Street-lights-turned-off-in-their-thousands-to-meet-carbon-emission-targets.html (accessed 30 July 2015).
- Massey R. Rising crash toll on unlit streets: lives being put at risk after figures show total number of accidents on roads without lights is 7% higher. Mail Online 2014. www.dailymail.co.uk/news/article-2861595/Rising-crash-toll-unlit-streets-Lives-risk-figures-total-number-accidents-roads-without-lights-7-higher.html (accessed 28 July 2015).
- Light Pollution and Astronomy. London: The Stationery Office; 2003.
- Falchi F, Cinzano P, Elvidge CD, Keith DM, Haim A. Limiting the impact of light pollution on human health, environment and stellar visibility. J Environ Manage 2011;92:2714-22. http://dx.doi.org/10.1016/j.jenvman.2011.06.029.
- Hölker F, Moss T, Griefahn B, Kloas W, Voigt CC, Henckel D, et al. The dark side of light: a transdisciplinary research agenda for light pollution policy. Ecol Soc 2010;15.
- Vohra S. Trafford LED Street Lighting Programme Health Impact Assessment. Edinburgh: IOM Centre for Health Impact Assessment; 2013.
- Shuboni D, Yan L. Nighttime dim light exposure alters the responses of the circadian system. Neuroscience 2010;170:1172-8. http://dx.doi.org/10.1016/j.neuroscience.2010.08.009.
- Navara KJ, Nelson RJ. The dark side of light at night: physiological, epidemiological, and ecological consequences. J Pineal Res 2007;43:215-24. http://dx.doi.org/10.1111/j.1600-079X.2007.00473.x.
- Fonken LK, Finy MS, Walton JC, Weil ZM, Workman JL, Ross J, et al. Influence of light at night on murine anxiety- and depressive-like responses. Behav Brain Res 2009;205:349-54. http://dx.doi.org/10.1016/j.bbr.2009.07.001.
- Pauley S. Lighting for the human circadian clock: recent research indicates that lighting has become a public health issue. Med Hypotheses 2004;63:588-96. http://dx.doi.org/10.1016/j.mehy.2004.03.020.
- Atkins S, Husain S, Storey A, Laycock G. Crime Prevention Unit Paper No 28. London: Home Office Crime Prevention Unit; 1991.
- Painter K, Farrington D, Clarke R. Situational Crime Prevention: Successful Case Studies. Guilderland, NY: Harrow & Heston; 1997.
- Trotter RT, Needle RH, Goosby E, Bates C, Singer M. A methodological model for Rapid Assessment, Response, and Evaluation: the RARE program in public health. Field Methods 2001;13:137-59. http://dx.doi.org/10.1177/1525822X0101300202.
- Beebe J. Basic concepts and techniques of rapid appraisal. Hum Organ 1995;54:42-51. http://dx.doi.org/10.17730/humo.54.1.k84tv883mr2756l3.
- Green J, Thorogood N. Qualitative Methods for Health Research. London: Sage; 2014.
- Letter to the Editor . The Comet 2012.
- Letter to the Editor . The Comet 2012.
- Letter to the Editor . The Comet 2012.
- Letter to the Editor . The Comet 2012.
- Jones A. On the South Bank: The Production of Public Space. Farnham: Ashgate Publishing Limited; 2014.
- Citizenship Survey: 2009–10. Department for Communities and Local Government; 2010.
- Survey of English Housing, 2004–2005. London: Office of the Deputy Prime Minister; 2006.
- Home Office . British Crime Survey, 2007–2008 2009.
- Derges J, Lynch R, Clow A, Petticrew M, Draper A. Complaints about dog faeces as a symbolic representation of incivility in London, UK: a qualitative study. Crit Public Health 2012;22:419-25. http://dx.doi.org/10.1080/09581596.2012.710738.
- Helmore E. ‘Detroit Will Rise Again’: Glimmers Of Defiance After City’s Bankruptcy. Observer 2013. www.theguardian.com/world/2013/jul/20/bankrupt-detroit-pins-hopes-creative-hub (accessed 24 August 2015).
- Shaw R. Controlling darkness: self, dark and the domestic night. Cult Geogr 2014. http://dx.doi.org/10.1177/1474474014539250.
- von Elm E, Altman DG, Egger M, Pocock SJ, Gøtzsche PC, Vandenbroucke JP. STROBE Initiative . The Strengthening the Reporting of Observational Studies in Epidemology (STROBE) guidelines for reporting observational studies. BMJ 2007;335:806-8. http://dx.doi.org/10.1136/bmj.39335.541782.AD.
- Welsh B, Farrington D. Effects of improved street lighting on crime. Campbell Syst Rev 2008;13:1-51.
- Beyer F, Ker K. Street lighting for preventing road traffic injuries. Cochrane Database Syst Rev 2009;1. http://dx.doi.org/10.1002/14651858.cd004728.pub2.
- Department for Transport . STATS19 n.d. http://data.gov.uk/dataset/road-accidents-safety-data (accessed 24 August 2015).
- Home Office . Police.Uk: Crime and Policing in England, Wales and Northern Ireland n.d. data.police.uk (accessed 24 August 2015).
- Tompson L, Johnson S, Ashby M, Perkins C, Edwards P. UK open source crime data: accuracy and possibilities for research. Cartogr Geogr Inf Sci 2015;42:97-111. http://dx.doi.org/10.1080/15230406.2014.972456.
- Ordnance Survey . MasterMap Integrated Transport Network Layer 2013.
- Armstrong B, Gasparrini A, Tobias A. Conditional Poisson models: a flexible alternative to conditional logistic case cross-over analysis. BMC Med Res Methodol 2014;14. http://dx.doi.org/10.1186/1471-2288-14-122.
- Grundy C, Steinbach R, Edwards P, Green J, Armstrong B, Wilkinson P. The effect of 20 mph traffic speed zones on road injuries in London, 1986–2006: a controlled interrupted time series analysis. BMJ 2009;339. http://dx.doi.org/10.1136/bmj.b4469.
- Index of Multiple Deprivation 2010. London: Department for Communities and Local Government; 2010.
- Wilson JQ, Kelling GL. Broken windows. Atl Mon 1982;249:29-38.
- Zimbardo P, Ward C. Vandalism. London: The Architectural Press; 1972.
- Shiell A, Hawe P, Gold L. Complex interventions or complex systems? Implications for health economic evaluation. BMJ 2008;336:1281-3. http://dx.doi.org/10.1136/bmj.39569.510521.AD.
- Weatherly H, Drummond M, Claxton K, Cookson R, Ferguson B, Godfrey C, et al. Methods for assessing the cost-effectiveness of public health interventions: key challenges and recommendations. Health Policy 2009;93:85-92. http://dx.doi.org/10.1016/j.healthpol.2009.07.012.
- Smith RD, Petticrew M. Public health evaluation in the twenty-first century: time to see the wood as well as the trees. J Public Health 2010;32:2-7. http://dx.doi.org/10.1093/pubmed/fdp122.
- Carande-Kulis VG, Getzen TE, Thacker SB. Public goods and externalities: a research agenda for public health economics. J Public Health Manag Pract 2007;13:227-32. http://dx.doi.org/10.1097/00124784-200703000-00024.
- Sacks G, Veerman JL, Moodie M, Swinburn B. ‘Traffic-light’ nutrition labelling and ‘junk-food’ tax: a modelled comparison of cost-effectiveness for obesity prevention. Int J Obes (Lond) 2011;35:1001-9. http://dx.doi.org/10.1038/ijo.2010.228.
- Payne K, McAllister M, Davies LM. Valuing the economic benefits of complex interventions: when maximising health is not sufficient. Health Econ 2013;22:258-71. http://dx.doi.org/10.1002/hec.2795.
- Kelly MP, McDaid D, Ludbrook A, Powell A. Economic Appraisal of Public Health Interventions. London: Health Development Agency; 2005.
- Trueman P, Anokye NK. Applying economic evaluation to public health interventions: the case of interventions to promote physical activity. J Public Health 2013;35:32-9. http://dx.doi.org/10.1093/pubmed/fds050.
- Elvik R, Kolbenstvedt M, Elvebakk B, Hervik A, Bræin L. Costs and benefits to Sweden of Swedish road safety research. Accid Anal Prev 2009;41:387-92. http://dx.doi.org/10.1016/j.aap.2008.12.009.
- Mendivil J, García-Altés A, Pérez K, Marí-Dell’Olmo M, Tobías A. Speed cameras in an urban setting: a cost–benefit analysis. Inj Prev 2012;18:75-80. http://dx.doi.org/10.1136/ip.2010.030882.
- Steinbach R, Cairns J, Grundy C, Edwards P. Cost benefit analysis of 20 mph zones in London. Inj Prev 2013;19:211-13. http://dx.doi.org/10.1136/injuryprev-2012-040347.
- Edwards P, Steinbach R, Green J, Petticrew M, Goodman A, Jones A, et al. Health impacts of free bus travel for young people: evaluation of a natural experiment in London. J Epidemiol Community Health 2013;67:641-7. http://dx.doi.org/10.1136/jech-2012-202156.
