Notes
Article history
The research reported in this issue of the journal was funded by the PHR programme as project number 09/3001/06. The contractual start date was in January 2010. The final report began editorial review in March 2015 and was accepted for publication in August 2015. The authors have been wholly responsible for all data collection, analysis and interpretation, and for writing up their work. The PHR editors and production house have tried to ensure the accuracy of the authors’ report and would like to thank the reviewers for their constructive comments on the final report document. However, they do not accept liability for damages or losses arising from material published in this report.
Declared competing interests of authors
David Ogilvie, Jenna Panter, Cornelia Guell, Andy Jones and Simon Griffin report additional funding from the Medical Research Council. David Ogilvie, Jenna Panter and Simon Griffin report additional funding from the National Institute for Health Research Trainees Coordinating Centre. David Ogilvie, Andy Jones and Simon Griffin report additional funding from the UK Clinical Research Collaboration. David Ogilvie reports additional funding from the Wellcome Trust. Andy Jones is a member of the Research Funding Board of the National Institute for Health Research Public Health Research programme.
Permissions
Copyright statement
© Queen’s Printer and Controller of HMSO 2016. This work was produced by Ogilvie et al. under the terms of a commissioning contract issued by the Secretary of State for Health. This issue may be freely reproduced for the purposes of private research and study and extracts (or indeed, the full report) may be included in professional journals provided that suitable acknowledgement is made and the reproduction is not associated with any form of advertising. Applications for commercial reproduction should be addressed to: NIHR Journals Library, National Institute for Health Research, Evaluation, Trials and Studies Coordinating Centre, Alpha House, University of Southampton Science Park, Southampton SO16 7NS, UK.
Chapter 1 Background
Active travel, physical activity and health
Physical inactivity increases the risk of coronary heart disease, type 2 diabetes and many other preventable chronic diseases, and is estimated to account for 9% of premature mortality in the world. 1–3 Epidemiological evidence suggests that as little as 20–30 minutes of moderate-intensity physical activity on most days is enough to confer substantial health benefits,4 but many adults in the UK are comparatively inactive and would benefit from a more active lifestyle. 5,6 Increasing the level of physical activity in the population has, therefore, been described as the ‘best buy’ for improving public health,7 and is the subject of a new national framework for action in England. 8
Efforts to promote physical activity have traditionally been directed at ‘high risk’ individuals and focused on promoting sport, recreation or health-directed exercise. 9 However, there is limited evidence that such approaches have been effective in increasing and maintaining levels of physical activity in the medium to long term. 10 Greater public health benefits may, therefore, accrue from a population strategy to shift the distribution of physical activity in the population as a whole, rather than from further efforts to target high-risk groups, and to promote an increase in physical activity as part of people’s daily routines rather than as ‘exercise’ to be incorporated into leisure time. 11
One reason for the limited success of previous strategies may be that health-related behaviours are enabled or constrained by the environments in which people live. A growing body of evidence suggests a relationship between physical activity and the physical environment, exemplified by numerous observational studies showing that people who live in more ‘walkable’ neighbourhoods tend to walk more. However, much of this evidence is derived from research in North America or Australia and may, therefore, be of limited relevance to the UK. 12 Most studies have also been limited to the analysis of data collected at a single point in time, and many have tended to examine the general attributes of the neighbourhoods in which people live rather than more specific environmental exposures. 12–14 Although further observational epidemiological research can contribute to advancing our understanding of how the environment shapes behaviour, this is likely to be most useful if it focuses on more specific exposure–outcome relationships linking particular environmental attributes with particular forms of activity, and if it involves longitudinal study designs capable of supporting more robust causal inference. 15,16
Notwithstanding the contribution of observational studies, the most useful new evidence to support policy and practice in this field is likely to come from intervention studies. Programme guidance on physical activity and the environment issued by the National Institute for Health and Care Excellence (NICE) in 2008 drew attention to the lack of studies examining whether or not changing the physical environment leads to changes in physical activity, and identified a need for more – and more rigorous – studies involving longitudinal designs, controlled comparisons, and robust measures of physical activity outcomes. 17 The evidence update published by NICE in April 2014 identified few new studies of this kind that had appeared in the global research literature since the publication of the original guidance. 18 Among other things, this reflects the broader challenges of evaluating complex public health interventions, which often include studying the effects of ‘natural experiments’ in which interventions and their assignment are out of the control of researchers. 14,19,20
Most people need to travel, and one way of increasing levels of physical activity is to encourage the use of active modes of transport such as walking and cycling. Modifying transport infrastructure to support walking and cycling for transport (active travel), for example by constructing cycle routes or redesigning roads to discourage car use, is one way of changing the physical environment that was identified in the Foresight report as one of the top five recommendations for tackling obesity in the UK. 21 The potential to enable people to incorporate walking or cycling more readily into their daily routines, for example on the journey to and from work (active commuting), makes this an attractive strategy for increasing population levels of physical activity, particularly as it need not entail replacing entire journeys with active travel. For example, travelling by public transport can involve a substantial daily quantity of walking, and commuters who use public transport tend to walk more than those who commute by car. 22–24 These may be particularly important considerations in a country such as the UK, where many people live too far from their workplace to walk or cycle the entire journey. Nevertheless, several systematic reviews16,25–30 and the recent NICE guidance on walking and cycling31 have highlighted the lack of robust public health intervention studies in this area, and when the present study was being designed no study had convincingly demonstrated that investing in new transport infrastructure led to an increase in active travel in the local population.
The Cambridgeshire Guided Busway
We therefore took the opportunity presented by the opening of the Cambridgeshire Guided Busway – a major new transport infrastructure project serving the city and environs of Cambridge, in the east of England – to establish a quasi-experimental study of the effects of providing new transport infrastructure on travel behaviour, physical activity and related wider health outcomes.
Cambridge is a city of approximately 124,000 residents which lies 80 km north of London. The city is comparatively flat and has a large university as well as a number of other major biomedical, scientific and technological employers, all of which may contribute to the fact that it is the city with the highest prevalence of cycling in the UK. The surrounding area includes market towns such as Ely, Huntingdon, Newmarket, Royston and St Ives as well as many smaller villages, from which substantial numbers of commuters travel into Cambridge each day. The historic centre of Cambridge is relatively inaccessible by car and, as is the case in many other cities, the main arterial roads into the city become congested during peak times.
This formed the background to the plan to construct a guided busway, in order to provide a high-quality, fast and reliable alternative to driving for commuters travelling into Cambridge along the A14 trunk road from the north-west (Figure 1a). The busway comprises a new bus network and a traffic-free walking and cycling route (Figure 1b). Buses run on a guideway (concrete track) completely segregated from other traffic along much of the route, and a new high-quality surfaced path for pedestrians and cyclists runs alongside the guideway. The longest section of guideway runs for 19 km from the market town of St Ives to the Science Park on the northern fringe of the city. Buses then run on the existing road network through the city centre before joining a further section of guideway. This runs south for 3 km from the city’s railway station to the southern fringe of the city at Trumpington via the Biomedical Campus, which includes Addenbrooke’s Hospital and is a major generator of traffic in the region. Two new park-and-ride sites on the northern section of the busway provide car parking and interchange facilities, and the southern section of the busway terminates at an existing park-and-ride site.
FIGURE 1.
The Cambridgeshire Guided Busway. (a) Map of the busway; and (b) view of the busway. Map reproduced with kind permission of Cambridgeshire County Council. Photograph © Eva Heinen and reproduced with permission.

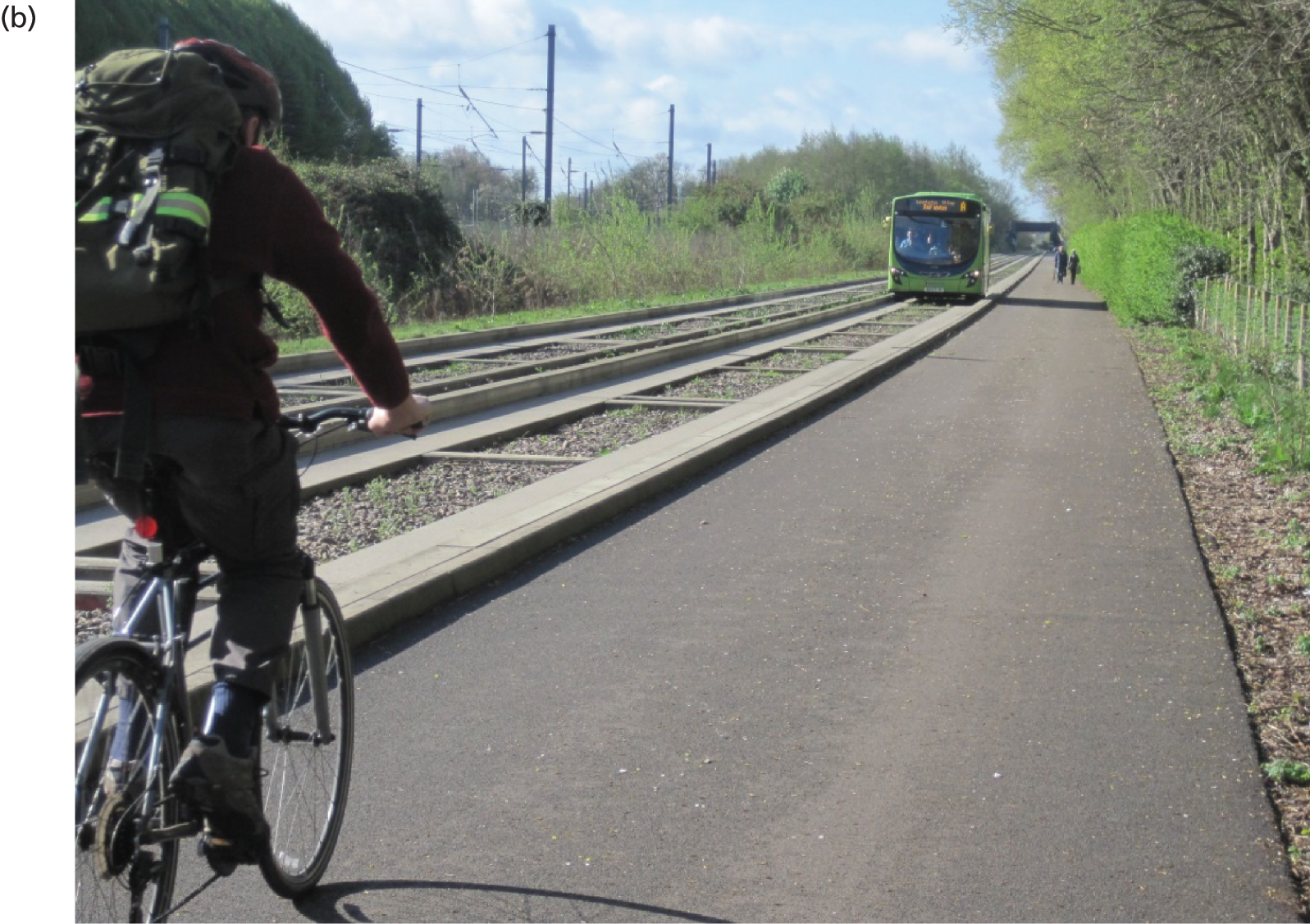
Construction of the busway began in March 2007 and was scheduled for completion in spring 2009, but following a series of complications in construction and contractual disputes the busway was finally opened more than 2 years late on 7 August 2011 at an eventual cost of approximately £150M.
A full description of the busway, including routes, times and fares, can be found at www.thebusway.info.
Although the guided busway had the potential to support and promote the use of active travel and public transport for a variety of journey purposes, the primary motivation for its construction was to improve conditions for commuting into Cambridge. Our study was, therefore, designed with a focus on active commuting as the main outcome of public health interest. Our aim was not to evaluate the busway against a comprehensive set of outcomes, but rather to use the opportunity presented by this natural experiment to investigate a more specific set of research questions linking environmental change to physical activity behaviour change and related health outcomes.
Main research questions
The aim of the study, which was named the Commuting and Health in Cambridge study, was to address the following primary research question:
-
Is investment in new high-quality transport infrastructure associated with an increase in the use of active modes of travel (walking and cycling) on the journey to and from work?
We also aimed to address the following secondary research questions:
-
What are the wider health impacts of changes in travel behaviour in terms of overall physical activity, well-being, sickness absence and carbon emissions?
-
What are the determinants of the use and uptake of active modes of travel?
-
How are any changes in travel behaviour distributed in the population?
-
How are travel behaviour and changes in travel behaviour embedded in and shaped by the wider social context?
-
Are changes in travel behaviour sustained over time?
It was not possible to address the fifth secondary research question directly because of the delay in the completion of the busway and subsequent reconfiguration of the study (see Chapter 2, Overall research design).
This report
This report represents an original summary and synthesis of a large body of research, most of which has already been published – or submitted or prepared for publication – in other open-access academic journals, and to which an extensive study team has contributed in various ways (see Acknowledgements). Further details of the methods and results of the various analyses summarised in the report can be found in these publications, which are referred to in callout boxes in the text and are available via the study website at www.cambridgecommutingstudy.org.uk.
Chapter 2 Methods
Introduction
In this chapter, we describe the methods for the study in terms of the overall research design, participant recruitment and data collection, key variables derived from the data, and the general approach to quantitative and qualitative analysis.
Overall research design
The main aim of the study was to investigate the effect of changing the environment, in this case by providing new transport infrastructure, on commuter travel behaviour. However, we also aimed to address a broader set of research questions relating to active commuting, its environmental and social context and its health benefits. The study was, therefore, originally conceptualised as a cohort study of adults living within a radius of approximately 30 km of Cambridge city centre and working in areas of the city to be served by the busway, supplemented with in-depth quantitative and qualitative studies in subsamples of the cohort.
A core questionnaire was administered to all members of the cohort in each year of the study. This was designed to capture day-to-day variation in commuting trips over a 7-day period, allowing us to derive a simple estimate of self-reported time spent in active commuting – the primary outcome for the study – as well as ascertaining other forms of physical activity. The questionnaire also captured participants’ perceptions of the supportiveness of the route between their home and their workplace for active travel.
When we embarked on the study, construction of the busway was under way and its opening was scheduled for spring 2009. The original study design envisaged three annual phases of survey data collection from the cohort: one before the intervention (phase 1, in 2009) and two after (phase 2, in 2010, and phase 3, in 2011). However, the completion of the busway was significantly delayed, and by the time it was formally opened our third annual phase of data collection was already more than half complete. We kept the evolving situation under review, reflecting on the scientific and operational options for managing the unpredictable implementation timetable in our regular progress reports to the Public Health Research programme and at the regular meetings of our independent study steering committee. With the support of the steering committee, once the busway was opened we sought a funded extension to the original study design, which was approved by the Public Health Research programme. We thereby extended the study to a fourth year (phase 4, in 2012) to obtain post-intervention data to support the main evaluation of the busway. The core questionnaire was reissued to all participants who had taken part in previous phases, and phase 4 thereby effectively replaced the original phase 2 as the phase of the study in which the main outcomes of the intervention were ascertained.
We also recruited subgroups of participants for in-depth studies of travel and physical activity behaviour in each annual phase of the study, using objective recording of physical activity and locational data as well as a detailed household travel diary. We thus aimed to offset the relative strengths and weaknesses of subjective and objective measurement of physical activity. Self-reported measures are relatively easy and inexpensive to administer and can readily differentiate between behaviours such as walking and cycling, but their main disadvantage is that they may be susceptible to error or bias in reporting, and no validated self-reported measure of physical activity as part of commuting had been published at the outset of our study. Devices such as accelerometers, in contrast, can provide more accurate estimates of the intensity, frequency and duration of physical activity, but they record only certain aspects of movement and do not readily distinguish between behaviours and the contexts in which they occur. During phase 1 of the study, when we tested the feasibility of rapidly recruiting and obtaining objective baseline physical activity measures in a large sample, a subsample of participants was asked to wear an accelerometer for 1 week. In subsequent phases, those participants who had successfully completed accelerometry in phase 1 were invited to take part in more detailed activity monitoring, using combined heart rate and movement sensors in combination with global positioning system (GPS) receivers. We used these data in combination to objectively ascertain the modes of transport used by commuters and the physical activity energy expenditure (PAEE) involved in using different modes (or combinations of modes) of transport.
The delay in completing the intervention provided an opportunity to further develop and apply our methods of studying commuter travel behaviour. We validated our core questionnaire estimate of weekly time spent in active commuting by comparing it with those derived from household travel diaries and from combined objective measurement, and further explored the implications of using different methods for assessing commute distance and overall physical activity. We also conducted a variety of quantitative, qualitative and mixed-method analyses to improve our understanding of active commuting and its environmental and social context, the busway, and the factors that might determine its acceptability and uptake. Drawing on the insights from all these analyses, we then proceeded to the main quantitative outcome evaluation. We studied the impact of the busway on travel behaviour, first through a supplementary intercept survey of busway users and then in terms of mode of travel to and from work (hereafter referred to simply as ‘to work’), carbon emissions attributable to commuting, and time spent in active commuting and in physical activity overall in our main study cohort. We then investigated selected causal pathways by which exposure to the busway was hypothesised to affect commuting behaviour and explored the reasons why people may or may not have used the busway. Finally, we investigated the longitudinal associations between active commuting and changes in overall physical activity and selected health outcomes.
For further details, see Ogilvie et al. 32
Samples
We recruited two participant samples for this study. The main cohort of commuters was recruited in the months before the anticipated opening of the busway in 2009. A supplementary sample of busway users, not restricted to commuters, was recruited in 2012, approximately 1 year after the commencement of guided bus services.
Cohort study
Inclusion criteria
In keeping with the aims of the study, we focused on recruiting participants who regularly commuted between home and work, specifically adults aged 16 years and over who lived within a radius of approximately 30 km of the city centre and worked in areas of Cambridge that were to be served by the busway. Participants were eligible for inclusion irrespective of their employer, workplace, type or grade of occupation, length of employment contract or working hours; whether or not they also worked at other locations; and whether or not they had any disability that may have limited their mobility. However, interested individuals were excluded if they lived in the same immediate area of the city as their workplace, including in on-site staff accommodation, or if they were taking part in other research that involved measuring their physical activity.
Recruitment
Because the study was focused on travel to work, participants were recruited primarily through workplaces rather than using a general population sampling frame such as an electoral or primary care register. The workplaces were distributed across Cambridge, in locations ranging from the city centre to the urban fringe locations, and included a range of types of organisation including local authorities, health-care providers, higher and further education institutions and retail outlets. In the interests of data protection and to assure participants of the independence of the study from their employers, we did not use corporate staff databases as a sampling frame and, therefore, could not compute a population response rate as such. We used a range of other methods for approaching potential participants between March and October 2009, including recruitment stands, newspaper and magazine advertisements, posters, fliers, and announcements distributed through corporate e-mail distribution lists, intranets and staff newsletters. E-mail distribution via employers’ mailing lists proved to be a particularly effective method for soliciting expressions of interest. A total of 2163 expressions of interest to participate were received, almost all via a web form, of which 1582 met the inclusion criteria.
Data collection
Core questionnaire
People who met the inclusion criteria were sent the core phase 1 questionnaire and consent form by post. The core questionnaire collected information related to the main outcomes of interest, namely participants’ usual physical activity and travel behaviour, and other information of interest. Briefly, it incorporated:
-
The Recent Physical Activity Questionnaire (RPAQ), which is based on a previously-validated physical activity questionnaire but takes the past 4 weeks rather than the past year as its reference period. 33 PAEE estimates derived from RPAQ information have been shown to have good test–retest reliability (with an intraclass correlation coefficient of 0.76) and strong criterion validity (with a correlation coefficient of 0.39) against PAEE objectively assessed using the doubly-labelled water technique. 34
-
A retrospective 7-day travel record focusing on the journey to and from work, based on an instrument used (and shown to have acceptable test–retest reliability) in a previous study of active commuting. 35 For each day of the previous week, participants reported whether or not they travelled to work, the times at which they started and finished work, and the modes of travel they used for each trip to and from work. Participants were also asked if they ever walked or cycled part or all of the journey to or from work. If they did, participants were asked to report the usual duration in minutes of walking or cycling involved in their journey.
-
A 1-day record of all trips made on the previous day, adapted and simplified from the travel diary used in the UK National Travel Survey. 36 This questionnaire captured the purpose, the modes of travel and the duration in minutes of each stage of each trip of the participant during the previous day.
-
Items ascertaining participants’ perceptions of the route of their journey to and from work. The questions were derived from those previously applied to participants’ residential environments in the M74 study in Glasgow, where they showed acceptable test–retest reliability. 37
-
Items to assess personal attitudes and beliefs corresponding to four Theory of Planned Behaviour constructs hypothesised to mediate choice of mode of transport, adapted from those used in a previous intervention study and applied to car use. 38
-
Items from the Self-Report Index of Habit Strength applied to car use. These items provide a psychological assessment of the extent to which a behaviour, such as using a car, is a personal habit. 39
-
An item on self-reported sickness absence in the past year, previously shown to be strongly correlated with sickness absence objectively verified from employment records in the Whitehall study. 40
-
The Medical Outcomes Study Short Form 8 (SF-8) for assessing general physical and mental health and well-being. 41
-
Items to capture key demographic, socioeconomic and other health-related characteristics, including age, gender, postcode of home and of workplace, level of educational attainment, presence of a long-term limiting illness or disability (hereafter referred to as a ‘health condition’), difficulty walking, and self-reported height and weight; as well as information on access to a bicycle, possession of a driving licence, the fuel type and engine size of the car most often used for commuting (if any), and whether or not car parking was available to them at work and, if so, whether or not they had to pay for it.
-
Follow-up versions of the questionnaire included additional items on awareness and use of the guided busway (including walking and cycling along the path) and reasons for using it or not using it, as well as any recent changes in household circumstances likely to influence travel behaviour, such as pregnancy, childbirth, children starting or moving school, becoming a carer, taking on new responsibilities at work or changes in household income.
The full questionnaires issued in phases 1 and 4, comprising all survey questions, are reproduced in Appendices 1 and 2.
Household travel diary
When participants were contacted again in 2010 and 2011, they were invited to complete a detailed day-by-day travel diary in addition to the core questionnaire. The diary captured the purpose, the start and end times and the locations of each trip, and the mode of transport and duration in minutes of each stage of each trip, of all members of the household, over a 7-day period. The main study participant in each household was asked to complete the diary day by day during the monitoring period with, or on behalf of, the other members of their household. The main purpose was to provide a detailed travel diary for the main participant that could be compared with the contemporaneous objective measures of travel and physical activity behaviour (if collected). The diary also allowed us to explore the inter-relationships of travel behaviour within households, for example between parents and children.
The travel diary is reproduced in Appendix 3.
Basic activity monitoring
The first year of the study presented an opportunity to explore the feasibility of collecting objective physical activity data using accelerometers in a large number of working adults in a free-living, natural experimental setting. In this section, we report our experience in doing this prior to the anticipated opening of the busway, which was originally scheduled for spring 2009 but was progressively rescheduled every few weeks during the early months of the study. 42
As part of recruitment to the study, participants were asked if they would be willing to wear a physical activity monitor for 1 week, and more than 90% of those who expressed an interest in taking part in the study indicated that they were willing to do so. This by far exceeded the number of devices available. We therefore selected a quasi-random sample of 714 willing participants to receive an ActiGraph accelerometer (ActiGraph Corp., Pensacola, FL, USA) in a series of weekly batches of survey packs posted to participants.
The ActiGraph is a small, lightweight accelerometer that provides detailed information about the intensity, frequency and duration of physical activity. It has been extensively validated in both laboratory and free-living conditions. 43,44 Outputs produced from these devices are significantly correlated with estimates of energy expenditure from studies using doubly-labelled water. 45 Monitors were drawn from a pool of ActiGraph GT1M and GT3X models, both set to record activity counts in 5-second epochs in the biaxial mode.
Participants received an accelerometer, an elastic waist belt and an instruction and log sheet in a survey pack that also contained the core questionnaire and consent form. They were asked to wear the accelerometer over the right hip using the belt provided during waking hours for 7 days, removing it for bathing or swimming and logging any such removals. Participants were asked to return their accelerometer and completed questionnaire in person or by (prepaid) postal delivery.
We found that considerable follow-up was required to retrieve accelerometers with sufficient data for analysis. Only 22% of the accelerometers originally issued were returned with sufficient data within 2 weeks, and we sent a total of 694 reminder letters to retrieve the devices. Two rounds of reminder letters or e-mails were sufficient to retrieve 90% of the devices, leaving a comparatively small number of participants requiring more prolonged follow-up. Some accelerometers were returned with insufficient data, for example because participants were on holiday or were unaware that they needed to begin using the accelerometer promptly because of limited battery life. In 109 such cases, we invited the participants to wear the accelerometer again. Learning from experience, we altered our study procedures after the initial batches to include advance e-mails alerting participants to the imminent arrival of their device and including more explicit instructions.
Although we had estimated that we could issue an accelerometer to a new participant every 4 weeks, accounting for posting, wear time and processing of the device and data, delays due to the need to issue reminders as well as device loss or failure or the capture of insufficient data meant that the effective recycling time was twice as long as anticipated. In total, a pool of 221 devices was required to service the 714 participants. We retrieved all but 45 of the devices, while three devices failed. A total of 550 (77%) of the participants returned any accelerometer data, and 486 returned data for at least 10 hours on each of 4 days. Our experience demonstrated that it is feasible to use accelerometers to collect objective physical activity data by post from a large number of participants in a limited time period. However, researchers should plan carefully for the recycling of devices during data collection to reach the target sample size.
For further details, see Yang et al. 42
Enhanced activity monitoring
In the second and subsequent years of the study, all participants who had successfully completed accelerometer monitoring at baseline were invited to opt in to enhanced objective measurement. They were invited to undertake 7 days of monitoring using both Actiheart combined heart rate and movement sensors (CamNtech Ltd, Cambridge, UK) and QStarz BT-Q1000X GPS receivers (QStarz International Co. Ltd, Taipei, Taiwan, Province of China). Those who did not wish to ‘step up’ to this enhanced measurement were given the opportunity to repeat their activity monitoring using the accelerometers used at baseline.
The Actiheart combined monitor is a lightweight, waterproof device. It clips onto standard electrocardiogram (ECG) electrodes on the chest and measures acceleration, heart rate, heart rate variability and ECG amplitude. It has been shown to be a valid and reliable tool for measuring both acceleration and heart rate,46 which we recorded in 60-second epochs in 2010 (using Actiheart devices) and in 15-second epochs in 2011 and 2012 (using Actiheart 4 devices). While providing a form of follow-up accelerometer data, this offered a more accurate objective assessment of physical activity and PAEE than accelerometry alone, particularly for activities such as cycling, which are not optimally detected using hip-worn accelerometers. Individual calibration of the combined heart rate and movement sensor data was not required because a simple calibration protocol based on sleeping heart rate and gender has been shown to be adequate for free-living studies. 47
The QStarz BT-1000X GPS receiver is a small portable device without display that records the spatial co-ordinates of participants at 5-second intervals and has been used in previous studies. 48,49 The device was worn on an elastic waist belt during waking hours, and participants were provided with a charger and asked to recharge the battery each night.
For this part of the study, participants attended one-to-one appointments during which a research assistant fitted the devices and obtained their consent. Participants were asked to wear both devices simultaneously, to complete the travel diary at the end of each day, and to complete the questionnaire at the end of the 7-day measurement period. They returned all questionnaires and devices to the research institute by post.
Sample achieved and follow-up
A total of 1164 individuals participated in baseline data collection between May and October 2009 and thus joined our study cohort, of whom 1143 (72% of the 1582 who met the eligibility criteria for entry to the study) provided valid baseline data on active commuting. The characteristics of the baseline sample are summarised in Table 1. Our sample contained more women than men. Participants’ mean age was 42.3 [standard deviation (SD) 11.4] years. The sample was relatively high in socioeconomic status and educational attainment. Four-fifths of participants lived in areas that rank in the top 50% nationally in terms of income deprivation, almost three-quarters had a university degree or higher educational qualification and 84% defined themselves as working in sedentary occupations. Approximately 10% of participants reported a health condition. Most participants had access to one or more cars in their household. At the same time, the majority of participants had access to a bicycle. Approximately two-thirds of participants lived in urban locations, that is continuous built-up areas with a population of more than 10,000, with one-third living in rural towns and villages (see Derivation of key variables) and a median distance from work of 8.0 km [interquartile range (IQR) 4–20 km] for the sample as a whole.
Characteristic | % (n) |
---|---|
Individual characteristics | |
Gender (n = 1143) | |
Male | 31.5 (360) |
Female | 68.5 (783) |
Age (years) (n = 1143) | |
Under 30 | 16.4 (188) |
30–39 | 28.6 (327) |
40–49 | 25.9 (297) |
50–59 | 21.3 (244) |
Over 60 | 7.6 (87) |
Household characteristics | |
Number of children in the household (n = 1142)a | |
None | 80.0 (913) |
One or more | 20.0 (229) |
Socioeconomic characteristics | |
Highest educational qualification (n = 1136) | |
Less than degree | 28.1 (319) |
Degree or higher | 71.9 (817) |
Home ownership (n = 1139) | |
Not home owner | 27.2 (310) |
Home owner | 72.8 (829) |
Number of cars in household (n = 1143) | |
None | 14.7 (169) |
One or more | 85.3 (974) |
Driving licence (n = 1142) | |
No | 9.6 (110) |
Yes | 90.4 (1032) |
Health characteristics | |
Weight status (n = 1125) | |
Normal or underweight | 62.8 (707) |
Overweight | 27.6 (310) |
Obese | 9.6 (108) |
Difficulty walking (n = 1141) | |
No | 98.6 (1123) |
Yes | 1.5 (18) |
Health condition (n = 1139) | |
No | 89.9 (1024) |
Yes | 10.1 (115) |
Geographic characteristics | |
Home location (n = 1142) | |
Urban | 65.8 (752) |
Non-urban | 34.2 (390) |
Distance from home to work (km) (n = 1142) | |
0–3.0 | 12.4 (142) |
3.1–5.0 | 26.5 (302) |
5.1–10.0 | 19.0 (216) |
> 10.0 | 42.1 (482) |
Members of the cohort were contacted in subsequent years for repeat data collection. Wherever possible, follow-up was matched to the same week of the year as closely as possible in order to eliminate seasonal fluctuations in commuting behaviour. We recruited small numbers of additional participants to the cohort in 2010 (n = 72) and 2011 (n = 178) to partly offset the effect of attrition over time. Some participants also rejoined the study in later phases after dropping out for 1 or more years. The number of participants who completed the core questionnaire and participated in the various in-depth quantitative studies during each phase of the project is summarised in Table 2. In the fourth annual phase of data collection in 2012, after the completion of the intervention, 665 participants completed the core questionnaire, of whom 501 (43% of the baseline sample) had taken part in phase 1 and 470 (40% of the baseline sample) provided valid data on active commuting in phase 1 and phase 4. Further information about the impact of attrition on the composition of the 3-year longitudinal sample is given as part of the account of the main outcome analyses (see Chapter 5, Linking environmental change with travel behaviour change in commuters).
Element of the study | Phase 1 | Phase 2 | Phase 3 | Phase 4 |
---|---|---|---|---|
Core questionnaire | 1168 | 774 | 770 | 665 |
Household travel diary | N/A | 491 | 365 | N/A |
ActiGraph | 501 | 142 | 120 | 73 |
Actiheart | N/A | 201 | 141 | 131 |
GPS | N/A | 196 | 132 | 131 |
Intercept survey
The aims of the intercept survey were to obtain a random and more socioeconomically heterogeneous sample of busway users, which would enable comparisons with the main study cohort and partly offset the volunteer bias in recruitment to the cohort, and to study the perceptions of the busway and its impact on travel behaviour in this sample.
Inclusion criteria
People were eligible for inclusion in this face-to-face survey if they were aged 16 years or over and travelling on the busway, either using a guided bus or walking or cycling on the path.
Recruitment
The intercept survey was conducted in two phases, each lasting 14 consecutive days, in July and September 2012. The survey was carried out at each of five sites along the busway with two interviewers present, one assigned to path users and one assigned to bus users at each site. To ensure recruitment of a quasi-random sample of users, each interviewer was instructed to approach the next adult walking or cycling on the path, or waiting for or alighting from a guided bus, after completing data collection from the previous participant. Users who were unable to complete the survey were provided with a date-stamped, credit-card-sized survey card giving details of how they could later complete a web-based version of the survey. If a user declined to participate in the survey, this was recorded along with details about the size of the group in which they were travelling (if any) and the number of children in the group. When there were no potential users on either the bus or the path, interviewers sampled from the other user group. Data collection commenced at 06.30 daily and ceased at 19.00. Interviewers worked in shifts between these times.
Data collection
The intercept survey questionnaire comprised four sections focusing on the purpose and nature of the trip; the perceived impact of the busway; the participant’s health and physical activity; and his or her demographic and household characteristics. Although shorter than the core questionnaire, the intercept survey questionnaire also contained items to facilitate comparisons in terms of changes in physical activity and travel behaviour with the main cohort (and vice versa). The full intercept survey questionnaire is reproduced in Appendix 4.
Sample achieved and follow-up
We describe the composition of the achieved sample of busway users later (see Chapter 5, Patterns and predictors of busway use). Respondents were not invited to become part of the main study cohort, but some of those who completed the survey and gave consent to be reapproached were subsequently invited to take part in a qualitative interview.
Qualitative investigations
We also explored the commuting contexts, decisions and experiences of participants in a series of qualitative substudies. This entailed more than 120 semistructured interviews, as well as a photo-elicitation study, ethnographic participant observation, media analysis and a qualitative study of the final stakeholder forum for the project.
Semistructured interviews
Our study involved three phases of semistructured interviews. During each phase, we purposively invited participants with a view to achieving balanced samples in terms of age, gender and home location relative to the busway. Interviews were conducted at a place of each participant’s choice, at his or her home or workplace or at the research institute.
In 2009 and 2010, participants in the main study cohort who had reported using different usual modes of transport for commuting were interviewed about their travel to and from work, typical journeys, routes and modes of transport, and time and other factors that shaped their commuting choices and possible alternatives. 50 A total of 49 participants were interviewed in two phases: 19 between March and August 2009 and 30 between March and September 2010.
In 2011, interviews focused on a purposive subsample of commuters who had moved home, work or both (i.e. between different UK postcode sectors, as ascertained in the core questionnaire) between phases 1 and 2 of the study. 51 Interviews took place in spring 2011 – between 1 and 2 years after relocation – and explored participants’ reasons for and experiences of relocating, their priorities in choosing their new location, and how they travelled to and from work before and after relocation. A total of 26 participants were interviewed.
In 2013 we recruited a further group of 38 interviewees, from both the main study cohort and the intercept survey sample. Using information provided in at least two annual core questionnaires, we recruited a mixture of cohort participants who had changed and cohort participants who had maintained their usual mode of travel to work. In addition, we purposively recruited participants from lower social groups from the intercept survey sample. Interviewees were loosely classified into ‘intervention’ and ‘control’ groups, based on their home location relative to the busway (see Chapter 5, Contextualising and interpreting the effects). These post-intervention interviews also explored the perceived impact (if any) of the busway on participants’ travel behaviour. For these interviews we employed two vignettes, systematically constructed using the quantitative predictors of active travel identified elsewhere within the study, to facilitate discussion. One vignette depicted active travel and the use of public transport, while the second focused on car use.
The vignettes are reproduced in Appendix 5.
