Notes
Article history
The research reported in this issue of the journal was funded by the PHR programme as project number 09/3007/02. The contractual start date was in July 2011. The final report began editorial review in November 2014 and was accepted for publication in April 2015. The authors have been wholly responsible for all data collection, analysis and interpretation, and for writing up their work. The PHR editors and production house have tried to ensure the accuracy of the authors’ report and would like to thank the reviewers for their constructive comments on the final report document. However, they do not accept liability for damages or losses arising from material published in this report.
Declared competing interests of authors
none
Permissions
Copyright statement
© Queen’s Printer and Controller of HMSO 2016. This work was produced by Fone et al. under the terms of a commissioning contract issued by the Secretary of State for Health. This issue may be freely reproduced for the purposes of private research and study and extracts (or indeed, the full report) may be included in professional journals provided that suitable acknowledgement is made and the reproduction is not associated with any form of advertising. Applications for commercial reproduction should be addressed to: NIHR Journals Library, National Institute for Health Research, Evaluation, Trials and Studies Coordinating Centre, Alpha House, University of Southampton Science Park, Southampton SO16 7NS, UK.
Chapter 1 Alcohol outlet density and harm to population health: literature review
Introduction
Excess alcohol consumption has many adverse effects on health including liver cirrhosis,1 cancer of the oral cavity, pharynx, larynx, oesophagus, liver2 and breast,3 high blood pressure4 and stroke. 5 There is also an increased risk of harm resulting from violence, including homicide,6 suicide,7 road traffic accidents,8 domestic violence9 and attendance at emergency departments and minor injuries units for treatment of violence-related injuries. 10
Binge drinking is typically defined as consuming double the guideline limits in a single day during the previous week. 11 The prevalence is highest in the 16- to 24-year age group for both women and men12,13 and alcohol misuse is associated with antisocial behaviour. 14 Around half of all violent crimes in the UK are alcohol related. 15 Binge drinking places an acute burden on the NHS, as up to 40% of all attendances at accident and emergency (A&E) departments are alcohol related. 16 The majority of these patients are males under 40 years who most commonly present at nights and weekends. Epidemiological evidence also suggests that binge drinking may reduce the benefits of moderate alcohol consumption for coronary heart disease risk. 17,18
Recent data from 2014 show that around 37% of men and 25% of women exceeded UK guidelines for safe levels of alcohol consumption (defined in women as more than three units per day and in men as more than four units per day). This included 18% of men who exceed recommended amounts (four to eight units on any day) and 19% who binge drink (more than eight units on any day) and 14% of women who exceed recommended amounts (three to six units on any day) and 11% who binge drink (more than six units on any day). 13 Given the wide range of harm resulting from this substantial level of excess consumption, the potential impact on health at the population level from a reduction in consumption is considerable. One of the principal evidence-based policies recently recommended by the British Medical Association to reduce alcohol consumption is to reduce easy access to alcohol through controls on hours of sale and outlet density. 19,20 This policy depends on the ‘availability theory’, which states that adverse outcomes are linked directly or indirectly to a greater availability of alcohol. Thus, a higher outlet density leads to higher consumption of alcohol, which leads to negative health and/or social outcomes. 21
Methods
Against a background of a known large number of cross-sectional studies,22 our primary objective was to identify and discuss longitudinal analyses of associations between outlet density and health-related outcomes. We therefore updated the scoping review performed for the project proposal in response to the National Institute for Health Research (NIHR) Public Health Research (PHR) commissioning brief.
A search strategy was developed in Ovid MEDLINE using a range of keywords and indexed terms and adapted to other databases (see Appendix 1 for the search strategies used for each database, including the dates of searches). The search strategy was verified using a range of papers identified in the scoping search carried out for the project proposal.
The following databases were searched for papers published in English to August 2014 that considered the relationship between alcohol-related harms and alcohol outlet density: ASSIA (Applied Social Sciences Index and Abstracts), CINAHL (Cumulative Index to Nursing and Allied Health Literature), EMBASE, HMIC (Health Management Information Consortium), MEDLINE, MEDLINE In-Process & Other Non-Indexed Citations, PsycINFO, Scopus and Web of Science (Science Citation Index and Social Science Citation Index). Papers were included or excluded if they did, or did not, contain a measure of outlet density and a measure of population-based harm in a longitudinal analysis, respectively.
Results
A total of 1142 citations were identified from the database searches. After removal of duplicates, we reviewed 601 unique records by title and abstract, of which 18 longitudinal studies23–40 and one review article41 of studies investigating a change in outlet density met the broad inclusion criteria.
Summary of cross-sectional studies
A 2009 systematic review of 44 studies of alcohol outlet density found that the most frequently investigated alcohol-related harm was violent crime, especially assault. 22 The majority of these studies were from the USA. High outlet densities were associated with higher rates of assault, self-reported injuries, motor vehicle accidents and high rates of pedestrian collisions. Higher outlet density was also shown to be associated with increased domestic violence and child abuse.
Of two additional American studies not included in the review,22 one found a cross-sectional association between higher outlet density and an increased rate of alcohol-related hospital admissions during 1 year (1996) in San Diego County, CA, USA,42 and the other, set in California and Louisiana, found an association with increased self-reported liver disease, sexually transmitted infections (STIs) and violence. 43 Very few studies have investigated alcohol consumption. In this review, two out of three studies found an association between outlet density and high levels of consumption. 22
Longitudinal studies of outlet density and alcohol-related harm
The review article of longitudinal studies, which included papers published up to 2006, discussed the results of studies investigating change in outlet density in three groups of study designs: (1) interrupted time series; (2) natural experiments from alcohol bans; and (3) changes in licensing arrangements. 41 None was set in the UK. Many of these studies were old and few accounted for spatial autocorrelation in their analyses (i.e. the lack of independence of proximate small areas). This review did not include any methodological assessment of the measurement of outlet density. The overall conclusion was that an increase in outlet density was associated with increased consumption and interpersonal violence, but the evidence was weaker for an association with motor vehicle crashes.
We found a further 18 papers23–40 that investigated associations between change in outlet density and a limited range of outcomes, namely alcohol consumption,24–27 violence,23,28–33,36,37 STIs,34,35 suicide38 and other causes of death. 39,40 Of these, seven23,24–26,33,39,40 were published after this study [change in alcohol outlet density and alcohol-related harm to population health (CHALICE)] was funded.
Alcohol consumption
Three papers from Finland have investigated proximity to alcohol outlets and alcohol consumption. 24–26 The first study investigated within-individual change in proximity to an outlet allowing on-premises consumption, defined as a bar or restaurant. 24 Using data from the Finnish Public Sector Study cohort, the preliminary cross-sectional analysis suggested that both heavy drinking (above Finnish weekly guidelines) and ‘extreme drinking occasion’ (i.e. passing out, which was not defined clinically) were significantly associated with residence < 1 km from a defined outlet compared with residence ≥ 1 km from a defined outlet. Longitudinally (n = 54,778 participants who responded at least twice), a small increase in the odds of becoming a heavy user [odds ratio (OR) 1.17, 95% confidence interval (CI) 1.02 to 1.34 per 1-km log-transformed distance], but not in the odds of engaging in extreme drinking occasions (OR 1.03, 95% CI 0.89 to 1.18), was associated with a decrease in proximity. Using the same data set, a companion study found some evidence that change to closer proximity to an off-premises outlet was associated with higher odds of incident heavy alcohol use in women but not in men. 25 The associations varied with the measure of proximity and between ‘beer’ outlets and ‘liquor’ outlets and, overall, the study sample was probably too small to estimate the models with sufficient precision. The third study, using data from the same data set, followed up 6639 men and 28,074 women for 4 years and found that an increase in wine on- and off-premises outlets (as defined by the Finnish licensing system – different from that in the UK) was associated with an increase in wine consumption in women but not in men. 26 There were no significant associations between change in beer and liquor outlets and subsequent consumption.
The final study of 5115 subjects aged 18–30 years at baseline and followed up for 15 years, set in four US cities, found little evidence of an association between an increase in the number of bars within a 0.5-km radius and alcohol consumption. 27
Violence
A 6-year longitudinal study of change in outlet density (counts per zip code) set in California found that increases in bar and off-licensed outlet densities were positively related to an increase in the rate of violence, defined as a hospital admission with an overnight stay with an International Classification of Diseases, Ninth Edition (ICD-9) ‘E-code’ of E960–969 for injuries resulting from interpersonal violence, which are not alcohol specific. 28 Using the same data set a second paper reported that change in each measure of outlet density was positively related to accident rates using police-recorded crashes judged to include alcohol as well as hospital admissions resulting from motor vehicle crashes identified from ICD-9 codes E810–E825 (which are not alcohol specific). 29 A smaller study set in New Mexico reported a non-significant reduction in alcohol-related motor vehicle crashes following the legislative closure of drive-up alcohol outlets. 30 A particularly thorough paper set in California showed a significant association between bar density and admissions to hospital for assault injuries. 23
A time series analysis of Norwegian data from 1960 to 1995 found that outlet density was significantly associated with violent crime. 31 A study set in Melbourne found that an increase in the number of outlets over a 9-year period was positively related to an increase in the number of violent assaults recorded by the police as taking place between 20.00 and 06.00 (used as a proxy for alcohol-related assaults, for which specific data were not recorded). 32 The fixed-effects analyses, which controlled for spatial autocorrelation, modelled numbers of assaults and outlets rather than rates or density. This analysis further categorised postcodes into five clusters based on sociodemographic factors to investigate more detailed relationships and found the strongest associations in central and inner-city suburbs. A further analysis from Melbourne was the first to investigate change in outlet density and domestic violence and found that an increase of one outlet per 1000 residents was associated with an increase in the domestic violence rate of 0.08 per 1000 residents. 33
Sexually transmitted infections
A study set in Los Angeles, CA, reported that a reduction in alcohol availability within census tracts (resulting from a riot in 1992) was associated with a significant reduction in gonococcal infection rates,34 partially mediated by neighbourhood social capital. 35 A significant reduction was also found for violent assaults36 but a more sophisticated statistical analysis published 1 year later suggested that the association reported for violent assaults was no longer significant after using a different statistical modelling method. 37
Suicide and mortality
A study of suicide outcomes using the Californian data set found that changes in bar and off-premise outlet densities were positively related, and restaurant densities negatively related, to attempted and completed suicide rates. 38 Two studies have investigated longitudinal associations between outlet density and alcohol-related mortality. 39,40 The first, set in British Columbia, found that the number of ‘liquor stores’ per capita in 89 local health areas was significantly associated with population rates of alcohol-related death (n = 11,966) over a 6-year period. 39 A very wide definition of alcohol-related death was used. The second study was set in Switzerland (n = 4,376,873 adults aged 30–94 years in the Swiss National Cohort) and linked census records to mortality data from 2001 2008 (n = 8602). 40 A gradient of decreasing hazard ratios (HRs) of alcohol-related mortality was found with decreasing outlet density. No individual measure of alcohol consumption was known.
Outlet density, neighbourhood deprivation and population migration
Two US papers investigated the association between outlet density and neighbourhood deprivation. 44,45 Higher outlet densities were found in more deprived areas but paradoxically one study suggested that levels of consumption were highest in less deprived areas, suggesting that the mismatch between supply and demand could result in alcohol-related harm being disproportionately higher in people living in deprived neighbourhoods in proximity to alcohol outlets. 44
A study set in New Zealand also found an association between higher outlet density and areas of deprivation. 46 A cross-sectional study set in Glasgow found that the spatial association between outlet density and deprivation did not vary systematically, highlighting the importance of local context in the design of future studies. 47 Two studies, set in Australia32 and the USA,23 have found evidence that outlet density is more important in areas with high pre-existing outlet numbers and in areas of high neighbourhood deprivation.
Although it is clear from published research that selective population migration may account for a spurious widening of health inequalities over time,48,49 there are no studies that explicitly investigate this possibility for alcohol-related harm outcomes.
Measurement of outlet density
In the longitudinal studies, the primary measure of outlet density was estimated as the number of outlets per resident population in 331,33,39 of the 10 studies that estimated a density. 23,30,31,33–37,39,40 One study modelled the number of outlets with the resident population as an offset. 32 Five studies estimated outlet density as number of outlets per road-mile30,34–37 and one study estimated density per square mile. 23 Two studies compared densities estimated per road-mile with densities estimated per population and per square mile and reported no difference between the methods. 35,36 Another variant was using the distance from each residence of study participants to the nearest outlet,24–27,40 or the number of outlets per administrative area. 28,29,38
Although most cross-sectional papers used resident population as the denominator, one study set in California noted that there is no standard for measurement of outlet density and estimated outlet density using several different measures for the denominator, including resident population, geographical area (e.g. square mileage) and other more spatial measures of exposure to outlets including Euclidean distance from home to nearest outlet and number of outlets per 0.5-mile ‘buffer zone’, that is, the geographical area defined by 10–15 minutes’ walking time. 44 In this analysis there was little difference in the results for these measures. A New Zealand study used 10 minutes’ travelling time by car to define a neighbourhood area with the density measure as the number of outlets within each neighbourhood. 46 The only published UK research, from Glasgow, estimated outlet density using two different measures: outlets per 1000 residents and the mean network distance from the centroid of each small area of interest to the nearest outlet. 47
Summary
Overall, there is cross-sectional evidence from mainly US studies to suggest that higher outlet density is associated with alcohol-related harm, particularly involving violence. The evidence from longitudinal studies for associations between harm and a change in outlet density is less well established. Some evidence has been published after the CHALICE study was funded which suggests that a decrease in proximity to outlets is associated with a small increase in alcohol consumption. No longitudinal studies of admissions to hospital have been published for non-violent outcomes. Many methodological questions remain over the best way to measure outlet density. Little is known about the effect of a change in outlet density on inequalities in alcohol-related health and the role of population migration. Interestingly, there is a suggestion from two (non-UK) papers published after the CHALICE study was funded that mortality is associated with outlet density, but investigating this is outside the scope of this project.
Chapter 2 Research questions
National Institute for Health Research call
This project was a response to the NIHR PHR (number 09/3007/02) commissioning brief research question: ‘What is the impact of a reduction in the availability of alcohol on community alcohol health-related harm and/or consumption in the local community?’
We proposed to measure alcohol availability as outlet density. It is acknowledged that availability may change in either direction. This change in outlet density is a complex intervention that takes the form of a natural experiment as is common in public health contexts:50 here, for example, in changes to policy on the availability of alcohol. These are not amenable to randomisation but have a natural non-random variability with regard to geographical location and time.
Primary research question
The primary research question was:
-
What is the impact of a change in the density of alcohol outlets on alcohol consumption and alcohol-related harms to health in the community?
This study investigated the effect of change in alcohol outlet density on important alcohol-related health outcomes in Wales. The four groups of outcomes investigated were (1) alcohol consumption, including number of units and binge drinking; (2) hospital admissions; (3) A&E department attendances; and (4) violence against the person (VAP), as recorded by police forces. The record-linked hospital admission and A&E attendance outcomes used in this study exploited the in-house potential of record-linked routine data sets.
Secondary research questions
Our secondary research questions were:
-
Does a health selection effect from population migration at small-area level explain any observed associations between outlet density and alcohol-related harm?
-
What effect does change in outlet density have on population inequalities in alcohol-related health?
Here, we directly addressed the NIHR commissioning brief to consider the impact on health inequalities. We suggested that this had two important methodological components. First, there is some evidence that selective population migration may account for a spurious widening of health inequalities over time. 48,49 We estimated the effect of population migration on the estimates of outlet density and hence on the associations with alcohol-related harm. Second, we assessed the impact of change in outlet density on alcohol-related health inequalities, measured by differences in outcomes between different levels of small-area or neighbourhood social deprivation.
Research design
This project was designed to include four separate studies, one for each outcome. We investigated health-related outcomes modelled as a function of alcohol availability, measured by outlet density, during the time period of each study:
-
Study 1: alcohol consumption. This study included residents of Wales aged ≥ 16 years who responded to the five annual Welsh Health Surveys (WHSs) carried out between 2008 and 2012. The WHSs include data on units of alcohol consumed.
-
Study 2: hospital admissions. This study included residents of Wales aged ≥ 16 years admitted to hospital from 2006 to 2011 inclusive for an alcohol-related cause.
-
Study 3: A&E department attendances. This study included residents of Wales aged ≥ 16 years who attended an A&E department between midnight and 0600 from April 2009 to 2011 inclusive.
-
Study 4: violent crime against the person. This study included small-area aggregated data on residents of Wales aged ≥ 16 years who were victims of alcohol-related VAP from 2008 to 2011 inclusive.
This was an ambitious project that was dependent on the quality of the proposed data sets. Although the WHS and hospital admission data have been well utilised in research, the robustness of A&E data and police-recorded crime data for epidemiological studies was not known and we employed a pragmatic approach to assessing their use.
Chapter 3 Study setting
Introduction
The setting was Wales, UK, with a total population of 3,063,456 in the 2011 census [Office for National Statistics (ONS)]. 51 Of the total population, 2,507,917 were aged ≥ 16 years. Wales comprises 22 local authorities, the administrative areas of local government (Table 1).
Local authority | Population | Population aged ≥ 16 years |
---|---|---|
Isle of Anglesey | 69,751 | 58,023 |
Gwynedd | 121,874 | 100,655 |
Conwy | 115,228 | 96,263 |
Denbighshire | 93,734 | 76,899 |
Flintshire | 152,506 | 124,082 |
Wrexham | 134,844 | 109,228 |
Powys | 132,976 | 110,310 |
Ceredigion | 75,922 | 64,128 |
Pembrokeshire | 122,439 | 100,611 |
Carmarthenshire | 183,777 | 150,935 |
Swansea | 239,023 | 197,369 |
Neath Port Talbot | 139,812 | 115,206 |
Bridgend | 139,178 | 114,052 |
Vale of Glamorgan | 126,336 | 102,903 |
Cardiff | 346,090 | 282,368 |
Rhondda Cynon Taf | 234,410 | 190,048 |
Merthyr Tydfil | 58,802 | 47,882 |
Caerphilly | 178,806 | 143,951 |
Blaenau Gwent | 69,814 | 57,338 |
Torfaen | 91,075 | 73,986 |
Monmouthshire | 91,323 | 75,295 |
Newport | 145,736 | 116,385 |
Total | 3,063,456 | 2,507,917 |
Lower Layer Super Output Areas
The 2001 census geography defined 1896 Lower Layer Super Output Areas (LSOAs) in Wales. 52 LSOAs contain a mean population of approximately 1300 people aged ≥ 16 years. In Wales, LSOAs are the most commonly used small geographical level at which most administrative data can be analysed and they were used in this study as the best proxy for the residential neighbourhood.
Welsh Index of Multiple Deprivation 2008
We used the Welsh Index of Multiple Deprivation (WIMD) 2008 calculated at LSOA level as the measure of neighbourhood deprivation in this study. 53 The WIMD is available for 2005, 2008 and 2011. Because each version includes a different set of variables and there are therefore differences in the index construction, the three scores are not directly comparable. We therefore chose the WIMD 2008 version as it was the version that was closest in date to the start of the study time periods. The WIMD 2008 contains eight weighted domains of deprivation (Table 2). The overall index is constructed by ranking the LSOAs in each domain from 1 to 1896, exponentiating the [transformed (0,1)] ranks, which are then summed and weighted as in Table 2 to give the final LSOA WIMD score, with high scores indicating high levels of deprivation.
Domain | Weighting (%) |
---|---|
Income | 23.5 |
Employment | 23.5 |
Health | 14 |
Education | 14 |
Housing | 5 |
Access to services | 10 |
Environment | 5 |
Community safety | 5 |
The main criticism of the WIMD is that it is largely driven by data availability and subjective assessment of its quality and utility rather than any by a priori considerations (although income and employment account for nearly one-half of the final score). The inclusion of a health domain, which might be considered as outcome data and subject to circularity, in fact makes little difference in analyses of the WIMD and health outcomes. 54 The WIMD is very highly correlated with other deprivation indices such as the Townsend score55 and has the advantage of being updated regularly in the intercensal period.
Because the final WIMD scores have little intuitive meaning and their distribution is highly skewed (Figure 1), we chose to create five groups, or quintiles, of deprivation with an equal count of LSOAs in each group (Table 3). This was also necessary as an anonymised categorisation in quintiles is permissible in the Secure Anonymised Information Linkage (SAIL) Databank (see Chapter 4).
FIGURE 1.
Distribution of WIMD 2008 scores. SD, standard deviation.

Quintile | Frequency | % |
---|---|---|
Least deprived | 380 | 20 |
Less deprived | 379 | 20 |
Mid deprived | 379 | 20 |
More deprived | 379 | 20 |
Most deprived | 379 | 20 |
Total | 1896 | 100 |
Figure 2 shows a map of Wales and the LSOA WIMD 2008 quintiles.
FIGURE 2.
Map of Wales showing the LSOA WIMD 2008 quintiles. © Crown copyright and database rights 2013 Ordnance Survey 100019153.

Rural–urban settlement type classification
We also used the 2004 rural–urban classification published by the ONS56 as the measure of settlement type for 2001 census LSOAs.
For LSOAs there are six settlement types, categorised into three groups:
-
urban (population > 10,000)
-
town and fringe
-
village, hamlet and isolated dwellings.
Figure 3 shows a map of LSOAs in Wales with the three settlement types.
FIGURE 3.
Settlement type classification. © Crown copyright and database rights 2013 Ordnance Survey 100019153.

Chapter 4 The Secure Anonymised Information Linkage Databank
Introduction
This chapter will discuss the use of the SAIL Databank at Swansea University. The SAIL Databank contains demographic, health, social and education data for the resident population of Wales. 57 Within the SAIL Databank is the NHS Welsh Demographic Service (WDS) data set, containing address information linked anonymously at the individual level. 58
We have recently been funded as the Centre for Improvement of Population Health through E-records Research (CIPHER), one of four UK e-health informatics research centres funded by a joint investment from Arthritis Research UK, the British Heart Foundation, Cancer Research UK, the Chief Scientist Office (Scottish Government Health Directorates), the Economic and Social Research Council, the Engineering and Physical Sciences Research Council, the Medical Research Council, the NIHR, the National Institute for Social Care and Health Research (Welsh Government) and the Wellcome Trust.
The Centre for Improvement of Population Health through E-records Research is part of the Farr Institute of Health Informatics Research, which consists of four nodes in the UK: University College London (Farr Institute @ London), University of Manchester (Farr Institute @ HeRC N8), Swansea University (Farr Institute @ CIPHER) and the University of Dundee (Farr Institute @ Scotland) [see www.farrinstitute.org/ (accessed 25 February 2016)]. With a £17.5M research award from the 10-funder consortium, plus an additional £20M of capital funds from the Medical Research Council, the Farr Institute aims to deliver high-quality, cutting-edge research linking electronic health data with other forms of research and routinely collected data, as well as build capacity in health informatics research. The Farr Institute aims to provide the physical and electronic infrastructure to facilitate collaboration across the four nodes, support their safe use of patient and research data for medical research and enable partnerships by providing a physical structure to co-locate NHS organisations, industry and other UK academic centres.
This project has also been adopted by DECIPHer, the Centre for the Development and Evaluation of Complex Interventions for Public Health Improvement, a UK Clinical Research Collaboration (UKCRC) Public Health Research Centre of Excellence [see www.decipher.uk.net/ (accessed 25 February 2016)].
The Centre for the Development and Evaluation of Complex Interventions for Public Health Improvement brings together researchers from a range of disciplines to tackle public health issues such as diet and nutrition, physical activity, and alcohol, tobacco and drug use, with a particular focus on developing and evaluating multilevel interventions that will have an impact on the health and well-being of children and young people. The centre engages strongly with policy, practice and public user communities, as our stakeholders, to translate the research results into practical outcomes.
Welsh Demographic Service and Anonymised Linking Fields
The WDS data set is held and managed by the NHS Wales Informatics Service (NWIS), the NHS organisation in Wales mandated to hold personally identifiable data. It contains addresses for all individuals who register with a general practitioner (GP). The accuracy of matching between the GP data set and the WDS data set in 2009 was > 99.99%. 58 The WDS data set contains demographic data including age and sex and is used as the population register within the SAIL Databank. These data may be used to create population subgroups for required date(s) or duration(s).
The ‘split-file’ method is used in the SAIL Databank to anonymously link data sets (Figure 4). 57,58 Each individual in the WDS data set is assigned a unique Anonymised Linking Field (ALF) for use as the primary key variable for record linkage. Each NHS Wales data set held by the NWIS [in this project the Patient Episode Database for Wales (PEDW) and A&E department attendance data] is split into part 1, identifiable name and address data, and part 2, the clinical data. A system-linking field is added so that data may be later relinked within the SAIL Databank. Part 1 is replaced with the ALF for each person by the NWIS and part 2 of the data set is delivered to the SAIL Databank where the clinical data are relinked using the system-linking field. The ALF is further encrypted to the Anonymised Linking Field_encrypted (ALF_E), which is visible to external project statisticians. The dates of each change of address are recorded, providing duration of residency and the ability to link time-varying exposures to each residence and LSOA as individuals move home. The WDS data set also includes a field for date of death.
FIGURE 4.
The split-file approach to building the SAIL Databank. ALF, Anonymised Linking Field.

Welsh Demographic Service study cohorts
For the secondary outcome of hospital admissions, the full WDS data set for all persons aged ≥ 16 years living in Wales on 1 January 2006 was used to define the cohort for analysis. To account for moves within Wales, each individual’s LSOA code of residence was recorded at the start of each quarterly time period.
We created a field for people who left the WDS data set, that is, moved to England or elsewhere, with time in days from 1 January 2006 to the first day of the quarter of migration. This was to allow censoring for migration, that is, left the study. Because of the complexities of making assumptions about exposure to alcohol outlets while resident out of Wales in the absence of any information on this, we made the simplifying assumption that no individuals moved back into Wales. To censor for death, each ALF_E was linked to the ONS mortality files to link on the date of death and the underlying cause of death code was defined by the International Statistical Classification of Diseases and Related Health Problems, 10th Revision (ICD-10). 59
We derived population data for those aged ≥ 16 years for each LSOA per year, per quarter and by age group and sex. These population data were used to calculate simple rates per 1000 people per LSOA. For the secondary outcome of A&E attendances the start date of the cohort was 1 April 2009.
Residential Anonymous Linking Fields
A particular strength of the SAIL system is the development of a system for anonymising all households in Wales and linking household-level data from local authorities and others with individual health-related data while protecting anonymity, using individual linking fields (ALF_Es) and Residential Anonymous Linking Fields_Encrypted (RALF_Es). The environment Geographic Information System (eGIS) (see Environment Geographic Information System) may be used to create high-resolution spatial metrics surrounding each residence. Geocoded address data are matched by our trusted third party, NWIS, who replace identifiable addresses with a consistent Residential Anonymous Linking Field (RALF) using the split-file method. 60 The residence-based metrics may then be fully incorporated into the SAIL Databank by linking RALF_Es to ALF_Es.
Patient Episode Database for Wales
The SAIL Databank includes the complete PEDW, which includes demographic and clinical data on all inpatient and day case admissions in NHS Wales hospitals and on all Welsh residents treated in England from 1 January 1999. Each record of an admission contains a wealth of fields including, inter alia, date of admission, admission method (e.g. emergency or elective), episode and spell number, provider unit code, specialty code, patient classification (inpatient or day case), 14 diagnosis (ICD-10) and six procedure code fields using the Office of Population, Censuses and Surveys Classification of Surgical Operations and Procedures version 4.6 (OPCS-4.6),61 discharge destination (to identify interhospital transfers), discharge method (to identify death in hospital) and date of discharge. Full details of all available fields are given in the NHS Wales Data Dictionary published by the NWIS [see www.datadictionary.wales.nhs.uk/ (accessed 3 June 2015)].
The pseudonymisation process results in the encryption of a unique, unidentifiable ALF_E that enables a patient-based analysis rather than an admissions-based analysis to be carried out, as all admission rows can be linked for each anonymised patient. Each PEDW record is also linked to the LSOA of residence. This LSOA code is then linked to a deprivation score, which in this study is quintiles of the WIMD 2008,53 and the ONS settlement type classification. 56 We can then attribute admissions to a LSOA deprivation quintile and rural–urban settlement type for analysis.
Accident and emergency department attendances
The Emergency Department Dataset (EDD) is a new data set within the SAIL Databank, also provided by the NWIS. This is a source of patient-level data on attendances at emergency care facilities in Wales that tends mainly to be used for performance targets. There is no systematic clinical coding in this data set. Patient-level data are collected in the EDD for all ‘major accident and emergency departments’. The first wave of data for the 13 A&E departments in Wales was received for 2009 and data for subsequent years are entered into the SAIL Databank as they are received, on a monthly basis. Attendances are recorded with the date and time of day. This study uses data between 2009 quarter 2 and 2011 quarter 4. This data set is linked to individual and residence information, allowing us to summarise A&E attendances per LSOA, per yearly quarter, by age group and by sex.
Environment Geographic Information System
In parallel with the SAIL Databank, but completely separate, is the eGIS. The eGIS is a databank containing project-specific map data from the UK Ordnance Survey (OS) at high spatial resolution and locally sourced data sets, for example from local government. The OS MasterMap® (OSMM) AddressBase® Premium (ABP) data set62 contains point data for all residences and the OSMM Integrated Transport Network™ (ITN) data set. 63 The ABP data layer contains a point for each residence, which is located within the footprint of the residence. The buildings are surveyed with a spatial accuracy of ±1–2 m, providing georeferences at a high spatial resolution. It is possible to create many useful environment data sets, which can be anonymously joined with health data. The household-level links to individual health data mean that environmental metrics are created based on the dwellings identified by Unique Property Reference Numbers (UPRNs). 60 We have used the word ‘environment’ to distinguish the eGIS from the SAIL Databank containing health and other types of individual-level anonymised data. For the purpose of maintaining anonymous individual-level health data it is not possible to make direct links between the two databanks.
Research ethics and information governance
As this study involves the analysis of retrospective routinely collected anonymised health service data it is not possible to obtain individual consent. This is not required because the study data are anonymised within the SAIL Databank. We received advice from the National Research Ethics Service (NRES) that NHS Research Ethics Committee review was not required for the study, as it did not involve NHS patients or staff but was classified under the category of an anonymised research database. 64
The design of the anonymisation process involves NWIS carrying out identity matching and subsequent encryption of NHS numbers and address identities without access to any clinical content. Subsequent second-stage encryption and linkage of anonymised data sets at Swansea University mean that the research team cannot identify individuals or addresses. Multistage preparation of data for analysis by trained health analysts working to ONS guidance ensures that individuals cannot be identified through small numbers.
Georeferenced point data are not available within the SAIL Databank because they would contravene the SAIL Databank rules that ensure health data privacy. As the description of a rare outcome may have produced unique and identifiable data, the data are checked by an experienced information governance reviewer to ensure k-anonymisation standards60 before release into a secure area (the SAIL Gateway) for viewing and analysis by trusted researchers. 57 All such researchers complete Safe Researcher Training.
The SAIL Databank was designed following consultation with the Information Commissioner’s Office in Wales, extensively reviewed by NHS Information Governance bodies (including Informing Healthcare and NWIS – the Welsh equivalents of Connecting for Health and the NHS Information Centre) and externally assessed before such organisations agreed to provide data to the system. An Information Governance Review Panel (IGRP) assesses whether or not all proposals for analysis meet the strict information governance arrangements set out in the multiple data access agreements, ensures anonymity and does not require referral to the NRES. The IGRP includes members from the British Medical Association, the NRES, the Public Health Wales NHS Trust and Informing Healthcare as well as lay members. A successful application was made to the IGRP for this study.
Chapter 5 Alcohol outlet data
Data collation
Since November 2005, all local authorities have been required under the Licensing Act 200365 to issue and maintain public registers of licences for all premises and clubs selling/providing alcohol for consumption on and/or off the premises. All local authorities require outlets to apply for a licence using the same UK government-issued application form, in compliance with the Licensing Act 2003,65 but the information held varies considerably between local authorities. From this information we were able, after overcoming some difficulties, to build a georeferenced database for every recorded alcohol outlet in the 22 local authorities in Wales between 2005 and 2011.
We contacted every local authority by telephone and e-mail to make the important contact with the Licensing Department and Licensing Officers. Following a full explanation of the study we made an identical request to each local authority. We received a wide range of responses, from a rapid willingness to prepare the data to a situation in which no data were forthcoming after a series of informal and subsequent freedom of information requests. This situation was undoubtedly multifactorial, given the number of requests that local authority officers regularly have to deal with and the time that they were able to give to each request.
Although all local authorities are required by law to maintain a public register of licensed outlets using the same licence application process, it became apparent throughout the data collection process that different local authorities stored different amounts of information on each outlet. Despite the standard information collection process, we found that a range of methods was used to store and extract the information. The systems used by the local authorities are focused on maintaining existing records, making the extraction of historical information difficult or impossible. We did not find a standard method of digital information storage that could be easily searched. A number of local authorities were unsure how to obtain the information requested from their systems and how to provide it electronically. A particular problem, given the proposed small-area analysis in this project, was the lack of standardised ways of recording outlet addresses with validated postcodes. The research team were given the impression that the licensing systems appeared to be input focused: they were set up for data input rather than for summarising and extracting data.
Throughout the data collection process, several local authorities changed their record-keeping systems or how they made the information available to the public. Many local authorities now make licensing information widely available through their websites, either via an online database or with regularly updated reports of their public register. This enabled data to be systematically collected by the research team and reduced or eliminated the workload on local authority staff from our request for outlet data. A concern about workload was raised by two of the local authorities that did not have a website with outlet data accessible by the public. The input-focused system design was also present in the online systems as it was still not possible for the researchers to obtain a simple overview of outlet types or on/off licence status.
Table 4 summarises the number of requests for information, the data variables provided and the format of the data for each of the 22 local authorities.
Local authority | Number of requests | Start date | End date | On/off | Opening times | Dates available | Format | Source | Class of licence | Outlets | |||
---|---|---|---|---|---|---|---|---|---|---|---|---|---|
Club | Premise | TENa | n (closures) | % closure | |||||||||
1 | 6 in total (2 up to 2010, 4 for 2011 data) | Yes | Yes | Yes | No | November 2005–April 12 | 6 × text files | Mailed in two batches | ✓ | ✓ | – | 298 (32) | 10.7 |
2 | 2 in total (1 up to 2010, 1 for 2011 data) | Yes | Yes | No | No | November 2005–March 2012 | 2 × spreadsheets | 2011–12 online, 2005–10 by e-mail | ✓ | ✓ | – | 491 (6) | 1.2 |
3 | 13+ in total (5 requests between March 2010 and February 2012, then continued dialogue with alternative contact) | Yes | Yes | No | No | Only current register – alternative source for historical data identified | 305 × printed sheets | Collected in person from council (printed from a database) | ✓ | ✓ | – | 553 (16) | 2.9 |
4 | 2 in total (1 up to 2010, 1 for 2011 data) | Yes | Yes | Yes | Yes – until July 2010 | November 2005–January 2012 | Multiple PDF files and spreadsheets | Files e-mailed | ✓ | ✓ | – | 1319 (193) | 14.6 |
5 | 4 in total (3 up to 2010, 1 for 2011 data) | Yes | Yes | Yes | Yes | June 2005–December 2012 | 2 × spreadsheets and 1 × PDF file | Files e-mailed | ✓ | ✓ | – | 912 (114) | 12.5 |
6 | 2 in total (1 up to 2010, 1 for 2011 data) | Yes | Yes | Yes | Yes | November 2005–January 2012 | Multiple text documents | E-mailed spreadsheet, then available online | – | ✓ | – | 490 (110) | 22.4 |
7 | 2 in total (1 up to 2010, 1 for 2011 data) | Yes | Yes | No | No | January 2005 – February 2012 | 1 × spreadsheet and 59 PDF files | E-mailed spreadsheet, PDFs available online | ✓ | ✓ | – | 685 (129) | 18.8 |
8 | 3 in total (2 up to 2010, 1 for 2011 data) | Yes | Yes | No | No | January 2005–January 2012 | 11 × spreadsheets and multiple PDF files | E-mailed spreadsheets, then PDFs available online | ✓ | ✓ | – | 578 (174) | 30.1 |
9 | 1 in total (all available online) | Yes | Yes | Yes | Yes | January 2005–January 2012 | 1 × spreadsheet | Gathered online | ✓ | ✓ | ✓ | 667 (271) | 40.6 |
10 | 9 by this study with no success; another NIHR project shared the data | Yes | Yes | No | No | April 2005–June 2012 | 1 × spreadsheet | File e-mailed | Unknown | 797 (58) | 7.3 | ||
11 | 6 in total (3 to receive current 2012 register data), historical data became available online following contact | Yes | Yes | No | No | November 2005–January 2012 | 71 × printed sheets (current), 2 × PDF files (historical) | Received through the post (printed from a database), then PDFs available online | ✓ | ✓ | – | 327 (95) | 29.1 |
12 | 4 in total (1 up to 2010, 3 for 2011 data) | Yes | Yes | No | No | November 2005–April 2012 | 3 × text files and 1 × spreadsheet | Files e-mailed | ✓ | ✓ | – | 243 (34) | 14.0 |
13 | 3 in total (1 up to 2010, 2 for 2011 data) | Yes | Yes | Yes | Yes | November 2005–January 2012 | 16 × text documents and multiple Word documents | Files e-mailed (2005–10) and sourced online (2010+) | ✓ | ✓ | – | 455 (10) | 2.2 |
14 | 2 in total (1 up to 2010, 1 for 2011 data) | Yes | Yes | Yes | Yes | June 2005–February 2012 | 3 × PDF files and multiple PDF files from the web | Files e-mailed (2005–10) and sourced online (2010+) | ✓ | ✓ | – | 532 (34) | 6.4 |
15 | 4 in total (swift once request acknowledged) | Yes | Yes | Yes | No | April 2004–January 2012 | 1 × spreadsheet | File e-mailed | ✓ | ✓ | – | 512 (42) | 8.2 |
16 | 3 in total (2 up to 2010, 1 for 2011 data) | Yes | Yes | Yes | Yes | November 2005–January 2012 | 600 × PDF files | Available online | ✓ | ✓ | – | 972 (121) | 12.4 |
17 | 2 in total (1 up to 2010, 1 for 2011 data) | Yes | Yes | No | No | June 2005–January 2012 | 2 × spreadsheets | Files e-mailed | ✓ | ✓ | – | 1027 (165) | 16.1 |
18 | 9 in total (2 up to 2010, 7 for 2011 data) | Yes | Yes | Yes | Yes | April 2005–December 2011 | 2 × spreadsheets | Files e-mailed | ✓ | ✓ | – | 658 (22) | 3.3 |
19 | 2 in total (1 up to 2010, 1 for 2011 data) | Yes | Yes | Yes | No | March 2005–January 2012 | 1 × spreadsheet | File e-mailed | ✓ | ✓ | – | 1147 (234) | 20.4 |
20 | 1 in total (2011 data sourced online) | Yes | Yes | No | Yes | November 2005–January 2012 | 6 × PDF files with two different structures | Gathered online | ✓ | ✓ | – | 358 (187) | 52.2 |
21 | 2 in total (1 up to 2010, 1 for 2011 data) | Yes | Yes | No | No | April 2005–December 2011 | 1 × spreadsheet | Gathered online | – | ✓ | – | 459 (64) | 13.9 |
22 | 2 in total (1 up to 2010, 1 for 2011 data) | Yes | Yes | No | No | April 2001–February 2012 | 1 × spreadsheet + 100 × printed sheets | Files e-mailed | ✓ | ✓ | – | 448 (65) | 14.5 |
Overall, the collation of an alcohol outlet data set was a hugely time-consuming, difficult process. Our project coincided with a substantial amount of internal local authority changes in the prevailing economic climate, which meant that anything other than maintaining a public register of current premises to comply with the Licensing Act 200365 was not a priority. It required a substantial amount of time to be able to create a verified and validated data set of outlets for further analysis.
Table 4 shows that only 11 of the 22 local authorities provided some form of on-/off-sales information. The analysis of on-/off-sales separately would therefore necessarily be restricted to these 11 local authorities, which included 1006 LSOAs. However, two problems meant that it was not possible to use the on-/off-sales data to compute a valid measure of on-/off-sales outlet density. The first is that on inspection of the data it was found that around 500 of the 1006 LSOAs had no recorded outlets. This seems implausible and suggests that the data were of poor quality. The second is that even if the on-/off-sales data were valid and complete, there would be a major problem from boundary effects, with the measures of density for LSOAs bordering a local authority that did not supply data being severely underestimated.
We were, therefore, unable to analyse the impact of a change in on-sales or off-sales in this project.
Geographic Information System data processing of alcohol outlet data
Ordnance Survey MasterMap Integrated Transport Network™ layer
The ITN layer is a data set representing the road network and road routing information for Great Britain. 66 It was launched in November 2001 as part of OSMM, the OS flagship digital mapping product. The road network element of the ITN data set contains all classifications of roads, from motorways down to local streets. The road routing information contains information such as height, width and weight restrictions, traffic calming information, turn restrictions and one-way roads. Although precise details on how the software is derived are scarce because of the sensitive commercial nature, the OS website suggests that a combination of ground surveys and photogrammetry has been used. 66 Overall OSMM accuracy is quoted as being 1.0 m or better with a 99% confidence level for urban areas at a scale of 1 : 1250 and 2.5 m or better with a 99% confidence level for rural areas at a scale of 1 : 2500. 66 The ITN data in this project have been used to model the shortest routes between residences and alcohol outlet locations for two different scenarios: walking and driving.
Integrated Transport Network™ driving network
The ITN data, as supplied by the OS, contain information on road types and route restrictions, which enables the modelling of a route between two locations in a Geographic Information System (GIS). Unfortunately, average road speeds and rural–urban designation were not provided as part of the data set. To model road speeds for the network a broad estimate of road speeds was developed using a nominal road speed applied to a road section based on road classification, for example motorway [57 miles per hour (mph)], A road (57 mph or 27 mph) and B road (27 mph).
The final step was to assign a travel time to each section of road based on the estimated road speed using the road length. This was achieved by using the following conversion equation:
Refining the road network to better represent drive times in urban and rural areas
During data validation, the default drive time estimations were found to be too generous. The default network model assumes that there are no time penalties when performing turns on the network or when crossing junctions where there may be traffic lights. As a result, the distances travelled by car in 10 minutes far exceeded what was reasonable in the given time. To address this issue the underlying network model was adjusted to better reflect reality, using local knowledge of driving in rural and urban areas. Time delays at different points in the road network (e.g. road junctions, right turns and left turns) were introduced to the model to better represent a typical car journey through the network. Figure 5 (Cardiff, urban area) and Figure 6 (Pembrokeshire, rural area) illustrate how time penalties for certain road manoeuvres (e.g. turning right onto a road) and crossing junctions reduce the service area for a fixed point. As a result there was a 90% reduction in the modelled alcohol outlet availability for both urban and rural test locations.
FIGURE 5.
Drive time of 10 minutes for urban areas, showing increasing time penalty adjustments and decreasing range. © Crown copyright and database rights 2013 Ordnance Survey 100019153.

FIGURE 6.
Drive time of 10 minutes for rural areas, showing increasing time penalty adjustments and decreasing range. © Crown copyright and database rights 2013 Ordnance Survey 100019153.

Integrated Transport Network™ walking network
The ITN walking model primarily used the same data as the driving model except for the addition of urban paths. As the name suggests, this models urban-based footpaths in major conurbations, thus allowing the calculation of non-vehicular routes for pedestrians. The addition of these data improved the modelling for urban areas for walking, as illustrated in Figure 7, by reducing the travel time and distance from a household to an outlet through the use of footpaths. A universal speed of 5 km per hour (kph) was assigned to the walking model, as this is widely accepted as the average walking speed for an adult.
FIGURE 7.
Integrated Transport NetworkTM urban paths. © Crown copyright and database rights 2013 Ordnance Survey 100050829.
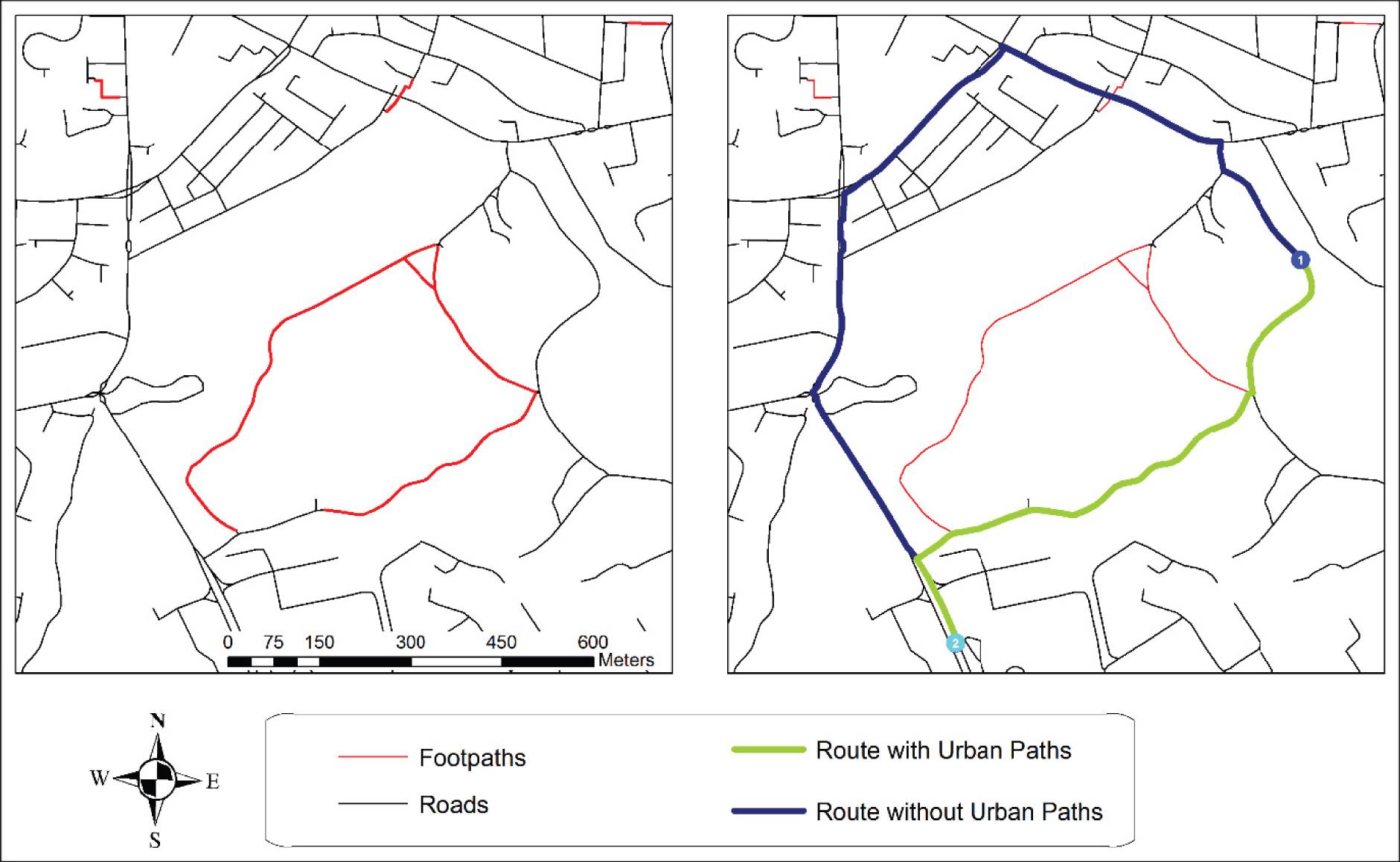
AddressBase Premium
AddressBase Premium62 is the most comprehensive address data set for Great Britain and is based on three data products: the local government’s National Land and Property Gazetteer (NLPG), OSMM Address Layer 2 and the Royal Mail Postcode Address File® (PAF). Collectively this is known as the National Address Gazetteer, from which the ABP products are extracted. ABP provides a complete view of an address or property, including its full lifecycle from build to retirement. It contains all current addresses, alternative addresses, provisional properties and historical property information when available. The data were received in comma-separated value (CSV) text file format and were processed into a database for (1) the geocoding of outlet data and (2) identifying the precise location of residential properties for the density modelling process.
This level of detail was essential so that both outlet availability and residential housing availability and locations could be modelled during the study period. By selecting residential property types, using the supplied ABP classification lookup table (which includes type and start and end dates for each address) and the start and end dates for the quarterly time periods defined for the project, we were able to model almost all of the residential housing availability for each of the quarters during the study period. There were a couple of limitations found within the data with regard to the start dates for properties when the local authority had batch processed a number of residential properties, giving them the same start date. As many of these properties as possible were investigated and when the date was found to be inaccurate it was adjusted to a more appropriate date. For example, a large number of residences in a unitary authority had a start date in late 2007 (possibly at the inception of the NLPG and ABP). On investigation it became clear from alternative data sources [e.g. Google Maps and Google Street View (Google Inc., Mountain View, CA, USA)] that the housing stock predated this date by decades and, therefore, these records were reassigned a start date prior to the study period so that these residences were included in the density calculations.
Points of Interest database
Points of Interest (POI) is a database covering all of Great Britain and containing over 4 million different geographical features. 67 The POI database includes a number of data sets. An indicative summary is supplied by the OS (Table 5).
Source | Contribution (%) |
---|---|
OS | 42.4 |
118 Information | 33.6 |
Department for Transport | 9.0 |
Local Data Company | 3.6 |
UK Payphone Directory | 2.1 |
LINK Interchange Network Ltd | 1.6 |
Visit Britain (England) | 1.0 |
PointX | 0.8 |
Education Direct® | 0.7 |
Edubase | 0.6 |
Each record is georeferenced and classified according to the following main classifications:
-
accommodation, eating and drinking
-
commercial services
-
attractions
-
sport and entertainment
-
education and health
-
public infrastructure
-
manufacturing and production
-
retail
-
transport.
Although the POI database contains information on the location of alcohol outlets we could not use it systematically because no information is provided on opening and closing dates. In addition, we found that there was under-reporting of outlets in urban areas and over-reporting of outlets in rural areas.
Georeferencing outlets
Each alcohol outlet had to be geographically located so that (1) a simple count of outlets per LSOA could be derived and (2) the distance between each residence and each outlet could be computed using a GIS for the walking and driving outlet density calculations. We had to assign an accurate (x,y) co-ordinate to each outlet by geocoding the outlet address. Geocoding is the process of matching text-based address data to known geographical co-ordinates, typically via a GIS. A number of studies have assessed geocoding techniques, positional accuracy68–70 and the impacts of match rates and positional accuracy on resulting analyses. 71–73 In particular it has been shown that, whenever possible, high-resolution address data (building level) should be employed as the reference data set, particularly when fine-scale analysis is being performed. 73 To estimate the alcohol availability for 10-minute walking and driving buffers around a residence, it was important to use the best-quality address data available for the study area.
The text-based address data received from the local authorities were parsed and broken down into address components (organisation name, building name, street number, thoroughfare, post town and postcode) as defined by the Royal Mail. 74 Each category was indexed before the reference data were searched and the best match was recorded using software written for this particular project. The ABP data set was used as the reference data set. Each successfully fully matched record was allocated the corresponding UPRN from the ABP data, as well as the corresponding (x,y) co-ordinates. The ABP data set contains a point for each residence, which is located within the footprint of the residence. The buildings are surveyed with a spatial accuracy of ±1–2 m in urban areas. For records that were not fully matched, a probabilistic matching algorithm was implemented to identify addresses based on partial address matches. For example, premises were identified from street numbers and names when a premise name could not be matched. The final automatic geocoding step filtered for matches based on the postcode provided in the licence data. In Great Britain there are, on average, 18 properties per postcode. Filtering identified the premise or estimated the location.
The results of the geocoding process varied across the 21 local authorities that supplied historical licence data. One local authority was unable to provide us with historical data – the current licence register was provided with the required geographical references. Table 6 provides an overview of the geocoding process and the percentage of outlets that we were able to geocode using the geocoding software. The average automatic ABP match rate for the local authorities in Wales was 50.5%.
Geocoding | Record characteristics | ||||
---|---|---|---|---|---|
Local authority | Geocoded, n (%) | Manual matched, n (%) | Approximately matched, n (%) | Missing postcode, n (%) | Single field address, n (%) |
1 | 94 (31.6) | 203 (68.4) | 0 (0) | 8 (2.7) | 3 (1.0) |
2 | 251 (50.7) | 242 (48.9) | 2 (0.4) | 0 (0) | 1 (0.2) |
3 | 0 (0) | 536 (93.7) | 36 (6.3) | 572 (100) | 11 (1.9) |
4 | 901 (68.2) | 416 (31.5) | 5 (0.4) | 4 (0.3) | 799 (60.4) |
5 | 614 (67.8) | 280 (30.9) | 11 (1.2) | 0 (0) | 0 (0) |
6 | 290 (58.5) | 165 (33.3) | 41 (8.3) | 17 (3.4) | 11 (2.2) |
7 | 339 (49.2) | 292 (42.4) | 58 (8.4) | 103 (14.9) | 1 (0.1) |
8 | 288 (49.0) | 248 (42.2) | 52 (8.8) | 25 (4.3) | 0 (0) |
9 | 500 (34.9) | 876 (61.2) | 55 (3.8) | 121 (8.5) | 1 (0.1) |
10 | 451 (56.1) | 303 (37.7) | 50 (6.2) | 10 (1.2) | 2 (0.2) |
11 | 140 (37.5) | 224 (60.1) | 9 (2.4) | 5 (1.3) | 16 (4.3) |
12 | 136 (55.5) | 103 (42.0) | 6 (2.4) | 0 (0) | 0 (0) |
13 | 130 (27.8) | 325 (69.6) | 12 (2.6) | 23 (4.9) | 0 (0) |
14 | 263 (49.5) | 244 (46.0) | 24 (4.5) | 531 (100) | 0 (0) |
15 | 1035 (64.1) | 557 (34.5) | 22 (1.4) | 29 (1.8) | 0 (0) |
16 | 431 (44.8) | 486 (50.5) | 45 (4.7) | 10 (1.0) | 18 (1.9) |
17 | 438 (42.6) | 528 (51.4) | 61 (5.9) | 60 (5.8) | 0 (0) |
18 | 378 (57.9) | 271 (41.5) | 4 (0.6) | 1 (0.2) | 1 (0.2) |
19 | 608 (64.7) | 322 (34.3) | 9 (1.0) | 4 (0.4) | 6 (0.6) |
20 | 432 (55.0) | 351 (44.7) | 3 (0.4) | 52 (6.6) | 0 (0) |
21 | 199 (43.1) | 246 (53.2) | 17 (3.7) | 96 (20.8) | 0 (0) |
22 | 209 (46.7) | 220 (49.1) | 19 (4.2) | 42 (9.4) | 2 (0.4) |
Total | 8127 (50.5) | 7438 (46.2) | 541 (3.3) | 1713 (10.6) | 872 (5.4) |
The main reasons for a failure to match an address record were an absent or inaccurate premise name or street name or an absent or inaccurate postcode. For example, a premise was recorded with the following address: ‘Market Square, NPXX 4XX’; however, the correct address was in fact ‘Market Street, NPXX 4XX’. Small errors in the main address elements make it difficult for a machine to disambiguate possible matches. This is particularly true when the error in the address could partially match to an alternative address. This example illustrates this because both Market Square and Market Street exist in the locality, making identifying the correct address impossible for an automated system.
The geocoding match rate was markedly higher for records obtained from more urbanised local authorities than for records obtained from those local authorities in a more rural setting. The match rate generally improved as local authorities became more urbanised (Figure 8).
FIGURE 8.
Comparison of geocoding match rates between local authorities.