- Green J, Steinbach R, Jones A, Edwards P, Kelly C, Nellthorp J, et al. On the buses: a mixed-method evaluation of the impact of free bus travel for young people on the public health. Public Health Res 2014;2.
- Smith RD, Olsen JA, Harris A. A Review of Methodological Issues in the Conduct of Willingness-to-Pay Studies in Health Care I: Construction and Specification of the Contingent Market. Heidelberg West, VIC: Centre for Health Program Evaluation; 1999.
- Willis KG, Powe NA, Garrod GD. Estimating the value of improved street lighting: a factor analytical discrete choice approach. Urban Stud 2005;42:2289-303. http://dx.doi.org/10.1080/00420980500332106.
- Powe NA, Willis KG, Garrod GG. Difficulties in valuing street light improvement: trust, surprise and bound effects. Appl Econ 2006;38:371-81. http://dx.doi.org/10.1080/00036840500369274.
- Heald D. Why is transparency about public expenditure so elusive?. Int Rev Admin Sci 2012;78:30-49. http://dx.doi.org/10.1177/0020852311429931.
- Subramanian S, Tangka FK, Hoover S, Beebe MC, DeGroff A, Royalty J, et al. Costs of planning and implementing the CDC’s colorectal cancer screening demonstration program. Cancer 2013;119:S2855-62. http://dx.doi.org/10.1002/cncr.28158.
- Kelly MP, Stewart E, Morgan A, Killoran A, Fischer A, Threlfall A, et al. A conceptual framework for public health: NICE’s emerging approach. Public Health 2009;123:e14-20. http://dx.doi.org/10.1016/j.puhe.2008.10.031.
- Baxter S, Killoran A, Kelly MP, Goyder E. Synthesizing diverse evidence: the use of primary qualitative data analysis methods and logic models in public health reviews. Public Health 2010;124:99-106. http://dx.doi.org/10.1016/j.puhe.2010.01.002.
- Oliver S, Bagnall A, Thomas J, Shepherd J, Sowden A, White I, et al. Randomised controlled trials for policy interventions: a review of reviews and meta-regression. Health Technol Assess 2010;14. http://dx.doi.org/10.3310/hta14160.
- Pridemore WA, Snowden AJ. Reduction in suicide mortality following a new national alcohol policy in Slovenia: an interrupted time-series analysis. Am J Public Health 2009;99:915-20. http://dx.doi.org/10.2105/AJPH.2008.146183.
- Cummins S, Petticrew M, Higgins C, Findlay A, Sparks L. Large scale food retailing as an intervention for diet and health: quasi-experimental evaluation of a natural experiment. J Epidemiol Community Health 2005;59:1035-40. http://dx.doi.org/10.1136/jech.2004.029843.
- Craig P, Cooper C, Gunnell D, Haw S, Lawson K, Macintyre S, et al. Using natural experiments to evaluate population health interventions: new Medical Research Council guidance. J Epidemiol Community Health 2012;66:1182-6. http://dx.doi.org/10.1136/jech-2011-200375.
- Home Office . The Economic and Social Costs of Crime Against Individuals and Households 2003 04 2005.
- Reported Road Casualties in Great Britain: 2011 Annual Report, A Valuation of Road Accidents and Casualties in Great Britain in 2011. London: Department for Transport; 2012.
- Barbier EB, Acreman MC, Knowler D. Economic Valuation of Wetlands: A Guide for Policy Makers and Planners. Gland: Ramsar Convention Bureau; 1997.
- Riera P, Signorello G, Thiene M, Mahieu P-A, Navrud S, Kaval P, et al. Non-market valuation of forest goods and services: good practice guidelines. J For Econ 2012;18:259-70. http://dx.doi.org/10.1016/j.jfe.2012.07.001.
- Reported Road Casualties in Great Britain: 2012 Annual Report: A Valuation of Road Accidents and Casualties in Great Britain in 2012. London: Department for Transport; 2013.
- Retail Prices Index 2015. London: ONS; 2015.
- Brand S, Price R. The Economic and Social Costs of Crime. London: Home Office; 2000.
- Greenhouse Gas Conversion Factor Repository 2015. Defra; 2015.
- Updated Short-Term Traded Carbon Values Used for UK Public Policy Appraisal. London: Department of Energy and Climate Change; 2014.
- PLG 08: Guidance on the Application of Adaptive Lighting within the Public Realm. Rugby: ILP; 2013.
- Forecasts for the UK Economy: A Comparison of Independent Forecasters. London: HM Treasury; 2014.
- Appraisal of New and Replacement Lighting on the Strategic Motorway and All Purpose Trunk Road Network: TA49/07. London: The Highways Agency; 2007.
- Low Energy Streetlighting: Making the Switch. Edinburgh: Green Investment Bank; 2014.
- The Green Book: Appraisal and Evaluation in Central Government. London: The Stationery Office; 2011.
- McFadden E, Jones ME, Schoemaker MJ, Ashworth A, Swerdlow AJ. The relationship between obesity and exposure to light at night: cross-sectional analyses of over 100,000 women in the breakthrough generations study. Am J Epidemiol 2014;180:245-50. http://dx.doi.org/10.1093/aje/kwu117.
- Zhong C-B, Bohns V, Gino F. Good lamps are the best police: darkness increases dishonesty and self-interested behaviour. Psychol Sci 2010;21:311-14. http://dx.doi.org/10.1177/0956797609360754.
- Phillips G, Green J. Working for the public health: politics, localism and epistemologies of practice. Sociol Health Ill 2015;37:491-505. http://dx.doi.org/10.1111/1467-9566.12214.
- Green J, Edwards P. The limitations of targeting to address inequalities in health: a case study of road traffic injury prevention from the UK. Crit Public Health 2008;18:175-87. http://dx.doi.org/10.1080/09581590701697300.
- Guide to the Intelligent Lifetime Management of Public Lighting Life Safety Engineering Systems. Ferring: HEA; 2013.
- Green J, Roberts H, Petticrew M, Steinbach R, Goodman A, Jones A, et al. Integrating quasi-experimental and inductive designs in evaluation: a case study of the impact of free bus travel on public health. Evaluation 2015.
Appendix 1 Rapid appraisal summary of participants
Area | Formal interviews | Informal fieldwork interviews | Stakeholders |
---|---|---|---|
Buckinghamshire | 11 | 7 | 1 |
Hertfordshire | 27 | 15 | 1 |
Swansea | 0 | 19 | 3 |
Wakefield | 12 | 4 | 2 |
Shropshire | 2 | 13 | 2 |
Southwark | 0 | 3 | 1 |
Westminster | 0 | 2 | 2 |
Hackney | 7 | 0 | 1 |
National organisations | 3 | ||
Total | 59 | 63 | 16 |
Categories | Count, n |
---|---|
Age range (years) | |
75+ | 3 |
66–75 | 12 |
56–65 | 12 |
46 –55 | 11 |
36–45 | 4 |
26–35 | 4 |
18–25 | 5 |
< 18 | 2 |
Not stated | 6 |
Gender | |
Male | 23 |
Female | 36 |
Ethnicity | |
White British | 42 |
White other | 2 |
Black/black British | 3 |
Mixed/other | 3 |
Not stated | 9 |
Occupation/last occupation | |
Higher professional/managerial | 10 |
Teacher/police office/nurse | 14 |
Clerical | 10 |
Manual | 4 |
Own business | 3 |
Unemployed | 3 |
School/college | 3 |
Homemaker | 1 |
None/none stated | 11 |
Appendix 2 Content coding framework
Main code | Subcodes | Short form |
---|---|---|
Normative expectations of Street Lighting provision | Exceptions | NormExpSL |
Rural/Urban | ||
Time of day | ||
Expectations of LA provision of Street Lighting | ExptLAProv | |
Expectations of LA informing/consulting on SL change | Surprise of change | ExptLAInf |
Accepting different views of SL provision | DiffView | |
Descriptions of part and unlit streets | Experienced | DescPNL |
Imagined | ||
Describing sources of light at night | SrcLAN | |
Describing negative consequences of reduced SL for mobility | For self | NegConsq |
For others | ||
Experiences of incidents and near misses | ||
Fear of injury | ||
Fear of tripping | ||
Describing positive consequences of reduced SL | For self | PosConsq |
For others | ||
Strategies for dealing with reduced street lighting | For self | Strat |
For others | ||
Noticing change | Notice | |
Engaging with the rationality of assessments or reductions | Legitimising views | Engage |
Sources of evidence | ||
Strategies of engagement | ||
Accepting the rationale | ||
Symbolic associations of lighting/darkness | Lighting levels | Symb |
On/off | ||
Fear of dark | ||
Colour of light | ||
Expressing views to LA | Own experiences | Expr |
Reported of others |
Appendix 3 Household survey coded text responses
An open question at the end of the questionnaire invited ‘other comments about your street or neighbourhood after dark’. These were coded into broad themes. More respondents from part-night lighting streets made comments, made negative comments about reduction (though many in the ‘no reduction’ group also thought theirs had gone off/did not have any anyway), mentioned negative effects and made negative comments about their neighbourhoods. More in streets with no intervention made negative comments about the amount of street lighting at night. Around half of all respondents made at least one comment; some made more than one comment.