Photo-elicitation interviews
All 30 participants who were interviewed in 2010 were also invited to take part in additional photo-elicitation interviews, a method we adapted to study commuting behaviour (see Chapter 4, Eliciting motivations and contextual factors). 52 Participants were given minimal directions to take photographs of either their actual commutes or their ‘ideal’ (desired) commutes. The photographs were digitised and treated as data in their own right, but also served as the interview guide in subsequent interviews. Nineteen participants (with three of their children) chose to take part in this project, and we conducted their subsequent photo-elicitation interviews between May and September 2010.
Media analysis
Our evaluation of the intervention included an analysis of discourse about the busway in print and social media. 53 Newspaper articles dating from 21 October 2004 to 21 November 2012 were retrieved from the LexisNexis database (LexisNexis Group, London, UK), which includes national newspapers of various formats as well as local titles. Tweets were identified from the online archive Topsy (www.topsy.com; Topsy Labs, Inc., San Francisco, CA, USA). In addition, the media analysis incorporated data from the semistructured interviews conducted in 2013, as described above.
Participant observation
We also undertook an ethnographic study of people on the busway between September and December 2011, in the first few months after the commencement of guided bus services. 54 An experienced researcher travelled on the busway on weekdays – when most commuting journeys take place – throughout this period, observing and interacting with passengers at bus stops and on the guided buses themselves along the entire route. Sampling was opportunistic in that participants were recruited as they were encountered and found to be amenable to interaction. There was no formal topic guide, but passengers were encouraged to discuss their experience of the guided busway and how it compared with their previous commuting experiences. All observations were recorded in ethnographic field notes.
Ethnographic stakeholder study
The final piece of qualitative research was attached to the forum that was held at the end of the study in January 2015 to discuss the findings and implications of the study with relevant stakeholders. The event was designed around an interactive ‘marketplace’ and open plenary format to encourage knowledge translation and coproduction of insights rather than merely the didactic presentation of ‘results’, and provided the opportunity for extensive ethnographic observations. Field notes recorded during the event provided the background information for follow-up interviews with attendees, which were conducted either in person or by telephone.
Incentives and feedback for participants
Participants were entered into a prize draw to win one of eight £50 gift vouchers as an incentive to participate in each phase of the cohort study and in the intercept survey. The study team produced an annual newsletter to inform participants of the emerging study findings. In addition, those who completed basic activity monitoring were offered brief individualised feedback in the form of a bar chart comparing their recorded daily minutes of moderate to vigorous physical activity (MVPA) with the average for their age group and gender in the sample, and with current physical activity guidelines. Those who completed data collection with combined heart rate and movement sensors were offered more detailed individualised feedback on their activity data.
Derivation of key variables
In this section, we introduce the key variables we used in our quantitative analyses.
Active commuting
The primary outcome for the study was the time participants spent in active commuting, that is the time they spent walking or cycling to and from work each week.
Time spent in active commuting
We used information from the 7-day travel record in the core questionnaire to calculate an estimate of ‘weekly time spent walking to and from work’ and ‘weekly time spent cycling to and from work’ by multiplying the number of occasions on which the respective mode of transport had been used during the past week by the self-reported usual duration of that part of the journey. These were summed to produce an estimate of ‘weekly time spent in active commuting’.
Changes in active commuting
In longitudinal analyses, we investigated changes in active commuting in several ways. Simple change scores in the weekly time spent walking, cycling or in active commuting were computed by subtracting the value for each participant at baseline from the value at follow-up. For some analyses changes were also categorised in terms of an increase or decrease, as compared with no change, and in terms of whether participants ‘maintained’ or ‘took up’ active commuting. If participants reported spending no time walking (or cycling) to and from work at baseline, but reported spending some time doing so at follow-up, this outcome was denoted as their having ‘taken up’ walking’ (or cycling). Similarly, if participants reported spending some time walking or cycling to and from work at baseline and continued to do so at follow-up, this outcome was denoted as their having ‘maintained’ walking (or cycling).
Mode of travel to work
We used information on mode of travel to work from the core questionnaire to summarise and categorise the travel behaviour of our sample in other ways.
Usual mode of travel to work
We determined participants’ usual mode of transport from two sources of information in the core questionnaire. In the RPAQ, most participants indicated that they ‘always’ used a single mode of transport to travel to work in the past 4 weeks, and their ‘usual mode’ was classified accordingly. For those who selected more than one mode of transport, additional rules were established to identify the mode that was presumed to constitute the main part of their trip and to assign them to one of four main modes of transport: walking, cycling, car or public transport. 55 Alternatively, participants’ usual mode of travel to work could be determined using the disaggregated information in the 7-day travel record, in which the modes of transport used on each trip to and from work were reported. Participants were also classified according to their most frequently reported mode (or combination of modes) of transport in the 7-day travel record, using the following overarching categories to differentiate between commuting patterns involving more or less active travel:56
-
car or motorbike only
-
walking only
-
cycling only
-
bus or train in combination with walking or cycling
-
bus or train only
-
car in combination with walking or cycling
-
bus or train and car in combination with walking or cycling
-
bus or train in combination with car.
Changes in mode of travel to work
In longitudinal analyses, we used these data to investigate changes in travel behaviour. In particular, we studied changes in the proportions of commuting trips made entirely by car, involving any active travel and involving any public transport. We also used the categories derived above to study shifts in participants’ most frequently reported mode (or combination of modes) of travel. If participants shifted from ‘car or motorbike only’ to one of the other categories as their most frequently reported mode or combination of modes, this outcome was denoted as their having ‘taken up an alternative to the car’. If participants most frequently reported a mode or combination of modes other than ‘car or motorbike only’ at both time points, this outcome was denoted as their having ‘maintained an alternative to the car’.
Use of the busway
Use of the busway was summarised using three binary variables indicating whether participants had ever used the guided bus service, ever used the path for walking or ever used the path for cycling. These were derived from the responses to two questions in the core questionnaire: ‘Have you ever travelled on a guided bus in Cambridgeshire?’ (response options: ‘yes’ or ‘no’) and ‘Have you ever walked or cycled along any part of the footpath or cycle path beside the guided busway?’ (response options, of which more than one could be selected: ‘yes – I have walked beside the busway’, ‘yes – I have cycled beside the busway,’ or ‘no – I have not walked or cycled along the path beside the busway at all’).
Physical activity
Estimates of time spent in MVPA were derived either from the information in the core questionnaire (‘reported’) or from objective measurement (‘recorded’), while estimates of PAEE were derived from the combined heart rate and movement sensor data.
Reported physical activity
In the RPAQ, participants reported their physical activity at home, at work, for transport and for recreation. Home-based physical activity was estimated using the frequency of stair climbing and the duration of sedentary behaviours such as television viewing on typical weekdays and weekend days. Physical activity at work was estimated using a categorical measure (from ‘sedentary occupation’ to ‘heavy manual work’) and the number of hours worked per week. Physical activity for transport was estimated using information on the frequency with which different modes of travel were used for commuting (reported on an ordinal scale from ‘never or rarely’ to ‘always’), the number of trips per week and the self-reported commute distance. We converted measures of activity for each domain to a common weekly metric and used this information to derive three outcome measures. Total weekly time spent walking (or cycling) for commuting and recreation was derived by summing the time spent walking (or cycling) for recreation (reported in RPAQ) and the time spent walking (or cycling) for commuting derived from the 7-day travel record. Total weekly time spent in recreational MVPA was derived by summing the time spent in all recreational activities reported in RPAQ and associated with a metabolic equivalent (MET) of at least 3 METs according to the physical activity compendium, where 1 MET represents the basal metabolic rate and 3 METs represents the threshold used to define moderate intensity physical activity. 57 Total (‘reported’) weekly time in MVPA was derived by summing the time spent in activities classified above the MVPA threshold across the four domains. 34
Recorded physical activity
We defined ‘recorded’ time spent in MVPA as the average number of minutes per day in which ≥ 1952 accelerations were counted by the hip-worn accelerometers. This corresponds to the physiological intensity cut-point of 3 METs during treadmill locomotion. 58
Physical activity energy expenditure
We used combined heart rate and movement data in conjunction with GPS data and summarised PAEE according to the mode or combinations of modes of transport reported in the travel diary for each trip. We later report how we also interrogated the combined heart rate and movement sensor and GPS data for other purposes (see Chapter 3, Validating a self-reported measure of time spent in active commuting).
We visually inspected the GPS data in the geographic information system (GIS) ArcGIS (Esri, Redlands, CA, USA) to identify the start and end times for each trip to or from work. The start time was defined as the first 5-second epoch after which participants left either their home or the outline of their workplace building, and the end time as the last 5-second epoch before they reached the corresponding destination. We summarised the combined heart rate and movement data in 1-minute epochs and annotated each trace with the trip start and end times ascertained from the GPS data. As trips did not always begin at the start of a ‘clock minute’ (e.g. precisely at 10:00:00), the first and last clock minutes of each trip were excluded to avoid misclassifying non-commuting energy expenditure as part of the trip. For example, in a trip starting at 10:00:30, the first 30 seconds of the minute from 10:00 to 10:01 would not be part of the commuting trip but would still be included in the calculation of energy expenditure for that clock minute. Excluding the first and last clock minute therefore ensured that all energy expenditure data included in our calculations were drawn from the trip itself. We also identified whether participants had travelled to or from work via an intermediate destination such as a school or shop, and excluded any time spent at such intermediate destinations from our computation. PAEE was derived following a published method and translated to standard METs for each minute. 46
Carbon emissions
We also investigated the impact of the busway on changes in carbon dioxide emissions attributable to commuting in our cohort between 2009 and 2012. We estimated the carbon emissions for each commuting trip reported by each participant and summed these across the week to produce a total for each participant at each time point. Carbon emissions were estimated by multiplying the distance travelled by each mode of transport by a published emissions factor specific to each mode of transport, and specific to each vehicle where possible. 59
The distance travelled on each trip to and from work was estimated using the Google Directions API (application programming interface) (Google™, Google Inc., Mountain View, CA, USA) with the origin and destination of the trip. These were derived from unit postcodes but shifted by 1 minute of longitude or latitude to prevent the release of potentially identifiable pairs of information (i.e. linked home and workplace locations) to Google. Distance calculations took into account the mode or modes of transport used. For participants who reported a park-and-ride commute, for example, the trip was divided into two parts, and the Google option for mode of transport was set to ‘driving’ for the first part of the trip and ‘transit’ (i.e. public transport) for the second.
For guided bus trips, we used the emission factor for trams (87.61 gCO2/km), on the basis that the environmental performance of a guided bus is broadly comparable with that of light rail, and that if the greater flexibility for timetable optimisation is taken into account a guided bus system can match the carbon emissions of trams. 60 For bus trips, we also estimated the speed for each trip based on the distance travelled, time travelled and number of stops. Trips with an average speed of > 50 miles per hour were defined as ‘coach’ trips, and others as ‘local bus’ trips, and emission factors were assigned accordingly. For car travel, we drew on the information from our core questionnaire to distinguish between fuel type (petrol, diesel or hybrid) and engine size (< 1.4 l, 1.4–2.0 l or > 2.0 l) of cars used at each time point to apply the appropriate emission factors.
Indicators of health and well-being
Physical and mental well-being
Responses to the SF-8 were used to create physical component summary (PCS-8) and mental component summary (MCS-8) scores, as well as change scores in these measures representing the difference between the values at baseline and follow-up for each participant. 41
Sickness absence
Sickness absence was self-reported as the total number of days absent from work in the past year.
Body mass index
Body mass index (BMI) was calculated by dividing self-reported weight in kilograms by the square of the self-reported height in metres. If reported height differed between years, baseline height measures were used to replace implausible follow-up values attributable to obvious data entry errors in five cases. In two participants, large computed changes in BMI (of ≥ 25 units) were found to be the result of their having reported weight in imperial units in the metric response option on the questionnaire. These were converted to metric values, which were confirmed to be similar to their baseline weights. For some analyses, BMI was summarised using categories of weight status according to international cut-offs. 61
Exposure to the intervention
Evaluation studies, especially in health research, are often carried out by comparing outcomes in intervention and control groups, preferably created by the random allocation of individuals or groups to the treatment conditions. In evaluations of place-based interventions such as new transport infrastructure, however, randomisation is generally not possible and it is not necessarily meaningful to treat exposure to the intervention as a binary variable. In the light of this, a number of approaches for modelling ‘exposure’ in quasi-experimental studies of place-based interventions have been developed. Exposure may be modelled in terms of coincidence or adjacency, for example by denoting a particular administrative area or a buffer zone the size of a ‘walkable’ area around the intervention. Alternatively, exposure may be modelled in terms of varying intensities, either ordinal or continuous, derived from the proximity of a given participant’s location to the intervention. Measures of exposure may be further refined by using additional information on the characteristics of individual participants, such as their travel patterns.
Exploratory analysis of the characteristics of our cohort showed that comparable intervention and control groups could not be created based on area of residence. For example, the distribution of participants who reported incorporating walking or cycling in their commute at baseline appeared neither random nor associated in any singular way with the planned route of the busway. Participants living closer to the planned route were more likely to report cycling for commuting, but less likely to report walking for commuting, than those living further away (Figure 2). This can be explained in part by the fact that distance from the busway was correlated with distance from the city centre and that local commuting patterns were inevitably reflected in our achieved sample (see Chapter 4, Patterns of active commuting). For example, the tendency of commuters to combine walking with using a car or public transport meant that reporting some walking for commuting remained relatively common even among those living furthest from Cambridge, while the prevalence of cycling for commuting declined sharply at distances > 10 km. 62 Similar location effects could be seen in other characteristics of our sample, for example in the tendency for younger and more mobile participants to live closer to the planned route of the busway.
FIGURE 2.
Distribution of active commuting at baseline by proximity to the route of the busway. Proportion of participants within each distance interval reporting any walking or cycling to and from work at baseline (for walking, n = 456; for cycling, n = 468).
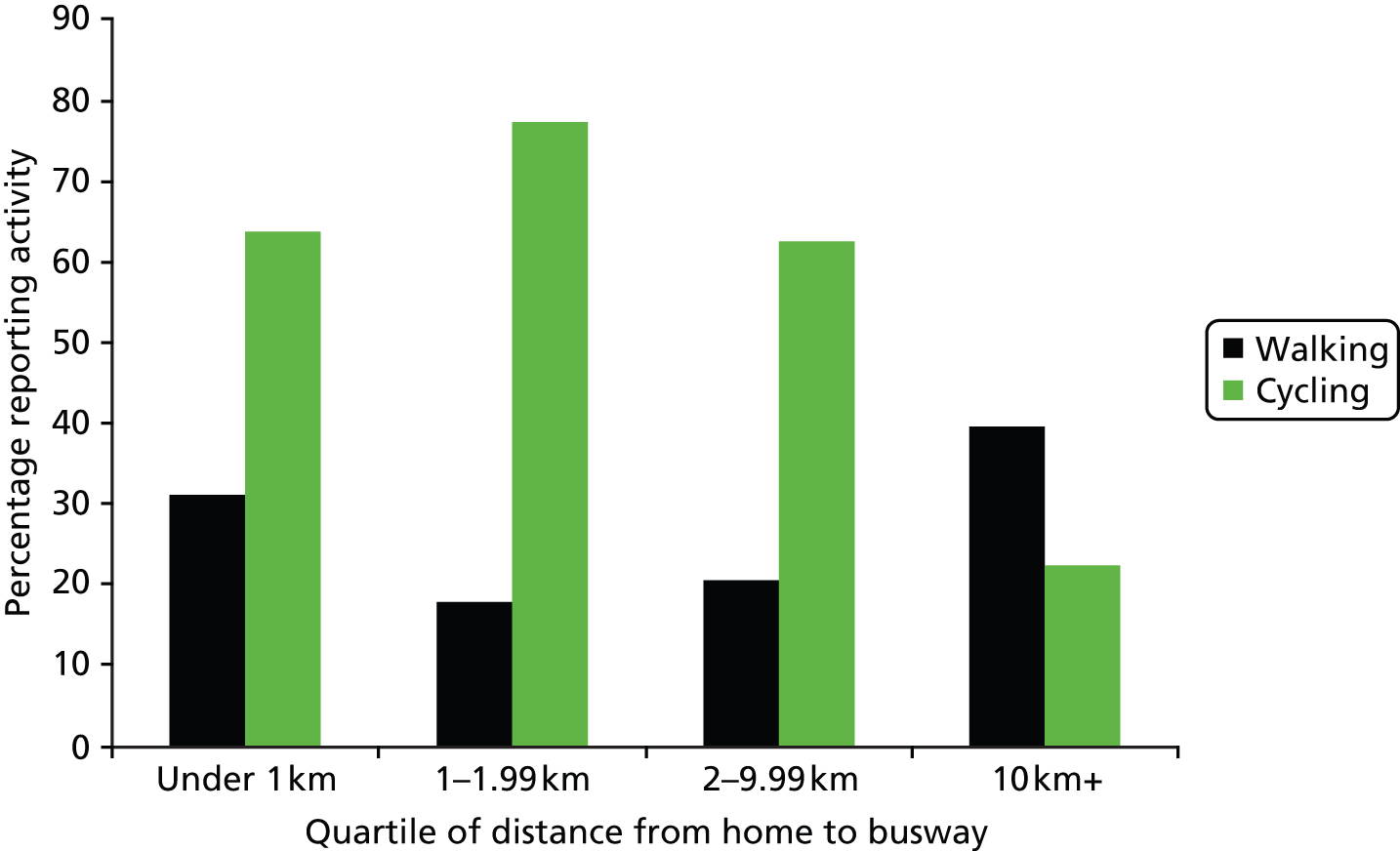
Rather than assigning participants to simple ‘intervention’ and ‘control’ areas, which would have been unbalanced on the baseline distribution of active commuting and other socioeconomic covariates, we therefore computed graded, participant-level measures of intervention exposure. Our graded measure of exposure was based on the distance from each participant’s home to the nearest access point to the busway. We calculated this distance using route networks created in the GIS (see Derivation of key variables).
In studying the predictors of using the busway for a given mode of transport (bus, walking or cycling) the graded measure of exposure was adapted to take account of the mode of transport in question (see Chapter 5, Patterns and predictors of busway use). For the outcome of guided bus use, we computed the distance from each participant’s home to the nearest busway stop using any combination of the road and pedestrian and cyclist route networks (including the path along the busway). This reflected the fact that, in order to travel on a guided bus, participants would first have to travel to a bus stop using either a car or an active mode of transport, and in the latter case could use the path along the busway to do so. For the outcomes of walking or cycling on the path, we computed the distance from each participant’s home to the nearest access point to the path, using the same route networks.
In studying the effect of the busway on time spent in active commuting and physical activity, we hypothesised that the busway could promote walking and cycling either as modes of travel along the path or as feeder modes to the guided bus service (see Chapter 5, Linking environmental change with travel behaviour change in commuters). We therefore used the distance from each participant’s home to either the nearest busway stop or the nearest access point to the path, whichever was closer.
Exploratory analysis further showed that a given increment in distance had a smaller effect on use of the busway as distance increased, which suggested that it would be inappropriate to model exposure to the intervention as a linear function of distance. Although the log transformation is commonly used in studies of distance decay in transport studies, we selected a square root transformation because the estimates produced were slightly more conservative and more easily interpretable. For ease of interpretation, we defined exposure to the intervention in terms of ‘proximity’ rather than distance, proximity being defined as the negative square root of the distance. For example, for a pair of participants living 9 km and 4 km, respectively, from the busway, the measures of proximity were –2 and –1, respectively. Because –1 is larger than –2, this enabled us to report the effects of ‘increasing proximity’ to the busway.
In studying the predictors of using the busway for a given mode of transport, we also explored the application of more detailed participant-level measures of intervention exposure (see Chapter 5, Predictors of busway use). We hypothesised that individuals would be more ‘exposed’ to the intervention if the new infrastructure were to reduce the time taken to travel to work. This reduction in travel time could result from a reduction in either the bus journey time, or the walking or cycling distance from home to work, and would vary according to the work location as well as the home location of the participant. For example, a participant living close to the busway and working at a location close to the route might experience a reduction in the time it would take to walk or cycle to work, whereas a neighbour living next door but working at a location further from the busway might not benefit to the same extent. These ‘individually calibrated’ measures of intervention exposure were calculated using each participant’s home and workplace addresses. We computed changes in estimated travel time to work by bus using Accession, a software package that uses road networks, road speed data and public transport timetable information to calculate travel times (Citilabs Inc., Lafayette, CA, USA). We used this to estimate the quickest potential bus journey for each participant on the networks that existed before and after the completion of the busway on a Wednesday, with the requirement that the journey should commence after 06.00 and end before 10.00 to correspond to a typical morning commute. The change in the modelled walking or cycling distance to work induced by the busway was calculated using the GIS by comparing the distances in the pedestrian and cyclist route network before and after addition of the path along the busway.
Other environmental variables
We also studied the influence of subjective and objective factors on active commuting. Our core questionnaire contained questions on participants’ perceptions of the environment on their route to work. We also used the GIS to derive variables corresponding to objective features of the environment.
Perceptions of the route environment
Our core questionnaire included seven items on participants’ perceptions of the environment with respect to their journey to and from work (Box 1). Participants reported their level of agreement with each statement on a 5-point Likert scale. When responses to these items were entered into regression analyses individually, responses were collapsed such that those who ‘strongly agreed’ or ‘agreed’ with an item were compared with those who ‘strongly disagreed’, ‘disagreed’ or ‘neither disagreed or agreed’ (and vice versa for negatively worded items). We also used responses to all seven items to generate a total score representing the overall perceived supportiveness of the route environment for active commuting and summarised this using tertiles. In longitudinal analyses, we created change scores for each item by subtracting the baseline value from the follow-up value for each participant.
It is pleasant to walk.
There is convenient public transport.
There are convenient routes for cycling.
There is little traffic.
It is safe to cross the road.
The roads are dangerous for cyclists.
There are no convenient routes for walking.
Objective features of the environment
We further used the GIS to derive variables corresponding to objective features of the environment, such as the urban–rural context of participants’ home location, the distance to work specific to a given mode of transport, and other attributes of the route environment between home and work.
Home and workplace postcodes reported by participants were georeferenced to a location using the Ordnance Survey CodePoint® database (Ordnance Survey, Southampton, UK). Each participant’s home location was also categorised according to an urban–rural classification using the Census Output Area of the home postcode. 63 According to this classification, ‘urban’ areas are connected built-up areas that have resident populations of more than 10,000 people. ‘Rural’ areas are those that are not urban, and are further divided into ‘town and fringe’ areas and ‘villages’ or ‘hamlets’ and ‘isolated dwellings’ based on dwelling densities. In our results we refer to town and fringe areas as ‘towns’ and to villages or hamlets and isolated dwellings as ‘villages’.
We created route networks for travel by car or by walking and cycling. These were used to calculate distances specific to a given mode of transport, for example between home and work or for accessing the busway from a participant’s home (see Derivation of key variables). The car route network was created using the Ordnance Survey MasterMap® Integrated Transport Network road network data set (Ordnance Survey, Southampton, UK), from which the guided busway (which cannot be used by cars) was manually removed. 64 A pedestrian and cyclist route network was created by removing motorways from the car network and by adding footpaths and cycle routes from local authority data on rights-of-way (public footpaths, bridleways and byways), cycle route information from the UK charity Sustrans, and any additional paths from OpenStreetMap.com, a collaborative, open-source database of informal pathways (OpenStreetMap Foundation, Sutton Coldfield, UK). 65
We also derived a series of variables reflecting objective features of the environment in the area around the home, in the work neighbourhood and on the route between home and work using the GIS in conjunction with the Ordnance Survey Address Layer 2® database (Ordnance Survey, Southampton, UK). For this analysis, the GIS was used to identify sections of the pedestrian and cyclist route network that constituted the shortest route between home and work for each participant.
The variables derived included the length of the shortest possible route between each participant’s home and work locations, a categorical indicator of the direction of travel based on the locations of home (either in Cambridge or in the surrounding areas) and workplace (either in the city or on the outskirts) and the proportion of the route that followed ‘A’ or ‘B’ (‘busy’) roads. In addition, measures of the ‘walkability’ of the streets in the home neighbourhood were calculated, including road density, junction density, road connectivity, the presence of ‘A’ (major) roads and the proportion of foot or cycle paths.
We further derived public transport variables using the pedestrian and cyclist route network in combination with the National Public Transport Data Repository and Access Nodes data sets. 66,67 The first variable reflected whether or not there was a bus service passing through the home neighbourhood that would take the participant to work, either directly or with one or more changes. The other public transport variables, calculated for both home and work locations, reflected the distance to the nearest bus stop (again using the pedestrian route network), bus service frequency, the number of bus stops, the number of bus routes served and the distance to the nearest railway station.
Land use variables included a count of the number of destinations of interest (schools, eating and drinking establishments, stage and screen venues, sports complexes and retail units) within 100 m of the route to work and in the home and work neighbourhoods,68 and indicators of land use mix and building density at the home location and along the route to work.
For further details, including more information about the sourcing of environmental data used in these analyses, see Dalton et al. 55
Psychological measures
Theory of Planned Behaviour constructs
Our core questionnaire included eight statements of attitudes and beliefs relating to car use, corresponding to four constructs of the Theory of Planned Behaviour hypothesised to mediate choice of mode of transport (Table 3). As it was considered unreasonable to ask participants to repeat these measures in respect of all modes of transport, we decided to frame them in relation to car use because the overarching aim of the intervention was to promote the use of alternatives to the car. Participants reported their agreement with each statement using a 5-point Likert scale. Mean scores for each construct (pair of statements) were calculated, and classified either in tertiles or as above or below the median (analysis of incorporating walking or cycling into car journeys to and from work), and for longitudinal analysis, change scores for each construct were created by subtracting the baseline value from the follow-up value for each participant.
Construct | Questionnaire item |
---|---|
Affective attitude | Overall, it would be good to use a car |
It would be pleasant to use a car | |
Subjective norm | Most people who are important to me would support my using a car |
Most people who are important to me think I should use a car | |
Perceived behavioural control | It would be easy for me to use a car |
I would be able to use a car | |
Intention | I intend to use a car |
I am likely to use a car |
Habit strength
We derived a summary score for habit strength using five items from the Self-Report Index of Habit Strength applied to car use. 39 These items referred to the behaviour being automatic, requiring effort not to do, belonging to a daily routine, being something the participant found it hard not to do and being ‘typically me’. We excluded other items representing the frequency and history of past behaviour because, in the context of a daily activity such as commuting, these could have artificially strengthened any apparent associations between habit strength and behavioural outcomes. The distribution of our summary habit score was skewed, with 40% of participants reporting a mean score of 1 (the lowest possible score). A binary summary variable was therefore created to distinguish those with a mean score of 1 from those with a mean score > 1.
Overall approach to analysis
Sample size estimation
In our original study design we aimed to achieve a sample of 788 participants at 1-year follow-up, which would have provided 80% power to detect a standardised mean difference between intervention and control groups of 0.2, equivalent to a mean increase of 2 minutes per day in our primary outcome of time spent in active commuting. 32 The study was not designed to have comparable statistical power to detect changes in the secondary outcomes. The delayed completion of the busway resulted in an enforced follow-up period of 3 years rather than 1 year and a concomitant reduction in the final sample size for analysis. This smaller achieved sample would have provided 80% power to detect a mean difference of 4 minutes per day, which, although larger than the original target effect size, is still commensurate with the effect sizes observed for comparable interventions in previous studies. 27,69 In the event, the distribution of the primary outcome data precluded the use of a continuous outcome measure to model the effect of the intervention (see Chapter 5, Linking environmental change with travel behaviour change in commuters).
Quantitative analysis
Many different quantitative analyses are summarised in this report. Further details of specific analyses can be found in subsequent chapters and in the relevant publications, but our general approach was to use multivariable regression modelling to estimate adjusted associations between dependent (‘outcome’) and independent (‘exposure’) variables. Most analyses involved the use of linear, logistic or multinomial regression to model continuous, binary and categorical outcomes, respectively. We built up these models in stages by progressively adjusting them for various sets of individual, household, geographic and other covariates that were hypothesised to be potential confounders of the relationships of interest. We generally selected such covariates to be taken forward into multivariable models if they met the generous statistical criterion of being associated with the dependent variable at p < 0.25 in univariable analysis. We stratified some models by prespecified effect modifiers, for example by gender in the analysis of the correlates of physical activity and by baseline commuting behaviour in the main outcome analyses. We mostly did not impute missing data for covariates, and we never imputed missing data for behavioural or health outcome variables.
Qualitative analysis
The qualitative substudies produced a range of qualitative data, most notably interview transcripts but also participant-produced photos, ethnographic field notes, and documents (newspaper articles and tweets). All qualitative data were analysed using variations of thematic content analysis in which data were categorised and synthesised into emerging themes. The initial coding was usually carried out by the researcher who collected the data in the context of a particular substudy, with subsequent refinement and interpretation in collaboration with other members of the research team. Various theoretical frameworks informed particular analyses, for example practice theory and normalisation process theory from the fields of medical sociology and anthropology, respectively (see Chapter 4, Active commuting in context, and Chapter 5, Understanding the intervention).
Ethical approval
Ethical approval for the different components of this study is summarised in Table 4.
Element of study | Year of data collection | Body granting ethical approval | |
---|---|---|---|
Hertfordshire Research Ethics Committee | Cambridge Psychology Research Ethics Committee | ||
Phase 1 cohort study | 2009 | 08/H0311/208 | |
Phase 2 cohort study | 2010 | 09/H0311/116 | |
Phase 3 cohort study | 2011 | 10/H0311/65 | |
Participant observation study | 2011 | 2011.50 | |
Phase 4 cohort study | 2012 | 2012.14 | |
Intercept survey | 2012 | 2012.31 | |
Stakeholder engagement study | 2014–15 | 2014.97 |
Chapter 3 Methodological investigation and development
Introduction
Methodological challenges exist in relation to the accurate assessment of commuting and physical activity behaviours and characterising their social and physical context. In this chapter we illustrate the ways in which we addressed these challenges in this study. We first report the validation of the primary outcome measure used in the study, a self-reported measure of time spent walking and cycling for commuting. This required the development of new methods to provide an objective estimate with which our self-reported estimates could be compared. Following this, we summarise a series of papers using different methods to assess overall physical activity and the distance from home to work. Finally, we report how we adapted a method of qualitative investigation to obtain more insight into the individual and social dimensions of commuting behaviour.
Validating a self-reported measure of time spent in active commuting
Rationale
Accurate measurement of active commuting and associated physical activity in free-living conditions is important for describing patterns in these behaviours and studying their determinants in populations. It is also essential if we are to have confidence in the effect sizes from intervention studies and for the attribution of subsequent health impacts. A validated, short, self-reported measure of time spent walking or cycling for transport was not available when data collection for our study began in 2009. Many studies have used comparatively simple measures of travel behaviour. These have often focused on either the main mode of transport, that is the mode used for the longest part of a trip (if more than one mode is used), or on the usual mode of transport, that is the mode used most frequently over a specified period of time. Neither approach is entirely satisfactory for estimating the physical activity associated with commuting because each entails simplifying the detail and complexity of travel behaviour. Focusing on the main mode of transport means that active travel may be underascertained, because actual commuting journeys, especially those involving public transport, often involve more than one mode of transport (‘multimodal’ trips) and may involve walking or cycling at either end of the trip. Focusing on the usual mode of transport, on the other hand, may fail to capture day-to-day variation in the mode, distance or duration of trips. Although some validation of the modes and frequency of trips recorded in detailed day-by-day travel diaries has been reported, it remains important to validate measures of time spent in active commuting because this information can be used to quantify the health impacts of commuting. 70
The selection of a ‘gold standard’ method for validating self-reported measures in this field is challenging. Direct observation of participants, as well as other methods such as wearable cameras, have been used in other, smaller studies, but it is unlikely that these would have been acceptable to participants or practical to implement in a study of our size. 71 Likewise, the identification of walking and cycling from the data captured by physical activity monitors is not sufficiently advanced to enable the time spent in active travel to be confidently determined. 72 A similar problem exists with the use of GPS data alone. These have been used to infer mode of transport from either the maximum or the average speed recorded in a trip, whereas in our study many participants commuted into city centre locations at low average speeds, often using a combination of modes of transport, which would have increased the risk of misclassification. 73,74
We therefore developed a new method for obtaining objective estimates of the time spent using active and motorised modes of transport, by combining reported data on mode of transport with georeferenced combined heart rate and movement sensor data in a subsample of our study cohort. We then used these objective estimates to assess the convergent validity of self-reported estimates of time spent in active commuting derived from our core questionnaire data. 75
Methods
In order to generate objective estimates of time spent using active and motorised modes of travel, and total commuting travel time, it was first necessary to process the GPS data to identify commuting trips and stages within trips. Because the processing and cleaning of trip-level GPS data is a labour-intensive task, we selected a quasi-random sample of commuters from the larger subsample who provided combined heart rate and movement sensor and GPS data, as well as information about their commuting trips in the past week in both the travel diary and the core questionnaire, in either 2010 or 2011. We aimed to include at least 50 commuting trips for each of six combinations of mode of transport found in the cohort study: (1) walking, (2) cycling, (3) car or motorcycle only, (4) car in combination with walking, (5) car in combination with cycling and (6) bus with or without walking or cycling (see Chapter 4, Patterns of active commuting). Because relatively few participants had recorded walking all the way to or from work, reflecting our recruitment strategy and local commuting patterns, we achieved only 42 such trips for analysis.
We used the GIS to project the GPS data onto mapping information and to locate the home and workplace of each participant. We then inspected the GPS tracks to obtain an objective estimate of the total duration of each commuting trip by calculating the difference between the time at which participants left home or entered the building outline of their workplace. If the trip involved only one mode of transport (a ‘unimodal’ trip), it was added to the data set for analysis at this stage.
If a trip involved a combination of active and motorised travel, we needed to obtain objective estimates of the duration of each stage of the trip. For trips that combined walking or cycling with car travel, we were able to identify the time at which an active stage of the trip began from the combined heart rate and movement sensor data alone, but this proved impossible for trips that involved walking or cycling in combination with bus travel. We therefore cross-referenced the GPS data with the locations of bus stops, which enabled us to more clearly identify the start and end times of the bus stages of trips. Waiting times at bus stops before or after boarding were identified by GPS points with non-regular speeds in close geographic proximity. These waiting periods were excluded from analysis, along with any periods of longer than 5 minutes spent at a location en route (such as a school or shop) if no change in mode of transport was reported.
Once the different stages of commuting trips had been identified, we calculated our objective estimates of time spent using active and motorised modes of travel, as well as the total duration of the commuting trip. We then carried out three comparisons to assess the convergent validity of our self-reported measures of active commuting:
-
We compared estimates of the duration of commuting trips, and stages of trips, spent using active and motorised modes of transport, as well as total trip duration, all derived from the detailed travel diary, with the corresponding objective estimates.
-
We compared estimates of usual time spent using active modes of transport derived from the core questionnaire with the corresponding objective estimates.
-
We compared the two self-reported estimates, namely the estimates of usual time spent using active modes of transport derived from the core questionnaire with those derived from the detailed travel diary.
If data were of insufficient quality to allow modal transitions and destinations en route to be confidently identified, trips were included only in the analysis of total trip duration and excluded from the analyses of time spent using active or motorised modes.