Manual georeferencing
We developed a manual procedure using Google Maps for those outlets not successfully automatically geocoded. There is a precedent for using web-based mapping technologies to fill gaps and perform neighbourhood audits. 75–77 Google Maps and Google Street View were examined for the outlet location using the information contained in the licence record, for example outlet name, street and locality. The outlet was identified as a point on Google Maps and the latitude and longitude of the outlet location were extracted using the information for the point contained in the URL. When the record was unclear because of differences in urban and rural geocoding, the appropriate street location was identified and an approximate outlet location was assigned by assessing the buildings along the street.
Location difference errors, introduced as part of the manual match process, were calculated from the two sets of co-ordinates using a Euclidean distance difference measure. The final data set contained the match type (geocoded, manually matched and approximated) recorded against each outlet. This enabled purely spatial research focused on urban areas to omit the approximated locations.
Verification of manually matched locations
As a large proportion of the licence files had to be geocoded manually it was decided to validate the methodology using known addresses that had been successfully matched as part of the geocoding process. A sample of 1604 known outlet locations – those that had been successfully matched to an ABP record – was matched manually. This resulted in a data set with two sets of co-ordinates: the known outlet location and the manually matched location. These two sets of co-ordinates allowed us to perform some basic analyses on any errors introduced as part of the manual match process. Table 7 provides a summary of the verification results and shows that the manual matching process was a reasonable method to fill in the gaps in the licensed premise locations.
Distance | % manually matched locations within distance |
---|---|
100 m | 79 |
500 m | 91 |
800 m | 93 |
We also compared the assignment of the correct LSOA to an outlet using the manually matched method and the geocoded method. Figure 9 shows where the manual matching process resulted in a different LSOA being assigned to the outlet. The maps show that there is a spread across urban and rural areas, with no particular error pattern being attributable to either an urban or a rural setting. The error rate for incorrect LSOA assignment was 6%.
FIGURE 9.
Comparison of geocoded with manually matched LSOA assignment. © Crown copyright and database rights 2013 Ordnance Survey 100019153.

Opening and closure date calculations
Local authority licensing officers noted a number of factors that may result in inaccuracies in the dates of opening and closure of outlets in the public register. First, licensees can opt to surrender a licence. Less commonly, when a business goes bankrupt, a licensee may fail to pay an annual fee and so the local authority will cancel a licence. Thus, there may be a lag between a business appearing in a public register and a licence being surrendered. Although no data were available to explore the potential implications of this lag, the maximum duration can be estimated to be around 1 year. Local authorities impose steep fines for missed payments for the renewal of licences and actively chase fees. This suggests that it is likely that local authorities would identify bankrupt licensed premises within a few months of the date of the expected receipt of the annual renewal fee.
A second limitation concerns the renewal of the annual licence when a premise is not open to the public. In rare cases, licensees may pay the annual fee even when the business is closed. For example, some bars and shops may close but the annual fee is paid as the owners assume that a sale is more likely when a new owner can avoid the bureaucracy of gaining a new licence. Licensing officers in Cardiff informed us that, if a premise is closed at the annual inspection, the public register would not be amended to show that it was not currently trading. Thus, the register in Cardiff records all active licences but not necessarily all licensed premises open to the public. Again, data on the frequency with which this occurs are not available but licensing officers at Cardiff noted that this situation is rare.
Calculation of alcohol outlet density required an opening and a closure date for every outlet record. Several local authorities provided monthly, yearly or sporadic summaries in their public registers. These documents did not contain actual dates of licence issue or closure and so the dates that the summaries were produced were used as proxies. Outlets with actual opening/issue dates but no closure date were taken to be still open and assigned an arbitrarily distant closure date (1 January 2029) to facilitate identification of such records. Some local authorities provided all changes to licences, including the opening and closure of a single outlet. In several instances, outlets would open and close on the same day, indicating a temporary change in the licensing conditions.
To allow for opening and closure dates we calculated the proportion of the quarter that each outlet was open and hence we could estimate a ‘weighted’ number of outlets open as well as whether or not an outlet was open on the first day of a quarter.
Estimation of outlet density: accessibility of the population to alcohol outlets
Our scoping literature review found four main approaches to the problem of estimating outlet density: (1) the number of outlets in an administrative area per capita or per unit geographical area; (2) the number of outlets per network miles per area; (3) the number of outlets per buffer zone defined by walking or drive time; and (4) the closest Euclidian distance from a residence to an outlet.
We aimed to estimate a measure of outlet density that also included accessibility as a measure of availability. We therefore extended methods (3) and (4) to estimate a combined summary statistic for distance from a residence to all outlets defined within a meaningful network range, which included network distance/travel time as a more accurate measure of exposure than Euclidian distance. This spatial methodology to estimate outlet density requires each residence to be identifiable and so we could not carry out the analysis using the RALFs within the SAIL Databank. Instead, we used the ABP data set in the eGIS (see Chapter 4, Environment Geographic Information System). However, it is possible through the anonymised encrypted linking method to link the computed residence metrics to the SAIL Databank once completed. The general method relating to the use of network distances and anonymous linkage to the SAIL Databank was previously applied to network distance calculations from homes to parks and playgrounds. 78
-
We calculated the mean of the values of the weighted network distance for each residence to each outlet within a meaningful network range (10 minutes’ walking time and 10 minutes’ drive time) for each residence. Weights were determined using the Butterworth function79 for distance decay (see Formulae for the outlet density computation), rescaled to 1. This estimated a household-level measure of outlet density exposure.
-
The LSOA density was derived by averaging the values for each residence in the LSOA.
The ABP data set identified 1,420,354 residences in Wales. We produced a residence–outlet matrix with a cut-off at our defined threshold network distances of 10 minutes’ walking time (defined as a distance of 833 m) and 10 minutes’ drive time. Software was written in the Python (www.python.org) and Cython (www.cython.org) programming languages, which enabled us to produce a range of metrics for each residence in Wales for each quarter over the study period. Box 1 lists the metrics produced by the software for each LSOA.
-
LSOA code.
-
Median nearest outlet distance.
-
Median number of outlets within a 10-minute walk.
-
Median proportion of quarter nearest outlet is open.
-
Year.
-
Quarter.
-
Density value for all outlets.
-
Mean nearest outlet distance.
-
Number of residences in an LSOA for quarters.
Methodology
The methodological steps used to create a network outlet distance measure for each residence were as follows:
-
We created the network data, residence data and outlet data GIS feature layers using the ABP data set (Figure 10a–c).
-
We loaded the residence and outlet data onto their computed network locations [the nearest network feature to the (x,y) co-ordinate of the point feature] (Figure 10d).
-
We calculated a residence–outlet matrix for each residence within the study. This calculates the distance between two network locations within a given threshold (Figure 10e).
-
Some residences and outlets were located a short distance from the official road network, for example along a private road or a long driveway. We added the distance that a residence or outlet was located from the road network to the residence–outlet matrix network distance. We then removed from the calculation any outlets that had moved beyond the 10-minute network threshold.
-
We calculated metrics required to compute the density scores for each residence and took the LSOA mean score.
FIGURE 10.
Steps in the outlet density calculation. (a) Network; (b) residential properties; (c) outlets; (d) residences and outlets; (e) residence–outlet matrix; and (f) outlets in residence–outlet matrix. © Crown copyright and database rights 2013 Ordnance Survey 100050829. Local Government Information House Limited copyright and database rights 2013 100050829.
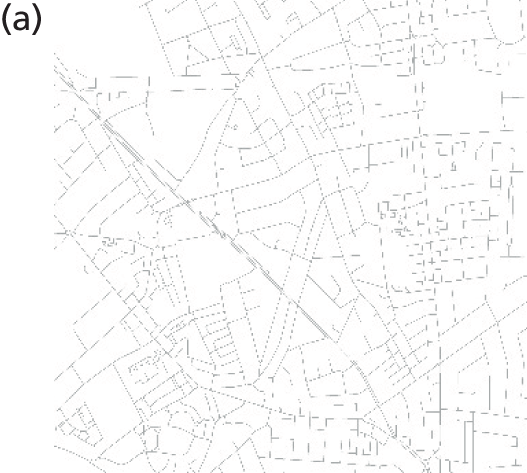



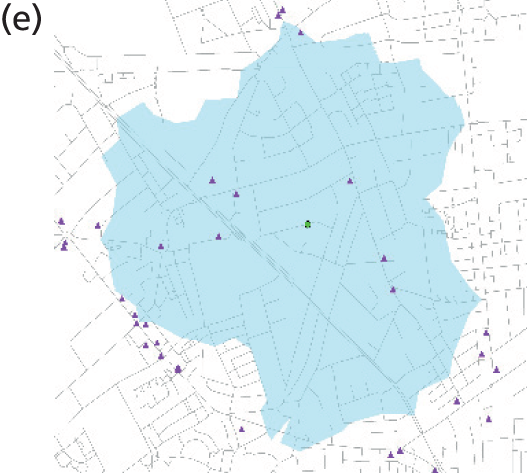
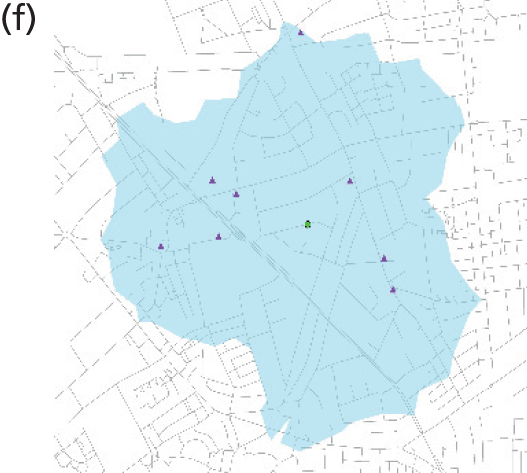
Formulae for the outlet density computation
The method can be written as follows:
-
For each residence, j, calculate the distance (dij) to each outlet (Oi).
-
Define the size of the buffer in terms of a distance (β) (depending on speeds and whether walking or driving).
-
Define the access to Oi from the residence, j, as:(2)11+γdij 2k
-
if dij < β and 0 otherwise.
-
Define the accessibility of outlets from RALF, j, as:(3)aj=∑dij<β11+γdij 2k.
The parameters in Equations 2 and 3 can be specified as follows.
Cut-off distance (β)
The buffer was defined by a 10-minute travel time radius. This was converted into a distance based on walking speed or driving speed. For walking, a buffer of 833 m (based on a standard speed of 5 kph) was calculated. For driving 10 miles was used (assuming a speed of 60 mph) as the cut-off point.
Butterworth filter (γ and κ)
The Butterworth filter has its origins in signal processing but has been used before as a filter in gravity models in accessibility research. 79 The shape of the Butterworth filter lends itself to accessibility modelling as it allows closer locations to have similar weightings up to a threshold distance before a distance decay is encountered. It was hypothesised that an individual would be prepared to travel to any outlet within about 200 m of his or her origin before distance became a motivational factor. The Butterworth filter models this initial distance more closely than other more commonly used filters (Figure 11).
FIGURE 11.
Plots of the reciprocal function, exponential function and Butterworth function with β = 2, α = 0.5 and κ = 3 respectively (for illustration).

To derive the weighting scores the distance decay was modelled at two given distances and then γ and κ were determined from Equations 4 and 5:
where the accessibility was set to be ε at the edge of the buffer, at a distance β, and ϕ at some other distance, α. The values were set as 0.1 at β = 833 m and 0.6 at a distance α to obtain the values κ = 1.81 and γ = 2.577 × 10–9 for walking calculations. For the 10 minutes of driving time, with a buffer at 10 miles, the accessibility could be set to be 0.1 when β = 10 miles and to be 0.8 when α = 1 mile. The values of κ and γ are 1.123 and 0.5625, respectively.
Calculation of outlet density
To compute a LSOA score for each quarter in the study period the mean of the density scores for every residence in a LSOA was taken. An (x,y) co-ordinate within the LSOA was then calculated based on the average density location within the LSOA to produce a density-weighted centroid to which the density scores could be attached. For the other non-density metrics shown in Table 8 (e.g. nearest outlet distance) it was decided to use a median score to aggregate to the LSOA. The median value was chosen as the method of aggregation to reduce the impact of the outliers on the variables being calculated and to give a more representative score.
Local authority | Outlet density matrix pairs: walking | Outlet density matrix pairs: driving |
---|---|---|
Cardiff | 2,727,339 | 26,280,880 |
Swansea | 1,342,427 | 14,851,125 |
Powys | 493,640 | 1,984,474 |
Ceredigion | 343,658 | 1,337,771 |
Optimisation of computing power
The calculation of the density scores and other variables required a substantial amount of computing power. To efficiently manage the process and produce the results in a reasonable amount of time it was necessary to employ some advanced computing techniques. Parallel computing is the process of breaking a computational problem into chunks for processing, either on one of the processor’s cores or through the distribution of data and code across a network of computers.
It was found to be necessary to use each core of a quad core machine to achieve the required efficiency gains. The data were easily split into manageable chunks, first by geography in the form of local authorities and second by time in the form of years and quarters. Splitting the residential data resulted in two major benefits. First, the smaller chunks of data made the processing of the density scores much more manageable given the system resources and stability and, second, the chunks acted as a failsafe so that if the system crashed then it would require only the last chunk to be reprocessed rather than the whole data set.
Figure 12 illustrates the conceptual parallel processing framework that was employed. Each local authority was processed by splitting the data into yearly chunks, with each quarter for that year being processed in parallel. The parallel processing framework and refinement of the algorithms resulted in massive efficiency savings and cut the processing time down from approximately several days to 8 hours for the walking calculations and from several weeks to 3 days for the driving calculations. The difference in processing time between driving and walking scenarios can be accounted for by examining the size of the data that each outlet density matrix produced for the different scenarios. Table 8 illustrates the differences for four local authorities and how the increase in the number of calculations required in urban areas is substantially more than in rural areas.
FIGURE 12.
Parallel processing framework. Q, quarter.

When the data shown in Table 8 are aggregated for the whole study period it shows the amount of computing power required to complete the density calculations. For Cardiff alone the number of residence–outlet pairs was approximately 600 million for the whole study period. Twenty variables were calculated for each residence–outlet pair resulting in approximately 12 billion calculations for driving density and 1.3 billion calculations for walking density. For rural areas this figure dropped to a more modest 950 million and 236 million calculations respectively. The final output was a CSV text file.
Interpretation of the density score
The density scores model the impact of localised geography on potential access to alcohol outlets by taking into account the ‘attractiveness’ (the likelihood that someone would visit) of an outlet at household level. This attractiveness is modelled using a geographical gravity model, which uses the road and footpath network distances between a residence and an outlet and weights the distance using the Butterworth filter. However, variations in local geography mean that it is not straightforward to interpret the density score. Tables 9 and 10 are reference tables showing the required change in the number of outlets at a distance interval that gives a unit change in density score. The tables were created using 1000 iterations for each distance interval (e.g. 0–150 m) and averaging the score to simulate the variety of possible distances between a household and an outlet and aggregating to LSOAs. These tables are indicative only but can be used as reference tables to interpret a density score change.
Distance (m) | Number of outlets within buffer | ||||||
---|---|---|---|---|---|---|---|
0 | 10 | 20 | 30 | 40 | 50 | Median | |
50 | 3.1 | 3.2 | 3.1 | 3.1 | 3.1 | 3.1 | 3.1 |
100 | 3.1 | 3.2 | 3.1 | 3.1 | 3.1 | 3.1 | 3.1 |
150 | 3.1 | 3.2 | 3.1 | 3.1 | 3.1 | 3.1 | 3.1 |
200 | 3.1 | 3.3 | 3.1 | 3.1 | 3.1 | 3.1 | 3.1 |
250 | 3.2 | 3.4 | 3.3 | 3.1 | 3.2 | 3.1 | 3.2 |
300 | 3.3 | 3.5 | 3.4 | 3.3 | 3.3 | 3.2 | 3.3 |
350 | 3.5 | 3.6 | 3.4 | 3.6 | 3.6 | 3.6 | 3.6 |
400 | 3.7 | 3.7 | 3.7 | 3.5 | 3.7 | 3.4 | 3.7 |
450 | 3.8 | 4.2 | 3.9 | 3.7 | 3.9 | 3.9 | 3.9 |
500 | 4.1 | 4.4 | 4.1 | 4.1 | 3.7 | 4.3 | 4.1 |
550 | 4.3 | 4.2 | 4.2 | 4.1 | 4.2 | 4.2 | 4.2 |
600 | 4.4 | 4.8 | 4.3 | 4.4 | 4.8 | 4.3 | 4.4 |
650 | 4.7 | 4.7 | 4.7 | 4.9 | 4.8 | 4.6 | 4.7 |
700 | 4.9 | 5.1 | 4.7 | 4.3 | 4.5 | 4.7 | 4.7 |
750 | 5.1 | 5.2 | 4.8 | 4.8 | 5.2 | 5.1 | 5.1 |
800 | 5.3 | 5.6 | 4.8 | 5.2 | 4.9 | 4.8 | 5.05 |
833 | 5.4 | 5.4 | 5.6 | 5.6 | 5.2 | 5.7 | 5.5 |
Distance (m) | Number of outlets within buffer | ||||||||||||||||
---|---|---|---|---|---|---|---|---|---|---|---|---|---|---|---|---|---|
0 | 10 | 20 | 30 | 40 | 50 | 60 | 70 | 80 | 90 | 100 | 110 | 120 | 130 | 140 | 150 | Median | |
1609 | 4 | 4 | 4 | 4 | 4 | 4 | 4 | 4 | 4 | 4 | 4 | 4 | 4 | 4 | 4 | 4 | 4 |
3219 | 4 | 4 | 4 | 4 | 4 | 4 | 4 | 4 | 4 | 4 | 4 | 4 | 4 | 4 | 4 | 4 | 4 |
4828 | 4 | 5 | 4 | 4 | 5 | 5 | 4 | 5 | 5 | 5 | 4 | 5 | 5 | 4 | 4 | 5 | 5 |
6437 | 5 | 5 | 5 | 5 | 5 | 5 | 5 | 5 | 5 | 5 | 5 | 5 | 5 | 5 | 5 | 5 | 5 |
8047 | 5 | 5 | 5 | 6 | 6 | 5 | 5 | 6 | 5 | 6 | 6 | 5 | 6 | 5 | 5 | 5 | 5 |
9656 | 6 | 6 | 6 | 6 | 6 | 6 | 6 | 6 | 6 | 7 | 6 | 6 | 6 | 6 | 6 | 6 | 6 |
11,265 | 6 | 6 | 6 | 6 | 6 | 6 | 7 | 6 | 6 | 6 | 7 | 6 | 6 | 7 | 7 | 6 | 6 |
12,874 | 7 | 7 | 6 | 7 | 7 | 7 | 7 | 7 | 7 | 6 | 7 | 7 | 7 | 7 | 8 | 7 | 7 |
14,484 | 7 | 7 | 7 | 7 | 7 | 7 | 7 | 7 | 7 | 7 | 7 | 8 | 6 | 7 | 7 | 6 | 7 |
16,093 | 8 | 8 | 7 | 7 | 7 | 8 | 8 | 8 | 7 | 8 | 8 | 7 | 7 | 8 | 7 | 7 | 7.5 |
Results: outlet density
Number of outlets
The total number of outlets open in Wales on 1 January 2006 was 9610, rising to 11,732 by 2011 quarter 4 (Figure 13).
FIGURE 13.
Quarterly trend in count of outlets open on the first day of the quarter, 2006–11. Q, quarter.

Figure 14 shows the highly skewed nature of the LSOA distribution of counts in 2006 quarter 1.
FIGURE 14.
Distribution of LSOA numbers of outlets open on the first day of 2006 quarter 1. SD, standard deviation.

The descriptive statistics for the number of outlets per LSOA open on the first day of a quarter are shown in Table 11 for quarter 1 of each year and Table 12 for quarter 4 of each year. Both show a similar trend of an increasing maximum number of outlets and mean number of outlets per LSOA over the time period.
Summary | 2006 Q1 | 2007 Q1 | 2008 Q1 | 2009 Q1 | 2010 Q1 | 2011 Q1 |
---|---|---|---|---|---|---|
Mean | 5.07 | 5.65 | 5.87 | 5.99 | 6.03 | 6.11 |
SD | 8.14 | 8.85 | 9.02 | 9.14 | 9.42 | 9.72 |
Minimum | 0 | 0 | 0 | 0 | 0 | 0 |
Maximum | 215 | 228 | 229 | 233 | 251 | 268 |
25th centile | 1 | 2 | 2 | 2 | 2 | 2 |
Median | 3 | 4 | 4 | 4 | 4 | 4 |
75th centile | 6 | 7 | 7 | 7 | 7 | 7 |
Summary | 2006 Q4 | 2007 Q4 | 2008 Q4 | 2009 Q4 | 2010 Q4 | 2011Q4 |
---|---|---|---|---|---|---|
Mean | 5.56 | 5.81 | 5.97 | 6.05 | 6.08 | 6.19 |
SD | 8.71 | 8.97 | 9.08 | 9.39 | 9.62 | 10.04 |
Minimum | 0 | 0 | 0 | 0 | 0 | 0 |
Maximum | 223 | 227 | 228 | 248 | 262 | 286 |
25th centile | 2 | 2 | 2 | 2 | 2 | 2 |
Median | 4 | 4 | 4 | 4 | 4 | 4 |
75th centile | 7 | 7 | 7 | 7 | 7 | 7 |
Table 13 shows the descriptive statistics for the weighted number of outlets per LSOA open during quarter 4.
Summary | 2006 Q4 | 2007 Q4 | 2008 Q4 | 2009 Q4 | 2010 Q4 | 2011 Q4 |
---|---|---|---|---|---|---|
Mean | 5.59 | 5.84 | 5.99 | 6.05 | 6.10 | 6.19 |
SD | 8.76 | 8.98 | 9.12 | 9.40 | 9.69 | 10.1 |
Minimum | 0.00 | 0.00 | 0.00 | 0.00 | 0.00 | 0.00 |
Maximum | 225.4 | 227.2 | 231.1 | 249.1 | 266.1 | 286.2 |
25th centile | 2.00 | 2.00 | 2.00 | 2.00 | 2.00 | 2.00 |
Median | 3.92 | 4.00 | 4.00 | 4.00 | 4.00 | 4.00 |
75th centile | 7.00 | 7.00 | 7.00 | 7.00 | 7.00 | 7.00 |
In 2006 quarter 4 there were 201 LSOAs with no outlets, falling to 164 by 2011 quarter 4. Around 90% of LSOAs had ≤ 10 outlets at the start of 2006 quarter 4, falling to 85% at the end of the study period. This also suggests a small increase overall in the number of outlets (Table 14).
Number of outlets | 2006 Q1 | 2011 Q4 | ||
---|---|---|---|---|
Frequency | % | Frequency | % | |
0 | 201 | 10.6 | 164 | 8.6 |
1 | 282 | 14.9 | 219 | 11.6 |
2 | 267 | 14.1 | 254 | 13.4 |
3 | 243 | 12.8 | 242 | 12.8 |
4 | 200 | 10.5 | 177 | 9.3 |
5 | 163 | 8.6 | 151 | 8.0 |
6 | 104 | 5.5 | 129 | 6.8 |
7 | 94 | 5.0 | 102 | 5.4 |
8 | 59 | 3.1 | 69 | 3.6 |
9 | 57 | 3.0 | 64 | 3.4 |
10 | 39 | 2.1 | 47 | 2.5 |
Total | 1709 | 90.2 | 1618 | 85.4 |
Simple outlet density: static population and changing population
Simple outlet density was calculated as the weighted number of outlets time open ‘at risk’ (i.e. days open during the quarter or year) divided by the LSOA population aged ≥ 16 years. For ‘static population’, the denominator was the 2006 quarter 1 population, whereas for ‘changing population’ the population estimate for each successive quarter was used as the denominator. Tables 15 and 16 show the annual descriptive statistics for the static population changing population respectively. The overall trend is for greater alcohol availability over time with increasing LSOA variability.
Summary | 2006 | 2007 | 2008 | 2009 | 2010 | 2011 |
---|---|---|---|---|---|---|
Mean | 3.89 | 4.13 | 4.27 | 4.33 | 4.36 | 4.42 |
SD | 4.87 | 5.14 | 5.26 | 5.33 | 5.40 | 5.49 |
Minimum | 0.00 | 0.00 | 0.00 | 0.00 | 0.00 | 0.00 |
Maximum | 59.9 | 64.7 | 66.8 | 65.3 | 69.0 | 74.4 |
25th centile | 1.24 | 1.37 | 1.41 | 1.42 | 1.43 | 1.47 |
Median | 2.63 | 2.81 | 2.87 | 2.87 | 2.87 | 2.93 |
75th centile | 4.84 | 5.12 | 5.26 | 5.28 | 5.32 | 5.43 |
Summary | 2006 | 2007 | 2008 | 2009 | 2010 | 2011 |
---|---|---|---|---|---|---|
Mean | 3.88 | 4.09 | 4.19 | 4.23 | 4.25 | 4.33 |
SD | 4.88 | 5.04 | 5.07 | 5.10 | 5.15 | 5.25 |
Minimum | 0.00 | 0.00 | 0.00 | 0.00 | 0.00 | 0.00 |
Maximum | 62.7 | 59.1 | 57.8 | 60.4 | 64.2 | 67.6 |
25th centile | 1.24 | 1.34 | 1.38 | 1.41 | 1.40 | 1.42 |
Median | 2.63 | 2.76 | 2.85 | 2.83 | 2.81 | 2.87 |
75th centile | 4.83 | 5.05 | 5.14 | 5.16 | 5.20 | 5.36 |
Figures 15 and 16 show the distribution of values for the 2011 static population outlet densities and the 2011 changing population outlet densities, respectively, confirming the negative skew described in Tables 15 and 16.
FIGURE 15.
Histogram of 2011 static population outlet densities.

FIGURE 16.
Histogram of 2011 changing population outlet densities.

Figure 17 shows a map of the simple outlet density for the static population for 2011 quarter 4.
FIGURE 17.
Map of simple outlet density: static population, 2011 quarter 4. © Crown copyright and database rights 2013 Ordnance Survey 100019153.

Figure 18 shows a map of the simple outlet density for the changing population for 2011 quarter 4.
FIGURE 18.
Map of simple outlet density: changing population, 2011 quarter 4. © Crown copyright and database rights 2013 Ordnance Survey 100019153.
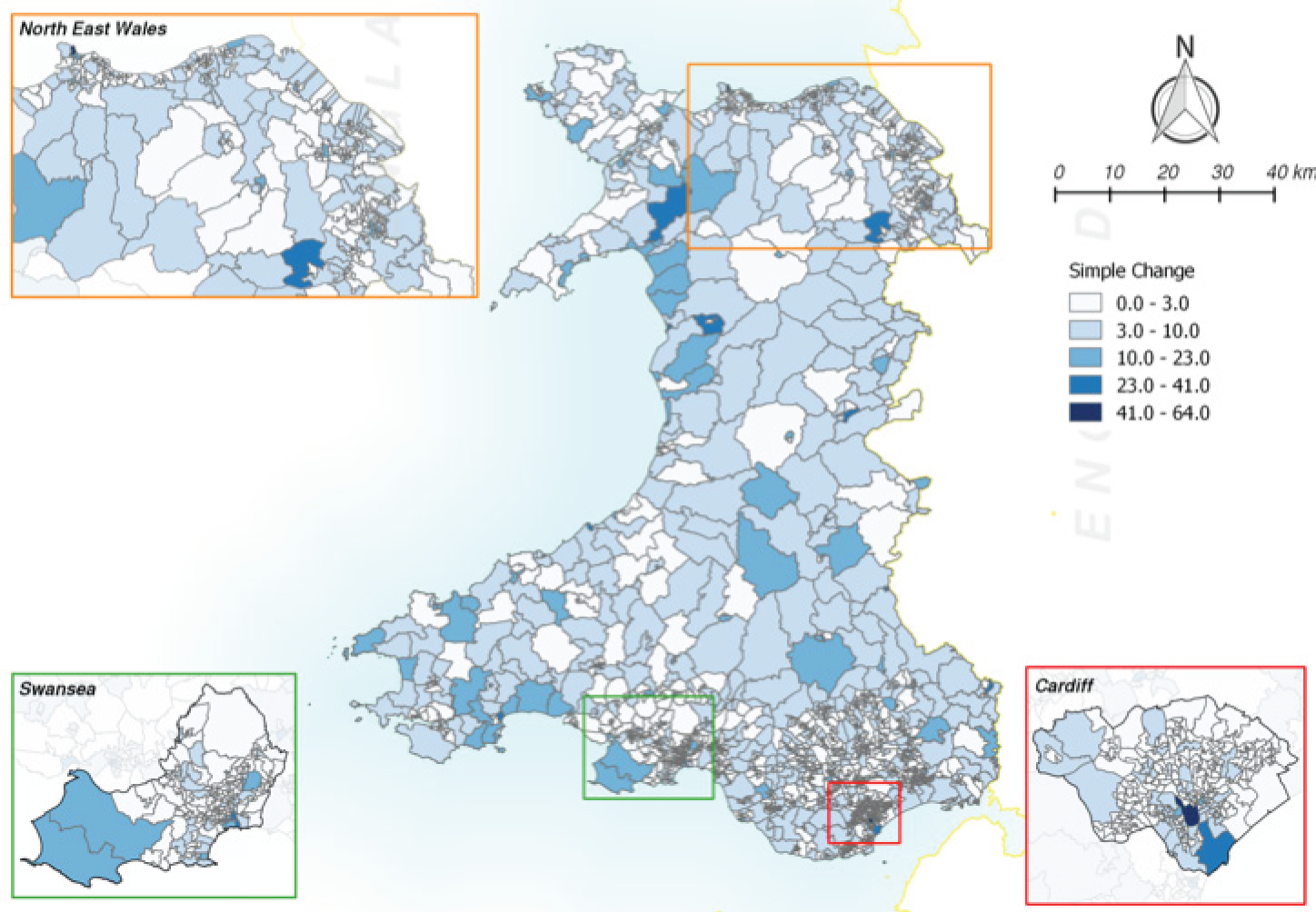
The correlation matrix in Table 17 shows a high degree of correlation between the annual static population and the changing population outlet densities.
Static population | Changing population | |||||
---|---|---|---|---|---|---|
2006 | 2007 | 2008 | 2009 | 2010 | 2011 | |
2006 | 1 | 0.987 | 0.972 | 0.962 | 0.953 | 0.946 |
2007 | 0.987 | 1 | 0.990 | 0.980 | 0.972 | 0.964 |
2008 | 0.972 | 0.990 | 0.999 | 0.993 | 0.985 | 0.977 |
2009 | 0.962 | 0.981 | 0.993 | 0.999 | 0.993 | 0.985 |
2010 | 0.953 | 0.972 | 0.986 | 0.993 | 0.998 | 0.992 |
2011 | 0.947 | 0.965 | 0.978 | 0.985 | 0.992 | 0.990 |
Figure 19 shows a scatterplot of the static and changing population outlet densities for 2011. The scatterplot suggests that two of the 1896 LSOAs have an outlying difference between the static population and the changing population outlet densities. These two LSOAs are W01000743 (Castle 2, Swansea, difference = 24.2 per 1000 population) and W01001701 (Butetown 3, Cardiff, difference = 17.6 per 1000 population). The difference in values is explained by large population increases, from 1627 to 2604 in Castle 2 and from 1683 to 2917 in Butetown 3.
FIGURE 19.
Scatterplot of static and changing population outlet densities, 2011.

Overall, the differences between the static population and the changing population were small (Table 18).
Summary | 2011 |
---|---|
Mean | 0.09 |
SD | 0.80 |
Minimum | –2.88 |
Maximum | 24.2 |
25th centile | –0.03 |
Median | 0.01 |
75th centile | 0.10 |
Nineteen LSOAs showed a difference of more than ±2 per 1000 population between 2001 and 2006 (Table 19) and this accounts for the differences between the static population and the changing population.
LSOA | LSOA name | Local authority | Difference in outlet density per 1000 population for static – changing population |
---|---|---|---|
W01000743 | Castle 2 | Swansea | 24.2 |
W01001701 | Butetown 3 | Cardiff | 17.6 |
W01001723 | Cathays 6 | Cardiff | 6.84 |
W01000372 | Grosvenor 2 | Wrexham | 4.56 |
W01001763 | Grangetown 5 | Cardiff | 4.40 |
W01001695 | Adamsdown 2 | Cardiff | 3.00 |
W01000350 | Brynyffynnon 2 | Wrexham | 2.90 |
W01001700 | Butetown 2 | Cardiff | 2.74 |
W01000773 | Gorseinon 1 | Swansea | 2.71 |
W01001656 | Marshfield 1 | Newport | 2.51 |
W01000745 | Castle 4 | Swansea | 2.49 |
W01001685 | Stow Hill 1 | Newport | 2.46 |
W01001661 | Pillgwenlly 3 | Newport | 2.40 |
W01001398 | Penyrheol 6 | Caerphilly | 2.21 |
W01000748 | Castle 7 | Cardiff | 2.16 |
W01000939 | Neath North 2 | Neath Port Talbot | 2.10 |
W01001646 | Liswerry 5 | Newport | –2.45 |
W01000049 | Abermaw 2 | Gwynedd | –2.72 |
W01000616 | Saundersfoot 2 | Pembrokeshire | –2.88 |
Overall, there was no justifiable reason not to use the changing population in the main analyses. Comparison with the static outlet density values will provide some measure of the impact of population migration but this will effectively be limited to the effect of very few LSOAs and is unlikely to be important.
Walking outlet density
Table 20 shows the descriptive quarterly data for variation in the LSOA measure of walking outlet density.
Summary | 2006 | 2007 | 2008 | 2009 | 2010 | 2011 | ||||||||||||||||||
---|---|---|---|---|---|---|---|---|---|---|---|---|---|---|---|---|---|---|---|---|---|---|---|---|
Q1 | Q2 | Q3 | Q4 | Q1 | Q2 | Q3 | Q4 | Q1 | Q2 | Q3 | Q4 | Q1 | Q2 | Q3 | Q4 | Q1 | Q2 | Q3 | Q4 | Q1 | Q2 | Q3 | Q4 | |
Mean | 2.60 | 2.65 | 2.70 | 2.74 | 2.76 | 2.78 | 2.81 | 2.84 | 2.85 | 2.86 | 2.87 | 2.89 | 2.88 | 2.90 | 2.91 | 2.92 | 2.91 | 2.90 | 2.93 | 2.93 | 2.92 | 2.94 | 2.95 | 2.96 |
SD | 3.71 | 3.76 | 3.83 | 3.88 | 3.94 | 3.97 | 4.01 | 4.02 | 4.02 | 4.04 | 4.06 | 4.09 | 4.07 | 4.12 | 4.13 | 4.15 | 4.12 | 4.11 | 4.13 | 4.12 | 4.11 | 4.15 | 4.18 | 4.20 |
Minimum | 0.00 | 0.00 | 0.00 | 0.00 | 0.00 | 0.00 | 0.00 | 0.00 | 0.00 | 0.00 | 0.00 | 0.00 | 0.00 | 0.00 | 0.00 | 0.00 | 0.00 | 0.00 | 0.00 | 0.00 | 0.00 | 0.00 | 0.00 | 0.00 |
Maximum | 47.0 | 47.5 | 47.5 | 47.9 | 46.4 | 44.7 | 44.4 | 43.7 | 43.8 | 45.4 | 46.5 | 46.1 | 47.1 | 48.2 | 49.5 | 50.1 | 51.1 | 51.6 | 51.6 | 52.8 | 54.2 | 54.6 | 56.6 | 57.1 |
25th centile | 0.69 | 0.70 | 0.72 | 0.73 | 0.73 | 0.74 | 0.74 | 0.76 | 0.76 | 0.76 | 0.77 | 0.77 | 0.77 | 0.77 | 0.78 | 0.79 | 0.77 | 0.77 | 0.78 | 0.78 | 0.78 | 0.78 | 0.78 | 0.78 |
Median | 1.39 | 1.42 | 1.42 | 1.45 | 1.46 | 1.47 | 1.49 | 1.52 | 1.52 | 1.52 | 1.53 | 1.54 | 1.53 | 1.52 | 1.54 | 1.53 | 1.53 | 1.53 | 1.54 | 1.54 | 1.54 | 1.54 | 1.56 | 1.55 |
75th centile | 2.88 | 2.91 | 2.93 | 3.01 | 3.01 | 3.02 | 3.06 | 3.09 | 3.10 | 3.09 | 3.12 | 3.14 | 3.16 | 3.23 | 3.22 | 3.19 | 3.18 | 3.16 | 3.20 | 3.18 | 3.20 | 3.20 | 3.17 | 3.19 |
Figure 20 shows the distribution of 2011 quarter 4 walking outlet density values.
FIGURE 20.
Histogram of 2011 quarter 4 walking outlet density values. Q, quarter; SD, standard deviation.
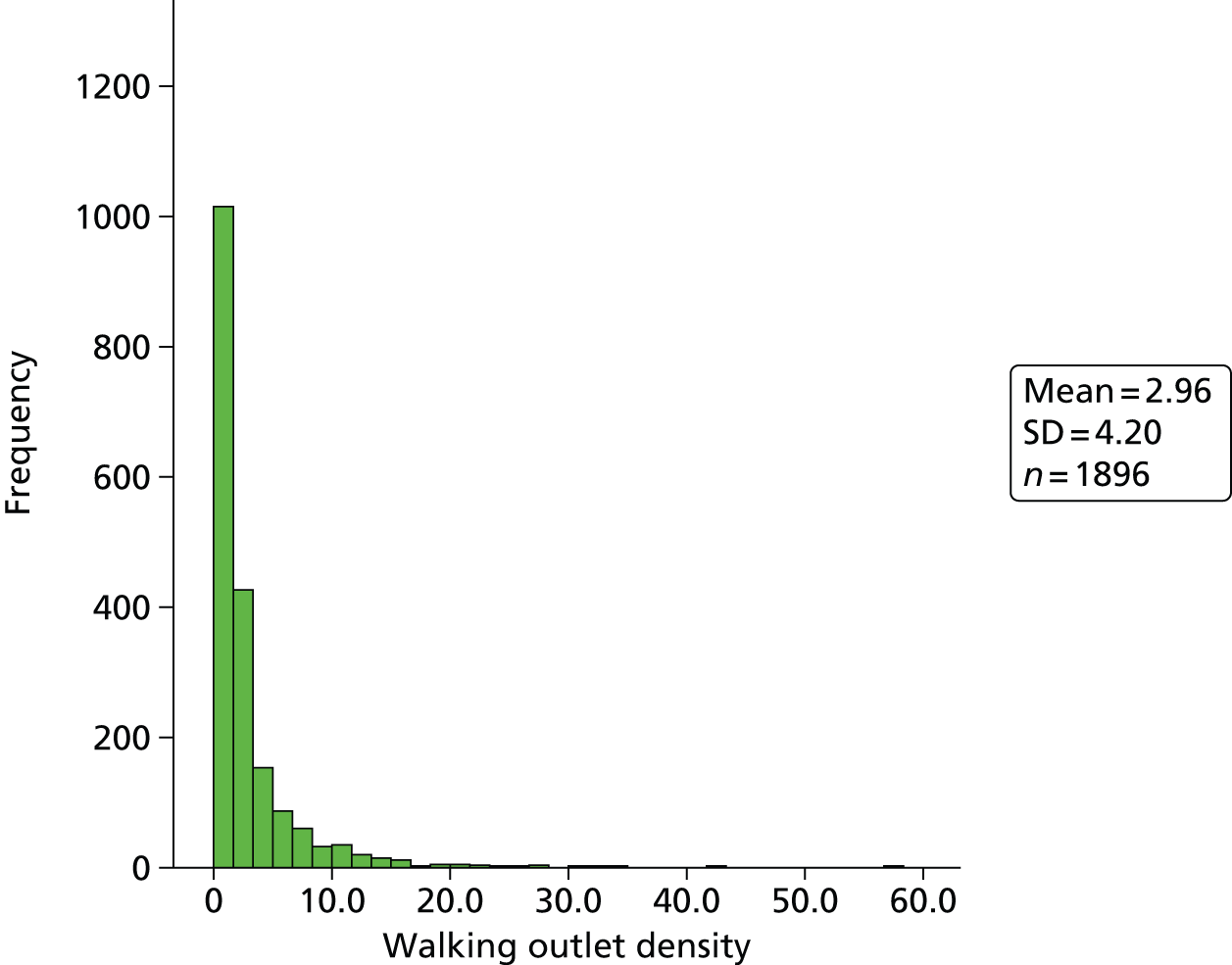
Figure 21 shows the LSOA spatial variation in walking outlet density.
FIGURE 21.
Map of Wales showing the LSOA spatial variation in walking outlet density. © Crown copyright and database rights 2013 Ordnance Survey 100019153.
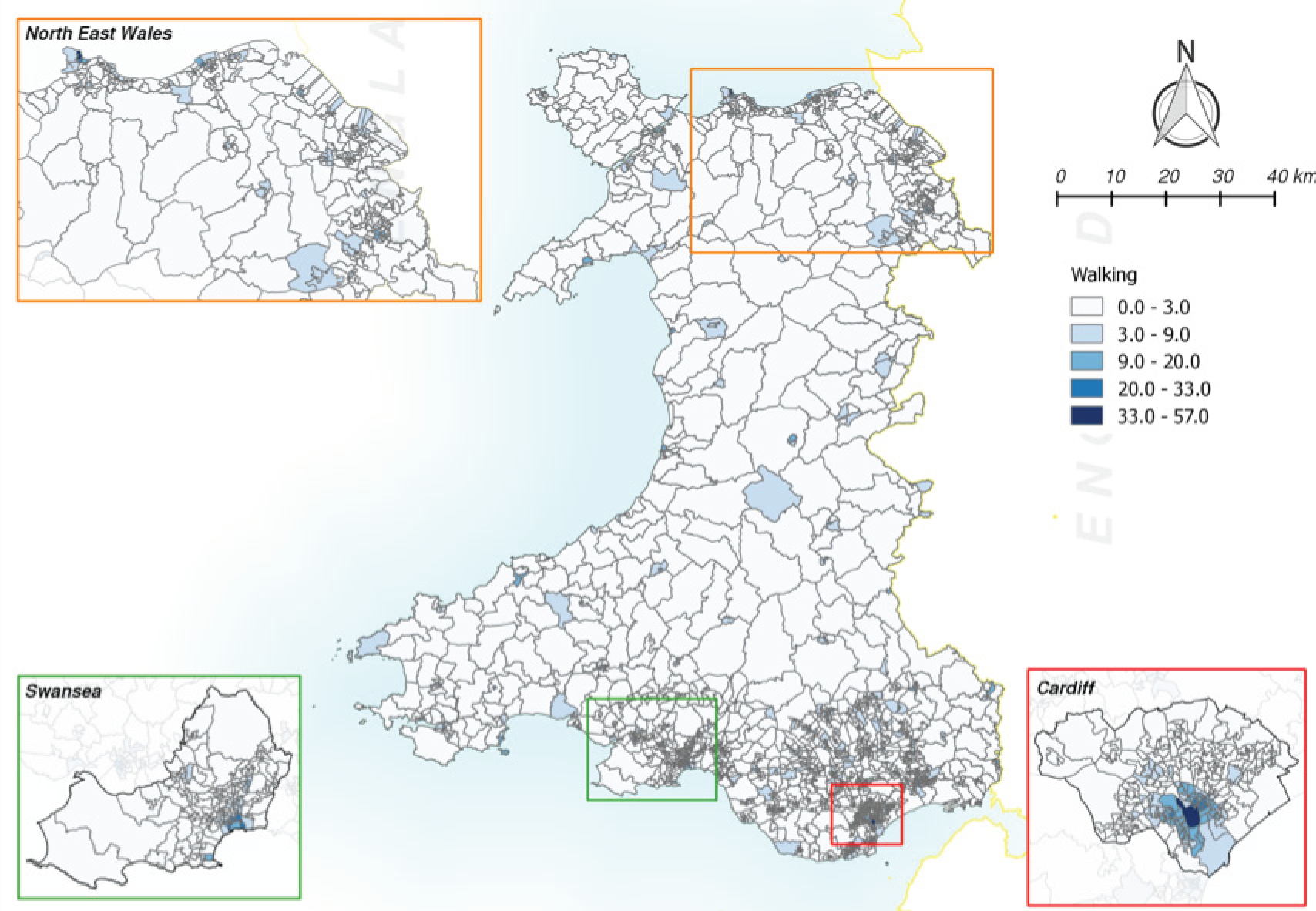
Driving outlet density
Table 21 shows the descriptive quarterly data for variation in the LSOA measure of driving outlet density.
Summary | 2006 | 2007 | 2008 | 2009 | 2010 | 2011 | ||||||||||||||||||
---|---|---|---|---|---|---|---|---|---|---|---|---|---|---|---|---|---|---|---|---|---|---|---|---|
Q1 | Q2 | Q3 | Q4 | Q1 | Q2 | Q3 | Q4 | Q1 | Q2 | Q3 | Q4 | Q1 | Q2 | Q3 | Q4 | Q1 | Q2 | Q3 | Q4 | Q1 | Q2 | Q3 | Q4 | |
Mean | 47.3 | 48.4 | 49.0 | 49.9 | 50.3 | 50.7 | 51.3 | 52.2 | 51.6 | 51.8 | 52.0 | 52.7 | 52.2 | 52.3 | 52.7 | 52.9 | 52.7 | 52.8 | 53.2 | 53.2 | 53.2 | 53.7 | 53.9 | 54.2 |
SD | 54.2 | 54.9 | 55.6 | 56.0 | 56.3 | 56.4 | 57.0 | 57.1 | 57.4 | 57.1 | 57.4 | 57.7 | 57.3 | 57.9 | 58.3 | 59.0 | 59.0 | 59.3 | 59.9 | 60.0 | 60.3 | 60.8 | 61.3 | 61.4 |
Minimum | 1.4 | 1.4 | 1.4 | 1.5 | 1.5 | 1.5 | 1.5 | 1.5 | 1.4 | 1.4 | 1.4 | 1.4 | 1.4 | 1.4 | 1.4 | 1.4 | 1.7 | 1.7 | 1.7 | 1.7 | 1.5 | 1.5 | 1.4 | 1.4 |
Maximum | 364.3 | 368.6 | 374.6 | 378.1 | 380.4 | 380.2 | 383.3 | 382.7 | 385.5 | 386.4 | 389.4 | 388.2 | 388.9 | 394.4 | 398.3 | 402.7 | 404.4 | 409.2 | 413.1 | 419.6 | 424.4 | 429.7 | 435.5 | 438.7 |
25th centile | 17.2 | 17.4 | 18.0 | 18.5 | 18.4 | 18.7 | 19.0 | 19.6 | 19.2 | 19.4 | 19.7 | 19.8 | 19.8 | 19.8 | 20.1 | 20.3 | 20.1 | 20.3 | 20.4 | 20.4 | 20.3 | 20.5 | 20.6 | 20.9 |
Median | 32.0 | 33.2 | 33.7 | 34.6 | 34.9 | 34.9 | 35.2 | 36.4 | 35.9 | 36.4 | 36.4 | 36.7 | 36.5 | 36.4 | 37.0 | 36.8 | 36.5 | 36.5 | 37.0 | 37.0 | 36.8 | 37.5 | 37.6 | 37.6 |
75th centile | 51.2 | 52.9 | 53.5 | 54.7 | 55.8 | 55.9 | 56.6 | 58.3 | 56.7 | 57.7 | 57.9 | 59.8 | 58.1 | 57.7 | 58.3 | 58.8 | 58.0 | 57.7 | 57.9 | 58.0 | 57.9 | 58.3 | 58.9 | 59.5 |
Figure 22 shows the distribution of 2011 quarter 4 driving outlet density values.
FIGURE 22.
Histogram of 2011 quarter 4 driving outlet density values. Q, quarter.