Topic | PNL (N = 250), n | No reduction in street lighting (N = 233), n |
---|---|---|
Factual information only | ||
On street light provision | 24 | 24 |
On home improvements | 12 | 19 |
On local environment/services | 2 | 3 |
Other | 2 | 1 |
Total | 40 | 47 |
Negative opinions of street lighting reduction | ||
General/want more lights | 13 | 10 |
Increase crime/danger/decrease in security | 14 | 5 |
Change in habits | 1 | 1 |
Problem for shift workers | 1 | 2 |
Can’t see house number | 1 | 0 |
Total | 30 | 18 |
Positive opinions of street lighting reduction | ||
Generally a good thing | 5 | 4 |
No change in crime | 1 | 2 |
Better sleep | 1 | 0 |
See stars | 1 | 0 |
Total | 9 | 6 |
Experienced negative effects of reduced street lighting | ||
Crime | 3 | 0 |
Reduced mobility | 2 | 0 |
RTI | 1 | 0 |
Total | 6 | 0 |
Negative opinions of street light at night | ||
Affects sleep | 1 | 0 |
Too much/should switch off | 0 | 7 |
Like no lights | 0 | 2 |
Total | 1 | 9 |
Seeing the night sky | ||
Too much light to see | 2 | 0 |
Can see stars | 5 | 6 |
Don’t care about seeing stars | 2 | 0 |
Total | 9 | 6 |
Views of local authority/government | ||
Doing it to save money (negative) | 6 | 0 |
Saving money (positive) | 1 | 0 |
Good for environment | 1 | 0 |
The local authority don’t care/are incompetent | 1 | 6 |
Total | 9 | |
General views on neighbourhood | ||
Negative | 6 | 0 |
Positive | 6 | 8 |
Mixed | 4 | 2 |
Total | 16 | 10 |
Opinions on neighbours’ lighting | ||
Negative (light pollution, sleep) | 2 | 2 |
Positive (security) | 0 | 2 |
Total | 2 | 4 |
Other comments on street lighting | ||
Doesn’t concern us | 1 | 1 |
Suggestions of alternatives to current provision | 2 | 4 |
Mixed views of street lighting | 0 | 0 |
Total | 3 | 5 |
Other comments: why not applicable | ||
Too old/disabled to go out anyway | 3 | |
Tenant – no opinion | 1 | |
Recently arrived/other | 2 | |
Total | 6 | |
Total number of comments | 131 | 119 |
Appendix 4 Stata commands
Night-time traffic collisions (road segment level)
We estimated the association between night-time collisions and street light adaptation strategies at the road segment level adjusting for changes in daytime collisions and trends over time using conditional Poisson regression models. To run these analyses we stacked two time series data sets on top of each other. Data set 1 contained the count of night-time collisions on each road segment in each month of each year. Data set 2 contained the count of daytime collisions on each road segment in each month of each year. We created a binary variable in our stacked data set (night) to indicate whether collision counts referred to daytime or night-time.
The code used to run the models is shown below:
Crime (middle super output area level)
We estimated the association between crime and the proportion of road km in a MSOA that had received street lighting adaptions. Our data set was a time series with one observation per month and year, with variables describing counts of different crime types (burglary, etc.) and the proportion of road km in the area which had received lighting interventions.
We created a time variable indicating the month of the study (1–36). Analyses used conditional Poisson regression models and accounted for changes over time using an autoregressive function allowing for counts to depend on counts in the previous 3 months.
We found through initial explorations that residual variance was greater than that expected (i.e. overdispersion) in the Poisson statistical distribution. We allowed for this overdispersion in standard errors using a scale factor estimated from the Pearson chi-squared statistic divided by the degrees of freedom of the residuals. We used an add-on ado to correct estimates for over dispersion after running Poisson models from Armstrong et al. 41
The code we used to run the models is shown below:
Appendix 5 Quantitative analysis tables
Local authority | Year | |||||||||||||
---|---|---|---|---|---|---|---|---|---|---|---|---|---|---|
2000 | 2001 | 2002 | 2003 | 2004 | 2005 | 2006 | 2007 | 2008 | 2009 | 2010 | 2011 | 2012 | 2013 | |
East Midlands | ||||||||||||||
Derby | 109 | 131 | 124 | 100 | 114 | 98 | 110 | 103 | 111 | 116 | 91 | 92 | 81 | 76 |
Derbyshire | 625 | 582 | 603 | 578 | 557 | 482 | 476 | 498 | 424 | 458 | 317 | 329 | 333 | 304 |
Leicester | 100 | 97 | 71 | 78 | 92 | 69 | 88 | 88 | 94 | 87 | 86 | 95 | 89 | 92 |
Leicestershire | 341 | 369 | 353 | 292 | 296 | 305 | 300 | 265 | 254 | 261 | 239 | 237 | 194 | 186 |
Lincolnshire | 459 | 537 | 719 | 607 | 497 | 430 | 389 | 420 | 339 | 453 | 465 | 487 | 426 | 416 |
Northamptonshire | 635 | 533 | 541 | 526 | 498 | 511 | 446 | 453 | 435 | 395 | 296 | 309 | 352 | 329 |
Nottinghamshire | 738 | 698 | 693 | 668 | 670 | 589 | 542 | 512 | 476 | 453 | 415 | 450 | 445 | 346 |
Nottingham City | 269 | 251 | 219 | 222 | 178 | 197 | 183 | 176 | 156 | 148 | 139 | 152 | 132 | 117 |
East of England | ||||||||||||||
Essex | 1075 | 1068 | 1066 | 1141 | 1066 | 945 | 981 | 881 | 710 | 654 | 663 | 628 | 599 | 570 |
Hertfordshire | 912 | 886 | 817 | 677 | 679 | 576 | 515 | 559 | 479 | 432 | 423 | 362 | 428 | 399 |
Norfolk | 686 | 674 | 600 | 535 | 542 | 566 | 499 | 455 | 380 | 386 | 353 | 355 | 351 | 391 |
Suffolk | 519 | 464 | 408 | 449 | 423 | 382 | 365 | 315 | 374 | 364 | 301 | 330 | 366 | 299 |
London | ||||||||||||||
Barnet | 253 | 247 | 258 | 220 | 173 | 147 | 146 | 151 | 135 | 138 | 129 | 135 | 110 | 129 |