Results
We carried out these comparisons separately for commuting trips that involved different combinations of modes of transport. In the first comparison these showed that in the travel diary, participants overestimated the time they spent walking or cycling to work by 1.13 minutes per trip on average [95% limit of agreement (LOA) −7.67 to 9.95; p = 0.001]. The median duration of these trips was 38 minutes. The mean overestimation of active travel time was slightly larger in the subset of trips made by walking or cycling alone (2.39 minutes per trip, 95% LOA −6.78 to 11.55), even though these trips tended to be shorter, with a median duration of 17 minutes. However, the difference between objective and self-reported estimates in this subset of trips was not significant (p = 0.25). For the subset of trips involving active travel in combination with any motorised mode of transport, the magnitude of the difference was smaller (mean +0.40 minutes per trip) but statistically significant (p = 0.001) (Table 5).
Quantity estimated | Number of trips | Mean difference (SD) | 95% LOA | p-value |
---|---|---|---|---|
Time spent in active travel for trip or stagea | ||||
All active trips | 136 | 1.13 (4.49) | –7.67 to 9.95 | ** |
Walking or cycling only | 50 | 2.39 (4.68) | –6.78 to 11.55 | NS |
Walking or cycling in combination with other modes | 86 | 0.40 (4.23) | –7.90 to 8.69 | ** |
Time spent in motorised travel for trip or stagea | ||||
All motorised trips | 139 | 10.48 (12.30) | –13.62 to 34.58 | *** |
Car only | 40 | 0.56 (6.04) | –11.27 to 12.40 | *** |
Car or bus in combination with other modes | 99 | 14.49 (11.91) | –8.86 to 37.84 | * |
Total trip duration | ||||
All trips | 204 | 1.85 (8.75) | –15.31 to 19.01 | *** |
Single mode used | 90 | 1.40 (6.05) | –10.46 to 13.26 | *** |
More than one mode used | 114 | 2.21 (10.42) | –18.21 to 22.62 | *** |
As we discuss in more detail below (see Interpretation), this suggests that participants are able to provide acceptably accurate estimates of their active travel time for trips that also involve other modes of transport in a travel diary. In contrast, we found that participants’ estimates of time spent using motorised modes of travel were subject to a substantially larger overestimation than were their estimates of active travel time, with an average overestimation of 10.48 minutes per trip (95% LOA −13.62 to 34.58). Trips involving motorised modes in combination with active modes appear to be responsible for this finding (mean difference 14.49 minutes per trip, 95% LOA −8.86 to 37.84; median total trip duration 45 minutes).
When we compared estimates of usual active commuting time derived from the core questionnaire with the corresponding objective estimates, we found differences of a similar magnitude to those observed in the travel diary comparisons. The difference was smaller for cycling, with an average underestimation of 1.12 minutes per trip compared with a median reported usual time of 17 minutes (95% LOA –8.67 to 6.44, n = 11 participants), than for walking, with an average overestimation of 2.37 minutes per trip compared with a median reported usual time of 13 minutes (95% LOA –10.91 to 15.64, n = 16 participants); neither difference was statistically significant (p > 0.35). The questionnaire and objective measures showed stronger agreement for time spent cycling (with a concordance coefficient of 0.96) than for walking (with a concordance coefficient of 0.84).
In our third comparison, we found that the questionnaire estimates of usual active travel time were not significantly different from the mean duration derived from the trips reported in the travel diary (p > 0.1), with an average difference of less than 1 minute per trip for both cycling and walking.
Interpretation
When we compared estimates of active and total travel time from day-by-day travel diaries and from retrospective questionnaires to objective estimates, both self-reported measures performed acceptably well for our purposes. The mean differences between self-reported and objective estimates were relatively small, both for the detailed travel diary (an overestimation of approximately 1 minute per trip) and for the retrospective travel record. These findings are similar to those of previous validation studies. 71 The results of the travel diary comparison further suggest that where trips involved a combination of active and motorised modes of transport, participants tended to report the duration of the active stages more accurately than the duration of the motorised stages. The small mean overestimation in the self-reported measures indicates that such methods can provide a reasonably accurate estimate of aggregate active commuting time in a population. Nevertheless, the wide LOAs indicate a risk of substantial over- or underestimation at an individual level, which means that self-reported measures should be used with caution to infer aggregate weekly quantities of physical activity as part of commuting. For example, a 2-minute overestimation per trip corresponds to a 20-minute difference over a 5-day working week. The effect sizes of the most promising interventions to promote walking for transport are of the order of 15–30 minutes per week, which is directly comparable with the size of the measurement error suggested by our analysis. 27 This suggests that evaluations of interventions that are anticipated to have relatively modest effect sizes may have limited power to detect effects on time spent in active commuting if they rely on self-reported measures alone, because the potentially large measurement error may mask small but important real changes in behaviour.
For further details, see Panter et al. 75
Comparing methods for measuring physical activity
Rationale
Although the measurement of physical activity is an area of investigation in its own right and our study was not designed to compare different measures of physical activity, we explored the possible consequences of using different measures of physical activity in two papers.
In the first paper, we investigated which of a range of sociodemographic, health and geographic characteristics were associated with either self-reported (‘reported’) or accelerometer-measured (‘recorded’) estimates of daily time spent in MVPA. 76 We did this because our knowledge about the extent to which the correlates of physical activity vary according to the approach to measurement is limited. 77 If different measures were to identify different correlates, this would in turn suggest different determinants of change in physical activity and the possibility that different intervention effects might be detected with different measurement methods. In the second paper, we set out to understand the characteristics of those whose physical activity level was ranked differently according to the approach to measurement. 78 This is important because such mismatches may complicate the assessment of the effects of interventions.
Methods
Both papers used baseline cohort data from 2009. In the first paper, ‘recorded’ daily time in MVPA was derived as previously described (see Chapter 2, Derivation of key variables). 76 In the second paper, we processed the accelerometer data to obtain average daily accelerometer counts per minute for each participant. 78 Participants were further assigned to one of three groups according to whether their average level of physical activity was (1) ranked equally (i.e. categorised in the same quartile) by self-report and accelerometer, (2) ranked higher (i.e. categorised in a higher quartile) by self-report than by accelerometer, or (3) ranked higher by accelerometer than by self-report. For both papers, participants needed to provide accelerometer data on at least 3 days to be included in analysis, and the analysis was carried out separately for men and women because previous research suggests that the correlates of physical activity differ by gender. Multiple regression models of recorded daily time in MVPA were adjusted for accelerometer wear time, because participants who wore the accelerometer for longer periods tended to accumulate more minutes of physical activity.
Results
The first paper showed that different correlates were associated with ‘reported’ and ‘recorded’ daily time spent in MVPA, and few variables were associated with both measures in either men or women. 76
In men, four individual characteristics were significantly associated with reported MVPA. Men who had a standing or manual occupation as opposed to a sedentary occupation, who did not have at least degree-level education, who had access to a bicycle and who reported a mental component summary score above the lowest quartile reported substantially more MVPA. Different variables were significantly associated with men’s recorded MVPA. Men who lived with additional adults in the same household, or in areas with higher proportions of greenspace, achieved lower levels of recorded MVPA.
In women, three variables were significantly associated with reported MVPA. Women with access to a bicycle and with degree-level education reported more time spent in MVPA per day, while women in households with at least one child reported less. In contrast, one variable – having at least degree-level education – was associated with higher recorded MVPA. Both having access to a car and being overweight or obese were associated with lower recorded MVPA. Although the directions of associations tended to be consistent across both measures for variables such as age, access to a bicycle and weight status, especially for women, not all of these associations were statistically significant.
In the second paper, we found that approximately one-third of participants were ranked in the same quartile with both physical activity measures, while one-third were ranked lower, and one-third ranked higher, on one measure than the other. 78 In adjusted analyses, the physical activity of overweight or obese individuals was less likely to be ranked in a higher quartile by an accelerometer than that of normal-weight individuals [odds ratio (OR) 0.54, 95% confidence interval (CI) 0.32 to 0.92] and twice as likely as that of normal-weight individuals to be ranked in a higher quartile by self-report (OR 2.07, 95% CI 1.28 to 3.34). These overall results were replicated in the separate analysis for women, but not in that for men. This suggests that individual characteristics such as gender and weight status may be associated with mismatches in estimates of physical activity between different measures.
Both analyses included a smaller number of men than women, which may have further reduced the power to detect associations in this group. In addition, the estimates of reported and recorded MVPA used in these analyses were neither synchronous nor of matching duration, in that ‘recorded’ activity was measured over 7 days, whereas ‘reported’ activity was captured in terms of weekly frequency and average duration over the past 4 weeks and transformed to daily MVPA. However, it is unlikely that levels of activity differed systematically between these two time periods.
Interpretation
The results from these two papers illustrate some common themes. The first paper indicated that few of the sociodemographic characteristics investigated were associated with both recorded and reported measures of daily time spent in MVPA, and the second paper showed that it was common for people’s activity to be ranked differently according to the measurement method. These findings probably reflect differences in the way activity is ascertained by questionnaires and activity monitors. Activity of ‘moderate to vigorous’ intensity covers a very wide range of behaviours, such as cycling, swimming and team sports, which are undertaken in a variety of settings and may be differentially related to sociodemographic, health and contextual characteristics. Questionnaires collect information on physical activity behaviours, while devices measure physical movement. Although our experience, as well as that of others, suggests that pedometers or accelerometers capture walking adequately for most purposes, other devices or, indeed, self-reported measures may be required to capture a wider range of activities. At the same time, the different specificities of measurement techniques need to be weighed against the practical implications of using them in different situations. In some cases, it may be helpful to use a combination of reported and recorded measures to establish a more accurate and comprehensive assessment of context-specific behaviours.
Comparing methods for estimating distance to work
Rationale
Previous research suggests that the physical environment is an important influence on active commuting, and the distance of the daily commuting journey has been shown to have a strong and consistent influence on travel behaviour. 79 Distance between home and work can be assessed in several ways: by asking people to report the length of the route, by modelling a likely route or by measuring the length of the actual route. Although distance to work may be relatively accurately recalled because the journey to work is made regularly, self-reported distance may still be subject to recall bias. When modelling routes using a GIS, it is often assumed that the shortest available route is used with the aim of minimising travel time,80 but this may be different from the actual route used, for example if commuters take children to school on the way to work or go shopping on the way home. The choice of route may depend on several factors, including the social context in which household decisions are made as well as individual preferences and physical constraints. One way of capturing data on the actual routes followed by commuters is to use GPS devices. Although these are increasingly small, unobtrusive and affordable, the participant has to remember to wear the device and recharge it each night, and considerable effort is required to clean, process and analyse the resulting data. To explore the implications of relying on modelled routes instead of collecting GPS data from a large number of participants, we investigated the differences between distances estimated on the basis of GIS-modelled routes and those derived from actual routes recorded using GPS. 81
Methods
We derived distances representing the shortest route available between participants’ home and work locations with the help of the route networks created in the GIS. For participants who reported having used the car, we used the car route network to model the shortest possible route from their home to their workplace. For participants who reported having walked or cycled, we used the pedestrian and cyclist route network to model the shortest possible route. Distances estimated on the basis of GIS-modelled routes thus reflected the actual modes of transport used by each participant.
Actual routes were obtained from the GPS receivers worn by a subsample of participants in 2010 and 2011. Briefly, this involved extracting GPS data for the relevant trip in the GIS, as well as any intermediate destinations that were visited en route, with the help of background mapping and aerial photography. Scattering of data points resulting from signal error or reflection from buildings was removed manually. We used multilevel mixed-effects generalised linear regression models to assess the concordance of GIS-modelled and actual commuting routes; in the published paper, we also compared other features of the modelled and actual routes travelled between home and work. 81
Results
We found that the actual routes taken by commuters often diverged from modelled routes, sometimes substantially. On average the actual routes were 27% or 4.3 km longer than modelled routes, but this summary result conceals important differences depending on which mode of transport was used. Actual routes were even longer among those who travelled to work by car, either singly (35% or 6.5 km) or in combination with walking (25% or 6.2 km) or cycling (26% or 5.0 km). In contrast, the lengths of actual routes followed on cycling trips were closer to those of the modelled routes, with an average difference of 23% or 0.9 km. Actual walking trips were 13% or 0.2 km shorter than modelled routes, suggesting that participants who walked to work may have used cut-throughs and other paths not present in the route networks used to model routes. Agreement between route lengths was highest for walking and cycling, with concordance coefficients above 0.93, and lowest for trips made entirely by car, with a concordance coefficient of 0.44.
Taking as an example one participant who travelled 20 km from work to home by car, the shortest route modelled using the GIS underestimated the actual distance travelled by 79% and overlapped the actual route for only 15% of its length. For a second participant who walked 2.2 km from work to home, the route was more similar in length to that modelled using the GIS but only 19.2% of the route overlapped the modelled route. Figure 3 provides a graphical example of this type of divergence between actual and modelled routes.
FIGURE 3.
Comparison of actual and modelled commuting routes. The actual route taken – by bicycle in this example – is longer than the modelled route and overlaps only in parts. In practice the hypothetical participant takes a less direct route, travels along quieter roads and passes fewer destinations than the modelled route would suggest.

Interpretation
Modelled routes and actual routes can differ substantially. Many commuting journeys are not made with the aim of minimising travel time by choosing the shortest distance, but instead often incorporate other destinations. The next chapter offers additional insights into the motivations for route choice, either to avoid undesirable features of the shortest available route or to experience desirable features of the alternative route. Nevertheless, the shortest modelled route to a destination – whether this is a workplace, or the nearest bus stop or access point to the path on the busway – may be a reasonably accurate estimate of the actual distance followed, particularly for walking or cycling trips, and can serve as an indicator of the accessibility of that destination for a given participant (see Chapter 5, Linking environmental change with travel behaviour change in commuters).
For further details, see Dalton et al. 81
Eliciting motivations and contextual factors
Rationale
In the previous section we noted that the actual routes taken by commuters often diverged from the shortest route available, in some cases substantially. This provides a clue that commuting decisions may be more complex than commonly assumed. From the outset, we had envisaged using qualitative methods to explore, and arrive at an ethnographically ‘thick description’ of, individual everyday commuting decisions. 82 The semistructured interviews we conducted with this end in mind tended to revolve around the practicalities of commuting, that is how people made commuting decisions to fit their social lives and any structural barriers that prevented them from making their preferred choices, but it was difficult to get people to reflect on their commuting behaviour beyond these concerns. We therefore decided to adopt ‘photo-elicitation’ techniques – using participant-produced photographs as an interview guide – to gather unstructured, open-ended information on participants’ experiences and the meanings they gave to them. In this section, we describe the ‘photovoice’ procedure we developed to address this challenge and the methodological questions it helped us to address. 83
Methods
Qualitative research that aims to capture experiences that are not easily verbalised usually adopts an ethnographic method, in which the researcher observes and participates in the behaviour or event of interest, and engages the participant in informal conversations about the meanings given to these. The difficulties of getting participants to reflect on their regular commuting behaviour raised the question of how we would be able to obtain observational ethnographic insights into commuting experiences and decision-making without physically joining each participant on their commute. So-called ‘walk-along’ interviews may have been successfully adapted as ‘bus-alongs’ in other studies but seemed less than ideal for cycle commuting, which was prevalent in our sample. Instead, we decided to attempt to capture commuting experiences ethnographically with the help of the visual method of photo-elicitation.
Participants were given low-tech, unintimidating disposable cameras; others chose to use their own digital cameras or mobile phone cameras. With minimal direction, they were asked to take some time on their way to or from work to pause on their journeys and reflect on aspects of their behaviour that they might take for granted or undertake habitually. They could document their actual commuting journeys and aspects or experiences of the journeys that were significant to them, or an ‘ideal’ (desired) commute. We subsequently met participants for follow-up interviews, which lasted between 20 and 60 minutes. These elicitation interviews were purely driven by the discussion of the photographs produced by participants or the subset of photographs that the participants had selected for discussion.
Participants sorted their photographs into a particular order, usually to correspond with the stages of their usual journey. Many had prepared the narrative, or purpose, of their photo story before the interview. Some also provided written ‘memos’ with their photographs, which were also analysed. At the end of the interview, participants drew the journey they had documented on a map; some pointed out where the photographs had been taken along the route. In addition to the photographs and subsequent interviews, which were audio-recorded and transcribed, ethnographic data collection also included general observation of travel practices in the local area and local public discourses around travelling, in order to further contextualise participants’ representations of travel behaviour. 84 We framed our interpretations within anthropological theories of well-being,85 visual anthropology86 and social practice theories,87 eventually funnelling our analytical findings towards three themes. 88
Results
Participants produced over 500 photographs in total. Most strikingly, when applied to commuting the photovoice method brought to light a range of experiences that had been absent in semistructured interviews focusing on the practicalities of commuting. In particular, many of the photovoice accounts focused on positive experiences of what we termed ‘well-being’ in commuting. For example, a number of participants produced accounts of themselves enjoying pleasant scenery. Others appreciated commuting as a relaxing or otherwise valuable transitional time between home and work life. Still others supplied photographs of unorthodox use of the busway, even before its opening (Figure 4). The insights gained by this method are described in more detail in the following chapter.
FIGURE 4.
Participant-produced photographs of well-being in commuting. Photographs reproduced with permission of participants.
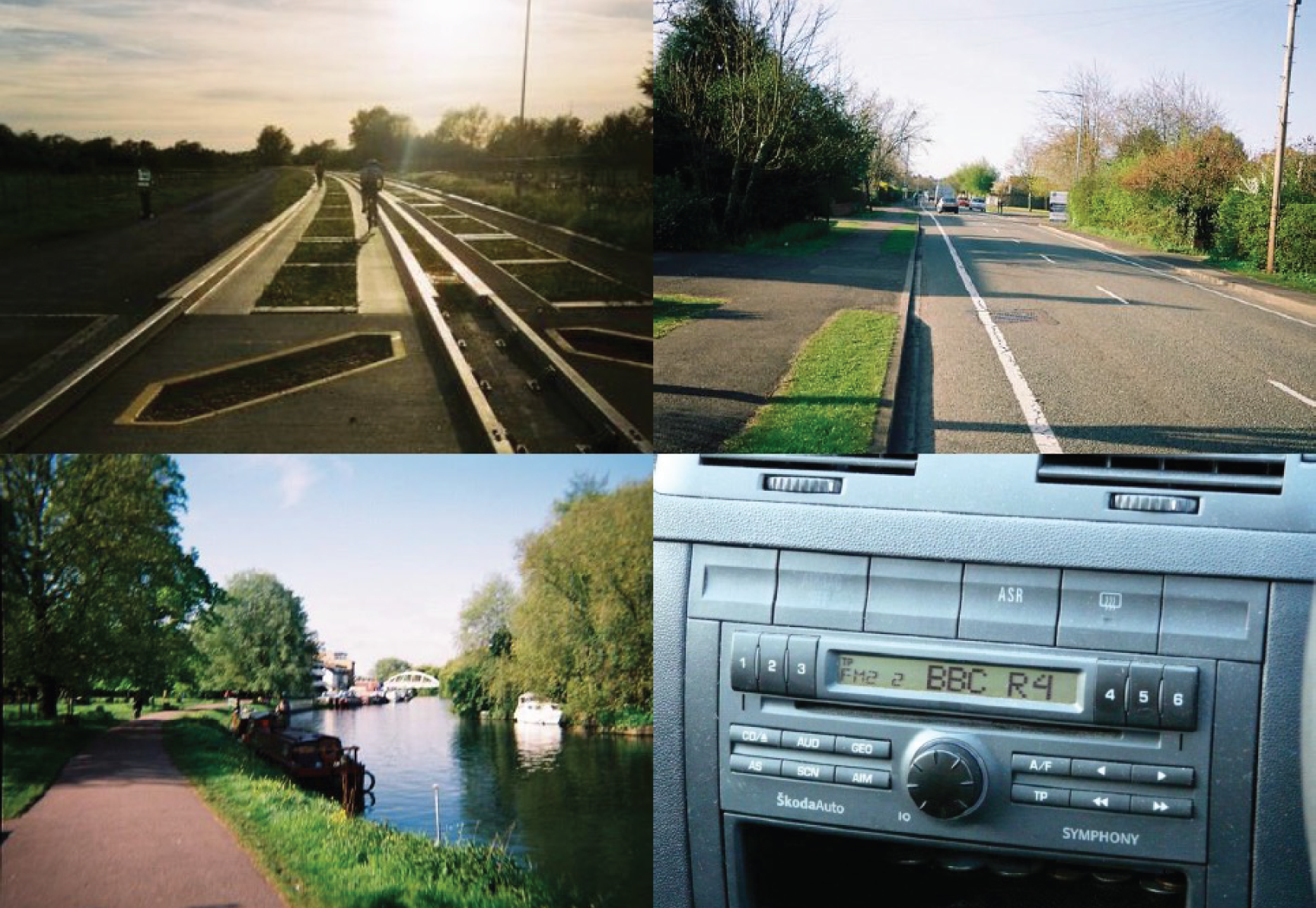
Interpretation
Although our approach produced data that were rich and strikingly different from those produced from our other quantitative and qualitative methods, the question then arose as to how these data should be treated analytically. Did these photovoice narratives produce credible insights into the actual experiences, or at least aspirations, of Cambridge commuters, or did the participant-produced photographs simply represent the subjects of relatively easy photo opportunities? We suggest that the photovoice procedure allowed participants to uncover and subsequently describe different, but no less real, experiences and emotions related to commuting. These experiences were captured in photographs and richly described during the elicitation interview. Moreover, many used the opportunity of the elicitation interview to explain how they had failed to capture all of their experiences with their cameras, narrating these instead as ‘would-be’ pictures – still continuing the same themes as they relayed through their photo-stories. This suggests that their photovoice reflected more than simple ‘Kodak moments’ and provided insights into the actual, in part inner, experiences of commuters. Finally, not all participants documented or described positive experiences of commuting, and narratives of stress and distress could also be explored in greater detail with the help of participants’ photographs.
The different accounts produced in the initial and photo-elicitation interviews thus did not discredit each other’s findings, but provided different kinds of insights into commuting experiences, the latter providing insights suitable for a ‘thick description’ even if these might have been difficult to obtain by standard ethnographic methods such as participant observation. We conclude that photography can produce rich observational data in addition to traditional methods of interviewing and focus groups, and thereby help to document and understand a more complete and complex picture of commuting-related experiences. 89–91
For further details, see Guell and Ogilvie. 83
Conclusion
In this chapter, we have shown how we investigated, adapted or developed a variety of methods for studying commuting and physical activity behaviour. Above all, our comparison of self-reported and objective estimates of time spent walking and cycling to and from work suggests that the estimate of weekly time spent in active commuting derived from our core questionnaire was fit for purpose – albeit far from perfect – for the evaluation of the busway. We have also presented our exploration of different ways of capturing key constructs of importance to the study. This illustrates how the choice of method can influence not only the practicality of measurement but also the inferences that may be drawn, for example about the correlates of physical activity or the characteristics of commuting routes. Our subsequent evaluation of the busway, therefore, drew on findings using different types of data, analysed from a variety of perspectives, to draw robust and meaningful conclusions about its effects.
Chapter 4 Understanding active commuting
Introduction
In the previous two chapters we described the general approach and methods for the study, the data collected, and the investigation, adaptation and development of methods using these data. In this chapter, we describe how we used a variety of methods to improve our understanding of active commuting. We focused on understanding the baseline patterns of commuting behaviour in our cohort, the relationships between active commuting and aspects of health and well-being, and the ways in which the social and physical environment shape active commuting. This understanding was important for the subsequent evaluation of the busway, an intervention that involved changing the social and physical environment for commuting.
Patterns of active commuting
When participants were asked about their usual mode of travel to work at entry to our study cohort in 2009, 43% reported using the bicycle, 39% reported using the car and 11% reported using public transport, while only 7% reported walking. Cambridge is known for its high prevalence of cycling, which is well above the national average, and as such these mode shares were broadly representative of the patterns in the local population. 92 The small proportion of participants who reported usually walking to or from work reflects our decision not to recruit commuters who lived in the same immediate area of the city as their workplace. 32
The retrospective 7-day commuting data showed that when participants were asked to report all modes of travel used for commuting, more than one-quarter reported using combinations of modes within a trip, for example by combining walking with public transport or by driving part of the way and cycling the remainder. 62 Over 50 unique modes or combinations of modes of travel were reported, suggesting that simple classifications such as ‘main’ or ‘usual’ mode of transport may fail to adequately capture the complexity of commuting behaviour.
Although it is widely acknowledged that public transport users incorporate walking or cycling into their journeys,24 we found that some car commuters did the same. Among those for whom the car was the most frequently reported mode of transport in the past 7 days, 31% reported usually incorporating substantial (of at least 5 minutes’ duration) walking or cycling into their commuting trips. 93 Of those who reported ‘always’ or ‘usually’ commuting by car, similar-sized minorities had combined this with walking (14%), cycling (9%), public transport (5%) or a combination thereof (3%) during the past week, or had made some commuting trips without using the car at all (8%). 94 This suggests that car users also have the potential to accrue substantial amounts of physical activity as part of their journey to and from work.
Alongside our identification of modal combinations, we computed the duration of walking and cycling within commuting trips. The median time spent in active commuting at baseline was 120 minutes per week (IQR 33–200 minutes per week), but this concealed differences in the distribution of weekly time spent walking and cycling. Only 30% of participants reported spending any time spent walking for commuting, and in most analyses it was, therefore, appropriate only to distinguish between those participants reporting ‘no walking’ and those reporting ‘any walking’ for commuting. In contrast, more than half (53%) of participants reported spending any time cycling for commuting. In subsequent analyses, we therefore distinguished between ‘no cycling in the last week’, ‘1–149 minutes per week’ and ‘150 minutes per week or more’. 62
Between 2009 and 2010, the modes of travel used for commuting in our sample were relatively stable. Based on the data from the retrospective 7-day travel record, the majority of participants did not change their most frequently reported mode (or combination of modes) of travel. Twenty-one per cent used the car and 68% used an alternative to the car as their most frequent mode of transport at both time points, while 6% switched to the car and 6% switched away from the car. 56 Consistent with these results, we found small average changes in weekly time spent walking or cycling for commuting over the first year of the study. However, those who switched away from the car reported substantial and statistically significant mean increases in time spent walking and cycling, whereas those switching to the car reported substantial mean decreases. Changes in time spent walking and cycling for commuting were significantly different between the four groups of participants categorised in this way (p < 0.001 for both walking and cycling; Figure 5).
FIGURE 5.
Changes in time spent in active commuting. Mean changes in time spent walking and cycling to and from work between 2009 and 2010 for four categories of participant, defined according to their most frequently reported mode or combination of modes of travel to work in 2009 and 2010 (n = 655).
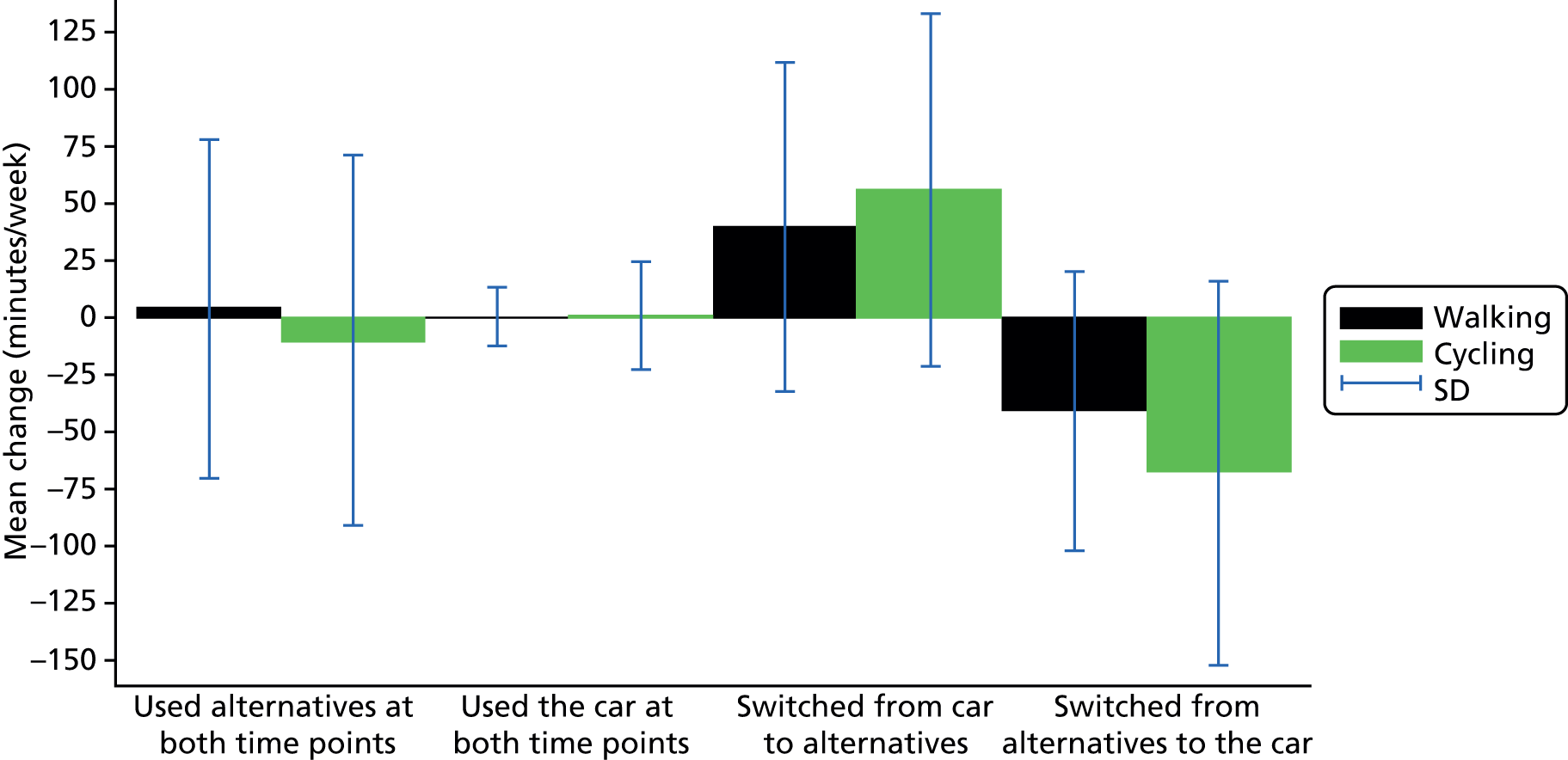
However, we found a larger and statistically significant decrease in time spent in active commuting in the overall sample between 2009 and 2012, the period covered by the main evaluation. The median time spent in active commuting fell from 120 minutes per week (IQR 33–200 minutes per week) in 2009 to 100 minutes per week (IQR 0–170 minutes per week) in 2012 (p = 0.001). This decrease in active commuting was mostly due to a decrease in time spent cycling (medians 70 vs. 40 minutes per week; p = 0.016), and was accompanied by an increase of two percentage points in the proportion of commuting trips made entirely by car.
In summary, despite 85% of the sample having access to a car, active commuting was a highly prevalent behaviour at baseline, which declined somewhat over the duration of the study in the overall sample. Among other things, this pointed to the value of investigating why people chose to walk or cycle to work despite having access to a car (see Active commuting in context) as a step to understand the propensity to change commuting behaviour.
Relationships with physical activity, health and well-being
Physical activity
Previous research suggests that active commuting is associated with higher levels of physical activity. However, most studies of this relationship have considered only the main mode of transport as the independent variable in their analyses. 23,95–97 Having found that a substantial proportion of commuters used a combination of active and motorised modes of transport, we deduced that using a measure of time spent in active commuting would more accurately capture all relevant activity on the journey to and from work.
Using objective measures of daily time spent in MVPA collected with accelerometers in 2009 (see Chapter 2, Derivation of key variables),98 we found that time spent in active commuting was associated with time spent in total physical activity, even in a sample in which participants recorded a relatively high mean of 55 minutes of daily MVPA (Table 6). Compared with those who reported no walking for commuting, those who reported spending 1–149 minutes per week walking for commuting recorded an additional 6.5 minutes of daily MVPA, and those who reported spending more than 150 minutes per week recorded an additional 14.3 minutes of daily MVPA (p = 0.01). We did not observe statistically significant associations between time spent cycling for commuting and total physical activity in men or women, possibly in part due to the underascertainment of physical activity associated with cycling by hip-worn accelerometers. Total time spent in active commuting was not associated with MVPA in men; but for women, spending 150 minutes or more in active commuting per week was associated with an additional 8.5 minutes of daily MVPA (95% CI 1.8 to 15.3; p = 0.01). The lack of an association in men may have reflected the smaller sample of men available for analysis and their tendency to cycle more and walk less (see Active commuting in context). 62
Main exposure | Linear regression coefficients (95% CI) | |||||
---|---|---|---|---|---|---|
Men (n = 141) | Women (n = 320) | All (n = 453) | ||||
Unadjusted | Adjusteda | Unadjusted | Adjustedb | Unadjusted | Adjustedc | |
Time spent walking for commuting (reference: none) | ||||||
1–149 minutes per week | 12.28 (–0.7 to 25.3) | 13.97 (0.5 to 27.45)* | 5.74 (–0.3 to 11.8) | 5. 72 (–0.1 to 11.5)* | 5. 35 (0.2 to 10.9)* | 6.50 (1.1 to 11.9)* |
≥ 150 minutes per week | 11.73 (–3.4 to 26.9) | 13.27 (–1.7 to 28.2) | 15.74 (6.3 to 25.1)* | 15.64 (6.6 to 24.7)** | 14.22 (6.2 to 22.2)** | 14.33 (6.5 to 22.2)** |
Time spent cycling for commuting (reference: none) | ||||||
1–149 minutes per week | –3.69 (–14.2 to 6.8) | –0.65 (–11.0 to 9.7) | –0.97 (–7.7 to 5.8) | –4.78 (–11.8 to 2.3) | –0.26 (–6.0 to 5.5) | –3.04 (–8.7 to 2.7) |
≥ 150 minutes per week | –6.83 (–15.6 to 2.0) | –7.51 (–16.6 to 1.56) | 4.12 (–2.1 to 10.3) | –1.05 (–8.1 to 6.0) | 1.65 (–3.4 to 6.7) | –2.85 (–8.0 to 12.3) |
Time spent in active commuting (reference: none) | ||||||
1–149 minutes per week | 1.40 (–9.3 to 12.1) | 3.54 (–6.6 to 13.7) | 5.36 (–0.6 to 11.3) | 4.51 (–1.8 to 10.8) | 4.68 (–0.6 to 10.0) | 3.08 (–2.2 to 8.4) |
≥ 150 minutes per week | –1.80 (–11.4 to 7.9) | –2.07 (–11.5 to 7.4) | 10.39 (4.5 to 16.3)* | 8.50 (1.8 to 15.3)** | 7.45 (2.4 to 12.5)** | 4.1 (–1.2 to 9.4) |
For further details, see Yang et al. 98
We also investigated the relationship between active commuting and physical activity using the enhanced activity monitoring data collected in 2010 and 2011, because the combined heart rate and movement sensor data allow for a more accurate assessment of physical activity than hip-worn accelerometers. We analysed 181 commuting trips made by 41 participants, objectively deriving the trips by inspecting the GPS data to identify when participants left home and arrived at work, and using the georeferenced combined heart rate and movement sensor data to estimate the energy expenditure associated with different modes or combinations of modes of transport (see Chapter 2, Derivation of key variables).
Although walking or cycling all the way to work involved substantial energy expenditure as expected, their incorporation into longer motor-vehicle journeys also made an important contribution in this respect. Compared with those who drove all the way to and from work, participants using any other mode or combinations of modes expended significantly more energy per minute (Table 7). Adjusting for other factors, regression analysis showed that those who commuted by bus expended an additional 0.7 METs each minute on average. Combining car use with walking and cycling was associated with additional energy expenditure of approximately 0.6 and 1.6 METs per minute, respectively, while those who walked or cycled all the way to work expended an additional 2.5 and 3.9 METs, respectively.