Figure 23 shows the LSOA spatial variation in driving outlet density.
FIGURE 23.
Map of Wales showing LSOA spatial variation in driving outlet density. © Crown copyright and database rights 2013 Ordnance Survey 100019153.
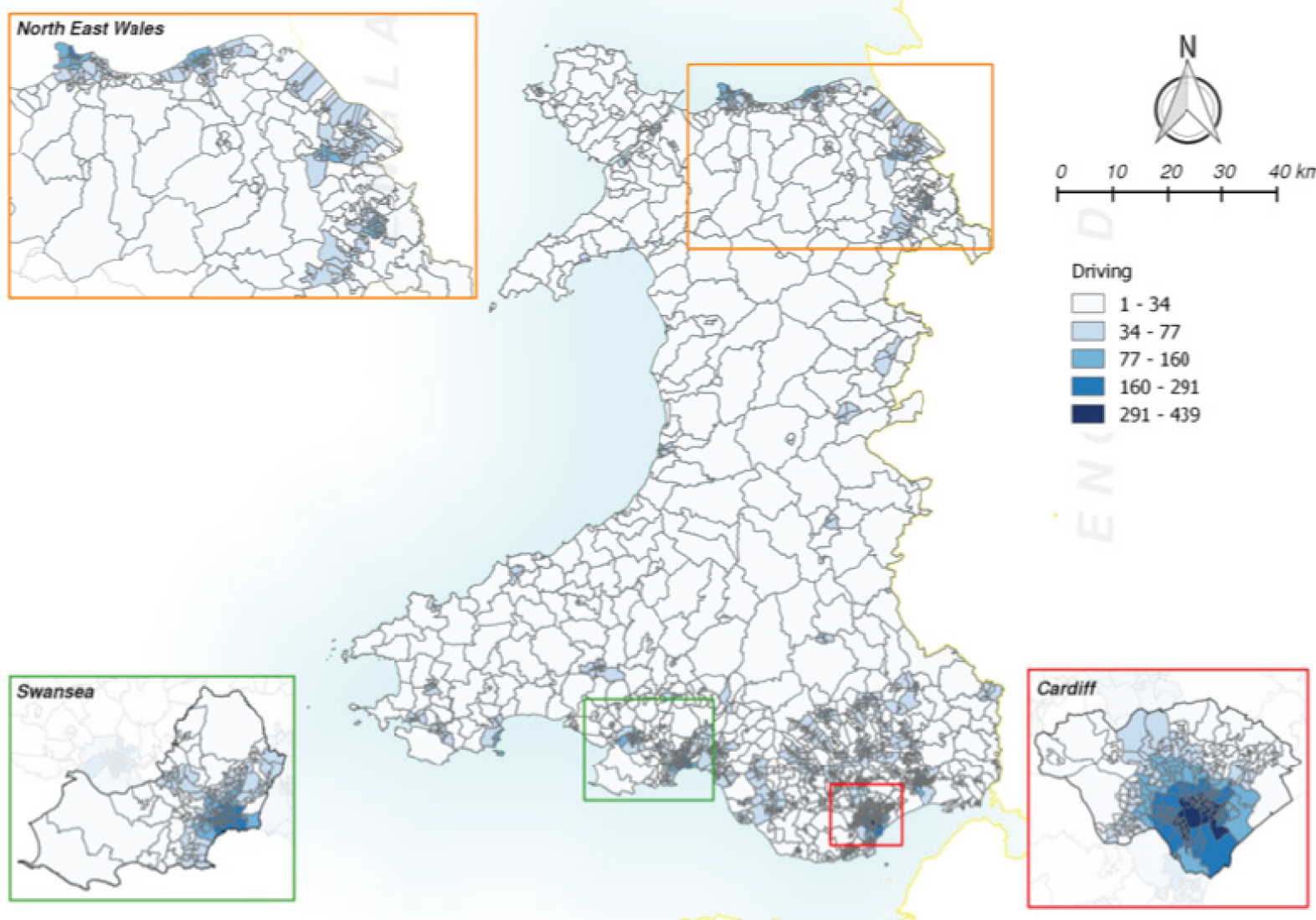
Table 22 shows the correlations between the measures of outlet density for the start and end density values. Clearly the three measures are measuring different aspects of alcohol availability.
Outlet density | 2006 Q1 S | 2011 Q4 S | 2006 Q1 W | 2011 Q4 W | 2006 Q1 D | 2011 Q4 D |
---|---|---|---|---|---|---|
2006 Q1 simple | 1 | 0.930 | 0.435 | 0.410 | –0.034 | –0.044 |
2011 Q4 simple | 0.930 | 1 | 0.384 | 0.425 | –0.085 | –0.055 |
2006 Q1 walking | 0.435 | 0.384 | 1 | 0.957 | 0.583 | 0.574 |
2011 Q4 walking | 0.410 | 0.425 | 0.957 | 1 | 0.550 | 0.580 |
2006 Q1 driving | –0.034 | –0.085 | 0.583 | 0.550 | 1 | 0.972 |
2011 Q4 driving | –0.044 | –0.055 | 0.574 | 0.580 | 0.972 | 1 |
Figure 24 shows the linear association between the walking and the driving outlet density measures.
FIGURE 24.
Linear association between the walking and driving outlet density measures. Q, quarter.

The essential difference between simple and walking outlet density is that urban areas tend to have more dense networks and hence higher values of walking outlet density. Figure 25 shows the difference between the mean simple and the mean walking density for each local authority and the differences are smaller, or negative, in the urban authorities. The pattern is the same for driving outlet density (not shown).
FIGURE 25.
Differences between mean simple and walking density for local authorities.
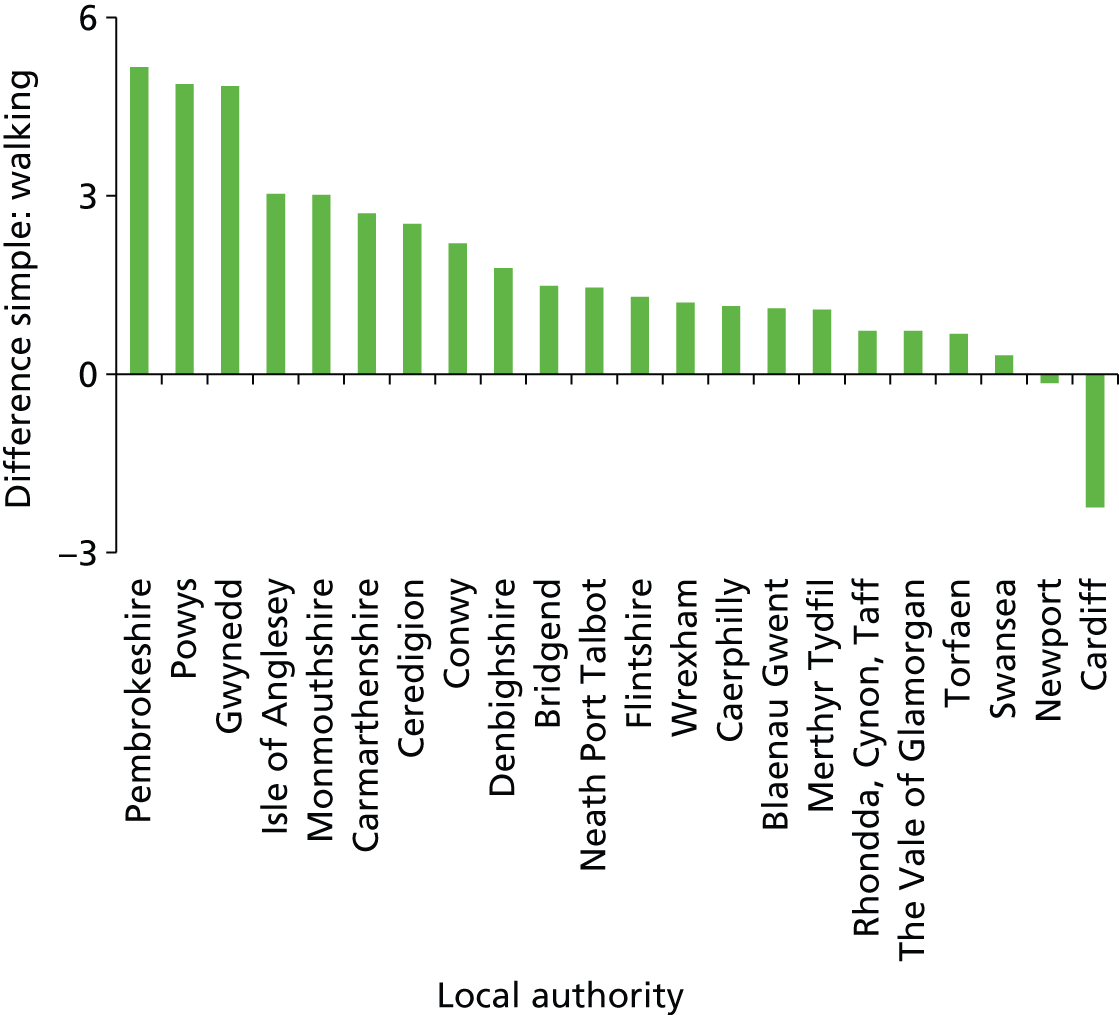
Associations of outlet density with social deprivation and rural–urban settlement type
Tables 23–26 show the mean walking and driving outlet density scores by deprivation quintile and rural–urban settlement type for 2011 quarter 4.
Deprivation quintile | n | Mean |
---|---|---|
Least deprived | 380 | 1.90 |
Less deprived | 379 | 2.32 |
Mid deprived | 379 | 2.99 |
More deprived | 379 | 3.92 |
Most deprived | 379 | 3.69 |
Total | 1896 | 2.96 |
Settlement type | n | Mean |
---|---|---|
Urban | 1238 | 347.5 |
Town and fringe | 337 | 351.4 |
Village and hamlet | 321 | 613.6 |
Total | 1896 | 393.3 |
Deprivation quintile | n | Mean |
---|---|---|
Least deprived | 380 | 51.2 |
Less deprived | 379 | 42.3 |
Mid deprived | 379 | 48.7 |
More deprived | 379 | 62.0 |
Most deprived | 379 | 66.8 |
Total | 1896 | 54.2 |
Settlement type | n | Mean |
---|---|---|
Urban | 1238 | 71.6 |
Town and fringe | 337 | 29.1 |
Village and hamlet | 321 | 13.3 |
Total | 1896 | 54.2 |
Measuring change in alcohol outlet density
The fundamental question is, ‘What is a change in outlet density and how best should it be modelled to reflect the shape of any dependence between the outcome measure and the availability of alcohol’. Change in outlet density is one component of the overall alcohol availability process, which we conclude has three main components: (1) a measure of current or historical availability; (2) a measure of change; and (3) a measure of temporal volatility.
Alcohol availability process: current and historical availability
If the alcohol availability data are thought of as a vector A(t) = [a(t),. . ., a(t – 7)] (say, the LSOA outlet density over the current and previous seven quarters), we are interested in determining what function of this vector best explains alcohol consumption C(t + 1) at the next measurement occasion. It might be something simple such as ‘consumption depends only on most recent past availability’:
that is, the value for the previous quarter.
Alternatively, ‘consumption depends on the most recent and earlier availability’, perhaps through a linear combination of all elements of A(t – 1):
It can also be hypothesised that ‘consumption depends on the maximum historical availability’, which is very non-linear (and non-differentiable in places) and could be written as:
Equivalently, ‘consumption depends on the minimum historical availability’.
Finally, it can be hypothesised that ‘consumption depends on the maximum or minimum availability within the previous four quarters’.
All of these cases are functions of the history of the availability process. It will then be possible to compare the various possible models above using the above measures by assessing the best fit of the availability process.
Change in outlet density
Change in outlet density can be estimated simply by subtracting an earlier quarterly value from a later quarterly value. As the degree of change will be some function of the absolute starting values, it is sensible to calculate change relative to the baseline value. However, as inevitably there will be densities that do not change between quarters, this will provide a surfeit of zeros.
Relative change was, therefore, estimated as the absolute annual change between two successive years’ quarter 4 values divided by the mean of the five quarterly values. This resulted in a stable set of values for change. Two separate variables for change in outlet density were then derived: one variable for a zero or positive change and a second variable for a zero or negative change.
Because this method will constrain the variance to be small where the baseline and final outlet density values are large, the variance was stabilised by dividing the absolute change by the square root of the mean of the five quarterly values.
Volatility
The next question was how to derive a variable for the volatility (or equivalently the inverse of stability) of the five quarterly values. The general shapes might be a regular increase or decrease, shown as series 2 (green) and series 3 (blue) in Figure 26, or no change, shown as series 1 (black).
FIGURE 26.
Hypothetical change in quarterly outlet densities. Q, quarter.

A more complex pattern of change between quarters may exist, summarised in Figure 27, in which densities display a ‘V’ or inverse ‘V’ pattern but the change is zero in both cases.
FIGURE 27.
Hypothetical zero change in quarterly outlet densities. Q, quarter.

The pattern may also be more complex, such as a ‘M’ shape (Figure 28). The previous quarter values are 1 for black and 0 for green. Here, the relative change for the series 1 black densities is 1/2 = +0.5 and for the series 2 green densities = –1/2 = –0.5, but these values ignore the variability in quarterly measurements, given that they are equally volatile. The same would hold for an inverse ‘M’ shape.
FIGURE 28.
Hypothetical complex change in quarterly outlet densities. Q, quarter.

A ‘volatility’ variable was therefore estimated, equal to the sum of the absolute differences between the five quarters/the mean of the five quarters. So, for the black densities, volatility = (4 + 2 + 1 + 2)/2 = 4.5, and for the green densities, volatility = (2 + 1 + 2 + 4)/2 = 4.5. The variance of the volatility measure was stabilised by dividing by the square root of the mean of the five quarterly values.
This combination of three measures attempts to capture as much as possible a complex, real-world process based on administrative data.
Descriptive statistics for the alcohol availability process
Change in alcohol outlet density
The difference in annual outlet density was computed between 2011 quarter 4 and 2010 quarter 4, between 2010 quarter 4 and 2009 quarter 4, and so on up to between 2007 quarter 4 and 2006 quarter 4, for each of the simple, walking and driving outlet densities.
Change in simple outlet density
A number of LSOAs will have zero change as all five quarterly values are zero. For example, for the change between 2011 quarter 4 and 2010 quarter 4 there are 158 LSOAs with a zero value for each of the five quarterly values. Clearly dividing by a mean of zero is unhelpful and these LSOAs will be recoded as having a zero change. Tables 27 and 28 show the descriptive statistics for the last period of change (2010 quarter 4 to 2011 quarter 4) for the static and changing populations respectively.
Summary | Previous quarter | Absolute change | Relative change | Total change | Volatility |
---|---|---|---|---|---|
Mean | 4.43 | 0.066 | 0.027 | 0.219 | 0.092 |
SD | 5.51 | 0.461 | 0.203 | 0.500 | 0.196 |
Minimum | 0 | –3.947 | –1.768 | 0 | 0 |
25th centile | 1.47 | 0 | 0 | 0 | 0 |
Median | 2.94 | 0 | 0 | 0 | 0 |
75th centile | 5.41 | 0 | 0 | 0.076 | 0.040 |
Maximum | 75.3 | 5.33 | 1.22 | 5.79 | 1.77 |
Summary | Previous quarter | Absolute change | Relative change | Total change | Volatility |
---|---|---|---|---|---|
Mean | 4.34 | 0.078 | 0.032 | 0.311 | 0.132 |
SD | 5.28 | 0.471 | 0.206 | 0.59 | 0.199 |
Minimum | 0 | –3.924 | –1.764 | 0 | 0 |
25th centile | 1.42 | –0.011 | –0.011 | 0.029 | 0.022 |
Median | 2.88 | 0.008 | 0.009 | 0.077 | 0.04 |
75th centile | 5.35 | 0.062 | 0.036 | 0.335 | 0.136 |
Maximum | 68.38 | 4.79 | 1.191 | 11.67 | 1.76 |
Change in walking outlet density
Table 29 shows the descriptive statistics for the last period of change (2010 quarter 4 to 2011 quarter 4).
Summary | Previous quarter | Absolute change | Relative change | Total change | Volatility |
---|---|---|---|---|---|
Mean | 2.93 | 0.047 | 0.021 | 0.274 | 0.149 |
SD | 4.15 | 0.370 | 0.172 | 0.652 | 0.311 |
Minimum | 0 | –1.634 | –0.747 | 0 | 0 |
25th centile | 0.77 | –0.003 | –0.003 | 0.002 | 0.002 |
Median | 1.55 | 0 | 0 | 0.029 | 0.027 |
75th centile | 3.15 | 0.04 | 0.033 | 0.279 | 0.181 |
Maximum | 56.2 | 7.61 | 2.68 | 13.6 | 3.8 |
Figures 29–31 map the walking outlet density positive and negative change and volatility respectively.
FIGURE 29.
Walking outlet density, positive change, 2011 quarter 4. © Crown copyright and database rights 2013 Ordnance Survey 100019153.

FIGURE 30.
Walking outlet density, negative change, 2011 quarter 4. © Crown copyright and database rights 2013 Ordnance Survey 100019153.
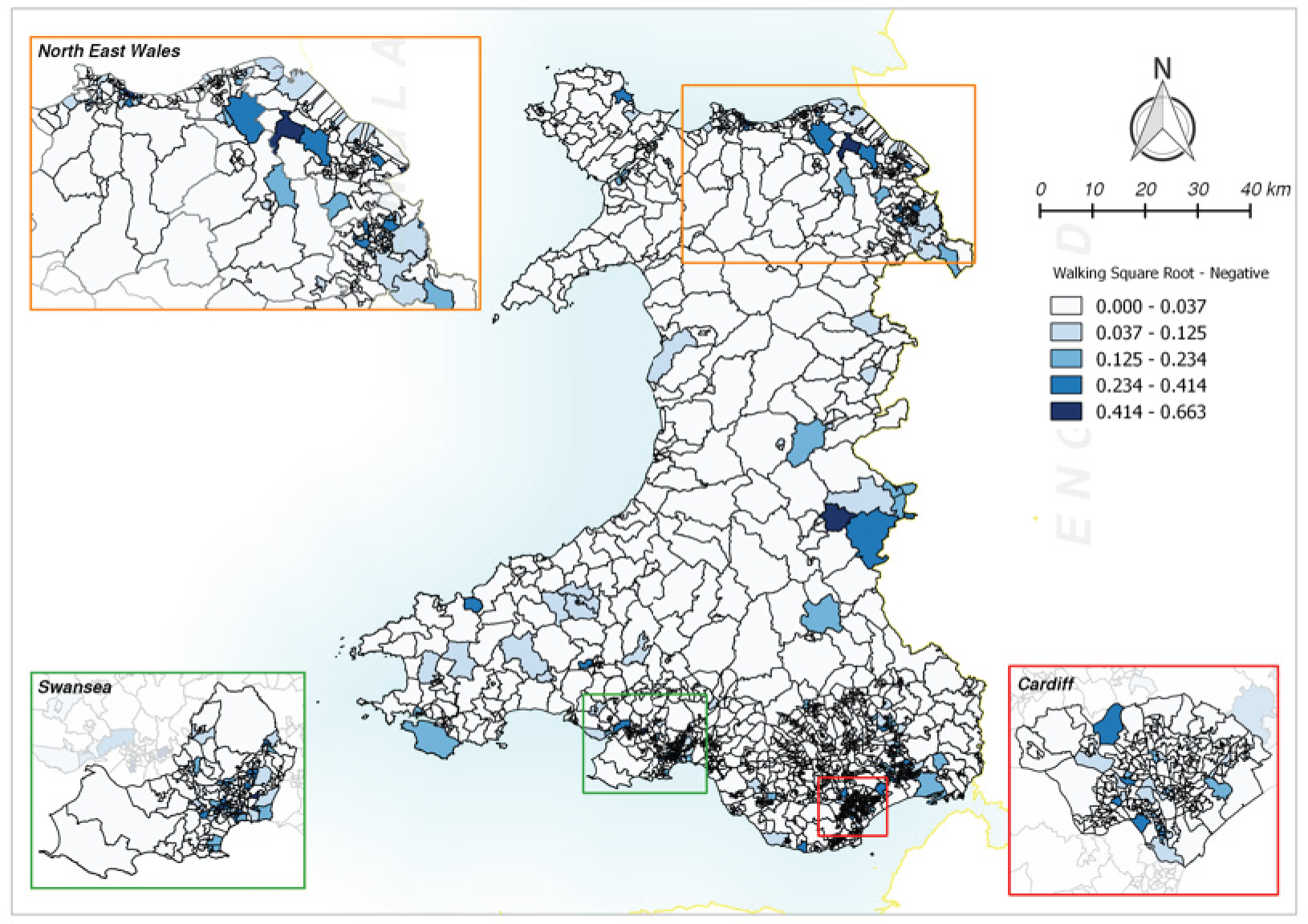
FIGURE 31.
Walking outlet density, volatility, 2011 quarter 4. © Crown copyright and database rights 2013 Ordnance Survey 100019153.
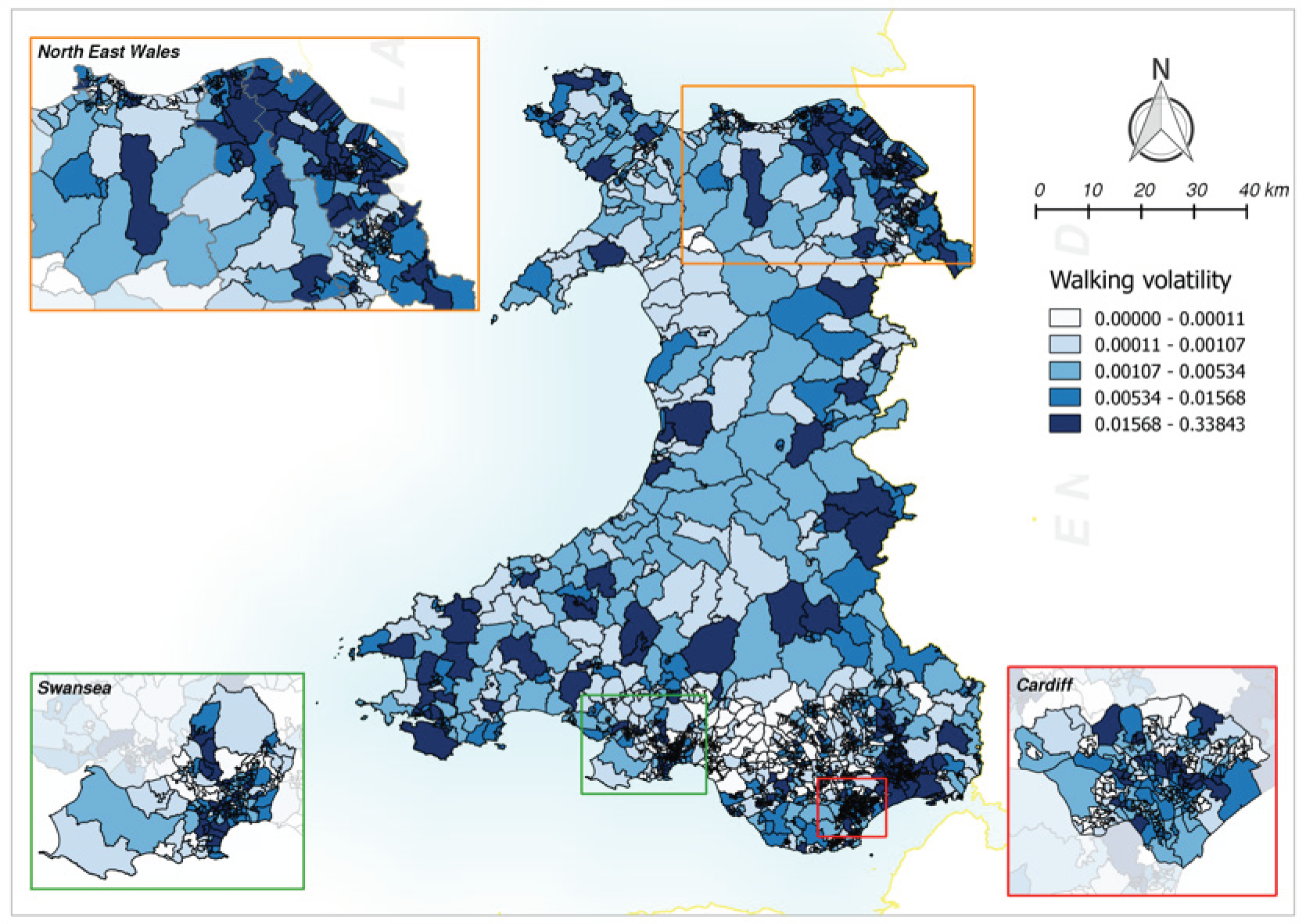
Change in driving outlet density
Table 30 shows the descriptive statistics for the last period of change (2010 quarter 4 to 2011 quarter 4).
Summary | Previous quarter | Absolute change | Relative change | Total change | Volatility |
---|---|---|---|---|---|
Mean | 53.90 | 0.963 | 0.120 | 3.570 | 0.476 |
SD | 61.30 | 3.490 | 0.364 | 5.020 | 0.609 |
Minimum | 1.375 | –8.704 | –1.098 | 0 | 0 |
25th centile | 20.60 | –0.101 | –0.022 | 0.696 | 0.143 |
Median | 37.60 | 0.424 | 0.085 | 1.746 | 0.285 |
75th centile | 58.70 | 1.450 | 0.229 | 4.340 | 0.562 |
Maximum | 435.5 | 22.70 | 2.660 | 34.50 | 6.920 |
Associations between alcohol availability and social deprivation and rural–urban settlement type
Tables 31–34 show the mean walking and driving outlet density change and volatility scores for 2010 quarter 4 to 2011 quarter 4 by deprivation quintile and rural–urban settlement type. In general, the highest change and volatility were in the least deprived urban and town and fringe areas, compared with the most deprived rural village areas.
Deprivation quintile | n | Mean positive change | Mean negative change | Mean volatility |
---|---|---|---|---|
Least deprived | 380 | 0.060 | 0.031 | 0.174 |
Less deprived | 379 | 0.047 | 0.030 | 0.158 |
Mid deprived | 379 | 0.042 | 0.026 | 0.126 |
More deprived | 379 | 0.058 | 0.028 | 0.154 |
Most deprived | 379 | 0.040 | 0.028 | 0.134 |
Total | 1896 | 0.049 | 0.028 | 0.149 |
Settlement type | n | Mean positive change | Mean negative change | Mean volatility |
---|---|---|---|---|
Urban | 1238 | 0.049 | 0.032 | 0.160 |
Town and fringe | 337 | 0.068 | 0.022 | 0.148 |
Village and hamlet | 321 | 0.031 | 0.021 | 0.108 |
Total | 1896 | 0.049 | 0.028 | 0.149 |
Deprivation quintile | n | Mean positive change | Mean negative change | Mean volatility |
---|---|---|---|---|
Least deprived | 380 | 0.209 | 0.078 | 0.566 |
Less deprived | 379 | 0.188 | 0.059 | 0.519 |
Mid deprived | 379 | 0.164 | 0.047 | 0.419 |
More deprived | 379 | 0.174 | 0.052 | 0.461 |
Most deprived | 379 | 0.158 | 0.057 | 0.419 |
Total | 1896 | 0.178 | 0.059 | 0.477 |
Settlement type | n | Mean positive change | Mean negative change | Mean volatility |
---|---|---|---|---|
Urban | 1238 | 0.189 | 0.068 | 0.531 |
Town and fringe | 337 | 0.208 | 0.040 | 0.432 |
Village and hamlet | 321 | 0.108 | 0.041 | 0.316 |
Total | 1896 | 0.178 | 0.059 | 0.477 |
Chapter 6 Study 1: alcohol consumption
Introduction
The WHS data sets were supplied to us by the Health Statistics and Analysis Unit of the Welsh Government under a formal data access agreement with Cardiff University. The WHS does not have the necessary consents and permissions to link into the SAIL Databank.
The WHS is an annual cross-sectional survey of the resident adult population of Wales, described elsewhere in more detail. 80 Briefly, a random sample of private households from the PAF is selected each year using a multistage probability sampling design with stratification. Data are collected from around 15,000 adults, using a household interview and self-completion questionnaires by all adults in the household. The survey includes questions on a wide range of demographic, socioeconomic and health and lifestyle factors. The survey year (e.g. 2008) refers to the calendar year of data collection (January–December 2008) and the data set and the Welsh government’s report are published in the following year (i.e. 2009).
The WHS has the strength of a large sampling fraction and high response rate resulting in a representative data set. The response each year equates to around 1 in 160 of a socially diverse population living in a geographically defined area, with detailed exposure data linked to the 2001 census LSOA of residence. Because the primary sampling unit for the data set is the household, we were able to include the household level in the multilevel analysis.
The general limitations of the WHS are the cross-sectional design and the likelihood of non-response bias. This is always a possibility in population surveys but successive surveys had a consistently good overall response to the interviewer-led method, from 74% of sampled households and 85% of individuals within responding households in 2003–481 to 78% and 81%, respectively, in 2012. 82 Lower response rates from some population subgroups are inevitable and can lead to bias in either direction if the relationships between the variables are substantially different in those subgroups from the rest of the population.
Alcohol questions
Participants were asked to state the highest number of units that they had drunk on any 1 day in the previous 7 days, using a standard prompt to convert different types and quantities of alcoholic drinks into units (2005–7) or by reporting measures of consumption translated into units by the data set coders (2008–12).
We originally planned to use data on consumption from the seven annual consecutive WHSs from 2005 to 2011. The data set for 2012 became available during the course of the study. We found two problems with the data sets that restricted the analysis to data from the five consecutive annual surveys from 2008 to 2012. 82–86 The problems were (1) changes in the alcohol consumption questions and (2) the under-representation of LSOAs in the surveys before 2008.
Changes to the Welsh Health Survey alcohol questions
Between the 2007 and 2008 surveys, the questions on alcohol consumption and the method of conversion of drinks to units changed. This was to achieve consistency with the new ONS methodology first introduced in 2006. 87 These changes and their impact on the survey estimates are discussed in this section.
In response to the question included in the 2005/6 and 2007 surveys (Table 35),88 the participant may have under- or overestimated their consumption of alcohol due to the lack of inclusion of wine glass size, advice to not include cans and bottles and the onus being on the participants to calculate their own total units consumed. This may have resulted in an underestimate of consumption from calculation error and from reporting consumption at a desirable level, that is, social desirability bias. 89–91 Additionally, wine consumption was defined only as a small glass. This is no longer the standard measure provided in pubs and restaurants and may also have resulted in an underestimate of consumption. Alternatively, respondents may have overestimated their consumption from counting all bottles as two units.
Please use the following table to answer questions 34a and 34b | ||||
1 pint of normal strength beer, lager, stout, cider or shandy (excluding cans or bottles of shandy) = | 1 pint of strong beer, lager, stout or cider = | 1 small glass of wine, sherry or vermouth = | Single spirit measure (whisky, gin, vodka, etc.) = | 1 alcopop = |
2 units | 3 units | 1 unit | 1 unit | 1.5 units |
Q34a On a day when you drink alcohol, on average how many units do you drink? Q34b In the last 7 days, what is the most units of alcohol you have drunk in any one day? |
The new alcohol question, first used in the 2008 survey83 and shown in Table 36, removes the responsibility to complete the calculations from the respondent. Respondents are now required only to enter the number of each size of each drink type consumed on their maximal drinking day and the calculations are completed by the survey coders. This allows the respondent to navigate the question quickly and provides space for the respondent to document/itemise the drinks consumed, reducing the calculation burden on respondents, which may lead to a more accurate number of units being recorded. However, a respondent may still under- or overestimate the numbers of drinks consumed.
Q39a Please think about the day in the last week on which you drank the most alcohol Please write in day ▭ Q39b Write in how much of each type of alcohol you drank on that day |
|||||
Normal strength beer, lager, stout, cider or shandy (less than 6% alcohol). Exclude bottles/cans of shandy. You can include half-pints under pints, e.g. ‘1½‘ | Pints □□ |
Large cans or bottles □□ |
Small cans or bottles □□ |
||
Strong beer, lager, stout or cider (6% alcohol or more): Tennants Super, Special Brew, Diamond White. You can include half-pints under pints, e.g. ‘1½‘ | Pints □□ |
Large cans or bottles □□ |
Small cans or bottles □□ |
||
Wine including champagne and Babycham. You can write in parts of a bottle, e.g. ‘½‘ | Large glasses (250 ml) □□ |
Standard glasses (175 ml) □□ |
Small glasses (125 ml) □□ |
Bottles (750 ml) □□ |
|
Spirits or liqueurs such as gin, whisky, rum, brandy, vodka, tequila, Baileys, Archers | Measures or shots (count doubles as 2 singles) □□ |
||||
Fortified wines such as sherry, port, vermouth, Martini, Cinzano, Dubonnet | Small glasses (count doubles as 2 singles) □□ |
||||
Alcopops (alcoholic soft drink) such as Hooch, WKD, Bacardi Breezer, Smirnoff Ice, Archers Aqua, Reef | Small cans or bottles □□ |
||||
Other kinds of alcoholic drink. Write in name of drink ▭ ▭ |
Glasses (count doubles as two singles) □□ □□ |
Pints □□ □□ |
Large cans or bottles □□ □□ |
Small cans or bottles □□ □□ |
Welsh Health Survey alcohol conversion factor
In addition to the change in question, the conversion factors assigned to each alcoholic drink were altered. Table 37 shows the conversion of volumes using the original method (before the change) and the updated method (after the change).
Type of drink | Measure | Volume (ml) | Estimate of average ABV (%) | Actual units | Units using original method | Units using updated method |
---|---|---|---|---|---|---|
Normal strength beer, lager or cider | Half-pint | 284 | 4.0 | 1.1 | 1.0 | 1.0 |
Strong beer, larger, stout or cider (ABV > 6%) | Half-pint | 284 | 6.5 | 1.8 | 1.5 | 2.0 |
Table wine | Glass | 125 | 12.5 | 1.6 | 1.0 | 1.5 |
175 | 12.5 | 2.2 | 1.0 | 2.0 | ||
250 | 12.5 | 3.1 | 1.0 | 3.0 | ||
Fortified wines | Glass | 50 | 17.0 | 0.9 | 1.0 | 1.0 |
Spirits or liqueurs | Single | 25 | 37.5 | 0.9 | 1.0 | 1.0 |
Alcopops | Bottle | 275 | 5.0 | 1.4 | 1.5 | 1.5 |
The inclusion of glass sizes in the updated method resulted in a significant change for wine consumption, with the previous method possibly resulting in women underestimating consumption. It also changed the assumed alcohol by volume (ABV) of wine from 9% to 12%. For example, a respondent to the 2007 survey who consumed three glasses of wine on their heaviest drinking day in the previous 7 days would enter three units. However, if these glasses were 250 ml then the actual units consumed would be 9.3, recorded as nine units in the 2008 survey. This changes the respondent’s drinking categorisation from ‘sensible’ to ‘binge’, defined by the classification of units into ordinal categories of consumption coded in the WHS data set using the Department of Health92 definitions (Table 38).
Category | Maximum units drunk on any day in the last week |
---|---|
Never | Never drinks |
None | Did not drink in the last 7 days |
Sensible | Men drinking no more than 4 units, women no more than 3 units |
Above guidelines, less than binge | Men drinking > 4 and up to and including 8 units, women > 3 and up to and including 6 units |
Binge | Men drinking > 8 units, women > 6 units |
The substantial effects of the changes on consumption estimates are shown in Table 39. In particular, the proportion of binge drinkers increased by almost a factor of 2 for women and by a factor of 1.5 for men.
Year | None | Within | Exceed | Binge | Total | ||||
---|---|---|---|---|---|---|---|---|---|
Count | % within WHS year | Count | % within WHS year | Count | % within WHS year | Count | % within WHS year | Count | |
Women | |||||||||
2005/6 | 3427 | 46.9 | 1992 | 27.3 | 1072 | 14.7 | 812 | 11.1 | 7303 |
2007 | 3345 | 46.3 | 2021 | 28.0 | 1079 | 15.0 | 773 | 10.7 | 7218 |
2008 | 3281 | 47.5 | 1108 | 16.1 | 1110 | 16.1 | 1406 | 20.4 | 6905 |
2009 | 3803 | 46.6 | 1280 | 15.7 | 1406 | 17.2 | 1679 | 20.6 | 8168 |
2010 | 3996 | 48.6 | 1208 | 14.7 | 1376 | 16.8 | 1636 | 19.9 | 8216 |
2011 | 3938 | 47.7 | 1261 | 15.3 | 1328 | 16.1 | 1726 | 20.9 | 8253 |
Men | |||||||||
2005/6 | 1944 | 30.4 | 1676 | 26.2 | 1156 | 18.1 | 1620 | 25.3 | 6396 |
2007 | 1837 | 29.6 | 1662 | 26.8 | 1151 | 18.5 | 1560 | 25.1 | 6210 |
2008 | 1846 | 31.2 | 1084 | 18.3 | 1038 | 17.6 | 1943 | 32.9 | 5911 |
2009 | 2212 | 31.1 | 1253 | 17.6 | 1291 | 18.2 | 2349 | 33.1 | 7105 |
2010 | 2257 | 31.6 | 1275 | 17.8 | 1309 | 18.3 | 2311 | 32.3 | 7152 |
2011 | 2414 | 33.6 | 1206 | 16.8 | 1278 | 17.8 | 2295 | 31.9 | 7193 |
Under-representation of Lower Layer Super Output Areas
The 2005/6 and 2007 surveys included respondents from fewer than 61% of the LSOAs, in contrast to the surveys from 2008 onwards (Table 40).
Survey year | Number of LSOAs with at least one respondent | % of LSOAs with at least one respondent |
---|---|---|
2005/6 | 1114 | 58.8 |
2007 | 1146 | 60.4 |
2008 | 1844 | 97.3 |
2009 | 1872 | 98.7 |
2010 | 1881 | 99.2 |
2011 | 1876 | 98.9 |
2012 | 1875 | 98.9 |
It is clear that, because of the effects of the question change and the under-representation of LSOAs before 2008, the analysis should use survey data from 2008 to 2012.
Analysis strategy
The WHS data consisted of five sequential cross-sectional data sets. Two separate modelling strategies were planned.
Modelling the number of units consumed
Here we used a multilevel Poisson model, in the family of generalised linear mixed models (GLMMs), fitting the count of units consumed by each individual as a function of the alcohol availability process, adjusting for individual- and LSOA-level covariates. This model predicted consumption in the year following the alcohol availability process measured in the preceding year.
The multilevel structure was year at level 1 with individuals nested within households, LSOAs and unitary authorities. We first estimated a null model of the unexplained random variation at each level. We then fitted the alcohol availability process in four separate models as the (1) previous quarter for current availability; (2) the historical maximum; (3) the historical minimum; and (4) the highest of the four previous quarters. We compared the model fits using the Akaike information criterion (AIC) and selected the model with the best fit. To this best-fitting model we added terms for positive and negative change in outlet density and volatility.
We then added individual-level covariates to adjust for age group in 10-year bands, sex, and the head of household NS-SEC3 (3-part National Statistics Socio-Economic Classification), the three-class variable defined by the NE-SEC as a measure of socioeconomic position. 93 We also fitted a term to allow for different linear trends in consumption by year. We fitted terms for LSOA deprivation quintiles and the three-category rural–urban settlement type. In our previous analysis of this data set94 we found significant interactions between age group, sex and deprivation quintile and we also assessed this in these models. We then explored whether or not the effect of a change in outlet density on consumption varied with deprivation by modelling the interaction between change in outlet density and deprivation quintiles. We also explored a stratification approach in which the data set was divided into deprivation quintiles 1 and 2 (low deprivation) and deprivation quintiles 4 and 5 (high deprivation).
We accounted for missing NS-SEC data using multiple imputation with chained equations,95 based on all data set variables in the imputation specification and five imputation models. In total, we assessed four models: two for simple outlet density with static and changing populations and two for the measures of walking and driving outlet density. All models were fitted in R software (version 3.0.2, The R Foundation for Statistical Computing, Vienna, Austria).
Geographically Weighted Regression spatial analysis of binge drinking
We then proceeded to a Geographically Weighted Regression (GWR) model with binge drinking as the outcome, also adjusting for the same individual-level covariates associated with alcohol consumption. We used a pragmatic approach to bandwidth selection as the size of the data set was too large for an adaptive process to run, deciding on 150 nearest LSOA neighbours. We adjusted for multiple comparisons using the false discovery rate of Benjamini and Hochberg96 (a conservative approach to multiple significance testing). We presented the model outputs in thematic maps for the intercept model-predicted binge drinking coefficients followed by the measures of alcohol availability that showed geographical variability.
Descriptive analyses
Overall, there were 77,074 respondents to the WHSs from 2008 to 2012 (Table 41).
Year | n | % of total data set 2008–12 | % household response rate | % response for individuals within responding households |
---|---|---|---|---|
2008 | 13,313 | 17.3 | 73.8 | 78.1 |
2009 | 16,017 | 20.8 | 78.2 | 82.2 |
2010 | 15,999 | 20.8 | 79.1 | 82.9 |
2011 | 16,058 | 20.8 | 78.4 | 82.6 |
2012 | 15,687 | 20.4 | 77.6 | 81.4 |
Total | 77,074 | 100 | – | – |
The response by LSOA is summarised in Table 42.
LSOA data | 2008 | 2009 | 2010 | 2011 | 2012 |
---|---|---|---|---|---|
Number of LSOAs with respondents | 1844 | 1872 | 1881 | 1876 | 1875 |
Number of LSOAs with no respondents | 52 | 24 | 15 | 20 | 21 |
Range of number of respondents per LSOA | 1–34 | 1–29 | 1–32 | 1–39 | 1–31 |
Table 43 shows the response by local authority and the response as a proportion of the resident population aged ≥ 16 years according to the 2011 census. The final 5-year response represented 3.1%, or 1 in 32, of the adult population of Wales aged ≥ 16 years.
Local authority | Number of respondents | Percentage of total respondents | Population aged ≥ 16 years in 2011 | Response as % of 2011 population |
---|---|---|---|---|
Isle of Anglesey | 3131 | 4.1 | 58,023 | 5.4 |
Gwynedd | 3232 | 4.2 | 100,655 | 3.2 |
Conwy | 3488 | 4.5 | 96,263 | 3.6 |
Denbighshire | 3379 | 4.4 | 76,899 | 4.4 |
Flintshire | 3553 | 4.6 | 124,082 | 2.9 |
Wrexham | 3538 | 4.6 | 109,228 | 3.2 |
Powys | 3199 | 4.2 | 110,310 | 2.9 |
Ceredigion | 3277 | 4.3 | 64,128 | 5.1 |
Pembrokeshire | 3152 | 4.1 | 100,611 | 3.1 |
Carmarthenshire | 3594 | 4.7 | 150,935 | 2.4 |
Swansea | 4310 | 5.6 | 197,369 | 2.2 |
Neath Port Talbot | 3450 | 4.5 | 115,206 | 3.0 |
Bridgend | 3332 | 4.3 | 114,052 | 2.9 |
Vale of Glamorgan | 3191 | 4.1 | 102,903 | 3.1 |
Cardiff | 5312 | 6.9 | 282,368 | 1.9 |
Rhondda Cynon Taf | 4291 | 5.6 | 190,048 | 2.3 |
Merthyr Tydfil | 3271 | 4.2 | 47,882 | 6.8 |
Caerphilly | 3599 | 4.7 | 143,951 | 2.5 |
Blaenau Gwent | 3091 | 4 | 57,338 | 5.4 |
Torfaen | 3203 | 4.2 | 73,986 | 4.3 |
Monmouthshire | 3236 | 4.2 | 75,295 | 4.3 |
Newport | 3245 | 4.2 | 116,385 | 2.8 |
Total | 77,074 | 100 | 2,507,917 | 3.1 |
Alcohol consumption outcome measures
A total of 74,069 (96.1%) respondents responded to the questions on alcohol consumption. The mean number of units consumed, including the never drinks/none categories, was 5.2 [standard deviation (SD) 7.7, range 0–306]. The median number of units consumed was 2.5 (interquartile range 0–8). The distribution was negatively skewed as expected (Figure 32).
FIGURE 32.
Distribution of units consumed on the heaviest drinking day in the last week. SD, standard deviation.