Camden | 255 | 249 | 243 | 210 | 154 | 145 | 132 | 128 | 145 | 156 | 119 | 94 | 125 | 105 |
Enfield | 246 | 240 | 212 | 197 | 170 | 125 | 134 | 98 | 82 | 97 | 98 | 99 | 86 | 71 |
Hackney | 188 | 250 | 174 | 156 | 156 | 124 | 119 | 131 | 159 | 107 | 105 | 110 | 147 | 87 |
Hammersmith and Fulham | 153 | 153 | 130 | 123 | 118 | 121 | 141 | 101 | 94 | 92 | 76 | 81 | 81 | 53 |
Islington | 233 | 210 | 177 | 160 | 108 | 92 | 82 | 101 | 75 | 79 | 78 | 109 | 124 | 70 |
Kingston | 76 | 96 | 86 | 86 | 65 | 63 | 77 | 49 | 65 | 53 | 46 | 44 | 36 | 38 |
Richmond | 105 | 85 | 113 | 128 | 81 | 74 | 102 | 75 | 65 | 59 | 69 | 70 | 52 | 50 |
Southwark | 272 | 271 | 247 | 222 | 132 | 145 | 163 | 156 | 176 | 151 | 174 | 144 | 134 | 111 |
Sutton | 132 | 122 | 100 | 122 | 67 | 66 | 84 | 71 | 75 | 58 | 50 | 49 | 43 | 33 |
Waltham Forest | 196 | 186 | 145 | 129 | 100 | 93 | 99 | 84 | 109 | 59 | 72 | 67 | 67 | 54 |
North East | ||||||||||||||
Hartlepool | 37 | 50 | 45 | 57 | 47 | 40 | 41 | 47 | 28 | 28 | 34 | 35 | 34 | 23 |
Sunderland | 115 | 128 | 114 | 122 | 100 | 89 | 111 | 119 | 88 | 98 | 88 | 75 | 98 | 72 |
North West | ||||||||||||||
Knowsley | 93 | 78 | 57 | 65 | 73 | 78 | 65 | 59 | 78 | 67 | 63 | 48 | 73 | 70 |
Sefton | 113 | 94 | 98 | 102 | 119 | 87 | 94 | 90 | 96 | 86 | 74 | 84 | 89 | 92 |
Wigan | 155 | 126 | 130 | 123 | 127 | 138 | 102 | 111 | 103 | 100 | 68 | 86 | 78 | 69 |
South East | ||||||||||||||
Buckinghamshire | 389 | 432 | 391 | 405 | 410 | 325 | 297 | 325 | 221 | 246 | 223 | 213 | 224 | 228 |
East Sussex | 413 | 339 | 404 | 376 | 349 | 356 | 393 | 379 | 395 | 372 | 319 | 304 | 304 | 346 |
Hampshire | 938 | 841 | 910 | 842 | 652 | 590 | 614 | 715 | 580 | 648 | 632 | 765 | 683 | 714 |
Oxfordshire | 516 | 521 | 498 | 474 | 381 | 339 | 371 | 374 | 339 | 344 | 393 | 353 | 303 | 326 |
Reading | 74 | 61 | 78 | 50 | 57 | 31 | 47 | 38 | 46 | 52 | 39 | 51 | 41 | 52 |
Surrey | 665 | 710 | 695 | 548 | 642 | 575 | 577 | 577 | 516 | 564 | 519 | 580 | 571 | 599 |
West Berkshire | 128 | 136 | 123 | 112 | 100 | 78 | 73 | 105 | 50 | 63 | 60 | 77 | 79 | 54 |
West Sussex | 499 | 575 | 500 | 485 | 381 | 478 | 464 | 469 | 480 | 448 | 371 | 455 | 417 | 438 |
Wokingham | 85 | 85 | 88 | 60 | 72 | 47 | 68 | 60 | 51 | 50 | 35 | 50 | 38 | 58 |
South West | ||||||||||||||
Bath | 80 | 64 | 73 | 90 | 78 | 80 | 69 | 54 | 37 | 50 | 42 | 26 | 41 | 62 |
Cornwall | 315 | 318 | 324 | 326 | 251 | 249 | 265 | 265 | 206 | 191 | 189 | 223 | 213 | 220 |
Devon | 380 | 404 | 412 | 333 | 227 | 309 | 309 | 338 | 268 | 185 | 298 | 239 | 322 | 322 |
Dorset | 263 | 268 | 314 | 289 | 271 | 270 | 260 | 274 | 293 | 266 | 211 | 201 | 222 | 223 |
Gloucestershire | 390 | 390 | 337 | 346 | 275 | 274 | 268 | 261 | 259 | 242 | 190 | 247 | 257 | 217 |
Plymouth | 95 | 94 | 103 | 83 | 77 | 53 | 61 | 65 | 61 | 44 | 60 | 74 | 61 | 64 |
Poole | 54 | 50 | 62 | 72 | 58 | 68 | 43 | 64 | 64 | 48 | 56 | 53 | 70 | 68 |
South Gloucestershire | 126 | 131 | 130 | 112 | 115 | 103 | 92 | 93 | 76 | 65 | 87 | 33 | 52 | 60 |
West Midlands | ||||||||||||||
Birmingham | 600 | 550 | 534 | 492 | 481 | 506 | 480 | 505 | 428 | 420 | 421 | 471 | 398 | 403 |
Dudley | 142 | 102 | 119 | 107 | 88 | 131 | 112 | 142 | 121 | 116 | 92 | 93 | 102 | 102 |
Herefordshire | 182 | 188 | 150 | 147 | 150 | 152 | 125 | 135 | 96 | 105 | 66 | 79 | 82 | 63 |
Sandwell | 199 | 192 | 169 | 137 | 115 | 108 | 134 | 126 | 98 | 110 | 102 | 113 | 111 | 96 |
Shropshire | 360 | 273 | 301 | 209 | 213 | 229 | 168 | 176 | 159 | 149 | 130 | 130 | 151 | 123 |
Solihull | 121 | 104 | 78 | 84 | 70 | 92 | 84 | 80 | 92 | 88 | 63 | 64 | 61 | 44 |
Staffordshire | 368 | 403 | 369 | 412 | 379 | 319 | 353 | 318 | 285 | 265 | 216 | 202 | 228 | 169 |
Telford | 97 | 83 | 63 | 81 | 84 | 58 | 50 | 48 | 37 | 61 | 37 | 45 | 33 | 36 |
Walsall | 123 | 114 | 79 | 92 | 86 | 75 | 96 | 121 | 95 | 79 | 75 | 67 | 64 | 83 |
Warwickshire | 624 | 636 | 567 | 527 | 519 | 407 | 403 | 394 | 348 | 307 | 305 | 315 | 297 | 292 |
Wales | ||||||||||||||
Carmarthenshire | 187 | 178 | 171 | 153 | 159 | 106 | 110 | 92 | 98 | 98 | 85 | 84 | 115 | 101 |
Denbighshire | 81 | 66 | 34 | 57 | 57 | 29 | 35 | 59 | 56 | 43 | 50 | 58 | 46 | 51 |
Swansea | 64 | 68 | 61 | 71 | 73 | 87 | 107 | 82 | 76 | 80 | 73 | 78 | 50 | 63 |
Yorkshire and the Humber | ||||||||||||||
Kirklees | 254 | 244 | 238 | 196 | 212 | 220 | 232 | 215 | 206 | 161 | 141 | 165 | 156 | 141 |
Leeds | 517 | 457 | 521 | 468 | 461 | 368 | 392 | 387 | 389 | 337 | 323 | 314 | 317 | 302 |
Rotherham | 142 | 112 | 92 | 123 | 151 | 90 | 97 | 118 | 100 | 98 | 61 | 87 | 88 | 113 |
Sheffield | 244 | 277 | 311 | 297 | 252 | 292 | 262 | 260 | 201 | 206 | 160 | 160 | 180 | 169 |
Totals (199,817) | 18,805 | 18,341 | 17,842 | 16,751 | 15,348 | 14,271 | 14,097 | 14,010 | 12,632 | 12,236 | 11,255 | 11,625 | 11,550 | 11,054 |
Year | Type of intervention | |||||
---|---|---|---|---|---|---|
![]() |
![]() |
![]() |
![]() |
![]() |
![]() |
|
Night-time casualties | ||||||
2000 | 156 | 738 | 109 | 1739 | 493 | 1371 |
2001 | 171 | 834 | 129 | 1709 | 516 | 1382 |
2002 | 182 | 809 | 115 | 1585 | 636 | 1412 |
2003 | 169 | 714 | 106 | 1684 | 508 | 1332 |
2004 | 215 | 739 | 116 | 1478 | 475 | 1251 |
2005 | 170 | 716 | 115 | 1457 | 509 | 1265 |
2006 | 167 | 720 | 117 | 1395 | 467 | 1224 |
2007 | 162 | 690 | 121 | 1343 | 443 | 1168 |
2008 | 128 | 589 | 103 | 1152 | 379 | 1093 |
2009 | 139 | 496 | 85 | 1242 | 429 | 1064 |