Mode or combination of modes of transport | Sample size (trips/individuals) | Trip duration, median (IQR) (minutes) | Trip distance, median (IQR) (km) | PAEE, median (IQR) (METs per minute) |
---|---|---|---|---|
Car | 35/11 | 31 (22–38) | 25.88 (20.50–29.63) | 1.28 (1.16–1.78) |
Bus | 38/10 | 45.5 (38–65) | 12.41 (8.21–22.85) | 1.67 (1.16–2.02) |
Car and walking combined | 34/12 | 47 (33–65) | 35.87 (28.67–40.26) | 1.78 (1.56–2.57) |
Car and cycling combined | 28/15 | 35 (33–41) | 20.71 (19.01–23.55) | 2.21 (1.67–3.03) |
Walking | 15/5 | 14 (2–22) | 1.41 (0.30–2.16) | 4.61 (4.29–4.95) |
Cycling | 32/8 | 27 (14–42) | 7.24 (3.71–12.13) | 6.44 (4.40–7.00) |
For trips in which walking and cycling were reported in combination with other modes, the median fraction of the total duration of the trip spent in MVPA was 20%. This equates to around 8 minutes for an average commuting trip of around 40 minutes (see Chapter 3, Validating a self-reported measure of time spent in active commuting), and over the course of 1 week this could amount to over half the recommended weekly levels of physical activity for adults.
For further details, see Costa et al. 99
In summary, we found evidence of an association between time spent in active commuting and total physical activity, regardless of the main mode of travel to work. Engaging in 150 minutes or more of active commuting per week was associated with achieving an additional 8.5 minutes of daily MVPA among women, which corresponds to about 16% of the achieved daily MVPA in this population and about 30% of the recommended minimum level of MVPA. We further showed that active commuting was associated with substantial additional energy expenditure, and that incorporating even modest quantities of walking and cycling into the journey to and from work could make a substantial contribution to meeting physical activity guidelines.
Health and well-being
Previous quantitative research has found consistent associations between physical activity and physical and mental well-being. However, this evidence comes mainly from studies examining relationships with recreational physical activity. 100–103 Although active commuting may be associated with higher levels of physical activity, an association between active commuting and physical or mental well-being cannot be assumed, and therefore we investigated this association using the summary scores of both physical and mental well-being derived from the SF-8 instrument included in the core questionnaire (see Chapter 2, Derivation of key variables). The analysis was adjusted for known correlates of active commuting (gender, age, educational attainment and distance between home and work) and in particular for time spent in recreational and work-related physical activity (see Chapter 2, Derivation of key variables). Given that being overweight or obese may confound or mediate any relationship between active commuting and well-being, we also tested the effect of excluding weight status from these models.
In this cross-sectional analysis of data collected in 2009, we found that time spent in active commuting was positively associated with self-rated physical well-being and that the largest benefit was associated with participating in at least 45 minutes of active commuting per day. Those with ‘very high’ levels (> 225 minutes per week) of active commuting reported PCS-8 scores that were on average 1.21 points higher than those with ‘very low’ levels (0–29 minutes per week). Including weight status in the analysis slightly attenuated the relationship between active commuting and physical well-being. Although the effect size was lower than the threshold for ‘clinical significance’ in SF-8 summary scores, it may nevertheless correspond to some degree of ‘population significance’ in settings in which active commuting is common.
For further details, see Humphreys et al. 104
Although we found no association between active commuting and mental well-being in this quantitative analysis, our qualitative findings suggested that participants articulated well-being in a variety of ways (see Chapter 3, Eliciting motivations and contextual factors). 83 In the photo-elicitation study, many participants produced images of the landscape or of nature, and follow-up interviews contained stories of relaxation and ‘me time’ on the commute. Photovoice showed that people can take pleasure in commuting: ‘I really enjoy my cycle to work . . . [the scenery is] absolutely glorious’ (woman, 34, who commuted by bicycle). Commuters did not necessarily regard travel time as ‘wasted’ time. 50 This well-being in commuting was experienced not only by those who walked or cycled but also by car drivers. One participant, who had spent many years cycling in Cambridge, reflected on the tensions involved in sharing the road with others as a more vulnerable road user and reported: ‘I would say I’m much calmer now I drive and I don’t cycle’ (woman, 57, who commuted by car and parked on-site).
Some photovoice accounts also focused on negative experiences of commuting, such as traffic, stress, danger and boredom. For example, one cyclist photographed a sign that lights up to alert drivers to the fact that they are travelling faster than the speed limit, to document that ‘90% of the cars are breaking the speed limit’ (man, 62, who commuted by bicycle). However, those who depicted negative aspects sometimes also mentioned more positive experiences. Appreciating joyful, positive aspects of commuting may serve as a tactic to make everyday travel routines habitable; however, it seems that a sense of choice is important in experiencing ‘happy’ commutes. 83 For example, one participant reported how he chose a route that he found pleasant: ‘All along my route is . . . it’s all green, there are huge houses with nice gardens and I’ve started to choose the route now for its pleasantness at the start of the morning . . .’ (man, 44, who commuted to by car to a park-and-ride site and continued by bicycle).
For further details, see Guell and Ogilvie. 83
In summary, time spent in active commuting was positively associated with a summary measure of physical well-being, independent of recreational and work-related physical activity. It is possible that associations between active commuting and physical well-being would be less favourable in other contexts, for example where active travel may be imposed rather than chosen; in our qualitative analysis, positive experiences of commuting appeared to be associated with having choices. We found no association between time spent in active commuting and mental well-being. However, our qualitative research suggested that well-being during commuting might be experienced and articulated in different ways to those captured in the survey, and not necessarily only in relation to active commuting.
Active commuting in context
This section begins by discussing the role of attitudes and beliefs in active commuting, before moving on to consider a range of broader contextual factors relating to the social and physical environment.
Attitudes and beliefs
Some of the attitudes and beliefs assessed through the psychological measures included in our core questionnaire were associated with time spent in active commuting. For example, we found that participants who reported the highest level of perceived behavioural control with respect to car use – that is, those who agreed that ‘it would be easy’ for them to use a car or that they ‘would be able’ to use a car – were approximately half as likely to report any time spent walking as those who did not. Other findings were not entirely as expected. For example, the most favourable affective attitudes towards car use – that is, broad agreement that ‘it would be pleasant’ or ‘it would be good’ to use a car – were negatively associated with time spent cycling for commuting but positively associated with time reporting walking for commuting. This may in part reflect the prevalence of a pattern of behaviour in which some commuters drove part of the way to work and walked the remainder of the journey, and thus might report both a favourable attitude towards driving and spending time walking to work. 62
For further details, see Panter et al. 76
The remainder of the psychological measures included in our core questionnaire revealed no associations with the uptake or maintenance of walking, cycling or alternatives to the car in longitudinal analysis, except that participants with less favourable affective attitudes towards car use were five times more likely to maintain an alternative to the car as their most frequently used mode of transport. 56
The limited and sometimes confusing patterns of associations of these psychological constructs with active commuting may in part reflect the facts that the questionnaire items permitted different interpretations and the responses might have been sensitive to unmeasured contextual factors. Our qualitative research illustrated that attitudes relating to commuting behaviour were complex and ambiguous, or involved factors that were outside the scope of our core questionnaire. As we have seen above, participants appreciated having choices about their commute because this gave them control over their overall journey experience. We described the opportunity to control interactions with other commuters and to have personal space on the journey as ‘the choreography of avoidance’. 94 Interviewees often elaborated how they achieved this; it affected all modes of travel, and appeared primarily to be about avoiding unpleasant or stressful interactions with other commuters. Cyclists explained how they chose routes away not only from cars, but also from pedestrians. Others avoided groups of school children on public transport, and still others preferred cars to public transport in order to have their own space, as illustrated by the interview excerpt below. Car and public transport commuters frequently described leaving early to avoid the delay and unpredictability of travelling in rush hour or – less frequently – to find a parking space. 94
I was getting very stressed . . . because of the behaviour of others on the road. Yeah, I think it’s important to have some sort of me-time and I actually find that works very well now I’m in the car for, well, I suppose on average an hour a day.
Woman, 57 years, who commuted by car and parked on site
Finding quick, reliable and convenient commuting routes was described as an important factor in decision-making, but pleasantness or opportunities for relaxation and ‘me time’ were equally valued – often by the same participants. 50
Convenience was a particularly prominent theme. Some participants cited the frequency of public transport or the time when they had to be at work to explain why a particular mode of transport was more convenient. Convenience could trump personal dislike of cycling, and sometimes it appeared to capture the overall burden or ease associated with commuting, as the following interview excerpts illustrate in turn. 51
The buses were really convenient, they would go maybe every 5 minutes.
Woman, 48 years, who commuted by car to a park-and-ride site and continued on foot
I don’t actually enjoy cycling really, I do it purely because it’s convenient.
Woman, 30 years, who commuted by bus and sometimes by car
Because parking is difficult in the centre of Cambridge, on this site in particular, it would be difficult to bring the car in and it’s slower and more expensive so yeah, that’s the main reason . . . Before I lived there I would come in on the motorbike . . . That was quite good but really it’s just more convenient on the train . . .
Man, 55 years, who commuted by motorbike
For further details, see Guell et al. 50
The complex reasoning behind commuting choices and the importance of convenience were echoed in our mixed-method analyses that explored why participants who reported non-conducive route environments nonetheless walked or cycled to work, and in the experiences and motivations of participants regarding commuting around the period of moving home, which we report below. 51,52
Social factors
Individual sociodemographic factors
A number of individual and household characteristics were associated with mode choice and active commuting. It became apparent early in the study that active commuting, and cycling in particular, was patterned by gender. Men were four times more likely than women to report cycling rather than driving, and two and a half times more likely to report walking rather than driving, as their usual mode of transport to work. 55 Further, men were twice as likely to report spending any time cycling for commuting but half as likely to spend any time walking,62 and analysis of the more detailed 1-day travel record in our core questionnaire confirmed this. 105 Men thus had higher odds of reporting an active usual mode of commuting, and in particular were more likely to incorporate cycling, while women were more likely to report some walking for commuting.
We also found that markers of individual socioeconomic position – such as having access to a car, living in a more affluent area, or possessing a university degree – were associated with commuting choices. Not surprisingly, participants living in households with one or more cars were less likely to walk, cycle or use public transport than those without access to a car. 55 Other indicators of socioeconomic position exhibited less intuitive relationships with active commuting. For example, living in areas of relative income deprivation appeared to reduce the likelihood of walking as the usual mode of transport. 55 Similarly, participants with at least degree-level education were twice as likely as those without degree-level education to report cycling rather than driving as their usual mode of travel to work, and were also more likely than those without degree-level education to report spending any time cycling to and from work. 62 These associations were echoed in our analysis of shopping and leisure trips reported in the 1-day travel record. 105 Nevertheless, when sociodemographic variables were combined with environmental factors in quantitative models, these explained only a relatively small amount of the variation in commuting behaviours. Greater understanding of the interaction between sociodemographic factors and the particular social and environmental contexts in which these play out could help to clarify these associations. 55,62,105 We explored this further in a mixed-method analysis of the social lifeworlds of Cambridge residents (described in Linking individual sociodemographic factors and social lifeworlds), in which lower socioeconomic positions were more likely to require longer commutes and, therefore, car driving. 94
For further details, see Carse et al. 105
Social ties and commitments
The importance of social ties and commitments in shaping active commuting became apparent through quantitative evidence that having children appeared to influence commuting choices. For example, we found that those with at least one child in the household were more likely to report cycling as their usual mode of travel to work, and more likely to report spending any time cycling to and from work than those without children. 55,62 In longitudinal analysis, we found that commuters with children in the household were more likely to take up walking than those without children. 56 We also found that parents’ mode of travel to work and children’s mode of travel to school were related: children were more likely to walk or cycle to school if their parent reported walking or cycling as their usual mode of travel to work.
Our qualitative research further highlighted that commuting practices were embedded in and therefore shaped by constantly changing social lifeworlds, including through children and other family members. 50 This changeability was related to minor, inevitable day-to-day changes due to social commitments or major life events such as moving home. At the day-to-day level, participants had different arrangements depending on work schedules, children’s school and nursery hours, and other commitments. One participant explained that starting to care for a friend had affected her commuting choices.
I’ve had quite a complicated pattern because I was in fact driving . . . to their house first of all to give them their medication and then driving in to work.
Woman, 63 years, who commuted by car
I have to take my son to nursery . . . so I drop him off first . . . I tend to cycle if I’m going from home to work . . . But I do work out of the office quite often . . . so yesterday I drove . . . So it’s a bit complicated . . .
Woman, 34 years, who commuted by bicycle or car
Another explained that her commuting decisions varied, depending on whether she worked in or away from the office. Sometimes a decision about the mode of transport for commuting might even be made by a family member on the participant’s behalf. For example, one participant reported that, after he had suffered a fall, his wife was concerned about him cycling, as she worried it would be ‘too dangerous’. 50
Commuting patterns could also be influenced by major changes in participants’ lives. One mother explained that she and her partner, despite living a considerable distance from work, used to cycle together some days of the week. The birth of their child had changed this routine.
Our son hadn’t started school, our child minder was too far away to cycle . . . So for that period of time when my son was 6 months old till . . . school starting, it was really necessary to take the car.
Woman, 40 years, who commuted by bus
For further details, see Guell et al. 50
Another life change, often regarded as a time when commuting habits are reassessed, is that of relocation. Of the 690 participants who completed our core questionnaire in both 2009 and 2010, 126 (18%) moved over the course of the year: 76 of them moved home, 43 moved workplace and 7 did both. We explored the influence of moving home on commuting behaviour in a qualitative interview study. 51 These interviews revealed that although the journey to work was not the main consideration, it was nonetheless influential in deciding where to relocate. Distance to family and friends, shopping and leisure amenities, schools and other services, as well as the relative cost of housing, were traded off against one another.
I think with every move there’s a sacrifice along the way and it’s the how I get to work that’s sacrificed really.
Woman, 37 years, who commuted by bicycle before moving and by car after moving
I’ve lost the cycling . . . I think it was the right thing for me to do in terms of my career, that side of it, and I think that’s slightly more important than the cycling, the commute.
Woman, 29 years, who commuted by bicycle before moving and by car after moving
Interviews further revealed that even those participants who had considered how they would travel to work prior to relocation continued to revise their commute in the light of subsequent experience and day-to-day commitments.
I still like for the first few months just got the bus. I mean I kind of knew the bus route . . . so no I didn’t think about cycle routes or anything like that . . . but . . . then realising it’s not that far away . . . that probably influenced me, yeah, to start thinking a bit more about getting a bike.
Woman, 30 years, who commuted by bus before and initially after moving, later changing to commuting by bicycle
Many of the participants who were interviewed had moved further away from work and had changed their usual mode of commuting following relocation. When asked why a particular mode of travel to work was ultimately adopted, participants often mentioned a combination of cost (e.g. of car parking), speed, reliability and convenience as the main factors that influenced their choice. Increases in physical activity were reported to be incidental, although increases in physical activity and consequent increases in physical fitness were perceived as beneficial and worth maintaining in future.
For further details, see Jones and Ogilvie. 51
Linking individual sociodemographic factors and social lifeworlds
We further explored the socioeconomic patterning of active commuting through a mixed-method investigation focused on regular car commuters. 94 Initial analysis of interview data suggested that age, health and socioeconomic factors might be associated with distance between home and work. For example, it suggested that younger and more highly educated workers were likely to live in privately rented accommodation in the city and close to work. In contrast, those who sought to settle in the area more permanently tended to own their home outside Cambridge – owing to high housing costs in the city – and therefore tended to travel longer distances to work unless they were affluent enough to continue to live within cycling distance, in which case they might have no need of regular car commuting. In this interview excerpt, a participant explains that, in Cambridge, not owning and using a car to commute may be a marker of relative wealth.
To be honest, not having a car in Cambridge and being able to survive, it’s indicative of quite a wealthy lifestyle because house prices are so expensive. It is an odd factor about Cambridge that you’ll find, if you can afford to, you’re only cycling 3 miles . . .
Man, 44 years, who commuted by car to a park-and-ride site and continued by bicycle
We developed a series of hypotheses based on the qualitative data that could be tested using the quantitative data. 94 We hypothesised that demographic, health and socioeconomic characteristics were associated with commute distance, and that these associations might mediate the associations of those individual characteristics with regular car commuting. We also hypothesised that following relocation away from Cambridge, participants might be likely to buy a car, with the consequence that the associations between commute distance and regular car commuting would be partly mediated by car availability in the household.
As expected, we found that lower levels of education and home ownership increased the likelihood of regular car commuting, while area income deprivation showed an inverted U-shaped association. In other words, while the most affluent and the least affluent were relatively unlikely to regularly use a car for commuting, those living in areas of income deprivation between these extremes were more likely to regularly drive to work. As expected, these socioeconomic factors ceased to have a significant effect on regular car commuting once distance between home and workplace was included in the model; however, car ownership, possession of a driving licence and availability of workplace car parking did not greatly affect the association between distance and regular car commuting. The model thus supported a general interpretation to the effect that regular car commuting was apparently affordable for most participants in our sample and became a way of reconciling life goals, such as home ownership and children, with their daily work commitments.
For further details, see Goodman et al. 94
In summary, while some social factors were associated with commuting behaviour in our sample, indicators of socioeconomic position were sometimes counterintuitively related to active commuting. Qualitative research highlighted that commuting practices were complex, as a result of being shaped by a multitude of social commitments which were liable to change, and the mode of transport or commuting pattern that was most compatible with these commitments and least burdensome on the commuter and their families was ultimately adopted. Nevertheless, both qualitative and quantitative analyses corroborated the hypothesis that socioeconomic circumstances and participants’ life stage influenced how far they lived from Cambridge, which in turn affected their commuting behaviour. Social context thus shaped commuting practices not only through changeable social ties, but also through broader socioeconomic patterns.
Environmental factors
Distance
Previous research has shown that people who live closer to work are more likely to walk or cycle to work. 79 In line with this finding, our analysis showed that each additional km between a participant’s home and workplace reduced the likelihood of their walking or cycling by a factor of 3.9 or 1.3, respectively. 55,105
Distance between home and work was especially important for time spent walking for commuting. In households without a car, those who lived less than 3 km from work were more likely to report any time walking for commuting, but the effect of distance on walking was weaker among those with access to a car. 62 A possible explanation is that commuters with access to a car may choose to walk part of the way to work, and this behaviour is less sensitive to distance (see below), whereas people without access to a car may walk all the way if the distance is short enough. Similarly, those who lived closer to work were more likely to spend time cycling to and from work. Compared with participants who reported living more than 5 km from their workplace, those who lived closer were 10 times more likely to cycle if a car was available, and four times more likely if no car was available in the household. 62
Although distance was strongly associated with the usual mode of travel to work and with time spent walking or cycling for commuting, we found that distance was not associated with the likelihood of incorporating walking (of at least 5 minutes) or cycling stages into car journeys to work. 93 This is important because it suggests that even commuters with a long journey may be able to integrate incidental physical activity into their commute. We found that those participants who reported using active modes in combination with the car reported an average of 12 minutes of walking and 17 minutes of cycling per day on their journey to and from work.
For further details, see Panter et al. 93
Perceptions and characteristics of the commuting environment
Perceptions of the route to work
Few previous studies have examined associations between active commuting and perceptions of the route between home and work. We investigated the associations between time spent in active commuting and individual route perception items, as well as an overall composite score assessing the perceived supportiveness of the route environment for walking and cycling. 62,93
Participants who agreed that it was pleasant to walk for commuting and reported convenient public transport were more likely to report spending time walking for commuting (OR 4.18, 95% CI 3.02 to 5.78, and OR 1.46, 95% CI 1.10 to 1.96, respectively), and those who reported convenient routes for cycling were more likely to report spending 1–149 minutes per week cycling for commuting (OR 3.51, 95% CI 2.26 to 5.47). Somewhat surprisingly however, participants who reported ‘little traffic’ on the route to work were less likely to report spending time walking for commuting than those who disagreed with this statement. 62 Those who reported their routes to be most supportive were also three times more likely to incorporate active travel into their car journeys to work. 93
For further details, see Panter et al. 62
Routes and resilience
Some participants reported unsupportive route environments but, nonetheless, reported active commuting. We examined interview data from a sample of 15 participants to gain a deeper understanding of these initially counterintuitive quantitative results and identified three reasons for their behaviour and reporting. 52 First, participants may have developed mechanisms for coping with unsupportive route environments. For example, knowledge of the local route network may have provided them with options for circumnavigating unfavourable conditions. Important in this negotiation of routes to work was the existence of opportunity for route choices. In interviews, several participants reported using different routes, depending on traffic conditions and time of day, and we have seen earlier that the actual routes taken by participants often diverged from modelled routes (see Chapter 3, Comparing methods for estimating distance to work). Experience, often gained through years of cycling, was another factor that enabled commuters to engage in active travel despite unsupportive conditions, as illustrated by the following interview excerpt.
I do know all the little snickets [shortcuts] and sideways and I tend to use those . . . I’ll use the funny little footpaths, the roads that are shut off to traffic . . . because I know most of the routes I can duck and dive a bit.
Man, 61 years, who commuted by bicycle
Second, we found that some participants found the route environment unsupportive for active travel but decided to walk or cycle to work for other reasons. These included the lack of a car or driving licence, and a preference for active travel, for example to gain more control over the duration of the journey. For example, one participant reported that she cycled simply because it was quicker than the alternatives.
Because it’s quicker, I think that’s my primary reason actually. I mean if it was quicker for me to drive, if I wasn’t sitting in traffic queues then I might be tempted to drive more often.
Woman, 52 years, who commuted by bicycle
Finally, some participants who reported unsupportive conditions may have done so to reflect public opinion or a political statement. In interviews they talked confidently about their own active commuting, albeit in unsupportive environments, but they also highlighted that others around them struggled with or were discouraged by these same environments. In other words, they were not so much reporting their own experience but (also) trying to reflect how the routes were perceived by others, or how they thought the supportiveness of the route should be reported in order to engender political action to improve provision.
You see I’m, what obsesses me is all the things that motorists do to me. [Laughs] . . . I’m not alone in that . . . everybody has experiences . . . it’s a series of frightening experiences and yes it’s certainly put both of my daughters off.
Man, 61 years, who commuted by bicycle
For further details, see Guell et al. 52
Objective attributes of the surroundings of the home and workplace and the route to work
While route perceptions appeared to influence commuting behaviour, at least one surprising association was found. Most studies examining associations between the physical environment and physical activity behaviours have used perceptions which are, by definition, self-reported. However, such perceptions are a function of the actual environmental conditions and how those are experienced, and examining the role of the environment itself in determining behaviour is also important. 106 We therefore investigated whether or not objectively assessed characteristics of the environment were associated with the usual mode of travel to work. 55 As described earlier, we used the GIS to calculate 30 environmental variables pertaining to the home, route and work environment that might influence active commuting (see Chapter 2, Derivation of key variables).
We found that only some of the environmental measures were associated with the usual mode of travel to work in multivariable analyses. Four measures of the home neighbourhood were found to be associated: low junction density (indicating low road connectivity) and a longer distance to the nearest railway station were associated with lower odds of walking, cycling and public transport use. As we have seen earlier, poorly connected areas with indirect routes may prompt some commuters to use shortcuts that are available only by walking (see Chapter 3, Comparing methods for estimating distance to work). Longer distance to the nearest bus stop and less frequent bus service provision were associated with lower odds of public transport use, and the odds of cycling were also reduced among those living in neighbourhoods with fewer bus services. One explanation may be that good public transport provision reduces the reliance on the car for transport more generally. Only two measures of the work neighbourhood were associated with the usual mode of travel to work: those with free car parking at work or working in areas with fewer amenities were less likely to cycle or use public transport. 55
For further details, see Dalton et al. 55
The cost and availability of workplace car parking was a recurring influence on commuting behaviour in both qualitative and quantitative analyses. 93,94 Car parking availability at work was a strong influence on incorporating walking or cycling into car journeys to work; those who reported having no parking at work were 26 times more likely to include a walking or cycling stage as part of their car journey than those with free parking at work. 93 Interviews also provided insights into how walking and cycling were combined with car use. 52,94 Some reported parking for free on streets within walking distance of their workplace, while others used park-and-ride sites creatively. Rather than using the latter to access (and pay for) dedicated bus services, as intended, they used them as free car parks and continued their journeys on foot or by bicycle.
Environmental factors and changes in active commuting over time
In longitudinal analyses, we were also able to show that perceptions of the route to work and objectively assessed characteristics of the environment predicted changes in active commuting between 2009 and 2010. We first examined the associations of perceptions measured in 2009, and objective features of the environment ascertained from existing data available in 2009, with changes in commuting behaviour over the following year. 56 We then investigated whether or not changes in participants’ perceptions of the route between 2009 and 2010 were associated with changes in active commuting over the same period. 107 Although neither approach can be regarded as establishing proof of a causal relationship, in combination they offer a significant analytical advance on the predominantly cross-sectional evidence available to date. 16
The first analysis showed that perceptions of supportive routes for active travel at baseline also predicted uptake or maintenance of active commuting 1 year later. Those who agreed that there was convenient public transport on the route to work were twice as likely to have taken up walking for commuting as those who did not. Similarly, those who reported convenient cycle routes were three times more likely to take up cycling, and four times more likely to take up alternatives to the car in general, than those who did not. Finally, those who agreed that the route was pleasant to walk were approximately twice as likely to maintain walking to work as those who did not. In terms of objective characteristics of the environment, we found that those who had more frequent bus services in their home neighbourhood were four times more likely to take up alternatives to the car than those who did not. Our analysis also showed that participants without car parking at work, or who had to pay for parking, were twice as likely to take up walking, and alternatives to the car in general, as those with free parking at work. 56
The second analysis showed that average overall changes in route perceptions between 2009 and 2010 were generally small and not statistically significant, which is plausible in the light of the fact that the busway was not formally opened until 2011. The only significant change in mean route perceptions over time was that women, but not men, reported that it was less pleasant to walk in 2010 (mean score 3.47) than in 2009 (mean score 3.35; p = 0.03). We also observed that participants who perceived their route to work as less favourable at baseline tended to report improved perceptions at follow-up, while those with initially more positive perceptions tended to report stable or less favourable perceptions at follow-up – although adjustment of the analysis for baseline perceptions did not materially affect the results. 107
Changes in a few route perceptions predicted changes in time spent in active commuting and the proportion of trips made entirely by car, as well as uptake of walking, cycling and alternatives to the car. Those who reported that the route had become less pleasant to walk reported a decrease in time spent walking of 12 minutes per week (95% CI –24 to –1) and a 6% increase (95% CI 1 to 11) in the proportion of car trips. Those who reported that it became safer to cycle over time and who reported more convenient public transport were more likely to take up alternatives to the car. Increases in the perceived danger of cycling or of crossing the road were also associated with increases in the proportion of car trips. These results were robust to adjustment for individual and household characteristics, as well changes in family composition or home or work location, car parking or work responsibilities, all of which might have been expected to influence changes in route perceptions or behaviour. 107
Although the determinants of the different outcomes modelled – changes in time spent in active commuting, the proportion of car trips and the uptake of active commuting – were not identical, the results were generally consistent across the different analyses. We found some examples in which negative changes in route perceptions were associated with a decrease in time spent walking, but significant associations were not observed for positive changes. It may be that negative changes in attributes of the route to work can act as a barrier to active commuting, but the converse perceived improvements were not sufficient to encourage uptake of active commuting.
In summary, our investigation of environmental factors thus confirmed that distance between home and work was an important influence on commuting behaviour, but need present no barrier to the incorporation of substantial walking or cycling stages into car commutes. We further found that both perceived and objective features of the environment were associated with active commuting in cross-sectional analyses and with the uptake or maintenance of active commuting in complementary longitudinal analyses. The latter provide important new empirical support for the hypothesis that interventions aiming to provide safe, convenient routes for walking and cycling and convenient public transport may be expected to promote active commuting and should be evaluated on this basis.
Conclusion
Although most people in our study cohort could afford a car and had access to one in their household, active commuting was common and walking and cycling were often combined with car use on the journey to and from work. We have demonstrated relationships between time spent in active commuting and total physical activity and physical well-being, and estimated more precisely than before the energy expenditure associated with different forms of active commuting. We have gone on to show how commuting behaviour is shaped by the social and physical environment, as well as by people’s attitudes and beliefs. Although people appreciate their commute as a time for themselves, they also tactically accommodate their commute within a broader set of social commitments and responsibilities, both at the day-to-day level and in order to achieve their life goals in a socioeconomic context. Our analysis confirms distance as a strong influence on mode choice, but also shows how commuters can incorporate active travel into their commute irrespective of the overall distance. We have also shown how changes in the perceived safety and pleasantness of walking and cycling routes and the convenience of public transport were associated with changes in active commuting, which suggests that improving these attributes of the environment may be an effective strategy for promoting walking and cycling to work.
Chapter 5 Evaluating new transport infrastructure
Introduction
In the preceding chapters, we outlined the methods we developed for studying active commuting and the insights we gained from applying these methods to investigating the patterns and contexts of commuting in our sample before the opening of the busway. In this chapter, we report our evaluation of the impacts of the busway.
Our aim was not to evaluate the busway against a comprehensive set of outcomes, but rather to use the opportunity presented by this natural experiment to investigate a more specific set of research questions linking environmental change with physical activity behaviour change and related health outcomes. As discussed in previous chapters, we were not able to randomise exposure to the intervention, compare its effects in discrete ‘intervention’ and ‘control’ groups, or follow the members of our study cohort for long enough after the completion of the intervention to be able to directly observe some of the health outcomes of interest. We therefore sought to build an evidential case for causal inference, using multiple sources of data and types of analysis to shed light on complementary aspects of causal estimation and causal explanation. 108
We began with an analysis of the discourse about the busway in the media before and after its completion, and an ethnographic study of the use of busway in the first few months after its opening. We then used the results of our intercept survey to describe the users of the busway and their subjective evaluation of the busway and its effect on their travel behaviour. Turning to our main study cohort, we treated exposure to the intervention as a continuous variable defined in terms of proximity to the busway (see Chapter 2, Derivation of key variables) and confirmed its face validity by showing that it predicted use of the busway for all three possible modes of travel in cross-sectional analysis. We then used this measure to model the effects of the busway on changes in commuting behaviour in our cohort over time, focusing on changes in mode share for commuting and time spent in active commuting as our primary outcomes and changes in carbon emissions and time spent in overall physical activity as our secondary outcomes. Because the distributions of these outcome variables rendered them unsuitable for linear modelling, it was necessary to express the findings in terms of the likelihood of various categorical outcomes, rather than in the neater terms of a simple ‘effect size’ such as an increase in the number of minutes spent cycling per week. We adjusted these analyses for a suite of key covariates, including distance to work, baseline commuting behaviour and major life changes such as moving home or workplace, which might have confounded any observed changes in our behavioural outcomes. We then investigated selected causal pathways by which exposure to the busway was hypothesised to affect commuting behaviour, before returning to qualitative research methods to explore the reasons why participants may or may not have taken up the opportunity to use the busway. Finally, we investigated the longitudinal associations between active commuting and changes in overall physical activity and selected health outcomes.
Understanding the intervention
Our evaluation began with an analysis of the discourses about the busway in print and social media and an ethnographic participant observation study of the use of the busway in the first few months after the guided bus services commenced in August 2011. 53,54 These complementary qualitative research methods allowed us to better understand the nature of the intervention, the ways in which it interacted with its context and the factors that might determine its acceptability and uptake. We postulated that when users first experienced the busway, their responses would partly reflect the expectations generated by – and thus be framed by – the media discourse, and would in turn give rise to further media discourse. In this way, such discourse might act as both a component of and a contributor to the context of the intervention. The media analysis also provided important insights into exposure and access to the intervention. The ethnographic study was informed by normalisation process theory. This emphasises that an important aspect of users’ experience of an intervention may be as a continuation or disruption of what had gone before, and, therefore, provided a useful framework for analysing how individual user experiences collected in ethnographic field notes might represent a wider set of responses across a population. 109 The theory also draws attention to users’ reflexive monitoring of their use of a new intervention, which may have collective dimensions.
Prior to the formal opening of the busway, media coverage (in the pre-Twitter era) revealed tensions about the design of the busway and conflicts related to the costs and delays associated with the project. The promotional discourse emphasised that the guided bus services would be frequent and reliable, by virtue of running on a segregated guideway and additional measures by which the buses shared the road with normal traffic, and would offer a high-quality, comfortable alternative to driving. Although there was also wide consensus about the need for increased transport capacity along the A14 corridor, the media analysis highlighted that the actual implementation of the busway might have had limited local acceptability.
Once the busway opened, initial experiences and responses to it were framed by the preceding media messages. Initially, bus services were met with high levels of demand, which led to overcrowding and meant that services were not as reliable as expected. Print media and Twitter users further emphasised that, for many journeys, the guided buses would not be any faster than pre-existing bus services, in part because the buses shared normal roads with regular traffic on parts of their route and in part owing to the buses making additional stops. This was illustrated by an article in a local newspaper.
[This] represents the beginning of the public’s realisation that the guided busway does not improve journey times or reliability for the vast majority of typical journeys.
Hunts Post, 5 January 2012110
The contrast between idealised and realised experiences, which was common in the media, provided scope for negative evaluations of the busway that could discourage some potential users from trialling the intervention, or prevent initial use of the busway from being translated into continuing use.
Nevertheless, these initial negative experiences did not lead to a major shift of passengers away from the new service, and overcrowding was addressed through the addition of buses and stops and the modification of routes. These measures and their discussion in the media further illustrated how exposure and access to the busway could vary in time and space. In local news coverage, the introduction of an additional stop was noted to have multiple effects: it would facilitate access to the service, increase the capacity of the system and reduce some journey times. This showed how the provision of bus stops played an important role in defining the exposure of the local population to the intervention.
Local news also illustrated that the timing of exposure to the ‘maintenance track’ (path) was difficult to determine because walkers and cyclists reportedly began to use it before construction had finished, as illustrated in the following quote.
Cambridgeshire’s guided bus track is proving a hit even before it is up and running with cyclists. Bike enthusiasts have been taking to the smooth concrete roadway to explore the countryside near their homes, and some have even been using it as a cycleway to get to and from work.
Cambridge Evening News, 30 May 2009111
The phased introduction of the busway together with subsequent modifications clarified that, in studying its impact on travel behaviour, the implementation of the intervention could not necessarily be represented by a singular date.
The media analysis also showed how different stakeholders prioritised different aspects of the intervention as critical for success. While the local authority was focusing on completion and usage of the busway, the media amplified user criticism about attention to the path, notably about a lack of lighting, which reportedly led to cycling accidents and safety concerns among women and elderly users. In response, the local authority installed lighting, but initially only at junctions and bus stops in order to minimise any light pollution. Other media users, however, continued to question the implicit prioritisation of the busway over the path, as illustrated in the first tweet below. Others perceived the path as a positive alternative to the busway itself, particularly after the rural section received a smooth tarmac surface thanks to additional funding from the transport charity Sustrans.
Almost 56,000 trips made on the Cambridge #busway in the first 7 days. I wonder how many cyclists use the path?
@OutspokenDeliv, 16 August 2011112
I could almost be in the Netherlands . . . lovely wide cycle path alongside the Cambridgeshire guided busway . . .