Clearly it is not possible to consume 306 units in 1 day and so a ceiling had to be applied to define a plausible level of consumption. Previous research on occasion-based drinking patterns has considered a maximum threshold of 450 g of ethanol per occasion, which is approximately equivalent to 56 units (at 8 g per unit). This also equates approximately to a maximum blood alcohol content of 0.5%, estimated using the Widmark formula,97 which is usually incompatible with life. 98
We therefore excluded the 141 (0.19%) respondents reporting a consumption of > 56 units, leaving 73,928 respondents in the analysis. Of these 73,928 respondents, 9800 (13.3%) were ‘never drinkers’. The full categorisation according to the Department of Health definition92 is shown in Table 44.
Category | n | % |
---|---|---|
Never drinks | 9800 | 13.3 |
None | 20,412 | 27.6 |
Within guidelines | 12,120 | 16.4 |
Exceeding guidelines but less than binge drinking | 12,609 | 17.1 |
Binge drinking | 18,987 | 25.7 |
Total | 73,928 | 100 |
Of the 64,128 respondents who were not ‘never drinkers’, the mean number of units consumed was 5.8 (SD 6.9), ranging from 0 to 56. The median number of units consumed was 4 (interquartile range 0–9). Table 45 shows the descriptive statistics by survey year.
Summary | 2008 | 2009 | 2010 | 2011 | 2012 |
---|---|---|---|---|---|
Respondents, n | 11,106 | 13,333 | 13,241 | 13,334 | 13,114 |
Mean | 5.94 | 5.88 | 5.8 | 5.87 | 5.53 |
SD | 7.11 | 6.91 | 6.86 | 7.1 | 6.73 |
Minimum | 0 | 0 | 0 | 0 | 0 |
Maximum | 56 | 56 | 56 | 56 | 56 |
25th centile | 0 | 0 | 0 | 0 | 0 |
Median | 4 | 4 | 4 | 4 | 4 |
75th centile | 9 | 9 | 9 | 9 | 9 |
Around 32% of ‘ever drinkers’ did not report drinking in the previous week and a further 65% consumed between 1 and 20 units. Table 46 shows the number of respondents reporting consumption of each successive possible number of units from 0 to 20 units.
Number of units | Number of respondents | % of respondents (n = 64,128) |
---|---|---|
0.0 | 20,412 | 31.8 |
1.0 | 1226 | 1.91 |
1.5 | 1870 | 2.92 |
2.0 | 3710 | 5.79 |
2.5 | 204 | 0.32 |
3.0 | 2719 | 4.24 |
3.5 | 230 | 0.36 |
4.0 | 4755 | 7.41 |
4.5 | 1125 | 1.75 |
5.0 | 888 | 1.38 |
5.5 | 254 | 0.40 |
6.0 | 4826 | 7.53 |
6.5 | 189 | 0.29 |
7.0 | 762 | 1.19 |
7.5 | 442 | 0.69 |
8.0 | 3499 | 5.46 |
8.5 | 131 | 0.20 |
9.0 | 2485 | 3.88 |
9.5 | 137 | 0.21 |
10.0 | 2296 | 3.58 |
10.5 | 225 | 0.35 |
11.0 | 574 | 0.90 |
11.5 | 95 | 0.15 |
12.0 | 2723 | 4.25 |
12.5 | 103 | 0.16 |
13.0 | 516 | 0.80 |
13.5 | 216 | 0.34 |
14.0 | 991 | 1.55 |
14.5 | 57 | 0.09 |
15.0 | 504 | 0.79 |
15.5 | 49 | 0.08 |
16.0 | 1352 | 2.11 |
16.5 | 75 | 0.12 |
17.0 | 243 | 0.38 |
17.5 | 59 | 0.09 |
18.0 | 818 | 1.28 |
18.5 | 30 | 0.05 |
19.0 | 152 | 0.24 |
19.5 | 45 | 0.07 |
20.0 | 796 | 1.24 |
Total | 61,783 | 96.3 |
Table 47 shows the descriptive statistics for the categories of consumption by survey year.
Year | Never drinks | None | Within guidelines | Exceeding guidelines but less than binge drinking | Binge drinking | |||||
---|---|---|---|---|---|---|---|---|---|---|
n | % | n | % | n | % | n | % | n | % | |
2008 | 1687 | 13.2 | 3440 | 26.9 | 2192 | 17.1 | 2148 | 16.8 | 3326 | 26.0 |
2009 | 1910 | 12.5 | 4105 | 26.9 | 2532 | 16.6 | 2697 | 17.7 | 3999 | 26.2 |
2010 | 2103 | 13.7 | 4150 | 27.0 | 2483 | 16.2 | 2685 | 17.5 | 3923 | 25.6 |
2011 | 2072 | 13.4 | 4280 | 27.8 | 2467 | 16.0 | 2606 | 16.9 | 3981 | 25.8 |
2012 | 2028 | 13.4 | 4437 | 29.3 | 2446 | 16.2 | 2473 | 16.3 | 3758 | 24.8 |
Response by demographic and socioeconomic variables
Overall, 39,561 (53.5%) respondents were female and 34,367 were male. The proportions of respondents by sex varied little by year. The overall response by age group is shown in Table 48. There was little variation by year and so the data are presented aggregated across the six surveys.
Age group (years) | n | % |
---|---|---|
16–24 | 8672 | 11.7 |
25–34 | 8825 | 11.9 |
35–44 | 11,614 | 15.7 |
45–54 | 12,627 | 17.1 |
55–64 | 13,505 | 18.3 |
65–74 | 10,619 | 14.4 |
75–84 | 6159 | 8.3 |
85+ | 1907 | 2.6 |
Total | 73,928 | 100 |
The breakdown of respondents into the categories of socioeconomic position is shown in Table 49.
NS-SEC3 group | n | % |
---|---|---|
Professional and managerial | 26,268 | 35.5 |
Intermediate | 14,419 | 19.5 |
Routine | 29,253 | 39.6 |
Never worked | 1648 | 2.2 |
Missing | 2340 | 3.2 |
Total | 73,928 | 100 |
The breakdown of respondents into deprivation quintiles is shown in Table 50.
Deprivation quintile | n | % |
---|---|---|
Least deprived | 14,589 | 19.7 |
Less deprived | 15,807 | 21.4 |
Mid deprived | 16,339 | 22.1 |
More deprived | 14,208 | 19.2 |
Most deprived | 12,985 | 17.6 |
Total | 73,928 | 100 |
The breakdown of respondents into the categories of LSOA settlement type is shown in Table 51.
Settlement type | n | % |
---|---|---|
Urban | 45,046 | 60.9 |
Town and fringe | 14,165 | 19.2 |
Village and hamlet | 14,717 | 19.9 |
Total | 73,928 | 100 |
Table 52 shows the descriptive statistics for the mean number of units consumed on the heaviest drinking day and the categories of consumption for the demographic and socioeconomic individual variables. Table 53 shows the descriptive statistics by LSOA deprivation quintile and settlement type and Table 54 shows the descriptive statistics by local authority.
Variable | Category | Mean number of units | SD | Never drinks | None | Within guidelines | Exceeding guidelines but less than binge drinking | Binge drinking | Total N | |||||
---|---|---|---|---|---|---|---|---|---|---|---|---|---|---|
n | % | n | % | n | % | n | % | n | % | |||||
Sex | Female | 3.6 | 5.3 | 6403 | 16.2 | 12,600 | 31.8 | 6055 | 15.3 | 6457 | 16.3 | 8046 | 20.3 | 39,561 |
Male | 6.7 | 7.7 | 3397 | 9.9 | 7812 | 22.7 | 6065 | 17.6 | 6152 | 17.9 | 10,941 | 31.8 | 34,367 | |
Age group (years) | 16–24 | 6.3 | 8.8 | 1057 | 12.2 | 3018 | 34.8 | 824 | 9.5 | 947 | 10.9 | 2826 | 32.6 | 8672 |
25–34 | 6.5 | 8.2 | 710 | 8.0 | 2793 | 31.6 | 1067 | 12.1 | 1190 | 13.5 | 3065 | 34.7 | 8825 | |
35–44 | 6.3 | 7.3 | 925 | 8.0 | 3083 | 26.5 | 1504 | 12.9 | 2059 | 17.7 | 4043 | 34.8 | 11,614 | |
45–54 | 5.9 | 6.8 | 1192 | 9.4 | 3063 | 24.3 | 1798 | 14.2 | 2479 | 19.6 | 4095 | 32.4 | 12,627 | |
55–64 | 4.7 | 5.5 | 1574 | 11.7 | 3321 | 24.6 | 2425 | 18.0 | 2991 | 22.1 | 3194 | 23.7 | 13,505 | |
65–74 | 3.3 | 4.6 | 1900 | 17.9 | 2783 | 26.2 | 2460 | 23.2 | 2068 | 19.5 | 1408 | 13.3 | 10,619 | |
75–84 | 1.9 | 3.4 | 1708 | 27.7 | 1781 | 28.9 | 1590 | 25.8 | 754 | 12.2 | 326 | 5.3 | 6159 | |
85+ | 1.0 | 2.4 | 734 | 38.5 | 570 | 29.9 | 452 | 23.7 | 121 | 6.3 | 30 | 1.6 | 1907 | |
NS-SEC3 group | Professional and managerial | 5.4 | 6.3 | 2264 | 8.6 | 5987 | 22.8 | 5321 | 20.3 | 5435 | 20.7 | 7261 | 27.6 | 26,268 |
Intermediate | 4.9 | 6.7 | 1878 | 13.0 | 4105 | 28.5 | 2405 | 16.7 | 2430 | 16.9 | 3601 | 25.0 | 14,419 | |
Routine | 4.7 | 7.0 | 4917 | 16.8 | 9136 | 31.2 | 3903 | 13.3 | 4246 | 14.5 | 7051 | 24.1 | 29,253 | |
Never worked | 3.5 | 7.4 | 500 | 30.3 | 585 | 35.5 | 151 | 9.2 | 143 | 8.7 | 269 | 16.3 | 1648 | |
Missing | 6.5 | 8.1 | 241 | 10.3 | 599 | 25.6 | 340 | 14.5 | 355 | 15.2 | 805 | 34.4 | 2340 |
Variable | Category | Mean number of units | SD | Never drinks | None | Within guidelines | Exceeding guidelines but less than binge drinking | Binge drinking | Total N | |||||
---|---|---|---|---|---|---|---|---|---|---|---|---|---|---|
n | % | n | % | n | % | n | % | n | % | |||||
Deprivation quintile | Least deprived | 5.2 | 6.2 | 1411 | 9.7 | 3226 | 22.1 | 3001 | 20.6 | 3035 | 20.8 | 3916 | 26.8 | 14,589 |
Less deprived | 5.0 | 6.4 | 1740 | 11.0 | 4227 | 26.7 | 2915 | 18.4 | 2987 | 18.9 | 3938 | 24.9 | 15,807 | |
Mid deprived | 4.8 | 6.7 | 2212 | 13.5 | 4671 | 28.6 | 2803 | 17.2 | 2703 | 16.5 | 3950 | 24.2 | 16,339 | |
More deprived | 5.2 | 7.2 | 1977 | 13.9 | 4250 | 29.9 | 1955 | 13.8 | 2172 | 15.3 | 3854 | 27.1 | 14,208 | |
Most deprived | 4.9 | 7.4 | 2460 | 18.9 | 4038 | 31.1 | 1446 | 11.1 | 1712 | 13.2 | 3329 | 25.6 | 12,985 | |
Settlement type | Urban | 5.2 | 7.0 | 6144 | 13.6 | 12,350 | 27.4 | 6968 | 15.5 | 7440 | 16.5 | 12,144 | 27.0 | 45,046 |
Town and fringe | 5.0 | 6.7 | 1862 | 13.1 | 3988 | 28.2 | 2283 | 16.1 | 2403 | 17.0 | 3629 | 25.6 | 14,165 | |
Village and hamlet | 4.5 | 6.0 | 1794 | 12.2 | 4074 | 27.7 | 2869 | 19.5 | 2766 | 18.8 | 3214 | 21.8 | 14,717 |
Local authority | Mean number of units | SD | Never drinks | None | Within guidelines | Exceeding guidelines but less than binge drinking | Binge drinking | Total N | |||||
---|---|---|---|---|---|---|---|---|---|---|---|---|---|
n | % | n | % | n | % | n | % | n | % | ||||
Isle of Anglesey | 4.7 | 6.6 | 425 | 14.1 | 848 | 28.2 | 527 | 17.5 | 507 | 16.8 | 702 | 23.3 | 3009 |
Gwynedd | 4.8 | 6.4 | 410 | 13.3 | 871 | 28.3 | 485 | 15.7 | 546 | 17.7 | 768 | 24.9 | 3080 |
Conwy | 4.7 | 6.2 | 437 | 13.2 | 896 | 27.0 | 613 | 18.5 | 575 | 17.3 | 801 | 24.1 | 3322 |
Denbighshire | 4.8 | 6.6 | 412 | 12.7 | 915 | 28.3 | 567 | 17.5 | 580 | 17.9 | 762 | 23.5 | 3236 |
Flintshire | 5.2 | 6.6 | 389 | 11.4 | 864 | 25.4 | 622 | 18.3 | 608 | 17.9 | 918 | 27.0 | 3401 |
Wrexham | 5.0 | 6.7 | 473 | 14.0 | 935 | 27.6 | 512 | 15.1 | 575 | 17.0 | 888 | 26.2 | 3383 |
Powys | 4.4 | 6.1 | 365 | 11.9 | 904 | 29.4 | 630 | 20.5 | 522 | 17.0 | 656 | 21.3 | 3077 |
Ceredigion | 4.5 | 6.5 | 419 | 13.4 | 918 | 29.3 | 569 | 18.1 | 544 | 17.4 | 685 | 21.9 | 3135 |
Pembrokeshire | 4.2 | 6.0 | 377 | 12.5 | 923 | 30.7 | 577 | 19.2 | 531 | 17.6 | 603 | 20.0 | 3011 |
Carmarthenshire | 4.4 | 6.4 | 508 | 14.7 | 1101 | 31.9 | 566 | 16.4 | 542 | 15.7 | 739 | 21.4 | 3456 |
Swansea | 5.3 | 6.8 | 530 | 12.7 | 1078 | 25.9 | 666 | 16.0 | 738 | 17.8 | 1145 | 27.5 | 4157 |
Neath Port Talbot | 5.5 | 7.3 | 467 | 14.0 | 943 | 28.3 | 425 | 12.8 | 529 | 15.9 | 965 | 29.0 | 3329 |
Bridgend | 5.6 | 7.2 | 371 | 11.5 | 836 | 25.9 | 531 | 16.5 | 549 | 17.0 | 939 | 29.1 | 3226 |
Vale of Glamorgan | 5.2 | 6.2 | 300 | 9.8 | 751 | 24.4 | 611 | 19.9 | 600 | 19.5 | 810 | 26.4 | 3072 |
Cardiff | 5.3 | 7.0 | 739 | 14.5 | 1203 | 23.7 | 845 | 16.6 | 907 | 17.8 | 1390 | 27.3 | 5084 |
Rhondda Cynon Taf | 5.5 | 7.4 | 555 | 13.5 | 1159 | 28.2 | 574 | 14.0 | 635 | 15.4 | 1191 | 28.9 | 4114 |
Merthyr Tydfil | 5.3 | 7.4 | 502 | 16.0 | 937 | 30.0 | 359 | 11.5 | 435 | 13.9 | 895 | 28.6 | 3128 |
Caerphilly | 5.3 | 7.1 | 464 | 13.5 | 1000 | 29.1 | 473 | 13.8 | 545 | 15.9 | 952 | 27.7 | 3434 |
Blaenau Gwent | 5.3 | 7.6 | 466 | 15.7 | 891 | 30.0 | 365 | 12.3 | 453 | 15.3 | 794 | 26.7 | 2969 |
Torfaen | 5.2 | 7.0 | 411 | 13.3 | 855 | 27.8 | 517 | 16.8 | 492 | 16.0 | 804 | 26.1 | 3079 |
Monmouthshire | 4.9 | 6.0 | 335 | 10.8 | 749 | 24.1 | 615 | 19.8 | 660 | 21.2 | 753 | 24.2 | 3112 |
Newport | 5.1 | 6.6 | 445 | 14.3 | 835 | 26.8 | 471 | 15.1 | 536 | 17.2 | 827 | 26.6 | 3114 |
Distribution of consumption by Lower Layer Super Output Area
Table 55 shows the descriptive statistics for the mean number of units per LSOA for 2008 to 2012, for all respondents consuming ≤ 56 units.
Summary | 2008 | 2009 | 2010 | 2011 | 2012 |
---|---|---|---|---|---|
Number of LSOAs | 1844 | 1872 | 1881 | 1875 | 1875 |
Missing | 52 | 24 | 15 | 21 | 21 |
Mean | 5.14 | 5.05 | 4.94 | 5.06 | 4.77 |
SD | 3.78 | 3.29 | 3.15 | 3.30 | 3.06 |
Minimum | 0 | 0 | 0 | 0 | 0 |
Maximum | 48.5 | 32.0 | 32.0 | 34.0 | 30.2 |
25th centile | 2.60 | 2.97 | 2.88 | 2.80 | 2.67 |
Median | 4.55 | 4.55 | 4.50 | 4.60 | 4.42 |
75th centile | 6.83 | 6.70 | 6.50 | 6.67 | 6.33 |
In total, 1768 LSOAs had survey respondents for each year. Of the 128 missing LSOAs, one LSOA had three missing years and four LSOAs had two missing years. The other 123 LSOAs were missing data for 1 of the 5 years.
Generalised linear mixed models of counts of alcohol units
We found that the ‘previous quarter’ measure of current alcohol availability gave the best model fit, although the differences between models were not substantial (the AIC ranged from 490,568 to 490,610). The models with the best fits included ‘previous quarter’ and ‘historical maximum’. Ultimately, we chose to adopt ‘previous quarter’ over ‘historical maximum’ because the latter has the unappealing property that the date range over which the maximum is defined grows larger as we move through the study period. The results are given in the following sections for (1) simple outlet density to compare the effect of the changing population, (2) walking outlet density and (3) driving outlet density.
Simple outlet density: static population
Table 56 shows the parameter estimates and standard errors (SEs) with p-values for the final model of consumption and simple outlet density, static population. The previous quarter outlet density was significantly and positively associated with consumption in the following year. Change and volatility were not significant in the model. The patterns of association with the other model covariates were as expected.
Variable | Parameter estimate | SE | p-value | Rate ratio | 95% CI |
---|---|---|---|---|---|
Intercept | 1.416 | 0.034 | < 0.001 | ||
Yearly linear trend since 2008 | –0.020 | 0.005 | < 0.001 | 0.980 | 0.971 to 0.990 |
Alcohol availability | |||||
Previous quarter | 0.004 | 0.001 | 0.003 | 1.004 | 1.002 to 1.006 |
Change positive | 0.105 | 0.086 | 0.221 | 1.111 | 0.938 to 1.315 |
Change negative | 0.112 | 0.096 | 0.242 | 1.119 | 0.927 to 1.350 |
Volatility | –0.119 | 0.077 | 0.123 | 0.888 | 0.763 to 1.032 |
LSOA deprivation quintile | |||||
Least deprived | Reference | ||||
Less deprived | –0.077 | 0.024 | 0.001 | 0.926 | 0.883 to 0.970 |
Mid deprived | –0.117 | 0.025 | < 0.001 | 0.890 | 0.847 to 0.934 |
More deprived | –0.109 | 0.026 | < 0.001 | 0.897 | 0.852 to 0.944 |
Most deprived | –0.169 | 0.028 | < 0.001 | 0.845 | 0.799 to 0.892 |
Settlement type | |||||
Town and fringe | Reference | ||||
Urban | 0.022 | 0.02 | 0.276 | 1.022 | 0.983 to 1.063 |
Village and hamlet | –0.069 | 0.025 | 0.005 | 0.933 | 0.889 to 0.980 |
Individual covariates | |||||
Age group (years) | |||||
18–24 | Reference | ||||
25–34 | –0.087 | 0.015 | < 0.001 | 0.917 | 0.890 to 0.944 |
35–44 | –0.023 | 0.013 | 0.065 | 0.977 | 0.953 to 1.002 |
45–54 | –0.119 | 0.012 | < 0.001 | 0.888 | 0.867 to 0.909 |
55–64 | –0.362 | 0.014 | < 0.001 | 0.696 | 0.677 to 0.716 |
65–74 | –0.659 | 0.019 | < 0.001 | 0.517 | 0.498 to 0.537 |
75–84 | –1.054 | 0.029 | < 0.001 | 0.349 | 0.329 to 0.369 |
85+ | –1.385 | 0.058 | < 0.001 | 0.250 | 0.223 to 0.280 |
Sex | |||||
Female | Reference | ||||
Male | 0.486 | 0.012 | < 0.001 | 1.626 | 1.588 to 1.664 |
Sex × age group | |||||
Male*18–24 years | Reference | ||||
Male*25–34 years | 0.178 | 0.017 | < 0.001 | 1.195 | 1.156 to 1.235 |
Male*35–44 years | 0.067 | 0.016 | < 0.001 | 1.069 | 1.036 to 1.103 |
Male*45–54 years | 0.047 | 0.015 | 0.002 | 1.048 | 1.018 to 1.079 |
Male*55–64 years | 0.168 | 0.016 | < 0.001 | 1.183 | 1.146 to 1.221 |
Male*65–74 years | 0.238 | 0.019 | < 0.001 | 1.269 | 1.222 to 1.317 |
Male*75–84 years | 0.210 | 0.031 | < 0.001 | 1.234 | 1.161 to 1.311 |
Male*85+ years | 0.194 | 0.073 | 0.008 | 1.214 | 1.052 to 1.401 |
NS-SEC3 | |||||
Professional/managerial | Reference | ||||
Intermediate occupations | –0.085 | 0.032 | 0.028 | 0.919 | 0.863 to 0.978 |
Routine/manual | –0.132 | 0.025 | 0.001 | 0.876 | 0.834 to 0.920 |
Never worked/long-term unemployed | –0.358 | 0.073 | 0.001 | 0.699 | 0.606 to 0.807 |
We found that the interaction of age group*sex*deprivation quintile was not significant. Table 57 shows the random-effects variance (as the SD for direct comparison with the fixed effects) in the null and final models. Clearly the majority of the unexplained variation in alcohol consumption is attributable to the household and its magnitude was large in comparison with the fixed-effect model estimates. There was little difference between the null model and the final model. The individual-level variance in a Poisson model is constrained to be the mean and is therefore not estimated as a separate parameter.
Level | Null | Final | ||
---|---|---|---|---|
SD | Intraclass correlation (%) | SD | Intraclass correlation (%) | |
Household | 1.255 | 84.3 | 1.158 | 86.9 |
LSOA | 0.128 | 8.6 | 0.098 | 7.4 |
Local authority | 0.105 | 7.1 | 0.077 | 5.8 |
Simple outlet density: changing population
Table 58 shows the results for the final model of consumption and simple outlet density, changing population. As in the static population model, the previous quarter outlet density was significantly and positively associated with consumption in the following year. Change and volatility were not significant.
Variable | Parameter estimate | SE | p-value | Rate ratio | 95% CI |
---|---|---|---|---|---|
Intercept | 1.416 | 0.034 | < 0.001 | ||
Yearly linear trend since 2008 | –0.020 | 0.005 | < 0.001 | 0.980 | 0.971 to 0.990 |
Alcohol availability | |||||
Previous quarter | 0.005 | 0.002 | 0.001 | 1.005 | 1.001 to 1.009 |
Change positive | 0.128 | 0.081 | 0.117 | 1.137 | 0.97 to 1.332 |
Change negative | 0.126 | 0.09 | 0.162 | 1.134 | 0.951 to 1.353 |
Volatility | –0.136 | 0.072 | 0.06 | 0.873 | 0.756 to 1.005 |
LSOA deprivation quintiles | |||||
Least deprived | Reference | ||||
Less deprived | –0.077 | 0.024 | 0.001 | 0.926 | 0.883 to 0.970 |
Mid deprived | –0.117 | 0.025 | < 0.001 | 0.890 | 0.847 to 0.934 |
More deprived | –0.109 | 0.026 | < 0.001 | 0.897 | 0.852 to 0.944 |
Most deprived | –0.169 | 0.028 | < 0.001 | 0.845 | 0.799 to 0.892 |
Settlement type | |||||
Town and fringe | Reference | ||||
Urban | 0.023 | 0.020 | 0.263 | 1.023 | 0.984 to 1.064 |
Village and hamlet | –0.069 | 0.025 | 0.005 | 0.933 | 0.889 to 0.980 |
Individual covariates | |||||
Age group (years) | |||||
18–24 | Reference | ||||
25–34 | –0.087 | 0.015 | < 0.001 | 0.917 | 0.890 to 0.944 |
35–44 | –0.023 | 0.013 | 0.065 | 0.977 | 0.953 to 1.002 |
45–54 | –0.119 | 0.012 | < 0.001 | 0.888 | 0.867 to 0.909 |
55–64 | –0.362 | 0.014 | < 0.001 | 0.696 | 0.677 to 0.716 |
65–74 | –0.659 | 0.019 | < 0.001 | 0.517 | 0.498 to 0.537 |
75–84 | –1.054 | 0.029 | < 0.001 | 0.349 | 0.329 to 0.369 |
85+ | –1.385 | 0.058 | < 0.001 | 0.250 | 0.223 to 0.280 |
Sex | |||||
Female | Reference | ||||
Male | 0.486 | 0.012 | < 0.001 | 1.626 | 1.588 to 1.664 |
Sex × age group | |||||
Male*18–24 years | Reference | ||||
Male*25–34 years | 0.178 | 0.017 | < 0.001 | 1.195 | 1.156 to 1.235 |
Male*35–44 years | 0.067 | 0.016 | < 0.001 | 1.195 | 1.156 to 1.235 |
Male*45–54 years | 0.047 | 0.015 | 0.002 | 1.069 | 1.036 to 1.103 |
Male*55–64 years | 0.168 | 0.016 | < 0.001 | 1.048 | 1.018 to 1.079 |
Male*65–74 years | 0.238 | 0.019 | < 0.001 | 1.183 | 1.146 to 1.221 |
Male*75–84 years | 0.211 | 0.031 | < 0.001 | 1.269 | 1.222 to 1.317 |
Male*85+ years | 0.193 | 0.073 | 0.008 | 1.235 | 1.162 to 1.312 |
NS-SEC3 | |||||
Professional/managerial | Reference | ||||
Intermediate occupations | –0.086 | 0.032 | 0.027 | 0.918 | 0.862 to 0.977 |
Routine/manual | –0.132 | 0.025 | 0.001 | 0.876 | 0.834 to 0.920 |
Never worked/long-term unemployed | –0.358 | 0.073 | 0.001 | 0.699 | 0.606 to 0.807 |
Table 59 shows the random-effects variance in the null and final models. As with the static population model, the majority of the unexplained variation in alcohol consumption was attributable to the household and its magnitude was large in comparison with the fixed-effect model estimates.
Level | Null | Final | ||
---|---|---|---|---|
SD | Intraclass correlation (%) | SD | Intraclass correlation (%) | |
Household | 1.255 | 84.3 | 1.158 | 86.9 |
LSOA | 0.128 | 8.6 | 0.098 | 7.3 |
Local authority | 0.106 | 7.1 | 0.077 | 5.8 |
There were no substantive differences in the estimates for the measures of outlet density with and without correcting for population migration.
Walking outlet density
Table 60 shows the results of the final model of consumption and walking outlet density. The previous quarter outlet density was significantly and positively associated with consumption in the following year. The positive and negative change parameters were not significant in the model, although the direction of association of these main effects was plausible. Volatility was not significantly associated with consumption in the following year.
Variable | Parameter estimate | SE | p-value | Rate ratio | 95% CI |
---|---|---|---|---|---|
Intercept | 1.398 | 0.033 | < 0.001 | ||
Yearly linear trend since 2008 | –0.021 | 0.005 | < 0.001 | 0.979 | 0.970 to 0.989 |
Alcohol availability | |||||
Previous quarter | 0.010 | 0.002 | < 0.001 | 1.010 | 1.006 to 1.014 |
Change positive | 0.001 | 0.053 | 0.984 | 1.001 | 0.902 to 1.111 |
Change negative | –0.048 | 0.056 | 0.389 | 0.953 | 0.854 to 1.064 |
Volatility | –0.026 | 0.029 | 0.38 | 0.974 | 0.920 to 1.031 |
LSOA deprivation quintile | |||||
Least deprived | Reference | ||||
Less deprived | –0.068 | 0.023 | 0.003 | 0.934 | 0.893 to 0.977 |
Mid deprived | –0.116 | 0.024 | < 0.001 | 0.890 | 0.850 to 0.933 |
More deprived | –0.113 | 0.025 | < 0.001 | 0.893 | 0.850 to 0.938 |
Most deprived | –0.173 | 0.026 | < 0.001 | 0.841 | 0.799 to 0.885 |
Settlement type | |||||
Town and fringe | Reference | ||||
Urban | 0.019 | 0.02 | 0.344 | 1.019 | 0.980 to 1.060 |
Village and hamlet | –0.044 | 0.025 | 0.076 | 0.957 | 0.911 to 1.005 |
Individual covariates | |||||
Age group (years) | |||||
18–24 | Reference | ||||
25–34 | –0.077 | 0.014 | < 0.001 | 0.926 | 0.901 to 0.952 |
35–44 | –0.015 | 0.012 | 0.223 | 0.985 | 0.962 to 1.009 |
45–54 | –0.107 | 0.011 | < 0.001 | 0.899 | 0.879 to 0.918 |
55–64 | –0.348 | 0.014 | < 0.001 | 0.706 | 0.687 to 0.726 |
65–74 | –0.643 | 0.018 | < 0.001 | 0.526 | 0.507 to 0.545 |
75–84 | –1.033 | 0.028 | < 0.001 | 0.356 | 0.337 to 0.376 |
85+ | –1.391 | 0.057 | < 0.001 | 0.249 | 0.223 to 0.278 |
Sex | |||||
Female | Reference | ||||
Male | 0.492 | 0.012 | < 0.001 | 1.636 | 1.598 to 1.675 |
Sex × age group | |||||
Male*18–24 years | Reference | ||||
Male*25–34 years | 0.163 | 0.016 | < 0.001 | 1.177 | 1.141 to 1.215 |
Male*35–44 years | 0.062 | 0.015 | < 0.001 | 1.064 | 1.033 to 1.096 |
Male*45–54 years | 0.041 | 0.015 | 0.006 | 1.042 | 1.012 to 1.073 |
Male*55–64 years | 0.153 | 0.015 | < 0.001 | 1.165 | 1.132 to 1.200 |
Male*65–74 years | 0.237 | 0.019 | < 0.001 | 1.267 | 1.221 to 1.316 |
Male*75–84 years | 0.205 | 0.029 | < 0.001 | 1.228 | 1.160 to 1.299 |
Male*85+ years | 0.195 | 0.071 | 0.006 | 1.215 | 1.057 to 1.397 |
NS-SEC3 | |||||
Professional/managerial | Reference | ||||
Intermediate occupations | –0.088 | 0.032 | 0.029 | 0.916 | 0.860 to 0.975 |
Routine/manual | –0.135 | 0.022 | < 0.001 | 0.874 | 0.837 to 0.912 |
Never worked/long-term unemployed | –0.373 | 0.063 | < 0.001 | 0.689 | 0.609 to 0.779 |
Table 61 shows the random-effects variance in the null and final models. As with the simple outlet density models, the majority of the unexplained variation in alcohol consumption was attributable to the household and its magnitude was large in comparison with the fixed-effect model estimates.
Level | Null | Final | ||
---|---|---|---|---|
SD | Intraclass correlation (%) | SD | Intraclass correlation (%) | |
Household | 1.256 | 84.5 | 1.160 | 86.8 |
LSOA | 0.132 | 8.9 | 0.101 | 7.6 |
Local authority | 0.098 | 6.6 | 0.076 | 5.7 |
We also fitted the walking density model excluding the 109 LSOAs with an English border to investigate the possibility of bias from boundary effects, as an English alcohol outlet might lie within a 10-minute buffer zone but would not be counted. The estimates were very similar (data not shown).
Table 62 shows the alcohol availability estimates for the final model of consumption and walking outlet density for deprivation quintiles 1 and 2 (least deprived LSOAs).
Variable | Parameter estimate | SE | p-value | Rate ratio | 95% CI |
---|---|---|---|---|---|
Intercept | 1.357 | 0.038 | < 0.001 | ||
Yearly linear trend since 2008 | –0.007 | 0.007 | 0.256 | 0.993 | 0.979 to 1.007 |
Alcohol availability | |||||
Previous quarter | 0.009 | 0.004 | 0.019 | 1.009 | 1.001 to 1.017 |
Change positive | –0.001 | 0.069 | 0.989 | 0.999 | 0.873 to 1.144 |
Change negative | –0.046 | 0.074 | 0.531 | 0.955 | 0.826 to 1.104 |
Volatility | –0.035 | 0.039 | 0.368 | 0.966 | 0.895 to 1.042 |
Table 63 shows the alcohol availability estimates for the final model of consumption and walking outlet density for deprivation quintiles 4 and 5 (most deprived LSOAs). There was little difference between the estimates in the deprivation-stratified models.
Variable | Parameter estimate | SE | p-value | Rate ratio | 95% CI |
---|---|---|---|---|---|
Intercept | 1.254 | 0.058 | < 0.001 | ||
Yearly linear trend since 2008 | –0.032 | 0.009 | < 0.001 | 0.969 | 0.952 to 0.986 |
Alcohol availability | |||||
Previous quarter | 0.007 | 0.003 | 0.019 | 1.007 | 1.001 to 1.013 |
Change positive | 0.011 | 0.091 | 0.908 | 1.011 | 0.846 to 1.208 |
Change negative | –0.069 | 0.097 | 0.473 | 0.933 | 0.772 to 1.129 |
Volatility | –0.006 | 0.049 | 0.905 | 0.994 | 0.903 to 1.094 |
Driving outlet density
Table 64 shows the results of the final model of consumption and driving outlet density. The previous quarter outlet density was significantly and positively associated with consumption in the following year. Change and volatility were not significant.
Variable | Parameter estimate | SE | p-value | Rate ratio | 95% CI |
---|---|---|---|---|---|
Intercept | 1.396 | 0.033 | < 0.001 | ||
Yearly linear trend since 2008 | –0.020 | 0.005 | < 0.001 | 0.980 | 0.971 to 0.990 |
Alcohol availability | |||||
Previous quarter | 0.001 | 0.000 | 0.003 | 1.001 | 1.001 to 1.001 |
Change positive | 0.020 | 0.025 | 0.412 | 1.020 | 0.971 to 1.071 |
Change negative | 0.022 | 0.027 | 0.412 | 1.022 | 0.970 to 1.078 |
Volatility | –0.011 | 0.015 | 0.483 | 0.989 | 0.960 to 1.019 |
LSOA deprivation quintile | |||||
Least deprived | Reference | ||||
Less deprived | –0.063 | 0.023 | 0.007 | 0.939 | 0.898 to 0.982 |
Mid deprived | –0.107 | 0.024 | < 0.001 | 0.899 | 0.857 to 0.942 |
More deprived | –0.102 | 0.025 | < 0.001 | 0.903 | 0.860 to 0.948 |
Most deprived | –0.161 | 0.026 | < 0.001 | 0.851 | 0.809 to 0.896 |
Settlement type | |||||
Town and fringe | Reference | ||||
Urban | 0.007 | 0.020 | 0.746 | 1.007 | 0.968 to 1.047 |
Village and hamlet | –0.062 | 0.025 | 0.012 | 0.940 | 0.895 to 0.987 |
Individual covariates | |||||
Age group (years) | |||||
18–24 | Reference | ||||
25–34 | –0.076 | 0.014 | < 0.001 | 0.927 | 0.902 to 0.953 |
35–44 | –0.015 | 0.012 | 0.219 | 0.985 | 0.962 to 1.009 |
45–54 | –0.107 | 0.011 | < 0.001 | 0.899 | 0.879 to 0.918 |
55–64 | –0.349 | 0.014 | < 0.001 | 0.705 | 0.686 to 0.725 |
65–74 | –0.643 | 0.018 | < 0.001 | 0.526 | 0.507 to 0.545 |
75–84 | –1.032 | 0.028 | < 0.001 | 0.356 | 0.337 to 0.376 |
85+ | –1.389 | 0.057 | < 0.001 | 0.249 | 0.223 to 0.279 |
Sex | |||||
Female | Reference | ||||
Male | 0.492 | 0.012 | < 0.001 | 1.636 | 1.598 to 1.675 |
Sex × age group | |||||
Male*18–24 years | Reference | ||||
Male*25–34 years | 0.162 | 0.016 | < 0.001 | 1.176 | 1.140 to 1.213 |
Male*35–44 years | 0.062 | 0.015 | < 0.001 | 1.064 | 1.033 to 1.096 |
Male*45–54 years | 0.041 | 0.015 | 0.006 | 1.042 | 1.012 to 1.073 |
Male*55–64 years | 0.153 | 0.015 | < 0.001 | 1.165 | 1.132 to 1.200 |
Male*65–74 years | 0.237 | 0.019 | < 0.001 | 1.267 | 1.221 to 1.316 |
Male*75–84 years | 0.205 | 0.029 | < 0.001 | 1.228 | 1.160 to 1.299 |
Male*85+ years | 0.193 | 0.071 | 0.007 | 1.213 | 1.055 to 1.394 |
NS-SEC3 | |||||
Professional/managerial | Reference | ||||
Intermediate occupations | –0.088 | 0.032 | 0.030 | 0.916 | 0.860 to 0.975 |
Routine/manual | –0.135 | 0.022 | < 0.001 | 0.874 | 0.837 to 0.912 |
Never worked/long-term unemployed | –0.374 | 0.063 | < 0.001 | 0.688 | 0.608 to 0.778 |
Table 65 shows the random-effects variance in the null and final models. As with the previous models, the majority of the unexplained variation in alcohol consumption was attributable to the household and its magnitude was large in comparison with the fixed-effect model estimates.
Level | Null | Final | ||
---|---|---|---|---|
SD | Intraclass correlation (%) | SD | Intraclass correlation (%) | |
Household | 1.256 | 84.8 | 1.160 | 86.8 |
LSOA | 0.135 | 9.1 | 0.106 | 7.9 |
Local authority | 0.091 | 6.1 | 0.071 | 5.3 |
Walking outlet density: non-linear dependence
We further explored the shape of the relationship between the previous quarter walking outlet density and consumption by fitting a cubic spline with three degrees of freedom. 99 Three degrees of freedom allowed sufficient flexibility in the shape of the relationship without overfitting the models. The shape of the relationship is shown in Figure 33, with five plots, one for each imputation model. The slope is steeper at outlet density values between 0 and 1, which includes 712 (37.5%) LSOAs, and is much shallower at values > 1. We therefore refitted the walking density model with a separate term for above and below a natural cut-point of outlet density = 1 and the estimates are shown in Table 66.
FIGURE 33.
Shape of the relationship between the previous quarter walking outlet density and consumption. Pred, predicted consumption; prevqrt, previous quarter.
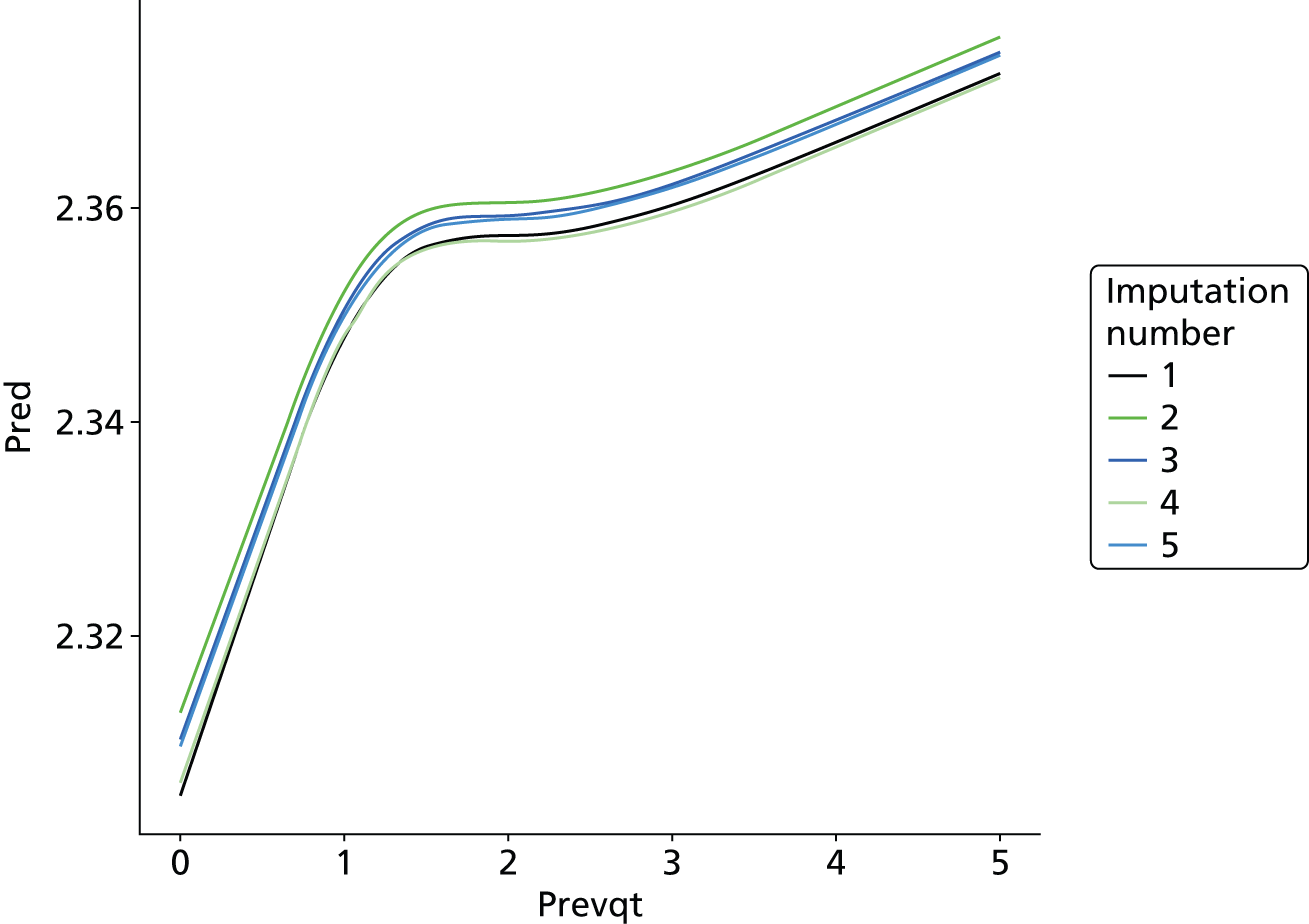
Variable | Parameter estimate | SE | p-value | Rate ratio | 95% CI |
---|---|---|---|---|---|
Intercept | 1.284 | 0.042 | < 0.001 | ||
Yearly linear trend since 2008 | –0.021 | 0.005 | < 0.001 | 0.979 | 0.970 to 0.989 |
Alcohol availability | |||||
Previous quarter (0–1) | 0.059 | 0.032 | 0.068 | 1.061 | 0.996 to 1.129 |
Previous quarter (> 1) | 0.007 | 0.002 | 0.001 | 1.007 | 1.003 to 1.011 |
Change positive | 0.009 | 0.053 | 0.869 | 1.009 | 0.909 to 1.119 |
Change negative | –0.045 | 0.056 | 0.427 | 0.956 | 0.857 to 1.067 |
Volatility | –0.030 | 0.029 | 0.307 | 0.970 | 0.917 to 1.027 |
The rate ratio for the values of outlet density between 0 and 1 was larger than that for higher values and suggests that in these less dense LSOAs a unit change in outlet density was associated with a 6% increase in consumption. A unit change in outlet density at values > 1 was associated with an additional 0.7% change in consumption. The combined terms for previous quarter were globally significant (p < 0.001).
Geographically Weighted Regression models
Binge drinking: walking outlet density
In the initial global model we fitted the probability of individual binge drinking as a function of alcohol availability, adjusting for age group, sex, the interaction between age group*sex, WIMD 2008 deprivation quintile and settlement type (Table 67).
Parameter | Estimate | SE | p-value |
---|---|---|---|
Intercept | –0.624 | 0.046 | < 0.001 |
Alcohol availability | |||
Previous quarter | 0.007 | 0.003 | 0.007 |
Change positive | 0.040 | 0.072 | 0.578 |
Change negative | –0.093 | 0.081 | 0.248 |
Volatility | –0.034 | 0.037 | 0.366 |
LSOA deprivation quintile | |||
Least deprived | Reference | ||
Less deprived | –0.054 | 0.029 | 0.065 |
Mid deprived | –0.076 | 0.029 | 0.009 |
More deprived | 0.002 | 0.030 | 0.940 |
Most deprived | –0.006 | 0.032 | 0.853 |
Settlement type | |||
Town and fringe | Reference | ||
Urban | 0.042 | 0.024 | 0.079 |
Village and hamlet | –0.209 | 0.031 | < 0.001 |
Individual covariates | |||
Age group (years) | |||
18–24 | Reference | ||
25–34 | –0.064 | 0.049 | 0.185 |
35–44 | –0.048 | 0.046 | 0.295 |
45–54 | –0.176 | 0.046 | < 0.001 |
55–64 | –0.751 | 0.049 | < 0.001 |
65–74 | –1.578 | 0.064 | < 0.001 |
75–84 | –2.355 | 0.112 | < 0.001 |
85+ | –3.803 | 0.381 | < 0.001 |
Sex | |||
Female | Reference | ||
Male | 0.245 | 0.051 | < 0.001 |
Sex × age group | |||
Male*18–24 years | Reference | ||
Male*25–34 years | 0.301 | 0.069 | < 0.001 |
Male*35–44 years | 0.276 | 0.065 | < 0.001 |
Male*45–54 years | 0.351 | 0.065 | < 0.001 |
Male*55–64 years | 0.592 | 0.067 | < 0.001 |
Male*65–74 years | 0.798 | 0.082 | < 0.001 |
Male*75–84 years | 0.594 | 0.136 | < 0.001 |
Male*85+ years | 1.189 | 0.438 | 0.007 |
NS-SEC3 | |||
Professional/managerial | Reference | ||
Intermediate occupations | –0.044 | 0.025 | 0.085 |
Routine/manual | –0.076 | 0.022 | < 0.001 |
Never worked/long-term unemployed | –0.423 | 0.074 | < 0.001 |
The GWR model omitted the terms for WIMD 2008 deprivation quintile and settlement type to assess the spatial variation in the association between binge drinking and alcohol availability by LSOA type. Figure 34 shows the parameter estimates of binge drinking for each LSOA derived from the intercept of the GWR. For illustration, the mean probability (on the log odds scale) is shown in grey, with higher probabilities in red and lower probabilities in green.
FIGURE 34.
Model-predicted estimates for binge drinking. © Crown copyright and database rights 2013 Ordnance Survey 100019153.
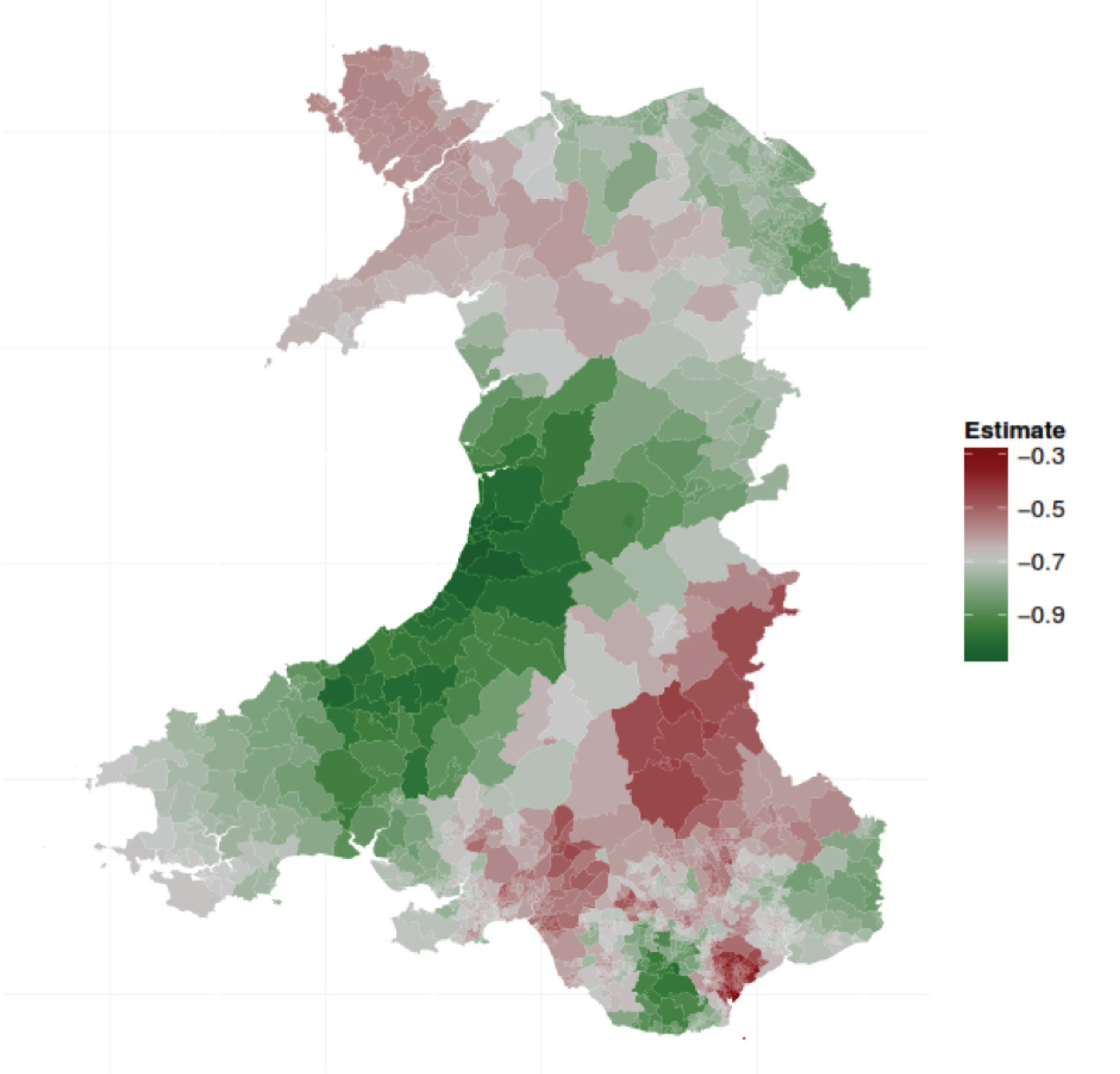
Figures 35–38 show the Benjamini–Hochberg-adjusted p-values that were significant in any LSOA over the full study period, that is, the consistent findings. These figures show evidence that there is important spatial variation in the associations between binge drinking and alcohol availability through the time period of the study. The maps have to be interpreted with care as sampling variation in the binge drinking outcome when there were few respondents in some more sparsely populated LSOAs may account for some of the effects in the rural areas of Wales. Figure 35 may well show this effect in sparsely populated areas of mid-Wales. Because the output will also depend on the bandwidth chosen, the effect shown in the Isle of Anglesey may well be a result of the bandwidth including the Llŷn Peninsula.
FIGURE 35.
Walking outlet density, previous quarter. © Crown copyright and database rights 2013 Ordnance Survey 100019153.

FIGURE 36.
Walking outlet density, negative change. © Crown copyright and database rights 2013 Ordnance Survey 100019153.

FIGURE 37.
Walking outlet density, positive change. © Crown copyright and database rights 2013 Ordnance Survey 100019153.

FIGURE 38.
Walking outlet density, volatility. © Crown copyright and database rights 2013 Ordnance Survey 100019153.

Very little spatial variation in negative change, positive change or volatility was found (see Figures 36–38).
Chapter 7 Study 2: hospital admissions
Data request
The PEDW is the database of all inpatient and day case admissions for residents of Wales (see Chapter 4, Patient Episode Database for Wales). A request was made for the full record (including all 14 diagnosis codes and all 12 OPCS-4.6 procedure codes) for every episode in a spell with an admission date between 1 January 2006 and 31 December 2011 that contained any of the alcohol-related codes (as defined in Definition of an alcohol-related admission) at any point in the spell. Figure 39 shows all of the required information for each spell given that at least one ICD-10 code in any episode is alcohol related. The extract included all episodes within each spell, not just those episodes with alcohol-related diagnoses. This allowed examination of the entire hospital admission record and enabled the development of appropriate inclusion/exclusion rules for the analysis data set.
FIGURE 39.
Patient Episode Database for Wales criteria and records required.

Data consisting of almost 1.7 million rows of alcohol-related PEDW records, with 32 variables, were received. Processing the PEDW data was a substantial challenge with a massive amount of cleaning and restructuring required. The format of the extracts received was such that one patient admission consisted of multiple rows. Data were checked for possible errors and to identify variables that formed the unique information of each spell. Data were then transposed such that one row equated to a single episode, then to a single spell, and then again such that one row equated to a single admission. The process undertaken is described in the following sections.
Cleaning the data
Our exploration of the data and PEDW information implied that the hierarchy within the data should be as shown in Figure 40.
FIGURE 40.
Patient Episode Database for Wales data structure. ADMIS_DT, admission date; DISCH_DT, discharge date; GNDR_CD, gender code; PAT_CLASS_CD, patient class code; Prov., provider (text description); Prov_Unit_CD, provider unit code (numeric); SPELL_NUM_E, spell number encrypted.
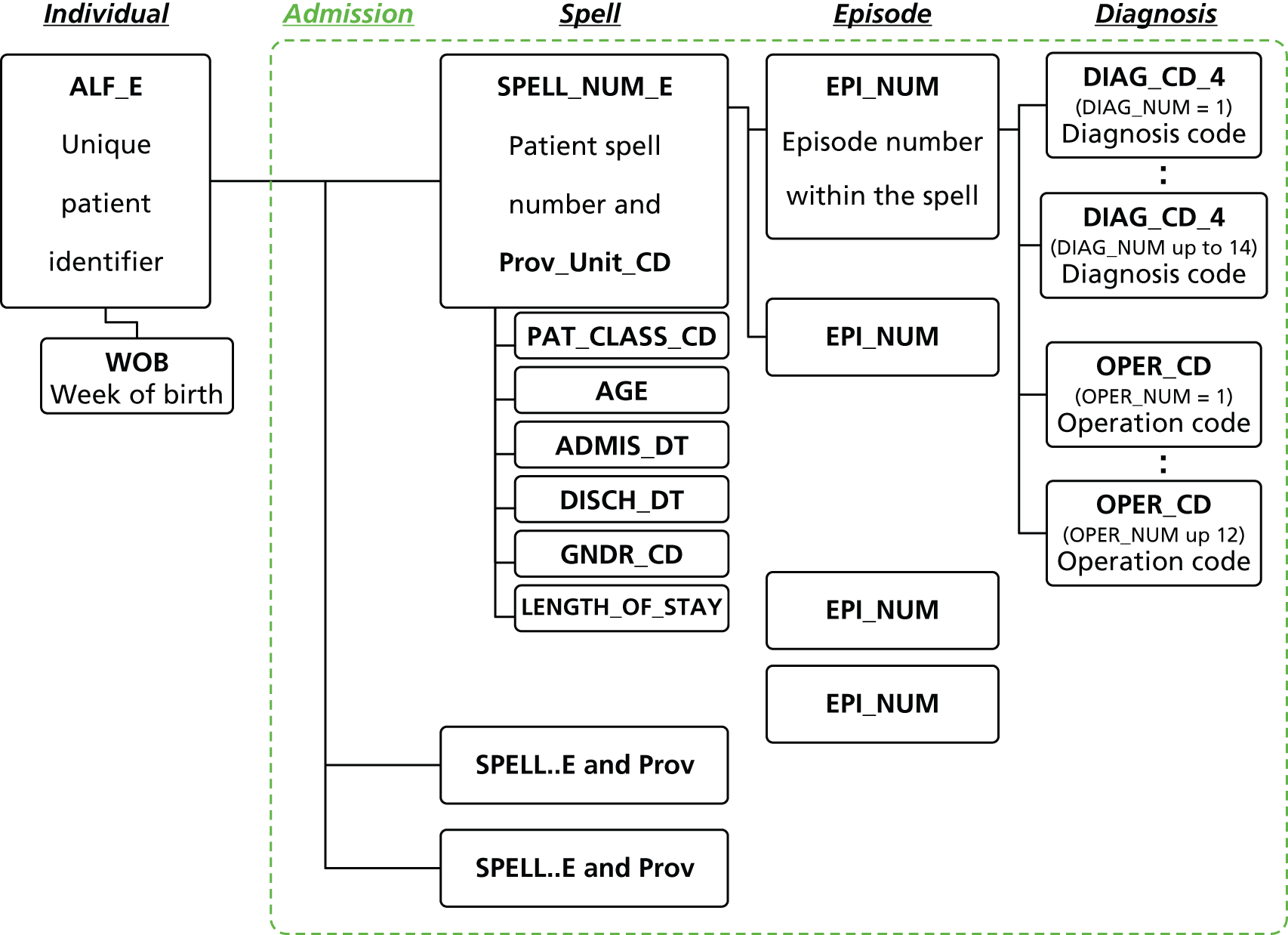
It was expected that a single spell would relate to a single pair of admission and discharge dates. However, cases were present in which these rules did not hold – when admission dates changed within a spell and because discharge dates were not always present. Five issues were identified that required a solution before implementing the several procedures to transpose the data and provide the required admission information:
-
mismatch – spells with equal spell numbers (IDs) but non-equal information such as different admission dates
-
repetition – spells with identical information
-
partial repetition – spells with identical admission and discharge dates but non-equal episode information
-
transfer – linked spells as indicated by a discharge destination with codes 51–57
-
frequent spells – spells that occur in a short space of time and which are of a short duration (such as radiotherapy) are flagged.
Cleaning diagnosis-level data
Mismatch
In total, 120 records (equating to 91 episodes) from the original data were identified as being possible errors with equal spell numbers but non-equal admission dates. To manage these exceptions to the database structure a new spell number was generated consisting of the original spell number plus a flag to denote each apparent spell within the records.
Several spells with different admission dates were located in the extract. A single spell was defined as a unique combination of ALF_E, spell number, date of admission, number of episodes and diagnosis code in the first position of the first patient episode. Identical spells with identical episode information were assumed to be repetition. Data were transposed such that a row represented a single episode, with individual variables used to represent the 14 diagnosis code positions and 12 operation code positions.
Cleaning episode-level data
Repetition
We identified individuals with different spell numbers but with the same admission and discharge dates. The time of admission was not provided and so it was assumed that if two spells had identical admission and discharge dates they should be part of the same spell.
Relabelling episodes
Table 68 shows the distribution of episode numbers in the raw data and the new frequency of the new episode numbers (up to 13 in a single spell). The data containing a single case per episode were reconfigured into a wide format such that a single row or case represented a single spell.
Episode number: SAIL Databank (as received) | Frequency | % | Episode number: renumbered spells | Frequency | % |
---|---|---|---|---|---|
1 | 133,316 | 74.8 | 1 | 135,177 | 75.8 |
2 | 32,077 | 18.0 | 2 | 31,369 | 17.6 |
3 | 8436 | 4.7 | 3 | 7960 | 4.5 |
4 | 2868 | 1.6 | 4 | 2532 | 1.4 |
5 | 973 | 0.5 | 5 | 816 | 0.5 |
6 | 375 | 0.2 | 6 | 294 | 0.2 |
7 | 160 | 0.1 | 7 | 113 | 0.1 |
8 | 66 | < 0.0 | 8 | 46 | < 0.0 |
9 | 28 | < 0.0 | 9 | 19 | < 0.0 |
10 | 14 | < 0.0 | 10 | 8 | < 0.0 |
11 | 10 | < 0.0 | 11 | 5 | < 0.0 |
12 | 4 | < 0.0 | 12 | 2 | < 0.0 |
13 | 4 | < 0.0 | 13 | 2 | < 0.0 |
14 | 2 | < 0.0 | |||
15 | 3 | < 0.0 | |||
17 | 1 | < 0.0 | |||
19 | 1 | < 0.0 | |||
31 | 2 | < 0.0 | |||
33 | 1 | < 0.0 | |||
75 | 2 | < 0.0 | |||
Total | 178,343 | 100 | Total | 178,343 | 100 |
Cleaning spell-level data
Repetition
As with the previous checks, spells were compared to ensure that repetition was not present in the data. Spells with different operation information but an identical diagnosis, admission date and discharge date were assumed to imply a single admission with multiple operations. This is likely to occur because of the spell number being used for administrative purposes and/or because more than one spell was used to represent the procedures undertaken.
Partial repetition
Spells with the same admission and discharge date but different spell numbers were flagged as potentially being part of the same admission (such as concurrent episodes in a spell). These were examined to ensure that episodes within a spell were identified. A single admission required that no two spells had the same admission and discharge date as this would imply that the spell was duplicated or part of the same spell.
Definition of a single admission
We required an admission to account for transfers of patients between hospitals within 24 hours to best represent one single presentation of illness. It was therefore necessary to separate transfers from genuine new admissions. If the discharge method (DISCH_MTHD_CD) was coded 8 (not applicable – transfer) or the discharge destination (DISCH_DESTINATION_CD) was coded 51–57 (to another NHS hospital) it was interpreted to be the same period of admission at the new site. Transfers occurred in 6691 spells, with up to three transfers in an admission, and matched to 3737 same-day and 231 next-day spells. These transfers were attached to the end of the admission record to enable identification of transfer spells. As an example, an individual may have multiple spells but some have transfer codes and some appear to be part of the same spell. Some of these spells may be combined to represent unique admissions to hospital (Figure 41).
FIGURE 41.
Example of conversion of spells to admissions. Asterisks denote the renumbering of an episode within a spell. #-## is used to illustrate spell number (#) and episode number (##) within an admission.