2010 | 121 | 501 | 85 | 962 | 373 | 950 |
2011 | 99 | 454 | 60 | 976 | 378 | 884 |
2012 | 93 | 436 | 70 | 892 | 298 | 898 |
2013 | 106 | 390 | 60 | 890 | 252 | 762 |
Daytime casualties | ||||||
2000 | 777 | 3451 | 531 | 6027 | 2276 | 5950 |
2001 | 738 | 3398 | 532 | 6129 | 2307 | 5848 |
2002 | 712 | 3419 | 529 | 5715 | 2052 | 5514 |
2003 | 714 | 3209 | 488 | 5283 | 1904 | 5338 |
2004 | 652 | 3109 | 483 | 5205 | 2070 | 4951 |
2005 | 780 | 3106 | 480 | 4936 | 2075 | 4987 |
2006 | 674 | 2958 | 462 | 4838 | 2051 | 4897 |
2007 | 631 | 2913 | 462 | 4800 | 1924 | 4694 |
2008 | 654 | 2639 | 460 | 4339 | 1739 | 4236 |
2009 | 571 | 2589 | 347 | 4388 | 1751 | 4159 |
2010 | 528 | 2444 | 340 | 4221 | 1654 | 4217 |
2011 | 513 | 2200 | 344 | 4041 | 1641 | 4247 |
2012 | 429 | 2155 | 306 | 3815 | 1517 | 3623 |
2013 | 463 | 2111 | 342 | 3557 | 1468 | 3480 |
Night-time KSI casualties | ||||||
2000 | 46 | 117 | 17 | 218 | 56 | 227 |
2001 | 35 | 141 | 23 | 249 | 76 | 203 |
2002 | 42 | 146 | 18 | 231 | 83 | 238 |
2003 | 39 | 112 | 12 | 221 | 81 | 208 |
2004 | 43 | 115 | 19 | 209 | 57 | 194 |
2005 | 30 | 116 | 23 | 180 | 59 | 165 |
2006 | 32 | 108 | 14 | 195 | 70 | 170 |
2007 | 42 | 120 | 19 | 186 | 73 | 135 |
2008 | 27 | 89 | 15 | 180 | 63 | 118 |
2009 | 25 | 85 | 13 | 182 | 54 | 134 |
2010 | 31 | 70 | 14 | 124 | 31 | 111 |
2011 | 27 | 58 | 9 | 140 | 46 | 137 |
2012 | 20 | 87 | 16 | 108 | 51 | 112 |
2013 | 17 | 64 | 11 | 132 | 43 | 120 |
Daytime KSI casualties | ||||||
2000 | 121 | 486 | 66 | 665 | 244 | 663 |
2001 | 105 | 424 | 77 | 657 | 247 | 555 |
2002 | 104 | 413 | 59 | 638 | 217 | 579 |
2003 | 115 | 344 | 48 | 517 | 175 | 533 |
2004 | 114 | 336 | 48 | 527 | 209 | 448 |
2005 | 118 | 324 | 55 | 486 | 203 | 418 |
2006 | 92 | 333 | 49 | 527 | 186 | 451 |
2007 | 103 | 311 | 50 | 463 | 189 | 453 |
2008 | 87 | 290 | 40 | 453 | 156 | 392 |
2009 | 96 | 261 | 33 | 441 | 181 | 390 |
2010 | 70 | 286 | 43 | 440 | 202 | 384 |
2011 | 83 | 241 | 47 | 423 | 167 | 435 |
2012 | 63 | 285 | 42 | 429 | 191 | 380 |
2013 | 87 | 249 | 43 | 400 | 211 | 392 |
Provider | Type of crime | ||||||||||||||
---|---|---|---|---|---|---|---|---|---|---|---|---|---|---|---|
ASB | Burglary | Criminal damage | Robbery | Vehicle theft | Bicycle theft | Drugs | Other crime | Other theft | Weapon | Public disorder | Public order | Shoplifting | Theft from person | Violence | |
East Midlands | |||||||||||||||
Derby | 47,080 | 6347 | 5246 | 827 | 4550 | 126 | 1595 | 11,062 | 5712 | 23 | 1023 | 65 | 3277 | 52 | 11,211 |
Derbyshire | 101,496 | 15,140 | 14,772 | 692 | 11,116 | 337 | 4289 | 19,491 | 12,334 | 124 | 1576 | 452 | 6258 | 214 | 21,831 |
Leicester | 41,757 | 10,330 | 10,363 | 1776 | 8523 | 1021 | 2989 | 15,857 | 11,523 | 139 | 2274 | 809 | 5909 | 689 | 19,258 |
Leicestershire | 37,672 | 13,292 | 11,900 | 558 | 11,031 | 670 | 2218 | 17,082 | 11,830 | 71 | 2275 | 664 | 5962 | 231 | 17,338 |
Lincolnshire | 83,504 | 17,164 | 14,560 | 628 | 10,246 | 0 | 0 | 0 | 0 | 0 | 0 | 0 | 0 | 0 | 0 |
Northamptonshire | 105,241 | 18,266 | 17,520 | 1890 | 14,561 | 587 | 3882 | 26,037 | 14,692 | 158 | 1986 | 595 | 8807 | 500 | 27,047 |
Nottinghamshire | 70,282 | 14,314 | 12,414 | 842 | 11,735 | 199 | 3058 | 21,450 | 11,119 | 39 | 1766 | 133 | 5813 | 49 | 19,020 |
Nottingham City | 50,444 | 9469 | 8096 | 1989 | 7292 | 275 | 3595 | 17,289 | 10,994 | 68 | 2104 | 247 | 6298 | 289 | 16,063 |
East of England | |||||||||||||||
Essex | 124,903 | 30,831 | 23,617 | 2103 | 26,586 | 958 | 6266 | 34,172 | 25,664 | 216 | 4364 | 1314 | 11,514 | 613 | 42,092 |
Hertfordshire | 114,430 | 19,854 | 19,024 | 1527 | 18,682 | 799 | 10,851 | 36,273 | 19,767 | 187 | 457 | 1380 | 10,709 | 759 | 29,969 |
Norfolk | 90,566 | 10,198 | 13,141 | 494 | 6776 | 220 | 4056 | 21,356 | 11,620 | 40 | 2123 | 255 | 6159 | 40 | 22,798 |
Suffolk | 88,527 | 14,445 | 16,171 | 841 | 11,677 | 0 | 0 | 0 | 0 | 0 | 0 | 0 | 0 | 0 | 0 |
London | |||||||||||||||
Barnet | 35,911 | 14,151 | 4365 | 3103 | 12,468 | 168 | 2139 | 9547 | 8925 | 57 | 1209 | 469 | 2149 | 436 | 11,686 |
Camden | 33,421 | 8105 | 3236 | 3038 | 7541 | 173 | 3961 | 13,178 | 23,139 | 18 | 2081 | 161 | 2220 | 609 | 11,625 |
Enfield | 36,695 | 11,136 | 4690 | 3207 | 12,454 | 144 | 2892 | 7511 | 6612 | 79 | 1102 | 463 | 1790 | 377 | 12,207 |
Hackney | 33,699 | 6728 | 3506 | 3167 | 8220 | 574 | 4062 | 9627 | 16,199 | 53 | 1741 | 313 | 1182 | 998 | 13,521 |
Hammersmith and Fulham | 23,732 | 5266 | 2590 | 1802 | 7769 | 213 | 2968 | 8511 | 9554 | 16 | 1974 | 168 | 3121 | 136 | 9054 |
Islington | 31,473 | 6641 | 3231 | 2873 | 6621 | 152 | 2530 | 9976 | 16,178 | 11 | 2319 | 177 | 2016 | 697 | 11,211 |
Kingston | 14,235 | 3405 | 1839 | 554 | 2133 | 68 | 870 | 4079 | 4386 | 10 | 644 | 59 | 1798 | 73 | 5101 |
Richmond | 13,861 | 5261 | 2174 | 520 | 4208 | 137 | 852 | 3809 | 4305 | 6 | 732 | 77 | 1072 | 52 | 4485 |
Southwark | 34,631 | 7685 | 3809 | 5693 | 7203 | 0 | 3704 | 11,919 | 14,019 | 0 | 2059 | 0 | 1652 | 0 | 13,546 |
Sutton | 14,788 | 3952 | 2351 | 741 | 4367 | 28 | 805 | 3931 | 2798 | 4 | 657 | 74 | 1495 | 31 | 5832 |
Waltham Forest | 28,121 | 7976 | 3434 | 3705 | 10,813 | 64 | 2305 | 8120 | 7320 | 13 | 1381 | 132 | 1517 | 124 | 11,454 |
North East England | |||||||||||||||
Hartlepool | 21,217 | 1812 | 2723 | 90 | 1124 | 38 | 796 | 3108 | 1895 | 13 | 415 | 58 | 1232 | 7 | 3600 |
Sunderland | 45,950 | 4382 | 6347 | 182 | 3482 | 96 | 1880 | 9727 | 3919 | 52 | 1233 | 180 | 3331 | 21 | 6616 |