@harryrutter, 11 December 2012113
Our ethnographic research further illustrated how ‘uptake’ of the intervention was a haphazard process. For some individuals, the initial experience of the busway could be decisive. We encountered some users who had used the busway for an exceptional, one-off trip but found it so convenient that they began to use it for their regular commuting, for example instead of driving. For other users, their initial experience was important because it fell short of the promotional discourse or idealised media representations, thus jeopardising the prospect of their using the service in future.
The majority of users we observed and interacted with were still getting used to the busway during the ethnographic observation period, for example in relation to the different routes and ticketing requirements and procedures. In this respect, the adoption of the busway had a collective dimension. Groups travelling together, as well as passengers and drivers, shared information and collectively ‘evaluated’ the busway. These collective experiences of the busway were sometimes also shared more broadly via the media, for example in a local magazine which reported: ‘On the first day everyone was beaming which was nice to see’. 114 In the interviews we subsequently conducted, participants also sometimes spontaneously raised themes from the media, which confirmed that the media contributed to the framing of expectations of the busway.
Our ethnographic fieldwork also showed that previous experience was crucial in framing user evaluations of the busway. Conversations with passengers highlighted that bus users could be divided into two distinct groups: those who prior to the opening of the busway had regularly travelled by bus, and those who had mainly travelled by car. The former group tended to compare the guided bus services with the bus services they had used previously, some of which had been discontinued after the opening of the busway. They generally noted that journey times had not necessarily decreased and passenger numbers had increased, which meant that the ability to obtain a seat was no longer guaranteed. The latter group tended to compare the guided bus services with the car journey they used to make. Car journeys sometimes took even longer than the bus, driving was less relaxing and more expensive, and the busway was less burdensome for them overall. This highlighted that the busway could have a differential impact on different groups of commuters, depending on their previous travel patterns.
In summary, the media analysis clarified the potential for exposure and access to the busway and the fidelity of its implementation to vary in time and space. In particular, it confirmed that the path began to be used long before the commencement of guided bus services and that the location of bus stops was important in determining exposure. These insights contributed to our quantitative analytical strategy, notably the selection of our primary measure of intervention exposure and the decision to focus on changes between the first (2009) and final (2012) phases of cohort questionnaire data in defining the main outcomes of the study. Participant observation further showed the importance of previous experience in determining people’s perceptions and use of the busway, identifying an important contrast between the more positive appraisals of former car users and the more dissatisfied appraisals of former bus users. This suggested the possibility of differential effects on behavioural outcomes depending on baseline travel behaviour.
Patterns and predictors of busway use
We then carried out an intercept survey on the busway in 2012, about 1 year after its official opening, to describe its users and study their perceptions of the busway and its effect on their travel behaviour. We also used our 2012 cohort data to study the predictors of use of the busway, with a view to testing the face validity of our graded, participant-level measures of intervention exposure.
Patterns of busway use
We were able to recruit 1710 eligible busway users for the intercept survey, of whom 178 were unable to stop when approached but later completed the survey via the internet. In total, 57% of participants were intercepted while using the guided bus, 31% were intercepted while cycling on the path and 12% were intercepted while walking on the path.
The characteristics of users intercepted on the busway are summarised in Table 8. In comparison with the main study cohort (see Table 1), the gender composition of the sample of busway users was more balanced (51% male). Fifty-seven per cent of participants described themselves as in full-time employment, and 54% had a university degree or higher educational qualification; although this was higher than the average for Cambridgeshire (36%), it was considerably lower than in the cohort sample. Although the home addresses of the participants were concentrated along and near the ends of the busway, as expected, we intercepted considerable numbers of users who lived in locations more widely scattered over the study area, and 12% lived outside the study area altogether (Figure 6). In other respects, the intercept survey and cohort samples were similar.
Characteristics | Overall (%) | Male (%) | Female (%) |
---|---|---|---|
Individual characteristics | |||
Gender (n = 1693) | 50.5 | 49.5 | |
Age (years) (n = 1684) | |||
16–19 | 6.5 | 6.5 | 6.6 |
20–29 | 14.9 | 14.4 | 15.4 |
30–39 | 17.5 | 17.5 | 17.6 |
40–49 | 17.9 | 19.8 | 16.2 |
50–59 | 18.1 | 17.7 | 18.5 |
60–69 | 15.4 | 13.9 | 16.8 |
70+ | 9.8 | 10.4 | 9.0 |
Household characteristics | |||
Bicycle access (n = 1671) | |||
Yes | 77.6 | 82.7 | 72.4 |
Socioeconomic characteristics | |||
Car access (n = 1663) | |||
None | 12.1 | 13.3 | 10.9 |
One | 43.3 | 42.6 | 44.5 |
Two | 33.3 | 33.9 | 32.1 |
More than two | 11.3 | 10.2 | 12.6 |
Education (n = 1674) | |||
Degree or higher | 53.9 | 57.6 | 50.9 |
Housing tenure (n = 1667) | |||
Rented | 23.5 | 24.7 | 22.6 |
Owned outright or in part | 76.5 | 75.3 | 77.5 |
Employment status (n = 1680) | |||
Full-time | 57.3 | 65.1 | 46.8 |
Part-time | 11.2 | 7.1 | 15.4 |
Retired | 20.6 | 18.9 | 22.1 |
Not employed, studying or other | 10.9 | 9.0 | 12.7 |
Health characteristics | |||
Self-reported health (n = 1684) | |||
Excellent | 27.1 | 28.0 | 26.2 |
Very good | 36.6 | 35.1 | 38.8 |
Good | 26.9 | 27.9 | 25.1 |
Fair, poor or very poor | 9.4 | 9.1 | 9.9 |
Limited mobility (n = 1666) | |||
Yes | 13.1 | 13.7 | 12.7 |
FIGURE 6.
Places of residence of users intercepted on the busway. Twelve per cent of users intercepted on the busway lived outside the study area denoted by the circle, some of them outside the border of the map. Contains Ordanance Survey MasterMap® Integrated Transport Network Layer™ and Address Layer 2 data © Crown Copyright and Database Right 2015.
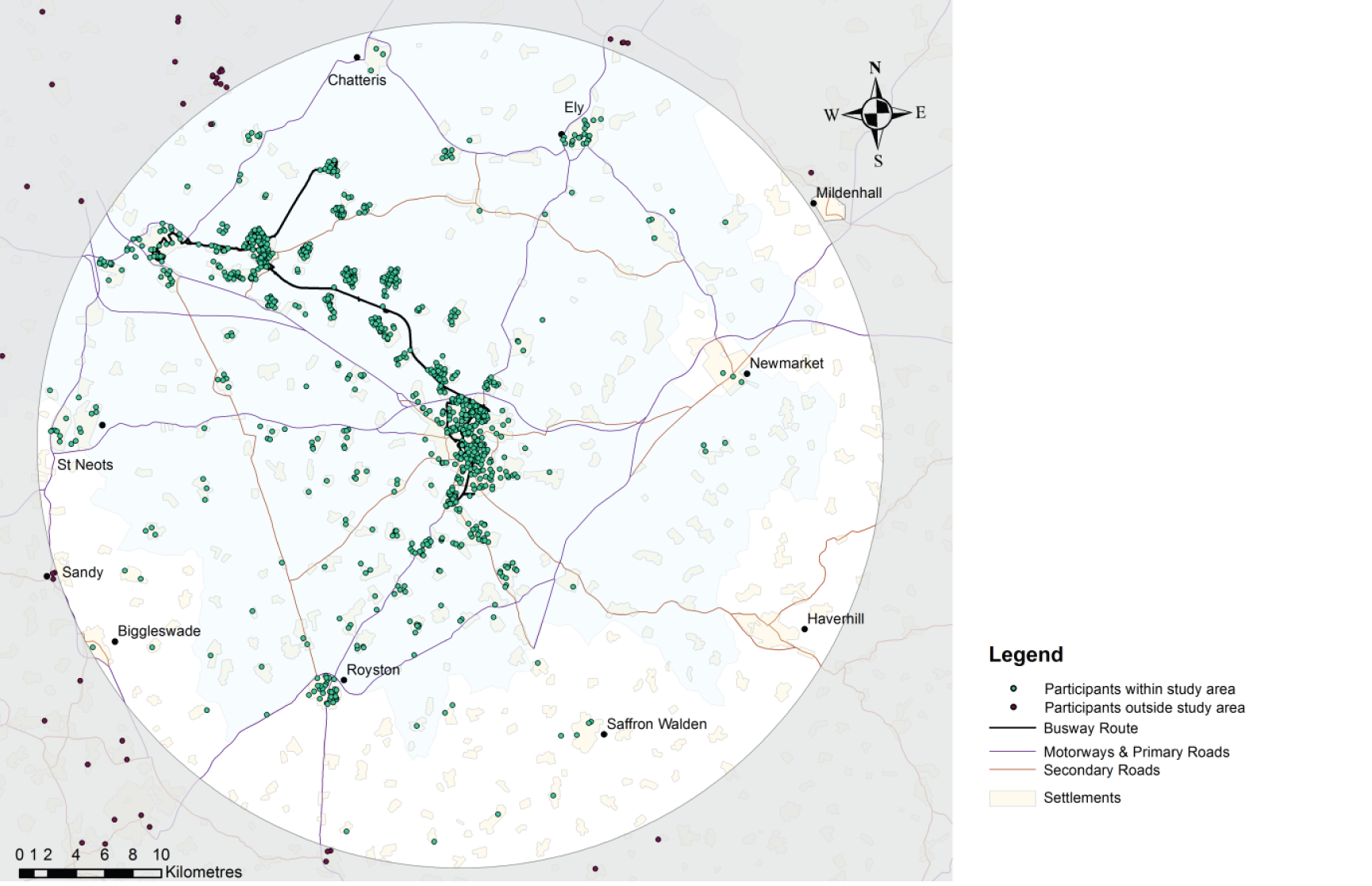
The sample of busway users revealed statistically significant gender differences in use of the busway, in terms of both mode of transport and trip purpose (Table 9). Men were more likely than women to have been using the path for cycling when intercepted, consistent with our general observation that cycling as a mode of transport was more common for men than for women in and around Cambridge (see Chapter 4, Active commuting in context). Correspondingly, more women than men were intercepted while using the guided bus service. Nearly the same number of men and women were intercepted while walking along the path. Just over half of the users surveyed were travelling to or from work, on business or for study, and men and women were approximately equally likely to use the busway for these purposes. Men were more likely to use the busway for leisure trips, while women were more likely to use it for shopping trips.
How the busway was used | Men (%) | Women (%) |
---|---|---|
Mode of transport when approached (n = 1664) | ||
Bus | 49.7 | 63.9 |
Walking | 12.1 | 11.9 |
Cycling | 37.5 | 23.0 |
Other | 1.0 | 1.0 |
Trip purpose (n = 1683) | ||
To or from work or study or on business | 49.5 | 52.0 |
On holiday or day out or for leisure | 21.9 | 11.5 |
Shopping | 7.9 | 16.2 |
Visiting friends or relatives, personal business or other | 20.7 | 20.3 |
Fifty-one per cent of path users reported that they had undertaken moderate physical activity on ≥ 4 of the past 7 days, while 16% reported that they had not engaged in moderate physical activity on any of the past 7 days. In contrast, 29% of guided bus users reported that they had undertaken moderate physical activity on ≥ 4 of the past 7 days, while 31% reported that they had not engaged in physical activity on any of the past 7 days.
The median trip distance reported by busway users was 19.3 km (IQR 9.7–32.2 km), which is more than the average distance from home to work for the participants in our main study cohort (see Chapter 2, Samples). Trip distance varied according to the mode of use of the busway, with median (IQR) trip distances of 24.1 km (16.1–33.8 km), 14.5 km (6.4–27.4 km) and 6.4 km (3.2–17.7 km) for guided bus users, cyclists and walkers, respectively. The median reported trip frequency on the busway in the previous month was 6 (IQR 2–20), which suggested that many participants were using the busway regularly, including for daily commuting.
Perceptions of busway users
Overall, the busway was experienced as having improved transport options and the quality of foot and cycle paths, with weaker overall agreement with statements that it had improved the regularity or reliability of transport services, access to local services or personal safety. Among guided bus users, the most frequently reported benefits were in the range of transport options (83% of participants), the regularity of transport (77%) and the quality of foot and cycle paths (76%). Among path users, the most frequently reported benefits were in the quality of foot and cycle paths (93% of participants), the range of transport options (87%) and personal safety (69%).
A key element of the intercept survey was to capture users’ perceptions of the impact of the busway on their travel behaviour. We asked them what mode of transport they would have used in the absence of the busway for the trip they were currently making, and how the availability of the busway had affected the extent to which they used each of the four main modes of travel.
According to user perceptions, trips on the busway had replaced many car trips, but also some trips that would previously have been walked or cycled, as well as generating some new trips. This pattern of modal substitution differed depending on the mode of transport being used by participants when they were approached for the survey (Table 10). More than 50% of guided bus users indicated that their current trip had replaced a car trip, with much lower proportions reporting that it had replaced a walking (4%) or cycling (3%) trip or that it represented a new trip (8%). Cycle trips were more likely to be described as new trips (15%), and a substantial minority of cycle trips were also said to have replaced car trips (24%).
Mode of transport when intercepted | Number | How users would have made their journey in the absence of the busway (%) | ||||
---|---|---|---|---|---|---|
Bus | Car | Walking | Cycling | No trip | ||
Bus | 960 | 46.0 | 50.2 | 4.0 | 3.4 | 7.7 |
Walking | 200 | 8.0 | 14.0 | 73.5 | 10.0 | 9.0 |
Cycling | 506 | 4.5 | 23.5 | 2.6 | 62.3 | 15.2 |
According to user perceptions, the availability of the guided bus and the path had also affected the overall travel behaviour of the sample. For example, 48% of path users reported that the availability of the path meant that they now cycled more. Substantial minorities of path users also reported that the availability of the bus service meant that they now used the bus more often (43%), cycled more (30%) and used the car less (29%). Similarly, many bus users reported that the availability of guided bus services now meant they used the bus more (58%) and the car less (44%), but bus users also reported that the availability of the path meant that they now walked more (41%), cycled more (41%) and drove less (25%).
The findings of the intercept survey, therefore, indicated a favourable assessment of the busway among many of its users, and provided preliminary quantitative support for the hypothesis that the busway may have influenced travel behaviour in the local population and contributed to increased active commuting or physical activity among some users.
Predictors of busway use
The busway was widely discussed in local media, and walking and cycling on the path began long before the formal opening of the busway and was an important part of the uptake of the intervention. Our core questionnaire data likewise showed that almost all members of our study cohort (more than 99%) were aware of the busway. We went on to study the predictors of the three different modes of use of the busway in the final (2012) phase of our cohort study, with a view to testing the face validity of our measures of intervention exposure (see Chapter 2, Derivation of key variables). 115
People who have recently moved may be more likely to reconsider their commuting behaviour, notwithstanding the results reported earlier (see Chapter 4, Active commuting in context). For this analysis we therefore excluded the 212 members of our cohort who had moved home or workplace at any time between 2009 and 2012. The remaining 453 participants included in this analysis lived on average 6.9 km (SD 8.1 km) from the busway, and their average distance from home to work was 11.4 km (SD 9.5 km). Of these participants, 31% reported having used a guided bus, 40% having cycled on the path and 32% having walked on the path by 2012, albeit not necessarily for commuting.
To account for alternative explanations of busway use in this analysis, we included as covariates the availability of car parking at each participant’s workplace and the shortest distance between each participant’s home and workplace using the pedestrian and cyclist route network, as both of these variables have been shown to influence commuting behaviour (see Chapter 4, Active commuting in context). We found that the availability of the busway made only a small difference to the route lengths and estimated travel times to work for participants. On average, the path shortened the travel distance for participants who had used the path for walking or cycling by approximately 50 m (SD 190 m), and on average the guided bus service reduced the estimated travel time to work for those who had used the guided bus service by just under 1 minute (SD 4 minutes). We found no evidence that these reductions in distance or estimated travel time to work were associated with use of the busway for walking or cycling or for guided bus travel, respectively. Testing the predictive validity of these additional putative exposure measures, while blinded to their relationship with the main travel behaviour-change outcome measures, enabled us to select the most appropriate exposure measure for the subsequent main outcome analyses.
In multivariable logistic regression, we found that residential proximity to the busway was associated with use of the busway for each of the three outcomes studied (Table 11). The strength of the relationship meant that, compared with those living 9 km away, participants living 4 km along the pedestrian and cyclist route network from the nearest guided bus stop were 53% more likely to have used the guided bus service, and those living 4 km from the nearest access point to the path were 34% more likely to have walked along the path and over twice as likely to have cycled on the path.
Outcome | Adjusted OR (95% CI) | ||
---|---|---|---|
Bus usea | Walkingb | Cyclingc | |
Predictor | |||
Proximity to bus stop | 1.53 (1.15 to 2.02) | N/A | N/A |
Proximity to path | N/A | 1.34 (1.05 to 1.70) | 2.18 (1.58 to 3.00) |
Few of the other covariates were associated with any of the three outcomes. Women were less likely than men to report having cycled on the path (OR 0.41, 95% CI 0.24 to 0.69). Living in a rural area increased the odds of having used a guided bus service or having cycled on the path by between two and three times compared with living in an urban area. The association between proximity and use of the guided bus service was also strengthened among those who lived in towns, and that for walking on the path was strengthened among those who lived in towns and villages, compared with those who lived in an urban area. This may partly reflect the fact that in rural areas both the population and the transport infrastructure tend to be more clustered (in and around villages).
The association of use with proximity was stronger for cycling than for walking or bus use. One possible explanation is that the strength of the associations reflected the different ways in which the busway and path were used. It has been proposed that the time for which people are willing to travel to access public transport infrastructure increases with the total trip duration. The data from our intercept survey suggest that, on average, guided bus users may have travelled further than cyclists on each trip. Bus users would, therefore, be expected to be less sensitive than cycle users to a given increment in access distance. Walking on the path as captured by our core questionnaire could reflect a mixture of walking to access the bus service and walking in its own right, which may explain why the association of walking with proximity was intermediate between those for cycling and bus use.
These results, therefore, confirmed the face validity of our graded measure of intervention exposure based on the shortest network distance between each participant’s home and the nearest access point to the busway, and that we should use this – rather than the alternative measures of change in distance or travel time to work induced by the busway – as our exposure measure in the subsequent main outcome analyses. The results further show little association between the sociodemographic factors included in our study and use of the busway, apart from whether participants lived in urban or rural contexts and a further confirmation that women were less likely to cycle than men (see Chapter 4, Active commuting in context).
For further details, see Heinen et al. 115
Linking environmental change with travel behaviour change in commuters
The intercept survey suggested that a substantial minority of busway users had changed their travel patterns in response to the busway. Having shown that residential proximity to the busway was a good measure of exposure to the intervention, we went on to assess the impact of the busway on travel patterns more formally, using information on changes in commuter travel behaviour over time in our study cohort. We examined effects on the proportion of commuting trips undertaken by different modes of transport and time spent in active commuting, and then went on to examine effects on overall physical activity, controlling our analyses for a number of alternative potential explanations of behaviour change. The participants included in these analyses were those who had provided valid data on active commuting in both 2009 and 2012 (see Chapter 2, Samples). In a dropout analysis comparing the 470 members of this longitudinal sample with the 673 participants who had provided valid data only at baseline, those with valid data at follow-up tended to be older (mean age 44.3 vs. 40.9 years; p = 0.001) and more likely to own their home (78.2% vs. 69.2%; p = 0.001) than those without, but there were no significant differences in other sociodemographic characteristics or in time spent in active commuting or physical activity at baseline.
Changes in mode of travel to work
Initially, we used information from the 7-day travel record – in which we ascertained the modes of travel used on each trip to and from work during the past week at baseline in 2009 and at follow-up in 2012 – to study the impact of exposure to the intervention on three outcomes: the proportion of trips that involved any active travel, the proportion of trips that were made entirely by car and the proportion of trips that involved any public transport.
We categorised changes in the first two outcomes in five groups representing a large increase (between 30% and 100%), a small increase (between 0% and 30%), no change, a small decrease (between 0% and 30%) and a large decrease (between 30% and 100%). For example, a participant who previously drove all the way to and from work every day of the week, and now walked to the busway and took a guided bus (and the reverse on the way home) on 1 day each week, would be categorised as having experienced a small (20%) increase in the proportion of trips involving active travel and a small (20%) decrease in the proportion made entirely by car. Another participant who made a similar change on 2 days each week would be categorised as having experienced a large (40%) increase and a large (40%) decrease, respectively. The change in the proportion of trips that involved any public transport was categorised in only three groups representing increase, no change and decrease, to reflect the distribution of this variable, particularly the smaller number of cases in which the proportion had changed.
We took steps to account for a range of alternative explanations of changes in commuting patterns in our analyses. Moving home or workplace may lead not only to a reassessment of commuting options, but also to changes in commute distance, public transport commute travel time or distance from home to the busway, any of which could have prompted changes in commuting patterns. We therefore included continuous variables representing these covariates in our models (see Chapter 2, Derivation of key variables), along with binary variables to account for changes in parking provision at work and the number of children in the household.
The analysis was adjusted sequentially. Initially, we adjusted for distance between home and workplace and for the characteristics of the commute that may change with relocation. Subsequent adjustments included sociodemographic characteristics and the urban–rural context in which participants lived.
We found that a substantial minority of participants had experienced a change in mode share at follow-up (cf. Chapter 4, Patterns of active commuting), with 39% reporting a change in active mode share (with an average change of –1.3%), 37% a change in car mode share (average change +3.4%) and 24% a change in public transport mode share (average change –1.0%). As noted earlier, it is possible that these overall trends, away from active travel and towards the use of the car, reflect the effects of an ageing cohort who tend to move further from work over time (see Chapter 4, Active commuting in context). They contrast with the changes reported in the intercept survey of busway users, who tended to report a shift away from the car and towards more active travel and public transport.
Against this background, we found that living closer to the busway was consistently associated with changes in the commuting outcomes we examined (Table 12). Proximity to the busway was associated with a large increase in active mode share and a large decrease in car mode share. Taking the square root transformation of the exposure variable into account, the results correspond – for example – to participants living 4 km from the nearest access point to the busway being nearly twice as likely to experience a large increase in the proportion of commuting trips that involved any walking or cycling [relative risk ratio (RRR) 1.80, 95% CI 1.27 to 2.55] as those living 9 km away, and approximately twice as likely to experience a large decrease in the proportion of commuting trips made entirely by car (RRR 2.09, 95% CI 1.35 to 3.21). These effect sizes could equally be expressed in terms of any pair of distances representing a one-unit increase in the square root of the distance in km, such as the difference between 1 km and 4 km. Proximity to the busway also protected against a small decrease in active mode share, but it was not associated with changes in public transport mode share (data not shown).
Outcome | Unadjusted model | Adjusted for commute characteristics | Adjusted for commute and sociodemographic characteristics | Maximally adjusted modela |
---|---|---|---|---|
Change in active mode share (RRR and 95% CI) | ||||
Large decrease | 0.94 (0.77 to 1.14) | 1.09 (0.80 to 1.47) | 1.04 (0.75 to 1.44) | 1.08 (0.77 to 1.50) |
Small decrease | 0.77 (0.62 to 0.97)* | 0.57 (0.35 to 0.92)* | 0.47 (0.28 to 0.81)** | 0.47 (0.28 to 0.81)** |
Small increase | 0.99 (0.77 to 1.27) | 0.63 (0.37 to 1.06) | 0.64 (0.35 to 1.15) | 0.69 (0.38 to 1.26) |
Large increase | 1.15 (0.90 to 1.46) | 1.53 (1.14 to 2.06)** | 1.57 (1.14 to 2.15)** | 1.80 (1.27 to 2.55)** |
Change in car mode share (RRR and 95% CI) | ||||
Large decrease | 1.16 (0.86 to 1.57) | 1.71 (1.20 to 2.44)** | 1.71 (1.17 to 2.49)** | 2.09 (1.35 to 3.21)** |
Small decrease | 0.85 (0.68 to 1.07) | 0.60 (0.37 to 0.97)* | 0.59 (0.36 to 0.99)* | 0.64 (0.38 to 1.08) |
Small increase | 0.82 (0.66 to 1.01) | 0.70 (0.45 to 1.07) | 0.65 (0.41 to 1.03) | 0.71 (0.44 to 1.14) |
Large increase | 0.86 (0.70 to 1.05) | 1.13 (0.85 to 1.51) | 1.08 (0.79 to 1.48) | 1.10 (0.80 to 1.52) |
Table 12 shows that most effects of proximity to the busway on mode of travel to work became statistically significant only after adjustment for commute distance and were further strengthened by adjustment for sociodemographic characteristics. The statistical relationship between exposure and behaviour change was, therefore, masked by associations between exposure and some of these covariates, as illustrated for example by the observation that younger and more mobile participants tended to live closer to where the busway was built, as discussed earlier (see Chapter 2, Derivation of key variables). Further adjustment for the relevant baseline commuting pattern of each participant or their car ownership or workplace parking provision did not materially change the results, nor did restricting the analysis to individuals who did not move home or workplace.
We also investigated whether or not the intervention had an effect on the weekly number of commuting trips. This may be important because changes in physical activity and carbon emissions due to the busway would depend not only on changes in the proportion of trips made by different modes of transport, but also on changes in the absolute quantity of travel. However, we found that proximity to the intervention was not associated with a change in the weekly number of commuting trips in our sample.
In summary, against a background of increasing car use and shift away from active travel in our cohort, we found that exposure to the intervention was significantly associated with changes in mode of travel to work after adjustment for multiple potential confounders. Proximity to the busway was associated with a large increase in active travel commute share and a large decrease in car mode share, a pattern consistent in part with the perceptions of busway users found in the intercept survey. The lack of an effect on public transport mode share in the cohort analysis may reflect two factors that reduced statistical power to detect such an effect. The first is that the average change in public transport mode share was much smaller than the average changes in the other mode shares, and the second is the comparatively small proportion of trips (15%) that involved any public transport at baseline.
For further details, see Heinen et al. 116
Changes in time spent in active commuting
We used the same measure of intervention exposure to estimate the effect of the intervention on time spent in active commuting. As noted earlier, this was in the context of a significant decrease in time spent in active commuting in our cohort between 2009 and 2012 (see Chapter 4, Patterns of active commuting). For this analysis changes in time spent in active commuting were categorised in three groups representing increase, no change and decrease, given that a substantial number of participants reported no active commuting at baseline or follow-up. We also conducted a sensitivity analysis using a more stringent definition of change, comparing participants whose active commuting increased or decreased by 50 minutes per week or more with those reporting changes of less than 50 minutes per week (including no change). We also stratified our analysis to compare the effects of the intervention among participants who had reported any active commuting at baseline and those who had not.
As with the previous analyses, we made progressive adjustments to our models to account for potential alternative explanations of changes in active commuting. We adjusted first for age and gender, and then for further sociodemographic characteristics that we had previously found to be associated with active commuting and changes in active commuting (see Chapter 4, Active commuting in context). At this stage we also adjusted for participants’ active commuting at baseline, to account for the possibility that high (or low) levels at the outset might have been associated with particular changes in active commuting at follow-up. We included further adjustments for major changes in life circumstances ascertained at follow-up, in particular whether a participant had moved home or work during the study (see Chapter 2, Samples).
In initial multivariable multinomial regression models adjusted only for age and gender, exposure to the busway was associated with change (both increase and decrease) in total weekly time spent in active commuting and change (both increase and decrease) in time spent cycling for commuting (Table 13). After further adjustment, the effects of exposure to the busway on overall time spent in active commuting ceased to be statistically significant, but the association with an increase in weekly cycle commuting time persisted, and participants living 4 km from the busway were one-third more likely to have increased their cycle commuting time than those living 9 km away (RRR 1.34, 95% CI 1.03 to 1.76). Among those who had increased their cycle commuting time, the increase was often substantial, with a mean increase in this group of 87 minutes per week. The effect of exposure to the busway was somewhat strengthened in the sensitivity analysis restricted to larger changes, in which participants living 4 km from the busway were 44% more likely to have increased their cycle commuting by 50 minutes per week or more than those living 9 km away (RRR 1.44, 95% CI 1.03 to 2.03).
Outcome: change in activity (minutes per week) | N | Mean (SD) | RRR (95% CI) | ||
---|---|---|---|---|---|
Model 1a | Model 2b | Model 3c | |||
Active commuting | 454 | ||||
No change | 122 | 0 (0) | (Reference) | ||
Increase | 136 | 80.7 (70.9) | 1.31 (1.11 to 1.56)*** | 1.14 (0.90 to 1.45) | 1.14 (0.90 to 1.46) |
Decrease | 196 | –81.8 (69.0) | 1.34 (1.14 to 1.57)*** | 1.06 (0.83 to 1.37) | 1.07 (0.83 to 1.37) |
Walking for commuting | 456 | ||||
No change | 297 | 0 (0) | (Reference) | ||
Increase | 76 | 73.4 (66.6) | 0.84 (0.71 to 1.00) | 0.90 (0.69 to 1.19) | 0.90 (0.69 to 1.18) |
Decrease | 83 | –84.7 (70.8) | 0.90 (0.76 to 1.07) | 1.13 (0.83 to 1.55) | 1.13 (0.83 to 1.55) |
Cycling for commuting | 468 | ||||
No change | 214 | 0 (0) | (Reference) | ||
Increase | 108 | 86.6 (74.0) | 1.66 (1.37 to 2.03)*** | 1.34 (1.03 to 1.76)* | 1.34 (1.03 to 1.76)* |
Decrease | 146 | –85.9 (67.6) | 1.53 (1.30 to 1.81)*** | 1.00 (0.73 to 1.36) | 1.00 (0.73 to 1.37) |
Although there was no evidence of a statistically significant effect of exposure to the intervention on active commuting in the sample as a whole, there was a significant effect among those who did not report any active commuting at baseline. Among this subgroup of participants, those living 4 km from the busway were 76% more likely to have increased their active commuting time than those living 9 km away (RRR 1.76, 95% CI 1.16 to 2.67), whereas there was no effect on overall time spent in active commuting among the subgroups of participants who reported either 1–149 minutes per week or 150 minutes per week or more of active commuting at baseline.
In summary, exposure to the intervention was associated with an increase in time spent cycling to and from work, and with an increase in overall time spent in active commuting among those who reported no active commuting at baseline. We found no evidence of an effect of proximity to the busway on changes in time spent walking on the way to and from work. This may reflect the lower level of walking, relative to cycling, for commuting in our sample, and that changes in walking were reported by fewer participants than changes in cycling (see Chapter 4, Patterns of active commuting).
For further details, see Panter et al. 117
Changes in time spent in physical activity
We went on to investigate whether or not proximity to the busway was associated with changes in three outcomes reflecting broader impacts on physical activity (see Chapter 2, Derivation of key variables). Our analysis followed the same approach, except that the outcomes were categorised into tertiles (corresponding approximately to an increase, no change and a decrease) because no participants reported exactly the same quantity of physical activity at both time points.
In contrast to the background changes in time spent in active commuting, we found that time spent in recreational and total physical activity changed little in our cohort between 2009 and 2012. The median total time spent in physical activity was 423 minutes per week (IQR 232–675 minutes per week) at baseline and 407 minutes per week (IQR 240–631 minutes per week) at follow-up, and the difference was not statistically significant (p = 0.12). A similar pattern was observed for time spent in recreational physical activity (baseline: median 282 minutes per week, IQR 150–523 minutes per week; follow-up: median 279 minutes per week, IQR 146–480 minutes per week; p = 0.28).
In multivariable multinomial regression models, we found no evidence of a significant effect of proximity to the busway on the time spent in recreational or total physical activity. In the minimally adjusted model, proximity to the busway was associated with both an increase and a decrease in time spent cycling for commuting and recreation combined (shown in the table as ‘total cycling’). The association with a decrease in cycling ceased to be statistically significant after further adjustment, but the association with an increase persisted. In the maximally adjusted model, those living 4 km from the busway were 32% more likely to report an increase in time spent cycling for commuting and recreation combined than those living 9 km from the busway (RRR 1.32, 95% CI 1.04 to 1.68). No such association was observed for ‘total walking’ or ‘total walking and cycling’ (Table 14).
Outcome: change in activity (minutes per week, tertiles) | N | Mean (SD) | RRR (95% CI) | ||
---|---|---|---|---|---|
Model 1a | Model 2b | Model 3c | |||
Total walking and cycling | 469 | ||||
Middle tertile (≈no change) | 156 | –7.7 (28.7) | (Reference) | ||
Highest tertile (≈increase) | 156 | 223.9 (264.2) | 1.11 (0.95 to 1.30) | 1.21 (0.96 to 1.52) | 1.21 (0.96 to 1.52) |
Lowest tertile (≈decrease) | 157 | –246.1 (297) | 1.02 (0.88 to 1.19) | 1.17 (0.92 to 1.47) | 1.17 (0.92 to 1.47) |
Total walking | |||||
Middle tertile (≈no change) | 158 | 2.4 (20.6) | (Reference) | ||
Highest tertile (≈increase) | 157 | 179.9 (213.6) | 1.01 (0.86 to 1.18) | 1.00 (0.80 to 1.25) | 1.00 (0.80 to 1.25) |
Lowest tertile (≈decrease) | 154 | –188.3 (256.8) | 0.99 (0.85 to 1.16) | 0.93 (0.74 to 1.18) | 0.94 (0.74 to 1.18) |
Total cycling | |||||
Middle tertile (≈no change) | 168 | –1.5 (4.5) | (Reference) | ||
Highest tertile (≈increase) | 158 | 113.5 (151) | 1.65 (1.38 to 1.96)*** | 1.32 (1.04 to 1.68)* | 1.32 (1.04 to 1.68)* |
Lowest tertile (≈decrease) | 143 | –123.3 (184) | 1.56 (1.33 to 1.84)*** | 1.20 (0.94 to 1.53) | 1.20 (0.94 to 1.54) |
Total recreational physical activity | |||||
Middle tertile (≈no change) | 157 | –5.5 (41.2) | (Reference) | ||
Highest tertile (≈increase) | 156 | 323.4 (455.0) | 0.95 (0.81 to 1.12) | 0.98 (0.78 to 1.22) | 0.98 (0.78 to 1.22) |
Lowest tertile (≈decrease) | 156 | –370.16 (439.0) | 0.95 (0.81 to 1.12) | 0.88 (0.69 to 1.13) | 0.88 (0.69 to 1.13) |
Total physical activity | |||||
Middle tertile (≈no change) | 156 | –14.5 (51.4) | (Reference) | ||
Highest tertile (≈increase) | 157 | 390.4 (475.7) | 1.02 (0.88 to 1.20) | 1.06 (0.85 to 1.32) | 1.06 (0.84 to 1.32) |
Lowest tertile (≈decrease) | 156 | –494.6 (596.4) | 1.09 (0.93 to 1.28) | 1.00 (0.77 to 1.29) | 1.00 (0.77 to 1.29) |
In summary, we found that exposure to the intervention was associated with an increase in time spent cycling for commuting and recreation combined, with an effect size very similar to that for time spent in cycling for commuting alone. We found no evidence of an association with an increase in recreational or total physical activity.
For further details, see Panter et al. 117
Changes in carbon emissions attributable to commuting
While the busway had complex effects on commuting patterns, exposure to the intervention was associated with a shift away from the car and towards active modes of travel for the journey to and from work and a significantly greater likelihood of an increase in weekly time spent cycling. We went on to assess the impact of the busway on carbon emissions, using the same general approach as in the preceding outcome analyses. Specifically, we investigated whether proximity to the busway was associated with an increase or a decrease in carbon emissions attributable to commuting trips in our cohort.