Definition of an alcohol-related admission
The ONS has published a list of ICD-10 codes (shown in Table 69) to define causes of death that are exclusively alcohol related, that is, not just those for which alcohol could be a contributory aetiological factor. 100 This definition has previously been used in Wales to quantify the annual number of alcohol-related admissions. 101 However, the classification does not include non-fatal alcohol codes.
Description | ICD-10 code |
---|---|
Mental and behavioural disorders due to alcohol | F10 |
Degeneration of the nervous system from use of alcohol | G31.2 |
Alcoholic polyneuropathy | G62.1 |
Alcoholic cardiomyopathy | I42.6 |
Alcoholic gastritis | K29.2 |
Alcoholic liver disease | K70 |
Chronic hepatitis, not elsewhere classified | K73 |
Fibrosis and cirrhosis of liver (excluding K74.3–K74.5 – biliary cirrhosis) | K74 |
Alcohol-induced chronic pancreatitis | K86.0 |
Accidental poisoning by and exposure to alcohol | X45 |
Intentional self-poisoning by and exposure to alcohol | X65 |
Poisoning by and exposure to alcohol, undetermined intent | Y15 |
There are other published definitions of ‘exclusively’ alcohol-related conditions that overlap. Additional definitions published by the Centers for Disease Control and Prevention are shown in Table 70. 102 The differences from the ONS definitions largely relate to non-fatal conditions being excluded from the ONS cause of death list.
Description | ICD-10 code |
---|---|
Alcoholic myopathy | G72.1 |
Excessive blood level of alcohol | R78.0 |
Toxic effect of alcohol | T51.0, T51.9 |
Maternal care for (suspected) damage to fetus from alcohol | O35.4 |
The complete list of wholly attributable alcohol ICD-10 codes we defined for use in this study is shown in Table 71. Our aim was to define a precise list of codes that were by definition wholly related to alcohol. We judged that it was preferable to exclude some codes that may be partially attributable to alcohol rather than include non-alcohol-related admissions.
ICD-10 | Definition | Title |
---|---|---|
E24.4 | Alcohol-induced pseudo-Cushing syndrome | E24 – Cushing syndrome |
E51.2 | Wernicke’s encephalopathy | E51 – thiamine deficiency |
F10.0 | Acute intoxication | F10 – mental and behavioural disorders due to alcohol (F10.0–F10.9) |
F10.1 | Harmful use | |
F10.2 | Dependence syndrome | |
F10.3 | Withdrawal state | |
F10.4 | Withdrawal state with delirium | |
F10.5 | Psychotic disorder | |
F10.6 | Amnesic syndrome | |
F10.7 | Residual and late-onset psychotic disorder | |
F10.8 | Other mental and behavioural disorders | |
F10.9 | Unspecified mental and behavioural disorder | |
G31.2 | Degeneration of the nervous system from use of alcohol | G31 – other degenerative diseases of the nervous system, not elsewhere classified |
G40.5 | Special epileptic syndromes – if paired with other alcohol code | G40 – epilepsy |
G62.1 | Alcoholic polyneuropathy | G62 – other polyneuropathies |
G72.1 | Alcoholic myopathy | G72 – other myopathies |
I42.6 | Alcoholic cardiomyopathy | I42 – cardiomyopathy |
K29.2 | Alcoholic gastritis | K29 – gastritis and duodenitis |
K70.0 | Alcoholic fatty liver | K70 – alcoholic liver disease (K70.0–K70.4, K70.9) |
K70.1 | Alcoholic hepatitis | |
K70.2 | Alcoholic fibrosis and sclerosis of the liver | |
K70.3 | Alcoholic cirrhosis of the liver | |
K70.4 | Alcoholic hepatic failure | |
K70.9 | Alcoholic liver disease, unspecified | |
K85.2 | Alcohol-induced acute pancreatitis | K85 – acute pancreatitis |
K86.0 | Alcohol-induced chronic pancreatitis | K86 – other diseases of the pancreas |
O35.4 | Maternal care for (suspected) damage to the fetus from alcohol | O35 – maternal care for known or suspected fetal abnormality and damage |
R78.0 | Finding of alcohol in the blood | R78 – findings of drugs and other substances not normally found in blood |
T51.0 | Toxic effect: ethanol [excluding acute alcohol intoxication or ‘hangover’ effects (F10.0), drunkenness (F10.0), pathological alcohol intoxication (F10.0)] | T51 – toxic effect of alcohol |
Chapter XX: external causes of morbidity and mortality (V01–Y98) | ||
X45.0 | Occurrence at home | X45 – accidental poisoning by and exposure to alcohol (X45.0–X45.2, X45.4–X45.6, X45.8, X45.9) |
X45.1 | Occurrence in residential institution | |
X45.2 | Occurrence at school, other institution/public administration area | |
X45.4 | Occurrence on street/highway | |
X45.5 | Occurrence at trade/service area | |
X45.6 | Occurrence at industrial/construction area | |
X45.8 | Occurrence at other specified place | |
X45.9 | Occurrence at unspecified place | |
X65.0 | Occurrence at home | X65 – intentional self-poisoning by and exposure to alcohol (X65.0–X65.2, X65.4–X65.6, X65.8, X65.9) |
X65.1 | Occurrence in residential institution | |
X65.2 | Occurrence at school, other institution/public administration area | |
X65.4 | Occurrence on street/highway | |
X65.5 | Occurrence at trade/service area | |
X65.6 | Occurrence at industrial/construction area | |
X65.8 | Occurrence at other specified place | |
X65.9 | Occurrence at unspecified place | |
Y15.0 | Occurrence at home | Y15 – poisoning by and exposure to alcohol, undetermined intent (Y15.0, Y15.2, Y15.4, Y15.8, Y15.9) |
Y15.2 | Occurrence at school, other institution/public administration area | |
Y15.4 | Occurrence on street/highway | |
Y15.8 | Occurrence at other specified place | |
Y15.9 | Occurrence at unspecified place | |
Y90.0 | Blood alcohol level of < 20 mg/100 ml | Y90 – evidence of alcohol involvement determined by blood alcohol level (Y90.0–Y90.9) |
Y90.1 | Blood alcohol level of 20–39 mg/100 ml | |
Y90.2 | Blood alcohol level of 40–59 mg/100 ml | |
Y90.3 | Blood alcohol level of 60–79 mg/100 ml | |
Y90.4 | Blood alcohol level of 80–99 mg/100 ml | |
Y90.5 | Blood alcohol level of 100–119 mg/100 ml | |
Y90.6 | Blood alcohol level of 120–199 mg/100 ml | |
Y90.7 | Blood alcohol level of 200–239 mg/100 ml | |
Y90.8 | Blood alcohol level of ≥ 240 mg/100 ml | |
Y90.9 | Presence of alcohol in blood, level not specified | |
Y91.0 | Mild alcohol intoxication | Y91 – evidence of alcohol involvement determined by level of intoxication (Y91.0–Y90.3, Y90.9) |
Y91.1 | Moderate alcohol intoxication | |
Y91.2 | Severe alcohol intoxication | |
Y91.3 | Very severe alcohol intoxication | |
Y91.9 | Alcohol involvement, not otherwise specified | |
Chapter XXI: factors influencing health status and contact with health services (Z00–Z99) | ||
Z50.2 | Alcohol rehabilitation | Z50 – care involving use of rehabilitation procedures |
Z71.4 | Alcohol abuse counselling and surveillance | Z71 – persons encountering health services for other counselling and medical advice, not elsewhere classified |
Z72.1 | Alcohol use | Z72 – problems related to lifestyle |
The ICD-10 codes K73 and K74 from the ONS (see Table 69) and T51.9 from the Centers for Disease Control and Prevention list (see Table 70) were not included in our classification. ICD-10 codes K73 and K74 are chronic conditions, not wholly attributable to alcohol:59
-
K73 – chronic hepatitis, not elsewhere classified. Excludes:
-
hepatitis (chronic): alcoholic (K70.1)
-
-
K74 – fibrosis and cirrhosis of the liver. Excludes:
-
alcoholic fibrosis of liver (K70.2)
-
cirrhosis (of liver): alcoholic (K70.3).
-
ICD-10 code T51.9 was excluded, as this refers to alcohol (unspecified), which may or may not be ethanol and which would not necessarily indicate consumption at or from licensed alcohol outlets. Two diagnostic ICD-10 codes were also included following consultation with the study steering committee:59
-
G40.5 – special epileptic syndromes (only if paired with another alcohol code):
-
epilepsia partialis continua (Kozhevnikof)
-
epileptic seizures related to:
-
alcohol
-
drugs
-
hormonal changes
-
sleep deprivation
-
stress
-
-
-
K85.2 – alcohol-induced acute pancreatitis.
We further defined three ‘Z’ codes (Chapter XXI: factors influencing health status and contact with health services):
-
Z50.2 – alcohol rehabilitation
-
Z71.4 – alcohol abuse counselling and surveillance
-
Z72.1 – alcohol use.
Our defined list of ICD-10 codes59 was similar to the list of ‘wholly attributable conditions’ published by Public Health England103 in April 2014, after we had completed our work on definitions. Here, Public Health England distinguished between a ‘narrow’ measure of wholly attributable conditions (or alcohol specific), which was closest to our aim, and a ‘broad’ measure of conditions in which alcohol may be implicated in their aetiology. As stated, we did not want to assess a broad measure; our aim was to define a precise list of wholly alcohol-related ICD-10 codes.
The differences between our definitions are that Public Health England included methanol poisoning (T51.1) and the toxic effect of alcohol, unspecified (T51.9) (which we did not consider were specific to ethanol) and fetal alcohol syndrome (dysmorphic) (Q86.0), which is not relevant to adults. Public Health England excluded Wernicke’s encephalopathy (E51.2), special epileptic syndromes – if paired with another alcohol code (G40.5), maternal care for (suspected) damage to the fetus from alcohol (O35.4) and the three non-diagnostic ‘Z’ codes: alcohol rehabilitation (Z50.2), alcohol abuse counselling and surveillance (Z71.4) and alcohol use (Z72.1).
Our definition of codes was larger than the set used by McDonald et al. 104 in a study carried out in Scotland, using hospital admission data from the Scottish Morbidity Record (SMR01), which is the equivalent of the PEDW in Wales. McDonald et al. 102 excluded the following codes: E24.4, E51.2, G72.1, K85.2, O35.4, Y15 and Z71.4. These codes were included in this current study for the reasons given earlier.
The following ICD-10 codes59 for injury and external causes were also included when they were paired with an alcohol-defined code:
-
Chapter XIX: injury, poisoning and certain other consequences of external causes:
-
S00–S09 – injuries to the head
-
S10–S19 – injuries to the neck
-
S20–S29 – injuries to the thorax
-
S30–S39 – injuries to the abdomen, lower back, lumbar spine and pelvis
-
S40–S49 – injuries to the shoulder and upper arm
-
S50–S59 – injuries to the elbow and forearm
-
S60–S69 – injuries to the wrist and hand
-
S70–S79 – injuries to the hip and thigh
-
S80–S89 – Injuries to the knee and lower leg
-
S90–S99 – injuries to the ankle and foot
-
T00–T07 – injuries involving multiple body regions
-
T08–T14 – injuries to unspecified part of trunk, limb or body region
-
T15–T19 – effects of foreign body entering through natural orifice.
-
-
Chapter XX: external causes of morbidity and mortality:
-
V01–V99 – transport accidents
-
V01–V09 – pedestrian injured in transport accident
-
V10–V19 – pedal cyclist injured in transport accident
-
V20–V29 – motorcycle rider injured in transport accident
-
V30–V39 – occupant of three-wheeled motor vehicle injured in transport accident
-
V40–V49 – car occupant injured in transport accident
-
V50–V59 – occupant of pick-up truck or van injured in transport accident
-
V60–V69 – occupant of heavy transport vehicle injured in transport accident
-
V70–V79 – bus occupant injured in transport accident
-
V80–V89 – other land transport accidents
-
V90–V94 – water transport accidents
-
V95–V97 – air and space transport accidents
-
V98–V99 – other and unspecified transport accidents.
-
Data preparation
Subjects in the PEDW extract were found with dates of birth as long ago as 1890, and 4486 were born before 1 January 1914. It was decided to exclude all subjects aged ≥ 100 years as a first plausible attempt at improving the quality of this administrative database. The initial data set processing achieved a file of one complete admission per row with 133,680 admissions. After populating missing sex and LSOA fields from the WDS data set, it was found that 194 records had a completely missing LSOA code, 1937 records had an English LSOA and 10 records had a Scottish LSOA, leaving 131,539 admissions with a Welsh resident LSOA code. The age and sex coding was complete.
From these 131,539 admission records we excluded 80 admissions in which the alcohol code was in the second episode and therefore probably not the reason for admission. We further excluded 3699 admissions for chemotherapy or radiotherapy and 463 in which the G40.5 code was not paired with an alcohol code in another position. This left 127,297 admissions. Finally, we removed 2715 admissions in children aged < 16 years and 15,578 admissions occurring before the start of the study period (1 January 2006), leaving 109,004 admissions for patients aged 16–99 years in the completed data set.
Each first admission spell for each individual patient was then linked to the WDS study cohort with the following fields:
-
date of admission and discharge
-
age on admission
-
LSOA on admission, that is, on the first day of that quarter
-
admission type: emergency, elective, maternity, other or not known
-
provider site
-
primary diagnosis code
-
the first defined alcohol code in any position
-
the position of the first alcohol code
-
time to admission in days from 1 January 2006 (i.e. from the first outlet density measure)
-
length of stay
-
whether or not died in hospital.
The WDS data set therefore included information on each of the 2.5 million subjects on whether or not an admission took place and, if so, the above fields were available for the analysis. The WDS PEDW cohort also included the date of death, time in days to death from 1 January 2006 and the underlying cause of death ICD-10 code; the anonymised LSOA code on 1 January 2006 with attached deprivation quintile and three-part settlement type; the anonymised LSOA code for each quarter to link the time-varying outlet density covariates; and the quarter date of migration (leaving Wales) with time in days to migration from 1 January 2006 to the first day of the migration quarter.
Final definition of an admission
The data set was extracted on the basis of a defined alcohol-related ICD-10 code in any of the 14 coding position fields. Deciding how many coding positions to use to define an admission is an inexact science and clearly involves a trade-off between sensitivity (all 14 positions) and specificity (first position only). The intention was to be as specific as possible (i.e. fewest false positives admitted for a non-alcohol reason who have a long-standing alcohol code in their hospital notes) without losing too much sensitivity (thereby missing alcohol-related admissions). Problems arise from the use of non-diagnostic Chapter XVIII codes (symptoms, signs; ‘R’ codes) and Chapter XXI codes (factors influencing health status and contact with health services; ‘Z’ codes). These non-diagnostic ‘R’ and ‘Z’ codes commonly appear in the first coding position in the PEDW and many records do not have a linked diagnostic code in a lower coding position. Thus, the commonly used method of choosing only the first position underestimates the number of admissions and loses sensitivity.
Figure 42 shows the number of admissions in each of the 14 coding positions and provides some evidence to justify our definition. It is clear that the subsidiary position (second position) is not always populated and that the third position is well populated with alcohol codes.
FIGURE 42.
Coding position of first alcohol-related ICD-10 code.
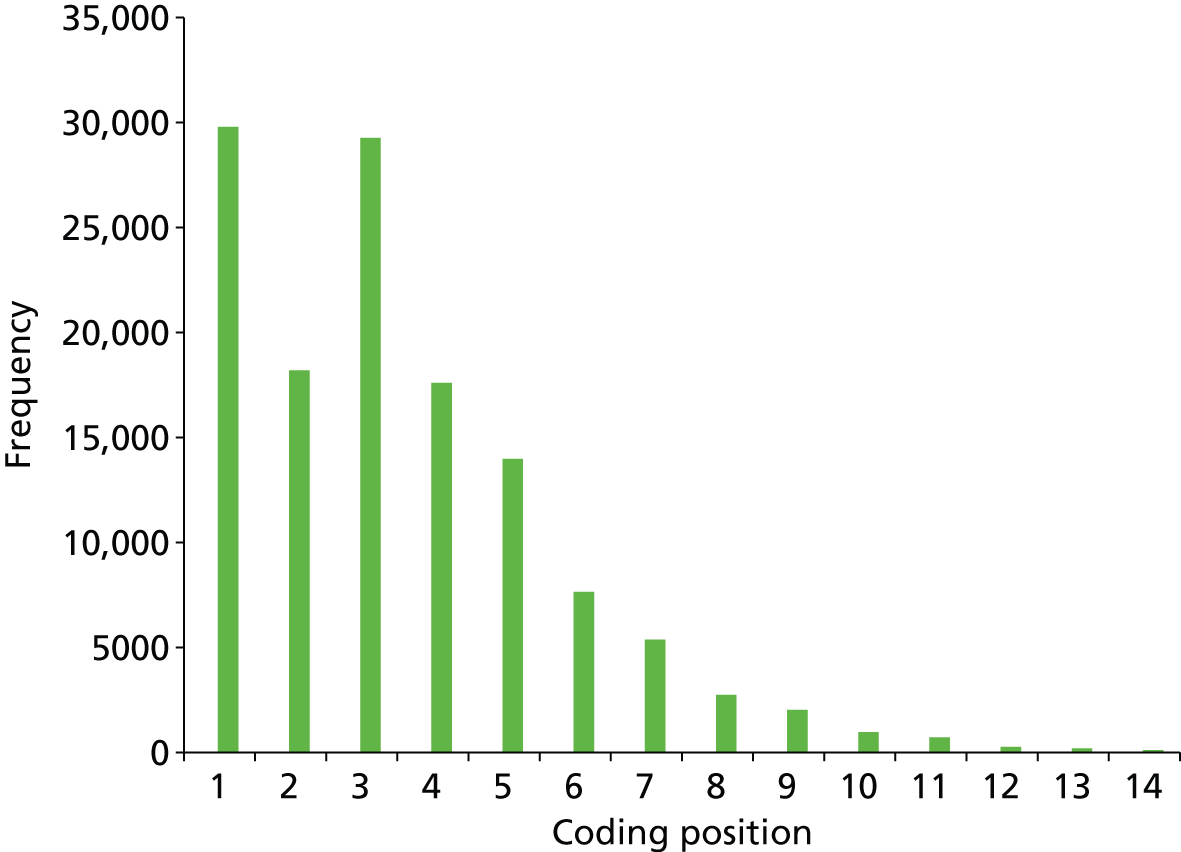
An admission was defined as alcohol related if there was an alcohol-related code in the first, second subsidiary or third coding position or in position 4 when positions 1, 2 and 3 contained successive non-diagnostic ‘R’ or ‘Z’ codes (excluding our defined ‘Z’ codes: Z50.2, Z71.4 or Z72.1).
The PEDW admission fields for the second admission for each patient were added to the WDS analysis file when the first admission was an alcohol code in positions 4 downwards and when the second admission coding position was 123 non-‘R’ non-‘Z’.
Analysis strategy
Baseline cohort analysis
A Cox regression was planned for all 2.5 million adult residents of Wales in the WDS data set on 1 January 2006, with the event defined as the first emergency admission with an alcohol code in positions 1, 2 or 3 or position 4 when positions 1, 2, and 3 all contained a ‘R’ or ‘Z’ code.
The baseline outlet density exposure measured by the previous quarter value for 2006 quarter 1 was fitted and HRs with 95% CIs were estimated for the first defined emergency admission. For ease of interpretation of the HRs we fitted the outlet density in quintiles, with equal counts of LSOAs in each quintile. We fitted individual-level terms for age group in 10-year bands at baseline and sex. Information on socioeconomic variables (e.g. NS-SEC3 and employment status) is not available in the PEDW. We fitted terms for LSOA deprivation quintiles and rural–urban settlement type.
It was explored whether or not the effect of baseline outlet density on consumption varied with deprivation by modelling the interaction between previous quarter outlet density and deprivation quintile.
Migration out of Wales and death were censored in all models. Multiple imputation was not required as there were no missing covariate data in the PEDW when linked to the WDS data set. This analysis was carried out using IBM SPSS statistics version 20 (IBM Corporation, Armonk, NY, USA) through the SAIL Gateway.
Hierarchical Cox regression with time-dependent covariates
A hierarchical Cox regression was planned with time-dependent covariates for all 2.5 million adult residents of Wales in the WDS data set on 1 January 2006, with the event defined as the first emergency admission as per the simple cohort analysis.
The ideal multilevel structure is individuals nested within LSOAs and unitary authorities, cross-classified within admitting hospitals. It was first assessed whether or not the data on hospital of admission were sufficiently completely coded and whether or not it was possible to reasonably assign a nearest hospital to those subjects not admitted. This can easily be carried out by geographical proximity but will not necessarily reflect the real potential hospital of admission.
First, a null model of the unexplained random variation at each level was estimated. Then the alcohol availability process was fitted as per the primary outcome, that is, the previous quarter, positive and negative change in outlet density and volatility. To fit the alcohol availability process in the five quarters preceding the date of first admission the outlet density variables were fitted as time-dependent covariates in the Cox model [see http://cran.r-project.org/web/packages/survival/vignettes/timedep.pdf (accessed 6 June 2015)].
Individual-level terms were then fitted for age group in 10-year bands at baseline and sex. Information on socioeconomic variables (e.g. NS-SEC3 and employment status) is not available in the PEDW. Finally, terms for LSOA deprivation quintile and rural–urban settlement type were fitted. As people move LSOAs within Wales these covariates were also time-dependent and these were fitted accordingly.
Migration out of Wales and death in all models were censored. Multiple imputation was not required as there were no missing covariate data in the PEDW when linked to the WDS data set.
Generalised linear mixed model analysis
A GLMM Poisson model for the annual counts of emergency admissions for the 5-year period 2007–11 was fitted, as a function of the alcohol availability process, adjusting for individual- and LSOA-level covariates. This model predicted emergency admission in the year following the alcohol availability process measured in the preceding year.
The multilevel structure was year at level 1 with individuals nested within households, LSOAs and unitary authorities. We first estimated a null model of the unexplained random variation at each level. The alcohol availability process was fitted as the previous quarter for current availability, positive and negative change in outlet density and volatility.
Terms for LSOA deprivation quintiles and the three category rural–urban settlement type were added. We then explored whether or not the effect of a change in outlet density on consumption varied with deprivation by modelling the interaction between change in previous quarter outlet density and deprivation quintiles.
This analysis was carried out using the repeated measures functionality in MLwiN version 2.31 (MLwiN, Centre for Multilevel Modelling, Bristol, UK) after extracting the admission counts from the SAIL Gateway.
Descriptive analyses
Overview of the data set
During the 6-year study period there were 109,004 admissions with an alcohol code in any coding position. This descriptive analysis is for the 64,150 admissions defined by an alcohol code in positions 1, 2 or 3 or an alcohol code in position 4 preceded by three non-diagnostic ‘R’ or ‘Z’ codes. Table 72 shows the breakdown of admissions by year.
Year | Number of admissions |
---|---|
2006 | 10,797 |
2007 | 10,539 |
2008 | 10,508 |
2009 | 10,714 |
2010 | 10,592 |
2011 | 11,000 |
Total | 64,150 |
Table 73 shows the breakdown of admissions by age group and sex for the total study period and Table 74 shows the mean annual admission rates per 1000 census 2011 population.
Age group (years) | Male | Female | Total |
---|---|---|---|
16–24 | 3639 | 2204 | 5843 |
25–34 | 5838 | 2470 | 8308 |
35–44 | 10,097 | 4748 | 14,845 |
45–54 | 10,841 | 4973 | 15,814 |
55–64 | 8254 | 3325 | 11,579 |
65–74 | 3914 | 1401 | 5315 |
75–84 | 1431 | 650 | 2081 |
85+ | 218 | 147 | 365 |
Total | 44,232 | 19,918 | 64,150 |
Age group (years) | Male | Female | Total |
---|---|---|---|
16–24 | 3.19 | 2.02 | 2.61 |
25–34 | 5.36 | 2.29 | 3.84 |
35–44 | 8.69 | 3.96 | 6.29 |
45–54 | 8.69 | 3.85 | 6.23 |
55–64 | 7.15 | 2.78 | 4.93 |
65–74 | 4.45 | 1.50 | 2.93 |
75–84 | 2.89 | 1.02 | 1.84 |
85+ | 1.50 | 0.48 | 0.81 |
Total | 6.05 | 2.58 | 4.26 |
Table 75 shows the number of admissions by local authority and the mean annual admission rate, using the 2011 census population aged ≥ 16 years as the denominator.
Local authority | Number of admissions | Population aged ≥ 16 years, 2011 | Mean annual rate per 1000 population |
---|---|---|---|
Isle of Anglesey | 1084 | 58,023 | 3.11 |
Gwynedd | 1837 | 100,655 | 3.04 |
Conwy | 2767 | 96,263 | 4.79 |
Denbighshire | 2378 | 76,899 | 5.15 |
Flintshire | 3241 | 124,082 | 4.35 |
Wrexham | 2966 | 109,228 | 4.53 |
Powys | 1798 | 110,310 | 2.72 |
Ceredigion | 1054 | 64,128 | 2.74 |
Pembrokeshire | 2553 | 100,611 | 4.23 |
Carmarthenshire | 3183 | 150,935 | 3.51 |
Swansea | 4519 | 197,369 | 3.82 |
Neath Port Talbot | 3181 | 115,206 | 4.60 |
Bridgend | 2859 | 114,052 | 4.18 |
Vale of Glamorgan | 2950 | 102,903 | 4.78 |
Cardiff | 6970 | 282,368 | 4.11 |
Rhondda Cynon Taf | 4979 | 190,048 | 4.37 |
Merthyr Tydfil | 1634 | 47,882 | 5.69 |
Caerphilly | 4015 | 143,951 | 4.65 |
Blaenau Gwent | 2474 | 57,338 | 7.19 |
Torfaen | 2302 | 73,986 | 5.19 |
Monmouthshire | 1476 | 75,295 | 3.27 |
Newport | 3930 | 116,385 | 5.63 |
Total | 64,150 | 2,507,917 | 4.26 |
Of the 64,150 admissions, 29,567 patients were admitted once in the study period and 34,583 at least twice. A total of 2099 admissions resulted in death in hospital. Of these, 1154 had the underlying cause of death coded with one of our alcohol-related set of codes. Table 76 shows the number of deaths by the number of admissions by age group and sex and Table 77 summarises the underlying cause of death for the 1154 alcohol-related deaths.
Age group (years) | Male | Female | Total | |||
---|---|---|---|---|---|---|
Number of admissions | Number of deaths | Number of admissions | Number of deaths | Number of admissions | Number of deaths | |
16–24 | 3639 | 0 | 2204 | 0 | 5843 | 0 |
25–34 | 5838 | 33 | 2470 | 27 | 8308 | 60 |
35–44 | 10,097 | 152 | 4748 | 86 | 14,845 | 238 |
45–54 | 10,841 | 342 | 4973 | 198 | 15,814 | 540 |
55–64 | 8254 | 447 | 3325 | 213 | 11,579 | 660 |
65–74 | 3914 | 286 | 1401 | 102 | 5315 | 388 |
75–84 | 1431 | 115 | 650 | 58 | 2081 | 173 |
85+ | 218 | 31 | 147 | 9 | 365 | 40 |
Total | 44,232 | 1406 | 19,918 | 693 | 64,150 | 2099 |
ICD-10 code | Description | Number of deaths |
---|---|---|
F10 | Mental and behavioural disorders due to alcohol | 32 |
I426 | Alcoholic cardiomyopathy | 5 |
K700 | Alcoholic fatty liver | < 5 |
K701 | Alcoholic hepatitis | 39 |
K703 | Alcoholic cirrhosis of liver | 235 |
K704 | Alcoholic hepatic failure | 226 |
K709 | Alcoholic liver disease, unspecified | 606 |
K852 | Alcohol-induced acute pancreatitis | < 5 |
K86 | Alcohol-induced chronic pancreatitis | 5 |
X45 | Accidental poisoning by and exposure to alcohol | < 5 |
Total | 1154 |
Of the other causes of death, the highest single cause was coded K746 – other and unspecified cirrhosis of the liver (n = 111), followed by J449 – chronic obstructive pulmonary disease, unspecified (n = 26), J189 – pneumonia, unspecified (n = 24), J690 – pneumonitis due to food and vomit (n = 21) and I219 (n = 23) and I25 (n = 25) – ischaemic heart disease.
Table 78 shows the frequency of the first alcohol ICD-10 code in each admission.
ICD-10 code | Description | n | % |
---|---|---|---|
F102 | Dependence syndrome | 11,001 | 17.1 |
F100 | Acute intoxication | 10,178 | 15.9 |
F101 | Harmful use | 7407 | 11.5 |
K709 | Alcoholic liver disease, unspecified | 7265 | 11.3 |
T510 | Toxic effect: ethanol | 5528 | 8.6 |
Z721 | Alcohol use | 5321 | 8.3 |
F103 | Withdrawal state | 5129 | 8.0 |
K703 | Alcoholic cirrhosis of the liver | 4883 | 7.6 |
K860 | Alcohol-induced chronic pancreatitis | 2179 | 3.4 |
K292 | Alcoholic gastritis | 784 | 1.2 |
K701 | Alcoholic hepatitis | 758 | 1.2 |
K704 | Alcoholic hepatic failure | 674 | 1.1 |
F104 | Withdrawal state with delirium | 447 | 0.7 |
G405 | Special epileptic syndromes – alcohol | 278 | 0.4 |
F106 | Amnesic syndrome | 260 | 0.4 |
Y919 | Alcohol involvement, not otherwise specified | 260 | 0.4 |
I426 | Alcoholic cardiomyopathy | 259 | 0.4 |
F109 | Unspecified mental and behavioural disorder | 207 | 0.3 |
F105 | Psychotic disorder | 169 | 0.3 |
K700 | Alcoholic fatty liver | 164 | 0.3 |
F107 | Residual and late-onset psychotic disorder | 151 | 0.2 |
G312 | Degeneration of the nervous system from use of alcohol | 135 | 0.2 |
Z502 | Alcohol rehabilitation | 102 | 0.2 |
All other codes | 611 | 1.0 | |
Total | 64,150 | 100 |
Table 79 shows the breakdown of admissions by elective and emergency admission type and year.
Year | Emergency | Elective | Othera |
---|---|---|---|
2006 | 9488 | 1257 | 52 |
2007 | 9345 | 1138 | 56 |
2008 | 9192 | 1259 | 57 |
2009 | 9331 | 1316 | 67 |
2010 | 9332 | 1224 | 36 |
2011 | 9712 | 1195 | 93 |
Total | 56,400 | 7389 | 361 |
The main ICD-10 codes for emergency and elective admissions are shown in Table 80. Tables 81 and 82 show the frequency of the first ICD-10 code in each emergency admission and elective admission respectively.
ICD-10 code | Description | Emergency | Elective |
---|---|---|---|
F10 | Mental and behavioural disorders due to alcohol | 32,262 | 2471 |
K70 | Alcoholic liver disease | 10,499 | 3215 |
K860 | Alcohol-induced chronic pancreatitis | 2044 | 131 |
T510 | Toxic effect: ethanol | 5519 | 5 |
Z721 | Alcohol use | 3885 | 1398 |
All other codes | 2191 | 169 | |
Total | 56,400 | 7389 |
ICD-10 code | Description | n | % |
---|---|---|---|
F102 | Dependence syndrome | 9373 | 16.6 |
F100 | Acute intoxication | 9975 | 17.7 |
F101 | Harmful use | 6734 | 11.9 |
K709 | Alcoholic liver disease, unspecified | 5740 | 10.2 |
T510 | Toxic effect: ethanol | 5519 | 9.8 |
Z721 | Alcohol use | 3885 | 6.9 |
F103 | Withdrawal state | 5042 | 8.9 |
K703 | Alcoholic cirrhosis of liver | 3366 | 6.0 |
K860 | Alcohol-induced chronic pancreatitis | 2044 | 3.6 |
All other codes | 4722 | 8.4 | |
Total | 56,400 | 100 |
ICD-10 code | Description | n | % |
---|---|---|---|
F102 | Dependence syndrome | 1533 | 20.7 |
F100 | Acute intoxication | 178 | 2.4 |
F101 | Harmful use | 593 | 8.0 |
K709 | Alcoholic liver disease, unspecified | 1489 | 20.2 |
Z721 | Alcohol use | 1398 | 18.9 |
K703 | Alcoholic cirrhosis of liver | 1501 | 20.3 |
K860 | Alcohol-induced chronic pancreatitis | 131 | 1.8 |
All other codes | 566 | 7.7 | |
Total | 7389 | 100 |
Table 83 shows the frequency of the first procedure code in each admission. A total of 15,323 admissions had a procedure code.
OPCS-4.6 code | Description | n | % |
---|---|---|---|
No procedure | 48,827 | 76.1 | |
T46 | Paracentesis abdominis for ascites | 2337 | 3.6 |
U05 | Computed tomography of head | 2171 | 3.4 |
G45 | Fibreoptic endoscopic examination of upper gastrointestinal tract | 1990 | 3.1 |
G43 | Fibreoptic endoscopic examination of upper gastrointestinal tract with snare/resection/ligation | 620 | 1.0 |
U21 | Magnetic resonance imaging | 500 | 0.8 |
W20 | Primary open reduction of fracture of long bone and extramedullary fixation using plate | 397 | 0.6 |
U08 | Imaging of abdomen | 271 | 0.4 |
X36 | Venesection | 247 | 0.4 |
X33 | Blood transfusion | 209 | 0.3 |
W24 | Closed reduction of fracture and internal fixation | 207 | 0.3 |
X29 | Intravenous infusion | 201 | 0.3 |
T12 | Drainage of pleural cavity | 168 | 0.3 |
E85 | Ventilation | 167 | 0.3 |
W19 | Primary open reduction of fracture | 159 | 0.2 |
J13 | Percutaneous biopsy of lesion of liver | 153 | 0.2 |
H25 | Diagnostic endoscopic examination of lower bowel using fibreoptic sigmoidoscope | 124 | 0.2 |
V15 | Reduction of fracture of alveolus of mandible | 111 | 0.2 |
K63 | Coronary arteriography | 107 | 0.2 |
A55 | Radiculography | 105 | 0.2 |
H22 | Diagnostic fibreoptic endoscopic examination of colon | 102 | 0.2 |
All other | 4977 | 7.8 | |
Total | 64,150 | 100 |
Tables 84 and 85 show admission rates by deprivation quintile and settlement type respectively.
Deprivation quintile | Number of admissions | WDS population aged ≥ 16 years | Mean annual admission rate per 1000 population |
---|---|---|---|
Least deprived | 6669 | 526,687 | 2.1 |
Less deprived | 8562 | 532,434 | 2.7 |
Mid deprived | 10,877 | 531,165 | 3.4 |
More deprived | 15,605 | 516,087 | 5.0 |
Most deprived | 22,437 | 501,854 | 7.5 |
Total | 64,150 | 2,608,227 | 4.1 |
Settlement type | Number of admissions | WDS population aged ≥ 16 years | Mean annual admission rate per 1000 population |
---|---|---|---|
Urban | 47,700 | 1,711,595 | 4.6 |
Town and fringe | 10,960 | 453,997 | 4.0 |
Village and hamlet | 5490 | 442,635 | 2.1 |
Total | 64,150 | 2,608,227 | 4.1 |
Emergency admissions with injury
A total of 6699 (11.9%) of the 56,400 emergency admissions had an associated injury code within the 123 non-‘RZ’ definition (Table 86). By far the most common injury was head injury.
ICD-10 code | Description | n | % |
---|---|---|---|
S00–S09 | Injuries to the head | 3940 | 58.8 |
S10–S19 | Injuries to the neck | 80 | 1.2 |
S20–S29 | Injuries to the thorax | 168 | 2.5 |
S30–S39 | Injuries to the abdomen, lower back, lumbar spine and pelvis | 192 | 2.9 |
S40–S49 | Injuries to the shoulder and upper arm | 382 | 5.7 |
S50–S59 | Injuries to the elbow and forearm | 386 | 5.8 |
S60–S69 | Injuries to the wrist and hand | 341 | 5.1 |
S70–S79 | Injuries to the hip and thigh | 298 | 4.4 |
S80–S89 | Injuries to the knee and lower leg | 672 | 10.0 |
S90–S99 | Injuries to the ankle and foot | 136 | 2.0 |
T00–T07 | Injuries involving multiple body regions | 29 | 0.4 |
T08–T14 | Injuries to unspecified part of trunk, limb or body region | 52 | 0.8 |
T15–T19 | Effects of foreign body entering through natural orifice | 23 | 0.3 |
Total | 6699 | 100 |
Table 87 shows the number of injury admissions by age group and sex. Fifty patients had more than one injury code and so the total number of patients was 6649.
Age group (years) | Male | Female | Total |
---|---|---|---|
16–24 | 951 | 249 | 1200 |
25–34 | 821 | 225 | 1046 |
35–44 | 928 | 304 | 1232 |
45–54 | 962 | 349 | 1311 |
55–64 | 713 | 307 | 1020 |
65–74 | 401 | 149 | 550 |
75–84 | 152 | 87 | 239 |
85+ | 33 | 18 | 51 |
Total | 4961 | 1688 | 6649 |
Table 88 shows the rates of injury admissions per 1000 population by age group and sex.
Age group (years) | Male | Female | Total |
---|---|---|---|
16–24 | 5.00 | 1.37 | 3.22 |
25–34 | 4.52 | 1.25 | 2.90 |
35–44 | 4.79 | 1.52 | 3.13 |
45–54 | 4.63 | 1.62 | 3.10 |
55–64 | 3.70 | 1.54 | 2.61 |
65–74 | 2.73 | 0.95 | 1.82 |
75–84 | 1.84 | 0.82 | 1.27 |
85+ | 1.37 | 0.35 | 0.68 |
Total | 4.07 | 1.31 | 2.65 |
We also considered the ICD-10 codes V01–V99 – transport accidents in Chapter XX (‘External causes of morbidity and mortality’) and found that there were an additional eight admissions without an associated injury code.
Further consideration of the data set for inferential analysis
Table 89 shows the breakdown of admissions by the patient class variable.
Patient class | Admission type | n a | ||
---|---|---|---|---|
Emergency | Elective | Other | ||
Inpatient | 56,397 | 3307 | 354 | 60,058 |
Day case | 1841 | 1841 | ||
Regular day attender | 252 | 252 | ||
Regular night attender | 5 | |||
Mother and babies | 6 | 6 | ||
Invalid | ||||
Diagnostic endoscopy | 1568 | 1568 | ||
Ear syringing | ||||
Outpatient | 306 | 306 | ||
Invalid or blank day case | 109 | |||
Total | 56,400 | 7389 | 361 | 64,051 |
All but three of the 56,400 emergency admissions were inpatient admissions. However, all of the other patient class types were elective admissions or other admissions. In view of these coding issues it seems sensible to restrict the main inferential analyses to emergency admissions only. It is quite likely that elective admissions occur as the result of NHS supply-side factors, such as waiting lists for endoscopies and other procedures or outpatient attendances for venesection/blood transfusion and so on. Elective admissions are therefore less likely to be influenced by community alcohol availability.
The provider site (i.e. hospital) code was found to be poorly populated, with around 40% of records missing this code. This, therefore, could not be used as the higher level in a hierarchical analysis.
Cox regression analyses
Definition of final cohort for analysis
A total of 23,753 individual patients who were aged between 16 and 99 years on the inception date of the cohort (1 January 2006) had their first emergency admission using our definition of admission during the 6-year period of the study. Of these, 13,733 were coded as F10 (mental and behavioural conditions due to alcohol). A further 1969 patients had a first admission with an alcohol code not in our coding position definition but subsequently had a second admission qualifying under our definition. These patients were included in the analysis, giving a total of 25,722 patients with a defined emergency admission. Of these, a total of 4300 patients were admitted with a paired injury code. The extra eight transport accident patients without an injury code were also included in the cohort.
The cohort size on 1 January 2006 was 2,566,749. During the time period of the study 175,590 patients died, with 2113 of these deaths being alcohol related using our set of codes. The overall crude death rate was 11.4 per 1000 population (aged 16–99 years), which is consistent with the expected death rate. A total of 184,809 patients were lost to migration out of Wales during the 6-year follow-up period (around 31,000 or 1.2% of the population per year).
Incidence rates
The 2,566,749 subjects contributed 14,104,088 person-years at risk, censoring for death and migration over the 6-year study period. The incidence rate for a defined first emergency admission (n = 25,722) for those aged 16–99 years was therefore 1.824 per 1000 person-years at risk.
Walking outlet density: baseline cohort analysis
Table 90 shows the HRs for the baseline cohort analysis walking outlet density survival model. The risk of emergency admission associated with outlet density appears not to be linear, suggesting the possibility of a threshold effect. Men had twice the risk of women and a deprivation gradient was observed.
Variable | HR | 95% CI | p-value |
---|---|---|---|
Alcohol availability: previous quarter | |||
Lowest availability | Reference | ||
Low availability | 1.10 | 1.05 to 1.15 | < 0.001 |
Middle availability | 1.12 | 1.07 to 1.18 | < 0.001 |
High availability | 1.10 | 1.05 to 1.16 | < 0.001 |
Highest availability | 1.21 | 1.15 to 1.26 | < 0.001 |
Deprivation quintile | |||
Least deprived | Reference | ||
Less deprived | 1.37 | 1.31 to 1.44 | < 0.001 |
Mid deprived | 1.65 | 1.57 to 1.73 | < 0.001 |
More deprived | 2.04 | 1.95 to 2.14 | < 0.001 |
Most deprived | 2.83 | 2.71 to 2.95 | < 0.001 |
Settlement type | |||
Urban | Reference | ||
Town and fringe | 0.98 | 0.95 to 1.02 | 0.29 |
Village and hamlet | 0.71 | 0.68 to 0.75 | < 0.001 |
Individual covariates | |||
Age group (years) | |||
18–24 | Reference | ||
25–34 | 1.01 | 0.97 to 1.05 | 0.70 |
35–44 | 1.24 | 1.19 to 1.29 | < 0.001 |
45–54 | 1.23 | 1.18 to 1.28 | < 0.001 |
55–64 | 1.00 | 0.96 to 1.05 | 0.88 |
65–74 | 0.67 | 0.64 to 0.71 | < 0.001 |
75–84 | 0.49 | 0.46 to 0.53 | < 0.001 |
85+ | 0.32 | 0.27 to 0.38 | < 0.001 |
Sex | |||
Female | Reference | ||
Male | 2.03 | 1.98 to 2.09 | < 0.001 |
Figure 43 shows the survival plot for walking outlet density in quintiles.
FIGURE 43.
Cumulative survival plot for walking outlet density, 2006 quarter 1, in quintiles.
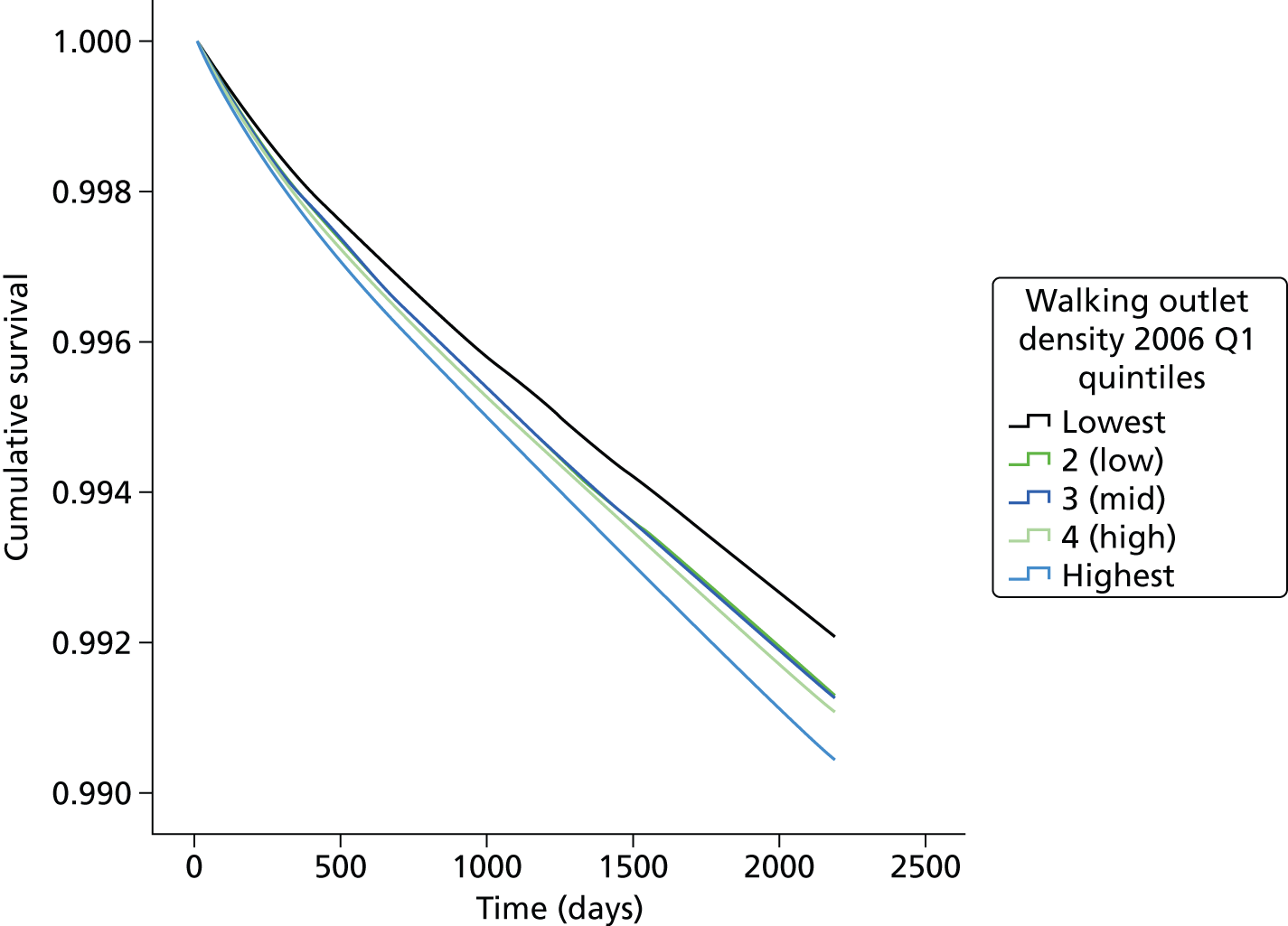
The interaction term for walking outlet density*sex suggested that the risk of emergency admission associated with walking outlet density was significantly higher in men than in women (Table 91).
Interaction term | HR | 95% CI | p-value |
---|---|---|---|
Lowest availability female | Reference | ||
Low availability*male | 1.10 | 1.01 to 1.21 | 0.04 |
Middle availability*male | 1.09 | 1.00 to 1.19 | 0.06 |
High availability*male | 1.13 | 1.03 to 1.24 | 0.01 |
Highest availability*male | 1.15 | 1.05 to 1.25 | 0.002 |
The interaction term for deprivation*outlet density was not significant (p = 0.2), suggesting that the effect of walking outlet density on the risk of admission did not vary with deprivation quintile.
The risk of emergency admission was little changed after excluding admissions occurring in the first year (Table 92).
Variable | HR | 95% CI | p-value |
---|---|---|---|
Alcohol availability: previous quarter | |||
Lowest availability | Reference | ||
Low availability | 1.06 | 1.01 to 1.12 | 0.031 |
Middle availability | 1.13 | 1.07 to 1.19 | < 0.001 |
High availability | 1.10 | 1.04 to 1.16 | 0.001 |
Highest availability | 1.17 | 1.11 to 1.23 | < 0.001 |
Walking outlet density: emergency admissions from mental and behavioural disorders due to use of alcohol
Compared with all emergency admissions, the fully adjusted HRs associated with walking outlet density were a little higher for the ICD-10 code F10 (mental and behavioural disorders due to use of alcohol) (n = 14,909) (Table 93).
Variable | HR | 95% CI | p-value |
---|---|---|---|
Alcohol availability: previous quarter | |||
Lowest availability | Reference | ||
Low availability | 1.14 | 1.07 to 1.21 | < 0.001 |
Middle availability | 1.17 | 1.10 to 1.24 | < 0.001 |
High availability | 1.16 | 1.09 to 1.23 | < 0.001 |
Highest availability | 1.27 | 1.19 to 1.35 | < 0.001 |
Walking outlet density: emergency admissions from injuries and external causes
The fully adjusted HRs associated with walking outlet density for the injury and external causes emergency admissions associated with alcohol (n = 4308) were similar to the mental and behavioural disorder estimates (Table 94).
Variable | HR | 95% CI | p-value |
---|---|---|---|
Alcohol availability: previous quarter | |||
Lowest availability | Reference | ||
Low availability | 1.16 | 1.03 to 1.30 | < 0.001 |
Middle availability | 1.19 | 1.06 to 1.34 | < 0.001 |
High availability | 1.18 | 1.05 to 1.32 | < 0.001 |
Highest availability | 1.27 | 1.13 to 1.42 | < 0.001 |
Walking outlet density: time-dependent Cox multilevel analysis
This class of models was computationally intensive. We were not able at first to fit a model to the whole data set of 2.5 million subjects and so we explored a case–cohort approach in which we included all of the 25,722 admission events and a 10% random sample of the subjects with no event. 105
Table 95 shows the HRs with 95% CIs for the final model of walking outlet density on the full data set. Previous quarter was fitted as the lower and upper parameter to account for the non-linear dependence (see Chapter 6, Walking outlet density: non-linear dependence). The largest association was for the lower range of the previous quarter variable. In this model, negative change was associated with a significantly higher risk: that is, a significantly slower decrease in the risk of emergency admission in the quarter following the decrease in outlet density.
Variable | HR | 95% CI | p-value | |
---|---|---|---|---|
Alcohol availability | ||||
Previous quarter: lower (0–1) | 1.24 | 1.13 to 1.35 | < 0.001 | |
Previous quarter: upper (> 1) | 1.01 | 1.01 to 1.02 | < 0.001 | |
Change positive | 1.03 | 0.93 to 1.13 | 0.601 | |
Change negative | 1.13 | 1.01 to 1.26 | 0.027 | |
Volatility | 0.97 | 0.92 to 1.01 | 0.123 | |
Deprivation quintile | ||||
Least deprived | Reference | |||
Less deprived | 1.34 | 1.25 to 1.43 | < 0.001 | |
Mid deprived | 1.57 | 1.47 to 1.68 | < 0.001 | |
More deprived | 1.96 | 1.84 to 2.09 | < 0.001 | |
Most deprived | 2.78 | 2.61 to 2.96 | < 0.001 | |
Settlement type | ||||
Urban | Reference | |||
Town and fringe | 0.97 | 0.92 to 1.02 | 0.216 | |
Village and hamlet | 0.70 | 0.66 to 0.75 | < 0.001 | |
Individual covariates: | ||||
Age group (years) | ||||
18–24 | Reference | |||
25–34 | 0.99 | 0.94 to 1.04 | 0.653 | |
35–44 | 1.17 | 1.12 to 1.23 | < 0.001 | |
45–54 | 1.13 | 1.08 to 1.19 | < 0.001 | |
55–64 | 0.93 | 0.88 to 0.98 | 0.004 | |
65–74 | 0.64 | 0.60 to 0.68 | < 0.001 | |
75–84 | 0.47 | 0.43 to 0.52 | < 0.001 | |
85+ | 0.31 | 0.25 to 0.39 | < 0.001 | |
Sex | ||||
Female | Reference | |||
Male | 2.04 | 1.98 to 2.10 | < 0.001 | |
Random effects | SD | Intraclass correlation (%) | ||
LSOA | 0.21 | 0.58 | ||
Local authority | 0.16 | 0.42 |
Driving outlet density: baseline cohort analysis
Table 96 shows the HRs for the baseline cohort analysis driving outlet density survival model. The risks were very similar to those in the walking model.
Variable | HR | 95% CI | p-value |
---|---|---|---|
Alcohol availability: previous quarter | |||
Lowest availability | Reference | ||
Low availability | 1.09 | 1.04 to 1.15 | < 0.001 |
Middle availability | 1.15 | 1.09 to 1.21 | < 0.001 |
High availability | 1.19 | 1.13 to 1.26 | < 0.001 |
Highest availability | 1.20 | 1.14 to 1.26 | < 0.001 |
Deprivation quintile | |||
Least deprived | Reference | ||
Less deprived | 1.39 | 1.32 to 1.46 | < 0.001 |
Mid deprived | 1.70 | 1.62 to 1.78 | < 0.001 |
More deprived | 2.10 | 2.00 to 2.19 | < 0.001 |
Most deprived | 2.91 | 2.79 to 3.04 | < 0.001 |
Settlement type | |||
Urban | Reference | ||
Town and fringe | 1.05 | 1.01 to 1.09 | 0.022 |
Village and hamlet | 0.76 | 0.71 to 0.80 | < 0.001 |
Individual covariates | |||
Age group (years) | |||
18–24 | Reference | ||
25–34 | 1.01 | 0.97 to 1.05 | 0.673 |
35–44 | 1.24 | 1.19 to 1.29 | < 0.001 |
45–54 | 1.23 | 1.18 to 1.28 | < 0.001 |
55–64 | 1.00 | 0.96 to 1.05 | 0.941 |
65–74 | 0.67 | 0.64 to 0.71 | < 0.001 |
75–84 | 0.49 | 0.46 to 0.53 | < 0.001 |
85+ | 0.32 | 0.27 to 0.38 | < 0.001 |
Sex | |||
Female | Reference | ||
Male | 2.03 | 1.98 to 2.09 | < 0.001 |
The interaction term for driving outlet density*sex was not statistically significant (p = 0.21). The interaction term for deprivation*outlet density was also not significant (p = 0.20), suggesting that the effect of driving outlet density on the risk of admission did not vary with deprivation quintile.
The risk of admission was little changed after excluding admissions occurring in the first year (Table 97).
Variable | HR | 95% CI | p-value |
---|---|---|---|
Alcohol availability: previous quarter | |||
Lowest availability | Reference | ||
Low availability | 1.08 | 1.02 to 1.14 | 0.006 |
Middle availability | 1.12 | 1.06 to 1.19 | < 0.001 |
High availability | 1.14 | 1.08 to 1.22 | < 0.001 |
Highest availability | 1.15 | 1.08 to 1.22 | < 0.001 |
Driving outlet density: emergency admissions from mental and behavioural disorders due to use of alcohol
Compared with the walking density model, the driving density model showed a generally weaker association with emergency admissions for the ICD-10-coded mental and behavioural disorders due to use of alcohol (F10; n = 14,909) (Table 98).
Variable | HR | 95% CI | p-value |
---|---|---|---|
Alcohol availability: previous quarter | |||
Lowest availability | Reference | ||
Low availability | 1.08 | 1.02 to 1.15 | 0.015 |
Middle availability | 1.11 | 1.04 to 1.18 | 0.003 |
High availability | 1.19 | 1.11 to 1.28 | < 0.001 |
Highest availability | 1.15 | 1.08 to 1.24 | < 0.001 |
Driving outlet density: emergency admissions from injuries and external causes
The fully adjusted HRs associated with driving outlet density for the injury and external causes emergency admissions associated with alcohol (n = 4308) were less consistent and did not suggest a particularly strong association (Table 99).
Variable | HR | 95% CI | p-value |
---|---|---|---|
Alcohol availability: previous quarter | |||
Lowest availability | Reference | ||
Low availability | 1.09 | 0.97 to 1.22 | 0.152 |
Middle availability | 1.21 | 1.07 to 1.37 | 0.002 |
High availability | 1.11 | 0.97 to 1.26 | 0.122 |
Highest availability | 1.10 | 0.97 to 1.25 | 0.151 |
Generalised linear mixed model analysis
We modelled the counts of emergency admissions per LSOA as a function of the walking alcohol availability process, deprivation and settlement type in a GLMM Poisson multilevel analysis with year at level 1, LSOA at level 2 and local authority at level 3. We specified the 5 years from 2007 to 2011 in the model to enable the preceding years’ walking alcohol exposure to be fitted, that is, 2006 alcohol availability associated with 2007 admissions and so on. The model random variances are shown in Table 100. The majority of the unexplained random variation in the risk of an emergency admission to hospital is attributable to the local authority, although the magnitude of this variation is small in comparison to the fixed-effect estimates in Table 101.
Model | Level | Variance | SD | Intraclass correlation (%) |
---|---|---|---|---|
Null model | LSOA | 0.0652 | 0.138 | 10.3 |
Local authority | 0.5672 | 0.105 | 89.7 | |
Final model | LSOA | 0.0278 | 0.096 | 9.5 |
Local authority | 0.2646 | 0.087 | 90.5 |
Variable | Risk ratio | 95% CI |
---|---|---|
Alcohol availability: previous quarter | ||
Lowest availability | Reference | |
Low availability | 1.08 | 1.02 to 1.13 |
Middle availability | 1.11 | 1.06 to 1.17 |
High availability | 1.16 | 1.10 to 1.23 |
Highest availability | 1.41 | 1.33 to 1.49 |
Positive change (z-score) | 1.005 | 0.990 to 1.019 |
Negative change (z-score) | 1.004 | 0.990 to 1.019 |
Volatility (z-score) | 0.995 | 0.980 to 1.010 |
Deprivation quintile | ||
Least deprived | Reference | |
Less deprived | 1.41 | 1.34 to 1.49 |
Mid deprived | 1.68 | 1.60 to 1.77 |
More deprived | 2.33 | 2.22 to 2.45 |
Most deprived | 3.44 | 3.27 to 3.61 |
Settlement type | ||
Urban | Reference | |
Town and fringe | 0.93 | 0.89 to 0.97 |
Village and hamlet | 0.67 | 0.63 to 0.71 |
The final model estimates (see Table 101) show a significantly increased risk of emergency hospital admission with higher previous quarter outlet density. There was little difference between the risks for the middle quintiles of outlet density and a stronger association for the highest quintile of outlet density. No significant associations were found for positive and negative change or for volatility in outlet density. As expected, there was a strong association with increasing area deprivation, and the risk of emergency admission was significantly lower in town and country areas than in urban areas. The interaction between previous quarter walking outlet density and area deprivation was not significant (p = 0.42).
Chapter 8 Study 3: accident and emergency department attendances
Definition of an alcohol-related attendance
There was no definitive uniformly applied clinical coding to identify alcohol-related attendances in this data set. The strongest indicator of alcohol involvement in A&E attendance is time of attendance. 106 Previous research suggests that the majority of alcohol attendances take place at night. Studies utilising alcohol breath test data have found that the majority of positive tests occur in those arriving at A&E departments after midnight. Holt et al. 107 found that 70% of attendances after midnight had a positive breath test and Walsh and Macleod108 took breath tests throughout the day and found that 33.3% of patients had a positive breath test between 21.00 and midnight, with 62.6% positive between midnight and 03.00. In a study of six major A&E departments in Ireland, Hope et al. 109 found that a higher proportion of patients were attending for non-alcohol-related injuries between 06.00 and midnight and a higher proportion of patients were attending for alcohol-related injuries between midnight and 06.00. Time lag effects between consumption, injury and arrival at A&E will occur, but Hope et al. ’s109 study found that 52% of patients presented to A&E within an hour of sustaining an alcohol-related injury.
We therefore chose attendance between the hours of midnight and 06.00 to define attendance due to alcohol as a proxy for alcohol-related attendance, as the majority of injury attendances at night (particularly in those aged 16–39 years) are likely to be related to alcohol. As these data are held within the SAIL Databank, it was possible to link A&E attendances with admissions to hospital in the PEDW using the ALF_E.
We received a file of 142,690 attendances between January 2009 and March 2012. Of these, 678 had a completely missing LSOA code, 2960 attendances had an English LSOA code and nine had a Scottish LSOA code, and these were excluded. Five attendances found to be coded as April–December 2008 were excluded. It was then found that the data for the quarters at the start and end of the study time period were clearly incomplete and 1304 attendances for January–March 2009 and 8343 for January–March were excluded. Applying the same rule as for the PEDW, 90 attendances of patients aged ≥ 100 years and 11,632 attendances of patients aged < 16 years were excluded leaving a final data set of 117,669 aged 16–99 years.
The A&E data set included the following fields, which were linked to the WDS data set:
-
ALF_E
-
age on attendance
-
sex
-
LSOA and local authority on attendance
-
date, day and time
-
quarter and year
-
time to attendance from 1 April 2009.
The WDS A&E cohort also included the date of death, time in days to death from 1 April 2009 and the underlying cause of death ICD-10 code; the anonymised LSOA code on 1 April 2009 with attached deprivation quintile and settlement type; the anonymised LSOA codes for each quarter to link the time-varying outlet density covariates; and the quarter date of migration (leaving Wales) with time in days to migration from 1 April 2009 to the first day of the migration quarter.
Analysis strategy
We carried out a baseline Cox regression analysis as per the PEDW analysis except that the cohort was defined on 1 April 2009 and the event was the first A&E attendance during the period 1 April 2009 to 31 December 2011.
Descriptive analyses
During the 2.75-year study period (1 March 2009 to 31 December 2011) there were 117,669 A&E attendances between midnight and 06.00 in patients aged 16–99 years. Table 102 shows the number of attendances by year.
Year | Number of attendances |
---|---|
2009 (from quarter 2) | 31,523 |
2010 | 42,343 |
2011 | 43,803 |
Total | 117,669 |
Table 103 shows the numbers and mean annual rates of A&E attendance by local authority.
Local authority | Number of attendances | % | 2011 census population aged ≥ 16 years | Mean annual rate per 1000 population |
---|---|---|---|---|
Isle of Anglesey | 3292 | 2.8 | 58,023 | 20.6 |
Gwynedd | 5767 | 4.9 | 100,655 | 20.8 |
Conwy | 5960 | 5.1 | 96,263 | 22.5 |
Denbighshire | 5589 | 4.7 | 76,899 | 26.4 |
Flintshire | 3325 | 2.8 | 124,082 | 9.7 |
Wrexham | 4775 | 4.1 | 109,228 | 15.9 |
Powys | 1514 | 1.3 | 110,310 | 5.0 |
Ceredigion | 3604 | 3.1 | 64,128 | 20.4 |
Pembrokeshire | 3843 | 3.3 | 100,611 | 13.9 |
Carmarthenshire | 5108 | 4.3 | 150,935 | 12.3 |
Swansea | 13,296 | 11.3 | 197,369 | 24.5 |
Neath Port Talbot | 5180 | 4.4 | 115,206 | 16.4 |
Bridgend | 7085 | 6.0 | 114,052 | 22.6 |
Vale of Glamorgan | 4195 | 3.6 | 102,903 | 14.8 |
Rhondda Cynon Taf | 4256 | 3.6 | 190,048 | 8.1 |
Merthyr Tydfil | 3051 | 2.6 | 47,882 | 23.2 |
Caerphilly | 6449 | 5.5 | 143,951 | 16.3 |
Blaenau Gwent | 2163 | 1.8 | 57,338 | 13.7 |
Torfaen | 2646 | 2.2 | 73,986 | 13.0 |
Monmouthshire | 1615 | 1.4 | 75,295 | 7.8 |
Newport | 4611 | 3.9 | 116,385 | 14.4 |
Cardiff | 20,345 | 17.3 | 282,368 | 26.2 |
Total | 117,669 | 100 | 2,507,917 | 17.1 |
Table 104 shows the number of A&E attendances by age group and sex for the total study period and Table 105 shows the mean annual A&E attendance rate by age group and sex.
Age group (years) | Male | Female | Total |
---|---|---|---|
16–24 | 19,699 | 15,445 | 35,144 |
25–34 | 11,594 | 8323 | 19,917 |
35–44 | 8787 | 6773 | 15,560 |
45–54 | 7403 | 5706 | 13,109 |
55–64 | 5142 | 4036 | 9178 |
65–74 | 4781 | 3706 | 8487 |
75–84 | 4499 | 4716 | 9215 |
85+ | 2422 | 4637 | 7059 |
Total | 64,327 | 53,342 | 117,669 |
Age group (years) | Male | Female | Total |
---|---|---|---|
16–24 | 37.7 | 30.8 | 34.3 |
25–34 | 23.2 | 16.9 | 20.1 |
35–44 | 16.5 | 12.3 | 14.4 |
45–54 | 13.0 | 9.6 | 11.3 |
55–64 | 9.7 | 7.4 | 8.5 |
65–74 | 11.9 | 8.6 | 10.2 |
75–84 | 19.8 | 16.2 | 17.8 |
85+ | 36.4 | 33.0 | 34.1 |
Total | 19.2 | 15.0 | 17.1 |
Our data showed an expected pattern of attendance by day of the week (Table 106). The highest number of attendances occurred on Sunday morning from midnight to 06.00, which equates to ‘Saturday night’ and so on.
Day of the week | Frequency | % |
---|---|---|
Monday | 15,585 | 13.2 |
Tuesday | 13,701 | 11.6 |
Wednesday | 13,040 | 11.1 |
Thursday | 14,629 | 12.4 |
Friday | 14,405 | 12.2 |
Saturday | 21,463 | 18.2 |
Sunday | 24,846 | 21.1 |
Total | 117,669 | 100 |
We also found a suggestion of a seasonal pattern, shown in Figure 44.
FIGURE 44.
Quarterly trend in A&E attendances. Q, quarter.