North West England | |||||||||||||||
Knowsley | 17,662 | 2757 | 3742 | 240 | 2395 | 17 | 2465 | 5145 | 1809 | 21 | 740 | 45 | 1028 | 14 | 2431 |
Sefton | 26,536 | 6900 | 5197 | 428 | 3862 | 104 | 3151 | 8239 | 3718 | 30 | 887 | 97 | 2702 | 43 | 5233 |
Wigan | 44,201 | 7953 | 7800 | 500 | 6544 | 233 | 1519 | 8484 | 5127 | 58 | 963 | 382 | 2730 | 73 | 8823 |
South East England | |||||||||||||||
Buckinghamshire | 27,870 | 10,502 | 7916 | 701 | 8877 | 274 | 2172 | 12,520 | 8835 | 75 | 1383 | 467 | 3417 | 236 | 11,772 |
East Sussex | 56,631 | 7775 | 8598 | 509 | 6502 | 101 | 2391 | 15,268 | 8049 | 35 | 1412 | 158 | 3770 | 88 | 13,114 |
Hampshire | 107,149 | 17,659 | 17,747 | 740 | 13,047 | 0 | 5220 | 34,587 | 16,768 | 0 | 4393 | 0 | 8731 | 0 | 27,607 |
Oxfordshire | 35,672 | 9760 | 9572 | 665 | 7216 | 1173 | 4264 | 19,274 | 17,104 | 87 | 2808 | 784 | 5863 | 357 | 14,360 |
Reading | 13,593 | 5049 | 3156 | 555 | 3849 | 264 | 1215 | 7465 | 5749 | 41 | 833 | 172 | 3183 | 199 | 6589 |
Surrey | 113,643 | 19,670 | 16,757 | 798 | 15,210 | 223 | 5472 | 30,311 | 15,301 | 43 | 3217 | 268 | 6832 | 53 | 26,215 |
West Berkshire | 8039 | 3580 | 2495 | 133 | 2383 | 55 | 739 | 4660 | 2606 | 15 | 450 | 86 | 1292 | 25 | 3827 |
West Sussex | 89,552 | 13,942 | 15,293 | 742 | 12,095 | 881 | 4749 | 23,777 | 16,466 | 182 | 2558 | 811 | 8748 | 402 | 23,570 |
Wokingham | 5173 | 2223 | 1413 | 90 | 1339 | 39 | 436 | 2809 | 1527 | 6 | 229 | 19 | 445 | 9 | 1815 |
South West England | |||||||||||||||
Bath | 19,540 | 2553 | 3189 | 116 | 1789 | 155 | 915 | 4850 | 3192 | 12 | 51 | 352 | 2230 | 85 | 6021 |
Cornwall | 43,548 | 6866 | 9097 | 231 | 3959 | 162 | 2297 | 10,813 | 7516 | 65 | 1724 | 870 | 4071 | 130 | 15,340 |
Devon | 56,066 | 9631 | 12,016 | 301 | 6297 | 491 | 3159 | 14,295 | 11,300 | 132 | 3116 | 1516 | 6036 | 201 | 21,672 |
Dorset | 35,480 | 5088 | 5286 | 155 | 4064 | 0 | 925 | 8401 | 5227 | 0 | 715 | 0 | 1928 | 0 | 6473 |
Gloucestershire | 65,640 | 13,479 | 9090 | 666 | 9003 | 191 | 2761 | 19,126 | 9882 | 17 | 1466 | 105 | 5176 | 53 | 10,643 |
Plymouth | 28,527 | 4251 | 5384 | 287 | 3264 | 39 | 1706 | 8563 | 4465 | 20 | 1866 | 258 | 3055 | 46 | 11,091 |
South West England | |||||||||||||||
Poole | 18,622 | 3245 | 3196 | 87 | 3296 | 260 | 467 | 4136 | 3158 | 19 | 332 | 123 | 1478 | 34 | 3904 |
South Gloucestershire | 25,615 | 5649 | 4322 | 230 | 3305 | 151 | 1071 | 6716 | 3769 | 17 | 32 | 254 | 2190 | 34 | 6284 |
West Midlands | |||||||||||||||
Birmingham | 37,367 | 9790 | 9530 | 3226 | 10,115 | 860 | 3432 | 4375 | 8670 | 385 | 1012 | 2107 | 5569 | 1268 | 17,432 |
Dudley | 24,454 | 5503 | 4543 | 866 | 4960 | 17 | 1076 | 7549 | 2926 | 4 | 623 | 32 | 1807 | 6 | 6141 |
Herefordshire | 22,951 | 2810 | 2784 | 100 | 2025 | 88 | 895 | 5023 | 3392 | 17 | 670 | 149 | 1805 | 30 | 5642 |
Sandwell | 25,525 | 9237 | 6575 | 2391 | 8969 | 238 | 2139 | 10,002 | 4964 | 109 | 954 | 373 | 2820 | 137 | 10,368 |
Shropshire | 31,245 | 4693 | 3591 | 168 | 2923 | 56 | 973 | 6561 | 3741 | 12 | 685 | 57 | 1842 | 11 | 6148 |
Solihull | 15,168 | 4582 | 2803 | 735 | 4793 | 33 | 883 | 6677 | 2786 | 8 | 595 | 67 | 1504 | 17 | 4816 |
Staffordshire | 53,425 | 12,699 | 12,886 | 674 | 8675 | 156 | 4361 | 20,062 | 11,481 | 37 | 2314 | 306 | 4407 | 59 | 22,731 |
Telford | 26,524 | 3659 | 3324 | 260 | 2981 | 31 | 772 | 5080 | 2523 | 8 | 507 | 64 | 1617 | 9 | 5244 |
Walsall | 23,690 | 8236 | 6117 | 1122 | 6336 | 183 | 1347 | 8900 | 4416 | 44 | 886 | 347 | 4100 | 121 | 9645 |
Warwickshire | 62,866 | 14,445 | 10,374 | 824 | 12,509 | 503 | 2586 | 14,689 | 13,279 | 89 | 1398 | 368 | 4947 | 209 | 13,682 |
Wales | |||||||||||||||
Carmarthenshire | 27,832 | 1644 | 2703 | 36 | 1174 | 64 | 3477 | 3805 | 1859 | 41 | 979 | 373 | 1375 | 19 | 4370 |
Denbighshire | 14,424 | 1978 | 2333 | 103 | 991 | 41 | 537 | 2965 | 1813 | 16 | 606 | 151 | 978 | 19 | 4831 |
Swansea | 30,874 | 5187 | 6009 | 200 | 5616 | 236 | 2180 | 7761 | 5491 | 39 | 1260 | 506 | 2778 | 250 | 8775 |
Yorkshire and the Humber | |||||||||||||||
Kirklees | 45,247 | 13,764 | 9324 | 1051 | 9686 | 135 | 2043 | 14,288 | 9593 | 62 | 1509 | 385 | 4301 | 179 | 11,953 |
Leeds | 90,931 | 30,315 | 17,357 | 2655 | 20,788 | 398 | 5599 | 33,743 | 25,371 | 58 | 3355 | 468 | 9061 | 613 | 25,153 |
Rotherham | 55,297 | 8160 | 7538 | 359 | 6353 | 0 | 0 | 0 | 0 | 0 | 0 | 0 | 0 | 0 | 0 |
Sheffield | 132,543 | 20,453 | 16,191 | 1684 | 17,289 | 0 | 0 | 0 | 0 | 0 | 0 | 0 | 0 | 0 | 0 |
Totals | |||||||||||||||
Total | 2,882,758 | 581,837 | 486,367 | 67,470 | 475,657 | 14,899 | 151,957 | 724,031 | 508,377 | 3201 | 84,023 | 20,765 | 219,097 | 12,026 | 730,280 |
000s | 2883 | 582 | 486 | 67 | 476 | 15 | 152 | 724 | 508 | 3 | 84 | 21 | 219 | 12 | 730 |
Rank | 1 | 4 | 6 | 11 | 7 | 13 | 9 | 3 | 5 | 15 | 10 | 12 | 8 | 14 | 2 |
Appendix 6 Associations between street lighting adaptation strategies and crime, showing estimates for each police force
FIGURE 8.
Aggregate count of crime. (a) Switch-off; (b) part-night lighting; (c) dimming; and (d) white light. PNL, part-night lighting.




FIGURE 9.
Burglary. (a) Switch-off; (b) part-night lighting; (c) dimming; and (d) white light. PNL, part-night lighting.




FIGURE 10.
Robbery. (a) Switch-off; (b) part-night lighting; (c) dimming; and (d) white light. PNL, part-night lighting.
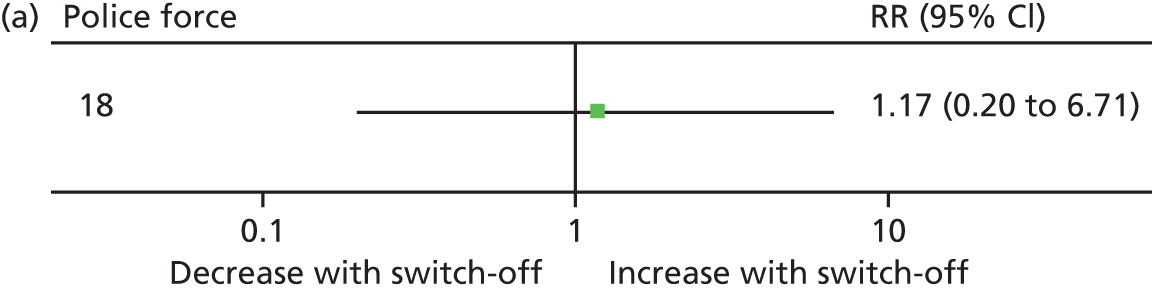



FIGURE 11.
Vehicle crime. (a) Switch-off; (b) part-night lighting; (c) dimming; and (d) white light. PNL, part-night lighting.