We found that, for commuting trips made by public transport and not involving use of a car, the mean estimated carbon emissions were 1.38 kg per trip in 2009 and 1.78 kg per trip in 2012. For commuting trips involving any use of a car (with or without public transport), the mean of the estimated carbon emissions ranged from 2.70 kg per trip for car-only trips in 2009 to 3.32 kg per trip for trips made by car plus public transport in 2012. We therefore defined participants with a change in their estimated carbon emissions of < 3 kg per week – approximating to the emissions attributable to one ‘average’ one-way car-based commuting trip in our sample – as having made only a small change, and included them with participants who reported no use of motor vehicles and thus no carbon emissions at either time point in our reference outcome category of ‘no change’.
In unadjusted multinomial models, we found that proximity to the busway was associated with a reduced likelihood of both a decrease and an increase in carbon emissions over time. This pattern remained when the models were adjusted for age and gender. After further adjustment for all other covariates, however, the counterintuitive association between proximity and a reduced likelihood of a decrease in carbon emissions ceased to be statistically significant, and proximity to the busway remained associated only with a reduced likelihood of an increase in carbon emissions (RRR 0.57, 95% CI 0.43 to 0.75). In other words, for those living 4 km from the busway, the likelihood of an increase in commuting carbon emissions was approximately half that for those living 9 km from the busway. The other covariates associated with either a decrease or an increase in carbon emissions in maximally adjusted models were education, car ownership, baseline carbon emissions, urban–rural status and moving, all with effects in the expected direction. We further found that the effect estimate for the intervention was only minimally affected by removing workplace car parking, urban–rural status or baseline carbon emissions from the models. Excluding those who had moved home from the analysis led to a strengthening of the effect (albeit with a wider CI owing to the smaller sample size: RRR 0.44, 95% CI 0.26 to 0.73), which may reflect the fact that moving home tended to be associated with a lengthening of the commuting journey in our cohort.
Investigating causal mechanisms
Having estimated the size of the effect of the busway on commuter travel behaviour, we went on to investigate the mechanisms by which the intervention may have led to behaviour change. Identifying plausible mechanisms in this way can increase understanding of an intervention and provide further support for causal inference. 118 As we found no evidence of a significant effect on walking, this analysis was focused on elucidating mechanisms leading to an increase or decrease in time spent cycling for commuting.
We identified three groups of mediating factors that could be involved in this causal pathway: self-reported use of the path along the busway, changes in perceptions of the route environment between home and work and changes in attitudes and beliefs relating to car use (see Chapter 2, Derivation of key variables). We further hypothesised that the most likely mechanisms would be those that included only significant associations between variables (p < 0.05 when adjusted for covariates but not the other mediators). On this basis, we first calculated all statistically significant associations between the various exposure, mediator and outcome variables. We then reduced the complexity of the model by excluding any mediators that were associated only with variables from within the same group of mediators (e.g. perceptions of the route environment), as well mediators that were not on a pathway between exposure and outcome, and eliminated mechanisms that contained more than two mediators from within the same group of mediators.
The resulting model identified one direct association between proximity to the busway and an increase in time spent cycling, as well as several indirect pathways (Figure 7). The direct association was equivalent to that reported in the previous section. The model further showed that although there was no direct association between exposure and a decrease in time spent cycling, there were two indirect pathways leading to this outcome.
FIGURE 7.
Mediators of changes in time spent in cycle commuting. This is a reduced model showing only those associations significant at p < 0.05. The dashed line is unidirectional towards more cycling.
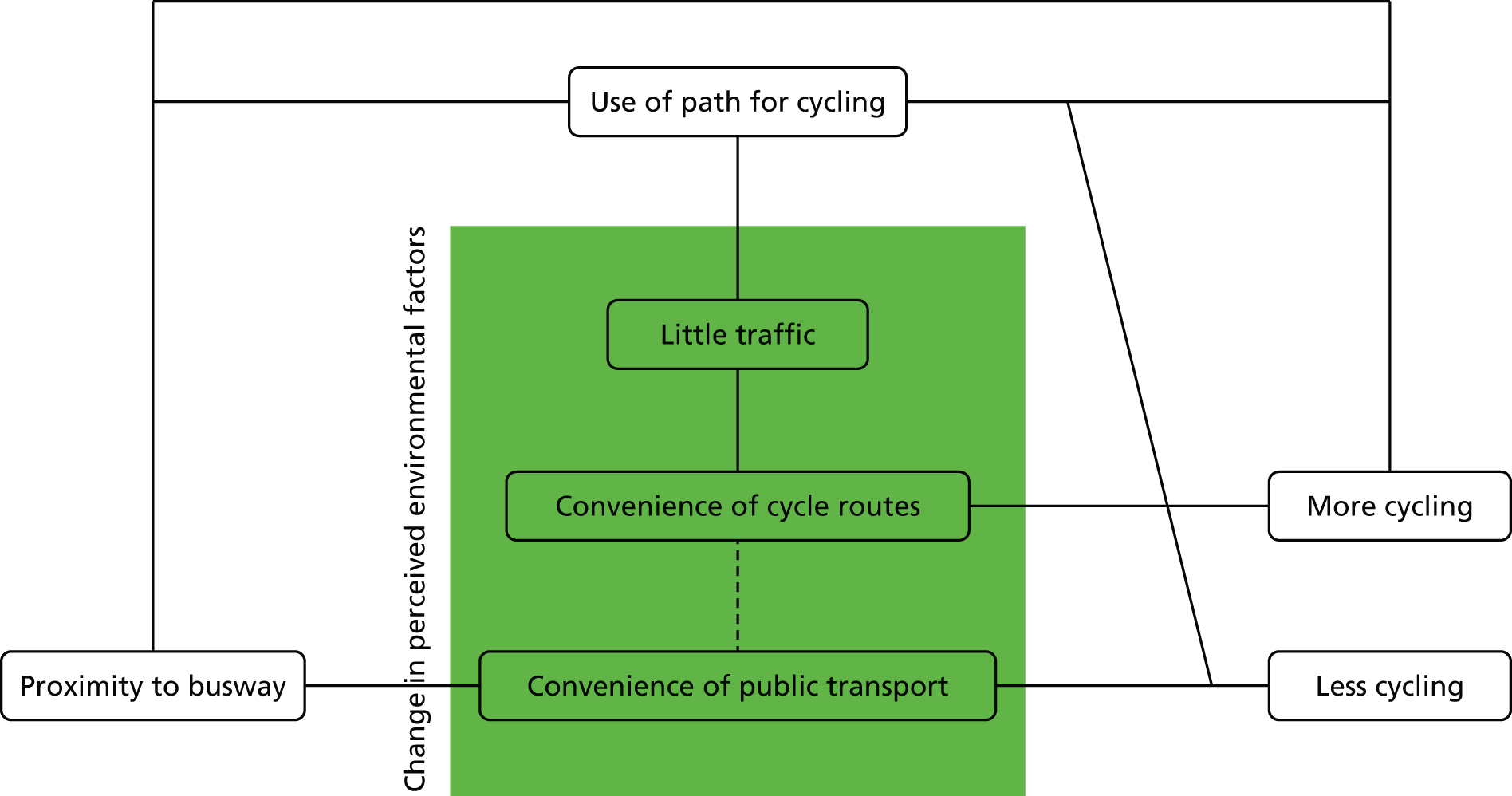
We then used path regression analysis to test which of these pathways were statistically significant overall. We found that the indirect pathways via use of the path leading to either an increase or a decrease in cycle commuting time were statistically significant and explained most of the effect of the intervention. The indirect pathway towards an increase in cycling explained 86% of the overall effect, while the direct pathway between exposure and outcome explained only 13% of the effect. None of the other pathways towards an increase in cycle commuting time via perceptions of the route environment was statistically significant. Similarly, the indirect pathway towards a decrease in cycling explained 96% of the overall effect, while the indirect pathway via convenient public transport was not statistically significant.
We further divided our sample and repeated the analysis separately for those reporting (a) no active commuting and (b) any active commuting at baseline. This led to the identification of six possible pathways linking proximity to the busway to changes in cycling. In the group reporting no active commuting at baseline, only the indirect pathway linking proximity with an increase in cycling via use of the path was statistically significant, and this explained 80% of the overall effect. Among those who already reported some active commuting at baseline, proximity to the busway was associated both with an increase and a decrease in cycling, and only the indirect pathways via use of the path were statistically significant.
These results showed that of a number of plausible mechanisms tested, self-reported use of the path explained the majority of the association between proximity to the busway and an increase in time spent cycling for commuting, while changes in perceptions of the environment or changes in Theory of Planned Behaviour constructs were not significant in mediating the observed changes in commuting behaviour. It should be noted that that if the questionnaire items used to ascertain these cognitive constructs had been framed differently, the analysis might have suggested stronger support for their role as mediators. Nevertheless, showing that the effect of proximity to the busway on commuting behaviour was largely explained by use of the busway provides further evidence against the possibility that the effects observed in the main outcome analyses could have been attributable to confounding, and indicates a plausible mechanism by which the busway may have changed commuting behaviour: where people live near new infrastructure that supports active commuting and begin to use it (for any purpose), this may lead to changes in their overall commuting behaviour. Contextual factors may have been important in bringing about such an outcome. In particular, the intervention we studied was implemented in a context in which cycle commuting was already common and compatible with high social status, while car commuting was comparatively burdensome owing to congestion and difficulties with parking. All of these factors might have encouraged participants to trial the path along the busway and then to use it more consistently for cycling. In addition, these results suggest that the association between proximity to the pathway and decreases in weekly cycle commuting time in the minimally adjusted models reported earlier represented a real effect of the busway among some people, particularly those with higher levels of active commuting at baseline.
Contextualising and interpreting the effects
Although exposure to the intervention affected commuting patterns, not all participants used the guided bus service or walked or cycled on the path. In this section, we explore why commuters may or may not have used the busway through an analysis of qualitative interview data collected after the formal opening of the busway.
Given that the main study cohort of commuters was more educated and more affluent on average than the local population, we widened recruitment for these interviews to include people who had taken part in our intercept survey of busway users, and we purposively recruited participants from lower socioeconomic groups. The group of people who took part in this interview study comprised 15 intercept survey participants and 23 members of the main cohort, and was relatively gender-balanced, with 55% women. Four participants were not in paid employment. During the interviews, we explored participants’ commuting experiences and choices, before asking them about the impact of the busway on their travel behaviour. In addition, we used two ‘vignettes’ constructed from the quantitative predictors of maintenance and uptake of active travel (see Chapter 4, Patterns of active commuting) to elicit reactions about active commuting and its alternatives. The first vignette focused on positive attitudes towards active commuting and public transport, while the second contrasting vignette depicted the experience of car use as it emerged from our study (see Appendix 5).
Consistent with some of our observations above, interviewees reported that the busway affected their choices and opportunities differently depending on where they lived and worked. Those who were able to take advantage of the quicker, off-road sections of the guided bus service rather than the slower, on-road sections, or who were able to use the relatively junction-free cycle path, described the busway as ‘efficient’ or ‘convenient’. An added benefit of the busway was that it eliminated the stresses and costs associated with parking a car. The busway was thus particularly relevant to commuters if it was located on their commuting route and able to replace a previous mode of transport altogether. In contrast, those who had cause to assess the performance of the bus service within the city on the normal road network reported that it was not necessarily faster than driving or using regular buses. In other words, what was regarded as a novel, viable, quasi-tramway service by some was experienced as no different from an ordinary bus service by others.
Even so, we found that the mechanisms by which the busway could lead to changes in commuting behaviour were sometimes more complex. Some commuters had trialled using parts of the busway as they became available to see if it could serve their needs. This could also lead to a trial of active commuting. One participant reported that she had tried parking at the new park-and-ride site and then cycling along the busway to avoid having to park at her workplace.
In 2009 I would have driven to work and parked on site, because that’s really the only option. I live about 23–25 miles away, and the buses would have taken me quite a long time to get to work, so I would have driven and parked on site. Then there was the opportunity to park at the Trumpington Park-and-Ride and cycle along the guided busway, so I opted to try some of those.
Woman, 60s, who used to drive to work and now commuted by car to a park-and-ride site and then cycled along the busway in summer, or used the guided bus in winter
We saw earlier that the experience of commuting, or the space in which commuting takes place, was important to people. These factors were also mentioned in assessments of the busway. Crowding and the proximity of other people on buses was described by some interviewees as unappealing and as a reason not to use public transport, especially in the light of the higher price of tickets. The availability of more comfortable seating, free internet access or power sockets was seen as inconsequential to those users who considered the busway to be no different from regular bus services. However, other participants for whom the busway presented a viable travel option rated the space created by the busway positively, for example because it provided more generous seating and avoided traffic congestion.
It’s somewhere you can relax and sort of not get stressed by driving and things like that, which I find has been a real difference.
Man, 50s, who used to share a car journey to work and now commuted by guided bus four times per week and by car on other days
Variation in how participants experienced the space created by the busway was particularly pronounced in respect of the path. Some cyclists rated the ‘off-road’ and remote nature of the path negatively, while others, particularly those with less confidence or experience, appreciated the path because it involved fewer safety concerns than when cycling on the road.
There is just almost no chance of impact, the buses can’t get you because they’re in the busway and there are no cars allowed anywhere near.
Man, 50s, who commuted by car or occasionally by cycling along the busway
Participants trialled the busway in part because the busway was ‘there’, and our interview data indicated that active commuting could be taken up as a result of such trials. One participant reported that she tried the busway because it had been provided. Another had tried walking 1 or 2 days per week, but then discovered her ability to do so more often. This provides contextual elaboration of our account of exposure to the intervention, but also ties in with the observation that the uptake of a new mode of transport is best understood as a haphazard process, which often starts with a trial and, subsequently, may be incorporated into more habitual travel behaviour over time.
I thought it’s being provided, it’s been a long time coming, I really should give it a try, and I did find that it suited me, both time, frequency, cost. [. . .] and then it’s successful so I’ve go[ne] with it, yes.
Woman, 60s, who used to drive to work and now commuted by car to a park-and-ride site and then cycled along the busway in summer, or used the guided bus in winter
I know when I first started doing it [walking along the busway to the park-and-ride site] I thought, ‘Oh, I’ll do it once a week, twice a week,’ which is what I did, actually. [. . .] And then I thought, ‘Well actually, I can do it every day,’ but it was a question of building up to it.
Woman, 50s, who used to drive to work and now commuted by car to a park-and-ride site and then walked or used the guided bus
In summary, our post-intervention interviews suggested that although people were unlikely to use the new infrastructure unless it closely matched the journeys they needed to make, a range of other salient factors informed travel behaviour, and these were dependent on the value individuals attributed to different aspects of their journey experience. Generally, these involved considerations of comfort, ambience or pleasantness, and considerations of feeling safe, which could trump considerations of reliability and speed. Responses to the busway were diverse; what was described as a barrier by some, for example the discomfort of overcrowded bus travel or cycling on a remote path, was experienced as a facilitator by others, such as not having to negotiate traffic congestion as a bus passenger or enjoying car-free off-road cycling. These experiences, albeit complex and ambiguous, culminated in meaningful travel behaviour change for some, through a process of shifts in the balance between influential factors, planning and adopting the environmental change over time.
For further details, see Kesten et al. 119
Linking active commuting with health benefits
Exposure to the intervention was associated with an increase in time spent cycling, but we found no evidence of a direct association with an increase in total physical activity. However, the study lacked statistical power to detect such an effect, and active commuting is associated with health benefits in its own right. 120 We therefore went on to explore the longitudinal associations between active commuting and overall physical activity and selected short-term health outcomes in our study cohort. We first investigated whether or not changes in active commuting were associated with changes in self-reported recreational and total physical activity. We then investigated the extent to which active commuting was associated with sickness absence from work, physical and mental well-being and BMI at follow-up.
Changes in time spent in physical activity
The distribution of time spent in active commuting was skewed at baseline and follow-up, as discussed earlier (see Chapter 4, Patterns of active commuting). In our analysis of longitudinal associations between active commuting and self-reported physical activity in the sample used for the main outcome analyses reported above, we therefore categorised exposure to active commuting as an increase, no change or a decrease in weekly time spent walking, cycling or in active commuting over time, and examined associations with changes in physical activity over the same time period categorised as tertiles. The highest tertile represented a large increase (mean +390 minutes per week for total physical activity and +323 minutes per week for recreational physical activity), and the lowest tertile represented a large decrease in physical activity (mean –495 minutes per week for total physical activity and –370 minutes per week for recreational physical activity). Covariates were added to the analysis in steps and the final model was adjusted for age, gender, car ownership, access to a bicycle, distance from home to work, baseline BMI and baseline total physical activity.
In maximally adjusted multinomial regression models, a decrease in active commuting was associated with a greater likelihood of a large decrease in total physical activity (RRR 2.1, 95% CI 1.1 to 4.1). In other words, participants who reported a decrease in active commuting between 2009 and 2012 were approximately twice as likely to report a large decrease in their total physical activity as those who reported an increase in active commuting. Conversely, an increase in active commuting was associated with a greater likelihood of a large increase in total physical activity (RRR 1.8, 95% CI 1.0 to 3.4), although this result was of borderline statistical significance (p = 0.06).
We further found that an increase in cycle commuting time was associated with a greater likelihood of both a large increase (RRR 2.5, 95% CI 1.2 to 5.0) and a large decrease (RRR 3.0, 95% CI 1.4 to 6.3) in total physical activity. A post-hoc t-test indicated that participants whose cycle commuting increased and total physical activity decreased reported significantly higher baseline physical activity than those whose commuting and total physical activity both increased (930 vs. 471 minutes per week; p = 0.001). Finally, we found no associations between changes in time spent walking for commuting and total physical activity, or between any of the active commuting exposures and self-reported recreational physical activity.
For further details, see Foley et al. 121
Changes in health and well-being
We also investigated whether or not walking and cycling for commuting predicted sickness absence from work, physical and mental well-being, and BMI at follow-up.
We analysed these longitudinal associations separately for time spent walking for commuting and time spent cycling for commuting. We categorised each of these exposures in respect of whether it was reported at all at both baseline and 1-year follow-up (yes or no) and whether or not it had changed over that time period (increased, decreased or not changed). We then tested whether or not maintenance of these behaviours predicted each of the four health indicators ascertained at 1-year follow-up, and if changes in the behaviours predicted changes in each of the four health indicators over the same time period. We also adjusted each analysis for the value of the respective outcome indicator at baseline. To increase our sample size, and because this analysis was not focused on the effects of the busway, we pooled data across all three 1-year transitions available in the study, that is those between 2009 and 2010, between 2010 and 2011 and between 2011 and 2012. Each participant was included only once in each analysis, based on the first year of the study in which they had returned a questionnaire with a completed travel-to-work record. The regression analyses were adjusted for potential confounders, as detailed below.
We found that maintenance of cycle commuting predicted three of the four health indicators 1 year later (Table 15). On average, those who reported maintaining cycle commuting reported lower sickness absence, higher mental well-being and lower BMI at 1-year follow-up than those who reported no cycle commuting. The effect size was equivalent to 1.2 (p = 0.005) or 1.1 (adjusted for baseline sickness absence; p = 0.004) fewer days of sickness absence over 1 year. This compares with a mean of 3.3 self-reported sick days per year (SD 11.1 days) in the cohort. The effect sizes for physical and mental well-being were of a similar order of magnitude as those we found in our earlier cross-sectional analysis (see Chapter 4, Relationships with physical activity, health and well-being), although the findings for physical well-being were non-significant and the findings for mental well-being became non-significant after adjustment for baseline mental well-being. The difference in BMI was also attenuated and became non-significant after adjustment for baseline BMI.
Exposure | Cycling for commuting | Walking for commuting | ||
---|---|---|---|---|
Analysis | Baseline as predictor of follow-up (680 < n < 691) | Change as predictor of change (n = 801) | Baseline as predictor of follow-up (649 < n < 659) | Change as predictor of change (788 < n < 801) |
Outcome | ||||
Physical well-being: model 1 | 1.08 (95% CI –0.07 to 2.23) | I: 0.94 (95% CI –0.22 to 2.11) | –0.18 (95% CI –1.39 to 1.03) | I: –0.07 (95% CI –1.26 to 1.13) |
D: 0.89 (95% CI –0.89 to 1.07) | D: –0.07 (95% CI –1.31 to 1.15) | |||
Physical well-being: model 2 | 0.87 (95% CI –0.17 to 1.93) | I: 1.01 (95% CI –0.47 to 2.07) | –0.15 (95% CI –1.27 to 0.97) | I: –0.21 (95% CI –1.29 to 0.88) |
D: –0.31 (95% CI –1.33 to 0.69) | D: –0.22 (95% CI –1.34 to 0.90) | |||
Mental well-being: model 1 | 1.50 (95% CI 0.10 to 2.97)* | I: 0.20 (95% CI –1.26 to 1.65) | –1.36 (95% CI –2.86 to 0.14) | I: 0.93 (95% CI –0.56 to 2.42) |
D: –0.11 (95% CI –1.51 to 1.29) | D: –0.14 (95% CI –1.68 to 1.39) | |||
Mental well-being: model 2 | 0.82 (95% CI –0.42 to 2.08) | I: 0.69 (95% CI –0.59 to 1.97) | –0.65 (95% CI –1.99 to 0.68) | I: 0.38 (95% CI –0.93 to 1.69) |
D: –0.18 (95% CI –1.41 to 1.05) | D: –0.44 (95% CI –1.78 to 0.91) | |||
Sickness absence: model 1 | –0.46 (95% CI –0.80 to –0.14)** | I: –0.40 (95% CI –1.51 to 0.72) | 0.20 (95% CI –0.13 to 0.53) | I: –0.65 (95% CI –1.79 to 0.48) |
D: 0.03 (95% CI –1.03 to 1.10) | D: –0.41 (95% CI –1.58 to 0.76) | |||
Sickness absence: model 2 | –0.46 (95% CI –0.77 to –0.14)** | I: –0.37 (95% CI –1.33 to 0.59) | 0.12 (95% CI –0.19 to 0.43) | I: –0.33 (95% CI –1.31 to 0.66) |
D: –0.14 (95% CI –1.06 to 0.79) | D: 0.20 (95% CI –0.81 to 1.22) | |||
BMI: model 1 | –0.77 (95% CI –1.47 to –0.07)* | I: 0.11 (95% CI –0.14 to 0.36) | –0.38 (95% CI –1.13 to 0.37) | I: –0.24 (95% CI –0.49 to 0.02) |
D: 0.12 (95% CI –0.12 to 0.36) | D: 0.24 (95% CI –0.02 to 0.51) | |||
BMI: model 2 | 0.02 (95% CI –0.39 to 0.43) | I: 0.09 (95% CI –0.16 to 0.34) | –0.20 (95% CI –0.64 to 0.23) | I: –0.25 (95% CI –0.51 to 0.00) |
D: 0.11 (95% CI –0.13 to 0.35) | D: 0.24 (95% CI –0.02 to 0.51) |
Changes in active commuting were associated with changes in the health indicators in two ways. First, an increase in time spent cycling for commuting was associated with a net increase of approximately one unit in the PCS-8 score after adjustment for potential confounders and baseline PCS-8. Second, an increase in time spent walking to work predicted a net decrease in BMI of 0.2 kg/m2, with a reduction in time spent walking to work predicting a net increase of 0.2 kg/m2. Although not statistically significant, these associations are consistent with the hypothesis that active commuting has positive effects on health and well-being.
In summary, changes in active commuting were associated with commensurate changes in total self-reported physical activity over a 3-year period of observation and we found no evidence of a compensatory change in self-reported recreational physical activity. We further found that active commuting was associated with lower sickness absence at work and improved indicators of health and well-being in some analyses, albeit with modest effects at the individual level.
For further details, see: Mytton et al. 122
Conclusion
Using a variety of approaches to better understand both the intervention and its effects, and by accounting for a range of other possible explanations, we have been able to isolate the effect of the intervention on commuting behaviour change. We were able to show that proximity to the busway was associated with an increased likelihood of a large increase in commuting trips involving any active travel and of a large decrease in the proportion of trips made only by car. Proximity to the busway was also associated with a reduced likelihood of an increase in carbon emissions. Proximity to the busway was further associated with an increased likelihood of an increase in weekly cycle commuting time, and of an increase in weekly active commuting time among those who reported no active commuting at baseline. These effects were not just a result of small changes in individual behaviour, but reflected substantial average increases in time spent cycling to and from work. We found no evidence that proximity to the busway was associated with an increase in overall physical activity – or with a decrease in overall physical activity – but we found that proximity to the busway was associated with an increased likelihood of an increase in total cycling time, that is for commuting and recreation combined. Our investigation of the mechanisms by which the busway had an effect on commuting behaviour suggested that the majority of the effect on time spent cycling was through the use of the path. Finally, we showed that active commuting was associated with favourable physical activity and health and well-being outcomes in longitudinal observational analyses.
Chapter 6 Discussion
Introduction
Promoting physical activity in the population at large forms a key part of national and international strategies to reduce the burden of non-communicable disease. Active travel, in particular active commuting, can provide a feasible way for people to incorporate more physical activity in their daily lives. We took the opportunity presented by the construction of the Cambridgeshire Guided Busway to conduct a natural experimental study, the Commuting and Health in Cambridge study, which used a combination of quantitative and qualitative research methods to investigate active commuting and assess the effects of improving transport infrastructure to support walking, cycling and public transport. In this chapter, we begin by summarising the principal findings of the study. We go on to discuss the contributions and implications of our findings for three aspects of public health science: our understanding of active commuting as a source of health and well-being and a target for public health intervention; the evidence linking environmental change with travel and physical activity behaviour change; and the methods for public health research in this field. We then give a brief account of our experience of engaging with key stakeholder groups in the course of the study. Last, we summarise the conceptual and methodological challenges we faced, the strengths and limitations of the ways in which we addressed these, and the implications of our findings and experience for future research. Our discussion is necessarily focused on a few selected issues arising from the large body of research summarised in the report. Further discussion of many of the more specific or technical issues can be found in the relevant publications (see Acknowledgements).
Principal findings
Understanding active commuting
Despite 85% of the sample having access to a car, active commuting was a highly prevalent behaviour in our cohort, reflecting the background prevalence of cycling in Cambridge and the selection of largely healthy workers into the cohort. Although time spent in physical activity overall changed little over the duration of the study, time spent in active commuting declined and this was mostly explained by a decrease in time spent cycling. Commuters reported using a great variety of modes and combinations of modes of transport on the journey to and from work, and walking and cycling were often incorporated into longer journeys by car or public transport. On average, 20% of the duration of these multimodal trips was spent above the moderate physical activity intensity threshold, indicating their substantial potential contribution to commuters’ weekly volume of physical activity. Those who spent more time in active commuting were more likely to record higher levels of overall physical activity as measured by accelerometer, and changes in active commuting were associated with commensurate changes in overall physical activity over a 3-year period of observation. Cycle commuting in particular was associated with lower sickness-related absence at work and improved well-being indicators 1 year later, albeit with modest effects at the individual level, and qualitative narratives also indicated experiences of well-being related to active commuting.
Understanding commuting as a complex behaviour led us to explore how it was shaped by complex motivations and circumstances, with a particular focus on social and environmental factors. The convenience, pleasantness, speed, reliability and safety of the transport options available were important considerations in making commuting choices, but these choices were often not straightforward. For example, cycling might have been chosen as the fastest mode of transport, but detours could be incorporated to make a given journey safer and more pleasant away from traffic. Similarly, commuters could have positive attitudes towards one mode of transport, such as the car, while also using another, such as walking or cycling. Commuters tactically accommodated their commute within a broader set of social and physical constraints and contexts. Commuting practices differed between men and women and were shaped by relationships and responsibilities within and beyond the immediate household, for example the need to care for children or elderly relatives. The ability to live closer to work or to be flexible about the timing of the commute was related to higher socioeconomic position, and changing life circumstances such as becoming parents or home owners often required commuters to accept longer journeys from more affordable residential locations outside the city. Although distance between home and work was an important influence on commuting behaviour, it did not necessarily present an obstacle to incorporating walking or cycling into longer journeys made by car or public transport. The likelihood of active commuting was also related to the availability of parking at work, safe, convenient routes for walking and cycling, and convenient public transport.
Evaluating new transport infrastructure
Our evaluation of the busway highlighted the complexity of the intervention in providing a new guided bus service as well as a traffic-free path for walking and cycling. While the guided bus service provided an obvious alternative to car travel, it also had the potential to replace trips previously made by walking or cycling and to generate new trips. Our survey of busway users found that more than half of guided bus trips and one-quarter of cycle trips intercepted on the busway were said to have replaced a car trip, and nearly half of users reported that they now walked or cycled more than they had before the opening of the busway. In cross-sectional analysis of our cohort data, exposure to the intervention – defined in terms of proximity, modelled as the negative square root of the shortest network distance from home to busway – was associated with a greater likelihood of using the busway for walking, cycling and bus travel. Of these, cycling was the mode of transport most sensitive to proximity. The effect of proximity was strengthened in towns for bus use, and in towns and villages for walking, compared with urban areas. Men were more likely than women to have cycled on the busway, whereas individual socioeconomic characteristics did not predict guided bus use or walking.
Against the background of a general decline in active commuting in our sample over time, and using a robust quasi-experimental study design, we went on to show that the provision of the busway was associated with a shift away from using the car and an increase in time spent in physical activity on the journey to and from work. Longitudinal analysis of our cohort data found that exposure to the intervention was associated with nearly a doubling of the likelihood of a large increase in the proportion of commuting trips involving any active travel, and of a large decrease in the proportion of trips made entirely by car, as well as with a correspondingly lower likelihood of an increase in estimated carbon emissions attributable to commuting. Exposure to the intervention was also associated with a greater likelihood of an increase in weekly time spent cycling on the journey to and from work, to the effect that participants living 4 km from the busway were one-third more likely to have increased their cycle commuting time between 2009 and 2012 than those living 9 km away. This effect was maintained in analysis of time spent cycling for commuting and recreation combined, suggesting that an increase in commuter cycling was not offset by a compensatory decrease in recreational cycling, but we found no effect on time spent in physical activity overall. Use of the busway explained the large majority of the observed effect on active commuting, while changes in cognitions relating to the route environment or to car use were not significant in mediating the observed effect. The effect was moderated by baseline commuting behaviour, with a significant effect on overall time spent in active commuting among those who reported no active commuting at baseline. Interviews suggested that although people were unlikely to use the new infrastructure unless it closely matched the journeys they needed to make, a range of other factors informed travel behaviour, and these were dependent on the value attributed to different aspects of the journey experience. These generally involved considerations of comfort, ambience or pleasantness and of feeling safe, which could trump considerations of reliability and speed. Although experiences of the busway were complex and ambiguous, they culminated in meaningful travel behaviour change for some users, through shifts in the balance between influential factors and planning, trialling and adopting new practices over time.
Principal contributions and implications of the study
Understanding active commuting
The contribution of active commuting to health and well-being
Our study has shown that active commuting can make a substantial contribution to overall physical activity and to health and well-being more generally.
We found that time spent in active commuting was associated with time spent in overall physical activity in cross-sectional analysis, and that changes in time spent in active commuting were associated with commensurate changes in time spent in overall physical activity over a 3-year period of observation. These findings build on a limited body of previous cross-sectional studies reporting similar associations, and a single longitudinal study which reported a comparable relationship between changes in active travel (not limited to commuting) and overall physical activity in adults. 123–125 Although these observational analyses are consistent with a hypothesis that an increase in active travel in a given population is likely to be translated into an increase in overall physical activity, they are not proof of a causal relationship in that direction, being equally consistent with a hypothesis that active travel may increase as a consequence of factors driving a more general increase in physical activity. Nevertheless, they can be interpreted as providing some evidence against the substitution hypothesis that increases in one domain of physical activity (such as transport) may be counteracted by decreases in another domain (such as recreation), and our subsequent analysis of changes in physical activity attributable to the busway also found no evidence of a decrease in recreational physical activity. Our findings, therefore, lend support to the inference from health impact modelling studies that a shift towards active commuting in the population is likely to be associated with overall population health gain. 126
A particular contribution of our study is the demonstration of the potential contribution of multimodal journeys to overall PAEE. The incorporation of walking or cycling into longer car or public transport journeys was associated with significant additional energy expenditure. On average, 20% of the duration of these trips was spent above the MVPA threshold, and because many of the commuters in our cohort travelled a considerable distance to work, this equates to approximately 8 minutes per trip or 80 minutes per week on average for a full-time worker. Efforts to promote active commuting have sometimes focused on encouraging people to walk or cycle the entire journey to and from work. Although this may be a desirable and feasible goal for some commuters, the UK has the longest average commuting journeys in Europe, and Cambridge is not unique among UK cities in having a large rural commuting hinterland. Our findings show that active travel can be incorporated into commuting irrespective of the total length of the journey, and therefore illustrate the public health potential of promoting more of this form of ‘incidental’ walking and cycling, which may be more accessible and achievable for a considerable proportion of the population than replacing entire motor vehicle trips with active travel.
Aside from their contributions to overall physical activity, walking and cycling in general, and active commuting in particular, have also been shown to be associated with certain health benefits in adults in their own right. The most convincing longitudinal epidemiological evidence comes from systematic reviews showing reductions in the risk of mortality127 and adverse cardiovascular outcomes,120 respectively, independently of other domains of physical activity, although the studies contributing to the latter review were few in number and investigated a variety of specific outcomes. Studying ‘hard’ clinical end points was beyond the scope of our study, but we were able to exploit our cohort data to investigate associations between active commuting and sickness absence, well-being and BMI. Although the attrition of our cohort over time undoubtedly reduced the statistical power of our analyses to detect significant associations, the pattern of our findings can be regarded as providing preliminary support for the hypothesis that active commuting – and, in particular, cycle commuting – may be associated with beneficial effects on each of these outcomes, and our qualitative data provide some corroboration. The point estimates for the effect sizes on sickness absence and well-being are consistent with effects of ‘population significance’. For example, the difference we observed in sickness absence (1.1 days per year) is one-quarter of the mean sickness absence in the UK working population (4.4 days). 128 For well-being, the difference we observed in PCS-8 was comparable with those observed in our cohort between adults in their twenties and those aged 50 years and above (1.1 units), or between adults with a BMI < 25 kg/m2 and those with a BMI > 30 kg/m2 (1.3 units). Similarly, the difference we observed in MCS-8 was greater than that between men and women in our cohort (1.1 units), although less than the increase observed with advancing age (4–5 units between adults in their twenties and those aged 50 years and above). However, the effects were attenuated when the analyses were adjusted for baseline values of PCS-8 and MCS-8, respectively, that is when effectively comparing cyclists and non-cyclists with the same level of well-being at baseline. A recent analysis of a much larger cross-sectional dataset found a clear association between mode of travel to work and BMI. 129 Although that analysis provides no basis for the direct inference of a causal relationship, taken together with our findings it indicates the value of seeking to replicate our longitudinal analyses in larger cohorts with a longer duration of follow-up and, where possible, objective ascertainment of key outcomes, such as body composition, and of potential confounders such as dietary intake.