A small number of attendances [n = 2299 (2.0%)] resulted in a defined alcohol-related admission to hospital within 1 day. (Early) middle-aged patients were more likely to be admitted to hospital (Table 107).
Age group (years) | Not admitted | Admitted | Total | % admitted |
---|---|---|---|---|
16–24 | 34,581 | 563 | 35,144 | 1.6 |
25–34 | 19,494 | 423 | 19,917 | 2.1 |
35–44 | 15,128 | 432 | 15,560 | 2.8 |
45–54 | 12,632 | 477 | 13,109 | 3.6 |
55–64 | 8928 | 250 | 9178 | 2.7 |
65–74 | 8381 | 106 | 8487 | 1.2 |
75–84 | 9175 | 40 | 9215 | 0.4 |
85+ | 7051 | 8 | 7059 | 0.1 |
Total | 115,370 | 2299 | 117,669 | 2.0 |
The 2299 linked attendances included 1701 individual patients. The majority of attendances that were subsequently admitted were coded as ICD-10 F10 (60%); this was followed by the codes T510 (17%) and Z721 (9%).
Tables 108 and 109 show attendance rates by LSOA deprivation quintile and settlement type respectively.
Deprivation quintile | Number of attendances | WDS population aged ≥ 16 years | Mean annual rate per 1000 population |
---|---|---|---|
Least deprived | 18,786 | 526,687 | 13.0 |
Less deprived | 18,241 | 532,434 | 12.5 |
Mid deprived | 22,324 | 531,165 | 15.3 |
More deprived | 25,655 | 516,087 | 18.1 |
Most deprived | 32,663 | 501,854 | 23.7 |
Total | 117,669 | 2,608,227 | 16.4 |
Settlement type | Number of attendances | Population aged ≥ 16 years | Mean annual rate per 1000 population |
---|---|---|---|
Urban | 85,742 | 1,711,595 | 18.2 |
Town and fringe | 18,028 | 453,997 | 14.4 |
Village and hamlet | 13,899 | 442,635 | 11.4 |
Total | 117,669 | 2,608,227 | 16.4 |
Cox regression analyses
Definition of the final cohort for analysis
We linked the 117,669 A&E attendances to the WDS data set. A total of 87,704 individual patients aged between 16 and 99 years on the inception date of the cohort (1 April 2009) had a first A&E attendance during the 2.75-year time period of the study.
The 2,478,468 subjects contributed 6,479,578 person-years at risk, censoring for death and migration over the 2.75-year study period. The incidence rate for a first attendance (n = 87,704) for those aged 16–99 years was therefore 13.5 per 1000 person-years at risk.
Walking outlet density
Table 110 shows the HRs for the baseline cohort analysis walking outlet density survival model. There is little association between the risk of A&E attendance and outlet density. Men had twice the risk of A&E attendance as women and a deprivation gradient is observed.
Variable | HR | 95% CI | p-value |
---|---|---|---|
Alcohol availability: previous quarter | |||
Lowest availability | Reference | ||
Low availability | 0.99 | 0.97 to 1.01 | 0.376 |
Middle availability | 0.96 | 0.94 to 0.98 | < 0.001 |
High availability | 0.90 | 0.88 to 0.92 | < 0.001 |
Highest availability | 0.98 | 0.96 to 1.01 | 0.157 |
Deprivation quintile | |||
Least deprived | Reference | ||
Less deprived | 1.03 | 1.01 to 1.06 | 0.006 |
Mid deprived | 1.26 | 1.24 to 1.29 | < 0.001 |
More deprived | 1.35 | 1.32 to 1.38 | < 0.001 |
Most deprived | 1.66 | 1.63 to 1.70 | < 0.001 |
Settlement type | |||
Urban | Reference | ||
Town and fringe | 0.91 | 0.89 to 0.92 | < 0.001 |
Village and hamlet | 0.84 | 0.82 to 0.86 | < 0.001 |
Individual covariates | |||
Age group (years) | |||
18–24 | Reference | ||
25–34 | 0.52 | 0.51 to 0.53 | < 0.001 |
35–44 | 0.38 | 0.37 to 0.39 | < 0.001 |
45–54 | 0.32 | 0.32 to 0.33 | < 0.001 |
55–64 | 0.27 | 0.26 to 0.28 | < 0.001 |
65–74 | 0.36 | 0.35 to 0.37 | < 0.001 |
75–84 | 0.63 | 0.61 to 0.65 | < 0.001 |
85+ | 1.21 | 1.17 to 1.24 | < 0.001 |
Sex | |||
Female | Reference | ||
Male | 1.28 | 1.26 to 1.29 | < 0.001 |
The interaction terms for walking outlet density*sex and deprivation*outlet density were not statistically significant.
Driving outlet density
Table 111 shows the HRs for the baseline cohort analysis driving outlet density survival model. The risks appear to exhibit a threshold effect.
Variable | HR | 95% CI | p-value |
---|---|---|---|
Alcohol availability: previous quarter | |||
Lowest availability | Reference | ||
Low availability | 1.06 | 1.03 to 1.08 | < 0.001 |
Middle availability | 1.06 | 1.03 to 1.08 | < 0.001 |
High availability | 1.05 | 1.02 to 1.08 | < 0.001 |
Highest availability | 1.28 | 1.24 to 1.31 | < 0.001 |
Deprivation quintile | |||
Least deprived | Reference | ||
Less deprived | 1.04 | 1.01 to 1.06 | 0.002 |
Mid deprived | 1.25 | 1.22 to 1.28 | < 0.001 |
More deprived | 1.34 | 1.31 to 1.37 | < 0.001 |
Most deprived | 1.64 | 1.61 to 1.68 | < 0.001 |
Settlement type | |||
Urban | Reference | ||
Town and fringe | 0.96 | 0.94 to 0.98 | < 0.001 |
Village and hamlet | 0.94 | 0.91 to 0.96 | < 0.001 |
Individual covariates | |||
Age group (years) | |||
18–24 | Reference | ||
25–34 | 0.51 | 0.50 to 0.53 | < 0.001 |
35–44 | 0.38 | 0.37 to 0.39 | < 0.001 |
45–54 | 0.33 | 0.32 to 0.33 | < 0.001 |
55–64 | 0.27 | 0.27 to 0.28 | < 0.001 |
65–74 | 0.36 | 0.35 to 0.37 | < 0.001 |
75–84 | 0.63 | 0.61 to 0.65 | < 0.001 |
85+ | 1.20 | 1.16 to 1.24 | < 0.001 |
Sex | |||
Female | Reference | ||
Male | 1.27 | 1.26 to 1.29 | < 0.001 |
The interaction terms for driving outlet density*sex and deprivation*outlet density were not statistically significant.
Chapter 9 Study 4: violence against the person
Recorded violent crime
Levels and trends in community violence in England and Wales have traditionally been measured using two official Home Office sources of data: the Crime Survey for England and Wales (CSEW; formerly the British Crime Survey) and police records. 110 Police crime records are sensitive to reporting and recording practices. Decisions to report violence to the police depend on the location and context of the incident, being able to identify an offender, whether or not the incident is regarded as a crime, willingness to have one’s own conduct investigated, fear of reprisals and confidence in the police to deliver justice. Historically, once a violent crime was reported, the decision to record the incident as a crime was influenced by the circumstances of the incident as well as local and national policies. In 1998, the Home Office Counting Rules (HOCR) changed to counting victims rather than offences so that one victim equates to one crime. Since 2002, National Crime Recording Standards (NCRS) and Home Office Counting Rules have been amended to increase the likelihood that all reported violent incidents appear in police records across all forces in England and Wales. 111 Audits undertaken by the Audit Commission on behalf of the Home Office indicate substantial improvements in crime recording across forces in the 2–3 years following the introduction of the NCRS. 112
Police forces in Wales and the sharing of crime data
The population of Wales is served by four police forces (Figure 45). A standard letter and e-mail detailing the objectives of the study were sent to each Chief Constable, who were then contacted by telephone 2 weeks later to ascertain the availability of computerised crime data and their agreement to participate in the study. All four police forces had retrievable computerised records on violent crimes. To access and use data, separate Data Sharing Agreements between Cardiff University and each police force were signed following the national Data Sharing Code of Practice published by the Information Commissioner. 113
FIGURE 45.
Police forces in Wales. (a) Dyfed Powys; (b) Gwent; (c) North Wales; and (d) South Wales.
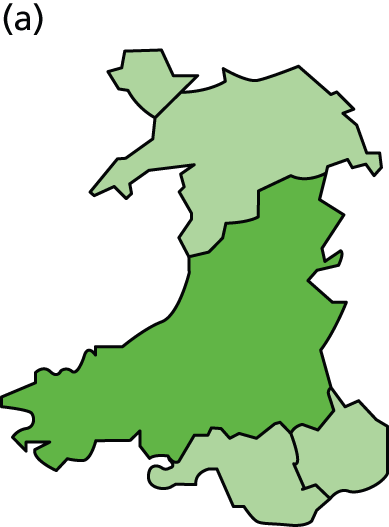

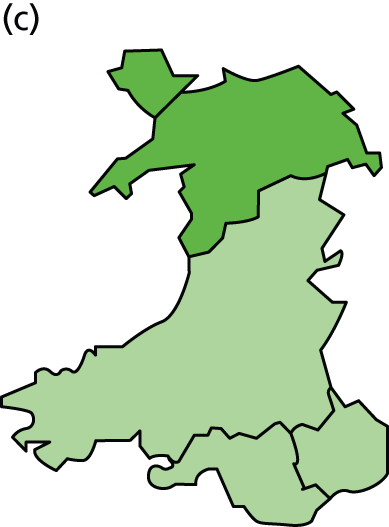

Violent crimes are defined as all crimes within the group ‘VAP’ (Table 112) rather than those in the global category of violent crime, which includes sexual crimes as well as VAP.
Code | Description |
---|---|
VAP with injury | |
1 | Murder |
2 | Attempted murder |
4.1 | Manslaughter |
4.2 | Infanticide – applies to infants aged < 12 months killed by the mother while of disturbed mind |
4.3 | Intentional destruction of a viable unborn – applies to the unborn child ‘capable of being born alive’ |
4.4 | Causing death by dangerous driving |
4.6 | Causing death by careless driving when under the influence of drink or drugs |
4.7 | Causing or allowing death of a child or vulnerable person |
4.8 | Causing death by careless or inconsiderate driving |
4.9 | Causing death by driving: unlicensed drivers, etc. |
4.10 | Corporate manslaughter |
5A | Inflicting grievous bodily harm with intent |
5B | Use of substance or object to endanger life |
5C | Possession of items to endanger life |
8F | Inflicting grievous bodily harm without intent |
8G | Actual bodily harm and other injury |
8H | Racially or religiously aggravated inflicting grievous bodily harm without intent |
8J | Racially or religiously aggravated actual bodily harm or other injury |
8K | Poisoning or female genital mutilation |
37.1 | Causing death by aggravated vehicle taking |
VAP without injury | |
3A | Conspiracy to murder |
3B | Threats to kill |
6 | Endangering railway passengers |
7 | Endangering life at sea |
8L | Harassment |
9A | Public fear, alarm or distress |
8M | Racially or religiously aggravated harassment |
9B | Racially or religiously aggravated public fear, alarm or distress |
10A | Possession of firearms with intent |
10C | Possession of other weapons |
10D | Possession of article with blade or point |
11 | Cruelty to and neglect of children |
12 | Abandoning a child under the age of 2 years |
13 | Child abduction |
14 | Procuring illegal abortion |
104 | Assault without injury on a constable – summary offences, closely associated with actual bodily harm (see classification 8G) |
105A | Assault without injury – Summary offences, closely associated with actual bodily harm (see classification 8G). Includes, among other offences, common assault and battery (section 39 of the Criminal Justice Act 1988114). From 1 April 2002 includes only assaults involving no injury |
105B | Racially or religiously aggravated assault without injury (see classification 105A) |
The VAP crime data were requested as counts per LSOA from the four police forces:
-
all VAP crime recorded for calendar years 2005–11, by quarter
-
all VAP crime recorded as being ‘alcohol related’ for calendar years 2005–11, by quarter
-
the above VAP data by sex and age group (specifically 16–24, 25–44 and 45+ years)
-
victim/offender flags for VAP crime.
Data were requested in electronic format either by e-mail or on digital media.
Data availability and consistency
Data were received from all four Welsh police forces, as summarised in Table 113. Two provided data on other ‘violent crimes’ not within the VAP category and these were excluded to ensure consistency between the data sets. This standardised record keeping allows crimes to be comparable across forces and offence codes to be coherently categorised into VAP.
Field | Police force | Count or % populated | ||||||
---|---|---|---|---|---|---|---|---|
Dyfed-Powys | Gwent | North Wales | South Walesa | Dyfed-Powys | Gwent | North Wales | South Wales | |
Crime reference number | Y | Y | Y | Y | 45,867 | 65,502 | 81,012 | 100,676 |
HOCR or NCRS code | Y | Y | Y | Y | – | – | – | – |
Year, quarter | Y | Y | Y | Y | – | – | – | – |
LSOA code | Y | Y | Y | Y | 99.1% | 92.1% | 94.7% | 98.6% |
LSOA manually assigned | – | Y | – | – | – | 6.8% | – | – |
Alcohol – offender | – | Y | – | Y | – | 18.2% | – | 11.4% |
Alcohol – aggrieved | – | Y | – | Y | – | 9.2% | – | 12.8% |
Alcohol related | Y | Y | Y | Y | 54.5% | 20.5% | 31.9% | 11.4% |
Domestic related | Y | Y | Y | Y | 21.3% | 23.5% | 23.8% | 12.8% |
Victim age | Y | Y | Y | Y | 75.4% | 15.0% | 67.1% | 63.0% |
Victim sex | Y | Y | Y | Y | 89.2% | 15.5% | 67.1% | 64.5% |
Number of offenders, average (maximum) | Y | Y | Y | Y | 1.036 (5) | 1.031 (6) | 1 (1) | 1.046 (6) |
Offender_1_age | Y | Y | Y | Y | 64.8% | 12.3% | 52.5% | 45.3% |
Offender_1_gender | Y | Y | Y | Y | 64.9% | 12.3% | 52.5% | 45.2% |
As requested, a field indicating whether or not a crime was alcohol related was provided by each force. However, this flag is not standardised and may be under- or overpopulated. Victim and offender details were provided when available, but this differed substantially between forces. Although under the recording standard a single crime equates to a single victim, up to six offenders per crime were observed in the data received (from Gwent, Dyfed-Powys and South Wales). North Wales police provided offender details for the first offender of a crime but the total number of offenders for each crime was unknown.
South Wales police force
The first extract of data received from South Wales police force contained a high-level categorisation of crime (VAP) with no subcategories to enable separation of crimes into subgroups based on the level of harm. The location of incidents was initially provided at ward level. Wards are larger than LSOAs and so a ward may contain several LSOAs. We then requested that subclass crime codes – either the HOCR code or the NCRS grouping – and LSOAs be assigned to each crime. Data were received with all VAP crimes assigned a HOCR code and > 98% were assigned a LSOA.
Gwent police force
The location of incidents was initially provided for electoral wards. Gwent police force was able to provide this information with LSOA codes after the CHALICE team provided suitable shapefiles for its GIS. The LSOA was provided when it could be easily computed by the force, but the force had trouble mapping a proportion of crimes to LSOAs. Instead, they provided the street name as an approximate location to calculate a LSOA. We assigned every address located in the Gwent police force area to a LSOA and summarised the proportion of addresses in the street that fell within a particular LSOA if a street crossed LSOA boundaries.
The first data set received contained only a single field (crime force tag) containing either an alcohol-related flag or a domestic-related flag. This format did not allow both flags to be present on a single crime. A further request to the force revealed that this was not a feature of the database but a result of the output format used. A further extract was received containing all crime reference numbers for the period with all crime force tags assigned to them (up to three: ‘alcohol – aggrieved’, ‘alcohol – likely offender’, ‘domestic’).
Dyfed-Powys police force
Records were available only from quarter 1 of financial year 2007–8 (March 2007, 2007 quarter 2). Data were provided as two separate files for records of offenders and victims. Location information was assigned to both data files. A large proportion of VAP crimes were flagged as alcohol related (54.5% of all VAP crimes in the data period available), but details of which party (the offender and/or the victim) the alcohol-related flag referred to could not be provided.
North Wales police force
The data extract received was accompanied by comprehensive descriptive notes. The LSOA code was assigned from Eastings and Northings data, but geographical information was not always available. Information on victims and offenders depended on the classification on data entry. Victim and offender ages were based on the date of birth and the date the crime was recorded and so these data and gender were dependent on correct data entry. Alcohol-related and domestic-related crimes could be extracted only if the relevant flags had been applied to the Modus Operandi section of the record. The notes confirmed that, in theory, the data set recorded one crime per victim but that persons may be recorded as ‘victims’ when they were merely present during the commission of the offence. This would result in a higher number of victims.
Collating police data
The format of the data following stage 1 is shown in Table 114. The resulting data set consisted of a single record (row) per crime incident and offender/victim information (to allow for multiple victims and/or offenders). The data within this table maintained much of the formatting of the data as provided by the forces, with official Home Office offence codes used to ensure consistency between the forces.
Field | Variable | Descriptor |
---|---|---|
1 | Unique_ID | As provided by force |
2 | Actual_Date | In the same format as provided by force |
3 | Date_Grouped | Grouped into year quarters |
4 | Crime_Code | Refined (using official Home Office counting rules) from those provided by force to ensure consistency between forces |
5 | Crime_Description | As provided by force |
6 | Crime_Class | VAP and others for initial filtering of data |
7 | With_Without_Injury | All VAP classified into with or without injury according to Home Office Counting Rules for recorded crime |
8 | Alcohol_Flag | 1 if present, 0 if not, –1 if information not provided |
9 | Domestic_Flag | 1 if present, 0 if not, –1 if information not provided |
10 | LSOA | Long LSOA code |
11 | LSOA_name | Descriptive name of LSOA |
12 | Offender_Age | As provided by force |
13 | Offender_Gender | As provided by force |
14 | Victim_Age | As provided by force |
15 | Victim_Gender | As provided by force |
Police force areas
Each police force georeferenced each crime to a LSOA (aside from a proportion of the Gwent data as discussed earlier). All LSOAs were expected to nest within police force areas but in 34 cases two forces provided crime data relating to the same LSOA. In these cases the force with the largest number of crimes assigned to that LSOA was identified as the force covering that neighbourhood (Table 115).
LSOA number | Number of crimes reported for each police force within each LSOA | Number of sources | |||
---|---|---|---|---|---|
Dyfed-Powys | Gwent | North Wales | South Wales | ||
60 | 2 | 0 | 36 | 0 | 2 |
802 | 2 | 0 | 0 | 78 | 2 |
928 | 3 | 0 | 0 | 59 | 2 |
929 | 1 | 0 | 0 | 47 | 2 |
931 | 2 | 0 | 0 | 59 | 2 |
972 | 6 | 0 | 0 | 71 | 2 |
973 | 6 | 0 | 0 | 64 | 2 |
1323 | 1 | 0 | 0 | 69 | 2 |
1474 | 2 | 180 | 0 | 0 | 2 |
1563 | 1 | 37 | 0 | 0 | 2 |
1564 | 1 | 185 | 0 | 0 | 2 |
1694 | 0 | 1 | 0 | 313 | 2 |
1695 | 0 | 1 | 0 | 396 | 2 |
1696 | 0 | 1 | 0 | 292 | 2 |
1697 | 0 | 1 | 0 | 211 | 2 |
1698 | 0 | 1 | 0 | 163 | 2 |
1699 | 0 | 1 | 0 | 160 | 2 |
1700 | 0 | 1 | 0 | 579 | 2 |
1701 | 0 | 1 | 0 | 472 | 2 |
1702 | 0 | 1 | 0 | 131 | 2 |
1703 | 0 | 1 | 0 | 127 | 2 |
1704 | 0 | 1 | 0 | 267 | 2 |
1705 | 0 | 1 | 0 | 155 | 2 |
1706 | 0 | 1 | 0 | 201 | 2 |
1707 | 0 | 1 | 0 | 253 | 2 |
1708 | 0 | 1 | 0 | 192 | 2 |
1709 | 0 | 1 | 0 | 169 | 2 |
1710 | 0 | 1 | 0 | 129 | 2 |
1711 | 0 | 1 | 0 | 217 | 2 |
1712 | 1 | 1 | 0 | 99 | 3 |
1713 | 0 | 1 | 0 | 172 | 2 |
1714 | 0 | 1 | 0 | 44 | 2 |
1715 | 0 | 1 | 0 | 49 | 2 |
1723 | 1 | 0 | 0 | 8365 | 2 |
Summarising police data for analysis
The second stage of data preparation was to tabulate the data into a format suitable for analysis (Table 116). This required counts of crime for each date in each LSOA given a range of conditions.
Field(s) | Variable | Descriptor |
---|---|---|
1 | Unique_ID | (Created) |
2 | Date_grouped | Grouped into year quarters |
3 | LSOA | Long LSOA code |
4 | LSOA_name | Descriptive name of LSOA |
Count of VAP crimes subject to the following | ||
5 | All_VAP_CrimeCount | Count of all VAP crimes |
6–10 | Crime_Group | (Maximum 5) Labels of VAP classified into with or without injury and other classes of crime |
11–13 | Alcohol_ | Present, not present or missing (no information provided) |
14–16 | Domestic_ | Yes, no or missing (no information provided) |
17–25 | Offender_Age_ | < 18, 18–24, 25–34, . . ., 75–84, 85+ years, missing (nine groups if 10-year bands used) |
26–28 | Offender_Gender_ | Male, female or missing (three groups) |
29–37 | Victim_Age | < 18, 18–24, 25–34, . . ., 75–84, 85+ years, missing (nine groups if 10-year bands used) |
38–40 | Victim_Gender | Male, female or missing (three groups) |
Conclusion
The completion of the best available data set of violent crime against the person for analysis, given the data received, was clearly a painstaking and time-consuming task. However, the final data set was of sufficient coverage and geographical accuracy to use in the analysis.
Analysis strategy
The crime outcome data were available as counts aggregated at LSOA level by quarter from 2007 quarter 2 to 2011 quarter 4. The modelling method that we used was GWR.
We had four possible outcome measures: (1) all violent crime against the person; (2) violent crime against the person with an injury code; (3) violent crime against the person with an alcohol code; and (4) violent crime against the person with an injury and an alcohol code.
We first assessed the validity of each outcome and proceeded to inferential models only when we considered the crime data to be sufficiently robust. In view of the potentially large number of models that could be fitted we aggregated crime counts into the complete years 2008, 2009, 2010 and 2011. We first fitted models for the 2011 crime outcomes associated with alcohol availability from 2010 quarter 4 and compared the results with models for crime data from 2008 associated with alcohol availability from 2007 quarter 4, that is, for the start and end of the study period.
We fitted three sequential models, A, B and C, for walking and driving outlet density. Model A fitted the alcohol availability process (previous quarter, positive and negative change, volatility) and model B added terms for deprivation quintile, settlement type, local authority and police force. In model C the models were stratified by deprivation quintile to compare quintiles 1 and 2 (least deprived) against quintiles 4 and 5 (most deprived), thus addressing the study’s secondary hypothesis on health inequalities.
The model outputs first presented the overall parameter estimates with SEs and p-values. Second, the numbers of LSOAs with significant associations were mapped and interpreted. Third, the final model residuals were mapped with Moran’s test for spatial autocorrelation115 and any clustering/patterns of significant LSOAs and residuals were commented on.
Finally, it was assessed whether or not it was possible to fit a spatiotemporal GWR count model for all violent crime and walking outlet density with the 16 quarters as the time variable.
Descriptive analyses
Data were received from all four forces from 2007 quarter 2 until 2011 quarter 4. The numbers of violent crimes against the person reported by category of crime and year are shown in Table 117.
Category of crime | 2007 Q2–Q4 | 2008 | 2009 | 2010 | 2011 |
---|---|---|---|---|---|
All violent crime | 36,334 | 47,413 | 46,004 | 41,992 | 39,871 |
With alcohol | 6508 | 12,146 | 13,298 | 11,633 | 9660 |
With injury | 16,982 | 22,564 | 21,498 | 19,668 | 17,955 |
With alcohol and injury | 2717 | 5745 | 6277 | 5574 | 4475 |
Table 118 shows the equivalent crime rates per 1000 population aged ≥ 16 years.
Category of crime | 2007 Q2–Q4 | 2008 | 2009 | 2010 | 2011 |
---|---|---|---|---|---|
All violent crime | 14.0 | 18.2 | 17.6 | 16.0 | 15.2 |
With alcohol | 2.5 | 4.7 | 5.1 | 4.4 | 3.7 |
With injury | 6.6 | 8.7 | 8.2 | 7.5 | 6.9 |
With alcohol and injury | 1.0 | 2.2 | 2.4 | 2.1 | 1.7 |
Figure 46 shows the quarterly trends in the numbers of violent crimes against the person.
FIGURE 46.
Numbers of violent crimes againts the person by quarter. Q, quarter.
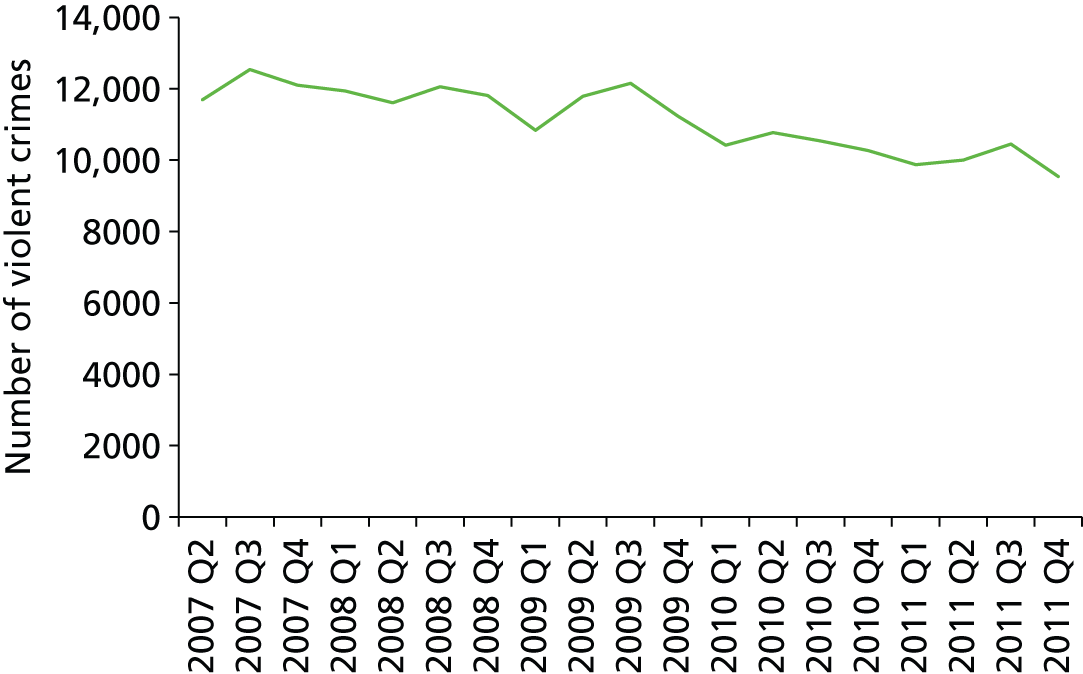
Reported violent crime against the person by local authority
The overall rates of all violent crime against the person by local authority and year are shown in Table 119.
Local authority | 2007 Q2–Q4 | 2008 | 2009 | 2010 | 2011 |
---|---|---|---|---|---|
Isle of Anglesey | 12.5 | 15.6 | 17.4 | 15.4 | 14.4 |
Gwynedd | 15.0 | 17.2 | 18.9 | 16.8 | 16.9 |
Conwy | 13.0 | 16.9 | 18.5 | 16.6 | 16.6 |
Denbighshire | 15.8 | 20.8 | 24.4 | 22.3 | 21.4 |
Flintshire | 11.2 | 13.8 | 15.2 | 13.1 | 12.8 |
Wrexham | 16.1 | 21.9 | 23.9 | 20.7 | 21.9 |
Powys | 9.08 | 11.7 | 12.1 | 11.3 | 11.0 |
Ceredigion | 10.0 | 11.4 | 11.0 | 10.7 | 11.4 |
Pembrokeshire | 11.9 | 13.5 | 13.0 | 13.5 | 11.9 |
Carmarthenshire | 11.2 | 13.5 | 12.3 | 11.3 | 11.9 |
Swansea | 14.1 | 21.5 | 17.8 | 16.2 | 14.9 |
Neath Port Talbot | 11.9 | 16.8 | 15.5 | 14.9 | 11.9 |
Bridgend | 13.0 | 17.7 | 16.9 | 14.4 | 15.0 |
Vale of Glamorgan | 12.4 | 17.2 | 14.9 | 13.2 | 13.2 |
Cardiff | 18.0 | 23.5 | 21.0 | 20.6 | 19.0 |
Rhondda Cynon Taf | 14.7 | 19.8 | 17.9 | 16.9 | 15.4 |
Merthyr Tydfil | 17.4 | 26.7 | 24.4 | 19.6 | 16.3 |
Caerphilly | 14.1 | 17.2 | 16.8 | 15.4 | 13.9 |
Blaenau Gwent | 13.7 | 16.3 | 20.8 | 18.3 | 16.2 |
Torfaen | 13.7 | 16.9 | 16.6 | 15.3 | 15.0 |
Monmouthshire | 8.64 | 10.2 | 9.92 | 8.20 | 8.40 |
Newport | 22.6 | 26.4 | 25.0 | 20.6 | 19.8 |
Total | 14.0 | 18.2 | 17.6 | 16.0 | 15.2 |
Reported violent crime against the person by Lower Layer Super Output Area
The descriptive statistics for LSOA rates of all violent crime are shown in Table 120.
Quarter | Mean | SD | Minimum | Maximum | 25th centile | Median | 75th centile |
---|---|---|---|---|---|---|---|
2007 Q2 | 4.40 | 6.72 | 0.00 | 113.0 | 1.24 | 2.81 | 5.41 |
2007 Q3 | 4.71 | 6.99 | 0.00 | 122.5 | 1.37 | 3.09 | 6.00 |
2007 Q4 | 4.48 | 7.31 | 0.00 | 124.9 | 1.21 | 2.75 | 5.61 |
2008 Q1 | 4.45 | 6.51 | 0.00 | 103.4 | 1.12 | 2.90 | 5.58 |
2008 Q2 | 4.34 | 5.90 | 0.00 | 89.8 | 1.23 | 2.93 | 5.65 |
2008 Q3 | 4.49 | 6.67 | 0.00 | 114.5 | 1.25 | 2.97 | 5.68 |
2008 Q4 | 4.35 | 7.09 | 0.00 | 123.6 | 1.10 | 2.80 | 5.30 |
2009 Q1 | 4.00 | 5.96 | 0.00 | 92.6 | 0.98 | 2.54 | 5.00 |
2009 Q2 | 4.35 | 6.71 | 0.00 | 114.1 | 1.16 | 2.89 | 5.46 |
2009 Q3 | 4.49 | 6.77 | 0.00 | 106.4 | 1.30 | 2.89 | 5.65 |
2009 Q4 | 4.10 | 6.66 | 0.00 | 111.3 | 1.01 | 2.66 | 4.94 |
2010 Q1 | 3.83 | 5.95 | 0.00 | 100.1 | 0.93 | 2.41 | 4.86 |
2010 Q2 | 3.96 | 5.64 | 0.00 | 98.6 | 1.06 | 2.62 | 5.12 |
2010 Q3 | 3.88 | 5.50 | 0.00 | 101.1 | 0.92 | 2.56 | 5.06 |
2010 Q4 | 3.74 | 5.78 | 0.00 | 114.5 | 0.90 | 2.36 | 4.80 |
2011 Q1 | 3.61 | 5.49 | 0.00 | 93.1 | 0.91 | 2.33 | 4.49 |
2011 Q2 | 3.69 | 5.49 | 0.00 | 103.6 | 0.93 | 2.40 | 4.74 |
2011 Q3 | 3.86 | 5.66 | 0.00 | 96.8 | 1.05 | 2.46 | 4.87 |
2011 Q4 | 3.45 | 5.74 | 0.00 | 102.6 | 0.86 | 2.22 | 4.19 |
Reported crimes by police force
Tables 121–124 show the numbers of LSOAs with no reported crimes by the first quarter of each year for each police force.
Police force | Number of LSOAs | 2007 Q2 | 2008 Q1 | 2009 Q1 | 2010 Q1 | 2011 Q1 |
---|---|---|---|---|---|---|
Dyfed Powys | 310 | 38 | 66 | 60 | 67 | 68 |
Gwent | 369 | 29 | 37 | 42 | 36 | 38 |
North Wales | 425 | 39 | 56 | 53 | 53 | 47 |
South Wales | 792 | 62 | 47 | 70 | 77 | 90 |
Police force | Number of LSOAs | 2007 Q2 | 2008 Q1 | 2009 Q1 | 2010 Q1 | 2011 Q1 |
---|---|---|---|---|---|---|
Dyfed Powys | 310 | 98 | 115 | 121 | 126 | 174 |
Gwent | 369 | 367 | 156 | 131 | 136 | 148 |
North Wales | 425 | 167 | 205 | 189 | 208 | 207 |
South Wales | 792 | 716 | 592 | 493 | 520 | 514 |
Police force | Number of LSOAs | 2007 Q2 | 2008 Q1 | 2009 Q1 | 2010 Q1 | 2011 Q1 |
---|---|---|---|---|---|---|
Dyfed Powys | 310 | 88 | 108 | 118 | 141 | 123 |
Gwent | 369 | 55 | 76 | 68 | 72 | 81 |
North Wales | 425 | 113 | 133 | 126 | 122 | 140 |
South Wales | 792 | 167 | 149 | 190 | 198 | 216 |
Police force | Number of LSOAs | 2007 Q2 | 2008 Q1 | 2009 Q1 | 2010 Q1 | 2011 Q1 |
---|---|---|---|---|---|---|
Dyfed Powys | 310 | 150 | 164 | 159 | 188 | 221 |
Gwent | 369 | 369 | 200 | 159 | 178 | 193 |
North Wales | 425 | 258 | 302 | 277 | 281 | 271 |
South Wales | 792 | 748 | 642 | 586 | 625 | 599 |
The numbers of LSOAs with no reported crimes by category of crime and year are shown in Table 125.
Year | All crime | With alcohol | With injury | With alcohol and injury |
---|---|---|---|---|
2007 | 17 | 519 | 91 | 636 |
2008 | 11 | 330 | 50 | 554 |
2009 | 14 | 312 | 66 | 544 |
2010 | 17 | 345 | 88 | 598 |
2011 | 21 | 406 | 90 | 659 |
Missing all years | 0 | 53 | 0 | 119 |
It is clear that there are systematic differences in the way that violent crime with alcohol is reported and there are too many LSOAs with no such reported crimes. The most robust analyses will therefore be for all violent crime against the person, from 2008 to 2011.
Associations between crime rates and Lower Layer Super Output Area deprivation and settlement type
Tables 126 and 127 show the mean rates of all violent crime per 1000 population aged ≥ 16 years by LSOA deprivation quintile and settlement type.
Deprivation quintile | 2008 | 2009 | 2010 | 2011 |
---|---|---|---|---|
Least deprived | 7.42 | 6.91 | 6.13 | 5.86 |
Less deprived | 9.40 | 9.47 | 8.32 | 8.21 |
Mid deprived | 14.1 | 13.6 | 12.8 | 12.5 |
More deprived | 24.1 | 23.3 | 21.1 | 19.9 |
Most deprived | 33.3 | 31.6 | 28.9 | 26.8 |
Total | 17.6 | 17.0 | 15.4 | 14.6 |
Settlement type | 2008 | 2009 | 2010 | 2011 |
---|---|---|---|---|
Urban | 21.3 | 20.2 | 18.2 | 17.2 |
Town and fringe | 15.2 | 15.3 | 14.4 | 13.8 |
Village and hamlet | 6.0 | 6.2 | 5.6 | 5.7 |
Spatial analyses: Geographically Weighted Regression
All violent crime against the person: walking outlet density
We show first the model for 2011 crime data, the most recent data collection period. Table 128 shows the parameter estimates (SEs) with p-values for models A and B for the analysis of rates of all violent crime against the person 2011 and walking outlet density. The parameter estimates can be directly interpreted as crimes per 1000 people.
Variable | Model A | Model B | Benjamini–Hochberg correctiona | ||||
---|---|---|---|---|---|---|---|
Parameter estimate | SE | p-value | Parameter estimate | SE | p-value | ||
Intercept | 6.22 | 0.53 | < 0.001 | 0.74 | 0.96 | 0.44 | 0 |
Alcohol availability | |||||||
Previous quarter | 2.82 | 0.10 | < 0.001 | 2.59 | 0.10 | < 0.001 | 1896 |
Change positive | –5.96 | 3.77 | 0.11 | –4.45 | 3.57 | 0.21 | 134 |
Change negative | –5.55 | 5.71 | 0.33 | –4.64 | 5.39 | 0.39 | 0 |
Volatility | 3.82 | 1.78 | 0.03 | 4.58 | 1.69 | 0.01 | 32 |
Deprivation quintile | |||||||
Least deprived | Reference | ||||||
Less deprived | 1.44 | 1.25 | 0.25 | 0 | |||
Mid deprived | 3.89 | 1.24 | < 0.001 | 0 | |||
More deprived | 8.97 | 1.23 | < 0.001 | 644 | |||
Most deprived | 16.4 | 1.24 | < 0.001 | 1614 | |||
Settlement type | |||||||
Urban | Reference | ||||||
Town and fringe | –0.96 | 1.02 | 0.35 | 0 | |||
Village and hamlet | –0.06 | 1.13 | 0.96 | 0 | |||
Moran | 0.06 | < 0.001 | 0.06 | < 0.001 | |||
R2 (adjusted) | 0.54 | 0.44 |
Violent crime against the person was significantly and positively associated with previous quarter walking outlet density and volatility. The R2 value of 0.54 shows that 54% of the variation in crime is explained by the final model.
Including police force area as a term in the GWR model resulted in a lack of spatial variation (as 1896 LSOAs are grouped into four large areas). This resulted in severe spatial collinearity in the model, which could not be estimated. Police force area was, therefore, excluded from the analyses. All of the 1896 LSOAs showed significant associations with previous quarter outlet density using the Benjamini–Hochberg correction for multiple testing. The geographical variation in these LSOA estimates is shown in Figure 47.
FIGURE 47.
Previous quarter significant LSOA estimates: all crime, walking outlet density. © Crown copyright and database rights 2013 Ordnance Survey 100019153.