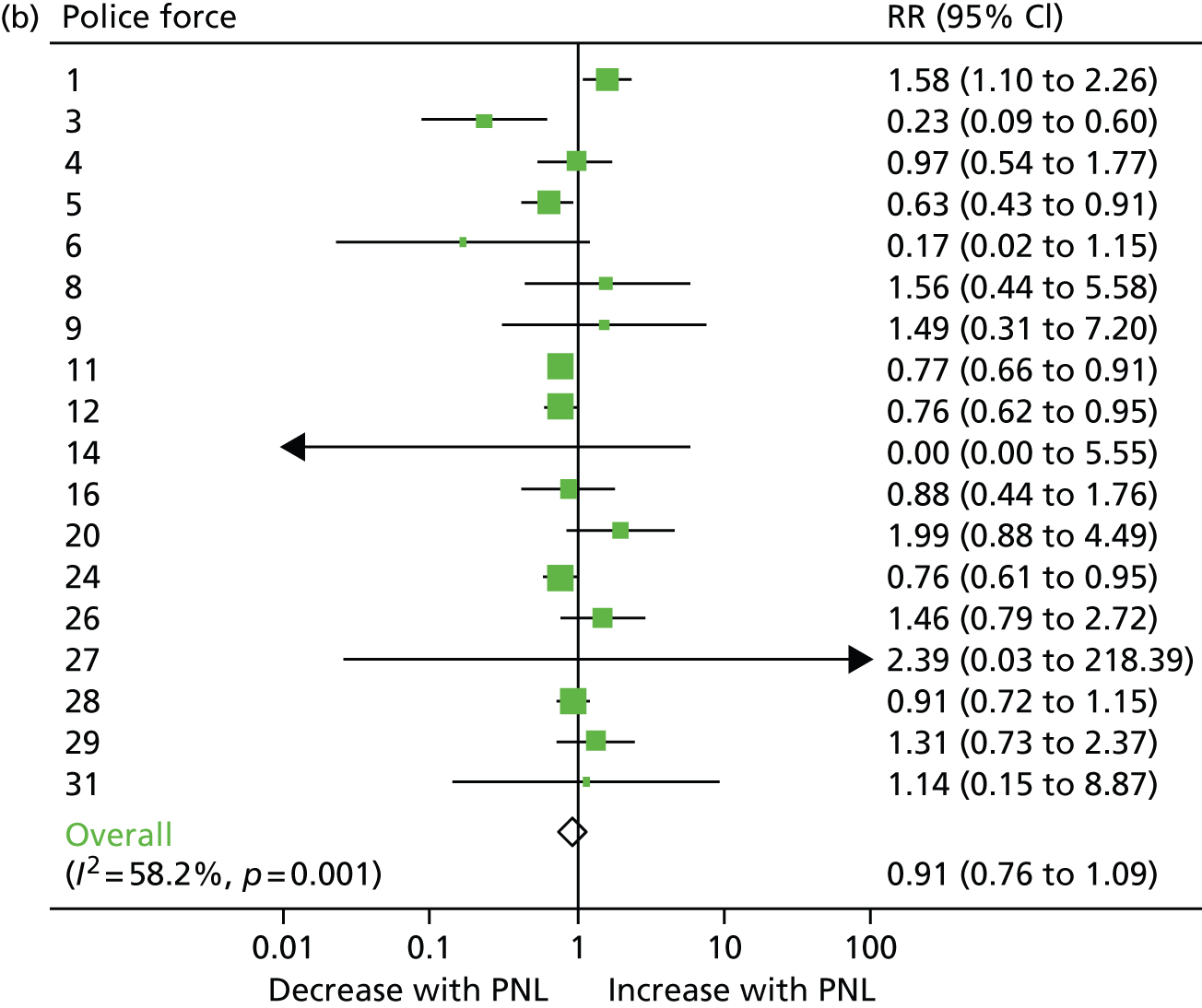
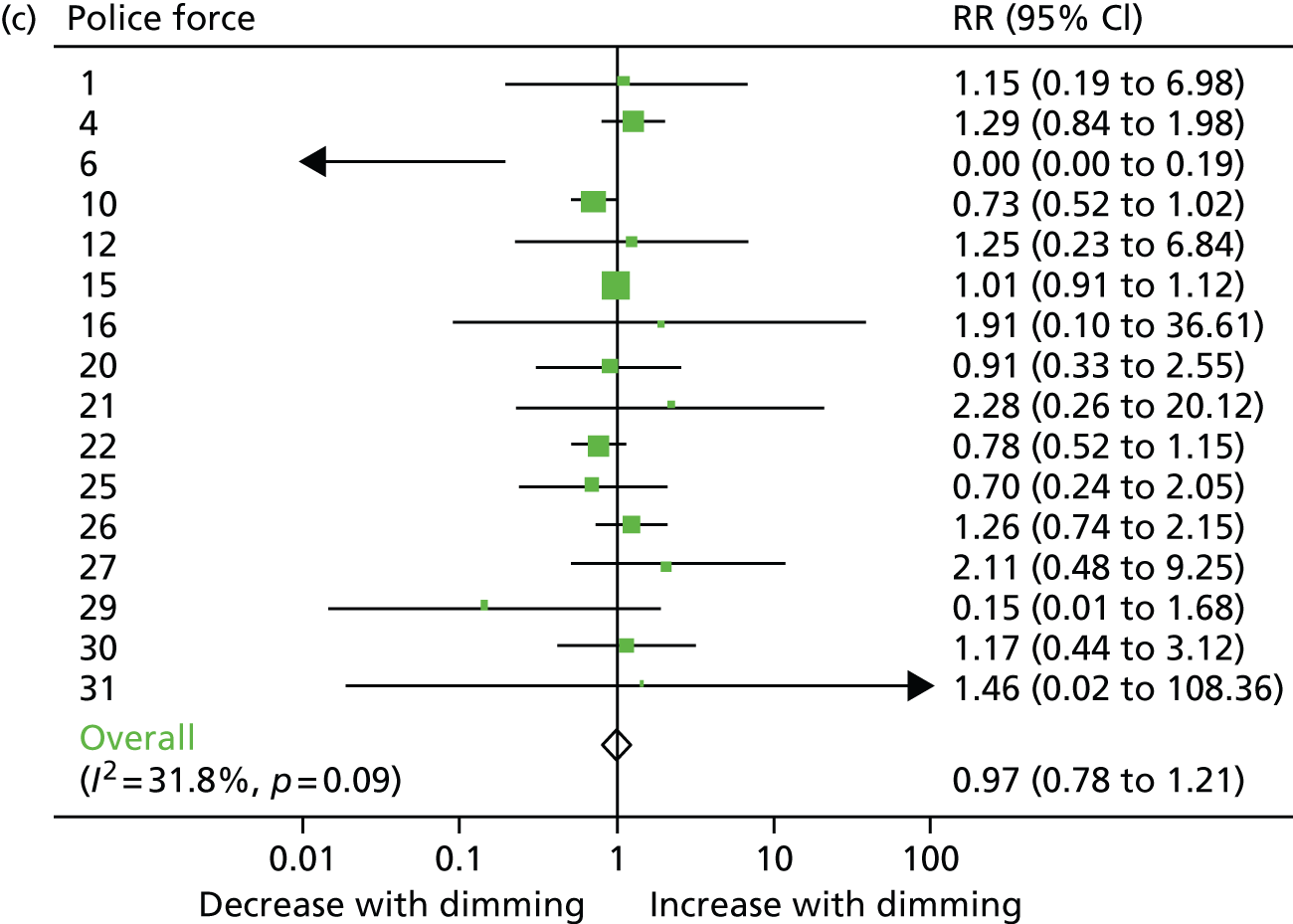

FIGURE 12.
Violence. (a) Switch-off; (b) part-night lighting; (c) dimming; and (d) white light. PNL, part-night lighting.
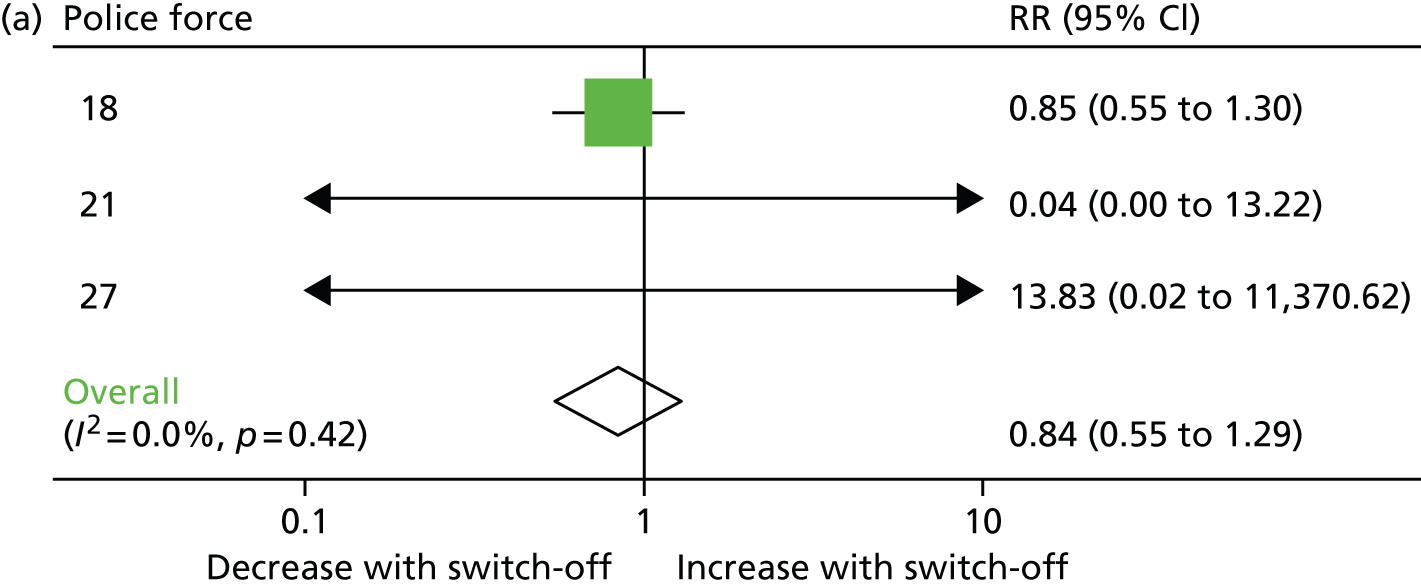



Appendix 7 Mapping of Police.uk codes to Home Office categories for burglary and vehicle theft
Police.uk code | Home Office code |
---|---|
Burglary | Burglary – indictable |
Burglary – violence | |
Burglary in a dwelling – either way | |
Artifice burglary in a dwelling | |
Aggravated burglary in a dwelling | |
Burglary in a building other than a dwelling – indictable only | |
Artifice burglary in a building other than a dwelling | |
Burglary in a building other than a dwelling – either way | |
Aggravated burglary in building other than a dwelling | |
Vehicle crime | Interference with a motor vehicle |
Unauthorised taking of a motor vehicle (does not include being carried knowing motor vehicle has been taken) | |
Aggravated vehicle taking where the only aggravating factor is criminal damage of £5000 or under | |
Aggravated vehicle taking | |
Theft from a motor vehicle | |
Theft from vehicle other than a motor vehicle | |
Theft of a motor vehicle | |
Tampering with motor vehicles |
Appendix 8 Summary of discussion at stakeholder meeting, Tuesday 23 September 2014
Participants were lighting professionals from 27 local authorities, public health professionals, energy provider organisations, one representative from ILP and the LANTERNS project team. A half-day meeting reviewed the provisional findings from the project, discussed the logic and costing models, and participants took part in a discussion and survey of the role of evidence in decision-making about reduced street lighting.