Active commuting as a target for public health intervention
One way of thinking about the promotion of physical activity is to regard people’s time as being divided into different time budgets, such as Sleep, Leisure, Occupation, Transportation and Home-based activities (the SLOTH model), and to encourage people to redistribute the time they spend in in more and less active behaviours over the course of a day or week. 130 Part of the case for focusing on active commuting is that people who travel to work have already assigned a time budget to the activity. Many people may find it easier to change the way in which they use that existing time budget than to find a new time budget for physical activity, for example by replacing time spent watching television with a more active form of recreation such as swimming. Our quantitative and qualitative findings illustrate the myriad ways in which commuters already divide their commuting time between multiple modes of transport, either within a single trip or over the course of a week. They also show how making comparatively small or marginal initial changes to travel patterns – for example, cycling instead of driving on 1 or 2 days each week, or driving to a park-and-ride site and walking the remainder of the journey – can substantially increase the weekly volume of physical activity, while also leading in some cases to larger and more sustained changes in travel behaviour over time.
Our observational analyses corroborate and extend existing, mostly cross-sectional, evidence suggesting that attributes of the environment such as the presence of convenient public transport and walking and cycling routes are important for promoting and maintaining active commuting. 16 In keeping with the notion of commuting as a complex set of behaviours and practices, however, the specific pattern of associations differed according to the specific outcome studied, suggesting that some environmental changes (such as improving pedestrian routes) may be more effective in promoting walking without necessarily reducing car trips, whereas others (such as changes in parking provision) may be more effective in reducing car trips. While these findings clearly provide additional support for a general strategy of improving the environment to support active travel,8 when taken together with our findings on the importance of multimodal journeys they also suggest a particular line of intervention development and evaluation relating to the ‘last leg’ of the journey to work. This would entail encouraging the more widespread use of park-and-ride or other facilities to park away from the workplace, which might be achieved by a variety of economic or infrastructural measures, coupled with improving the quality and safety of routes for completing the journey to work on foot or by bicycle.
At the same time, our qualitative and quantitative findings clearly show that how people travel to work is shaped by numerous other factors. These range from day-to-day changes in their social commitments to socioeconomic factors completely outside of their control, such as the geography of the provision and cost of housing and of major sites of employment such as business parks, hospitals and universities. These economic and social considerations will inevitably influence the outcomes of any specific intervention to promote active commuting, whether that is directed at individual behaviour change or at improving particular attributes of the environment. The implication is that a comprehensive public health strategy to promote physical activity through active travel would need to address the wider determinants of health in sectors such as employment, housing and planning policy. 131
Linking environmental change with travel and physical activity behaviour change
In reflecting on the meaning of our evaluative findings, it is important to bear in mind two aspects of the way in which the study was conceptualised. The first is that we did not set out to evaluate whether the busway was ‘effective’ in any overall or comprehensive sense, and certainly not to evaluate its value for money. While similar transport projects exist around the world and more are planned, a guided busway is only one of many specific ways in which central or local government might seek to improve infrastructure to promote the use of more sustainable modes of transport. Instead, we took the opportunity presented by this natural experiment to investigate a more specific set of research questions focused on linking environmental change with travel and physical activity behaviour change in commuters. The second aspect is that we were not able to conduct this research using a straightforward parallel-group study design, for the reasons outlined in previous chapters. We therefore sought to build an evidential case for causal inference using multiple sources of data and types of analysis. With these considerations in mind, in this section we discuss the meaning of our findings as they relate to the complementary scientific goals of causal estimation, causal explanation and generalisable causal inference. 108
Estimating the effects of the intervention
Using a robust quasi-experimental study design, and against a background of decreasing active commuting in our study cohort, we were able to show that exposure to the intervention was associated with an increase in the proportion of commuting trips involving any active travel, a decrease in the proportion of trips made entirely by car and an increase in time spent cycling on the journey to and from work.
Although the best available evidence at the inception of the study suggested that changing the environment in this way was likely to lead to an increase in active travel or physical activity, that evidence was limited in quantity, internal validity and external validity with respect to the UK. For example, the guidance on physical activity and the environment published by NICE in 2008 identified three studies of ‘multi-use trails’ and seven studies of ‘cycle infrastructure’ suggesting a positive effect on walking or cycling. 17 However, NICE also highlighted a lack of good quality studies that used valid pre- and post-intervention measures of the duration or volume of activities, which are important for estimating their health impacts; or that provided evidence on impacts on physical activity (as opposed to more proximate impacts, such as on the use of infrastructure or of specific modes of transport), impacts over sufficient follow-up periods, impacts in the UK or impacts in populations living in rural areas. 17 A widely cited review of infrastructure, programmes and policies to promote cycling published in 2010 concluded that ‘Most of the evidence examined in this review supports the crucial role of public policy in encouraging bicycling’. 132 However, that conclusion depended substantially on inferences from uncontrolled, cross-sectional or case studies, and the authors noted that ‘most studies fall far short of the ideal research design for evaluating interventions [. . .] As a result, these studies do not adequately address the direction of causality’. 132 Our own systematic review of controlled studies of interventions to promote cycling published in the same year found only a handful of studies involving any environmental change, none of which included any measure of behaviour change over time in the same individuals or any form of controlled analysis of physical activity outcomes; we described the available evidence as ‘of limited quantity and validity’. 29 Our study is one of a number of studies that have been developed in the UK in recent years to address some of these limitations of the existing evidence base.
An evidence update issued by NICE in 2014 found no relevant studies of transport projects published in the global literature since the original 2008 guidance, and only one in the category of community design;18 this was the RESIDE natural experimental study from Perth, Western Australia. RESIDE showed that among people moving into newly built neighbourhoods, walking for transport (but not for recreation) was associated with the degree to which their new residential environment was compliant with design guidance, particularly that relating to the movement network. 133 The strengths of RESIDE include its controlled design, the collection of repeated measures of environmental exposures and behavioural outcomes for a substantial cohort at up to four time points over a period of 6–8 years, and the development and use of detailed and specific objective measures of exposure to relevant attributes of the physical environment. Indeed, the use of these more specific measures of exposure has been important for demonstrating an association between the degree of environmental change and an increase in walking for transport133 that was not observed in an analysis based on a simpler comparison of ‘intervention’ and ‘control’ groups134 – a finding that reinforces our own decision to use a graded measure of intervention exposure for our main outcome analyses. However, the transferability of the findings of RESIDE from a sprawling Australian city to the UK is open to question. 18
As we have shown in previous systematic reviews, few studies have reported the equity or other distributional impacts of interventions to promote active travel. 25,27,29,135 Our cohort lacked sufficient socioeconomic heterogeneity to permit a meaningful evaluation of the equity impact of the busway, but we have provided some evidence that the effect of the busway on active commuting was most pronounced among those who reported no active commuting at baseline. This is an important contribution in view of the findings of the iConnect study, which was published shortly after the evidence update issued by NICE. The core of the iConnect study comprised an evaluation of new walking and cycling routes in Cardiff, Kenilworth and Southampton, and found that living closer to the new routes predicted net increases in time spent walking and cycling, and in overall physical activity, among local adult residents at 2-year follow-up. The estimated effect size was an additional 15 minutes of walking and cycling per week, and an additional 13 minutes of overall MVPA per week, for each additional km of proximity to the new routes. 69 However, the iConnect study also showed that baseline activity levels strongly predicted the use of the new walking and cycling routes,136 and some previous studies have indicated that a substantial proportion of new cycle trips generated by infrastructural or promotional interventions were attributable to existing cyclists making more trips rather than to new cyclists. 25,29 Those findings raise the question of whether new infrastructure might be most influential among those who are already more active, at least initially. In contrast, our results suggest that the busway might have been successful in encouraging the uptake of active commuting among the less active, rather than merely encouraging those who were already active to do a little more. The former is likely to lead to greater population health gain than the latter.
Despite the fact that the busway was intended to increase public transport use mainly by attracting car users, we did not find a significant effect of the intervention on the proportion of commuting trips involving public transport. That is not to say that the busway was not effective in promoting the use of public transport, because patronage of the guided buses exceeded initial expectations and our intercept survey confirmed that a substantial proportion of busway users were intercepted while using the guided bus rather than the path. We may have failed to detect an effect on public transport mode share for several reasons. First, a shift from a conventional bus service to a guided bus service would not have been captured by our measure. Second, the uptake of bus travel for purposes other than commuting would not have been captured by this measure either. Third, our cohort sample may in any case have lacked sufficient statistical power to detect a change in public transport mode share, which was not the primary outcome measure for the study. It is also possible that in another setting a guided busway might have resulted in a more prominent shift from the car to public transport, whereas the high prevalence of cycling in our study population at baseline might have encouraged a greater proportion of users to cycle on the path instead of using the bus.
We also found no evidence of an effect of the busway on time spent walking, either for commuting or in combination with recreational walking. As with the use of public transport, this may reflect any of several aspects of our study design and study population. For example, the infrastructure projects evaluated in the iConnect study were constructed in areas in which cycling was much less prevalent than in Cambridge, and local residents who reported using the new routes at follow-up were much more likely to report using them for walking than for cycling, and for recreation than for transport. 136 In this study, in contrast, we focused on walking or cycling for commuting, and deliberately sampled participants who did not live in the same immediate area of the city as their workplace in order to focus on those making somewhat longer journeys to and from work. For this reason walking as the sole mode of transport was not a prevalent commuting pattern in our sample, and although walking was often undertaken as part of longer car or public transport journeys, short ‘incidental’ walks of this kind are notoriously difficult to capture using self-reported survey instruments and may simply have been under-reported. Unlike walking to and from work, recreational walking was highly prevalent in our sample and remained comparatively stable over the course of the study.
We sought, but did not find, evidence of an increase in overall physical activity attributable to exposure to the busway. Again, this may reflect at least two potential explanations. The first is a lack of statistical power to detect a significant change in this secondary outcome measure, given the substantial additional attrition of the cohort consequent on the extension of the study. Estimates of overall physical activity derived from self-reported measures are subject to large measurement error, and our analysis may simply have been unable to detect the signal of an effect against the background noise of this measurement error. The second is that in the iConnect study the significant effects on time spent walking, cycling or in overall physical activity were observed at 2-year follow-up but not at 1-year follow-up, suggesting that a longer period of follow-up might have been required to detect an effect on overall physical activity attributable to the busway. 69 Having said that, it is reassuring that the effect of the intervention on time spent cycling for commuting was not attenuated when included with cycling for recreation, and that we found no evidence of a compensatory decrease in time spent in overall recreational physical activity.
Explaining the effects of the intervention
Although quantifying the effect of interventions forms an important part of causal inference to support evidence-based public health, it is also important to elucidate a causal understanding of the mechanisms linking interventions with their observed effects – partly because this understanding can contribute to an assessment of the generalisability of the findings, and partly because it can provide additional support for causal inference in studies, such as this one, in which the possibility of confounding cannot be eliminated from the estimate of the main effect. 20,108
Our path regression analysis showed that a large majority of the association between exposure to the busway and change in cycle commuting time was explained by self-reported use of the path, mirroring the findings of the iConnect study to the effect that changes in physical activity were limited to the subgroup of the study cohort reporting use of the new infrastructure69 and largely explained by such use rather than by more complex causal pathways involving cognitive constructs. 137 Although at first sight this may appear an obvious finding, it provides an important buttress to the main outcome analyses because it confirms the most obvious causal pathway linking the provision of new infrastructure with behaviour change. Despite our best efforts to control for a variety of potential confounders of that relationship, we cannot be completely confident that the observed association was not accounted for by some other factor, particularly an unmeasured confounder that may have been unequally distributed in the study population at baseline. At the same time, the findings of the path regression analysis challenge an assumption that changes in cognitions relating to the route environment or to car use necessarily form part of the mechanism linking environmental change with behaviour change. A full discussion of this issue is beyond the scope of this report, but, in brief, the findings might be regarded as consistent with – although certainly not proof of – a hypothesis that the changing of actual environmental cues might be at least as important in bringing about behaviour change than the changing of cognitions or perceptions about the environment. In other words, at least some of the effect of this type of intervention might be mediated through unconscious processes, rather than through the rational decision-making processes described by some of our participants. 138
Our cross-sectional cohort analysis showed that living closer to the busway was associated with use of the busway for walking and cycling, as well as for bus use. This is important because it shows that high-quality infrastructure can attract pedestrians and cyclists even when high-quality public transport is also provided, suggesting that public transport and active travel can co-exist in a sustainable and health-promoting transport system rather than necessarily competing with each other. Our qualitative findings provide additional support for this interpretation by showing how people used these modes of transport in combination, whether as part of the same trip or on different days of the week. At the same time, we should remain alert to the possibility that interventions of this kind may reduce active travel among some groups of users and potential users. This is well illustrated in some of our qualitative narratives, and also in our stratified path regression analysis. The latter found that among participants with a higher level of active commuting at baseline, use of the path was associated with both an increase and a decrease in weekly cycle commuting time. There are at least two potential explanations for this apparently counterintuitive finding. First, the provision of a high-quality bus service may have been sufficiently appealing to attract some commuters, who had previously cycled to work but for whom commuting by car was not attractive or not feasible. Our intercept survey data confirmed that a small but not inconsequential proportion of guided bus trips had indeed displaced trips previously made by walking or cycling. A second explanation is that the busway might have reduced the actual or perceived duration of cycle commuting trips for some people because the new route was more efficient for cycling, with fewer intersections and traffic lights and the opportunity to maintain a higher average speed. This could explain a reduction in cycle commuting time among some participants, even in the absence of a significant reduction in the average length of the journey to work.
Our cross-sectional cohort analysis also revealed that use of the busway was moderated by some contextual factors. In particular, the effect of proximity on use was strengthened in towns for bus use, and in towns and villages for walking. This indicates that the uptake of the intervention was moderated by characteristics of the settlements in which people lived, with people in rural settings apparently more sensitive to the provision of the new transport infrastructure. This may reflect the fact that people living in urban areas have more transport options available to them and shorter distances to travel, whereas an equivalent change to the transport infrastructure in a more rural area may constitute a more substantial ‘dose’ of change for the people living there. Given the potential contribution of multimodal journeys to overall physical activity, this is an important finding because it suggests that interventions of this kind may have greater potential for shifting travel behaviour among people living outside the urban areas that have tended to be the focus of most previous research in this field. 17
Generalising the effects of the intervention
At face value, there are three main threats to the external validity or generalisability of our findings. The first is that our study cohort was comparatively highly educated, healthy and physically active, both in general and as active commuters in particular. These characteristics reflect the results of a healthy cohort effect combined with a focus on adults of working age travelling to work at predominantly ‘white collar’ workplaces in a comparatively affluent part of the UK. The second is that the subject of our study was a somewhat unique intervention, in the form of a transport infrastructure project that improved provision for public transport, walking and cycling (which we could not entirely disentangle from each other in our evaluation) and may not be implemented in exactly the same form in other places in the UK or elsewhere. The third is that it is very likely that certain contextual factors were important in producing or moderating the outcomes of the intervention. For example, our study took place in a context where cycling was already prevalent and regarded as compatible with high social status, while car commuting was comparatively unattractive for many car-owning participants because of traffic congestion and difficulties with parking.
Of course, it cannot be assumed that an effect observed in Cambridge would necessarily be replicated in other parts of the UK. 139 However, the potential critique of ‘unrepresentativeness’ can be countered with a converse argument. If an effect of new transport infrastructure can be shown against the background of a comparatively active study population and a comparatively supportive environment – and shown to be greatest among the least active – then that is at least consistent with a hypothesis that similar environmental changes could also be effective, if not more effective, in other settings. Our reflection on the problem of generalisability is that no single intervention study in this field, or indeed in most areas of public health research, is likely to be capable of supporting generalisable causal inference. On the contrary, the only way to generate such evidence is to combine evidence from multiple studies of related interventions in different contexts, which together could form the basis of inferences about what works, for whom and in what circumstances. 140 To that end, our findings begin to corroborate and extend those of the iConnect, RESIDE and other studies in providing growing empirical support for a more fundamental underlying hypothesis that changing transport infrastructure can lead to travel and physical activity behaviour change, and, more specifically, that this can happen in the UK.
Methodological investigation and development
Although methodological research was not the primary aim of this study, we have made a number of contributions to methods for public health research in the course of its execution. Most importantly, we devised and subsequently validated a self-reported estimate of weekly time spent in active commuting. In doing so, we not only provided the first short, validated measure of this kind in a field that has tended to rely on measures that are either not specific or not validated, but also made a methodological contribution to the challenge of inferring activity patterns from georeferenced activity data, which may play an increasingly important role in future public health studies linking environment and behaviour. Alongside our investigation of different quantitative methods of capturing key constructs of importance to the study, we also explored the qualitative method of photo-elicitation as a means of gathering ethnographic observational data. This resulted in a change in participants’ narratives from more operational considerations of their transport options to more emotional and indirect reflections, for example in respect of commuting as a source of well-being. Perhaps the most important implication of this work is to highlight a more general observation that different elements of the study contributed to our understanding of the research topic in different ways – ranging from the testing of pre-specified statistical hypotheses about aggregate effects of the intervention, to the unstructured and flexible exploration of the detail of what the practice of ‘active commuting’ entails and means for different people.
Engagement with stakeholders
We aimed to engage with a variety of relevant stakeholders at all stages of the research process. At the planning stage of the study these included the director of planning and the head of delivery for the busway at the local authority; the local public health department; and representatives of several major local employers, particularly those on the Cambridge Biomedical Campus and Cambridge Science Park, from which many of the study participants were ultimately recruited. We went on to hold a forum for local stakeholders in January 2011, midway through data collection. This event was attended by a mixture of academic, public health and local government representatives from the region, and provided an opportunity to discuss preliminary findings, emerging research questions and strategies for knowledge translation. A number of changes were made to the core questionnaire used in 2011 and 2012 following the discussions at this event.
Drawing on our experience of this stakeholder forum and our wider knowledge translation activities within the Centre for Diet and Activity Research (CEDAR), we then held a second event in January 2015. At this event, the Commuting and Health Research and Policy Forum, we sought to engage existing and new stakeholders in a dialogue to coproduce some of the learning from the study. A larger number and variety of stakeholders were represented at this event, including some from the voluntary sector, from central government and from overseas. By this time the study had produced 22 scientific publications, with more in progress, using a variety of quantitative and qualitative research methods. The format was designed to encourage interaction relating to the study findings and related topics, both between the study team and the invited stakeholders and between different stakeholders. The event began with two plenary presentations, the first from the local authority introducing the busway and the second from the research team providing an overview of the key findings of the study. These findings and related materials subsequently formed the basis for more detailed discussion at a series of stations offered in a ‘marketplace’ or ‘open day’ format. At these stations, members of the research team facilitated discussions on the topics of ‘approaches to evaluation and measurement’, ‘active travel and health’, ‘the role of environment in shaping travel behaviour’, ‘mapping changes in travel behaviour’ and ‘drawing the threads together’ of the various aspects of the study. The event closed with a chaired plenary discussion of key learning points from the forum, including invited commentaries from external stakeholders and an open discussion of the implications for research, policy and practice.
As the event was designed to encourage the coproduction of insights rather than the didactic ‘dissemination’ of findings, this provided an opportunity to explore participants’ experiences and expectations of knowledge translation and the use of evidence. Using preliminary participant observation during the event itself in combination with in-depth follow-up interviews, we aimed to investigate stakeholders’ reflections on the process of knowledge translation and how they understood, interpreted and might or might not be able to apply the evidence. Preliminary findings suggested that attendees appreciated the interactive format of the forum and the opportunities to ask specific questions of particular members of the research team and to debate and network with colleagues. There was a consensus that physical activity and health were increasingly recognised as important for policy, including in the transport sector, but participants regarded the complexity of results and researchers’ uneasiness about producing ‘soundbites’ as a significant barrier to influencing key decision-makers. Follow-up interviews explored institutional constraints to the use of evidence and the ways in which competing interests, budgets and concerns shaped decision-making at the intersections of health, transport and other sectors, and in local and national government. Knowledge translation was described as a continuous and complex process involving hierarchies of decision-making and the translation of both findings and designs of research across sectors.
Challenges, strengths and limitations of the study
Challenges of the study
We faced a number of challenges in the design and execution of this study. We have chosen to highlight four of these here because they illustrate some of the difficulties inherent in addressing the research recommendations on physical activity and the environment published by NICE in 2008,17 which formed part of the background to the inception of the study. The first challenge was that of combining different disciplinary perspectives on the assessment of behaviour change in the context of the evaluation of a transport infrastructure project. The fields of physical activity research and transport research have tended to assess travel behaviour using different instruments and summary measures, and we aimed to establish some common methodological ground between these different approaches. This was important in order to be able to assess the effect of the intervention using a suite of complementary metrics to serve the needs of different groups of users of the findings, while also understanding the social and physical contexts of the target behaviours. The second challenge was that of implementing rapid and robust baseline measurement in the somewhat unpredictable setting of a natural experimental study of an intervention that was outside the control of the research team. This required us to make trade-offs between the competing merits of different approaches and technologies for capturing travel and physical activity behaviour change. The third challenge was that of finding a way of defining exposure to the intervention that would enable us to make controlled comparisons and thereby strengthen the basis for causal estimation of the effect of the intervention. This required us to deal rigorously and transparently with the emerging realisation that a simple parallel-group design was not suitable for the evaluation of the busway. The fourth challenge was that of designing and adapting an analytical strategy that was able to cope with the delayed implementation of the intervention. This required us to use different types of data and approaches to analysis to piece together a combination of evidence for causal estimation and evidence for causal explanation along an extended putative causal pathway linking environmental change with behaviour change and population health impacts. In the following sections, we briefly discuss our responses to these four challenges in terms of their implications for the strengths and limitations of the study and for future research, most which have been described elsewhere in the report but are summarised here for the sake of completeness.
Strengths of the study
Together with our funders, we demonstrated a flexible approach to the unpredictable realities of natural experimental research in two important ways: first by implementing rapid baseline data collection in what we believed to be a brief window of opportunity before the originally scheduled opening of the busway, and later by extending the study and endeavouring to make the best possible use of the available data for causal inference and understanding. We measured physical activity in general, and travel to and from work in particular, using a combination of simpler and more detailed, self-reported and objective measures. This enabled us to assess the main outcomes of the evaluation using a comparatively simple self-reported instrument that was readily administered to the entire study cohort in the core questionnaire at baseline – and could be used in future studies – while later adding more detailed measurement data to validate our primary outcome measure and investigate more detailed patterns and relationships. By combining disciplinary perspectives on the measurement of travel to and from work, we were able to collect disaggregated data at the level of the trip and, to some extent, the stages of each trip. This enabled us to identify more complex patterns of behaviour such as the use of different combinations of modes of transport or different ‘intensities’ of car commuting, and, therefore, to investigate their relationships with potential targets of interventions such as the provision and cost of parking at work. We also demonstrated a variety of ways of using qualitative data, separately and in combination with quantitative data, to understand travel behaviour and behaviour change in commuters. For example, we used mixed-method approaches to interrogate initially counterintuitive quantitative data with insights from qualitative data; and we used a variety of qualitative methods, including vignettes constructed from the results of quantitative analyses, to investigate the effects of the busway. Our detailed exploration of the target behaviours and their relationship with perceived and objective attributes of the environment – particularly in relation to the route to work – generated valuable original observational evidence in its own right as well as highlighting the spatial distribution of the target behaviours with respect to the busway, which had an important bearing on our ultimate analytical strategy for the evaluation. We built on the methods of analysis developed in this work to create a general graded measure of intervention exposure with demonstrable face validity that could be adapted to the causal analysis of different outcomes, and an example of how similar principles could be applied in future studies. In our ultimate estimation of the effects of exposure to the intervention, we systematically accounted for a set of confounders representing plausible alternative explanations, thereby providing a considerable increase in rigour over most previous evaluation studies in the field. 17,18,132 Finally, the study provides a rich observational data set that constitutes a valuable platform for further analyses, particularly using the intermediate years of the core questionnaire data and the objective measurement data, about which potential collaborators can find out more at our data sharing portal at http://epi-meta.medschl.cam.ac.uk.
Limitations of the study
Our rapid and rolling approach to the recruitment of participants in multiple workplaces meant that we had no defined sampling frame such as a population register, and, therefore, could not compute a population response rate as such. As is often the case with studies of this kind, our cohort sample included a predominance of women and people educated to degree level or above; although this was partly offset by the more heterogeneous intercept survey sample, it limited the statistical power of the study to detect associations in men and to examine socioeconomic gradients in the effects of the intervention. Our cohort also suffered considerable attrition over time; although this was not surprising, particularly in a city such as Cambridge with a high turnover of professionals, together with the need to extend the study this resulted in a loss of statistical power for comparisons between the first and last years of the cohort study. Although we validated our primary outcome measure, it was far from perfect, and self-reported outcome data are inevitably susceptible to potential bias as a result of intentional or unintentional misreporting. The multiplicity of ways of summarising travel behaviour change – and the distributions of these outcome variables, which rendered them unsuitable for linear modelling – meant that our evaluative findings were expressed in terms of the likelihood of various categorical outcomes, rather than in the neater terms of a simple ‘effect size’ such as an increase in the number of minutes spent cycling per week. We ultimately defined exposure to the intervention solely in terms of where participants lived. Using more detailed individual measures, for example including both the location of the workplace and the route followed between home and work, might have captured exposure more fully and produced stronger associations with behaviour change, but it might also have involved considerably more work for little additional causal understanding. 14,131,141 Our analysis of mediators of the effects of the intervention was limited, both in terms of the types of mediators considered and in terms of how those were measured. It is possible that if the questionnaire items used to ascertain the cognitive constructs had been framed differently, the analysis would have suggested stronger support for their role as mediators. Finally, owing to circumstances beyond our control we were unable to assess the maintenance of travel behaviour change after the intervention over a second year of follow-up as originally planned; and because we did not observe an effect of the busway on overall physical activity after 1 year, we did not attempt to model the direct impacts of the busway on the other health outcomes of interest, but instead investigated their longitudinal associations with active commuting by way of contributing a different piece of the ‘evidence jigsaw’.
Implications for future research
Future epidemiological research should seek to replicate our longitudinal observational analyses of the health benefits of active commuting in larger cohorts with a longer duration of follow-up and, where possible, objective ascertainment of key outcomes and potential confounders. Future evaluative research should seek to confirm and extend our findings by assessing the aggregate and equity impacts of related environmental and policy changes, in other socioeconomic, cultural and topographical contexts and over longer periods of observation, defining exposure to interventions in such a way as to enable controlled comparisons where possible. This is necessary for the gradual cumulation of evidence to support more generalisable causal inference linking environmental change with travel and physical activity behaviour change in populations. Such studies may entail recruiting larger, more representative and more socioeconomically diverse samples for the collection of original data, or making greater use of existing observational cohorts and national data sets. 20,139,142 They should seek to quantify travel and physical activity behaviour change using self-reported measures, objective measures or both, commensurate with their research questions, with careful consideration of what is and is not captured by different measures. They should also endeavour to understand the social and environmental context and mechanisms of behaviour change using qualitative methods, quantitative methods or both. While greater use could be made of objective measurement to assess intervention outcomes, researchers and funders should not underestimate the data processing and analytical requirements and the potential additional volunteer bias inherent in taking this approach. The success of future natural experimental studies of this kind is likely to depend on a flexible approach from researchers and funders to respond to changing intervention timetables and the need for an evolving analytical strategy. 20
Conclusion
Although rigorous studies in this field remain few in number, our findings, together with those of others, provide increasing empirical support for the argument that reconfiguring transport systems to support active travel is likely to improve population health. Further natural experimental studies of interventions in a wider range of contexts would help to strengthen the evidence to support more generalisable causal inference and intersectoral policy-making to improve health.
Acknowledgements
Contributions of authors
David Ogilvie (Programme Leader in Physical Activity and Public Health, Medical Research Council Epidemiology Unit and CEDAR) led the design and execution of the study, oversaw all study analyses and publications and led the preparation of the final report.
Jenna Panter (National Institute for Health Research post-doctoral fellow, Medical Research Council Epidemiology Unit and CEDAR) was the lead quantitative researcher on the study, led multiple study analyses and publications and contributed to others, and led the preparation of sections of the final report and contributed to all sections.
Cornelia Guell (Career Development Fellow, Medical Research Council Epidemiology Unit and CEDAR) was the lead qualitative researcher on the study, led multiple study analyses and publications and contributed to others, and led the preparation of sections of the final report and contributed to all sections.
Andy Jones (Professor of Public Health, Norwich Medical School) contributed to the design, execution and oversight of the study in general, and led or oversaw some study analyses and publications and contributed to others.
Roger Mackett (Emeritus Professor of Transport Studies, University College London) contributed to the design, execution and oversight of the study in general, and led or oversaw some study analyses and publications and contributed to others.
Simon Griffin (Professor of General Practice, University of Cambridge, and Assistant Director, Medical Research Council Epidemiology Unit and CEDAR) contributed to the design, execution and oversight of the study in general and to some study analyses and publications.
All authors contributed to the design of the final report and approved the final version.
Wider contributions
Adam Bostanci (scientific editor, Medical Research Council Epidemiology Unit and CEDAR) led the scientific and technical editing of the content of the final report.
This report represents an original summary and synthesis of a large body of research, most of which has already been published – or submitted or prepared for publication – in more detail in other open-access academic journals. Lena Alexander, Andrew Carse, Silvia Costa, Alice Dalton, Louise Foley, Anna Goodman, Eva Heinen, David Humphreys, Caroline Jones, Joanna Kesten, Calum Mattocks, Oliver Mytton, Richard Prins, Mark Tully and Lin Yang each led one or more of these analyses or publications, and Søren Brage, Simon Cohn, Cheryl Chapman, Carol Desouza, Amelia Harshfield, Natalia Jones and James Smith each contributed to one or more of these analyses or publications.
Baseline data collection was funded by CEDAR, a Public Health Research Centre of Excellence supported by the British Heart Foundation, Economic and Social Research Council, Medical Research Council, National Institute for Health Research and Wellcome Trust under the auspices of the UK Clinical Research Collaboration.
The authors also thank the study participants, many of whom contributed to multiple parts of the study in multiple years; their employers, whose co-operation and support enabled us to recruit participants through workplaces; the Cambridgeshire Travel for Work Partnership, for assistance with recruiting employers; Cambridgeshire County Council, whose co-operation, information and support made it possible for us to use the busway as a research laboratory; CTS Traffic and Transportation, for conducting the intercept survey; all staff from the Medical Research Council Epidemiology Unit Functional Group Team, in particular for study co-ordination and data collection (led by Cheryl Chapman, Fiona Whittle and Andrew Dymond), physical activity data processing (Kate Westgate and Stephanie Mayle) and data management (Lena Alexander, Ruhul Amin, Rupesh Ghelani, Amelia Harshfield and Wing Wong); Natalia Jones and Louise Foley, for their contributions to the collection of interview data and the preparation of survey data for analysis respectively; Stephen Sharp, for statistical advice; Mark Petticrew, Ashley Cooper and Harry Rutter, for their guidance and support as members of the independent study steering committee; and Oliver Francis, for his support with knowledge translation.
Publications
Ogilvie D, Giles-Corti B, Hooper P, Yang L, Bull F. Methods for researching the physical activity impacts of ‘natural experiments’ in modifying the built environment. J Phys Act Health 2010;7:S341–3.
Ogilvie D, Griffin S, Jones A, Mackett R, Guell C, Panter J, et al. Commuting and health in Cambridge: a study of a ‘natural experiment’ in the provision of new transport infrastructure. BMC Public Health 2010;10:703.
Panter J, Griffin S, Jones A, Mackett R, Ogilvie D. Correlates of time spent walking and cycling to and from work: baseline results from the commuting and health in Cambridge study. Int J Behav Nutr Phys Act 2011;8:124.
Goodman A, Guell C, Panter J, Jones N, Ogilvie D. Healthy travel and the socio-economic structure of car commuting in Cambridge, UK: a mixed-methods analysis. Soc Sci Med 2012;74:1929–38.
Guell C, Panter J, Jones N, Ogilvie D. Towards a differentiated understanding of active travel behaviour: using social theory to explore everyday commuting. Soc Sci Med 2012;75:233–9.
Jones C, Ogilvie D. Motivations for active commuting: a qualitative investigation of the period of home or work relocation. Int J Behav Nutr Phys Act 2012;9:109.
Panter J, Griffin S, Ogilvie D. Correlates of reported and recorded time spent in physical activity in working adults: results from the Commuting and Health in Cambridge study. PLOS ONE 2012;7:e42202.
Yang L, Griffin S, Chapman C, Ogilvie D. The feasibility of rapid baseline objective physical activity measurement in a natural experimental study of a commuting population. BMC Public Health 2012;12:841.
Yang L, Panter J, Griffin S, Ogilvie D. Associations between active commuting and physical activity in working adults: cross-sectional results from the Commuting and Health in Cambridge study. Prev Med 2012;55:453–7.
Carse A, Goodman A, Mackett R, Panter J, Ogilvie D. The factors influencing car use in a cycle-friendly city: the case of Cambridge. J Transport Geogr 2013;28:67–74.
Dalton A, Jones A, Panter J, Ogilvie D. Neighbourhood, route and workplace-related environmental characteristics predict adults’ mode of travel to work. PLOS ONE 2013;8:e67575.
Humphreys D, Goodman A, Ogilvie D. Associations between active commuting and physical and mental wellbeing. Prev Med 2013;57:135–9.
Jones C, Cohn S, Ogilvie D. Making sense of a new transport system: an ethnographic study of the Cambridgeshire Guided Busway. PLOS ONE 2013;8:e69254.
Ogilvie D, Panter J, Woodcock J, Francis O. Active travel promotion can help meet the public health challenge. Transportation Prof 2013;March:28–9.
Panter J, Desousa C, Ogilvie D. Incorporating walking or cycling into car journeys to and from work: the role of individual, workplace and environmental characteristics. Prev Med 2013;65:211–17.
Panter J, Griffin S, Dalton A, Ogilvie D. Patterns and predictors of changes in active commuting over 12 months. Prev Med 2013;57:776–84.
Guell C, Panter J, Ogilvie D. Walking and cycling to work despite reporting an unsupportive environment: insights from a mixed-method exploration of counterintuitive findings. BMC Public Health 2013;13:497.
Kesten J, Cohn S, Ogilvie D. The contribution of media analysis to the evaluation of environmental interventions: the Commuting and Health in Cambridge study. BMC Public Health 2014;14:482.
Panter J, Costa S, Dalton A, Jones A, Ogilvie D. Development of methods to objectively identify time spent using active and motorised modes of travel to work: how do self-reported measures compare? Int J Behav Nutr Phys Act 2014;11:116.
Panter J, Griffin S, Ogilvie D. Active commuting and perceptions of the route environment: a longitudinal analysis. Prev Med 2014;67:134–40.
Tully M, Panter J, Ogilvie D. Individual characteristics associated with mismatches between self-reported and accelerometer-measured physical activity. PLOS ONE 2014;9:e99636.
Dalton A, Jones A, Panter J, Ogilvie D. Are GIS-modelled routes a useful proxy for the actual routes followed by commuters? J Transport Health 2015;2:219–29.
Guell C, Ogilvie D. Guell C, Ogilvie D. Picturing commuting: photovoice and seeking wellbeing in everyday travel. Qual Res 2015;15:201–18.
Heinen E, Panter J, Dalton A, Jones A, Ogilvie D. Sociospatial patterning of the use of new transport infrastructure: walking, cycling and bus travel on the Cambridgeshire Guided Busway. J Transport Health 2015:2:199–211.
Heinen E, Panter J, Mackett R, Ogilvie D. Changes in mode of travel to work: a natural experimental study of new transport infrastructure. Int J Behav Nutr Phys Act 2015;12:81.
Kesten J, Guell C, Cohn S, Ogilvie D. From the concrete to the intangible: understanding diverse experiences of new transport infrastructure. Int J Behav Nutr Phys Act 2015;12:72.