Figures 48 and 49 show the significant LSOA estimates for positive change in walking outlet density and Figures 50 and 51 show the significant LSOA estimates for volatility in walking outlet density. Both positive change and volatility showed little geographical variation, and there was no variation in the negative change estimates.
FIGURE 48.
Significant positive change LSOA estimates: all crime, walking outlet density. © Crown copyright and database rights 2013 Ordnance Survey 100019153.

FIGURE 49.
Clustering of significant LSOA estimates for positive change in walking outlet density. © Crown copyright and database rights 2013 Ordnance Survey 100019153.

FIGURE 50.
Significant volatility LSOA estimates: all crime, walking outlet density. © Crown copyright and database rights 2013 Ordnance Survey 100019153.

FIGURE 51.
Clustering of significant LSOA estimates for volatility in walking outlet density. © Crown copyright and database rights 2013 Ordnance Survey 100019153.
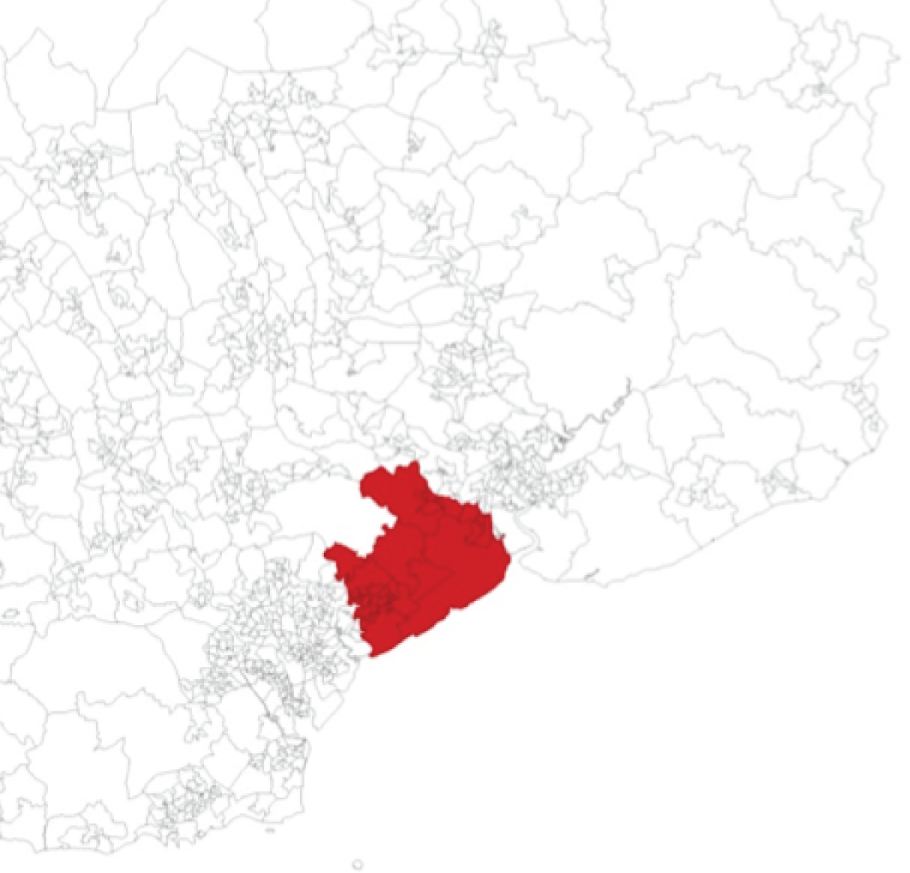
We found that 54 LSOAs had standardised residuals greater or less than ±2 SDs and they tended to be located in urban or built-up areas. Here we present maps for the main urban centre, Cardiff, as an example of an analysis of the residuals. Figure 52 shows the model LSOA residuals from model A2 for Wales.
FIGURE 52.
Lower Layer Super Output Area standardised residuals: all crime, walking outlet density. © Crown copyright and database rights 2013 Ordnance Survey 100019153.
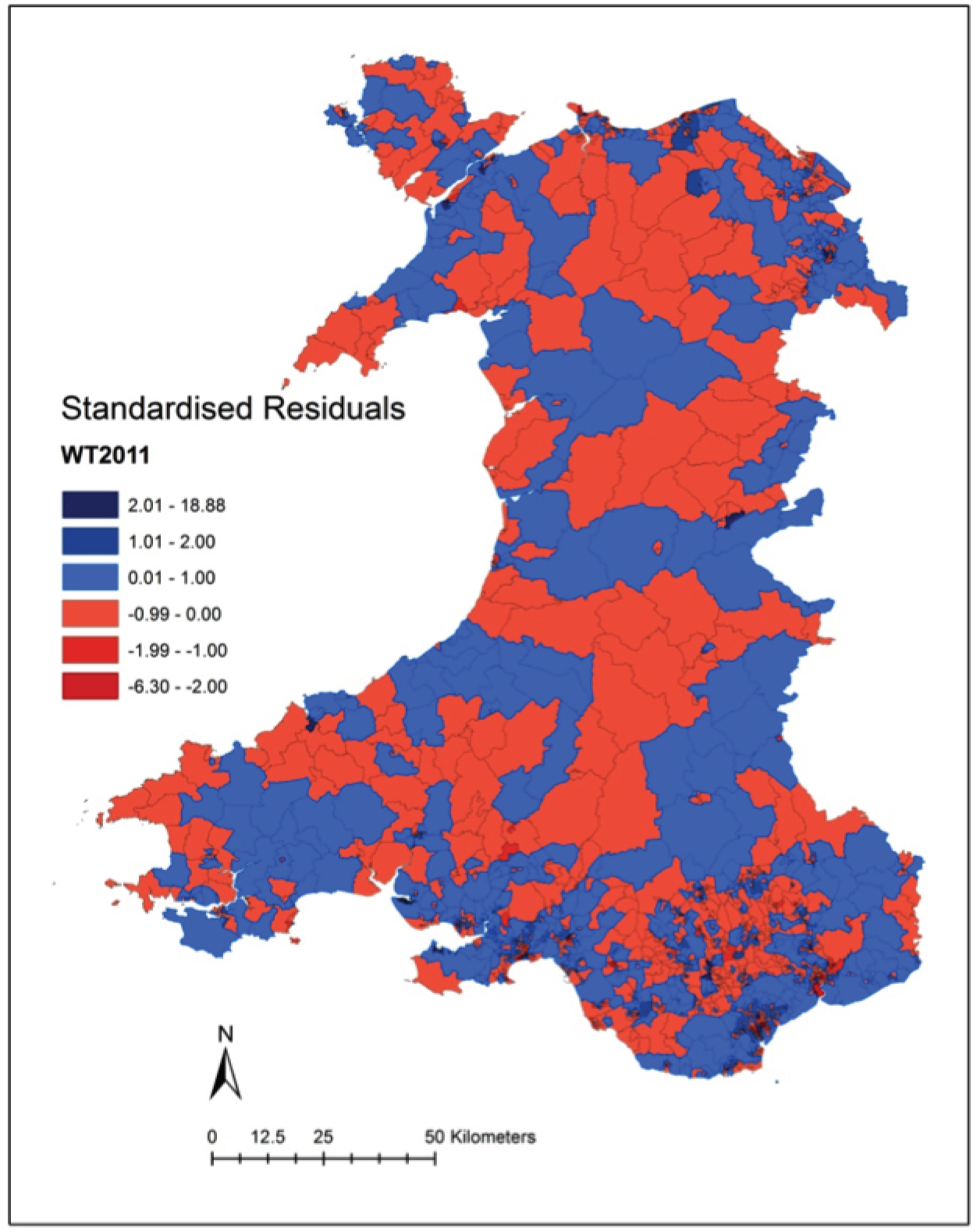
Figure 53 shows the Cardiff standardised residuals from Figure 52 and Figure 54 shows the Cardiff standardised residuals that are greater or smaller than 2 SDs.
FIGURE 53.
Cardiff standardised residuals: all crime, walking outlet density. © Crown copyright and database rights 2013 Ordnance Survey 100019153.

FIGURE 54.
Cardiff standardised residuals that are ±2 SDs: all crime, walking outlet density. © Crown copyright and database rights 2013 Ordnance Survey 100019153.

Figure 55 shows an analysis of the residuals using local measures of spatial autocorrelation (local Moran I) to see if any spatial clusters/outliers of residuals appear. Again, there were clusters in built-up areas and it was possible to classify them depending upon whether they were clusters of high positive residuals, low negative residuals or high positive residuals next to low negative residuals. We found a pattern of city-centre clusters and neighbouring LSOAs being in different cluster classifications. The city-centre LSOA was a high positive residual (the crime rate was higher than the model predicted) and the surrounding LSOAs were low negative residuals (the crime rate was lower than the model predicted); that is, the surrounding LSOAs had a high accessibility score due to their proximity to the city centre but the crime rate was lower because the crime also occurred in the city centre where people went to drink. There were some interesting suburban clusters of high positive residuals – the crime rate here was higher than the model predicted given the accessibility to alcohol outlets.
FIGURE 55.
Spatial clustering of residuals in Cardiff. © Crown copyright and database rights 2013 Ordnance Survey 100019153.

All violent crime against the person: walking outlet density, stratified by deprivation quintile
Table 129 shows the parameter estimates (SEs) for model C3, stratified by deprivation quintile. The previous quarter estimate was statistically significant in both models but was substantially larger in the least deprived quintile model. The volatility estimate was large in magnitude and statistically significant in the least deprived quintile model but was not important in the most deprived quintile model.
Variable | Quintiles 1 and 2 (least deprived) (n = 758) | Quintiles 4 and 5 (most deprived) (n = 758) | ||||
---|---|---|---|---|---|---|
Parameter estimate | SE | p-value | Parameter estimate | SE | p-value | |
Intercept | 10.31 | 2.82 | < 0.001 | 5.13 | 0.45 | < 0.001 |
Alcohol availability | ||||||
Previous quarter | 3.37 | 0.18 | < 0.001 | 0.86 | 0.08 | < 0.001 |
Change positive | –11.39 | 7.53 | 0.13 | 2.59 | 2.10 | 0.22 |
Change negative | –11.31 | 12.73 | 0.37 | –1.96 | 3.02 | 0.52 |
Volatility | 12.83 | 4.35 | 0.003 | –0.21 | 0.88 | 0.81 |
Settlement type | ||||||
Urban | Reference | |||||
Town and fringe | –2.57 | –2.29 | 0.26 | 1.16 | –0.59 | 0.051 |
Village and hamlet | –3.20 | 21.47 | 0.88 | –0.82 | 0.29 | 0.005 |
Moran | 0.04 | < 0.001 | 0.06 | < 0.001 | ||
R2 (adjusted) | 0.39 | 0.38 |
Table 130 shows the number of LSOAs in the two deprivation strata with Benjamini–Hochberg-adjusted significant estimates. There was more spatial variation in the least deprived LSOA quintiles 1 and 2 in Wales, with a significant effect of positive change in walking outlet density on higher violent crime in 71 of the 758 LSOAs in the least deprived areas.
Alcohol availability | Quintiles 1 and 2 (least deprived) | Quintiles 4 and 5 (most deprived) |
---|---|---|
Intercept | 0 | 410 |
Previous quarter | 758 | 408 |
Change positive | 71 | 1 |
Change negative | 0 | 0 |
Volatility | 0 | 0 |
Figure 56 shows that the significant estimates for positive change in the least deprived quintiles tended to be in the suburban areas of Cardiff and Newport.
FIGURE 56.
Clustering of significant LSOA positive change estimates in the least deprived quintile around Cardiff and Newport: all crime, walking outlet density. © Crown copyright and database rights 2013 Ordnance Survey 100019153.

Table 131 shows the alcohol availability estimates for each year from 2008 to 2011 in the walking outlet density models.
Alcohol availability | 2008 | 2009 | 2010 | 2011 | ||||
---|---|---|---|---|---|---|---|---|
Estimate | p-value | Estimate | p-value | Estimate | p-value | Estimate | p-value | |
Previous quarter | 2.93 | < 0.001 | 2.87 | < 0.001 | 2.67 | < 0.001 | 2.59 | < 0.001 |
Change positive | 6.17 | 0.09 | 16.1 | 0.0003 | 9.49 | 0.03 | –4.45 | 0.21 |
Change negative | 3.87 | 0.13 | 5.99 | 0.13 | –1.14 | 0.79 | –4.64 | 0.39 |
Volatility | –1.88 | 0.19 | –0.82 | 0.69 | –0.79 | 0.59 | 4.58 | 0.01 |
Table 132 shows the numbers of LSOAs from 2008 to 2011 with significant Benjamini–Hochberg-adjusted estimates for the alcohol availability variables.
Alcohol availability | 2008 | 2009 | 2010 | 2011 |
---|---|---|---|---|
Previous quarter | 1894 | 1881 | 1896 | 1896 |
Change positive | 0 | 412 | 311 | 134 |
Change negative | 133 | 0 | 0 | 0 |
Volatility | 0 | 487 | 482 | 32 |
Overall, the consistent pattern for walking outlet density and violent crime was that levels of crime were associated with the previous quarter outlet density. We found that the positive change in outlet density variable was of statistical significance and associated with higher crime in 2009 and 2010, with a degree of spatial variation. The estimates for positive and negative change and volatility were inconsistent between years. Tables 131 and 132 suggest that this variability in the estimates could be due to collinearity between the change and volatility variables. We proceeded to fit a spatiotemporal model for walking outlet density and assess model fit and validity.
All violent crime against the person: driving outlet density
Table 133 shows the parameter estimates (SE) with p-values for models A and B for the analysis of all violent crime against the person 2011 and driving outlet density. Violent crime against the person was significantly and positively associated with previous quarter walking outlet density and volatility.
Variable | Model A | Model B | Benjamini–Hochberg correctiona | ||||
---|---|---|---|---|---|---|---|
Parameter estimate | SE | p-value | Parameter estimate | SE | p-value | ||
Intercept | 10.6 | 0.71 | < 0.001 | 2.85 | 1.26 | 0.02 | 0 |
Alcohol availability | |||||||
Previous quarter | 0.09 | 0.01 | < 0.001 | 0.06 | 0.01 | < 0.001 | 1896 |
Change positive | –5.15 | 2.11 | 0.01 | –2.73 | 2.00 | 0.17 | 0 |
Change negative | –12.4 | 4.31 | 0.004 | –7.32 | 4.08 | 0.07 | 0 |
Volatility | 2.02 | 1.02 | 0.047 | 2.26 | 0.96 | 0.02 | 0 |
Deprivation quintile | |||||||
Least deprived | Reference | ||||||
Less deprived | 3.71 | 1.44 | 0.01 | 0 | |||
Mid deprived | 7.28 | 1.43 | < 0.001 | 1148 | |||
More deprived | 13.5 | 1.42 | < 0.001 | 1892 | |||
Most deprived | 19.4 | 1.44 | < 0.001 | 1896 | |||
Settlement type | |||||||
Urban | Reference | ||||||
Town and fringe | 0.04 | 1.24 | 0.97 | 0 | |||
Village and hamlet | –3.17 | 1.36 | 0.02 | 0 | |||
Moran | 0.03 | < 0.001 | 0.02 | < 0.001 | |||
R2 (adjusted) | 0.12 | 0.21 |
All of the 1896 LSOAs showed significant associations with previous quarter outlet density using the Benjamini–Hochberg correction for multiple testing. Figure 57 shows the estimated coefficients that had significant adjusted p-values in the local LSOA models.
FIGURE 57.
Previous quarter significant LSOA estimates: all crime, driving outlet density. © Crown copyright and database rights 2013 Ordnance Survey 100019153.

Figure 58 shows the model LSOA residuals. Thirty-five LSOAs had standardised residuals greater than 2. None had standardised residuals smaller than −2. As with the walking outlet density analysis, the most interesting effects were in the city centres where the crime rate was higher than the model prediction. Figure 59 shows the standardised residuals +2 SDs in Cardiff. The pattern was similar to the walking density pattern.
FIGURE 58.
Lower Layer Super Output Area standardised residuals: all crime, driving outlet density. © Crown copyright and database rights 2013 Ordnance Survey 100019153.

FIGURE 59.
Cardiff standardised residuals that are +2 SDs: all crime, driving outlet density. © Crown copyright and database rights 2013 Ordnance Survey 100019153.

All violent crime against the person: driving outlet density, stratified by deprivation quintile
Table 134 shows the parameter estimates (SEs) for model C3, stratified by deprivation quintile. The previous quarter estimate was small in magnitude and statistically significant in the least deprived quintile model but not in the most deprived quintile model. The volatility estimate was large in magnitude and statistically significant in the least deprived quintile model, but not in the most deprived quintile model.
Variable | Quintiles 1 and 2 (least deprived) (n = 758) | Quintiles 4 and 5 (most deprived) (n = 758) | ||||
---|---|---|---|---|---|---|
Parameter estimate | SE | p-value | Parameter estimate | SE | p-value | |
Alcohol availability | ||||||
Previous quarter | 0.10 | 0.02 | < 0.001 | 0.01 | 0.01 | 0.34 |
Change positive | –9.28 | 5.18 | 0.07 | 0.77 | 0.97 | 0.43 |
Change negative | –14.8 | 10.04 | 0.14 | –3.50 | 2.05 | 0.09 |
Volatility | 6.71 | 2.56 | 0.01 | –0.33 | 0.48 | 0.49 |
Settlement type | ||||||
Urban | Reference | |||||
Town and fringe | –1.80 | 2.85 | 0.53 | 1.26 | 0.67 | 0.06 |
Village and hamlet | –6.88 | 6.79 | 0.31 | –2.10 | 0.47 | < 0.001 |
Table 135 shows the number of LSOAs in the two deprivation strata with Benjamini–Hochberg-adjusted significant estimates. There was more spatial variation in the least deprived LSOAs in Wales, with a significant effect of previous quarter in driving outlet density on higher violent crime in all of the 758 LSOAs in the least deprived areas but in only 31 of the 758 most deprived LSOAs. No spatial variation in the significance of the estimates was found for the change variables.
Alcohol availability | Quintiles 1 and 2 (least deprived) | Quintiles 4 and 5 (most deprived) |
---|---|---|
Intercept | 641 | 751 |
Previous quarter | 758 | 31 |
Change positive | 0 | 0 |
Change negative | 0 | 0 |
Volatility | 0 | 0 |
Table 136 shows the alcohol availability estimates for each year from 2008 to 2011 in the driving outlet density models.
Alcohol availability | 2008 | 2009 | 2010 | 2011 | ||||
---|---|---|---|---|---|---|---|---|
Estimate | p-value | Estimate | p-value | Estimate | p-value | Estimate | p-value | |
Previous quarter | 0.09 | < 0.001 | 0.07 | < 0.001 | 0.07 | < 0.001 | 0.06 | < 0.001 |
Change positive | 0.97 | 0.68 | –1.50 | 0.61 | –1.32 | 0.62 | –2.73 | 0.17 |
Change negative | 0.24 | 0.86 | –0.55 | 0.81 | 4.70 | 0.07 | –7.32 | 0.07 |
Volatility | 0.11 | 0.86 | 2.28 | 0.07 | 0.59 | 0.48 | 2.26 | 0.02 |
Overall, the consistent pattern for driving outlet density and violent crime was that levels of crime were associated with the previous quarter outlet density. There was evidence of spatial variation for the previous quarter outlet density, particularly in the least deprived areas, but no spatial variation in the other parts of the alcohol availability process.
Spatiotemporal Geographically Weighted Regression models
This analysis fitted quarterly crime counts in a global Poisson model as a function of quarterly walking outlet density with a quarterly population offset. The exponent of the parameter estimates can therefore be directly interpreted as the relative risk of crime. Including the settlement type variable in the GWR model led to estimation difficulties from geographical grouping of LSOAs in the same classification. This variable was, therefore, also omitted from the global model. All four measures of alcohol availability were significantly associated with crime in the following quarter (Table 137). The largest effect was found for previous quarter.
Variable | Estimate | SE | z-value | p-value | RR | 95% CI |
---|---|---|---|---|---|---|
Intercept | –6.583 | 0.009 | –769.0 | < 0.001 | ||
Alcohol availability | ||||||
Previous quarter | 0.064 | 0.000 | 297.2 | < 0.001 | 1.066 | 1.065 to 1.066 |
Change positive | 0.313 | 0.014 | 21.6 | < 0.001 | 1.368 | 1.330 to 1.407 |
Change negative | 0.117 | 0.016 | 7.4 | < 0.001 | 1.124 | 1.089 to 1.159 |
Volatility | 0.038 | 0.005 | 7.2 | < 0.001 | 1.039 | 1.028 to 1.050 |
Deprivation quintile | ||||||
Least deprived | Reference | |||||
Less deprived | 0.254 | 0.011 | 22.7 | < 0.001 | 1.290 | 1.262 to 1.318 |
Mid deprived | 0.614 | 0.010 | 59.3 | < 0.001 | 1.848 | 1.811 to 1.886 |
More deprived | 0.993 | 0.010 | 101.1 | < 0.001 | 2.700 | 2.649 to 2.753 |
Most deprived | 1.370 | 0.009 | 145.6 | < 0.001 | 3.937 | 3.865 to 4.010 |
In the GWR model there was substantial spatial variation in the alcohol availability estimates. A total of 1888 LSOAs showed significant Benjamini–Hochberg-adjusted p-value estimates for previous quarter outlet density across all 16 quarterly time periods. The number of LSOAs was 1202 for positive change, 986 for negative change and 1413 for volatility.
Figure 60 shows the z-values associated with the minimum absolute size of the estimates for previous quarter walking outlet density across the 16 quarterly time periods. The strongest associations between violent crime and previous quarter walking outlet density are found in the most urban areas of Wales. Dark grey shading in Figures 60–63 denotes non-significant estimates for each quarter over the 4-year study period.
FIGURE 60.
Spatiotemporal model: all crime, previous quarter walking outlet density. © Crown copyright and database rights 2013 Ordnance Survey 100019153.

FIGURE 61.
Spatiotemporal model: all crime, positive change walking outlet density. © Crown copyright and database rights 2013 Ordnance Survey 100019153.
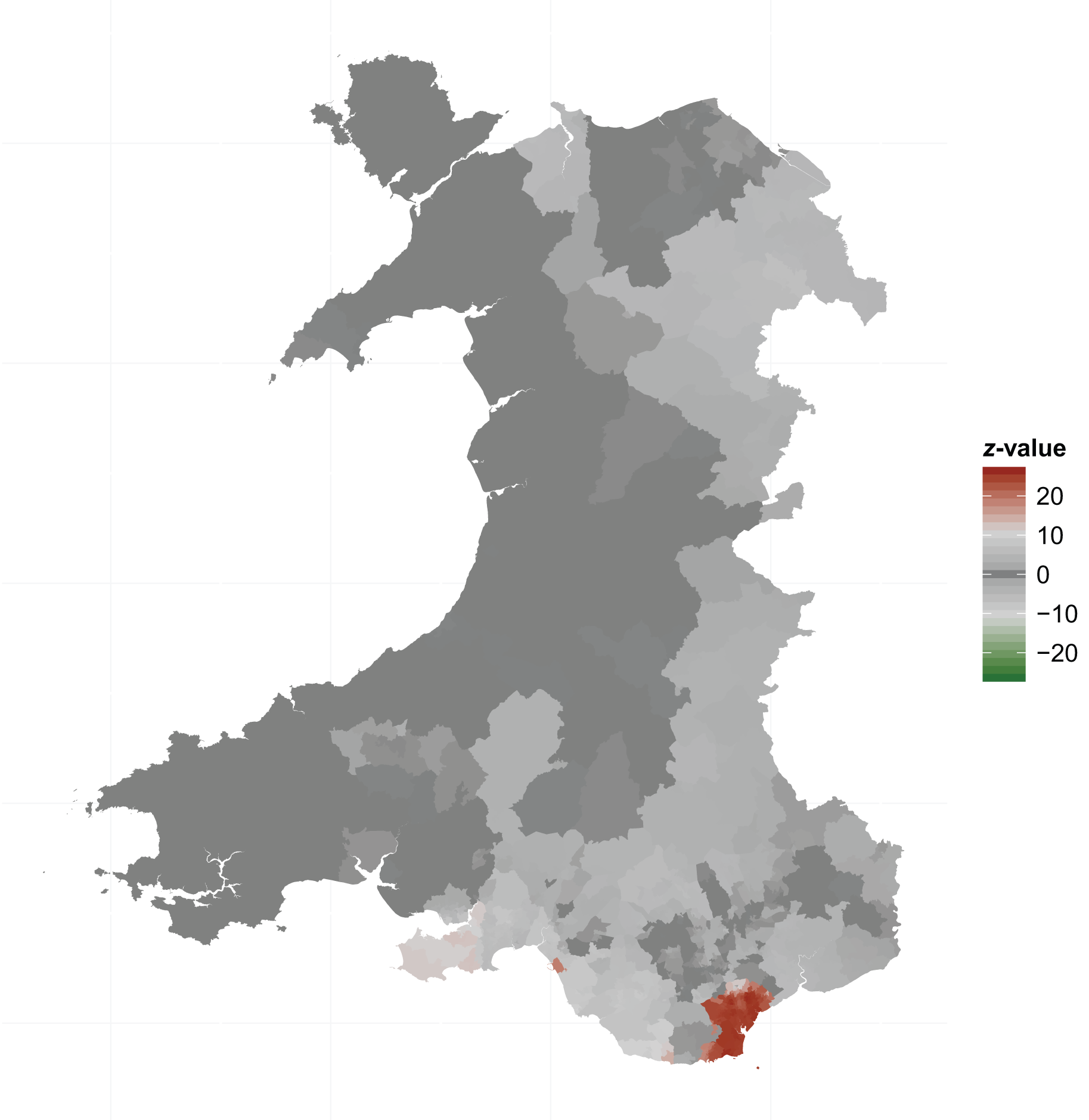
FIGURE 62.
Spatiotemporal model: all crime, negative change walking outlet density. © Crown copyright and database rights 2013 Ordnance Survey 100019153.

FIGURE 63.
Spatiotemporal model: all crime, volatility walking outlet density. © Crown copyright and database rights 2013 Ordnance Survey 100019153.