Comments on the logic model
Content: context is important – street lighting decisions driven by cost reduction etc., but in the context of broader issues faced by local authorities (schools, potholes, etc.). This focuses on reduced street lighting – should another pathway be for different spectrum lighting? Health outcomes also include mental health, impacts for people with disabilities and inequalities. ‘Feelings about neighbourhood’ should include impact on antisocial behaviour and local economy. Visibility of houses/pedestrians, etc.: should split into what local authority have responsibility for (street) and houses (not their responsibility). Also split between visibility to people and on CCTV. Link between reduced energy use and costs mediated by tariff issues: also add in other savings (see economic points).
Format: should be weighted so that most important issues (costs, crime, injuries) are at top. Colour coding could reflect positive and negative impacts.
Key points from break-out groups on decision-making
The rationale for decisions may not be known by those implementing them; and those making decisions may not know enough about local contexts to make decisions about street lighting. ‘No two roads are the same’: detailed knowledge, by those who are familiar with locality, is needed. Is the driver saving money, or saving the planet? Saving money is the key issue – politically more important than planet, crime or injury.
Key issue is context of budget cuts. There is loss of ‘lighting champions’ in authorities; and the right person may not be at meetings deciding on strategies. ‘Need Professionals to make professional decisions’. Street light reductions may be (seem) an easy hit – even if savings are not clear. Others are national (and increasingly) European standards. (Lighting standards currently used may be out of date.)
For evidence from the LANTERNS project to be useful in decision-making, it should have clear findings (yes or no) and clear guidance on how certain the causal inferences are, and be relevant to local context. Will we be able to pick up context such as efforts made to mitigate some of negative effects? Or age and replacement of street lighting stock? Can we tease out why there are different effects across different local authorities? Particularly rural/urban settings. Can we pick out other effects and costs of central management systems?
ILP and other organisations have a role in disseminating and endorsing the findings.
Key points from discussion of economic evaluation model
Division between capital and revenue costs important. Issues to add in: age/replacement of stock; levy to PFI if you do change your mind; scouting; consultation costs; may be income from columns (e.g. WiFi); bat surveys; court and financial costs (liabilities); maintenance costs (and how these are rolled into routine rolling programmes); uncertainties of costs; issues of agreeing tariffs/collective bargaining over energy.
Appendix 9 Household survey questionnaire
Appendix 10 Household survey results
Survey item | PNL (N = 250), n (%) | Non-PNL (N = 233), n (%) | p-value (chi-squared test excluding missing) |
---|---|---|---|
Thinking about your neighbourhood in general, would you say that people can rely on each other for help? | |||
Always | 37 (14.8) | 46 (19.7) | p = 0.305 |
Usually | 160 (64.0) | 144 (61.8) | |
Rarely | 42 (16.8) | 35 (15.0) | |
Never | 9 (3.6) | 4 (1.7) | |
Missing | 2 (0.8) | 4 (1.7) | |
Do you trust your local council to do its best for your neighbourhood? | |||
Always | 9 (3.6) | 9 (3.9) | p = 0.457 |
Usually | 148 (59.2) | 149 (63.9) | |
Rarely | 77 (30.8) | 56 (24.0) | |
Never | 14 (5.6) | 15 (6.4) | |
Missing | 2 (0.8) | 4 (1.7) | |
Thinking about the spring and autumn periods, how often do you usually walk alone in your neighbourhood after dark? | |||
At least once a week | 73 (29.2) | 79 (33.9) | p = 0.596 |
At least once a fortnight | 20 (8.0) | 17 (7.3) | |
At least once a month | 39 (15.6) | 26 (11.2) | |
Less than once a month | 64 (25.6) | 57 (24.5) | |
Missing | 0 (0.0) | 2 (0.9) | |
Thinking about the spring and autumn periods, how safe do you feel walking alone in your neighbourhood after dark? | |||
Very safe | 39 (15.6) | 61 (26.2) | p = 0.043 |
Fairly safe | 136 (54.4) | 109 (46.8) | |
A bit unsafe | 50 (20.0) | 42 (18.0) | |
Very unsafe | 16 (6.4) | 14 (6.0) | |
Missing | 9 (3.6) | 7 (3.0) | |
Thinking about the spring and autumn periods, how safe do you feel driving home after dark? | |||
Very safe | 115 (46.0) | 117 (50.2) | p = 0.110 |
Fairly safe | 77 (30.8) | 79 (33.9) | |
A bit unsafe | 16 (6.4) | 6 (2.6) | |
Very unsafe | 115 (46.0) | 117 (50.2) | |
I don’t drive | 39 (15.65) | 24 (10.3) | |
Missing | 1 (0.4) | 4 (1.7) | |
How worried are you about having your car stolen or broken into after dark in your neighbourhood? | |||
Very worried | 7 (2.8) | 7 (3.0) | p = 0.040 |
Fairly worried | 29 (11.6) | 15 (6.4) | |
Not very worried | 121 (48.4) | 118 (50.6) | |
Not at all worried | 56 (22.4) | 71 (30.5) | |
I don’t have a car | 37 (14.8) | 21 (9.0) | |
Missing | 0 (0.0) | 1 (0.4) | |
Please indicate how much you agree with the following statements | |||
‘There is enough street lighting to see clearly at night in my street’ | |||
Strongly disagree | 69 (27.6) | 63 (27.0) | p = 0.155 |
Slightly disagree | 49 (19.6) | 32 (13.7) | |
Somewhat agree | 83 (33.2) | 68 (29.2) | |
Strongly agree | 48 (19.2) | 60 (25.8) | |
Missing | 1 (0.4) | 10 (4.3) | |
‘Street lights outside my home keep me awake at night’ | |||
Strongly disagree | 186 (74.4) | 162 (69.5) | p = 0.756 |
Slightly disagree | 35 (14.0) | 26 (11.2) | |
Somewhat agree | 19 (7.6) | 19 (8.2) | |
Strongly agree | 6 (2.4) | 8 (3.4) | |
Missing | 4 (1.6) | 18 (7.7) | |
‘On a clear night, I can see the stars if I stand outside in my street’ | |||
Strongly agree | 141 (56.4) | 135 (57.9) | p = 0.985 |
Somewhat agree | 85 (34.0) | 77 (33.0) | |
Slightly disagree | 15 (6.0) | 13 (5.6) | |
Strongly disagree | 6 (2.4) | 5 (2.2) | |
Missing | 3 (1.2) | 3 (1.3) | |
‘It’s important to me to be able to see the stars at night’ | |||
Strongly agree | 105 (42.0) | 116 (49.8) | p = 0.110 |
Somewhat agree | 96 (38.4) | 88 (37.8) | |
Slightly disagree | 29 (11.6) | 21 (9.0) | |
Strongly disagree | 14 (5.6) | 5 (2.2) | |
Missing | 6 (2.4) | 3 (1.3) | |
Thinking about your house or flat, have you or the owner done any of the following within the last 2 years? | |||
Installed any lights at the front or garden to improve visibility or security? | |||
Yes | 79 (31.6) | 74 (31.8) | p = 0.888 |
No | |||
Missing | |||
Installed any lights at the back or garden to improve visibility or security? | |||
Yes | 84 (33.6) | 75 (32.2) | p = 0.634 |
No | |||
Missing | |||
Installed a burglar alarm? | |||
Yes | 24 (9.6) | 24 (10.3) | p = 0.863 |
No | |||
Missing | |||
Made other improvements to the visibility of your front entrance? | |||
Yes | 33 (13.2) | 34 (14.6) | p = 0.647 |
No | |||
Missing | |||
Has the number or brightness of street lights in your neighbourhood been reduced within the last few years? | |||
Yes in my street | 126 (50.4) | 56 (24.0) | p < 0.001 |
Yes in streets nearby | 52 (20.8) | 37 (15.9) | |
No | 41 (16.4) | 88 (37.8) | |
Don’t know | 26 (10.4) | 37 (15.9) | |
Missing | 5 (2.0) | 15 (6.4) | |
Do you carry a torch with you when you go out at night? | |||
Always | 33 (13.2) | 28 (12.0) | p = 0.105 |
Usually | 39 (15.6) | 57 (24.5) | |
Rarely | 71 (28.4) | 57 (24.5) | |
Never | 97 (38.8) | 81 (34.8) | |
Missing | 10 (4.0) | 10 (4.3) |
List of abbreviations
- CBA
- cost–benefit analysis
- CCA
- cost–consequence analysis
- CEA
- cost-effectiveness analysis
- CI
- confidence interval
- CMS
- central management system
- CUA
- cost–utility analysis
- GIS
- geographic information system
- ILP
- Institution of Lighting Professionals
- IMD
- Index of Multiple Deprivation
- IQR
- interquartile range
- LANTERNS
- Local Authority collaborators’ National Evaluation of Reduced Night-time Streetlight
- LED
- light-emitting diode
- LSOA
- lower super output area
- MSOA
- middle super output area
- NIHR
- National Institute for Health Research
- NPV
- net present value
- PFI
- Private Finance Initiative
- PHR
- Public Health Research
- QALY
- quality-adjusted life-year
- RCT
- randomised controlled trial
- RR
- rate ratio