Costa S, Ogilvie D, Dalton A, Westgate K, Brage S, Panter J. Quantifying the physical activity energy expenditure of commuters using a combination of global positioning system and combined heart rate and movement sensors. Prev Med 2015;81:339–44.
Foley L, Panter J, Heinen E, Ogilvie D. Changes in active commuting and changes in self-reported and objectively measured physical activity in adults: a cohort study. Int J Behav Nutr Phys Act 2015;12:161.
Mytton O, Panter J, Ogilvie D. Longitudinal associations of active commuting with sickness absence and wellbeing. Prev Med 2016; in press.
Panter J, Heinen E, Mackett R, Ogilvie D. Impact of new transport infrastructure on walking, cycling and physical activity [published online ahead of print November 5 2015]. Am J Prev Med 2015.
Data sharing statement
The data set used in this study is managed by the Medical Research Council Epidemiology Unit at the University of Cambridge. The access policy for sharing is based on the Medical Research Council Policy and Guidance on Sharing of Research Data from Population and Patient Studies. All data sharing must meet the terms of existing participants’ consent and study ethical approvals. The authors’ Data Access and Sharing Policy defines the principles and processes for accessing and sharing our data. They welcome proposals for projects and aim to make data as widely available as possible while safeguarding the privacy of our participants, protecting confidential data and maintaining the reputations of our studies and participants. All data sharing is dependent on the project being approved by the study team, a data sharing agreement being in place with the University of Cambridge and resources being available to support the request. For further information, please refer to the Medical Research Council Epidemiology Unit data sharing portal at http://epi-meta.medschl.cam.ac.uk.
Disclaimers
This report presents independent research funded by the National Institute for Health Research (NIHR). The views and opinions expressed by authors in this publication are those of the authors and do not necessarily reflect those of the NHS, the NIHR, NETSCC, the PHR programme or the Department of Health. If there are verbatim quotations included in this publication the views and opinions expressed by the interviewees are those of the interviewees and do not necessarily reflect those of the authors, those of the NHS, the NIHR, NETSCC, the PHR programme or the Department of Health.
References
- Start Active, Stay Active: A Report on Physical Activity for Health from the Four Home Countries’ Chief Medical Officers. London: DH; 2011.
- Global Health Risks: Mortality and Burden of Disease Attributable to Selected Major Risks. Geneva: WHO Press; 2009.
- Lee IM, Shiroma EJ, Lobelo F, Puska P, Blair SN, Katzmarzyk PT. Effect of physical inactivity on major non-communicable diseases worldwide: an analysis of burden of disease and life expectancy. Lancet 2012;380:219-29. http://dx.doi.org/10.1016/S0140-6736(12)61031-9.
- Pate R, Pratt M, Blair S, Haskell W, Macera C, Bouchard C, et al. Physical activity and public health: a recommendation from the Centers for Disease Control and Prevention and the American College of Sports Medicine. JAMA 1995;273:402-7. http://dx.doi.org/10.1001/jama.1995.03520290054029.
- At Least Five a Week: Evidence on the Impact of Physical Activity and its Relationship to Health. London: Department of Health; 2004.
- Sproston K, Primatesta P. Health Survey for England 2003. London: The Stationery Office; 2004.
- Morris J. Exercise in the prevention of coronary heart disease: today’s best buy in public health. Med Sci Sports Exerc 1994;26:807-14. http://dx.doi.org/10.1249/00005768-199407000-00001.
- Everybody Active, Every Day: A Framework to Embed Physical Activity into Daily Life. London: Public Health England; 2014.
- Dora C. A different route to health: implications of transport policies. BMJ 1999;318:1686-9. http://dx.doi.org/10.1136/bmj.318.7199.1686.
- Foster C, Hillsdon M, Thorogood M, Kaur A, Wedatilake T. Interventions for promoting physical activity. Cochrane Database Syst Rev 2005;1. http://dx.doi.org/10.1002/14651858.cd003180.pub2.
- Rose G. The Strategy of Preventive Medicine. New York, NY: Oxford University Press; 1992.
- Gebel K, Bauman AE, Petticrew M. The physical environment and physical activity: a critical appraisal of review articles. Am J Prev Med 2007;32:361-9. http://dx.doi.org/10.1016/j.amepre.2007.01.020.
- Bauman AE, Reis RS, Sallis JF, Wells JC, Loos RJF, Martin BW. Correlates of physical activity: why are some people physically active and others not?. Lancet 2012;380:258-71. http://dx.doi.org/10.1016/S0140-6736(12)60735-1.
- Bauman A. The physical environment and physical activity: moving from ecological associations to intervention evidence. J Epidemiol Community Health 2005;59:535-6. http://dx.doi.org/10.1136/jech.2004.032342.
- Giles-Corti B, Timperio A, Bull F, Pikora T. Understanding physical activity environmental correlates: increased specificity for ecological models. Exerc Sport Sci Rev 2005;33:175-81. http://dx.doi.org/10.1097/00003677-200510000-00005.
- McCormack G, Shiell A. In search of causality: a systematic review of the relationship between the built environment and physical activity among adults. Int J Behav Nutr Phys Act 2011;8. http://dx.doi.org/10.1186/1479-5868-8-125.
- Promoting and Creating Built or Natural Environments that Encourage and Support Physical Activity. London: NICE; 2008.
- Physical Activity and the Environment: A Summary of Selected New Evidence Relevant to NICE Public Health Guidance 8 ‘Physical Activity And The Environment’ (2008). London: NICE; 2014.
- Petticrew M, Cummins S, Ferrell C, Findlay A, Higgins C, Hoy C, et al. Natural experiments: an underused tool for public health?. Public Health 2005;119:751-7. http://dx.doi.org/10.1016/j.puhe.2004.11.008.
- Craig P, Cooper C, Gunnell D, Haw S, Lawson K, Macintyre S, et al. Using natural experiments to evaluate population health interventions: new Medical Research Council guidance. J Epidemiol Community Health 2012;66:1182-6. http://dx.doi.org/10.1136/jech-2011-200375.
- Tackling Obesities: Future Choices. London: Government Office for Science; 2007.
- Besser L, Dannenberg A. Walking to public transit: steps to help meet physical activity recommendations. Am J Prev Med 2005;29:273-80. http://dx.doi.org/10.1016/j.amepre.2005.06.010.
- Villanueva K, Giles-Corti B, McCormack G. Achieving 10,000 steps: a comparison of public transport users and drivers in a university setting. Prev Med 2008;47:338-41. http://dx.doi.org/10.1016/j.ypmed.2008.03.005.
- Rissel C, Curac N, Greenaway M, Bauman A. Physical activity associated with public transport use – a review and modelling of potential benefits. Int J Environ Res Public Health 2012;9:2454-78. http://dx.doi.org/10.3390/ijerph9072454.
- Ogilvie D, Egan M, Hamilton V, Petticrew M. Promoting walking and cycling as an alternative to using cars: systematic review. BMJ 2004;329:763-6. http://dx.doi.org/10.1136/bmj.38216.714560.55.
- Heath G, Brownson R, Kruger J, Miles R, Powell K, Ramsey L, et al. The effectiveness of urban design and land use and transport policies and practices to increase physical activity: a systematic review. J Phys Act Health 2006;3:55-76.
- Ogilvie D, Foster C, Rothnie H, Cavill N, Hamilton V, Fitzsimons C, et al. Interventions to promote walking: systematic review. BMJ 2007;334:1204-7. http://dx.doi.org/10.1136/bmj.39198.722720.BE.
- Saunders LE, Green JM, Petticrew MP, Steinbach R, Roberts H. What are the health benefits of active travel? A systematic review of trials and cohort studies. PLOS ONE 2013;8. http://dx.doi.org/10.1371/journal.pone.0069912.
- Yang L, Sahlqvist S, McMinn A, Griffin S, Ogilvie D. Interventions to promote cycling: systematic review. BMJ 2010;341. http://dx.doi.org/10.1136/bmj.c5293.
- Heath GW, Parra DC, Sarmiento OL, Andersen LB, Owen N, Goenka S, et al. Evidence-based intervention in physical activity: lessons from around the world. Lancet 2012;380:272-81. http://dx.doi.org/10.1016/S0140-6736(12)60816-2.
- Walking and Cycling: Local Measures to Promote Walking and Cycling as Forms of Travel or Recreation. London: NICE; 2012.
- Ogilvie D, Griffin S, Jones A, Mackett R, Guell C, Panter J, et al. Commuting and health in Cambridge: a study of a ‘natural experiment’ in the provision of new transport infrastructure. BMC Public Health 2010;10. http://dx.doi.org/10.1186/1471-2458-10-703.
- Wareham NJ, Jakes RW, Rennie KL, Mitchell J, Hennings S, Day NE. Validity and repeatability of the EPIC-Norfolk Physical Activity Questionnaire. Int J Epidemiol 2002;31:168-74. http://dx.doi.org/10.1093/ije/31.1.168.
- Besson H, Brage S, Jakes RW, Ekelund U, Wareham NJ. Estimating physical activity energy expenditure, sedentary time, and physical activity intensity by self-report in adults. Am J Clin Nutr 2010;91:106-14. http://dx.doi.org/10.3945/ajcn.2009.28432.
- Shannon T, Giles-Corti B, Pikora T, Bulsara M, Shilton T, Bull F. Active commuting in a university setting: Assessing commuting habits and potential for modal change. Transport Pol 2006;13:240-53. http://dx.doi.org/10.1016/j.tranpol.2005.11.002.
- Stratford N, Simmonds N, Nicolaas G, Costigan P. National Travel Survey 2002. London: Department for Transport; 2003.
- Ogilvie D, Mitchell R, Mutrie N, Petticrew M, Platt S. Perceived characteristics of the environment associated with active travel: development and testing of a new scale. Int J Behav Nutr Phys Act 2008;5. http://dx.doi.org/10.1186/1479-5868-5-32.
- Bamberg S, Ajzen I, Schmidt P. Choice of travel mode in the Theory of Planned Behavior: the roles of past behavior, habit, and reasoned action. Basic Appl Soc Psychol 2003;25:175-87. http://dx.doi.org/10.1207/S15324834BASP2503_01.
- Verplanken B, Orbell S. Reflections on past behavior: a self-report index of habit strength. J Appl Soc Psychol 2003;33:1313-30. http://dx.doi.org/10.1111/j.1559-1816.2003.tb01951.x.
- Ferrie J, Kivimaki M, Head J, Shipley M, Vahtera J, Marmot M. A comparison of self-reported sickness absence with absences recorded in employers’ registers: evidence from the Whitehall II study. Occup Environ Med 2005;62:74-9. http://dx.doi.org/10.1136/oem.2004.013896.
- Ware J, Kosinski M, Dewey J, Gandek B. How to Score and Interpret Single-Item Health Status Measures: A Manual for Users of the SF-8 (TM) Health Survey. Lincoln, RI: QualityMetric Incorporated; 2001.
- Yang L, Griffin S, Chapman C, Ogilvie D. The feasibility of rapid baseline objective physical activity measurement in a natural experimental study of a commuting population. BMC Public Health 2012;12. http://dx.doi.org/10.1186/1471-2458-12-841.
- Hendelman D, Miller K, Baggett C, Debold E, Freedson P. Validity of accelerometry for the assessment of moderate intensity physical activity in the field. Med Sci Sports Exerc 2000;32:442-9. http://dx.doi.org/10.1097/00005768-200009001-00002.
- Trost SG, Owen N, Bauman A, Sallis J, Brown W. Correlates of adults’ participation in physical activity: review and update. Med Sci Sports Exerc 2002;34:1996-2001. http://dx.doi.org/10.1097/00005768-200212000-00020.
- Plasqui G, Westerterp KR. Physical activity assessment with accelerometers: an evaluation against doubly labeled water. Obesity 2007;15:2371-9. http://dx.doi.org/10.1038/oby.2007.281.
- Brage S, Brage N, Franks PW, Ekelund U, Wareham NJ. Reliability and validity of the combined heart rate and movement sensor Actiheart. Eur J Clin Nutr 2005;59:561-70. http://dx.doi.org/10.1038/sj.ejcn.1602118.
- Brage S, Ekelund U, Brage N, Hennings MA, Froberg K, Franks PW, et al. Hierarchy of individual calibration levels for heart rate and accelerometry to measure physical activity. J Appl Physiol 2007;103:682-92. http://dx.doi.org/10.1152/japplphysiol.00092.2006.
- Evenson KR, Wen F, Hillier A, Cohen DA. Assessing the contribution of parks to physical activity using GPS and accelerometry. Med Sci Sports Exerc 2013;45:1981-7. http://dx.doi.org/10.1249/MSS.0b013e318293330e.
- O’Connor TM, Cerin E, Robles J, Lee RE, Kerr J, Butte N, et al. Feasibility study to objectively assess activity and location of Hispanic preschoolers: a short communication. Geospatial Health 2013;7:375-80. http://dx.doi.org/10.4081/gh.2013.94.
- Guell C, Panter J, Jones NR, Ogilvie D. Towards a differentiated understanding of active travel behaviour: using social theory to explore everyday commuting. Soc Sci Med 2012;75:233-9. http://dx.doi.org/10.1016/j.socscimed.2012.01.038.
- Jones C, Ogilvie D. Motivations for active commuting: a qualitative investigation of the period of home or work relocation. Int J Behav Nutr Phys Act 2012;9. http://dx.doi.org/10.1186/1479-5868-9-109.
- Guell C, Panter J, Ogilvie D. Walking and cycling to work despite reporting an unsupportive environment: insights from a mixed-method exploration of counterintuitive findings. BMC Public Health 2013;13. http://dx.doi.org/10.1186/1471-2458-13-497.
- Kesten J, Cohn S, Ogilvie D. The contribution of media analysis to the evaluation of environmental interventions: the commuting and health in Cambridge study. BMC Public Health 2014;14. http://dx.doi.org/10.1186/1471-2458-14-482.
- Jones CHD, Cohn S, Ogilvie D. Making sense of a new transport system: an ethnographic study of the Cambridgeshire Guided Busway. PLOS ONE 2013;8. http://dx.doi.org/10.1371/journal.pone.0069254.
- Dalton AM, Jones A, Panter JR, Ogilvie D. Neighbourhood, route and workplace-related environmental characteristics predict adults’ mode of travel to work. PLOS ONE 2013;8. http://dx.doi.org/10.1371/journal.pone.0067575.
- Panter J, Griffin S, Dalton AM, Ogilvie D. Patterns and predictors of changes in active commuting over 12 months. Prev Med 2013;57:776-84. http://dx.doi.org/10.1016/j.ypmed.2013.07.020.
- Ainsworth B, Haskell W, Whitt M, Irwin M, Swartz A, Strath S, et al. Compendium of physical activities: an update of activity codes and MET intensities. Med Sci Sports Exerc 2000;32:S498-504. http://dx.doi.org/10.1097/00005768-200009001-00009.
- Freedson PS, Melanson E, Sirard J. Calibration of the Computer Science and Applications, Inc. accelerometer. Med Sci Sports Exerc 1998;30:777-81. http://dx.doi.org/10.1097/00005768-199805000-00021.
- 2010 Guidelines to Defra/DECC’s G Conversion Factors for Company Reporting: Methodology Paper for Emissions Factors. London: Department for Environment, Food and Rural Affairs; 2010.
- Hodgson P, Potter S, Warren J, Gillingwater D. Can bus really be the new tram?. Res Transport Econ 2013;39:158-66. http://dx.doi.org/10.1016/j.retrec.2012.06.009.
- Obesity: Preventing and Managing the Global Epidemic. Report of a WHO Consultation. Geneva: WHO; 2000.
- Panter J, Griffin S, Jones A, Mackett R, Ogilvie D. Correlates of time spent walking and cycling to and from work: baseline results from the commuting and health in Cambridge study. Int J Behav Nutr Phys Act 2011;8. http://dx.doi.org/10.1186/1479-5868-8-124.
- Bibby P, Shepherd J. Developing a New Classification of Urban and Rural Areas for Policy Purposes – The Methodology. London: Office for National Statistics; 2004.
- OS MasterMap Integrated Transport Network (ITN) Layer. Southampton: OS; 2013.
- OpenStreetMap . Openstreetmap: The Free Wiki World Map n.d. www.openstreetmap.org (accessed December 2010).
- Department for Transport . Thales National Public Transport Data Repository (NPTDR) n.d. https://data.gov.uk/dataset/nptdr (accessed June 2011).
- Department for Transport . Thales National Public Transport Access Nodes (NaPTAN) n.d. https://data.gov.uk/dataset/naptan (accessed August 2010).
- Forsyth A. NEAT-GIS (Neighborhood Environment for Active Transport-Geographic Information Systems) Protocols 2010 n.d. http://208.106.193.160/pdfs/GIS_Protocols/NEAT_GIS_V5_0_26Nov2010FIN.pdf (accessed 4 January 2011).
- Goodman A, Sahlqvist S, Ogilvie D. on behalf of the iConnect consortium. New walking and cycling routes and increased physical activity: one- and 2-year findings from the UK iConnect study. Am J Public Health 2014;104:e38-46. http://dx.doi.org/10.2105/AJPH.2014.302059.
- Kelly P, Krenn P, Titze S, Stopher P, Foster C. Quantifying the difference between self-reported and global positioning systems-measured journey durations: a systematic review. Transport Rev 2013;33:443-59. http://dx.doi.org/10.1080/01441647.2013.815288.
- Kelly P, Doherty A, Berry E, Hodges S, Batterham A, Foster C. Can we use digital life-log images to investigate active and sedentary travel behaviour? Results from a pilot study. Int J Behav Nutr Phys Act 2011;8. http://dx.doi.org/10.1186/1479-5868-8-44.
- van Hees VT, Golubic R, Ekelund U, Brage S. Impact of study design on development and evaluation of an activity-type classifier. J Appl Physiol 2013;114:1042-51. http://dx.doi.org/10.1152/japplphysiol.00984.2012.
- Troped PJ, Oliveira MS, Matthews CE, Cromley EK, Melly SJ, Craig BA. Prediction of activity mode with global positioning system and accelerometer data. Med Sci Sports Exerc 2008;40:972-8. http://dx.doi.org/10.1249/MSS.0b013e318164c407.
- Kang B, Moudon AV, Hurvitz PM, Reichley L, Saelens BE. Walking objectively measured: classifying accelerometer data with GPS and travel diaries. Med Sci Sports Exerc 2013;45:1419-28. http://dx.doi.org/10.1249/MSS.0b013e318285f202.
- Panter J, Costa S, Dalton A, Jones A, Ogilvie D. Development of methods to objectively identify time spent using active and motorised modes of travel to work: how do self-reported measures compare?. Int J Behav Nutr Phys Act 2014;11. http://dx.doi.org/10.1186/s12966-014-0116-x.
- Panter J, Griffin S, Ogilvie D. Correlates of reported and recorded time spent in physical activity: results from the Commuting and Health in Cambridge study. PLOS ONE 2012;7. http://dx.doi.org/10.1371/journal.pone.0042202.
- Cleland VJ, Schmidt MD, Salmon J, Dwyer T, Venn A. Correlates of pedometer-measured and self-reported physical activity among young Australian adults. J Sci Med Sport 2011;14:496-503. http://dx.doi.org/10.1016/j.jsams.2011.04.006.
- Tully MA, Panter J, Ogilvie D. Individual characteristics associated with mismatches between self-reported and accelerometer-measured physical activity. PLOS ONE 2014;9. http://dx.doi.org/10.1371/journal.pone.0099636.
- Panter J, Jones A. Attitudes and the environment as determinants of active travel in adults: what do we know and don’t we know?. J Phys Act Health 2010;7:551-61.
- Rodríguez DA, Joo J. The relationship between non-motorized mode choice and the local physical environment. Transport Res D 2004;9:151-73. http://dx.doi.org/10.1016/j.trd.2003.11.001.
- Dalton AM, Jones AP, Pater J, Ogilvie D. Are GIS-modelled routes a useful proxy for the actual routes followed by commuters?. J Transport Health 2015;2:219-29. http://dx.doi.org/10.1016/j.jth.2014.10.001.
- Geertz C. Thick Description: Toward an Interpretive Theory of Culture. New York, NY: Basic Books; 1973.
- Guell C, Ogilvie D. Picturing commuting: photovoice and seeking wellbeing in everyday travel. Qual Res 2015;15:201-18. http://dx.doi.org/10.1177/1468794112468472.
- Atkinson P, Delamont S, Housley W. Contours of Culture: Complex Ethnography and the Ethnography of Complexity. Walnut Creek, CA: Alta Mira Press; 2008.
- Mathews G, Izquierdo C. Pursuits of Happiness: Well-Being in Anthropological Perspective. New York, NY, and Oxford: Berghahn; 2009.
- Chalfen R, Prosser J. Image-Based Research: A Sourcebook for Qualitative Researchers. London: Falmer Press Ltd; 1998.
- de Certeau M. The Practice of Everyday Life. Berkeley, CA: California University Press; 1984.
- Silverman D. Interpreting Qualitative Data. London: Sage; 2006.
- Cannuscio CC, Weiss EE, Fruchtman H, Schroeder J, Weiner J, Asch DA. Visual epidemiology: photographs as tools for probing street-level etiologies. Soc Sci Med 2009;69:553-64. http://dx.doi.org/10.1016/j.socscimed.2009.06.013.
- Fleury J, Keller C, Perez A. Exploring resources for physical activity in hispanic women, using photo elicitation. Qual Health Res 2009;19:677-86. http://dx.doi.org/10.1177/1049732309334471.
- Oliffe J, Bottorff J. Further than the eye can see? Photo elicitation and research with men. Qual Health Res 2007;17:850-8. http://dx.doi.org/10.1177/1049732306298756.
- 2011 Census: Aggregate Data (England and Wales). Office for National Statistics; 2011.
- Panter J, Desousa C, Ogilvie D. Incorporating walking or cycling into car journeys to and from work: the role of individual, workplace and environmental characteristics. Prev Med 2013;565:211-7. http://dx.doi.org/10.1016/j.ypmed.2013.01.014.
- Goodman A, Guell C, Panter J, Jones NR, Ogilvie D. Healthy travel and the socio-economic structure of car commuting in Cambridge, UK: a mixed-methods analysis. Soc Sci Med 2012;74:1929-38. http://dx.doi.org/10.1016/j.socscimed.2012.01.042.
- Sisson SB, Tudor-Locke C. Comparison of cyclists’ and motorists’ utilitarian physical activity at an urban university. Prev Med 2008;46:77-9. http://dx.doi.org/10.1016/j.ypmed.2007.07.004.
- Sirard J, Riner W, McIver K, Pate R. Physical activity and active commuting to elementary school. Med Sci Sports Exerc 2005;37:2062-9. http://dx.doi.org/10.1249/01.mss.0000179102.17183.6b.
- Wener RE, Evans GW. A morning stroll: levels of physical activity in car and mass transit commuting. Environ Behav 2007;39:62-74. http://dx.doi.org/10.1177/0013916506295571.
- Yang L, Panter J, Griffin SJ, Ogilvie D. Associations between active commuting and physical activity in working adults: cross-sectional results from the Commuting and Health in Cambridge study. Prev Med 2012;55:453-7. http://dx.doi.org/10.1016/j.ypmed.2012.08.019.
- Costa S, Ogilvie D, Dalton A, Westgate K, Brage S, Panter J. Quantifiying the physical activity energy expenditure of commuters using a combination of global positioning system and combined heart rate and movement sensors. Prev Med 2015;81:339-44. http://dx.doi.org/10.1016/j.ypmed.2015.09.022.
- Dunn AL, Trivedi MH, Kampert JB, Clark CG, Chambliss HO. Exercise treatment for depression: efficacy and dose response. Am J Prev Med 2005;28:1-8. http://dx.doi.org/10.1016/j.amepre.2004.09.003.
- Anokye NK, Trueman P, Green C, Pavey TG, Taylor RS. Physical activity and health related quality of life. BMC Public Health 2012;12. http://dx.doi.org/10.1186/1471-2458-12-624.
- Bize R, Johnson JA, Plotnikoff RC. Physical activity level and health-related quality of life in the general adult population: a systematic review. Prev Med 2007;45:401-15. http://dx.doi.org/10.1016/j.ypmed.2007.07.017.
- Hamer M, Stamatakis E. Objectively assessed physical activity, fitness and subjective wellbeing. Mental Health Phys Act 2010;3:67-71. http://dx.doi.org/10.1016/j.mhpa.2010.09.001.
- Humphreys D, Goodman A, Ogilvie D. Associations between active commuting and physical and mental wellbeing. Prev Med 2013;57:135-9. http://dx.doi.org/10.1016/j.ypmed.2013.04.008.
- Carse A, Goodman A, Mackett RL, Panter J, Ogilvie D. The factors influencing car use in a cycle-friendly city: the case of Cambridge. J Transport Geogr 2013;28:67-74. http://dx.doi.org/10.1016/j.jtrangeo.2012.10.013.
- Ball K, Jeffery RW, Crawford DA, Roberts RJ, Salmon J, Timperio AF. Mismatch between perceived and objective measures of physical activity environments. Prev Med 2008;47:294-8. http://dx.doi.org/10.1016/j.ypmed.2008.05.001.
- Panter J, Griffin S, Ogilvie D. Active commuting and perceptions of the route environment: a longitudinal analysis. Prev Med 2014;67:134-40. http://dx.doi.org/10.1016/j.ypmed.2014.06.033.
- Victora C, Habicht J-P, Bryce J. Evidence-based public health: moving beyond randomized trials. Am J Public Health 2004;94:400-5. http://dx.doi.org/10.2105/AJPH.94.3.400.
- Murray MC, Finch T, Mair F, Treweek S. Normalization Process Theory On-Line User’s Manual and Toolkit n.d. www.normalizationprocess.org (accessed 22 April 2012).
- Phillips T. Guided Busway Was Sheer Folly. Hunts Post 2012.
- Eliott C. Cyclists Make Tracks Along Guided Busway 2009.
- Outspoken Delivery . Almost 56,000 Trips Made on the Cambridge #busway in the First 7 Days. I Wonder How Many Cyclists Use the Path? 2011. https://twitter.com/OutspokenDeliv/status/103381814474833920.
- Rutter H. I Could Almost Be in the Netherlands . . . Lovely Wide Cycle Path Alongside the Cambridgeshire Guided Busway 2012. https://twitter.com/harryrutter/status/267991729406357504.
- Anon . Cambridge First 2011.
- Heinen E, Panter J, Dalton A, Jones A, Ogilvie D. Sociospatial patterning of the use of new transport infrastructure: walking, cycling and bus travel on the Cambridgeshire guided busway. J Transport Health 2015;2:199-211. http://dx.doi.org/10.1016/j.jth.2014.10.006.
- Heinen E, Panter J, Mackett R, Ogilvie D. Changes in mode of travel to work: a natural experimental study of new transport infrastructure. Int J Behav Nutr Phys Act 2015;12. http://dx.doi.org/10.1186/s12966-015-0239-8.
- Panter J, Heinen E, Mackett R, Ogilvie D. Impact of new transport infrastructure on walking, cycling and physical activity. Am J Prev Med 2015.
- Broadbent A, Illari PM, Russo F, Williamson J. Causality in the Sciences. Oxford: Oxford University Press; 2011.
- Kesten J, Guell C, Cohn S, Ogilvie D. From the concrete to the intangible: understanding diverse experiences of new transport infrastructure. Int J Behav Nutr Phys Act 2015;12. http://dx.doi.org/10.1186/s12966-015-0230-4.
- Hamer M, Chida Y. Active commuting and cardiovascular risk: a meta-analytic review. Prev Med 2008;46:9-13. http://dx.doi.org/10.1016/j.ypmed.2007.03.006.
- Foley L, Panter J, Heinen E, Prins R, Ogiluie D. Changes in active commuting and changes in physical activity in adults: a cohort study. Int J Behav Nutr Phys Act 2015;12.
- Mytton O, Panter J, Ogiluie D. Longitudinal associations of active commuting with sickness absence and wellbeing. Prev Med 2016.
- Badland H, Schofield G, Garrett N. Travel behaviour and objectively measured urban design variables: associations for adults traveling to work. Health Place 2008;14:85-9. http://dx.doi.org/10.1016/j.healthplace.2007.05.002.
- Sahlqvist S, Song Y, Ogilvie D. on behalf of the iConnect consortium . Is active travel associated with greater physical activity? The contribution of commuting and non-commuting active travel to total physical activity in adults. Prev Med 2012;55:206-11. http://dx.doi.org/10.1016/j.ypmed.2012.06.028.
- Sahlqvist S, Goodman A, Cooper A, Ogilvie D. on behalf of the iConnect consortium. Change in active travel and changes in recreational and total physical activity in adults: longitudinal findings from the iConnect study. Int J Behav Nutr Phys Act 2013;10. http://dx.doi.org/10.1186/1479-5868-10-28.
- Woodcock J, Edwards P, Tonne C, Armstrong B, Ashiru O, Banister D, et al. Public health benefits of strategies to reduce greenhouse-gas emissions: urban land transport. Lancet 2009;274:1930-43. http://dx.doi.org/10.1016/S0140-6736(09)61714-1.
- Kelly P, Kahlmeier S, Götschi T, Orsini N, Richards J, Roberts N, et al. Systematic review and meta-analysis of reduction in all-cause mortality from walking and cycling and shape of dose response relationship. Int J Behav Nutr Phys Act 2014;11. http://dx.doi.org/10.1186/s12966-014-0132-x.
- Sickness Absence in the Labour Market, February 2014. Office for National Statistics; 2014.
- Flint E, Cummins S, Sacker A. Associations between active commuting, body fat, and body mass index: population based, cross sectional study in the United Kingdom. BMJ 2014;349. http://dx.doi.org/10.1136/bmj.g4887.
- Pratt M, Macera C, Sallis J, O’Donnell M, Frank L. Economic interventions to promote physical activity: application of the SLOTH model. Am J Prev Med 2004;27:136-45. http://dx.doi.org/10.1016/j.amepre.2004.06.015.
- Sallis J, Cervero R, Ascher W, Henderson K, Kraft M, Kerr J. An ecological approach to creating active living communities. Annu Rev Public Health 2006;27:297-322. http://dx.doi.org/10.1146/annurev.publhealth.27.021405.102100.
- Pucher J, Dill J, Handy S. Infrastructure, programs, and policies to increase bicycling: an international review. Prev Med 2009;50:106-25. http://dx.doi.org/10.1016/j.ypmed.2009.07.028.
- Hooper P, Giles-Corti B, Knuiman M. Evaluating the implementation and active living impacts of a state government planning policy designed to create walkable neighborhoods in Perth, Western Australia. Am J Health Promot 2014;28:5-18. http://dx.doi.org/10.4278/ajhp.130503-QUAN-226.
- Christian H, Knuiman M, Bull F, Timperio A, Foster S, Divitini M, et al. A new urban planning code’s impact on walking: the Residential Environments project. Am J Public Health 2013;103:1219-28. http://dx.doi.org/10.2105/AJPH.2013.301230.
- Humphreys D, Ogilvie D. Synthesising evidence for equity impacts of population-based physical activity interventions: a pilot study. Int J Behav Nutr Phys Act 2013;10. http://dx.doi.org/10.1186/1479-5868-10-76.
- Goodman A, Sahlqvist S, Ogilvie D. on behalf of the iConnect consortium . Who uses new walking and cycling infrastructure and how? Longitudinal results from the UK iConnect study. Prev Med 2013;57:518-24. http://dx.doi.org/10.1016/j.ypmed.2013.07.007.
- Panter J, Ogilvie D. on behalf of the iConnect consortium . Theorising and testing environmental pathways to behaviour change: natural experimental study of the perception and use of new infrastructure to promote walking and cycling in local communities. BMJ Open 2015;5. http://dx.doi.org/10.1136/bmjopen-2015-007593.
- Marteau T, Hollands G, Fletcher P. Changing human behavior to prevent disease: the importance of targeting automatic processes. Science 2012;337:1492-5. http://dx.doi.org/10.1126/science.1226918.
- Goodman A, Panter J, Sharp S, Ogilvie D. Effectiveness and equity impacts of town-wide cycling initiatives in England: a longitudinal, controlled natural experimental study. Soc Sci Med 2013;97:228-37. http://dx.doi.org/10.1016/j.socscimed.2013.08.030.
- Pawson R, Tilley N. Realistic Evaluation. London: Sage; 1997.
- Chaix B, Méline J, Duncan S, Merrien C, Karusisi N, Perchoux C, et al. GPS tracking in neighborhood and health studies: a step forward for environmental exposure assessment, a step backward for causal inference?. Health Place 2013;21:46-51. http://dx.doi.org/10.1016/j.healthplace.2013.01.003.
- Green J, Steinbach R, Jones A, Edwards P, Kelly C, Nellthorp J, et al. On the buses: a mixed-method evaluation of the impact of free bus travel for young people on the public health. Public Health Res 2014;2.
Appendix 1 Core questionnaire 2009
Appendix 2 Core questionnaire 2012
Appendix 3 Household travel diary
Only the instructions, preliminary questions and example daily diary page are reproduced here.
Appendix 4 Intercept survey
Appendix 5 Vignettes for qualitative interviews
Vignette 1
I mean it’s really in the last five years my attitude’s changed to public transport. There’s a very good bus route, coming into the centre of Cambridge so I either take the bus or cycle in to work, depending on how the weather is. When the weather is not nice, the bus that I take leaves at about five past nine and arrives here at half past nine-ish, depending on the traffic. And I do that so that I can take my son to school for nine o’clock and then take that bus. It was a real change in my behaviour when they introduced these buses. The old buses were a lot less comfortable, they didn’t have any air conditioning, so I often felt sick when I was reading on the bus, these buses have air conditioning, they’re very well kitted out. They’ve got lots of leg space and plug sockets for you to plug your laptop in. And by having access to the internet, I basically start work as soon as I get on the bus. I opt for the bike in the summer because I’ve always enjoyed cycling. I’m not someone who will exercise for the sake of exercising, I don’t enjoy it and don’t tend to stick to it so doing it this way, as the commute to work, it means that I’m doing exercise consistently for a reason and I’ll stick to it.
Vignette 2
The last few years I’ve been driving and I love driving, I drive everywhere. Having the car gives you much more freedom, especially with a child. One reason for driving is a lack of ideal public transport. Deciding between driving and other options is like a balance between the convenience of a car which can literally get you from door to door, with trying to do the green thing and taking public transport or cycling. I didn’t always drive everywhere, I used to cycle to work from a park-and-ride, until a few years back when I fell off my bicycle and now my wife won’t let me cycle in town. Comparatively cycling is not actually much different to the car because I’d still leave the same time and I’d probably arrive at the same time because of that last bit coming into Cambridge the traffic is probably comparable. It’s just the slight inconvenience of cycling and having to change when you come to work.
List of abbreviations
- BMI
- body mass index
- CEDAR
- Centre for Diet and Activity Research
- CI
- confidence interval
- ECG
- electrocardiogram
- GIS
- geographic information system
- GPS
- global positioning system
- IQR
- interquartile range
- LOA
- limit of agreement
- MCS-8
- mental component summary
- MET
- metabolic equivalent
- MVPA
- moderate to vigorous physical activity
- NICE
- National Institute for Health and Care Excellence
- OR
- odds ratio
- PAEE
- physical activity energy expenditure
- PCS-8
- physical component summary
- RPAQ
- Recent Physical Activity Questionnaire
- RRR
- relative risk ratio
- SD
- standard deviation
- SF-8
- Medical Outcomes Study Short Form 8