The geographical patterns shown in Figures 61–63 for the change and volatility estimates show that the strongest association of crime with positive and negative change over each quarter of the study period was in Cardiff. Here, the model suggested that an increase in outlet density was associated with an additional ‘spike’ in crime, over and above the association with previous quarter. The positive coefficient for the negative change variable also in Cardiff suggested that any reduction in crime associated with a decrease in outlet density would be slower than expected. The negative coefficient for the volatility variable suggested that this measure of alcohol availability would attenuate the association between previous quarter and crime. The influence of these changes in outlet density variables was, however, small, by a factor of 10, in relation to the size of the association between crime and previous quarter outlet density.
Chapter 10 Discussion
Overview
In this substantial and complex project we have addressed the research questions as far as possible given the dependence on external sources of data and the huge amount of computational time and processing power necessary to prepare the data sets for analysis.
We investigated the effect of change in alcohol outlet density on important alcohol-related health outcomes in Wales. This was a natural experiment of the effect of change in alcohol outlet density measured in the 1896 LSOA small areas within 22 local government areas. The primary research question was, ‘What is the impact of a change in the density of alcohol outlets on alcohol consumption and alcohol-related harms to health in the community’?
The secondary research questions were as follows:
-
Does a health selection effect from population migration at small-area level explain any observed associations between outlet density and alcohol-related harm?
-
What effect does change in outlet density have on population inequalities in alcohol-related health?
Overall, the trend in numbers of outlets and density was increasing, from 9000 to 11,000 outlets across Wales over 6 years, with only 1 of 22 local authorities showing an overall decrease. However, roughly equal numbers of LSOAs increased and decreased their numbers of outlets and so we had both positive and negative change to investigate. We developed a new network-based method of measuring outlet density for alcohol availability by walking and driving and formulated measures of the local alcohol availability process including the current measure of density, change over the preceding year and the degree of volatility in that change.
We found a consistent pattern of associations between alcohol-related harms and alcohol availability, which were stronger for walking outlet density. Change in walking outlet density was associated with change in alcohol-related harms: consumption of alcohol, emergency hospital admissions and violent crime against the person all tracked the changes in the previous quarter measure of small-area outlet density, that is, the outcome measure for each successive time period was associated with the outlet density exposure in the preceding time period. The highest level of alcohol availability was significantly associated with around a 20–25% increased risk of an emergency admission to hospital and less convincingly associated with an A&E attendance, for which the lack of clinical coding meant that it was not possible to distinguish an alcohol-related attendance. The models for both consumption and hospital admissions suggested that change in outlet density had a greater effect on men than women. Clearly, an increased burden to the NHS is associated with higher levels of outlet density. We found no evidence for an important effect of population migration. Social deprivation was in general strongly associated with our outcome measures but did not substantially modify the associations between the outcomes and alcohol availability, except that positive change in walking outlet density had a significant association with violent crime in the least deprived areas of Wales.
We were able to carry out a spatial analysis for the alcohol binge drinking and violent crime outcomes. Thus, we were able to distinguish localised effects and geographical variation from the overall effects of all the within-area changes over time for Wales from the non-spatial analysis.
The strength of our findings is the consistency of these associations over a range of adverse outcomes explored using a range of statistical methods. The main limitation of the research is the dependence on external sources of data and associated data quality concerns, particularly in the absence of any standardised methods of alcohol outlet data collation, processing and validation.
Alcohol outlet density
We developed a new network-based measure of outlet density in this project in which we considered alcohol availability to be related to accessibility. We computed the walking or driving network (not Euclidian) distance from each of the 1.3 million households in Wales to each of the approximately 10,000 outlets within defined buffer zones, or service areas, of 10 minutes’ walking and 10 minutes’ driving. This was clearly an intensive computational undertaking but it was achievable and produced plausible distributions of values.
Collation of alcohol outlet data
The major difficulty that we had was obtaining data on alcohol outlets from the 22 local authorities in Wales. The Licensing Act 200365 requires local authorities to maintain public registers of current licences, populated using licence application forms that include (1) the date that permission was granted or the date that the licence became active (referred to as start date); (2) the licence expiry date or an indicated date of outlet closure (end date); (3) whether the premise is licensed for on- and/or off-premise sales (on/off status); and (4) the hours during which it is permissible to sell alcohol or the general opening hours of the outlet (opening hours)
We found that current and historical alcohol outlet licence data were collected and retained within most local authorities. However, there were large differences between the unitary authorities in the way that the same data items were stored. Each local authority presented its own unique challenges for data gathering, such as the need to collect records in person, collation from multiple PDF files, text files without consistent formatting or printouts that had to be converted into a useable format. As a result, extensive processing was required to gather all files, transpose the information into useable outlet records and collate the data into a single database of outlets. The tools and techniques used included web scraping, string manipulation and optical character recognition. Several of the local authorities were unable to provide precise outlet closure dates and so the date of the last interaction with the outlet by the local authority was used to generate an approximate end date. Only half of the local authorities were able to provide the on/off status of outlets and nine were able to provide opening hours. These data were not sufficiently complete, or were only partially available in free text, and could not be analysed in this project. This was a major limitation but it was not possible to make any further progress.
The data collation stage of the project took more than 18 months to complete, much longer than expected. A total of six authorities required as many as 10 follow-up calls or up to 3 months’ data-checking time. One local authority could not provide historical data because it deleted old records. We had to resort to freedom of information requests after repeated attempts to contact several of the licensing departments. Numerous follow-up requests had to be made to ensure that historical, rather than only current, licensing data were provided, with many local authorities unclear how to obtain such information from their systems.
The final database consisted of 21,137 outlets. Of these, 16,106 records remained after removing those that were not alcohol licences, that were temporary event notices or that were duplicates. Of these, 8127 (50.5%) local authority records could be matched using geocoding software. There were substantial differences in the quality and accuracy of the address details captured, which impacted on the geocoding process and required the introduction of a three-stage matching process (geocoded, manually matched and approximate).
The bilingual status of Wales had an impact on geocoding because licences may be applied for and records may be administered and maintained in either language, leading to different formatting and spelling in licence records. This is particularly true of address fields, which contained misspelled outlet names and streets, resulting in no matches found during the geocoding process. It also made the manual matching process more challenging.
Delays were incurred from difficulties in identifying an accurate location for many outlets, as reflected in the relatively poor overall 50.5% geocoding rate. This resulted in the subsequent development of a manual matching method to locate premises that could not be geocoded. The ABP data set, used as the geocoding data source, is in part generated and maintained by the same local authorities approving licence applications. From the low match rate, we concluded that basic checks on vital address components, such as postcodes or building names and numbers, had not taken place. These are essential to achieve accurate and fast geocoding.
The most complete, highest-quality data were received from three main urban areas in Wales (Cardiff, Swansea and Newport) and resulted in an average geocoding rate of 66%. The urban outlet geocoding rate from this study is lower than geocoding rates found in other research, which focuses on residential properties and suggests that 85% is an acceptable rate. 71
We suspect that the lack of structure in the commercial premise addresses that were received contributed to the lower geocoding success rate. The more sparsely populated rural unitary authorities were further investigated. In most of these, fewer than half of the addresses provided for licensed premises could be geocoded. Given that there are statutory requirements to record these data and supply summary statistics to the Home Office, it was expected that these licence data would be accurately recorded.
This study has used the most accurate geocoding standards to produce a complete spatial data set of outlet locations at the highest resolution possible. Given that the quality of addresses varied systematically by rural and urban areas, the 97% combined geocoding and manual match rate that was achieved was the best solution for aggregation into small-area geographies because it limited urban–rural bias.
It is suspected that the larger night-time economies in the urban areas require urban local authorities to record accurate data on the location of premises from a planning and policing perspective. It is likely that this requirement has led to the manual adjustment of addresses by the local authority to match records when the licence application form is submitted for consideration in urban areas. Moreover, there are more likely to be checks on licensed premises in urban areas by licensing officers who update the records. The local authorities do not necessarily know if an outlet has temporarily or permanently ceased trading unless the licence expires and payment is due or the owner informs them. The annual licensing inspection process means that we would expect to have licensing information valid to within 1 year.
The use of a manual matching process to find the spatial location of licensed outlets is a relatively straightforward, if time-consuming, process. Limitations of the manual matching process include human error through misidentification of outlets from the address and data errors remaining unresolved in Google Maps. However, the validation process has tested the introduction of errors with the reassuring result that nearly 80% of outlets were located within 100 m of the geocoded location. However, the spatial accuracy of the approximated locations is unknown. The geocoded, manually matched and approximated outlets have the greatest utility for subsequent data linkage at household level.
Longitudinal data were required to assess changes through time and the impact of outlet density on alcohol-related harm. The requirement to retrospectively collect historical data was challenging. First, older premises were more likely to have closed or changed name. The final data set covered a 6-year period; for longer-term studies the concurrency of address data would likely result in poor match rates. Second, the ABP data set used in the geocoding process retains historical properties but we suspect that historical records may have been batch processed, resulting in poor-quality data, as thousands of properties with the same start date appeared in the address record. Additionally, local knowledge or colloquialisms had been incorporated into some alcohol outlet address data, probably by the landlord or licensee completing the form. We also found that, occasionally, the home or work address of the licensee or club secretary was recorded rather than the address of the outlet. These addresses are now ambiguous, leading to inaccuracies when trying to map an outlet, especially once it has closed.
The Licensing Act 200365 was designed to help the government monitor the impact of, among other things, the sale of alcohol in relation to alcohol-related crime. The potential of administrative licensing data as a resource for public health surveillance and associated data access issues have already been highlighted. 116 We faced similar problems trying to access alcohol licence data and largely agree with these conclusions. Through the process of geocoding a longitudinal data set we have identified further issues surrounding the variation in the number of data items stored, the accuracy of the address data and historical preservation of the licence data.
A standardised system of recording and maintenance of records between all local authorities with a standardised address-checking service at the point of capture or at data entry would overcome these problems. Such a standardised system of recording data on alcohol outlets is required for research purposes. A minimum data set should be defined and then used by each licensing authority that includes validated and reliable complete data on geographical location, opening and closing dates, type of outlet (e.g. on-sales and off-sales, pub, club, restaurant, shop, supermarket) and opening hours.
Computation of alcohol outlet density
The strength of our network approach to the computation of outlet density is that it is derived from an underlying theoretical model of geographical access and so is an improvement over a simple method of outlet counts per population that does not take accessibility into account. Small population denominators will bias upwards simple measures of density in rural areas, despite accessibility being lower. Ten-minute buffer zones, or service areas, for walking and driving access to alcohol outlets seem to have face validity and the resulting measures behave as expected between urban, town and rural areas of Wales.
The main limitations of the approach are the computational effort involved, the necessary inclusion of assumptions (although widely used in geographical research) of distance decay and the problem of boundary effects. Boundary, or edge, effects are primarily a problem for walking and driving density at the Wales–England border. Here, the 10-minute buffer zone for residences that are located within 10 minutes of the border will exclude the measurement of distance to alcohol outlets in England that are within a 10-minute walk but are not counted as the outlet data were held by an English local authority, not available to this project. Thus, the density for border LSOAs will tend to be underestimated. The problem is larger for driving density as many more than just the border LSOAs will lie within a 10-minute driving buffer. This was also a substantial problem for any attempt at measuring an outlet density for on- or off-sales as the border problem was manifested across local authorities that did and did not supply on–off outlet data. It was not possible to compute walking and driving densities to account for population migration as the only feasible distance matrix was based on distance from each residence, rather than each individual, to each outlet.
Finally, these network measures are less easy to interpret than a simple method based on per-capita counts or a single absolute distance. The statistical model outputs in general will give a change in the outcome for a unit change in outlet density and so this unit change must also be expressed in a countable form. It is not possible to have a single interpretation of a unit change as this can result equally from change in a larger number of outlets at a greater distance or a smaller change in the number of outlets at a shorter distance.
To achieve a unit change in density score requires a change in three to five outlets within 833 m. This is 833 m in any direction and so effectively this is a three- to five-outlet change within 0.7 km2. The actual number of outlets required to effect a unit density change will vary from household to household (because of the local geographies) and this will therefore affect the aggregated density score at LSOA level. Because of this variability in localised density scores, the number of outlets required to make a unit change was modelled from a simulation and we computed a matrix to show the number of outlets that would need to change at a range of distances within the buffer zones.
An important outcome for future work from our development of a new method of estimating outlet density using network distances is that it is generalisable to other areas of public health concern in which geolocated exposure data are available. For example, a density for fast-food outlets and green spaces could be estimated in the same way and would offer a different perspective to a measure of simple density or nearest distance. Our measure of density can be conceptualised as a measure of exposure, in contrast to a measure of nearest distance that is best conceptualised as accessibility.
Alcohol availability
We considered the alcohol availability process in some detail and concluded that a simple measurement of ‘change’ would be too simplistic an approximation to a hugely complex real-world process. Our interpretation of the overall availability process was that three measures were needed. The first was some measure of the historical or current availability, which would change quarter by quarter but that would provide a basis for the baseline assessment of availability. We tested a range of potential measures, including historical maxima and minima over successive time periods, but found that the previous quarter value was the best-fitting measure in the statistical models.
To assess change, the time lag for exposure–outcome had to be defined for each outcome. We considered that a 1-year (i.e. five successive quarters from quarter 4 in one year to quarter 4 in the next year preceding the outcome measurement) lag would represent a realistic exposure period. Change is arithmetically easy to calculate but absolute change will represent a different process in LSOAs with widely different numbers of outlets. We computed a simple measure of relative change, adjusting for the five time-period mean quarterly values and further divided by the square root to stabilise the variance. Necessarily, the degree of change will still be small in comparison to the baseline (previous quarter) starting value.
Finally, we observed that many LSOAs showed some volatility in outlet densities quarter by quarter that was not captured by a simple measure of change between two density values five quarters apart. To try and capture this volatility we computed the amount of absolute change in quarter-by-quarter density and expressed this as a relative quantity as per the change variables.
We have not found any previous research attempting to derive measures of alcohol availability that can be investigated in statistical analysis. One problem worthy of further research is the extent to which the measures of change and volatility are independent. We found in the crime GWR analyses, in which a substantial number of local geographical associations were apparent, that negative change and volatility were probably not optimal measures.
Alcohol consumption
Main results
We first modelled units of alcohol consumption from the WHS in a non-spatial model as a function of the alcohol availability process, adjusting for individual covariates and LSOA measures of multiple deprivation and settlement type. We first compared model results for the simple measures of outlet density (outlets per population) and found no essential differences between the two measures, suggesting that population migration has only a very limited effect on the alcohol availability–harm relationship.
Our two main models used walking and driving outlet density. We found a significant, but small, association between the previous quarter walking outlet density and consumption in the following year. A 1-point increase in outlet density was associated with a 1.0% increase in units consumed in the following year, with a 1-point increase in outlet density being equivalent to an extra four outlets within a 500-m radius of a residence. This association did not vary importantly between areas of low deprivation and areas of high deprivation. The association between walking outlet density and consumption was not linear and followed a ‘broken stick’ shape, with the natural break at an outlet density of 1. The association with consumption was stronger in LSOAs with densities < 1 (representing 35% of LSOAs). Here, we found that the unit change in walking density was associated with a 6% increase in consumption. For LSOAs with densities > 1, we found that the unit change in walking density was associated with an additional 0.7% significant increase in consumption. The models suggested that change in previous quarter outlet density had a greater effect on men than women.
We found that the availability measures of positive and negative change and volatility were not statistically significantly associated with consumption. However, it is of interest that the signs of the estimates were in the expected direction.
In the driving outlet density models the previous quarter remained significant although the strength of the association was weaker, with a 0.1% increase in units consumed in the following year for a 1-point increase in driving outlet density. Change and volatility in driving density were not significant.
We then proceeded to model the probability of an individual being a binge drinker, as defined by the Department of Health, in a GWR spatiotemporal model for walking outlet density. In the global model we found that the probability of binge drinking was positively associated with the previous quarter values of density. This association was tracked through the 5 years of the study and is evidence that change in outlet density is associated with binge drinking.
In a spatial analysis we found that the associations between binge drinking and all four measures of outlet density varied significantly across the 1896 LSOAs in Wales, with some potentially important local geographical effects. The geographical output has to be interpreted with care as the results will vary with choice of bandwidth, p-value adjustment for multiple testing and sampling variation in the binge drinking outcome where there are few respondents in some more sparsely populated LSOAs. However, the general patterns can be interpreted and the evidence is clear that there is important spatial variation.
Strengths and limitations
In general, the WHS is considered to be a good-quality data set. The sampling fraction is large and the household and individuals-within-household response rates are high. The number of missing data items rarely exceeds 5% on each variable. The change in the questions asked about alcohol consumption in 2008 meant that we were unable to use the 2006 or 2007 data but we were able to use five consecutive survey years from 2008 to 2012. Through a special data access agreement we were able to obtain 2001 LSOA codes to facilitate the small-area and geographical analysis.
The main limitations are that each year’s data set is a new cross-sectional data set, which limited the analytical possibilities. As with all survey data we expect that the self-reporting of consumption is biased (downwards). There were clearly errors with regard to the small percentage of respondents with implausibly high levels of reported consumption and we excluded these on a pragmatic basis aligned to approximate measures of blood alcohol concentration compatible with life. We had no information on duration of residence and so the problem of endogeneity or reverse ‘cause’ arises as participants with high levels of consumption may have selectively migrated into areas of high outlet density. However, the three Finnish longitudinal analyses were able to assess the impact of endogeneity and found that it did not make a substantial contribution. 24–26
Permission to anonymously record-link WHS data into the SAIL Databank has recently been granted for 2012 data onwards and so unfortunately was not available for this project. A future analysis will therefore be able to investigate the relationships between alcohol consumption, outlet density and the risk of A&E attendance and hospital admission, taking endogeneity into account.
Fit with previous research
There have been few papers published modelling consumption and outlet density. Three studies set in Finland found some evidence that proximity to bars/restaurants, off-premises and wine outlets was associated with heavy drinking, ‘extreme drinking occasions’ and wine consumption. 24–26 A study set in the USA found little evidence of an association between an increase in the proximity of bars and alcohol consumption. 27 Our results using a total walking network availability model of outlet density rather than simple proximity adds to the evidence that higher levels of consumption and binge drinking are associated with high outlet density and that the levels of consumption and binge drinking track the availability process through time. The association of decreased consumption with higher volatility is interesting and it is possible that higher volatility, representing the opening and closure of alcohol outlets, is a general marker of the prevailing economic climate. Overall, we have found evidence to support the alcohol availability–harm model, in which it is theorised that a higher level of consumption is a mediator of that relationship. 21
Hospital admissions
Main results
Although the incidence risk of our narrow definition of alcohol-related emergency admissions appears to be small (at 1.8 per 1000 person-years), this resulted in 56,400 emergency admissions over the 6-year period and > 25,000 patients admitted in our total population cohort aged 16–99 years at baseline on 1 January 2006. Over half of these admissions were attributable to ‘mental and behavioural disorders due to use of alcohol’, which includes alcohol dependence, acute intoxication and harmful use as the three single largest categories. Around one-fifth of patients were coded with alcoholic liver disease.
We carried out three groups of models. In the baseline cohort analyses we found that the highest quintile of both walking and driving previous quarter outlet density was significantly associated with a 20% increased risk of an alcohol-related emergency admission over the 6-year study period. The effect was stronger for walking density and for emergency admissions coded as ‘mental and behavioural disorders due to use of alcohol’ and ‘injury and external causes’. The effect was also stronger in men than in women in the highest-density quintile. Although LSOA deprivation was also strongly associated with the risk of admission, there was little evidence that the effect of outlet density varied consistently between areas of high deprivation and areas of low deprivation.
The pattern of associations suggested a non-linear effect of walking outlet density, similar to the consumption associations. We found that, compared with the reference quintile, the elevated risk of admission was similar in outlet density quintiles 2–4, with a larger increase in the highest quintile. We then fitted a Cox multilevel model of individuals nested within LSOAs and local authorities and fitted the alcohol availability process as time-dependent covariates. To explore the non-linearity and possibility of threshold effects further we fitted the previous quarter variable using the same ‘broken stick’ method as in the consumption analysis. We also fitted LSOA deprivation and settlement type as time-dependent covariates to allow for migration within Wales. We found that the previous quarter measure of walking density was associated with a significantly higher risk of emergency admission. The risk of admission associated with unit change in outlet density at values < 1 was 24%, with an additional 1% for each unit change in density at values > 1. The negative change variable was associated with a higher risk: that is, a significantly slower decrease in the risk of admission following a reduction in outlet density.
In the Poisson multilevel models of counts of emergency admissions per LSOA we found that the LSOA admission rate tracked the previous quarter walking density (in quintiles) through the 6-year study period, but there was no significant effect of positive or negative change, nor of volatility, in outlet density.
Strengths and limitations
We were able to prepare a 6-year data set of > 100,000 admissions defined by an alcohol code in any position for the population of Wales. In the absence of any UK-wide or international consensus, at the time of carrying out the work we defined a narrow ICD-10 code definition of ‘alcohol related’ and have been able to determine a reasonable definition of how many coding positions should make up an alcohol cause for admission. Given the apparently undifferentiated structure of the raw data we consider this to be a strength of the study. However, a limitation is that comparisons with other published data on alcohol-related admissions to hospital are not straightforward because of the variety of different definitions used. For example, Public Health England101 has recently published a slightly different set of ICD-10 codes with a definition for the ‘narrow’ wholly attributable admission that uses the first coding position only plus any external cause code in all other positions, does not include ‘Z’ codes, appears to search across all episodes and makes no reference to hospital transfers.
Future work would benefit from a more precise agreed definition of an alcohol-related admission, to include, inter alia, ICD-10 codes, coding positions, episode numbers, super spells and interhospital transfers.
Some limitations arose that were outside of our control and which were related to the validity of the raw data. For example, the hospital of admission should be considered as a random effect but we could not fit this as the variable was only 40% completed. Migration data for censoring could be computed only by quarter when daily would have been preferable.
Although it may appear to be a trivial process, matching each patient and his or her potentially multiple admissions to the baseline population cohort and then computing migration dates and time periods was computationally intensive and for some time exceeded the memory capacity of our facility. The merging data set required to model time-dependent covariates required a minimum of 60 million rows (2.5 million population × 24 quarterly time periods) with a large number of columns. Running the analysis therefore required huge matrices and it necessarily took a substantial amount of time to achieve our objectives. At first, we were unable to fit this model to the full data set and had to use a case–cohort approach. We found that we could use all of the events but only sample a maximum of 10% of the full non-admitted cohort, which was less efficient. However, the experience gained and lessons learned in this process will be invaluable for future work using large record-linked hospital admission data sets.
Fit with previous research
We have not found any previous research investigating longitudinal associations between hospital admissions and alcohol outlet density. One US paper published in 2000 found a cross-sectional association between a simple measure of outlet density and alcohol-related hospital admissions. 42
Accident and emergency attendances
Main results
In the A&E attendance analysis we found that the baseline cohort adjusted HR for walking density was best interpreted as non-significant, with a stronger main effect for the highest quintile of driving density (HR 1.28, 95% CI 1.24 to 1.31). We did not find any evidence that the associations varied with deprivation. We cannot explain why walking density was unimportant and driving density appeared to show an association with A&E attendance. The most likely explanation is that a substantial proportion of attendances were not caused by alcohol and so these findings are spurious.
Strengths and limitations
The main strength of the analysis is that it is the first attempt to quantify the risk of an A&E attendance related to alcohol outlet density. However, the major limitation is the absence of any systematic approach to clinical coding of the reason for attendance and so it likely that a large proportion of attendances were not related to alcohol, even though we investigated only night-time attendances, which previous research has suggested is the best proxy for alcohol-related attendance. 106–109 We found, through record linkage, that 2% of attendees were admitted to hospital within 24 hours as an alcohol-related admission. No such data have been published previously, but it suggests that only a few night-time attendances reach an admission threshold. Many will of course be for minor injuries but we simply did not have any clinical data to analyse.
As a result, the major unavoidable limitation is that, because it is not possible to confidently ascribe these attendances to an alcohol-related cause, the associations that we found may be biased in any particular direction, depending on whether or not attendances for any particular cause were associated with outlet density, either acting directly or as a measurement proxy for some other unmeasured factor associated with attendance.
Fit with previous research
We have not found any published research investigating associations between A&E attendances and outlet density and so, despite the limitations of this part of the project, we have been able to make a new contribution.
Violent crime against the person
Main results
We found that higher levels of crime were consistently associated with the previous quarter walking outlet density for each of the 4 years of the study. The estimates for positive and negative change and volatility in outlet density were inconsistent between years.
In GWR we found that the associations between violent crime and all four measures of outlet density varied significantly across the 1896 LSOAs in Wales, with some potentially important localised spatial effects. The geographical output has to be interpreted with care as the results will vary with choice of bandwidth, p-value adjustment for multiple testing and sampling variation in the crime outcome when there are few respondents in some more sparsely populated LSOAs. However, the general patterns can be interpreted and the evidence is clear that there is important spatial variation.
Associations between violent crime and driving outlet density were generally weaker with no spatial variation other than for the previous quarter measure.
We were able to fit a spatiotemporal model to the full data set with time measured in 16 quarterly periods for walking outlet density. This model confirmed that higher levels of crime tracked the previous quarter walking outlet density through time. The local geographical associations between crime and change and volatility in outlet density were also confirmed as consistent patterns over the time period. The strongest association of crime with positive and negative quarterly change in outlet density was in Cardiff. Here, the model suggested that an increase in outlet density was associated with an additional ‘spike’ in crime, over and above the association with previous quarter and any reduction in crime associated with a decrease in outlet density would be slower than expected. Volatility in outlet density would attenuate the association between previous quarter and crime. The influence of these changes in outlet density variables was, however, small, by a factor of 10, in relation to the size of the association between crime and previous quarter outlet density.
Strengths and limitations
We were able to collate a data set of all violent crime recorded in Wales for 2008–11 by successful collaboration with the four police forces in Wales and implementation of data-sharing agreements. This substantial task built on positive and successful working collaborations in previous work by members of the research team. The overall levels of violent crime, secular trends and relationships with social deprivation and urban areas are in broad agreement with data reported by the CSEW. Using the most accurate geocoding methods available we were able to locate the vast majority of crimes to the LSOA of occurrence and proceed to a spatial analysis.
In contrast to all previously published GWR analyses we were able to model both space and time simultaneously. All previous analyses, except that by Yu et al. ,36 have aggregated data over time and therefore have not been able to model change. In our models we have been able to show that the levels of violent crime track measures of alcohol availability through time. Our model differs in that GWR is conceptually simpler because all parameters vary across space and time; in contrast, Yu et al. 36 used a spatiotemporal model with some terms being common across all areas and times but with correlated space/time residuals. The limitation of GWR is that it is an exploratory technique, so that local geographical outputs should not be overinterpreted.
The main limitation of our analysis was its dependence on the quality of the data provided. Although the overall reporting of violent crime was as good as it probably could be, the quality of the coding of alcohol-related crime was variable and incomplete. This is because the recording of alcohol involvement is not a requirement for the police. The coding of injuries was more complete but ultimately the most robust analysis was just to consider all reported violent crime against the person. This outcome measure of all violent crime against the person included crimes that were not alcohol related. One possibility to improve the outcome measure is that alcohol-attributable fractions (AAFs) could be used to weight the number of police-recorded violent incidents to reflect only alcohol-related violence. Although this is possible, some features of the AAFs make their use problematic in this case. First, the estimates of how much violence is attributable to alcohol are really estimates of the proportion of people arrested immediately after perpetrating a violent offence who were drunk at the time. 117 Given that victims of violence who completed the CSEW 2013/14 reported that approximately 51% of assailants were drunk,118 the AAF methodology would result in a considerable underestimate of the AAF for violence. Additionally, the AAF methodology does not consider the intoxication of the victim. At a theoretical level, the inclusion of AAFs in the analysis would make the assumption that alcohol outlets affect the likelihood of violence only through the intoxication of one of the parties. This would be an unnecessarily narrow interpretation of the role of alcohol outlets in community violence.
Fit with previous research
Although there is much published research showing cross-sectional associations between outlet density measured in different ways and a range of violent outcomes, there have been few longitudinal studies and each has used a different measure of both outlet density and crime. The Californian studies investigated bar and off-licensed outlet densities and found associations with different measures of violence: hospital admissions for injuries resulting from interpersonal violence and police-recorded motor vehicle crashes. 28,29 Our results tend to add to the evidence from the 35-year time series analysis from Norway31 and the 9-year study set in Melbourne,32 which found that outlet density was significantly associated with violent crime. The Melbourne study32 found that the strongest associations were in central and inner-city suburbs, which is in line with our results, but we were further able to show important spatial effects of change in outlet density over time.
Public and patient involvement: dissemination of findings
As discussed in the project protocol, the scope for patient involvement was limited as patients were included only in an anonymised secondary analysis of record-linked data sets. The victims of violent crime could also not be identified.
We will work with the Farr Institute Consumer Panel for Data Linkage Research to advocate our work [see www.farrinstitute.org/centre/CIPHER/36_Public-Engagement.html (accessed 7 June 2015)]
We have worked closely with the charity Alcohol Concern Cymru, represented on the Study Steering Committee by the Director. We also were able to recruit a member of the Clinical Research Collaboration Cymru Involving People network [see www.involvingpeople.org.uk/ (accessed 7 June 2015)] onto the SSC to give a public perspective.
Our dissemination strategy is focused on working closely with Alcohol Concern Cymru and the Director of Policy and Research at the Public Health Wales NHS Trust. We will, in the first instance, plan to present the results to and work with:
-
Alcohol Health Alliance UK, a grouping of health organisations facilitated by the Royal College of Physicians
-
the Institute of Alcohol Studies
-
the Public Health England Licensing Forum, which includes the Home Office, the Association of Chief Police Officers (ACPO) and the National Association of Licensing and Enforcement Officers (NALEO)
-
Alcohol Research UK
-
Wales Heads of Trading Standards (WHoTS)
-
local authority trading standards officers
-
the Chartered Institute of Environmental Health, including its Wales regional network
-
local authority environmental health officers
-
the Institute of Licensing, including Wales region
-
local authority licensing officers
-
the Public Health England annual conference
-
the Public Health Wales annual conference
-
the Alcohol Concern annual conference
-
Alcohol Concern Cymru – newsletter and networks in Wales
-
Alcohol Focus Scotland licensing training and conferences
-
Drink Wise North West
-
Balance North East
-
the Kettil Bruun Society for Social and Epidemiological Research on Alcohol.
Chapter 11 Conclusions
A consistent but complex pattern of associations was found between the alcohol-related harm outcomes and alcohol availability, measured as walking outlet density. Change in outlet density was associated with change in alcohol-related harms: consumption of alcohol, emergency admissions to hospital and violent crime against the person all tracked the changes in the previous quarter measure of small-area outlet density. Using methods of spatial analysis it has been shown that these associations showed different degrees of important localised geographical variability. It was also found that the shape of the relationships between outlet density and the outcomes were non-linear, with complex threshold effects.
The models for both consumption and hospital admissions suggested that change in outlet density had a greater effect on men than on women. Clearly, an increased burden to the NHS is associated with higher levels of outlet density. No evidence was found for an important effect of population migration. Social deprivation was in general strongly associated with the outcome measures but did not modify the associations between the outcomes and alcohol availability.
The strength of the findings was the consistency of these associations over a range of adverse outcomes explored using a range of statistical methods. The main limitation of the research was the dependence on external sources of data and associated data quality concerns, particularly in the absence of any standardised methods of alcohol outlet data collation, processing and validation. As a result of the data quality the incomplete data on on-sales and off-sales could not be used. The limitations of survey data are well known, while the hospital admission data set was too large for detailed clinical interpretation. The study was dependent on the quality of clinical coding and administrative records. The A&E data set did not contain systematic levels of clinical coding and so alcohol attendances could not be identified other than by proxy. Police-recorded crime data were the best available and a valuable data source.
The method of estimating outlet density is generalisable to all geolocated data, including food and gambling outlets, and also to larger geographical areas such as green and natural recreational spaces.
Every possible step was taken to explore and work with the strengths and limitations of these data sets and conservative conclusions have been drawn from these findings.
The following are recommendations for future work to underpin the operation and management of future projects:
-
A standard system of recording data on alcohol outlets is required for research purposes. A minimum data set should be defined and then used by each licensing authority, including validated and reliable complete data on geographical location, opening and closing dates, type of outlet (e.g. on-sales and off-sales, pub, club, restaurant, shop, supermarket) and opening hours. Specifically, geographical location should include complete address data and the National Land and Property Gazetteer (NLPG) generated Unique Property Reference Number (UPRN) to facilitate data linkage.
-
A more precise agreed definition of an alcohol-related admission, to include, inter alia, ICD-10 codes, coding positions, episode numbers, super spells and interhospital transfers, would be of benefit.
The following recommendations for future research, subject to funding, have been made based on experience from this project:
-
A further analysis of the outlet data to classify by type of outlet would be possible using existing GIS software. A second analysis of the outcome measures could then be carried out by outlet type. Further investigation of possible threshold effects by type would then be possible.
-
An analysis of hospital admissions and A&E attendances should be carried out for children and young people. These data have been collated and could be processed and analysed for this important population group.
-
Further methodological work on the estimation of network-based measures of outlet density, including further work on the impact of ‘edge’ effects caused by national borders and islands, is required.
-
A density value for each household residence linked anonymously to individuals, rather than an arbitrary buffer zone or administrative boundary such as the LSOA, can be computed. This finer-grained approach would remove the modifiable areal unit problem and associated bias and so it would be possible in further research to estimate a more accurate risk of hospital admission as a function of outlet density.
-
A formal health economic analysis is needed to estimate the population impact and economic cost of our model-predicted alcohol harms arising from outlet density.
-
Qualitative research is needed to investigate alternative consumer patterns in different types of neighbourhood, using, for example, in-depth case studies and participant observation.
Acknowledgements
This project was funded by the NIHR PHR programme (project number 09/3007/02). The views and opinions expressed therein are those of the authors and do not necessarily reflect those of the NIHR PHR programme or the Department of Health.
The work was undertaken at the Centre for the Development and Evaluation of Complex Interventions for Public Health Improvement (DECIPHer), a UKCRC Public Health Research Centre of Excellence. Funding from the British Heart Foundation, Cancer Research UK, the Economic and Social Research Council (RES-590–28–0005), the Medical Research Council, the Welsh Government and the Wellcome Trust (WT087640MA), under the auspices of the UKCRC, is gratefully acknowledged.
This study makes use of anonymised data held in the SAIL system, which is part of the national e-health records research infrastructure for Wales. We would like to acknowledge all of the data providers who make anonymised data available for research.
We acknowledge the support from the Farr Institute @ CIPHER. The Farr Institute @ CIPHER is supported by a 10-funder consortium: Arthritis Research UK, the British Heart Foundation, Cancer Research UK, the Economic and Social Research Council, the Engineering and Physical Sciences Research Council, the Medical Research Council, the NIHR, the National Institute for Social Care and Health Research (Welsh Government), the Chief Scientist Office (Scottish Government Health Directorates) and the Wellcome Trust (Medical Research Council grant number MR/K006525/1).
We are grateful to Dr Christine Dobbs, Research Fellow in Information Governance and Public Engagement @ CIPHER, for writing the first draft of the plain English summary.
Contributions of authors
All authors made substantial contributions to the conception or design of the work; the acquisition, analysis or interpretation of data; drafting of the report or revising it critically for important intellectual content; and final approval of the version to be published.
In addition, the specific role of each author was as follows:
Professor David Fone (Professor of Health Sciences Research) is principal investigator of the CHALICE project and has led and been involved in every aspect of the research. He carried out the baseline cohort analyses of hospital admissions and A&E attendances and the GLMMs of hospital admissions.
Dr Jennifer Morgan (Research Associate) was responsible for the day-to-day running of the project, liaison with the 22 local authorities and collation and processing of the outlet data, preparation, coding and analysis of the WHS, WDS, PEDW and A&E data sets, co-developed the methodology for the walking and driving outlet densities, and drafted the report.
Dr Richard Fry (Senior Research Associate) contributed to the preparation of the outlet data set and geocoding and validation, co-developed the methodology for the walking and driving outlet densities and carried out the GIS computation of the network outlet densities.
Dr Sarah Rodgers (Associate Professor in Spatial Epidemiology) led the development and tested the methodology for the walking and driving outlet densities.
Dr Scott Orford (Senior Lecturer in Spatial Analysis and GIS) contributed to the geocoding and validation of the outlet data set, co-developed the methodology for the walking and driving outlet densities and carried out the single-year GWR crime models.
Dr Daniel Farewell (Senior Lecturer in Statistics) contributed to the preparation and coding of the PEDW and EDD data sets, carried out the GLMMs of consumption, developed the code for and fitted the spatiotemporal GWR models and carried out these GWR models and carried out the Cox models with time-dependent covariates over the SAIL Gateway.
Professor Frank Dunstan (Professor of Medical Statistics) advised on the technical aspects of the statistical analyses.
Dr James White (Research Fellow) contributed to the literature review.
Mr Vas Sivarajasingam (Reader in Oral and Maxillofacial Surgery) liaised with the police forces in Wales, assured the data sharing agreements and obtained the crime data for analysis.
Dr Laszlo Trefan (Statistician) contributed to the preparation, coding and analyses of the PEDW and EDD data sets.
Dr Iain Brennan (Senior Lecturer in Criminology and Psychology, School of Social Sciences, University of Hull) contributed to the interpretation of the crime data.
Dr Shin Lee (Associate Professor, International School of Urban Sciences, University of Seoul, South Korea) contributed to the contextualisation of the measurement and interpretation of outlet density within urban environments.
Dr Narushige Shiode (Associate Professor in Urban Studies Centre for Interdisciplinary Methodologies, University of Warwick) contributed to the development and understanding of advanced GIS methods.
Dr Alison Weightman [Director, Support Unit for Research Evidence (SURE), Cardiff University Library Service] carried out the original scoping literature review and supervised the final literature review for the report.
Professor Chris Webster (Dean, Faculty of Architecture, University of Hong Kong) contributed to the measurement and understanding of outlet density within the built environment.
Professor Ronan Lyons (Professor of Public Health) contributed to the use of the record-linked data sets and the definitions of alcohol-related admissions and clinical coding used in the project.
Publication
Fone D, Dunstan F, White J, Webster C, Rodgers S, Lee S, et al. Change in alcohol outlet density and alcohol-related harm to population health (CHALICE). BMC Public Health 2012;12:428.
Data sharing statement
Requests for access to the data should be addressed to the data custodian.
Disclaimers
This report presents independent research funded by the National Institute for Health Research (NIHR). The views and opinions expressed by authors in this publication are those of the authors and do not necessarily reflect those of the NHS, the NIHR, NETSCC, the PHR programme or the Department of Health. If there are verbatim quotations included in this publication the views and opinions expressed by the interviewees are those of the interviewees and do not necessarily reflect those of the authors, those of the NHS, the NIHR, NETSCC, the PHR programme or the Department of Health.
References
- Leon DA, McCambridge J. Liver cirrhosis mortality rates in Britain from 1950 to 2002: an analysis of routine data. Lancet 2006;367:52-6. http://dx.doi.org/10.1016/S0140-6736(06)67924-5.
- Alcohol Drinking. IARC Monographs on the Evaluation of Carcinogenic Risks to Humans 44. Lyon: IARC; 1998.
- Narod SA. Alcohol and risk of breast cancer (editorial). JAMA 2011;306:1920-1. http://dx.doi.org/10.1001/jama.2011.1589.
- Klatsky AL, Gunderson E. Alcohol and hypertension: a review. J Am Soc Hypertens 2008;2:307-17. http://dx.doi.org/10.1016/j.jash.2008.03.010.
- Reynolds K, Lewis LB, Nolen JDL, Kinney GL, Sathya B, He J. Alcohol consumption and risk of stroke: a meta-analysis. JAMA 2003;289:579-88. http://dx.doi.org/10.1001/jama.289.5.579.
- Parker RN. Alcohol, homicide, and cultural context: a cross-national analysis of gender-specific homicide victimization. Homicide Stud 1998;2. http://dx.doi.org/10.1177/1088767998002001002.
- Ramstedt M. Alcohol and suicide in 14 European countries. Addiction 2001;96:59-75. http://dx.doi.org/10.1080/09652140020021189.
- del Rio MC, Gomez J, Sancho M, Alvarez F. Alcohol, illicit drugs and medicinal drugs in fatally injured drivers in Spain between 1991 and 2000. Forensic Sci Int 2002;127:63-70. http://dx.doi.org/10.1016/S0379-0738(02)00116-0.
- Abramsky T, Watts CH, Garcia-Moreno C, Devries K, Kiss L, Ellsberg M, et al. What factors are associated with recent intimate partner violence? Findings from the WHO multi-country study on women’s health and domestic violence. BMC Public Health 2011;11. http://dx.doi.org/10.1186/1471-2458-11-109.
- Sivarajasingam V, Morgan P, Matthews K, Shepherd JP, Walker R. Trends in violence in England and Wales 2000–2004: an accident and emergency perspective. Injury 2009;40:820-5. http://dx.doi.org/10.1016/j.injury.2008.08.017.
- McAlaney J, McMahon J. Establishing rates of binge drinking in the UK: anomalies in the data. Alcohol Alcohol 2006;41:355-7. http://dx.doi.org/10.1093/alcalc/agl025.
- Smith L, Foxcroft D. Drinking in the UK. An Exploration of Trends. London: Joseph Rowntree Foundation; 2009.
- Health and Social Care Information Centre . Health Survey for England – 2014 2015. www.hscic.gov.uk/catalogue/PUB19295 (accessed 25 February 2016).
- Hayward R, Sharp C. Young People, Crime and Anti-social Behaviour: Findings from the 2003 Crime and Justice Survey. London: Home Office, Research, Development and Statistics Directorate; 2005.
- Flatley J, Kershaw C, Smith K, Chaplin R, Moon D. Crime in England and Wales 2009/10. London: Home Office; 2010.
- Charalambous MP. Alcohol and the accident and emergency department: a current review. Alcohol Alcohol 2002;37:307-12.
- Pletcher MJ, Varosy P, Kiefe CI, Lewis CE, Sidney S, Hulley SB. Alcohol consumption, binge drinking, and early coronary calcification: findings from the Coronary Artery Risk Development In young Adults (CARDIA) study. Am J Epidemiol 2005;161:423-33. http://dx.doi.org/10.1093/aje/kwi062.
- Bagnardi V, Zatonski W, Scotti L, La Vecchia C, Corrao G. Does drinking pattern modify the effect of alcohol on the risk of coronary heart disease? Evidence from a meta-analysis. J Epidemiol Community Health 2008;62:615-19. http://dx.doi.org/10.1136/jech.2007.065607.
- Alcohol Misuse: Tackling the UK Epidemic. London: BMA; 2008.
- Babor TF. Tackling alcohol misuse in the UK (editorial). BMJ 2008;336. http://dx.doi.org/10.1136/bmj.39496.556435.80.
- Stockwell T, Gruenewald P, Heather N, Stockwell T. The Essential Handbook of Treatment and Prevention of Alcohol Problems. Chichester: John Wiley; 2004.
- Popova S, Giesbrecht N, Bekmuradov D, Patra J. Hours and days of sale and density of alcohol outlets: impacts on alcohol consumption and damage: a systematic review. Alcohol Alcohol 2009;44:500-16. http://dx.doi.org/10.1093/alcalc/agp054.
- Mair C, Gruenewald PJ, Ponicki WR, Remer L. Varying impacts of alcohol outlet densities on violent assaults: explaining differences across neighborhoods. J Stud Alcohol Drugs 2013;74:50-8. http://dx.doi.org/10.15288/jsad.2013.74.50.
- Halonen JI, Kivimaki M, Virtanen M, Pentti J, Subramanian SV, Kawachi I, et al. Living in proximity of a bar and risky alcohol behaviours: a longitudinal study. Addiction 2013;108:320-8. http://dx.doi.org/10.1111/j.1360-0443.2012.04053.x.
- Halonen JI, Kivimaki M, Virtanen M, Pentti J, Subramanian SV, Kawachi I, et al. Proximity of off-premise alcohol outlets and heavy alcohol consumption: a cohort study. Drug Alcohol Depend 2013;132:295-300. http://dx.doi.org/10.1016/j.drugalcdep.2013.02.022.
- Halonen JI, Kivimaki M, Pentti J, Virtanen M, Subramanian SV, Kawachi I, et al. Association of the availability of beer, wine, and liquor outlets with beverage-specific alcohol consumption: a cohort study. Alcohol Clin Exp Res 2014;38:1086-93. http://dx.doi.org/10.1111/acer.12350.
- Picone G, MacDougald J, Sloan F, Platt A, Kertesz S. The effects of residential proximity to bars on alcohol consumption. Int J Health Care Finance Econ 2010;10:347-67. http://dx.doi.org/10.1007/s10754-010-9084-0.
- Gruenewald PJ, Remer L. Changes in outlet densities affect violence rates. Alcohol Clin Exp Res 2006;30:1184-93. http://dx.doi.org/10.1111/j.1530-0277.2006.00141.x.
- Treno AJ, Johnson FW, Remer LG, Gruenewald P. The impact of outlet densities on alcohol-related crashes: a spatial panel approach. Accid Anal Prev 2007;39:894-901. http://dx.doi.org/10.1016/j.aap.2006.12.011.
- Lapham SC, Gruenwald PJ, Remer L, Layne L. New Mexico’s 1998 drive-up liquor window closure. Study 1: effect on alcohol-involved crashes. Addiction 2004;99:598-606. http://dx.doi.org/10.1111/j.1360-0443.2004.00708.x.
- Norstrom T. Outlet density and criminal violence in Norway, 1960–1995. J Stud Alcohol 2000;61:907-11. http://dx.doi.org/10.15288/jsa.2000.61.907.
- Livingston M. A longitudinal analysis of alcohol outlet density and assault. Alcohol Clin Exp Res 2008;32:1074-9. http://dx.doi.org/10.1111/j.1530-0277.2008.00669.x.
- Livingston M. A longitudinal analysis of alcohol outlet density and domestic violence. Addiction 2011;106. http://dx.doi.org/10.1111/j.1360-0443.2010.03333.x.
- Cohen DA, Ghosh-Dastidar B, Scribner R, Miu A, Scott M, Robinson P, et al. Alcohol outlets, gonorrhea, and the Los Angeles civil unrest: a longitudinal analysis. Soc Sci Med 2006;62:3062-71. http://dx.doi.org/10.1016/j.socscimed.2005.11.060.
- Theall KP, Scribner R, Ghosh-Dastidar B, Cohen D, Mason K, Simonsen N. Neighbourhood alcohol availability and gonorrhea rates: impact of social capital. Geospat Health 2009;3:241-55. http://dx.doi.org/10.4081/gh.2009.224.
- Yu QZ, Scribner R, Carlin B, Theall K, Simonsen N, Ghosh-Dastidar B, et al. Multilevel spatio-temporal dual changepoint models for relating alcohol outlet destruction and changes in neighbourhood rates of assaultive violence. Geospat Health 2008;2:161-72. http://dx.doi.org/10.4081/gh.2008.240.
- Yu QZ, Li B, Scribner RA. Hierarchical additive modeling of nonlinear association with spatial correlations – an application to relate alcohol outlet density and neighborhood assault rates. Stat Med 2009;28:1896-912. http://dx.doi.org/10.1002/sim.3600.
- Johnson FW, Gruenewald PJ, Remer LG. Suicide and alcohol: do outlets play a role?. Alcohol Clin Exp Res 2009;33:2124-33. http://dx.doi.org/10.1111/j.1530-0277.2009.01052.x.
- Stockwell T, Zhao J, Macdonald S, Vallance K, Gruenewald PJ, Ponicki W, et al. Impact on alcohol-related mortality of a rapid rise in the density of private liquor outlets in British Columbia: a local area multi-level analysis. Addiction 2011;106:768-76. http://dx.doi.org/10.1111/j.1360-0443.2010.03331.x.
- Spoerri A, Zwahlen M, Panczak R, Egger M, Huss A. Swiss National Cohort . Alcohol-selling outlets and mortality in Switzerland – the Swiss National Cohort. Addiction 2013;108:1603-11. http://dx.doi.org/10.1111/add.12218.
- Campbell CA, Hahn RA, Elder R, Brewer R, Chattopadhyay S, Fielding J, et al. Guide to community preventive services: the effectiveness of limiting alcohol outlet density as a means of reducing excessive alcohol consumption and alcohol-related harms. Am J Prev Med 2009;6:556-69. http://dx.doi.org/10.1016/j.amepre.2009.09.028.
- Tatlow JR, Clapp JD, Hohman MM. The relationship between the geographic density of alcohol outlets and alcohol-related hospital admissions in San Diego County. J Epidemiol Community Health 2000;25:79-88.
- Theall KP, Scribner R, Cohen D, Bluthenthal RN, Schonlau M, Lynch S, et al. The neighborhood alcohol environment and alcohol-related morbidity. Alcohol Alcohol 2009;44:491-9. http://dx.doi.org/10.1093/alcalc/agp042.
- Pollack CE, Cubbin C, Ahn D, Winkleby M. Neighbourhood deprivation and alcohol consumption: does the availability of alcohol play a role?. Int J Epidemiol 2005;34:772-80. http://dx.doi.org/10.1093/ije/dyi026.
- Berke EM, Tanski SE, Demidenko E, Alford-Teaster J, Shi X, Sargent JD. Alcohol retail density and demographic predictors of health disparities: a geographic analysis. Am J Public Health 2010;10:1967-71. http://dx.doi.org/10.2105/AJPH.2009.170464.
- Huckle T, Huakau J, Sweetsur P, Huisman O, Casswell S. Density of alcohol outlets and teenage drinking: living in an alcogenic environment is associated with higher consumption in a metropolitan setting. Addiction 2008;103:1614-21. http://dx.doi.org/10.1111/j.1360-0443.2008.02318.x.
- Ellaway A, Macdonald L, Forsyth A, Macintyre S. The socio-spatial distribution of alcohol outlets in Glasgow city. Health Place 2010;16:167-72. http://dx.doi.org/10.1016/j.healthplace.2009.08.007.
- Norman P, Boyle P, Rees P. Selective migration, health and deprivation: a longitudinal analysis. Soc Sci Med 2005;60:2755-71. http://dx.doi.org/10.1016/j.socscimed.2004.11.008.
- Connolly S, O’Reilly D, Rosato M. Increasing inequalities in health: is it an artefact caused by the selective movement of people?. Soc Sci Med 2007;64:2008-15. http://dx.doi.org/10.1016/j.socscimed.2007.02.021.
- Petticrew M, Cummins S, Ferrell C, Findlay A, Higgins C, Hoy C, et al. Natural experiments: an underused tool for public health?. Public Health 2005;119:751-7. http://dx.doi.org/10.1016/j.puhe.2004.11.008.
- Office for National Statistics . 2011 Census: Usual Resident Population by Single Year of Age, Unrounded Estimates, Local Authorities in England and Wales 2012. www.ons.gov.uk/ons/publications/re-reference-tables.html?edition=tcm%3A77-274670 (accessed 25 February 2016).
- Office for National Statistics . Super Output Areas (SOAs) n.d. www.ons.gov.uk/ons/guide-method/geography/beginner-s-guide/census/super-output-areas--soas-/index.html (accessed 25 February 2016).
- Welsh Assembly Statistical Directorate and Local Government Data Unit . Welsh Index of Multiple Deprivation 2008 n.d. http://wales.gov.uk/docs/statistics/2008/080609wimd2008leafleten.pdf (accessed 25 February 2016).
- Adams J, White M. Removing the health domain from the Index of Multiple Deprivation 2004 – effect on measured inequalities in census measure of health. J Public Health 2006;28:379-83. http://dx.doi.org/10.1093/pubmed/fdl061.
- Townsend P, Phillimore P, Beattie A. Health and Deprivation: Inequality and the North. London: Croom Helm; 1988.
- Office for National Statistics Geography . Rural/Urban/Definition/(England/and/Wales) n.d. www.ons.gov.uk/ons/guide-method/geography/products/area-classifications/rural-urban-definition-and-la/rural-urban-definition--england-and-wales-/index.html (accessed 25 February 2016).
- Ford D, Jones K, Verplancke J-P, Lyons R, John G, Brown G, et al. The SAIL Databank: building a national architecture for e-health research and evaluation. BMC Health Serv Res 2009;9. http://dx.doi.org/10.1186/1472-6963-9-157.
- Lyons RA, Jones KH, John G, Brooks CJ, Verplancke JP, Ford DV, et al. The SAIL Databank: linking multiple health and social care datasets. BMC Med Inform Decis Mak 2009;9. http://dx.doi.org/10.1186/1472-6947-9-3.
- International Classification of Diseases and Related Health Problems, Tenth Revision. Geneva: World Health Organization; n.d.
- Rodgers SE, Lyons RA, Dsilva R, Jones KH, Brooks CJ, Ford DV, et al. Residential Anonymous Linking Fields (RALFs): a novel information infrastructure to study the interaction between the environment and individuals’ health. J Public Health 2009;31:582-8. http://dx.doi.org/10.1093/pubmed/fdp041.
- Health and Social Care Information Centre . OPCS-4 Classification Version 4.6 n.d. http://systems.hscic.gov.uk/data/clinicalcoding/codingstandards/opcs4 (accessed 25 February 2016).
- Ordnance Survey . AddressBase® Premium – CSV. Technical Specification. V1.3–08 2013 n.d. www.ordnancesurvey.co.uk/oswebsite/docs/technical-specifications/addressbase-premium-technical-specification-csv.pdf (accessed 25 February 2016).
- Ordnance Survey . OS MasterMap® Integrated Transport Network™ Layer. User Guide and Technical Specification. V2.0–12 2010 n.d. www.ordnancesurvey.co.uk/docs/technical-specifications/os-mastermap-itn-layer-technical-specification.pdf (accessed 25 February 2016).
- National Research Ethics Service (NRES) . Ethical Review of Research Databases 2010. www.hra.nhs.uk/documents/2013/09/ethical-review-of-research-databases.pdf (accessed 25 February 2016).
- Licensing Act 2003. London: The Stationery Office; 2003.
- Ordnance Survey . OS MasterMap® Topography Layer. User Guide and Technical Specification. V1.12 – 03 2014 n.d. www.ordnancesurvey.co.uk/docs/user-guides/os-mastermap-topography-layer-user-guide.pdf (accessed 25 February 2016).
- Ordnance Survey . Points of Interest Database. User Guide and Technical Specification. V3.2 – 03 2012 n.d. www.ordnancesurvey.co.uk/docs/user-guides/points-of-interest-user-guide.pdf (accessed 25 February 2016).
- Duncan DT, Castro MC, Blossom JC, Bennett GG, Gortmaker SL. Evaluation of the positional difference between two common geocoding methods. Geospatial Health 2011;5:265-73. http://dx.doi.org/10.4081/gh.2011.179.
- Ward MH, Nuckols JR, Giglierano J, Bonner MR, Wolter C, Airola M, et al. Positional accuracy of two methods of geocoding. Epidemiology 2005;16:542-7. http://dx.doi.org/10.1097/01.ede.0000165364.54925.f3.
- Zandbergen PA. A comparison of address point, parcel and street geocoding techniques. Compute Environ Urban Syst 2008;32:214-32. http://dx.doi.org/10.1016/j.compenvurbsys.2007.11.006.
- Krieger N, Waterman P, Lemieux K, Zierler S, Hogan JW. On the wrong side of the tracts? Evaluating the accuracy of geocoding in public health research. Am J Public Health 2001;91:1114-16. http://dx.doi.org/10.2105/AJPH.91.7.1114.
- Ratcliffe JH. Geocoding crime and a first estimate of a minimum acceptable hit rate. Int J Geogr Inf Sci 2004;18:61-2. http://dx.doi.org/10.1080/13658810310001596076.
- Zandbergen PA. Influence of geocoding quality on environmental exposure assessment of children living near high traffic roads. BMC Public Health 2007;7. http://dx.doi.org/10.1186/1471-2458-7-37.
- Royal Mail . Royal Mail Programmers’ Guide Edition 7, Version 5 2009. www.royalmail.com/sites/default/files/docs/pdf/programmers_guide_edition_7_v5.pdf (accessed 25 February 2016).
- Clarke P, Ailshire J, Melendez R, Bader M, Morenoff J. Google Earth to conduct a neighborhood audit: reliability of a virtual audit instrument. Health Place 2010;16:1224-9. http://dx.doi.org/10.1016/j.healthplace.2010.08.007.
- Rossen LM, Pollack KM, Curriero FC. Verification of retail food outlet location data from a local health department using ground-truthing and remote-sensing technology: assessing differences by neighborhood characteristics. Health Place 2012;18:956-62. http://dx.doi.org/10.1016/j.healthplace.2012.06.012.
- Rundle AG, Bader MDM, Richards CA, Neckerman KM, Teitler JO. Using Google Street View to audit neighborhood environments. Am J Prev Med 2011;40:94-100. http://dx.doi.org/10.1016/j.amepre.2010.09.034.
- Rodgers SE, Demmler JC, Dsilva R, Lyons RA. Protecting health data privacy while using residence-based environment and demographic data. Health Place 2012;18:209-17. http://dx.doi.org/10.1016/j.healthplace.2011.09.006.
- Langford M, Fry R, Higgs G. Measuring transit system accessibility using a modified two-step floating catchment technique. Int J Geogr Inf Sci 2012;26:193-214. http://dx.doi.org/10.1080/13658816.2011.574140.
- Doyle-Francis M, Sadler K, Kingdon A, Roberts C, Walters L. Welsh Health Survey User Guide. National Centre for Social Research; 2011.
- McGee A, Jotangia D, Prescott A, Pickering K, Sproston K. Welsh Health Survey – Year One. Technical Report 2005. http://wales.gov.uk/docs/statistics/2005/050701healthsurvey0304techen.pdf (accessed 25 February 2016).
- Sadler K, Doyle M, Hussey D, Stafford R. Welsh Health Survey 2012. Technical Report 2013. http://wales.gov.uk/docs/statistics/2013/130911-welsh-health-survey-2012-technical-report-en.pdf (accessed 25 February 2016).
- Welsh Assembly Government . Welsh Health Survey 2008 User Guide n.d. www.esds.ac.uk/doc/6372/mrdoc/pdf/6372userguide.pdf (accessed 25 February 2016).
- Welsh Assembly Government . Welsh Health Survey 2009 User Guide n.d. http://doc.ukdataservice.ac.uk/doc/6589/mrdoc/pdf/6589userguide.pdf (accessed 25 February 2016).
- Sadler K, Doyle M, Hussey D, Stafford R. Welsh Health Survey 2010. Technical Report n.d. www.esds.ac.uk/doc/6895/mrdoc/pdf/6895technical_report.pdf (accessed 25 February 2016).
- Sadler K, Doyle M, Hussey D, Pickering K, Stafford R. Welsh Health Survey 2011. Technical Report 2012. http://wales.gov.uk/docs/statistics/2012/120919technicalreporten.pdf (accessed 25 February 2016).
- Goddard E. Estimating Alcohol Consumption from Survey Data: Updated Method of Converting Volumes to Units. Newport: ONS; 2007.
- Dolman R, Kingdon A, Roberts C. Welsh Health Survey 2007 n.d. http://wales.gov.uk/docs/statistics/2007/070104-whs-questionnaire-adult-en.pdf (accessed 25 February 2016).
- van de Mortel Thea F. Faking it: social desirability response bias in self-report research. Aust J Adv Nurs 2008;25:40-8.
- Embree BG, Whitehead PC. Validity and reliability of self-reported drinking behavior: dealing with the problem of response bias. J Stud Alcohol 1993;54:334-44. http://dx.doi.org/10.15288/jsa.1993.54.334.
- Stockwell T, Donath S, Cooper-Stanbury M, Chikritzhs T, Catalano P, Mateo C. Under-reporting of alcohol consumption in household surveys: a comparison of quantity-frequency, graduated-frequency and recent recall. Addiction 2004;99:1024-33. http://dx.doi.org/10.1111/j.1360-0443.2004.00815.x.
- Safe. Sensible. Social. The Next Steps in the National Alcohol Strategy. London: Department of Health Publications; 2007.
- Office for National Statistics . SOC2010 Volume 3: The National Statistics Socio-Economic Classification (NS-SEC Rebased on SOC2010) n.d. www.ons.gov.uk/ons/guide-method/classifications/current-standard-classifications/soc2010/soc2010-volume-3-ns-sec--rebased-on-soc2010--user-manual/index.html (accessed 25 February 2016).
- Fone DL, Farewell D, White J, Lyons R, Dunstan F. Social and spatial patterning of excess alcohol consumption and binge drinking: multilevel associations with neighbourhood deprivation. BMJ Open 2013;3. http://dx.doi.org/10.1136/bmjopen-2012-002337.
- White IR, Royston P, Wood AM. Multiple imputation using chained equations: issues and guidance for practice. Stat Med 2010;30:377-99. http://dx.doi.org/10.1002/sim.4067.
- Benjamini Y, Hochberg Y. Controlling the false discovery rate: a practical and powerful approach to multiple testing. J R Stat Soc Series B Methodol 1995;57:289-300.
- Watson PE, Watson ID, Batt RD. Prediction of blood alcohol concentrations in human subjects. Updating the widmark equation. J Stud Alcohol 1981;42:547-56.
- Hill-McManus D, Angus C, Meng Y, Holmes J, Brennan A, Sylvia Meier P. Estimation of usual occasion-based individual drinking patterns using diary survey data. Drug Alcohol Depend 2014;134:136-43. http://dx.doi.org/10.1016/j.drugalcdep.2013.09.022.
- Hastie TJ, Tibshirani RJ, Hastie TJ, Tibshirani RJ. Generalized Additive Models. Boca Raton, FL: Chapman & Hall; 1990.
- Office for National Statistics . Alcohol-Related Deaths in the United Kingdom 2010 n.d. www.ons.gov.uk/ons/dcp171778_254061.pdf (accessed 25 February 2016).
- Gartner A, Cosh H, Gibbon R, Lester N. A Profile of Alcohol and Health in Wales. Cardiff: National Public Health Service for Wales/Wales Centre for Health; 2009.
- Centers for Disease Control and Prevention . Alcohol-Related Disease Impact (ARDI) n.d. http://nccd.cdc.gov/DPH_ARDI/Info/ICDCodes.aspx (accessed 25 February 2016).
- Public Health England . User Guide: Local Alcohol Profiles for England 2014 n.d. www.lape.org.uk/downloads/LAPE%20User%20Guide_Final.pdf (accessed 25 February 2016).
- McDonald SA, Hutchinson SJ, Bird SM, Graham L, Robertson C, Mills PR, et al. Association of self-reported alcohol use and hospitalization for an alcohol-related cause in Scotland: a record-linkage study of 23,183 individuals. Addiction 2009;104:593-602. http://dx.doi.org/10.1111/j.1360-0443.2009.02497.x.
- Therneau TM, Li H. Computing the Cox model for case cohort designs. Lifetime Data Anal 1999;5:99-112. http://dx.doi.org/10.1023/A:1009691327335.
- Young DJ, Stockwell T, Cherpitel C, Ye Y, Macdonald S, Borges G, et al. Emergency room injury presentations as an indicator of alcohol-related problems in the community: a multilevel analysis of an international study. J Stud Alcohol 2004;65:605-12. http://dx.doi.org/10.15288/jsa.2004.65.605.
- Holt S, Stewart IC, Dixon JM, Elton RA, Taylor TV, Little K. Alcohol and the emergency service patient. BMJ 1980;281:638-40. http://dx.doi.org/10.1136/bmj.281.6241.638.
- Walsh ME, Macleod AD. Breath alcohol analysis in the accident and emergency department. Injury 1983;15:62-6. http://dx.doi.org/10.1016/0020-1383(83)90165-1.
- Hope A, Gill A, Costello G, Sheehan J, Brazil E, Reid V. Alcohol and Injuries in the Accident and Emergency Department: A National Perspective. Dublin: Health Promotion Unit, Department of Health and Children; 2005.
- Chaplin R, Lately J, Smith K. Crime in England and Wales 2010 11. Findings from the British Crime Survey and Police Recorded Crime (2nd Edition) 2011. www.gov.uk/government/uploads/system/uploads/attachment_data/file/116417/hosb1011.pdf (accessed 25 February 2016).
- The National Crime Recording Standard (NCRS): What You Need to Know. Londion: Home Office; 2011.
- Simmons J, Legg C, Hosking R. National Crime Recording Standard (NCRS): An Analysis of the Impact on Recorded Crime. Companion Volume to Crime in England and Wales 2002 2003. Part One: The National Picture 2003. http://image.guardian.co.uk/sys-files/Guardian/documents/2003/07/17/NCRS1.pdf (accessed 25 February 2016).
- Information Commissioner’s Office . Data Sharing Code of Practice 2011. https://ico.org.uk/media/for-organisations/documents/1068/data_sharing_code_of_practice.pdf (accessed 25 February 2016).
- Criminal Justice Act 1988. London: The Stationery Office; 1988.
- Moran PAP. Notes on continuous stochastic phenomena. Biometrika 1950;37:17-23.
- Humphreys DK, Smith DM. Alcohol licensing data: why is it an underused resource in public health?. Health Place 2013;24:110-14. http://dx.doi.org/10.1016/j.healthplace.2013.07.006.
- UK Prime Minister’s Strategy Unit . Alcohol-Attributable Fractions n.d. webarchive.nationalarchives.gov.uk/20100407182953/http://www.cabinetoffice.gov.uk/media/cabinetoffice/strategy/assets/econ_annexes.pdf (accessed 25 February 2016).
- Office for National Statistics . Crime Survey for England and Wales. Crime Statistics, Focus on Violent Crime and Sexual Offences, 2013 14 Release. 5 Facts about Alcohol-Related Violence 2015. www.ons.gov.uk/ons/rel/crime-stats/crime-statistics/focus-on-violent-crime-and-sexual-offences--2013-14/sty-facts-about-alcohol-related-violence.html (accessed 25 February 2016).
Appendix 1 Literature review search strategies by database
Ovid MEDLINE
Date range searched: 1996 to week 4, July 2014.
-
binge drinking/
-
Alcoholic Intoxication/pc [Prevention & Control]
-
Alcoholic Beverages/ec, sn, sd [Economics, Statistics & Numerical Data, Supply & Distribution]
-
Beer/ec, sn, sd [Economics, Statistics & Numerical Data, Supply & Distribution]
-
Wine/ec, sn, sd [Economics, Statistics & Numerical Data, Supply & Distribution]
-
Ethanol/ec, sd [Economics, Supply & Distribution]
-
Alcohol Drinking/ep, lj, pc, px [Epidemiology, Legislation & Jurisprudence, Prevention & Control, Psychology]
-
((alcohol or beer or wine or spirit) adj3 (drink* or intoxicat* or consum*)).tw.
-
(drunk or intoxicat* or inebriat* or drunkenness or binge drinking).tw.
-
or/1–9
-
((high or low) adj (percentage* or proportion* or rate)).tw.
-
(density or dense or densities or volume or closeness or crowding or crowdedness or intensity or concentration).tw.
-
11 or 12
-
(outlet* or liquor store* or off-licence* or off-license* or public house* or licensed premises or licenced premises or club*).tw.
-
13 and 14
-
10 and 15
Ovid MEDLINE In-Process & Other Non-Indexed Citations
Date range searched: 1946 to 4 August 2014.
-
((alcohol or beer or wine or spirit) adj3 (drink* or intoxicat* or consum*)).tw.
-
(drunk or intoxicat* or inebriat* or drunkenness or binge drinking).tw.
-
1 or 2
-
((high or low) adj (percentage* or proportion* or rate)).tw.
-
(density or dense or densities or volume or closeness or crowding or crowdedness or intensity or concentration).tw.
-
4 or 5
-
(outlet* or liquor store* or off-licence* or off-license* or public house* or licensed premises or licenced premises or club*).tw.
-
6 and 7
-
3 and 8
Ovid EMBASE
Date range searched: 1996 to 4 August 2014.
-
binge drinking/
-
alcohol intoxication/
-
alcoholic beverage/
-
beer/
-
wine/
-
alcohol/ae, an, to [Adverse Drug Reaction, Drug Analysis, Drug Toxicity]
-
drinking behaviour/
-
((alcohol or beer or wine or spirit) adj3 (drink* or intoxicat* or consum*)).tw.
-
(drunk or intoxicat* or inebriat* or drunkenness or binge drinking).tw.
-
or/1–9
-
((high or low) adj (percentage* or proportion* or rate)).tw.
-
(density or dense or densities or volume or closeness or crowding or crowdedness or intensity or concentration).tw.
-
11 or 12
-
(outlet* or liquor store* or off-licence* or off-license* or public house* or licensed premises or licenced premises or club*).tw.
-
13 and 14
-
10 and 15
Ovid PsycINFO
Date range searched: 1806 to week 5, July 2014.
-
Binge drinking/
-
Alcohol intoxication/
-
Alcoholic beverage/
-
Beer/
-
Wine/
-
Alcohol Drinking Patterns/
-
Alcohols/
-
((alcohol or beer* or wine* or spirit*) adj3 (drink* or intoxicat* or consum*)).tw.
-
(drunk or intoxicat* or inebriat* or drunkenness or binge drinking).tw.
-
or/1–9
-
((high or low) adj (percentage* or proportion* or rate)).tw.
-
(density or dense or densities or volume or closeness or crowding or crowdedness or intensity or concentration).tw.
-
11 or 12
-
(outlet* or liquor store* or off-licence* or off-license* or public house* or licensed premises or licenced premises or club*).tw.
-
13 and 14
-
10 and 15
Ovid Health Management Information Consortium
Date range searched: 1979 to 6 August 2014.
-
Alcohol/
-
Beer/
-
Wine/
-
Alcohol consumption/
-
Alcohol related problems/
-
Alcohol supply & suppliers/
-
((alcohol or beer* or wine* or spirit*) adj3 (drink* or intoxicat* or consum*)).tw.
-
(drunk or intoxicat* or inebriat* or drunkenness or binge drinking).tw.
-
or/1–8
-
((high or low) adj (percentage* or proportion* or rate)).tw.
-
(density or dense or densities or volume or closeness or crowding or crowdedness or intensity or concentration).tw.
-
10 or 11
-
(outlet* or liquor store* or off-licence* or off-license* or public house* or licensed premises or licenced premises or club*).tw.
-
12 and 13
-
9 and 14
Proquest Applied Social Sciences Index and Abstracts
Date range searched: 1987 to 7 August 2014.
ti,ab((alcohol OR beer* OR wine* OR spirit*) N3 (drink* OR intoxicat* OR consum*)) OR ti,ab(drunk OR intoxicat* OR inebriat* OR drunkenness OR binge drinking) AND ti,ab(density OR dense OR densities OR volume OR closeness OR crowding OR crowdedness OR intensity OR concentration AND outlet* OR liquor store* OR off-licence* OR off-license* OR public house* OR licensed premises OR licenced premises OR club*)
Web of Science (Science Citation Index and Social Science Citation Index) (7 August 2014)
#9 | (#7 NOT #8) AND LANGUAGE: (English) Indexes = SCI-EXPANDED, SSCI Timespan = All years |
313 |
#8 | (TS = (‘drinking water’)) AND LANGUAGE: (English) Indexes = SCI-EXPANDED, SSCI Timespan = All years |
49,185 |
#7 | #6 AND #3 Indexes = SCI-EXPANDED, SSCI Timespan = All years |
436 |
#6 | #5 AND #4 Indexes = SCI-EXPANDED, SSCI Timespan = All years |
10,144 |
#5 | (TS = (outlet* OR ‘liquor store’ or ‘liquor stores’ OR off-licence* OR off-license* OR ‘public house’ OR ‘public houses’* OR ‘licensed premises’ OR ‘licenced premises’ OR club*)) AND LANGUAGE: (English) Indexes = SCI-EXPANDED, SSCI Timespan = All years |
48,998 |
#4 | (TS = (density OR dense OR densities OR volume OR closeness OR crowding OR crowdedness OR intensity OR concentration)) AND LANGUAGE: (English) Indexes = SCI-EXPANDED, SSCI Timespan = All years |
4,093,705 |
#3 | OR #1 Indexes = SCI-EXPANDED, SSCI Timespan = All years |
182,477 |
#2 | (TS = (drunk OR intoxicat* OR inebriat* OR drunkenness OR ‘binge drinking’)) AND LANGUAGE: (English) Indexes = SCI-EXPANDED, SSCI Timespan = All years |
147,813 |
#1 | (TS = ((alcohol OR beer* OR wine* OR spirit*) SAME (drink* OR intoxicat* OR consum*))) AND LANGUAGE: (English) | 73,649 |
SCOPUS
Date range searched: 1823 to 6 August 2014.
TITLE-ABS-KEY(alcohol AND drinking OR use OR abuse OR consumption) AND TITLE-ABS-KEY(density OR dense OR densities OR VOLUME OR closeness OR crowding OR crowdedness OR intensity OR concentration AND outlet* OR liquor store* OR off-licence* OR off-license* OR public house* OR licensed premises OR licenced premises OR club*))
EBSCOhost Cumulative Index to Nursing and Allied Health Literature
Date range searched: 1937 to 7 August 2014.
S12 S8 AND S11
S11 S9 AND S10
S10 AB (outlet* or ‘liquor store’ or ‘liquor stores’ or off-licence or off-license or off-licenses or off-licenses or ‘licensed premises’ or ‘licenced premises’ or club or clubs)
S9 AB (density or dense or densities or volume or concentration)
S8 S1 OR S2 OR S3 OR S4 OR S5 OR S6 OR S7
S7 AB (drunk or intoxicat* or inebriat* or drunkenness or ‘binge drinking’)
S6 AB (alcohol or beer* or wine* or spirit*) AND TX (drink* or intoxicat* or consum*)
S5 (MH ‘Alcohol Drinking/PC/EP/LJ’)
S4 (MH ‘Ethanol/SD/EC’)
S3 (MH ‘Alcoholic Beverages/SD/EC’)
S2 (MH ‘Alcoholic Intoxication/PC’)
S1 (MH ‘Binge Drinking’)
List of abbreviations
- A&E
- accident and emergency
- AAF
- alcohol-attributable fraction
- ABP
- AddressBase® Premium
- AIC
- Akaike information criterion
- ALF
- Anonymised Linking Field
- ALF_E
- Anonymised Linking Field_encrypted
- CHALICE
- change in alcohol outlet density and alcohol-related harm to population health
- CI
- confidence interval
- CIPHER
- Centre for Improvement in Population Health through E-records Research
- CSEW
- Crime Survey for England and Wales
- CSV
- comma-separated value
- EDD
- Emergency Department Dataset
- eGIS
- environment Geographic Information System
- GIS
- Geographical Information System
- GLMM
- generalised linear mixed model
- GP
- general practitioner
- GWR
- Geographically Weighted Regression
- HOCR
- Home Office Counting Rules
- HR
- hazard ratio
- ICD-9
- International Classification of Diseases, Ninth Edition
- ICD-10
- International Classification of Diseases, Tenth Edition
- IGRP
- Information Governance Review Panel
- ITN
- Integrated Transport NetworkTM
- kph
- kilometre per hour
- LSOA
- Lower Layer Super Output Area
- mph
- miles per hour
- NCRS
- National Crime Recording Standards
- NIHR
- National Institute for Health Research
- NLPG
- National Land and Property Gazetteer
- NRES
- National Research Ethics Service
- NS-SEC3
- 3-part National Statistics Socio-Economic Classification
- NWIS
- NHS Wales Informatics Service
- ONS
- Office for National Statistics
- OPCS-4.6
- Office of Population Censuses and Surveys Classification of Surgical Operations and Procedures version 4.6
- OR
- odds ratio
- OS
- Ordnance Survey
- OSMM
- Ordnance Survey MasterMap®
- PAF
- Royal Mail Postcode Address File
- PEDW
- Patient Episode Database for Wales
- PHR
- Public Health Research
- POI
- Points of Interest
- RALF
- Residential Anonymous Linking Field
- RALF_E
- Residential Anonymous Linking Field_Encrypted
- RR
- relative risk
- SAIL
- Secure Anonymised Information Linkage
- SD
- standard deviation
- SE
- standard error
- STI
- sexually transmitted infection
- UKCRC
- UK Clinical Research Collaboration
- UPRN
- Unique Property Reference Number
- VAP
- violence against the person
- WDS
- Welsh Demographic Service
- WHS
- Welsh Health Survey
- WIMD
- Welsh Index of Multiple Deprivation